hexsha
stringlengths 40
40
| size
int64 6
14.9M
| ext
stringclasses 1
value | lang
stringclasses 1
value | max_stars_repo_path
stringlengths 6
260
| max_stars_repo_name
stringlengths 6
119
| max_stars_repo_head_hexsha
stringlengths 40
41
| max_stars_repo_licenses
sequence | max_stars_count
int64 1
191k
⌀ | max_stars_repo_stars_event_min_datetime
stringlengths 24
24
⌀ | max_stars_repo_stars_event_max_datetime
stringlengths 24
24
⌀ | max_issues_repo_path
stringlengths 6
260
| max_issues_repo_name
stringlengths 6
119
| max_issues_repo_head_hexsha
stringlengths 40
41
| max_issues_repo_licenses
sequence | max_issues_count
int64 1
67k
⌀ | max_issues_repo_issues_event_min_datetime
stringlengths 24
24
⌀ | max_issues_repo_issues_event_max_datetime
stringlengths 24
24
⌀ | max_forks_repo_path
stringlengths 6
260
| max_forks_repo_name
stringlengths 6
119
| max_forks_repo_head_hexsha
stringlengths 40
41
| max_forks_repo_licenses
sequence | max_forks_count
int64 1
105k
⌀ | max_forks_repo_forks_event_min_datetime
stringlengths 24
24
⌀ | max_forks_repo_forks_event_max_datetime
stringlengths 24
24
⌀ | avg_line_length
float64 2
1.04M
| max_line_length
int64 2
11.2M
| alphanum_fraction
float64 0
1
| cells
sequence | cell_types
sequence | cell_type_groups
sequence |
---|---|---|---|---|---|---|---|---|---|---|---|---|---|---|---|---|---|---|---|---|---|---|---|---|---|---|---|---|---|---|
e7834a89ee66cde83ed4458aab6b93b6970454a4 | 16,727 | ipynb | Jupyter Notebook | notebooks/Miscellaneous/Reshaping an Excel table.ipynb | xgrg/alfa | 50ca84428a53288f27ba39c9b8b51650a0a9f100 | [
"MIT"
] | null | null | null | notebooks/Miscellaneous/Reshaping an Excel table.ipynb | xgrg/alfa | 50ca84428a53288f27ba39c9b8b51650a0a9f100 | [
"MIT"
] | null | null | null | notebooks/Miscellaneous/Reshaping an Excel table.ipynb | xgrg/alfa | 50ca84428a53288f27ba39c9b8b51650a0a9f100 | [
"MIT"
] | null | null | null | 32.798039 | 123 | 0.343935 | [
[
[
"import pandas as pd",
"_____no_output_____"
]
],
[
[
"Show me the first lines of the original file",
"_____no_output_____"
]
],
[
[
"df = pd.read_excel('/tmp/gonzalo_test/aseg.xls')\ndf.head()",
"_____no_output_____"
]
],
[
[
"Show me the region names containing 'Vent' or 'WM' or 'Hippo'",
"_____no_output_____"
]
],
[
[
"names = set([each for each in df['StructName'].tolist() \\\n if 'WM' in each \n or 'Vent' in each \n or 'Hippo' in each])\nnames",
"_____no_output_____"
]
],
[
[
"Reshape the table and show me the first lines",
"_____no_output_____"
]
],
[
[
"df = pd.DataFrame(df[df['StructName'].isin(names)], columns=['subject', 'StructName', 'Volume_mm3'])\ndf = df.pivot(index='subject', columns='StructName', values='Volume_mm3')\ndf.head()",
"_____no_output_____"
]
],
[
[
"Save it and success !",
"_____no_output_____"
]
],
[
[
"df.to_excel('/tmp/gonzalo_test/aseg_pivot.xls')",
"_____no_output_____"
],
[
"from IPython.display import Image\nImage(url='http://s2.quickmeme.com/img/c3/c37a6cc5f88867e5387b8787aaf67afc350b3f37f357ed0a3088241488063bce.jpg')",
"_____no_output_____"
]
]
] | [
"code",
"markdown",
"code",
"markdown",
"code",
"markdown",
"code",
"markdown",
"code"
] | [
[
"code"
],
[
"markdown"
],
[
"code"
],
[
"markdown"
],
[
"code"
],
[
"markdown"
],
[
"code"
],
[
"markdown"
],
[
"code",
"code"
]
] |
e7836b88d3c4895484e8a199fb25bd73f2185dbd | 85,045 | ipynb | Jupyter Notebook | Regression_Analysis_Chemical_Process.ipynb | mohan-mj/Regression_Analysis | 783d086cb6fa9a3f1d40297b75e859bbb85ac6cf | [
"Apache-2.0"
] | 2 | 2018-04-19T01:40:28.000Z | 2018-04-25T13:47:35.000Z | Regression_Analysis_Chemical_Process.ipynb | mohan-mj/Regression_Analysis | 783d086cb6fa9a3f1d40297b75e859bbb85ac6cf | [
"Apache-2.0"
] | null | null | null | Regression_Analysis_Chemical_Process.ipynb | mohan-mj/Regression_Analysis | 783d086cb6fa9a3f1d40297b75e859bbb85ac6cf | [
"Apache-2.0"
] | 4 | 2019-04-02T16:30:22.000Z | 2019-11-10T04:13:35.000Z | 96.862187 | 18,474 | 0.730766 | [
[
[
"<a href=\"https://colab.research.google.com/github/mohan-mj/Regression_Analysis/blob/master/Regression_Analysis_Chemical_Process.ipynb\" target=\"_parent\"><img src=\"https://colab.research.google.com/assets/colab-badge.svg\" alt=\"Open In Colab\"/></a>",
"_____no_output_____"
],
[
"### The effect of temperature and reaction time affects the %yield. Develop a model for %yield in terms of temperature and time",
"_____no_output_____"
]
],
[
[
"import pandas as mypanda\nimport numpy as np\nfrom scipy import stats as mystats\nimport matplotlib.pyplot as myplot\nfrom pandas.plotting import scatter_matrix\nfrom statsmodels.formula.api import ols as myols\nfrom statsmodels.stats.anova import anova_lm",
"_____no_output_____"
],
[
"myData=mypanda.read_csv('datasets/Mult_Reg_Yield.csv')\nmyData",
"_____no_output_____"
],
[
"tmp=myData.Temperature\nyld =myData.Yield\ntime=myData.Time",
"_____no_output_____"
]
],
[
[
"##### check for relationship now",
"_____no_output_____"
]
],
[
[
"scatter_matrix(myData)\nmyplot.show()",
"C:\\ProgramData\\Anaconda3\\lib\\site-packages\\ipykernel_launcher.py:1: FutureWarning: 'pandas.tools.plotting.scatter_matrix' is deprecated, import 'pandas.plotting.scatter_matrix' instead.\n \"\"\"Entry point for launching an IPython kernel.\n"
]
],
[
[
"##### correlation between xs and y should be high",
"_____no_output_____"
]
],
[
[
"np.corrcoef(tmp,yld)",
"_____no_output_____"
],
[
"np.corrcoef(time,yld)",
"_____no_output_____"
],
[
"np.corrcoef(time,tmp)",
"_____no_output_____"
],
[
"mymodel=myols(\"yld ~ time + tmp\",myData)\nmymodel=mymodel.fit()\nmymodel.summary()",
"C:\\ProgramData\\Anaconda3\\lib\\site-packages\\scipy\\stats\\stats.py:1334: UserWarning: kurtosistest only valid for n>=20 ... continuing anyway, n=16\n \"anyway, n=%i\" % int(n))\n"
]
],
[
[
"##### check p value ==> only time is related to yield",
"_____no_output_____"
]
],
[
[
"mymodel=myols(\"yld ~ time \",myData).fit()\nmymodel.summary()",
"C:\\ProgramData\\Anaconda3\\lib\\site-packages\\scipy\\stats\\stats.py:1334: UserWarning: kurtosistest only valid for n>=20 ... continuing anyway, n=16\n \"anyway, n=%i\" % int(n))\n"
],
[
"pred=mymodel.predict()\nres=yld-pred\nres",
"_____no_output_____"
],
[
"#print(yld, res)",
"_____no_output_____"
],
[
"myplot.scatter(yld,pred)\nmyplot.show()",
"_____no_output_____"
],
[
"mystats.probplot(res,plot=myplot)\nmyplot.show()",
"_____no_output_____"
],
[
"mystats.normaltest(res)",
"C:\\ProgramData\\Anaconda3\\lib\\site-packages\\scipy\\stats\\stats.py:1334: UserWarning: kurtosistest only valid for n>=20 ... continuing anyway, n=16\n \"anyway, n=%i\" % int(n))\n"
]
],
[
[
"##### Implies it is normal",
"_____no_output_____"
]
],
[
[
"myplot.scatter(time,res)\nmyplot.show()",
"_____no_output_____"
],
[
"myplot.scatter(pred,res)\nmyplot.show()",
"_____no_output_____"
]
],
[
[
"##### random values /scattered plot means that the model is good.\nthere should not be any pattern in the plot. if pattern then there exists a better prediction using the method",
"_____no_output_____"
]
]
] | [
"markdown",
"code",
"markdown",
"code",
"markdown",
"code",
"markdown",
"code",
"markdown",
"code",
"markdown"
] | [
[
"markdown",
"markdown"
],
[
"code",
"code",
"code"
],
[
"markdown"
],
[
"code"
],
[
"markdown"
],
[
"code",
"code",
"code",
"code"
],
[
"markdown"
],
[
"code",
"code",
"code",
"code",
"code",
"code"
],
[
"markdown"
],
[
"code",
"code"
],
[
"markdown"
]
] |
e7837268c71241e21233ba3e25fa5a2ea7fcb03a | 438,691 | ipynb | Jupyter Notebook | pandasProjectCognizant/project_python_Pandas.ipynb | luizpavanello/cognizant_bootcamp_DIO | 89a50e2c57de3f55f87f2ae205c20f53882e581a | [
"MIT"
] | null | null | null | pandasProjectCognizant/project_python_Pandas.ipynb | luizpavanello/cognizant_bootcamp_DIO | 89a50e2c57de3f55f87f2ae205c20f53882e581a | [
"MIT"
] | null | null | null | pandasProjectCognizant/project_python_Pandas.ipynb | luizpavanello/cognizant_bootcamp_DIO | 89a50e2c57de3f55f87f2ae205c20f53882e581a | [
"MIT"
] | null | null | null | 76.121985 | 47,678 | 0.692857 | [
[
[
"<a href=\"https://colab.research.google.com/github/luizpavanello/cognizant_bootcamp_DIO/blob/master/project_python_Pandas.ipynb\" target=\"_parent\"><img src=\"https://colab.research.google.com/assets/colab-badge.svg\" alt=\"Open In Colab\"/></a>",
"_____no_output_____"
],
[
"#**Análise de Dados com Python e Pandas**",
"_____no_output_____"
]
],
[
[
"# Monta o drive no ambiente virtual permitindo acesso aos arquivos do drive\nfrom google.colab import drive\ndrive.mount('/content/drive')\n\n# Permite escolher um arquivo da máquina para upload no colab\nfrom google.colab import files\narq = files.upload()",
"_____no_output_____"
],
[
"from google.colab import drive\ndrive.mount('/content/drive')",
"Drive already mounted at /content/drive; to attempt to forcibly remount, call drive.mount(\"/content/drive\", force_remount=True).\n"
]
],
[
[
"###*Importando a biblioteca Pandas*",
"_____no_output_____"
]
],
[
[
"#importando a biblioteca Pandas\nimport pandas as pd",
"_____no_output_____"
]
],
[
[
"###*Lendo arquivos*",
"_____no_output_____"
]
],
[
[
"#Lendo CSV\ndf = pd.read_csv(\"/content/drive/MyDrive/Datasets/Gapminder.csv\", error_bad_lines=False, sep=\";\")",
"_____no_output_____"
],
[
"#Visualizando as 5 primeiras linhas\ndf.head()",
"_____no_output_____"
]
],
[
[
"###*Renomeando Colunas*",
"_____no_output_____"
]
],
[
[
"df = df.rename(columns={'country':'Country', 'continent':'Continent', 'year':'Year', 'lifeExp':'LifeExp', 'pop':'Population', 'gdpPercap':'PIB'})",
"_____no_output_____"
],
[
"df.head()",
"_____no_output_____"
]
],
[
[
"###*Trabalhando com Linhas e Colunas do arquivo*",
"_____no_output_____"
]
],
[
[
"#Quantidade de linhas e colunas dentro do arquivo\ndf.shape",
"_____no_output_____"
],
[
"#Nome das colunas\ndf.columns",
"_____no_output_____"
]
],
[
[
"",
"_____no_output_____"
]
],
[
[
"#Tipo de dado em ccada coluna\ndf.dtypes",
"_____no_output_____"
],
[
"#Últimas cindo linhas por padrao do arquivo (df.tail(10) → Últimas 10 linhas...)\ndf.tail()",
"_____no_output_____"
],
[
"#Média entre os dados das respectivas linhas e colunas\ndf.describe()",
"_____no_output_____"
]
],
[
[
"###*Trabalhando com Filtros*",
"_____no_output_____"
]
],
[
[
"df['Continent'].unique()",
"_____no_output_____"
],
[
"Oceania = df.loc[df['Continent'] == 'Oceania']\nOceania.head()",
"_____no_output_____"
],
[
"Oceania['Continent'].unique()",
"_____no_output_____"
],
[
"df.groupby('Continent')['Country'].nunique()",
"_____no_output_____"
],
[
"df.groupby('Year')['LifeExp'].mean()",
"_____no_output_____"
],
[
"df['PIB'].mean()",
"_____no_output_____"
],
[
"df['PIB'].sum()",
"_____no_output_____"
]
],
[
[
"# **Trabalhando com Planilhas de Excel**",
"_____no_output_____"
],
[
"### *Leitura dos Arquivos*",
"_____no_output_____"
]
],
[
[
"df1 = pd.read_excel(\"/content/drive/MyDrive/Datasets/Aracaju.xlsx\")\ndf2 = pd.read_excel(\"/content/drive/MyDrive/Datasets/Fortaleza.xlsx\")\ndf3 = pd.read_excel(\"/content/drive/MyDrive/Datasets/Natal.xlsx\")\ndf4 = pd.read_excel(\"/content/drive/MyDrive/Datasets/Recife.xlsx\")\ndf5 = pd.read_excel(\"/content/drive/MyDrive/Datasets/Salvador.xlsx\")",
"_____no_output_____"
],
[
"#Juntado todos os arquivos\ndf = pd.concat([df1, df2, df3, df4, df5])",
"_____no_output_____"
],
[
"#Exibindo as 5 primeiras linhas\ndf.head()",
"_____no_output_____"
],
[
"#Exibindo as 5 últimas linhas\ndf.tail()",
"_____no_output_____"
],
[
"df.sample(5)",
"_____no_output_____"
],
[
"#Verifincado o tipo de dado de cada coluna\ndf.dtypes",
"_____no_output_____"
],
[
"#Alterando o tipo de dado da coluna LojaID [int64 → object]\ndf['LojaID'] = df['LojaID'].astype('object')",
"_____no_output_____"
],
[
"df.dtypes",
"_____no_output_____"
]
],
[
[
"##***Tratando valores faltantes***",
"_____no_output_____"
]
],
[
[
"#Consultando linhas com valores faltantes\ndf.isnull().sum()",
"_____no_output_____"
],
[
"#Apagando as linhas com valores nulos\ndf.dropna(inplace=True)",
"_____no_output_____"
],
[
"#Apagando as linhas com valores nulos com base apenas em 1 coluna\ndf.dropna(subset=['Vendas'], inplace=True)",
"_____no_output_____"
],
[
"#Removendo linhas que estejam com valores faltantes em todas as colunas\ndf.dropna(how='all', inplace=True)",
"_____no_output_____"
]
],
[
[
"### ***Criando novas colunas***",
"_____no_output_____"
]
],
[
[
"#Criando a coluna de receita\ndf['Receita'] = df['Vendas'].mul(df['Qtde'])",
"_____no_output_____"
],
[
"df.head()",
"_____no_output_____"
],
[
"df.tail()",
"_____no_output_____"
],
[
"df['Receita/Venda'] = df['Receita'] / df['Vendas']",
"_____no_output_____"
],
[
"df.head()",
"_____no_output_____"
],
[
"#Retornando maior receita\ndf['Receita'].max()",
"_____no_output_____"
],
[
"#Retornando a menor receita\ndf['Receita'].min()",
"_____no_output_____"
],
[
"#nlargest\ndf.nlargest(3,'Receita')",
"_____no_output_____"
],
[
"#nsmallest\ndf.nsmallest(3, 'Receita')",
"_____no_output_____"
],
[
"#Agrupamento por cidade\ndf.groupby('Cidade')['Receita'].sum()",
"_____no_output_____"
],
[
"#Ordenando o conjunto de dados\ndf.sort_values('Receita', ascending=False).head(8)",
"_____no_output_____"
]
],
[
[
"# ***Trabalhando com datas***",
"_____no_output_____"
]
],
[
[
"#Transfomando a coluna de dataa em tipo inteiro\ndf['Data'] = df['Data'].astype('int64')",
"_____no_output_____"
],
[
"#Verificando o tipo de dado de cada coluna\ndf.dtypes",
"_____no_output_____"
],
[
"#Transformando a coluna de Data em Data\ndf['Data'] = pd.to_datetime(df['Data'])",
"_____no_output_____"
],
[
"df.dtypes",
"_____no_output_____"
],
[
"#Agrupamento por ano\ndf.groupby(df['Data'].dt.year)['Receita'].sum()",
"_____no_output_____"
],
[
"#Criado uma nova coluna com o ano\ndf['Ano_Venda'] = df['Data'].dt.year",
"_____no_output_____"
],
[
"df.sample(5)",
"_____no_output_____"
],
[
"#Extraindo o mes e o dia\ndf['mes_venda'], df['dia_venda'] = (df['Data'].dt.month, df['Data'].dt.day)",
"_____no_output_____"
],
[
"df.sample(5)",
"_____no_output_____"
],
[
"#Retornando a data mais antiga\ndf['Data'].min()",
"_____no_output_____"
],
[
"#Retornanoa data mais nova\ndf['Data'].max()",
"_____no_output_____"
],
[
"#Calculando a diferenca de dias\ndf['Diferenca_dias'] = df['Data'] - df['Data'].min()",
"_____no_output_____"
],
[
"df.sample(5)",
"_____no_output_____"
],
[
"#Criando a coluna de trimestre\ndf['Trimestre'] = df['Data'].dt.quarter",
"_____no_output_____"
],
[
"df.sample(5)",
"_____no_output_____"
],
[
"#Filtrando as vendas de 2019 do mes de janeiro\nvendas_jan_19 = df.loc[(df['Data'].dt.year == 2019) & (df['Data'].dt.month == 1)]",
"_____no_output_____"
],
[
"vendas_jan_19",
"_____no_output_____"
]
],
[
[
"# **Visualizacao de Dados**",
"_____no_output_____"
]
],
[
[
"df['LojaID'].value_counts(ascending=False)",
"_____no_output_____"
]
],
[
[
"### ***Gráficos***",
"_____no_output_____"
]
],
[
[
"#Gráfico de barras\ndf['LojaID'].value_counts(ascending=False).plot.bar();",
"_____no_output_____"
],
[
"#Gráfico de barras horizontais\ndf['LojaID'].value_counts().plot.barh();",
"_____no_output_____"
],
[
"#Gráfco de barras horizonatal\ndf['LojaID'].value_counts(ascending=True).plot.barh();",
"_____no_output_____"
],
[
"#Gráfico de Pizza\ndf.groupby(df['Data'].dt.year)['Receita'].sum().plot.pie();",
"_____no_output_____"
],
[
"#Total de vendas por cidade\ndf['Cidade'].value_counts()",
"_____no_output_____"
],
[
"#Adicionando um título e alterando o nome dos eixos\nimport matplotlib.pyplot as plt\ndf['Cidade'].value_counts().plot.bar(title='Total de vendas por Cidade')\nplt.xlabel('Cidade')\nplt.ylabel('Total de vendas');",
"_____no_output_____"
],
[
"",
"_____no_output_____"
],
[
"#Alterando a cor do gráfico\nimport matplotlib.pyplot as plt\ndf['Cidade'].value_counts().plot.bar(title='Total de vendas por Cidade', color='green')\nplt.xlabel('Cidade')\nplt.ylabel('Total de vendas');",
"_____no_output_____"
],
[
"#Editando o Estilo\nplt.style.use('ggplot')",
"_____no_output_____"
],
[
"df.groupby(df['mes_venda'])['Qtde'].sum().plot(title = 'Total de Vendas')\nplt.xlabel('Mes')\nplt.ylabel('Venda')\nplt.legend();",
"_____no_output_____"
],
[
"df.groupby(df['mes_venda'])['Qtde'].sum()",
"_____no_output_____"
],
[
"#Selecionando apenas as vendas de 2019\ndf_2019 = df[df['Ano_Venda'] == 2019]",
"_____no_output_____"
],
[
"df_2019",
"_____no_output_____"
],
[
"#Total vendidos por mes\ndf_2019.groupby(df_2019['mes_venda'])['Qtde'].sum().plot(marker = 'v')\nplt.xlabel('Mes')\nplt.ylabel('Total de Produtos Vendidos')\nplt.legend();",
"_____no_output_____"
],
[
"#Histograma\nplt.hist(df['Qtde'], color='darkturquoise');",
"_____no_output_____"
],
[
"plt.scatter(x=df_2019['dia_venda'], y = df_2019['Receita']);",
"_____no_output_____"
],
[
"#Salvando em png\ndf_2019.groupby(df_2019['mes_venda'])['Qtde'].sum().plot(marker = 'v')\nplt.title('Quantidade de produtos vendidos x mes')\nplt.xlabel('Mes')\nplt.ylabel('Total de Produtos Vendidos')\nplt.legend()\nplt.savefig('grafico Qtde x mes.png');",
"_____no_output_____"
]
],
[
[
"# **Análise Exploratória**",
"_____no_output_____"
]
],
[
[
"plt.style.use('seaborn')",
"_____no_output_____"
],
[
"#Upload de arquivo\nfrom google.colab import files\narq = files.upload()",
"_____no_output_____"
],
[
"#Criando nosso DataFrame\ndf = pd.read_excel(\"/content/drive/MyDrive/Datasets/AdventureWorks.xlsx\")",
"_____no_output_____"
],
[
"df.head()",
"_____no_output_____"
],
[
"#Quantidade de linhas e colunas\ndf.shape",
"_____no_output_____"
],
[
"#Verificando os tipos de dados\ndf.dtypes",
"_____no_output_____"
],
[
"#Qual a Receita total?\ndf['Valor Venda'].sum()",
"_____no_output_____"
],
[
"#Qual o Custo Total?\ndf['Custo'] = df['Custo Unitário'].mul(df['Quantidade']) #Criando a coluna de custo",
"_____no_output_____"
],
[
"df.head(1)",
"_____no_output_____"
],
[
"#Qual o custo Total?\nround(df['Custo'].sum(), 2)",
"_____no_output_____"
],
[
"#Agora que temos a receita, custo e total, podemos achar o Lucro Toal\n#Vamos criar uma coluna de Lucro que será Receia -Custo\ndf['Lucro'] = df['Valor Venda'] - df['Custo']",
"_____no_output_____"
],
[
"df.head(1)",
"_____no_output_____"
],
[
"#Total Lucro\nround(df['Lucro'].sum(), 2)",
"_____no_output_____"
],
[
"#Criando uma coluna com o total de dias para enviar o produto\ndf['Tempo_envio'] = df['Data Envio'] - df['Data Venda']",
"_____no_output_____"
],
[
"df.head(1)",
"_____no_output_____"
],
[
"#extraindo apenas os dias\ndf['Tempo_envio'] = (df['Data Envio'] - df['Data Venda']).dt.days",
"_____no_output_____"
],
[
"df.head(1)",
"_____no_output_____"
],
[
"#Verificando o tipo de coluna Tempo_envio\ndf['Tempo_envio'].dtype",
"_____no_output_____"
],
[
"#Média de tempo de envio por Marca\ndf.groupby('Marca')['Tempo_envio'].mean()",
"_____no_output_____"
],
[
"#Vaerificando se temos dados faltantes\ndf.isnull().sum()",
"_____no_output_____"
],
[
"#Agrupar por ano e Marca\ndf.groupby([df['Data Venda'].dt.year, 'Marca'])['Lucro'].sum()",
"_____no_output_____"
],
[
"#resetando o index\nlucro_ano = df.groupby([df['Data Venda'].dt.year, 'Marca'])['Lucro'].sum().reset_index()\nlucro_ano",
"_____no_output_____"
],
[
"#Qual o total de produtos vendidos\ndf.groupby('Produto')['Quantidade'].sum().sort_values(ascending=False)",
"_____no_output_____"
],
[
"#Gráfico Total de Produtos vendidos\ndf.groupby('Produto')['Quantidade'].sum().sort_values(ascending=True).plot.barh(title='Total Produtos Vendidos')\nplt.xlabel('Total')\nplt.ylabel('Produto');",
"_____no_output_____"
],
[
"#Selecionando apenas as vendas de 2009\ndf_2009 = df[df['Data Venda'].dt.year == 2009]",
"_____no_output_____"
],
[
"df_2009.head()",
"_____no_output_____"
],
[
"df_2009.groupby(df_2009[\"Data Venda\"].dt.month)[\"Lucro\"].sum().plot(title=\"Lucro x Mês\")\nplt.xlabel(\"Mês\")\nplt.ylabel(\"Lucro\");",
"_____no_output_____"
],
[
"df_2009.groupby(\"Marca\")[\"Lucro\"].sum().plot.bar(title=\"Lucro x Marca\")\nplt.xlabel(\"Marca\")\nplt.ylabel(\"Lucro\")\nplt.xticks(rotation='horizontal');",
"_____no_output_____"
],
[
"df[\"Tempo_envio\"].describe()",
"_____no_output_____"
],
[
"#Gráfico de Boxplot\nplt.boxplot(df[\"Tempo_envio\"]);",
"_____no_output_____"
],
[
"#Histograma\nplt.hist(df[\"Tempo_envio\"]);",
"_____no_output_____"
],
[
"#Tempo mínimo de envio\ndf[\"Tempo_envio\"].min()",
"_____no_output_____"
],
[
"#Tempo máximo de envio\ndf['Tempo_envio'].max()",
"_____no_output_____"
],
[
"#Identificando o Outlier\ndf[df[\"Tempo_envio\"] == 20]",
"_____no_output_____"
],
[
"df.to_csv('Project Python_Pandas.csv', index=False)",
"_____no_output_____"
],
[
"",
"_____no_output_____"
]
]
] | [
"markdown",
"code",
"markdown",
"code",
"markdown",
"code",
"markdown",
"code",
"markdown",
"code",
"markdown",
"code",
"markdown",
"code",
"markdown",
"code",
"markdown",
"code",
"markdown",
"code",
"markdown",
"code",
"markdown",
"code",
"markdown",
"code",
"markdown",
"code"
] | [
[
"markdown",
"markdown"
],
[
"code",
"code"
],
[
"markdown"
],
[
"code"
],
[
"markdown"
],
[
"code",
"code"
],
[
"markdown"
],
[
"code",
"code"
],
[
"markdown"
],
[
"code",
"code"
],
[
"markdown"
],
[
"code",
"code",
"code"
],
[
"markdown"
],
[
"code",
"code",
"code",
"code",
"code",
"code",
"code"
],
[
"markdown",
"markdown"
],
[
"code",
"code",
"code",
"code",
"code",
"code",
"code",
"code"
],
[
"markdown"
],
[
"code",
"code",
"code",
"code"
],
[
"markdown"
],
[
"code",
"code",
"code",
"code",
"code",
"code",
"code",
"code",
"code",
"code",
"code"
],
[
"markdown"
],
[
"code",
"code",
"code",
"code",
"code",
"code",
"code",
"code",
"code",
"code",
"code",
"code",
"code",
"code",
"code",
"code",
"code"
],
[
"markdown"
],
[
"code"
],
[
"markdown"
],
[
"code",
"code",
"code",
"code",
"code",
"code",
"code",
"code",
"code",
"code",
"code",
"code",
"code",
"code",
"code",
"code",
"code"
],
[
"markdown"
],
[
"code",
"code",
"code",
"code",
"code",
"code",
"code",
"code",
"code",
"code",
"code",
"code",
"code",
"code",
"code",
"code",
"code",
"code",
"code",
"code",
"code",
"code",
"code",
"code",
"code",
"code",
"code",
"code",
"code",
"code",
"code",
"code",
"code",
"code",
"code",
"code"
]
] |
e783bcc1f598e13c3d3d5401d171719558e1f0e0 | 1,014 | ipynb | Jupyter Notebook | hacker-rank/Data Structures/Linked Lists/Compare two linked lists.ipynb | izan-majeed/archives | 89af2a24f4a6f07bda8ee38d99ae8667d42727f4 | [
"Apache-2.0"
] | null | null | null | hacker-rank/Data Structures/Linked Lists/Compare two linked lists.ipynb | izan-majeed/archives | 89af2a24f4a6f07bda8ee38d99ae8667d42727f4 | [
"Apache-2.0"
] | null | null | null | hacker-rank/Data Structures/Linked Lists/Compare two linked lists.ipynb | izan-majeed/archives | 89af2a24f4a6f07bda8ee38d99ae8667d42727f4 | [
"Apache-2.0"
] | null | null | null | 19.882353 | 54 | 0.499014 | [
[
[
"def compare_lists(head1, head2):\n temp1, temp2 = head1, head2\n \n while temp1 or temp2:\n if not temp1 or not temp2: return 0\n if temp1.data != temp2.data: return 0\n temp1 = temp1.next\n temp2 = temp2.next\n\n return 1",
"_____no_output_____"
]
]
] | [
"code"
] | [
[
"code"
]
] |
e783bfc779b2f6deeef5c74e5eb7913d5be4987a | 63,125 | ipynb | Jupyter Notebook | notebooks/java/java-advanced_collection_data_types.ipynb | markprincely/interactive-notebooks | 934312074fa62099e0d0d23deffd55f31914550f | [
"MIT"
] | 1 | 2021-09-27T16:58:21.000Z | 2021-09-27T16:58:21.000Z | notebooks/java/java-advanced_collection_data_types.ipynb | markprincely/interactive-notebooks | 934312074fa62099e0d0d23deffd55f31914550f | [
"MIT"
] | null | null | null | notebooks/java/java-advanced_collection_data_types.ipynb | markprincely/interactive-notebooks | 934312074fa62099e0d0d23deffd55f31914550f | [
"MIT"
] | null | null | null | 44.579802 | 489 | 0.637624 | [
[
[
"# Aerospike Java Client – Advanced Collection Data Types\n*Last updated: June 22, 2021*\n\nThe goal of this tutorial is to highlight the power of working with [collection data types (CDTs)](\"https://docs.aerospike.com/docs/guide/cdt.html\") in Aerospike. It covers the following topics:\n1. Setting [contexts (CTXs)](\"https://docs.aerospike.com/docs/guide/cdt-context.html\") to apply operations to nested Maps and Lists.\n2. Showing the return type options provided by CDT get/read operations.\n3. Highlighting how policies shape application transactions.\n\nThis [Jupyter Notebook](https://jupyter-notebook.readthedocs.io/en/stable/notebook.html) requires the Aerospike Database running locally with Java kernel and Aerospike Java Client. To create a Docker container that satisfies the requirements and holds a copy of these notebooks, visit the [Aerospike Notebooks Repo](https://github.com/aerospike-examples/interactive-notebooks).",
"_____no_output_____"
],
[
"## Prerequisites\n\nThis Notebook builds on the material in the following notebooks:\n1. [Working with Lists](\"./java-working_with_lists.ipynb\") \n2. [Working with Maps](\"./java-working_with_lists.ipynb\")\n3. [Introduction to Transactions](\"./java-intro_to_transactions.ipynb\")\n\nIt uses examples based on those from [Modeling Using Lists](./java-modeling_using_lists.ipynb) and Working with Maps. If any of the following is confusing, please refer to a relevant notebook. ",
"_____no_output_____"
],
[
"# Notebook Setup",
"_____no_output_____"
],
[
"### Import Jupyter Java Integration \n\nMake it easier to work with Java in Jupyter.",
"_____no_output_____"
]
],
[
[
"import io.github.spencerpark.ijava.IJava;\nimport io.github.spencerpark.jupyter.kernel.magic.common.Shell;\n\nIJava.getKernelInstance().getMagics().registerMagics(Shell.class);",
"_____no_output_____"
]
],
[
[
"### Start Aerospike\n\nEnsure Aerospike Database is running locally.",
"_____no_output_____"
]
],
[
[
"%sh asd",
"_____no_output_____"
]
],
[
[
"### Download the Aerospike Java Client\n\nAsk Maven to download and install the project object model (POM) of the Aerospike Java Client.",
"_____no_output_____"
]
],
[
[
"%%loadFromPOM\n<dependencies>\n <dependency>\n <groupId>com.aerospike</groupId>\n <artifactId>aerospike-client</artifactId>\n <version>5.0.0</version>\n </dependency>\n</dependencies>",
"_____no_output_____"
]
],
[
[
"### Start the Aerospike Java Client and Connect\n\nCreate an instance of the Aerospike Java Client, and connect to the demo cluster.\n\nThe default cluster location for the Docker container is *localhost* port *3000*. If your cluster is not running on your local machine, modify *localhost* and *3000* to the values for your Aerospike cluster.",
"_____no_output_____"
]
],
[
[
"import com.aerospike.client.AerospikeClient;\n\nAerospikeClient client = new AerospikeClient(\"localhost\", 3000);\nSystem.out.println(\"Initialized the client and connected to the cluster.\");",
"Initialized the client and connected to the cluster.\n"
]
],
[
[
"# Create CDT Data, Put into Aerospike, and Print It",
"_____no_output_____"
]
],
[
[
"import com.aerospike.client.Key;\nimport com.aerospike.client.Bin;\nimport com.aerospike.client.policy.ClientPolicy;\nimport com.aerospike.client.Record;\nimport com.aerospike.client.Operation;\nimport com.aerospike.client.Value;\nimport com.aerospike.client.cdt.ListOperation;\nimport com.aerospike.client.cdt.ListPolicy;\nimport com.aerospike.client.cdt.ListOrder;\nimport com.aerospike.client.cdt.ListWriteFlags;\nimport com.aerospike.client.cdt.MapOperation;\nimport com.aerospike.client.cdt.MapPolicy;\nimport com.aerospike.client.cdt.MapOrder;\nimport com.aerospike.client.cdt.MapWriteFlags;\n\n\nimport java.util.ArrayList;\nimport java.util.Arrays;\nimport java.util.HashMap;\nimport java.util.List;\nimport java.util.Map;\n\n\n// Create whale migration list of tuples. \n\nArrayList<Value> whaleMigration0 = new ArrayList<Value>();\nwhaleMigration0.add(Value.get(1420));\nwhaleMigration0.add(Value.get(\"beluga whale\"));\nwhaleMigration0.add(Value.get(\"Beaufort Sea\"));\nwhaleMigration0.add(Value.get(\"Bering Sea\"));\n\nArrayList<Value> whaleMigration1 = new ArrayList<Value>();\nwhaleMigration1.add(Value.get(13988));\nwhaleMigration1.add(Value.get(\"gray whale\"));\nwhaleMigration1.add(Value.get(\"Baja California\"));\nwhaleMigration1.add(Value.get(\"Chukchi Sea\"));\n\nArrayList<Value> whaleMigration2 = new ArrayList<Value>();\nwhaleMigration2.add(Value.get(1278));\nwhaleMigration2.add(Value.get(\"north pacific right whale\"));\nwhaleMigration2.add(Value.get(\"Japan\"));\nwhaleMigration2.add(Value.get(\"Sea of Okhotsk\"));\n\nArrayList<Value> whaleMigration3 = new ArrayList<Value>();\nwhaleMigration3.add(Value.get(5100));\nwhaleMigration3.add(Value.get(\"humpback whale\"));\nwhaleMigration3.add(Value.get(\"Columbia\"));\nwhaleMigration3.add(Value.get(\"Antarctic Peninsula\"));\n\nArrayList<Value> whaleMigration4 = new ArrayList<Value>();\nwhaleMigration4.add(Value.get(3100));\nwhaleMigration4.add(Value.get(\"southern hemisphere blue whale\"));\nwhaleMigration4.add(Value.get(\"Corcovado Gulf\"));\nwhaleMigration4.add(Value.get(\"The Galapagos\"));\n\n\n\nArrayList<Value> whaleMigration = new ArrayList<Value>();\nwhaleMigration.add(Value.get(whaleMigration0));\nwhaleMigration.add(Value.get(whaleMigration1));\nwhaleMigration.add(Value.get(whaleMigration2));\nwhaleMigration.add(Value.get(whaleMigration3));\nwhaleMigration.add(Value.get(whaleMigration4));\n\n\n// Create Map of Whale Observations\n\nHashMap <Value, Value> mapObs = new HashMap <Value, Value>();\nHashMap <String, Integer> mapCoords0 = new HashMap <String, Integer>();\nmapCoords0.put(\"lat\", -85);\nmapCoords0.put(\"long\", -130);\nHashMap <String, Integer> mapCoords1 = new HashMap <String, Integer>();\nmapCoords1.put(\"lat\", -25);\nmapCoords1.put(\"long\", -50);\nHashMap <String, Integer> mapCoords2 = new HashMap <String, Integer>();\nmapCoords2.put(\"lat\", 35);\nmapCoords2.put(\"long\", 30);\n\n\nmapObs.put(Value.get(13456), Value.get(mapCoords1));\nmapObs.put(Value.get(14567), Value.get(mapCoords2));\nmapObs.put(Value.get(12345), Value.get(mapCoords0));\n\n\n// Put data in Aerospike, get the data, and print it\n\nString nestedCDTSetName = \"nestedset1\";\nString nestedCDTNamespaceName = \"test\";\n\nInteger whaleMigrationWriteFlags = ListWriteFlags.ADD_UNIQUE \n | ListWriteFlags.NO_FAIL \n | ListWriteFlags.PARTIAL;\nListPolicy whaleMigrationPolicy = new ListPolicy(ListOrder.UNORDERED, whaleMigrationWriteFlags);\nMapPolicy mapObsPolicy = new MapPolicy(MapOrder.KEY_ORDERED, MapWriteFlags.DEFAULT);\n\nInteger whaleKeyName = 2;\nString listWhaleBinName = \"listwhalebin\";\nString mapObsBinName = \"mapobsbin\";\n\nBin bin1 = new Bin(listWhaleBinName, whaleMigration);\n\nKey whaleKey = new Key(nestedCDTNamespaceName, nestedCDTSetName, whaleKeyName);\n\nRecord putDataIn = client.operate(client.writePolicyDefault, whaleKey,\n Operation.put(bin1),\n MapOperation.putItems(mapObsPolicy, mapObsBinName, mapObs)\n );\n\nSystem.out.println(listWhaleBinName + \": \" + whaleMigration + \"\\n\\n\" + \n mapObsBinName + \": \" + mapObs );",
"listwhalebin: [[1420, beluga whale, Beaufort Sea, Bering Sea], [13988, gray whale, Baja California, Chukchi Sea], [1278, north pacific right whale, Japan, Sea of Okhotsk], [5100, humpback whale, Columbia, Antarctic Peninsula], [3100, southern hemisphere blue whale, Corcovado Gulf, The Galapagos]]\n\nmapobsbin: {13456={lat=-25, long=-50}, 14567={lat=35, long=30}, 12345={lat=-85, long=-130}}\n"
]
],
[
[
"# Using Contexts (CTXs) to work with Nested CDTs\nWhat are Nested CDTs and CTXs?\n",
"_____no_output_____"
],
[
"## What is a Nested CDT?\nThe primary use case of Key-Value Stores, like Aerospike Database, is to store document-oriented data, like a JSON map. As document-oriented data grows organically, it is common for one CDT (list or map) to contain another CDT. Does the application need a list in a map in a list in a map? Aerospike fully supports nesting CDTs, so that’s no problem. ",
"_____no_output_____"
],
[
"## What is a Context?\n\nA Context (CTX) is a reference to a nested CDT, a List or Map that is stored in a List or Map somewhere in an Aerospike Bin. All [List](https://docs.aerospike.com/apidocs/java/com/aerospike/client/cdt/ListOperation.html) and [Map Operations](https://docs.aerospike.com/apidocs/java/com/aerospike/client/cdt/MapOperation.html) accept an optional CTX argument. Any CTX argument must refer to data of the type supported by the operation. \n\nThe most common ways to access a CTX are to look up a Map CTX directly by its key within the Bin and to drill down within a List or Map by index, rank or value. A CTX can also be created within a List or Map. For more details, see the [CTX APIs](https://docs.aerospike.com/apidocs/java/com/aerospike/client/cdt/CDT.html). ",
"_____no_output_____"
],
[
"## Look up a Map CTX in a Bin by Mapkey\n\nUse the `mapKey` method to look up a CTX in a Map directly by mapkey. This works for a Map anywhere in a Bin.\n\nThe following is an example of finding a Map CTX in a Bin by Mapkey:",
"_____no_output_____"
]
],
[
[
"import com.aerospike.client.cdt.CTX;\nimport com.aerospike.client.cdt.MapReturnType;\n\nInteger lookupMapKey = 14567;\nString latKeyName = \"lat\";\n\nRecord whaleSightings = client.operate(client.writePolicyDefault, whaleKey, \n MapOperation.getByKey(mapObsBinName, Value.get(latKeyName), MapReturnType.VALUE, CTX.mapKey(Value.get(lookupMapKey)))\n );\n\nSystem.out.println(mapObsBinName + \": \" + mapObs );\nSystem.out.println(\"The \" + latKeyName + \" of sighting at timestamp \" + lookupMapKey + \": \" + whaleSightings.getValue(mapObsBinName));",
"mapobsbin: {13456={lat=-25, long=-50}, 14567={lat=35, long=30}, 12345={lat=-85, long=-130}}\nThe lat of sighting at timestamp 14567: 35\n"
]
],
[
[
"## Drill down into a List or Map\nHere are the options to drill down into a CDT.\n\nDrilling down to a CTX in a List:\n* `listIndex`: Lookup list by index offset.\n* `listRank`: Lookup list by rank.\n* `listValue`: Lookup list by value.\n\nDrilling down to a CTX in a Map: \n* `mapIndex`: Lookup map by index offset.\n* `mapRank`: Lookup map by rank.\n* `mapValue`: Lookup map by value.\n\n\nThe following is an example of drilling down within a List and Map CTX:",
"_____no_output_____"
]
],
[
[
"import com.aerospike.client.cdt.ListReturnType;\n\n// CDT Drilldown Values\n\nInteger drilldownIndex = 2;\nInteger drilldownRank = 1;\nValue listDrilldownValue = Value.get(whaleMigration1);\nValue mapDrilldownValue = Value.get(mapCoords0);\n\n// Variables to access parts of the selected CDT.\n\nInteger getIndex = 1;\n\nRecord theRecord = client.get(null, whaleKey);\nRecord drilldown = client.operate(client.writePolicyDefault, whaleKey, \n ListOperation.getByIndex(listWhaleBinName, getIndex, MapReturnType.VALUE, CTX.listIndex(drilldownIndex)),\n ListOperation.getByIndex(listWhaleBinName, getIndex, MapReturnType.VALUE, CTX.listRank(drilldownRank)),\n ListOperation.getByIndex(listWhaleBinName, getIndex, MapReturnType.VALUE, CTX.listValue(listDrilldownValue)),\n MapOperation.getByIndex(mapObsBinName, getIndex, MapReturnType.VALUE, CTX.mapIndex(drilldownIndex)),\n MapOperation.getByIndex(mapObsBinName, getIndex, MapReturnType.VALUE, CTX.mapRank(drilldownRank)),\n MapOperation.getByIndex(mapObsBinName, getIndex, MapReturnType.VALUE, CTX.mapValue(mapDrilldownValue))\n );\n\nList<?> returnWhaleList = drilldown.getList(listWhaleBinName);\nList<?> returnObsList = drilldown.getList(mapObsBinName); \n\nSystem.out.println(\"The whale migration list is: \" + theRecord.getValue(listWhaleBinName) + \"\\n\");\nSystem.out.println(\"The whale name from the CTX selected by index \" + drilldownIndex + \": \" + returnWhaleList.get(0));\nSystem.out.println(\"The whale name from the CTX selected by rank \" + drilldownRank + \": \" + returnWhaleList.get(1));\nSystem.out.println(\"The whale name from the CTX selected by value \" + listDrilldownValue + \": \" + returnWhaleList.get(2) + \"\\n\\n\");\n\n\nSystem.out.println(\"The observation map is: \" + theRecord.getValue(mapObsBinName) + \"\\n\");\nSystem.out.println(\"The longitude of the observation from the CTX selected by index \" + drilldownIndex + \": \" + returnObsList.get(0));\nSystem.out.println(\"The longitude of the observation from the CTX selected by rank \" + drilldownRank + \": \" + returnObsList.get(1));\nSystem.out.println(\"The longitude of the observation from the CTX selected by value \" + mapDrilldownValue + \": \" + returnObsList.get(2));\n",
"The whale migration list is: [[1420, beluga whale, Beaufort Sea, Bering Sea], [13988, gray whale, Baja California, Chukchi Sea], [1278, north pacific right whale, Japan, Sea of Okhotsk], [5100, humpback whale, Columbia, Antarctic Peninsula], [3100, southern hemisphere blue whale, Corcovado Gulf, The Galapagos]]\n\nThe whale name from the CTX selected by index 2: north pacific right whale\nThe whale name from the CTX selected by rank 1: beluga whale\nThe whale name from the CTX selected by value [13988, gray whale, Baja California, Chukchi Sea]: gray whale\n\n\nThe observation map is: {12345={lat=-85, long=-130}, 13456={lat=-25, long=-50}, 14567={lat=35, long=30}}\n\nThe longitude of the observation from the CTX selected by index 2: 30\nThe longitude of the observation from the CTX selected by rank 1: -50\nThe longitude of the observation from the CTX selected by value {lat=-85, long=-130}: -130\n"
]
],
[
[
"## Create a CTX Example\nIf the context for the operation does not yet exist, it can be created using the following methods.\n\nCreating a CTX in a List or Map:\n* `listIndexCreate`: Create list by base list's index offset.\n* `mapKeyCreate`: Create map by base map's key.\n\nThe following are examples of creating a list and map CTX and then writing data to the new CTX. ",
"_____no_output_____"
]
],
[
[
"ArrayList<Value> newWhaleMigration = new ArrayList<Value>();\nnewWhaleMigration.add(Value.get(1449));\nnewWhaleMigration.add(Value.get(\"sei whale\"));\nnewWhaleMigration.add(Value.get(\"Greenland\"));\nnewWhaleMigration.add(Value.get(\"Gulf of Maine\"));\n\nInteger whaleIndex = 5;\n\nHashMap <Value, Value> mapCoords3 = new HashMap <Value, Value>();\nmapCoords3.put(Value.get(\"lat\"), Value.get(95));\nmapCoords3.put(Value.get(\"long\"), Value.get(110));\n\n\nInteger newObsKey = 15678;\n\n\nRecord createCTX = client.operate(client.writePolicyDefault, whaleKey, \n ListOperation.insertItems(listWhaleBinName, 0, newWhaleMigration, CTX.listIndexCreate(whaleIndex, ListOrder.UNORDERED, true)),\n MapOperation.putItems(mapObsPolicy, mapObsBinName, mapCoords3, CTX.mapKeyCreate(Value.get(newObsKey), MapOrder.KEY_ORDERED))\n );\n\nRecord postCreate = client.get(null, whaleKey);\n\nSystem.out.println(\"Before, the whale migration list was: \" + theRecord.getValue(listWhaleBinName) + \"\\n\");\nSystem.out.println(\"After the addition, it is:\" + postCreate.getValue(listWhaleBinName) + \"\\n\\n\");\n\nSystem.out.println(\"Before, the observation map was: \" + theRecord.getValue(mapObsBinName) + \"\\n\");\nSystem.out.println(\"After the addition, it is: \" + postCreate.getValue(mapObsBinName));",
"Before, the whale migration list was: [[1420, beluga whale, Beaufort Sea, Bering Sea], [13988, gray whale, Baja California, Chukchi Sea], [1278, north pacific right whale, Japan, Sea of Okhotsk], [5100, humpback whale, Columbia, Antarctic Peninsula], [3100, southern hemisphere blue whale, Corcovado Gulf, The Galapagos]]\n\nAfter the addition, it is:[[1420, beluga whale, Beaufort Sea, Bering Sea], [13988, gray whale, Baja California, Chukchi Sea], [1278, north pacific right whale, Japan, Sea of Okhotsk], [5100, humpback whale, Columbia, Antarctic Peninsula], [3100, southern hemisphere blue whale, Corcovado Gulf, The Galapagos], [1449, sei whale, Greenland, Gulf of Maine]]\n\n\nBefore, the observation map was: {12345={lat=-85, long=-130}, 13456={lat=-25, long=-50}, 14567={lat=35, long=30}}\n\nAfter the addition, it is: {12345={lat=-85, long=-130}, 13456={lat=-25, long=-50}, 14567={lat=35, long=30}, 15678={lat=95, long=110}}\n"
]
],
[
[
"# Choosing the Return Type Options for CDTs\nOperations on CDTs can return different types of data, depending on the return type value specified. A return type can be combined with the INVERTED flag to return all data from the CDT that was not selected by the operation. The following are the [Return Types for Lists](https://docs.aerospike.com/apidocs/java/com/aerospike/client/cdt/ListReturnType.html) and [Maps](https://docs.aerospike.com/apidocs/java/com/aerospike/client/cdt/MapReturnType.html).",
"_____no_output_____"
],
[
"## Standard Return Type Options for CDTs\nAerospike Lists and Maps both provide the following return type options.\n\n* `COUNT`: Return count of items selected.\n* `INDEX`: Return index offset order.\n* `NONE`: Do not return a result.\n* `RANK`: Return value order. If the list/map is not ordered, Aerospike will JIT-sort the list/map.\n* `REVERSE_INDEX`: Return reverse index offset order.\n* `REVERSE_RANK`: Return value order from a version of the list sorted from maximum to minimum value. If the list is not ordered, Aerospike will JIT-sort the list. \n* `VALUE`: Return value for single item read and list of values from a range read.\n\nAll indexes are 0-based, with the last element accessible by index -1. \n\nThe following is an example demonstrating each possible return type from the same operation.",
"_____no_output_____"
]
],
[
[
"ArrayList<Value> lowTuple = new ArrayList<Value>();\nlowTuple.add(Value.get(1400));\nlowTuple.add(Value.NULL);\n\nArrayList<Value> highTuple = new ArrayList<Value>();\nhighTuple.add(Value.get(3500));\nhighTuple.add(Value.NULL);\n\nRecord between1400and3500 = client.operate(client.writePolicyDefault, whaleKey, \n ListOperation.getByValueRange(listWhaleBinName, Value.get(lowTuple), Value.get(highTuple), \n ListReturnType.COUNT),\n ListOperation.getByValueRange(listWhaleBinName, Value.get(lowTuple), Value.get(highTuple), \n ListReturnType.INDEX), \n ListOperation.getByValueRange(listWhaleBinName, Value.get(lowTuple), Value.get(highTuple), \n ListReturnType.NONE), \n ListOperation.getByValueRange(listWhaleBinName, Value.get(lowTuple), Value.get(highTuple), \n ListReturnType.RANK), \n ListOperation.getByValueRange(listWhaleBinName, Value.get(lowTuple), Value.get(highTuple), \n ListReturnType.REVERSE_INDEX), \n ListOperation.getByValueRange(listWhaleBinName, Value.get(lowTuple), Value.get(highTuple), \n ListReturnType.REVERSE_RANK), \n ListOperation.getByValueRange(listWhaleBinName, Value.get(lowTuple), Value.get(highTuple), \n ListReturnType.VALUE) \n );\n\nList<?> returnWhaleRange = between1400and3500.getList(listWhaleBinName);\n\nSystem.out.println(\"The current whale migration list is: \" + postCreate.getValue(listWhaleBinName) + \"\\n\");\nSystem.out.println(\"For the whales who migrate between 1400 and 3500 miles...\");\nSystem.out.println(\"Return COUNT: \" + returnWhaleRange.get(0));\nSystem.out.println(\"Return INDEX: \" + returnWhaleRange.get(1));\nSystem.out.println(\"Return NONE: has no return value.\");\nSystem.out.println(\"Return RANK: \" + returnWhaleRange.get(2));\nSystem.out.println(\"Return REVERSE_INDEX: \" + returnWhaleRange.get(3));\nSystem.out.println(\"Return REVERSE_RANK: \" + returnWhaleRange.get(4));\nSystem.out.println(\"Return Values: \" + returnWhaleRange.get(5));",
"The current whale migration list is: [[1420, beluga whale, Beaufort Sea, Bering Sea], [13988, gray whale, Baja California, Chukchi Sea], [1278, north pacific right whale, Japan, Sea of Okhotsk], [5100, humpback whale, Columbia, Antarctic Peninsula], [3100, southern hemisphere blue whale, Corcovado Gulf, The Galapagos], [1449, sei whale, Greenland, Gulf of Maine]]\n\nFor the whales who migrate between 1400 and 3500 miles...\nReturn COUNT: 3\nReturn INDEX: [0, 4, 5]\nReturn NONE: has no return value.\nReturn RANK: [1, 2, 3]\nReturn REVERSE_INDEX: [5, 1, 0]\nReturn REVERSE_RANK: [2, 3, 4]\nReturn Values: [[1420, beluga whale, Beaufort Sea, Bering Sea], [3100, southern hemisphere blue whale, Corcovado Gulf, The Galapagos], [1449, sei whale, Greenland, Gulf of Maine]]\n"
]
],
[
[
"## Additional Return Type Options for Maps\nBecause Maps have a replicable key/value structure, Aerospike provides options to return mapkeys or key/value pairs, in addition to value.\n\n* `KEY`: Return key for single key read and key list for range read.\n* `KEY_VALUE`: Return key/value pairs for items.\n\nThe following is an example demonstrating returning a key or key/value pair.",
"_____no_output_____"
]
],
[
[
"Integer latestObsRank = -1;\n\nRecord latestWhaleObs = client.operate(client.writePolicyDefault, whaleKey, \n MapOperation.getByRank(mapObsBinName, latestObsRank, MapReturnType.KEY),\n MapOperation.getByRank(mapObsBinName, latestObsRank, MapReturnType.KEY_VALUE)\n );\n\nList<?> latestObs = latestWhaleObs.getList(mapObsBinName);\n\nSystem.out.println(\"The current whale observations map is: \" + postCreate.getValue(mapObsBinName) + \"\\n\");\nSystem.out.println(\"For the most recent observation...\");\nSystem.out.println(\"Return the key: \" + latestObs.get(0));\nSystem.out.println(\"Return key/value pair: \" + latestObs.get(1));",
"The current whale observations map is: {12345={lat=-85, long=-130}, 13456={lat=-25, long=-50}, 14567={lat=35, long=30}, 15678={lat=95, long=110}}\n\nFor the most recent observation...\nReturn the key: 15678\nReturn key/value pair: [15678={lat=95, long=110}]\n"
]
],
[
[
"## Invert the Operation Results for CDT Operations \nAerospike also provides the `INVERTED` flag for CDT operations. When `INVERTED` is “logical or”-ed to the return type, the flag instructs a list or map operation to return the return type data for list or Map elements that were not selected by the operation. This flag instructs an operation to act as though a logical NOT operator was applied to the entire operation. \n\nThe following is an example demonstrating inverted return values.\n",
"_____no_output_____"
]
],
[
[
"ArrayList<Value> lowTuple = new ArrayList<Value>();\nlowTuple.add(Value.get(1400));\nlowTuple.add(Value.NULL);\n\nArrayList<Value> highTuple = new ArrayList<Value>();\nhighTuple.add(Value.get(3500));\nhighTuple.add(Value.NULL);\n\nRecord between1400and3500 = client.operate(client.writePolicyDefault, whaleKey, \n ListOperation.getByValueRange(listWhaleBinName, Value.get(lowTuple), Value.get(highTuple), \n ListReturnType.COUNT | ListReturnType.INVERTED),\n ListOperation.getByValueRange(listWhaleBinName, Value.get(lowTuple), Value.get(highTuple), \n ListReturnType.INDEX | ListReturnType.INVERTED), \n ListOperation.getByValueRange(listWhaleBinName, Value.get(lowTuple), Value.get(highTuple), \n ListReturnType.NONE | ListReturnType.INVERTED), \n ListOperation.getByValueRange(listWhaleBinName, Value.get(lowTuple), Value.get(highTuple), \n ListReturnType.RANK | ListReturnType.INVERTED), \n ListOperation.getByValueRange(listWhaleBinName, Value.get(lowTuple), Value.get(highTuple), \n ListReturnType.REVERSE_INDEX | ListReturnType.INVERTED), \n ListOperation.getByValueRange(listWhaleBinName, Value.get(lowTuple), Value.get(highTuple), \n ListReturnType.REVERSE_RANK | ListReturnType.INVERTED), \n ListOperation.getByValueRange(listWhaleBinName, Value.get(lowTuple), Value.get(highTuple), \n ListReturnType.VALUE | ListReturnType.INVERTED) \n );\n\nList<?> returnWhaleRange = between1400and3500.getList(listWhaleBinName);\n\nSystem.out.println(\"The current whale migration list is: \" + postCreate.getValue(listWhaleBinName) + \"\\n\");\nSystem.out.println(\"For the whales who migrate between 1400 and 3500 miles...\");\nSystem.out.println(\"Return INVERTED COUNT: \" + returnWhaleRange.get(0));\nSystem.out.println(\"Return INVERTED INDEX: \" + returnWhaleRange.get(1));\nSystem.out.println(\"Return INVERTED NONE: has no return value.\");\nSystem.out.println(\"Return INVERTED RANK: \" + returnWhaleRange.get(2));\nSystem.out.println(\"Return INVERTED REVERSE_INDEX: \" + returnWhaleRange.get(3));\nSystem.out.println(\"Return INVERTED REVERSE_RANK: \" + returnWhaleRange.get(4));\nSystem.out.println(\"Return INVERTED Values: \" + returnWhaleRange.get(5));",
"The current whale migration list is: [[1420, beluga whale, Beaufort Sea, Bering Sea], [13988, gray whale, Baja California, Chukchi Sea], [1278, north pacific right whale, Japan, Sea of Okhotsk], [5100, humpback whale, Columbia, Antarctic Peninsula], [3100, southern hemisphere blue whale, Corcovado Gulf, The Galapagos], [1449, sei whale, Greenland, Gulf of Maine]]\n\nFor the whales who migrate between 1400 and 3500 miles...\nReturn INVERTED COUNT: 3\nReturn INVERTED INDEX: [1, 2, 3]\nReturn INVERTED NONE: has no return value.\nReturn INVERTED RANK: [0, 4, 5]\nReturn INVERTED REVERSE_INDEX: [4, 3, 2]\nReturn INVERTED REVERSE_RANK: [5, 0, 1]\nReturn INVERTED Values: [[13988, gray whale, Baja California, Chukchi Sea], [1278, north pacific right whale, Japan, Sea of Okhotsk], [5100, humpback whale, Columbia, Antarctic Peninsula]]\n"
]
],
[
[
"# Highlighting how policies shape application transactions\nEach data type operation has a write policy which can be set per CDT write/put operation to optionally:\n* Just-in-time sort the data being operated on. \n* Apply flags that instruct Aerospike’s transaction write behavior.\n\nCreate and set a MapPolicy or ListPolicy with the proper sort and write flags to change how Aerospike processes a transaction. ",
"_____no_output_____"
],
[
"## MapOrder and ListOrder, Just-in-time Sorting for an Operation \nBy default, Maps and Lists are stored unordered. There are explicit techniques to store a list or map in order. The Map data in this notebook is key sorted. Please refer to the code snippet creating the map data (above) for an example of this. There are examples of ordering lists in the notebook [Modeling Using Lists](./java-modeling_using_lists.ipynb). \n\nApplying a MapOrder or ListOrder has performance implications on operation performance. This can be a reason to apply a MapOrder or ListOrder when working with data. To understand the relative worst-case time complexity of Aerospike operations go [here for lists](https://docs.aerospike.com/docs/guide/cdt-list-performance.html) and [here for maps](https://docs.aerospike.com/docs/guide/cdt-map-performance.html). \n\nWhether to allow duplicates in a list is a function of ListOrder.\n\n**Note:** Aerospike finds that worst-case performance can be helpful in determining how to prioritize application use-cases against one another, but do not set realistic performance expectations for Aerospike Database. An example where they help is asking tough questions, like, “the worst case time complexity for operation A is X, is operation A important enough to do daily or just monthly in light of the other workloads that are more time sensitive?”\n",
"_____no_output_____"
],
[
"## Write Flags\nThe following are lists of [write flags for Lists](https://docs.aerospike.com/apidocs/java/com/aerospike/client/cdt/ListWriteFlags.html) and [Maps](https://docs.aerospike.com/apidocs/java/com/aerospike/client/cdt/MapWriteFlags.html). Beneath each are example transactions. \n\nA powerful use case for Aerospike is to group operations together into single-record atomic transactions using the `Operate` method. This technique is used above in this notebook. When applying transactions to data, there are common circumstances where:\n* All possible operations should be executed in a fault tolerant manner \n* Specific operation failure should cause all operations to fail\n\nWrite flags can be used in any combination, as appropriate to the application and Aerospike operation being applied.",
"_____no_output_____"
],
[
"### Write Flags for all CDTs\n* `DEFAULT`\n * For Lists, allow duplicate values and insertions at any index. \n * For Maps, allow map create or updates.\n* `NO_FAIL`: Do not raise an error if a CDT item is denied due to write flag constraints.\n* `PARTIAL`: Allow other valid CDT items to be committed if a CDT item is denied due to write flag constraints.\n\nThese flags provide fault tolerance to transactions. Apply some combination of the above three flags–`DEFAULT`, `NO_FAIL`, and `PARTIAL`–to operations by using “logical or” as demonstrated below. All other write flags set conditions for operations. \n\n**Note:** Without `NO_FAIL`, operations that fail due to the below policies will throw [either error code 24 or 26](https://docs.aerospike.com/docs/dev_reference/error_codes.html).",
"_____no_output_____"
],
[
"#### Default Examples",
"_____no_output_____"
],
[
"All of the above code snippets use a Default write flag policy. These operations are unrestricted by write policies.",
"_____no_output_____"
],
[
"#### No Fail Examples",
"_____no_output_____"
],
[
"All of the examples in the following sections show both an exception caused by a write flag, and then pair the demonstrated write flag with No Fail to show how the same operation can fail silently.",
"_____no_output_____"
],
[
"#### Partial Flag Example",
"_____no_output_____"
],
[
"Partial is generally used only in a transaction containing operations using the No Fail write flag. Otherwise, the transaction would contain no failures to overlook. The following example are a list and map transaction combining both failing and successful map and list operations. ",
"_____no_output_____"
]
],
[
[
"// create policy to apply and data to trigger operation failure\nInteger inBoundsIndex = 0;\nInteger outOfBoundsIndex = 20;\n\nHashMap <Value, Value> mapCoords4 = new HashMap <Value, Value>();\nmapCoords4.put(Value.get(\"lat\"), Value.get(0));\nmapCoords4.put(Value.get(\"long\"), Value.get(0));\n\nInteger existingObsKey = 13456;\n\nInteger listPartialWriteFlags = ListWriteFlags.INSERT_BOUNDED \n | ListWriteFlags.NO_FAIL \n | ListWriteFlags.PARTIAL;\nListPolicy listPartialWritePolicy = new ListPolicy(ListOrder.UNORDERED, listPartialWriteFlags);\n\nInteger mapPartialWriteFlags = MapWriteFlags.CREATE_ONLY \n | MapWriteFlags.NO_FAIL \n | MapWriteFlags.PARTIAL;\nMapPolicy mapPartialWritePolicy = new MapPolicy(MapOrder.KEY_ORDERED, mapPartialWriteFlags);\n\n\n// create fresh record\nInteger partialFlagKeyName = 6;\nKey partialFlagKey = new Key(nestedCDTNamespaceName, nestedCDTSetName, partialFlagKeyName);\n\nBin bin1 = new Bin(listWhaleBinName, whaleMigration);\nRecord putDataIn = client.operate(null, partialFlagKey,\n Operation.put(bin1),\n MapOperation.putItems(mapObsPolicy, mapObsBinName, mapObs)\n );\nRecord partialDataPutIn = client.get(client.writePolicyDefault, partialFlagKey);\n\n\n// one failed and one successful operation for both list and map\nRecord partialSuccessOp = client.operate(null, partialFlagKey,\n ListOperation.insert(listPartialWritePolicy, listWhaleBinName, outOfBoundsIndex, Value.get(newWhaleMigration)),\n ListOperation.set(listPartialWritePolicy, listWhaleBinName, inBoundsIndex, Value.get(newWhaleMigration)), \n MapOperation.put(mapPartialWritePolicy, mapObsBinName, Value.get(existingObsKey), Value.get(mapCoords4)),\n MapOperation.put(mapPartialWritePolicy, mapObsBinName, Value.get(newObsKey), Value.get(mapCoords3))\n );\nRecord partialSuccessData = client.get(client.writePolicyDefault, partialFlagKey);\nSystem.out.println (\"Failed to add a 5th item.\\nSucceeded at changing the first item.\\n\");\nSystem.out.println (\"Original List: \" + partialDataPutIn.getValue(listWhaleBinName) + \"\\n\");\nSystem.out.println (\"Updated List: \" + partialSuccessData.getValue(listWhaleBinName) + \"\\n\\n\"); \nSystem.out.println (\"Failed to modify an exiting observation.\\nSucceeded at adding a new observation.\\n\");\nSystem.out.println (\"Original Map: \" + partialDataPutIn.getValue(mapObsBinName) + \"\\n\");\nSystem.out.println (\"Updated Map: \" + partialSuccessData.getValue(mapObsBinName) + \"\\n\\nFor more about the failed operations, see the examples below.\");\n\nBoolean partialExampleRecordDeleted=client.delete(null, partialFlagKey);\n",
"Failed to add a 5th item.\nSucceeded at changing the first item.\n\nOriginal List: [[1420, beluga whale, Beaufort Sea, Bering Sea], [13988, gray whale, Baja California, Chukchi Sea], [1278, north pacific right whale, Japan, Sea of Okhotsk], [5100, humpback whale, Columbia, Antarctic Peninsula], [3100, southern hemisphere blue whale, Corcovado Gulf, The Galapagos]]\n\nUpdated List: [[1449, sei whale, Greenland, Gulf of Maine], [13988, gray whale, Baja California, Chukchi Sea], [1278, north pacific right whale, Japan, Sea of Okhotsk], [5100, humpback whale, Columbia, Antarctic Peninsula], [3100, southern hemisphere blue whale, Corcovado Gulf, The Galapagos]]\n\n\nFailed to modify an exiting observation.\nSucceeded at adding a new observation.\n\nOriginal Map: {12345={lat=-85, long=-130}, 13456={lat=-25, long=-50}, 14567={lat=35, long=30}}\n\nUpdated Map: {12345={lat=-85, long=-130}, 13456={lat=-25, long=-50}, 14567={lat=35, long=30}, 15678={lat=95, long=110}}\n\nFor more about the failed operations, see the examples below.\n"
]
],
[
[
"### Write Flags for Lists Only:\n* `INSERT_BOUNDED`: Enforce list boundaries when inserting. Do not allow values to be inserted at index outside current list boundaries.\n* `ADD_UNIQUE`: Only add unique values. ",
"_____no_output_____"
],
[
"#### Insert Bounded Example",
"_____no_output_____"
]
],
[
[
"// create policy to apply and data to break policy\nInteger outOfBoundsIndex = 20;\n\nListPolicy listInsertBoundedPolicy = new ListPolicy(ListOrder.UNORDERED, ListWriteFlags.INSERT_BOUNDED);\nListPolicy listBoundedNoFailPolicy = new ListPolicy(ListOrder.UNORDERED, ListWriteFlags.INSERT_BOUNDED \n | ListWriteFlags.NO_FAIL);\n\n// create fresh record\nInteger whaleBoundedKeyName = 7;\n\nBin bin1 = new Bin(listWhaleBinName, whaleMigration);\n\nKey whaleBoundedKey = new Key(nestedCDTNamespaceName, nestedCDTSetName, whaleBoundedKeyName);\n\nclient.put(client.writePolicyDefault, whaleBoundedKey, bin1);\nRecord ibDataPutIn = client.get(null, whaleBoundedKey);\nSystem.out.println(\"Data in the record: \" + ibDataPutIn.getValue(listWhaleBinName) + \"\\n\");\n\n\n// fail for INSERT_BOUNDED\ntry {\n Record ibFail = client.operate(client.writePolicyDefault, whaleBoundedKey,\n ListOperation.insert(listInsertBoundedPolicy, listWhaleBinName, outOfBoundsIndex, Value.get(newWhaleMigration))\n );\n System.out.println(\"The code does not get here.\");\n} \ncatch(Exception e) {\n System.out.println(\"Out of Bounds Attempt 1: Exception caught.\");\n Record ibNoFail = client.operate(client.writePolicyDefault, whaleBoundedKey,\n ListOperation.insert(listBoundedNoFailPolicy, listWhaleBinName, outOfBoundsIndex, Value.get(newWhaleMigration))\n );\n Record ibNoFailData = client.get(client.writePolicyDefault, whaleBoundedKey);\n if(ibNoFailData.getValue(listWhaleBinName).equals(ibDataPutIn.getValue(listWhaleBinName))) {\n System.out.println(\"Out of Bounds Attempt 2: No operation was executed. Error was suppressed by NO_FAIL.\\n\");\n }\n}\n\nRecord noIB = client.operate(client.writePolicyDefault, whaleBoundedKey,\n ListOperation.insert(listWhaleBinName, outOfBoundsIndex, Value.get(newWhaleMigration))\n);\nRecord noIBData = client.get(null, whaleBoundedKey);\nSystem.out.println(\"Without Insert Bounded, a series of nulls is inside the Bin: \" + noIBData.getValue(listWhaleBinName));",
"Data in the record: [[1420, beluga whale, Beaufort Sea, Bering Sea], [13988, gray whale, Baja California, Chukchi Sea], [1278, north pacific right whale, Japan, Sea of Okhotsk], [5100, humpback whale, Columbia, Antarctic Peninsula], [3100, southern hemisphere blue whale, Corcovado Gulf, The Galapagos]]\n\nOut of Bounds Attempt 1: Exception caught.\nOut of Bounds Attempt 2: No operation was executed. Error was suppressed by NO_FAIL.\n\nWithout Insert Bounded, a series of nulls is insein the Bin: [[1420, beluga whale, Beaufort Sea, Bering Sea], [13988, gray whale, Baja California, Chukchi Sea], [1278, north pacific right whale, Japan, Sea of Okhotsk], [5100, humpback whale, Columbia, Antarctic Peninsula], [3100, southern hemisphere blue whale, Corcovado Gulf, The Galapagos], null, null, null, null, null, null, null, null, null, null, null, null, null, null, null, [1449, sei whale, Greenland, Gulf of Maine]]\n"
]
],
[
[
"#### Add Unique Example",
"_____no_output_____"
]
],
[
[
"// create policy to apply\nListPolicy listAddUniquePolicy = new ListPolicy(ListOrder.UNORDERED, ListWriteFlags.ADD_UNIQUE);\nListPolicy listAddUniqueNoFailPolicy = new ListPolicy(ListOrder.UNORDERED, ListWriteFlags.ADD_UNIQUE \n | ListWriteFlags.NO_FAIL);\n\n// create fresh record\nInteger whaleAddUniqueKeyName = 8;\nBin bin1 = new Bin(listWhaleBinName, whaleMigration);\nKey whaleAddUniqueKey = new Key(nestedCDTNamespaceName, nestedCDTSetName, whaleAddUniqueKeyName);\nclient.put(client.writePolicyDefault, whaleAddUniqueKey, bin1);\nRecord auDataPutIn = client.get(null, whaleAddUniqueKey);\n\n\n// successful ADD_UNIQUE operation\nRecord auSuccess = client.operate(client.writePolicyDefault, whaleAddUniqueKey,\n ListOperation.append(listAddUniquePolicy, listWhaleBinName, Value.get(newWhaleMigration))\n);\nRecord auSuccessData = client.get(null, whaleAddUniqueKey);\n\nSystem.out.println(\"Data after the unique add of \" + newWhaleMigration + \": \" + auSuccessData.getValue(listWhaleBinName) + \"\\n\");\n\n\n// fail for 2nd ADD_UNIQUE\ntry {\n Record auFail = client.operate(client.writePolicyDefault, whaleAddUniqueKey,\n ListOperation.append(listAddUniquePolicy, listWhaleBinName, Value.get(newWhaleMigration))\n );\n System.out.println(\"The code does not get here.\");\n}\ncatch(Exception e) {\n System.out.println(\"Non-Unique Add 1: Exception caught.\");\n Record auNoFail = client.operate(client.writePolicyDefault, whaleAddUniqueKey,\n ListOperation.append(listAddUniqueNoFailPolicy, listWhaleBinName, Value.get(newWhaleMigration))\n );\n Record auNoFailData = client.get(null, whaleAddUniqueKey);\n if(auNoFailData.getValue(listWhaleBinName).equals(auSuccessData.getValue(listWhaleBinName))) {\n System.out.println(\"Non-Unique Add 2: No operation was executed. Error was suppressed by NO_FAIL.\\n\");\n }\n}\n\nRecord noAU = client.operate(client.writePolicyDefault, whaleAddUniqueKey,\n ListOperation.append(listWhaleBinName, Value.get(newWhaleMigration))\n);\nRecord noAUData = client.get(null, whaleAddUniqueKey);\nSystem.out.println(\"Without Add Unique here, the tuple for a sei whale is there 2x: \" + noAUData.getValue(listWhaleBinName));",
"Data after the unique add of [1449, sei whale, Greenland, Gulf of Maine]: [[1420, beluga whale, Beaufort Sea, Bering Sea], [13988, gray whale, Baja California, Chukchi Sea], [1278, north pacific right whale, Japan, Sea of Okhotsk], [5100, humpback whale, Columbia, Antarctic Peninsula], [3100, southern hemisphere blue whale, Corcovado Gulf, The Galapagos], [1449, sei whale, Greenland, Gulf of Maine]]\n\nNon-Unique Add 1: Exception caught.\nNon-Unique Add 2: No operation was executed. Error was suppressed by NO_FAIL.\n\nWithout Add Unique here, the tuple for a sei whale is there 2x: [[1420, beluga whale, Beaufort Sea, Bering Sea], [13988, gray whale, Baja California, Chukchi Sea], [1278, north pacific right whale, Japan, Sea of Okhotsk], [5100, humpback whale, Columbia, Antarctic Peninsula], [3100, southern hemisphere blue whale, Corcovado Gulf, The Galapagos], [1449, sei whale, Greenland, Gulf of Maine], [1449, sei whale, Greenland, Gulf of Maine]]\n"
]
],
[
[
"### Write Flags for Maps Only:\n* `CREATE_ONLY`: If the key already exists, the item will be denied.\n* `UPDATE_ONLY`: If the key already exists, the item will be overwritten. If the key does not exist, the item will be denied.",
"_____no_output_____"
],
[
"#### Create Only Example",
"_____no_output_____"
]
],
[
[
"// create modify data and policy to apply\nHashMap <Value, Value> mapCoords4 = new HashMap <Value, Value>();\nmapCoords4.put(Value.get(\"lat\"), Value.get(0));\nmapCoords4.put(Value.get(\"long\"), Value.get(0));\n\nMapPolicy mapCreateOnlyPolicy = new MapPolicy(MapOrder.KEY_ORDERED, MapWriteFlags.CREATE_ONLY);\nMapPolicy mapCreateOnlyNoFailPolicy = new MapPolicy(MapOrder.KEY_ORDERED, MapWriteFlags.CREATE_ONLY \n | MapWriteFlags.NO_FAIL);\n\n// create fresh record\nInteger obsCreateOnlyKeyName = 9;\nKey obsCreateOnlyKey = new Key(nestedCDTNamespaceName, nestedCDTSetName, obsCreateOnlyKeyName);\nRecord putDataIn = client.operate(client.writePolicyDefault, obsCreateOnlyKey,\n MapOperation.putItems(mapObsPolicy, mapObsBinName, mapObs)\n );\nRecord coDataPutIn = client.get(null, obsCreateOnlyKey);\n\n\n// success for CREATE_ONLY\nRecord coSuccess = client.operate(client.writePolicyDefault, obsCreateOnlyKey,\n MapOperation.put(mapCreateOnlyPolicy, mapObsBinName, Value.get(newObsKey), Value.get(mapCoords3))\n);\nRecord coSuccessData = client.get(null, obsCreateOnlyKey);\nSystem.out.println(\"Created record and new key \" + newObsKey + \". The data is now: \" + coSuccessData.getValue(mapObsBinName) + \"\\n\");\n\n\n// fail for CREATE_ONLY\ntry {\n Record coFail = client.operate(client.writePolicyDefault, obsCreateOnlyKey,\n MapOperation.put(mapCreateOnlyPolicy, mapObsBinName, Value.get(newObsKey), Value.get(mapCoords4))\n );\n System.out.println(\"The code does not get here.\");\n}\ncatch(Exception e) {\n System.out.println(\"Update attempt 1: Exception caught.\");\n Record coNoFail = client.operate(client.writePolicyDefault, obsCreateOnlyKey,\n MapOperation.put(mapCreateOnlyNoFailPolicy, mapObsBinName, Value.get(newObsKey), Value.get(mapCoords4))\n );\n Record coNoFailData = client.get(null, obsCreateOnlyKey);\n if(coNoFailData.getValue(mapObsBinName).equals(coSuccessData.getValue(mapObsBinName))) {\n System.out.println(\"Update attempt 2: No operation was executed. Error was suppressed by NO_FAIL.\\n\");\n }\n}\n\nRecord noCO = client.operate(client.writePolicyDefault, obsCreateOnlyKey, \n MapOperation.put(mapObsPolicy, mapObsBinName, Value.get(newObsKey), Value.get(mapCoords4))\n);\nRecord noCOData = client.get(null, obsCreateOnlyKey);\nSystem.out.println(\"Without Create Only, the observation at 15678 is overwritten: \" + noCOData.getValue(mapObsBinName));\n\nBoolean createOnlyExampleRecordDeleted=client.delete(null, obsCreateOnlyKey);",
"Created record and new key 15678. The data is now: {12345={lat=-85, long=-130}, 13456={lat=-25, long=-50}, 14567={lat=35, long=30}, 15678={lat=95, long=110}}\n\nUpdate attempt 1: Exception caught.\nUpdate attempt 2: No operation was executed. Error was suppressed by NO_FAIL.\n\nWithout Create Only, the observation at 15678 is overwritten: {12345={lat=-85, long=-130}, 13456={lat=-25, long=-50}, 14567={lat=35, long=30}, 15678={lat=0, long=0}}\n"
]
],
[
[
"#### Update Only Example",
"_____no_output_____"
]
],
[
[
"// create policy to apply\nMapPolicy mapUpdateOnlyPolicy = new MapPolicy(MapOrder.KEY_ORDERED, MapWriteFlags.UPDATE_ONLY);\nMapPolicy mapUpdateOnlyNoFailPolicy = new MapPolicy(MapOrder.KEY_ORDERED, MapWriteFlags.UPDATE_ONLY \n | MapWriteFlags.NO_FAIL);\n\n// create Aerospike data elements for a fresh record\nInteger obsUpdateOnlyKeyName = 10;\nKey obsUpdateOnlyKey = new Key(nestedCDTNamespaceName, nestedCDTSetName, obsUpdateOnlyKeyName);\n\nRecord uoPutDataIn = client.operate(client.writePolicyDefault, obsUpdateOnlyKey,\n MapOperation.putItems(mapObsPolicy, mapObsBinName, mapObs)\n );\nRecord uoDataPutIn = client.get(null, obsUpdateOnlyKey);\nSystem.out.println(\"Created record: \" + uoDataPutIn.getValue(mapObsBinName) + \"\\n\");\n\n\n// fail for UPDATE_ONLY\ntry {\n Record uoFail = client.operate(client.writePolicyDefault, obsUpdateOnlyKey,\n MapOperation.put(mapUpdateOnlyPolicy, mapObsBinName, Value.get(newObsKey), Value.get(mapCoords3))\n );\n System.out.println(\"The code does not get here.\");\n}\ncatch(Exception e) {\n System.out.println(\"Create Attempt 1: Exception caught.\");\n Record uoNoFail = client.operate(client.writePolicyDefault, obsUpdateOnlyKey,\n MapOperation.put(mapUpdateOnlyNoFailPolicy, mapObsBinName, Value.get(newObsKey), Value.get(mapCoords3))\n );\n Record uoNoFailData = client.get(null, obsUpdateOnlyKey);\n if(uoNoFailData.getValue(mapObsBinName).equals(uoDataPutIn.getValue(mapObsBinName))){\n System.out.println(\"Create Attempt 2: No operation was executed. Error was suppressed by NO_FAIL.\\n\");\n }\n}\n\nRecord noUO = client.operate(client.writePolicyDefault, obsUpdateOnlyKey, \n MapOperation.put(mapObsPolicy, mapObsBinName, Value.get(newObsKey), Value.get(mapCoords3))\n);\nRecord noUOData = client.get(null, obsUpdateOnlyKey);\n\n// success for UPDATE_ONLY\nRecord uoSuccess = client.operate(client.writePolicyDefault, obsUpdateOnlyKey,\n MapOperation.put(mapUpdateOnlyPolicy, mapObsBinName, Value.get(existingObsKey), Value.get(mapCoords4))\n);\nRecord uoSuccessData = client.get(null, obsUpdateOnlyKey);\nSystem.out.println(\"Using update only, the value of an existing key \" + existingObsKey + \" can be updated: \" + uoSuccessData.getValue(mapObsBinName) + \"\\n\");\n\nBoolean uoExampleRecordDeleted=client.delete(null, obsUpdateOnlyKey);",
"Created record: {12345={lat=-85, long=-130}, 13456={lat=-25, long=-50}, 14567={lat=35, long=30}}\n\nCreate Attempt 1: Exception caught.\nCreate Attempt 2: No operation was executed. Error was suppressed by NO_FAIL.\n\nUsing update only, the value of an existing key 13456 can be updated: {12345={lat=-85, long=-130}, 13456={lat=0, long=0}, 14567={lat=35, long=30}, 15678={lat=95, long=110}}\n\n"
]
],
[
[
"# Notebook Cleanup",
"_____no_output_____"
],
[
"### Truncate the Set\nTruncate the set from the Aerospike Database.",
"_____no_output_____"
]
],
[
[
"import com.aerospike.client.policy.InfoPolicy;\nInfoPolicy infoPolicy = new InfoPolicy();\n\nclient.truncate(infoPolicy, nestedCDTNamespaceName, nestedCDTSetName, null);\nSystem.out.println(\"Set Truncated.\");",
"Set Truncated.\n"
]
],
[
[
"### Close the Client connections to Aerospike",
"_____no_output_____"
]
],
[
[
"client.close();\nSystem.out.println(\"Server connection(s) closed.\");",
"Server connection(s) closed.\n"
]
],
[
[
"# Takeaways – CDTs Provide Flexible Document-Oriented Data Power\n\nAerospike Collection Data Types...\n1. facilitate complex data structures by supporting nesting through the use of contexts (CTXs)\n2. provide intuitive and flexible return types options from operations\n3. support policies that empower efficient and flexible transaction processing",
"_____no_output_____"
],
[
"# What's Next?",
"_____no_output_____"
],
[
"## Next Steps\n\nHave questions? Don't hesitate to reach out if you have additional questions about data modeling at https://discuss.aerospike.com/c/how-developers-are-using-aerospike/data-modeling/143.\n\nWant to check out other Java notebooks?\n1. [Intro to Transactions](./java-intro_to_transactions.ipynb)\n2. [Modeling Using Lists](./java-modeling_using_lists.ipynb)\n3. [Working with Maps](./java-working_with_maps.ipynb)\n4. [Aerospike Query and UDF](query_udf.ipynb)\n\n\nAre you running this from Binder? [Download the Aerospike Notebook Repo](https://github.com/aerospike-examples/interactive-notebooks) and work with Aerospike Database and Jupyter locally using a Docker container.",
"_____no_output_____"
],
[
"## Additional Resources\n\n* Want to get started with Java? [Download](https://www.aerospike.com/download/client/) or [install](https://github.com/aerospike/aerospike-client-java) the Aerospike Java Client. \n(https://www.aerospike.com/apidocs/java/com/aerospike/client/cdt/MapOperation.html).\n* What are Namespaces, Sets, and Bins? Check out the [Aerospike Data Model](https://www.aerospike.com/docs/architecture/data-model.html). \n* How robust is the Aerospike Database? Browses the [Aerospike Database Architecture](https://www.aerospike.com/docs/architecture/index.html).",
"_____no_output_____"
]
]
] | [
"markdown",
"code",
"markdown",
"code",
"markdown",
"code",
"markdown",
"code",
"markdown",
"code",
"markdown",
"code",
"markdown",
"code",
"markdown",
"code",
"markdown",
"code",
"markdown",
"code",
"markdown",
"code",
"markdown",
"code",
"markdown",
"code",
"markdown",
"code",
"markdown",
"code",
"markdown",
"code",
"markdown",
"code",
"markdown",
"code",
"markdown"
] | [
[
"markdown",
"markdown",
"markdown",
"markdown"
],
[
"code"
],
[
"markdown"
],
[
"code"
],
[
"markdown"
],
[
"code"
],
[
"markdown"
],
[
"code"
],
[
"markdown"
],
[
"code"
],
[
"markdown",
"markdown",
"markdown",
"markdown"
],
[
"code"
],
[
"markdown"
],
[
"code"
],
[
"markdown"
],
[
"code"
],
[
"markdown",
"markdown"
],
[
"code"
],
[
"markdown"
],
[
"code"
],
[
"markdown"
],
[
"code"
],
[
"markdown",
"markdown",
"markdown",
"markdown",
"markdown",
"markdown",
"markdown",
"markdown",
"markdown",
"markdown"
],
[
"code"
],
[
"markdown",
"markdown"
],
[
"code"
],
[
"markdown"
],
[
"code"
],
[
"markdown",
"markdown"
],
[
"code"
],
[
"markdown"
],
[
"code"
],
[
"markdown",
"markdown"
],
[
"code"
],
[
"markdown"
],
[
"code"
],
[
"markdown",
"markdown",
"markdown",
"markdown"
]
] |
e783cc69f98e72f8830e008fcdfc4ab730206fe5 | 874,368 | ipynb | Jupyter Notebook | analysis_archive/notebooks/final-cmp_merfish_v_10x.ipynb | nmarkari/BYVSTZP_2020 | e24df0a3906f65dcf7105481135cdf3ed033fbdc | [
"BSD-2-Clause"
] | null | null | null | analysis_archive/notebooks/final-cmp_merfish_v_10x.ipynb | nmarkari/BYVSTZP_2020 | e24df0a3906f65dcf7105481135cdf3ed033fbdc | [
"BSD-2-Clause"
] | null | null | null | analysis_archive/notebooks/final-cmp_merfish_v_10x.ipynb | nmarkari/BYVSTZP_2020 | e24df0a3906f65dcf7105481135cdf3ed033fbdc | [
"BSD-2-Clause"
] | null | null | null | 757.028571 | 664,180 | 0.945118 | [
[
[
"!date",
"Mon Nov 9 11:35:17 PST 2020\n"
]
],
[
[
"# Merfish 10x comparison",
"_____no_output_____"
]
],
[
[
"import anndata\nimport pandas as pd\nimport numpy as np\nimport matplotlib.pyplot as plt\nimport matplotlib as mpl\nimport matplotlib.patches as mpatches\nimport scanpy as scanp\nfrom scipy.stats import ks_2samp, ttest_ind\nfrom scipy.sparse import csr_matrix\nfrom scipy import stats\nfrom sklearn.decomposition import TruncatedSVD\nfrom sklearn.manifold import TSNE\nfrom umap import UMAP\nfrom sklearn.cluster import KMeans\nfrom sklearn.metrics import adjusted_rand_score\nfrom sklearn.preprocessing import LabelEncoder\nfrom sklearn.neighbors import NeighborhoodComponentsAnalysis\nfrom matplotlib import cm\nfrom scipy.spatial import ConvexHull\nfrom sklearn.preprocessing import LabelEncoder\nfrom sklearn.preprocessing import normalize\n\nimport warnings\nwarnings.filterwarnings('ignore')\n\nimport sys\nsys.path.append('/home/sina/projects/mop/BYVSTZP_2020/trackfig')\nfrom trackfig.utils import get_notebook_name\nfrom trackfig.trackfig import trackfig \n\nTRACKFIG = \"/home/sina/projects/mop/BYVSTZP_2020/trackfig.txt\"\nNB = get_notebook_name()\n\nfsize=20\n\nplt.rcParams.update({'font.size': fsize})\n%config InlineBackend.figure_format = 'retina'",
"_____no_output_____"
],
[
"unique_map = {'Astrocytes': \"Astro\",\n'Endothelial':\"Endo\",\n'SMC':\"SMC\",\n'L23_IT':\"L2/3 IT\",\n'VLMC': \"VLMC\",\n'L6_CT': \"L6 CT\",\n'L45_IT': \"L4/5 IT\",\n'L5_PT': \"L5 PT\",\n'L5_IT': \"L5 IT\",\n'Sst': \"Sst\",\n'L6_IT': \"L6 IT\",\n'Sncg': \"Sncg\",\n'L6_IT_Car3': \"L6 IT Car3\",\n'Vip': \"Vip\",\n'L56_NP': \"L5/6 NP\",\n'Pvalb': \"Pvalb\", \n'L6b': \"L6b\",\n'Lamp5': \"Lamp5\"}\n\ninv_map = {v: k for k, v in unique_map.items()}",
"_____no_output_____"
],
[
"cluster_cmap = {\n\"Astro\": (0.38823529411764707, 0.4745098039215686, 0.2235294117647059 ), # 637939,\n\"Endo\" : (0.5490196078431373, 0.6352941176470588, 0.3215686274509804 ), # 8ca252,\n\"SMC\" : (0.7098039215686275, 0.8117647058823529, 0.4196078431372549 ), # b5cf6b,\n\"VLMC\" : (0.807843137254902, 0.8588235294117647, 0.611764705882353 ), # cedb9c,\n\"Low Quality\" : (0,0,0),\n\"L2/3 IT\" : (0.9921568627450981, 0.6823529411764706, 0.4196078431372549 ), # fdae6b\n\"L5 PT\" : (0.9921568627450981, 0.8156862745098039, 0.6352941176470588 ), # fdd0a2\n\"L5 IT\" : (0.5176470588235295, 0.23529411764705882, 0.2235294117647059 ), # 843c39\n\"L5/6 NP\": \"#D43F3A\",\n\"L6 CT\" : (0.8392156862745098, 0.3803921568627451, 0.4196078431372549 ), # d6616b\n\"L6 IT\" : (0.9058823529411765, 0.5882352941176471, 0.611764705882353 ), # e7969c\n\"L6b\" : (1.0, 0.4980392156862745, 0.054901960784313725), # ff7f0e\n\"L6 IT Car3\" : (1.0, 0.7333333333333333, 0.47058823529411764 ), # ffbb78\n\"Lamp5\" : (0.19215686274509805, 0.5098039215686274, 0.7411764705882353 ), # 3182bd # blues\n\"Sncg\" : (0.4196078431372549, 0.6823529411764706, 0.8392156862745098 ), # 6baed6\n\"Vip\" : (0.6196078431372549, 0.792156862745098, 0.8823529411764706 ), # 9ecae1\n\"Sst\" : (0.7764705882352941, 0.8588235294117647, 0.9372549019607843 ), # c6dbef\n\"Pvalb\":(0.7372549019607844, 0.7411764705882353, 0.8627450980392157 ), # bcbddc\n}",
"_____no_output_____"
],
[
"def trim_axs(axs, N):\n \"\"\"little helper to massage the axs list to have correct length...\"\"\"\n axs = axs.flat\n for ax in axs[N:]:\n ax.remove()\n return axs[:N]",
"_____no_output_____"
],
[
"def split_by_target(mat, targets, target, axis=0):\n \"\"\"\n Split the rows of mat by the proper assignment\n \n mat = ndarray\n targets, length is equal to number of components (axis=0) or features (axis=1)\n target is a singular element from unique(assignments/features) \n \"\"\"\n if axis==0 and len(targets) != mat.shape[axis]: return -1\n if axis==1 and len(targets) != mat.shape[axis]: return -1\n \n mask = targets == target\n \n if axis==0:\n t_mat = mat[mask] # target matrix\n c_mat = mat[~mask] # complement matrix\n elif axis==1:\n t_mat = mat[:, mask] # target matrix\n c_mat = mat[:, ~mask] # complement matrix\n \n return (t_mat, c_mat)\n\ndef group_mtx_by_cluster(mtx, components, features, s2t, source_id=\"cell_id\", target_id=\"subclass_label\", by=\"components\"):\n \"\"\"\n mtx: ndarray components by features \n components: labels for rows of mtx\n features: labels for columns of mtx\n s2t: pandas dataframe mapping source (features or components) to a\n targets features(components) to group by\n target_id: column name in s2t to group by\n \"\"\"\n if target_id not in s2t.columns: return -1\n \n ncomp = components.shape[0]\n nfeat = features.shape[0]\n ntarget = s2t[target_id].nunique()\n \n if by ==\"features\": \n source = features\n elif by ==\"components\": \n source = components\n \n # Map the source to an index\n source2idx = dict(zip(source, range(len(source))))\n # Map the target to a list of source indices\n target2idx = (s2t.groupby(target_id)[source_id].apply(lambda x: [source2idx[i] for i in x])).to_dict()\n \n # array of unique targets\n unique = s2t[target_id].unique().astype(str)\n nuniq = unique.shape[0]\n X = np.zeros((nuniq, mtx.shape[1]))\n \n for tidx, t in enumerate(unique):\n # Grab the matrix indices corresponding to columns and source columns to group by\n source_indices = target2idx[t]\n #print(source_indices)\n \n # breaks generality\n sub_mtx = mtx[source_indices,:].mean(axis=0) # Sum on source indicies\n X[tidx,:] = sub_mtx # place summed vector in new matrix\n \n # Return matrix that is grouped by\n return (X, components, unique)",
"_____no_output_____"
],
[
"def nd(arr):\n return np.asarray(arr).reshape(-1)",
"_____no_output_____"
],
[
"mfish = anndata.read_h5ad(\"../../data/notebook/revision/merfish-updated.h5ad\")\n\nmfish.obs[\"tenx_subclass\"] = mfish.obs[\"subclass\"].apply(lambda x: unique_map.get(x, \"None\"))\nmfish = mfish[mfish.obs.tenx_subclass != \"None\"]",
"_____no_output_____"
],
[
"md = pd.read_csv(\"../../reference/10xv3_cluster_labels/sample_metadata.csv\", index_col = 0)\n\nmd[\"sex\"] = md[\"Gender\"].apply(lambda x: {\"Male\": \"M\", \"Female\":\"F\"}.get(x, \"X\"))\n\ntenx = anndata.read_h5ad(\"../../data/notebook/revision/10xv3_gene.h5ad\")\ntenx.obs[\"date\"] = tenx.obs.index.map(md[\"Amp_Date\"])\ntenx.obs[\"sex\"] = tenx.obs.index.map(md[\"sex\"])\n\ntenx = tenx[:,tenx.var.gene_short_name.isin(mfish.var.index)]\n\ntenx.var.index = tenx.var.gene_short_name.values\n#tenx = tenx[tenx.obs.eval(\"date == '11/29/2018'\").values] # males\n#tenx = tenx[tenx.obs.eval(\"date == '12/7/2018'\").values] # females\ntenx = tenx[tenx.obs.eval(\"date == '4/26/2019'\").values] # females and males\n#tenx = tenx[tenx.obs.subclass_label!=\"Low Quality\"]\n\n",
"_____no_output_____"
],
[
"md.groupby(\"Amp_Date\")[\"sex\"].value_counts()",
"_____no_output_____"
],
[
"print(tenx)\nprint(mfish)",
"View of AnnData object with n_obs × n_vars = 35370 × 254\n obs: 'batch', 'cluster_id', 'cluster_label', 'subclass_label', 'class_label', 'subclass_id', 'class_id', 'date', 'sex'\n var: 'gene_name', 'gene_id', 'gene_short_name'\nView of AnnData object with n_obs × n_vars = 228355 × 254\n obs: 'fovID', 'fov_x', 'fov_y', 'volume', 'center_x', 'center_y', 'slice_id', 'sample_id', 'label', 'subclass', 'class_label', 'cell_id', 'tenx_subclass'\n var: 'n_iso'\n layers: 'X', 'log1p', 'norm'\n"
],
[
"tenx.obs.subclass_label.value_counts()",
"_____no_output_____"
],
[
"mfish.obs.subclass.value_counts()",
"_____no_output_____"
]
],
[
[
"# Process",
"_____no_output_____"
]
],
[
[
"from sklearn.preprocessing import normalize",
"_____no_output_____"
],
[
"tenx.layers[\"X\"] = tenx.X\ntenx.layers[\"norm\"] = normalize(tenx.X, norm='l1', axis=1)*1000000\ntenx.layers[\"log1p\"] = csr_matrix(np.log1p(tenx.layers[\"norm\"]))",
"_____no_output_____"
],
[
"from sklearn.preprocessing import scale",
"_____no_output_____"
],
[
"%%time\nmat = tenx.layers[\"log1p\"].todense()\nmtx = scale(mat, axis=0, with_mean=True, with_std=True, copy=True)\ntenx.X = mtx",
"CPU times: user 272 ms, sys: 110 ms, total: 382 ms\nWall time: 381 ms\n"
],
[
"del mat",
"_____no_output_____"
]
],
[
[
"# Cluster comparisons",
"_____no_output_____"
]
],
[
[
"tenx = tenx[:,tenx.var.sort_index().index]\nmfish = mfish[:,mfish.var.sort_index().index]",
"_____no_output_____"
],
[
"tenx.var.head()",
"_____no_output_____"
],
[
"mfish.var.head()",
"_____no_output_____"
],
[
"mfish_mat = mfish.X\nmfish_ass = mfish.obs.tenx_subclass.values\n\ntenx_mat = tenx.X\ntenx_ass = tenx.obs.subclass_label.values",
"_____no_output_____"
],
[
"features = mfish.var.index.values\n\nunique = np.intersect1d(np.unique(mfish_ass), np.unique(tenx_ass))",
"_____no_output_____"
],
[
"%%time\nrvals = []\ntenx_x = []\nmfish_x = []\n\nfor uidx, u in enumerate(unique):\n mfish_t_mat, _ = split_by_target(mfish_mat, mfish_ass, u)\n tenx_t_mat, _ = split_by_target(tenx_mat, tenx_ass, u)\n \n \n mf = np.asarray(mfish_t_mat.mean(axis=0)).reshape(-1)\n t = np.asarray(tenx_t_mat.mean(axis=0)).reshape(-1)\n \n tenx_x.append(t)\n mfish_x.append(mf)\n \n r, p = stats.pearsonr(mf, t)\n rvals.append(r)\n print(\"[{} of {}] {:,.2f}: {}\".format(uidx+1, unique.shape[0],r, u) )",
"[1 of 17] 0.79: Astro\n[2 of 17] 0.76: Endo\n[3 of 17] 0.84: L2/3 IT\n[4 of 17] 0.44: L5 IT\n[5 of 17] 0.78: L5 PT\n[6 of 17] 0.89: L5/6 NP\n[7 of 17] 0.90: L6 CT\n[8 of 17] 0.85: L6 IT\n[9 of 17] 0.83: L6 IT Car3\n[10 of 17] 0.89: L6b\n[11 of 17] 0.90: Lamp5\n[12 of 17] 0.86: Pvalb\n[13 of 17] 0.62: SMC\n[14 of 17] 0.84: Sncg\n[15 of 17] 0.90: Sst\n[16 of 17] 0.32: VLMC\n[17 of 17] 0.86: Vip\nCPU times: user 1.76 s, sys: 1.95 s, total: 3.7 s\nWall time: 3.69 s\n"
],
[
"tenx_size = tenx.obs[\"subclass_label\"].value_counts()[unique]",
"_____no_output_____"
],
[
"fig, ax = plt.subplots(figsize=(10,7))\n\nx = tenx_size\ny = rvals\n\nfor i, txt in enumerate(unique):\n ax.annotate(i, (x[i], y[i]))\n ax.scatter(x[i], y[i], label=\"{}: {}\".format(i, txt), color=cluster_cmap[txt])\nax.set_ylim((0, 1))\nax.set_xscale(\"log\")\n \nax.set_xlabel(\"Number of 10xv3 cells\")\nax.set_ylabel(\"Pearson correlation\")\nax.legend(fontsize=15,loc='center left', bbox_to_anchor=(1, 0.5), markerscale=3)\nax.set_title(\"MERFISH v. 10xv3 gene subclass correlation\")\nplt.savefig(trackfig(\"../../figures/merfish-updated_10x_gene_subclass_size.png\", TRACKFIG, NB), bbox_inches='tight', dpi=300)\nplt.show()",
"_____no_output_____"
],
[
"# males\nmales = pd.DataFrame({\"subclass\": unique.tolist(), \n \"rvals\": rvals,\n \"size\": tenx.obs.subclass_label.value_counts()[unique]})",
"_____no_output_____"
],
[
"males",
"_____no_output_____"
],
[
"fig, ax = plt.subplots(figsize=(15,15), ncols=4, nrows=5)\nfig.subplots_adjust(hspace=0, wspace=0)\naxs = trim_axs(ax, len(unique))\nfig.suptitle('MERFISH v. 10xv3 gene subclass correlation', y=0.9)\n#fig.subplots_adjust(top=1)\n\nfor cidx, (ax, c) in enumerate(zip(axs, unique)):\n \n \n x = tenx_x[cidx]\n y = mfish_x[cidx]\n\n \n ax.scatter(x, y, label=\"{}: {:,}\".format(c, tenx_size[cidx]), color=\"k\", alpha=0.1)\n\n slope, intercept, r_value, p_value, std_err = stats.linregress(x, y)\n\n minx = min(x)\n maxx = max(x)\n x = np.linspace(minx, maxx, 10)\n y = slope*x+intercept\n ax.plot(x, y, label=\"corr : {:,.2f}\".format(r_value**2), color=\"red\", linewidth=3)\n ax.legend(fontsize=15)\n ax.xaxis.set_ticklabels([])\n ax.yaxis.set_ticklabels([])\n ax.set_axis_off()\nfig.text(0.5, 0.1, '10xv3 scaled $log(TPM+1)$', ha='center', va='center', fontsize=30)\nfig.text(0.1, 0.5, 'MERFISH scaled $log(CPM+1)$', ha='center', va='center', rotation='vertical', fontsize=30)\n\nplt.savefig(trackfig(\"../../figures/merfish-updated_10x_gene_subclass_correlation_scatter.png\", TRACKFIG, NB), bbox_inches='tight',dpi=300)\nplt.show()",
"_____no_output_____"
],
[
"tenx[tenx.obs.subclass_label==\"L5 IT\"].obs.cluster_label.value_counts()",
"_____no_output_____"
],
[
"mfish[mfish.obs.subclass==\"L5_IT\"].obs.label.value_counts()",
"_____no_output_____"
],
[
"rvals",
"_____no_output_____"
],
[
"unique.tolist()",
"_____no_output_____"
]
]
] | [
"code",
"markdown",
"code",
"markdown",
"code",
"markdown",
"code"
] | [
[
"code"
],
[
"markdown"
],
[
"code",
"code",
"code",
"code",
"code",
"code",
"code",
"code",
"code",
"code",
"code",
"code"
],
[
"markdown"
],
[
"code",
"code",
"code",
"code",
"code"
],
[
"markdown"
],
[
"code",
"code",
"code",
"code",
"code",
"code",
"code",
"code",
"code",
"code",
"code",
"code",
"code",
"code",
"code"
]
] |
e783ceb9cba6e4205f98d45bf0dc1080719dcb92 | 1,021 | ipynb | Jupyter Notebook | jupyter notebooks (explain codes)/persian to finlglish.ipynb | mmahdibarghi/tts-dataset-team | 3a4df1facc9547443522c7de2aaad65f78e48041 | [
"MIT"
] | null | null | null | jupyter notebooks (explain codes)/persian to finlglish.ipynb | mmahdibarghi/tts-dataset-team | 3a4df1facc9547443522c7de2aaad65f78e48041 | [
"MIT"
] | null | null | null | jupyter notebooks (explain codes)/persian to finlglish.ipynb | mmahdibarghi/tts-dataset-team | 3a4df1facc9547443522c7de2aaad65f78e48041 | [
"MIT"
] | 1 | 2022-02-04T16:17:55.000Z | 2022-02-04T16:17:55.000Z | 28.361111 | 291 | 0.622919 | [
[
[
"# تبدیل متن فارسی به فینگلیش\r\nدر طی این پروژه تیم نرم افزار برای کار با مدل تاکاترون 2 علاوه بر متن فارسی و صوت هر یک از جملات مورد بررسی در دیتاست به فینگلیش شده آن متن فارسی نیز نیاز دارند، بنابراین یکی از مراحل و کار هایی که باید انجام میشد تبدیل متن فارسی به فینگلیش بود برای این کار در طی دو مرحله کار پیش رفت.",
"_____no_output_____"
],
[
"## تبدیل دستی\r\nابتدا در حدود 5000 جمله فارسی به صورت دستی و در طی تلاش سه نفر کاملا به فینگلیش تبدیل شدند",
"_____no_output_____"
],
[
"## تبدیل اتوماتیک\r\nپس از تبدیل جملات طی پردازشی این جملات همگی به صورت دیتاستی از واژگان تبدیل شدند که شامل 38367 لغت فارسی و مطابق آنها به همین تعداد لغت فینگلش شده وجود داشت.\r\n",
"_____no_output_____"
]
]
] | [
"markdown"
] | [
[
"markdown",
"markdown",
"markdown"
]
] |
e783cf0b86bce489f403325d84bdc78b47997796 | 1,399 | ipynb | Jupyter Notebook | 475-music-festival.ipynb | arkeros/projecteuler | c95db97583034af8fc61d5786692d82eabe50c12 | [
"MIT"
] | 2 | 2017-02-19T12:37:13.000Z | 2021-01-19T04:58:09.000Z | 475-music-festival.ipynb | arkeros/projecteuler | c95db97583034af8fc61d5786692d82eabe50c12 | [
"MIT"
] | null | null | null | 475-music-festival.ipynb | arkeros/projecteuler | c95db97583034af8fc61d5786692d82eabe50c12 | [
"MIT"
] | 4 | 2018-01-05T14:29:09.000Z | 2020-01-27T13:37:40.000Z | 26.903846 | 147 | 0.551823 | [
[
[
"empty"
]
]
] | [
"empty"
] | [
[
"empty"
]
] |
e783dac5c66d34ab12eb3a4d1ec281a1d205e176 | 147,504 | ipynb | Jupyter Notebook | src/theory/sandbox/random_parameter_sampling.ipynb | RPGroup-PBoC/chann_cap | f2a826166fc2d47c424951c616c46d497ed74b39 | [
"MIT"
] | 2 | 2020-08-21T04:06:12.000Z | 2022-02-09T07:36:58.000Z | src/theory/sandbox/random_parameter_sampling.ipynb | RPGroup-PBoC/chann_cap | f2a826166fc2d47c424951c616c46d497ed74b39 | [
"MIT"
] | null | null | null | src/theory/sandbox/random_parameter_sampling.ipynb | RPGroup-PBoC/chann_cap | f2a826166fc2d47c424951c616c46d497ed74b39 | [
"MIT"
] | 2 | 2020-04-29T17:43:28.000Z | 2020-09-09T00:20:16.000Z | 164.808939 | 60,932 | 0.874844 | [
[
[
"# Random sampling of parameters",
"_____no_output_____"
],
[
"(c) 2019 Manuel Razo. This work is licensed under a [Creative Commons Attribution License CC-BY 4.0](https://creativecommons.org/licenses/by/4.0/). All code contained herein is licensed under an [MIT license](https://opensource.org/licenses/MIT). \n\n---",
"_____no_output_____"
]
],
[
[
"import os\nimport itertools\nimport pickle\nimport cloudpickle\nimport re\nimport glob\nimport git\n\n# Our numerical workhorses\nimport numpy as np\nimport pandas as pd\nimport scipy as sp\n\n# Import library to perform maximum entropy fits\nfrom maxentropy.skmaxent import FeatureTransformer, MinDivergenceModel\n\n# Import libraries to parallelize processes\nfrom joblib import Parallel, delayed\n\n# Import matplotlib stuff for plotting\nimport matplotlib.pyplot as plt\nimport matplotlib.cm as cm\nimport matplotlib as mpl\n\n# Seaborn, useful for graphics\nimport seaborn as sns\n# Increase DPI of displayed figures\n%config InlineBackend.figure_format = 'retina'\n\n# Import the project utils\nimport ccutils\n\n# Find home directory for repo\nrepo = git.Repo(\"./\", search_parent_directories=True)\nhomedir = repo.working_dir\n\n# Define directories for data and figure \nfigdir = f'{homedir}/fig/MaxEnt_approx_joint/'\ndatadir = f'{homedir}/data/csv_maxEnt_dist'",
"_____no_output_____"
],
[
"# Set PBoC plotting format\nccutils.viz.set_plotting_style()\n# Increase dpi\nmpl.rcParams['figure.dpi'] = 110",
"_____no_output_____"
]
],
[
[
"### $\\LaTeX$ macros\n\n$\\newcommand{kpon}{k^p_{\\text{on}}}$\n$\\newcommand{kpoff}{k^p_{\\text{off}}}$\n$\\newcommand{kron}{k^r_{\\text{on}}}$\n$\\newcommand{kroff}{k^r_{\\text{off}}}$\n$\\newcommand{rm}{r _m}$\n$\\newcommand{rp}{r _p}$\n$\\newcommand{gm}{\\gamma _m}$\n$\\newcommand{gp}{\\gamma _p}$\n$\\newcommand{mm}{\\left\\langle m \\right\\rangle}$\n$\\newcommand{foldchange}{\\text{fold-change}}$\n$\\newcommand{ee}[1]{\\left\\langle #1 \\right\\rangle}$\n$\\newcommand{var}[1]{\\text{Var}\\left( #1 \\right)}$\n$\\newcommand{bb}[1]{\\mathbf{#1}}$\n$\\newcommand{th}[1]{\\text{th}}$",
"_____no_output_____"
],
[
"## Variability in the kinetic parameters",
"_____no_output_____"
],
[
"An idea that could explain the systematic variation between our theoretical predictions and the data is the stochasticity that could be associated with random variation of the kinetic parameters. For example, if cells happen to stochastically have different number of ribosomes, how much would that affect the final distribution. Another good example is the variability in repressor copy number, which would affect the $\\kron$ rate.\n\nTo simplify things what we will do is sample random variations to some of the kinetic parameters, run the dynamics with such parameters, and then reconstruct the corresponding MaxEnt distribution. Then we will combine all of these distributions to see how different this is compared to the one with single parameter values.",
"_____no_output_____"
],
[
"### Unregulated promoter parameter variation",
"_____no_output_____"
],
[
"Let's begin with the unregulated promoter. The parameters here are $\\kpon, \\kpoff, r_m, \\gm, r_p$, and $\\gp$. The simplest scenario would be to sample variations out of a Gaussian distribution. We will set these distributions to be centered at the current value of the parameter we are using, and allow a variation of some defined percentage.\n\nLet's define a function that given an array of parameters, it samples random variations.",
"_____no_output_____"
]
],
[
[
"def param_normal_sample(param, n_samples, std=0.2):\n '''\n Function that samples variations to the parameter values out of a normal\n distribution.\n Parameters\n ----------\n param : array-like.\n List of parameters from which the samples will be generated.\n n_samples : int.\n Number or random samples to draw from the distribution\n std : float or array-like.\n Fractional standard deviations for each of the samples to be taken. \n If a single value is given, then all of the distributions will have \n the same standard deviation proportional to the mean.\n \n Returns\n -------\n samples : array-like. Shape = len(param) x n_samples\n Random samples of the parameters.\n '''\n # Initialize array to save output\n samples = np.zeros([n_samples, len(param)])\n \n # Loop through parameters\n for i, par in enumerate(param):\n if len(std) == len(param):\n samples[:, i] = np.random.normal(par, par * std[i], n_samples)\n elif len(std) == 1:\n samples[:, i] = np.random.normal(par, par * std[0], n_samples)\n \n return samples",
"_____no_output_____"
]
],
[
[
"Let's now load the parameters and generate random samples.",
"_____no_output_____"
]
],
[
[
"# Load parameter values\npar = ccutils.model.load_constants()\n\n# Define parametesr for unregulated promoter\npar_names = ['kp_on', 'kp_off', 'rm', 'gm', 'rp']\nparam = [par[x] for x in par_names]\n\n# Generate samples of all parameters with a 10% variability\nn_samples = 999 \nstd = [0.15]\nparam_sample = param_normal_sample(param, n_samples, std)\n# Add reference parameters to list\nparam_sample = np.append(np.array([[*param]]), param_sample, axis=0)",
"_____no_output_____"
]
],
[
[
"Having sampled the parameters let's go ahead and run the dynamics for each of these parameter sets. First we need to load the matrix to compute the moments of the distribution after the cell division as a function of the moments before the cell division.",
"_____no_output_____"
]
],
[
[
"# Read matrix into memory\nwith open(f'{homedir}/src/theory/pkl_files/binom_coeff_matrix.pkl', \n 'rb') as file:\n unpickler = pickle.Unpickler(file)\n Z_mat = unpickler.load()\n expo_binom = unpickler.load()",
"_____no_output_____"
]
],
[
[
"Now let's load the matrix to compute the dynamics of the unregualted two-state promoter",
"_____no_output_____"
]
],
[
[
"with open('../pkl_files/two_state_protein_dynamics_matrix.pkl',\n 'rb') as file:\n A_mat_unreg_lam = cloudpickle.load(file)\n expo_unreg = cloudpickle.load(file)",
"_____no_output_____"
]
],
[
[
"Next let's define all of the parameters that we will need for the integration.",
"_____no_output_____"
]
],
[
[
"# Define doubling time\ndoubling_time = 100\n# Define fraction of cell cycle spent with one copy\nt_single_frac = 0.6\n# Define time for single-promoter state\nt_single = 60 * t_single_frac * doubling_time # sec\nt_double = 60 * (1 - t_single_frac) * doubling_time # sec\nn_cycles = 6\n\n# Define names for dataframe columns\nnames = par_names + ['m' + str(m[0]) + 'p' + str(m[1]) for m in expo_unreg]\n\n# Initialize DataFrame to save constraints\ndf_moments = pd.DataFrame([], columns=names)",
"_____no_output_____"
]
],
[
[
"Now we are ready to run the dynamics in parallel, let's define the function so that we can perform this numerical integration in parallel",
"_____no_output_____"
]
],
[
[
"compute_dynamics = False\n\nif compute_dynamics:\n # Define function for parallel computation\n def constraints_parallel(par):\n kp_on = par[0]\n kp_off = par[1]\n rm = par[2]\n gm = par[3]\n rp = par[4]\n\n # Single promoter\n gp_init = 1 / (60 * 60)\n rp_init = 500 * gp_init\n\n # Generate matrices for dynamics\n # Single promoter\n par_unreg_s = [kp_on, kp_off, rm, gm, rp, 0]\n # Two promoters\n par_unreg_d = [kp_on, kp_off, 2 * rm, gm, rp, 0]\n\n # Initial conditions\n A_unreg_s_init = A_mat_unreg_lam(\n kp_on, kp_off, rm, gm, rp_init, gp_init\n )\n\n # Define initial conditions\n mom_init = np.zeros(len(expo_unreg) * 2)\n # Set initial condition for zero moment\n # Since this needs to add up to 1\n mom_init[0] = 1\n\n # Define time on which to perform integration\n t = np.linspace(0, 4000 * 60, 10000)\n # Numerically integrate equations\n m_init = sp.integrate.odeint(\n ccutils.model.rhs_dmomdt, mom_init, t, args=(A_unreg_s_init,)\n )\n # Keep last time point as initial condition\n m_init = m_init[-1, :]\n\n # Integrate moment equations\n df = ccutils.model.dmomdt_cycles(\n m_init,\n t_single,\n t_double,\n A_mat_unreg_lam,\n par_unreg_s,\n par_unreg_d,\n expo_unreg,\n n_cycles,\n Z_mat,\n states=[\"A\", \"I\"],\n n_steps=3000,\n )\n\n # Keep only last cycle\n df = df[df[\"cycle\"] == df[\"cycle\"].max()]\n\n # Extract time of last cell cycle\n time = np.sort(df[\"time\"].unique())\n\n # Compute the time differences\n time_diff = np.diff(time)\n # Compute the cumulative time difference\n time_cumsum = np.cumsum(time_diff)\n time_cumsum = time_cumsum / time_cumsum[-1]\n\n # Define array for spacing of cell cycle\n a_array = np.zeros(len(time))\n a_array[1:] = time_cumsum\n\n # Compute probability based on this array\n p_a_array = np.log(2) * 2 ** (1 - a_array)\n\n # Initialize list to append moments\n moms = list()\n # Loop through moments computing the average moment\n for i, mom in enumerate(expo_unreg):\n # Generate string that finds the moment\n mom_name = \"m\" + str(mom[0]) + \"p\" + str(mom[1])\n # List rows with moment\n mom_bool = [x for x in df.columns if mom_name in x]\n # Extract data for this particular moment\n df_mom = df.loc[:, mom_bool].sum(axis=1)\n\n # Average moment and append it to list\n moms.append(sp.integrate.simps(df_mom * p_a_array, a_array))\n\n # Save results into series in order to append it to data frame\n series = pd.Series(list(par) + moms, index=names)\n\n return series\n\n # Run function in parallel\n constraint_series = Parallel(n_jobs=6)(\n delayed(constraints_parallel)(par) for par in param_sample\n )\n\n # Initialize data frame to save list of pareters\n df_moments = pd.DataFrame([], columns=names)\n\n for s in constraint_series:\n df_moments = df_moments.append(s, ignore_index=True)\n df_moments.to_csv(\n f\"{homedir}/data/csv_maxEnt_dist/\" + \"MaxEnt_unreg_random.csv\",\n index=False,\n )\n\ndf_moments = pd.read_csv(\n f\"{homedir}/data/csv_maxEnt_dist/\" + \"MaxEnt_unreg_random.csv\"\n)\ndf_moments.head()",
"_____no_output_____"
]
],
[
[
"Let's look at the distribution of means and standard deviations in mRNA count for these variations in parameters.",
"_____no_output_____"
]
],
[
[
"# Compute mRNA standard deviations\nmRNA_std = np.sqrt(df_moments.m2p0 - df_moments.m1p0**2)\n\n# Initialize figure\nfig, ax = plt.subplots(1, 2, figsize=(7, 3))\n\n# Generate ECDF for mean\nx, y = ccutils.stats.ecdf(df_moments.m1p0)\nax[0].plot(x, y, lw=0, marker='.')\n# add reference line\nax[0].axvline(df_moments.m1p0[0], color='black',\n linestyle='--')\n# label axis\nax[0].set_xlabel(r'$\\left\\langle \\right.$mRNA/cell$\\left. \\right\\rangle$')\nax[0].set_ylabel('ECDF')\n\n# Generate ECDF for standard deviation \nx, y = ccutils.stats.ecdf(mRNA_std)\nax[1].plot(x, y, lw=0, marker='.')\n# add reference line\nax[1].axvline(mRNA_std[0], color='black', linestyle='--')\n# label axis\nax[1].set_xlabel('STD(mRNA/cell)')\nax[1].set_ylabel('ECDF');",
"_____no_output_____"
]
],
[
[
"There is quite a lot of variability compared to the reference value. Let's repeat these plots, but this time for the protein values",
"_____no_output_____"
]
],
[
[
"# Compute protein standard deviations\nprotein_std = np.sqrt(df_moments.m0p2 - df_moments.m0p1 ** 2)\n\n# Initialize figure\nfig, ax = plt.subplots(1, 2, figsize=(7, 3))\n\n# Generate ECDF for mean\nx, y = ccutils.stats.ecdf(df_moments.m0p1)\nax[0].plot(x, y, lw=0, marker=\".\")\n# add reference line\nax[0].axvline(df_moments.m0p1[0], color=\"black\", linestyle=\"--\")\n# label axis\nax[0].set_xlabel(r\"$\\left\\langle \\right.$protein/cell$\\left. \\right\\rangle$\")\nax[0].set_ylabel(\"ECDF\")\n\n# Generate ECDF for standard deviation\nx, y = ccutils.stats.ecdf(protein_std)\nax[1].plot(x, y, lw=0, marker=\".\")\n# add reference line\nax[1].axvline(protein_std[0], color=\"black\", linestyle=\"--\")\n# label axis\nax[1].set_xlabel(\"STD(protein/cell)\")\nax[1].set_ylabel(\"ECDF\")",
"_____no_output_____"
]
],
[
[
"### Moments of the conditional distribution",
"_____no_output_____"
],
[
"Let's now compare the mean, variance and skewness of the resulting distribution. For this all we have to use is the so-called [law of total expectation](https://en.wikipedia.org/wiki/Law_of_total_expectation) that states that\n$$\n\\ee{f(p)} = \\ee{\\ee{f(p) \\mid \\theta}_p}_\\theta,\n$$\ni.e. to compute the expected value of the function $f(p)$ (could be something like $f(p) p^2$) we first compute the average of the function for a parameter set $\\theta$, then we average the expected value of the function over all values of $\\theta$.",
"_____no_output_____"
],
[
"Let's for example first compare the resulting mean protein copy numbers for the original value and the one that includes the variability",
"_____no_output_____"
]
],
[
[
"mean_delta = df_moments.m0p1[0]\nmean_sample = df_moments.m0p1.mean()\n\nprint(f'mean delta: {np.round(mean_delta, 0)}')\nprint(f'mean sample: {np.round(mean_sample, 0)}')\nprint(f'fractional change: {(mean_sample - mean_delta) / mean_delta}')",
"mean delta: 7733.0\nmean sample: 8075.0\nfractional change: 0.04428981949722924\n"
]
],
[
[
"There is an increment of roughly a 4%, so that is pretty small. Let's now look at the variance",
"_____no_output_____"
]
],
[
[
"var_delta = df_moments.m0p2[0] - df_moments.m0p1[0]**2\nvar_sample = df_moments.m0p2.mean() - df_moments.m0p1.mean()**2\n\nprint(f'variance delta: {np.round(var_delta, 0)}')\nprint(f'variance sample: {np.round(var_sample, 0)}')\nprint(f'fractional change: {(var_sample - var_delta) / var_delta}')",
"variance delta: 2607605.0\nvariance sample: 10714739.0\nfractional change: 3.1090341346727075\n"
]
],
[
[
"The change in the variance is quite large! Let's see how this reflects to the change in the noise (std/mean).",
"_____no_output_____"
]
],
[
[
"noise_delta = np.sqrt(var_delta) / mean_delta\nnoise_sample = np.sqrt(var_sample) / mean_sample\n\nprint(f'noise delta: {np.round(noise_delta, 2)}')\nprint(f'noise sample: {np.round(noise_sample, 2)}')",
"noise delta: 0.21\nnoise sample: 0.41\n"
]
],
[
[
"There is a factor of two of difference when computing the noise. That is quite interesting since that is exactly what we saw the systematic deviation in the data was like. Let's see what the change in the skewness is then.",
"_____no_output_____"
]
],
[
[
"skew_delta = (\n df_moments.m0p3[0] - 3 * mean_delta * var_delta - mean_delta ** 3\n) / var_delta ** (3 / 2)\n\nskew_sample = (\n df_moments.m0p3.mean() - 3 * mean_sample * var_sample - mean_sample ** 3\n) / var_sample ** (3 / 2)\n\nprint(f\"skewness delta: {np.round(skew_delta, 2)}\")\nprint(f\"skewness sample: {np.round(skew_sample, 2)}\")",
"skewness delta: 0.71\nskewness sample: 1.26\n"
]
],
[
[
"This is quite suggestive. It seems that the random variability",
"_____no_output_____"
]
]
] | [
"markdown",
"code",
"markdown",
"code",
"markdown",
"code",
"markdown",
"code",
"markdown",
"code",
"markdown",
"code",
"markdown",
"code",
"markdown",
"code",
"markdown",
"code",
"markdown",
"code",
"markdown",
"code",
"markdown",
"code",
"markdown",
"code",
"markdown"
] | [
[
"markdown",
"markdown"
],
[
"code",
"code"
],
[
"markdown",
"markdown",
"markdown",
"markdown",
"markdown"
],
[
"code"
],
[
"markdown"
],
[
"code"
],
[
"markdown"
],
[
"code"
],
[
"markdown"
],
[
"code"
],
[
"markdown"
],
[
"code"
],
[
"markdown"
],
[
"code"
],
[
"markdown"
],
[
"code"
],
[
"markdown"
],
[
"code"
],
[
"markdown",
"markdown",
"markdown"
],
[
"code"
],
[
"markdown"
],
[
"code"
],
[
"markdown"
],
[
"code"
],
[
"markdown"
],
[
"code"
],
[
"markdown"
]
] |
e783dd0b46e518b7e27a5c7615ba49e661dd3436 | 7,080 | ipynb | Jupyter Notebook | .ipynb_checkpoints/Lunarlander-checkpoint.ipynb | ezztherose/notebooks | 47a68e217d35bc66cd34098a61a8b0e9de48f75d | [
"MIT"
] | null | null | null | .ipynb_checkpoints/Lunarlander-checkpoint.ipynb | ezztherose/notebooks | 47a68e217d35bc66cd34098a61a8b0e9de48f75d | [
"MIT"
] | null | null | null | .ipynb_checkpoints/Lunarlander-checkpoint.ipynb | ezztherose/notebooks | 47a68e217d35bc66cd34098a61a8b0e9de48f75d | [
"MIT"
] | null | null | null | 81.37931 | 1,491 | 0.667232 | [
[
[
"https://spinningup.openai.com/en/latest/algorithms/ppo.html",
"_____no_output_____"
]
],
[
[
"%pylab inline\nimport random\nimport time\nimport numpy as np\n\nimport tensorflow as tf\nimport tensorflow.keras.backend as K\nfrom tensorflow.keras.models import Model, clone_model\nfrom tensorflow.keras.optimizers import Adam, SGD\nfrom tensorflow.keras.layers import Input, Dense, Activation, Lambda\n\nimport gym",
"Populating the interactive namespace from numpy and matplotlib\n"
],
[
"#env = gym.make(\"CartPole-v1\")\nenv = gym.make(\"Lander\")\nenv.observation_space, env.action_space, type(env.action_space)",
"_____no_output_____"
]
]
] | [
"markdown",
"code"
] | [
[
"markdown"
],
[
"code",
"code"
]
] |
e783deb969f7d791cbe95294469a0c7251876862 | 67,787 | ipynb | Jupyter Notebook | analysis/model_selection/stage1/01_hw-tscv.ipynb | TomMonks/swast-benchmarking | 96964fb705a8b3cebbce8adcf03e42d4fc3dd05a | [
"MIT"
] | null | null | null | analysis/model_selection/stage1/01_hw-tscv.ipynb | TomMonks/swast-benchmarking | 96964fb705a8b3cebbce8adcf03e42d4fc3dd05a | [
"MIT"
] | null | null | null | analysis/model_selection/stage1/01_hw-tscv.ipynb | TomMonks/swast-benchmarking | 96964fb705a8b3cebbce8adcf03e42d4fc3dd05a | [
"MIT"
] | 1 | 2021-11-16T14:38:22.000Z | 2021-11-16T14:38:22.000Z | 32.715734 | 253 | 0.410521 | [
[
[
"# Time Series Cross Validation: Holt-Winters Exponential Smoothing with additive errors and seasonality.",
"_____no_output_____"
]
],
[
[
"import pandas as pd\nimport numpy as np\nimport matplotlib.pyplot as plt\nplt.style.use('ggplot')\n\n\n#forecast error metrics\nfrom forecast_tools.metrics import (mean_absolute_scaled_error, \n root_mean_squared_error,\n symmetric_mean_absolute_percentage_error)\n\nimport statsmodels as sm\nfrom statsmodels.tsa.statespace.exponential_smoothing import ExponentialSmoothing\n\nimport warnings\nwarnings.filterwarnings('ignore')",
"_____no_output_____"
],
[
"print(sm.__version__)",
"0.11.0\n"
],
[
"#ensemble learning\nfrom amb_forecast.ensemble import (Ensemble, UnweightedVote)",
"_____no_output_____"
]
],
[
[
"# Data Input\n\nThe constants `TOP_LEVEL`, `STAGE`, `REGION`,`TRUST` and `METHOD` are used to control data selection and the directory for outputting results. \n\n> Output file is `f'{TOP_LEVEL}/{STAGE}/{REGION}-{METHOD}_{metric}.csv'.csv`. where metric will be smape, rmse, mase, coverage_80 and coverage_95. Note: `REGION`: is also used to select the correct data from the input dataframe.",
"_____no_output_____"
]
],
[
[
"TOP_LEVEL = '../../../results/model_selection'\nSTAGE = 'stage1'\nREGION = 'Trust'\nMETHOD = 'hw'\n\nFILE_NAME = 'Daily_Responses_5_Years_2019_full.csv'\n\n#split training and test data.\nTEST_SPLIT_DATE = '2019-01-01'\n\n#second subdivide: train and val\nVAL_SPLIT_DATE = '2017-07-01'\n\n#discard data after 2020 due to coronavirus\n#this is the subject of a seperate study.\nDISCARD_DATE = '2020-01-01'",
"_____no_output_____"
],
[
"#read in path\npath = f'../../../data/{FILE_NAME}'",
"_____no_output_____"
],
[
"def pre_process_daily_data(path, index_col, by_col, \n values, dayfirst=False):\n '''\n Daily data is stored in long format. Read in \n and pivot to wide format so that there is a single \n colmumn for each regions time series.\n '''\n df = pd.read_csv(path, index_col=index_col, parse_dates=True, \n dayfirst=dayfirst)\n df.columns = map(str.lower, df.columns)\n df.index.rename(str(df.index.name).lower(), inplace=True)\n \n clean_table = pd.pivot_table(df, values=values.lower(), \n index=[index_col.lower()],\n columns=[by_col.lower()], aggfunc=np.sum)\n \n clean_table.index.freq = 'D'\n \n return clean_table",
"_____no_output_____"
],
[
"clean = pre_process_daily_data(path, 'Actual_dt', 'ORA', 'Actual_Value', \n dayfirst=False)\nclean.head()",
"_____no_output_____"
]
],
[
[
"## Train Test Split",
"_____no_output_____"
]
],
[
[
"def ts_train_test_split(data, split_date):\n '''\n Split time series into training and test data\n \n Parameters:\n -------\n data - pd.DataFrame - time series data. Index expected as datatimeindex\n split_date - the date on which to split the time series\n \n Returns:\n --------\n tuple (len=2) \n 0. pandas.DataFrame - training dataset\n 1. pandas.DataFrame - test dataset\n '''\n train = data.loc[data.index < split_date]\n test = data.loc[data.index >= split_date]\n return train, test",
"_____no_output_____"
],
[
"train, test = ts_train_test_split(clean, split_date=TEST_SPLIT_DATE)\n\n#exclude data after 2020 due to coronavirus.\ntest, discard = ts_train_test_split(test, split_date=DISCARD_DATE)\n\n#train split into train and validation\ntrain, val = ts_train_test_split(train, split_date=VAL_SPLIT_DATE)",
"_____no_output_____"
],
[
"train.shape",
"_____no_output_____"
],
[
"val.shape",
"_____no_output_____"
]
],
[
[
"# Test fitting and predicting with model.\n\nThe class below is a 'wrapper' class that provides the same interfacew for all methods and works in the time series cross valiation code. ",
"_____no_output_____"
]
],
[
[
"class ExponentialSmoothingWrapper:\n '''\n Facade for statsmodels exponential smoothing models. This wrapper\n provides a common interface for all models and allow interop with\n the custom time series cross validation code.\n '''\n def __init__(self, trend=False, damped_trend=False, seasonal=None):\n self._trend = trend\n self._seasonal= seasonal\n self._damped_trend = damped_trend\n\n def _get_resids(self):\n return self._fitted.resid\n\n def _get_preds(self):\n return self._fitted.fittedvalues\n\n def fit(self, train):\n '''\n Fit the model\n \n Parameters:\n train: array-like\n time series to fit.\n '''\n self._model = ExponentialSmoothing(endog=train,\n trend=self._trend, \n damped_trend=self._damped_trend,\n seasonal=self._seasonal)\n self._fitted = self._model.fit()\n self._t = len(train)\n \n def predict(self, horizon, return_conf_int=False, alpha=0.2):\n '''\n Forecast the time series from the final point in the fitted series.\n \n Parameters:\n ----------\n \n horizon: int\n steps ahead to forecast \n \n return_conf_int: bool, optional (default=False)\n Return prediction interval? \n \n alpha: float\n Used if return_conf_int=True. 100(1-alpha) interval.\n '''\n \n forecast = self._fitted.get_forecast(horizon)\n \n mean_forecast = forecast.summary_frame()['mean'].to_numpy()\n \n if return_conf_int:\n df = forecast.summary_frame(alpha=alpha)\n pi = df[['mean_ci_lower', 'mean_ci_upper']].to_numpy()\n return mean_forecast, pi \n else:\n return mean_forecast\n\n fittedvalues = property(_get_preds)\n resid = property(_get_resids)",
"_____no_output_____"
]
],
[
[
"# Example fitting and prediction with comb",
"_____no_output_____"
]
],
[
[
"model_1 = ExponentialSmoothingWrapper(trend=True, damped_trend=True, \n seasonal=7)",
"_____no_output_____"
],
[
"estimators = {'shw': model_1}\nens = Ensemble(estimators, UnweightedVote())",
"_____no_output_____"
],
[
"ens.fit(train[REGION])",
"_____no_output_____"
],
[
"H = 5\nens_preds = ens.predict(horizon=H)",
"_____no_output_____"
],
[
"ens_preds, pi = ens.predict(horizon=H, return_conf_int=True)",
"_____no_output_____"
],
[
"ens_preds",
"_____no_output_____"
],
[
"pi",
"_____no_output_____"
]
],
[
[
"## Time Series Cross Validation\n\n`time_series_cv` implements rolling forecast origin cross validation for time series. \nIt does not calculate forecast error, but instead returns the predictions, pred intervals and actuals in an array that can be passed to any forecast error function. (this is for efficiency and allows additional metrics to be calculated if needed).",
"_____no_output_____"
]
],
[
[
"def time_series_cv(model, train, val, horizons, alpha=0.2, step=1):\n '''\n Time series cross validation across multiple horizons for a single model.\n\n Incrementally adds additional training data to the model and tests\n across a provided list of forecast horizons. Note that function tests a\n model only against complete validation sets. E.g. if horizon = 15 and \n len(val) = 12 then no testing is done. In the case of multiple horizons\n e.g. [7, 14, 28] then the function will use the maximum forecast horizon\n to calculate the number of iterations i.e if len(val) = 365 and step = 1\n then no. iterations = len(val) - max(horizon) = 365 - 28 = 337.\n \n Parameters:\n --------\n model - forecasting model\n\n error_func - function to measure forecast error\n\n train - np.array - vector of training data\n\n val - np.array - vector of validation data\n\n horizon - list of ints, forecast horizon e.g. [7, 14, 28] days\n\n step -- step taken in cross validation \n e.g. 1 in next cross validation training data includes next point \n from the validation set.\n e.g. 7 in the next cross validation training data includes next 7 points\n (default=1)\n \n Returns:\n -------\n np.array - vector of forecast errors from the CVs.\n '''\n cv_preds = [] #mean forecast\n cv_actuals = [] # actuals \n cv_pis = [] #prediction intervals\n split = 0\n\n print('split => ', end=\"\")\n for i in range(0, len(val) - max(horizons) + 1, step):\n split += 1\n print(f'{split}, ', end=\"\")\n \n train_cv = np.concatenate([train, val[:i]], axis=0)\n model.fit(train_cv)\n \n #predict the maximum horizon \n preds, pis = model.predict(horizon=len(val[i:i+max(horizons)]), \n return_conf_int=True,\n alpha=alpha)\n \n cv_h_preds = []\n cv_test = []\n cv_h_pis = []\n \n for h in horizons:\n #store the h-step prediction\n cv_h_preds.append(preds[:h])\n #store the h-step actual value\n cv_test.append(val.iloc[i:i+h]) \n cv_h_pis.append(pis[:h])\n \n cv_preds.append(cv_h_preds)\n cv_actuals.append(cv_test)\n cv_pis.append(cv_h_pis)\n \n print('done.\\n') \n return cv_preds, cv_actuals, cv_pis",
"_____no_output_____"
]
],
[
[
"## Custom functions for calculating CV scores for point predictions and coverage.\n\nThese functions have been written to work with the output of `time_series_cv`",
"_____no_output_____"
]
],
[
[
"def split_cv_error(cv_preds, cv_test, error_func):\n '''\n Forecast error in the current split\n \n Params:\n -----\n cv_preds, np.array\n Split predictions\n \n \n cv_test: np.array\n acutal ground truth observations\n \n error_func: object\n function with signature (y_true, y_preds)\n \n Returns:\n -------\n np.ndarray\n cross validation errors for split\n '''\n n_splits = len(cv_preds)\n cv_errors = []\n \n for split in range(n_splits):\n pred_error = error_func(cv_test[split], cv_preds[split])\n cv_errors.append(pred_error)\n \n return np.array(cv_errors)\n\ndef forecast_errors_cv(cv_preds, cv_test, error_func):\n '''\n Forecast errors by forecast horizon\n \n Params:\n ------\n cv_preds: np.ndarray\n Array of arrays. Each array is of size h representing\n the forecast horizon specified.\n \n cv_test: np.ndarray\n Array of arrays. Each array is of size h representing\n the forecast horizon specified.\n \n error_func: object\n function with signature (y_true, y_preds)\n \n Returns:\n -------\n np.ndarray\n \n '''\n cv_test = np.array(cv_test)\n cv_preds = np.array(cv_preds)\n n_horizons = len(cv_test) \n \n horizon_errors = []\n for h in range(n_horizons):\n split_errors = split_cv_error(cv_preds[h], cv_test[h], error_func)\n horizon_errors.append(split_errors)\n\n return np.array(horizon_errors)\n\ndef split_coverage(cv_test, cv_intervals):\n n_splits = len(cv_test)\n cv_errors = []\n \n for split in range(n_splits):\n val = np.asarray(cv_test[split])\n lower = cv_intervals[split].T[0]\n upper = cv_intervals[split].T[1]\n \n coverage = len(np.where((val > lower) & (val < upper))[0])\n coverage = coverage / len(val)\n \n cv_errors.append(coverage)\n \n return np.array(cv_errors)\n \n \ndef prediction_int_coverage_cv(cv_test, cv_intervals):\n cv_test = np.array(cv_test)\n cv_intervals = np.array(cv_intervals)\n n_horizons = len(cv_test) \n \n horizon_coverage = []\n for h in range(n_horizons):\n split_coverages = split_coverage(cv_test[h], cv_intervals[h])\n horizon_coverage.append(split_coverages)\n\n return np.array(horizon_coverage) ",
"_____no_output_____"
],
[
"def split_cv_error_scaled(cv_preds, cv_test, y_train):\n n_splits = len(cv_preds)\n cv_errors = []\n \n for split in range(n_splits):\n pred_error = mean_absolute_scaled_error(cv_test[split], cv_preds[split], \n y_train, period=7)\n \n cv_errors.append(pred_error)\n \n return np.array(cv_errors)\n\ndef forecast_errors_cv_scaled(cv_preds, cv_test, y_train):\n cv_test = np.array(cv_test)\n cv_preds = np.array(cv_preds)\n n_horizons = len(cv_test) \n \n horizon_errors = []\n for h in range(n_horizons):\n split_errors = split_cv_error_scaled(cv_preds[h], cv_test[h], y_train)\n horizon_errors.append(split_errors)\n \n return np.array(horizon_errors)",
"_____no_output_____"
]
],
[
[
"### Get model and conduct tscv.",
"_____no_output_____"
]
],
[
[
"def get_model():\n '''\n Create ensemble model\n '''\n model_1 = ExponentialSmoothingWrapper(trend=True, damped_trend=True, \n seasonal=7)\n estimators = {'hw': model_1}\n return Ensemble(estimators, UnweightedVote())\n ",
"_____no_output_____"
],
[
"horizons = [7, 14, 21, 28, 35, 42, 49, 56, 63, 70, 77, 84, 365]\nmodel = get_model()\n\nresults = time_series_cv(model, train[REGION], val[REGION], horizons, \n alpha=0.2, step=7)",
"split => 1, 2, 3, 4, 5, 6, 7, 8, 9, 10, 11, 12, 13, 14, 15, 16, 17, 18, 19, 20, 21, 22, 23, 24, 25, 26, 27, done.\n\n"
],
[
"cv_preds, cv_test, cv_intervals = results\n#CV point predictions smape\ncv_errors = forecast_errors_cv(cv_preds, cv_test, \n symmetric_mean_absolute_percentage_error)\ndf = pd.DataFrame(cv_errors)\ndf.columns = horizons\ndf.describe()",
"_____no_output_____"
],
[
"#output sMAPE results to file\nmetric = 'smape'\nprint(f'{TOP_LEVEL}/{STAGE}/{REGION}-{METHOD}_{metric}.csv')\ndf.to_csv(f'{TOP_LEVEL}/{STAGE}/{REGION}-{METHOD}_{metric}.csv')",
"../../../results/model_selection/stage1/Trust-hw_smape.csv\n"
],
[
"#CV point predictions rmse\ncv_errors = forecast_errors_cv(cv_preds, cv_test, root_mean_squared_error)\ndf = pd.DataFrame(cv_errors)\ndf.columns = horizons\ndf.describe()",
"_____no_output_____"
],
[
"#output rmse\nmetric = 'rmse'\nprint(f'{TOP_LEVEL}/{STAGE}/{REGION}-{METHOD}_{metric}.csv')\ndf.to_csv(f'{TOP_LEVEL}/{STAGE}/{REGION}-{METHOD}_{metric}.csv')",
"../../../results/model_selection/stage1/Trust-hw_rmse.csv\n"
],
[
"#mase\ncv_errors = forecast_errors_cv_scaled(cv_preds, cv_test, train[REGION])\ndf = pd.DataFrame(cv_errors)\ndf.columns = horizons\ndf.describe()",
"_____no_output_____"
],
[
"#output rmse\nmetric = 'mase'\nprint(f'{TOP_LEVEL}/{STAGE}/{REGION}-{METHOD}_{metric}.csv')\ndf.to_csv(f'{TOP_LEVEL}/{STAGE}/{REGION}-{METHOD}_{metric}.csv')",
"../../../results/model_selection/stage1/Trust-hw_mase.csv\n"
],
[
"#80% PIs\ncv_coverage = prediction_int_coverage_cv(cv_test, cv_intervals)\ndf = pd.DataFrame(cv_coverage)\ndf.columns = horizons\ndf.describe()",
"_____no_output_____"
],
[
"#output 80% PI coverage\nmetric = 'coverage_80'\nprint(f'{TOP_LEVEL}/{STAGE}/{REGION}-{METHOD}_{metric}.csv')\ndf.to_csv(f'{TOP_LEVEL}/{STAGE}/{REGION}-{METHOD}_{metric}.csv')",
"../../../results/model_selection/stage1/Trust-hw_coverage_80.csv\n"
]
],
[
[
"### Rerun for 95% PI coverage",
"_____no_output_____"
]
],
[
[
"horizons = [7, 14, 21, 28, 35, 42, 49, 56, 63, 70, 77, 84, 365]\nmodel = get_model()\n\nresults = time_series_cv(model, train[REGION], val[REGION], horizons, \n alpha=0.05, step=7)",
"split => 1, 2, 3, 4, 5, 6, 7, 8, 9, 10, 11, 12, 13, 14, 15, 16, 17, 18, 19, 20, 21, 22, 23, 24, 25, 26, 27, done.\n\n"
],
[
"#95% PIs\ncv_preds, cv_test, cv_intervals = results\ncv_coverage = prediction_int_coverage_cv(cv_test, cv_intervals)\ndf = pd.DataFrame(cv_coverage)\ndf.columns = horizons\ndf.describe()",
"_____no_output_____"
],
[
"#output 95% PI coverage\nmetric = 'coverage_95'\nprint(f'{TOP_LEVEL}/{STAGE}/{REGION}-{METHOD}_{metric}.csv')\ndf.to_csv(f'{TOP_LEVEL}/{STAGE}/{REGION}-{METHOD}_{metric}.csv')",
"../../../results/model_selection/stage1/Trust-hw_coverage_95.csv\n"
]
],
[
[
"# End",
"_____no_output_____"
]
]
] | [
"markdown",
"code",
"markdown",
"code",
"markdown",
"code",
"markdown",
"code",
"markdown",
"code",
"markdown",
"code",
"markdown",
"code",
"markdown",
"code",
"markdown",
"code",
"markdown"
] | [
[
"markdown"
],
[
"code",
"code",
"code"
],
[
"markdown"
],
[
"code",
"code",
"code",
"code"
],
[
"markdown"
],
[
"code",
"code",
"code",
"code"
],
[
"markdown"
],
[
"code"
],
[
"markdown"
],
[
"code",
"code",
"code",
"code",
"code",
"code",
"code"
],
[
"markdown"
],
[
"code"
],
[
"markdown"
],
[
"code",
"code"
],
[
"markdown"
],
[
"code",
"code",
"code",
"code",
"code",
"code",
"code",
"code",
"code",
"code"
],
[
"markdown"
],
[
"code",
"code",
"code"
],
[
"markdown"
]
] |
e783e0e1f3f07ad808ef6250b42b8bd9aaad7876 | 453,803 | ipynb | Jupyter Notebook | human-activity-recognition.ipynb | varunsh20/Human-activity-recognition- | ffd7827e89e8c64050ba67303595157ca679cc04 | [
"MIT"
] | null | null | null | human-activity-recognition.ipynb | varunsh20/Human-activity-recognition- | ffd7827e89e8c64050ba67303595157ca679cc04 | [
"MIT"
] | 1 | 2021-06-13T18:44:10.000Z | 2021-06-13T18:44:10.000Z | human-activity-recognition.ipynb | varunsh20/Human-activity-recognition- | ffd7827e89e8c64050ba67303595157ca679cc04 | [
"MIT"
] | null | null | null | 453,803 | 453,803 | 0.933218 | [
[
[
"# This Python 3 environment comes with many helpful analytics libraries installed\n# It is defined by the kaggle/python Docker image: https://github.com/kaggle/docker-python\n# For example, here's several helpful packages to load\n\nimport numpy as np # linear algebra\nimport pandas as pd # data processing, CSV file I/O (e.g. pd.read_csv)\n\n# Input data files are available in the read-only \"../input/\" directory\n# For example, running this (by clicking run or pressing Shift+Enter) will list all files under the input directory\n\nimport os\nfor dirname, _, filenames in os.walk('/kaggle/input'):\n for filename in filenames:\n print(os.path.join(dirname, filename))\n\n# You can write up to 20GB to the current directory (/kaggle/working/) that gets preserved as output when you create a version using \"Save & Run All\" \n# You can also write temporary files to /kaggle/temp/, but they won't be saved outside of the current session",
"/kaggle/input/human-activity-recognition-with-smartphones/train.csv\n/kaggle/input/human-activity-recognition-with-smartphones/test.csv\n"
]
],
[
[
"**Preparing data**",
"_____no_output_____"
]
],
[
[
"train = pd.read_csv('../input/human-activity-recognition-with-smartphones/train.csv')\ntrain.head()",
"_____no_output_____"
],
[
"train.shape",
"_____no_output_____"
],
[
"train.isnull().values.any()",
"_____no_output_____"
],
[
"test = pd.read_csv('../input/human-activity-recognition-with-smartphones/test.csv')\ntest.head()",
"_____no_output_____"
],
[
"print(test.shape)\ntest.isnull().values.any()",
"(2947, 563)\n"
],
[
"X_train = train.iloc[:,:-2]\nY_train = train.iloc[:,-1]\n\nprint(X_train.shape)\nprint(Y_train.shape)",
"(7352, 561)\n(7352,)\n"
],
[
"X_test = test.iloc[:,:-2]\nY_test = test.iloc[:,-1]\n\nprint(X_test.shape)\nprint(Y_test.shape)",
"(2947, 561)\n(2947,)\n"
],
[
"Category_counts = np.array(Y_train.value_counts())\nCategory_counts",
"_____no_output_____"
]
],
[
[
"**There are five different activities i.e 'Standing','Sitting','Laying','Walking','Walking_downstairs','Walking_upstairs'.**",
"_____no_output_____"
],
[
"**Plotting a count plot of each activity in the training data.**",
"_____no_output_____"
]
],
[
[
"import matplotlib.pyplot as plt\nimport seaborn as sns\n\nplt.figure(figsize=(10,8))\nsns.countplot(train.Activity)\nplt.xticks(rotation=45)\n",
"_____no_output_____"
]
],
[
[
"**Creating a scatter plot using t-SNE**",
"_____no_output_____"
],
[
"Using t-SNE data can be visualized from a extremely high dimensional space to a low dimensional space and still it retains lots of actual information. Given training data has 562 unqiue features, using t-SNE let's visualize it to a 2D space.",
"_____no_output_____"
]
],
[
[
"from sklearn.manifold import TSNE\ntsne = TSNE(random_state = 42, n_components=2, verbose=1, perplexity=50, n_iter=1000).fit_transform(X_train)",
"[t-SNE] Computing 151 nearest neighbors...\n[t-SNE] Indexed 7352 samples in 0.518s...\n[t-SNE] Computed neighbors for 7352 samples in 44.519s...\n[t-SNE] Computed conditional probabilities for sample 1000 / 7352\n[t-SNE] Computed conditional probabilities for sample 2000 / 7352\n[t-SNE] Computed conditional probabilities for sample 3000 / 7352\n[t-SNE] Computed conditional probabilities for sample 4000 / 7352\n[t-SNE] Computed conditional probabilities for sample 5000 / 7352\n[t-SNE] Computed conditional probabilities for sample 6000 / 7352\n[t-SNE] Computed conditional probabilities for sample 7000 / 7352\n[t-SNE] Computed conditional probabilities for sample 7352 / 7352\n[t-SNE] Mean sigma: 1.437672\n[t-SNE] KL divergence after 250 iterations with early exaggeration: 74.125961\n[t-SNE] KL divergence after 1000 iterations: 1.282853\n"
],
[
"plt.figure(figsize=(12,8))\nsns.scatterplot(x =tsne[:, 0], y = tsne[:, 1],data = train,hue = train[\"Activity\"])\n",
"_____no_output_____"
],
[
"train['tBodyAcc-mean()-X'].hist()",
"_____no_output_____"
],
[
"train['tBodyAcc-mean()-Y'].hist()",
"_____no_output_____"
],
[
"train['tBodyAcc-mean()-Z'].hist()",
"_____no_output_____"
],
[
"#Y_train = Y_train.reshape((-1,1))\n#Y_test = Y_test.reshape((-1,1))\n\n#print(Y_train.shape)\n#print(Y_test.shape)",
"_____no_output_____"
]
],
[
[
"**Scaling the data**",
"_____no_output_____"
],
[
" **Creating labels for different classes**",
"_____no_output_____"
]
],
[
[
"from sklearn.preprocessing import LabelEncoder\nle = LabelEncoder()\n\nY_train = le.fit_transform(Y_train)\nY_test = le.transform(Y_test)\n\nle.classes_",
"_____no_output_____"
]
],
[
[
"**It is necessary to create a one-hot vector for classes to fit the data in the model.**",
"_____no_output_____"
]
],
[
[
"Y_train = pd.get_dummies(Y_train).values\nY_test = pd.get_dummies(Y_test).values",
"_____no_output_____"
],
[
"Y_train",
"_____no_output_____"
],
[
"Y_train.shape\n\n",
"_____no_output_____"
]
],
[
[
"**Creating our model**",
"_____no_output_____"
]
],
[
[
"from tensorflow.keras import models\nfrom tensorflow.keras.layers import Dense,Dropout\n\nmodel = models.Sequential()\n\nmodel.add(Dense(64,activation='relu',input_dim=X_train.shape[1]))\nmodel.add(Dropout(0.25))\nmodel.add(Dense(128,activation='relu'))\nmodel.add(Dense(64,activation='relu'))\nmodel.add(Dense(32,activation='relu'))\nmodel.add(Dropout(0.25))\nmodel.add(Dense(10,activation='relu'))\nmodel.add(Dense(6,activation='softmax'))\n\nmodel.summary()\n",
"Model: \"sequential\"\n_________________________________________________________________\nLayer (type) Output Shape Param # \n=================================================================\ndense (Dense) (None, 64) 35968 \n_________________________________________________________________\ndropout (Dropout) (None, 64) 0 \n_________________________________________________________________\ndense_1 (Dense) (None, 128) 8320 \n_________________________________________________________________\ndense_2 (Dense) (None, 64) 8256 \n_________________________________________________________________\ndense_3 (Dense) (None, 32) 2080 \n_________________________________________________________________\ndropout_1 (Dropout) (None, 32) 0 \n_________________________________________________________________\ndense_4 (Dense) (None, 10) 330 \n_________________________________________________________________\ndense_5 (Dense) (None, 6) 66 \n=================================================================\nTotal params: 55,020\nTrainable params: 55,020\nNon-trainable params: 0\n_________________________________________________________________\n"
]
],
[
[
"**Compiling and training the model.**",
"_____no_output_____"
]
],
[
[
"model.compile(optimizer='adam',loss='categorical_crossentropy',metrics=['accuracy'])",
"_____no_output_____"
],
[
"hist = model.fit(X_train,Y_train,epochs=30,batch_size = 128,validation_split=0.3)",
"Epoch 1/30\n41/41 [==============================] - 0s 9ms/step - loss: 1.3677 - accuracy: 0.4131 - val_loss: 0.8933 - val_accuracy: 0.6668\nEpoch 2/30\n41/41 [==============================] - 0s 4ms/step - loss: 0.7899 - accuracy: 0.6731 - val_loss: 0.5051 - val_accuracy: 0.8314\nEpoch 3/30\n41/41 [==============================] - 0s 4ms/step - loss: 0.5186 - accuracy: 0.7928 - val_loss: 0.3797 - val_accuracy: 0.8613\nEpoch 4/30\n41/41 [==============================] - 0s 4ms/step - loss: 0.3697 - accuracy: 0.8581 - val_loss: 0.3890 - val_accuracy: 0.8708\nEpoch 5/30\n41/41 [==============================] - 0s 4ms/step - loss: 0.2890 - accuracy: 0.8877 - val_loss: 0.2865 - val_accuracy: 0.9130\nEpoch 6/30\n41/41 [==============================] - 0s 4ms/step - loss: 0.2318 - accuracy: 0.9162 - val_loss: 0.2936 - val_accuracy: 0.8867\nEpoch 7/30\n41/41 [==============================] - 0s 4ms/step - loss: 0.2054 - accuracy: 0.9217 - val_loss: 0.2423 - val_accuracy: 0.9229\nEpoch 8/30\n41/41 [==============================] - 0s 4ms/step - loss: 0.1832 - accuracy: 0.9339 - val_loss: 0.2686 - val_accuracy: 0.9180\nEpoch 9/30\n41/41 [==============================] - 0s 4ms/step - loss: 0.1609 - accuracy: 0.9403 - val_loss: 0.2350 - val_accuracy: 0.9234\nEpoch 10/30\n41/41 [==============================] - 0s 4ms/step - loss: 0.1368 - accuracy: 0.9491 - val_loss: 0.2061 - val_accuracy: 0.9302\nEpoch 11/30\n41/41 [==============================] - 0s 4ms/step - loss: 0.1432 - accuracy: 0.9493 - val_loss: 0.2020 - val_accuracy: 0.9311\nEpoch 12/30\n41/41 [==============================] - 0s 4ms/step - loss: 0.1283 - accuracy: 0.9532 - val_loss: 0.1948 - val_accuracy: 0.9284\nEpoch 13/30\n41/41 [==============================] - 0s 4ms/step - loss: 0.1163 - accuracy: 0.9571 - val_loss: 0.2416 - val_accuracy: 0.9374\nEpoch 14/30\n41/41 [==============================] - 0s 4ms/step - loss: 0.1073 - accuracy: 0.9594 - val_loss: 0.2372 - val_accuracy: 0.9329\nEpoch 15/30\n41/41 [==============================] - 0s 4ms/step - loss: 0.1043 - accuracy: 0.9637 - val_loss: 0.1879 - val_accuracy: 0.9306\nEpoch 16/30\n41/41 [==============================] - 0s 4ms/step - loss: 0.1036 - accuracy: 0.9619 - val_loss: 0.3256 - val_accuracy: 0.9093\nEpoch 17/30\n41/41 [==============================] - 0s 4ms/step - loss: 0.1034 - accuracy: 0.9633 - val_loss: 0.2749 - val_accuracy: 0.9248\nEpoch 18/30\n41/41 [==============================] - 0s 4ms/step - loss: 0.0881 - accuracy: 0.9689 - val_loss: 0.2850 - val_accuracy: 0.9352\nEpoch 19/30\n41/41 [==============================] - 0s 4ms/step - loss: 0.0807 - accuracy: 0.9695 - val_loss: 0.2876 - val_accuracy: 0.9266\nEpoch 20/30\n41/41 [==============================] - 0s 4ms/step - loss: 0.1229 - accuracy: 0.9536 - val_loss: 0.2350 - val_accuracy: 0.9361\nEpoch 21/30\n41/41 [==============================] - 0s 4ms/step - loss: 0.0986 - accuracy: 0.9660 - val_loss: 0.2541 - val_accuracy: 0.9306\nEpoch 22/30\n41/41 [==============================] - 0s 4ms/step - loss: 0.0909 - accuracy: 0.9666 - val_loss: 0.2134 - val_accuracy: 0.9343\nEpoch 23/30\n41/41 [==============================] - 0s 4ms/step - loss: 0.0816 - accuracy: 0.9699 - val_loss: 0.2951 - val_accuracy: 0.9275\nEpoch 24/30\n41/41 [==============================] - 0s 4ms/step - loss: 0.0827 - accuracy: 0.9709 - val_loss: 0.1860 - val_accuracy: 0.9406\nEpoch 25/30\n41/41 [==============================] - 0s 4ms/step - loss: 0.0900 - accuracy: 0.9648 - val_loss: 0.2276 - val_accuracy: 0.9388\nEpoch 26/30\n41/41 [==============================] - 0s 4ms/step - loss: 0.0711 - accuracy: 0.9738 - val_loss: 0.2106 - val_accuracy: 0.9393\nEpoch 27/30\n41/41 [==============================] - 0s 4ms/step - loss: 0.0739 - accuracy: 0.9749 - val_loss: 0.2445 - val_accuracy: 0.9329\nEpoch 28/30\n41/41 [==============================] - 0s 4ms/step - loss: 0.0848 - accuracy: 0.9675 - val_loss: 0.2974 - val_accuracy: 0.9288\nEpoch 29/30\n41/41 [==============================] - 0s 4ms/step - loss: 0.0686 - accuracy: 0.9755 - val_loss: 0.1919 - val_accuracy: 0.9415\nEpoch 30/30\n41/41 [==============================] - 0s 4ms/step - loss: 0.0589 - accuracy: 0.9788 - val_loss: 0.2649 - val_accuracy: 0.9356\n"
]
],
[
[
"**Visualising loss and accuracy curve of the model.**",
"_____no_output_____"
]
],
[
[
"plt.plot(hist.history['loss'],label='train_loss')\nplt.plot(hist.history['val_loss'],label='val_loss')\nplt.xlabel('Epochs',fontsize=18)\nplt.ylabel('Loss',fontsize=18)\nplt.legend()\nplt.title('Loss Curve',fontsize=22)\nplt.show()",
"_____no_output_____"
],
[
"plt.plot(hist.history['accuracy'],label='train_accuracy')\nplt.plot(hist.history['val_accuracy'],label='val_accuracy')\nplt.xlabel('Epochs',fontsize=18)\nplt.ylabel('Accuracy',fontsize=18)\nplt.legend()\nplt.title('Accuracy Curve',fontsize=22)\nplt.show()",
"_____no_output_____"
],
[
"model.save('my_model.h5')",
"_____no_output_____"
]
],
[
[
"**Making predictions on test data**",
"_____no_output_____"
]
],
[
[
"predict = model.predict(X_test)\n\npredictions = np.argmax(predict,axis=1)\n\n",
"_____no_output_____"
],
[
"predictions",
"_____no_output_____"
],
[
"Y_test = np.argmax(Y_test,axis=1)",
"_____no_output_____"
]
],
[
[
"**Calculating accuracy**",
"_____no_output_____"
]
],
[
[
"from sklearn.metrics import accuracy_score,precision_score,recall_score,confusion_matrix\nfrom mlxtend.plotting import plot_confusion_matrix\n\nconf_matrix = confusion_matrix(Y_test,predictions)\nplot_confusion_matrix(conf_matrix)\n\nprecision = precision_score(Y_test,predictions,average='weighted')\nrecall = recall_score(Y_test, predictions,average='weighted')\naccuracy = accuracy_score(Y_test,predictions)\n\nprint(\"Accuracy = \"+str(accuracy))\nprint(\"Precision = \"+str(precision))\nprint(\"Recall = \"+str(recall))",
"Accuracy = 0.9216152019002375\nPrecision = 0.9282570445597496\nRecall = 0.9216152019002375\n"
]
],
[
[
"**The model was able to produce 93% accurate results.**",
"_____no_output_____"
]
]
] | [
"code",
"markdown",
"code",
"markdown",
"code",
"markdown",
"code",
"markdown",
"code",
"markdown",
"code",
"markdown",
"code",
"markdown",
"code",
"markdown",
"code",
"markdown",
"code",
"markdown",
"code",
"markdown"
] | [
[
"code"
],
[
"markdown"
],
[
"code",
"code",
"code",
"code",
"code",
"code",
"code",
"code"
],
[
"markdown",
"markdown"
],
[
"code"
],
[
"markdown",
"markdown"
],
[
"code",
"code",
"code",
"code",
"code",
"code"
],
[
"markdown",
"markdown"
],
[
"code"
],
[
"markdown"
],
[
"code",
"code",
"code"
],
[
"markdown"
],
[
"code"
],
[
"markdown"
],
[
"code",
"code"
],
[
"markdown"
],
[
"code",
"code",
"code"
],
[
"markdown"
],
[
"code",
"code",
"code"
],
[
"markdown"
],
[
"code"
],
[
"markdown"
]
] |
e783e3c644477d2c8b4aa913cc1e899169b2de9a | 317,654 | ipynb | Jupyter Notebook | 16_nlp_with_rnns_and_attention.ipynb | otamilocintra/ml2gh | 917bd7c7576dff094ea493f85e178e2ed5ef6e24 | [
"Apache-2.0"
] | null | null | null | 16_nlp_with_rnns_and_attention.ipynb | otamilocintra/ml2gh | 917bd7c7576dff094ea493f85e178e2ed5ef6e24 | [
"Apache-2.0"
] | null | null | null | 16_nlp_with_rnns_and_attention.ipynb | otamilocintra/ml2gh | 917bd7c7576dff094ea493f85e178e2ed5ef6e24 | [
"Apache-2.0"
] | null | null | null | 75.255627 | 105,909 | 0.716097 | [
[
[
"**Chapter 16 – Natural Language Processing with RNNs and Attention**",
"_____no_output_____"
],
[
"_This notebook contains all the sample code in chapter 16._",
"_____no_output_____"
],
[
"<table align=\"left\">\n <td>\n <a target=\"_blank\" href=\"https://colab.research.google.com/github/ageron/handson-ml2/blob/master/16_nlp_with_rnns_and_attention.ipynb\"><img src=\"https://www.tensorflow.org/images/colab_logo_32px.png\" />Run in Google Colab</a>\n </td>\n</table>",
"_____no_output_____"
],
[
"# Setup",
"_____no_output_____"
],
[
"First, let's import a few common modules, ensure MatplotLib plots figures inline and prepare a function to save the figures. We also check that Python 3.5 or later is installed (although Python 2.x may work, it is deprecated so we strongly recommend you use Python 3 instead), as well as Scikit-Learn ≥0.20 and TensorFlow ≥2.0.",
"_____no_output_____"
]
],
[
[
"# Python ≥3.5 is required\nimport sys\nassert sys.version_info >= (3, 5)\n\n# Scikit-Learn ≥0.20 is required\nimport sklearn\nassert sklearn.__version__ >= \"0.20\"\n\ntry:\n # %tensorflow_version only exists in Colab.\n %tensorflow_version 2.x\n !pip install -q -U tensorflow-addons\n IS_COLAB = True\nexcept Exception:\n IS_COLAB = False\n\n# TensorFlow ≥2.0 is required\nimport tensorflow as tf\nfrom tensorflow import keras\nassert tf.__version__ >= \"2.0\"\n\nif not tf.config.list_physical_devices('GPU'):\n print(\"No GPU was detected. LSTMs and CNNs can be very slow without a GPU.\")\n if IS_COLAB:\n print(\"Go to Runtime > Change runtime and select a GPU hardware accelerator.\")\n\n# Common imports\nimport numpy as np\nimport os\n\n# to make this notebook's output stable across runs\nnp.random.seed(42)\ntf.random.set_seed(42)\n\n# To plot pretty figures\n%matplotlib inline\nimport matplotlib as mpl\nimport matplotlib.pyplot as plt\nmpl.rc('axes', labelsize=14)\nmpl.rc('xtick', labelsize=12)\nmpl.rc('ytick', labelsize=12)\n\n# Where to save the figures\nPROJECT_ROOT_DIR = \".\"\nCHAPTER_ID = \"nlp\"\nIMAGES_PATH = os.path.join(PROJECT_ROOT_DIR, \"images\", CHAPTER_ID)\nos.makedirs(IMAGES_PATH, exist_ok=True)\n\ndef save_fig(fig_id, tight_layout=True, fig_extension=\"png\", resolution=300):\n path = os.path.join(IMAGES_PATH, fig_id + \".\" + fig_extension)\n print(\"Saving figure\", fig_id)\n if tight_layout:\n plt.tight_layout()\n plt.savefig(path, format=fig_extension, dpi=resolution)",
"No GPU was detected. LSTMs and CNNs can be very slow without a GPU.\n"
]
],
[
[
"# Char-RNN",
"_____no_output_____"
],
[
"## Splitting a sequence into batches of shuffled windows",
"_____no_output_____"
],
[
"For example, let's split the sequence 0 to 14 into windows of length 5, each shifted by 2 (e.g.,`[0, 1, 2, 3, 4]`, `[2, 3, 4, 5, 6]`, etc.), then shuffle them, and split them into inputs (the first 4 steps) and targets (the last 4 steps) (e.g., `[2, 3, 4, 5, 6]` would be split into `[[2, 3, 4, 5], [3, 4, 5, 6]]`), then create batches of 3 such input/target pairs:",
"_____no_output_____"
]
],
[
[
"np.random.seed(42)\ntf.random.set_seed(42)\n\nn_steps = 5\ndataset = tf.data.Dataset.from_tensor_slices(tf.range(15))\ndataset = dataset.window(n_steps, shift=2, drop_remainder=True)\ndataset = dataset.flat_map(lambda window: window.batch(n_steps))\ndataset = dataset.shuffle(10).map(lambda window: (window[:-1], window[1:]))\ndataset = dataset.batch(3).prefetch(1)\nfor index, (X_batch, Y_batch) in enumerate(dataset):\n print(\"_\" * 20, \"Batch\", index, \"\\nX_batch\")\n print(X_batch.numpy())\n print(\"=\" * 5, \"\\nY_batch\")\n print(Y_batch.numpy())",
"____________________ Batch 0 \nX_batch\n[[6 7 8 9]\n [2 3 4 5]\n [4 5 6 7]]\n===== \nY_batch\n[[ 7 8 9 10]\n [ 3 4 5 6]\n [ 5 6 7 8]]\n____________________ Batch 1 \nX_batch\n[[ 0 1 2 3]\n [ 8 9 10 11]\n [10 11 12 13]]\n===== \nY_batch\n[[ 1 2 3 4]\n [ 9 10 11 12]\n [11 12 13 14]]\n"
]
],
[
[
"## Loading the Data and Preparing the Dataset",
"_____no_output_____"
]
],
[
[
"shakespeare_url = \"https://raw.githubusercontent.com/karpathy/char-rnn/master/data/tinyshakespeare/input.txt\"\nfilepath = keras.utils.get_file(\"shakespeare.txt\", shakespeare_url)\nwith open(filepath) as f:\n shakespeare_text = f.read()",
"Downloading data from https://raw.githubusercontent.com/karpathy/char-rnn/master/data/tinyshakespeare/input.txt\n1122304/1115394 [==============================] - 0s 0us/step\n"
],
[
"print(shakespeare_text[:148])",
"First Citizen:\nBefore we proceed any further, hear me speak.\n\nAll:\nSpeak, speak.\n\nFirst Citizen:\nYou are all resolved rather to die than to famish?\n\n"
],
[
"\"\".join(sorted(set(shakespeare_text.lower())))",
"_____no_output_____"
],
[
"tokenizer = keras.preprocessing.text.Tokenizer(char_level=True)\ntokenizer.fit_on_texts(shakespeare_text)",
"_____no_output_____"
],
[
"tokenizer.texts_to_sequences([\"First\"])",
"_____no_output_____"
],
[
"tokenizer.sequences_to_texts([[20, 6, 9, 8, 3]])",
"_____no_output_____"
],
[
"max_id = len(tokenizer.word_index) # number of distinct characters\ndataset_size = tokenizer.document_count # total number of characters",
"_____no_output_____"
],
[
"[encoded] = np.array(tokenizer.texts_to_sequences([shakespeare_text])) - 1\ntrain_size = dataset_size * 90 // 100\ndataset = tf.data.Dataset.from_tensor_slices(encoded[:train_size])",
"_____no_output_____"
],
[
"n_steps = 100\nwindow_length = n_steps + 1 # target = input shifted 1 character ahead\ndataset = dataset.repeat().window(window_length, shift=1, drop_remainder=True)",
"_____no_output_____"
],
[
"dataset = dataset.flat_map(lambda window: window.batch(window_length))",
"_____no_output_____"
],
[
"np.random.seed(42)\ntf.random.set_seed(42)",
"_____no_output_____"
],
[
"batch_size = 32\ndataset = dataset.shuffle(10000).batch(batch_size)\ndataset = dataset.map(lambda windows: (windows[:, :-1], windows[:, 1:]))",
"_____no_output_____"
],
[
"dataset = dataset.map(\n lambda X_batch, Y_batch: (tf.one_hot(X_batch, depth=max_id), Y_batch))",
"_____no_output_____"
],
[
"dataset = dataset.prefetch(1)",
"_____no_output_____"
],
[
"for X_batch, Y_batch in dataset.take(1):\n print(X_batch.shape, Y_batch.shape)",
"(32, 100, 39) (32, 100)\n"
]
],
[
[
"## Creating and Training the Model",
"_____no_output_____"
]
],
[
[
"model = keras.models.Sequential([\n keras.layers.GRU(128, return_sequences=True, input_shape=[None, max_id],\n dropout=0.2, recurrent_dropout=0.2),\n keras.layers.GRU(128, return_sequences=True,\n dropout=0.2, recurrent_dropout=0.2),\n keras.layers.TimeDistributed(keras.layers.Dense(max_id,\n activation=\"softmax\"))\n])\nmodel.compile(loss=\"sparse_categorical_crossentropy\", optimizer=\"adam\")\nhistory = model.fit(dataset, steps_per_epoch=train_size // batch_size,\n epochs=10)",
"Train for 31370 steps\nEpoch 1/10\n31370/31370 [==============================] - 7150s 228ms/step - loss: 1.4671\nEpoch 2/10\n31370/31370 [==============================] - 7094s 226ms/step - loss: 1.3614\nEpoch 3/10\n31370/31370 [==============================] - 7063s 225ms/step - loss: 1.3404\nEpoch 4/10\n31370/31370 [==============================] - 7039s 224ms/step - loss: 1.3311\nEpoch 5/10\n31370/31370 [==============================] - 7056s 225ms/step - loss: 1.3256\nEpoch 6/10\n31370/31370 [==============================] - 7049s 225ms/step - loss: 1.3209\nEpoch 7/10\n31370/31370 [==============================] - 7068s 225ms/step - loss: 1.3166\nEpoch 8/10\n31370/31370 [==============================] - 7030s 224ms/step - loss: 1.3138\nEpoch 9/10\n31370/31370 [==============================] - 7061s 225ms/step - loss: 1.3120\nEpoch 10/10\n31370/31370 [==============================] - 7177s 229ms/step - loss: 1.3105\n"
]
],
[
[
"## Using the Model to Generate Text",
"_____no_output_____"
]
],
[
[
"def preprocess(texts):\n X = np.array(tokenizer.texts_to_sequences(texts)) - 1\n return tf.one_hot(X, max_id)",
"_____no_output_____"
],
[
"X_new = preprocess([\"How are yo\"])\nY_pred = model.predict_classes(X_new)\ntokenizer.sequences_to_texts(Y_pred + 1)[0][-1] # 1st sentence, last char",
"_____no_output_____"
],
[
"tf.random.set_seed(42)\n\ntf.random.categorical([[np.log(0.5), np.log(0.4), np.log(0.1)]], num_samples=40).numpy()",
"_____no_output_____"
],
[
"def next_char(text, temperature=1):\n X_new = preprocess([text])\n y_proba = model.predict(X_new)[0, -1:, :]\n rescaled_logits = tf.math.log(y_proba) / temperature\n char_id = tf.random.categorical(rescaled_logits, num_samples=1) + 1\n return tokenizer.sequences_to_texts(char_id.numpy())[0]",
"_____no_output_____"
],
[
"tf.random.set_seed(42)\n\nnext_char(\"How are yo\", temperature=1)",
"_____no_output_____"
],
[
"def complete_text(text, n_chars=50, temperature=1):\n for _ in range(n_chars):\n text += next_char(text, temperature)\n return text",
"_____no_output_____"
],
[
"tf.random.set_seed(42)\n\nprint(complete_text(\"t\", temperature=0.2))",
"WARNING:tensorflow:5 out of the last 6 calls to <function _make_execution_function.<locals>.distributed_function at 0x7f8d44616830> triggered tf.function retracing. Tracing is expensive and the excessive number of tracings is likely due to passing python objects instead of tensors. Also, tf.function has experimental_relax_shapes=True option that relaxes argument shapes that can avoid unnecessary retracing. Please refer to https://www.tensorflow.org/tutorials/customization/performance#python_or_tensor_args and https://www.tensorflow.org/api_docs/python/tf/function for more details.\nWARNING:tensorflow:6 out of the last 7 calls to <function _make_execution_function.<locals>.distributed_function at 0x7f8d44616830> triggered tf.function retracing. Tracing is expensive and the excessive number of tracings is likely due to passing python objects instead of tensors. Also, tf.function has experimental_relax_shapes=True option that relaxes argument shapes that can avoid unnecessary retracing. Please refer to https://www.tensorflow.org/tutorials/customization/performance#python_or_tensor_args and https://www.tensorflow.org/api_docs/python/tf/function for more details.\nWARNING:tensorflow:7 out of the last 8 calls to <function _make_execution_function.<locals>.distributed_function at 0x7f8d44616830> triggered tf.function retracing. Tracing is expensive and the excessive number of tracings is likely due to passing python objects instead of tensors. Also, tf.function has experimental_relax_shapes=True option that relaxes argument shapes that can avoid unnecessary retracing. Please refer to https://www.tensorflow.org/tutorials/customization/performance#python_or_tensor_args and https://www.tensorflow.org/api_docs/python/tf/function for more details.\nWARNING:tensorflow:8 out of the last 9 calls to <function _make_execution_function.<locals>.distributed_function at 0x7f8d44616830> triggered tf.function retracing. Tracing is expensive and the excessive number of tracings is likely due to passing python objects instead of tensors. Also, tf.function has experimental_relax_shapes=True option that relaxes argument shapes that can avoid unnecessary retracing. Please refer to https://www.tensorflow.org/tutorials/customization/performance#python_or_tensor_args and https://www.tensorflow.org/api_docs/python/tf/function for more details.\nWARNING:tensorflow:9 out of the last 10 calls to <function _make_execution_function.<locals>.distributed_function at 0x7f8d44616830> triggered tf.function retracing. Tracing is expensive and the excessive number of tracings is likely due to passing python objects instead of tensors. Also, tf.function has experimental_relax_shapes=True option that relaxes argument shapes that can avoid unnecessary retracing. Please refer to https://www.tensorflow.org/tutorials/customization/performance#python_or_tensor_args and https://www.tensorflow.org/api_docs/python/tf/function for more details.\nWARNING:tensorflow:10 out of the last 11 calls to <function _make_execution_function.<locals>.distributed_function at 0x7f8d44616830> triggered tf.function retracing. Tracing is expensive and the excessive number of tracings is likely due to passing python objects instead of tensors. Also, tf.function has experimental_relax_shapes=True option that relaxes argument shapes that can avoid unnecessary retracing. Please refer to https://www.tensorflow.org/tutorials/customization/performance#python_or_tensor_args and https://www.tensorflow.org/api_docs/python/tf/function for more details.\nWARNING:tensorflow:10 out of the last 11 calls to <function _make_execution_function.<locals>.distributed_function at 0x7f8d44616830> triggered tf.function retracing. Tracing is expensive and the excessive number of tracings is likely due to passing python objects instead of tensors. Also, tf.function has experimental_relax_shapes=True option that relaxes argument shapes that can avoid unnecessary retracing. Please refer to https://www.tensorflow.org/tutorials/customization/performance#python_or_tensor_args and https://www.tensorflow.org/api_docs/python/tf/function for more details.\nWARNING:tensorflow:10 out of the last 11 calls to <function _make_execution_function.<locals>.distributed_function at 0x7f8d44616830> triggered tf.function retracing. Tracing is expensive and the excessive number of tracings is likely due to passing python objects instead of tensors. Also, tf.function has experimental_relax_shapes=True option that relaxes argument shapes that can avoid unnecessary retracing. Please refer to https://www.tensorflow.org/tutorials/customization/performance#python_or_tensor_args and https://www.tensorflow.org/api_docs/python/tf/function for more details.\nWARNING:tensorflow:10 out of the last 11 calls to <function _make_execution_function.<locals>.distributed_function at 0x7f8d44616830> triggered tf.function retracing. Tracing is expensive and the excessive number of tracings is likely due to passing python objects instead of tensors. Also, tf.function has experimental_relax_shapes=True option that relaxes argument shapes that can avoid unnecessary retracing. Please refer to https://www.tensorflow.org/tutorials/customization/performance#python_or_tensor_args and https://www.tensorflow.org/api_docs/python/tf/function for more details.\nWARNING:tensorflow:10 out of the last 11 calls to <function _make_execution_function.<locals>.distributed_function at 0x7f8d44616830> triggered tf.function retracing. Tracing is expensive and the excessive number of tracings is likely due to passing python objects instead of tensors. Also, tf.function has experimental_relax_shapes=True option that relaxes argument shapes that can avoid unnecessary retracing. Please refer to https://www.tensorflow.org/tutorials/customization/performance#python_or_tensor_args and https://www.tensorflow.org/api_docs/python/tf/function for more details.\nWARNING:tensorflow:10 out of the last 11 calls to <function _make_execution_function.<locals>.distributed_function at 0x7f8d44616830> triggered tf.function retracing. Tracing is expensive and the excessive number of tracings is likely due to passing python objects instead of tensors. Also, tf.function has experimental_relax_shapes=True option that relaxes argument shapes that can avoid unnecessary retracing. Please refer to https://www.tensorflow.org/tutorials/customization/performance#python_or_tensor_args and https://www.tensorflow.org/api_docs/python/tf/function for more details.\nWARNING:tensorflow:10 out of the last 11 calls to <function _make_execution_function.<locals>.distributed_function at 0x7f8d44616830> triggered tf.function retracing. Tracing is expensive and the excessive number of tracings is likely due to passing python objects instead of tensors. Also, tf.function has experimental_relax_shapes=True option that relaxes argument shapes that can avoid unnecessary retracing. Please refer to https://www.tensorflow.org/tutorials/customization/performance#python_or_tensor_args and https://www.tensorflow.org/api_docs/python/tf/function for more details.\nWARNING:tensorflow:10 out of the last 11 calls to <function _make_execution_function.<locals>.distributed_function at 0x7f8d44616830> triggered tf.function retracing. Tracing is expensive and the excessive number of tracings is likely due to passing python objects instead of tensors. Also, tf.function has experimental_relax_shapes=True option that relaxes argument shapes that can avoid unnecessary retracing. Please refer to https://www.tensorflow.org/tutorials/customization/performance#python_or_tensor_args and https://www.tensorflow.org/api_docs/python/tf/function for more details.\nWARNING:tensorflow:10 out of the last 11 calls to <function _make_execution_function.<locals>.distributed_function at 0x7f8d44616830> triggered tf.function retracing. Tracing is expensive and the excessive number of tracings is likely due to passing python objects instead of tensors. Also, tf.function has experimental_relax_shapes=True option that relaxes argument shapes that can avoid unnecessary retracing. Please refer to https://www.tensorflow.org/tutorials/customization/performance#python_or_tensor_args and https://www.tensorflow.org/api_docs/python/tf/function for more details.\nWARNING:tensorflow:10 out of the last 11 calls to <function _make_execution_function.<locals>.distributed_function at 0x7f8d44616830> triggered tf.function retracing. Tracing is expensive and the excessive number of tracings is likely due to passing python objects instead of tensors. Also, tf.function has experimental_relax_shapes=True option that relaxes argument shapes that can avoid unnecessary retracing. Please refer to https://www.tensorflow.org/tutorials/customization/performance#python_or_tensor_args and https://www.tensorflow.org/api_docs/python/tf/function for more details.\nWARNING:tensorflow:11 out of the last 12 calls to <function _make_execution_function.<locals>.distributed_function at 0x7f8d44616830> triggered tf.function retracing. Tracing is expensive and the excessive number of tracings is likely due to passing python objects instead of tensors. Also, tf.function has experimental_relax_shapes=True option that relaxes argument shapes that can avoid unnecessary retracing. Please refer to https://www.tensorflow.org/tutorials/customization/performance#python_or_tensor_args and https://www.tensorflow.org/api_docs/python/tf/function for more details.\nWARNING:tensorflow:11 out of the last 11 calls to <function _make_execution_function.<locals>.distributed_function at 0x7f8d44616830> triggered tf.function retracing. Tracing is expensive and the excessive number of tracings is likely due to passing python objects instead of tensors. Also, tf.function has experimental_relax_shapes=True option that relaxes argument shapes that can avoid unnecessary retracing. Please refer to https://www.tensorflow.org/tutorials/customization/performance#python_or_tensor_args and https://www.tensorflow.org/api_docs/python/tf/function for more details.\nWARNING:tensorflow:11 out of the last 11 calls to <function _make_execution_function.<locals>.distributed_function at 0x7f8d44616830> triggered tf.function retracing. Tracing is expensive and the excessive number of tracings is likely due to passing python objects instead of tensors. Also, tf.function has experimental_relax_shapes=True option that relaxes argument shapes that can avoid unnecessary retracing. Please refer to https://www.tensorflow.org/tutorials/customization/performance#python_or_tensor_args and https://www.tensorflow.org/api_docs/python/tf/function for more details.\nWARNING:tensorflow:11 out of the last 11 calls to <function _make_execution_function.<locals>.distributed_function at 0x7f8d44616830> triggered tf.function retracing. Tracing is expensive and the excessive number of tracings is likely due to passing python objects instead of tensors. Also, tf.function has experimental_relax_shapes=True option that relaxes argument shapes that can avoid unnecessary retracing. Please refer to https://www.tensorflow.org/tutorials/customization/performance#python_or_tensor_args and https://www.tensorflow.org/api_docs/python/tf/function for more details.\nWARNING:tensorflow:11 out of the last 11 calls to <function _make_execution_function.<locals>.distributed_function at 0x7f8d44616830> triggered tf.function retracing. Tracing is expensive and the excessive number of tracings is likely due to passing python objects instead of tensors. Also, tf.function has experimental_relax_shapes=True option that relaxes argument shapes that can avoid unnecessary retracing. Please refer to https://www.tensorflow.org/tutorials/customization/performance#python_or_tensor_args and https://www.tensorflow.org/api_docs/python/tf/function for more details.\nWARNING:tensorflow:11 out of the last 11 calls to <function _make_execution_function.<locals>.distributed_function at 0x7f8d44616830> triggered tf.function retracing. Tracing is expensive and the excessive number of tracings is likely due to passing python objects instead of tensors. Also, tf.function has experimental_relax_shapes=True option that relaxes argument shapes that can avoid unnecessary retracing. Please refer to https://www.tensorflow.org/tutorials/customization/performance#python_or_tensor_args and https://www.tensorflow.org/api_docs/python/tf/function for more details.\nWARNING:tensorflow:11 out of the last 11 calls to <function _make_execution_function.<locals>.distributed_function at 0x7f8d44616830> triggered tf.function retracing. Tracing is expensive and the excessive number of tracings is likely due to passing python objects instead of tensors. Also, tf.function has experimental_relax_shapes=True option that relaxes argument shapes that can avoid unnecessary retracing. Please refer to https://www.tensorflow.org/tutorials/customization/performance#python_or_tensor_args and https://www.tensorflow.org/api_docs/python/tf/function for more details.\nWARNING:tensorflow:11 out of the last 11 calls to <function _make_execution_function.<locals>.distributed_function at 0x7f8d44616830> triggered tf.function retracing. Tracing is expensive and the excessive number of tracings is likely due to passing python objects instead of tensors. Also, tf.function has experimental_relax_shapes=True option that relaxes argument shapes that can avoid unnecessary retracing. Please refer to https://www.tensorflow.org/tutorials/customization/performance#python_or_tensor_args and https://www.tensorflow.org/api_docs/python/tf/function for more details.\nWARNING:tensorflow:11 out of the last 11 calls to <function _make_execution_function.<locals>.distributed_function at 0x7f8d44616830> triggered tf.function retracing. Tracing is expensive and the excessive number of tracings is likely due to passing python objects instead of tensors. Also, tf.function has experimental_relax_shapes=True option that relaxes argument shapes that can avoid unnecessary retracing. Please refer to https://www.tensorflow.org/tutorials/customization/performance#python_or_tensor_args and https://www.tensorflow.org/api_docs/python/tf/function for more details.\nWARNING:tensorflow:11 out of the last 11 calls to <function _make_execution_function.<locals>.distributed_function at 0x7f8d44616830> triggered tf.function retracing. Tracing is expensive and the excessive number of tracings is likely due to passing python objects instead of tensors. Also, tf.function has experimental_relax_shapes=True option that relaxes argument shapes that can avoid unnecessary retracing. Please refer to https://www.tensorflow.org/tutorials/customization/performance#python_or_tensor_args and https://www.tensorflow.org/api_docs/python/tf/function for more details.\nWARNING:tensorflow:11 out of the last 11 calls to <function _make_execution_function.<locals>.distributed_function at 0x7f8d44616830> triggered tf.function retracing. Tracing is expensive and the excessive number of tracings is likely due to passing python objects instead of tensors. Also, tf.function has experimental_relax_shapes=True option that relaxes argument shapes that can avoid unnecessary retracing. Please refer to https://www.tensorflow.org/tutorials/customization/performance#python_or_tensor_args and https://www.tensorflow.org/api_docs/python/tf/function for more details.\nWARNING:tensorflow:11 out of the last 11 calls to <function _make_execution_function.<locals>.distributed_function at 0x7f8d44616830> triggered tf.function retracing. Tracing is expensive and the excessive number of tracings is likely due to passing python objects instead of tensors. Also, tf.function has experimental_relax_shapes=True option that relaxes argument shapes that can avoid unnecessary retracing. Please refer to https://www.tensorflow.org/tutorials/customization/performance#python_or_tensor_args and https://www.tensorflow.org/api_docs/python/tf/function for more details.\nWARNING:tensorflow:11 out of the last 11 calls to <function _make_execution_function.<locals>.distributed_function at 0x7f8d44616830> triggered tf.function retracing. Tracing is expensive and the excessive number of tracings is likely due to passing python objects instead of tensors. Also, tf.function has experimental_relax_shapes=True option that relaxes argument shapes that can avoid unnecessary retracing. Please refer to https://www.tensorflow.org/tutorials/customization/performance#python_or_tensor_args and https://www.tensorflow.org/api_docs/python/tf/function for more details.\nWARNING:tensorflow:11 out of the last 11 calls to <function _make_execution_function.<locals>.distributed_function at 0x7f8d44616830> triggered tf.function retracing. Tracing is expensive and the excessive number of tracings is likely due to passing python objects instead of tensors. Also, tf.function has experimental_relax_shapes=True option that relaxes argument shapes that can avoid unnecessary retracing. Please refer to https://www.tensorflow.org/tutorials/customization/performance#python_or_tensor_args and https://www.tensorflow.org/api_docs/python/tf/function for more details.\nWARNING:tensorflow:11 out of the last 11 calls to <function _make_execution_function.<locals>.distributed_function at 0x7f8d44616830> triggered tf.function retracing. Tracing is expensive and the excessive number of tracings is likely due to passing python objects instead of tensors. Also, tf.function has experimental_relax_shapes=True option that relaxes argument shapes that can avoid unnecessary retracing. Please refer to https://www.tensorflow.org/tutorials/customization/performance#python_or_tensor_args and https://www.tensorflow.org/api_docs/python/tf/function for more details.\nWARNING:tensorflow:11 out of the last 11 calls to <function _make_execution_function.<locals>.distributed_function at 0x7f8d44616830> triggered tf.function retracing. Tracing is expensive and the excessive number of tracings is likely due to passing python objects instead of tensors. Also, tf.function has experimental_relax_shapes=True option that relaxes argument shapes that can avoid unnecessary retracing. Please refer to https://www.tensorflow.org/tutorials/customization/performance#python_or_tensor_args and https://www.tensorflow.org/api_docs/python/tf/function for more details.\nWARNING:tensorflow:11 out of the last 11 calls to <function _make_execution_function.<locals>.distributed_function at 0x7f8d44616830> triggered tf.function retracing. Tracing is expensive and the excessive number of tracings is likely due to passing python objects instead of tensors. Also, tf.function has experimental_relax_shapes=True option that relaxes argument shapes that can avoid unnecessary retracing. Please refer to https://www.tensorflow.org/tutorials/customization/performance#python_or_tensor_args and https://www.tensorflow.org/api_docs/python/tf/function for more details.\nWARNING:tensorflow:11 out of the last 11 calls to <function _make_execution_function.<locals>.distributed_function at 0x7f8d44616830> triggered tf.function retracing. Tracing is expensive and the excessive number of tracings is likely due to passing python objects instead of tensors. Also, tf.function has experimental_relax_shapes=True option that relaxes argument shapes that can avoid unnecessary retracing. Please refer to https://www.tensorflow.org/tutorials/customization/performance#python_or_tensor_args and https://www.tensorflow.org/api_docs/python/tf/function for more details.\nWARNING:tensorflow:11 out of the last 11 calls to <function _make_execution_function.<locals>.distributed_function at 0x7f8d44616830> triggered tf.function retracing. Tracing is expensive and the excessive number of tracings is likely due to passing python objects instead of tensors. Also, tf.function has experimental_relax_shapes=True option that relaxes argument shapes that can avoid unnecessary retracing. Please refer to https://www.tensorflow.org/tutorials/customization/performance#python_or_tensor_args and https://www.tensorflow.org/api_docs/python/tf/function for more details.\nWARNING:tensorflow:11 out of the last 11 calls to <function _make_execution_function.<locals>.distributed_function at 0x7f8d44616830> triggered tf.function retracing. Tracing is expensive and the excessive number of tracings is likely due to passing python objects instead of tensors. Also, tf.function has experimental_relax_shapes=True option that relaxes argument shapes that can avoid unnecessary retracing. Please refer to https://www.tensorflow.org/tutorials/customization/performance#python_or_tensor_args and https://www.tensorflow.org/api_docs/python/tf/function for more details.\nWARNING:tensorflow:11 out of the last 11 calls to <function _make_execution_function.<locals>.distributed_function at 0x7f8d44616830> triggered tf.function retracing. Tracing is expensive and the excessive number of tracings is likely due to passing python objects instead of tensors. Also, tf.function has experimental_relax_shapes=True option that relaxes argument shapes that can avoid unnecessary retracing. Please refer to https://www.tensorflow.org/tutorials/customization/performance#python_or_tensor_args and https://www.tensorflow.org/api_docs/python/tf/function for more details.\nWARNING:tensorflow:11 out of the last 11 calls to <function _make_execution_function.<locals>.distributed_function at 0x7f8d44616830> triggered tf.function retracing. Tracing is expensive and the excessive number of tracings is likely due to passing python objects instead of tensors. Also, tf.function has experimental_relax_shapes=True option that relaxes argument shapes that can avoid unnecessary retracing. Please refer to https://www.tensorflow.org/tutorials/customization/performance#python_or_tensor_args and https://www.tensorflow.org/api_docs/python/tf/function for more details.\nWARNING:tensorflow:11 out of the last 11 calls to <function _make_execution_function.<locals>.distributed_function at 0x7f8d44616830> triggered tf.function retracing. Tracing is expensive and the excessive number of tracings is likely due to passing python objects instead of tensors. Also, tf.function has experimental_relax_shapes=True option that relaxes argument shapes that can avoid unnecessary retracing. Please refer to https://www.tensorflow.org/tutorials/customization/performance#python_or_tensor_args and https://www.tensorflow.org/api_docs/python/tf/function for more details.\nWARNING:tensorflow:11 out of the last 11 calls to <function _make_execution_function.<locals>.distributed_function at 0x7f8d44616830> triggered tf.function retracing. Tracing is expensive and the excessive number of tracings is likely due to passing python objects instead of tensors. Also, tf.function has experimental_relax_shapes=True option that relaxes argument shapes that can avoid unnecessary retracing. Please refer to https://www.tensorflow.org/tutorials/customization/performance#python_or_tensor_args and https://www.tensorflow.org/api_docs/python/tf/function for more details.\nWARNING:tensorflow:11 out of the last 11 calls to <function _make_execution_function.<locals>.distributed_function at 0x7f8d44616830> triggered tf.function retracing. Tracing is expensive and the excessive number of tracings is likely due to passing python objects instead of tensors. Also, tf.function has experimental_relax_shapes=True option that relaxes argument shapes that can avoid unnecessary retracing. Please refer to https://www.tensorflow.org/tutorials/customization/performance#python_or_tensor_args and https://www.tensorflow.org/api_docs/python/tf/function for more details.\nWARNING:tensorflow:11 out of the last 11 calls to <function _make_execution_function.<locals>.distributed_function at 0x7f8d44616830> triggered tf.function retracing. Tracing is expensive and the excessive number of tracings is likely due to passing python objects instead of tensors. Also, tf.function has experimental_relax_shapes=True option that relaxes argument shapes that can avoid unnecessary retracing. Please refer to https://www.tensorflow.org/tutorials/customization/performance#python_or_tensor_args and https://www.tensorflow.org/api_docs/python/tf/function for more details.\nWARNING:tensorflow:11 out of the last 11 calls to <function _make_execution_function.<locals>.distributed_function at 0x7f8d44616830> triggered tf.function retracing. Tracing is expensive and the excessive number of tracings is likely due to passing python objects instead of tensors. Also, tf.function has experimental_relax_shapes=True option that relaxes argument shapes that can avoid unnecessary retracing. Please refer to https://www.tensorflow.org/tutorials/customization/performance#python_or_tensor_args and https://www.tensorflow.org/api_docs/python/tf/function for more details.\nWARNING:tensorflow:11 out of the last 11 calls to <function _make_execution_function.<locals>.distributed_function at 0x7f8d44616830> triggered tf.function retracing. Tracing is expensive and the excessive number of tracings is likely due to passing python objects instead of tensors. Also, tf.function has experimental_relax_shapes=True option that relaxes argument shapes that can avoid unnecessary retracing. Please refer to https://www.tensorflow.org/tutorials/customization/performance#python_or_tensor_args and https://www.tensorflow.org/api_docs/python/tf/function for more details.\nWARNING:tensorflow:11 out of the last 11 calls to <function _make_execution_function.<locals>.distributed_function at 0x7f8d44616830> triggered tf.function retracing. Tracing is expensive and the excessive number of tracings is likely due to passing python objects instead of tensors. Also, tf.function has experimental_relax_shapes=True option that relaxes argument shapes that can avoid unnecessary retracing. Please refer to https://www.tensorflow.org/tutorials/customization/performance#python_or_tensor_args and https://www.tensorflow.org/api_docs/python/tf/function for more details.\nWARNING:tensorflow:11 out of the last 11 calls to <function _make_execution_function.<locals>.distributed_function at 0x7f8d44616830> triggered tf.function retracing. Tracing is expensive and the excessive number of tracings is likely due to passing python objects instead of tensors. Also, tf.function has experimental_relax_shapes=True option that relaxes argument shapes that can avoid unnecessary retracing. Please refer to https://www.tensorflow.org/tutorials/customization/performance#python_or_tensor_args and https://www.tensorflow.org/api_docs/python/tf/function for more details.\nWARNING:tensorflow:11 out of the last 11 calls to <function _make_execution_function.<locals>.distributed_function at 0x7f8d44616830> triggered tf.function retracing. Tracing is expensive and the excessive number of tracings is likely due to passing python objects instead of tensors. Also, tf.function has experimental_relax_shapes=True option that relaxes argument shapes that can avoid unnecessary retracing. Please refer to https://www.tensorflow.org/tutorials/customization/performance#python_or_tensor_args and https://www.tensorflow.org/api_docs/python/tf/function for more details.\nthe belly the charges of the other words\nand belly \n"
],
[
"print(complete_text(\"t\", temperature=1))",
"thing! they know't.\n\nbiondello:\nfor you are the own\n"
],
[
"print(complete_text(\"t\", temperature=2))",
"th no cyty\nuse ffor was firive this toighingaber; b\n"
]
],
[
[
"## Stateful RNN",
"_____no_output_____"
]
],
[
[
"tf.random.set_seed(42)",
"_____no_output_____"
],
[
"dataset = tf.data.Dataset.from_tensor_slices(encoded[:train_size])\ndataset = dataset.window(window_length, shift=n_steps, drop_remainder=True)\ndataset = dataset.flat_map(lambda window: window.batch(window_length))\ndataset = dataset.repeat().batch(1)\ndataset = dataset.map(lambda windows: (windows[:, :-1], windows[:, 1:]))\ndataset = dataset.map(\n lambda X_batch, Y_batch: (tf.one_hot(X_batch, depth=max_id), Y_batch))\ndataset = dataset.prefetch(1)",
"_____no_output_____"
],
[
"batch_size = 32\nencoded_parts = np.array_split(encoded[:train_size], batch_size)\ndatasets = []\nfor encoded_part in encoded_parts:\n dataset = tf.data.Dataset.from_tensor_slices(encoded_part)\n dataset = dataset.window(window_length, shift=n_steps, drop_remainder=True)\n dataset = dataset.flat_map(lambda window: window.batch(window_length))\n datasets.append(dataset)\ndataset = tf.data.Dataset.zip(tuple(datasets)).map(lambda *windows: tf.stack(windows))\ndataset = dataset.repeat().map(lambda windows: (windows[:, :-1], windows[:, 1:]))\ndataset = dataset.map(\n lambda X_batch, Y_batch: (tf.one_hot(X_batch, depth=max_id), Y_batch))\ndataset = dataset.prefetch(1)",
"_____no_output_____"
],
[
"model = keras.models.Sequential([\n keras.layers.GRU(128, return_sequences=True, stateful=True,\n dropout=0.2, recurrent_dropout=0.2,\n batch_input_shape=[batch_size, None, max_id]),\n keras.layers.GRU(128, return_sequences=True, stateful=True,\n dropout=0.2, recurrent_dropout=0.2),\n keras.layers.TimeDistributed(keras.layers.Dense(max_id,\n activation=\"softmax\"))\n])",
"_____no_output_____"
],
[
"class ResetStatesCallback(keras.callbacks.Callback):\n def on_epoch_begin(self, epoch, logs):\n self.model.reset_states()",
"_____no_output_____"
],
[
"model.compile(loss=\"sparse_categorical_crossentropy\", optimizer=\"adam\")\nsteps_per_epoch = train_size // batch_size // n_steps\nhistory = model.fit(dataset, steps_per_epoch=steps_per_epoch, epochs=50,\n callbacks=[ResetStatesCallback()])",
"Train for 313 steps\nEpoch 1/50\n313/313 [==============================] - 62s 198ms/step - loss: 2.6189\nEpoch 2/50\n313/313 [==============================] - 58s 187ms/step - loss: 2.2091\nEpoch 3/50\n313/313 [==============================] - 56s 178ms/step - loss: 2.0775\nEpoch 4/50\n313/313 [==============================] - 56s 179ms/step - loss: 2.4689\nEpoch 5/50\n313/313 [==============================] - 56s 179ms/step - loss: 2.3274\nEpoch 6/50\n313/313 [==============================] - 57s 183ms/step - loss: 2.1412\nEpoch 7/50\n313/313 [==============================] - 57s 183ms/step - loss: 2.0748\nEpoch 8/50\n313/313 [==============================] - 56s 179ms/step - loss: 1.9850\nEpoch 9/50\n313/313 [==============================] - 56s 179ms/step - loss: 1.9465\nEpoch 10/50\n313/313 [==============================] - 56s 179ms/step - loss: 1.8995\nEpoch 11/50\n313/313 [==============================] - 57s 182ms/step - loss: 1.8576\nEpoch 12/50\n313/313 [==============================] - 56s 179ms/step - loss: 1.8510\nEpoch 13/50\n313/313 [==============================] - 57s 184ms/step - loss: 1.8038\nEpoch 14/50\n313/313 [==============================] - 56s 178ms/step - loss: 1.7867\nEpoch 15/50\n313/313 [==============================] - 56s 180ms/step - loss: 1.7635\nEpoch 16/50\n313/313 [==============================] - 56s 179ms/step - loss: 1.7270\nEpoch 17/50\n313/313 [==============================] - 58s 184ms/step - loss: 1.7097\n<<31 more lines>>\n313/313 [==============================] - 58s 185ms/step - loss: 1.5998\nEpoch 34/50\n313/313 [==============================] - 58s 184ms/step - loss: 1.5954\nEpoch 35/50\n313/313 [==============================] - 58s 185ms/step - loss: 1.5944\nEpoch 36/50\n313/313 [==============================] - 57s 183ms/step - loss: 1.5902\nEpoch 37/50\n313/313 [==============================] - 57s 183ms/step - loss: 1.5893\nEpoch 38/50\n313/313 [==============================] - 59s 187ms/step - loss: 1.5845\nEpoch 39/50\n313/313 [==============================] - 57s 183ms/step - loss: 1.5821\nEpoch 40/50\n313/313 [==============================] - 59s 187ms/step - loss: 1.5798\nEpoch 41/50\n313/313 [==============================] - 57s 181ms/step - loss: 1.5794\nEpoch 42/50\n313/313 [==============================] - 57s 182ms/step - loss: 1.5774\nEpoch 43/50\n313/313 [==============================] - 57s 182ms/step - loss: 1.5755\nEpoch 44/50\n313/313 [==============================] - 58s 186ms/step - loss: 1.5735\nEpoch 45/50\n313/313 [==============================] - 58s 186ms/step - loss: 1.5714\nEpoch 46/50\n313/313 [==============================] - 57s 181ms/step - loss: 1.5686\nEpoch 47/50\n313/313 [==============================] - 57s 181ms/step - loss: 1.5675\nEpoch 48/50\n313/313 [==============================] - 56s 180ms/step - loss: 1.5657\nEpoch 49/50\n313/313 [==============================] - 58s 185ms/step - loss: 1.5654\nEpoch 50/50\n313/313 [==============================] - 57s 182ms/step - loss: 1.5620\n"
]
],
[
[
"To use the model with different batch sizes, we need to create a stateless copy. We can get rid of dropout since it is only used during training:",
"_____no_output_____"
]
],
[
[
"stateless_model = keras.models.Sequential([\n keras.layers.GRU(128, return_sequences=True, input_shape=[None, max_id]),\n keras.layers.GRU(128, return_sequences=True),\n keras.layers.TimeDistributed(keras.layers.Dense(max_id,\n activation=\"softmax\"))\n])",
"_____no_output_____"
]
],
[
[
"To set the weights, we first need to build the model (so the weights get created):",
"_____no_output_____"
]
],
[
[
"stateless_model.build(tf.TensorShape([None, None, max_id]))",
"_____no_output_____"
],
[
"stateless_model.set_weights(model.get_weights())\nmodel = stateless_model",
"_____no_output_____"
],
[
"tf.random.set_seed(42)\n\nprint(complete_text(\"t\"))",
