File size: 16,916 Bytes
c0af20c |
1 2 3 4 5 6 7 8 9 10 11 12 13 14 15 16 17 18 19 20 21 22 23 24 25 26 27 28 29 30 31 32 33 34 35 36 37 38 39 40 41 42 43 44 45 46 47 48 49 50 51 52 53 54 55 56 57 58 59 60 61 62 63 64 65 66 67 68 69 70 71 72 73 74 75 76 77 78 79 80 81 82 83 84 85 86 87 88 89 90 91 92 93 94 95 96 97 98 99 100 101 102 103 104 105 106 107 108 109 110 111 112 113 114 115 116 117 118 119 120 121 122 123 124 125 126 127 128 129 130 131 132 133 134 135 136 137 138 139 140 141 142 143 144 145 146 147 148 149 150 151 152 153 154 155 156 157 158 159 160 161 162 163 164 165 166 167 168 169 170 171 172 173 174 175 176 177 178 179 180 181 182 183 184 185 186 187 188 189 190 191 192 193 194 195 196 197 198 199 200 201 202 203 204 205 206 207 208 209 210 211 212 213 214 215 216 217 218 219 220 221 222 223 224 225 226 227 228 229 230 231 232 233 234 235 236 237 238 239 240 241 242 243 244 245 246 247 248 249 250 251 252 253 254 255 256 257 258 259 260 261 262 263 264 265 266 267 268 269 270 271 272 273 274 275 276 277 278 279 280 281 282 283 284 285 286 287 288 289 290 291 292 293 294 295 296 297 298 299 300 301 302 303 304 305 306 307 308 309 310 311 312 313 314 315 316 317 318 319 320 321 322 323 324 325 326 327 328 329 330 331 332 333 334 335 336 337 338 339 340 341 342 343 344 345 346 347 348 349 350 351 352 353 354 355 356 357 358 359 360 361 362 363 364 365 366 367 368 369 370 371 372 373 374 375 376 377 378 379 380 381 382 383 384 385 386 387 388 389 390 391 392 393 394 395 396 397 398 399 400 401 402 403 404 405 406 407 408 409 410 411 412 |
<!--Copyright 2023 The HuggingFace Team. All rights reserved.
Licensed under the Apache License, Version 2.0 (the "License"); you may not use this file except in compliance with
the License. You may obtain a copy of the License at
http://www.apache.org/licenses/LICENSE-2.0
Unless required by applicable law or agreed to in writing, software distributed under the License is distributed on
an "AS IS" BASIS, WITHOUT WARRANTIES OR CONDITIONS OF ANY KIND, either express or implied. See the License for the
specific language governing permissions and limitations under the License.
-->
# Stable diffusion XL
Stable Diffusion XL was proposed in [SDXL: Improving Latent Diffusion Models for High-Resolution Image Synthesis](https://arxiv.org/abs/2307.01952) by Dustin Podell, Zion English, Kyle Lacey, Andreas Blattmann, Tim Dockhorn, Jonas Müller, Joe Penna, Robin Rombach
The abstract of the paper is the following:
*We present SDXL, a latent diffusion model for text-to-image synthesis. Compared to previous versions of Stable Diffusion, SDXL leverages a three times larger UNet backbone: The increase of model parameters is mainly due to more attention blocks and a larger cross-attention context as SDXL uses a second text encoder. We design multiple novel conditioning schemes and train SDXL on multiple aspect ratios. We also introduce a refinement model which is used to improve the visual fidelity of samples generated by SDXL using a post-hoc image-to-image technique. We demonstrate that SDXL shows drastically improved performance compared the previous versions of Stable Diffusion and achieves results competitive with those of black-box state-of-the-art image generators.*
## Tips
- Stable Diffusion XL works especially well with images between 768 and 1024.
- Stable Diffusion XL can pass a different prompt for each of the text encoders it was trained on as shown below. We can even pass different parts of the same prompt to the text encoders.
- Stable Diffusion XL output image can be improved by making use of a refiner as shown below.
