Upload 14 files
Browse files- .flake8 +4 -0
- .gitattributes +5 -34
- .gitignore +11 -0
- .pre-commit-config.yaml +28 -0
- CHANGELOG.md +69 -0
- LICENSE +21 -0
- MANIFEST.in +5 -0
- README.md +147 -3
- approach.png +0 -0
- language-breakdown.svg +0 -0
- model-card.md +69 -0
- pyproject.toml +8 -0
- requirements.txt +6 -0
- setup.py +43 -0
.flake8
ADDED
@@ -0,0 +1,4 @@
|
|
|
|
|
|
|
|
|
|
|
1 |
+
[flake8]
|
2 |
+
per-file-ignores =
|
3 |
+
*/__init__.py: F401
|
4 |
+
|
.gitattributes
CHANGED
@@ -1,35 +1,6 @@
|
|
1 |
-
|
2 |
-
|
|
|
3 |
*.bin filter=lfs diff=lfs merge=lfs -text
|
4 |
-
*.
|
5 |
-
*.
|
6 |
-
*.ftz filter=lfs diff=lfs merge=lfs -text
|
7 |
-
*.gz filter=lfs diff=lfs merge=lfs -text
|
8 |
-
*.h5 filter=lfs diff=lfs merge=lfs -text
|
9 |
-
*.joblib filter=lfs diff=lfs merge=lfs -text
|
10 |
-
*.lfs.* filter=lfs diff=lfs merge=lfs -text
|
11 |
-
*.mlmodel filter=lfs diff=lfs merge=lfs -text
|
12 |
-
*.model filter=lfs diff=lfs merge=lfs -text
|
13 |
-
*.msgpack filter=lfs diff=lfs merge=lfs -text
|
14 |
-
*.npy filter=lfs diff=lfs merge=lfs -text
|
15 |
-
*.npz filter=lfs diff=lfs merge=lfs -text
|
16 |
-
*.onnx filter=lfs diff=lfs merge=lfs -text
|
17 |
-
*.ot filter=lfs diff=lfs merge=lfs -text
|
18 |
-
*.parquet filter=lfs diff=lfs merge=lfs -text
|
19 |
-
*.pb filter=lfs diff=lfs merge=lfs -text
|
20 |
-
*.pickle filter=lfs diff=lfs merge=lfs -text
|
21 |
-
*.pkl filter=lfs diff=lfs merge=lfs -text
|
22 |
-
*.pt filter=lfs diff=lfs merge=lfs -text
|
23 |
-
*.pth filter=lfs diff=lfs merge=lfs -text
|
24 |
-
*.rar filter=lfs diff=lfs merge=lfs -text
|
25 |
-
*.safetensors filter=lfs diff=lfs merge=lfs -text
|
26 |
-
saved_model/**/* filter=lfs diff=lfs merge=lfs -text
|
27 |
-
*.tar.* filter=lfs diff=lfs merge=lfs -text
|
28 |
-
*.tar filter=lfs diff=lfs merge=lfs -text
|
29 |
-
*.tflite filter=lfs diff=lfs merge=lfs -text
|
30 |
-
*.tgz filter=lfs diff=lfs merge=lfs -text
|
31 |
-
*.wasm filter=lfs diff=lfs merge=lfs -text
|
32 |
-
*.xz filter=lfs diff=lfs merge=lfs -text
|
33 |
-
*.zip filter=lfs diff=lfs merge=lfs -text
|
34 |
-
*.zst filter=lfs diff=lfs merge=lfs -text
|
35 |
-
*tfevents* filter=lfs diff=lfs merge=lfs -text
|
|
|
1 |
+
# Override jupyter in Github language stats for more accurate estimate of repo code languages
|
2 |
+
# reference: https://github.com/github/linguist/blob/master/docs/overrides.md#generated-code
|
3 |
+
*.ipynb linguist-generated
|
4 |
*.bin filter=lfs diff=lfs merge=lfs -text
|
5 |
+
*.json filter=lfs diff=lfs merge=lfs -text
|
6 |
+
*.flac filter=lfs diff=lfs merge=lfs -text
|
|
|
|
|
|
|
|
|
|
|
|
|
|
|
|
|
|
|
|
|
|
|
|
|
|
|
|
|
|
|
|
|
|
|
|
|
|
|
|
|
|
|
|
|
|
|
|
|
|
|
|
|
|
|
|
|
|
|
|
.gitignore
ADDED
@@ -0,0 +1,11 @@
|
|
|
|
|
|
|
|
|
|
|
|
|
|
|
|
|
|
|
|
|
|
|
|
|
1 |
+
__pycache__/
|
2 |
+
*.py[cod]
|
3 |
+
*$py.class
|
4 |
+
*.egg-info
|
5 |
+
.pytest_cache
|
6 |
+
.ipynb_checkpoints
|
7 |
+
|
8 |
+
thumbs.db
|
9 |
+
.DS_Store
|
10 |
+
.idea
|
11 |
+
|
.pre-commit-config.yaml
ADDED
@@ -0,0 +1,28 @@
|
|
|
|
|
|
|
|
|
|
|
|
|
|
|
|
|
|
|
|
|
|
|
|
|
|
|
|
|
|
|
|
|
|
|
|
|
|
|
|
|
|
|
|
|
|
|
|
|
|
|
|
|
|
|
|
|
|
|
1 |
+
repos:
|
2 |
+
- repo: https://github.com/pre-commit/pre-commit-hooks
|
3 |
+
rev: v4.0.1
|
4 |
+
hooks:
|
5 |
+
- id: check-json
|
6 |
+
- id: end-of-file-fixer
|
7 |
+
types: [file, python]
|
8 |
+
- id: trailing-whitespace
|
9 |
+
types: [file, python]
|
10 |
+
- id: mixed-line-ending
|
11 |
+
- id: check-added-large-files
|
12 |
+
args: [--maxkb=4096]
|
13 |
+
- repo: https://github.com/psf/black
|
14 |
+
rev: 23.7.0
|
15 |
+
hooks:
|
16 |
+
- id: black
|
17 |
+