"WARNING:tensorflow:5 out of the last 5 calls to <function _make_execution_function.<locals>.distributed_function at 0x7f8d44bc53b0> triggered tf.function retracing. Tracing is expensive and the excessive number of tracings is likely due to passing python objects instead of tensors. Also, tf.function has experimental_relax_shapes=True option that relaxes argument shapes that can avoid unnecessary retracing. Please refer to https://www.tensorflow.org/tutorials/customization/performance#python_or_tensor_args and https://www.tensorflow.org/api_docs/python/tf/function for more details.\nWARNING:tensorflow:6 out of the last 6 calls to <function _make_execution_function.<locals>.distributed_function at 0x7f8d44bc53b0> triggered tf.function retracing. Tracing is expensive and the excessive number of tracings is likely due to passing python objects instead of tensors. Also, tf.function has experimental_relax_shapes=True option that relaxes argument shapes that can avoid unnecessary retracing. Please refer to https://www.tensorflow.org/tutorials/customization/performance#python_or_tensor_args and https://www.tensorflow.org/api_docs/python/tf/function for more details.\nWARNING:tensorflow:7 out of the last 7 calls to <function _make_execution_function.<locals>.distributed_function at 0x7f8d44bc53b0> triggered tf.function retracing. Tracing is expensive and the excessive number of tracings is likely due to passing python objects instead of tensors. Also, tf.function has experimental_relax_shapes=True option that relaxes argument shapes that can avoid unnecessary retracing. Please refer to https://www.tensorflow.org/tutorials/customization/performance#python_or_tensor_args and https://www.tensorflow.org/api_docs/python/tf/function for more details.\nWARNING:tensorflow:8 out of the last 8 calls to <function _make_execution_function.<locals>.distributed_function at 0x7f8d44bc53b0> triggered tf.function retracing. Tracing is expensive and the excessive number of tracings is likely due to passing python objects instead of tensors. Also, tf.function has experimental_relax_shapes=True option that relaxes argument shapes that can avoid unnecessary retracing. Please refer to https://www.tensorflow.org/tutorials/customization/performance#python_or_tensor_args and https://www.tensorflow.org/api_docs/python/tf/function for more details.\nWARNING:tensorflow:9 out of the last 9 calls to <function _make_execution_function.<locals>.distributed_function at 0x7f8d44bc53b0> triggered tf.function retracing. Tracing is expensive and the excessive number of tracings is likely due to passing python objects instead of tensors. Also, tf.function has experimental_relax_shapes=True option that relaxes argument shapes that can avoid unnecessary retracing. Please refer to https://www.tensorflow.org/tutorials/customization/performance#python_or_tensor_args and https://www.tensorflow.org/api_docs/python/tf/function for more details.\nWARNING:tensorflow:10 out of the last 10 calls to <function _make_execution_function.<locals>.distributed_function at 0x7f8d44bc53b0> triggered tf.function retracing. Tracing is expensive and the excessive number of tracings is likely due to passing python objects instead of tensors. Also, tf.function has experimental_relax_shapes=True option that relaxes argument shapes that can avoid unnecessary retracing. Please refer to https://www.tensorflow.org/tutorials/customization/performance#python_or_tensor_args and https://www.tensorflow.org/api_docs/python/tf/function for more details.\nWARNING:tensorflow:11 out of the last 11 calls to <function _make_execution_function.<locals>.distributed_function at 0x7f8d44bc53b0> triggered tf.function retracing. Tracing is expensive and the excessive number of tracings is likely due to passing python objects instead of tensors. Also, tf.function has experimental_relax_shapes=True option that relaxes argument shapes that can avoid unnecessary retracing. Please refer to https://www.tensorflow.org/tutorials/customization/performance#python_or_tensor_args and https://www.tensorflow.org/api_docs/python/tf/function for more details.\nWARNING:tensorflow:11 out of the last 11 calls to <function _make_execution_function.<locals>.distributed_function at 0x7f8d44bc53b0> triggered tf.function retracing. Tracing is expensive and the excessive number of tracings is likely due to passing python objects instead of tensors. Also, tf.function has experimental_relax_shapes=True option that relaxes argument shapes that can avoid unnecessary retracing. Please refer to https://www.tensorflow.org/tutorials/customization/performance#python_or_tensor_args and https://www.tensorflow.org/api_docs/python/tf/function for more details.\nWARNING:tensorflow:11 out of the last 11 calls to <function _make_execution_function.<locals>.distributed_function at 0x7f8d44bc53b0> triggered tf.function retracing. Tracing is expensive and the excessive number of tracings is likely due to passing python objects instead of tensors. Also, tf.function has experimental_relax_shapes=True option that relaxes argument shapes that can avoid unnecessary retracing. Please refer to https://www.tensorflow.org/tutorials/customization/performance#python_or_tensor_args and https://www.tensorflow.org/api_docs/python/tf/function for more details.\nWARNING:tensorflow:11 out of the last 11 calls to <function _make_execution_function.<locals>.distributed_function at 0x7f8d44bc53b0> triggered tf.function retracing. Tracing is expensive and the excessive number of tracings is likely due to passing python objects instead of tensors. Also, tf.function has experimental_relax_shapes=True option that relaxes argument shapes that can avoid unnecessary retracing. Please refer to https://www.tensorflow.org/tutorials/customization/performance#python_or_tensor_args and https://www.tensorflow.org/api_docs/python/tf/function for more details.\nWARNING:tensorflow:11 out of the last 11 calls to <function _make_execution_function.<locals>.distributed_function at 0x7f8d44bc53b0> triggered tf.function retracing. Tracing is expensive and the excessive number of tracings is likely due to passing python objects instead of tensors. Also, tf.function has experimental_relax_shapes=True option that relaxes argument shapes that can avoid unnecessary retracing. Please refer to https://www.tensorflow.org/tutorials/customization/performance#python_or_tensor_args and https://www.tensorflow.org/api_docs/python/tf/function for more details.\nWARNING:tensorflow:11 out of the last 11 calls to <function _make_execution_function.<locals>.distributed_function at 0x7f8d44bc53b0> triggered tf.function retracing. Tracing is expensive and the excessive number of tracings is likely due to passing python objects instead of tensors. Also, tf.function has experimental_relax_shapes=True option that relaxes argument shapes that can avoid unnecessary retracing. Please refer to https://www.tensorflow.org/tutorials/customization/performance#python_or_tensor_args and https://www.tensorflow.org/api_docs/python/tf/function for more details.\nWARNING:tensorflow:11 out of the last 11 calls to <function _make_execution_function.<locals>.distributed_function at 0x7f8d44bc53b0> triggered tf.function retracing. Tracing is expensive and the excessive number of tracings is likely due to passing python objects instead of tensors. Also, tf.function has experimental_relax_shapes=True option that relaxes argument shapes that can avoid unnecessary retracing. Please refer to https://www.tensorflow.org/tutorials/customization/performance#python_or_tensor_args and https://www.tensorflow.org/api_docs/python/tf/function for more details.\nWARNING:tensorflow:11 out of the last 11 calls to <function _make_execution_function.<locals>.distributed_function at 0x7f8d44bc53b0> triggered tf.function retracing. Tracing is expensive and the excessive number of tracings is likely due to passing python objects instead of tensors. Also, tf.function has experimental_relax_shapes=True option that relaxes argument shapes that can avoid unnecessary retracing. Please refer to https://www.tensorflow.org/tutorials/customization/performance#python_or_tensor_args and https://www.tensorflow.org/api_docs/python/tf/function for more details.\nWARNING:tensorflow:11 out of the last 11 calls to <function _make_execution_function.<locals>.distributed_function at 0x7f8d44bc53b0> triggered tf.function retracing. Tracing is expensive and the excessive number of tracings is likely due to passing python objects instead of tensors. Also, tf.function has experimental_relax_shapes=True option that relaxes argument shapes that can avoid unnecessary retracing. Please refer to https://www.tensorflow.org/tutorials/customization/performance#python_or_tensor_args and https://www.tensorflow.org/api_docs/python/tf/function for more details.\nWARNING:tensorflow:11 out of the last 11 calls to <function _make_execution_function.<locals>.distributed_function at 0x7f8d44bc53b0> triggered tf.function retracing. Tracing is expensive and the excessive number of tracings is likely due to passing python objects instead of tensors. Also, tf.function has experimental_relax_shapes=True option that relaxes argument shapes that can avoid unnecessary retracing. Please refer to https://www.tensorflow.org/tutorials/customization/performance#python_or_tensor_args and https://www.tensorflow.org/api_docs/python/tf/function for more details.\nWARNING:tensorflow:11 out of the last 11 calls to <function _make_execution_function.<locals>.distributed_function at 0x7f8d44bc53b0> triggered tf.function retracing. Tracing is expensive and the excessive number of tracings is likely due to passing python objects instead of tensors. Also, tf.function has experimental_relax_shapes=True option that relaxes argument shapes that can avoid unnecessary retracing. Please refer to https://www.tensorflow.org/tutorials/customization/performance#python_or_tensor_args and https://www.tensorflow.org/api_docs/python/tf/function for more details.\nWARNING:tensorflow:11 out of the last 11 calls to <function _make_execution_function.<locals>.distributed_function at 0x7f8d44bc53b0> triggered tf.function retracing. Tracing is expensive and the excessive number of tracings is likely due to passing python objects instead of tensors. Also, tf.function has experimental_relax_shapes=True option that relaxes argument shapes that can avoid unnecessary retracing. Please refer to https://www.tensorflow.org/tutorials/customization/performance#python_or_tensor_args and https://www.tensorflow.org/api_docs/python/tf/function for more details.\nWARNING:tensorflow:11 out of the last 11 calls to <function _make_execution_function.<locals>.distributed_function at 0x7f8d44bc53b0> triggered tf.function retracing. Tracing is expensive and the excessive number of tracings is likely due to passing python objects instead of tensors. Also, tf.function has experimental_relax_shapes=True option that relaxes argument shapes that can avoid unnecessary retracing. Please refer to https://www.tensorflow.org/tutorials/customization/performance#python_or_tensor_args and https://www.tensorflow.org/api_docs/python/tf/function for more details.\nWARNING:tensorflow:11 out of the last 11 calls to <function _make_execution_function.<locals>.distributed_function at 0x7f8d44bc53b0> triggered tf.function retracing. Tracing is expensive and the excessive number of tracings is likely due to passing python objects instead of tensors. Also, tf.function has experimental_relax_shapes=True option that relaxes argument shapes that can avoid unnecessary retracing. Please refer to https://www.tensorflow.org/tutorials/customization/performance#python_or_tensor_args and https://www.tensorflow.org/api_docs/python/tf/function for more details.\nWARNING:tensorflow:11 out of the last 11 calls to <function _make_execution_function.<locals>.distributed_function at 0x7f8d44bc53b0> triggered tf.function retracing. Tracing is expensive and the excessive number of tracings is likely due to passing python objects instead of tensors. Also, tf.function has experimental_relax_shapes=True option that relaxes argument shapes that can avoid unnecessary retracing. Please refer to https://www.tensorflow.org/tutorials/customization/performance#python_or_tensor_args and https://www.tensorflow.org/api_docs/python/tf/function for more details.\nWARNING:tensorflow:11 out of the last 11 calls to <function _make_execution_function.<locals>.distributed_function at 0x7f8d44bc53b0> triggered tf.function retracing. Tracing is expensive and the excessive number of tracings is likely due to passing python objects instead of tensors. Also, tf.function has experimental_relax_shapes=True option that relaxes argument shapes that can avoid unnecessary retracing. Please refer to https://www.tensorflow.org/tutorials/customization/performance#python_or_tensor_args and https://www.tensorflow.org/api_docs/python/tf/function for more details.\nWARNING:tensorflow:11 out of the last 11 calls to <function _make_execution_function.<locals>.distributed_function at 0x7f8d44bc53b0> triggered tf.function retracing. Tracing is expensive and the excessive number of tracings is likely due to passing python objects instead of tensors. Also, tf.function has experimental_relax_shapes=True option that relaxes argument shapes that can avoid unnecessary retracing. Please refer to https://www.tensorflow.org/tutorials/customization/performance#python_or_tensor_args and https://www.tensorflow.org/api_docs/python/tf/function for more details.\nWARNING:tensorflow:11 out of the last 11 calls to <function _make_execution_function.<locals>.distributed_function at 0x7f8d44bc53b0> triggered tf.function retracing. Tracing is expensive and the excessive number of tracings is likely due to passing python objects instead of tensors. Also, tf.function has experimental_relax_shapes=True option that relaxes argument shapes that can avoid unnecessary retracing. Please refer to https://www.tensorflow.org/tutorials/customization/performance#python_or_tensor_args and https://www.tensorflow.org/api_docs/python/tf/function for more details.\nWARNING:tensorflow:11 out of the last 11 calls to <function _make_execution_function.<locals>.distributed_function at 0x7f8d44bc53b0> triggered tf.function retracing. Tracing is expensive and the excessive number of tracings is likely due to passing python objects instead of tensors. Also, tf.function has experimental_relax_shapes=True option that relaxes argument shapes that can avoid unnecessary retracing. Please refer to https://www.tensorflow.org/tutorials/customization/performance#python_or_tensor_args and https://www.tensorflow.org/api_docs/python/tf/function for more details.\nWARNING:tensorflow:11 out of the last 11 calls to <function _make_execution_function.<locals>.distributed_function at 0x7f8d44bc53b0> triggered tf.function retracing. Tracing is expensive and the excessive number of tracings is likely due to passing python objects instead of tensors. Also, tf.function has experimental_relax_shapes=True option that relaxes argument shapes that can avoid unnecessary retracing. Please refer to https://www.tensorflow.org/tutorials/customization/performance#python_or_tensor_args and https://www.tensorflow.org/api_docs/python/tf/function for more details.\nWARNING:tensorflow:11 out of the last 11 calls to <function _make_execution_function.<locals>.distributed_function at 0x7f8d44bc53b0> triggered tf.function retracing. Tracing is expensive and the excessive number of tracings is likely due to passing python objects instead of tensors. Also, tf.function has experimental_relax_shapes=True option that relaxes argument shapes that can avoid unnecessary retracing. Please refer to https://www.tensorflow.org/tutorials/customization/performance#python_or_tensor_args and https://www.tensorflow.org/api_docs/python/tf/function for more details.\nWARNING:tensorflow:11 out of the last 11 calls to <function _make_execution_function.<locals>.distributed_function at 0x7f8d44bc53b0> triggered tf.function retracing. Tracing is expensive and the excessive number of tracings is likely due to passing python objects instead of tensors. Also, tf.function has experimental_relax_shapes=True option that relaxes argument shapes that can avoid unnecessary retracing. Please refer to https://www.tensorflow.org/tutorials/customization/performance#python_or_tensor_args and https://www.tensorflow.org/api_docs/python/tf/function for more details.\nWARNING:tensorflow:11 out of the last 11 calls to <function _make_execution_function.<locals>.distributed_function at 0x7f8d44bc53b0> triggered tf.function retracing. Tracing is expensive and the excessive number of tracings is likely due to passing python objects instead of tensors. Also, tf.function has experimental_relax_shapes=True option that relaxes argument shapes that can avoid unnecessary retracing. Please refer to https://www.tensorflow.org/tutorials/customization/performance#python_or_tensor_args and https://www.tensorflow.org/api_docs/python/tf/function for more details.\nWARNING:tensorflow:11 out of the last 11 calls to <function _make_execution_function.<locals>.distributed_function at 0x7f8d44bc53b0> triggered tf.function retracing. Tracing is expensive and the excessive number of tracings is likely due to passing python objects instead of tensors. Also, tf.function has experimental_relax_shapes=True option that relaxes argument shapes that can avoid unnecessary retracing. Please refer to https://www.tensorflow.org/tutorials/customization/performance#python_or_tensor_args and https://www.tensorflow.org/api_docs/python/tf/function for more details.\nWARNING:tensorflow:11 out of the last 11 calls to <function _make_execution_function.<locals>.distributed_function at 0x7f8d44bc53b0> triggered tf.function retracing. Tracing is expensive and the excessive number of tracings is likely due to passing python objects instead of tensors. Also, tf.function has experimental_relax_shapes=True option that relaxes argument shapes that can avoid unnecessary retracing. Please refer to https://www.tensorflow.org/tutorials/customization/performance#python_or_tensor_args and https://www.tensorflow.org/api_docs/python/tf/function for more details.\nWARNING:tensorflow:11 out of the last 11 calls to <function _make_execution_function.<locals>.distributed_function at 0x7f8d44bc53b0> triggered tf.function retracing. Tracing is expensive and the excessive number of tracings is likely due to passing python objects instead of tensors. Also, tf.function has experimental_relax_shapes=True option that relaxes argument shapes that can avoid unnecessary retracing. Please refer to https://www.tensorflow.org/tutorials/customization/performance#python_or_tensor_args and https://www.tensorflow.org/api_docs/python/tf/function for more details.\nWARNING:tensorflow:11 out of the last 11 calls to <function _make_execution_function.<locals>.distributed_function at 0x7f8d44bc53b0> triggered tf.function retracing. Tracing is expensive and the excessive number of tracings is likely due to passing python objects instead of tensors. Also, tf.function has experimental_relax_shapes=True option that relaxes argument shapes that can avoid unnecessary retracing. Please refer to https://www.tensorflow.org/tutorials/customization/performance#python_or_tensor_args and https://www.tensorflow.org/api_docs/python/tf/function for more details.\nWARNING:tensorflow:11 out of the last 11 calls to <function _make_execution_function.<locals>.distributed_function at 0x7f8d44bc53b0> triggered tf.function retracing. Tracing is expensive and the excessive number of tracings is likely due to passing python objects instead of tensors. Also, tf.function has experimental_relax_shapes=True option that relaxes argument shapes that can avoid unnecessary retracing. Please refer to https://www.tensorflow.org/tutorials/customization/performance#python_or_tensor_args and https://www.tensorflow.org/api_docs/python/tf/function for more details.\nWARNING:tensorflow:11 out of the last 11 calls to <function _make_execution_function.<locals>.distributed_function at 0x7f8d44bc53b0> triggered tf.function retracing. Tracing is expensive and the excessive number of tracings is likely due to passing python objects instead of tensors. Also, tf.function has experimental_relax_shapes=True option that relaxes argument shapes that can avoid unnecessary retracing. Please refer to https://www.tensorflow.org/tutorials/customization/performance#python_or_tensor_args and https://www.tensorflow.org/api_docs/python/tf/function for more details.\nWARNING:tensorflow:11 out of the last 11 calls to <function _make_execution_function.<locals>.distributed_function at 0x7f8d44bc53b0> triggered tf.function retracing. Tracing is expensive and the excessive number of tracings is likely due to passing python objects instead of tensors. Also, tf.function has experimental_relax_shapes=True option that relaxes argument shapes that can avoid unnecessary retracing. Please refer to https://www.tensorflow.org/tutorials/customization/performance#python_or_tensor_args and https://www.tensorflow.org/api_docs/python/tf/function for more details.\nWARNING:tensorflow:11 out of the last 11 calls to <function _make_execution_function.<locals>.distributed_function at 0x7f8d44bc53b0> triggered tf.function retracing. Tracing is expensive and the excessive number of tracings is likely due to passing python objects instead of tensors. Also, tf.function has experimental_relax_shapes=True option that relaxes argument shapes that can avoid unnecessary retracing. Please refer to https://www.tensorflow.org/tutorials/customization/performance#python_or_tensor_args and https://www.tensorflow.org/api_docs/python/tf/function for more details.\nWARNING:tensorflow:11 out of the last 11 calls to <function _make_execution_function.<locals>.distributed_function at 0x7f8d44bc53b0> triggered tf.function retracing. Tracing is expensive and the excessive number of tracings is likely due to passing python objects instead of tensors. Also, tf.function has experimental_relax_shapes=True option that relaxes argument shapes that can avoid unnecessary retracing. Please refer to https://www.tensorflow.org/tutorials/customization/performance#python_or_tensor_args and https://www.tensorflow.org/api_docs/python/tf/function for more details.\nWARNING:tensorflow:11 out of the last 11 calls to <function _make_execution_function.<locals>.distributed_function at 0x7f8d44bc53b0> triggered tf.function retracing. Tracing is expensive and the excessive number of tracings is likely due to passing python objects instead of tensors. Also, tf.function has experimental_relax_shapes=True option that relaxes argument shapes that can avoid unnecessary retracing. Please refer to https://www.tensorflow.org/tutorials/customization/performance#python_or_tensor_args and https://www.tensorflow.org/api_docs/python/tf/function for more details.\nWARNING:tensorflow:11 out of the last 11 calls to <function _make_execution_function.<locals>.distributed_function at 0x7f8d44bc53b0> triggered tf.function retracing. Tracing is expensive and the excessive number of tracings is likely due to passing python objects instead of tensors. Also, tf.function has experimental_relax_shapes=True option that relaxes argument shapes that can avoid unnecessary retracing. Please refer to https://www.tensorflow.org/tutorials/customization/performance#python_or_tensor_args and https://www.tensorflow.org/api_docs/python/tf/function for more details.\nWARNING:tensorflow:11 out of the last 11 calls to <function _make_execution_function.<locals>.distributed_function at 0x7f8d44bc53b0> triggered tf.function retracing. Tracing is expensive and the excessive number of tracings is likely due to passing python objects instead of tensors. Also, tf.function has experimental_relax_shapes=True option that relaxes argument shapes that can avoid unnecessary retracing. Please refer to https://www.tensorflow.org/tutorials/customization/performance#python_or_tensor_args and https://www.tensorflow.org/api_docs/python/tf/function for more details.\nWARNING:tensorflow:11 out of the last 11 calls to <function _make_execution_function.<locals>.distributed_function at 0x7f8d44bc53b0> triggered tf.function retracing. Tracing is expensive and the excessive number of tracings is likely due to passing python objects instead of tensors. Also, tf.function has experimental_relax_shapes=True option that relaxes argument shapes that can avoid unnecessary retracing. Please refer to https://www.tensorflow.org/tutorials/customization/performance#python_or_tensor_args and https://www.tensorflow.org/api_docs/python/tf/function for more details.\nWARNING:tensorflow:11 out of the last 11 calls to <function _make_execution_function.<locals>.distributed_function at 0x7f8d44bc53b0> triggered tf.function retracing. Tracing is expensive and the excessive number of tracings is likely due to passing python objects instead of tensors. Also, tf.function has experimental_relax_shapes=True option that relaxes argument shapes that can avoid unnecessary retracing. Please refer to https://www.tensorflow.org/tutorials/customization/performance#python_or_tensor_args and https://www.tensorflow.org/api_docs/python/tf/function for more details.\nWARNING:tensorflow:11 out of the last 11 calls to <function _make_execution_function.<locals>.distributed_function at 0x7f8d44bc53b0> triggered tf.function retracing. Tracing is expensive and the excessive number of tracings is likely due to passing python objects instead of tensors. Also, tf.function has experimental_relax_shapes=True option that relaxes argument shapes that can avoid unnecessary retracing. Please refer to https://www.tensorflow.org/tutorials/customization/performance#python_or_tensor_args and https://www.tensorflow.org/api_docs/python/tf/function for more details.\nWARNING:tensorflow:11 out of the last 11 calls to <function _make_execution_function.<locals>.distributed_function at 0x7f8d44bc53b0> triggered tf.function retracing. Tracing is expensive and the excessive number of tracings is likely due to passing python objects instead of tensors. Also, tf.function has experimental_relax_shapes=True option that relaxes argument shapes that can avoid unnecessary retracing. Please refer to https://www.tensorflow.org/tutorials/customization/performance#python_or_tensor_args and https://www.tensorflow.org/api_docs/python/tf/function for more details.\nWARNING:tensorflow:11 out of the last 11 calls to <function _make_execution_function.<locals>.distributed_function at 0x7f8d44bc53b0> triggered tf.function retracing. Tracing is expensive and the excessive number of tracings is likely due to passing python objects instead of tensors. Also, tf.function has experimental_relax_shapes=True option that relaxes argument shapes that can avoid unnecessary retracing. Please refer to https://www.tensorflow.org/tutorials/customization/performance#python_or_tensor_args and https://www.tensorflow.org/api_docs/python/tf/function for more details.\ntor:\nin the negver up how it thou like him;\nwhen it\n"
]
],
[
[
"# Sentiment Analysis",
"_____no_output_____"
]
],
[
[
"tf.random.set_seed(42)",
"_____no_output_____"
]
],
[
[
"You can load the IMDB dataset easily:",
"_____no_output_____"
]
],
[
[
"(X_train, y_test), (X_valid, y_test) = keras.datasets.imdb.load_data()",
"Downloading data from https://storage.googleapis.com/tensorflow/tf-keras-datasets/imdb.npz\n17465344/17464789 [==============================] - 0s 0us/step\n"
],
[
"X_train[0][:10]",
"_____no_output_____"
],
[
"word_index = keras.datasets.imdb.get_word_index()\nid_to_word = {id_ + 3: word for word, id_ in word_index.items()}\nfor id_, token in enumerate((\"<pad>\", \"<sos>\", \"<unk>\")):\n id_to_word[id_] = token\n\" \".join([id_to_word[id_] for id_ in X_train[0][:10]])",
"Downloading data from https://storage.googleapis.com/tensorflow/tf-keras-datasets/imdb_word_index.json\n1646592/1641221 [==============================] - 0s 0us/step\n"
],
[
"import tensorflow_datasets as tfds\n\ndatasets, info = tfds.load(\"imdb_reviews\", as_supervised=True, with_info=True)",
"\u001b[1mDownloading and preparing dataset imdb_reviews/plain_text/1.0.0 (download: 80.23 MiB, generated: Unknown size, total: 80.23 MiB) to /home/aurelien_geron_kiwisoft_io/tensorflow_datasets/imdb_reviews/plain_text/1.0.0...\u001b[0m\n"
],
[
"datasets.keys()",
"_____no_output_____"
],
[
"train_size = info.splits[\"train\"].num_examples\ntest_size = info.splits[\"test\"].num_examples",
"_____no_output_____"
],
[
"train_size, test_size",
"_____no_output_____"
],
[
"for X_batch, y_batch in datasets[\"train\"].batch(2).take(1):\n for review, label in zip(X_batch.numpy(), y_batch.numpy()):\n print(\"Review:\", review.decode(\"utf-8\")[:200], \"...\")\n print(\"Label:\", label, \"= Positive\" if label else \"= Negative\")\n print()",
"Review: This was an absolutely terrible movie. Don't be lured in by Christopher Walken or Michael Ironside. Both are great actors, but this must simply be their worst role in history. Even their great acting ...\nLabel: 0 = Negative\n\nReview: I have been known to fall asleep during films, but this is usually due to a combination of things including, really tired, being warm and comfortable on the sette and having just eaten a lot. However ...\nLabel: 0 = Negative\n\n"
],
[
"def preprocess(X_batch, y_batch):\n X_batch = tf.strings.substr(X_batch, 0, 300)\n X_batch = tf.strings.regex_replace(X_batch, rb\"<br\\s*/?>\", b\" \")\n X_batch = tf.strings.regex_replace(X_batch, b\"[^a-zA-Z']\", b\" \")\n X_batch = tf.strings.split(X_batch)\n return X_batch.to_tensor(default_value=b\"<pad>\"), y_batch",
"_____no_output_____"
],
[
"preprocess(X_batch, y_batch)",
"_____no_output_____"
],
[
"from collections import Counter\n\nvocabulary = Counter()\nfor X_batch, y_batch in datasets[\"train\"].batch(32).map(preprocess):\n for review in X_batch:\n vocabulary.update(list(review.numpy()))",
"_____no_output_____"
],
[
"vocabulary.most_common()[:3]",
"_____no_output_____"
],
[
"len(vocabulary)",
"_____no_output_____"
],
[
"vocab_size = 10000\ntruncated_vocabulary = [\n word for word, count in vocabulary.most_common()[:vocab_size]]",
"_____no_output_____"
],
[
"word_to_id = {word: index for index, word in enumerate(truncated_vocabulary)}\nfor word in b\"This movie was faaaaaantastic\".split():\n print(word_to_id.get(word) or vocab_size)",
"22\n12\n11\n10000\n"
],
[
"words = tf.constant(truncated_vocabulary)\nword_ids = tf.range(len(truncated_vocabulary), dtype=tf.int64)\nvocab_init = tf.lookup.KeyValueTensorInitializer(words, word_ids)\nnum_oov_buckets = 1000\ntable = tf.lookup.StaticVocabularyTable(vocab_init, num_oov_buckets)",
"_____no_output_____"
],
[
"table.lookup(tf.constant([b\"This movie was faaaaaantastic\".split()]))",
"_____no_output_____"
],
[
"def encode_words(X_batch, y_batch):\n return table.lookup(X_batch), y_batch\n\ntrain_set = datasets[\"train\"].repeat().batch(32).map(preprocess)\ntrain_set = train_set.map(encode_words).prefetch(1)",
"_____no_output_____"
],
[
"for X_batch, y_batch in train_set.take(1):\n print(X_batch)\n print(y_batch)",
"tf.Tensor(\n[[ 22 11 28 ... 0 0 0]\n [ 6 21 70 ... 0 0 0]\n [4099 6881 1 ... 0 0 0]\n ...\n [ 22 12 118 ... 331 1047 0]\n [1757 4101 451 ... 0 0 0]\n [3365 4392 6 ... 0 0 0]], shape=(32, 60), dtype=int64)\ntf.Tensor([0 0 0 1 1 1 0 0 0 0 0 1 1 0 1 0 1 1 1 0 1 1 1 1 1 0 0 0 1 0 0 0], shape=(32,), dtype=int64)\n"
],
[
"embed_size = 128\nmodel = keras.models.Sequential([\n keras.layers.Embedding(vocab_size + num_oov_buckets, embed_size,\n mask_zero=True, # not shown in the book\n input_shape=[None]),\n keras.layers.GRU(128, return_sequences=True),\n keras.layers.GRU(128),\n keras.layers.Dense(1, activation=\"sigmoid\")\n])\nmodel.compile(loss=\"binary_crossentropy\", optimizer=\"adam\", metrics=[\"accuracy\"])\nhistory = model.fit(train_set, steps_per_epoch=train_size // 32, epochs=5)",
"Train for 781 steps\nEpoch 1/5\n781/781 [==============================] - 118s 152ms/step - loss: 0.5305 - accuracy: 0.7282\nEpoch 2/5\n781/781 [==============================] - 113s 145ms/step - loss: 0.3459 - accuracy: 0.8554\nEpoch 3/5\n781/781 [==============================] - 113s 145ms/step - loss: 0.1913 - accuracy: 0.9319\nEpoch 4/5\n781/781 [==============================] - 114s 146ms/step - loss: 0.1341 - accuracy: 0.9535\nEpoch 5/5\n781/781 [==============================] - 116s 148ms/step - loss: 0.1011 - accuracy: 0.9624\n"
]
],
[
[
"Or using manual masking:",
"_____no_output_____"
]
],
[
[
"K = keras.backend\nembed_size = 128\ninputs = keras.layers.Input(shape=[None])\nmask = keras.layers.Lambda(lambda inputs: K.not_equal(inputs, 0))(inputs)\nz = keras.layers.Embedding(vocab_size + num_oov_buckets, embed_size)(inputs)\nz = keras.layers.GRU(128, return_sequences=True)(z, mask=mask)\nz = keras.layers.GRU(128)(z, mask=mask)\noutputs = keras.layers.Dense(1, activation=\"sigmoid\")(z)\nmodel = keras.models.Model(inputs=[inputs], outputs=[outputs])\nmodel.compile(loss=\"binary_crossentropy\", optimizer=\"adam\", metrics=[\"accuracy\"])\nhistory = model.fit(train_set, steps_per_epoch=train_size // 32, epochs=5)",
"Train for 781 steps\nEpoch 1/5\n781/781 [==============================] - 118s 152ms/step - loss: 0.5425 - accuracy: 0.7155\nEpoch 2/5\n781/781 [==============================] - 112s 143ms/step - loss: 0.3479 - accuracy: 0.8558\nEpoch 3/5\n781/781 [==============================] - 112s 144ms/step - loss: 0.1761 - accuracy: 0.9388\nEpoch 4/5\n781/781 [==============================] - 115s 147ms/step - loss: 0.1281 - accuracy: 0.9531\nEpoch 5/5\n781/781 [==============================] - 116s 148ms/step - loss: 0.1088 - accuracy: 0.9603\n"
]
],
[
[
"## Reusing Pretrained Embeddings",
"_____no_output_____"
]
],
[
[
"tf.random.set_seed(42)",
"_____no_output_____"
],
[
"TFHUB_CACHE_DIR = os.path.join(os.curdir, \"my_tfhub_cache\")\nos.environ[\"TFHUB_CACHE_DIR\"] = TFHUB_CACHE_DIR",
"_____no_output_____"
],
[
"import tensorflow_hub as hub\n\nmodel = keras.Sequential([\n hub.KerasLayer(\"https://tfhub.dev/google/tf2-preview/nnlm-en-dim50/1\",\n dtype=tf.string, input_shape=[], output_shape=[50]),\n keras.layers.Dense(128, activation=\"relu\"),\n keras.layers.Dense(1, activation=\"sigmoid\")\n])\nmodel.compile(loss=\"binary_crossentropy\", optimizer=\"adam\",\n metrics=[\"accuracy\"])",
"_____no_output_____"
],
[
"for dirpath, dirnames, filenames in os.walk(TFHUB_CACHE_DIR):\n for filename in filenames:\n print(os.path.join(dirpath, filename))",
"./my_tfhub_cache/82c4aaf4250ffb09088bd48368ee7fd00e5464fe.descriptor.txt\n./my_tfhub_cache/82c4aaf4250ffb09088bd48368ee7fd00e5464fe/saved_model.pb\n./my_tfhub_cache/82c4aaf4250ffb09088bd48368ee7fd00e5464fe/variables/variables.data-00000-of-00001\n./my_tfhub_cache/82c4aaf4250ffb09088bd48368ee7fd00e5464fe/variables/variables.index\n./my_tfhub_cache/82c4aaf4250ffb09088bd48368ee7fd00e5464fe/assets/tokens.txt\n"
],
[
"import tensorflow_datasets as tfds\n\ndatasets, info = tfds.load(\"imdb_reviews\", as_supervised=True, with_info=True)\ntrain_size = info.splits[\"train\"].num_examples\nbatch_size = 32\ntrain_set = datasets[\"train\"].repeat().batch(batch_size).prefetch(1)\nhistory = model.fit(train_set, steps_per_epoch=train_size // batch_size, epochs=5)",
"Train for 781 steps\nEpoch 1/5\n781/781 [==============================] - 128s 164ms/step - loss: 0.5460 - accuracy: 0.7267\nEpoch 2/5\n781/781 [==============================] - 128s 164ms/step - loss: 0.5129 - accuracy: 0.7495\nEpoch 3/5\n781/781 [==============================] - 129s 165ms/step - loss: 0.5082 - accuracy: 0.7530\nEpoch 4/5\n781/781 [==============================] - 128s 164ms/step - loss: 0.5047 - accuracy: 0.7533\nEpoch 5/5\n781/781 [==============================] - 128s 164ms/step - loss: 0.5015 - accuracy: 0.7560\n"
]
],
[
[
"## Automatic Translation",
"_____no_output_____"
]
],
[
[
"tf.random.set_seed(42)",
"_____no_output_____"
],
[
"vocab_size = 100\nembed_size = 10",
"_____no_output_____"
],
[
"import tensorflow_addons as tfa\n\nencoder_inputs = keras.layers.Input(shape=[None], dtype=np.int32)\ndecoder_inputs = keras.layers.Input(shape=[None], dtype=np.int32)\nsequence_lengths = keras.layers.Input(shape=[], dtype=np.int32)\n\nembeddings = keras.layers.Embedding(vocab_size, embed_size)\nencoder_embeddings = embeddings(encoder_inputs)\ndecoder_embeddings = embeddings(decoder_inputs)\n\nencoder = keras.layers.LSTM(512, return_state=True)\nencoder_outputs, state_h, state_c = encoder(encoder_embeddings)\nencoder_state = [state_h, state_c]\n\nsampler = tfa.seq2seq.sampler.TrainingSampler()\n\ndecoder_cell = keras.layers.LSTMCell(512)\noutput_layer = keras.layers.Dense(vocab_size)\ndecoder = tfa.seq2seq.basic_decoder.BasicDecoder(decoder_cell, sampler,\n output_layer=output_layer)\nfinal_outputs, final_state, final_sequence_lengths = decoder(\n decoder_embeddings, initial_state=encoder_state,\n sequence_length=sequence_lengths)\nY_proba = tf.nn.softmax(final_outputs.rnn_output)\n\nmodel = keras.models.Model(\n inputs=[encoder_inputs, decoder_inputs, sequence_lengths],\n outputs=[Y_proba])",
"_____no_output_____"
],
[
"model.compile(loss=\"sparse_categorical_crossentropy\", optimizer=\"adam\")",
"_____no_output_____"
],
[
"X = np.random.randint(100, size=10*1000).reshape(1000, 10)\nY = np.random.randint(100, size=15*1000).reshape(1000, 15)\nX_decoder = np.c_[np.zeros((1000, 1)), Y[:, :-1]]\nseq_lengths = np.full([1000], 15)\n\nhistory = model.fit([X, X_decoder, seq_lengths], Y, epochs=2)",
"Train on 1000 samples\nEpoch 1/2\n1000/1000 [==============================] - 6s 6ms/sample - loss: 4.6053\nEpoch 2/2\n1000/1000 [==============================] - 3s 3ms/sample - loss: 4.6031\n"
]
],
[
[
"### Bidirectional Recurrent Layers",
"_____no_output_____"
]
],
[
[
"model = keras.models.Sequential([\n keras.layers.GRU(10, return_sequences=True, input_shape=[None, 10]),\n keras.layers.Bidirectional(keras.layers.GRU(10, return_sequences=True))\n])\n\nmodel.summary()",
"Model: \"sequential_5\"\n_________________________________________________________________\nLayer (type) Output Shape Param # \n=================================================================\ngru_10 (GRU) (None, None, 10) 660 \n_________________________________________________________________\nbidirectional (Bidirectional (None, None, 20) 1320 \n=================================================================\nTotal params: 1,980\nTrainable params: 1,980\nNon-trainable params: 0\n_________________________________________________________________\n"
]
],
[
[
"### Positional Encoding",
"_____no_output_____"
]
],
[
[
"class PositionalEncoding(keras.layers.Layer):\n def __init__(self, max_steps, max_dims, dtype=tf.float32, **kwargs):\n super().__init__(dtype=dtype, **kwargs)\n if max_dims % 2 == 1: max_dims += 1 # max_dims must be even\n p, i = np.meshgrid(np.arange(max_steps), np.arange(max_dims // 2))\n pos_emb = np.empty((1, max_steps, max_dims))\n pos_emb[0, :, ::2] = np.sin(p / 10000**(2 * i / max_dims)).T\n pos_emb[0, :, 1::2] = np.cos(p / 10000**(2 * i / max_dims)).T\n self.positional_embedding = tf.constant(pos_emb.astype(self.dtype))\n def call(self, inputs):\n shape = tf.shape(inputs)\n return inputs + self.positional_embedding[:, :shape[-2], :shape[-1]]",
"_____no_output_____"
],
[
"max_steps = 201\nmax_dims = 512\npos_emb = PositionalEncoding(max_steps, max_dims)\nPE = pos_emb(np.zeros((1, max_steps, max_dims), np.float32))[0].numpy()",
"_____no_output_____"
],
[
"i1, i2, crop_i = 100, 101, 150\np1, p2, p3 = 22, 60, 35\nfig, (ax1, ax2) = plt.subplots(nrows=2, ncols=1, sharex=True, figsize=(9, 5))\nax1.plot([p1, p1], [-1, 1], \"k--\", label=\"$p = {}$\".format(p1))\nax1.plot([p2, p2], [-1, 1], \"k--\", label=\"$p = {}$\".format(p2), alpha=0.5)\nax1.plot(p3, PE[p3, i1], \"bx\", label=\"$p = {}$\".format(p3))\nax1.plot(PE[:,i1], \"b-\", label=\"$i = {}$\".format(i1))\nax1.plot(PE[:,i2], \"r-\", label=\"$i = {}$\".format(i2))\nax1.plot([p1, p2], [PE[p1, i1], PE[p2, i1]], \"bo\")\nax1.plot([p1, p2], [PE[p1, i2], PE[p2, i2]], \"ro\")\nax1.legend(loc=\"center right\", fontsize=14, framealpha=0.95)\nax1.set_ylabel(\"$P_{(p,i)}$\", rotation=0, fontsize=16)\nax1.grid(True, alpha=0.3)\nax1.hlines(0, 0, max_steps - 1, color=\"k\", linewidth=1, alpha=0.3)\nax1.axis([0, max_steps - 1, -1, 1])\nax2.imshow(PE.T[:crop_i], cmap=\"gray\", interpolation=\"bilinear\", aspect=\"auto\")\nax2.hlines(i1, 0, max_steps - 1, color=\"b\")\ncheat = 2 # need to raise the red line a bit, or else it hides the blue one\nax2.hlines(i2+cheat, 0, max_steps - 1, color=\"r\")\nax2.plot([p1, p1], [0, crop_i], \"k--\")\nax2.plot([p2, p2], [0, crop_i], \"k--\", alpha=0.5)\nax2.plot([p1, p2], [i2+cheat, i2+cheat], \"ro\")\nax2.plot([p1, p2], [i1, i1], \"bo\")\nax2.axis([0, max_steps - 1, 0, crop_i])\nax2.set_xlabel(\"$p$\", fontsize=16)\nax2.set_ylabel(\"$i$\", rotation=0, fontsize=16)\nplt.savefig(\"positional_embedding_plot\")\nplt.show()",
"_____no_output_____"
],
[
"embed_size = 512; max_steps = 500; vocab_size = 10000\nencoder_inputs = keras.layers.Input(shape=[None], dtype=np.int32)\ndecoder_inputs = keras.layers.Input(shape=[None], dtype=np.int32)\nembeddings = keras.layers.Embedding(vocab_size, embed_size)\nencoder_embeddings = embeddings(encoder_inputs)\ndecoder_embeddings = embeddings(decoder_inputs)\npositional_encoding = PositionalEncoding(max_steps, max_dims=embed_size)\nencoder_in = positional_encoding(encoder_embeddings)\ndecoder_in = positional_encoding(decoder_embeddings)",
"_____no_output_____"
]
],
[
[
"Here is a (very) simplified Transformer (the actual architecture has skip connections, layer norm, dense nets, and most importantly it uses Multi-Head Attention instead of regular Attention):",
"_____no_output_____"
]
],
[
[
"Z = encoder_in\nfor N in range(6):\n Z = keras.layers.Attention(use_scale=True)([Z, Z])\n\nencoder_outputs = Z\nZ = decoder_in\nfor N in range(6):\n Z = keras.layers.Attention(use_scale=True, causal=True)([Z, Z])\n Z = keras.layers.Attention(use_scale=True)([Z, encoder_outputs])\n\noutputs = keras.layers.TimeDistributed(\n keras.layers.Dense(vocab_size, activation=\"softmax\"))(Z)",
"_____no_output_____"
]
],
[
[
"Here's a basic implementation of the `MultiHeadAttention` layer. One will likely be added to `keras.layers` in the near future. Note that `Conv1D` layers with `kernel_size=1` (and the default `padding=\"valid\"` and `strides=1`) is equivalent to a `TimeDistributed(Dense(...))` layer.",
"_____no_output_____"
]
],
[
[
"K = keras.backend\n\nclass MultiHeadAttention(keras.layers.Layer):\n def __init__(self, n_heads, causal=False, use_scale=False, **kwargs):\n self.n_heads = n_heads\n self.causal = causal\n self.use_scale = use_scale\n super().__init__(**kwargs)\n def build(self, batch_input_shape):\n self.dims = batch_input_shape[0][-1]\n self.q_dims, self.v_dims, self.k_dims = [self.dims // self.n_heads] * 3 # could be hyperparameters instead\n self.q_linear = keras.layers.Conv1D(self.n_heads * self.q_dims, kernel_size=1, use_bias=False)\n self.v_linear = keras.layers.Conv1D(self.n_heads * self.v_dims, kernel_size=1, use_bias=False)\n self.k_linear = keras.layers.Conv1D(self.n_heads * self.k_dims, kernel_size=1, use_bias=False)\n self.attention = keras.layers.Attention(causal=self.causal, use_scale=self.use_scale)\n self.out_linear = keras.layers.Conv1D(self.dims, kernel_size=1, use_bias=False)\n super().build(batch_input_shape)\n def _multi_head_linear(self, inputs, linear):\n shape = K.concatenate([K.shape(inputs)[:-1], [self.n_heads, -1]])\n projected = K.reshape(linear(inputs), shape)\n perm = K.permute_dimensions(projected, [0, 2, 1, 3])\n return K.reshape(perm, [shape[0] * self.n_heads, shape[1], -1])\n def call(self, inputs):\n q = inputs[0]\n v = inputs[1]\n k = inputs[2] if len(inputs) > 2 else v\n shape = K.shape(q)\n q_proj = self._multi_head_linear(q, self.q_linear)\n v_proj = self._multi_head_linear(v, self.v_linear)\n k_proj = self._multi_head_linear(k, self.k_linear)\n multi_attended = self.attention([q_proj, v_proj, k_proj])\n shape_attended = K.shape(multi_attended)\n reshaped_attended = K.reshape(multi_attended, [shape[0], self.n_heads, shape_attended[1], shape_attended[2]])\n perm = K.permute_dimensions(reshaped_attended, [0, 2, 1, 3])\n concat = K.reshape(perm, [shape[0], shape_attended[1], -1])\n return self.out_linear(concat)",
"_____no_output_____"
],
[
"Q = np.random.rand(2, 50, 512)\nV = np.random.rand(2, 80, 512)\nmulti_attn = MultiHeadAttention(8)\nmulti_attn([Q, V]).shape",
"WARNING:tensorflow:Layer multi_head_attention is casting an input tensor from dtype float64 to the layer's dtype of float32, which is new behavior in TensorFlow 2. The layer has dtype float32 because it's dtype defaults to floatx.\n\nIf you intended to run this layer in float32, you can safely ignore this warning. If in doubt, this warning is likely only an issue if you are porting a TensorFlow 1.X model to TensorFlow 2.\n\nTo change all layers to have dtype float64 by default, call `tf.keras.backend.set_floatx('float64')`. To change just this layer, pass dtype='float64' to the layer constructor. If you are the author of this layer, you can disable autocasting by passing autocast=False to the base Layer constructor.\n\n"
]
],
[
[
"# Exercise solutions",
"_____no_output_____"
],
[
"## 1. to 7.",
"_____no_output_____"
],
[
"See Appendix A.",
"_____no_output_____"
],
[
"## 8.\n_Exercise:_ Embedded Reber grammars _were used by Hochreiter and Schmidhuber in [their paper](https://homl.info/93) about LSTMs. They are artificial grammars that produce strings such as \"BPBTSXXVPSEPE.\" Check out Jenny Orr's [nice introduction](https://homl.info/108) to this topic. Choose a particular embedded Reber grammar (such as the one represented on Jenny Orr's page), then train an RNN to identify whether a string respects that grammar or not. You will first need to write a function capable of generating a training batch containing about 50% strings that respect the grammar, and 50% that don't._",
"_____no_output_____"
],
[
"First we need to build a function that generates strings based on a grammar. The grammar will be represented as a list of possible transitions for each state. A transition specifies the string to output (or a grammar to generate it) and the next state.",
"_____no_output_____"
]
],
[
[
"default_reber_grammar = [\n [(\"B\", 1)], # (state 0) =B=>(state 1)\n [(\"T\", 2), (\"P\", 3)], # (state 1) =T=>(state 2) or =P=>(state 3)\n [(\"S\", 2), (\"X\", 4)], # (state 2) =S=>(state 2) or =X=>(state 4)\n [(\"T\", 3), (\"V\", 5)], # and so on...\n [(\"X\", 3), (\"S\", 6)],\n [(\"P\", 4), (\"V\", 6)],\n [(\"E\", None)]] # (state 6) =E=>(terminal state)\n\nembedded_reber_grammar = [\n [(\"B\", 1)],\n [(\"T\", 2), (\"P\", 3)],\n [(default_reber_grammar, 4)],\n [(default_reber_grammar, 5)],\n [(\"T\", 6)],\n [(\"P\", 6)],\n [(\"E\", None)]]\n\ndef generate_string(grammar):\n state = 0\n output = []\n while state is not None:\n index = np.random.randint(len(grammar[state]))\n production, state = grammar[state][index]\n if isinstance(production, list):\n production = generate_string(grammar=production)\n output.append(production)\n return \"\".join(output)",
"_____no_output_____"
]
],
[
[
"Let's generate a few strings based on the default Reber grammar:",
"_____no_output_____"
]
],
[
[
"np.random.seed(42)\n\nfor _ in range(25):\n print(generate_string(default_reber_grammar), end=\" \")",
"BTXXTTVPXTVPXTTVPSE BPVPSE BTXSE BPVVE BPVVE BTSXSE BPTVPXTTTVVE BPVVE BTXSE BTXXVPSE BPTTTTTTTTVVE BTXSE BPVPSE BTXSE BPTVPSE BTXXTVPSE BPVVE BPVVE BPVVE BPTTVVE BPVVE BPVVE BTXXVVE BTXXVVE BTXXVPXVVE "
]
],
[
[
"Looks good. Now let's generate a few strings based on the embedded Reber grammar:",
"_____no_output_____"
]
],
[
[
"np.random.seed(42)\n\nfor _ in range(25):\n print(generate_string(embedded_reber_grammar), end=\" \")",
"BTBPTTTVPXTVPXTTVPSETE BPBPTVPSEPE BPBPVVEPE BPBPVPXVVEPE BPBTXXTTTTVVEPE BPBPVPSEPE BPBTXXVPSEPE BPBTSSSSSSSXSEPE BTBPVVETE BPBTXXVVEPE BPBTXXVPSEPE BTBTXXVVETE BPBPVVEPE BPBPVVEPE BPBTSXSEPE BPBPVVEPE BPBPTVPSEPE BPBTXXVVEPE BTBPTVPXVVETE BTBPVVETE BTBTSSSSSSSXXVVETE BPBTSSSXXTTTTVPSEPE BTBPTTVVETE BPBTXXTVVEPE BTBTXSETE "
]
],
[
[
"Okay, now we need a function to generate strings that do not respect the grammar. We could generate a random string, but the task would be a bit too easy, so instead we will generate a string that respects the grammar, and we will corrupt it by changing just one character:",
"_____no_output_____"
]
],
[
[
"POSSIBLE_CHARS = \"BEPSTVX\"\n\ndef generate_corrupted_string(grammar, chars=POSSIBLE_CHARS):\n good_string = generate_string(grammar)\n index = np.random.randint(len(good_string))\n good_char = good_string[index]\n bad_char = np.random.choice(sorted(set(chars) - set(good_char)))\n return good_string[:index] + bad_char + good_string[index + 1:]",
"_____no_output_____"
]
],
[
[
"Let's look at a few corrupted strings:",
"_____no_output_____"
]
],
[
[
"np.random.seed(42)\n\nfor _ in range(25):\n print(generate_corrupted_string(embedded_reber_grammar), end=\" \")",
"BTBPTTTPPXTVPXTTVPSETE BPBTXEEPE BPBPTVVVEPE BPBTSSSSXSETE BPTTXSEPE BTBPVPXTTTTTTEVETE BPBTXXSVEPE BSBPTTVPSETE BPBXVVEPE BEBTXSETE BPBPVPSXPE BTBPVVVETE BPBTSXSETE BPBPTTTPTTTTTVPSEPE BTBTXXTTSTVPSETE BBBTXSETE BPBTPXSEPE BPBPVPXTTTTVPXTVPXVPXTTTVVEVE BTBXXXTVPSETE BEBTSSSSSXXVPXTVVETE BTBXTTVVETE BPBTXSTPE BTBTXXTTTVPSBTE BTBTXSETX BTBTSXSSTE "
]
],
[
[
"We cannot feed strings directly to an RNN, so we need to encode them somehow. One option would be to one-hot encode each character. Another option is to use embeddings. Let's go for the second option (but since there are just a handful of characters, one-hot encoding would probably be a good option as well). For embeddings to work, we need to convert each string into a sequence of character IDs. Let's write a function for that, using each character's index in the string of possible characters \"BEPSTVX\":",
"_____no_output_____"
]
],
[
[
"def string_to_ids(s, chars=POSSIBLE_CHARS):\n return [POSSIBLE_CHARS.index(c) for c in s]",
"_____no_output_____"
],
[
"string_to_ids(\"BTTTXXVVETE\")",
"_____no_output_____"
]
],
[
[
"We can now generate the dataset, with 50% good strings, and 50% bad strings:",
"_____no_output_____"
]
],
[
[
"def generate_dataset(size):\n good_strings = [string_to_ids(generate_string(embedded_reber_grammar))\n for _ in range(size // 2)]\n bad_strings = [string_to_ids(generate_corrupted_string(embedded_reber_grammar))\n for _ in range(size - size // 2)]\n all_strings = good_strings + bad_strings\n X = tf.ragged.constant(all_strings, ragged_rank=1)\n y = np.array([[1.] for _ in range(len(good_strings))] +\n [[0.] for _ in range(len(bad_strings))])\n return X, y",
"_____no_output_____"
],
[
"np.random.seed(42)\n\nX_train, y_train = generate_dataset(10000)\nX_valid, y_valid = generate_dataset(2000)",
"_____no_output_____"
]
],
[
[
"Let's take a look at the first training sequence:",
"_____no_output_____"
]
],
[
[
"X_train[0]",
"_____no_output_____"
]
],
[
[
"What classes does it belong to?",
"_____no_output_____"
]
],
[
[
"y_train[0]",
"_____no_output_____"
]
],
[
[
"Perfect! We are ready to create the RNN to identify good strings. We build a simple sequence binary classifier:",
"_____no_output_____"
]
],
[
[
"np.random.seed(42)\ntf.random.set_seed(42)\n\nembedding_size = 5\n\nmodel = keras.models.Sequential([\n keras.layers.InputLayer(input_shape=[None], dtype=tf.int32, ragged=True),\n keras.layers.Embedding(input_dim=len(POSSIBLE_CHARS), output_dim=embedding_size),\n keras.layers.GRU(30),\n keras.layers.Dense(1, activation=\"sigmoid\")\n])\noptimizer = keras.optimizers.SGD(lr=0.02, momentum = 0.95, nesterov=True)\nmodel.compile(loss=\"binary_crossentropy\", optimizer=optimizer, metrics=[\"accuracy\"])\nhistory = model.fit(X_train, y_train, epochs=20, validation_data=(X_valid, y_valid))",
"Train on 10000 samples, validate on 2000 samples\nEpoch 1/20\n"
]
],
[
[
"Now let's test our RNN on two tricky strings: the first one is bad while the second one is good. They only differ by the second to last character. If the RNN gets this right, it shows that it managed to notice the pattern that the second letter should always be equal to the second to last letter. That requires a fairly long short-term memory (which is the reason why we used a GRU cell).",
"_____no_output_____"
]
],
[
[
"test_strings = [\"BPBTSSSSSSSXXTTVPXVPXTTTTTVVETE\",\n \"BPBTSSSSSSSXXTTVPXVPXTTTTTVVEPE\"]\nX_test = tf.ragged.constant([string_to_ids(s) for s in test_strings], ragged_rank=1)\n\ny_proba = model.predict(X_test)\nprint()\nprint(\"Estimated probability that these are Reber strings:\")\nfor index, string in enumerate(test_strings):\n print(\"{}: {:.2f}%\".format(string, 100 * y_proba[index][0]))",
"\nEstimated probability that these are Reber strings:\nBPBTSSSSSSSXXTTVPXVPXTTTTTVVETE: 0.40%\nBPBTSSSSSSSXXTTVPXVPXTTTTTVVEPE: 99.96%\n"
]
],
[
[
"Ta-da! It worked fine. The RNN found the correct answers with very high confidence. :)",
"_____no_output_____"
],
[
"## 9.\n_Exercise: Train an Encoder–Decoder model that can convert a date string from one format to another (e.g., from \"April 22, 2019\" to \"2019-04-22\")._",
"_____no_output_____"
],
[
"Let's start by creating the dataset. We will use random days between 1000-01-01 and 9999-12-31:",
"_____no_output_____"
]
],
[
[
"from datetime import date\n\n# cannot use strftime()'s %B format since it depends on the locale\nMONTHS = [\"January\", \"February\", \"March\", \"April\", \"May\", \"June\",\n \"July\", \"August\", \"September\", \"October\", \"November\", \"December\"]\n\ndef random_dates(n_dates):\n min_date = date(1000, 1, 1).toordinal()\n max_date = date(9999, 12, 31).toordinal()\n\n ordinals = np.random.randint(max_date - min_date, size=n_dates) + min_date\n dates = [date.fromordinal(ordinal) for ordinal in ordinals]\n\n x = [MONTHS[dt.month - 1] + \" \" + dt.strftime(\"%d, %Y\") for dt in dates]\n y = [dt.isoformat() for dt in dates]\n return x, y",
"_____no_output_____"
]
],
[
[
"Here are a few random dates, displayed in both the input format and the target format:",
"_____no_output_____"
]
],
[
[
"np.random.seed(42)\n\nn_dates = 3\nx_example, y_example = random_dates(n_dates)\nprint(\"{:25s}{:25s}\".format(\"Input\", \"Target\"))\nprint(\"-\" * 50)\nfor idx in range(n_dates):\n print(\"{:25s}{:25s}\".format(x_example[idx], y_example[idx]))",
"Input Target \n--------------------------------------------------\nSeptember 20, 7075 7075-09-20 \nMay 15, 8579 8579-05-15 \nJanuary 11, 7103 7103-01-11 \n"
]
],
[
[
"Let's get the list of all possible characters in the inputs:",
"_____no_output_____"
]
],
[
[
"INPUT_CHARS = \"\".join(sorted(set(\"\".join(MONTHS)))) + \"01234567890, \"\nINPUT_CHARS",
"_____no_output_____"
]
],
[
[
"And here's the list of possible characters in the outputs:",
"_____no_output_____"
]
],
[
[
"OUTPUT_CHARS = \"0123456789-\"",
"_____no_output_____"
]
],
[
[
"Let's write a function to convert a string to a list of character IDs, as we did in the previous exercise:",
"_____no_output_____"
]
],
[
[
"def date_str_to_ids(date_str, chars=INPUT_CHARS):\n return [chars.index(c) for c in date_str]",
"_____no_output_____"
],
[
"date_str_to_ids(x_example[0], INPUT_CHARS)",
"_____no_output_____"
],
[
"date_str_to_ids(y_example[0], OUTPUT_CHARS)",
"_____no_output_____"
],
[
"def prepare_date_strs(date_strs, chars=INPUT_CHARS):\n X_ids = [date_str_to_ids(dt, chars) for dt in date_strs]\n X = tf.ragged.constant(X_ids, ragged_rank=1)\n return (X + 1).to_tensor() # using 0 as the padding token ID\n\ndef create_dataset(n_dates):\n x, y = random_dates(n_dates)\n return prepare_date_strs(x, INPUT_CHARS), prepare_date_strs(y, OUTPUT_CHARS)",
"_____no_output_____"
],
[
"np.random.seed(42)\n\nX_train, Y_train = create_dataset(10000)\nX_valid, Y_valid = create_dataset(2000)\nX_test, Y_test = create_dataset(2000)",
"_____no_output_____"
],
[
"Y_train[0]",
"_____no_output_____"
]
],
[
[
"### First version: a very basic seq2seq model",
"_____no_output_____"
],
[
"Let's first try the simplest possible model: we feed in the input sequence, which first goes through the encoder (an embedding layer followed by a single LSTM layer), which outputs a vector, then it goes through a decoder (a single LSTM layer, followed by a dense output layer), which outputs a sequence of vectors, each representing the estimated probabilities for all possible output character.\n\nSince the decoder expects a sequence as input, we repeat the vector (which is output by the decoder) as many times as the longest possible output sequence.",
"_____no_output_____"
]
],
[
[
"embedding_size = 32\nmax_output_length = Y_train.shape[1]\n\nnp.random.seed(42)\ntf.random.set_seed(42)\n\nencoder = keras.models.Sequential([\n keras.layers.Embedding(input_dim=len(INPUT_CHARS) + 1,\n output_dim=embedding_size,\n input_shape=[None]),\n keras.layers.LSTM(128)\n])\n\ndecoder = keras.models.Sequential([\n keras.layers.LSTM(128, return_sequences=True),\n keras.layers.Dense(len(OUTPUT_CHARS) + 1, activation=\"softmax\")\n])\n\nmodel = keras.models.Sequential([\n encoder,\n keras.layers.RepeatVector(max_output_length),\n decoder\n])\n\noptimizer = keras.optimizers.Nadam()\nmodel.compile(loss=\"sparse_categorical_crossentropy\", optimizer=optimizer,\n metrics=[\"accuracy\"])\nhistory = model.fit(X_train, Y_train, epochs=20,\n validation_data=(X_valid, Y_valid))",
"Epoch 1/20\n313/313 [==============================] - 6s 18ms/step - loss: 1.8111 - accuracy: 0.3533 - val_loss: 1.3581 - val_accuracy: 0.4965\nEpoch 2/20\n313/313 [==============================] - 5s 15ms/step - loss: 1.3518 - accuracy: 0.5103 - val_loss: 1.1915 - val_accuracy: 0.5694\nEpoch 3/20\n313/313 [==============================] - 5s 15ms/step - loss: 1.1706 - accuracy: 0.5908 - val_loss: 0.9983 - val_accuracy: 0.6398\nEpoch 4/20\n313/313 [==============================] - 5s 15ms/step - loss: 0.9158 - accuracy: 0.6686 - val_loss: 0.8012 - val_accuracy: 0.6987\nEpoch 5/20\n313/313 [==============================] - 5s 15ms/step - loss: 0.7058 - accuracy: 0.7308 - val_loss: 0.6224 - val_accuracy: 0.7599\nEpoch 6/20\n313/313 [==============================] - 5s 15ms/step - loss: 0.7756 - accuracy: 0.7203 - val_loss: 0.6541 - val_accuracy: 0.7599\nEpoch 7/20\n313/313 [==============================] - 5s 16ms/step - loss: 0.5379 - accuracy: 0.8034 - val_loss: 0.4174 - val_accuracy: 0.8440\nEpoch 8/20\n313/313 [==============================] - 5s 15ms/step - loss: 0.4867 - accuracy: 0.8262 - val_loss: 0.4188 - val_accuracy: 0.8480\nEpoch 9/20\n313/313 [==============================] - 5s 15ms/step - loss: 0.2979 - accuracy: 0.8951 - val_loss: 0.2549 - val_accuracy: 0.9126\nEpoch 10/20\n313/313 [==============================] - 5s 14ms/step - loss: 0.1785 - accuracy: 0.9479 - val_loss: 0.1461 - val_accuracy: 0.9594\nEpoch 11/20\n313/313 [==============================] - 5s 15ms/step - loss: 0.1830 - accuracy: 0.9557 - val_loss: 0.1644 - val_accuracy: 0.9550\nEpoch 12/20\n313/313 [==============================] - 5s 15ms/step - loss: 0.0775 - accuracy: 0.9857 - val_loss: 0.0595 - val_accuracy: 0.9901\nEpoch 13/20\n313/313 [==============================] - 5s 15ms/step - loss: 0.0400 - accuracy: 0.9953 - val_loss: 0.0342 - val_accuracy: 0.9957\nEpoch 14/20\n313/313 [==============================] - 5s 15ms/step - loss: 0.0248 - accuracy: 0.9979 - val_loss: 0.0231 - val_accuracy: 0.9983\nEpoch 15/20\n313/313 [==============================] - 5s 15ms/step - loss: 0.0161 - accuracy: 0.9991 - val_loss: 0.0149 - val_accuracy: 0.9995\nEpoch 16/20\n313/313 [==============================] - 5s 15ms/step - loss: 0.0108 - accuracy: 0.9997 - val_loss: 0.0106 - val_accuracy: 0.9996\nEpoch 17/20\n313/313 [==============================] - 5s 15ms/step - loss: 0.0074 - accuracy: 0.9999 - val_loss: 0.0077 - val_accuracy: 0.9999\nEpoch 18/20\n313/313 [==============================] - 5s 15ms/step - loss: 0.0053 - accuracy: 1.0000 - val_loss: 0.0054 - val_accuracy: 0.9999\nEpoch 19/20\n313/313 [==============================] - 5s 15ms/step - loss: 0.0039 - accuracy: 1.0000 - val_loss: 0.0041 - val_accuracy: 1.0000\nEpoch 20/20\n313/313 [==============================] - 5s 15ms/step - loss: 0.0029 - accuracy: 1.0000 - val_loss: 0.0032 - val_accuracy: 1.0000\n"
]
],
[
[
"Looks great, we reach 100% validation accuracy! Let's use the model to make some predictions. We will need to be able to convert a sequence of character IDs to a readable string:",
"_____no_output_____"
]
],
[
[
"def ids_to_date_strs(ids, chars=OUTPUT_CHARS):\n return [\"\".join([(\"?\" + chars)[index] for index in sequence])\n for sequence in ids]",
"_____no_output_____"
]
],
[
[
"Now we can use the model to convert some dates",
"_____no_output_____"
]
],
[
[
"X_new = prepare_date_strs([\"September 17, 2009\", \"July 14, 1789\"])",
"_____no_output_____"
],
[
"ids = model.predict_classes(X_new)\nfor date_str in ids_to_date_strs(ids):\n print(date_str)",
"WARNING:tensorflow:From <ipython-input-15-472ea7c41409>:1: Sequential.predict_classes (from tensorflow.python.keras.engine.sequential) is deprecated and will be removed after 2021-01-01.\nInstructions for updating:\nPlease use instead:* `np.argmax(model.predict(x), axis=-1)`, if your model does multi-class classification (e.g. if it uses a `softmax` last-layer activation).* `(model.predict(x) > 0.5).astype(\"int32\")`, if your model does binary classification (e.g. if it uses a `sigmoid` last-layer activation).\n2009-09-17\n1789-07-14\n"
]
],
[
[
"Perfect! :)",
"_____no_output_____"
],
[
"However, since the model was only trained on input strings of length 18 (which is the length of the longest date), it does not perform well if we try to use it to make predictions on shorter sequences:",
"_____no_output_____"
]
],
[
[
"X_new = prepare_date_strs([\"May 02, 2020\", \"July 14, 1789\"])",
"_____no_output_____"
],
[
"ids = model.predict_classes(X_new)\nfor date_str in ids_to_date_strs(ids):\n print(date_str)",
"2020-01-02\n1789-02-14\n"
]
],
[
[
"Oops! We need to ensure that we always pass sequences of the same length as during training, using padding if necessary. Let's write a little helper function for that:",
"_____no_output_____"
]
],
[
[
"max_input_length = X_train.shape[1]\n\ndef prepare_date_strs_padded(date_strs):\n X = prepare_date_strs(date_strs)\n if X.shape[1] < max_input_length:\n X = tf.pad(X, [[0, 0], [0, max_input_length - X.shape[1]]])\n return X\n\ndef convert_date_strs(date_strs):\n X = prepare_date_strs_padded(date_strs)\n ids = model.predict_classes(X)\n return ids_to_date_strs(ids)",
"_____no_output_____"
],
[
"convert_date_strs([\"May 02, 2020\", \"July 14, 1789\"])",
"_____no_output_____"
]
],
[
[
"Cool! Granted, there are certainly much easier ways to write a date conversion tool (e.g., using regular expressions or even basic string manipulation), but you have to admit that using neural networks is way cooler. ;-)",
"_____no_output_____"
],
[
"However, real-life sequence-to-sequence problems will usually be harder, so for the sake of completeness, let's build a more powerful model.",
"_____no_output_____"
],
[
"### Second version: feeding the shifted targets to the decoder (teacher forcing)",
"_____no_output_____"
],
[
"Instead of feeding the decoder a simple repetition of the encoder's output vector, we can feed it the target sequence, shifted by one time step to the right. This way, at each time step the decoder will know what the previous target character was. This should help is tackle more complex sequence-to-sequence problems.\n\nSince the first output character of each target sequence has no previous character, we will need a new token to represent the start-of-sequence (sos).\n\nDuring inference, we won't know the target, so what will we feed the decoder? We can just predict one character at a time, starting with an sos token, then feeding the decoder all the characters that were predicted so far (we will look at this in more details later in this notebook).\n\nBut if the decoder's LSTM expects to get the previous target as input at each step, how shall we pass it it the vector output by the encoder? Well, one option is to ignore the output vector, and instead use the encoder's LSTM state as the initial state of the decoder's LSTM (which requires that encoder's LSTM must have the same number of units as the decoder's LSTM).\n\nNow let's create the decoder's inputs (for training, validation and testing). The sos token will be represented using the last possible output character's ID + 1.",
"_____no_output_____"
]
],
[
[
"sos_id = len(OUTPUT_CHARS) + 1\n\ndef shifted_output_sequences(Y):\n sos_tokens = tf.fill(dims=(len(Y), 1), value=sos_id)\n return tf.concat([sos_tokens, Y[:, :-1]], axis=1)\n\nX_train_decoder = shifted_output_sequences(Y_train)\nX_valid_decoder = shifted_output_sequences(Y_valid)\nX_test_decoder = shifted_output_sequences(Y_test)",
"_____no_output_____"
]
],
[
[
"Let's take a look at the decoder's training inputs:",
"_____no_output_____"
]
],
[
[
"X_train_decoder",
"_____no_output_____"
]
],
[
[
"Now let's build the model. It's not a simple sequential model anymore, so let's use the functional API:",
"_____no_output_____"
]
],
[
[
"encoder_embedding_size = 32\ndecoder_embedding_size = 32\nlstm_units = 128\n\nnp.random.seed(42)\ntf.random.set_seed(42)\n\nencoder_input = keras.layers.Input(shape=[None], dtype=tf.int32)\nencoder_embedding = keras.layers.Embedding(\n input_dim=len(INPUT_CHARS) + 1,\n output_dim=encoder_embedding_size)(encoder_input)\n_, encoder_state_h, encoder_state_c = keras.layers.LSTM(\n lstm_units, return_state=True)(encoder_embedding)\nencoder_state = [encoder_state_h, encoder_state_c]\n\ndecoder_input = keras.layers.Input(shape=[None], dtype=tf.int32)\ndecoder_embedding = keras.layers.Embedding(\n input_dim=len(OUTPUT_CHARS) + 2,\n output_dim=decoder_embedding_size)(decoder_input)\ndecoder_lstm_output = keras.layers.LSTM(lstm_units, return_sequences=True)(\n decoder_embedding, initial_state=encoder_state)\ndecoder_output = keras.layers.Dense(len(OUTPUT_CHARS) + 1,\n activation=\"softmax\")(decoder_lstm_output)\n\nmodel = keras.models.Model(inputs=[encoder_input, decoder_input],\n outputs=[decoder_output])\n\noptimizer = keras.optimizers.Nadam()\nmodel.compile(loss=\"sparse_categorical_crossentropy\", optimizer=optimizer,\n metrics=[\"accuracy\"])\nhistory = model.fit([X_train, X_train_decoder], Y_train, epochs=10,\n validation_data=([X_valid, X_valid_decoder], Y_valid))",
"Epoch 1/10\n313/313 [==============================] - 5s 17ms/step - loss: 1.6898 - accuracy: 0.3714 - val_loss: 1.4141 - val_accuracy: 0.4603\nEpoch 2/10\n313/313 [==============================] - 5s 15ms/step - loss: 1.2118 - accuracy: 0.5541 - val_loss: 0.9360 - val_accuracy: 0.6653\nEpoch 3/10\n313/313 [==============================] - 5s 15ms/step - loss: 0.6399 - accuracy: 0.7766 - val_loss: 0.4054 - val_accuracy: 0.8631\nEpoch 4/10\n313/313 [==============================] - 5s 15ms/step - loss: 0.2207 - accuracy: 0.9463 - val_loss: 0.1069 - val_accuracy: 0.9869\nEpoch 5/10\n313/313 [==============================] - 5s 15ms/step - loss: 0.0805 - accuracy: 0.9910 - val_loss: 0.0445 - val_accuracy: 0.9976\nEpoch 6/10\n313/313 [==============================] - 5s 15ms/step - loss: 0.0297 - accuracy: 0.9993 - val_loss: 0.0237 - val_accuracy: 0.9992\nEpoch 7/10\n313/313 [==============================] - 5s 15ms/step - loss: 0.0743 - accuracy: 0.9857 - val_loss: 0.0702 - val_accuracy: 0.9889\nEpoch 8/10\n313/313 [==============================] - 5s 15ms/step - loss: 0.0187 - accuracy: 0.9995 - val_loss: 0.0112 - val_accuracy: 0.9999\nEpoch 9/10\n313/313 [==============================] - 5s 15ms/step - loss: 0.0084 - accuracy: 1.0000 - val_loss: 0.0072 - val_accuracy: 1.0000\nEpoch 10/10\n313/313 [==============================] - 5s 15ms/step - loss: 0.0057 - accuracy: 1.0000 - val_loss: 0.0053 - val_accuracy: 1.0000\n"
]
],
[
[
"This model also reaches 100% validation accuracy, but it does so even faster.",
"_____no_output_____"
],
[
"Let's once again use the model to make some predictions. This time we need to predict characters one by one.",
"_____no_output_____"
]
],
[
[
"sos_id = len(OUTPUT_CHARS) + 1\n\ndef predict_date_strs(date_strs):\n X = prepare_date_strs_padded(date_strs)\n Y_pred = tf.fill(dims=(len(X), 1), value=sos_id)\n for index in range(max_output_length):\n pad_size = max_output_length - Y_pred.shape[1]\n X_decoder = tf.pad(Y_pred, [[0, 0], [0, pad_size]])\n Y_probas_next = model.predict([X, X_decoder])[:, index:index+1]\n Y_pred_next = tf.argmax(Y_probas_next, axis=-1, output_type=tf.int32)\n Y_pred = tf.concat([Y_pred, Y_pred_next], axis=1)\n return ids_to_date_strs(Y_pred[:, 1:])",
"_____no_output_____"
],
[
"predict_date_strs([\"July 14, 1789\", \"May 01, 2020\"])",
"_____no_output_____"
]
],
[
[
"Works fine! :)",
"_____no_output_____"
],
[
"### Third version: using TF-Addons's seq2seq implementation",
"_____no_output_____"
],
[
"Let's build exactly the same model, but using TF-Addon's seq2seq API. The implementation below is almost very similar to the TFA example higher in this notebook, except without the model input to specify the output sequence length, for simplicity (but you can easily add it back in if you need it for your projects, when the output sequences have very different lengths).",
"_____no_output_____"
]
],
[
[
"import tensorflow_addons as tfa\n\nnp.random.seed(42)\ntf.random.set_seed(42)\n\nencoder_embedding_size = 32\ndecoder_embedding_size = 32\nunits = 128\n\nencoder_inputs = keras.layers.Input(shape=[None], dtype=np.int32)\ndecoder_inputs = keras.layers.Input(shape=[None], dtype=np.int32)\nsequence_lengths = keras.layers.Input(shape=[], dtype=np.int32)\n\nencoder_embeddings = keras.layers.Embedding(\n len(INPUT_CHARS) + 1, encoder_embedding_size)(encoder_inputs)\n\ndecoder_embedding_layer = keras.layers.Embedding(\n len(INPUT_CHARS) + 2, decoder_embedding_size)\ndecoder_embeddings = decoder_embedding_layer(decoder_inputs)\n\nencoder = keras.layers.LSTM(units, return_state=True)\nencoder_outputs, state_h, state_c = encoder(encoder_embeddings)\nencoder_state = [state_h, state_c]\n\nsampler = tfa.seq2seq.sampler.TrainingSampler()\n\ndecoder_cell = keras.layers.LSTMCell(units)\noutput_layer = keras.layers.Dense(len(OUTPUT_CHARS) + 1)\n\ndecoder = tfa.seq2seq.basic_decoder.BasicDecoder(decoder_cell,\n sampler,\n output_layer=output_layer)\nfinal_outputs, final_state, final_sequence_lengths = decoder(\n decoder_embeddings,\n initial_state=encoder_state)\nY_proba = keras.layers.Activation(\"softmax\")(final_outputs.rnn_output)\n\nmodel = keras.models.Model(inputs=[encoder_inputs, decoder_inputs],\n outputs=[Y_proba])\noptimizer = keras.optimizers.Nadam()\nmodel.compile(loss=\"sparse_categorical_crossentropy\", optimizer=optimizer,\n metrics=[\"accuracy\"])\nhistory = model.fit([X_train, X_train_decoder], Y_train, epochs=15,\n validation_data=([X_valid, X_valid_decoder], Y_valid))",
"Epoch 1/15\n313/313 [==============================] - 5s 17ms/step - loss: 1.6757 - accuracy: 0.3683 - val_loss: 1.4602 - val_accuracy: 0.4214\nEpoch 2/15\n313/313 [==============================] - 5s 15ms/step - loss: 1.3873 - accuracy: 0.4566 - val_loss: 1.2904 - val_accuracy: 0.4957\nEpoch 3/15\n313/313 [==============================] - 5s 15ms/step - loss: 1.0471 - accuracy: 0.6109 - val_loss: 0.7737 - val_accuracy: 0.7276\nEpoch 4/15\n313/313 [==============================] - 5s 15ms/step - loss: 0.5056 - accuracy: 0.8296 - val_loss: 0.2695 - val_accuracy: 0.9305\nEpoch 5/15\n313/313 [==============================] - 5s 15ms/step - loss: 0.1677 - accuracy: 0.9657 - val_loss: 0.0870 - val_accuracy: 0.9912\nEpoch 6/15\n313/313 [==============================] - 5s 15ms/step - loss: 0.1007 - accuracy: 0.9850 - val_loss: 0.0492 - val_accuracy: 0.9975\nEpoch 7/15\n313/313 [==============================] - 5s 15ms/step - loss: 0.0308 - accuracy: 0.9993 - val_loss: 0.0228 - val_accuracy: 0.9996\nEpoch 8/15\n313/313 [==============================] - 5s 15ms/step - loss: 0.0168 - accuracy: 0.9999 - val_loss: 0.0144 - val_accuracy: 0.9999\nEpoch 9/15\n313/313 [==============================] - 5s 15ms/step - loss: 0.0107 - accuracy: 1.0000 - val_loss: 0.0095 - val_accuracy: 0.9999\nEpoch 10/15\n313/313 [==============================] - 5s 15ms/step - loss: 0.0074 - accuracy: 1.0000 - val_loss: 0.0066 - val_accuracy: 0.9999\nEpoch 11/15\n313/313 [==============================] - 5s 15ms/step - loss: 0.0053 - accuracy: 1.0000 - val_loss: 0.0051 - val_accuracy: 0.9999\nEpoch 12/15\n313/313 [==============================] - 5s 15ms/step - loss: 0.0039 - accuracy: 1.0000 - val_loss: 0.0037 - val_accuracy: 1.0000\nEpoch 13/15\n313/313 [==============================] - 5s 15ms/step - loss: 0.0029 - accuracy: 1.0000 - val_loss: 0.0030 - val_accuracy: 1.0000\nEpoch 14/15\n313/313 [==============================] - 5s 15ms/step - loss: 0.0023 - accuracy: 1.0000 - val_loss: 0.0022 - val_accuracy: 1.0000\nEpoch 15/15\n313/313 [==============================] - 5s 15ms/step - loss: 0.0018 - accuracy: 1.0000 - val_loss: 0.0018 - val_accuracy: 1.0000\n"
]
],
[
[
"And once again, 100% validation accuracy! To use the model, we can just reuse the `predict_date_strs()` function:",
"_____no_output_____"
]
],
[
[
"predict_date_strs([\"July 14, 1789\", \"May 01, 2020\"])",
"_____no_output_____"
]
],
[
[
"However, there's a much more efficient way to perform inference. Until now, during inference, we've run the model once for each new character. Instead, we can create a new decoder, based on the previously trained layers, but using a `GreedyEmbeddingSampler` instead of a `TrainingSampler`.\n\nAt each time step, the `GreedyEmbeddingSampler` will compute the argmax of the decoder's outputs, and run the resulting token IDs through the decoder's embedding layer. Then it will feed the resulting embeddings to the decoder's LSTM cell at the next time step. This way, we only need to run the decoder once to get the full prediction.",
"_____no_output_____"
]
],
[
[
"inference_sampler = tfa.seq2seq.sampler.GreedyEmbeddingSampler(\n embedding_fn=decoder_embedding_layer)\ninference_decoder = tfa.seq2seq.basic_decoder.BasicDecoder(\n decoder_cell, inference_sampler, output_layer=output_layer,\n maximum_iterations=max_output_length)\nbatch_size = tf.shape(encoder_inputs)[:1]\nstart_tokens = tf.fill(dims=batch_size, value=sos_id)\nfinal_outputs, final_state, final_sequence_lengths = inference_decoder(\n start_tokens,\n initial_state=encoder_state,\n start_tokens=start_tokens,\n end_token=0)\n\ninference_model = keras.models.Model(inputs=[encoder_inputs],\n outputs=[final_outputs.sample_id])",
"_____no_output_____"
]
],
[
[
"A few notes:\n* The `GreedyEmbeddingSampler` needs the `start_tokens` (a vector containing the start-of-sequence ID for each decoder sequence), and the `end_token` (the decoder will stop decoding a sequence once the model outputs this token).\n* We must set `maximum_iterations` when creating the `BasicDecoder`, or else it may run into an infinite loop (if the model never outputs the end token for at least one of the sequences). This would force you would to restart the Jupyter kernel.\n* The decoder inputs are not needed anymore, since all the decoder inputs are generated dynamically based on the outputs from the previous time step.\n* The model's outputs are `final_outputs.sample_id` instead of the softmax of `final_outputs.rnn_outputs`. This allows us to directly get the argmax of the model's outputs. If you prefer to have access to the logits, you can replace `final_outputs.sample_id` with `final_outputs.rnn_outputs`.",
"_____no_output_____"
],
[
"Now we can write a simple function that uses the model to perform the date format conversion:",
"_____no_output_____"
]
],
[
[
"def fast_predict_date_strs(date_strs):\n X = prepare_date_strs_padded(date_strs)\n Y_pred = inference_model.predict(X)\n return ids_to_date_strs(Y_pred)",
"_____no_output_____"
],
[
"fast_predict_date_strs([\"July 14, 1789\", \"May 01, 2020\"])",
"_____no_output_____"
]
],
[
[
"Let's check that it really is faster:",
"_____no_output_____"
]
],
[
[
"%timeit predict_date_strs([\"July 14, 1789\", \"May 01, 2020\"])",
"199 ms ± 3.94 ms per loop (mean ± std. dev. of 7 runs, 1 loop each)\n"
],
[
"%timeit fast_predict_date_strs([\"July 14, 1789\", \"May 01, 2020\"])",
"18.3 ms ± 366 µs per loop (mean ± std. dev. of 7 runs, 10 loops each)\n"
]
],
[
[
"That's more than a 10x speedup! And it would be even more if we were handling longer sequences.",
"_____no_output_____"
],
[
"### Fourth version: using TF-Addons's seq2seq implementation with a scheduled sampler",
"_____no_output_____"
],
[
"**Warning**: due to a TF bug, this version only works using TensorFlow 2.2.",
"_____no_output_____"
],
[
"When we trained the previous model, at each time step _t_ we gave the model the target token for time step _t_ - 1. However, at inference time, the model did not get the previous target at each time step. Instead, it got the previous prediction. So there is a discrepancy between training and inference, which may lead to disappointing performance. To alleviate this, we can gradually replace the targets with the predictions, during training. For this, we just need to replace the `TrainingSampler` with a `ScheduledEmbeddingTrainingSampler`, and use a Keras callback to gradually increase the `sampling_probability` (i.e., the probability that the decoder will use the prediction from the previous time step rather than the target for the previous time step).",
"_____no_output_____"
]
],
[
[
"import tensorflow_addons as tfa\n\nnp.random.seed(42)\ntf.random.set_seed(42)\n\nn_epochs = 20\nencoder_embedding_size = 32\ndecoder_embedding_size = 32\nunits = 128\n\nencoder_inputs = keras.layers.Input(shape=[None], dtype=np.int32)\ndecoder_inputs = keras.layers.Input(shape=[None], dtype=np.int32)\nsequence_lengths = keras.layers.Input(shape=[], dtype=np.int32)\n\nencoder_embeddings = keras.layers.Embedding(\n len(INPUT_CHARS) + 1, encoder_embedding_size)(encoder_inputs)\n\ndecoder_embedding_layer = keras.layers.Embedding(\n len(INPUT_CHARS) + 2, decoder_embedding_size)\ndecoder_embeddings = decoder_embedding_layer(decoder_inputs)\n\nencoder = keras.layers.LSTM(units, return_state=True)\nencoder_outputs, state_h, state_c = encoder(encoder_embeddings)\nencoder_state = [state_h, state_c]\n\nsampler = tfa.seq2seq.sampler.ScheduledEmbeddingTrainingSampler(\n sampling_probability=0.,\n embedding_fn=decoder_embedding_layer)\n# we must set the sampling_probability after creating the sampler\n# (see https://github.com/tensorflow/addons/pull/1714)\nsampler.sampling_probability = tf.Variable(0.)\n\ndecoder_cell = keras.layers.LSTMCell(units)\noutput_layer = keras.layers.Dense(len(OUTPUT_CHARS) + 1)\n\ndecoder = tfa.seq2seq.basic_decoder.BasicDecoder(decoder_cell,\n sampler,\n output_layer=output_layer)\nfinal_outputs, final_state, final_sequence_lengths = decoder(\n decoder_embeddings,\n initial_state=encoder_state)\nY_proba = keras.layers.Activation(\"softmax\")(final_outputs.rnn_output)\n\nmodel = keras.models.Model(inputs=[encoder_inputs, decoder_inputs],\n outputs=[Y_proba])\noptimizer = keras.optimizers.Nadam()\nmodel.compile(loss=\"sparse_categorical_crossentropy\", optimizer=optimizer,\n metrics=[\"accuracy\"])\n\ndef update_sampling_probability(epoch, logs):\n proba = min(1.0, epoch / (n_epochs - 10))\n sampler.sampling_probability.assign(proba)\n\nsampling_probability_cb = keras.callbacks.LambdaCallback(\n on_epoch_begin=update_sampling_probability)\nhistory = model.fit([X_train, X_train_decoder], Y_train, epochs=n_epochs,\n validation_data=([X_valid, X_valid_decoder], Y_valid),\n callbacks=[sampling_probability_cb])",
"Epoch 1/20\n"
]
],
[
[
"Not quite 100% validation accuracy, but close enough!",
"_____no_output_____"
],
[
"For inference, we could do the exact same thing as earlier, using a `GreedyEmbeddingSampler`. However, just for the sake of completeness, let's use a `SampleEmbeddingSampler` instead. It's almost the same thing, except that instead of using the argmax of the model's output to find the token ID, it treats the outputs as logits and uses them to sample a token ID randomly. This can be useful when you want to generate text. The `softmax_temperature` argument serves the \nsame purpose as when we generated Shakespeare-like text (the higher this argument, the more random the generated text will be).",
"_____no_output_____"
]
],
[
[
"softmax_temperature = tf.Variable(1.)\n\ninference_sampler = tfa.seq2seq.sampler.SampleEmbeddingSampler(\n embedding_fn=decoder_embedding_layer,\n softmax_temperature=softmax_temperature)\ninference_decoder = tfa.seq2seq.basic_decoder.BasicDecoder(\n decoder_cell, inference_sampler, output_layer=output_layer,\n maximum_iterations=max_output_length)\nbatch_size = tf.shape(encoder_inputs)[:1]\nstart_tokens = tf.fill(dims=batch_size, value=sos_id)\nfinal_outputs, final_state, final_sequence_lengths = inference_decoder(\n start_tokens,\n initial_state=encoder_state,\n start_tokens=start_tokens,\n end_token=0)\n\ninference_model = keras.models.Model(inputs=[encoder_inputs],\n outputs=[final_outputs.sample_id])",
"_____no_output_____"
],
[
"def creative_predict_date_strs(date_strs, temperature=1.0):\n softmax_temperature.assign(temperature)\n X = prepare_date_strs_padded(date_strs)\n Y_pred = inference_model.predict(X)\n return ids_to_date_strs(Y_pred)",
"_____no_output_____"
],
[
"tf.random.set_seed(42)\n\ncreative_predict_date_strs([\"July 14, 1789\", \"May 01, 2020\"])",
"_____no_output_____"
]
],
[
[
"Dates look good at room temperature. Now let's heat things up a bit:",
"_____no_output_____"
]
],
[
[
"tf.random.set_seed(42)\n\ncreative_predict_date_strs([\"July 14, 1789\", \"May 01, 2020\"],\n temperature=5.)",
"_____no_output_____"
]
],
[
[
"Oops, the dates are overcooked, now. Let's call them \"creative\" dates.",
"_____no_output_____"
],
[
"### Fifth version: using TFA seq2seq, the Keras subclassing API and attention mechanisms",
"_____no_output_____"
],
[
"The sequences in this problem are pretty short, but if we wanted to tackle longer sequences, we would probably have to use attention mechanisms. While it's possible to code our own implementation, it's simpler and more efficient to use TF-Addons's implementation instead. Let's do that now, this time using Keras' subclassing API.\n\n**Warning**: due to a TensorFlow bug (see [this issue](https://github.com/tensorflow/addons/issues/1153) for details), the `get_initial_state()` method fails in eager mode, so for now we have to use the subclassing API, as Keras automatically calls `tf.function()` on the `call()` method (so it runs in graph mode).",
"_____no_output_____"
],
[
"In this implementation, we've reverted back to using the `TrainingSampler`, for simplicity (but you can easily tweak it to use a `ScheduledEmbeddingTrainingSampler` instead). We also use a `GreedyEmbeddingSampler` during inference, so this class is pretty easy to use:",
"_____no_output_____"
]
],
[
[
"class DateTranslation(keras.models.Model):\n def __init__(self, units=128, encoder_embedding_size=32,\n decoder_embedding_size=32, **kwargs):\n super().__init__(**kwargs)\n self.encoder_embedding = keras.layers.Embedding(\n input_dim=len(INPUT_CHARS) + 1,\n output_dim=encoder_embedding_size)\n self.encoder = keras.layers.LSTM(units,\n return_sequences=True,\n return_state=True)\n self.decoder_embedding = keras.layers.Embedding(\n input_dim=len(OUTPUT_CHARS) + 2,\n output_dim=decoder_embedding_size)\n self.attention = tfa.seq2seq.LuongAttention(units)\n decoder_inner_cell = keras.layers.LSTMCell(units)\n self.decoder_cell = tfa.seq2seq.AttentionWrapper(\n cell=decoder_inner_cell,\n attention_mechanism=self.attention)\n output_layer = keras.layers.Dense(len(OUTPUT_CHARS) + 1)\n self.decoder = tfa.seq2seq.BasicDecoder(\n cell=self.decoder_cell,\n sampler=tfa.seq2seq.sampler.TrainingSampler(),\n output_layer=output_layer)\n self.inference_decoder = tfa.seq2seq.BasicDecoder(\n cell=self.decoder_cell,\n sampler=tfa.seq2seq.sampler.GreedyEmbeddingSampler(\n embedding_fn=self.decoder_embedding),\n output_layer=output_layer,\n maximum_iterations=max_output_length)\n\n def call(self, inputs, training=None):\n encoder_input, decoder_input = inputs\n encoder_embeddings = self.encoder_embedding(encoder_input)\n encoder_outputs, encoder_state_h, encoder_state_c = self.encoder(\n encoder_embeddings,\n training=training)\n encoder_state = [encoder_state_h, encoder_state_c]\n\n self.attention(encoder_outputs,\n setup_memory=True)\n \n decoder_embeddings = self.decoder_embedding(decoder_input)\n\n decoder_initial_state = self.decoder_cell.get_initial_state(\n decoder_embeddings)\n decoder_initial_state = decoder_initial_state.clone(\n cell_state=encoder_state)\n \n if training:\n decoder_outputs, _, _ = self.decoder(\n decoder_embeddings,\n initial_state=decoder_initial_state,\n training=training)\n else:\n start_tokens = tf.zeros_like(encoder_input[:, 0]) + sos_id\n decoder_outputs, _, _ = self.inference_decoder(\n decoder_embeddings,\n initial_state=decoder_initial_state,\n start_tokens=start_tokens,\n end_token=0)\n\n return tf.nn.softmax(decoder_outputs.rnn_output)",
"_____no_output_____"
],
[
"np.random.seed(42)\ntf.random.set_seed(42)\n\nmodel = DateTranslation()\noptimizer = keras.optimizers.Nadam()\nmodel.compile(loss=\"sparse_categorical_crossentropy\", optimizer=optimizer,\n metrics=[\"accuracy\"])\nhistory = model.fit([X_train, X_train_decoder], Y_train, epochs=25,\n validation_data=([X_valid, X_valid_decoder], Y_valid))",
"Epoch 1/25\n313/313 [==============================] - 7s 21ms/step - loss: 2.1549 - accuracy: 0.2295 - val_loss: 2.1450 - val_accuracy: 0.2239\nEpoch 2/25\n313/313 [==============================] - 6s 19ms/step - loss: 1.8147 - accuracy: 0.3492 - val_loss: 1.4931 - val_accuracy: 0.4476\nEpoch 3/25\n313/313 [==============================] - 6s 18ms/step - loss: 1.3585 - accuracy: 0.4909 - val_loss: 1.3168 - val_accuracy: 0.5100\nEpoch 4/25\n313/313 [==============================] - 6s 18ms/step - loss: 1.2787 - accuracy: 0.5293 - val_loss: 1.1767 - val_accuracy: 0.5624\nEpoch 5/25\n313/313 [==============================] - 6s 18ms/step - loss: 1.1236 - accuracy: 0.5776 - val_loss: 1.0769 - val_accuracy: 0.5907\nEpoch 6/25\n313/313 [==============================] - 6s 18ms/step - loss: 1.0369 - accuracy: 0.6073 - val_loss: 1.0159 - val_accuracy: 0.6199\nEpoch 7/25\n313/313 [==============================] - 6s 18ms/step - loss: 0.9752 - accuracy: 0.6295 - val_loss: 0.9723 - val_accuracy: 0.6346\nEpoch 8/25\n313/313 [==============================] - 6s 18ms/step - loss: 0.9794 - accuracy: 0.6315 - val_loss: 0.9444 - val_accuracy: 0.6371\nEpoch 9/25\n313/313 [==============================] - 6s 18ms/step - loss: 0.9338 - accuracy: 0.6415 - val_loss: 0.9296 - val_accuracy: 0.6381\nEpoch 10/25\n313/313 [==============================] - 6s 19ms/step - loss: 0.9439 - accuracy: 0.6418 - val_loss: 0.9028 - val_accuracy: 0.6574\nEpoch 11/25\n313/313 [==============================] - 6s 19ms/step - loss: 0.8807 - accuracy: 0.6637 - val_loss: 0.9835 - val_accuracy: 0.6369\nEpoch 12/25\n313/313 [==============================] - 6s 19ms/step - loss: 0.7307 - accuracy: 0.6953 - val_loss: 0.8942 - val_accuracy: 0.6873\nEpoch 13/25\n313/313 [==============================] - 6s 19ms/step - loss: 0.5833 - accuracy: 0.7327 - val_loss: 0.6944 - val_accuracy: 0.7391\nEpoch 14/25\n313/313 [==============================] - 6s 19ms/step - loss: 0.4664 - accuracy: 0.7940 - val_loss: 0.6228 - val_accuracy: 0.7885\nEpoch 15/25\n313/313 [==============================] - 6s 19ms/step - loss: 0.3205 - accuracy: 0.8740 - val_loss: 0.4825 - val_accuracy: 0.8780\nEpoch 16/25\n313/313 [==============================] - 6s 19ms/step - loss: 0.2329 - accuracy: 0.9216 - val_loss: 0.3851 - val_accuracy: 0.9118\nEpoch 17/25\n313/313 [==============================] - 7s 21ms/step - loss: 0.2480 - accuracy: 0.9372 - val_loss: 0.2785 - val_accuracy: 0.9111\nEpoch 18/25\n313/313 [==============================] - 7s 22ms/step - loss: 0.1182 - accuracy: 0.9801 - val_loss: 0.1372 - val_accuracy: 0.9786\nEpoch 19/25\n313/313 [==============================] - 7s 22ms/step - loss: 0.0643 - accuracy: 0.9937 - val_loss: 0.0681 - val_accuracy: 0.9909\nEpoch 20/25\n313/313 [==============================] - 6s 18ms/step - loss: 0.0446 - accuracy: 0.9952 - val_loss: 0.0487 - val_accuracy: 0.9934\nEpoch 21/25\n313/313 [==============================] - 6s 18ms/step - loss: 0.0247 - accuracy: 0.9987 - val_loss: 0.0228 - val_accuracy: 0.9987\nEpoch 22/25\n313/313 [==============================] - 6s 18ms/step - loss: 0.0456 - accuracy: 0.9918 - val_loss: 0.0207 - val_accuracy: 0.9985\nEpoch 23/25\n313/313 [==============================] - 6s 18ms/step - loss: 0.0131 - accuracy: 0.9997 - val_loss: 0.0127 - val_accuracy: 0.9993\nEpoch 24/25\n313/313 [==============================] - 6s 19ms/step - loss: 0.0360 - accuracy: 0.9933 - val_loss: 0.0146 - val_accuracy: 0.9990\nEpoch 25/25\n313/313 [==============================] - 6s 19ms/step - loss: 0.0092 - accuracy: 0.9998 - val_loss: 0.0089 - val_accuracy: 0.9992\n"
]
],
[
[
"Not quite 100% validation accuracy, but close. It took a bit longer to converge this time, but there were also more parameters and more computations per iteration. And we did not use a scheduled sampler.\n\nTo use the model, we can write yet another little function:",
"_____no_output_____"
]
],
[
[
"def fast_predict_date_strs_v2(date_strs):\n X = prepare_date_strs_padded(date_strs)\n X_decoder = tf.zeros(shape=(len(X), max_output_length), dtype=tf.int32)\n Y_probas = model.predict([X, X_decoder])\n Y_pred = tf.argmax(Y_probas, axis=-1)\n return ids_to_date_strs(Y_pred)",
"_____no_output_____"
],
[
"fast_predict_date_strs_v2([\"July 14, 1789\", \"May 01, 2020\"])",
"_____no_output_____"
]
],
[
[
"There are still a few interesting features from TF-Addons that you may want to look at:\n* Using a `BeamSearchDecoder` rather than a `BasicDecoder` for inference. Instead of outputing the character with the highest probability, this decoder keeps track of the several candidates, and keeps only the most likely sequences of candidates (see chapter 16 in the book for more details).\n* Setting masks or specifying `sequence_length` if the input or target sequences may have very different lengths.\n* Using a `ScheduledOutputTrainingSampler`, which gives you more flexibility than the `ScheduledEmbeddingTrainingSampler` to decide how to feed the output at time _t_ to the cell at time _t_+1. By default it feeds the outputs directly to cell, without computing the argmax ID and passing it through an embedding layer. Alternatively, you specify a `next_inputs_fn` function that will be used to convert the cell outputs to inputs at the next step.",
"_____no_output_____"
],
[
"## 10.\n_Exercise: Go through TensorFlow's [Neural Machine Translation with Attention tutorial](https://homl.info/nmttuto)._",
"_____no_output_____"
],
[
"Simply open the Colab and follow its instructions. Alternatively, if you want a simpler example of using TF-Addons's seq2seq implementation for Neural Machine Translation (NMT), look at the solution to the previous question. The last model implementation will give you a simpler example of using TF-Addons to build an NMT model using attention mechanisms.",
"_____no_output_____"
],
[
"## 11.\n_Exercise: Use one of the recent language models (e.g., GPT) to generate more convincing Shakespearean text._",
"_____no_output_____"
],
[
"The simplest way to use recent language models is to use the excellent [transformers library](https://huggingface.co/transformers/), open sourced by Hugging Face. It provides many modern neural net architectures (including BERT, GPT-2, RoBERTa, XLM, DistilBert, XLNet and more) for Natural Language Processing (NLP), including many pretrained models. It relies on either TensorFlow or PyTorch. Best of all: it's amazingly simple to use.",
"_____no_output_____"
],
[
"First, let's load a pretrained model. In this example, we will use OpenAI's GPT model, with an additional Language Model on top (just a linear layer with weights tied to the input embeddings). Let's import it and load the pretrained weights (this will download about 445MB of data to `~/.cache/torch/transformers`):",
"_____no_output_____"
]
],
[
[
"from transformers import TFOpenAIGPTLMHeadModel\n\nmodel = TFOpenAIGPTLMHeadModel.from_pretrained(\"openai-gpt\")",
"_____no_output_____"
]
],
[
[
"Next we will need a specialized tokenizer for this model. This one will try to use the [spaCy](https://spacy.io/) and [ftfy](https://pypi.org/project/ftfy/) libraries if they are installed, or else it will fall back to BERT's `BasicTokenizer` followed by Byte-Pair Encoding (which should be fine for most use cases).",
"_____no_output_____"
]
],
[
[
"from transformers import OpenAIGPTTokenizer\n\ntokenizer = OpenAIGPTTokenizer.from_pretrained(\"openai-gpt\")",
"_____no_output_____"
]
],
[
[
"Now let's use the tokenizer to tokenize and encode the prompt text:",
"_____no_output_____"
]
],
[
[
"prompt_text = \"This royal throne of kings, this sceptred isle\"\nencoded_prompt = tokenizer.encode(prompt_text,\n add_special_tokens=False,\n return_tensors=\"tf\")\nencoded_prompt",
"_____no_output_____"
]
],
[
[
"Easy! Next, let's use the model to generate text after the prompt. We will generate 5 different sentences, each starting with the prompt text, followed by 40 additional tokens. For an explanation of what all the hyperparameters do, make sure to check out this great [blog post](https://huggingface.co/blog/how-to-generate) by Patrick von Platen (from Hugging Face). You can play around with the hyperparameters to try to obtain better results.",
"_____no_output_____"
]
],
[
[
"num_sequences = 5\nlength = 40\n\ngenerated_sequences = model.generate(\n input_ids=encoded_prompt,\n do_sample=True,\n max_length=length + len(encoded_prompt[0]),\n temperature=1.0,\n top_k=0,\n top_p=0.9,\n repetition_penalty=1.0,\n num_return_sequences=num_sequences,\n)\n\ngenerated_sequences",
"_____no_output_____"
]
],
[
[
"Now let's decode the generated sequences and print them:",
"_____no_output_____"
]
],
[
[
"for sequence in generated_sequences:\n text = tokenizer.decode(sequence, clean_up_tokenization_spaces=True)\n print(text)\n print(\"-\" * 80)",
"this royal throne of kings, this sceptred isle. even if someone had given them permission, even if it were required, they would never have been allowed to live through the hell they've survived.'\n'they couldn't have known that.\n--------------------------------------------------------------------------------\nthis royal throne of kings, this sceptred isle and these people are royalty.'\n then the mute prince and prince edward broke off and went to their rooms. \n the talk passed again between the princes and the guards and the princess was of great\n--------------------------------------------------------------------------------\nthis royal throne of kings, this sceptred isle has its own highness, an alatte that waits to save you. in this kingdom your people must emulate the kings of the realm. in this kingdom your kin should be saved from this pit and\n--------------------------------------------------------------------------------\nthis royal throne of kings, this sceptred isle belongs to me. \" \n \" the great throne of penvynne? \" \n \" indeed, \" said the king with a nod of his head. \" this world was once composed of a magical\n--------------------------------------------------------------------------------\nthis royal throne of kings, this sceptred isle is empty. this is a modern - day fedaykin court, a place where kings are governed, not emperors and judges. i don't see any sign of life that is not their own\n--------------------------------------------------------------------------------\n"
]
],
[
[
"You can try more recent (and larger) models, such as GPT-2, CTRL, Transformer-XL or XLNet, which are all available as pretrained models in the transformers library, including variants with Language Models on top. The preprocessing steps vary slightly between models, so make sure to check out this [generation example](https://github.com/huggingface/transformers/blob/master/examples/run_generation.py) from the transformers documentation (this example uses PyTorch, but it will work with very little tweaks, such as adding `TF` at the beginning of the model class name, removing the `.to()` method calls, and using `return_tensors=\"tf\"` instead of `\"pt\"`.",
"_____no_output_____"
],
[
"Hope you enjoyed this chapter! :)",
"_____no_output_____"
]
]
] | [
"markdown",
"code",
"markdown",
"code",
"markdown",
"code",
"markdown",
"code",
"markdown",
"code",
"markdown",
"code",
"markdown",
"code",
"markdown",
"code",
"markdown",
"code",
"markdown",
"code",
"markdown",
"code",
"markdown",
"code",
"markdown",
"code",
"markdown",
"code",
"markdown",
"code",
"markdown",
"code",
"markdown",
"code",
"markdown",
"code",
"markdown",
"code",
"markdown",
"code",
"markdown",
"code",
"markdown",
"code",
"markdown",
"code",
"markdown",
"code",
"markdown",
"code",
"markdown",
"code",
"markdown",
"code",
"markdown",
"code",
"markdown",
"code",
"markdown",
"code",
"markdown",
"code",
"markdown",
"code",
"markdown",
"code",
"markdown",
"code",
"markdown",
"code",
"markdown",
"code",
"markdown",
"code",
"markdown",
"code",
"markdown",
"code",
"markdown",
"code",
"markdown",
"code",
"markdown",
"code",
"markdown",
"code",
"markdown",
"code",
"markdown",
"code",
"markdown",
"code",
"markdown",
"code",
"markdown",
"code",
"markdown",
"code",
"markdown",
"code",
"markdown",
"code",
"markdown",
"code",
"markdown",
"code",
"markdown",
"code",
"markdown",
"code",
"markdown",
"code",
"markdown",
"code",
"markdown"
] | [
[
"markdown",
"markdown",
"markdown",
"markdown",
"markdown"
],
[
"code"
],
[
"markdown",
"markdown",
"markdown"
],
[
"code"
],
[
"markdown"
],
[
"code",
"code",
"code",
"code",
"code",
"code",
"code",
"code",
"code",
"code",
"code",
"code",
"code",
"code",
"code"
],
[
"markdown"
],
[
"code"
],
[
"markdown"
],
[
"code",
"code",
"code",
"code",
"code",
"code",
"code",
"code",
"code"
],
[
"markdown"
],
[
"code",
"code",
"code",
"code",
"code",
"code"
],
[
"markdown"
],
[
"code"
],
[
"markdown"
],
[
"code",
"code",
"code"
],
[
"markdown"
],
[
"code"
],
[
"markdown"
],
[
"code",
"code",
"code",
"code",
"code",
"code",
"code",
"code",
"code",
"code",
"code",
"code",
"code",
"code",
"code",
"code",
"code",
"code",
"code",
"code"
],
[
"markdown"
],
[
"code"
],
[
"markdown"
],
[
"code",
"code",
"code",
"code",
"code"
],
[
"markdown"
],
[
"code",
"code",
"code",
"code",
"code"
],
[
"markdown"
],
[
"code"
],
[
"markdown"
],
[
"code",
"code",
"code",
"code"
],
[
"markdown"
],
[
"code"
],
[
"markdown"
],
[
"code",
"code"
],
[
"markdown",
"markdown",
"markdown",
"markdown",
"markdown"
],
[
"code"
],
[
"markdown"
],
[
"code"
],
[
"markdown"
],
[
"code"
],
[
"markdown"
],
[
"code"
],
[
"markdown"
],
[
"code"
],
[
"markdown"
],
[
"code",
"code"
],
[
"markdown"
],
[
"code",
"code"
],
[
"markdown"
],
[
"code"
],
[
"markdown"
],
[
"code"
],
[
"markdown"
],
[
"code"
],
[
"markdown"
],
[
"code"
],
[
"markdown",
"markdown",
"markdown"
],
[
"code"
],
[
"markdown"
],
[
"code"
],
[
"markdown"
],
[
"code"
],
[
"markdown"
],
[
"code"
],
[
"markdown"
],
[
"code",
"code",
"code",
"code",
"code",
"code"
],
[
"markdown",
"markdown"
],
[
"code"
],
[
"markdown"
],
[
"code"
],
[
"markdown"
],
[
"code",
"code"
],
[
"markdown",
"markdown"
],
[
"code",
"code"
],
[
"markdown"
],
[
"code",
"code"
],
[
"markdown",
"markdown",
"markdown",
"markdown"
],
[
"code"
],
[
"markdown"
],
[
"code"
],
[
"markdown"
],
[
"code"
],
[
"markdown",
"markdown"
],
[
"code",
"code"
],
[
"markdown",
"markdown",
"markdown"
],
[
"code"
],
[
"markdown"
],
[
"code"
],
[
"markdown"
],
[
"code"
],
[
"markdown",
"markdown"
],
[
"code",
"code"
],
[
"markdown"
],
[
"code",
"code"
],
[
"markdown",
"markdown",
"markdown",
"markdown"
],
[
"code"
],
[
"markdown",
"markdown"
],
[
"code",
"code",
"code"
],
[
"markdown"
],
[
"code"
],
[
"markdown",
"markdown",
"markdown",
"markdown"
],
[
"code",
"code"
],
[
"markdown"
],
[
"code",
"code"
],
[
"markdown",
"markdown",
"markdown",
"markdown",
"markdown",
"markdown"
],
[
"code"
],
[
"markdown"
],
[
"code"
],
[
"markdown"
],
[
"code"
],
[
"markdown"
],
[
"code"
],
[
"markdown"
],
[
"code"
],
[
"markdown",
"markdown"
]
] |
e783ea9055b57243b9677cff0fd6ab2ca8653fe4 | 296,095 | ipynb | Jupyter Notebook | content/week5/C9_sistemaecuaciones.ipynb | andresgm/ua-imec2001-hc-202210 | caa64731c55f2a78740025923513aec8e81ee75b | [
"MIT"
] | null | null | null | content/week5/C9_sistemaecuaciones.ipynb | andresgm/ua-imec2001-hc-202210 | caa64731c55f2a78740025923513aec8e81ee75b | [
"MIT"
] | null | null | null | content/week5/C9_sistemaecuaciones.ipynb | andresgm/ua-imec2001-hc-202210 | caa64731c55f2a78740025923513aec8e81ee75b | [
"MIT"
] | 2 | 2022-02-24T16:35:26.000Z | 2022-03-28T19:44:54.000Z | 145.429764 | 11,907 | 0.672727 | [
[
[
"empty"
]
]
] | [
"empty"
] | [
[
"empty"
]
] |
e783f6e65c8db6de147e92a759f391db4f437ac7 | 161,161 | ipynb | Jupyter Notebook | simulation/verification.ipynb | rajibchakravorty/QDataSet | 8eb21b8c7dad5654358021dd73b93ab90443f6d0 | [
"MIT"
] | null | null | null | simulation/verification.ipynb | rajibchakravorty/QDataSet | 8eb21b8c7dad5654358021dd73b93ab90443f6d0 | [
"MIT"
] | null | null | null | simulation/verification.ipynb | rajibchakravorty/QDataSet | 8eb21b8c7dad5654358021dd73b93ab90443f6d0 | [
"MIT"
] | null | null | null | 269.5 | 32,496 | 0.881404 | [
[
[
"<H1> Notebook to verify the calculations of our simulator </H1>",
"_____no_output_____"
],
[
"## Importing required libraries",
"_____no_output_____"
]
],
[
[
"# importaing standard libraries\nimport matplotlib.pyplot as plt\nimport matplotlib.ticker as ticker\nfrom scipy.signal import freqs,periodogram,cheby1\nimport numpy as np",
"_____no_output_____"
],
[
"# import quantum libraries\nimport qutip\nfrom itertools import product\nfrom numpy import array, kron\nfrom qmldataset import pauli_operators, create_custom_simulator, run_experiment",
"2021-09-26 16:34:01.309496: I tensorflow/stream_executor/platform/default/dso_loader.cc:49] Successfully opened dynamic library libcudart.so.11.0\n"
]
],
[
[
"## Step 1: Create a simulator\n\nWe supply the parameters and create a simulator. Here we will create a 1-qubit experiment with Control on X-Axis, Type 1 noise on Z-Axis",
"_____no_output_____"
]
],
[
[
"dimension = 2\nevolution_time = 1\nnum_time_steps = 1024\nomega = 12\ndynamic_operators = [0.5*pauli_operators[1]]\nstatic_operators = [0.5*pauli_operators[3]*omega]\nnoise_operators = [0.5*pauli_operators[3]]\nmeasurement_operators = pauli_operators[1:]\ninitial_states = [\n np.array([[0.5, 0.5], [0.5, 0.5]]), np.array([[0.5, -0.5], [-0.5, 0.5]]),\n np.array([[0.5, -0.5j], [0.5j, 0.5]]), np.array([[0.5, 0.5j], [-0.5j, 0.5]]),\n np.array([[1, 0], [0, 0]]), np.array([[0, 0], [0, 1]])\n]\nnum_realizations = 200\nnum_pulses = 5\nnoise_profile = ['Type 1']\ndistortion = True\n\nsimulator_with_distortion = create_custom_simulator(\n evolution_time=evolution_time,\n num_time_steps=num_time_steps,\n dimension=dimension,\n dynamic_operators=dynamic_operators,\n static_operators=static_operators,\n noise_operators=noise_operators,\n measurement_operators=measurement_operators,\n initial_states=initial_states,\n num_realizations=num_realizations,\n num_pulses=num_pulses,\n noise_profile=noise_profile,\n distortion=distortion,\n pulse_shape=\"Square\"\n)",
"2021-09-26 16:34:05.687838: I tensorflow/compiler/jit/xla_cpu_device.cc:41] Not creating XLA devices, tf_xla_enable_xla_devices not set\n2021-09-26 16:34:05.689143: I tensorflow/stream_executor/platform/default/dso_loader.cc:49] Successfully opened dynamic library libcuda.so.1\n2021-09-26 16:34:05.737996: I tensorflow/stream_executor/cuda/cuda_gpu_executor.cc:941] successful NUMA node read from SysFS had negative value (-1), but there must be at least one NUMA node, so returning NUMA node zero\n2021-09-26 16:34:05.738543: I tensorflow/core/common_runtime/gpu/gpu_device.cc:1720] Found device 0 with properties: \npciBusID: 0000:0e:00.0 name: GeForce GTX 1050 Ti computeCapability: 6.1\ncoreClock: 1.43GHz coreCount: 6 deviceMemorySize: 3.94GiB deviceMemoryBandwidth: 104.43GiB/s\n2021-09-26 16:34:05.738610: I tensorflow/stream_executor/platform/default/dso_loader.cc:49] Successfully opened dynamic library libcudart.so.11.0\n2021-09-26 16:34:05.741933: I tensorflow/stream_executor/platform/default/dso_loader.cc:49] Successfully opened dynamic library libcublas.so.11\n2021-09-26 16:34:05.742095: I tensorflow/stream_executor/platform/default/dso_loader.cc:49] Successfully opened dynamic library libcublasLt.so.11\n2021-09-26 16:34:05.743695: I tensorflow/stream_executor/platform/default/dso_loader.cc:49] Successfully opened dynamic library libcufft.so.10\n2021-09-26 16:34:05.744247: I tensorflow/stream_executor/platform/default/dso_loader.cc:49] Successfully opened dynamic library libcurand.so.10\n2021-09-26 16:34:05.746528: I tensorflow/stream_executor/platform/default/dso_loader.cc:49] Successfully opened dynamic library libcusolver.so.10\n2021-09-26 16:34:05.747524: I tensorflow/stream_executor/platform/default/dso_loader.cc:49] Successfully opened dynamic library libcusparse.so.11\n2021-09-26 16:34:05.747858: I tensorflow/stream_executor/platform/default/dso_loader.cc:49] Successfully opened dynamic library libcudnn.so.8\n2021-09-26 16:34:05.748020: I tensorflow/stream_executor/cuda/cuda_gpu_executor.cc:941] successful NUMA node read from SysFS had negative value (-1), but there must be at least one NUMA node, so returning NUMA node zero\n2021-09-26 16:34:05.748524: I tensorflow/stream_executor/cuda/cuda_gpu_executor.cc:941] successful NUMA node read from SysFS had negative value (-1), but there must be at least one NUMA node, so returning NUMA node zero\n2021-09-26 16:34:05.748866: I tensorflow/core/common_runtime/gpu/gpu_device.cc:1862] Adding visible gpu devices: 0\n2021-09-26 16:34:05.749988: I tensorflow/compiler/jit/xla_gpu_device.cc:99] Not creating XLA devices, tf_xla_enable_xla_devices not set\n2021-09-26 16:34:05.750242: I tensorflow/stream_executor/cuda/cuda_gpu_executor.cc:941] successful NUMA node read from SysFS had negative value (-1), but there must be at least one NUMA node, so returning NUMA node zero\n2021-09-26 16:34:05.750682: I tensorflow/core/common_runtime/gpu/gpu_device.cc:1720] Found device 0 with properties: \npciBusID: 0000:0e:00.0 name: GeForce GTX 1050 Ti computeCapability: 6.1\ncoreClock: 1.43GHz coreCount: 6 deviceMemorySize: 3.94GiB deviceMemoryBandwidth: 104.43GiB/s\n2021-09-26 16:34:05.750765: I tensorflow/stream_executor/platform/default/dso_loader.cc:49] Successfully opened dynamic library libcudart.so.11.0\n2021-09-26 16:34:05.750804: I tensorflow/stream_executor/platform/default/dso_loader.cc:49] Successfully opened dynamic library libcublas.so.11\n2021-09-26 16:34:05.750829: I tensorflow/stream_executor/platform/default/dso_loader.cc:49] Successfully opened dynamic library libcublasLt.so.11\n2021-09-26 16:34:05.750852: I tensorflow/stream_executor/platform/default/dso_loader.cc:49] Successfully opened dynamic library libcufft.so.10\n2021-09-26 16:34:05.750874: I tensorflow/stream_executor/platform/default/dso_loader.cc:49] Successfully opened dynamic library libcurand.so.10\n2021-09-26 16:34:05.750896: I tensorflow/stream_executor/platform/default/dso_loader.cc:49] Successfully opened dynamic library libcusolver.so.10\n2021-09-26 16:34:05.750918: I tensorflow/stream_executor/platform/default/dso_loader.cc:49] Successfully opened dynamic library libcusparse.so.11\n2021-09-26 16:34:05.750941: I tensorflow/stream_executor/platform/default/dso_loader.cc:49] Successfully opened dynamic library libcudnn.so.8\n2021-09-26 16:34:05.751068: I tensorflow/stream_executor/cuda/cuda_gpu_executor.cc:941] successful NUMA node read from SysFS had negative value (-1), but there must be at least one NUMA node, so returning NUMA node zero\n2021-09-26 16:34:05.751499: I tensorflow/stream_executor/cuda/cuda_gpu_executor.cc:941] successful NUMA node read from SysFS had negative value (-1), but there must be at least one NUMA node, so returning NUMA node zero\n2021-09-26 16:34:05.751834: I tensorflow/core/common_runtime/gpu/gpu_device.cc:1862] Adding visible gpu devices: 0\n2021-09-26 16:34:05.751902: I tensorflow/stream_executor/platform/default/dso_loader.cc:49] Successfully opened dynamic library libcudart.so.11.0\n2021-09-26 16:34:06.554192: I tensorflow/core/common_runtime/gpu/gpu_device.cc:1261] Device interconnect StreamExecutor with strength 1 edge matrix:\n2021-09-26 16:34:06.554242: I tensorflow/core/common_runtime/gpu/gpu_device.cc:1267] 0 \n2021-09-26 16:34:06.554252: I tensorflow/core/common_runtime/gpu/gpu_device.cc:1280] 0: N \n2021-09-26 16:34:06.554522: I tensorflow/stream_executor/cuda/cuda_gpu_executor.cc:941] successful NUMA node read from SysFS had negative value (-1), but there must be at least one NUMA node, so returning NUMA node zero\n2021-09-26 16:34:06.555210: I tensorflow/stream_executor/cuda/cuda_gpu_executor.cc:941] successful NUMA node read from SysFS had negative value (-1), but there must be at least one NUMA node, so returning NUMA node zero\n2021-09-26 16:34:06.555616: I tensorflow/stream_executor/cuda/cuda_gpu_executor.cc:941] successful NUMA node read from SysFS had negative value (-1), but there must be at least one NUMA node, so returning NUMA node zero\n2021-09-26 16:34:06.555966: I tensorflow/core/common_runtime/gpu/gpu_device.cc:1406] Created TensorFlow device (/job:localhost/replica:0/task:0/device:GPU:0 with 3250 MB memory) -> physical GPU (device: 0, name: GeForce GTX 1050 Ti, pci bus id: 0000:0e:00.0, compute capability: 6.1)\n"
]
],
[
[
"## Now we run a single experiment\n\nThe experiment will produce a result by simulating `num_realizations` number of noise realizations.",
"_____no_output_____"
]
],
[
[
"experiment_result = run_experiment(simulator=simulator_with_distortion)",
"2021-09-26 16:34:09.738690: I tensorflow/compiler/mlir/mlir_graph_optimization_pass.cc:116] None of the MLIR optimization passes are enabled (registered 2)\n2021-09-26 16:34:09.761987: I tensorflow/core/platform/profile_utils/cpu_utils.cc:112] CPU Frequency: 3094175000 Hz\n2021-09-26 16:34:13.227801: I tensorflow/stream_executor/platform/default/dso_loader.cc:49] Successfully opened dynamic library libcublas.so.11\n2021-09-26 16:34:13.612214: I tensorflow/stream_executor/platform/default/dso_loader.cc:49] Successfully opened dynamic library libcublasLt.so.11\n2021-09-26 16:34:13.642600: I tensorflow/stream_executor/platform/default/dso_loader.cc:49] Successfully opened dynamic library libcufft.so.10\n2021-09-26 16:34:13.917894: I tensorflow/core/util/cuda_solvers.cc:180] Creating CudaSolver handles for stream 0x5646df642640\n2021-09-26 16:34:13.918105: I tensorflow/stream_executor/platform/default/dso_loader.cc:49] Successfully opened dynamic library libcusolver.so.10\n2021-09-26 16:34:14.231919: I tensorflow/stream_executor/platform/default/dso_loader.cc:49] Successfully opened dynamic library libcublas.so.11\n"
]
],
[
[
"## Once run, let us read the experiment outcome",
"_____no_output_____"
]
],
[
[
"# plot the pulse\nplt.figure()\nnum_controls = len(experiment_result[\"sim_parameters\"][\"dynamic_operators\"])\nfor idx in range(num_controls):\n plt.subplot(num_controls , 1, idx+1 )\n plt.plot(experiment_result[\"time_range\"], experiment_result[\"pulses\"][:,0,idx], label=\"undistorted\")\n plt.plot(experiment_result[\"time_range\"], experiment_result[\"distorted_pulses\"][:,0,idx], label=\"distorted\")\n plt.xlabel('t')\n plt.ylabel('f(t)')\n plt.grid()\n plt.legend()\nprint(experiment_result[\"pulse_parameters\"])",
"[[-20.345783 0.12233578 0.1 ]\n [ 58.95591 0.27380085 0.1 ]\n [ 38.14025 0.4457677 0.1 ]\n [ 29.669308 0.61551726 0.1 ]\n [-74.14498 0.7660476 0.1 ]]\n"
]
],
[
[
"## Display the distortion if exists",
"_____no_output_____"
]
],
[
[
"if distortion:\n # display distortion filter if exists\n distortion = cheby1(4,0.1,2*np.pi*20, analog=True)\n # evaluate frequency response of the filter\n w, Hw = freqs(distortion[0], distortion[1])\n plt.figure(figsize=[15,4])\n plt.subplot(1,2,1)\n plt.semilogx(w, 20*np.log(np.abs(Hw)))\n plt.xlabel(r'$\\Omega$')\n plt.ylabel(r'$|H(\\Omega)|$')\n plt.grid()\n plt.subplot(1,2,2)\n plt.semilogx(w, np.angle(Hw))\n plt.xlabel(r'$\\Omega$')\n plt.ylabel(r'arg $H(\\Omega)$')\n plt.grid()",
"_____no_output_____"
]
],
[
[
"## Display the noise",
"_____no_output_____"
]
],
[
[
"# display noise if exists\nfor idx_profile,profile in enumerate(experiment_result[\"sim_parameters\"][\"noise_profile\"]): \n if profile in ['Type 2','Type 3','Type 4'] or (profile=='Type 6' and p==0): \n # estimate the correlation matrix of the noise\n correlation = 0\n for k in range(experiment_result[\"sim_parameters\"][\"num_realizations\"]):\n correlation = correlation + experiment_result[\"noise\"][:,k:k+1,idx_profile]@experiment_result[\"noise\"][:,k:k+1,idx_profile].T\n correlation = correlation/data[\"sim_parameters\"][\"num_realizations\"]\n # plot correlation matrix\n plt.figure()\n plt.matshow(correlation,0)\n plt.colorbar()\n p = 0\n elif profile in ['Type 1','Type 5']:\n # estimate the PSD of the noise\n psd = 0\n for k in range(experiment_result[\"sim_parameters\"][\"num_realizations\"]):\n f, Pxx = periodogram(experiment_result[\"noise\"][:,k,idx_profile], experiment_result[\"sim_parameters\"][\"num_time_steps\"]/experiment_result[\"sim_parameters\"][\"evolution_time\"]) \n psd = psd + Pxx\n psd = psd/experiment_result[\"sim_parameters\"][\"num_realizations\"]\n plt.figure()\n plt.plot(f[f>0], psd[1:])\n plt.xlabel('f')\n plt.ylabel('psd')\n plt.grid()\n p = 1",
"_____no_output_____"
]
],
[
[
"## Comparing the output with `qutip`\n\nHint: They should be same !!",
"_____no_output_____"
]
],
[
[
"# load initial states, measurement operators, and control Hamilotonian\ninitial_states = [qutip.Qobj(state) for state in experiment_result[\"sim_parameters\"][\"initial_states\"] ] \nmeasurements = [qutip.Qobj(op) for op in experiment_result[\"sim_parameters\"][\"measurement_operators\"] ]\n\nH0 = [ [qutip.Qobj(op), np.ones((len(experiment_result[\"sim_parameters\"][\"time_range\"])))] \n for op in experiment_result[\"sim_parameters\"][\"static_operators\"] ] + [\n [qutip.Qobj(op), experiment_result[\"distorted_pulses\"][:,0,idx]] \n for idx, op in enumerate(experiment_result[\"sim_parameters\"][\"dynamic_operators\"])]\n\nexpectations = np.zeros(\n (1,experiment_result[\"sim_parameters\"][\"num_realizations\"], \n len(initial_states)*len(measurements))) \n\nfor idx_K in range(experiment_result[\"sim_parameters\"][\"num_realizations\"]): \n H1 = [ \n [qutip.Qobj(op), experiment_result[\"noise\"][:, idx_K, idx]] \n for idx, op in enumerate(experiment_result[\"sim_parameters\"][\"noise_operators\"]) ]\n results = [ qutip.mesolve(H0 + H1, rho, np.array(experiment_result[\"sim_parameters\"][\"time_range\"]),\n e_ops=measurements).expect for rho in initial_states]\n expectations [0, idx_K, :] = np.concatenate(\n [np.array([results[idx_rho][idx_M][-1] \n for idx_M in range(len(measurements))]) for idx_rho in range(len(initial_states))])\n print(idx_K+1, end=\"\\r\")",
"200\r"
],
[
"# plot the average expectation over all noise realizations for every observable\nplt.figure()\nplt.plot(np.average(expectations, 1)[0], label=\"qutip\")\nplt.plot(experiment_result[\"average_expectation\"][0], label = \"tf\")\nplt.ylabel(\"Average observable value\")\nplt.xlabel(\"observable Index\")\nplt.gca().xaxis.set_major_locator(ticker.MaxNLocator(integer=True))\nplt.legend()\nplt.grid()",
"_____no_output_____"
],
[
"# plot all possible observables for a particular noise realization\nidx_K = 10\nplt.figure()\nplt.plot(expectations[0, idx_K,:], label=\"qutip\")\nplt.plot(experiment_result[\"expectations\"][idx_K,:], label = \"tf\")\nplt.ylabel(\"Observable Value for realization %d\"%idx_K)\nplt.xlabel(\"Observable Index\")\nplt.gca().xaxis.set_major_locator(ticker.MaxNLocator(integer=True))\nplt.legend()\nplt.grid()",
"_____no_output_____"
]
]
] | [
"markdown",
"code",
"markdown",
"code",
"markdown",
"code",
"markdown",
"code",
"markdown",
"code",
"markdown",
"code",
"markdown",
"code"
] | [
[
"markdown",
"markdown"
],
[
"code",
"code"
],
[
"markdown"
],
[
"code"
],
[
"markdown"
],
[
"code"
],
[
"markdown"
],
[
"code"
],
[
"markdown"
],
[
"code"
],
[
"markdown"
],
[
"code"
],
[
"markdown"
],
[
"code",
"code",
"code"
]
] |
e783f80d749b054bd32782f4dc1910dc65300c02 | 256,910 | ipynb | Jupyter Notebook | TEMA-2/Clase10_MetodoAceptacionRechazo.ipynb | AndresHdzJmz/SPF-2021-I | 2e2b25b0bfb9e3716ceea4253741a6c364f2a579 | [
"MIT"
] | null | null | null | TEMA-2/Clase10_MetodoAceptacionRechazo.ipynb | AndresHdzJmz/SPF-2021-I | 2e2b25b0bfb9e3716ceea4253741a6c364f2a579 | [
"MIT"
] | null | null | null | TEMA-2/Clase10_MetodoAceptacionRechazo.ipynb | AndresHdzJmz/SPF-2021-I | 2e2b25b0bfb9e3716ceea4253741a6c364f2a579 | [
"MIT"
] | null | null | null | 427.470882 | 83,460 | 0.935771 | [
[
[
"# Continuación clase método de la transformada inversa",
"_____no_output_____"
]
],
[
[
"# Librería de optimización \nfrom scipy import optimize\nfrom scipy.stats import beta\nimport matplotlib.pyplot as plt\nimport numpy as np\nimport pandas as pd\n# %matplotlib notebook\n%matplotlib inline",
"_____no_output_____"
]
],
[
[
"### Función para crear histograma de distribuciones discretas",
"_____no_output_____"
]
],
[
[
"def Gen_distr_discreta(p_acum: 'P.Acumulada de la distribución a generar',\n indices: 'valores reales a generar aleatoriamente',\n N: 'cantidad de números aleatorios a generar'):\n \n U =np.random.rand(N)\n # Diccionario de valores aleatorios\n rand2reales = {i: idx for i, idx in enumerate(indices)}\n\n # Series de los valores aletorios\n y = pd.Series([sum([1 for p in p_acum if p < ui]) for ui in U]).map(rand2reales)\n\n return y",
"_____no_output_____"
],
[
"def plot_histogram_discrete(distribucion:'señal de varibles aleatorias de un distribución DISCRETA dada',\n label:'label del legend a aparecer en el gráfica',\n densidad:'por defecto regresa el histograma en densidad'=True):\n # len(set(distribucion)) cuenta la cantidad de elementos distintos de la variable 'distribucion'\n plt.figure(figsize=[10,4])\n y, x = np.histogram(distribucion, bins=len(set(distribucion)), density=densidad) \n plt.bar(x[1:], y, label=label)\n plt.legend()\n plt.show()",
"_____no_output_____"
]
],
[
[
"### Ejemplo binomial: \nLa distribución binomial modela el número de éxitos de n ensayos independientes donde hay una probabilidad p de éxito en cada ensayo.\n\nGenerar una variable aletoria binomial con parámetros $n=10$ y $p=0.7$. Recordar que\n$$X\\sim binomial(n,p) \\longrightarrow p_i=P(X=i)=\\frac{n!}{i!(n-i)!}p^i(1-p)^{n-i},\\quad i=0,1,\\cdots,n$$\n> ## <font color ='red'>Tarea: Demostrar la validez de la siguiente ecuación\n>$$p_{i+1}=\\frac{n-i}{i+1}\\frac{p}{1-p} p_i \\longrightarrow \\text{Hablar de las ventajas que sea recursiva}$$",
"_____no_output_____"
],
[
"**El Algoritmo que debemos realizar:**\n 1. Generar $U$.\n 2. Si $U<p_0$, poner $X=0$ y detenerse.\n 3. Si $p_0<U<p_0+p_1$, poner $X=1$ y detenerse.\n $$ \\vdots$$\n 4. Si $p_0+\\cdots+p_{n-1}<U<p_0+\\cdots+p_{n}$, poner $X=n$ y detenerse.",
"_____no_output_____"
]
],
[
[
"# Función que calcula la probabilidad acumulada optimizada\ndef P_acum_Binomial_o(n,p):\n Pr = np.zeros(n)\n Pr[0] = (1-p)**n\n def pr(i):\n nonlocal Pr\n c = p/(1-p)\n Pr[i+1]=(c*(n-i)/(i+1))*Pr[i]\n \n # Lleno el vector Pr usando compresión de listas\n [pr(i) for i in range(n-1)]\n return np.cumsum(Pr)",
"_____no_output_____"
],
[
"# def D_binomial_intermedia(n,p,N):\nn = 10; p = 0.7; N = 10**5\n\np_acum = P_acum_Binomial_o(n,p)\n\n# Usando el método de la transformada inversa\nd_binomial = Gen_distr_discreta(p_acum, np.arange(0, n+1), N)\nplot_histogram_discrete(d_binomial, 'función creada con tran. Inversa')\n\n# Usando numpy\nd_bino_numpy = np.random.binomial(n,p,N)\nplot_histogram_discrete(d_bino_numpy, 'función creada con numpy')\n",
"_____no_output_____"
]
],
[
[
"Explore el funcionamiento del siguiente comando",
"_____no_output_____"
]
],
[
[
"list(set(d_binomial))",
"_____no_output_____"
]
],
[
[
"> ## <font color ='red'>Tarea\nSeguir un procedimiento similar al mostrado cuando se generó una distribución binomial, pero en esta caso genere un código que genere variables aletorias Poisson cuya función de distribución de probabilidad esta dada por:\n>$$P(k,\\lambda)=\\frac{e^{-\\lambda}(\\lambda)^k}{k!}$$\n \n> Demuestre matemáticamente que \n> $$P(k+1)=\\frac{\\lambda}{k+1}P(k)$$\n> y a partir de esta relación genere variables aletorias que distribuyen poisson usando el método de la transformada inversa.\n\nEnlace: https://es.wikipedia.org/wiki/Distribuci%C3%B3n_de_Poisson",
"_____no_output_____"
],
[
"$\n\\begin{aligned}\n\\frac{p_{k+1}}{p_k}& = \\frac{e^{-\\lambda}(\\lambda)^k}{k!} \\\\\n& = \\frac{e^{-\\lambda}(\\lambda)^k}{k!}\n\\end{aligned}\n$",
"_____no_output_____"
],
[
"# Método de aceptación rechazo\n\nEste método surgió debido a que muchas distribuciones continuas, no era factible aplicar el método de transformación inversa porque $x= F^{-1}(U)$ no se puede calcular (o al menos no es computacionalmente eficientemente).Con frecuencia, estos métodos son considerablemente más rápidos que el método de transformación inversa. Ahora ilustramos el **método de aceptación y rechazo** en un ejemplo simple.",
"_____no_output_____"
],
[
"Suponga que tenemos una función de densidad de probabilidad (PDF) de una distribución beta, la cual viene dada:\n$$f(x)=\\frac{x^{\\alpha_1-1}(1-x)^{\\alpha_2-1}}{B(\\alpha_1,\\alpha_2)} \\quad x\\in[0,1] \\longrightarrow B(\\alpha_1,\\alpha_2)\\equiv \\int_{0}^{1}x^{\\alpha_1-1}(1-x)^{\\alpha_2-1}, \\ \\alpha_1,\\alpha_2>1$$\n\n**Hablar de las desventajas**",
"_____no_output_____"
],
[
"Ahora definiremos formalmente el método:\n\nNote que $f(x)$ debe ser una **función acotada y con dominio finito** $a\\leq x \\leq b$ como se muestra a continuación:\n\n\nDe acuerdo a esta función $f(x)$ el método propone los siguientes pasos. Asuma que podemos encontrar una función $t(x)$ tal que\n$$t(x)\\geq f(x), \\quad \\forall x$$\nNote que la función $t(x)\\geq 0$ no es una PDF debido a \n$$\\int_{-\\infty}^{\\infty}t(x)dx\\geq \\int_{-\\infty}^{\\infty}f(x)dx =1$$\nTomemos\n$$c=\\int_{-\\infty}^{\\infty}t(x)\\geq 1$$\nDefinamos la función $g(x)=t(x)/c \\rightarrow g(x)$ **es una densidad**. Resultando entonces \n$$\\frac{f(x)}{g(x)}\\leq c,\\quad \\forall x$$\nEl siguiente algoritmo genera una variable aleatoria $X$, distribuida de acuerdo a la densidad $f(x)$\n 1. Generar $R_1$ teniendo densidad $g(x)$ \n 2. Generar $R_2 \\rightarrow U \\sim U(0,1)$ independiente de $R_1$ del paso 1 .\n 3. Evaluar la función de probabilidad en $R_1$.\n 4. Determinar si la siguiente desigualdad se cumple: $$R_2\\leq \\frac{f(R_1)}{t(R_1)}$$\n Si la respuesta es afirmativa se utiliza $X=R_1$, de lo contrario es necesario pasar nuevamente al paso 1, tantas veces como sea necesario.\n\n> Se puede demostrar que la $P(aceptar)=1/c$",
"_____no_output_____"
],
[
"### Ejemplo 1: Función beta\n\n$$f(x; a,b) = \\frac{1}{B(\\alpha, \\beta)} x^{\\alpha - 1}\n (1 - x)^{\\beta - 1}$$",
"_____no_output_____"
],
[
"### a). Caso particular: $\\alpha=\\beta=3$\nCon estos valores la PDF es \n$$f(x)=30(x^2-2x^3+x^4)$$",
"_____no_output_____"
]
],
[
[
"# Función de aceptación y rechazo usando for\ndef Acep_rechazo2(R2:'Variables distruidas U~U(0,1)',\n R1:'Variables distribuidas como g(x)',\n f:'función objetivo a generar',\n t:'función que mayora a f'):\n# R1 = np.random.rand(N)\n f_x = f(R1)\n t_x = t(R1)\n condition = R2*t_x <=f_x\n for i in range(len(R1)):\n if condition[i]:\n plt.plot(R1[i],R2[i]*t_x[i],'ob')\n else:\n plt.plot(R1[i],R2[i]*t_x[i],'o')\n plt.show()",
"_____no_output_____"
],
[
"# Función de aceptación y rechazo usando compresión de listas\ndef Acep_rechazo(R2:'Variables distruidas U~U(0,1)',\n R1:'Variables distribuidas como g(x)',\n f:'función objetivo a generar',\n t:'función que mayora a f'):\n# R1 = np.random.rand(N)\n f_x = f(R1)\n t_x = t(R1)\n condition = R2*t_x <=f_x\n# [plt.plot(R1[i],R2[i]*t_x[i],'ob') if condition[i] else plt.plot(R1[i],R2[i]*t_x[i],'o') \\\n# for i in range(len(R1))] \n# plt.show()\n \n x = [R1[i] for i in range(len(R1)) if condition[i]]\n \n return x",
"_____no_output_____"
],
[
"# Ilustración del método de aceptación y rechazo cuando se toma t(x) constante\nN = 100\n\n# Función objetivo \nf = lambda x: 30 * (x**2 -2 * x**3 + x**4) \n# Máximo de la función f\nmax_f = f(optimize.fmin(lambda x:-f(x), 0, disp=False))\n# Función t -> Función constante\nt = lambda x: max_f * np.ones([len(x)])\n\n# Rango donde se graficará las funciones\nx = np.arange(0, 1, 0.01)\nprint('El máximo de f es:',max_f)\n\n# Gráficas de las funciones\nplt.plot(x,f(x),label='f(x)')\nplt.plot(x,t(x),label='t(x)')\nplt.legend()\n\n# Validación del método\nN = 20000 # número de puntos a simular\n# Como estoy tomando t(x) constante solo es necesario generar valores aleatorios U~(0,1)\nR2 = np.random.rand(N)\nR1 = np.random.uniform(0, 1, size=N)\n\nx_r = Acep_rechazo(R2, R1, f, t)\n\ny,x_n, _ = plt.hist(x_r, bins=50, density=True)",
"El máximo de f es: [1.875]\n"
],
[
"np.cumsum(y)[-1]",
"_____no_output_____"
]
],
[
[
"### b). Caso general: $\\alpha,\\beta>0$ ",
"_____no_output_____"
]
],
[
[
"# Parámetros de la función beta\na =10; b=3\nN = 500 # número de puntos\n# Función objetivo\nf = lambda x: beta.pdf(x,a,b)\nx = np.arange(0,1,0.01)\nplt.plot(x,f(x),'k')\n# Encuentro el máximo de la función f\nc = float(f(optimize.fmin(lambda x:-f(x),0,disp=False)))\nprint('El máximo de la función es:',c)\n\nt = lambda x: c*np.ones(len(x))\nplt.plot(x,f(x),'k')\nplt.plot(x,t(x),'b')\nR2 = np.random.rand(N)\nR1 = np.random.rand(N)\n\nAcep_rechazo(R2,R1,f,t)\n\nplt.show()",
"El máximo de la función es: 3.5848168690361635\n"
]
],
[
[
"# Tarea 6\nPartiendo que se desea generar variables aleatorias para la siguiente función de densidad\n$$f(x)=30(x^2-2x^3+x^4)$$\nResponda los siguientes literales:\n1. Usar como función que mayora a $f(x)$ a $t(x)=a \\sin(\\pi x)$ donde a es el máximo de la función $f(x)$ y graficarlas en una misma gráfica, para validar que en realidad si cumple la condición $t(x)\\geq f(x)$.\n2. Encontrar la función de densidad $g(x)$ según lo visto en clase. Reportar todos los cálculos realizados para encontrar dicha función usando Markdown (Latex).\n3. Usar la función encontrada en el punto 2 y utilizar el método de la transformada inversa visto en la clase 9, para generar variables aleatorias que sigan la distribución $g(x)$. **Nota:** Recuerde que el método de la transformada inversa funciona con la distribución de probabilidad acumulada y no con su densidad. Nuevamente similar al punto anterior reportar todos los cálculos usando Markdown (Latex). \n4. Según el punto 3, generar 10000 puntos aleatorios que sigan la distribución $g(x)$ y comparar con su histograma para validar que los puntos generados siguen la distribución deseada. El resultado debe ser como sigue:\n",
"_____no_output_____"
],
[
"5. Genere 500 puntos aleatorios usando el método de aceptación y rechazo y las funciones $f(x)$ y $t(x)$ para validar que todos los cálculos anteriores están correctamente realizados. El resultado debe de ser como sigue:\n",
"_____no_output_____"
],
[
"6. Comparar el porcentaje de puntos de aceptación cuando se usa $t(x)$ constante y $t(x)$ un pulso senoidal. Concluir",
"_____no_output_____"
],
[
"7. Genere una variable aleatoria $X$ a partir de la siguiente PDF\n$$f(x)=20x(1-x)^3$$ \nusando el método de aceptación y rechazo",
"_____no_output_____"
],
[
"8. Seguir un procedimiento similar al mostrado cuando se generó una distribución binomial, pero en esta caso genere un código que genere variables aletorias Poisson cuya función de distribución de probabilidad esta dada por:\n>$$P(k,\\lambda)=\\frac{e^{-\\lambda}(\\lambda)^k}{k!}$$\n \n> Demuestre matemáticamente que \n> $$P(k+1)=\\frac{\\lambda}{k+1}P(k)$$\n> y a partir de esta relación genere variables aletorias que distribuyen poisson usando el método de la transformada inversa.\n\nEnlace: https://es.wikipedia.org/wiki/Distribuci%C3%B3n_de_Poisson",
"_____no_output_____"
],
[
"## Parámetros de entrega\nVoy a habilitar un link en Canvas donde deben de subir su cuaderno de python con la sulución de los problemas planteados en parejas. La podrán entregar a mas tardar el martes 6 de octubre a las 6pm. Como será en parejas, deben de crear un proyecto conjunto en github y realizar los ejercicios de manera conjunta, de manera similar a como realizaron los ejercicios en la tarea 1. **Deben de poner en la solución de la tarea el enlace de github de el administrador del repositorio**, del cuál me basaré para poner la calificación.",
"_____no_output_____"
],
[
"# Solución Tarea",
"_____no_output_____"
]
],
[
[
"import numpy as np\nimport matplotlib.pyplot as plt",
"_____no_output_____"
],
[
"\n",
"_____no_output_____"
]
],
[
[
"<script>\n $(document).ready(function(){\n $('div.prompt').hide();\n $('div.back-to-top').hide();\n $('nav#menubar').hide();\n $('.breadcrumb').hide();\n $('.hidden-print').hide();\n });\n</script>\n\n<footer id=\"attribution\" style=\"float:right; color:#808080; background:#fff;\">\nCreated with Jupyter by Oscar David Jaramillo Z.\n</footer>",
"_____no_output_____"
]
]
] | [
"markdown",
"code",
"markdown",
"code",
"markdown",
"code",
"markdown",
"code",
"markdown",
"code",
"markdown",
"code",
"markdown",
"code",
"markdown"
] | [
[
"markdown"
],
[
"code"
],
[
"markdown"
],
[
"code",
"code"
],
[
"markdown",
"markdown"
],
[
"code",
"code"
],
[
"markdown"
],
[
"code"
],
[
"markdown",
"markdown",
"markdown",
"markdown",
"markdown",
"markdown",
"markdown"
],
[
"code",
"code",
"code",
"code"
],
[
"markdown"
],
[
"code"
],
[
"markdown",
"markdown",
"markdown",
"markdown",
"markdown",
"markdown",
"markdown"
],
[
"code",
"code"
],
[
"markdown"
]
] |
e783fd3d7a5a00063bf619b9a6a7b5e4d2af0444 | 58,304 | ipynb | Jupyter Notebook | 2.Model_code/Linear/ridge_grid_search.ipynb | PpangPpang93/Main_project_police | ce5d8829ebbd981ad56225a254c384fcef9f99f7 | [
"MIT"
] | 1 | 2022-02-22T03:57:07.000Z | 2022-02-22T03:57:07.000Z | 2.Model_code/Linear/ridge_grid_search.ipynb | taeyang1224/Main_project_police | ce5d8829ebbd981ad56225a254c384fcef9f99f7 | [
"MIT"
] | 30 | 2021-11-26T07:58:02.000Z | 2021-12-12T23:42:25.000Z | 2.Model_code/Linear/ridge_grid_search.ipynb | taeyang1224/Main_project_police | ce5d8829ebbd981ad56225a254c384fcef9f99f7 | [
"MIT"
] | 15 | 2021-11-26T07:59:11.000Z | 2021-12-10T05:18:56.000Z | 31.195292 | 149 | 0.426437 | [
[
[
"import pandas as pd\nimport numpy as np\n#import matplotlib.pyplot as plt\n#import seaborn as sns",
"_____no_output_____"
],
[
"df = pd.read_csv('total_data1.csv')",
"_____no_output_____"
]
],
[
[
"### xdata로 상관계수가 높은 column을 넣어서 Ridge\n- elasticnet으로 상관계수가 높은 feature를 넣어 모델생성",
"_____no_output_____"
]
],
[
[
"from sklearn.metrics import mean_squared_error",
"_____no_output_____"
],
[
"# 필요 패키지 로드\n#from sklearn.preprocessing import MinMaxScaler\nfrom sklearn.preprocessing import StandardScaler\nfrom sklearn.linear_model import Ridge\nfrom sklearn.metrics import mean_absolute_error",
"_____no_output_____"
],
[
"# y값인 q1-q5가 결측인 2020년 데이터 제거\na = df[0:-82]\na",
"_____no_output_____"
],
[
"# 경찰서와 연도 데이터 제거\na.drop(columns = ['jur_stn', 'year'], inplace = True)",
"C:\\Anaconda3\\lib\\site-packages\\pandas\\core\\frame.py:3990: SettingWithCopyWarning: \nA value is trying to be set on a copy of a slice from a DataFrame\n\nSee the caveats in the documentation: https://pandas.pydata.org/pandas-docs/stable/user_guide/indexing.html#returning-a-view-versus-a-copy\n return super().drop(\n"
],
[
"a_ = a.drop(columns = ['q1', 'q2', 'q3', 'q4', 'q5'])",
"_____no_output_____"
],
[
"a_",
"_____no_output_____"
],
[
"a_.columns",
"_____no_output_____"
],
[
"a_1 = a_",
"_____no_output_____"
],
[
"a_2 = a_",
"_____no_output_____"
],
[
"a_3 = a_",
"_____no_output_____"
],
[
"a_4 = a_",
"_____no_output_____"
],
[
"a_5 = a_",
"_____no_output_____"
],
[
"# StandardScaling\nscaler1 = StandardScaler()\nscaler1.fit(a_1) # scaler에 xdata 학습\na_s1 = scaler1.transform(a_1)\na_s1",
"_____no_output_____"
],
[
"# StandardScaling\nscaler2 = StandardScaler()\nscaler2.fit(a_2) # scaler에 xdata 학습\na_s2 = scaler2.transform(a_2)\na_s2",
"_____no_output_____"
],
[
"# StandardScaling\nscaler3 = StandardScaler()\nscaler3.fit(a_3) # scaler에 xdata 학습\na_s3 = scaler3.transform(a_3)\na_s3",
"_____no_output_____"
],
[
"# StandardScaling\nscaler4 = StandardScaler()\nscaler4.fit(a_4) # scaler에 xdata 학습\na_s4 = scaler4.transform(a_4)\na_s4",
"_____no_output_____"
],
[
"# StandardScaling\nscaler5 = StandardScaler()\nscaler5.fit(a_5) # scaler에 xdata 학습\na_s5 = scaler5.transform(a_5)\na_s5",
"_____no_output_____"
],
[
"# x데이터 설정 2017, 2018 데이터를 학습용, 2019년 데이터를 검증용 데이터셋으로 설정\n\nxtrain1 = a_s1[:-82]\nxtest1 = a_s1[-82:]\nxtrain2 = a_s2[:-82]\nxtest2 = a_s2[-82:]\nxtrain3 = a_s3[:-82]\nxtest3 = a_s3[-82:]\nxtrain4 = a_s4[:-82]\nxtest4 = a_s4[-82:]\nxtrain5 = a_s5[:-82]\nxtest5 = a_s5[-82:]",
"_____no_output_____"
],
[
"# y데이터 설정 2017, 2018 데이터를 학습용, 2019년 데이터를 검증용 데이터셋으로 설정\n\ntrain = a[:-82]\ntest = a[-82:]\n\nytrain1 = train['q1']\nytrain2 = train['q2']\nytrain3 = train['q3']\nytrain4 = train['q4']\nytrain5 = train['q5']\n\nytest1 = test['q1']\nytest2 = test['q2']\nytest3 = test['q3']\nytest4 = test['q4']\nytest5 = test['q5']",
"_____no_output_____"
],
[
"a_1.columns",
"_____no_output_____"
],
[
"# 그리드 서치 패키지\nfrom sklearn.model_selection import GridSearchCV",
"_____no_output_____"
],
[
"# 최적 성능을 내는 lasso의 alpha값을 얻기 위해 param_grid 생성\n\nparam_grid = {'alpha' : np.linspace(0.001, 10.0, 10000)}\n\n#그리드 서치 설정\ngrid_search = GridSearchCV(Ridge(), param_grid = param_grid, cv = 10, n_jobs = -1, scoring ='r2')\n#grid_search = GridSearchCV(Ridge(), param_grid = param_grid, cv = 10, n_jobs = -1, scoring ='neg_mean_absolute_error')",
"_____no_output_____"
]
],
[
[
"#### q1 절도폭력 ",
"_____no_output_____"
]
],
[
[
"# 그리드 서치 후 최고 성능의 모델을 ridge1에 저장\n\ngrid_search.fit(xtrain1, ytrain1)\nridge1 = grid_search.best_estimator_",
"_____no_output_____"
],
[
"# MAE 출력\n\ny_pred1 = ridge1.predict(xtest1)\nmean_absolute_error(ytest1, y_pred1)",
"_____no_output_____"
],
[
"# 결과\n\nprint('alpha =', ridge1.alpha)\nprint(ridge1.coef_) # Ridge 회귀분석으로 나온 weghit값\nprint('가장 강한 양의 상관관계: ',a_1.columns[ridge1.coef_.argmax()], '\\n가장 강한 음의 상관관계: ', a_1.columns[ridge1.coef_.argmin()])",
"alpha = 10.0\n[ 0.1858718 0.35944705 -0.18654878 -0.88752003 0.12550527 -0.00879849\n 0.23483285 -0.25640454 0.46469126 0.50380436 0.85031836 -0.29439753\n -0.17669475 0.36080588 0.12274043 -0.78509441 -0.35994638 0.30965558\n 0.10691769 0.51217057 -0.14159903 0.05857899 0.07225416 -0.33213259\n -0.30612817 -0.28521751 -0.02244433 0.04295547 0.23212191 -0.43095751\n 0.99679932 0.67407008 0.04719795 0.02266245 -0.26957157 0.1635311\n 0.27777131 0.18326995 -0.26235237 -0.253049 0.18470932 -0.32227054\n -0.11586935 0.20246951 0.00619761 -0.08666845 -0.39903773 0.31214314\n 0.26027898 -0.34774913 -0.043771 0.18120353 0.03499807 -0.3080387\n -0.20039405 0.26735254 -0.12913279 0.02064122 -0.08359852 0.24854472]\n가장 강한 양의 상관관계: 외국인인구수대비검거수 \n가장 강한 음의 상관관계: vio_cnt\n"
]
],
[
[
"#### q2 강도살인",
"_____no_output_____"
]
],
[
[
"# 그리드 서치 후 최고 성능의 모델을 ela2에 저장\n\ngrid_search.fit(xtrain2, ytrain2)\nridge2 = grid_search.best_estimator_",
"_____no_output_____"
],
[
"# MAE 출력\n\ny_pred2 = ridge2.predict(xtest2)\nmean_absolute_error(ytest2, y_pred2)",
"_____no_output_____"
],
[
"# 결과\n\nprint('alpha =', ridge2.alpha)\nprint(ridge2.coef_) # Ridge 회귀분석으로 나온 weghit값\nprint('가장 강한 양의 상관관계: ',a_2.columns[ridge2.coef_.argmax()], '\\n가장 강한 음의 상관관계: ', a_2.columns[ridge2.coef_.argmin()])",
"alpha = 4.566000000000001\n[ 0.0860792 0.4554509 -0.42882456 -1.39474717 -0.04082773 0.67245513\n 0.44065367 -0.35757788 0.36512942 1.13101206 1.47174792 -0.50713043\n -0.21119051 0.64726755 0.26600496 -1.10801628 -0.61302412 0.37013122\n -0.29569089 0.61392221 -0.10098044 0.05371732 0.21285102 -0.44627423\n -0.21074038 -0.11088239 -0.02215864 0.05477171 0.20860851 -0.79054475\n 0.7222386 1.1018597 -0.29250057 0.16025184 -0.52723943 0.31317932\n 0.42472581 0.10930088 -0.19559189 -0.25294844 0.02556162 -0.55658869\n -0.10951789 0.24595558 0.02282998 -0.02243589 -0.49783467 0.394488\n 0.30491444 -0.03552448 -0.15389 0.13197577 0.11347984 -0.57586571\n 0.02578398 0.08091628 -0.29398064 0.16571328 -0.32548941 0.66159982]\n가장 강한 양의 상관관계: for_u20 \n가장 강한 음의 상관관계: vio_cnt\n"
]
],
[
[
"#### q3 교통안전",
"_____no_output_____"
]
],
[
[
"# 그리드 서치 후 최고 성능의 모델을 lasso3에 저장\n\ngrid_search.fit(xtrain3, ytrain3)\nridge3 = grid_search.best_estimator_",
"_____no_output_____"
],
[
"ridge3 = Ridge(alpha = 23)\nridge3.fit(xtrain3, ytrain3)",
"_____no_output_____"
],
[
"# MAE 출력\n\ny_pred3 = ridge3.predict(xtest3)\nmean_absolute_error(ytest3, y_pred3)",
"_____no_output_____"
],
[
"# 결과\n\nprint('alpha =', ridge3.alpha)\nprint(ridge3.coef_) # Ridge 회귀분석으로 나온 weghit값\nprint('가장 강한 양의 상관관계: ',a_3.columns[ridge3.coef_.argmax()], '\\n가장 강한 음의 상관관계: ', a_3.columns[ridge3.coef_.argmin()])",
"alpha = 23\n[ 0.60462781 -0.01836216 0.33480332 0.07597766 0.05094331 -0.01801049\n 0.12955529 -0.03098228 -0.02389251 0.32231312 0.36155864 -0.06357061\n -0.17338605 0.10758736 -0.13071408 -0.23385295 -0.16651811 0.02234594\n 0.22738254 0.10056028 -0.08911714 -0.08948507 0.10180638 -0.05404081\n 0.32308556 -0.05319068 -0.00210497 0.03723783 0.19267206 0.11423388\n 0.02766457 0.52296002 -0.01345592 0.01568679 0.13478935 -0.21232877\n -0.05575848 0.2075885 -0.04617672 -0.14361172 0.12973644 -0.55315098\n -0.43058753 0.13637724 0.26248718 0.09187102 -0.28458975 0.21837938\n 0.20221084 0.26698087 -0.06346565 -0.14705863 0.1048014 -0.05481377\n -0.00895966 0.02854603 -0.05046488 -0.08276508 0.03386703 0.16616376]\n가장 강한 양의 상관관계: child \n가장 강한 음의 상관관계: ofn_10\n"
]
],
[
[
"#### q4 법질서 준수도",
"_____no_output_____"
]
],
[
[
"# 그리드 서치 후 최고 성능의 모델을 lasso4에 저장\n\ngrid_search.fit(xtrain4, ytrain4)\nridge4 = grid_search.best_estimator_",
"_____no_output_____"
],
[
"# MAE 출력\n\ny_pred4 = ridge4.predict(xtest4)\nmean_absolute_error(ytest4, y_pred4)",
"_____no_output_____"
],
[
"# 결과\n\nprint('alpha =', ridge4.alpha)\nprint(ridge4.coef_) # Ridge 회귀분석으로 나온 weghit값\nprint('가장 강한 양의 상관관계: ',a_4.columns[ridge4.coef_.argmax()], '\\n가장 강한 음의 상관관계: ', a_4.columns[ridge4.coef_.argmin()])",
"alpha = 10.0\n[ 0.08422014 0.47496439 0.14834264 -0.31512474 0.90924745 0.72152184\n -0.16558919 -0.19975831 -0.11015924 0.08660646 0.65342416 -0.33030785\n -0.23131614 0.15442808 -0.02441021 -0.79282277 -0.16042672 0.17084599\n 0.28093741 0.34898235 -0.48400998 0.13322148 -0.25607675 -0.2081495\n 0.36480096 -0.54529326 -0.28543694 0.0490413 0.16311427 -0.4195897\n 0.58055968 -0.99569428 -0.04666948 0.45819622 -0.02352386 0.81593753\n 0.04091217 0.1235867 0.20385689 -0.3854837 0.20093322 -0.4193185\n -0.48252598 0.45797532 0.03029416 0.08614095 -0.38301169 0.23971484\n 0.48417109 0.48881207 -0.05618829 -0.22196673 0.16491527 -0.3411742\n 0.12608131 -0.01440109 -0.33302747 -0.25247363 0.13361622 0.38994271]\n가장 강한 양의 상관관계: mur_rob_cnt \n가장 강한 음의 상관관계: 인구수대비경찰수\n"
]
],
[
[
"#### q5 전반적 안전도",
"_____no_output_____"
]
],
[
[
"# 그리드 서치 후 최고 성능의 모델을 lasso4에 저장\n\ngrid_search.fit(xtrain5, ytrain5)\nridge5 = grid_search.best_estimator_",
"_____no_output_____"
],
[
"ridge5 = Ridge(alpha = 6.25)\nridge5.fit(xtrain5, ytrain5)",
"_____no_output_____"
],
[
"# MAE 출력\n\ny_pred5 = ridge5.predict(xtest5)\nmean_absolute_error(ytest5, y_pred5)",
"_____no_output_____"
],
[
"# 결과\n\nprint('alpha =', ridge5.alpha)\nprint(ridge5.coef_) # Ridge 회귀분석으로 나온 weghit값\nprint('가장 강한 양의 상관관계: ',a_5.columns[ridge5.coef_.argmax()], '\\n가장 강한 음의 상관관계: ', a_5.columns[ridge5.coef_.argmin()])",
"alpha = 6.25\n[ 0.06421938 0.4187727 0.01456397 -0.98143189 0.27599427 0.34433021\n 0.25962214 -0.1884639 0.36799516 0.6268941 0.78630458 -0.33307267\n -0.33674456 0.32468536 -0.07203347 -0.87313847 -0.42267696 0.30238462\n 0.04100536 0.52735428 -0.07934469 -0.02891441 0.096653 -0.20399747\n 0.23988935 -0.51472116 -0.10890164 -0.01168908 0.09871749 -0.28613236\n 0.67575661 0.2864437 -0.0625666 0.13997026 -0.18723721 0.13303888\n 0.25390993 0.1604691 -0.01088797 -0.19593194 0.06767935 -0.38309175\n -0.24771206 0.3046604 0.00549987 0.06127319 -0.37182862 0.27782696\n 0.29352346 -0.01387247 -0.08819971 -0.00617579 0.13260857 -0.30891844\n 0.00401649 0.11547854 -0.32287934 -0.00472153 -0.06880729 0.2824365 ]\n가장 강한 양의 상관관계: for_u20 \n가장 강한 음의 상관관계: vio_cnt\n"
]
]
] | [
"code",
"markdown",
"code",
"markdown",
"code",
"markdown",
"code",
"markdown",
"code",
"markdown",
"code",
"markdown",
"code"
] | [
[
"code",
"code"
],
[
"markdown"
],
[
"code",
"code",
"code",
"code",
"code",
"code",
"code",
"code",
"code",
"code",
"code",
"code",
"code",
"code",
"code",
"code",
"code",
"code",
"code",
"code",
"code",
"code"
],
[
"markdown"
],
[
"code",
"code",
"code"
],
[
"markdown"
],
[
"code",
"code",
"code"
],
[
"markdown"
],
[
"code",
"code",
"code",
"code"
],
[
"markdown"
],
[
"code",
"code",
"code"
],
[
"markdown"
],
[
"code",
"code",
"code",
"code"
]
] |
e784049b0590d203afc42f9ccc840381c39aa91f | 9,139 | ipynb | Jupyter Notebook | exercise_2/3_Dropout-optional.ipynb | nazmicancalik/i2dl | 35580b2fb5d10ab33e3b1aedd1bdad328784050d | [
"RSA-MD"
] | null | null | null | exercise_2/3_Dropout-optional.ipynb | nazmicancalik/i2dl | 35580b2fb5d10ab33e3b1aedd1bdad328784050d | [
"RSA-MD"
] | 6 | 2020-03-24T18:20:03.000Z | 2022-01-13T02:26:05.000Z | exercise_2/3_Dropout-optional.ipynb | enisimsar/i2dl-exercises | 0bb808f0096ae7ac0454ba8296896d1fcc8c9e7c | [
"RSA-MD"
] | null | null | null | 33.599265 | 359 | 0.571069 | [
[
[
"# Optional: Dropout\n\n**Note**: This exercise is optional and using dropout is not required to pass beyond the linear regime of the scoring function for your fully connected network.\n\nDropout [1] is a technique for regularizing neural networks by randomly setting some features to zero during the forward pass. In this exercise you will implement a dropout layer and modify your fully-connected network to optionally use dropout.\n\n[1] Geoffrey E. Hinton et al, \"Improving neural networks by preventing co-adaptation of feature detectors\", arXiv 2012",
"_____no_output_____"
]
],
[
[
"# As usual, a bit of setup\n\nimport time\nimport numpy as np\nimport matplotlib.pyplot as plt\nfrom exercise_code.classifiers.fc_net import *\nfrom exercise_code.data_utils import get_CIFAR10_data\nfrom exercise_code.gradient_check import eval_numerical_gradient, eval_numerical_gradient_array\nfrom exercise_code.solver import Solver\n\n%matplotlib inline\nplt.rcParams['figure.figsize'] = (10.0, 8.0) # set default size of plots\nplt.rcParams['image.interpolation'] = 'nearest'\nplt.rcParams['image.cmap'] = 'gray'\n\n# for auto-reloading external modules\n# see http://stackoverflow.com/questions/1907993/autoreload-of-modules-in-ipython\n%load_ext autoreload\n%autoreload 2\n\n# supress cluttering warnings in solutions\nimport warnings\nwarnings.filterwarnings('ignore')\n\ndef rel_error(x, y):\n \"\"\" returns relative error \"\"\"\n return np.max(np.abs(x - y) / (np.maximum(1e-8, np.abs(x) + np.abs(y))))",
"_____no_output_____"
],
[
"# Load the (preprocessed) CIFAR10 data.\n\ndata = get_CIFAR10_data()\nfor k, v in data.items():\n print('%s: ' % k, v.shape)",
"_____no_output_____"
]
],
[
[
"# Dropout forward pass\nIn the file `exercise_code/layers.py`, implement the forward pass for dropout. Since dropout behaves differently during training and testing, make sure to implement the operation for both modes.\n\nOnce you have done so, run the cell below to test your implementation.",
"_____no_output_____"
]
],
[
[
"x = np.random.randn(500, 500) + 10\n\nfor p in [0.3, 0.6, 0.75]:\n out, _ = dropout_forward(x, {'mode': 'train', 'p': p})\n out_test, _ = dropout_forward(x, {'mode': 'test', 'p': p})\n\n print('Running tests with p = ', p)\n print('Mean of input: ', x.mean())\n print('Mean of train-time output: ', out.mean())\n print('Mean of test-time output: ', out_test.mean())\n print('Fraction of train-time output set to zero: ', (out == 0).mean())\n print('Fraction of test-time output set to zero: ', (out_test == 0).mean())\n print()",
"_____no_output_____"
]
],
[
[
"# Dropout backward pass\nIn the file `exercise_code/layers.py`, implement the backward pass for dropout. After doing so, run the following cell to numerically gradient-check your implementation.",
"_____no_output_____"
]
],
[
[
"x = np.random.randn(10, 10) + 10\ndout = np.random.randn(*x.shape)\n\ndropout_param = {'mode': 'train', 'p': 0.8, 'seed': 123}\nout, cache = dropout_forward(x, dropout_param)\ndx = dropout_backward(dout, cache)\ndx_num = eval_numerical_gradient_array(lambda xx: dropout_forward(xx, dropout_param)[0], x, dout)\n\nprint('dx relative error: ', rel_error(dx, dx_num))",
"_____no_output_____"
]
],
[
[
"# Fully-connected nets with Dropout\nIn the file `exercise_code/classifiers/fc_net.py`, modify your implementation to use dropout. Specificially, if the constructor the the net receives a nonzero value for the `dropout` parameter, then the net should add dropout immediately after every ReLU nonlinearity. After doing so, run the following to numerically gradient-check your implementation.",
"_____no_output_____"
]
],
[
[
"N, D, H1, H2, C = 2, 15, 20, 30, 10\nX = np.random.randn(N, D)\ny = np.random.randint(C, size=(N,))\n\nfor dropout in [0, 0.25, 0.5]:\n print('Running check with dropout = ', dropout)\n model = FullyConnectedNet([H1, H2], input_dim=D, num_classes=C,\n weight_scale=5e-2, dtype=np.float64,\n dropout=dropout, seed=123)\n\n loss, grads = model.loss(X, y)\n print('Initial loss: ', loss)\n\n for name in sorted(grads):\n f = lambda _: model.loss(X, y)[0]\n grad_num = eval_numerical_gradient(f, model.params[name], verbose=False, h=1e-5)\n print('%s relative error: %.2e' % (name, rel_error(grad_num, grads[name])))\n print()",
"_____no_output_____"
]
],
[
[
"# Regularization experiment\nAs an experiment, we will train a pair of two-layer networks on 500 training examples: one will use no dropout, and one will use a dropout probability of 0.75. We will then visualize the training and validation accuracies of the two networks over time.",
"_____no_output_____"
]
],
[
[
"# Train two identical nets, one with dropout and one without\n\nnum_train = 500\nsmall_data = {\n 'X_train': data['X_train'][:num_train],\n 'y_train': data['y_train'][:num_train],\n 'X_val': data['X_val'],\n 'y_val': data['y_val'],\n}\n\nsolvers = {}\ndropout_choices = [0, 0.75]\nfor dropout in dropout_choices:\n model = FullyConnectedNet([500], dropout=dropout)\n print(\"dropout = \", dropout)\n\n solver = Solver(model, small_data,\n num_epochs=25, batch_size=100,\n update_rule='adam',\n optim_config={\n 'learning_rate': 5e-4,\n },\n verbose=True, print_every=100)\n solver.train()\n solvers[dropout] = solver",
"_____no_output_____"
],
[
"# Plot train and validation accuracies of the two models\n\ntrain_accs = []\nval_accs = []\nfor dropout in dropout_choices:\n solver = solvers[dropout]\n train_accs.append(solver.train_acc_history[-1])\n val_accs.append(solver.val_acc_history[-1])\n\nplt.subplot(3, 1, 1)\nfor dropout in dropout_choices:\n plt.plot(solvers[dropout].train_acc_history, 'o', label='%.2f dropout' % dropout)\nplt.title('Train accuracy')\nplt.xlabel('Epoch')\nplt.ylabel('Accuracy')\nplt.legend(ncol=2, loc='lower right')\n \nplt.subplot(3, 1, 2)\nfor dropout in dropout_choices:\n plt.plot(solvers[dropout].val_acc_history, 'o', label='%.2f dropout' % dropout)\nplt.title('Val accuracy')\nplt.xlabel('Epoch')\nplt.ylabel('Accuracy')\nplt.legend(ncol=2, loc='lower right')\n\nplt.gcf().set_size_inches(15, 15)\nplt.show()",
"_____no_output_____"
]
],
[
[
"<div class=\"alert alert-info\">\n <h3>Inline Question</h3>\n <p>Describe the results of this experiment and try to reason why you got these results.</p>\n</div>",
"_____no_output_____"
]
]
] | [
"markdown",
"code",
"markdown",
"code",
"markdown",
"code",
"markdown",
"code",
"markdown",
"code",
"markdown"
] | [
[
"markdown"
],
[
"code",
"code"
],
[
"markdown"
],
[
"code"
],
[
"markdown"
],
[
"code"
],
[
"markdown"
],
[
"code"
],
[
"markdown"
],
[
"code",
"code"
],
[
"markdown"
]
] |
e78404a26476f6af61c24ca76362474275dddbdf | 2,001 | ipynb | Jupyter Notebook | ReproducingMLpipelines/Paper6/ModelQDAPCA.ipynb | CompareML/AIM-Manuscript | 4cf118c1f06e8a1843d56e1f7f8f3d1698aac248 | [
"MIT"
] | null | null | null | ReproducingMLpipelines/Paper6/ModelQDAPCA.ipynb | CompareML/AIM-Manuscript | 4cf118c1f06e8a1843d56e1f7f8f3d1698aac248 | [
"MIT"
] | null | null | null | ReproducingMLpipelines/Paper6/ModelQDAPCA.ipynb | CompareML/AIM-Manuscript | 4cf118c1f06e8a1843d56e1f7f8f3d1698aac248 | [
"MIT"
] | null | null | null | 20.212121 | 120 | 0.477261 | [
[
[
"### QDA",
"_____no_output_____"
]
],
[
[
"load(\"PCA.rda\")\nload(\"DP.rda\")\nsuppressMessages(library(caret))\nset.seed(201703)",
"_____no_output_____"
],
[
"options(warn=-1)\n# QDA\npca_qda_s = train(response~., data = pca_train, method = \"qda\", trControl = trainControl(method = \"LOOCV\"))\npca_qda_te = predict(pca_qda_s, data.frame(pca_test_s))\npca_qda_ac = mean(pca_qda_te == golub_test_r)\npca_qda_re = c(LOOCV = pca_qda_s$results$Accuracy, Test = pca_qda_ac)",
"_____no_output_____"
],
[
"pca_qda_re",
"_____no_output_____"
]
]
] | [
"markdown",
"code"
] | [
[
"markdown"
],
[
"code",
"code",
"code"
]
] |
e784062e6efd78177bc2906f27920be7caa4446d | 2,179 | ipynb | Jupyter Notebook | Udacity Course.ipynb | jtkrohm/jt | 16df8d5dd92c594db0b60d389f314aa60fa61a7a | [
"MIT"
] | 1 | 2020-06-11T16:28:39.000Z | 2020-06-11T16:28:39.000Z | Udacity Course.ipynb | jtkrohm/jt | 16df8d5dd92c594db0b60d389f314aa60fa61a7a | [
"MIT"
] | null | null | null | Udacity Course.ipynb | jtkrohm/jt | 16df8d5dd92c594db0b60d389f314aa60fa61a7a | [
"MIT"
] | null | null | null | 21.574257 | 225 | 0.425883 | [
[
[
"<a href=\"https://colab.research.google.com/github/jtkrohm/jt/blob/master/Udacity%20Course.ipynb\" target=\"_parent\"><img src=\"https://colab.research.google.com/assets/colab-badge.svg\" alt=\"Open In Colab\"/></a>",
"_____no_output_____"
]
],
[
[
"!pwd",
"/content\n"
]
],
[
[
"",
"_____no_output_____"
]
],
[
[
"",
"_____no_output_____"
],
[
"print(\"JT\")",
"JT\n"
]
]
] | [
"markdown",
"code",
"markdown",
"code"
] | [
[
"markdown"
],
[
"code"
],
[
"markdown"
],
[
"code",
"code"
]
] |
e7841c3afc5d43949f82d6e531e1e217582e813e | 791,439 | ipynb | Jupyter Notebook | Model backlog/Models/41-openvaccine-weighted-samples.ipynb | dimitreOliveira/COVID-19-Vaccine-Degradation-Prediction | c726c1a87aefa5dca8d1df3be79ee06ff4da99e4 | [
"MIT"
] | null | null | null | Model backlog/Models/41-openvaccine-weighted-samples.ipynb | dimitreOliveira/COVID-19-Vaccine-Degradation-Prediction | c726c1a87aefa5dca8d1df3be79ee06ff4da99e4 | [
"MIT"
] | null | null | null | Model backlog/Models/41-openvaccine-weighted-samples.ipynb | dimitreOliveira/COVID-19-Vaccine-Degradation-Prediction | c726c1a87aefa5dca8d1df3be79ee06ff4da99e4 | [
"MIT"
] | 1 | 2020-11-08T14:43:09.000Z | 2020-11-08T14:43:09.000Z | 306.759302 | 573,092 | 0.876912 | [
[
[
"## Dependencies",
"_____no_output_____"
]
],
[
[
"from openvaccine_scripts import *\nimport warnings, json\nfrom sklearn.model_selection import KFold, StratifiedKFold\nimport tensorflow.keras.layers as L\nimport tensorflow.keras.backend as K\nfrom tensorflow.keras import optimizers, losses, Model\nfrom tensorflow.keras.callbacks import EarlyStopping, ReduceLROnPlateau\n\n\nSEED = 0\nseed_everything(SEED)\nwarnings.filterwarnings('ignore')",
"_____no_output_____"
]
],
[
[
"# Model parameters",
"_____no_output_____"
]
],
[
[
"config = {\n \"BATCH_SIZE\": 64,\n \"EPOCHS\": 120,\n \"LEARNING_RATE\": 1e-3,\n \"ES_PATIENCE\": 10,\n \"N_FOLDS\": 5,\n \"N_USED_FOLDS\": 5,\n \"PB_SEQ_LEN\": 107,\n \"PV_SEQ_LEN\": 130,\n}\n\nwith open('config.json', 'w') as json_file:\n json.dump(json.loads(json.dumps(config)), json_file)\n \nconfig",
"_____no_output_____"
]
],
[
[
"# Load data",
"_____no_output_____"
]
],
[
[
"database_base_path = '/kaggle/input/stanford-covid-vaccine/'\ntrain = pd.read_json(database_base_path + 'train.json', lines=True)\ntest = pd.read_json(database_base_path + 'test.json', lines=True)\n\nprint('Train samples: %d' % len(train))\ndisplay(train.head())\nprint(f'Test samples: {len(test)}')\ndisplay(test.head())",
"Train samples: 2400\n"
]
],
[
[
"## Auxiliary functions",
"_____no_output_____"
]
],
[
[
"def get_dataset(x, y=None, sample_weights=None, labeled=True, shuffled=True, batch_size=32, buffer_size=-1, seed=0):\n input_map = {'inputs_seq': x['sequence'], \n 'inputs_struct': x['structure'], \n 'inputs_loop': x['predicted_loop_type'], \n 'inputs_bpps_max': x['bpps_max'], \n 'inputs_bpps_sum': x['bpps_sum'], \n 'inputs_bpps_mean': x['bpps_mean'], \n 'inputs_bpps_scaled': x['bpps_scaled']}\n \n if labeled:\n output_map = {'output_react': y['reactivity'], \n 'output_bg_ph': y['deg_Mg_pH10'], \n 'output_ph': y['deg_pH10'], \n 'output_mg_c': y['deg_Mg_50C'], \n 'output_c': y['deg_50C']}\n if sample_weights is not None:\n dataset = tf.data.Dataset.from_tensor_slices((input_map, output_map, sample_weights))\n else:\n dataset = tf.data.Dataset.from_tensor_slices((input_map, output_map))\n else:\n dataset = tf.data.Dataset.from_tensor_slices((input_map))\n \n if shuffled:\n dataset = dataset.shuffle(2048, seed=seed)\n dataset = dataset.batch(batch_size)\n dataset = dataset.prefetch(buffer_size)\n \n return dataset",
"_____no_output_____"
]
],
[
[
"# Model",
"_____no_output_____"
]
],
[
[
"def model_fn(hidden_dim=384, dropout=.5, pred_len=68, n_outputs=5):\n inputs_seq = L.Input(shape=(None, 1), name='inputs_seq') \n inputs_struct = L.Input(shape=(None, 1), name='inputs_struct') \n inputs_loop = L.Input(shape=(None, 1), name='inputs_loop')\n inputs_bpps_max = L.Input(shape=(None, 1), name='inputs_bpps_max')\n inputs_bpps_sum = L.Input(shape=(None, 1), name='inputs_bpps_sum')\n inputs_bpps_mean = L.Input(shape=(None, 1), name='inputs_bpps_mean')\n inputs_bpps_scaled = L.Input(shape=(None, 1), name='inputs_bpps_scaled')\n\n def _one_hot(x, num_classes):\n return K.squeeze(K.one_hot(K.cast(x, 'uint8'), num_classes=num_classes), axis=2)\n\n ohe_seq = L.Lambda(_one_hot, arguments={'num_classes': len(token2int_seq)}, input_shape=(None, 1))(inputs_seq)\n ohe_struct = L.Lambda(_one_hot, arguments={'num_classes': len(token2int_struct)}, input_shape=(None, 1))(inputs_struct)\n ohe_loop = L.Lambda(_one_hot, arguments={'num_classes': len(token2int_loop)}, input_shape=(None, 1))(inputs_loop)\n \n # Conv block\n conv_seq = L.Conv1D(filters=64, \n kernel_size=5, \n strides=1, \n padding='same')(ohe_seq)\n conv_struct = L.Conv1D(filters=64, \n kernel_size=5, \n strides=1, \n padding='same')(ohe_struct)\n conv_loop = L.Conv1D(filters=64, \n kernel_size=5, \n strides=1, \n padding='same')(ohe_loop)\n conv_bpps_max = L.Conv1D(filters=64, \n kernel_size=5, \n strides=1, \n padding='same')(inputs_bpps_max)\n conv_bpps_sum = L.Conv1D(filters=64, \n kernel_size=5, \n strides=1, \n padding='same')(inputs_bpps_sum)\n conv_bpps_mean = L.Conv1D(filters=64, \n kernel_size=5, \n strides=1, \n padding='same')(inputs_bpps_mean)\n conv_bpps_scaled = L.Conv1D(filters=64, \n kernel_size=5, \n strides=1, \n padding='same')(inputs_bpps_scaled)\n \n x_concat = L.concatenate([conv_seq, conv_struct, conv_loop, conv_bpps_max, \n conv_bpps_sum, conv_bpps_mean, conv_bpps_scaled], axis=-1, name='conv_concatenate')\n\n # Recurrent block\n x = L.Bidirectional(L.GRU(hidden_dim, dropout=dropout, return_sequences=True, kernel_initializer='orthogonal'))(x_concat)\n \n x_rec = L.Bidirectional(L.GRU(hidden_dim, dropout=dropout, return_sequences=True, kernel_initializer='orthogonal'))(x)\n x = L.Add()([x_rec, x])\n \n x_rec = L.Bidirectional(L.GRU(hidden_dim, dropout=dropout, return_sequences=True, kernel_initializer='orthogonal'))(x)\n x = L.Add()([x_rec, x])\n \n \n # Since we are only making predictions on the first part of each sequence, we have to truncate it\n x_truncated = x[:, :pred_len]\n \n output_react = L.Dense(1, activation='linear', name='output_react')(x_truncated)\n output_bg_ph = L.Dense(1, activation='linear', name='output_bg_ph')(x_truncated)\n output_ph = L.Dense(1, activation='linear', name='output_ph')(x_truncated)\n output_mg_c = L.Dense(1, activation='linear', name='output_mg_c')(x_truncated)\n output_c = L.Dense(1, activation='linear', name='output_c')(x_truncated)\n \n \n model = Model(inputs=[inputs_seq, inputs_struct, inputs_loop, inputs_bpps_max, \n inputs_bpps_sum, inputs_bpps_mean, inputs_bpps_scaled], \n outputs=[output_react, output_bg_ph, output_ph, output_mg_c, output_c])\n\n opt = optimizers.Adam(learning_rate=config['LEARNING_RATE'])\n model.compile(optimizer=opt, loss={'output_react': MCRMSE, \n 'output_bg_ph': MCRMSE, \n 'output_ph': MCRMSE, \n 'output_mg_c': MCRMSE, \n 'output_c': MCRMSE},\n loss_weights={'output_react': 2., \n 'output_bg_ph': 2., \n 'output_ph': 1., \n 'output_mg_c': 2., \n 'output_c': 1.})\n\n return model\n\nmodel = model_fn()\nmodel.summary()",
"Model: \"functional_1\"\n__________________________________________________________________________________________________\nLayer (type) Output Shape Param # Connected to \n==================================================================================================\ninputs_seq (InputLayer) [(None, None, 1)] 0 \n__________________________________________________________________________________________________\ninputs_struct (InputLayer) [(None, None, 1)] 0 \n__________________________________________________________________________________________________\ninputs_loop (InputLayer) [(None, None, 1)] 0 \n__________________________________________________________________________________________________\nlambda (Lambda) (None, None, 4) 0 inputs_seq[0][0] \n__________________________________________________________________________________________________\nlambda_1 (Lambda) (None, None, 3) 0 inputs_struct[0][0] \n__________________________________________________________________________________________________\nlambda_2 (Lambda) (None, None, 7) 0 inputs_loop[0][0] \n__________________________________________________________________________________________________\ninputs_bpps_max (InputLayer) [(None, None, 1)] 0 \n__________________________________________________________________________________________________\ninputs_bpps_sum (InputLayer) [(None, None, 1)] 0 \n__________________________________________________________________________________________________\ninputs_bpps_mean (InputLayer) [(None, None, 1)] 0 \n__________________________________________________________________________________________________\ninputs_bpps_scaled (InputLayer) [(None, None, 1)] 0 \n__________________________________________________________________________________________________\nconv1d (Conv1D) (None, None, 64) 1344 lambda[0][0] \n__________________________________________________________________________________________________\nconv1d_1 (Conv1D) (None, None, 64) 1024 lambda_1[0][0] \n__________________________________________________________________________________________________\nconv1d_2 (Conv1D) (None, None, 64) 2304 lambda_2[0][0] \n__________________________________________________________________________________________________\nconv1d_3 (Conv1D) (None, None, 64) 384 inputs_bpps_max[0][0] \n__________________________________________________________________________________________________\nconv1d_4 (Conv1D) (None, None, 64) 384 inputs_bpps_sum[0][0] \n__________________________________________________________________________________________________\nconv1d_5 (Conv1D) (None, None, 64) 384 inputs_bpps_mean[0][0] \n__________________________________________________________________________________________________\nconv1d_6 (Conv1D) (None, None, 64) 384 inputs_bpps_scaled[0][0] \n__________________________________________________________________________________________________\nconv_concatenate (Concatenate) (None, None, 448) 0 conv1d[0][0] \n conv1d_1[0][0] \n conv1d_2[0][0] \n conv1d_3[0][0] \n conv1d_4[0][0] \n conv1d_5[0][0] \n conv1d_6[0][0] \n__________________________________________________________________________________________________\nbidirectional (Bidirectional) (None, None, 768) 1921536 conv_concatenate[0][0] \n__________________________________________________________________________________________________\nbidirectional_1 (Bidirectional) (None, None, 768) 2658816 bidirectional[0][0] \n__________________________________________________________________________________________________\nadd (Add) (None, None, 768) 0 bidirectional_1[0][0] \n bidirectional[0][0] \n__________________________________________________________________________________________________\nbidirectional_2 (Bidirectional) (None, None, 768) 2658816 add[0][0] \n__________________________________________________________________________________________________\nadd_1 (Add) (None, None, 768) 0 bidirectional_2[0][0] \n add[0][0] \n__________________________________________________________________________________________________\ntf_op_layer_strided_slice (Tens [(None, None, 768)] 0 add_1[0][0] \n__________________________________________________________________________________________________\noutput_react (Dense) (None, None, 1) 769 tf_op_layer_strided_slice[0][0] \n__________________________________________________________________________________________________\noutput_bg_ph (Dense) (None, None, 1) 769 tf_op_layer_strided_slice[0][0] \n__________________________________________________________________________________________________\noutput_ph (Dense) (None, None, 1) 769 tf_op_layer_strided_slice[0][0] \n__________________________________________________________________________________________________\noutput_mg_c (Dense) (None, None, 1) 769 tf_op_layer_strided_slice[0][0] \n__________________________________________________________________________________________________\noutput_c (Dense) (None, None, 1) 769 tf_op_layer_strided_slice[0][0] \n==================================================================================================\nTotal params: 7,249,221\nTrainable params: 7,249,221\nNon-trainable params: 0\n__________________________________________________________________________________________________\n"
]
],
[
[
"# Pre-process",
"_____no_output_____"
]
],
[
[
"# Add bpps as features\nbpps_max = []\nbpps_sum = []\nbpps_mean = []\nbpps_scaled = []\nbpps_nb_mean = 0.077522 # mean of bpps_nb across all training data\nbpps_nb_std = 0.08914 # std of bpps_nb across all training data\nfor row in train.itertuples():\n probability = np.load(f'{database_base_path}/bpps/{row.id}.npy')\n bpps_max.append(probability.max(-1).tolist())\n bpps_sum.append((1-probability.sum(-1)).tolist())\n bpps_mean.append((1-probability.mean(-1)).tolist())\n # bpps nb\n bpps_nb = (probability > 0).sum(axis=0) / probability.shape[0]\n bpps_nb = (bpps_nb - bpps_nb_mean) / bpps_nb_std\n bpps_scaled.append(bpps_nb)\ntrain = train.assign(bpps_max=bpps_max, bpps_sum=bpps_sum, bpps_mean=bpps_mean, bpps_scaled=bpps_scaled)\n\nbpps_max = []\nbpps_sum = []\nbpps_mean = []\nbpps_scaled = []\nfor row in test.itertuples():\n probability = np.load(f'{database_base_path}/bpps/{row.id}.npy')\n bpps_max.append(probability.max(-1).tolist())\n bpps_sum.append((1-probability.sum(-1)).tolist())\n bpps_mean.append((1-probability.mean(-1)).tolist())\n # bpps nb\n bpps_nb = (probability > 0).sum(axis=0) / probability.shape[0]\n bpps_nb = (bpps_nb - bpps_nb_mean) / bpps_nb_std\n bpps_scaled.append(bpps_nb)\ntest = test.assign(bpps_max=bpps_max, bpps_sum=bpps_sum, bpps_mean=bpps_mean, bpps_scaled=bpps_scaled)\n\n\nfeature_cols = ['sequence', 'structure', 'predicted_loop_type', 'bpps_max', 'bpps_sum', 'bpps_mean', 'bpps_scaled']\npred_cols = ['reactivity', 'deg_Mg_pH10', 'deg_pH10', 'deg_Mg_50C', 'deg_50C']\nencoder_list = [token2int_seq, token2int_struct, token2int_loop, None, None, None, None]\n\npublic_test = test.query(\"seq_length == 107\").copy()\nprivate_test = test.query(\"seq_length == 130\").copy()\n\nx_test_public = get_features_dict(public_test, feature_cols, encoder_list, public_test.index)\nx_test_private = get_features_dict(private_test, feature_cols, encoder_list, private_test.index)\n\n# To use as stratified col\ntrain['signal_to_noise_int'] = train['signal_to_noise'].astype(int)",
"_____no_output_____"
]
],
[
[
"# Training",
"_____no_output_____"
]
],
[
[
"AUTO = tf.data.experimental.AUTOTUNE\nskf = KFold(n_splits=config['N_USED_FOLDS'], shuffle=True, random_state=SEED)\nhistory_list = []\n\noof = train[['id', 'SN_filter', 'signal_to_noise'] + pred_cols].copy()\noof_preds = np.zeros((len(train), 68, len(pred_cols)))\ntest_public_preds = np.zeros((len(public_test), config['PB_SEQ_LEN'], len(pred_cols)))\ntest_private_preds = np.zeros((len(private_test), config['PV_SEQ_LEN'], len(pred_cols)))\n\nfor fold,(train_idx, valid_idx) in enumerate(skf.split(train['signal_to_noise_int'])):\n if fold >= config['N_USED_FOLDS']:\n break\n print(f'\\nFOLD: {fold+1}')\n \n ### Create datasets\n x_train = get_features_dict(train, feature_cols, encoder_list, train_idx)\n x_valid = get_features_dict(train, feature_cols, encoder_list, valid_idx)\n y_train = get_targets_dict(train, pred_cols, train_idx)\n y_valid = get_targets_dict(train, pred_cols, valid_idx)\n w_train = np.log(train.iloc[train_idx]['signal_to_noise'].values+1.1)/2\n w_valid = np.log(train.iloc[valid_idx]['signal_to_noise'].values+1.1)/2\n \n \n train_ds = get_dataset(x_train, y_train, w_train, labeled=True, shuffled=True, batch_size=config['BATCH_SIZE'], buffer_size=AUTO, seed=SEED)\n valid_ds = get_dataset(x_valid, y_valid, w_valid, labeled=True, shuffled=False, batch_size=config['BATCH_SIZE'], buffer_size=AUTO, seed=SEED)\n oof_ds = get_dataset(x_valid, labeled=False, shuffled=False, batch_size=config['BATCH_SIZE'], buffer_size=AUTO, seed=SEED)\n test_public_ds = get_dataset(x_test_public, labeled=False, shuffled=False, batch_size=config['BATCH_SIZE'], buffer_size=AUTO, seed=SEED)\n test_private_ds = get_dataset(x_test_private, labeled=False, shuffled=False, batch_size=config['BATCH_SIZE'], buffer_size=AUTO, seed=SEED)\n\n\n ### Model\n K.clear_session()\n model = model_fn()\n\n model_path = f'model_{fold}.h5'\n es = EarlyStopping(monitor='val_loss', mode='min', patience=config['ES_PATIENCE'], restore_best_weights=True, verbose=1)\n rlrp = ReduceLROnPlateau(monitor='val_loss', mode='min', factor=0.1, patience=5, verbose=1)\n \n ### Train\n history = model.fit(train_ds,\n validation_data=valid_ds,\n callbacks=[es, rlrp],\n epochs=config['EPOCHS'],\n batch_size=config['BATCH_SIZE'],\n verbose=2).history\n \n history_list.append(history)\n # Save last model weights\n model.save_weights(model_path)\n\n ### Inference\n oof_ds_preds = np.array(model.predict(oof_ds)).reshape((len(pred_cols), len(valid_idx), 68)).transpose((1, 2, 0))\n oof_preds[valid_idx] = oof_ds_preds\n \n # Short sequence (public test)\n model = model_fn(pred_len=config['PB_SEQ_LEN'])\n model.load_weights(model_path)\n test_public_ds_preds = np.array(model.predict(test_public_ds)).reshape((len(pred_cols), len(public_test), \n config['PB_SEQ_LEN'])).transpose((1, 2, 0))\n test_public_preds += test_public_ds_preds * (1 / config['N_USED_FOLDS'])\n \n # Long sequence (private test)\n model = model_fn(pred_len=config['PV_SEQ_LEN'])\n model.load_weights(model_path)\n test_private_ds_preds = np.array(model.predict(test_private_ds)).reshape((len(pred_cols), len(private_test), \n config['PV_SEQ_LEN'])).transpose((1, 2, 0))\n test_private_preds += test_private_ds_preds * (1 / config['N_USED_FOLDS'])",
