Upload README.md with huggingface_hub
Browse files
README.md
ADDED
@@ -0,0 +1,1425 @@
|
|
|
|
|
|
|
|
|
|
|
|
|
|
|
|
|
|
|
|
|
|
|
|
|
|
|
|
|
|
|
|
|
|
|
|
|
|
|
|
|
|
|
|
|
|
|
|
|
|
|
|
|
|
|
|
|
|
|
|
|
|
|
|
|
|
|
|
|
|
|
|
|
|
|
|
|
|
|
|
|
|
|
|
|
|
|
|
|
|
|
|
|
|
|
|
|
|
|
|
|
|
|
|
|
|
|
|
|
|
|
|
|
|
|
|
|
|
|
|
|
|
|
|
|
|
|
|
|
|
|
|
|
|
|
|
|
|
|
|
|
|
|
|
|
|
|
|
|
|
|
|
|
|
|
|
|
|
|
|
|
|
|
|
|
|
|
|
|
|
|
|
|
|
|
|
|
|
|
|
|
|
|
|
|
|
|
|
|
|
|
|
|
|
|
|
|
|
|
|
|
|
|
|
|
|
|
|
|
|
|
|
|
|
|
|
|
|
|
|
|
|
|
|
|
|
|
|
|
|
|
|
|
|
|
|
|
|
|
|
|
|
|
|
|
|
|
|
|
|
|
|
|
|
|
|
|
|
|
|
|
|
|
|
|
|
|
|
|
|
|
|
|
|
|
|
|
|
|
|
|
|
|
|
|
|
|
|
|
|
|
|
|
|
|
|
|
|
|
|
|
|
|
|
|
|
|
|
|
|
|
|
|
|
|
|
|
|
|
|
|
|
|
|
|
|
|
|
|
|
|
|
|
|
|
|
|
|
|
|
|
|
|
|
|
|
|
|
|
|
|
|
|
|
|
|
|
|
|
|
|
|
|
|
|
|
|
|
|
|
|
|
|
|
|
|
|
|
|
|
|
|
|
|
|
|
|
|
|
|
|
|
|
|
|
|
|
|
|
|
|
|
|
|
|
|
|
|
|
|
|
|
|
|
|
|
|
|
|
|
|
|
|
|
|
|
|
|
|
|
|
|
|
|
|
|
|
|
|
|
|
|
|
|
|
|
|
|
|
|
|
|
|
|
|
|
|
|
|
|
|
|
|
|
|
|
|
|
|
|
|
|
|
|
|
|
|
|
|
|
|
|
|
|
|
|
|
|
|
|
|
|
|
|
|
|
|
|
|
|
|
|
|
|
|
|
|
|
|
|
|
|
|
|
|
|
|
|
|
|
|
|
|
|
|
|
|
|
|
|
|
|
|
|
|
|
|
|
|
|
|
|
|
|
|
|
|
|
|
|
|
|
|
|
|
|
|
|
|
|
|
|
|
|
|
|
|
|
|
|
|
|
|
|
|
|
|
|
|
|
|
|
|
|
|
|
|
|
|
|
|
|
|
|
|
|
|
|
|
|
|
|
|
|
|
|
|
|
|
|
|
|
|
|
|
|
|
|
|
|
|
|
|
|
|
|
|
|
|
|
|
|
|
|
|
|
|
|
|
|
|
|
|
|
|
|
|
|
|
|
|
|
|
|
|
|
|
|
|
|
|
|
|
|
|
|
|
|
|
|
|
|
|
|
|
|
|
|
|
|
|
|
|
|
|
|
|
|
|
|
|
|
|
|
|
|
|
|
|
|
|
|
|
|
|
|
|
|
|
|
|
|
|
|
|
|
|
|
|
|
|
|
|
|
|
|
|
|
|
|
|
|
|
|
|
|
|
|
|
|
|
|
|
|
|
|
|
|
|
|
|
|
|
|
|
|
|
|
|
|
|
|
|
|
|
|
|
|
|
|
|
|
|
|
|
|
|
|
|
|
|
|
|
|
|
|
|
|
|
|
|
|
|
|
|
|
|
|
|
|
|
|
|
|
|
|
|
|
|
|
|
|
|
|
|
|
|
|
|
|
|
|
|
|
|
|
|
|
|
|
|
|
|
|
|
|
|
|
|
|
|
|
|
|
|
|
|
|
|
|
|
|
|
|
|
|
|
|
|
|
|
|
|
|
|
|
|
|
|
|
|
|
|
|
|
|
|
|
|
|
|
|
|
|
|
|
|
|
|
|
|
|
|
|
|
|
|
|
|
|
|
|
|
|
|
|
|
|
|
|
|
|
|
|
|
|
|
|
|
|
|
|
|
|
|
|
|
|
|
|
|
|
|
|
|
|
|
|
|
|
|
|
|
|
|
|
|
|
|
|
|
|
|
|
|
|
|
|
|
|
|
|
|
|
|
|
|
|
|
|
|
|
|
|
|
|
|
|
|
|
|
|
|
|
|
|
|
|
|
|
|
|
|
|
|
|
|
|
|
|
|
|
|
|
|
|
|
|
|
|
|
|
|
|
|
|
|
|
|
|
|
|
|
|
|
|
|
|
|
|
|
|
|
|
|
|
|
|
|
|
|
|
|
|
|
|
|
|
|
|
|
|
|
|
|
|
|
|
|
|
|
|
|
|
|
|
|
|
|
|
|
|
|
|
|
|
|
|
|
|
|
|
|
|
|
|
|
|
|
|
|
|
|
|
|
|
|
|
|
|
|
|
|
|
|
|
|
|
|
|
|
|
|
|
|
|
|
|
|
|
|
|
|
|
|
|
|
|
|
|
|
|
|
|
|
|
|
|
|
|
|
|
|
|
|
|
|
|
|
|
|
|
|
|
|
|
|
|
|
|
|
|
|
|
|
|
|
|
|
|
|
|
|
|
|
|
|
|
|
|
|
|
|
|
|
|
|
|
|
|
|
|
|
|
|
|
|
|
|
|
|
|
|
|
|
|
|
|
|
|
|
|
|
|
|
|
|
|
|
|
|
|
|
|
|
|
|
|
|
|
|
|
|
|
|
|
|
|
|
|
|
|
|
|
|
|
|
|
|
|
|
|
|
|
|
|
|
|
|
|
|
|
|
|
|
|
|
|
|
|
|
|
|
|
|
|
|
|
|
|
|
|
|
|
|
|
|
|
|
|
|
|
|
|
|
|
|
|
|
|
|
|
|
|
|
|
|
|
|
|
|
|
|
|
|
|
|
|
|
|
|
|
|
|
|
|
|
|
|
|
|
|
|
|
|
|
|
|
|
|
|
|
|
|
|
|
|
|
|
|
|
|
|
|
|
|
|
|
|
|
|
|
|
|
|
|
|
|
|
|
|
|
|
|
|
|
|
|
|
|
|
|
|
|
|
|
|
|
|
|
|
|
|
|
|
|
|
|
|
|
|
|
|
|
|
|
|
|
|
|
|
|
|
|
|
|
|
|
|
|
|
|
|
|
|
|
|
|
|
|
|
|
|
|
|
|
|
|
|
|
|
|
|
|
|
|
|
|
|
|
|
|
|
|
|
|
|
|
|
|
|
|
|
|
|
|
|
|
|
|
|
|
|
|
|
|
|
|
|
|
|
|
|
|
|
|
|
|
|
|
|
|
|
|
|
|
|
|
|
|
|
|
|
|
|
|
|
|
|
|
|
|
|
|
|
|
|
|
|
|
|
|
|
|
|
|
|
|
|
|
|
|
|
|
|
|
|
|
|
|
|
|
|
|
|
|
|
|
|
|
|
|
|
|
|
|
|
|
|
|
|
|
|
|
|
|
|
|
|
|
|
|
|
|
|
|
|
|
|
|
|
|
|
|
|
|
|
|
|
|
|
|
|
|
|
|
|
|
|
|
|
|
|
|
|
|
|
|
|
|
|
|
|
|
|
|
|
|
|
|
|
|
|
|
|
|
|
|
|
|
|
|
|
|
|
|
|
|
|
|
|
|
|
|
|
|
|
|
|
|
|
|
|
|
|
|
|
|
|
|
|
|
|
|
|
|
|
|
|
|
|
|
|
|
|
|
|
|
|
|
|
|
|
|
|
|
|
|
|
|
|
|
|
|
|
|
|
|
|
|
|
|
|
|
|
|
|
|
|
|
|
|
|
|
|
|
|
|
|
|
|
|
|
|
|
|
|
|
|
|
|
|
|
|
|
|
|
|
|
|
|
|
|
|
|
|
|
|
|
|
|
|
|
|
|
|
|
|
|
|
|
|
|
|
|
|
|
|
|
|
|
|
|
|
|
|
|
|
|
|
|
|
|
|
|
|
|
|
|
|
|
|
|
|
|
|
|
|
|
|
|
|
|
|
|
|
|
|
|
|
|
|
|
|
|
|
|
|
|
|
|
|
|
|
|
|
|
|
|
|
|
|
|
|
|
|
|
|
|
|
|
|
|
|
|
|
|
|
|
|
|
|
|
|
|
|
|
|
|
|
|
|
|
|
|
|
|
|
|
|
|
|
|
|
|
|
|
|
|
|
|
|
|
|
|
|
|
|
|
|
|
|
|
|
|
|
|
|
|
|
|
|
|
|
|
|
|
|
|
|
|
|
|
|
|
|
|
|
|
|
|
|
|
|
|
|
|
|
|
|
|
|
|
|
|
|
|
|
|
|
|
|
|
|
|
|
|
|
|
|
|
|
|
|
|
|
|
|
|
|
|
|
|
|
|
|
|
|
|
|
|
|
|
|
|
|
|
|
|
|
|
|
|
|
|
|
|
|
|
|
|
|
|
|
|
|
|
|
|
|
|
|
|
|
|
|
|
|
|
|
|
|
|
|
|
|
|
|
|
|
|
|
|
|
|
|
|
|
|
|
|
|
|
|
|
|
|
|
|
|
|
|
|
|
|
|
|
|
|
|
|
|
|
|
|
|
|
|
|
|
|
|
|
|
|
|
|
|
|
|
|
|
|
|
|
|
|
|
|
|
|
|
|
|
|
|
|
|
|
|
|
|
|
|
|
|
|
|
|
|
|
|
|
|
|
|
|
|
|
|
|
|
|
|
|
|
|
|
|
|
|
|
|
|
|
|
|
|
|
|
|
|
|
|
|
|
|
|
|
|
|
|
|
|
|
|
|
|
|
|
|
|
|
|
|
|
|
|
|
|
|
|
|
|
|
|
|
|
|
|
|
|
|
|
|
|
|
|
|
|
|
|
|
|
|
|
|
|
|
|
|
|
|
|
|
|
|
|
|
|
|
|
|
|
|
|
|
|
|
|
|
|
|
|
|
|
|
|
|
|
|
|
|
|
|
|
|
|
|
|
|
|
|
|
|
|
|
|
|
|
|
|
|
|
|
|
|
|
|
|
|
|
|
|
|
|
|
|
|
|
|
|
|
|
|
|
|
|
|
|
|
|
|
|
|
|
|
|
|
|
|
|
|
|
|
|
|
|
|
|
|
|
|
|
|
|
|
|
|
|
|
|
|
|
|
|
|
|
|
|
|
|
|
|
|
|
|
|
|
|
|
|
|
|
|
|
|
|
|
|
|
|
|
|
|
|
|
|
|
|
|
|
|
|
|
|
|
|
|
|
|
|
|
|
|
|
|
|
|
|
|
|
|
|
|
|
|
|
|
|
|
|
|
|
|
|
|
|
|
|
|
|
|
|
|
|
|
|
|
|
|
|
|
|
|
|
|
|
|
|
|
|
|
|
|
|
|
|
|
|
|
|
|
|
|
|
|
|
|
|
|
|
|
|
|
|
|
|
|
|
|
|
|
|
|
|
|
|
|
|
|
|
|
|
|
|
|
|
|
|
|
|
|
|
|
|
|
|
|
|
|
|
|
|
|
|
|
|
|
|
|
|
|
|
|
|
|
|
|
|
|
|
|
|
|
|
|
|
|
|
|
|
|
|
|
|
|
|
|
|
|
|
|
|
|
|
|
|
|
|
|
|
|
|
|
|
|
|
|
|
|
|
|
|
|
|
|
|
|
|
|
|
|
|
|
|
|
|
|
|
|
|
|
|
|
|
|
|
|
|
|
|
|
|
|
|
|
|
|
|
|
|
|
|
|
|
|
|
|
|
|
|
|
|
|
|
|
|
|
|
|
|
|
|
|
|
|
|
|
|
|
|
|
|
|
|
|
|
|
|
|
|
|
|
|
|
|
|
|
|
|
|
|
|
|
|
|
|
|
|
|
|
|
|
|
|
|
|
|
|
|
|
|
|
|
|
|
|
|
|
|
|
|
|
|
|
|
|
|
|
|
|
|
|
|
|
|
|
|
|
|
|
|
|
|
|
|
|
|
|
|
|
|
|
|
|
|
|
|
|
|
|
|
|
|
|
|
|
|
|
|
|
|
|
|
|
|
|
|
|
|
|
|
|
|
|
|
|
|
|
|
|
|
|
|
|
|
|
|
|
|
|
|
|
|
|
|
|
|
|
|
|
|
|
|
|
|
|
|
|
|
|
|
|
|
|
|
|
|
|
|
|
|
|
|
|
|
|
|
|
|
|
|
|
|
|
|
|
|
|
|
|
|
|
|
|
|
|
|
|
|
|
|
|
|
|
|
|
|
|
|
|
|
|
|
|
|
|
|
|
|
|
|
|
|
|
|
|
|
|
|
|
|
|
|
|
|
|
|
|
|
|
|
|
|
|
|
|
|
|
|
|
|
|
|
|
|
|
|
|
|
|
|
|
|
|
|
|
|
|
|
|
|
1 |
+
|
2 |
+
---
|
3 |
+
|
4 |
+
license: llama3.2
|
5 |
+
language:
|
6 |
+
- zh
|
7 |
+
- en
|
8 |
+
- it
|
9 |
+
- de
|
10 |
+
- fr
|
11 |
+
- ja
|
12 |
+
- ko
|
13 |
+
base_model:
|
14 |
+
- meta-llama/Llama-3.2-3B
|
15 |
+
- lianghsun/Llama-3.2-Taiwan-3B
|
16 |
+
datasets:
|
17 |
+
- lianghsun/tw-emergency-medicine-bench
|
18 |
+
- lianghsun/tw-legal-nlp
|
19 |
+
- lianghsun/tw-legal-synthetic-qa
|
20 |
+
- lianghsun/tw-law-article-qa
|
21 |
+
- lianghsun/tw-judgment-qa
|
22 |
+
- lianghsun/tw-judgment-gist-chat
|
23 |
+
- lianghsun/tw-bar-examination-2020-chat
|
24 |
+
- lianghsun/tw-structured-law-article
|
25 |
+
- lianghsun/tw-judgment-gist-chat
|
26 |
+
- lianghsun/tw-contract-review-chat
|
27 |
+
- lianghsun/reasoning-base-20k-chat
|
28 |
+
- lianghsun/vulnerability-mitigation-qa-zh_tw
|
29 |
+
- lianghsun/tw-instruct
|
30 |
+
- rombodawg/Everything_Instruct_Multilingual
|
31 |
+
- xzuyn/manythings-translations-alpaca
|
32 |
+
- neural-bridge/rag-dataset-12000
|
33 |
+
- minyichen/glaive_toolcall_zh_tw
|
34 |
+
pipeline_tag: text-generation
|
35 |
+
library_name: transformers
|
36 |
+
tags:
|
37 |
+
- Taiwan
|
38 |
+
- ROC
|
39 |
+
- zh-tw
|
40 |
+
- instruct
|
41 |
+
- chat
|
42 |
+
- llama3.2
|
43 |
+
- SLM
|
44 |
+
model-index:
|
45 |
+
- name: Llama-3.2-Taiwan-3B-Instruct
|
46 |
+
results:
|
47 |
+
- task:
|
48 |
+
type: text-generation
|
49 |
+
name: Single Choice Question
|
50 |
+
dataset:
|
51 |
+
type: lianghsun/tw-legal-benchmark-v1
|
52 |
+
name: tw-legal-benchmark-v1
|
53 |
+
metrics:
|
54 |
+
- name: single choice
|
55 |
+
type: accuracy
|
56 |
+
value: 31.1
|
57 |
+
- task:
|
58 |
+
type: text-generation
|
59 |
+
name: Single Choice Question
|
60 |
+
dataset:
|
61 |
+
type: lianghsun/Formosa-bench
|
62 |
+
name: (Society) Formosa Taiwan Knowledge Bench
|
63 |
+
config: society
|
64 |
+
split: test
|
65 |
+
revision: v2024.11.27
|
66 |
+
metrics:
|
67 |
+
- name: single choice
|
68 |
+
type: accuracy
|
69 |
+
value: 60.42
|
70 |
+
- task:
|
71 |
+
type: text-generation