- repo: https://github.com/pycqa/isort
|
18 |
+
rev: 5.12.0
|
19 |
+
hooks:
|
20 |
+
- id: isort
|
21 |
+
name: isort (python)
|
22 |
+
args: ["--profile", "black", "-l", "88", "--trailing-comma", "--multi-line", "3"]
|
23 |
+
- repo: https://github.com/pycqa/flake8.git
|
24 |
+
rev: 6.0.0
|
25 |
+
hooks:
|
26 |
+
- id: flake8
|
27 |
+
types: [python]
|
28 |
+
args: ["--max-line-length", "88", "--ignore", "E203,E501,W503,W504"]
|
CHANGELOG.md
ADDED
@@ -0,0 +1,69 @@
|
|
|
|
|
|
|
|
|
|
|
|
|
|
|
|
|
|
|
|
|
|
|
|
|
|
|
|
|
|
|
|
|
|
|
|
|
|
|
|
|
|
|
|
|
|
|
|
|
|
|
|
|
|
|
|
|
|
|
|
|
|
|
|
|
|
|
|
|
|
|
|
|
|
|
|
|
|
|
|
|
|
|
|
|
|
|
|
|
|
|
|
|
|
|
|
|
|
|
|
|
|
|
|
|
|
|
|
|
|
|
|
|
|
|
|
|
|
|
|
|
|
|
|
|
|
|
|
|
|
|
|
|
|
|
|
|
|
|
|
|
1 |
+
# CHANGELOG
|
2 |
+
|
3 |
+
## [v20230918](https://github.com/openai/whisper/releases/tag/v20230918)
|
4 |
+
|
5 |
+
* Add .pre-commit-config.yaml ([#1528](https://github.com/openai/whisper/pull/1528))
|
6 |
+
* fix doc of TextDecoder ([#1526](https://github.com/openai/whisper/pull/1526))
|
7 |
+
* Update model-card.md ([#1643](https://github.com/openai/whisper/pull/1643))
|
8 |
+
* word timing tweaks ([#1559](https://github.com/openai/whisper/pull/1559))
|
9 |
+
* Avoid rearranging all caches ([#1483](https://github.com/openai/whisper/pull/1483))
|
10 |
+
* Improve timestamp heuristics. ([#1461](https://github.com/openai/whisper/pull/1461))
|
11 |
+
* fix condition_on_previous_text ([#1224](https://github.com/openai/whisper/pull/1224))
|
12 |
+
* Fix numba depreceation notice ([#1233](https://github.com/openai/whisper/pull/1233))
|
13 |
+
* Updated README.md to provide more insight on BLEU and specific appendices ([#1236](https://github.com/openai/whisper/pull/1236))
|
14 |
+
* Avoid computing higher temperatures on no_speech segments ([#1279](https://github.com/openai/whisper/pull/1279))
|
15 |
+
* Dropped unused execute bit from mel_filters.npz. ([#1254](https://github.com/openai/whisper/pull/1254))
|
16 |
+
* Drop ffmpeg-python dependency and call ffmpeg directly. ([#1242](https://github.com/openai/whisper/pull/1242))
|
17 |
+
* Python 3.11 ([#1171](https://github.com/openai/whisper/pull/1171))
|
18 |
+
* Update decoding.py ([#1219](https://github.com/openai/whisper/pull/1219))
|
19 |
+
* Update decoding.py ([#1155](https://github.com/openai/whisper/pull/1155))
|
20 |
+
* Update README.md to reference tiktoken ([#1105](https://github.com/openai/whisper/pull/1105))
|
21 |
+
* Implement max line width and max line count, and make word highlighting optional ([#1184](https://github.com/openai/whisper/pull/1184))
|
22 |
+
* Squash long words at window and sentence boundaries. ([#1114](https://github.com/openai/whisper/pull/1114))
|
23 |
+
* python-publish.yml: bump actions version to fix node warning ([#1211](https://github.com/openai/whisper/pull/1211))
|
24 |
+
* Update tokenizer.py ([#1163](https://github.com/openai/whisper/pull/1163))
|
25 |
+
|
26 |
+
## [v20230314](https://github.com/openai/whisper/releases/tag/v20230314)
|
27 |
+
|
28 |
+
* abort find_alignment on empty input ([#1090](https://github.com/openai/whisper/pull/1090))
|
29 |
+
* Fix truncated words list when the replacement character is decoded ([#1089](https://github.com/openai/whisper/pull/1089))
|
30 |
+
* fix github language stats getting dominated by jupyter notebook ([#1076](https://github.com/openai/whisper/pull/1076))
|
31 |
+
* Fix alignment between the segments and the list of words ([#1087](https://github.com/openai/whisper/pull/1087))
|
32 |
+
* Use tiktoken ([#1044](https://github.com/openai/whisper/pull/1044))
|
33 |
+
|
34 |
+