### Available checkpoints:
- *Text-to-Image (1024x1024 resolution)*: [stabilityai/stable-diffusion-xl-base-1.0](https://huggingface.co/stabilityai/stable-diffusion-xl-base-1.0) with [`StableDiffusionXLPipeline`]
- *Image-to-Image / Refiner (1024x1024 resolution)*: [stabilityai/stable-diffusion-xl-refiner-1.0](https://huggingface.co/stabilityai/stable-diffusion-xl-refiner-1.0) with [`StableDiffusionXLImg2ImgPipeline`]
## Usage Example
Before using SDXL make sure to have `transformers`, `accelerate`, `safetensors` and `invisible_watermark` installed.
You can install the libraries as follows:
```
pip install transformers
pip install accelerate
pip install safetensors
pip install invisible-watermark>=0.2.0
```
### Text-to-Image
You can use SDXL as follows for *text-to-image*:
```py
from diffusers import StableDiffusionXLPipeline
import torch
pipe = StableDiffusionXLPipeline.from_pretrained(
"stabilityai/stable-diffusion-xl-base-1.0", torch_dtype=torch.float16, variant="fp16", use_safetensors=True
)
pipe.to("cuda")
prompt = "Astronaut in a jungle, cold color palette, muted colors, detailed, 8k"
image = pipe(prompt=prompt).images[0]
```
### Image-to-image
You can use SDXL as follows for *image-to-image*:
```py
import torch
from diffusers import StableDiffusionXLImg2ImgPipeline
from diffusers.utils import load_image
pipe = StableDiffusionXLImg2ImgPipeline.from_pretrained(
"stabilityai/stable-diffusion-xl-refiner-1.0", torch_dtype=torch.float16, variant="fp16", use_safetensors=True
)
pipe = pipe.to("cuda")
url = "https://huggingface.co/datasets/patrickvonplaten/images/resolve/main/aa_xl/000000009.png"
init_image = load_image(url).convert("RGB")
prompt = "a photo of an astronaut riding a horse on mars"
image = pipe(prompt, image=init_image).images[0]
```
### Inpainting
You can use SDXL as follows for *inpainting*
```py
import torch
from diffusers import StableDiffusionXLInpaintPipeline
from diffusers.utils import load_image
pipe = StableDiffusionXLInpaintPipeline.from_pretrained(
"stabilityai/stable-diffusion-xl-base-1.0", torch_dtype=torch.float16, variant="fp16", use_safetensors=True
)
pipe.to("cuda")
img_url = "https://raw.githubusercontent.com/CompVis/latent-diffusion/main/data/inpainting_examples/overture-creations-5sI6fQgYIuo.png"
mask_url = "https://raw.githubusercontent.com/CompVis/latent-diffusion/main/data/inpainting_examples/overture-creations-5sI6fQgYIuo_mask.png"
init_image = load_image(img_url).convert("RGB")
mask_image = load_image(mask_url).convert("RGB")
prompt = "A majestic tiger sitting on a bench"
image = pipe(prompt=prompt, image=init_image, mask_image=mask_image, num_inference_steps=50, strength=0.80).images[0]
```
### Refining the image output
In addition to the [base model checkpoint](https://huggingface.co/stabilityai/stable-diffusion-xl-base-1.0),
StableDiffusion-XL also includes a [refiner checkpoint](huggingface.co/stabilityai/stable-diffusion-xl-refiner-1.0)
that is specialized in denoising low-noise stage images to generate images of improved high-frequency quality.
This refiner checkpoint can be used as a "second-step" pipeline after having run the base checkpoint to improve
image quality.
When using the refiner, one can easily
- 1.) employ the base model and refiner as an *Ensemble of Expert Denoisers* as first proposed in [eDiff-I](https://research.nvidia.com/labs/dir/eDiff-I/) or
- 2.) simply run the refiner in [SDEdit](https://arxiv.org/abs/2108.01073) fashion after the base model.
**Note**: The idea of using SD-XL base & refiner as an ensemble of experts was first brought forward by
a couple community contributors which also helped shape the following `diffusers` implementation, namely:
- [SytanSD](https://github.com/SytanSD)
- [bghira](https://github.com/bghira)
- [Birch-san](https://github.com/Birch-san)
- [AmericanPresidentJimmyCarter](https://github.com/AmericanPresidentJimmyCarter)
#### 1.) Ensemble of Expert Denoisers
When using the base and refiner model as an ensemble of expert of denoisers, the base model should serve as the
expert for the high-noise diffusion stage and the refiner serves as the expert for the low-noise diffusion stage.