"\nFOLD: 1\nEpoch 1/120\n30/30 - 7s - loss: 3.5876 - output_react_loss: 0.4014 - output_bg_ph_loss: 0.5248 - output_ph_loss: 0.5226 - output_mg_c_loss: 0.4188 - output_c_loss: 0.3750 - val_loss: 2.3896 - val_output_react_loss: 0.2423 - val_output_bg_ph_loss: 0.3301 - val_output_ph_loss: 0.3582 - val_output_mg_c_loss: 0.3026 - val_output_c_loss: 0.2814\nEpoch 2/120\n30/30 - 4s - loss: 2.3368 - output_react_loss: 0.2458 - output_bg_ph_loss: 0.3183 - output_ph_loss: 0.3391 - output_mg_c_loss: 0.2968 - output_c_loss: 0.2759 - val_loss: 2.1912 - val_output_react_loss: 0.2287 - val_output_bg_ph_loss: 0.2984 - val_output_ph_loss: 0.3103 - val_output_mg_c_loss: 0.2815 - val_output_c_loss: 0.2637\nEpoch 3/120\n30/30 - 4s - loss: 2.1930 - output_react_loss: 0.2331 - output_bg_ph_loss: 0.2995 - output_ph_loss: 0.3077 - output_mg_c_loss: 0.2797 - output_c_loss: 0.2607 - val_loss: 2.1003 - val_output_react_loss: 0.2207 - val_output_bg_ph_loss: 0.2852 - val_output_ph_loss: 0.2954 - val_output_mg_c_loss: 0.2694 - val_output_c_loss: 0.2543\nEpoch 4/120\n30/30 - 4s - loss: 2.1113 - output_react_loss: 0.2256 - output_bg_ph_loss: 0.2875 - output_ph_loss: 0.2958 - output_mg_c_loss: 0.2688 - output_c_loss: 0.2517 - val_loss: 2.0628 - val_output_react_loss: 0.2158 - val_output_bg_ph_loss: 0.2790 - val_output_ph_loss: 0.2873 - val_output_mg_c_loss: 0.2689 - val_output_c_loss: 0.2481\nEpoch 5/120\n30/30 - 4s - loss: 2.0656 - output_react_loss: 0.2222 - output_bg_ph_loss: 0.2797 - output_ph_loss: 0.2893 - output_mg_c_loss: 0.2634 - output_c_loss: 0.2458 - val_loss: 2.0033 - val_output_react_loss: 0.2101 - val_output_bg_ph_loss: 0.2707 - val_output_ph_loss: 0.2806 - val_output_mg_c_loss: 0.2582 - val_output_c_loss: 0.2447\nEpoch 6/120\n30/30 - 4s - loss: 2.0200 - output_react_loss: 0.2169 - output_bg_ph_loss: 0.2731 - output_ph_loss: 0.2834 - output_mg_c_loss: 0.2571 - output_c_loss: 0.2423 - val_loss: 1.9670 - val_output_react_loss: 0.2091 - val_output_bg_ph_loss: 0.2643 - val_output_ph_loss: 0.2761 - val_output_mg_c_loss: 0.2527 - val_output_c_loss: 0.2388\nEpoch 7/120\n30/30 - 4s - loss: 1.9784 - output_react_loss: 0.2111 - output_bg_ph_loss: 0.2672 - output_ph_loss: 0.2794 - output_mg_c_loss: 0.2514 - output_c_loss: 0.2395 - val_loss: 1.9392 - val_output_react_loss: 0.2027 - val_output_bg_ph_loss: 0.2598 - val_output_ph_loss: 0.2728 - val_output_mg_c_loss: 0.2518 - val_output_c_loss: 0.2378\nEpoch 8/120\n30/30 - 4s - loss: 1.9386 - output_react_loss: 0.2071 - output_bg_ph_loss: 0.2625 - output_ph_loss: 0.2737 - output_mg_c_loss: 0.2455 - output_c_loss: 0.2344 - val_loss: 1.8829 - val_output_react_loss: 0.1985 - val_output_bg_ph_loss: 0.2537 - val_output_ph_loss: 0.2657 - val_output_mg_c_loss: 0.2406 - val_output_c_loss: 0.2314\nEpoch 9/120\n30/30 - 4s - loss: 1.8977 - output_react_loss: 0.2042 - output_bg_ph_loss: 0.2566 - output_ph_loss: 0.2687 - output_mg_c_loss: 0.2393 - output_c_loss: 0.2288 - val_loss: 1.8674 - val_output_react_loss: 0.2007 - val_output_bg_ph_loss: 0.2505 - val_output_ph_loss: 0.2620 - val_output_mg_c_loss: 0.2378 - val_output_c_loss: 0.2274\nEpoch 10/120\n30/30 - 4s - loss: 1.8620 - output_react_loss: 0.2009 - output_bg_ph_loss: 0.2526 - output_ph_loss: 0.2634 - output_mg_c_loss: 0.2335 - output_c_loss: 0.2246 - val_loss: 1.7981 - val_output_react_loss: 0.1917 - val_output_bg_ph_loss: 0.2430 - val_output_ph_loss: 0.2556 - val_output_mg_c_loss: 0.2259 - val_output_c_loss: 0.2214\nEpoch 11/120\n30/30 - 4s - loss: 1.8257 - output_react_loss: 0.1971 - output_bg_ph_loss: 0.2474 - output_ph_loss: 0.2592 - output_mg_c_loss: 0.2282 - output_c_loss: 0.2212 - val_loss: 1.7811 - val_output_react_loss: 0.1907 - val_output_bg_ph_loss: 0.2395 - val_output_ph_loss: 0.2524 - val_output_mg_c_loss: 0.2241 - val_output_c_loss: 0.2202\nEpoch 12/120\n30/30 - 4s - loss: 1.7962 - output_react_loss: 0.1954 - output_bg_ph_loss: 0.2432 - output_ph_loss: 0.2553 - output_mg_c_loss: 0.2234 - output_c_loss: 0.2169 - val_loss: 1.7353 - val_output_react_loss: 0.1875 - val_output_bg_ph_loss: 0.2335 - val_output_ph_loss: 0.2477 - val_output_mg_c_loss: 0.2163 - val_output_c_loss: 0.2132\nEpoch 13/120\n30/30 - 4s - loss: 1.7619 - output_react_loss: 0.1927 - output_bg_ph_loss: 0.2374 - output_ph_loss: 0.2505 - output_mg_c_loss: 0.2186 - output_c_loss: 0.2140 - val_loss: 1.6985 - val_output_react_loss: 0.1841 - val_output_bg_ph_loss: 0.2283 - val_output_ph_loss: 0.2429 - val_output_mg_c_loss: 0.2094 - val_output_c_loss: 0.2120\nEpoch 14/120\n30/30 - 4s - loss: 1.7308 - output_react_loss: 0.1895 - output_bg_ph_loss: 0.2336 - output_ph_loss: 0.2465 - output_mg_c_loss: 0.2141 - output_c_loss: 0.2101 - val_loss: 1.6867 - val_output_react_loss: 0.1823 - val_output_bg_ph_loss: 0.2246 - val_output_ph_loss: 0.2381 - val_output_mg_c_loss: 0.2142 - val_output_c_loss: 0.2063\nEpoch 15/120\n30/30 - 4s - loss: 1.7042 - output_react_loss: 0.1884 - output_bg_ph_loss: 0.2298 - output_ph_loss: 0.2409 - output_mg_c_loss: 0.2103 - output_c_loss: 0.2064 - val_loss: 1.6697 - val_output_react_loss: 0.1855 - val_output_bg_ph_loss: 0.2197 - val_output_ph_loss: 0.2369 - val_output_mg_c_loss: 0.2079 - val_output_c_loss: 0.2067\nEpoch 16/120\n30/30 - 4s - loss: 1.6806 - output_react_loss: 0.1866 - output_bg_ph_loss: 0.2256 - output_ph_loss: 0.2385 - output_mg_c_loss: 0.2068 - output_c_loss: 0.2041 - val_loss: 1.6219 - val_output_react_loss: 0.1798 - val_output_bg_ph_loss: 0.2175 - val_output_ph_loss: 0.2300 - val_output_mg_c_loss: 0.1986 - val_output_c_loss: 0.2002\nEpoch 17/120\n30/30 - 4s - loss: 1.6525 - output_react_loss: 0.1837 - output_bg_ph_loss: 0.2234 - output_ph_loss: 0.2343 - output_mg_c_loss: 0.2017 - output_c_loss: 0.2007 - val_loss: 1.6066 - val_output_react_loss: 0.1761 - val_output_bg_ph_loss: 0.2152 - val_output_ph_loss: 0.2272 - val_output_mg_c_loss: 0.1986 - val_output_c_loss: 0.1997\nEpoch 18/120\n30/30 - 4s - loss: 1.6309 - output_react_loss: 0.1818 - output_bg_ph_loss: 0.2197 - output_ph_loss: 0.2312 - output_mg_c_loss: 0.1988 - output_c_loss: 0.1988 - val_loss: 1.5870 - val_output_react_loss: 0.1748 - val_output_bg_ph_loss: 0.2136 - val_output_ph_loss: 0.2283 - val_output_mg_c_loss: 0.1931 - val_output_c_loss: 0.1955\nEpoch 19/120\n30/30 - 4s - loss: 1.6094 - output_react_loss: 0.1798 - output_bg_ph_loss: 0.2166 - output_ph_loss: 0.2287 - output_mg_c_loss: 0.1956 - output_c_loss: 0.1968 - val_loss: 1.5582 - val_output_react_loss: 0.1740 - val_output_bg_ph_loss: 0.2089 - val_output_ph_loss: 0.2212 - val_output_mg_c_loss: 0.1884 - val_output_c_loss: 0.1945\nEpoch 20/120\n30/30 - 4s - loss: 1.5891 - output_react_loss: 0.1787 - output_bg_ph_loss: 0.2138 - output_ph_loss: 0.2258 - output_mg_c_loss: 0.1922 - output_c_loss: 0.1938 - val_loss: 1.5465 - val_output_react_loss: 0.1723 - val_output_bg_ph_loss: 0.2074 - val_output_ph_loss: 0.2199 - val_output_mg_c_loss: 0.1876 - val_output_c_loss: 0.1918\nEpoch 21/120\n30/30 - 4s - loss: 1.5734 - output_react_loss: 0.1770 - output_bg_ph_loss: 0.2115 - output_ph_loss: 0.2227 - output_mg_c_loss: 0.1901 - output_c_loss: 0.1935 - val_loss: 1.5534 - val_output_react_loss: 0.1733 - val_output_bg_ph_loss: 0.2077 - val_output_ph_loss: 0.2189 - val_output_mg_c_loss: 0.1901 - val_output_c_loss: 0.1923\nEpoch 22/120\n30/30 - 4s - loss: 1.5669 - output_react_loss: 0.1770 - output_bg_ph_loss: 0.2107 - output_ph_loss: 0.2226 - output_mg_c_loss: 0.1891 - output_c_loss: 0.1906 - val_loss: 1.5548 - val_output_react_loss: 0.1749 - val_output_bg_ph_loss: 0.2074 - val_output_ph_loss: 0.2198 - val_output_mg_c_loss: 0.1889 - val_output_c_loss: 0.1926\nEpoch 23/120\n30/30 - 4s - loss: 1.5423 - output_react_loss: 0.1747 - output_bg_ph_loss: 0.2067 - output_ph_loss: 0.2198 - output_mg_c_loss: 0.1855 - output_c_loss: 0.1887 - val_loss: 1.5106 - val_output_react_loss: 0.1702 - val_output_bg_ph_loss: 0.2019 - val_output_ph_loss: 0.2144 - val_output_mg_c_loss: 0.1827 - val_output_c_loss: 0.1866\nEpoch 24/120\n30/30 - 4s - loss: 1.5258 - output_react_loss: 0.1735 - output_bg_ph_loss: 0.2046 - output_ph_loss: 0.2168 - output_mg_c_loss: 0.1829 - output_c_loss: 0.1871 - val_loss: 1.5220 - val_output_react_loss: 0.1725 - val_output_bg_ph_loss: 0.2026 - val_output_ph_loss: 0.2141 - val_output_mg_c_loss: 0.1829 - val_output_c_loss: 0.1918\nEpoch 25/120\n30/30 - 4s - loss: 1.5203 - output_react_loss: 0.1732 - output_bg_ph_loss: 0.2036 - output_ph_loss: 0.2154 - output_mg_c_loss: 0.1820 - output_c_loss: 0.1872 - val_loss: 1.5117 - val_output_react_loss: 0.1699 - val_output_bg_ph_loss: 0.2024 - val_output_ph_loss: 0.2127 - val_output_mg_c_loss: 0.1838 - val_output_c_loss: 0.1870\nEpoch 26/120\n30/30 - 4s - loss: 1.5020 - output_react_loss: 0.1709 - output_bg_ph_loss: 0.2012 - output_ph_loss: 0.2140 - output_mg_c_loss: 0.1795 - output_c_loss: 0.1846 - val_loss: 1.4843 - val_output_react_loss: 0.1663 - val_output_bg_ph_loss: 0.1983 - val_output_ph_loss: 0.2096 - val_output_mg_c_loss: 0.1803 - val_output_c_loss: 0.1847\nEpoch 27/120\n30/30 - 4s - loss: 1.4868 - output_react_loss: 0.1695 - output_bg_ph_loss: 0.1987 - output_ph_loss: 0.2121 - output_mg_c_loss: 0.1775 - output_c_loss: 0.1832 - val_loss: 1.4843 - val_output_react_loss: 0.1678 - val_output_bg_ph_loss: 0.1985 - val_output_ph_loss: 0.2095 - val_output_mg_c_loss: 0.1787 - val_output_c_loss: 0.1849\nEpoch 28/120\n30/30 - 4s - loss: 1.4696 - output_react_loss: 0.1672 - output_bg_ph_loss: 0.1963 - output_ph_loss: 0.2095 - output_mg_c_loss: 0.1760 - output_c_loss: 0.1810 - val_loss: 1.4791 - val_output_react_loss: 0.1653 - val_output_bg_ph_loss: 0.1986 - val_output_ph_loss: 0.2102 - val_output_mg_c_loss: 0.1790 - val_output_c_loss: 0.1831\nEpoch 29/120\n30/30 - 4s - loss: 1.4549 - output_react_loss: 0.1656 - output_bg_ph_loss: 0.1946 - output_ph_loss: 0.2077 - output_mg_c_loss: 0.1733 - output_c_loss: 0.1801 - val_loss: 1.4733 - val_output_react_loss: 0.1647 - val_output_bg_ph_loss: 0.1971 - val_output_ph_loss: 0.2090 - val_output_mg_c_loss: 0.1777 - val_output_c_loss: 0.1851\nEpoch 30/120\n30/30 - 4s - loss: 1.4524 - output_react_loss: 0.1648 - output_bg_ph_loss: 0.1944 - output_ph_loss: 0.2081 - output_mg_c_loss: 0.1729 - output_c_loss: 0.1800 - val_loss: 1.4779 - val_output_react_loss: 0.1646 - val_output_bg_ph_loss: 0.1985 - val_output_ph_loss: 0.2102 - val_output_mg_c_loss: 0.1793 - val_output_c_loss: 0.1831\nEpoch 31/120\n30/30 - 4s - loss: 1.4463 - output_react_loss: 0.1650 - output_bg_ph_loss: 0.1932 - output_ph_loss: 0.2056 - output_mg_c_loss: 0.1727 - output_c_loss: 0.1788 - val_loss: 1.4795 - val_output_react_loss: 0.1642 - val_output_bg_ph_loss: 0.1987 - val_output_ph_loss: 0.2091 - val_output_mg_c_loss: 0.1810 - val_output_c_loss: 0.1825\nEpoch 32/120\n30/30 - 4s - loss: 1.4305 - output_react_loss: 0.1628 - output_bg_ph_loss: 0.1910 - output_ph_loss: 0.2042 - output_mg_c_loss: 0.1709 - output_c_loss: 0.1770 - val_loss: 1.4637 - val_output_react_loss: 0.1654 - val_output_bg_ph_loss: 0.1962 - val_output_ph_loss: 0.2053 - val_output_mg_c_loss: 0.1771 - val_output_c_loss: 0.1809\nEpoch 33/120\n30/30 - 4s - loss: 1.4080 - output_react_loss: 0.1612 - output_bg_ph_loss: 0.1875 - output_ph_loss: 0.2017 - output_mg_c_loss: 0.1669 - output_c_loss: 0.1751 - val_loss: 1.4503 - val_output_react_loss: 0.1617 - val_output_bg_ph_loss: 0.1945 - val_output_ph_loss: 0.2064 - val_output_mg_c_loss: 0.1753 - val_output_c_loss: 0.1808\nEpoch 34/120\n30/30 - 4s - loss: 1.3964 - output_react_loss: 0.1589 - output_bg_ph_loss: 0.1861 - output_ph_loss: 0.2005 - output_mg_c_loss: 0.1656 - output_c_loss: 0.1746 - val_loss: 1.4477 - val_output_react_loss: 0.1612 - val_output_bg_ph_loss: 0.1934 - val_output_ph_loss: 0.2053 - val_output_mg_c_loss: 0.1769 - val_output_c_loss: 0.1796\nEpoch 35/120\n30/30 - 4s - loss: 1.3855 - output_react_loss: 0.1582 - output_bg_ph_loss: 0.1841 - output_ph_loss: 0.1993 - output_mg_c_loss: 0.1642 - output_c_loss: 0.1730 - val_loss: 1.4545 - val_output_react_loss: 0.1636 - val_output_bg_ph_loss: 0.1967 - val_output_ph_loss: 0.2045 - val_output_mg_c_loss: 0.1745 - val_output_c_loss: 0.1804\nEpoch 36/120\n30/30 - 4s - loss: 1.3699 - output_react_loss: 0.1563 - output_bg_ph_loss: 0.1831 - output_ph_loss: 0.1966 - output_mg_c_loss: 0.1612 - output_c_loss: 0.1720 - val_loss: 1.4480 - val_output_react_loss: 0.1625 - val_output_bg_ph_loss: 0.1951 - val_output_ph_loss: 0.2040 - val_output_mg_c_loss: 0.1748 - val_output_c_loss: 0.1791\nEpoch 37/120\n30/30 - 4s - loss: 1.3578 - output_react_loss: 0.1550 - output_bg_ph_loss: 0.1809 - output_ph_loss: 0.1955 - output_mg_c_loss: 0.1600 - output_c_loss: 0.1706 - val_loss: 1.4404 - val_output_react_loss: 0.1603 - val_output_bg_ph_loss: 0.1939 - val_output_ph_loss: 0.2032 - val_output_mg_c_loss: 0.1751 - val_output_c_loss: 0.1785\nEpoch 38/120\n30/30 - 4s - loss: 1.3499 - output_react_loss: 0.1540 - output_bg_ph_loss: 0.1800 - output_ph_loss: 0.1946 - output_mg_c_loss: 0.1585 - output_c_loss: 0.1702 - val_loss: 1.4460 - val_output_react_loss: 0.1627 - val_output_bg_ph_loss: 0.1938 - val_output_ph_loss: 0.2036 - val_output_mg_c_loss: 0.1748 - val_output_c_loss: 0.1797\nEpoch 39/120\n30/30 - 4s - loss: 1.3364 - output_react_loss: 0.1533 - output_bg_ph_loss: 0.1777 - output_ph_loss: 0.1922 - output_mg_c_loss: 0.1568 - output_c_loss: 0.1686 - val_loss: 1.4498 - val_output_react_loss: 0.1634 - val_output_bg_ph_loss: 0.1934 - val_output_ph_loss: 0.2049 - val_output_mg_c_loss: 0.1760 - val_output_c_loss: 0.1794\nEpoch 40/120\n30/30 - 4s - loss: 1.3255 - output_react_loss: 0.1515 - output_bg_ph_loss: 0.1755 - output_ph_loss: 0.1918 - output_mg_c_loss: 0.1559 - output_c_loss: 0.1679 - val_loss: 1.4271 - val_output_react_loss: 0.1605 - val_output_bg_ph_loss: 0.1918 - val_output_ph_loss: 0.2000 - val_output_mg_c_loss: 0.1724 - val_output_c_loss: 0.1777\nEpoch 41/120\n30/30 - 4s - loss: 1.3098 - output_react_loss: 0.1499 - output_bg_ph_loss: 0.1738 - output_ph_loss: 0.1895 - output_mg_c_loss: 0.1530 - output_c_loss: 0.1670 - val_loss: 1.4364 - val_output_react_loss: 0.1606 - val_output_bg_ph_loss: 0.1939 - val_output_ph_loss: 0.2019 - val_output_mg_c_loss: 0.1734 - val_output_c_loss: 0.1787\nEpoch 42/120\n30/30 - 4s - loss: 1.2943 - output_react_loss: 0.1479 - output_bg_ph_loss: 0.1708 - output_ph_loss: 0.1874 - output_mg_c_loss: 0.1519 - output_c_loss: 0.1658 - val_loss: 1.4241 - val_output_react_loss: 0.1592 - val_output_bg_ph_loss: 0.1919 - val_output_ph_loss: 0.1998 - val_output_mg_c_loss: 0.1728 - val_output_c_loss: 0.1764\nEpoch 43/120\n30/30 - 4s - loss: 1.2860 - output_react_loss: 0.1469 - output_bg_ph_loss: 0.1700 - output_ph_loss: 0.1867 - output_mg_c_loss: 0.1503 - output_c_loss: 0.1649 - val_loss: 1.4232 - val_output_react_loss: 0.1588 - val_output_bg_ph_loss: 0.1908 - val_output_ph_loss: 0.2020 - val_output_mg_c_loss: 0.1719 - val_output_c_loss: 0.1782\nEpoch 44/120\n30/30 - 4s - loss: 1.2816 - output_react_loss: 0.1464 - output_bg_ph_loss: 0.1691 - output_ph_loss: 0.1864 - output_mg_c_loss: 0.1499 - output_c_loss: 0.1644 - val_loss: 1.4265 - val_output_react_loss: 0.1592 - val_output_bg_ph_loss: 0.1931 - val_output_ph_loss: 0.2009 - val_output_mg_c_loss: 0.1721 - val_output_c_loss: 0.1766\nEpoch 45/120\n30/30 - 4s - loss: 1.2646 - output_react_loss: 0.1461 - output_bg_ph_loss: 0.1662 - output_ph_loss: 0.1838 - output_mg_c_loss: 0.1468 - output_c_loss: 0.1625 - val_loss: 1.4248 - val_output_react_loss: 0.1617 - val_output_bg_ph_loss: 0.1898 - val_output_ph_loss: 0.2020 - val_output_mg_c_loss: 0.1713 - val_output_c_loss: 0.1771\nEpoch 46/120\n30/30 - 4s - loss: 1.2535 - output_react_loss: 0.1443 - output_bg_ph_loss: 0.1645 - output_ph_loss: 0.1822 - output_mg_c_loss: 0.1457 - output_c_loss: 0.1623 - val_loss: 1.4157 - val_output_react_loss: 0.1588 - val_output_bg_ph_loss: 0.1907 - val_output_ph_loss: 0.1993 - val_output_mg_c_loss: 0.1713 - val_output_c_loss: 0.1747\nEpoch 47/120\n30/30 - 4s - loss: 1.2434 - output_react_loss: 0.1428 - output_bg_ph_loss: 0.1631 - output_ph_loss: 0.1813 - output_mg_c_loss: 0.1444 - output_c_loss: 0.1613 - val_loss: 1.4238 - val_output_react_loss: 0.1591 - val_output_bg_ph_loss: 0.1909 - val_output_ph_loss: 0.1999 - val_output_mg_c_loss: 0.1731 - val_output_c_loss: 0.1779\nEpoch 48/120\n30/30 - 4s - loss: 1.2313 - output_react_loss: 0.1413 - output_bg_ph_loss: 0.1612 - output_ph_loss: 0.1800 - output_mg_c_loss: 0.1430 - output_c_loss: 0.1604 - val_loss: 1.4135 - val_output_react_loss: 0.1573 - val_output_bg_ph_loss: 0.1904 - val_output_ph_loss: 0.1980 - val_output_mg_c_loss: 0.1719 - val_output_c_loss: 0.1762\nEpoch 49/120\n30/30 - 4s - loss: 1.2177 - output_react_loss: 0.1402 - output_bg_ph_loss: 0.1592 - output_ph_loss: 0.1783 - output_mg_c_loss: 0.1408 - output_c_loss: 0.1592 - val_loss: 1.4164 - val_output_react_loss: 0.1576 - val_output_bg_ph_loss: 0.1905 - val_output_ph_loss: 0.2003 - val_output_mg_c_loss: 0.1717 - val_output_c_loss: 0.1764\nEpoch 50/120\n30/30 - 4s - loss: 1.2093 - output_react_loss: 0.1388 - output_bg_ph_loss: 0.1581 - output_ph_loss: 0.1777 - output_mg_c_loss: 0.1397 - output_c_loss: 0.1583 - val_loss: 1.4258 - val_output_react_loss: 0.1585 - val_output_bg_ph_loss: 0.1924 - val_output_ph_loss: 0.2019 - val_output_mg_c_loss: 0.1731 - val_output_c_loss: 0.1760\nEpoch 51/120\n30/30 - 4s - loss: 1.1991 - output_react_loss: 0.1378 - output_bg_ph_loss: 0.1561 - output_ph_loss: 0.1764 - output_mg_c_loss: 0.1387 - output_c_loss: 0.1578 - val_loss: 1.4040 - val_output_react_loss: 0.1570 - val_output_bg_ph_loss: 0.1893 - val_output_ph_loss: 0.1959 - val_output_mg_c_loss: 0.1699 - val_output_c_loss: 0.1756\nEpoch 52/120\n30/30 - 4s - loss: 1.1923 - output_react_loss: 0.1369 - output_bg_ph_loss: 0.1546 - output_ph_loss: 0.1757 - output_mg_c_loss: 0.1380 - output_c_loss: 0.1574 - val_loss: 1.4119 - val_output_react_loss: 0.1568 - val_output_bg_ph_loss: 0.1897 - val_output_ph_loss: 0.1990 - val_output_mg_c_loss: 0.1722 - val_output_c_loss: 0.1756\nEpoch 53/120\n30/30 - 4s - loss: 1.1826 - output_react_loss: 0.1350 - output_bg_ph_loss: 0.1534 - output_ph_loss: 0.1749 - output_mg_c_loss: 0.1371 - output_c_loss: 0.1566 - val_loss: 1.3991 - val_output_react_loss: 0.1555 - val_output_bg_ph_loss: 0.1890 - val_output_ph_loss: 0.1962 - val_output_mg_c_loss: 0.1698 - val_output_c_loss: 0.1744\nEpoch 54/120\n30/30 - 4s - loss: 1.1733 - output_react_loss: 0.1351 - output_bg_ph_loss: 0.1520 - output_ph_loss: 0.1738 - output_mg_c_loss: 0.1352 - output_c_loss: 0.1550 - val_loss: 1.3966 - val_output_react_loss: 0.1555 - val_output_bg_ph_loss: 0.1883 - val_output_ph_loss: 0.1963 - val_output_mg_c_loss: 0.1695 - val_output_c_loss: 0.1737\nEpoch 55/120\n30/30 - 4s - loss: 1.1656 - output_react_loss: 0.1341 - output_bg_ph_loss: 0.1510 - output_ph_loss: 0.1722 - output_mg_c_loss: 0.1341 - output_c_loss: 0.1552 - val_loss: 1.4028 - val_output_react_loss: 0.1571 - val_output_bg_ph_loss: 0.1893 - val_output_ph_loss: 0.1979 - val_output_mg_c_loss: 0.1684 - val_output_c_loss: 0.1754\nEpoch 56/120\n30/30 - 4s - loss: 1.1554 - output_react_loss: 0.1320 - output_bg_ph_loss: 0.1492 - output_ph_loss: 0.1718 - output_mg_c_loss: 0.1333 - output_c_loss: 0.1546 - val_loss: 1.4014 - val_output_react_loss: 0.1566 - val_output_bg_ph_loss: 0.1881 - val_output_ph_loss: 0.1978 - val_output_mg_c_loss: 0.1700 - val_output_c_loss: 0.1743\nEpoch 57/120\n30/30 - 4s - loss: 1.1445 - output_react_loss: 0.1312 - output_bg_ph_loss: 0.1472 - output_ph_loss: 0.1705 - output_mg_c_loss: 0.1321 - output_c_loss: 0.1531 - val_loss: 1.3987 - val_output_react_loss: 0.1584 - val_output_bg_ph_loss: 0.1880 - val_output_ph_loss: 0.1964 - val_output_mg_c_loss: 0.1679 - val_output_c_loss: 0.1737\nEpoch 58/120\n30/30 - 4s - loss: 1.1385 - output_react_loss: 0.1304 - output_bg_ph_loss: 0.1464 - output_ph_loss: 0.1695 - output_mg_c_loss: 0.1314 - output_c_loss: 0.1527 - val_loss: 1.3989 - val_output_react_loss: 0.1554 - val_output_bg_ph_loss: 0.1891 - val_output_ph_loss: 0.1963 - val_output_mg_c_loss: 0.1695 - val_output_c_loss: 0.1748\nEpoch 59/120\n\nEpoch 00059: ReduceLROnPlateau reducing learning rate to 0.00010000000474974513.\n30/30 - 4s - loss: 1.1291 - output_react_loss: 0.1290 - output_bg_ph_loss: 0.1454 - output_ph_loss: 0.1685 - output_mg_c_loss: 0.1298 - output_c_loss: 0.1523 - val_loss: 1.4020 - val_output_react_loss: 0.1566 - val_output_bg_ph_loss: 0.1908 - val_output_ph_loss: 0.1954 - val_output_mg_c_loss: 0.1697 - val_output_c_loss: 0.1724\nEpoch 60/120\n30/30 - 4s - loss: 1.0961 - output_react_loss: 0.1250 - output_bg_ph_loss: 0.1409 - output_ph_loss: 0.1642 - output_mg_c_loss: 0.1256 - output_c_loss: 0.1490 - val_loss: 1.3785 - val_output_react_loss: 0.1536 - val_output_bg_ph_loss: 0.1865 - val_output_ph_loss: 0.1942 - val_output_mg_c_loss: 0.1664 - val_output_c_loss: 0.1715\nEpoch 61/120\n30/30 - 4s - loss: 1.0803 - output_react_loss: 0.1238 - output_bg_ph_loss: 0.1385 - output_ph_loss: 0.1622 - output_mg_c_loss: 0.1229 - output_c_loss: 0.1477 - val_loss: 1.3770 - val_output_react_loss: 0.1537 - val_output_bg_ph_loss: 0.1859 - val_output_ph_loss: 0.1939 - val_output_mg_c_loss: 0.1665 - val_output_c_loss: 0.1710\nEpoch 62/120\n30/30 - 4s - loss: 1.0757 - output_react_loss: 0.1226 - output_bg_ph_loss: 0.1379 - output_ph_loss: 0.1620 - output_mg_c_loss: 0.1226 - output_c_loss: 0.1474 - val_loss: 1.3769 - val_output_react_loss: 0.1540 - val_output_bg_ph_loss: 0.1861 - val_output_ph_loss: 0.1931 - val_output_mg_c_loss: 0.1663 - val_output_c_loss: 0.1710\nEpoch 63/120\n30/30 - 4s - loss: 1.0726 - output_react_loss: 0.1226 - output_bg_ph_loss: 0.1371 - output_ph_loss: 0.1613 - output_mg_c_loss: 0.1224 - output_c_loss: 0.1471 - val_loss: 1.3742 - val_output_react_loss: 0.1535 - val_output_bg_ph_loss: 0.1855 - val_output_ph_loss: 0.1931 - val_output_mg_c_loss: 0.1660 - val_output_c_loss: 0.1710\nEpoch 64/120\n30/30 - 4s - loss: 1.0701 - output_react_loss: 0.1222 - output_bg_ph_loss: 0.1370 - output_ph_loss: 0.1612 - output_mg_c_loss: 0.1219 - output_c_loss: 0.1469 - val_loss: 1.3771 - val_output_react_loss: 0.1539 - val_output_bg_ph_loss: 0.1862 - val_output_ph_loss: 0.1932 - val_output_mg_c_loss: 0.1664 - val_output_c_loss: 0.1710\nEpoch 65/120\n30/30 - 4s - loss: 1.0683 - output_react_loss: 0.1217 - output_bg_ph_loss: 0.1365 - output_ph_loss: 0.1614 - output_mg_c_loss: 0.1218 - output_c_loss: 0.1469 - val_loss: 1.3770 - val_output_react_loss: 0.1540 - val_output_bg_ph_loss: 0.1861 - val_output_ph_loss: 0.1932 - val_output_mg_c_loss: 0.1663 - val_output_c_loss: 0.1708\nEpoch 66/120\n30/30 - 4s - loss: 1.0662 - output_react_loss: 0.1215 - output_bg_ph_loss: 0.1363 - output_ph_loss: 0.1608 - output_mg_c_loss: 0.1215 - output_c_loss: 0.1467 - val_loss: 1.3724 - val_output_react_loss: 0.1534 - val_output_bg_ph_loss: 0.1858 - val_output_ph_loss: 0.1926 - val_output_mg_c_loss: 0.1655 - val_output_c_loss: 0.1705\nEpoch 67/120\n30/30 - 4s - loss: 1.0620 - output_react_loss: 0.1211 - output_bg_ph_loss: 0.1360 - output_ph_loss: 0.1600 - output_mg_c_loss: 0.1208 - output_c_loss: 0.1462 - val_loss: 1.3752 - val_output_react_loss: 0.1537 - val_output_bg_ph_loss: 0.1860 - val_output_ph_loss: 0.1928 - val_output_mg_c_loss: 0.1661 - val_output_c_loss: 0.1707\nEpoch 68/120\n30/30 - 4s - loss: 1.0630 - output_react_loss: 0.1213 - output_bg_ph_loss: 0.1362 - output_ph_loss: 0.1605 - output_mg_c_loss: 0.1208 - output_c_loss: 0.1459 - val_loss: 1.3735 - val_output_react_loss: 0.1538 - val_output_bg_ph_loss: 0.1854 - val_output_ph_loss: 0.1928 - val_output_mg_c_loss: 0.1658 - val_output_c_loss: 0.1707\nEpoch 69/120\n30/30 - 4s - loss: 1.0598 - output_react_loss: 0.1211 - output_bg_ph_loss: 0.1353 - output_ph_loss: 0.1602 - output_mg_c_loss: 0.1203 - output_c_loss: 0.1461 - val_loss: 1.3737 - val_output_react_loss: 0.1537 - val_output_bg_ph_loss: 0.1856 - val_output_ph_loss: 0.1925 - val_output_mg_c_loss: 0.1661 - val_output_c_loss: 0.1706\nEpoch 70/120\n30/30 - 4s - loss: 1.0572 - output_react_loss: 0.1203 - output_bg_ph_loss: 0.1349 - output_ph_loss: 0.1597 - output_mg_c_loss: 0.1204 - output_c_loss: 0.1462 - val_loss: 1.3752 - val_output_react_loss: 0.1540 - val_output_bg_ph_loss: 0.1860 - val_output_ph_loss: 0.1928 - val_output_mg_c_loss: 0.1658 - val_output_c_loss: 0.1709\nEpoch 71/120\n\nEpoch 00071: ReduceLROnPlateau reducing learning rate to 1.0000000474974514e-05.\n30/30 - 4s - loss: 1.0552 - output_react_loss: 0.1202 - output_bg_ph_loss: 0.1348 - output_ph_loss: 0.1597 - output_mg_c_loss: 0.1198 - output_c_loss: 0.1457 - val_loss: 1.3739 - val_output_react_loss: 0.1537 - val_output_bg_ph_loss: 0.1856 - val_output_ph_loss: 0.1925 - val_output_mg_c_loss: 0.1660 - val_output_c_loss: 0.1707\nEpoch 72/120\n30/30 - 4s - loss: 1.0525 - output_react_loss: 0.1202 - output_bg_ph_loss: 0.1344 - output_ph_loss: 0.1588 - output_mg_c_loss: 0.1196 - output_c_loss: 0.1453 - val_loss: 1.3732 - val_output_react_loss: 0.1535 - val_output_bg_ph_loss: 0.1856 - val_output_ph_loss: 0.1926 - val_output_mg_c_loss: 0.1658 - val_output_c_loss: 0.1707\nEpoch 73/120\n30/30 - 4s - loss: 1.0532 - output_react_loss: 0.1202 - output_bg_ph_loss: 0.1344 - output_ph_loss: 0.1589 - output_mg_c_loss: 0.1198 - output_c_loss: 0.1455 - val_loss: 1.3745 - val_output_react_loss: 0.1537 - val_output_bg_ph_loss: 0.1859 - val_output_ph_loss: 0.1927 - val_output_mg_c_loss: 0.1659 - val_output_c_loss: 0.1707\nEpoch 74/120\n30/30 - 4s - loss: 1.0519 - output_react_loss: 0.1197 - output_bg_ph_loss: 0.1340 - output_ph_loss: 0.1591 - output_mg_c_loss: 0.1198 - output_c_loss: 0.1458 - val_loss: 1.3735 - val_output_react_loss: 0.1536 - val_output_bg_ph_loss: 0.1857 - val_output_ph_loss: 0.1927 - val_output_mg_c_loss: 0.1657 - val_output_c_loss: 0.1706\nEpoch 75/120\n30/30 - 4s - loss: 1.0519 - output_react_loss: 0.1201 - output_bg_ph_loss: 0.1342 - output_ph_loss: 0.1590 - output_mg_c_loss: 0.1194 - output_c_loss: 0.1454 - val_loss: 1.3752 - val_output_react_loss: 0.1538 - val_output_bg_ph_loss: 0.1861 - val_output_ph_loss: 0.1929 - val_output_mg_c_loss: 0.1660 - val_output_c_loss: 0.1706\nEpoch 76/120\nRestoring model weights from the end of the best epoch.\n\nEpoch 00076: ReduceLROnPlateau reducing learning rate to 1.0000000656873453e-06.\n30/30 - 4s - loss: 1.0535 - output_react_loss: 0.1202 - output_bg_ph_loss: 0.1344 - output_ph_loss: 0.1595 - output_mg_c_loss: 0.1198 - output_c_loss: 0.1453 - val_loss: 1.3733 - val_output_react_loss: 0.1535 - val_output_bg_ph_loss: 0.1857 - val_output_ph_loss: 0.1926 - val_output_mg_c_loss: 0.1658 - val_output_c_loss: 0.1706\nEpoch 00076: early stopping\n\nFOLD: 2\nEpoch 1/120\n30/30 - 6s - loss: 3.6145 - output_react_loss: 0.3859 - output_bg_ph_loss: 0.5921 - output_ph_loss: 0.4493 - output_mg_c_loss: 0.4325 - output_c_loss: 0.3441 - val_loss: 2.3399 - val_output_react_loss: 0.2505 - val_output_bg_ph_loss: 0.3187 - val_output_ph_loss: 0.3453 - val_output_mg_c_loss: 0.2940 - val_output_c_loss: 0.2685\nEpoch 2/120\n30/30 - 4s - loss: 2.3576 - output_react_loss: 0.2459 - output_bg_ph_loss: 0.3224 - output_ph_loss: 0.3355 - output_mg_c_loss: 0.3020 - output_c_loss: 0.2814 - val_loss: 2.2172 - val_output_react_loss: 0.2371 - val_output_bg_ph_loss: 0.3000 - val_output_ph_loss: 0.3191 - val_output_mg_c_loss: 0.2854 - val_output_c_loss: 0.2530\nEpoch 3/120\n30/30 - 4s - loss: 2.2149 - output_react_loss: 0.2330 - output_bg_ph_loss: 0.3027 - output_ph_loss: 0.3102 - output_mg_c_loss: 0.2846 - output_c_loss: 0.2642 - val_loss: 2.0746 - val_output_react_loss: 0.2288 - val_output_bg_ph_loss: 0.2810 - val_output_ph_loss: 0.2896 - val_output_mg_c_loss: 0.2628 - val_output_c_loss: 0.2399\nEpoch 4/120\n30/30 - 4s - loss: 2.1275 - output_react_loss: 0.2246 - output_bg_ph_loss: 0.2904 - output_ph_loss: 0.2974 - output_mg_c_loss: 0.2725 - output_c_loss: 0.2550 - val_loss: 2.0056 - val_output_react_loss: 0.2222 - val_output_bg_ph_loss: 0.2705 - val_output_ph_loss: 0.2807 - val_output_mg_c_loss: 0.2531 - val_output_c_loss: 0.2333\nEpoch 5/120\n30/30 - 4s - loss: 2.0735 - output_react_loss: 0.2192 - output_bg_ph_loss: 0.2818 - output_ph_loss: 0.2905 - output_mg_c_loss: 0.2655 - output_c_loss: 0.2498 - val_loss: 1.9704 - val_output_react_loss: 0.2194 - val_output_bg_ph_loss: 0.2644 - val_output_ph_loss: 0.2763 - val_output_mg_c_loss: 0.2475 - val_output_c_loss: 0.2314\nEpoch 6/120\n30/30 - 4s - loss: 2.0289 - output_react_loss: 0.2151 - output_bg_ph_loss: 0.2754 - output_ph_loss: 0.2853 - output_mg_c_loss: 0.2589 - output_c_loss: 0.2449 - val_loss: 1.9270 - val_output_react_loss: 0.2155 - val_output_bg_ph_loss: 0.2589 - val_output_ph_loss: 0.2696 - val_output_mg_c_loss: 0.2413 - val_output_c_loss: 0.2260\nEpoch 7/120\n30/30 - 4s - loss: 1.9888 - output_react_loss: 0.2102 - output_bg_ph_loss: 0.2702 - output_ph_loss: 0.2806 - output_mg_c_loss: 0.2530 - output_c_loss: 0.2415 - val_loss: 1.8879 - val_output_react_loss: 0.2115 - val_output_bg_ph_loss: 0.2536 - val_output_ph_loss: 0.2647 - val_output_mg_c_loss: 0.2361 - val_output_c_loss: 0.2208\nEpoch 8/120\n30/30 - 4s - loss: 1.9514 - output_react_loss: 0.2075 - output_bg_ph_loss: 0.2642 - output_ph_loss: 0.2749 - output_mg_c_loss: 0.2480 - output_c_loss: 0.2371 - val_loss: 1.8471 - val_output_react_loss: 0.2068 - val_output_bg_ph_loss: 0.2480 - val_output_ph_loss: 0.2605 - val_output_mg_c_loss: 0.2298 - val_output_c_loss: 0.2174\nEpoch 9/120\n30/30 - 4s - loss: 1.9122 - output_react_loss: 0.2040 - output_bg_ph_loss: 0.2591 - output_ph_loss: 0.2702 - output_mg_c_loss: 0.2419 - output_c_loss: 0.2320 - val_loss: 1.8044 - val_output_react_loss: 0.2034 - val_output_bg_ph_loss: 0.2428 - val_output_ph_loss: 0.2542 - val_output_mg_c_loss: 0.2228 - val_output_c_loss: 0.2123\nEpoch 10/120\n30/30 - 4s - loss: 1.8711 - output_react_loss: 0.2001 - output_bg_ph_loss: 0.2537 - output_ph_loss: 0.2650 - output_mg_c_loss: 0.2357 - output_c_loss: 0.2270 - val_loss: 1.7675 - val_output_react_loss: 0.1992 - val_output_bg_ph_loss: 0.2380 - val_output_ph_loss: 0.2501 - val_output_mg_c_loss: 0.2171 - val_output_c_loss: 0.2087\nEpoch 11/120\n30/30 - 4s - loss: 1.8335 - output_react_loss: 0.1971 - output_bg_ph_loss: 0.2484 - output_ph_loss: 0.2608 - output_mg_c_loss: 0.2294 - output_c_loss: 0.2228 - val_loss: 1.7336 - val_output_react_loss: 0.1977 - val_output_bg_ph_loss: 0.2323 - val_output_ph_loss: 0.2453 - val_output_mg_c_loss: 0.2118 - val_output_c_loss: 0.2047\nEpoch 12/120\n30/30 - 4s - loss: 1.8107 - output_react_loss: 0.1965 - output_bg_ph_loss: 0.2439 - output_ph_loss: 0.2586 - output_mg_c_loss: 0.2258 - output_c_loss: 0.2198 - val_loss: 1.7074 - val_output_react_loss: 0.1958 - val_output_bg_ph_loss: 0.2284 - val_output_ph_loss: 0.2408 - val_output_mg_c_loss: 0.2082 - val_output_c_loss: 0.2018\nEpoch 13/120\n30/30 - 4s - loss: 1.7743 - output_react_loss: 0.1928 - output_bg_ph_loss: 0.2393 - output_ph_loss: 0.2523 - output_mg_c_loss: 0.2205 - output_c_loss: 0.2166 - val_loss: 1.6761 - val_output_react_loss: 0.1924 - val_output_bg_ph_loss: 0.2229 - val_output_ph_loss: 0.2397 - val_output_mg_c_loss: 0.2029 - val_output_c_loss: 0.1999\nEpoch 14/120\n30/30 - 4s - loss: 1.7513 - output_react_loss: 0.1912 - output_bg_ph_loss: 0.2354 - output_ph_loss: 0.2497 - output_mg_c_loss: 0.2166 - output_c_loss: 0.2151 - val_loss: 1.6811 - val_output_react_loss: 0.1942 - val_output_bg_ph_loss: 0.2250 - val_output_ph_loss: 0.2379 - val_output_mg_c_loss: 0.2030 - val_output_c_loss: 0.1988\nEpoch 15/120\n30/30 - 4s - loss: 1.7119 - output_react_loss: 0.1879 - output_bg_ph_loss: 0.2308 - output_ph_loss: 0.2437 - output_mg_c_loss: 0.2109 - output_c_loss: 0.2090 - val_loss: 1.6297 - val_output_react_loss: 0.1884 - val_output_bg_ph_loss: 0.2167 - val_output_ph_loss: 0.2328 - val_output_mg_c_loss: 0.1967 - val_output_c_loss: 0.1935\nEpoch 16/120\n30/30 - 4s - loss: 1.6810 - output_react_loss: 0.1860 - output_bg_ph_loss: 0.2267 - output_ph_loss: 0.2385 - output_mg_c_loss: 0.2058 - output_c_loss: 0.2055 - val_loss: 1.6170 - val_output_react_loss: 0.1875 - val_output_bg_ph_loss: 0.2155 - val_output_ph_loss: 0.2305 - val_output_mg_c_loss: 0.1937 - val_output_c_loss: 0.1930\nEpoch 17/120\n30/30 - 4s - loss: 1.6523 - output_react_loss: 0.1832 - output_bg_ph_loss: 0.2224 - output_ph_loss: 0.2354 - output_mg_c_loss: 0.2018 - output_c_loss: 0.2021 - val_loss: 1.5968 - val_output_react_loss: 0.1862 - val_output_bg_ph_loss: 0.2149 - val_output_ph_loss: 0.2259 - val_output_mg_c_loss: 0.1904 - val_output_c_loss: 0.1879\nEpoch 18/120\n30/30 - 4s - loss: 1.6380 - output_react_loss: 0.1828 - output_bg_ph_loss: 0.2208 - output_ph_loss: 0.2325 - output_mg_c_loss: 0.1994 - output_c_loss: 0.1995 - val_loss: 1.5862 - val_output_react_loss: 0.1854 - val_output_bg_ph_loss: 0.2106 - val_output_ph_loss: 0.2241 - val_output_mg_c_loss: 0.1908 - val_output_c_loss: 0.1885\nEpoch 19/120\n30/30 - 4s - loss: 1.6157 - output_react_loss: 0.1806 - output_bg_ph_loss: 0.2169 - output_ph_loss: 0.2290 - output_mg_c_loss: 0.1973 - output_c_loss: 0.1972 - val_loss: 1.5765 - val_output_react_loss: 0.1847 - val_output_bg_ph_loss: 0.2082 - val_output_ph_loss: 0.2216 - val_output_mg_c_loss: 0.1914 - val_output_c_loss: 0.1865\nEpoch 20/120\n30/30 - 4s - loss: 1.6029 - output_react_loss: 0.1791 - output_bg_ph_loss: 0.2152 - output_ph_loss: 0.2268 - output_mg_c_loss: 0.1956 - output_c_loss: 0.1964 - val_loss: 1.5573 - val_output_react_loss: 0.1836 - val_output_bg_ph_loss: 0.2079 - val_output_ph_loss: 0.2193 - val_output_mg_c_loss: 0.1852 - val_output_c_loss: 0.1847\nEpoch 21/120\n30/30 - 4s - loss: 1.5803 - output_react_loss: 0.1772 - output_bg_ph_loss: 0.2124 - output_ph_loss: 0.2248 - output_mg_c_loss: 0.1916 - output_c_loss: 0.1931 - val_loss: 1.5394 - val_output_react_loss: 0.1816 - val_output_bg_ph_loss: 0.2045 - val_output_ph_loss: 0.2176 - val_output_mg_c_loss: 0.1823 - val_output_c_loss: 0.1849\nEpoch 22/120\n30/30 - 4s - loss: 1.5563 - output_react_loss: 0.1744 - output_bg_ph_loss: 0.2090 - output_ph_loss: 0.2214 - output_mg_c_loss: 0.1885 - output_c_loss: 0.1912 - val_loss: 1.5260 - val_output_react_loss: 0.1777 - val_output_bg_ph_loss: 0.2033 - val_output_ph_loss: 0.2172 - val_output_mg_c_loss: 0.1819 - val_output_c_loss: 0.1830\nEpoch 23/120\n30/30 - 4s - loss: 1.5434 - output_react_loss: 0.1736 - output_bg_ph_loss: 0.2071 - output_ph_loss: 0.2185 - output_mg_c_loss: 0.1869 - output_c_loss: 0.1897 - val_loss: 1.5234 - val_output_react_loss: 0.1809 - val_output_bg_ph_loss: 0.2025 - val_output_ph_loss: 0.2137 - val_output_mg_c_loss: 0.1805 - val_output_c_loss: 0.1819\nEpoch 24/120\n30/30 - 4s - loss: 1.5343 - output_react_loss: 0.1722 - output_bg_ph_loss: 0.2054 - output_ph_loss: 0.2185 - output_mg_c_loss: 0.1856 - output_c_loss: 0.1893 - val_loss: 1.5283 - val_output_react_loss: 0.1780 - val_output_bg_ph_loss: 0.2032 - val_output_ph_loss: 0.2157 - val_output_mg_c_loss: 0.1841 - val_output_c_loss: 0.1821\nEpoch 25/120\n30/30 - 4s - loss: 1.5174 - output_react_loss: 0.1716 - output_bg_ph_loss: 0.2034 - output_ph_loss: 0.2156 - output_mg_c_loss: 0.1826 - output_c_loss: 0.1867 - val_loss: 1.5109 - val_output_react_loss: 0.1774 - val_output_bg_ph_loss: 0.2005 - val_output_ph_loss: 0.2153 - val_output_mg_c_loss: 0.1783 - val_output_c_loss: 0.1831\nEpoch 26/120\n30/30 - 4s - loss: 1.5015 - output_react_loss: 0.1694 - output_bg_ph_loss: 0.2014 - output_ph_loss: 0.2131 - output_mg_c_loss: 0.1808 - output_c_loss: 0.1853 - val_loss: 1.5142 - val_output_react_loss: 0.1784 - val_output_bg_ph_loss: 0.2008 - val_output_ph_loss: 0.2152 - val_output_mg_c_loss: 0.1790 - val_output_c_loss: 0.1829\nEpoch 27/120\n30/30 - 4s - loss: 1.4996 - output_react_loss: 0.1689 - output_bg_ph_loss: 0.2007 - output_ph_loss: 0.2131 - output_mg_c_loss: 0.1809 - output_c_loss: 0.1855 - val_loss: 1.4946 - val_output_react_loss: 0.1759 - val_output_bg_ph_loss: 0.1988 - val_output_ph_loss: 0.2121 - val_output_mg_c_loss: 0.1765 - val_output_c_loss: 0.1802\nEpoch 28/120\n30/30 - 4s - loss: 1.4816 - output_react_loss: 0.1671 - output_bg_ph_loss: 0.1989 - output_ph_loss: 0.2108 - output_mg_c_loss: 0.1780 - output_c_loss: 0.1829 - val_loss: 1.4931 - val_output_react_loss: 0.1736 - val_output_bg_ph_loss: 0.1997 - val_output_ph_loss: 0.2120 - val_output_mg_c_loss: 0.1778 - val_output_c_loss: 0.1788\nEpoch 29/120\n30/30 - 4s - loss: 1.4568 - output_react_loss: 0.1649 - output_bg_ph_loss: 0.1953 - output_ph_loss: 0.2080 - output_mg_c_loss: 0.1738 - output_c_loss: 0.1806 - val_loss: 1.4792 - val_output_react_loss: 0.1718 - val_output_bg_ph_loss: 0.1966 - val_output_ph_loss: 0.2116 - val_output_mg_c_loss: 0.1762 - val_output_c_loss: 0.1785\nEpoch 30/120\n30/30 - 4s - loss: 1.4500 - output_react_loss: 0.1651 - output_bg_ph_loss: 0.1939 - output_ph_loss: 0.2071 - output_mg_c_loss: 0.1727 - output_c_loss: 0.1796 - val_loss: 1.4692 - val_output_react_loss: 0.1729 - val_output_bg_ph_loss: 0.1954 - val_output_ph_loss: 0.2076 - val_output_mg_c_loss: 0.1738 - val_output_c_loss: 0.1774\nEpoch 31/120\n30/30 - 4s - loss: 1.4315 - output_react_loss: 0.1628 - output_bg_ph_loss: 0.1913 - output_ph_loss: 0.2046 - output_mg_c_loss: 0.1702 - output_c_loss: 0.1783 - val_loss: 1.4725 - val_output_react_loss: 0.1725 - val_output_bg_ph_loss: 0.1965 - val_output_ph_loss: 0.2093 - val_output_mg_c_loss: 0.1739 - val_output_c_loss: 0.1775\nEpoch 32/120\n30/30 - 4s - loss: 1.4301 - output_react_loss: 0.1631 - output_bg_ph_loss: 0.1913 - output_ph_loss: 0.2036 - output_mg_c_loss: 0.1701 - output_c_loss: 0.1775 - val_loss: 1.4742 - val_output_react_loss: 0.1708 - val_output_bg_ph_loss: 0.1988 - val_output_ph_loss: 0.2076 - val_output_mg_c_loss: 0.1744 - val_output_c_loss: 0.1785\nEpoch 33/120\n30/30 - 4s - loss: 1.4128 - output_react_loss: 0.1607 - output_bg_ph_loss: 0.1888 - output_ph_loss: 0.2019 - output_mg_c_loss: 0.1677 - output_c_loss: 0.1768 - val_loss: 1.4512 - val_output_react_loss: 0.1699 - val_output_bg_ph_loss: 0.1930 - val_output_ph_loss: 0.2068 - val_output_mg_c_loss: 0.1718 - val_output_c_loss: 0.1749\nEpoch 34/120\n30/30 - 4s - loss: 1.4018 - output_react_loss: 0.1591 - output_bg_ph_loss: 0.1874 - output_ph_loss: 0.1999 - output_mg_c_loss: 0.1671 - output_c_loss: 0.1747 - val_loss: 1.4551 - val_output_react_loss: 0.1690 - val_output_bg_ph_loss: 0.1959 - val_output_ph_loss: 0.2054 - val_output_mg_c_loss: 0.1725 - val_output_c_loss: 0.1748\nEpoch 35/120\n30/30 - 4s - loss: 1.3803 - output_react_loss: 0.1570 - output_bg_ph_loss: 0.1846 - output_ph_loss: 0.1977 - output_mg_c_loss: 0.1634 - output_c_loss: 0.1726 - val_loss: 1.4474 - val_output_react_loss: 0.1691 - val_output_bg_ph_loss: 0.1930 - val_output_ph_loss: 0.2054 - val_output_mg_c_loss: 0.1712 - val_output_c_loss: 0.1755\nEpoch 36/120\n30/30 - 4s - loss: 1.3753 - output_react_loss: 0.1562 - output_bg_ph_loss: 0.1836 - output_ph_loss: 0.1969 - output_mg_c_loss: 0.1628 - output_c_loss: 0.1732 - val_loss: 1.4415 - val_output_react_loss: 0.1677 - val_output_bg_ph_loss: 0.1933 - val_output_ph_loss: 0.2050 - val_output_mg_c_loss: 0.1693 - val_output_c_loss: 0.1759\nEpoch 37/120\n30/30 - 4s - loss: 1.3664 - output_react_loss: 0.1550 - output_bg_ph_loss: 0.1825 - output_ph_loss: 0.1963 - output_mg_c_loss: 0.1618 - output_c_loss: 0.1714 - val_loss: 1.4405 - val_output_react_loss: 0.1660 - val_output_bg_ph_loss: 0.1931 - val_output_ph_loss: 0.2038 - val_output_mg_c_loss: 0.1717 - val_output_c_loss: 0.1752\nEpoch 38/120\n30/30 - 4s - loss: 1.3563 - output_react_loss: 0.1542 - output_bg_ph_loss: 0.1803 - output_ph_loss: 0.1948 - output_mg_c_loss: 0.1611 - output_c_loss: 0.1705 - val_loss: 1.4525 - val_output_react_loss: 0.1674 - val_output_bg_ph_loss: 0.1941 - val_output_ph_loss: 0.2042 - val_output_mg_c_loss: 0.1751 - val_output_c_loss: 0.1751\nEpoch 39/120\n30/30 - 4s - loss: 1.3425 - output_react_loss: 0.1530 - output_bg_ph_loss: 0.1785 - output_ph_loss: 0.1926 - output_mg_c_loss: 0.1587 - output_c_loss: 0.1695 - val_loss: 1.4482 - val_output_react_loss: 0.1660 - val_output_bg_ph_loss: 0.1944 - val_output_ph_loss: 0.2048 - val_output_mg_c_loss: 0.1745 - val_output_c_loss: 0.1739\nEpoch 40/120\n30/30 - 4s - loss: 1.3267 - output_react_loss: 0.1514 - output_bg_ph_loss: 0.1765 - output_ph_loss: 0.1906 - output_mg_c_loss: 0.1561 - output_c_loss: 0.1680 - val_loss: 1.4371 - val_output_react_loss: 0.1674 - val_output_bg_ph_loss: 0.1924 - val_output_ph_loss: 0.2034 - val_output_mg_c_loss: 0.1701 - val_output_c_loss: 0.1740\nEpoch 41/120\n30/30 - 4s - loss: 1.3195 - output_react_loss: 0.1513 - output_bg_ph_loss: 0.1748 - output_ph_loss: 0.1901 - output_mg_c_loss: 0.1548 - output_c_loss: 0.1675 - val_loss: 1.4320 - val_output_react_loss: 0.1677 - val_output_bg_ph_loss: 0.1907 - val_output_ph_loss: 0.2030 - val_output_mg_c_loss: 0.1695 - val_output_c_loss: 0.1732\nEpoch 42/120\n30/30 - 4s - loss: 1.3130 - output_react_loss: 0.1507 - output_bg_ph_loss: 0.1737 - output_ph_loss: 0.1888 - output_mg_c_loss: 0.1543 - output_c_loss: 0.1668 - val_loss: 1.4384 - val_output_react_loss: 0.1667 - val_output_bg_ph_loss: 0.1920 - val_output_ph_loss: 0.2024 - val_output_mg_c_loss: 0.1724 - val_output_c_loss: 0.1740\nEpoch 43/120\n30/30 - 4s - loss: 1.2921 - output_react_loss: 0.1475 - output_bg_ph_loss: 0.1710 - output_ph_loss: 0.1871 - output_mg_c_loss: 0.1514 - output_c_loss: 0.1653 - val_loss: 1.4282 - val_output_react_loss: 0.1652 - val_output_bg_ph_loss: 0.1922 - val_output_ph_loss: 0.2021 - val_output_mg_c_loss: 0.1695 - val_output_c_loss: 0.1723\nEpoch 44/120\n30/30 - 4s - loss: 1.2815 - output_react_loss: 0.1466 - output_bg_ph_loss: 0.1692 - output_ph_loss: 0.1856 - output_mg_c_loss: 0.1500 - output_c_loss: 0.1642 - val_loss: 1.4339 - val_output_react_loss: 0.1656 - val_output_bg_ph_loss: 0.1940 - val_output_ph_loss: 0.2022 - val_output_mg_c_loss: 0.1696 - val_output_c_loss: 0.1732\nEpoch 45/120\n30/30 - 4s - loss: 1.2732 - output_react_loss: 0.1459 - output_bg_ph_loss: 0.1680 - output_ph_loss: 0.1845 - output_mg_c_loss: 0.1482 - output_c_loss: 0.1642 - val_loss: 1.4221 - val_output_react_loss: 0.1641 - val_output_bg_ph_loss: 0.1924 - val_output_ph_loss: 0.2002 - val_output_mg_c_loss: 0.1686 - val_output_c_loss: 0.1718\nEpoch 46/120\n30/30 - 4s - loss: 1.2570 - output_react_loss: 0.1437 - output_bg_ph_loss: 0.1657 - output_ph_loss: 0.1829 - output_mg_c_loss: 0.1467 - output_c_loss: 0.1619 - val_loss: 1.4303 - val_output_react_loss: 0.1656 - val_output_bg_ph_loss: 0.1914 - val_output_ph_loss: 0.2012 - val_output_mg_c_loss: 0.1709 - val_output_c_loss: 0.1733\nEpoch 47/120\n30/30 - 4s - loss: 1.2501 - output_react_loss: 0.1438 - output_bg_ph_loss: 0.1645 - output_ph_loss: 0.1816 - output_mg_c_loss: 0.1453 - output_c_loss: 0.1614 - val_loss: 1.4307 - val_output_react_loss: 0.1647 - val_output_bg_ph_loss: 0.1925 - val_output_ph_loss: 0.2022 - val_output_mg_c_loss: 0.1704 - val_output_c_loss: 0.1734\nEpoch 48/120\n30/30 - 4s - loss: 1.2440 - output_react_loss: 0.1427 - output_bg_ph_loss: 0.1635 - output_ph_loss: 0.1804 - output_mg_c_loss: 0.1451 - output_c_loss: 0.1610 - val_loss: 1.4270 - val_output_react_loss: 0.1643 - val_output_bg_ph_loss: 0.1927 - val_output_ph_loss: 0.2016 - val_output_mg_c_loss: 0.1691 - val_output_c_loss: 0.1731\nEpoch 49/120\n30/30 - 4s - loss: 1.2308 - output_react_loss: 0.1412 - output_bg_ph_loss: 0.1621 - output_ph_loss: 0.1796 - output_mg_c_loss: 0.1426 - output_c_loss: 0.1595 - val_loss: 1.4181 - val_output_react_loss: 0.1639 - val_output_bg_ph_loss: 0.1909 - val_output_ph_loss: 0.2019 - val_output_mg_c_loss: 0.1673 - val_output_c_loss: 0.1721\nEpoch 50/120\n30/30 - 4s - loss: 1.2201 - output_react_loss: 0.1394 - output_bg_ph_loss: 0.1601 - output_ph_loss: 0.1780 - output_mg_c_loss: 0.1418 - output_c_loss: 0.1596 - val_loss: 1.4202 - val_output_react_loss: 0.1648 - val_output_bg_ph_loss: 0.1908 - val_output_ph_loss: 0.1992 - val_output_mg_c_loss: 0.1688 - val_output_c_loss: 0.1722\nEpoch 51/120\n30/30 - 4s - loss: 1.2128 - output_react_loss: 0.1396 - output_bg_ph_loss: 0.1582 - output_ph_loss: 0.1767 - output_mg_c_loss: 0.1410 - output_c_loss: 0.1586 - val_loss: 1.4129 - val_output_react_loss: 0.1630 - val_output_bg_ph_loss: 0.1901 - val_output_ph_loss: 0.1986 - val_output_mg_c_loss: 0.1676 - val_output_c_loss: 0.1728\nEpoch 52/120\n30/30 - 4s - loss: 1.1992 - output_react_loss: 0.1369 - output_bg_ph_loss: 0.1572 - output_ph_loss: 0.1754 - output_mg_c_loss: 0.1391 - output_c_loss: 0.1575 - val_loss: 1.4104 - val_output_react_loss: 0.1624 - val_output_bg_ph_loss: 0.1908 - val_output_ph_loss: 0.1987 - val_output_mg_c_loss: 0.1671 - val_output_c_loss: 0.1710\nEpoch 53/120\n30/30 - 4s - loss: 1.1897 - output_react_loss: 0.1354 - output_bg_ph_loss: 0.1559 - output_ph_loss: 0.1739 - output_mg_c_loss: 0.1382 - output_c_loss: 0.1568 - val_loss: 1.4089 - val_output_react_loss: 0.1623 - val_output_bg_ph_loss: 0.1895 - val_output_ph_loss: 0.1988 - val_output_mg_c_loss: 0.1672 - val_output_c_loss: 0.1720\nEpoch 54/120\n30/30 - 4s - loss: 1.1787 - output_react_loss: 0.1347 - output_bg_ph_loss: 0.1537 - output_ph_loss: 0.1731 - output_mg_c_loss: 0.1364 - output_c_loss: 0.1561 - val_loss: 1.4114 - val_output_react_loss: 0.1631 - val_output_bg_ph_loss: 0.1891 - val_output_ph_loss: 0.1992 - val_output_mg_c_loss: 0.1684 - val_output_c_loss: 0.1711\nEpoch 55/120\n30/30 - 4s - loss: 1.1707 - output_react_loss: 0.1338 - output_bg_ph_loss: 0.1526 - output_ph_loss: 0.1727 - output_mg_c_loss: 0.1350 - output_c_loss: 0.1553 - val_loss: 1.4131 - val_output_react_loss: 0.1642 - val_output_bg_ph_loss: 0.1899 - val_output_ph_loss: 0.1999 - val_output_mg_c_loss: 0.1669 - val_output_c_loss: 0.1713\nEpoch 56/120\n30/30 - 4s - loss: 1.1640 - output_react_loss: 0.1334 - output_bg_ph_loss: 0.1515 - output_ph_loss: 0.1714 - output_mg_c_loss: 0.1341 - output_c_loss: 0.1547 - val_loss: 1.4110 - val_output_react_loss: 0.1626 - val_output_bg_ph_loss: 0.1892 - val_output_ph_loss: 0.2002 - val_output_mg_c_loss: 0.1675 - val_output_c_loss: 0.1722\nEpoch 57/120\n30/30 - 4s - loss: 1.1600 - output_react_loss: 0.1331 - output_bg_ph_loss: 0.1498 - output_ph_loss: 0.1712 - output_mg_c_loss: 0.1343 - output_c_loss: 0.1544 - val_loss: 1.4093 - val_output_react_loss: 0.1622 - val_output_bg_ph_loss: 0.1895 - val_output_ph_loss: 0.2006 - val_output_mg_c_loss: 0.1668 - val_output_c_loss: 0.1716\nEpoch 58/120\n\nEpoch 00058: ReduceLROnPlateau reducing learning rate to 0.00010000000474974513.\n30/30 - 4s - loss: 1.1474 - output_react_loss: 0.1314 - output_bg_ph_loss: 0.1481 - output_ph_loss: 0.1701 - output_mg_c_loss: 0.1322 - output_c_loss: 0.1538 - val_loss: 1.4093 - val_output_react_loss: 0.1614 - val_output_bg_ph_loss: 0.1886 - val_output_ph_loss: 0.1999 - val_output_mg_c_loss: 0.1685 - val_output_c_loss: 0.1724\nEpoch 59/120\n30/30 - 4s - loss: 1.1176 - output_react_loss: 0.1275 - output_bg_ph_loss: 0.1443 - output_ph_loss: 0.1662 - output_mg_c_loss: 0.1284 - output_c_loss: 0.1510 - val_loss: 1.3902 - val_output_react_loss: 0.1602 - val_output_bg_ph_loss: 0.1875 - val_output_ph_loss: 0.1962 - val_output_mg_c_loss: 0.1648 - val_output_c_loss: 0.1690\nEpoch 60/120\n30/30 - 4s - loss: 1.1001 - output_react_loss: 0.1252 - output_bg_ph_loss: 0.1424 - output_ph_loss: 0.1636 - output_mg_c_loss: 0.1260 - output_c_loss: 0.1493 - val_loss: 1.3827 - val_output_react_loss: 0.1596 - val_output_bg_ph_loss: 0.1860 - val_output_ph_loss: 0.1955 - val_output_mg_c_loss: 0.1637 - val_output_c_loss: 0.1687\nEpoch 61/120\n30/30 - 4s - loss: 1.0945 - output_react_loss: 0.1250 - output_bg_ph_loss: 0.1411 - output_ph_loss: 0.1633 - output_mg_c_loss: 0.1252 - output_c_loss: 0.1487 - val_loss: 1.3811 - val_output_react_loss: 0.1592 - val_output_bg_ph_loss: 0.1861 - val_output_ph_loss: 0.1953 - val_output_mg_c_loss: 0.1634 - val_output_c_loss: 0.1685\nEpoch 62/120\n30/30 - 4s - loss: 1.0906 - output_react_loss: 0.1246 - output_bg_ph_loss: 0.1407 - output_ph_loss: 0.1625 - output_mg_c_loss: 0.1246 - output_c_loss: 0.1482 - val_loss: 1.3849 - val_output_react_loss: 0.1597 - val_output_bg_ph_loss: 0.1867 - val_output_ph_loss: 0.1955 - val_output_mg_c_loss: 0.1638 - val_output_c_loss: 0.1688\nEpoch 63/120\n30/30 - 4s - loss: 1.0896 - output_react_loss: 0.1246 - output_bg_ph_loss: 0.1402 - output_ph_loss: 0.1625 - output_mg_c_loss: 0.1246 - output_c_loss: 0.1482 - val_loss: 1.3850 - val_output_react_loss: 0.1597 - val_output_bg_ph_loss: 0.1867 - val_output_ph_loss: 0.1955 - val_output_mg_c_loss: 0.1639 - val_output_c_loss: 0.1689\nEpoch 64/120\n30/30 - 4s - loss: 1.0856 - output_react_loss: 0.1240 - output_bg_ph_loss: 0.1397 - output_ph_loss: 0.1618 - output_mg_c_loss: 0.1241 - output_c_loss: 0.1481 - val_loss: 1.3833 - val_output_react_loss: 0.1593 - val_output_bg_ph_loss: 0.1866 - val_output_ph_loss: 0.1956 - val_output_mg_c_loss: 0.1636 - val_output_c_loss: 0.1688\nEpoch 65/120\n30/30 - 4s - loss: 1.0841 - output_react_loss: 0.1239 - output_bg_ph_loss: 0.1396 - output_ph_loss: 0.1618 - output_mg_c_loss: 0.1237 - output_c_loss: 0.1477 - val_loss: 1.3844 - val_output_react_loss: 0.1594 - val_output_bg_ph_loss: 0.1868 - val_output_ph_loss: 0.1957 - val_output_mg_c_loss: 0.1638 - val_output_c_loss: 0.1688\nEpoch 66/120\n\nEpoch 00066: ReduceLROnPlateau reducing learning rate to 1.0000000474974514e-05.\n30/30 - 4s - loss: 1.0825 - output_react_loss: 0.1236 - output_bg_ph_loss: 0.1392 - output_ph_loss: 0.1619 - output_mg_c_loss: 0.1238 - output_c_loss: 0.1474 - val_loss: 1.3842 - val_output_react_loss: 0.1594 - val_output_bg_ph_loss: 0.1869 - val_output_ph_loss: 0.1953 - val_output_mg_c_loss: 0.1638 - val_output_c_loss: 0.1688\nEpoch 67/120\n30/30 - 4s - loss: 1.0786 - output_react_loss: 0.1230 - output_bg_ph_loss: 0.1389 - output_ph_loss: 0.1613 - output_mg_c_loss: 0.1230 - output_c_loss: 0.1475 - val_loss: 1.3829 - val_output_react_loss: 0.1594 - val_output_bg_ph_loss: 0.1866 - val_output_ph_loss: 0.1953 - val_output_mg_c_loss: 0.1635 - val_output_c_loss: 0.1686\nEpoch 68/120\n30/30 - 4s - loss: 1.0785 - output_react_loss: 0.1231 - output_bg_ph_loss: 0.1389 - output_ph_loss: 0.1613 - output_mg_c_loss: 0.1231 - output_c_loss: 0.1472 - val_loss: 1.3836 - val_output_react_loss: 0.1594 - val_output_bg_ph_loss: 0.1867 - val_output_ph_loss: 0.1954 - val_output_mg_c_loss: 0.1637 - val_output_c_loss: 0.1686\nEpoch 69/120\n30/30 - 4s - loss: 1.0787 - output_react_loss: 0.1231 - output_bg_ph_loss: 0.1390 - output_ph_loss: 0.1613 - output_mg_c_loss: 0.1230 - output_c_loss: 0.1471 - val_loss: 1.3834 - val_output_react_loss: 0.1594 - val_output_bg_ph_loss: 0.1867 - val_output_ph_loss: 0.1954 - val_output_mg_c_loss: 0.1636 - val_output_c_loss: 0.1686\nEpoch 70/120\n30/30 - 4s - loss: 1.0777 - output_react_loss: 0.1229 - output_bg_ph_loss: 0.1388 - output_ph_loss: 0.1613 - output_mg_c_loss: 0.1229 - output_c_loss: 0.1471 - val_loss: 1.3826 - val_output_react_loss: 0.1593 - val_output_bg_ph_loss: 0.1865 - val_output_ph_loss: 0.1952 - val_output_mg_c_loss: 0.1635 - val_output_c_loss: 0.1686\nEpoch 71/120\nRestoring model weights from the end of the best epoch.\n\nEpoch 00071: ReduceLROnPlateau reducing learning rate to 1.0000000656873453e-06.\n30/30 - 4s - loss: 1.0761 - output_react_loss: 0.1230 - output_bg_ph_loss: 0.1383 - output_ph_loss: 0.1610 - output_mg_c_loss: 0.1226 - output_c_loss: 0.1472 - val_loss: 1.3837 - val_output_react_loss: 0.1595 - val_output_bg_ph_loss: 0.1867 - val_output_ph_loss: 0.1953 - val_output_mg_c_loss: 0.1636 - val_output_c_loss: 0.1686\nEpoch 00071: early stopping\n\nFOLD: 3\nEpoch 1/120\n30/30 - 6s - loss: 3.6279 - output_react_loss: 0.4708 - output_bg_ph_loss: 0.4401 - output_ph_loss: 0.5027 - output_mg_c_loss: 0.4080 - output_c_loss: 0.4872 - val_loss: 2.3493 - val_output_react_loss: 0.2399 - val_output_bg_ph_loss: 0.3151 - val_output_ph_loss: 0.3559 - val_output_mg_c_loss: 0.2959 - val_output_c_loss: 0.2916\nEpoch 2/120\n30/30 - 4s - loss: 2.3306 - output_react_loss: 0.2492 - output_bg_ph_loss: 0.3149 - output_ph_loss: 0.3309 - output_mg_c_loss: 0.2956 - output_c_loss: 0.2803 - val_loss: 2.1497 - val_output_react_loss: 0.2243 - val_output_bg_ph_loss: 0.2951 - val_output_ph_loss: 0.3007 - val_output_mg_c_loss: 0.2761 - val_output_c_loss: 0.2581\nEpoch 3/120\n30/30 - 4s - loss: 2.1989 - output_react_loss: 0.2361 - output_bg_ph_loss: 0.2977 - output_ph_loss: 0.3080 - output_mg_c_loss: 0.2802 - output_c_loss: 0.2626 - val_loss: 2.0834 - val_output_react_loss: 0.2165 - val_output_bg_ph_loss: 0.2847 - val_output_ph_loss: 0.2912 - val_output_mg_c_loss: 0.2693 - val_output_c_loss: 0.2512\nEpoch 4/120\n30/30 - 4s - loss: 2.1209 - output_react_loss: 0.2280 - output_bg_ph_loss: 0.2863 - output_ph_loss: 0.2969 - output_mg_c_loss: 0.2705 - output_c_loss: 0.2542 - val_loss: 2.0157 - val_output_react_loss: 0.2112 - val_output_bg_ph_loss: 0.2745 - val_output_ph_loss: 0.2839 - val_output_mg_c_loss: 0.2586 - val_output_c_loss: 0.2433\nEpoch 5/120\n30/30 - 4s - loss: 2.0648 - output_react_loss: 0.2220 - output_bg_ph_loss: 0.2786 - output_ph_loss: 0.2894 - output_mg_c_loss: 0.2631 - output_c_loss: 0.2480 - val_loss: 1.9764 - val_output_react_loss: 0.2073 - val_output_bg_ph_loss: 0.2704 - val_output_ph_loss: 0.2772 - val_output_mg_c_loss: 0.2526 - val_output_c_loss: 0.2388\nEpoch 6/120\n30/30 - 4s - loss: 2.0171 - output_react_loss: 0.2174 - output_bg_ph_loss: 0.2718 - output_ph_loss: 0.2831 - output_mg_c_loss: 0.2561 - output_c_loss: 0.2433 - val_loss: 1.9254 - val_output_react_loss: 0.2017 - val_output_bg_ph_loss: 0.2617 - val_output_ph_loss: 0.2729 - val_output_mg_c_loss: 0.2456 - val_output_c_loss: 0.2346\nEpoch 7/120\n30/30 - 4s - loss: 1.9804 - output_react_loss: 0.2134 - output_bg_ph_loss: 0.2666 - output_ph_loss: 0.2790 - output_mg_c_loss: 0.2510 - output_c_loss: 0.2393 - val_loss: 1.8904 - val_output_react_loss: 0.1986 - val_output_bg_ph_loss: 0.2558 - val_output_ph_loss: 0.2685 - val_output_mg_c_loss: 0.2407 - val_output_c_loss: 0.2317\nEpoch 8/120\n30/30 - 4s - loss: 1.9470 - output_react_loss: 0.2095 - output_bg_ph_loss: 0.2620 - output_ph_loss: 0.2744 - output_mg_c_loss: 0.2469 - output_c_loss: 0.2359 - val_loss: 1.8707 - val_output_react_loss: 0.1958 - val_output_bg_ph_loss: 0.2540 - val_output_ph_loss: 0.2636 - val_output_mg_c_loss: 0.2396 - val_output_c_loss: 0.2282\nEpoch 9/120\n30/30 - 4s - loss: 1.9133 - output_react_loss: 0.2058 - output_bg_ph_loss: 0.2575 - output_ph_loss: 0.2693 - output_mg_c_loss: 0.2427 - output_c_loss: 0.2320 - val_loss: 1.8330 - val_output_react_loss: 0.1929 - val_output_bg_ph_loss: 0.2480 - val_output_ph_loss: 0.2612 - val_output_mg_c_loss: 0.2325 - val_output_c_loss: 0.2251\nEpoch 10/120\n30/30 - 4s - loss: 1.8769 - output_react_loss: 0.2033 - output_bg_ph_loss: 0.2529 - output_ph_loss: 0.2644 - output_mg_c_loss: 0.2360 - output_c_loss: 0.2282 - val_loss: 1.7937 - val_output_react_loss: 0.1910 - val_output_bg_ph_loss: 0.2442 - val_output_ph_loss: 0.2535 - val_output_mg_c_loss: 0.2252 - val_output_c_loss: 0.2194\nEpoch 11/120\n30/30 - 4s - loss: 1.8367 - output_react_loss: 0.1997 - output_bg_ph_loss: 0.2481 - output_ph_loss: 0.2597 - output_mg_c_loss: 0.2289 - output_c_loss: 0.2235 - val_loss: 1.7648 - val_output_react_loss: 0.1890 - val_output_bg_ph_loss: 0.2392 - val_output_ph_loss: 0.2512 - val_output_mg_c_loss: 0.2202 - val_output_c_loss: 0.2167\nEpoch 12/120\n30/30 - 4s - loss: 1.8048 - output_react_loss: 0.1977 - output_bg_ph_loss: 0.2433 - output_ph_loss: 0.2558 - output_mg_c_loss: 0.2239 - output_c_loss: 0.2192 - val_loss: 1.7376 - val_output_react_loss: 0.1860 - val_output_bg_ph_loss: 0.2374 - val_output_ph_loss: 0.2471 - val_output_mg_c_loss: 0.2156 - val_output_c_loss: 0.2126\nEpoch 13/120\n30/30 - 4s - loss: 1.7767 - output_react_loss: 0.1948 - output_bg_ph_loss: 0.2394 - output_ph_loss: 0.2518 - output_mg_c_loss: 0.2202 - output_c_loss: 0.2162 - val_loss: 1.7016 - val_output_react_loss: 0.1839 - val_output_bg_ph_loss: 0.2292 - val_output_ph_loss: 0.2427 - val_output_mg_c_loss: 0.2118 - val_output_c_loss: 0.2093\nEpoch 14/120\n30/30 - 4s - loss: 1.7474 - output_react_loss: 0.1928 - output_bg_ph_loss: 0.2342 - output_ph_loss: 0.2482 - output_mg_c_loss: 0.2160 - output_c_loss: 0.2130 - val_loss: 1.6829 - val_output_react_loss: 0.1834 - val_output_bg_ph_loss: 0.2253 - val_output_ph_loss: 0.2390 - val_output_mg_c_loss: 0.2099 - val_output_c_loss: 0.2067\nEpoch 15/120\n30/30 - 4s - loss: 1.7325 - output_react_loss: 0.1918 - output_bg_ph_loss: 0.2336 - output_ph_loss: 0.2449 - output_mg_c_loss: 0.2133 - output_c_loss: 0.2102 - val_loss: 1.6513 - val_output_react_loss: 0.1831 - val_output_bg_ph_loss: 0.2217 - val_output_ph_loss: 0.2346 - val_output_mg_c_loss: 0.2026 - val_output_c_loss: 0.2020\nEpoch 16/120\n30/30 - 4s - loss: 1.6941 - output_react_loss: 0.1881 - output_bg_ph_loss: 0.2277 - output_ph_loss: 0.2409 - output_mg_c_loss: 0.2077 - output_c_loss: 0.2060 - val_loss: 1.6195 - val_output_react_loss: 0.1785 - val_output_bg_ph_loss: 0.2184 - val_output_ph_loss: 0.2301 - val_output_mg_c_loss: 0.1983 - val_output_c_loss: 0.1989\nEpoch 17/120\n30/30 - 4s - loss: 1.6725 - output_react_loss: 0.1860 - output_bg_ph_loss: 0.2254 - output_ph_loss: 0.2373 - output_mg_c_loss: 0.2044 - output_c_loss: 0.2035 - val_loss: 1.6132 - val_output_react_loss: 0.1794 - val_output_bg_ph_loss: 0.2200 - val_output_ph_loss: 0.2279 - val_output_mg_c_loss: 0.1947 - val_output_c_loss: 0.1969\nEpoch 18/120\n30/30 - 4s - loss: 1.6494 - output_react_loss: 0.1849 - output_bg_ph_loss: 0.2210 - output_ph_loss: 0.2333 - output_mg_c_loss: 0.2015 - output_c_loss: 0.2011 - val_loss: 1.5786 - val_output_react_loss: 0.1762 - val_output_bg_ph_loss: 0.2117 - val_output_ph_loss: 0.2239 - val_output_mg_c_loss: 0.1929 - val_output_c_loss: 0.1933\nEpoch 19/120\n30/30 - 4s - loss: 1.6229 - output_react_loss: 0.1826 - output_bg_ph_loss: 0.2178 - output_ph_loss: 0.2306 - output_mg_c_loss: 0.1967 - output_c_loss: 0.1980 - val_loss: 1.5810 - val_output_react_loss: 0.1745 - val_output_bg_ph_loss: 0.2117 - val_output_ph_loss: 0.2241 - val_output_mg_c_loss: 0.1959 - val_output_c_loss: 0.1928\nEpoch 20/120\n30/30 - 4s - loss: 1.6070 - output_react_loss: 0.1805 - output_bg_ph_loss: 0.2163 - output_ph_loss: 0.2276 - output_mg_c_loss: 0.1949 - output_c_loss: 0.1959 - val_loss: 1.5546 - val_output_react_loss: 0.1742 - val_output_bg_ph_loss: 0.2108 - val_output_ph_loss: 0.2193 - val_output_mg_c_loss: 0.1880 - val_output_c_loss: 0.1892\nEpoch 21/120\n30/30 - 4s - loss: 1.5869 - output_react_loss: 0.1796 - output_bg_ph_loss: 0.2125 - output_ph_loss: 0.2249 - output_mg_c_loss: 0.1919 - output_c_loss: 0.1940 - val_loss: 1.5329 - val_output_react_loss: 0.1726 - val_output_bg_ph_loss: 0.2073 - val_output_ph_loss: 0.2173 - val_output_mg_c_loss: 0.1846 - val_output_c_loss: 0.1866\nEpoch 22/120\n30/30 - 4s - loss: 1.5733 - output_react_loss: 0.1779 - output_bg_ph_loss: 0.2106 - output_ph_loss: 0.2236 - output_mg_c_loss: 0.1904 - output_c_loss: 0.1919 - val_loss: 1.5222 - val_output_react_loss: 0.1710 - val_output_bg_ph_loss: 0.2052 - val_output_ph_loss: 0.2158 - val_output_mg_c_loss: 0.1834 - val_output_c_loss: 0.1872\nEpoch 23/120\n30/30 - 4s - loss: 1.5579 - output_react_loss: 0.1772 - output_bg_ph_loss: 0.2084 - output_ph_loss: 0.2210 - output_mg_c_loss: 0.1876 - output_c_loss: 0.1904 - val_loss: 1.5246 - val_output_react_loss: 0.1722 - val_output_bg_ph_loss: 0.2057 - val_output_ph_loss: 0.2136 - val_output_mg_c_loss: 0.1846 - val_output_c_loss: 0.1860\nEpoch 24/120\n30/30 - 4s - loss: 1.5364 - output_react_loss: 0.1741 - output_bg_ph_loss: 0.2062 - output_ph_loss: 0.2176 - output_mg_c_loss: 0.1850 - output_c_loss: 0.1881 - val_loss: 1.5003 - val_output_react_loss: 0.1682 - val_output_bg_ph_loss: 0.2027 - val_output_ph_loss: 0.2137 - val_output_mg_c_loss: 0.1802 - val_output_c_loss: 0.1843\nEpoch 25/120\n30/30 - 4s - loss: 1.5273 - output_react_loss: 0.1735 - output_bg_ph_loss: 0.2043 - output_ph_loss: 0.2179 - output_mg_c_loss: 0.1834 - output_c_loss: 0.1871 - val_loss: 1.4845 - val_output_react_loss: 0.1662 - val_output_bg_ph_loss: 0.1994 - val_output_ph_loss: 0.2108 - val_output_mg_c_loss: 0.1792 - val_output_c_loss: 0.1840\nEpoch 26/120\n30/30 - 4s - loss: 1.5129 - output_react_loss: 0.1714 - output_bg_ph_loss: 0.2027 - output_ph_loss: 0.2159 - output_mg_c_loss: 0.1817 - output_c_loss: 0.1855 - val_loss: 1.4768 - val_output_react_loss: 0.1652 - val_output_bg_ph_loss: 0.1994 - val_output_ph_loss: 0.2103 - val_output_mg_c_loss: 0.1780 - val_output_c_loss: 0.1812\nEpoch 27/120\n30/30 - 4s - loss: 1.4976 - output_react_loss: 0.1711 - output_bg_ph_loss: 0.2004 - output_ph_loss: 0.2128 - output_mg_c_loss: 0.1791 - output_c_loss: 0.1837 - val_loss: 1.4699 - val_output_react_loss: 0.1654 - val_output_bg_ph_loss: 0.1987 - val_output_ph_loss: 0.2090 - val_output_mg_c_loss: 0.1759 - val_output_c_loss: 0.1810\nEpoch 28/120\n30/30 - 4s - loss: 1.4888 - output_react_loss: 0.1696 - output_bg_ph_loss: 0.1992 - output_ph_loss: 0.2127 - output_mg_c_loss: 0.1777 - output_c_loss: 0.1830 - val_loss: 1.4813 - val_output_react_loss: 0.1656 - val_output_bg_ph_loss: 0.2007 - val_output_ph_loss: 0.2127 - val_output_mg_c_loss: 0.1770 - val_output_c_loss: 0.1819\nEpoch 29/120\n30/30 - 4s - loss: 1.4731 - output_react_loss: 0.1675 - output_bg_ph_loss: 0.1969 - output_ph_loss: 0.2094 - output_mg_c_loss: 0.1768 - output_c_loss: 0.1813 - val_loss: 1.4557 - val_output_react_loss: 0.1649 - val_output_bg_ph_loss: 0.1959 - val_output_ph_loss: 0.2065 - val_output_mg_c_loss: 0.1743 - val_output_c_loss: 0.1790\nEpoch 30/120\n30/30 - 4s - loss: 1.4539 - output_react_loss: 0.1659 - output_bg_ph_loss: 0.1936 - output_ph_loss: 0.2075 - output_mg_c_loss: 0.1740 - output_c_loss: 0.1794 - val_loss: 1.4551 - val_output_react_loss: 0.1638 - val_output_bg_ph_loss: 0.1959 - val_output_ph_loss: 0.2085 - val_output_mg_c_loss: 0.1741 - val_output_c_loss: 0.1788\nEpoch 31/120\n30/30 - 4s - loss: 1.4469 - output_react_loss: 0.1637 - output_bg_ph_loss: 0.1931 - output_ph_loss: 0.2079 - output_mg_c_loss: 0.1732 - output_c_loss: 0.1790 - val_loss: 1.4536 - val_output_react_loss: 0.1646 - val_output_bg_ph_loss: 0.1944 - val_output_ph_loss: 0.2072 - val_output_mg_c_loss: 0.1746 - val_output_c_loss: 0.1792\nEpoch 32/120\n30/30 - 4s - loss: 1.4325 - output_react_loss: 0.1638 - output_bg_ph_loss: 0.1906 - output_ph_loss: 0.2051 - output_mg_c_loss: 0.1706 - output_c_loss: 0.1775 - val_loss: 1.4452 - val_output_react_loss: 0.1623 - val_output_bg_ph_loss: 0.1946 - val_output_ph_loss: 0.2037 - val_output_mg_c_loss: 0.1747 - val_output_c_loss: 0.1784\nEpoch 33/120\n30/30 - 4s - loss: 1.4220 - output_react_loss: 0.1622 - output_bg_ph_loss: 0.1893 - output_ph_loss: 0.2040 - output_mg_c_loss: 0.1692 - output_c_loss: 0.1767 - val_loss: 1.4594 - val_output_react_loss: 0.1629 - val_output_bg_ph_loss: 0.1978 - val_output_ph_loss: 0.2033 - val_output_mg_c_loss: 0.1769 - val_output_c_loss: 0.1812\nEpoch 34/120\n30/30 - 4s - loss: 1.4184 - output_react_loss: 0.1625 - output_bg_ph_loss: 0.1887 - output_ph_loss: 0.2031 - output_mg_c_loss: 0.1680 - output_c_loss: 0.1769 - val_loss: 1.4377 - val_output_react_loss: 0.1619 - val_output_bg_ph_loss: 0.1934 - val_output_ph_loss: 0.2034 - val_output_mg_c_loss: 0.1733 - val_output_c_loss: 0.1773\nEpoch 35/120\n30/30 - 4s - loss: 1.4003 - output_react_loss: 0.1603 - output_bg_ph_loss: 0.1857 - output_ph_loss: 0.2006 - output_mg_c_loss: 0.1664 - output_c_loss: 0.1748 - val_loss: 1.4235 - val_output_react_loss: 0.1610 - val_output_bg_ph_loss: 0.1923 - val_output_ph_loss: 0.2016 - val_output_mg_c_loss: 0.1700 - val_output_c_loss: 0.1751\nEpoch 36/120\n30/30 - 4s - loss: 1.3808 - output_react_loss: 0.1586 - output_bg_ph_loss: 0.1832 - output_ph_loss: 0.1980 - output_mg_c_loss: 0.1633 - output_c_loss: 0.1724 - val_loss: 1.4229 - val_output_react_loss: 0.1599 - val_output_bg_ph_loss: 0.1926 - val_output_ph_loss: 0.2031 - val_output_mg_c_loss: 0.1697 - val_output_c_loss: 0.1755\nEpoch 37/120\n30/30 - 4s - loss: 1.3685 - output_react_loss: 0.1564 - output_bg_ph_loss: 0.1817 - output_ph_loss: 0.1970 - output_mg_c_loss: 0.1615 - output_c_loss: 0.1722 - val_loss: 1.4198 - val_output_react_loss: 0.1599 - val_output_bg_ph_loss: 0.1915 - val_output_ph_loss: 0.2013 - val_output_mg_c_loss: 0.1707 - val_output_c_loss: 0.1743\nEpoch 38/120\n30/30 - 4s - loss: 1.3600 - output_react_loss: 0.1562 - output_bg_ph_loss: 0.1804 - output_ph_loss: 0.1961 - output_mg_c_loss: 0.1599 - output_c_loss: 0.1710 - val_loss: 1.4149 - val_output_react_loss: 0.1596 - val_output_bg_ph_loss: 0.1908 - val_output_ph_loss: 0.1992 - val_output_mg_c_loss: 0.1703 - val_output_c_loss: 0.1743\nEpoch 39/120\n30/30 - 4s - loss: 1.3497 - output_react_loss: 0.1552 - output_bg_ph_loss: 0.1786 - output_ph_loss: 0.1941 - output_mg_c_loss: 0.1589 - output_c_loss: 0.1702 - val_loss: 1.4254 - val_output_react_loss: 0.1590 - val_output_bg_ph_loss: 0.1934 - val_output_ph_loss: 0.2004 - val_output_mg_c_loss: 0.1725 - val_output_c_loss: 0.1750\nEpoch 40/120\n30/30 - 4s - loss: 1.3433 - output_react_loss: 0.1548 - output_bg_ph_loss: 0.1776 - output_ph_loss: 0.1930 - output_mg_c_loss: 0.1579 - output_c_loss: 0.1695 - val_loss: 1.4085 - val_output_react_loss: 0.1595 - val_output_bg_ph_loss: 0.1896 - val_output_ph_loss: 0.1986 - val_output_mg_c_loss: 0.1694 - val_output_c_loss: 0.1730\nEpoch 41/120\n30/30 - 4s - loss: 1.3233 - output_react_loss: 0.1523 - output_bg_ph_loss: 0.1749 - output_ph_loss: 0.1909 - output_mg_c_loss: 0.1550 - output_c_loss: 0.1679 - val_loss: 1.4082 - val_output_react_loss: 0.1584 - val_output_bg_ph_loss: 0.1920 - val_output_ph_loss: 0.1984 - val_output_mg_c_loss: 0.1675 - val_output_c_loss: 0.1739\nEpoch 42/120\n30/30 - 4s - loss: 1.3142 - output_react_loss: 0.1512 - output_bg_ph_loss: 0.1739 - output_ph_loss: 0.1907 - output_mg_c_loss: 0.1533 - output_c_loss: 0.1668 - val_loss: 1.4005 - val_output_react_loss: 0.1576 - val_output_bg_ph_loss: 0.1896 - val_output_ph_loss: 0.1986 - val_output_mg_c_loss: 0.1675 - val_output_c_loss: 0.1725\nEpoch 43/120\n30/30 - 4s - loss: 1.3001 - output_react_loss: 0.1491 - output_bg_ph_loss: 0.1718 - output_ph_loss: 0.1889 - output_mg_c_loss: 0.1516 - output_c_loss: 0.1661 - val_loss: 1.3872 - val_output_react_loss: 0.1562 - val_output_bg_ph_loss: 0.1884 - val_output_ph_loss: 0.1952 - val_output_mg_c_loss: 0.1655 - val_output_c_loss: 0.1716\nEpoch 44/120\n30/30 - 4s - loss: 1.2919 - output_react_loss: 0.1484 - output_bg_ph_loss: 0.1699 - output_ph_loss: 0.1882 - output_mg_c_loss: 0.1510 - output_c_loss: 0.1651 - val_loss: 1.3897 - val_output_react_loss: 0.1564 - val_output_bg_ph_loss: 0.1880 - val_output_ph_loss: 0.1962 - val_output_mg_c_loss: 0.1666 - val_output_c_loss: 0.1716\nEpoch 45/120\n30/30 - 4s - loss: 1.2778 - output_react_loss: 0.1472 - output_bg_ph_loss: 0.1683 - output_ph_loss: 0.1851 - output_mg_c_loss: 0.1490 - output_c_loss: 0.1635 - val_loss: 1.3925 - val_output_react_loss: 0.1570 - val_output_bg_ph_loss: 0.1884 - val_output_ph_loss: 0.1960 - val_output_mg_c_loss: 0.1669 - val_output_c_loss: 0.1719\nEpoch 46/120\n30/30 - 4s - loss: 1.2677 - output_react_loss: 0.1463 - output_bg_ph_loss: 0.1662 - output_ph_loss: 0.1843 - output_mg_c_loss: 0.1479 - output_c_loss: 0.1627 - val_loss: 1.3833 - val_output_react_loss: 0.1568 - val_output_bg_ph_loss: 0.1867 - val_output_ph_loss: 0.1960 - val_output_mg_c_loss: 0.1646 - val_output_c_loss: 0.1712\nEpoch 47/120\n30/30 - 4s - loss: 1.2556 - output_react_loss: 0.1445 - output_bg_ph_loss: 0.1647 - output_ph_loss: 0.1827 - output_mg_c_loss: 0.1461 - output_c_loss: 0.1622 - val_loss: 1.4004 - val_output_react_loss: 0.1552 - val_output_bg_ph_loss: 0.1895 - val_output_ph_loss: 0.1976 - val_output_mg_c_loss: 0.1695 - val_output_c_loss: 0.1743\nEpoch 48/120\n30/30 - 4s - loss: 1.2482 - output_react_loss: 0.1434 - output_bg_ph_loss: 0.1634 - output_ph_loss: 0.1821 - output_mg_c_loss: 0.1456 - output_c_loss: 0.1614 - val_loss: 1.3820 - val_output_react_loss: 0.1557 - val_output_bg_ph_loss: 0.1876 - val_output_ph_loss: 0.1940 - val_output_mg_c_loss: 0.1656 - val_output_c_loss: 0.1703\nEpoch 49/120\n30/30 - 4s - loss: 1.2309 - output_react_loss: 0.1416 - output_bg_ph_loss: 0.1612 - output_ph_loss: 0.1799 - output_mg_c_loss: 0.1428 - output_c_loss: 0.1598 - val_loss: 1.3827 - val_output_react_loss: 0.1567 - val_output_bg_ph_loss: 0.1871 - val_output_ph_loss: 0.1941 - val_output_mg_c_loss: 0.1650 - val_output_c_loss: 0.1711\nEpoch 50/120\n30/30 - 4s - loss: 1.2231 - output_react_loss: 0.1412 - output_bg_ph_loss: 0.1596 - output_ph_loss: 0.1790 - output_mg_c_loss: 0.1418 - output_c_loss: 0.1589 - val_loss: 1.3795 - val_output_react_loss: 0.1544 - val_output_bg_ph_loss: 0.1885 - val_output_ph_loss: 0.1933 - val_output_mg_c_loss: 0.1649 - val_output_c_loss: 0.1706\nEpoch 51/120\n30/30 - 4s - loss: 1.2174 - output_react_loss: 0.1405 - output_bg_ph_loss: 0.1583 - output_ph_loss: 0.1784 - output_mg_c_loss: 0.1411 - output_c_loss: 0.1593 - val_loss: 1.3675 - val_output_react_loss: 0.1533 - val_output_bg_ph_loss: 0.1867 - val_output_ph_loss: 0.1930 - val_output_mg_c_loss: 0.1627 - val_output_c_loss: 0.1690\nEpoch 52/120\n30/30 - 4s - loss: 1.2036 - output_react_loss: 0.1388 - output_bg_ph_loss: 0.1565 - output_ph_loss: 0.1764 - output_mg_c_loss: 0.1395 - output_c_loss: 0.1576 - val_loss: 1.3853 - val_output_react_loss: 0.1563 - val_output_bg_ph_loss: 0.1880 - val_output_ph_loss: 0.1951 - val_output_mg_c_loss: 0.1656 - val_output_c_loss: 0.1704\nEpoch 53/120\n30/30 - 4s - loss: 1.1960 - output_react_loss: 0.1374 - output_bg_ph_loss: 0.1554 - output_ph_loss: 0.1759 - output_mg_c_loss: 0.1384 - output_c_loss: 0.1575 - val_loss: 1.3918 - val_output_react_loss: 0.1545 - val_output_bg_ph_loss: 0.1888 - val_output_ph_loss: 0.1959 - val_output_mg_c_loss: 0.1683 - val_output_c_loss: 0.1728\nEpoch 54/120\n30/30 - 4s - loss: 1.1874 - output_react_loss: 0.1364 - output_bg_ph_loss: 0.1540 - output_ph_loss: 0.1747 - output_mg_c_loss: 0.1374 - output_c_loss: 0.1571 - val_loss: 1.3927 - val_output_react_loss: 0.1559 - val_output_bg_ph_loss: 0.1898 - val_output_ph_loss: 0.1962 - val_output_mg_c_loss: 0.1667 - val_output_c_loss: 0.1717\nEpoch 55/120\n30/30 - 4s - loss: 1.1849 - output_react_loss: 0.1362 - output_bg_ph_loss: 0.1539 - output_ph_loss: 0.1743 - output_mg_c_loss: 0.1369 - output_c_loss: 0.1565 - val_loss: 1.3862 - val_output_react_loss: 0.1550 - val_output_bg_ph_loss: 0.1889 - val_output_ph_loss: 0.1968 - val_output_mg_c_loss: 0.1655 - val_output_c_loss: 0.1707\nEpoch 56/120\n30/30 - 4s - loss: 1.1682 - output_react_loss: 0.1345 - output_bg_ph_loss: 0.1513 - output_ph_loss: 0.1728 - output_mg_c_loss: 0.1344 - output_c_loss: 0.1550 - val_loss: 1.3599 - val_output_react_loss: 0.1527 - val_output_bg_ph_loss: 0.1842 - val_output_ph_loss: 0.1922 - val_output_mg_c_loss: 0.1627 - val_output_c_loss: 0.1685\nEpoch 57/120\n30/30 - 4s - loss: 1.1616 - output_react_loss: 0.1333 - output_bg_ph_loss: 0.1503 - output_ph_loss: 0.1717 - output_mg_c_loss: 0.1338 - output_c_loss: 0.1550 - val_loss: 1.3782 - val_output_react_loss: 0.1538 - val_output_bg_ph_loss: 0.1884 - val_output_ph_loss: 0.1950 - val_output_mg_c_loss: 0.1645 - val_output_c_loss: 0.1698\nEpoch 58/120\n30/30 - 4s - loss: 1.1523 - output_react_loss: 0.1327 - output_bg_ph_loss: 0.1490 - output_ph_loss: 0.1706 - output_mg_c_loss: 0.1324 - output_c_loss: 0.1536 - val_loss: 1.3716 - val_output_react_loss: 0.1548 - val_output_bg_ph_loss: 0.1865 - val_output_ph_loss: 0.1927 - val_output_mg_c_loss: 0.1632 - val_output_c_loss: 0.1698\nEpoch 59/120\n30/30 - 4s - loss: 1.1425 - output_react_loss: 0.1312 - output_bg_ph_loss: 0.1474 - output_ph_loss: 0.1693 - output_mg_c_loss: 0.1314 - output_c_loss: 0.1531 - val_loss: 1.3839 - val_output_react_loss: 0.1552 - val_output_bg_ph_loss: 0.1883 - val_output_ph_loss: 0.1946 - val_output_mg_c_loss: 0.1658 - val_output_c_loss: 0.1708\nEpoch 60/120\n30/30 - 4s - loss: 1.1355 - output_react_loss: 0.1303 - output_bg_ph_loss: 0.1460 - output_ph_loss: 0.1680 - output_mg_c_loss: 0.1314 - output_c_loss: 0.1522 - val_loss: 1.3736 - val_output_react_loss: 0.1523 - val_output_bg_ph_loss: 0.1877 - val_output_ph_loss: 0.1945 - val_output_mg_c_loss: 0.1644 - val_output_c_loss: 0.1702\nEpoch 61/120\n\nEpoch 00061: ReduceLROnPlateau reducing learning rate to 0.00010000000474974513.\n30/30 - 4s - loss: 1.1305 - output_react_loss: 0.1289 - output_bg_ph_loss: 0.1459 - output_ph_loss: 0.1683 - output_mg_c_loss: 0.1298 - output_c_loss: 0.1527 - val_loss: 1.3654 - val_output_react_loss: 0.1529 - val_output_bg_ph_loss: 0.1861 - val_output_ph_loss: 0.1922 - val_output_mg_c_loss: 0.1635 - val_output_c_loss: 0.1682\nEpoch 62/120\n30/30 - 4s - loss: 1.0979 - output_react_loss: 0.1258 - output_bg_ph_loss: 0.1411 - output_ph_loss: 0.1641 - output_mg_c_loss: 0.1255 - output_c_loss: 0.1490 - val_loss: 1.3404 - val_output_react_loss: 0.1499 - val_output_bg_ph_loss: 0.1825 - val_output_ph_loss: 0.1894 - val_output_mg_c_loss: 0.1597 - val_output_c_loss: 0.1668\nEpoch 63/120\n30/30 - 4s - loss: 1.0789 - output_react_loss: 0.1238 - output_bg_ph_loss: 0.1381 - output_ph_loss: 0.1619 - output_mg_c_loss: 0.1229 - output_c_loss: 0.1476 - val_loss: 1.3418 - val_output_react_loss: 0.1497 - val_output_bg_ph_loss: 0.1827 - val_output_ph_loss: 0.1899 - val_output_mg_c_loss: 0.1600 - val_output_c_loss: 0.1671\nEpoch 64/120\n30/30 - 4s - loss: 1.0765 - output_react_loss: 0.1233 - output_bg_ph_loss: 0.1380 - output_ph_loss: 0.1616 - output_mg_c_loss: 0.1225 - output_c_loss: 0.1472 - val_loss: 1.3376 - val_output_react_loss: 0.1495 - val_output_bg_ph_loss: 0.1822 - val_output_ph_loss: 0.1888 - val_output_mg_c_loss: 0.1595 - val_output_c_loss: 0.1664\nEpoch 65/120\n30/30 - 4s - loss: 1.0710 - output_react_loss: 0.1227 - output_bg_ph_loss: 0.1369 - output_ph_loss: 0.1608 - output_mg_c_loss: 0.1220 - output_c_loss: 0.1470 - val_loss: 1.3407 - val_output_react_loss: 0.1497 - val_output_bg_ph_loss: 0.1825 - val_output_ph_loss: 0.1895 - val_output_mg_c_loss: 0.1601 - val_output_c_loss: 0.1666\nEpoch 66/120\n30/30 - 4s - loss: 1.0704 - output_react_loss: 0.1227 - output_bg_ph_loss: 0.1370 - output_ph_loss: 0.1607 - output_mg_c_loss: 0.1218 - output_c_loss: 0.1467 - val_loss: 1.3403 - val_output_react_loss: 0.1492 - val_output_bg_ph_loss: 0.1826 - val_output_ph_loss: 0.1891 - val_output_mg_c_loss: 0.1604 - val_output_c_loss: 0.1669\nEpoch 67/120\n30/30 - 4s - loss: 1.0669 - output_react_loss: 0.1224 - output_bg_ph_loss: 0.1365 - output_ph_loss: 0.1602 - output_mg_c_loss: 0.1214 - output_c_loss: 0.1462 - val_loss: 1.3418 - val_output_react_loss: 0.1498 - val_output_bg_ph_loss: 0.1827 - val_output_ph_loss: 0.1895 - val_output_mg_c_loss: 0.1603 - val_output_c_loss: 0.1667\nEpoch 68/120\n30/30 - 4s - loss: 1.0631 - output_react_loss: 0.1218 - output_bg_ph_loss: 0.1359 - output_ph_loss: 0.1593 - output_mg_c_loss: 0.1212 - output_c_loss: 0.1460 - val_loss: 1.3403 - val_output_react_loss: 0.1498 - val_output_bg_ph_loss: 0.1825 - val_output_ph_loss: 0.1890 - val_output_mg_c_loss: 0.1600 - val_output_c_loss: 0.1666\nEpoch 69/120\n\nEpoch 00069: ReduceLROnPlateau reducing learning rate to 1.0000000474974514e-05.\n30/30 - 4s - loss: 1.0639 - output_react_loss: 0.1221 - output_bg_ph_loss: 0.1357 - output_ph_loss: 0.1603 - output_mg_c_loss: 0.1210 - output_c_loss: 0.1461 - val_loss: 1.3406 - val_output_react_loss: 0.1498 - val_output_bg_ph_loss: 0.1826 - val_output_ph_loss: 0.1890 - val_output_mg_c_loss: 0.1600 - val_output_c_loss: 0.1666\nEpoch 70/120\n30/30 - 4s - loss: 1.0592 - output_react_loss: 0.1215 - output_bg_ph_loss: 0.1353 - output_ph_loss: 0.1594 - output_mg_c_loss: 0.1203 - output_c_loss: 0.1456 - val_loss: 1.3397 - val_output_react_loss: 0.1497 - val_output_bg_ph_loss: 0.1824 - val_output_ph_loss: 0.1890 - val_output_mg_c_loss: 0.1599 - val_output_c_loss: 0.1666\nEpoch 71/120\n30/30 - 4s - loss: 1.0588 - output_react_loss: 0.1216 - output_bg_ph_loss: 0.1351 - output_ph_loss: 0.1593 - output_mg_c_loss: 0.1203 - output_c_loss: 0.1453 - val_loss: 1.3392 - val_output_react_loss: 0.1496 - val_output_bg_ph_loss: 0.1824 - val_output_ph_loss: 0.1890 - val_output_mg_c_loss: 0.1599 - val_output_c_loss: 0.1666\nEpoch 72/120\n30/30 - 4s - loss: 1.0593 - output_react_loss: 0.1213 - output_bg_ph_loss: 0.1353 - output_ph_loss: 0.1589 - output_mg_c_loss: 0.1208 - output_c_loss: 0.1456 - val_loss: 1.3390 - val_output_react_loss: 0.1496 - val_output_bg_ph_loss: 0.1823 - val_output_ph_loss: 0.1889 - val_output_mg_c_loss: 0.1599 - val_output_c_loss: 0.1666\nEpoch 73/120\n30/30 - 4s - loss: 1.0578 - output_react_loss: 0.1212 - output_bg_ph_loss: 0.1352 - output_ph_loss: 0.1589 - output_mg_c_loss: 0.1201 - output_c_loss: 0.1458 - val_loss: 1.3388 - val_output_react_loss: 0.1496 - val_output_bg_ph_loss: 0.1823 - val_output_ph_loss: 0.1890 - val_output_mg_c_loss: 0.1598 - val_output_c_loss: 0.1665\nEpoch 74/120\nRestoring model weights from the end of the best epoch.\n\nEpoch 00074: ReduceLROnPlateau reducing learning rate to 1.0000000656873453e-06.\n30/30 - 4s - loss: 1.0578 - output_react_loss: 0.1212 - output_bg_ph_loss: 0.1350 - output_ph_loss: 0.1591 - output_mg_c_loss: 0.1203 - output_c_loss: 0.1457 - val_loss: 1.3391 - val_output_react_loss: 0.1496 - val_output_bg_ph_loss: 0.1824 - val_output_ph_loss: 0.1890 - val_output_mg_c_loss: 0.1598 - val_output_c_loss: 0.1666\nEpoch 00074: early stopping\n\nFOLD: 4\nEpoch 1/120\n30/30 - 6s - loss: 3.5823 - output_react_loss: 0.4021 - output_bg_ph_loss: 0.4325 - output_ph_loss: 0.4183 - output_mg_c_loss: 0.5652 - output_c_loss: 0.3643 - val_loss: 2.3387 - val_output_react_loss: 0.2429 - val_output_bg_ph_loss: 0.3170 - val_output_ph_loss: 0.3297 - val_output_mg_c_loss: 0.3079 - val_output_c_loss: 0.2732\nEpoch 2/120\n30/30 - 4s - loss: 2.3416 - output_react_loss: 0.2468 - output_bg_ph_loss: 0.3150 - output_ph_loss: 0.3305 - output_mg_c_loss: 0.3056 - output_c_loss: 0.2763 - val_loss: 2.1665 - val_output_react_loss: 0.2286 - val_output_bg_ph_loss: 0.2981 - val_output_ph_loss: 0.3018 - val_output_mg_c_loss: 0.2791 - val_output_c_loss: 0.2531\nEpoch 3/120\n30/30 - 4s - loss: 2.2095 - output_react_loss: 0.2356 - output_bg_ph_loss: 0.2987 - output_ph_loss: 0.3096 - output_mg_c_loss: 0.2841 - output_c_loss: 0.2631 - val_loss: 2.0789 - val_output_react_loss: 0.2214 - val_output_bg_ph_loss: 0.2849 - val_output_ph_loss: 0.2868 - val_output_mg_c_loss: 0.2673 - val_output_c_loss: 0.2448\nEpoch 4/120\n30/30 - 4s - loss: 2.1361 - output_react_loss: 0.2284 - output_bg_ph_loss: 0.2880 - output_ph_loss: 0.3003 - output_mg_c_loss: 0.2736 - output_c_loss: 0.2558 - val_loss: 2.0266 - val_output_react_loss: 0.2151 - val_output_bg_ph_loss: 0.2761 - val_output_ph_loss: 0.2838 - val_output_mg_c_loss: 0.2601 - val_output_c_loss: 0.2403\nEpoch 5/120\n30/30 - 4s - loss: 2.0682 - output_react_loss: 0.2218 - output_bg_ph_loss: 0.2780 - output_ph_loss: 0.2908 - output_mg_c_loss: 0.2647 - output_c_loss: 0.2484 - val_loss: 1.9716 - val_output_react_loss: 0.2101 - val_output_bg_ph_loss: 0.2693 - val_output_ph_loss: 0.2742 - val_output_mg_c_loss: 0.2521 - val_output_c_loss: 0.2345\nEpoch 6/120\n30/30 - 4s - loss: 2.0186 - output_react_loss: 0.2167 - output_bg_ph_loss: 0.2715 - output_ph_loss: 0.2837 - output_mg_c_loss: 0.2577 - output_c_loss: 0.2432 - val_loss: 1.9286 - val_output_react_loss: 0.2043 - val_output_bg_ph_loss: 0.2636 - val_output_ph_loss: 0.2702 - val_output_mg_c_loss: 0.2461 - val_output_c_loss: 0.2306\nEpoch 7/120\n30/30 - 4s - loss: 1.9794 - output_react_loss: 0.2131 - output_bg_ph_loss: 0.2661 - output_ph_loss: 0.2788 - output_mg_c_loss: 0.2519 - output_c_loss: 0.2383 - val_loss: 1.8964 - val_output_react_loss: 0.2028 - val_output_bg_ph_loss: 0.2595 - val_output_ph_loss: 0.2648 - val_output_mg_c_loss: 0.2403 - val_output_c_loss: 0.2263\nEpoch 8/120\n30/30 - 4s - loss: 1.9333 - output_react_loss: 0.2085 - output_bg_ph_loss: 0.2602 - output_ph_loss: 0.2725 - output_mg_c_loss: 0.2448 - output_c_loss: 0.2339 - val_loss: 1.8370 - val_output_react_loss: 0.1947 - val_output_bg_ph_loss: 0.2520 - val_output_ph_loss: 0.2572 - val_output_mg_c_loss: 0.2333 - val_output_c_loss: 0.2198\nEpoch 9/120\n30/30 - 4s - loss: 1.8834 - output_react_loss: 0.2045 - output_bg_ph_loss: 0.2542 - output_ph_loss: 0.2659 - output_mg_c_loss: 0.2361 - output_c_loss: 0.2281 - val_loss: 1.8088 - val_output_react_loss: 0.1929 - val_output_bg_ph_loss: 0.2510 - val_output_ph_loss: 0.2530 - val_output_mg_c_loss: 0.2263 - val_output_c_loss: 0.2153\nEpoch 10/120\n30/30 - 4s - loss: 1.8425 - output_react_loss: 0.2013 - output_bg_ph_loss: 0.2478 - output_ph_loss: 0.2609 - output_mg_c_loss: 0.2304 - output_c_loss: 0.2228 - val_loss: 1.7647 - val_output_react_loss: 0.1912 - val_output_bg_ph_loss: 0.2408 - val_output_ph_loss: 0.2485 - val_output_mg_c_loss: 0.2200 - val_output_c_loss: 0.2122\nEpoch 11/120\n30/30 - 4s - loss: 1.8167 - output_react_loss: 0.1992 - output_bg_ph_loss: 0.2440 - output_ph_loss: 0.2577 - output_mg_c_loss: 0.2260 - output_c_loss: 0.2206 - val_loss: 1.7501 - val_output_react_loss: 0.1888 - val_output_bg_ph_loss: 0.2405 - val_output_ph_loss: 0.2455 - val_output_mg_c_loss: 0.2174 - val_output_c_loss: 0.2113\nEpoch 12/120\n30/30 - 4s - loss: 1.7783 - output_react_loss: 0.1968 - output_bg_ph_loss: 0.2379 - output_ph_loss: 0.2527 - output_mg_c_loss: 0.2198 - output_c_loss: 0.2167 - val_loss: 1.7254 - val_output_react_loss: 0.1857 - val_output_bg_ph_loss: 0.2370 - val_output_ph_loss: 0.2433 - val_output_mg_c_loss: 0.2153 - val_output_c_loss: 0.2064\nEpoch 13/120\n30/30 - 4s - loss: 1.7545 - output_react_loss: 0.1948 - output_bg_ph_loss: 0.2343 - output_ph_loss: 0.2489 - output_mg_c_loss: 0.2165 - output_c_loss: 0.2144 - val_loss: 1.6945 - val_output_react_loss: 0.1844 - val_output_bg_ph_loss: 0.2308 - val_output_ph_loss: 0.2409 - val_output_mg_c_loss: 0.2091 - val_output_c_loss: 0.2049\nEpoch 14/120\n30/30 - 4s - loss: 1.7199 - output_react_loss: 0.1912 - output_bg_ph_loss: 0.2298 - output_ph_loss: 0.2453 - output_mg_c_loss: 0.2111 - output_c_loss: 0.2104 - val_loss: 1.6693 - val_output_react_loss: 0.1828 - val_output_bg_ph_loss: 0.2273 - val_output_ph_loss: 0.2347 - val_output_mg_c_loss: 0.2062 - val_output_c_loss: 0.2020\nEpoch 15/120\n30/30 - 4s - loss: 1.7001 - output_react_loss: 0.1908 - output_bg_ph_loss: 0.2265 - output_ph_loss: 0.2412 - output_mg_c_loss: 0.2083 - output_c_loss: 0.2076 - val_loss: 1.6376 - val_output_react_loss: 0.1790 - val_output_bg_ph_loss: 0.2223 - val_output_ph_loss: 0.2338 - val_output_mg_c_loss: 0.2018 - val_output_c_loss: 0.1975\nEpoch 16/120\n30/30 - 4s - loss: 1.6763 - output_react_loss: 0.1888 - output_bg_ph_loss: 0.2231 - output_ph_loss: 0.2393 - output_mg_c_loss: 0.2040 - output_c_loss: 0.2052 - val_loss: 1.6350 - val_output_react_loss: 0.1826 - val_output_bg_ph_loss: 0.2237 - val_output_ph_loss: 0.2268 - val_output_mg_c_loss: 0.1991 - val_output_c_loss: 0.1974\nEpoch 17/120\n30/30 - 4s - loss: 1.6524 - output_react_loss: 0.1862 - output_bg_ph_loss: 0.2214 - output_ph_loss: 0.2341 - output_mg_c_loss: 0.2005 - output_c_loss: 0.2021 - val_loss: 1.5882 - val_output_react_loss: 0.1756 - val_output_bg_ph_loss: 0.2165 - val_output_ph_loss: 0.2255 - val_output_mg_c_loss: 0.1940 - val_output_c_loss: 0.1905\nEpoch 18/120\n30/30 - 4s - loss: 1.6277 - output_react_loss: 0.1833 - output_bg_ph_loss: 0.2173 - output_ph_loss: 0.2318 - output_mg_c_loss: 0.1976 - output_c_loss: 0.1996 - val_loss: 1.5811 - val_output_react_loss: 0.1760 - val_output_bg_ph_loss: 0.2158 - val_output_ph_loss: 0.2202 - val_output_mg_c_loss: 0.1939 - val_output_c_loss: 0.1894\nEpoch 19/120\n30/30 - 4s - loss: 1.6048 - output_react_loss: 0.1822 - output_bg_ph_loss: 0.2142 - output_ph_loss: 0.2282 - output_mg_c_loss: 0.1936 - output_c_loss: 0.1967 - val_loss: 1.5823 - val_output_react_loss: 0.1775 - val_output_bg_ph_loss: 0.2169 - val_output_ph_loss: 0.2204 - val_output_mg_c_loss: 0.1927 - val_output_c_loss: 0.1878\nEpoch 20/120\n30/30 - 4s - loss: 1.5862 - output_react_loss: 0.1800 - output_bg_ph_loss: 0.2113 - output_ph_loss: 0.2259 - output_mg_c_loss: 0.1914 - output_c_loss: 0.1948 - val_loss: 1.5650 - val_output_react_loss: 0.1768 - val_output_bg_ph_loss: 0.2115 - val_output_ph_loss: 0.2230 - val_output_mg_c_loss: 0.1889 - val_output_c_loss: 0.1875\nEpoch 21/120\n30/30 - 4s - loss: 1.5777 - output_react_loss: 0.1791 - output_bg_ph_loss: 0.2095 - output_ph_loss: 0.2254 - output_mg_c_loss: 0.1905 - output_c_loss: 0.1941 - val_loss: 1.5429 - val_output_react_loss: 0.1721 - val_output_bg_ph_loss: 0.2116 - val_output_ph_loss: 0.2152 - val_output_mg_c_loss: 0.1877 - val_output_c_loss: 0.1849\nEpoch 22/120\n30/30 - 4s - loss: 1.5588 - output_react_loss: 0.1763 - output_bg_ph_loss: 0.2085 - output_ph_loss: 0.2219 - output_mg_c_loss: 0.1877 - output_c_loss: 0.1920 - val_loss: 1.5415 - val_output_react_loss: 0.1723 - val_output_bg_ph_loss: 0.2125 - val_output_ph_loss: 0.2138 - val_output_mg_c_loss: 0.1864 - val_output_c_loss: 0.1854\nEpoch 23/120\n30/30 - 4s - loss: 1.5387 - output_react_loss: 0.1756 - output_bg_ph_loss: 0.2049 - output_ph_loss: 0.2187 - output_mg_c_loss: 0.1848 - output_c_loss: 0.1894 - val_loss: 1.5317 - val_output_react_loss: 0.1734 - val_output_bg_ph_loss: 0.2069 - val_output_ph_loss: 0.2141 - val_output_mg_c_loss: 0.1860 - val_output_c_loss: 0.1850\nEpoch 24/120\n30/30 - 4s - loss: 1.5313 - output_react_loss: 0.1749 - output_bg_ph_loss: 0.2038 - output_ph_loss: 0.2174 - output_mg_c_loss: 0.1841 - output_c_loss: 0.1884 - val_loss: 1.5225 - val_output_react_loss: 0.1708 - val_output_bg_ph_loss: 0.2102 - val_output_ph_loss: 0.2109 - val_output_mg_c_loss: 0.1831 - val_output_c_loss: 0.1832\nEpoch 25/120\n30/30 - 4s - loss: 1.5198 - output_react_loss: 0.1732 - output_bg_ph_loss: 0.2032 - output_ph_loss: 0.2165 - output_mg_c_loss: 0.1820 - output_c_loss: 0.1865 - val_loss: 1.5092 - val_output_react_loss: 0.1707 - val_output_bg_ph_loss: 0.2060 - val_output_ph_loss: 0.2100 - val_output_mg_c_loss: 0.1819 - val_output_c_loss: 0.1819\nEpoch 26/120\n30/30 - 4s - loss: 1.4993 - output_react_loss: 0.1707 - output_bg_ph_loss: 0.1994 - output_ph_loss: 0.2137 - output_mg_c_loss: 0.1800 - output_c_loss: 0.1853 - val_loss: 1.5011 - val_output_react_loss: 0.1676 - val_output_bg_ph_loss: 0.2052 - val_output_ph_loss: 0.2101 - val_output_mg_c_loss: 0.1827 - val_output_c_loss: 0.1802\nEpoch 27/120\n30/30 - 4s - loss: 1.4854 - output_react_loss: 0.1697 - output_bg_ph_loss: 0.1975 - output_ph_loss: 0.2121 - output_mg_c_loss: 0.1775 - output_c_loss: 0.1838 - val_loss: 1.4949 - val_output_react_loss: 0.1661 - val_output_bg_ph_loss: 0.2053 - val_output_ph_loss: 0.2086 - val_output_mg_c_loss: 0.1820 - val_output_c_loss: 0.1796\nEpoch 28/120\n30/30 - 4s - loss: 1.4652 - output_react_loss: 0.1671 - output_bg_ph_loss: 0.1949 - output_ph_loss: 0.2095 - output_mg_c_loss: 0.1749 - output_c_loss: 0.1820 - val_loss: 1.4817 - val_output_react_loss: 0.1649 - val_output_bg_ph_loss: 0.2028 - val_output_ph_loss: 0.2067 - val_output_mg_c_loss: 0.1799 - val_output_c_loss: 0.1797\nEpoch 29/120\n30/30 - 4s - loss: 1.4570 - output_react_loss: 0.1660 - output_bg_ph_loss: 0.1937 - output_ph_loss: 0.2082 - output_mg_c_loss: 0.1741 - output_c_loss: 0.1812 - val_loss: 1.4784 - val_output_react_loss: 0.1650 - val_output_bg_ph_loss: 0.2023 - val_output_ph_loss: 0.2065 - val_output_mg_c_loss: 0.1798 - val_output_c_loss: 0.1777\nEpoch 30/120\n30/30 - 4s - loss: 1.4460 - output_react_loss: 0.1661 - output_bg_ph_loss: 0.1917 - output_ph_loss: 0.2062 - output_mg_c_loss: 0.1721 - output_c_loss: 0.1802 - val_loss: 1.4758 - val_output_react_loss: 0.1670 - val_output_bg_ph_loss: 0.2029 - val_output_ph_loss: 0.2043 - val_output_mg_c_loss: 0.1774 - val_output_c_loss: 0.1770\nEpoch 31/120\n30/30 - 4s - loss: 1.4358 - output_react_loss: 0.1645 - output_bg_ph_loss: 0.1911 - output_ph_loss: 0.2049 - output_mg_c_loss: 0.1706 - output_c_loss: 0.1785 - val_loss: 1.4832 - val_output_react_loss: 0.1686 - val_output_bg_ph_loss: 0.2021 - val_output_ph_loss: 0.2041 - val_output_mg_c_loss: 0.1796 - val_output_c_loss: 0.1785\nEpoch 32/120\n30/30 - 4s - loss: 1.4263 - output_react_loss: 0.1634 - output_bg_ph_loss: 0.1897 - output_ph_loss: 0.2040 - output_mg_c_loss: 0.1691 - output_c_loss: 0.1779 - val_loss: 1.4731 - val_output_react_loss: 0.1646 - val_output_bg_ph_loss: 0.2030 - val_output_ph_loss: 0.2041 - val_output_mg_c_loss: 0.1781 - val_output_c_loss: 0.1774\nEpoch 33/120\n30/30 - 4s - loss: 1.4067 - output_react_loss: 0.1617 - output_bg_ph_loss: 0.1863 - output_ph_loss: 0.2020 - output_mg_c_loss: 0.1664 - output_c_loss: 0.1758 - val_loss: 1.4670 - val_output_react_loss: 0.1635 - val_output_bg_ph_loss: 0.2002 - val_output_ph_loss: 0.2062 - val_output_mg_c_loss: 0.1782 - val_output_c_loss: 0.1769\nEpoch 34/120\n30/30 - 4s - loss: 1.3967 - output_react_loss: 0.1602 - output_bg_ph_loss: 0.1851 - output_ph_loss: 0.2008 - output_mg_c_loss: 0.1653 - output_c_loss: 0.1748 - val_loss: 1.4740 - val_output_react_loss: 0.1648 - val_output_bg_ph_loss: 0.2007 - val_output_ph_loss: 0.2066 - val_output_mg_c_loss: 0.1789 - val_output_c_loss: 0.1787\nEpoch 35/120\n30/30 - 4s - loss: 1.3834 - output_react_loss: 0.1589 - output_bg_ph_loss: 0.1827 - output_ph_loss: 0.1988 - output_mg_c_loss: 0.1639 - output_c_loss: 0.1735 - val_loss: 1.4549 - val_output_react_loss: 0.1615 - val_output_bg_ph_loss: 0.2006 - val_output_ph_loss: 0.2023 - val_output_mg_c_loss: 0.1769 - val_output_c_loss: 0.1745\nEpoch 36/120\n30/30 - 4s - loss: 1.3745 - output_react_loss: 0.1570 - output_bg_ph_loss: 0.1814 - output_ph_loss: 0.1975 - output_mg_c_loss: 0.1635 - output_c_loss: 0.1733 - val_loss: 1.4527 - val_output_react_loss: 0.1617 - val_output_bg_ph_loss: 0.1999 - val_output_ph_loss: 0.2023 - val_output_mg_c_loss: 0.1758 - val_output_c_loss: 0.1757\nEpoch 37/120\n30/30 - 4s - loss: 1.3622 - output_react_loss: 0.1562 - output_bg_ph_loss: 0.1804 - output_ph_loss: 0.1964 - output_mg_c_loss: 0.1608 - output_c_loss: 0.1711 - val_loss: 1.4521 - val_output_react_loss: 0.1622 - val_output_bg_ph_loss: 0.1991 - val_output_ph_loss: 0.2038 - val_output_mg_c_loss: 0.1743 - val_output_c_loss: 0.1769\nEpoch 38/120\n30/30 - 4s - loss: 1.3482 - output_react_loss: 0.1548 - output_bg_ph_loss: 0.1783 - output_ph_loss: 0.1951 - output_mg_c_loss: 0.1582 - output_c_loss: 0.1707 - val_loss: 1.4610 - val_output_react_loss: 0.1620 - val_output_bg_ph_loss: 0.2001 - val_output_ph_loss: 0.2033 - val_output_mg_c_loss: 0.1781 - val_output_c_loss: 0.1773\nEpoch 39/120\n30/30 - 4s - loss: 1.3435 - output_react_loss: 0.1556 - output_bg_ph_loss: 0.1775 - output_ph_loss: 0.1932 - output_mg_c_loss: 0.1570 - output_c_loss: 0.1701 - val_loss: 1.4591 - val_output_react_loss: 0.1601 - val_output_bg_ph_loss: 0.1998 - val_output_ph_loss: 0.2049 - val_output_mg_c_loss: 0.1774 - val_output_c_loss: 0.1796\nEpoch 40/120\n30/30 - 4s - loss: 1.3337 - output_react_loss: 0.1529 - output_bg_ph_loss: 0.1768 - output_ph_loss: 0.1918 - output_mg_c_loss: 0.1566 - output_c_loss: 0.1694 - val_loss: 1.4426 - val_output_react_loss: 0.1603 - val_output_bg_ph_loss: 0.1975 - val_output_ph_loss: 0.2000 - val_output_mg_c_loss: 0.1764 - val_output_c_loss: 0.1741\nEpoch 41/120\n30/30 - 4s - loss: 1.3097 - output_react_loss: 0.1507 - output_bg_ph_loss: 0.1727 - output_ph_loss: 0.1900 - output_mg_c_loss: 0.1529 - output_c_loss: 0.1671 - val_loss: 1.4403 - val_output_react_loss: 0.1614 - val_output_bg_ph_loss: 0.1966 - val_output_ph_loss: 0.2001 - val_output_mg_c_loss: 0.1746 - val_output_c_loss: 0.1748\nEpoch 42/120\n30/30 - 4s - loss: 1.2990 - output_react_loss: 0.1496 - output_bg_ph_loss: 0.1711 - output_ph_loss: 0.1881 - output_mg_c_loss: 0.1516 - output_c_loss: 0.1663 - val_loss: 1.4326 - val_output_react_loss: 0.1595 - val_output_bg_ph_loss: 0.1977 - val_output_ph_loss: 0.1992 - val_output_mg_c_loss: 0.1733 - val_output_c_loss: 0.1722\nEpoch 43/120\n30/30 - 4s - loss: 1.2873 - output_react_loss: 0.1487 - output_bg_ph_loss: 0.1690 - output_ph_loss: 0.1869 - output_mg_c_loss: 0.1500 - output_c_loss: 0.1650 - val_loss: 1.4424 - val_output_react_loss: 0.1615 - val_output_bg_ph_loss: 0.1981 - val_output_ph_loss: 0.2005 - val_output_mg_c_loss: 0.1741 - val_output_c_loss: 0.1744\nEpoch 44/120\n30/30 - 4s - loss: 1.2752 - output_react_loss: 0.1478 - output_bg_ph_loss: 0.1677 - output_ph_loss: 0.1849 - output_mg_c_loss: 0.1478 - output_c_loss: 0.1637 - val_loss: 1.4444 - val_output_react_loss: 0.1597 - val_output_bg_ph_loss: 0.1990 - val_output_ph_loss: 0.2009 - val_output_mg_c_loss: 0.1753 - val_output_c_loss: 0.1754\nEpoch 45/120\n30/30 - 4s - loss: 1.2712 - output_react_loss: 0.1466 - output_bg_ph_loss: 0.1671 - output_ph_loss: 0.1844 - output_mg_c_loss: 0.1477 - output_c_loss: 0.1639 - val_loss: 1.4365 - val_output_react_loss: 0.1613 - val_output_bg_ph_loss: 0.1957 - val_output_ph_loss: 0.1989 - val_output_mg_c_loss: 0.1747 - val_output_c_loss: 0.1741\nEpoch 46/120\n30/30 - 4s - loss: 1.2567 - output_react_loss: 0.1453 - output_bg_ph_loss: 0.1646 - output_ph_loss: 0.1829 - output_mg_c_loss: 0.1460 - output_c_loss: 0.1621 - val_loss: 1.4306 - val_output_react_loss: 0.1597 - val_output_bg_ph_loss: 0.1965 - val_output_ph_loss: 0.1983 - val_output_mg_c_loss: 0.1734 - val_output_c_loss: 0.1732\nEpoch 47/120\n30/30 - 4s - loss: 1.2402 - output_react_loss: 0.1428 - output_bg_ph_loss: 0.1625 - output_ph_loss: 0.1811 - output_mg_c_loss: 0.1436 - output_c_loss: 0.1612 - val_loss: 1.4266 - val_output_react_loss: 0.1595 - val_output_bg_ph_loss: 0.1955 - val_output_ph_loss: 0.1983 - val_output_mg_c_loss: 0.1724 - val_output_c_loss: 0.1735\nEpoch 48/120\n30/30 - 4s - loss: 1.2358 - output_react_loss: 0.1429 - output_bg_ph_loss: 0.1610 - output_ph_loss: 0.1803 - output_mg_c_loss: 0.1435 - output_c_loss: 0.1607 - val_loss: 1.4273 - val_output_react_loss: 0.1578 - val_output_bg_ph_loss: 0.1974 - val_output_ph_loss: 0.1983 - val_output_mg_c_loss: 0.1730 - val_output_c_loss: 0.1726\nEpoch 49/120\n30/30 - 4s - loss: 1.2235 - output_react_loss: 0.1419 - output_bg_ph_loss: 0.1597 - output_ph_loss: 0.1784 - output_mg_c_loss: 0.1414 - output_c_loss: 0.1592 - val_loss: 1.4392 - val_output_react_loss: 0.1610 - val_output_bg_ph_loss: 0.1981 - val_output_ph_loss: 0.1990 - val_output_mg_c_loss: 0.1740 - val_output_c_loss: 0.1740\nEpoch 50/120\n30/30 - 4s - loss: 1.2135 - output_react_loss: 0.1402 - output_bg_ph_loss: 0.1583 - output_ph_loss: 0.1773 - output_mg_c_loss: 0.1404 - output_c_loss: 0.1585 - val_loss: 1.4262 - val_output_react_loss: 0.1578 - val_output_bg_ph_loss: 0.1959 - val_output_ph_loss: 0.1997 - val_output_mg_c_loss: 0.1728 - val_output_c_loss: 0.1736\nEpoch 51/120\n30/30 - 4s - loss: 1.2031 - output_react_loss: 0.1393 - output_bg_ph_loss: 0.1566 - output_ph_loss: 0.1761 - output_mg_c_loss: 0.1388 - output_c_loss: 0.1576 - val_loss: 1.4383 - val_output_react_loss: 0.1601 - val_output_bg_ph_loss: 0.1985 - val_output_ph_loss: 0.1987 - val_output_mg_c_loss: 0.1738 - val_output_c_loss: 0.1747\nEpoch 52/120\n30/30 - 4s - loss: 1.1939 - output_react_loss: 0.1373 - output_bg_ph_loss: 0.1555 - output_ph_loss: 0.1759 - output_mg_c_loss: 0.1377 - output_c_loss: 0.1572 - val_loss: 1.4197 - val_output_react_loss: 0.1575 - val_output_bg_ph_loss: 0.1939 - val_output_ph_loss: 0.1999 - val_output_mg_c_loss: 0.1721 - val_output_c_loss: 0.1728\nEpoch 53/120\n30/30 - 4s - loss: 1.1848 - output_react_loss: 0.1372 - output_bg_ph_loss: 0.1532 - output_ph_loss: 0.1748 - output_mg_c_loss: 0.1361 - output_c_loss: 0.1570 - val_loss: 1.4191 - val_output_react_loss: 0.1578 - val_output_bg_ph_loss: 0.1945 - val_output_ph_loss: 0.1976 - val_output_mg_c_loss: 0.1723 - val_output_c_loss: 0.1721\nEpoch 54/120\n30/30 - 4s - loss: 1.1797 - output_react_loss: 0.1367 - output_bg_ph_loss: 0.1527 - output_ph_loss: 0.1738 - output_mg_c_loss: 0.1354 - output_c_loss: 0.1562 - val_loss: 1.4224 - val_output_react_loss: 0.1571 - val_output_bg_ph_loss: 0.1954 - val_output_ph_loss: 0.2002 - val_output_mg_c_loss: 0.1720 - val_output_c_loss: 0.1730\nEpoch 55/120\n30/30 - 4s - loss: 1.1625 - output_react_loss: 0.1344 - output_bg_ph_loss: 0.1498 - output_ph_loss: 0.1721 - output_mg_c_loss: 0.1335 - output_c_loss: 0.1550 - val_loss: 1.4158 - val_output_react_loss: 0.1557 - val_output_bg_ph_loss: 0.1957 - val_output_ph_loss: 0.1979 - val_output_mg_c_loss: 0.1713 - val_output_c_loss: 0.1724\nEpoch 56/120\n30/30 - 4s - loss: 1.1535 - output_react_loss: 0.1331 - output_bg_ph_loss: 0.1486 - output_ph_loss: 0.1706 - output_mg_c_loss: 0.1326 - output_c_loss: 0.1542 - val_loss: 1.4149 - val_output_react_loss: 0.1578 - val_output_bg_ph_loss: 0.1943 - val_output_ph_loss: 0.1963 - val_output_mg_c_loss: 0.1714 - val_output_c_loss: 0.1717\nEpoch 57/120\n30/30 - 4s - loss: 1.1467 - output_react_loss: 0.1326 - output_bg_ph_loss: 0.1476 - output_ph_loss: 0.1699 - output_mg_c_loss: 0.1313 - output_c_loss: 0.1537 - val_loss: 1.4238 - val_output_react_loss: 0.1579 - val_output_bg_ph_loss: 0.1965 - val_output_ph_loss: 0.1971 - val_output_mg_c_loss: 0.1729 - val_output_c_loss: 0.1721\nEpoch 58/120\n30/30 - 4s - loss: 1.1412 - output_react_loss: 0.1322 - output_bg_ph_loss: 0.1468 - output_ph_loss: 0.1691 - output_mg_c_loss: 0.1307 - output_c_loss: 0.1528 - val_loss: 1.4103 - val_output_react_loss: 0.1573 - val_output_bg_ph_loss: 0.1933 - val_output_ph_loss: 0.1966 - val_output_mg_c_loss: 0.1702 - val_output_c_loss: 0.1722\nEpoch 59/120\n30/30 - 4s - loss: 1.1307 - output_react_loss: 0.1303 - output_bg_ph_loss: 0.1454 - output_ph_loss: 0.1680 - output_mg_c_loss: 0.1297 - output_c_loss: 0.1521 - val_loss: 1.4202 - val_output_react_loss: 0.1564 - val_output_bg_ph_loss: 0.1952 - val_output_ph_loss: 0.1973 - val_output_mg_c_loss: 0.1730 - val_output_c_loss: 0.1736\nEpoch 60/120\n30/30 - 4s - loss: 1.1223 - output_react_loss: 0.1289 - output_bg_ph_loss: 0.1443 - output_ph_loss: 0.1668 - output_mg_c_loss: 0.1285 - output_c_loss: 0.1520 - val_loss: 1.4188 - val_output_react_loss: 0.1562 - val_output_bg_ph_loss: 0.1951 - val_output_ph_loss: 0.1973 - val_output_mg_c_loss: 0.1725 - val_output_c_loss: 0.1740\nEpoch 61/120\n30/30 - 4s - loss: 1.1185 - output_react_loss: 0.1289 - output_bg_ph_loss: 0.1435 - output_ph_loss: 0.1667 - output_mg_c_loss: 0.1277 - output_c_loss: 0.1516 - val_loss: 1.4116 - val_output_react_loss: 0.1573 - val_output_bg_ph_loss: 0.1939 - val_output_ph_loss: 0.1964 - val_output_mg_c_loss: 0.1706 - val_output_c_loss: 0.1716\nEpoch 62/120\n30/30 - 4s - loss: 1.1087 - output_react_loss: 0.1275 - output_bg_ph_loss: 0.1422 - output_ph_loss: 0.1657 - output_mg_c_loss: 0.1266 - output_c_loss: 0.1504 - val_loss: 1.4122 - val_output_react_loss: 0.1556 - val_output_bg_ph_loss: 0.1954 - val_output_ph_loss: 0.1955 - val_output_mg_c_loss: 0.1718 - val_output_c_loss: 0.1710\nEpoch 63/120\n\nEpoch 00063: ReduceLROnPlateau reducing learning rate to 0.00010000000474974513.\n30/30 - 4s - loss: 1.1008 - output_react_loss: 0.1265 - output_bg_ph_loss: 0.1410 - output_ph_loss: 0.1645 - output_mg_c_loss: 0.1257 - output_c_loss: 0.1499 - val_loss: 1.4111 - val_output_react_loss: 0.1567 - val_output_bg_ph_loss: 0.1946 - val_output_ph_loss: 0.1957 - val_output_mg_c_loss: 0.1706 - val_output_c_loss: 0.1715\nEpoch 64/120\n30/30 - 4s - loss: 1.0687 - output_react_loss: 0.1228 - output_bg_ph_loss: 0.1365 - output_ph_loss: 0.1606 - output_mg_c_loss: 0.1214 - output_c_loss: 0.1468 - val_loss: 1.3944 - val_output_react_loss: 0.1544 - val_output_bg_ph_loss: 0.1922 - val_output_ph_loss: 0.1940 - val_output_mg_c_loss: 0.1689 - val_output_c_loss: 0.1695\nEpoch 65/120\n30/30 - 4s - loss: 1.0562 - output_react_loss: 0.1207 - output_bg_ph_loss: 0.1348 - output_ph_loss: 0.1592 - output_mg_c_loss: 0.1202 - output_c_loss: 0.1455 - val_loss: 1.3915 - val_output_react_loss: 0.1541 - val_output_bg_ph_loss: 0.1915 - val_output_ph_loss: 0.1938 - val_output_mg_c_loss: 0.1686 - val_output_c_loss: 0.1694\nEpoch 66/120\n30/30 - 4s - loss: 1.0498 - output_react_loss: 0.1208 - output_bg_ph_loss: 0.1336 - output_ph_loss: 0.1583 - output_mg_c_loss: 0.1187 - output_c_loss: 0.1452 - val_loss: 1.3897 - val_output_react_loss: 0.1537 - val_output_bg_ph_loss: 0.1915 - val_output_ph_loss: 0.1934 - val_output_mg_c_loss: 0.1682 - val_output_c_loss: 0.1693\nEpoch 67/120\n30/30 - 4s - loss: 1.0466 - output_react_loss: 0.1199 - output_bg_ph_loss: 0.1331 - output_ph_loss: 0.1581 - output_mg_c_loss: 0.1187 - output_c_loss: 0.1452 - val_loss: 1.3895 - val_output_react_loss: 0.1536 - val_output_bg_ph_loss: 0.1916 - val_output_ph_loss: 0.1930 - val_output_mg_c_loss: 0.1682 - val_output_c_loss: 0.1694\nEpoch 68/120\n30/30 - 4s - loss: 1.0450 - output_react_loss: 0.1201 - output_bg_ph_loss: 0.1331 - output_ph_loss: 0.1574 - output_mg_c_loss: 0.1183 - output_c_loss: 0.1448 - val_loss: 1.3906 - val_output_react_loss: 0.1537 - val_output_bg_ph_loss: 0.1917 - val_output_ph_loss: 0.1935 - val_output_mg_c_loss: 0.1683 - val_output_c_loss: 0.1696\nEpoch 69/120\n30/30 - 4s - loss: 1.0420 - output_react_loss: 0.1199 - output_bg_ph_loss: 0.1325 - output_ph_loss: 0.1573 - output_mg_c_loss: 0.1176 - output_c_loss: 0.1446 - val_loss: 1.3891 - val_output_react_loss: 0.1538 - val_output_bg_ph_loss: 0.1914 - val_output_ph_loss: 0.1934 - val_output_mg_c_loss: 0.1679 - val_output_c_loss: 0.1694\nEpoch 70/120\n30/30 - 4s - loss: 1.0388 - output_react_loss: 0.1191 - output_bg_ph_loss: 0.1323 - output_ph_loss: 0.1571 - output_mg_c_loss: 0.1173 - output_c_loss: 0.1443 - val_loss: 1.3896 - val_output_react_loss: 0.1537 - val_output_bg_ph_loss: 0.1915 - val_output_ph_loss: 0.1931 - val_output_mg_c_loss: 0.1683 - val_output_c_loss: 0.1695\nEpoch 71/120\n30/30 - 4s - loss: 1.0374 - output_react_loss: 0.1192 - output_bg_ph_loss: 0.1318 - output_ph_loss: 0.1571 - output_mg_c_loss: 0.1171 - output_c_loss: 0.1441 - val_loss: 1.3843 - val_output_react_loss: 0.1532 - val_output_bg_ph_loss: 0.1906 - val_output_ph_loss: 0.1926 - val_output_mg_c_loss: 0.1674 - val_output_c_loss: 0.1692\nEpoch 72/120\n30/30 - 4s - loss: 1.0367 - output_react_loss: 0.1191 - output_bg_ph_loss: 0.1315 - output_ph_loss: 0.1569 - output_mg_c_loss: 0.1171 - output_c_loss: 0.1444 - val_loss: 1.3886 - val_output_react_loss: 0.1538 - val_output_bg_ph_loss: 0.1913 - val_output_ph_loss: 0.1931 - val_output_mg_c_loss: 0.1679 - val_output_c_loss: 0.1694\nEpoch 73/120\n30/30 - 4s - loss: 1.0347 - output_react_loss: 0.1186 - output_bg_ph_loss: 0.1316 - output_ph_loss: 0.1566 - output_mg_c_loss: 0.1169 - output_c_loss: 0.1438 - val_loss: 1.3895 - val_output_react_loss: 0.1537 - val_output_bg_ph_loss: 0.1915 - val_output_ph_loss: 0.1933 - val_output_mg_c_loss: 0.1680 - val_output_c_loss: 0.1696\nEpoch 74/120\n30/30 - 4s - loss: 1.0339 - output_react_loss: 0.1185 - output_bg_ph_loss: 0.1315 - output_ph_loss: 0.1565 - output_mg_c_loss: 0.1167 - output_c_loss: 0.1441 - val_loss: 1.3882 - val_output_react_loss: 0.1536 - val_output_bg_ph_loss: 0.1915 - val_output_ph_loss: 0.1930 - val_output_mg_c_loss: 0.1678 - val_output_c_loss: 0.1692\nEpoch 75/120\n30/30 - 4s - loss: 1.0325 - output_react_loss: 0.1184 - output_bg_ph_loss: 0.1313 - output_ph_loss: 0.1565 - output_mg_c_loss: 0.1164 - output_c_loss: 0.1438 - val_loss: 1.3885 - val_output_react_loss: 0.1540 - val_output_bg_ph_loss: 0.1912 - val_output_ph_loss: 0.1935 - val_output_mg_c_loss: 0.1677 - val_output_c_loss: 0.1694\nEpoch 76/120\n\nEpoch 00076: ReduceLROnPlateau reducing learning rate to 1.0000000474974514e-05.\n30/30 - 4s - loss: 1.0312 - output_react_loss: 0.1178 - output_bg_ph_loss: 0.1312 - output_ph_loss: 0.1562 - output_mg_c_loss: 0.1166 - output_c_loss: 0.1436 - val_loss: 1.3896 - val_output_react_loss: 0.1540 - val_output_bg_ph_loss: 0.1918 - val_output_ph_loss: 0.1930 - val_output_mg_c_loss: 0.1679 - val_output_c_loss: 0.1693\nEpoch 77/120\n30/30 - 4s - loss: 1.0297 - output_react_loss: 0.1183 - output_bg_ph_loss: 0.1305 - output_ph_loss: 0.1558 - output_mg_c_loss: 0.1164 - output_c_loss: 0.1435 - val_loss: 1.3870 - val_output_react_loss: 0.1537 - val_output_bg_ph_loss: 0.1912 - val_output_ph_loss: 0.1928 - val_output_mg_c_loss: 0.1676 - val_output_c_loss: 0.1691\nEpoch 78/120\n30/30 - 4s - loss: 1.0273 - output_react_loss: 0.1175 - output_bg_ph_loss: 0.1306 - output_ph_loss: 0.1558 - output_mg_c_loss: 0.1160 - output_c_loss: 0.1434 - val_loss: 1.3881 - val_output_react_loss: 0.1538 - val_output_bg_ph_loss: 0.1914 - val_output_ph_loss: 0.1929 - val_output_mg_c_loss: 0.1678 - val_output_c_loss: 0.1693\nEpoch 79/120\n30/30 - 4s - loss: 1.0267 - output_react_loss: 0.1175 - output_bg_ph_loss: 0.1307 - output_ph_loss: 0.1554 - output_mg_c_loss: 0.1159 - output_c_loss: 0.1432 - val_loss: 1.3860 - val_output_react_loss: 0.1536 - val_output_bg_ph_loss: 0.1910 - val_output_ph_loss: 0.1927 - val_output_mg_c_loss: 0.1675 - val_output_c_loss: 0.1690\nEpoch 80/120\n30/30 - 4s - loss: 1.0253 - output_react_loss: 0.1177 - output_bg_ph_loss: 0.1301 - output_ph_loss: 0.1553 - output_mg_c_loss: 0.1157 - output_c_loss: 0.1430 - val_loss: 1.3869 - val_output_react_loss: 0.1537 - val_output_bg_ph_loss: 0.1911 - val_output_ph_loss: 0.1928 - val_output_mg_c_loss: 0.1677 - val_output_c_loss: 0.1691\nEpoch 81/120\nRestoring model weights from the end of the best epoch.\n\nEpoch 00081: ReduceLROnPlateau reducing learning rate to 1.0000000656873453e-06.\n30/30 - 4s - loss: 1.0248 - output_react_loss: 0.1175 - output_bg_ph_loss: 0.1302 - output_ph_loss: 0.1550 - output_mg_c_loss: 0.1157 - output_c_loss: 0.1430 - val_loss: 1.3866 - val_output_react_loss: 0.1537 - val_output_bg_ph_loss: 0.1911 - val_output_ph_loss: 0.1927 - val_output_mg_c_loss: 0.1676 - val_output_c_loss: 0.1691\nEpoch 00081: early stopping\n\nFOLD: 5\nEpoch 1/120\n30/30 - 7s - loss: 3.4458 - output_react_loss: 0.3593 - output_bg_ph_loss: 0.4657 - output_ph_loss: 0.4703 - output_mg_c_loss: 0.4055 - output_c_loss: 0.5145 - val_loss: 2.3242 - val_output_react_loss: 0.2370 - val_output_bg_ph_loss: 0.3207 - val_output_ph_loss: 0.3390 - val_output_mg_c_loss: 0.2940 - val_output_c_loss: 0.2819\nEpoch 2/120\n30/30 - 4s - loss: 2.3412 - output_react_loss: 0.2486 - output_bg_ph_loss: 0.3172 - output_ph_loss: 0.3346 - output_mg_c_loss: 0.2977 - output_c_loss: 0.2796 - val_loss: 2.1392 - val_output_react_loss: 0.2231 - val_output_bg_ph_loss: 0.2903 - val_output_ph_loss: 0.3000 - val_output_mg_c_loss: 0.2757 - val_output_c_loss: 0.2611\nEpoch 3/120\n30/30 - 4s - loss: 2.1906 - output_react_loss: 0.2336 - output_bg_ph_loss: 0.2973 - output_ph_loss: 0.3080 - output_mg_c_loss: 0.2799 - output_c_loss: 0.2610 - val_loss: 2.0496 - val_output_react_loss: 0.2155 - val_output_bg_ph_loss: 0.2775 - val_output_ph_loss: 0.2863 - val_output_mg_c_loss: 0.2639 - val_output_c_loss: 0.2495\nEpoch 4/120\n30/30 - 4s - loss: 2.1081 - output_react_loss: 0.2254 - output_bg_ph_loss: 0.2860 - output_ph_loss: 0.2960 - output_mg_c_loss: 0.2687 - output_c_loss: 0.2519 - val_loss: 1.9834 - val_output_react_loss: 0.2082 - val_output_bg_ph_loss: 0.2697 - val_output_ph_loss: 0.2792 - val_output_mg_c_loss: 0.2532 - val_output_c_loss: 0.2422\nEpoch 5/120\n30/30 - 4s - loss: 2.0613 - output_react_loss: 0.2210 - output_bg_ph_loss: 0.2797 - output_ph_loss: 0.2892 - output_mg_c_loss: 0.2618 - output_c_loss: 0.2471 - val_loss: 1.9542 - val_output_react_loss: 0.2049 - val_output_bg_ph_loss: 0.2635 - val_output_ph_loss: 0.2762 - val_output_mg_c_loss: 0.2509 - val_output_c_loss: 0.2396\nEpoch 6/120\n30/30 - 4s - loss: 2.0134 - output_react_loss: 0.2154 - output_bg_ph_loss: 0.2728 - output_ph_loss: 0.2841 - output_mg_c_loss: 0.2554 - output_c_loss: 0.2420 - val_loss: 1.9134 - val_output_react_loss: 0.2021 - val_output_bg_ph_loss: 0.2574 - val_output_ph_loss: 0.2724 - val_output_mg_c_loss: 0.2428 - val_output_c_loss: 0.2362\nEpoch 7/120\n30/30 - 4s - loss: 1.9820 - output_react_loss: 0.2123 - output_bg_ph_loss: 0.2671 - output_ph_loss: 0.2796 - output_mg_c_loss: 0.2520 - output_c_loss: 0.2396 - val_loss: 1.8820 - val_output_react_loss: 0.1984 - val_output_bg_ph_loss: 0.2530 - val_output_ph_loss: 0.2684 - val_output_mg_c_loss: 0.2393 - val_output_c_loss: 0.2321\nEpoch 8/120\n30/30 - 4s - loss: 1.9454 - output_react_loss: 0.2090 - output_bg_ph_loss: 0.2620 - output_ph_loss: 0.2747 - output_mg_c_loss: 0.2467 - output_c_loss: 0.2352 - val_loss: 1.8977 - val_output_react_loss: 0.2002 - val_output_bg_ph_loss: 0.2519 - val_output_ph_loss: 0.2695 - val_output_mg_c_loss: 0.2451 - val_output_c_loss: 0.2338\nEpoch 9/120\n30/30 - 4s - loss: 1.9095 - output_react_loss: 0.2074 - output_bg_ph_loss: 0.2577 - output_ph_loss: 0.2687 - output_mg_c_loss: 0.2401 - output_c_loss: 0.2304 - val_loss: 1.8009 - val_output_react_loss: 0.1922 - val_output_bg_ph_loss: 0.2415 - val_output_ph_loss: 0.2581 - val_output_mg_c_loss: 0.2264 - val_output_c_loss: 0.2226\nEpoch 10/120\n30/30 - 4s - loss: 1.8745 - output_react_loss: 0.2030 - output_bg_ph_loss: 0.2535 - output_ph_loss: 0.2633 - output_mg_c_loss: 0.2359 - output_c_loss: 0.2263 - val_loss: 1.7623 - val_output_react_loss: 0.1896 - val_output_bg_ph_loss: 0.2361 - val_output_ph_loss: 0.2520 - val_output_mg_c_loss: 0.2206 - val_output_c_loss: 0.2178\nEpoch 11/120\n30/30 - 4s - loss: 1.8198 - output_react_loss: 0.1991 - output_bg_ph_loss: 0.2455 - output_ph_loss: 0.2571 - output_mg_c_loss: 0.2266 - output_c_loss: 0.2203 - val_loss: 1.7228 - val_output_react_loss: 0.1851 - val_output_bg_ph_loss: 0.2301 - val_output_ph_loss: 0.2478 - val_output_mg_c_loss: 0.2159 - val_output_c_loss: 0.2128\nEpoch 12/120\n30/30 - 4s - loss: 1.7833 - output_react_loss: 0.1955 - output_bg_ph_loss: 0.2410 - output_ph_loss: 0.2523 - output_mg_c_loss: 0.2210 - output_c_loss: 0.2160 - val_loss: 1.7021 - val_output_react_loss: 0.1849 - val_output_bg_ph_loss: 0.2294 - val_output_ph_loss: 0.2438 - val_output_mg_c_loss: 0.2097 - val_output_c_loss: 0.2104\nEpoch 13/120\n30/30 - 4s - loss: 1.7582 - output_react_loss: 0.1937 - output_bg_ph_loss: 0.2375 - output_ph_loss: 0.2488 - output_mg_c_loss: 0.2169 - output_c_loss: 0.2132 - val_loss: 1.6732 - val_output_react_loss: 0.1831 - val_output_bg_ph_loss: 0.2242 - val_output_ph_loss: 0.2397 - val_output_mg_c_loss: 0.2050 - val_output_c_loss: 0.2091\nEpoch 14/120\n30/30 - 4s - loss: 1.7339 - output_react_loss: 0.1927 - output_bg_ph_loss: 0.2336 - output_ph_loss: 0.2440 - output_mg_c_loss: 0.2130 - output_c_loss: 0.2112 - val_loss: 1.6394 - val_output_react_loss: 0.1817 - val_output_bg_ph_loss: 0.2180 - val_output_ph_loss: 0.2364 - val_output_mg_c_loss: 0.1997 - val_output_c_loss: 0.2042\nEpoch 15/120\n30/30 - 4s - loss: 1.7064 - output_react_loss: 0.1901 - output_bg_ph_loss: 0.2288 - output_ph_loss: 0.2406 - output_mg_c_loss: 0.2105 - output_c_loss: 0.2070 - val_loss: 1.6321 - val_output_react_loss: 0.1809 - val_output_bg_ph_loss: 0.2161 - val_output_ph_loss: 0.2334 - val_output_mg_c_loss: 0.2008 - val_output_c_loss: 0.2030\nEpoch 16/120\n30/30 - 4s - loss: 1.6720 - output_react_loss: 0.1868 - output_bg_ph_loss: 0.2251 - output_ph_loss: 0.2358 - output_mg_c_loss: 0.2047 - output_c_loss: 0.2028 - val_loss: 1.5972 - val_output_react_loss: 0.1764 - val_output_bg_ph_loss: 0.2135 - val_output_ph_loss: 0.2312 - val_output_mg_c_loss: 0.1941 - val_output_c_loss: 0.1979\nEpoch 17/120\n30/30 - 4s - loss: 1.6563 - output_react_loss: 0.1854 - output_bg_ph_loss: 0.2229 - output_ph_loss: 0.2326 - output_mg_c_loss: 0.2035 - output_c_loss: 0.2002 - val_loss: 1.5762 - val_output_react_loss: 0.1759 - val_output_bg_ph_loss: 0.2097 - val_output_ph_loss: 0.2266 - val_output_mg_c_loss: 0.1917 - val_output_c_loss: 0.1948\nEpoch 18/120\n30/30 - 4s - loss: 1.6257 - output_react_loss: 0.1831 - output_bg_ph_loss: 0.2185 - output_ph_loss: 0.2303 - output_mg_c_loss: 0.1976 - output_c_loss: 0.1968 - val_loss: 1.5659 - val_output_react_loss: 0.1721 - val_output_bg_ph_loss: 0.2082 - val_output_ph_loss: 0.2277 - val_output_mg_c_loss: 0.1911 - val_output_c_loss: 0.1953\nEpoch 19/120\n30/30 - 4s - loss: 1.6121 - output_react_loss: 0.1813 - output_bg_ph_loss: 0.2168 - output_ph_loss: 0.2274 - output_mg_c_loss: 0.1960 - output_c_loss: 0.1966 - val_loss: 1.5488 - val_output_react_loss: 0.1719 - val_output_bg_ph_loss: 0.2071 - val_output_ph_loss: 0.2247 - val_output_mg_c_loss: 0.1867 - val_output_c_loss: 0.1927\nEpoch 20/120\n30/30 - 4s - loss: 1.5916 - output_react_loss: 0.1797 - output_bg_ph_loss: 0.2138 - output_ph_loss: 0.2248 - output_mg_c_loss: 0.1932 - output_c_loss: 0.1934 - val_loss: 1.5293 - val_output_react_loss: 0.1700 - val_output_bg_ph_loss: 0.2039 - val_output_ph_loss: 0.2205 - val_output_mg_c_loss: 0.1851 - val_output_c_loss: 0.1908\nEpoch 21/120\n30/30 - 4s - loss: 1.5736 - output_react_loss: 0.1787 - output_bg_ph_loss: 0.2109 - output_ph_loss: 0.2210 - output_mg_c_loss: 0.1913 - output_c_loss: 0.1907 - val_loss: 1.5306 - val_output_react_loss: 0.1701 - val_output_bg_ph_loss: 0.2029 - val_output_ph_loss: 0.2201 - val_output_mg_c_loss: 0.1869 - val_output_c_loss: 0.1908\nEpoch 22/120\n30/30 - 4s - loss: 1.5604 - output_react_loss: 0.1762 - output_bg_ph_loss: 0.2097 - output_ph_loss: 0.2204 - output_mg_c_loss: 0.1891 - output_c_loss: 0.1900 - val_loss: 1.5215 - val_output_react_loss: 0.1683 - val_output_bg_ph_loss: 0.2035 - val_output_ph_loss: 0.2204 - val_output_mg_c_loss: 0.1841 - val_output_c_loss: 0.1895\nEpoch 23/120\n30/30 - 4s - loss: 1.5393 - output_react_loss: 0.1746 - output_bg_ph_loss: 0.2069 - output_ph_loss: 0.2178 - output_mg_c_loss: 0.1855 - output_c_loss: 0.1875 - val_loss: 1.5298 - val_output_react_loss: 0.1689 - val_output_bg_ph_loss: 0.2016 - val_output_ph_loss: 0.2216 - val_output_mg_c_loss: 0.1889 - val_output_c_loss: 0.1893\nEpoch 24/120\n30/30 - 4s - loss: 1.5338 - output_react_loss: 0.1746 - output_bg_ph_loss: 0.2053 - output_ph_loss: 0.2184 - output_mg_c_loss: 0.1847 - output_c_loss: 0.1863 - val_loss: 1.4955 - val_output_react_loss: 0.1678 - val_output_bg_ph_loss: 0.1998 - val_output_ph_loss: 0.2164 - val_output_mg_c_loss: 0.1794 - val_output_c_loss: 0.1852\nEpoch 25/120\n30/30 - 4s - loss: 1.5171 - output_react_loss: 0.1725 - output_bg_ph_loss: 0.2039 - output_ph_loss: 0.2148 - output_mg_c_loss: 0.1823 - output_c_loss: 0.1851 - val_loss: 1.4934 - val_output_react_loss: 0.1669 - val_output_bg_ph_loss: 0.2001 - val_output_ph_loss: 0.2154 - val_output_mg_c_loss: 0.1795 - val_output_c_loss: 0.1852\nEpoch 26/120\n30/30 - 4s - loss: 1.5046 - output_react_loss: 0.1709 - output_bg_ph_loss: 0.2015 - output_ph_loss: 0.2142 - output_mg_c_loss: 0.1805 - output_c_loss: 0.1846 - val_loss: 1.5036 - val_output_react_loss: 0.1680 - val_output_bg_ph_loss: 0.1997 - val_output_ph_loss: 0.2164 - val_output_mg_c_loss: 0.1818 - val_output_c_loss: 0.1882\nEpoch 27/120\n30/30 - 4s - loss: 1.4908 - output_react_loss: 0.1699 - output_bg_ph_loss: 0.1989 - output_ph_loss: 0.2112 - output_mg_c_loss: 0.1796 - output_c_loss: 0.1827 - val_loss: 1.4716 - val_output_react_loss: 0.1648 - val_output_bg_ph_loss: 0.1954 - val_output_ph_loss: 0.2138 - val_output_mg_c_loss: 0.1767 - val_output_c_loss: 0.1838\nEpoch 28/120\n30/30 - 4s - loss: 1.4667 - output_react_loss: 0.1673 - output_bg_ph_loss: 0.1962 - output_ph_loss: 0.2087 - output_mg_c_loss: 0.1754 - output_c_loss: 0.1803 - val_loss: 1.4610 - val_output_react_loss: 0.1630 - val_output_bg_ph_loss: 0.1939 - val_output_ph_loss: 0.2120 - val_output_mg_c_loss: 0.1761 - val_output_c_loss: 0.1832\nEpoch 29/120\n30/30 - 4s - loss: 1.4574 - output_react_loss: 0.1658 - output_bg_ph_loss: 0.1956 - output_ph_loss: 0.2075 - output_mg_c_loss: 0.1738 - output_c_loss: 0.1796 - val_loss: 1.4602 - val_output_react_loss: 0.1624 - val_output_bg_ph_loss: 0.1954 - val_output_ph_loss: 0.2102 - val_output_mg_c_loss: 0.1756 - val_output_c_loss: 0.1832\nEpoch 30/120\n30/30 - 4s - loss: 1.4417 - output_react_loss: 0.1647 - output_bg_ph_loss: 0.1932 - output_ph_loss: 0.2046 - output_mg_c_loss: 0.1720 - output_c_loss: 0.1773 - val_loss: 1.4464 - val_output_react_loss: 0.1611 - val_output_bg_ph_loss: 0.1924 - val_output_ph_loss: 0.2095 - val_output_mg_c_loss: 0.1743 - val_output_c_loss: 0.1814\nEpoch 31/120\n30/30 - 4s - loss: 1.4285 - output_react_loss: 0.1642 - output_bg_ph_loss: 0.1907 - output_ph_loss: 0.2035 - output_mg_c_loss: 0.1696 - output_c_loss: 0.1760 - val_loss: 1.4446 - val_output_react_loss: 0.1618 - val_output_bg_ph_loss: 0.1919 - val_output_ph_loss: 0.2100 - val_output_mg_c_loss: 0.1734 - val_output_c_loss: 0.1803\nEpoch 32/120\n30/30 - 4s - loss: 1.4184 - output_react_loss: 0.1622 - output_bg_ph_loss: 0.1895 - output_ph_loss: 0.2019 - output_mg_c_loss: 0.1689 - output_c_loss: 0.1754 - val_loss: 1.4534 - val_output_react_loss: 0.1609 - val_output_bg_ph_loss: 0.1953 - val_output_ph_loss: 0.2112 - val_output_mg_c_loss: 0.1741 - val_output_c_loss: 0.1815\nEpoch 33/120\n30/30 - 4s - loss: 1.4091 - output_react_loss: 0.1610 - output_bg_ph_loss: 0.1895 - output_ph_loss: 0.2008 - output_mg_c_loss: 0.1665 - output_c_loss: 0.1745 - val_loss: 1.4502 - val_output_react_loss: 0.1623 - val_output_bg_ph_loss: 0.1925 - val_output_ph_loss: 0.2104 - val_output_mg_c_loss: 0.1750 - val_output_c_loss: 0.1802\nEpoch 34/120\n30/30 - 4s - loss: 1.3896 - output_react_loss: 0.1586 - output_bg_ph_loss: 0.1855 - output_ph_loss: 0.1991 - output_mg_c_loss: 0.1648 - output_c_loss: 0.1725 - val_loss: 1.4296 - val_output_react_loss: 0.1595 - val_output_bg_ph_loss: 0.1910 - val_output_ph_loss: 0.2064 - val_output_mg_c_loss: 0.1716 - val_output_c_loss: 0.1789\nEpoch 35/120\n30/30 - 4s - loss: 1.3768 - output_react_loss: 0.1578 - output_bg_ph_loss: 0.1833 - output_ph_loss: 0.1967 - output_mg_c_loss: 0.1630 - output_c_loss: 0.1719 - val_loss: 1.4474 - val_output_react_loss: 0.1614 - val_output_bg_ph_loss: 0.1937 - val_output_ph_loss: 0.2085 - val_output_mg_c_loss: 0.1749 - val_output_c_loss: 0.1790\nEpoch 36/120\n30/30 - 4s - loss: 1.3706 - output_react_loss: 0.1572 - output_bg_ph_loss: 0.1834 - output_ph_loss: 0.1951 - output_mg_c_loss: 0.1617 - output_c_loss: 0.1709 - val_loss: 1.4299 - val_output_react_loss: 0.1599 - val_output_bg_ph_loss: 0.1896 - val_output_ph_loss: 0.2079 - val_output_mg_c_loss: 0.1724 - val_output_c_loss: 0.1781\nEpoch 37/120\n30/30 - 4s - loss: 1.3548 - output_react_loss: 0.1558 - output_bg_ph_loss: 0.1795 - output_ph_loss: 0.1946 - output_mg_c_loss: 0.1597 - output_c_loss: 0.1702 - val_loss: 1.4276 - val_output_react_loss: 0.1587 - val_output_bg_ph_loss: 0.1909 - val_output_ph_loss: 0.2072 - val_output_mg_c_loss: 0.1711 - val_output_c_loss: 0.1792\nEpoch 38/120\n30/30 - 4s - loss: 1.3451 - output_react_loss: 0.1547 - output_bg_ph_loss: 0.1786 - output_ph_loss: 0.1928 - output_mg_c_loss: 0.1586 - output_c_loss: 0.1685 - val_loss: 1.4262 - val_output_react_loss: 0.1580 - val_output_bg_ph_loss: 0.1890 - val_output_ph_loss: 0.2086 - val_output_mg_c_loss: 0.1729 - val_output_c_loss: 0.1779\nEpoch 39/120\n30/30 - 4s - loss: 1.3280 - output_react_loss: 0.1532 - output_bg_ph_loss: 0.1765 - output_ph_loss: 0.1897 - output_mg_c_loss: 0.1559 - output_c_loss: 0.1670 - val_loss: 1.4240 - val_output_react_loss: 0.1572 - val_output_bg_ph_loss: 0.1906 - val_output_ph_loss: 0.2071 - val_output_mg_c_loss: 0.1718 - val_output_c_loss: 0.1777\nEpoch 40/120\n30/30 - 4s - loss: 1.3176 - output_react_loss: 0.1509 - output_bg_ph_loss: 0.1751 - output_ph_loss: 0.1895 - output_mg_c_loss: 0.1548 - output_c_loss: 0.1665 - val_loss: 1.4150 - val_output_react_loss: 0.1572 - val_output_bg_ph_loss: 0.1895 - val_output_ph_loss: 0.2054 - val_output_mg_c_loss: 0.1696 - val_output_c_loss: 0.1771\nEpoch 41/120\n30/30 - 4s - loss: 1.2996 - output_react_loss: 0.1494 - output_bg_ph_loss: 0.1720 - output_ph_loss: 0.1880 - output_mg_c_loss: 0.1517 - output_c_loss: 0.1655 - val_loss: 1.4102 - val_output_react_loss: 0.1566 - val_output_bg_ph_loss: 0.1882 - val_output_ph_loss: 0.2034 - val_output_mg_c_loss: 0.1698 - val_output_c_loss: 0.1775\nEpoch 42/120\n30/30 - 4s - loss: 1.2954 - output_react_loss: 0.1483 - output_bg_ph_loss: 0.1717 - output_ph_loss: 0.1868 - output_mg_c_loss: 0.1519 - output_c_loss: 0.1649 - val_loss: 1.4034 - val_output_react_loss: 0.1561 - val_output_bg_ph_loss: 0.1871 - val_output_ph_loss: 0.2030 - val_output_mg_c_loss: 0.1694 - val_output_c_loss: 0.1751\nEpoch 43/120\n30/30 - 4s - loss: 1.2840 - output_react_loss: 0.1478 - output_bg_ph_loss: 0.1697 - output_ph_loss: 0.1851 - output_mg_c_loss: 0.1500 - output_c_loss: 0.1639 - val_loss: 1.4042 - val_output_react_loss: 0.1552 - val_output_bg_ph_loss: 0.1871 - val_output_ph_loss: 0.2044 - val_output_mg_c_loss: 0.1698 - val_output_c_loss: 0.1757\nEpoch 44/120\n30/30 - 4s - loss: 1.2650 - output_react_loss: 0.1461 - output_bg_ph_loss: 0.1671 - output_ph_loss: 0.1829 - output_mg_c_loss: 0.1472 - output_c_loss: 0.1614 - val_loss: 1.4036 - val_output_react_loss: 0.1555 - val_output_bg_ph_loss: 0.1871 - val_output_ph_loss: 0.2035 - val_output_mg_c_loss: 0.1695 - val_output_c_loss: 0.1760\nEpoch 45/120\n30/30 - 4s - loss: 1.2563 - output_react_loss: 0.1449 - output_bg_ph_loss: 0.1655 - output_ph_loss: 0.1821 - output_mg_c_loss: 0.1463 - output_c_loss: 0.1609 - val_loss: 1.4015 - val_output_react_loss: 0.1549 - val_output_bg_ph_loss: 0.1872 - val_output_ph_loss: 0.2050 - val_output_mg_c_loss: 0.1680 - val_output_c_loss: 0.1765\nEpoch 46/120\n30/30 - 4s - loss: 1.2468 - output_react_loss: 0.1436 - output_bg_ph_loss: 0.1643 - output_ph_loss: 0.1808 - output_mg_c_loss: 0.1448 - output_c_loss: 0.1605 - val_loss: 1.4169 - val_output_react_loss: 0.1565 - val_output_bg_ph_loss: 0.1893 - val_output_ph_loss: 0.2080 - val_output_mg_c_loss: 0.1704 - val_output_c_loss: 0.1765\nEpoch 47/120\n30/30 - 4s - loss: 1.2330 - output_react_loss: 0.1423 - output_bg_ph_loss: 0.1624 - output_ph_loss: 0.1792 - output_mg_c_loss: 0.1425 - output_c_loss: 0.1592 - val_loss: 1.4140 - val_output_react_loss: 0.1580 - val_output_bg_ph_loss: 0.1892 - val_output_ph_loss: 0.2049 - val_output_mg_c_loss: 0.1693 - val_output_c_loss: 0.1761\nEpoch 48/120\n30/30 - 4s - loss: 1.2217 - output_react_loss: 0.1412 - output_bg_ph_loss: 0.1608 - output_ph_loss: 0.1776 - output_mg_c_loss: 0.1410 - output_c_loss: 0.1582 - val_loss: 1.4001 - val_output_react_loss: 0.1567 - val_output_bg_ph_loss: 0.1860 - val_output_ph_loss: 0.2021 - val_output_mg_c_loss: 0.1687 - val_output_c_loss: 0.1752\nEpoch 49/120\n30/30 - 4s - loss: 1.2181 - output_react_loss: 0.1397 - output_bg_ph_loss: 0.1599 - output_ph_loss: 0.1769 - output_mg_c_loss: 0.1415 - output_c_loss: 0.1589 - val_loss: 1.4031 - val_output_react_loss: 0.1582 - val_output_bg_ph_loss: 0.1863 - val_output_ph_loss: 0.2025 - val_output_mg_c_loss: 0.1677 - val_output_c_loss: 0.1762\nEpoch 50/120\n30/30 - 4s - loss: 1.2070 - output_react_loss: 0.1389 - output_bg_ph_loss: 0.1581 - output_ph_loss: 0.1765 - output_mg_c_loss: 0.1397 - output_c_loss: 0.1572 - val_loss: 1.4027 - val_output_react_loss: 0.1563 - val_output_bg_ph_loss: 0.1855 - val_output_ph_loss: 0.2055 - val_output_mg_c_loss: 0.1693 - val_output_c_loss: 0.1751\nEpoch 51/120\n30/30 - 4s - loss: 1.1981 - output_react_loss: 0.1384 - output_bg_ph_loss: 0.1564 - output_ph_loss: 0.1750 - output_mg_c_loss: 0.1385 - output_c_loss: 0.1565 - val_loss: 1.3961 - val_output_react_loss: 0.1568 - val_output_bg_ph_loss: 0.1855 - val_output_ph_loss: 0.2011 - val_output_mg_c_loss: 0.1673 - val_output_c_loss: 0.1756\nEpoch 52/120\n30/30 - 4s - loss: 1.1854 - output_react_loss: 0.1367 - output_bg_ph_loss: 0.1551 - output_ph_loss: 0.1732 - output_mg_c_loss: 0.1366 - output_c_loss: 0.1556 - val_loss: 1.3965 - val_output_react_loss: 0.1543 - val_output_bg_ph_loss: 0.1869 - val_output_ph_loss: 0.2033 - val_output_mg_c_loss: 0.1681 - val_output_c_loss: 0.1746\nEpoch 53/120\n30/30 - 4s - loss: 1.1750 - output_react_loss: 0.1346 - output_bg_ph_loss: 0.1535 - output_ph_loss: 0.1729 - output_mg_c_loss: 0.1355 - output_c_loss: 0.1548 - val_loss: 1.3956 - val_output_react_loss: 0.1550 - val_output_bg_ph_loss: 0.1876 - val_output_ph_loss: 0.2023 - val_output_mg_c_loss: 0.1670 - val_output_c_loss: 0.1740\nEpoch 54/120\n30/30 - 4s - loss: 1.1608 - output_react_loss: 0.1338 - output_bg_ph_loss: 0.1511 - output_ph_loss: 0.1706 - output_mg_c_loss: 0.1334 - output_c_loss: 0.1535 - val_loss: 1.3888 - val_output_react_loss: 0.1533 - val_output_bg_ph_loss: 0.1854 - val_output_ph_loss: 0.2016 - val_output_mg_c_loss: 0.1672 - val_output_c_loss: 0.1752\nEpoch 55/120\n30/30 - 4s - loss: 1.1588 - output_react_loss: 0.1331 - output_bg_ph_loss: 0.1512 - output_ph_loss: 0.1709 - output_mg_c_loss: 0.1330 - output_c_loss: 0.1533 - val_loss: 1.3910 - val_output_react_loss: 0.1560 - val_output_bg_ph_loss: 0.1858 - val_output_ph_loss: 0.2017 - val_output_mg_c_loss: 0.1661 - val_output_c_loss: 0.1734\nEpoch 56/120\n30/30 - 4s - loss: 1.1483 - output_react_loss: 0.1316 - output_bg_ph_loss: 0.1495 - output_ph_loss: 0.1692 - output_mg_c_loss: 0.1323 - output_c_loss: 0.1525 - val_loss: 1.3862 - val_output_react_loss: 0.1553 - val_output_bg_ph_loss: 0.1846 - val_output_ph_loss: 0.2018 - val_output_mg_c_loss: 0.1654 - val_output_c_loss: 0.1739\nEpoch 57/120\n30/30 - 4s - loss: 1.1401 - output_react_loss: 0.1306 - output_bg_ph_loss: 0.1481 - output_ph_loss: 0.1686 - output_mg_c_loss: 0.1311 - output_c_loss: 0.1519 - val_loss: 1.3812 - val_output_react_loss: 0.1548 - val_output_bg_ph_loss: 0.1853 - val_output_ph_loss: 0.1993 - val_output_mg_c_loss: 0.1645 - val_output_c_loss: 0.1728\nEpoch 58/120\n30/30 - 4s - loss: 1.1335 - output_react_loss: 0.1299 - output_bg_ph_loss: 0.1469 - output_ph_loss: 0.1677 - output_mg_c_loss: 0.1300 - output_c_loss: 0.1522 - val_loss: 1.3878 - val_output_react_loss: 0.1552 - val_output_bg_ph_loss: 0.1857 - val_output_ph_loss: 0.2006 - val_output_mg_c_loss: 0.1659 - val_output_c_loss: 0.1736\nEpoch 59/120\n30/30 - 4s - loss: 1.1235 - output_react_loss: 0.1286 - output_bg_ph_loss: 0.1459 - output_ph_loss: 0.1659 - output_mg_c_loss: 0.1292 - output_c_loss: 0.1503 - val_loss: 1.3851 - val_output_react_loss: 0.1539 - val_output_bg_ph_loss: 0.1864 - val_output_ph_loss: 0.2004 - val_output_mg_c_loss: 0.1656 - val_output_c_loss: 0.1731\nEpoch 60/120\n30/30 - 4s - loss: 1.1176 - output_react_loss: 0.1273 - output_bg_ph_loss: 0.1444 - output_ph_loss: 0.1662 - output_mg_c_loss: 0.1286 - output_c_loss: 0.1509 - val_loss: 1.3796 - val_output_react_loss: 0.1534 - val_output_bg_ph_loss: 0.1850 - val_output_ph_loss: 0.1988 - val_output_mg_c_loss: 0.1654 - val_output_c_loss: 0.1733\nEpoch 61/120\n30/30 - 4s - loss: 1.1088 - output_react_loss: 0.1266 - output_bg_ph_loss: 0.1433 - output_ph_loss: 0.1646 - output_mg_c_loss: 0.1273 - output_c_loss: 0.1497 - val_loss: 1.3802 - val_output_react_loss: 0.1539 - val_output_bg_ph_loss: 0.1847 - val_output_ph_loss: 0.1986 - val_output_mg_c_loss: 0.1658 - val_output_c_loss: 0.1728\nEpoch 62/120\n30/30 - 4s - loss: 1.0994 - output_react_loss: 0.1260 - output_bg_ph_loss: 0.1420 - output_ph_loss: 0.1630 - output_mg_c_loss: 0.1258 - output_c_loss: 0.1487 - val_loss: 1.3924 - val_output_react_loss: 0.1541 - val_output_bg_ph_loss: 0.1875 - val_output_ph_loss: 0.1997 - val_output_mg_c_loss: 0.1679 - val_output_c_loss: 0.1738\nEpoch 63/120\n30/30 - 4s - loss: 1.0950 - output_react_loss: 0.1249 - output_bg_ph_loss: 0.1416 - output_ph_loss: 0.1630 - output_mg_c_loss: 0.1253 - output_c_loss: 0.1485 - val_loss: 1.3826 - val_output_react_loss: 0.1545 - val_output_bg_ph_loss: 0.1859 - val_output_ph_loss: 0.1999 - val_output_mg_c_loss: 0.1645 - val_output_c_loss: 0.1728\nEpoch 64/120\n30/30 - 4s - loss: 1.0861 - output_react_loss: 0.1235 - output_bg_ph_loss: 0.1400 - output_ph_loss: 0.1621 - output_mg_c_loss: 0.1244 - output_c_loss: 0.1480 - val_loss: 1.3814 - val_output_react_loss: 0.1538 - val_output_bg_ph_loss: 0.1852 - val_output_ph_loss: 0.1987 - val_output_mg_c_loss: 0.1660 - val_output_c_loss: 0.1728\nEpoch 65/120\n30/30 - 4s - loss: 1.0824 - output_react_loss: 0.1231 - output_bg_ph_loss: 0.1395 - output_ph_loss: 0.1610 - output_mg_c_loss: 0.1243 - output_c_loss: 0.1475 - val_loss: 1.3740 - val_output_react_loss: 0.1532 - val_output_bg_ph_loss: 0.1842 - val_output_ph_loss: 0.1975 - val_output_mg_c_loss: 0.1643 - val_output_c_loss: 0.1731\nEpoch 66/120\n30/30 - 4s - loss: 1.0727 - output_react_loss: 0.1223 - output_bg_ph_loss: 0.1380 - output_ph_loss: 0.1603 - output_mg_c_loss: 0.1224 - output_c_loss: 0.1472 - val_loss: 1.3818 - val_output_react_loss: 0.1545 - val_output_bg_ph_loss: 0.1845 - val_output_ph_loss: 0.1992 - val_output_mg_c_loss: 0.1656 - val_output_c_loss: 0.1732\nEpoch 67/120\n30/30 - 4s - loss: 1.0669 - output_react_loss: 0.1214 - output_bg_ph_loss: 0.1374 - output_ph_loss: 0.1591 - output_mg_c_loss: 0.1220 - output_c_loss: 0.1462 - val_loss: 1.3793 - val_output_react_loss: 0.1535 - val_output_bg_ph_loss: 0.1842 - val_output_ph_loss: 0.1990 - val_output_mg_c_loss: 0.1658 - val_output_c_loss: 0.1735\nEpoch 68/120\n30/30 - 4s - loss: 1.0648 - output_react_loss: 0.1207 - output_bg_ph_loss: 0.1369 - output_ph_loss: 0.1597 - output_mg_c_loss: 0.1217 - output_c_loss: 0.1466 - val_loss: 1.3731 - val_output_react_loss: 0.1524 - val_output_bg_ph_loss: 0.1842 - val_output_ph_loss: 0.1980 - val_output_mg_c_loss: 0.1649 - val_output_c_loss: 0.1720\nEpoch 69/120\n30/30 - 4s - loss: 1.0554 - output_react_loss: 0.1203 - output_bg_ph_loss: 0.1356 - output_ph_loss: 0.1577 - output_mg_c_loss: 0.1203 - output_c_loss: 0.1453 - val_loss: 1.3766 - val_output_react_loss: 0.1543 - val_output_bg_ph_loss: 0.1839 - val_output_ph_loss: 0.1981 - val_output_mg_c_loss: 0.1648 - val_output_c_loss: 0.1726\nEpoch 70/120\n30/30 - 4s - loss: 1.0462 - output_react_loss: 0.1189 - output_bg_ph_loss: 0.1337 - output_ph_loss: 0.1576 - output_mg_c_loss: 0.1193 - output_c_loss: 0.1447 - val_loss: 1.3739 - val_output_react_loss: 0.1541 - val_output_bg_ph_loss: 0.1839 - val_output_ph_loss: 0.1983 - val_output_mg_c_loss: 0.1638 - val_output_c_loss: 0.1721\nEpoch 71/120\n30/30 - 4s - loss: 1.0415 - output_react_loss: 0.1181 - output_bg_ph_loss: 0.1331 - output_ph_loss: 0.1571 - output_mg_c_loss: 0.1190 - output_c_loss: 0.1442 - val_loss: 1.3765 - val_output_react_loss: 0.1533 - val_output_bg_ph_loss: 0.1847 - val_output_ph_loss: 0.1975 - val_output_mg_c_loss: 0.1651 - val_output_c_loss: 0.1727\nEpoch 72/120\n30/30 - 4s - loss: 1.0342 - output_react_loss: 0.1173 - output_bg_ph_loss: 0.1322 - output_ph_loss: 0.1552 - output_mg_c_loss: 0.1180 - output_c_loss: 0.1442 - val_loss: 1.3744 - val_output_react_loss: 0.1531 - val_output_bg_ph_loss: 0.1840 - val_output_ph_loss: 0.1988 - val_output_mg_c_loss: 0.1643 - val_output_c_loss: 0.1729\nEpoch 73/120\n30/30 - 4s - loss: 1.0332 - output_react_loss: 0.1169 - output_bg_ph_loss: 0.1318 - output_ph_loss: 0.1559 - output_mg_c_loss: 0.1183 - output_c_loss: 0.1433 - val_loss: 1.3703 - val_output_react_loss: 0.1526 - val_output_bg_ph_loss: 0.1837 - val_output_ph_loss: 0.1982 - val_output_mg_c_loss: 0.1635 - val_output_c_loss: 0.1725\nEpoch 74/120\n30/30 - 4s - loss: 1.0244 - output_react_loss: 0.1163 - output_bg_ph_loss: 0.1304 - output_ph_loss: 0.1541 - output_mg_c_loss: 0.1171 - output_c_loss: 0.1429 - val_loss: 1.3742 - val_output_react_loss: 0.1532 - val_output_bg_ph_loss: 0.1841 - val_output_ph_loss: 0.1984 - val_output_mg_c_loss: 0.1645 - val_output_c_loss: 0.1723\nEpoch 75/120\n30/30 - 4s - loss: 1.0191 - output_react_loss: 0.1154 - output_bg_ph_loss: 0.1299 - output_ph_loss: 0.1534 - output_mg_c_loss: 0.1162 - output_c_loss: 0.1426 - val_loss: 1.3764 - val_output_react_loss: 0.1536 - val_output_bg_ph_loss: 0.1844 - val_output_ph_loss: 0.1981 - val_output_mg_c_loss: 0.1650 - val_output_c_loss: 0.1723\nEpoch 76/120\n30/30 - 4s - loss: 1.0117 - output_react_loss: 0.1140 - output_bg_ph_loss: 0.1289 - output_ph_loss: 0.1533 - output_mg_c_loss: 0.1153 - output_c_loss: 0.1419 - val_loss: 1.3760 - val_output_react_loss: 0.1528 - val_output_bg_ph_loss: 0.1840 - val_output_ph_loss: 0.1975 - val_output_mg_c_loss: 0.1663 - val_output_c_loss: 0.1724\nEpoch 77/120\n30/30 - 4s - loss: 1.0066 - output_react_loss: 0.1138 - output_bg_ph_loss: 0.1276 - output_ph_loss: 0.1524 - output_mg_c_loss: 0.1151 - output_c_loss: 0.1414 - val_loss: 1.3641 - val_output_react_loss: 0.1525 - val_output_bg_ph_loss: 0.1827 - val_output_ph_loss: 0.1961 - val_output_mg_c_loss: 0.1630 - val_output_c_loss: 0.1715\nEpoch 78/120\n30/30 - 4s - loss: 1.0015 - output_react_loss: 0.1136 - output_bg_ph_loss: 0.1269 - output_ph_loss: 0.1516 - output_mg_c_loss: 0.1137 - output_c_loss: 0.1415 - val_loss: 1.3709 - val_output_react_loss: 0.1526 - val_output_bg_ph_loss: 0.1836 - val_output_ph_loss: 0.1965 - val_output_mg_c_loss: 0.1649 - val_output_c_loss: 0.1722\nEpoch 79/120\n30/30 - 4s - loss: 1.0020 - output_react_loss: 0.1129 - output_bg_ph_loss: 0.1273 - output_ph_loss: 0.1513 - output_mg_c_loss: 0.1145 - output_c_loss: 0.1413 - val_loss: 1.3770 - val_output_react_loss: 0.1553 - val_output_bg_ph_loss: 0.1840 - val_output_ph_loss: 0.1985 - val_output_mg_c_loss: 0.1636 - val_output_c_loss: 0.1727\nEpoch 80/120\n30/30 - 4s - loss: 0.9949 - output_react_loss: 0.1124 - output_bg_ph_loss: 0.1257 - output_ph_loss: 0.1514 - output_mg_c_loss: 0.1134 - output_c_loss: 0.1405 - val_loss: 1.3778 - val_output_react_loss: 0.1536 - val_output_bg_ph_loss: 0.1844 - val_output_ph_loss: 0.1979 - val_output_mg_c_loss: 0.1655 - val_output_c_loss: 0.1729\nEpoch 81/120\n30/30 - 4s - loss: 0.9892 - output_react_loss: 0.1110 - output_bg_ph_loss: 0.1255 - output_ph_loss: 0.1500 - output_mg_c_loss: 0.1128 - output_c_loss: 0.1405 - val_loss: 1.3727 - val_output_react_loss: 0.1537 - val_output_bg_ph_loss: 0.1836 - val_output_ph_loss: 0.1973 - val_output_mg_c_loss: 0.1645 - val_output_c_loss: 0.1719\nEpoch 82/120\n\nEpoch 00082: ReduceLROnPlateau reducing learning rate to 0.00010000000474974513.\n30/30 - 4s - loss: 0.9845 - output_react_loss: 0.1109 - output_bg_ph_loss: 0.1248 - output_ph_loss: 0.1497 - output_mg_c_loss: 0.1119 - output_c_loss: 0.1398 - val_loss: 1.3714 - val_output_react_loss: 0.1526 - val_output_bg_ph_loss: 0.1843 - val_output_ph_loss: 0.1971 - val_output_mg_c_loss: 0.1645 - val_output_c_loss: 0.1716\nEpoch 83/120\n30/30 - 4s - loss: 0.9578 - output_react_loss: 0.1073 - output_bg_ph_loss: 0.1210 - output_ph_loss: 0.1464 - output_mg_c_loss: 0.1087 - output_c_loss: 0.1373 - val_loss: 1.3552 - val_output_react_loss: 0.1510 - val_output_bg_ph_loss: 0.1816 - val_output_ph_loss: 0.1953 - val_output_mg_c_loss: 0.1623 - val_output_c_loss: 0.1701\nEpoch 84/120\n30/30 - 4s - loss: 0.9430 - output_react_loss: 0.1058 - output_bg_ph_loss: 0.1188 - output_ph_loss: 0.1444 - output_mg_c_loss: 0.1066 - output_c_loss: 0.1360 - val_loss: 1.3524 - val_output_react_loss: 0.1510 - val_output_bg_ph_loss: 0.1808 - val_output_ph_loss: 0.1954 - val_output_mg_c_loss: 0.1617 - val_output_c_loss: 0.1699\nEpoch 85/120\n30/30 - 4s - loss: 0.9379 - output_react_loss: 0.1051 - output_bg_ph_loss: 0.1180 - output_ph_loss: 0.1442 - output_mg_c_loss: 0.1060 - output_c_loss: 0.1355 - val_loss: 1.3515 - val_output_react_loss: 0.1510 - val_output_bg_ph_loss: 0.1808 - val_output_ph_loss: 0.1951 - val_output_mg_c_loss: 0.1615 - val_output_c_loss: 0.1697\nEpoch 86/120\n30/30 - 4s - loss: 0.9328 - output_react_loss: 0.1049 - output_bg_ph_loss: 0.1170 - output_ph_loss: 0.1433 - output_mg_c_loss: 0.1053 - output_c_loss: 0.1352 - val_loss: 1.3519 - val_output_react_loss: 0.1511 - val_output_bg_ph_loss: 0.1806 - val_output_ph_loss: 0.1954 - val_output_mg_c_loss: 0.1617 - val_output_c_loss: 0.1698\nEpoch 87/120\n30/30 - 4s - loss: 0.9294 - output_react_loss: 0.1041 - output_bg_ph_loss: 0.1172 - output_ph_loss: 0.1429 - output_mg_c_loss: 0.1045 - output_c_loss: 0.1348 - val_loss: 1.3500 - val_output_react_loss: 0.1509 - val_output_bg_ph_loss: 0.1803 - val_output_ph_loss: 0.1952 - val_output_mg_c_loss: 0.1614 - val_output_c_loss: 0.1695\nEpoch 88/120\n30/30 - 4s - loss: 0.9272 - output_react_loss: 0.1036 - output_bg_ph_loss: 0.1168 - output_ph_loss: 0.1426 - output_mg_c_loss: 0.1046 - output_c_loss: 0.1347 - val_loss: 1.3502 - val_output_react_loss: 0.1509 - val_output_bg_ph_loss: 0.1807 - val_output_ph_loss: 0.1948 - val_output_mg_c_loss: 0.1614 - val_output_c_loss: 0.1696\nEpoch 89/120\n30/30 - 4s - loss: 0.9260 - output_react_loss: 0.1035 - output_bg_ph_loss: 0.1166 - output_ph_loss: 0.1427 - output_mg_c_loss: 0.1044 - output_c_loss: 0.1343 - val_loss: 1.3490 - val_output_react_loss: 0.1507 - val_output_bg_ph_loss: 0.1802 - val_output_ph_loss: 0.1949 - val_output_mg_c_loss: 0.1613 - val_output_c_loss: 0.1697\nEpoch 90/120\n30/30 - 4s - loss: 0.9232 - output_react_loss: 0.1035 - output_bg_ph_loss: 0.1159 - output_ph_loss: 0.1420 - output_mg_c_loss: 0.1041 - output_c_loss: 0.1342 - val_loss: 1.3500 - val_output_react_loss: 0.1507 - val_output_bg_ph_loss: 0.1807 - val_output_ph_loss: 0.1948 - val_output_mg_c_loss: 0.1614 - val_output_c_loss: 0.1696\nEpoch 91/120\n30/30 - 4s - loss: 0.9227 - output_react_loss: 0.1034 - output_bg_ph_loss: 0.1157 - output_ph_loss: 0.1421 - output_mg_c_loss: 0.1040 - output_c_loss: 0.1344 - val_loss: 1.3476 - val_output_react_loss: 0.1507 - val_output_bg_ph_loss: 0.1802 - val_output_ph_loss: 0.1944 - val_output_mg_c_loss: 0.1611 - val_output_c_loss: 0.1694\nEpoch 92/120\n30/30 - 4s - loss: 0.9214 - output_react_loss: 0.1029 - output_bg_ph_loss: 0.1158 - output_ph_loss: 0.1416 - output_mg_c_loss: 0.1042 - output_c_loss: 0.1341 - val_loss: 1.3497 - val_output_react_loss: 0.1510 - val_output_bg_ph_loss: 0.1804 - val_output_ph_loss: 0.1947 - val_output_mg_c_loss: 0.1613 - val_output_c_loss: 0.1696\nEpoch 93/120\n30/30 - 4s - loss: 0.9200 - output_react_loss: 0.1031 - output_bg_ph_loss: 0.1155 - output_ph_loss: 0.1419 - output_mg_c_loss: 0.1036 - output_c_loss: 0.1338 - val_loss: 1.3500 - val_output_react_loss: 0.1506 - val_output_bg_ph_loss: 0.1807 - val_output_ph_loss: 0.1948 - val_output_mg_c_loss: 0.1615 - val_output_c_loss: 0.1696\nEpoch 94/120\n30/30 - 4s - loss: 0.9215 - output_react_loss: 0.1032 - output_bg_ph_loss: 0.1155 - output_ph_loss: 0.1419 - output_mg_c_loss: 0.1039 - output_c_loss: 0.1342 - val_loss: 1.3506 - val_output_react_loss: 0.1507 - val_output_bg_ph_loss: 0.1808 - val_output_ph_loss: 0.1950 - val_output_mg_c_loss: 0.1615 - val_output_c_loss: 0.1697\nEpoch 95/120\n30/30 - 4s - loss: 0.9169 - output_react_loss: 0.1029 - output_bg_ph_loss: 0.1148 - output_ph_loss: 0.1411 - output_mg_c_loss: 0.1032 - output_c_loss: 0.1339 - val_loss: 1.3491 - val_output_react_loss: 0.1506 - val_output_bg_ph_loss: 0.1804 - val_output_ph_loss: 0.1946 - val_output_mg_c_loss: 0.1614 - val_output_c_loss: 0.1697\nEpoch 96/120\n\nEpoch 00096: ReduceLROnPlateau reducing learning rate to 1.0000000474974514e-05.\n30/30 - 4s - loss: 0.9155 - output_react_loss: 0.1027 - output_bg_ph_loss: 0.1143 - output_ph_loss: 0.1413 - output_mg_c_loss: 0.1032 - output_c_loss: 0.1337 - val_loss: 1.3490 - val_output_react_loss: 0.1506 - val_output_bg_ph_loss: 0.1805 - val_output_ph_loss: 0.1945 - val_output_mg_c_loss: 0.1613 - val_output_c_loss: 0.1697\nEpoch 97/120\n30/30 - 4s - loss: 0.9136 - output_react_loss: 0.1022 - output_bg_ph_loss: 0.1145 - output_ph_loss: 0.1411 - output_mg_c_loss: 0.1028 - output_c_loss: 0.1336 - val_loss: 1.3496 - val_output_react_loss: 0.1507 - val_output_bg_ph_loss: 0.1806 - val_output_ph_loss: 0.1946 - val_output_mg_c_loss: 0.1614 - val_output_c_loss: 0.1697\nEpoch 98/120\n30/30 - 4s - loss: 0.9124 - output_react_loss: 0.1021 - output_bg_ph_loss: 0.1139 - output_ph_loss: 0.1412 - output_mg_c_loss: 0.1027 - output_c_loss: 0.1338 - val_loss: 1.3495 - val_output_react_loss: 0.1507 - val_output_bg_ph_loss: 0.1805 - val_output_ph_loss: 0.1946 - val_output_mg_c_loss: 0.1614 - val_output_c_loss: 0.1697\nEpoch 99/120\n30/30 - 4s - loss: 0.9112 - output_react_loss: 0.1018 - output_bg_ph_loss: 0.1140 - output_ph_loss: 0.1411 - output_mg_c_loss: 0.1025 - output_c_loss: 0.1334 - val_loss: 1.3486 - val_output_react_loss: 0.1506 - val_output_bg_ph_loss: 0.1804 - val_output_ph_loss: 0.1945 - val_output_mg_c_loss: 0.1613 - val_output_c_loss: 0.1696\nEpoch 100/120\n30/30 - 4s - loss: 0.9126 - output_react_loss: 0.1022 - output_bg_ph_loss: 0.1142 - output_ph_loss: 0.1408 - output_mg_c_loss: 0.1027 - output_c_loss: 0.1336 - val_loss: 1.3490 - val_output_react_loss: 0.1506 - val_output_bg_ph_loss: 0.1805 - val_output_ph_loss: 0.1945 - val_output_mg_c_loss: 0.1613 - val_output_c_loss: 0.1696\nEpoch 101/120\nRestoring model weights from the end of the best epoch.\n\nEpoch 00101: ReduceLROnPlateau reducing learning rate to 1.0000000656873453e-06.\n30/30 - 4s - loss: 0.9104 - output_react_loss: 0.1018 - output_bg_ph_loss: 0.1138 - output_ph_loss: 0.1407 - output_mg_c_loss: 0.1026 - output_c_loss: 0.1335 - val_loss: 1.3486 - val_output_react_loss: 0.1506 - val_output_bg_ph_loss: 0.1804 - val_output_ph_loss: 0.1945 - val_output_mg_c_loss: 0.1613 - val_output_c_loss: 0.1696\nEpoch 00101: early stopping\n"
]
],
[
[
"## Model loss graph",
"_____no_output_____"
]
],
[
[
"for fold, history in enumerate(history_list):\n print(f'\\nFOLD: {fold+1}')\n print(f\"Train {np.array(history['loss']).min():.5f} Validation {np.array(history['val_loss']).min():.5f}\")\n\nplot_metrics_agg(history_list)",
"\nFOLD: 1\nTrain 1.05189 Validation 1.37240\n\nFOLD: 2\nTrain 1.07609 Validation 1.38107\n\nFOLD: 3\nTrain 1.05777 Validation 1.33757\n\nFOLD: 4\nTrain 1.02478 Validation 1.38429\n\nFOLD: 5\nTrain 0.91044 Validation 1.34764\n"
]
],
[
[
"# Post-processing",
"_____no_output_____"
]
],
[
[
"# Assign preds to OOF set\nfor idx, col in enumerate(pred_cols):\n val = oof_preds[:, :, idx]\n oof = oof.assign(**{f'{col}_pred': list(val)})\n \noof.to_csv('oof.csv', index=False)\n\noof_preds_dict = {}\nfor col in pred_cols:\n oof_preds_dict[col] = oof_preds[:, :, idx]\n\n# Assign values to test set\npreds_ls = []\n\nfor df, preds in [(public_test, test_public_preds), (private_test, test_private_preds)]:\n for i, uid in enumerate(df.id):\n single_pred = preds[i]\n\n single_df = pd.DataFrame(single_pred, columns=pred_cols)\n single_df['id_seqpos'] = [f'{uid}_{x}' for x in range(single_df.shape[0])]\n\n preds_ls.append(single_df)\n\npreds_df = pd.concat(preds_ls)",
"_____no_output_____"
]
],
[
[
"# Model evaluation",
"_____no_output_____"
]
],
[
[
"y_true_dict = get_targets_dict(train, pred_cols, train.index)\ny_true = np.array([y_true_dict[col] for col in pred_cols]).transpose((1, 2, 0, 3)).reshape(oof_preds.shape)\n\ndisplay(evaluate_model(train, y_true, oof_preds, pred_cols))",
"_____no_output_____"
]
],
[
[
"# Visualize test predictions",
"_____no_output_____"
]
],
[
[
"submission = pd.read_csv(database_base_path + 'sample_submission.csv')\nsubmission = submission[['id_seqpos']].merge(preds_df, on=['id_seqpos'])",
"_____no_output_____"
]
],
[
[
"# Test set predictions",
"_____no_output_____"
]
],
[
[
"display(submission.head(10))\ndisplay(submission.describe())\n\nsubmission.to_csv('submission.csv', index=False)",
"_____no_output_____"
]
]
] | [
"markdown",
"code",
"markdown",
"code",
"markdown",
"code",
"markdown",
"code",
"markdown",
"code",
"markdown",
"code",
"markdown",
"code",
"markdown",
"code",
"markdown",
"code",
"markdown",
"code",
"markdown",
"code",
"markdown",
"code"
] | [
[
"markdown"
],
[
"code"
],
[
"markdown"
],
[
"code"
],
[
"markdown"
],
[
"code"
],
[
"markdown"
],
[
"code"
],
[
"markdown"
],
[
"code"
],
[
"markdown"
],
[
"code"
],
[
"markdown"
],
[
"code"
],
[
"markdown"
],
[
"code"
],
[
"markdown"
],
[
"code"
],
[
"markdown"
],
[
"code"
],
[
"markdown"
],
[
"code"
],
[
"markdown"
],
[
"code"
]
] |
e78423c59f93a634982bffeb617bf6f64dae0526 | 668,323 | ipynb | Jupyter Notebook | week3/1- HW1 Hand Keypoint Detection Data Preprocess.ipynb | onurboyar/Applied-AI-Study-Group-2020-June | d4c006bfacf35c352db9320d16019873932e9229 | [
"MIT"
] | 40 | 2020-11-23T11:35:15.000Z | 2022-02-11T21:09:47.000Z | week3/1- HW1 Hand Keypoint Detection Data Preprocess.ipynb | onurboyar/Applied-AI-Study-Group-2020-June | d4c006bfacf35c352db9320d16019873932e9229 | [
"MIT"
] | 3 | 2021-07-29T08:40:20.000Z | 2022-02-26T10:04:18.000Z | week3/1- HW1 Hand Keypoint Detection Data Preprocess.ipynb | onurboyar/Applied-AI-Study-Group-2020-June | d4c006bfacf35c352db9320d16019873932e9229 | [
"MIT"
] | 34 | 2020-12-27T13:13:41.000Z | 2022-03-29T15:09:46.000Z | 1,071.030449 | 145,068 | 0.95763 | [
[
[
"import matplotlib.pyplot as plt\nimport pickle\nimport numpy as np\nimport glob\nimport os\nfrom PIL import Image\nimport pandas as pd\nimport copy\nfrom PIL import Image, ImageOps",
"_____no_output_____"
],
[
"sample_num = 0\n\nroot = '/home/electron/Desktop/rovit dataset/amme dataset/'\npath = os.path.join(root,'annotated_frames')\nlpath = os.path.join(root,'projections_2d')\nbpath = os.path.join(root,'bounding_boxes')\npath_save = root",
"_____no_output_____"
],
[
"sample_num = 0\nfor folder in sorted(glob.glob(os.path.join(path,'data_'+'[1-2]'))):\n sample_num += len(glob.glob(os.path.join(folder,'*.jpg')))\n\nimages = []*sample_num\nprint(sample_num)\n\nfor foldername in sorted(glob.glob(os.path.join(path,'data_'+'[1-2]'))):\n print(foldername)\n for i,filename in enumerate(sorted(glob.glob(os.path.join(foldername,'*.jpg')))):\n if i%250 == 0:\n print(i)\n #image = np.array(Image.open(filename))\n image = Image.open(filename)\n images.append(copy.copy(image))\n image.close()\n \n\nprint(np.shape(images[0]))\nplt.imshow(images[0])",
"5636\n/home/electron/Desktop/rovit dataset/amme dataset/annotated_frames/data_1\n0\n500\n1000\n1500\n2000\n2500\n/home/electron/Desktop/rovit dataset/amme dataset/annotated_frames/data_2\n0\n500\n1000\n1500\n2000\n2500\n(480, 640, 3)\n"
],
[
"sample_num = 0\nfor folder in sorted(glob.glob(os.path.join(lpath,'data_'+'[1-2]'))):\n sample_num += len(glob.glob(os.path.join(folder,'*.txt')))\n\nlabels = []*sample_num\nprint(sample_num)\n\nfor foldername in sorted(glob.glob(os.path.join(lpath,'data_'+'[1-2]'))):\n print(foldername)\n for i,filename in enumerate(sorted(glob.glob(os.path.join(foldername,'*.txt')))):\n if i%500 == 0:\n print(i) \n label=pd.read_csv(filename,delimiter =' ', header = None, usecols = [1,2]).values\n labels.append(label)",
"5636\n/home/electron/Desktop/rovit dataset/amme dataset/projections_2d/data_1\n0\n500\n1000\n1500\n2000\n2500\n/home/electron/Desktop/rovit dataset/amme dataset/projections_2d/data_2\n0\n500\n1000\n1500\n2000\n2500\n"
],
[
"sample_num = 0\nfor folder in sorted(glob.glob(os.path.join(bpath,'data_'+'[1-2]'))):\n sample_num += len(glob.glob(os.path.join(folder,'*.txt')))\n\nblabels = []*sample_num\nprint(sample_num)\n\nfor foldername in sorted(glob.glob(os.path.join(bpath,'data_'+'[1-2]'))):\n print(foldername)\n for i,filename in enumerate(sorted(glob.glob(os.path.join(foldername,'*.txt')))):\n if i%500 == 0:\n print(i) \n blabel=pd.read_csv(filename,delimiter =' ' , header = None, usecols = [1]).values\n blabels.append(blabel)",
"5636\n/home/electron/Desktop/rovit dataset/amme dataset/bounding_boxes/data_1\n0\n500\n1000\n1500\n2000\n2500\n/home/electron/Desktop/rovit dataset/amme dataset/bounding_boxes/data_2\n0\n500\n1000\n1500\n2000\n2500\n"
],
[
"from matplotlib.patches import Circle\nimport random\ni = random.randint(1,8000)\ni = 0\nprint(i)\nfig,ax = plt.subplots(1)\nax.set_aspect('equal')\nax.imshow(images[i])\n\nfor xx,yy in labels[i]:\n circ = Circle((xx,yy),3, color = 'red')\n ax.add_patch(circ)",
"0\n"
],
[
"print(len(images))\nprint(len(labels))\nprint(len(blabels))",
"5636\n5636\n5636\n"
],
[
"myrandom=random.randint(1,30)\nfor i,_ in enumerate(images):\n t, l, b, r = blabels[i]\n t,l,b,r = int(t),int(l),int(b),int(r)\n plt.imshow(images[i].crop((l,t,r,b)))\n \n if(i==myrandom):\n break\n \n \n ",
"_____no_output_____"
],
[
"mh,mw = 0,0\nfor i,_ in enumerate(blabels):\n t, l, b, r = blabels[i]\n t,l,b,r = int(t),int(l),int(b),int(r)\n \n if(b-t>mh):\n mh=b-t\n if(r-l>mw):\n mw=r-l\n \nprint(mh,mw)",
"282 334\n"
],
[
"cropped = sample_num * []\n\n\nfor i,_ in enumerate(images):\n t, l, b, r = blabels[i]\n t,l,b,r = int(t),int(l),int(b),int(r)\n cropped.append(images[i].crop((l,t,r,b)))\n ",
"_____no_output_____"
],
[
"plt.imshow(cropped[0])",
"_____no_output_____"
],
[
"images = None\npadded = sample_num * []\n\n\nfor i,item in enumerate(cropped):\n t, l, b, r = blabels[i]\n t,l,b,r = int(t),int(l),int(b),int(r)\n right_pad = mw-item.size[0]\n bottom_pad = mh-item.size[1]\n padded.append(ImageOps.expand(cropped[i], (0,0,right_pad,bottom_pad)))",
"_____no_output_____"
],
[
"plt.imshow(padded[100])",
"_____no_output_____"
],
[
"labels = np.array(labels)",
"_____no_output_____"
],
[
"for i,item in enumerate(labels):\n t, l, b, r = blabels[i]\n t,l,b,r = int(t),int(l),int(b),int(r)\n labels[i,:,0] = labels[i,:,0] - l \n labels[i,:,1] = labels[i,:,1] - t\n ",
"_____no_output_____"
],
[
"np.amin(np.array(labels)[:,:,1])",
"_____no_output_____"
],
[
"print(np.shape(labels))\nprint(np.shape(blabels))",
"(5636, 21, 2)\n(5636, 4, 1)\n"
],
[
"jo = random.randint(1,8000)\nfig,ax = plt.subplots(1)\nax.set_aspect('equal')\n\nax.imshow(padded[jo])\n\nfor xx,yy in labels[jo]:\n circ = Circle((xx,yy),2, color = 'red')\n ax.add_patch(circ)",
"_____no_output_____"
],
[
"cropped = None\npadded_pickle = sample_num * []\nlabels_pickle = sample_num * []\nblabels_pickle = sample_num * []\n\n\nfor i,item in enumerate(padded):\n padded_pickle.append(np.array(padded[i]))\npadded_pickle=np.array(padded_pickle) \n\nfor i,item in enumerate(labels):\n labels_pickle.append(np.array(labels[i]))\nlabels_pickle=np.array(labels_pickle) \n\n\nfor i,item in enumerate(blabels):\n blabels_pickle.append(np.array(blabels[i]))\nblabels_pickle=np.array(blabels_pickle) ",
"_____no_output_____"
],
[
"print(padded_pickle.shape)\nprint(labels_pickle.shape)\nprint(blabels_pickle.shape)\n\ndel padded,labels, blabels",
"(5636, 282, 334, 3)\n(5636, 21, 2)\n(5636, 4, 1)\n"
],
[
"my_dict = {'padded':padded_pickle,'labels':labels_pickle,'blabels':blabels_pickle}\n\nfor key in my_dict.keys():\n print(my_dict[key].shape)\n",
"(5636, 282, 334, 3)\n(5636, 21, 2)\n(5636, 4, 1)\n"
],
[
"with open('data_hand_pose.pickle','wb') as file_to_dump:\n pickle.dump(my_dict,file_to_dump)\n\n ",
"_____no_output_____"
],
[
"# file_to_dump=open('data_hand_pose3.pickle','wb')\n# pickle.dump(my_dict,file_to_dump)\n\n# file_to_dump.close()",
"_____no_output_____"
]
]
] | [
"code"
] | [
[
"code",
"code",
"code",
"code",
"code",
"code",
"code",
"code",
"code",
"code",
"code",
"code",
"code",
"code",
"code",
"code",
"code",
"code",
"code",
"code",
"code",
"code",
"code"
]
] |
e7842695d88b29e610a3ef567b4bb5eb47b63521 | 3,510 | ipynb | Jupyter Notebook | enem-4/main.ipynb | arnaldoneto01/aceleracao-data-science | bb86feae802e6f2d136e8e157a0bea0653275c96 | [
"MIT"
] | null | null | null | enem-4/main.ipynb | arnaldoneto01/aceleracao-data-science | bb86feae802e6f2d136e8e157a0bea0653275c96 | [
"MIT"
] | null | null | null | enem-4/main.ipynb | arnaldoneto01/aceleracao-data-science | bb86feae802e6f2d136e8e157a0bea0653275c96 | [
"MIT"
] | null | null | null | 29.495798 | 101 | 0.578917 | [
[
[
"import pandas as pd\nfrom collections import Counter\nimport numpy as np\nfrom sklearn.ensemble import RandomForestClassifier",
"_____no_output_____"
],
[
"df_test = pd.read_csv('test.csv')\ndf_train = pd.read_csv('train.csv')\n\n#Counter(df_train.IN_TREINEIRO)\n\nID = 'NU_INSCRICAO'\ntarget = 'IN_TREINEIRO'\ndf = df_train[list(df_test.columns)].fillna(0)\ndf = df.drop(columns=[ID])\ntrain_features = pd.get_dummies(df)\n\n\n# Labels are the values we want to predict\ntrain_labels = np.array(df_train[target].to_list())\n# Saving feature names for later use\nfeature_list = list(train_features.columns)\n# Convert to numpy array\nfeatures_nparray = np.array(train_features)\n\nprint('Training Features Shape:', train_features.shape)\nprint('Training Labels Shape:', train_labels.shape)\n\n\n# Instantiate model with 1000 decision trees\nrf = RandomForestClassifier(n_estimators = 200, random_state = 42, n_jobs = -1, verbose = 1)\n# Train the model on training data\nrf.fit(train_features, train_labels);\n\n#predictions = rf.predict(train_features)\n\n#sum(predictions-train_labels)\n\ndf_answer = pd.DataFrame()\n\ndf_answer[ID] = df_test[ID]\ndf_test = df_test.drop(columns=[ID]).fillna(0)\ntest_features = pd.get_dummies(df_test)\npredictions = rf.predict(test_features)\n\ndf_answer[target] = list(predictions)\n#df_answer.head()\n\ndf_answer.to_csv('answer.csv', index=False)",
"Training Features Shape: (13730, 123)\nTraining Labels Shape: (13730,)\n"
]
]
] | [
"code"
] | [
[
"code",
"code"
]
] |
e78432d627a6f7286736bb60f1c69faf16938d01 | 971,774 | ipynb | Jupyter Notebook | deprecated/.ipynb_checkpoints/lfads_demo-checkpoint.ipynb | lyprince/hierarchical_lfads | cc897286a3ade52038840900bc0313fe7195f871 | [
"MIT"
] | 18 | 2019-11-13T11:32:08.000Z | 2022-02-15T17:46:55.000Z | deprecated/.ipynb_checkpoints/lfads_demo-checkpoint.ipynb | lyprince/hierarchical_lfads | cc897286a3ade52038840900bc0313fe7195f871 | [
"MIT"
] | 2 | 2020-01-20T20:20:15.000Z | 2021-12-02T10:45:35.000Z | deprecated/.ipynb_checkpoints/lfads_demo-checkpoint.ipynb | lyprince/hierarchical_lfads | cc897286a3ade52038840900bc0313fe7195f871 | [
"MIT"
] | 10 | 2019-11-19T15:41:34.000Z | 2021-11-15T08:42:39.000Z | 365.741061 | 148,748 | 0.892964 | [
[
[
"import torch\nimport torchvision\n\nfrom models import LFADS, LadderLFADS\nfrom utils import read_data, load_parameters, save_parameters, batchify_random_sample\n\nnp = torch._np\nimport matplotlib.pyplot as plt\nimport yaml\nimport os",
"_____no_output_____"
],
[
"device = 'cuda' if torch.cuda.is_available() else 'cpu'; print('Using device: %s'%device)",
"Using device: cuda\n"
],
[
"seed = 700\nif os.path.exists('./synth_data/lorenz_%s'%seed):\n data_dict = read_data('./synth_data/lorenz_%s'%seed)\nelse:\n from synthetic_data import generate_lorenz_data\n data_dict = generate_lorenz_data(N_cells=30, N_inits=65, N_trials=20, N_steps=200, N_stepsinbin=2, dt_lorenz=0.015, dt_spike = 1./20, base_firing_rate= 1.0, save=True, seed=250)\n\n# For spike data\ntrain_data = torch.Tensor(data_dict['train_fluor']).to(device)\nvalid_data = torch.Tensor(data_dict['valid_fluor']).to(device)\n\ntrain_truth = {'spikes' : data_dict['train_spikes'],\n 'rates' : data_dict['train_rates'],\n 'latent' : data_dict['train_latent']}\n\nvalid_truth = {'spikes' : data_dict['valid_spikes'],\n 'rates' : data_dict['valid_rates'],\n 'latent' : data_dict['valid_latent']}\n\ntrain_ds = torch.utils.data.TensorDataset(train_data)\nvalid_ds = torch.utils.data.TensorDataset(valid_data)\n\nnum_trials, num_steps, num_cells = train_data.shape;\nprint(train_data.shape);\nprint('Number of datapoints = %s'%train_data.numel())",
"torch.Size([1040, 100, 30])\nNumber of datapoints = 3120000\n"
],
[
"hyperparams = load_parameters('./parameters/parameters_lorenz_spikes.yaml')\nhyperparams['run_name'] += '_localtest'\nsave_parameters(hyperparams, path=None)\n\nhyperparams",
"_____no_output_____"
],
[
"model = LFADS(inputs_dim = num_cells, T = num_steps, dt = float(data_dict['dt']), device=device,\n model_hyperparams=hyperparams).to(device)",
"Random seed: 7629\n"
],
[
"total_params = 0\nfor ix, (name, param) in enumerate(model.named_parameters()):\n print(ix, name, list(param.shape), param.numel(), param.requires_grad)\n total_params += param.numel()\n \nprint('Total parameters: %i'%total_params)",
"0 efgen_g0_init [64] 64 True\n1 ebgen_g0_init [64] 64 True\n2 g0_prior_mu [64] 64 True\n3 gru_Egen_g0.weight_ih_l0 [192, 30] 5760 True\n4 gru_Egen_g0.weight_hh_l0 [192, 64] 12288 True\n5 gru_Egen_g0.bias_ih_l0 [192] 192 True\n6 gru_Egen_g0.bias_hh_l0 [192] 192 True\n7 gru_Egen_g0.weight_ih_l0_reverse [192, 30] 5760 True\n8 gru_Egen_g0.weight_hh_l0_reverse [192, 64] 12288 True\n9 gru_Egen_g0.bias_ih_l0_reverse [192] 192 True\n10 gru_Egen_g0.bias_hh_l0_reverse [192] 192 True\n11 fc_g0mean.weight [64, 128] 8192 True\n12 fc_g0mean.bias [64] 64 True\n13 fc_g0logvar.weight [64, 128] 8192 True\n14 fc_g0logvar.bias [64] 64 True\n15 gru_generator.fc_h_ru.weight [128, 64] 8192 True\n16 gru_generator.fc_h_ru.bias [128] 128 True\n17 gru_generator.fc_rh_c.weight [64, 64] 4096 True\n18 gru_generator.fc_rh_c.bias [64] 64 True\n19 fc_factors.weight [3, 64] 192 True\n20 fc_logrates.weight [30, 3] 90 True\n21 fc_logrates.bias [30] 30 True\nTotal parameters: 66360\n"
],
[
"model.load_checkpoint('recent')",
"_____no_output_____"
],
[
"model.fit(train_ds, valid_ds, train_truth=train_truth, valid_truth=valid_truth,\n max_epochs=2000, batch_size=65, use_tensorboard=False, health_check=False)",
"Beginning training...\nEpoch: 1, Step: 16, Losses [Train, Valid]: Total [1223.56, 1429.92], Recon [1222.28, 1211.00], KL [242.74, 214.96], L2 3.96, Runtime: 3.3538 secs\nEpoch: 2, Step: 32, Losses [Train, Valid]: Total [1208.90, 1341.27], Recon [1206.05, 1199.39], KL [186.39, 137.93], L2 3.95, Runtime: 4.0260 secs\nEpoch: 3, Step: 48, Losses [Train, Valid]: Total [1200.50, 1233.25], Recon [1198.18, 1195.49], KL [91.16, 33.82], L2 3.94, Runtime: 3.2413 secs\nEpoch: 4, Step: 64, Losses [Train, Valid]: Total [1196.92, 1209.23], Recon [1196.01, 1194.70], KL [22.53, 10.60], L2 3.93, Runtime: 3.2689 secs\nEpoch: 5, Step: 80, Losses [Train, Valid]: Total [1196.49, 1209.41], Recon [1195.55, 1194.39], KL [16.84, 11.12], L2 3.91, Runtime: 3.2406 secs\nEpoch: 6, Step: 96, Losses [Train, Valid]: Total [1196.17, 1209.18], Recon [1195.08, 1193.67], KL [15.82, 11.61], L2 3.90, Runtime: 3.2648 secs\nEpoch: 7, Step: 112, Losses [Train, Valid]: Total [1195.84, 1209.29], Recon [1194.53, 1193.10], KL [16.05, 12.31], L2 3.88, Runtime: 3.2407 secs\nEpoch: 8, Step: 128, Losses [Train, Valid]: Total [1195.19, 1210.67], Recon [1193.63, 1191.98], KL [16.90, 14.81], L2 3.87, Runtime: 4.9034 secs\nEpoch: 9, Step: 144, Losses [Train, Valid]: Total [1194.16, 1212.68], Recon [1192.13, 1190.18], KL [19.87, 18.65], L2 3.85, Runtime: 3.3241 secs\nEpoch: 10, Step: 160, Losses [Train, Valid]: Total [1191.64, 1208.77], Recon [1189.08, 1184.34], KL [22.98, 20.60], L2 3.83, Runtime: 3.2432 secs\nEpoch: 11, Step: 176, Losses [Train, Valid]: Total [1188.27, 1214.20], Recon [1185.22, 1182.64], KL [25.12, 27.74], L2 3.81, Runtime: 3.3212 secs\nEpoch: 12, Step: 192, Losses [Train, Valid]: Total [1185.66, 1205.84], Recon [1181.82, 1175.49], KL [29.51, 26.51], L2 3.84, Runtime: 6.4502 secs\nEpoch: 13, Step: 208, Losses [Train, Valid]: Total [1181.76, 1202.69], Recon [1177.74, 1175.28], KL [28.25, 23.56], L2 3.85, Runtime: 3.2918 secs\nEpoch: 14, Step: 224, Losses [Train, Valid]: Total [1178.20, 1195.75], Recon [1174.41, 1168.98], KL [24.11, 22.90], L2 3.88, Runtime: 4.1914 secs\nEpoch: 15, Step: 240, Losses [Train, Valid]: Total [1174.72, 1195.80], Recon [1170.39, 1166.75], KL [25.86, 25.14], L2 3.92, Runtime: 4.0741 secs\nEpoch: 16, Step: 256, Losses [Train, Valid]: Total [1174.95, 1198.30], Recon [1169.91, 1165.38], KL [28.48, 28.98], L2 3.93, Runtime: 4.0882 secs\nEpoch: 17, Step: 272, Losses [Train, Valid]: Total [1170.92, 1191.69], Recon [1165.25, 1159.09], KL [30.38, 28.63], L2 3.97, Runtime: 5.8759 secs\nEpoch: 18, Step: 288, Losses [Train, Valid]: Total [1165.61, 1187.43], Recon [1159.73, 1154.96], KL [29.57, 28.47], L2 4.01, Runtime: 3.3429 secs\nEpoch: 19, Step: 304, Losses [Train, Valid]: Total [1161.83, 1182.92], Recon [1155.55, 1150.21], KL [29.85, 28.65], L2 4.05, Runtime: 4.2123 secs\nEpoch: 20, Step: 320, Losses [Train, Valid]: Total [1160.93, 1185.23], Recon [1153.50, 1149.66], KL [33.93, 31.51], L2 4.07, Runtime: 4.1309 secs\nEpoch: 21, Step: 336, Losses [Train, Valid]: Total [1158.46, 1187.02], Recon [1151.09, 1149.74], KL [31.76, 33.17], L2 4.12, Runtime: 3.4619 secs\nEpoch: 22, Step: 352, Losses [Train, Valid]: Total [1156.51, 1179.28], Recon [1148.17, 1143.13], KL [34.62, 31.99], L2 4.15, Runtime: 3.2580 secs\nEpoch: 23, Step: 368, Losses [Train, Valid]: Total [1152.17, 1186.00], Recon [1143.84, 1147.34], KL [32.77, 34.48], L2 4.18, Runtime: 3.2593 secs\nEpoch: 24, Step: 384, Losses [Train, Valid]: Total [1154.21, 1173.13], Recon [1145.72, 1140.99], KL [31.92, 27.94], L2 4.20, Runtime: 3.2593 secs\nEpoch: 25, Step: 400, Losses [Train, Valid]: Total [1148.81, 1170.54], Recon [1140.34, 1137.06], KL [30.34, 29.26], L2 4.22, Runtime: 3.2382 secs\nEpoch: 26, Step: 416, Losses [Train, Valid]: Total [1147.33, 1175.81], Recon [1138.69, 1139.24], KL [29.61, 32.33], L2 4.24, Runtime: 5.7616 secs\nEpoch: 27, Step: 432, Losses [Train, Valid]: Total [1148.16, 1171.31], Recon [1138.70, 1135.65], KL [31.39, 31.40], L2 4.26, Runtime: 3.2619 secs\nEpoch: 28, Step: 448, Losses [Train, Valid]: Total [1146.03, 1168.33], Recon [1136.19, 1135.31], KL [31.47, 28.72], L2 4.30, Runtime: 3.3002 secs\nEpoch: 29, Step: 464, Losses [Train, Valid]: Total [1146.17, 1169.16], Recon [1136.71, 1136.01], KL [28.85, 28.82], L2 4.33, Runtime: 3.2639 secs\nEpoch: 30, Step: 480, Losses [Train, Valid]: Total [1146.24, 1167.56], Recon [1135.92, 1133.74], KL [30.60, 29.48], L2 4.35, Runtime: 4.0749 secs\nEpoch: 31, Step: 496, Losses [Train, Valid]: Total [1143.69, 1158.40], Recon [1133.77, 1127.10], KL [28.10, 26.93], L2 4.38, Runtime: 3.2785 secs\nEpoch: 32, Step: 512, Losses [Train, Valid]: Total [1141.11, 1156.92], Recon [1130.80, 1125.71], KL [28.28, 26.81], L2 4.41, Runtime: 4.9470 secs\nEpoch: 33, Step: 528, Losses [Train, Valid]: Total [1140.28, 1158.23], Recon [1130.45, 1129.67], KL [25.82, 24.12], L2 4.43, Runtime: 4.0175 secs\nEpoch: 34, Step: 544, Losses [Train, Valid]: Total [1142.77, 1159.81], Recon [1132.54, 1129.55], KL [26.08, 25.81], L2 4.45, Runtime: 3.2377 secs\nEpoch: 35, Step: 560, Losses [Train, Valid]: Total [1141.07, 1158.03], Recon [1130.36, 1127.37], KL [26.55, 26.18], L2 4.47, Runtime: 3.9893 secs\nEpoch: 36, Step: 576, Losses [Train, Valid]: Total [1139.38, 1153.71], Recon [1128.72, 1123.54], KL [25.53, 25.67], L2 4.51, Runtime: 3.1807 secs\nEpoch: 37, Step: 592, Losses [Train, Valid]: Total [1138.49, 1151.20], Recon [1127.59, 1122.08], KL [25.36, 24.61], L2 4.51, Runtime: 4.7887 secs\nEpoch: 38, Step: 608, Losses [Train, Valid]: Total [1141.19, 1163.81], Recon [1129.46, 1134.86], KL [26.73, 24.41], L2 4.54, Runtime: 3.2046 secs\nEpoch: 39, Step: 624, Losses [Train, Valid]: Total [1140.81, 1153.15], Recon [1129.02, 1122.18], KL [26.05, 26.40], L2 4.57, Runtime: 3.2497 secs\nEpoch: 40, Step: 640, Losses [Train, Valid]: Total [1140.31, 1159.85], Recon [1128.08, 1130.73], KL [26.36, 24.52], L2 4.59, Runtime: 3.2250 secs\nEpoch: 41, Step: 656, Losses [Train, Valid]: Total [1139.98, 1156.80], Recon [1127.57, 1127.72], KL [26.02, 24.46], L2 4.62, Runtime: 3.3206 secs\nEpoch: 42, Step: 672, Losses [Train, Valid]: Total [1142.88, 1156.18], Recon [1130.95, 1127.73], KL [24.12, 23.84], L2 4.61, Runtime: 3.2998 secs\nLearning rate decreased to 0.009500\nEpoch: 43, Step: 688, Losses [Train, Valid]: Total [1141.09, 1154.91], Recon [1128.83, 1127.54], KL [24.21, 22.72], L2 4.64, Runtime: 4.0649 secs\nEpoch: 44, Step: 704, Losses [Train, Valid]: Total [1138.97, 1156.69], Recon [1126.12, 1129.13], KL [24.88, 22.91], L2 4.65, Runtime: 3.2075 secs\nEpoch: 45, Step: 720, Losses [Train, Valid]: Total [1141.58, 1150.89], Recon [1128.99, 1122.46], KL [23.62, 23.79], L2 4.64, Runtime: 3.3005 secs\nEpoch: 46, Step: 736, Losses [Train, Valid]: Total [1137.10, 1152.35], Recon [1124.28, 1125.68], KL [23.53, 22.02], L2 4.66, Runtime: 5.6883 secs\nEpoch: 47, Step: 752, Losses [Train, Valid]: Total [1137.74, 1151.94], Recon [1125.46, 1125.00], KL [21.74, 22.28], L2 4.66, Runtime: 4.0759 secs\nEpoch: 48, Step: 768, Losses [Train, Valid]: Total [1138.97, 1150.46], Recon [1125.33, 1122.28], KL [24.02, 23.49], L2 4.68, Runtime: 4.0757 secs\nEpoch: 49, Step: 784, Losses [Train, Valid]: Total [1138.71, 1149.83], Recon [1125.19, 1124.35], KL [23.18, 20.79], L2 4.69, Runtime: 3.2572 secs\nEpoch: 50, Step: 800, Losses [Train, Valid]: Total [1136.08, 1146.38], Recon [1123.26, 1119.53], KL [21.19, 22.14], L2 4.71, Runtime: 3.3566 secs\nEpoch: 51, Step: 816, Losses [Train, Valid]: Total [1134.70, 1144.84], Recon [1121.38, 1120.51], KL [21.65, 19.61], L2 4.72, Runtime: 4.1092 secs\nEpoch: 52, Step: 832, Losses [Train, Valid]: Total [1135.87, 1144.78], Recon [1122.39, 1120.09], KL [21.42, 19.96], L2 4.73, Runtime: 3.2566 secs\nEpoch: 53, Step: 848, Losses [Train, Valid]: Total [1133.87, 1146.80], Recon [1120.84, 1122.91], KL [20.08, 19.15], L2 4.75, Runtime: 4.1511 secs\nEpoch: 54, Step: 864, Losses [Train, Valid]: Total [1136.18, 1148.14], Recon [1122.32, 1122.60], KL [21.12, 20.77], L2 4.77, Runtime: 4.2592 secs\nEpoch: 55, Step: 880, Losses [Train, Valid]: Total [1135.13, 1145.06], Recon [1121.39, 1121.20], KL [20.41, 19.08], L2 4.78, Runtime: 4.1454 secs\nEpoch: 56, Step: 896, Losses [Train, Valid]: Total [1135.71, 1144.15], Recon [1122.07, 1119.84], KL [19.79, 19.53], L2 4.78, Runtime: 4.7441 secs\nEpoch: 57, Step: 912, Losses [Train, Valid]: Total [1133.51, 1141.99], Recon [1119.58, 1117.79], KL [19.85, 19.41], L2 4.80, Runtime: 6.6054 secs\nEpoch: 58, Step: 928, Losses [Train, Valid]: Total [1136.61, 1141.60], Recon [1122.55, 1118.04], KL [19.64, 18.77], L2 4.80, Runtime: 3.2627 secs\nLearning rate decreased to 0.009025\nEpoch: 59, Step: 944, Losses [Train, Valid]: Total [1134.37, 1141.22], Recon [1120.16, 1117.40], KL [19.48, 19.00], L2 4.81, Runtime: 3.2770 secs\nEpoch: 60, Step: 960, Losses [Train, Valid]: Total [1135.98, 1143.95], Recon [1121.13, 1120.59], KL [20.13, 18.54], L2 4.82, Runtime: 3.2584 secs\nEpoch: 61, Step: 976, Losses [Train, Valid]: Total [1136.12, 1143.58], Recon [1121.17, 1119.38], KL [19.87, 19.36], L2 4.84, Runtime: 4.0300 secs\nEpoch: 62, Step: 992, Losses [Train, Valid]: Total [1133.64, 1138.54], Recon [1118.71, 1116.19], KL [19.42, 17.48], L2 4.87, Runtime: 3.2744 secs\nEpoch: 63, Step: 1008, Losses [Train, Valid]: Total [1134.92, 1142.03], Recon [1120.06, 1117.47], KL [18.90, 19.69], L2 4.88, Runtime: 5.0180 secs\nEpoch: 64, Step: 1024, Losses [Train, Valid]: Total [1136.03, 1142.93], Recon [1120.54, 1118.74], KL [19.51, 19.31], L2 4.88, Runtime: 7.4739 secs\nEpoch: 65, Step: 1040, Losses [Train, Valid]: Total [1133.65, 1139.81], Recon [1118.25, 1117.89], KL [18.99, 17.04], L2 4.88, Runtime: 4.8514 secs\nEpoch: 66, Step: 1056, Losses [Train, Valid]: Total [1132.42, 1138.22], Recon [1117.70, 1115.85], KL [17.58, 17.49], L2 4.89, Runtime: 4.9008 secs\nEpoch: 67, Step: 1072, Losses [Train, Valid]: Total [1133.02, 1141.19], Recon [1118.00, 1119.49], KL [17.68, 16.82], L2 4.89, Runtime: 4.1459 secs\nEpoch: 68, Step: 1088, Losses [Train, Valid]: Total [1133.83, 1139.92], Recon [1119.09, 1117.70], KL [16.93, 17.32], L2 4.90, Runtime: 5.8687 secs\nEpoch: 69, Step: 1104, Losses [Train, Valid]: Total [1135.62, 1143.28], Recon [1119.90, 1120.82], KL [18.04, 17.56], L2 4.90, Runtime: 4.1210 secs\nEpoch: 70, Step: 1120, Losses [Train, Valid]: Total [1133.20, 1138.72], Recon [1117.57, 1117.48], KL [17.56, 16.34], L2 4.90, Runtime: 5.7058 secs\nEpoch: 71, Step: 1136, Losses [Train, Valid]: Total [1132.51, 1136.60], Recon [1117.03, 1114.66], KL [17.04, 17.03], L2 4.91, Runtime: 4.2924 secs\nEpoch: 72, Step: 1152, Losses [Train, Valid]: Total [1132.82, 1141.01], Recon [1117.27, 1119.84], KL [16.83, 16.26], L2 4.91, Runtime: 4.6844 secs\nEpoch: 73, Step: 1168, Losses [Train, Valid]: Total [1134.18, 1142.42], Recon [1117.49, 1120.67], KL [18.09, 16.82], L2 4.92, Runtime: 5.3389 secs\nEpoch: 74, Step: 1184, Losses [Train, Valid]: Total [1134.73, 1138.50], Recon [1118.71, 1116.75], KL [16.87, 16.84], L2 4.92, Runtime: 5.7647 secs\nEpoch: 75, Step: 1200, Losses [Train, Valid]: Total [1135.46, 1138.98], Recon [1119.17, 1117.67], KL [16.93, 16.36], L2 4.95, Runtime: 4.5398 secs\nEpoch: 76, Step: 1216, Losses [Train, Valid]: Total [1137.68, 1138.91], Recon [1120.35, 1116.39], KL [17.99, 17.59], L2 4.94, Runtime: 4.1875 secs\nLearning rate decreased to 0.008574\nEpoch: 77, Step: 1232, Losses [Train, Valid]: Total [1135.21, 1137.07], Recon [1118.54, 1116.34], KL [16.85, 15.79], L2 4.94, Runtime: 3.2931 secs\nEpoch: 78, Step: 1248, Losses [Train, Valid]: Total [1133.14, 1138.99], Recon [1116.90, 1118.86], KL [16.01, 15.17], L2 4.96, Runtime: 7.0967 secs\nEpoch: 79, Step: 1264, Losses [Train, Valid]: Total [1132.75, 1135.72], Recon [1116.53, 1115.33], KL [15.70, 15.42], L2 4.97, Runtime: 4.0180 secs\nEpoch: 80, Step: 1280, Losses [Train, Valid]: Total [1134.55, 1135.89], Recon [1117.47, 1114.58], KL [16.51, 16.34], L2 4.97, Runtime: 3.2372 secs\nEpoch: 81, Step: 1296, Losses [Train, Valid]: Total [1134.34, 1138.12], Recon [1117.02, 1117.76], KL [16.53, 15.39], L2 4.97, Runtime: 4.0390 secs\nEpoch: 82, Step: 1312, Losses [Train, Valid]: Total [1132.69, 1134.93], Recon [1115.98, 1115.28], KL [15.52, 14.66], L2 4.99, Runtime: 7.3063 secs\nEpoch: 83, Step: 1328, Losses [Train, Valid]: Total [1133.12, 1136.29], Recon [1116.31, 1116.02], KL [15.37, 15.27], L2 4.99, Runtime: 4.0403 secs\nEpoch: 84, Step: 1344, Losses [Train, Valid]: Total [1133.72, 1137.16], Recon [1116.08, 1117.15], KL [16.13, 15.02], L2 4.98, Runtime: 4.0337 secs\nEpoch: 85, Step: 1360, Losses [Train, Valid]: Total [1132.65, 1133.04], Recon [1115.57, 1113.82], KL [15.22, 14.22], L2 5.00, Runtime: 3.8663 secs\nEpoch: 86, Step: 1376, Losses [Train, Valid]: Total [1132.49, 1133.94], Recon [1115.48, 1114.78], KL [14.89, 14.18], L2 4.99, Runtime: 3.5105 secs\nEpoch: 87, Step: 1392, Losses [Train, Valid]: Total [1131.25, 1132.99], Recon [1113.98, 1113.93], KL [14.98, 14.08], L2 4.98, Runtime: 3.2784 secs\nEpoch: 88, Step: 1408, Losses [Train, Valid]: Total [1132.19, 1134.75], Recon [1115.16, 1115.53], KL [14.49, 14.25], L2 4.97, Runtime: 4.1551 secs\nEpoch: 89, Step: 1424, Losses [Train, Valid]: Total [1133.63, 1137.60], Recon [1115.69, 1118.53], KL [15.29, 14.11], L2 4.96, Runtime: 3.3313 secs\nEpoch: 90, Step: 1440, Losses [Train, Valid]: Total [1134.76, 1135.91], Recon [1117.34, 1116.18], KL [14.49, 14.77], L2 4.96, Runtime: 4.9523 secs\nLearning rate decreased to 0.008145\nEpoch: 91, Step: 1456, Losses [Train, Valid]: Total [1134.12, 1134.44], Recon [1116.26, 1115.04], KL [14.78, 14.45], L2 4.95, Runtime: 4.1994 secs\nEpoch: 92, Step: 1472, Losses [Train, Valid]: Total [1134.65, 1134.52], Recon [1116.39, 1115.27], KL [14.99, 14.29], L2 4.96, Runtime: 4.9495 secs\nEpoch: 93, Step: 1488, Losses [Train, Valid]: Total [1135.53, 1137.67], Recon [1117.07, 1118.46], KL [14.98, 14.23], L2 4.97, Runtime: 6.5290 secs\nEpoch: 94, Step: 1504, Losses [Train, Valid]: Total [1136.74, 1137.71], Recon [1118.15, 1117.94], KL [14.90, 14.80], L2 4.98, Runtime: 4.1493 secs\nEpoch: 95, Step: 1520, Losses [Train, Valid]: Total [1135.88, 1135.23], Recon [1117.38, 1116.09], KL [14.60, 14.17], L2 4.98, Runtime: 3.3552 secs\nEpoch: 96, Step: 1536, Losses [Train, Valid]: Total [1135.25, 1133.37], Recon [1116.24, 1114.34], KL [14.92, 14.04], L2 4.99, Runtime: 4.1896 secs\nEpoch: 97, Step: 1552, Losses [Train, Valid]: Total [1133.80, 1136.38], Recon [1115.18, 1117.59], KL [14.29, 13.79], L2 5.00, Runtime: 5.8199 secs\nEpoch: 98, Step: 1568, Losses [Train, Valid]: Total [1136.22, 1135.96], Recon [1116.70, 1116.19], KL [15.01, 14.79], L2 4.99, Runtime: 4.0600 secs\nEpoch: 99, Step: 1584, Losses [Train, Valid]: Total [1136.62, 1136.65], Recon [1116.89, 1117.55], KL [15.04, 14.11], L2 4.99, Runtime: 4.0681 secs\n"
],
[
"model.load_checkpoint('best')",
"_____no_output_____"
],
[
"fig_dict = model.plot_summary(data=valid_data, truth=valid_truth)",
"_____no_output_____"
],
[
"results_dict = model.plot_recon_rsquared(valid_data, valid_truth, train_data, train_truth)",
"_____no_output_____"
],
[
"fig, axs = plt.subplots(figsize=(8,8), nrows=3, ncols=1, sharex=True, sharey=True)\nfor ix in range(3):\n plt.sca(axs[ix])\n plt.plot(data_dict['valid_latent'][0, :100, ix])\n plt.plot(results_dict['factors']['aligned'][:100, ix])\n \nfig.suptitle('Aligned factors')\nfig.legend(['data', 'reconstruction'])",
"_____no_output_____"
]
],
[
[
"## Inspecting trained model ",
"_____no_output_____"
]
],
[
[
"seed = 600\nsystem = 'chaotic-rnn'\nif os.path.exists('./synth_data/%s_%s'%(system, seed)):\n data_dict = read_data('./synth_data/%s_%s'%(system, seed))\nelse:\n from synthetic_data import generate_chaotic_rnn_data\n param_dict = yaml.load(open('./synth_data/%s_params.yaml'%system, 'r'), Loader=yaml.FullLoader)\n data_dict = generate_chaotic_rnn_data(Ncells=param_dict['cells'],\n Ninits=param_dict['inits'],\n Ntrial=param_dict['trials'],\n Nsteps=param_dict['steps'],\n# Nstepsinbin=param_dict['steps_in_bin'],\n dt_rnn=param_dict['dt_sys'],\n dt_spike = param_dict['dt_spike'],\n maxRate= param_dict['rate_scale'],\n save=False,\n seed=seed)\n\n \n \n# For spike data\ntrain_data = torch.Tensor(data_dict['train_spikes']).to(device)\nvalid_data = torch.Tensor(data_dict['valid_spikes']).to(device)\n\ntrain_truth = {'rates' : data_dict['train_rates']}\n\nvalid_truth = {'rates' : data_dict['valid_rates']}\n\ntrain_ds = torch.utils.data.TensorDataset(train_data)\nvalid_ds = torch.utils.data.TensorDataset(valid_data)\n\nnum_trials, num_steps, num_cells = train_data.shape;\nprint(train_data.shape);\nprint('Number of datapoints = %s'%train_data.numel())",
"torch.Size([3200, 100, 50])\nNumber of datapoints = 16000000\n"
],
[
"hyperparams = load_parameters('parameters/parameters_%s_spikes.yaml'%system)\nhyperparams['run_name'] = 'poisson_%s%i_f20_g1200_eg1128_u1_c1128_ec1128_191125_localtest'%(system, seed)",
"_____no_output_____"
],
[
"model = LFADS(inputs_dim = num_cells, T = num_steps, dt = float(data_dict['dt']), device=device,\n model_hyperparams=hyperparams).to(device)\n# model.load_checkpoint('best')\n# model.epochs",
"Random seed: 392\n"
],
[
"model.gru_generator.fc_h_ru.weight.std()",
"_____no_output_____"
],
[
"1/(np.sqrt(400))",
"_____no_output_____"
],
[
"total_params = 0\nfor ix, (name, param) in enumerate(model.named_parameters()):\n print(ix, name, list(param.shape), param.numel(), param.requires_grad)\n total_params += param.numel()\n \nprint('Total parameters: %i'%total_params)",
"0 efgen_g0_init [128] 128 True\n1 ebgen_g0_init [128] 128 True\n2 efcon_c_init [128] 128 True\n3 ebcon_c_init [128] 128 True\n4 c_init [128] 128 True\n5 g0_prior_mu [64] 64 True\n6 u_prior_logtau [1] 1 True\n7 u_prior_logkappa [1] 1 True\n8 gru_Egen_g0.weight_ih_l0 [384, 50] 19200 True\n9 gru_Egen_g0.weight_hh_l0 [384, 128] 49152 True\n10 gru_Egen_g0.bias_ih_l0 [384] 384 True\n11 gru_Egen_g0.bias_hh_l0 [384] 384 True\n12 gru_Egen_g0.weight_ih_l0_reverse [384, 50] 19200 True\n13 gru_Egen_g0.weight_hh_l0_reverse [384, 128] 49152 True\n14 gru_Egen_g0.bias_ih_l0_reverse [384] 384 True\n15 gru_Egen_g0.bias_hh_l0_reverse [384] 384 True\n16 gru_Econ_c.weight_ih_l0 [384, 50] 19200 True\n17 gru_Econ_c.weight_hh_l0 [384, 128] 49152 True\n18 gru_Econ_c.bias_ih_l0 [384] 384 True\n19 gru_Econ_c.bias_hh_l0 [384] 384 True\n20 gru_Econ_c.weight_ih_l0_reverse [384, 50] 19200 True\n21 gru_Econ_c.weight_hh_l0_reverse [384, 128] 49152 True\n22 gru_Econ_c.bias_ih_l0_reverse [384] 384 True\n23 gru_Econ_c.bias_hh_l0_reverse [384] 384 True\n24 fc_g0mean.weight [64, 256] 16384 True\n25 fc_g0mean.bias [64] 64 True\n26 fc_g0logvar.weight [64, 256] 16384 True\n27 fc_g0logvar.bias [64] 64 True\n28 gru_controller_c.fc_x_ru.weight [256, 276] 70656 True\n29 gru_controller_c.fc_x_c.weight [128, 276] 35328 True\n30 gru_controller_c.fc_h_ru.weight [256, 128] 32768 True\n31 gru_controller_c.fc_h_ru.bias [256] 256 True\n32 gru_controller_c.fc_rh_c.weight [128, 128] 16384 True\n33 gru_controller_c.fc_rh_c.bias [128] 128 True\n34 fc_umean.weight [1, 128] 128 True\n35 fc_umean.bias [1] 1 True\n36 fc_ulogvar.weight [1, 128] 128 True\n37 fc_ulogvar.bias [1] 1 True\n38 fc_icgen.weight [200, 64] 12800 True\n39 fc_icgen.bias [200] 200 True\n40 gru_generator.fc_x_ru.weight [400, 1] 400 True\n41 gru_generator.fc_x_c.weight [200, 1] 200 True\n42 gru_generator.fc_h_ru.weight [400, 200] 80000 True\n43 gru_generator.fc_h_ru.bias [400] 400 True\n44 gru_generator.fc_rh_c.weight [200, 200] 40000 True\n45 gru_generator.fc_rh_c.bias [200] 200 True\n46 fc_factors.weight [20, 200] 4000 True\n47 fc_logrates.weight [50, 20] 1000 True\n48 fc_logrates.bias [50] 50 True\nTotal parameters: 605110\n"
],
[
"model.fit(train_ds, valid_ds, train_truth=train_truth, valid_truth=valid_truth,\n max_epochs=2000, batch_size=128, use_tensorboard=True, health_check=True)",
