# SD3 Controlnet softedge
The softedge controlnet is finetuned based on SD3-medium. It is trained using 12M open source and internal e-commerce dataset, and achieve good performance on both general and e-commerce image generation. It supports preprocessors such as pidinet, hed as well as their safe mode.
## Examples
From left to right: pidinet preprocessor, ours with pidinet, hed preprocessor, ours with hed.
`pidinet` |`controlnet`|`hed` |`controlnet`
:--:|:--:|:--:|:--:
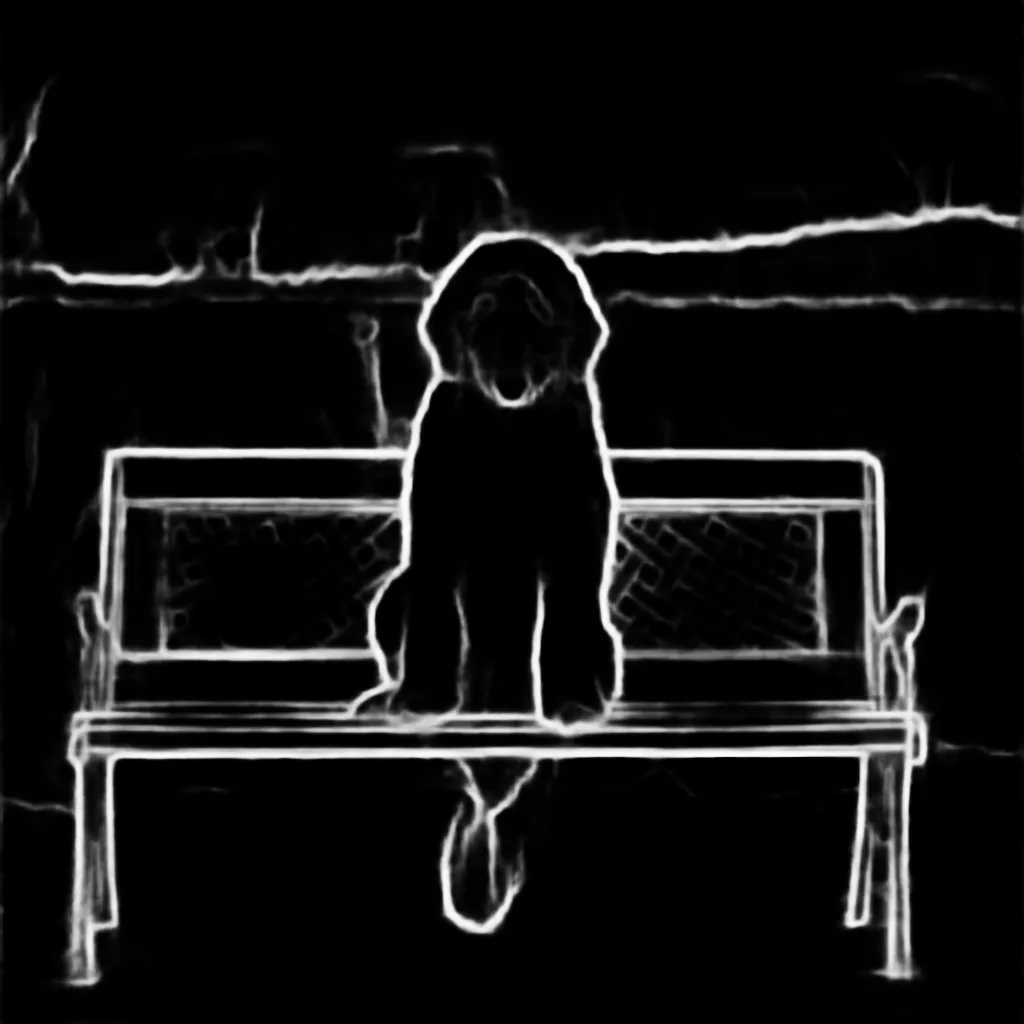 | 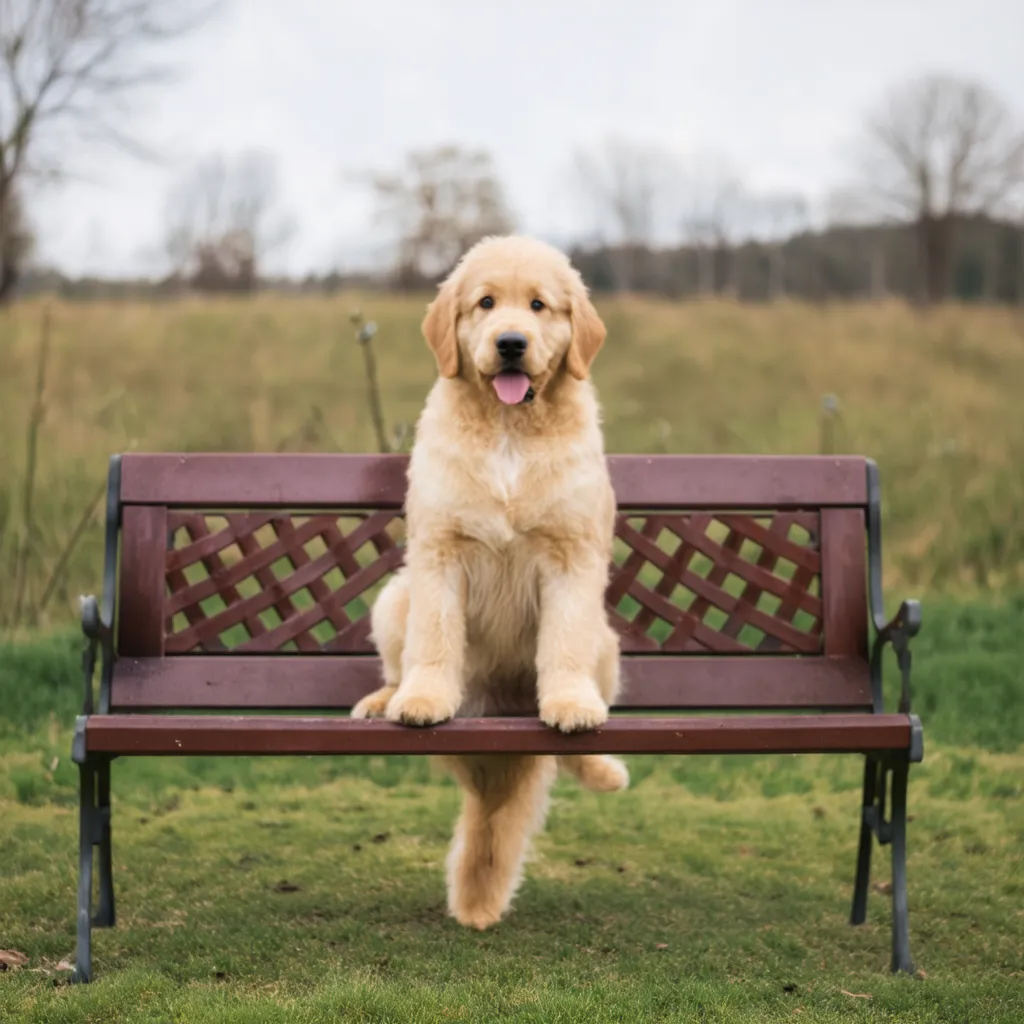 | 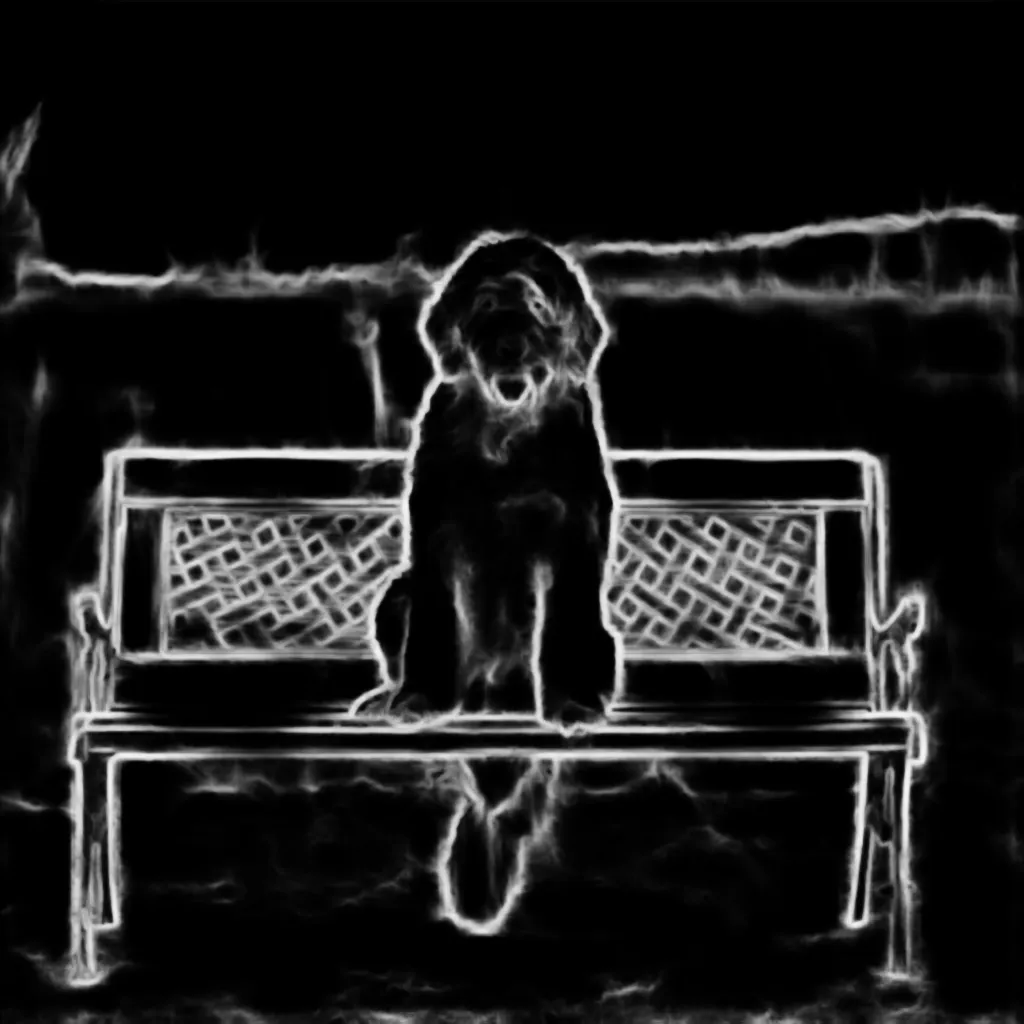 | 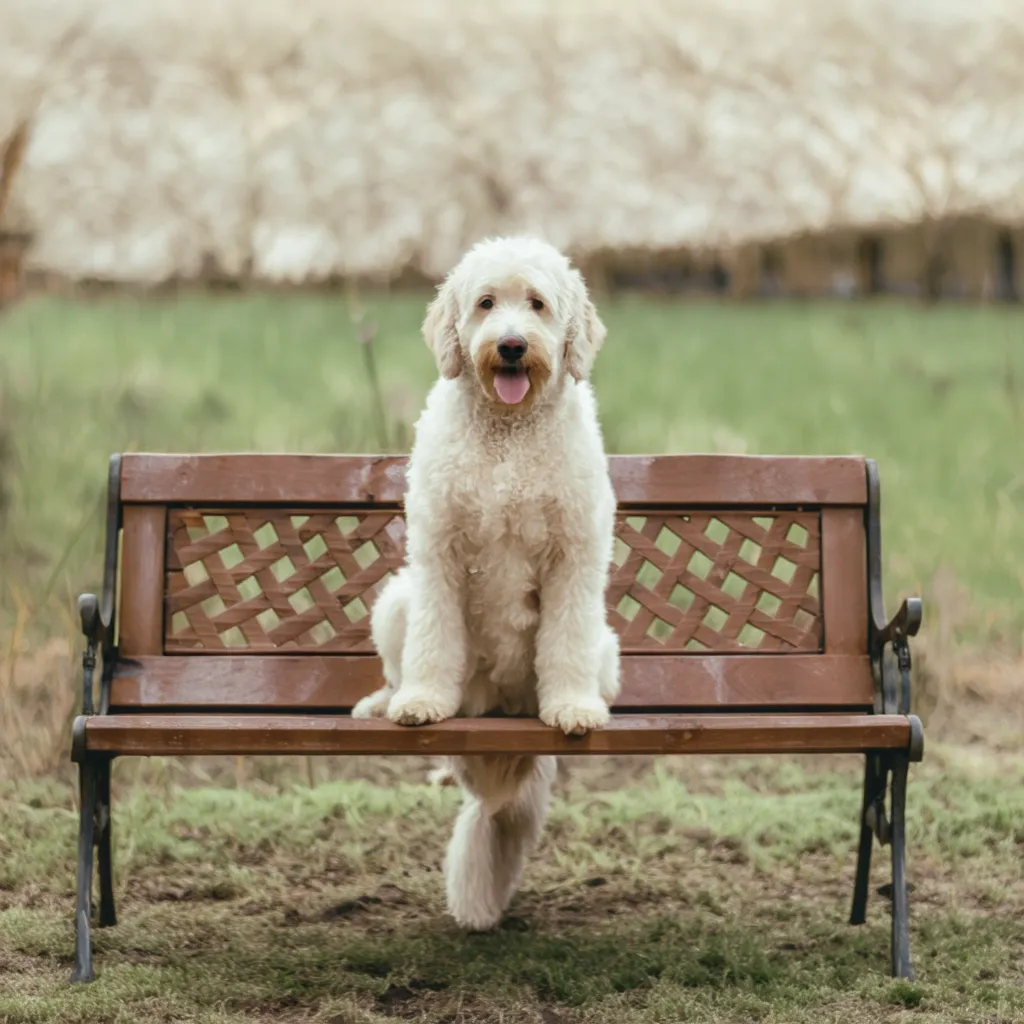
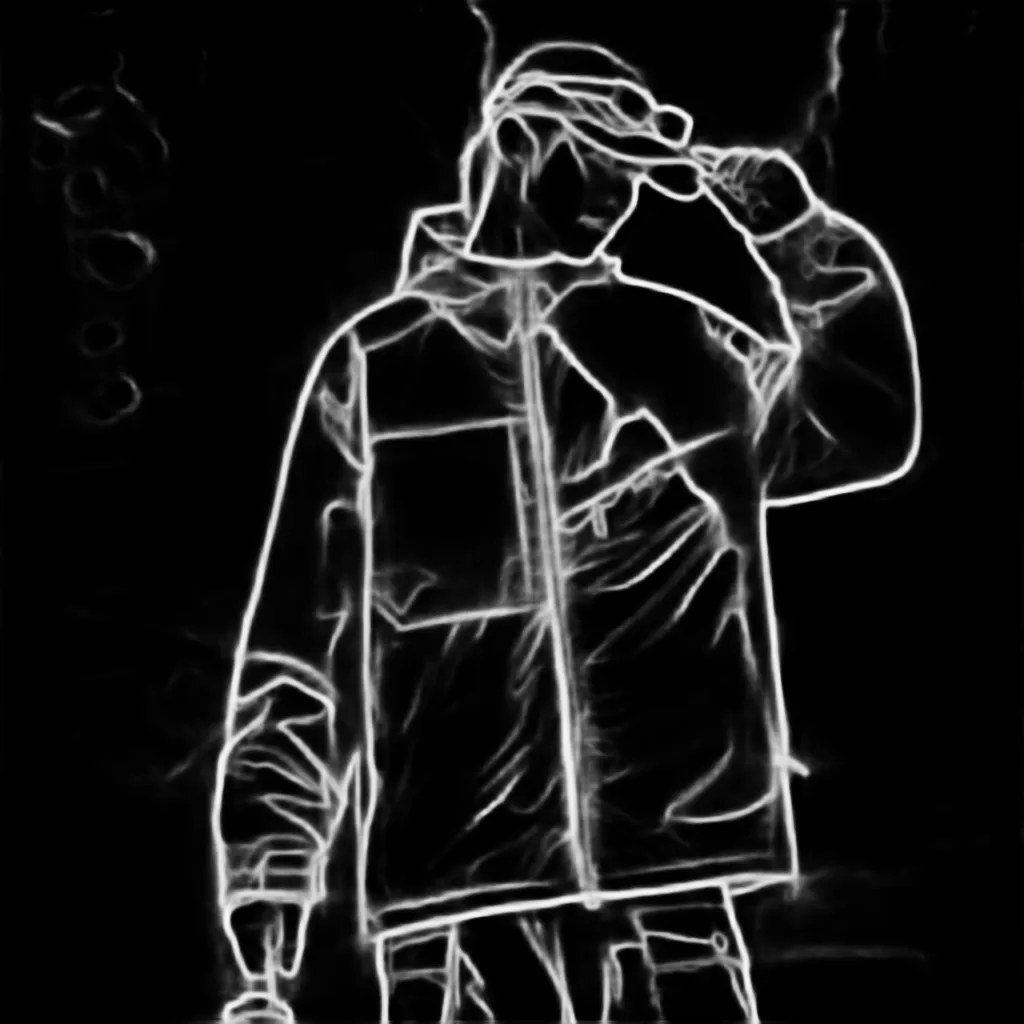 | 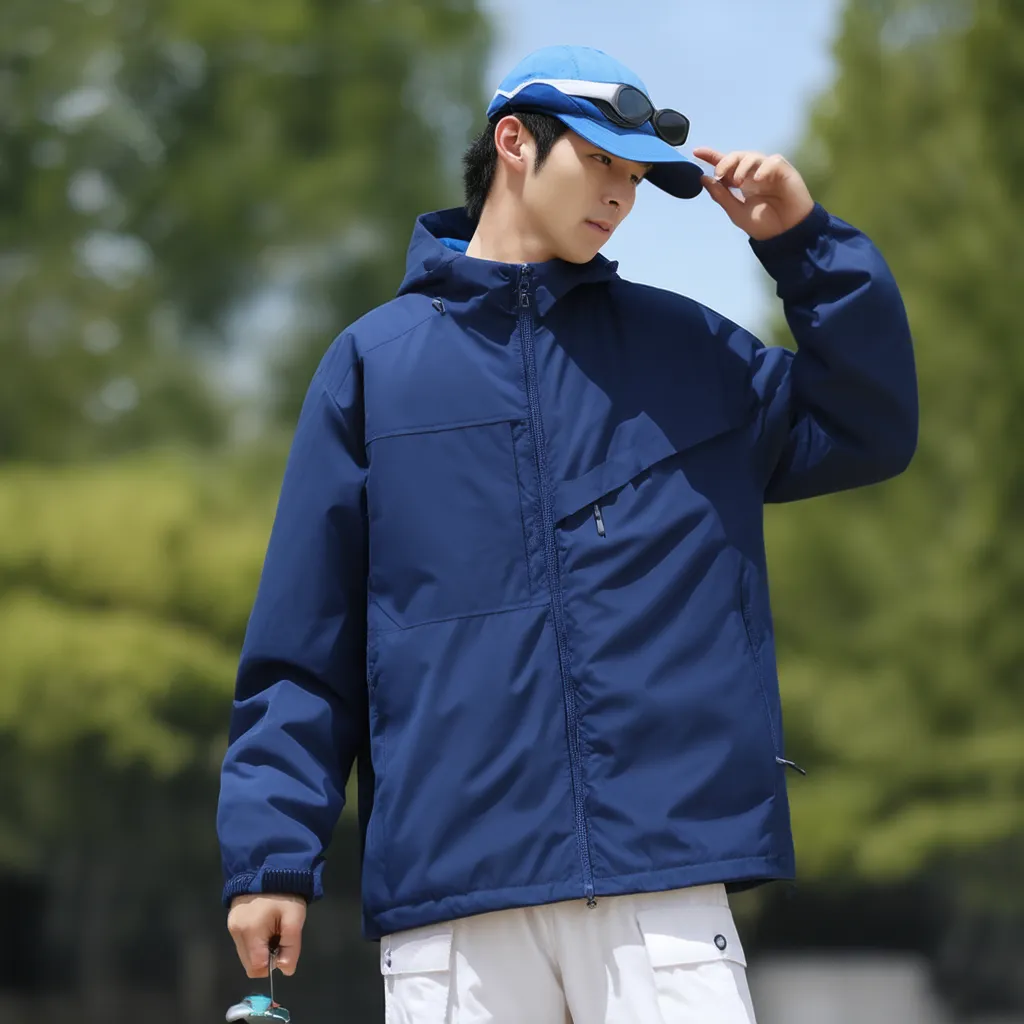 |  | 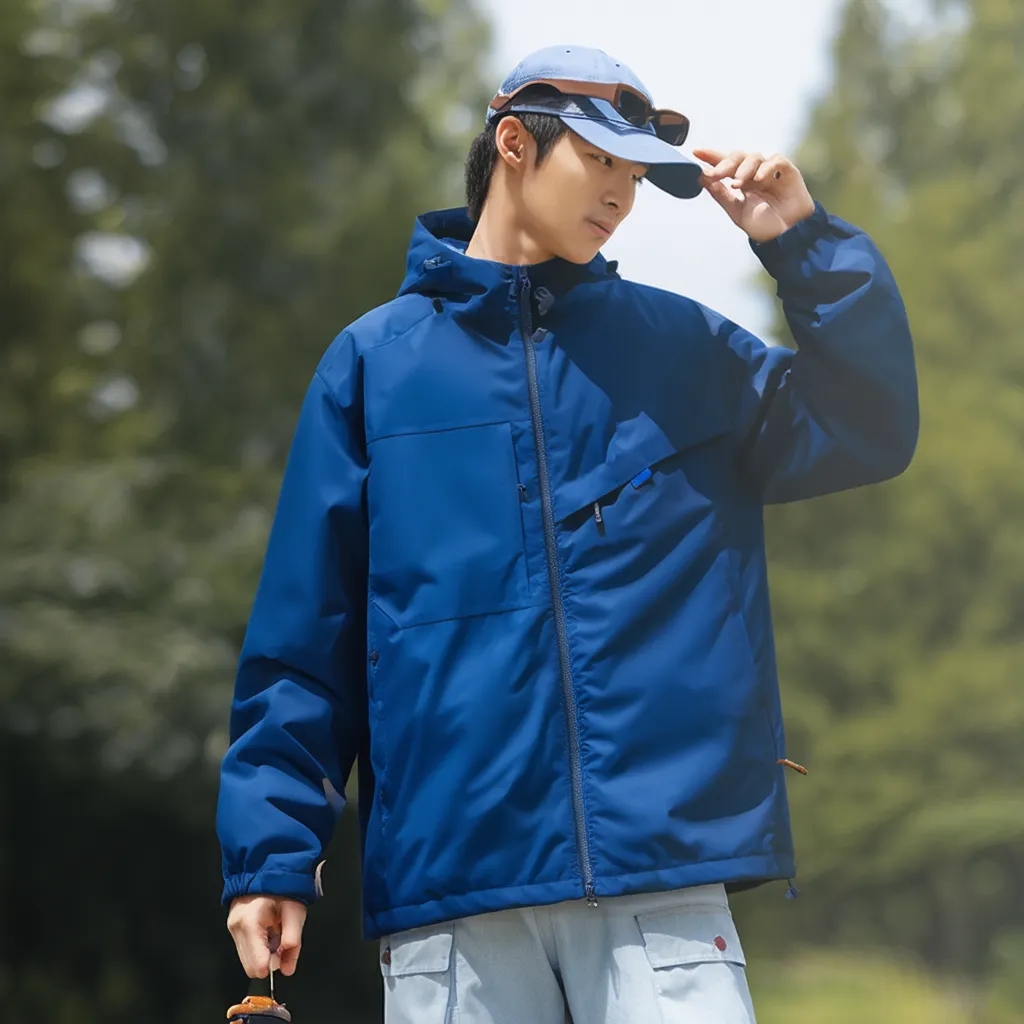