|
72 |
+
name: Single Choice Question
|
73 |
+
dataset:
|
74 |
+
type: lianghsun/Formosa-bench
|
75 |
+
name: (Governmnt) Formosa Taiwan Knowledge Bench
|
76 |
+
config: governmnt
|
77 |
+
split: test
|
78 |
+
revision: v2024.11.27
|
79 |
+
metrics:
|
80 |
+
- name: single choice
|
81 |
+
type: accuracy
|
82 |
+
value: 44.25
|
83 |
+
- task:
|
84 |
+
type: text-generation
|
85 |
+
name: Single Choice Question
|
86 |
+
dataset:
|
87 |
+
type: lianghsun/Formosa-bench
|
88 |
+
name: (Geography) Formosa Taiwan Knowledge Bench
|
89 |
+
config: geography
|
90 |
+
split: test
|
91 |
+
revision: v2024.11.27
|
92 |
+
metrics:
|
93 |
+
- name: single choice
|
94 |
+
type: accuracy
|
95 |
+
value: 47.54
|
96 |
+
- task:
|
97 |
+
type: text-generation
|
98 |
+
name: Single Choice Question
|
99 |
+
dataset:
|
100 |
+
type: lianghsun/Formosa-bench
|
101 |
+
name: (History) Formosa Taiwan Knowledge Bench
|
102 |
+
config: history
|
103 |
+
split: test
|
104 |
+
revision: v2024.11.27
|
105 |
+
metrics:
|
106 |
+
- name: single choice
|
107 |
+
type: accuracy
|
108 |
+
value: 60
|
109 |
+
- task:
|
110 |
+
type: question-answering
|
111 |
+
name: Single Choice Question
|
112 |
+
dataset:
|
113 |
+
type: ikala/tmmluplus
|
114 |
+
name: (geography_of_taiwan) tmmlu++
|
115 |
+
config: geography_of_taiwan
|
116 |
+
split: test
|
117 |
+
revision: c0e8ae955997300d5dbf0e382bf0ba5115f85e8c
|
118 |
+
metrics:
|
119 |
+
- name: single choice
|
120 |
+
type: accuracy
|
121 |
+
value: 36.2
|
122 |
+
- task:
|
123 |
+
type: question-answering
|
124 |
+
name: Single Choice Question
|
125 |
+
dataset:
|
126 |
+
type: ikala/tmmluplus
|
127 |
+
name: (dentistry) tmmlu++
|
128 |
+
config: dentistry
|
129 |
+
split: test
|
130 |
+
revision: c0e8ae955997300d5dbf0e382bf0ba5115f85e8c
|
131 |
+
metrics:
|
132 |
+
- name: single choice
|
133 |
+
type: accuracy
|
134 |
+
value: 33.83
|
135 |
+
- task:
|
136 |
+
type: question-answering
|
137 |
+
name: Single Choice Question
|
138 |
+
dataset:
|
139 |
+
type: ikala/tmmluplus
|
140 |
+
name: (technical) tmmlu++
|
141 |
+
config: technical
|
142 |
+
split: test
|
143 |
+
revision: c0e8ae955997300d5dbf0e382bf0ba5115f85e8c
|
144 |
+
metrics:
|
145 |
+
- name: single choice
|
146 |
+
type: accuracy
|
147 |
+
value: 35.07
|
148 |
+
- task:
|
149 |
+
type: question-answering
|
150 |
+
name: Single Choice Question
|
151 |
+
dataset:
|
152 |
+
type: ikala/tmmluplus
|
153 |
+
name: (statistics_and_machine_learning) tmmlu++
|
154 |
+
config: statistics_and_machine_learning
|
155 |
+
split: test
|
156 |
+
revision: c0e8ae955997300d5dbf0e382bf0ba5115f85e8c
|
157 |
+
metrics:
|
158 |
+
- name: single choice
|
159 |
+
type: accuracy
|
160 |
+
value: 28.57
|
161 |
+
- task:
|
162 |
+
type: question-answering
|
163 |
+
name: Single Choice Question
|
164 |
+
dataset:
|
165 |
+
type: ikala/tmmluplus
|
166 |
+
name: (clinical_psychology) tmmlu++
|
167 |
+
config: clinical_psychology
|
168 |
+
split: test
|
169 |
+
revision: c0e8ae955997300d5dbf0e382bf0ba5115f85e8c
|
170 |
+
metrics:
|
171 |
+
- name: single choice
|
172 |
+
type: accuracy
|
173 |
+
value: 29.6
|
174 |
+
- task:
|
175 |
+
type: question-answering
|
176 |
+
name: Single Choice Question
|
177 |
+
dataset:
|
178 |
+
type: ikala/tmmluplus
|
179 |
+
name: (tve_design) tmmlu++
|
180 |
+
config: tve_design
|
181 |
+
split: test
|
182 |
+
revision: c0e8ae955997300d5dbf0e382bf0ba5115f85e8c
|
183 |
+
metrics:
|
184 |
+
- name: single choice
|
185 |
+
type: accuracy
|
186 |
+
value: 38.54
|
187 |
+
- task:
|
188 |
+
type: question-answering
|
189 |
+
name: Single Choice Question
|
190 |
+
dataset:
|
191 |
+
type: ikala/tmmluplus
|
192 |
+
name: (three_principles_of_people) tmmlu++
|
193 |
+
config: three_principles_of_people
|
194 |
+
split: test
|
195 |
+
revision: c0e8ae955997300d5dbf0e382bf0ba5115f85e8c
|
196 |
+
metrics:
|
197 |
+
- name: single choice
|
198 |
+
type: accuracy
|
199 |
+
value: 48.2
|
200 |
+
- task:
|
201 |
+
type: question-answering
|
202 |
+
name: Single Choice Question
|
203 |
+
dataset:
|
204 |
+
type: ikala/tmmluplus
|
205 |
+
name: (introduction_to_law) tmmlu++
|
206 |
+
config: introduction_to_law
|
207 |
+
split: test
|
208 |
+
revision: c0e8ae955997300d5dbf0e382bf0ba5115f85e8c
|
209 |
+
metrics:
|
210 |
+
- name: single choice
|
211 |
+
type: accuracy
|
212 |
+
value: 29.96
|
213 |
+
- task:
|
214 |
+
type: question-answering
|
215 |
+
name: Single Choice Question
|
216 |
+
dataset:
|
217 |
+
type: ikala/tmmluplus
|
218 |
+
name: (linear_algebra) tmmlu++
|
219 |
+
config: linear_algebra
|
220 |
+
split: test
|
221 |
+
revision: c0e8ae955997300d5dbf0e382bf0ba5115f85e8c
|
222 |
+
metrics:
|
223 |
+
- name: single choice
|
224 |
+
type: accuracy
|
225 |
+
value: 21.43
|
226 |
+
- task:
|
227 |
+
type: question-answering
|
228 |
+
name: Single Choice Question
|
229 |
+
dataset:
|
230 |
+
type: ikala/tmmluplus
|
231 |
+
name: (agriculture) tmmlu++
|
232 |
+
config: agriculture
|
233 |
+
split: test
|
234 |
+
revision: c0e8ae955997300d5dbf0e382bf0ba5115f85e8c
|
235 |
+
metrics:
|
236 |
+
- name: single choice
|
237 |
+
type: accuracy
|
238 |
+
value: 24.5
|
239 |
+
- task:
|
240 |
+
type: question-answering
|
241 |
+
name: Single Choice Question
|
242 |
+
dataset:
|
243 |
+
type: ikala/tmmluplus
|
244 |
+
name: (jce_humanities) tmmlu++
|
245 |
+
config: jce_humanities
|
246 |
+
split: test
|
247 |
+
revision: c0e8ae955997300d5dbf0e382bf0ba5115f85e8c
|
248 |
+
metrics:
|
249 |
+
- name: single choice
|
250 |
+
type: accuracy
|
251 |
+
value: 38.89
|
252 |
+
- task:
|
253 |
+
type: question-answering
|
254 |
+
name: Single Choice Question
|
255 |
+
dataset:
|
256 |
+
type: ikala/tmmluplus
|
257 |
+
name: (music) tmmlu++
|
258 |
+
config: music
|
259 |
+
split: test
|
260 |
+
revision: c0e8ae955997300d5dbf0e382bf0ba5115f85e8c
|
261 |
+
metrics:
|
262 |
+
- name: single choice
|
263 |
+
type: accuracy
|
264 |
+
value: 25.9
|
265 |
+
- task:
|
266 |
+
type: question-answering
|
267 |
+
name: Single Choice Question
|
268 |
+
dataset:
|
269 |
+
type: ikala/tmmluplus
|
270 |
+
name: (secondary_physics) tmmlu++
|
271 |
+
config: secondary_physics
|
272 |
+
split: test
|
273 |
+
revision: c0e8ae955997300d5dbf0e382bf0ba5115f85e8c
|
274 |
+
metrics:
|
275 |
+
- name: single choice
|
276 |
+
type: accuracy
|
277 |
+
value: 33.04
|
278 |
+
- task:
|
279 |
+
type: question-answering
|
280 |
+
name: Single Choice Question
|
281 |
+
dataset:
|
282 |
+
type: ikala/tmmluplus
|
283 |
+
name: (physics) tmmlu++
|
284 |
+
config: physics
|
285 |
+
split: test
|
286 |
+
revision: c0e8ae955997300d5dbf0e382bf0ba5115f85e8c
|
287 |
+
metrics:
|
288 |
+
- name: single choice
|
289 |
+
type: accuracy
|
290 |
+
value: 27.84
|
291 |
+
- task:
|
292 |
+
type: question-answering
|
293 |
+
name: Single Choice Question
|
294 |
+
dataset:
|
295 |
+
type: ikala/tmmluplus
|
296 |
+
name: (advance_chemistry) tmmlu++
|
297 |
+
config: advance_chemistry
|
298 |
+
split: test
|
299 |
+
revision: c0e8ae955997300d5dbf0e382bf0ba5115f85e8c
|
300 |
+
metrics:
|
301 |
+
- name: single choice
|
302 |
+
type: accuracy
|
303 |
+
value: 27.64
|
304 |
+
- task:
|
305 |
+
type: question-answering
|
306 |
+
name: Single Choice Question
|
307 |
+
dataset:
|
308 |
+
type: ikala/tmmluplus
|
309 |
+
name: (junior_science_exam) tmmlu++
|
310 |
+
config: junior_science_exam
|
311 |
+
split: test
|
312 |
+
revision: c0e8ae955997300d5dbf0e382bf0ba5115f85e8c
|
313 |
+
metrics:
|
314 |
+
- name: single choice
|
315 |
+
type: accuracy
|
316 |
+
value: 30.05
|
317 |
+
- task:
|
318 |
+
type: question-answering
|
319 |
+
name: Single Choice Question
|
320 |
+
dataset:
|
321 |
+
type: ikala/tmmluplus
|
322 |
+
name: (veterinary_pathology) tmmlu++
|
323 |
+
config: veterinary_pathology
|
324 |
+
split: test
|
325 |
+
revision: c0e8ae955997300d5dbf0e382bf0ba5115f85e8c
|
