File size: 8,098 Bytes
c419b54 c92905b c419b54 c92905b c419b54 ea393b4 7e62d1c c419b54 7e62d1c c419b54 7e62d1c 8ea1d65 7e62d1c c419b54 8fcf92b c419b54 c92905b |
1 2 3 4 5 6 7 8 9 10 11 12 13 14 15 16 17 18 19 20 21 22 23 24 25 26 27 28 29 30 31 32 33 34 35 36 37 38 39 40 41 42 43 44 45 46 47 48 49 50 51 52 53 54 55 56 57 58 59 60 61 62 63 64 65 66 67 68 69 70 71 72 73 74 75 76 77 78 79 80 81 82 83 84 85 86 87 88 89 90 91 92 93 94 95 96 97 98 99 100 101 102 103 104 105 106 107 108 109 110 111 112 113 114 115 116 117 118 119 120 121 122 123 124 125 126 127 128 129 130 131 132 133 134 135 136 137 138 139 140 141 142 143 144 145 146 147 148 149 150 151 152 153 154 155 156 157 158 159 160 161 162 163 164 165 166 167 168 169 170 171 172 173 174 175 176 177 178 179 180 181 182 183 184 185 186 187 188 189 190 191 192 193 194 195 196 197 198 199 200 201 202 203 204 205 206 207 208 209 210 211 212 213 214 215 216 217 218 219 220 221 222 223 224 225 226 227 228 229 230 |
---
language:
- en
license: other
library_name: transformers
tags:
- chat
base_model: Qwen/Qwen2.5-Math-72B
license_name: qwen
license_link: https://huggingface.co/Qwen/Qwen2.5-Math-72B-Instruct/blob/main/LICENSE
pipeline_tag: text-generation
model-index:
- name: Qwen2.5-Math-72B-Instruct
results:
- task:
type: text-generation
name: Text Generation
dataset:
name: IFEval (0-Shot)
type: HuggingFaceH4/ifeval
args:
num_few_shot: 0
metrics:
- type: inst_level_strict_acc and prompt_level_strict_acc
value: 40.03
name: strict accuracy
source:
url: https://huggingface.co/spaces/open-llm-leaderboard/open_llm_leaderboard?query=Qwen/Qwen2.5-Math-72B-Instruct
name: Open LLM Leaderboard
- task:
type: text-generation
name: Text Generation
dataset:
name: BBH (3-Shot)
type: BBH
args:
num_few_shot: 3
metrics:
- type: acc_norm
value: 48.97
name: normalized accuracy
source:
url: https://huggingface.co/spaces/open-llm-leaderboard/open_llm_leaderboard?query=Qwen/Qwen2.5-Math-72B-Instruct
name: Open LLM Leaderboard
- task:
type: text-generation
name: Text Generation
dataset:
name: MATH Lvl 5 (4-Shot)
type: hendrycks/competition_math
args:
num_few_shot: 4
metrics:
- type: exact_match
value: 18.5
name: exact match
source:
url: https://huggingface.co/spaces/open-llm-leaderboard/open_llm_leaderboard?query=Qwen/Qwen2.5-Math-72B-Instruct
name: Open LLM Leaderboard
- task:
type: text-generation
name: Text Generation
dataset:
name: GPQA (0-shot)
type: Idavidrein/gpqa
args:
num_few_shot: 0
metrics:
- type: acc_norm
value: 10.85
name: acc_norm
source:
url: https://huggingface.co/spaces/open-llm-leaderboard/open_llm_leaderboard?query=Qwen/Qwen2.5-Math-72B-Instruct
name: Open LLM Leaderboard
- task:
type: text-generation
name: Text Generation
dataset:
name: MuSR (0-shot)
type: TAUR-Lab/MuSR
args:
num_few_shot: 0
metrics:
- type: acc_norm
value: 16.34
name: acc_norm
source:
url: https://huggingface.co/spaces/open-llm-leaderboard/open_llm_leaderboard?query=Qwen/Qwen2.5-Math-72B-Instruct
name: Open LLM Leaderboard
- task:
type: text-generation
name: Text Generation
dataset:
name: MMLU-PRO (5-shot)
type: TIGER-Lab/MMLU-Pro
config: main
split: test
args:
num_few_shot: 5
metrics:
- type: acc
value: 42.36
name: accuracy
source:
url: https://huggingface.co/spaces/open-llm-leaderboard/open_llm_leaderboard?query=Qwen/Qwen2.5-Math-72B-Instruct
name: Open LLM Leaderboard
---
# Qwen2.5-Math-72B-Instruct
> [!Warning]
> <div align="center">
> <b>
> 🚨 Qwen2.5-Math mainly supports solving English and Chinese math problems through CoT and TIR. We do not recommend using this series of models for other tasks.