"Beginning training...\nEpoch: 1, Step: 25, training loss: 2557.217, validation loss: 2610.053, Runtime: 17.2304 secs\nEpoch: 2, Step: 50, training loss: 2208.789, validation loss: 2479.728, Runtime: 17.0755 secs\nEpoch: 3, Step: 75, training loss: 2140.418, validation loss: 2327.982, Runtime: 17.3312 secs\nEpoch: 4, Step: 100, training loss: 2118.082, validation loss: 2299.716, Runtime: 18.5046 secs\nEpoch: 5, Step: 125, training loss: 2106.918, validation loss: 2264.256, Runtime: 17.5414 secs\nEpoch: 6, Step: 150, training loss: 2101.052, validation loss: 2238.768, Runtime: 17.4161 secs\nEpoch: 7, Step: 175, training loss: 2094.568, validation loss: 2230.944, Runtime: 17.3690 secs\nEpoch: 8, Step: 200, training loss: 2086.985, validation loss: 2213.271, Runtime: 16.8770 secs\nEpoch: 9, Step: 225, training loss: 2083.442, validation loss: 2219.212, Runtime: 16.9674 secs\nEpoch: 10, Step: 250, training loss: 2083.308, validation loss: 2194.288, Runtime: 17.6247 secs\nEpoch: 11, Step: 275, training loss: 2077.380, validation loss: 2191.504, Runtime: 17.2168 secs\nEpoch: 12, Step: 300, training loss: 2073.728, validation loss: 2186.133, Runtime: 17.1301 secs\nEpoch: 13, Step: 325, training loss: 2071.191, validation loss: 2174.103, Runtime: 19.4337 secs\nEpoch: 14, Step: 350, training loss: 2067.840, validation loss: 2169.330, Runtime: 17.3926 secs\nEpoch: 15, Step: 375, training loss: 2071.379, validation loss: 2168.049, Runtime: 17.1836 secs\nEpoch: 16, Step: 400, training loss: 2067.823, validation loss: 2174.122, Runtime: 17.1792 secs\nEpoch: 17, Step: 425, training loss: 2067.137, validation loss: 2164.121, Runtime: 17.0217 secs\nEpoch: 18, Step: 450, training loss: 2065.873, validation loss: 2158.567, Runtime: 17.2118 secs\nEpoch: 19, Step: 475, training loss: 2065.072, validation loss: 2155.914, Runtime: 17.0723 secs\nEpoch: 20, Step: 500, training loss: 2064.417, validation loss: 2149.900, Runtime: 17.0730 secs\nEpoch: 21, Step: 525, training loss: 2065.707, validation loss: 2149.442, Runtime: 17.1612 secs\nEpoch: 22, Step: 550, training loss: 2066.400, validation loss: 2142.000, Runtime: 17.0136 secs\nEpoch: 23, Step: 575, training loss: 2066.134, validation loss: 2143.854, Runtime: 16.9664 secs\nEpoch: 24, Step: 600, training loss: 2066.398, validation loss: 2140.450, Runtime: 16.9461 secs\nEpoch: 25, Step: 625, training loss: 2067.836, validation loss: 2133.832, Runtime: 17.0684 secs\nLearning rate decreased to 0.009500\nEpoch: 26, Step: 650, training loss: 2068.568, validation loss: 2135.610, Runtime: 17.1019 secs\nEpoch: 27, Step: 675, training loss: 2069.200, validation loss: 2137.359, Runtime: 16.9644 secs\nEpoch: 28, Step: 700, training loss: 2070.060, validation loss: 2131.551, Runtime: 16.9092 secs\nEpoch: 29, Step: 725, training loss: 2069.614, validation loss: 2123.320, Runtime: 16.7581 secs\nEpoch: 30, Step: 750, training loss: 2070.496, validation loss: 2127.988, Runtime: 16.9421 secs\nEpoch: 31, Step: 775, training loss: 2070.842, validation loss: 2129.290, Runtime: 16.9420 secs\nLearning rate decreased to 0.009025\nEpoch: 32, Step: 800, training loss: 2071.292, validation loss: 2122.950, Runtime: 16.9435 secs\nEpoch: 33, Step: 825, training loss: 2071.658, validation loss: 2116.812, Runtime: 16.8053 secs\nEpoch: 34, Step: 850, training loss: 2072.536, validation loss: 2119.495, Runtime: 17.3354 secs\nEpoch: 35, Step: 875, training loss: 2073.963, validation loss: 2120.222, Runtime: 16.9053 secs\nEpoch: 36, Step: 900, training loss: 2073.641, validation loss: 2115.243, Runtime: 17.0454 secs\nEpoch: 37, Step: 925, training loss: 2074.630, validation loss: 2114.853, Runtime: 16.9358 secs\nLearning rate decreased to 0.008574\nEpoch: 38, Step: 950, training loss: 2074.569, validation loss: 2111.541, Runtime: 16.8864 secs\nEpoch: 39, Step: 975, training loss: 2074.638, validation loss: 2110.571, Runtime: 17.0627 secs\nEpoch: 40, Step: 1000, training loss: 2075.544, validation loss: 2107.673, Runtime: 17.2504 secs\nEpoch: 41, Step: 1025, training loss: 2076.550, validation loss: 2106.699, Runtime: 17.0304 secs\nEpoch: 42, Step: 1050, training loss: 2079.190, validation loss: 2109.195, Runtime: 17.0670 secs\nEpoch: 43, Step: 1075, training loss: 2078.244, validation loss: 2107.376, Runtime: 17.0391 secs\nEpoch: 44, Step: 1100, training loss: 2078.474, validation loss: 2105.810, Runtime: 16.8592 secs\nEpoch: 45, Step: 1125, training loss: 2078.417, validation loss: 2105.702, Runtime: 17.0339 secs\nEpoch: 46, Step: 1150, training loss: 2079.082, validation loss: 2104.099, Runtime: 17.0431 secs\nEpoch: 47, Step: 1175, training loss: 2081.112, validation loss: 2103.929, Runtime: 17.7244 secs\nLearning rate decreased to 0.008145\nEpoch: 48, Step: 1200, training loss: 2080.164, validation loss: 2102.307, Runtime: 17.7893 secs\nEpoch: 49, Step: 1225, training loss: 2080.680, validation loss: 2100.925, Runtime: 18.4226 secs\nEpoch: 50, Step: 1250, training loss: 2083.177, validation loss: 2103.238, Runtime: 18.3050 secs\nEpoch: 51, Step: 1275, training loss: 2082.651, validation loss: 2103.636, Runtime: 17.5654 secs\nEpoch: 52, Step: 1300, training loss: 2083.261, validation loss: 2099.311, Runtime: 17.0221 secs\nEpoch: 53, Step: 1325, training loss: 2084.113, validation loss: 2100.873, Runtime: 16.9593 secs\nLearning rate decreased to 0.007738\nEpoch: 54, Step: 1350, training loss: 2083.778, validation loss: 2099.234, Runtime: 16.9570 secs\nEpoch: 55, Step: 1375, training loss: 2083.756, validation loss: 2098.243, Runtime: 16.7348 secs\nEpoch: 56, Step: 1400, training loss: 2084.866, validation loss: 2096.655, Runtime: 16.8157 secs\nEpoch: 57, Step: 1425, training loss: 2084.759, validation loss: 2095.695, Runtime: 16.6578 secs\nEpoch: 58, Step: 1450, training loss: 2084.947, validation loss: 2096.219, Runtime: 18.2158 secs\nEpoch: 59, Step: 1475, training loss: 2086.287, validation loss: 2096.567, Runtime: 17.3117 secs\nLearning rate decreased to 0.007351\nEpoch: 60, Step: 1500, training loss: 2086.724, validation loss: 2095.279, Runtime: 17.7393 secs\nEpoch: 61, Step: 1525, training loss: 2087.409, validation loss: 2095.054, Runtime: 17.0783 secs\nEpoch: 62, Step: 1550, training loss: 2086.950, validation loss: 2095.240, Runtime: 16.8750 secs\nEpoch: 63, Step: 1575, training loss: 2087.802, validation loss: 2094.493, Runtime: 16.8748 secs\nEpoch: 64, Step: 1600, training loss: 2088.250, validation loss: 2095.209, Runtime: 16.8424 secs\nEpoch: 65, Step: 1625, training loss: 2092.006, validation loss: 2094.851, Runtime: 16.8836 secs\nLearning rate decreased to 0.006983\nEpoch: 66, Step: 1650, training loss: 2089.890, validation loss: 2094.803, Runtime: 16.9112 secs\nEpoch: 67, Step: 1675, training loss: 2091.322, validation loss: 2096.738, Runtime: 16.7898 secs\nEpoch: 68, Step: 1700, training loss: 2091.398, validation loss: 2093.709, Runtime: 16.7823 secs\nEpoch: 69, Step: 1725, training loss: 2090.719, validation loss: 2093.071, Runtime: 16.8302 secs\nEpoch: 70, Step: 1750, training loss: 2090.991, validation loss: 2092.824, Runtime: 16.7782 secs\nEpoch: 71, Step: 1775, training loss: 2091.716, validation loss: 2093.909, Runtime: 18.4659 secs\nEpoch: 72, Step: 1800, training loss: 2092.196, validation loss: 2094.285, Runtime: 18.0281 secs\nLearning rate decreased to 0.006634\nEpoch: 73, Step: 1825, training loss: 2092.286, validation loss: 2092.443, Runtime: 18.9930 secs\nEpoch: 74, Step: 1850, training loss: 2092.779, validation loss: 2094.164, Runtime: 17.1668 secs\nEpoch: 75, Step: 1875, training loss: 2093.282, validation loss: 2093.334, Runtime: 17.1332 secs\nEpoch: 76, Step: 1900, training loss: 2095.317, validation loss: 2092.685, Runtime: 17.1204 secs\nEpoch: 77, Step: 1925, training loss: 2093.348, validation loss: 2091.717, Runtime: 17.3353 secs\nEpoch: 78, Step: 1950, training loss: 2093.715, validation loss: 2091.104, Runtime: 17.0682 secs\nEpoch: 79, Step: 1975, training loss: 2094.113, validation loss: 2091.806, Runtime: 16.9667 secs\nEpoch: 80, Step: 2000, training loss: 2095.139, validation loss: 2093.281, Runtime: 16.9700 secs\nSaving checkpoint: 0.0114 s taken\nEpoch: 81, Step: 2025, training loss: 2094.763, validation loss: 2092.162, Runtime: 18.3518 secs\nSaving checkpoint: 0.0123 s taken\nEpoch: 82, Step: 2050, training loss: 2094.215, validation loss: 2090.937, Runtime: 18.8124 secs\nSaving checkpoint: 0.0123 s taken\nEpoch: 83, Step: 2075, training loss: 2094.516, validation loss: 2091.899, Runtime: 18.3377 secs\nEpoch: 84, Step: 2100, training loss: 2094.275, validation loss: 2093.546, Runtime: 17.6807 secs\nEpoch: 85, Step: 2125, training loss: 2094.466, validation loss: 2091.580, Runtime: 17.5125 secs\nEpoch: 86, Step: 2150, training loss: 2093.487, validation loss: 2092.417, Runtime: 17.2390 secs\nEpoch: 87, Step: 2175, training loss: 2096.345, validation loss: 2094.923, Runtime: 17.7086 secs\nLearning rate decreased to 0.006302\nEpoch: 88, Step: 2200, training loss: 2094.774, validation loss: 2090.439, Runtime: 17.5883 secs\nSaving checkpoint: 0.0122 s taken\nEpoch: 89, Step: 2225, training loss: 2093.257, validation loss: 2090.267, Runtime: 17.2987 secs\nSaving checkpoint: 0.0125 s taken\nEpoch: 90, Step: 2250, training loss: 2094.950, validation loss: 2091.810, Runtime: 18.8502 secs\nEpoch: 91, Step: 2275, training loss: 2093.756, validation loss: 2089.801, Runtime: 17.4153 secs\nSaving checkpoint: 0.0118 s taken\nEpoch: 92, Step: 2300, training loss: 2093.240, validation loss: 2090.921, Runtime: 18.5797 secs\nEpoch: 93, Step: 2325, training loss: 2094.063, validation loss: 2092.502, Runtime: 18.6665 secs\nEpoch: 94, Step: 2350, training loss: 2093.097, validation loss: 2091.004, Runtime: 18.6146 secs\nEpoch: 95, Step: 2375, training loss: 2092.921, validation loss: 2090.645, Runtime: 17.7936 secs\nEpoch: 96, Step: 2400, training loss: 2092.869, validation loss: 2090.207, Runtime: 17.4161 secs\nEpoch: 97, Step: 2425, training loss: 2092.778, validation loss: 2090.204, Runtime: 17.8503 secs\nEpoch: 98, Step: 2450, training loss: 2092.721, validation loss: 2089.999, Runtime: 18.5511 secs\nEpoch: 99, Step: 2475, training loss: 2092.169, validation loss: 2090.886, Runtime: 17.5230 secs\nEpoch: 100, Step: 2500, training loss: 2092.364, validation loss: 2089.582, Runtime: 17.5571 secs\nSaving checkpoint: 0.0125 s taken\nEpoch: 101, Step: 2525, training loss: 2092.410, validation loss: 2093.627, Runtime: 19.4166 secs\nEpoch: 102, Step: 2550, training loss: 2094.083, validation loss: 2090.050, Runtime: 17.6604 secs\nLearning rate decreased to 0.005987\nEpoch: 103, Step: 2575, training loss: 2092.059, validation loss: 2088.891, Runtime: 18.6726 secs\nSaving checkpoint: 0.0125 s taken\nEpoch: 104, Step: 2600, training loss: 2091.418, validation loss: 2089.193, Runtime: 19.1972 secs\nEpoch: 105, Step: 2625, training loss: 2091.610, validation loss: 2090.213, Runtime: 18.8939 secs\nEpoch: 106, Step: 2650, training loss: 2091.648, validation loss: 2090.257, Runtime: 18.0404 secs\nEpoch: 107, Step: 2675, training loss: 2091.624, validation loss: 2088.615, Runtime: 17.9147 secs\nSaving checkpoint: 0.0125 s taken\nEpoch: 108, Step: 2700, training loss: 2091.148, validation loss: 2090.734, Runtime: 18.1776 secs\nEpoch: 109, Step: 2725, training loss: 2093.523, validation loss: 2090.241, Runtime: 17.7027 secs\nLearning rate decreased to 0.005688\nEpoch: 110, Step: 2750, training loss: 2091.603, validation loss: 2088.875, Runtime: 17.7850 secs\nEpoch: 111, Step: 2775, training loss: 2090.695, validation loss: 2089.929, Runtime: 17.6940 secs\nEpoch: 112, Step: 2800, training loss: 2091.062, validation loss: 2088.886, Runtime: 17.6880 secs\nEpoch: 113, Step: 2825, training loss: 2090.546, validation loss: 2089.800, Runtime: 17.7293 secs\nEpoch: 114, Step: 2850, training loss: 2092.872, validation loss: 2089.582, Runtime: 17.6147 secs\nEpoch: 115, Step: 2875, training loss: 2091.223, validation loss: 2088.700, Runtime: 17.6011 secs\nEpoch: 116, Step: 2900, training loss: 2091.355, validation loss: 2092.352, Runtime: 17.5403 secs\nEpoch: 117, Step: 2925, training loss: 2091.161, validation loss: 2088.697, Runtime: 17.5880 secs\nEpoch: 118, Step: 2950, training loss: 2090.829, validation loss: 2089.151, Runtime: 17.1864 secs\nEpoch: 119, Step: 2975, training loss: 2091.971, validation loss: 2090.777, Runtime: 17.2890 secs\nEpoch: 120, Step: 3000, training loss: 2090.681, validation loss: 2089.204, Runtime: 17.2815 secs\nEpoch: 121, Step: 3025, training loss: 2090.094, validation loss: 2089.403, Runtime: 17.2736 secs\nEpoch: 122, Step: 3050, training loss: 2090.743, validation loss: 2088.776, Runtime: 17.1933 secs\nEpoch: 123, Step: 3075, training loss: 2090.178, validation loss: 2088.152, Runtime: 17.1438 secs\nSaving checkpoint: 0.0120 s taken\nEpoch: 124, Step: 3100, training loss: 2090.269, validation loss: 2090.168, Runtime: 17.2830 secs\nEpoch: 125, Step: 3125, training loss: 2090.022, validation loss: 2088.736, Runtime: 17.2406 secs\nEpoch: 126, Step: 3150, training loss: 2089.945, validation loss: 2088.403, Runtime: 17.2731 secs\nEpoch: 127, Step: 3175, training loss: 2090.107, validation loss: 2088.738, Runtime: 17.3145 secs\nEpoch: 128, Step: 3200, training loss: 2089.872, validation loss: 2088.277, Runtime: 17.2772 secs\nEpoch: 129, Step: 3225, training loss: 2089.760, validation loss: 2091.068, Runtime: 17.0515 secs\nEpoch: 130, Step: 3250, training loss: 2090.951, validation loss: 2087.813, Runtime: 17.2143 secs\nLearning rate decreased to 0.005404\nSaving checkpoint: 0.0122 s taken\nEpoch: 131, Step: 3275, training loss: 2089.330, validation loss: 2088.217, Runtime: 17.3209 secs\nEpoch: 132, Step: 3300, training loss: 2089.707, validation loss: 2088.515, Runtime: 17.2765 secs\nEpoch: 133, Step: 3325, training loss: 2089.926, validation loss: 2088.532, Runtime: 17.2861 secs\nEpoch: 134, Step: 3350, training loss: 2089.162, validation loss: 2088.852, Runtime: 17.1830 secs\nEpoch: 135, Step: 3375, training loss: 2089.409, validation loss: 2087.770, Runtime: 17.4957 secs\nSaving checkpoint: 0.0126 s taken\nEpoch: 136, Step: 3400, training loss: 2088.823, validation loss: 2089.170, Runtime: 17.2138 secs\nEpoch: 137, Step: 3425, training loss: 2089.726, validation loss: 2088.773, Runtime: 17.2521 secs\nEpoch: 138, Step: 3450, training loss: 2089.493, validation loss: 2087.706, Runtime: 17.2729 secs\nSaving checkpoint: 0.0120 s taken\nEpoch: 139, Step: 3475, training loss: 2089.084, validation loss: 2088.140, Runtime: 17.2735 secs\nEpoch: 140, Step: 3500, training loss: 2089.281, validation loss: 2088.184, Runtime: 17.5229 secs\nEpoch: 141, Step: 3525, training loss: 2089.173, validation loss: 2088.621, Runtime: 17.2021 secs\nEpoch: 142, Step: 3550, training loss: 2089.331, validation loss: 2088.189, Runtime: 17.2656 secs\nEpoch: 143, Step: 3575, training loss: 2089.893, validation loss: 2090.813, Runtime: 17.2831 secs\nLearning rate decreased to 0.005133\nEpoch: 144, Step: 3600, training loss: 2089.574, validation loss: 2088.197, Runtime: 17.2496 secs\nEpoch: 145, Step: 3625, training loss: 2088.185, validation loss: 2087.163, Runtime: 17.2435 secs\nSaving checkpoint: 0.0119 s taken\nEpoch: 146, Step: 3650, training loss: 2088.306, validation loss: 2087.844, Runtime: 17.2380 secs\nEpoch: 147, Step: 3675, training loss: 2088.323, validation loss: 2087.980, Runtime: 17.2756 secs\nEpoch: 148, Step: 3700, training loss: 2088.221, validation loss: 2087.567, Runtime: 17.1709 secs\nEpoch: 149, Step: 3725, training loss: 2089.204, validation loss: 2088.667, Runtime: 17.3133 secs\nEpoch: 150, Step: 3750, training loss: 2088.540, validation loss: 2087.231, Runtime: 17.2668 secs\nEpoch: 151, Step: 3775, training loss: 2089.352, validation loss: 2088.374, Runtime: 17.1433 secs\nLearning rate decreased to 0.004877\nEpoch: 152, Step: 3800, training loss: 2088.277, validation loss: 2087.791, Runtime: 17.1756 secs\nEpoch: 153, Step: 3825, training loss: 2088.238, validation loss: 2087.448, Runtime: 17.1852 secs\nEpoch: 154, Step: 3850, training loss: 2088.124, validation loss: 2087.645, Runtime: 17.6555 secs\nEpoch: 155, Step: 3875, training loss: 2088.001, validation loss: 2086.973, Runtime: 17.2430 secs\nSaving checkpoint: 0.0124 s taken\nEpoch: 156, Step: 3900, training loss: 2087.852, validation loss: 2086.883, Runtime: 17.2762 secs\nSaving checkpoint: 0.0119 s taken\nEpoch: 157, Step: 3925, training loss: 2087.797, validation loss: 2087.191, Runtime: 17.2783 secs\nEpoch: 158, Step: 3950, training loss: 2088.032, validation loss: 2087.229, Runtime: 17.1849 secs\nEpoch: 159, Step: 3975, training loss: 2087.671, validation loss: 2087.547, Runtime: 17.5071 secs\nEpoch: 160, Step: 4000, training loss: 2088.077, validation loss: 2087.109, Runtime: 17.1807 secs\nEpoch: 161, Step: 4025, training loss: 2087.662, validation loss: 2086.691, Runtime: 17.3615 secs\nSaving checkpoint: 0.0126 s taken\nEpoch: 162, Step: 4050, training loss: 2087.688, validation loss: 2086.468, Runtime: 17.4640 secs\nSaving checkpoint: 0.0122 s taken\nEpoch: 163, Step: 4075, training loss: 2087.851, validation loss: 2087.604, Runtime: 17.4069 secs\nEpoch: 164, Step: 4100, training loss: 2087.475, validation loss: 2086.868, Runtime: 17.3153 secs\nEpoch: 165, Step: 4125, training loss: 2087.576, validation loss: 2087.004, Runtime: 17.1360 secs\nEpoch: 166, Step: 4150, training loss: 2087.731, validation loss: 2087.134, Runtime: 17.2306 secs\nEpoch: 167, Step: 4175, training loss: 2087.316, validation loss: 2086.410, Runtime: 17.3174 secs\nSaving checkpoint: 0.0124 s taken\nEpoch: 168, Step: 4200, training loss: 2087.553, validation loss: 2087.152, Runtime: 17.2569 secs\nEpoch: 169, Step: 4225, training loss: 2087.156, validation loss: 2086.566, Runtime: 17.2512 secs\nEpoch: 170, Step: 4250, training loss: 2087.121, validation loss: 2086.875, Runtime: 17.0890 secs\nEpoch: 171, Step: 4275, training loss: 2087.990, validation loss: 2089.144, Runtime: 17.1245 secs\nLearning rate decreased to 0.004633\nEpoch: 172, Step: 4300, training loss: 2087.625, validation loss: 2087.084, Runtime: 17.3916 secs\nEpoch: 173, Step: 4325, training loss: 2087.594, validation loss: 2088.207, Runtime: 17.3392 secs\nEpoch: 174, Step: 4350, training loss: 2087.408, validation loss: 2086.192, Runtime: 17.2900 secs\nSaving checkpoint: 0.0123 s taken\nEpoch: 175, Step: 4375, training loss: 2086.760, validation loss: 2086.352, Runtime: 17.3438 secs\nEpoch: 176, Step: 4400, training loss: 2086.773, validation loss: 2086.994, Runtime: 17.3175 secs\nEpoch: 177, Step: 4425, training loss: 2087.526, validation loss: 2086.160, Runtime: 17.2179 secs\nSaving checkpoint: 0.0124 s taken\nEpoch: 178, Step: 4450, training loss: 2086.867, validation loss: 2087.184, Runtime: 17.3915 secs\nEpoch: 179, Step: 4475, training loss: 2087.004, validation loss: 2087.292, Runtime: 17.2580 secs\nEpoch: 180, Step: 4500, training loss: 2086.840, validation loss: 2087.277, Runtime: 17.4833 secs\nEpoch: 181, Step: 4525, training loss: 2086.998, validation loss: 2086.753, Runtime: 17.3611 secs\nEpoch: 182, Step: 4550, training loss: 2086.882, validation loss: 2087.314, Runtime: 17.3764 secs\nEpoch: 183, Step: 4575, training loss: 2086.773, validation loss: 2085.969, Runtime: 17.3662 secs\nSaving checkpoint: 0.0119 s taken\nEpoch: 184, Step: 4600, training loss: 2086.482, validation loss: 2087.134, Runtime: 17.1217 secs\nEpoch: 185, Step: 4625, training loss: 2086.625, validation loss: 2087.222, Runtime: 17.0685 secs\nEpoch: 186, Step: 4650, training loss: 2086.786, validation loss: 2086.500, Runtime: 17.3040 secs\nEpoch: 187, Step: 4675, training loss: 2086.871, validation loss: 2086.711, Runtime: 17.3649 secs\nEpoch: 188, Step: 4700, training loss: 2087.088, validation loss: 2087.094, Runtime: 17.3635 secs\nLearning rate decreased to 0.004401\nEpoch: 189, Step: 4725, training loss: 2086.966, validation loss: 2088.581, Runtime: 17.2786 secs\nEpoch: 190, Step: 4750, training loss: 2086.689, validation loss: 2087.201, Runtime: 17.2264 secs\nEpoch: 191, Step: 4775, training loss: 2086.466, validation loss: 2086.854, Runtime: 17.1603 secs\nEpoch: 192, Step: 4800, training loss: 2086.654, validation loss: 2086.980, Runtime: 17.1964 secs\nEpoch: 193, Step: 4825, training loss: 2086.562, validation loss: 2087.721, Runtime: 17.0258 secs\nEpoch: 194, Step: 4850, training loss: 2086.908, validation loss: 2086.041, Runtime: 17.3135 secs\nEpoch: 195, Step: 4875, training loss: 2086.501, validation loss: 2087.321, Runtime: 17.1562 secs\nEpoch: 196, Step: 4900, training loss: 2086.138, validation loss: 2086.646, Runtime: 17.7375 secs\nEpoch: 197, Step: 4925, training loss: 2086.178, validation loss: 2086.050, Runtime: 17.6936 secs\nEpoch: 198, Step: 4950, training loss: 2086.246, validation loss: 2085.987, Runtime: 17.1806 secs\nEpoch: 199, Step: 4975, training loss: 2086.368, validation loss: 2086.245, Runtime: 17.4140 secs\nEpoch: 200, Step: 5000, training loss: 2085.867, validation loss: 2086.665, Runtime: 17.4369 secs\nEpoch: 201, Step: 5025, training loss: 2086.334, validation loss: 2086.649, Runtime: 17.3802 secs\nEpoch: 202, Step: 5050, training loss: 2085.952, validation loss: 2087.130, Runtime: 17.2247 secs\nEpoch: 203, Step: 5075, training loss: 2086.135, validation loss: 2086.370, Runtime: 17.4259 secs\nEpoch: 204, Step: 5100, training loss: 2086.846, validation loss: 2089.112, Runtime: 18.1112 secs\nLearning rate decreased to 0.004181\nEpoch: 205, Step: 5125, training loss: 2086.423, validation loss: 2086.894, Runtime: 17.2761 secs\nEpoch: 206, Step: 5150, training loss: 2086.302, validation loss: 2086.469, Runtime: 17.3358 secs\nEpoch: 207, Step: 5175, training loss: 2085.644, validation loss: 2086.401, Runtime: 17.4700 secs\nEpoch: 208, Step: 5200, training loss: 2085.258, validation loss: 2086.404, Runtime: 17.4251 secs\nEpoch: 209, Step: 5225, training loss: 2085.722, validation loss: 2088.842, Runtime: 17.3783 secs\nEpoch: 210, Step: 5250, training loss: 2086.933, validation loss: 2087.909, Runtime: 17.4375 secs\nLearning rate decreased to 0.003972\nEpoch: 211, Step: 5275, training loss: 2085.754, validation loss: 2085.928, Runtime: 17.3851 secs\nSaving checkpoint: 0.0123 s taken\nEpoch: 212, Step: 5300, training loss: 2085.492, validation loss: 2086.887, Runtime: 17.4158 secs\nEpoch: 213, Step: 5325, training loss: 2085.843, validation loss: 2086.955, Runtime: 17.3761 secs\nEpoch: 214, Step: 5350, training loss: 2085.433, validation loss: 2086.859, Runtime: 17.3186 secs\nEpoch: 215, Step: 5375, training loss: 2085.216, validation loss: 2085.859, Runtime: 17.1612 secs\nSaving checkpoint: 0.0126 s taken\nEpoch: 216, Step: 5400, training loss: 2085.358, validation loss: 2086.396, Runtime: 17.5248 secs\nEpoch: 217, Step: 5425, training loss: 2085.291, validation loss: 2085.998, Runtime: 17.3326 secs\nEpoch: 218, Step: 5450, training loss: 2085.043, validation loss: 2086.322, Runtime: 17.4176 secs\nEpoch: 219, Step: 5475, training loss: 2084.884, validation loss: 2086.243, Runtime: 17.3587 secs\nEpoch: 220, Step: 5500, training loss: 2085.550, validation loss: 2087.989, Runtime: 17.2265 secs\nLearning rate decreased to 0.003774\nEpoch: 221, Step: 5525, training loss: 2085.451, validation loss: 2085.695, Runtime: 17.3055 secs\nSaving checkpoint: 0.0124 s taken\nEpoch: 222, Step: 5550, training loss: 2085.442, validation loss: 2086.258, Runtime: 17.3763 secs\nEpoch: 223, Step: 5575, training loss: 2084.674, validation loss: 2085.641, Runtime: 17.2749 secs\nSaving checkpoint: 0.0125 s taken\nEpoch: 224, Step: 5600, training loss: 2084.752, validation loss: 2086.279, Runtime: 17.3453 secs\nEpoch: 225, Step: 5625, training loss: 2085.175, validation loss: 2085.552, Runtime: 17.2529 secs\nSaving checkpoint: 0.0145 s taken\nEpoch: 226, Step: 5650, training loss: 2084.658, validation loss: 2086.028, Runtime: 17.3257 secs\nEpoch: 227, Step: 5675, training loss: 2084.695, validation loss: 2085.877, Runtime: 17.7774 secs\nEpoch: 228, Step: 5700, training loss: 2084.962, validation loss: 2086.155, Runtime: 17.4118 secs\nEpoch: 229, Step: 5725, training loss: 2085.450, validation loss: 2086.354, Runtime: 17.3958 secs\nLearning rate decreased to 0.003585\nEpoch: 230, Step: 5750, training loss: 2084.917, validation loss: 2085.941, Runtime: 17.2994 secs\nEpoch: 231, Step: 5775, training loss: 2084.984, validation loss: 2085.947, Runtime: 17.3319 secs\nEpoch: 232, Step: 5800, training loss: 2084.439, validation loss: 2085.642, Runtime: 17.4672 secs\nEpoch: 233, Step: 5825, training loss: 2084.359, validation loss: 2086.044, Runtime: 17.4045 secs\nEpoch: 234, Step: 5850, training loss: 2084.576, validation loss: 2086.512, Runtime: 17.3132 secs\nEpoch: 235, Step: 5875, training loss: 2084.798, validation loss: 2085.927, Runtime: 17.4211 secs\nEpoch: 236, Step: 5900, training loss: 2084.393, validation loss: 2085.760, Runtime: 17.4050 secs\nEpoch: 237, Step: 5925, training loss: 2084.706, validation loss: 2085.530, Runtime: 17.3877 secs\nSaving checkpoint: 0.0132 s taken\nEpoch: 238, Step: 5950, training loss: 2084.273, validation loss: 2085.751, Runtime: 17.4115 secs\nEpoch: 239, Step: 5975, training loss: 2084.092, validation loss: 2086.116, Runtime: 17.2992 secs\nEpoch: 240, Step: 6000, training loss: 2084.437, validation loss: 2085.452, Runtime: 17.3304 secs\nSaving checkpoint: 0.0126 s taken\nEpoch: 241, Step: 6025, training loss: 2084.655, validation loss: 2085.819, Runtime: 17.3661 secs\nEpoch: 242, Step: 6050, training loss: 2084.343, validation loss: 2085.901, Runtime: 17.2353 secs\nEpoch: 243, Step: 6075, training loss: 2084.656, validation loss: 2085.640, Runtime: 17.4373 secs\nEpoch: 244, Step: 6100, training loss: 2084.085, validation loss: 2085.472, Runtime: 17.2923 secs\nEpoch: 245, Step: 6125, training loss: 2084.452, validation loss: 2086.590, Runtime: 17.3759 secs\nEpoch: 246, Step: 6150, training loss: 2084.839, validation loss: 2086.319, Runtime: 17.4653 secs\nLearning rate decreased to 0.003406\nEpoch: 247, Step: 6175, training loss: 2084.153, validation loss: 2085.692, Runtime: 17.4638 secs\nEpoch: 248, Step: 6200, training loss: 2083.812, validation loss: 2085.811, Runtime: 17.6271 secs\nEpoch: 249, Step: 6225, training loss: 2084.373, validation loss: 2085.303, Runtime: 17.2694 secs\nSaving checkpoint: 0.0125 s taken\nEpoch: 250, Step: 6250, training loss: 2084.273, validation loss: 2085.787, Runtime: 17.4027 secs\nEpoch: 251, Step: 6275, training loss: 2083.569, validation loss: 2085.365, Runtime: 17.4463 secs\nEpoch: 252, Step: 6300, training loss: 2083.994, validation loss: 2085.291, Runtime: 17.2883 secs\nSaving checkpoint: 0.0128 s taken\nEpoch: 253, Step: 6325, training loss: 2083.970, validation loss: 2085.545, Runtime: 17.4676 secs\nEpoch: 254, Step: 6350, training loss: 2083.948, validation loss: 2084.905, Runtime: 17.2826 secs\nSaving checkpoint: 0.0126 s taken\nEpoch: 255, Step: 6375, training loss: 2083.916, validation loss: 2085.267, Runtime: 17.3050 secs\nEpoch: 256, Step: 6400, training loss: 2083.534, validation loss: 2085.440, Runtime: 17.4014 secs\nEpoch: 257, Step: 6425, training loss: 2083.695, validation loss: 2085.386, Runtime: 17.4143 secs\nEpoch: 258, Step: 6450, training loss: 2083.827, validation loss: 2085.291, Runtime: 17.4052 secs\nEpoch: 259, Step: 6475, training loss: 2084.006, validation loss: 2086.559, Runtime: 17.4340 secs\nLearning rate decreased to 0.003235\nEpoch: 260, Step: 6500, training loss: 2083.629, validation loss: 2085.435, Runtime: 17.6401 secs\nEpoch: 261, Step: 6525, training loss: 2083.797, validation loss: 2085.190, Runtime: 17.4169 secs\nEpoch: 262, Step: 6550, training loss: 2083.571, validation loss: 2085.343, Runtime: 17.4104 secs\nEpoch: 263, Step: 6575, training loss: 2083.601, validation loss: 2085.712, Runtime: 17.2439 secs\nEpoch: 264, Step: 6600, training loss: 2083.736, validation loss: 2085.860, Runtime: 17.3231 secs\nEpoch: 265, Step: 6625, training loss: 2083.264, validation loss: 2085.426, Runtime: 17.4012 secs\nEpoch: 266, Step: 6650, training loss: 2083.207, validation loss: 2086.681, Runtime: 17.2680 secs\nEpoch: 267, Step: 6675, training loss: 2083.938, validation loss: 2085.295, Runtime: 17.4537 secs\nLearning rate decreased to 0.003074\nEpoch: 268, Step: 6700, training loss: 2083.356, validation loss: 2085.674, Runtime: 17.8439 secs\nEpoch: 269, Step: 6725, training loss: 2083.215, validation loss: 2085.275, Runtime: 17.2070 secs\nEpoch: 270, Step: 6750, training loss: 2083.416, validation loss: 2085.668, Runtime: 17.5627 secs\nEpoch: 271, Step: 6775, training loss: 2083.383, validation loss: 2085.717, Runtime: 17.4310 secs\nEpoch: 272, Step: 6800, training loss: 2083.093, validation loss: 2084.944, Runtime: 17.4457 secs\nEpoch: 273, Step: 6825, training loss: 2083.266, validation loss: 2085.338, Runtime: 17.3978 secs\nEpoch: 274, Step: 6850, training loss: 2083.223, validation loss: 2085.747, Runtime: 17.3445 secs\nEpoch: 275, Step: 6875, training loss: 2083.270, validation loss: 2085.205, Runtime: 17.3241 secs\nEpoch: 276, Step: 6900, training loss: 2083.073, validation loss: 2084.955, Runtime: 17.3937 secs\nEpoch: 277, Step: 6925, training loss: 2083.200, validation loss: 2086.093, Runtime: 17.4876 secs\nEpoch: 278, Step: 6950, training loss: 2083.354, validation loss: 2085.623, Runtime: 17.4250 secs\nLearning rate decreased to 0.002920\nEpoch: 279, Step: 6975, training loss: 2083.153, validation loss: 2086.289, Runtime: 17.4744 secs\nEpoch: 280, Step: 7000, training loss: 2082.597, validation loss: 2085.365, Runtime: 17.3977 secs\nEpoch: 281, Step: 7025, training loss: 2082.764, validation loss: 2084.969, Runtime: 17.2332 secs\nEpoch: 282, Step: 7050, training loss: 2083.312, validation loss: 2085.633, Runtime: 17.4483 secs\nEpoch: 283, Step: 7075, training loss: 2083.199, validation loss: 2085.469, Runtime: 17.3845 secs\nEpoch: 284, Step: 7100, training loss: 2082.970, validation loss: 2085.715, Runtime: 17.5090 secs\nEpoch: 285, Step: 7125, training loss: 2082.655, validation loss: 2085.106, Runtime: 17.5767 secs\nEpoch: 286, Step: 7150, training loss: 2082.778, validation loss: 2084.761, Runtime: 17.4798 secs\nSaving checkpoint: 0.0129 s taken\nEpoch: 287, Step: 7175, training loss: 2082.651, validation loss: 2085.166, Runtime: 17.4062 secs\nEpoch: 288, Step: 7200, training loss: 2083.041, validation loss: 2086.179, Runtime: 17.3023 secs\nEpoch: 289, Step: 7225, training loss: 2082.868, validation loss: 2085.720, Runtime: 17.3575 secs\nEpoch: 290, Step: 7250, training loss: 2082.602, validation loss: 2085.611, Runtime: 17.4047 secs\nEpoch: 291, Step: 7275, training loss: 2083.178, validation loss: 2085.080, Runtime: 17.3754 secs\nLearning rate decreased to 0.002774\nEpoch: 292, Step: 7300, training loss: 2082.759, validation loss: 2085.615, Runtime: 17.3619 secs\nEpoch: 293, Step: 7325, training loss: 2082.528, validation loss: 2085.469, Runtime: 17.2605 secs\nEpoch: 294, Step: 7350, training loss: 2082.599, validation loss: 2085.126, Runtime: 17.3832 secs\nEpoch: 295, Step: 7375, training loss: 2082.252, validation loss: 2085.410, Runtime: 17.2327 secs\nEpoch: 296, Step: 7400, training loss: 2082.368, validation loss: 2084.516, Runtime: 17.8021 secs\nSaving checkpoint: 0.0132 s taken\nEpoch: 297, Step: 7425, training loss: 2082.523, validation loss: 2085.124, Runtime: 17.4754 secs\nEpoch: 298, Step: 7450, training loss: 2082.692, validation loss: 2085.760, Runtime: 17.2666 secs\nEpoch: 299, Step: 7475, training loss: 2082.460, validation loss: 2085.246, Runtime: 17.3421 secs\nEpoch: 300, Step: 7500, training loss: 2082.548, validation loss: 2085.464, Runtime: 17.3970 secs\nEpoch: 301, Step: 7525, training loss: 2082.348, validation loss: 2085.351, Runtime: 17.4238 secs\nEpoch: 302, Step: 7550, training loss: 2082.332, validation loss: 2085.346, Runtime: 17.4198 secs\nEpoch: 303, Step: 7575, training loss: 2082.597, validation loss: 2085.133, Runtime: 17.2757 secs\nEpoch: 304, Step: 7600, training loss: 2082.353, validation loss: 2085.222, Runtime: 17.1473 secs\nEpoch: 305, Step: 7625, training loss: 2082.326, validation loss: 2085.467, Runtime: 17.3821 secs\nEpoch: 306, Step: 7650, training loss: 2082.367, validation loss: 2085.428, Runtime: 17.3402 secs\nEpoch: 307, Step: 7675, training loss: 2082.218, validation loss: 2084.735, Runtime: 17.3484 secs\nEpoch: 308, Step: 7700, training loss: 2082.220, validation loss: 2085.173, Runtime: 17.6708 secs\nEpoch: 309, Step: 7725, training loss: 2082.304, validation loss: 2085.266, Runtime: 17.5108 secs\nEpoch: 310, Step: 7750, training loss: 2082.162, validation loss: 2085.511, Runtime: 17.4043 secs\nEpoch: 311, Step: 7775, training loss: 2082.149, validation loss: 2085.124, Runtime: 17.2998 secs\nEpoch: 312, Step: 7800, training loss: 2082.319, validation loss: 2085.040, Runtime: 17.2150 secs\nEpoch: 313, Step: 7825, training loss: 2082.222, validation loss: 2085.511, Runtime: 17.4901 secs\nEpoch: 314, Step: 7850, training loss: 2082.283, validation loss: 2085.437, Runtime: 17.3623 secs\nEpoch: 315, Step: 7875, training loss: 2082.411, validation loss: 2085.661, Runtime: 17.2986 secs\nLearning rate decreased to 0.002635\nEpoch: 316, Step: 7900, training loss: 2082.214, validation loss: 2085.583, Runtime: 17.2870 secs\nEpoch: 317, Step: 7925, training loss: 2081.914, validation loss: 2085.167, Runtime: 17.3622 secs\nEpoch: 318, Step: 7950, training loss: 2082.126, validation loss: 2085.363, Runtime: 17.4624 secs\nEpoch: 319, Step: 7975, training loss: 2082.203, validation loss: 2084.302, Runtime: 17.3563 secs\nSaving checkpoint: 0.0133 s taken\nEpoch: 320, Step: 8000, training loss: 2082.077, validation loss: 2085.670, Runtime: 17.3627 secs\nEpoch: 321, Step: 8025, training loss: 2082.073, validation loss: 2085.230, Runtime: 17.4459 secs\nEpoch: 322, Step: 8050, training loss: 2082.270, validation loss: 2085.594, Runtime: 17.3989 secs\nLearning rate decreased to 0.002503\nEpoch: 323, Step: 8075, training loss: 2081.964, validation loss: 2086.282, Runtime: 17.4532 secs\nEpoch: 324, Step: 8100, training loss: 2081.978, validation loss: 2085.487, Runtime: 17.3470 secs\nEpoch: 325, Step: 8125, training loss: 2081.902, validation loss: 2086.038, Runtime: 17.2792 secs\nEpoch: 326, Step: 8150, training loss: 2081.632, validation loss: 2085.507, Runtime: 17.2168 secs\nEpoch: 327, Step: 8175, training loss: 2081.846, validation loss: 2084.861, Runtime: 17.3472 secs\nEpoch: 328, Step: 8200, training loss: 2081.828, validation loss: 2085.014, Runtime: 17.4539 secs\nEpoch: 329, Step: 8225, training loss: 2081.750, validation loss: 2085.386, Runtime: 17.3339 secs\nEpoch: 330, Step: 8250, training loss: 2081.775, validation loss: 2085.493, Runtime: 17.3618 secs\nEpoch: 331, Step: 8275, training loss: 2081.734, validation loss: 2085.192, Runtime: 17.2099 secs\nEpoch: 332, Step: 8300, training loss: 2081.373, validation loss: 2085.703, Runtime: 17.2939 secs\nEpoch: 333, Step: 8325, training loss: 2081.576, validation loss: 2084.927, Runtime: 17.3546 secs\nEpoch: 334, Step: 8350, training loss: 2081.427, validation loss: 2084.689, Runtime: 17.4806 secs\nEpoch: 335, Step: 8375, training loss: 2081.874, validation loss: 2085.482, Runtime: 17.5387 secs\nLearning rate decreased to 0.002378\nEpoch: 336, Step: 8400, training loss: 2081.344, validation loss: 2085.152, Runtime: 17.4123 secs\nEpoch: 337, Step: 8425, training loss: 2081.340, validation loss: 2085.823, Runtime: 17.9299 secs\nEpoch: 338, Step: 8450, training loss: 2081.989, validation loss: 2084.847, Runtime: 17.1671 secs\nEpoch: 339, Step: 8475, training loss: 2081.342, validation loss: 2085.253, Runtime: 17.4217 secs\nEpoch: 340, Step: 8500, training loss: 2081.403, validation loss: 2085.388, Runtime: 17.3611 secs\nEpoch: 341, Step: 8525, training loss: 2081.700, validation loss: 2085.640, Runtime: 17.5225 secs\nEpoch: 342, Step: 8550, training loss: 2081.662, validation loss: 2085.437, Runtime: 17.4949 secs\nEpoch: 343, Step: 8575, training loss: 2081.414, validation loss: 2085.335, Runtime: 17.5190 secs\nEpoch: 344, Step: 8600, training loss: 2081.695, validation loss: 2084.933, Runtime: 17.4322 secs\nEpoch: 345, Step: 8625, training loss: 2081.274, validation loss: 2084.771, Runtime: 17.2270 secs\nEpoch: 346, Step: 8650, training loss: 2081.465, validation loss: 2084.812, Runtime: 17.2981 secs\nEpoch: 347, Step: 8675, training loss: 2081.527, validation loss: 2085.214, Runtime: 17.3667 secs\nEpoch: 348, Step: 8700, training loss: 2081.278, validation loss: 2084.152, Runtime: 17.3398 secs\nSaving checkpoint: 0.0146 s taken\nEpoch: 349, Step: 8725, training loss: 2081.214, validation loss: 2085.321, Runtime: 17.3625 secs\nEpoch: 350, Step: 8750, training loss: 2081.249, validation loss: 2085.734, Runtime: 17.3519 secs\nEpoch: 351, Step: 8775, training loss: 2081.330, validation loss: 2085.230, Runtime: 17.3261 secs\nEpoch: 352, Step: 8800, training loss: 2081.605, validation loss: 2085.198, Runtime: 17.4698 secs\nLearning rate decreased to 0.002259\nEpoch: 353, Step: 8825, training loss: 2081.032, validation loss: 2085.615, Runtime: 17.3887 secs\nEpoch: 354, Step: 8850, training loss: 2081.177, validation loss: 2085.081, Runtime: 17.4038 secs\nEpoch: 355, Step: 8875, training loss: 2081.049, validation loss: 2085.027, Runtime: 17.3571 secs\nEpoch: 356, Step: 8900, training loss: 2081.175, validation loss: 2085.316, Runtime: 17.2807 secs\nEpoch: 357, Step: 8925, training loss: 2081.078, validation loss: 2084.960, Runtime: 17.3260 secs\nEpoch: 358, Step: 8950, training loss: 2081.129, validation loss: 2084.909, Runtime: 17.2837 secs\nEpoch: 359, Step: 8975, training loss: 2080.939, validation loss: 2084.892, Runtime: 17.4044 secs\nEpoch: 360, Step: 9000, training loss: 2080.972, validation loss: 2085.876, Runtime: 17.2574 secs\nEpoch: 361, Step: 9025, training loss: 2081.303, validation loss: 2084.543, Runtime: 17.2149 secs\nLearning rate decreased to 0.002146\nEpoch: 362, Step: 9050, training loss: 2080.560, validation loss: 2084.640, Runtime: 17.1153 secs\nEpoch: 363, Step: 9075, training loss: 2080.830, validation loss: 2084.806, Runtime: 17.2889 secs\nEpoch: 364, Step: 9100, training loss: 2080.966, validation loss: 2085.051, Runtime: 17.3882 secs\nEpoch: 365, Step: 9125, training loss: 2080.790, validation loss: 2085.370, Runtime: 17.2125 secs\nEpoch: 366, Step: 9150, training loss: 2080.901, validation loss: 2085.248, Runtime: 17.3404 secs\nEpoch: 367, Step: 9175, training loss: 2080.915, validation loss: 2085.455, Runtime: 17.3109 secs\nEpoch: 368, Step: 9200, training loss: 2080.795, validation loss: 2085.479, Runtime: 17.4264 secs\nEpoch: 369, Step: 9225, training loss: 2080.642, validation loss: 2084.879, Runtime: 17.4932 secs\nEpoch: 370, Step: 9250, training loss: 2080.931, validation loss: 2085.400, Runtime: 17.3950 secs\nEpoch: 371, Step: 9275, training loss: 2080.868, validation loss: 2085.394, Runtime: 17.3292 secs\nEpoch: 372, Step: 9300, training loss: 2080.731, validation loss: 2085.266, Runtime: 17.3159 secs\nEpoch: 373, Step: 9325, training loss: 2080.549, validation loss: 2084.781, Runtime: 17.4224 secs\nEpoch: 374, Step: 9350, training loss: 2080.735, validation loss: 2085.533, Runtime: 17.6613 secs\nEpoch: 375, Step: 9375, training loss: 2080.637, validation loss: 2085.507, Runtime: 17.4038 secs\nEpoch: 376, Step: 9400, training loss: 2081.009, validation loss: 2085.815, Runtime: 17.3260 secs\nLearning rate decreased to 0.002039\nEpoch: 377, Step: 9425, training loss: 2080.565, validation loss: 2085.008, Runtime: 17.4012 secs\nEpoch: 378, Step: 9450, training loss: 2080.648, validation loss: 2084.794, Runtime: 17.8411 secs\nEpoch: 379, Step: 9475, training loss: 2080.811, validation loss: 2084.953, Runtime: 17.4202 secs\nEpoch: 380, Step: 9500, training loss: 2080.445, validation loss: 2084.706, Runtime: 17.3149 secs\nEpoch: 381, Step: 9525, training loss: 2080.860, validation loss: 2085.349, Runtime: 17.3068 secs\nEpoch: 382, Step: 9550, training loss: 2080.778, validation loss: 2085.283, Runtime: 17.2569 secs\nEpoch: 383, Step: 9575, training loss: 2080.678, validation loss: 2085.996, Runtime: 17.4913 secs\nEpoch: 384, Step: 9600, training loss: 2080.970, validation loss: 2085.381, Runtime: 17.4390 secs\nLearning rate decreased to 0.001937\nEpoch: 385, Step: 9625, training loss: 2080.502, validation loss: 2084.421, Runtime: 17.3588 secs\nEpoch: 386, Step: 9650, training loss: 2080.390, validation loss: 2085.660, Runtime: 17.3450 secs\nEpoch: 387, Step: 9675, training loss: 2080.449, validation loss: 2084.825, Runtime: 17.4770 secs\nEpoch: 388, Step: 9700, training loss: 2080.507, validation loss: 2084.734, Runtime: 17.4325 secs\nEpoch: 389, Step: 9725, training loss: 2080.079, validation loss: 2085.518, Runtime: 17.4187 secs\nEpoch: 390, Step: 9750, training loss: 2080.611, validation loss: 2085.228, Runtime: 17.4313 secs\nEpoch: 391, Step: 9775, training loss: 2080.372, validation loss: 2085.340, Runtime: 17.3752 secs\nEpoch: 392, Step: 9800, training loss: 2080.418, validation loss: 2085.221, Runtime: 17.5405 secs\nEpoch: 393, Step: 9825, training loss: 2080.375, validation loss: 2085.379, Runtime: 17.3003 secs\nEpoch: 394, Step: 9850, training loss: 2080.478, validation loss: 2084.902, Runtime: 17.3757 secs\nEpoch: 395, Step: 9875, training loss: 2080.258, validation loss: 2084.728, Runtime: 17.2632 secs\nEpoch: 396, Step: 9900, training loss: 2080.461, validation loss: 2084.850, Runtime: 17.2542 secs\nEpoch: 397, Step: 9925, training loss: 2080.388, validation loss: 2084.858, Runtime: 17.2859 secs\nEpoch: 398, Step: 9950, training loss: 2079.959, validation loss: 2085.109, Runtime: 17.2827 secs\nEpoch: 399, Step: 9975, training loss: 2080.454, validation loss: 2084.521, Runtime: 17.3774 secs\nEpoch: 400, Step: 10000, training loss: 2080.302, validation loss: 2086.081, Runtime: 17.3412 secs\nEpoch: 401, Step: 10025, training loss: 2080.048, validation loss: 2084.601, Runtime: 17.3592 secs\nEpoch: 402, Step: 10050, training loss: 2080.454, validation loss: 2085.162, Runtime: 17.3909 secs\nEpoch: 403, Step: 10075, training loss: 2079.902, validation loss: 2085.023, Runtime: 17.4737 secs\nEpoch: 404, Step: 10100, training loss: 2080.463, validation loss: 2085.226, Runtime: 17.5336 secs\nLearning rate decreased to 0.001840\nEpoch: 405, Step: 10125, training loss: 2080.243, validation loss: 2085.052, Runtime: 17.3537 secs\nEpoch: 406, Step: 10150, training loss: 2080.441, validation loss: 2084.992, Runtime: 17.4125 secs\nEpoch: 407, Step: 10175, training loss: 2080.180, validation loss: 2084.990, Runtime: 17.3331 secs\nEpoch: 408, Step: 10200, training loss: 2079.803, validation loss: 2084.796, Runtime: 17.4092 secs\nEpoch: 409, Step: 10225, training loss: 2080.121, validation loss: 2085.029, Runtime: 17.3961 secs\nEpoch: 410, Step: 10250, training loss: 2080.217, validation loss: 2084.570, Runtime: 17.2754 secs\nEpoch: 411, Step: 10275, training loss: 2080.004, validation loss: 2084.688, Runtime: 17.4704 secs\nEpoch: 412, Step: 10300, training loss: 2079.759, validation loss: 2084.324, Runtime: 17.3561 secs\nEpoch: 413, Step: 10325, training loss: 2079.897, validation loss: 2085.207, Runtime: 17.4386 secs\nEpoch: 414, Step: 10350, training loss: 2079.932, validation loss: 2084.856, Runtime: 17.2792 secs\nEpoch: 415, Step: 10375, training loss: 2080.000, validation loss: 2084.353, Runtime: 17.3099 secs\nEpoch: 416, Step: 10400, training loss: 2079.917, validation loss: 2085.354, Runtime: 17.2875 secs\nEpoch: 417, Step: 10425, training loss: 2080.140, validation loss: 2085.221, Runtime: 17.3147 secs\nLearning rate decreased to 0.001748\nEpoch: 418, Step: 10450, training loss: 2080.245, validation loss: 2085.123, Runtime: 17.3971 secs\nEpoch: 419, Step: 10475, training loss: 2079.853, validation loss: 2084.967, Runtime: 17.3609 secs\nEpoch: 420, Step: 10500, training loss: 2079.942, validation loss: 2085.469, Runtime: 17.3123 secs\nEpoch: 421, Step: 10525, training loss: 2079.596, validation loss: 2085.071, Runtime: 17.3532 secs\nEpoch: 422, Step: 10550, training loss: 2079.712, validation loss: 2084.989, Runtime: 17.3488 secs\nEpoch: 423, Step: 10575, training loss: 2080.089, validation loss: 2085.103, Runtime: 17.3875 secs\nEpoch: 424, Step: 10600, training loss: 2079.811, validation loss: 2085.053, Runtime: 17.4778 secs\nEpoch: 425, Step: 10625, training loss: 2079.974, validation loss: 2085.368, Runtime: 17.3920 secs\nEpoch: 426, Step: 10650, training loss: 2079.843, validation loss: 2085.303, Runtime: 17.4598 secs\nEpoch: 427, Step: 10675, training loss: 2079.933, validation loss: 2084.529, Runtime: 17.3172 secs\nEpoch: 428, Step: 10700, training loss: 2079.946, validation loss: 2085.638, Runtime: 17.4026 secs\nEpoch: 429, Step: 10725, training loss: 2079.748, validation loss: 2084.540, Runtime: 17.2962 secs\nEpoch: 430, Step: 10750, training loss: 2079.707, validation loss: 2084.938, Runtime: 17.3379 secs\nEpoch: 431, Step: 10775, training loss: 2079.907, validation loss: 2084.966, Runtime: 17.4987 secs\nEpoch: 432, Step: 10800, training loss: 2079.721, validation loss: 2084.717, Runtime: 17.5183 secs\nEpoch: 433, Step: 10825, training loss: 2079.766, validation loss: 2085.978, Runtime: 17.5385 secs\nEpoch: 434, Step: 10850, training loss: 2079.816, validation loss: 2085.578, Runtime: 17.4218 secs\nEpoch: 435, Step: 10875, training loss: 2079.845, validation loss: 2085.161, Runtime: 17.9002 secs\nEpoch: 436, Step: 10900, training loss: 2079.633, validation loss: 2084.726, Runtime: 17.4327 secs\nEpoch: 437, Step: 10925, training loss: 2079.454, validation loss: 2084.859, Runtime: 17.5965 secs\nEpoch: 438, Step: 10950, training loss: 2079.760, validation loss: 2085.111, Runtime: 17.4530 secs\nEpoch: 439, Step: 10975, training loss: 2079.678, validation loss: 2084.636, Runtime: 17.3814 secs\nEpoch: 440, Step: 11000, training loss: 2079.646, validation loss: 2084.965, Runtime: 17.4234 secs\nEpoch: 441, Step: 11025, training loss: 2079.653, validation loss: 2085.218, Runtime: 17.4785 secs\nEpoch: 442, Step: 11050, training loss: 2079.911, validation loss: 2085.427, Runtime: 17.5559 secs\nLearning rate decreased to 0.001661\nEpoch: 443, Step: 11075, training loss: 2079.724, validation loss: 2084.649, Runtime: 17.4892 secs\nEpoch: 444, Step: 11100, training loss: 2079.554, validation loss: 2085.557, Runtime: 17.4829 secs\nEpoch: 445, Step: 11125, training loss: 2079.280, validation loss: 2085.169, Runtime: 17.4378 secs\nEpoch: 446, Step: 11150, training loss: 2079.608, validation loss: 2085.146, Runtime: 17.4361 secs\nEpoch: 447, Step: 11175, training loss: 2079.211, validation loss: 2085.143, Runtime: 17.4961 secs\nEpoch: 448, Step: 11200, training loss: 2079.696, validation loss: 2085.375, Runtime: 17.3641 secs\nEpoch: 449, Step: 11225, training loss: 2079.698, validation loss: 2085.152, Runtime: 17.3912 secs\nEpoch: 450, Step: 11250, training loss: 2079.291, validation loss: 2085.142, Runtime: 17.5164 secs\nEpoch: 451, Step: 11275, training loss: 2079.473, validation loss: 2085.224, Runtime: 17.4672 secs\nEpoch: 452, Step: 11300, training loss: 2079.223, validation loss: 2085.296, Runtime: 17.3564 secs\nEpoch: 453, Step: 11325, training loss: 2079.571, validation loss: 2084.892, Runtime: 17.5968 secs\nEpoch: 454, Step: 11350, training loss: 2079.335, validation loss: 2084.808, Runtime: 17.3346 secs\nEpoch: 455, Step: 11375, training loss: 2079.344, validation loss: 2084.839, Runtime: 17.3394 secs\nEpoch: 456, Step: 11400, training loss: 2079.429, validation loss: 2084.742, Runtime: 17.4895 secs\nEpoch: 457, Step: 11425, training loss: 2079.494, validation loss: 2085.061, Runtime: 17.8189 secs\nEpoch: 458, Step: 11450, training loss: 2079.367, validation loss: 2085.038, Runtime: 17.2024 secs\nEpoch: 459, Step: 11475, training loss: 2079.150, validation loss: 2085.079, Runtime: 17.4597 secs\nEpoch: 460, Step: 11500, training loss: 2079.494, validation loss: 2084.816, Runtime: 17.5079 secs\nEpoch: 461, Step: 11525, training loss: 2079.404, validation loss: 2085.425, Runtime: 17.4806 secs\nEpoch: 462, Step: 11550, training loss: 2079.428, validation loss: 2085.264, Runtime: 17.3274 secs\nEpoch: 463, Step: 11575, training loss: 2079.447, validation loss: 2085.227, Runtime: 17.3898 secs\nEpoch: 464, Step: 11600, training loss: 2079.334, validation loss: 2085.240, Runtime: 17.3822 secs\nEpoch: 465, Step: 11625, training loss: 2079.442, validation loss: 2085.489, Runtime: 17.4329 secs\nEpoch: 466, Step: 11650, training loss: 2079.226, validation loss: 2084.399, Runtime: 17.6466 secs\nEpoch: 467, Step: 11675, training loss: 2079.153, validation loss: 2085.402, Runtime: 17.3967 secs\nEpoch: 468, Step: 11700, training loss: 2079.461, validation loss: 2085.271, Runtime: 17.4329 secs\nLearning rate decreased to 0.001578\nEpoch: 469, Step: 11725, training loss: 2079.132, validation loss: 2084.999, Runtime: 17.4073 secs\nEpoch: 470, Step: 11750, training loss: 2079.449, validation loss: 2084.592, Runtime: 17.3623 secs\nEpoch: 471, Step: 11775, training loss: 2079.079, validation loss: 2084.704, Runtime: 17.4021 secs\nEpoch: 472, Step: 11800, training loss: 2079.131, validation loss: 2084.935, Runtime: 17.3859 secs\nEpoch: 473, Step: 11825, training loss: 2079.107, validation loss: 2085.156, Runtime: 17.5299 secs\nEpoch: 474, Step: 11850, training loss: 2079.230, validation loss: 2084.877, Runtime: 17.3433 secs\nEpoch: 475, Step: 11875, training loss: 2078.972, validation loss: 2084.882, Runtime: 17.3984 secs\nEpoch: 476, Step: 11900, training loss: 2079.204, validation loss: 2085.548, Runtime: 17.2669 secs\nEpoch: 477, Step: 11925, training loss: 2079.226, validation loss: 2085.219, Runtime: 17.4029 secs\nEpoch: 478, Step: 11950, training loss: 2078.970, validation loss: 2085.441, Runtime: 17.5248 secs\nEpoch: 479, Step: 11975, training loss: 2079.020, validation loss: 2085.321, Runtime: 17.3394 secs\nEpoch: 480, Step: 12000, training loss: 2079.308, validation loss: 2084.734, Runtime: 17.2683 secs\nLearning rate decreased to 0.001499\nEpoch: 481, Step: 12025, training loss: 2078.746, validation loss: 2085.402, Runtime: 17.2861 secs\nEpoch: 482, Step: 12050, training loss: 2079.108, validation loss: 2084.853, Runtime: 17.3627 secs\nEpoch: 483, Step: 12075, training loss: 2079.212, validation loss: 2085.214, Runtime: 17.4834 secs\nEpoch: 484, Step: 12100, training loss: 2078.893, validation loss: 2084.181, Runtime: 17.4727 secs\nEpoch: 485, Step: 12125, training loss: 2078.995, validation loss: 2085.533, Runtime: 17.4893 secs\nEpoch: 486, Step: 12150, training loss: 2079.199, validation loss: 2085.055, Runtime: 17.4219 secs\nEpoch: 487, Step: 12175, training loss: 2079.160, validation loss: 2084.697, Runtime: 17.3692 secs\nEpoch: 488, Step: 12200, training loss: 2078.899, validation loss: 2085.394, Runtime: 17.2669 secs\nEpoch: 489, Step: 12225, training loss: 2078.839, validation loss: 2085.381, Runtime: 17.3461 secs\nEpoch: 490, Step: 12250, training loss: 2078.901, validation loss: 2085.196, Runtime: 17.6438 secs\nEpoch: 491, Step: 12275, training loss: 2079.003, validation loss: 2085.125, Runtime: 17.3198 secs\nEpoch: 492, Step: 12300, training loss: 2079.058, validation loss: 2085.572, Runtime: 17.3759 secs\nEpoch: 493, Step: 12325, training loss: 2078.882, validation loss: 2085.020, Runtime: 17.3348 secs\nEpoch: 494, Step: 12350, training loss: 2079.024, validation loss: 2084.741, Runtime: 17.4109 secs\nEpoch: 495, Step: 12375, training loss: 2078.814, validation loss: 2085.148, Runtime: 17.4258 secs\nEpoch: 496, Step: 12400, training loss: 2079.003, validation loss: 2085.422, Runtime: 17.5519 secs\nEpoch: 497, Step: 12425, training loss: 2079.012, validation loss: 2085.005, Runtime: 17.4554 secs\nEpoch: 498, Step: 12450, training loss: 2079.004, validation loss: 2085.201, Runtime: 17.2492 secs\nEpoch: 499, Step: 12475, training loss: 2078.928, validation loss: 2085.009, Runtime: 17.5561 secs\nEpoch: 500, Step: 12500, training loss: 2078.737, validation loss: 2084.823, Runtime: 17.4496 secs\nEpoch: 501, Step: 12525, training loss: 2079.055, validation loss: 2085.295, Runtime: 17.5068 secs\nLearning rate decreased to 0.001424\nEpoch: 502, Step: 12550, training loss: 2078.853, validation loss: 2085.036, Runtime: 17.3897 secs\nEpoch: 503, Step: 12575, training loss: 2078.682, validation loss: 2085.476, Runtime: 17.4234 secs\nEpoch: 504, Step: 12600, training loss: 2078.911, validation loss: 2084.944, Runtime: 17.3570 secs\nEpoch: 505, Step: 12625, training loss: 2078.667, validation loss: 2084.762, Runtime: 17.3885 secs\nEpoch: 506, Step: 12650, training loss: 2078.670, validation loss: 2084.836, Runtime: 17.5369 secs\nEpoch: 507, Step: 12675, training loss: 2078.381, validation loss: 2085.440, Runtime: 17.3908 secs\nEpoch: 508, Step: 12700, training loss: 2078.471, validation loss: 2084.947, Runtime: 17.4832 secs\nEpoch: 509, Step: 12725, training loss: 2078.774, validation loss: 2085.788, Runtime: 17.3979 secs\nEpoch: 510, Step: 12750, training loss: 2078.698, validation loss: 2084.682, Runtime: 17.4578 secs\nEpoch: 511, Step: 12775, training loss: 2078.760, validation loss: 2085.196, Runtime: 17.4298 secs\nEpoch: 512, Step: 12800, training loss: 2078.742, validation loss: 2085.256, Runtime: 17.2816 secs\nEpoch: 513, Step: 12825, training loss: 2078.670, validation loss: 2084.743, Runtime: 17.3047 secs\nEpoch: 514, Step: 12850, training loss: 2078.366, validation loss: 2085.388, Runtime: 17.3358 secs\nEpoch: 515, Step: 12875, training loss: 2078.644, validation loss: 2085.020, Runtime: 17.3112 secs\nEpoch: 516, Step: 12900, training loss: 2078.663, validation loss: 2085.246, Runtime: 17.4432 secs\nEpoch: 517, Step: 12925, training loss: 2079.000, validation loss: 2084.933, Runtime: 17.3523 secs\nLearning rate decreased to 0.001353\nEpoch: 518, Step: 12950, training loss: 2078.623, validation loss: 2085.423, Runtime: 17.4018 secs\nEpoch: 519, Step: 12975, training loss: 2078.858, validation loss: 2085.154, Runtime: 17.4153 secs\nEpoch: 520, Step: 13000, training loss: 2078.329, validation loss: 2084.772, Runtime: 17.4028 secs\nEpoch: 521, Step: 13025, training loss: 2078.681, validation loss: 2085.056, Runtime: 17.2752 secs\nEpoch: 522, Step: 13050, training loss: 2078.538, validation loss: 2085.309, Runtime: 17.5368 secs\nEpoch: 523, Step: 13075, training loss: 2078.587, validation loss: 2085.239, Runtime: 17.4391 secs\nEpoch: 524, Step: 13100, training loss: 2078.608, validation loss: 2085.761, Runtime: 17.2646 secs\nEpoch: 525, Step: 13125, training loss: 2078.679, validation loss: 2085.325, Runtime: 17.3909 secs\nEpoch: 526, Step: 13150, training loss: 2078.737, validation loss: 2085.428, Runtime: 17.3359 secs\nLearning rate decreased to 0.001285\nEpoch: 527, Step: 13175, training loss: 2078.414, validation loss: 2085.096, Runtime: 17.4078 secs\nEpoch: 528, Step: 13200, training loss: 2078.320, validation loss: 2085.096, Runtime: 17.4098 secs\nEpoch: 529, Step: 13225, training loss: 2078.309, validation loss: 2084.352, Runtime: 17.3707 secs\nEpoch: 530, Step: 13250, training loss: 2078.373, validation loss: 2084.606, Runtime: 17.4923 secs\nEpoch: 531, Step: 13275, training loss: 2078.101, validation loss: 2085.382, Runtime: 17.3506 secs\nEpoch: 532, Step: 13300, training loss: 2078.405, validation loss: 2085.176, Runtime: 17.2746 secs\nEpoch: 533, Step: 13325, training loss: 2078.394, validation loss: 2085.233, Runtime: 17.3632 secs\nEpoch: 534, Step: 13350, training loss: 2078.518, validation loss: 2085.312, Runtime: 17.4210 secs\nLearning rate decreased to 0.001221\nEpoch: 535, Step: 13375, training loss: 2078.127, validation loss: 2085.346, Runtime: 17.4312 secs\nEpoch: 536, Step: 13400, training loss: 2078.276, validation loss: 2085.306, Runtime: 17.3820 secs\nEpoch: 537, Step: 13425, training loss: 2078.511, validation loss: 2085.207, Runtime: 17.4246 secs\nEpoch: 538, Step: 13450, training loss: 2078.134, validation loss: 2084.985, Runtime: 17.0895 secs\nEpoch: 539, Step: 13475, training loss: 2078.360, validation loss: 2085.653, Runtime: 17.2275 secs\nEpoch: 540, Step: 13500, training loss: 2078.215, validation loss: 2084.879, Runtime: 17.2542 secs\nEpoch: 541, Step: 13525, training loss: 2078.142, validation loss: 2085.448, Runtime: 17.3280 secs\nEpoch: 542, Step: 13550, training loss: 2078.322, validation loss: 2085.038, Runtime: 17.4475 secs\nEpoch: 543, Step: 13575, training loss: 2078.177, validation loss: 2085.428, Runtime: 17.4187 secs\nEpoch: 544, Step: 13600, training loss: 2078.152, validation loss: 2085.133, Runtime: 17.3816 secs\nEpoch: 545, Step: 13625, training loss: 2078.271, validation loss: 2085.369, Runtime: 17.4425 secs\nEpoch: 546, Step: 13650, training loss: 2078.020, validation loss: 2084.981, Runtime: 17.6624 secs\nEpoch: 547, Step: 13675, training loss: 2078.220, validation loss: 2084.750, Runtime: 17.4128 secs\nEpoch: 548, Step: 13700, training loss: 2078.122, validation loss: 2085.270, Runtime: 17.5214 secs\nEpoch: 549, Step: 13725, training loss: 2078.291, validation loss: 2085.513, Runtime: 17.4854 secs\nLearning rate decreased to 0.001160\nEpoch: 550, Step: 13750, training loss: 2078.110, validation loss: 2085.079, Runtime: 17.4074 secs\nEpoch: 551, Step: 13775, training loss: 2078.210, validation loss: 2084.824, Runtime: 17.3552 secs\nEpoch: 552, Step: 13800, training loss: 2078.148, validation loss: 2085.490, Runtime: 17.2295 secs\nEpoch: 553, Step: 13825, training loss: 2078.131, validation loss: 2085.322, Runtime: 17.4317 secs\nEpoch: 554, Step: 13850, training loss: 2078.260, validation loss: 2085.518, Runtime: 17.3137 secs\nEpoch: 555, Step: 13875, training loss: 2078.036, validation loss: 2085.378, Runtime: 17.3775 secs\nEpoch: 556, Step: 13900, training loss: 2078.239, validation loss: 2085.290, Runtime: 17.9813 secs\nEpoch: 557, Step: 13925, training loss: 2078.034, validation loss: 2084.551, Runtime: 17.3629 secs\nEpoch: 558, Step: 13950, training loss: 2078.031, validation loss: 2084.960, Runtime: 17.4641 secs\nEpoch: 559, Step: 13975, training loss: 2078.023, validation loss: 2085.361, Runtime: 17.4052 secs\nEpoch: 560, Step: 14000, training loss: 2078.244, validation loss: 2085.307, Runtime: 17.4638 secs\nEpoch: 561, Step: 14025, training loss: 2077.814, validation loss: 2085.154, Runtime: 17.4382 secs\nEpoch: 562, Step: 14050, training loss: 2078.000, validation loss: 2085.441, Runtime: 17.4989 secs\nEpoch: 563, Step: 14075, training loss: 2077.587, validation loss: 2085.224, Runtime: 17.4278 secs\nEpoch: 564, Step: 14100, training loss: 2078.071, validation loss: 2085.427, Runtime: 17.3547 secs\nEpoch: 565, Step: 14125, training loss: 2078.211, validation loss: 2085.016, Runtime: 17.3325 secs\nEpoch: 566, Step: 14150, training loss: 2077.913, validation loss: 2085.214, Runtime: 17.3539 secs\nEpoch: 567, Step: 14175, training loss: 2077.733, validation loss: 2085.291, Runtime: 17.2996 secs\nEpoch: 568, Step: 14200, training loss: 2077.926, validation loss: 2085.290, Runtime: 17.4623 secs\nEpoch: 569, Step: 14225, training loss: 2077.845, validation loss: 2085.188, Runtime: 17.3832 secs\nEpoch: 570, Step: 14250, training loss: 2077.728, validation loss: 2085.602, Runtime: 17.2423 secs\nEpoch: 571, Step: 14275, training loss: 2077.890, validation loss: 2085.451, Runtime: 17.1520 secs\nEpoch: 572, Step: 14300, training loss: 2078.047, validation loss: 2085.211, Runtime: 17.3906 secs\nLearning rate decreased to 0.001102\nEpoch: 573, Step: 14325, training loss: 2077.626, validation loss: 2085.372, Runtime: 17.5362 secs\nEpoch: 574, Step: 14350, training loss: 2077.712, validation loss: 2085.230, Runtime: 17.6373 secs\nEpoch: 575, Step: 14375, training loss: 2077.767, validation loss: 2085.285, Runtime: 17.3396 secs\nEpoch: 576, Step: 14400, training loss: 2078.131, validation loss: 2085.587, Runtime: 17.2316 secs\nEpoch: 577, Step: 14425, training loss: 2077.925, validation loss: 2085.046, Runtime: 17.3391 secs\nEpoch: 578, Step: 14450, training loss: 2077.924, validation loss: 2085.859, Runtime: 17.3738 secs\nEpoch: 579, Step: 14475, training loss: 2077.616, validation loss: 2085.103, Runtime: 17.2986 secs\nEpoch: 580, Step: 14500, training loss: 2077.744, validation loss: 2084.672, Runtime: 17.4952 secs\nEpoch: 581, Step: 14525, training loss: 2077.842, validation loss: 2085.042, Runtime: 17.5082 secs\nEpoch: 582, Step: 14550, training loss: 2077.948, validation loss: 2085.102, Runtime: 17.4321 secs\nEpoch: 583, Step: 14575, training loss: 2077.990, validation loss: 2085.119, Runtime: 17.2743 secs\nLearning rate decreased to 0.001047\nEpoch: 584, Step: 14600, training loss: 2077.680, validation loss: 2085.249, Runtime: 17.3303 secs\nEpoch: 585, Step: 14625, training loss: 2077.693, validation loss: 2085.399, Runtime: 17.2857 secs\nEpoch: 586, Step: 14650, training loss: 2077.745, validation loss: 2085.400, Runtime: 17.3081 secs\nEpoch: 587, Step: 14675, training loss: 2077.752, validation loss: 2084.633, Runtime: 17.3155 secs\nEpoch: 588, Step: 14700, training loss: 2077.571, validation loss: 2085.680, Runtime: 17.3801 secs\nEpoch: 589, Step: 14725, training loss: 2077.717, validation loss: 2085.075, Runtime: 17.3438 secs\nEpoch: 590, Step: 14750, training loss: 2077.432, validation loss: 2084.931, Runtime: 17.3301 secs\nEpoch: 591, Step: 14775, training loss: 2077.685, validation loss: 2085.592, Runtime: 17.3034 secs\nEpoch: 592, Step: 14800, training loss: 2077.462, validation loss: 2085.510, Runtime: 17.2299 secs\nEpoch: 593, Step: 14825, training loss: 2077.681, validation loss: 2085.300, Runtime: 17.3964 secs\nEpoch: 594, Step: 14850, training loss: 2077.506, validation loss: 2085.077, Runtime: 17.4641 secs\nEpoch: 595, Step: 14875, training loss: 2077.427, validation loss: 2085.615, Runtime: 17.2106 secs\nEpoch: 596, Step: 14900, training loss: 2077.747, validation loss: 2084.653, Runtime: 17.3673 secs\nLearning rate decreased to 0.000994\nEpoch: 597, Step: 14925, training loss: 2077.565, validation loss: 2085.230, Runtime: 17.2453 secs\nEpoch: 598, Step: 14950, training loss: 2077.545, validation loss: 2085.438, Runtime: 17.4056 secs\nEpoch: 599, Step: 14975, training loss: 2077.505, validation loss: 2085.680, Runtime: 17.4072 secs\nEpoch: 600, Step: 15000, training loss: 2077.743, validation loss: 2084.978, Runtime: 17.3449 secs\nEpoch: 601, Step: 15025, training loss: 2077.259, validation loss: 2084.958, Runtime: 17.4189 secs\nEpoch: 602, Step: 15050, training loss: 2077.263, validation loss: 2085.304, Runtime: 17.3758 secs\nEpoch: 603, Step: 15075, training loss: 2077.396, validation loss: 2085.747, Runtime: 17.5947 secs\nEpoch: 604, Step: 15100, training loss: 2077.611, validation loss: 2085.358, Runtime: 17.2404 secs\nEpoch: 605, Step: 15125, training loss: 2077.401, validation loss: 2085.383, Runtime: 17.3774 secs\nEpoch: 606, Step: 15150, training loss: 2077.184, validation loss: 2084.747, Runtime: 17.3810 secs\nEpoch: 607, Step: 15175, training loss: 2077.719, validation loss: 2085.313, Runtime: 17.4498 secs\nLearning rate decreased to 0.000945\nEpoch: 608, Step: 15200, training loss: 2077.800, validation loss: 2085.279, Runtime: 17.3417 secs\nEpoch: 609, Step: 15225, training loss: 2077.275, validation loss: 2085.242, Runtime: 17.7734 secs\nEpoch: 610, Step: 15250, training loss: 2077.242, validation loss: 2085.067, Runtime: 17.4167 secs\nEpoch: 611, Step: 15275, training loss: 2077.430, validation loss: 2084.994, Runtime: 17.3533 secs\nEpoch: 612, Step: 15300, training loss: 2077.450, validation loss: 2085.772, Runtime: 17.3522 secs\nEpoch: 613, Step: 15325, training loss: 2077.497, validation loss: 2085.627, Runtime: 17.3484 secs\nEpoch: 614, Step: 15350, training loss: 2077.712, validation loss: 2085.960, Runtime: 17.2829 secs\nEpoch: 615, Step: 15375, training loss: 2077.356, validation loss: 2085.229, Runtime: 17.4100 secs\nEpoch: 616, Step: 15400, training loss: 2077.180, validation loss: 2085.625, Runtime: 17.3313 secs\nEpoch: 617, Step: 15425, training loss: 2077.180, validation loss: 2085.373, Runtime: 17.3239 secs\nEpoch: 618, Step: 15450, training loss: 2077.365, validation loss: 2085.194, Runtime: 17.4427 secs\nEpoch: 619, Step: 15475, training loss: 2077.575, validation loss: 2085.825, Runtime: 17.3786 secs\nEpoch: 620, Step: 15500, training loss: 2077.175, validation loss: 2085.710, Runtime: 17.5891 secs\nEpoch: 621, Step: 15525, training loss: 2077.383, validation loss: 2085.556, Runtime: 17.2500 secs\nEpoch: 622, Step: 15550, training loss: 2077.239, validation loss: 2085.102, Runtime: 17.3343 secs\nEpoch: 623, Step: 15575, training loss: 2077.293, validation loss: 2085.403, Runtime: 17.4117 secs\nEpoch: 624, Step: 15600, training loss: 2077.409, validation loss: 2084.966, Runtime: 17.1967 secs\nEpoch: 625, Step: 15625, training loss: 2077.405, validation loss: 2085.168, Runtime: 17.3367 secs\nEpoch: 626, Step: 15650, training loss: 2077.265, validation loss: 2085.423, Runtime: 17.2208 secs\nEpoch: 627, Step: 15675, training loss: 2077.252, validation loss: 2085.522, Runtime: 17.3711 secs\nEpoch: 628, Step: 15700, training loss: 2077.378, validation loss: 2085.199, Runtime: 17.4143 secs\nEpoch: 629, Step: 15725, training loss: 2077.565, validation loss: 2085.647, Runtime: 17.4225 secs\nLearning rate decreased to 0.000897\nEpoch: 630, Step: 15750, training loss: 2077.243, validation loss: 2085.434, Runtime: 17.2654 secs\nEpoch: 631, Step: 15775, training loss: 2077.227, validation loss: 2085.462, Runtime: 17.3596 secs\nEpoch: 632, Step: 15800, training loss: 2077.044, validation loss: 2085.275, Runtime: 17.4099 secs\nEpoch: 633, Step: 15825, training loss: 2077.214, validation loss: 2085.401, Runtime: 17.4202 secs\nEpoch: 634, Step: 15850, training loss: 2077.446, validation loss: 2085.110, Runtime: 17.2972 secs\nEpoch: 635, Step: 15875, training loss: 2077.101, validation loss: 2085.831, Runtime: 17.8322 secs\nEpoch: 636, Step: 15900, training loss: 2077.274, validation loss: 2085.172, Runtime: 17.4851 secs\nEpoch: 637, Step: 15925, training loss: 2077.300, validation loss: 2086.066, Runtime: 17.2272 secs\nEpoch: 638, Step: 15950, training loss: 2077.017, validation loss: 2085.237, Runtime: 17.4308 secs\nEpoch: 639, Step: 15975, training loss: 2077.079, validation loss: 2085.553, Runtime: 17.2510 secs\nEpoch: 640, Step: 16000, training loss: 2077.414, validation loss: 2085.456, Runtime: 17.2102 secs\nEpoch: 641, Step: 16025, training loss: 2077.148, validation loss: 2085.420, Runtime: 17.3250 secs\nEpoch: 642, Step: 16050, training loss: 2077.296, validation loss: 2085.392, Runtime: 17.3562 secs\nEpoch: 643, Step: 16075, training loss: 2077.132, validation loss: 2085.409, Runtime: 17.5163 secs\nEpoch: 644, Step: 16100, training loss: 2077.312, validation loss: 2085.456, Runtime: 17.4498 secs\nEpoch: 645, Step: 16125, training loss: 2077.151, validation loss: 2085.714, Runtime: 17.6465 secs\nEpoch: 646, Step: 16150, training loss: 2077.114, validation loss: 2085.154, Runtime: 17.8054 secs\nEpoch: 647, Step: 16175, training loss: 2077.410, validation loss: 2085.177, Runtime: 17.3432 secs\nLearning rate decreased to 0.000853\nEpoch: 648, Step: 16200, training loss: 2077.305, validation loss: 2085.245, Runtime: 17.3694 secs\nEpoch: 649, Step: 16225, training loss: 2076.806, validation loss: 2085.078, Runtime: 17.2740 secs\nEpoch: 650, Step: 16250, training loss: 2076.978, validation loss: 2085.572, Runtime: 17.4010 secs\nEpoch: 651, Step: 16275, training loss: 2077.098, validation loss: 2085.465, Runtime: 17.3422 secs\nEpoch: 652, Step: 16300, training loss: 2077.017, validation loss: 2085.341, Runtime: 17.4453 secs\nEpoch: 653, Step: 16325, training loss: 2076.950, validation loss: 2085.265, Runtime: 17.4024 secs\nEpoch: 654, Step: 16350, training loss: 2076.912, validation loss: 2085.501, Runtime: 17.3677 secs\nEpoch: 655, Step: 16375, training loss: 2077.057, validation loss: 2085.806, Runtime: 17.4707 secs\nEpoch: 656, Step: 16400, training loss: 2077.022, validation loss: 2085.437, Runtime: 17.8765 secs\nEpoch: 657, Step: 16425, training loss: 2077.242, validation loss: 2084.945, Runtime: 17.4638 secs\nLearning rate decreased to 0.000810\nEpoch: 658, Step: 16450, training loss: 2077.088, validation loss: 2085.619, Runtime: 17.3948 secs\nEpoch: 659, Step: 16475, training loss: 2076.655, validation loss: 2085.152, Runtime: 17.4683 secs\nEpoch: 660, Step: 16500, training loss: 2076.979, validation loss: 2085.072, Runtime: 17.5307 secs\nEpoch: 661, Step: 16525, training loss: 2077.054, validation loss: 2085.258, Runtime: 17.4083 secs\nEpoch: 662, Step: 16550, training loss: 2076.928, validation loss: 2084.896, Runtime: 17.4629 secs\nEpoch: 663, Step: 16575, training loss: 2076.859, validation loss: 2085.386, Runtime: 17.3719 secs\nEpoch: 664, Step: 16600, training loss: 2076.930, validation loss: 2085.496, Runtime: 17.3659 secs\nEpoch: 665, Step: 16625, training loss: 2076.807, validation loss: 2084.979, Runtime: 17.3998 secs\nEpoch: 666, Step: 16650, training loss: 2076.958, validation loss: 2085.811, Runtime: 17.3891 secs\nEpoch: 667, Step: 16675, training loss: 2076.901, validation loss: 2085.005, Runtime: 17.4396 secs\nEpoch: 668, Step: 16700, training loss: 2077.093, validation loss: 2085.569, Runtime: 17.4250 secs\nLearning rate decreased to 0.000769\nEpoch: 669, Step: 16725, training loss: 2076.816, validation loss: 2085.569, Runtime: 17.5988 secs\nEpoch: 670, Step: 16750, training loss: 2076.752, validation loss: 2085.823, Runtime: 17.3776 secs\nEpoch: 671, Step: 16775, training loss: 2076.791, validation loss: 2085.476, Runtime: 17.5423 secs\nEpoch: 672, Step: 16800, training loss: 2076.722, validation loss: 2085.261, Runtime: 17.6595 secs\nEpoch: 673, Step: 16825, training loss: 2076.822, validation loss: 2085.309, Runtime: 17.6376 secs\nEpoch: 674, Step: 16850, training loss: 2076.751, validation loss: 2085.510, Runtime: 17.4300 secs\nEpoch: 675, Step: 16875, training loss: 2076.744, validation loss: 2085.687, Runtime: 17.4395 secs\nEpoch: 676, Step: 16900, training loss: 2076.843, validation loss: 2085.275, Runtime: 17.2661 secs\nLearning rate decreased to 0.000731\nEpoch: 677, Step: 16925, training loss: 2076.943, validation loss: 2086.125, Runtime: 17.8852 secs\nEpoch: 678, Step: 16950, training loss: 2076.453, validation loss: 2085.421, Runtime: 17.4508 secs\nEpoch: 679, Step: 16975, training loss: 2076.533, validation loss: 2085.663, Runtime: 17.3911 secs\nEpoch: 680, Step: 17000, training loss: 2076.933, validation loss: 2084.841, Runtime: 17.4102 secs\nEpoch: 681, Step: 17025, training loss: 2076.820, validation loss: 2085.075, Runtime: 17.4731 secs\nEpoch: 682, Step: 17050, training loss: 2076.607, validation loss: 2085.141, Runtime: 17.5682 secs\nEpoch: 683, Step: 17075, training loss: 2076.902, validation loss: 2085.750, Runtime: 17.3830 secs\nEpoch: 684, Step: 17100, training loss: 2076.695, validation loss: 2085.259, Runtime: 17.4081 secs\nEpoch: 685, Step: 17125, training loss: 2076.703, validation loss: 2085.684, Runtime: 17.3508 secs\nEpoch: 686, Step: 17150, training loss: 2076.874, validation loss: 2085.396, Runtime: 17.4252 secs\nEpoch: 687, Step: 17175, training loss: 2076.744, validation loss: 2085.163, Runtime: 17.5462 secs\nEpoch: 688, Step: 17200, training loss: 2076.630, validation loss: 2085.327, Runtime: 17.4314 secs\nEpoch: 689, Step: 17225, training loss: 2076.754, validation loss: 2085.277, Runtime: 17.3788 secs\nEpoch: 690, Step: 17250, training loss: 2076.521, validation loss: 2085.575, Runtime: 17.3919 secs\nEpoch: 691, Step: 17275, training loss: 2076.552, validation loss: 2085.537, Runtime: 17.3631 secs\nEpoch: 692, Step: 17300, training loss: 2076.646, validation loss: 2085.637, Runtime: 17.3602 secs\nEpoch: 693, Step: 17325, training loss: 2076.620, validation loss: 2085.419, Runtime: 17.5109 secs\nEpoch: 694, Step: 17350, training loss: 2076.708, validation loss: 2085.169, Runtime: 17.4409 secs\nEpoch: 695, Step: 17375, training loss: 2076.613, validation loss: 2084.898, Runtime: 17.4578 secs\nEpoch: 696, Step: 17400, training loss: 2076.439, validation loss: 2085.327, Runtime: 17.4239 secs\nEpoch: 697, Step: 17425, training loss: 2076.527, validation loss: 2085.626, Runtime: 17.4123 secs\nEpoch: 698, Step: 17450, training loss: 2076.546, validation loss: 2085.298, Runtime: 17.3343 secs\nEpoch: 699, Step: 17475, training loss: 2076.583, validation loss: 2085.922, Runtime: 17.2821 secs\nEpoch: 700, Step: 17500, training loss: 2076.431, validation loss: 2085.971, Runtime: 17.6071 secs\nEpoch: 701, Step: 17525, training loss: 2076.595, validation loss: 2085.741, Runtime: 17.4290 secs\nEpoch: 702, Step: 17550, training loss: 2076.718, validation loss: 2085.146, Runtime: 17.3735 secs\nLearning rate decreased to 0.000694\nEpoch: 703, Step: 17575, training loss: 2076.347, validation loss: 2084.885, Runtime: 17.4737 secs\nEpoch: 704, Step: 17600, training loss: 2076.596, validation loss: 2085.863, Runtime: 17.3297 secs\nEpoch: 705, Step: 17625, training loss: 2076.646, validation loss: 2085.958, Runtime: 17.3614 secs\nEpoch: 706, Step: 17650, training loss: 2076.748, validation loss: 2085.593, Runtime: 17.4260 secs\nEpoch: 707, Step: 17675, training loss: 2076.660, validation loss: 2085.222, Runtime: 17.4396 secs\nEpoch: 708, Step: 17700, training loss: 2076.736, validation loss: 2085.232, Runtime: 17.3295 secs\nEpoch: 709, Step: 17725, training loss: 2076.571, validation loss: 2085.692, Runtime: 17.2700 secs\nEpoch: 710, Step: 17750, training loss: 2076.436, validation loss: 2085.666, Runtime: 17.3678 secs\nEpoch: 711, Step: 17775, training loss: 2076.359, validation loss: 2085.547, Runtime: 17.3182 secs\nEpoch: 712, Step: 17800, training loss: 2076.477, validation loss: 2085.010, Runtime: 17.4070 secs\nEpoch: 713, Step: 17825, training loss: 2076.406, validation loss: 2085.365, Runtime: 17.5629 secs\nEpoch: 714, Step: 17850, training loss: 2076.479, validation loss: 2086.005, Runtime: 17.5275 secs\nEpoch: 715, Step: 17875, training loss: 2076.652, validation loss: 2085.130, Runtime: 17.5154 secs\nLearning rate decreased to 0.000660\nEpoch: 716, Step: 17900, training loss: 2076.614, validation loss: 2085.776, Runtime: 17.4676 secs\nEpoch: 717, Step: 17925, training loss: 2076.584, validation loss: 2085.796, Runtime: 17.5148 secs\nEpoch: 718, Step: 17950, training loss: 2076.337, validation loss: 2085.467, Runtime: 17.5257 secs\nEpoch: 719, Step: 17975, training loss: 2076.576, validation loss: 2085.510, Runtime: 17.7023 secs\nEpoch: 720, Step: 18000, training loss: 2076.558, validation loss: 2086.140, Runtime: 17.4078 secs\nEpoch: 721, Step: 18025, training loss: 2076.668, validation loss: 2085.572, Runtime: 17.4972 secs\nLearning rate decreased to 0.000627\nEpoch: 722, Step: 18050, training loss: 2076.383, validation loss: 2086.094, Runtime: 17.4236 secs\nEpoch: 723, Step: 18075, training loss: 2076.444, validation loss: 2084.936, Runtime: 17.3359 secs\nEpoch: 724, Step: 18100, training loss: 2076.404, validation loss: 2085.624, Runtime: 17.2653 secs\nEpoch: 725, Step: 18125, training loss: 2076.345, validation loss: 2085.280, Runtime: 17.3553 secs\nEpoch: 726, Step: 18150, training loss: 2076.097, validation loss: 2085.595, Runtime: 17.2678 secs\nEpoch: 727, Step: 18175, training loss: 2076.272, validation loss: 2084.960, Runtime: 17.4715 secs\nEpoch: 728, Step: 18200, training loss: 2076.321, validation loss: 2085.388, Runtime: 17.4663 secs\nEpoch: 729, Step: 18225, training loss: 2076.198, validation loss: 2085.648, Runtime: 17.3235 secs\nEpoch: 730, Step: 18250, training loss: 2076.587, validation loss: 2085.415, Runtime: 17.4665 secs\nLearning rate decreased to 0.000595\nEpoch: 731, Step: 18275, training loss: 2076.339, validation loss: 2085.831, Runtime: 17.8644 secs\nEpoch: 732, Step: 18300, training loss: 2076.407, validation loss: 2086.341, Runtime: 17.4843 secs\nEpoch: 733, Step: 18325, training loss: 2076.335, validation loss: 2085.816, Runtime: 17.3450 secs\nEpoch: 734, Step: 18350, training loss: 2076.484, validation loss: 2085.339, Runtime: 17.3323 secs\nEpoch: 735, Step: 18375, training loss: 2076.077, validation loss: 2086.175, Runtime: 17.3088 secs\nEpoch: 736, Step: 18400, training loss: 2076.239, validation loss: 2086.016, Runtime: 17.4115 secs\nEpoch: 737, Step: 18425, training loss: 2076.441, validation loss: 2085.954, Runtime: 17.3480 secs\nEpoch: 738, Step: 18450, training loss: 2076.173, validation loss: 2084.893, Runtime: 17.4765 secs\nEpoch: 739, Step: 18475, training loss: 2076.219, validation loss: 2085.517, Runtime: 17.2841 secs\nEpoch: 740, Step: 18500, training loss: 2076.185, validation loss: 2085.359, Runtime: 17.3962 secs\nEpoch: 741, Step: 18525, training loss: 2076.499, validation loss: 2086.475, Runtime: 17.3861 secs\nLearning rate decreased to 0.000566\nEpoch: 742, Step: 18550, training loss: 2076.111, validation loss: 2085.211, Runtime: 17.4488 secs\nEpoch: 743, Step: 18575, training loss: 2076.177, validation loss: 2085.428, Runtime: 17.4312 secs\nEpoch: 744, Step: 18600, training loss: 2076.336, validation loss: 2085.340, Runtime: 17.5233 secs\nEpoch: 745, Step: 18625, training loss: 2076.312, validation loss: 2085.628, Runtime: 17.2938 secs\nEpoch: 746, Step: 18650, training loss: 2076.375, validation loss: 2086.366, Runtime: 17.2071 secs\nEpoch: 747, Step: 18675, training loss: 2076.149, validation loss: 2085.910, Runtime: 17.3372 secs\nEpoch: 748, Step: 18700, training loss: 2076.184, validation loss: 2085.146, Runtime: 17.4312 secs\nEpoch: 749, Step: 18725, training loss: 2076.127, validation loss: 2085.748, Runtime: 17.5125 secs\nEpoch: 750, Step: 18750, training loss: 2076.056, validation loss: 2085.565, Runtime: 17.4285 secs\nEpoch: 751, Step: 18775, training loss: 2076.078, validation loss: 2085.570, Runtime: 17.4908 secs\nEpoch: 752, Step: 18800, training loss: 2076.069, validation loss: 2085.623, Runtime: 17.3951 secs\nEpoch: 753, Step: 18825, training loss: 2076.289, validation loss: 2085.367, Runtime: 17.4365 secs\nLearning rate decreased to 0.000537\nEpoch: 754, Step: 18850, training loss: 2076.323, validation loss: 2085.574, Runtime: 17.3122 secs\nEpoch: 755, Step: 18875, training loss: 2076.075, validation loss: 2085.779, Runtime: 17.4337 secs\nEpoch: 756, Step: 18900, training loss: 2076.100, validation loss: 2085.219, Runtime: 17.4872 secs\nEpoch: 757, Step: 18925, training loss: 2076.304, validation loss: 2085.146, Runtime: 17.3890 secs\nEpoch: 758, Step: 18950, training loss: 2076.057, validation loss: 2085.583, Runtime: 17.3766 secs\nEpoch: 759, Step: 18975, training loss: 2075.885, validation loss: 2086.243, Runtime: 17.4474 secs\nEpoch: 760, Step: 19000, training loss: 2076.065, validation loss: 2085.765, Runtime: 17.5078 secs\nEpoch: 761, Step: 19025, training loss: 2076.012, validation loss: 2085.991, Runtime: 17.3071 secs\nEpoch: 762, Step: 19050, training loss: 2076.205, validation loss: 2085.883, Runtime: 17.4803 secs\nEpoch: 763, Step: 19075, training loss: 2076.009, validation loss: 2085.876, Runtime: 17.4627 secs\nEpoch: 764, Step: 19100, training loss: 2076.061, validation loss: 2085.952, Runtime: 17.3479 secs\nEpoch: 765, Step: 19125, training loss: 2076.170, validation loss: 2085.856, Runtime: 17.5807 secs\nEpoch: 766, Step: 19150, training loss: 2076.172, validation loss: 2085.523, Runtime: 17.5672 secs\nEpoch: 767, Step: 19175, training loss: 2076.244, validation loss: 2085.823, Runtime: 17.5112 secs\nLearning rate decreased to 0.000510\nEpoch: 768, Step: 19200, training loss: 2075.891, validation loss: 2085.481, Runtime: 17.3093 secs\nEpoch: 769, Step: 19225, training loss: 2075.883, validation loss: 2085.793, Runtime: 17.4069 secs\nEpoch: 770, Step: 19250, training loss: 2076.231, validation loss: 2085.385, Runtime: 17.4820 secs\nEpoch: 771, Step: 19275, training loss: 2076.271, validation loss: 2085.747, Runtime: 17.3268 secs\nEpoch: 772, Step: 19300, training loss: 2076.146, validation loss: 2085.386, Runtime: 17.4357 secs\nEpoch: 773, Step: 19325, training loss: 2076.155, validation loss: 2085.674, Runtime: 17.3520 secs\nEpoch: 774, Step: 19350, training loss: 2076.095, validation loss: 2085.353, Runtime: 17.3606 secs\nEpoch: 775, Step: 19375, training loss: 2076.163, validation loss: 2085.653, Runtime: 17.2359 secs\nEpoch: 776, Step: 19400, training loss: 2076.047, validation loss: 2085.656, Runtime: 17.3990 secs\nEpoch: 777, Step: 19425, training loss: 2076.067, validation loss: 2085.586, Runtime: 17.4656 secs\nEpoch: 778, Step: 19450, training loss: 2076.095, validation loss: 2085.711, Runtime: 17.3578 secs\nEpoch: 779, Step: 19475, training loss: 2075.945, validation loss: 2085.086, Runtime: 17.3948 secs\nEpoch: 780, Step: 19500, training loss: 2076.151, validation loss: 2086.125, Runtime: 17.3746 secs\nEpoch: 781, Step: 19525, training loss: 2076.117, validation loss: 2085.459, Runtime: 17.4544 secs\nEpoch: 782, Step: 19550, training loss: 2076.061, validation loss: 2085.771, Runtime: 17.3938 secs\nEpoch: 783, Step: 19575, training loss: 2076.271, validation loss: 2085.579, Runtime: 17.3062 secs\nLearning rate decreased to 0.000485\nEpoch: 784, Step: 19600, training loss: 2075.931, validation loss: 2085.562, Runtime: 17.3609 secs\nEpoch: 785, Step: 19625, training loss: 2075.792, validation loss: 2085.721, Runtime: 17.3811 secs\nEpoch: 786, Step: 19650, training loss: 2075.697, validation loss: 2085.206, Runtime: 17.2651 secs\nEpoch: 787, Step: 19675, training loss: 2075.911, validation loss: 2085.658, Runtime: 17.5258 secs\nEpoch: 788, Step: 19700, training loss: 2076.096, validation loss: 2086.042, Runtime: 17.6211 secs\nEpoch: 789, Step: 19725, training loss: 2076.021, validation loss: 2085.887, Runtime: 17.6424 secs\nEpoch: 790, Step: 19750, training loss: 2075.958, validation loss: 2085.384, Runtime: 17.2753 secs\nEpoch: 791, Step: 19775, training loss: 2076.024, validation loss: 2085.735, Runtime: 17.4720 secs\nEpoch: 792, Step: 19800, training loss: 2075.779, validation loss: 2085.596, Runtime: 17.2455 secs\nEpoch: 793, Step: 19825, training loss: 2075.839, validation loss: 2085.599, Runtime: 17.5369 secs\nEpoch: 794, Step: 19850, training loss: 2075.915, validation loss: 2085.653, Runtime: 17.3590 secs\nEpoch: 795, Step: 19875, training loss: 2075.958, validation loss: 2086.394, Runtime: 17.5098 secs\nEpoch: 796, Step: 19900, training loss: 2075.892, validation loss: 2085.486, Runtime: 17.4073 secs\nEpoch: 797, Step: 19925, training loss: 2075.946, validation loss: 2084.788, Runtime: 17.4299 secs\nEpoch: 798, Step: 19950, training loss: 2076.192, validation loss: 2085.255, Runtime: 17.4424 secs\nLearning rate decreased to 0.000461\nEpoch: 799, Step: 19975, training loss: 2075.808, validation loss: 2085.231, Runtime: 17.4458 secs\nEpoch: 800, Step: 20000, training loss: 2075.874, validation loss: 2085.376, Runtime: 17.5100 secs\nEpoch: 801, Step: 20025, training loss: 2075.846, validation loss: 2085.975, Runtime: 17.3635 secs\nEpoch: 802, Step: 20050, training loss: 2076.014, validation loss: 2085.511, Runtime: 17.3601 secs\nEpoch: 803, Step: 20075, training loss: 2075.931, validation loss: 2085.923, Runtime: 17.4244 secs\nEpoch: 804, Step: 20100, training loss: 2075.540, validation loss: 2086.352, Runtime: 17.4421 secs\nEpoch: 805, Step: 20125, training loss: 2075.713, validation loss: 2085.686, Runtime: 17.4301 secs\nEpoch: 806, Step: 20150, training loss: 2075.889, validation loss: 2085.368, Runtime: 17.3970 secs\nEpoch: 807, Step: 20175, training loss: 2076.069, validation loss: 2085.906, Runtime: 17.2879 secs\nLearning rate decreased to 0.000438\nEpoch: 808, Step: 20200, training loss: 2075.856, validation loss: 2085.683, Runtime: 17.3948 secs\nEpoch: 809, Step: 20225, training loss: 2075.757, validation loss: 2085.015, Runtime: 17.3777 secs\nEpoch: 810, Step: 20250, training loss: 2075.735, validation loss: 2085.696, Runtime: 17.4616 secs\nEpoch: 811, Step: 20275, training loss: 2075.546, validation loss: 2085.625, Runtime: 17.3573 secs\nEpoch: 812, Step: 20300, training loss: 2075.755, validation loss: 2085.543, Runtime: 17.3958 secs\nEpoch: 813, Step: 20325, training loss: 2075.526, validation loss: 2085.472, Runtime: 17.3139 secs\nEpoch: 814, Step: 20350, training loss: 2075.564, validation loss: 2085.681, Runtime: 17.4993 secs\nEpoch: 815, Step: 20375, training loss: 2075.763, validation loss: 2085.499, Runtime: 17.5001 secs\nLearning rate decreased to 0.000416\nEpoch: 816, Step: 20400, training loss: 2075.358, validation loss: 2085.664, Runtime: 17.4280 secs\nEpoch: 817, Step: 20425, training loss: 2075.756, validation loss: 2085.616, Runtime: 17.4123 secs\nEpoch: 818, Step: 20450, training loss: 2075.664, validation loss: 2085.784, Runtime: 17.4057 secs\nEpoch: 819, Step: 20475, training loss: 2075.761, validation loss: 2085.108, Runtime: 17.3550 secs\nEpoch: 820, Step: 20500, training loss: 2075.875, validation loss: 2085.683, Runtime: 17.9248 secs\nEpoch: 821, Step: 20525, training loss: 2075.912, validation loss: 2085.728, Runtime: 17.2927 secs\nLearning rate decreased to 0.000395\nEpoch: 822, Step: 20550, training loss: 2075.584, validation loss: 2085.441, Runtime: 17.4596 secs\nEpoch: 823, Step: 20575, training loss: 2075.763, validation loss: 2085.684, Runtime: 17.3920 secs\nEpoch: 824, Step: 20600, training loss: 2075.726, validation loss: 2085.930, Runtime: 17.2376 secs\nEpoch: 825, Step: 20625, training loss: 2075.770, validation loss: 2085.555, Runtime: 17.2349 secs\nEpoch: 826, Step: 20650, training loss: 2075.618, validation loss: 2085.835, Runtime: 17.2862 secs\nEpoch: 827, Step: 20675, training loss: 2075.840, validation loss: 2085.790, Runtime: 17.4213 secs\nEpoch: 828, Step: 20700, training loss: 2075.880, validation loss: 2085.613, Runtime: 17.4905 secs\nLearning rate decreased to 0.000375\nEpoch: 829, Step: 20725, training loss: 2075.637, validation loss: 2085.647, Runtime: 17.5623 secs\nEpoch: 830, Step: 20750, training loss: 2075.203, validation loss: 2085.618, Runtime: 17.2595 secs\nEpoch: 831, Step: 20775, training loss: 2075.445, validation loss: 2085.633, Runtime: 17.4690 secs\nEpoch: 832, Step: 20800, training loss: 2075.764, validation loss: 2085.264, Runtime: 17.5627 secs\nEpoch: 833, Step: 20825, training loss: 2075.530, validation loss: 2085.996, Runtime: 17.6230 secs\nEpoch: 834, Step: 20850, training loss: 2075.618, validation loss: 2085.870, Runtime: 17.2889 secs\nEpoch: 835, Step: 20875, training loss: 2075.628, validation loss: 2085.485, Runtime: 17.1887 secs\nEpoch: 836, Step: 20900, training loss: 2075.719, validation loss: 2085.491, Runtime: 17.2916 secs\nEpoch: 837, Step: 20925, training loss: 2075.571, validation loss: 2085.667, Runtime: 17.4470 secs\nEpoch: 838, Step: 20950, training loss: 2075.665, validation loss: 2085.984, Runtime: 17.4414 secs\nEpoch: 839, Step: 20975, training loss: 2075.639, validation loss: 2086.456, Runtime: 17.4017 secs\nEpoch: 840, Step: 21000, training loss: 2075.549, validation loss: 2086.077, Runtime: 17.4372 secs\nEpoch: 841, Step: 21025, training loss: 2075.575, validation loss: 2085.464, Runtime: 17.4712 secs\nEpoch: 842, Step: 21050, training loss: 2075.695, validation loss: 2084.902, Runtime: 17.3599 secs\nEpoch: 843, Step: 21075, training loss: 2075.590, validation loss: 2085.894, Runtime: 17.4655 secs\nEpoch: 844, Step: 21100, training loss: 2075.685, validation loss: 2085.896, Runtime: 17.3892 secs\nEpoch: 845, Step: 21125, training loss: 2075.406, validation loss: 2085.810, Runtime: 17.3165 secs\nEpoch: 846, Step: 21150, training loss: 2075.604, validation loss: 2085.216, Runtime: 17.3661 secs\nEpoch: 847, Step: 21175, training loss: 2075.671, validation loss: 2085.837, Runtime: 17.2671 secs\nEpoch: 848, Step: 21200, training loss: 2075.519, validation loss: 2085.605, Runtime: 17.3854 secs\nEpoch: 849, Step: 21225, training loss: 2075.699, validation loss: 2085.978, Runtime: 17.3879 secs\nLearning rate decreased to 0.000356\nEpoch: 850, Step: 21250, training loss: 2075.591, validation loss: 2086.074, Runtime: 17.5110 secs\nEpoch: 851, Step: 21275, training loss: 2075.926, validation loss: 2085.308, Runtime: 17.6822 secs\nEpoch: 852, Step: 21300, training loss: 2075.673, validation loss: 2085.746, Runtime: 17.4931 secs\nEpoch: 853, Step: 21325, training loss: 2075.762, validation loss: 2085.523, Runtime: 17.3524 secs\nEpoch: 854, Step: 21350, training loss: 2075.450, validation loss: 2085.883, Runtime: 17.2301 secs\nEpoch: 855, Step: 21375, training loss: 2075.520, validation loss: 2085.737, Runtime: 17.2962 secs\nEpoch: 856, Step: 21400, training loss: 2075.716, validation loss: 2085.783, Runtime: 17.4326 secs\nEpoch: 857, Step: 21425, training loss: 2075.775, validation loss: 2085.486, Runtime: 17.4099 secs\nEpoch: 858, Step: 21450, training loss: 2075.501, validation loss: 2086.500, Runtime: 17.8878 secs\nEpoch: 859, Step: 21475, training loss: 2075.728, validation loss: 2085.866, Runtime: 17.3710 secs\nEpoch: 860, Step: 21500, training loss: 2075.535, validation loss: 2085.322, Runtime: 17.4379 secs\nEpoch: 861, Step: 21525, training loss: 2075.442, validation loss: 2085.813, Runtime: 17.4988 secs\nEpoch: 862, Step: 21550, training loss: 2075.562, validation loss: 2085.842, Runtime: 17.4660 secs\nEpoch: 863, Step: 21575, training loss: 2075.266, validation loss: 2085.491, Runtime: 17.3982 secs\nEpoch: 864, Step: 21600, training loss: 2075.352, validation loss: 2085.532, Runtime: 17.4687 secs\nEpoch: 865, Step: 21625, training loss: 2075.313, validation loss: 2085.757, Runtime: 17.4346 secs\nEpoch: 866, Step: 21650, training loss: 2075.523, validation loss: 2085.446, Runtime: 17.3564 secs\nEpoch: 867, Step: 21675, training loss: 2075.664, validation loss: 2085.637, Runtime: 17.5335 secs\nLearning rate decreased to 0.000339\nEpoch: 868, Step: 21700, training loss: 2075.601, validation loss: 2085.906, Runtime: 17.4927 secs\nEpoch: 869, Step: 21725, training loss: 2075.565, validation loss: 2085.912, Runtime: 17.4713 secs\nEpoch: 870, Step: 21750, training loss: 2075.459, validation loss: 2086.004, Runtime: 17.4064 secs\nEpoch: 871, Step: 21775, training loss: 2075.289, validation loss: 2085.407, Runtime: 17.3773 secs\nEpoch: 872, Step: 21800, training loss: 2075.567, validation loss: 2086.105, Runtime: 17.5273 secs\nEpoch: 873, Step: 21825, training loss: 2075.359, validation loss: 2085.802, Runtime: 17.3610 secs\nEpoch: 874, Step: 21850, training loss: 2075.531, validation loss: 2086.000, Runtime: 17.4013 secs\nEpoch: 875, Step: 21875, training loss: 2075.532, validation loss: 2085.388, Runtime: 17.5071 secs\nEpoch: 876, Step: 21900, training loss: 2075.556, validation loss: 2086.382, Runtime: 17.4612 secs\nEpoch: 877, Step: 21925, training loss: 2075.518, validation loss: 2085.625, Runtime: 17.4280 secs\nEpoch: 878, Step: 21950, training loss: 2075.588, validation loss: 2086.203, Runtime: 17.4005 secs\nLearning rate decreased to 0.000322\nEpoch: 879, Step: 21975, training loss: 2075.526, validation loss: 2086.171, Runtime: 17.4384 secs\nEpoch: 880, Step: 22000, training loss: 2075.885, validation loss: 2085.845, Runtime: 17.5261 secs\nEpoch: 881, Step: 22025, training loss: 2075.471, validation loss: 2085.815, Runtime: 17.3874 secs\nEpoch: 882, Step: 22050, training loss: 2075.216, validation loss: 2085.186, Runtime: 17.4139 secs\nEpoch: 883, Step: 22075, training loss: 2075.186, validation loss: 2085.606, Runtime: 17.5676 secs\nEpoch: 884, Step: 22100, training loss: 2075.478, validation loss: 2086.266, Runtime: 17.4049 secs\nEpoch: 885, Step: 22125, training loss: 2075.365, validation loss: 2085.815, Runtime: 17.3638 secs\nEpoch: 886, Step: 22150, training loss: 2075.225, validation loss: 2085.901, Runtime: 17.5597 secs\nEpoch: 887, Step: 22175, training loss: 2075.499, validation loss: 2085.562, Runtime: 17.2036 secs\nLearning rate decreased to 0.000306\nEpoch: 888, Step: 22200, training loss: 2075.371, validation loss: 2085.874, Runtime: 17.3723 secs\nEpoch: 889, Step: 22225, training loss: 2075.369, validation loss: 2085.678, Runtime: 17.4198 secs\nEpoch: 890, Step: 22250, training loss: 2075.369, validation loss: 2085.666, Runtime: 17.4794 secs\nEpoch: 891, Step: 22275, training loss: 2075.460, validation loss: 2085.766, Runtime: 17.4714 secs\nEpoch: 892, Step: 22300, training loss: 2075.459, validation loss: 2085.425, Runtime: 17.1427 secs\nEpoch: 893, Step: 22325, training loss: 2075.540, validation loss: 2085.315, Runtime: 17.3859 secs\nLearning rate decreased to 0.000290\nEpoch: 894, Step: 22350, training loss: 2075.294, validation loss: 2086.199, Runtime: 17.3989 secs\nEpoch: 895, Step: 22375, training loss: 2075.327, validation loss: 2085.869, Runtime: 17.5053 secs\nEpoch: 896, Step: 22400, training loss: 2075.254, validation loss: 2085.774, Runtime: 17.4725 secs\nEpoch: 897, Step: 22425, training loss: 2075.279, validation loss: 2085.866, Runtime: 17.4827 secs\nEpoch: 898, Step: 22450, training loss: 2075.219, validation loss: 2085.805, Runtime: 17.5849 secs\nEpoch: 899, Step: 22475, training loss: 2075.384, validation loss: 2085.492, Runtime: 17.4619 secs\nEpoch: 900, Step: 22500, training loss: 2075.134, validation loss: 2085.840, Runtime: 17.3840 secs\nEpoch: 901, Step: 22525, training loss: 2075.222, validation loss: 2086.265, Runtime: 17.3600 secs\nEpoch: 902, Step: 22550, training loss: 2075.183, validation loss: 2085.774, Runtime: 17.4392 secs\nEpoch: 903, Step: 22575, training loss: 2075.302, validation loss: 2085.738, Runtime: 17.5777 secs\nEpoch: 904, Step: 22600, training loss: 2075.137, validation loss: 2085.977, Runtime: 17.3802 secs\nEpoch: 905, Step: 22625, training loss: 2075.369, validation loss: 2086.141, Runtime: 17.4196 secs\nEpoch: 906, Step: 22650, training loss: 2075.098, validation loss: 2086.028, Runtime: 17.3317 secs\nEpoch: 907, Step: 22675, training loss: 2075.456, validation loss: 2085.975, Runtime: 17.7799 secs\nLearning rate decreased to 0.000276\nEpoch: 908, Step: 22700, training loss: 2075.281, validation loss: 2085.515, Runtime: 17.8206 secs\nEpoch: 909, Step: 22725, training loss: 2075.278, validation loss: 2086.132, Runtime: 17.2556 secs\nEpoch: 910, Step: 22750, training loss: 2075.352, validation loss: 2085.865, Runtime: 17.5098 secs\nEpoch: 911, Step: 22775, training loss: 2075.171, validation loss: 2085.366, Runtime: 17.3368 secs\nEpoch: 912, Step: 22800, training loss: 2075.172, validation loss: 2085.143, Runtime: 17.5010 secs\nEpoch: 913, Step: 22825, training loss: 2075.208, validation loss: 2085.662, Runtime: 17.3132 secs\nEpoch: 914, Step: 22850, training loss: 2075.222, validation loss: 2086.679, Runtime: 17.5483 secs\nEpoch: 915, Step: 22875, training loss: 2075.043, validation loss: 2086.089, Runtime: 17.3891 secs\nEpoch: 916, Step: 22900, training loss: 2075.234, validation loss: 2085.833, Runtime: 17.4720 secs\nEpoch: 917, Step: 22925, training loss: 2075.444, validation loss: 2085.294, Runtime: 17.4054 secs\nLearning rate decreased to 0.000262\nEpoch: 918, Step: 22950, training loss: 2075.254, validation loss: 2085.870, Runtime: 17.3936 secs\nEpoch: 919, Step: 22975, training loss: 2075.272, validation loss: 2086.142, Runtime: 17.4623 secs\nEpoch: 920, Step: 23000, training loss: 2075.368, validation loss: 2085.765, Runtime: 17.3294 secs\nEpoch: 921, Step: 23025, training loss: 2075.267, validation loss: 2085.945, Runtime: 17.3000 secs\nEpoch: 922, Step: 23050, training loss: 2075.053, validation loss: 2085.702, Runtime: 17.5126 secs\nEpoch: 923, Step: 23075, training loss: 2075.297, validation loss: 2085.940, Runtime: 17.3764 secs\nEpoch: 924, Step: 23100, training loss: 2075.474, validation loss: 2085.468, Runtime: 17.4334 secs\nLearning rate decreased to 0.000249\nEpoch: 925, Step: 23125, training loss: 2075.114, validation loss: 2085.783, Runtime: 17.3410 secs\nEpoch: 926, Step: 23150, training loss: 2075.217, validation loss: 2086.658, Runtime: 17.5377 secs\nEpoch: 927, Step: 23175, training loss: 2075.199, validation loss: 2085.872, Runtime: 17.6217 secs\nEpoch: 928, Step: 23200, training loss: 2075.324, validation loss: 2086.021, Runtime: 17.5363 secs\nEpoch: 929, Step: 23225, training loss: 2075.084, validation loss: 2085.906, Runtime: 17.4457 secs\nEpoch: 930, Step: 23250, training loss: 2075.403, validation loss: 2085.789, Runtime: 17.5056 secs\nEpoch: 931, Step: 23275, training loss: 2075.262, validation loss: 2085.612, Runtime: 17.4592 secs\nEpoch: 932, Step: 23300, training loss: 2075.323, validation loss: 2086.337, Runtime: 17.3344 secs\nEpoch: 933, Step: 23325, training loss: 2075.206, validation loss: 2085.690, Runtime: 17.3934 secs\nEpoch: 934, Step: 23350, training loss: 2075.224, validation loss: 2085.205, Runtime: 17.4391 secs\nEpoch: 935, Step: 23375, training loss: 2075.117, validation loss: 2085.797, Runtime: 17.5343 secs\nEpoch: 936, Step: 23400, training loss: 2075.250, validation loss: 2085.974, Runtime: 17.5543 secs\nEpoch: 937, Step: 23425, training loss: 2075.072, validation loss: 2085.899, Runtime: 17.3106 secs\nEpoch: 938, Step: 23450, training loss: 2075.150, validation loss: 2086.071, Runtime: 17.5575 secs\nEpoch: 939, Step: 23475, training loss: 2075.311, validation loss: 2085.998, Runtime: 17.4680 secs\nLearning rate decreased to 0.000236\nEpoch: 940, Step: 23500, training loss: 2074.835, validation loss: 2086.041, Runtime: 17.2728 secs\nEpoch: 941, Step: 23525, training loss: 2075.011, validation loss: 2085.911, Runtime: 17.4113 secs\nEpoch: 942, Step: 23550, training loss: 2075.195, validation loss: 2085.599, Runtime: 17.4266 secs\nEpoch: 943, Step: 23575, training loss: 2075.187, validation loss: 2085.736, Runtime: 17.4938 secs\nEpoch: 944, Step: 23600, training loss: 2075.126, validation loss: 2085.350, Runtime: 17.4511 secs\nEpoch: 945, Step: 23625, training loss: 2075.133, validation loss: 2086.073, Runtime: 17.4164 secs\nEpoch: 946, Step: 23650, training loss: 2075.004, validation loss: 2085.696, Runtime: 17.5031 secs\nEpoch: 947, Step: 23675, training loss: 2075.254, validation loss: 2085.476, Runtime: 17.5978 secs\nLearning rate decreased to 0.000225\nEpoch: 948, Step: 23700, training loss: 2075.158, validation loss: 2085.628, Runtime: 17.2494 secs\nEpoch: 949, Step: 23725, training loss: 2075.321, validation loss: 2085.845, Runtime: 17.4197 secs\nEpoch: 950, Step: 23750, training loss: 2074.825, validation loss: 2085.998, Runtime: 17.5258 secs\nEpoch: 951, Step: 23775, training loss: 2075.235, validation loss: 2085.762, Runtime: 17.3288 secs\nEpoch: 952, Step: 23800, training loss: 2075.081, validation loss: 2085.771, Runtime: 17.5218 secs\nEpoch: 953, Step: 23825, training loss: 2075.152, validation loss: 2085.762, Runtime: 17.4128 secs\nEpoch: 954, Step: 23850, training loss: 2075.188, validation loss: 2085.917, Runtime: 17.3098 secs\nEpoch: 955, Step: 23875, training loss: 2075.309, validation loss: 2085.675, Runtime: 17.4832 secs\nEpoch: 956, Step: 23900, training loss: 2074.952, validation loss: 2086.111, Runtime: 17.3366 secs\nEpoch: 957, Step: 23925, training loss: 2075.045, validation loss: 2085.734, Runtime: 17.3646 secs\nEpoch: 958, Step: 23950, training loss: 2075.110, validation loss: 2085.988, Runtime: 17.4440 secs\nEpoch: 959, Step: 23975, training loss: 2075.167, validation loss: 2085.772, Runtime: 17.3252 secs\nEpoch: 960, Step: 24000, training loss: 2075.000, validation loss: 2086.154, Runtime: 17.5126 secs\nEpoch: 961, Step: 24025, training loss: 2075.417, validation loss: 2086.059, Runtime: 17.4213 secs\nLearning rate decreased to 0.000213\nEpoch: 962, Step: 24050, training loss: 2075.147, validation loss: 2085.969, Runtime: 17.4703 secs\nEpoch: 963, Step: 24075, training loss: 2075.082, validation loss: 2086.099, Runtime: 17.3285 secs\nEpoch: 964, Step: 24100, training loss: 2075.022, validation loss: 2085.933, Runtime: 17.4780 secs\nEpoch: 965, Step: 24125, training loss: 2075.167, validation loss: 2086.380, Runtime: 17.2920 secs\nEpoch: 966, Step: 24150, training loss: 2075.111, validation loss: 2086.195, Runtime: 17.2385 secs\nEpoch: 967, Step: 24175, training loss: 2075.274, validation loss: 2086.097, Runtime: 17.5335 secs\nEpoch: 968, Step: 24200, training loss: 2074.828, validation loss: 2085.634, Runtime: 17.2363 secs\nEpoch: 969, Step: 24225, training loss: 2075.263, validation loss: 2085.919, Runtime: 17.2896 secs\nEpoch: 970, Step: 24250, training loss: 2075.219, validation loss: 2085.870, Runtime: 17.2633 secs\nEpoch: 971, Step: 24275, training loss: 2075.098, validation loss: 2086.251, Runtime: 17.3234 secs\nEpoch: 972, Step: 24300, training loss: 2075.098, validation loss: 2086.332, Runtime: 17.4634 secs\nEpoch: 973, Step: 24325, training loss: 2074.962, validation loss: 2085.603, Runtime: 17.3684 secs\nEpoch: 974, Step: 24350, training loss: 2074.894, validation loss: 2086.403, Runtime: 17.4056 secs\nEpoch: 975, Step: 24375, training loss: 2075.162, validation loss: 2085.802, Runtime: 17.4313 secs\nEpoch: 976, Step: 24400, training loss: 2075.245, validation loss: 2086.259, Runtime: 17.4528 secs\nLearning rate decreased to 0.000203\nEpoch: 977, Step: 24425, training loss: 2075.212, validation loss: 2085.564, Runtime: 17.3666 secs\nEpoch: 978, Step: 24450, training loss: 2075.310, validation loss: 2085.385, Runtime: 17.6306 secs\nEpoch: 979, Step: 24475, training loss: 2075.137, validation loss: 2085.802, Runtime: 17.9376 secs\nEpoch: 980, Step: 24500, training loss: 2075.121, validation loss: 2085.869, Runtime: 17.5492 secs\nEpoch: 981, Step: 24525, training loss: 2074.970, validation loss: 2085.732, Runtime: 17.5477 secs\nEpoch: 982, Step: 24550, training loss: 2075.033, validation loss: 2086.608, Runtime: 17.2729 secs\nEpoch: 983, Step: 24575, training loss: 2075.036, validation loss: 2085.763, Runtime: 17.2641 secs\nEpoch: 984, Step: 24600, training loss: 2075.247, validation loss: 2086.247, Runtime: 17.3933 secs\nEpoch: 985, Step: 24625, training loss: 2074.962, validation loss: 2086.044, Runtime: 17.4278 secs\nEpoch: 986, Step: 24650, training loss: 2074.898, validation loss: 2086.184, Runtime: 17.4062 secs\nEpoch: 987, Step: 24675, training loss: 2075.101, validation loss: 2085.316, Runtime: 17.4010 secs\nEpoch: 988, Step: 24700, training loss: 2075.217, validation loss: 2085.754, Runtime: 17.4474 secs\nEpoch: 989, Step: 24725, training loss: 2074.974, validation loss: 2085.924, Runtime: 17.4960 secs\nEpoch: 990, Step: 24750, training loss: 2075.161, validation loss: 2085.729, Runtime: 17.6180 secs\nEpoch: 991, Step: 24775, training loss: 2074.826, validation loss: 2085.637, Runtime: 17.5796 secs\nEpoch: 992, Step: 24800, training loss: 2074.859, validation loss: 2086.342, Runtime: 17.4604 secs\nEpoch: 993, Step: 24825, training loss: 2075.291, validation loss: 2085.925, Runtime: 17.4314 secs\nLearning rate decreased to 0.000193\nEpoch: 994, Step: 24850, training loss: 2074.966, validation loss: 2085.510, Runtime: 17.3955 secs\nEpoch: 995, Step: 24875, training loss: 2075.118, validation loss: 2085.814, Runtime: 17.4168 secs\nEpoch: 996, Step: 24900, training loss: 2075.121, validation loss: 2085.447, Runtime: 17.4161 secs\nEpoch: 997, Step: 24925, training loss: 2075.019, validation loss: 2085.850, Runtime: 17.3483 secs\nEpoch: 998, Step: 24950, training loss: 2074.876, validation loss: 2085.645, Runtime: 17.4385 secs\nEpoch: 999, Step: 24975, training loss: 2075.097, validation loss: 2086.390, Runtime: 17.4161 secs\nEpoch: 1000, Step: 25000, training loss: 2075.021, validation loss: 2085.924, Runtime: 17.3863 secs\nEpoch: 1001, Step: 25025, training loss: 2074.908, validation loss: 2085.331, Runtime: 17.4042 secs\nEpoch: 1002, Step: 25050, training loss: 2074.826, validation loss: 2085.784, Runtime: 17.3146 secs\nEpoch: 1003, Step: 25075, training loss: 2075.253, validation loss: 2085.474, Runtime: 17.3083 secs\nLearning rate decreased to 0.000183\nEpoch: 1004, Step: 25100, training loss: 2074.809, validation loss: 2086.452, Runtime: 17.2120 secs\nEpoch: 1005, Step: 25125, training loss: 2074.787, validation loss: 2085.907, Runtime: 17.4503 secs\nEpoch: 1006, Step: 25150, training loss: 2074.788, validation loss: 2086.273, Runtime: 17.2808 secs\nEpoch: 1007, Step: 25175, training loss: 2075.022, validation loss: 2085.975, Runtime: 17.3685 secs\nEpoch: 1008, Step: 25200, training loss: 2075.000, validation loss: 2086.039, Runtime: 17.3506 secs\nEpoch: 1009, Step: 25225, training loss: 2074.897, validation loss: 2085.945, Runtime: 17.4306 secs\nEpoch: 1010, Step: 25250, training loss: 2074.858, validation loss: 2086.248, Runtime: 17.2876 secs\nEpoch: 1011, Step: 25275, training loss: 2074.857, validation loss: 2086.149, Runtime: 17.4400 secs\nEpoch: 1012, Step: 25300, training loss: 2075.090, validation loss: 2086.353, Runtime: 17.3057 secs\nLearning rate decreased to 0.000174\nEpoch: 1013, Step: 25325, training loss: 2074.971, validation loss: 2086.084, Runtime: 17.3441 secs\nEpoch: 1014, Step: 25350, training loss: 2074.860, validation loss: 2086.076, Runtime: 17.8802 secs\nEpoch: 1015, Step: 25375, training loss: 2074.788, validation loss: 2086.124, Runtime: 17.3560 secs\nEpoch: 1016, Step: 25400, training loss: 2075.058, validation loss: 2085.937, Runtime: 17.3865 secs\nEpoch: 1017, Step: 25425, training loss: 2075.133, validation loss: 2086.056, Runtime: 17.4132 secs\nEpoch: 1018, Step: 25450, training loss: 2074.936, validation loss: 2086.374, Runtime: 17.3065 secs\nEpoch: 1019, Step: 25475, training loss: 2075.078, validation loss: 2085.704, Runtime: 17.3105 secs\nEpoch: 1020, Step: 25500, training loss: 2074.926, validation loss: 2086.245, Runtime: 17.4453 secs\nEpoch: 1021, Step: 25525, training loss: 2074.847, validation loss: 2086.317, Runtime: 17.3614 secs\nEpoch: 1022, Step: 25550, training loss: 2075.184, validation loss: 2086.069, Runtime: 17.4484 secs\nLearning rate decreased to 0.000165\nEpoch: 1023, Step: 25575, training loss: 2075.169, validation loss: 2086.113, Runtime: 17.3033 secs\nEpoch: 1024, Step: 25600, training loss: 2074.909, validation loss: 2085.811, Runtime: 17.4407 secs\nEpoch: 1025, Step: 25625, training loss: 2075.074, validation loss: 2086.131, Runtime: 17.5841 secs\nEpoch: 1026, Step: 25650, training loss: 2074.868, validation loss: 2085.794, Runtime: 17.7026 secs\nEpoch: 1027, Step: 25675, training loss: 2074.961, validation loss: 2086.459, Runtime: 17.4151 secs\nEpoch: 1028, Step: 25700, training loss: 2074.705, validation loss: 2086.081, Runtime: 17.4018 secs\nEpoch: 1029, Step: 25725, training loss: 2074.937, validation loss: 2086.573, Runtime: 17.4374 secs\nEpoch: 1030, Step: 25750, training loss: 2074.827, validation loss: 2085.862, Runtime: 17.4217 secs\nEpoch: 1031, Step: 25775, training loss: 2074.882, validation loss: 2085.929, Runtime: 17.4324 secs\nEpoch: 1032, Step: 25800, training loss: 2074.766, validation loss: 2085.601, Runtime: 17.4311 secs\nEpoch: 1033, Step: 25825, training loss: 2074.987, validation loss: 2086.900, Runtime: 17.4260 secs\nLearning rate decreased to 0.000157\nEpoch: 1034, Step: 25850, training loss: 2074.913, validation loss: 2085.623, Runtime: 17.4292 secs\nEpoch: 1035, Step: 25875, training loss: 2074.844, validation loss: 2085.782, Runtime: 17.4321 secs\nEpoch: 1036, Step: 25900, training loss: 2074.630, validation loss: 2086.220, Runtime: 17.5064 secs\nEpoch: 1037, Step: 25925, training loss: 2074.753, validation loss: 2086.338, Runtime: 17.3647 secs\nEpoch: 1038, Step: 25950, training loss: 2074.873, validation loss: 2086.007, Runtime: 17.5290 secs\nEpoch: 1039, Step: 25975, training loss: 2074.702, validation loss: 2086.456, Runtime: 17.4306 secs\nEpoch: 1040, Step: 26000, training loss: 2075.077, validation loss: 2086.356, Runtime: 17.4636 secs\nLearning rate decreased to 0.000149\nEpoch: 1041, Step: 26025, training loss: 2074.579, validation loss: 2085.520, Runtime: 17.4370 secs\nEpoch: 1042, Step: 26050, training loss: 2074.901, validation loss: 2086.264, Runtime: 17.2904 secs\nEpoch: 1043, Step: 26075, training loss: 2074.721, validation loss: 2086.188, Runtime: 17.3319 secs\nEpoch: 1044, Step: 26100, training loss: 2074.847, validation loss: 2085.844, Runtime: 17.3120 secs\nEpoch: 1045, Step: 26125, training loss: 2074.656, validation loss: 2086.124, Runtime: 17.5072 secs\nEpoch: 1046, Step: 26150, training loss: 2074.690, validation loss: 2085.898, Runtime: 17.4314 secs\nEpoch: 1047, Step: 26175, training loss: 2074.726, validation loss: 2086.621, Runtime: 17.4054 secs\nEpoch: 1048, Step: 26200, training loss: 2074.792, validation loss: 2086.078, Runtime: 17.5218 secs\nEpoch: 1049, Step: 26225, training loss: 2074.946, validation loss: 2085.871, Runtime: 17.3935 secs\nLearning rate decreased to 0.000142\nEpoch: 1050, Step: 26250, training loss: 2074.822, validation loss: 2085.709, Runtime: 17.4247 secs\nEpoch: 1051, Step: 26275, training loss: 2075.064, validation loss: 2085.838, Runtime: 17.3713 secs\nEpoch: 1052, Step: 26300, training loss: 2074.943, validation loss: 2086.004, Runtime: 17.3385 secs\nEpoch: 1053, Step: 26325, training loss: 2074.894, validation loss: 2086.286, Runtime: 17.4922 secs\nEpoch: 1054, Step: 26350, training loss: 2074.721, validation loss: 2085.994, Runtime: 17.3899 secs\nEpoch: 1055, Step: 26375, training loss: 2074.749, validation loss: 2086.053, Runtime: 17.7061 secs\nEpoch: 1056, Step: 26400, training loss: 2074.932, validation loss: 2085.974, Runtime: 17.5916 secs\nEpoch: 1057, Step: 26425, training loss: 2074.707, validation loss: 2086.079, Runtime: 17.8829 secs\nEpoch: 1058, Step: 26450, training loss: 2074.807, validation loss: 2086.084, Runtime: 17.3259 secs\nEpoch: 1059, Step: 26475, training loss: 2074.833, validation loss: 2085.738, Runtime: 17.4459 secs\nEpoch: 1060, Step: 26500, training loss: 2074.841, validation loss: 2086.035, Runtime: 17.5085 secs\nEpoch: 1061, Step: 26525, training loss: 2074.849, validation loss: 2086.104, Runtime: 17.4559 secs\nEpoch: 1062, Step: 26550, training loss: 2074.515, validation loss: 2085.703, Runtime: 17.4400 secs\nEpoch: 1063, Step: 26575, training loss: 2074.698, validation loss: 2085.963, Runtime: 17.4102 secs\nEpoch: 1064, Step: 26600, training loss: 2074.862, validation loss: 2086.115, Runtime: 17.3568 secs\nLearning rate decreased to 0.000135\nEpoch: 1065, Step: 26625, training loss: 2074.938, validation loss: 2086.278, Runtime: 17.4339 secs\nEpoch: 1066, Step: 26650, training loss: 2074.814, validation loss: 2085.776, Runtime: 17.2190 secs\nEpoch: 1067, Step: 26675, training loss: 2074.958, validation loss: 2086.063, Runtime: 17.2602 secs\nEpoch: 1068, Step: 26700, training loss: 2074.693, validation loss: 2086.014, Runtime: 17.2680 secs\nEpoch: 1069, Step: 26725, training loss: 2074.794, validation loss: 2086.137, Runtime: 17.3617 secs\nEpoch: 1070, Step: 26750, training loss: 2074.857, validation loss: 2086.089, Runtime: 17.3232 secs\nEpoch: 1071, Step: 26775, training loss: 2075.129, validation loss: 2085.332, Runtime: 17.1384 secs\nLearning rate decreased to 0.000128\nEpoch: 1072, Step: 26800, training loss: 2074.611, validation loss: 2085.606, Runtime: 17.3348 secs\nEpoch: 1073, Step: 26825, training loss: 2074.615, validation loss: 2086.510, Runtime: 17.4466 secs\nEpoch: 1074, Step: 26850, training loss: 2074.794, validation loss: 2085.814, Runtime: 17.4212 secs\nEpoch: 1075, Step: 26875, training loss: 2074.825, validation loss: 2086.700, Runtime: 17.3427 secs\nEpoch: 1076, Step: 26900, training loss: 2074.775, validation loss: 2086.118, Runtime: 17.5599 secs\nEpoch: 1077, Step: 26925, training loss: 2074.888, validation loss: 2086.293, Runtime: 17.5273 secs\nEpoch: 1078, Step: 26950, training loss: 2074.805, validation loss: 2085.858, Runtime: 17.3381 secs\nEpoch: 1079, Step: 26975, training loss: 2074.787, validation loss: 2086.289, Runtime: 17.4264 secs\nEpoch: 1080, Step: 27000, training loss: 2074.852, validation loss: 2086.368, Runtime: 17.2803 secs\nEpoch: 1081, Step: 27025, training loss: 2074.736, validation loss: 2085.748, Runtime: 17.5274 secs\nEpoch: 1082, Step: 27050, training loss: 2074.933, validation loss: 2085.971, Runtime: 17.4327 secs\nLearning rate decreased to 0.000121\nEpoch: 1083, Step: 27075, training loss: 2074.848, validation loss: 2085.526, Runtime: 17.3743 secs\nEpoch: 1084, Step: 27100, training loss: 2074.725, validation loss: 2086.249, Runtime: 17.3650 secs\nEpoch: 1085, Step: 27125, training loss: 2074.686, validation loss: 2085.968, Runtime: 17.3724 secs\nEpoch: 1086, Step: 27150, training loss: 2074.665, validation loss: 2086.592, Runtime: 17.7533 secs\nEpoch: 1087, Step: 27175, training loss: 2074.562, validation loss: 2086.016, Runtime: 17.3260 secs\nEpoch: 1088, Step: 27200, training loss: 2074.748, validation loss: 2086.358, Runtime: 17.5767 secs\nEpoch: 1089, Step: 27225, training loss: 2074.749, validation loss: 2085.325, Runtime: 17.5893 secs\nEpoch: 1090, Step: 27250, training loss: 2074.765, validation loss: 2086.099, Runtime: 17.4301 secs\nLearning rate decreased to 0.000115\nEpoch: 1091, Step: 27275, training loss: 2074.994, validation loss: 2085.575, Runtime: 17.3921 secs\nEpoch: 1092, Step: 27300, training loss: 2074.617, validation loss: 2086.187, Runtime: 17.4713 secs\nEpoch: 1093, Step: 27325, training loss: 2074.782, validation loss: 2085.802, Runtime: 17.4974 secs\nEpoch: 1094, Step: 27350, training loss: 2074.656, validation loss: 2086.265, Runtime: 17.3455 secs\nEpoch: 1095, Step: 27375, training loss: 2074.704, validation loss: 2086.368, Runtime: 17.4143 secs\nEpoch: 1096, Step: 27400, training loss: 2074.765, validation loss: 2086.413, Runtime: 17.5121 secs\nEpoch: 1097, Step: 27425, training loss: 2074.876, validation loss: 2086.222, Runtime: 17.2081 secs\nEpoch: 1098, Step: 27450, training loss: 2074.705, validation loss: 2085.636, Runtime: 17.3991 secs\nEpoch: 1099, Step: 27475, training loss: 2074.734, validation loss: 2085.812, Runtime: 17.3809 secs\nEpoch: 1100, Step: 27500, training loss: 2074.632, validation loss: 2085.924, Runtime: 17.6541 secs\nEpoch: 1101, Step: 27525, training loss: 2074.726, validation loss: 2085.919, Runtime: 17.4984 secs\nEpoch: 1102, Step: 27550, training loss: 2074.702, validation loss: 2085.820, Runtime: 17.6566 secs\nEpoch: 1103, Step: 27575, training loss: 2074.911, validation loss: 2085.743, Runtime: 17.5479 secs\nLearning rate decreased to 0.000110\nEpoch: 1104, Step: 27600, training loss: 2074.610, validation loss: 2085.843, Runtime: 17.9597 secs\nEpoch: 1105, Step: 27625, training loss: 2074.750, validation loss: 2086.333, Runtime: 17.4771 secs\nEpoch: 1106, Step: 27650, training loss: 2074.776, validation loss: 2085.849, Runtime: 17.3429 secs\nEpoch: 1107, Step: 27675, training loss: 2074.647, validation loss: 2086.360, Runtime: 17.3002 secs\nEpoch: 1108, Step: 27700, training loss: 2074.710, validation loss: 2085.783, Runtime: 17.2358 secs\nEpoch: 1109, Step: 27725, training loss: 2074.791, validation loss: 2085.951, Runtime: 17.3902 secs\nEpoch: 1110, Step: 27750, training loss: 2074.740, validation loss: 2086.250, Runtime: 17.4648 secs\nEpoch: 1111, Step: 27775, training loss: 2074.626, validation loss: 2086.379, Runtime: 17.3709 secs\nEpoch: 1112, Step: 27800, training loss: 2074.480, validation loss: 2085.885, Runtime: 17.5185 secs\nEpoch: 1113, Step: 27825, training loss: 2074.727, validation loss: 2086.111, Runtime: 17.4905 secs\nEpoch: 1114, Step: 27850, training loss: 2074.585, validation loss: 2086.170, Runtime: 17.4020 secs\nEpoch: 1115, Step: 27875, training loss: 2075.094, validation loss: 2086.154, Runtime: 17.4450 secs\nLearning rate decreased to 0.000104\nEpoch: 1116, Step: 27900, training loss: 2074.391, validation loss: 2086.452, Runtime: 17.4850 secs\nEpoch: 1117, Step: 27925, training loss: 2074.506, validation loss: 2085.505, Runtime: 17.5494 secs\nEpoch: 1118, Step: 27950, training loss: 2074.691, validation loss: 2085.676, Runtime: 17.4137 secs\nEpoch: 1119, Step: 27975, training loss: 2074.675, validation loss: 2086.129, Runtime: 17.3997 secs\nEpoch: 1120, Step: 28000, training loss: 2074.770, validation loss: 2085.673, Runtime: 17.3645 secs\nEpoch: 1121, Step: 28025, training loss: 2074.811, validation loss: 2086.383, Runtime: 17.4349 secs\nEpoch: 1122, Step: 28050, training loss: 2074.781, validation loss: 2085.776, Runtime: 17.3871 secs\nEpoch: 1123, Step: 28075, training loss: 2074.627, validation loss: 2086.220, Runtime: 17.2820 secs\nEpoch: 1124, Step: 28100, training loss: 2074.482, validation loss: 2085.743, Runtime: 17.3236 secs\nEpoch: 1125, Step: 28125, training loss: 2074.661, validation loss: 2086.203, Runtime: 17.4280 secs\nEpoch: 1126, Step: 28150, training loss: 2074.742, validation loss: 2086.057, Runtime: 17.5172 secs\nEpoch: 1127, Step: 28175, training loss: 2074.611, validation loss: 2085.985, Runtime: 17.4261 secs\nEpoch: 1128, Step: 28200, training loss: 2074.652, validation loss: 2085.811, Runtime: 17.7220 secs\nEpoch: 1129, Step: 28225, training loss: 2074.737, validation loss: 2086.690, Runtime: 17.3222 secs\nEpoch: 1130, Step: 28250, training loss: 2074.475, validation loss: 2085.887, Runtime: 17.2786 secs\nEpoch: 1131, Step: 28275, training loss: 2074.724, validation loss: 2085.784, Runtime: 17.3274 secs\nEpoch: 1132, Step: 28300, training loss: 2074.744, validation loss: 2086.470, Runtime: 17.4087 secs\nLearning rate decreased to 0.000099\nEpoch: 1133, Step: 28325, training loss: 2074.768, validation loss: 2085.958, Runtime: 17.6448 secs\nEpoch: 1134, Step: 28350, training loss: 2074.689, validation loss: 2085.870, Runtime: 17.3092 secs\nEpoch: 1135, Step: 28375, training loss: 2074.719, validation loss: 2086.275, Runtime: 17.2635 secs\nEpoch: 1136, Step: 28400, training loss: 2074.808, validation loss: 2085.847, Runtime: 17.4449 secs\nEpoch: 1137, Step: 28425, training loss: 2074.573, validation loss: 2086.399, Runtime: 17.4479 secs\nEpoch: 1138, Step: 28450, training loss: 2074.570, validation loss: 2086.118, Runtime: 17.4628 secs\nEpoch: 1139, Step: 28475, training loss: 2074.690, validation loss: 2086.599, Runtime: 17.3566 secs\nEpoch: 1140, Step: 28500, training loss: 2074.833, validation loss: 2086.312, Runtime: 17.4730 secs\nLearning rate decreased to 0.000094\nEpoch: 1141, Step: 28525, training loss: 2074.859, validation loss: 2085.938, Runtime: 17.3842 secs\nEpoch: 1142, Step: 28550, training loss: 2074.582, validation loss: 2086.267, Runtime: 17.3405 secs\nEpoch: 1143, Step: 28575, training loss: 2074.782, validation loss: 2085.844, Runtime: 17.3838 secs\nEpoch: 1144, Step: 28600, training loss: 2074.480, validation loss: 2086.050, Runtime: 17.4861 secs\nEpoch: 1145, Step: 28625, training loss: 2075.000, validation loss: 2086.394, Runtime: 17.3385 secs\nEpoch: 1146, Step: 28650, training loss: 2074.763, validation loss: 2086.272, Runtime: 17.4070 secs\nEpoch: 1147, Step: 28675, training loss: 2074.663, validation loss: 2086.067, Runtime: 17.3638 secs\nEpoch: 1148, Step: 28700, training loss: 2074.930, validation loss: 2085.699, Runtime: 17.4491 secs\nEpoch: 1149, Step: 28725, training loss: 2074.599, validation loss: 2086.241, Runtime: 17.4291 secs\nEpoch: 1150, Step: 28750, training loss: 2074.667, validation loss: 2086.023, Runtime: 17.3900 secs\nEpoch: 1151, Step: 28775, training loss: 2074.907, validation loss: 2086.015, Runtime: 17.3230 secs\nEpoch: 1152, Step: 28800, training loss: 2074.677, validation loss: 2086.424, Runtime: 17.3544 secs\nEpoch: 1153, Step: 28825, training loss: 2074.566, validation loss: 2086.331, Runtime: 17.3084 secs\nEpoch: 1154, Step: 28850, training loss: 2074.529, validation loss: 2086.374, Runtime: 17.4010 secs\nEpoch: 1155, Step: 28875, training loss: 2074.784, validation loss: 2086.285, Runtime: 17.5508 secs\nEpoch: 1156, Step: 28900, training loss: 2074.621, validation loss: 2085.963, Runtime: 17.4366 secs\nEpoch: 1157, Step: 28925, training loss: 2074.559, validation loss: 2086.212, Runtime: 17.3546 secs\nEpoch: 1158, Step: 28950, training loss: 2074.522, validation loss: 2086.469, Runtime: 17.3723 secs\nEpoch: 1159, Step: 28975, training loss: 2074.672, validation loss: 2086.109, Runtime: 17.4711 secs\nEpoch: 1160, Step: 29000, training loss: 2074.591, validation loss: 2086.211, Runtime: 17.4798 secs\nEpoch: 1161, Step: 29025, training loss: 2074.563, validation loss: 2086.085, Runtime: 17.3094 secs\nEpoch: 1162, Step: 29050, training loss: 2074.906, validation loss: 2085.544, Runtime: 17.4548 secs\nLearning rate decreased to 0.000089\nEpoch: 1163, Step: 29075, training loss: 2074.360, validation loss: 2085.838, Runtime: 17.4395 secs\nEpoch: 1164, Step: 29100, training loss: 2074.570, validation loss: 2085.897, Runtime: 17.4305 secs\nEpoch: 1165, Step: 29125, training loss: 2074.835, validation loss: 2086.338, Runtime: 17.3309 secs\nEpoch: 1166, Step: 29150, training loss: 2074.669, validation loss: 2085.949, Runtime: 17.4389 secs\nEpoch: 1167, Step: 29175, training loss: 2074.640, validation loss: 2086.031, Runtime: 17.4507 secs\nEpoch: 1168, Step: 29200, training loss: 2074.715, validation loss: 2085.759, Runtime: 17.2789 secs\nEpoch: 1169, Step: 29225, training loss: 2074.679, validation loss: 2085.487, Runtime: 17.2982 secs\nEpoch: 1170, Step: 29250, training loss: 2074.716, validation loss: 2085.988, Runtime: 17.4991 secs\nEpoch: 1171, Step: 29275, training loss: 2074.720, validation loss: 2086.619, Runtime: 17.5066 secs\nEpoch: 1172, Step: 29300, training loss: 2074.506, validation loss: 2086.029, Runtime: 17.4566 secs\nEpoch: 1173, Step: 29325, training loss: 2074.676, validation loss: 2085.786, Runtime: 17.3791 secs\nEpoch: 1174, Step: 29350, training loss: 2074.526, validation loss: 2085.882, Runtime: 17.4323 secs\nEpoch: 1175, Step: 29375, training loss: 2074.645, validation loss: 2086.131, Runtime: 17.2612 secs\nEpoch: 1176, Step: 29400, training loss: 2074.661, validation loss: 2086.158, Runtime: 17.2730 secs\nEpoch: 1177, Step: 29425, training loss: 2074.558, validation loss: 2085.556, Runtime: 17.3511 secs\nEpoch: 1178, Step: 29450, training loss: 2074.641, validation loss: 2086.111, Runtime: 17.4123 secs\nEpoch: 1179, Step: 29475, training loss: 2074.742, validation loss: 2086.005, Runtime: 17.4611 secs\nLearning rate decreased to 0.000085\nEpoch: 1180, Step: 29500, training loss: 2074.786, validation loss: 2086.616, Runtime: 17.3850 secs\nEpoch: 1181, Step: 29525, training loss: 2074.704, validation loss: 2085.902, Runtime: 17.4977 secs\nEpoch: 1182, Step: 29550, training loss: 2074.720, validation loss: 2085.651, Runtime: 17.4816 secs\nEpoch: 1183, Step: 29575, training loss: 2074.611, validation loss: 2086.943, Runtime: 17.3657 secs\nEpoch: 1184, Step: 29600, training loss: 2074.929, validation loss: 2086.375, Runtime: 17.4041 secs\nEpoch: 1185, Step: 29625, training loss: 2074.697, validation loss: 2086.160, Runtime: 17.3874 secs\nEpoch: 1186, Step: 29650, training loss: 2074.546, validation loss: 2086.078, Runtime: 17.3794 secs\nEpoch: 1187, Step: 29675, training loss: 2074.651, validation loss: 2085.943, Runtime: 17.1615 secs\nEpoch: 1188, Step: 29700, training loss: 2074.453, validation loss: 2085.617, Runtime: 17.3632 secs\nEpoch: 1189, Step: 29725, training loss: 2074.747, validation loss: 2085.706, Runtime: 17.3629 secs\nEpoch: 1190, Step: 29750, training loss: 2074.778, validation loss: 2085.846, Runtime: 17.4561 secs\nEpoch: 1191, Step: 29775, training loss: 2074.603, validation loss: 2086.305, Runtime: 17.6105 secs\nEpoch: 1192, Step: 29800, training loss: 2074.626, validation loss: 2086.303, Runtime: 17.3531 secs\nEpoch: 1193, Step: 29825, training loss: 2074.533, validation loss: 2086.333, Runtime: 17.5217 secs\nEpoch: 1194, Step: 29850, training loss: 2074.804, validation loss: 2086.030, Runtime: 17.3378 secs\nLearning rate decreased to 0.000081\nEpoch: 1195, Step: 29875, training loss: 2074.632, validation loss: 2085.842, Runtime: 17.4468 secs\nEpoch: 1196, Step: 29900, training loss: 2074.648, validation loss: 2086.039, Runtime: 17.4975 secs\nEpoch: 1197, Step: 29925, training loss: 2074.485, validation loss: 2086.054, Runtime: 17.8603 secs\nEpoch: 1198, Step: 29950, training loss: 2074.612, validation loss: 2085.807, Runtime: 17.4684 secs\nEpoch: 1199, Step: 29975, training loss: 2074.471, validation loss: 2086.936, Runtime: 17.2944 secs\nEpoch: 1200, Step: 30000, training loss: 2074.756, validation loss: 2086.266, Runtime: 17.3082 secs\nEpoch: 1201, Step: 30025, training loss: 2074.423, validation loss: 2085.567, Runtime: 17.1715 secs\nEpoch: 1202, Step: 30050, training loss: 2074.410, validation loss: 2085.639, Runtime: 17.4557 secs\nEpoch: 1203, Step: 30075, training loss: 2074.676, validation loss: 2086.144, Runtime: 17.2663 secs\nEpoch: 1204, Step: 30100, training loss: 2074.417, validation loss: 2086.624, Runtime: 17.4467 secs\nEpoch: 1205, Step: 30125, training loss: 2074.796, validation loss: 2086.178, Runtime: 17.4860 secs\nLearning rate decreased to 0.000077\nEpoch: 1206, Step: 30150, training loss: 2074.452, validation loss: 2086.467, Runtime: 17.3671 secs\nEpoch: 1207, Step: 30175, training loss: 2074.556, validation loss: 2085.857, Runtime: 17.5267 secs\nEpoch: 1208, Step: 30200, training loss: 2074.455, validation loss: 2086.250, Runtime: 17.4203 secs\nEpoch: 1209, Step: 30225, training loss: 2074.487, validation loss: 2086.133, Runtime: 17.5326 secs\nEpoch: 1210, Step: 30250, training loss: 2074.580, validation loss: 2086.167, Runtime: 17.5059 secs\nEpoch: 1211, Step: 30275, training loss: 2074.366, validation loss: 2085.877, Runtime: 17.3910 secs\nEpoch: 1212, Step: 30300, training loss: 2074.500, validation loss: 2086.774, Runtime: 17.4234 secs\nEpoch: 1213, Step: 30325, training loss: 2074.449, validation loss: 2086.021, Runtime: 17.4563 secs\nEpoch: 1214, Step: 30350, training loss: 2074.483, validation loss: 2086.247, Runtime: 17.4905 secs\nEpoch: 1215, Step: 30375, training loss: 2074.575, validation loss: 2086.065, Runtime: 17.5094 secs\nEpoch: 1216, Step: 30400, training loss: 2074.504, validation loss: 2086.871, Runtime: 17.6058 secs\nEpoch: 1217, Step: 30425, training loss: 2074.731, validation loss: 2086.091, Runtime: 17.5643 secs\nLearning rate decreased to 0.000073\nEpoch: 1218, Step: 30450, training loss: 2074.600, validation loss: 2085.819, Runtime: 17.6851 secs\nEpoch: 1219, Step: 30475, training loss: 2074.517, validation loss: 2086.146, Runtime: 17.7522 secs\nEpoch: 1220, Step: 30500, training loss: 2074.470, validation loss: 2086.020, Runtime: 17.4519 secs\nEpoch: 1221, Step: 30525, training loss: 2074.656, validation loss: 2085.689, Runtime: 17.4845 secs\nEpoch: 1222, Step: 30550, training loss: 2074.615, validation loss: 2086.360, Runtime: 17.4294 secs\nEpoch: 1223, Step: 30575, training loss: 2074.715, validation loss: 2086.069, Runtime: 17.3887 secs\nEpoch: 1224, Step: 30600, training loss: 2074.445, validation loss: 2086.319, Runtime: 17.4867 secs\nEpoch: 1225, Step: 30625, training loss: 2074.389, validation loss: 2085.573, Runtime: 17.2766 secs\nEpoch: 1226, Step: 30650, training loss: 2074.523, validation loss: 2085.913, Runtime: 17.4370 secs\nEpoch: 1227, Step: 30675, training loss: 2074.329, validation loss: 2086.818, Runtime: 17.3510 secs\nEpoch: 1228, Step: 30700, training loss: 2074.460, validation loss: 2086.082, Runtime: 17.5247 secs\nEpoch: 1229, Step: 30725, training loss: 2074.596, validation loss: 2086.378, Runtime: 17.4612 secs\nEpoch: 1230, Step: 30750, training loss: 2074.557, validation loss: 2085.875, Runtime: 17.4154 secs\nEpoch: 1231, Step: 30775, training loss: 2074.777, validation loss: 2085.854, Runtime: 17.5793 secs\nLearning rate decreased to 0.000069\nEpoch: 1232, Step: 30800, training loss: 2074.449, validation loss: 2085.531, Runtime: 17.4273 secs\nEpoch: 1233, Step: 30825, training loss: 2074.424, validation loss: 2086.380, Runtime: 17.3130 secs\nEpoch: 1234, Step: 30850, training loss: 2074.733, validation loss: 2085.526, Runtime: 17.1719 secs\nEpoch: 1235, Step: 30875, training loss: 2074.433, validation loss: 2085.842, Runtime: 17.2833 secs\nEpoch: 1236, Step: 30900, training loss: 2074.735, validation loss: 2085.932, Runtime: 17.4434 secs\nEpoch: 1237, Step: 30925, training loss: 2074.526, validation loss: 2085.756, Runtime: 17.4875 secs\nEpoch: 1238, Step: 30950, training loss: 2074.563, validation loss: 2086.063, Runtime: 17.4141 secs\nEpoch: 1239, Step: 30975, training loss: 2074.652, validation loss: 2086.813, Runtime: 17.4574 secs\nEpoch: 1240, Step: 31000, training loss: 2074.597, validation loss: 2086.491, Runtime: 17.4703 secs\nEpoch: 1241, Step: 31025, training loss: 2074.729, validation loss: 2086.225, Runtime: 17.4350 secs\nEpoch: 1242, Step: 31050, training loss: 2074.520, validation loss: 2085.904, Runtime: 17.3822 secs\nEpoch: 1243, Step: 31075, training loss: 2074.526, validation loss: 2085.810, Runtime: 18.1746 secs\nEpoch: 1244, Step: 31100, training loss: 2074.527, validation loss: 2085.867, Runtime: 17.2761 secs\nEpoch: 1245, Step: 31125, training loss: 2074.530, validation loss: 2085.913, Runtime: 17.3640 secs\nEpoch: 1246, Step: 31150, training loss: 2074.609, validation loss: 2085.679, Runtime: 17.4319 secs\nEpoch: 1247, Step: 31175, training loss: 2074.425, validation loss: 2086.044, Runtime: 17.4517 secs\nEpoch: 1248, Step: 31200, training loss: 2074.649, validation loss: 2086.312, Runtime: 17.4854 secs\nLearning rate decreased to 0.000066\nEpoch: 1249, Step: 31225, training loss: 2074.502, validation loss: 2086.577, Runtime: 17.4995 secs\nEpoch: 1250, Step: 31250, training loss: 2074.650, validation loss: 2086.908, Runtime: 17.4703 secs\nEpoch: 1251, Step: 31275, training loss: 2074.610, validation loss: 2086.113, Runtime: 17.3602 secs\nEpoch: 1252, Step: 31300, training loss: 2074.853, validation loss: 2085.950, Runtime: 17.4139 secs\nEpoch: 1253, Step: 31325, training loss: 2074.630, validation loss: 2086.366, Runtime: 17.4087 secs\nEpoch: 1254, Step: 31350, training loss: 2074.442, validation loss: 2086.013, Runtime: 17.3527 secs\nEpoch: 1255, Step: 31375, training loss: 2074.558, validation loss: 2086.422, Runtime: 17.3937 secs\nEpoch: 1256, Step: 31400, training loss: 2074.298, validation loss: 2085.599, Runtime: 17.3725 secs\nEpoch: 1257, Step: 31425, training loss: 2074.353, validation loss: 2086.015, Runtime: 17.4509 secs\nEpoch: 1258, Step: 31450, training loss: 2074.282, validation loss: 2086.245, Runtime: 17.3427 secs\nEpoch: 1259, Step: 31475, training loss: 2074.653, validation loss: 2085.361, Runtime: 17.3628 secs\nLearning rate decreased to 0.000062\nEpoch: 1260, Step: 31500, training loss: 2074.619, validation loss: 2086.620, Runtime: 17.4215 secs\nEpoch: 1261, Step: 31525, training loss: 2074.390, validation loss: 2086.029, Runtime: 17.3668 secs\nEpoch: 1262, Step: 31550, training loss: 2074.669, validation loss: 2086.524, Runtime: 17.3814 secs\nEpoch: 1263, Step: 31575, training loss: 2074.533, validation loss: 2086.793, Runtime: 17.1203 secs\nEpoch: 1264, Step: 31600, training loss: 2074.446, validation loss: 2086.071, Runtime: 17.2340 secs\nEpoch: 1265, Step: 31625, training loss: 2074.488, validation loss: 2086.211, Runtime: 17.2795 secs\nEpoch: 1266, Step: 31650, training loss: 2074.276, validation loss: 2086.491, Runtime: 17.3065 secs\nEpoch: 1267, Step: 31675, training loss: 2074.336, validation loss: 2085.742, Runtime: 17.4619 secs\nEpoch: 1268, Step: 31700, training loss: 2074.572, validation loss: 2085.899, Runtime: 17.4130 secs\nEpoch: 1269, Step: 31725, training loss: 2074.683, validation loss: 2086.195, Runtime: 17.4493 secs\nLearning rate decreased to 0.000059\nEpoch: 1270, Step: 31750, training loss: 2074.351, validation loss: 2086.342, Runtime: 17.5724 secs\nEpoch: 1271, Step: 31775, training loss: 2074.431, validation loss: 2085.723, Runtime: 17.6353 secs\nEpoch: 1272, Step: 31800, training loss: 2074.427, validation loss: 2085.891, Runtime: 17.5526 secs\nEpoch: 1273, Step: 31825, training loss: 2074.493, validation loss: 2085.550, Runtime: 17.7220 secs\nEpoch: 1274, Step: 31850, training loss: 2074.435, validation loss: 2086.209, Runtime: 17.3520 secs\nEpoch: 1275, Step: 31875, training loss: 2074.581, validation loss: 2086.363, Runtime: 17.3599 secs\nEpoch: 1276, Step: 31900, training loss: 2074.427, validation loss: 2085.846, Runtime: 17.4758 secs\nEpoch: 1277, Step: 31925, training loss: 2074.435, validation loss: 2086.190, Runtime: 17.4788 secs\nEpoch: 1278, Step: 31950, training loss: 2074.506, validation loss: 2086.345, Runtime: 17.5477 secs\nEpoch: 1279, Step: 31975, training loss: 2074.465, validation loss: 2086.142, Runtime: 17.3526 secs\nEpoch: 1280, Step: 32000, training loss: 2074.545, validation loss: 2086.124, Runtime: 17.3602 secs\nEpoch: 1281, Step: 32025, training loss: 2074.438, validation loss: 2085.883, Runtime: 17.4288 secs\nEpoch: 1282, Step: 32050, training loss: 2074.437, validation loss: 2086.497, Runtime: 17.5002 secs\nEpoch: 1283, Step: 32075, training loss: 2074.471, validation loss: 2086.058, Runtime: 17.4262 secs\nEpoch: 1284, Step: 32100, training loss: 2074.375, validation loss: 2085.761, Runtime: 17.4184 secs\nEpoch: 1285, Step: 32125, training loss: 2074.525, validation loss: 2085.947, Runtime: 17.5244 secs\nEpoch: 1286, Step: 32150, training loss: 2074.593, validation loss: 2085.712, Runtime: 17.4749 secs\nLearning rate decreased to 0.000056\nEpoch: 1287, Step: 32175, training loss: 2074.387, validation loss: 2085.994, Runtime: 17.3340 secs\nEpoch: 1288, Step: 32200, training loss: 2074.549, validation loss: 2085.837, Runtime: 17.4643 secs\nEpoch: 1289, Step: 32225, training loss: 2074.607, validation loss: 2086.286, Runtime: 17.3494 secs\nEpoch: 1290, Step: 32250, training loss: 2074.576, validation loss: 2086.409, Runtime: 17.2228 secs\nEpoch: 1291, Step: 32275, training loss: 2074.304, validation loss: 2086.211, Runtime: 17.2845 secs\nEpoch: 1292, Step: 32300, training loss: 2074.603, validation loss: 2086.089, Runtime: 17.3873 secs\nEpoch: 1293, Step: 32325, training loss: 2074.562, validation loss: 2086.410, Runtime: 17.6033 secs\nEpoch: 1294, Step: 32350, training loss: 2074.504, validation loss: 2085.879, Runtime: 17.3969 secs\nEpoch: 1295, Step: 32375, training loss: 2074.511, validation loss: 2086.305, Runtime: 17.4834 secs\nEpoch: 1296, Step: 32400, training loss: 2074.688, validation loss: 2086.013, Runtime: 17.4969 secs\nLearning rate decreased to 0.000053\nEpoch: 1297, Step: 32425, training loss: 2074.241, validation loss: 2086.168, Runtime: 17.4596 secs\nEpoch: 1298, Step: 32450, training loss: 2074.411, validation loss: 2086.123, Runtime: 17.4590 secs\nEpoch: 1299, Step: 32475, training loss: 2074.404, validation loss: 2086.378, Runtime: 17.3811 secs\nEpoch: 1300, Step: 32500, training loss: 2074.498, validation loss: 2086.308, Runtime: 17.3756 secs\nEpoch: 1301, Step: 32525, training loss: 2074.474, validation loss: 2085.933, Runtime: 17.4724 secs\nEpoch: 1302, Step: 32550, training loss: 2074.515, validation loss: 2086.364, Runtime: 17.4316 secs\nEpoch: 1303, Step: 32575, training loss: 2074.593, validation loss: 2085.974, Runtime: 17.2909 secs\nLearning rate decreased to 0.000051\nEpoch: 1304, Step: 32600, training loss: 2074.708, validation loss: 2086.358, Runtime: 17.4591 secs\nEpoch: 1305, Step: 32625, training loss: 2074.491, validation loss: 2086.879, Runtime: 17.4053 secs\nEpoch: 1306, Step: 32650, training loss: 2074.301, validation loss: 2086.619, Runtime: 17.3930 secs\nEpoch: 1307, Step: 32675, training loss: 2074.422, validation loss: 2086.163, Runtime: 17.4518 secs\nEpoch: 1308, Step: 32700, training loss: 2074.637, validation loss: 2086.657, Runtime: 17.4699 secs\nEpoch: 1309, Step: 32725, training loss: 2074.493, validation loss: 2086.799, Runtime: 17.4835 secs\nEpoch: 1310, Step: 32750, training loss: 2074.383, validation loss: 2086.146, Runtime: 17.3809 secs\nEpoch: 1311, Step: 32775, training loss: 2074.456, validation loss: 2085.935, Runtime: 17.3026 secs\nEpoch: 1312, Step: 32800, training loss: 2074.494, validation loss: 2086.208, Runtime: 17.4367 secs\nEpoch: 1313, Step: 32825, training loss: 2074.293, validation loss: 2086.248, Runtime: 17.3537 secs\nEpoch: 1314, Step: 32850, training loss: 2074.421, validation loss: 2086.386, Runtime: 17.2441 secs\nEpoch: 1315, Step: 32875, training loss: 2074.419, validation loss: 2085.948, Runtime: 17.3234 secs\nEpoch: 1316, Step: 32900, training loss: 2074.648, validation loss: 2086.325, Runtime: 17.4192 secs\nLearning rate decreased to 0.000048\nEpoch: 1317, Step: 32925, training loss: 2074.551, validation loss: 2086.760, Runtime: 17.4783 secs\nEpoch: 1318, Step: 32950, training loss: 2074.503, validation loss: 2086.678, Runtime: 17.4391 secs\nEpoch: 1319, Step: 32975, training loss: 2074.576, validation loss: 2086.250, Runtime: 17.6676 secs\nEpoch: 1320, Step: 33000, training loss: 2074.255, validation loss: 2086.120, Runtime: 17.3831 secs\nEpoch: 1321, Step: 33025, training loss: 2074.462, validation loss: 2086.340, Runtime: 17.4207 secs\nEpoch: 1322, Step: 33050, training loss: 2074.513, validation loss: 2085.997, Runtime: 17.4383 secs\nEpoch: 1323, Step: 33075, training loss: 2074.313, validation loss: 2086.285, Runtime: 17.4128 secs\nEpoch: 1324, Step: 33100, training loss: 2074.500, validation loss: 2086.068, Runtime: 17.4193 secs\nEpoch: 1325, Step: 33125, training loss: 2074.773, validation loss: 2085.913, Runtime: 17.3555 secs\nLearning rate decreased to 0.000046\nEpoch: 1326, Step: 33150, training loss: 2074.477, validation loss: 2086.763, Runtime: 17.9397 secs\nEpoch: 1327, Step: 33175, training loss: 2074.577, validation loss: 2086.630, Runtime: 17.3100 secs\nEpoch: 1328, Step: 33200, training loss: 2074.306, validation loss: 2085.751, Runtime: 17.5260 secs\nEpoch: 1329, Step: 33225, training loss: 2074.247, validation loss: 2085.777, Runtime: 17.5340 secs\nEpoch: 1330, Step: 33250, training loss: 2074.567, validation loss: 2085.627, Runtime: 17.3199 secs\nEpoch: 1331, Step: 33275, training loss: 2074.595, validation loss: 2085.652, Runtime: 17.3550 secs\nEpoch: 1332, Step: 33300, training loss: 2074.450, validation loss: 2086.663, Runtime: 17.4537 secs\nEpoch: 1333, Step: 33325, training loss: 2074.195, validation loss: 2086.326, Runtime: 17.3383 secs\nEpoch: 1334, Step: 33350, training loss: 2074.486, validation loss: 2086.472, Runtime: 17.5018 secs\nEpoch: 1335, Step: 33375, training loss: 2074.490, validation loss: 2086.329, Runtime: 17.3990 secs\nEpoch: 1336, Step: 33400, training loss: 2074.585, validation loss: 2086.591, Runtime: 17.4995 secs\nEpoch: 1337, Step: 33425, training loss: 2074.464, validation loss: 2085.714, Runtime: 17.3756 secs\nEpoch: 1338, Step: 33450, training loss: 2074.372, validation loss: 2086.089, Runtime: 17.3908 secs\nEpoch: 1339, Step: 33475, training loss: 2074.525, validation loss: 2085.961, Runtime: 17.3363 secs\nEpoch: 1340, Step: 33500, training loss: 2074.396, validation loss: 2085.785, Runtime: 17.3407 secs\nEpoch: 1341, Step: 33525, training loss: 2074.289, validation loss: 2085.918, Runtime: 17.2970 secs\nEpoch: 1342, Step: 33550, training loss: 2074.562, validation loss: 2086.033, Runtime: 17.4277 secs\nEpoch: 1343, Step: 33575, training loss: 2074.785, validation loss: 2086.146, Runtime: 17.3246 secs\nLearning rate decreased to 0.000044\nEpoch: 1344, Step: 33600, training loss: 2074.421, validation loss: 2085.810, Runtime: 17.3888 secs\nEpoch: 1345, Step: 33625, training loss: 2074.517, validation loss: 2085.696, Runtime: 17.3742 secs\nEpoch: 1346, Step: 33650, training loss: 2074.490, validation loss: 2085.764, Runtime: 17.2726 secs\nEpoch: 1347, Step: 33675, training loss: 2074.340, validation loss: 2086.259, Runtime: 17.3815 secs\nEpoch: 1348, Step: 33700, training loss: 2074.306, validation loss: 2085.854, Runtime: 17.4537 secs\nEpoch: 1349, Step: 33725, training loss: 2074.495, validation loss: 2086.108, Runtime: 17.5157 secs\nEpoch: 1350, Step: 33750, training loss: 2074.703, validation loss: 2086.481, Runtime: 17.5185 secs\nLearning rate decreased to 0.000041\nEpoch: 1351, Step: 33775, training loss: 2074.723, validation loss: 2085.944, Runtime: 17.8124 secs\nEpoch: 1352, Step: 33800, training loss: 2074.391, validation loss: 2086.053, Runtime: 17.3504 secs\nEpoch: 1353, Step: 33825, training loss: 2074.565, validation loss: 2085.616, Runtime: 17.3072 secs\nEpoch: 1354, Step: 33850, training loss: 2074.346, validation loss: 2086.301, Runtime: 17.5379 secs\nEpoch: 1355, Step: 33875, training loss: 2074.660, validation loss: 2086.141, Runtime: 17.4617 secs\nEpoch: 1356, Step: 33900, training loss: 2074.254, validation loss: 2086.182, Runtime: 17.6225 secs\nEpoch: 1357, Step: 33925, training loss: 2074.424, validation loss: 2086.238, Runtime: 17.4120 secs\nEpoch: 1358, Step: 33950, training loss: 2074.576, validation loss: 2086.615, Runtime: 17.3764 secs\nEpoch: 1359, Step: 33975, training loss: 2074.385, validation loss: 2086.482, Runtime: 17.3601 secs\nEpoch: 1360, Step: 34000, training loss: 2074.474, validation loss: 2086.087, Runtime: 17.2942 secs\nEpoch: 1361, Step: 34025, training loss: 2074.480, validation loss: 2086.375, Runtime: 17.2550 secs\nEpoch: 1362, Step: 34050, training loss: 2074.533, validation loss: 2086.827, Runtime: 17.1645 secs\nEpoch: 1363, Step: 34075, training loss: 2074.490, validation loss: 2086.507, Runtime: 17.2628 secs\nEpoch: 1364, Step: 34100, training loss: 2074.656, validation loss: 2086.173, Runtime: 17.5462 secs\nLearning rate decreased to 0.000039\nEpoch: 1365, Step: 34125, training loss: 2074.598, validation loss: 2085.748, Runtime: 17.3049 secs\nEpoch: 1366, Step: 34150, training loss: 2074.171, validation loss: 2086.336, Runtime: 17.2874 secs\nEpoch: 1367, Step: 34175, training loss: 2074.489, validation loss: 2086.155, Runtime: 17.4991 secs\nEpoch: 1368, Step: 34200, training loss: 2074.339, validation loss: 2086.615, Runtime: 17.3259 secs\nEpoch: 1369, Step: 34225, training loss: 2074.480, validation loss: 2085.985, Runtime: 17.4223 secs\nEpoch: 1370, Step: 34250, training loss: 2074.415, validation loss: 2086.475, Runtime: 17.4507 secs\nEpoch: 1371, Step: 34275, training loss: 2074.500, validation loss: 2086.431, Runtime: 17.3256 secs\nEpoch: 1372, Step: 34300, training loss: 2074.251, validation loss: 2086.085, Runtime: 17.6242 secs\nEpoch: 1373, Step: 34325, training loss: 2074.697, validation loss: 2086.489, Runtime: 17.4789 secs\nLearning rate decreased to 0.000037\nEpoch: 1374, Step: 34350, training loss: 2074.595, validation loss: 2086.044, Runtime: 17.4947 secs\nEpoch: 1375, Step: 34375, training loss: 2074.335, validation loss: 2086.135, Runtime: 17.3620 secs\nEpoch: 1376, Step: 34400, training loss: 2074.397, validation loss: 2086.075, Runtime: 17.3678 secs\nEpoch: 1377, Step: 34425, training loss: 2074.407, validation loss: 2086.381, Runtime: 17.4078 secs\nEpoch: 1378, Step: 34450, training loss: 2074.539, validation loss: 2086.252, Runtime: 17.2584 secs\nEpoch: 1379, Step: 34475, training loss: 2074.393, validation loss: 2086.476, Runtime: 17.4170 secs\nEpoch: 1380, Step: 34500, training loss: 2074.441, validation loss: 2086.104, Runtime: 17.5655 secs\nEpoch: 1381, Step: 34525, training loss: 2074.498, validation loss: 2085.923, Runtime: 17.4033 secs\nEpoch: 1382, Step: 34550, training loss: 2074.688, validation loss: 2086.178, Runtime: 17.3640 secs\nLearning rate decreased to 0.000035\nEpoch: 1383, Step: 34575, training loss: 2074.383, validation loss: 2086.787, Runtime: 17.3856 secs\nEpoch: 1384, Step: 34600, training loss: 2074.487, validation loss: 2085.702, Runtime: 17.4425 secs\nEpoch: 1385, Step: 34625, training loss: 2074.370, validation loss: 2086.233, Runtime: 17.4148 secs\nEpoch: 1386, Step: 34650, training loss: 2074.511, validation loss: 2086.333, Runtime: 17.5276 secs\nEpoch: 1387, Step: 34675, training loss: 2074.519, validation loss: 2086.270, Runtime: 17.3011 secs\nEpoch: 1388, Step: 34700, training loss: 2074.460, validation loss: 2086.248, Runtime: 17.3984 secs\nEpoch: 1389, Step: 34725, training loss: 2074.645, validation loss: 2085.394, Runtime: 17.3350 secs\nLearning rate decreased to 0.000034\nEpoch: 1390, Step: 34750, training loss: 2074.263, validation loss: 2085.955, Runtime: 17.4378 secs\nEpoch: 1391, Step: 34775, training loss: 2074.401, validation loss: 2086.529, Runtime: 17.4089 secs\nEpoch: 1392, Step: 34800, training loss: 2074.432, validation loss: 2086.209, Runtime: 17.4760 secs\nEpoch: 1393, Step: 34825, training loss: 2074.432, validation loss: 2086.394, Runtime: 17.3825 secs\nEpoch: 1394, Step: 34850, training loss: 2074.327, validation loss: 2086.241, Runtime: 17.4112 secs\nEpoch: 1395, Step: 34875, training loss: 2074.382, validation loss: 2086.325, Runtime: 17.3638 secs\nEpoch: 1396, Step: 34900, training loss: 2074.599, validation loss: 2086.115, Runtime: 17.4238 secs\nLearning rate decreased to 0.000032\nEpoch: 1397, Step: 34925, training loss: 2074.449, validation loss: 2085.743, Runtime: 17.4546 secs\nEpoch: 1398, Step: 34950, training loss: 2074.543, validation loss: 2085.616, Runtime: 17.3588 secs\nEpoch: 1399, Step: 34975, training loss: 2074.531, validation loss: 2085.678, Runtime: 17.3806 secs\nEpoch: 1400, Step: 35000, training loss: 2074.368, validation loss: 2085.867, Runtime: 17.6352 secs\nEpoch: 1401, Step: 35025, training loss: 2074.353, validation loss: 2085.797, Runtime: 17.5601 secs\nEpoch: 1402, Step: 35050, training loss: 2074.465, validation loss: 2086.352, Runtime: 17.5046 secs\nEpoch: 1403, Step: 35075, training loss: 2074.027, validation loss: 2086.542, Runtime: 17.4633 secs\nEpoch: 1404, Step: 35100, training loss: 2074.448, validation loss: 2086.547, Runtime: 17.6105 secs\nEpoch: 1405, Step: 35125, training loss: 2074.266, validation loss: 2086.430, Runtime: 17.3539 secs\nEpoch: 1406, Step: 35150, training loss: 2074.283, validation loss: 2085.671, Runtime: 17.4082 secs\nEpoch: 1407, Step: 35175, training loss: 2074.250, validation loss: 2086.395, Runtime: 17.3249 secs\nEpoch: 1408, Step: 35200, training loss: 2074.461, validation loss: 2085.695, Runtime: 17.3045 secs\nEpoch: 1409, Step: 35225, training loss: 2074.425, validation loss: 2085.641, Runtime: 17.3848 secs\nEpoch: 1410, Step: 35250, training loss: 2074.500, validation loss: 2085.708, Runtime: 17.3790 secs\nLearning rate decreased to 0.000030\nEpoch: 1411, Step: 35275, training loss: 2074.471, validation loss: 2086.335, Runtime: 17.4913 secs\nEpoch: 1412, Step: 35300, training loss: 2074.374, validation loss: 2086.342, Runtime: 17.4841 secs\nEpoch: 1413, Step: 35325, training loss: 2074.354, validation loss: 2086.904, Runtime: 17.3383 secs\nEpoch: 1414, Step: 35350, training loss: 2074.299, validation loss: 2086.666, Runtime: 17.3846 secs\nEpoch: 1415, Step: 35375, training loss: 2074.690, validation loss: 2086.345, Runtime: 17.4153 secs\nEpoch: 1416, Step: 35400, training loss: 2074.314, validation loss: 2086.316, Runtime: 17.5065 secs\nEpoch: 1417, Step: 35425, training loss: 2074.412, validation loss: 2085.943, Runtime: 17.4264 secs\nEpoch: 1418, Step: 35450, training loss: 2074.453, validation loss: 2086.364, Runtime: 17.4097 secs\nEpoch: 1419, Step: 35475, training loss: 2074.337, validation loss: 2086.235, Runtime: 17.3838 secs\nEpoch: 1420, Step: 35500, training loss: 2074.472, validation loss: 2086.164, Runtime: 17.3781 secs\nEpoch: 1421, Step: 35525, training loss: 2074.517, validation loss: 2086.143, Runtime: 17.6832 secs\nEpoch: 1422, Step: 35550, training loss: 2074.287, validation loss: 2086.103, Runtime: 17.3970 secs\nEpoch: 1423, Step: 35575, training loss: 2074.261, validation loss: 2086.238, Runtime: 17.4776 secs\nEpoch: 1424, Step: 35600, training loss: 2074.611, validation loss: 2086.429, Runtime: 17.3373 secs\nLearning rate decreased to 0.000029\nEpoch: 1425, Step: 35625, training loss: 2074.379, validation loss: 2086.171, Runtime: 17.4657 secs\nEpoch: 1426, Step: 35650, training loss: 2074.446, validation loss: 2085.521, Runtime: 17.4427 secs\nEpoch: 1427, Step: 35675, training loss: 2074.502, validation loss: 2085.759, Runtime: 17.3732 secs\nEpoch: 1428, Step: 35700, training loss: 2074.398, validation loss: 2085.852, Runtime: 17.3163 secs\nEpoch: 1429, Step: 35725, training loss: 2074.320, validation loss: 2086.222, Runtime: 17.3495 secs\nEpoch: 1430, Step: 35750, training loss: 2074.244, validation loss: 2085.861, Runtime: 17.3255 secs\nEpoch: 1431, Step: 35775, training loss: 2074.355, validation loss: 2086.153, Runtime: 17.4824 secs\nEpoch: 1432, Step: 35800, training loss: 2074.101, validation loss: 2086.256, Runtime: 17.4608 secs\nEpoch: 1433, Step: 35825, training loss: 2074.184, validation loss: 2086.104, Runtime: 17.5219 secs\nEpoch: 1434, Step: 35850, training loss: 2074.392, validation loss: 2086.523, Runtime: 17.3037 secs\nEpoch: 1435, Step: 35875, training loss: 2074.507, validation loss: 2086.324, Runtime: 17.3654 secs\nLearning rate decreased to 0.000027\nEpoch: 1436, Step: 35900, training loss: 2074.194, validation loss: 2085.670, Runtime: 17.3521 secs\nEpoch: 1437, Step: 35925, training loss: 2074.374, validation loss: 2086.671, Runtime: 17.2922 secs\nEpoch: 1438, Step: 35950, training loss: 2074.445, validation loss: 2086.967, Runtime: 17.3667 secs\nEpoch: 1439, Step: 35975, training loss: 2074.403, validation loss: 2085.959, Runtime: 17.4394 secs\nEpoch: 1440, Step: 36000, training loss: 2074.661, validation loss: 2086.343, Runtime: 17.4065 secs\nEpoch: 1441, Step: 36025, training loss: 2074.562, validation loss: 2086.535, Runtime: 17.3387 secs\nEpoch: 1442, Step: 36050, training loss: 2074.442, validation loss: 2086.580, Runtime: 17.4270 secs\nEpoch: 1443, Step: 36075, training loss: 2074.084, validation loss: 2085.608, Runtime: 17.4030 secs\nEpoch: 1444, Step: 36100, training loss: 2074.218, validation loss: 2086.345, Runtime: 17.4292 secs\nEpoch: 1445, Step: 36125, training loss: 2074.414, validation loss: 2086.453, Runtime: 17.2932 secs\nEpoch: 1446, Step: 36150, training loss: 2074.351, validation loss: 2086.421, Runtime: 17.4109 secs\nEpoch: 1447, Step: 36175, training loss: 2074.491, validation loss: 2086.501, Runtime: 17.4089 secs\nEpoch: 1448, Step: 36200, training loss: 2074.183, validation loss: 2086.561, Runtime: 17.4256 secs\nEpoch: 1449, Step: 36225, training loss: 2074.341, validation loss: 2086.038, Runtime: 17.4234 secs\nEpoch: 1450, Step: 36250, training loss: 2074.680, validation loss: 2086.632, Runtime: 17.4215 secs\nLearning rate decreased to 0.000026\nEpoch: 1451, Step: 36275, training loss: 2074.440, validation loss: 2085.998, Runtime: 17.3779 secs\nEpoch: 1452, Step: 36300, training loss: 2074.476, validation loss: 2086.349, Runtime: 17.3710 secs\nEpoch: 1453, Step: 36325, training loss: 2074.301, validation loss: 2085.913, Runtime: 17.5104 secs\nEpoch: 1454, Step: 36350, training loss: 2074.325, validation loss: 2086.339, Runtime: 17.5382 secs\nEpoch: 1455, Step: 36375, training loss: 2074.207, validation loss: 2086.062, Runtime: 17.3388 secs\nEpoch: 1456, Step: 36400, training loss: 2074.580, validation loss: 2086.486, Runtime: 17.4128 secs\nEpoch: 1457, Step: 36425, training loss: 2074.326, validation loss: 2085.881, Runtime: 17.4783 secs\nEpoch: 1458, Step: 36450, training loss: 2074.308, validation loss: 2086.546, Runtime: 17.7863 secs\nEpoch: 1459, Step: 36475, training loss: 2074.188, validation loss: 2086.378, Runtime: 17.4499 secs\nEpoch: 1460, Step: 36500, training loss: 2074.371, validation loss: 2085.441, Runtime: 17.3922 secs\nEpoch: 1461, Step: 36525, training loss: 2074.271, validation loss: 2086.255, Runtime: 17.3875 secs\nEpoch: 1462, Step: 36550, training loss: 2074.481, validation loss: 2086.336, Runtime: 17.3947 secs\nEpoch: 1463, Step: 36575, training loss: 2074.375, validation loss: 2086.001, Runtime: 17.3987 secs\nEpoch: 1464, Step: 36600, training loss: 2074.334, validation loss: 2086.562, Runtime: 17.4441 secs\nEpoch: 1465, Step: 36625, training loss: 2074.485, validation loss: 2086.291, Runtime: 17.3038 secs\nLearning rate decreased to 0.000025\nEpoch: 1466, Step: 36650, training loss: 2074.350, validation loss: 2086.478, Runtime: 17.4614 secs\nEpoch: 1467, Step: 36675, training loss: 2074.219, validation loss: 2085.610, Runtime: 17.2729 secs\nEpoch: 1468, Step: 36700, training loss: 2074.211, validation loss: 2086.569, Runtime: 17.4914 secs\nEpoch: 1469, Step: 36725, training loss: 2074.319, validation loss: 2086.185, Runtime: 17.4116 secs\nEpoch: 1470, Step: 36750, training loss: 2074.326, validation loss: 2085.881, Runtime: 17.4354 secs\nEpoch: 1471, Step: 36775, training loss: 2074.274, validation loss: 2086.396, Runtime: 17.4444 secs\nEpoch: 1472, Step: 36800, training loss: 2074.459, validation loss: 2086.202, Runtime: 17.8119 secs\nLearning rate decreased to 0.000024\nEpoch: 1473, Step: 36825, training loss: 2074.435, validation loss: 2085.802, Runtime: 17.4320 secs\nEpoch: 1474, Step: 36850, training loss: 2074.296, validation loss: 2085.964, Runtime: 17.4800 secs\nEpoch: 1475, Step: 36875, training loss: 2074.167, validation loss: 2086.351, Runtime: 17.4642 secs\nEpoch: 1476, Step: 36900, training loss: 2074.163, validation loss: 2085.663, Runtime: 17.4478 secs\nEpoch: 1477, Step: 36925, training loss: 2074.398, validation loss: 2086.172, Runtime: 17.3876 secs\nEpoch: 1478, Step: 36950, training loss: 2074.211, validation loss: 2086.250, Runtime: 17.2816 secs\nEpoch: 1479, Step: 36975, training loss: 2074.362, validation loss: 2086.447, Runtime: 17.3200 secs\nEpoch: 1480, Step: 37000, training loss: 2074.516, validation loss: 2085.988, Runtime: 17.4575 secs\nLearning rate decreased to 0.000022\nEpoch: 1481, Step: 37025, training loss: 2074.399, validation loss: 2086.607, Runtime: 17.4498 secs\nEpoch: 1482, Step: 37050, training loss: 2074.540, validation loss: 2085.771, Runtime: 17.3599 secs\nEpoch: 1483, Step: 37075, training loss: 2074.499, validation loss: 2085.756, Runtime: 17.4331 secs\nEpoch: 1484, Step: 37100, training loss: 2074.460, validation loss: 2085.912, Runtime: 17.3680 secs\nEpoch: 1485, Step: 37125, training loss: 2074.472, validation loss: 2086.073, Runtime: 17.4234 secs\nEpoch: 1486, Step: 37150, training loss: 2074.445, validation loss: 2086.298, Runtime: 17.2297 secs\nEpoch: 1487, Step: 37175, training loss: 2074.175, validation loss: 2085.605, Runtime: 17.4351 secs\nEpoch: 1488, Step: 37200, training loss: 2074.441, validation loss: 2086.281, Runtime: 17.4687 secs\nEpoch: 1489, Step: 37225, training loss: 2074.103, validation loss: 2086.321, Runtime: 17.4958 secs\nEpoch: 1490, Step: 37250, training loss: 2074.492, validation loss: 2085.842, Runtime: 17.3997 secs\nLearning rate decreased to 0.000021\nEpoch: 1491, Step: 37275, training loss: 2074.536, validation loss: 2086.412, Runtime: 17.3781 secs\nEpoch: 1492, Step: 37300, training loss: 2074.472, validation loss: 2085.987, Runtime: 17.4314 secs\nEpoch: 1493, Step: 37325, training loss: 2074.230, validation loss: 2086.035, Runtime: 17.5429 secs\nEpoch: 1494, Step: 37350, training loss: 2074.149, validation loss: 2086.453, Runtime: 17.4607 secs\nEpoch: 1495, Step: 37375, training loss: 2074.381, validation loss: 2086.500, Runtime: 17.4338 secs\nEpoch: 1496, Step: 37400, training loss: 2074.542, validation loss: 2086.009, Runtime: 17.4127 secs\nLearning rate decreased to 0.000020\nEpoch: 1497, Step: 37425, training loss: 2074.527, validation loss: 2086.089, Runtime: 17.9187 secs\nEpoch: 1498, Step: 37450, training loss: 2074.203, validation loss: 2086.107, Runtime: 17.5474 secs\nEpoch: 1499, Step: 37475, training loss: 2074.615, validation loss: 2086.153, Runtime: 17.8948 secs\nEpoch: 1500, Step: 37500, training loss: 2074.391, validation loss: 2085.671, Runtime: 17.5449 secs\nEpoch: 1501, Step: 37525, training loss: 2074.395, validation loss: 2086.334, Runtime: 17.3675 secs\nEpoch: 1502, Step: 37550, training loss: 2074.147, validation loss: 2086.360, Runtime: 17.4619 secs\nEpoch: 1503, Step: 37575, training loss: 2074.337, validation loss: 2085.932, Runtime: 17.3765 secs\nEpoch: 1504, Step: 37600, training loss: 2074.158, validation loss: 2086.096, Runtime: 17.4277 secs\nEpoch: 1505, Step: 37625, training loss: 2074.347, validation loss: 2086.390, Runtime: 17.3212 secs\nEpoch: 1506, Step: 37650, training loss: 2074.074, validation loss: 2086.419, Runtime: 17.3485 secs\nEpoch: 1507, Step: 37675, training loss: 2074.348, validation loss: 2086.344, Runtime: 17.5845 secs\nEpoch: 1508, Step: 37700, training loss: 2074.419, validation loss: 2086.272, Runtime: 17.7339 secs\nLearning rate decreased to 0.000019\nEpoch: 1509, Step: 37725, training loss: 2074.455, validation loss: 2086.030, Runtime: 17.4286 secs\nEpoch: 1510, Step: 37750, training loss: 2074.344, validation loss: 2086.362, Runtime: 17.4799 secs\nEpoch: 1511, Step: 37775, training loss: 2074.467, validation loss: 2085.782, Runtime: 17.5073 secs\nEpoch: 1512, Step: 37800, training loss: 2074.595, validation loss: 2086.005, Runtime: 17.4655 secs\nEpoch: 1513, Step: 37825, training loss: 2074.301, validation loss: 2086.551, Runtime: 17.3644 secs\nEpoch: 1514, Step: 37850, training loss: 2074.314, validation loss: 2086.359, Runtime: 17.6791 secs\nEpoch: 1515, Step: 37875, training loss: 2074.397, validation loss: 2086.148, Runtime: 17.4197 secs\nEpoch: 1516, Step: 37900, training loss: 2074.446, validation loss: 2086.408, Runtime: 17.4700 secs\nEpoch: 1517, Step: 37925, training loss: 2074.396, validation loss: 2086.383, Runtime: 17.3329 secs\nEpoch: 1518, Step: 37950, training loss: 2074.505, validation loss: 2086.626, Runtime: 17.2747 secs\nEpoch: 1519, Step: 37975, training loss: 2074.371, validation loss: 2086.188, Runtime: 17.3665 secs\nEpoch: 1520, Step: 38000, training loss: 2074.424, validation loss: 2086.345, Runtime: 17.4076 secs\nEpoch: 1521, Step: 38025, training loss: 2074.591, validation loss: 2085.875, Runtime: 17.3627 secs\nLearning rate decreased to 0.000018\nEpoch: 1522, Step: 38050, training loss: 2074.461, validation loss: 2086.297, Runtime: 17.3233 secs\nEpoch: 1523, Step: 38075, training loss: 2074.474, validation loss: 2086.621, Runtime: 17.3718 secs\nEpoch: 1524, Step: 38100, training loss: 2074.354, validation loss: 2086.145, Runtime: 17.5394 secs\nEpoch: 1525, Step: 38125, training loss: 2074.427, validation loss: 2086.780, Runtime: 17.4402 secs\nEpoch: 1526, Step: 38150, training loss: 2074.447, validation loss: 2086.319, Runtime: 17.4488 secs\nEpoch: 1527, Step: 38175, training loss: 2074.706, validation loss: 2086.371, Runtime: 17.2469 secs\nLearning rate decreased to 0.000017\nEpoch: 1528, Step: 38200, training loss: 2074.482, validation loss: 2086.018, Runtime: 17.3231 secs\nEpoch: 1529, Step: 38225, training loss: 2074.240, validation loss: 2085.916, Runtime: 17.4615 secs\nEpoch: 1530, Step: 38250, training loss: 2074.260, validation loss: 2086.034, Runtime: 17.7167 secs\nEpoch: 1531, Step: 38275, training loss: 2074.372, validation loss: 2085.892, Runtime: 17.2659 secs\nEpoch: 1532, Step: 38300, training loss: 2074.279, validation loss: 2086.672, Runtime: 17.3324 secs\nEpoch: 1533, Step: 38325, training loss: 2074.645, validation loss: 2086.251, Runtime: 17.4606 secs\nEpoch: 1534, Step: 38350, training loss: 2074.291, validation loss: 2086.011, Runtime: 17.4458 secs\nEpoch: 1535, Step: 38375, training loss: 2074.220, validation loss: 2086.608, Runtime: 17.5106 secs\nEpoch: 1536, Step: 38400, training loss: 2074.468, validation loss: 2086.399, Runtime: 17.3145 secs\nEpoch: 1537, Step: 38425, training loss: 2074.445, validation loss: 2085.440, Runtime: 17.4322 secs\nEpoch: 1538, Step: 38450, training loss: 2074.302, validation loss: 2085.956, Runtime: 17.4742 secs\nEpoch: 1539, Step: 38475, training loss: 2074.645, validation loss: 2086.269, Runtime: 17.3779 secs\nLearning rate decreased to 0.000016\nEpoch: 1540, Step: 38500, training loss: 2074.257, validation loss: 2086.322, Runtime: 17.3593 secs\nEpoch: 1541, Step: 38525, training loss: 2074.287, validation loss: 2086.102, Runtime: 17.2546 secs\nEpoch: 1542, Step: 38550, training loss: 2074.401, validation loss: 2086.450, Runtime: 17.2534 secs\nEpoch: 1543, Step: 38575, training loss: 2074.395, validation loss: 2086.313, Runtime: 17.4693 secs\nEpoch: 1544, Step: 38600, training loss: 2074.473, validation loss: 2086.168, Runtime: 17.9017 secs\nEpoch: 1545, Step: 38625, training loss: 2074.319, validation loss: 2085.571, Runtime: 17.3560 secs\nEpoch: 1546, Step: 38650, training loss: 2074.391, validation loss: 2086.009, Runtime: 17.2662 secs\nEpoch: 1547, Step: 38675, training loss: 2074.304, validation loss: 2086.477, Runtime: 17.3674 secs\nEpoch: 1548, Step: 38700, training loss: 2074.161, validation loss: 2086.499, Runtime: 17.2969 secs\nEpoch: 1549, Step: 38725, training loss: 2074.352, validation loss: 2086.099, Runtime: 17.4227 secs\nEpoch: 1550, Step: 38750, training loss: 2074.493, validation loss: 2086.158, Runtime: 17.2588 secs\nLearning rate decreased to 0.000016\nEpoch: 1551, Step: 38775, training loss: 2074.345, validation loss: 2086.165, Runtime: 17.3836 secs\nEpoch: 1552, Step: 38800, training loss: 2074.215, validation loss: 2086.435, Runtime: 17.4677 secs\nEpoch: 1553, Step: 38825, training loss: 2074.380, validation loss: 2086.578, Runtime: 17.1095 secs\nEpoch: 1554, Step: 38850, training loss: 2074.275, validation loss: 2086.090, Runtime: 17.4037 secs\nEpoch: 1555, Step: 38875, training loss: 2074.302, validation loss: 2085.655, Runtime: 17.3527 secs\nEpoch: 1556, Step: 38900, training loss: 2074.433, validation loss: 2085.714, Runtime: 17.4477 secs\nEpoch: 1557, Step: 38925, training loss: 2074.186, validation loss: 2086.406, Runtime: 17.4458 secs\nEpoch: 1558, Step: 38950, training loss: 2074.349, validation loss: 2086.726, Runtime: 17.5001 secs\nEpoch: 1559, Step: 38975, training loss: 2074.240, validation loss: 2086.103, Runtime: 17.4267 secs\nEpoch: 1560, Step: 39000, training loss: 2074.327, validation loss: 2086.407, Runtime: 17.3985 secs\nEpoch: 1561, Step: 39025, training loss: 2074.407, validation loss: 2085.735, Runtime: 17.4439 secs\nEpoch: 1562, Step: 39050, training loss: 2074.364, validation loss: 2086.332, Runtime: 17.4884 secs\nEpoch: 1563, Step: 39075, training loss: 2074.078, validation loss: 2086.109, Runtime: 17.4180 secs\nEpoch: 1564, Step: 39100, training loss: 2074.190, validation loss: 2085.903, Runtime: 17.4465 secs\nEpoch: 1565, Step: 39125, training loss: 2074.490, validation loss: 2085.890, Runtime: 17.4140 secs\nLearning rate decreased to 0.000015\nEpoch: 1566, Step: 39150, training loss: 2074.228, validation loss: 2086.879, Runtime: 17.4493 secs\nEpoch: 1567, Step: 39175, training loss: 2074.130, validation loss: 2086.191, Runtime: 17.3191 secs\nEpoch: 1568, Step: 39200, training loss: 2074.466, validation loss: 2085.827, Runtime: 17.3071 secs\nEpoch: 1569, Step: 39225, training loss: 2074.291, validation loss: 2086.189, Runtime: 17.4314 secs\nEpoch: 1570, Step: 39250, training loss: 2074.425, validation loss: 2086.075, Runtime: 17.3825 secs\nEpoch: 1571, Step: 39275, training loss: 2074.303, validation loss: 2086.488, Runtime: 17.3309 secs\nEpoch: 1572, Step: 39300, training loss: 2074.382, validation loss: 2085.802, Runtime: 17.2732 secs\nEpoch: 1573, Step: 39325, training loss: 2074.293, validation loss: 2086.226, Runtime: 17.3971 secs\nEpoch: 1574, Step: 39350, training loss: 2074.328, validation loss: 2086.410, Runtime: 17.3574 secs\nEpoch: 1575, Step: 39375, training loss: 2074.322, validation loss: 2086.366, Runtime: 17.4705 secs\nEpoch: 1576, Step: 39400, training loss: 2074.138, validation loss: 2086.010, Runtime: 17.4283 secs\nEpoch: 1577, Step: 39425, training loss: 2074.431, validation loss: 2086.583, Runtime: 17.3656 secs\nLearning rate decreased to 0.000014\nEpoch: 1578, Step: 39450, training loss: 2074.427, validation loss: 2086.371, Runtime: 17.4468 secs\nEpoch: 1579, Step: 39475, training loss: 2074.301, validation loss: 2085.808, Runtime: 17.2613 secs\nEpoch: 1580, Step: 39500, training loss: 2074.121, validation loss: 2086.492, Runtime: 17.3124 secs\nEpoch: 1581, Step: 39525, training loss: 2074.237, validation loss: 2086.295, Runtime: 17.3967 secs\nEpoch: 1582, Step: 39550, training loss: 2074.488, validation loss: 2086.566, Runtime: 17.3536 secs\nEpoch: 1583, Step: 39575, training loss: 2074.292, validation loss: 2085.915, Runtime: 17.4623 secs\nEpoch: 1584, Step: 39600, training loss: 2074.380, validation loss: 2085.856, Runtime: 17.2739 secs\nEpoch: 1585, Step: 39625, training loss: 2074.293, validation loss: 2086.331, Runtime: 17.2905 secs\nEpoch: 1586, Step: 39650, training loss: 2074.513, validation loss: 2086.426, Runtime: 17.4721 secs\nLearning rate decreased to 0.000013\nEpoch: 1587, Step: 39675, training loss: 2074.577, validation loss: 2086.476, Runtime: 17.4157 secs\nEpoch: 1588, Step: 39700, training loss: 2074.333, validation loss: 2086.589, Runtime: 17.4989 secs\nEpoch: 1589, Step: 39725, training loss: 2074.287, validation loss: 2085.946, Runtime: 17.5389 secs\nEpoch: 1590, Step: 39750, training loss: 2074.217, validation loss: 2086.161, Runtime: 17.4990 secs\nEpoch: 1591, Step: 39775, training loss: 2074.364, validation loss: 2086.063, Runtime: 17.3773 secs\nEpoch: 1592, Step: 39800, training loss: 2074.337, validation loss: 2086.112, Runtime: 17.4747 secs\nEpoch: 1593, Step: 39825, training loss: 2074.093, validation loss: 2085.966, Runtime: 17.3852 secs\nEpoch: 1594, Step: 39850, training loss: 2074.445, validation loss: 2086.272, Runtime: 17.4213 secs\nLearning rate decreased to 0.000013\nEpoch: 1595, Step: 39875, training loss: 2074.309, validation loss: 2086.282, Runtime: 17.3996 secs\nEpoch: 1596, Step: 39900, training loss: 2074.399, validation loss: 2086.072, Runtime: 17.4565 secs\nEpoch: 1597, Step: 39925, training loss: 2074.302, validation loss: 2086.535, Runtime: 17.3022 secs\nEpoch: 1598, Step: 39950, training loss: 2074.168, validation loss: 2086.311, Runtime: 17.2455 secs\nEpoch: 1599, Step: 39975, training loss: 2074.642, validation loss: 2085.980, Runtime: 17.4064 secs\nEpoch: 1600, Step: 40000, training loss: 2074.355, validation loss: 2086.024, Runtime: 17.5482 secs\nEpoch: 1601, Step: 40025, training loss: 2074.278, validation loss: 2086.457, Runtime: 17.3656 secs\nEpoch: 1602, Step: 40050, training loss: 2074.388, validation loss: 2086.023, Runtime: 17.3825 secs\nEpoch: 1603, Step: 40075, training loss: 2074.397, validation loss: 2086.394, Runtime: 17.3484 secs\nEpoch: 1604, Step: 40100, training loss: 2074.263, validation loss: 2086.995, Runtime: 17.3358 secs\nEpoch: 1605, Step: 40125, training loss: 2074.183, validation loss: 2086.322, Runtime: 17.2805 secs\nEpoch: 1606, Step: 40150, training loss: 2074.273, validation loss: 2086.330, Runtime: 17.8128 secs\nEpoch: 1607, Step: 40175, training loss: 2074.376, validation loss: 2086.484, Runtime: 17.3262 secs\nEpoch: 1608, Step: 40200, training loss: 2074.509, validation loss: 2086.434, Runtime: 17.2976 secs\nLearning rate decreased to 0.000012\nEpoch: 1609, Step: 40225, training loss: 2074.330, validation loss: 2085.792, Runtime: 17.2698 secs\nEpoch: 1610, Step: 40250, training loss: 2074.494, validation loss: 2086.082, Runtime: 17.4054 secs\nEpoch: 1611, Step: 40275, training loss: 2074.144, validation loss: 2086.337, Runtime: 17.5179 secs\nEpoch: 1612, Step: 40300, training loss: 2074.291, validation loss: 2086.753, Runtime: 17.2929 secs\nEpoch: 1613, Step: 40325, training loss: 2074.688, validation loss: 2086.297, Runtime: 17.3270 secs\nEpoch: 1614, Step: 40350, training loss: 2074.287, validation loss: 2086.196, Runtime: 17.4414 secs\nEpoch: 1615, Step: 40375, training loss: 2074.491, validation loss: 2086.376, Runtime: 17.3211 secs\nEpoch: 1616, Step: 40400, training loss: 2074.392, validation loss: 2085.629, Runtime: 17.3692 secs\nEpoch: 1617, Step: 40425, training loss: 2074.379, validation loss: 2085.968, Runtime: 17.4159 secs\nEpoch: 1618, Step: 40450, training loss: 2074.454, validation loss: 2086.378, Runtime: 17.5482 secs\nEpoch: 1619, Step: 40475, training loss: 2074.369, validation loss: 2085.552, Runtime: 17.2738 secs\nEpoch: 1620, Step: 40500, training loss: 2074.336, validation loss: 2086.583, Runtime: 17.4051 secs\nEpoch: 1621, Step: 40525, training loss: 2074.336, validation loss: 2086.455, Runtime: 17.4310 secs\nEpoch: 1622, Step: 40550, training loss: 2074.305, validation loss: 2086.107, Runtime: 17.4193 secs\nEpoch: 1623, Step: 40575, training loss: 2074.320, validation loss: 2086.314, Runtime: 17.4164 secs\nEpoch: 1624, Step: 40600, training loss: 2074.443, validation loss: 2085.669, Runtime: 17.3420 secs\nEpoch: 1625, Step: 40625, training loss: 2074.312, validation loss: 2085.735, Runtime: 17.4259 secs\nEpoch: 1626, Step: 40650, training loss: 2074.286, validation loss: 2086.301, Runtime: 17.3187 secs\nEpoch: 1627, Step: 40675, training loss: 2074.664, validation loss: 2086.794, Runtime: 17.4514 secs\nLearning rate decreased to 0.000011\nEpoch: 1628, Step: 40700, training loss: 2074.535, validation loss: 2086.569, Runtime: 17.4684 secs\nEpoch: 1629, Step: 40725, training loss: 2074.368, validation loss: 2086.290, Runtime: 17.3113 secs\nEpoch: 1630, Step: 40750, training loss: 2074.311, validation loss: 2086.350, Runtime: 17.4251 secs\nEpoch: 1631, Step: 40775, training loss: 2074.076, validation loss: 2086.391, Runtime: 17.7905 secs\nEpoch: 1632, Step: 40800, training loss: 2074.025, validation loss: 2086.100, Runtime: 17.4608 secs\nEpoch: 1633, Step: 40825, training loss: 2074.306, validation loss: 2086.035, Runtime: 17.4149 secs\nEpoch: 1634, Step: 40850, training loss: 2074.114, validation loss: 2086.462, Runtime: 17.5210 secs\nEpoch: 1635, Step: 40875, training loss: 2074.266, validation loss: 2086.376, Runtime: 17.6115 secs\nEpoch: 1636, Step: 40900, training loss: 2074.408, validation loss: 2086.402, Runtime: 17.3915 secs\nLearning rate decreased to 0.000011\nEpoch: 1637, Step: 40925, training loss: 2074.456, validation loss: 2085.869, Runtime: 17.4546 secs\nEpoch: 1638, Step: 40950, training loss: 2074.213, validation loss: 2086.060, Runtime: 17.4931 secs\nEpoch: 1639, Step: 40975, training loss: 2074.180, validation loss: 2086.115, Runtime: 17.3166 secs\nEpoch: 1640, Step: 41000, training loss: 2074.101, validation loss: 2086.024, Runtime: 17.7589 secs\nEpoch: 1641, Step: 41025, training loss: 2074.423, validation loss: 2086.085, Runtime: 17.3371 secs\nEpoch: 1642, Step: 41050, training loss: 2074.310, validation loss: 2086.052, Runtime: 17.4618 secs\nEpoch: 1643, Step: 41075, training loss: 2074.392, validation loss: 2085.971, Runtime: 17.3652 secs\nEpoch: 1644, Step: 41100, training loss: 2074.341, validation loss: 2086.062, Runtime: 17.4821 secs\nEpoch: 1645, Step: 41125, training loss: 2074.391, validation loss: 2085.951, Runtime: 17.4468 secs\nEpoch: 1646, Step: 41150, training loss: 2074.345, validation loss: 2086.271, Runtime: 17.4131 secs\nEpoch: 1647, Step: 41175, training loss: 2074.331, validation loss: 2086.177, Runtime: 17.3760 secs\nEpoch: 1648, Step: 41200, training loss: 2074.400, validation loss: 2086.010, Runtime: 17.3875 secs\nLearning rate decreased to 0.000010\nEpoch: 1649, Step: 41225, training loss: 2074.094, validation loss: 2086.418, Runtime: 17.4398 secs\nEpoch: 1650, Step: 41250, training loss: 2074.300, validation loss: 2085.857, Runtime: 17.3888 secs\nEpoch: 1651, Step: 41275, training loss: 2074.212, validation loss: 2086.157, Runtime: 17.6161 secs\nEpoch: 1652, Step: 41300, training loss: 2074.065, validation loss: 2086.751, Runtime: 17.3781 secs\nEpoch: 1653, Step: 41325, training loss: 2074.400, validation loss: 2085.960, Runtime: 17.3265 secs\nEpoch: 1654, Step: 41350, training loss: 2074.352, validation loss: 2086.264, Runtime: 17.4073 secs\nEpoch: 1655, Step: 41375, training loss: 2074.246, validation loss: 2086.205, Runtime: 17.2920 secs\nEpoch: 1656, Step: 41400, training loss: 2074.187, validation loss: 2086.447, Runtime: 17.4270 secs\nEpoch: 1657, Step: 41425, training loss: 2074.355, validation loss: 2085.937, Runtime: 17.4316 secs\nEpoch: 1658, Step: 41450, training loss: 2074.370, validation loss: 2086.950, Runtime: 17.4762 secs\nEpoch: 1659, Step: 41475, training loss: 2074.316, validation loss: 2085.911, Runtime: 17.4606 secs\nEpoch: 1660, Step: 41500, training loss: 2074.166, validation loss: 2086.148, Runtime: 17.3242 secs\nEpoch: 1661, Step: 41525, training loss: 2074.163, validation loss: 2085.881, Runtime: 17.5342 secs\nEpoch: 1662, Step: 41550, training loss: 2074.400, validation loss: 2086.326, Runtime: 17.4161 secs\nLearning rate decreased to 0.000010\n...training complete.\n"
],
[
"model.load_checkpoint('best')",
"_____no_output_____"
],
[
"model.plot_summary(valid_data, valid_truth)",
"_____no_output_____"
],
[
"results_dict = model.plot_recon_rsquared(valid_data, valid_truth, train_data, train_truth)",
"_____no_output_____"
]
]
] | [
"code",
"markdown",
"code"
] | [
[
"code",
"code",
"code",
"code",
"code",
"code",
"code",
"code",
"code",
"code",
"code",
"code"
],
[
"markdown"
],
[
"code",
"code",
"code",
"code",
"code",
"code",
"code",
"code",
"code",
"code"
]
] |
e78433bd342b0353176c8bbdfb9ce5047e931bc8 | 89,268 | ipynb | Jupyter Notebook | courses/machine_learning/deepdive2/how_google_does_ml/bigquery/solution/analyze_with_bigquery_solution.ipynb | Glairly/introduction_to_tensorflow | aa0a44d9c428a6eb86d1f79d73f54c0861b6358d | [
"Apache-2.0"
] | 2 | 2022-01-06T11:52:57.000Z | 2022-01-09T01:53:56.000Z | courses/machine_learning/deepdive2/how_google_does_ml/bigquery/solution/analyze_with_bigquery_solution.ipynb | Glairly/introduction_to_tensorflow | aa0a44d9c428a6eb86d1f79d73f54c0861b6358d | [
"Apache-2.0"
] | null | null | null | courses/machine_learning/deepdive2/how_google_does_ml/bigquery/solution/analyze_with_bigquery_solution.ipynb | Glairly/introduction_to_tensorflow | aa0a44d9c428a6eb86d1f79d73f54c0861b6358d | [
"Apache-2.0"
] | null | null | null | 38.998689 | 494 | 0.381066 | [
[
[
"# Analyze a large dataset with Google BigQuery\n\n**Learning Objectives**\n\n1. Access an ecommerce dataset\n1. Look at the dataset metadata\n1. Remove duplicate entries\n1. Write and execute queries\n\n\n## Introduction \nBigQuery is Google's fully managed, NoOps, low cost analytics database. With BigQuery you can query terabytes and terabytes of data without having any infrastructure to manage or needing a database administrator. BigQuery uses SQL and can take advantage of the pay-as-you-go model. BigQuery allows you to focus on analyzing data to find meaningful insights.\n\nWe have a publicly available ecommerce dataset that has millions of Google Analytics records for the Google Merchandise Store loaded into a table in BigQuery. In this lab, you use a copy of that dataset. Sample scenarios are provided, from which you look at the data and ways to remove duplicate information. The lab then steps you through further analysis the data.\n\nBigQuery can be accessed by its own browser-based interface, Google Data Studio, and many third party tools. In this lab you will use the BigQuery directly in notebook cells using the iPython magic command `%%bigquery`.\n\nThe steps you will follow in the lab are analogous to what you would do to prepare data for use in advanced ML operations. You will follow the notebook to experiment with the BigQuery queries provided to analyze the data.",
"_____no_output_____"
],
[
"### Set up the notebook environment\n\n__VERY IMPORTANT__: In the cell below you must replace the text `<YOUR PROJECT>` with you GCP project id.",
"_____no_output_____"
]
],
[
[
"import os\n\nimport pandas as pd\n\nPROJECT = \"<YOUR PROJECT>\" #TODO Replace with your project id\n\nos.environ[\"PROJECT\"] = PROJECT\n\npd.options.display.max_columns = 50",
"_____no_output_____"
]
],
[
[
"## Explore eCommerce data and identify duplicate records\n\nScenario: You were provided with Google Analytics logs for an eCommerce website in a BigQuery dataset. The data analyst team created a new BigQuery table of all the raw eCommerce visitor session data. This data tracks user interactions, location, device types, time on page, and details of any transaction. Your ultimate plan is to use this data in an ML capacity to create a model that delivers highly accurate predictions of user behavior to support tailored marketing campaigns.\n\nFirst, a few notes on BigQuery within a python notebook context. Any cell that starts with `%%bigquery` (the BigQuery Magic) will be interpreted as a SQL query that is executed on BigQuery, and the result is printed to our notebook.\n\nBigQuery supports [two flavors](https://cloud.google.com/bigquery/docs/reference/standard-sql/migrating-from-legacy-sql#comparison_of_legacy_and_standard_sql) of SQL syntax: legacy SQL and standard SQL. The preferred is standard SQL because it complies with the official SQL:2011 standard. To instruct BigQuery to interpret our syntax as such we start the query with `#standardSQL`.\n\nOur first query is accessing the BigQuery Information Schema which stores all object-related metadata. In this case we want to see metadata details for the \"all_sessions_raw\" table. \n\nTip: To run the current cell you can click the cell and hit **shift enter**",
"_____no_output_____"
],
[
"TODO 2",
"_____no_output_____"
]
],
[
[
"%%bigquery --project $PROJECT\n#standardsql\nSELECT * \nEXCEPT \n (table_catalog, table_schema, is_generated, generation_expression, is_stored, \n is_updatable, is_hidden, is_system_defined, is_partitioning_column, clustering_ordinal_position)\nFROM `data-to-insights.ecommerce.INFORMATION_SCHEMA.COLUMNS`\nWHERE table_name=\"all_sessions_raw\"",
"_____no_output_____"
]
],
[
[
"Next examine how many rows are in the table.",
"_____no_output_____"
],
[
"TODO 1",
"_____no_output_____"
]
],
[
[
"%%bigquery --project $PROJECT \n#standardSQL\nSELECT count(*)\nFROM `data-to-insights.ecommerce.all_sessions_raw`",
"_____no_output_____"
]
],
[
[
"Now take a quick at few rows of data in the table.",
"_____no_output_____"
]
],
[
[
"%%bigquery --project $PROJECT \n#standardSQL\nSELECT *\nFROM `data-to-insights.ecommerce.all_sessions_raw`\nLIMIT 7",
"_____no_output_____"
]
],
[
[
"### Identify duplicate rows\n\nSeeing a sample amount of data may give you greater intuition for what is included in the dataset. But since the table is quite large, a preview is not likely to render meaningful results. As you scan and scroll through the sample rows you see there is no singular field that uniquely identifies a row, so you need advanced logic to identify duplicate rows.\n\nThe query below uses the SQL GROUP BY function on every field and counts (COUNT) where there are rows that have the same values across every field.\n\nIf every field is unique, the COUNT will return 1 as there are no other groupings of rows with the exact same value for all fields.\nIf there is a row with the same values for all fields, they will be grouped together and the COUNT will be greater than 1. The last part of the query is an aggregation filter using HAVING to only show the results that have a COUNT of duplicates greater than 1.\nRun the following query to find duplicate records across all columns.",
"_____no_output_____"
],
[
"TODO 3",
"_____no_output_____"
]
],
[
[
"%%bigquery --project $PROJECT \n#standardSQL\nSELECT count(*) AS num_duplicate_rows, \n * \nFROM `data-to-insights.ecommerce.all_sessions_raw` \nGROUP BY fullvisitorid, \n channelgrouping, \n time, \n country, \n city, \n totaltransactionrevenue, \n transactions, \n timeonsite, \n pageviews, \n sessionqualitydim, \n date, \n visitid, \n type, \n productrefundamount, \n productquantity, \n productprice, \n productrevenue, \n productsku, \n v2productname, \n v2productcategory, \n productvariant, \n currencycode, \n itemquantity, \n itemrevenue, \n transactionrevenue, \n transactionid, \n pagetitle, \n searchkeyword, \n pagepathlevel1, \n ecommerceaction_type, \n ecommerceaction_step, \n ecommerceaction_option \nHAVING num_duplicate_rows > 1; ",
"_____no_output_____"
]
],
[
[
"As you can see there are quite a few \"duplicate\" records (615) when analyzed with these parameters.\n\nIn your own datasets, even if you have a unique key, it is still beneficial to confirm the uniqueness of the rows with COUNT, GROUP BY, and HAVING before you begin your analysis.",
"_____no_output_____"
],
[
"## Analyze the new all_sessions table\n\nIn this section you use a deduplicated table called all_sessions.\n\nScenario: Your data analyst team has provided you with a relevant query, and your schema experts have identified the key fields that must be unique for each record per your schema.\n\nRun the query to confirm that no duplicates exist, this time against the \"all_sessions\" table:",
"_____no_output_____"
]
],
[
[
"%%bigquery --project $PROJECT\n#standardSQL\nSELECT fullvisitorid, # the unique visitor ID \n visitid, # a visitor can have multiple visits \n date, # session date stored as string YYYYMMDD \n time, # time of the individual site hit (can be 0 or more) \n v2productname, # not unique since a product can have variants like Color \n productsku, # unique for each product \n type, # visit and/or event trigger \n ecommerceaction_type, # maps to ‘add to cart', ‘completed checkout' \n ecommerceaction_step, \n ecommerceaction_option, \n transactionrevenue, # revenue of the order \n transactionid, # unique identifier for revenue bearing transaction \n count(*) AS row_count \nFROM `data-to-insights.ecommerce.all_sessions` \nGROUP BY 1, \n 2, \n 3, \n 4, \n 5, \n 6, \n 7, \n 8, \n 9, \n 10, \n 11, \n 12 \nHAVING row_count > 1 # find duplicates \n",
"_____no_output_____"
]
],
[
[
"The query returns zero records indicating no duplicates exist.",
"_____no_output_____"
],
[
"## Write basic SQL against the eCommerce data (TODO 4)\n\nIn this section, you query for insights on the ecommerce dataset.\n\nA good first path of analysis is to find the total unique visitors\nThe query below determines the total views by counting product_views and the number of unique visitors by counting fullVisitorID.",
"_____no_output_____"
]
],
[
[
"%%bigquery --project $PROJECT\n#standardSQL\nSELECT count(*) AS product_views, \n count(DISTINCT fullvisitorid) AS unique_visitors \nFROM `data-to-insights.ecommerce.all_sessions`; ",
"_____no_output_____"
]
],
[
[
"The next query shows total unique visitors(fullVisitorID) by the referring site (channelGrouping):",
"_____no_output_____"
]
],
[
[
"%%bigquery --project $PROJECT\n#standardSQL\nSELECT count(DISTINCT fullvisitorid) AS unique_visitors, \n channelgrouping \nFROM `data-to-insights.ecommerce.all_sessions` \nGROUP BY 2 \nORDER BY 2 DESC;",
"_____no_output_____"
]
],
[
[
"To find deeper insights in the data, the next query lists the five products with the most views (product_views) from unique visitors. The query counts number of times a product (v2ProductName) was viewed (product_views), puts the list in descending order, and lists the top 5 entries:",
"_____no_output_____"
]
],
[
[
"%%bigquery --project $PROJECT\n#standardSQL\nSELECT count(*) AS product_views, \n ( v2productname ) AS ProductName \nFROM `data-to-insights.ecommerce.all_sessions` \nWHERE type = 'PAGE' \nGROUP BY v2productname \nORDER BY product_views DESC \nLIMIT 5;",
"_____no_output_____"
]
],
[
[
"Now expand your previous query to include the total number of distinct products ordered and the total number of total units ordered (productQuantity):",
"_____no_output_____"
]
],
[
[
"%%bigquery --project $PROJECT\n#standardSQL\nSELECT count(*) AS product_views, \n count(productquantity) AS orders, \n sum(productquantity) AS quantity_product_ordered, \n v2productname \nFROM `data-to-insights.ecommerce.all_sessions` \nWHERE type = 'PAGE' \nGROUP BY v2productname \nORDER BY product_views DESC \nLIMIT 5; ",
"_____no_output_____"
]
],
[
[
"Lastly, expand the query to include the average amount of product per order (total number of units ordered/total number of orders, or `SUM(productQuantity)/COUNT(productQuantity)`).",
"_____no_output_____"
]
],
[
[
"%%bigquery --project $PROJECT\n#standardSQL\nSELECT count(*) AS product_views, \n count(productquantity) AS orders, \n sum(productquantity) AS quantity_product_ordered, \n sum(productquantity) / Count(productquantity) AS avg_per_order, \n v2productname AS productName \nFROM `data-to-insights.ecommerce.all_sessions` \nWHERE type = 'PAGE' \nGROUP BY v2productname \nORDER BY product_views DESC \nLIMIT 5; ",
"_____no_output_____"
]
],
[
[
"You can see that among these top 5 products by product views that the 22 oz YouTube Bottle Infuser had the highest avg_per_order with 9.38 units per order.",
"_____no_output_____"
],
[
"You have completed this lab exercise. In this situation the \"all_sessions\" was provided to you with the deduplicated records. In the course of your own future analysis you may have to create this on your own using BigQuery and the `create table DATASET.TABLE2 as select * from DATASET.TABLE1` syntax.",
"_____no_output_____"
],
[
"Copyright 2019 Google Inc.\nLicensed under the Apache License, Version 2.0 (the \"License\"); you may not use this file except in compliance with the License. You may obtain a copy of the License at\nhttp://www.apache.org/licenses/LICENSE-2.0\nUnless required by applicable law or agreed to in writing, software distributed under the License is distributed on an \"AS IS\" BASIS, WITHOUT WARRANTIES OR CONDITIONS OF ANY KIND, either express or implied. See the License for the specific language governing permissions and limitations under the License.",
"_____no_output_____"
]
]
] | [
"markdown",
"code",
"markdown",
"code",
"markdown",
"code",
"markdown",
"code",
"markdown",
"code",
"markdown",
"code",
"markdown",
"code",
"markdown",
"code",
"markdown",
"code",
"markdown",
"code",
"markdown",
"code",
"markdown"
] | [
[
"markdown",
"markdown"
],
[
"code"
],
[
"markdown",
"markdown"
],
[
"code"
],
[
"markdown",
"markdown"
],
[
"code"
],
[
"markdown"
],
[
"code"
],
[
"markdown",
"markdown"
],
[
"code"
],
[
"markdown",
"markdown"
],
[
"code"
],
[
"markdown",
"markdown"
],
[
"code"
],
[
"markdown"
],
[
"code"
],
[
"markdown"
],
[
"code"
],
[
"markdown"
],
[
"code"
],
[
"markdown"
],
[
"code"
],
[
"markdown",
"markdown",
"markdown"
]
] |
e784351e4d4a0916b61a46768ccd101c3d3ba97a | 792,714 | ipynb | Jupyter Notebook | Climate.ipynb | Deyang-Li/tidy-beauty | 8e74041173bed2bae666aae77447b404eb548833 | [
"Apache-2.0"
] | 5 | 2019-08-30T12:16:54.000Z | 2019-10-16T02:20:06.000Z | Climate.ipynb | Deyang-Li/tidy-beauty | 8e74041173bed2bae666aae77447b404eb548833 | [
"Apache-2.0"
] | null | null | null | Climate.ipynb | Deyang-Li/tidy-beauty | 8e74041173bed2bae666aae77447b404eb548833 | [
"Apache-2.0"
] | null | null | null | 976.248768 | 253,064 | 0.938721 | [
[
[
"library(tidyverse)\nlibrary(skimr)",
"── \u001b[1mAttaching packages\u001b[22m ─────────────────────────────────────── tidyverse 1.2.1 ──\n\u001b[32m✔\u001b[39m \u001b[34mggplot2\u001b[39m 3.2.1 \u001b[32m✔\u001b[39m \u001b[34mpurrr \u001b[39m 0.3.2\n\u001b[32m✔\u001b[39m \u001b[34mtibble \u001b[39m 2.1.3 \u001b[32m✔\u001b[39m \u001b[34mdplyr \u001b[39m 0.8.3\n\u001b[32m✔\u001b[39m \u001b[34mtidyr \u001b[39m 1.0.0 \u001b[32m✔\u001b[39m \u001b[34mstringr\u001b[39m 1.4.0\n\u001b[32m✔\u001b[39m \u001b[34mreadr \u001b[39m 1.3.1 \u001b[32m✔\u001b[39m \u001b[34mforcats\u001b[39m 0.4.0\n── \u001b[1mConflicts\u001b[22m ────────────────────────────────────────── tidyverse_conflicts() ──\n\u001b[31m✖\u001b[39m \u001b[34mdplyr\u001b[39m::\u001b[32mfilter()\u001b[39m masks \u001b[34mstats\u001b[39m::filter()\n\u001b[31m✖\u001b[39m \u001b[34mdplyr\u001b[39m::\u001b[32mlag()\u001b[39m masks \u001b[34mstats\u001b[39m::lag()\n\nAttaching package: ‘skimr’\n\nThe following object is masked from ‘package:stats’:\n\n filter\n\n"
]
],
[
[
"download the dataset from https://www.ncdc.noaa.gov/cag/global/time-series",
"_____no_output_____"
],
[
"# Data wrangling #",
"_____no_output_____"
],
[
"Normalize the column name :change the `value` to `Surface Temperature in Africa` in each dataframe",
"_____no_output_____"
]
],
[
[
"Africa1 <- read_csv(file = \"Africa.csv\")",
"Parsed with column specification:\ncols(\n Year = \u001b[32mcol_double()\u001b[39m,\n Value = \u001b[32mcol_double()\u001b[39m\n)\n"
],
[
"Africa <- Africa1 %>% rename(\"SurfaceTemperature\" = \"Value\")\nAfrica2 <- Africa %>% rename(\"Surface Temperature in Africa\" = \"SurfaceTemperature\")",
"_____no_output_____"
],
[
"North_America1 <- read_csv(file = \"North America.csv\")",
"Parsed with column specification:\ncols(\n Year = \u001b[32mcol_double()\u001b[39m,\n Value = \u001b[32mcol_double()\u001b[39m\n)\n"
],
[
"North_America <- North_America1 %>% rename(\"SurfaceTemperature\" = \"Value\")\nNorth_America2 <- North_America %>% rename(\"Surface Temperature in North America\" = \"SurfaceTemperature\")",
"_____no_output_____"
],
[
"South_America1 <- read_csv(file = \"South America.csv\")",
"Parsed with column specification:\ncols(\n Year = \u001b[32mcol_double()\u001b[39m,\n Value = \u001b[32mcol_double()\u001b[39m\n)\n"
],
[
"South_America <- South_America1 %>% rename(\"SurfaceTemperature\" = \"Value\")\nSouth_America2 <- South_America %>% rename(\"Surface Temperature in South America\" = \"SurfaceTemperature\")",
"_____no_output_____"
],
[
"Europe1 <- read_csv(file = \"Europe.csv\")",
"Parsed with column specification:\ncols(\n Year = \u001b[32mcol_double()\u001b[39m,\n Value = \u001b[32mcol_double()\u001b[39m\n)\n"
],
[
"Europe <- Europe1 %>% rename(\"SurfaceTemperature\" = \"Value\")\nEurope2 <- Europe %>% rename(\"Surface Temperature in Europe\" = \"SurfaceTemperature\")",
"_____no_output_____"
],
[
"Asia1 <- read_csv(file = \"Asia.csv\")",
"Parsed with column specification:\ncols(\n Year = \u001b[32mcol_double()\u001b[39m,\n Value = \u001b[32mcol_double()\u001b[39m\n)\n"
],
[
"Asia <- Asia1 %>% rename(\"SurfaceTemperature\" = \"Value\")\nAsia2 <- Asia %>% rename(\"Surface Temperature in Asia\" = \"SurfaceTemperature\")",
"_____no_output_____"
],
[
"Oceania1 <- read_csv(file = \"Oceania.csv\")",
"Parsed with column specification:\ncols(\n Year = \u001b[32mcol_double()\u001b[39m,\n Value = \u001b[32mcol_double()\u001b[39m\n)\n"
],
[
"Oceania <- Oceania1 %>% rename(\"SurfaceTemperature\" = \"Value\")\nOceania2 <- Oceania %>% rename(\"Surface Temperature in Oceania\" = \"SurfaceTemperature\")",
"_____no_output_____"
]
],
[
[
"Join together!",
"_____no_output_____"
]
],
[
[
"climate_df <- Africa2 %>% \n full_join(North_America2) %>%\n full_join(South_America2) %>%\n full_join(Europe2) %>%\n full_join(Asia2) %>%\n full_join(Oceania2)",
"Joining, by = \"Year\"\nJoining, by = \"Year\"\nJoining, by = \"Year\"\nJoining, by = \"Year\"\nJoining, by = \"Year\"\n"
]
],
[
[
"check about the types of the columns, the missing values, and output a quick summary of the dataset.",
"_____no_output_____"
]
],
[
[
"glimpse(climate_df)",
"Observations: 109\nVariables: 7\n$ Year \u001b[3m\u001b[38;5;246m<dbl>\u001b[39m\u001b[23m 1910, 1911, 1912, 1913, 1914, …\n$ `Surface Temperature in Africa` \u001b[3m\u001b[38;5;246m<dbl>\u001b[39m\u001b[23m -0.38, -0.57, -0.24, -0.11, -0…\n$ `Surface Temperature in North America` \u001b[3m\u001b[38;5;246m<dbl>\u001b[39m\u001b[23m -0.13, -0.40, -0.73, -0.67, -0…\n$ `Surface Temperature in South America` \u001b[3m\u001b[38;5;246m<dbl>\u001b[39m\u001b[23m -0.43, -0.57, -0.25, -0.16, -0…\n$ `Surface Temperature in Europe` \u001b[3m\u001b[38;5;246m<dbl>\u001b[39m\u001b[23m 0.01, -0.16, -0.71, -0.14, -0.…\n$ `Surface Temperature in Asia` \u001b[3m\u001b[38;5;246m<dbl>\u001b[39m\u001b[23m -0.59, -0.58, -0.88, -0.42, 0.…\n$ `Surface Temperature in Oceania` \u001b[3m\u001b[38;5;246m<dbl>\u001b[39m\u001b[23m -0.28, -0.47, -0.05, -0.60, 0.…\n"
],
[
"summary(climate_df) ",
"_____no_output_____"
],
[
"climate_df %>%\n skim() %>%\n kable()",
"Skim summary statistics \n n obs: 109 \n n variables: 7 \n\nVariable type: numeric\n\n| variable | missing | complete | n | mean | sd | p0 | p25 | p50 | p75 | p100 | hist |\n|--------------------------------------|---------|----------|-----|------|-------|-------|-------|------|------|------|----------|\n| Surface Temperature in Africa | 0 | 109 | 109 | 0.17 | 0.49 | -0.68 | -0.19 | 0.02 | 0.43 | 1.52 | ▂▇▇▅▂▃▂▁ |\n| Surface Temperature in Asia | 0 | 109 | 109 | 0.2 | 0.61 | -0.88 | -0.26 | 0.05 | 0.67 | 1.7 | ▂▇▇▆▂▃▃▁ |\n| Surface Temperature in Europe | 0 | 109 | 109 | 0.19 | 0.64 | -1.07 | -0.16 | 0.06 | 0.58 | 1.87 | ▂▅▇▆▃▃▂▁ |\n| Surface Temperature in North America | 0 | 109 | 109 | 0.17 | 0.61 | -1.3 | -0.29 | 0.1 | 0.5 | 1.92 | ▁▂▇▇▆▂▂▁ |\n| Surface Temperature in Oceania | 0 | 109 | 109 | 0.14 | 0.49 | -0.89 | -0.25 | 0.11 | 0.44 | 1.33 | ▁▃▇▆▆▃▂▂ |\n| Surface Temperature in South America | 0 | 109 | 109 | 0.16 | 0.5 | -0.87 | -0.19 | 0.07 | 0.47 | 1.48 | ▂▃▇▆▃▃▂▁ |\n| Year | 0 | 109 | 109 | 1964 | 31.61 | 1910 | 1937 | 1964 | 1991 | 2018 | ▇▇▇▇▇▇▇▇ |\n"
],
[
"write_csv(climate_df,\"Climate.csv\")",
"_____no_output_____"
]
],
[
[
"# Data analysis#",
"_____no_output_____"
],
[
"choose the data from 1950 to 2018 for ploting",
"_____no_output_____"
]
],
[
[
"Africa$pos = Africa$SurfaceTemperature >= 0",
"_____no_output_____"
],
[
"Africa_climate_plot <- Africa %>% \n filter( Year >= 1950) %>%\n ggplot(aes( \n x = Year, \n y = SurfaceTemperature,\n fill = pos)) + \n labs(title = \"Time Series of Surface Temperature Anomalies in Africa\") +\n scale_x_continuous(breaks=seq(1950, 2020, 10)) +\n scale_y_continuous(breaks=seq(-1, 1.8, 0.2)) +\n geom_bar(stat = \"identity\",position = \"identity\", colour = \"black\", size = 0.05) +\n xlab(\"Year\") + ylab (\"Surface Temperature ( ºC )\") +\n theme_light()+\n theme(plot.title = element_text(hjust = 0.5)) +\n scale_fill_manual(values = c(\"#CCEEFF\", \"#FFDDDD\"), guide = FALSE) \nAfrica_climate_plot",
"_____no_output_____"
],
[
"ggsave(Africa_climate_plot,filename = \"Africa climate plot.jpg\",width = 12,height = 9)",
"_____no_output_____"
],
[
"North_America$pos = North_America$SurfaceTemperature >= 0",
"_____no_output_____"
],
[
"North_America_climate_plot <- North_America %>% \n filter( Year >= 1950) %>%\n ggplot(aes( \n x = Year, \n y = SurfaceTemperature,\n fill = pos)) + \n labs(title = \"Time Series of Surface Temperature Anomalies in North America\") +\n scale_x_continuous(breaks=seq(1950, 2020, 10)) +\n scale_y_continuous(breaks=seq(-1, 1.8, 0.2)) +\n geom_bar(stat = \"identity\",position = \"identity\", colour = \"black\", size = 0.05) +\n xlab(\"Year\") + ylab (\"Surface Temperature ( ºC )\") +\n theme_light()+\n theme(plot.title = element_text(hjust = 0.5)) +\n scale_fill_manual(values = c(\"#CCEEFF\", \"#FFDDDD\"), guide = FALSE) \nNorth_America_climate_plot",
"_____no_output_____"
],
[
"ggsave(North_America_climate_plot,filename = \"North America climate plot.jpg\",width = 12,height = 9)",
"_____no_output_____"
],
[
"South_America$pos = South_America$SurfaceTemperature >= 0",
"_____no_output_____"
],
[
"South_America_climate_plot <- South_America %>% \n filter( Year >= 1950) %>%\n ggplot(aes( \n x = Year, \n y = SurfaceTemperature,\n fill = pos)) + \n labs(title = \"Time Series of Surface Temperature Anomalies in South America\") +\n scale_x_continuous(breaks=seq(1950, 2020, 10)) +\n scale_y_continuous(breaks=seq(-1, 1.8, 0.2)) +\n geom_bar(stat = \"identity\",position = \"identity\", colour = \"black\", size = 0.05) +\n xlab(\"Year\") + ylab (\"Surface Temperature ( ºC )\") +\n theme_light()+\n theme(plot.title = element_text(hjust = 0.5)) +\n scale_fill_manual(values = c(\"#CCEEFF\", \"#FFDDDD\"), guide = FALSE) \nSouth_America_climate_plot",
"_____no_output_____"
],
[
"ggsave(South_America_climate_plot,filename = \"South America climate plot.jpg\",width = 12,height = 9)",
"_____no_output_____"
],
[
"Europe$pos = Europe$SurfaceTemperature >= 0",
"_____no_output_____"
],
[
"Europe_climate_plot <- Europe %>% \n filter( Year >= 1950) %>%\n ggplot(aes( \n x = Year, \n y = SurfaceTemperature,\n fill = pos)) + \n labs(title = \"Time Series of Surface Temperature Anomalies in Europe\") +\n scale_x_continuous(breaks=seq(1950, 2020, 10)) +\n scale_y_continuous(breaks=seq(-1, 1.8, 0.2)) +\n geom_bar(stat = \"identity\",position = \"identity\", colour = \"black\", size = 0.05) +\n xlab(\"Year\") + ylab (\"Surface Temperature ( ºC )\") +\n theme_light()+\n theme(plot.title = element_text(hjust = 0.5)) +\n scale_fill_manual(values = c(\"#CCEEFF\", \"#FFDDDD\"), guide = FALSE) \nEurope_climate_plot",
"_____no_output_____"
],
[
"ggsave(Europe_climate_plot,filename = \"Europe climate plot.jpg\",width = 12,height = 9)",
"_____no_output_____"
],
[
"Asia$pos = Asia$SurfaceTemperature >= 0",
"_____no_output_____"
],
[
"Asia_climate_plot <- Asia %>% \n filter( Year >= 1950) %>%\n ggplot(aes( \n x = Year, \n y = SurfaceTemperature,\n fill = pos)) + \n labs(title = \"Time Series of Surface Temperature Anomalies in Asia\") +\n scale_x_continuous(breaks=seq(1950, 2020, 10)) +\n scale_y_continuous(breaks=seq(-1, 1.8, 0.2)) +\n geom_bar(stat = \"identity\",position = \"identity\", colour = \"black\", size = 0.05) +\n xlab(\"Year\") + ylab (\"Surface Temperature ( ºC )\") +\n theme_light()+\n theme(plot.title = element_text(hjust = 0.5)) +\n scale_fill_manual(values = c(\"#CCEEFF\", \"#FFDDDD\"), guide = FALSE) \nAsia_climate_plot",
"_____no_output_____"
],
[
"ggsave(Asia_climate_plot,filename = \"Asia climate plot.jpg\",width = 12,height = 9)",
"_____no_output_____"
],
[
"Oceania$pos = Oceania$SurfaceTemperature >= 0",
"_____no_output_____"
],
[
"Oceania_climate_plot <- Oceania %>% \n filter( Year >= 1950) %>%\n ggplot(aes( \n x = Year, \n y = SurfaceTemperature,\n fill = pos)) + \n labs(title = \"Time Series of Surface Temperature Anomalies in Oceania\") +\n scale_x_continuous(breaks=seq(1950, 2020, 10)) +\n scale_y_continuous(breaks=seq(-1, 1.8, 0.2)) +\n geom_bar(stat = \"identity\",position = \"identity\", colour = \"black\", size = 0.05) +\n xlab(\"Year\") + ylab (\"Surface Temperature ( ºC )\") +\n theme_light()+\n theme(plot.title = element_text(hjust = 0.5)) +\n scale_fill_manual(values = c(\"#CCEEFF\", \"#FFDDDD\"), guide = FALSE) \nOceania_climate_plot",
"_____no_output_____"
],
[
"ggsave(Oceania_climate_plot,filename = \"Oceania climate plot.jpg\",width = 12,height = 9)",
"_____no_output_____"
]
],
[
[
"Put all plots together",
"_____no_output_____"
]
],
[
[
"library(ggpubr)",
"Loading required package: magrittr\n\nAttaching package: ‘magrittr’\n\nThe following object is masked from ‘package:purrr’:\n\n set_names\n\nThe following object is masked from ‘package:tidyr’:\n\n extract\n\n"
],
[
"general_plot <- ggarrange(Africa_climate_plot, Asia_climate_plot, \n Europe_climate_plot, South_America_climate_plot, \n North_America_climate_plot, Oceania_climate_plot, ncol = 2, nrow = 3)\ngeneral_plot",
"_____no_output_____"
],
[
"ggsave(general_plot,filename = \"Climate general plot.jpg\",width = 12,height = 9)",
"_____no_output_____"
]
]
] | [
"code",
"markdown",
"code",
"markdown",
"code",
"markdown",
"code",
"markdown",
"code",
"markdown",
"code"
] | [
[
"code"
],
[
"markdown",
"markdown",
"markdown"
],
[
"code",
"code",
"code",
"code",
"code",
"code",
"code",
"code",
"code",
"code",
"code",
"code"
],
[
"markdown"
],
[
"code"
],
[
"markdown"
],
[
"code",
"code",
"code",
"code"
],
[
"markdown",
"markdown"
],
[
"code",
"code",
"code",
"code",
"code",
"code",
"code",
"code",
"code",
"code",
"code",
"code",
"code",
"code",
"code",
"code",
"code",
"code"
],
[
"markdown"
],
[
"code",
"code",
"code"
]
] |
e784582be1a374dc47c72dd08c76871845ee37ec | 129,290 | ipynb | Jupyter Notebook | brownian/ex/Brownian Workup.ipynb | ryanpdwyer/brownian | 26fe50ecda001fc3ea59ea03fd7d0274095e88b6 | [
"MIT"
] | null | null | null | brownian/ex/Brownian Workup.ipynb | ryanpdwyer/brownian | 26fe50ecda001fc3ea59ea03fd7d0274095e88b6 | [
"MIT"
] | null | null | null | brownian/ex/Brownian Workup.ipynb | ryanpdwyer/brownian | 26fe50ecda001fc3ea59ea03fd7d0274095e88b6 | [
"MIT"
] | null | null | null | 395.382263 | 26,552 | 0.928587 | [
[
[
"import h5py\nimport numpy as np\nimport matplotlib as mpl\nimport matplotlib.pyplot as plt\nimport brownian\nfrom brownian import u\nfrom brownian import Cantilever\n\n%matplotlib inline",
"_____no_output_____"
],
[
"f = h5py.File('brownian173033.h5', 'r') ",
"_____no_output_____"
],
[
"freq = f['x'][()]\nPSD = f['y'][()]\nPSD_std = f['y_std'][()]\nPSD_err = PSD_std / (f['y'].attrs['n_avg']**0.5) # Standard error",
"_____no_output_____"
],
[
"m = brownian.make_mask(freq, 70000, 71000)\nplt.semilogy(freq[m], PSD[m])",
"_____no_output_____"
],
[
"cantilever = Cantilever(f_c=70.5*u.kHz,\n Q=28000*u.dimensionless,\n k_c=3.5*u.N/u.m)\nbmf = brownian.BrownianMotionFitter(freq, PSD, PSD_err, 298, cantilever)",
"_____no_output_____"
],
[
"bmf.calc_fit(70300, 70800)",
"\nResiduals\n-------------------------------------\n Mean: -8.21e-02\n Std. dev.: 2.06e-01\n"
],
[
"fig, ax = bmf.plot_fit()",
"_____no_output_____"
],
[
"ax.set_xlim(70540, 70580)\nax.set_ylim(1e-7, 1e-4)\nfig",
"_____no_output_____"
],
[
"bmf.plot_residuals()",
"_____no_output_____"
],
[
"bmf.plot_reduced_residuals()",
"_____no_output_____"
],
[
"bmf.plot_cdf()",
"_____no_output_____"
],
[
"print(bmf.report())",
"\n Input\n -----------------------------------------------\n Temperature T: 298 kelvin\n\n Estimates\n -----------------------------------------------\n Spring constant k_c: 3.5 newton/meter\n Resonance frequency f_c: 70.5 kilohertz\n Quality factor Q: 28000 dimensionless\n \n Fitting\n -----------------------------------------------\n Fit frequency min f_min: 70300 hertz\n Fit frequency max f_max: 70800 hertz\n\n Results\n -----------------------------------------------\n Resonance frequency f_c: 70561.35(4) hertz\n Spring constant k_c: 22.3(8) newton/meter\n Quality Factor Q: 2.9(1)×10⁴ dimensionless\n Detector Noise : 7.38(7)×10⁻⁹ nanometer²/hertz\n \n"
]
]
] | [
"code"
] | [
[
"code",
"code",
"code",
"code",
"code",
"code",
"code",
"code",
"code",
"code",
"code",
"code"
]
] |
e7849512da2e28eaf73e79e71c341e233d21e2aa | 8,383 | ipynb | Jupyter Notebook | hw-4.ipynb | anspears/astr-119-hw-4 | 300a39e4298ee3deb0ee8945d23e936107b177cb | [
"MIT"
] | null | null | null | hw-4.ipynb | anspears/astr-119-hw-4 | 300a39e4298ee3deb0ee8945d23e936107b177cb | [
"MIT"
] | null | null | null | hw-4.ipynb | anspears/astr-119-hw-4 | 300a39e4298ee3deb0ee8945d23e936107b177cb | [
"MIT"
] | null | null | null | 29.517606 | 103 | 0.443517 | [
[
[
"%matplotlib inline\nimport numpy as np\nimport matplotlib.pyplot as plt",
"_____no_output_____"
],
[
"#define the function to find the roots of\ndef function_for_roots(x):\n a = 1.01\n b = -3.04\n c = 2.07\n return a*x**2 +b*x +c",
"_____no_output_____"
],
[
"#define the derivative\ndef derivative_for_root(x):\n a = 1.01\n b = -3.04\n return 2*a*x +b",
"_____no_output_____"
],
[
"# define to check our initial guesses\ndef check_initial_values(f, x_min, x_max, tol):\n \n y_min = f(x_min)\n y_max = f(x_max)\n \n #check that x_min and x_max contain a zero crossing\n if(y_min*y_max>=0.0):\n print(\"No zero crossing found in the range = \",x_min, x_max)\n s = \"f(%f) = %f, f(%f) = %f\" % (x_min, y_min, x_max, y_max)\n print(s)\n return 0\n \n #if x_min is a root, then return flag ==1\n if(np.fabs(y_min)<tol):\n return 1\n \n #if x_max is a root, then return flag ==2\n if(np.fabs(y_max)<tol):\n return 2\n \n #if we reach this point, the bracket is valid and we will return 3\n return 3",
"_____no_output_____"
],
[
"#define function that uses bisection search to find a root\ndef bisection_root_finding(f, x_min_start, x_max_start, tol):\n \n x_min = x_min_start #min x\n x_max = x_max_start #max x\n x_mid = 0.0 #mid point\n \n y_min = f(x_min) #function value at x_min\n y_max = f(x_max) #...x_max\n y_mid = 0.0 #...mid point\n \n imax = 10000 #set a maximum number of iterations\n i = 0 #iteration counter\n \n #check the initial values\n flag = check_initial_values(f,x_min,x_max,tol)\n if(flag==0):\n print(\"Error in bisection_root_finding().\")\n raise ValueError('Initial values invalid',x_min,x_max)\n elif(flag==1):\n return x_min\n elif(flag==2):\n return x_max\n \n #if we reach here, then we need to conduct the search\n \n #set a flag\n flag = 1\n \n #enter a while loop\n while(flag):\n x_mid = 0.5*(x_min+x_max) #mid point\n y_mid = f(x_mid) #function value at x_mid\n \n #check if x_mid is a root\n if(np.fabs(y_mid)<tol):\n flag = 0\n else:\n #x_mid is not a root\n \n #if the product of the function at the midpoint\n #and at one of the end points is greater than\n #zero, replace this end point\n if(f(x_min)*f(x_mid)>0):\n #replace x_min with x_mid\n x_min = x_mid\n else:\n #replace x_max with x_mid\n x_max = x_mid\n \n \n #print out the iteration\n print(x_min,f(x_min),x_max,f(x_max))\n \n #count the iteration\n i += 1\n \n #if we have exceeded the max number\n #of iterations, exit\n if(i>=imax):\n print(\"Exceeded max number of iterations = \",i)\n s = \"Min bracket f(%f) = %f\" % (x_min, f(x_min))\n print(s)\n s = \"Max bracket f(%f) = %f\" % (x_max, f(x_max))\n print(s)\n s = \"Mid bracket f(%f) = %f\" % (x_mid, f(x_mid))\n print(s)\n raise StopIteration('Stopping iterations after ',i)\n \n #we are done!\n return x_mid",