326 |
+
metrics:
|
327 |
+
- name: single choice
|
328 |
+
type: accuracy
|
329 |
+
value: 25.09
|
330 |
+
- task:
|
331 |
+
type: question-answering
|
332 |
+
name: Single Choice Question
|
333 |
+
dataset:
|
334 |
+
type: ikala/tmmluplus
|
335 |
+
name: (financial_analysis) tmmlu++
|
336 |
+
config: financial_analysis
|
337 |
+
split: test
|
338 |
+
revision: c0e8ae955997300d5dbf0e382bf0ba5115f85e8c
|
339 |
+
metrics:
|
340 |
+
- name: single choice
|
341 |
+
type: accuracy
|
342 |
+
value: 25.13
|
343 |
+
- task:
|
344 |
+
type: question-answering
|
345 |
+
name: Single Choice Question
|
346 |
+
dataset:
|
347 |
+
type: ikala/tmmluplus
|
348 |
+
name: (national_protection) tmmlu++
|
349 |
+
config: national_protection
|
350 |
+
split: test
|
351 |
+
revision: c0e8ae955997300d5dbf0e382bf0ba5115f85e8c
|
352 |
+
metrics:
|
353 |
+
- name: single choice
|
354 |
+
type: accuracy
|
355 |
+
value: 42.65
|
356 |
+
- task:
|
357 |
+
type: question-answering
|
358 |
+
name: Single Choice Question
|
359 |
+
dataset:
|
360 |
+
type: ikala/tmmluplus
|
361 |
+
name: (macroeconomics) tmmlu++
|
362 |
+
config: macroeconomics
|
363 |
+
split: test
|
364 |
+
revision: c0e8ae955997300d5dbf0e382bf0ba5115f85e8c
|
365 |
+
metrics:
|
366 |
+
- name: single choice
|
367 |
+
type: accuracy
|
368 |
+
value: 26.76
|
369 |
+
- task:
|
370 |
+
type: question-answering
|
371 |
+
name: Single Choice Question
|
372 |
+
dataset:
|
373 |
+
type: ikala/tmmluplus
|
374 |
+
name: (politic_science) tmmlu++
|
375 |
+
config: politic_science
|
376 |
+
split: test
|
377 |
+
revision: c0e8ae955997300d5dbf0e382bf0ba5115f85e8c
|
378 |
+
metrics:
|
379 |
+
- name: single choice
|
380 |
+
type: accuracy
|
381 |
+
value: 27.44
|
382 |
+
- task:
|
383 |
+
type: question-answering
|
384 |
+
name: Single Choice Question
|
385 |
+
dataset:
|
386 |
+
type: ikala/tmmluplus
|
387 |
+
name: (ttqav2) tmmlu++
|
388 |
+
config: ttqav2
|
389 |
+
split: test
|
390 |
+
revision: c0e8ae955997300d5dbf0e382bf0ba5115f85e8c
|
391 |
+
metrics:
|
392 |
+
- name: single choice
|
393 |
+
type: accuracy
|
394 |
+
value: 61.06
|
395 |
+
- task:
|
396 |
+
type: question-answering
|
397 |
+
name: Single Choice Question
|
398 |
+
dataset:
|
399 |
+
type: ikala/tmmluplus
|
400 |
+
name: (junior_chinese_exam) tmmlu++
|
401 |
+
config: junior_chinese_exam
|
402 |
+
split: test
|
403 |
+
revision: c0e8ae955997300d5dbf0e382bf0ba5115f85e8c
|
404 |
+
metrics:
|
405 |
+
- name: single choice
|
406 |
+
type: accuracy
|
407 |
+
value: 30.86
|
408 |
+
- task:
|
409 |
+
type: question-answering
|
410 |
+
name: Single Choice Question
|
411 |
+
dataset:
|
412 |
+
type: ikala/tmmluplus
|
413 |
+
name: (traditional_chinese_medicine_clinical_medicine) tmmlu++
|
414 |
+
config: traditional_chinese_medicine_clinical_medicine
|
415 |
+
split: test
|
416 |
+
revision: c0e8ae955997300d5dbf0e382bf0ba5115f85e8c
|
417 |
+
metrics:
|
418 |
+
- name: single choice
|
419 |
+
type: accuracy
|
420 |
+
value: 25.9
|
421 |
+
- task:
|
422 |
+
type: question-answering
|
423 |
+
name: Single Choice Question
|
424 |
+
dataset:
|
425 |
+
type: ikala/tmmluplus
|
426 |
+
name: (junior_math_exam) tmmlu++
|
427 |
+
config: junior_math_exam
|
428 |
+
split: test
|
429 |
+
revision: c0e8ae955997300d5dbf0e382bf0ba5115f85e8c
|
430 |
+
metrics:
|
431 |
+
- name: single choice
|
432 |
+
type: accuracy
|
433 |
+
value: 21.71
|
434 |
+
- task:
|
435 |
+
type: question-answering
|
436 |
+
name: Single Choice Question
|
437 |
+
dataset:
|
438 |
+
type: ikala/tmmluplus
|
439 |
+
name: (auditing) tmmlu++
|
440 |
+
config: auditing
|
441 |
+
split: test
|
442 |
+
revision: c0e8ae955997300d5dbf0e382bf0ba5115f85e8c
|
443 |
+
metrics:
|
444 |
+
- name: single choice
|
445 |
+
type: accuracy
|
446 |
+
value: 21.82
|
447 |
+
- task:
|
448 |
+
type: question-answering
|
449 |
+
name: Single Choice Question
|
450 |
+
dataset:
|
451 |
+
type: ikala/tmmluplus
|
452 |
+
name: (anti_money_laundering) tmmlu++
|
453 |
+
config: anti_money_laundering
|
454 |
+
split: test
|
455 |
+
revision: c0e8ae955997300d5dbf0e382bf0ba5115f85e8c
|
456 |
+
metrics:
|
457 |
+
- name: single choice
|
458 |
+
type: accuracy
|
459 |
+
value: 37.31
|
460 |
+
- task:
|
461 |
+
type: question-answering
|
462 |
+
name: Single Choice Question
|
463 |
+
dataset:
|
464 |
+
type: ikala/tmmluplus
|
465 |
+
name: (pharmacology) tmmlu++
|
466 |
+
config: pharmacology
|
467 |
+
split: test
|
468 |
+
revision: c0e8ae955997300d5dbf0e382bf0ba5115f85e8c
|
469 |
+
metrics:
|
470 |
+
- name: single choice
|
471 |
+
type: accuracy
|
472 |
+
value: 30.68
|
473 |
+
- task:
|
474 |
+
type: question-answering
|
475 |
+
name: Single Choice Question
|
476 |
+
dataset:
|
477 |
+
type: ikala/tmmluplus
|
478 |
+
name: (trust_practice) tmmlu++
|
479 |
+
config: trust_practice
|
480 |
+
split: test
|
481 |
+
revision: c0e8ae955997300d5dbf0e382bf0ba5115f85e8c
|
482 |
+
metrics:
|
483 |
+
- name: single choice
|
484 |
+
type: accuracy
|
485 |
+
value: 28.18
|
486 |
+
- task:
|
487 |
+
type: question-answering
|
488 |
+
name: Single Choice Question
|
489 |
+
dataset:
|
490 |
+
type: ikala/tmmluplus
|
491 |
+
name: (tve_mathematics) tmmlu++
|
492 |
+
config: tve_mathematics
|
493 |
+
split: test
|
494 |
+
revision: c0e8ae955997300d5dbf0e382bf0ba5115f85e8c
|
495 |
+
metrics:
|
496 |
+
- name: single choice
|
497 |
+
type: accuracy
|
498 |
+
value: 18.67
|
499 |
+
- task:
|
500 |
+
type: question-answering
|
501 |
+
name: Single Choice Question
|
502 |
+
dataset:
|
503 |
+
type: ikala/tmmluplus
|
504 |
+
name: (human_behavior) tmmlu++
|
505 |
+
config: human_behavior
|
506 |
+
split: test
|
507 |
+
revision: c0e8ae955997300d5dbf0e382bf0ba5115f85e8c
|
508 |
+
metrics:
|
509 |
+
- name: single choice
|
510 |
+
type: accuracy
|
511 |
+
value: 32.04
|
512 |
+
- task:
|
513 |
+
type: question-answering
|
514 |
+
name: Single Choice Question
|
515 |
+
dataset:
|
516 |
+
type: ikala/tmmluplus
|
517 |
+
name: (pharmacy) tmmlu++
|
518 |
+
config: pharmacy
|
519 |
+
split: test
|
520 |
+
revision: c0e8ae955997300d5dbf0e382bf0ba5115f85e8c
|
521 |
+
metrics:
|
522 |
+
- name: single choice
|
523 |
+
type: accuracy
|
524 |
+
value: 22.76
|
525 |
+
- task:
|
526 |
+
type: question-answering
|
527 |
+
name: Single Choice Question
|
528 |
+
dataset:
|
529 |
+
type: ikala/tmmluplus
|
530 |
+
name: (tve_chinese_language) tmmlu++
|
531 |
+
config: tve_chinese_language
|
532 |
+
split: test
|
533 |
+
revision: c0e8ae955997300d5dbf0e382bf0ba5115f85e8c
|
534 |
+
metrics:
|
535 |
+
- name: single choice
|
536 |
+
type: accuracy
|
537 |
+
value: 36.65
|
538 |
+
- task:
|
539 |
+
type: question-answering
|
540 |
+
name: Single Choice Question
|
541 |
+
dataset:
|
542 |
+
type: ikala/tmmluplus
|
543 |
+
name: (optometry) tmmlu++
|
544 |
+
config: optometry
|
545 |
+
split: test
|
546 |
+
revision: c0e8ae955997300d5dbf0e382bf0ba5115f85e8c
|
547 |
+
metrics:
|
548 |
+
- name: single choice
|
549 |
+
type: accuracy
|
550 |
+
value: 25.11
|
551 |
+
- task:
|
552 |
+
type: question-answering
|
553 |
+
name: Single Choice Question
|
554 |
+
dataset:
|
555 |
+
type: ikala/tmmluplus
|
556 |
+
name: (physical_education) tmmlu++
|
557 |
+
config: physical_education
|
558 |
+
split: test
|
559 |
+
revision: c0e8ae955997300d5dbf0e382bf0ba5115f85e8c
|
560 |
+
metrics:
|
561 |
+
- name: single choice
|
562 |
+
type: accuracy
|
563 |
+
value: 30.73
|
564 |
+
- task:
|
565 |
+
type: question-answering
|
566 |
+
name: Single Choice Question
|
567 |
+
dataset:
|
568 |
+
type: ikala/tmmluplus
|
569 |
+
name: (organic_chemistry) tmmlu++
|
570 |
+
config: organic_chemistry
|
571 |
+
split: test
|
572 |
+
revision: c0e8ae955997300d5dbf0e382bf0ba5115f85e8c
|
573 |
+
metrics:
|
574 |
+
- name: single choice
|
575 |
+
type: accuracy
|
576 |
+
value: 35.78
|
577 |
+
- task:
|
578 |
+
type: question-answering
|