## [v20230308](https://github.com/openai/whisper/releases/tag/v20230308)
|
35 |
+
|
36 |
+
* kwargs in decode() for convenience ([#1061](https://github.com/openai/whisper/pull/1061))
|
37 |
+
* fix all_tokens handling that caused more repetitions and discrepancy in JSON ([#1060](https://github.com/openai/whisper/pull/1060))
|
38 |
+
* fix typo in CHANGELOG.md
|
39 |
+
|
40 |
+
## [v20230307](https://github.com/openai/whisper/releases/tag/v20230307)
|
41 |
+
|
42 |
+
* Fix the repetition/hallucination issue identified in #1046 ([#1052](https://github.com/openai/whisper/pull/1052))
|
43 |
+
* Use triton==2.0.0 ([#1053](https://github.com/openai/whisper/pull/1053))
|
44 |
+
* Install triton in x86_64 linux only ([#1051](https://github.com/openai/whisper/pull/1051))
|
45 |
+
* update setup.py to specify python >= 3.8 requirement
|
46 |
+
|
47 |
+
## [v20230306](https://github.com/openai/whisper/releases/tag/v20230306)
|
48 |
+
|
49 |
+
* remove auxiliary audio extension ([#1021](https://github.com/openai/whisper/pull/1021))
|
50 |
+
* apply formatting with `black`, `isort`, and `flake8` ([#1038](https://github.com/openai/whisper/pull/1038))
|
51 |
+
* word-level timestamps in `transcribe()` ([#869](https://github.com/openai/whisper/pull/869))
|
52 |
+
* Decoding improvements ([#1033](https://github.com/openai/whisper/pull/1033))
|
53 |
+
* Update README.md ([#894](https://github.com/openai/whisper/pull/894))
|
54 |
+
* Fix infinite loop caused by incorrect timestamp tokens prediction ([#914](https://github.com/openai/whisper/pull/914))
|
55 |
+
* drop python 3.7 support ([#889](https://github.com/openai/whisper/pull/889))
|
56 |
+
|
57 |
+
## [v20230124](https://github.com/openai/whisper/releases/tag/v20230124)
|
58 |
+
|
59 |
+
* handle printing even if sys.stdout.buffer is not available ([#887](https://github.com/openai/whisper/pull/887))
|
60 |
+
* Add TSV formatted output in transcript, using integer start/end time in milliseconds ([#228](https://github.com/openai/whisper/pull/228))
|
61 |
+
* Added `--output_format` option ([#333](https://github.com/openai/whisper/pull/333))
|
62 |
+
* Handle `XDG_CACHE_HOME` properly for `download_root` ([#864](https://github.com/openai/whisper/pull/864))
|
63 |
+
* use stdout for printing transcription progress ([#867](https://github.com/openai/whisper/pull/867))
|
64 |
+
* Fix bug where mm is mistakenly replaced with hmm in e.g. 20mm ([#659](https://github.com/openai/whisper/pull/659))
|
65 |
+
* print '?' if a letter can't be encoded using the system default encoding ([#859](https://github.com/openai/whisper/pull/859))
|
66 |
+
|
67 |
+
## [v20230117](https://github.com/openai/whisper/releases/tag/v20230117)
|
68 |
+
|
69 |
+
The first versioned release available on [PyPI](https://pypi.org/project/openai-whisper/)
|
LICENSE
ADDED
@@ -0,0 +1,21 @@
|
|
|
|
|
|
|
|
|
|
|
|
|
|
|
|
|
|
|
|
|
|
|
|
|
|
|
|
|
|
|
|
|
|
|
|
|
|
|
|
|
|
|
|
|
1 |
+
MIT License
|
2 |
+
|
3 |
+
Copyright (c) 2022 OpenAI
|
4 |
+
|
5 |
+
Permission is hereby granted, free of charge, to any person obtaining a copy
|
6 |
+
of this software and associated documentation files (the "Software"), to deal
|
7 |
+
in the Software without restriction, including without limitation the rights
|
8 |
+
to use, copy, modify, merge, publish, distribute, sublicense, and/or sell
|
9 |
+
copies of the Software, and to permit persons to whom the Software is
|
10 |
+
furnished to do so, subject to the following conditions:
|
11 |
+
|
12 |
+
The above copyright notice and this permission notice shall be included in all
|
13 |
+
copies or substantial portions of the Software.
