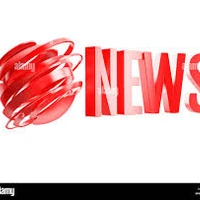
HermesPenn/LSTM-CNN-Res_CNN
Updated
•
7
None defined yet.
!pip install datasets > delete.txt
import pandas as pd
df_test = pd.read_csv('/content/test_data.csv',index_col="Unnamed: 0")
df_test.head()
from huggingface_hub import snapshot_download
import keras
# Download model from hugging face
local_path = snapshot_download(repo_id="HermesPenn/athena_model")
# Load model from local
model = keras.saving.load_model(local_path)
from datasets import load_dataset
dataset = load_dataset("HermesPenn/athena_data")
dataset = dataset['train']
data = dataset.to_pandas()
data.head()
from sklearn.preprocessing import LabelEncoder
from tensorflow.keras.preprocessing.sequence import pad_sequences
from tensorflow.keras.preprocessing.text import Tokenizer
# Data preprocessing
le = LabelEncoder()
data['label'] = le.fit_transform(data['source'])
X = data['title']
y = data['label']
# Tokenize and pad text data
tokenizer = Tokenizer(num_words=20000, oov_token="<OOV>")
tokenizer.fit_on_texts(X)
X_seq = tokenizer.texts_to_sequences(X)
X_padded = pad_sequences(X_seq, maxlen=200, padding='post', truncating='post')
from sklearn.metrics import classification_report
X_test = df_test['title']
y_test = df_test['label']
X_test_seq = tokenizer.texts_to_sequences(X_test)
X_test_padded = pad_sequences(X_test_seq, maxlen=200, padding='post', truncating='post')
# Predict the labels using the model
y_pred_probs = model.predict(X_test_padded)
y_pred = (y_pred_probs > 0.5).astype(int)
# Evaluate the model
print("Classification Report:")
print(classification_report(y_test, y_pred))
try:
news_outlets = le.inverse_transform(y_pred.flatten()) # le must be pre-fitted
df_test['Predicted News Outlet'] = news_outlets
except NameError:
df_test['Predicted News Outlet'] = y_pred.flatten()
# Display test set with predictions
print("\nTest Set with Predictions:")
df_test[['title', 'News Outlet', 'Predicted News Outlet']]