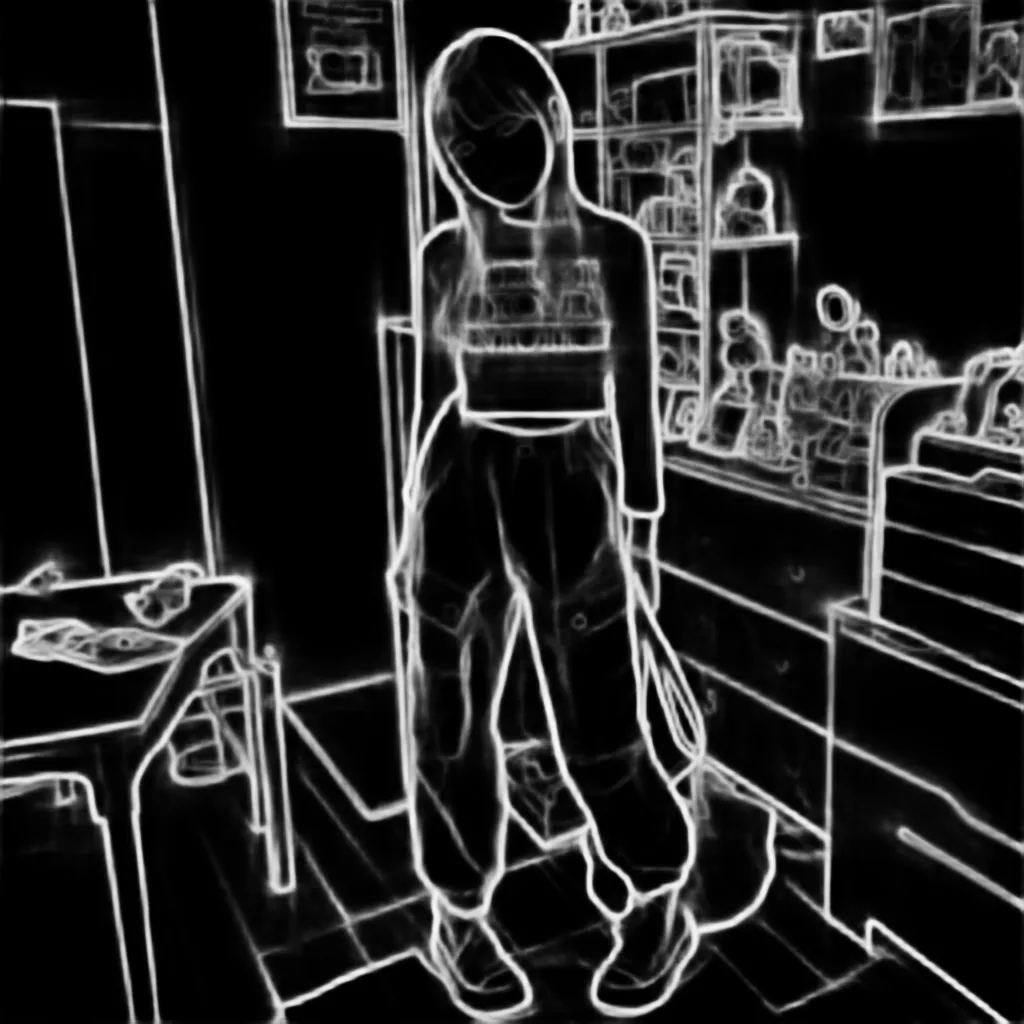 | 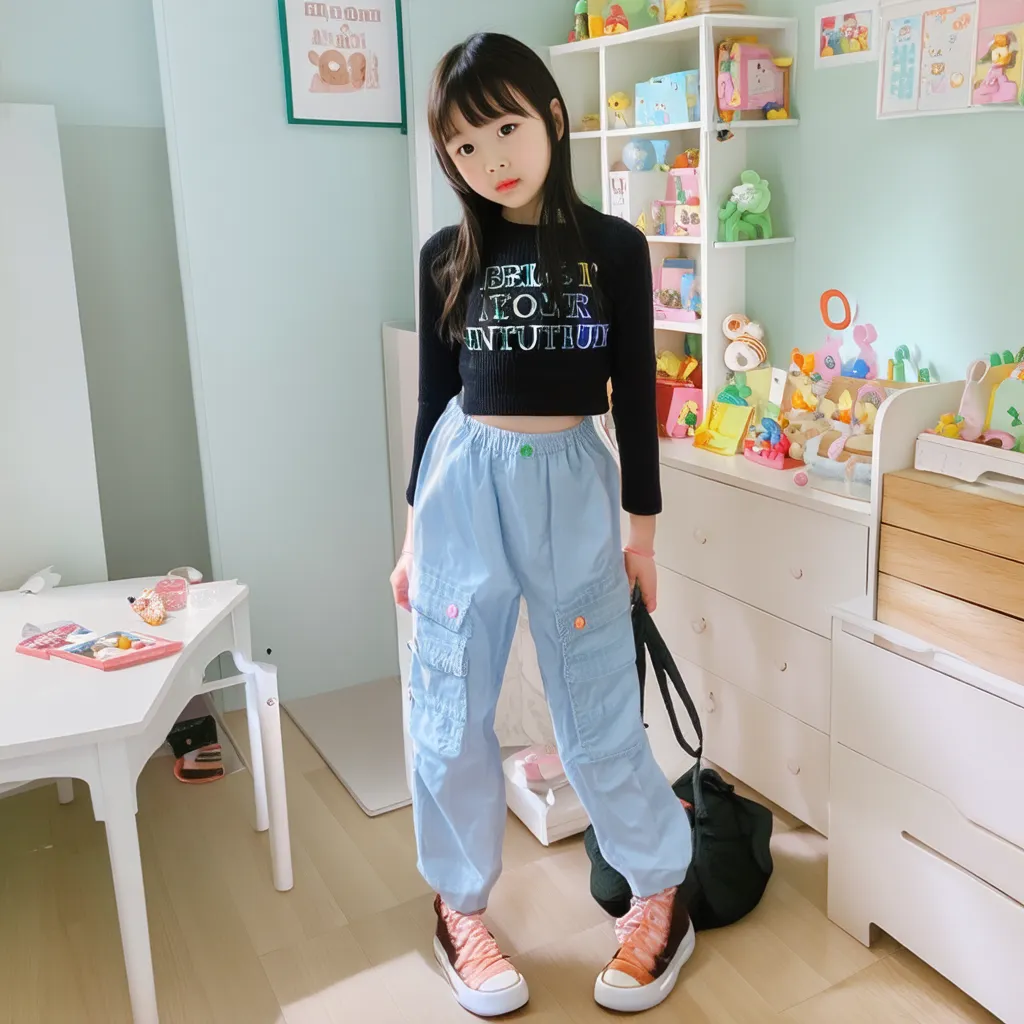 | 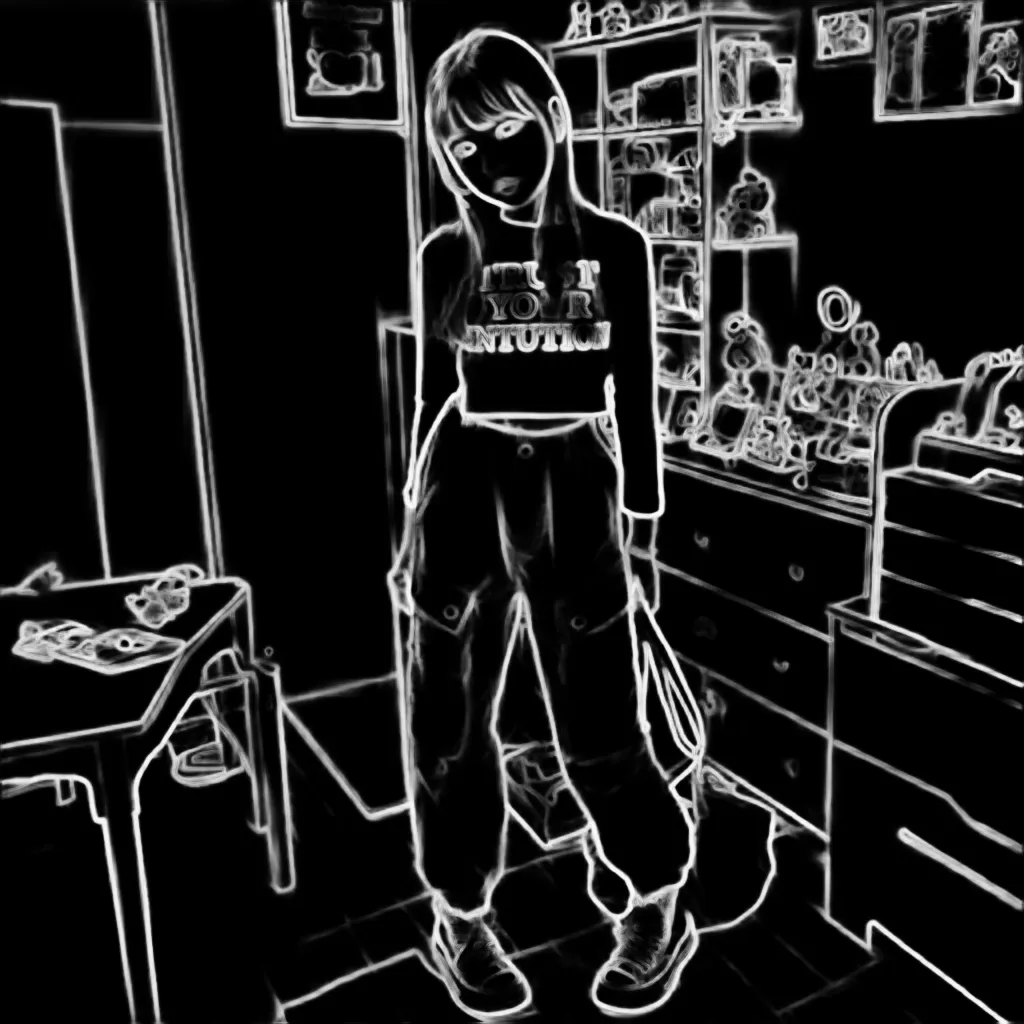 | 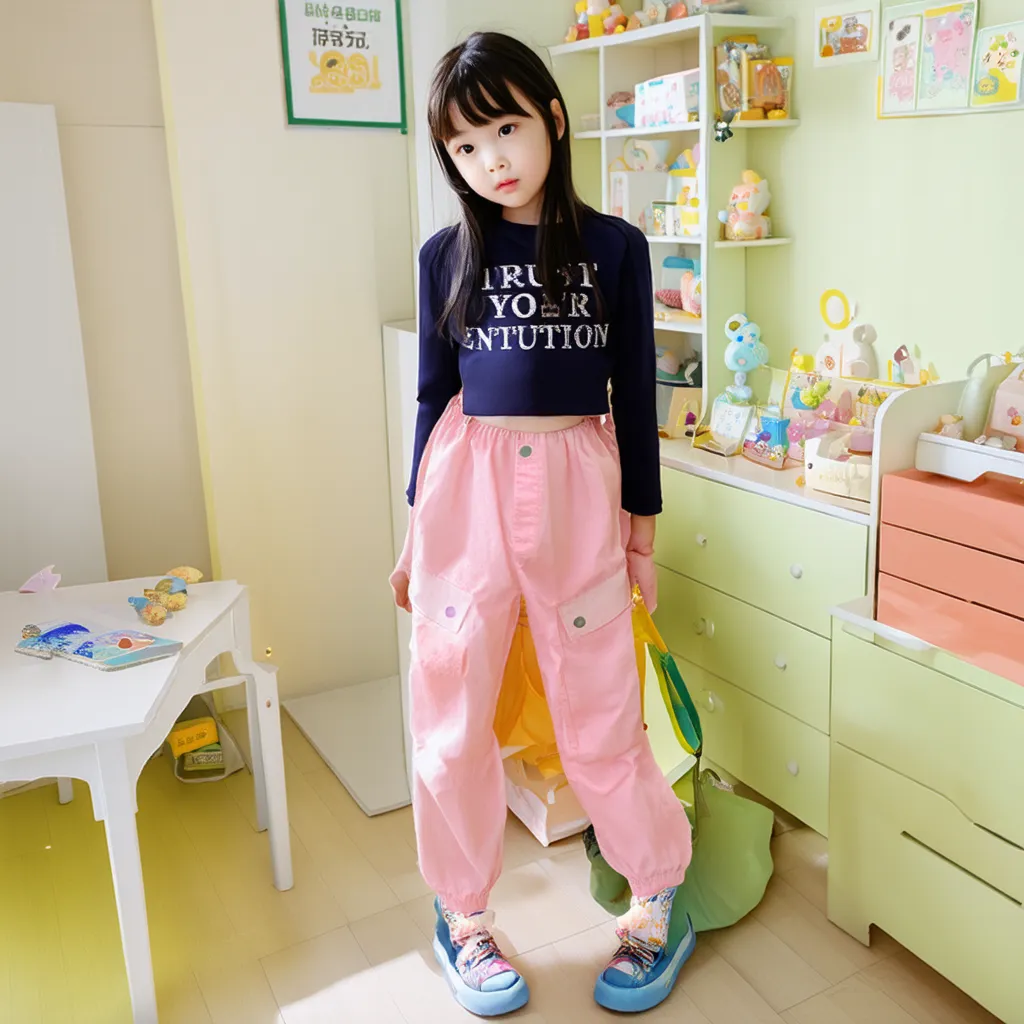
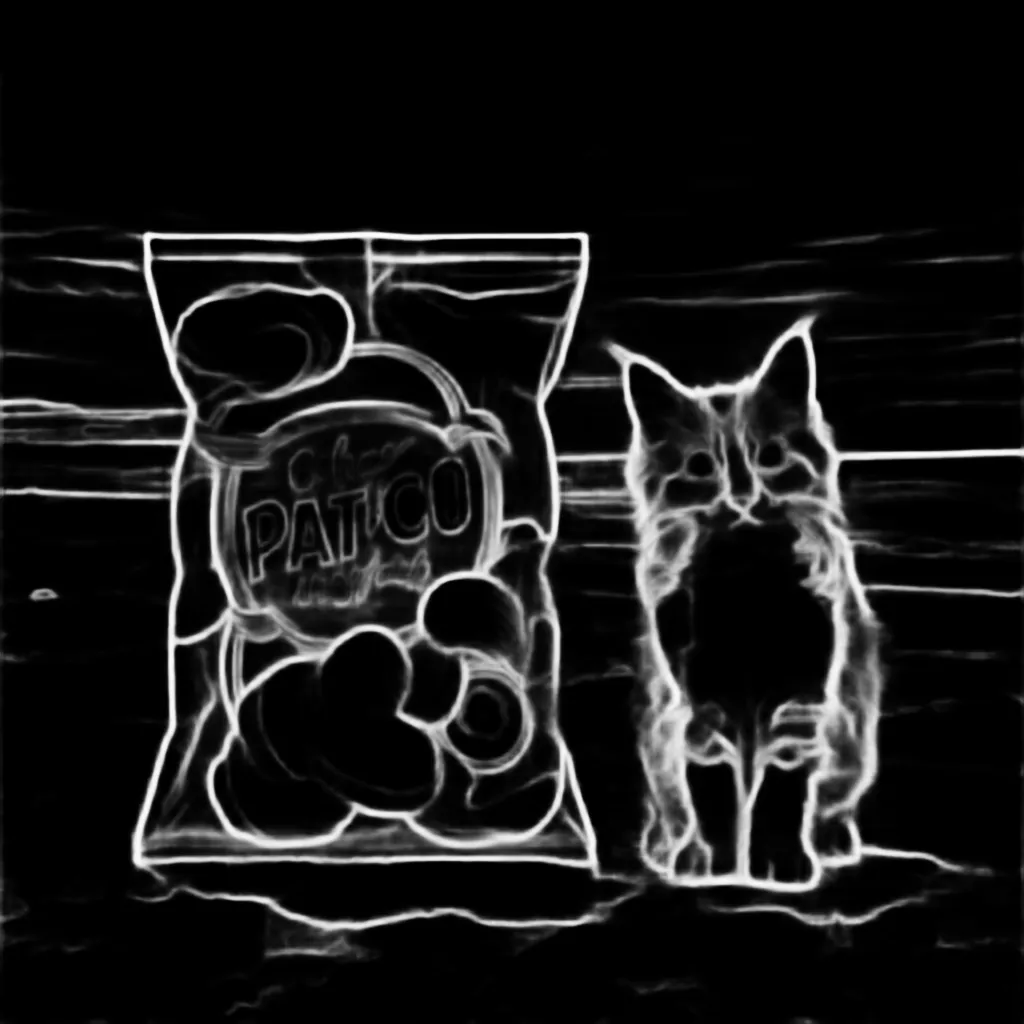 | 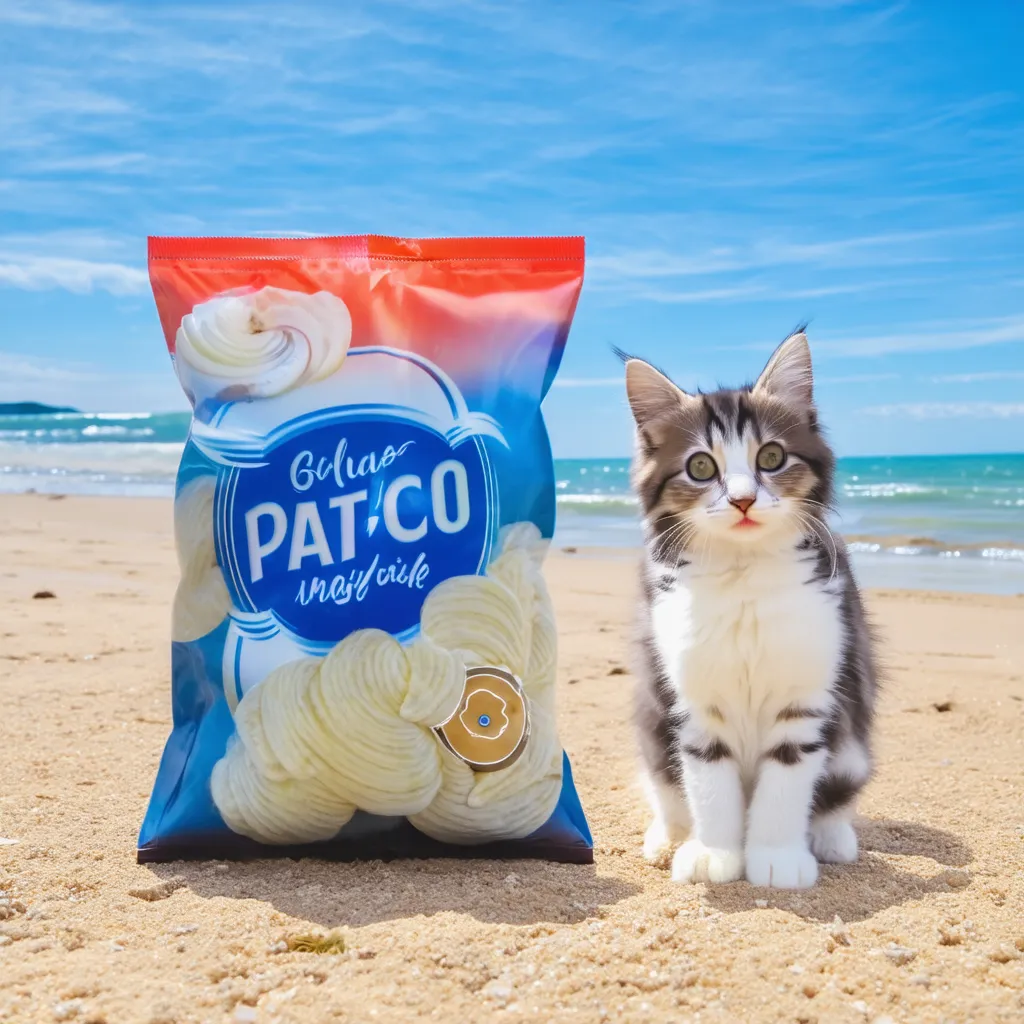 | 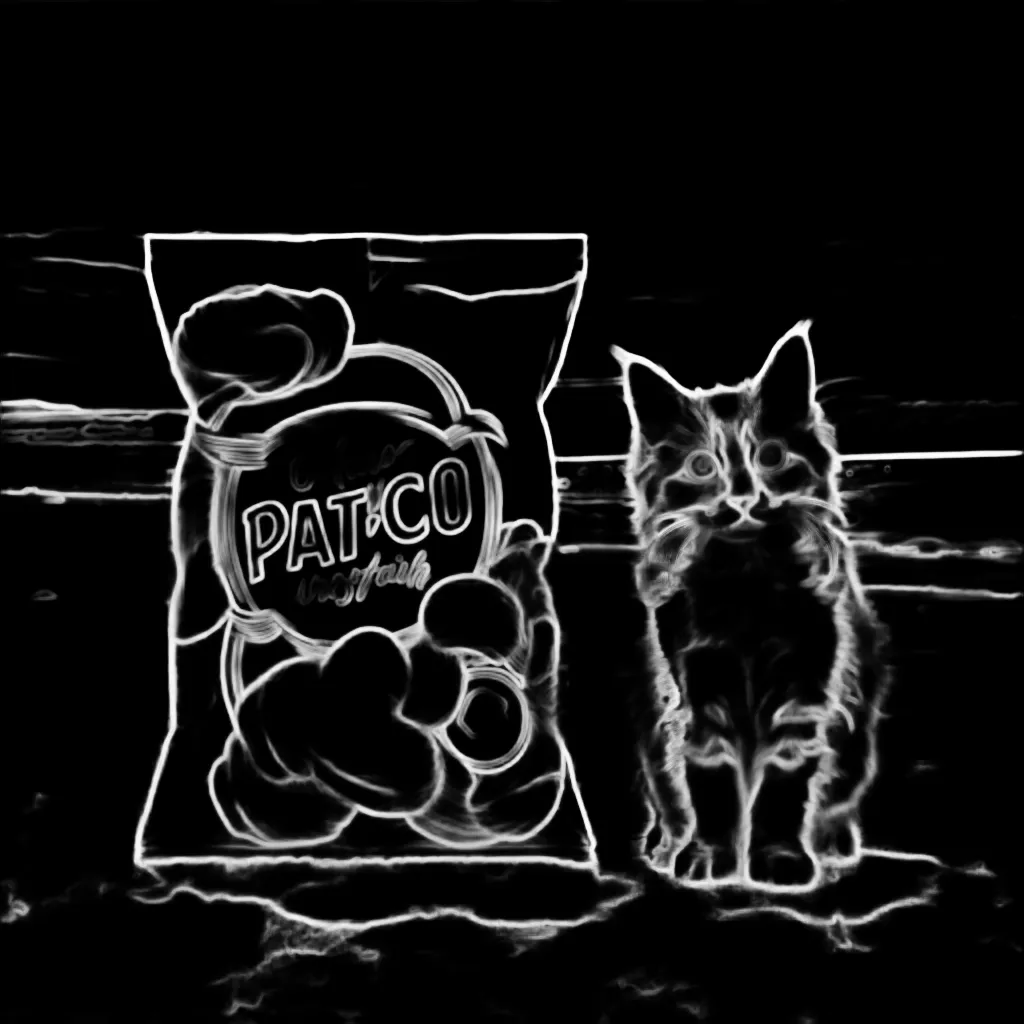 | 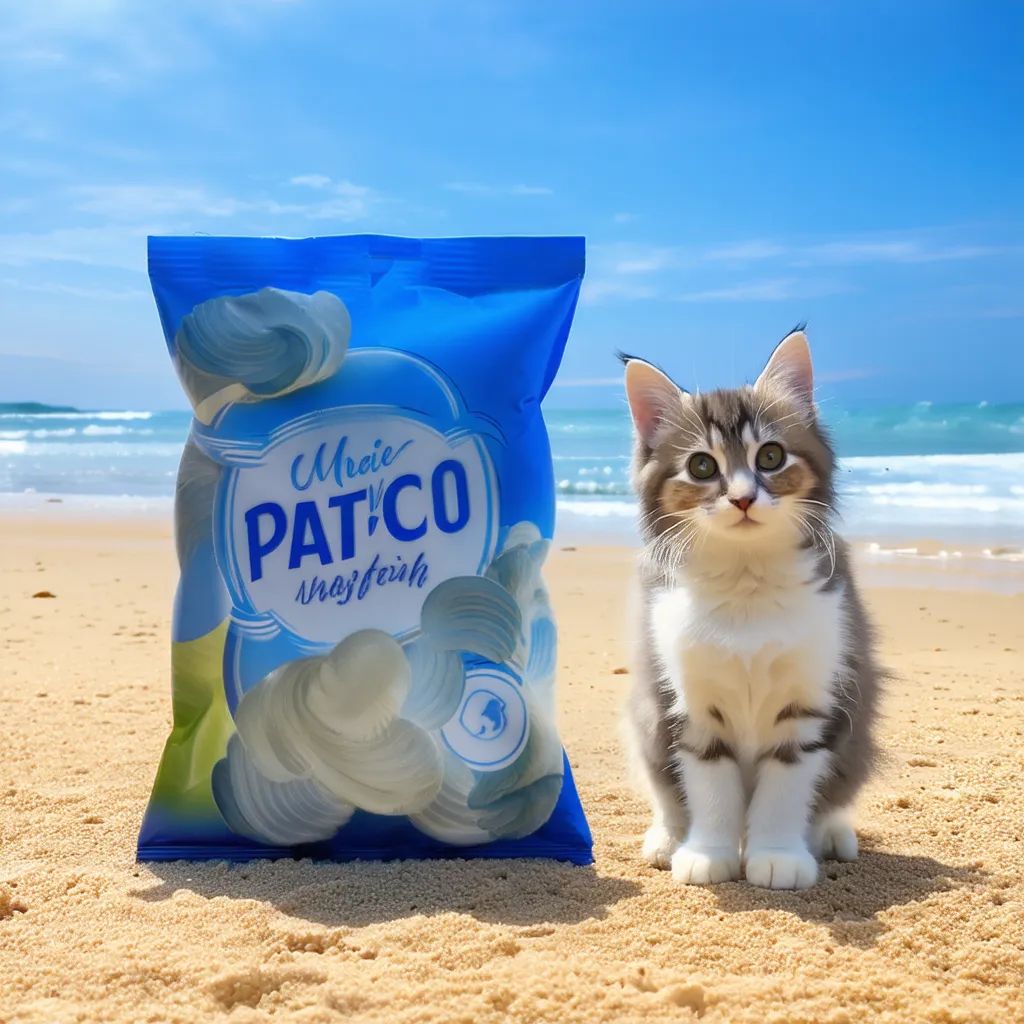
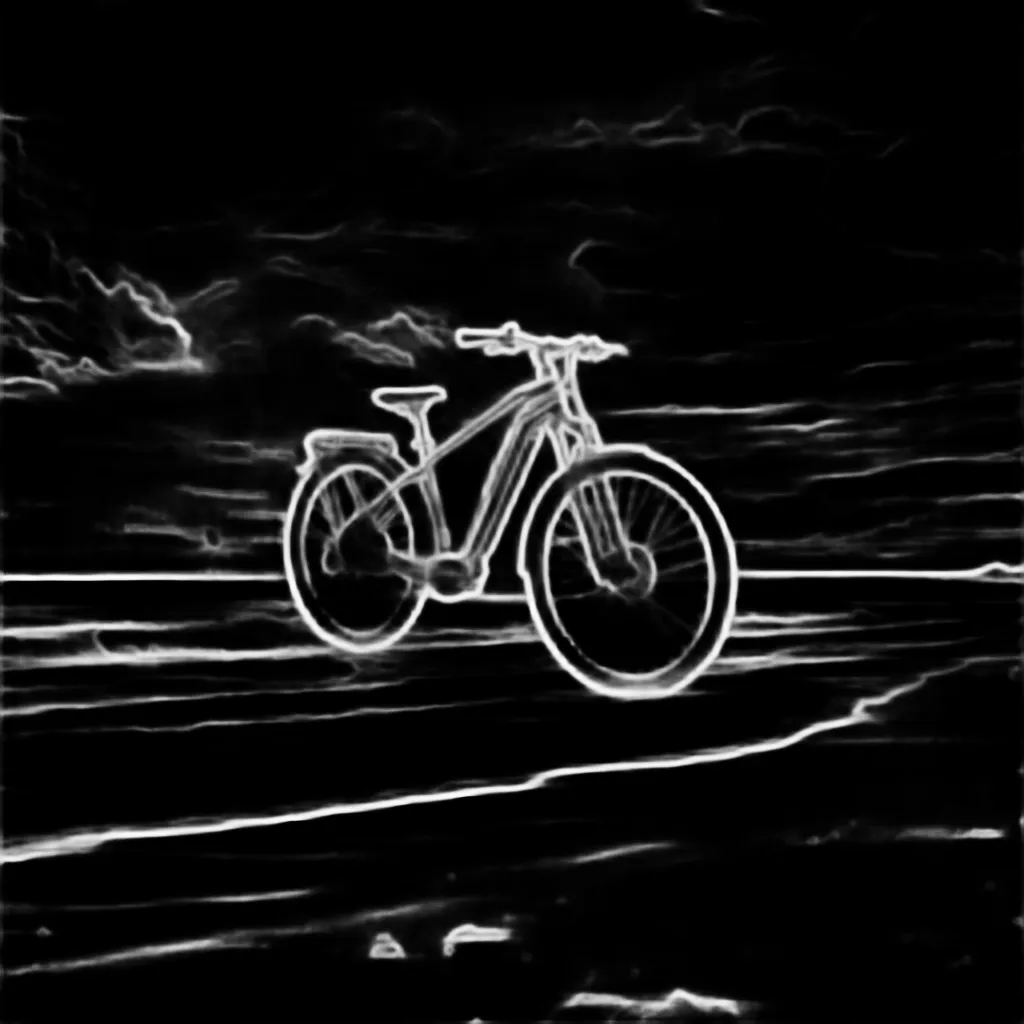 | 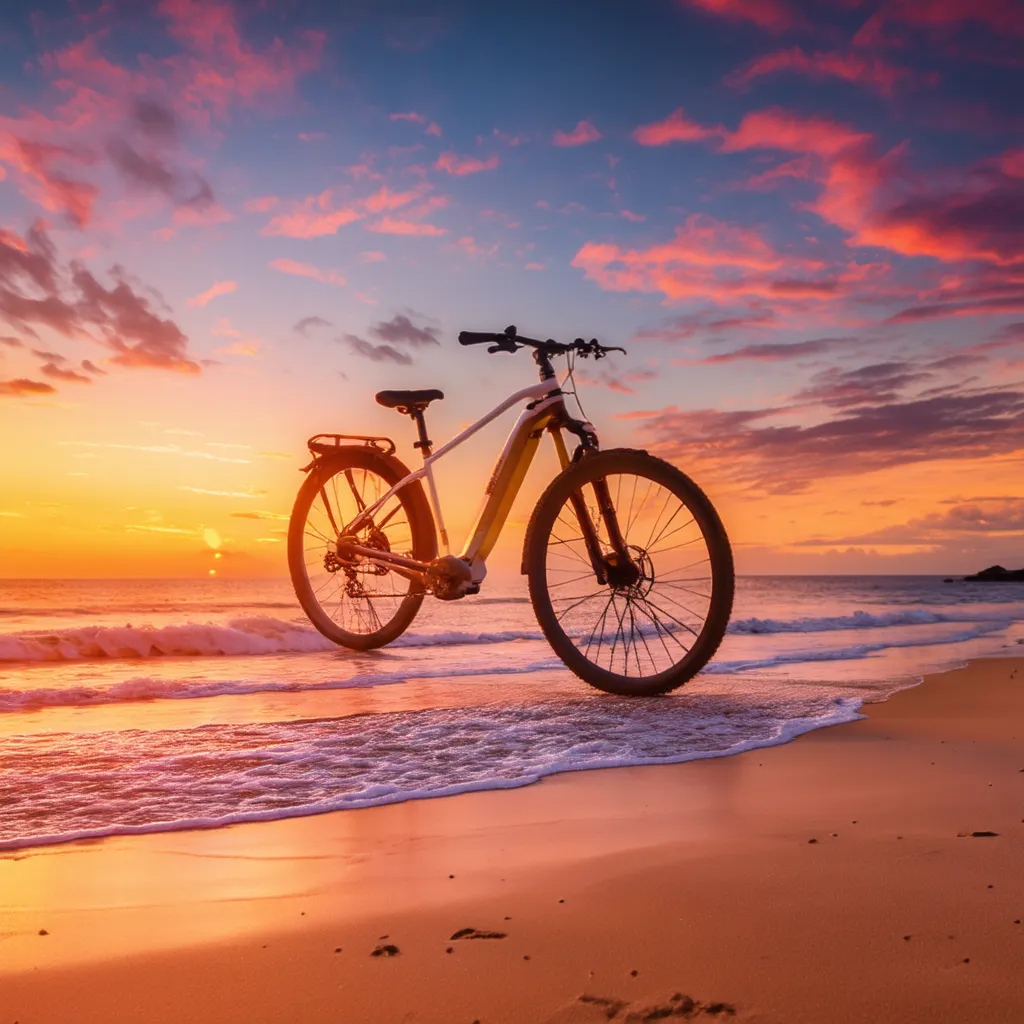 | 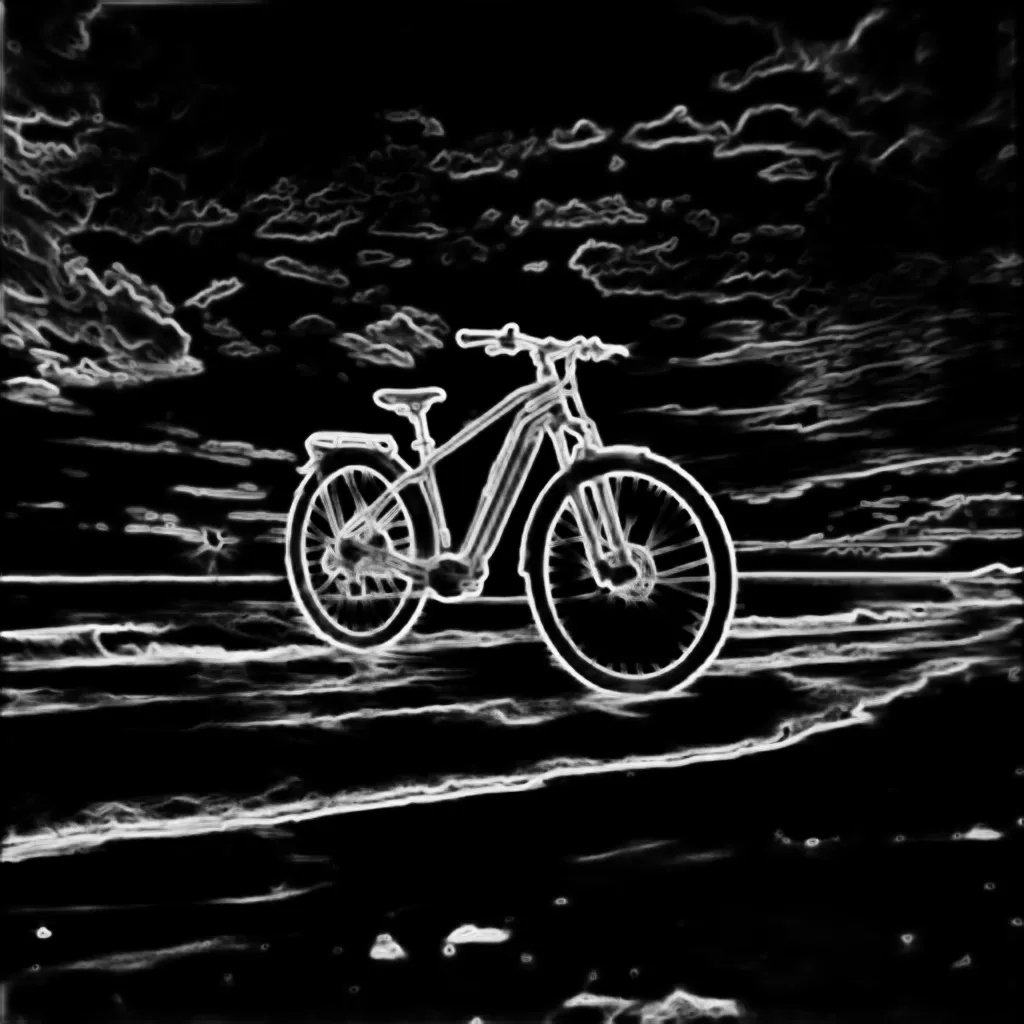 | 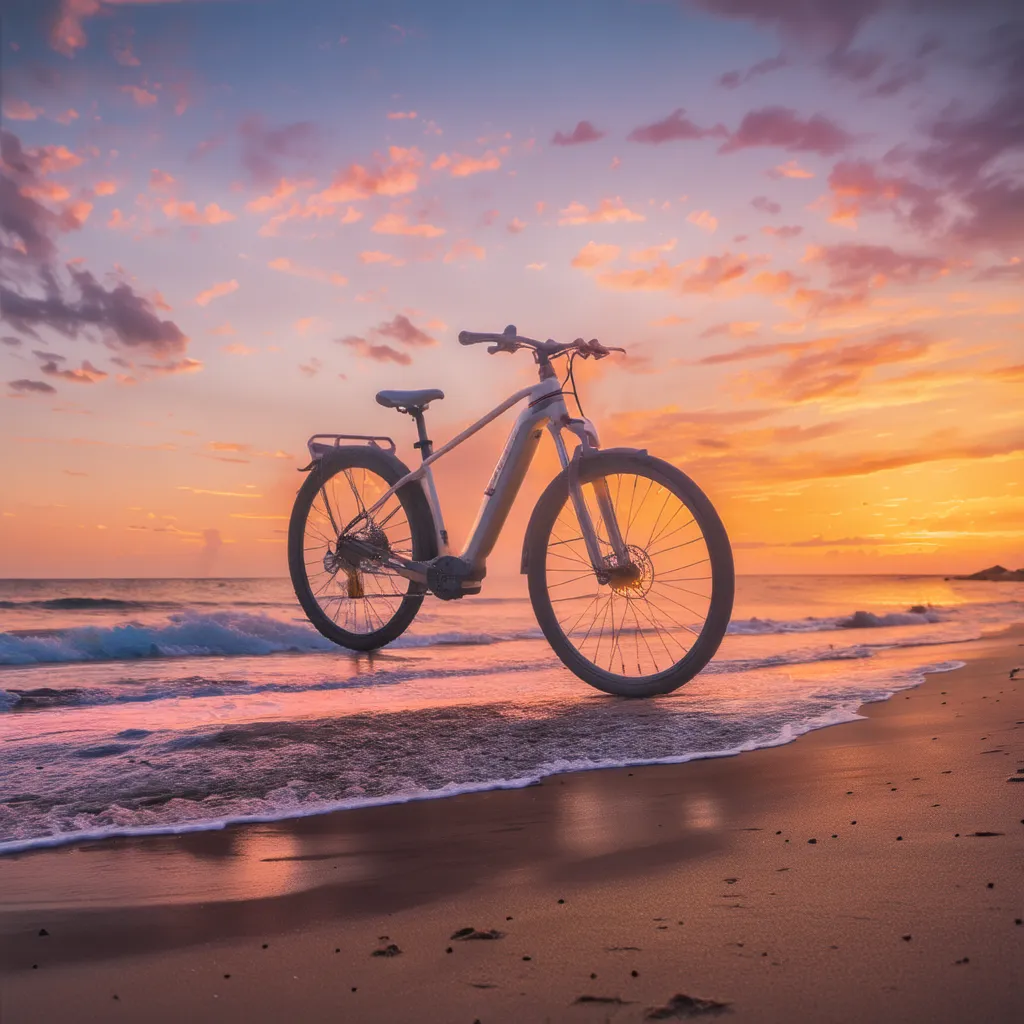
## Usage with Diffusers
```python
import torch
from diffusers.utils import load_image, check_min_version
from diffusers.models import SD3ControlNetModel
from diffusers import StableDiffusion3ControlNetPipeline
from controlnet_aux import PidiNetDetector
controlnet = SD3ControlNetModel.from_pretrained(
"alimama-creative/SD3-Controlnet-Softedge",torch_dtype=torch.float16