579 |
+
name: Single Choice Question
|
580 |
+
dataset:
|
581 |
+
type: ikala/tmmluplus
|
582 |
+
name: (tve_natural_sciences) tmmlu++
|
583 |
+
config: tve_natural_sciences
|
584 |
+
split: test
|
585 |
+
revision: c0e8ae955997300d5dbf0e382bf0ba5115f85e8c
|
586 |
+
metrics:
|
587 |
+
- name: single choice
|
588 |
+
type: accuracy
|
589 |
+
value: 33.73
|
590 |
+
- task:
|
591 |
+
type: question-answering
|
592 |
+
name: Single Choice Question
|
593 |
+
dataset:
|
594 |
+
type: ikala/tmmluplus
|
595 |
+
name: (education) tmmlu++
|
596 |
+
config: education
|
597 |
+
split: test
|
598 |
+
revision: c0e8ae955997300d5dbf0e382bf0ba5115f85e8c
|
599 |
+
metrics:
|
600 |
+
- name: single choice
|
601 |
+
type: accuracy
|
602 |
+
value: 37.9
|
603 |
+
- task:
|
604 |
+
type: question-answering
|
605 |
+
name: Single Choice Question
|
606 |
+
dataset:
|
607 |
+
type: ikala/tmmluplus
|
608 |
+
name: (mechanical) tmmlu++
|
609 |
+
config: mechanical
|
610 |
+
split: test
|
611 |
+
revision: c0e8ae955997300d5dbf0e382bf0ba5115f85e8c
|
612 |
+
metrics:
|
613 |
+
- name: single choice
|
614 |
+
type: accuracy
|
615 |
+
value: 42.37
|
616 |
+
- task:
|
617 |
+
type: question-answering
|
618 |
+
name: Single Choice Question
|
619 |
+
dataset:
|
620 |
+
type: ikala/tmmluplus
|
621 |
+
name: (taiwanese_hokkien) tmmlu++
|
622 |
+
config: taiwanese_hokkien
|
623 |
+
split: test
|
624 |
+
revision: c0e8ae955997300d5dbf0e382bf0ba5115f85e8c
|
625 |
+
metrics:
|
626 |
+
- name: single choice
|
627 |
+
type: accuracy
|
628 |
+
value: 14.73
|
629 |
+
- task:
|
630 |
+
type: question-answering
|
631 |
+
name: Single Choice Question
|
632 |
+
dataset:
|
633 |
+
type: ikala/tmmluplus
|
634 |
+
name: (nautical_science) tmmlu++
|
635 |
+
config: nautical_science
|
636 |
+
split: test
|
637 |
+
revision: c0e8ae955997300d5dbf0e382bf0ba5115f85e8c
|
638 |
+
metrics:
|
639 |
+
- name: single choice
|
640 |
+
type: accuracy
|
641 |
+
value: 30.49
|
642 |
+
- task:
|
643 |
+
type: question-answering
|
644 |
+
name: Single Choice Question
|
645 |
+
dataset:
|
646 |
+
type: ikala/tmmluplus
|
647 |
+
name: (business_management) tmmlu++
|
648 |
+
config: business_management
|
649 |
+
split: test
|
650 |
+
revision: c0e8ae955997300d5dbf0e382bf0ba5115f85e8c
|
651 |
+
metrics:
|
652 |
+
- name: single choice
|
653 |
+
type: accuracy
|
654 |
+
value: 39.57
|
655 |
+
- task:
|
656 |
+
type: question-answering
|
657 |
+
name: Single Choice Question
|
658 |
+
dataset:
|
659 |
+
type: ikala/tmmluplus
|
660 |
+
name: (logic_reasoning) tmmlu++
|
661 |
+
config: logic_reasoning
|
662 |
+
split: test
|
663 |
+
revision: c0e8ae955997300d5dbf0e382bf0ba5115f85e8c
|
664 |
+
metrics:
|
665 |
+
- name: single choice
|
666 |
+
type: accuracy
|
667 |
+
value: 27.34
|
668 |
+
- task:
|
669 |
+
type: question-answering
|
670 |
+
name: Single Choice Question
|
671 |
+
dataset:
|
672 |
+
type: ikala/tmmluplus
|
673 |
+
name: (marketing_management) tmmlu++
|
674 |
+
config: marketing_management
|
675 |
+
split: test
|
676 |
+
revision: c0e8ae955997300d5dbf0e382bf0ba5115f85e8c
|
677 |
+
metrics:
|
678 |
+
- name: single choice
|
679 |
+
type: accuracy
|
680 |
+
value: 39.78
|
681 |
+
- task:
|
682 |
+
type: question-answering
|
683 |
+
name: Single Choice Question
|
684 |
+
dataset:
|
685 |
+
type: ikala/tmmluplus
|
686 |
+
name: (economics) tmmlu++
|
687 |
+
config: economics
|
688 |
+
split: test
|
689 |
+
revision: c0e8ae955997300d5dbf0e382bf0ba5115f85e8c
|
690 |
+
metrics:
|
691 |
+
- name: single choice
|
692 |
+
type: accuracy
|
693 |
+
value: 25.95
|
694 |
+
- task:
|
695 |
+
type: question-answering
|
696 |
+
name: Single Choice Question
|
697 |
+
dataset:
|
698 |
+
type: ikala/tmmluplus
|
699 |
+
name: (basic_medical_science) tmmlu++
|
700 |
+
config: basic_medical_science
|
701 |
+
split: test
|
702 |
+
revision: c0e8ae955997300d5dbf0e382bf0ba5115f85e8c
|
703 |
+
metrics:
|
704 |
+
- name: single choice
|
705 |
+
type: accuracy
|
706 |
+
value: 28.41
|
707 |
+
- task:
|
708 |
+
type: question-answering
|
709 |
+
name: Single Choice Question
|
710 |
+
dataset:
|
711 |
+
type: ikala/tmmluplus
|
712 |
+
name: (occupational_therapy_for_psychological_disorders) tmmlu++
|
713 |
+
config: occupational_therapy_for_psychological_disorders
|
714 |
+
split: test
|
715 |
+
revision: c0e8ae955997300d5dbf0e382bf0ba5115f85e8c
|
716 |
+
metrics:
|
717 |
+
- name: single choice
|
718 |
+
type: accuracy
|
719 |
+
value: 35.73
|
720 |
+
- task:
|
721 |
+
type: question-answering
|
722 |
+
name: Single Choice Question
|
723 |
+
dataset:
|
724 |
+
type: ikala/tmmluplus
|
725 |
+
name: (general_principles_of_law) tmmlu++
|
726 |
+
config: general_principles_of_law
|
727 |
+
split: test
|
728 |
+
revision: c0e8ae955997300d5dbf0e382bf0ba5115f85e8c
|
729 |
+
metrics:
|
730 |
+
- name: single choice
|
731 |
+
type: accuracy
|
732 |
+
value: 31.13
|
733 |
+
- task:
|
734 |
+
type: question-answering
|
735 |
+
name: Single Choice Question
|
736 |
+
dataset:
|
737 |
+
type: ikala/tmmluplus
|
738 |
+
name: (junior_chemistry) tmmlu++
|
739 |
+
config: junior_chemistry
|
740 |
+
split: test
|
741 |
+
revision: c0e8ae955997300d5dbf0e382bf0ba5115f85e8c
|
742 |
+
metrics:
|
743 |
+
- name: single choice
|
744 |
+
type: accuracy
|
745 |
+
value: 24.88
|
746 |
+
- task:
|
747 |
+
type: question-answering
|
748 |
+
name: Single Choice Question
|
749 |
+
dataset:
|
750 |
+
type: ikala/tmmluplus
|
751 |
+
name: (veterinary_pharmacology) tmmlu++
|
752 |
+
config: veterinary_pharmacology
|
753 |
+
split: test
|
754 |
+
revision: c0e8ae955997300d5dbf0e382bf0ba5115f85e8c
|
755 |
+
metrics:
|
756 |
+
- name: single choice
|
757 |
+
type: accuracy
|
758 |
+
value: 36.3
|
759 |
+
- task:
|
760 |
+
type: question-answering
|
761 |
+
name: Single Choice Question
|
762 |
+
dataset:
|
763 |
+
type: ikala/tmmluplus
|
764 |
+
name: (educational_psychology) tmmlu++
|
765 |
+
config: educational_psychology
|
766 |
+
split: test
|
767 |
+
revision: c0e8ae955997300d5dbf0e382bf0ba5115f85e8c
|
768 |
+
metrics:
|
769 |
+
- name: single choice
|
770 |
+
type: accuracy
|
771 |
+
value: 33.52
|
772 |
+
- task:
|
773 |
+
type: question-answering
|
774 |
+
name: Single Choice Question
|
775 |
+
dataset:
|
776 |
+
type: ikala/tmmluplus
|
777 |
+
name: (finance_banking) tmmlu++
|
778 |
+
config: finance_banking
|
779 |
+
split: test
|
780 |
+
revision: c0e8ae955997300d5dbf0e382bf0ba5115f85e8c
|
781 |
+
metrics:
|
782 |
+
- name: single choice
|
783 |
+
type: accuracy
|
784 |
+
value: 32.59
|
785 |
+
- task:
|
786 |
+
type: question-answering
|
787 |
+
name: Single Choice Question
|
788 |
+
dataset:
|
789 |
+
type: ikala/tmmluplus
|
790 |
+
name: (official_document_management) tmmlu++
|
791 |
+
config: official_document_management
|
792 |
+
split: test
|
793 |
+
revision: c0e8ae955997300d5dbf0e382bf0ba5115f85e8c
|
794 |
+
metrics:
|
795 |
+
- name: single choice
|
796 |
+
type: accuracy
|
797 |
+
value: 32.43
|
798 |
+
- task:
|
799 |
+
type: question-answering
|
800 |
+
name: Single Choice Question
|
801 |
+
dataset:
|
802 |
+
type: ikala/tmmluplus
|
803 |
+
name: (fire_science) tmmlu++
|
804 |
+
config: fire_science
|
805 |
+
split: test
|
806 |
+
revision: c0e8ae955997300d5dbf0e382bf0ba5115f85e8c
|
807 |
+
metrics:
|
808 |
+
- name: single choice
|
809 |
+
type: accuracy
|
810 |
+
value: 30.65
|
811 |
+
- task:
|
812 |
+
type: question-answering
|
813 |
+
name: Single Choice Question
|
814 |
+
dataset:
|
815 |
+
type: ikala/tmmluplus
|
816 |
+
name: (junior_social_studies) tmmlu++
|
817 |
+
config: junior_social_studies
|
818 |
+
split: test
|
819 |
+
revision: c0e8ae955997300d5dbf0e382bf0ba5115f85e8c
|
820 |
+
metrics:
|
821 |
+
- name: single choice
|
822 |
+
type: accuracy
|
823 |
+
value: 47.62
|
824 |
+
- task:
|
825 |
+
type: question-answering
|
826 |
+
name: Single Choice Question
|