|
14 |
+
|
15 |
+
THE SOFTWARE IS PROVIDED "AS IS", WITHOUT WARRANTY OF ANY KIND, EXPRESS OR
|
16 |
+
IMPLIED, INCLUDING BUT NOT LIMITED TO THE WARRANTIES OF MERCHANTABILITY,
|
17 |
+
FITNESS FOR A PARTICULAR PURPOSE AND NONINFRINGEMENT. IN NO EVENT SHALL THE
|
18 |
+
AUTHORS OR COPYRIGHT HOLDERS BE LIABLE FOR ANY CLAIM, DAMAGES OR OTHER
|
19 |
+
LIABILITY, WHETHER IN AN ACTION OF CONTRACT, TORT OR OTHERWISE, ARISING FROM,
|
20 |
+
OUT OF OR IN CONNECTION WITH THE SOFTWARE OR THE USE OR OTHER DEALINGS IN THE
|
21 |
+
SOFTWARE.
|
MANIFEST.in
ADDED
@@ -0,0 +1,5 @@
|
|
|
|
|
|
|
|
|
|
|
|
|
1 |
+
include requirements.txt
|
2 |
+
include README.md
|
3 |
+
include LICENSE
|
4 |
+
include whisper/assets/*
|
5 |
+
include whisper/normalizers/english.json
|
README.md
CHANGED
@@ -1,3 +1,147 @@
|
|
1 |
-
|
2 |
-
|
3 |
-
|
|
|
|
|
|
|
|
|
|
|
|
|
|
|
|
|
|
|
|
|
|
|
|
|
|
|
|
|
|
|
|
|
|
|
|
|
|
|
|
|
|
|
|
|
|
|
|
|
|
|
|
|
|
|
|
|
|
|
|
|
|
|
|
|
|
|
|
|
|
|
|
|
|
|
|
|
|
|
|
|
|
|
|
|
|
|
|
|
|
|
|
|
|
|
|
|
|
|
|
|
|
|
|
|
|
|
|
|
|
|
|
|
|
|
|
|
|
|
|
|
|
|
|
|
|
|
|
|
|
|
|
|
|
|
|
|
|
|
|
|
|
|
|
|
|
|
|
|
|
|
|
|
|
|
|
|
|
|
|
|
|
|
|
|
|
|
|
|
|
|
|
|
|
|
|
|
|
|
|
|
|
|
|
|
|
|
|
|
|
|
|
|
|
|
|
|
|
|
|
|
|
|
|
|
|
|
|
|
|
|
|
|
|
|
|
|
|
|
|
|
|
|
|
|
|
|
|
|
|
|
|
|
|
|
|
|
|
|
|
|
|
|
|
|
|
|
|
|
|
|
|
|
|
|
|
|
|
|
|
|
|
|
|
|
|
|
|
|
|
|
|
|
|
|
|
|
|
|
|
|
|
|
|
|
|
|
|
|
|
1 |
+
# Whisper
|
2 |
+
|
3 |
+
[[Blog]](https://openai.com/blog/whisper)
|
4 |
+
[[Paper]](https://arxiv.org/abs/2212.04356)
|
5 |
+
[[Model card]](https://github.com/openai/whisper/blob/main/model-card.md)
|
6 |
+
[[Colab example]](https://colab.research.google.com/github/openai/whisper/blob/master/notebooks/LibriSpeech.ipynb)
|
7 |
+
|
8 |
+
Whisper is a general-purpose speech recognition model. It is trained on a large dataset of diverse audio and is also a multitasking model that can perform multilingual speech recognition, speech translation, and language identification.
|
9 |
+
|
10 |
+
|
11 |
+
## Approach
|
12 |
+
|
13 |
+
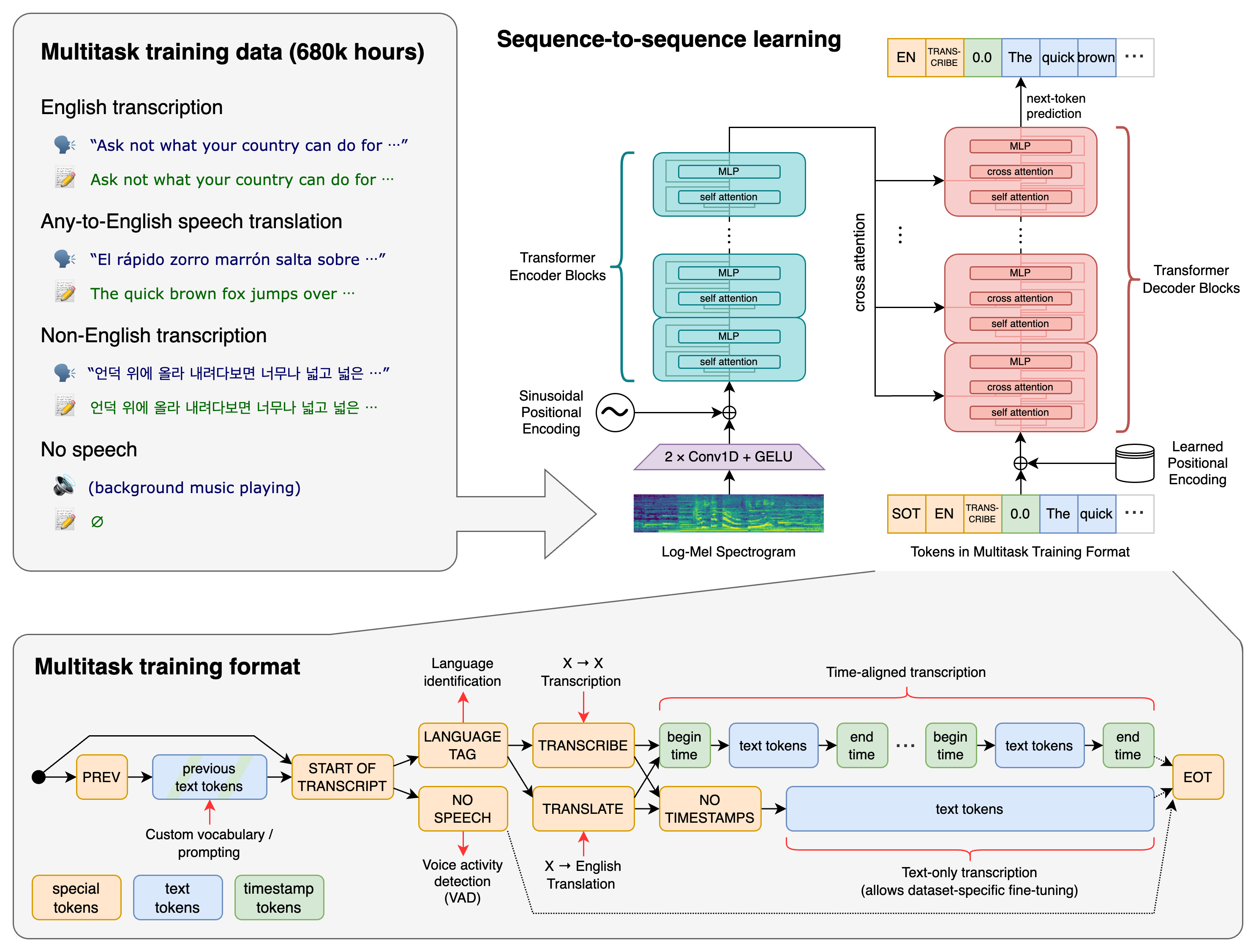
|
14 |
+
|
15 |
+
A Transformer sequence-to-sequence model is trained on various speech processing tasks, including multilingual speech recognition, speech translation, spoken language identification, and voice activity detection. These tasks are jointly represented as a sequence of tokens to be predicted by the decoder, allowing a single model to replace many stages of a traditional speech-processing pipeline. The multitask training format uses a set of special tokens that serve as task specifiers or classification targets.
|