The advantage of 1.) over 2.) is that it requires less overall denoising steps and therefore should be significantly
faster. The drawback is that one cannot really inspect the output of the base model; it will still be heavily denoised.
To use the base model and refiner as an ensemble of expert denoisers, make sure to define the span
of timesteps which should be run through the high-noise denoising stage (*i.e.* the base model) and the low-noise
denoising stage (*i.e.* the refiner model) respectively. We can set the intervals using the [`denoising_end`](https://huggingface.co/docs/diffusers/main/en/api/pipelines/stable_diffusion/stable_diffusion_xl#diffusers.StableDiffusionXLPipeline.__call__.denoising_end) of the base model
and [`denoising_start`](https://huggingface.co/docs/diffusers/main/en/api/pipelines/stable_diffusion/stable_diffusion_xl#diffusers.StableDiffusionXLImg2ImgPipeline.__call__.denoising_start) of the refiner model.
For both `denoising_end` and `denoising_start` a float value between 0 and 1 should be passed.
When passed, the end and start of denoising will be defined by proportions of discrete timesteps as
defined by the model schedule.
Note that this will override `strength` if it is also declared, since the number of denoising steps
is determined by the discrete timesteps the model was trained on and the declared fractional cutoff.
Let's look at an example.
First, we import the two pipelines. Since the text encoders and variational autoencoder are the same
you don't have to load those again for the refiner.
```py
from diffusers import DiffusionPipeline
import torch
base = DiffusionPipeline.from_pretrained(
"stabilityai/stable-diffusion-xl-base-1.0", torch_dtype=torch.float16, variant="fp16", use_safetensors=True
)
base.to("cuda")
refiner = DiffusionPipeline.from_pretrained(
"stabilityai/stable-diffusion-xl-refiner-1.0",
text_encoder_2=base.text_encoder_2,
vae=base.vae,
torch_dtype=torch.float16,
use_safetensors=True,
variant="fp16",
)
refiner.to("cuda")
```
Now we define the number of inference steps and the point at which the model shall be run through the
high-noise denoising stage (*i.e.* the base model).
```py
n_steps = 40
high_noise_frac = 0.8
```
Stable Diffusion XL base is trained on timesteps 0-999 and Stable Diffusion XL refiner is finetuned
from the base model on low noise timesteps 0-199 inclusive, so we use the base model for the first
800 timesteps (high noise) and the refiner for the last 200 timesteps (low noise). Hence, `high_noise_frac`
is set to 0.8, so that all steps 200-999 (the first 80% of denoising timesteps) are performed by the
base model and steps 0-199 (the last 20% of denoising timesteps) are performed by the refiner model.
Remember, the denoising process starts at **high value** (high noise) timesteps and ends at