827 |
+
dataset:
|
828 |
+
type: ikala/tmmluplus
|
829 |
+
name: (accounting) tmmlu++
|
830 |
+
config: accounting
|
831 |
+
split: test
|
832 |
+
revision: c0e8ae955997300d5dbf0e382bf0ba5115f85e8c
|
833 |
+
metrics:
|
834 |
+
- name: single choice
|
835 |
+
type: accuracy
|
836 |
+
value: 20.94
|
837 |
+
- task:
|
838 |
+
type: question-answering
|
839 |
+
name: Single Choice Question
|
840 |
+
dataset:
|
841 |
+
type: ikala/tmmluplus
|
842 |
+
name: (engineering_math) tmmlu++
|
843 |
+
config: engineering_math
|
844 |
+
split: test
|
845 |
+
revision: c0e8ae955997300d5dbf0e382bf0ba5115f85e8c
|
846 |
+
metrics:
|
847 |
+
- name: single choice
|
848 |
+
type: accuracy
|
849 |
+
value: 27.18
|
850 |
+
- task:
|
851 |
+
type: question-answering
|
852 |
+
name: Single Choice Question
|
853 |
+
dataset:
|
854 |
+
type: ikala/tmmluplus
|
855 |
+
name: (education_(profession_level)) tmmlu++
|
856 |
+
config: education_(profession_level)
|
857 |
+
split: test
|
858 |
+
revision: c0e8ae955997300d5dbf0e382bf0ba5115f85e8c
|
859 |
+
metrics:
|
860 |
+
- name: single choice
|
861 |
+
type: accuracy
|
862 |
+
value: 24.07
|
863 |
+
- task:
|
864 |
+
type: question-answering
|
865 |
+
name: Single Choice Question
|
866 |
+
dataset:
|
867 |
+
type: ikala/tmmluplus
|
868 |
+
name: (chinese_language_and_literature) tmmlu++
|
869 |
+
config: chinese_language_and_literature
|
870 |
+
split: test
|
871 |
+
revision: c0e8ae955997300d5dbf0e382bf0ba5115f85e8c
|
872 |
+
metrics:
|
873 |
+
- name: single choice
|
874 |
+
type: accuracy
|
875 |
+
value: 27.64
|
876 |
+
- task:
|
877 |
+
type: question-answering
|
878 |
+
name: Single Choice Question
|
879 |
+
dataset:
|
880 |
+
type: ikala/tmmluplus
|
881 |
+
name: (management_accounting) tmmlu++
|
882 |
+
config: management_accounting
|
883 |
+
split: test
|
884 |
+
revision: c0e8ae955997300d5dbf0e382bf0ba5115f85e8c
|
885 |
+
metrics:
|
886 |
+
- name: single choice
|
887 |
+
type: accuracy
|
888 |
+
value: 24.19
|
889 |
+
- task:
|
890 |
+
type: question-answering
|
891 |
+
name: Single Choice Question
|
892 |
+
dataset:
|
893 |
+
type: ikala/tmmluplus
|
894 |
+
name: (culinary_skills) tmmlu++
|
895 |
+
config: culinary_skills
|
896 |
+
split: test
|
897 |
+
revision: c0e8ae955997300d5dbf0e382bf0ba5115f85e8c
|
898 |
+
metrics:
|
899 |
+
- name: single choice
|
900 |
+
type: accuracy
|
901 |
+
value: 39.38
|
902 |
+
- task:
|
903 |
+
type: question-answering
|
904 |
+
name: Single Choice Question
|
905 |
+
dataset:
|
906 |
+
type: ikala/tmmluplus
|
907 |
+
name: (administrative_law) tmmlu++
|
908 |
+
config: administrative_law
|
909 |
+
split: test
|
910 |
+
revision: c0e8ae955997300d5dbf0e382bf0ba5115f85e8c
|
911 |
+
metrics:
|
912 |
+
- name: single choice
|
913 |
+
type: accuracy
|
914 |
+
value: 25.71
|
915 |
+
- task:
|
916 |
+
type: question-answering
|
917 |
+
name: Single Choice Question
|
918 |
+
dataset:
|
919 |
+
type: ikala/tmmluplus
|
920 |
+
name: (insurance_studies) tmmlu++
|
921 |
+
config: insurance_studies
|
922 |
+
split: test
|
923 |
+
revision: c0e8ae955997300d5dbf0e382bf0ba5115f85e8c
|
924 |
+
metrics:
|
925 |
+
- name: single choice
|
926 |
+
type: accuracy
|
927 |
+
value: 33.42
|
928 |
+
- task:
|
929 |
+
type: question-answering
|
930 |
+
name: Single Choice Question
|
931 |
+
dataset:
|
932 |
+
type: ikala/tmmluplus
|
933 |
+
name: (real_estate) tmmlu++
|
934 |
+
config: real_estate
|
935 |
+
split: test
|
936 |
+
revision: c0e8ae955997300d5dbf0e382bf0ba5115f85e8c
|
937 |
+
metrics:
|
938 |
+
- name: single choice
|
939 |
+
type: accuracy
|
940 |
+
value: 22.83
|
941 |
+
- task:
|
942 |
+
type: question-answering
|
943 |
+
name: Single Choice Question
|
944 |
+
dataset:
|
945 |
+
type: ikala/tmmluplus
|
946 |
+
name: (computer_science) tmmlu++
|
947 |
+
config: computer_science
|
948 |
+
split: test
|
949 |
+
revision: c0e8ae955997300d5dbf0e382bf0ba5115f85e8c
|
950 |
+
metrics:
|
951 |
+
- name: single choice
|
952 |
+
type: accuracy
|
953 |
+
value: 31.61
|
954 |
+
- task:
|
955 |
+
type: question-answering
|
956 |
+
name: Single Choice Question
|
957 |
+
dataset:
|
958 |
+
type: ikala/tmmluplus
|
959 |
+
name: (taxation) tmmlu++
|
960 |
+
config: taxation
|
961 |
+
split: test
|
962 |
+
revision: c0e8ae955997300d5dbf0e382bf0ba5115f85e8c
|
963 |
+
metrics:
|
964 |
+
- name: single choice
|
965 |
+
type: accuracy
|
966 |
+
value: 27.47
|
967 |
+
- task:
|
968 |
+
type: question-answering
|
969 |
+
name: Single Choice Question
|
970 |
+
dataset:
|
971 |
+
type: ikala/tmmluplus
|
972 |
+
name: (trade) tmmlu++
|
973 |
+
config: trade
|
974 |
+
split: test
|
975 |
+
revision: c0e8ae955997300d5dbf0e382bf0ba5115f85e8c
|
976 |
+
metrics:
|
977 |
+
- name: single choice
|
978 |
+
type: accuracy
|
979 |
+
value: 20.32
|
980 |
+
widget:
|
981 |
+
- text: 中華民國憲法第一條
|
982 |
+
metrics:
|
983 |
+
- accuracy
|
984 |
+
|
985 |
+
---
|
986 |
+
|
987 |
+
[](https://hf.co/QuantFactory)
|
988 |
+
|
989 |
+
|
990 |
+
# QuantFactory/Llama-3.2-Taiwan-3B-Instruct-GGUF
|
991 |
+
This is quantized version of [lianghsun/Llama-3.2-Taiwan-3B-Instruct](https://huggingface.co/lianghsun/Llama-3.2-Taiwan-3B-Instruct) created using llama.cpp
|
992 |
+
|
993 |
+
# Original Model Card
|
994 |
+
|
995 |
+
|
996 |
+
# Model Card for lianghsun/Llama-3.2-Taiwan-3B-Instruct
|
997 |
+
|
998 |
+
<!-- Provide a quick summary of what the model is/does. -->
|
999 |
+
<a href="https://discord.gg/fj6WbHMvfs" target="_blank">[Discord]</a>
|
1000 |
+
|
1001 |
+
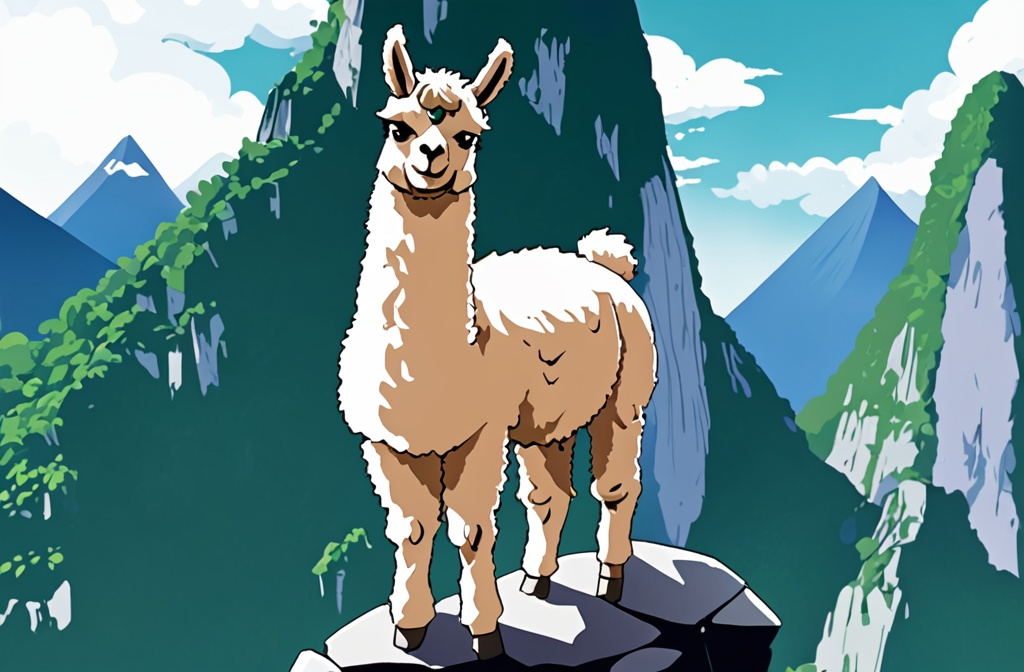
|
1002 |
+
*圖像生成來自 [OpenArt](https://openart.ai/home):An anime-style 🦙 standing proudly atop the summit of Taiwan’s [Yushan (Jade Mountain)](https://zh.wikipedia.org/wiki/%E7%8E%89%E5%B1%B1), gazing forward.*
|
1003 |
+
|
1004 |
+
採用 [lianghsun/Llama-3.2-Taiwan-3B](https://huggingface.co/lianghsun/Llama-3.2-Taiwan-3B) 為[基礎模型(foundation model)](https://en.wikipedia.org/wiki/Foundation_model),使用大量[中華民國台灣](https://zh.wikipedia.org/zh-tw/%E8%87%BA%E7%81%A3)的繁體中文對話集和多國語言對話集進行模型[指令微調(instruction fine-tuning)](https://www.ibm.com/topics/instruction-tuning)和多輪迭代[直接偏好優化(direct preference optimization, DPO)](https://arxiv.org/abs/2305.18290),旨在訓練出具有中華民國台灣知識及風格的[小語言模型(small langugae model, SLM)](https://www.ibm.com/think/topics/small-language-models)之對話模型。
|
1005 |
+
|
1006 |
+
<details>
|
1007 |
+
<summary><b>Model Change Log</b></summary>
|
1008 |
+
|
1009 |
+
| Update Date | Model Version | Key Changes |
|
1010 |
+
|--------------|-----------------------|-------------------------------------|
|
1011 |
+
| 2025/01/01 | v2025.01.01 | Fine-tuning is based on the [foundation model](https://huggingface.co/lianghsun/Llama-3.2-Taiwan-3B) version v2024.12.28, and it uses self-prepared instruction datasets for this round of fine-tuning. |
|
1012 |
+
| 2024/12/13 | v2024.12.13 | Completed 1st round DPO training (10/10 epochs). Preparing for next round DPO training. |
|
1013 |
+
| 2024/11/27 | v2024.11.27 | Completed SFT training (5/5 epochs). Preparing for multi-round DPO training. |
|
1014 |
+
| 2024/11/25 | v2024.11.25 | Updated model version to v2024.11.25, training progressed to (3/5) epochs. Still in SFT stage, DPO training remains pending. |
|
1015 |
+
| 2024/11/22 | v2024.11.22 | Initial upload: Model version v2024.11.22, training completed up to (1/5) epochs. Currently trained only on SFT, DPO training not yet performed. |
|
1016 |
+
|
1017 |
+
</details>
|
1018 |
+
|
1019 |
+
## Model Details
|
1020 |
+
|
1021 |
+
### Model Description
|
1022 |
+
|
1023 |
+
<!-- Provide a longer summary of what this model is. -->
|
1024 |
+
- **Developed by:** [Huang Liang Hsun](https://www.linkedin.com/in/lianghsunhuang)
|
1025 |
+
- **Model type:** LlamaForCausalLM
|
1026 |
+
- **Language(s) (NLP):** Tranditional Chinese (zh-tw), English
|
1027 |
+