16 |
+
|
17 |
+
|
18 |
+
## Setup
|
19 |
+
|
20 |
+
We used Python 3.9.9 and [PyTorch](https://pytorch.org/) 1.10.1 to train and test our models, but the codebase is expected to be compatible with Python 3.8-3.11 and recent PyTorch versions. The codebase also depends on a few Python packages, most notably [OpenAI's tiktoken](https://github.com/openai/tiktoken) for their fast tokenizer implementation. You can download and install (or update to) the latest release of Whisper with the following command:
|
21 |
+
|
22 |
+
pip install -U openai-whisper
|
23 |
+
|
24 |
+
Alternatively, the following command will pull and install the latest commit from this repository, along with its Python dependencies:
|
25 |
+
|
26 |
+
pip install git+https://github.com/openai/whisper.git
|
27 |
+
|
28 |
+
To update the package to the latest version of this repository, please run:
|
29 |
+
|
30 |
+
pip install --upgrade --no-deps --force-reinstall git+https://github.com/openai/whisper.git
|
31 |
+
|
32 |
+
It also requires the command-line tool [`ffmpeg`](https://ffmpeg.org/) to be installed on your system, which is available from most package managers:
|
33 |
+
|
34 |
+
```bash
|
35 |
+
# on Ubuntu or Debian
|
36 |
+
sudo apt update && sudo apt install ffmpeg
|
37 |
+
|
38 |
+
# on Arch Linux
|
39 |
+
sudo pacman -S ffmpeg
|
40 |
+
|
41 |
+
# on MacOS using Homebrew (https://brew.sh/)
|
42 |
+
brew install ffmpeg
|
43 |
+
|
44 |
+
# on Windows using Chocolatey (https://chocolatey.org/)
|
45 |
+
choco install ffmpeg
|
46 |
+
|
47 |
+
# on Windows using Scoop (https://scoop.sh/)
|
48 |
+
scoop install ffmpeg
|
49 |
+
```
|
50 |
+
|
51 |
+
You may need [`rust`](http://rust-lang.org) installed as well, in case [tiktoken](https://github.com/openai/tiktoken) does not provide a pre-built wheel for your platform. If you see installation errors during the `pip install` command above, please follow the [Getting started page](https://www.rust-lang.org/learn/get-started) to install Rust development environment. Additionally, you may need to configure the `PATH` environment variable, e.g. `export PATH="$HOME/.cargo/bin:$PATH"`. If the installation fails with `No module named 'setuptools_rust'`, you need to install `setuptools_rust`, e.g. by running:
|
52 |
+
|
53 |
+
```bash
|
54 |
+
pip install setuptools-rust
|
55 |
+
```
|
56 |
+
|
57 |
+
|
58 |
+
## Available models and languages
|
59 |
+
|
60 |
+
There are five model sizes, four with English-only versions, offering speed and accuracy tradeoffs. Below are the names of the available models and their approximate memory requirements and relative speed.