**low value** (low noise) timesteps.
Let's run the two pipelines now. Make sure to set `denoising_end` and
`denoising_start` to the same values and keep `num_inference_steps` constant. Also remember that
the output of the base model should be in latent space:
```py
prompt = "A majestic lion jumping from a big stone at night"
image = base(
prompt=prompt,
num_inference_steps=n_steps,
denoising_end=high_noise_frac,
output_type="latent",
).images
image = refiner(
prompt=prompt,
num_inference_steps=n_steps,
denoising_start=high_noise_frac,
image=image,
).images[0]
```
Let's have a look at the images
| Original Image | Ensemble of Denoisers Experts |
|---|---|
| 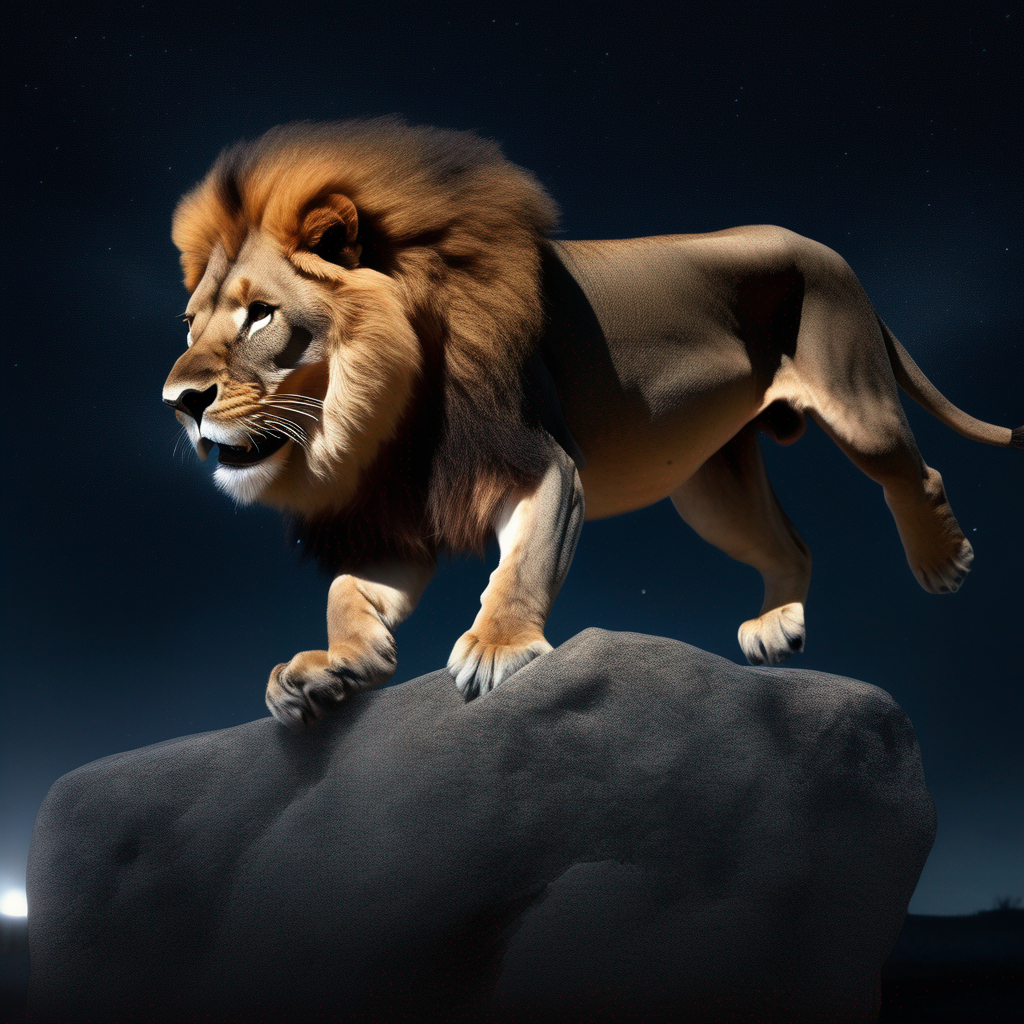 | 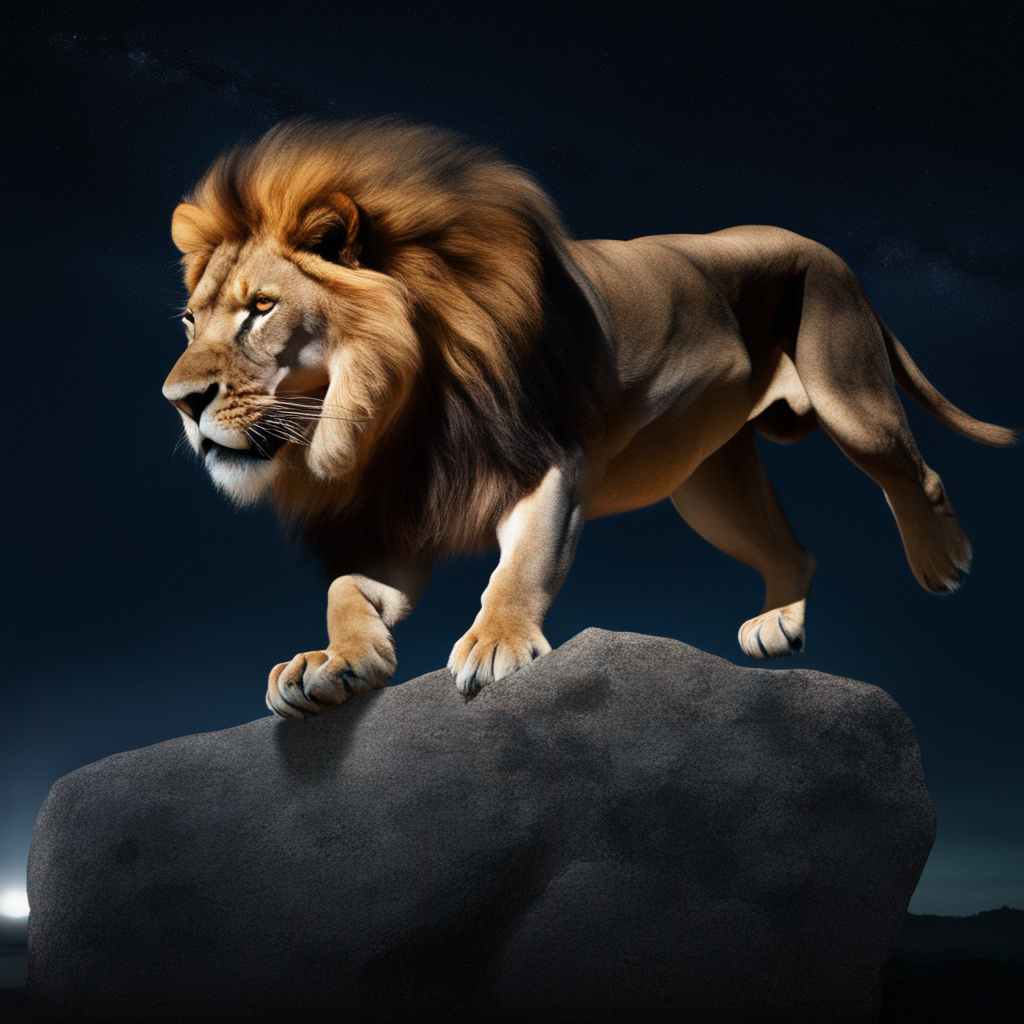
If we would have just run the base model on the same 40 steps, the image would have been arguably less detailed (e.g. the lion eyes and nose):
<Tip>
The ensemble-of-experts method works well on all available schedulers!
</Tip>
#### 2.) Refining the image output from fully denoised base image
In standard [`StableDiffusionImg2ImgPipeline`]-fashion, the fully-denoised image generated of the base model
can be further improved using the [refiner checkpoint](huggingface.co/stabilityai/stable-diffusion-xl-refiner-1.0).
For this, you simply run the refiner as a normal image-to-image pipeline after the "base" text-to-image
pipeline. You can leave the outputs of the base model in latent space.