> </b>
> </div>
## Introduction
In August 2024, we released the first series of mathematical LLMs - [Qwen2-Math](https://qwenlm.github.io/blog/qwen2-math/) - of our Qwen family. A month later, we have upgraded it and open-sourced **Qwen2.5-Math** series, including base models **Qwen2.5-Math-1.5B/7B/72B**, instruction-tuned models **Qwen2.5-Math-1.5B/7B/72B-Instruct**, and mathematical reward model **Qwen2.5-Math-RM-72B**.
Unlike Qwen2-Math series which only supports using Chain-of-Thught (CoT) to solve English math problems, Qwen2.5-Math series is expanded to support using both CoT and Tool-integrated Reasoning (TIR) to solve math problems in both Chinese and English. The Qwen2.5-Math series models have achieved significant performance improvements compared to the Qwen2-Math series models on the Chinese and English mathematics benchmarks with CoT.
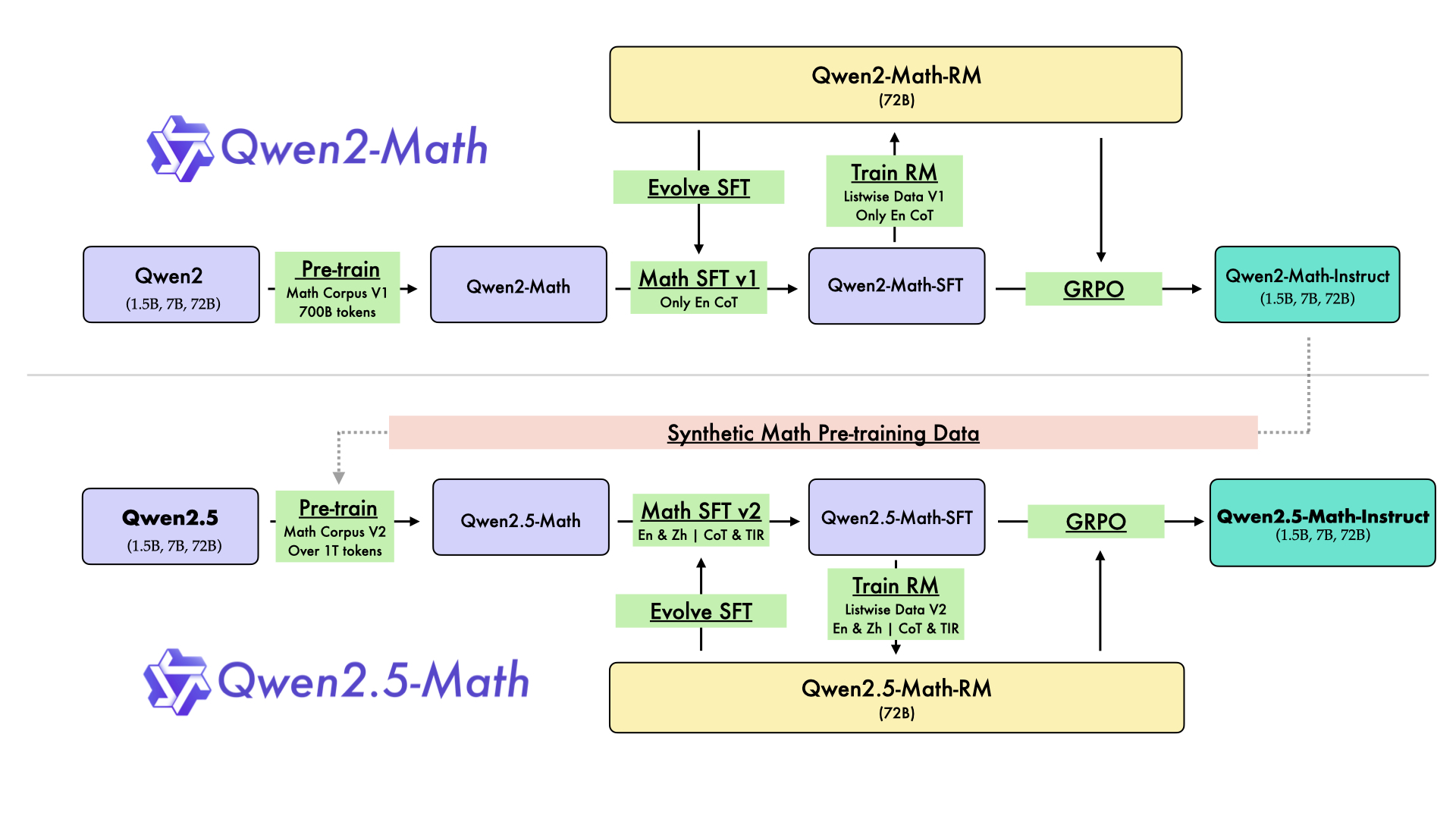
While CoT plays a vital role in enhancing the reasoning capabilities of LLMs, it faces challenges in achieving computational accuracy and handling complex mathematical or algorithmic reasoning tasks, such as finding the roots of a quadratic equation or computing the eigenvalues of a matrix. TIR can further improve the model's proficiency in precise computation, symbolic manipulation, and algorithmic manipulation. Qwen2.5-Math-1.5B/7B/72B-Instruct achieve 79.7, 85.3, and 87.8 respectively on the MATH benchmark using TIR.