"_____no_output_____"
],
[
"x_min = 0.0\nx_max = 1.5\ntolerance = 1.0e-6\n\n#print the initial guess\nprint(x_min,function_for_roots(x_min))\nprint(x_max,function_for_roots(x_max))\n\nx_root = bisection_root_finding(function_for_roots,x_min,x_max,tolerance)\ny_root = function_for_roots(x_root)\n\ns = \"Root found with y(%f) = %f\" % (x_root,y_root)\nprint(s)",
"_____no_output_____"
],
[
"def newton_raphson_root_finding(f, dfdx, x_start, tol):\n\n \n flag = 1\n \n imax = 10000\n \n i = 0\n \n #define the new and old guesses\n x_old = x_start\n x_new = 0.0\n y_new = 0.0\n \n #start the loop\n while(flag):\n \n #make a new guess\n x_new = x_old - f(x_old)/dfdx(x_old)\n \n print(x_new,x_old,f(x_old),dfdx(x_old))\n \n #if the abs value of the new function value\n #is < tol, then stop\n y_new = f(x_new)\n if(np.fabs(y_new)<tol):\n flag = 0 \n else:\n \n #plot\n fig = plt.figure(figsize =(7,7))\n xarr = function_for_roots(x)\n yarr = f(xarr)\n plt.plot(xarr,yarr, color = \"orange\")\n plt.axis([x_min,x_max,f(x_min),f(x_max)])\n plt.xlim([0,3])\n plt.ylim([-0.5,2.1])\n \n xapprox = np.linspace(0, 3, 1000)\n plt.plot(xapprox, color = \"black\")\n plt.suptitle('f(x) vs. x')\n plt.show()\n \n #save the result\n x_old = x_new\n #increment the iteration\n i += 1\n \n if(i>=imax):\n print(\"Max iterations reached.\")\n raise StopIteration('Stopping iterations after ',i)\n \n return x_new",
"_____no_output_____"
],
[
"x_start = 0.5\ntolerance = 1.0e-6\n\n#print the initial guess\nprint(x_start,function_for_roots(x_start))\n\nx_root = newton_raphson_root_finding(function_for_roots,derivative_for_root,x_start,tolerance)\ny_root = function_for_roots(x_root)\n \ns = \"Root found with y(%f) = %f\" % (x_root,y_root)\nprint(s)",
"_____no_output_____"
]
]
] | [
"code"
] | [
[
"code",
"code",
"code",
"code",
"code",
"code",
"code",
"code"
]
] |
e784a5a9793a45240bb6ca5ccb633055b8e5b25e | 16,079 | ipynb | Jupyter Notebook | tests/test-knowledge-graph.ipynb | AetherPrior/malaya | 45d37b171dff9e92c5d30bd7260b282cd0912a7d | [
"MIT"
] | 88 | 2021-01-06T10:01:31.000Z | 2022-03-30T17:34:09.000Z | tests/test-knowledge-graph.ipynb | AetherPrior/malaya | 45d37b171dff9e92c5d30bd7260b282cd0912a7d | [
"MIT"
] | 43 | 2021-01-14T02:44:41.000Z | 2022-03-31T19:47:42.000Z | tests/test-knowledge-graph.ipynb | AetherPrior/malaya | 45d37b171dff9e92c5d30bd7260b282cd0912a7d | [
"MIT"
] | 38 | 2021-01-06T07:15:03.000Z | 2022-03-19T05:07:50.000Z | 40.603535 | 459 | 0.628459 | [
[
[
"import sys\nimport os\n\nSOURCE_DIR = os.path.dirname(os.path.dirname(os.path.abspath(__name__)))\nsys.path.insert(0, SOURCE_DIR)",
"_____no_output_____"
],
[
"import malaya\nimport logging\n\nlogging.basicConfig(level=logging.DEBUG)",
"_____no_output_____"
],
[
"malaya.__home__",
"_____no_output_____"
],
[
"string1 = \"Yang Berhormat Dato Sri Haji Mohammad Najib bin Tun Haji Abdul Razak ialah ahli politik Malaysia dan merupakan bekas Perdana Menteri Malaysia ke-6 yang mana beliau menjawat jawatan dari 3 April 2009 hingga 9 Mei 2018. Beliau juga pernah berkhidmat sebagai bekas Menteri Kewangan dan merupakan Ahli Parlimen Pekan Pahang\"\nstring2 = \"Pahang ialah negeri yang ketiga terbesar di Malaysia Terletak di lembangan Sungai Pahang yang amat luas negeri Pahang bersempadan dengan Kelantan di utara Perak Selangor serta Negeri Sembilan di barat Johor di selatan dan Terengganu dan Laut China Selatan di timur.\"",
"_____no_output_____"
],
[
"model = malaya.knowledge_graph.transformer()\nquantized_model = malaya.knowledge_graph.transformer(quantized = True)",
"DEBUG:urllib3.connectionpool:Starting new HTTPS connection (1): f000.backblazeb2.com:443\nDEBUG:urllib3.connectionpool:https://f000.backblazeb2.com:443 \"HEAD /file/malaya-model/knowledge-graph-generator/base/model.pb HTTP/1.1\" 200 0\nDEBUG:urllib3.connectionpool:Starting new HTTPS connection (1): f000.backblazeb2.com:443\nDEBUG:urllib3.connectionpool:https://f000.backblazeb2.com:443 \"HEAD /file/malaya-model/bpe/sp10m.cased.ms-en.model HTTP/1.1\" 200 0\nDEBUG:urllib3.connectionpool:Starting new HTTPS connection (1): f000.backblazeb2.com:443\nDEBUG:urllib3.connectionpool:https://f000.backblazeb2.com:443 \"HEAD /file/malaya-model/knowledge-graph-generator/base/version HTTP/1.1\" 404 0\nDEBUG:urllib3.connectionpool:Starting new HTTPS connection (1): f000.backblazeb2.com:443\n"
],
[
"r = model.greedy_decoder([string1, string2])\nr[0]",
"WARNING:root:1\n"
],
[
"model = malaya.knowledge_graph.transformer(model = 'large')\nquantized_model = malaya.knowledge_graph.transformer(model = 'large', quantized = True)",
"DEBUG:urllib3.connectionpool:Starting new HTTPS connection (1): f000.backblazeb2.com:443\nDEBUG:urllib3.connectionpool:https://f000.backblazeb2.com:443 \"HEAD /file/malaya-model/knowledge-graph-generator/large/model.pb HTTP/1.1\" 200 0\nDEBUG:urllib3.connectionpool:Starting new HTTPS connection (1): f000.backblazeb2.com:443\nDEBUG:urllib3.connectionpool:https://f000.backblazeb2.com:443 \"HEAD /file/malaya-model/bpe/sp10m.cased.ms-en.model HTTP/1.1\" 200 0\nDEBUG:urllib3.connectionpool:Starting new HTTPS connection (1): f000.backblazeb2.com:443\nDEBUG:urllib3.connectionpool:https://f000.backblazeb2.com:443 \"HEAD /file/malaya-model/knowledge-graph-generator/large/version HTTP/1.1\" 404 0\nDEBUG:urllib3.connectionpool:Starting new HTTPS connection (1): f000.backblazeb2.com:443\n"
],
[
"r = model.greedy_decoder([string1, string2])\nr[0]",
"WARNING:root:1\n"
],
[
"malaya.utils.delete_cache('knowledge-graph-generator')",
"_____no_output_____"
]
]
] | [
"code"
] | [
[
"code",
"code",
"code",
"code",
"code",
"code",
"code",
"code",
"code"
]
] |
e784ab349becc02783badd7ec52e551d6f65c2d6 | 29,055 | ipynb | Jupyter Notebook | ecco_LPsstvarbudget_load.ipynb | cpatrizio88/pangeo_binder_example | 8f61ac1ed0fea3b01c9c40b3b0c11e42b8cc4f9b | [
"BSD-3-Clause"
] | 1 | 2022-03-08T12:27:08.000Z | 2022-03-08T12:27:08.000Z | ecco_LPsstvarbudget_load.ipynb | cpatrizio88/pangeo_binder_example | 8f61ac1ed0fea3b01c9c40b3b0c11e42b8cc4f9b | [
"BSD-3-Clause"
] | null | null | null | ecco_LPsstvarbudget_load.ipynb | cpatrizio88/pangeo_binder_example | 8f61ac1ed0fea3b01c9c40b3b0c11e42b8cc4f9b | [
"BSD-3-Clause"
] | 1 | 2020-05-09T01:07:03.000Z | 2020-05-09T01:07:03.000Z | 27.566414 | 264 | 0.527827 | [
[
[
"import xarray as xr\nimport gcsfs\nimport intake\nimport numpy as np\nimport matplotlib\n#import cmocean\nimport stats\nimport stats as st\nfrom matplotlib import pyplot as plt\n%matplotlib inline\nimport ecco_v4_tools as ecco\n# Import plotting libraries\nimport importlib\nimport llcmapping\nimportlib.reload(llcmapping)\nfrom llcmapping import LLCMapper\nimportlib.reload(ecco)\nimportlib.reload(ecco.tile_plot_proj)\nimport glob",
"/glade/u/home/patrizio/miniconda3/envs/pangeo/lib/python3.7/site-packages/tqdm/autonotebook/__init__.py:18: TqdmExperimentalWarning: Using `tqdm.autonotebook.tqdm` in notebook mode. Use `tqdm.tqdm` instead to force console mode (e.g. in jupyter console)\n \" (e.g. in jupyter console)\", TqdmExperimentalWarning)\n"
],
[
"matplotlib.rcParams.update({'font.size': 16})\nmatplotlib.rcParams.update({'axes.titlesize': 16})\nmatplotlib.rcParams.update({'figure.figsize': (10,8)})\nmatplotlib.rcParams.update({'lines.linewidth': 2})\nmatplotlib.rcParams.update({'legend.fontsize': 18})\nmatplotlib.rcParams.update({'mathtext.fontset': 'cm'})\nmatplotlib.rcParams.update({'ytick.major.size': 3})\nmatplotlib.rcParams.update({'axes.labelsize': 16})\nmatplotlib.rcParams.update({'ytick.labelsize': 16})\nmatplotlib.rcParams.update({'xtick.labelsize': 16})",
"_____no_output_____"
],
[
"fin = '/glade/work/patrizio/ECCO/' \nfout = '/glade/scratch/patrizio/figs/'",
"_____no_output_____"
],
[
"fcoords = glob.glob(fin + 'fields/*coords.nc')[0]\nfsnp = glob.glob(fin + 'fields/*snp.nc')\nfvars = set(glob.glob(fin + 'fields/*.nc')) - set(glob.glob(fin + 'fields/*coords.nc')) - set(glob.glob(fin + 'fields/*snp.nc'))\nfvars = list(fvars)\nfvars ",
"_____no_output_____"
],
[
"coords = xr.open_dataset(fcoords)\ncoords",
"_____no_output_____"
],
[
"ntchunk=288\n\nds_snp = xr.open_mfdataset(fsnp,concat_dim=None)\nds_snp = ds_snp.chunk({'time':ntchunk-1})",
"_____no_output_____"
],
[
"ds = xr.open_mfdataset(fvars,concat_dim=None)\nds",
"_____no_output_____"
],
[
"ds_MXLDEPTH = xr.open_zarr(fin + 'ecco-data/MXLDEPTH', chunks={'time':ntchunk})\nmxldepth = ds_MXLDEPTH.MXLDEPTH",
"_____no_output_____"
],
[
"mxldepth",
"_____no_output_____"
]
],
[
[
"For some reason the mixed layer depth coordinate indices are displaced by +1 in relation to the ECCO data stored on Pangeo. The coordinates need to be matched for future calculations. ",
"_____no_output_____"
]
],
[
[
"mxldepth.coords['i'] = coords['i']\nmxldepth.coords['j'] = coords['j']",
"_____no_output_____"
]
],
[
[
"Calculate climatological mean mixed layer depth. We will be using this later to mask grid points outside of the mixed layer. ",
"_____no_output_____"
]
],
[
[
"mxldepth_clim=mxldepth.mean(dim='time').load()\n#mxldepth_clim=mxldepth.mean(dim='time').persist()",
"_____no_output_____"
]
],
[
[
"Make a mask of points outside the ocean mixed layer:",
"_____no_output_____"
]
],
[
[
"mxlpoints = np.abs(coords['Z']) <= mxldepth_clim",
"_____no_output_____"
],
[
"# Flag for low-pass filtering\nlowpass=True\n\n# Filter requirements\norder = 5\nfs = 1 # sample rate, (cycles per month)\nTn = 12*3.\ncutoff = 1/Tn # desired cutoff frequency of the filter (cycles per month)\n\n# Face numbers to analyze\n# 0: Southern Ocean (Atlantic)\n# 1: South Atlantic Ocean / Africa \n# 2: East North Atlantic / Europe\n# 3: Southern Ocean (Indian)\n# 4: Indian Ocean\n# 5: Asia\n# 6: Arctic\n# 7: North Pacific (central)\n# 8: West South Pacific\n# 9: Southern Ocean (West Pacific)\n# 10: North America / West North Atlantic\n# 11: East South Pacific / South America\n# 12: Southern Ocean(East Pacific)\n#facen = [5,7]\n\n#Note: longitude bounds can either be 0 < bounds < 360, or -180 < bounds < 180. \n#The only requirement is that the left longitude bound is less than the right bound \n#(along date line must use 0 < bounds < 360).\n#(along prime meridian must use -180 < bounds < 180)\n\n# Complete global \n#facen=[0,1,2,3,4,5,6,7,8,9,10,11,12]\n#bnds = [0,359.9,-90,90]\n\n#facen=[]\n#bnds = [0,359.9,-90,90]\n\n# Global (excluding polar regions)\n#facen=[1,2,4,5,7,8,10,11]\n#bnds = [0,359.9,-58,70]\n\n#Southern Ocean (Atlantic)\n#facen=[0]\n#bnds = [-20,20,-58,-90]\n\n#1: South Atlantic Ocean / Africa\n#facen=[1]\n#bnds = [-38,30,-58,10]\n\n#2: East North Atlantic \n#facen=[2]\n#bnds = [-38,30,10,70]\n\n#3: Southern Ocean (Indian)\n#facen=[3]\n#bnds = [60,143,-58,-90]\n\n#4: Indian Ocean\n#facen=[4]\n#bnds = [60,143,-58,10]\n\n#7: North Pacific (central)\n#facen=[7]\n#bnds = [145,230,10,70]\n\n#8: West South Pacific\n#facen=[8]\n#bnds = [145,230,-58,10]\n\n#11: East South Pacific\n#facen=[11]\n#bnds = [-128,-38,-58,10]\n\n#2, 10: North Atlantic\nfacen=[2,10]\nbnds = [-80,0,10,70]\n\n#5,7,10: North Pacific\n#facen=[5,7,10]\n#bnds = [100,270,10,70]\n\n#4,5,7,8,10,11: Pacific\n#facen=[4,5,7,8,10,11]\n#bnds = [100,300,-70,70]\n\n#5,7,8,10,11: Tropical Pacific\n#facen=[5,7,8,10,11]\n#bnds = [145,290,-15,15]\n\n#5,7: KOE\n#facen=[5,7]\n#bnds = [120,180,15,60]",
"_____no_output_____"
],
[
"rho0 = 1029 #sea-water density (kg/m^3)\nc_p = 3994 #sea-water heat capacity (J/kg/K)",
"_____no_output_____"
],
[
"coords=coords.isel(face=facen)",
"_____no_output_____"
],
[
"# Vertical grid spacing\ndrF = coords.drF\nhFacC = coords.hFacC\n#rA = coords.rA.isel(face=facen).load()\n#vol = drF*hFacC*rA.load()",
"_____no_output_____"
],
[
"c_o = rho0*c_p*drF*hFacC",
"_____no_output_____"
],
[
"T = ds_snp.T.isel(face=facen)\nadv_ConvH = ds.adv_ConvH.isel(face=facen)\ndif_ConvH = ds.dif_ConvH.isel(face=facen)\nforcH = ds.forcH.isel(face=facen)",
"_____no_output_____"
],
[
"dt = coords.time_snp[1:].load()\ndt = dt.rename({'time_snp': 'time'})\n# delta t in seconds. Note: divide by 10**9 to convert nanoseconds to seconds\ndt.values = [float(t)/10**9 for t in np.diff(coords.time_snp)]\n\n# time axis of dt should be the same as of the monthly averages\ndt.time.values = coords.time[1:-1].values",
"_____no_output_____"
],
[
"lons = coords.XC\nlats = coords.YC",
"_____no_output_____"
],
[
"T_anom, T_clim = st.anom(T) \nC_adv_anom, C_adv_clim = st.anom(adv_ConvH)\nC_dif_anom, C_dif_clim = st.anom(dif_ConvH)\nC_forc_anom, C_forc_clim = st.anom(forcH)\ntotalH_anom = C_adv_anom + C_dif_anom + C_forc_anom",
"_____no_output_____"
],
[
"T_anom = T_anom.chunk({'time':ntchunk-1})\nC_adv_anom = C_adv_anom.chunk({'time':ntchunk})\nC_dif_anom = C_dif_anom.chunk({'time':ntchunk})\nC_forc_anom = C_forc_anom.chunk({'time':ntchunk})",
"_____no_output_____"
],
[
"if lowpass:\n \n T_anom = T_anom.chunk({'time':288, 'j':10, 'i':10})\n \n C_adv_anom = C_adv_anom.chunk({'time':288, 'j':10, 'i':10})\n C_dif_anom = C_dif_anom.chunk({'time':288, 'j':10, 'i':10})\n C_forc_anom = C_forc_anom.chunk({'time':288, 'j':10, 'i':10})\n \n T_anom = stats.butter_lowpass_filter_xr(T_anom, cutoff, fs, order)\n \n C_adv_anom = stats.butter_lowpass_filter_xr(C_adv_anom, cutoff, fs, order)\n C_dif_anom = stats.butter_lowpass_filter_xr(C_dif_anom, cutoff, fs, order)\n C_forc_anom = stats.butter_lowpass_filter_xr(C_forc_anom, cutoff, fs, order)\n \n totalH_anom = C_adv_anom + C_dif_anom + C_forc_anom",
"_____no_output_____"
],
[
"%time T_anom.load()\n%time C_adv_anom.load()\n%time C_dif_anom.load()\n%time C_forc_anom.load()",
"_____no_output_____"
],
[
"tendH_perMonth = (T_anom.shift(time=-1)-T_anom)[:-1]",
"_____no_output_____"
],
[
"# Make sure time axis is the same as for the monthly variables\ntendH_perMonth.time.values = coords.time[1:-1].values\n\n# Convert tendency from 1/month to 1/s\ntendH_perSec = tendH_perMonth/dt\ntendH_perSec = tendH_perSec.transpose('face','time', 'k', 'j', 'i')",
"_____no_output_____"
],
[
"# Define tendH array with correct dimensions\ntendH_anom = xr.DataArray(np.nan*np.zeros([len(facen),np.shape(tendH_perSec)[1]+2,50,90,90]),\n coords={'face': facen, 'time': range(np.shape(tendH_perSec)[1]+2),'k': np.array(range(0,50)),\n 'j': np.array(range(0,90)),'i': np.array(range(0,90))},dims=['face', 'time','k', 'j','i'])\n\ntendH_anom.time.values = coords.time.values",
"_____no_output_____"
],
[
"tendH_anom",
"_____no_output_____"
],
[
"tendH_anom.nbytes/1e9",
"_____no_output_____"
],
[
"# Add coordinates#\ntendH_anom['XC'] = lons\ntendH_anom['YC'] = lats\ntendH_anom['Z'] = coords.Z\n\n# Total tendency (degC/s)\ntendH_anom.values[:,1:-1,:] = tendH_perSec.values\n%time tendH_anom.load()\n#%time tendH.persist()",
"_____no_output_____"
],
[
"# Convert from degC/s to W/m^2\ntendH_anom = c_o*tendH_anom\ntendH_anom = tendH_anom.transpose('time','face', 'k', 'j', 'i')",
"_____no_output_____"
],
[
"face=0\nk = 0\nj = 15\ni = 15\n\nplt.figure(figsize=(14,10))\nplt.subplot(2, 1, 1)\nplt.plot(tendH_anom.time, tendH_anom.isel(face=face,k=k,j=j,i=i), lw=4, color='K', marker='.',label='total tendency')\nplt.plot(C_forc_anom.time, C_forc_anom.isel(face=face,k=k,j=j,i=i), lw=2, color='C0', marker='.',label='forcing')\nplt.plot(C_adv_anom.time, C_adv_anom.isel(face=face,k=k,j=j,i=i), lw=2, color='C1', marker='.',label='advection')\nplt.axhline(0,color='k',lw=1)\nplt.plot(C_dif_anom.time, C_dif_anom.isel(face=face,k=k,j=j,i=i), lw=2, color='C2',label='diffusion')\nplt.setp(plt.gca(), 'xticklabels',[])\nplt.legend(loc='best',frameon=False,fontsize=14)\n\nplt.subplot(2, 1, 2)\nplt.plot(totalH_anom.time, totalH_anom.isel(face=face,k=k,j=j,i=i), lw=4, color='red', marker='.',label='RHS')\nplt.plot(tendH_anom.time, tendH_anom.isel(face=face,k=k,j=j,i=i), lw=2, color='blue', marker='.',label='LHS')\nplt.plot(tendH_anom.time, (totalH_anom-tendH_anom).isel(face=face,k=k,j=j,i=i), lw=2, color='k', marker='.',label='RHS - LHS')\nplt.legend(loc='best',frameon=False,fontsize=14)\nplt.savefig(fout + 'sstbudget_anom_ts.png')",
"_____no_output_____"
],
[
"T_var = T_anom.var(dim='time')\n%time T_var.load()\n#%time T_var.persist()",
"_____no_output_____"
],
[
"tendH_anom = tendH_anom/c_o",
"_____no_output_____"
],
[
"#tendH_anom = tendH_anom.transpose('time','face', 'k', 'j', 'i')\ncov_adv = st.cov(tendH_anom, C_adv_anom)\ncov_dif = st.cov(tendH_anom, C_dif_anom)\ncov_forc = st.cov(tendH_anom, C_forc_anom)",
"_____no_output_____"
],
[
"cov_adv.nbytes/1e9",
"_____no_output_____"
],
[
"%time cov_adv.load()\n%time cov_dif.load()\n%time cov_forc.load()",
"_____no_output_____"
],
[
"deltat = dt.mean()\ndeltat.compute()",
"_____no_output_____"
],
[
"r_1 = st.cor(T_anom, T_anom,lagx=1).compute()\nr_1",
"_____no_output_____"
],
[
"fac = (deltat**2/(2*c_o*(1-r_1)))\nfac.load()",
"_____no_output_____"
],
[
"T_var_sum = fac*(cov_adv + cov_dif + cov_forc)",
"_____no_output_____"
],
[
"%time T_var_sum.load()\n#%time T_var_sum.persist()",
"_____no_output_____"
],
[
"mapper = LLCMapper(coords)",
"_____no_output_____"
],
[
"k=0\nmapper(T_var.isel(k=k), bnds=bnds, cmap='cubehelix_r', vmin=0,vmax=1.0)\nmapper(T_var_sum.isel(k=k), bnds=bnds, cmap='cubehelix_r', vmin=0,vmax=1.0)",
"_____no_output_____"
]
],
[
[
"The temperature variance budget is clearly balanced! Let's take a look at the contribution due to each term.",
"_____no_output_____"
]
],
[
[
"T_var_adv = fac*cov_adv\nT_var_dif = fac*cov_dif\nT_var_forc = fac*cov_forc",
"_____no_output_____"
],
[
"vmin=-1.0\nvmax=1.0\nsstmax=1.6\nif lowpass:\n sstmax=0.5\n vmin=-0.5\n vmax=0.5",
"_____no_output_____"
]
],
[
[
"### Contributions to temperature variance from advection, diffusion and surface forcing",
"_____no_output_____"
]
],
[
[
"k=0\nmapper(T_var_sum.isel(k=k), bnds=bnds, cmap='cubehelix_r', vmin=0,vmax=sstmax)\nplt.title(r'temperature variance (K$^2$)')\nplt.savefig(fout + 'Tvar_sum.png')\nmapper(T_var_adv.isel(k=k), bnds=bnds, cmap='RdBu_r', vmin=vmin,vmax=vmax)\nplt.title(r'advective contribution (K$^2$)')\nplt.savefig(fout + 'Tvar_adv.png')\nmapper(T_var_dif.isel(k=k), bnds=bnds, cmap='RdBu_r', vmin=vmin,vmax=vmax)\nplt.title(r'diffusive contribution (K$^2$)')\nplt.savefig(fout + 'Tvar_dif.png')\nmapper(T_var_forc.isel(k=k), bnds=bnds, cmap='RdBu_r', vmin=vmin,vmax=vmax)\nplt.title(r'surface forcing contribution (K$^2$)')\nplt.savefig(fout + 'Tvar_forc.png')",
"_____no_output_____"
]
],
[
[
"### Contributions to ocean mixed layer temperature variance from advection, diffusion and surface forcing",
"_____no_output_____"
]
],
[
[
"mxlpoints = mxlpoints.isel(face=facen)\ndelz = drF*hFacC\ndelz=delz.where(mxlpoints)\ndelz_sum = delz.sum(dim='k')",
"_____no_output_____"
],
[
"mxlpoints",
"_____no_output_____"
],
[
"weights = delz/delz_sum",
"_____no_output_____"
],
[
"T_var_mxl = (weights*T_var).where(mxlpoints).sum(dim='k')",
"_____no_output_____"
],
[
"T_var_adv_mxl = (weights*T_var_adv).where(mxlpoints).sum(dim='k')\nT_var_dif_mxl = (weights*T_var_dif).where(mxlpoints).sum(dim='k')\nT_var_forc_mxl = (weights*T_var_forc).where(mxlpoints).sum(dim='k')",
"_____no_output_____"
],
[
"T_var_sum_mxl = T_var_adv_mxl + T_var_dif_mxl + T_var_forc_mxl",
"_____no_output_____"
],
[
"#f, axes = plt.subplots(2,2,figsize=(16,12))\n#f.tight_layout()\nmapper(T_var_sum_mxl, bnds=bnds, cmap='cubehelix_r', vmin=0,vmax=sstmax)\nplt.title(r'temperature variance (K$^2$)')\nplt.savefig(fout + 'Tmxlvar_sum.png')\n\nmapper(T_var_adv_mxl, bnds=bnds, cmap='RdBu_r', vmin=vmin,vmax=vmax)\nplt.title(r'advective contribution (K$^2$)')\nplt.savefig(fout + 'Tmxlvar_adv.png')\n\nmapper(T_var_dif_mxl, bnds=bnds, cmap='RdBu_r', vmin=vmin,vmax=vmax)\nplt.title(r'diffusive contribution (K$^2$)')\nplt.savefig(fout + 'Tmxlvar_dif.png')\n\nmapper(T_var_forc_mxl, bnds=bnds, cmap='RdBu_r', vmin=vmin,vmax=vmax)\nplt.title(r'surface forcing contribution (K$^2$)')\nplt.savefig(fout + 'Tmxlvar_forc.png')",
"_____no_output_____"
],
[
"#mapper(T_var_sum_mxl, bnds=bnds, cmap='cubehelix_r', vmin=0,vmax=1.0)\n#plt.title(r'temperature variance (K$^2$)')\n#plt.savefig(fout + 'Tmxlvar_sum.png')\nmapper(T_var_adv_mxl + T_var_dif_mxl, bnds=bnds, cmap='RdBu_r', vmin=vmin,vmax=vmax)\nplt.title(r'ocean dynamics (advective + diffusive) contribution (K$^2$)')\nplt.savefig(fout + 'Tmxlvar_ocndyn.png')\n#mapper(T_var_forc_mxl, bnds=bnds, cmap='RdBu_r', vmin=-1.0,vmax=1.0)\n#plt.title(r'surface forcing contribution (K$^2$)')\n#plt.savefig(fout + 'Tmxlvar_forc.png')",
"_____no_output_____"
]
]
] | [
"code",
"markdown",
"code",
"markdown",
"code",
"markdown",
"code",
"markdown",
"code",
"markdown",
"code",
"markdown",
"code"
] | [
[
"code",
"code",
"code",
"code",
"code",
"code",
"code",
"code",
"code"
],
[
"markdown"
],
[
"code"
],
[
"markdown"
],
[
"code"
],
[
"markdown"
],
[
"code",
"code",
"code",
"code",
"code",
"code",
"code",
"code",
"code",
"code",
"code",
"code",
"code",
"code",
"code",
"code",
"code",
"code",
"code",
"code",
"code",
"code",
"code",
"code",
"code",
"code",
"code",
"code",
"code",
"code",
"code",
"code",
"code"
],
[
"markdown"
],
[
"code",
"code"
],
[
"markdown"
],
[
"code"
],
[
"markdown"
],
[
"code",
"code",
"code",
"code",
"code",
"code",
"code",
"code"
]
] |
e784b400e029887a8b2c7c6d940eb19af2ee952d | 36,162 | ipynb | Jupyter Notebook | Speaker_predictions.ipynb | aakaashjois/Dense-Recurrent-Net-For-Speech-Command-Classification | 3e4d581c0ad3594756e405e8c9a1b45f04ed2179 | [
"Apache-2.0"
] | 1 | 2020-12-29T19:04:51.000Z | 2020-12-29T19:04:51.000Z | Speaker_predictions.ipynb | aakaashjois/Dense-Recurrent-Net-For-Speech-Command-Classification | 3e4d581c0ad3594756e405e8c9a1b45f04ed2179 | [
"Apache-2.0"
] | null | null | null | Speaker_predictions.ipynb | aakaashjois/Dense-Recurrent-Net-For-Speech-Command-Classification | 3e4d581c0ad3594756e405e8c9a1b45f04ed2179 | [
"Apache-2.0"
] | null | null | null | 48.474531 | 6,452 | 0.661551 | [
[
[
"import pickle\nimport tensorflow as tf\nimport os\nimport numpy as np\nfrom src.utils import DatasetUtils\nfrom sklearn.metrics import confusion_matrix\nimport matplotlib.pyplot as plt\nimport plotly.offline as ply\nimport plotly.graph_objs as go\nkeras = tf.keras\nply.init_notebook_mode(connected=True)",
"c:\\users\\aakaas~1\\docume~1\\nyu\\projects\\speech~1\\env\\lib\\site-packages\\h5py\\__init__.py:36: FutureWarning: Conversion of the second argument of issubdtype from `float` to `np.floating` is deprecated. In future, it will be treated as `np.float64 == np.dtype(float).type`.\n from ._conv import register_converters as _register_converters\n"
],
[
"model1 = keras.models.load_model('./models/noisy/convnet.h5')",
"_____no_output_____"
],
[
"model2 = keras.models.load_model('./models/noisy/resnet.h5')",
"_____no_output_____"
],
[
"model3 = keras.models.load_model('./models/noisy/residualresnet.h5')",
"_____no_output_____"
],
[
"utils = DatasetUtils()",
"_____no_output_____"
],
[
"classes = utils.get_label_encoder().classes_",
"_____no_output_____"
],
[
"test_data, test_labels = utils.get_dataset_and_encoded_labels('test_data.npy', 'test_labels.npy')",
"_____no_output_____"
],
[
"all_predictions1 = model1.predict(test_data)\nall_predictions2 = model2.predict(test_data)\nall_predictions3 = model3.predict(test_data)",
"_____no_output_____"
],
[
"test_labels = classes[np.argmax(test_labels, axis=-1)]",
"_____no_output_____"
],
[
"all_pred_labels1 = classes[np.argmax(all_predictions1, axis=-1)]\nall_pred_labels2 = classes[np.argmax(all_predictions2, axis=-1)]\nall_pred_labels3 = classes[np.argmax(all_predictions3, axis=-1)]",
"_____no_output_____"
],
[
"cm1 = confusion_matrix(test_labels, all_pred_labels1, labels=classes)\ncm1 = cm1.astype('float') / cm1.sum(axis=1)[:, np.newaxis]\ncm2 = confusion_matrix(test_labels, all_pred_labels2, labels=classes)\ncm2 = cm2.astype('float') / cm2.sum(axis=1)[:, np.newaxis]\ncm3 = confusion_matrix(test_labels, all_pred_labels3, labels=classes)\ncm3 = cm3.astype('float') / cm3.sum(axis=1)[:, np.newaxis]",
"_____no_output_____"
],
[
"per_class_accuracy1 = dict()\nfor i, label in enumerate(classes):\n per_class_accuracy1[label] = cm1[i, i] * 100\nper_class_accuracy2 = dict()\nfor i, label in enumerate(classes):\n per_class_accuracy2[label] = cm2[i, i] * 100\nper_class_accuracy3 = dict()\nfor i, label in enumerate(classes):\n per_class_accuracy3[label] = cm3[i, i] * 100",
"_____no_output_____"
],
[
"sorted_per_class_accuracy1 = sorted(per_class_accuracy1.items(), key=lambda x: x[1], reverse=True)\nsorted_per_class_accuracy2 = sorted(per_class_accuracy2.items(), key=lambda x: x[1], reverse=True)\nsorted_per_class_accuracy3 = sorted(per_class_accuracy3.items(), key=lambda x: x[1], reverse=True)",
"_____no_output_____"
],
[
"sorted_per_class_accuracy3",
"_____no_output_____"
],
[
"trace1 = go.Histogram(x=list(per_class_accuracy1.values()),\n name='ConvNet',\n opacity=0.8)\ntrace2 = go.Histogram(x=list(per_class_accuracy2.values()),\n name='DenseNet',\n opacity=0.8)\ntrace3 = go.Histogram(x=list(per_class_accuracy3.values()),\n name='Recurrent-DenseNet',\n opacity=0.8)\ndata = [trace1, trace2, trace3]\nlayout = go.Layout()\nply.iplot(dict(data=data, layout=layout))",
"_____no_output_____"
],
[
"sorted_per_class_acc = sorted(per_class_accuracy.items(), key=lambda x: x[1], reverse=True)",
"_____no_output_____"
]
],
[
[
"speakers = os.listdir('./speaker_spectrograms/')\nspeaker_pred = dict()\nfor speaker in speakers:\n spects = np.load('./speaker_spectrograms/' + speaker)\n spects = spects.reshape(spects.shape+(1,))\n pred = model.predict(spects)\n pred = np.argmax(pred, axis=-1)\n pred_labels = classes[pred]\n speaker_pred[speaker.split('.')[0]] = pred_labels\nwith open('./per_speaker_pred.pkl', 'wb') as handle:\n pickle.dump(speaker_pred, handle, protocol=pickle.HIGHEST_PROTOCOL)",
"_____no_output_____"
]
],
[
[
"speaker_pred = pickle.load(open('./per_speaker_pred.pkl', 'rb'))",
"_____no_output_____"
],
[
"speaker_gt = pickle.load(open('./per_speaker_gt.pkl', 'rb'))",
"_____no_output_____"
],
[
"per_speaker = dict()\nfor speaker in os.listdir('./speaker_spectrograms/'):\n speaker = speaker.split('.')[0]\n pred = np.array(speaker_pred[speaker])\n gt = np.array(speaker_gt[speaker])\n per_label = dict()\n for label in np.unique(gt):\n label_idx = np.where(gt == label)\n acc = np.sum(np.core.defchararray.equal(pred[label_idx], gt[label_idx])) / len(label_idx[0])\n per_label[label] = acc * 100\n per_speaker[speaker] = per_label",
"_____no_output_____"
],
[
"list(per_speaker.values())[0]",
"_____no_output_____"
],
[
"per_speaker_acc = dict()\nfor speaker in os.listdir('./speaker_spectrograms/'):\n speaker = speaker.split('.')[0]\n pred = speaker_pred[speaker]\n gt = speaker_gt[speaker]\n acc = np.sum(np.core.defchararray.equal(pred, gt)) / len(pred)\n per_speaker_acc[speaker] = acc * 100\nsorted_per_speaker_acc = sorted(per_speaker_acc.items(), key=lambda x: x[1], reverse=True)",
"_____no_output_____"
],
[
"class_names = []\nclass_accs = []\nper_class_accuracy_list = np.full((len(classes), len(per_speaker)), np.nan)\nfor index, item in enumerate(sorted_per_class_acc):\n class_names.append(item[0])\n class_accs.append(item[1])\n for i, speaker in enumerate(list(per_speaker.values())):\n if item[0] in speaker.keys():\n per_class_accuracy_list[index, i] = speaker[item[0]]",
"_____no_output_____"
],
[
"boxprops = dict(linestyle='-', linewidth=1.0, color='k')\nmedianprops = dict(linestyle='-', linewidth=1.0, color='k')\nwhiskerprops = dict(linestyle='-', linewidth=1.0, color='k')\ncapprops = dict(linestyle='-', linewidth=1.0, color='k')\nplt.boxplot(per_class_accuracy_list.T,\n patch_artist = True,\n boxprops=boxprops,\n capprops=capprops,\n medianprops=medianprops,\n whiskerprops=whiskerprops,\n whis=\"range\")",
"c:\\users\\aakaas~1\\docume~1\\nyu\\projects\\speech~1\\env\\lib\\site-packages\\numpy\\lib\\function_base.py:4291: RuntimeWarning: Invalid value encountered in percentile\n interpolation=interpolation)\nc:\\users\\aakaas~1\\docume~1\\nyu\\projects\\speech~1\\env\\lib\\site-packages\\numpy\\core\\_methods.py:29: RuntimeWarning: invalid value encountered in reduce\n return umr_minimum(a, axis, None, out, keepdims)\nc:\\users\\aakaas~1\\docume~1\\nyu\\projects\\speech~1\\env\\lib\\site-packages\\numpy\\core\\_methods.py:26: RuntimeWarning: invalid value encountered in reduce\n return umr_maximum(a, axis, None, out, keepdims)\nc:\\users\\aakaas~1\\docume~1\\nyu\\projects\\speech~1\\env\\lib\\site-packages\\matplotlib\\cbook\\__init__.py:1872: RuntimeWarning: invalid value encountered in less_equal\n wiskhi = np.compress(x <= hival, x)\nc:\\users\\aakaas~1\\docume~1\\nyu\\projects\\speech~1\\env\\lib\\site-packages\\matplotlib\\cbook\\__init__.py:1879: RuntimeWarning: invalid value encountered in greater_equal\n wisklo = np.compress(x >= loval, x)\nc:\\users\\aakaas~1\\docume~1\\nyu\\projects\\speech~1\\env\\lib\\site-packages\\matplotlib\\cbook\\__init__.py:1887: RuntimeWarning: invalid value encountered in less\n np.compress(x < stats['whislo'], x),\nc:\\users\\aakaas~1\\docume~1\\nyu\\projects\\speech~1\\env\\lib\\site-packages\\matplotlib\\cbook\\__init__.py:1888: RuntimeWarning: invalid value encountered in greater\n np.compress(x > stats['whishi'], x)\n"
],
[
"class_names",
"_____no_output_____"
],
[
"fig, ax = plt.subplots(1, 1, sharex=True, figsize=(5, 3))\n\nboxprops = dict(linestyle='-', linewidth=1.0, color='k')\nmedianprops = dict(linestyle='-', linewidth=1.0, color='k')\nwhiskerprops = dict(linestyle='-', linewidth=1.0, color='k')\ncapprops = dict(linestyle='-', linewidth=1.0, color='k')\nbplot = ax.boxplot(\n [100 * ,\n 100 * none_accs,\n 100 * all_accs],\n patch_artist = True,\n boxprops=boxprops,\n capprops=capprops,\n medianprops=medianprops,\n whiskerprops=whiskerprops,\n whis=\"range\");",
"_____no_output_____"
]
]
] | [
"code",
"markdown",
"code"
] | [
[
"code",
"code",
"code",
"code",
"code",
"code",
"code",
"code",
"code",
"code",
"code",
"code",
"code",
"code",
"code",
"code"
],
[
"markdown"
],
[
"code",
"code",
"code",
"code",
"code",
"code",
"code",
"code",
"code"
]
] |
e784bf374b03e950ab5dbdfccdeeebeba8f7f3d0 | 11,349 | ipynb | Jupyter Notebook | Dalon_4_RTD_MiniPro_Tweepy_Q5.ipynb | intellect82/venkateswarlu_SVAP_Asmt_R3 | ed6a9fffd0510d9ee31ad2189ea04f4ae274043a | [
"Apache-2.0",
"MIT"
] | null | null | null | Dalon_4_RTD_MiniPro_Tweepy_Q5.ipynb | intellect82/venkateswarlu_SVAP_Asmt_R3 | ed6a9fffd0510d9ee31ad2189ea04f4ae274043a | [
"Apache-2.0",
"MIT"
] | null | null | null | Dalon_4_RTD_MiniPro_Tweepy_Q5.ipynb | intellect82/venkateswarlu_SVAP_Asmt_R3 | ed6a9fffd0510d9ee31ad2189ea04f4ae274043a | [
"Apache-2.0",
"MIT"
] | null | null | null | 38.212121 | 783 | 0.565248 | [
[
[
"# Tweepy streamer\n",
"_____no_output_____"
],
[
" ## Find Top tweeting user:\n\n - Find User who is tweeting a lot.\n - Find top 50 across the world.\n",
"_____no_output_____"
],
[
"Since this is streaming application, we will use python logging module to log. [Further read.](https://www.webcodegeeks.com/python/python-logging-example/)",
"_____no_output_____"
]
],
[
[
"import logging # python logging module\n\n# basic format for logging\nlogFormat = \"%(asctime)s - [%(levelname)s] (%(funcName)s:%(lineno)d) %(message)s\"\n\n# logs will be stored in tweepy.log\nlogging.basicConfig(filename='tweepytopuser.log', level=logging.INFO, \n format=logFormat, datefmt=\"%Y-%m-%d %H:%M:%S\")\n",
"_____no_output_____"
]
],
[
[
"## Authentication and Authorisation\n\nCreate an app in twitter [here](https://apps.twitter.com/). Copy the necessary keys and access tokens, which will be used here in our code. \n\nThe authorization is done using Oauth, An open protocol to allow secure authorization in a simple and standard method from web, mobile and desktop applications. [Further read](https://oauth.net/). \n\nWe will use Tweepy a python module. Tweepy is open-sourced, hosted on [GitHub](https://github.com/tweepy/tweepy) and enables Python to communicate with Twitter platform and use its API. Tweepy supports oauth authentication. Authentication is handled by the tweepy.AuthHandler class.",
"_____no_output_____"
]
],
[
[
"import tweepy # importing all the modules required\nimport socket # will be used to create sockets\nimport json # manipulate json\n\nfrom httplib import IncompleteRead",
"_____no_output_____"
],
[
"# Keep these tokens secret, as anyone can have full access to your\n# twitter account, using these tokens\n\nconsumerKey = \"#\"\nconsumerSecret = \"#\"\n\naccessToken = \"#-#\"\naccessTokenSecret = \"#\"\n",
"_____no_output_____"
]
],
[
[
"Post this step, we will have full access to twitter api's",
"_____no_output_____"
]
],
[
[
"# Performing the authentication and authorization, post this step \n# we will have full access to twitter api's\ndef connectToTwitter():\n \"\"\"Connect to twitter.\"\"\"\n try:\n auth = tweepy.OAuthHandler(consumerKey, consumerSecret)\n auth.set_access_token(accessToken, accessTokenSecret)\n\n api = tweepy.API(auth)\n logging.info(\"Successfully logged in to twitter.\")\n return api, auth\n except Exception as e:\n logging.info(\"Something went wrong in oauth, please check your tokens.\")\n logging.error(e)\n",
"_____no_output_____"
]
],
[
[
"## Streaming with tweepy\n\nThe Twitter streaming API is used to download twitter messages in real time. We use streaming api instead of rest api because, the REST api is used to pull data from twitter but the streaming api pushes messages to a persistent session. This allows the streaming api to download more data in real time than could be done using the REST API.\n\nIn Tweepy, an instance of tweepy.Stream establishes a streaming session and routes messages to StreamListener instance. The on_data method of a stream listener receives all messages and calls functions according to the message type. \n\nBut the on_data method is only a stub, so we need to implement the functionality by subclassing StreamListener. \n\nUsing the streaming api has three steps.\n\n1. Create a class inheriting from StreamListener\n2. Using that class create a Stream object\n3. Connect to the Twitter API using the Stream.\n\n",
"_____no_output_____"
]
],
[
[
"# Tweet listner class which subclasses from tweepy.StreamListener\nclass TweetListner(tweepy.StreamListener):\n \"\"\"Twitter stream listner\"\"\"\n \n def __init__(self, csocket):\n self.clientSocket = csocket\n \n def dataProcessing(self, data):\n \"\"\"Process the data, before sending to spark streaming\n \"\"\"\n sendData = {} # data that is sent to spark streamer\n user = data.get(\"user\", {})\n name = user.get(\"name\", \"undefined\").encode('utf-8')\n sendData[\"name\"] = name\n #data_string = \"{}:{}\".format(name, followersCount) \n self.clientSocket.send(json.dumps(sendData) + u\"\\n\") # append new line character, so that spark recognizes it\n logging.debug(json.dumps(sendData))\n \n def on_data(self, raw_data):\n \"\"\" Called when raw data is received from connection.\n return False to stop stream and close connection.\n \"\"\"\n try:\n data = json.loads(raw_data)\n self.dataProcessing(data)\n #self.clientSocket.send(json.dumps(sendData) + u\"\\n\") # Because the connection was breaking\n return True\n except Exception as e:\n logging.error(\"An unhandled exception has occured, check your data processing\")\n logging.error(e)\n raise e\n \n def on_error(self, status_code):\n \"\"\"Called when a non-200 status code is returned\"\"\"\n logging.error(\"A non-200 status code is returned\")\n return True\n ",
"_____no_output_____"
],
[
"# Creating a proxy socket\ndef createProxySocket(host, port):\n \"\"\" Returns a socket which can be used to connect\n to spark.\n \"\"\"\n try:\n s = socket.socket() # initialize socket instance\n s.bind((host, port)) # bind to the given host and port \n s.listen(5) # Enable a server to accept connections.\n logging.info(\"Listening on the port {}\".format(port))\n cSocket, address = s.accept() # waiting for a connection\n logging.info(\"Received Request from: {}\".format(address))\n return cSocket\n except socket.error as e: \n if e.errno == socket.errno.EADDRINUSE: # Address in use\n logging.error(\"The given host:port {}:{} is already in use\"\\\n .format(host, port))\n logging.info(\"Trying on port: {}\".format(port + 1))\n return createProxySocket(host, port + 1)\n",
"_____no_output_____"
]
],
[
[
"## Drawbacks of twitter streaming API\n\nThe major drawback of the Streaming API is that Twitter’s Steaming API provides only a sample of tweets that are occurring. The actual percentage of total tweets users receive with Twitter’s Streaming API varies heavily based on the criteria users request and the current traffic. Studies have estimated that using Twitter’s Streaming API users can expect to receive anywhere from 1% of the tweets to over 40% of tweets in near real-time. The reason that you do not receive all of the tweets from the Twitter Streaming API is simply because Twitter doesn’t have the current infrastructure to support it, and they don’t want to; hence, the Twitter Firehose. [Ref](https://brightplanet.com/2013/06/twitter-firehose-vs-twitter-api-whats-the-difference-and-why-should-you-care/)\n\nSo we will use a hack i.e. get the top trending topics and use that to filter data.\n",
"_____no_output_____"
]
],
[
[
"if __name__ == \"__main__\":\n try:\n api, auth = connectToTwitter() # connecting to twitter\n # Global information is available by using 1 as the WOEID\n # woeid = getWOEIDForTrendsAvailable(api, \"Worldwide\") # get the woeid of the worldwide\n \n host = \"localhost\"\n port = 8600\n cSocket = createProxySocket(host, port) # Creating a socket\n \n while True:\n try:\n # Connect/reconnect the stream\n tweetStream = tweepy.Stream(auth, TweetListner(cSocket)) # Stream the twitter data\n # DON'T run this approach async or you'll just create a ton of streams!\n tweetStream.filter(track=\"iphone\") # Filter on trending topics\n except IncompleteRead:\n # Oh well, reconnect and keep trucking\n continue\n except KeyboardInterrupt:\n # Or however you want to exit this loop\n tweetStream.disconnect()\n break\n except Exception as e:\n logging.error(\"Unhandled exception has occured\")\n logging.error(e)\n continue\n \n except KeyboardInterrupt: # Keyboard interrupt called\n logging.error(\"KeyboardInterrupt was hit\")\n except Exception as e:\n logging.error(\"Unhandled exception has occured\")\n logging.error(e)\n",
"_____no_output_____"
]
]
] | [
"markdown",
"code",
"markdown",
"code",
"markdown",
"code",
"markdown",
"code",
"markdown",
"code"
] | [
[
"markdown",
"markdown",
"markdown"
],
[
"code"
],
[
"markdown"
],
[
"code",
"code"
],
[
"markdown"
],
[
"code"
],
[
"markdown"
],
[
"code",
"code"
],
[
"markdown"
],
[
"code"
]
] |
e784cc98f07693b4ce8605744e78f5e4976a6791 | 11,088 | ipynb | Jupyter Notebook | 2.IMDB.ipynb | Krax7/master-data-ai | 856e2b7b9ed0b5007aebf2d979adbf831bcb746f | [
"MIT"
] | 14 | 2021-02-12T00:40:17.000Z | 2021-07-05T23:34:29.000Z | 2.IMDB.ipynb | Krax7/master-data-ai | 856e2b7b9ed0b5007aebf2d979adbf831bcb746f | [
"MIT"
] | 10 | 2022-02-11T19:50:01.000Z | 2022-03-04T04:16:02.000Z | 2.IMDB.ipynb | Krax7/master-data-ai | 856e2b7b9ed0b5007aebf2d979adbf831bcb746f | [
"MIT"
] | 22 | 2021-02-12T02:34:26.000Z | 2021-07-22T01:30:23.000Z | 20.686567 | 324 | 0.530483 | [
[
[
"# Analizando información de IMDB con Keras\n\nYa aprendiste cómo se construye una red neuronal. ¡Ahora es tu turno! En este reto, vas a construir una red neuronal que logra predecir si hay un sentimiento positivo o negativo en un review.",
"_____no_output_____"
]
],
[
[
"import numpy as np\nimport keras\nfrom keras.datasets import imdb\nfrom keras.models import Sequential\nfrom keras.layers import Dense, Dropout, Activation\nfrom keras.preprocessing.text import Tokenizer\nimport matplotlib.pyplot as plt\n%matplotlib inline\n\nnp.random.seed(42)",
"_____no_output_____"
]
],
[
[
"## Paso 1. Cargar la información",
"_____no_output_____"
]
],
[
[
"# IMDB ya es un dataset que es parte de Keras, así que lo tenemos fácil!\n(x_train, y_train), (x_test, y_test) = imdb.load_data(num_words=1000)\n\nprint(x_train.shape)\nprint(x_test.shape)",
"_____no_output_____"
]
],
[
[
"## Paso 2. Comprender la información\n\nEsta vez la información ya esta preprocesada, por lo cuál es mucho más fácil trabajar con ella. Todas las palabras han sido transformadas a números, y cada review es un vector con las palabras que contine. El output es el sentimiento, donde 1 es un sentimiento positivo y 0 un sentimiento negativo",
"_____no_output_____"
]
],
[
[
"print(x_train[0])\nprint(y_train[0])",
"_____no_output_____"
]
],
[
[
"## Paso 3. Modificar la información para la red neuronal\n\n### One-hot encoding\n\nTenemos un vector con números, pero queremos convertirlo en muchos vectores con valor 0 ó 1. Por ejemplo, si el vector preprocesado contiene el número 14, entonces el vector procesado, en la entrada 14, será 1. Haremos lo mismo para la salida. Estamos trabajando con 50mil datos, así que se puede tardar unos segundos.",
"_____no_output_____"
]
],
[
[
"# One-hot encoding the output into vector mode, each of length 1000\ntokenizer = Tokenizer(num_words=1000)\nx_train = tokenizer.sequences_to_matrix(x_train, mode='binary')\nx_test = tokenizer.sequences_to_matrix(x_test, mode='binary')\nprint(x_train[0])",
"_____no_output_____"
],
[
"# One-hot encoding the output\nnum_classes = 2\ny_train = keras.utils.to_categorical(y_train, num_classes)\ny_test = keras.utils.to_categorical(y_test, num_classes)\nprint(y_train.shape)\nprint(y_test.shape)",
"_____no_output_____"
]
],
[
[
"## Paso 4. Construimos Arquitectura del Modelo\n\nConstruye un modelo secuencial. Siéntete libre de explorar y experimentar.",
"_____no_output_____"
]
],
[
[
"## TODO: Construye un modelo secuencial\n\n\n## TODO: Compila el modelo con un optimizador y una función de pérdida",
"_____no_output_____"
]
],
[
[
"## Paso 5. Entrenamos el modelo",
"_____no_output_____"
]
],
[
[
"## TODO: Corre el modelo. Experimenta con diferentes tamaños de batch y número de epochs. \n# Usa verbose=2 para ver cómo va progresando el modelo",
"_____no_output_____"
]
],
[
[
"## Paso 6. Evaluamos el modelo\n\n¿Crees poder llegar a más de 80%? ¿Qué tal arriba de 85%?",
"_____no_output_____"
]
],
[
[
"score = model.evaluate(x_test, y_test, verbose=0)\nprint(\"Accuracy: \", score[1])",
"_____no_output_____"
]
],
[
[
"# SOLUCIONES",
"_____no_output_____"
],
[
"No las veas antes de intentar tú primero",
"_____no_output_____"
],
[
"Ya intentaste tú primero?",
"_____no_output_____"
],
[
"# Intenta primero",
"_____no_output_____"
]
],
[
[
"## TODO: Construye un modelo secuencial\nmodel = Sequential()\nmodel.add(Dense(512, activation='relu', input_dim=1000))\nmodel.add(Dropout(0.5))\nmodel.add(Dense(num_classes, activation='softmax'))\nmodel.summary()\n\n## TODO: Compila el modelo con un optimizador y una función de pérdida\nmodel.compile(loss='categorical_crossentropy',\n optimizer='rmsprop',\n metrics=['accuracy'])\n\n## TODO: Corre el modelo. Experimenta con diferentes tamaños de batch y número de epochs. \n# Usa verbose=2 para ver cómo va progresando el modelo\nmodel.fit(x_train, y_train,\n batch_size=32,\n epochs=10,\n validation_data=(x_test, y_test), \n verbose=2)",
"_____no_output_____"
]
]
] | [
"markdown",
"code",
"markdown",
"code",
"markdown",
"code",
"markdown",
"code",
"markdown",
"code",
"markdown",
"code",
"markdown",
"code",
"markdown",
"code"
] | [
[
"markdown"
],
[
"code"
],
[
"markdown"
],
[
"code"
],
[
"markdown"
],
[
"code"
],
[
"markdown"
],
[
"code",
"code"
],
[
"markdown"
],
[
"code"
],
[
"markdown"
],
[
"code"
],
[
"markdown"
],
[
"code"
],
[
"markdown",
"markdown",
"markdown",
"markdown"
],
[
"code"
]
] |
e784da07931c26b28b23e1796b253f8a5d5b20c5 | 11,839 | ipynb | Jupyter Notebook | notebooks/Pre-trained_Models.ipynb | zuhairah87/ztdl-masterclasses | 424a63ec6736a7ac566575de87d9a2dc4b093786 | [
"Apache-2.0"
] | 78 | 2020-05-24T09:39:54.000Z | 2022-01-08T21:06:11.000Z | notebooks/Pre-trained_Models.ipynb | zuhairah87/ztdl-masterclasses | 424a63ec6736a7ac566575de87d9a2dc4b093786 | [
"Apache-2.0"
] | null | null | null | notebooks/Pre-trained_Models.ipynb | zuhairah87/ztdl-masterclasses | 424a63ec6736a7ac566575de87d9a2dc4b093786 | [
"Apache-2.0"
] | 9 | 2020-04-05T03:39:18.000Z | 2021-09-13T16:15:09.000Z | 24.063008 | 260 | 0.542782 | [
[
[
"<a href=\"https://colab.research.google.com/github/zerotodeeplearning/ztdl-masterclasses/blob/master/notebooks/Pre-trained_Models.ipynb\" target=\"_parent\"><img src=\"https://colab.research.google.com/assets/colab-badge.svg\" alt=\"Open In Colab\"/></a>",
"_____no_output_____"
],
[
"## Learn with us: www.zerotodeeplearning.com\n\nCopyright © 2021: Zero to Deep Learning ® Catalit LLC.",
"_____no_output_____"
]
],
[
[
"# Licensed under the Apache License, Version 2.0 (the \"License\");\n# you may not use this file except in compliance with the License.\n# You may obtain a copy of the License at\n#\n# https://www.apache.org/licenses/LICENSE-2.0\n#\n# Unless required by applicable law or agreed to in writing, software\n# distributed under the License is distributed on an \"AS IS\" BASIS,\n# WITHOUT WARRANTIES OR CONDITIONS OF ANY KIND, either express or implied.\n# See the License for the specific language governing permissions and\n# limitations under the License.",
"_____no_output_____"
]
],
[
[
"# Pre-trained Models",
"_____no_output_____"
]
],
[
[
"import numpy as np\nimport pandas as pd\nimport matplotlib.pyplot as plt\nimport seaborn as sns\nimport tensorflow as tf\nimport os\nfrom tensorflow.keras.preprocessing.image import ImageDataGenerator",
"_____no_output_____"
],
[
"# sports_images_path = tf.keras.utils.get_file(\n# 'sports_images',\n# 'https://archive.org/download/ztdl_sports_images/sports_images.tgz',\n# untar=True)",
"_____no_output_____"
],
[
"![[ ! -f sports_images.tar.gz ]] && gsutil cp gs://ztdl-datasets/sports_images.tar.gz .\n![[ ! -d sports_images ]] && echo \"Extracting images...\" && tar zxf sports_images.tar.gz\nsports_images_path = './sports_images'",
"_____no_output_____"
],
[
"train_path = os.path.join(sports_images_path, 'train')\ntest_path = os.path.join(sports_images_path, 'test')",
"_____no_output_____"
],
[
"batch_size = 16\nimg_size = 224",
"_____no_output_____"
],
[
"train_datagen = ImageDataGenerator() \\\n .flow_from_directory(train_path, \n target_size = (img_size, img_size),\n batch_size = batch_size,\n class_mode = 'sparse')",
"_____no_output_____"
],
[
"try:\n assert(train_datagen.samples == 11414)\nexcept:\n raise Exception(\"Found less images than expected. Please remove the files and download again.\")",
"_____no_output_____"
],
[
"classes_dict = train_datagen.class_indices\nclasses = list(classes_dict.keys())\nclasses",
"_____no_output_____"
],
[
"batch, labels = train_datagen.next()",
"_____no_output_____"
],
[
"batch.shape",
"_____no_output_____"
],
[
"labels.shape",
"_____no_output_____"
],
[
"plt.figure(figsize=(10, 10))\nfor i in range(len(batch)):\n plt.subplot(4, 4, i+1)\n plt.imshow(batch[i].astype('int'))\n plt.title(classes[int(labels[i])])\n plt.axis('off')\n\nplt.tight_layout()",
"_____no_output_____"
]
],
[
[
"### Pre-trained model\n\nLet's use a Resnet50 model to classify the images without any training.",
"_____no_output_____"
]
],
[
[
"from PIL import Image\nfrom io import BytesIO\nfrom IPython.display import HTML\nimport base64",
"_____no_output_____"
],
[
"from tensorflow.keras.applications.resnet50 import ResNet50\nfrom tensorflow.keras.applications.resnet50 import preprocess_input as preprocess_input_resnet50\nfrom tensorflow.keras.applications.resnet50 import decode_predictions as decode_predictions_resnet50",
"_____no_output_____"
],
[
"model = ResNet50(weights='imagenet')",
"_____no_output_____"
],
[
"batch_preprocessed = preprocess_input_resnet50(batch.copy())",
"_____no_output_____"
],
[
"predictions = model.predict(batch_preprocessed)",
"_____no_output_____"
],
[
"decoded_top_3 = decode_predictions_resnet50(predictions, top=3)",
"_____no_output_____"
],
[
"def image_formatter(a):\n im = Image.fromarray(a)\n im.thumbnail((28, 28), Image.LANCZOS)\n with BytesIO() as buffer:\n im.save(buffer, 'jpeg')\n im_base64 = base64.b64encode(buffer.getvalue()).decode()\n return f'<img src=\"data:image/jpeg;base64,{im_base64}\">'",
"_____no_output_____"
],
[
"def display_batch(batch, decoded_top_3):\n res = []\n for i, top3 in enumerate(decoded_top_3):\n im = image_formatter(batch[i].astype('uint8'))\n cl = classes[int(labels[i])]\n line = [im, cl]\n for item in top3:\n line = line + list(item[1:])\n res.append(line)\n\n\n res_df = pd.DataFrame(res,\n columns=['image', 'ground_truth',\n 'top_1', 'prob_1',\n 'top_2', 'prob_2',\n 'top_3', 'prob_3'])\n\n \n return res_df.style.bar(color='lightgreen', vmin=0, vmax=1)",
"_____no_output_____"
],
[
"display_batch(batch, decoded_top_3)",
"_____no_output_____"
]
],
[
[
"### Exercise 1:\n\nUse a different pre-trained model from the ones provided at: https://keras.io/applications/\n\nDo the predictions match?\n\nYou will need to:\n- import the pre-trained model\n- import the corresponding `preprocess_input` and `decode_predictions`\n- check the correct imput shape for your chosen model and possibly re-load a new batch with updated image size\n- pipe the batch through the predict function and display the results",
"_____no_output_____"
]
]
] | [
"markdown",
"code",
"markdown",
"code",
"markdown",
"code",
"markdown"
] | [
[
"markdown",
"markdown"
],
[
"code"
],
[
"markdown"
],
[
"code",
"code",
"code",
"code",
"code",
"code",
"code",
"code",
"code",
"code",
"code",
"code"
],
[
"markdown"
],
[
"code",
"code",
"code",
"code",
"code",
"code",
"code",
"code",
"code"
],
[
"markdown"
]
] |
e784e11691baae963e1708676acc0ed7027ff4a4 | 47,543 | ipynb | Jupyter Notebook | notebooks/plot_stochastic.ipynb | vfdev-5/POT | e757b75976ece1e6e53e655852b9f8863e7b6f5a | [
"MIT"
] | null | null | null | notebooks/plot_stochastic.ipynb | vfdev-5/POT | e757b75976ece1e6e53e655852b9f8863e7b6f5a | [
"MIT"
] | null | null | null | notebooks/plot_stochastic.ipynb | vfdev-5/POT | e757b75976ece1e6e53e655852b9f8863e7b6f5a | [
"MIT"
] | null | null | null | 77.811784 | 6,888 | 0.822498 | [
[
[
"%matplotlib inline",
"_____no_output_____"
]
],
[
[
"\n# Stochastic examples\n\n\nThis example is designed to show how to use the stochatic optimization\nalgorithms for descrete and semicontinous measures from the POT library.\n\n\n",
"_____no_output_____"
]
],
[
[
"# Author: Kilian Fatras <[email protected]>\n#\n# License: MIT License\n\nimport matplotlib.pylab as pl\nimport numpy as np\nimport ot\nimport ot.plot",
"_____no_output_____"
]
],
[
[
"COMPUTE TRANSPORTATION MATRIX FOR SEMI-DUAL PROBLEM\n############################################################################\n\n",
"_____no_output_____"
]
],
[
[
"print(\"------------SEMI-DUAL PROBLEM------------\")",
"------------SEMI-DUAL PROBLEM------------\n"
]
],
[
[
"DISCRETE CASE\nSample two discrete measures for the discrete case\n---------------------------------------------\n\nDefine 2 discrete measures a and b, the points where are defined the source\nand the target measures and finally the cost matrix c.\n\n",
"_____no_output_____"
]
],
[
[
"n_source = 7\nn_target = 4\nreg = 1\nnumItermax = 1000\n\na = ot.utils.unif(n_source)\nb = ot.utils.unif(n_target)\n\nrng = np.random.RandomState(0)\nX_source = rng.randn(n_source, 2)\nY_target = rng.randn(n_target, 2)\nM = ot.dist(X_source, Y_target)",
"_____no_output_____"
]
],
[
[
"Call the \"SAG\" method to find the transportation matrix in the discrete case\n---------------------------------------------\n\nDefine the method \"SAG\", call ot.solve_semi_dual_entropic and plot the\nresults.\n\n",
"_____no_output_____"
]
],
[
[
"method = \"SAG\"\nsag_pi = ot.stochastic.solve_semi_dual_entropic(a, b, M, reg, method,\n numItermax)\nprint(sag_pi)",
"[[2.55553509e-02 9.96395660e-02 1.76579142e-02 4.31178196e-06]\n [1.21640234e-01 1.25357448e-02 1.30225078e-03 7.37891338e-03]\n [3.56123975e-03 7.61451746e-02 6.31505947e-02 1.33831456e-07]\n [2.61515202e-02 3.34246014e-02 8.28734709e-02 4.07550428e-04]\n [9.85500870e-03 7.52288517e-04 1.08262628e-02 1.21423583e-01]\n [2.16904253e-02 9.03825797e-04 1.87178503e-03 1.18391107e-01]\n [4.15462212e-02 2.65987989e-02 7.23177216e-02 2.39440107e-03]]\n"
]
],
[
[
"SEMICONTINOUS CASE\nSample one general measure a, one discrete measures b for the semicontinous\ncase\n---------------------------------------------\n\nDefine one general measure a, one discrete measures b, the points where\nare defined the source and the target measures and finally the cost matrix c.\n\n",
"_____no_output_____"
]
],
[
[
"n_source = 7\nn_target = 4\nreg = 1\nnumItermax = 1000\nlog = True\n\na = ot.utils.unif(n_source)\nb = ot.utils.unif(n_target)\n\nrng = np.random.RandomState(0)\nX_source = rng.randn(n_source, 2)\nY_target = rng.randn(n_target, 2)\nM = ot.dist(X_source, Y_target)",
"_____no_output_____"
]
],
[
[
"Call the \"ASGD\" method to find the transportation matrix in the semicontinous\ncase\n---------------------------------------------\n\nDefine the method \"ASGD\", call ot.solve_semi_dual_entropic and plot the\nresults.\n\n",
"_____no_output_____"
]
],
[
[
"method = \"ASGD\"\nasgd_pi, log_asgd = ot.stochastic.solve_semi_dual_entropic(a, b, M, reg, method,\n numItermax, log=log)\nprint(log_asgd['alpha'], log_asgd['beta'])\nprint(asgd_pi)",
"[3.75309361 7.63288278 3.76418767 2.53747778 1.70389504 3.53981297\n 2.67663944] [-2.49164966 -2.25281897 -0.77666675 5.52113539]\n[[2.19699465e-02 1.03185982e-01 1.76983379e-02 2.87611188e-06]\n [1.20688044e-01 1.49823131e-02 1.50635578e-03 5.68043045e-03]\n [3.01194583e-03 7.75764779e-02 6.22686313e-02 8.78225379e-08]\n [2.28707628e-02 3.52120795e-02 8.44977549e-02 2.76545693e-04]\n [1.19721129e-02 1.10087991e-03 1.53333937e-02 1.14450756e-01]\n [2.65247890e-02 1.33140544e-03 2.66861405e-03 1.12332334e-01]\n [3.71512413e-02 2.86513804e-02 7.53932500e-02 1.66127118e-03]]\n"
]
],
[
[
"Compare the results with the Sinkhorn algorithm\n---------------------------------------------\n\nCall the Sinkhorn algorithm from POT\n\n",
"_____no_output_____"
]
],
[
[
"sinkhorn_pi = ot.sinkhorn(a, b, M, reg)\nprint(sinkhorn_pi)",
"[[2.55535622e-02 9.96413843e-02 1.76578860e-02 4.31043335e-06]\n [1.21640742e-01 1.25369034e-02 1.30234529e-03 7.37715259e-03]\n [3.56096458e-03 7.61460101e-02 6.31500344e-02 1.33788624e-07]\n [2.61499607e-02 3.34255577e-02 8.28741973e-02 4.07427179e-04]\n [9.85698720e-03 7.52505948e-04 1.08291770e-02 1.21418473e-01]\n [2.16947591e-02 9.04086158e-04 1.87228707e-03 1.18386011e-01]\n [4.15442692e-02 2.65998963e-02 7.23192701e-02 2.39370724e-03]]\n"
]
],
[
[
"PLOT TRANSPORTATION MATRIX\n#############################################################################\n\n",
"_____no_output_____"
],
[
"Plot SAG results\n----------------\n\n",
"_____no_output_____"
]
],
[
[
"pl.figure(4, figsize=(5, 5))\not.plot.plot1D_mat(a, b, sag_pi, 'semi-dual : OT matrix SAG')\npl.show()",
"_____no_output_____"
]
],
[
[
"Plot ASGD results\n-----------------\n\n",
"_____no_output_____"
]
],
[
[
"pl.figure(4, figsize=(5, 5))\not.plot.plot1D_mat(a, b, asgd_pi, 'semi-dual : OT matrix ASGD')\npl.show()",
"_____no_output_____"
]
],
[
[
"Plot Sinkhorn results\n---------------------\n\n",
"_____no_output_____"
]
],
[
[
"pl.figure(4, figsize=(5, 5))\not.plot.plot1D_mat(a, b, sinkhorn_pi, 'OT matrix Sinkhorn')\npl.show()",
"_____no_output_____"
]
],
[
[
"COMPUTE TRANSPORTATION MATRIX FOR DUAL PROBLEM\n############################################################################\n\n",
"_____no_output_____"
]
],
[
[
"print(\"------------DUAL PROBLEM------------\")",
"------------DUAL PROBLEM------------\n"
]
],
[
[
"SEMICONTINOUS CASE\nSample one general measure a, one discrete measures b for the semicontinous\ncase\n---------------------------------------------\n\nDefine one general measure a, one discrete measures b, the points where\nare defined the source and the target measures and finally the cost matrix c.\n\n",
"_____no_output_____"
]
],
[
[
"n_source = 7\nn_target = 4\nreg = 1\nnumItermax = 100000\nlr = 0.1\nbatch_size = 3\nlog = True\n\na = ot.utils.unif(n_source)\nb = ot.utils.unif(n_target)\n\nrng = np.random.RandomState(0)\nX_source = rng.randn(n_source, 2)\nY_target = rng.randn(n_target, 2)\nM = ot.dist(X_source, Y_target)",
"_____no_output_____"
]
],
[
[
"Call the \"SGD\" dual method to find the transportation matrix in the\nsemicontinous case\n---------------------------------------------\n\nCall ot.solve_dual_entropic and plot the results.\n\n",
"_____no_output_____"
]
],
[
[
"sgd_dual_pi, log_sgd = ot.stochastic.solve_dual_entropic(a, b, M, reg,\n batch_size, numItermax,\n lr, log=log)\nprint(log_sgd['alpha'], log_sgd['beta'])\nprint(sgd_dual_pi)",
"[ 1.67648902 5.3770004 1.70385554 0.4276547 -0.77206786 1.0474898\n 0.54202203] [-0.23723788 -0.20259434 1.30855788 8.06179985]\n[[2.62451875e-02 1.00499531e-01 1.78515577e-02 4.57450829e-06]\n [1.20510690e-01 1.21972758e-02 1.27002374e-03 7.55197481e-03]\n [3.65708350e-03 7.67963231e-02 6.38381061e-02 1.41974930e-07]\n [2.64286344e-02 3.31748063e-02 8.24445965e-02 4.25479786e-04]\n [9.59295422e-03 7.19190875e-04 1.03739180e-02 1.22100712e-01]\n [2.09087627e-02 8.55676046e-04 1.77617241e-03 1.17896019e-01]\n [4.18792948e-02 2.63326297e-02 7.17598381e-02 2.49335733e-03]]\n"
]
],
[
[
"Compare the results with the Sinkhorn algorithm\n---------------------------------------------\n\nCall the Sinkhorn algorithm from POT\n\n",
"_____no_output_____"
]
],
[
[
"sinkhorn_pi = ot.sinkhorn(a, b, M, reg)\nprint(sinkhorn_pi)",
"[[2.55535622e-02 9.96413843e-02 1.76578860e-02 4.31043335e-06]\n [1.21640742e-01 1.25369034e-02 1.30234529e-03 7.37715259e-03]\n [3.56096458e-03 7.61460101e-02 6.31500344e-02 1.33788624e-07]\n [2.61499607e-02 3.34255577e-02 8.28741973e-02 4.07427179e-04]\n [9.85698720e-03 7.52505948e-04 1.08291770e-02 1.21418473e-01]\n [2.16947591e-02 9.04086158e-04 1.87228707e-03 1.18386011e-01]\n [4.15442692e-02 2.65998963e-02 7.23192701e-02 2.39370724e-03]]\n"
]
],
[
[
"Plot SGD results\n-----------------\n\n",
"_____no_output_____"
]
],
[
[
"pl.figure(4, figsize=(5, 5))\not.plot.plot1D_mat(a, b, sgd_dual_pi, 'dual : OT matrix SGD')\npl.show()",
"_____no_output_____"
]
],
[
[
"Plot Sinkhorn results\n---------------------\n\n",
"_____no_output_____"
]
],
[
[
"pl.figure(4, figsize=(5, 5))\not.plot.plot1D_mat(a, b, sinkhorn_pi, 'OT matrix Sinkhorn')\npl.show()",
"_____no_output_____"
]
]
] | [
"code",
"markdown",
"code",
"markdown",
"code",
"markdown",
"code",
"markdown",
"code",
"markdown",
"code",
"markdown",
"code",
"markdown",
"code",
"markdown",
"code",
"markdown",
"code",
"markdown",
"code",
"markdown",
"code",
"markdown",
"code",
"markdown",
"code",
"markdown",
"code",
"markdown",
"code",
"markdown",
"code"
] | [
[
"code"
],
[
"markdown"
],
[
"code"
],
[
"markdown"
],
[
"code"
],
[
"markdown"
],
[
"code"
],
[
"markdown"
],
[
"code"
],
[
"markdown"
],
[
"code"
],
[
"markdown"
],
[
"code"
],
[
"markdown"
],
[
"code"
],
[
"markdown",
"markdown"
],
[
"code"
],
[
"markdown"
],
[
"code"
],
[
"markdown"
],
[
"code"
],
[
"markdown"
],
[
"code"
],
[
"markdown"
],
[
"code"
],
[
"markdown"
],
[
"code"
],
[
"markdown"
],
[
"code"
],
[
"markdown"
],
[
"code"
],
[
"markdown"
],
[
"code"
]
] |
e784e601e32045e48b69b9abe7caaead15b305a6 | 731,995 | ipynb | Jupyter Notebook | Chapter 4/Python/discriminant analysis/QDA visualization from outside.ipynb | borisgarbuzov/schulich_data_science_1 | fd05ec2bbbe35408f90ebfcf10bb4ca588e7871c | [
"MIT"
] | null | null | null | Chapter 4/Python/discriminant analysis/QDA visualization from outside.ipynb | borisgarbuzov/schulich_data_science_1 | fd05ec2bbbe35408f90ebfcf10bb4ca588e7871c | [
"MIT"
] | null | null | null | Chapter 4/Python/discriminant analysis/QDA visualization from outside.ipynb | borisgarbuzov/schulich_data_science_1 | fd05ec2bbbe35408f90ebfcf10bb4ca588e7871c | [
"MIT"
] | 6 | 2020-10-25T05:26:50.000Z | 2021-07-07T08:25:58.000Z | 731,995 | 731,995 | 0.960707 | [
[
[
"https://xavierbourretsicotte.github.io/LDA_QDA.html ",
"_____no_output_____"
]
],
[
[
"import numpy as np\nimport pandas as pd\nfrom matplotlib import pyplot as plt\nimport matplotlib.colors as colors\n# from mpl_toolkits.mplot3d import Axes3D\n# from mpl_toolkits import mplot3d\nfrom sklearn import linear_model, datasets\nimport seaborn as sns\nimport itertools\n\n%matplotlib inline\nsns.set()\n#plt.style.use('seaborn-white')",
"_____no_output_____"
],
[
"def multivariate_gaussian_pdf(X,MU,SIGMA):\n '''Returns the pdf of a nultivariate gaussian distribution\n - X, MU are p x 1 vectors\n - SIGMA is a p x p matrix'''\n #Initialize and reshape\n X = X.reshape(-1,1)\n MU = MU.reshape(-1,1)\n p,_ = SIGMA.shape\n\n #Compute values\n SIGMA_inv = np.linalg.inv(SIGMA)\n denominator = np.sqrt((2 * np.pi)**p * np.linalg.det(SIGMA))\n exponent = -(1/2) * ((X - MU).T @ SIGMA_inv @ (X - MU))\n \n #Return result\n return float((1. / denominator) * np.exp(exponent) ) \n\ndef calculate_boundary(X,MU_k,MU_l, SIGMA,pi_k,pi_l): \n return (np.log(pi_k / pi_l) - 1/2 * (MU_k + MU_l).T @ np.linalg.inv(SIGMA)@(MU_k - MU_l) + X.T @ np.linalg.inv(SIGMA)@ (MU_k - MU_l)).flatten()[0] \n\n\n\ndef QDA_score(X,MU_k,SIGMA,pi_k): \n #Returns the value of the linear discriminant score function for a given class \"k\" and \n # a given x value X\n \n SIGMA_inv = np.linalg.inv(SIGMA)\n \n return (np.log(pi_k) - 1/2 * np.log(np.linalg.det(SIGMA_inv)) - 1/2 * (X - MU_k).T @ SIGMA_inv @ (X - MU_k)).flatten()[0] \n\ndef predict_QDA_class(X,MU_list,SIGMA_list,pi_list): \n #Returns the class for which the the linear discriminant score function is largest\n scores_list = []\n classes = len(MU_list)\n \n for p in range(classes):\n score = QDA_score(X.reshape(-1,1),MU_list[p].reshape(-1,1),SIGMA_list[p],pi_list[p]) \n scores_list.append(score)\n \n return np.argmax(scores_list)",
"_____no_output_____"
],
[
"iris = sns.load_dataset(\"iris\")\nsns.pairplot(iris, hue=\"species\")",
"_____no_output_____"
],
[
"iris = iris.rename(index = str, columns = {'sepal_length':'1_sepal_length','sepal_width':'2_sepal_width', 'petal_length':'3_petal_length', 'petal_width':'4_petal_width'})\nsns.FacetGrid(iris, hue=\"species\", size=6) .map(plt.scatter,\"1_sepal_length\", \"2_sepal_width\", ) .add_legend()\nplt.title('Scatter plot')\ndf1 = iris[[\"1_sepal_length\", \"2_sepal_width\",'species']]",
"/usr/local/lib/python3.7/dist-packages/seaborn/axisgrid.py:316: UserWarning: The `size` parameter has been renamed to `height`; please update your code.\n warnings.warn(msg, UserWarning)\n"
]
],
[
[
"Visualizing the gaussian estimations and the boundary lines",
"_____no_output_____"
]
],
[
[
"#Estimating the parameters\nmu_list = np.split(df1.groupby('species').mean().values,[1,2])\nsigma = df1.cov().values\npi_list = df1.iloc[:,2].value_counts().values / len(df1)\n\n# Our 2-dimensional distribution will be over variables X and Y\nN = 100\nX = np.linspace(3, 8, N)\nY = np.linspace(1.5, 5, N)\nX, Y = np.meshgrid(X, Y)\n\n#fig = plt.figure(figsize = (10,10))\n#ax = fig.gca()\ncolor_list = ['Blues','Greens','Reds']\nmy_norm = colors.Normalize(vmin=-1.,vmax=1.)\n\ng = sns.FacetGrid(iris, hue=\"species\", size=10, palette = 'colorblind') .map(plt.scatter,\"1_sepal_length\", \"2_sepal_width\", ) .add_legend()\nmy_ax = g.ax\n\nfor i,v in enumerate(itertools.combinations([0,1,2],2)):\n mu = mu_list[i]\n Sigma = sigma\n\n#Computing the cost function for each theta combination\n zz = np.array( [multivariate_gaussian_pdf( np.array([xx,yy]).reshape(-1,1), mu, Sigma) \n for xx, yy in zip(np.ravel(X), np.ravel(Y)) ] )\n \n bb = np.array( [ calculate_boundary(np.array([xx,yy]).reshape(-1,1),mu_list[v[0]].reshape(-1,1),mu_list[v[1]].reshape(-1,1), sigma , .33,.33)\n for xx, yy in zip(np.ravel(X), np.ravel(Y)) ] )\n \n#Reshaping the cost values \n Z = zz.reshape(X.shape)\n B = bb.reshape(X.shape)\n\n#Plot the result in 3D\n my_ax.contour( X, Y, Z, 3,cmap = color_list[i] , norm = my_norm, alpha = .3)\n\n my_ax.contour( X, Y, B , levels = [0] ,cmap = color_list[i] , norm = my_norm)\n\n \n\n# Adjust the limits, ticks and view angle\nmy_ax.set_xlabel('X')\nmy_ax.set_ylabel('Y')\nmy_ax.set_title('LDA: gaussians of each class and boundary lines')\n\nplt.show()",
"/usr/local/lib/python3.7/dist-packages/seaborn/axisgrid.py:316: UserWarning: The `size` parameter has been renamed to `height`; please update your code.\n warnings.warn(msg, UserWarning)\n"
]
],
[
[
"Visualizing the Gaussian estimations with different covariance matrices",
"_____no_output_____"
]
],
[
[
"#Estimating the parameters\nmu_list = np.split(df1.groupby('species').mean().values,[1,2])\nsigma_list = np.split(df1.groupby('species').cov().values,[2,4], axis = 0)\npi_list = df1.iloc[:,2].value_counts().values / len(df1)\n\n# Our 2-dimensional distribution will be over variables X and Y\nN = 100\nX = np.linspace(3, 8, N)\nY = np.linspace(1.5, 5, N)\nX, Y = np.meshgrid(X, Y)\n\n#fig = plt.figure(figsize = (10,10))\n#ax = fig.gca()\ncolor_list = ['Blues','Greens','Reds']\nmy_norm = colors.Normalize(vmin=-1.,vmax=1.)\n\ng = sns.FacetGrid(iris, hue=\"species\", size=10, palette = 'colorblind') .map(plt.scatter, \"1_sepal_length\", \"2_sepal_width\",) .add_legend()\nmy_ax = g.ax\n\nfor i in range(3):\n mu = mu_list[i]\n Sigma = sigma_list[i]\n\n#Computing the cost function for each theta combination\n zz = np.array( [multivariate_gaussian_pdf( np.array([xx,yy]).reshape(-1,1), mu, Sigma) \n for xx, yy in zip(np.ravel(X), np.ravel(Y)) ] )\n#Reshaping the cost values \n Z = zz.reshape(X.shape)\n Zm = np.ma.masked_array(Z, Z < 0.15)\n\n#Plot the result in 3D\n my_ax.contour( X, Y, Z, 15, alpha = .3 ,cmap = color_list[i], norm = my_norm)\n my_ax.pcolor(X,Y,Zm, alpha = .1, cmap = color_list[i], norm = my_norm)\n\n\n# Adjust the limits, ticks and view angle\nmy_ax.set_xlabel('X')\nmy_ax.set_ylabel('Y')\nmy_ax.set_title('Multivariate Gaussians with different Sigma ')\n\nplt.show()",
"/usr/local/lib/python3.7/dist-packages/seaborn/axisgrid.py:316: UserWarning: The `size` parameter has been renamed to `height`; please update your code.\n warnings.warn(msg, UserWarning)\n"
]
],
[
[
"Visualizing the quadratic boundary curves",
"_____no_output_____"
]
],
[
[
"#Estimating the parameters\nmu_list = np.split(df1.groupby('species').mean().values,[1,2])\nsigma_list = np.split(df1.groupby('species').cov().values,[2,4], axis = 0)\npi_list = df1.iloc[:,2].value_counts().values / len(df1)\n\n# Our 2-dimensional distribution will be over variables X and Y\nN = 200\nX = np.linspace(4, 8, N)\nY = np.linspace(1.5, 5, N)\nX, Y = np.meshgrid(X, Y)\n\n#fig = plt.figure(figsize = (10,10))\n#ax = fig.gca()\ncolor_list = ['Blues','Greens','Reds']\nmy_norm = colors.Normalize(vmin=-1.,vmax=1.)\n\ng = sns.FacetGrid(iris, hue=\"species\", size=10, palette = 'colorblind') .map(plt.scatter, \"1_sepal_length\", \"2_sepal_width\",) .add_legend()\nmy_ax = g.ax\n\n\n#Computing the predicted class function for each value on the grid\nzz = np.array( [predict_QDA_class( np.array([xx,yy]).reshape(-1,1), mu_list, sigma_list, pi_list) \n for xx, yy in zip(np.ravel(X), np.ravel(Y)) ] )\n \n#Reshaping the predicted class into the meshgrid shape\nZ = zz.reshape(X.shape)\n\n\n#Plot the filled and boundary contours\nmy_ax.contourf( X, Y, Z, 2, alpha = .1, colors = ('blue','green','red'))\nmy_ax.contour( X, Y, Z, 2, alpha = 1, colors = ('blue','green','red'))\n\n# Addd axis and title\nmy_ax.set_xlabel('X')\nmy_ax.set_ylabel('Y')\nmy_ax.set_title('QDA and boundaries')\n\nplt.show()",
"/usr/local/lib/python3.7/dist-packages/seaborn/axisgrid.py:316: UserWarning: The `size` parameter has been renamed to `height`; please update your code.\n warnings.warn(msg, UserWarning)\n"
]
],
[
[
"QDA Accuracy",
"_____no_output_____"
]
],
[
[
"from sklearn.discriminant_analysis import QuadraticDiscriminantAnalysis\nX_data = df1.iloc[:,0:2]\ny_labels = df1.iloc[:,2].replace({'setosa':0,'versicolor':1,'virginica':2}).copy()\n\nqda = QuadraticDiscriminantAnalysis(store_covariance=True)\nqda.fit(X_data,y_labels)\n\n#Numpy accuracy\ny_pred = np.array( [predict_QDA_class( np.array([xx,yy]).reshape(-1,1), mu_list, sigma_list, pi_list) \n for xx, yy in zip(np.ravel(X_data.values[:,0]), np.ravel(X_data.values[:,1])) ] )\ndisplay(np.mean(y_pred == y_labels))\n\n#predict_QDA_class( np.array([xx,yy]).reshape(-1,1), mu_list, sigma_list, pi_list) \n\n#Sklearn accuracy\ndisplay(qda.score(X_data,y_labels))",
"_____no_output_____"
]
]
] | [
"markdown",
"code",
"markdown",
"code",
"markdown",
"code",
"markdown",
"code",
"markdown",
"code"
] | [
[
"markdown"
],
[
"code",
"code",
"code",
"code"
],
[
"markdown"
],
[
"code"
],
[
"markdown"
],
[
"code"
],
[
"markdown"
],
[
"code"
],
[
"markdown"
],
[
"code"
]
] |
e784e9f90eba7351831ee799331da1dcc9f5319b | 207,215 | ipynb | Jupyter Notebook | lectures/03_e_dimension_reduction.ipynb | bioshape-lab/ece594n | 01ab65108ee6bdff0e88fd2a0b4dcc6e0d2cf580 | [
"MIT"
] | 2 | 2022-03-30T00:47:45.000Z | 2022-03-31T18:22:16.000Z | lectures/03_e_dimension_reduction.ipynb | bioshape-lab/ece594n | 01ab65108ee6bdff0e88fd2a0b4dcc6e0d2cf580 | [
"MIT"
] | null | null | null | lectures/03_e_dimension_reduction.ipynb | bioshape-lab/ece594n | 01ab65108ee6bdff0e88fd2a0b4dcc6e0d2cf580 | [
"MIT"
] | null | null | null | 165.3751 | 78,832 | 0.897792 | [
[
[
"###### 03 Geometric Machine Learning for Shape Analysis\n\n## E) Unsupervised Learning: Dimension Reduction\n\n$\\color{#003660}{\\text{Nina Miolane - Assistant Professor}}$ @ BioShape Lab @ UCSB ECE",
"_____no_output_____"
],
[
"<center><img src=\"figs/03_dimred.png\" width=750px alt=\"default\"/></center>",
"_____no_output_____"
],
[
"# This Unit\n\n- **Unit 1 (Geometry - Math!)**: Differential Geometry for Engineers\n- **Unit 2 (Shapes)**: Computational Representations of Biomedical Shapes\n- **Unit 3 (Machine Learning)**: **Geometric Machine Learning for Shape Analysis**\n - A) Mean and Covariance\n - B) Supervised Learning: Classification\n - C) Supervised Learning: Regression\n - D) Unsupervised Learning: Clustering\n - **E) Unsupervised Learning: Dimension Reduction**\n - Motivation: Dimension Reduction on Optic Nerve Heads (5 landmarks)\n - Traditional Principal Component Analysis (PCA)\n - Dimension Reduction Method 1: Tangent PCA\n - Dimension Reduction Method 2: Principal Geodesic Analysis\n- **Unit 4 (Deep Learning)**: Geometric Deep Learning for Shape Analysis\n\n$\\rightarrow$ We explain the machine learning algorithms and statistics used in these real-world scenarios.",
"_____no_output_____"
],
[
"# Overview of Machine Learning (ML)\n\nMachine Learning is divided into two principal categories of algorithms: supervised and unsupervised learning algorithms. Both learn from data.\n\n$\\color{#EF5645}{\\text{Definition}}$: **Unsupervised learning** refers to the task of discovering any naturally occuring patterns in a dataset of data points $x$. We say that the model is:\n- a clustering: if we want to find groups (clusters),\n- a dimension reduction: if we want to find the main sources of variations.\n\n",
"_____no_output_____"
],
[
"# Why Dimension Reduction\n\n- Some data are (very) high dimensional\n- Dimension reduction: Extract a low dimensional structure for:\n - Visualization\n - More efficient use of resources (memory)\n - Downstream tasks: fewer dimensions -> better generalization.\n \n<center><img src=\"figs/03_dimred.png\" width=850px alt=\"default\"/></center>",
"_____no_output_____"
],
[
"# Dimension Reduction\n\n\n\n$\\color{#EF5645}{\\text{Given}}$:\n- dataset $X_1, . . . , X_n$ in a data space of dimension $D$\n- integer $d < D$,\n\n$\\color{#EF5645}{\\text{Goal}}$:\n - Find representations $z_1, ..., z_n$ of the data points,\n - that belong to a lower-dimensional space of dimension $d < D$,\n - that are \"representative\" of the $X_1, ..., X_n$.\n\n\n<center><img src=\"figs/03_pca.png\" width=250px alt=\"default\"/></center>",
"_____no_output_____"
],
[
"# Motivation: Dimension Reduction for Optical Nerve Heads",
"_____no_output_____"
],
[
"$\\color{#EF5645}{\\text{Question}}$: Are the shapes of optic nerve heads split into two clusters: healthy versus glaucoma? --> _Can we visualize the dataset?_\n\nData acquired with a Heidelberg Retina Tomograph - Patrangenaru and Ellingson (2015):\n- 11 Rhesus monkeys\n- 22 images of monkeys’ eyes:\n - an experimental glaucoma was introduced in one eye, \n - while the second eye was kept as control.\n \n$\\rightarrow$ On each image, 5 anatomical \"landmarks\" were recorded.\n\n<center><img src=\"figs/01_optic_nerves.png\" width=400px alt=\"default\"/></center>\n<center>Comparison of optic nerve heads in monkeys with and without glaucoma.</center>",
"_____no_output_____"
]
],
[
[
"import numpy as np\nimport matplotlib.pyplot as plt\nimport matplotlib.colors as colors\nfrom mpl_toolkits.mplot3d import Axes3D\nfrom mpl_toolkits.mplot3d.art3d import Poly3DCollection\nimport matplotlib.patches as mpatches\n\nimport warnings\nwarnings.filterwarnings(\"ignore\")",
"_____no_output_____"
],
[
"import geomstats.datasets.utils as data_utils\n\nnerves, labels, monkeys = data_utils.load_optical_nerves()\n# Keep the 5 landmarks\nprint(nerves.shape)\nprint(labels)\nprint(monkeys)",
"(22, 5, 3)\n[0 1 0 1 0 1 0 1 0 1 0 1 0 1 0 1 0 1 0 1 0 1]\n[ 0 0 1 1 2 2 3 3 4 4 5 5 6 6 7 7 8 8 9 9 10 10]\n"
]
],
[
[
"Plot two optical shapes: ",
"_____no_output_____"
]
],
[
[
"two_nerves = nerves[monkeys == 0]\nprint(two_nerves.shape)\n\ntwo_labels = labels[monkeys == 0]\nprint(two_labels)",
"(2, 5, 3)\n[0 1]\n"
],
[
"label_to_str = {0: \"Normal nerve\", 1: \"Glaucoma nerve\"}\nlabel_to_color = {\n 0: (102 / 255, 178 / 255, 255 / 255, 1.0),\n 1: (255 / 255, 178 / 255, 102 / 255, 1.0),\n}",
"_____no_output_____"
],
[
"fig = plt.figure(); ax = Axes3D(fig); ax.set_xlim((2000, 4000)); ax.set_ylim((1000, 5000)); ax.set_zlim((-600, 200))\n\nfor nerve, label in zip(two_nerves, two_labels):\n x = nerve[:, 0]\n y = nerve[:, 1]\n z = nerve[:, 2]\n\n verts = [list(zip(x, y, z))]\n\n poly = Poly3DCollection(verts, alpha=0.5)\n color = label_to_color[int(label)]\n poly.set_color(colors.rgb2hex(color))\n poly.set_edgecolor(\"k\")\n ax.add_collection3d(poly)\n\npatch_0 = mpatches.Patch(color=label_to_color[0], label=label_to_str[0], alpha=0.5)\npatch_1 = mpatches.Patch(color=label_to_color[1], label=label_to_str[1], alpha=0.5)\nplt.legend(handles=[patch_0, patch_1], prop={\"size\": 20})\nplt.show()",
"_____no_output_____"
]
],
[
[
"## Refresher: Traditional Principal Component Analysis",
"_____no_output_____"
],
[
"$\\color{#EF5645}{\\text{Principal Component Analysis (PCA)}}$ is an:\n- orthogonal projection of the data (belonging to a vector space $\\mathbb{R}^D$),\n- into a (lower dimensional) linear subspace $\\mathbb{R}^d$, $d < D$, \n- so that the variance of the projected data is maximized.",
"_____no_output_____"
],
[
"<center><img src=\"figs/03_pcadims.png\" width=800px alt=\"default\"/></center>\n\n$\\color{#EF5645}{\\text{Notations}}$: $D$ original dimension, $d$ dimension of lower-dimensional subspace.",
"_____no_output_____"
],
[
"## PCA: Intuition",
"_____no_output_____"
],
[
"What is the 1-dimensional linear subspace that maximizes the variance of the projected data?\n\n<center><img src=\"figs/03_apca1.png\" width=400px alt=\"default\"/></center>",
"_____no_output_____"
],
[
"<center><img src=\"figs/03_apca2.png\" width=400px alt=\"default\"/></center>",
"_____no_output_____"
],
[
"<center><img src=\"figs/03_apca3.png\" width=400px alt=\"default\"/></center>",
"_____no_output_____"
],
[
"## PCA: Mathematical Notations\n\nFind a orthonormal basis $\\left\\{v_{1}, \\ldots, v_{D}\\right\\}$ of $\\mathbb{R}^{D}$, which satisfies the recursive relationship\n$$\n\\begin{gathered}\nv_{1}=\\underset{\\|v\\|=1}{\\arg \\max } \\sum_{i=1}^{n}\\left(v \\cdot x_{i}\\right)^{2} \\\\\nv_{k}=\\underset{\\|v\\|=1}{\\arg \\max } \\sum_{i=1}^{n} \\sum_{j=1}^{k-1}\\left(v_{j} \\cdot x_{i}\\right)^{2}+\\left(v \\cdot x_{i}\\right)^{2}\n\\end{gathered}\n$$\n\nThe $x_i$ are centered at the mean in the equations above.\n\n<center><img src=\"figs/03_apca3.png\" width=300px alt=\"default\"/></center>\n\n",
"_____no_output_____"
],
[
"- The subspace $V_{k}=\\operatorname{span}\\left(\\left\\{v_{1}, \\ldots, v_{k}\\right\\}\\right)$ is:\n - the $k$-dimensional subspace \n - that maximizes the variance \n - of the data projected to that subspace: $\\pi_{V_1}(x_i) = v \\cdot x_{i}$\n ",
"_____no_output_____"
],
[
"$\\color{#047C91}{\\text{Exercise}}$: Consider the two projections below. Which maximizes the variance?\n\n\n<center><img src=\"figs/03_var.png\" width=700px alt=\"default\"/></center>",
"_____no_output_____"
],
[
"## PCA: Method\n\nThe basis $\\left\\{v_{k}\\right\\}$ is computed as the set of ordered eigenvectors of the sample covariance matrix of the data.\n \n$\\color{#6D7D33}{\\text{Method}}$: Given data $\\left\\{X_{1}, \\ldots, X_n\\right\\}$:\n- Compute covariance matrix $\\Sigma$, where $\\quad \\overline{\\mathbf{x}}=\\frac{1}{n} \\sum_{i=1}^{n} X_{i}$:\n\n$$\\Sigma=\\frac{1}{n} \\sum_{i=1}^{n}\\left(\\mathbf{x}_{i}-\\overline{\\mathbf{x}}\\right)(\\mathbf{x}_i-\\overline{\\mathbf{x}})^{T} \\quad$$ \n- Compute eigenvectors, eigenvalues of $\\Sigma$:\n - Eigenvectors: principal components (PCs)\n - Eigenvalues: orders PCs",
"_____no_output_____"
],
[
"## PCA: Explanation\n\n$\\color{#EF5645}{\\text{Goal (Rewritten)}}$: Maximize $\\quad \\mathbf{u}^{\\top} \\mathbf{X X}^{\\top} \\mathbf{u}$\ns.t $\\quad \\mathbf{u}^{\\top} \\mathbf{u}=1$, where $\\Sigma = \\mathbf{X X}^{\\top}$\n\n$\\color{#6D7D33}{\\text{Method}}$:\n- Construct Lagrangian $\\mathbf{u}^{\\top} \\mathbf{X X}^{\\top} \\mathbf{u}-\\lambda \\mathbf{u}^{\\top} \\mathbf{u}$\n- Set partial derivatives to zero\n$$\n\\mathbf{X X}^{\\top} \\mathbf{u}-\\lambda \\mathbf{u}=\\left(\\mathbf{X X}^{\\top}-\\lambda \\mathrm{I}\\right) \\mathbf{u}=\\mathbf{0}\n$$\n\nAs $\\mathbf{u} \\neq \\mathbf{0}$ then $\\mathbf{u}$ must be an eigenvector of $XX^{\\top}$ with eigenvalue $\\lambda$",
"_____no_output_____"
],
[
"# How Many Principal Components (PCs) ?\n\nMaximum number of PCs:\n- For $D$ original dimensions, sample covariance matrix is $D \\times D$, and has up to $D$ eigenvectors.\n- Maximum number: $D$ PCs.\n\nInteresting number of PCs:\n- Ignore the components of lesser significance, i.e. small eigenvalues.\n- Interesting number: $d$ PCs.\n\n<center><img src=\"figs/03_pcs.png\" width=400px alt=\"default\"/></center>\n",
"_____no_output_____"
],
[
"## PCA: Two Interpretations\n\n$\\color{#EF5645}{\\text{Maximum Variance Direction:}}$\n projection captures maximum variance in the data\n$$\n\\frac{1}{n} \\sum_{i=1}^{n}\\left(\\mathbf{v}^{T} \\mathbf{x}_{i}\\right)^{2}=\\mathbf{v}^{T} \\mathbf{X X}^{T} \\mathbf{v}\n$$\n\n$\\color{#EF5645}{\\text{Minimum Reconstruction Error:}}$ projection yields minimum mean square error\n$$\n\\frac{1}{n} \\sum_{i=1}^{n}\\left\\|\\mathbf{x}_{i}-\\left(\\mathbf{v}^{T} \\mathbf{x}_{i}\\right) \\mathbf{v}\\right\\|^{2}\n$$\n\n<center><img src=\"figs/03_orthogonal.png\" width=400px alt=\"default\"/></center>\n",
"_____no_output_____"
],
[
"## Dimension Reduction Method 1: Tangent Principal Component Analysis",
"_____no_output_____"
],
[
"### Recall: \"Trick\": Tangent Space at the Fréchet Mean\n\nThe Fréchet mean gives us a way of transforming our non-linear data into vectors!\n1. Compute the Fréchet mean $\\bar{x}$ of the data points\n2. Consider the tangent space $T_\\bar{x}M$of the manifold $M$ at $\\bar{x}$\n3. Compute the Logarithms of the data points at $\\bar{x}$\n\n$\\rightarrow$ Get a dataset on a vector space, and apply classical machine learning on it.",
"_____no_output_____"
],
[
"## Tangent Principal Component Analysis\n\n= Apply PCA on the tangent space at the Fréchet mean.\n\nThe next slides illustrate the use of tangent PCA on:\n- the hyperbolic space (synthetic data)\n- Kendall shape space (optical nerve head data)",
"_____no_output_____"
],
[
"## On the Hyperbolic Space",
"_____no_output_____"
]
],
[
[
"from geomstats.geometry.hyperboloid import Hyperboloid\nfrom geomstats.learning.frechet_mean import FrechetMean\nfrom geomstats.learning.pca import TangentPCA\n\n\nimport matplotlib.pyplot as plt\nimport numpy as np\n\nimport geomstats.visualization as viz",
"_____no_output_____"
]
],
[
[
"1. Set-up\n\n- $\\color{#EF5645}{\\text{Decide on the model:}}$ We use tangent PCA\n- $\\color{#EF5645}{\\text{Decide on a loss function:}}$ Minimize -variance",
"_____no_output_____"
]
],
[
[
"# Synthetic data\nhyperbolic_plane = Hyperboloid(dim=2)\ndata = hyperbolic_plane.random_point(n_samples=140)\n\n# Set-up\nmean = FrechetMean(metric=hyperbolic_plane.metric)\ntpca = TangentPCA(metric=hyperbolic_plane.metric, n_components=2)",
"_____no_output_____"
]
],
[
[
"2. $\\color{#EF5645}{\\text{Split dataset into train / test sets:}}$ \n - Train $X_1, ..., X_{n_\\text{train}}$: build the algorithm\n - Test $X_{n_\\text{train}+1}, ..., X_n$: assess its performances.",
"_____no_output_____"
]
],
[
[
"from sklearn.model_selection import train_test_split\n\ntrain, test = train_test_split(data)\nprint(train.shape)\nprint(test.shape)",
"(105, 3)\n(35, 3)\n"
]
],
[
[
"3. $\\color{#EF5645}{\\text{Train:}}$ Build the algorithm",
"_____no_output_____"
]
],
[
[
"mean.fit(train)\nmean_estimate = mean.estimate_\n\ntpca = tpca.fit(train, base_point=mean_estimate)\ntangent_projected_data = tpca.transform(train)",
"_____no_output_____"
]
],
[
[
"4. $\\color{#EF5645}{\\text{Test:}}$ Assess its performances",
"_____no_output_____"
]
],
[
[
"geodesic_0 = hyperbolic_plane.metric.geodesic(\n initial_point=mean_estimate, initial_tangent_vec=tpca.components_[0]\n )\ngeodesic_1 = hyperbolic_plane.metric.geodesic(\n initial_point=mean_estimate, initial_tangent_vec=tpca.components_[1]\n )\n\nn_steps = 100\nt = np.linspace(-1, 1, n_steps)\ngeodesic_points_0 = geodesic_0(t)\ngeodesic_points_1 = geodesic_1(t)",
"_____no_output_____"
],
[
"fig = plt.figure(figsize=(15, 5))\nax_var = fig.add_subplot(121)\nxticks = np.arange(1, 2 + 1, 1); ax_var.xaxis.set_ticks(xticks)\nax_var.set_title(\"Explained variance\"); ax_var.set_xlabel(\"Number of Principal Components\")\nax_var.set_ylim((0, 1))\nax_var.plot(xticks, tpca.explained_variance_ratio_)\n\nax = fig.add_subplot(122)\n\nviz.plot(\n mean_estimate, ax, space=\"H2_poincare_disk\", color=\"darkgreen\", s=10\n)\nviz.plot(geodesic_points_0, ax, space=\"H2_poincare_disk\", linewidth=2)\nviz.plot(geodesic_points_1, ax, space=\"H2_poincare_disk\", linewidth=2)\nviz.plot(data, ax, space=\"H2_poincare_disk\", color=\"black\", alpha=0.7)\n\nax.set_aspect(\"equal\")\nplt.show()",
"_____no_output_____"
]
],
[
[
"## On Kendall Shape Spaces",
"_____no_output_____"
]
],
[
[
"from geomstats.geometry.pre_shape import PreShapeSpace, KendallShapeMetric\n\nm_ambient = 3\nk_landmarks = 5\n\npreshape = PreShapeSpace(m_ambient=m_ambient, k_landmarks=k_landmarks)\nmatrices_metric = preshape.embedding_metric\n\n\nnerves_preshape = preshape.projection(nerves)\nprint(nerves_preshape.shape)\nprint(preshape.belongs(nerves_preshape))\nprint(np.isclose(matrices_metric.norm(nerves_preshape), 1.0))",
"(22, 5, 3)\n[ True True True True True True True True True True True True\n True True True True True True True True True True]\n[ True True True True True True True True True True True True\n True True True True True True True True True True]\n"
],
[
"base_point = nerves_preshape[0]\n\nnerves_shape = preshape.align(point=nerves_preshape, base_point=base_point)",
"_____no_output_____"
]
],
[
[
"1. Set-up\n\n- $\\color{#EF5645}{\\text{Decide on the model:}}$ We use tangent PCA\n- $\\color{#EF5645}{\\text{Decide on a loss function:}}$ Minimize -variance",
"_____no_output_____"
]
],
[
[
"kendall_metric = KendallShapeMetric(m_ambient=m_ambient, k_landmarks=k_landmarks)\n\ntpca = TangentPCA(kendall_metric)",
"_____no_output_____"
]
],
[
[
"2. $\\color{#EF5645}{\\text{Split dataset into train / test sets:}}$ \n - Train $X_1, ..., X_{n_\\text{train}}$: build the algorithm\n - Test $X_{n_\\text{train}+1}, ..., X_n$: assess its performances.",
"_____no_output_____"
]
],
[
[
"from sklearn.model_selection import train_test_split\n\ntrain_nerves_shape = nerves_shape[:18]\ntest_nerves_shape = nerves_shape[18:]\n\nprint(train_nerves_shape.shape)\nprint(test_nerves_shape.shape)\n",
"(18, 5, 3)\n(4, 5, 3)\n"
]
],
[
[
"3. $\\color{#EF5645}{\\text{Train:}}$ Build the algorithm",
"_____no_output_____"
]
],
[
[
"tpca.fit(train_nerves_shape)\n\nplt.plot(tpca.explained_variance_ratio_)\nplt.xlabel(\"Number of principal tangent components\", size=14)\nplt.ylabel(\"Fraction of explained variance\", size=14);",
"_____no_output_____"
]
],
[
[
"Two principal components describe around 60% of the variance. We plot the data projected in the tangent space defined by these two principal components.",
"_____no_output_____"
],
[
"4. $\\color{#EF5645}{\\text{Test:}}$ Assess its performances\n\n- We project the whole dataset on the principal components.",
"_____no_output_____"
]
],
[
[
"X = tpca.transform(nerves_shape)\nplt.figure(figsize=(11, 11))\nfor label, col in label_to_color.items():\n mask = labels == label\n plt.scatter(X[mask, 0], X[mask, 1], color=col, s=100, label=label_to_str[label])\nplt.legend(fontsize=14)\nfor label, x, y in zip(monkeys, X[:, 0], X[:, 1]):\n plt.annotate(label, xy=(x, y), xytext=(-20, 20), textcoords=\"offset points\", ha=\"right\", va=\"bottom\", bbox=dict(boxstyle=\"round,pad=0.5\", fc=\"white\", alpha=0.5), arrowprops=dict(arrowstyle=\"->\", connectionstyle=\"arc3,rad=0\"))\nplt.show()",
"_____no_output_____"
]
],
[
[
"## Dimension Reduction Method 2: Principal Geodesic Analysis\n",
"_____no_output_____"
],
[
"- Variance. Following the work of Fréchet, we define the sample variance of the data as the expected value of the squared Riemannian distance from the mean.\n- Geodesic subspaces. The lower-dimensional subspaces in PCA are linear subspaces. For general manifolds we extend the concept of a linear subspace to that of a geodesic submanifold.\n- Projection. In PCA the data is projected onto linear subspaces. We define a projection operator for geodesic submanifolds, and show how it may be efficiently approximated.",
"_____no_output_____"
],
[
"$\\color{#EF5645}{\\text{Principal Geodesic Analysis (PGA)}}$ is an:\n- $\\color{#EF5645}{\\text{orthogonal projection}}$ of the data\n- into a (lower dimensional) $\\color{#EF5645}{\\text{geodesic subspace}}$, \n- so that the variance of the projected data is maximized.",
"_____no_output_____"
],
[
"## Geodesic Subspace\n\n$\\color{#EF5645}{\\text{A submanifold $N$ of a manifold $M$}}$ is a subset of $M$ that is also a manifold.\n\nIn general, if $N$ is a submanifold of a manifold $M$, geodesics of $N$ are not necessarily geodesics of $M$.\n\n- $\\color{#047C91}{\\text{Example}}$: $S^2$ as a submanifold of $\\mathbb{R}^3$.",
"_____no_output_____"
],
[
"$\\color{#EF5645}{\\text{A submanifold $H$ of $M$ is said to be geodesic at $x \\in H$}}$ if all geodesics of $H$ _passing through $x$_ are also geodesics of $M$. \n\n- $\\color{#047C91}{\\text{Example}}$: A linear subspace of $\\mathbb{R}^{D}$ is a submanifold geodesic at 0.\n\n$\\color{#EF5645}{\\text{Remark}}$: Submanifolds geodesic at $x$ preserve distances to $x.$ This is an essential property for PGA because variance is defined as the average squared distance to the mean. Thus submanifolds geodesic at the mean will be the generalizations of the linear subspaces of PCA.",
"_____no_output_____"
],
[
"## Projection\n\n$\\color{#EF5645}{\\text{The projection of a point $x \\in M$}}$ onto a geodesic submanifold $H$ of $M$ is defined as the point on $H$ that is nearest to $x$ in Riemannian distance. Thus we define the projection operator $\\pi_{H}: M \\rightarrow H$ as\n$$\n\\pi_{H}(x)=\\underset{y \\in H}{\\arg \\min } d(x, y)^{2}\n$$\n\n\n<center><img src=\"figs/03_proj.png\" width=400px alt=\"default\"/></center>",
"_____no_output_____"
],
[
"## PGA: Mathematical Notations\n\nThe principal geodesic submanifolds are the images of the $V_{k}$ under the exponential map: $H_{k}=\\operatorname{Exp}_{\\mu}\\left(V_{k}\\right)$. The first principal direction is chosen to maximize the projected variance along the corresponding geodesic:\n$$\nv_{1}=\\underset{\\|v\\|=1}{\\arg \\max } \\sum_{i=1}^{n}\\left\\|\\log _{\\mu}\\left(\\pi_{H}\\left(x_{i}\\right)\\right)\\right\\|^{2},\n$$\nwhere $H=\\operatorname{Exp}_{\\mu}(\\operatorname{span}(\\{v\\}) \\cap U)$.\nThe remaining principal directions are then defined recursively as\n$$\n\\begin{aligned}\n&v_{k}=\\underset{\\|v\\|=1}{\\arg \\max } \\sum_{i=1}^{n}\\left\\|\\log _{\\mu}\\left(\\pi_{H}\\left(x_{i}\\right)\\right)\\right\\|^{2} \\\\\n&\\text { where } H=\\operatorname{Exp}_{\\mu}\\left(\\operatorname{span}\\left(\\left\\{v_{1}, \\ldots, v_{k-1}, v\\right\\}\\right) \\cap U\\right) .\n\\end{aligned}\n$$",
"_____no_output_____"
]
],
[
[
"- The subspace $V_{k}=\\operatorname{span}\\left(\\left\\{v_{1}, \\ldots, v_{k}\\right\\}\\right)$ is:\n - the $k$-dimensional subspace \n - that maximizes the variance \n - of the data projected to that subspace: $\\pi_{V_1}(x_i) = v \\cdot x_{i}$",
"_____no_output_____"
]
],
[
[
"<center><img src=\"figs/03_pga_alg.png\" width=900px alt=\"default\"/></center>\n",
"_____no_output_____"
],
[
"## Example on the Sphere\n\nThe following code runs PGA on the sphere. Note that you need to specify pytorch backend for automatic differentiation.\n\nhttps://github.com/nguigs/geomstats/blob/nguigs-pga/examples/pga-s2.py",
"_____no_output_____"
],
[
"# This Unit\n\n- **Unit 1 (Geometry - Math!)**: Differential Geometry for Engineers\n- **Unit 2 (Shapes)**: Computational Representations of Biomedical Shapes\n- **Unit 3 (Machine Learning)**: **Geometric Machine Learning for Shape Analysis**\n - A) Mean and Covariance\n - B) Supervised Learning: Classification\n - C) Supervised Learning: Regression\n - D) Unsupervised Learning: Clustering**\n - **E) Unsupervised Learning: Dimension Reduction**\n - Motivation: Dimension Reduction on Optic Nerve Heads (5 landmarks)\n - Traditional Principal Component Analysis (PCA)\n - Dimension Reduction Method 1: Tangent PCA\n - Dimension Reduction Method 2: Principal Geodesic Analysis\n- **Unit 4 (Deep Learning)**: Geometric Deep Learning for Shape Analysis\n\n$\\rightarrow$ We explain the machine learning algorithms and statistics used in these real-world scenarios.",
"_____no_output_____"
]
]
] | [
"markdown",
"code",
"markdown",
"code",
"markdown",
"code",
"markdown",
"code",
"markdown",
"code",
"markdown",
"code",
"markdown",
"code",
"markdown",
"code",
"markdown",
"code",
"markdown",
"code",
"markdown",
"code",
"markdown",
"code",
"markdown",
"code",
"markdown"
] | [
[
"markdown",
"markdown",
"markdown",
"markdown",
"markdown",
"markdown",
"markdown",
"markdown"
],
[
"code",
"code"
],
[
"markdown"
],
[
"code",
"code",
"code"
],
[
"markdown",
"markdown",
"markdown",
"markdown",
"markdown",
"markdown",
"markdown",
"markdown",
"markdown",
"markdown",
"markdown",
"markdown",
"markdown",
"markdown",
"markdown",
"markdown",
"markdown",
"markdown"
],
[
"code"
],
[
"markdown"
],
[
"code"
],
[
"markdown"
],
[
"code"
],
[
"markdown"
],
[
"code"
],
[
"markdown"
],
[
"code",
"code"
],
[
"markdown"
],
[
"code",
"code"
],
[
"markdown"
],
[
"code"
],
[
"markdown"
],
[
"code"
],
[
"markdown"
],
[
"code"
],
[
"markdown",
"markdown"
],
[
"code"
],
[
"markdown",
"markdown",
"markdown",
"markdown",
"markdown",
"markdown",
"markdown"
],
[
"code"
],
[
"markdown",
"markdown",
"markdown"
]
] |
e784ecabf57e4ca1c8130968a23a183363609ffd | 821,140 | ipynb | Jupyter Notebook | Rekognition.ipynb | jsalomon-mdsol/medihack-aws-code | 7df90ece41eb93524ad3e60b5a8165bbb0143645 | [
"MIT"
] | null | null | null | Rekognition.ipynb | jsalomon-mdsol/medihack-aws-code | 7df90ece41eb93524ad3e60b5a8165bbb0143645 | [
"MIT"
] | null | null | null | Rekognition.ipynb | jsalomon-mdsol/medihack-aws-code | 7df90ece41eb93524ad3e60b5a8165bbb0143645 | [
"MIT"
] | null | null | null | 821.961962 | 224,488 | 0.94205 | [
[
[
"Label Detection. Face Detection and Comparison, Celebrity Recognition, Image moderation, Text in image detection",
"_____no_output_____"
]
],
[
[
"import cv2\nimport boto3\nimport numpy as np\nimport os\nimport matplotlib.pyplot as plt",
"_____no_output_____"
],
[
"# Helpers\ndef show_image(filename):\n image = cv2.imread(filename)\n plt.imshow(image)\n plt.show()\n \n# Change color channels\ndef show_image_rgb(filename):\n image = cv2.imread(filename)\n plt.imshow(cv2.cvtColor(image, cv2.COLOR_BGR2RGB))\n plt.show()\n \ndef image_encoder(image_array):\n #image_uint8 = image_array.astype(np.uint8)\n ret, buf = cv2.imencode('.jpg', image_array)\n encoded = {\n 'Bytes':buf.tobytes()\n }\n return encoded",
"_____no_output_____"
],
[
"def detect_image_entities(filename):\n rekognition = boto3.client('rekognition')\n # Read image array\n image = cv2.imread(filename)\n # Encode Image\n encoded = image_encoder(image)\n # Send to rekognition\n response = rekognition.detect_labels(\n Image = encoded\n )\n return response['Labels']",
"_____no_output_____"
],
[
"filename = 'new-york-city.jpg'\nshow_image(filename)\ndetect_image_entities(filename)",
"_____no_output_____"
],
[
"def detect_image_text(filename):\n rekognition = boto3.client('rekognition')\n # Read image array\n image = cv2.imread(filename)\n # Encode Image\n encoded = image_encoder(image)\n # Send to rekognition\n response = rekognition.detect_text(\n Image = encoded\n )\n return response['TextDetections']",
"_____no_output_____"
],
[
"filename = 'innovation.jpg'\nshow_image(filename)\ndetect_image_text(filename)",
"_____no_output_____"
],
[
"def analyze_face(filename):\n rekognition = boto3.client('rekognition')\n # Read image array\n image = cv2.imread(filename)\n # Encode Image\n encoded = image_encoder(image)\n # Send to rekognition\n response = rekognition.detect_faces(\n Image = encoded,\n Attributes=[\n 'ALL',\n ]\n )\n return response['FaceDetails']",
"_____no_output_____"
],
[
"filename = 'harry_megan.JPG'\nshow_image_rgb(filename)\nanalyze_face(filename)",
"_____no_output_____"
],
[
"def detect_celebrity(filename):\n rekognition = boto3.client('rekognition')\n # Read image array\n image = cv2.imread(filename)\n # Encode Image\n encoded = image_encoder(image)\n # Send to rekognition\n response = rekognition.recognize_celebrities(\n Image = encoded\n )\n return response['CelebrityFaces']",
"_____no_output_____"
],
[
"filename = 'elon.jpg'\nshow_image_rgb(filename)\ndetect_celebrity(filename)",
"_____no_output_____"
],
[
"def compare_faces(filename1,filename2):\n rekognition = boto3.client('rekognition')\n # Read image array\n image1 = cv2.imread(filename1)\n image2 = cv2.imread(filename2)\n # Encode Image\n encoded1 = image_encoder(image1)\n encoded2 = image_encoder(image2)\n # Send to rekognition\n response = rekognition.compare_faces(\n SourceImage = encoded1,\n TargetImage = encoded2\n )\n return response['SourceImageFace'], response['FaceMatches'], response['UnmatchedFaces']",
"_____no_output_____"
],
[
"filename1 = 'obama1.jpg'\nfilename2 = 'obama2.jpg'\n\nshow_image_rgb(filename1)\nshow_image_rgb(filename2)\n\ncompare_faces(filename1,filename2)",
"_____no_output_____"
]
]
] | [
"markdown",
"code"
] | [
[
"markdown"
],
[
"code",
"code",
"code",
"code",
"code",
"code",
"code",
"code",
"code",
"code",
"code",
"code"
]
] |
e784eea12a40c4457ef33a4720fb3684c906628e | 24,296 | ipynb | Jupyter Notebook | 2DofArm_simulation_data_generator_and_physics.ipynb | ricardodeazambuja/IJCNN2017 | 817165185de6152041bbaf21cbad6d12fb58f064 | [
"MIT"
] | 3 | 2020-07-18T14:30:15.000Z | 2021-07-29T12:52:51.000Z | 2DofArm_simulation_data_generator_and_physics.ipynb | ricardodeazambuja/IJCNN2017 | 817165185de6152041bbaf21cbad6d12fb58f064 | [
"MIT"
] | 1 | 2021-01-18T06:06:02.000Z | 2021-01-18T09:39:56.000Z | 2DofArm_simulation_data_generator_and_physics.ipynb | ricardodeazambuja/IJCNN2017 | 817165185de6152041bbaf21cbad6d12fb58f064 | [
"MIT"
] | null | null | null | 39.634584 | 176 | 0.528112 | [
[
[
"# In this notebook I'm generating the movements and the states variables (torques and angles) in order to produce this movement using the 2dof simulator.\n\n### Some of the algorithms (or inspiration) to simulate the 2dof arm came from: http://www.gribblelab.org/compneuro/",
"_____no_output_____"
],
[
"# Here starts the 2 joint arm study",
"_____no_output_____"
],
[
"# Main functions to the 2 joint arm simulation",
"_____no_output_____"
]
],
[
[
"# Makes possible to show the output from matplotlib inline\n%matplotlib inline\nimport matplotlib.pyplot as plt\n\n# Makes the figures in the PNG format:\n# For more information see %config InlineBackend\n%config InlineBackend.figure_formats=set([u'png'])\n\nplt.rcParams['figure.figsize'] = 20, 10\n\nimport numpy\nimport sys\nimport save_load_file as slf",
"_____no_output_____"
],
[
"# Loads the modules and starts the object to be used with the parallel processing iPython stuff...\n\n# Remember to execute at the shell: ipcluster start -n 4\n# or from the iPython notebook interface!\n# from IPython.parallel import Client\nfrom ipyparallel import Client\n\n# When using the ipython in my desktop, launch the cluster in the right profile :)\ncli = Client()\n\n# lbview = cli.load_balanced_view()\ndview = cli[:]",
"_____no_output_____"
],
[
"%%file simulation_2DoF_Arm_physics.py\n\n# 2 DOF Simulator module\n# Strongly based on http://www.gribblelab.org/compneuro/index.html\n\nimport numpy\n\n# forward kinematics\ndef joints_to_hand(A,aparams):\n \"\"\"\n Given joint angles A=(a1,a2) and anthropometric params aparams,\n returns hand position H=(hx,hy) and elbow position E=(ex,ey)\n Note1: A must be type matrix (or array([[a1,a2],...]))\n Note2: If A has multiple lines, H and E will have the same number of lines.\n \"\"\"\n l1 = aparams['l1']\n l2 = aparams['l2']\n n = numpy.shape(A)[0]\n E = numpy.zeros((n,2))\n H = numpy.zeros((n,2))\n for i in range(n):\n E[i,0] = l1 * numpy.cos(A[i,0])\n E[i,1] = l1 * numpy.sin(A[i,0])\n H[i,0] = E[i,0] + (l2 * numpy.cos(A[i,0]+A[i,1]))\n H[i,1] = E[i,1] + (l2 * numpy.sin(A[i,0]+A[i,1]))\n return H,E\n\n\n# I could do the inverse kinematics using all the possible values (workspace) of the arm and creating a numpy array. Then I \n# could use the argmin trick to find the value.\n# In order to solve the problem when multiple solutions appear I could use the minimum jerk criterion. The user should enter\n# the actual position and the next one, then the system solves according to the one that uses mininum energy.\n# One curious thing about inverse kinematics is that as human beings we cannot do a inverse kinematic of our hand position\n# without taking in account the actual position. The function, below, doesn't care about the actual position, and that is why \n# more than one solution appears.\n# So, I don't think the brain solves the problem of multiple solutions. Who solves this problem is the morphology of the limbs.\n# It is impossible to change trajectories instantaneously, therefore the continuity of the movements is guaranteed.\n# Summary: there are no positions, but trajectories :)\n\n# inverse kinematics\ndef hand_to_joints(H,aparams):\n \"\"\"\n Given hand position H=(hx,hy) and anthropometric params aparams,\n returns joint angles A=(a1,a2)\n Note1: H must be type matrix (or array([[hx,hy],...]))\n Note2: If H has multiple lines, A will have the same number of lines.\n \"\"\"\n l1 = aparams['l1']\n l2 = aparams['l2']\n n = numpy.shape(H)[0]\n A = numpy.zeros((n,2))\n for i in range(n):\n A[i,1] = numpy.arccos(((H[i,0]*H[i,0])+(H[i,1]*H[i,1])-(l1*l1)-(l2*l2))/(2.0*l1*l2))\n A[i,0] = numpy.arctan2(H[i,1],H[i,0]) - numpy.arctan2((l2*numpy.sin(A[i,1])),(l1+(l2*numpy.cos(A[i,1]))))\n# if A[i,0] < 0:\n# print \"<0:\",A[i,0]\n# A[i,0] = A[i,0] + pi\n# elif A[i,0] > pi:\n# print \">0:\",A[i,0]\n# A[i,0] = A[i,0] - pi\n return A\n\n\n# inverse kinematics\ndef hand_to_joints(H,aparams,ang_error=0.01):\n \"\"\"\n Given hand position H=(hx,hy) and anthropometric params aparams,\n returns joint angles A=(a1,a2)\n Note1: H must be type matrix (or array([[hx,hy],...]))\n Note2: If H has multiple lines, A will have the same number of lines.\n \"\"\"\n l1 = aparams['l1']\n l2 = aparams['l2']\n n = numpy.shape(H)[0]\n A = numpy.zeros((n,2))\n t_bias=[0,0] \n for i in range(n):\n A[i,1] = numpy.arccos(((H[i,0]*H[i,0])+(H[i,1]*H[i,1])-(l1*l1)-(l2*l2))/(2.0*l1*l2)) + t_bias[1]\n A[i,0] = numpy.arctan2(H[i,1],H[i,0]) - numpy.arctan2((l2*numpy.sin(A[i,1])),(l1+(l2*numpy.cos(A[i,1])))) + t_bias[0]\n if i>0:\n # Here I'm trying to avoid descontinuity problems when there's a 2pi difference between them!\n if 0<=abs(abs((A[i,1]-A[i-1,1])/numpy.pi)-2)<=ang_error:\n print \"Correction on Joint 2:\",(A[i,1],A[i-1,1])\n if (A[i,1]-A[i-1,1])>0:\n A[i,1]-=2*numpy.pi\n t_bias[1]-=2*numpy.pi\n else:\n A[i,1]+=2*numpy.pi\n t_bias[1]+=2*numpy.pi \n \n if 0<=abs(abs((A[i,0]-A[i-1,0])/numpy.pi)-2)<=ang_error:\n print \"Correction on Joint 1:\",(A[i,0],A[i-1,0])\n if (A[i,0]-A[i-1,0])>0:\n A[i,0]-=2*numpy.pi\n t_bias[0]-=2*numpy.pi\n else:\n A[i,0]+=2*numpy.pi\n t_bias[0]+=2*numpy.pi\n return A\n\n\n# Generates the movements according to:\n# Flash, Tamar and Neville Hogan. 1985. The Coordination of Arm Movements: An Experimentally Confirmed Mathematical Model. The Journal of Neuroscience 5 (7): 1688-1703\ndef cartesian_movement_generation_training(xstart,ystart,xdest,ydest,MT,t):\n '''\n xstart,ystart: initial position of the trajectory\n xdest,ydest: final position of the trajectory\n MT: total time spent doing the trajectory\n t: current time\n \n returns a matrix: [[x0,y0],[x1,y1],...]\n '''\n x_t=xstart+(xstart-xdest)*(15*(t/MT)**4-6*(t/MT)**5-10*(t/MT)**3)\n y_t=ystart+(ystart-ydest)*(15*(t/MT)**4-6*(t/MT)**5-10*(t/MT)**3) \n return numpy.array([x_t,y_t]).T\n\n\n# Used to generate the velocities and the accelerations using the position and time vectors\ndef derivator(v,t):\n return numpy.array([(v[i+1]-v[i])/(t[i+1]-t[i]) for i in range(len(t)-1)])\n\n\ndef twojointarm_torques(state, t, aparams):\n \"\"\"\n Calculates the necessaries torques to generate the accelerations\n \"\"\"\n import numpy\n\n a1,a2,a1d,a2d,a1dd,a2dd = state # joint_angle_a1,joint_angle_a2,joint_vel_a1,joint_vel_a2,joint_acc_a1,joint_acc_a2\n\n l1,l2 = aparams['l1'], aparams['l2'] # lenght link 1 and 2\n m1,m2 = aparams['m1'], aparams['m2'] # mass link 1 and 2\n i1,i2 = aparams['i1'], aparams['i2'] # moment of inertia link 1 and 2\n lc1,lc2 = aparams['lc1'], aparams['lc2'] # distance to the center of mass of link 1 and 2\n\n M11 = i1 + i2 + (m1*lc1*lc1) + (m2*((l1*l1) + (lc2*lc2) + (2*l1*lc2*numpy.cos(a2))))\n M12 = i2 + (m2*((lc2*lc2) + (l1*lc2*numpy.cos(a2))))\n M21 = M12\n M22 = i2 + (m2*lc2*lc2)\n M = numpy.matrix([[M11,M12],[M21,M22]]) # H matrix\n\n C1 = -(m2*l1*a2d*a2d*lc2*numpy.sin(a2)) - (2*m2*l1*a1d*a2d*lc2*numpy.sin(a2))\n C2 = m2*l1*a1d*a1d*lc2*numpy.sin(a2)\n C = numpy.matrix([[C1],[C2]])\n\n ACC = numpy.array([[a1dd],[a2dd]])\n\n T = M*ACC + C\n\n return numpy.array([T[0,0],T[1,0]])\n\n\n# forward dynamics equations of our two-joint arm\ndef twojointarm(state, t, aparams, torque):\n import numpy\n \n \"\"\"\n two-joint arm in plane\n X is fwd(+) and back(-)\n Y is up(+) and down(-)\n shoulder angle a1 relative to Y vert, +ve counter-clockwise\n elbow angle a2 relative to upper arm, +ve counter-clockwise\n \"\"\"\n a1,a2,a1d,a2d = state # joint_angle_a1, joint_angle_a2, joint_velocity_a1, joint_velocity_a2\n\n l1,l2 = aparams['l1'], aparams['l2'] # lenght link 1 and 2\n m1,m2 = aparams['m1'], aparams['m2'] # mass link 1 and 2\n i1,i2 = aparams['i1'], aparams['i2'] # moment of inertia link 1 and 2\n lc1,lc2 = aparams['lc1'], aparams['lc2'] # distance to the center of mass of link 1 and 2\n\n M11 = i1 + i2 + (m1*lc1*lc1) + (m2*((l1*l1) + (lc2*lc2) + (2*l1*lc2*numpy.cos(a2))))\n M12 = i2 + (m2*((lc2*lc2) + (l1*lc2*numpy.cos(a2))))\n M21 = M12\n M22 = i2 + (m2*lc2*lc2)\n M = numpy.matrix([[M11,M12],[M21,M22]]) # H matrix\n\n C1 = -(m2*l1*a2d*a2d*lc2*numpy.sin(a2)) - (2*m2*l1*a1d*a2d*lc2*numpy.sin(a2))\n C2 = m2*l1*a1d*a1d*lc2*numpy.sin(a2)\n C = numpy.matrix([[C1],[C2]])\n\n T = numpy.matrix([[torque[0]],[torque[1]]])\n\n ACC = numpy.linalg.inv(M) * (T-C) # calculates the accelerations of joints 1 and 2\n\n a1dd,a2dd = ACC[0,0], ACC[1,0]\n\n return [a1d, a2d, a1dd, a2dd] # It returns the first and second derivatives of the joints\n\n\ndef animatearm(state,t,aparams):\n \"\"\"\n animate the twojointarm\n \"\"\"\n import matplotlib.pyplot as plt\n import numpy\n import time\n\n A = state[:,[0,1]] # Gets the angles a1 and a2 from the states matrix\n A[:,0] = A[:,0]\n H,E = joints_to_hand(A,aparams)\n l1,l2 = aparams['l1'], aparams['l2']\n plt.figure()\n plt.plot(0,0,'b.')\n plt.plot(H[:,0],H[:,1],'g.-');\n p1, = plt.plot(E[0,0],E[0,1],'b.')\n p2, = plt.plot(H[0,0],H[0,1],'b.')\n p3, = plt.plot((0,E[0,0],H[0,0]),(0,E[0,1],H[0,1]),'b-')\n plt.xlim([-l1-l2, l1+l2])\n plt.ylim([-l1-l2, l1+l2])\n dt = t[1]-t[0]\n tt = plt.title(\"Click on this plot to continue...\")\n plt.ginput(1)\n for i in xrange(0,numpy.shape(state)[0]):\n time.sleep(0.05)\n p1.set_xdata((E[i,0]))\n p1.set_ydata((E[i,1]))\n p2.set_xdata((H[i,0]))\n p2.set_ydata((H[i,1]))\n p3.set_xdata((0,E[i,0],H[i,0]))\n p3.set_ydata((0,E[i,1],H[i,1]))\n tt.set_text(\"Current time:%4.2f sec - click to next slide!\" % (i*dt))\n plt.draw()\n tt.set_text(\"Current time:%4.2f sec - finished!\" % ((numpy.shape(state)[0]-1)*dt))\n plt.draw() \n\n \ndef animatearm_JS(state,t,aparams):\n \"\"\"\n animate the twojointarm\n \"\"\"\n import matplotlib.pyplot as plt\n import numpy\n from JSAnimation import IPython_display\n from matplotlib import animation\n\n\n A = state[:,[0,1]] # Gets the angles a1 and a2 from the states matrix\n A[:,0] = A[:,0]\n H,E = joints_to_hand(A,aparams)\n l1,l2 = aparams['l1'], aparams['l2']\n\n # Set up the axes, making sure the axis ratio is equal\n# ax = fig.add_axes([0, 0, 1, 1], xlim=(-0.02, 13.02), ylim=(-0.02, 5.02),\n# xticks=range(14), yticks=range(6), aspect='equal', frameon=False)\n\n fig = plt.figure(figsize=(6, 6),dpi=100)\n ax = plt.axes(xlim=(-1, 1), ylim=(-1, 1), aspect='equal')\n ax.plot(0,0,'b.')\n ax.plot(H[:,0],H[:,1],'g.-');\n p1, = ax.plot(E[0,0],E[0,1],'b.')\n p2, = ax.plot(H[0,0],H[0,1],'b.')\n p3, = ax.plot((0,E[0,0],H[0,0]),(0,E[0,1],H[0,1]),'b-') \n \n def init():\n p1.set_data([],[])\n p2.set_data([],[])\n p3.set_data([],[]) \n return p1,p2,p3\n \n def animate(i):\n p1.set_data([E[i,0]],[E[i,1]])\n p2.set_data(H[i,0],H[i,1])\n p3.set_data((0,E[i,0],H[i,0]),(0,E[i,1],H[i,1]))\n return p1,p2,p3\n anim = animation.FuncAnimation(fig, animate, init_func=init, frames=len(E[:,0]), interval=20, blit=True)\n return anim\n # In order to make the JSAnimation to work it is necessary that the function returns the animation object!\n \n \ndef odeint_arms(twojointarm, state, t, aparams, torque):\n '''\n twojointarm: function object. Must receive (state,t,aparams,torque) and return [a1d,a2d,a1dd,a2dd]\n state: current states => [a1(t),a2(t),a1d(t),a2d(t)]\n t: array([t,t+1]) => current time step and next (t+1)\n returns next states [a1(t+1),a2(t+1),a1d(t+1),a2d(t+1)]\n '''\n from scipy.integrate import odeint\n\n return odeint(twojointarm, state, t, args=(aparams,torque))\n\n\ndef moving_average (values,window=6):\n weights = numpy.repeat(1.0, window)/window\n sma = numpy.convolve(numpy.concatenate((numpy.zeros(int((window-1)/2.0)),values,numpy.zeros((window-1)-int((window-1)/2.0)))), weights, 'valid')\n # I should try the function numpy.lib.pad instead of concatenating manually\n return sma\n\n\ndef moving_average (values, window=6):\n weights = numpy.repeat(1.0, window)/window\n sma = numpy.convolve(values, weights, 'valid')\n # I should try the function numpy.lib.pad instead of concatenating manually\n return numpy.lib.pad(sma, (int((window-1)/2.0),(window-1)-int((window-1)/2.0)), 'edge')",
"Overwriting simulation_2DoF_Arm_physics.py\n"
],
[
"from simulation_2DoF_Arm_physics import *",
"_____no_output_____"
],
[
"@dview.parallel(block=True)\ndef generate_trajectories(sim_inputs):\n import numpy\n import sys\n import save_load_file as slf\n \n import simulation_2DoF_Arm_physics\n reload(sys.modules['simulation_2DoF_Arm_physics']) # Makes sure the interpreter is going to reload the module\n s2ap = simulation_2DoF_Arm_physics\n\n tji,positions,sim_params = sim_inputs\n \n xstart,ystart = positions[0]\n xdest,ydest = positions[1]\n\n sim_set,base_dir,MT,time_step,Ninput,aparams = sim_params\n \n t_mov=numpy.arange(0, MT, time_step) # t starts in 0s and steps time_step(s) until reaches MT(s)\n\n # Generates the movements according to:\n # Flash, Tamar, and Neville Hogan. 1985\n H_path=s2ap.cartesian_movement_generation_training(xstart, ystart, xdest, ydest, MT, t_mov)\n\n # These are the values teta1 and teta2 can have because the system limits the resolution.\n # According to Joshi/Maass paper there are 50 neurons to code the positions of each variable.\n #\n #\n teta1=numpy.linspace(-numpy.pi/6,numpy.pi,num=Ninput)\n teta2=numpy.linspace(0,numpy.pi,num=Ninput)\n teta1_teta2 = numpy.array([teta1,teta2]).T # This is the matrix to use with the function\n # to generate the x,y values of the workspace\n\n # Joint's workspace: all the possible combinations between teta1 and teta2.\n teta_workspace = numpy.array([[t1,t2] for t1 in teta1 for t2 in teta2])\n\n # Arm's workspace: x,y points that the arm (endpoint) can reach\n H_workspace = s2ap.joints_to_hand(teta_workspace,aparams)[0] # I'm getting the first because it returns the elbow's positions too.\n\n # Generate the joint's positions according to the ORIGINAL (X,Y) values.\n # I'm using the traditional geometric way to do the inverse kinematics here. I need to\n # implement the minimum jerk way to generate the joint's positions taking into account the movement's dynamics.\n\n Joints=s2ap.hand_to_joints(H_path, aparams,ang_error=0.1)\n\n # Here I'm extending the Joints matrix because I need two extra positions to calculate the accelerations.\n # Consequently, because the trajectory always finish with velocity ZERO, keeping the same position seems a good choice. \n Joints_extended=numpy.concatenate((Joints,[Joints[-1],Joints[-1]]))\n\n # But the time array (t_mov) must be extended too:\n t_mov_extended=numpy.concatenate((t_mov,[t_mov[-1]+time_step],[t_mov[-1]+2*time_step]))\n\n\n # Joint's velocities\n teta1_d=s2ap.derivator(Joints_extended[:,0],t_mov_extended)\n teta2_d=s2ap.derivator(Joints_extended[:,1],t_mov_extended)\n \n\n # Joint's accelerations\n teta1_dd=s2ap.derivator(teta1_d,t_mov_extended[:251])\n teta2_dd=s2ap.derivator(teta2_d,t_mov_extended[:251])\n\n #\n # WITH ORIGINAL JOINT'S VELOCITIES\n #\n\n # And generates a matrix with [teta1,teta2,teta1d,teta2d,teta1dd,teta2dd]\n # Using this matrix I will generate the torques.\n states_mov = numpy.array([[Joints[:,0][i],Joints[:,1][i],teta1_d[i],teta2_d[i],teta1_dd[i],teta2_dd[i]] for i in range(len(Joints[:,0]))])\n\n\n # Applying the function to all lines of the states_mov matrix, I generate the torques matrix\n T_mov=numpy.array([s2ap.twojointarm_torques(states_mov[i], t_mov, aparams) for i in range(numpy.shape(states_mov)[0])])\n\n # Here I calculate the states using the calculated torques, just to make sure it is working!\n state = states_mov[0,[0,1,2,3]] # This is the initial state. The rest of the states will be generated dynamically \n # according to the input torques.\n state_v2 = [state]\n t = t_mov\n torque = T_mov\n for i in range(len(t)-1):\n print \"state:\",state\n state = s2ap.odeint_arms(s2ap.twojointarm, state, [t[i], t[i+1]], aparams, torque[i])[1]\n state_v2.append(state)\n\n state_v2=numpy.array(state_v2)\n\n # slf.save_to_file_gz([numpy.array(state_v2),numpy.array(torque)],\"./\"+base_dir+\"/\"+sim_set+\"/States_Torques_movement\"+str(tji)+\".gzpickle\")\n \n return tji",
"_____no_output_____"
]
],
[
[
"## End of the main functions!",
"_____no_output_____"
],
[
"# Adjusting the parameters:",
"_____no_output_____"
]
],
[
[
"# Experiment identifier\nsim_sets = [\"set_A\", \"set_B\", \"set_C\", \"set_D\"]\n\nsim_set = sim_sets[0]\n\n# Base dir to save / access\nbase_dir = \"2DofArm_simulation_data\"\n\n# List with all trajectories to be generated\n# [[[start_x,start_y],[final_x,final_y]],...]\ntrajectories = [[[0.75,0.25],[0.0,0.5]], [[0.25,0.60],[-0.25,0.60]], [[-0.10,0.75],[-0.10,0.25]],[[-0.75,0.50],[-0.40,0.00]]]\n\n\n# The values below must match the ones used with the SNN simulation:\n\n# Total time spent during the movement (in seconds)\nMT = 0.5\n\n# Simulation time step (in seconds)\ntime_step = 2/1000.0\n\n# Number of neurons at the input layer (defines the resolution of the system)\nNinput = 50\n\n\n# Arm parametres used with the 2 dof arm simulator\n# (according to Joshi/Maass 2006 paper)\naparams = {\n 'l1' : 0.5, # metres\n 'l2' : 0.5,\n 'lc1' : 0.25,\n 'lc2' : 0.25,\n 'm1' : 1.0, # kg\n 'm2' : 1.0,\n 'i1' : 0.03, # kg*m*m\n 'i2' : 0.03\n}\n\n# Variable only used to pass the parameters\nsim_params = sim_set,base_dir,MT,time_step,Ninput,aparams",
"_____no_output_____"
],
[
"%time results = generate_trajectories.map([(tji,positions,sim_params) for tji,positions in zip(range(1,len(trajectories)+1),trajectories)])",
"CPU times: user 15.6 ms, sys: 6.15 ms, total: 21.7 ms\nWall time: 1.69 s\n"
]
]
] | [
"markdown",
"code",
"markdown",
"code"
] | [
[
"markdown",
"markdown",
"markdown"
],
[
"code",
"code",
"code",
"code",
"code"
],
[
"markdown",
"markdown"
],
[
"code",
"code"
]
] |
e7850142188e9cba46dcd40519c905a981c3d536 | 6,398 | ipynb | Jupyter Notebook | python/ipynb/functions.ipynb | joaodartora/challenges-and-studies | da831f0f4ed9d7609f94f2b70a05b98ddaccde9f | [
"MIT"
] | null | null | null | python/ipynb/functions.ipynb | joaodartora/challenges-and-studies | da831f0f4ed9d7609f94f2b70a05b98ddaccde9f | [
"MIT"
] | null | null | null | python/ipynb/functions.ipynb | joaodartora/challenges-and-studies | da831f0f4ed9d7609f94f2b70a05b98ddaccde9f | [
"MIT"
] | null | null | null | 20.63871 | 67 | 0.457174 | [
[
[
"# Simple function to print the sum of 2 numbers\n\ndef simple_sum(a,b):\n print(a + b)\n \nsimple_sum(2,9)\nsimple_sum(7,8)\nsimple_sum(10,15)",
"_____no_output_____"
],
[
"# Simple function to sum 2 numbers\n\ndef simple_sum(a,b):\n return(a + b)\n\nprint(simple_sum(2,9))",
"_____no_output_____"
],
[
"# Function to verify if a number is pair\n\ndef is_pair(x):\n return (x % 2 == 0)\n \nprint(is_pair(2))\nprint(is_pair(3))",
"_____no_output_____"
],
[
"# Using a function inside another function\n\ndef is_pair(x):\n return (x % 2 == 0)\n\ndef even_or_odd(x):\n if is_pair(x):\n return \"pair\"\n else:\n return \"odd\"\n \nprint(even_or_odd(4))\nprint(even_or_odd(5))",
"_____no_output_____"
],
[
"# Function to search for a value into a list\n\ndef search(list, value):\n for x,e in enumerate(list):\n if e == value:\n return x\n return None\n \nL = [10,20,25,30]\n\nprint(search(L,25))\nprint(search(L,27))\n",
"_____no_output_____"
],
[
"# Function to calculate the mean of all elements on a list\n\nL = [10,20,25,30]\ndef simple_sum(L):\n total = 0\n for e in L:\n total += e\n return total\n \ndef mean(L):\n return (simple_sum(L) / len(L))\n\nprint(mean(L))",
"_____no_output_____"
],
[
"# Function to print traces below the name of the course\n\ncourse = \"biomedical informatics\"\ndef print_course():\n print(course)\n print(\"-\" * len(course))\nprint_course()",
"_____no_output_____"
],
[
"# Function to change a value locally on a function\n\na = 5\n\ndef change_and_print():\n a = 7\n print (\"'A' inside function: %d\" %a)\n \nprint (\"'A' before changing: %d\" %a)\nchange_and_print()\nprint (\"'A' after changing: %d\" %a)",
"_____no_output_____"
],
[
"# Function to change a value globally on a function\n\na = 5\ndef change_and_print():\n global a\n a = 7\n print (\"'A' inside function: %d\" %a)\n \nprint (\"'A' before changing: %d\" %a)\nchange_and_print()\nprint (\"'A' after changing: %d\" %a)",
"_____no_output_____"
],
[
"# Function to print the factorial of a number\n\ndef factorial(n):\n if n == 0 or n == 1:\n return 1\n else:\n return n * factorial(n - 1)\n \nprint(factorial(5))",
"_____no_output_____"
],
[
"# Function passing default parameters\n\ndef trace(n = 40, character = \"-\"):\n print(character * n)\n \ntrace()\ntrace(character = \"*\")\ntrace(10)\ntrace(character = \"?\")",
"_____no_output_____"
],
[
"# Packing parameters\n\ndef simple_sum(a,b):\n print(a + b)\n \nL = [2,3]\nsimple_sum(*L)",
"_____no_output_____"
],
[
"# Unpacking parameters\n\ndef simple_sum(*args):\n s = 0\n for x in args:\n s += x\n return s\nprint(simple_sum(1,2))\nprint(simple_sum(2))\nprint(simple_sum(5,6,7,8))\nprint(simple_sum(9,10,20,30,40))",
"_____no_output_____"
]
]
] | [
"code"
] | [
[
"code",
"code",
"code",
"code",
"code",
"code",
"code",
"code",
"code",
"code",
"code",
"code",
"code"
]
] |
e7850ad2af16bfc7038c0edae802484b052719bc | 7,200 | ipynb | Jupyter Notebook | Plotly/Create Waterfall chart (Advanced).ipynb | Charles-de-Montigny/awesome-notebooks | 79485142ba557e9c20e6f6dca4fdc12a3443813e | [
"BSD-3-Clause"
] | 1,114 | 2020-09-28T07:32:23.000Z | 2022-03-31T22:35:50.000Z | Plotly/Create Waterfall chart (Advanced).ipynb | mmcfer/awesome-notebooks | 8d2892e40db480a323049e04decfefac45904af4 | [
"BSD-3-Clause"
] | 298 | 2020-10-29T09:39:17.000Z | 2022-03-31T15:24:44.000Z | Plotly/Create Waterfall chart (Advanced).ipynb | mmcfer/awesome-notebooks | 8d2892e40db480a323049e04decfefac45904af4 | [
"BSD-3-Clause"
] | 153 | 2020-09-29T06:07:39.000Z | 2022-03-31T17:41:16.000Z | 27.692308 | 1,015 | 0.581389 | [
[
[
"<img width=\"10%\" alt=\"Naas\" src=\"https://landen.imgix.net/jtci2pxwjczr/assets/5ice39g4.png?w=160\"/>\n",
"_____no_output_____"
],
[
"# Plotly - Create Waterfall chart (Advanced)\n<a href=\"https://app.naas.ai/user-redirect/naas/downloader?url=https://raw.githubusercontent.com/jupyter-naas/awesome-notebooks/master/Plotly/Create%20Waterfall%20chart%20%28Advanced%29.ipynb\" target=\"_parent\"><img src=\"https://img.shields.io/badge/-Open%20in%20Naas-success?labelColor=000000&logo=data:image/svg+xml;base64,PD94bWwgdmVyc2lvbj0iMS4wIiBlbmNvZGluZz0iVVRGLTgiPz4KPHN2ZyB3aWR0aD0iMTAyNHB4IiBoZWlnaHQ9IjEwMjRweCIgdmlld0JveD0iMCAwIDEwMjQgMTAyNCIgeG1sbnM9Imh0dHA6Ly93d3cudzMub3JnLzIwMDAvc3ZnIiB4bWxuczp4bGluaz0iaHR0cDovL3d3dy53My5vcmcvMTk5OS94bGluayIgdmVyc2lvbj0iMS4xIj4KIDwhLS0gR2VuZXJhdGVkIGJ5IFBpeGVsbWF0b3IgUHJvIDIuMC41IC0tPgogPGRlZnM+CiAgPHRleHQgaWQ9InN0cmluZyIgdHJhbnNmb3JtPSJtYXRyaXgoMS4wIDAuMCAwLjAgMS4wIDIyOC4wIDU0LjUpIiBmb250LWZhbWlseT0iQ29tZm9ydGFhLVJlZ3VsYXIsIENvbWZvcnRhYSIgZm9udC1zaXplPSI4MDAiIHRleHQtZGVjb3JhdGlvbj0ibm9uZSIgZmlsbD0iI2ZmZmZmZiIgeD0iMS4xOTk5OTk5OTk5OTk5ODg2IiB5PSI3MDUuMCI+bjwvdGV4dD4KIDwvZGVmcz4KIDx1c2UgaWQ9Im4iIHhsaW5rOmhyZWY9IiNzdHJpbmciLz4KPC9zdmc+Cg==\"/></a>",
"_____no_output_____"
],
[
"**Tags:** #plotly #chart #warterfall #dataviz",
"_____no_output_____"
],
[
"## Input",
"_____no_output_____"
],
[
"### Install packages",
"_____no_output_____"
]
],
[
[
"!pip install numpy\n!pip install matplotlib",
"_____no_output_____"
]
],
[
[
"### Import library",
"_____no_output_____"
]
],
[
[
"import numpy as np\nimport pandas as pd\nimport matplotlib.pyplot as plt\nfrom matplotlib.ticker import FuncFormatter",
"_____no_output_____"
]
],
[
[
"## Model",
"_____no_output_____"
],
[
"### Create the waterfall chart",
"_____no_output_____"
]
],
[
[
"#Use python 2.7+ syntax to format currency\ndef money(x, pos):\n 'The two args are the value and tick position'\n return \"${:,.0f}\".format(x)\nformatter = FuncFormatter(money)\n\n#Data to plot. Do not include a total, it will be calculated\nindex = ['sales','returns','credit fees','rebates','late charges','shipping']\ndata = {'amount': [350000,-30000,-7500,-25000,95000,-7000]}\n\n#Store data and create a blank series to use for the waterfall\ntrans = pd.DataFrame(data=data,index=index)\nblank = trans.amount.cumsum().shift(1).fillna(0)\n\n#Get the net total number for the final element in the waterfall\ntotal = trans.sum().amount\ntrans.loc[\"net\"]= total\nblank.loc[\"net\"] = total\n\n#The steps graphically show the levels as well as used for label placement\nstep = blank.reset_index(drop=True).repeat(3).shift(-1)\nstep[1::3] = np.nan\n\n#When plotting the last element, we want to show the full bar,\n#Set the blank to 0\nblank.loc[\"net\"] = 0\n\n#Plot and label\nmy_plot = trans.plot(kind='bar', stacked=True, bottom=blank,legend=None, figsize=(10, 5), title=\"2014 Sales Waterfall\")\nmy_plot.plot(step.index, step.values,'k')\nmy_plot.set_xlabel(\"Transaction Types\")\n\n#Format the axis for dollars\nmy_plot.yaxis.set_major_formatter(formatter)\n\n#Get the y-axis position for the labels\ny_height = trans.amount.cumsum().shift(1).fillna(0)\n\n#Get an offset so labels don't sit right on top of the bar\nmax = trans.max()\nneg_offset = max / 25\npos_offset = max / 50\nplot_offset = int(max / 15)\n\n#Start label loop\nloop = 0\nfor index, row in trans.iterrows():\n # For the last item in the list, we don't want to double count\n if row['amount'] == total:\n y = y_height[loop]\n else:\n y = y_height[loop] + row['amount']\n # Determine if we want a neg or pos offset\n if row['amount'] > 0:\n y += pos_offset\n else:\n y -= neg_offset\n my_plot.annotate(\"{:,.0f}\".format(row['amount']),(loop,y),ha=\"center\")\n loop+=1",
"_____no_output_____"
]
],
[
[
"## Output",
"_____no_output_____"
],
[
"### Display result",
"_____no_output_____"
]
],
[
[
"#Scale up the y axis so there is room for the labels\nmy_plot.set_ylim(0,blank.max()+int(plot_offset))\n#Rotate the labels\nmy_plot.set_xticklabels(trans.index,rotation=0)\nmy_plot.get_figure().savefig(\"waterfall.png\",dpi=200,bbox_inches='tight')",
"_____no_output_____"
]
]
] | [
"markdown",
"code",
"markdown",
"code",
"markdown",
"code",
"markdown",
"code"
] | [
[
"markdown",
"markdown",
"markdown",
"markdown",
"markdown"
],
[
"code"
],
[
"markdown"
],
[
"code"
],
[
"markdown",
"markdown"
],
[
"code"
],
[
"markdown",
"markdown"
],
[
"code"
]
] |
e78517dcd1342c1ab963773da1f9204c3d2b881c | 20,863 | ipynb | Jupyter Notebook | ICCT_en/examples/04/SS-33_State_feedback_control_for_the_mass-spring-damper_system.ipynb | ICCTerasmus/ICCT | fcd56ab6b5fddc00f72521cc87accfdbec6068f6 | [
"BSD-3-Clause"
] | 6 | 2021-05-22T18:42:14.000Z | 2021-10-03T14:10:22.000Z | ICCT/ENG/examples/04/SS-33_State_feedback_control_for_the_mass-spring-damper_system.ipynb | tuxsaurus/ICCT | 30d1aea4fb056c9736c9b4c5a0f50fff14fa6382 | [
"BSD-3-Clause"
] | null | null | null | ICCT/ENG/examples/04/SS-33_State_feedback_control_for_the_mass-spring-damper_system.ipynb | tuxsaurus/ICCT | 30d1aea4fb056c9736c9b4c5a0f50fff14fa6382 | [
"BSD-3-Clause"
] | 2 | 2021-05-24T11:40:09.000Z | 2021-08-29T16:36:18.000Z | 36.032815 | 266 | 0.472751 | [
[
[
"# Erasmus+ ICCT project (2018-1-SI01-KA203-047081)\n\n# Toggle cell visibility\n\nfrom IPython.display import HTML\ntag = HTML('''<script>\ncode_show=true; \nfunction code_toggle() {\n if (code_show){\n $('div.input').hide()\n } else {\n $('div.input').show()\n }\n code_show = !code_show\n} \n$( document ).ready(code_toggle);\n</script>\nToggle cell visibility <a href=\"javascript:code_toggle()\">here</a>.''')\ndisplay(tag)\n\n# Hide the code completely\n\n# from IPython.display import HTML\n# tag = HTML('''<style>\n# div.input {\n# display:none;\n# }\n# </style>''')\n# display(tag)\n",
"_____no_output_____"
]
],
[
[
"## State feedback control for the mass-spring-damper system\n\nGiven the mass-spring-damper system, we want to control it in order to have a step response with zero error at steady state and a settling time for 5% tolerance band of less than 6 s.\n\nThe system's equations written in state space form are:\n\n$$\n\\begin{bmatrix}\n\\dot{x_1} \\\\\n\\dot{x_2}\n\\end{bmatrix}=\\underbrace{\\begin{bmatrix}\n0 && 1 \\\\\n-\\frac{k}{m} && -\\frac{c}{m}\n\\end{bmatrix}}_{A}\\begin{bmatrix}\nx_1 \\\\\nx_2\n\\end{bmatrix}+\\underbrace{\\begin{bmatrix}\n0 \\\\\n\\frac{1}{m}\n\\end{bmatrix}}_{B}u,\n$$\n\nwith $m=5$ kg, $k=2$ N/m, $c=1$ Ns/m, $x_1$ representing the position and $x_2$ the velocity. By defining the gain matrix of the state feedback as $K=\\begin{bmatrix}k_1&k_2\\end{bmatrix}^T$ and substituting it in $A-BK$ we obtain:\n\n$$\nA-BK = \\begin{bmatrix}0&1\\\\-\\frac{2}{5}-\\frac{k_1}{5}&-\\frac{1}{5}-\\frac{k_2}{5}\\end{bmatrix}\\,.\n$$\n\nNote that the system is in canonical controllability form, the characteristic polynomial is\n\n$$\n\\lambda^2+(\\frac{k_2}{5}+\\frac{1}{5})\\lambda+(\\frac{k_1}{5}+\\frac{2}{5})\n$$\n\nand imposing the roots to be equal to $\\lambda_{1,2}=-1$ rad/s $\\left((\\lambda+1)^2=\\lambda^2+2\\lambda+1\\right)$ we find the values $k_1 = 3$ and $k_2=9$.\n\nIn order to reach zero steady-state error, it is possible to simply adjust the closed-loop gain: we multiply the reference input $u_{ref}$ by the inverse of the closed-loop gain to have the closed-loop transfer function staying at $0$ dB at low frequencies.\n\nThe static gain is calculated as $G(0)=C(-A+BK)^{-1}B$ with $C=\\begin{bmatrix}1&0\\end{bmatrix}$.\n\nThe final controlled system, that is still SISO from the input $u_{ref}$ to the position $x_1$, is:\n\n$$\n\\begin{cases}\n\\begin{bmatrix}\n\\dot{x_1} \\\\\n\\dot{x_2}\n\\end{bmatrix}=\\underbrace{\\begin{bmatrix}\n0 && 1 \\\\\n-1 && -2\n\\end{bmatrix}}_{A-BK}\\begin{bmatrix}\nx_1 \\\\\nx_2\n\\end{bmatrix}+\\underbrace{\\begin{bmatrix}\n0 \\\\\n\\frac{1}{5}\n\\end{bmatrix}}_{B}\\frac{1}{0.2}u_{\\text{ref}} \\\\\ny = \\begin{bmatrix}1&0\\end{bmatrix}\\begin{bmatrix}\nx_1 \\\\\nx_2\n\\end{bmatrix}\n\\end{cases}\n$$\n\n### How to use this notebook?\nTry to change the eigenvalues and adjust the reference signal gain to achieve zero steady-state error. ",
"_____no_output_____"
]
],
[
[
"%matplotlib inline\nimport control as control\nimport numpy\nimport sympy as sym\nfrom IPython.display import display, Markdown\nimport ipywidgets as widgets\nimport matplotlib.pyplot as plt\n\n\n#print a matrix latex-like\ndef bmatrix(a):\n \"\"\"Returns a LaTeX bmatrix - by Damir Arbula (ICCT project)\n\n :a: numpy array\n :returns: LaTeX bmatrix as a string\n \"\"\"\n if len(a.shape) > 2:\n raise ValueError('bmatrix can at most display two dimensions')\n lines = str(a).replace('[', '').replace(']', '').splitlines()\n rv = [r'\\begin{bmatrix}']\n rv += [' ' + ' & '.join(l.split()) + r'\\\\' for l in lines]\n rv += [r'\\end{bmatrix}']\n return '\\n'.join(rv)\n\n\n# Display formatted matrix: \ndef vmatrix(a):\n if len(a.shape) > 2:\n raise ValueError('bmatrix can at most display two dimensions')\n lines = str(a).replace('[', '').replace(']', '').splitlines()\n rv = [r'\\begin{vmatrix}']\n rv += [' ' + ' & '.join(l.split()) + r'\\\\' for l in lines]\n rv += [r'\\end{vmatrix}']\n return '\\n'.join(rv)\n\n\n#matrixWidget is a matrix looking widget built with a VBox of HBox(es) that returns a numPy array as value !\nclass matrixWidget(widgets.VBox):\n def updateM(self,change):\n for irow in range(0,self.n):\n for icol in range(0,self.m):\n self.M_[irow,icol] = self.children[irow].children[icol].value\n #print(self.M_[irow,icol])\n self.value = self.M_\n\n def dummychangecallback(self,change):\n pass\n \n \n def __init__(self,n,m):\n self.n = n\n self.m = m\n self.M_ = numpy.matrix(numpy.zeros((self.n,self.m)))\n self.value = self.M_\n widgets.VBox.__init__(self,\n children = [\n widgets.HBox(children = \n [widgets.FloatText(value=0.0, layout=widgets.Layout(width='90px')) for i in range(m)]\n ) \n for j in range(n)\n ])\n \n #fill in widgets and tell interact to call updateM each time a children changes value\n for irow in range(0,self.n):\n for icol in range(0,self.m):\n self.children[irow].children[icol].value = self.M_[irow,icol]\n self.children[irow].children[icol].observe(self.updateM, names='value')\n #value = Unicode('[email protected]', help=\"The email value.\").tag(sync=True)\n self.observe(self.updateM, names='value', type= 'All')\n \n def setM(self, newM):\n #disable callbacks, change values, and reenable\n self.unobserve(self.updateM, names='value', type= 'All')\n for irow in range(0,self.n):\n for icol in range(0,self.m):\n self.children[irow].children[icol].unobserve(self.updateM, names='value')\n self.M_ = newM\n self.value = self.M_\n for irow in range(0,self.n):\n for icol in range(0,self.m):\n self.children[irow].children[icol].value = self.M_[irow,icol]\n for irow in range(0,self.n):\n for icol in range(0,self.m):\n self.children[irow].children[icol].observe(self.updateM, names='value')\n self.observe(self.updateM, names='value', type= 'All') \n\n #self.children[irow].children[icol].observe(self.updateM, names='value')\n\n \n#overlaod class for state space systems that DO NOT remove \"useless\" states (what \"professor\" of automatic control would do this?)\nclass sss(control.StateSpace):\n def __init__(self,*args):\n #call base class init constructor\n control.StateSpace.__init__(self,*args)\n #disable function below in base class\n def _remove_useless_states(self):\n pass",
"_____no_output_____"
],
[
"# Preparatory cell\n\nA = numpy.matrix([[0,1],[-2/5,-1/5]])\nB = numpy.matrix('0; 1')\nC = numpy.matrix('1 0')\nX0 = numpy.matrix('0; 0')\nK = numpy.matrix([3,9])\n\nAw = matrixWidget(2,2)\nAw.setM(A)\nBw = matrixWidget(2,1)\nBw.setM(B)\nCw = matrixWidget(1,2)\nCw.setM(C)\nX0w = matrixWidget(2,1)\nX0w.setM(X0)\nKw = matrixWidget(1,2)\nKw.setM(K)\n\n\neig1c = matrixWidget(1,1)\neig2c = matrixWidget(2,1)\neig1c.setM(numpy.matrix([-1])) \neig2c.setM(numpy.matrix([[-1],[0]]))",
"_____no_output_____"
],
[
"# Misc\n\n#create dummy widget \nDW = widgets.FloatText(layout=widgets.Layout(width='0px', height='0px'))\n\n#create button widget\nSTART = widgets.Button(\n description='Test',\n disabled=False,\n button_style='', # 'success', 'info', 'warning', 'danger' or ''\n tooltip='Test',\n icon='check'\n)\n \ndef on_start_button_clicked(b):\n #This is a workaround to have intreactive_output call the callback:\n # force the value of the dummy widget to change\n if DW.value> 0 :\n DW.value = -1\n else: \n DW.value = 1\n pass\nSTART.on_click(on_start_button_clicked)\n\n# Define type of method \nselm = widgets.Dropdown(\n options= ['Set K', 'Set the eigenvalues'],\n value= 'Set the eigenvalues',\n description='',\n disabled=False\n)\n\n# Define the number of complex eigenvalues for the observer\nselc = widgets.Dropdown(\n options= ['0 complex eigenvalues', '2 complex eigenvalues'],\n value= '0 complex eigenvalues',\n description='Eigenvalues:',\n disabled=False\n)\n\n#define type of ipout \nselu = widgets.Dropdown(\n options=['impulse', 'step', 'sinusoid', 'square wave'],\n value='step',\n description='Type of reference:',\n style = {'description_width': 'initial'},\n disabled=False\n)\n# Define the values of the input\nu = widgets.FloatSlider(\n value=1,\n min=0,\n max=20.0,\n step=0.1,\n description='Input reference:',\n style = {'description_width': 'initial'},\n disabled=False,\n continuous_update=False,\n orientation='horizontal',\n readout=True,\n readout_format='.1f',\n)\nperiod = widgets.FloatSlider(\n value=1,\n min=0.01,\n max=4,\n step=0.01,\n description='Period: ',\n disabled=False,\n continuous_update=False,\n orientation='horizontal',\n readout=True,\n readout_format='.2f',\n)\ngain = widgets.FloatText(\n value=0.2,\n description='Inverse reference gain:',\n style = {'description_width': 'initial'},\n disabled=False\n)\nm = widgets.FloatSlider(\n value=5,\n min=0.1,\n max=10.0,\n step=0.1,\n description='m [kg]:',\n disabled=False,\n continuous_update=False,\n orientation='horizontal',\n readout=True,\n readout_format='.1f',\n)\nk = widgets.FloatSlider(\n value=2,\n min=0,\n max=10.0,\n step=0.1,\n description='k [N/m]:',\n disabled=False,\n continuous_update=False,\n orientation='horizontal',\n readout=True,\n readout_format='.1f',\n)\nc = widgets.FloatSlider(\n value=1,\n min=0,\n max=10.0,\n step=0.1,\n description='c [Ns/m]:',\n disabled=False,\n continuous_update=False,\n orientation='horizontal',\n readout=True,\n readout_format='.1f',\n)",
"_____no_output_____"
],
[
"# Support functions\n\ndef eigen_choice(selc):\n if selc == '0 complex eigenvalues':\n eig1c.children[0].children[0].disabled = False\n eig2c.children[1].children[0].disabled = True\n eigc = 0\n if selc == '2 complex eigenvalues':\n eig1c.children[0].children[0].disabled = True\n eig2c.children[1].children[0].disabled = False\n eigc = 2\n return eigc\n\ndef method_choice(selm):\n if selm == 'Set K':\n method = 1\n selc.disabled = True\n if selm == 'Set the eigenvalues':\n method = 2\n selc.disabled = False\n return method",
"_____no_output_____"
],
[
"def main_callback(m, k, c, gain, X0w, K, eig1c, eig2c, u, period, selm, selc, selu, DW):\n A, B = numpy.matrix([[0,1],[-k/m,-c/m]]), numpy.matrix([[0],[1/m]])\n sols = numpy.linalg.eig(A)\n eigc = eigen_choice(selc)\n method = method_choice(selm)\n \n if method == 1:\n sol = numpy.linalg.eig(A-B*K)\n if method == 2:\n if eigc == 0:\n K = control.acker(A, B, [eig1c[0,0], eig2c[0,0]])\n Kw.setM(K) \n if eigc == 2:\n K = control.acker(A, B, [numpy.complex(eig2c[0,0],eig2c[1,0]), \n numpy.complex(eig2c[0,0],-eig2c[1,0])])\n Kw.setM(K)\n sol = numpy.linalg.eig(A-B*K)\n print('The system\\'s eigenvalues are:',round(sols[0][0],4),'and',round(sols[0][1],4))\n print('The controlled system\\'s eigenvalues are:',round(sol[0][0],4),'and',round(sol[0][1],4))\n \n sys1 = sss(A-B*K,B,C,0)\n sg = control.evalfr(sys1,0)\n print('The static gain of the controlled system is: %f' %sg)\n if gain != 0:\n sys = sss(A-B*K,B*1/gain,C,0)\n else:\n print('The inverse gain setted is 0 and it is changed to 1')\n sys = sss(A-B*K,B,C,0)\n T = numpy.linspace(0, 10, 1000)\n \n if selu == 'impulse': #selu\n U = [0 for t in range(0,len(T))]\n U[0] = u\n T, yout, xout = control.forced_response(sys,T,U,X0w)\n if selu == 'step':\n U = [u for t in range(0,len(T))]\n T, yout, xout = control.forced_response(sys,T,U,X0w)\n if selu == 'sinusoid':\n U = u*numpy.sin(2*numpy.pi/period*T)\n T, yout, xout = control.forced_response(sys,T,U,X0w)\n if selu == 'square wave':\n U = u*numpy.sign(numpy.sin(2*numpy.pi/period*T))\n T, yout, xout = control.forced_response(sys,T,U,X0w)\n \n fig = plt.figure(num='Bode plot', figsize=(16,10))\n control.bode_plot(sys)\n fig.suptitle('Bode plot', fontsize=16)\n \n plt.figure(num='Simulation', figsize=(16,4))\n plt.title('Position input response')\n plt.ylabel('position vs ref')\n plt.plot(T,xout[0],T,U,'r--')\n plt.xlabel('$t$ [s]')\n plt.axvline(x=0,color='black',linewidth=0.8)\n plt.axhline(y=0,color='black',linewidth=0.8)\n plt.legend(['position','Reference'])\n plt.grid()\n\n \nalltogether = widgets.VBox([widgets.HBox([selm, \n selc, \n selu]),\n widgets.Label(' ',border=3),\n widgets.HBox([widgets.Label('K:',border=3), Kw, \n widgets.Label(' ',border=3),\n widgets.Label(' ',border=3),\n widgets.Label('Eigenvalues:',border=3), \n eig1c, \n eig2c, \n widgets.Label(' ',border=3),\n widgets.Label(' ',border=3),\n widgets.Label('X0:',border=3), X0w]),\n widgets.Label(' ',border=3),\n widgets.HBox([u, \n period, \n START]),\n widgets.Label(' ',border=3),\n widgets.HBox([m,\n k,\n c,\n gain])])\nout = widgets.interactive_output(main_callback, {'m':m, 'k':k, 'c':c, 'gain':gain, 'X0w':X0w, 'K':Kw, 'eig1c':eig1c, 'eig2c':eig2c, \n 'u':u, 'period':period, 'selm':selm, 'selc':selc, 'selu':selu, 'DW':DW})\nout.layout.height = '1050px'\ndisplay(out, alltogether)",
"_____no_output_____"
]
]
] | [
"code",
"markdown",
"code"
] | [
[
"code"
],
[
"markdown"
],
[
"code",
"code",
"code",
"code",
"code"
]
] |
e785187a65205f1b08d44202f4a6c234680c093d | 36,974 | ipynb | Jupyter Notebook | Chapter_01.Operations_numpy_and_pandas/Numpy_operations.Indexing_slicing_splitting_iterator_sorting_combining_reshaping.ipynb | Eduardo0697/DataVisualizationWorkshop | 3da39d34102d42ae18c7f7b2738f207117ee1a0f | [
"MIT"
] | null | null | null | Chapter_01.Operations_numpy_and_pandas/Numpy_operations.Indexing_slicing_splitting_iterator_sorting_combining_reshaping.ipynb | Eduardo0697/DataVisualizationWorkshop | 3da39d34102d42ae18c7f7b2738f207117ee1a0f | [
"MIT"
] | null | null | null | Chapter_01.Operations_numpy_and_pandas/Numpy_operations.Indexing_slicing_splitting_iterator_sorting_combining_reshaping.ipynb | Eduardo0697/DataVisualizationWorkshop | 3da39d34102d42ae18c7f7b2738f207117ee1a0f | [
"MIT"
] | null | null | null | 29.697992 | 131 | 0.498053 | [
[
[
"## Operations for indexing, splitting, slicing and iterating over a dataset",
"_____no_output_____"
]
],
[
[
"import numpy as np",
"_____no_output_____"
]
],
[
[
"#### Indexing",
"_____no_output_____"
]
],
[
[
"dataset = np.genfromtxt('../Datasets/normal_distribution_splittable.csv', delimiter=',')",
"_____no_output_____"
],
[
"# Mean of the second row\nsecond_row = dataset[1]\nnp.mean(second_row)",
"_____no_output_____"
],
[
"# Mean of the last row\nlast_row = dataset[-1]\nnp.mean(last_row)",
"_____no_output_____"
],
[
"# Mean of the first value of the first row\nfirst_val_first_row = dataset[0][0]\nprint(np.mean(first_val_first_row))\nprint(first_val_first_row)",
"99.14931546\n99.14931546\n"
],
[
"# Index the value of the last element in the second last row\nlast_val_second_last_row = dataset[-2, -1]\nnp.mean(last_val_second_last_row)",
"_____no_output_____"
]
],
[
[
"#### Slicing",
"_____no_output_____"
]
],
[
[
"# Create a 2x2 matrix that starts in the second row and second column\nsubsection_2x2 = dataset[1:3, 1:3]\nnp.mean(subsection_2x2)",
"_____no_output_____"
],
[
"# Get every element in the 5th row, but only get every second element of that row\nevery_other_elem = dataset[4, ::2]\nprint(dataset[4])\nprint(every_other_elem)\nprint(np.mean(every_other_elem))",
"[101.20862522 103.5730309 100.28690912 105.85269352 93.37126331\n 108.57980357 100.79478953 94.20019732 96.10020311]\n[101.20862522 100.28690912 93.37126331 100.79478953 96.10020311]\n98.35235805800001\n"
],
[
"# Revesed last row of the dataset\nreversed_last_row = dataset[-1, ::-1]\nprint(dataset[-1])\nprint(reversed_last_row)\nprint(np.mean(reversed_last_row))",
"[ 94.11176915 99.62387832 104.51786419 97.62787811 93.97853495\n 98.75108352 106.05042487 100.07721494 106.89005002]\n[106.89005002 100.07721494 106.05042487 98.75108352 93.97853495\n 97.62787811 104.51786419 99.62387832 94.11176915]\n100.18096645222222\n"
]
],
[
[
"#### Splitting",
"_____no_output_____"
]
],
[
[
"# Split horizontally the dataset in three equal subsets\nhor_splits = np.hsplit(dataset,(3))",
"_____no_output_____"
],
[
"# Split the first third in 2 equal vertically parts\nver_splits = np.vsplit(hor_splits[0],(2))",
"_____no_output_____"
],
[
"print(\"Dataset\", dataset.shape)\nprint(\"Subset\", ver_splits[0].shape)",
"Dataset (24, 9)\nSubset (12, 3)\n"
]
],
[
[
"#### Iterating",
"_____no_output_____"
]
],
[
[
"# Iterate over the whole dataset using nditer\ncurr_index = 0\nfor x in np.nditer(dataset):\n print(x, curr_index)\n curr_index += 1",
"99.14931546 0\n104.03852715 1\n107.43534677 2\n97.85230675 3\n98.74986914 4\n98.80833412 5\n96.81964892 6\n98.56783189 7\n101.34745901 8\n92.02628776 9\n97.10439252 10\n99.32066924 11\n97.24584816 12\n92.9267508 13\n92.65657752 14\n105.7197853 15\n101.23162942 16\n93.87155456 17\n95.66253664 18\n95.17750125 19\n90.93318132 20\n110.18889465 21\n98.80084371 22\n105.95297652 23\n98.37481387 24\n106.54654286 25\n107.22482426 26\n91.37294597 27\n100.96781394 28\n100.40118279 29\n113.42090475 30\n105.48508838 31\n91.6604946 32\n106.1472841 33\n95.08715803 34\n103.40412146 35\n101.20862522 36\n103.5730309 37\n100.28690912 38\n105.85269352 39\n93.37126331 40\n108.57980357 41\n100.79478953 42\n94.20019732 43\n96.10020311 44\n102.80387079 45\n98.29687616 46\n93.24376389 47\n97.24130034 48\n89.03452725 49\n96.2832753 50\n104.60344836 51\n101.13442416 52\n97.62787811 53\n106.71751618 54\n102.97585605 55\n98.45723272 56\n100.72418901 57\n106.39798503 58\n95.46493436 59\n94.35373179 60\n106.83273763 61\n100.07721494 62\n96.02548256 63\n102.82360856 64\n106.47551845 65\n101.34745901 66\n102.45651798 67\n98.74767493 68\n97.57544275 69\n92.5748759 70\n91.37294597 71\n105.30350449 72\n92.87730812 73\n103.19258339 74\n104.40518318 75\n101.29326772 76\n100.85447132 77\n101.2226037 78\n106.03868807 79\n97.85230675 80\n110.44484313 81\n93.87155456 82\n101.5363647 83\n97.65393524 84\n92.75048583 85\n101.72074646 86\n96.96851209 87\n103.29147111 88\n99.14931546 89\n101.3514185 90\n100.37372248 91\n106.6471081 92\n100.61742813 93\n105.0320535 94\n99.35999981 95\n98.87007532 96\n95.85284217 97\n93.97853495 98\n97.21315663 99\n107.02874163 100\n102.17642112 101\n96.74630281 102\n95.93799169 103\n102.62384733 104\n105.07475277 105\n97.59572169 106\n106.57364584 107\n95.65982034 108\n107.22482426 109\n107.19119932 110\n102.93039474 111\n85.98839623 112\n95.19184343 113\n91.32093303 114\n102.35313953 115\n100.39303522 116\n100.39303522 117\n92.0108226 118\n97.75887636 119\n93.18884302 120\n100.44940274 121\n108.09423367 122\n96.50342927 123\n99.58664719 124\n95.19184343 125\n103.1521596 126\n109.40523174 127\n93.83969256 128\n99.95827854 129\n101.83462816 130\n99.69982772 131\n103.05289628 132\n103.93383957 133\n104.15899829 134\n106.11454989 135\n88.80221141 136\n94.5081787 137\n94.59300658 138\n101.08830521 139\n96.34622848 140\n96.89244283 141\n98.07122664 142\n100.28690912 143\n96.78266211 144\n99.84251605 145\n104.03478031 146\n106.57052697 147\n105.13668343 148\n105.37011896 149\n99.07551254 150\n104.15899829 151\n98.75108352 152\n101.86186193 153\n103.61720152 154\n99.57859892 155\n99.4889538 156\n103.05541444 157\n98.65912661 158\n98.72774132 159\n104.70526438 160\n110.44484313 161\n97.49594839 162\n96.59385486 163\n104.63817694 164\n102.55198606 165\n105.86078488 166\n96.5937781 167\n93.04610867 168\n99.92159953 169\n100.96781394 170\n96.76814836 171\n91.6779221 172\n101.79132774 173\n101.20773355 174\n98.29243952 175\n101.83845792 176\n97.94046856 177\n102.20618501 178\n91.37294597 179\n106.89005002 180\n106.57364584 181\n102.26648279 182\n107.40064604 183\n99.94318168 184\n103.40412146 185\n106.38276709 186\n98.00253006 187\n97.10439252 188\n99.80873105 189\n101.63973121 190\n106.46476468 191\n110.43976681 192\n100.69156231 193\n99.99579473 194\n101.32113654 195\n94.76253572 196\n97.24130034 197\n96.10020311 198\n94.57421727 199\n100.80409326 200\n105.02389857 201\n98.61325194 202\n95.62359311 203\n97.99762409 204\n103.83852459 205\n101.2226037 206\n94.11176915 207\n99.62387832 208\n104.51786419 209\n97.62787811 210\n93.97853495 211\n98.75108352 212\n106.05042487 213\n100.07721494 214\n106.89005002 215\n"
],
[
"# Iterate over the whole dataset using ndenumerate\nfor index, value in np.ndenumerate(dataset):\n print(index, value)",
"(0, 0) 99.14931546\n(0, 1) 104.03852715\n(0, 2) 107.43534677\n(0, 3) 97.85230675\n(0, 4) 98.74986914\n(0, 5) 98.80833412\n(0, 6) 96.81964892\n(0, 7) 98.56783189\n(0, 8) 101.34745901\n(1, 0) 92.02628776\n(1, 1) 97.10439252\n(1, 2) 99.32066924\n(1, 3) 97.24584816\n(1, 4) 92.9267508\n(1, 5) 92.65657752\n(1, 6) 105.7197853\n(1, 7) 101.23162942\n(1, 8) 93.87155456\n(2, 0) 95.66253664\n(2, 1) 95.17750125\n(2, 2) 90.93318132\n(2, 3) 110.18889465\n(2, 4) 98.80084371\n(2, 5) 105.95297652\n(2, 6) 98.37481387\n(2, 7) 106.54654286\n(2, 8) 107.22482426\n(3, 0) 91.37294597\n(3, 1) 100.96781394\n(3, 2) 100.40118279\n(3, 3) 113.42090475\n(3, 4) 105.48508838\n(3, 5) 91.6604946\n(3, 6) 106.1472841\n(3, 7) 95.08715803\n(3, 8) 103.40412146\n(4, 0) 101.20862522\n(4, 1) 103.5730309\n(4, 2) 100.28690912\n(4, 3) 105.85269352\n(4, 4) 93.37126331\n(4, 5) 108.57980357\n(4, 6) 100.79478953\n(4, 7) 94.20019732\n(4, 8) 96.10020311\n(5, 0) 102.80387079\n(5, 1) 98.29687616\n(5, 2) 93.24376389\n(5, 3) 97.24130034\n(5, 4) 89.03452725\n(5, 5) 96.2832753\n(5, 6) 104.60344836\n(5, 7) 101.13442416\n(5, 8) 97.62787811\n(6, 0) 106.71751618\n(6, 1) 102.97585605\n(6, 2) 98.45723272\n(6, 3) 100.72418901\n(6, 4) 106.39798503\n(6, 5) 95.46493436\n(6, 6) 94.35373179\n(6, 7) 106.83273763\n(6, 8) 100.07721494\n(7, 0) 96.02548256\n(7, 1) 102.82360856\n(7, 2) 106.47551845\n(7, 3) 101.34745901\n(7, 4) 102.45651798\n(7, 5) 98.74767493\n(7, 6) 97.57544275\n(7, 7) 92.5748759\n(7, 8) 91.37294597\n(8, 0) 105.30350449\n(8, 1) 92.87730812\n(8, 2) 103.19258339\n(8, 3) 104.40518318\n(8, 4) 101.29326772\n(8, 5) 100.85447132\n(8, 6) 101.2226037\n(8, 7) 106.03868807\n(8, 8) 97.85230675\n(9, 0) 110.44484313\n(9, 1) 93.87155456\n(9, 2) 101.5363647\n(9, 3) 97.65393524\n(9, 4) 92.75048583\n(9, 5) 101.72074646\n(9, 6) 96.96851209\n(9, 7) 103.29147111\n(9, 8) 99.14931546\n(10, 0) 101.3514185\n(10, 1) 100.37372248\n(10, 2) 106.6471081\n(10, 3) 100.61742813\n(10, 4) 105.0320535\n(10, 5) 99.35999981\n(10, 6) 98.87007532\n(10, 7) 95.85284217\n(10, 8) 93.97853495\n(11, 0) 97.21315663\n(11, 1) 107.02874163\n(11, 2) 102.17642112\n(11, 3) 96.74630281\n(11, 4) 95.93799169\n(11, 5) 102.62384733\n(11, 6) 105.07475277\n(11, 7) 97.59572169\n(11, 8) 106.57364584\n(12, 0) 95.65982034\n(12, 1) 107.22482426\n(12, 2) 107.19119932\n(12, 3) 102.93039474\n(12, 4) 85.98839623\n(12, 5) 95.19184343\n(12, 6) 91.32093303\n(12, 7) 102.35313953\n(12, 8) 100.39303522\n(13, 0) 100.39303522\n(13, 1) 92.0108226\n(13, 2) 97.75887636\n(13, 3) 93.18884302\n(13, 4) 100.44940274\n(13, 5) 108.09423367\n(13, 6) 96.50342927\n(13, 7) 99.58664719\n(13, 8) 95.19184343\n(14, 0) 103.1521596\n(14, 1) 109.40523174\n(14, 2) 93.83969256\n(14, 3) 99.95827854\n(14, 4) 101.83462816\n(14, 5) 99.69982772\n(14, 6) 103.05289628\n(14, 7) 103.93383957\n(14, 8) 104.15899829\n(15, 0) 106.11454989\n(15, 1) 88.80221141\n(15, 2) 94.5081787\n(15, 3) 94.59300658\n(15, 4) 101.08830521\n(15, 5) 96.34622848\n(15, 6) 96.89244283\n(15, 7) 98.07122664\n(15, 8) 100.28690912\n(16, 0) 96.78266211\n(16, 1) 99.84251605\n(16, 2) 104.03478031\n(16, 3) 106.57052697\n(16, 4) 105.13668343\n(16, 5) 105.37011896\n(16, 6) 99.07551254\n(16, 7) 104.15899829\n(16, 8) 98.75108352\n(17, 0) 101.86186193\n(17, 1) 103.61720152\n(17, 2) 99.57859892\n(17, 3) 99.4889538\n(17, 4) 103.05541444\n(17, 5) 98.65912661\n(17, 6) 98.72774132\n(17, 7) 104.70526438\n(17, 8) 110.44484313\n(18, 0) 97.49594839\n(18, 1) 96.59385486\n(18, 2) 104.63817694\n(18, 3) 102.55198606\n(18, 4) 105.86078488\n(18, 5) 96.5937781\n(18, 6) 93.04610867\n(18, 7) 99.92159953\n(18, 8) 100.96781394\n(19, 0) 96.76814836\n(19, 1) 91.6779221\n(19, 2) 101.79132774\n(19, 3) 101.20773355\n(19, 4) 98.29243952\n(19, 5) 101.83845792\n(19, 6) 97.94046856\n(19, 7) 102.20618501\n(19, 8) 91.37294597\n(20, 0) 106.89005002\n(20, 1) 106.57364584\n(20, 2) 102.26648279\n(20, 3) 107.40064604\n(20, 4) 99.94318168\n(20, 5) 103.40412146\n(20, 6) 106.38276709\n(20, 7) 98.00253006\n(20, 8) 97.10439252\n(21, 0) 99.80873105\n(21, 1) 101.63973121\n(21, 2) 106.46476468\n(21, 3) 110.43976681\n(21, 4) 100.69156231\n(21, 5) 99.99579473\n(21, 6) 101.32113654\n(21, 7) 94.76253572\n(21, 8) 97.24130034\n(22, 0) 96.10020311\n(22, 1) 94.57421727\n(22, 2) 100.80409326\n(22, 3) 105.02389857\n(22, 4) 98.61325194\n(22, 5) 95.62359311\n(22, 6) 97.99762409\n(22, 7) 103.83852459\n(22, 8) 101.2226037\n(23, 0) 94.11176915\n(23, 1) 99.62387832\n(23, 2) 104.51786419\n(23, 3) 97.62787811\n(23, 4) 93.97853495\n(23, 5) 98.75108352\n(23, 6) 106.05042487\n(23, 7) 100.07721494\n(23, 8) 106.89005002\n"
]
],
[
[
"#### Filtering",
"_____no_output_____"
]
],
[
[
"vals_greater_five = dataset[dataset > 105]\nvals_greater_five",
"_____no_output_____"
],
[
"vals_between_90_95 = np.extract((dataset > 90) & (dataset < 95), dataset)\nvals_between_90_95",
"_____no_output_____"
],
[
"rows, cols = np.where(abs(dataset - 100) < 1)\n# Create a list comprehension\none_away_indices = [[rows[index], cols[index]] for (index, _) in np.ndenumerate(rows)]\none_away_indices",
"_____no_output_____"
]
],
[
[
"#### Sorting",
"_____no_output_____"
]
],
[
[
"# Each row will be sorted\nrow_sorted = np.sort(dataset)\nrow_sorted",
"_____no_output_____"
],
[
"# Sort each column\ncol_sorted = np.sort(dataset, axis=0)\ncol_sorted",
"_____no_output_____"
],
[
"# create a sorted index list using a fancy indexing to keep the order of the dataset and only obtain the values of index\nindex_sorted = np.argsort(dataset[0])\ndataset[0][index_sorted]",
"_____no_output_____"
]
],
[
[
"#### Combining",
"_____no_output_____"
]
],
[
[
"# Dividimos horizontalmente en 3 partes nuestro dataset es decir si son 12 columnas serian 3 bloques de 4 columnas\nthirds = np.hsplit(dataset, (3))\nprint(dataset.shape)\nprint(thirds[0].shape)\n#Dividimos verticalmente el primer bloque de los 3, en 2 partes , es decir si son 10 filas serian 2 bloques de 5 filas c/u\nhalfed_first = np.vsplit(thirds[0], (2))\nprint(halfed_first[0].shape)\n# Imprimimos el primer bloque de esta mitad\nhalfed_first[0]",
"(24, 9)\n(24, 3)\n(12, 3)\n"
],
[
"# Apilamos verticalmente las 2 mitades, esto nos deberia devolver el primer tercio thirds[0]\nfirst_col = np.vstack([halfed_first[0], halfed_first[1]])\nprint(thirds[0] == first_col)",
"[[ True True True]\n [ True True True]\n [ True True True]\n [ True True True]\n [ True True True]\n [ True True True]\n [ True True True]\n [ True True True]\n [ True True True]\n [ True True True]\n [ True True True]\n [ True True True]\n [ True True True]\n [ True True True]\n [ True True True]\n [ True True True]\n [ True True True]\n [ True True True]\n [ True True True]\n [ True True True]\n [ True True True]\n [ True True True]\n [ True True True]\n [ True True True]]\n"
],
[
"# Combinamos los 3 tercios de nuestros datos que serian igual a dataset\nfirst_second_col = np.hstack([first_col, thirds[1]])\nfull_data = np.hstack([first_second_col, thirds[2]])",
"_____no_output_____"
]
],
[
[
"#### Reshaping",
"_____no_output_____"
]
],
[
[
"# Reshape the dataset in to a single list\nsingle_list = np.reshape(dataset, (1, -1))\nprint(dataset.shape)\nprint(single_list.shape)",
"(24, 9)\n(1, 216)\n"
],
[
"# reshaping to a matrix with two columns\n# -1 Tells python to figure oyt the dimension out itself\ntwo_col_dataset = dataset.reshape(-1, 2)\nprint(two_col_dataset.shape)",
"(108, 2)\n"
]
]
] | [
"markdown",
"code",
"markdown",
"code",
"markdown",
"code",
"markdown",
"code",
"markdown",
"code",
"markdown",
"code",
"markdown",
"code",
"markdown",
"code",
"markdown",
"code"
] | [
[
"markdown"
],
[
"code"
],
[
"markdown"
],
[
"code",
"code",
"code",
"code",
"code"
],
[
"markdown"
],
[
"code",
"code",
"code"
],
[
"markdown"
],
[
"code",
"code",
"code"
],
[
"markdown"
],
[
"code",
"code"
],
[
"markdown"
],
[
"code",
"code",
"code"
],
[
"markdown"
],
[
"code",
"code",
"code"
],
[
"markdown"
],
[
"code",
"code",
"code"
],
[
"markdown"
],
[
"code",
"code"
]
] |
e7851988d698a211c6adf8b9cf135beb14a9700c | 8,488 | ipynb | Jupyter Notebook | docs/source/user_guide/clean/clean_co_nit.ipynb | jwa345/dataprep | cb386934b0e151ef538c3873ae8fa37bb8bd1513 | [
"MIT"
] | 1 | 2022-02-04T00:58:04.000Z | 2022-02-04T00:58:04.000Z | docs/source/user_guide/clean/clean_co_nit.ipynb | jwa345/dataprep | cb386934b0e151ef538c3873ae8fa37bb8bd1513 | [
"MIT"
] | null | null | null | docs/source/user_guide/clean/clean_co_nit.ipynb | jwa345/dataprep | cb386934b0e151ef538c3873ae8fa37bb8bd1513 | [
"MIT"
] | null | null | null | 24.251429 | 361 | 0.538525 | [
[
[
"# Colombian Identity Codes",
"_____no_output_____"
],
[
"## Introduction",
"_____no_output_____"
],
[
"The function `clean_co_nit()` cleans a column containing Colombian identity code (NIT) strings, and standardizes them in a given format. The function `validate_co_nit()` validates either a single NIT strings, a column of NIT strings or a DataFrame of NIT strings, returning `True` if the value is valid, and `False` otherwise.",
"_____no_output_____"
],
[
"NIT strings can be converted to the following formats via the `output_format` parameter:\n\n* `compact`: only number strings without any seperators or whitespace, like \"2131234321\"\n* `standard`: NIT strings with proper whitespace in the proper places, like \"213.123.432-1\"\n\nInvalid parsing is handled with the `errors` parameter:\n\n* `coerce` (default): invalid parsing will be set to NaN\n* `ignore`: invalid parsing will return the input\n* `raise`: invalid parsing will raise an exception\n\nThe following sections demonstrate the functionality of `clean_co_nit()` and `validate_co_nit()`. ",
"_____no_output_____"
],
[
"### An example dataset containing NIT strings",
"_____no_output_____"
]
],
[
[
"import pandas as pd\nimport numpy as np\ndf = pd.DataFrame(\n {\n \"nit\": [\n \"2131234321\",\n \"2131234325\",\n \"51824753556\",\n \"51 824 753 556\",\n \"hello\",\n np.nan,\n \"NULL\"\n ], \n \"address\": [\n \"123 Pine Ave.\",\n \"main st\",\n \"1234 west main heights 57033\",\n \"apt 1 789 s maple rd manhattan\",\n \"robie house, 789 north main street\",\n \"(staples center) 1111 S Figueroa St, Los Angeles\",\n \"hello\",\n ]\n }\n)\ndf",
"_____no_output_____"
]
],
[
[
"## 1. Default `clean_co_nit`\n\nBy default, `clean_co_nit` will clean nit strings and output them in the standard format with proper separators.",
"_____no_output_____"
]
],
[
[
"from dataprep.clean import clean_co_nit\nclean_co_nit(df, column = \"nit\")",
"_____no_output_____"
]
],
[
[
"## 2. Output formats",
"_____no_output_____"
],
[
"This section demonstrates the output parameter.",
"_____no_output_____"
],
[
"### `standard` (default)",
"_____no_output_____"
]
],
[
[
"clean_co_nit(df, column = \"nit\", output_format=\"standard\")",
"_____no_output_____"
]
],
[
[
"### `compact`",
"_____no_output_____"
]
],
[
[
"clean_co_nit(df, column = \"nit\", output_format=\"compact\")",
"_____no_output_____"
]
],
[
[
"## 3. `inplace` parameter\n\nThis deletes the given column from the returned DataFrame. \nA new column containing cleaned NIT strings is added with a title in the format `\"{original title}_clean\"`.",
"_____no_output_____"
]
],
[
[
"clean_co_nit(df, column=\"nit\", inplace=True)",
"_____no_output_____"
]
],
[
[
"## 4. `errors` parameter",
"_____no_output_____"
],
[
"### `coerce` (default)",
"_____no_output_____"
]
],
[
[
"clean_co_nit(df, \"nit\", errors=\"coerce\")",
"_____no_output_____"
]
],
[
[
"### `ignore`",
"_____no_output_____"
]
],
[
[
"clean_co_nit(df, \"nit\", errors=\"ignore\")",
"_____no_output_____"
]
],
[
[
"## 4. `validate_co_nit()`",
"_____no_output_____"
],
[
"`validate_co_nit()` returns `True` when the input is a valid NIT. Otherwise it returns `False`.\n\nThe input of `validate_co_nit()` can be a string, a Pandas DataSeries, a Dask DataSeries, a Pandas DataFrame and a dask DataFrame.\n\nWhen the input is a string, a Pandas DataSeries or a Dask DataSeries, user doesn't need to specify a column name to be validated. \n\nWhen the input is a Pandas DataFrame or a dask DataFrame, user can both specify or not specify a column name to be validated. If user specify the column name, `validate_co_nit()` only returns the validation result for the specified column. If user doesn't specify the column name, `validate_co_nit()` returns the validation result for the whole DataFrame.",
"_____no_output_____"
]
],
[
[
"from dataprep.clean import validate_co_nit\nprint(validate_co_nit(\"2131234321\"))\nprint(validate_co_nit(\"2131234325\"))\nprint(validate_co_nit(\"51824753556\"))\nprint(validate_co_nit(\"51 824 753 556\"))\nprint(validate_co_nit(\"hello\"))\nprint(validate_co_nit(np.nan))\nprint(validate_co_nit(\"NULL\"))",
"_____no_output_____"
]
],
[
[
"### Series",
"_____no_output_____"
]
],
[
[
"validate_co_nit(df[\"nit\"])",
"_____no_output_____"
]
],
[
[
"### DataFrame + Specify Column",
"_____no_output_____"
]
],
[
[
"validate_co_nit(df, column=\"nit\")",
"_____no_output_____"
]
],
[
[
"### Only DataFrame",
"_____no_output_____"
]
],
[
[
"validate_co_nit(df)",
"_____no_output_____"
]
]
] | [
"markdown",
"code",
"markdown",
"code",
"markdown",
"code",
"markdown",
"code",
"markdown",
"code",
"markdown",
"code",
"markdown",
"code",
"markdown",
"code",
"markdown",
"code",
"markdown",
"code",
"markdown",
"code"
] | [
[
"markdown",
"markdown",
"markdown",
"markdown",
"markdown"
],
[
"code"
],
[
"markdown"
],
[
"code"
],
[
"markdown",
"markdown",
"markdown"
],
[
"code"
],
[
"markdown"
],
[
"code"
],
[
"markdown"
],
[
"code"
],
[
"markdown",
"markdown"
],
[
"code"
],
[
"markdown"
],
[
"code"
],
[
"markdown",
"markdown"
],
[
"code"
],
[
"markdown"
],
[
"code"
],
[
"markdown"
],
[
"code"
],
[
"markdown"
],
[
"code"
]
] |
e78519d604f7c77ca582540101463f8a7df11019 | 20,146 | ipynb | Jupyter Notebook | AICA_v2.ipynb | Mayner0220/AICA | 1318b8af7c3fef04ff3654480dc7c5ac6c50eeb2 | [
"Apache-2.0"
] | 1 | 2021-04-01T06:19:05.000Z | 2021-04-01T06:19:05.000Z | AICA_v2.ipynb | Mayner0220/AICA | 1318b8af7c3fef04ff3654480dc7c5ac6c50eeb2 | [
"Apache-2.0"
] | null | null | null | AICA_v2.ipynb | Mayner0220/AICA | 1318b8af7c3fef04ff3654480dc7c5ac6c50eeb2 | [
"Apache-2.0"
] | null | null | null | 26.612946 | 119 | 0.539561 | [
[
[
"### Setup",
"_____no_output_____"
]
],
[
[
"import os\nos.environ['TF_CPP_MIN_LOG_LEVEL'] = \"3\"",
"_____no_output_____"
]
],
[
[
"To prevent elements such as Tensorflow import logs, perform these tasks.",
"_____no_output_____"
]
],
[
[
"import glob\nimport numpy as np\nimport tensorflow as tf\nimport matplotlib.pyplot as plt",
"_____no_output_____"
],
[
"try:\n tpu = tf.distribute.cluster_resolver.TPUClusterResolver()\n print(\"Device:\", tpu.master())\n tf.config.experimental_connect_to_cluster(tpu)\n tf.tpu.experimental.initialize_tpu_system(tpu)\n strategy = tf.distribute.experimental.TPUStrategy(tpu)\nexcept:\n strategy = tf.distribute.get_strategy()\nprint(\"Number of replicas:\", strategy.num_replicas_in_sync)",
"Number of replicas: 1\n"
],
[
"AUTOTUNE = tf.data.experimental.AUTOTUNE\nBATCH_SIZE = 16 * strategy.num_replicas_in_sync\nIMAGE_SIZE = [176, 208]\nEPOCHS = 100",
"_____no_output_____"
]
],
[
[
"### Convert the data",
"_____no_output_____"
]
],
[
[
"def _bytes_feature(value: [str, bytes]) -> tf.train.Feature:\n \"\"\"string / byte를 byte_list로 반환합니다.\"\"\"\n if isinstance(value, type(tf.constant(0))):\n value = value.numpy() # BytesList는 EagerTensor에서 문자열을 풀지 않습니다.\n \n return tf.train.Feature(bytes_list=tf.train.BytesList(value=[value]))",
"_____no_output_____"
],
[
"def _float_feature(value: float) -> tf.train.Feature:\n \"\"\"float / double를 float_list로 반환합니다.\"\"\"\n return tf.train.Feature(float_list=tf.train.FloatList(value=[value]))",
"_____no_output_____"
],
[
"def _int64_feature(value: [bool, int]) -> tf.train.Feature:\n \"\"\"bool / enum / int / uint를 int64_list로 반환합니다.\"\"\"\n return tf.train.Feature(int64_list=tf.train.Int64List(value=[value]))",
"_____no_output_____"
],
[
"def serialize_example(image: bytes, label: int) -> tf.train.Example.SerializeToString:\n \"\"\"\n 파일을 만들기 위해서 tf.train.Example 메시지를 만듭니다.\n \"\"\"\n feature = {\n \"raw_image\": _bytes_feature(image),\n \"label\": _int64_feature(label),\n }\n \n return tf.train.Example(features=tf.train.Features(feature=feature))",
"_____no_output_____"
],
[
"def write_tfrecord(main_path: str) -> None:\n \"\"\"\n datset의 위치를 입력 받아, 이미지와 라벨 등을 구하여 반환한다.\n \"\"\"\n train_paths = glob.glob(main_path + \"/train/*/*.jpg\")\n test_paths = glob.glob(main_path + \"/test/*/*.jpg\")\n image_labels = {\"NonDemented\": 0, \"VeryMildDemented\": 1, \"MildDemented\": 2, \"ModerateDemented\": 3}\n train_file = \"./tfrecord/train.tfrecord\"\n test_file = \"./tfrecord/test.tfrecord\"\n \n # train TFRecord file\n with tf.io.TFRecordWriter(train_file) as writer:\n for path in train_paths:\n image_string = open(path, \"rb\").read()\n \n label_str = path.split(\"\\\\\")[1]\n label = image_labels[label_str]\n \n tf_example = serialize_example(image_string, label)\n writer.write(tf_example.SerializeToString())\n \n print(\"Train TFRecord Converting Done!\")\n \n # test TFRecord file\n with tf.io.TFRecordWriter(test_file) as writer:\n for path in test_paths:\n image_string = open(path, \"rb\").read()\n \n label_str = path.split(\"\\\\\")[1]\n label = image_labels[label_str]\n \n tf_example = serialize_example(image_string, label)\n writer.write(tf_example.SerializeToString())\n \n print(\"Test TFRecord Converting Done!\")",
"_____no_output_____"
],
[
"dataset_path = \"./dataset\"\nwrite_tfrecord(dataset_path)",
"_____no_output_____"
]
],
[
[
"### Load the data",
"_____no_output_____"
]
],
[
[
"train_dataset = tf.data.TFRecordDataset(\"./tfrecord/train.tfrecord\")\ntest_dataset = tf.data.TFRecordDataset(\"./tfrecord/test.tfrecord\")",
"_____no_output_____"
],
[
"TRAIN_DATA_SIZE = len(list(train_dataset))\ntrain_size = int(0.75 * TRAIN_DATA_SIZE)\n\ntrain_dataset = train_dataset.shuffle(1000)\ntest_dataset = test_dataset.shuffle(1000)\n\nvalidation_dataset = train_dataset.skip(train_size)\ntrain_dataset = train_dataset.take(train_size)",
"_____no_output_____"
],
[
"train_len = len(list(train_dataset))\nvalidation_len = len(list(validation_dataset))\ntest_len = len(list(test_dataset))\n\nprint(\"Train dataset:\", train_len)\nprint(\"Validation dataset:\", validation_len)\nprint(\"Test dataset:\", test_len)",
"_____no_output_____"
],
[
"image_feature_description = {\n \"raw_image\": tf.io.FixedLenFeature([], tf.string),\n \"label\": tf.io.FixedLenFeature([], tf.int64),\n}",
"_____no_output_____"
],
[
"@tf.autograph.experimental.do_not_convert\ndef _parse_image_function(example_proto):\n features = tf.io.parse_single_example(example_proto, image_feature_description)\n \n for feature in features: \n image = tf.io.decode_raw(feature['image'], tf.uint8)\n image.set_shape([3 * 176 * 208])\n image = tf.reshape(image, [176, 208, 3])\n\n label = tf.cast(feature[\"label\"].numpy(), tf.int64)\n label = tf.one_hot(label, 4)\n\n return image, label",
"_____no_output_____"
],
[
"def read_dataset(epochs, batch_size, dataset):\n dataset = dataset.map(_parse_image_function)\n dataset = dataset.prefetch(10)\n dataset = dataset.repeat(epochs)\n dataset = dataset.shuffle(buffer_size=10 * batch_size)\n dataset = dataset.batch(batch_size, drop_remainder=True)\n\n return dataset",
"_____no_output_____"
],
[
"train_dataset = read_dataset(EPOCHS, BATCH_SIZE, train_dataset)\nvalidation_dataset = read_dataset(EPOCHS, BATCH_SIZE, validation_dataset)\ntest_dataset = read_dataset(EPOCHS, BATCH_SIZE, test_dataset)",
"_____no_output_____"
],
[
"parsed_train_dataset.take(train_len)",
"_____no_output_____"
]
],
[
[
"### Visualize dataset",
"_____no_output_____"
]
],
[
[
"# train TFRecord\nfor image_features in parsed_train_dataset.take(1):\n image_raw = image_features[\"raw_image\"].numpy()\n image_label = image_features[\"label\"].numpy()\n display.display(display.Image(data=image_raw))\n print(\"Label:\", image_label)",
"_____no_output_____"
],
[
"# test TFRecord\nfor image_features in parsed_test_dataset.take(1):\n image_raw = image_features[\"raw_image\"].numpy()\n image_label = image_features[\"label\"].numpy()\n display.display(display.Image(data=image_raw))\n print(\"Label:\", image_label)",
"_____no_output_____"
]
],
[
[
"### Build Model",
"_____no_output_____"
]
],
[
[
"# 경증 치매, 중증도 치매, 비 치매, 매우 경미한 치매\nCLASS_NAMES = ['MildDementia', 'ModerateDementia', 'NonDementia', 'VeryMildDementia']\nNUM_CLASSES = len(CLASS_NAMES)",
"_____no_output_____"
],
[
"TRAIN_DATA_SIZE = len(list(parsed_train_dataset))\ntrain_size = int(0.75 * TRAIN_DATA_SIZE)\n# val_size = int(0.25 * TRAIN_DATA_SIZE)\n# 테스트용 데이터셋은 따로 존재하기에 분할하지 않는다.\n# test_size = ",
"_____no_output_____"
],
[
"# train / validation data split\ntrain_dataset = parsed_train_dataset.shuffle(100)\ntrain_dataset = train_dataset.take(train_size)\nvalidation_dataset = train_dataset.skip(train_size)\n\ntrain_dataset = train_dataset.batch(BATCH_SIZE)\nvalidation_dataset = validation_dataset.batch(BATCH_SIZE)",
"_____no_output_____"
],
[
"def conv_block(filters):\n block = tf.keras.Sequential([\n tf.keras.layers.SeparableConv2D(filters, 3, activation='relu', padding='same'),\n tf.keras.layers.SeparableConv2D(filters, 3, activation='relu', padding='same'),\n tf.keras.layers.BatchNormalization(),\n tf.keras.layers.MaxPool2D()\n ])\n \n return block",
"_____no_output_____"
],
[
"def dense_block(units, dropout_rate):\n block = tf.keras.Sequential([\n tf.keras.layers.Dense(units, activation='relu'),\n tf.keras.layers.BatchNormalization(),\n tf.keras.layers.Dropout(dropout_rate)\n ])\n \n return block",
"_____no_output_____"
],
[
"def build_model():\n model = tf.keras.Sequential([\n tf.keras.Input(shape=(*IMAGE_SIZE, 3)),\n \n tf.keras.layers.Conv2D(16, 3, activation='relu', padding='same'),\n tf.keras.layers.Conv2D(16, 3, activation='relu', padding='same'),\n tf.keras.layers.MaxPool2D(),\n \n conv_block(32),\n conv_block(64),\n \n conv_block(128),\n tf.keras.layers.Dropout(0.2),\n \n conv_block(256),\n tf.keras.layers.Dropout(0.2),\n \n tf.keras.layers.Flatten(),\n dense_block(512, 0.7),\n dense_block(128, 0.5),\n dense_block(64, 0.3),\n \n tf.keras.layers.Dense(NUM_CLASSES, activation='softmax')\n ])\n \n return model",
"_____no_output_____"
],
[
"with strategy.scope():\n model = build_model()\n\n METRICS = [tf.keras.metrics.AUC(name='auc')]\n \n model.compile(\n optimizer='adam',\n loss=tf.losses.CategoricalCrossentropy(),\n metrics=METRICS\n )\n \n model.summary()",
"_____no_output_____"
]
],
[
[
"### Train Model",
"_____no_output_____"
]
],
[
[
"@tf.autograph.experimental.do_not_convert\ndef exponential_decay(lr0, s):\n def exponential_decay_fn(epoch):\n return lr0 * 0.1 **(epoch / s)\n return exponential_decay_fn\n\nexponential_decay_fn = exponential_decay(0.01, 20)\n\nlr_scheduler = tf.keras.callbacks.LearningRateScheduler(exponential_decay_fn)\n\ncheckpoint_cb = tf.keras.callbacks.ModelCheckpoint(\"AICAv2.h5\",\n save_best_only=True)\n\nearly_stopping_cb = tf.keras.callbacks.EarlyStopping(patience=10,\n restore_best_weights=True)",
"_____no_output_____"
],
[
"history = model.fit(\n train_dataset,\n validation_data=validation_dataset,\n callbacks=[checkpoint_cb, early_stopping_cb, lr_scheduler],\n epochs=EPOCHS\n)",
"_____no_output_____"
]
]
] | [
"markdown",
"code",
"markdown",
"code",
"markdown",
"code",
"markdown",
"code",
"markdown",
"code",
"markdown",
"code",
"markdown",
"code"
] | [
[
"markdown"
],
[
"code"
],
[
"markdown"
],
[
"code",
"code",
"code"
],
[
"markdown"
],
[
"code",
"code",
"code",
"code",
"code",
"code"
],
[
"markdown"
],
[
"code",
"code",
"code",
"code",
"code",
"code",
"code",
"code"
],
[
"markdown"
],
[
"code",
"code"
],
[
"markdown"
],
[
"code",
"code",
"code",
"code",
"code",
"code",
"code"
],
[
"markdown"
],
[
"code",
"code"
]
] |
e7851b204896343eaf15c4f6387b77e0abc5aa55 | 3,266 | ipynb | Jupyter Notebook | contador.ipynb | anapsantos1/Desafio_dataproc | 99eef19041b425c41fd22508106dfae1ac55f1f1 | [
"Apache-2.0"
] | null | null | null | contador.ipynb | anapsantos1/Desafio_dataproc | 99eef19041b425c41fd22508106dfae1ac55f1f1 | [
"Apache-2.0"
] | null | null | null | contador.ipynb | anapsantos1/Desafio_dataproc | 99eef19041b425c41fd22508106dfae1ac55f1f1 | [
"Apache-2.0"
] | null | null | null | 52.677419 | 1,617 | 0.632272 | [
[
[
"import sys\nfrom pyspark import SparkContext, SparkConf\nif __name__ == \"__main__\":\n sc = SparkContext(\"local\",\"PySpark Exemplo - Desafio Dataproc\")\n words = sc.textFile(\"gs://{SEU_BUCKET}/livro.txt\").flatMap(lambda line: line.split(\" \"))\n wordCounts = words.map(lambda word: (word, 1)).reduceByKey(lambda a,b:a +b).sortBy(lambda a:a[1], ascending=False)\n wordCounts.saveAsTextFile(\"gs://{SEU_BUCKET}/resultado\")",
"_____no_output_____"
]
]
] | [
"code"
] | [
[
"code"
]
] |
e7851c74700494befdf88f2483e3b691887fc0ef | 9,258 | ipynb | Jupyter Notebook | notebooks/2-DataPreparation/1-SelectData/3-DB-SONAR-ISSUES.ipynb | chus-chus/softwareDevTypes | b2c0f4824eb2af4b0c410c0fcc998cecc166ebce | [
"MIT"
] | null | null | null | notebooks/2-DataPreparation/1-SelectData/3-DB-SONAR-ISSUES.ipynb | chus-chus/softwareDevTypes | b2c0f4824eb2af4b0c410c0fcc998cecc166ebce | [
"MIT"
] | null | null | null | notebooks/2-DataPreparation/1-SelectData/3-DB-SONAR-ISSUES.ipynb | chus-chus/softwareDevTypes | b2c0f4824eb2af4b0c410c0fcc998cecc166ebce | [
"MIT"
] | null | null | null | 28.22561 | 125 | 0.471592 | [
[
[
"# **SONAR_ISSUES**\n\nThis notebook the selection of the rellevant attributes of the table `SONAR_ISSUES`.\n\nFirst, we import the libraries we need and, then, we read the corresponding csv.",
"_____no_output_____"
]
],
[
[
"import pandas as pd",
"_____no_output_____"
],
[
"sonarIssues = pd.read_csv(\"../../../data/raw/SONAR_ISSUES.csv\")\nprint(sonarIssues.shape)\nlist(sonarIssues)",
"(1941508, 18)\n"
]
],
[
[
"We select the desired attributes of the table.",
"_____no_output_____"
]
],
[
[
"attributes = ['projectID', 'creationDate', 'closeDate', 'creationCommitHash', 'closeCommitHash', 'type', 'severity',\n 'debt', 'author']\nsonarIssues = sonarIssues[attributes]",
"_____no_output_____"
],
[
"print(sonarIssues.shape)\nsonarIssues.head()",
"(1941508, 9)\n"
]
],
[
[
"We save this new table into a csv.",
"_____no_output_____"
]
],
[
[
"sonarIssues.to_csv('../../../data/interim/DataPreparation/SelectData/SONAR_ISSUES_select.csv', header=True)",
"_____no_output_____"
]
]
] | [
"markdown",
"code",
"markdown",
"code",
"markdown",
"code"
] | [
[
"markdown"
],
[
"code",
"code"
],
[
"markdown"
],
[
"code",
"code"
],
[
"markdown"
],
[
"code"
]
] |
e7851d5d9e7909b3daf8cf220d6b109561736c24 | 70,369 | ipynb | Jupyter Notebook | Applied Math/Y1S4/Data Science/.ipynb_checkpoints/R - Week 2 (exercises)-checkpoint.ipynb | darkeclipz/jupyter-notebooks | 5de784244ad9db12cfacbbec3053b11f10456d7e | [
"Unlicense"
] | 1 | 2018-08-28T12:16:12.000Z | 2018-08-28T12:16:12.000Z | Applied Math/Y1S4/Data Science/.ipynb_checkpoints/R - Week 2 (exercises)-checkpoint.ipynb | darkeclipz/jupyter-notebooks | 5de784244ad9db12cfacbbec3053b11f10456d7e | [
"Unlicense"
] | null | null | null | Applied Math/Y1S4/Data Science/.ipynb_checkpoints/R - Week 2 (exercises)-checkpoint.ipynb | darkeclipz/jupyter-notebooks | 5de784244ad9db12cfacbbec3053b11f10456d7e | [
"Unlicense"
] | null | null | null | 42.960317 | 10,382 | 0.679333 | [
[
[
"# R - Week 2 (exercises)",
"_____no_output_____"
],
[
"## R-code on solving equations with inverse matrix",
"_____no_output_____"
],
[
"Solve the following system of equations:",
"_____no_output_____"
],
[
"1. $2x+y+2z=3$\n2. $x-3z=-5$\n3. $2y+5z=4$",
"_____no_output_____"
],
[
"$$ \\begin{bmatrix} 2 & 1 & 2 \\\\ 1 & 6 & -3 \\\\ 0 & 2 & 5 \\end{bmatrix} \\cdot \\begin{bmatrix} x \\\\ y \\\\ z \\end{bmatrix} = \\begin{bmatrix} 3 & -5 & 4 \\end{bmatrix} $$",
"_____no_output_____"
],
[
"$ A \\vec{x} = \\vec{b}$\n\n$A^{-1}\\cdot A \\vec{x} = A^{-1} \\vec{b}$\n\n$I \\vec{x} = A^{-1} \\vec{b}$",
"_____no_output_____"
],
[
"Define matrix $A$:",
"_____no_output_____"
]
],
[
[
"A = matrix(c(2,1,2,1,6,-3,0,2,5), nrow=3)\nA",
"_____no_output_____"
]
],
[
[
"The inverse $A^{-1}$ is:",
"_____no_output_____"
]
],
[
[
"solve(A)",
"_____no_output_____"
]
],
[
[
"Define vector $\\vec{b}$:",
"_____no_output_____"
]
],
[
[
"b = c(3, -5, 4)",
"_____no_output_____"
]
],
[
[
"Solve the system with R functions `solve(A,b)`:",
"_____no_output_____"
]
],
[
[
"solve(A,b)",
"_____no_output_____"
]
],
[
[
"Solve the system with $\\vec{x}=A^{-1}\\vec{b}$:",
"_____no_output_____"
]
],
[
[
"solve(A) %*% b",
"_____no_output_____"
]
],
[
[
"## R-code on least square method",
"_____no_output_____"
],
[
"$y=ax+b$",
"_____no_output_____"
],
[
"$A\\cdot \\vec{x} = \\vec{b}$\n\n$A^T\\cdot A \\vec{x} = A^T \\vec{b}$\n\n$(A^T A)^{-1}A^T A \\vec{x} = A^T \\vec{b}$\n\n$(A^T A)^{-1} ...$",
"_____no_output_____"
],
[
"Define $\\vec{x}=\\begin{bmatrix}12 & 2 & 3 & 5 & 10 & 9 & 8 \\end{bmatrix}$:",
"_____no_output_____"
]
],
[
[
"x = c(12, 2, 3, 5, 10, 9, 8)",
"_____no_output_____"
],
[
"x",
"_____no_output_____"
]
],
[
[
"Define $\\vec{y} = \\begin{bmatrix}125 & 30 & 43 & 62 & 108 & 102 & 90 \\end{bmatrix}$:",
"_____no_output_____"
]
],
[
[
"y = c(125, 30, 43, 62, 108, 102, 90)",
"_____no_output_____"
],
[
"y",
"_____no_output_____"
],
[
"length(x)==length(y)",
"_____no_output_____"
],
[
"A = matrix(union(x,y), nrow=length(x))",
"_____no_output_____"
],
[
"A",
"_____no_output_____"
],
[
"lm(y~x)",
"_____no_output_____"
],
[
"fit <- function(x) 9.488*x+13.583",
"_____no_output_____"
],
[
"fit(5)",
"_____no_output_____"
],
[
"plot(x,sapply(x, fit), 'l', col='blue')\npoints(x,y)",
"_____no_output_____"
],
[
"x",
"_____no_output_____"
],
[
"y",
"_____no_output_____"
]
],
[
[
"$y=ax+b$",
"_____no_output_____"
]
],
[
[
"X = matrix(c(y, rep(1, length(y))), ncol=2)",
"_____no_output_____"
],
[
"X",
"_____no_output_____"
],
[
"b = matrix(y)",
"_____no_output_____"
],
[
"b",
"_____no_output_____"
]
],
[
[
"Dit is de vorm $A\\cdot\\vec{x} = \\vec{b}$. Wat we op willen lossen met $A^{-1}A\\vec{x}=A^{-1}\\vec{b}$, maar dit werkt niet omdat $A$ geen vierkante matrix is en daardoor geen inverse kan bepalen voor $A$.\n\nDoor gebruik te maken van de getransponeerde $A^T$ kunnen we een vierkante matrix krijgen. Dus matrix-vermenigvuldigen van $A\\vec{x}=\\vec{b}$ met $A^T$ geeft $A^T\\cdot A\\vec{x} = A^T\\cdot\\vec{b}$.",
"_____no_output_____"
]
],
[
[
"t(A) %*% A",
"_____no_output_____"
]
],
[
[
"Wat inderdaad een vierkant matrix geeft. Vervolgens is deze op te lossen door de inverse te bepalen. De hele formule wordt dan:\n\n$$ (A^T \\cdot A)^{-1}\\cdot(A^T \\cdot A)\\cdot\\vec{x} = (A^T \\cdot A)^{-1} \\cdot A^T \\cdot \\vec{b} $$\n\nLaat $B = (A^T \\cdot A)^{-1}$ zijn. Substitueren en vereenvoudigen geeft: \n\n$$ I\\cdot\\vec{x} = B \\cdot A^T \\cdot \\vec{b} $$",
"_____no_output_____"
]
],
[
[
"B = solve(t(A) %*% A)\nB",
"_____no_output_____"
],
[
"B %*% t(A) %*% b",
"_____no_output_____"
],
[
"lm(y~x)",
"_____no_output_____"
]
],
[
[
"**Versimpeld voorbeeld lreg**",
"_____no_output_____"
]
],
[
[
"A = matrix(c(-1,0,2,3,1,1,1,1),ncol=2)",
"_____no_output_____"
],
[
"A",
"_____no_output_____"
],
[
"b = c(-1,2,1,2)",
"_____no_output_____"
],
[
"b",
"_____no_output_____"
],
[
"x = A[0:4]",
"_____no_output_____"
],
[
"solve(t(A) %*% A) %*% t(A) %*% b",
"_____no_output_____"
],
[
"lm(b~x)",
"_____no_output_____"
],
[
"fit <- function(x) 0.5*x+0.5",
"_____no_output_____"
],
[
"plot(-5:5, sapply(-5:5, fit), 'l')\npoints(x,b)",
"_____no_output_____"
],
[
"lreg <- function(x, y) {\n A = cbind(x, rep(1, length(x)))\n s = solve(t(A) %*% A) %*% t(A) %*% y\n function(x) s[1] * x + s[2] # Return f(x)=ax+b\n}",
"_____no_output_____"
],
[
"x = c(12,2,3,5,10,9,8)\ny = c(125,30,43,62,108,102,90)",
"_____no_output_____"
],
[
"fit <- lreg(x, y)",
"_____no_output_____"
],
[
"plot(0:15, sapply(0:15, fit), 'l')\npoints(x,y)",
"_____no_output_____"
]
],
[
[
"With other functionality:",
"_____no_output_____"
],
[
"### Least squares regression model",
"_____no_output_____"
],
[
"Find the best fitting line $y=ax+b$ for the following data points:",
"_____no_output_____"
]
],
[
[
"x <- c(12,2,3,5,10,9,8)\nb <- c(125,30,43,62,108,102,90)",
"_____no_output_____"
]
],
[
[
"We can do this by solving the equation $A\\vec{x}=\\vec{b}$. Constructing the equation with the matrices for our data points yields:\n\n$$ \\begin{bmatrix} 12 & 1 \\\\ 2 & 1 \\\\ 3 & 1 \\\\ 5 & 1 \\\\ 10 & 1 \\\\ 9 & 1 \\\\ 8 & 1 \\end{bmatrix} \\cdot \\begin{bmatrix}a \\\\ b \\end{bmatrix} = \\begin{bmatrix} 125 \\\\ 30 \\\\ 43 \\\\ 62 \\\\ 108 \\\\ 102 \\\\ 90 \\end{bmatrix} $$",
"_____no_output_____"
],
[
"First we will construct our matrix $A$:",
"_____no_output_____"
]
],
[
[
"ones <- rep(1, length(x))",
"_____no_output_____"
],
[
"A <- cbind(x, ones)",
"_____no_output_____"
],
[
"A",
"_____no_output_____"
]
],
[
[
"If we want to solve the equation $A\\vec{x}=\\vec{b}$ we can multiply both sides $A^{-1}$ to get:\n\n$$ \\begin{align} A\\vec{x}&=\\vec{b} \\\\ (A^{-1}\\cdot A)\\vec{x}&=A^{-1}\\vec{b} \\\\ I\\vec{x}&=A^{-1}\\vec{b} \\end{align} $$",
"_____no_output_____"
],
[
"However, we need to calculate the inverse of $A$, but $A$ is not a square matrix. To solve this problem we multiply $A$ with $A^T$ to get a square matrix.",
"_____no_output_____"
],
[
"$$ \\begin{align} A\\vec{x}&=\\vec{b} \\\\ (A^T \\cdot A) \\cdot \\vec{x} &= A^T \\cdot \\vec{b} \\\\ (A^T \\cdot A)^{-1} \\cdot (A^T \\cdot A) \\cdot \\vec{x} &= (A^T \\cdot A)^{-1} \\cdot A^T \\cdot \\vec{b} \\\\ I \\cdot \\vec{x} &= (A^T \\cdot A)^{-1} \\cdot A^T \\cdot \\vec{b} \\end{align} $$",
"_____no_output_____"
]
],
[
[
"S = solve(t(A) %*% A) %*% t(A) %*% b",
"_____no_output_____"
]
],
[
[
"The resulting matrix $S$ will have our coefficients $a$ and $b$ to construct the line:",
"_____no_output_____"
]
],
[
[
"lsm = c(S[2], S[1])",
"_____no_output_____"
],
[
"lsm",
"_____no_output_____"
]
],
[
[
"If we verify the coefficients with built-in R functionality for least-squares regression, we can see that our solution is correct.",
"_____no_output_____"
]
],
[
[
"lm(b~x)",
"_____no_output_____"
]
],
[
[
"Plotting our values yields:",
"_____no_output_____"
]
],
[
[
"plot(x, b)\nabline(lsm)",
"_____no_output_____"
]
],
[
[
"Tada.",
"_____no_output_____"
]
]
] | [
"markdown",
"code",
"markdown",
"code",
"markdown",
"code",
"markdown",
"code",
"markdown",
"code",
"markdown",
"code",
"markdown",
"code",
"markdown",
"code",
"markdown",
"code",
"markdown",
"code",
"markdown",
"code",
"markdown",
"code",
"markdown",
"code",
"markdown",
"code",
"markdown",
"code",
"markdown",
"code",
"markdown",
"code",
"markdown"
] | [
[
"markdown",
"markdown",
"markdown",
"markdown",
"markdown",
"markdown",
"markdown"
],
[
"code"
],
[
"markdown"
],
[
"code"
],
[
"markdown"
],
[
"code"
],
[
"markdown"
],
[
"code"
],
[
"markdown"
],
[
"code"
],
[
"markdown",
"markdown",
"markdown",
"markdown"
],
[
"code",
"code"
],
[
"markdown"
],
[
"code",
"code",
"code",
"code",
"code",
"code",
"code",
"code",
"code",
"code",
"code"
],
[
"markdown"
],
[
"code",
"code",
"code",
"code"
],
[
"markdown"
],
[
"code"
],
[
"markdown"
],
[
"code",
"code",
"code"
],
[
"markdown"
],
[
"code",
"code",
"code",
"code",
"code",
"code",
"code",
"code",
"code",
"code",
"code",
"code",
"code"
],
[
"markdown",
"markdown",
"markdown"
],
[
"code"
],
[
"markdown",
"markdown"
],
[
"code",
"code",
"code"
],
[
"markdown",
"markdown",
"markdown"
],
[
"code"
],
[
"markdown"
],
[
"code",
"code"
],
[
"markdown"
],
[
"code"
],
[
"markdown"
],
[
"code"
],
[
"markdown"
]
] |
e785262302f9c2f6c7720b37b3aac8f76f6bdcf1 | 32,761 | ipynb | Jupyter Notebook | docs/site/tutorials/custom_differentiation.ipynb | sendilkumarn/swift | 60d3c495c96b3de791caf2eb2daa50a0949e7842 | [
"CC-BY-4.0"
] | 1 | 2019-10-02T06:13:47.000Z | 2019-10-02T06:13:47.000Z | docs/site/tutorials/custom_differentiation.ipynb | sendilkumarn/swift | 60d3c495c96b3de791caf2eb2daa50a0949e7842 | [
"CC-BY-4.0"
] | null | null | null | docs/site/tutorials/custom_differentiation.ipynb | sendilkumarn/swift | 60d3c495c96b3de791caf2eb2daa50a0949e7842 | [
"CC-BY-4.0"
] | null | null | null | 43.681333 | 700 | 0.548121 | [
[
[
"##### Copyright 2018 The TensorFlow Authors.",
"_____no_output_____"
]
],
[
[
"#@title Licensed under the Apache License, Version 2.0 (the \"License\"); { display-mode: \"form\" }\n// Licensed under the Apache License, Version 2.0 (the \"License\");\n// you may not use this file except in compliance with the License.\n// You may obtain a copy of the License at\n//\n// https://www.apache.org/licenses/LICENSE-2.0\n//\n// Unless required by applicable law or agreed to in writing, software\n// distributed under the License is distributed on an \"AS IS\" BASIS,\n// WITHOUT WARRANTIES OR CONDITIONS OF ANY KIND, either express or implied.\n// See the License for the specific language governing permissions and\n// limitations under the License.",
"_____no_output_____"
]
],
[
[
"# Custom differentiation\n\nThis tutorial will show you how to define your own custom derivatives, perform derivative surgery, and implement your own gradient checkpointing API in just 5 lines of Swift.",
"_____no_output_____"
],
[
"## Declaring custom derivatives",
"_____no_output_____"
],
[
"You can define custom derivatives for any Swift function that has differentiable parameters and results. By doing that, you can even import a C function and make it differentiable.",
"_____no_output_____"
]
],
[
[
"import Glibc\n\nfunc sillyExp(_ x: Float) -> Float {\n let 𝑒 = Float(M_E)\n print(\"Taking 𝑒(\\(𝑒)) to the power of \\(x)!\")\n return pow(𝑒, x)\n}\n\n@differentiating(sillyExp)\nfunc sillyDerivative(_ x: Float) -> (value: Float, pullback: (Float) -> Float) {\n let y = sillyExp(x)\n return (value: y, pullback: { v in v * y })\n}\n\nprint(\"exp(3) =\", sillyExp(3))\nprint(\"𝛁exp(3) =\", gradient(of: sillyExp)(3))",
"Taking 𝑒(2.7182817) to the power of 3.0!\r\nexp(3) = 20.085535\r\nTaking 𝑒(2.7182817) to the power of 3.0!\r\n𝛁exp(3) = 20.085535\r\n"
]
],
[
[