```py
from diffusers import DiffusionPipeline
import torch
pipe = DiffusionPipeline.from_pretrained(
"stabilityai/stable-diffusion-xl-base-1.0", torch_dtype=torch.float16, variant="fp16", use_safetensors=True
)
pipe.to("cuda")
refiner = DiffusionPipeline.from_pretrained(
"stabilityai/stable-diffusion-xl-refiner-1.0",
text_encoder_2=pipe.text_encoder_2,
vae=pipe.vae,
torch_dtype=torch.float16,
use_safetensors=True,
variant="fp16",
)
refiner.to("cuda")
prompt = "Astronaut in a jungle, cold color palette, muted colors, detailed, 8k"
image = pipe(prompt=prompt, output_type="latent" if use_refiner else "pil").images[0]
image = refiner(prompt=prompt, image=image[None, :]).images[0]
```
| Original Image | Refined Image |
|---|---|
| 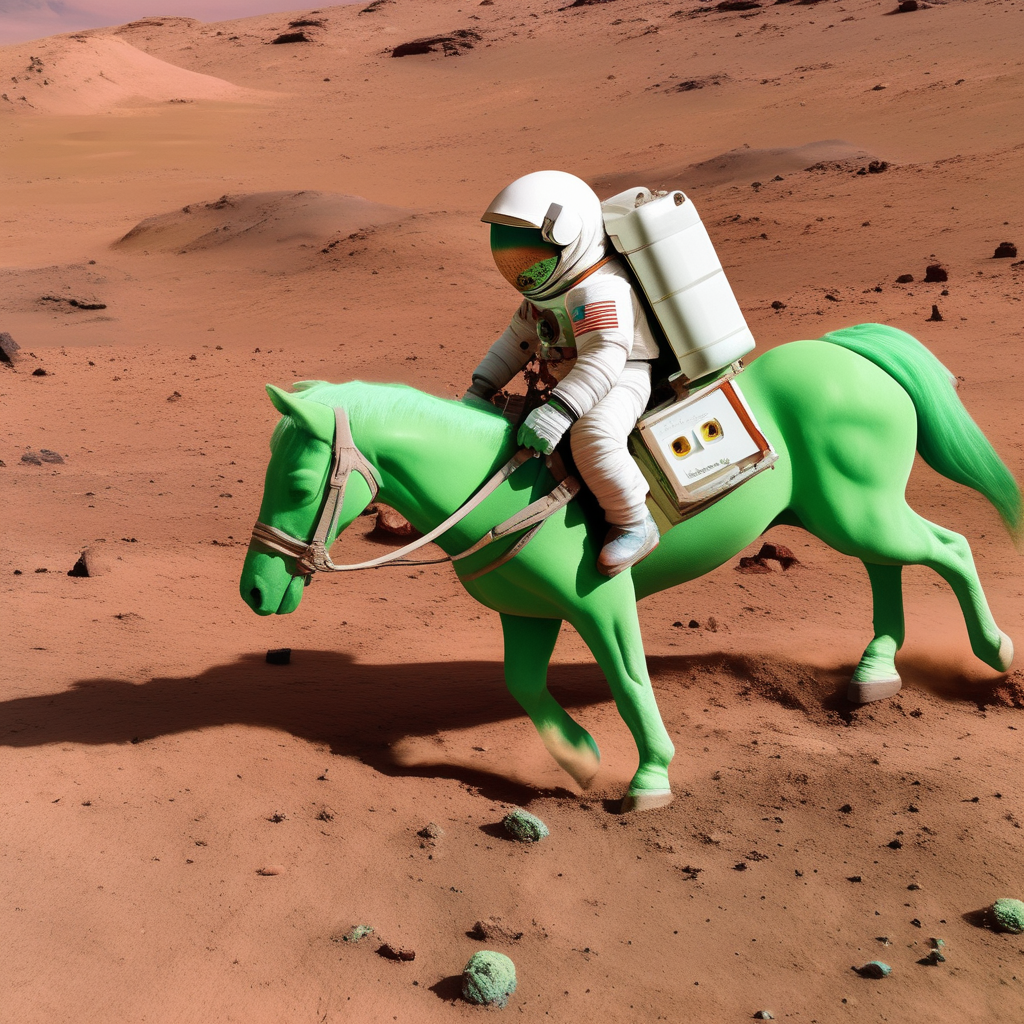 | 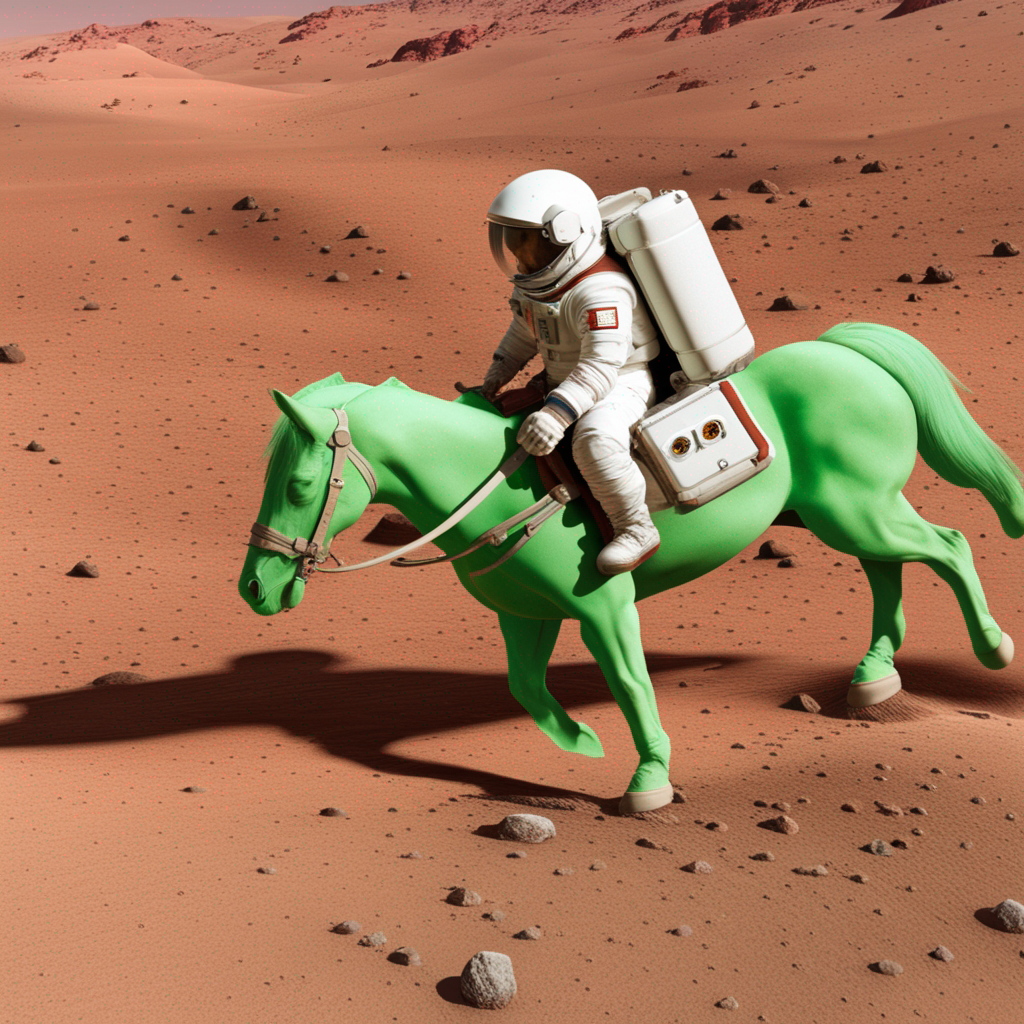 |
<Tip>
The refiner can also very well be used in an in-painting setting. To do so just make
sure you use the [`StableDiffusionXLInpaintPipeline`] classes as shown below
</Tip>
To use the refiner for inpainting in the Ensemble of Expert Denoisers setting you can do the following:
```py
from diffusers import StableDiffusionXLInpaintPipeline
from diffusers.utils import load_image
pipe = StableDiffusionXLInpaintPipeline.from_pretrained(