|
61 |
+
|
62 |
+
|
63 |
+
| Size | Parameters | English-only model | Multilingual model | Required VRAM | Relative speed |
|
64 |
+
|:------:|:----------:|:------------------:|:------------------:|:-------------:|:--------------:|
|
65 |
+
| tiny | 39 M | `tiny.en` | `tiny` | ~1 GB | ~32x |
|
66 |
+
| base | 74 M | `base.en` | `base` | ~1 GB | ~16x |
|
67 |
+
| small | 244 M | `small.en` | `small` | ~2 GB | ~6x |
|
68 |
+
| medium | 769 M | `medium.en` | `medium` | ~5 GB | ~2x |
|
69 |
+
| large | 1550 M | N/A | `large` | ~10 GB | 1x |
|
70 |
+
|
71 |
+
The `.en` models for English-only applications tend to perform better, especially for the `tiny.en` and `base.en` models. We observed that the difference becomes less significant for the `small.en` and `medium.en` models.
|
72 |
+
|
73 |
+
Whisper's performance varies widely depending on the language. The figure below shows a WER (Word Error Rate) breakdown by languages of the Fleurs dataset using the `large-v2` model (The smaller the numbers, the better the performance). Additional WER scores corresponding to the other models and datasets can be found in Appendix D.1, D.2, and D.4. Meanwhile, more BLEU (Bilingual Evaluation Understudy) scores can be found in Appendix D.3. Both are found in [the paper](https://arxiv.org/abs/2212.04356).
|
74 |
+
|
75 |
+

|
76 |
+
|
77 |
+
|
78 |
+
|
79 |
+
## Command-line usage
|
80 |
+
|
81 |
+
The following command will transcribe speech in audio files, using the `medium` model:
|
82 |
+
|
83 |
+
whisper audio.flac audio.mp3 audio.wav --model medium
|
84 |
+
|
85 |
+
The default setting (which selects the `small` model) works well for transcribing English. To transcribe an audio file containing non-English speech, you can specify the language using the `--language` option:
|
86 |
+
|
87 |
+
whisper japanese.wav --language Japanese
|
88 |
+
|
89 |
+
Adding `--task translate` will translate the speech into English:
|
90 |
+
|
91 |
+
whisper japanese.wav --language Japanese --task translate
|
92 |
+
|
93 |
+
Run the following to view all available options:
|
94 |
+
|
95 |
+
whisper --help
|
96 |
+
|
97 |
+
See [tokenizer.py](https://github.com/openai/whisper/blob/main/whisper/tokenizer.py) for the list of all available languages.
|
98 |
+
|
99 |
+
|
100 |
+
## Python usage
|
101 |
+
|
102 |
+
Transcription can also be performed within Python:
|
103 |
+
|
104 |
+
```python
|
105 |
+
import whisper
|
106 |
+
|
107 |
+
model = whisper.load_model("base")
|
108 |
+
result = model.transcribe("audio.mp3")
|
109 |
+
print(result["text"])
|
110 |
+
```
|
111 |
+
|
112 |
+
Internally, the `transcribe()` method reads the entire file and processes the audio with a sliding 30-second window, performing autoregressive sequence-to-sequence predictions on each window.
|
113 |
+
|
114 |
+
Below is an example usage of `whisper.detect_language()` and `whisper.decode()` which provide lower-level access to the model.
|
115 |
+
|
116 |
+
```python
|
117 |
+
import whisper
|
118 |
+
|
119 |
+
model = whisper.load_model("base")
|
120 |
+
|
121 |
+
# load audio and pad/trim it to fit 30 seconds
|
122 |
+
audio = whisper.load_audio("audio.mp3")
|
123 |
+
audio = whisper.pad_or_trim(audio)
|
124 |
+
|
125 |
+
# make log-Mel spectrogram and move to the same device as the model
|
126 |
+
mel = whisper.log_mel_spectrogram(audio).to(model.device)
|
127 |
+
|
128 |
+
# detect the spoken language
|
129 |
+
_, probs = model.detect_language(mel)
|
130 |
+
print(f"Detected language: {max(probs, key=probs.get)}")
|
131 |
+
|
132 |
+
# decode the audio
|
133 |
+
options = whisper.DecodingOptions()
|
134 |
+
result = whisper.decode(model, mel, options)
|
135 |
+
|
136 |
+
# print the recognized text
|
137 |
+
print(result.text)
|
138 |
+
```
|
139 |
+
|
140 |
+
## More examples
|
141 |
+
|
142 |
+
Please use the [🙌 Show and tell](https://github.com/openai/whisper/discussions/categories/show-and-tell) category in Discussions for sharing more example usages of Whisper and third-party extensions such as web demos, integrations with other tools, ports for different platforms, etc.
|
143 |
+
|
144 |
+
|
145 |
+
## License
|
146 |
+
|
147 |
+
Whisper's code and model weights are released under the MIT License. See [LICENSE](https://github.com/openai/whisper/blob/main/LICENSE) for further details.
