File size: 9,242 Bytes
ef3dd32 4c7ab2f 2466802 4c7ab2f ef3dd32 86c77fb 3705fa7 86c77fb cbb73b8 aefb768 86c77fb 82dd418 86c77fb 6506e5f 86c77fb 6506e5f 86c77fb 6506e5f 86c77fb 6506e5f 86c77fb 6506e5f 86c77fb 6506e5f 86c77fb 6506e5f 86c77fb 6506e5f |
1 2 3 4 5 6 7 8 9 10 11 12 13 14 15 16 17 18 19 20 21 22 23 24 25 26 27 28 29 30 31 32 33 34 35 36 37 38 39 40 41 42 43 44 45 46 47 48 49 50 51 52 53 54 55 56 57 58 59 60 61 62 63 64 65 66 67 68 69 70 71 72 73 74 75 76 77 78 79 80 81 82 83 84 85 86 87 88 89 90 91 92 93 94 95 96 97 98 99 100 101 102 103 104 105 106 107 108 109 110 111 112 113 114 115 116 117 118 119 120 121 122 123 124 125 126 127 128 129 130 131 132 133 134 135 136 137 138 139 140 141 142 143 144 145 146 147 148 149 150 151 152 153 154 155 156 157 158 159 160 161 162 163 164 165 166 167 168 169 170 171 172 173 174 175 176 177 178 179 180 181 182 183 184 185 186 187 188 189 190 191 192 193 194 195 196 197 198 199 200 201 202 203 204 205 206 207 208 209 210 211 212 213 214 215 216 217 218 219 220 221 222 223 224 225 226 227 228 229 230 231 232 233 234 235 236 237 238 239 240 241 242 243 244 245 246 247 248 249 250 251 252 253 254 255 256 257 258 259 260 261 262 263 264 265 266 267 268 269 270 271 272 273 274 275 276 |
---
language: zh
license: creativeml-openrail-m
tags:
- stable-diffusion
- stable-diffusion-diffusers
- text-to-image
- zh
- Chinese
inference: false
extra_gated_prompt: |-
One more step before getting this model.
This model is open access and available to all, with a CreativeML OpenRAIL-M license further specifying rights and usage.
The CreativeML OpenRAIL License specifies:
1. You can't use the model to deliberately produce nor share illegal or harmful outputs or content
2. BAAI claims no rights on the outputs you generate, you are free to use them and are accountable for their use which must not go against the provisions set in the license
3. You may re-distribute the weights and use the model commercially and/or as a service. If you do, please be aware you have to include the same use restrictions as the ones in the license and share a copy of the CreativeML OpenRAIL-M to all your users (please read the license entirely and carefully)
Please read the full license here: https://huggingface.co/spaces/CompVis/stable-diffusion-license
By clicking on "Access repository" below, you accept that your *contact information* (email address and username) can be shared with the model authors as well.
extra_gated_fields:
I have read the License and agree with its terms: checkbox
---
# AltCLIP
| 名称 Name | 任务 Task | 语言 Language(s) | 模型 Model | Github |
|:------------------:|:----------:|:-------------------:|:--------:|:------:|
| AltCLIP | text-image representation| 中英文 Chinese&English | CLIP | [FlagAI](https://github.com/FlagAI-Open/FlagAI) |
## 简介 Brief Introduction
我们提出了一个简单高效的方法去训练更加优秀的双语CLIP模型。命名为AltCLIP。AltCLIP基于 [Stable Diffusiosn](https://github.com/CompVis/stable-diffusion) 训练,训练数据来自 [WuDao数据集](https://data.baai.ac.cn/details/WuDaoCorporaText) 和 [LIAON](https://huggingface.co/datasets/ChristophSchuhmann/improved_aesthetics_6plus)
AltCLIP模型可以为本项目中的AltDiffusion模型提供支持,关于AltDiffusion模型的具体信息可查看[此教程](https://github.com/FlagAI-Open/FlagAI/tree/master/examples/AltDiffusion/README.md) 。
模型代码已经在 [FlagAI](https://github.com/FlagAI-Open/FlagAI/tree/master/examples/AltCLIP) 上开源,权重位于我们搭建的 [modelhub](https://model.baai.ac.cn/model-detail/100075) 上。我们还提供了微调,推理,验证的脚本,欢迎试用。
We propose a simple and efficient method to train a better bilingual CLIP model. Named AltCLIP. AltCLIP is trained based on [Stable Diffusiosn](https://github.com/CompVis/stable-diffusion) with training data from [WuDao dataset](https://data.baai.ac.cn/details/WuDaoCorporaText) and [Liaon](https://huggingface.co/datasets/laion/laion2B-en).
The AltCLIP model can provide support for the AltDiffusion model in this project. Specific information on the AltDiffusion model can be found in [this tutorial](https://github.com/FlagAI-Open/FlagAI/tree/master/examples/AltDiffusion/README.md).