"## Stop derivatives from propagating\n\nCommonly known as \"stop gradient\" in machine learning use cases, method [`withoutDerivative()`](https://www.tensorflow.org/swift/api_docs/Protocols/Differentiable#/s:10TensorFlow14DifferentiablePAAE17withoutDerivativexyF) stops derivatives from propagating.\n\nPlus, `withoutDerivative()` can sometimes help the Swift compiler with identifying what not to differentiate and producing more efficient derivaitves. When it is detectable that the derivative of a function will always be zero, the Swift compiler will produce a warning. Explicitly using `.withoutDerivative()` silences that warning.",
"_____no_output_____"
]
],
[
[
"let x: Float = 2.0\nlet y: Float = 3.0\ngradient(at: x, y) { x, y in\n sin(sin(sin(x))) + cos(cos(cos(y))).withoutDerivative()\n}",
"_____no_output_____"
]
],
[
[
"## Derivative surgery\n\nMethod [`withGradient(_:)`](https://www.tensorflow.org/swift/api_docs/Protocols/Differentiable#/s:10TensorFlow14DifferentiablePAAE12withGradientyxy15CotangentVectorQzzcF) makes arbitrary operations (including mutation) run on the gradient at a value during the enclosing function’s backpropagation. \n\nUse this to debug or make experimental tweaks to backpropagation.",
"_____no_output_____"
],
[
"### It works anywhere",
"_____no_output_____"
],
[
"All differentiation APIs provided by the standard library are defined generically over all types that conform to the `Differentiable` protocol: `Float`, `Double`, `Float80`, SIMD vectors, and even your own types!\n\nRead technical document [Differentiable Types](https://github.com/tensorflow/swift/blob/master/docs/DifferentiableTypes.md) for more insights on the `Differentiable` protocol.",
"_____no_output_____"
]
],
[
[
"var x: Float = 30\nx.gradient { x -> Float in\n // Print the partial derivative with respect to the result of `sin(x)`.\n let a = sin(x).withGradient { print(\"∂+/∂sin = \\($0)\") } \n // Force the partial derivative with respect to `x` to be `0.5`.\n let b = log(x.withGradient { (dx: inout Float) in\n print(\"∂log/∂x = \\(dx), but rewritten to 0.5\");\n dx = 0.5\n })\n return a + b\n}",
"∂log/∂x = 0.033333335, but rewritten to 0.5\r\n∂+/∂sin = 1.0\r\n"
]
],
[
[
"### Use it in a neural network module",
"_____no_output_____"
],
[
"Just like how we used it in a simple `Float` function, we can use it in any numerical application, like the following neural network built using the [Swift for TensorFlow Deep Learning Library](https://github.com/tensorflow/swift-apis).",
"_____no_output_____"
]
],
[
[
"import TensorFlow\n\nstruct MLP: Layer {\n var layer1 = Dense<Float>(inputSize: 2, outputSize: 10, activation: relu)\n var layer2 = Dense<Float>(inputSize: 10, outputSize: 1, activation: relu)\n \n @differentiable\n func applied(to input: Tensor<Float>, in context: Context) -> Tensor<Float> {\n let h0 = layer1.applied(to: input, in: context).withGradient { print(\"∂L/∂layer1 =\", $0) }\n return layer2.applied(to: h0, in: context)\n }\n}\n\nlet optimizer = SGD<MLP, Float>(learningRate: 0.02)\nvar classifier = MLP()\nlet context = Context(learningPhase: .training)\n\nlet x: Tensor<Float> = [[0, 0], [0, 1], [1, 0], [1, 1]]\nlet y: Tensor<Float> = [0, 1, 1, 0]\n\nfor _ in 0..<10 {\n let 𝛁model = classifier.gradient { classifier -> Tensor<Float> in\n let ŷ = classifier.applied(to: x, in: context).withGradient { print(\"∂L/∂ŷ =\", $0) }\n let loss = (ŷ - y).squared().mean()\n print(\"Loss: \\(loss)\")\n return loss\n }\n optimizer.update(&classifier.allDifferentiableVariables, along: 𝛁model)\n}",
"Loss: 0.33426732\n∂L/∂ŷ = [[-0.25], [-0.078446716], [-0.12092987], [0.031454742]]\n∂L/∂layer1 = [[0.0, 0.0, 0.0, 0.0, 0.0, 0.0, 0.0, 0.0, 0.0, 0.0], [-0.03357383, -0.027463656, 0.037523113, -0.002631738, -0.030937709, -0.014981618, -0.02623924, -0.026290288, 0.027446445, 0.01046889], [-0.051755875, -0.042336714, 0.057843916, -0.004056967, -0.047692157, -0.023094976, -0.040449213, -0.040527906, 0.042310182, 0.016138362], [0.013462082, 0.0110120885, -0.015045625, 0.0010552468, 0.012405078, 0.006007172, 0.010521135, 0.010541604, -0.011005187, -0.0041977055]]\nLoss: 0.33176333\n∂L/∂ŷ = [[-0.24746439], [-0.07523262], [-0.11674469], [0.03514868]]\n∂L/∂layer1 = [[-0.10602461, -0.08665162, 0.11829134, -0.008301959, -0.09804342, -0.04726032, -0.08280819, -0.082981184, 0.08658129, 0.032860693], [-0.032232955, -0.0263433, 0.035962217, -0.0025239112, -0.029806564, -0.014367796, -0.025174841, -0.025227433, 0.026321916, 0.009990108], [-0.050018553, -0.040879082, 0.05580555, -0.003916562, -0.046253316, -0.022295699, -0.039065886, -0.0391475, 0.0408459, 0.015502479], [0.015059238, 0.01230759, -0.016801547, 0.0011791714, 0.013925628, 0.006712634, 0.011761686, 0.011786258, -0.0122976, -0.0046673785]]\nLoss: 0.3263967\n∂L/∂ŷ = [[-0.24090183], [-0.068522125], [-0.10922298], [0.04169026]]\n∂L/∂layer1 = [[-0.10332261, -0.08436972, 0.115082964, -0.008081798, -0.09583634, -0.046007015, -0.08064418, -0.08082552, 0.08428522, 0.031835504], [-0.029389087, -0.023998126, 0.032734204, -0.002298787, -0.027259693, -0.013086237, -0.022938434, -0.022990014, 0.02397409, 0.009055292], [-0.046845652, -0.038252562, 0.052177705, -0.0036642232, -0.043451436, -0.020859215, -0.036563434, -0.03664565, 0.03821425, 0.014433966], [0.01788092, 0.01460095, -0.019916158, 0.0013986289, 0.016585354, 0.0079619335, 0.013956212, 0.013987594, -0.014586327, -0.005509425]]\nLoss: 0.32171223\n∂L/∂ŷ = [[-0.23473385], [-0.062339008], [-0.102276154], [0.047599167]]\n∂L/∂layer1 = [[-0.10078285, -0.08222727, 0.11207248, -0.007874874, -0.09368697, -0.044829067, -0.078606784, -0.07879931, 0.082127206, 0.030880556], [-0.026765218, -0.021837354, 0.029763442, -0.0020913552, -0.024880743, -0.011905396, -0.02087585, -0.02092698, 0.021810781, 0.008201047], [-0.04391221, -0.035827335, 0.04883123, -0.0034311705, -0.040820457, -0.019532524, -0.03424985, -0.034333736, 0.035783738, 0.013455003], [0.020436676, 0.016673988, -0.02272598, 0.0015968615, 0.018997777, 0.009090407, 0.015939828, 0.015978869, -0.016653698, -0.006261938]]\nLoss: 0.31760892\n∂L/∂ŷ = [[-0.22893232], [-0.056644887], [-0.0958622], [0.0529218]]\n∂L/∂layer1 = [[-0.09839373, -0.080213994, 0.109245166, -0.007680244, -0.0915977, -0.0437211, -0.0766867, -0.076893255, 0.0800974, 0.02998989], [-0.024345629, -0.019847406, 0.02703061, -0.0019003282, -0.022664083, -0.010817943, -0.018974645, -0.019025752, 0.019818557, 0.00742042], [-0.041200995, -0.033588488, 0.045744885, -0.0032159945, -0.038355254, -0.018307598, -0.03211148, -0.03219797, 0.033539664, 0.0125578465], [0.02274547, 0.0185429, -0.025253974, 0.0017754257, 0.021174446, 0.010106915, 0.017727504, 0.01777525, -0.018515948, -0.0069327]]\nLoss: 0.3140006\n∂L/∂ŷ = [[-0.22347087], [-0.051403634], [-0.08994151], [0.057702184]]\n∂L/∂layer1 = [[-0.09614439, -0.07832037, 0.106587306, -0.0074970224, -0.08956989, -0.04267808, -0.07487536, -0.07509866, 0.07818659, 0.029158076], [-0.022115506, -0.018015554, 0.024517624, -0.0017244942, -0.020603213, -0.009816977, -0.017223122, -0.017274486, 0.017984781, 0.0067070536], [-0.038695745, -0.031522017, 0.04289876, -0.0030173666, -0.03604967, -0.017176874, -0.030135486, -0.030225359, 0.03146817, 0.011735407], [0.024825346, 0.020223022, -0.027521798, 0.0019357986, 0.02312775, 0.011019863, 0.019333491, 0.01939115, -0.020188477, -0.0075288774]]\nLoss: 0.3108136\n∂L/∂ŷ = [[-0.21832475], [-0.046581082], [-0.084476836], [0.061981946]]\n∂L/∂layer1 = [[-0.094024695, -0.07653748, 0.10408614, -0.0073243803, -0.08760406, -0.041695286, -0.07316483, -0.07340741, 0.076386094, 0.02838015], [-0.020060813, -0.016329797, 0.022207491, -0.0015627067, -0.018690927, -0.008895975, -0.015610217, -0.015661974, 0.016297497, 0.0060551], [-0.036381166, -0.029614802, 0.04027426, -0.0028340372, -0.033896815, -0.01613324, -0.028309815, -0.028403677, 0.029556224, 0.010981189], [0.026693417, 0.021728832, -0.02954984, 0.0020793765, 0.024870614, 0.011837205, 0.020771343, 0.020840213, -0.021685854, -0.008057066]]\nLoss: 0.30798542\n∂L/∂ŷ = [[-0.2134709], [-0.042145163], [-0.07943327], [0.06580055]]\n∂L/∂layer1 = [[-0.092025176, -0.07485708, 0.10172984, -0.007161543, -0.08570006, -0.040768307, -0.07154774, -0.07181193, 0.07468786, 0.02765159], [-0.018168358, -0.0147788925, 0.020084333, -0.00141389, -0.016919604, -0.00804881, -0.014125537, -0.014177696, 0.014745485, 0.0054592015], [-0.034242887, -0.027854579, 0.03785403, -0.0026648352, -0.031889293, -0.015170028, -0.026623163, -0.02672147, 0.027791614, 0.010289253], [0.028365958, 0.023074042, -0.031357337, 0.0022074832, 0.026416298, 0.012566475, 0.022053968, 0.022135403, -0.023021882, -0.008523362]]\nLoss: 0.30546278\n∂L/∂ŷ = [[-0.20888776], [-0.03806588], [-0.07477814], [0.069194704]]\n∂L/∂layer1 = [[-0.09013698, -0.07327145, 0.099507414, -0.007007787, -0.08385716, -0.039893024, -0.07001727, -0.070305154, 0.07308434, 0.026968256], [-0.016425777, -0.013352349, 0.018133363, -0.0012770379, -0.015281396, -0.007269756, -0.012759335, -0.012811797, 0.013318251, 0.0049144593], [-0.03226745, -0.02622989, 0.035621904, -0.0025086643, -0.030019386, -0.014281, -0.025064949, -0.025168007, 0.026162906, 0.009654161], [0.02985815, 0.024271391, -0.032962132, 0.0023213506, 0.027777938, 0.013214685, 0.023193432, 0.023288796, -0.024209408, -0.008933316]]\nLoss: 0.30320063\n∂L/∂ŷ = [[-0.20455518], [-0.0343152], [-0.07048094], [0.07219905]]\n∂L/∂layer1 = [[-0.08835188, -0.07177346, 0.09740865, -0.0068624374, -0.082074195, -0.039065596, -0.068567075, -0.06888049, 0.07156848, 0.02632637], [-0.014821488, -0.012040373, 0.016340809, -0.0011512097, -0.013768374, -0.0065534576, -0.011502485, -0.011555062, 0.012005987, 0.0044163857], [-0.03044227, -0.024730057, 0.033562843, -0.0023645016, -0.028279249, -0.013460329, -0.023625273, -0.023733262, 0.02465943, 0.0090709375], [0.031184357, 0.025332898, -0.034381, 0.0024221407, 0.028968606, 0.01378845, 0.024201185, 0.024311805, -0.025260549, -0.009292059]]\n"
]
],
[
[
"## Recomputing activations during backpropagation to save memory (checkpointing)\n\nCheckpointing is a traditional technique in reverse-mode automatic differentiation to save memory when computing derivatives by making large intermediate values in the original computation not be saved in memory for backpropagation, but instead recomputed as needed during backpropagation. This technique has been realized in modern deep learning libraries as well. In Swift, API [`withComputationInPullbacks(_:)`](https://www.tensorflow.org/swift/api_docs/Protocols/Differentiable#/s:10TensorFlow14DifferentiablePAAE28withRecomputationInPullbacksyqd__qd__xcAaBRd__lF) makes you able to control what to recompute during backpropagation, and it is available on all `Differentiable` types.\n\nBut today, let us learn how to define our own gradient checkpointing APIs from scratch, in just a few lines of code.",
"_____no_output_____"
],
[
"### My gradient checkpointing API",
"_____no_output_____"
],
[
"We can define our own gradient checkpointing API, `makeRecomputedInGradient(_:)`, in terms of standard library function [`differentiableFunction(from:)`](https://www.tensorflow.org/swift/api_docs/Functions#/s:10TensorFlow22differentiableFunction4fromq0_x_q_tcq0_5value_15CotangentVectorQz_AEQy_tAEQy0_c8pullbacktx_q_tc_tAA14DifferentiableRzAaJR_AaJR0_r1_lF), which is a shorthand for creating a differentiable function directly from a derivative function (also called a \"vector-Jacobian products (VJP) function\").\n\nAs we have seen before, the derivative function returns a tuple of the original function's result and a pullback closure. We return `original(x)` in `value:`, and call `pullback(at:in:)` on `original` to evaluate the original function again and get a pullback.",
"_____no_output_____"
]
],
[
[
"/// Given a differentiable function, returns the same differentiable function except when\n/// derivatives of this function is being computed, values in the original function that are needed\n/// for computing the derivatives will be recomputed, instead of being captured by the differnetial\n/// or pullback.\n///\n/// - Parameter body: The body of the differentiable function.\n/// - Returns: The same differentiable function whose derivatives, when computed, will recompute\n/// some values from the original function.\nfunc makeRecomputedInGradient<T: Differentiable, U: Differentiable>(\n _ original: @escaping @differentiable (T) -> U\n) -> @differentiable (T) -> U {\n return differentiableFunction { x in\n (value: original(x), pullback: { v in pullback(at: x, in: original)(v) })\n }\n}",
"_____no_output_____"
]
],
[
[
"### Verify it works",
"_____no_output_____"
]
],
[
[
"let input: Float = 10.0\nprint(\"Running original computation...\")\n\n// Differentiable multiplication with checkpointing.\nlet square = makeRecomputedInGradient { (x: Float) -> Float in\n print(\" Computing square...\")\n return x * x\n}\n\n// Differentiate `f(x) = (cos(x))^2`.\nlet (output, backprop) = input.valueWithPullback { input -> Float in\n return square(cos(input))\n}\nprint(\"Running backpropagation...\")\nlet grad = backprop(1)\nprint(\"Gradient = \\(grad)\")",
"Running original computation...\r\n Computing square...\r\nRunning backpropagation...\r\n Computing square...\r\nGradient = -0.9129453\r\n"
]
],
[
[
"### Extend it to neural network modules\n\nIn this example, we define a simple convolutional neural network.\n\n```swift\nstruct Model: Layer {\n var conv = Conv2D<Float>(filterShape: (5, 5, 3, 6))\n var maxPool = MaxPool2D<Float>(poolSize: (2, 2), strides: (2, 2))\n var flatten = Flatten<Float>()\n var dense = Dense<Float>(inputSize: 36 * 6, outputSize: 10)\n\n @differentiable\n func applied(to input: Tensor<Float>, in context: Context) -> Tensor<Float> {\n return input.sequenced(in: context, through: conv, maxPool, flatten, dense)\n }\n}\n```\n\nWe want to make activations in the convolution layer (`conv`) be recomputed during backpropagation. However, using `makeRecomputedInGradient(_:)` could make the resulting code look cumbersome, especially when we want to apply layers sequentially using [`sequenced(in:through:_:_:_:_:)`](https://www.tensorflow.org/swift/api_docs/Protocols/Differentiable#/s:10TensorFlow14DifferentiablePAAE9sequenced2in7through____6OutputQyd_3_AA7ContextC_qd__qd_0_qd_1_qd_2_qd_3_t5InputQyd__RszAA5LayerRd__AaMRd_0_AaMRd_1_AaMRd_2_AaMRd_3_AKQyd_0_AGRtd__AKQyd_1_AGRtd_0_AKQyd_2_AGRtd_1_AKQyd_3_AGRtd_2_r3_lF).\n\n```swift\ninput.sequenced(in: context, through: conv, maxPool, flatten, dense)\n```\n\nSo, why don't we define a **special layer type** that wraps a layer and makes its activations be recomputed during backpropagation? Let's do it.",
"_____no_output_____"
],
[
"First, we define a `makeRecomputedInGradient(_:)` function that takes a binary function.",
"_____no_output_____"
]
],
[
[
"// Same as the previous `makeRecomputedInGradient(_:)`, except it's for binary functions.\nfunc makeRecomputedInGradient<T: Differentiable, U: Differentiable, V: Differentiable>(\n _ original: @escaping @differentiable (T, U) -> V\n) -> @differentiable (T, U) -> V {\n return differentiableFunction { x, y in\n (value: original(x, y), pullback: { v in pullback(at: x, y, in: original)(v) })\n }\n}",
"_____no_output_____"
]
],
[
[
"Then, we define a generic layer `ActivationRecomputing<Wrapped>`.",
"_____no_output_____"
]
],
[
[
"/// A layer wrapper that makes the underlying layer's activations be discarded during application\n/// and recomputed during backpropagation.\nstruct ActivationDiscarding<Wrapped: Layer>: Layer \n where Wrapped.AllDifferentiableVariables == Wrapped.CotangentVector {\n /// The wrapped layer.\n var wrapped: Wrapped\n\n @differentiable\n func applied(to input: Wrapped.Input, in context: Context) -> Wrapped.Output {\n let apply = makeRecomputedInGradient { (layer: Wrapped, input: Input) -> Wrapped.Output in\n print(\" Applying \\(Wrapped.self) layer...\")\n return layer.applied(to: input, in: context)\n }\n return apply(wrapped, input)\n }\n}",
"_____no_output_____"
]
],
[
[
"Finally, we can add a method on all layers that returns the same layer except its activations are discarded during application and recomputeed during backpropagation.",
"_____no_output_____"
]
],
[
[
"extension Layer where AllDifferentiableVariables == CotangentVector {\n func discardingActivations() -> ActivationDiscarding<Self> {\n return ActivationDiscarding(wrapped: self)\n }\n}",
"_____no_output_____"
]
],
[
[
"Back in the model, all we have to change is to wrap the convolution layer into the activation-recomputing layer.\n\n```swift\nvar conv = Conv2D<Float>(filterShape: (5, 5, 3, 6)).discardingActivations()\n```",
"_____no_output_____"
],
[
"Now, simply use it in the model!",
"_____no_output_____"
]
],
[
[
"struct Model: Layer {\n var conv = Conv2D<Float>(filterShape: (5, 5, 3, 6)).discardingActivations()\n var maxPool = MaxPool2D<Float>(poolSize: (2, 2), strides: (2, 2))\n var flatten = Flatten<Float>()\n var dense = Dense<Float>(inputSize: 36 * 6, outputSize: 10)\n\n @differentiable\n func applied(to input: Tensor<Float>, in context: Context) -> Tensor<Float> {\n return input.sequenced(in: context, through: conv, maxPool, flatten, dense)\n }\n}",
"_____no_output_____"
]
],
[
[
"When we run a training loop, we can see that the convolution layer's activations are computed twice: once during layer application, and once during backpropagation.",
"_____no_output_____"
]
],
[
[
"// Use random training data.\nlet x = Tensor<Float>(randomNormal: [10, 16, 16, 3])\nlet y = Tensor<Int32>(rangeFrom: 0, to: 10, stride: 1)\n\nvar model = Model()\nlet opt = SGD<Model, Float>()\nlet context = Context(learningPhase: .training)\n\nfor i in 1...5 {\n print(\"Starting training step \\(i)\")\n print(\" Running original computation...\")\n let (logits, backprop) = model.appliedForBackpropagation(to: x, in: context)\n let (loss, dL_dŷ) = logits.valueWithGradient { logits in\n softmaxCrossEntropy(logits: logits, labels: y)\n }\n print(\" Loss: \\(loss)\")\n print(\" Running backpropagation...\")\n let (dL_dθ, _) = backprop(dL_dŷ)\n \n opt.update(&model.allDifferentiableVariables, along: dL_dθ)\n}",
"Starting training step 1\r\n Running original computation...\r\n Applying Conv2D<Float> layer...\n Loss: 3.6660562\n Running backpropagation...\n Applying Conv2D<Float> layer...\nStarting training step 2\n Running original computation...\n Applying Conv2D<Float> layer...\n Loss: 3.1203392\n Running backpropagation...\n Applying Conv2D<Float> layer...\nStarting training step 3\n Running original computation...\n Applying Conv2D<Float> layer...\n Loss: 2.7324893\n Running backpropagation...\n Applying Conv2D<Float> layer...\nStarting training step 4\n Running original computation...\n Applying Conv2D<Float> layer...\n Loss: 2.4246051\n Running backpropagation...\n Applying Conv2D<Float> layer...\nStarting training step 5\n Running original computation...\n Applying Conv2D<Float> layer...\n Loss: 2.1656146\n Running backpropagation...\n Applying Conv2D<Float> layer...\n"
]
],
[
[
"Just like that, it is super easy to define generic differentiable programming libraries for different domains.",
"_____no_output_____"
]
]
] | [
"markdown",
"code",
"markdown",
"code",
"markdown",
"code",
"markdown",
"code",
"markdown",
"code",
"markdown",
"code",
"markdown",
"code",
"markdown",
"code",
"markdown",
"code",
"markdown",
"code",
"markdown",
"code",
"markdown",
"code",
"markdown"
] | [
[
"markdown"
],
[
"code"
],
[
"markdown",
"markdown",
"markdown"
],
[
"code"
],
[
"markdown"
],
[
"code"
],
[
"markdown",
"markdown",
"markdown"
],
[
"code"
],
[
"markdown",
"markdown"
],
[
"code"
],
[
"markdown",
"markdown",
"markdown"
],
[
"code"
],
[
"markdown"
],
[
"code"
],
[
"markdown",
"markdown"
],
[
"code"
],
[
"markdown"
],
[
"code"
],
[
"markdown"
],
[
"code"
],
[
"markdown",
"markdown"
],
[
"code"
],
[
"markdown"
],
[
"code"
],
[
"markdown"
]
] |
e78526e7fac7ef287f4126b8775852b363281e93 | 30,629 | ipynb | Jupyter Notebook | notebooks/10-2.alexnet_in_keras.ipynb | sunny191019/dl-illustrated | 6a7b004aeeaed52aee3bc08cf2969524017541b0 | [
"MIT"
] | 1 | 2021-02-06T09:27:46.000Z | 2021-02-06T09:27:46.000Z | notebooks/10-2.alexnet_in_keras.ipynb | sunny191019/dl-illustrated | 6a7b004aeeaed52aee3bc08cf2969524017541b0 | [
"MIT"
] | null | null | null | notebooks/10-2.alexnet_in_keras.ipynb | sunny191019/dl-illustrated | 6a7b004aeeaed52aee3bc08cf2969524017541b0 | [
"MIT"
] | null | null | null | 54.989228 | 323 | 0.474877 | [
[
[
"# 케라스로 AlexNet 만들기",
"_____no_output_____"
],
[
"이 노트북에서 [AlexNet](https://papers.nips.cc/paper/4824-imagenet-classification-with-deep-convolutional-neural-networks)과 비슷한 심층 합성곱 신경망으로 [Oxford Flowers](http://www.robots.ox.ac.uk/~vgg/data/flowers/17/) 데이터셋의 꽃을 17개의 카테고리로 분류하겠습니다.",
"_____no_output_____"
],
[
"[](https://colab.research.google.com/github/rickiepark/dl-illustrated/blob/master/notebooks/10-2.alexnet_in_keras.ipynb)",
"_____no_output_____"
],
[
"#### 라이브러리를 적재합니다.",
"_____no_output_____"
]
],
[
[
"from tensorflow import keras\nfrom tensorflow.keras.models import Sequential\nfrom tensorflow.keras.layers import Dense, Dropout, Flatten, Conv2D, MaxPooling2D\nfrom tensorflow.keras.layers import BatchNormalization",
"_____no_output_____"
]
],
[
[
"#### 데이터를 적재하고 전처리합니다.",
"_____no_output_____"
],
[
"원서 노트북은 tflearn을 사용해 oxflower17 데이터셋을 다운로드합니다. 이 라이브러리는 텐서플로 2와 호환되지 않습니다. 여기에서는 사전에 tflearn으로 다운받은 데이터를 다운로드하여 사용합니다.\n\n이 데이터셋에 대한 자세한 내용은 http://www.robots.ox.ac.uk/~vgg/data/flowers/17/ 을 참고하세요.",
"_____no_output_____"
]
],
[
[
"!rm oxflower17*\n!wget https://bit.ly/31IvwtD -O oxflower17.npz",
"rm: cannot remove 'oxflower17*': No such file or directory\n--2021-02-01 15:40:25-- https://bit.ly/31IvwtD\nResolving bit.ly (bit.ly)... 67.199.248.11, 67.199.248.10\nConnecting to bit.ly (bit.ly)|67.199.248.11|:443... connected.\nHTTP request sent, awaiting response... 301 Moved Permanently\nLocation: https://onedrive.live.com/download?cid=822579D69D2DC3B5&resid=822579D69D2DC3B5!597497&authkey=AGGo9IgYC0QZfXo [following]\n--2021-02-01 15:40:25-- https://onedrive.live.com/download?cid=822579D69D2DC3B5&resid=822579D69D2DC3B5!597497&authkey=AGGo9IgYC0QZfXo\nResolving onedrive.live.com (onedrive.live.com)... 13.107.42.13\nConnecting to onedrive.live.com (onedrive.live.com)|13.107.42.13|:443... connected.\nHTTP request sent, awaiting response... 302 Found\nLocation: https://evtwow.bl.files.1drv.com/y4mj6Q18QJugmPu4IyZM_bNTnaH6izWk_AI1kCIBS6QBpEg3m0m9RrFhbHGSHL7rOWZ491KhaXuB7gP3DSXh5eOWt2xNgUk7kcNyzhYIqhiOu3XiukEKWY1RxFqmFNbXsqnccyxPI_YKjALX3b2qxZ5so9LeW39Atyi_EvwuTjDj0fj7h6L3J1JwDFBVXUQcvoXYW4_wy_htpogQocLCErOKg/oxflower17.npz?download&psid=1 [following]\n--2021-02-01 15:40:26-- https://evtwow.bl.files.1drv.com/y4mj6Q18QJugmPu4IyZM_bNTnaH6izWk_AI1kCIBS6QBpEg3m0m9RrFhbHGSHL7rOWZ491KhaXuB7gP3DSXh5eOWt2xNgUk7kcNyzhYIqhiOu3XiukEKWY1RxFqmFNbXsqnccyxPI_YKjALX3b2qxZ5so9LeW39Atyi_EvwuTjDj0fj7h6L3J1JwDFBVXUQcvoXYW4_wy_htpogQocLCErOKg/oxflower17.npz?download&psid=1\nResolving evtwow.bl.files.1drv.com (evtwow.bl.files.1drv.com)... 13.107.42.12\nConnecting to evtwow.bl.files.1drv.com (evtwow.bl.files.1drv.com)|13.107.42.12|:443... connected.\nHTTP request sent, awaiting response... 200 OK\nLength: 252415092 (241M) [application/zip]\nSaving to: ‘oxflower17.npz’\n\noxflower17.npz 100%[===================>] 240.72M 18.9MB/s in 12s \n\n2021-02-01 15:40:39 (19.4 MB/s) - ‘oxflower17.npz’ saved [252415092/252415092]\n\n"
],
[
"import numpy as np\n\ndata = np.load('oxflower17.npz')\nX = data['X']\nY = data['Y']",
"_____no_output_____"
],
[
"X.shape, Y.shape",
"_____no_output_____"
]
],
[
[
"#### 신경망 모델을 만듭니다.",
"_____no_output_____"
]
],
[
[
"model = Sequential()\n\nmodel.add(Conv2D(96, kernel_size=(11, 11), strides=(4, 4), activation='relu', input_shape=(224, 224, 3)))\nmodel.add(MaxPooling2D(pool_size=(3, 3), strides=(2, 2)))\nmodel.add(BatchNormalization())\n\nmodel.add(Conv2D(256, kernel_size=(5, 5), activation='relu'))\nmodel.add(MaxPooling2D(pool_size=(3, 3), strides=(2, 2)))\nmodel.add(BatchNormalization())\n\nmodel.add(Conv2D(256, kernel_size=(3, 3), activation='relu'))\nmodel.add(Conv2D(384, kernel_size=(3, 3), activation='relu'))\nmodel.add(Conv2D(384, kernel_size=(3, 3), activation='relu'))\nmodel.add(MaxPooling2D(pool_size=(3, 3), strides=(2, 2)))\nmodel.add(BatchNormalization())\n\nmodel.add(Flatten())\nmodel.add(Dense(4096, activation='tanh'))\nmodel.add(Dropout(0.5))\nmodel.add(Dense(4096, activation='tanh'))\nmodel.add(Dropout(0.5))\n\nmodel.add(Dense(17, activation='softmax'))",
"_____no_output_____"
],
[
"model.summary()",
"Model: \"sequential\"\n_________________________________________________________________\nLayer (type) Output Shape Param # \n=================================================================\nconv2d (Conv2D) (None, 54, 54, 96) 34944 \n_________________________________________________________________\nmax_pooling2d (MaxPooling2D) (None, 26, 26, 96) 0 \n_________________________________________________________________\nbatch_normalization (BatchNo (None, 26, 26, 96) 384 \n_________________________________________________________________\nconv2d_1 (Conv2D) (None, 22, 22, 256) 614656 \n_________________________________________________________________\nmax_pooling2d_1 (MaxPooling2 (None, 10, 10, 256) 0 \n_________________________________________________________________\nbatch_normalization_1 (Batch (None, 10, 10, 256) 1024 \n_________________________________________________________________\nconv2d_2 (Conv2D) (None, 8, 8, 256) 590080 \n_________________________________________________________________\nconv2d_3 (Conv2D) (None, 6, 6, 384) 885120 \n_________________________________________________________________\nconv2d_4 (Conv2D) (None, 4, 4, 384) 1327488 \n_________________________________________________________________\nmax_pooling2d_2 (MaxPooling2 (None, 1, 1, 384) 0 \n_________________________________________________________________\nbatch_normalization_2 (Batch (None, 1, 1, 384) 1536 \n_________________________________________________________________\nflatten (Flatten) (None, 384) 0 \n_________________________________________________________________\ndense (Dense) (None, 4096) 1576960 \n_________________________________________________________________\ndropout (Dropout) (None, 4096) 0 \n_________________________________________________________________\ndense_1 (Dense) (None, 4096) 16781312 \n_________________________________________________________________\ndropout_1 (Dropout) (None, 4096) 0 \n_________________________________________________________________\ndense_2 (Dense) (None, 17) 69649 \n=================================================================\nTotal params: 21,883,153\nTrainable params: 21,881,681\nNon-trainable params: 1,472\n_________________________________________________________________\n"
]
],
[
[
"#### 모델을 설정합니다.",
"_____no_output_____"
]
],
[
[
"model.compile(loss='categorical_crossentropy', optimizer='adam', metrics=['accuracy'])",
"_____no_output_____"
]
],
[
[
"#### 훈련!",
"_____no_output_____"
]
],
[
[
"model.fit(X, Y, batch_size=64, epochs=100, verbose=1, validation_split=0.1, shuffle=True)",
"Epoch 1/100\n20/20 [==============================] - 9s 78ms/step - loss: 4.6429 - accuracy: 0.1772 - val_loss: 6.9113 - val_accuracy: 0.0662\nEpoch 2/100\n20/20 [==============================] - 1s 50ms/step - loss: 3.2046 - accuracy: 0.2823 - val_loss: 3.8402 - val_accuracy: 0.1838\nEpoch 3/100\n20/20 [==============================] - 1s 50ms/step - loss: 2.3982 - accuracy: 0.3301 - val_loss: 5.0392 - val_accuracy: 0.1250\nEpoch 4/100\n20/20 [==============================] - 1s 50ms/step - loss: 2.4482 - accuracy: 0.3819 - val_loss: 4.1104 - val_accuracy: 0.2279\nEpoch 5/100\n20/20 [==============================] - 1s 51ms/step - loss: 2.2152 - accuracy: 0.4310 - val_loss: 4.6752 - val_accuracy: 0.2206\nEpoch 6/100\n20/20 [==============================] - 1s 50ms/step - loss: 2.2077 - accuracy: 0.4286 - val_loss: 4.6224 - val_accuracy: 0.2279\nEpoch 7/100\n20/20 [==============================] - 1s 51ms/step - loss: 1.9991 - accuracy: 0.4783 - val_loss: 3.2980 - val_accuracy: 0.3015\nEpoch 8/100\n20/20 [==============================] - 1s 51ms/step - loss: 1.8836 - accuracy: 0.4758 - val_loss: 4.4707 - val_accuracy: 0.2721\nEpoch 9/100\n20/20 [==============================] - 1s 50ms/step - loss: 2.0022 - accuracy: 0.4745 - val_loss: 6.2244 - val_accuracy: 0.2059\nEpoch 10/100\n20/20 [==============================] - 1s 50ms/step - loss: 1.7743 - accuracy: 0.5284 - val_loss: 4.5157 - val_accuracy: 0.2574\nEpoch 11/100\n20/20 [==============================] - 1s 50ms/step - loss: 1.8367 - accuracy: 0.5051 - val_loss: 3.1568 - val_accuracy: 0.3750\nEpoch 12/100\n20/20 [==============================] - 1s 52ms/step - loss: 1.9101 - accuracy: 0.4916 - val_loss: 2.5058 - val_accuracy: 0.3971\nEpoch 13/100\n20/20 [==============================] - 1s 52ms/step - loss: 1.7524 - accuracy: 0.5305 - val_loss: 2.6367 - val_accuracy: 0.3824\nEpoch 14/100\n20/20 [==============================] - 1s 51ms/step - loss: 1.6340 - accuracy: 0.5884 - val_loss: 3.8476 - val_accuracy: 0.3382\nEpoch 15/100\n20/20 [==============================] - 1s 51ms/step - loss: 1.7423 - accuracy: 0.5187 - val_loss: 2.8836 - val_accuracy: 0.4412\nEpoch 16/100\n20/20 [==============================] - 1s 50ms/step - loss: 1.4916 - accuracy: 0.5700 - val_loss: 5.7071 - val_accuracy: 0.3382\nEpoch 17/100\n20/20 [==============================] - 1s 49ms/step - loss: 1.2675 - accuracy: 0.6554 - val_loss: 3.1568 - val_accuracy: 0.4118\nEpoch 18/100\n20/20 [==============================] - 1s 51ms/step - loss: 1.1675 - accuracy: 0.6507 - val_loss: 4.5942 - val_accuracy: 0.4191\nEpoch 19/100\n20/20 [==============================] - 1s 50ms/step - loss: 1.3940 - accuracy: 0.6306 - val_loss: 2.8359 - val_accuracy: 0.4706\nEpoch 20/100\n20/20 [==============================] - 1s 49ms/step - loss: 1.2050 - accuracy: 0.6855 - val_loss: 2.7855 - val_accuracy: 0.5515\nEpoch 21/100\n20/20 [==============================] - 1s 51ms/step - loss: 1.1311 - accuracy: 0.6832 - val_loss: 3.0645 - val_accuracy: 0.4853\nEpoch 22/100\n20/20 [==============================] - 1s 52ms/step - loss: 1.2334 - accuracy: 0.6764 - val_loss: 3.5505 - val_accuracy: 0.4706\nEpoch 23/100\n20/20 [==============================] - 1s 52ms/step - loss: 1.0275 - accuracy: 0.7142 - val_loss: 3.0626 - val_accuracy: 0.4706\nEpoch 24/100\n20/20 [==============================] - 1s 50ms/step - loss: 0.9573 - accuracy: 0.7388 - val_loss: 2.9081 - val_accuracy: 0.5221\nEpoch 25/100\n20/20 [==============================] - 1s 51ms/step - loss: 0.7289 - accuracy: 0.7712 - val_loss: 2.2599 - val_accuracy: 0.5809\nEpoch 26/100\n20/20 [==============================] - 1s 52ms/step - loss: 0.8660 - accuracy: 0.7556 - val_loss: 2.5860 - val_accuracy: 0.5809\nEpoch 27/100\n20/20 [==============================] - 1s 52ms/step - loss: 0.7782 - accuracy: 0.7686 - val_loss: 4.9205 - val_accuracy: 0.3676\nEpoch 28/100\n20/20 [==============================] - 1s 51ms/step - loss: 0.7287 - accuracy: 0.7853 - val_loss: 2.6654 - val_accuracy: 0.5368\nEpoch 29/100\n20/20 [==============================] - 1s 52ms/step - loss: 0.6768 - accuracy: 0.7977 - val_loss: 3.3202 - val_accuracy: 0.5294\nEpoch 30/100\n20/20 [==============================] - 1s 52ms/step - loss: 0.7499 - accuracy: 0.7763 - val_loss: 2.9776 - val_accuracy: 0.5368\nEpoch 31/100\n20/20 [==============================] - 1s 50ms/step - loss: 1.0794 - accuracy: 0.7134 - val_loss: 4.4612 - val_accuracy: 0.4559\nEpoch 32/100\n20/20 [==============================] - 1s 51ms/step - loss: 0.7277 - accuracy: 0.7920 - val_loss: 3.6071 - val_accuracy: 0.4632\nEpoch 33/100\n20/20 [==============================] - 1s 55ms/step - loss: 0.6720 - accuracy: 0.8274 - val_loss: 5.9109 - val_accuracy: 0.3309\nEpoch 34/100\n20/20 [==============================] - 1s 53ms/step - loss: 0.5504 - accuracy: 0.8461 - val_loss: 4.8567 - val_accuracy: 0.4338\nEpoch 35/100\n20/20 [==============================] - 1s 51ms/step - loss: 0.6322 - accuracy: 0.8097 - val_loss: 5.7461 - val_accuracy: 0.4485\nEpoch 36/100\n20/20 [==============================] - 1s 51ms/step - loss: 1.0962 - accuracy: 0.7786 - val_loss: 4.8283 - val_accuracy: 0.4338\nEpoch 37/100\n20/20 [==============================] - 1s 52ms/step - loss: 0.7069 - accuracy: 0.7959 - val_loss: 3.1211 - val_accuracy: 0.5441\nEpoch 38/100\n20/20 [==============================] - 1s 53ms/step - loss: 0.5671 - accuracy: 0.8275 - val_loss: 3.0753 - val_accuracy: 0.5809\nEpoch 39/100\n20/20 [==============================] - 1s 52ms/step - loss: 0.7584 - accuracy: 0.8193 - val_loss: 3.6496 - val_accuracy: 0.4412\nEpoch 40/100\n20/20 [==============================] - 1s 53ms/step - loss: 0.7445 - accuracy: 0.8087 - val_loss: 4.3113 - val_accuracy: 0.5000\nEpoch 41/100\n20/20 [==============================] - 1s 52ms/step - loss: 0.6313 - accuracy: 0.8254 - val_loss: 3.3609 - val_accuracy: 0.5515\nEpoch 42/100\n20/20 [==============================] - 1s 52ms/step - loss: 1.3299 - accuracy: 0.7039 - val_loss: 5.8714 - val_accuracy: 0.4118\nEpoch 43/100\n20/20 [==============================] - 1s 52ms/step - loss: 1.2052 - accuracy: 0.7081 - val_loss: 6.4298 - val_accuracy: 0.3382\nEpoch 44/100\n20/20 [==============================] - 1s 52ms/step - loss: 0.8349 - accuracy: 0.7821 - val_loss: 3.2248 - val_accuracy: 0.5368\nEpoch 45/100\n20/20 [==============================] - 1s 52ms/step - loss: 0.6766 - accuracy: 0.8154 - val_loss: 2.9413 - val_accuracy: 0.5735\nEpoch 46/100\n20/20 [==============================] - 1s 52ms/step - loss: 0.8999 - accuracy: 0.7800 - val_loss: 5.3587 - val_accuracy: 0.3529\nEpoch 47/100\n20/20 [==============================] - 1s 51ms/step - loss: 0.7444 - accuracy: 0.7849 - val_loss: 3.3938 - val_accuracy: 0.5147\nEpoch 48/100\n20/20 [==============================] - 1s 51ms/step - loss: 0.5099 - accuracy: 0.8597 - val_loss: 3.2823 - val_accuracy: 0.5882\nEpoch 49/100\n20/20 [==============================] - 1s 52ms/step - loss: 0.6309 - accuracy: 0.8320 - val_loss: 3.0612 - val_accuracy: 0.6471\nEpoch 50/100\n20/20 [==============================] - 1s 53ms/step - loss: 0.4649 - accuracy: 0.8590 - val_loss: 3.9522 - val_accuracy: 0.5441\nEpoch 51/100\n20/20 [==============================] - 1s 51ms/step - loss: 0.5488 - accuracy: 0.8589 - val_loss: 3.7538 - val_accuracy: 0.5662\nEpoch 52/100\n20/20 [==============================] - 1s 52ms/step - loss: 0.4251 - accuracy: 0.8835 - val_loss: 2.6496 - val_accuracy: 0.6544\nEpoch 53/100\n20/20 [==============================] - 1s 52ms/step - loss: 0.2903 - accuracy: 0.9169 - val_loss: 2.7501 - val_accuracy: 0.6618\nEpoch 54/100\n20/20 [==============================] - 1s 53ms/step - loss: 0.4575 - accuracy: 0.8965 - val_loss: 3.5671 - val_accuracy: 0.6324\nEpoch 55/100\n20/20 [==============================] - 1s 51ms/step - loss: 0.2682 - accuracy: 0.9198 - val_loss: 2.7003 - val_accuracy: 0.6765\nEpoch 56/100\n20/20 [==============================] - 1s 52ms/step - loss: 0.2174 - accuracy: 0.9196 - val_loss: 3.3102 - val_accuracy: 0.6029\nEpoch 57/100\n20/20 [==============================] - 1s 51ms/step - loss: 0.4567 - accuracy: 0.8849 - val_loss: 5.5328 - val_accuracy: 0.4632\nEpoch 58/100\n20/20 [==============================] - 1s 52ms/step - loss: 1.0789 - accuracy: 0.7738 - val_loss: 3.0945 - val_accuracy: 0.5515\nEpoch 59/100\n20/20 [==============================] - 1s 52ms/step - loss: 0.5780 - accuracy: 0.8461 - val_loss: 4.2850 - val_accuracy: 0.4926\nEpoch 60/100\n20/20 [==============================] - 1s 53ms/step - loss: 0.4107 - accuracy: 0.8884 - val_loss: 4.2642 - val_accuracy: 0.4853\nEpoch 61/100\n20/20 [==============================] - 1s 51ms/step - loss: 0.2438 - accuracy: 0.9170 - val_loss: 2.4405 - val_accuracy: 0.6691\nEpoch 62/100\n20/20 [==============================] - 1s 52ms/step - loss: 0.1929 - accuracy: 0.9441 - val_loss: 2.9831 - val_accuracy: 0.6912\nEpoch 63/100\n20/20 [==============================] - 1s 52ms/step - loss: 0.1585 - accuracy: 0.9387 - val_loss: 3.8142 - val_accuracy: 0.5956\nEpoch 64/100\n20/20 [==============================] - 1s 52ms/step - loss: 0.2168 - accuracy: 0.9435 - val_loss: 3.9020 - val_accuracy: 0.5735\nEpoch 65/100\n20/20 [==============================] - 1s 51ms/step - loss: 0.1878 - accuracy: 0.9484 - val_loss: 3.6544 - val_accuracy: 0.6029\nEpoch 66/100\n20/20 [==============================] - 1s 52ms/step - loss: 0.5521 - accuracy: 0.9019 - val_loss: 4.1064 - val_accuracy: 0.5294\nEpoch 67/100\n20/20 [==============================] - 1s 51ms/step - loss: 0.4251 - accuracy: 0.8947 - val_loss: 3.4000 - val_accuracy: 0.5956\nEpoch 68/100\n20/20 [==============================] - 1s 52ms/step - loss: 0.3610 - accuracy: 0.8991 - val_loss: 3.0546 - val_accuracy: 0.6324\nEpoch 69/100\n20/20 [==============================] - 1s 52ms/step - loss: 0.2694 - accuracy: 0.9378 - val_loss: 3.6128 - val_accuracy: 0.6544\nEpoch 70/100\n20/20 [==============================] - 1s 51ms/step - loss: 0.1124 - accuracy: 0.9633 - val_loss: 2.7719 - val_accuracy: 0.7132\nEpoch 71/100\n20/20 [==============================] - 1s 51ms/step - loss: 0.1700 - accuracy: 0.9529 - val_loss: 3.3141 - val_accuracy: 0.6912\nEpoch 72/100\n20/20 [==============================] - 1s 53ms/step - loss: 0.1228 - accuracy: 0.9611 - val_loss: 3.3079 - val_accuracy: 0.6838\nEpoch 73/100\n20/20 [==============================] - 1s 51ms/step - loss: 0.1909 - accuracy: 0.9535 - val_loss: 4.6782 - val_accuracy: 0.5662\nEpoch 74/100\n20/20 [==============================] - 1s 53ms/step - loss: 0.3665 - accuracy: 0.9193 - val_loss: 6.2701 - val_accuracy: 0.4779\nEpoch 75/100\n20/20 [==============================] - 1s 55ms/step - loss: 0.1703 - accuracy: 0.9522 - val_loss: 4.0180 - val_accuracy: 0.5809\nEpoch 76/100\n20/20 [==============================] - 1s 52ms/step - loss: 0.1623 - accuracy: 0.9538 - val_loss: 4.4584 - val_accuracy: 0.5956\nEpoch 77/100\n20/20 [==============================] - 1s 51ms/step - loss: 0.1211 - accuracy: 0.9786 - val_loss: 3.2625 - val_accuracy: 0.6618\nEpoch 78/100\n20/20 [==============================] - 1s 51ms/step - loss: 0.0457 - accuracy: 0.9852 - val_loss: 2.9800 - val_accuracy: 0.6691\nEpoch 79/100\n20/20 [==============================] - 1s 52ms/step - loss: 0.2802 - accuracy: 0.9531 - val_loss: 3.1548 - val_accuracy: 0.6838\nEpoch 80/100\n20/20 [==============================] - 1s 52ms/step - loss: 0.1678 - accuracy: 0.9532 - val_loss: 3.5188 - val_accuracy: 0.6544\nEpoch 81/100\n20/20 [==============================] - 1s 52ms/step - loss: 0.3620 - accuracy: 0.9322 - val_loss: 6.0728 - val_accuracy: 0.4338\nEpoch 82/100\n20/20 [==============================] - 1s 52ms/step - loss: 0.6820 - accuracy: 0.8755 - val_loss: 3.5640 - val_accuracy: 0.5809\nEpoch 83/100\n20/20 [==============================] - 1s 51ms/step - loss: 0.4729 - accuracy: 0.8956 - val_loss: 3.7106 - val_accuracy: 0.5882\nEpoch 84/100\n20/20 [==============================] - 1s 52ms/step - loss: 0.3984 - accuracy: 0.9084 - val_loss: 3.6485 - val_accuracy: 0.6324\nEpoch 85/100\n20/20 [==============================] - 1s 51ms/step - loss: 0.1942 - accuracy: 0.9470 - val_loss: 4.6554 - val_accuracy: 0.5441\nEpoch 86/100\n20/20 [==============================] - 1s 52ms/step - loss: 0.0973 - accuracy: 0.9677 - val_loss: 3.3203 - val_accuracy: 0.6691\nEpoch 87/100\n20/20 [==============================] - 1s 52ms/step - loss: 0.0920 - accuracy: 0.9700 - val_loss: 2.9472 - val_accuracy: 0.6765\nEpoch 88/100\n20/20 [==============================] - 1s 51ms/step - loss: 0.1521 - accuracy: 0.9595 - val_loss: 2.9696 - val_accuracy: 0.6985\nEpoch 89/100\n20/20 [==============================] - 1s 53ms/step - loss: 0.2279 - accuracy: 0.9486 - val_loss: 3.7965 - val_accuracy: 0.6324\nEpoch 90/100\n20/20 [==============================] - 1s 52ms/step - loss: 0.2021 - accuracy: 0.9517 - val_loss: 3.2243 - val_accuracy: 0.6691\nEpoch 91/100\n20/20 [==============================] - 1s 52ms/step - loss: 0.1544 - accuracy: 0.9627 - val_loss: 3.6171 - val_accuracy: 0.6985\nEpoch 92/100\n20/20 [==============================] - 1s 52ms/step - loss: 0.2457 - accuracy: 0.9491 - val_loss: 4.3328 - val_accuracy: 0.6103\nEpoch 93/100\n20/20 [==============================] - 1s 51ms/step - loss: 0.1223 - accuracy: 0.9587 - val_loss: 3.0888 - val_accuracy: 0.7132\nEpoch 94/100\n20/20 [==============================] - 1s 52ms/step - loss: 0.1074 - accuracy: 0.9683 - val_loss: 3.4378 - val_accuracy: 0.6765\nEpoch 95/100\n20/20 [==============================] - 1s 52ms/step - loss: 0.4015 - accuracy: 0.9229 - val_loss: 4.6498 - val_accuracy: 0.5882\nEpoch 96/100\n20/20 [==============================] - 1s 53ms/step - loss: 0.5772 - accuracy: 0.8908 - val_loss: 4.6537 - val_accuracy: 0.5735\nEpoch 97/100\n20/20 [==============================] - 1s 50ms/step - loss: 0.4412 - accuracy: 0.8848 - val_loss: 6.5095 - val_accuracy: 0.4632\nEpoch 98/100\n20/20 [==============================] - 1s 52ms/step - loss: 0.1980 - accuracy: 0.9405 - val_loss: 3.0124 - val_accuracy: 0.6618\nEpoch 99/100\n20/20 [==============================] - 1s 52ms/step - loss: 0.2180 - accuracy: 0.9522 - val_loss: 3.4620 - val_accuracy: 0.6250\nEpoch 100/100\n20/20 [==============================] - 1s 51ms/step - loss: 0.2533 - accuracy: 0.9323 - val_loss: 5.3905 - val_accuracy: 0.5294\n"
]
]
] | [
"markdown",
"code",
"markdown",
"code",
"markdown",
"code",
"markdown",
"code",
"markdown",
"code"
] | [
[
"markdown",
"markdown",
"markdown",
"markdown"
],
[
"code"
],
[
"markdown",
"markdown"
],
[
"code",
"code",
"code"
],
[
"markdown"
],
[
"code",
"code"
],
[
"markdown"
],
[
"code"
],
[
"markdown"
],
[
"code"
]
] |
e7852a251661b7efd79f6836a91fd5ff1dd35a55 | 40,427 | ipynb | Jupyter Notebook | Exercise_1_Cats_vs_Dogs_Question-FINAL.ipynb | Mostafa-wael/Convolutional-Neural-Networks-in-TensorFlow | 6d724dbd8d812945170c965ae742ecf39d59560b | [
"MIT"
] | null | null | null | Exercise_1_Cats_vs_Dogs_Question-FINAL.ipynb | Mostafa-wael/Convolutional-Neural-Networks-in-TensorFlow | 6d724dbd8d812945170c965ae742ecf39d59560b | [
"MIT"
] | null | null | null | Exercise_1_Cats_vs_Dogs_Question-FINAL.ipynb | Mostafa-wael/Convolutional-Neural-Networks-in-TensorFlow | 6d724dbd8d812945170c965ae742ecf39d59560b | [
"MIT"
] | null | null | null | 75.564486 | 12,896 | 0.776437 | [
[
[
"# ATTENTION: Please do not alter any of the provided code in the exercise. Only add your own code where indicated\n# ATTENTION: Please do not add or remove any cells in the exercise. The grader will check specific cells based on the cell position.\n# ATTENTION: Please use the provided epoch values when training.\n\n# In this exercise you will train a CNN on the FULL Cats-v-dogs dataset\n# This will require you doing a lot of data preprocessing because\n# the dataset isn't split into training and validation for you\n# This code block has all the required inputs\nimport os\nimport zipfile\nimport random\nimport tensorflow as tf\nimport shutil\nfrom tensorflow.keras.optimizers import RMSprop\nfrom tensorflow.keras.preprocessing.image import ImageDataGenerator\nfrom shutil import copyfile\nfrom os import getcwd",
"_____no_output_____"
],
[
"path_cats_and_dogs = f\"{getcwd()}/../tmp2/cats-and-dogs.zip\"\nshutil.rmtree('/tmp')\n\nlocal_zip = path_cats_and_dogs\nzip_ref = zipfile.ZipFile(local_zip, 'r')\nzip_ref.extractall('/tmp')\nzip_ref.close()\n",
"_____no_output_____"
],
[
"print(len(os.listdir('/tmp/PetImages/Cat/')))\nprint(len(os.listdir('/tmp/PetImages/Dog/')))\n\n# Expected Output:\n# 1500\n# 1500",
"1500\n1500\n"
],
[
"# Use os.mkdir to create your directories\n# You will need a directory for cats-v-dogs, and subdirectories for training\n# and testing. These in turn will need subdirectories for 'cats' and 'dogs'\ntry:\n print(len(os.listdir(\"/tmp/PetImages/\")))\n print(\"CAT_SOURCE_DIR = \", len(os.listdir('/tmp/PetImages/Cat/')))\n print(\"DOG_SOURCE_DIR = \", len(os.listdir('/tmp/PetImages/Dog/')))\n \n os.mkdir('/tmp/cats-v-dogs/')\n os.mkdir('/tmp/cats-v-dogs/training/'); os.mkdir('/tmp/cats-v-dogs/training/cats'); os.mkdir('/tmp/cats-v-dogs/training/dogs')\n os.mkdir('/tmp/cats-v-dogs/testing/'); os.mkdir('/tmp/cats-v-dogs/testing/cats'); os.mkdir('/tmp/cats-v-dogs/testing/dogs')\nexcept OSError:\n print(\"\\nDone\")\n print(\"/tmp/cats-v-dogs/ -> \", len(os.listdir(\"/tmp/cats-v-dogs/\")))\n print(\"TRAINING_CATS_DIR = \", len(os.listdir('/tmp/cats-v-dogs/training/cats')))\n print(\"TESTING_CATS_DIR = \", len(os.listdir('/tmp/cats-v-dogs/testing/cats')))\n \n print(\"TRAINING_DOGS_DIR = \", len(os.listdir('/tmp/cats-v-dogs/training/dogs')))\n print(\"TESTING_DOGS_DIR = \", len(os.listdir('/tmp/cats-v-dogs/testing/dogs')))\n \n pass",
"3\nCAT_SOURCE_DIR = 1500\nDOG_SOURCE_DIR = 1500\nDone\n/tmp/cats-v-dogs/ -> 2\nTRAINING_CATS_DIR = 0\nTESTING_CATS_DIR = 0\nTRAINING_DOGS_DIR = 0\nTESTING_DOGS_DIR = 0\n"
],
[
"CAT_SOURCE_DIR = \"/tmp/PetImages/Cat/\"\nTRAINING_CATS_DIR = \"/tmp/cats-v-dogs/training/cats/\"\nTESTING_CATS_DIR = \"/tmp/cats-v-dogs/testing/cats/\"\n\nDOG_SOURCE_DIR = \"/tmp/PetImages/Dog/\"\nTRAINING_DOGS_DIR = \"/tmp/cats-v-dogs/training/dogs/\"\nTESTING_DOGS_DIR = \"/tmp/cats-v-dogs/testing/dogs/\"\n# Write a python function called split_data which takes\n# a SOURCE directory containing the files\n# a TRAINING directory that a portion of the files will be copied to\n# a TESTING directory that a portion of the files will be copie to\n# a SPLIT SIZE to determine the portion\n# The files should also be randomized, so that the training set is a random\n# X% of the files, and the test set is the remaining files\n# SO, for example, if SOURCE is PetImages/Cat, and SPLIT SIZE is .9\n# Then 90% of the images in PetImages/Cat will be copied to the TRAINING dir\n# and 10% of the images will be copied to the TESTING dir\n# Also -- All images should be checked, and if they have a zero file length,\n# they will not be copied over\n#\n# os.listdir(DIRECTORY) gives you a listing of the contents of that directory\n# os.path.getsize(PATH) gives you the size of the file\n# copyfile(source, destination) copies a file from source to destination\n# random.sample(list, len(list)) shuffles a list\n\ndef split_data(SOURCE, TRAINING, TESTING, SPLIT_SIZE):\n good_images = []\n for filename in os.listdir(SOURCE):\n if os.path.getsize(SOURCE + filename) > 0:\n good_images.append(filename)\n\n num_train = int(len(good_images) * SPLIT_SIZE)\n num_test = int(len(good_images) - num_train)\n \n good_images = random.sample(good_images, len(good_images))\n \n train_list = good_images[0:num_train]\n test_list = good_images[ :num_test ]\n\n for filename in train_list:\n copyfile(SOURCE + filename, TRAINING + filename)\n\n for filename in test_list:\n copyfile(SOURCE + filename, TESTING + filename)\n\n\n\nsplit_size = .9\nsplit_data(CAT_SOURCE_DIR, TRAINING_CATS_DIR, TESTING_CATS_DIR, split_size)\nsplit_data(DOG_SOURCE_DIR, TRAINING_DOGS_DIR, TESTING_DOGS_DIR, split_size)",
"_____no_output_____"
],
[
"print(len(os.listdir('/tmp/cats-v-dogs/training/cats/')))\nprint(len(os.listdir('/tmp/cats-v-dogs/training/dogs/')))\nprint(len(os.listdir('/tmp/cats-v-dogs/testing/cats/')))\nprint(len(os.listdir('/tmp/cats-v-dogs/testing/dogs/')))\n\n# Expected output:\n# 1350\n# 1350\n# 150\n# 150",
"1350\n1350\n150\n150\n"
],
[
"# DEFINE A KERAS MODEL TO CLASSIFY CATS V DOGS\n# USE AT LEAST 3 CONVOLUTION LAYERS\nmodel = tf.keras.models.Sequential([\n # Note the input shape is the desired size of the image 150x150 with 3 bytes color\n tf.keras.layers.Conv2D(16, (3,3), activation='relu', input_shape=(150, 150, 3)),\n tf.keras.layers.MaxPooling2D(2,2),\n \n tf.keras.layers.Conv2D(32, (3,3), activation='relu'),\n tf.keras.layers.MaxPooling2D(2,2), \n \n tf.keras.layers.Conv2D(64, (3,3), activation='relu'), \n tf.keras.layers.MaxPooling2D(2,2),\n \n # Flatten the results to feed into a DNN\n tf.keras.layers.Flatten(), \n # 512 neuron hidden layer\n tf.keras.layers.Dense(512, activation='relu'), \n # Only 1 output neuron. It will contain a value from 0-1 where 0 for 1 class ('cats') and 1 for the other ('dogs')\n tf.keras.layers.Dense(1, activation='sigmoid') \n \n])\n\nmodel.compile(optimizer=RMSprop(lr=0.001), loss='binary_crossentropy', metrics=['acc'])\nmodel.summary()",
"Model: \"sequential_1\"\n_________________________________________________________________\nLayer (type) Output Shape Param # \n=================================================================\nconv2d_3 (Conv2D) (None, 148, 148, 16) 448 \n_________________________________________________________________\nmax_pooling2d_3 (MaxPooling2 (None, 74, 74, 16) 0 \n_________________________________________________________________\nconv2d_4 (Conv2D) (None, 72, 72, 32) 4640 \n_________________________________________________________________\nmax_pooling2d_4 (MaxPooling2 (None, 36, 36, 32) 0 \n_________________________________________________________________\nconv2d_5 (Conv2D) (None, 34, 34, 64) 18496 \n_________________________________________________________________\nmax_pooling2d_5 (MaxPooling2 (None, 17, 17, 64) 0 \n_________________________________________________________________\nflatten_1 (Flatten) (None, 18496) 0 \n_________________________________________________________________\ndense_2 (Dense) (None, 512) 9470464 \n_________________________________________________________________\ndense_3 (Dense) (None, 1) 513 \n=================================================================\nTotal params: 9,494,561\nTrainable params: 9,494,561\nNon-trainable params: 0\n_________________________________________________________________\n"
]
],
[
[
"# NOTE:\n\nIn the cell below you **MUST** use a batch size of 10 (`batch_size=10`) for the `train_generator` and the `validation_generator`. Using a batch size greater than 10 will exceed memory limits on the Coursera platform.",
"_____no_output_____"
]
],
[
[
"TRAINING_DIR = '/tmp/cats-v-dogs/training/'\ntrain_datagen = ImageDataGenerator( rescale = 1.0/255. )\n\n# NOTE: YOU MUST USE A BATCH SIZE OF 10 (batch_size=10) FOR THE \n# TRAIN GENERATOR.\ntrain_generator = train_datagen.flow_from_directory(TRAINING_DIR,\n batch_size=10,\n class_mode='binary',\n target_size=(150, 150)) \n\nVALIDATION_DIR = '/tmp/cats-v-dogs/testing/'\nvalidation_datagen = ImageDataGenerator( rescale = 1.0/255. )\n\n# NOTE: YOU MUST USE A BACTH SIZE OF 10 (batch_size=10) FOR THE \n# VALIDATION GENERATOR.\nvalidation_generator = validation_datagen.flow_from_directory(VALIDATION_DIR,\n batch_size=10,\n class_mode='binary',\n target_size=(150, 150)) \n\n\n\n# Expected Output:\n# Found 2700 images belonging to 2 classes.\n# Found 300 images belonging to 2 classes.",
"Found 2700 images belonging to 2 classes.\nFound 300 images belonging to 2 classes.\n"
],
[
"history = model.fit_generator(train_generator,\n epochs=2,\n verbose=1,\n validation_data=validation_generator)\n",
"Epoch 1/2\n270/270 [==============================] - 37s 136ms/step - loss: 3.9129 - acc: 0.5211 - val_loss: 0.7052 - val_acc: 0.5433\nEpoch 2/2\n270/270 [==============================] - 33s 123ms/step - loss: 0.6467 - acc: 0.6430 - val_loss: 0.5377 - val_acc: 0.7300\n"
],
[
"# PLOT LOSS AND ACCURACY\n%matplotlib inline\n\nimport matplotlib.image as mpimg\nimport matplotlib.pyplot as plt\n\n#-----------------------------------------------------------\n# Retrieve a list of list results on training and test data\n# sets for each training epoch\n#-----------------------------------------------------------\nacc=history.history['acc']\nval_acc=history.history['val_acc']\nloss=history.history['loss']\nval_loss=history.history['val_loss']\n\nepochs=range(len(acc)) # Get number of epochs\n\n#------------------------------------------------\n# Plot training and validation accuracy per epoch\n#------------------------------------------------\nplt.plot(epochs, acc, 'r', \"Training Accuracy\")\nplt.plot(epochs, val_acc, 'b', \"Validation Accuracy\")\nplt.title('Training and validation accuracy')\nplt.figure()\n\n#------------------------------------------------\n# Plot training and validation loss per epoch\n#------------------------------------------------\nplt.plot(epochs, loss, 'r', \"Training Loss\")\nplt.plot(epochs, val_loss, 'b', \"Validation Loss\")\n\n\nplt.title('Training and validation loss')\n\n# Desired output. Charts with training and validation metrics. No crash :)",
"_____no_output_____"
]
],
[
[
"# Submission Instructions",
"_____no_output_____"
]
],
[
[
"# Now click the 'Submit Assignment' button above.",
"_____no_output_____"
]
],
[
[
"# When you're done or would like to take a break, please run the two cells below to save your work and close the Notebook. This will free up resources for your fellow learners. ",
"_____no_output_____"
]
],
[
[
"%%javascript\n<!-- Save the notebook -->\nIPython.notebook.save_checkpoint();",
"_____no_output_____"
],
[
"%%javascript\nIPython.notebook.session.delete();\nwindow.onbeforeunload = null\nsetTimeout(function() { window.close(); }, 1000);",
"_____no_output_____"
]
]
] | [
"code",
"markdown",
"code",
"markdown",
"code",
"markdown",
"code"
] | [
[
"code",
"code",
"code",
"code",
"code",
"code",
"code"
],
[
"markdown"
],
[
"code",
"code",
"code"
],
[
"markdown"
],
[
"code"
],
[
"markdown"
],
[
"code",
"code"
]
] |
e7852a2b83968059bee7e40f3417ca368f32ae60 | 3,597 | ipynb | Jupyter Notebook | notebooks/read_prepare_data.ipynb | uw-edsi/cnns4qspr | 409238e581f16efe7731c15c7eb022eee37d219b | [
"MIT"
] | 2 | 2021-04-05T17:28:23.000Z | 2021-04-06T17:52:31.000Z | notebooks/read_prepare_data.ipynb | uw-edsi/cnns4qspr | 409238e581f16efe7731c15c7eb022eee37d219b | [
"MIT"
] | null | null | null | notebooks/read_prepare_data.ipynb | uw-edsi/cnns4qspr | 409238e581f16efe7731c15c7eb022eee37d219b | [
"MIT"
] | null | null | null | 23.057692 | 107 | 0.495413 | [
[
[
"from silx.io.dictdump import h5todict\nimport os\nimport numpy as np\n#vox = h5todict(name + \".h5\", path='.')\n#vox = h5todict('/Users/prguser/Documents/cnns4qspr_trial/notebooks/test-output/1a4r.h5')\n",
"_____no_output_____"
],
[
"channels = ['all_C', 'all_O', 'all_N', 'acidic', 'basic', 'polar', 'nonpolar',\\\n 'charged', 'amphipathic','hydrophobic', 'aromatic', 'acceptor', 'donor',\\\n 'ring', 'hyb', 'heavyvalence', 'heterovalence', 'partialcharge','protein', 'ligand']",
"_____no_output_____"
],
[
"path = '/Users/prguser/Documents/cnns4qspr_trial/notebooks/small_set/'\n\ndef convert_h5_data(file_path, channels_considered):\n\n file_names = os.listdir(file_path)\n data_for_cnn = []\n target = []\n\n for file in file_names:\n input_file = os.path.join(file_path, file)\n vox = h5todict(input_file)\n data_structure = []\n for channel in channels_considered:\n data_structure.append(vox[channel])\n data_for_cnn.append(data_structure)\n target.append(vox['affinity'])\n\n return np.array(data_for_cnn), np.array(target)",
"_____no_output_____"
],
[
"input_data, affinity = convert_h5_data(path, channels)\n",
"_____no_output_____"
],
[
"from sys import getsizeof\ngetsizeof(input_data)",
"_____no_output_____"
],
[
"affinity",
"_____no_output_____"
],
[
"input_data.shape[1]",
"_____no_output_____"
]
]
] | [
"code"
] | [
[
"code",
"code",
"code",
"code",
"code",
"code",
"code"
]
] |
e785321198b84928e3b60f1d5cbe7e8019a60785 | 200,133 | ipynb | Jupyter Notebook | notebooks/plotTrainingGraphs.ipynb | CleonWong/Can-You-Find-The-Tumour | 2376238e97c5ac7396fca157fe14e1a51c859f06 | [
"MIT"
] | 23 | 2021-02-25T13:59:13.000Z | 2022-03-18T17:41:57.000Z | notebooks/plotTrainingGraphs.ipynb | CleonWong/Can-You-Find-The-Tumour | 2376238e97c5ac7396fca157fe14e1a51c859f06 | [
"MIT"
] | 2 | 2021-09-13T14:43:54.000Z | 2022-02-03T23:54:21.000Z | notebooks/plotTrainingGraphs.ipynb | CleonWong/Can-You-Find-The-Tumour | 2376238e97c5ac7396fca157fe14e1a51c859f06 | [
"MIT"
] | 8 | 2021-07-19T12:23:00.000Z | 2022-03-18T17:42:05.000Z | 159.978417 | 47,000 | 0.836394 | [
[
[
"import pandas as pd\nimport numpy as np\nimport matplotlib.pyplot as plt\nfrom matplotlib.ticker import MultipleLocator\nimport os",
"_____no_output_____"
]
],
[
[
"# Helper function to plot",
"_____no_output_____"
]
],
[
[
"def plot_graph(axis_title, x, y_train, y_val, xlabel, ylabel, xtick_range, ytick_range, save_path=None):\n\n fig, ax = plt.subplots(nrows=1, ncols=1, figsize=(9, 6))\n\n line1 = ax.plot(x, y_train, color=\"blue\", label=\"train\")\n line2 = ax.plot(x, y_val, color=\"red\", label=\"val\")\n\n # Nicer visuals.\n ax.set_title(axis_title)\n ax.spines['right'].set_visible(False)\n ax.spines['top'].set_visible(False)\n ax.grid(b=True, which=\"major\", axis=\"both\", color=\"#d3d3d3\", linestyle=\"-\")\n ax.grid(b=True, which=\"minor\", axis=\"both\", color=\"#e7e7e7\", linestyle=\"dashed\")\n ax.set_xlabel(xlabel)\n ax.set_ylabel(ylabel)\n ax.xaxis.set_ticks(np.arange(xtick_range[0], xtick_range[1], xtick_range[2]))\n ax.xaxis.set_minor_locator(MultipleLocator(5))\n ax.set_xlim(left=0)\n ax.yaxis.set_ticks(np.arange(ytick_range[0], ytick_range[1], ytick_range[2]))\n ax.yaxis.set_minor_locator(MultipleLocator(0.01))\n ax.patch.set_alpha(0)\n \n handles, labels = ax.get_legend_handles_labels()\n ax.legend(handles=handles, labels=labels, loc=\"best\")\n \n # Save graph\n plt.tight_layout()\n if save_path:\n plt.savefig(fname=save_path, dpi=300)",
"_____no_output_____"
]
],
[
[
"# Batch-size = 64",
"_____no_output_____"
]
],
[
[
"df_64 = pd.read_csv(\"../results/fit/20201203_040325___INSTANCE/csv_logger/csv_logger.csv\")",
"_____no_output_____"
],
[
"df_64.head()",
"_____no_output_____"
],
[
"plot_graph(axis_title=\"Batch Size = 64\",\n x=df_64[\"epoch\"],\n y_train=df_64[\"loss\"],\n y_val=df_64[\"val_loss\"],\n xlabel=\"Epoch\",\n ylabel=\"Binary crossentropy loss\",\n xtick_range=(0, 50, 10),\n ytick_range=(0, 0.16, 0.02),\n save_path=\"../results/fit/20201203_040325___INSTANCE/csv_logger/loss.png\")",
"_____no_output_____"
],
[
"plot_graph(axis_title=\"Batch Size = 64\",\n x=df_64[\"epoch\"],\n y_train=df_64[\"iouMetric\"],\n y_val=df_64[\"val_iouMetric\"],\n xlabel=\"Epoch\",\n ylabel=\"iouMetric\",\n xtick_range=(0, 50, 10),\n ytick_range=(0, 0.26, 0.02),\n save_path=\"../results/fit/20201203_040325___INSTANCE/csv_logger/iouMetric.png\")",
"_____no_output_____"
]
],
[
[
"# Batch-size = 10",
"_____no_output_____"
]
],
[
[
"df_10 = pd.read_csv(\"../results/fit/20201203_013807___INSTANCE/csv_logger/csv_logger.csv\")",
"_____no_output_____"
],
[
"df_10.head()",
"_____no_output_____"
],
[
"plot_graph(axis_title=\"Batch Size = 10\",\n x=df_10[\"epoch\"],\n y_train=df_10[\"loss\"],\n y_val=df_10[\"val_loss\"],\n xlabel=\"Epoch\",\n ylabel=\"Binary crossentropy loss\",\n xtick_range=(0, 50, 10),\n ytick_range=(0, 0.16, 0.02),\n save_path=\"../results/fit/20201203_013807___INSTANCE/csv_logger/loss.png\")",
"_____no_output_____"
],
[
"plot_graph(axis_title=\"Batch Size = 10\",\n x=df_10[\"epoch\"],\n y_train=df_10[\"iouMetric\"],\n y_val=df_10[\"val_iouMetric\"],\n xlabel=\"Epoch\",\n ylabel=\"iouMetric\",\n xtick_range=(0, 50, 10),\n ytick_range=(0, 0.26, 0.02),\n save_path=\"../results/fit/20201203_013807___INSTANCE/csv_logger/iouMetric.png\")",
"_____no_output_____"
]
],
[
[
"---\n\n# Combine .csv",
"_____no_output_____"
]
],
[
[
"mass_train_df = pd.read_csv(\"../data/raw_data/csv-description-updated/Mass-Training-Description-UPDATED.csv\")\nmass_test_df = pd.read_csv(\"../data/raw_data/csv-description-updated/Mass-Test-Description-UPDATED.csv\")\n\nmass_df = pd.concat([mass_train_df, mass_test_df])\n\nmass_df",
"_____no_output_____"
],
[
"# Create identifier column.\nmass_df.insert(loc=0, column=\"identifier\", value=np.nan)\nmass_df[\"identifier\"] = mass_df.apply(lambda x: \"_\".join([x[\"patient_id\"], x[\"left_or_right_breast\"], x[\"image_view\"]]), axis=1)\n\n# Drop filepath columns, they are useless because the filepaths always change.\nmass_df.drop([\"image_file_path\", \"cropped_image_file_path\", \"ROI_mask_file_path\", \"full_path\", \"mask_path\", \"crop_path\"], axis=1, inplace=True)\n\n# Sort by identifier column.\nmass_df.sort_values(by=[\"identifier\"])",
"_____no_output_____"
],
[
"mass_df.to_csv(\"../data/csv/Mass_all.csv\", index=False)",
"_____no_output_____"
],
[
"# Get list of test and train image identifiers.\ntrain_identifiers = []\ntest_identifiers = []\n\ntrain_path = \"../data/preprocessed/Mass/Train_FULL\"\ntest_path = \"../data/preprocessed/Mass/Test_FULL\"\n\n# Train images.\nfor curdir, dirs, files in os.walk(train_path):\n \n files.sort()\n \n for f in files:\n if f.endswith(\".png\"):\n f = f.replace(\"_FULL___PRE.png\", \"\")\n train_identifiers.append(f)\n \n# Test images.\nfor curdir, dirs, files in os.walk(test_path):\n \n files.sort()\n \n for f in files:\n if f.endswith(\".png\"):\n f = f.replace(\"_FULL___PRE.png\", \"\")\n test_identifiers.append(f)\n \nprint(len(train_identifiers))\nprint(train_identifiers[:5])\nprint(len(test_identifiers))\nprint(test_identifiers[:5])\n\n# Create dataframe for train images.\nmass_train_df_new = mass_df[mass_df[\"identifier\"].isin(train_identifiers)]\n\n# Create dataframe for test images.\nmass_test_df_new = mass_df[mass_df[\"identifier\"].isin(test_identifiers)]",
"1433\n['P_00001_LEFT_CC', 'P_00004_LEFT_CC', 'P_00004_LEFT_MLO', 'P_00009_RIGHT_CC', 'P_00009_RIGHT_MLO']\n159\n['P_00001_LEFT_MLO', 'P_00004_RIGHT_MLO', 'P_00018_RIGHT_CC', 'P_00021_RIGHT_CC', 'P_00023_RIGHT_CC']\n"
],
[
"print(mass_train_df_new.shape)\nprint(mass_test_df_new.shape)",
"(1528, 12)\n(168, 12)\n"
],
[
"mass_train_df_new.to_csv(\"../data/csv/Mass_train.csv\", index=False)\nmass_test_df_new.to_csv(\"../data/csv/Mass_test.csv\", index=False)",
"_____no_output_____"
]
]
] | [
"code",
"markdown",
"code",
"markdown",
"code",
"markdown",
"code",
"markdown",
"code"
] | [
[
"code"
],
[
"markdown"
],
[
"code"
],
[
"markdown"
],
[
"code",
"code",
"code",
"code"
],
[
"markdown"
],
[
"code",
"code",
"code",
"code"
],
[
"markdown"
],
[
"code",
"code",
"code",
"code",
"code",
"code"
]
] |
e78533849d487aacb74d4d0b6d6ceefdc3b6d56e | 8,732 | ipynb | Jupyter Notebook | 01_gene_train_dataset/discard/gandatamask3_test.ipynb | tony92151/pedestrian_generator | 7c01594a32f271687a49c89dec396b89775bec32 | [
"Apache-2.0"
] | null | null | null | 01_gene_train_dataset/discard/gandatamask3_test.ipynb | tony92151/pedestrian_generator | 7c01594a32f271687a49c89dec396b89775bec32 | [
"Apache-2.0"
] | null | null | null | 01_gene_train_dataset/discard/gandatamask3_test.ipynb | tony92151/pedestrian_generator | 7c01594a32f271687a49c89dec396b89775bec32 | [
"Apache-2.0"
] | 2 | 2020-03-31T03:17:52.000Z | 2020-08-18T15:09:58.000Z | 30.638596 | 142 | 0.529432 | [
[
[
"import matplotlib.pyplot as plt\nfrom PIL import Image\nimport numpy as np\nimport json\nimport os\nimport random\nimport glob\n#from detectron_pro import detectron_mask_img,detectron_mask_img_composite\nimport shutil\nimport cv2",
"_____no_output_____"
],
[
"\n# to get location that stickimg will sticked on jpg_dir center or random\ndef center_location(jpg_dir,stickimg_dir):\n im = np.array(Image.open(jpg_dir), dtype=np.uint8)\n x_center,y_center = im.shape[1]/2,im.shape[0]/2\n im_stick = np.array(Image.open(stickimg_dir), dtype=np.uint8)\n im_stick_shape = im_stick.shape\n bd_box_x,bd_box_y = x_center-(im_stick_shape[1]/2),y_center-(im_stick_shape[0]/2)\n bd_box_length,bd_box_height =im_stick_shape[1],im_stick_shape[0]\n return bd_box_x,bd_box_y,bd_box_length,bd_box_height\n\ndef random_location(jpg_dir,stickimg_dir):\n im = np.array(Image.open(jpg_dir), dtype=np.uint8)\n # x boundary\n rangeX = 128\n x_left_bound,x_right_bound = rangeX, im.shape[1]-rangeX\n x_center = random.randrange(x_left_bound, x_right_bound)\n seq = [350,300,250,200]\n y_center = random.choice(seq)\n i_shape1 = [128,64]\n i_shape2 = [128*7/8,64*7/8]\n i_shape3 = [128*3/4,64*3/4]\n i_shape4 = [128*2/4,64*2/4]\n dict = {350:i_shape1,300:i_shape2 ,250:i_shape3,200:i_shape4}\n image_shape = dict[y_center]\n bd_box_x,bd_box_y = x_center-(image_shape[1]/2),y_center-(image_shape[0]/2)\n bd_box_length,bd_box_height =image_shape[1],image_shape[0]\n return bd_box_x,bd_box_y,bd_box_length,bd_box_height",
"_____no_output_____"
],
[
"#it can generate json file defined by your street_jpg and people_jpg \n\ndef create_json_file(jpg_dir,street_json,stickimg_dir,results_dir,function='center'):\n if function == 'center':\n bd_box_x,bd_box_y,bd_box_length,bd_box_height = center_location(jpg_dir,stickimg_dir)\n elif function == 'random':\n bd_box_x,bd_box_y,bd_box_length,bd_box_height = random_location(jpg_dir,stickimg_dir)\n \n input_file = open (street_json)\n json_array = json.load(input_file)\n \n data = []\n data.append({\n 'end':0,\n 'hide':0,\n 'id':0,\n 'init':0,\n 'lbl':\"person\",\n 'lock':0,\n 'occl':0,\n 'pos':[\n bd_box_x,\n bd_box_y,\n bd_box_length,\n bd_box_height], \n 'posv':[\n 0,\n 0,\n 0,\n 0],\n 'str':0\n })\n \n if json_array != []:\n for item in json_array:\n data.append(item)\n \n with open(results_dir, 'w') as outfile:\n json.dump(data, outfile)",
"_____no_output_____"
]
],
[
[
"# Start create",
"_____no_output_____"
]
],
[
[
"# Image read dir\nstreet_dir = '/root/notebooks/0858611-2/final_project/caltech_pedestrian_extractor/video_extractor/*'\n\npeople_dir = '/root/notebooks/0858611-2/final_project/caltech_pedestrian_extractor/js_on_image/people_img/Market-1501-v15.09.15'\n\n# Image save dir\nsave_dir = '/root/notebooks/0858611-2/final_project/caltech_pedestrian_extractor/0603_result'\n\nnum_imgs = 10",
"_____no_output_____"
],
[
"# Check dir folder exit\n# If not, create one\nif os.path.exists(save_dir) == False:\n os.makedirs(save_dir)\n\nfor s in ['people', 'mask', 'street', 'street_json','json']:\n if os.path.exists(os.path.join(save_dir, s)) == False:\n os.makedirs(os.path.join(save_dir, s))",
"_____no_output_____"
],
[
"street_imgs = glob.glob(street_dir+'/**/*.jpg', recursive=True)\n\n#street_imgs = random.shuffle(random.sample(street_imgs, 5000))\nstreet_imgs = random.sample(street_imgs, num_imgs)\n\nrandom.shuffle(street_imgs)",
"_____no_output_____"
],
[
"people_imgs = glob.glob(people_dir+'/bounding_box_train/*.jpg', recursive=True)\n\npeople_imgs = random.sample(people_imgs, num_imgs)\n\nrandom.shuffle(people_imgs)",
"_____no_output_____"
],
[
"for i in range(num_imgs):\n \n if (i%100==0):\n print(\"Process (\",i,\"/\",num_imgs,\") \",\"{:.2f}\".format(100*i/num_imgs),\" %\")\n \n # create mask and save\n try:\n mask_img = detectron_mask_img(people_imgs[i],(64,128))\n mask_img = Image.fromarray(mask_img)\n except Exception as e:\n print(\"Skip image :\",i)\n continue\n \n mask_img.save(save_dir+'/mask/'+str('{0:06}'.format(i))+'.jpg')\n \n # save street img\n street_img = cv2.imread(street_imgs[i])\n street_img = cv2.resize(street_img,(640,480))\n cv2.imwrite(save_dir+'/street/'+str('{0:06}'.format(i))+'.jpg', street_img)\n \n ################################################################\n img_path = street_imgs[i]\n json_dir = img_path.replace('images', 'annotations')\n json_dir = json_dir.replace('jpg', 'json')\n shutil.copyfile(json_dir, save_dir+'/street_json/'+str('{0:06}'.format(count))+'.json')\n ################################################################\n \n # save poeple img\n people_img = cv2.imread(people_imgs[i])\n people_img = cv2.resize(people_img,(64,128))\n cv2.imwrite(save_dir+'/people/'+str('{0:06}'.format(i))+'.jpg', people_img)\n \n # create json file and save\n create_json_file(save_dir+'/street/'+str('{0:06}'.format(i))+'.jpg',\n save_dir+'/street_json/'+str('{0:06}'.format(i))+'.json',\n save_dir+'/people/'+str('{0:06}'.format(i))+'.jpg',\n save_dir+'/json/'+str('{0:06}'.format(i))+'.json',\n function=\"random\")",
"_____no_output_____"
],
[
"import json\n\njson_path = '/root/notebooks/0858611-2/final_project/caltech_pedestrian_extractor/video_extractor/set00/V009/annotations/I00018.json'\n\ninput_file = open (json_path)\njson_array = json.load(input_file)",
"_____no_output_____"
],
[
"json_array\nprint(type(json_array[0]))",
"_____no_output_____"
]
]
] | [
"code",
"markdown",
"code"
] | [
[
"code",
"code",
"code"
],
[
"markdown"
],
[
"code",
"code",
"code",
"code",
"code",
"code",
"code"
]
] |
e785630b91212928e6e34374897742b5bf288236 | 163,828 | ipynb | Jupyter Notebook | day2_visualation.ipynb | klaruszek/dw_matrix_car | 38b2c3441b283ae93d6992a89e3819b3d10638a0 | [
"MIT"
] | null | null | null | day2_visualation.ipynb | klaruszek/dw_matrix_car | 38b2c3441b283ae93d6992a89e3819b3d10638a0 | [
"MIT"
] | null | null | null | day2_visualation.ipynb | klaruszek/dw_matrix_car | 38b2c3441b283ae93d6992a89e3819b3d10638a0 | [
"MIT"
] | null | null | null | 163,828 | 163,828 | 0.928394 | [
[
[
"import pandas as pd\nimport numpy as np\nimport matplotlib.pyplot as plt\nimport seaborn as sns",
"_____no_output_____"
],
[
"cd \"/content/drive/My Drive/Colab Notebook/dw_matrix/matrix_2/dw_matrix_car\"",
"/content/drive/My Drive/Colab Notebook/dw_matrix/matrix_2/dw_matrix_car\n"
],
[
"ls data/car.h5",
"data/car.h5\n"
],
[
"!pip install --upgrade tables #instalacja tables",
"Collecting tables\n\u001b[?25l Downloading https://files.pythonhosted.org/packages/ed/c3/8fd9e3bb21872f9d69eb93b3014c86479864cca94e625fd03713ccacec80/tables-3.6.1-cp36-cp36m-manylinux1_x86_64.whl (4.3MB)\n\u001b[K |████████████████████████████████| 4.3MB 4.7MB/s \n\u001b[?25hRequirement already satisfied, skipping upgrade: numexpr>=2.6.2 in /usr/local/lib/python3.6/dist-packages (from tables) (2.7.1)\nRequirement already satisfied, skipping upgrade: numpy>=1.9.3 in /usr/local/lib/python3.6/dist-packages (from tables) (1.17.5)\nInstalling collected packages: tables\n Found existing installation: tables 3.4.4\n Uninstalling tables-3.4.4:\n Successfully uninstalled tables-3.4.4\nSuccessfully installed tables-3.6.1\n"
],
[
"df = pd.read_hdf('data/car.h5')\ndf.shape\n",
"_____no_output_____"
],
[
"df.columns.values",
"_____no_output_____"
],
[
"df['price_value'].hist(bins=100);",
"_____no_output_____"
],
[
"df['price_value'].max() #cena max samochodu",
"_____no_output_____"
],
[
"df['price_value'].describe()",
"_____no_output_____"
],
[
"df['param_marka-pojazdu'].unique() #unikalne wartości",
"_____no_output_____"
],
[
"df.groupby('param_marka-pojazdu')['price_value'].mean()",
"_____no_output_____"
],
[
"df.groupby('param_marka-pojazdu')['price_value'].agg(np.mean).plot(kind='bar')",
"_____no_output_____"
],
[
"def group_and_barplot(feat_groupby, feat_agg='price_value', agg_funcs=[np.mean, np.median, np.size], feat_sort='mean', top=50, subplots=True):\n return(\n df\n .groupby(feat_groupby)[feat_agg]\n .agg(agg_funcs)\n .sort_values(by = feat_sort, ascending=False) #sortowanie wartości (odwrócenie początku)\n .head(top) #pozostawienie 50 początkowych\n\n ).plot(kind='bar',figsize=(15,5), subplots=subplots)",
"_____no_output_____"
],
[
"group_and_barplot('param_marka-pojazdu');",
"_____no_output_____"
],
[
"group_and_barplot('param_kraj-pochodzenia', feat_sort='size');",
"_____no_output_____"
],
[
"group_and_barplot('param_kolor', feat_sort='mean');",
"_____no_output_____"
],
[
"",
"_____no_output_____"
]
]
] | [
"code"
] | [
[
"code",
"code",
"code",
"code",
"code",
"code",
"code",
"code",
"code",
"code",
"code",
"code",
"code",
"code",
"code",
"code",
"code"
]
] |
e78563e32b5c98ea3fe6918e0da64ce7cff635d9 | 57,697 | ipynb | Jupyter Notebook | __Project Files/.ipynb_checkpoints/Data Cleaning_merge all data together_backup-checkpoint.ipynb | joannasys/Predictions-of-ICU-Mortality | a138139d944f127f25e0ce5f153800e40a8da27e | [
"CC-BY-3.0"
] | null | null | null | __Project Files/.ipynb_checkpoints/Data Cleaning_merge all data together_backup-checkpoint.ipynb | joannasys/Predictions-of-ICU-Mortality | a138139d944f127f25e0ce5f153800e40a8da27e | [
"CC-BY-3.0"
] | null | null | null | __Project Files/.ipynb_checkpoints/Data Cleaning_merge all data together_backup-checkpoint.ipynb | joannasys/Predictions-of-ICU-Mortality | a138139d944f127f25e0ce5f153800e40a8da27e | [
"CC-BY-3.0"
] | 1 | 2020-12-30T16:49:25.000Z | 2020-12-30T16:49:25.000Z | 32.359506 | 982 | 0.401095 | [
[
[
"### Import Packages",
"_____no_output_____"
]
],
[
[
"# Import packages\n\nimport glob\nimport csv\nimport pandas as pd\nimport numpy as np\nfrom sqlalchemy import create_engine\nimport psycopg2\n",
"_____no_output_____"
]
],
[
[
"### Append each .txt file into a DataFrame\nEach txt file is a row",
"_____no_output_____"
]
],
[
[
"# Iterate through each file name\n\nmain = pd.DataFrame()\n\nfor filename in glob.iglob('./training_set_a/*.txt'):\n \n # Open each file as data\n with open(filename) as inputfile:\n \n data = list(csv.reader(inputfile)) # list of list\n data = pd.DataFrame(data[1:],columns=data[0]) # Convert list of list to DataFrame\n data.Value = data.Value.astype(float) # Change Value to float\n \n \n \n # Pivot_table to convert from long to wide dataset\n\n # Creation of new features - aggregate across the time series to find mean, min, max values\n # mean is chosen rather than median because we want to take into the account of 'outlier values'\n\n wide_data = pd.pivot_table(data,values=['Value'],columns='Parameter',aggfunc=[np.mean,np.min,np.max])\n wide_data.columns = wide_data.columns.droplevel(level=0)\n \n \n \n # rename new columns & lower capitalise\n new_columns = []\n\n for ind, col in enumerate(wide_data.columns):\n \n if ind < wide_data.columns.shape[0]/3:\n col = 'mean_'+col \n new_columns.append(col)\n\n elif ind >= wide_data.columns.shape[0]/3 and ind < 2*wide_data.columns.shape[0]/3:\n col = 'min_'+col\n new_columns.append(col)\n\n else:\n col = 'max_'+col\n new_columns.append(col)\n \n wide_data.columns = new_columns\n wide_data.columns = wide_data.columns.str.lower()\n \n \n # rename descriptor row\n wide_data.rename(columns={'mean_age':'age','mean_gender':'gender','mean_height':'height',\n 'mean_icutype':'icutype','mean_recordid':'recordid'},inplace=True)\n \n # drop min/max descriptor rows\n wide_data.drop(['min_age','max_age','min_gender','max_gender','min_height','max_height',\n 'min_icutype','max_icutype','min_recordid','max_recordid'],axis=1,inplace=True)\n \n # set recordid as index\n wide_data.set_index(['recordid'],inplace = True)\n \n main = main.append(wide_data)",
"_____no_output_____"
],
[
"# Open set a outcomes file as dataframe\nwith open('training_outcomes_a.txt') as outcomesfile:\n \n label = list(csv.reader(outcomesfile)) # list of list\n label = pd.DataFrame(label[1:],columns=label[0]) # Convert list of list to DataFrame\n \n label = label.astype(float) # Change all values to float\n label.columns = label.columns.str.lower() # Change all column to lowercase\n \n \n label.set_index(['recordid'],inplace = True) # set recordid as index",
"_____no_output_____"
],
[
"# merge main data and label data\nmortality = main.merge(label,how='outer',left_index=True,right_index=True)",
"_____no_output_____"
],
[
"mortality.head(5)",
"_____no_output_____"
]
],
[
[
"RecordID (a unique integer for each ICU stay)\n\nAge (years)<br>\nGender (0: female, or 1: male)<br>\nHeight (cm)<br>\nICUType (1: Coronary Care Unit, 2: Cardiac Surgery Recovery Unit, 3: Medical ICU, or 4: Surgical ICU)<br>\nWeight (kg)",
"_____no_output_____"
],
[
"Variables Description \n\nALB Albumin (g/dL) <br>\nALP Alkaline phosphatase (IU/L) <br>\nALT Alanine transaminase (IU/L) <br>\nAST Aspartate transaminase (IU/L) <br>\nBIL Bilirubin (mg/dL) <br>\nBUN Blood urea nitrogen (mg/dL) <br>\nCHO Cholesterol (mg/dL) <br>\nCREA Serum creatinine (mg/dL) <br>\nDBP Invasive diastolic arterial blood pressure (mmHg) <br>\nFIO Fractional inspired O2 (0-1) <br>\nGCS Glasgow Coma Score (3-15) <br>\nGLU Serum glucose (mg/dL) <br>\nHCO Serum bicarbonate (mmol/L) <br> \nHCT Hematocrit (%) <br>\nHR Heart rate (bpm) <br>\nK Serum potassium (mEq/L) <br>\nLAC Lactate (mmol/L) <br>\nMG Serum magnesium (mmol/L) <br>\nMAP Invasive mean arterial blood pressure (mmHg) <br>\nMEVE Mechanical ventilation respiration <br>\nNA Serum sodium (mEq/L) <br>\nNBP Non-invasive diastolic arterial blood pressure (mmHg) <br>\nNAP Non-invasive mean arterial blood pressure (mmHg) <br>\nNSP Non-invasive systolic arterial blood pressure (mmHg) <br>\nPCO partial pressure of arterial <br>\nCO2 (mmHg) <br>\nPO2 Partial pressure of arterial <br>\nO2 (mmHg) <br>\nPH Arterial pH (0-14) <br>\nPLA cells/nL RRA Respiration rate (bpm) <br>\nSO2 O2 saturation in hemoglobin (%) <br>\nSBP Invasive systolic arterial blood pressure (mmHg) <br>\nTEM Temperature (°C) <br>\nTRI Troponin-I (μg/L) <br>\nTRT Troponin-T (μg/L) <br>\nURI Urine output (mL) <br>\nWBC White blood cell count (cells/nL) <br>\nWEI kg <br>",
"_____no_output_____"
]
],
[
[
"# Open file\n\nwith open('./training_set_a/132539.txt') as inputfile:\n \n results = list(csv.reader(inputfile)) # Open file in list of list\n results = pd.DataFrame(results[1:],columns=results[0]) # Convert list of list to DataFrame\n results.Value = results.Value.astype(float) # Change Value to float\n \nresults.head(8)",
"_____no_output_____"
],
[
"# Pivot_table to convert from long to wide dataset\n\n# Creation of new features - aggregate across the time series to find mean, min, max values\n# mean is chosen rather than median because we want to take into the account of 'outlier values'\n\nwide_result = pd.pivot_table(results,values=['Value'],columns='Parameter',aggfunc=[np.mean,np.min,np.max])\n\nwide_result.columns = wide_result.columns.droplevel(level=0)",
"_____no_output_____"
],
[
"new_columns = []\n\nfor ind, col in enumerate(wide_result.columns):\n \n if ind < wide_result.columns.shape[0]/3:\n col = 'mean_'+col \n new_columns.append(col)\n\n elif ind >= wide_result.columns.shape[0]/3 and ind < 2*wide_result.columns.shape[0]/3:\n col = 'min_'+col\n new_columns.append(col)\n\n else:\n col = 'max_'+col\n new_columns.append(col)\n \nprint new_columns",
"['mean_Age', 'mean_BUN', 'mean_Creatinine', 'mean_GCS', 'mean_Gender', 'mean_Glucose', 'mean_HCO3', 'mean_HCT', 'mean_HR', 'mean_Height', 'mean_ICUType', 'mean_K', 'mean_Mg', 'mean_NIDiasABP', 'mean_NIMAP', 'mean_NISysABP', 'mean_Na', 'mean_Platelets', 'mean_RecordID', 'mean_RespRate', 'mean_Temp', 'mean_Urine', 'mean_WBC', 'mean_Weight', 'min_Age', 'min_BUN', 'min_Creatinine', 'min_GCS', 'min_Gender', 'min_Glucose', 'min_HCO3', 'min_HCT', 'min_HR', 'min_Height', 'min_ICUType', 'min_K', 'min_Mg', 'min_NIDiasABP', 'min_NIMAP', 'min_NISysABP', 'min_Na', 'min_Platelets', 'min_RecordID', 'min_RespRate', 'min_Temp', 'min_Urine', 'min_WBC', 'min_Weight', 'max_Age', 'max_BUN', 'max_Creatinine', 'max_GCS', 'max_Gender', 'max_Glucose', 'max_HCO3', 'max_HCT', 'max_HR', 'max_Height', 'max_ICUType', 'max_K', 'max_Mg', 'max_NIDiasABP', 'max_NIMAP', 'max_NISysABP', 'max_Na', 'max_Platelets', 'max_RecordID', 'max_RespRate', 'max_Temp', 'max_Urine', 'max_WBC', 'max_Weight']\n"
],
[
"# rename the columns and lower capitalise\n\n#new_columns = [u'Age', u'mean_BUN', u'mean_Creatinine', u'mean_GCS', u'Gender', u'mean_Glucose', u'mean_HCO3',\n # u'mean_HCT', u'mean_HR', u'Height', u'ICUType', u'mean_K', u'mean_Mg', u'mean_NIDiasABP',\n # u'mean_NIMAP', u'mean_NISysABP', u'mean_Na', u'mean_Platelets', u'RecordID', u'mean_RespRate',\n # u'mean_Temp', u'mean_Urine', u'mean_WBC', u'mean_Weight', u'min_Age', u'min_BUN', u'min_Creatinine',\n # u'min_GCS', u'min_Gender', u'min_Glucose', u'min_HCO3', u'min_HCT', u'min_HR', u'min_Height',\n # u'min_ICUType', u'min_K', u'min_Mg', u'min_NIDiasABP', u'min_NIMAP', u'min_NISysABP', u'min_Na',\n # u'min_Platelets', u'min_RecordID', u'min_RespRate', u'min_Temp', u'min_Urine', u'min_WBC',\n #u'min_Weight', u'max_Age', u'max_BUN', u'max_Creatinine', u'max_GCS', u'max_Gender', u'max_Glucose',\n #u'max_HCO3', u'max_HCT', u'max_HR', u'max_Height', u'max_ICUType', u'max_K', u'max_Mg',\n #u'max_NIDiasABP', u'max_NIMAP', u'max_NISysABP', u'max_Na', u'max_Platelets', u'max_RecordID',\n #u'max_RespRate', u'max_Temp', u'max_Urine', u'max_WBC', u'max_Weight']\nwide_result.columns = new_columns\nwide_result.columns = wide_result.columns.str.lower()",
"_____no_output_____"
],
[
"wide_result.head()",
"_____no_output_____"
],
[
"# rename descriptor row\nwide_result.rename(columns={'mean_age':'age','mean_gender':'gender','mean_height':'height',\n 'mean_icutype':'icutype','mean_recordid':'recordid'},inplace=True)",
"_____no_output_____"
],
[
"wide_result.columns",
"_____no_output_____"
],
[
"# drop descriptor rows\n\nwide_result.drop(['min_age','max_age','min_gender','max_gender','min_height','max_height'\n ,'min_icutype','max_icutype','min_recordid','max_recordid'],axis=1,inplace=True)",
"_____no_output_____"
],
[
"wide_result.set_index(['recordid'],inplace = True)",
"_____no_output_____"
],
[
"wide_result",
"_____no_output_____"
],
[
"main = pd.DataFrame()",
"_____no_output_____"
],
[
"main = main.append(wide_result)",
"_____no_output_____"
],
[
"main",
"_____no_output_____"
],
[
"# Open each file as result\nwith open('./training_set_a/132599.txt') as inputfile:\n \n data = list(csv.reader(inputfile)) # list of list\n data = pd.DataFrame(data[1:],columns=data[0]) # Convert list of list to DataFrame\n data.Value = data.Value.astype(float) # Change Value to float\n \n \n \n # Pivot_table to convert from long to wide dataset\n\n # Creation of new features - aggregate across the time series to find mean, min, max values\n # mean is chosen rather than median because we want to take into the account of 'outlier values'\n\n wide_data = pd.pivot_table(data,values=['Value'],columns='Parameter',aggfunc=[np.mean,np.min,np.max])\n wide_data.columns = wide_data.columns.droplevel(level=0)\n \n \n \n # rename new columns & lower capitalise\n new_columns = []\n\n for ind, col in enumerate(wide_data.columns):\n \n if ind < wide_data.columns.shape[0]/3: \n col = 'mean_'+col \n new_columns.append(col)\n\n elif ind >= wide_data.columns.shape[0]/3 and ind < 2*wide_data.columns.shape[0]/3:\n col = 'min_'+col\n new_columns.append(col)\n\n else:\n col = 'max_'+col\n new_columns.append(col)\n \n wide_data.columns = new_columns\n wide_data.columns = wide_data.columns.str.lower()\n \n \n # rename descriptor row\n wide_data.rename(columns={'mean_age':'age','mean_gender':'gender','mean_height':'height',\n 'mean_icutype':'icutype','mean_recordid':'recordid'},inplace=True)\n \n # drop min/max descriptor rows\n wide_data.drop(['min_age','max_age','min_gender','max_gender','min_height','max_height',\n 'min_icutype','max_icutype','min_recordid','max_recordid'],axis=1,inplace=True)\n \n # set recordid as index\n wide_data.set_index(['recordid'],inplace = True)",
"_____no_output_____"
],
[
"main = main.append(wide_data)",
"_____no_output_____"
],
[
"main",
"_____no_output_____"
],
[
"for col in main.columns:\n print col",
"age\ngender\nheight\nicutype\nmax_albumin\nmax_alp\nmax_alt\nmax_ast\nmax_bilirubin\nmax_bun\nmax_creatinine\nmax_diasabp\nmax_fio2\nmax_gcs\nmax_glucose\nmax_hco3\nmax_hct\nmax_hr\nmax_k\nmax_lactate\nmax_map\nmax_mechvent\nmax_mg\nmax_na\nmax_nidiasabp\nmax_nimap\nmax_nisysabp\nmax_paco2\nmax_pao2\nmax_ph\nmax_platelets\nmax_resprate\nmax_sao2\nmax_sysabp\nmax_temp\nmax_troponint\nmax_urine\nmax_wbc\nmax_weight\nmean_albumin\nmean_alp\nmean_alt\nmean_ast\nmean_bilirubin\nmean_bun\nmean_creatinine\nmean_diasabp\nmean_fio2\nmean_gcs\nmean_glucose\nmean_hco3\nmean_hct\nmean_hr\nmean_k\nmean_lactate\nmean_map\nmean_mechvent\nmean_mg\nmean_na\nmean_nidiasabp\nmean_nimap\nmean_nisysabp\nmean_paco2\nmean_pao2\nmean_ph\nmean_platelets\nmean_resprate\nmean_sao2\nmean_sysabp\nmean_temp\nmean_troponint\nmean_urine\nmean_wbc\nmean_weight\nmin_albumin\nmin_alp\nmin_alt\nmin_ast\nmin_bilirubin\nmin_bun\nmin_creatinine\nmin_diasabp\nmin_fio2\nmin_gcs\nmin_glucose\nmin_hco3\nmin_hct\nmin_hr\nmin_k\nmin_lactate\nmin_map\nmin_mechvent\nmin_mg\nmin_na\nmin_nidiasabp\nmin_nimap\nmin_nisysabp\nmin_paco2\nmin_pao2\nmin_ph\nmin_platelets\nmin_resprate\nmin_sao2\nmin_sysabp\nmin_temp\nmin_troponint\nmin_urine\nmin_wbc\nmin_weight\n"
],
[
"#wide_result.reset_index(inplace=True)\n#wide_result.drop('index',axis=1,inplace=True)",
"_____no_output_____"
],
[
"# Pivot_table to convert from long to wide dataset\n\n#wide_result = pd.pivot_table(results,values=['Value'],columns='Parameter',index=['Time'])\n\n#wide_result.columns = wide_result.columns.droplevel(level=0)\n#wide_result.reset_index(inplace=True)",
"_____no_output_____"
],
[
"# Trying to convert time to an 'aggreable' data type\n\n#def str_time2(time):\n # hours, minutes = map(int, time.split(':'))\n # time = (hours,minutes)\n \n # return time\n\n#def str_time(time):\n # hours, minutes = map(int, time.split(':'))\n # time = time.format(int(hours),int(minutes))\n # return time\n\n#for time in wide_result.index:\n # hours, minutes = map(int, time.split(':'))\n # time = (hours,minutes)\n # print time\n \n#wide_result.Time = wide_result.Time.apply(str_time)",
"_____no_output_____"
],
[
"#class patient_details(object):\n # \"\"\"Run description of the patient when admitted on the 48th hour\"\"\"\n \n # def __init__(self,df = wide_result):\n \n # self.record_id = df[df.Time == '00:00']['RecordID'][0]\n # self.age = df[df.Time == '00:00']['Age'][0]\n # self.gender = df[df.Time == '00:00']['Gender'][0]\n # self.height = df[df.Time == '00:00']['Height'][0]\n # self.ICUtype = df[df.Time == '00:00']['ICUType'][0]\n\n \n \n # def fill(self,df = wide_result,details='RecordID'):\n # \"\"\"Filling of the NaN values with patient's details can be automated \n # by specifying the descriptor(column) in **kwargs \"\"\"\n # \"\"\"Default set as RecordID\"\"\"\n \n # wide_result[details].fillna(value=df[df.Time == '00:00'][details][0],inplace=True)\n ",
"_____no_output_____"
],
[
"# Initiate the class patient_details\n#patient = patient_details()\n\n# Fill NaN values in respective descriptor columns\n#patient.fill()\n#patient.fill(details='Age')\n#patient.fill(details='Gender')\n#patient.fill(details='Height')\n#patient.fill(details='ICUType')",
"_____no_output_____"
],
[
"# change all column names to lower key\n#wide_result.columns = wide_result.columns.str.lower()",
"_____no_output_____"
],
[
"# Connect to database\n\nconn = psycopg2.connect(host=\"localhost\",dbname=\"mortality\")\ncur = conn.cursor()",
"_____no_output_____"
]
],
[
[
"## EDA\n\n### 1. Check if the data is unbalanced",
"_____no_output_____"
]
],
[
[
"# Open outcomes file\n\nwith open('./training_outcomes_a.txt') as outcomefile:\n \n # Open file in list of list\n \n outcome = list(csv.reader(outcomefile))\n \noutcome = pd.DataFrame(outcome[1:],columns=outcome[0]) # Convert list of list to DataFrame\noutcome = outcome.astype(float,'ignore') # Change values to float",
"_____no_output_____"
],
[
"# Count the number of positives in dataset\n# Positives = 1 = Death, Negative = 0 = Survived\n\ndef imbalance_check(column,labels):\n \"\"\"labels can be a list or a tuple.\"\"\"\n \n for x in labels:\n label = float(column[column == x].count())\n total = float(column.count())\n \n percentage = float((label/total)*100)\n \n print 'percentage of',x,'in dataset:',percentage,'%'\n",
"_____no_output_____"
],
[
"imbalance_check(outcome['In-hospital_death'],[0,1]) # Conclude that this is an imbalanced dataset",
"percentage of 0 in dataset: 86.15 %\npercentage of 1 in dataset: 13.85 %\n"
]
],
[
[
"### 2. Create outcomes table in database",
"_____no_output_____"
]
],
[
[
"outcome.head(5)",
"_____no_output_____"
],
[
"pd.to_sql()",
"_____no_output_____"
]
]
] | [
"markdown",
"code",
"markdown",
"code",
"markdown",
"code",
"markdown",
"code",
"markdown",
"code"
] | [
[
"markdown"
],
[
"code"
],
[
"markdown"
],
[
"code",
"code",
"code",
"code"
],
[
"markdown",
"markdown"
],
[
"code",
"code",
"code",
"code",
"code",
"code",
"code",
"code",
"code",
"code",
"code",
"code",
"code",
"code",
"code",
"code",
"code",
"code",
"code",
"code",
"code",
"code",
"code",
"code"
],
[
"markdown"
],
[
"code",
"code",
"code"
],
[
"markdown"
],
[
"code",
"code"
]
] |
e7856b67a7789c90cd913029a87805a0ffb95b64 | 31,941 | ipynb | Jupyter Notebook | notebooks/eager/nmt_w_attention.ipynb | cnodadiaz/tf-workshop | b5bf332d3771ec2474613e0ce15f419acecdeb3c | [
"Apache-2.0"
] | null | null | null | notebooks/eager/nmt_w_attention.ipynb | cnodadiaz/tf-workshop | b5bf332d3771ec2474613e0ce15f419acecdeb3c | [
"Apache-2.0"
] | null | null | null | notebooks/eager/nmt_w_attention.ipynb | cnodadiaz/tf-workshop | b5bf332d3771ec2474613e0ce15f419acecdeb3c | [
"Apache-2.0"
] | null | null | null | 40.380531 | 593 | 0.514449 | [
[
[
"##### Copyright 2018 The TensorFlow Authors.\n\nLicensed under the Apache License, Version 2.0 (the \"License\").\n\n# Neural Machine Translation with Attention\n\n<table class=\"tfo-notebook-buttons\" align=\"left\"><td>\n<a target=\"_blank\" href=\"https://colab.research.google.com/github/tensorflow/tensorflow/blob/master/tensorflow/contrib/eager/python/examples/nmt_with_attention/nmt_with_attention.ipynb\">\n <img src=\"https://www.tensorflow.org/images/colab_logo_32px.png\" />Run in Google Colab</a> \n</td><td>\n<a target=\"_blank\" href=\"https://github.com/tensorflow/tensorflow/tree/master/tensorflow/contrib/eager/python/examples/nmt_with_attention/nmt_with_attention.ipynb\"><img width=32px src=\"https://www.tensorflow.org/images/GitHub-Mark-32px.png\" />View source on GitHub</a></td></table>",
"_____no_output_____"
],
[
"This notebook trains a sequence to sequence (seq2seq) model for Spanish to English translation using [tf.keras](https://www.tensorflow.org/programmers_guide/keras) and [eager execution](https://www.tensorflow.org/programmers_guide/eager). This is an advanced example that assumes some knowledge of sequence to sequence models.\n\nAfter training the model in this notebook, you will be able to input a Spanish sentence, such as *\"¿todavia estan en casa?\"*, and return the English translation: *\"are you still at home?\"*\n\nThe translation quality is reasonable for a toy example, but the generated attention plot is perhaps more interesting. This shows which parts of the input sentence has the model's attention while translating:\n\n<img src=\"https://tensorflow.org/images/spanish-english.png\" alt=\"spanish-english attention plot\">\n\nNote: This example takes approximately 10 mintues to run on a single P100 GPU.",
"_____no_output_____"
]
],
[
[
"from __future__ import absolute_import, division, print_function\n\n# Import TensorFlow >= 1.9 and enable eager execution\nimport tensorflow as tf\n\ntf.enable_eager_execution()\n\nimport matplotlib.pyplot as plt\nfrom sklearn.model_selection import train_test_split\n\nimport unicodedata\nimport re\nimport numpy as np\nimport os\nimport time\n\nprint(tf.__version__)",
"_____no_output_____"
]
],
[
[
"## Download and prepare the dataset\n\nWe'll use a language dataset provided by http://www.manythings.org/anki/. This dataset contains language translation pairs in the format:\n\n```\nMay I borrow this book?\t¿Puedo tomar prestado este libro?\n```\n\nThere are a variety of languages available, but we'll use the English-Spanish dataset. For convenience, we've hosted a copy of this dataset on Google Cloud, but you can also download your own copy. After downloading the dataset, here are the steps we'll take to prepare the data:\n\n1. Add a *start* and *end* token to each sentence.\n2. Clean the sentences by removing special characters.\n3. Create a word index and reverse word index (dictionaries mapping from word → id and id → word).\n4. Pad each sentence to a maximum length.",
"_____no_output_____"
]
],
[
[
"# Download the file\npath_to_zip = tf.keras.utils.get_file(\n 'spa-eng.zip', origin='http://download.tensorflow.org/data/spa-eng.zip', \n extract=True)\n\npath_to_file = os.path.dirname(path_to_zip)+\"/spa-eng/spa.txt\"",
"_____no_output_____"
],
[
"# Converts the unicode file to ascii\ndef unicode_to_ascii(s):\n return ''.join(c for c in unicodedata.normalize('NFD', s)\n if unicodedata.category(c) != 'Mn')\n\n\ndef preprocess_sentence(w):\n w = unicode_to_ascii(w.lower().strip())\n \n # creating a space between a word and the punctuation following it\n # eg: \"he is a boy.\" => \"he is a boy .\" \n # Reference:- https://stackoverflow.com/questions/3645931/python-padding-punctuation-with-white-spaces-keeping-punctuation\n w = re.sub(r\"([?.!,¿])\", r\" \\1 \", w)\n w = re.sub(r'[\" \"]+', \" \", w)\n \n # replacing everything with space except (a-z, A-Z, \".\", \"?\", \"!\", \",\")\n w = re.sub(r\"[^a-zA-Z?.!,¿]+\", \" \", w)\n \n w = w.rstrip().strip()\n \n # adding a start and an end token to the sentence\n # so that the model know when to start and stop predicting.\n w = '<start> ' + w + ' <end>'\n return w",
"_____no_output_____"
],
[
"# 1. Remove the accents\n# 2. Clean the sentences\n# 3. Return word pairs in the format: [ENGLISH, SPANISH]\ndef create_dataset(path, num_examples):\n lines = open(path, encoding='UTF-8').read().strip().split('\\n')\n \n word_pairs = [[preprocess_sentence(w) for w in l.split('\\t')] for l in lines[:num_examples]]\n \n return word_pairs",
"_____no_output_____"
],
[
"# This class creates a word -> index mapping (e.g,. \"dad\" -> 5) and vice-versa \n# (e.g., 5 -> \"dad\") for each language,\nclass LanguageIndex():\n def __init__(self, lang):\n self.lang = lang\n self.word2idx = {}\n self.idx2word = {}\n self.vocab = set()\n \n self.create_index()\n \n def create_index(self):\n for phrase in self.lang:\n self.vocab.update(phrase.split(' '))\n \n self.vocab = sorted(self.vocab)\n \n self.word2idx['<pad>'] = 0\n for index, word in enumerate(self.vocab):\n self.word2idx[word] = index + 1\n \n for word, index in self.word2idx.items():\n self.idx2word[index] = word",
"_____no_output_____"
],
[
"def max_length(tensor):\n return max(len(t) for t in tensor)\n\n\ndef load_dataset(path, num_examples):\n # creating cleaned input, output pairs\n pairs = create_dataset(path, num_examples)\n\n # index language using the class defined above \n inp_lang = LanguageIndex(sp for en, sp in pairs)\n targ_lang = LanguageIndex(en for en, sp in pairs)\n \n # Vectorize the input and target languages\n \n # Spanish sentences\n input_tensor = [[inp_lang.word2idx[s] for s in sp.split(' ')] for en, sp in pairs]\n \n # English sentences\n target_tensor = [[targ_lang.word2idx[s] for s in en.split(' ')] for en, sp in pairs]\n \n # Calculate max_length of input and output tensor\n # Here, we'll set those to the longest sentence in the dataset\n max_length_inp, max_length_tar = max_length(input_tensor), max_length(target_tensor)\n \n # Padding the input and output tensor to the maximum length\n input_tensor = tf.keras.preprocessing.sequence.pad_sequences(input_tensor, \n maxlen=max_length_inp,\n padding='post')\n \n target_tensor = tf.keras.preprocessing.sequence.pad_sequences(target_tensor, \n maxlen=max_length_tar, \n padding='post')\n \n return input_tensor, target_tensor, inp_lang, targ_lang, max_length_inp, max_length_tar",
"_____no_output_____"
]
],
[
[
"### Limit the size of the dataset to experiment faster (optional)\n\nTraining on the complete dataset of >100,000 sentences will take a long time. To train faster, we can limit the size of the dataset to 30,000 sentences (of course, translation quality degrades with less data):",
"_____no_output_____"
]
],
[
[
"# Try experimenting with the size of that dataset\nnum_examples = 30000\ninput_tensor, target_tensor, inp_lang, targ_lang, max_length_inp, max_length_targ = load_dataset(path_to_file, num_examples)",
"_____no_output_____"
],
[
"# Creating training and validation sets using an 80-20 split\ninput_tensor_train, input_tensor_val, target_tensor_train, target_tensor_val = train_test_split(input_tensor, target_tensor, test_size=0.2)\n\n# Show length\nlen(input_tensor_train), len(target_tensor_train), len(input_tensor_val), len(target_tensor_val)",
"_____no_output_____"
]
],
[
[
"### Create a tf.data dataset",
"_____no_output_____"
]
],
[
[
"BUFFER_SIZE = len(input_tensor_train)\nBATCH_SIZE = 64\nN_BATCH = BUFFER_SIZE//BATCH_SIZE\nembedding_dim = 256\nunits = 1024\nvocab_inp_size = len(inp_lang.word2idx)\nvocab_tar_size = len(targ_lang.word2idx)\n\ndataset = tf.data.Dataset.from_tensor_slices((input_tensor_train, target_tensor_train)).shuffle(BUFFER_SIZE)\ndataset = dataset.apply(tf.contrib.data.batch_and_drop_remainder(BATCH_SIZE))",
"_____no_output_____"
]
],
[
[
"## Write the encoder and decoder model\n\nHere, we'll implement an encoder-decoder model with attention which you can read about in the TensorFlow [Neural Machine Translation (seq2seq) tutorial](https://www.tensorflow.org/tutorials/seq2seq). This example uses a more recent set of APIs. This notebook implements the [attention equations](https://www.tensorflow.org/tutorials/seq2seq#background_on_the_attention_mechanism) from the seq2seq tutorial. The following diagram shows that each input words is assigned a weight by the attention mechanism which is then used by the decoder to predict the next word in the sentence.\n\n<img src=\"https://www.tensorflow.org/images/seq2seq/attention_mechanism.jpg\" width=\"500\" alt=\"attention mechanism\">\n\nThe input is put through an encoder model which gives us the encoder output of shape *(batch_size, max_length, hidden_size)* and the encoder hidden state of shape *(batch_size, hidden_size)*. \n\nHere are the equations that are implemented:\n\n<img src=\"https://www.tensorflow.org/images/seq2seq/attention_equation_0.jpg\" alt=\"attention equation 0\" width=\"800\">\n<img src=\"https://www.tensorflow.org/images/seq2seq/attention_equation_1.jpg\" alt=\"attention equation 1\" width=\"800\">\n\nWe're using *Bahdanau attention*. Lets decide on notation before writing the simplified form:\n\n* FC = Fully connected (dense) layer\n* EO = Encoder output\n* H = hidden state\n* X = input to the decoder\n\nAnd the pseudo-code:\n\n* `score = FC(tanh(FC(EO) + FC(H)))`\n* `attention weights = softmax(score, axis = 1)`. Softmax by default is applied on the last axis but here we want to apply it on the *1st axis*, since the shape of score is *(batch_size, max_length, hidden_size)*. `Max_length` is the length of our input. Since we are trying to assign a weight to each input, softmax should be applied on that axis.\n* `context vector = sum(attention weights * EO, axis = 1)`. Same reason as above for choosing axis as 1.\n* `embedding output` = The input to the decoder X is passed through an embedding layer.\n* `merged vector = concat(embedding output, context vector)`\n* This merged vector is then given to the GRU\n \nThe shapes of all the vectors at each step have been specified in the comments in the code:",
"_____no_output_____"
]
],
[
[
"def gru(units):\n # If you have a GPU, we recommend using CuDNNGRU(provides a 3x speedup than GRU)\n # the code automatically does that.\n if tf.test.is_gpu_available():\n return tf.keras.layers.CuDNNGRU(units, \n return_sequences=True, \n return_state=True, \n recurrent_initializer='glorot_uniform')\n else:\n return tf.keras.layers.GRU(units, \n return_sequences=True, \n return_state=True, \n recurrent_activation='sigmoid', \n recurrent_initializer='glorot_uniform')",
"_____no_output_____"
],
[
"class Encoder(tf.keras.Model):\n def __init__(self, vocab_size, embedding_dim, enc_units, batch_sz):\n super(Encoder, self).__init__()\n self.batch_sz = batch_sz\n self.enc_units = enc_units\n self.embedding = tf.keras.layers.Embedding(vocab_size, embedding_dim)\n self.gru = gru(self.enc_units)\n \n def call(self, x, hidden):\n x = self.embedding(x)\n output, state = self.gru(x, initial_state = hidden) \n return output, state\n \n def initialize_hidden_state(self):\n return tf.zeros((self.batch_sz, self.enc_units))",
"_____no_output_____"
],
[
"class Decoder(tf.keras.Model):\n def __init__(self, vocab_size, embedding_dim, dec_units, batch_sz):\n super(Decoder, self).__init__()\n self.batch_sz = batch_sz\n self.dec_units = dec_units\n self.embedding = tf.keras.layers.Embedding(vocab_size, embedding_dim)\n self.gru = gru(self.dec_units)\n self.fc = tf.keras.layers.Dense(vocab_size)\n \n # used for attention\n self.W1 = tf.keras.layers.Dense(self.dec_units)\n self.W2 = tf.keras.layers.Dense(self.dec_units)\n self.V = tf.keras.layers.Dense(1)\n \n def call(self, x, hidden, enc_output):\n # enc_output shape == (batch_size, max_length, hidden_size)\n \n # hidden shape == (batch_size, hidden size)\n # hidden_with_time_axis shape == (batch_size, 1, hidden size)\n # we are doing this to perform addition to calculate the score\n hidden_with_time_axis = tf.expand_dims(hidden, 1)\n \n # score shape == (batch_size, max_length, hidden_size)\n score = tf.nn.tanh(self.W1(enc_output) + self.W2(hidden_with_time_axis))\n \n # attention_weights shape == (batch_size, max_length, 1)\n # we get 1 at the last axis because we are applying score to self.V\n attention_weights = tf.nn.softmax(self.V(score), axis=1)\n \n # context_vector shape after sum == (batch_size, hidden_size)\n context_vector = attention_weights * enc_output\n context_vector = tf.reduce_sum(context_vector, axis=1)\n \n # x shape after passing through embedding == (batch_size, 1, embedding_dim)\n x = self.embedding(x)\n \n # x shape after concatenation == (batch_size, 1, embedding_dim + hidden_size)\n x = tf.concat([tf.expand_dims(context_vector, 1), x], axis=-1)\n \n # passing the concatenated vector to the GRU\n output, state = self.gru(x)\n \n # output shape == (batch_size * max_length, hidden_size)\n output = tf.reshape(output, (-1, output.shape[2]))\n \n # output shape == (batch_size * max_length, vocab)\n x = self.fc(output)\n \n return x, state, attention_weights\n \n def initialize_hidden_state(self):\n return tf.zeros((self.batch_sz, self.dec_units))",
"_____no_output_____"
],
[
"encoder = Encoder(vocab_inp_size, embedding_dim, units, BATCH_SIZE)\ndecoder = Decoder(vocab_tar_size, embedding_dim, units, BATCH_SIZE)",
"_____no_output_____"
]
],
[
[
"## Define the optimizer and the loss function",
"_____no_output_____"
]
],
[
[
"optimizer = tf.train.AdamOptimizer()\n\n\ndef loss_function(real, pred):\n mask = 1 - np.equal(real, 0)\n loss_ = tf.nn.sparse_softmax_cross_entropy_with_logits(labels=real, logits=pred) * mask\n return tf.reduce_mean(loss_)",
"_____no_output_____"
]
],
[
[
"## Training\n\n1. Pass the *input* through the *encoder* which return *encoder output* and the *encoder hidden state*.\n2. The encoder output, encoder hidden state and the decoder input (which is the *start token*) is passed to the decoder.\n3. The decoder returns the *predictions* and the *decoder hidden state*.\n4. The decoder hidden state is then passed back into the model and the predictions are used to calculate the loss.\n5. Use *teacher forcing* to decide the next input to the decoder.\n6. *Teacher forcing* is the technique where the *target word* is passed as the *next input* to the decoder.\n7. The final step is to calculate the gradients and apply it to the optimizer and backpropagate.",
"_____no_output_____"
]
],
[
[
"EPOCHS = 10\n\nfor epoch in range(EPOCHS):\n start = time.time()\n \n hidden = encoder.initialize_hidden_state()\n total_loss = 0\n \n for (batch, (inp, targ)) in enumerate(dataset):\n loss = 0\n \n with tf.GradientTape() as tape:\n enc_output, enc_hidden = encoder(inp, hidden)\n \n dec_hidden = enc_hidden\n \n dec_input = tf.expand_dims([targ_lang.word2idx['<start>']] * BATCH_SIZE, 1) \n \n # Teacher forcing - feeding the target as the next input\n for t in range(1, targ.shape[1]):\n # passing enc_output to the decoder\n predictions, dec_hidden, _ = decoder(dec_input, dec_hidden, enc_output)\n \n loss += loss_function(targ[:, t], predictions)\n \n # using teacher forcing\n dec_input = tf.expand_dims(targ[:, t], 1)\n \n batch_loss = (loss / int(targ.shape[1]))\n \n total_loss += batch_loss\n \n variables = encoder.variables + decoder.variables\n \n gradients = tape.gradient(loss, variables)\n \n optimizer.apply_gradients(zip(gradients, variables), tf.train.get_or_create_global_step())\n \n if batch % 100 == 0:\n print('Epoch {} Batch {} Loss {:.4f}'.format(epoch + 1,\n batch,\n batch_loss.numpy()))\n \n print('Epoch {} Loss {:.4f}'.format(epoch + 1,\n total_loss / N_BATCH))\n print('Time taken for 1 epoch {} sec\\n'.format(time.time() - start))",
"_____no_output_____"
]
],
[
[
"## Translate\n\n* The evaluate function is similar to the training loop, except we don't use *teacher forcing* here. The input to the decoder at each time step is its previous predictions along with the hidden state and the encoder output.\n* Stop predicting when the model predicts the *end token*.\n* And store the *attention weights for every time step*.\n\nNote: The encoder output is calculated only once for one input.",
"_____no_output_____"
]
],
[
[
"def evaluate(sentence, encoder, decoder, inp_lang, targ_lang, max_length_inp, max_length_targ):\n attention_plot = np.zeros((max_length_targ, max_length_inp))\n \n sentence = preprocess_sentence(sentence)\n\n inputs = [inp_lang.word2idx[i] for i in sentence.split(' ')]\n inputs = tf.keras.preprocessing.sequence.pad_sequences([inputs], maxlen=max_length_inp, padding='post')\n inputs = tf.convert_to_tensor(inputs)\n \n result = ''\n\n hidden = [tf.zeros((1, units))]\n enc_out, enc_hidden = encoder(inputs, hidden)\n\n dec_hidden = enc_hidden\n dec_input = tf.expand_dims([targ_lang.word2idx['<start>']], 0)\n\n for t in range(max_length_targ):\n predictions, dec_hidden, attention_weights = decoder(dec_input, dec_hidden, enc_out)\n \n # storing the attention weigths to plot later on\n attention_weights = tf.reshape(attention_weights, (-1, ))\n attention_plot[t] = attention_weights.numpy()\n\n predicted_id = tf.multinomial(tf.exp(predictions), num_samples=1)[0][0].numpy()\n\n result += targ_lang.idx2word[predicted_id] + ' '\n\n if targ_lang.idx2word[predicted_id] == '<end>':\n return result, sentence, attention_plot\n \n # the predicted ID is fed back into the model\n dec_input = tf.expand_dims([predicted_id], 0)\n\n return result, sentence, attention_plot",
"_____no_output_____"
],
[
"# function for plotting the attention weights\ndef plot_attention(attention, sentence, predicted_sentence):\n fig = plt.figure(figsize=(10,10))\n ax = fig.add_subplot(1, 1, 1)\n ax.matshow(attention, cmap='viridis')\n \n fontdict = {'fontsize': 14}\n \n ax.set_xticklabels([''] + sentence, fontdict=fontdict, rotation=90)\n ax.set_yticklabels([''] + predicted_sentence, fontdict=fontdict)\n\n plt.show()",
"_____no_output_____"
],
[
"def translate(sentence, encoder, decoder, inp_lang, targ_lang, max_length_inp, max_length_targ):\n result, sentence, attention_plot = evaluate(sentence, encoder, decoder, inp_lang, targ_lang, max_length_inp, max_length_targ)\n \n print('Input: {}'.format(sentence))\n print('Predicted translation: {}'.format(result))\n \n attention_plot = attention_plot[:len(result.split(' ')), :len(sentence.split(' '))]\n plot_attention(attention_plot, sentence.split(' '), result.split(' '))",
"_____no_output_____"
],
[
"translate('hace mucho frio aqui.', encoder, decoder, inp_lang, targ_lang, max_length_inp, max_length_targ)",
"_____no_output_____"
],
[
"translate('esta es mi vida.', encoder, decoder, inp_lang, targ_lang, max_length_inp, max_length_targ)",
"_____no_output_____"
],
[
"translate('¿todavia estan en casa?', encoder, decoder, inp_lang, targ_lang, max_length_inp, max_length_targ)",
"_____no_output_____"
],
[
"# wrong translation\ntranslate('trata de averiguarlo.', encoder, decoder, inp_lang, targ_lang, max_length_inp, max_length_targ)",
"_____no_output_____"
]
],
[
[
"## Next steps\n\n* [Download a different dataset](http://www.manythings.org/anki/) to experiment with translations, for example, English to German, or English to French.\n* Experiment with training on a larger dataset, or using more epochs\n",
"_____no_output_____"
]
]
] | [
"markdown",
"code",
"markdown",
"code",
"markdown",
"code",
"markdown",
"code",
"markdown",
"code",
"markdown",
"code",
"markdown",
"code",
"markdown",
"code",
"markdown"
] | [
[
"markdown",
"markdown"
],
[
"code"
],
[
"markdown"
],
[
"code",
"code",
"code",
"code",
"code"
],
[
"markdown"
],
[
"code",
"code"
],
[
"markdown"
],
[
"code"
],
[
"markdown"
],
[
"code",
"code",
"code",
"code"
],
[
"markdown"
],
[
"code"
],
[
"markdown"
],
[
"code"
],
[
"markdown"
],
[
"code",
"code",
"code",
"code",
"code",
"code",
"code"
],
[
"markdown"
]
] |
e7856c7105455e6ec725162cba6e325d02d290e6 | 22,579 | ipynb | Jupyter Notebook | data-prep/04_create_ground_truth_job.ipynb | jonslo/amazon-sagemaker-aws-greengrass-custom-object-detection-model | 4b45f34b4c92cbfc3633938136366ac729155396 | [
"MIT-0"
] | 36 | 2019-07-23T12:32:40.000Z | 2022-03-15T16:32:02.000Z | data-prep/04_create_ground_truth_job.ipynb | jonslo/amazon-sagemaker-aws-greengrass-custom-object-detection-model | 4b45f34b4c92cbfc3633938136366ac729155396 | [
"MIT-0"
] | 4 | 2019-10-10T16:16:39.000Z | 2021-12-01T15:17:19.000Z | data-prep/04_create_ground_truth_job.ipynb | jonslo/amazon-sagemaker-aws-greengrass-custom-object-detection-model | 4b45f34b4c92cbfc3633938136366ac729155396 | [
"MIT-0"
] | 28 | 2019-08-30T20:05:03.000Z | 2022-02-24T20:45:05.000Z | 36.713821 | 222 | 0.562868 | [
[
[
"%matplotlib inline\nimport os\nfrom collections import namedtuple\nfrom collections import defaultdict\nfrom collections import Counter\nfrom datetime import datetime\nimport itertools\nimport base64\nimport glob\nimport json\nimport random\nimport time\nimport imageio\nimport numpy as np\nimport matplotlib\nimport matplotlib.pyplot as plt\nimport shutil\nfrom matplotlib.backends.backend_pdf import PdfPages\nfrom sklearn.metrics import confusion_matrix\nimport boto3\nimport botocore\nimport sagemaker\nfrom urllib.parse import urlparse",
"_____no_output_____"
],
[
"role = sagemaker.get_execution_role()\nregion = boto3.session.Session().region_name\ns3 = boto3.client('s3')",
"_____no_output_____"
]
],
[
[
"## Job parameters",
"_____no_output_____"
]
],
[
[
"BUCKET=\"tanmcrae-greengrass-blog\"",
"_____no_output_____"
],
[
"bucket_region = s3.head_bucket(Bucket=BUCKET)['ResponseMetadata']['HTTPHeaders']['x-amz-bucket-region']\nassert bucket_region == region, \"Your S3 bucket {} and this notebook need to be in the same region.\".format(BUCKET)",
"_____no_output_____"
],
[
"MANIFEST = \"blue_box_large_job.json\"\nJOB_NAME = \"blue-box-large-job-public\"\nEXP_NAME = 'blue-box'\nprint(JOB_NAME)\n",
"blue-box-large-job-public\n"
],
[
"USE_AUTO_LABELING = False\nRUN_FULL_AL_DEMO = False \nUSE_PRIVATE_WORKFORCE = False",
"_____no_output_____"
]
],
[
[
"## specifying categories",
"_____no_output_____"
]
],
[
[
"CLASS_NAME = \"storage box\"",
"_____no_output_____"
],
[
"CLASS_LIST = [CLASS_NAME]\nprint(\"Label space is {}\".format(CLASS_LIST))\n\njson_body = {\n 'labels': [{'label': label} for label in CLASS_LIST]\n}\nwith open('class_labels.json', 'w') as f:\n json.dump(json_body, f)\n\nLABEL_KEY = \"ground-truth/{}/class_labels.json\".format(EXP_NAME)\ns3.upload_file('class_labels.json', BUCKET, LABEL_KEY)\nprint (\"uploaded s3://{}/{}\".format(BUCKET, LABEL_KEY))",
"Label space is ['storage box']\nuploaded s3://tanmcrae-greengrass-blog/ground-truth/blue-box/class_labels.json\n"
]
],
[
[
"## Create the instruction template\n",
"_____no_output_____"
]
],
[
[
"def make_template(test_template=False, save_fname='instructions.template'):\n template = r\"\"\"<script src=\"https://assets.crowd.aws/crowd-html-elements.js\"></script>\n\n<crowd-form>\n <crowd-bounding-box\n name=\"boundingBox\"\n src=\"{{ task.input.taskObject | grant_read_access }}\"\n header=\"Draw bounding box for the storage boxes in the picture (blue). Each bounding box should fit tight around the box. Only draw one bounding box per storage box, even if part of the box may be occluded.\"\n labels=\"['storage box']\"\n >\n <full-instructions header=\"Please annotate storage boxes in the picture\">\n <ol>\n <li><strong>Inspect</strong> the image</li>\n <li><strong>Determine</strong> if there are visible blue storage box in the picture.</li>\n <li><strong>Outline</strong> the storage box in the image using the provided “Box” tool. </li>\n </ol>\n\n <h2><span style=\"color: rgb(0, 138, 0);\">Good Example</span></h2>\n <p><img src=\" https://s3.amazonaws.com/angelaw-workshop/groundtruth/greengrass-blog/good-exmaples/good-example-arm.png \" style=\"max-width:450\"></p>\n <p><img src=\" https://s3.amazonaws.com/angelaw-workshop/groundtruth/greengrass-blog/good-exmaples/good-example-occlusion.png \" style=\"max-width:450\"></p>\n <p><img src=\" https://s3.amazonaws.com/angelaw-workshop/groundtruth/greengrass-blog/good-exmaples/good-example-partial.png \" style=\"max-width:450\"></p>\n <p><img src=\" https://s3.amazonaws.com/angelaw-workshop/groundtruth/greengrass-blog/good-exmaples/good-example-standard.png \" style=\"max-width:450\"></p>\n <h2><span style=\"color: rgb(230, 0, 0);\">Bad Example</span></h2>\n\n <p>The bounding boxes below are bad as it didn't cover the entire box. </p>\n <p><img src=\"https://s3.amazonaws.com/angelaw-workshop/groundtruth/greengrass-blog/bad-examples/bad-example-not-full.png\" style=\"max-width:450\"></p>\n <p><img src=\"https://s3.amazonaws.com/angelaw-workshop/groundtruth/greengrass-blog/bad-examples/bad-example-not-full-2.png\" style=\"max-width:450\"></p>\n <p>The bounding boxes below are bad as it's not tight around storage box. </p>\n <p><img src=\"https://s3.amazonaws.com/angelaw-workshop/groundtruth/greengrass-blog/bad-examples/bad-example-not-tight.png\" style=\"max-width:450\"></p>\n <p><img src=\"https://s3.amazonaws.com/angelaw-workshop/groundtruth/greengrass-blog/bad-examples/bad-example-not-tight-2.png\" style=\"max-width:450\"></p>\n <p>The labeling below are bad as it didn't cover the full </p>\n <p><img src=\"https://s3.amazonaws.com/angelaw-workshop/groundtruth/greengrass-blog/bad-examples/bad-example-occlusion-partial.png\" style=\"max-width:450\"></p>\n <p><img src=\"https://s3.amazonaws.com/angelaw-workshop/groundtruth/greengrass-blog/bad-examples/bad-example-occlusion-partial-2.png\" style=\"max-width:450\"></p>\n\n </full-instructions>\n\n <short-instructions>\n <p>Label every blue storage box in the picture. Boxes should fit tight. If the target goes off the screen, label up to the edge of the image. Do not label if it completely cannot be seen. </p>\n <p><img src=\" https://s3.amazonaws.com/angelaw-workshop/groundtruth/greengrass-blog/good-exmaples/good-example-arm.png \" style=\"max-width:100%\"/></p>\n <p><img src=\" https://s3.amazonaws.com/angelaw-workshop/groundtruth/greengrass-blog/good-exmaples/good-example-occlusion.png \" style=\"max-width:100%\"/></p>\n <p><img src=\" https://s3.amazonaws.com/angelaw-workshop/groundtruth/greengrass-blog/good-exmaples/good-example-partial.png \" style=\"max-width:100%\"/></p>\n <p><img src=\" https://s3.amazonaws.com/angelaw-workshop/groundtruth/greengrass-blog/good-exmaples/good-example-standard.png \" style=\"max-width:100%\"/></p>\n <p><br/></p>\n <h2><span style=\"color: rgb(230, 0, 0);\">Bad examples</span></h2>\n <p>The bounding boxes below are bad as it didn't cover the entire box. </p>\n <p><img src=\"https://s3.amazonaws.com/angelaw-workshop/groundtruth/greengrass-blog/bad-examples/bad-example-not-full.png\" style=\"max-width:100%\"></p>\n <p><img src=\"https://s3.amazonaws.com/angelaw-workshop/groundtruth/greengrass-blog/bad-examples/bad-example-not-full-2.png\" style=\"max-width:100%\"></p>\n <p>The bounding boxes below are bad as it's not tight around storage box. </p>\n <p><img src=\"https://s3.amazonaws.com/angelaw-workshop/groundtruth/greengrass-blog/bad-examples/bad-example-not-tight.png\" style=\"max-width:100%\"></p>\n <p><img src=\"https://s3.amazonaws.com/angelaw-workshop/groundtruth/greengrass-blog/bad-examples/bad-example-not-tight-2.png\" style=\"max-width:100%\"></p>\n <p>The labeling below are bad as it only labeled part of the storage box </p>\n <p><img src=\"https://s3.amazonaws.com/angelaw-workshop/groundtruth/greengrass-blog/bad-examples/bad-example-occlusion-partial.png\" style=\"max-width:100%\"></p>\n <p><img src=\"https://s3.amazonaws.com/angelaw-workshop/groundtruth/greengrass-blog/bad-examples/bad-example-occlusion-partial-2.png\" style=\"max-width:100%\"></p>\n\n </short-instructions>\n </crowd-bounding-box>\n</crowd-form>\n \"\"\"\n with open(save_fname, 'w') as f:\n f.write(template)\n \ntemplate_name = 'instructions.template'\n# make_template(test_template=True, save_fname='instructions.html')\nmake_template(test_template=False, save_fname=template_name)\ns3.upload_file(template_name, BUCKET, EXP_NAME + '/' + template_name)\n\nprint(\"uploaded template to s3://{}/ground-truth/{}/{}\".format(BUCKET, EXP_NAME, template_name))",
"uploaded template to s3://tanmcrae-greengrass-blog/ground-truth/blue-box/instructions.template\n"
],
[
"private_workteam_arn = \"arn:aws:sagemaker:us-east-1:854681337758:workteam/private-crowd/greengrass-blog\"\n",
"_____no_output_____"
]
],
[
[
"## Create job",
"_____no_output_____"
]
],
[
[
"task_description = 'Dear Annotator, please draw a box around the yellow or blue storage box in the picture. Thank you!'\ntask_keywords = ['image', 'object', 'detection', CLASS_NAME]\ntask_title = 'Draw a box around storage box in the picture'\n\nprint(\"task_title: {}\".format(task_title))\nprint(\"JOB_NAME: {}\".format(JOB_NAME))\ntask_keywords",
"task_title: Draw a box around storage box in the picture\nJOB_NAME: blue-box-large-job-public\n"
],
[
"# Specify ARNs for resources needed to run an object detection job.\nac_arn_map = {'us-west-2': '081040173940',\n 'us-east-1': '432418664414',\n 'us-east-2': '266458841044',\n 'eu-west-1': '568282634449',\n 'ap-northeast-1': '477331159723'}\n\nprehuman_arn = 'arn:aws:lambda:{}:{}:function:PRE-BoundingBox'.format(region, ac_arn_map[region])\nacs_arn = 'arn:aws:lambda:{}:{}:function:ACS-BoundingBox'.format(region, ac_arn_map[region]) \nlabeling_algorithm_specification_arn = 'arn:aws:sagemaker:{}:027400017018:labeling-job-algorithm-specification/object-detection'.format(region)\npublic_workteam_arn = 'arn:aws:sagemaker:{}:394669845002:workteam/public-crowd/default'.format(region)",
"_____no_output_____"
],
[
"human_task_config = {\n \"AnnotationConsolidationConfig\": {\n \"AnnotationConsolidationLambdaArn\": acs_arn,\n },\n \"PreHumanTaskLambdaArn\": prehuman_arn,\n \"MaxConcurrentTaskCount\": 300, # 200 images will be sent at a time to the workteam.\n \"NumberOfHumanWorkersPerDataObject\": 1, # We will obtain and consolidate just 1 human annotation for each image.\n \"TaskAvailabilityLifetimeInSeconds\": 43200, #864000, #43200 # Your workteam has 10 days to complete all pending tasks.\n \"TaskDescription\": task_description,\n \"TaskKeywords\": task_keywords,\n \"TaskTimeLimitInSeconds\": 600, # Each image must be labeled within 10 minutes.\n \"TaskTitle\": task_title,\n \"UiConfig\": {\n \"UiTemplateS3Uri\": 's3://{}/{}/{}'.format(BUCKET, EXP_NAME, template_name),\n }\n }",
"_____no_output_____"
],
[
"if not USE_PRIVATE_WORKFORCE:\n human_task_config[\"PublicWorkforceTaskPrice\"] = {\n \"AmountInUsd\": {\n \"Dollars\": 0,\n \"Cents\": 3,\n \"TenthFractionsOfACent\": 6,\n }\n } \n human_task_config[\"WorkteamArn\"] = public_workteam_arn\nelse:\n human_task_config[\"WorkteamArn\"] = private_workteam_arn",
"_____no_output_____"
],
[
"print(json.dumps (human_task_config, indent =2 ))",
"{\n \"AnnotationConsolidationConfig\": {\n \"AnnotationConsolidationLambdaArn\": \"arn:aws:lambda:us-east-1:432418664414:function:ACS-BoundingBox\"\n },\n \"PreHumanTaskLambdaArn\": \"arn:aws:lambda:us-east-1:432418664414:function:PRE-BoundingBox\",\n \"MaxConcurrentTaskCount\": 300,\n \"NumberOfHumanWorkersPerDataObject\": 1,\n \"TaskAvailabilityLifetimeInSeconds\": 43200,\n \"TaskDescription\": \"Dear Annotator, please draw a box around the yellow or blue storage box in the picture. Thank you!\",\n \"TaskKeywords\": [\n \"image\",\n \"object\",\n \"detection\",\n \"storage box\"\n ],\n \"TaskTimeLimitInSeconds\": 600,\n \"TaskTitle\": \"Draw a box around storage box in the picture\",\n \"UiConfig\": {\n \"UiTemplateS3Uri\": \"s3://tanmcrae-greengrass-blog/blue-box/instructions.template\"\n },\n \"PublicWorkforceTaskPrice\": {\n \"AmountInUsd\": {\n \"Dollars\": 0,\n \"Cents\": 3,\n \"TenthFractionsOfACent\": 6\n }\n },\n \"WorkteamArn\": \"arn:aws:sagemaker:us-east-1:394669845002:workteam/public-crowd/default\"\n}\n"
],
[
"ground_truth_request = {\n \"InputConfig\" : {\n \"DataSource\": {\n \"S3DataSource\": {\n \"ManifestS3Uri\": 's3://{}/{}/{}'.format(BUCKET, 'manifests', MANIFEST),\n }\n },\n \"DataAttributes\": {\n \"ContentClassifiers\": [\n \"FreeOfPersonallyIdentifiableInformation\",\n \"FreeOfAdultContent\"\n ]\n }, \n },\n \"OutputConfig\" : {\n \"S3OutputPath\": 's3://{}/ground-truth-output/'.format(BUCKET),\n },\n \"HumanTaskConfig\" : human_task_config,\n \"LabelingJobName\": JOB_NAME,\n \"RoleArn\": role, \n \"LabelAttributeName\": \"bb\",\n \"LabelCategoryConfigS3Uri\": 's3://{}/{}'.format(BUCKET, LABEL_KEY),\n }\n\n\nif USE_AUTO_LABELING and RUN_FULL_AL_DEMO:\n ground_truth_request[ \"LabelingJobAlgorithmsConfig\"] = {\n \"LabelingJobAlgorithmSpecificationArn\": labeling_algorithm_specification_arn\n }",
"_____no_output_____"
],
[
"print(json.dumps (ground_truth_request, indent =2 ))",
"{\n \"InputConfig\": {\n \"DataSource\": {\n \"S3DataSource\": {\n \"ManifestS3Uri\": \"s3://tanmcrae-greengrass-blog/manifests/blue_box_large_job.json\"\n }\n },\n \"DataAttributes\": {\n \"ContentClassifiers\": [\n \"FreeOfPersonallyIdentifiableInformation\",\n \"FreeOfAdultContent\"\n ]\n }\n },\n \"OutputConfig\": {\n \"S3OutputPath\": \"s3://tanmcrae-greengrass-blog/ground-truth-output/\"\n },\n \"HumanTaskConfig\": {\n \"AnnotationConsolidationConfig\": {\n \"AnnotationConsolidationLambdaArn\": \"arn:aws:lambda:us-east-1:432418664414:function:ACS-BoundingBox\"\n },\n \"PreHumanTaskLambdaArn\": \"arn:aws:lambda:us-east-1:432418664414:function:PRE-BoundingBox\",\n \"MaxConcurrentTaskCount\": 300,\n \"NumberOfHumanWorkersPerDataObject\": 1,\n \"TaskAvailabilityLifetimeInSeconds\": 43200,\n \"TaskDescription\": \"Dear Annotator, please draw a box around the yellow or blue storage box in the picture. Thank you!\",\n \"TaskKeywords\": [\n \"image\",\n \"object\",\n \"detection\",\n \"storage box\"\n ],\n \"TaskTimeLimitInSeconds\": 600,\n \"TaskTitle\": \"Draw a box around storage box in the picture\",\n \"UiConfig\": {\n \"UiTemplateS3Uri\": \"s3://tanmcrae-greengrass-blog/blue-box/instructions.template\"\n },\n \"PublicWorkforceTaskPrice\": {\n \"AmountInUsd\": {\n \"Dollars\": 0,\n \"Cents\": 3,\n \"TenthFractionsOfACent\": 6\n }\n },\n \"WorkteamArn\": \"arn:aws:sagemaker:us-east-1:394669845002:workteam/public-crowd/default\"\n },\n \"LabelingJobName\": \"blue-box-large-job-public\",\n \"RoleArn\": \"arn:aws:iam::854681337758:role/service-role/AmazonSageMaker-ExecutionRole-20190521T132559\",\n \"LabelAttributeName\": \"bb\",\n \"LabelCategoryConfigS3Uri\": \"s3://tanmcrae-greengrass-blog/ground-truth/blue-box/class_labels.json\"\n}\n"
],
[
"sagemaker_client = boto3.client('sagemaker')\nsagemaker_client.create_labeling_job(**ground_truth_request)",
"_____no_output_____"
]
],
[
[
"## look at output manifest",
"_____no_output_____"
]
],
[
[
"job_name = 'yellow-box-small-job-public'\nOUTPUT_MANIFEST = 's3://{}/ground-truth-output/{}/manifests/output/output.manifest'.format(BUCKET, job_name)\n\noutput_file = job_name+'.output.manifest'\n!aws s3 cp {OUTPUT_MANIFEST} {output_file}",
"Completed 15.7 KiB/15.7 KiB (261.1 KiB/s) with 1 file(s) remaining\rdownload: s3://tanmcrae-greengrass-blog/ground-truth-output/yellow-box-small-job-public/manifests/output/output.manifest to ./yellow-box-small-job-public.output.manifest\r\n"
],
[
"with open(output_file, 'r') as f:\n output = [json.loads(line.strip()) for line in f.readlines()]",
"_____no_output_____"
],
[
"len(output)",
"_____no_output_____"
]
]
] | [
"code",
"markdown",
"code",
"markdown",
"code",
"markdown",
"code",
"markdown",
"code",
"markdown",
"code"
] | [
[
"code",
"code"
],
[
"markdown"
],
[
"code",
"code",
"code",
"code"
],
[
"markdown"
],
[
"code",
"code"
],
[
"markdown"
],
[
"code",
"code"
],
[
"markdown"
],
[
"code",
"code",
"code",
"code",
"code",
"code",
"code",
"code"
],
[
"markdown"
],
[
"code",
"code",
"code"
]
] |
e78585299188063dd3ebbddd33a50ff787399174 | 9,983 | ipynb | Jupyter Notebook | emmet-cli/emmet/cli/driver_scripts/visualization.ipynb | wuxiaohua1011/emmet | 49ae8f67fe42a00e1c3157667a23b4ce2d86a729 | [
"BSD-3-Clause-LBNL"
] | null | null | null | emmet-cli/emmet/cli/driver_scripts/visualization.ipynb | wuxiaohua1011/emmet | 49ae8f67fe42a00e1c3157667a23b4ce2d86a729 | [
"BSD-3-Clause-LBNL"
] | 29 | 2021-05-31T07:05:46.000Z | 2022-03-28T15:08:24.000Z | emmet-cli/emmet/cli/driver_scripts/visualization.ipynb | wuxiaohua1011/emmet | 49ae8f67fe42a00e1c3157667a23b4ce2d86a729 | [
"BSD-3-Clause-LBNL"
] | null | null | null | 35.151408 | 142 | 0.521487 | [
[
[
"from maggma.stores.advanced_stores import MongograntStore\nfrom maggma.stores.advanced_stores import Sort\nfrom typing import List\nfrom pathlib import Path\nimport plotly.graph_objects as go\nimport plotly.express as px\nimport pandas as pd\nimport numpy as np\nfrom datetime import timedelta, date, datetime\nfrom monty.json import MontyDecoder\nfrom datetime import timedelta\n# configuration stuff\nfrom sys import platform\nimport maggma\nif platform == \"linux\" or platform == \"linux2\":\n import plotly.io as pio\n pio.orca.config.use_xvfb = True\nimport plotly.graph_objs as go\nimport plotly.offline as py\nimport plotly.express as px\nimport plotly.graph_objects as go\nimport plotly",
"_____no_output_____"
],
[
"renderer = \"notebook\" # change to pdf for live viewing",
"_____no_output_____"
],
[
"gdrive_mongo_store = MongograntStore(mongogrant_spec=\"rw:knowhere.lbl.gov/mp_core_mwu\",\n collection_name=\"gdrive\")\ngdrive_mongo_store.connect()\n\ntasks_mongo_store = MongograntStore(mongogrant_spec=\"ro:mongodb04.nersc.gov/mp_emmet_prod\",\n collection_name=\"tasks\")\ntasks_mongo_store.connect()",
"_____no_output_____"
],
[
"import plotly.graph_objects as go\n\ndf = pd.DataFrame()\ndf[\"title\"] = np.array([\"Total in Gdrive\", \"Total Tasks\"])\ndf[\"count\"] = np.array([gdrive_mongo_store.count(), tasks_mongo_store.count()])\nfig = px.pie(df, values='count', names='title', title=\"Tasks & GDrive\")\nfig.show(renderer=renderer)\n\nprint(\"WARNING: This Pie chart might not reflect the actual progress since there are tasks that belong to an deprecated material\")",
"_____no_output_____"
],
[
"df = pd.DataFrame()\ndf[\"type\"] = np.array([\"Total in Gdrive\",\"Total in NOMAD\" ])\ndf[\"count\"] = np.array([gdrive_mongo_store.count(criteria={\"error\": {\"$eq\": None}}), \n gdrive_mongo_store.count(criteria={\"nomad_updated\": {\"$ne\": None}})])\nfig = px.bar(df,\n x=\"type\",\n y=\"count\", title=\"Num uploaded to Gdrive and NOMAD\", color=\"type\")\nfig.show(renderer=renderer)\n\n\n\n",
"_____no_output_____"
],
[
"all_content_gdrive = gdrive_mongo_store.query(criteria={\"error\": None},properties={\"file_size\":1})\ngdrive_size = 0\nfor c in all_content_gdrive:\n gdrive_size += c[\"file_size\"]\nprint(f\"GDrive: {gdrive_size} bytes = {gdrive_size*1e-6} mb = {gdrive_size*1e-9} gb\")\n\n\nall_content_nomad = gdrive_mongo_store.query(criteria={\"$and\": \n [{\"error\": None}, \n {\"nomad_updated\": {\"$ne\":None}}]\n },\n properties={\"file_size\":1})\nnomad_size = 0\nfor c in all_content_nomad:\n nomad_size += c[\"file_size\"]\nprint(f\"Nomad: {nomad_size} bytes = {nomad_size*1e-6} mb = {nomad_size*1e-9} gb\")\n\n\ndf = pd.DataFrame()\ndf[\"title\"] = np.array([\"GDrive Upload GB\",\"Nomad Upload GB\" ])\ndf[\"bytes\"] = np.array([gdrive_size*1e-9, nomad_size*1e-9])\nfig = px.bar(df, y='bytes', x='title', color='title', title=\"GDrive & NOMAD by bytes\")\nfig.show(renderer=renderer)",
"_____no_output_____"
],
[
"df = pd.DataFrame()\ndf[\"title\"] = np.array([\"Success\",\"Failed\" ])\ndf[\"count\"] = np.array([gdrive_mongo_store.count(criteria={\"error\": {\"$eq\": None}}), \n gdrive_mongo_store.count(criteria={\"error\": {\"$ne\": None}})])\nfig = px.pie(df, values='count', names='title', title=\"GDrive Upload Status\")\nfig.show(renderer=renderer)\n\n",
"_____no_output_____"
],
[
"def find_dates_btw(start_dt, end_dt):\n \"\"\"\n find the number of dates between start date and end date\n \"\"\"\n def daterange(date1, date2):\n if date1 is None: date1 = date2\n if date2 is None: date2 = date1\n for n in range(int((date2 - date1).days)+1):\n yield date1 + timedelta(n)\n dates = []\n for dt in daterange(start_dt, end_dt+timedelta(days=1)):\n date_format = dt.date()\n dates.append(datetime(date_format.year, date_format.month, date_format.day))\n return dates\n\ndef find_earliest_date(store, field):\n \"\"\"\n find the earliest record date\n \"\"\"\n return list(store.query(criteria={\"error\": {\"$eq\": None}}, sort={field:maggma.core.store.Sort.Ascending}, limit=1))[0][field]\n\ndef find_latest_date(store, field):\n \"\"\"\n find the latest_record date\n \"\"\"\n return list(store.query(criteria={\"error\": {\"$eq\": None}}, sort={field:maggma.core.store.Sort.Descending}, limit=1))[0][field]",
"_____no_output_____"
],
[
"def make_time_series_data(field_name):\n \"\"\"\n Find all time series data for that field, put them in buckets of dates.\n \"\"\"\n dates = find_dates_btw(find_earliest_date(gdrive_mongo_store, field_name), \n find_latest_date(gdrive_mongo_store, field_name))\n # last_updated \n result = dict()\n for i in range(len(dates)):\n if i == 0:\n result[dates[i]] = 0\n else:\n c = gdrive_mongo_store.count(criteria={field_name: {\"$lte\": dates[i]}})\n result[dates[i]] = c\n return result\ndef make_time_series_data_nomad(field_name=\"nomad_updated\"):\n \"\"\"\n Find all time series data for that field, put them in buckets of dates.\n \"\"\"\n start = list(gdrive_mongo_store.query(criteria={field_name: {\"$ne\": None}}, \n sort={field_name:maggma.core.store.Sort.Ascending}, limit=1))[0][field_name]\n \n end = list(gdrive_mongo_store.query(criteria={field_name: {\"$ne\": None}}, \n sort={field_name:maggma.core.store.Sort.Descending}, limit=1))[0][field_name]\n dates = find_dates_btw(start, end)\n # last_updated \n result = dict()\n for i in range(len(dates)):\n if i == 0:\n result[dates[i]] = 0\n else:\n c = gdrive_mongo_store.count(criteria={field_name: {\"$lte\": dates[i]}})\n result[dates[i]] = c\n return result",
"_____no_output_____"
],
[
"last_updated_data = make_time_series_data(\"last_updated\")\nnomad_updated_data = make_time_series_data_nomad()",
"_____no_output_____"
],
[
"Xs = set(last_updated_data.keys()).union(set(nomad_updated_data.keys()))\nfig = go.Figure()\nfig.add_trace(go.Scatter(x=list(last_updated_data.keys()), y=list(last_updated_data.values()),\n mode='lines+markers',\n name='last_updated'))\nfig.add_trace(go.Scatter(x=list(nomad_updated_data.keys()), y=list(nomad_updated_data.values()),\n mode='lines+markers',\n name='nomad_updated'))\n\n# add features\nfig.update_layout(\n title=\"GDrive Upload Status\",\n xaxis_title=\"Time\",\n yaxis_title=\"# Submission\",\n font=dict(\n family=\"Franklin Gothic\",\n size=14,\n color=\"#0d0d0d\"\n ), \n yaxis_type=\"log\",\n)\nfig.show(renderer=renderer)",
"_____no_output_____"
]
]
] | [
"code"
] | [
[
"code",
"code",
"code",
"code",
"code",
"code",
"code",
"code",
"code",
"code",
"code"
]
] |
e78586b6f80a578870447a7be0c9cc261ba9e2a6 | 77,299 | ipynb | Jupyter Notebook | 10_synchro.io.ipynb | wiessall/theonerig | b90f9a631d696c8fd31ad602c40c0723e58bbbb0 | [
"Apache-2.0"
] | null | null | null | 10_synchro.io.ipynb | wiessall/theonerig | b90f9a631d696c8fd31ad602c40c0723e58bbbb0 | [
"Apache-2.0"
] | null | null | null | 10_synchro.io.ipynb | wiessall/theonerig | b90f9a631d696c8fd31ad602c40c0723e58bbbb0 | [
"Apache-2.0"
] | null | null | null | 46.259126 | 245 | 0.50914 | [
[
[
"#default_exp synchro.io",
"_____no_output_____"
],
[
"%load_ext autoreload\n%autoreload 2",
"_____no_output_____"
]
],
[
[
"# synchro.io\n> IO classes to read files in the different format Asari lab is acquiring from: hdf5, rhd, raw, npy, all adapted from the SpykingCircus project by Pierre Yger and Olivier Marre https://spyking-circus.readthedocs.io/en/latest/",
"_____no_output_____"
]
],
[
[
"#export\nimport numpy as np\nimport re, sys, os, logging, struct\nimport h5py\nfrom colorama import Fore\n\nlogger = logging.getLogger(__name__)\n\ndef atoi(text):\n return int(text) if text.isdigit() else text\n\ndef natural_keys(text):\n '''\n alist.sort(key=natural_keys) sorts in human order\n http://nedbatchelder.com/blog/200712/human_sorting.html\n (See Toothy's implementation in the comments)\n '''\n return [atoi(c) for c in re.split('(\\d+)', text) ]\n\ndef filter_per_extension(files, extension):\n results = []\n for file in files:\n fn, ext = os.path.splitext(file)\n if ext == extension:\n results += [file]\n return results\n\ndef print_and_log(to_print, level='info', logger=None, display=True):\n if display:\n if level == 'default':\n for line in to_print:\n print(Fore.WHITE + line + '\\n')\n if level == 'info':\n print_info(to_print)\n elif level == 'error':\n print_error(to_print)\n sys.stdout.flush()\n\ndef print_info(lines):\n \"\"\"Prints informations messages, enhanced graphical aspects.\"\"\"\n print(Fore.YELLOW + \"------------------------- Informations -------------------------\\n\")\n for line in lines:\n print(Fore.YELLOW + \"| \" + line + '\\n')\n print(Fore.YELLOW + \"------------------------------------------------------------------\\n\" + Fore.WHITE)\n\ndef print_error(lines):\n \"\"\"Prints errors messages, enhanced graphical aspects.\"\"\"\n print(Fore.RED + \"---------------------------- Error -----------------------------\\n\")\n for line in lines:\n print(Fore.RED + \"| \" + line + '\\n')\n print(Fore.RED + \"------------------------------------------------------------------\\n\" + Fore.WHITE)\n\n\ndef get_offset(data_dtype, dtype_offset):\n\n if dtype_offset == 'auto':\n if data_dtype in ['uint16', np.uint16]:\n dtype_offset = 32768\n elif data_dtype in ['int16', np.int16]:\n dtype_offset = 0\n elif data_dtype in ['int32', np.int32]:\n dtype_offset = 0\n elif data_dtype in ['int64', np.int64]:\n dtype_offset = 0\n elif data_dtype in ['float32', np.float32]:\n dtype_offset = 0\n elif data_dtype in ['int8', np.int8]:\n dtype_offset = 0\n elif data_dtype in ['uint8', np.uint8]:\n dtype_offset = 127\n elif data_dtype in ['float64', np.float64]:\n dtype_offset = 0\n elif data_dtype==\">d\":\n dtype_offset = 0\n else:\n try:\n dtype_offset = int(dtype_offset)\n except:\n print(\"Offset %s is not valid\" %dtype_offset)\n\n return dtype_offset",
"_____no_output_____"
],
[
"#export\nclass DataFile(object):\n\n '''\n A generic class that will represent how the program interacts with the data. Such an abstraction\n layer should allow people to write their own wrappers, for several file formats, with or without\n parallel write, streams, and so on. Note that depending on the complexity of the datastructure,\n this extra layer can slow down the code.\n '''\n\n description = \"mydatafile\" # Description of the file format\n extension = [\".myextension\"] # extensions\n parallel_write = False # can be written in parallel (using the comm object)\n is_writable = False # can be written\n is_streamable = ['multi-files'] # If the file format can support streams of data ['multi-files' is a default, but can be something else]\n _shape = None # The total shape of the data (nb time steps, nb channels) accross streams if any\n _t_start = None # The global t_start of the data\n _t_stop = None # The final t_stop of the data, accross all streams if any\n\n # This is a dictionary of values that need to be provided to the constructor, with the corresponding type\n _required_fields = {}\n\n # This is a dictionary of values that may have a default value, if not provided to the constructor\n _default_values = {}\n\n _params = {}\n\n def __init__(self, file_name, params, is_empty=False, stream_mode=None):\n '''\n The constructor that will create the DataFile object. Note that by default, values are read from the header\n of the file. If not found in the header, they are read from the parameter file. If no values are found, the\n code will trigger an error\n\n What you need to specify at a generic level (for a given file format)\n - parallel_write : can the file be safely written in parallel ?\n - is_writable : if the file can be written\n - is_streamable : if the file format can support streaming data\n - required_fields : what parameter must be specified for the file format, along with the type\n - default_values : parameters that may have default values if not provided\n\n What you need to specify at a low level (maybe by getting specific values with _read_from_header)\n - _shape : the size of the data, should be a tuple (duration in time bins, nb_channels)\n - _t_start : the time (in time steps) of the recording (0 by default)\n '''\n\n self.params = {}\n self.params.update(self._params)\n\n if not is_empty:\n self._check_filename(file_name)\n\n if stream_mode is not None:\n self.is_stream = True\n if not stream_mode in self.is_streamable:\n if self.is_master:\n print_and_log([\"The file format %s does not support stream mode %s\" %(self.description, stream_mode)], 'error', logger)\n sys.exit(1)\n if is_empty:\n sys.exit(1)\n else:\n self.is_stream = False\n\n self.file_name = file_name\n self.is_empty = is_empty\n self.stream_mode = stream_mode\n\n f_next, extension = os.path.splitext(self.file_name)\n\n self._check_extension(extension)\n self._fill_from_params(params)\n\n if not self.is_empty:\n try:\n self._fill_from_header(self._read_from_header())\n except Exception as ex:\n print_and_log([\"There is an error in the _read_from_header method of the wrapper\\n\" + str(ex)], 'error', logger)\n else:\n self._shape = (0, 0)\n\n if self._shape is None:\n sys.exit(1)\n\n self.params['dtype_offset'] = get_offset(self.data_dtype, self.dtype_offset)\n\n if self.stream_mode:\n self._sources = self.set_streams(self.stream_mode)\n self._times = []\n for source in self._sources:\n self._times += [source.t_start]\n print_and_log(['The file is composed of %d streams' %len(self._sources),\n 'Times are between %d and %d' %(self._sources[0].t_start, self._sources[-1].t_stop)], 'debug',logger)\n\n ##################################################################################################################\n ##################################################################################################################\n ######### Methods that need to be overwritten for a given fileformat #######\n ##################################################################################################################\n ##################################################################################################################\n\n\n def _read_from_header(self):\n '''\n This function is called only if the file is not empty, and should fill the values in the constructor\n such as _shape. It returns a dictionnary, that will be added to self._params based on the constrains given by\n required_fields and default_values\n '''\n raise NotImplementedError('The _read_from_header method needs to be implemented for file format %s' %self.description)\n\n\n def _open(self, mode=''):\n '''\n This function should open the file\n - mode can be to read only 'r', or to write 'w'\n '''\n raise NotImplementedError('The open method needs to be implemented for file format %s' %self.description)\n\n\n def _close(self):\n '''\n This function closes the file\n '''\n raise NotImplementedError('The close method needs to be implemented for file format %s' %self.description)\n\n\n def read_chunk(self, idx, chunk_size, padding=(0, 0), nodes=None):\n '''\n Assuming the analyze function has been called before, this is the main function\n used by the code, in all steps, to get data chunks. More precisely, assuming your\n dataset can be divided in nb_chunks (see analyze) of temporal size (chunk_size),\n\n - idx is the index of the chunk you want to load\n - chunk_size is the time of those chunks, in time steps\n - if the data loaded are data[idx:idx+1], padding should add some offsets,\n in time steps, such that we can load data[idx+padding[0]:idx+padding[1]]\n - nodes is a list of nodes, between 0 and nb_channels\n '''\n\n raise NotImplementedError('The read_chunk method needs to be implemented for file format %s' %self.description)\n\n def read_chunk_adc(self, idx, chunk_size, padding=(0, 0), nodes=None):\n '''\n Same as read_chunk, but for the analog channel of the file.\n\n - idx is the index of the chunk you want to load\n - chunk_size is the time of those chunks, in time steps\n - if the data loaded are data[idx:idx+1], padding should add some offsets,\n in time steps, such that we can load data[idx+padding[0]:idx+padding[1]]\n - nodes is a list of nodes, between 0 and nb_channels\n '''\n\n raise NotImplementedError('The read_chunk_adc method needs to be implemented for file format %s' %self.description)\n\n\n def write_chunk(self, time, data):\n '''\n This function writes data at a given time.\n - time is expressed in timestep\n - data must be a 2D matrix of size time_length x nb_channels\n '''\n raise NotImplementedError('The set_data method needs to be implemented for file format %s' %self.description)\n\n\n def set_streams(self, stream_mode):\n '''\n This function is only used for file format supporting streams, and need to return a list of datafiles, with\n appropriate t_start for each of them. Note that the results will be using the times defined by the streams.\n You can do anything regarding the keyword used for the stream mode, but multi-files is immplemented by default\n This will allow every file format to be streamed from multiple sources, and processed as a single file.\n '''\n\n if stream_mode == 'multi-files':\n dirname = os.path.abspath(os.path.dirname(self.file_name))\n fname = os.path.basename(self.file_name)\n fn, ext = os.path.splitext(fname)\n all_files = os.listdir(dirname)\n all_files = filter_per_extension(all_files, ext)\n all_files.sort(key=natural_keys)\n\n sources = []\n to_write = []\n global_time = 0\n params = self.get_description()\n\n for fname in all_files:\n new_data = type(self)(os.path.join(os.path.abspath(dirname), fname), params)\n new_data._t_start = global_time\n global_time += new_data.duration\n sources += [new_data]\n to_write += ['We found the datafile %s with t_start %s and duration %s' %(new_data.file_name, new_data.t_start, new_data.duration)]\n print_and_log(to_write, 'debug', logger)\n return sources\n\n ################################## Optional, only if internal names are changed ##################################\n\n @property\n def sampling_rate(self):\n return self.params['sampling_rate']\n\n @property\n def data_dtype(self):\n return self.params['data_dtype']\n\n @property\n def dtype_offset(self):\n return self.params['dtype_offset']\n\n @property\n def data_offset(self):\n return self.params['data_offset']\n\n @property\n def nb_channels(self):\n return int(self.params['nb_channels'])\n\n @property\n def gain(self):\n return self.params['gain']\n\n ##################################################################################################################\n ##################################################################################################################\n ######### End of methods that need to be overwritten for a given fileformat #######\n ##################################################################################################################\n ##################################################################################################################\n\n\n def get_file_names(self):\n res = []\n if self.stream_mode == 'multi-files':\n for source in self._sources:\n res += [source.file_name]\n return res\n\n def _check_filename(self, file_name):\n if not os.path.exists(file_name):\n sys.exit(1)\n\n\n def _check_extension(self, extension):\n if len(self.extension) > 0:\n if not extension in self.extension + [item.upper() for item in self.extension]:\n sys.exit(1)\n\n\n def _fill_from_params(self, params):\n\n for key in self._required_fields:\n if key not in params:\n self._check_requirements_(params)\n else:\n self.params[key] = self._required_fields[key](params[key])\n\n for key in self._default_values:\n if key not in params:\n self.params[key] = self._default_values[key]\n else:\n self.params[key] = type(self._default_values[key])(params[key])\n\n def _fill_from_header(self, header):\n\n for key in list(header.keys()):\n self.params[key] = header[key]\n\n def _check_requirements_(self, params):\n\n missing = {}\n\n for key, value in list(self._required_fields.items()):\n if key not in list(params.keys()):\n missing[key] = value\n\n if len(missing) > 0:\n self._display_requirements_()\n sys.exit(1)\n\n\n def _display_requirements_(self):\n\n to_write = ['The parameters for %s file format are:' %self.description.upper(), '']\n nb_params = 0\n\n for key, value in list(self._required_fields.items()):\n mystring = '-- %s -- %s' %(key, str(value))\n mystring += ' [** mandatory **]'\n to_write += [mystring]\n nb_params += 1\n\n to_write += ['']\n\n for key, value in list(self._default_values.items()):\n mystring = '-- %s -- %s' %(key, str(type(value)))\n mystring += ' [default is %s]' %value\n to_write += [mystring]\n nb_params += 1\n\n def _scale_data_to_float32(self, data):\n '''\n This function will convert data from local data dtype into float32, the default format of the algorithm\n '''\n if self.data_dtype != np.float32:\n data = data.astype(np.float32)\n\n if self.dtype_offset != 0:\n data -= self.dtype_offset\n\n if np.any(self.gain != 1):\n data *= self.gain\n\n return np.ascontiguousarray(data)\n\n\n def _unscale_data_from_float32(self, data):\n '''\n This function will convert data from float32 back to the original format of the file\n '''\n\n if np.any(self.gain != 1):\n data /= self.gain\n\n if self.dtype_offset != 0:\n data += self.dtype_offset\n\n if (data.dtype != self.data_dtype) and (self.data_dtype != np.float32):\n data = data.astype(self.data_dtype)\n\n return data\n\n\n def _count_chunks(self, chunk_size, duration, strict=False):\n '''\n This function will count how many block of size chunk_size can be found within a certain duration\n This returns the number of blocks, plus the remaining part\n '''\n nb_chunks = duration // chunk_size\n last_chunk_len = duration - nb_chunks * chunk_size\n\n if not strict and last_chunk_len > 0:\n nb_chunks += 1\n\n return nb_chunks, last_chunk_len\n\n\n def _get_t_start_t_stop(self, idx, chunk_size, padding=(0,0)):\n\n t_start = idx*np.int64(chunk_size)+padding[0]\n t_stop = (idx+1)*np.int64(chunk_size)+padding[1]\n\n if t_stop > self.duration:\n t_stop = self.duration\n\n if t_start < 0:\n t_start = 0\n\n return t_start, t_stop\n\n\n def _get_streams_index_by_time(self, local_time):\n if self.is_stream:\n cidx = np.searchsorted(self._times, local_time, 'right') - 1\n return cidx\n\n def is_first_chunk(self, idx, nb_chunks):\n\n if self.is_stream:\n cidx = np.searchsorted(self._chunks_in_sources, idx, 'right') - 1\n idx -= self._chunks_in_sources[cidx]\n if idx == 0:\n return True\n else:\n if idx == 0:\n return True\n return False\n\n def is_last_chunk(self, idx, nb_chunks):\n\n if self.is_stream:\n if (idx > 0) and (idx in self._chunks_in_sources - 1):\n return True\n else:\n if idx == nb_chunks:\n return True\n return False\n\n def get_snippet(self, global_time, length, nodes=None):\n '''\n This function should return a time snippet of size length x nodes\n - time is in timestep\n - length is in timestep\n - nodes is a list of nodes, between 0 and nb_channels\n '''\n if self.is_stream:\n cidx = self._get_streams_index_by_time(global_time)\n return self._sources[cidx].get_snippet(global_time, length, nodes)\n else:\n local_time = global_time - self.t_start\n return self.get_data(0, chunk_size=length, padding=(local_time, local_time), nodes=nodes)[0]\n \n def get_snippet_adc(self, global_time, length, nodes=None):\n '''\n This function should return a time snippet of size length x nodes\n - time is in timestep\n - length is in timestep\n - nodes is a list of nodes, between 0 and nb_channels\n '''\n if self.is_stream:\n cidx = self._get_streams_index_by_time(global_time)\n return self._sources[cidx].get_snippet_adc(global_time, length, nodes)\n else:\n local_time = global_time - self.t_start\n return self.get_data_adc(0, chunk_size=length, padding=(local_time, local_time), nodes=nodes)[0]\n\n\n def get_data(self, idx, chunk_size, padding=(0, 0), nodes=None):\n\n if self.is_stream:\n cidx = np.searchsorted(self._chunks_in_sources, idx, 'right') - 1\n idx -= self._chunks_in_sources[cidx]\n return self._sources[cidx].read_chunk(idx, chunk_size, padding, nodes), self._sources[cidx].t_start + idx*chunk_size\n else:\n return self.read_chunk(idx, chunk_size, padding, nodes), self.t_start + idx*chunk_size\n \n def get_data_adc(self, idx, chunk_size, padding=(0, 0), nodes=None):\n\n if self.is_stream:\n cidx = np.searchsorted(self._chunks_in_sources, idx, 'right') - 1\n idx -= self._chunks_in_sources[cidx]\n return self._sources[cidx].read_chunk_adc(idx, chunk_size, padding, nodes), self._sources[cidx].t_start + idx*chunk_size\n else:\n return self.read_chunk_adc(idx, chunk_size, padding, nodes), self.t_start + idx*chunk_size\n \n def get_data_dig_in(self, idx, chunk_size, padding=(0, 0), nodes=None):\n\n if self.is_stream:\n cidx = np.searchsorted(self._chunks_in_sources, idx, 'right') - 1\n idx -= self._chunks_in_sources[cidx]\n return self._sources[cidx].read_chunk_dig_in(idx, chunk_size, padding, nodes), self._sources[cidx].t_start + idx*chunk_size\n else:\n return self.read_chunk_dig_in(idx, chunk_size, padding, nodes), self.t_start + idx*chunk_size\n \n def get_data_both(self, idx, chunk_size, padding=(0, 0), nodes=None):\n\n if self.is_stream:\n cidx = np.searchsorted(self._chunks_in_sources, idx, 'right') - 1\n idx -= self._chunks_in_sources[cidx]\n return (*self._sources[cidx].read_chunk_both(idx, chunk_size, padding, nodes), self._sources[cidx].t_start + idx*chunk_size)\n else:\n return (*self.read_chunk_both(idx, chunk_size, padding, nodes), self.t_start + idx*chunk_size)\n\n def set_data(self, global_time, data):\n\n if self.is_stream:\n cidx = self._get_streams_index_by_time(global_time)\n local_time = global_time - self._sources[cidx].t_start\n return self._sources[cidx].write_chunk(local_time, data)\n else:\n local_time = global_time - self.t_start\n return self.write_chunk(local_time, data)\n\n\n def analyze(self, chunk_size, strict=False):\n '''\n This function should return two values:\n - the number of temporal chunks of temporal size chunk_size that can be found\n in the data. Note that even if the last chunk is not complete, it has to be\n counted. chunk_size is expressed in time steps\n - the length of the last uncomplete chunk, in time steps\n '''\n if self.is_stream:\n nb_chunks = 0\n last_chunk_len = 0\n self._chunks_in_sources = [0]\n\n for source in self._sources:\n a, b = self._count_chunks(chunk_size, source.duration, strict)\n nb_chunks += a\n last_chunk_len += b\n\n self._chunks_in_sources += [nb_chunks]\n\n self._chunks_in_sources = np.array(self._chunks_in_sources)\n\n return nb_chunks, last_chunk_len\n else:\n return self._count_chunks(chunk_size, self.duration, strict)\n\n\n def get_description(self):\n result = {}\n for key in ['sampling_rate', 'data_dtype', 'gain', 'nb_channels', 'dtype_offset'] + list(self._default_values.keys()) + list(self._required_fields.keys()):\n result[key] = self.params[key]\n return result\n\n\n @property\n def shape(self):\n return (self.duration, int(self.nb_channels))\n\n\n @property\n def duration(self):\n if self.is_stream:\n duration = 0\n for source in self._sources:\n duration += source.duration\n return duration\n else:\n return np.int64(self._shape[0])\n\n\n @property\n def is_master(self):\n return True#comm.rank == 0\n\n\n @property\n def t_start(self):\n if self.is_stream:\n return self._sources[0].t_start\n else:\n if self._t_start is None:\n self._t_start = 0\n return self._t_start\n\n\n @property\n def t_stop(self):\n if self.is_stream:\n return self._sources[-1].t_stop\n else:\n if self._t_stop is None:\n self._t_stop = self.t_start + self.duration\n return self._t_stop\n\n\n @property\n def nb_streams(self):\n if self.is_stream:\n return len(self._sources)\n else:\n return 1\n\n def open(self, mode='r'):\n if self.is_stream:\n for source in self._sources:\n source._open(mode)\n else:\n self._open(mode)\n\n\n def close(self):\n if self.is_stream:\n for source in self._sources:\n source._close()\n else:\n self._close()",
"_____no_output_____"
],
[
"#export\ndef read_header(fid):\n \"\"\"Reads the Intan File Format header from the given file.\"\"\"\n\n # Check 'magic number' at beginning of file to make sure this is an Intan\n # Technologies RHD2000 data file.\n magic_number, = struct.unpack('<I', fid.read(4))\n if magic_number != int('c6912702', 16): raise Exception('Unrecognized file type.')\n\n header = {}\n # Read version number.\n version = {}\n (version['major'], version['minor']) = struct.unpack('<hh', fid.read(4))\n header['version'] = version\n\n freq = {}\n\n # Read information of sampling rate and amplifier frequency settings.\n header['sample_rate'], = struct.unpack('<f', fid.read(4))\n (freq['dsp_enabled'], freq['actual_dsp_cutoff_frequency'], freq['actual_lower_bandwidth'], freq['actual_upper_bandwidth'],\n freq['desired_dsp_cutoff_frequency'], freq['desired_lower_bandwidth'], freq['desired_upper_bandwidth']) = struct.unpack('<hffffff', fid.read(26))\n\n\n # This tells us if a software 50/60 Hz notch filter was enabled during\n # the data acquisition.\n notch_filter_mode, = struct.unpack('<h', fid.read(2))\n header['notch_filter_frequency'] = 0\n if notch_filter_mode == 1:\n header['notch_filter_frequency'] = 50\n elif notch_filter_mode == 2:\n header['notch_filter_frequency'] = 60\n freq['notch_filter_frequency'] = header['notch_filter_frequency']\n\n (freq['desired_impedance_test_frequency'], freq['actual_impedance_test_frequency']) = struct.unpack('<ff', fid.read(8))\n\n note1 = read_qstring(fid)\n note2 = read_qstring(fid)\n note3 = read_qstring(fid)\n header['notes'] = { 'note1' : note1, 'note2' : note2, 'note3' : note3}\n\n # If data file is from GUI v1.1 or later, see if temperature sensor data was saved.\n header['num_temp_sensor_channels'] = 0\n if (version['major'] == 1 and version['minor'] >= 1) or (version['major'] > 1) :\n header['num_temp_sensor_channels'], = struct.unpack('<h', fid.read(2))\n\n\n # If data file is from GUI v1.3 or later, load eval board mode.\n header['eval_board_mode'] = 0\n if ((version['major'] == 1) and (version['minor'] >= 3)) or (version['major'] > 1) :\n header['eval_board_mode'], = struct.unpack('<h', fid.read(2))\n\n # Place frequency-related information in data structure. (Note: much of this structure is set above)\n freq['amplifier_sample_rate'] = header['sample_rate']\n freq['aux_input_sample_rate'] = header['sample_rate'] / 4\n freq['supply_voltage_sample_rate'] = header['sample_rate'] / 60\n freq['board_adc_sample_rate'] = header['sample_rate']\n freq['board_dig_in_sample_rate'] = header['sample_rate']\n\n header['frequency_parameters'] = freq\n\n # Create structure arrays for each type of data channel.\n header['spike_triggers'] = []\n header['amplifier_channels'] = []\n header['aux_input_channels'] = []\n header['supply_voltage_channels'] = []\n header['board_adc_channels'] = []\n header['board_dig_in_channels'] = []\n header['board_dig_out_channels'] = []\n\n # Read signal summary from data file header.\n\n if (header['version']['major'] > 1):\n header['reference_channel'] = read_qstring(fid)\n\n number_of_signal_groups, = struct.unpack('<h', fid.read(2))\n\n for signal_group in range(0, number_of_signal_groups):\n signal_group_name = read_qstring(fid)\n signal_group_prefix = read_qstring(fid)\n (signal_group_enabled, signal_group_num_channels, signal_group_num_amp_channels) = struct.unpack('<hhh', fid.read(6))\n\n if (signal_group_num_channels > 0) and (signal_group_enabled > 0):\n for signal_channel in range(0, signal_group_num_channels):\n new_channel = {'port_name' : signal_group_name, 'port_prefix' : signal_group_prefix, 'port_number' : signal_group}\n new_channel['native_channel_name'] = read_qstring(fid)\n new_channel['custom_channel_name'] = read_qstring(fid)\n (new_channel['native_order'], new_channel['custom_order'], signal_type, channel_enabled, new_channel['chip_channel'], new_channel['board_stream']) = struct.unpack('<hhhhhh', fid.read(12))\n new_trigger_channel = {}\n (new_trigger_channel['voltage_trigger_mode'], new_trigger_channel['voltage_threshold'], new_trigger_channel['digital_trigger_channel'], new_trigger_channel['digital_edge_polarity']) = struct.unpack('<hhhh', fid.read(8))\n (new_channel['electrode_impedance_magnitude'], new_channel['electrode_impedance_phase']) = struct.unpack('<ff', fid.read(8))\n\n if channel_enabled:\n if signal_type == 0:\n header['amplifier_channels'].append(new_channel)\n header['spike_triggers'].append(new_trigger_channel)\n elif signal_type == 1:\n header['aux_input_channels'].append(new_channel)\n elif signal_type == 2:\n header['supply_voltage_channels'].append(new_channel)\n elif signal_type == 3:\n header['board_adc_channels'].append(new_channel)\n elif signal_type == 4:\n header['board_dig_in_channels'].append(new_channel)\n elif signal_type == 5:\n header['board_dig_out_channels'].append(new_channel)\n else:\n raise Exception('Unknown channel type.')\n\n\n # Summarize contents of data file.\n header['num_amplifier_channels'] = len(header['amplifier_channels'])\n header['num_aux_input_channels'] = len(header['aux_input_channels'])\n header['num_supply_voltage_channels'] = len(header['supply_voltage_channels'])\n header['num_board_adc_channels'] = len(header['board_adc_channels'])\n header['num_board_dig_in_channels'] = len(header['board_dig_in_channels'])\n header['num_board_dig_out_channels'] = len(header['board_dig_out_channels'])\n\n return header\n\n\ndef get_bytes_per_data_block(header):\n \"\"\"Calculates the number of bytes in each 60-sample datablock.\"\"\"\n\n if (header['version']['major'] == 1):\n num_samples_per_data_block = 60\n else:\n num_samples_per_data_block = 128\n\n # Each data block contains 60 amplifier samples.\n bytes_per_block = num_samples_per_data_block * 4 # timestamp data\n bytes_per_block = bytes_per_block + num_samples_per_data_block * 2 * header['num_amplifier_channels']\n\n # Auxiliary inputs are sampled 4x slower than amplifiers\n bytes_per_block = bytes_per_block + (num_samples_per_data_block / 4) * 2 * header['num_aux_input_channels']\n\n # Supply voltage is sampled 60x slower than amplifiers\n bytes_per_block = bytes_per_block + 1 * 2 * header['num_supply_voltage_channels']\n\n # Board analog inputs are sampled at same rate as amplifiers\n bytes_per_block = bytes_per_block + num_samples_per_data_block * 2 * header['num_board_adc_channels']\n\n # Board digital inputs are sampled at same rate as amplifiers\n if header['num_board_dig_in_channels'] > 0:\n bytes_per_block = bytes_per_block + num_samples_per_data_block * 2\n\n # Board digital outputs are sampled at same rate as amplifiers\n if header['num_board_dig_out_channels'] > 0:\n bytes_per_block = bytes_per_block + num_samples_per_data_block * 2\n\n # Temp sensor is sampled 60x slower than amplifiers\n if header['num_temp_sensor_channels'] > 0:\n bytes_per_block = bytes_per_block + 1 * 2 * header['num_temp_sensor_channels']\n\n return bytes_per_block\n\n\n\ndef read_qstring(fid):\n \"\"\"Read Qt style QString.\n\n The first 32-bit unsigned number indicates the length of the string (in bytes).\n If this number equals 0xFFFFFFFF, the string is null.\n\n Strings are stored as unicode.\n \"\"\"\n\n length, = struct.unpack('<I', fid.read(4))\n if length == int('ffffffff', 16): return \"\"\n\n if length > (os.fstat(fid.fileno()).st_size - fid.tell() + 1) :\n print(length)\n raise Exception('Length too long.')\n\n # convert length from bytes to 16-bit Unicode words\n length = int(length / 2)\n\n data = []\n for i in range(0, length):\n c, = struct.unpack('<H', fid.read(2))\n data.append(c)\n\n if sys.version_info >= (3,0):\n a = ''.join([chr(c) for c in data])\n else:\n a = ''.join([chr(c) for c in data])\n\n return a\n\n\nclass RHDFile(DataFile):\n\n description = \"rhd\"\n extension = [\".rhd\"]\n parallel_write = True\n is_writable = True\n is_streamable = ['multi-files']\n\n _required_fields = {}\n _default_values = {}\n\n _params = {'dtype_offset' : 'auto',\n 'data_dtype' : 'uint16',\n 'gain' : 0.195}\n\n def _read_from_header(self):\n\n header = {}\n\n self.file = open(self.file_name, 'rb')\n full_header = read_header(self.file)\n self.header = full_header\n header['nb_channels'] = full_header['num_amplifier_channels']\n header['sampling_rate'] = full_header['sample_rate']\n\n if full_header['version']['major'] == 1:\n self.SAMPLES_PER_RECORD = 60\n else:\n self.SAMPLES_PER_RECORD = 128\n self.nb_channels_adc = full_header['num_board_adc_channels']\n self.nb_channels_dig_in = full_header['num_board_dig_in_channels']\n header['data_offset'] = self.file.tell()\n \n data_present = False\n filesize = os.path.getsize(self.file_name)\n self.bytes_per_block = get_bytes_per_data_block(full_header)\n self.block_offset = self.SAMPLES_PER_RECORD * 4\n self.block_size = 2 * self.SAMPLES_PER_RECORD * header['nb_channels']\n self.block_offset_adc = (self.block_offset + self.block_size +\n (self.SAMPLES_PER_RECORD/4) * full_header['num_aux_input_channels'] * 2 +\n 2 * full_header['num_supply_voltage_channels'])\n self.block_size_adc = 2 * self.SAMPLES_PER_RECORD * self.nb_channels_adc\n self.block_offset_dig_in = self.block_offset_adc + self.block_size_adc\n self.block_size_dig_in = 2 * self.SAMPLES_PER_RECORD\n bytes_remaining = filesize - self.file.tell()\n\n self.bytes_per_block_div = self.bytes_per_block / 2\n self.block_offset_div = self.block_offset / 2\n self.block_offset_div_adc = self.block_offset_adc / 2\n self.block_offset_div_dig_in = self.block_offset_dig_in / 2\n self.block_size_div = self.block_size / 2\n self.block_size_div_adc = self.block_size_adc / 2\n self.block_size_div_dig_in = self.block_size_dig_in / 2\n\n if bytes_remaining > 0:\n data_present = True\n if bytes_remaining % self.bytes_per_block != 0:\n print_and_log(['Something is wrong with file size : should have a whole number of data blocks'], 'error', logger)\n\n num_data_blocks = int(bytes_remaining / self.bytes_per_block)\n self.num_amplifier_samples = self.SAMPLES_PER_RECORD * num_data_blocks\n\n self.size = self.num_amplifier_samples\n self._shape = (self.size, header['nb_channels'])\n self.file.close()\n\n return header\n\n def _get_slice_(self, t_start, t_stop):\n\n x_beg = np.int64(t_start // self.SAMPLES_PER_RECORD)\n r_beg = np.mod(t_start, self.SAMPLES_PER_RECORD)\n x_end = np.int64(t_stop // self.SAMPLES_PER_RECORD)\n r_end = np.mod(t_stop, self.SAMPLES_PER_RECORD)\n\n if x_beg == x_end:\n g_offset = x_beg * self.bytes_per_block_div + self.block_offset_div\n data_slice = np.arange(g_offset + r_beg * self.nb_channels, g_offset + r_end * self.nb_channels, dtype=np.int64)\n yield data_slice\n else:\n for count, nb_blocks in enumerate(np.arange(x_beg, x_end + 1, dtype=np.int64)):\n g_offset = nb_blocks * self.bytes_per_block_div + self.block_offset_div\n if count == 0:\n data_slice = np.arange(g_offset + r_beg * self.nb_channels, g_offset + self.block_size_div, dtype=np.int64)\n elif (count == (x_end - x_beg)):\n data_slice = np.arange(g_offset, g_offset + r_end * self.nb_channels, dtype=np.int64)\n else:\n data_slice = np.arange(g_offset, g_offset + self.block_size_div, dtype=np.int64)\n yield data_slice\n\n def _get_slice_adc_(self, t_start, t_stop):\n\n x_beg = np.int64(t_start // self.SAMPLES_PER_RECORD)\n r_beg = np.mod(t_start, self.SAMPLES_PER_RECORD)\n x_end = np.int64(t_stop // self.SAMPLES_PER_RECORD)\n r_end = np.mod(t_stop, self.SAMPLES_PER_RECORD)\n\n if x_beg == x_end:\n g_offset = x_beg * self.bytes_per_block_div + self.block_offset_div_adc\n data_slice = np.arange(g_offset + r_beg * self.nb_channels_adc, g_offset + r_end * self.nb_channels_adc, dtype=np.int64)\n yield data_slice\n else:\n for count, nb_blocks in enumerate(np.arange(x_beg, x_end + 1, dtype=np.int64)):\n g_offset = nb_blocks * self.bytes_per_block_div + self.block_offset_div_adc\n if count == 0:\n data_slice = np.arange(g_offset + r_beg * self.nb_channels_adc, g_offset + self.block_size_div_adc, dtype=np.int64)\n elif (count == (x_end - x_beg)):\n data_slice = np.arange(g_offset, g_offset + r_end * self.nb_channels_adc, dtype=np.int64)\n else:\n data_slice = np.arange(g_offset, g_offset + self.block_size_div_adc, dtype=np.int64)\n yield data_slice\n \n def _get_slice_dig_in_(self, t_start, t_stop):\n\n x_beg = np.int64(t_start // self.SAMPLES_PER_RECORD)\n r_beg = np.mod(t_start, self.SAMPLES_PER_RECORD)\n x_end = np.int64(t_stop // self.SAMPLES_PER_RECORD)\n r_end = np.mod(t_stop, self.SAMPLES_PER_RECORD)\n\n if x_beg == x_end:\n g_offset = x_beg * self.bytes_per_block_div + self.block_offset_div_dig_in\n data_slice = np.arange(g_offset + r_beg, g_offset + r_end, dtype=np.int64)\n yield data_slice\n else:\n for count, nb_blocks in enumerate(np.arange(x_beg, x_end + 1, dtype=np.int64)):\n g_offset = nb_blocks * self.bytes_per_block_div + self.block_offset_div_dig_in\n if count == 0:\n data_slice = np.arange(g_offset + r_beg, g_offset + self.block_size_div_dig_in, dtype=np.int64)\n elif (count == (x_end - x_beg)):\n data_slice = np.arange(g_offset, g_offset + r_end, dtype=np.int64)\n else:\n data_slice = np.arange(g_offset, g_offset + self.block_size_div_dig_in, dtype=np.int64)\n yield data_slice\n\n\n def read_chunk(self, idx, chunk_size, padding=(0, 0), nodes=None):\n\n t_start, t_stop = self._get_t_start_t_stop(idx, chunk_size, padding)\n local_shape = t_stop - t_start\n\n local_chunk = np.zeros((self.nb_channels, local_shape), dtype=self.data_dtype)\n data_slice = self._get_slice_(t_start, t_stop)\n\n self._open()\n count = 0\n\n for s in data_slice:\n t_slice = len(s)//self.nb_channels\n local_chunk[:, count:count + t_slice] = self.data[s].reshape(self.nb_channels, len(s)//self.nb_channels)\n count += t_slice\n\n local_chunk = local_chunk.T\n self._close()\n\n if nodes is not None:\n if not np.all(nodes == np.arange(self.nb_channels)):\n local_chunk = np.take(local_chunk, nodes, axis=1)\n\n return self._scale_data_to_float32(local_chunk)\n\n def read_chunk_adc(self, idx, chunk_size, padding=(0, 0), nodes=None):\n\n t_start, t_stop = self._get_t_start_t_stop(idx, chunk_size, padding)\n local_shape = t_stop - t_start\n\n local_chunk = np.zeros((self.nb_channels_adc, local_shape), dtype=self.data_dtype)\n data_slice = self._get_slice_adc_(t_start, t_stop)\n\n self._open()\n count = 0\n\n for s in data_slice:\n t_slice = len(s)//self.nb_channels_adc\n local_chunk[:, count:count + t_slice] = self.data[s].reshape(self.nb_channels_adc, len(s)//self.nb_channels_adc)\n count += t_slice\n\n local_chunk = local_chunk.T\n self._close()\n\n if nodes is not None:\n if not np.all(nodes == np.arange(self.nb_channels_adc)):\n local_chunk = np.take(local_chunk, nodes, axis=1)\n\n return self._scale_data_to_float32(local_chunk)\n \n def read_chunk_dig_in(self, idx, chunk_size, padding=(0, 0), nodes=None):\n\n t_start, t_stop = self._get_t_start_t_stop(idx, chunk_size, padding)\n local_shape = t_stop - t_start\n\n tmp_chunk = np.zeros((local_shape,), dtype=np.uint64)\n local_chunk = np.zeros((self.nb_channels_dig_in, local_shape), dtype=np.uint8)\n data_slice = self._get_slice_dig_in_(t_start, t_stop)\n\n self._open()\n count = 0\n for s in data_slice:\n t_slice = len(s)\n tmp_chunk[count:count + t_slice] = self.data[s] #Putting all data in channel 1, then masking\n count += t_slice\n for i in range(self.nb_channels_dig_in):\n chann_bitmask = 1 << self.header['board_dig_in_channels'][i]['native_order']\n local_chunk[i] = np.not_equal(np.bitwise_and(tmp_chunk, \n chann_bitmask), \n 0)\n local_chunk = local_chunk.T\n self._close()\n \n if nodes is not None:\n if not np.all(nodes == np.arange(self.nb_channels_dig_in)):\n local_chunk = np.take(local_chunk, nodes, axis=1)\n\n return local_chunk\n \n def read_chunk_both(self, idx, chunk_size, padding=(0, 0), nodes=None):\n\n t_start, t_stop = self._get_t_start_t_stop(idx, chunk_size, padding)\n local_shape = t_stop - t_start\n\n local_chunk_adc = np.zeros((self.nb_channels_adc, local_shape), dtype=self.data_dtype)\n local_chunk = np.zeros((self.nb_channels, local_shape), dtype=self.data_dtype)\n data_slice_adc = self._get_slice_adc_(t_start, t_stop)\n data_slice = self._get_slice_(t_start, t_stop)\n\n self._open()\n count = 0\n for s in data_slice_adc:\n t_slice = len(s)//self.nb_channels_adc\n local_chunk_adc[:, count:count + t_slice] = self.data[s].reshape(self.nb_channels_adc, len(s)//self.nb_channels_adc)\n count += t_slice\n \n count = 0\n for s in data_slice:\n t_slice = len(s)//self.nb_channels\n local_chunk[:, count:count + t_slice] = self.data[s].reshape(self.nb_channels, len(s)//self.nb_channels)\n count += t_slice\n\n local_chunk = local_chunk.T\n local_chunk_adc = local_chunk_adc.T\n self._close()\n\n if nodes is not None:\n if not np.all(nodes == np.arange(self.nb_channels_adc)):\n local_chunk_adc = np.take(local_chunk_adc, nodes, axis=1)\n if not np.all(nodes == np.arange(self.nb_channels)):\n local_chunk = np.take(local_chunk, nodes, axis=1)\n\n return self._scale_data_to_float32(local_chunk), self._scale_data_to_float32(local_chunk_adc)\n\n def write_chunk(self, time, data):\n\n t_start = time\n t_stop = time + data.shape[0]\n\n if t_stop > self.duration:\n t_stop = self.duration\n\n data = self._unscale_data_from_float32(data)\n data_slice = self._get_slice_(t_start, t_stop)\n\n self._open(mode='r+')\n count = 0\n for s in data_slice:\n t_slice = len(s)//self.nb_channels\n self.data[s] = data[count:count + t_slice, :].T.ravel()\n count += t_slice\n\n self._close()\n\n def _open(self, mode='r'):\n self.data = np.memmap(self.file_name, offset=self.data_offset, dtype=self.data_dtype, mode=mode)\n\n def _close(self):\n self.data = None",
"_____no_output_____"
],
[
"#export\nclass H5File(DataFile):\n\n description = \"hdf5\"\n extension = [\".h5\", \".hdf5\"]\n parallel_write = h5py.get_config().mpi\n is_writable = True\n\n _required_fields = {'h5_key' : str,\n 'sampling_rate' : float}\n\n _default_values = {'dtype_offset' : 'auto',\n 'h5_key_adc' : \"Data/Recording_0/AnalogStream/Stream_1/ChannelData\",\n 'gain' : 1.,\n 'data_dtype' : 'uint8',\n 'nb_channels' : 1}\n\n\n def _check_compression(self):\n # HDF5 does not support parallel writes with compression\n if self.compression != '':\n self.parallel_write = False\n if self.is_master:\n print_and_log(['Data are compressed thus parallel writing is disabled'], 'debug', logger)\n\n def __check_valid_key__(self, key):\n file = h5py.File(self.file_name, mode='r')\n all_fields = []\n file.visit(all_fields.append)\n if not key in all_fields:\n print_and_log(['The key %s can not be found in the dataset! Keys found are:' %key,\n \", \".join(all_fields)], 'error', logger)\n sys.exit(1)\n file.close()\n\n def _read_from_header(self):\n\n self.__check_valid_key__(self.h5_key)\n self._open()\n\n header = {}\n header['data_dtype'] = self.my_file.get(self.h5_key).dtype\n self.compression = self.my_file.get(self.h5_key).compression\n\n self._check_compression()\n\n self.size = self.my_file.get(self.h5_key).shape\n\n if self.size[0] > self.size[1]:\n self.time_axis = 0\n self._shape = (self.size[0], self.size[1])\n else:\n self.time_axis = 1\n self._shape = (self.size[1], self.size[0])\n\n header['nb_channels'] = self._shape[1]\n self._close()\n\n return header\n\n def read_chunk(self, idx, chunk_size, padding=(0, 0), nodes=None):\n\n t_start, t_stop = self._get_t_start_t_stop(idx, chunk_size, padding)\n\n if nodes is None:\n if self.time_axis == 0:\n local_chunk = self.data[t_start:t_stop, :]\n elif self.time_axis == 1:\n local_chunk = self.data[:, t_start:t_stop].T\n else:\n if self.time_axis == 0:\n local_chunk = self.data[t_start:t_stop, nodes]\n elif self.time_axis == 1:\n local_chunk = self.data[nodes, t_start:t_stop].T\n\n return self._scale_data_to_float32(local_chunk)\n\n def read_chunk_adc(self, idx, chunk_size, padding=(0, 0), nodes=None):\n\n t_start, t_stop = self._get_t_start_t_stop(idx, chunk_size, padding)\n\n if nodes is None:\n local_chunk = self.data_adc[0,t_start:t_stop]\n else:\n local_chunk = self.data_adc[0,t_start:t_stop]\n\n return self._scale_data_to_float32(local_chunk)\n\n def write_chunk(self, time, data):\n\n data = self._unscale_data_from_float32(data)\n\n if self.time_axis == 0:\n self.data[time:time+data.shape[0], :] = data\n elif self.time_axis == 1:\n self.data[:, time:time+data.shape[0]] = data.T\n\n def _open(self, mode='r'):\n# if mode in ['r+', 'w'] and self.parallel_write:\n# self.my_file = h5py.File(self.file_name, mode=mode, driver='mpio', comm=comm)\n# else:\n self.my_file = h5py.File(self.file_name, mode=mode)\n\n self.data = self.my_file.get(self.h5_key)\n self.data_adc = self.my_file.get(self.h5_key_adc)\n\n def _close(self):\n self.my_file.close()\n del self.data\n del self.data_adc\n\n @property\n def h5_key(self):\n return self.params['h5_key']\n\n @property\n def h5_key_adc(self):\n return self.params['h5_key_adc']",
"_____no_output_____"
],
[
"#export\nclass RawBinaryFile(DataFile):\n\n description = \"raw_binary\"\n extension = []\n parallel_write = True\n is_writable = True\n\n _required_fields = {'data_dtype' : str,\n 'sampling_rate' : float,\n 'nb_channels' : int}\n\n _default_values = {'dtype_offset' : 'auto',\n 'data_offset' : 0,\n 'gain' : 1.}\n\n def _read_from_header(self):\n self._open()\n self.size = len(self.data)\n self._shape = (self.size//self.nb_channels, int(self.nb_channels))\n self._close()\n return {}\n\n def allocate(self, shape, data_dtype=None):\n if data_dtype is None:\n data_dtype = self.data_dtype\n\n if self.is_master:\n self.data = np.memmap(self.file_name, offset=self.data_offset, dtype=data_dtype, mode='w+', shape=shape)\n# comm.Barrier()\n\n self._read_from_header()\n del self.data\n\n def read_chunk(self, idx, chunk_size, padding=(0, 0), nodes=None):\n\n t_start, t_stop = self._get_t_start_t_stop(idx, chunk_size, padding)\n local_shape = t_stop - t_start\n\n self._open()\n local_chunk = self.data[t_start*self.nb_channels:t_stop*self.nb_channels]\n local_chunk = local_chunk.reshape(local_shape, self.nb_channels)\n self._close()\n\n if nodes is not None:\n if not np.all(nodes == np.arange(self.nb_channels)):\n local_chunk = np.take(local_chunk, nodes, axis=1)\n\n return self._scale_data_to_float32(local_chunk)\n\n def read_chunk_adc(self, idx, chunk_size, padding=(0, 0), nodes=None):\n return self.read_chunk(idx, chunk_size, padding=padding, nodes=nodes)\n\n def write_chunk(self, time, data):\n self._open(mode='r+')\n\n data = self._unscale_data_from_float32(data)\n data = data.ravel()\n self.data[self.nb_channels*time:self.nb_channels*time+len(data)] = data\n self._close()\n\n\n def _open(self, mode='r'):\n self.data = np.memmap(self.file_name, offset=self.data_offset, dtype=self.data_dtype, mode=mode)\n\n def _close(self):\n self.data = None\n",
"_____no_output_____"
],
[
"#export\nfrom numpy.lib.format import open_memmap\n\nclass NumpyFile(RawBinaryFile):\n\n description = \"numpy\"\n extension = [\".npy\"]\n parallel_write = True\n is_writable = True\n\n _required_fields = {'sampling_rate' : float}\n\n _default_values = {'dtype_offset' : 'auto',\n 'gain' : 1.}\n\n def _read_from_header(self):\n \n header = {}\n\n self._open()\n self.size = self.data.shape\n\n if self.size[0] > self.size[1]:\n self.time_axis = 0\n self._shape = (self.size[0], self.size[1])\n else:\n self.time_axis = 1\n self._shape = (self.size[1], self.size[0])\n\n header['nb_channels'] = self._shape[1]\n header['data_dtype'] = self.data.dtype\n self.size = len(self.data)\n self._close()\n\n return header\n\n\n def read_chunk(self, idx, chunk_size, padding=(0, 0), nodes=None):\n \n self._open()\n\n t_start, t_stop = self._get_t_start_t_stop(idx, chunk_size, padding)\n\n if self.time_axis == 0:\n local_chunk = self.data[t_start:t_stop, :].copy()\n elif self.time_axis == 1:\n local_chunk = self.data[:, t_start:t_stop].copy().T\n self._close()\n\n if nodes is not None:\n if not np.all(nodes == np.arange(self.nb_channels)):\n local_chunk = np.take(local_chunk, nodes, axis=1)\n\n return self._scale_data_to_float32(local_chunk)\n\n def read_chunk_adc(self, idx, chunk_size, padding=(0, 0), nodes=None):\n return self.read_chunk(idx, chunk_size, padding=padding, nodes=nodes)\n\n def write_chunk(self, time, data):\n self._open(mode='r+')\n data = self._unscale_data_from_float32(data)\n if self.time_axis == 0:\n self.data[time:time+len(data)] = data\n elif self.time_axis == 1:\n self.data[:, time:time+len(data)] = data.T\n self._close()\n\n\n def _open(self, mode='r'):\n self.data = open_memmap(self.file_name, mode=mode)\n\n\n def _close(self):\n self.data = None",
"_____no_output_____"
],
[
"#export\ndef load_all_data(datafile:DataFile):\n \"\"\"Read all the data contained by a file. For rhd and hdf5, correspond to the ephy channels. To read the ADC\n data, see `load_all_data_adc`\"\"\"\n datafile.open()\n if isinstance(datafile, RHDFile):\n chunk_size = 1800960\n else:\n chunk_size = datafile.duration\n n_chunks, _ = datafile.analyze(chunk_size)\n data = np.zeros((datafile.duration, datafile._shape[1]))\n print(\"Loading the data... \"+str(round(0,2))+\"% \",end='\\n',flush=True)\n for idx in range(n_chunks):\n data_tmp, t_offset = datafile.get_data(idx, chunk_size)\n data[t_offset:t_offset+len(data_tmp)] = data_tmp\n print(\"Loading the data... \"+str(round(100*(idx+1)/n_chunks,2))+\"% \",end='\\n',flush=True)\n print(\"Loading the data... \"+str(round(100,2))+\"% \",end='\\n',flush=True)\n datafile.close()\n return data\n \ndef load_all_data_adc(datafile:DataFile, channel_idx=0):\n \"\"\"Read all the data contained by a file. For rhd and hdf5, correspond to the adc channels. To read the ephy\n data, see `load_all_data`\"\"\"\n datafile.open()\n if isinstance(datafile, RHDFile):\n chunk_size = 1800960\n else:\n chunk_size = datafile.duration\n n_chunks, _ = datafile.analyze(chunk_size)\n data = np.zeros(datafile.duration)\n print(\"Loading the data... \"+str(round(0,2))+\"% \",end='\\n',flush=True)\n for idx in range(n_chunks):\n data_tmp, t_offset = datafile.get_data_adc(idx, chunk_size)\n if data_tmp.ndim == 2:\n data_tmp = data_tmp[:,channel_idx]\n data[t_offset:t_offset+len(data_tmp)] = data_tmp\n print(\"Loading the data... \"+str(round(100*(idx+1)/n_chunks,2))+\"% \",end='\\n',flush=True)\n print(\"Loading the data... \"+str(round(100,2))+\"% \",end='\\n',flush=True)\n datafile.close()\n return data\n\ndef load_all_data_dig_in(datafile:DataFile, channel_idx=0):\n \"\"\"Read all the data contained by a file. For rhd and hdf5, correspond to the adc channels. To read the ephy\n data, see `load_all_data`\"\"\"\n datafile.open()\n if isinstance(datafile, RHDFile):\n chunk_size = 1800960\n else:\n chunk_size = datafile.duration\n n_chunks, _ = datafile.analyze(chunk_size)\n data = np.zeros(datafile.duration, dtype=float)\n print(\"Loading the data... \"+str(round(0,2))+\"% \",end='\\r',flush=True)\n for idx in range(n_chunks):\n data_tmp, t_offset = datafile.get_data_dig_in(idx, chunk_size)\n if data_tmp.ndim == 2:\n data_tmp = data_tmp[:,channel_idx]\n data[t_offset:t_offset+len(data_tmp)] = data_tmp\n print(\"Loading the data... \"+str(round(100*(idx+1)/n_chunks,2))+\"% \",end='\\n',flush=True)\n print(\"Loading the data... \"+str(round(100,2))+\"% \",end='\\n',flush=True)\n datafile.close()\n return data\n\ndef load_all_data_both(datafile:DataFile):\n \"\"\"Read all the data contained by a file. For rhd and hdf5, correspond to the adc channels. To read the ephy\n data, see `load_all_data`\"\"\"\n datafile.open()\n if isinstance(datafile, RHDFile):\n chunk_size = 1800960\n else:\n chunk_size = datafile.duration\n n_chunks, _ = datafile.analyze(chunk_size)\n data_adc = np.zeros(datafile.duration)\n data = np.zeros((datafile.duration, datafile._shape[1]))\n print(\"Loading the data... \"+str(round(0,2))+\"% \",end='\\n',flush=True)\n for idx in range(n_chunks):\n data_tmp, data_tmp_adc, t_offset = datafile.get_data_both(idx, chunk_size)\n data[t_offset:t_offset+len(data_tmp)] = data_tmp\n if data_tmp_adc.ndim == 2:\n data_tmp_adc = data_tmp_adc[:,0]\n data_adc[t_offset:t_offset+len(data_tmp)] = data_tmp_adc\n print(\"Loading the data... \"+str(round(100*(idx+1)/n_chunks,2))+\"% \",end='\\n',flush=True)\n print(\"Loading the data... \"+str(round(100,2))+\"% \",end='\\n',flush=True)\n datafile.close()\n return data, data_adc\n\ndef export_adc_raw(datafile:DataFile, output_fn=\"\", channel_idx=0):\n \"\"\"Exports a datafile adc channel to a single raw binary file. Useful to reduce disk usage after that\n spike sorting is done.\"\"\"\n data = load_all_data_adc(datafile, channel_idx=channel_idx)\n if output_fn==\"\":\n raw_fn = os.path.splitext(datafile.file_name)[0]+\".dat\"\n else:\n raw_fn = os.path.split(datafile.file_name)[0]+\"/\"+output_fn\n param_d = {'sampling_rate': datafile.sampling_rate,\n 'data_dtype': 'uint16',\n 'gain': 0.195,\n 'nb_channels': 1,\n 'dtype_offset': 32768}\n raw_file = RawBinaryFile(raw_fn, param_d, is_empty=True)\n raw_file.allocate(datafile.shape[0])\n raw_file.set_data(0, data)\n raw_file.close()\n \ndef export_dig_in_raw(datafile:DataFile, output_fn=\"\", channel_idx=0):\n \"\"\"Exports a datafile adc channel to a single raw binary file. Useful to reduce disk usage after that\n spike sorting is done.\"\"\"\n data = load_all_data_dig_in(datafile, channel_idx=channel_idx)\n if output_fn==\"\":\n raw_fn = os.path.splitext(datafile.file_name)[0]+\".dat\"\n else:\n raw_fn = os.path.split(datafile.file_name)[0]+\"/\"+output_fn\n param_d = {'sampling_rate': datafile.sampling_rate,\n 'data_dtype': 'uint8',\n 'gain': 1,\n 'nb_channels': 1,\n 'dtype_offset': 127}\n raw_file = RawBinaryFile(raw_fn, param_d, is_empty=True)\n raw_file.allocate(datafile.shape[0])\n raw_file.set_data(0, data)\n raw_file.close()\n \ndef export_raw(datafile:DataFile, output_fn=\"\"):\n \"\"\"Exports a datafile adc channel to a single raw binary file. Useful to reduce disk usage after that\n spike sorting is done.\"\"\"\n data = load_all_data(datafile)\n if output_fn==\"\":\n raw_fn = os.path.splitext(datafile.file_name)[0]+\".dat\"\n else:\n raw_fn = os.path.split(datafile.file_name)[0]+\"/\"+output_fn\n param_d = datafile.get_description()\n raw_file = RawBinaryFile(raw_fn, param_d, is_empty=True)\n raw_file.allocate(datafile.shape)\n raw_file.set_data(0, data)\n raw_file.close()\n \ndef export_both_raw(datafile:DataFile):\n \"\"\"Exports a both raw data, adc and ephy.\"\"\"\n data, data_adc = load_all_data_both(datafile)\n raw_fn = os.path.splitext(datafile.file_name)[0]+\".dat\"\n param_d = {'sampling_rate': datafile.sampling_rate,\n 'data_dtype': 'uint16',\n 'gain': 0.195,\n 'nb_channels': 1,\n 'dtype_offset': 32768}\n raw_file = RawBinaryFile(raw_fn, param_d, is_empty=True)\n raw_file.allocate(datafile.shape[0])\n raw_file.set_data(0, data_adc)\n raw_file.close()\n \n os.rename(raw_fn, os.path.splitext(datafile.file_name)[0]+\".data\")\n \n param_d = datafile.get_description()\n raw_file = RawBinaryFile(raw_fn, param_d, is_empty=True)\n raw_file.allocate(datafile.shape)\n raw_file.set_data(0, data)\n raw_file.close()\n \n \ndef load_adc_raw(filepath, sampling_rate=30000):\n \"\"\"Loads adc raw data, in the format exported by `export_adc_raw`\"\"\"\n param_d = {'sampling_rate': sampling_rate,\n 'data_dtype': 'uint16',\n 'gain': 0.195,\n 'nb_channels': 1,\n 'dtype_offset': 32768}\n raw_file = RawBinaryFile(filepath, param_d)\n return load_all_data_adc(raw_file)\n\ndef load_digin_raw(filepath, sampling_rate=30000):\n \"\"\"Loads adc raw data, in the format exported by `export_adc_raw`\"\"\"\n param_d = {'sampling_rate': sampling_rate,\n 'data_dtype': 'uint8',\n 'gain': 1,\n 'nb_channels': 1,\n 'dtype_offset': 127}\n raw_file = RawBinaryFile(filepath, param_d)\n return load_all_data_adc(raw_file)\n\ndef load_sync_raw(filepath, sampling_rate=10000):\n \"\"\"Loads the sync files made by labview for Asari Lab 2P setup\"\"\"\n param_d = {'sampling_rate': sampling_rate,\n 'data_dtype': '>d',\n 'gain': 1,\n 'nb_channels': 1,\n 'dtype_offset': 0}\n raw_file = RawBinaryFile(filepath, param_d)\n return load_all_data_adc(raw_file)",