"stabilityai/stable-diffusion-xl-base-1.0", torch_dtype=torch.float16, variant="fp16", use_safetensors=True
)
pipe.to("cuda")
refiner = StableDiffusionXLInpaintPipeline.from_pretrained(
"stabilityai/stable-diffusion-xl-refiner-1.0",
text_encoder_2=pipe.text_encoder_2,
vae=pipe.vae,
torch_dtype=torch.float16,
use_safetensors=True,
variant="fp16",
)
refiner.to("cuda")
img_url = "https://raw.githubusercontent.com/CompVis/latent-diffusion/main/data/inpainting_examples/overture-creations-5sI6fQgYIuo.png"
mask_url = "https://raw.githubusercontent.com/CompVis/latent-diffusion/main/data/inpainting_examples/overture-creations-5sI6fQgYIuo_mask.png"
init_image = load_image(img_url).convert("RGB")
mask_image = load_image(mask_url).convert("RGB")
prompt = "A majestic tiger sitting on a bench"
num_inference_steps = 75
high_noise_frac = 0.7
image = pipe(
prompt=prompt,
image=init_image,
mask_image=mask_image,
num_inference_steps=num_inference_steps,
denoising_start=high_noise_frac,
output_type="latent",
).images
image = refiner(
prompt=prompt,
image=image,
mask_image=mask_image,
num_inference_steps=num_inference_steps,
denoising_start=high_noise_frac,
).images[0]
```
To use the refiner for inpainting in the standard SDE-style setting, simply remove `denoising_end` and `denoising_start` and choose a smaller
number of inference steps for the refiner.