- **License:** [llama3.2](https://huggingface.co/meta-llama/Llama-3.2-1B/blob/main/LICENSE.txt)
|
1028 |
+
- **Fine-tuned from model:** [lianghsun/Llama-3.2-Taiwan-3B](https://huggingface.co/lianghsun/Llama-3.2-Taiwan-3B)
|
1029 |
+
|
1030 |
+
### Model Sources
|
1031 |
+
|
1032 |
+
<!-- Provide the basic links for the model. -->
|
1033 |
+
- **Repository:** [lianghsun/Llama-3.2-Taiwan-3B](https://huggingface.co/lianghsun/Llama-3.2-Taiwan-3B)
|
1034 |
+
- **Paper:** (WIP, show me the time)
|
1035 |
+
- **Playground:** [🦉 Tawian SmolLM Chat](https://huggingface.co/spaces/lianghsun/tw-smol-chat) 👈🏼 來玩看看 😻
|
1036 |
+
- **Demo:**
|
1037 |
+
```yaml
|
1038 |
+
user: 請介紹台灣
|
1039 |
+
assistant: 台灣,位於亞洲東部,地處太平洋與菲律賓海之間,面積約36,000平方公里,人口約2,300萬,是民主自由的國家,經濟實力強勁,擁有世界第10大經濟體。台灣以美食、文化、自然美景著稱,還有豐富的歷史與傳統,吸引全球遊客。台灣語為官方語言,但中文也廣為使用,英語也常用於國際交流。台灣政治多元,執政黨為民進黨,��灣是全球科技產業的重鎮,擁有先進的製造業與服務業。台灣氣候溫暖潮濕,四季分明,夏季炎熱,冬季涼爽,雨季則在5月至10月。台灣的美食以小吃為主,如滷肉飯、珍珠
|
1040 |
+
```
|
1041 |
+
|
1042 |
+
## Uses
|
1043 |
+
|
1044 |
+
<!-- Address questions around how the model is intended to be used, including the foreseeable users of the model and those affected by the model. -->
|
1045 |
+
|
1046 |
+
### Direct Use
|
1047 |
+
|
1048 |
+
<!-- This section is for the model use without fine-tuning or plugging into a larger ecosystem/app. -->
|
1049 |
+
本模型已經具備有繁體中文對話能力,使用者可以直接部署推論端點使用。
|
1050 |
+
|
1051 |
+
### Downstream Use
|
1052 |
+
|
1053 |
+
<!-- This section is for the model use when fine-tuned for a task, or when plugged into a larger ecosystem/app -->
|
1054 |
+
若需強化模型在特定領域的知識,可透過微調進一步提升其性能與專業能力。
|
1055 |
+
|
1056 |
+
### Out-of-Scope Use
|
1057 |
+
|
1058 |
+
<!-- This section addresses misuse, malicious use, and uses that the model will not work well for. -->
|
1059 |
+
本模型旨在提供資訊,不參與任何政治或法律問題的評斷或立場表達。
|
1060 |
+
|
1061 |
+
## Bias, Risks, and Limitations
|
1062 |
+
|
1063 |
+
<!-- This section is meant to convey both technical and sociotechnical limitations. -->
|
1064 |
+
語言模型的生成內容可能因訓練集的多樣性而帶有偏見、特定立場,或包含與事實不符的言論,請使用者務必在使用過程中仔細確認內容的準確性與中立性。
|
1065 |
+
|
1066 |
+
### Recommendations
|
1067 |
+
|
1068 |
+
<!-- This section is meant to convey recommendations with respect to the bias, risk, and technical limitations. -->
|
1069 |
+
|
1070 |
+
Users (both direct and downstream) should be made aware of the risks, biases and limitations of the model. More information needed for further recommendations.
|
1071 |
+
|
1072 |
+
## How to Get Started with the Model
|
1073 |
+
|
1074 |
+
要使用 [vLLM Docker image](https://docs.vllm.ai/en/latest/serving/deploying_with_docker.html) 來啟動此模型,您可以按照以下操作:
|
1075 |
+
```bash
|
1076 |
+
docker run --runtime nvidia --gpus all \
|
1077 |
+
-v ~/.cache/huggingface:/root/.cache/huggingface \
|
1078 |
+
--env "HUGGING_FACE_HUB_TOKEN=<secret>" \
|
1079 |
+
-p 8000:8000 \
|
1080 |
+
--ipc=host \
|
1081 |
+
vllm/vllm-openai:latest \
|
1082 |
+
--model lianghsun/Llama-3.2-Taiwan-3B-Instruct
|
1083 |
+
```
|
1084 |
+
|
1085 |
+
請注意,如果想要使用不同版本的 checkpoint,請加上 `--revision <tag_name>`
|
1086 |
+
```bash
|
1087 |
+
docker run --runtime nvidia --gpus all \
|
1088 |
+
-v ~/.cache/huggingface:/root/.cache/huggingface \
|
1089 |
+
--env "HUGGING_FACE_HUB_TOKEN=<secret>" \
|
1090 |
+
-p 8000:8000 \
|
1091 |
+
--ipc=host \
|
1092 |
+
vllm/vllm-openai:latest \
|
1093 |
+
--model lianghsun/Llama-3.2-Taiwan-3B-Instruct --revision <tag_name>
|
1094 |
+
```
|
1095 |
+
|
1096 |
+
## Training Details
|
1097 |
+
|
1098 |
+
### Training Data
|
1099 |
+
|
1100 |
+
<!-- This should link to a Dataset Card, perhaps with a short stub of information on what the training data is all about as well as documentation related to data pre-processing or additional filtering. -->
|
1101 |
+
|
1102 |
+
<details>
|
1103 |
+
<summary><b>繁體中文對話資料集</b></summary>
|
1104 |
+
|
1105 |
+
- [lianghsun/tw-legal-nlp](https://huggingface.co/datasets/lianghsun/tw-legal-nlp)
|
1106 |
+
- [lianghsun/tw-legal-synthetic-qa](https://huggingface.co/datasets/lianghsun/tw-legal-synthetic-qa)
|
1107 |
+
- [lianghsun/tw-law-article-qa](https://huggingface.co/datasets/lianghsun/tw-law-article-qa)
|
1108 |
+
- [lianghsun/tw-judgment-qa](https://huggingface.co/datasets/lianghsun/tw-judgment-qa)
|
1109 |
+
- [lianghsun/tw-bar-examination-2020-chat](https://huggingface.co/datasets/lianghsun/tw-bar-examination-2020-chat)
|
1110 |
+
- [lianghsun/tw-structured-law-article](https://huggingface.co/datasets/lianghsun/tw-structured-law-article)
|
1111 |
+
- [lianghsun/tw-judgment-gist-chat](https://huggingface.co/datasets/lianghsun/tw-judgment-gist-chat)
|
1112 |
+
- [lianghsun/vulnerability-mitigation-qa-zh_tw](https://huggingface.co/datasets/lianghsun/vulnerability-mitigation-qa-zh_tw)
|
1113 |
+
- [lianghsun/tw-legal-qa-chat](https://huggingface.co/datasets/lianghsun/tw-legal-qa-chat)
|
1114 |
+
- [lianghsun/reasoning-base-20k-chat](https://huggingface.co/datasets/lianghsun/reasoning-base-20k-chat)
|
1115 |
+
- [lianghsun/tw-contract-review-chat](https://huggingface.co/datasets/lianghsun/tw-contract-review-chat)
|
1116 |
+
- [lianghsun/tw-legal-methodology-chat](https://huggingface.co/datasets/lianghsun/tw-legal-methodology-chat)
|
1117 |
+
- [minyichen/glaive_toolcall_zh_tw](https://huggingface.co/datasets/minyichen/glaive_toolcall_zh_tw)
|
1118 |
+
|
1119 |
+
</details>
|
1120 |
+
|
1121 |
+
<details>
|
1122 |
+
<summary><b>多國語系對話資料集</b></summary>
|
1123 |
+
|
1124 |
+
- [rombodawg/Everything_Instruct_Multilingual](https://huggingface.co/datasets/rombodawg/Everything_Instruct_Multilingual)
|
1125 |
+
- [xzuyn/manythings-translations-alpaca](https://huggingface.co/datasets/xzuyn/manythings-translations-alpaca)
|
1126 |
+
- [neural-bridge/rag-dataset-12000](https://huggingface.co/datasets/neural-bridge/rag-dataset-12000)
|
1127 |
+
|
1128 |
+
</details>
|
1129 |
+
|
1130 |
+
### Training Procedure
|
1131 |
+
|
1132 |
+
<!-- This relates heavily to the Technical Specifications. Content here should link to that section when it is relevant to the training procedure. -->
|
1133 |
+
|
1134 |
+
#### Preprocessing
|
1135 |
+
|
1136 |
+
(WIP)
|
1137 |
+
|
1138 |
+
#### Training Hyperparameters
|
1139 |
+
|
1140 |
+
<details>
|
1141 |
+
<summary><b>SFT stage for v2024.11.27</b></summary>
|
1142 |
+
|
1143 |
+
**Note:** 以下包含 `v2024.11.22` 和 `v2025.11.25` 的���參數設定
|
1144 |
+
- **learning_rate:** 5e-05
|
1145 |
+
- **min_learning_rate:** 5e-07
|
1146 |
+
- **train_batch_size:** 105
|
1147 |
+
- **seed:** 42
|
1148 |
+
- **distributed_type:** multi-GPU
|
1149 |
+
- **num_devices:** 4
|
1150 |
+
- **gradient_accumulation_steps:** 50
|
1151 |
+
- **total_train_batch_size:** 21,000
|
1152 |
+
- **optimizer:** Adam with betas=(0.9,0.999) and epsilon=1e-08
|
1153 |
+
- **lr_scheduler_type:** cosine
|
1154 |
+
- **lr_scheduler_warmup_ratio:** 0.01
|
1155 |
+
- **num_epochs:** 5.0
|
1156 |
+
- **global_step:** 590
|
1157 |
+
</details>
|
1158 |
+
|
1159 |
+
#### Speeds, Sizes, Times
|
1160 |
+
|
1161 |
+
<!-- This section provides information about throughput, start/end time, checkpoint size if relevant, etc. -->
|
1162 |
+
<details>
|
1163 |
+
<summary><b>SFT stage for v2024.11.27</b></summary>
|
1164 |
+
|
1165 |
+
**Note:** 以下包含 `v2024.11.22` 和 `v2025.11.25` 的超參數設定
|
1166 |
+
- **Duration**: 5 days, 16:15:11.17
|
1167 |
+
- **Train runtime**: 490,511.1789
|
1168 |
+
- **Train samples per second**: 25.37
|
1169 |
+
- **Train steps per second**: 0.001
|
1170 |
+
- **Total training FLOPs**: 26,658,386,120,540,160
|
1171 |
+
- **Train loss**: 0.8533
|
1172 |
+
</details>
|
1173 |
+
|
1174 |
+
## Evaluation
|
1175 |
+
|
1176 |
+
<!-- This section describes the evaluation protocols and provides the results. -->
|
1177 |
+
|
1178 |
+
### Testing Data, Factors & Metrics
|
1179 |
+
|
1180 |
+
<details>
|
1181 |
+
<summary><b>Formosa Taiwan Knowledge Bench</b></summary>
|
1182 |
+
|
1183 |
+
#### Testing Data
|
1184 |
+
|
1185 |
+