## Model Details
For more details, please refer to our [blog post](https://qwenlm.github.io/blog/qwen2.5-math/) and [GitHub repo](https://github.com/QwenLM/Qwen2.5-Math).
## Requirements
* `transformers>=4.37.0` for Qwen2.5-Math models. The latest version is recommended.
> [!Warning]
> <div align="center">
> <b>
> 🚨 This is a must because <code>transformers</code> integrated Qwen2 codes since <code>4.37.0</code>.
> </b>
> </div>
For requirements on GPU memory and the respective throughput, see similar results of Qwen2 [here](https://qwen.readthedocs.io/en/latest/benchmark/speed_benchmark.html).
## Quick Start
> [!Important]
>
> **Qwen2.5-Math-72B-Instruct** is an instruction model for chatting;
>
> **Qwen2.5-Math-72B** is a base model typically used for completion and few-shot inference, serving as a better starting point for fine-tuning.
>
### 🤗 Hugging Face Transformers
Qwen2.5-Math can be deployed and infered in the same way as [Qwen2.5](https://github.com/QwenLM/Qwen2.5). Here we show a code snippet to show you how to use the chat model with `transformers`:
```python
from transformers import AutoModelForCausalLM, AutoTokenizer
model_name = "Qwen/Qwen2.5-Math-72B-Instruct"
device = "cuda" # the device to load the model onto
model = AutoModelForCausalLM.from_pretrained(
model_name,
torch_dtype="auto",
device_map="auto"
)
tokenizer = AutoTokenizer.from_pretrained(model_name)
prompt = "Find the value of $x$ that satisfies the equation $4x+5 = 6x+7$."
# CoT
messages = [
{"role": "system", "content": "Please reason step by step, and put your final answer within \\boxed{}."},
{"role": "user", "content": prompt}
]
# TIR
messages = [
{"role": "system", "content": "Please integrate natural language reasoning with programs to solve the problem above, and put your final answer within \\boxed{}."},
{"role": "user", "content": prompt}
]
text = tokenizer.apply_chat_template(
messages,
tokenize=False,
add_generation_prompt=True
)
model_inputs = tokenizer([text], return_tensors="pt").to(device)
generated_ids = model.generate(
**model_inputs,
max_new_tokens=512
)
generated_ids = [
output_ids[len(input_ids):] for input_ids, output_ids in zip(model_inputs.input_ids, generated_ids)
]
response = tokenizer.batch_decode(generated_ids, skip_special_tokens=True)[0]
```
## Citation
If you find our work helpful, feel free to give us a citation.
```
@article{yang2024qwen25mathtechnicalreportmathematical,
title={Qwen2.5-Math Technical Report: Toward Mathematical Expert Model via Self-Improvement},
author={An Yang and Beichen Zhang and Binyuan Hui and Bofei Gao and Bowen Yu and Chengpeng Li and Dayiheng Liu and Jianhong Tu and Jingren Zhou and Junyang Lin and Keming Lu and Mingfeng Xue and Runji Lin and Tianyu Liu and Xingzhang Ren and Zhenru Zhang},
journal={arXiv preprint arXiv:2409.12122},
year={2024}
}
```
# [Open LLM Leaderboard Evaluation Results](https://huggingface.co/spaces/open-llm-leaderboard/open_llm_leaderboard)
Detailed results can be found [here](https://huggingface.co/datasets/open-llm-leaderboard/details_Qwen__Qwen2.5-Math-72B-Instruct)
| Metric |Value|
|-------------------|----:|
|Avg. |29.51|
|IFEval (0-Shot) |40.03|
|BBH (3-Shot) |48.97|
|MATH Lvl 5 (4-Shot)|18.50|
|GPQA (0-shot) |10.85|
|MuSR (0-shot) |16.34|
|MMLU-PRO (5-shot) |42.36|
|