|
approach.png
ADDED
![]() |
language-breakdown.svg
ADDED
|
model-card.md
ADDED
@@ -0,0 +1,69 @@
|
|
|
|
|
|
|
|
|
|
|
|
|
|
|
|
|
|
|
|
|
|
|
|
|
|
|
|
|
|
|
|
|
|
|
|
|
|
|
|
|
|
|
|
|
|
|
|
|
|
|
|
|
|
|
|
|
|
|
|
|
|
|
|
|
|
|
|
|
|
|
|
|
|
|
|
|
|
|
|
|
|
|
|
|
|
|
|
|
|
|
|
|
|
|
|
|
|
|
|
|
|
|
|
|
|
|
|
|
|
|
|
|
|
|
|
|
|
|
|
|
|
|
|
|
|
|
|
|
|
|
|
|
|
|
|
|
|
|
|
|
1 |
+
# Model Card: Whisper
|
2 |
+
|
3 |
+
This is the official codebase for running the automatic speech recognition (ASR) models (Whisper models) trained and released by OpenAI.
|
4 |
+
|
5 |
+
Following [Model Cards for Model Reporting (Mitchell et al.)](https://arxiv.org/abs/1810.03993), we're providing some information about the automatic speech recognition model. More information on how these models were trained and evaluated can be found [in the paper](https://arxiv.org/abs/2212.04356).
|
6 |
+
|
7 |
+
|
8 |
+
## Model Details
|
9 |
+
|
10 |
+
The Whisper models are trained for speech recognition and translation tasks, capable of transcribing speech audio into the text in the language it is spoken (ASR) as well as translated into English (speech translation). Researchers at OpenAI developed the models to study the robustness of speech processing systems trained under large-scale weak supervision. There are 9 models of different sizes and capabilities, summarized in the following table.
|
11 |
+
|
12 |
+
| Size | Parameters | English-only model | Multilingual model |
|
13 |
+
|:------:|:----------:|:------------------:|:------------------:|
|
14 |
+
| tiny | 39 M | ✓ | ✓ |
|
15 |
+
| base | 74 M | ✓ | ✓ |
|
16 |
+
| small | 244 M | ✓ | ✓ |
|
17 |
+
| medium | 769 M | ✓ | ✓ |
|
18 |
+
| large | 1550 M | | ✓ |
|
19 |
+
|
20 |
+
In December 2022, we [released an improved large model named `large-v2`](https://github.com/openai/whisper/discussions/661).
|
21 |
+
|
22 |
+
|
23 |
+
### Release date
|
24 |
+
|
25 |
+
September 2022 (original series) and December 2022 (`large-v2`)
|
26 |
+
|
27 |
+
### Model type
|
28 |
+
|
29 |
+
Sequence-to-sequence ASR (automatic speech recognition) and speech translation model
|
30 |
+
|
31 |
+
### Paper & samples
|
32 |
+
|
33 |
+
[Paper](https://arxiv.org/abs/2212.04356) / [Blog](https://openai.com/blog/whisper)
|
34 |
+
|
35 |
+
|
36 |
+
## Model Use
|
37 |
+
|
38 |
+
### Evaluated Use
|
39 |
+
|
40 |
+
The primary intended users of these models are AI researchers studying the robustness, generalization, capabilities, biases, and constraints of the current model. However, Whisper is also potentially quite useful as an ASR solution for developers, especially for English speech recognition. We recognize that once models are released, it is impossible to restrict access to only “intended” uses or to draw reasonable guidelines around what is or is not research.
|
41 |
+
|
42 |
+
The models are primarily trained and evaluated on ASR and speech translation to English tasks. They show strong ASR results in ~10 languages. They may exhibit additional capabilities, particularly if fine-tuned on certain tasks like voice activity detection, speaker classification, or speaker diarization but have not been robustly evaluated in these areas. We strongly recommend that users perform robust evaluations of the models in a particular context and domain before deploying them.
|
43 |
+
|
44 |
+
In particular, we caution against using Whisper models to transcribe recordings of individuals taken without their consent or purporting to use these models for any kind of subjective classification. We recommend against use in high-risk domains like decision-making contexts, where flaws in accuracy can lead to pronounced flaws in outcomes. The models are intended to transcribe and translate speech, use of the model for classification is not only not evaluated but also not appropriate, particularly to infer human attributes.
|
45 |
+
|
46 |
+
|
47 |
+
## Training Data
|
48 |
+
|
49 |
+
The models are trained on 680,000 hours of audio and the corresponding transcripts collected from the internet. 65% of this data (or 438,000 hours) represents English-language audio and matched English transcripts, roughly 18% (or 126,000 hours) represents non-English audio and English transcripts, while the final 17% (or 117,000 hours) represents non-English audio and the corresponding transcript. This non-English data represents 98 different languages.
|
50 |
+
|
51 |
+
As discussed in [the accompanying paper](https://arxiv.org/abs/2212.04356), we see that performance on transcription in a given language is directly correlated with the amount of training data we employ in that language.
|
52 |
+
|
53 |
+
|
54 |
+
## Performance and Limitations
|
55 |
+
|
56 |
+
Our studies show that, over many existing ASR systems, the models exhibit improved robustness to accents, background noise, and technical language, as well as zero-shot translation from multiple languages into English; and that accuracy on speech recognition and translation is near the state-of-the-art level.
|
57 |
+
|
58 |
+
However, because the models are trained in a weakly supervised manner using large-scale noisy data, the predictions may include texts that are not actually spoken in the audio input (i.e. hallucination). We hypothesize that this happens because, given their general knowledge of language, the models combine trying to predict the next word in audio with trying to transcribe the audio itself.