"_____no_output_____"
],
[
"#hide\nfrom nbdev.export import *\nnotebook2script()",
"Converted 00_core.ipynb.\nConverted 01_utils.ipynb.\nConverted 02_processing.ipynb.\nConverted 03_modelling.ipynb.\nConverted 04_plotting.ipynb.\nConverted 05_database.ipynb.\nConverted 06_eyetrack.ipynb.\nConverted 10_synchro.io.ipynb.\nConverted 11_synchro.extracting.ipynb.\nConverted 12_synchro.processing.ipynb.\nConverted 13_leddome.ipynb.\nConverted 99_testdata.ipynb.\nConverted index.ipynb.\n"
]
]
] | [
"code",
"markdown",
"code"
] | [
[
"code",
"code"
],
[
"markdown"
],
[
"code",
"code",
"code",
"code",
"code",
"code",
"code",
"code"
]
] |
e78591848f36b18347a72eec76eec5fa71d58189 | 16,120 | ipynb | Jupyter Notebook | Mission_to_Mars-Starter.ipynb | ptlhrs7/Web-Scraping-and-Mongo-Homework | 58f6273d72d35bfee098c543c7ea87eb91afd0d2 | [
"ADSL"
] | null | null | null | Mission_to_Mars-Starter.ipynb | ptlhrs7/Web-Scraping-and-Mongo-Homework | 58f6273d72d35bfee098c543c7ea87eb91afd0d2 | [
"ADSL"
] | null | null | null | Mission_to_Mars-Starter.ipynb | ptlhrs7/Web-Scraping-and-Mongo-Homework | 58f6273d72d35bfee098c543c7ea87eb91afd0d2 | [
"ADSL"
] | null | null | null | 27.138047 | 1,076 | 0.455149 | [
[
[
"# Import Splinter, BeautifulSoup, and Pandas\nfrom splinter import Browser\nfrom bs4 import BeautifulSoup as soup\nimport pandas as pd\nfrom webdriver_manager.chrome import ChromeDriverManager",
"_____no_output_____"
],
[
"# Set up Splinter\nexecutable_path = {'executable_path': ChromeDriverManager().install()}\nbrowser = Browser('chrome', **executable_path, headless=False)",
"\n\n====== WebDriver manager ======\nCurrent google-chrome version is 98.0.4758\nGet LATEST chromedriver version for 98.0.4758 google-chrome\nDriver [/Users/harshpatel/.wdm/drivers/chromedriver/mac64/98.0.4758.80/chromedriver] found in cache\n"
]
],
[
[
"## Visit the NASA mars news site",
"_____no_output_____"
]
],
[
[
"# Visit the Mars news site\nurl = 'https://redplanetscience.com/'\nbrowser.visit(url)\n\n# Optional delay for loading the page\nbrowser.is_element_present_by_css('div.list_text', wait_time=1)",
"_____no_output_____"
],
[
"# Convert the browser html to a soup object\nhtml = browser.html\nnews_soup = soup(html, 'html.parser')\n\nslide_elem = news_soup.select_one('div.list_text')\n#print(news_soup.prettify())",
"_____no_output_____"
],
[
"#display the current title content\nslide_elem.find('div', class_='content_title')",
"_____no_output_____"
],
[
"# Use the parent element to find the first a tag and save it as `news_title`\nnews_title = slide_elem.find('div', class_='content_title').get_text()\nnews_title",
"_____no_output_____"
],
[
"# Use the parent element to find the paragraph text\nnews_p = slide_elem.find('div', class_='article_teaser_body').get_text()\nnews_p",
"_____no_output_____"
]
],
[
[
"## JPL Space Images Featured Image",
"_____no_output_____"
]
],
[
[
"# Visit URL\nurl = 'https://spaceimages-mars.com'\nbrowser.visit(url)",
"_____no_output_____"
],
[
"# Find and click the full image button\nfull_image_link = browser.find_by_tag('button')[1]\nfull_image_link.click()",
"_____no_output_____"
],
[
"# Parse the resulting html with soup\nhtml = browser.html\nimg_soup = soup(html, 'html.parser')\n#print(news_soup.prettify())",
"_____no_output_____"
],
[
"img_url_rel = img_soup.find('img', class_='fancybox-image').get('src')",
"_____no_output_____"
],
[
"# find the relative image url\nimg_url_rel",
"_____no_output_____"
],
[
"# Use the base url to create an absolute url\nimg_url = f'https://spaceimages-mars.com/{img_url_rel}'\nimg_url",
"_____no_output_____"
]
],
[
[
"## Mars Facts",
"_____no_output_____"
]
],
[
[
"# Use `pd.read_html` to pull the data from the Mars-Earth Comparison section\n# hint use index 0 to find the table\ndf = pd.read_html(\"https://galaxyfacts-mars.com/\")[0]\ndf.head()",
"_____no_output_____"
],
[
"df.columns = ['Description', 'Mars', 'Earth']\ndf",
"_____no_output_____"
],
[
"df.set_index('Description', inplace=True)",
"_____no_output_____"
],
[
"df.to_html()",
"_____no_output_____"
]
],
[
[
"## Hemispheres",
"_____no_output_____"
]
],
[
[
"url = 'https://marshemispheres.com/'\n\nbrowser.visit(url)",
"_____no_output_____"
],
[
"# Create a list to hold the images and titles.\nhemisphere_image_urls = []\n\n\n# Get a list of all of the hemispheres\nlinks = browser.find_by_css('a.product-item img')\n\n\n# Next, loop through those links, click the link, find the sample anchor, return the href\nfor i in range(len(links)):\n \n #hemisphere info dictionary\n hemisphereInfo = {}\n \n # We have to find the elements on each loop to avoid a stale element exception\n browser.find_by_css('a.product-item img')[i].click()\n \n # Next, we find the Sample image anchor tag and extract the href\n sample = browser.links.find_by_text('Sample').first\n hemisphereInfo[\"img_url\"] = sample['href']\n \n # Get Hemisphere title\n hemisphereInfo['title'] = browser.find_by_css('h2.title').text\n \n # Append hemisphere object to list\n hemisphere_image_urls.append(hemisphereInfo)\n \n # Finally, we navigate backwards\n browser.back()",
"_____no_output_____"
],
[
"hemisphere_image_urls",
"_____no_output_____"
],
[
"browser.quit()\n\n# refence code used from Dr.A's Videos",
"_____no_output_____"
]
]
] | [
"code",
"markdown",
"code",
"markdown",
"code",
"markdown",
"code",
"markdown",
"code"
] | [
[
"code",
"code"
],
[
"markdown"
],
[
"code",
"code",
"code",
"code",
"code"
],
[
"markdown"
],
[
"code",
"code",
"code",
"code",
"code",
"code"
],
[
"markdown"
],
[
"code",
"code",
"code",
"code"
],
[
"markdown"
],
[
"code",
"code",
"code",
"code"
]
] |
e785964a79f9691f8428bf89a344c53a3753fdc6 | 17,440 | ipynb | Jupyter Notebook | examples/factrueval.ipynb | sloth2012/ner-bert | 52097650ffad0d2ee964d4bc8a64a901d6045cdd | [
"MIT"
] | 1 | 2019-09-24T04:23:50.000Z | 2019-09-24T04:23:50.000Z | examples/factrueval.ipynb | 672425265/ner-bert | 730cd1700513dbd51dc9736290db36855f27a3e0 | [
"MIT"
] | null | null | null | examples/factrueval.ipynb | 672425265/ner-bert | 730cd1700513dbd51dc9736290db36855f27a3e0 | [
"MIT"
] | 1 | 2019-11-07T07:48:08.000Z | 2019-11-07T07:48:08.000Z | 22.04804 | 154 | 0.488303 | [
[
[
"### FactRuEval example (Cased model), MutiHeadAttention",
"_____no_output_____"
]
],
[
[
"%reload_ext autoreload\n%autoreload 2\n%matplotlib inline\n\nimport warnings\nimport sys\n\nsys.path.append(\"../\")\n\nwarnings.filterwarnings(\"ignore\")",
"_____no_output_____"
],
[
"import os\n\n\ndata_path = \"/home/lis/ner/ulmfit/data/factrueval/\"\ntrain_path = os.path.join(data_path, \"train_with_pos.csv\")\nvalid_path = os.path.join(data_path, \"valid_with_pos.csv\")\nmodel_dir = \" /datadrive/models/multi_cased_L-12_H-768_A-12/\"\ninit_checkpoint_pt = os.path.join(\"/datadrive/models/multi_cased_L-12_H-768_A-12/\", \"pytorch_model.bin\")\nbert_config_file = os.path.join(\"/datadrive/bert/multi_cased_L-12_H-768_A-12/\", \"bert_config.json\")\nvocab_file = os.path.join(\"/datadrive/bert/multi_cased_L-12_H-768_A-12/\", \"vocab.txt\")",
"_____no_output_____"
],
[
"import torch\ntorch.cuda.set_device(1)\ntorch.cuda.is_available(), torch.cuda.current_device()",
"_____no_output_____"
]
],
[
[
"### 1. Create dataloaders",
"_____no_output_____"
]
],
[
[
"from modules import BertNerData as NerData",
"INFO:summarizer.preprocessing.cleaner:'pattern' package not found; tag filters are not available for English\n"
],
[
"data = NerData.create(train_path, valid_path, vocab_file)",
"_____no_output_____"
]
],
[
[
"For factrueval we use the following sample of labels:",
"_____no_output_____"
]
],
[
[
"print(data.label2idx)",
"{'<pad>': 0, '[CLS]': 1, '[SEP]': 2, 'B_O': 3, 'I_O': 4, 'B_ORG': 5, 'I_ORG': 6, 'B_LOC': 7, 'I_LOC': 8, 'B_PER': 9, 'I_PER': 10}\n"
]
],
[
[
"### 2. Create model\nFor creating pytorch model we need to create `NerModel` object.",
"_____no_output_____"
]
],
[
[
"from modules.models.bert_models import BertBiLSTMAttnCRF",
"_____no_output_____"
],
[
"model = BertBiLSTMAttnCRF.create(len(data.label2idx), bert_config_file, init_checkpoint_pt, enc_hidden_dim=256)",
"_____no_output_____"
],
[
"model.decoder",
"_____no_output_____"
],
[
"model.get_n_trainable_params()",
"_____no_output_____"
]
],
[
[
"### 3. Create learner\n\nFor training our pytorch model we need to create `NerLearner` object.",
"_____no_output_____"
]
],
[
[
"from modules import NerLearner",
"_____no_output_____"
],
[
"num_epochs = 100\nlearner = NerLearner(model, data,\n best_model_path=\"/datadrive/models/factrueval/exp_final_attn_cased1.cpt\",\n lr=0.001, clip=1.0, sup_labels=data.id2label[5:],\n t_total=num_epochs * len(data.train_dl))",
"_____no_output_____"
]
],
[
[
"### 4. Learn your NER model\nCall `learner.fit`",
"_____no_output_____"
]
],
[
[
"learner.fit(num_epochs, target_metric='f1')",
"_____no_output_____"
]
],
[
[
"### 5. Evaluate\nCreate new data loader from existing path.",
"_____no_output_____"
]
],
[
[
"from modules.data.bert_data import get_bert_data_loader_for_predict",
"_____no_output_____"
],
[
"dl = get_bert_data_loader_for_predict(data_path + \"valid.csv\", learner)",
"_____no_output_____"
],
[
"learner.load_model()",
"_____no_output_____"
],
[
"preds = learner.predict(dl)",
"_____no_output_____"
]
],
[
[
"IOB precision",
"_____no_output_____"
]
],
[
[
"from modules.train.train import validate_step\nprint(validate_step(learner.data.valid_dl, learner.model, learner.data.id2label, learner.sup_labels))",
"_____no_output_____"
]
],
[
[
"Tokens report",
"_____no_output_____"
]
],
[
[
"from sklearn_crfsuite.metrics import flat_classification_report",
"_____no_output_____"
],
[
"from modules.utils.utils import bert_labels2tokens",
"_____no_output_____"
],
[
"pred_tokens, pred_labels = bert_labels2tokens(dl, preds)\ntrue_tokens, true_labels = bert_labels2tokens(dl, [x.labels for x in dl.dataset])",
"_____no_output_____"
],
[
"assert pred_tokens == true_tokens\ntokens_report = flat_classification_report(true_labels, pred_labels)",
"_____no_output_____"
],
[
"print(tokens_report)",
" precision recall f1-score support\n\n I_LOC 0.93 0.90 0.92 230\n I_O 0.99 0.99 0.99 7203\n I_ORG 0.92 0.87 0.89 543\n I_PER 0.98 0.98 0.98 321\n\n micro avg 0.98 0.98 0.98 8297\n macro avg 0.96 0.94 0.95 8297\nweighted avg 0.98 0.98 0.98 8297\n\n"
],
[
"from modules.utils.plot_metrics import analyze_bert_errors",
"_____no_output_____"
],
[
"res_tokens, res_labels, errors = analyze_bert_errors(dl, preds)",
"_____no_output_____"
],
[
"len([error for error in errors if error])",
"_____no_output_____"
]
],
[
[
"Span precision",
"_____no_output_____"
]
],
[
[
"from modules.utils.utils import voting_choicer",
"_____no_output_____"
],
[
"print(get_bert_span_report(dl, preds, fn=voting_choicer))",
" precision recall f1-score support\n\n ORG 0.809 0.834 0.821 259\n LOC 0.851 0.859 0.855 192\n PER 0.936 0.936 0.936 188\n\n micro avg 0.858 0.872 0.865 639\n macro avg 0.865 0.877 0.871 639\nweighted avg 0.859 0.872 0.865 639\n\n"
]
],
[
[
"### 6. Get mean and stdv on 10 runs",
"_____no_output_____"
]
],
[
[
"from modules.utils.plot_metrics import *\n\n\nnum_runs = 10\nbest_reports = []\ntry:\n for i in range(num_runs):\n model = BertBiLSTMAttnCRF.create(len(data.label2idx), bert_config_file, init_checkpoint_pt, enc_hidden_dim=256)\n best_model_path = \"/datadrive/models/factrueval/exp_{}_attn_cased.cpt\".format(i)\n learner = NerLearner(model, data,\n best_model_path=best_model_path, verbose=False,\n base_lr=0.0001, lr_max=0.001, clip=5.0, use_lr_scheduler=True, sup_labels=data.id2label[5:])\n learner.fit(100, target_metric='prec')\n idx, res = get_mean_max_metric(learner.history, \"f1\", True)\n best_reports.append(learner.history[idx])\nexcept KeyboardInterrupt:\n print(\"End of exp\")",
"_____no_output_____"
],
[
"import numpy as np",
"_____no_output_____"
]
],
[
[
"#### f1",
"_____no_output_____"
],
[
"Mean and std",
"_____no_output_____"
]
],
[
[
"np.mean([get_mean_max_metric([r]) for r in best_reports]), np.round(np.std([get_mean_max_metric([r]) for r in best_reports]), 3)",
"_____no_output_____"
]
],
[
[
"Best",
"_____no_output_____"
]
],
[
[
"get_mean_max_metric(best_reports)",
"_____no_output_____"
]
],
[
[
"#### precision",
"_____no_output_____"
],
[
"Mean and std",
"_____no_output_____"
]
],
[
[
"np.mean([get_mean_max_metric([r], \"prec\") for r in best_reports]), np.round(np.std([get_mean_max_metric([r], \"prec\") for r in best_reports]), 3)",
"_____no_output_____"
]
],
[
[
"Best",
"_____no_output_____"
]
],
[
[
"get_mean_max_metric(best_reports, \"prec\")",
"_____no_output_____"
]
]
] | [
"markdown",
"code",
"markdown",
"code",
"markdown",
"code",
"markdown",
"code",
"markdown",
"code",
"markdown",
"code",
"markdown",
"code",
"markdown",
"code",
"markdown",
"code",
"markdown",
"code",
"markdown",
"code",
"markdown",
"code",
"markdown",
"code",
"markdown",
"code",
"markdown",
"code"
] | [
[
"markdown"
],
[
"code",
"code",
"code"
],
[
"markdown"
],
[
"code",
"code"
],
[
"markdown"
],
[
"code"
],
[
"markdown"
],
[
"code",
"code",
"code",
"code"
],
[
"markdown"
],
[
"code",
"code"
],
[
"markdown"
],
[
"code"
],
[
"markdown"
],
[
"code",
"code",
"code",
"code"
],
[
"markdown"
],
[
"code"
],
[
"markdown"
],
[
"code",
"code",
"code",
"code",
"code",
"code",
"code",
"code"
],
[
"markdown"
],
[
"code",
"code"
],
[
"markdown"
],
[
"code",
"code"
],
[
"markdown",
"markdown"
],
[
"code"
],
[
"markdown"
],
[
"code"
],
[
"markdown",
"markdown"
],
[
"code"
],
[
"markdown"
],
[
"code"
]
] |
e785afd67c8cfebe00e37a12abb507b5fb16f73d | 48,986 | ipynb | Jupyter Notebook | course_assignments/BLT Classifier/Untitled.ipynb | Maxalmina/maru | 986505765d6829f6a74ea85134d492af3d9ee45e | [
"MIT"
] | null | null | null | course_assignments/BLT Classifier/Untitled.ipynb | Maxalmina/maru | 986505765d6829f6a74ea85134d492af3d9ee45e | [
"MIT"
] | null | null | null | course_assignments/BLT Classifier/Untitled.ipynb | Maxalmina/maru | 986505765d6829f6a74ea85134d492af3d9ee45e | [
"MIT"
] | 1 | 2020-04-01T14:52:18.000Z | 2020-04-01T14:52:18.000Z | 193.620553 | 23,560 | 0.871147 | [
[
[
"import pandas as pd\nimport seaborn as sns\nimport matplotlib.pyplot as plt\n\nfrom sklearn.cluster import DBSCAN",
"_____no_output_____"
],
[
"blt = pd.read_csv(\"DataTugas2.csv\")\nblt.head()",
"_____no_output_____"
],
[
"print(blt.dtypes)\nprint(blt.columns)",
"pendapatan float64\nhutang float64\ndtype: object\nIndex(['pendapatan', 'hutang'], dtype='object')\n"
],
[
"blt.rename(columns={\" Pendapatan\": \"pendapatan\", \" Hutang\": \"hutang\"}, inplace=True)\nblt.drop(columns='No', inplace=True)",
"_____no_output_____"
],
[
"sns.scatterplot(x=\"hutang\", y=\"pendapatan\", data=blt)",
"_____no_output_____"
],
[
"y_pred = DBSCAN(eps=0.2, min_samples=3).fit_predict(blt)",
"_____no_output_____"
],
[
"sns.scatterplot(x=\"hutang\", y=\"pendapatan\",hue=y_pred, data=blt)",
"_____no_output_____"
]
]
] | [
"code"
] | [
[
"code",
"code",
"code",
"code",
"code",
"code",
"code"
]
] |
e785bb2287f235e7a9970885a9f965121d74bdf5 | 82,957 | ipynb | Jupyter Notebook | jupyter_notebooks/kath/encd-3132.ipynb | caseylitton/pyencoded-tools | 850d011f1ce8a8f12e9454befe078a4139ca610b | [
"MIT"
] | 9 | 2016-08-23T15:59:12.000Z | 2021-07-16T00:54:54.000Z | jupyter_notebooks/kath/encd-3132.ipynb | caseylitton/pyencoded-tools | 850d011f1ce8a8f12e9454befe078a4139ca610b | [
"MIT"
] | 12 | 2016-11-18T18:56:42.000Z | 2021-03-11T20:25:14.000Z | jupyter_notebooks/kath/encd-3132.ipynb | caseylitton/pyencoded-tools | 850d011f1ce8a8f12e9454befe078a4139ca610b | [
"MIT"
] | 14 | 2016-02-17T04:24:07.000Z | 2020-02-28T21:36:19.000Z | 39.260293 | 137 | 0.542594 | [
[
[
"import qancode",
"_____no_output_____"
],
[
"qa = qancode.QANCODE(prod_url=\"https://encd-3132-delete.demo.encodedcc.org/\", rc_url=\"https://delete-test.demo.encodedcc.org/\")",
"_____no_output_____"
],
[
"#comparison as public user\nqa.compare_facets(users=['Public'], browsers=['Safari'], browser_comparison=False)",
"Opening https://encd-3132-delete.demo.encodedcc.org/ in Safari\nGetting type: /search/?type=Experiment\nSearch page detected\nOpening https://encd-3132-delete.demo.encodedcc.org/ in Safari\nGetting type: /search/?type=File\nSearch page detected\nOpening https://encd-3132-delete.demo.encodedcc.org/ in Safari\nGetting type: /search/?type=Library\nSearch page detected\nOpening https://encd-3132-delete.demo.encodedcc.org/ in Safari\nGetting type: /search/?type=AntibodyLot\nSearch page detected\nOpening https://encd-3132-delete.demo.encodedcc.org/ in Safari\nGetting type: /search/?type=Biosample\nSearch page detected\nOpening https://encd-3132-delete.demo.encodedcc.org/ in Safari\nGetting type: /search/?type=Donor\nSearch page detected\nOpening https://encd-3132-delete.demo.encodedcc.org/ in Safari\nGetting type: search/?type=GeneticModification\nSearch page detected\nOpening https://encd-3132-delete.demo.encodedcc.org/ in Safari\nGetting type: /search/?type=FileSet\nSearch page detected\nOpening https://encd-3132-delete.demo.encodedcc.org/ in Safari\nGetting type: /search/?type=Annotation\nSearch page detected\nOpening https://encd-3132-delete.demo.encodedcc.org/ in Safari\nGetting type: /search/?type=Series\nSearch page detected\nOpening https://encd-3132-delete.demo.encodedcc.org/ in Safari\nGetting type: /search/?type=OrganismDevelopmentSeries\nSearch page detected\nOpening https://encd-3132-delete.demo.encodedcc.org/ in Safari\nGetting type: /search/?type=UcscBrowserComposite\nSearch page detected\nOpening https://encd-3132-delete.demo.encodedcc.org/ in Safari\nGetting type: /search/?type=ReferenceEpigenome\nSearch page detected\nOpening https://encd-3132-delete.demo.encodedcc.org/ in Safari\nGetting type: /search/?type=Project\nSearch page detected\nOpening https://encd-3132-delete.demo.encodedcc.org/ in Safari\nGetting type: /search/?type=ReplicationTimingSeries\nSearch page detected\nOpening https://encd-3132-delete.demo.encodedcc.org/ in Safari\nGetting type: /search/?type=PublicationData\nSearch page detected\nOpening https://encd-3132-delete.demo.encodedcc.org/ in Safari\nGetting type: /search/?type=MatchedSet\nSearch page detected\nOpening https://encd-3132-delete.demo.encodedcc.org/ in Safari\nGetting type: /search/?type=TreatmentConcentrationSeries\nSearch page detected\nOpening https://encd-3132-delete.demo.encodedcc.org/ in Safari\nGetting type: /search/?type=TreatmentTimeSeries\nSearch page detected\nOpening https://encd-3132-delete.demo.encodedcc.org/ in Safari\nGetting type: /search/?type=Target\nSearch page detected\nOpening https://encd-3132-delete.demo.encodedcc.org/ in Safari\nGetting type: /search/?type=Pipeline\nSearch page detected\nOpening https://encd-3132-delete.demo.encodedcc.org/ in Safari\nGetting type: /search/?type=Publication\nSearch page detected\nOpening https://encd-3132-delete.demo.encodedcc.org/ in Safari\nGetting type: /search/?type=Software\nSearch page detected\nOpening https://encd-3132-delete.demo.encodedcc.org/ in Safari\nGetting type: /matrix/?type=Experiment\nMatrix page detected\nOpening https://encd-3132-delete.demo.encodedcc.org/ in Safari\nGetting type: /matrix/?type=Annotation\nMatrix page detected\nOpening https://delete-test.demo.encodedcc.org/ in Safari\nGetting type: /search/?type=Experiment\nSearch page detected\nOpening https://delete-test.demo.encodedcc.org/ in Safari\nGetting type: /search/?type=File\nSearch page detected\nOpening https://delete-test.demo.encodedcc.org/ in Safari\nGetting type: /search/?type=Library\nSearch page detected\nOpening https://delete-test.demo.encodedcc.org/ in Safari\nGetting type: /search/?type=AntibodyLot\nSearch page detected\nOpening https://delete-test.demo.encodedcc.org/ in Safari\nGetting type: /search/?type=Biosample\nSearch page detected\nOpening https://delete-test.demo.encodedcc.org/ in Safari\nGetting type: /search/?type=Donor\nSearch page detected\nOpening https://delete-test.demo.encodedcc.org/ in Safari\nGetting type: search/?type=GeneticModification\nSearch page detected\nOpening https://delete-test.demo.encodedcc.org/ in Safari\nGetting type: /search/?type=FileSet\nSearch page detected\nOpening https://delete-test.demo.encodedcc.org/ in Safari\nGetting type: /search/?type=Annotation\nSearch page detected\nOpening https://delete-test.demo.encodedcc.org/ in Safari\nGetting type: /search/?type=Series\nSearch page detected\nOpening https://delete-test.demo.encodedcc.org/ in Safari\nGetting type: /search/?type=OrganismDevelopmentSeries\nSearch page detected\nOpening https://delete-test.demo.encodedcc.org/ in Safari\nGetting type: /search/?type=UcscBrowserComposite\nSearch page detected\nOpening https://delete-test.demo.encodedcc.org/ in Safari\nGetting type: /search/?type=ReferenceEpigenome\nSearch page detected\nOpening https://delete-test.demo.encodedcc.org/ in Safari\nGetting type: /search/?type=Project\nSearch page detected\nOpening https://delete-test.demo.encodedcc.org/ in Safari\nGetting type: /search/?type=ReplicationTimingSeries\nSearch page detected\nOpening https://delete-test.demo.encodedcc.org/ in Safari\nGetting type: /search/?type=PublicationData\nSearch page detected\nOpening https://delete-test.demo.encodedcc.org/ in Safari\nGetting type: /search/?type=MatchedSet\nSearch page detected\nOpening https://delete-test.demo.encodedcc.org/ in Safari\nGetting type: /search/?type=TreatmentConcentrationSeries\nSearch page detected\nOpening https://delete-test.demo.encodedcc.org/ in Safari\nGetting type: /search/?type=TreatmentTimeSeries\nSearch page detected\nOpening https://delete-test.demo.encodedcc.org/ in Safari\nGetting type: /search/?type=Target\nSearch page detected\nOpening https://delete-test.demo.encodedcc.org/ in Safari\nGetting type: /search/?type=Pipeline\nSearch page detected\nOpening https://delete-test.demo.encodedcc.org/ in Safari\nGetting type: /search/?type=Publication\nSearch page detected\nOpening https://delete-test.demo.encodedcc.org/ in Safari\nGetting type: /search/?type=Software\nSearch page detected\nOpening https://delete-test.demo.encodedcc.org/ in Safari\nGetting type: /matrix/?type=Experiment\nMatrix page detected\nOpening https://delete-test.demo.encodedcc.org/ in Safari\nGetting type: /matrix/?type=Annotation\nMatrix page detected\n\n---------------------------------- Experiment ----------------------------------\nComparing data between URLs.\nAs user: Public\nBrowser: Safari\nFirst URL: https://encd-3132-delete.demo.encodedcc.org/\nSecond URL: https://delete-test.demo.encodedcc.org/\nItem type: \u001b[1;32m/search/?type=Experiment\u001b[0m\nASSAY\n \u001b[33mprod: [('polyA RNA-seq', '776')]\u001b[0m\n \u001b[33mrc: [('polyA mRNA RNA-seq', '776')]\u001b[0m\nASSAY CATEGORY\n \u001b[33mrc: [('DNA sequencing', '512')]\u001b[0m\nAudit category\n \u001b[36mMATCH\u001b[0m\nAudit category2\n \u001b[36mMATCH\u001b[0m\nAudit category3\n \u001b[36mMATCH\u001b[0m\nAvailable data\n \u001b[36mMATCH\u001b[0m\nBiosample treatment\n \u001b[36mMATCH\u001b[0m\nBiosample type\n \u001b[36mMATCH\u001b[0m\nDate Submitted\n \u001b[36mMATCH\u001b[0m\nDate released\n \u001b[36mMATCH\u001b[0m\nExperiment status\n \u001b[36mMATCH\u001b[0m\nGENOME ASSEMBLY (VISUALIZATION)\n \u001b[31mGRCh38: 5981 (prod), 5904 (rc)\u001b[0m\n \u001b[31mce10: 598 (prod), 550 (rc)\u001b[0m\n \u001b[31mce11: 595 (prod), 547 (rc)\u001b[0m\n \u001b[31mhg19: 6251 (prod), 6174 (rc)\u001b[0m\n \u001b[31mmm10: 1551 (prod), 1504 (rc)\u001b[0m\nLab\n \u001b[36mMATCH\u001b[0m\nLibrary depleted in\n \u001b[36mMATCH\u001b[0m\nLibrary insert size (nt)\n \u001b[36mMATCH\u001b[0m\nLibrary made from\n \u001b[36mMATCH\u001b[0m\nLibrary treatment\n \u001b[36mMATCH\u001b[0m\nLife stage\n \u001b[36mMATCH\u001b[0m\nMapped read length (nt)\n \u001b[36mMATCH\u001b[0m\nORGAN\n \u001b[31mbrain: 922 (prod), 887 (rc)\u001b[0m\nOrganism\n \u001b[36mMATCH\u001b[0m\nProject\n \u001b[36mMATCH\u001b[0m\nRFA\n \u001b[36mMATCH\u001b[0m\nRead length (nt)\n \u001b[36mMATCH\u001b[0m\nReplication type\n \u001b[36mMATCH\u001b[0m\nRun type\n \u001b[36mMATCH\u001b[0m\nTarget of assay\n \u001b[36mMATCH\u001b[0m\n\n------------------------------------- File -------------------------------------\nComparing data between URLs.\nAs user: Public\nBrowser: Safari\nFirst URL: https://encd-3132-delete.demo.encodedcc.org/\nSecond URL: https://delete-test.demo.encodedcc.org/\nItem type: \u001b[1;32m/search/?type=File\u001b[0m\nAudit category\n \u001b[36mMATCH\u001b[0m\nAudit category2\n \u001b[36mMATCH\u001b[0m\nAudit category3\n \u001b[36mMATCH\u001b[0m\nContent category\n \u001b[36mMATCH\u001b[0m\nContent type\n \u001b[36mMATCH\u001b[0m\nFile format\n \u001b[36mMATCH\u001b[0m\nFile status\n \u001b[36mMATCH\u001b[0m\nLab\n \u001b[36mMATCH\u001b[0m\nMapping assembly\n \u001b[36mMATCH\u001b[0m\nProject\n \u001b[36mMATCH\u001b[0m\nSpecific file format type\n \u001b[36mMATCH\u001b[0m\n\n----------------------------------- Library ------------------------------------\nComparing data between URLs.\nAs user: Public\nBrowser: Safari\nFirst URL: https://encd-3132-delete.demo.encodedcc.org/\nSecond URL: https://delete-test.demo.encodedcc.org/\nItem type: \u001b[1;32m/search/?type=Library\u001b[0m\nAudit category\n \u001b[36mMATCH\u001b[0m\nAudit category2\n \u001b[36mMATCH\u001b[0m\nLibrary status\n \u001b[36mMATCH\u001b[0m\n\n--------------------------------- AntibodyLot ----------------------------------\nComparing data between URLs.\nAs user: Public\nBrowser: Safari\nFirst URL: https://encd-3132-delete.demo.encodedcc.org/\nSecond URL: https://delete-test.demo.encodedcc.org/\nItem type: \u001b[1;32m/search/?type=AntibodyLot\u001b[0m\nAudit category\n \u001b[36mMATCH\u001b[0m\nCharacterization method\n \u001b[36mMATCH\u001b[0m\nClonality\n \u001b[36mMATCH\u001b[0m\nEligibility status\n \u001b[36mMATCH\u001b[0m\nHost organism\n \u001b[36mMATCH\u001b[0m\nLab\n \u001b[36mMATCH\u001b[0m\nSource\n \u001b[36mMATCH\u001b[0m\nTarget Organism\n \u001b[36mMATCH\u001b[0m\nTarget of antibody\n \u001b[36mMATCH\u001b[0m\n\n---------------------------------- Biosample -----------------------------------\nComparing data between URLs.\nAs user: Public\nBrowser: Safari\nFirst URL: https://encd-3132-delete.demo.encodedcc.org/\nSecond URL: https://delete-test.demo.encodedcc.org/\nItem type: \u001b[1;32m/search/?type=Biosample\u001b[0m\nAudit category\n \u001b[36mMATCH\u001b[0m\nAudit category2\n \u001b[36mMATCH\u001b[0m\nBiosample status\n \u001b[36mMATCH\u001b[0m\nBiosample treatment\n \u001b[36mMATCH\u001b[0m\nBiosample type\n \u001b[36mMATCH\u001b[0m\nCell cycle phase\n \u001b[36mMATCH\u001b[0m\nLab\n \u001b[36mMATCH\u001b[0m\nLife stage\n \u001b[36mMATCH\u001b[0m\nORGAN\n \u001b[31mbrain: 485 (prod), 479 (rc)\u001b[0m\nOrganism\n \u001b[36mMATCH\u001b[0m\nProject\n \u001b[36mMATCH\u001b[0m\nRFA\n \u001b[36mMATCH\u001b[0m\nSex\n \u001b[36mMATCH\u001b[0m\nSource\n \u001b[36mMATCH\u001b[0m\nSubcellular fraction\n \u001b[36mMATCH\u001b[0m\n\n------------------------------------ Donor -------------------------------------\nComparing data between URLs.\nAs user: Public\nBrowser: Safari\nFirst URL: https://encd-3132-delete.demo.encodedcc.org/\nSecond URL: https://delete-test.demo.encodedcc.org/\nItem type: \u001b[1;32m/search/?type=Donor\u001b[0m\nAudit category\n \u001b[36mMATCH\u001b[0m\nData Type\n \u001b[36mMATCH\u001b[0m\n\n----------------------------- GeneticModification ------------------------------\nComparing data between URLs.\nAs user: Public\nBrowser: Safari\nFirst URL: https://encd-3132-delete.demo.encodedcc.org/\nSecond URL: https://delete-test.demo.encodedcc.org/\nItem type: \u001b[1;32msearch/?type=GeneticModification\u001b[0m\nCategory\n \u001b[36mMATCH\u001b[0m\nGenetic modification status\n \u001b[36mMATCH\u001b[0m\nLab\n \u001b[36mMATCH\u001b[0m\nMethod\n \u001b[36mMATCH\u001b[0m\nPurpose\n \u001b[36mMATCH\u001b[0m\n\n----------------------------------- FileSet ------------------------------------\nComparing data between URLs.\nAs user: Public\nBrowser: Safari\nFirst URL: https://encd-3132-delete.demo.encodedcc.org/\nSecond URL: https://delete-test.demo.encodedcc.org/\nItem type: \u001b[1;32m/search/?type=FileSet\u001b[0m\nAudit category\n \u001b[36mMATCH\u001b[0m\nData Type\n \u001b[36mMATCH\u001b[0m\n\n---------------------------------- Annotation ----------------------------------\nComparing data between URLs.\nAs user: Public\nBrowser: Safari\nFirst URL: https://encd-3132-delete.demo.encodedcc.org/\nSecond URL: https://delete-test.demo.encodedcc.org/\nItem type: \u001b[1;32m/search/?type=Annotation\u001b[0m\nAnnotation type\n \u001b[36mMATCH\u001b[0m\nAssay\n \u001b[36mMATCH\u001b[0m\nAvailable data\n \u001b[36mMATCH\u001b[0m\nBiosample type\n \u001b[36mMATCH\u001b[0m\nDate released\n \u001b[36mMATCH\u001b[0m\nEncyclopedia version\n \u001b[36mMATCH\u001b[0m\nFile set status\n \u001b[36mMATCH\u001b[0m\nGENOME ASSEMBLY (VISUALIZATION)\n \u001b[31mhg19: 1216 (prod), 1215 (rc)\u001b[0m\n \u001b[31mmm10: 455 (prod), 446 (rc)\u001b[0m\n \u001b[31mmm9: 17 (prod), 14 (rc)\u001b[0m\nLab\n \u001b[36mMATCH\u001b[0m\nLife stage\n \u001b[36mMATCH\u001b[0m\nORGAN\n \u001b[31mbrain: 329 (prod), 315 (rc)\u001b[0m\nOrganism\n \u001b[36mMATCH\u001b[0m\nProject\n \u001b[36mMATCH\u001b[0m\nRFA\n \u001b[36mMATCH\u001b[0m\nRead length (nt)\n \u001b[36mMATCH\u001b[0m\nRun type\n \u001b[36mMATCH\u001b[0m\nSoftware used\n \u001b[36mMATCH\u001b[0m\nTarget(s) of assay\n \u001b[36mMATCH\u001b[0m\nTarget(s) of assay2\n \u001b[36mMATCH\u001b[0m\n\n------------------------------------ Series ------------------------------------\nComparing data between URLs.\nAs user: Public\nBrowser: Safari\nFirst URL: https://encd-3132-delete.demo.encodedcc.org/\nSecond URL: https://delete-test.demo.encodedcc.org/\nItem type: \u001b[1;32m/search/?type=Series\u001b[0m\nAudit category\n \u001b[36mMATCH\u001b[0m\nData Type\n \u001b[36mMATCH\u001b[0m\n\n-------------------------- OrganismDevelopmentSeries ---------------------------\nComparing data between URLs.\nAs user: Public\nBrowser: Safari\nFirst URL: https://encd-3132-delete.demo.encodedcc.org/\nSecond URL: https://delete-test.demo.encodedcc.org/\nItem type: \u001b[1;32m/search/?type=OrganismDevelopmentSeries\u001b[0m\nAssay\n \u001b[36mMATCH\u001b[0m\nAvailable data\n \u001b[36mMATCH\u001b[0m\nBiosample type\n \u001b[36mMATCH\u001b[0m\nDate released\n \u001b[36mMATCH\u001b[0m\nGenome assembly (visualization)\n \u001b[36mMATCH\u001b[0m\nLab\n \u001b[36mMATCH\u001b[0m\nLibrary depleted in\n \u001b[36mMATCH\u001b[0m\nLibrary insert size (nt)\n \u001b[36mMATCH\u001b[0m\nLibrary made from\n \u001b[36mMATCH\u001b[0m\nLife stage\n \u001b[36mMATCH\u001b[0m\nMapped read length\n \u001b[36mMATCH\u001b[0m\nOrgan\n \u001b[36mMATCH\u001b[0m\nProject\n \u001b[36mMATCH\u001b[0m\nRead length\n \u001b[36mMATCH\u001b[0m\nSeries status\n \u001b[36mMATCH\u001b[0m\nTarget of assay\n \u001b[36mMATCH\u001b[0m\n\n----------------------------- UcscBrowserComposite -----------------------------\nComparing data between URLs.\nAs user: Public\nBrowser: Safari\nFirst URL: https://encd-3132-delete.demo.encodedcc.org/\nSecond URL: https://delete-test.demo.encodedcc.org/\nItem type: \u001b[1;32m/search/?type=UcscBrowserComposite\u001b[0m\nAvailable data\n \u001b[36mMATCH\u001b[0m\nComposite status\n \u001b[36mMATCH\u001b[0m\nDate released\n \u001b[36mMATCH\u001b[0m\nGenome assembly (visualization)\n \u001b[36mMATCH\u001b[0m\nLab\n \u001b[36mMATCH\u001b[0m\nProject\n \u001b[36mMATCH\u001b[0m\nRFA\n \u001b[36mMATCH\u001b[0m\n\n------------------------------ ReferenceEpigenome ------------------------------\nComparing data between URLs.\nAs user: Public\nBrowser: Safari\nFirst URL: https://encd-3132-delete.demo.encodedcc.org/\nSecond URL: https://delete-test.demo.encodedcc.org/\nItem type: \u001b[1;32m/search/?type=ReferenceEpigenome\u001b[0m\nAssay\n \u001b[36mMATCH\u001b[0m\nAudit category\n \u001b[36mMATCH\u001b[0m\nAvailable data\n \u001b[36mMATCH\u001b[0m\nBiosample treatment\n \u001b[36mMATCH\u001b[0m\nBiosample type\n \u001b[36mMATCH\u001b[0m\nDate released\n \u001b[36mMATCH\u001b[0m\nGENOME ASSEMBLY (VISUALIZATION)\n \u001b[31mGRCh38: 120 (prod), 119 (rc)\u001b[0m\nLab\n \u001b[36mMATCH\u001b[0m\nLibrary depleted in\n \u001b[36mMATCH\u001b[0m\nLibrary insert size (nt)\n \u001b[36mMATCH\u001b[0m\nLibrary made from\n \u001b[36mMATCH\u001b[0m\nLife stage\n \u001b[36mMATCH\u001b[0m\nMapped read length\n \u001b[36mMATCH\u001b[0m\nORGAN\n \u001b[31mbrain: 37 (prod), 35 (rc)\u001b[0m\nOrganism\n \u001b[36mMATCH\u001b[0m\nProject\n \u001b[36mMATCH\u001b[0m\nRFA\n \u001b[36mMATCH\u001b[0m\nRead length\n \u001b[36mMATCH\u001b[0m\nSeries status\n \u001b[36mMATCH\u001b[0m\nTarget of assay\n \u001b[36mMATCH\u001b[0m\n\n----------------------------------- Project ------------------------------------\nComparing data between URLs.\nAs user: Public\nBrowser: Safari\nFirst URL: https://encd-3132-delete.demo.encodedcc.org/\nSecond URL: https://delete-test.demo.encodedcc.org/\nItem type: \u001b[1;32m/search/?type=Project\u001b[0m\nAssay\n \u001b[36mMATCH\u001b[0m\nAudit category\n \u001b[36mMATCH\u001b[0m\nAvailable data\n \u001b[36mMATCH\u001b[0m\nBiosample type\n \u001b[36mMATCH\u001b[0m\nDate released\n \u001b[36mMATCH\u001b[0m\nGenome assembly (visualization)\n \u001b[36mMATCH\u001b[0m\nLab\n \u001b[36mMATCH\u001b[0m\nOrgan\n \u001b[36mMATCH\u001b[0m\nOrganism\n \u001b[36mMATCH\u001b[0m\nProject\n \u001b[36mMATCH\u001b[0m\nProject status\n \u001b[36mMATCH\u001b[0m\nProject type\n \u001b[36mMATCH\u001b[0m\nRFA\n \u001b[36mMATCH\u001b[0m\nRead length\n \u001b[36mMATCH\u001b[0m\nRun type\n \u001b[36mMATCH\u001b[0m\nTarget of assay\n \u001b[36mMATCH\u001b[0m\n\n--------------------------- ReplicationTimingSeries ----------------------------\nComparing data between URLs.\nAs user: Public\nBrowser: Safari\nFirst URL: https://encd-3132-delete.demo.encodedcc.org/\nSecond URL: https://delete-test.demo.encodedcc.org/\nItem type: \u001b[1;32m/search/?type=ReplicationTimingSeries\u001b[0m\nAssay\n \u001b[36mMATCH\u001b[0m\nAvailable data\n \u001b[36mMATCH\u001b[0m\nBiosample type\n \u001b[36mMATCH\u001b[0m\nDate released\n \u001b[36mMATCH\u001b[0m\nGenome assembly (visualization)\n \u001b[36mMATCH\u001b[0m\nLab\n \u001b[36mMATCH\u001b[0m\nLibrary insert size (nt)\n \u001b[36mMATCH\u001b[0m\nLibrary made from\n \u001b[36mMATCH\u001b[0m\nLife stage\n \u001b[36mMATCH\u001b[0m\nOrgan\n \u001b[36mMATCH\u001b[0m\nOrganism\n \u001b[36mMATCH\u001b[0m\nProject\n \u001b[36mMATCH\u001b[0m\nRFA\n \u001b[36mMATCH\u001b[0m\nRead length\n \u001b[36mMATCH\u001b[0m\nSeries status\n \u001b[36mMATCH\u001b[0m\nTarget of assay\n \u001b[36mMATCH\u001b[0m\n\n------------------------------- PublicationData --------------------------------\nComparing data between URLs.\nAs user: Public\nBrowser: Safari\nFirst URL: https://encd-3132-delete.demo.encodedcc.org/\nSecond URL: https://delete-test.demo.encodedcc.org/\nItem type: \u001b[1;32m/search/?type=PublicationData\u001b[0m\nAssay\n \u001b[36mMATCH\u001b[0m\nBiosample type\n \u001b[36mMATCH\u001b[0m\nDate released\n \u001b[36mMATCH\u001b[0m\nGenome assembly (visualization)\n \u001b[36mMATCH\u001b[0m\nLab\n \u001b[36mMATCH\u001b[0m\nOrgan\n \u001b[36mMATCH\u001b[0m\nOrganism\n \u001b[36mMATCH\u001b[0m\nProject\n \u001b[36mMATCH\u001b[0m\nPublication data status\n \u001b[36mMATCH\u001b[0m\nRFA\n \u001b[36mMATCH\u001b[0m\n\n---------------------------------- MatchedSet ----------------------------------\nComparing data between URLs.\nAs user: Public\nBrowser: Safari\nFirst URL: https://encd-3132-delete.demo.encodedcc.org/\nSecond URL: https://delete-test.demo.encodedcc.org/\nItem type: \u001b[1;32m/search/?type=MatchedSet\u001b[0m\nAssay\n \u001b[36mMATCH\u001b[0m\nAvailable data\n \u001b[36mMATCH\u001b[0m\nBiosample term name\n \u001b[36mMATCH\u001b[0m\nBiosample type\n \u001b[36mMATCH\u001b[0m\nDate released\n \u001b[36mMATCH\u001b[0m\nGenome assembly (visualization)\n \u001b[36mMATCH\u001b[0m\nLab\n \u001b[36mMATCH\u001b[0m\nLibrary insert size (nt)\n \u001b[36mMATCH\u001b[0m\nLibrary made from\n \u001b[36mMATCH\u001b[0m\nLife stage\n \u001b[36mMATCH\u001b[0m\nMapped read length\n \u001b[36mMATCH\u001b[0m\nOrgan\n \u001b[36mMATCH\u001b[0m\nOrgan3\n \u001b[36mMATCH\u001b[0m\nOrganism\n \u001b[36mMATCH\u001b[0m\nProject\n \u001b[36mMATCH\u001b[0m\nRFA\n \u001b[36mMATCH\u001b[0m\nRead length\n \u001b[36mMATCH\u001b[0m\nSeries status\n \u001b[36mMATCH\u001b[0m\nTarget of assay\n \u001b[36mMATCH\u001b[0m\n\n------------------------- TreatmentConcentrationSeries -------------------------\nComparing data between URLs.\nAs user: Public\nBrowser: Safari\nFirst URL: https://encd-3132-delete.demo.encodedcc.org/\nSecond URL: https://delete-test.demo.encodedcc.org/\nItem type: \u001b[1;32m/search/?type=TreatmentConcentrationSeries\u001b[0m\nAssay\n \u001b[36mMATCH\u001b[0m\nAvailable data\n \u001b[36mMATCH\u001b[0m\nBiosample treatment\n \u001b[36mMATCH\u001b[0m\nDate released\n \u001b[36mMATCH\u001b[0m\nGenome assembly (visualization)\n \u001b[36mMATCH\u001b[0m\nLab\n \u001b[36mMATCH\u001b[0m\nLibrary insert size (nt)\n \u001b[36mMATCH\u001b[0m\nLibrary made from\n \u001b[36mMATCH\u001b[0m\nLife stage\n \u001b[36mMATCH\u001b[0m\nMapped read length\n \u001b[36mMATCH\u001b[0m\nOrgan\n \u001b[36mMATCH\u001b[0m\nOrganism\n \u001b[36mMATCH\u001b[0m\nProject\n \u001b[36mMATCH\u001b[0m\nRFA\n \u001b[36mMATCH\u001b[0m\nRead length\n \u001b[36mMATCH\u001b[0m\nSeries status\n \u001b[36mMATCH\u001b[0m\nTarget\n \u001b[36mMATCH\u001b[0m\nTarget of assay\n \u001b[36mMATCH\u001b[0m\n\n----------------------------- TreatmentTimeSeries ------------------------------\nComparing data between URLs.\nAs user: Public\nBrowser: Safari\nFirst URL: https://encd-3132-delete.demo.encodedcc.org/\nSecond URL: https://delete-test.demo.encodedcc.org/\nItem type: \u001b[1;32m/search/?type=TreatmentTimeSeries\u001b[0m\nAssay\n \u001b[36mMATCH\u001b[0m\nAvailable data\n \u001b[36mMATCH\u001b[0m\nBiosample treatment\n \u001b[36mMATCH\u001b[0m\nBiosample type\n \u001b[36mMATCH\u001b[0m\nDate released\n \u001b[36mMATCH\u001b[0m\nGenome assembly (visualization)\n \u001b[36mMATCH\u001b[0m\nLab\n \u001b[36mMATCH\u001b[0m\nLibrary insert size (nt)\n \u001b[36mMATCH\u001b[0m\nLibrary made from\n \u001b[36mMATCH\u001b[0m\nLife stage\n \u001b[36mMATCH\u001b[0m\nMapped read length\n \u001b[36mMATCH\u001b[0m\nOrgan\n \u001b[36mMATCH\u001b[0m\nOrganism\n \u001b[36mMATCH\u001b[0m\nProject\n \u001b[36mMATCH\u001b[0m\nRFA\n \u001b[36mMATCH\u001b[0m\nRead length\n \u001b[36mMATCH\u001b[0m\nSeries status\n \u001b[36mMATCH\u001b[0m\nTarget of assay\n \u001b[36mMATCH\u001b[0m\n\n------------------------------------ Target ------------------------------------\nComparing data between URLs.\nAs user: Public\nBrowser: Safari\nFirst URL: https://encd-3132-delete.demo.encodedcc.org/\nSecond URL: https://delete-test.demo.encodedcc.org/\nItem type: \u001b[1;32m/search/?type=Target\u001b[0m\nOrganism\n \u001b[36mMATCH\u001b[0m\nTarget of assay\n \u001b[36mMATCH\u001b[0m\n\n----------------------------------- Pipeline -----------------------------------\nComparing data between URLs.\nAs user: Public\nBrowser: Safari\nFirst URL: https://encd-3132-delete.demo.encodedcc.org/\nSecond URL: https://delete-test.demo.encodedcc.org/\nItem type: \u001b[1;32m/search/?type=Pipeline\u001b[0m\nAssay\n \u001b[36mMATCH\u001b[0m\nDeveloped by\n \u001b[36mMATCH\u001b[0m\nPipeline groups\n \u001b[36mMATCH\u001b[0m\nPipeline status\n \u001b[36mMATCH\u001b[0m\n\n--------------------------------- Publication ----------------------------------\nComparing data between URLs.\nAs user: Public\nBrowser: Safari\nFirst URL: https://encd-3132-delete.demo.encodedcc.org/\nSecond URL: https://delete-test.demo.encodedcc.org/\nItem type: \u001b[1;32m/search/?type=Publication\u001b[0m\nAvailable supplemental data\n \u001b[36mMATCH\u001b[0m\nCategory\n \u001b[36mMATCH\u001b[0m\nHas dataset\n \u001b[36mMATCH\u001b[0m\nJournal\n \u001b[36mMATCH\u001b[0m\nPublication status\n \u001b[36mMATCH\u001b[0m\nPublication year\n \u001b[36mMATCH\u001b[0m\nPublished by\n \u001b[36mMATCH\u001b[0m\n\n----------------------------------- Software -----------------------------------\nComparing data between URLs.\nAs user: Public\nBrowser: Safari\nFirst URL: https://encd-3132-delete.demo.encodedcc.org/\nSecond URL: https://delete-test.demo.encodedcc.org/\nItem type: \u001b[1;32m/search/?type=Software\u001b[0m\nCreated by\n \u001b[36mMATCH\u001b[0m\nPurpose in project\n \u001b[36mMATCH\u001b[0m\nSoftware type\n \u001b[36mMATCH\u001b[0m\n\n---------------------------------- Experiment ----------------------------------\nComparing data between URLs.\nAs user: Public\nBrowser: Safari\nFirst URL: https://encd-3132-delete.demo.encodedcc.org/\nSecond URL: https://delete-test.demo.encodedcc.org/\nItem type: \u001b[1;32m/matrix/?type=Experiment\u001b[0m\nASSAY\n \u001b[33mprod: [('polyA RNA-seq', '776')]\u001b[0m\n \u001b[33mrc: [('polyA mRNA RNA-seq', '776')]\u001b[0m\nASSAY CATEGORY\n \u001b[33mrc: [('DNA sequencing', '512')]\u001b[0m\nAudit category\n \u001b[36mMATCH\u001b[0m\nAudit category2\n \u001b[36mMATCH\u001b[0m\nAudit category3\n \u001b[36mMATCH\u001b[0m\nAvailable data\n \u001b[36mMATCH\u001b[0m\nBiosample type\n \u001b[36mMATCH\u001b[0m\nDate released\n \u001b[36mMATCH\u001b[0m\nGENOME ASSEMBLY (VISUALIZATION)\n \u001b[31mGRCh38: 5981 (prod), 5904 (rc)\u001b[0m\n \u001b[31mce10: 598 (prod), 550 (rc)\u001b[0m\n \u001b[31mce11: 595 (prod), 547 (rc)\u001b[0m\n \u001b[31mhg19: 6251 (prod), 6174 (rc)\u001b[0m\n \u001b[31mmm10: 1551 (prod), 1504 (rc)\u001b[0m\nLab\n \u001b[36mMATCH\u001b[0m\nORGAN\n \u001b[31mbrain: 922 (prod), 887 (rc)\u001b[0m\nOrganism\n \u001b[36mMATCH\u001b[0m\nProject\n \u001b[36mMATCH\u001b[0m\nTarget of assay\n \u001b[36mMATCH\u001b[0m\n\n---------------------------------- Annotation ----------------------------------\nComparing data between URLs.\nAs user: Public\nBrowser: Safari\nFirst URL: https://encd-3132-delete.demo.encodedcc.org/\nSecond URL: https://delete-test.demo.encodedcc.org/\nItem type: \u001b[1;32m/matrix/?type=Annotation\u001b[0m\nAnnotation type\n \u001b[36mMATCH\u001b[0m\nAvailable data\n \u001b[36mMATCH\u001b[0m\nBiosample type\n \u001b[36mMATCH\u001b[0m\nDate released\n \u001b[36mMATCH\u001b[0m\nEncyclopedia version\n \u001b[36mMATCH\u001b[0m\nGENOME ASSEMBLY (VISUALIZATION)\n \u001b[31mhg19: 1216 (prod), 1215 (rc)\u001b[0m\n \u001b[31mmm10: 455 (prod), 446 (rc)\u001b[0m\n \u001b[31mmm9: 17 (prod), 14 (rc)\u001b[0m\nORGAN\n \u001b[31mbrain: 329 (prod), 315 (rc)\u001b[0m\nOrganism\n \u001b[36mMATCH\u001b[0m\nProject\n \u001b[36mMATCH\u001b[0m\nTarget(s) of assay\n \u001b[36mMATCH\u001b[0m\n"
],
[
"#comparison as admin user\nqa.compare_facets(users=['[email protected]'], browsers=['Safari'], browser_comparison=False)",
"Opening https://encd-3132-delete.demo.encodedcc.org/ in Safari\nLogging in as [email protected]\nLogin successful\nRefreshing.\nGetting type: /search/?type=Experiment\nSearch page detected\nOpening https://encd-3132-delete.demo.encodedcc.org/ in Safari\nLogging in as [email protected]\nLogin successful\nRefreshing.\nGetting type: /search/?type=File\nSearch page detected\nOpening https://encd-3132-delete.demo.encodedcc.org/ in Safari\nLogging in as [email protected]\nLogin successful\nRefreshing.\nGetting type: /search/?type=Library\nSearch page detected\nOpening https://encd-3132-delete.demo.encodedcc.org/ in Safari\nLogging in as [email protected]\nLogin successful\nRefreshing.\nGetting type: /search/?type=AntibodyLot\nSearch page detected\nOpening https://encd-3132-delete.demo.encodedcc.org/ in Safari\nLogging in as [email protected]\nLogin successful\nRefreshing.\nGetting type: /search/?type=Biosample\nSearch page detected\nOpening https://encd-3132-delete.demo.encodedcc.org/ in Safari\nLogging in as [email protected]\nLogin successful\nRefreshing.\nGetting type: /search/?type=Donor\nSearch page detected\nOpening https://encd-3132-delete.demo.encodedcc.org/ in Safari\nLogging in as [email protected]\nLogin successful\nRefreshing.\nGetting type: search/?type=GeneticModification\nSearch page detected\nOpening https://encd-3132-delete.demo.encodedcc.org/ in Safari\nLogging in as [email protected]\nLogin successful\nRefreshing.\nGetting type: /search/?type=FileSet\nSearch page detected\nOpening https://encd-3132-delete.demo.encodedcc.org/ in Safari\nLogging in as [email protected]\nLogin successful\nRefreshing.\nGetting type: /search/?type=Annotation\nSearch page detected\nOpening https://encd-3132-delete.demo.encodedcc.org/ in Safari\nLogging in as [email protected]\nLogin successful\nRefreshing.\nGetting type: /search/?type=Series\nSearch page detected\nOpening https://encd-3132-delete.demo.encodedcc.org/ in Safari\nLogging in as [email protected]\nLogin successful\nRefreshing.\nGetting type: /search/?type=OrganismDevelopmentSeries\nSearch page detected\nOpening https://encd-3132-delete.demo.encodedcc.org/ in Safari\nLogging in as [email protected]\nLogin successful\nRefreshing.\nGetting type: /search/?type=UcscBrowserComposite\nSearch page detected\nOpening https://encd-3132-delete.demo.encodedcc.org/ in Safari\nLogging in as [email protected]\nLogin successful\nRefreshing.\nGetting type: /search/?type=ReferenceEpigenome\nSearch page detected\nOpening https://encd-3132-delete.demo.encodedcc.org/ in Safari\nLogging in as [email protected]\nLogin successful\nRefreshing.\nGetting type: /search/?type=Project\nSearch page detected\nOpening https://encd-3132-delete.demo.encodedcc.org/ in Safari\nLogging in as [email protected]\nLogin successful\nRefreshing.\nGetting type: /search/?type=ReplicationTimingSeries\nSearch page detected\nOpening https://encd-3132-delete.demo.encodedcc.org/ in Safari\nLogging in as [email protected]\nLogin successful\nRefreshing.\nGetting type: /search/?type=PublicationData\nSearch page detected\nOpening https://encd-3132-delete.demo.encodedcc.org/ in Safari\nLogging in as [email protected]\nLogin successful\nRefreshing.\nGetting type: /search/?type=MatchedSet\nSearch page detected\nOpening https://encd-3132-delete.demo.encodedcc.org/ in Safari\nLogging in as [email protected]\nLogin successful\nRefreshing.\nGetting type: /search/?type=TreatmentConcentrationSeries\nSearch page detected\nOpening https://encd-3132-delete.demo.encodedcc.org/ in Safari\nLogging in as [email protected]\nLogin successful\nRefreshing.\nGetting type: /search/?type=TreatmentTimeSeries\nSearch page detected\nOpening https://encd-3132-delete.demo.encodedcc.org/ in Safari\nLogging in as [email protected]\nLogin successful\nRefreshing.\nGetting type: /search/?type=Target\nSearch page detected\nOpening https://encd-3132-delete.demo.encodedcc.org/ in Safari\nLogging in as [email protected]\nLogin successful\nRefreshing.\nGetting type: /search/?type=Pipeline\nSearch page detected\nOpening https://encd-3132-delete.demo.encodedcc.org/ in Safari\nLogging in as [email protected]\nLogin successful\nRefreshing.\nGetting type: /search/?type=Publication\nSearch page detected\nOpening https://encd-3132-delete.demo.encodedcc.org/ in Safari\nLogging in as [email protected]\nLogin successful\nRefreshing.\nGetting type: /search/?type=Software\nSearch page detected\nOpening https://encd-3132-delete.demo.encodedcc.org/ in Safari\nLogging in as [email protected]\nLogin successful\nRefreshing.\nGetting type: /matrix/?type=Experiment\nMatrix page detected\nOpening https://encd-3132-delete.demo.encodedcc.org/ in Safari\nLogging in as [email protected]\nLogin successful\nRefreshing.\nGetting type: /matrix/?type=Annotation\nMatrix page detected\nOpening https://delete-test.demo.encodedcc.org/ in Safari\nLogging in as [email protected]\nLogin successful\nRefreshing.\nGetting type: /search/?type=Experiment\nSearch page detected\nOpening https://delete-test.demo.encodedcc.org/ in Safari\nLogging in as [email protected]\nLogin successful\nRefreshing.\nGetting type: /search/?type=File\nSearch page detected\nOpening https://delete-test.demo.encodedcc.org/ in Safari\nLogging in as [email protected]\nLogin successful\nRefreshing.\nGetting type: /search/?type=Library\nSearch page detected\nOpening https://delete-test.demo.encodedcc.org/ in Safari\nLogging in as [email protected]\nLogin successful\nRefreshing.\nGetting type: /search/?type=AntibodyLot\nSearch page detected\nOpening https://delete-test.demo.encodedcc.org/ in Safari\nLogging in as [email protected]\nLogin successful\nRefreshing.\nGetting type: /search/?type=Biosample\nSearch page detected\nOpening https://delete-test.demo.encodedcc.org/ in Safari\nLogging in as [email protected]\nLogin successful\nRefreshing.\nGetting type: /search/?type=Donor\nSearch page detected\nOpening https://delete-test.demo.encodedcc.org/ in Safari\nLogging in as [email protected]\nLogin successful\nRefreshing.\nGetting type: search/?type=GeneticModification\nSearch page detected\nOpening https://delete-test.demo.encodedcc.org/ in Safari\nLogging in as [email protected]\nLogin successful\nRefreshing.\nGetting type: /search/?type=FileSet\nSearch page detected\nOpening https://delete-test.demo.encodedcc.org/ in Safari\nLogging in as [email protected]\nLogin successful\nRefreshing.\nGetting type: /search/?type=Annotation\nSearch page detected\nOpening https://delete-test.demo.encodedcc.org/ in Safari\nLogging in as [email protected]\nLogin successful\nRefreshing.\nGetting type: /search/?type=Series\nSearch page detected\nOpening https://delete-test.demo.encodedcc.org/ in Safari\nLogging in as [email protected]\nLogin successful\nRefreshing.\nGetting type: /search/?type=OrganismDevelopmentSeries\nSearch page detected\nOpening https://delete-test.demo.encodedcc.org/ in Safari\nLogging in as [email protected]\nLogin successful\nRefreshing.\nGetting type: /search/?type=UcscBrowserComposite\nSearch page detected\nOpening https://delete-test.demo.encodedcc.org/ in Safari\nLogging in as [email protected]\nLogin successful\nRefreshing.\nGetting type: /search/?type=ReferenceEpigenome\nSearch page detected\nOpening https://delete-test.demo.encodedcc.org/ in Safari\nLogging in as [email protected]\nLogin successful\nRefreshing.\nGetting type: /search/?type=Project\nSearch page detected\nOpening https://delete-test.demo.encodedcc.org/ in Safari\nLogging in as [email protected]\nLogin successful\nRefreshing.\nGetting type: /search/?type=ReplicationTimingSeries\nSearch page detected\nOpening https://delete-test.demo.encodedcc.org/ in Safari\nLogging in as [email protected]\nLogin successful\nRefreshing.\nGetting type: /search/?type=PublicationData\nSearch page detected\nOpening https://delete-test.demo.encodedcc.org/ in Safari\nLogging in as [email protected]\nLogin successful\nRefreshing.\nGetting type: /search/?type=MatchedSet\nSearch page detected\nOpening https://delete-test.demo.encodedcc.org/ in Safari\nLogging in as [email protected]\nLogin successful\nRefreshing.\nGetting type: /search/?type=TreatmentConcentrationSeries\nSearch page detected\nOpening https://delete-test.demo.encodedcc.org/ in Safari\n"
]
]
] | [
"code"
] | [
[
"code",
"code",
"code",
"code"
]
] |
e785d32a1b9cf27727a91c2db86faba17f3fd293 | 441,109 | ipynb | Jupyter Notebook | AmazonReviews_Example/NLP_Session_2_AmazonReviews_Example.ipynb | drshyamsundaram/nlp | 27b334b6e3f31fe9854b794906d876216ef6afba | [
"MIT"
] | 4 | 2021-10-03T10:21:16.000Z | 2021-11-01T13:04:25.000Z | AmazonReviews_Example/NLP_Session_2_AmazonReviews_Example.ipynb | drshyamsundaram/nlp | 27b334b6e3f31fe9854b794906d876216ef6afba | [
"MIT"
] | null | null | null | AmazonReviews_Example/NLP_Session_2_AmazonReviews_Example.ipynb | drshyamsundaram/nlp | 27b334b6e3f31fe9854b794906d876216ef6afba | [
"MIT"
] | null | null | null | 369.438023 | 384,426 | 0.921883 | [
[
[
"# NLP_Session_2_AmazonReviews_Example\n\n## Amazon Review Polarity Dataset\n\n## DOWNLOAD DATA FROM HERE\nhttps://www.kaggle.com/kritanjalijain/amazon-reviews/download \nExtract the train and test CSV files to your google drive location and configure that in the code\n\n### OVERVIEW\nContains 34,686,770 Amazon reviews from 6,643,669 users on 2,441,053 products, from the Stanford Network Analysis Project (SNAP). This subset contains 1,800,000 training samples and 200,000 testing samples in each polarity sentiment.\n\n### ORIGIN\nThe Amazon reviews dataset consists of reviews from amazon. The data span a period of 18 years, including ~35 million reviews up to March 2013. Reviews include product and user information, ratings, and a plaintext review. For more information, please refer to the following paper: J. McAuley and J. Leskovec. Hidden factors and hidden topics: understanding rating dimensions with review text. RecSys, 2013.\n\n### DESCRIPTION\nThe Amazon reviews polarity dataset is constructed by taking review score 1 and 2 as negative, and 4 and 5 as positive. Samples of score 3 is ignored. In the dataset, class 1 is the negative and class 2 is the positive. Each class has 1,800,000 training samples and 200,000 testing samples.\n\nIf you need help extracting the train.csv and test.csv files check out the starter code.\n\nThe files train.csv and test.csv contain all the training samples as comma-separated values.\n\nThe CSVs contain polarity, title, text. These 3 columns in them, correspond to class index (1 or 2), review title and review text.\n\npolarity - 1 for negative and 2 for positive\ntitle - review heading\ntext - review body\nThe review title and text are escaped using double quotes (\"), and any internal double quote is escaped by 2 double quotes (\"\"). New lines are escaped by a backslash followed with an \"n\" character, that is \"\\n\".\n\n### CITATION\nThe Amazon reviews polarity dataset is constructed by Xiang Zhang ([email protected]). It is used as a text classification benchmark in the following paper: Xiang Zhang, Junbo Zhao, Yann LeCun. Character-level Convolutional Networks for Text Classification. Advances in Neural Information Processing Systems 28 (NIPS 2015).\n\n# About TextBLOB library\nTextBlob is a Python (2 and 3) library for processing textual data. It provides a consistent API for diving into common natural language processing (NLP) tasks such as part-of-speech tagging, noun phrase extraction, sentiment analysis, and more.\n\nReference : https://textblob.readthedocs.io/en/dev/ \n\n## Reference\n1. https://www.kaggle.com/kritanjalijain/amazon-reviews\n2. https://www.kaggle.com/sindhuguttal/amazon-review-using-nlp/data\n2. https://textblob.readthedocs.io/en/dev/\n",
"_____no_output_____"
],
[
"# Importing the libraries",
"_____no_output_____"
]
],
[
[
"import numpy as np\nimport pandas as pd\nimport matplotlib.pyplot as plt\nimport tarfile \nimport seaborn as sns\nimport matplotlib.style as style\nimport matplotlib as mpl\nimport re\nimport string\nimport itertools\nimport collections\nfrom wordcloud import WordCloud\nimport nltk \nfrom nltk.util import ngrams\nfrom nltk.corpus import stopwords,RegexpTokenizer\nfrom textblob import TextBlob\nfrom nltk.stem import WordNetLemmatizer",
"_____no_output_____"
]
],
[
[
"# Setting up NLP Libraries and corpus",
"_____no_output_____"
]
],
[
[
"nltk.download('stopwords')\nnltk.download('punkt')\nnltk.download('averaged_perceptron_tagger')",
"[nltk_data] Downloading package stopwords to /root/nltk_data...\n[nltk_data] Package stopwords is already up-to-date!\n[nltk_data] Downloading package punkt to /root/nltk_data...\n[nltk_data] Package punkt is already up-to-date!\n[nltk_data] Downloading package averaged_perceptron_tagger to\n[nltk_data] /root/nltk_data...\n[nltk_data] Package averaged_perceptron_tagger is already up-to-\n[nltk_data] date!\n"
]
],
[
[
"# Mounting the data source",
"_____no_output_____"
]
],
[
[
"from google.colab import drive\ndrive.mount('/content/drive',force_remount=True)",
"Mounted at /content/drive\n"
]
],
[
[
"# Configuring the input, output and process folders",
"_____no_output_____"
]
],
[
[
"# Model Input and output folders\n# Setup in Google drive\n# '/content/drive/MyDrive/yourlocation/input/'\nmodel_input_folder='/content/drive/MyDrive/yourlocation/input/'\nmodel_output_folder='/content/drive/MyDrive/yourlocation/output/'\ninput_train_file=model_input_folder+'train.csv'\ninput_test_file=model_input_folder+'test.csv'\noutput_nlp_detail=model_output_folder+'nlp_details.csv'",
"_____no_output_____"
]
],
[
[
"# Loading the training dataset",
"_____no_output_____"
]
],
[
[
"# check out what the data looks like before you get started\n# look at the training data set\ntrain_df = pd.read_csv(input_train_file, header=None)\nprint(train_df.head())",
" 0 ... 2\n0 2 ... This sound track was beautiful! It paints the ...\n1 2 ... I'm reading a lot of reviews saying that this ...\n2 2 ... This soundtrack is my favorite music of all ti...\n3 2 ... I truly like this soundtrack and I enjoy video...\n4 2 ... If you've played the game, you know how divine...\n\n[5 rows x 3 columns]\n"
],
[
"train_df.shape",
"_____no_output_____"
]
],
[
[
"## Reducing the size of the dataframe for demonstration purposes",
"_____no_output_____"
]
],
[
[
"# Reducing the size of the dataframe\ntrain_df=train_df.loc[1:10000]",
"_____no_output_____"
],
[
"for col in train_df.columns:\n print(col)",
"0\n1\n2\n"
],
[
"#checking a null values\ntrain_df.isnull().sum()",
"_____no_output_____"
],
[
"#droping null vlaues\ntrain_df.dropna()",
"_____no_output_____"
],
[
"train_df.isnull().count()",
"_____no_output_____"
]
],
[
[
"# Quick look at the dataset loaded",
"_____no_output_____"
]
],
[
[
"train_df.info()",
"<class 'pandas.core.frame.DataFrame'>\nRangeIndex: 10000 entries, 1 to 10000\nData columns (total 3 columns):\n # Column Non-Null Count Dtype \n--- ------ -------------- ----- \n 0 0 10000 non-null int64 \n 1 1 10000 non-null object\n 2 2 10000 non-null object\ndtypes: int64(1), object(2)\nmemory usage: 234.5+ KB\n"
],
[
"train_df.drop([0],axis=1,inplace=True)",
"_____no_output_____"
],
[
"for col in train_df.columns:\n print(col)",
"1\n2\n"
],
[
"train_df.drop([1],axis=1,inplace=True)",
"_____no_output_____"
],
[
"train_df.shape",
"_____no_output_____"
],
[
"train_df.sample(5)",
"_____no_output_____"
],
[
"# Checking for Null Values\ntrain_df[train_df[2].isnull()]",
"_____no_output_____"
]
],
[
[
"# Obtaining the review lengths",
"_____no_output_____"
]
],
[
[
"train = train_df.copy()\ntrain[2].apply(str)",
"_____no_output_____"
],
[
"train[\"review_length\"] = train[2].apply(lambda w : len(re.findall(r'\\w+', w)))",
"_____no_output_____"
]
],
[
[
"# Getting some statistics around the review length",
"_____no_output_____"
]
],
[
[
"train['review_length'].describe()",
"_____no_output_____"
]
],
[
[
"## Doing some graphical plots",
"_____no_output_____"
]
],
[
[
"sns.boxplot(data = train , x=\"review_length\")\nplt.xlabel('Number of Words')\nplt.title('Review Length, Including Stop Words')\nplt.show()",
"_____no_output_____"
],
[
"sns.distplot(train['review_length'], kde = False)\nplt.xlabel('Distribution of Review Length')\nplt.title('Review Length, Including Stop Words')\nplt.show()",
"/usr/local/lib/python3.7/dist-packages/seaborn/distributions.py:2619: FutureWarning: `distplot` is a deprecated function and will be removed in a future version. Please adapt your code to use either `displot` (a figure-level function with similar flexibility) or `histplot` (an axes-level function for histograms).\n warnings.warn(msg, FutureWarning)\n"
]
],
[
[
"# If we want to do better we must pre-process data like\n1. Converting to lower case\n2. Removing punctuation\n3. Removing Numbers\n4. Removing trailing spaces\n5. Removing extra whitespaces",
"_____no_output_____"
]
],
[
[
"train_clean = train.copy()\nstop_words = stopwords.words(\"english\")",
"_____no_output_____"
]
],
[
[
"## Function to clean text",
"_____no_output_____"
]
],
[
[
"# Function for cleaning text\ndef clean(s):\n s = s.lower() #Converting to lower case\n s = re.sub(r'[^\\w\\s]', ' ', s) #Removing punctuation\n s = re.sub(r'[\\d+]', ' ', s) #Removing Numbers\n s = s.strip() #Removing trailing spaces\n s = re.sub(' +', ' ', s) #Removing extra whitespaces\n return s",
"_____no_output_____"
],
[
"train_clean[\"Reviews\"] = train_clean[2].apply(lambda x: clean(x))",
"_____no_output_____"
],
[
"train_clean.sample(2)",
"_____no_output_____"
]
],
[
[
"## 1. Removal of STOP WORDS",
"_____no_output_____"
]
],
[
[
"# Removal of Stop Words\ntrain_clean[\"Reviews\"] = train_clean[\"Reviews\"].apply(lambda x: \" \".join(x for x in x.split() if x not in stop_words))",
"_____no_output_____"
],
[
"import pandas as pd\nreviews = pd.Series(train_clean[\"Reviews\"].tolist()).astype(str)\nplt.figure(figsize = (9, 9))\nrev_wcloud_all = WordCloud(width = 900, height = 900, colormap = 'plasma', max_words = 150).generate(''.join(reviews))\nplt.imshow(rev_wcloud_all)\nplt.tight_layout(pad = 0.2)\nplt.axis('off')\nplt.show()",
"_____no_output_____"
]
],
[
[
"# Detailed NLP Analysis",
"_____no_output_____"
]
],
[
[
"tokenizer = RegexpTokenizer(r'\\w+')\ntrain_clean[\"review_token\"] = train_clean[\"Reviews\"].apply(lambda x: tokenizer.tokenize(x))\n# Sentiment analysis\ntrain_clean[\"sentiment_polarity\"] = train_clean[\"Reviews\"].apply(lambda x: TextBlob(x).sentiment.polarity)\ntrain_clean[\"sentiment_subjectivity\"] = train_clean[\"Reviews\"].apply(lambda x: TextBlob(x).sentiment.subjectivity)\n# Pos breakdown\ntrain_clean[\"pos\"] = train_clean[\"Reviews\"].apply(lambda x: TextBlob(x).tags)\ntrain_clean[\"words\"] = train_clean[\"Reviews\"].apply(lambda x: TextBlob(x).words)\ntrain_clean[\"sentences\"] = train_clean[\"Reviews\"].apply(lambda x: TextBlob(x).sentences)\n#returns a list of tuples of n successive words\ntrain_clean[\"ngrams_3\"] = train_clean[\"Reviews\"].apply(lambda x: TextBlob(x).ngrams(n=3))\ntrain_clean[\"ngrams_5\"] = train_clean[\"Reviews\"].apply(lambda x: TextBlob(x).ngrams(n=5))\n#writing the detailed analysis\ntrain_clean.to_csv()",
"_____no_output_____"
]
]
] | [
"markdown",
"code",
"markdown",
"code",
"markdown",
"code",
"markdown",
"code",
"markdown",
"code",
"markdown",
"code",
"markdown",
"code",
"markdown",
"code",
"markdown",
"code",
"markdown",
"code",
"markdown",
"code",
"markdown",
"code",
"markdown",
"code",
"markdown",
"code"
] | [
[
"markdown",
"markdown"
],
[
"code"
],
[
"markdown"
],
[
"code"
],
[
"markdown"
],
[
"code"
],
[
"markdown"
],
[
"code"
],
[
"markdown"
],
[
"code",
"code"
],
[
"markdown"
],
[
"code",
"code",
"code",
"code",
"code"
],
[
"markdown"
],
[
"code",
"code",
"code",
"code",
"code",
"code",
"code"
],
[
"markdown"
],
[
"code",
"code"
],
[
"markdown"
],
[
"code"
],
[
"markdown"
],
[
"code",
"code"
],
[
"markdown"
],
[
"code"
],
[
"markdown"
],
[
"code",
"code",
"code"
],
[
"markdown"
],
[
"code",
"code"
],
[
"markdown"
],
[
"code"
]
] |
e785f33279ac0d3120acdd8e224c7daa3b94d208 | 203,504 | ipynb | Jupyter Notebook | tutorials/Certification_Trainings/Public/databricks_notebooks/2.4/2.Text_Preprocessing_with_SparkNLP_Annotators_Transformers.ipynb | hatrungduc/spark-nlp-workshop | 4a4ec0195d1d3d847261df9ef2df7aa5f95bbaec | [
"Apache-2.0"
] | 687 | 2018-09-07T03:45:39.000Z | 2022-03-20T17:11:20.000Z | tutorials/Certification_Trainings/Public/databricks_notebooks/2.4/2.Text_Preprocessing_with_SparkNLP_Annotators_Transformers.ipynb | hatrungduc/spark-nlp-workshop | 4a4ec0195d1d3d847261df9ef2df7aa5f95bbaec | [
"Apache-2.0"
] | 89 | 2018-09-18T02:04:42.000Z | 2022-02-24T18:22:27.000Z | tutorials/Certification_Trainings/Public/databricks_notebooks/2.4/2.Text_Preprocessing_with_SparkNLP_Annotators_Transformers.ipynb | hatrungduc/spark-nlp-workshop | 4a4ec0195d1d3d847261df9ef2df7aa5f95bbaec | [
"Apache-2.0"
] | 407 | 2018-09-07T03:45:44.000Z | 2022-03-20T05:12:25.000Z | 101,752 | 203,503 | 0.540913 | [
[
[
"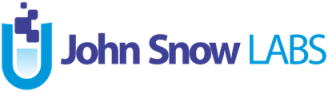",
"_____no_output_____"
],
[
"# 2. Text Preprocessing with Spark NLP",
"_____no_output_____"
],
[
"**Note** Read this article if you want to understand the basic concepts in Spark NLP.\n\nhttps://towardsdatascience.com/introduction-to-spark-nlp-foundations-and-basic-components-part-i-c83b7629ed59",
"_____no_output_____"
],
[
"## 1. Annotators and Transformer Concepts",
"_____no_output_____"
],
[
"In Spark NLP, all Annotators are either Estimators or Transformers as we see in Spark ML. An Estimator in Spark ML is an algorithm which can be fit on a DataFrame to produce a Transformer. E.g., a learning algorithm is an Estimator which trains on a DataFrame and produces a model. A Transformer is an algorithm which can transform one DataFrame into another DataFrame. E.g., an ML model is a Transformer that transforms a DataFrame with features into a DataFrame with predictions.\nIn Spark NLP, there are two types of annotators: AnnotatorApproach and AnnotatorModel\nAnnotatorApproach extends Estimators from Spark ML, which are meant to be trained through fit(), and AnnotatorModel extends Transformers which are meant to transform data frames through transform().\nSome of Spark NLP annotators have a Model suffix and some do not. The model suffix is explicitly stated when the annotator is the result of a training process. Some annotators, such as Tokenizer are transformers but do not contain the suffix Model since they are not trained, annotators. Model annotators have a pre-trained() on its static object, to retrieve the public pre-trained version of a model.\nLong story short, if it trains on a DataFrame and produces a model, it’s an AnnotatorApproach; and if it transforms one DataFrame into another DataFrame through some models, it’s an AnnotatorModel (e.g. WordEmbeddingsModel) and it doesn’t take Model suffix if it doesn’t rely on a pre-trained annotator while transforming a DataFrame (e.g. Tokenizer).\n\nBy convention, there are three possible names:\n\nApproach — Trainable annotator\n\nModel — Trained annotator\n\nnothing — Either a non-trainable annotator with pre-processing step or shorthand for a model\n\nSo for example, Stemmer doesn’t say Approach nor Model, however, it is a Model. On the other hand, Tokenizer doesn’t say Approach nor Model, but it has a TokenizerModel(). Because it is not “training” anything, but it is doing some preprocessing before converting into a Model. When in doubt, please refer to official documentation and API reference. Even though we will do many hands-on practices in the following articles, let us give you a glimpse to let you understand the difference between AnnotatorApproach and AnnotatorModel. As stated above, Tokenizer is an AnnotatorModel. So we need to call fit() and then transform().\n\nNow let’s see how this can be done in Spark NLP using Annotators and Transformers. Assume that we have the following steps that need to be applied one by one on a data frame.\n\nSplit text into sentences\nTokenize\nNormalize\nGet word embeddings\nimage.png\n\nWhat’s actually happening under the hood?\n\nWhen we fit() on the pipeline with Spark data frame (df), its text column is fed into DocumentAssembler() transformer at first and then a new column “document” is created in Document type (AnnotatorType). As we mentioned before, this transformer is basically the initial entry point to Spark NLP for any Spark data frame. Then its document column is fed into SentenceDetector() (AnnotatorApproach) and the text is split into an array of sentences and a new column “sentences” in Document type is created. Then “sentences” column is fed into Tokenizer() (AnnotatorModel) and each sentence is tokenized and a new column “token” in Token type is created. And so on.",
"_____no_output_____"
]
],
[
[
"import sparknlp\n\nspark = sparknlp.start()\n\nprint(\"Spark NLP version\", sparknlp.version())\n\nprint(\"Apache Spark version:\", spark.version)",
"_____no_output_____"
]
],
[
[
"## Create Spark Dataframe",
"_____no_output_____"
]
],
[
[
"text = 'Peter Parker is a nice guy and lives in New York'\n\nspark_df = spark.createDataFrame([[text]]).toDF(\"text\")\n\nspark_df.show(truncate=False)",
"_____no_output_____"
],
[
"# if you want to create a spark datafarme from a list of strings\nfrom pyspark.sql.types import StringType\ntext_list = ['Peter Parker is a nice guy and lives in New York.', 'Bruce Wayne is also a nice guy and lives in Gotham City.']\n\nspark.createDataFrame(text_list, StringType()).toDF(\"text\").show(truncate=80)",
"_____no_output_____"
],
[
"from pyspark.sql import Row\n\nspark.createDataFrame(list(map(lambda x: Row(text=x), text_list))).show(truncate=80)",
"_____no_output_____"
],
[
"!wget https://raw.githubusercontent.com/JohnSnowLabs/spark-nlp-workshop/master/jupyter/annotation/english/spark-nlp-basics/sample-sentences-en.txt",
"_____no_output_____"
],
[
"with open('sample-sentences-en.txt') as f:\n print (f.read())",
"_____no_output_____"
],
[
"spark_df = spark.read.text('sample-sentences-en.txt').toDF('text')\n\nspark_df.show(truncate=False)",
"_____no_output_____"
],
[
"spark_df.select('text').show(truncate=False)",
"_____no_output_____"
],
[
"textFiles = spark.sparkContext.wholeTextFiles(\"./*.txt\",4)\n \nspark_df = textFiles.toDF(schema=['path','text'])\n\nspark_df.show(truncate=30)",
"_____no_output_____"
],
[
"spark_df.select('text').take(1)",
"_____no_output_____"
]
],
[
[
"### Transformers",
"_____no_output_____"
],
[
"what are we going to do if our DataFrame doesn’t have columns in those type? Here comes transformers. In Spark NLP, we have five different transformers that are mainly used for getting the data in or transform the data from one AnnotatorType to another. Here is the list of transformers:\n\n`DocumentAssembler`: To get through the NLP process, we need to get raw data annotated. This is a special transformer that does this for us; it creates the first annotation of type Document which may be used by annotators down the road.\n\n`TokenAssembler`: This transformer reconstructs a Document type annotation from tokens, usually after these have been normalized, lemmatized, normalized, spell checked, etc, to use this document annotation in further annotators.\n\n`Doc2Chunk`: Converts DOCUMENT type annotations into CHUNK type with the contents of a chunkCol.\n\n`Chunk2Doc` : Converts a CHUNK type column back into DOCUMENT. Useful when trying to re-tokenize or do further analysis on a CHUNK result.\n\n`Finisher`: Once we have our NLP pipeline ready to go, we might want to use our annotation results somewhere else where it is easy to use. The Finisher outputs annotation(s) values into a string.",
"_____no_output_____"
],
[
"each annotator accepts certain types of columns and outputs new columns in another type (we call this AnnotatorType).\n\nIn Spark NLP, we have the following types: \n\n`Document`, `token`, `chunk`, `pos`, `word_embeddings`, `date`, `entity`, `sentiment`, `named_entity`, `dependency`, `labeled_dependency`. \n\nThat is, the DataFrame you have needs to have a column from one of these types if that column will be fed into an annotator; otherwise, you’d need to use one of the Spark NLP transformers.",
"_____no_output_____"
],
[
"## 2. Document Assembler",
"_____no_output_____"
],
[
"In Spark NLP, we have five different transformers that are mainly used for getting the data in or transform the data from one AnnotatorType to another.",
"_____no_output_____"
],
[
"That is, the DataFrame you have needs to have a column from one of these types if that column will be fed into an annotator; otherwise, you’d need to use one of the Spark NLP transformers. Here is the list of transformers: DocumentAssembler, TokenAssembler, Doc2Chunk, Chunk2Doc, and the Finisher.\n\nSo, let’s start with DocumentAssembler(), an entry point to Spark NLP annotators.",
"_____no_output_____"
],
[
"To get through the process in Spark NLP, we need to get raw data transformed into Document type at first. \n\nDocumentAssembler() is a special transformer that does this for us; it creates the first annotation of type Document which may be used by annotators down the road.\n\nDocumentAssembler() comes from sparknlp.base class and has the following settable parameters. See the full list here and the source code here.\n\n`setInputCol()` -> the name of the column that will be converted. We can specify only one column here. It can read either a String column or an Array[String]\n\n`setOutputCol()` -> optional : the name of the column in Document type that is generated. We can specify only one column here. Default is ‘document’\n\n`setIdCol()` -> optional: String type column with id information\n\n`setMetadataCol()` -> optional: Map type column with metadata information\n\n`setCleanupMode()` -> optional: Cleaning up options, \n\npossible values:\n```\ndisabled: Source kept as original. This is a default.\ninplace: removes new lines and tabs.\ninplace_full: removes new lines and tabs but also those which were converted to strings (i.e. \\n)\nshrink: removes new lines and tabs, plus merging multiple spaces and blank lines to a single space.\nshrink_full: remove new lines and tabs, including stringified values, plus shrinking spaces and blank lines.\n```",
"_____no_output_____"
]
],
[
[
"from sparknlp.base import *\n\ndocumentAssembler = DocumentAssembler()\\\n.setInputCol(\"text\")\\\n.setOutputCol(\"document\")\\\n.setCleanupMode(\"shrink\")\n\ndoc_df = documentAssembler.transform(spark_df)\n\ndoc_df.show(truncate=30)",
"_____no_output_____"
]
],
[
[
"At first, we define DocumentAssembler with desired parameters and then transform the data frame with it. The most important point to pay attention to here is that you need to use a String or String[Array] type column in .setInputCol(). So it doesn’t have to be named as text. You just use the column name as it is.",
"_____no_output_____"
]
],
[
[
"doc_df.printSchema()",
"_____no_output_____"
],
[
"doc_df.select('document.result','document.begin','document.end').show(truncate=False)",
"_____no_output_____"
],
[
"",
"_____no_output_____"
]
],
[
[
"The new column is in an array of struct type and has the parameters shown above. The annotators and transformers all come with universal metadata that would be filled down the road depending on the annotators being used. Unless you want to append other Spark NLP annotators to DocumentAssembler(), you don’t need to know what all these parameters mean for now. So we will talk about them in the following articles. You can access all these parameters with {column name}.{parameter name}.\n\nLet’s print out the first item’s result.",
"_____no_output_____"
]
],
[
[
"doc_df.select(\"document.result\").take(1)",
"_____no_output_____"
]
],
[
[
"If we would like to flatten the document column, we can do as follows.",
"_____no_output_____"
]
],
[
[
"import pyspark.sql.functions as F\n\ndoc_df.withColumn(\n \"tmp\", \n F.explode(\"document\"))\\\n .select(\"tmp.*\")\\\n .show(truncate=False)",
"_____no_output_____"
]
],
[
[
"## 3. Sentence Detector",
"_____no_output_____"
],
[
"Finds sentence bounds in raw text.",
"_____no_output_____"
],
[
"`setCustomBounds(string)`: Custom sentence separator text\n\n`setUseCustomOnly(bool)`: Use only custom bounds without considering those of Pragmatic Segmenter. Defaults to false. Needs customBounds.\n\n`setUseAbbreviations(bool)`: Whether to consider abbreviation strategies for better accuracy but slower performance. Defaults to true.\n\n`setExplodeSentences(bool)`: Whether to split sentences into different Dataset rows. Useful for higher parallelism in fat rows. Defaults to false.",
"_____no_output_____"
]
],
[
[
"from sparknlp.annotator import *\n\n# we feed the document column coming from Document Assembler\n\nsentenceDetector = SentenceDetector().\\\nsetInputCols(['document']).\\\nsetOutputCol('sentences')\n",
"_____no_output_____"
],
[
"sent_df = sentenceDetector.transform(doc_df)\n\nsent_df.show(truncate=False)",
"_____no_output_____"
],
[
"sent_df.select('sentences').take(3)",
"_____no_output_____"
],
[
"text ='The patient was prescribed 1 capsule of Advil for 5 days . He was seen by the endocrinology service and she was discharged on 40 units of insulin glargine at night , 12 units of insulin lispro with meals , and metformin 1000 mg two times a day . It was determined that all SGLT2 inhibitors should be discontinued indefinitely fro 3 months .'\ntext\n",
"_____no_output_____"
],
[
"spark_df = spark.createDataFrame([[text]]).toDF(\"text\")\n\nspark_df.show(truncate=False)",
"_____no_output_____"
],
[
"spark_df.show(truncate=50)",
"_____no_output_____"
],
[
"doc_df = documentAssembler.transform(spark_df)\n\nsent_df = sentenceDetector.transform(doc_df)\n\nsent_df.show(truncate=True)",
"_____no_output_____"
],
[
"sent_df.select('sentences.result').take(1)",
"_____no_output_____"
],
[
"sentenceDetector.setExplodeSentences(True)\n",
"_____no_output_____"
],
[
"sent_df = sentenceDetector.transform(doc_df)\n\nsent_df.show(truncate=50)",
"_____no_output_____"
],
[
"sent_df.select('sentences.result').show(truncate=False)",
"_____no_output_____"
],
[
"from pyspark.sql import functions as F\n\nsent_df.select(F.explode('sentences.result')).show(truncate=False)",
"_____no_output_____"
]
],
[
[
"## Tokenizer",
"_____no_output_____"
],
[
"Identifies tokens with tokenization open standards. It is an **Annotator Approach, so it requires .fit()**.\n\nA few rules will help customizing it if defaults do not fit user needs.\n\nsetExceptions(StringArray): List of tokens to not alter at all. Allows composite tokens like two worded tokens that the user may not want to split.\n\n`addException(String)`: Add a single exception\n\n`setExceptionsPath(String)`: Path to txt file with list of token exceptions\n\n`caseSensitiveExceptions(bool)`: Whether to follow case sensitiveness for matching exceptions in text\n\n`contextChars(StringArray)`: List of 1 character string to rip off from tokens, such as parenthesis or question marks. Ignored if using prefix, infix or suffix patterns.\n\n`splitChars(StringArray)`: List of 1 character string to split tokens inside, such as hyphens. Ignored if using infix, prefix or suffix patterns.\n\n`splitPattern (String)`: pattern to separate from the inside of tokens. takes priority over splitChars.\nsetTargetPattern: Basic regex rule to identify a candidate for tokenization. Defaults to \\\\S+ which means anything not a space\n\n`setSuffixPattern`: Regex to identify subtokens that are in the end of the token. Regex has to end with \\\\z and must contain groups (). Each group will become a separate token within the prefix. Defaults to non-letter characters. e.g. quotes or parenthesis\n\n`setPrefixPattern`: Regex to identify subtokens that come in the beginning of the token. Regex has to start with \\\\A and must contain groups (). Each group will become a separate token within the prefix. Defaults to non-letter characters. e.g. quotes or parenthesis\n\n`addInfixPattern`: Add an extension pattern regex with groups to the top of the rules (will target first, from more specific to the more general).\n\n`minLength`: Set the minimum allowed legth for each token\n\n`maxLength`: Set the maximum allowed legth for each token",
"_____no_output_____"
]
],
[
[
"tokenizer = Tokenizer() \\\n .setInputCols([\"document\"]) \\\n .setOutputCol(\"token\")",
"_____no_output_____"
],
[
"text = 'Peter Parker (Spiderman) is a nice guy and lives in New York but has no e-mail!'\n\nspark_df = spark.createDataFrame([[text]]).toDF(\"text\")\n",
"_____no_output_____"
],
[
"doc_df = documentAssembler.transform(spark_df)\n\ntoken_df = tokenizer.fit(doc_df).transform(doc_df)\n\ntoken_df.show(truncate=100)",
"_____no_output_____"
],
[
"token_df.select('token.result').take(1)",
"_____no_output_____"
],
[
"tokenizer = Tokenizer() \\\n .setInputCols([\"document\"]) \\\n .setOutputCol(\"token\") \\\n .setSplitChars(['-']) \\\n .setContextChars(['(', ')', '?', '!']) \\\n .addException(\"New York\") \\\n",
"_____no_output_____"
],
[
"token_df = tokenizer.fit(doc_df).transform(doc_df)\n\ntoken_df.select('token.result').take(1)",
"_____no_output_____"
]
],
[
[
"## Stacking Spark NLP Annotators in Spark ML Pipeline",
"_____no_output_____"
],
[
"Spark NLP provides an easy API to integrate with Spark ML Pipelines and all the Spark NLP annotators and transformers can be used within Spark ML Pipelines. So, it’s better to explain Pipeline concept through Spark ML official documentation.\n\nWhat is a Pipeline anyway? In machine learning, it is common to run a sequence of algorithms to process and learn from data. \n\nApache Spark ML represents such a workflow as a Pipeline, which consists of a sequence of PipelineStages (Transformers and Estimators) to be run in a specific order.\n\nIn simple terms, a pipeline chains multiple Transformers and Estimators together to specify an ML workflow. We use Pipeline to chain multiple Transformers and Estimators together to specify our machine learning workflow.\n\nThe figure below is for the training time usage of a Pipeline.",
"_____no_output_____"
],
[
"<img src=\"https://raw.githubusercontent.com/JohnSnowLabs/spark-nlp-workshop/master/tutorials/Certification_Trainings/Public/databricks_notebooks/images/pipeline.png\" style=\"float: left;\">",
"_____no_output_____"
],
[
"A Pipeline is specified as a sequence of stages, and each stage is either a Transformer or an Estimator. These stages are run in order, and the input DataFrame is transformed as it passes through each stage. That is, the data are passed through the fitted pipeline in order. Each stage’s transform() method updates the dataset and passes it to the next stage. With the help of Pipelines, we can ensure that training and test data go through identical feature processing steps.\n\nNow let’s see how this can be done in Spark NLP using Annotators and Transformers. Assume that we have the following steps that need to be applied one by one on a data frame.\n\n- Split text into sentences\n- Tokenize\n\nAnd here is how we code this pipeline up in Spark NLP.",
"_____no_output_____"
]
],
[
[
"from pyspark.ml import Pipeline\n\ndocumentAssembler = DocumentAssembler()\\\n.setInputCol(\"text\")\\\n.setOutputCol(\"document\")\n\nsentenceDetector = SentenceDetector().\\\nsetInputCols(['document']).\\\nsetOutputCol('sentences')\n\ntokenizer = Tokenizer() \\\n .setInputCols([\"sentences\"]) \\\n .setOutputCol(\"token\")\n\nnlpPipeline = Pipeline(stages=[\n documentAssembler, \n sentenceDetector,\n tokenizer\n ])\n\nempty_df = spark.createDataFrame([['']]).toDF(\"text\")\n\npipelineModel = nlpPipeline.fit(empty_df)",
"_____no_output_____"
],
[
"spark_df = spark.read.text('./sample-sentences-en.txt').toDF('text')\n\nspark_df.show(truncate=False)",
"_____no_output_____"
],
[
"result = pipelineModel.transform(spark_df)",
"_____no_output_____"
],
[
"result.show(truncate=20)",
"_____no_output_____"
],
[
"result.printSchema()",
"_____no_output_____"
],
[
"result.select('sentences.result').take(3)",
"_____no_output_____"
],
[
"result.select('token').take(3)[2]",
"_____no_output_____"
]
],
[
[
"## Normalizer",
"_____no_output_____"
],
[
"Removes all dirty characters from text following a regex pattern and transforms words based on a provided dictionary\n\n`setCleanupPatterns(patterns)`: Regular expressions list for normalization, defaults [^A-Za-z]\n\n`setLowercase(value)`: lowercase tokens, default false\n\n`setSlangDictionary(path)`: txt file with delimited words to be transformed into something else",
"_____no_output_____"
]
],
[
[
"import string\nstring.punctuation",
"_____no_output_____"
],
[
"from sparknlp.annotator import *\nfrom sparknlp.base import *\n\ndocumentAssembler = DocumentAssembler()\\\n.setInputCol(\"text\")\\\n.setOutputCol(\"document\")\n\ntokenizer = Tokenizer() \\\n .setInputCols([\"document\"]) \\\n .setOutputCol(\"token\")\n\nnormalizer = Normalizer() \\\n .setInputCols([\"token\"]) \\\n .setOutputCol(\"normalized\")\\\n .setLowercase(True)\\\n .setCleanupPatterns([\"[^\\w\\d\\s]\"]) # remove punctuations (keep alphanumeric chars)\n # if we don't set CleanupPatterns, it will only keep alphabet letters ([^A-Za-z])\n",
"_____no_output_____"
],
[
"\nnlpPipeline = Pipeline(stages=[\n documentAssembler, \n tokenizer,\n normalizer\n ])\n\nempty_df = spark.createDataFrame([['']]).toDF(\"text\")\n\npipelineModel = nlpPipeline.fit(empty_df)",
"_____no_output_____"
],
[
"result = pipelineModel.transform(spark_df)",
"_____no_output_____"
],
[
"result.show(truncate=20)",
"_____no_output_____"
],
[
"result.select('normalized.result').take(3)",
"_____no_output_____"
],
[
"result.select('normalized').take(3)",
"_____no_output_____"
]
],
[
[
"## Stopwords Cleaner",
"_____no_output_____"
],
[
"This annotator excludes from a sequence of strings (e.g. the output of a Tokenizer, Normalizer, Lemmatizer, and Stemmer) and drops all the stop words from the input sequences.",
"_____no_output_____"
],
[
"Functions:\n\n`setStopWords`: The words to be filtered out. Array[String]\n\n`setCaseSensitive`: Whether to do a case sensitive comparison over the stop words.",
"_____no_output_____"
]
],
[
[
"stopwords_cleaner = StopWordsCleaner()\\\n .setInputCols(\"token\")\\\n .setOutputCol(\"cleanTokens\")\\\n .setCaseSensitive(False)\\\n #.setStopWords([\"no\", \"without\"]) (e.g. read a list of words from a txt)\n",
"_____no_output_____"
],
[
"stopwords_cleaner.getStopWords()",
"_____no_output_____"
],
[
"documentAssembler = DocumentAssembler()\\\n.setInputCol(\"text\")\\\n.setOutputCol(\"document\")\n\ntokenizer = Tokenizer() \\\n .setInputCols([\"document\"]) \\\n .setOutputCol(\"token\")\n\nnlpPipeline = Pipeline(stages=[\n documentAssembler, \n tokenizer,\n stopwords_cleaner\n ])\n\nempty_df = spark.createDataFrame([['']]).toDF(\"text\")\n\npipelineModel = nlpPipeline.fit(empty_df)",
"_____no_output_____"
],
[
"spark_df = spark.read.text('./sample-sentences-en.txt').toDF('text')\n\nresult = pipelineModel.transform(spark_df)\n\nresult.show()",
"_____no_output_____"
],
[
"result.select('cleanTokens.result').take(1)",
"_____no_output_____"
]
],
[
[
"## Token Assembler",
"_____no_output_____"
]
],
[
[
"documentAssembler = DocumentAssembler()\\\n.setInputCol(\"text\")\\\n.setOutputCol(\"document\")\n\nsentenceDetector = SentenceDetector().\\\n setInputCols(['document']).\\\n setOutputCol('sentences')\n\ntokenizer = Tokenizer() \\\n .setInputCols([\"sentences\"]) \\\n .setOutputCol(\"token\")\n\nnormalizer = Normalizer() \\\n .setInputCols([\"token\"]) \\\n .setOutputCol(\"normalized\")\\\n .setLowercase(False)\\\n\nstopwords_cleaner = StopWordsCleaner()\\\n .setInputCols(\"normalized\")\\\n .setOutputCol(\"cleanTokens\")\\\n .setCaseSensitive(False)\\\n\ntokenassembler = TokenAssembler()\\\n .setInputCols([\"sentences\", \"cleanTokens\"]) \\\n .setOutputCol(\"clean_text\")\n\n\nnlpPipeline = Pipeline(stages=[\n documentAssembler,\n sentenceDetector,\n tokenizer,\n normalizer,\n stopwords_cleaner,\n tokenassembler\n ])\n\n\nempty_df = spark.createDataFrame([['']]).toDF(\"text\")\n\npipelineModel = nlpPipeline.fit(empty_df)\n\nresult = pipelineModel.transform(spark_df)\n\nresult.show()",
"_____no_output_____"
],
[
"result.select('text', 'clean_text.result').take(1)",
"_____no_output_____"
],
[
"# if we use TokenAssembler().setPreservePosition(True), the original borders will be preserved (dropped & unwanted chars will be replaced by spaces)\ntokenassembler.setPreservePosition(True)\nresult2 = pipelineModel.transform(spark_df)\nresult2.select('clean_text.result').take(1)",
"_____no_output_____"
],
[
"result.select('text', F.explode('clean_text.result').alias('clean_text')).show(truncate=False)",
"_____no_output_____"
],
[
"import pyspark.sql.functions as F\n\nresult.withColumn(\n \"tmp\", \n F.explode(\"clean_text\")) \\\n .select(\"tmp.*\").select(\"begin\",\"end\",\"result\",\"metadata.sentence\").show(truncate = False)",
"_____no_output_____"
],
[
"# if we hadn't used Sentence Detector, this would be what we got. (tokenizer gets document instead of sentences column)\n\ntokenizer = Tokenizer() \\\n .setInputCols([\"document\"]) \\\n .setOutputCol(\"token\")\n\ntokenassembler = TokenAssembler()\\\n .setInputCols([\"document\", \"cleanTokens\"]) \\\n .setOutputCol(\"clean_text\")\n\nnlpPipeline = Pipeline(stages=[\n documentAssembler,\n tokenizer,\n normalizer,\n stopwords_cleaner,\n tokenassembler\n ])\n\n\nempty_df = spark.createDataFrame([['']]).toDF(\"text\")\n\npipelineModel = nlpPipeline.fit(empty_df)\n\nresult = pipelineModel.transform(spark_df)\n\nresult.select('text', 'clean_text.result').show(truncate=False)",
"_____no_output_____"
],
[
"\nresult.withColumn(\n \"tmp\", \n F.explode(\"clean_text\")) \\\n .select(\"tmp.*\").select(\"begin\",\"end\",\"result\",\"metadata.sentence\").show(truncate = False)",
"_____no_output_____"
]
],
[
[
"## Stemmer",
"_____no_output_____"
],
[
"Returns hard-stems out of words with the objective of retrieving the meaningful part of the word",
"_____no_output_____"
]
],
[
[
"stemmer = Stemmer() \\\n .setInputCols([\"token\"]) \\\n .setOutputCol(\"stem\")",
"_____no_output_____"
],
[
"documentAssembler = DocumentAssembler()\\\n.setInputCol(\"text\")\\\n.setOutputCol(\"document\")\n\ntokenizer = Tokenizer() \\\n .setInputCols([\"document\"]) \\\n .setOutputCol(\"token\")\n\nnlpPipeline = Pipeline(stages=[\n documentAssembler, \n tokenizer,\n stemmer\n ])\n\nempty_df = spark.createDataFrame([['']]).toDF(\"text\")\n\npipelineModel = nlpPipeline.fit(empty_df)",
"_____no_output_____"
],
[
"result = pipelineModel.transform(spark_df)\n\nresult.show()",
"_____no_output_____"
],
[
"result.select('stem.result').show(truncate=False)",
"_____no_output_____"
],
[
"import pyspark.sql.functions as F\n\nresult_df = result.select(F.explode(F.arrays_zip('token.result', 'stem.result')).alias(\"cols\")) \\\n.select(F.expr(\"cols['0']\").alias(\"token\"),\n F.expr(\"cols['1']\").alias(\"stem\")).toPandas()\n\nresult_df.head(10)",
"_____no_output_____"
]
],
[
[
"## Lemmatizer",
"_____no_output_____"
],
[
"Retrieves lemmas out of words with the objective of returning a base dictionary word",
"_____no_output_____"
]
],
[
[
"!wget https://raw.githubusercontent.com/mahavivo/vocabulary/master/lemmas/AntBNC_lemmas_ver_001.txt -P /FileStore/",
"_____no_output_____"
],
[
"lemmatizer = Lemmatizer() \\\n .setInputCols([\"token\"]) \\\n .setOutputCol(\"lemma\") \\\n .setDictionary(\"/FileStore/AntBNC_lemmas_ver_001.txt\", value_delimiter =\"\\t\", key_delimiter = \"->\")",
"_____no_output_____"
],
[
"documentAssembler = DocumentAssembler()\\\n.setInputCol(\"text\")\\\n.setOutputCol(\"document\")\n\ntokenizer = Tokenizer() \\\n .setInputCols([\"document\"]) \\\n .setOutputCol(\"token\")\n\nstemmer = Stemmer() \\\n .setInputCols([\"token\"]) \\\n .setOutputCol(\"stem\")\n\nnlpPipeline = Pipeline(stages=[\n documentAssembler, \n tokenizer,\n stemmer,\n lemmatizer\n ])\n\nempty_df = spark.createDataFrame([['']]).toDF(\"text\")\n\npipelineModel = nlpPipeline.fit(empty_df)",
"_____no_output_____"
],
[
"result = pipelineModel.transform(spark_df)\n\nresult.show()",
"_____no_output_____"
],
[
"result.select('lemma.result').show(truncate=False)",
"_____no_output_____"
],
[
"result_df = result.select(F.explode(F.arrays_zip('token.result', 'stem.result', 'lemma.result')).alias(\"cols\")) \\\n.select(F.expr(\"cols['0']\").alias(\"token\"),\n F.expr(\"cols['1']\").alias(\"stem\"),\n F.expr(\"cols['2']\").alias(\"lemma\")).toPandas()\n\nresult_df.head(10)",
"_____no_output_____"
]
],
[
[
"## NGram Generator",
"_____no_output_____"
],
[
"NGramGenerator annotator takes as input a sequence of strings (e.g. the output of a `Tokenizer`, `Normalizer`, `Stemmer`, `Lemmatizer`, and `StopWordsCleaner`). \n\nThe parameter n is used to determine the number of terms in each n-gram. The output will consist of a sequence of n-grams where each n-gram is represented by a space-delimited string of n consecutive words with annotatorType `CHUNK` same as the Chunker annotator.\n\nFunctions:\n\n`setN:` number elements per n-gram (>=1)\n\n`setEnableCumulative:` whether to calculate just the actual n-grams or all n-grams from 1 through n\n\n`setDelimiter:` Glue character used to join the tokens",
"_____no_output_____"
]
],
[
[
"ngrams_cum = NGramGenerator() \\\n .setInputCols([\"token\"]) \\\n .setOutputCol(\"ngrams\") \\\n .setN(3) \\\n .setEnableCumulative(True)\\\n .setDelimiter(\"_\") # Default is space\n \n# .setN(3) means, take bigrams and trigrams.\n\nnlpPipeline = Pipeline(stages=[\n documentAssembler, \n tokenizer,\n ngrams_cum\n ])\n\nempty_df = spark.createDataFrame([['']]).toDF(\"text\")\n\npipelineModel = nlpPipeline.fit(empty_df)\n\nresult = pipelineModel.transform(spark_df)\n\nresult.select('ngrams.result').show(truncate=200)",
"_____no_output_____"
],
[
"ngrams_nonCum = NGramGenerator() \\\n .setInputCols([\"token\"]) \\\n .setOutputCol(\"ngrams_v2\") \\\n .setN(3) \\\n .setEnableCumulative(False)\\\n .setDelimiter(\"_\") # Default is space\n \nngrams_nonCum.transform(result).select('ngrams_v2.result').show(truncate=200)",
"_____no_output_____"
]
],
[
[
"## TextMatcher",
"_____no_output_____"
],
[
"Annotator to match entire phrases (by token) provided in a file against a Document\n\nFunctions:\n\nsetEntities(path, format, options): Provides a file with phrases to match. Default: Looks up path in configuration.\n\npath: a path to a file that contains the entities in the specified format.\n\nreadAs: the format of the file, can be one of {ReadAs.LINE_BY_LINE, ReadAs.SPARK_DATASET}. Defaults to LINE_BY_LINE.\n\noptions: a map of additional parameters. Defaults to {“format”: “text”}.\n\nentityValue : Value for the entity metadata field to indicate which chunk comes from which textMatcher when there are multiple textMatchers.\n\nmergeOverlapping : whether to merge overlapping matched chunks. Defaults false\n\ncaseSensitive : whether to match regardless of case. Defaults true",
"_____no_output_____"
]
],
[
[
"# first method for doing this, second option below\nimport urllib.request\nwith urllib.request.urlopen('https://s3.amazonaws.com/auxdata.johnsnowlabs.com/public/resources/en/pubmed/pubmed-sample.csv') as f: \n content = f.read().decode('utf-8')\n dbutils.fs.put(\"/dbfs/tmp/pubmed/pubmed-sample.csv\", content)",
"_____no_output_____"
],
[
"%sh\nTMP=/dbfs/tmp/pubmed\nif [ ! -d \"$TMP\" ]; then\n mkdir $TMP\n cd $TMP\n wget https://s3.amazonaws.com/auxdata.johnsnowlabs.com/public/resources/en/pubmed/pubmed-sample.csv\nfi",
"_____no_output_____"
],
[
"import pyspark.sql.functions as F\n\npubMedDF = spark.read\\\n .option(\"header\", \"true\")\\\n .csv(\"/dbfs/tmp/pubmed/pubmed-sample.csv\")\\\n .filter(\"AB IS NOT null\")\\\n .withColumnRenamed(\"AB\", \"text\")\\\n .drop(\"TI\")\n\npubMedDF.show(truncate=50)",
"_____no_output_____"
],
[
"pubMedDF.select('text').take(2)",
"_____no_output_____"
],
[
"%sh\nTMP=/FileStore/entities\nif [ ! -d \"$TMP\" ]; then\n mkdir $TMP\nfi",
"_____no_output_____"
],
[
"# write the target entities to txt file \n\nentities = ['KCNJ9', 'GIRK', 'diabetes mellitus', 'nucleotide polymorphisms']\ncontent = ''\nfor e in entities:\n content = content + \"\\n\" + e\ndbutils.fs.put(\"dbfs:/tmp/pubmed/clinical_entities.txt\", content)\n\n\nentities = ['breast cancer', 'colon cancer', 'lung cancer', 'monotherapy', 'therapy']\ncontent=''\nfor e in entities:\n content = content + \"\\n\" + e\ndbutils.fs.put(\"dbfs:/tmp/pubmed/cancer_entities.txt\", content)",
"_____no_output_____"
],
[
"clinical_entity_extractor = TextMatcher() \\\n .setInputCols([\"document\",'token'])\\\n .setOutputCol(\"clinical_entities\")\\\n .setEntities(\"dbfs:/tmp/pubmed/clinical_entities.txt\")\\\n .setCaseSensitive(False)\\\n .setEntityValue('clinical_entity')\n\ncancer_entity_extractor = TextMatcher() \\\n .setInputCols([\"document\",'token'])\\\n .setOutputCol(\"cancer_entities\")\\\n .setEntities(\"dbfs:/tmp/entities/cancer_entities.txt\")\\\n .setCaseSensitive(False)\\\n .setEntityValue('cancer_entity')\n\n\nnlpPipeline = Pipeline(stages=[\n documentAssembler, \n tokenizer,\n clinical_entity_extractor,\n cancer_entity_extractor\n ])\n\nempty_df = spark.createDataFrame([['']]).toDF(\"text\")\n\npipelineModel = nlpPipeline.fit(empty_df)\n",
"_____no_output_____"
],
[
"result = pipelineModel.transform(pubMedDF.limit(30))",
"_____no_output_____"
],
[
"result.select('clinical_entities.result','cancer_entities.result').take(2)",
"_____no_output_____"
],
[
"result.select('clinical_entities','cancer_entities').take(2)",
"_____no_output_____"
],
[
"result_df = result.select(F.explode(F.arrays_zip('clinical_entities.result', 'clinical_entities.begin', 'clinical_entities.end')).alias(\"cols\")) \\\n.select(F.expr(\"cols['0']\").alias(\"clinical_entities\"),\n F.expr(\"cols['1']\").alias(\"begin\"),\n F.expr(\"cols['2']\").alias(\"end\")).toPandas()\nresult_df.head(10)",
"_____no_output_____"
],
[
"result_df = result.select(F.explode(F.arrays_zip('cancer_entities.result', 'cancer_entities.begin', 'cancer_entities.end')).alias(\"cols\")) \\\n.select(F.expr(\"cols['0']\").alias(\"cancer_entities\"),\n F.expr(\"cols['1']\").alias(\"begin\"),\n F.expr(\"cols['2']\").alias(\"end\")).toPandas()\n\nresult_df.head(10)",
"_____no_output_____"
]
],
[
[
"## RegexMatcher",
"_____no_output_____"
]
],
[
[
"rules = '''\nrenal\\s\\w+, started with 'renal'\ncardiac\\s\\w+, started with 'cardiac'\n\\w*ly\\b, ending with 'ly'\n\\S*\\d+\\S*, match any word that contains numbers\n(\\d+).?(\\d*)\\s*(mg|ml|g), match medication metrics\n'''\n\ndbutils.fs.put(\"dbfs:/tmp/pubmed/regex_rules.txt\", rules)",
"_____no_output_____"
],
[
"import os\n\ndocumentAssembler = DocumentAssembler()\\\n.setInputCol(\"text\")\\\n.setOutputCol(\"document\")\n\nregex_matcher = RegexMatcher()\\\n .setInputCols('document')\\\n .setStrategy(\"MATCH_ALL\")\\\n .setOutputCol(\"regex_matches\")\\\n .setExternalRules(path='dbfs:/tmp/pubmed/regex_rules.txt', delimiter=',')\n \n\nnlpPipeline = Pipeline(stages=[\n documentAssembler, \n regex_matcher\n ])\n\nempty_df = spark.createDataFrame([['']]).toDF(\"text\")\n\npipelineModel = nlpPipeline.fit(empty_df)\n\nmatch_df = pipelineModel.transform(pubMedDF)\n\nmatch_df.select('regex_matches.result').take(3)",
"_____no_output_____"
],
[
"match_df.select('text','regex_matches.result')\\\n.toDF('text','matches').filter(F.size('matches')>1)\\\n.show(truncate=50)\n",
"_____no_output_____"
]
],
[
[
"## Text Cleaning with UDF",
"_____no_output_____"
]
],
[
[
"text = '<h1 style=\"color: #5e9ca0;\">Have a great <span style=\"color: #2b2301;\">birth</span> day!</h1>'\n\ntext_df = spark.createDataFrame([[text]]).toDF(\"text\")\n\nimport re\nfrom pyspark.sql.functions import udf\nfrom pyspark.sql.types import StringType, IntegerType\n\nclean_text = lambda s: re.sub(r'<[^>]*>', '', s)\n\ntext_df.withColumn('cleaned', udf(clean_text, StringType())('text')).select('text','cleaned').show(truncate= False)\n\n",
"_____no_output_____"
],
[
"find_not_alnum_count = lambda s: len([i for i in s if not i.isalnum() and i!=' '])\n\nfind_not_alnum_count(\"it's your birth day!\")",
"_____no_output_____"
],
[
"find_not_alnum_count = lambda s: len([i for i in s if not i.isalnum() and i!=' '])\n\nfind_not_alnum_count(\"it's your birth day!\")",
"_____no_output_____"
],
[
"text = '<h1 style=\"color: #5e9ca0;\">Have a great <span style=\"color: #2b2301;\">birth</span> day!</h1>'\n\nfind_not_alnum_count(text)",
"_____no_output_____"
],
[
"text_df.withColumn('cleaned', udf(find_not_alnum_count, IntegerType())('text')).select('text','cleaned').show(truncate= False)",
"_____no_output_____"
]
],
[
[
"## Finisher",
"_____no_output_____"
],
[
"***Finisher:*** Once we have our NLP pipeline ready to go, we might want to use our annotation results somewhere else where it is easy to use. The Finisher outputs annotation(s) values into a string.\n\nIf we just want the desired output column in the final dataframe, we can use Finisher to drop previous stages in the final output and gte the `result` from the process.\n\nThis is very handy when you want to use the output from Spark NLP annotator as an input to another Spark ML transformer.\n\nSettable parameters are:\n\n`setInputCols()`\n\n`setOutputCols()`\n\n`setCleanAnnotations(True)` -> Whether to remove intermediate annotations\n\n`setValueSplitSymbol(“#”)` -> split values within an annotation character\n\n`setAnnotationSplitSymbol(“@”)` -> split values between annotations character\n\n`setIncludeMetadata(False)` -> Whether to include metadata keys. Sometimes useful in some annotations.\n\n`setOutputAsArray(False)` -> Whether to output as Array. Useful as input for other Spark transformers.",
"_____no_output_____"
]
],
[
[
"finisher = Finisher() \\\n .setInputCols([\"regex_matches\"]) \\\n .setIncludeMetadata(False) # set to False to remove metadata\n\nnlpPipeline = Pipeline(stages=[\n documentAssembler, \n regex_matcher,\n finisher\n ])\n\nempty_df = spark.createDataFrame([['']]).toDF(\"text\")\n\npipelineModel = nlpPipeline.fit(empty_df)\n\nmatch_df = pipelineModel.transform(pubMedDF)\n\nmatch_df.show(truncate = 50)",
"_____no_output_____"
],
[
"match_df.printSchema()",
"_____no_output_____"
],
[
"match_df.filter(F.size('finished_regex_matches')>1).show(truncate = 50)",
"_____no_output_____"
]
],
[
[
"## LightPipeline",
"_____no_output_____"
],
[
"LightPipelines are Spark NLP specific Pipelines, equivalent to Spark ML Pipeline, but meant to deal with smaller amounts of data. They’re useful working with small datasets, debugging results, or when running either training or prediction from an API that serves one-off requests.\n\nSpark NLP LightPipelines are Spark ML pipelines converted into a single machine but the multi-threaded task, becoming more than 10x times faster for smaller amounts of data (small is relative, but 50k sentences are roughly a good maximum). To use them, we simply plug in a trained (fitted) pipeline and then annotate a plain text. We don't even need to convert the input text to DataFrame in order to feed it into a pipeline that's accepting DataFrame as an input in the first place. This feature would be quite useful when it comes to getting a prediction for a few lines of text from a trained ML model.\n\n **It is nearly 20x faster than using Spark ML Pipeline**\n\n`LightPipeline(someTrainedPipeline).annotate(someStringOrArray)`",
"_____no_output_____"
]
],
[
[
"documentAssembler = DocumentAssembler()\\\n.setInputCol(\"text\")\\\n.setOutputCol(\"document\")\n\ntokenizer = Tokenizer() \\\n .setInputCols([\"document\"]) \\\n .setOutputCol(\"token\")\n\nstemmer = Stemmer() \\\n .setInputCols([\"token\"]) \\\n .setOutputCol(\"stem\")\n\nnlpPipeline = Pipeline(stages=[\n documentAssembler, \n tokenizer,\n stemmer,\n lemmatizer\n ])\n\nempty_df = spark.createDataFrame([['']]).toDF(\"text\")\n\npipelineModel = nlpPipeline.fit(empty_df)\n\npipelineModel.transform(spark_df).show()\n",
"_____no_output_____"
],
[
"from sparknlp.base import LightPipeline\n\nlight_model = LightPipeline(pipelineModel)\n\nlight_result = light_model.annotate(\"John and Peter are brothers. However they don't support each other that much.\")",
"_____no_output_____"
],
[
"light_result.keys()",
"_____no_output_____"
],
[
"list(zip(light_result['token'], light_result['stem'], light_result['lemma']))",
"_____no_output_____"
],
[
"light_result = light_model.fullAnnotate(\"John and Peter are brothers. However they don't support each other that much.\")",
"_____no_output_____"
],
[
"light_result",
"_____no_output_____"
],
[
"text_list= [\"How did serfdom develop in and then leave Russia ?\",\n\"There will be some exciting breakthroughs in NLP this year.\"]\n\nlight_model.annotate(text_list)",
"_____no_output_____"
],
[
"## important note: When you use Finisher in your pipeline, regardless of setting cleanAnnotations to False or True, LigtPipeline will only return the finished columns.",
"_____no_output_____"
]
]
] | [
"markdown",
"code",
"markdown",
"code",
"markdown",
"code",
"markdown",
"code",
"markdown",
"code",
"markdown",
"code",
"markdown",
"code",
"markdown",
"code",
"markdown",
"code",
"markdown",
"code",
"markdown",
"code",
"markdown",
"code",
"markdown",
"code",
"markdown",
"code",
"markdown",
"code",
"markdown",
"code",
"markdown",
"code",
"markdown",
"code",
"markdown",
"code",
"markdown",
"code"
] | [
[
"markdown",
"markdown",
"markdown",
"markdown",
"markdown"
],
[
"code"
],
[
"markdown"
],
[
"code",
"code",
"code",
"code",
"code",
"code",
"code",
"code",
"code"
],
[
"markdown",
"markdown",
"markdown",
"markdown",
"markdown",
"markdown",
"markdown"
],
[
"code"
],
[
"markdown"
],
[
"code",
"code",
"code"
],
[
"markdown"
],
[
"code"
],
[
"markdown"
],
[
"code"
],
[
"markdown",
"markdown",
"markdown"
],
[
"code",
"code",
"code",
"code",
"code",
"code",
"code",
"code",
"code",
"code",
"code",
"code"
],
[
"markdown",
"markdown"
],
[
"code",
"code",
"code",
"code",
"code",
"code"
],
[
"markdown",
"markdown",
"markdown",
"markdown"
],
[
"code",
"code",
"code",
"code",
"code",
"code",
"code"
],
[
"markdown",
"markdown"
],
[
"code",
"code",
"code",
"code",
"code",
"code",
"code"
],
[
"markdown",
"markdown",
"markdown"
],
[
"code",
"code",
"code",
"code",
"code"
],
[
"markdown"
],
[
"code",
"code",
"code",
"code",
"code",
"code",
"code"
],
[
"markdown",
"markdown"
],
[
"code",
"code",
"code",
"code",
"code"
],
[
"markdown",
"markdown"
],
[
"code",
"code",
"code",
"code",
"code",
"code"
],
[
"markdown",
"markdown"
],
[
"code",
"code"
],
[
"markdown",
"markdown"
],
[
"code",
"code",
"code",
"code",
"code",
"code",
"code",
"code",
"code",
"code",
"code",
"code"
],
[
"markdown"
],
[
"code",
"code",
"code"
],
[
"markdown"
],
[
"code",
"code",
"code",
"code",
"code"
],
[
"markdown",
"markdown"
],
[
"code",
"code",
"code"
],
[
"markdown",
"markdown"
],
[
"code",
"code",
"code",
"code",
"code",
"code",
"code",
"code"
]
] |
e785f351e8d8e8810b4ce53b730d7d303aa4ddb4 | 3,417 | ipynb | Jupyter Notebook | Javascript-integration.ipynb | Grant-Steinfeld/astronomy-notebooks | a10183740c5b3e3dc91122b62711991226236dae | [
"MIT"
] | 72 | 2015-04-20T01:00:31.000Z | 2022-03-20T19:19:56.000Z | Javascript-integration.ipynb | Grant-Steinfeld/astronomy-notebooks | a10183740c5b3e3dc91122b62711991226236dae | [
"MIT"
] | 14 | 2015-04-15T20:15:43.000Z | 2019-08-15T18:24:28.000Z | Javascript-integration.ipynb | Grant-Steinfeld/astronomy-notebooks | a10183740c5b3e3dc91122b62711991226236dae | [
"MIT"
] | 41 | 2015-04-15T20:02:50.000Z | 2022-02-01T02:25:46.000Z | 27.336 | 162 | 0.595552 | [
[
[
"# How to deliver JavaScript to the IPython Notebook Viewer\n\nAt first glance there appear to be at least four mechanisms\nfor adding JavaScript code to an IPython notebook:\n \n* A notebook cell marked `%%javascript`\n* A Markdown cell with a `<script>` inside\n* An `HTML()` display with a `<script>` inside\n* A `JavaScript()` display with code inside\n\nHere are examples of all four possibilities:",
"_____no_output_____"
]
],
[
[
"%%javascript\n\nconsole.log('Log message from the %%javascript cell')",
"_____no_output_____"
]
],
[
[
"*(Markdown cell with a `<script>` inside.)*\n<script>console.log('Log message from the Markdown cell')</script>",
"_____no_output_____"
]
],
[
[
"from IPython.display import HTML\nHTML('<script>console.log(\"Log message from an HTML display\")</script>')",
"_____no_output_____"
],
[
"from IPython.display import Javascript\nJavascript('console.log(\"Log message from a Javascript display\")')",
"_____no_output_____"
]
],
[
[
"By checking your JavaScript console\nwhile viewing this notebook,\nyou can determine which of these four mechanisms fires\nwhen the static notebook is displayed later.\nIn particular,\nwhen [this notebook is viewed\nat the official IPython Notebook Viewer](http://nbviewer.ipython.org/github/brandon-rhodes/astronomy-notebooks/blob/master/Javascript-integration.ipynb),\nyou will find that **only two** of the four mechanisms work:\n\n* The Markdown cell with a `<script>` inside **works**\n* The `HTML()` display with a `<script>` inside **works**\n\nWhy do these two mechanisms survive the transition to a static notebook?\nBecause the IPython Notebook Viewer passes HTML through unharmed\nto the static display of the notebook,\nbut it is *not* willing to invoke the JavaScript expressions\nthat are produced by both ``%%javascript`` cells\nand ``Javascript()`` display objects.\n\nSo embedding JavaScript inside of HTML is the safe choice\nif you want notebooks that can both be opened live\nbut that can also be viewed later in a viewer.",
"_____no_output_____"
]
]
] | [
"markdown",
"code",
"markdown",
"code",
"markdown"
] | [
[
"markdown"
],
[
"code"
],
[
"markdown"
],
[
"code",
"code"
],
[
"markdown"
]
] |
e785fd0c7d64651a40092d71a9e33f214ed99a91 | 790,704 | ipynb | Jupyter Notebook | toolbox/python/nltk.ipynb | martinapugliese/tales-science-data-notebooks | 60ea220e79aa15b5125a351882289bf89f39d54a | [
"MIT"
] | null | null | null | toolbox/python/nltk.ipynb | martinapugliese/tales-science-data-notebooks | 60ea220e79aa15b5125a351882289bf89f39d54a | [
"MIT"
] | null | null | null | toolbox/python/nltk.ipynb | martinapugliese/tales-science-data-notebooks | 60ea220e79aa15b5125a351882289bf89f39d54a | [
"MIT"
] | null | null | null | 705.355932 | 142,960 | 0.944995 | [
[
[
"%run ../../common_functions/import_all.py\n\nfrom scipy import optimize\nfrom scipy.integrate import quad, odeint\nfrom scipy.interpolate import interp1d\nfrom scipy.signal import detrend\nfrom scipy.spatial import distance\nfrom matplotlib.legend_handler import HandlerLine2D\n\nfrom nltk.book import * # will print a list of books (texts) imported from here\nfrom nltk.text import Text\nfrom nltk.probability import FreqDist, ConditionalFreqDist\nfrom nltk.tokenize import WordPunctTokenizer \nfrom nltk.corpus import brown, inaugural\nfrom nltk import (word_tokenize, wordpunct_tokenize, sent_tokenize, pos_tag, bigrams, \n UnigramTagger, NgramTagger, PunktSentenceTokenizer, TreebankWordTokenizer)\nfrom nltk.corpus import treebank, wordnet\nfrom nltk.tag import pos_tag, UnigramTagger\nfrom nltk.tag.sequential import NgramTagger\nfrom nltk.corpus import brown\nfrom nltk.stem import (PorterStemmer,\n LancasterStemmer,\n SnowballStemmer, \n WordNetLemmatizer)\n\nfrom common_functions.nltk_helpers import measure_lexical_diversity, compute_perc_word_usage, plot_freqdist_freq\n\n%matplotlib inline\n\nfrom common_functions.setup_notebook import set_css_style, setup_matplotlib, config_ipython\nconfig_ipython()\nsetup_matplotlib()\nset_css_style()",
"_____no_output_____"
]
],
[
[
"# Playing around with NLTK\n\nSome material has been taken/adapted from the [NLTK book](http://www.nltk.org/book/)\n\n* Exploring NLTK books (Text instance)\n* Exploring NLTK corpora\n* Exploring NLTK Treebank\n* Exploring the WordNet corpus\n\nFor the linguistics concepts used here, refer to [the specific notebook](../nlp/concepts/linguistic-notions.ipynb).",
"_____no_output_____"
],
[
"## Books and corpora",
"_____no_output_____"
]
],
[
[
"## List of all the books and sents imported\ntexts()\nsents()\n\n# Choose the book to play with and some wordsText\nbook = text2\nword = 'love'\nword2 = 'him'\nwords = ['love', 'kiss', 'marriage', 'sense', 'children', 'house', 'hate']\n\n# Print first 100 token in book (book is an instance of nltk.text.Text, which behaves like a list of tokens)\n# Note that punctuation is included as tokens\nprint(book[0:100], type(book))\nprint(list(book)[0:100] == book[0:100])",
"text1: Moby Dick by Herman Melville 1851\ntext2: Sense and Sensibility by Jane Austen 1811\ntext3: The Book of Genesis\ntext4: Inaugural Address Corpus\ntext5: Chat Corpus\ntext6: Monty Python and the Holy Grail\ntext7: Wall Street Journal\ntext8: Personals Corpus\ntext9: The Man Who Was Thursday by G . K . Chesterton 1908\nsent1: Call me Ishmael .\nsent2: The family of Dashwood had long been settled in Sussex .\nsent3: In the beginning God created the heaven and the earth .\nsent4: Fellow - Citizens of the Senate and of the House of Representatives :\nsent5: I have a problem with people PMing me to lol JOIN\nsent6: SCENE 1 : [ wind ] [ clop clop clop ] KING ARTHUR : Whoa there !\nsent7: Pierre Vinken , 61 years old , will join the board as a nonexecutive director Nov. 29 .\nsent8: 25 SEXY MALE , seeks attrac older single lady , for discreet encounters .\nsent9: THE suburb of Saffron Park lay on the sunset side of London , as red and ragged as a cloud of sunset .\n['[', 'Sense', 'and', 'Sensibility', 'by', 'Jane', 'Austen', '1811', ']', 'CHAPTER', '1', 'The', 'family', 'of', 'Dashwood', 'had', 'long', 'been', 'settled', 'in', 'Sussex', '.', 'Their', 'estate', 'was', 'large', ',', 'and', 'their', 'residence', 'was', 'at', 'Norland', 'Park', ',', 'in', 'the', 'centre', 'of', 'their', 'property', ',', 'where', ',', 'for', 'many', 'generations', ',', 'they', 'had', 'lived', 'in', 'so', 'respectable', 'a', 'manner', 'as', 'to', 'engage', 'the', 'general', 'good', 'opinion', 'of', 'their', 'surrounding', 'acquaintance', '.', 'The', 'late', 'owner', 'of', 'this', 'estate', 'was', 'a', 'single', 'man', ',', 'who', 'lived', 'to', 'a', 'very', 'advanced', 'age', ',', 'and', 'who', 'for', 'many', 'years', 'of', 'his', 'life', ',', 'had', 'a', 'constant', 'companion'] <class 'nltk.text.Text'>\nTrue\n"
],
[
"## Counts and lexical diversity\nprint('Num of tokens', len(book))\nprint('Num of counts for given word', book.count(word))\nprint('Lexical diversity', measure_lexical_diversity(book))\nprint('Fraction of use of word in book', compute_perc_word_usage(word, book))",
"Num of tokens 141576\nNum of counts for given word 77\nLexical diversity 0.04826383002768831\nFraction of use of word in book 0.0005438774933604566\n"
],
[
"## Concordance and context\n\n# Choose a book and a word\nbook = text2\nword = 'love'\n\n# Concordance of chosen word in chosen book\nprint('Concordance: ')\nbook.concordance(word)\n\n# Words appearing in same contexts as chosen word in chosen book\nprint('Words in similar context as chosen word:')\n# given word w, this finds all contexts w_1 w w_2 and finds all words w' which appear in same context, \n#i.e., w_1 w' w-2\nbook.similar(word) \n\n# Choose two words and show the common contexts\nprint('Common contexts of two chosen words:')\nbook.common_contexts([word, word2])",
"Concordance: \nDisplaying 25 of 77 matches:\npriety of going , and her own tender love for all her three children determine\nes .\" \" I believe you are right , my love ; it will be better that there shoul\n . It implies everything amiable . I love him already .\" \" I think you will li\nsentiment of approbation inferior to love .\" \" You may esteem him .\" \" I have \nn what it was to separate esteem and love .\" Mrs . Dashwood now took pains to \noner did she perceive any symptom of love in his behaviour to Elinor , than sh\n how shall we do without her ?\" \" My love , it will be scarcely a separation .\nise . Edward is very amiable , and I love him tenderly . But yet -- he is not \nll never see a man whom I can really love . I require so much ! He must have a\nry possible charm .\" \" Remember , my love , that you are not seventeen . It is\nf I do not now . When you tell me to love him as a brother , I shall no more s\nhat Colonel Brandon was very much in love with Marianne Dashwood . She rather \ne were ever animated enough to be in love , must have long outlived every sens\nhirty - five anything near enough to love , to make him a desirable companion \nroach would have been spared .\" \" My love ,\" said her mother , \" you must not \npect that the misery of disappointed love had already been known to him . This\n most melancholy order of disastrous love . CHAPTER 12 As Elinor and Marianne \nhen she considered what Marianne ' s love for him was , a quarrel seemed almos\nctory way ;-- but you , Elinor , who love to doubt where you can -- it will no\n man whom we have all such reason to love , and no reason in the world to thin\nded as he must be of your sister ' s love , should leave her , and leave her p\ncannot think that . He must and does love her I am sure .\" \" But with a strang\n I believe not ,\" cried Elinor . \" I love Willoughby , sincerely love him ; an\nor . \" I love Willoughby , sincerely love him ; and suspicion of his integrity\ndeed a man could not very well be in love with either of her daughters , witho\nWords in similar context as chosen word:\naffection sister heart mother time see town life it dear elinor\nmarianne me word family her him do regard head\nCommon contexts of two chosen words:\nto_you of_in to_and in_by to_but\n"
],
[
"## Collocations\nprint('Collocations:')\nbook.collocations()",
"Collocations:\n"
],
[
"# Dispersion plot of text given some words (how far from the start word appears in text)\nplt.grid()\nbook.dispersion_plot(words)",
"_____no_output_____"
],
[
"## FreqDist for token counts \n\nfdist = FreqDist(book) # FreqDist needs a tokens list, gives dict {token: counts}\n\nword = 'love'\nprint('Num tokens for word %s: %f' %(word, fdist[word]))\nprint('Num tokens: ', fdist.N())\nprint('Num unique tokens', fdist.B())\nprint('Token with the highest count is %s with count %d' %(fdist.max(), fdist[fdist.max()]))\nprint('Hapaxes are (10 of them)', fdist.hapaxes()[:10])\n\n# Plot the 50 most frequent tokens and their token counts, normal and cumulative\nfdist.plot(50, title='Book token counts')\nfdist.plot(50, cumulative=True, title='Book token counts, cumulative')\n\n# Same distrib, normal but with frequency instead of counts\nplot_freqdist_freq(fdist, max_num=50, title='Book token frequencies')",
"_____no_output_____"
],
[
"## FreqDist for word lenghts\n\nfdist_wl = FreqDist([len(word) for word in book])\n\n# Plot and show as table\nfdist_wl.plot()\nfdist_wl.tabulate()",
"_____no_output_____"
],
[
"# Conditional freq distrib on Brown corpus genres\n\n# ConditionalFreqDist is a collection of freq dist, one per condition\n# requires tuples (condition, event)\n\n# print genres in corpus\nprint('All genres in Brown corpus: ', sorted(brown.categories()))\n\n# choosing some of the categories (genres) and get the words in each\ntuples = [(genre, word) for genre in ['romance', 'science_fiction'] for word in brown.words(categories=genre)]\n\n# Building the cfdist\ncfdist = ConditionalFreqDist(tuples)\n\n# Each cfdist[condition] will be a FreqDist\ntype(cfdist['romance'])\n\n# Tabulate selecting the conditions and the specific samples (no selection will give all)\ncfdist.tabulate(conditions=['romance'], samples=['the', 'love', 'hate'])\n\n# Plotting any of the dists on the condition\ncfdist['romance'].plot(50, title='Counts tokens in genre romance')\ncfdist['science_fiction'].plot(50, title='Counts tokens in genre science_fiction')",
"All genres in Brown corpus: ['adventure', 'belles_lettres', 'editorial', 'fiction', 'government', 'hobbies', 'humor', 'learned', 'lore', 'mystery', 'news', 'religion', 'reviews', 'romance', 'science_fiction']\n"
]
],
[
[
"## Treebank\n\n* Parsed sentences",
"_____no_output_____"
]
],
[
[
"# The Treebank corpus in NLTK contains 10% of the original Penn Treebank corpus\n\ntreebank.words()\n\ntreebank.parsed_sents()",
"_____no_output_____"
]
],
[
[
"## WordNet\n\n* Hypernyms and Hyponyms ",
"_____no_output_____"
]
],
[
[
"wn = wordnet\n\nsss = wn.synsets('dog')\n\ns1 = sss[0]\nprint(s1, s1.definition())\n\nprint(s1.hypernyms(), s1.hyponyms())",
"Synset('dog.n.01') a member of the genus Canis (probably descended from the common wolf) that has been domesticated by man since prehistoric times; occurs in many breeds\n[Synset('canine.n.02'), Synset('domestic_animal.n.01')] [Synset('basenji.n.01'), Synset('corgi.n.01'), Synset('cur.n.01'), Synset('dalmatian.n.02'), Synset('great_pyrenees.n.01'), Synset('griffon.n.02'), Synset('hunting_dog.n.01'), Synset('lapdog.n.01'), Synset('leonberg.n.01'), Synset('mexican_hairless.n.01'), Synset('newfoundland.n.01'), Synset('pooch.n.01'), Synset('poodle.n.01'), Synset('pug.n.01'), Synset('puppy.n.01'), Synset('spitz.n.01'), Synset('toy_dog.n.01'), Synset('working_dog.n.01')]\n"
]
],
[
[
"## Text manipulation\n\n* Tokenizing\n* POS tagging\n* Stemming/lemmatizing",
"_____no_output_____"
]
],
[
[
"# tagged sentences from Brown corpus\nbrown_tagged_sents = brown.tagged_sents(categories='news')\n\n# Separate tagged sents into train and test\ntrain_sents = brown_tagged_sents[:int(len(brown_tagged_sents) * 0.8)]\ntest_sents = brown_tagged_sents[int(len(brown_tagged_sents) * 0.8):]",
"_____no_output_____"
],
[
"# Tokenising\n# NOTE: obvs the easiest sentence tokenization (naive) is splitting on period with split('.'). \n# this won't understand \"Mr. Smith.\" though \n# similarly for tokenizing a sentence into tokens\n\ntext = \"\"\"What will you do? I go to the cinema this weekend. That's a test. I can't do better!\"\"\"\n\n# Standard methods are wrappers around the recommended tokenizers, so equivalent to \n# so equivalent to tokenizer = TreebankWordTokenizer(); tokenizer.tokenize(sentence)\n\n# Tokenizing text into sentences\n\n# sent_tokenize calls the PunktSentenceTokenizer (recommended)\nprint('* Docs of PunktSentenceTokenizer:')\nprint(PunktSentenceTokenizer.__doc__)\nst = sent_tokenize(text)\nprint('* Text tokenized', st)\n\n# To train tokenizer on a bespoke text:\n# import nltk.tokenize.punkt\n# tokenizer = PunktSentenceTokenizer()\n# text = open(\"someplain.txt\",\"r\").read()\n# tokenizer.train(text)\n\n# Tokenizing a sentence into tokens\n\n# word_tokenise calls the TreebankWordTokenizer (recommended)\nprint('* Docs of TreebankWordTokenizer:')\nprint(TreebankWordTokenizer.__doc__)\ntokens = word_tokenize(st[2])\nprint('* Sentence tokenized', tokens)\n# wordpunct_tokenise calls WordPunctTokenizer, it will separate all punctuation as tokens (uses a regexp)\nprint(WordPunctTokenizer.__doc__)\ntokens_punct = wordpunct_tokenize(st[2])\nprint('* Sentence tokenized with a regexp tokenizer', tokens_punct)",
"* Docs of PunktSentenceTokenizer:\n\n A sentence tokenizer which uses an unsupervised algorithm to build\n a model for abbreviation words, collocations, and words that start\n sentences; and then uses that model to find sentence boundaries.\n This approach has been shown to work well for many European\n languages.\n \n* Text tokenized ['What will you do?', 'I go to the cinema this weekend.', \"That's a test.\", \"I can't do better!\"]\n* Docs of TreebankWordTokenizer:\n\n The Treebank tokenizer uses regular expressions to tokenize text as in Penn Treebank.\n This is the method that is invoked by ``word_tokenize()``. It assumes that the\n text has already been segmented into sentences, e.g. using ``sent_tokenize()``.\n\n This tokenizer performs the following steps:\n\n - split standard contractions, e.g. ``don't`` -> ``do n't`` and ``they'll`` -> ``they 'll``\n - treat most punctuation characters as separate tokens\n - split off commas and single quotes, when followed by whitespace\n - separate periods that appear at the end of line\n\n >>> from nltk.tokenize import TreebankWordTokenizer\n >>> s = '''Good muffins cost $3.88\\nin New York. Please buy me\\ntwo of them.\\nThanks.'''\n >>> TreebankWordTokenizer().tokenize(s)\n ['Good', 'muffins', 'cost', '$', '3.88', 'in', 'New', 'York.', 'Please', 'buy', 'me', 'two', 'of', 'them.', 'Thanks', '.']\n >>> s = \"They'll save and invest more.\"\n >>> TreebankWordTokenizer().tokenize(s)\n ['They', \"'ll\", 'save', 'and', 'invest', 'more', '.']\n >>> s = \"hi, my name can't hello,\"\n >>> TreebankWordTokenizer().tokenize(s)\n ['hi', ',', 'my', 'name', 'ca', \"n't\", 'hello', ',']\n \n* Sentence tokenized ['That', \"'s\", 'a', 'test', '.']\n\n Tokenize a text into a sequence of alphabetic and\n non-alphabetic characters, using the regexp ``\\w+|[^\\w\\s]+``.\n\n >>> from nltk.tokenize import WordPunctTokenizer\n >>> s = \"Good muffins cost $3.88\\nin New York. Please buy me\\ntwo of them.\\n\\nThanks.\"\n >>> WordPunctTokenizer().tokenize(s)\n ['Good', 'muffins', 'cost', '$', '3', '.', '88', 'in', 'New', 'York',\n '.', 'Please', 'buy', 'me', 'two', 'of', 'them', '.', 'Thanks', '.']\n \n* Sentence tokenized with a regexp tokenizer ['That', \"'\", 's', 'a', 'test', '.']\n"
],
[
"# POS tagging\n\n# pos_tag uses the PerceptronTagger\nprint('* Tagged tokens from above', pos_tag(tokens))\n\n# Evaluate the performance of some taggers\n\n# The UnigramTagger will assign tag to token as the most probable for that token given a training set\nunigram_tagger = UnigramTagger(train_sents)\nprint('* Evaluation Unigram tagger:', unigram_tagger.evaluate(test_sents))\n\n# Repeat with an NGramTagger (assign the most probable tag given word and N - 1 previous context words)\nthreegram_tagger = NgramTagger(3, train_sents) # for n=2 there is already a BigramTagger\nprint('* Evaluation Ngram tagger with N=3:', threegram_tagger.evaluate(test_sents)) # slow due to sparsity: trained tagger hasn't seen many word-context combinations\n\n# Combining taggers: start with the Ngram one, if it can't find a tag for token fallback to the unigram one\nt0 = UnigramTagger(train_sents)\nt1 = NgramTagger(3, train_sents, backoff=t0)\nprint('* Evaluation combined tagger:', t1.evaluate(test_sents))",
"* Tagged tokens from above [('That', 'DT'), (\"'s\", 'VBZ'), ('a', 'DT'), ('test', 'NN'), ('.', '.')]\n* Evaluation Unigram tagger: 0.8026879907509996\n* Evaluation Ngram tagger with N=3: 0.05867334650031312\n* Evaluation combined tagger: 0.8053374440001927\n"
],
[
"# Stemming\n# Stemming some words with Porter, Lancaster and Snowball stemmers\n\nporter_stemmer = PorterStemmer()\nlancaster_stemmer = LancasterStemmer()\nsnowball_stemmer = SnowballStemmer('english')\n\nprint('* Stemming with (in order) Porter, Lancaster, Snowball')\nprint('multiply: ', \n porter_stemmer.stem('multiply'), \n lancaster_stemmer.stem('multiply'), \n snowball_stemmer.stem('multiply'))\nprint('mice: ', \n porter_stemmer.stem('mice'), \n lancaster_stemmer.stem('mice'), \n snowball_stemmer.stem('mice'))",
"* Stemming with (in order) Porter, Lancaster, Snowball\nmultiply: multipli multiply multipli\nmice: mice mic mice\n"
],
[
"# Lemmatizing with the WordNet lemmatizer\n\nwordnet_lemmatizer = WordNetLemmatizer()\n\nprint('mice: ', wordnet_lemmatizer.lemmatize('mice'))",
"mice: mouse\n"
]
],
[
[
"## Playing with frequency distributions",
"_____no_output_____"
]
],
[
[
"# Setting some sentences\n\nsentences = ['I go to school', 'I will go to the cinema', 'I like strawberries', 'I read books']",
"_____no_output_____"
],
[
"# FreqDist on the word length on some chosen sentences and on the last letter of words\n\nsplit_sentences = [sentence.split() for sentence in sentences]\nall_words = []\nfor sent in split_sentences:\n for word in sent:\n all_words.append(word)\n \nfdist = FreqDist([len(word) for word in all_words])\nfdist.plot(title='Counts word lengths')\n\nfdist = FreqDist([word[-1:] for word in all_words])\nfdist.plot(title='Counts last letter')",
"_____no_output_____"
],
[
"# ConditionalFreqDist on the words per last letter of words\n\nsplit_sentences = [sentence.split() for sentence in sentences]\nall_words = []\nfor sent in split_sentences:\n for word in sent:\n all_words.append(word)\n \ntuples = [(word[-1:], word) for word in all_words]\ncfdist = ConditionalFreqDist(tuples)\n\n# Can plot both at same time\ncfdist.plot()\n\ncfdist",
"_____no_output_____"
],
[
"data = [('breakfast', 'cereal'),\n ('breakfast', 'water'),\n ('evening', 'meat'), \n ('evening', 'salad'), \n ('evening', 'wine'),\n ('lunch', 'sandwich'),\n ('lunch', 'fruit'),\n ('lunch', 'water'),\n ('lunch', 'chocolate'),\n ('breakfast', 'milk')\n ]\n\n# word counts per category\ncfdist = ConditionalFreqDist(data)\ncfdist.plot()",
"_____no_output_____"
],
[
"# Conditional freq dist to see how words have been used in time, inaugural corpus\n\ncfdist = ConditionalFreqDist(\n (target, fileid[:4])\n for fileid in inaugural.fileids()\n for w in inaugural.words(fileid)\n for target in ['america', 'citizen']\n if w.lower().startswith(target))\ncfdist.plot()\n\n# Conditional freq dist to see words which end with chosen letters in Brown corpus\ncfdist = ConditionalFreqDist(\n (target, w)\n for w in brown.words()\n for target in ['zz']\n if w.lower().endswith(target))\ncfdist.plot()",
"_____no_output_____"
]
]
] | [
"code",
"markdown",
"code",
"markdown",
"code",
"markdown",
"code",
"markdown",
"code",
"markdown",
"code"
] | [
[
"code"
],
[
"markdown",
"markdown"
],
[
"code",
"code",
"code",
"code",
"code",
"code",
"code",
"code"
],
[
"markdown"
],
[
"code"
],
[
"markdown"
],
[
"code"
],
[
"markdown"
],
[
"code",
"code",
"code",
"code",
"code"
],
[
"markdown"
],
[
"code",
"code",
"code",
"code",
"code"
]
] |
e786080097809c9b6a0f394f27b9a1d4dab23ede | 8,020 | ipynb | Jupyter Notebook | book/assignments/geostrophy_ekman.ipynb | monocilindro/intro_to_physical_oceanography | 1cd76829d94dcbd13e5e81c923db924ff0798c1b | [
"MIT"
] | 82 | 2015-09-18T02:01:53.000Z | 2022-02-28T01:43:48.000Z | book/assignments/geostrophy_ekman.ipynb | monocilindro/intro_to_physical_oceanography | 1cd76829d94dcbd13e5e81c923db924ff0798c1b | [
"MIT"
] | 5 | 2015-09-19T01:35:28.000Z | 2022-02-28T17:23:53.000Z | book/assignments/geostrophy_ekman.ipynb | monocilindro/intro_to_physical_oceanography | 1cd76829d94dcbd13e5e81c923db924ff0798c1b | [
"MIT"
] | 51 | 2015-09-12T00:30:33.000Z | 2022-02-08T19:37:51.000Z | 35.486726 | 194 | 0.615087 | [
[
[
"# Homework 3: Thermal Wind and Ekman Transports #\n\nIn this problem set you will apply the concepts of geostrophic balance, thermal wind balance, and Ekman transport to simple calculations.",
"_____no_output_____"
],
[
"## 1) Surface Geostrophy and Thermal Wind in Drake Passage\n\nConsider the cartoon shown here which represents a simplified meridional cross section across the Drake Passage in the Southern Ocean.\nA major simplification is that $\\eta$ and $b$ are assumed to vary _linearly_ in the y direction, and that the geostrophic velocity $u_g$ varies _linearly_ in depth.\n\n\n\n### 1a) What is the strength of the sea surface height gradient $\\partial \\eta / \\partial y$ in terms of the given parameters.",
"_____no_output_____"
],
[
"### 1b) Using surface geostrophic balance, estimate the speed of the zonal surface current.\n\nUse the following parameters: $L = 850 km$, $\\eta_{max} = 1$ m.",
"_____no_output_____"
],
[
"### 1c) Calculate the effective depth $D$ over which the zonal current falls to zero\n\n...assuming a total transport of 180 Sv. Also assume the following:\n- The surface current speed is 0.1 m/s (should roughly match answer from part b)\n- The current decays linearly with depth until from the surface to $z=-D$\n- The current is uniform in the y direction\n\nNote that this part of the problem does not involve geostrophy or anything relating to equations of motion.\nIt's just a volume transport calculation.\n\n",
"_____no_output_____"
],
[
"### 1d) Use thermal wind to estimate the North-South temperature difference across the drake passage based on the geostrophic shear\n\nAssume:\n- The equation of state is linear and only contains temperature, with $b = g \\alpha \\Theta$, with $\\alpha = 1.6 \\times 10^{-4}$ K$^{-1}$.\n- The geostrophic shear is uniform over depth $D$ and zero below.\n- $D = 4000$ m (should roughly match the answer from part c).\n\nCompare you estimate the the potential temperature field from the [WOCE Southern Ocean Atlas Drake Passage section](http://woceatlas.tamu.edu/printed/SOA_S1.html).\nIs your number reasonable?",
"_____no_output_____"
],
[
"## 2) Surface Geostrophy and Thermal Wind in the North Atlantic\n\nThe cartoon below shows a zonal cross section across the North Atlantic passing through the Gulf Stream and the subtropical gyre.\nGoing from West to East, the sea-surface height $\\eta$ quickly increases to $\\eta_{max}$ and then decays more slowly back to zero on the Eastern boundary.\nAssume all these changes are linear; this is a highly simplified representation that makes it easier to make calculations.\nThis SSH pattern is qualitatively similar to the real SSH pattern off the Eastern US.\n\n\n\n",
"_____no_output_____"
],
[
"### 2a) What is the strength of the sea surface height gradient $\\partial \\eta / \\partial x$ in terms of the given parameters in the Northward Flow Region and the Southward Flow Region.",
"_____no_output_____"
],
[
"### 2b) Using surface geostrophic balance, estimate the speed of the meridional surface current in each of the two regions.\n\nUse the following parameters: $L_1 = 500 km$, $L_2 = 3000 km$, $\\eta_{max} = 0.5$ m.",
"_____no_output_____"
],
[
"### 2c) Calculate the effective depth $D$ over which the meridional current $v_g$ falls to zero\n\nAssume the total northward transport in the Northward Flow region (Gulf Stream) is 50 Sv.\nAssume equal and opposite transport in the Southward Flow region.\n\nAlso assume:\n- The meridional surface current speed in the gulf stream region is 0.1 m/s (should roughly match part b).\n- The current decays linearly with depth until from the surface to $z=-D$\n- The current is uniform in the x direction\n\nNote that this part of the problem does not involve geostrophy or anything relating to equations of motion.\nIt's just a volume transport calculation.\nIt does not matter whether you do the calculation for the Northward Flow region or the Southward Flow region.\nYou should get the same answer.",
"_____no_output_____"
],
[
"### 2d) Use thermal wind to calculate the implied temperature at the following locations\n\n- $z=-1000$ m, $x = L_1$\n- $z=-1000$ m, $x = L_1 + L_2$ (the Eastern boundary)\n\nAssume the temperature on the Western boundary at 1000 m depth is 5$^\\circ$ C.\nAssume the depth scale $D = 2000$ m (should match the answer from part c.)\n\nUse all the other same assumptions as part 1d.",
"_____no_output_____"
],
[
"## 3) Ekman Transport and Pumping\n\nConsider a region of the subtropical ocean shown in the cartoon, described using cartesian coordinates.\n\n\n\nThe region is of size ($L_x$, $L_y$).\nThe zonal wind stress increases linearly from $\\tau_x = 0$ at $y = 0$ to $\\tau_x = \\tau_0$ at $y = L_y$.\nThere is no meridional stress.\nThis is qualitatively similar to the mid-latitude South Atlantic or South Pacific.\n\nYou may assume that $f$ = $f_0$ = const.",
"_____no_output_____"
],
[
"### 3a) Calculate the Ekman transport velocity as a function of $y$\n\nI'm looking for a formula in terms of the provided parameters.",
"_____no_output_____"
],
[
"### 3b) Now plug in numbers and calculate the _net Ekman transport_ across the Southern ($y=0$) and Northern ($y=L_y$) borders of the region.\n\n- $L_x = 2000$ km\n- $L_y = 1000$ km\n- $\\tau_0 = 0.1$ N m$^{-2}$\n- $f = 0.8 \\times 10^{-4}$ s$^{-1}$\n- $\\rho_0 = 1025$ kg m$^{-3}$\n\nBy _net Ekman transport_ I mean $V_Ek$ integrated over the $x$ dimension. Give you answer in Sv.\n",
"_____no_output_____"
],
[
"### 3c) Calculate the Ekman pumping velocity over the region\n\nI'm looking for a formula in terms of the provided parameters",
"_____no_output_____"
],
[
"### 3d) Now plug in numbers and calculate the _new Ekman upwelling / downwelling_ over the entire region.\n\nUse the same numbers as above.\n\nGive you answer in Sv.",
"_____no_output_____"
]
]
] | [
"markdown"
] | [
[
"markdown",
"markdown",
"markdown",
"markdown",
"markdown",
"markdown",
"markdown",
"markdown",
"markdown",
"markdown",
"markdown",
"markdown",
"markdown",
"markdown",
"markdown"
]
] |
e7860d062ff43001ca0742e57f0720664769f9e6 | 110,532 | ipynb | Jupyter Notebook | l8/Lecture 50 - Combining Plot Styles.ipynb | thatguyandy27/python-sandbox | 33dffa286aee942c93c75303ab463313020bbd7a | [
"MIT"
] | null | null | null | l8/Lecture 50 - Combining Plot Styles.ipynb | thatguyandy27/python-sandbox | 33dffa286aee942c93c75303ab463313020bbd7a | [
"MIT"
] | null | null | null | l8/Lecture 50 - Combining Plot Styles.ipynb | thatguyandy27/python-sandbox | 33dffa286aee942c93c75303ab463313020bbd7a | [
"MIT"
] | null | null | null | 367.215947 | 28,374 | 0.927189 | [
[
[
"import numpy as np\nfrom numpy.random import randn\nimport pandas as pd\n\nfrom scipy import stats\n\nimport matplotlib as mpl\nimport matplotlib.pyplot as plt\nimport seaborn as sns\n\n%matplotlib inline",
"_____no_output_____"
],
[
"dataset = randn(100)",
"_____no_output_____"
],
[
"sns.distplot(dataset, bins=25)",
"_____no_output_____"
],
[
"sns.distplot(dataset, bins=25, rug=True, hist=False)",
"_____no_output_____"
],
[
"sns.distplot(dataset, bins=25, kde_kws={'color': 'indianred', 'label': 'KDE PLOT'},\n hist_kws={'color': 'blue', 'label': 'HIST'})",
"_____no_output_____"
],
[
"from pandas import Series",
"_____no_output_____"
],
[
"ser1 = Series(dataset, name='my_data')",
"_____no_output_____"
],
[
"ser1",
"_____no_output_____"
],
[
"sns.distplot(ser1, bins=25)",
"_____no_output_____"
]
]
] | [
"code"
] | [
[
"code",
"code",
"code",
"code",
"code",
"code",
"code",
"code",
"code"
]
] |
e7860fbab0742e86c828890ee48d597ed01bd95e | 2,660 | ipynb | Jupyter Notebook | docs/target/extension-guide/README.ipynb | aborruso/frictionless-py | 2b27d87ac042119d93320051c5be78d9ebf954b6 | [
"MIT"
] | null | null | null | docs/target/extension-guide/README.ipynb | aborruso/frictionless-py | 2b27d87ac042119d93320051c5be78d9ebf954b6 | [
"MIT"
] | null | null | null | docs/target/extension-guide/README.ipynb | aborruso/frictionless-py | 2b27d87ac042119d93320051c5be78d9ebf954b6 | [
"MIT"
] | null | null | null | 2,660 | 2,660 | 0.713534 | [
[
[
"# Extension Guide\n\nFrictionless is built on top of a powerful plugins system which is used internally and allows to extend the framework.\n",
"_____no_output_____"
],
[
"\n## Plugin Interface\n\nHere is a list of available hooks that you can implement in your plugin:\n- `create_check`\n- `create_control`\n- `create_dialect`\n- `create_loader`\n- `create_parser`\n- `create_server`\n\nTo create a plugin you need:\n- create a module called `frictionless_<name>` available in PYTHONPATH\n- subclass the Plugin class and override one of the methods above\n\nPlease consult with \"API Reference\" for in-detail information about the Plugin interface and how these methods can be implemented.\n",
"_____no_output_____"
],
[
"\n## Plugin Example\n\nLet's say we're interested in supporting the `csv2k` format that we have just invented. For simplicity, let's use a format that is exactly the same with CSV.\n\nFirst of all, we need to create a `frictionless_csv2k` module containing a Plugin implementation and a Parser implementation but we're going to re-use the CsvParser as our new format is the same:\n\n> frictionless_csv2k.py\n\n```python\nfrom frictionless import Plugin, parsers\n\nclass Csv2kPlugin(Plugin):\n def create_parser(self, file):\n if file.format == \"csv2k\":\n return Csv2kParser(file)\n\nclass Csv2kParser(parsers.CsvParser):\n pass\n```\n\nNow, we can just use our new format in any of Frctionless functions that accept a table source, for example, `extract` or `Table`:\n\n```python\nfrom frictionless import extract\n\nrows = extract('data/table.csv2k')\nprint(rows)\n```\n\nThis example is over-simplified to show the high-level mechanics but actually writing Frctionless Plugins is really easy. For inspiration, you can checks the `frictionless/plugins` directory and learn from real-life examples. Also, in the Frictionless codebase there are many `Check`, `Control`, `Dialect`, `Loader`, `Parser`, and `Server` implementations - you can read their code for better understanding of how to write your own subclass.\n",
"_____no_output_____"
]
]
] | [
"markdown"
] | [
[
"markdown",
"markdown",
"markdown"
]
] |
e7861a1977cc790b079b5a03d54bb993831f4c98 | 2,812 | ipynb | Jupyter Notebook | S01 - Bootcamp and Binary Classification/SLU15 - Hyperparameter Tuning/Examples notebook.ipynb | FarhadManiCodes/batch5-students | 3a147145dc4f4ac65a851542987cf687b9915d5b | [
"MIT"
] | 2 | 2022-02-04T17:40:04.000Z | 2022-03-26T18:03:12.000Z | S01 - Bootcamp and Binary Classification/SLU15 - Hyperparameter Tuning/Examples notebook.ipynb | FarhadManiCodes/batch5-students | 3a147145dc4f4ac65a851542987cf687b9915d5b | [
"MIT"
] | null | null | null | S01 - Bootcamp and Binary Classification/SLU15 - Hyperparameter Tuning/Examples notebook.ipynb | FarhadManiCodes/batch5-students | 3a147145dc4f4ac65a851542987cf687b9915d5b | [
"MIT"
] | 2 | 2021-10-30T16:20:13.000Z | 2021-11-25T12:09:31.000Z | 24.884956 | 90 | 0.541607 | [
[
[
"# SLU15 - Hyperparameter tunning: Examples notebook\n---",
"_____no_output_____"
],
[
"## 1 Load and the prepare the data",
"_____no_output_____"
]
],
[
[
"import pandas as pd\nfrom sklearn.datasets import load_breast_cancer\nfrom sklearn.model_selection import train_test_split\nfrom sklearn.tree import DecisionTreeClassifier\n\ncancer_data = load_breast_cancer()\nX = pd.DataFrame(cancer_data[\"data\"], columns=cancer_data[\"feature_names\"])\ny = cancer_data.target\n\nX_train, X_test, y_train, y_test = train_test_split(X, y, test_size=0.2, \n random_state=0)\nestimator = DecisionTreeClassifier()",
"_____no_output_____"
]
],
[
[
"## 2 Grid search",
"_____no_output_____"
]
],
[
[
"from sklearn.model_selection import GridSearchCV\n\nparameters = {'max_depth': range(1, 10),\n 'max_features': range(1, X.shape[1])}\n\ngrid_search = GridSearchCV(estimator, parameters, cv=5, scoring=\"roc_auc\")\ngrid_search.fit(X_train, y_train)\n\ny_pred = grid_search.predict(X_test)",
"_____no_output_____"
]
],
[
[
"## 2 Random search",
"_____no_output_____"
]
],
[
[
"from scipy.stats import randint\nfrom sklearn.model_selection import RandomizedSearchCV\n\nparameters_dist = {\"max_depth\": randint(1, 100),\n \"max_features\": randint(1, X.shape[1]),\n \"class_weight\": [\"balanced\", None]}\n\nrandom_search = RandomizedSearchCV(estimator, parameters_dist, cv=5, n_iter=250, \n random_state=0)\n\nrandom_search.fit(X_train, y_train)\ny_pred = random_search.predict(X_test)",
"_____no_output_____"
]
]
] | [
"markdown",
"code",
"markdown",
"code",
"markdown",
"code"
] | [
[
"markdown",
"markdown"
],
[
"code"
],
[
"markdown"
],
[
"code"
],
[
"markdown"
],
[
"code"
]
] |
e7861b8a5966944c2c6c1d1cbb0732bbc685ecfa | 354,083 | ipynb | Jupyter Notebook | ottilliani/project2_ottilliani.ipynb | pezLyfe/applied_ds | 269fe6a991241a92a25b55567e7ef6a51858d5e3 | [
"BSD-3-Clause"
] | 2 | 2019-04-03T00:15:02.000Z | 2020-09-14T17:32:45.000Z | ottilliani/project2_ottilliani.ipynb | pezLyfe/applied_ds | 269fe6a991241a92a25b55567e7ef6a51858d5e3 | [
"BSD-3-Clause"
] | 40 | 2019-03-11T00:32:02.000Z | 2019-05-12T01:26:05.000Z | ottilliani/project2_ottilliani.ipynb | pezLyfe/applied_ds | 269fe6a991241a92a25b55567e7ef6a51858d5e3 | [
"BSD-3-Clause"
] | 54 | 2019-03-01T00:02:23.000Z | 2019-05-09T22:31:58.000Z | 171.718235 | 37,812 | 0.846143 | [
[
[
"# The analysis of the equality of rights between gender using the Human Freedom Index",
"_____no_output_____"
],
[
"### author: Ottillia Ni\n### Project Report 2 (EM212: Applied Data Science)",
"_____no_output_____"
],
[
"#### Content:\nIntroduction\n<br>\nDatasheet\n<br>\nExploratory Data Anaylsis\n<br>",
"_____no_output_____"
],
[
"## Introduction",
"_____no_output_____"
],
[
"Throughout the world, people strive for freedom. Freedom is a means of human progression and growth within the self and amongst society. But how can someone measure a person’s freedom? According to the Cato Institute, the Human Freedom Index is a global measurement of human freedom based on personal, economic, and civil freedom. Since 2008, the Human Freedom Index has collected enough data to rank countries and their levels of freedom based upon various qualities on a scale of 0 to 10, with 10 being the greatest amount of freedom. There are 79 distinct indicators of personal and economic freedom, which are found in the areas of the Rule of Law, Security and Safety, Movement, Religion, Association, Assembly, and Civil Society, Expression and Information, Identity and Relationships, Size and Government, Legal System and Property Rights, Access to Sound Money, Freedom to Trade Internationally, and Regulation of Credit Labor, and Business.\n\nThe indicators within this dataset were evaluated by credible external sources, not by the creator of the index, to ensure objectivity. The intended use of the index is to fill in the gaps in literature and to look at how a range of different freedoms can be interconnected, such as economic freedom and personal freedom. When scoring the index, economic freedom takes up half the weight of the score while all other Personal freedoms complete the rest of the scored weight. Economic freedom takes half the weight because it has a central importance and affects all other freedoms. This data set has been used by researchers for a variety of reasons. Countries have developed news reports to discuss improvements in levels of freedom. Within the Personal and Economic Freedom, the data provides subcategories to listed areas. It has also been used to target and analyze more specific structures within the index such as women’s personal freedom. \n\nGiven that this data set has a variety of subcomponents, for this project, I will focus on the data of identity and relationships, specifically same-sex relationships. I will be observing the different levels of tolerance amongst the listed countries. This would incorporate further research to observe and understand what social events might have caused a significant shift in the score of same-sex relationships. Doing a quick skim through the data, I noticed that the scores are either 0 or 10, with a few 5 scattered in between. My initial reaction to this is the indication of legality of same sex relations in these countries. Given that data has been collected over the past decade, I will be using this information to potentially determine how a specific country’s score has fluctuated or stayed the same over the years. \n\nThe questions I will be asking through the use of this dataset are: what common trends do the countries with accepting of same-sex relationship have in common? How do these top countries rank of same-sex relationships compare to the other subcategories of identity and relationships? Are there any standout differences? An initial data issue I might uncover as I compare a country’s rank over the progression of the past decade is missing data. This potential issue might arise as the Human Freedom Index incorporates new countries with each given year. The analysis of this data can be utilized by companies advocating for global freedom such as Amnesty International, Human Rights Action Center, UNESCO (United Nations Educational, Scientific, and Cultural Organization), and other human rights organizations. Ideally, this data analysis would be funded on a donation basis as many of the organizations that would best benefit from this information are non-profits. \n\n---\n\nIn this analysis, I am looking to compare gender equality between men and women around the world using the data from the Human Freedom Index. Given that this index has a variety of variables, I will looking at personal freedom variables. I will be using the variable topic of identity and relationships to better understand the level of female equality around the world as compared to males. \n\n",
"_____no_output_____"
],
[
"## Datasheet",
"_____no_output_____"
],
[
"#### Motivation for Dataset Creation \nWhy was the dataset created? (e.g., were there specific tasks in mind, or a specific gap that needed to be filled?) \nThe Human Freedom Index is a global measurement of human freedom based on personal, economic, and civil freedom that can more objectively regulate these freedom relationships. Since 2008, the Human Freedom Index has collected enough data to rank countries and their levels of freedom based upon various qualities on a scale of 0 to 10, with 10 being the greatest amount of freedom. According to Fred McMahon of the Fraser Institute, the dataset “provides crucial and objective information on the state and evolution of freedom globally.” \n\nWhat (other) tasks could the dataset be used for? Are there obvious tasks for which it should not be used? \nAs the years progress and with each report, analysts can also use this data to compare how global freedom has changed over the years.\n\nHas the dataset been used for any tasks already? If so, where are the results so others can compare (e.g., links to published papers)? \nThose who compiled the dataset writes a report each year to accompany their data and findings. The central purpose of the report is to show a broad, yet accurate freedom of the overall world and have the larger purpose be for defining what they mean by freedom and to understand the relationships between the different existing forms. The link to the 2018 report can be found here: https://object.cato.org/sites/cato.org/files/human-freedom-index-files/human-freedom-index-2018-revised.pdf.\n\nWho funded the creation of the dataset? If there is an associated grant, provide the grant number. \nThis dataset was developed by the Cato Institute and was funded and sponsored by the Lotte & John Hecht Memorial Foundation, Liberty Fund, Liberales Institut, Fraser Institute, and Cato Institute. \n\n#### Dataset Composition \nWhat are the instances? (that is, examples; e.g., documents, images, people, countries) Are there multiple types of instances? (e.g., movies, users, ratings; people, interactions between them; nodes, edges) \nThe instances are countries willing to report their level of freedom and contribute to the Human Freedom Index.\n \nAre relationships between instances made explicit in the data (e.g., social network links, user/movie ratings, etc.)? \nI’m not certain, but I believe the instances are determined various forms of freedom this data is expressing.\n\nHow many instances of each type are there? \nThe instances consist of 162 countries with varying repetition as the data incorporates all its results starting 2008. \n\nWhat data does each instance consist of? “Raw” data (e.g., unprocessed text or images)? Features/attributes? Is there a label/target associated with instances? If the instances are related to people, are subpopulations identified (e.g., by age, gender, etc.) and what is their distribution? \nThe data consists of 79 distinct indicators of personal and economic freedom, which are found in the areas of the Rule of Law, Security and Safety, Movement, Religion, Association, Assembly, and Civil Society, Expression and Information, Identity and Relationships, Size and Government, Legal System and Property Rights, Access to Sound Money, Freedom to Trade Internationally, and Regulation of Credit Labor, and Business. \n\nIs everything included or does the data rely on external resources? (e.g., websites, tweets, datasets) If external resources, a) are there guarantees that they will exist, and remain constant, over time; b) is there an official archival version. Are there licenses, fees or rights associated with any of the data?\nGiven that the scoring of the freedom is based on other global indices, such as the Rule of Law Index and Social Institutions and Gender index, it is likely that these external sources will remain constant over time as many are forms of public government-based data.\n \nAre there recommended data splits or evaluation measures? (e.g., training, development, testing; accuracy/AUC) \nTo compare the specific instances, it is recommended to compare with at least one same variable, such as year or type of freedom.\n\nWhat experiments were initially run on this dataset? \nHave a summary of those results and, if available, provide the link to a paper with more information here. \nThe report has developed country profiles given the findings of the different specific factors of freedom.\n\n#### Data Collection Process \nHow was the data collected? (e.g., hardware apparatus/sensor, manual human curation, software program, software interface/API; how were these constructs/measures/methods validated?) \nThe Human Freedom Index is based upon sub-indexes, compiled and determined by government reporting. \n\nWho was involved in the data collection process? (e.g., students, crowd workers) How were they compensated? (e.g., how much were crowd workers paid?) \nThis data set is a collection of government and university-based indexes.\n\nOver what time-frame was the data collected? Does the collection time-frame match the creation time-frame? \nThe data is collected on an annual basis. \n\nHow was the data associated with each instance acquired? Was the data directly observable (e.g., raw text, movie ratings), reported by subjects (e.g., survey responses), or indirectly inferred/derived from other data (e.g., part of speech tags; model-based guesses for age or language)? If the latter two, were they validated/verified and if so how? \nThe data is derived from other data, more specifically specific based on various subfactors from the collection of government and university-based indexes.\n\nDoes the dataset contain all possible instances? Or is it, for instance, a sample (not necessarily random) from a larger set of instances? \nThe dataset contains all possible instances.\n\nIf the dataset is a sample, then what is the population? \nWhat was the sampling strategy (e.g., deterministic, probabilistic with specific sampling probabilities)? Is the sample representative of the larger set (e.g., geographic coverage)? If not, why not (e.g., to cover a more diverse range of in- stances)? How does this affect possible uses? \nThe dataset I am using is not a sample.\n\nIs there information missing from the dataset and why? \n(this does not include intentionally dropped instances; it might include, e.g., redacted text, withheld documents) Is this data missing because it was unavailable? \nYes, some countries have missing scores given that the Human Freedom Index incorporates new countries with each given year or that specific distinct indicators might not have data recorded for that year\n\nAre there any known errors, sources of noise, or redundancies in the data? \nUNKNOWN\n\n#### Data Preprocessing \nWhat preprocessing/cleaning was done? (e.g., discretization or bucketing, tokenization, part-of-speech tagging, SIFT feature extraction, removal of instances, processing of missing values, etc.) \nIt appears likely.\n\nWas the “raw” data saved in addition to the preprocessed/cleaned data? (e.g., to support unanticipated future uses) \nUNKNOWN\n\nIs the preprocessing software available? \nUNKNOWN \n\nDoes this dataset collection/processing procedure achieve the motivation for creating the dataset stated in the first section of this datasheet? \nUNKNOWN\n\n#### Dataset Distribution \nHow is the dataset distributed? (e.g., website, API, etc.; does the data have a DOI; is it archived redundantly?) \nThe data can be downloaded from https://www.kaggle.com/gsutters/the-human-freedom-index or https://object.cato.org/sites/cato.org/files/human-freedom-index-files/hfi2018web-revised3.xlsx.\n\nWhen will the dataset be released/first distributed? (Is there a canonical paper/reference for this dataset?) \nThe dataset was first released in 2008.\n\nWhat license (if any) is it distributed under? Are there any copyrights on the data? \nThe Human Freedom Index Report is copyrighted by the Cato Institute, Fraser Institute, and the Friedrich Naumann Foundation for Freedom. There is no clear indication of copyright to the data as it a collection of information from other index as well.\n\nAre there any fees or access/export restrictions? \nNo. \n\n#### Dataset Maintenance\nWho is supporting/hosting/maintaining the dataset? \nHow does one contact the owner/curator/manager of the dataset (e.g. email address, or other contact info)? \nThe data is maintained by Ian Vásquez and Tanja Porcnik, the Cato Institute, Fraser Institute, and the Friedrich Naumann Foundation for Freedom.\n\nWill the dataset be updated? How often and by whom? How will updates/revisions be documented and communicated (e.g., mailing list, GitHub)? Is there an erratum? \nThis dataset is updated annual.\n\nIf the dataset becomes obsolete how will this be communicated? \nThe Cato Institute would probably report on their webpage the discontinuation of this dataset. \n\nIs there a repository to link to any/all papers/systems that use this dataset? \nIf others want to extend/augment/build on this dataset, is there a mechanism for them to do so? If so, is there a process for tracking/assessing the quality of those contributions. What is the process for communicating/distributing these contributions to users? \nIf others wanted to extend or build on this dataset, they should contact the Cato Institute, but more specifically the authors Ian Vásquez and Tanja Porcnik.\n\n\n#### Legal and Ethical Considerations\nIf the dataset relates to people (e.g., their attributes) or was generated by people, were they informed about the data collection? (e.g., datasets that collect writing, photos, interactions, transactions, etc.) \nThere is uncertainty given that dataset is based upon a collection of people not all might have known their data was being collected\n\nIf it relates to other ethically protected subjects, have appropriate obligations been met? (e.g., medical data might include information collected from animals) \nUNKNOWN\n\nIf it relates to people, were there any ethical review applications/reviews/approvals? (e.g. Institutional Review Board applications) \nUNKNOWN\n\nIf it relates to people, were they told what the dataset would be used for and did they consent? What community norms exist for data collected from human communications? If consent was obtained, how? Were the people provided with any mechanism to revoke their consent in the future or for certain uses? \nUNKNOWN\n\nIf it relates to people, could this dataset expose people to harm or legal action? (e.g., financial social or otherwise) What was done to mitigate or reduce the potential for harm? \nUnlikely, since this data was a government-based collection.\n\nIf it relates to people, does it unfairly advantage or disadvantage a particular social group? In what ways? How was this mitigated? \nNo.\n\nIf it relates to people, were they provided with privacy guarantees? If so, what guarantees and how are these ensured? \nSince this was based on reports, it is highly likely they were provided with privacy guarantees.\n\nDoes the dataset comply with the EU General Data Protection Regulation (GDPR)? Does it comply with any other standards, such as the US Equal Employment Opportunity Act? \nYes.\n\nDoes the dataset contain information that might be considered sensitive or confidential? (e.g., personally identifying information) \nNo, since the data is a collection of already published data and a study of a collective group of people.\n\nDoes the dataset contain information that might be considered inappropriate or offensive? \nIf used or interpreted incorrectly, analyst could potentially make inappropriate or offensive accusations of freedom within a specific country.\n",
"_____no_output_____"
],
[
"## Exploratory Data Anaylsis\n",
"_____no_output_____"
]
],
[
[
"import matplotlib.pyplot as plt\nimport pandas as pd\nimport numpy as np\nimport seaborn as sns\nimport pdb\nimport matplotlib.pyplot as plt",
"_____no_output_____"
]
],
[
[
"### Importing Dataset\n\nTo begin, I will be using python to analyize my data. (This data of the Human Freedom Index is downloaded off Kaggle.)",
"_____no_output_____"
]
],
[
[
"# read Human Freedom Index data\nhfi = pd.read_csv('https://tufts.box.com/shared/static/7iwsgxhffhfs87v209scqihq57pnmev0.csv')\nhfi.head()",
"_____no_output_____"
]
],
[
[
"### Cleaning Data",
"_____no_output_____"
],
[
"Given that I am focusing specifically on the equality between female and male, I want to clean my data so that the variables printed give me the information relavent to my work. In addition, to make understanding the data easier, I will also rename various columns to be more comprehensive of what each variable actually means.",
"_____no_output_____"
]
],
[
[
"select_cols = [\"year\",\"countries\",\"region\",\"pf_ss_women\",\"pf_ss_women_fgm\", \"pf_ss_women_missing\",\"pf_ss_women_inheritance\",\"pf_ss_women_inheritance_widows\",\"pf_ss_women_inheritance_daughters\",\"pf_movement_women\",\"pf_identity_legal\",\"pf_identity_parental\",\"pf_identity_parental_marriage\",\"pf_identity_parental_divorce\",\"pf_identity_sex\",\"pf_identity_sex_male\",\"pf_identity_sex_female\",\"pf_identity_divorce\"]\nhfi_select = hfi[select_cols]\n#hfi_select.head()",
"_____no_output_____"
],
[
"hfi_select1 = hfi_select.rename(columns={'pf_ss_women': 'Women_Safety_Security', 'pf_ss_women_fgm': 'Female_Genital_Mutilation', 'pf_ss_women_missing': 'Missing_Women','pf_ss_women_inheritance':'Inheritance_Rights','pf_ss_women_inheritance_widows': 'Inheritance_Rights_Widows','pf_ss_women_inheritance_daughters':'Inheritance_Rights_Daughters','pf_movement_women':'Womens_Movement','pf_identity_legal':'Legal_Gender','pf_identity_parental':'Parental_rights','pf_identity_parental_marriage': 'Parental_rights_marriage','pf_identity_parental_divorce':'Parental_rights_after_divorce','pf_identity_sex':'Same_sex-relationship','pf_identity_sex_male':'Same_sex_males','pf_identity_sex_female':'Same_sex_female','pf_identity_divorce':'Divorce'})\nhfi_select1.head()",
"_____no_output_____"
],
[
"hfi_select1.dtypes",
"_____no_output_____"
]
],
[
[
"Printing the data types objects makes it immediately evident that the data is mostly in forms of numbers, thus indicating much of the data has already been cleaned. ",
"_____no_output_____"
]
],
[
[
"#Let us determine the percent of data missing per variable.",
"_____no_output_____"
],
[
"#f, ax = plt.subplots(figsize=(50,20))\n#((hfi.isnull().sum()/len(hfi)) * 100).plot(kind='bar')\n#plt.xticks(rotation=45, horizontalalignment='right')\n#plt.title('Percent Missing by Variable')",
"_____no_output_____"
],
[
"# a simple scatterplot \n\n#hfi.plot.scatter('pf_score', 'ef_score')",
"_____no_output_____"
],
[
"#hfi.plot.scatter('pf_identity_sex_male', 'pf_identity_sex')",
"_____no_output_____"
]
],
[
[
"To start off we can first lay out the number of countries represented by region through this bar plot from 2008-2016.",
"_____no_output_____"
]
],
[
[
"#hfi.region.value_counts().plot(kind='bar') \n#plt.xticks()",
"_____no_output_____"
],
[
"#hfi[''].value_counts().plot(kind='bar')",
"_____no_output_____"
],
[
"#filter to only focus on 2016 data\nfilter1 = hfi_select1.year == 2016\nhfi2016 = hfi_select1[filter1]\nhfi2016.sample(5)",
"_____no_output_____"
],
[
"#filter to only focuus on 2016 data\nfilter1 = hfi_select1.year == 2016\nfilter2 = hfi_select1.region == 'Sub-Saharan Africa'\nhfi2016Af = hfi_select1[filter1 & filter2]\nhfi2016Af.sample(5)",
"_____no_output_____"
]
],
[
[
"Diving into gender equality, let us understand observe the equality of parental rights between males and females in various regions around the world. ",
"_____no_output_____"
]
],
[
[
"#hfi2016Af.plot.scatter('pf_ss_women', 'pf_movement_women')",
"_____no_output_____"
],
[
"#sub['mean'] = sub['density'].mean()\n#plt.plot(sub['name'], sub['density'], 'ro')\n#plt.plot(sub['name'], sub['mean'], linestyle = '--')\n#plt.xticks(fontsize = 8, rotation = 'vertical')",
"_____no_output_____"
],
[
"hfi2016.region.value_counts().plot(kind='bar') \nplt.xticks()",
"_____no_output_____"
],
[
"sns.heatmap(hfi_select1.groupby(['year', 'region'])['Parental_rights'].mean().unstack(),\nannot=True, cbar=False, fmt='.0f', cmap='RdBu_r')",
"_____no_output_____"
]
],
[
[
"According to the Human Freedom Report, \"Parental rights refers to the extent to which women have equal rights based in law and custom regarding “legal guardianship of a child during a marriage and custody rights over a child after divorce.”\"",
"_____no_output_____"
],
[
"That being said, we can divide the rights parental rights in regards to legal guardianship of a child during a marriiage as compared to after a divorce. This heat map below shows that in most regions, females equal rights to guardianship of their children during marriage. However, in regions such as the Middle East & North Africa, South Asia, and Sub Saharuan Africa, there represents more of an imbalance.",
"_____no_output_____"
]
],
[
[
"sns.heatmap(hfi_select1.groupby(['year', 'region'])['Parental_rights_marriage'].mean().unstack(),\nannot=True, cbar=False, fmt='.0f', cmap='RdBu_r')",
"_____no_output_____"
]
],
[
[
"The same analysis can also be done with parental rights after divorces. The trend appears to be similar to that of parental rights during marriages, but what is most surprising is found in the Middle East & North Africa catagory. The ranking drops from a 5 to a 2 between 2012 and 2013 indicating a setback with progression toward equality in this region.",
"_____no_output_____"
]
],
[
[
"sns.heatmap(hfi_select1.groupby(['year', 'region'])['Parental_rights_after_divorce'].mean().unstack(),\nannot=True, cbar=False, fmt='.0f', cmap='RdBu_r')",
"_____no_output_____"
]
],
[
[
"Question: Which regions have the greatest amount of freedom in regards to same sex marriage?",
"_____no_output_____"
]
],
[
[
"sns.heatmap( hfi_select1.groupby(['year', 'region'])['Same_sex-relationship'].mean().unstack(),\nannot=True, cbar=False, fmt='.0f', cmap='RdBu_r')",
"_____no_output_____"
]
],
[
[
"From this heat map, we can see that the regions struggle with equality ",
"_____no_output_____"
]
],
[
[
"sns.heatmap(hfi_select1.groupby(['year', 'region'])['Same_sex_males'].mean().unstack(),\nannot=True, cbar=False, fmt='.0f', cmap='RdBu_r')",
"_____no_output_____"
],
[
"sns.heatmap(hfi_select1.groupby(['year', 'region'])['Same_sex_female'].mean().unstack(),\nannot=True, cbar=False, fmt='.0f', cmap='RdBu_r')",
"_____no_output_____"
]
],
[
[
"#### Merging Data",
"_____no_output_____"
]
],
[
[
"# read women purchasing power from https://ourworldindata.org/economic-inequality-by-gender\nge = pd.read_csv('https://tufts.box.com/shared/static/ikc9nsb0red47dv5ldc0rcsv5rml681l.csv')\nge.head()",
"_____no_output_____"
],
[
"#ge.dtypes",
"_____no_output_____"
],
[
"mergedata = hfi_select1.merge(ge, left_on=[\"year\", \"countries\"], right_on=[\"Year\", \"Entity\"], suffixes=(False, False))\nmergedata.head()",
"_____no_output_____"
]
],
[
[
"By merging these two datasets, we can also compare how women in various countries are given the opportunity to participate in purchase descision within their marriages.",
"_____no_output_____"
]
],
[
[
"#mergedata.dtypes",
"_____no_output_____"
]
],
[
[
"supervised learning - Scikit.learn \n",
"_____no_output_____"
]
],
[
[
"#hfi2016.plot.scatter('Inheritance_Rights', 'Parental_rights')",
"_____no_output_____"
],
[
"f, ax = plt.subplots(figsize=(6.5,6.5))\nsns.boxplot(x=\"Inheritance_Rights\", y=\"Parental_rights\", data=hfi2016, fliersize=0.5, linewidth=0.75, ax=ax)\n\n#ax.set_title('axes title')\nax.set_xlabel('Women Inheritance')\nax.set_ylabel('Parental Rights')",
"_____no_output_____"
],
[
"#filter to only focuus on 2016 data in merged\n#filtermerge1 = mergedata.year == 2016\n#merge2016 = mergedata[filter1]\n#mergedata.sample(5)",
"_____no_output_____"
],
[
"sns.heatmap(hfi_select1.groupby(['year', 'region'])['Inheritance_Rights'].mean().unstack(),\nannot=True, cbar=False, fmt='.0f', cmap='RdBu_r')",
"_____no_output_____"
]
]
] | [
"markdown",
"code",
"markdown",
"code",
"markdown",
"code",
"markdown",
"code",
"markdown",
"code",
"markdown",
"code",
"markdown",
"code",
"markdown",
"code",
"markdown",
"code",
"markdown",
"code",
"markdown",
"code",
"markdown",
"code",
"markdown",
"code"
] | [
[
"markdown",
"markdown",
"markdown",
"markdown",
"markdown",
"markdown",
"markdown",
"markdown"
],
[
"code"
],
[
"markdown"
],
[
"code"
],
[
"markdown",
"markdown"
],
[
"code",
"code",
"code"
],
[
"markdown"
],
[
"code",
"code",
"code",
"code"
],
[
"markdown"
],
[
"code",
"code",
"code",
"code"
],
[
"markdown"
],
[
"code",
"code",
"code",
"code"
],
[
"markdown",
"markdown"
],
[
"code"
],
[
"markdown"
],
[
"code"
],
[
"markdown"
],
[
"code"
],
[
"markdown"
],
[
"code",
"code"
],
[
"markdown"
],
[
"code",
"code",
"code"
],
[
"markdown"
],
[
"code"
],
[
"markdown"
],
[
"code",
"code",
"code",
"code"
]
] |
e7862468713cb25cfaa560868580792223b33cdc | 128,036 | ipynb | Jupyter Notebook | 05-roc_curve.ipynb | RXV06021/test_ml4se_colab | 66111e47f8dbdc31e625e16f01d7f27dbb1139f9 | [
"Apache-2.0"
] | null | null | null | 05-roc_curve.ipynb | RXV06021/test_ml4se_colab | 66111e47f8dbdc31e625e16f01d7f27dbb1139f9 | [
"Apache-2.0"
] | null | null | null | 05-roc_curve.ipynb | RXV06021/test_ml4se_colab | 66111e47f8dbdc31e625e16f01d7f27dbb1139f9 | [
"Apache-2.0"
] | null | null | null | 128,036 | 128,036 | 0.93148 | [
[
[
"# 第5章 ロジスティック回帰とROC曲線:学習モデルの評価方法",
"_____no_output_____"
],
[
"## 「05-roc_curve」の解説",
"_____no_output_____"
],
[
"ITエンジニアための機械学習理論入門「第5章 ロジスティック回帰とROC曲線:学習モデルの評価方法」で使用しているサンプルコード「05-roc_curve.py」の解説です。\n\n※ 解説用にコードの内容は少し変更しています。",
"_____no_output_____"
],
[
"はじめに必要なモジュールをインポートしておきます。\n\n関数 multivariate_normal は、多次元の正規分布に従う乱数を生成するために利用します。",
"_____no_output_____"
]
],
[
[
"import numpy as np\nimport matplotlib.pyplot as plt\nimport pandas as pd\nfrom pandas import Series, DataFrame\n\nfrom numpy.random import multivariate_normal",
"_____no_output_____"
]
],
[
[
"トレーニング用データを生成する関数を用意します。\n\n平面上の○☓の2種類のデータについて、それぞれの「個数、中心座標、分散」を引数で指定します。",
"_____no_output_____"
]
],
[
[
"def prepare_dataset(n1, mu1, variance1, n2, mu2, variance2):\n df1 = DataFrame(multivariate_normal(mu1, np.eye(2)*variance1 ,n1),\n columns=['x','y'])\n df1['type'] = 1\n df2 = DataFrame(multivariate_normal(mu2, np.eye(2)*variance2, n2),\n columns=['x','y'])\n df2['type'] = -1\n df = pd.concat([df1,df2], ignore_index=True)\n df = df.reindex(np.random.permutation(df.index)).reset_index(drop=True)\n return df",
"_____no_output_____"
]
],
[
[
"ロジスティック回帰で分割線を決定する関数を用意します。\n\nここでは、得られた結果を用いて、トレーニングセットの各データに対して確率の値を付与したデータフレームも返却するようにしています。",
"_____no_output_____"
]
],
[
[
"# ロジスティック回帰\ndef run_logistic(train_set):\n pd.options.mode.chained_assignment = None\n w = np.array([[0],[0.1],[0.1]])\n phi = train_set[['x','y']]\n phi['bias'] = 1\n phi = phi.as_matrix(columns=['bias','x','y'])\n t = (train_set[['type']] + 1)*0.5 # type = 1, -1 を type = 1, 0 に変換\n t = t.as_matrix()\n\n # 最大100回のIterationを実施\n for i in range(100):\n # IRLS法によるパラメータの修正\n y = np.array([])\n for line in phi:\n a = np.dot(line, w)\n y = np.append(y, [1.0/(1.0+np.exp(-a))])\n r = np.diag(y*(1-y)) \n y = y[np.newaxis,:].T\n tmp1 = np.linalg.inv(np.dot(np.dot(phi.T, r),phi))\n tmp2 = np.dot(phi.T, (y-t))\n w_new = w - np.dot(tmp1, tmp2)\n # パラメータの変化が 0.1% 未満になったら終了\n if np.dot((w_new-w).T, (w_new-w)) < 0.001 * np.dot(w.T, w):\n w = w_new\n break\n w = w_new\n \n # 分類誤差の計算\n w0, w1, w2 = w[0], w[1], w[2]\n err = 0\n for index, line in train_set.iterrows():\n a = np.dot(np.array([1, line.x, line.y]), w)\n p = 1.0/(1.0+np.exp(-a))\n train_set.loc[index, 'probability'] = p\n if (p-0.5)*line.type < 0:\n err += 1\n err_rate = err * 100 / len(train_set)\n result = train_set.sort_values(by=['probability'], ascending=[False]).reset_index()\n \n return w0, w1, w2, err_rate, result",
"_____no_output_____"
]
],
[
[
"結果をグラフ、および、ROC曲線として表示する関数を用意します。",
"_____no_output_____"
]
],
[
[
"# 結果の表示\ndef show_result(subplot, train_set, w0, w1, w2, err_rate):\n train_set1 = train_set[train_set['type']==1]\n train_set2 = train_set[train_set['type']==-1]\n ymin, ymax = train_set.y.min()-5, train_set.y.max()+10\n xmin, xmax = train_set.x.min()-5, train_set.x.max()+10\n\n subplot.set_ylim([ymin-1, ymax+1])\n subplot.set_xlim([xmin-1, xmax+1])\n subplot.scatter(train_set1.x, train_set1.y, marker='o', label=None)\n subplot.scatter(train_set2.x, train_set2.y, marker='x', label=None)\n\n linex = np.arange(xmin-5, xmax+5)\n liney = - linex * w1 / w2 - w0 / w2\n label = \"ERR %.2f%%\" % err_rate\n subplot.plot(linex, liney, label=label, color='red')\n subplot.legend(loc=1)",
"_____no_output_____"
],
[
"# ROC曲線の表示\ndef draw_roc(subplot, result):\n positives = len(result[result['type']==1])\n negatives = len(result[result['type']==-1])\n tp = [0.0] * len(result)\n fp = [0.0] * len(result)\n for index, line in result.iterrows():\n for c in np.arange(0, len(result)):\n if index < c:\n if line.type == 1:\n tp[c] += 1\n else:\n fp[c] += 1\n tp_rate = np.array(tp) / positives\n fp_rate = np.array(fp) / negatives\n\n subplot.set_ylim([0, 1])\n subplot.set_xlim([0, 1])\n subplot.set_xlabel(\"False positive rate\")\n subplot.set_ylabel(\"True positive rate\")\n subplot.plot(fp_rate, tp_rate)",
"_____no_output_____"
]
],
[
[
"比較的分散が小さくて、分類が容易なトレーニングセットを用意します。",
"_____no_output_____"
]
],
[
[
"train_set = prepare_dataset(80, [9,9], 50, 200, [-3,-3], 50)",
"_____no_output_____"
]
],
[
[
"ロジスティック回帰を適用した結果を表示します。",
"_____no_output_____"
]
],
[
[
"w0, w1, w2, err_rate, result = run_logistic(train_set)\nfig = plt.figure(figsize=(6, 12))\nsubplot = fig.add_subplot(2,1,1)\nshow_result(subplot, train_set, w0, w1, w2, err_rate)\nsubplot = fig.add_subplot(2,1,2)\ndraw_roc(subplot, result)",
"_____no_output_____"
]
],
[
[
"分散が大きくて、分類が困難なトレーニングセットを用意します。",
"_____no_output_____"
]
],
[
[
"train_set = prepare_dataset(80, [9,9], 150, 200, [-3,-3], 150)",
"_____no_output_____"
]
],
[
[
"ロジスティック回帰を適用した結果を表示します。",
"_____no_output_____"
]
],
[
[
"w0, w1, w2, err_rate, result = run_logistic(train_set)\nfig = plt.figure(figsize=(6, 12))\nsubplot = fig.add_subplot(2,1,1)\nshow_result(subplot, train_set, w0, w1, w2, err_rate)\nsubplot = fig.add_subplot(2,1,2)\ndraw_roc(subplot, result)",
"_____no_output_____"
]
]
] | [
"markdown",
"code",
"markdown",
"code",
"markdown",
"code",
"markdown",
"code",
"markdown",
"code",
"markdown",
"code",
"markdown",
"code",
"markdown",
"code"
] | [
[
"markdown",
"markdown",
"markdown",
"markdown"
],
[
"code"
],
[
"markdown"
],
[
"code"
],
[
"markdown"
],
[
"code"
],
[
"markdown"
],
[
"code",
"code"
],
[
"markdown"
],
[
"code"
],
[
"markdown"
],
[
"code"
],
[
"markdown"
],
[
"code"
],
[
"markdown"
],
[
"code"
]
] |
e786331a4fa06c03fc3933271cb7f4a0d96f2060 | 877,096 | ipynb | Jupyter Notebook | python-machine-learning-book-2nd-edition/code/ch11/ch11.ipynb | gopala-kr/ds-notebooks | bc35430ecdd851f2ceab8f2437eec4d77cb59423 | [
"MIT"
] | 464 | 2017-09-16T00:46:21.000Z | 2022-02-17T03:44:54.000Z | Chapter11/ch11.ipynb | wanpong007/Python-Machine-Learning-Second-Edition | 14165d66b58736bba8d3c53110c81c619ad410b9 | [
"MIT"
] | 1 | 2019-11-11T14:48:37.000Z | 2020-08-19T05:33:01.000Z | Chapter11/ch11.ipynb | wanpong007/Python-Machine-Learning-Second-Edition | 14165d66b58736bba8d3c53110c81c619ad410b9 | [
"MIT"
] | 354 | 2017-09-07T18:44:35.000Z | 2022-03-29T10:42:26.000Z | 607.828136 | 493,906 | 0.935481 | [
[
[
"*Python Machine Learning 2nd Edition* by [Sebastian Raschka](https://sebastianraschka.com), Packt Publishing Ltd. 2017\n\nCode Repository: https://github.com/rasbt/python-machine-learning-book-2nd-edition\n\nCode License: [MIT License](https://github.com/rasbt/python-machine-learning-book-2nd-edition/blob/master/LICENSE.txt)",
"_____no_output_____"
],
[
"# Python Machine Learning - Code Examples",
"_____no_output_____"
],
[
"# Chapter 11 - Working with Unlabeled Data – Clustering Analysis",
"_____no_output_____"
],
[
"Note that the optional watermark extension is a small IPython notebook plugin that I developed to make the code reproducible. You can just skip the following line(s).",
"_____no_output_____"
]
],
[
[
"%load_ext watermark\n%watermark -a \"Sebastian Raschka\" -u -d -v -p numpy,pandas,matplotlib,scipy,sklearn",
"Sebastian Raschka \nlast updated: 2017-08-25 \n\nCPython 3.6.1\nIPython 6.1.0\n\nnumpy 1.12.1\npandas 0.20.3\nmatplotlib 2.0.2\nscipy 0.19.1\nsklearn 0.19.0\n"
]
],
[
[
"*The use of `watermark` is optional. You can install this IPython extension via \"`pip install watermark`\". For more information, please see: https://github.com/rasbt/watermark.*",
"_____no_output_____"
],
[
"<br>\n<br>",
"_____no_output_____"
],
[
"### Overview",
"_____no_output_____"
],
[
"- [Grouping objects by similarity using k-means](#Grouping-objects-by-similarity-using-k-means)\n - [K-means clustering using scikit-learn](#K-means-clustering-using-scikit-learn)\n - [A smarter way of placing the initial cluster centroids using k-means++](#A-smarter-way-of-placing-the-initial-cluster-centroids-using-k-means++)\n - [Hard versus soft clustering](#Hard-versus-soft-clustering)\n - [Using the elbow method to find the optimal number of clusters](#Using-the-elbow-method-to-find-the-optimal-number-of-clusters)\n - [Quantifying the quality of clustering via silhouette plots](#Quantifying-the-quality-of-clustering-via-silhouette-plots)\n- [Organizing clusters as a hierarchical tree](#Organizing-clusters-as-a-hierarchical-tree)\n - [Grouping clusters in bottom-up fashion](#Grouping-clusters-in-bottom-up-fashion)\n - [Performing hierarchical clustering on a distance matrix](#Performing-hierarchical-clustering-on-a-distance-matrix)\n - [Attaching dendrograms to a heat map](#Attaching-dendrograms-to-a-heat-map)\n - [Applying agglomerative clustering via scikit-learn](#Applying-agglomerative-clustering-via-scikit-learn)\n- [Locating regions of high density via DBSCAN](#Locating-regions-of-high-density-via-DBSCAN)\n- [Summary](#Summary)",
"_____no_output_____"
],
[
"<br>\n<br>",
"_____no_output_____"
]
],
[
[
"from IPython.display import Image\n%matplotlib inline",
"_____no_output_____"
]
],
[
[
"# Grouping objects by similarity using k-means",
"_____no_output_____"
],
[
"## K-means clustering using scikit-learn",
"_____no_output_____"
]
],
[
[
"from sklearn.datasets import make_blobs\n\nX, y = make_blobs(n_samples=150, \n n_features=2, \n centers=3, \n cluster_std=0.5, \n shuffle=True, \n random_state=0)",
"_____no_output_____"
],
[
"import matplotlib.pyplot as plt\n\nplt.scatter(X[:, 0], X[:, 1], \n c='white', marker='o', edgecolor='black', s=50)\nplt.grid()\nplt.tight_layout()\n#plt.savefig('images/11_01.png', dpi=300)\nplt.show()",
"_____no_output_____"
],
[
"from sklearn.cluster import KMeans\n\nkm = KMeans(n_clusters=3, \n init='random', \n n_init=10, \n max_iter=300,\n tol=1e-04,\n random_state=0)\n\ny_km = km.fit_predict(X)",
"_____no_output_____"
],
[
"plt.scatter(X[y_km == 0, 0],\n X[y_km == 0, 1],\n s=50, c='lightgreen',\n marker='s', edgecolor='black',\n label='cluster 1')\nplt.scatter(X[y_km == 1, 0],\n X[y_km == 1, 1],\n s=50, c='orange',\n marker='o', edgecolor='black',\n label='cluster 2')\nplt.scatter(X[y_km == 2, 0],\n X[y_km == 2, 1],\n s=50, c='lightblue',\n marker='v', edgecolor='black',\n label='cluster 3')\nplt.scatter(km.cluster_centers_[:, 0],\n km.cluster_centers_[:, 1],\n s=250, marker='*',\n c='red', edgecolor='black',\n label='centroids')\nplt.legend(scatterpoints=1)\nplt.grid()\nplt.tight_layout()\n#plt.savefig('images/11_02.png', dpi=300)\nplt.show()",
