File size: 5,259 Bytes
380240d 056ee6f 9b553ff 056ee6f 380240d 056ee6f 49dc99f 056ee6f |
1 2 3 4 5 6 7 8 9 10 11 12 13 14 15 16 17 18 19 20 21 22 23 24 25 26 27 28 29 30 31 32 33 34 35 36 37 38 39 40 41 42 43 44 45 46 47 48 49 50 51 52 53 54 55 56 57 58 59 60 61 62 63 64 65 66 67 68 69 70 71 72 73 74 75 76 77 78 79 80 81 82 83 84 |
---
license: apache-2.0
library_name: tfhub
language: en
tags:
- text
- sentence-similarity
- use
- universal-sentence-encoder
- dan
- tensorflow
---
## Model name: universal-sentence-encoder
## Description adapted from [TFHub](https://tfhub.dev/google/universal-sentence-encoder/4)
# Overview
The Universal Sentence Encoder encodes text into high-dimensional vectors that can be used for text classification, semantic similarity, clustering and other natural language tasks.
The model is trained and optimized for greater-than-word length text, such as sentences, phrases or short paragraphs. It is trained on a variety of data sources and a variety of tasks with the aim of dynamically accommodating a wide variety of natural language understanding tasks. The input is variable length English text and the output is a 512 dimensional vector. We apply this model to the [STS benchmark](https://ixa2.si.ehu.es/stswiki/index.php/STSbenchmark) for semantic similarity, and the results can be seen in the [example notebook](https://colab.research.google.com/github/tensorflow/hub/blob/master/examples/colab/semantic_similarity_with_tf_hub_universal_encoder.ipynb) made available. The universal-sentence-encoder model is trained with a deep averaging network (DAN) encoder.
To learn more about text embeddings, refer to the [TensorFlow Embeddings](https://www.tensorflow.org/tutorials/text/word_embeddings) documentation. Our encoder differs from word level embedding models in that we train on a number of natural language prediction tasks that require modeling the meaning of word sequences rather than just individual words. Details are available in the paper "Universal Sentence Encoder" [1].
## Universal Sentence Encoder family
There are several versions of universal sentence encoder models trained with different goals including size/performance multilingual, and fine-grained question answer retrieval.
- [Universal Sentence Encoder family](https://tfhub.dev/google/collections/universal-sentence-encoder/1)
### Example use
### Using TF Hub and HF Hub
```
model_path = snapshot_download(repo_id="Dimitre/universal-sentence-encoder")
model = KerasLayer(handle=model_path)
embeddings = model([
"The quick brown fox jumps over the lazy dog.",
"I am a sentence for which I would like to get its embedding"])
print(embeddings)
# The following are example embedding output of 512 dimensions per sentence
# Embedding for: The quick brown fox jumps over the lazy dog.
# [-0.03133016 -0.06338634 -0.01607501, ...]
# Embedding for: I am a sentence for which I would like to get its embedding.
# [0.05080863 -0.0165243 0.01573782, ...]
```
### Using [TF Hub fork](https://github.com/dimitreOliveira/hub)
```
model = pull_from_hub(repo_id="Dimitre/universal-sentence-encoder")
embeddings = model([
"The quick brown fox jumps over the lazy dog.",
"I am a sentence for which I would like to get its embedding"])
print(embeddings)
# The following are example embedding output of 512 dimensions per sentence
# Embedding for: The quick brown fox jumps over the lazy dog.
# [-0.03133016 -0.06338634 -0.01607501, ...]
# Embedding for: I am a sentence for which I would like to get its embedding.
# [0.05080863 -0.0165243 0.01573782, ...]
```
This module is about 1GB. Depending on your network speed, it might take a while to load the first time you run inference with it. After that, loading the model should be faster as modules are cached by default ([learn more about caching](https://www.tensorflow.org/hub/tf2_saved_model)). Further, once a module is loaded to memory, inference time should be relatively fast.
### Preprocessing
The module does not require preprocessing the data before applying the module, it performs best effort text input preprocessing inside the graph.
# Semantic Similarity
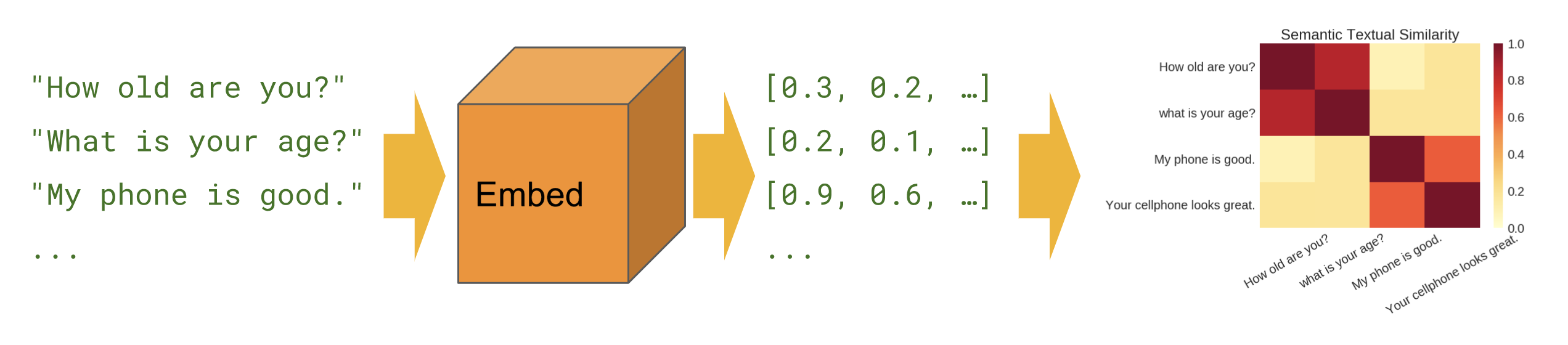
Semantic similarity is a measure of the degree to which two pieces of text carry the same meaning. This is broadly useful in obtaining good coverage over the numerous ways that a thought can be expressed using language without needing to manually enumerate them.
Simple applications include improving the coverage of systems that trigger behaviors on certain keywords, phrases or utterances. [This section of the notebook](https://colab.research.google.com/github/tensorflow/hub/blob/master/examples/colab/semantic_similarity_with_tf_hub_universal_encoder.ipynb#scrollTo=BnvjATdy64eR) shows how to encode text and compare encoding distances as a proxy for semantic similarity.
# Classification

[This notebook](https://colab.research.google.com/github/tensorflow/hub/blob/master/docs/tutorials/text_classification_with_tf_hub.ipynb) shows how to train a simple binary text classifier on top of any TF-Hub module that can embed sentences. The Universal Sentence Encoder was partially trained with custom text classification tasks in mind. These kinds of classifiers can be trained to perform a wide variety of classification tasks often with a very small amount of labeled examples. |