)
pipe = StableDiffusion3ControlNetPipeline.from_pretrained(
"stabilityai/stable-diffusion-3-medium-diffusers",
controlnet=controlnet,
variant="fp16",
torch_dtype=torch.float16,
)
pipe.text_encoder.to(torch.float16)
pipe.controlnet.to(torch.float16)
pipe.to("cuda")
image = load_image(
"https://huggingface.co/alimama-creative/SD3-Controlnet-Softedge/resolve/main/images/im1_0.png"
)
prompt = "A dog sitting on a park bench."
width = 1024
height = 1024
edge_processor = PidiNetDetector.from_pretrained('lllyasviel/Annotators')
edge_image = edge_processor(image, detect_resolution=width, image_resolution=width)
res_image = pipe(
prompt=prompt,
negative_prompt="deformed, distorted, disfigured, poorly drawn, bad anatomy, wrong anatomy, extra limb, missing limb, floating limbs, mutated hands and fingers, disconnected limbs, mutation, mutated, ugly, disgusting, blurry, amputation, NSFW",
height=height,
width=width,
control_image=edge_image,
num_inference_steps=25,
controlnet_conditioning_scale=0.95,
guidance_scale=5,
).images[0]
res_image.save("sd3.png")
```
## Training Detail
The model was trained on 12M laion2B and internal sources images with aesthetic 6+ for 20k steps at resolution 1024x1024. ControlNet with 6, 12 and 23 layers have been explored, and the 12-layer model achieves a good balance between performance and model size, so we release the 12-layer model.
Mixed precision : FP16
Learning rate : 1e-4
Batch size : 256
Timestep sampling mode : 'logit_normal'
Loss : Flow Matching
## LICENSE
The model is based on SD3 finetuning; therefore, the license follows the original SD3 license.