<!-- This should link to a Dataset Card if possible. -->
|
1186 |
+
|
1187 |
+
[lianghsun/Formosa-bench](https://huggingface.co/datasets/lianghsun/Formosa-bench)
|
1188 |
+
|
1189 |
+
#### Factors
|
1190 |
+
|
1191 |
+
<!-- These are the things the evaluation is disaggregating by, e.g., subpopulations or domains. -->
|
1192 |
+
|
1193 |
+
[More Information Needed]
|
1194 |
+
|
1195 |
+
#### Metrics
|
1196 |
+
|
1197 |
+
<!-- These are the evaluation metrics being used, ideally with a description of why. -->
|
1198 |
+
|
1199 |
+
[More Information Needed]
|
1200 |
+
|
1201 |
+
### Results
|
1202 |
+
|
1203 |
+
[More Information Needed]
|
1204 |
+
|
1205 |
+
#### Summary
|
1206 |
+
|
1207 |
+
</details>
|
1208 |
+
|
1209 |
+
<details>
|
1210 |
+
<summary><b>lianghsun/tw-legal-benchmark-v1</b></summary>
|
1211 |
+
|
1212 |
+
#### Testing Data
|
1213 |
+
|
1214 |
+
<!-- This should link to a Dataset Card if possible. -->
|
1215 |
+
|
1216 |
+
- **Dataset:** [lianghsun/tw-legal-benchmark-v1](https://huggingface.co/datasets/lianghsun/tw-legal-benchmark-v1)
|
1217 |
+
- **Revision:** 66c3a5f3ff2298f6a1cf23201070b5317bdd1893
|
1218 |
+
|
1219 |
+
#### Factors
|
1220 |
+
|
1221 |
+
<!-- These are the things the evaluation is disaggregating by, e.g., subpopulations or domains. -->
|
1222 |
+
|
1223 |
+
[More Information Needed]
|
1224 |
+
|
1225 |
+
#### Metrics
|
1226 |
+
|
1227 |
+
<!-- These are the evaluation metrics being used, ideally with a description of why. -->
|
1228 |
+
Accuracy
|
1229 |
+
|
1230 |
+
### Results
|
1231 |
+
|
1232 |
+
- **Model Revision:** v2024.11.27
|
1233 |
+
|
1234 |
+
| **Subset** | **Split** | **Score** |
|
1235 |
+
|--------------|-------|-------|
|
1236 |
+
| [lianghsun/tw-legal-benchmark-v1](https://huggingface.co/datasets/lianghsun/tw-legal-benchmark-v1/blob/main/benchmark.csv) | train | 31.1 |
|
1237 |
+
|
1238 |
+
#### Summary
|
1239 |
+
|
1240 |
+
</details>
|
1241 |
+
|
1242 |
+
<details>
|
1243 |
+
<summary><b>tmmlu++</b></summary>
|
1244 |
+
|
1245 |
+
#### Testing Data
|
1246 |
+
|
1247 |
+
<!-- This should link to a Dataset Card if possible. -->
|
1248 |
+
- **Dataset:** [ikala/tmmluplus](https://huggingface.co/datasets/ikala/tmmluplus)
|
1249 |
+
- **Revision:** c0e8ae955997300d5dbf0e382bf0ba5115f85e8c
|
1250 |
+
|
1251 |
+
#### Factors
|
1252 |
+
|
1253 |
+
<!-- These are the things the evaluation is disaggregating by, e.g., subpopulations or domains. -->
|
1254 |
+
[More Information Needed]
|
1255 |
+
|
1256 |
+
#### Metrics
|
1257 |
+
|
1258 |
+
<!-- These are the evaluation metrics being used, ideally with a description of why. -->
|
1259 |
+
Accuracy
|
1260 |
+
|
1261 |
+
### Results
|
1262 |
+
|
1263 |
+
- **Model Revision:** v2024.11.27
|
1264 |
+
|
1265 |
+
| **Subset** | **Split** | **Score** |
|
1266 |
+
|--------------|-------|-------|
|
1267 |
+
| [geography_of_taiwan](https://huggingface.co/datasets/ikala/tmmluplus/blob/main/data/geography_of_taiwan_test.csv) | test | 36.2 |
|
1268 |
+
| [dentistry](https://huggingface.co/datasets/ikala/tmmluplus/blob/main/data/dentistry_test.csv) | test | 33.83 |
|
1269 |
+
| [technical](https://huggingface.co/datasets/ikala/tmmluplus/blob/main/data/technical_test.csv) | test | 35.07 |
|
1270 |
+
| [statistics_and_machine_learning](https://huggingface.co/datasets/ikala/tmmluplus/blob/main/data/statistics_and_machine_learning_test.csv) | test | 28.57 |
|
1271 |
+
| [clinical_psychology](https://huggingface.co/datasets/ikala/tmmluplus/blob/main/data/clinical_psychology_test.csv) | test | 29.6 |
|
1272 |
+
| [tve_design](https://huggingface.co/datasets/ikala/tmmluplus/blob/main/data/tve_design_test.csv) | test | 38.54 |
|
1273 |
+
| [three_principles_of_people](https://huggingface.co/datasets/ikala/tmmluplus/blob/main/data/three_principles_of_people_test.csv) | test | 48.2 |
|
1274 |
+
| [introduction_to_law](https://huggingface.co/datasets/ikala/tmmluplus/blob/main/data/introduction_to_law_test.csv) | test | 29.96 |
|
1275 |
+
| [linear_algebra](https://huggingface.co/datasets/ikala/tmmluplus/blob/main/data/linear_algebra_test.csv) | test | 21.43 |
|
1276 |
+
| [agriculture](https://huggingface.co/datasets/ikala/tmmluplus/blob/main/data/agriculture_test.csv) | test | 24.5 |
|
1277 |
+
| [jce_humanities](https://huggingface.co/datasets/ikala/tmmluplus/blob/main/data/jce_humanities_test.csv) | test | 38.89 |
|
1278 |
+
| [music](https://huggingface.co/datasets/ikala/tmmluplus/blob/main/data/music_test.csv) | test | 25.9 |
|
1279 |
+
| [secondary_physics](https://huggingface.co/datasets/ikala/tmmluplus/blob/main/data/secondary_physics_test.csv) | test | 33.04 |
|
1280 |
+
| [physics](https://huggingface.co/datasets/ikala/tmmluplus/blob/main/data/physics_test.csv) | test | 27.84 |
|
1281 |
+
| [advance_chemistry](https://huggingface.co/datasets/ikala/tmmluplus/blob/main/data/advance_chemistry_test.csv) | test | 27.64 |
|
1282 |
+
| [junior_science_exam](https://huggingface.co/datasets/ikala/tmmluplus/blob/main/data/junior_science_exam_test.csv) | test | 30.05 |
|
1283 |
+
| [veterinary_pathology](https://huggingface.co/datasets/ikala/tmmluplus/blob/main/data/veterinary_pathology_test.csv) | test | 25.09 |
|
1284 |
+
| [financial_analysis](https://huggingface.co/datasets/ikala/tmmluplus/blob/main/data/financial_analysis_test.csv) | test | 25.13 |
|
1285 |
+
| [national_protection](https://huggingface.co/datasets/ikala/tmmluplus/blob/main/data/national_protection_test.csv) | test | 42.65 |
|
1286 |
+
| [macroeconomics](https://huggingface.co/datasets/ikala/tmmluplus/blob/main/data/macroeconomics_test.csv) | test | 26.76 |
|
1287 |
+
| [politic_science](https://huggingface.co/datasets/ikala/tmmluplus/blob/main/data/politic_science_test.csv) | test | 27.44 |
|
1288 |
+
| [ttqav2](https://huggingface.co/datasets/ikala/tmmluplus/blob/main/data/ttqav2_test.csv) | test | 61.06 |
|
1289 |
+
| [junior_chinese_exam](https://huggingface.co/datasets/ikala/tmmluplus/blob/main/data/junior_chinese_exam_test.csv) | test | 30.86 |
|
1290 |
+
| [traditional_chinese_medicine_clinical_medicine](https://huggingface.co/datasets/ikala/tmmluplus/blob/main/data/traditional_chinese_medicine_clinical_medicine_test.csv) | test | 25.9 |
|
1291 |
+
| [junior_math_exam](https://huggingface.co/datasets/ikala/tmmluplus/blob/main/data/junior_math_exam_test.csv) | test | 21.71 |
|
1292 |
+
| [auditing](https://huggingface.co/datasets/ikala/tmmluplus/blob/main/data/auditing_test.csv) | test | 21.82 |
|
1293 |
+
| [anti_money_laundering](https://huggingface.co/datasets/ikala/tmmluplus/blob/main/data/anti_money_laundering_test.csv) | test | 37.31 |
|
1294 |
+
| [pharmacology](https://huggingface.co/datasets/ikala/tmmluplus/blob/main/data/pharmacology_test.csv) | test | 30.68 |
|
1295 |
+
| [trust_practice](https://huggingface.co/datasets/ikala/tmmluplus/blob/main/data/trust_practice_test.csv) | test | 28.18 |
|
1296 |
+
| [tve_mathematics](https://huggingface.co/datasets/ikala/tmmluplus/blob/main/data/tve_mathematics_test.csv) | test | 18.67 |
|
1297 |
+
| [human_behavior](https://huggingface.co/datasets/ikala/tmmluplus/blob/main/data/human_behavior_test.csv) | test | 32.04 |
|
1298 |
+
| [pharmacy](https://huggingface.co/datasets/ikala/tmmluplus/blob/main/data/pharmacy_test.csv) | test | 22.76 |
|
1299 |
+
| [tve_chinese_language](https://huggingface.co/datasets/ikala/tmmluplus/blob/main/data/tve_chinese_language_test.csv) | test | 36.65 |
|
1300 |
+
| [optometry](https://huggingface.co/datasets/ikala/tmmluplus/blob/main/data/optometry_test.csv) | test | 25.11 |
|
1301 |
+
| [physical_education](https://huggingface.co/datasets/ikala/tmmluplus/blob/main/data/physical_education_test.csv) | test | 30.73 |
|
1302 |
+
| [organic_chemistry](https://huggingface.co/datasets/ikala/tmmluplus/blob/main/data/organic_chemistry_test.csv) | test | 35.78 |