The model code has been open sourced on [FlagAI](https://github.com/FlagAI-Open/FlagAI/tree/master/examples/AltCLIP) and the weights are located on [modelhub](https://model.baai.ac.cn/model-detail/100075). We also provide scripts for fine-tuning, inference, and validation, so feel free to try them out.
## 引用
关于AltCLIP,我们已经推出了相关报告,有更多细节可以查阅,如对您的工作有帮助,欢迎引用。
If you find this work helpful, please consider to cite
```
@article{https://doi.org/10.48550/arxiv.2211.06679,
doi = {10.48550/ARXIV.2211.06679},
url = {https://arxiv.org/abs/2211.06679},
author = {Chen, Zhongzhi and Liu, Guang and Zhang, Bo-Wen and Ye, Fulong and Yang, Qinghong and Wu, Ledell},
keywords = {Computation and Language (cs.CL), FOS: Computer and information sciences},
title = {AltCLIP: Altering the Language Encoder in CLIP for Extended Language Capabilities},
publisher = {arXiv},
year = {2022},
copyright = {arXiv.org perpetual, non-exclusive license}
}
```
## 训练 Training
训练共有两个阶段。
在平行知识蒸馏阶段,我们只是使用平行语料文本来进行蒸馏(平行语料相对于图文对更容易获取且数量更大)。在双语对比学习阶段,我们使用少量的中-英 图像-文本对(一共约2百万)来训练我们的文本编码器以更好地适应图像编码器。
There are two phases of training.
In the parallel knowledge distillation phase, we only use parallel corpus texts for distillation (parallel corpus is easier to obtain and larger in number compared to image text pairs). In the bilingual comparison learning phase, we use a small number of Chinese-English image-text pairs (about 2 million in total) to train our text encoder to better fit the image encoder.
## 下游效果 Performance
<table>
<tr>
<td rowspan=2>Language</td>
<td rowspan=2>Method</td>
<td colspan=3>Text-to-Image Retrival</td>
<td colspan=3>Image-to-Text Retrival</td>
<td rowspan=2>MR</td>
</tr>
<tr>
<td>R@1</td>
<td>R@5</td>
<td>R@10</td>
<td>R@1</td>
<td>R@5</td>
<td>R@10</td>
</tr>
<tr>
<td rowspan=7>English</td>
<td>CLIP</td>
<td>65.0 </td>
<td>87.1 </td>
<td>92.2 </td>
<td>85.1 </td>
<td>97.3 </td>
<td>99.2 </td>
<td>87.6 </td>
</tr>
<tr>
<td>Taiyi</td>
<td>25.3 </td>
<td>48.2 </td>
<td>59.2 </td>
<td>39.3 </td>
<td>68.1 </td>
<td>79.6 </td>
<td>53.3 </td>
</tr>
<tr>
<td>Wukong</td>
<td>-</td>
<td>-</td>
<td>-</td>
<td>-</td>
<td>-</td>
<td>-</td>
<td>-</td>
</tr>
<tr>
<td>R2D2</td>
<td>-</td>
<td>-</td>
<td>-</td>
<td>-</td>
<td>-</td>
<td>-</td>
<td>-</td>
</tr>
<tr>
<td>CN-CLIP</td>
<td>49.5 </td>
<td>76.9 </td>
<td>83.8 </td>
<td>66.5 </td>
<td>91.2 </td>
<td>96.0 </td>