|
59 |
+
|
60 |
+
Our models perform unevenly across languages, and we observe lower accuracy on low-resource and/or low-discoverability languages or languages where we have less training data. The models also exhibit disparate performance on different accents and dialects of particular languages, which may include a higher word error rate across speakers of different genders, races, ages, or other demographic criteria. Our full evaluation results are presented in [the paper accompanying this release](https://arxiv.org/abs/2212.04356).
|
61 |
+
|
62 |
+
In addition, the sequence-to-sequence architecture of the model makes it prone to generating repetitive texts, which can be mitigated to some degree by beam search and temperature scheduling but not perfectly. Further analysis of these limitations is provided in [the paper](https://arxiv.org/abs/2212.04356). It is likely that this behavior and hallucinations may be worse in lower-resource and/or lower-discoverability languages.
|
63 |
+
|
64 |
+
|
65 |
+
## Broader Implications
|
66 |
+
|
67 |
+
We anticipate that Whisper models’ transcription capabilities may be used for improving accessibility tools. While Whisper models cannot be used for real-time transcription out of the box – their speed and size suggest that others may be able to build applications on top of them that allow for near-real-time speech recognition and translation. The real value of beneficial applications built on top of Whisper models suggests that the disparate performance of these models may have real economic implications.
|
68 |
+
|
69 |
+
There are also potential dual-use concerns that come with releasing Whisper. While we hope the technology will be used primarily for beneficial purposes, making ASR technology more accessible could enable more actors to build capable surveillance technologies or scale up existing surveillance efforts, as the speed and accuracy allow for affordable automatic transcription and translation of large volumes of audio communication. Moreover, these models may have some capabilities to recognize specific individuals out of the box, which in turn presents safety concerns related both to dual use and disparate performance. In practice, we expect that the cost of transcription is not the limiting factor of scaling up surveillance projects.
|
pyproject.toml
ADDED
@@ -0,0 +1,8 @@
|
|
|
|
|
|
|
|
|
|
|
|
|
|
|
|
|
|
|
1 |
+
[tool.black]
|
2 |
+
|
3 |
+
[tool.isort]
|
4 |
+
profile = "black"
|
5 |
+
include_trailing_comma = true
|
6 |
+
line_length = 88
|
7 |
+
multi_line_output = 3
|
8 |
+
|
requirements.txt
ADDED
@@ -0,0 +1,6 @@
|
|
|
|
|
|
|
|
|
|
|
|
|
|
|
1 |
+
numba
|
2 |
+
numpy
|
3 |
+
torch
|
4 |
+
tqdm
|
5 |
+
more-itertools
|
6 |
+
tiktoken==0.3.3
|
setup.py
ADDED
@@ -0,0 +1,43 @@
|
|
|
|
|
|
|
|
|
|
|
|
|
|
|
|
|
|
|
|
|
|
|
|
|
|
|
|
|
|
|
|
|
|
|
|
|
|
|
|
|
|
|
|
|
|
|
|
|
|
|
|
|
|
|
|
|
|
|
|
|
|
|
|
|
|
|
|
|
|
|
|
|
|
|
|
|
|
|
|
|
|
|
|
|
|
|
|
|
1 |
+
import os
|
2 |
+
import platform
|
3 |
+
import sys
|
4 |
+
|
5 |
+
import pkg_resources
|
6 |
+
from setuptools import find_packages, setup
|
7 |
+
|
8 |
+
|
9 |
+
def read_version(fname="whisper/version.py"):
|
10 |
+
exec(compile(open(fname, encoding="utf-8").read(), fname, "exec"))
|
11 |
+
return locals()["__version__"]
|
12 |
+
|
13 |
+
|
14 |
+
requirements = []
|
15 |
+
if sys.platform.startswith("linux") and platform.machine() == "x86_64":
|
16 |
+
requirements.append("triton==2.0.0")
|
17 |
+
|
18 |
+
setup(
|
19 |
+
name="openai-whisper",
|
20 |
+
py_modules=["whisper"],
|
21 |
+
version=read_version(),
|
22 |
+
description="Robust Speech Recognition via Large-Scale Weak Supervision",
|
23 |
+
long_description=open("README.md", encoding="utf-8").read(),
|
24 |
+
long_description_content_type="text/markdown",
|
25 |
+
readme="README.md",
|
26 |
+
python_requires=">=3.8",
|
27 |
+
author="OpenAI",
|
28 |
+
url="https://github.com/openai/whisper",
|
29 |
+
license="MIT",
|
30 |
+
packages=find_packages(exclude=["tests*"]),
|
31 |
+
install_requires=requirements
|
32 |
+
+ [
|
33 |
+
str(r)
|
34 |
+
for r in pkg_resources.parse_requirements(
|
35 |
+
open(os.path.join(os.path.dirname(__file__), "requirements.txt"))
|
36 |
+
)
|
37 |
+
],
|
38 |
+
entry_points={
|
39 |
+
"console_scripts": ["whisper=whisper.transcribe:cli"],
|
40 |
+
},
|
41 |
+
include_package_data=True,
|
42 |
+
extras_require={"dev": ["pytest", "scipy", "black", "flake8", "isort"]},
|
43 |
+
)
|