|
1303 |
+
| [tve_natural_sciences](https://huggingface.co/datasets/ikala/tmmluplus/blob/main/data/tve_natural_sciences_test.csv) | test | 33.73 |
|
1304 |
+
| [education](https://huggingface.co/datasets/ikala/tmmluplus/blob/main/data/education_test.csv) | test | 37.9 |
|
1305 |
+
| [mechanical](https://huggingface.co/datasets/ikala/tmmluplus/blob/main/data/mechanical_test.csv) | test | 42.37 |
|
1306 |
+
| [taiwanese_hokkien](https://huggingface.co/datasets/ikala/tmmluplus/blob/main/data/taiwanese_hokkien_test.csv) | test | 14.73 |
|
1307 |
+
| [nautical_science](https://huggingface.co/datasets/ikala/tmmluplus/blob/main/data/nautical_science_test.csv) | test | 30.49 |
|
1308 |
+
| [business_management](https://huggingface.co/datasets/ikala/tmmluplus/blob/main/data/business_management_test.csv) | test | 39.57 |
|
1309 |
+
| [logic_reasoning](https://huggingface.co/datasets/ikala/tmmluplus/blob/main/data/logic_reasoning_test.csv) | test | 27.34 |
|
1310 |
+
| [marketing_management](https://huggingface.co/datasets/ikala/tmmluplus/blob/main/data/marketing_management_test.csv) | test | 39.78 |
|
1311 |
+
| [economics](https://huggingface.co/datasets/ikala/tmmluplus/blob/main/data/economics_test.csv) | test | 25.95 |
|
1312 |
+
| [basic_medical_science](https://huggingface.co/datasets/ikala/tmmluplus/blob/main/data/basic_medical_science_test.csv) | test | 28.41 |
|
1313 |
+
| [occupational_therapy_for_psychological_disorders](https://huggingface.co/datasets/ikala/tmmluplus/blob/main/data/occupational_therapy_for_psychological_disorders_test.csv) | test | 35.73 |
|
1314 |
+
| [general_principles_of_law](https://huggingface.co/datasets/ikala/tmmluplus/blob/main/data/general_principles_of_law_test.csv) | test | 31.13 |
|
1315 |
+
| [junior_chemistry](https://huggingface.co/datasets/ikala/tmmluplus/blob/main/data/junior_chemistry_test.csv) | test | 24.88 |
|
1316 |
+
| [veterinary_pharmacology](https://huggingface.co/datasets/ikala/tmmluplus/blob/main/data/veterinary_pharmacology_test.csv) | test | 36.3 |
|
1317 |
+
| [educational_psychology](https://huggingface.co/datasets/ikala/tmmluplus/blob/main/data/educational_psychology_test.csv) | test | 33.52 |
|
1318 |
+
| [finance_banking](https://huggingface.co/datasets/ikala/tmmluplus/blob/main/data/finance_banking_test.csv) | test | 32.59 |
|
1319 |
+
| [official_document_management](https://huggingface.co/datasets/ikala/tmmluplus/blob/main/data/official_document_management_test.csv) | test | 32.43 |
|
1320 |
+
| [fire_science](https://huggingface.co/datasets/ikala/tmmluplus/blob/main/data/fire_science_test.csv) | test | 30.65 |
|
1321 |
+
| [junior_social_studies](https://huggingface.co/datasets/ikala/tmmluplus/blob/main/data/junior_social_studies_test.csv) | test | 47.62 |
|
1322 |
+
| [accounting](https://huggingface.co/datasets/ikala/tmmluplus/blob/main/data/accounting_test.csv) | test | 20.94 |
|
1323 |
+
| [engineering_math](https://huggingface.co/datasets/ikala/tmmluplus/blob/main/data/engineering_math_test.csv) | test | 27.18 |
|
1324 |
+
| [education_(profession_level)](https://huggingface.co/datasets/ikala/tmmluplus/blob/main/data/education_(profession_level)_test.csv) | test | 24.07 |
|
1325 |
+
| [chinese_language_and_literature](https://huggingface.co/datasets/ikala/tmmluplus/blob/main/data/chinese_language_and_literature_test.csv) | test | 27.64 |
|
1326 |
+
| [management_accounting](https://huggingface.co/datasets/ikala/tmmluplus/blob/main/data/management_accounting_test.csv) | test | 24.19 |
|
1327 |
+
| [culinary_skills](https://huggingface.co/datasets/ikala/tmmluplus/blob/main/data/culinary_skills_test.csv) | test | 39.38 |
|
1328 |
+
| [administrative_law](https://huggingface.co/datasets/ikala/tmmluplus/blob/main/data/administrative_law_test.csv) | test | 25.71 |
|
1329 |
+
| [insurance_studies](https://huggingface.co/datasets/ikala/tmmluplus/blob/main/data/insurance_studies_test.csv) | test | 33.42 |
|
1330 |
+
| [real_estate](https://huggingface.co/datasets/ikala/tmmluplus/blob/main/data/real_estate_test.csv) | test | 22.83 |
|
1331 |
+
| [computer_science](https://huggingface.co/datasets/ikala/tmmluplus/blob/main/data/computer_science_test.csv) | test | 31.61 |
|
1332 |
+
| [taxation](https://huggingface.co/datasets/ikala/tmmluplus/blob/main/data/taxation_test.csv) | test | 27.47 |
|
1333 |
+
| [trade](https://huggingface.co/datasets/ikala/tmmluplus/blob/main/data/trade_test.csv) | test | 20.32 |
|
1334 |
+
|
1335 |
+
|
1336 |
+
#### Summary
|
1337 |
+
模型版號 `v2024.11.27`,無論是基礎模型([lianghsun/Llama-3.2-Taiwan-3B](https://huggingface.co/lianghsun/Llama-3.2-Taiwan-3B))還是指令微調模型([lianghsun/Llama-3.2-Taiwan-3B-Instruct](https://huggingface.co/lianghsun/Llama-3.2-Taiwan-3B-Instruct)),均未接受過 tmmlu++ 資料集的訓練,以確保測試的公平性。經測試,目前該模型在 tmmlu++ 上表現普遍不佳,未達及格分數,可能需要加入專業領域的資料集來強化基礎模型能力。
|
1338 |
+
|
1339 |
+
</details>
|
1340 |
+
|
1341 |
+
## Model Examination [optional]
|
1342 |
+
|
1343 |
+
<!-- Relevant interpretability work for the model goes here -->
|
1344 |
+
|
1345 |
+
[More Information Needed]
|
1346 |
+
|
1347 |
+
## Environmental Impact
|
1348 |
+
|
1349 |
+
<!-- Total emissions (in grams of CO2eq) and additional considerations, such as electricity usage, go here. Edit the suggested text below accordingly -->
|
1350 |
+
- **Hardware Type:** 🚀
|
1351 |
+
- **Hours used:** ⏳⏳⌛
|
1352 |
+
- **Cloud Provider:** [鴻鵠國際股份有限公司](https://www.honghutech.com/)
|
1353 |
+
- **Compute Region:** 🇹🇼
|
1354 |
+
- **Carbon Emitted:** ♻️
|
1355 |
+
|
1356 |
+
## Technical Specifications
|
1357 |
+
|
1358 |
+
### Model Architecture and Objective
|
1359 |
+
|
1360 |
+
[More Information Needed]
|
1361 |
+
|
1362 |
+
### Compute Infrastructure
|
1363 |
+
|
1364 |
+
[More Information Needed]
|
1365 |
+
|
1366 |
+
#### Hardware
|
1367 |
+
|
1368 |
+
- **CPU count:** 32
|
1369 |
+
- **Logical CPU count:** 64
|
1370 |
+
- **GPU count:** 4
|
1371 |
+
- **GPU type:** NVIDIA H100 NVL
|
1372 |
+
|
1373 |
+
#### Software
|
1374 |
+
|
1375 |
+
- **OS version:** Linux-5.15.0-124-generic-x86_64-with-glibc2.35
|
1376 |
+
- **Python version:** 3.12.7
|
1377 |
+
|
1378 |
+
## Citation
|
1379 |
+
|
1380 |
+
<!-- If there is a paper or blog post introducing the model, the APA and Bibtex information for that should go in this section. -->
|
1381 |
+
```bibtex
|
1382 |
+
@misc{lianghsun2024llama32taiwan3binstruct,
|
1383 |
+
author = {Huang, Liang Hsun},
|
1384 |
+
title = {Llama-3.2-Taiwan-3B-Instruct},
|
1385 |
+
year = {2024},
|
1386 |
+
publisher = {Hugging Face},
|
1387 |
+
howpublished = {\url{https://huggingface.co/lianghsun/Llama-3.2-Taiwan-3B-Instruct}},
|
1388 |
+
note = {Accessed: 2024-11-25}
|
1389 |
+
}
|
1390 |
+
```
|
1391 |
+
|
1392 |
+
## Glossary [optional]
|
1393 |
+
|
1394 |
+
<!-- If relevant, include terms and calculations in this section that can help readers understand the model or model card. -->
|
1395 |
+
N/A
|
1396 |
+
|
1397 |
+
## More Information
|
1398 |
+
|
1399 |
+
### Acknowledge
|
1400 |
+

|
1401 |
+
在此致謝[鴻鵠國際股份有限公司](https://www.honghutech.com/)蔡長明先生無償地贊助算力,以及曾經幫忙過:廖振翔、chweng、Ben、kevin、Maxxchu、Lam 和陳林彥…等朋友們,才能讓這個模型得以訓練完成,提供算力者乃人生父母。
|
1402 |
+
|
1403 |
+
### Usage
|
1404 |
+
如果你基於此指令模型進行微調,希望能不吝嗇在 **模型卡片(model card)** 裡標註 **基礎模型** 為:
|
1405 |
+
```yaml
|
1406 |
+
base_model: lianghsun/Llama-3.2-Taiwan-3B-Instruct
|
1407 |
+
```
|
1408 |
+
|
1409 |
+
標註和 ❤️ 是給予我們最大的鼓勵,謝謝。😀
|
1410 |
+
|
1411 |
+
## Model Card Authors
|
1412 |
+
|
1413 |
+
[Huang Liang Hsun](https://www.linkedin.com/in/lianghsunhuang)
|
1414 |
+
|
1415 |
+
## Model Card Contact
|
1416 |
+
|
1417 |
+
[Huang Liang Hsun](https://www.linkedin.com/in/lianghsunhuang)
|
1418 |
+
|
1419 |
+
### Framework versions
|
1420 |
+
|
1421 |
+
- Transformers 4.45.2
|
1422 |
+
- Pytorch 2.4.1+cu121
|
1423 |
+
- Datasets 2.21.0
|
1424 |
+
- Tokenizers 0.20.0
|
1425 |
+
|