### Loading single file checkpoints / original file format
By making use of [`~diffusers.loaders.FromSingleFileMixin.from_single_file`] you can also load the
original file format into `diffusers`:
```py
from diffusers import StableDiffusionXLPipeline, StableDiffusionXLImg2ImgPipeline
import torch
pipe = StableDiffusionXLPipeline.from_single_file(
"./sd_xl_base_1.0.safetensors", torch_dtype=torch.float16, variant="fp16", use_safetensors=True
)
pipe.to("cuda")
refiner = StableDiffusionXLImg2ImgPipeline.from_single_file(
"./sd_xl_refiner_1.0.safetensors", torch_dtype=torch.float16, use_safetensors=True, variant="fp16"
)
refiner.to("cuda")
```
### Memory optimization via model offloading
If you are seeing out-of-memory errors, we recommend making use of [`StableDiffusionXLPipeline.enable_model_cpu_offload`].
```diff
- pipe.to("cuda")
+ pipe.enable_model_cpu_offload()
```
and
```diff
- refiner.to("cuda")
+ refiner.enable_model_cpu_offload()
```
### Speed-up inference with `torch.compile`
You can speed up inference by making use of `torch.compile`. This should give you **ca.** 20% speed-up.
```diff
+ pipe.unet = torch.compile(pipe.unet, mode="reduce-overhead", fullgraph=True)
+ refiner.unet = torch.compile(refiner.unet, mode="reduce-overhead", fullgraph=True)
```
### Running with `torch < 2.0`
**Note** that if you want to run Stable Diffusion XL with `torch` < 2.0, please make sure to enable xformers
attention:
```
pip install xformers
```
```diff
+pipe.enable_xformers_memory_efficient_attention()
+refiner.enable_xformers_memory_efficient_attention()
```
## StableDiffusionXLPipeline
[[autodoc]] StableDiffusionXLPipeline
- all
- __call__
## StableDiffusionXLImg2ImgPipeline
[[autodoc]] StableDiffusionXLImg2ImgPipeline
- all
- __call__
## StableDiffusionXLInpaintPipeline
[[autodoc]] StableDiffusionXLInpaintPipeline
- all
- __call__
### Passing different prompts to each text-encoder
Stable Diffusion XL was trained on two text encoders. The default behavior is to pass the same prompt to each. But it is possible to pass a different prompt for each text-encoder, as [some users](https://github.com/huggingface/diffusers/issues/4004#issuecomment-1627764201) noted that it can boost quality.
To do so, you can pass `prompt_2` and `negative_prompt_2` in addition to `prompt` and `negative_prompt`. By doing that, you will pass the original prompts and negative prompts (as in `prompt` and `negative_prompt`) to `text_encoder` (in official SDXL 0.9/1.0 that is [OpenAI CLIP-ViT/L-14](https://huggingface.co/openai/clip-vit-large-patch14)),
and `prompt_2` and `negative_prompt_2` to `text_encoder_2` (in official SDXL 0.9/1.0 that is [OpenCLIP-ViT/bigG-14](https://huggingface.co/laion/CLIP-ViT-bigG-14-laion2B-39B-b160k)).
```py
from diffusers import StableDiffusionXLPipeline
import torch
pipe = StableDiffusionXLPipeline.from_pretrained(
"stabilityai/stable-diffusion-xl-base-1.0", torch_dtype=torch.float16, variant="fp16", use_safetensors=True
)
pipe.to("cuda")
# prompt will be passed to OAI CLIP-ViT/L-14
prompt = "Astronaut in a jungle, cold color palette, muted colors, detailed, 8k"
# prompt_2 will be passed to OpenCLIP-ViT/bigG-14
prompt_2 = "monet painting"
image = pipe(prompt=prompt, prompt_2=prompt_2).images[0]
```
|