<td>77.3 </td>
</tr>
<tr>
<td>AltCLIP</td>
<td>66.3 </td>
<td>87.8 </td>
<td>92.7 </td>
<td>85.9 </td>
<td>97.7 </td>
<td>99.1 </td>
<td>88.3 </td>
</tr>
<tr>
<td>AltCLIP∗</td>
<td>72.5 </td>
<td>91.6 </td>
<td>95.4 </td>
<td>86.0 </td>
<td>98.0 </td>
<td>99.1 </td>
<td>90.4 </td>
</tr>
<tr>
<td rowspan=7>Chinese</td>
<td>CLIP</td>
<td>0.0 </td>
<td>2.4 </td>
<td>4.0 </td>
<td>2.3 </td>
<td>8.1 </td>
<td>12.6 </td>
<td>5.0 </td>
</tr>
<tr>
<td>Taiyi</td>
<td>53.7 </td>
<td>79.8 </td>
<td>86.6 </td>
<td>63.8 </td>
<td>90.5 </td>
<td>95.9 </td>
<td>78.4 </td>
</tr>
<tr>
<td>Wukong</td>
<td>51.7 </td>
<td>78.9 </td>
<td>86.3 </td>
<td>76.1 </td>
<td>94.8 </td>
<td>97.5 </td>
<td>80.9 </td>
</tr>
<tr>
<td>R2D2</td>
<td>60.9 </td>
<td>86.8 </td>
<td>92.7 </td>
<td>77.6 </td>
<td>96.7 </td>
<td>98.9 </td>
<td>85.6 </td>
</tr>
<tr>
<td>CN-CLIP</td>
<td>68.0 </td>
<td>89.7 </td>
<td>94.4 </td>
<td>80.2 </td>
<td>96.6 </td>
<td>98.2 </td>
<td>87.9 </td>
</tr>
<tr>
<td>AltCLIP</td>
<td>63.7 </td>
<td>86.3 </td>
<td>92.1 </td>
<td>84.7 </td>
<td>97.4 </td>
<td>98.7 </td>
<td>87.2 </td>
</tr>
<tr>
<td>AltCLIP∗</td>
<td>69.8 </td>
<td>89.9 </td>
<td>94.7 </td>
<td>84.8 </td>
<td>97.4 </td>
<td>98.8 </td>
<td>89.2 </td>
</tr>
</table>
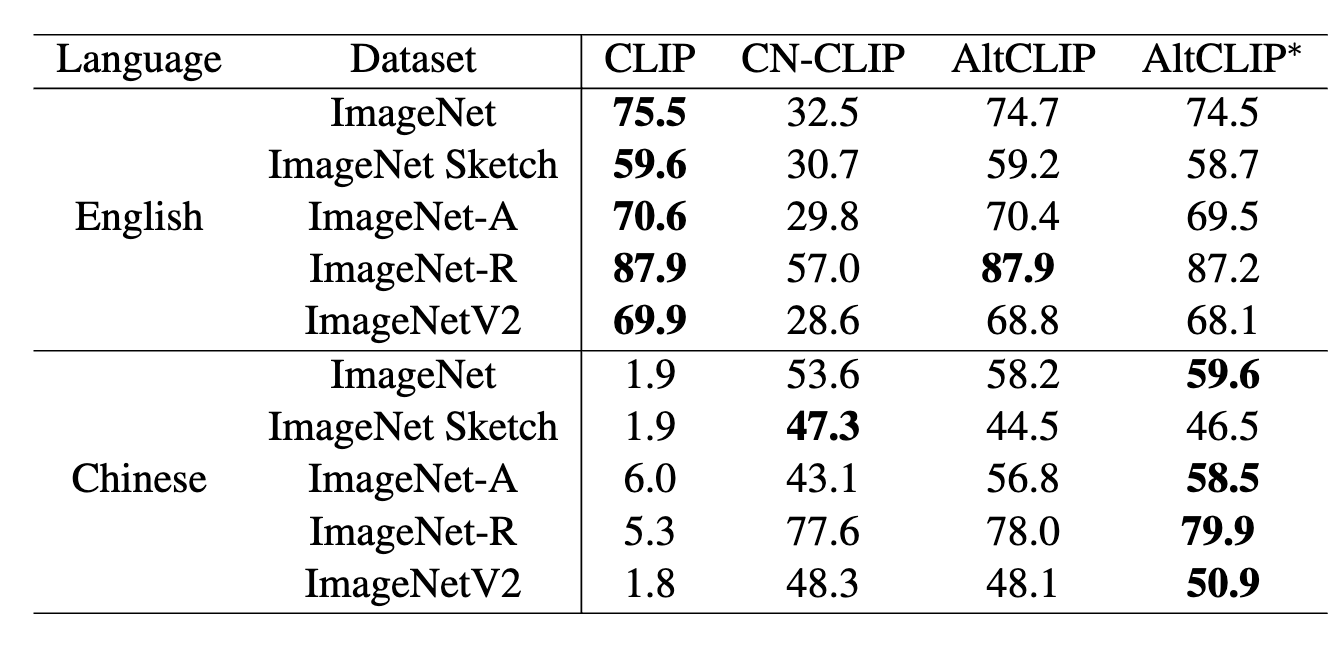
## 可视化效果 Visualization effects
基于AltCLIP,我们还开发了AltDiffusion模型,可视化效果如下。
Based on AltCLIP, we have also developed the AltDiffusion model, visualized as follows.

## 模型推理 Inference
Please download the code from [FlagAI AltCLIP](https://github.com/FlagAI-Open/FlagAI/tree/master/examples/AltCLIP)
```python
from PIL import Image
import requests
# transformers version >= 4.21.0
from modeling_altclip import AltCLIP
from processing_altclip import AltCLIPProcessor
# now our repo's in private, so we need `use_auth_token=True`
model = AltCLIP.from_pretrained("BAAI/AltCLIP")
processor = AltCLIPProcessor.from_pretrained("BAAI/AltCLIP")
url = "http://images.cocodataset.org/val2017/000000039769.jpg"
image = Image.open(requests.get(url, stream=True).raw)
inputs = processor(text=["a photo of a cat", "a photo of a dog"], images=image, return_tensors="pt", padding=True)
outputs = model(**inputs)
logits_per_image = outputs.logits_per_image # this is the image-text similarity score
probs = logits_per_image.softmax(dim=1) # we can take the softmax to get the label probabilities
```
|