"_____no_output_____"
]
],
[
[
"<br>",
"_____no_output_____"
],
[
"## A smarter way of placing the initial cluster centroids using k-means++",
"_____no_output_____"
],
[
"...",
"_____no_output_____"
],
[
"## Hard versus soft clustering",
"_____no_output_____"
],
[
"...",
"_____no_output_____"
],
[
"## Using the elbow method to find the optimal number of clusters ",
"_____no_output_____"
]
],
[
[
"print('Distortion: %.2f' % km.inertia_)",
"Distortion: 72.48\n"
],
[
"distortions = []\nfor i in range(1, 11):\n km = KMeans(n_clusters=i, \n init='k-means++', \n n_init=10, \n max_iter=300, \n random_state=0)\n km.fit(X)\n distortions.append(km.inertia_)\nplt.plot(range(1, 11), distortions, marker='o')\nplt.xlabel('Number of clusters')\nplt.ylabel('Distortion')\nplt.tight_layout()\n#plt.savefig('images/11_03.png', dpi=300)\nplt.show()",
"_____no_output_____"
]
],
[
[
"<br>",
"_____no_output_____"
],
[
"## Quantifying the quality of clustering via silhouette plots",
"_____no_output_____"
]
],
[
[
"import numpy as np\nfrom matplotlib import cm\nfrom sklearn.metrics import silhouette_samples\n\nkm = KMeans(n_clusters=3, \n init='k-means++', \n n_init=10, \n max_iter=300,\n tol=1e-04,\n random_state=0)\ny_km = km.fit_predict(X)\n\ncluster_labels = np.unique(y_km)\nn_clusters = cluster_labels.shape[0]\nsilhouette_vals = silhouette_samples(X, y_km, metric='euclidean')\ny_ax_lower, y_ax_upper = 0, 0\nyticks = []\nfor i, c in enumerate(cluster_labels):\n c_silhouette_vals = silhouette_vals[y_km == c]\n c_silhouette_vals.sort()\n y_ax_upper += len(c_silhouette_vals)\n color = cm.jet(float(i) / n_clusters)\n plt.barh(range(y_ax_lower, y_ax_upper), c_silhouette_vals, height=1.0, \n edgecolor='none', color=color)\n\n yticks.append((y_ax_lower + y_ax_upper) / 2.)\n y_ax_lower += len(c_silhouette_vals)\n \nsilhouette_avg = np.mean(silhouette_vals)\nplt.axvline(silhouette_avg, color=\"red\", linestyle=\"--\") \n\nplt.yticks(yticks, cluster_labels + 1)\nplt.ylabel('Cluster')\nplt.xlabel('Silhouette coefficient')\n\nplt.tight_layout()\n#plt.savefig('images/11_04.png', dpi=300)\nplt.show()",
"_____no_output_____"
]
],
[
[
"Comparison to \"bad\" clustering:",
"_____no_output_____"
]
],
[
[
"km = KMeans(n_clusters=2,\n init='k-means++',\n n_init=10,\n max_iter=300,\n tol=1e-04,\n random_state=0)\ny_km = km.fit_predict(X)\n\nplt.scatter(X[y_km == 0, 0],\n X[y_km == 0, 1],\n s=50,\n c='lightgreen',\n edgecolor='black',\n marker='s',\n label='cluster 1')\nplt.scatter(X[y_km == 1, 0],\n X[y_km == 1, 1],\n s=50,\n c='orange',\n edgecolor='black',\n marker='o',\n label='cluster 2')\n\nplt.scatter(km.cluster_centers_[:, 0], km.cluster_centers_[:, 1],\n s=250, marker='*', c='red', label='centroids')\nplt.legend()\nplt.grid()\nplt.tight_layout()\n#plt.savefig('images/11_05.png', dpi=300)\nplt.show()",
"_____no_output_____"
],
[
"cluster_labels = np.unique(y_km)\nn_clusters = cluster_labels.shape[0]\nsilhouette_vals = silhouette_samples(X, y_km, metric='euclidean')\ny_ax_lower, y_ax_upper = 0, 0\nyticks = []\nfor i, c in enumerate(cluster_labels):\n c_silhouette_vals = silhouette_vals[y_km == c]\n c_silhouette_vals.sort()\n y_ax_upper += len(c_silhouette_vals)\n color = cm.jet(float(i) / n_clusters)\n plt.barh(range(y_ax_lower, y_ax_upper), c_silhouette_vals, height=1.0, \n edgecolor='none', color=color)\n\n yticks.append((y_ax_lower + y_ax_upper) / 2.)\n y_ax_lower += len(c_silhouette_vals)\n \nsilhouette_avg = np.mean(silhouette_vals)\nplt.axvline(silhouette_avg, color=\"red\", linestyle=\"--\") \n\nplt.yticks(yticks, cluster_labels + 1)\nplt.ylabel('Cluster')\nplt.xlabel('Silhouette coefficient')\n\nplt.tight_layout()\n#plt.savefig('images/11_06.png', dpi=300)\nplt.show()",
"_____no_output_____"
]
],
[
[
"<br>\n<br>",
"_____no_output_____"
],
[
"# Organizing clusters as a hierarchical tree",
"_____no_output_____"
],
[
"## Grouping clusters in bottom-up fashion",
"_____no_output_____"
]
],
[
[
"Image(filename='./images/11_05.png', width=400) ",
"_____no_output_____"
],
[
"import pandas as pd\nimport numpy as np\n\nnp.random.seed(123)\n\nvariables = ['X', 'Y', 'Z']\nlabels = ['ID_0', 'ID_1', 'ID_2', 'ID_3', 'ID_4']\n\nX = np.random.random_sample([5, 3])*10\ndf = pd.DataFrame(X, columns=variables, index=labels)\ndf",
"_____no_output_____"
]
],
[
[
"<br>",
"_____no_output_____"
],
[
"## Performing hierarchical clustering on a distance matrix",
"_____no_output_____"
]
],
[
[
"from scipy.spatial.distance import pdist, squareform\n\nrow_dist = pd.DataFrame(squareform(pdist(df, metric='euclidean')),\n columns=labels,\n index=labels)\nrow_dist",
"_____no_output_____"
]
],
[
[
"We can either pass a condensed distance matrix (upper triangular) from the `pdist` function, or we can pass the \"original\" data array and define the `metric='euclidean'` argument in `linkage`. However, we should not pass the squareform distance matrix, which would yield different distance values although the overall clustering could be the same.",
"_____no_output_____"
]
],
[
[
"# 1. incorrect approach: Squareform distance matrix\n\nfrom scipy.cluster.hierarchy import linkage\n\nrow_clusters = linkage(row_dist, method='complete', metric='euclidean')\npd.DataFrame(row_clusters,\n columns=['row label 1', 'row label 2',\n 'distance', 'no. of items in clust.'],\n index=['cluster %d' % (i + 1)\n for i in range(row_clusters.shape[0])])",
"/Users/sebastian/miniconda3/lib/python3.6/site-packages/ipykernel_launcher.py:5: ClusterWarning: scipy.cluster: The symmetric non-negative hollow observation matrix looks suspiciously like an uncondensed distance matrix\n \"\"\"\n"
],
[
"# 2. correct approach: Condensed distance matrix\n\nrow_clusters = linkage(pdist(df, metric='euclidean'), method='complete')\npd.DataFrame(row_clusters,\n columns=['row label 1', 'row label 2',\n 'distance', 'no. of items in clust.'],\n index=['cluster %d' % (i + 1) \n for i in range(row_clusters.shape[0])])",
"_____no_output_____"
],
[
"# 3. correct approach: Input sample matrix\n\nrow_clusters = linkage(df.values, method='complete', metric='euclidean')\npd.DataFrame(row_clusters,\n columns=['row label 1', 'row label 2',\n 'distance', 'no. of items in clust.'],\n index=['cluster %d' % (i + 1)\n for i in range(row_clusters.shape[0])])",
"_____no_output_____"
],
[
"from scipy.cluster.hierarchy import dendrogram\n\n# make dendrogram black (part 1/2)\n# from scipy.cluster.hierarchy import set_link_color_palette\n# set_link_color_palette(['black'])\n\nrow_dendr = dendrogram(row_clusters, \n labels=labels,\n # make dendrogram black (part 2/2)\n # color_threshold=np.inf\n )\nplt.tight_layout()\nplt.ylabel('Euclidean distance')\n#plt.savefig('images/11_11.png', dpi=300, \n# bbox_inches='tight')\nplt.show()",
"_____no_output_____"
]
],
[
[
"<br>",
"_____no_output_____"
],
[
"## Attaching dendrograms to a heat map",
"_____no_output_____"
]
],
[
[
"# plot row dendrogram\nfig = plt.figure(figsize=(8, 8), facecolor='white')\naxd = fig.add_axes([0.09, 0.1, 0.2, 0.6])\n\n# note: for matplotlib < v1.5.1, please use orientation='right'\nrow_dendr = dendrogram(row_clusters, orientation='left')\n\n# reorder data with respect to clustering\ndf_rowclust = df.iloc[row_dendr['leaves'][::-1]]\n\naxd.set_xticks([])\naxd.set_yticks([])\n\n# remove axes spines from dendrogram\nfor i in axd.spines.values():\n i.set_visible(False)\n\n# plot heatmap\naxm = fig.add_axes([0.23, 0.1, 0.6, 0.6]) # x-pos, y-pos, width, height\ncax = axm.matshow(df_rowclust, interpolation='nearest', cmap='hot_r')\nfig.colorbar(cax)\naxm.set_xticklabels([''] + list(df_rowclust.columns))\naxm.set_yticklabels([''] + list(df_rowclust.index))\n\n#plt.savefig('images/11_12.png', dpi=300)\nplt.show()",
"_____no_output_____"
]
],
[
[
"<br>",
"_____no_output_____"
],
[
"## Applying agglomerative clustering via scikit-learn",
"_____no_output_____"
]
],
[
[
"from sklearn.cluster import AgglomerativeClustering\n\nac = AgglomerativeClustering(n_clusters=3, \n affinity='euclidean', \n linkage='complete')\nlabels = ac.fit_predict(X)\nprint('Cluster labels: %s' % labels)",
"Cluster labels: [1 0 0 2 1]\n"
],
[
"ac = AgglomerativeClustering(n_clusters=2, \n affinity='euclidean', \n linkage='complete')\nlabels = ac.fit_predict(X)\nprint('Cluster labels: %s' % labels)",
"Cluster labels: [0 1 1 0 0]\n"
]
],
[
[
"<br>\n<br>",
"_____no_output_____"
],
[
"# Locating regions of high density via DBSCAN",
"_____no_output_____"
]
],
[
[
"Image(filename='images/11_13.png', width=500) ",
"_____no_output_____"
],
[
"from sklearn.datasets import make_moons\n\nX, y = make_moons(n_samples=200, noise=0.05, random_state=0)\nplt.scatter(X[:, 0], X[:, 1])\nplt.tight_layout()\n#plt.savefig('images/11_14.png', dpi=300)\nplt.show()",
"_____no_output_____"
]
],
[
[
"K-means and hierarchical clustering:",
"_____no_output_____"
]
],
[
[
"f, (ax1, ax2) = plt.subplots(1, 2, figsize=(8, 3))\n\nkm = KMeans(n_clusters=2, random_state=0)\ny_km = km.fit_predict(X)\nax1.scatter(X[y_km == 0, 0], X[y_km == 0, 1],\n edgecolor='black',\n c='lightblue', marker='o', s=40, label='cluster 1')\nax1.scatter(X[y_km == 1, 0], X[y_km == 1, 1],\n edgecolor='black',\n c='red', marker='s', s=40, label='cluster 2')\nax1.set_title('K-means clustering')\n\nac = AgglomerativeClustering(n_clusters=2,\n affinity='euclidean',\n linkage='complete')\ny_ac = ac.fit_predict(X)\nax2.scatter(X[y_ac == 0, 0], X[y_ac == 0, 1], c='lightblue',\n edgecolor='black',\n marker='o', s=40, label='cluster 1')\nax2.scatter(X[y_ac == 1, 0], X[y_ac == 1, 1], c='red',\n edgecolor='black',\n marker='s', s=40, label='cluster 2')\nax2.set_title('Agglomerative clustering')\n\nplt.legend()\nplt.tight_layout()\n# plt.savefig('images/11_15.png', dpi=300)\nplt.show()",
"_____no_output_____"
]
],
[
[
"Density-based clustering:",
"_____no_output_____"
]
],
[
[
"from sklearn.cluster import DBSCAN\n\ndb = DBSCAN(eps=0.2, min_samples=5, metric='euclidean')\ny_db = db.fit_predict(X)\nplt.scatter(X[y_db == 0, 0], X[y_db == 0, 1],\n c='lightblue', marker='o', s=40,\n edgecolor='black', \n label='cluster 1')\nplt.scatter(X[y_db == 1, 0], X[y_db == 1, 1],\n c='red', marker='s', s=40,\n edgecolor='black', \n label='cluster 2')\nplt.legend()\nplt.tight_layout()\n#plt.savefig('images/11_16.png', dpi=300)\nplt.show()",
"_____no_output_____"
]
],
[
[
"<br>\n<br>",
"_____no_output_____"
],
[
"# Summary",
"_____no_output_____"
],
[
"...",
"_____no_output_____"
],
[
"---\n\nReaders may ignore the next cell.",
"_____no_output_____"
]
],
[
[
"! python ../.convert_notebook_to_script.py --input ch11.ipynb --output ch11.py",
"[NbConvertApp] Converting notebook ch11.ipynb to script\n[NbConvertApp] Writing 14002 bytes to ch11.py\n"
]
]
] | [
"markdown",
"code",
"markdown",
"code",
"markdown",
"code",
"markdown",
"code",
"markdown",
"code",
"markdown",
"code",
"markdown",
"code",
"markdown",
"code",
"markdown",
"code",
"markdown",
"code",
"markdown",
"code",
"markdown",
"code",
"markdown",
"code",
"markdown",
"code",
"markdown",
"code"
] | [
[
"markdown",
"markdown",
"markdown",
"markdown"
],
[
"code"
],
[
"markdown",
"markdown",
"markdown",
"markdown",
"markdown"
],
[
"code"
],
[
"markdown",
"markdown"
],
[
"code",
"code",
"code",
"code"
],
[
"markdown",
"markdown",
"markdown",
"markdown",
"markdown",
"markdown"
],
[
"code",
"code"
],
[
"markdown",
"markdown"
],
[
"code"
],
[
"markdown"
],
[
"code",
"code"
],
[
"markdown",
"markdown",
"markdown"
],
[
"code",
"code"
],
[
"markdown",
"markdown"
],
[
"code"
],
[
"markdown"
],
[
"code",
"code",
"code",
"code"
],
[
"markdown",
"markdown"
],
[
"code"
],
[
"markdown",
"markdown"
],
[
"code",
"code"
],
[
"markdown",
"markdown"
],
[
"code",
"code"
],
[
"markdown"
],
[
"code"
],
[
"markdown"
],
[
"code"
],
[
"markdown",
"markdown",
"markdown",
"markdown"
],
[
"code"
]
] |
e786423c4208833d6f4a8cc428707839d135ce3b | 19,808 | ipynb | Jupyter Notebook | OutOfStateCash.ipynb | NebFinance/out-of-state-cash | f96166496b9962993e11e2b78e8fc2f22c0462e0 | [
"MIT"
] | null | null | null | OutOfStateCash.ipynb | NebFinance/out-of-state-cash | f96166496b9962993e11e2b78e8fc2f22c0462e0 | [
"MIT"
] | null | null | null | OutOfStateCash.ipynb | NebFinance/out-of-state-cash | f96166496b9962993e11e2b78e8fc2f22c0462e0 | [
"MIT"
] | null | null | null | 50.920308 | 3,634 | 0.459865 | [
[
[
"import agate\nimport warnings\nwarnings.filterwarnings(\"ignore\")",
"_____no_output_____"
],
[
"donations = agate.Table.from_csv('formb1ab.csv')",
"_____no_output_____"
],
[
"print(donations)",
"| column | data_type |\n| ----------------------------- | --------- |\n| Committee Name | Text |\n| Committee ID | Text |\n| Date Received | Date |\n| Type of Contributor | Text |\n| Contributor ID | Text |\n| Contribution Date | Date |\n| Cash Contribution | Number |\n| In-Kind Contribution | Number |\n| Unpaid Pledges | Number |\n| Contributor Last Name | Text |\n| Contributor First Name | Text |\n| Contributor Middle Initial | Text |\n| Contributor Organization Name | Text |\n| Contributor Address | Text |\n| Contributor City | Text |\n| Contributor State | Text |\n| Contributor Zipcode | Text |\n\n"
],
[
"donations_since_2000 = donations.where(lambda row: 2000 <= row['Contribution Date'].year <= 2016)",
"_____no_output_____"
],
[
"by_state = donations_since_2000.group_by('Contributor State')",
"_____no_output_____"
],
[
"state_totals = by_state.aggregate([\n ('count', agate.Count()),\n ('average cash contribution', agate.Mean('Cash Contribution'))\n])\n\nsorted_totals = state_totals.order_by('count', reverse=True)\n\nsorted_totals.print_table(max_rows=25)\n",
"| Contributor State | count | average cash cont... |\n| ----------------- | ------ | -------------------- |\n| NE | 88,687 | 1,020.513… |\n| | 1,924 | 1,241.672… |\n| IA | 1,098 | 798.997… |\n| DC | 910 | 4,381.145… |\n| TX | 906 | 1,170.433… |\n| MO | 686 | 1,243.817… |\n| IL | 610 | 3,000.743… |\n| CO | 552 | 1,386.991… |\n| NY | 542 | 1,322.986… |\n| CA | 419 | 3,065.049… |\n| VA | 389 | 1,677.951… |\n| KS | 362 | 1,194.319… |\n| MN | 316 | 3,253.630… |\n| NC | 314 | 915.335… |\n| GA | 289 | 1,257.903… |\n| SD | 225 | 1,054.566… |\n| WI | 209 | 1,208.167… |\n| FL | 199 | 1,545.338… |\n| AZ | 198 | 1,667.354… |\n| AR | 161 | 1,241.887… |\n| MI | 146 | 1,901.954… |\n| OH | 134 | 1,776.880… |\n| PA | 128 | 1,076.838… |\n| NJ | 125 | 602.839… |\n| IN | 116 | 1,128.233… |\n| ... | ... | ... |\n"
],
[
"def in_state(row):\n if row['Contributor State'] == \"NE\":\n locale = \"In-state\"\n else:\n locale = \"Out-of-state\"\n return locale\n\ncontribs_by_locale = donations_since_2000.compute([\n ('locale', agate.Formula(agate.Text(), in_state)),\n ('year', agate.Formula(agate.Number(), lambda row: '%i' % row['Contribution Date'].year))\n])",
"_____no_output_____"
],
[
"print(contribs_by_locale)",
"| column | data_type |\n| ----------------------------- | --------- |\n| Committee Name | Text |\n| Committee ID | Text |\n| Date Received | Date |\n| Type of Contributor | Text |\n| Contributor ID | Text |\n| Contribution Date | Date |\n| Cash Contribution | Number |\n| In-Kind Contribution | Number |\n| Unpaid Pledges | Number |\n| Contributor Last Name | Text |\n| Contributor First Name | Text |\n| Contributor Middle Initial | Text |\n| Contributor Organization Name | Text |\n| Contributor Address | Text |\n| Contributor City | Text |\n| Contributor State | Text |\n| Contributor Zipcode | Text |\n| locale | Text |\n| year | Number |\n\n"
],
[
"contribs_by_locale.print_table(max_rows=5)",
"| Committee Name | Committee ID | Date Received | Type of Contributor | Contributor ID | Contribution Date | ... |\n| -------------------- | ------------ | ------------- | ------------------- | -------------- | ----------------- | --- |\n| CITIZENS FOR NORT... | 99BQC00037 | 2000-03-03 | I | 99CON02096 | 2000-02-29 | ... |\n| CITIZENS FOR NORT... | 99BQC00037 | 2000-03-03 | I | 99CON02093 | 2000-01-21 | ... |\n| CITIZENS FOR NORT... | 99BQC00037 | 2000-03-03 | I | 99CON01145 | 2000-01-26 | ... |\n| CITIZENS FOR NORT... | 99BQC00037 | 2000-03-03 | I | 99CON02094 | 2000-02-16 | ... |\n| CITIZENS FOR NORT... | 99BQC00037 | 2000-03-03 | I | 99CON02095 | 2000-02-16 | ... |\n| ... | ... | ... | ... | ... | ... | ... |\n"
],
[
"by_locale_by_year = contribs_by_locale.group_by('locale').group_by('year')",
"_____no_output_____"
],
[
"locale_totals = by_locale_by_year.aggregate([\n ('count', agate.Count()),\n ('average cash contribution', agate.Mean('Cash Contribution'))\n])",
"_____no_output_____"
],
[
"locale_totals.order_by(['locale', 'year']).print_table(max_rows=50)",
"| locale | year | count | average cash cont... |\n| ------------ | ----- | ------ | -------------------- |\n| In-state | 2,000 | 3,330 | 946.076… |\n| In-state | 2,001 | 3,972 | 922.352… |\n| In-state | 2,002 | 4,469 | 867.462… |\n| In-state | 2,003 | 1,956 | 671.867… |\n| In-state | 2,004 | 3,498 | 1,935.598… |\n| In-state | 2,005 | 4,698 | 870.634… |\n| In-state | 2,006 | 8,235 | 1,104.711… |\n| In-state | 2,007 | 3,439 | 734.352… |\n| In-state | 2,008 | 5,313 | 828.067… |\n| In-state | 2,009 | 5,485 | 846.334… |\n| In-state | 2,010 | 6,023 | 828.565… |\n| In-state | 2,011 | 2,367 | 809.634… |\n| In-state | 2,012 | 5,944 | 864.884… |\n| In-state | 2,013 | 6,151 | 1,140.225… |\n| In-state | 2,014 | 12,879 | 1,277.459… |\n| In-state | 2,015 | 4,096 | 1,044.081… |\n| In-state | 2,016 | 6,832 | 1,021.273… |\n| Out-of-state | 2,000 | 1,017 | 1,078.244… |\n| Out-of-state | 2,001 | 426 | 982.086… |\n| Out-of-state | 2,002 | 527 | 847.303… |\n| Out-of-state | 2,003 | 251 | 653.973… |\n| Out-of-state | 2,004 | 531 | 7,969.453… |\n| Out-of-state | 2,005 | 611 | 1,430.321… |\n| Out-of-state | 2,006 | 869 | 7,760.572… |\n| Out-of-state | 2,007 | 430 | 814.551… |\n| Out-of-state | 2,008 | 708 | 1,812.813… |\n| Out-of-state | 2,009 | 643 | 1,555.753… |\n| Out-of-state | 2,010 | 679 | 883.443… |\n| Out-of-state | 2,011 | 394 | 752.216… |\n| Out-of-state | 2,012 | 936 | 735.965… |\n| Out-of-state | 2,013 | 858 | 3,438.500… |\n| Out-of-state | 2,014 | 1,646 | 2,209.352… |\n| Out-of-state | 2,015 | 499 | 2,572.022… |\n| Out-of-state | 2,016 | 1,054 | 2,780.889… |\n"
],
[
"locale_totals.where(lambda row: row['locale'] == \"Out-of-state\").line_chart('year', 'count')",
"_____no_output_____"
],
[
"locale_totals.where(lambda row: row['locale'] == \"Out-of-state\").line_chart('year', 'average cash contribution')",
"_____no_output_____"
]
]
] | [
"code"
] | [
[
"code",
"code",
"code",
"code",
"code",
"code",
"code",
"code",
"code",
"code",
"code",
"code",
"code",
"code"
]
] |
e7864dba92e72eab040fdc06bfb195439325066e | 433,176 | ipynb | Jupyter Notebook | assignment2/BatchNormalization.ipynb | lalithnag/cs231n | ed540c4ed06a6ee01966314e4106b8c44f58546b | [
"MIT"
] | null | null | null | assignment2/BatchNormalization.ipynb | lalithnag/cs231n | ed540c4ed06a6ee01966314e4106b8c44f58546b | [
"MIT"
] | null | null | null | assignment2/BatchNormalization.ipynb | lalithnag/cs231n | ed540c4ed06a6ee01966314e4106b8c44f58546b | [
"MIT"
] | null | null | null | 388.150538 | 118,212 | 0.9291 | [
[
[
"# Batch Normalization\nOne way to make deep networks easier to train is to use more sophisticated optimization procedures such as SGD+momentum, RMSProp, or Adam. Another strategy is to change the architecture of the network to make it easier to train. \nOne idea along these lines is batch normalization which was proposed by [3] in 2015.\n\nThe idea is relatively straightforward. _Machine learning methods tend to work better when their input data consists of uncorrelated features with zero mean and unit variance._ When training a neural network, we can preprocess the data before feeding it to the network to explicitly decorrelate its features; this will ensure that the first layer of the network sees data that follows a nice distribution. However, even if we preprocess the input data, the activations at deeper layers of the network will likely no longer be decorrelated and will no longer have zero mean or unit variance since they are output from earlier layers in the network. Even worse, during the training process the distribution of features at each layer of the network will shift as the weights of each layer are updated.\n\nThe authors of [3] hypothesize that the shifting distribution of features inside deep neural networks may make training deep networks more difficult. To overcome this problem, [3] proposes to insert batch normalization layers into the network. At training time, a batch normalization layer uses a minibatch of data to estimate the mean and standard deviation of each feature. These estimated means and standard deviations are then used to center and normalize the features of the minibatch. A running average of these means and standard deviations is kept during training, and at test time these running averages are used to center and normalize features.\n\nIt is possible that this normalization strategy could reduce the representational power of the network, since it may sometimes be optimal for certain layers to have features that are not zero-mean or unit variance. To this end, the batch normalization layer includes learnable shift and scale parameters for each feature dimension.\n\n[3] [Sergey Ioffe and Christian Szegedy, \"Batch Normalization: Accelerating Deep Network Training by Reducing\nInternal Covariate Shift\", ICML 2015.](https://arxiv.org/abs/1502.03167)",
"_____no_output_____"
]
],
[
[
"# As usual, a bit of setup\nimport time\nimport numpy as np\nimport matplotlib.pyplot as plt\nfrom cs231n.classifiers.fc_net import *\nfrom cs231n.data_utils import get_CIFAR10_data\nfrom cs231n.gradient_check import eval_numerical_gradient, eval_numerical_gradient_array\nfrom cs231n.solver import Solver\n\n%matplotlib inline\nplt.rcParams['figure.figsize'] = (10.0, 8.0) # set default size of plots\nplt.rcParams['image.interpolation'] = 'nearest'\nplt.rcParams['image.cmap'] = 'gray'\n\n# for auto-reloading external modules\n# see http://stackoverflow.com/questions/1907993/autoreload-of-modules-in-ipython\n%load_ext autoreload\n%autoreload 2\n\ndef rel_error(x, y):\n \"\"\" returns relative error \"\"\"\n return np.max(np.abs(x - y) / (np.maximum(1e-8, np.abs(x) + np.abs(y))))\n\ndef print_mean_std(x,axis=0):\n print(' means: ', x.mean(axis=axis))\n print(' stds: ', x.std(axis=axis))\n print() ",
"The autoreload extension is already loaded. To reload it, use:\n %reload_ext autoreload\n"
],
[
"# Load the (preprocessed) CIFAR10 data.\ndata = get_CIFAR10_data()\nfor k, v in data.items():\n print('%s: ' % k, v.shape)",
"X_train: (49000, 3, 32, 32)\ny_train: (49000,)\nX_val: (1000, 3, 32, 32)\ny_val: (1000,)\nX_test: (1000, 3, 32, 32)\ny_test: (1000,)\n"
]
],
[
[
"## Batch normalization: forward\nIn the file `cs231n/layers.py`, implement the batch normalization forward pass in the function `batchnorm_forward`. Once you have done so, run the following to test your implementation.\n\nReferencing the paper linked to above would be helpful!",
"_____no_output_____"
]
],
[
[
"# Check the training-time forward pass by checking means and variances\n# of features both before and after batch normalization \n\n# Simulate the forward pass for a two-layer network\nnp.random.seed(231)\nN, D1, D2, D3 = 200, 50, 60, 3\nX = np.random.randn(N, D1)\nW1 = np.random.randn(D1, D2)\nW2 = np.random.randn(D2, D3)\na = np.maximum(0, X.dot(W1)).dot(W2)\n\nprint('Before batch normalization:')\nprint_mean_std(a,axis=0)\n\ngamma = np.ones((D3,))\nbeta = np.zeros((D3,))\n# Means should be close to zero and stds close to one\nprint('After batch normalization (gamma=1, beta=0)')\na_norm, _ = batchnorm_forward(a, gamma, beta, {'mode': 'train'})\nprint_mean_std(a_norm,axis=0)\n\ngamma = np.asarray([1.0, 2.0, 3.0])\nbeta = np.asarray([11.0, 12.0, 13.0])\n# Now means should be close to beta and stds close to gamma\nprint('After batch normalization (gamma=', gamma, ', beta=', beta, ')')\na_norm, _ = batchnorm_forward(a, gamma, beta, {'mode': 'train'})\nprint_mean_std(a_norm,axis=0)",
"Before batch normalization:\n means: [ -2.3814598 -13.18038246 1.91780462]\n stds: [27.18502186 34.21455511 37.68611762]\n\nAfter batch normalization (gamma=1, beta=0)\n means: [5.99520433e-17 6.93889390e-17 8.32667268e-19]\n stds: [0.99999999 1. 1. ]\n\nAfter batch normalization (gamma= [1. 2. 3.] , beta= [11. 12. 13.] )\n means: [11. 12. 13.]\n stds: [0.99999999 1.99999999 2.99999999]\n\n"
],
[
"# Check the test-time forward pass by running the training-time\n# forward pass many times to warm up the running averages, and then\n# checking the means and variances of activations after a test-time\n# forward pass.\n\nnp.random.seed(231)\nN, D1, D2, D3 = 200, 50, 60, 3\nW1 = np.random.randn(D1, D2)\nW2 = np.random.randn(D2, D3)\n\nbn_param = {'mode': 'train'}\ngamma = np.ones(D3)\nbeta = np.zeros(D3)\n\nfor t in range(50):\n X = np.random.randn(N, D1)\n a = np.maximum(0, X.dot(W1)).dot(W2)\n batchnorm_forward(a, gamma, beta, bn_param)\n\nbn_param['mode'] = 'test'\nX = np.random.randn(N, D1)\na = np.maximum(0, X.dot(W1)).dot(W2)\na_norm, _ = batchnorm_forward(a, gamma, beta, bn_param)\n\n# Means should be close to zero and stds close to one, but will be\n# noisier than training-time forward passes.\nprint('After batch normalization (test-time):')\nprint_mean_std(a_norm,axis=0)",
"After batch normalization (test-time):\n means: [-0.03927353 -0.04349151 -0.10452686]\n stds: [1.01531399 1.01238345 0.97819961]\n\n"
]
],
[
[
"## Batch normalization: backward\nNow implement the backward pass for batch normalization in the function `batchnorm_backward`.\n\nTo derive the backward pass you should write out the computation graph for batch normalization and backprop through each of the intermediate nodes. Some intermediates may have multiple outgoing branches; make sure to sum gradients across these branches in the backward pass.\n\nOnce you have finished, run the following to numerically check your backward pass.",
"_____no_output_____"
]
],
[
[
"# Gradient check batchnorm backward pass\nnp.random.seed(231)\nN, D = 4, 5\nx = 5 * np.random.randn(N, D) + 12\ngamma = np.random.randn(D)\nbeta = np.random.randn(D)\ndout = np.random.randn(N, D)\n\nbn_param = {'mode': 'train'}\nfx = lambda x: batchnorm_forward(x, gamma, beta, bn_param)[0]\nfg = lambda a: batchnorm_forward(x, a, beta, bn_param)[0]\nfb = lambda b: batchnorm_forward(x, gamma, b, bn_param)[0]\n\ndx_num = eval_numerical_gradient_array(fx, x, dout)\nda_num = eval_numerical_gradient_array(fg, gamma.copy(), dout)\ndb_num = eval_numerical_gradient_array(fb, beta.copy(), dout)\n\n_, cache = batchnorm_forward(x, gamma, beta, bn_param)\ndx, dgamma, dbeta = batchnorm_backward(dout, cache)\n#You should expect to see relative errors between 1e-13 and 1e-8\nprint('dx error: ', rel_error(dx_num, dx))\nprint('dgamma error: ', rel_error(da_num, dgamma))\nprint('dbeta error: ', rel_error(db_num, dbeta))",
"dx error: 1.6674604875341426e-09\ndgamma error: 7.417225040694815e-13\ndbeta error: 2.379446949959628e-12\n"
]
],
[
[
"## Batch normalization: alternative backward\nIn class we talked about two different implementations for the sigmoid backward pass. One strategy is to write out a computation graph composed of simple operations and backprop through all intermediate values. Another strategy is to work out the derivatives on paper. For example, you can derive a very simple formula for the sigmoid function's backward pass by simplifying gradients on paper.\n\nSurprisingly, it turns out that you can do a similar simplification for the batch normalization backward pass too. \nGiven a set of inputs $X=\\begin{bmatrix}x_1\\\\x_2\\\\...\\\\x_N\\end{bmatrix}$, \nwe first calculate the mean $\\mu=\\frac{1}{N}\\sum_{k=1}^N x_k$ and variance $v=\\frac{1}{N}\\sum_{k=1}^N (x_k-\\mu)^2.$ \nWith $\\mu$ and $v$ calculated, we can calculate the standard deviation $\\sigma=\\sqrt{v+\\epsilon}$ and normalized data $Y$ with $y_i=\\frac{x_i-\\mu}{\\sigma}.$\n\n\nThe meat of our problem is to get $\\frac{\\partial L}{\\partial X}$ from the upstream gradient $\\frac{\\partial L}{\\partial Y}.$ It might be challenging to directly reason about the gradients over $X$ and $Y$ - try reasoning about it in terms of $x_i$ and $y_i$ first.\n\nYou will need to come up with the derivations for $\\frac{\\partial L}{\\partial x_i}$, by relying on the Chain Rule to first calculate the intermediate $\\frac{\\partial \\mu}{\\partial x_i}, \\frac{\\partial v}{\\partial x_i}, \\frac{\\partial \\sigma}{\\partial x_i},$ then assemble these pieces to calculate $\\frac{\\partial y_i}{\\partial x_i}$. You should make sure each of the intermediary steps are all as simple as possible. \n\nAfter doing so, implement the simplified batch normalization backward pass in the function `batchnorm_backward_alt` and compare the two implementations by running the following. Your two implementations should compute nearly identical results, but the alternative implementation should be a bit faster.",
"_____no_output_____"
]
],
[
[
"np.random.seed(231)\nN, D = 100, 500\nx = 5 * np.random.randn(N, D) + 12\ngamma = np.random.randn(D)\nbeta = np.random.randn(D)\ndout = np.random.randn(N, D)\n\nbn_param = {'mode': 'train'}\nout, cache = batchnorm_forward(x, gamma, beta, bn_param)\n\nt1 = time.time()\ndx1, dgamma1, dbeta1 = batchnorm_backward(dout, cache)\nt2 = time.time()\ndx2, dgamma2, dbeta2 = batchnorm_backward_alt(dout, cache)\nt3 = time.time()\n\nprint('dx difference: ', rel_error(dx1, dx2))\nprint('dgamma difference: ', rel_error(dgamma1, dgamma2))\nprint('dbeta difference: ', rel_error(dbeta1, dbeta2))\nprint('speedup: %.2fx' % ((t2 - t1) / (t3 - t2)))",
"dx difference: 9.890497291190823e-13\ndgamma difference: 0.0\ndbeta difference: 0.0\nspeedup: 3.19x\n"
]
],
[
[
"## Fully Connected Nets with Batch Normalization\nNow that you have a working implementation for batch normalization, go back to your `FullyConnectedNet` in the file `cs231n/classifiers/fc_net.py`. Modify your implementation to add batch normalization.\n\nConcretely, when the `normalization` flag is set to `\"batchnorm\"` in the constructor, you should insert a batch normalization layer before each ReLU nonlinearity. The outputs from the last layer of the network should not be normalized. Once you are done, run the following to gradient-check your implementation.\n\nHINT: You might find it useful to define an additional helper layer similar to those in the file `cs231n/layer_utils.py`. If you decide to do so, do it in the file `cs231n/classifiers/fc_net.py`.",
"_____no_output_____"
]
],
[
[
"np.random.seed(231)\nN, D, H1, H2, C = 2, 15, 20, 30, 10\nX = np.random.randn(N, D)\ny = np.random.randint(C, size=(N,))\n\n# You should expect losses between 1e-4~1e-10 for W, \n# losses between 1e-08~1e-10 for b,\n# and losses between 1e-08~1e-09 for beta and gammas.\nfor reg in [0, 3.14]:\n print('Running check with reg = ', reg)\n model = FullyConnectedNet([H1, H2], input_dim=D, num_classes=C,\n reg=reg, weight_scale=5e-2, dtype=np.float64,\n normalization='batchnorm')\n\n loss, grads = model.loss(X, y)\n print('Initial loss: ', loss)\n\n for name in sorted(grads):\n f = lambda _: model.loss(X, y)[0]\n grad_num = eval_numerical_gradient(f, model.params[name], verbose=False, h=1e-5)\n print('%s relative error: %.2e' % (name, rel_error(grad_num, grads[name])))\n if reg == 0: print()",
"Running check with reg = 0\nInitial loss: 2.2611955101340957\nW1 relative error: 1.10e-04\nW2 relative error: 3.11e-06\nW3 relative error: 4.05e-10\nb1 relative error: 4.44e-08\nb2 relative error: 2.22e-08\nb3 relative error: 1.01e-10\nbeta1 relative error: 7.33e-09\nbeta2 relative error: 1.89e-09\ngamma1 relative error: 6.96e-09\ngamma2 relative error: 2.41e-09\n\nRunning check with reg = 3.14\nInitial loss: 5.884829928987633\nW1 relative error: 1.98e-06\nW2 relative error: 2.29e-06\nW3 relative error: 6.29e-10\nb1 relative error: 5.55e-09\nb2 relative error: 2.22e-08\nb3 relative error: 2.10e-10\nbeta1 relative error: 6.65e-09\nbeta2 relative error: 3.39e-09\ngamma1 relative error: 6.27e-09\ngamma2 relative error: 5.28e-09\n"
]
],
[
[
"# Batchnorm for deep networks\nRun the following to train a six-layer network on a subset of 1000 training examples both with and without batch normalization.",
"_____no_output_____"
]
],
[
[
"np.random.seed(231)\n# Try training a very deep net with batchnorm\nhidden_dims = [100, 100, 100, 100, 100]\n\nnum_train = 1000\nsmall_data = {\n 'X_train': data['X_train'][:num_train],\n 'y_train': data['y_train'][:num_train],\n 'X_val': data['X_val'],\n 'y_val': data['y_val'],\n}\n\nweight_scale = 2e-2\nbn_model = FullyConnectedNet(hidden_dims, weight_scale=weight_scale, normalization='batchnorm')\nmodel = FullyConnectedNet(hidden_dims, weight_scale=weight_scale, normalization=None)\n\nbn_solver = Solver(bn_model, small_data,\n num_epochs=10, batch_size=50,\n update_rule='adam',\n optim_config={\n 'learning_rate': 1e-3,\n },\n verbose=True,print_every=20)\nbn_solver.train()\n\nsolver = Solver(model, small_data,\n num_epochs=10, batch_size=50,\n update_rule='adam',\n optim_config={\n 'learning_rate': 1e-3,\n },\n verbose=True, print_every=20)\nsolver.train()",
"(Iteration 1 / 200) loss: 2.340974\n(Epoch 0 / 10) train acc: 0.107000; val_acc: 0.107000\n(Epoch 1 / 10) train acc: 0.324000; val_acc: 0.264000\n(Iteration 21 / 200) loss: 1.996679\n(Epoch 2 / 10) train acc: 0.426000; val_acc: 0.303000\n(Iteration 41 / 200) loss: 2.038482\n(Epoch 3 / 10) train acc: 0.483000; val_acc: 0.313000\n(Iteration 61 / 200) loss: 1.719336\n(Epoch 4 / 10) train acc: 0.599000; val_acc: 0.334000\n(Iteration 81 / 200) loss: 1.314874\n(Epoch 5 / 10) train acc: 0.633000; val_acc: 0.305000\n(Iteration 101 / 200) loss: 1.346061\n(Epoch 6 / 10) train acc: 0.723000; val_acc: 0.348000\n(Iteration 121 / 200) loss: 0.948622\n(Epoch 7 / 10) train acc: 0.751000; val_acc: 0.348000\n(Iteration 141 / 200) loss: 1.048444\n(Epoch 8 / 10) train acc: 0.764000; val_acc: 0.330000\n(Iteration 161 / 200) loss: 0.798128\n(Epoch 9 / 10) train acc: 0.847000; val_acc: 0.347000\n(Iteration 181 / 200) loss: 0.814854\n(Epoch 10 / 10) train acc: 0.847000; val_acc: 0.355000\n(Iteration 1 / 200) loss: 2.302332\n(Epoch 0 / 10) train acc: 0.114000; val_acc: 0.122000\n(Epoch 1 / 10) train acc: 0.263000; val_acc: 0.223000\n(Iteration 21 / 200) loss: 2.083578\n(Epoch 2 / 10) train acc: 0.305000; val_acc: 0.233000\n(Iteration 41 / 200) loss: 1.856229\n(Epoch 3 / 10) train acc: 0.367000; val_acc: 0.299000\n(Iteration 61 / 200) loss: 1.687658\n(Epoch 4 / 10) train acc: 0.410000; val_acc: 0.315000\n(Iteration 81 / 200) loss: 1.566294\n(Epoch 5 / 10) train acc: 0.446000; val_acc: 0.326000\n(Iteration 101 / 200) loss: 1.597560\n(Epoch 6 / 10) train acc: 0.500000; val_acc: 0.327000\n(Iteration 121 / 200) loss: 1.445800\n(Epoch 7 / 10) train acc: 0.558000; val_acc: 0.345000\n(Iteration 141 / 200) loss: 1.180650\n(Epoch 8 / 10) train acc: 0.601000; val_acc: 0.339000\n(Iteration 161 / 200) loss: 0.985692\n(Epoch 9 / 10) train acc: 0.644000; val_acc: 0.361000\n(Iteration 181 / 200) loss: 0.960458\n(Epoch 10 / 10) train acc: 0.711000; val_acc: 0.364000\n"
]
],
[
[
"Run the following to visualize the results from two networks trained above. You should find that using batch normalization helps the network to converge much faster.",
"_____no_output_____"
]
],
[
[
"def plot_training_history(title, label, baseline, bn_solvers, plot_fn, bl_marker='.', bn_marker='.', labels=None):\n \"\"\"utility function for plotting training history\"\"\"\n plt.title(title)\n plt.xlabel(label)\n bn_plots = [plot_fn(bn_solver) for bn_solver in bn_solvers]\n bl_plot = plot_fn(baseline)\n num_bn = len(bn_plots)\n for i in range(num_bn):\n label='with_norm'\n if labels is not None:\n label += str(labels[i])\n plt.plot(bn_plots[i], bn_marker, label=label)\n label='baseline'\n if labels is not None:\n label += str(labels[0])\n plt.plot(bl_plot, bl_marker, label=label)\n plt.legend(loc='lower center', ncol=num_bn+1) \n\n \nplt.subplot(3, 1, 1)\nplot_training_history('Training loss','Iteration', solver, [bn_solver], \\\n lambda x: x.loss_history, bl_marker='o', bn_marker='o')\nplt.subplot(3, 1, 2)\nplot_training_history('Training accuracy','Epoch', solver, [bn_solver], \\\n lambda x: x.train_acc_history, bl_marker='-o', bn_marker='-o')\nplt.subplot(3, 1, 3)\nplot_training_history('Validation accuracy','Epoch', solver, [bn_solver], \\\n lambda x: x.val_acc_history, bl_marker='-o', bn_marker='-o')\n\nplt.gcf().set_size_inches(15, 15)\nplt.show()",
"_____no_output_____"
]
],
[
[
"# Batch normalization and initialization\nWe will now run a small experiment to study the interaction of batch normalization and weight initialization.\n\nThe first cell will train 8-layer networks both with and without batch normalization using different scales for weight initialization. The second layer will plot training accuracy, validation set accuracy, and training loss as a function of the weight initialization scale.",
"_____no_output_____"
]
],
[
[
"np.random.seed(231)\n# Try training a very deep net with batchnorm\nhidden_dims = [50, 50, 50, 50, 50, 50, 50]\nnum_train = 1000\nsmall_data = {\n 'X_train': data['X_train'][:num_train],\n 'y_train': data['y_train'][:num_train],\n 'X_val': data['X_val'],\n 'y_val': data['y_val'],\n}\n\nbn_solvers_ws = {}\nsolvers_ws = {}\nweight_scales = np.logspace(-4, 0, num=20)\nfor i, weight_scale in enumerate(weight_scales):\n print('Running weight scale %d / %d' % (i + 1, len(weight_scales)))\n bn_model = FullyConnectedNet(hidden_dims, weight_scale=weight_scale, normalization='batchnorm')\n model = FullyConnectedNet(hidden_dims, weight_scale=weight_scale, normalization=None)\n\n bn_solver = Solver(bn_model, small_data,\n num_epochs=10, batch_size=50,\n update_rule='adam',\n optim_config={\n 'learning_rate': 1e-3,\n },\n verbose=False, print_every=200)\n bn_solver.train()\n bn_solvers_ws[weight_scale] = bn_solver\n\n solver = Solver(model, small_data,\n num_epochs=10, batch_size=50,\n update_rule='adam',\n optim_config={\n 'learning_rate': 1e-3,\n },\n verbose=False, print_every=200)\n solver.train()\n solvers_ws[weight_scale] = solver",
"Running weight scale 1 / 20\nRunning weight scale 2 / 20\nRunning weight scale 3 / 20\nRunning weight scale 4 / 20\nRunning weight scale 5 / 20\nRunning weight scale 6 / 20\nRunning weight scale 7 / 20\nRunning weight scale 8 / 20\nRunning weight scale 9 / 20\nRunning weight scale 10 / 20\nRunning weight scale 11 / 20\nRunning weight scale 12 / 20\nRunning weight scale 13 / 20\nRunning weight scale 14 / 20\nRunning weight scale 15 / 20\nRunning weight scale 16 / 20\nRunning weight scale 17 / 20\nRunning weight scale 18 / 20\nRunning weight scale 19 / 20\nRunning weight scale 20 / 20\n"
],
[
"# Plot results of weight scale experiment\nbest_train_accs, bn_best_train_accs = [], []\nbest_val_accs, bn_best_val_accs = [], []\nfinal_train_loss, bn_final_train_loss = [], []\n\nfor ws in weight_scales:\n best_train_accs.append(max(solvers_ws[ws].train_acc_history))\n bn_best_train_accs.append(max(bn_solvers_ws[ws].train_acc_history))\n \n best_val_accs.append(max(solvers_ws[ws].val_acc_history))\n bn_best_val_accs.append(max(bn_solvers_ws[ws].val_acc_history))\n \n final_train_loss.append(np.mean(solvers_ws[ws].loss_history[-100:]))\n bn_final_train_loss.append(np.mean(bn_solvers_ws[ws].loss_history[-100:]))\n \nplt.subplot(3, 1, 1)\nplt.title('Best val accuracy vs weight initialization scale')\nplt.xlabel('Weight initialization scale')\nplt.ylabel('Best val accuracy')\nplt.semilogx(weight_scales, best_val_accs, '-o', label='baseline')\nplt.semilogx(weight_scales, bn_best_val_accs, '-o', label='batchnorm')\nplt.legend(ncol=2, loc='lower right')\n\nplt.subplot(3, 1, 2)\nplt.title('Best train accuracy vs weight initialization scale')\nplt.xlabel('Weight initialization scale')\nplt.ylabel('Best training accuracy')\nplt.semilogx(weight_scales, best_train_accs, '-o', label='baseline')\nplt.semilogx(weight_scales, bn_best_train_accs, '-o', label='batchnorm')\nplt.legend()\n\nplt.subplot(3, 1, 3)\nplt.title('Final training loss vs weight initialization scale')\nplt.xlabel('Weight initialization scale')\nplt.ylabel('Final training loss')\nplt.semilogx(weight_scales, final_train_loss, '-o', label='baseline')\nplt.semilogx(weight_scales, bn_final_train_loss, '-o', label='batchnorm')\nplt.legend()\nplt.gca().set_ylim(1.0, 3.5)\n\nplt.gcf().set_size_inches(15, 15)\nplt.show()",
"_____no_output_____"
]
],
[
[
"## Inline Question 1:\nDescribe the results of this experiment. How does the scale of weight initialization affect models with/without batch normalization differently, and why?",
"_____no_output_____"
],
[
"## Answer:\nBacth norm is robust to weight initialisatio scale upto a point - after which both break.",
"_____no_output_____"
],
[
"# Batch normalization and batch size\nWe will now run a small experiment to study the interaction of batch normalization and batch size.\n\nThe first cell will train 6-layer networks both with and without batch normalization using different batch sizes. The second layer will plot training accuracy and validation set accuracy over time.",
"_____no_output_____"
]
],
[
[
"def run_batchsize_experiments(normalization_mode):\n np.random.seed(231)\n # Try training a very deep net with batchnorm\n hidden_dims = [100, 100, 100, 100, 100]\n num_train = 1000\n small_data = {\n 'X_train': data['X_train'][:num_train],\n 'y_train': data['y_train'][:num_train],\n 'X_val': data['X_val'],\n 'y_val': data['y_val'],\n }\n n_epochs=10\n weight_scale = 2e-2\n batch_sizes = [5,10,50]\n lr = 10**(-3.5)\n solver_bsize = batch_sizes[0]\n\n print('No normalization: batch size = ',solver_bsize)\n model = FullyConnectedNet(hidden_dims, weight_scale=weight_scale, normalization=None)\n solver = Solver(model, small_data,\n num_epochs=n_epochs, batch_size=solver_bsize,\n update_rule='adam',\n optim_config={\n 'learning_rate': lr,\n },\n verbose=False)\n solver.train()\n \n bn_solvers = []\n for i in range(len(batch_sizes)):\n b_size=batch_sizes[i]\n print('Normalization: batch size = ',b_size)\n bn_model = FullyConnectedNet(hidden_dims, weight_scale=weight_scale, normalization=normalization_mode)\n bn_solver = Solver(bn_model, small_data,\n num_epochs=n_epochs, batch_size=b_size,\n update_rule='adam',\n optim_config={\n 'learning_rate': lr,\n },\n verbose=False)\n bn_solver.train()\n bn_solvers.append(bn_solver)\n \n return bn_solvers, solver, batch_sizes\n\nbatch_sizes = [5,10,50]\nbn_solvers_bsize, solver_bsize, batch_sizes = run_batchsize_experiments('batchnorm')",
"No normalization: batch size = 5\nNormalization: batch size = 5\nNormalization: batch size = 10\nNormalization: batch size = 50\n"
],
[
"plt.subplot(2, 1, 1)\nplot_training_history('Training accuracy (Batch Normalization)','Epoch', solver_bsize, bn_solvers_bsize, \\\n lambda x: x.train_acc_history, bl_marker='-^', bn_marker='-o', labels=batch_sizes)\nplt.subplot(2, 1, 2)\nplot_training_history('Validation accuracy (Batch Normalization)','Epoch', solver_bsize, bn_solvers_bsize, \\\n lambda x: x.val_acc_history, bl_marker='-^', bn_marker='-o', labels=batch_sizes)\n\nplt.gcf().set_size_inches(15, 10)\nplt.show()",
"_____no_output_____"
]
],
[
[
"## Inline Question 2:\nDescribe the results of this experiment. What does this imply about the relationship between batch normalization and batch size? Why is this relationship observed?\n\n## Answer:\nBatchnorm is sensitive to batch size because mean and variance depend on these minibatches of data.",
"_____no_output_____"
],
[
"# Layer Normalization\nBatch normalization has proved to be effective in making networks easier to train, but the dependency on batch size makes it less useful in complex networks which have a cap on the input batch size due to hardware limitations. \n\nSeveral alternatives to batch normalization have been proposed to mitigate this problem; one such technique is Layer Normalization [4]. Instead of normalizing over the batch, we normalize over the features. In other words, when using Layer Normalization, each feature vector corresponding to a single datapoint is normalized based on the sum of all terms within that feature vector.\n\n[4] [Ba, Jimmy Lei, Jamie Ryan Kiros, and Geoffrey E. Hinton. \"Layer Normalization.\" stat 1050 (2016): 21.](https://arxiv.org/pdf/1607.06450.pdf)",
"_____no_output_____"
],
[
"## Inline Question 3:\nWhich of these data preprocessing steps is analogous to batch normalization, and which is analogous to layer normalization?\n\n1. Scaling each image in the dataset, so that the RGB channels for each row of pixels within an image sums up to 1.\n2. Scaling each image in the dataset, so that the RGB channels for all pixels within an image sums up to 1. \n3. Subtracting the mean image of the dataset from each image in the dataset.\n4. Setting all RGB values to either 0 or 1 depending on a given threshold.\n\n## Answer:\n",
"_____no_output_____"
],
[
"# Layer Normalization: Implementation\n\nNow you'll implement layer normalization. This step should be relatively straightforward, as conceptually the implementation is almost identical to that of batch normalization. One significant difference though is that for layer normalization, we do not keep track of the moving moments, and the testing phase is identical to the training phase, where the mean and variance are directly calculated per datapoint.\n\nHere's what you need to do:\n\n* In `cs231n/layers.py`, implement the forward pass for layer normalization in the function `layernorm_backward`. \n\nRun the cell below to check your results.\n* In `cs231n/layers.py`, implement the backward pass for layer normalization in the function `layernorm_backward`. \n\nRun the second cell below to check your results.\n* Modify `cs231n/classifiers/fc_net.py` to add layer normalization to the `FullyConnectedNet`. When the `normalization` flag is set to `\"layernorm\"` in the constructor, you should insert a layer normalization layer before each ReLU nonlinearity. \n\nRun the third cell below to run the batch size experiment on layer normalization.",
"_____no_output_____"
]
],
[
[
"N, D = x.shape\nprint('X-shape :', x.shape)\n\nmean = np.mean(x, axis = 1, keepdims = True)/D\nprint('mean-shape :', mean.shape)\n\n#xmu = (x.T - mu.T).T\n#print('xmu-shape :', xmu.shape)\n\ntemp = np.ones((1,D))\nmunew = np.matmul(mean, temp)\nxmu = x - munew\nprint('xmu-shape :', xmu.shape)",
"X-shape : (100, 500)\nmean-shape : (100, 1)\nxmu-shape : (100, 500)\n"
],
[
"# Check the training-time forward pass by checking means and variances\n# of features both before and after layer normalization \n\n# Simulate the forward pass for a two-layer network\nnp.random.seed(231)\nN, D1, D2, D3 =4, 50, 60, 3\nX = np.random.randn(N, D1)\nW1 = np.random.randn(D1, D2)\nW2 = np.random.randn(D2, D3)\na = np.maximum(0, X.dot(W1)).dot(W2)\n\nprint('Before layer normalization:')\nprint_mean_std(a,axis=1)\n\ngamma = np.ones(D3)\nbeta = np.zeros(D3)\n# Means should be close to zero and stds close to one\nprint('After layer normalization (gamma=1, beta=0)')\na_norm, _ = layernorm_forward(a, gamma, beta, {'mode': 'train'})\nprint_mean_std(a_norm,axis=1)\n\ngamma = np.asarray([3.0,3.0,3.0])\nbeta = np.asarray([5.0,5.0,5.0])\n# Now means should be close to beta and stds close to gamma\nprint('After layer normalization (gamma=', gamma, ', beta=', beta, ')')\na_norm, _ = layernorm_forward(a, gamma, beta, {'mode': 'train'})\nprint_mean_std(a_norm,axis=1)\n",
"Before layer normalization:\n means: [-59.06673243 -47.60782686 -43.31137368 -26.40991744]\n stds: [10.07429373 28.39478981 35.28360729 4.01831507]\n\nAfter layer normalization (gamma=1, beta=0)\nLayer norm in x shape (4, 3)\nLayer norm out shape (4, 3)\n means: [ 4.81096644e-16 -7.40148683e-17 2.22044605e-16 -5.92118946e-16]\n stds: [0.99999995 0.99999999 1. 0.99999969]\n\nAfter layer normalization (gamma= [3. 3. 3.] , beta= [5. 5. 5.] )\nLayer norm in x shape (4, 3)\nLayer norm out shape (4, 3)\n means: [5. 5. 5. 5.]\n stds: [2.99999985 2.99999998 2.99999999 2.99999907]\n\n"
],
[
"# Gradient check batchnorm backward pass\nnp.random.seed(231)\nN, D = 4, 5\nx = 5 * np.random.randn(N, D) + 12\ngamma = np.random.randn(D)\nbeta = np.random.randn(D)\ndout = np.random.randn(N, D)\n\nln_param = {}\nfx = lambda x: layernorm_forward(x, gamma, beta, ln_param)[0]\nfg = lambda a: layernorm_forward(x, a, beta, ln_param)[0]\nfb = lambda b: layernorm_forward(x, gamma, b, ln_param)[0]\n\ndx_num = eval_numerical_gradient_array(fx, x, dout)\nda_num = eval_numerical_gradient_array(fg, gamma.copy(), dout)\ndb_num = eval_numerical_gradient_array(fb, beta.copy(), dout)\n\n_, cache = layernorm_forward(x, gamma, beta, ln_param)\ndx, dgamma, dbeta = layernorm_backward(dout, cache)\n\n#You should expect to see relative errors between 1e-12 and 1e-8\nprint('dx error: ', rel_error(dx_num, dx))\nprint('dgamma error: ', rel_error(da_num, dgamma))\nprint('dbeta error: ', rel_error(db_num, dbeta))",
"dx error: 2.107277492956569e-09\ndgamma error: 4.519489546032799e-12\ndbeta error: 2.5842537629899423e-12\n"
]
],
[
[
"# Layer Normalization and batch size\n\nWe will now run the previous batch size experiment with layer normalization instead of batch normalization. Compared to the previous experiment, you should see a markedly smaller influence of batch size on the training history!",
"_____no_output_____"
]
],
[
[
"ln_solvers_bsize, solver_bsize, batch_sizes = run_batchsize_experiments('layernorm')\n\nplt.subplot(2, 1, 1)\nplot_training_history('Training accuracy (Layer Normalization)','Epoch', solver_bsize, ln_solvers_bsize, \\\n lambda x: x.train_acc_history, bl_marker='-^', bn_marker='-o', labels=batch_sizes)\nplt.subplot(2, 1, 2)\nplot_training_history('Validation accuracy (Layer Normalization)','Epoch', solver_bsize, ln_solvers_bsize, \\\n lambda x: x.val_acc_history, bl_marker='-^', bn_marker='-o', labels=batch_sizes)\n\nplt.gcf().set_size_inches(15, 10)\nplt.show()",
"No normalization: batch size = 5\nNormalization: batch size = 5\nNormalization: batch size = 10\nNormalization: batch size = 50\n"
]
],
[
[
"## Inline Question 4:\nWhen is layer normalization likely to not work well, and why?\n\n1. Using it in a very deep network\n2. Having a very small dimension of features\n3. Having a high regularization term\n\n\n## Answer:\nHaving a very small dimension of features\n",
"_____no_output_____"
]
]
] | [
"markdown",
"code",
"markdown",
"code",
"markdown",
"code",
"markdown",
"code",
"markdown",
"code",
"markdown",
"code",
"markdown",
"code",
"markdown",
"code",
"markdown",
"code",
"markdown",
"code",
"markdown",
"code",
"markdown"
] | [
[
"markdown"
],
[
"code",
"code"
],
[
"markdown"
],
[
"code",
"code"
],
[
"markdown"
],
[
"code"
],
[
"markdown"
],
[
"code"
],
[
"markdown"
],
[
"code"
],
[
"markdown"
],
[
"code"
],
[
"markdown"
],
[
"code"
],
[
"markdown"
],
[
"code",
"code"
],
[
"markdown",
"markdown",
"markdown"
],
[
"code",
"code"
],
[
"markdown",
"markdown",
"markdown",
"markdown"
],
[
"code",
"code",
"code"
],
[
"markdown"
],
[
"code"
],
[
"markdown"
]
] |
e78650dc3e6044d286fb5c863fd6474505704698 | 30,893 | ipynb | Jupyter Notebook | synestia-book/_build/jupyter_execute/docs/MoreInformation.ipynb | ststewart/synestiabook2 | 9c530cb7ed5a33c82bccccf828bb8969f9609b8b | [
"MIT"
] | null | null | null | synestia-book/_build/jupyter_execute/docs/MoreInformation.ipynb | ststewart/synestiabook2 | 9c530cb7ed5a33c82bccccf828bb8969f9609b8b | [
"MIT"
] | null | null | null | synestia-book/_build/jupyter_execute/docs/MoreInformation.ipynb | ststewart/synestiabook2 | 9c530cb7ed5a33c82bccccf828bb8969f9609b8b | [
"MIT"
] | null | null | null | 381.395062 | 28,881 | 0.947075 | [
[
[
"# More Information about Synestias\n\n[When Earth and the Moon Were One](https://www.scientificamerican.com/article/when-earth-and-the-moon-were-one/)\nby Simon J. Lock and Sarah T. Stewart<br>\n<i>Scientific American</i>, July 2019. Check your local library for an\nonline or print subscription.<p>\n\n[Where did the Moon come from? A New Theory](https://www.ted.com/talks/sarah_t_stewart_where_did_the_moon_come_from_a_new_theory?language=en)<br>\nSarah T. Stewart, TED Talk<p>\n\n",
"_____no_output_____"
]
],
[
[
"from IPython.display import YouTubeVideo\nYouTubeVideo('7uRPPaYuu44', width=640, height=360)",
"_____no_output_____"
]
]
] | [
"markdown",
"code"
] | [
[
"markdown"
],
[
"code"
]
] |
e78666549162ba9a558624c3aacbc236c2c8dd2e | 261,793 | ipynb | Jupyter Notebook | 0 -Web_Scraping_Selenium.ipynb | berkaycihan/mercedesprice_prediction | 857a388b47ee0fd26d152fa01a1ad02627059770 | [
"MIT"
] | 3 | 2021-03-31T14:32:16.000Z | 2021-04-13T06:38:29.000Z | 0 -Web_Scraping_Selenium.ipynb | berkaycihan/mercedesprice_prediction | 857a388b47ee0fd26d152fa01a1ad02627059770 | [
"MIT"
] | null | null | null | 0 -Web_Scraping_Selenium.ipynb | berkaycihan/mercedesprice_prediction | 857a388b47ee0fd26d152fa01a1ad02627059770 | [
"MIT"
] | null | null | null | 29.695213 | 1,890 | 0.431994 | [
[
[
"from selenium import webdriver\nimport time",
"_____no_output_____"
],
[
"import pandas as pd\ndriver_path='C:\\Program Files (x86)\\Google\\Chrome\\Application\\chromedriver.exe'\nbrowser=webdriver.Chrome(driver_path)\n\ncar1_url='https://www.araba.com/ilan/mercedes-benz-c-220-cdi-avantgarde-otomatik-full-full-1885379'\n\nbrowser.get(car1_url)\n\n\ncontent = browser.find_elements_by_css_selector('div.car-detail-container')\n\ncontlist=[]\nfor i in content:\n a=i.text\n contlist=a.split(\"\\n\")\ncontlist[::2]",
"_____no_output_____"
],
[
" \n# List1 \nlst = [ [ contlist[3],contlist[6],contlist[8],contlist[10],contlist[12],contlist[14],contlist[16],contlist[18],contlist[20],contlist[22],contlist[24],contlist[26],contlist[28],contlist[0] ] ] \n \ndf = pd.DataFrame(lst,columns=['city','advertisement_number', 'advertisement_date','brand','model','version','year','km','fuel','gear','engine_capacity','engine_power','from','price']) \ndf ",
"_____no_output_____"
],
[
"content[0].text[0:10]",
"_____no_output_____"
],
[
"\ndriver_path='C:\\Program Files (x86)\\Google\\Chrome\\Application\\chromedriver.exe'\nbrowser=webdriver.Chrome(driver_path)\nbrowser.get('https://www.araba.com/otomobil/mercedes')\n\n\n//*[@id=\"park_or_compare\"]/table/tbody/tr[1]\n\n//*[@id=\"park_or_compare\"]/table/tbody/tr[2]\n\n//*[@id=\"park_or_compare\"]/table/tbody/tr[22]\n",
"_____no_output_____"
],
[
"driver_path='C:\\Program Files (x86)\\Google\\Chrome\\Application\\chromedriver.exe'\nbrowser=webdriver.Chrome(driver_path)\n\ncar1_url='https://www.sahibinden.com/ilan/vasita-otomobil-mercedes-benz-c180-amg-2014-model-97000-km-hatasiz-boyasiz-tramersiz-915559307/detay'\n\nbrowser.get(car1_url)\n\ncontent = browser.find_elements_by_css_selector('div.classifiedInfo ')\nbaslik=browser.find_elements_by_css_selector('div.classifiedDetailTitle')\ncontlist1=[]\ncontlist2=[]\nfor i in content:\n a=i.text\n contlist1=a.split(\"\\n\")\nfor i in baslik:\n a=i.text\n contlist2=a.split(\"\\n\")\n \ncontlist=contlist1+contlist2\ncontlist[:]\n",
"_____no_output_____"
],
[
"driver_path='C:\\Program Files (x86)\\Google\\Chrome\\Application\\chromedriver.exe'\nbrowser=webdriver.Chrome(driver_path)\n\ncar1_url='https://www.arabam.com/ilan/galeriden-satilik-mercedes-benz-e-300-amg/levent-motors-2017-mercedes-e-300-coupe-command-hayalet-nvg-vkm/17188504'\n\nbrowser.get(car1_url)\n\ncontent=browser.find_elements_by_xpath('//*[@id=\"wrapper\"]/div[6]/div[3]/div/div[1]')\n\n#content\ncontlist=[]\nfor i in content:\n a=i.text\n contlist=a.split(\"\\n\")\ncontlist[:41]",
"_____no_output_____"
],
[
"import pandas as pd\nlst = [ [ contlist[9],contlist[13],contlist[17],contlist[15],contlist[19],contlist[29],contlist[6],contlist[11],contlist[23],contlist[21],contlist[25],contlist[27],contlist[39],contlist[0],contlist[7] ] ] \n \ndf_simple = pd.DataFrame(lst,columns=['advertisement_number','brand','model','series','year','km', 'price','advertisement_date','gear','fuel','engine_capacity','engine_power','from','title','city']) \ndf_simple",
"_____no_output_____"
],
[
"empty_list=[]\ndf = pd.DataFrame(empty_list,columns=['advertisement_number','brand','model','series','year','km', 'price','advertisement_date','gear','fuel','engine_capacity','engine_power','from','title','city']) \ndf",
"_____no_output_____"
],
[
"driver_path='C:\\Program Files (x86)\\Google\\Chrome\\Application\\chromedriver.exe'\nbrowser=webdriver.Chrome(driver_path)\nurl='https://www.arabam.com/ikinci-el/otomobil/mercedes-benz-e'\nbrowser.get(url)\n\nfor i in range(20):\n //*[@id=\"park_or_compare\"]/table/tbody/tr[2]\n //*[@id=\"park_or_compare\"]/table/tbody/tr[3]",
"_____no_output_____"
],
[
"driver_path='C:\\Program Files (x86)\\Google\\Chrome\\Application\\chromedriver.exe'\nbrowser=webdriver.Chrome(driver_path)\n\nurl='https://www.araba.com/ilan/lansman-rengi-2016-gla-180-amg-4244870'\n\nbrowser.get(url)\n\ncontent=browser.find_elements_by_xpath('/html/body/div[1]/div[7]/div[2]/div[3]/div[5]/div[1]/div[1]/div[2]')\n\n#content\ncontlist=[]\nfor i in content:\n a=i.text\n contlist=a.split(\"\\n\")\ncontlist[:]",
"_____no_output_____"
],
[
"import pandas as pd\nlst = [ [ contlist[12],contlist[16],contlist[18],contlist[20],contlist[22],contlist[24],contlist[6],contlist[14],contlist[28],contlist[26],contlist[30],contlist[32],contlist[34],contlist[9],contlist[3] ] ] \n \ndf_simple = pd.DataFrame(lst,columns=['advertisement_number','brand','series','version','year','km', 'price','advertisement_date','gear','fuel','engine_capacity','engine_power','from','city','title']) \ndf_simple",
"_____no_output_____"
],
[
"empty_list=[]\ndf = pd.DataFrame(empty_list,columns=['advertisement_number','brand','series','version','year','km', 'price','advertisement_date','gear','fuel','engine_capacity','engine_power','from','city']) \ndf",
"_____no_output_____"
],
[
"from selenium import webdriver\nimport time\nimport pandas as pd\n\ndriver_path='C:\\Program Files (x86)\\Google\\Chrome\\Application\\chromedriver.exe'\nbrowser=webdriver.Chrome(driver_path)\nurl='https://www.araba.com/otomobil/mercedes-e-serisi'\nbrowser.get(url)\n\nempty_list=[]\ndf_simple = pd.DataFrame(empty_list,columns=['advertisement_number','brand','series','version','year','km', 'price','advertisement_date','gear','fuel','engine_capacity','engine_power','from','city']) \ndf_simple.to_csv(r\"istihza3.csv\",encoding=\"utf-8\",index=False,mode=\"a\")\n\nfor k in range(2,50):\n try:\n for t in range(2,23):\n if(t==6 or t==11):\n continue\n box_ = browser.find_element_by_xpath('//*[@id=\"park_or_compare\"]/table/tbody/tr['+str(t)+ ']')\n box_.click()\n\n content=browser.find_elements_by_xpath('/html/body/div[1]/div[7]/div[2]/div[3]/div[5]/div[1]/div[1]/div[2]')\n\n #content\n contlist=[]\n for i in content:\n a=i.text\n contlist=a.split(\"\\n\")\n for f in contlist:\n if ( f.startswith('/')):\n contlist.remove(str(f)) \n try:\n lst = [ [ contlist[5],contlist[9],contlist[11],contlist[13],contlist[15],contlist[17],contlist[0],contlist[7],contlist[0],contlist[8],contlist[21],contlist[19],contlist[23],contlist[3] ] ] \n except:\n print('The error has occurred in this process')\n continue\n df = pd.DataFrame(lst) \n df.to_csv(r\"istihza3.csv\",encoding=\"utf-8\",index=False,mode=\"a\")\n\n browser.execute_script(\"window.history.go(-1)\")\n for j in contlist:\n print(j)\n\n print('***********************************************+1 '+str(t)+ '.araç verisi -------------------------------------------------')\n contlist=[]\n print('*-*-*-*-*-*-*-*-*************Sayfa '+str(k) + ' *-*-*-*-*-*-*-*-*-*-***********')\n\n #tıkla = browser.find_element_by_xpath('/html/body/div[1]/div[7]/div[2]/div[3]/div[2]/div[4]/div[1]/div/a['+str(k)+ ']')\n #tıkla.click()\n url='https://www.araba.com/otomobil/mercedes-e-serisi?sayfa='+str(k)\n browser.get(url)\n except:\n print('The error has occured in this page')\n url='https://www.araba.com/otomobil/mercedes-e-serisi?sayfa='+str(k)\n browser.get(url)\n \n\n \n \n \n \n \n \n \n",
"525.000 TL\n10.157 TL den başlayan taksitlerle »\nARABA DEĞERİ HESAPLA\nAnkara\nİlan No:\n#2798325\nİlan Tarihi:\n22/03/2021\nMarka:\nMercedes\nModel:\nE Serisi\nVaryant:\nE 250 BlueTEC\nModel Yılı:\n2015\nKilometre:\n150.000\nYakıt Türü:\nBenzin\nVites Tipi:\nOtomatik Vites\nMotor Hacmi:\n1801-2000 cc\nMotor Gücü:\n126-150 BG\nKimden:\nGaleriden\n***********************************************+1 2.araç verisi -------------------------------------------------\n389.000 TL\n7.526 TL den başlayan taksitlerle »\nARABA DEĞERİ HESAPLA\nAnkara\nİlan No:\n#4324911\nİlan Tarihi:\n21/03/2021\nMarka:\nMercedes\nModel:\nE Serisi\nVaryant:\nE 180 Elite\nModel Yılı:\n2013\nKilometre:\n80.200\nYakıt Türü:\nBenzin\nVites Tipi:\nYarı Otomatik Vites\nMotor Hacmi:\n1301-1600 cc\nMotor Gücü:\nBilmiyorum\nKimden:\nGaleriden\n***********************************************+1 3.araç verisi -------------------------------------------------\n310.000 TL\n5.997 TL den başlayan taksitlerle »\nARABA DEĞERİ HESAPLA\nKilis\nİlan No:\n#4590792\nİlan Tarihi:\n21/03/2021\nMarka:\nMercedes\nModel:\nE Serisi\nVaryant:\nE 250 CDI BlueEfficiency Avantgarde\nModel Yılı:\n2010\nKilometre:\n302.000\nYakıt Türü:\nDizel\nVites Tipi:\nYarı Otomatik Vites\nMotor Hacmi:\n2001-2500 cc\nMotor Gücü:\nBilmiyorum\nKimden:\nGaleriden\n***********************************************+1 4.araç verisi -------------------------------------------------\n215.000 TL\n4.159 TL den başlayan taksitlerle »\nARABA DEĞERİ HESAPLA\nKilis\nİlan No:\n#2743421\nİlan Tarihi:\n21/03/2021\nMarka:\nMercedes\nModel:\nE Serisi\nVaryant:\nE 220 CDI Elegance\nModel Yılı:\n2005\nKilometre:\n237.000\nYakıt Türü:\nDizel\nVites Tipi:\nYarı Otomatik Vites\nMotor Hacmi:\n2001-2500 cc\nMotor Gücü:\nBilmiyorum\nKimden:\nGaleriden\n***********************************************+1 5.araç verisi -------------------------------------------------\n695.000 TL\n13.445 TL den başlayan taksitlerle »\nARABA DEĞERİ HESAPLA\nİstanbul\nİlan No:\n#1184828\nİlan Tarihi:\n21/03/2021\nMarka:\nMercedes\nModel:\nE Serisi\nVaryant:\nE 180 Exclusive\nModel Yılı:\n2018\nKilometre:\n33.500\nYakıt Türü:\nBenzin\nVites Tipi:\nOtomatik Vites\nMotor Hacmi:\n1301-1600 cc\nMotor Gücü:\nBilmiyorum\nKimden:\nGaleriden\n***********************************************+1 7.araç verisi -------------------------------------------------\n555.000 TL\n10.737 TL den başlayan taksitlerle »\nARABA DEĞERİ HESAPLA\nAdana\nİlan No:\n#1371734\nİlan Tarihi:\n21/03/2021\nMarka:\nMercedes\nModel:\nE Serisi\nVaryant:\nE 250 CDI Edition\nModel Yılı:\n2015\nKilometre:\n184.000\nYakıt Türü:\nDizel\nVites Tipi:\nYarı Otomatik Vites\nMotor Hacmi:\n2001-2500 cc\nMotor Gücü:\nBilmiyorum\nKimden:\nGaleriden\n***********************************************+1 8.araç verisi -------------------------------------------------\n895.000 TL\n17.315 TL den başlayan taksitlerle »\nARABA DEĞERİ HESAPLA\nİstanbul\nİlan No:\n#4764206\nİlan Tarihi:\n21/03/2021\nMarka:\nMercedes\nModel:\nE Serisi\nVaryant:\nE 300 AMG\nModel Yılı:\n2017\nKilometre:\n104.000\nYakıt Türü:\nBenzin\nVites Tipi:\nOtomatik Vites\nMotor Hacmi:\n1801-2000 cc\nMotor Gücü:\nBilmiyorum\nKimden:\nGaleriden\n***********************************************+1 9.araç verisi -------------------------------------------------\n225.000 TL\n4.353 TL den başlayan taksitlerle »\nARABA DEĞERİ HESAPLA\nKonya\nİlan No:\n#1288254\nİlan Tarihi:\n21/03/2021\nMarka:\nMercedes\nModel:\nE Serisi\nVaryant:\nE 200 Komp. Avantgarde\nModel Yılı:\n2004\nKilometre:\n287.000\nYakıt Türü:\nBenzin\nVites Tipi:\nYarı Otomatik Vites\nMotor Hacmi:\n1601-1800 cc\nMotor Gücü:\nBilmiyorum\nKimden:\nGaleriden\n***********************************************+1 10.araç verisi -------------------------------------------------\n225.000 TL\n4.353 TL den başlayan taksitlerle »\nARABA DEĞERİ HESAPLA\nOrdu\nİlan No:\n#4732863\nİlan Tarihi:\n21/03/2021\nMarka:\nMercedes\nModel:\nE Serisi\nVaryant:\nE 200 Komp. Avantgarde\nModel Yılı:\n2004\nKilometre:\n219.000\nYakıt Türü:\nBenzin/LPG\nVites Tipi:\nYarı Otomatik Vites\nMotor Hacmi:\n1601-1800 cc\nMotor Gücü:\nBilmiyorum\nKimden:\nGaleriden\n***********************************************+1 12.araç verisi -------------------------------------------------\n529.000 TL\n10.234 TL den başlayan taksitlerle »\nARABA DEĞERİ HESAPLA\nOrdu\nİlan No:\n#4732869\nİlan Tarihi:\n21/03/2021\nMarka:\nMercedes\nModel:\nE Serisi\nVaryant:\nE 180 AMG Premium\nModel Yılı:\n2014\nKilometre:\n56.000\nYakıt Türü:\nBenzin\nVites Tipi:\nOtomatik Vites\nMotor Hacmi:\n1301-1600 cc\nMotor Gücü:\nBilmiyorum\nKimden:\nGaleriden\n***********************************************+1 13.araç verisi -------------------------------------------------\n549.000 TL\n10.621 TL den başlayan taksitlerle »\nARABA DEĞERİ HESAPLA\nDenizli\nİlan No:\n#379440\nİlan Tarihi:\n21/03/2021\nMarka:\nMercedes\nModel:\nE Serisi\nVaryant:\nE 200 AMG\nModel Yılı:\n2016\nKilometre:\n101.000\nYakıt Türü:\nBenzin\nVites Tipi:\nYarı Otomatik Vites\nMotor Hacmi:\n1801-2000 cc\nMotor Gücü:\nBilmiyorum\nKimden:\nGaleriden\n***********************************************+1 14.araç verisi -------------------------------------------------\n629.900 TL\n12.186 TL den başlayan taksitlerle »\nARABA DEĞERİ HESAPLA\nBursa\nİlan No:\n#2801737\nİlan Tarihi:\n21/03/2021\nMarka:\nMercedes\nModel:\nE Serisi\nVaryant:\nE 220 d Avantgarde\nModel Yılı:\n2016\nKilometre:\n167.000\nYakıt Türü:\nDizel\nVites Tipi:\nYarı Otomatik Vites\nMotor Hacmi:\n1801-2000 cc\nMotor Gücü:\nBilmiyorum\nKimden:\nGaleriden\n***********************************************+1 15.araç verisi -------------------------------------------------\n1.470.000 TL\nTL den başlayan taksitlerle »\nARABA DEĞERİ HESAPLA\nİstanbul\nİlan No:\n#3236666\nİlan Tarihi:\n21/03/2021\nMarka:\nMercedes\nModel:\nE Serisi\nVaryant:\nDiğer\nModel Yılı:\n2020\nKilometre:\n0\nYakıt Türü:\nDizel\nVites Tipi:\nOtomatik Vites\nMotor Hacmi:\n1300 cc ve altı\nMotor Gücü:\nBilmiyorum\nKimden:\nGaleriden\n***********************************************+1 16.araç verisi -------------------------------------------------\n920.000 TL\n17.798 TL den başlayan taksitlerle »\nARABA DEĞERİ HESAPLA\nSamsun\nİlan No:\n#3190405\nİlan Tarihi:\n21/03/2021\nMarka:\nMercedes\nModel:\nE Serisi\nVaryant:\nE 220 d Exclusive\nModel Yılı:\n2017\nKilometre:\n53.321\nYakıt Türü:\nDizel\nVites Tipi:\nOtomatik Vites\nMotor Hacmi:\n1801-2000 cc\nMotor Gücü:\nBilmiyorum\nKimden:\nGaleriden\n***********************************************+1 17.araç verisi -------------------------------------------------\n439.900 TL\n8.510 TL den başlayan taksitlerle »\nARABA DEĞERİ HESAPLA\nGiresun\nİlan No:\n#4674231\nİlan Tarihi:\n21/03/2021\nMarka:\nMercedes\nModel:\nE Serisi\nVaryant:\nDiğer\nModel Yılı:\n2016\nKilometre:\n138.000\nYakıt Türü:\nBenzin\nVites Tipi:\nYarı Otomatik Vites\nMotor Hacmi:\n1301-1600 cc\nMotor Gücü:\nBilmiyorum\nKimden:\nGaleriden\n***********************************************+1 18.araç verisi -------------------------------------------------\n323.000 TL\n6.249 TL den başlayan taksitlerle »\nARABA DEĞERİ HESAPLA\nİstanbul\nİlan No:\n#228489\nİlan Tarihi:\n21/03/2021\nMarka:\nMercedes\nModel:\nE Serisi\nVaryant:\nE 220 CDI BlueEfficiency\nModel Yılı:\n2011\nKilometre:\n199.000\nYakıt Türü:\nDizel\nVites Tipi:\nYarı Otomatik Vites\nMotor Hacmi:\n2001-2500 cc\nMotor Gücü:\nBilmiyorum\nKimden:\nGaleriden\n***********************************************+1 19.araç verisi -------------------------------------------------\nThe error has occurred in this process\nThe error has occured in this page\n460.000 TL\n8.899 TL den başlayan taksitlerle »\nARABA DEĞERİ HESAPLA\nMersin\nİlan No:\n#3437428\nİlan Tarihi:\n21/03/2021\nMarka:\nMercedes\nModel:\nE Serisi\nVaryant:\nE 250 AMG 7G-Tronic\nModel Yılı:\n2015\nKilometre:\n150.000\nYakıt Türü:\nBenzin\nVites Tipi:\nYarı Otomatik Vites\nMotor Hacmi:\n1801-2000 cc\nMotor Gücü:\nBilmiyorum\nKimden:\nGaleriden\n***********************************************+1 2.araç verisi -------------------------------------------------\n581.900 TL\n11.257 TL den başlayan taksitlerle »\nARABA DEĞERİ HESAPLA\nİstanbul\nİlan No:\n#1590897\nİlan Tarihi:\n21/03/2021\nMarka:\nMercedes\nModel:\nE Serisi\nVaryant:\nE 180 Exclusive\nModel Yılı:\n2018\nKilometre:\n34.000\nYakıt Türü:\nBenzin\nVites Tipi:\nOtomatik Vites\nMotor Hacmi:\n1301-1600 cc\nMotor Gücü:\nBilmiyorum\nKimden:\nGaleriden\n***********************************************+1 3.araç verisi -------------------------------------------------\n342.500 TL\n6.626 TL den başlayan taksitlerle »\nARABA DEĞERİ HESAPLA\nAnkara\nİlan No:\n#1229478\nİlan Tarihi:\n21/03/2021\nMarka:\nMercedes\nModel:\nE Serisi\nVaryant:\nE 250 CGI Premium\nModel Yılı:\n2012\nKilometre:\n127.000\nYakıt Türü:\nBenzin\nVites Tipi:\nYarı Otomatik Vites\nMotor Hacmi:\n1601-1800 cc\nMotor Gücü:\nBilmiyorum\nKimden:\nGaleriden\n***********************************************+1 4.araç verisi -------------------------------------------------\n655.000 TL\n12.672 TL den başlayan taksitlerle »\nARABA DEĞERİ HESAPLA\nİstanbul\nİlan No:\n#2751896\nİlan Tarihi:\n21/03/2021\nMarka:\nMercedes\nModel:\nE Serisi\nVaryant:\nE 180 Exclusive\nModel Yılı:\n2018\nKilometre:\n51.000\nYakıt Türü:\nBenzin\nVites Tipi:\nOtomatik Vites\nMotor Hacmi:\n1301-1600 cc\nMotor Gücü:\nBilmiyorum\nKimden:\nGaleriden\n***********************************************+1 5.araç verisi -------------------------------------------------\n217.900 TL\n4.215 TL den başlayan taksitlerle »\nARABA DEĞERİ HESAPLA\nAnkara\nİlan No:\n#1360112\nİlan Tarihi:\n21/03/2021\nMarka:\nMercedes\nModel:\nE Serisi\nVaryant:\nE 200 Komp. Elegance\nModel Yılı:\n2006\nKilometre:\n205.000\nYakıt Türü:\nBenzin/LPG\nVites Tipi:\nYarı Otomatik Vites\nMotor Hacmi:\n1601-1800 cc\nMotor Gücü:\nBilmiyorum\nKimden:\nGaleriden\n***********************************************+1 7.araç verisi -------------------------------------------------\n89.000 TL\n1.722 TL den başlayan taksitlerle »\nARABA DEĞERİ HESAPLA\nGaziantep\nİlan No:\n#2154935\nİlan Tarihi:\n21/03/2021\nMarka:\nMercedes\nModel:\nE Serisi\nVaryant:\nE 250 D 250 D\nModel Yılı:\n1993\nKilometre:\n550.000\nYakıt Türü:\nDizel\nVites Tipi:\nOtomatik Vites\nMotor Hacmi:\n1300 cc ve altı\nMotor Gücü:\nBilmiyorum\nKimden:\nGaleriden\n***********************************************+1 8.araç verisi -------------------------------------------------\nThe error has occured in this page\n105.000 TL\n2.031 TL den başlayan taksitlerle »\nARABA DEĞERİ HESAPLA\nGaziantep\nİlan No:\n#2275771\nİlan Tarihi:\n21/03/2021\nMarka:\nMercedes\nModel:\nE Serisi\nVaryant:\nE 220 CDI Elegance\nModel Yılı:\n1997\nKilometre:\n446.000\nYakıt Türü:\nDizel\nVites Tipi:\nDüz Vites\nMotor Hacmi:\n1300 cc ve altı\nMotor Gücü:\nBilmiyorum\nKimden:\nGaleriden\n***********************************************+1 2.araç verisi -------------------------------------------------\nThe error has occurred in this process\nThe error has occured in this page\n700.000 TL\n13.542 TL den başlayan taksitlerle »\nARABA DEĞERİ HESAPLA\nAntalya\nİlan No:\n#3834370\nİlan Tarihi:\n21/03/2021\nMarka:\nMercedes\nModel:\nE Serisi\nVaryant:\nE 180 AMG\nModel Yılı:\n2018\nKilometre:\n12.500\nYakıt Türü:\nBenzin\nVites Tipi:\nOtomatik Vites\nMotor Hacmi:\n1301-1600 cc\nMotor Gücü:\nBilmiyorum\nKimden:\nGaleriden\n***********************************************+1 2.araç verisi -------------------------------------------------\n77.000 TL\n1.490 TL den başlayan taksitlerle »\nARABA DEĞERİ HESAPLA\nDenizli\nİlan No:\n#4183304\nİlan Tarihi:\n21/03/2021\nMarka:\nMercedes\nModel:\nE Serisi\nVaryant:\nE 200 200\nModel Yılı:\n1993\nKilometre:\n400.000\nYakıt Türü:\nBenzin/LPG\nVites Tipi:\nDüz Vites\nMotor Hacmi:\n1801-2000 cc\nMotor Gücü:\nBilmiyorum\nKimden:\nGaleriden\n***********************************************+1 3.araç verisi -------------------------------------------------\n195.900 TL\n3.790 TL den başlayan taksitlerle »\nARABA DEĞERİ HESAPLA\nAnkara\nİlan No:\n#3584914\nİlan Tarihi:\n21/03/2021\nMarka:\nMercedes\nModel:\nE Serisi\nVaryant:\nE 200 Komp. Avantgarde\nModel Yılı:\n2004\nKilometre:\n340.000\nYakıt Türü:\nBenzin/LPG\nVites Tipi:\nYarı Otomatik Vites\nMotor Hacmi:\n1601-1800 cc\nMotor Gücü:\nBilmiyorum\nKimden:\nGaleriden\n***********************************************+1 4.araç verisi -------------------------------------------------\n325.000 TL\n6.287 TL den başlayan taksitlerle »\nARABA DEĞERİ HESAPLA\nAdıyaman\nİlan No:\n#4052363\nİlan Tarihi:\n21/03/2021\nMarka:\nMercedes\nModel:\nE Serisi\nVaryant:\nE 200 Komp. Avantgarde\nModel Yılı:\n2008\nKilometre:\n376.000\nYakıt Türü:\nBenzin/LPG\nVites Tipi:\nOtomatik Vites\nMotor Hacmi:\n1601-1800 cc\nMotor Gücü:\nBilmiyorum\nKimden:\nGaleriden\n***********************************************+1 5.araç verisi -------------------------------------------------\n420.000 TL\n8.125 TL den başlayan taksitlerle »\nARABA DEĞERİ HESAPLA\nMardin\nİlan No:\n#4727984\nİlan Tarihi:\n21/03/2021\nMarka:\nMercedes\nModel:\nE Serisi\nVaryant:\nE 180 Elite\nModel Yılı:\n2015\nKilometre:\n79.000\nYakıt Türü:\nBenzin\nVites Tipi:\nYarı Otomatik Vites\nMotor Hacmi:\n1301-1600 cc\nMotor Gücü:\nBilmiyorum\nKimden:\nGaleriden\n***********************************************+1 7.araç verisi -------------------------------------------------\n105.000 TL\n2.031 TL den başlayan taksitlerle »\nARABA DEĞERİ HESAPLA\nDenizli\nİlan No:\n#4798994\nİlan Tarihi:\n21/03/2021\nMarka:\nMercedes\nModel:\nE Serisi\nVaryant:\nE 200 Avantgarde\nModel Yılı:\n1997\nKilometre:\n425.000\nYakıt Türü:\nBenzin/LPG\nVites Tipi:\nOtomatik Vites\nMotor Hacmi:\n1801-2000 cc\nMotor Gücü:\nBilmiyorum\nKimden:\nGaleriden\n***********************************************+1 8.araç verisi -------------------------------------------------\n413.000 TL\n7.990 TL den başlayan taksitlerle »\nARABA DEĞERİ HESAPLA\nKayseri\nİlan No:\n#4785428\nİlan Tarihi:\n21/03/2021\nMarka:\nMercedes\nModel:\nE Serisi\nVaryant:\nE 250 Elite\nModel Yılı:\n2014\nKilometre:\n177.000\nYakıt Türü:\nBenzin\nVites Tipi:\nYarı Otomatik Vites\nMotor Hacmi:\n1801-2000 cc\nMotor Gücü:\nBilmiyorum\nKimden:\nGaleriden\n***********************************************+1 9.araç verisi -------------------------------------------------\n655.000 TL\n12.672 TL den başlayan taksitlerle »\nARABA DEĞERİ HESAPLA\nİstanbul\nİlan No:\n#4256629\nİlan Tarihi:\n21/03/2021\nMarka:\nMercedes\nModel:\nE Serisi\nVaryant:\nE 180 AMG\nModel Yılı:\n2017\nKilometre:\n25.000\nYakıt Türü:\nBenzin\nVites Tipi:\nOtomatik Vites\nMotor Hacmi:\n1301-1600 cc\nMotor Gücü:\nBilmiyorum\nKimden:\nGaleriden\n***********************************************+1 10.araç verisi -------------------------------------------------\n189.000 TL\n3.656 TL den başlayan taksitlerle »\nARABA DEĞERİ HESAPLA\nİstanbul\nİlan No:\n#2791021\nİlan Tarihi:\n21/03/2021\nMarka:\nMercedes\nModel:\nE Serisi\nVaryant:\nE 220 CDI Avantgarde\nModel Yılı:\n2007\nKilometre:\n410.000\nYakıt Türü:\nDizel\nVites Tipi:\nYarı Otomatik Vites\nMotor Hacmi:\n2001-2500 cc\nMotor Gücü:\nBilmiyorum\nKimden:\nGaleriden\n***********************************************+1 12.araç verisi -------------------------------------------------\n87.500 TL\n1.693 TL den başlayan taksitlerle »\nARABA DEĞERİ HESAPLA\nManisa\nİlan No:\n#1392873\nİlan Tarihi:\n21/03/2021\nMarka:\nMercedes\nModel:\nE Serisi\nVaryant:\nE 320 Avantgarde\nModel Yılı:\n1996\nKilometre:\n358.000\nYakıt Türü:\nBenzin/LPG\nVites Tipi:\nOtomatik Vites\nMotor Hacmi:\n3001-3500 cc\nMotor Gücü:\nBilmiyorum\nKimden:\nGaleriden\n***********************************************+1 13.araç verisi -------------------------------------------------\n459.900 TL\n8.897 TL den başlayan taksitlerle »\nARABA DEĞERİ HESAPLA\nBursa\nİlan No:\n#1894177\nİlan Tarihi:\n21/03/2021\nMarka:\nMercedes\nModel:\nE Serisi\nVaryant:\nE 250 CGI AMG\nModel Yılı:\n2013\nKilometre:\n154.000\nYakıt Türü:\nBenzin\nVites Tipi:\nOtomatik Vites\nMotor Hacmi:\n1300 cc ve altı\nMotor Gücü:\nBilmiyorum\nKimden:\nGaleriden\n***********************************************+1 14.araç verisi -------------------------------------------------\n487.500 TL\n9.431 TL den başlayan taksitlerle »\nARABA DEĞERİ HESAPLA\nİstanbul\nİlan No:\n#3337879\nİlan Tarihi:\n21/03/2021\nMarka:\nMercedes\nModel:\nE Serisi\nVaryant:\nE 180 Edition E\nModel Yılı:\n2015\nKilometre:\n43.575\nYakıt Türü:\nBenzin\nVites Tipi:\nYarı Otomatik Vites\nMotor Hacmi:\n1301-1600 cc\nMotor Gücü:\nBilmiyorum\nKimden:\nGaleriden\n***********************************************+1 15.araç verisi -------------------------------------------------\n43.500 TL\n842 TL den başlayan taksitlerle »\nARABA DEĞERİ HESAPLA\nGaziantep\nİlan No:\n#2233534\nİlan Tarihi:\n21/03/2021\nMarka:\nMercedes\nModel:\nE Serisi\nVaryant:\nE 300 300\nModel Yılı:\n1985\nKilometre:\n277.000\nYakıt Türü:\nBenzin/LPG\nVites Tipi:\nDüz Vites\nMotor Hacmi:\n1300 cc ve altı\nMotor Gücü:\nBilmiyorum\nKimden:\nGaleriden\n***********************************************+1 16.araç verisi -------------------------------------------------\n600.000 TL\n11.608 TL den başlayan taksitlerle »\nARABA DEĞERİ HESAPLA\nİstanbul\nİlan No:\n#4785009\nİlan Tarihi:\n21/03/2021\nMarka:\nMercedes\nModel:\nE Serisi\nVaryant:\nE 250 CDI Premium\nModel Yılı:\n2015\nKilometre:\n94.000\nYakıt Türü:\nDizel\nVites Tipi:\nOtomatik Vites\nMotor Hacmi:\n2001-2500 cc\nMotor Gücü:\nBilmiyorum\nKimden:\nGaleriden\n***********************************************+1 17.araç verisi -------------------------------------------------\n530.000 TL\n10.253 TL den başlayan taksitlerle »\nARABA DEĞERİ HESAPLA\nŞanlıurfa\nİlan No:\n#2369882\nİlan Tarihi:\n21/03/2021\nMarka:\nMercedes\nModel:\nE Serisi\nVaryant:\nE 250 CDI Premium\nModel Yılı:\n2014\nKilometre:\n145.000\nYakıt Türü:\nDizel\nVites Tipi:\nOtomatik Vites\nMotor Hacmi:\n2001-2500 cc\nMotor Gücü:\nBilmiyorum\nKimden:\nGaleriden\n***********************************************+1 18.araç verisi -------------------------------------------------\n390.000 TL\n7.545 TL den başlayan taksitlerle »\nARABA DEĞERİ HESAPLA\nİstanbul\nİlan No:\n#4607539\nİlan Tarihi:\n21/03/2021\nMarka:\nMercedes\nModel:\nE Serisi\nVaryant:\nE 180 Edition E\nModel Yılı:\n2015\nKilometre:\n103.000\nYakıt Türü:\nBenzin\nVites Tipi:\nYarı Otomatik Vites\nMotor Hacmi:\n1301-1600 cc\nMotor Gücü:\nBilmiyorum\nKimden:\nGaleriden\n***********************************************+1 19.araç verisi -------------------------------------------------\n390.000 TL\n7.545 TL den başlayan taksitlerle »\nARABA DEĞERİ HESAPLA\nİstanbul\nİlan No:\n#4607539\nİlan Tarihi:\n21/03/2021\nMarka:\nMercedes\nModel:\nE Serisi\nVaryant:\nE 180 Edition E\nModel Yılı:\n2015\nKilometre:\n103.000\nYakıt Türü:\nBenzin\nVites Tipi:\nYarı Otomatik Vites\nMotor Hacmi:\n1301-1600 cc\nMotor Gücü:\nBilmiyorum\nKimden:\nGaleriden\n***********************************************+1 20.araç verisi -------------------------------------------------\n448.000 TL\n8.667 TL den başlayan taksitlerle »\nARABA DEĞERİ HESAPLA\nİstanbul\nİlan No:\n#2578566\nİlan Tarihi:\n21/03/2021\nMarka:\nMercedes\nModel:\nE Serisi\nVaryant:\nE 180 Edition E\nModel Yılı:\n2016\nKilometre:\n56.000\nYakıt Türü:\nBenzin\nVites Tipi:\nYarı Otomatik Vites\nMotor Hacmi:\n1301-1600 cc\nMotor Gücü:\nBilmiyorum\nKimden:\nGaleriden\n***********************************************+1 21.araç verisi -------------------------------------------------\n89.750 TL\n1.736 TL den başlayan taksitlerle »\nARABA DEĞERİ HESAPLA\nAnkara\nİlan No:\n#1182690\nİlan Tarihi:\n21/03/2021\nMarka:\nMercedes\nModel:\nE Serisi\nVaryant:\nE 200 Elegance\nModel Yılı:\n1996\nKilometre:\n375.000\nYakıt Türü:\nBenzin/LPG\nVites Tipi:\nOtomatik Vites\nMotor Hacmi:\n1801-2000 cc\nMotor Gücü:\nBilmiyorum\nKimden:\nGaleriden\n***********************************************+1 22.araç verisi -------------------------------------------------\n*-*-*-*-*-*-*-*-*************Sayfa 5 *-*-*-*-*-*-*-*-*-*-***********\n485.000 TL\n9.383 TL den başlayan taksitlerle »\nARABA DEĞERİ HESAPLA\nHatay\nİlan No:\n#3103499\nİlan Tarihi:\n21/03/2021\nMarka:\nMercedes\nModel:\nE Serisi\nVaryant:\nE 250 AMG 7G-Tronic\nModel Yılı:\n2014\nKilometre:\n93.000\nYakıt Türü:\nBenzin\nVites Tipi:\nYarı Otomatik Vites\nMotor Hacmi:\n1801-2000 cc\nMotor Gücü:\nBilmiyorum\nKimden:\nGaleriden\n***********************************************+1 2.araç verisi -------------------------------------------------\n140.000 TL\n2.708 TL den başlayan taksitlerle »\nARABA DEĞERİ HESAPLA\nGaziantep\nİlan No:\n#940198\nİlan Tarihi:\n21/03/2021\nMarka:\nMercedes\nModel:\nE Serisi\nVaryant:\nE 320 Avantgarde\nModel Yılı:\n2001\nKilometre:\n278.000\nYakıt Türü:\nBenzin/LPG\nVites Tipi:\nYarı Otomatik Vites\nMotor Hacmi:\n3001-3500 cc\nMotor Gücü:\nBilmiyorum\nKimden:\nGaleriden\n***********************************************+1 3.araç verisi -------------------------------------------------\n569.950 TL\n11.026 TL den başlayan taksitlerle »\nARABA DEĞERİ HESAPLA\nİstanbul\nİlan No:\n#1753347\nİlan Tarihi:\n21/03/2021\nMarka:\nMercedes\nModel:\nE Serisi\nVaryant:\nE 180 Exclusive\nModel Yılı:\n2017\nKilometre:\n93.000\nYakıt Türü:\nBenzin\nVites Tipi:\nOtomatik Vites\nMotor Hacmi:\n1301-1600 cc\nMotor Gücü:\nBilmiyorum\nKimden:\nGaleriden\n***********************************************+1 4.araç verisi -------------------------------------------------\n176.900 TL\n3.422 TL den başlayan taksitlerle »\nARABA DEĞERİ HESAPLA\nDenizli\nİlan No:\n#1918831\nİlan Tarihi:\n21/03/2021\nMarka:\nMercedes\nModel:\nE Serisi\nVaryant:\nE 200 Komp. Avantgarde\nModel Yılı:\n2004\nKilometre:\n501.000\nYakıt Türü:\nBenzin/LPG\nVites Tipi:\nYarı Otomatik Vites\nMotor Hacmi:\n1601-1800 cc\nMotor Gücü:\nBilmiyorum\nKimden:\nGaleriden\n***********************************************+1 5.araç verisi -------------------------------------------------\n440.000 TL\n8.512 TL den başlayan taksitlerle »\nARABA DEĞERİ HESAPLA\nİstanbul\nİlan No:\n#4314454\nİlan Tarihi:\n21/03/2021\nMarka:\nMercedes\nModel:\nE Serisi\nVaryant:\nE 180 Elite\nModel Yılı:\n2013\nKilometre:\n75.000\nYakıt Türü:\nBenzin\nVites Tipi:\nYarı Otomatik Vites\nMotor Hacmi:\n1301-1600 cc\nMotor Gücü:\nBilmiyorum\nKimden:\nGaleriden\n***********************************************+1 7.araç verisi -------------------------------------------------\n330.000 TL\n6.384 TL den başlayan taksitlerle »\nARABA DEĞERİ HESAPLA\nİlan No:\n#4742299\nİlan Tarihi:\n21/03/2021\nMarka:\nMercedes\nModel:\nE Serisi\nVaryant:\nE 250 CGI Premium AMG\nModel Yılı:\n2011\nKilometre:\n257.000\nYakıt Türü:\nBenzin\nVites Tipi:\nOtomatik Vites\nMotor Hacmi:\n1601-1800 cc\nMotor Gücü:\nBilmiyorum\nKimden:\nGaleriden\n***********************************************+1 8.araç verisi -------------------------------------------------\n615.000 TL\n11.898 TL den başlayan taksitlerle »\nARABA DEĞERİ HESAPLA\nİstanbul\nİlan No:\n#2345373\nİlan Tarihi:\n21/03/2021\nMarka:\nMercedes\nModel:\nE Serisi\nVaryant:\nE 200 Exclusive\nModel Yılı:\n2016\nKilometre:\n76.000\nYakıt Türü:\nBenzin\nVites Tipi:\nYarı Otomatik Vites\nMotor Hacmi:\n1801-2000 cc\nMotor Gücü:\nBilmiyorum\nKimden:\nGaleriden\n***********************************************+1 9.araç verisi -------------------------------------------------\n680.000 TL\n13.155 TL den başlayan taksitlerle »\nARABA DEĞERİ HESAPLA\nİstanbul\nİlan No:\n#1764652\nİlan Tarihi:\n21/03/2021\nMarka:\nMercedes\nModel:\nE Serisi\nVaryant:\nE 220 d Avantgarde\nModel Yılı:\n2017\nKilometre:\n184.000\nYakıt Türü:\nDizel\nVites Tipi:\nOtomatik Vites\nMotor Hacmi:\n1801-2000 cc\nMotor Gücü:\nBilmiyorum\nKimden:\nGaleriden\n***********************************************+1 10.araç verisi -------------------------------------------------\n1.475.000 TL\nTL den başlayan taksitlerle »\nARABA DEĞERİ HESAPLA\nİstanbul\nİlan No:\n#3075357\nİlan Tarihi:\n21/03/2021\nMarka:\nMercedes\nModel:\nE Serisi\nVaryant:\nE 220 d AMG\nModel Yılı:\n2021\nKilometre:\n0\nYakıt Türü:\nDizel\nVites Tipi:\nOtomatik Vites\nMotor Hacmi:\n1801-2000 cc\nMotor Gücü:\nBilmiyorum\nKimden:\nGaleriden\n***********************************************+1 12.araç verisi -------------------------------------------------\n74.500 TL\n1.441 TL den başlayan taksitlerle »\nARABA DEĞERİ HESAPLA\nElazığ\nİlan No:\n#4051572\nİlan Tarihi:\n21/03/2021\nMarka:\nMercedes\nModel:\nE Serisi\nVaryant:\nE 200 Elegance\nModel Yılı:\n1997\nKilometre:\n329.000\nYakıt Türü:\nBenzin/LPG\nVites Tipi:\nOtomatik Vites\nMotor Hacmi:\n1801-2000 cc\nMotor Gücü:\nBilmiyorum\nKimden:\nGaleriden\n***********************************************+1 13.araç verisi -------------------------------------------------\n177.000 TL\n3.424 TL den başlayan taksitlerle »\nARABA DEĞERİ HESAPLA\nİstanbul\nİlan No:\n#1345314\nİlan Tarihi:\n21/03/2021\nMarka:\nMercedes\nModel:\nE Serisi\nVaryant:\nE 200 Komp. Avantgarde\nModel Yılı:\n2005\nKilometre:\n291.000\nYakıt Türü:\nBenzin\nVites Tipi:\nYarı Otomatik Vites\nMotor Hacmi:\n1601-1800 cc\nMotor Gücü:\nBilmiyorum\nKimden:\nGaleriden\n***********************************************+1 14.araç verisi -------------------------------------------------\n105.000 TL\n2.031 TL den başlayan taksitlerle »\nARABA DEĞERİ HESAPLA\nİstanbul\nİlan No:\n#1028713\nİlan Tarihi:\n21/03/2021\nMarka:\nMercedes\nModel:\nE Serisi\nVaryant:\nE 200 Avantgarde\nModel Yılı:\n1996\nKilometre:\n307.000\nYakıt Türü:\nBenzin/LPG\nVites Tipi:\nOtomatik Vites\nMotor Hacmi:\n1801-2000 cc\nMotor Gücü:\nBilmiyorum\nKimden:\nGaleriden\n***********************************************+1 15.araç verisi -------------------------------------------------\n345.000 TL\n6.674 TL den başlayan taksitlerle »\nARABA DEĞERİ HESAPLA\nHatay\nİlan No:\n#4364555\nİlan Tarihi:\n21/03/2021\nMarka:\nMercedes\nModel:\nE Serisi\nVaryant:\nE 220 CDI Start\nModel Yılı:\n2012\nKilometre:\n321.000\nYakıt Türü:\nDizel\nVites Tipi:\nOtomatik Vites\nMotor Hacmi:\n2001-2500 cc\nMotor Gücü:\nBilmiyorum\nKimden:\nGaleriden\n***********************************************+1 16.araç verisi -------------------------------------------------\nThe error has occured in this page\n650.000 TL\n12.575 TL den başlayan taksitlerle »\nARABA DEĞERİ HESAPLA\nŞanlıurfa\nİlan No:\n#4414787\nİlan Tarihi:\n21/03/2021\nMarka:\nMercedes\nModel:\nE Serisi\nVaryant:\nE 350 CDI BlueTEC Premium\nModel Yılı:\n2014\nKilometre:\n238.000\nYakıt Türü:\nDizel\nVites Tipi:\nOtomatik Vites\nMotor Hacmi:\n2501-3000 cc\nMotor Gücü:\nBilmiyorum\nKimden:\nGaleriden\n***********************************************+1 2.araç verisi -------------------------------------------------\n439.000 TL\n8.493 TL den başlayan taksitlerle »\nARABA DEĞERİ HESAPLA\nAnkara\nİlan No:\n#849611\nİlan Tarihi:\n21/03/2021\nMarka:\nMercedes\nModel:\nE Serisi\nVaryant:\nE 250 Elite\nModel Yılı:\n2015\nKilometre:\n107.000\nYakıt Türü:\nBenzin\nVites Tipi:\nYarı Otomatik Vites\nMotor Hacmi:\n1801-2000 cc\nMotor Gücü:\nBilmiyorum\nKimden:\nGaleriden\n***********************************************+1 3.araç verisi -------------------------------------------------\n298.000 TL\n5.765 TL den başlayan taksitlerle »\nARABA DEĞERİ HESAPLA\nBursa\nİlan No:\n#2741239\nİlan Tarihi:\n21/03/2021\nMarka:\nMercedes\nModel:\nE Serisi\nVaryant:\nE 200 CGI BlueEfficiency Prime\nModel Yılı:\n2010\nKilometre:\n235.000\nYakıt Türü:\nBenzin\nVites Tipi:\nYarı Otomatik Vites\nMotor Hacmi:\n1601-1800 cc\nMotor Gücü:\nBilmiyorum\nKimden:\nGaleriden\n***********************************************+1 4.araç verisi -------------------------------------------------\n258.000 TL\n4.991 TL den başlayan taksitlerle »\nARABA DEĞERİ HESAPLA\nİstanbul\nİlan No:\n#1516614\nİlan Tarihi:\n21/03/2021\nMarka:\nMercedes\nModel:\nE Serisi\nVaryant:\nE 220 CDI Avantgarde\nModel Yılı:\n2009\nKilometre:\n270.000\nYakıt Türü:\nDizel\nVites Tipi:\nYarı Otomatik Vites\nMotor Hacmi:\n2001-2500 cc\nMotor Gücü:\nBilmiyorum\nKimden:\nGaleriden\n***********************************************+1 5.araç verisi -------------------------------------------------\n780.000 TL\n15.090 TL den başlayan taksitlerle »\nARABA DEĞERİ HESAPLA\nZonguldak\nİlan No:\n#210654\nİlan Tarihi:\n21/03/2021\nMarka:\nMercedes\nModel:\nE Serisi\nVaryant:\nE 220 d Exclusive\nModel Yılı:\n2016\nKilometre:\n40.000\nYakıt Türü:\nDizel\nVites Tipi:\nYarı Otomatik Vites\nMotor Hacmi:\n1801-2000 cc\nMotor Gücü:\nBilmiyorum\nKimden:\nGaleriden\n***********************************************+1 7.araç verisi -------------------------------------------------\n590.000 TL\n11.414 TL den başlayan taksitlerle »\nARABA DEĞERİ HESAPLA\nKonya\nİlan No:\n#2363908\nİlan Tarihi:\n21/03/2021\nMarka:\nMercedes\nModel:\nE Serisi\nVaryant:\nE 250 EditionE\nModel Yılı:\n2015\nKilometre:\n42.000\nYakıt Türü:\nBenzin\nVites Tipi:\nYarı Otomatik Vites\nMotor Hacmi:\n1801-2000 cc\nMotor Gücü:\nBilmiyorum\nKimden:\nGaleriden\n***********************************************+1 8.araç verisi -------------------------------------------------\n495.000 TL\n9.576 TL den başlayan taksitlerle »\nARABA DEĞERİ HESAPLA\nKonya\nİlan No:\n#2304191\nİlan Tarihi:\n21/03/2021\nMarka:\nMercedes\nModel:\nE Serisi\nVaryant:\nE 250 CDI Premium\nModel Yılı:\n2013\nKilometre:\n127.000\nYakıt Türü:\nDizel\nVites Tipi:\nOtomatik Vites\nMotor Hacmi:\n2001-2500 cc\nMotor Gücü:\nBilmiyorum\nKimden:\nGaleriden\n***********************************************+1 9.araç verisi -------------------------------------------------\nThe error has occurred in this process\n145.000 TL\n2.805 TL den başlayan taksitlerle »\nARABA DEĞERİ HESAPLA\nİstanbul\nİlan No:\n#216669\nİlan Tarihi:\n21/03/2021\nMarka:\nMercedes\nModel:\nE Serisi\nVaryant:\nE 320 Avantgarde\nModel Yılı:\n2001\nKilometre:\n312.000\nYakıt Türü:\nBenzin\nVites Tipi:\nYarı Otomatik Vites\nMotor Hacmi:\n3001-3500 cc\nMotor Gücü:\nBilmiyorum\nKimden:\nGaleriden\n***********************************************+1 12.araç verisi -------------------------------------------------\n354.500 TL\n6.858 TL den başlayan taksitlerle »\nARABA DEĞERİ HESAPLA\nAydın\nİlan No:\n#2168540\nİlan Tarihi:\n21/03/2021\nMarka:\nMercedes\nModel:\nE Serisi\nVaryant:\nE 200 CGI BlueEfficiency Avantgarde\nModel Yılı:\n2010\nKilometre:\n205.000\nYakıt Türü:\nBenzin\nVites Tipi:\nOtomatik Vites\nMotor Hacmi:\n1300 cc ve altı\nMotor Gücü:\nBilmiyorum\nKimden:\nGaleriden\n***********************************************+1 13.araç verisi -------------------------------------------------\n649.000 TL\n12.556 TL den başlayan taksitlerle »\nARABA DEĞERİ HESAPLA\nİstanbul\nİlan No:\n#536822\nİlan Tarihi:\n21/03/2021\nMarka:\nMercedes\nModel:\nE Serisi\nVaryant:\nE 220 d Avantgarde\nModel Yılı:\n2017\nKilometre:\n109.000\nYakıt Türü:\nDizel\nVites Tipi:\nOtomatik Vites\nMotor Hacmi:\n1801-2000 cc\nMotor Gücü:\nBilmiyorum\nKimden:\nGaleriden\n***********************************************+1 14.araç verisi -------------------------------------------------\n950.000 TL\n18.379 TL den başlayan taksitlerle »\nARABA DEĞERİ HESAPLA\nAnkara\nİlan No:\n#4598418\nİlan Tarihi:\n21/03/2021\nMarka:\nMercedes\nModel:\nE Serisi\nVaryant:\nE 220 d AMG\nModel Yılı:\n2017\nKilometre:\n53.000\nYakıt Türü:\nDizel\nVites Tipi:\nOtomatik Vites\nMotor Hacmi:\n1801-2000 cc\nMotor Gücü:\nBilmiyorum\nKimden:\nGaleriden\n***********************************************+1 15.araç verisi -------------------------------------------------\n950.000 TL\n18.379 TL den başlayan taksitlerle »\nARABA DEĞERİ HESAPLA\nAnkara\nİlan No:\n#4598418\nİlan Tarihi:\n21/03/2021\nMarka:\nMercedes\nModel:\nE Serisi\nVaryant:\nE 220 d AMG\nModel Yılı:\n2017\nKilometre:\n53.000\nYakıt Türü:\nDizel\nVites Tipi:\nOtomatik Vites\nMotor Hacmi:\n1801-2000 cc\nMotor Gücü:\nBilmiyorum\nKimden:\nGaleriden\n***********************************************+1 16.araç verisi -------------------------------------------------\n369.000 TL\n7.139 TL den başlayan taksitlerle »\nARABA DEĞERİ HESAPLA\nİstanbul\nİlan No:\n#2164615\nİlan Tarihi:\n21/03/2021\nMarka:\nMercedes\nModel:\nE Serisi\nVaryant:\nE 200 CGI BlueEfficiency Avantgarde\nModel Yılı:\n2012\nKilometre:\n165.515\nYakıt Türü:\nBenzin\nVites Tipi:\nOtomatik Vites\nMotor Hacmi:\n1300 cc ve altı\nMotor Gücü:\nBilmiyorum\nKimden:\nGaleriden\n***********************************************+1 17.araç verisi -------------------------------------------------\n369.000 TL\n7.139 TL den başlayan taksitlerle »\nARABA DEĞERİ HESAPLA\nİstanbul\nİlan No:\n#2164615\nİlan Tarihi:\n21/03/2021\nMarka:\nMercedes\nModel:\nE Serisi\nVaryant:\nE 200 CGI BlueEfficiency Avantgarde\nModel Yılı:\n2012\nKilometre:\n165.515\nYakıt Türü:\nBenzin\nVites Tipi:\nOtomatik Vites\nMotor Hacmi:\n1300 cc ve altı\nMotor Gücü:\nBilmiyorum\nKimden:\nGaleriden\n***********************************************+1 18.araç verisi -------------------------------------------------\n232.000 TL\n4.488 TL den başlayan taksitlerle »\nARABA DEĞERİ HESAPLA\nManisa\nİlan No:\n#3839337\nİlan Tarihi:\n21/03/2021\nMarka:\nMercedes\nModel:\nE Serisi\nVaryant:\nE 200 Komp. Avantgarde\nModel Yılı:\n2006\nKilometre:\n250.000\nYakıt Türü:\nBenzin/LPG\nVites Tipi:\nYarı Otomatik Vites\nMotor Hacmi:\n1601-1800 cc\nMotor Gücü:\nBilmiyorum\nKimden:\nGaleriden\n***********************************************+1 19.araç verisi -------------------------------------------------\n204.750 TL\n3.961 TL den başlayan taksitlerle »\nARABA DEĞERİ HESAPLA\nAnkara\nİlan No:\n#4647775\nİlan Tarihi:\n21/03/2021\nMarka:\nMercedes\nModel:\nE Serisi\nVaryant:\nE 200 Komp. Avantgarde\nModel Yılı:\n2004\nKilometre:\n363.284\nYakıt Türü:\nBenzin/LPG\nVites Tipi:\nYarı Otomatik Vites\nMotor Hacmi:\n1601-1800 cc\nMotor Gücü:\nBilmiyorum\nKimden:\nGaleriden\n***********************************************+1 20.araç verisi -------------------------------------------------\n76.900 TL\n1.488 TL den başlayan taksitlerle »\nARABA DEĞERİ HESAPLA\nİstanbul\nİlan No:\n#2748310\nİlan Tarihi:\n21/03/2021\nMarka:\nMercedes\nModel:\nE Serisi\nVaryant:\nE 200 200\nModel Yılı:\n1996\nKilometre:\n256.000\nYakıt Türü:\nBenzin/LPG\nVites Tipi:\nDüz Vites\nMotor Hacmi:\n1300 cc ve altı\nMotor Gücü:\nBilmiyorum\nKimden:\nGaleriden\n***********************************************+1 21.araç verisi -------------------------------------------------\n97.000 TL\n1.877 TL den başlayan taksitlerle »\nARABA DEĞERİ HESAPLA\nİstanbul\nİlan No:\n#879272\nİlan Tarihi:\n21/03/2021\nMarka:\nMercedes\nModel:\nE Serisi\nVaryant:\nE 230 Elegance\nModel Yılı:\n1996\nKilometre:\n400.000\nYakıt Türü:\nBenzin/LPG\nVites Tipi:\nOtomatik Vites\nMotor Hacmi:\n1300 cc ve altı\nMotor Gücü:\nBilmiyorum\nKimden:\nGaleriden\n***********************************************+1 22.araç verisi -------------------------------------------------\n*-*-*-*-*-*-*-*-*************Sayfa 7 *-*-*-*-*-*-*-*-*-*-***********\n94.000 TL\n1.819 TL den başlayan taksitlerle »\nARABA DEĞERİ HESAPLA\nKonya\nİlan No:\n#317959\nİlan Tarihi:\n21/03/2021\nMarka:\nMercedes\nModel:\nE Serisi\nVaryant:\nE 200 Avantgarde\nModel Yılı:\n1996\nKilometre:\n420.000\nYakıt Türü:\nBenzin/LPG\nVites Tipi:\nOtomatik Vites\nMotor Hacmi:\n1801-2000 cc\nMotor Gücü:\nBilmiyorum\nKimden:\nGaleriden\n***********************************************+1 2.araç verisi -------------------------------------------------\n650.000 TL\n12.575 TL den başlayan taksitlerle »\nARABA DEĞERİ HESAPLA\nİstanbul\nİlan No:\n#4398784\nİlan Tarihi:\n21/03/2021\nMarka:\nMercedes\nModel:\nE Serisi\nVaryant:\nE 180 AMG\nModel Yılı:\n2018\nKilometre:\n31.000\nYakıt Türü:\nBenzin\nVites Tipi:\nOtomatik Vites\nMotor Hacmi:\n1301-1600 cc\nMotor Gücü:\nBilmiyorum\nKimden:\nGaleriden\n***********************************************+1 3.araç verisi -------------------------------------------------\n364.750 TL\n7.056 TL den başlayan taksitlerle »\nARABA DEĞERİ HESAPLA\nİstanbul\nİlan No:\n#2691912\nİlan Tarihi:\n21/03/2021\nMarka:\nMercedes\nModel:\nE Serisi\nVaryant:\nE 250 CDI Premium\nModel Yılı:\n2012\nKilometre:\n168.000\nYakıt Türü:\nDizel\nVites Tipi:\nYarı Otomatik Vites\nMotor Hacmi:\n2001-2500 cc\nMotor Gücü:\nBilmiyorum\nKimden:\nGaleriden\n***********************************************+1 4.araç verisi -------------------------------------------------\n298.950 TL\n5.783 TL den başlayan taksitlerle »\nARABA DEĞERİ HESAPLA\nİstanbul\nİlan No:\n#149410\nİlan Tarihi:\n21/03/2021\nMarka:\nMercedes\nModel:\nE Serisi\nVaryant:\nE 200 CGI AMG\nModel Yılı:\n2010\nKilometre:\n166.000\nYakıt Türü:\nBenzin\nVites Tipi:\nOtomatik Vites\nMotor Hacmi:\n1601-1800 cc\nMotor Gücü:\nBilmiyorum\nKimden:\nGaleriden\n***********************************************+1 5.araç verisi -------------------------------------------------\n137.000 TL\n2.650 TL den başlayan taksitlerle »\nARABA DEĞERİ HESAPLA\nDenizli\nİlan No:\n#1206490\nİlan Tarihi:\n21/03/2021\nMarka:\nMercedes\nModel:\nE Serisi\nVaryant:\nE 200 Komp. Avantgarde\nModel Yılı:\n2001\nKilometre:\n386.000\nYakıt Türü:\nBenzin/LPG\nVites Tipi:\nYarı Otomatik Vites\nMotor Hacmi:\n1801-2000 cc\nMotor Gücü:\nBilmiyorum\nKimden:\nGaleriden\n***********************************************+1 7.araç verisi -------------------------------------------------\n647.000 TL\n12.517 TL den başlayan taksitlerle »\nARABA DEĞERİ HESAPLA\nİstanbul\nİlan No:\n#327376\nİlan Tarihi:\n21/03/2021\nMarka:\nMercedes\nModel:\nE Serisi\nVaryant:\nE 180 Exclusive\nModel Yılı:\n2018\nKilometre:\n20.000\nYakıt Türü:\nBenzin\nVites Tipi:\nOtomatik Vites\nMotor Hacmi:\n1301-1600 cc\nMotor Gücü:\nBilmiyorum\nKimden:\nGaleriden\n***********************************************+1 8.araç verisi -------------------------------------------------\n86.750 TL\n1.678 TL den başlayan taksitlerle »\nARABA DEĞERİ HESAPLA\nAnkara\nİlan No:\n#386900\nİlan Tarihi:\n21/03/2021\nMarka:\nMercedes\nModel:\nE Serisi\nVaryant:\nE 220 d AMG\nModel Yılı:\n1996\nKilometre:\n343.000\nYakıt Türü:\nDizel\nVites Tipi:\nOtomatik Vites\nMotor Hacmi:\n1300 cc ve altı\nMotor Gücü:\nBilmiyorum\nKimden:\nGaleriden\n***********************************************+1 9.araç verisi -------------------------------------------------\nThe error has occurred in this process\n315.000 TL\n6.094 TL den başlayan taksitlerle »\nARABA DEĞERİ HESAPLA\nGiresun\nİlan No:\n#3612124\nİlan Tarihi:\n21/03/2021\nMarka:\nMercedes\nModel:\nE Serisi\nVaryant:\nE 250 CGI AMG\nModel Yılı:\n2011\nKilometre:\n187.000\nYakıt Türü:\nBenzin\nVites Tipi:\nOtomatik Vites\nMotor Hacmi:\n1300 cc ve altı\nMotor Gücü:\nBilmiyorum\nKimden:\nGaleriden\n***********************************************+1 12.araç verisi -------------------------------------------------\n98.000 TL\n1.896 TL den başlayan taksitlerle »\nARABA DEĞERİ HESAPLA\nKonya\nİlan No:\n#1484805\nİlan Tarihi:\n21/03/2021\nMarka:\nMercedes\nModel:\nE Serisi\nVaryant:\nE 200 200\nModel Yılı:\n1994\nKilometre:\n220.000\nYakıt Türü:\nBenzin/LPG\nVites Tipi:\nDüz Vites\nMotor Hacmi:\n1801-2000 cc\nMotor Gücü:\nBilmiyorum\nKimden:\nGaleriden\n***********************************************+1 13.araç verisi -------------------------------------------------\n499.900 TL\n9.671 TL den başlayan taksitlerle »\nARABA DEĞERİ HESAPLA\nİstanbul\nİlan No:\n#1499938\nİlan Tarihi:\n21/03/2021\nMarka:\nMercedes\nModel:\nE Serisi\nVaryant:\nE 250 CDI Elite\nModel Yılı:\n2014\nKilometre:\n200.000\nYakıt Türü:\nDizel\nVites Tipi:\nYarı Otomatik Vites\nMotor Hacmi:\n2001-2500 cc\nMotor Gücü:\nBilmiyorum\nKimden:\nGaleriden\n***********************************************+1 14.araç verisi -------------------------------------------------\n499.900 TL\n9.671 TL den başlayan taksitlerle »\nARABA DEĞERİ HESAPLA\nİstanbul\nİlan No:\n#1499938\nİlan Tarihi:\n21/03/2021\nMarka:\nMercedes\nModel:\nE Serisi\nVaryant:\nE 250 CDI Elite\nModel Yılı:\n2014\nKilometre:\n200.000\nYakıt Türü:\nDizel\nVites Tipi:\nYarı Otomatik Vites\nMotor Hacmi:\n2001-2500 cc\nMotor Gücü:\nBilmiyorum\nKimden:\nGaleriden\n***********************************************+1 15.araç verisi -------------------------------------------------\n188.750 TL\n3.652 TL den başlayan taksitlerle »\nARABA DEĞERİ HESAPLA\nGaziantep\nİlan No:\n#3860339\nİlan Tarihi:\n21/03/2021\nMarka:\nMercedes\nModel:\nE Serisi\nVaryant:\nE 200 Komp. Avantgarde\nModel Yılı:\n2005\nKilometre:\n307.000\nYakıt Türü:\nBenzin/LPG\nVites Tipi:\nYarı Otomatik Vites\nMotor Hacmi:\n1601-1800 cc\nMotor Gücü:\nBilmiyorum\nKimden:\nGaleriden\n***********************************************+1 16.araç verisi -------------------------------------------------\n500.000 TL\n9.673 TL den başlayan taksitlerle »\nARABA DEĞERİ HESAPLA\nVan\nİlan No:\n#1236873\nİlan Tarihi:\n18/03/2021\nMarka:\nMercedes\nModel:\nE Serisi\nVaryant:\nE 350 CDI Premium\nModel Yılı:\n2010\nKilometre:\n285.000\nYakıt Türü:\nDizel\nVites Tipi:\nYarı Otomatik Vites\nMotor Hacmi:\n2501-3000 cc\nMotor Gücü:\nBilmiyorum\nKimden:\nGaleriden\n***********************************************+1 17.araç verisi -------------------------------------------------\n105.750 TL\n2.046 TL den başlayan taksitlerle »\nARABA DEĞERİ HESAPLA\nElazığ\nİlan No:\n#707235\nİlan Tarihi:\n17/03/2021\nMarka:\nMercedes\nModel:\nE Serisi\nVaryant:\nE 200 Classic\nModel Yılı:\n1998\nKilometre:\n345.000\nYakıt Türü:\nBenzin/LPG\nVites Tipi:\nDüz Vites\nMotor Hacmi:\n1801-2000 cc\nMotor Gücü:\n101-125 BG\nKimden:\nGaleriden\n***********************************************+1 18.araç verisi -------------------------------------------------\n105.750 TL\n2.046 TL den başlayan taksitlerle »\nARABA DEĞERİ HESAPLA\nElazığ\nİlan No:\n#707235\nİlan Tarihi:\n17/03/2021\nMarka:\nMercedes\nModel:\nE Serisi\nVaryant:\nE 200 Classic\nModel Yılı:\n1998\nKilometre:\n345.000\nYakıt Türü:\nBenzin/LPG\nVites Tipi:\nDüz Vites\nMotor Hacmi:\n1801-2000 cc\nMotor Gücü:\n101-125 BG\nKimden:\nGaleriden\n***********************************************+1 19.araç verisi -------------------------------------------------\n389.000 TL\n7.526 TL den başlayan taksitlerle »\nARABA DEĞERİ HESAPLA\nAydın\nİlan No:\n#4160102\nİlan Tarihi:\n10/03/2021\nMarka:\nMercedes\nModel:\nE Serisi\nVaryant:\nE 250 Elite\nModel Yılı:\n2013\nKilometre:\n189.000\nYakıt Türü:\nBenzin\nVites Tipi:\nOtomatik Vites\nMotor Hacmi:\n2001-2500 cc\nMotor Gücü:\n151-175 BG\nKimden:\nSahibinden\n***********************************************+1 20.araç verisi -------------------------------------------------\n110.000 TL\n2.128 TL den başlayan taksitlerle »\nARABA DEĞERİ HESAPLA\nRize\nİlan No:\n#2535361\nİlan Tarihi:\n01/03/2021\nMarka:\nMercedes\nModel:\nE Serisi\nVaryant:\nE 200 Elegance\nModel Yılı:\n1997\nKilometre:\n41.500\nYakıt Türü:\nBenzin/LPG\nVites Tipi:\nOtomatik Vites\nMotor Hacmi:\n1801-2000 cc\nMotor Gücü:\nBilmiyorum\nKimden:\nGaleriden\n***********************************************+1 21.araç verisi -------------------------------------------------\n110.000 TL\n2.128 TL den başlayan taksitlerle »\nARABA DEĞERİ HESAPLA\nRize\nİlan No:\n#2535361\nİlan Tarihi:\n01/03/2021\nMarka:\nMercedes\nModel:\nE Serisi\nVaryant:\nE 200 Elegance\nModel Yılı:\n1997\nKilometre:\n41.500\nYakıt Türü:\nBenzin/LPG\nVites Tipi:\nOtomatik Vites\nMotor Hacmi:\n1801-2000 cc\nMotor Gücü:\nBilmiyorum\nKimden:\nGaleriden\n***********************************************+1 22.araç verisi -------------------------------------------------\n*-*-*-*-*-*-*-*-*************Sayfa 8 *-*-*-*-*-*-*-*-*-*-***********\n399.900 TL\n7.736 TL den başlayan taksitlerle »\nARABA DEĞERİ HESAPLA\nİstanbul\nİlan No:\n#2722123\nİlan Tarihi:\n24/02/2021\nMarka:\nMercedes\nModel:\nE Serisi\nVaryant:\nE 180 AMG Premium\nModel Yılı:\n2014\nKilometre:\n138.000\nYakıt Türü:\nBenzin\nVites Tipi:\nOtomatik Vites\nMotor Hacmi:\n1301-1600 cc\nMotor Gücü:\n151-175 BG\nKimden:\nGaleriden\n***********************************************+1 2.araç verisi -------------------------------------------------\n2.475.000 TL\nTL den başlayan taksitlerle »\nARABA DEĞERİ HESAPLA\nHatay\nİlan No:\n#3222257\nİlan Tarihi:\n16/02/2021\nMarka:\nMercedes\nModel:\nE Serisi\nVaryant:\nE 63 AMG\nModel Yılı:\n2018\nKilometre:\n24.000\nYakıt Türü:\nBenzin\nVites Tipi:\nOtomatik Vites\nMotor Hacmi:\n3501-4000 cc\nMotor Gücü:\n601 BG ve üzeri\nKimden:\nGaleriden\n***********************************************+1 3.araç verisi -------------------------------------------------\n290.000 TL\n5.610 TL den başlayan taksitlerle »\nARABA DEĞERİ HESAPLA\nYozgat\nİlan No:\n#3449286\nİlan Tarihi:\n16/02/2021\nMarka:\nMercedes\nModel:\nE Serisi\nVaryant:\nE 220 CDI Avantgarde\nModel Yılı:\n2009\nKilometre:\n270.000\nYakıt Türü:\nDizel\nVites Tipi:\nYarı Otomatik Vites\nMotor Hacmi:\n2001-2500 cc\nMotor Gücü:\nBilmiyorum\nKimden:\nGaleriden\n***********************************************+1 4.araç verisi -------------------------------------------------\n419.900 TL\n8.123 TL den başlayan taksitlerle »\nARABA DEĞERİ HESAPLA\nİstanbul\nİlan No:\n#2688657\nİlan Tarihi:\n15/02/2021\nMarka:\nMercedes\nModel:\nE Serisi\nVaryant:\nE 180 Edition E\nModel Yılı:\n2015\nKilometre:\n133.000\nYakıt Türü:\nBenzin\nVites Tipi:\nYarı Otomatik Vites\nMotor Hacmi:\n1301-1600 cc\nMotor Gücü:\nBilmiyorum\nKimden:\nGaleriden\n***********************************************+1 5.araç verisi -------------------------------------------------\n708.500 TL\n13.707 TL den başlayan taksitlerle »\nARABA DEĞERİ HESAPLA\nİstanbul\nİlan No:\n#297281\nİlan Tarihi:\n15/02/2021\nMarka:\nMercedes\nModel:\nE Serisi\nVaryant:\nE 180 AMG\nModel Yılı:\n2018\nKilometre:\n32.000\nYakıt Türü:\nBenzin\nVites Tipi:\nOtomatik Vites\nMotor Hacmi:\n1301-1600 cc\nMotor Gücü:\nBilmiyorum\nKimden:\nGaleriden\n***********************************************+1 7.araç verisi -------------------------------------------------\n325.000 TL\n6.287 TL den başlayan taksitlerle »\nARABA DEĞERİ HESAPLA\nErzincan\nİlan No:\n#2316769\nİlan Tarihi:\n15/02/2021\nMarka:\nMercedes\nModel:\nE Serisi\nVaryant:\nE 200 CGI BlueEfficiency Avantgarde\nModel Yılı:\n2010\nKilometre:\n188.000\nYakıt Türü:\nBenzin\nVites Tipi:\nOtomatik Vites\nMotor Hacmi:\n1300 cc ve altı\nMotor Gücü:\nBilmiyorum\nKimden:\nGaleriden\n***********************************************+1 8.araç verisi -------------------------------------------------\n84.500 TL\n1.635 TL den başlayan taksitlerle »\nARABA DEĞERİ HESAPLA\nAnkara\nİlan No:\n#4110574\nİlan Tarihi:\n15/02/2021\nMarka:\nMercedes\nModel:\nE Serisi\nVaryant:\nE 200 Elegance\nModel Yılı:\n1997\nKilometre:\n425.000\nYakıt Türü:\nBenzin/LPG\nVites Tipi:\nOtomatik Vites\nMotor Hacmi:\n1801-2000 cc\nMotor Gücü:\nBilmiyorum\nKimden:\nGaleriden\n***********************************************+1 9.araç verisi -------------------------------------------------\n386.500 TL\n7.477 TL den başlayan taksitlerle »\nARABA DEĞERİ HESAPLA\nİstanbul\nİlan No:\n#1329518\nİlan Tarihi:\n15/02/2021\nMarka:\nMercedes\nModel:\nE Serisi\nVaryant:\nE 180 Elite\nModel Yılı:\n2015\nKilometre:\n180.229\nYakıt Türü:\nBenzin\nVites Tipi:\nYarı Otomatik Vites\nMotor Hacmi:\n1301-1600 cc\nMotor Gücü:\nBilmiyorum\nKimden:\nGaleriden\n***********************************************+1 10.araç verisi -------------------------------------------------\n612.000 TL\n11.840 TL den başlayan taksitlerle »\nARABA DEĞERİ HESAPLA\nİstanbul\nİlan No:\n#4672364\nİlan Tarihi:\n15/02/2021\nMarka:\nMercedes\nModel:\nE Serisi\nVaryant:\nE 180 Exclusive\nModel Yılı:\n2018\nKilometre:\n35.000\nYakıt Türü:\nBenzin\nVites Tipi:\nOtomatik Vites\nMotor Hacmi:\n1301-1600 cc\nMotor Gücü:\nBilmiyorum\nKimden:\nGaleriden\n***********************************************+1 12.araç verisi -------------------------------------------------\n650.000 TL\n12.575 TL den başlayan taksitlerle »\nARABA DEĞERİ HESAPLA\nYalova\nİlan No:\n#4120326\nİlan Tarihi:\n15/02/2021\nMarka:\nMercedes\nModel:\nE Serisi\nVaryant:\nE 180 Exclusive\nModel Yılı:\n2018\nKilometre:\n59.000\nYakıt Türü:\nBenzin\nVites Tipi:\nOtomatik Vites\nMotor Hacmi:\n1301-1600 cc\nMotor Gücü:\nBilmiyorum\nKimden:\nGaleriden\n***********************************************+1 13.araç verisi -------------------------------------------------\n379.900 TL\n7.350 TL den başlayan taksitlerle »\nARABA DEĞERİ HESAPLA\nAydın\nİlan No:\n#1748166\nİlan Tarihi:\n15/02/2021\nMarka:\nMercedes\nModel:\nE Serisi\nVaryant:\nE 180 Elite\nModel Yılı:\n2013\nKilometre:\n117.000\nYakıt Türü:\nBenzin\nVites Tipi:\nYarı Otomatik Vites\nMotor Hacmi:\n1301-1600 cc\nMotor Gücü:\nBilmiyorum\nKimden:\nGaleriden\n***********************************************+1 14.araç verisi -------------------------------------------------\n450.000 TL\n8.706 TL den başlayan taksitlerle »\nARABA DEĞERİ HESAPLA\nAdana\nİlan No:\n#4493900\nİlan Tarihi:\n15/02/2021\nMarka:\nMercedes\nModel:\nE Serisi\nVaryant:\nE 180 Edition E\nModel Yılı:\n2015\nKilometre:\n95.000\nYakıt Türü:\nBenzin\nVites Tipi:\nYarı Otomatik Vites\nMotor Hacmi:\n1301-1600 cc\nMotor Gücü:\nBilmiyorum\nKimden:\nGaleriden\n***********************************************+1 15.araç verisi -------------------------------------------------\n62.500 TL\n1.209 TL den başlayan taksitlerle »\nARABA DEĞERİ HESAPLA\nMalatya\nİlan No:\n#1992300\nİlan Tarihi:\n15/02/2021\nMarka:\nMercedes\nModel:\nE Serisi\nVaryant:\nE 250 D Classic\nModel Yılı:\n1987\nKilometre:\n595.000\nYakıt Türü:\nDizel\nVites Tipi:\nDüz Vites\nMotor Hacmi:\n1300 cc ve altı\nMotor Gücü:\nBilmiyorum\nKimden:\nGaleriden\n***********************************************+1 16.araç verisi -------------------------------------------------\n495.900 TL\n9.594 TL den başlayan taksitlerle »\nARABA DEĞERİ HESAPLA\nAdana\nİlan No:\n#3650951\nİlan Tarihi:\n15/02/2021\nMarka:\nMercedes\nModel:\nE Serisi\nVaryant:\nE 350 CDI Premium\nModel Yılı:\n2011\nKilometre:\n169.000\nYakıt Türü:\nDizel\nVites Tipi:\nOtomatik Vites\nMotor Hacmi:\n1300 cc ve altı\nMotor Gücü:\nBilmiyorum\nKimden:\nGaleriden\n***********************************************+1 17.araç verisi -------------------------------------------------\n509.500 TL\n9.857 TL den başlayan taksitlerle »\nARABA DEĞERİ HESAPLA\nİstanbul\nİlan No:\n#4154400\nİlan Tarihi:\n15/02/2021\nMarka:\nMercedes\nModel:\nE Serisi\nVaryant:\nE 250 CDI AMG\nModel Yılı:\n2013\nKilometre:\n110.000\nYakıt Türü:\nDizel\nVites Tipi:\nOtomatik Vites\nMotor Hacmi:\n2001-2500 cc\nMotor Gücü:\nBilmiyorum\nKimden:\nGaleriden\n***********************************************+1 18.araç verisi -------------------------------------------------\n592.500 TL\n11.462 TL den başlayan taksitlerle »\nARABA DEĞERİ HESAPLA\nİstanbul\nİlan No:\n#2499145\nİlan Tarihi:\n15/02/2021\nMarka:\nMercedes\nModel:\nE Serisi\nVaryant:\nE 180 Exclusive\nModel Yılı:\n2017\nKilometre:\n94.000\nYakıt Türü:\nBenzin\nVites Tipi:\nOtomatik Vites\nMotor Hacmi:\n1301-1600 cc\nMotor Gücü:\nBilmiyorum\nKimden:\nGaleriden\n***********************************************+1 19.araç verisi -------------------------------------------------\n559.900 TL\n10.832 TL den başlayan taksitlerle »\nARABA DEĞERİ HESAPLA\nAnkara\nİlan No:\n#3055160\nİlan Tarihi:\n15/02/2021\nMarka:\nMercedes\nModel:\nE Serisi\nVaryant:\nE 180 Avantgarde\nModel Yılı:\n2017\nKilometre:\n50.000\nYakıt Türü:\nBenzin\nVites Tipi:\nOtomatik Vites\nMotor Hacmi:\n1301-1600 cc\nMotor Gücü:\nBilmiyorum\nKimden:\nGaleriden\n***********************************************+1 20.araç verisi -------------------------------------------------\n185.000 TL\n3.579 TL den başlayan taksitlerle »\nARABA DEĞERİ HESAPLA\nİzmir\nİlan No:\n#2295001\nİlan Tarihi:\n15/02/2021\nMarka:\nMercedes\nModel:\nE Serisi\nVaryant:\nE 200 Komp. Avantgarde\nModel Yılı:\n2005\nKilometre:\n360.000\nYakıt Türü:\nBenzin/LPG\nVites Tipi:\nYarı Otomatik Vites\nMotor Hacmi:\n1601-1800 cc\nMotor Gücü:\nBilmiyorum\nKimden:\nGaleriden\n***********************************************+1 21.araç verisi -------------------------------------------------\n405.000 TL\n7.835 TL den başlayan taksitlerle »\nARABA DEĞERİ HESAPLA\nİstanbul\nİlan No:\n#239688\nİlan Tarihi:\n15/02/2021\nMarka:\nMercedes\nModel:\nE Serisi\nVaryant:\nE 180 Elite\nModel Yılı:\n2015\nKilometre:\n145.000\nYakıt Türü:\nBenzin\nVites Tipi:\nYarı Otomatik Vites\nMotor Hacmi:\n1301-1600 cc\nMotor Gücü:\nBilmiyorum\nKimden:\nGaleriden\n***********************************************+1 22.araç verisi -------------------------------------------------\n*-*-*-*-*-*-*-*-*************Sayfa 9 *-*-*-*-*-*-*-*-*-*-***********\n193.000 TL\n3.734 TL den başlayan taksitlerle »\nARABA DEĞERİ HESAPLA\nDenizli\nİlan No:\n#1327296\nİlan Tarihi:\n15/02/2021\nMarka:\nMercedes\nModel:\nE Serisi\nVaryant:\nE 200 Komp. Elegance\nModel Yılı:\n2003\nKilometre:\n157.000\nYakıt Türü:\nBenzin\nVites Tipi:\nYarı Otomatik Vites\nMotor Hacmi:\n1601-1800 cc\nMotor Gücü:\nBilmiyorum\nKimden:\nGaleriden\n***********************************************+1 2.araç verisi -------------------------------------------------\n90.000 TL\n1.741 TL den başlayan taksitlerle »\nARABA DEĞERİ HESAPLA\nBurdur\nİlan No:\n#3132403\nİlan Tarihi:\n15/02/2021\nMarka:\nMercedes\nModel:\nE Serisi\nVaryant:\nE 200 Elegance\nModel Yılı:\n1997\nKilometre:\n266.000\nYakıt Türü:\nBenzin/LPG\nVites Tipi:\nOtomatik Vites\nMotor Hacmi:\n1801-2000 cc\nMotor Gücü:\nBilmiyorum\nKimden:\nGaleriden\n***********************************************+1 3.araç verisi -------------------------------------------------\n101.000 TL\n1.954 TL den başlayan taksitlerle »\nARABA DEĞERİ HESAPLA\nİlan No:\n#862681\nİlan Tarihi:\n15/02/2021\nMarka:\nMercedes\nModel:\nE Serisi\nVaryant:\nE 200 Elegance\nModel Yılı:\n1996\nKilometre:\n272.000\nYakıt Türü:\nBenzin/LPG\nVites Tipi:\nDüz Vites\nMotor Hacmi:\n1801-2000 cc\nMotor Gücü:\nBilmiyorum\nKimden:\nGaleriden\n***********************************************+1 4.araç verisi -------------------------------------------------\n284.000 TL\n5.494 TL den başlayan taksitlerle »\nARABA DEĞERİ HESAPLA\nHatay\nİlan No:\n#2992377\nİlan Tarihi:\n15/02/2021\nMarka:\nMercedes\nModel:\nE Serisi\nVaryant:\nE 200 CGI AMG\nModel Yılı:\n2010\nKilometre:\n298.000\nYakıt Türü:\nBenzin\nVites Tipi:\nOtomatik Vites\nMotor Hacmi:\n1601-1800 cc\nMotor Gücü:\nBilmiyorum\nKimden:\nGaleriden\n***********************************************+1 5.araç verisi -------------------------------------------------\n664.500 TL\n12.855 TL den başlayan taksitlerle »\nARABA DEĞERİ HESAPLA\nİstanbul\nİlan No:\n#4431571\nİlan Tarihi:\n15/02/2021\nMarka:\nMercedes\nModel:\nE Serisi\nVaryant:\nE 220 d Avantgarde\nModel Yılı:\n2016\nKilometre:\n140.000\nYakıt Türü:\nDizel\nVites Tipi:\nYarı Otomatik Vites\nMotor Hacmi:\n1801-2000 cc\nMotor Gücü:\nBilmiyorum\nKimden:\nGaleriden\n***********************************************+1 7.araç verisi -------------------------------------------------\n745.000 TL\n14.413 TL den başlayan taksitlerle »\nARABA DEĞERİ HESAPLA\nİstanbul\nİlan No:\n#4441510\nİlan Tarihi:\n15/02/2021\nMarka:\nMercedes\nModel:\nE Serisi\nVaryant:\nE 180 Exclusive\nModel Yılı:\n2018\nKilometre:\n44.000\nYakıt Türü:\nBenzin\nVites Tipi:\nOtomatik Vites\nMotor Hacmi:\n1301-1600 cc\nMotor Gücü:\nBilmiyorum\nKimden:\nGaleriden\n***********************************************+1 8.araç verisi -------------------------------------------------\n182.500 TL\n3.531 TL den başlayan taksitlerle »\nARABA DEĞERİ HESAPLA\nİstanbul\nİlan No:\n#4781201\nİlan Tarihi:\n15/02/2021\nMarka:\nMercedes\nModel:\nE Serisi\nVaryant:\nE 240 Elegance\nModel Yılı:\n2002\nKilometre:\n272.000\nYakıt Türü:\nBenzin/LPG\nVites Tipi:\nOtomatik Vites\nMotor Hacmi:\n2501-3000 cc\nMotor Gücü:\nBilmiyorum\nKimden:\nGaleriden\n***********************************************+1 9.araç verisi -------------------------------------------------\n425.000 TL\n8.222 TL den başlayan taksitlerle »\nARABA DEĞERİ HESAPLA\nGaziantep\nİlan No:\n#4411985\nİlan Tarihi:\n15/02/2021\nMarka:\nMercedes\nModel:\nE Serisi\nVaryant:\nE 180 Edition E\nModel Yılı:\n2015\nKilometre:\n137.000\nYakıt Türü:\nBenzin\nVites Tipi:\nYarı Otomatik Vites\nMotor Hacmi:\n1301-1600 cc\nMotor Gücü:\nBilmiyorum\nKimden:\nGaleriden\n***********************************************+1 10.araç verisi -------------------------------------------------\n456.000 TL\n8.822 TL den başlayan taksitlerle »\nARABA DEĞERİ HESAPLA\nİstanbul\nİlan No:\n#3400690\nİlan Tarihi:\n15/02/2021\nMarka:\nMercedes\nModel:\nE Serisi\nVaryant:\nE 250 CDI Elite\nModel Yılı:\n2013\nKilometre:\n187.000\nYakıt Türü:\nDizel\nVites Tipi:\nYarı Otomatik Vites\nMotor Hacmi:\n2001-2500 cc\nMotor Gücü:\nBilmiyorum\nKimden:\nGaleriden\n***********************************************+1 12.araç verisi -------------------------------------------------\n750.000 TL\n14.509 TL den başlayan taksitlerle »\nARABA DEĞERİ HESAPLA\nİzmir\nİlan No:\n#749766\nİlan Tarihi:\n15/02/2021\nMarka:\nMercedes\nModel:\nE Serisi\nVaryant:\nE 220 d AMG\nModel Yılı:\n2016\nKilometre:\n64.900\nYakıt Türü:\nDizel\nVites Tipi:\nYarı Otomatik Vites\nMotor Hacmi:\n1801-2000 cc\nMotor Gücü:\nBilmiyorum\nKimden:\nGaleriden\n***********************************************+1 13.araç verisi -------------------------------------------------\n415.000 TL\n8.029 TL den başlayan taksitlerle »\nARABA DEĞERİ HESAPLA\nİstanbul\nİlan No:\n#4014807\nİlan Tarihi:\n15/02/2021\nMarka:\nMercedes\nModel:\nE Serisi\nVaryant:\nE 180 Edition E\nModel Yılı:\n2015\nKilometre:\n110.000\nYakıt Türü:\nBenzin\nVites Tipi:\nYarı Otomatik Vites\nMotor Hacmi:\n1301-1600 cc\nMotor Gücü:\nBilmiyorum\nKimden:\nGaleriden\n***********************************************+1 14.araç verisi -------------------------------------------------\n187.019 TL\n3.618 TL den başlayan taksitlerle »\nARABA DEĞERİ HESAPLA\nİstanbul\nİlan No:\n#4167675\nİlan Tarihi:\n15/02/2021\nMarka:\nMercedes\nModel:\nE Serisi\nVaryant:\nE 200 Komp. Avantgarde\nModel Yılı:\n2005\nKilometre:\n291.000\nYakıt Türü:\nBenzin/LPG\nVites Tipi:\nYarı Otomatik Vites\nMotor Hacmi:\n1601-1800 cc\nMotor Gücü:\nBilmiyorum\nKimden:\nGaleriden\n***********************************************+1 15.araç verisi -------------------------------------------------\n372.750 TL\n7.211 TL den başlayan taksitlerle »\nARABA DEĞERİ HESAPLA\nİstanbul\nİlan No:\n#3686001\nİlan Tarihi:\n15/02/2021\nMarka:\nMercedes\nModel:\nE Serisi\nVaryant:\nE 180 Elite\nModel Yılı:\n2014\nKilometre:\n107.000\nYakıt Türü:\nBenzin\nVites Tipi:\nYarı Otomatik Vites\nMotor Hacmi:\n1301-1600 cc\nMotor Gücü:\nBilmiyorum\nKimden:\nGaleriden\n***********************************************+1 16.araç verisi -------------------------------------------------\n231.750 TL\n4.483 TL den başlayan taksitlerle »\nARABA DEĞERİ HESAPLA\nKocaeli\nİlan No:\n#4508892\nİlan Tarihi:\n15/02/2021\nMarka:\nMercedes\nModel:\nE Serisi\nVaryant:\nE 220 CDI Avantgarde\nModel Yılı:\n2008\nKilometre:\n230.000\nYakıt Türü:\nDizel\nVites Tipi:\nYarı Otomatik Vites\nMotor Hacmi:\n2001-2500 cc\nMotor Gücü:\nBilmiyorum\nKimden:\nGaleriden\n***********************************************+1 17.araç verisi -------------------------------------------------\n308.950 TL\n5.977 TL den başlayan taksitlerle »\nARABA DEĞERİ HESAPLA\nİstanbul\nİlan No:\n#2414109\nİlan Tarihi:\n15/02/2021\nMarka:\nMercedes\nModel:\nE Serisi\nVaryant:\nE 200 CGI AMG\nModel Yılı:\n2010\nKilometre:\n165.000\nYakıt Türü:\nBenzin\nVites Tipi:\nOtomatik Vites\nMotor Hacmi:\n1601-1800 cc\nMotor Gücü:\nBilmiyorum\nKimden:\nGaleriden\n***********************************************+1 18.araç verisi -------------------------------------------------\n309.950 TL\n5.996 TL den başlayan taksitlerle »\nARABA DEĞERİ HESAPLA\nİstanbul\nİlan No:\n#3226269\nİlan Tarihi:\n15/02/2021\nMarka:\nMercedes\nModel:\nE Serisi\nVaryant:\nE 250 CGI AMG\nModel Yılı:\n2011\nKilometre:\n113.000\nYakıt Türü:\nBenzin\nVites Tipi:\nYarı Otomatik Vites\nMotor Hacmi:\n1300 cc ve altı\nMotor Gücü:\nBilmiyorum\nKimden:\nGaleriden\n***********************************************+1 19.araç verisi -------------------------------------------------\n460.000 TL\n8.899 TL den başlayan taksitlerle »\nARABA DEĞERİ HESAPLA\nİstanbul\nİlan No:\n#1450995\nİlan Tarihi:\n15/02/2021\nMarka:\nMercedes\nModel:\nE Serisi\nVaryant:\nE 180 Elite\nModel Yılı:\n2014\nKilometre:\n97.000\nYakıt Türü:\nBenzin\nVites Tipi:\nYarı Otomatik Vites\nMotor Hacmi:\n1301-1600 cc\nMotor Gücü:\nBilmiyorum\nKimden:\nGaleriden\n***********************************************+1 20.araç verisi -------------------------------------------------\n285.000 TL\n5.514 TL den başlayan taksitlerle »\nARABA DEĞERİ HESAPLA\nKilis\nİlan No:\n#4004835\nİlan Tarihi:\n15/02/2021\nMarka:\nMercedes\nModel:\nE Serisi\nVaryant:\nE 200 CGI AMG\nModel Yılı:\n2010\nKilometre:\n184.000\nYakıt Türü:\nBenzin\nVites Tipi:\nOtomatik Vites\nMotor Hacmi:\n1601-1800 cc\nMotor Gücü:\nBilmiyorum\nKimden:\nGaleriden\n***********************************************+1 21.araç verisi -------------------------------------------------\n1.380.000 TL\nTL den başlayan taksitlerle »\nARABA DEĞERİ HESAPLA\nİstanbul\nİlan No:\n#440762\nİlan Tarihi:\n15/02/2021\nMarka:\nMercedes\nModel:\nE Serisi\nVaryant:\nE 220 d AMG\nModel Yılı:\n2020\nKilometre:\n10\nYakıt Türü:\nDizel\nVites Tipi:\nOtomatik Vites\nMotor Hacmi:\n1801-2000 cc\nMotor Gücü:\nBilmiyorum\nKimden:\nGaleriden\n***********************************************+1 22.araç verisi -------------------------------------------------\n*-*-*-*-*-*-*-*-*************Sayfa 10 *-*-*-*-*-*-*-*-*-*-***********\n545.000 TL\n10.544 TL den başlayan taksitlerle »\nARABA DEĞERİ HESAPLA\nİstanbul\nİlan No:\n#1768905\nİlan Tarihi:\n15/02/2021\nMarka:\nMercedes\nModel:\nE Serisi\nVaryant:\nE 250 AMG 7G-Tronic\nModel Yılı:\n2016\nKilometre:\n98.898\nYakıt Türü:\nBenzin\nVites Tipi:\nYarı Otomatik Vites\nMotor Hacmi:\n1801-2000 cc\nMotor Gücü:\nBilmiyorum\nKimden:\nGaleriden\n***********************************************+1 2.araç verisi -------------------------------------------------\n395.000 TL\n7.642 TL den başlayan taksitlerle »\nARABA DEĞERİ HESAPLA\nİstanbul\nİlan No:\n#999751\nİlan Tarihi:\n15/02/2021\nMarka:\nMercedes\nModel:\nE Serisi\nVaryant:\nE 350 CDI BlueEfficiency Elegance\nModel Yılı:\n2011\nKilometre:\n173.000\nYakıt Türü:\nDizel\nVites Tipi:\nYarı Otomatik Vites\nMotor Hacmi:\n2501-3000 cc\nMotor Gücü:\nBilmiyorum\nKimden:\nGaleriden\n***********************************************+1 3.araç verisi -------------------------------------------------\n1.475.000 TL\nTL den başlayan taksitlerle »\nARABA DEĞERİ HESAPLA\nİstanbul\nİlan No:\n#2673129\nİlan Tarihi:\n15/02/2021\nMarka:\nMercedes\nModel:\nE Serisi\nVaryant:\nE 300 BlueTEC Hybrid\nModel Yılı:\n2020\nKilometre:\n224\nYakıt Türü:\nBenzin\nVites Tipi:\nOtomatik Vites\nMotor Hacmi:\n1300 cc ve altı\nMotor Gücü:\nBilmiyorum\nKimden:\nGaleriden\n***********************************************+1 4.araç verisi -------------------------------------------------\n1.450.000 TL\nTL den başlayan taksitlerle »\nARABA DEĞERİ HESAPLA\nİstanbul\nİlan No:\n#2703516\nİlan Tarihi:\n15/02/2021\nMarka:\nMercedes\nModel:\nE Serisi\nVaryant:\nE 300 BlueTEC Hybrid\nModel Yılı:\n2020\nKilometre:\n2.850\nYakıt Türü:\nBenzin\nVites Tipi:\nOtomatik Vites\nMotor Hacmi:\n1300 cc ve altı\nMotor Gücü:\nBilmiyorum\nKimden:\nGaleriden\n***********************************************+1 5.araç verisi -------------------------------------------------\n293.500 TL\n5.678 TL den başlayan taksitlerle »\nARABA DEĞERİ HESAPLA\nİstanbul\nİlan No:\n#4064768\nİlan Tarihi:\n15/02/2021\nMarka:\nMercedes\nModel:\nE Serisi\nVaryant:\nE 250 CGI Premium\nModel Yılı:\n2011\nKilometre:\n157.237\nYakıt Türü:\nBenzin\nVites Tipi:\nOtomatik Vites\nMotor Hacmi:\n1601-1800 cc\nMotor Gücü:\nBilmiyorum\nKimden:\nGaleriden\n***********************************************+1 7.araç verisi -------------------------------------------------\n1.315.000 TL\nTL den başlayan taksitlerle »\nARABA DEĞERİ HESAPLA\nİstanbul\nİlan No:\n#225048\nİlan Tarihi:\n15/02/2021\nMarka:\nMercedes\nModel:\nE Serisi\nVaryant:\nE 300 CGI AMG\nModel Yılı:\n2018\nKilometre:\n3.741\nYakıt Türü:\nBenzin\nVites Tipi:\nOtomatik Vites\nMotor Hacmi:\n1801-2000 cc\nMotor Gücü:\nBilmiyorum\nKimden:\nGaleriden\n***********************************************+1 8.araç verisi -------------------------------------------------\n760.000 TL\n14.703 TL den başlayan taksitlerle »\nARABA DEĞERİ HESAPLA\nAnkara\nİlan No:\n#4092680\nİlan Tarihi:\n15/02/2021\nMarka:\nMercedes\nModel:\nE Serisi\nVaryant:\nE 180 AMG\nModel Yılı:\n2018\nKilometre:\n32.900\nYakıt Türü:\nBenzin\nVites Tipi:\nOtomatik Vites\nMotor Hacmi:\n1301-1600 cc\nMotor Gücü:\nBilmiyorum\nKimden:\nGaleriden\n***********************************************+1 9.araç verisi -------------------------------------------------\n449.000 TL\n8.686 TL den başlayan taksitlerle »\nARABA DEĞERİ HESAPLA\nİstanbul\nİlan No:\n#530308\nİlan Tarihi:\n09/02/2021\nMarka:\nMercedes\nModel:\nE Serisi\nVaryant:\nE 180 Elite\nModel Yılı:\n2016\nKilometre:\n93.500\nYakıt Türü:\nBenzin\nVites Tipi:\nYarı Otomatik Vites\nMotor Hacmi:\n1300 cc ve altı\nMotor Gücü:\nBilmiyorum\nKimden:\nGaleriden\n***********************************************+1 10.araç verisi -------------------------------------------------\n1.455.000 TL\nTL den başlayan taksitlerle »\nARABA DEĞERİ HESAPLA\nBursa\nİlan No:\n#3331132\nİlan Tarihi:\n08/02/2021\nMarka:\nMercedes\nModel:\nE Serisi\nVaryant:\nE 300 AMG\nModel Yılı:\n2020\nKilometre:\n1.700\nYakıt Türü:\nBenzin\nVites Tipi:\nOtomatik Vites\nMotor Hacmi:\n1300 cc ve altı\nMotor Gücü:\nBilmiyorum\nKimden:\nGaleriden\n***********************************************+1 12.araç verisi -------------------------------------------------\n496.000 TL\n9.596 TL den başlayan taksitlerle »\nARABA DEĞERİ HESAPLA\nİstanbul\nİlan No:\n#2124430\nİlan Tarihi:\n08/02/2021\nMarka:\nMercedes\nModel:\nE Serisi\nVaryant:\nE 180 Edition E\nModel Yılı:\n2015\nKilometre:\n48.180\nYakıt Türü:\nBenzin\nVites Tipi:\nYarı Otomatik Vites\nMotor Hacmi:\n1301-1600 cc\nMotor Gücü:\nBilmiyorum\nKimden:\nGaleriden\n***********************************************+1 13.araç verisi -------------------------------------------------\n359.000 TL\n6.945 TL den başlayan taksitlerle »\nARABA DEĞERİ HESAPLA\nVan\nİlan No:\n#3058914\nİlan Tarihi:\n08/02/2021\nMarka:\nMercedes\nModel:\nE Serisi\nVaryant:\nE 180 Elite\nModel Yılı:\n2013\nKilometre:\n232.000\nYakıt Türü:\nBenzin\nVites Tipi:\nYarı Otomatik Vites\nMotor Hacmi:\n1301-1600 cc\nMotor Gücü:\nBilmiyorum\nKimden:\nGaleriden\n***********************************************+1 14.araç verisi -------------------------------------------------\n749.000 TL\n14.490 TL den başlayan taksitlerle »\nARABA DEĞERİ HESAPLA\nİstanbul\nİlan No:\n#2510313\nİlan Tarihi:\n05/02/2021\nMarka:\nMercedes\nModel:\nE Serisi\nVaryant:\nE 220 d AMG\nModel Yılı:\n2016\nKilometre:\n66.300\nYakıt Türü:\nDizel\nVites Tipi:\nYarı Otomatik Vites\nMotor Hacmi:\n1801-2000 cc\nMotor Gücü:\nBilmiyorum\nKimden:\nGaleriden\n***********************************************+1 15.araç verisi -------------------------------------------------\n625.000 TL\n12.091 TL den başlayan taksitlerle »\nARABA DEĞERİ HESAPLA\nİstanbul\nİlan No:\n#3090310\nİlan Tarihi:\n05/02/2021\nMarka:\nMercedes\nModel:\nE Serisi\nVaryant:\nE 180 Exclusive\nModel Yılı:\n2018\nKilometre:\n22.289\nYakıt Türü:\nBenzin\nVites Tipi:\nOtomatik Vites\nMotor Hacmi:\n1301-1600 cc\nMotor Gücü:\nBilmiyorum\nKimden:\nGaleriden\n***********************************************+1 16.araç verisi -------------------------------------------------\n825.000 TL\n15.960 TL den başlayan taksitlerle »\nARABA DEĞERİ HESAPLA\nİzmir\nİlan No:\n#3278006\nİlan Tarihi:\n05/02/2021\nMarka:\nMercedes\nModel:\nE Serisi\nVaryant:\nE 220 d AMG\nModel Yılı:\n2017\nKilometre:\n81.000\nYakıt Türü:\nDizel\nVites Tipi:\nOtomatik Vites\nMotor Hacmi:\n1801-2000 cc\nMotor Gücü:\nBilmiyorum\nKimden:\nGaleriden\n***********************************************+1 17.araç verisi -------------------------------------------------\n620.000 TL\n11.995 TL den başlayan taksitlerle »\nARABA DEĞERİ HESAPLA\nYalova\nİlan No:\n#2056048\nİlan Tarihi:\n05/02/2021\nMarka:\nMercedes\nModel:\nE Serisi\nVaryant:\nE 180 Exclusive\nModel Yılı:\n2018\nKilometre:\n59.000\nYakıt Türü:\nBenzin\nVites Tipi:\nOtomatik Vites\nMotor Hacmi:\n1301-1600 cc\nMotor Gücü:\nBilmiyorum\nKimden:\nGaleriden\n***********************************************+1 18.araç verisi -------------------------------------------------\n400.000 TL\n7.738 TL den başlayan taksitlerle »\nARABA DEĞERİ HESAPLA\nİzmir\nİlan No:\n#1322308\nİlan Tarihi:\n05/02/2021\nMarka:\nMercedes\nModel:\nE Serisi\nVaryant:\nE 180 Elite\nModel Yılı:\n2014\nKilometre:\n117.000\nYakıt Türü:\nBenzin\nVites Tipi:\nYarı Otomatik Vites\nMotor Hacmi:\n1301-1600 cc\nMotor Gücü:\nBilmiyorum\nKimden:\nGaleriden\n***********************************************+1 19.araç verisi -------------------------------------------------\n729.000 TL\n14.103 TL den başlayan taksitlerle »\nARABA DEĞERİ HESAPLA\nUşak\nİlan No:\n#4668664\nİlan Tarihi:\n05/02/2021\nMarka:\nMercedes\nModel:\nE Serisi\nVaryant:\nE 220 d Exclusive\nModel Yılı:\n2016\nKilometre:\n84.000\nYakıt Türü:\nDizel\nVites Tipi:\nYarı Otomatik Vites\nMotor Hacmi:\n1801-2000 cc\nMotor Gücü:\nBilmiyorum\nKimden:\nGaleriden\n***********************************************+1 20.araç verisi -------------------------------------------------\n88.000 TL\n1.702 TL den başlayan taksitlerle »\nARABA DEĞERİ HESAPLA\nİstanbul\nİlan No:\n#1356212\nİlan Tarihi:\n05/02/2021\nMarka:\nMercedes\nModel:\nE Serisi\nVaryant:\nE 200 200\nModel Yılı:\n1994\nKilometre:\n358.000\nYakıt Türü:\nBenzin/LPG\nVites Tipi:\nDüz Vites\nMotor Hacmi:\n1300 cc ve altı\nMotor Gücü:\nBilmiyorum\nKimden:\nGaleriden\n***********************************************+1 21.araç verisi -------------------------------------------------\n86.500 TL\n1.673 TL den başlayan taksitlerle »\nARABA DEĞERİ HESAPLA\nAnkara\nİlan No:\n#255596\nİlan Tarihi:\n05/02/2021\nMarka:\nMercedes\nModel:\nE Serisi\nVaryant:\nE 200 Elegance\nModel Yılı:\n1998\nKilometre:\n361.000\nYakıt Türü:\nBenzin/LPG\nVites Tipi:\nOtomatik Vites\nMotor Hacmi:\n1801-2000 cc\nMotor Gücü:\nBilmiyorum\nKimden:\nGaleriden\n***********************************************+1 22.araç verisi -------------------------------------------------\n*-*-*-*-*-*-*-*-*************Sayfa 11 *-*-*-*-*-*-*-*-*-*-***********\n382.000 TL\n7.390 TL den başlayan taksitlerle »\nARABA DEĞERİ HESAPLA\nTekirdağ\nİlan No:\n#2877850\nİlan Tarihi:\n05/02/2021\nMarka:\nMercedes\nModel:\nE Serisi\nVaryant:\nE 180 Elite\nModel Yılı:\n2013\nKilometre:\n143.000\nYakıt Türü:\nBenzin\nVites Tipi:\nYarı Otomatik Vites\nMotor Hacmi:\n1301-1600 cc\nMotor Gücü:\nBilmiyorum\nKimden:\nGaleriden\n***********************************************+1 2.araç verisi -------------------------------------------------\n825.000 TL\n15.960 TL den başlayan taksitlerle »\nARABA DEĞERİ HESAPLA\nAnkara\nİlan No:\n#2722786\nİlan Tarihi:\n05/02/2021\nMarka:\nMercedes\nModel:\nE Serisi\nVaryant:\nE 180 Exclusive\nModel Yılı:\n2018\nKilometre:\n39.000\nYakıt Türü:\nBenzin\nVites Tipi:\nOtomatik Vites\nMotor Hacmi:\n1301-1600 cc\nMotor Gücü:\nBilmiyorum\nKimden:\nGaleriden\n***********************************************+1 3.araç verisi -------------------------------------------------\n417.500 TL\n8.077 TL den başlayan taksitlerle »\nARABA DEĞERİ HESAPLA\nİzmir\nİlan No:\n#4547963\nİlan Tarihi:\n05/02/2021\nMarka:\nMercedes\nModel:\nE Serisi\nVaryant:\nE 180 Premium\nModel Yılı:\n2014\nKilometre:\n102.854\nYakıt Türü:\nBenzin\nVites Tipi:\nYarı Otomatik Vites\nMotor Hacmi:\n1301-1600 cc\nMotor Gücü:\nBilmiyorum\nKimden:\nGaleriden\n***********************************************+1 4.araç verisi -------------------------------------------------\n589.000 TL\n11.395 TL den başlayan taksitlerle »\nARABA DEĞERİ HESAPLA\nKilis\nİlan No:\n#1628413\nİlan Tarihi:\n05/02/2021\nMarka:\nMercedes\nModel:\nE Serisi\nVaryant:\nE 180 AMG Premium\nModel Yılı:\n2015\nKilometre:\n24.000\nYakıt Türü:\nBenzin\nVites Tipi:\nOtomatik Vites\nMotor Hacmi:\n1301-1600 cc\nMotor Gücü:\nBilmiyorum\nKimden:\nGaleriden\n***********************************************+1 5.araç verisi -------------------------------------------------\n515.000 TL\n9.963 TL den başlayan taksitlerle »\nARABA DEĞERİ HESAPLA\nKilis\nİlan No:\n#2899087\nİlan Tarihi:\n05/02/2021\nMarka:\nMercedes\nModel:\nE Serisi\nVaryant:\nE 180 Edition E\nModel Yılı:\n2016\nKilometre:\n44.000\nYakıt Türü:\nBenzin\nVites Tipi:\nYarı Otomatik Vites\nMotor Hacmi:\n1301-1600 cc\nMotor Gücü:\nBilmiyorum\nKimden:\nGaleriden\n***********************************************+1 7.araç verisi -------------------------------------------------\n645.000 TL\n12.478 TL den başlayan taksitlerle »\nARABA DEĞERİ HESAPLA\nİstanbul\nİlan No:\n#3751088\nİlan Tarihi:\n05/02/2021\nMarka:\nMercedes\nModel:\nE Serisi\nVaryant:\nE 220 d Exclusive\nModel Yılı:\n2016\nKilometre:\n110.000\nYakıt Türü:\nDizel\nVites Tipi:\nYarı Otomatik Vites\nMotor Hacmi:\n1801-2000 cc\nMotor Gücü:\nBilmiyorum\nKimden:\nGaleriden\n***********************************************+1 8.araç verisi -------------------------------------------------\n535.900 TL\n10.368 TL den başlayan taksitlerle »\nARABA DEĞERİ HESAPLA\nİstanbul\nİlan No:\n#1671277\nİlan Tarihi:\n05/02/2021\nMarka:\nMercedes\nModel:\nE Serisi\nVaryant:\nE 250 AMG 7G-Tronic\nModel Yılı:\n2015\nKilometre:\n97.000\nYakıt Türü:\nBenzin\nVites Tipi:\nYarı Otomatik Vites\nMotor Hacmi:\n1801-2000 cc\nMotor Gücü:\nBilmiyorum\nKimden:\nGaleriden\n***********************************************+1 9.araç verisi -------------------------------------------------\nThe error has occurred in this process\n314.750 TL\n6.089 TL den başlayan taksitlerle »\nARABA DEĞERİ HESAPLA\nDiyarbakır\nİlan No:\n#557980\nİlan Tarihi:\n05/02/2021\nMarka:\nMercedes\nModel:\nE Serisi\nVaryant:\nE 180 Elite\nModel Yılı:\n2014\nKilometre:\n165.000\nYakıt Türü:\nBenzin\nVites Tipi:\nYarı Otomatik Vites\nMotor Hacmi:\n1301-1600 cc\nMotor Gücü:\nBilmiyorum\nKimden:\nGaleriden\n***********************************************+1 12.araç verisi -------------------------------------------------\n198.500 TL\n3.840 TL den başlayan taksitlerle »\nARABA DEĞERİ HESAPLA\nAnkara\nİlan No:\n#3571400\nİlan Tarihi:\n05/02/2021\nMarka:\nMercedes\nModel:\nE Serisi\nVaryant:\nE 200 Komp. Avantgarde\nModel Yılı:\n2004\nKilometre:\n340.000\nYakıt Türü:\nBenzin/LPG\nVites Tipi:\nYarı Otomatik Vites\nMotor Hacmi:\n1601-1800 cc\nMotor Gücü:\nBilmiyorum\nKimden:\nGaleriden\n***********************************************+1 13.araç verisi -------------------------------------------------\n185.000 TL\n3.579 TL den başlayan taksitlerle »\nARABA DEĞERİ HESAPLA\nOrdu\nİlan No:\n#3646960\nİlan Tarihi:\n05/02/2021\nMarka:\nMercedes\nModel:\nE Serisi\nVaryant:\nE 320 Avantgarde\nModel Yılı:\n2002\nKilometre:\n325.000\nYakıt Türü:\nBenzin\nVites Tipi:\nOtomatik Vites\nMotor Hacmi:\n3001-3500 cc\nMotor Gücü:\nBilmiyorum\nKimden:\nGaleriden\n***********************************************+1 14.araç verisi -------------------------------------------------\n413.000 TL\n7.990 TL den başlayan taksitlerle »\nARABA DEĞERİ HESAPLA\nKayseri\nİlan No:\n#2597119\nİlan Tarihi:\n05/02/2021\nMarka:\nMercedes\nModel:\nE Serisi\nVaryant:\nE 250 Elite\nModel Yılı:\n2014\nKilometre:\n177.000\nYakıt Türü:\nBenzin\nVites Tipi:\nYarı Otomatik Vites\nMotor Hacmi:\n1801-2000 cc\nMotor Gücü:\nBilmiyorum\nKimden:\nGaleriden\n***********************************************+1 15.araç verisi -------------------------------------------------\n530.000 TL\n10.253 TL den başlayan taksitlerle »\nARABA DEĞERİ HESAPLA\nManisa\nİlan No:\n#3629161\nİlan Tarihi:\n05/02/2021\nMarka:\nMercedes\nModel:\nE Serisi\nVaryant:\nE 250 Elite\nModel Yılı:\n2013\nKilometre:\n107.000\nYakıt Türü:\nBenzin\nVites Tipi:\nYarı Otomatik Vites\nMotor Hacmi:\n1801-2000 cc\nMotor Gücü:\nBilmiyorum\nKimden:\nGaleriden\n***********************************************+1 16.araç verisi -------------------------------------------------\n92.500 TL\n1.790 TL den başlayan taksitlerle »\nARABA DEĞERİ HESAPLA\nAnkara\nİlan No:\n#449857\nİlan Tarihi:\n05/02/2021\nMarka:\nMercedes\nModel:\nE Serisi\nVaryant:\nE 200 Elegance\nModel Yılı:\n1996\nKilometre:\n380.000\nYakıt Türü:\nBenzin/LPG\nVites Tipi:\nOtomatik Vites\nMotor Hacmi:\n1801-2000 cc\nMotor Gücü:\nBilmiyorum\nKimden:\nGaleriden\n***********************************************+1 17.araç verisi -------------------------------------------------\n92.500 TL\n1.790 TL den başlayan taksitlerle »\nARABA DEĞERİ HESAPLA\nAnkara\nİlan No:\n#449857\nİlan Tarihi:\n05/02/2021\nMarka:\nMercedes\nModel:\nE Serisi\nVaryant:\nE 200 Elegance\nModel Yılı:\n1996\nKilometre:\n380.000\nYakıt Türü:\nBenzin/LPG\nVites Tipi:\nOtomatik Vites\nMotor Hacmi:\n1801-2000 cc\nMotor Gücü:\nBilmiyorum\nKimden:\nGaleriden\n***********************************************+1 18.araç verisi -------------------------------------------------\nThe error has occurred in this process\nThe error has occured in this page\n439.900 TL\n8.510 TL den başlayan taksitlerle »\nARABA DEĞERİ HESAPLA\nGiresun\nİlan No:\n#4019097\nİlan Tarihi:\n05/02/2021\nMarka:\nMercedes\nModel:\nE Serisi\nVaryant:\nDiğer\nModel Yılı:\n2016\nKilometre:\n137.000\nYakıt Türü:\nBenzin\nVites Tipi:\nYarı Otomatik Vites\nMotor Hacmi:\n1301-1600 cc\nMotor Gücü:\nBilmiyorum\nKimden:\nGaleriden\n***********************************************+1 2.araç verisi -------------------------------------------------\n400.000 TL\n7.738 TL den başlayan taksitlerle »\nARABA DEĞERİ HESAPLA\nNevşehir\nİlan No:\n#1106368\nİlan Tarihi:\n05/02/2021\nMarka:\nMercedes\nModel:\nE Serisi\nVaryant:\nE 350 CDI BlueEfficiency Elegance\nModel Yılı:\n2010\nKilometre:\n235.000\nYakıt Türü:\nDizel\nVites Tipi:\nYarı Otomatik Vites\nMotor Hacmi:\n2501-3000 cc\nMotor Gücü:\nBilmiyorum\nKimden:\nGaleriden\n***********************************************+1 3.araç verisi -------------------------------------------------\n425.000 TL\n8.222 TL den başlayan taksitlerle »\nARABA DEĞERİ HESAPLA\nİstanbul\nİlan No:\n#690132\nİlan Tarihi:\n05/02/2021\nMarka:\nMercedes\nModel:\nE Serisi\nVaryant:\nE 250 CGI AMG\nModel Yılı:\n2013\nKilometre:\n102.000\nYakıt Türü:\nBenzin\nVites Tipi:\nOtomatik Vites\nMotor Hacmi:\n1300 cc ve altı\nMotor Gücü:\nBilmiyorum\nKimden:\nGaleriden\n***********************************************+1 4.araç verisi -------------------------------------------------\n234.000 TL\n4.527 TL den başlayan taksitlerle »\nARABA DEĞERİ HESAPLA\nİstanbul\nİlan No:\n#1999916\nİlan Tarihi:\n05/02/2021\nMarka:\nMercedes\nModel:\nE Serisi\nVaryant:\nE 200 Komp. Avantgarde\nModel Yılı:\n2005\nKilometre:\n198.000\nYakıt Türü:\nBenzin/LPG\nVites Tipi:\nYarı Otomatik Vites\nMotor Hacmi:\n1601-1800 cc\nMotor Gücü:\nBilmiyorum\nKimden:\nGaleriden\n***********************************************+1 5.araç verisi -------------------------------------------------\n622.500 TL\n12.043 TL den başlayan taksitlerle »\nARABA DEĞERİ HESAPLA\nİstanbul\nİlan No:\n#1411441\nİlan Tarihi:\n05/02/2021\nMarka:\nMercedes\nModel:\nE Serisi\nVaryant:\nE 180 Exclusive\nModel Yılı:\n2017\nKilometre:\n43.000\nYakıt Türü:\nBenzin\nVites Tipi:\nOtomatik Vites\nMotor Hacmi:\n1301-1600 cc\nMotor Gücü:\nBilmiyorum\nKimden:\nGaleriden\n***********************************************+1 7.araç verisi -------------------------------------------------\n559.000 TL\n10.814 TL den başlayan taksitlerle »\nARABA DEĞERİ HESAPLA\nİstanbul\nİlan No:\n#466545\nİlan Tarihi:\n05/02/2021\nMarka:\nMercedes\nModel:\nE Serisi\nVaryant:\nE 180 Avantgarde\nModel Yılı:\n2017\nKilometre:\n72.000\nYakıt Türü:\nBenzin\nVites Tipi:\nOtomatik Vites\nMotor Hacmi:\n1301-1600 cc\nMotor Gücü:\nBilmiyorum\nKimden:\nGaleriden\n***********************************************+1 8.araç verisi -------------------------------------------------\n545.000 TL\n10.544 TL den başlayan taksitlerle »\nARABA DEĞERİ HESAPLA\nİstanbul\nİlan No:\n#4142837\nİlan Tarihi:\n05/02/2021\nMarka:\nMercedes\nModel:\nE Serisi\nVaryant:\nE 250 CDI Edition\nModel Yılı:\n2015\nKilometre:\n167.000\nYakıt Türü:\nDizel\nVites Tipi:\nYarı Otomatik Vites\nMotor Hacmi:\n2001-2500 cc\nMotor Gücü:\nBilmiyorum\nKimden:\nGaleriden\n***********************************************+1 9.araç verisi -------------------------------------------------\n472.000 TL\n9.131 TL den başlayan taksitlerle »\nARABA DEĞERİ HESAPLA\nİstanbul\nİlan No:\n#2571859\nİlan Tarihi:\n05/02/2021\nMarka:\nMercedes\nModel:\nE Serisi\nVaryant:\nE 180 Edition E\nModel Yılı:\n2015\nKilometre:\n55.000\nYakıt Türü:\nBenzin\nVites Tipi:\nYarı Otomatik Vites\nMotor Hacmi:\n1301-1600 cc\nMotor Gücü:\nBilmiyorum\nKimden:\nGaleriden\n***********************************************+1 10.araç verisi -------------------------------------------------\n655.000 TL\n12.672 TL den başlayan taksitlerle »\nARABA DEĞERİ HESAPLA\nİstanbul\nİlan No:\n#2438193\nİlan Tarihi:\n05/02/2021\nMarka:\nMercedes\nModel:\nE Serisi\nVaryant:\nE 180 AMG\nModel Yılı:\n2018\nKilometre:\n30.000\nYakıt Türü:\nBenzin\nVites Tipi:\nOtomatik Vites\nMotor Hacmi:\n1301-1600 cc\nMotor Gücü:\nBilmiyorum\nKimden:\nGaleriden\n***********************************************+1 12.araç verisi -------------------------------------------------\nThe error has occured in this page\n1.105.000 TL\nTL den başlayan taksitlerle »\nARABA DEĞERİ HESAPLA\nİstanbul\nİlan No:\n#340445\nİlan Tarihi:\n05/02/2021\nMarka:\nMercedes\nModel:\nE Serisi\nVaryant:\nE 220 d Avantgarde\nModel Yılı:\n2020\nKilometre:\n15\nYakıt Türü:\nDizel\nVites Tipi:\nOtomatik Vites\nMotor Hacmi:\n1300 cc ve altı\nMotor Gücü:\nBilmiyorum\nKimden:\nGaleriden\n***********************************************+1 2.araç verisi -------------------------------------------------\n725.000 TL\n14.026 TL den başlayan taksitlerle »\nARABA DEĞERİ HESAPLA\nİstanbul\nİlan No:\n#4641759\nİlan Tarihi:\n05/02/2021\nMarka:\nMercedes\nModel:\nE Serisi\nVaryant:\nE 200 AMG\nModel Yılı:\n2016\nKilometre:\n62.459\nYakıt Türü:\nBenzin\nVites Tipi:\nYarı Otomatik Vites\nMotor Hacmi:\n1801-2000 cc\nMotor Gücü:\nBilmiyorum\nKimden:\nGaleriden\n***********************************************+1 3.araç verisi -------------------------------------------------\n1.475.000 TL\nTL den başlayan taksitlerle »\nARABA DEĞERİ HESAPLA\nİstanbul\nİlan No:\n#4248118\nİlan Tarihi:\n05/02/2021\nMarka:\nMercedes\nModel:\nE Serisi\nVaryant:\nE 300 AMG\nModel Yılı:\n2020\nKilometre:\n12\nYakıt Türü:\nBenzin\nVites Tipi:\nOtomatik Vites\nMotor Hacmi:\n1300 cc ve altı\nMotor Gücü:\nBilmiyorum\nKimden:\nGaleriden\n***********************************************+1 4.araç verisi -------------------------------------------------\n225.000 TL\n4.353 TL den başlayan taksitlerle »\nARABA DEĞERİ HESAPLA\nİstanbul\nİlan No:\n#2937093\nİlan Tarihi:\n05/02/2021\nMarka:\nMercedes\nModel:\nE Serisi\nVaryant:\nE 320 Avantgarde\nModel Yılı:\n2004\nKilometre:\n253.217\nYakıt Türü:\nBenzin/LPG\nVites Tipi:\nOtomatik Vites\nMotor Hacmi:\n3001-3500 cc\nMotor Gücü:\nBilmiyorum\nKimden:\nGaleriden\n***********************************************+1 5.araç verisi -------------------------------------------------\n134.900 TL\n2.610 TL den başlayan taksitlerle »\nARABA DEĞERİ HESAPLA\nKilis\nİlan No:\n#3216159\nİlan Tarihi:\n05/02/2021\nMarka:\nMercedes\nModel:\nE Serisi\nVaryant:\nE 320 CDI Avantgarde\nModel Yılı:\n2001\nKilometre:\n200.000\nYakıt Türü:\nDizel\nVites Tipi:\nYarı Otomatik Vites\nMotor Hacmi:\n3001-3500 cc\nMotor Gücü:\nBilmiyorum\nKimden:\nGaleriden\n***********************************************+1 7.araç verisi -------------------------------------------------\nThe error has occured in this page\n478.000 TL\n9.247 TL den başlayan taksitlerle »\nARABA DEĞERİ HESAPLA\nİstanbul\nİlan No:\n#2261094\nİlan Tarihi:\n24/01/2021\nMarka:\nMercedes\nModel:\nE Serisi\nVaryant:\nE 250 AMG 7G-Tronic\nModel Yılı:\n2013\nKilometre:\n60.845\nYakıt Türü:\nBenzin\nVites Tipi:\nOtomatik Vites\nMotor Hacmi:\n1300 cc ve altı\nMotor Gücü:\nBilmiyorum\nKimden:\nGaleriden\n***********************************************+1 2.araç verisi -------------------------------------------------\n82.500 TL\n1.596 TL den başlayan taksitlerle »\nARABA DEĞERİ HESAPLA\nGaziantep\nİlan No:\n#2077809\nİlan Tarihi:\n24/01/2021\nMarka:\nMercedes\nModel:\nE Serisi\nVaryant:\nE 320 Elegance\nModel Yılı:\n1996\nKilometre:\n230.000\nYakıt Türü:\nBenzin/LPG\nVites Tipi:\nOtomatik Vites\nMotor Hacmi:\n3001-3500 cc\nMotor Gücü:\nBilmiyorum\nKimden:\nGaleriden\n***********************************************+1 3.araç verisi -------------------------------------------------\n995.000 TL\n19.249 TL den başlayan taksitlerle »\nARABA DEĞERİ HESAPLA\nİlan No:\n#2465303\nİlan Tarihi:\n24/01/2021\nMarka:\nMercedes\nModel:\nE Serisi\nVaryant:\nE 300 Avantgarde\nModel Yılı:\n2017\nKilometre:\n26.000\nYakıt Türü:\nBenzin\nVites Tipi:\nOtomatik Vites\nMotor Hacmi:\n1801-2000 cc\nMotor Gücü:\nBilmiyorum\nKimden:\nGaleriden\n***********************************************+1 4.araç verisi -------------------------------------------------\nThe error has occurred in this process\nThe error has occured in this page\n574.900 TL\n11.122 TL den başlayan taksitlerle »\nARABA DEĞERİ HESAPLA\nAnkara\nİlan No:\n#1445750\nİlan Tarihi:\n24/01/2021\nMarka:\nMercedes\nModel:\nE Serisi\nVaryant:\nE 180 Avantgarde\nModel Yılı:\n2017\nKilometre:\n50.000\nYakıt Türü:\nBenzin\nVites Tipi:\nOtomatik Vites\nMotor Hacmi:\n1301-1600 cc\nMotor Gücü:\nBilmiyorum\nKimden:\nGaleriden\n***********************************************+1 2.araç verisi -------------------------------------------------\n278.000 TL\n5.378 TL den başlayan taksitlerle »\nARABA DEĞERİ HESAPLA\nİstanbul\nİlan No:\n#203188\nİlan Tarihi:\n24/01/2021\nMarka:\nMercedes\nModel:\nE Serisi\nVaryant:\nE 200 CGI BlueEfficiency Avantgarde\nModel Yılı:\n2011\nKilometre:\n198.000\nYakıt Türü:\nBenzin\nVites Tipi:\nYarı Otomatik Vites\nMotor Hacmi:\n1300 cc ve altı\nMotor Gücü:\nBilmiyorum\nKimden:\nGaleriden\n***********************************************+1 3.araç verisi -------------------------------------------------\n745.000 TL\n14.413 TL den başlayan taksitlerle »\nARABA DEĞERİ HESAPLA\nİstanbul\nİlan No:\n#3019458\nİlan Tarihi:\n24/01/2021\nMarka:\nMercedes\nModel:\nE Serisi\nVaryant:\nE 220 d Exclusive\nModel Yılı:\n2016\nKilometre:\n89.909\nYakıt Türü:\nDizel\nVites Tipi:\nYarı Otomatik Vites\nMotor Hacmi:\n1801-2000 cc\nMotor Gücü:\nBilmiyorum\nKimden:\nGaleriden\n***********************************************+1 4.araç verisi -------------------------------------------------\n352.900 TL\n6.827 TL den başlayan taksitlerle »\nARABA DEĞERİ HESAPLA\nİstanbul\nİlan No:\n#1430879\nİlan Tarihi:\n24/01/2021\nMarka:\nMercedes\nModel:\nE Serisi\nVaryant:\nE 200 CGI AMG\nModel Yılı:\n2011\nKilometre:\n132.000\nYakıt Türü:\nBenzin/LPG\nVites Tipi:\nYarı Otomatik Vites\nMotor Hacmi:\n1300 cc ve altı\nMotor Gücü:\nBilmiyorum\nKimden:\nGaleriden\n***********************************************+1 5.araç verisi -------------------------------------------------\n189.999 TL\n3.676 TL den başlayan taksitlerle »\nARABA DEĞERİ HESAPLA\nİstanbul\nİlan No:\n#3365549\nİlan Tarihi:\n24/01/2021\nMarka:\nMercedes\nModel:\nE Serisi\nVaryant:\nE 220 CDI Avantgarde\nModel Yılı:\n2007\nKilometre:\n412.000\nYakıt Türü:\nDizel\nVites Tipi:\nYarı Otomatik Vites\nMotor Hacmi:\n2001-2500 cc\nMotor Gücü:\nBilmiyorum\nKimden:\nGaleriden\n***********************************************+1 7.araç verisi -------------------------------------------------\n405.000 TL\n7.835 TL den başlayan taksitlerle »\nARABA DEĞERİ HESAPLA\nDenizli\nİlan No:\n#2828728\nİlan Tarihi:\n24/01/2021\nMarka:\nMercedes\nModel:\nE Serisi\nVaryant:\nE 180 Elite\nModel Yılı:\n2013\nKilometre:\n93.000\nYakıt Türü:\nBenzin\nVites Tipi:\nYarı Otomatik Vites\nMotor Hacmi:\n1301-1600 cc\nMotor Gücü:\nBilmiyorum\nKimden:\nGaleriden\n***********************************************+1 8.araç verisi -------------------------------------------------\n230.000 TL\n4.450 TL den başlayan taksitlerle »\nARABA DEĞERİ HESAPLA\nAdıyaman\nİlan No:\n#2158989\nİlan Tarihi:\n24/01/2021\nMarka:\nMercedes\nModel:\nE Serisi\nVaryant:\nE 220 CDI Avantgarde\nModel Yılı:\n2006\nKilometre:\n335.000\nYakıt Türü:\nDizel\nVites Tipi:\nYarı Otomatik Vites\nMotor Hacmi:\n2001-2500 cc\nMotor Gücü:\nBilmiyorum\nKimden:\nGaleriden\n***********************************************+1 9.araç verisi -------------------------------------------------\n686.000 TL\n13.271 TL den başlayan taksitlerle »\nARABA DEĞERİ HESAPLA\nİstanbul\nİlan No:\n#1392875\nİlan Tarihi:\n24/01/2021\nMarka:\nMercedes\nModel:\nE Serisi\nVaryant:\nE 180 Exclusive\nModel Yılı:\n2018\nKilometre:\n16.000\nYakıt Türü:\nBenzin\nVites Tipi:\nOtomatik Vites\nMotor Hacmi:\n1301-1600 cc\nMotor Gücü:\nBilmiyorum\nKimden:\nGaleriden\n***********************************************+1 10.araç verisi -------------------------------------------------\n705.000 TL\n13.639 TL den başlayan taksitlerle »\nARABA DEĞERİ HESAPLA\nAnkara\nİlan No:\n#1500003\nİlan Tarihi:\n24/01/2021\nMarka:\nMercedes\nModel:\nE Serisi\nVaryant:\nE 250 CDI Edition\nModel Yılı:\n2016\nKilometre:\n125.000\nYakıt Türü:\nDizel\nVites Tipi:\nYarı Otomatik Vites\nMotor Hacmi:\n2001-2500 cc\nMotor Gücü:\nBilmiyorum\nKimden:\nGaleriden\n***********************************************+1 12.araç verisi -------------------------------------------------\n335.000 TL\n6.481 TL den başlayan taksitlerle »\nARABA DEĞERİ HESAPLA\nKonya\nİlan No:\n#3597138\nİlan Tarihi:\n24/01/2021\nMarka:\nMercedes\nModel:\nE Serisi\nVaryant:\nE 280 CDI Avantgarde\nModel Yılı:\n2008\nKilometre:\n212.000\nYakıt Türü:\nDizel\nVites Tipi:\nOtomatik Vites\nMotor Hacmi:\n1300 cc ve altı\nMotor Gücü:\nBilmiyorum\nKimden:\nGaleriden\n***********************************************+1 13.araç verisi -------------------------------------------------\n504.950 TL\n9.769 TL den başlayan taksitlerle »\nARABA DEĞERİ HESAPLA\nİstanbul\nİlan No:\n#917791\nİlan Tarihi:\n24/01/2021\nMarka:\nMercedes\nModel:\nE Serisi\nVaryant:\nE 250 AMG 7G-Tronic\nModel Yılı:\n2015\nKilometre:\n80.000\nYakıt Türü:\nBenzin\nVites Tipi:\nYarı Otomatik Vites\nMotor Hacmi:\n1801-2000 cc\nMotor Gücü:\nBilmiyorum\nKimden:\nGaleriden\n***********************************************+1 14.araç verisi -------------------------------------------------\n94.750 TL\n1.833 TL den başlayan taksitlerle »\nARABA DEĞERİ HESAPLA\nİstanbul\nİlan No:\n#3384801\nİlan Tarihi:\n24/01/2021\nMarka:\nMercedes\nModel:\nE Serisi\nVaryant:\nDiğer\nModel Yılı:\n1996\nKilometre:\n197.000\nYakıt Türü:\nDizel\nVites Tipi:\nOtomatik Vites\nMotor Hacmi:\n1300 cc ve altı\nMotor Gücü:\nBilmiyorum\nKimden:\nGaleriden\n***********************************************+1 15.araç verisi -------------------------------------------------\n619.000 TL\n11.975 TL den başlayan taksitlerle »\nARABA DEĞERİ HESAPLA\nGaziantep\nİlan No:\n#2040193\nİlan Tarihi:\n24/01/2021\nMarka:\nMercedes\nModel:\nE Serisi\nVaryant:\nE 180 Exclusive\nModel Yılı:\n2017\nKilometre:\n127.000\nYakıt Türü:\nBenzin\nVites Tipi:\nOtomatik Vites\nMotor Hacmi:\n1301-1600 cc\nMotor Gücü:\nBilmiyorum\nKimden:\nGaleriden\n***********************************************+1 16.araç verisi -------------------------------------------------\n685.000 TL\n13.252 TL den başlayan taksitlerle »\nARABA DEĞERİ HESAPLA\nGaziantep\nİlan No:\n#4684029\nİlan Tarihi:\n24/01/2021\nMarka:\nMercedes\nModel:\nE Serisi\nVaryant:\nE 180 Exclusive\nModel Yılı:\n2018\nKilometre:\n56.000\nYakıt Türü:\nBenzin\nVites Tipi:\nOtomatik Vites\nMotor Hacmi:\n1301-1600 cc\nMotor Gücü:\nBilmiyorum\nKimden:\nGaleriden\n***********************************************+1 17.araç verisi -------------------------------------------------\n689.500 TL\n13.339 TL den başlayan taksitlerle »\nARABA DEĞERİ HESAPLA\nGaziantep\nİlan No:\n#3464446\nİlan Tarihi:\n24/01/2021\nMarka:\nMercedes\nModel:\nE Serisi\nVaryant:\nE 200 AMG\nModel Yılı:\n2016\nKilometre:\n72.000\nYakıt Türü:\nBenzin\nVites Tipi:\nYarı Otomatik Vites\nMotor Hacmi:\n1801-2000 cc\nMotor Gücü:\nBilmiyorum\nKimden:\nGaleriden\n***********************************************+1 18.araç verisi -------------------------------------------------\n429.900 TL\n8.317 TL den başlayan taksitlerle »\nARABA DEĞERİ HESAPLA\nHatay\nİlan No:\n#3775892\nİlan Tarihi:\n24/01/2021\nMarka:\nMercedes\nModel:\nE Serisi\nVaryant:\nE 250 CDI Elite Avantgarde\nModel Yılı:\n2012\nKilometre:\n177.000\nYakıt Türü:\nDizel\nVites Tipi:\nOtomatik Vites\nMotor Hacmi:\n2001-2500 cc\nMotor Gücü:\nBilmiyorum\nKimden:\nGaleriden\n***********************************************+1 19.araç verisi -------------------------------------------------\n613.000 TL\n11.859 TL den başlayan taksitlerle »\nARABA DEĞERİ HESAPLA\nGaziantep\nİlan No:\n#4445128\nİlan Tarihi:\n24/01/2021\nMarka:\nMercedes\nModel:\nE Serisi\nVaryant:\nE 200 AMG\nModel Yılı:\n2016\nKilometre:\n62.000\nYakıt Türü:\nBenzin\nVites Tipi:\nYarı Otomatik Vites\nMotor Hacmi:\n1801-2000 cc\nMotor Gücü:\nBilmiyorum\nKimden:\nGaleriden\n***********************************************+1 20.araç verisi -------------------------------------------------\n715.000 TL\n13.832 TL den başlayan taksitlerle »\nARABA DEĞERİ HESAPLA\nİstanbul\nİlan No:\n#4781951\nİlan Tarihi:\n24/01/2021\nMarka:\nMercedes\nModel:\nE Serisi\nVaryant:\nE 220 d Avantgarde\nModel Yılı:\n2017\nKilometre:\n184.000\nYakıt Türü:\nDizel\nVites Tipi:\nOtomatik Vites\nMotor Hacmi:\n1801-2000 cc\nMotor Gücü:\nBilmiyorum\nKimden:\nGaleriden\n***********************************************+1 21.araç verisi -------------------------------------------------\nThe error has occurred in this process\n*-*-*-*-*-*-*-*-*************Sayfa 16 *-*-*-*-*-*-*-*-*-*-***********\n356.950 TL\n6.906 TL den başlayan taksitlerle »\nARABA DEĞERİ HESAPLA\nİstanbul\nİlan No:\n#2514556\nİlan Tarihi:\n24/01/2021\nMarka:\nMercedes\nModel:\nE Serisi\nVaryant:\nE 180 Elite\nModel Yılı:\n2014\nKilometre:\n220.000\nYakıt Türü:\nBenzin\nVites Tipi:\nYarı Otomatik Vites\nMotor Hacmi:\n1301-1600 cc\nMotor Gücü:\nBilmiyorum\nKimden:\nGaleriden\n***********************************************+1 2.araç verisi -------------------------------------------------\n443.900 TL\n8.588 TL den başlayan taksitlerle »\nARABA DEĞERİ HESAPLA\nİstanbul\nİlan No:\n#511133\nİlan Tarihi:\n24/01/2021\nMarka:\nMercedes\nModel:\nE Serisi\nVaryant:\nDiğer\nModel Yılı:\n2016\nKilometre:\n107.000\nYakıt Türü:\nBenzin\nVites Tipi:\nYarı Otomatik Vites\nMotor Hacmi:\n1301-1600 cc\nMotor Gücü:\nBilmiyorum\nKimden:\nGaleriden\n***********************************************+1 3.araç verisi -------------------------------------------------\n489.900 TL\n9.478 TL den başlayan taksitlerle »\nARABA DEĞERİ HESAPLA\nİstanbul\nİlan No:\n#855231\nİlan Tarihi:\n24/01/2021\nMarka:\nMercedes\nModel:\nE Serisi\nVaryant:\nE 180 Elite\nModel Yılı:\n2015\nKilometre:\n51.000\nYakıt Türü:\nBenzin\nVites Tipi:\nYarı Otomatik Vites\nMotor Hacmi:\n1301-1600 cc\nMotor Gücü:\nBilmiyorum\nKimden:\nGaleriden\n***********************************************+1 4.araç verisi -------------------------------------------------\nThe error has occurred in this process\nThe error has occured in this page\n563.500 TL\n10.901 TL den başlayan taksitlerle »\nARABA DEĞERİ HESAPLA\nİstanbul\nİlan No:\n#1151862\nİlan Tarihi:\n24/01/2021\nMarka:\nMercedes\nModel:\nE Serisi\nVaryant:\nE 180 AMG\nModel Yılı:\n2017\nKilometre:\n91.942\nYakıt Türü:\nBenzin\nVites Tipi:\nOtomatik Vites\nMotor Hacmi:\n1301-1600 cc\nMotor Gücü:\nBilmiyorum\nKimden:\nGaleriden\n***********************************************+1 2.araç verisi -------------------------------------------------\n192.500 TL\n3.724 TL den başlayan taksitlerle »\nARABA DEĞERİ HESAPLA\nİstanbul\nİlan No:\n#1190253\nİlan Tarihi:\n24/01/2021\nMarka:\nMercedes\nModel:\nE Serisi\nVaryant:\nE 220 Classic\nModel Yılı:\n1995\nKilometre:\n318.405\nYakıt Türü:\nBenzin/LPG\nVites Tipi:\nOtomatik Vites\nMotor Hacmi:\n1300 cc ve altı\nMotor Gücü:\nBilmiyorum\nKimden:\nGaleriden\n***********************************************+1 3.araç verisi -------------------------------------------------\n399.500 TL\n7.729 TL den başlayan taksitlerle »\nARABA DEĞERİ HESAPLA\nİstanbul\nİlan No:\n#1201587\nİlan Tarihi:\n24/01/2021\nMarka:\nMercedes\nModel:\nE Serisi\nVaryant:\nE 350 CDI BlueEfficiency Elegance\nModel Yılı:\n2011\nKilometre:\n173.000\nYakıt Türü:\nDizel\nVites Tipi:\nYarı Otomatik Vites\nMotor Hacmi:\n2501-3000 cc\nMotor Gücü:\nBilmiyorum\nKimden:\nGaleriden\n***********************************************+1 4.araç verisi -------------------------------------------------\n545.000 TL\n10.544 TL den başlayan taksitlerle »\nARABA DEĞERİ HESAPLA\nİstanbul\nİlan No:\n#3688116\nİlan Tarihi:\n24/01/2021\nMarka:\nMercedes\nModel:\nE Serisi\nVaryant:\nE 250 AMG 7G-Tronic\nModel Yılı:\n2016\nKilometre:\n98.898\nYakıt Türü:\nBenzin\nVites Tipi:\nYarı Otomatik Vites\nMotor Hacmi:\n1801-2000 cc\nMotor Gücü:\nBilmiyorum\nKimden:\nGaleriden\n***********************************************+1 5.araç verisi -------------------------------------------------\n699.000 TL\n13.523 TL den başlayan taksitlerle »\nARABA DEĞERİ HESAPLA\nİstanbul\nİlan No:\n#3202318\nİlan Tarihi:\n24/01/2021\nMarka:\nMercedes\nModel:\nE Serisi\nVaryant:\nE 180 Exclusive\nModel Yılı:\n2017\nKilometre:\n10.480\nYakıt Türü:\nBenzin\nVites Tipi:\nOtomatik Vites\nMotor Hacmi:\n1301-1600 cc\nMotor Gücü:\nBilmiyorum\nKimden:\nGaleriden\n***********************************************+1 7.araç verisi -------------------------------------------------\n995.000 TL\n19.249 TL den başlayan taksitlerle »\nARABA DEĞERİ HESAPLA\nİstanbul\nİlan No:\n#4101418\nİlan Tarihi:\n24/01/2021\nMarka:\nMercedes\nModel:\nE Serisi\nVaryant:\nE 300 AMG\nModel Yılı:\n2017\nKilometre:\n32.162\nYakıt Türü:\nBenzin\nVites Tipi:\nOtomatik Vites\nMotor Hacmi:\n1801-2000 cc\nMotor Gücü:\nBilmiyorum\nKimden:\nGaleriden\n***********************************************+1 8.araç verisi -------------------------------------------------\n1.450.000 TL\nTL den başlayan taksitlerle »\nARABA DEĞERİ HESAPLA\nİstanbul\nİlan No:\n#4769155\nİlan Tarihi:\n24/01/2021\nMarka:\nMercedes\nModel:\nE Serisi\nVaryant:\nE 300 BlueTEC Hybrid\nModel Yılı:\n2020\nKilometre:\n2.850\nYakıt Türü:\nBenzin\nVites Tipi:\nOtomatik Vites\nMotor Hacmi:\n1300 cc ve altı\nMotor Gücü:\nBilmiyorum\nKimden:\nGaleriden\n***********************************************+1 9.araç verisi -------------------------------------------------\n1.315.000 TL\nTL den başlayan taksitlerle »\nARABA DEĞERİ HESAPLA\nİstanbul\nİlan No:\n#920893\nİlan Tarihi:\n24/01/2021\nMarka:\nMercedes\nModel:\nE Serisi\nVaryant:\nE 300 CGI AMG\nModel Yılı:\n2018\nKilometre:\n3.741\nYakıt Türü:\nBenzin\nVites Tipi:\nOtomatik Vites\nMotor Hacmi:\n1801-2000 cc\nMotor Gücü:\nBilmiyorum\nKimden:\nGaleriden\n***********************************************+1 10.araç verisi -------------------------------------------------\n450.000 TL\n8.706 TL den başlayan taksitlerle »\nARABA DEĞERİ HESAPLA\nİstanbul\nİlan No:\n#2089005\nİlan Tarihi:\n24/01/2021\nMarka:\nMercedes\nModel:\nE Serisi\nVaryant:\nE 220 CDI Avantgarde\nModel Yılı:\n2011\nKilometre:\n83.149\nYakıt Türü:\nDizel\nVites Tipi:\nOtomatik Vites\nMotor Hacmi:\n1300 cc ve altı\nMotor Gücü:\nBilmiyorum\nKimden:\nGaleriden\n***********************************************+1 12.araç verisi -------------------------------------------------\n688.000 TL\n13.310 TL den başlayan taksitlerle »\nARABA DEĞERİ HESAPLA\nİstanbul\nİlan No:\n#752206\nİlan Tarihi:\n24/01/2021\nMarka:\nMercedes\nModel:\nE Serisi\nVaryant:\nE 180 Exclusive\nModel Yılı:\n2018\nKilometre:\n47.451\nYakıt Türü:\nBenzin\nVites Tipi:\nOtomatik Vites\nMotor Hacmi:\n1301-1600 cc\nMotor Gücü:\nBilmiyorum\nKimden:\nGaleriden\n***********************************************+1 13.araç verisi -------------------------------------------------\n688.000 TL\n13.310 TL den başlayan taksitlerle »\nARABA DEĞERİ HESAPLA\nİstanbul\nİlan No:\n#752206\nİlan Tarihi:\n24/01/2021\nMarka:\nMercedes\nModel:\nE Serisi\nVaryant:\nE 180 Exclusive\nModel Yılı:\n2018\nKilometre:\n47.451\nYakıt Türü:\nBenzin\nVites Tipi:\nOtomatik Vites\nMotor Hacmi:\n1301-1600 cc\nMotor Gücü:\nBilmiyorum\nKimden:\nGaleriden\n***********************************************+1 14.araç verisi -------------------------------------------------\n698.000 TL\n13.503 TL den başlayan taksitlerle »\nARABA DEĞERİ HESAPLA\nİstanbul\nİlan No:\n#2398436\nİlan Tarihi:\n24/01/2021\nMarka:\nMercedes\nModel:\nE Serisi\nVaryant:\nE 180 AMG\nModel Yılı:\n2018\nKilometre:\n37.422\nYakıt Türü:\nBenzin\nVites Tipi:\nOtomatik Vites\nMotor Hacmi:\n1301-1600 cc\nMotor Gücü:\nBilmiyorum\nKimden:\nGaleriden\n***********************************************+1 15.araç verisi -------------------------------------------------\n609.500 TL\n11.791 TL den başlayan taksitlerle »\nARABA DEĞERİ HESAPLA\nİstanbul\nİlan No:\n#4281994\nİlan Tarihi:\n24/01/2021\nMarka:\nMercedes\nModel:\nE Serisi\nVaryant:\nE 180 Avantgarde\nModel Yılı:\n2018\nKilometre:\n40.000\nYakıt Türü:\nBenzin\nVites Tipi:\nOtomatik Vites\nMotor Hacmi:\n1301-1600 cc\nMotor Gücü:\nBilmiyorum\nKimden:\nGaleriden\n***********************************************+1 16.araç verisi -------------------------------------------------\n535.000 TL\n10.350 TL den başlayan taksitlerle »\nARABA DEĞERİ HESAPLA\nOrdu\nİlan No:\n#439121\nİlan Tarihi:\n24/01/2021\nMarka:\nMercedes\nModel:\nE Serisi\nVaryant:\nE 180 AMG Premium\nModel Yılı:\n2015\nKilometre:\n98.000\nYakıt Türü:\nBenzin\nVites Tipi:\nOtomatik Vites\nMotor Hacmi:\n1301-1600 cc\nMotor Gücü:\nBilmiyorum\nKimden:\nGaleriden\n***********************************************+1 17.araç verisi -------------------------------------------------\n240.000 TL\n4.643 TL den başlayan taksitlerle »\nARABA DEĞERİ HESAPLA\nİstanbul\nİlan No:\n#3674561\nİlan Tarihi:\n24/01/2021\nMarka:\nMercedes\nModel:\nE Serisi\nVaryant:\nE 200 Komp. Avantgarde\nModel Yılı:\n2003\nKilometre:\n60.443\nYakıt Türü:\nBenzin\nVites Tipi:\nYarı Otomatik Vites\nMotor Hacmi:\n1601-1800 cc\nMotor Gücü:\nBilmiyorum\nKimden:\nGaleriden\n***********************************************+1 18.araç verisi -------------------------------------------------\n625.000 TL\n12.091 TL den başlayan taksitlerle »\nARABA DEĞERİ HESAPLA\nİstanbul\nİlan No:\n#1063272\nİlan Tarihi:\n24/01/2021\nMarka:\nMercedes\nModel:\nE Serisi\nVaryant:\nE 180 Exclusive\nModel Yılı:\n2017\nKilometre:\n104.000\nYakıt Türü:\nBenzin\nVites Tipi:\nOtomatik Vites\nMotor Hacmi:\n1301-1600 cc\nMotor Gücü:\nBilmiyorum\nKimden:\nGaleriden\n***********************************************+1 19.araç verisi -------------------------------------------------\n470.000 TL\n9.093 TL den başlayan taksitlerle »\nARABA DEĞERİ HESAPLA\nAnkara\nİlan No:\n#1266059\nİlan Tarihi:\n24/01/2021\nMarka:\nMercedes\nModel:\nE Serisi\nVaryant:\nE 180 AMG Premium\nModel Yılı:\n2015\nKilometre:\n153.000\nYakıt Türü:\nBenzin\nVites Tipi:\nOtomatik Vites\nMotor Hacmi:\n1301-1600 cc\nMotor Gücü:\nBilmiyorum\nKimden:\nGaleriden\n***********************************************+1 20.araç verisi -------------------------------------------------\n62.500 TL\n1.209 TL den başlayan taksitlerle »\nARABA DEĞERİ HESAPLA\nMalatya\nİlan No:\n#3751843\nİlan Tarihi:\n13/01/2021\nMarka:\nMercedes\nModel:\nE Serisi\nVaryant:\nE 250 D Classic\nModel Yılı:\n1987\nKilometre:\n590.000\nYakıt Türü:\nDizel\nVites Tipi:\nDüz Vites\nMotor Hacmi:\n1300 cc ve altı\nMotor Gücü:\nBilmiyorum\nKimden:\nGaleriden\n***********************************************+1 21.araç verisi -------------------------------------------------\n539.900 TL\n10.445 TL den başlayan taksitlerle »\nARABA DEĞERİ HESAPLA\nİstanbul\nİlan No:\n#3683514\nİlan Tarihi:\n11/01/2021\nMarka:\nMercedes\nModel:\nE Serisi\nVaryant:\nE 250 CDI Edition\nModel Yılı:\n2015\nKilometre:\n120.000\nYakıt Türü:\nDizel\nVites Tipi:\nYarı Otomatik Vites\nMotor Hacmi:\n2001-2500 cc\nMotor Gücü:\nBilmiyorum\nKimden:\nGaleriden\n***********************************************+1 22.araç verisi -------------------------------------------------\n*-*-*-*-*-*-*-*-*************Sayfa 18 *-*-*-*-*-*-*-*-*-*-***********\n525.900 TL\n10.174 TL den başlayan taksitlerle »\nARABA DEĞERİ HESAPLA\nİstanbul\nİlan No:\n#1003358\nİlan Tarihi:\n11/01/2021\nMarka:\nMercedes\nModel:\nE Serisi\nVaryant:\nE 250 AMG 7G-Tronic\nModel Yılı:\n2015\nKilometre:\n74.000\nYakıt Türü:\nBenzin\nVites Tipi:\nYarı Otomatik Vites\nMotor Hacmi:\n1801-2000 cc\nMotor Gücü:\nBilmiyorum\nKimden:\nGaleriden\n***********************************************+1 2.araç verisi -------------------------------------------------\n473.000 TL\n9.151 TL den başlayan taksitlerle »\nARABA DEĞERİ HESAPLA\nKilis\nİlan No:\n#706074\nİlan Tarihi:\n06/01/2021\nMarka:\nMercedes\nModel:\nE Serisi\nVaryant:\nE 180 Edition E\nModel Yılı:\n2015\nKilometre:\n125.000\nYakıt Türü:\nBenzin\nVites Tipi:\nYarı Otomatik Vites\nMotor Hacmi:\n1301-1600 cc\nMotor Gücü:\nBilmiyorum\nKimden:\nGaleriden\n***********************************************+1 3.araç verisi -------------------------------------------------\n445.900 TL\n8.626 TL den başlayan taksitlerle »\nARABA DEĞERİ HESAPLA\nİstanbul\nİlan No:\n#3095235\nİlan Tarihi:\n06/01/2021\nMarka:\nMercedes\nModel:\nE Serisi\nVaryant:\nE 180 Edition E\nModel Yılı:\n2015\nKilometre:\n132.000\nYakıt Türü:\nBenzin\nVites Tipi:\nYarı Otomatik Vites\nMotor Hacmi:\n1301-1600 cc\nMotor Gücü:\nBilmiyorum\nKimden:\nGaleriden\n***********************************************+1 4.araç verisi -------------------------------------------------\n95.000 TL\n1.838 TL den başlayan taksitlerle »\nARABA DEĞERİ HESAPLA\nHatay\nİlan No:\n#2341645\nİlan Tarihi:\n06/01/2021\nMarka:\nMercedes\nModel:\nE Serisi\nVaryant:\nE 200 Elegance\nModel Yılı:\n1998\nKilometre:\n308.000\nYakıt Türü:\nBenzin/LPG\nVites Tipi:\nOtomatik Vites\nMotor Hacmi:\n1801-2000 cc\nMotor Gücü:\nBilmiyorum\nKimden:\nGaleriden\n***********************************************+1 5.araç verisi -------------------------------------------------\n382.000 TL\n7.390 TL den başlayan taksitlerle »\nARABA DEĞERİ HESAPLA\nUşak\nİlan No:\n#4504264\nİlan Tarihi:\n06/01/2021\nMarka:\nMercedes\nModel:\nE Serisi\nVaryant:\nE 180 Elite\nModel Yılı:\n2015\nKilometre:\n162.065\nYakıt Türü:\nBenzin\nVites Tipi:\nYarı Otomatik Vites\nMotor Hacmi:\n1301-1600 cc\nMotor Gücü:\nBilmiyorum\nKimden:\nGaleriden\n***********************************************+1 7.araç verisi -------------------------------------------------\n382.000 TL\n7.390 TL den başlayan taksitlerle »\nARABA DEĞERİ HESAPLA\nUşak\nİlan No:\n#4504264\nİlan Tarihi:\n06/01/2021\nMarka:\nMercedes\nModel:\nE Serisi\nVaryant:\nE 180 Elite\nModel Yılı:\n2015\nKilometre:\n162.065\nYakıt Türü:\nBenzin\nVites Tipi:\nYarı Otomatik Vites\nMotor Hacmi:\n1301-1600 cc\nMotor Gücü:\nBilmiyorum\nKimden:\nGaleriden\n***********************************************+1 8.araç verisi -------------------------------------------------\n1.070.000 TL\nTL den başlayan taksitlerle »\nARABA DEĞERİ HESAPLA\nİlan No:\n#2507836\nİlan Tarihi:\n06/01/2021\nMarka:\nMercedes\nModel:\nE Serisi\nVaryant:\nE 300 Avantgarde\nModel Yılı:\n2017\nKilometre:\n25.675\nYakıt Türü:\nBenzin\nVites Tipi:\nOtomatik Vites\nMotor Hacmi:\n1801-2000 cc\nMotor Gücü:\nBilmiyorum\nKimden:\nGaleriden\n***********************************************+1 9.araç verisi -------------------------------------------------\n176.500 TL\n3.415 TL den başlayan taksitlerle »\nARABA DEĞERİ HESAPLA\nAnkara\nİlan No:\n#3674372\nİlan Tarihi:\n06/01/2021\nMarka:\nMercedes\nModel:\nE Serisi\nVaryant:\nE 220 CDI Avantgarde\nModel Yılı:\n2003\nKilometre:\n398.000\nYakıt Türü:\nDizel\nVites Tipi:\nYarı Otomatik Vites\nMotor Hacmi:\n2001-2500 cc\nMotor Gücü:\nBilmiyorum\nKimden:\nGaleriden\n***********************************************+1 10.araç verisi -------------------------------------------------\n423.000 TL\n8.183 TL den başlayan taksitlerle »\nARABA DEĞERİ HESAPLA\nİzmir\nİlan No:\n#783826\nİlan Tarihi:\n06/01/2021\nMarka:\nMercedes\nModel:\nE Serisi\nVaryant:\nE 180 Premium\nModel Yılı:\n2014\nKilometre:\n102.854\nYakıt Türü:\nBenzin\nVites Tipi:\nYarı Otomatik Vites\nMotor Hacmi:\n1301-1600 cc\nMotor Gücü:\nBilmiyorum\nKimden:\nGaleriden\n***********************************************+1 12.araç verisi -------------------------------------------------\n600.000 TL\n11.608 TL den başlayan taksitlerle »\nARABA DEĞERİ HESAPLA\nKilis\nİlan No:\n#3421205\nİlan Tarihi:\n06/01/2021\nMarka:\nMercedes\nModel:\nE Serisi\nVaryant:\nE 180 AMG Premium\nModel Yılı:\n2015\nKilometre:\n24.000\nYakıt Türü:\nBenzin\nVites Tipi:\nOtomatik Vites\nMotor Hacmi:\n1301-1600 cc\nMotor Gücü:\nBilmiyorum\nKimden:\nGaleriden\n***********************************************+1 13.araç verisi -------------------------------------------------\n515.000 TL\n9.963 TL den başlayan taksitlerle »\nARABA DEĞERİ HESAPLA\nKilis\nİlan No:\n#2739906\nİlan Tarihi:\n06/01/2021\nMarka:\nMercedes\nModel:\nE Serisi\nVaryant:\nE 180 Edition E\nModel Yılı:\n2016\nKilometre:\n44.000\nYakıt Türü:\nBenzin\nVites Tipi:\nYarı Otomatik Vites\nMotor Hacmi:\n1301-1600 cc\nMotor Gücü:\nBilmiyorum\nKimden:\nGaleriden\n***********************************************+1 14.araç verisi -------------------------------------------------\n825.950 TL\n15.979 TL den başlayan taksitlerle »\nARABA DEĞERİ HESAPLA\nİstanbul\nİlan No:\n#4238195\nİlan Tarihi:\n06/01/2021\nMarka:\nMercedes\nModel:\nE Serisi\nVaryant:\nE 200 AMG\nModel Yılı:\n2016\nKilometre:\n85.000\nYakıt Türü:\nBenzin\nVites Tipi:\nYarı Otomatik Vites\nMotor Hacmi:\n1801-2000 cc\nMotor Gücü:\nBilmiyorum\nKimden:\nGaleriden\n***********************************************+1 15.araç verisi -------------------------------------------------\n199.500 TL\n3.860 TL den başlayan taksitlerle »\nARABA DEĞERİ HESAPLA\nAnkara\nİlan No:\n#628905\nİlan Tarihi:\n06/01/2021\nMarka:\nMercedes\nModel:\nE Serisi\nVaryant:\nE 200 Komp. Avantgarde\nModel Yılı:\n2004\nKilometre:\n340.000\nYakıt Türü:\nBenzin/LPG\nVites Tipi:\nYarı Otomatik Vites\nMotor Hacmi:\n1601-1800 cc\nMotor Gücü:\nBilmiyorum\nKimden:\nGaleriden\n***********************************************+1 16.araç verisi -------------------------------------------------\n294.000 TL\n5.688 TL den başlayan taksitlerle »\nARABA DEĞERİ HESAPLA\nİstanbul\nİlan No:\n#4434444\nİlan Tarihi:\n06/01/2021\nMarka:\nMercedes\nModel:\nE Serisi\nVaryant:\nE 250 CDI Avantgarde\nModel Yılı:\n2011\nKilometre:\n230.000\nYakıt Türü:\nDizel\nVites Tipi:\nYarı Otomatik Vites\nMotor Hacmi:\n2001-2500 cc\nMotor Gücü:\nBilmiyorum\nKimden:\nGaleriden\n***********************************************+1 17.araç verisi -------------------------------------------------\n439.000 TL\n8.493 TL den başlayan taksitlerle »\nARABA DEĞERİ HESAPLA\nKocaeli\nİlan No:\n#1826263\nİlan Tarihi:\n06/01/2021\nMarka:\nMercedes\nModel:\nE Serisi\nVaryant:\nE 250 CGI Premium\nModel Yılı:\n2013\nKilometre:\n149.000\nYakıt Türü:\nBenzin\nVites Tipi:\nOtomatik Vites\nMotor Hacmi:\n1801-2000 cc\nMotor Gücü:\nBilmiyorum\nKimden:\nGaleriden\n***********************************************+1 18.araç verisi -------------------------------------------------\n439.000 TL\n8.493 TL den başlayan taksitlerle »\nARABA DEĞERİ HESAPLA\nKocaeli\nİlan No:\n#1826263\nİlan Tarihi:\n06/01/2021\nMarka:\nMercedes\nModel:\nE Serisi\nVaryant:\nE 250 CGI Premium\nModel Yılı:\n2013\nKilometre:\n149.000\nYakıt Türü:\nBenzin\nVites Tipi:\nOtomatik Vites\nMotor Hacmi:\n1801-2000 cc\nMotor Gücü:\nBilmiyorum\nKimden:\nGaleriden\n***********************************************+1 19.araç verisi -------------------------------------------------\n1.400.000 TL\nTL den başlayan taksitlerle »\nARABA DEĞERİ HESAPLA\nİstanbul\nİlan No:\n#1232008\nİlan Tarihi:\n06/01/2021\nMarka:\nMercedes\nModel:\nE Serisi\nVaryant:\nE 220 d AMG\nModel Yılı:\n2020\nKilometre:\n0\nYakıt Türü:\nDizel\nVites Tipi:\nOtomatik Vites\nMotor Hacmi:\n1801-2000 cc\nMotor Gücü:\nBilmiyorum\nKimden:\nGaleriden\n***********************************************+1 20.araç verisi -------------------------------------------------\n1.400.000 TL\nTL den başlayan taksitlerle »\nARABA DEĞERİ HESAPLA\nİstanbul\nİlan No:\n#4004754\nİlan Tarihi:\n06/01/2021\nMarka:\nMercedes\nModel:\nE Serisi\nVaryant:\nE 220 d AMG\nModel Yılı:\n2020\nKilometre:\n0\nYakıt Türü:\nDizel\nVites Tipi:\nOtomatik Vites\nMotor Hacmi:\n1801-2000 cc\nMotor Gücü:\nBilmiyorum\nKimden:\nGaleriden\n***********************************************+1 21.araç verisi -------------------------------------------------\n619.500 TL\n11.985 TL den başlayan taksitlerle »\nARABA DEĞERİ HESAPLA\nGaziantep\nİlan No:\n#3686398\nİlan Tarihi:\n06/01/2021\nMarka:\nMercedes\nModel:\nE Serisi\nVaryant:\nE 180 AMG\nModel Yılı:\n2017\nKilometre:\n127.000\nYakıt Türü:\nBenzin\nVites Tipi:\nOtomatik Vites\nMotor Hacmi:\n1301-1600 cc\nMotor Gücü:\nBilmiyorum\nKimden:\nGaleriden\n***********************************************+1 22.araç verisi -------------------------------------------------\n*-*-*-*-*-*-*-*-*************Sayfa 19 *-*-*-*-*-*-*-*-*-*-***********\n735.000 TL\n14.219 TL den başlayan taksitlerle »\nARABA DEĞERİ HESAPLA\nAnkara\nİlan No:\n#2878633\nİlan Tarihi:\n06/01/2021\nMarka:\nMercedes\nModel:\nE Serisi\nVaryant:\nE 180 Exclusive\nModel Yılı:\n2018\nKilometre:\n80.000\nYakıt Türü:\nBenzin\nVites Tipi:\nOtomatik Vites\nMotor Hacmi:\n1301-1600 cc\nMotor Gücü:\nBilmiyorum\nKimden:\nGaleriden\n***********************************************+1 2.araç verisi -------------------------------------------------\n575.900 TL\n11.141 TL den başlayan taksitlerle »\nARABA DEĞERİ HESAPLA\nİstanbul\nİlan No:\n#4604190\nİlan Tarihi:\n06/01/2021\nMarka:\nMercedes\nModel:\nE Serisi\nVaryant:\nE 180 Exclusive\nModel Yılı:\n2017\nKilometre:\n120.000\nYakıt Türü:\nBenzin\nVites Tipi:\nOtomatik Vites\nMotor Hacmi:\n1301-1600 cc\nMotor Gücü:\nBilmiyorum\nKimden:\nGaleriden\n***********************************************+1 3.araç verisi -------------------------------------------------\n645.000 TL\n12.478 TL den başlayan taksitlerle »\nARABA DEĞERİ HESAPLA\nİzmir\nİlan No:\n#3144814\nİlan Tarihi:\n06/01/2021\nMarka:\nMercedes\nModel:\nE Serisi\nVaryant:\nE 220 d AMG\nModel Yılı:\n2016\nKilometre:\n99.800\nYakıt Türü:\nDizel\nVites Tipi:\nYarı Otomatik Vites\nMotor Hacmi:\n1801-2000 cc\nMotor Gücü:\nBilmiyorum\nKimden:\nGaleriden\n***********************************************+1 4.araç verisi -------------------------------------------------\nThe error has occurred in this process\nThe error has occured in this page\n405.000 TL\n7.835 TL den başlayan taksitlerle »\nARABA DEĞERİ HESAPLA\nİzmir\nİlan No:\n#1962284\nİlan Tarihi:\n27/12/2020\nMarka:\nMercedes\nModel:\nE Serisi\nVaryant:\nE 180 AMG\nModel Yılı:\n2014\nKilometre:\n110.000\nYakıt Türü:\nBenzin\nVites Tipi:\nOtomatik Vites\nMotor Hacmi:\n1301-1600 cc\nMotor Gücü:\nBilmiyorum\nKimden:\nGaleriden\n***********************************************+1 2.araç verisi -------------------------------------------------\n137.500 TL\n2.660 TL den başlayan taksitlerle »\nARABA DEĞERİ HESAPLA\nİstanbul\nİlan No:\n#4773068\nİlan Tarihi:\n27/12/2020\nMarka:\nMercedes\nModel:\nE Serisi\nVaryant:\nE 200 Komp. Avantgarde\nModel Yılı:\n1998\nKilometre:\n276.000\nYakıt Türü:\nBenzin/LPG\nVites Tipi:\nOtomatik Vites\nMotor Hacmi:\n1801-2000 cc\nMotor Gücü:\nBilmiyorum\nKimden:\nGaleriden\n***********************************************+1 3.araç verisi -------------------------------------------------\n410.000 TL\n7.932 TL den başlayan taksitlerle »\nARABA DEĞERİ HESAPLA\nGaziantep\nİlan No:\n#2701943\nİlan Tarihi:\n27/12/2020\nMarka:\nMercedes\nModel:\nE Serisi\nVaryant:\nE 250 CDI Premium\nModel Yılı:\n2012\nKilometre:\n210.000\nYakıt Türü:\nDizel\nVites Tipi:\nYarı Otomatik Vites\nMotor Hacmi:\n2001-2500 cc\nMotor Gücü:\nBilmiyorum\nKimden:\nGaleriden\n***********************************************+1 4.araç verisi -------------------------------------------------\n715.000 TL\n13.832 TL den başlayan taksitlerle »\nARABA DEĞERİ HESAPLA\nİlan No:\n#3805664\nİlan Tarihi:\n27/12/2020\nMarka:\nMercedes\nModel:\nE Serisi\nVaryant:\nE 220 d Exclusive\nModel Yılı:\n2016\nKilometre:\n56.000\nYakıt Türü:\nDizel\nVites Tipi:\nYarı Otomatik Vites\nMotor Hacmi:\n1801-2000 cc\nMotor Gücü:\nBilmiyorum\nKimden:\nGaleriden\n***********************************************+1 5.araç verisi -------------------------------------------------\n292.000 TL\n5.649 TL den başlayan taksitlerle »\nARABA DEĞERİ HESAPLA\nAdana\nİlan No:\n#4244326\nİlan Tarihi:\n27/12/2020\nMarka:\nMercedes\nModel:\nE Serisi\nVaryant:\nE 350 CDI Dynamic\nModel Yılı:\n2011\nKilometre:\n171.000\nYakıt Türü:\nDizel\nVites Tipi:\nYarı Otomatik Vites\nMotor Hacmi:\n2501-3000 cc\nMotor Gücü:\nBilmiyorum\nKimden:\nGaleriden\n***********************************************+1 7.araç verisi -------------------------------------------------\n429.000 TL\n8.299 TL den başlayan taksitlerle »\nARABA DEĞERİ HESAPLA\nİzmir\nİlan No:\n#3591304\nİlan Tarihi:\n27/12/2020\nMarka:\nMercedes\nModel:\nE Serisi\nVaryant:\nE 180 Premium\nModel Yılı:\n2014\nKilometre:\n102.854\nYakıt Türü:\nBenzin\nVites Tipi:\nYarı Otomatik Vites\nMotor Hacmi:\n1301-1600 cc\nMotor Gücü:\nBilmiyorum\nKimden:\nGaleriden\n***********************************************+1 8.araç verisi -------------------------------------------------\n480.000 TL\n9.286 TL den başlayan taksitlerle »\nARABA DEĞERİ HESAPLA\nAntalya\nİlan No:\n#3488835\nİlan Tarihi:\n27/12/2020\nMarka:\nMercedes\nModel:\nE Serisi\nVaryant:\nE 180 Edition E\nModel Yılı:\n2016\nKilometre:\n85.000\nYakıt Türü:\nBenzin\nVites Tipi:\nYarı Otomatik Vites\nMotor Hacmi:\n1301-1600 cc\nMotor Gücü:\nBilmiyorum\nKimden:\nGaleriden\n***********************************************+1 9.araç verisi -------------------------------------------------\n580.000 TL\n11.221 TL den başlayan taksitlerle »\nARABA DEĞERİ HESAPLA\nİstanbul\nİlan No:\n#549677\nİlan Tarihi:\n27/12/2020\nMarka:\nMercedes\nModel:\nE Serisi\nVaryant:\nE 250 CDI Elite\nModel Yılı:\n2015\nKilometre:\n178.000\nYakıt Türü:\nDizel\nVites Tipi:\nYarı Otomatik Vites\nMotor Hacmi:\n2001-2500 cc\nMotor Gücü:\nBilmiyorum\nKimden:\nGaleriden\n***********************************************+1 10.araç verisi -------------------------------------------------\nThe error has occurred in this process\n745.000 TL\n14.413 TL den başlayan taksitlerle »\nARABA DEĞERİ HESAPLA\nİstanbul\nİlan No:\n#2795625\nİlan Tarihi:\n27/12/2020\nMarka:\nMercedes\nModel:\nE Serisi\nVaryant:\nE 220 d Avantgarde\nModel Yılı:\n2016\nKilometre:\n140.000\nYakıt Türü:\nDizel\nVites Tipi:\nYarı Otomatik Vites\nMotor Hacmi:\n1801-2000 cc\nMotor Gücü:\nBilmiyorum\nKimden:\nGaleriden\n***********************************************+1 13.araç verisi -------------------------------------------------\nThe error has occurred in this process\nThe error has occured in this page\n465.000 TL\n8.996 TL den başlayan taksitlerle »\nARABA DEĞERİ HESAPLA\nİstanbul\nİlan No:\n#4546579\nİlan Tarihi:\n27/12/2020\nMarka:\nMercedes\nModel:\nE Serisi\nVaryant:\nE 250 CDI Elite\nModel Yılı:\n2013\nKilometre:\n185.000\nYakıt Türü:\nDizel\nVites Tipi:\nYarı Otomatik Vites\nMotor Hacmi:\n2001-2500 cc\nMotor Gücü:\nBilmiyorum\nKimden:\nGaleriden\n***********************************************+1 2.araç verisi -------------------------------------------------\n409.500 TL\n7.922 TL den başlayan taksitlerle »\nARABA DEĞERİ HESAPLA\nDenizli\nİlan No:\n#2124503\nİlan Tarihi:\n27/12/2020\nMarka:\nMercedes\nModel:\nE Serisi\nVaryant:\nE 180 Premium\nModel Yılı:\n2014\nKilometre:\n155.000\nYakıt Türü:\nBenzin\nVites Tipi:\nYarı Otomatik Vites\nMotor Hacmi:\n1301-1600 cc\nMotor Gücü:\nBilmiyorum\nKimden:\nGaleriden\n***********************************************+1 3.araç verisi -------------------------------------------------\n365.000 TL\n7.061 TL den başlayan taksitlerle »\nARABA DEĞERİ HESAPLA\nİstanbul\nİlan No:\n#3265552\nİlan Tarihi:\n27/12/2020\nMarka:\nMercedes\nModel:\nE Serisi\nVaryant:\nE 350 CDI Premium\nModel Yılı:\n2011\nKilometre:\n253.000\nYakıt Türü:\nDizel\nVites Tipi:\nOtomatik Vites\nMotor Hacmi:\n2501-3000 cc\nMotor Gücü:\nBilmiyorum\nKimden:\nGaleriden\n***********************************************+1 4.araç verisi -------------------------------------------------\n495.000 TL\n9.576 TL den başlayan taksitlerle »\nARABA DEĞERİ HESAPLA\nİstanbul\nİlan No:\n#2458033\nİlan Tarihi:\n27/12/2020\nMarka:\nMercedes\nModel:\nE Serisi\nVaryant:\nE 180 AMG\nModel Yılı:\n2015\nKilometre:\n77.900\nYakıt Türü:\nBenzin\nVites Tipi:\nOtomatik Vites\nMotor Hacmi:\n1301-1600 cc\nMotor Gücü:\nBilmiyorum\nKimden:\nGaleriden\n***********************************************+1 5.araç verisi -------------------------------------------------\n365.000 TL\n7.061 TL den başlayan taksitlerle »\nARABA DEĞERİ HESAPLA\nİstanbul\nİlan No:\n#2454502\nİlan Tarihi:\n27/12/2020\nMarka:\nMercedes\nModel:\nE Serisi\nVaryant:\nE 220 CDI Avantgarde\nModel Yılı:\n2011\nKilometre:\n245.000\nYakıt Türü:\nDizel\nVites Tipi:\nOtomatik Vites\nMotor Hacmi:\n1300 cc ve altı\nMotor Gücü:\nBilmiyorum\nKimden:\nGaleriden\n***********************************************+1 7.araç verisi -------------------------------------------------\n1.540.000 TL\nTL den başlayan taksitlerle »\nARABA DEĞERİ HESAPLA\nİstanbul\nİlan No:\n#2979444\nİlan Tarihi:\n27/12/2020\nMarka:\nMercedes\nModel:\nE Serisi\nVaryant:\nDiğer\nModel Yılı:\n2020\nKilometre:\n1\nYakıt Türü:\nDizel\nVites Tipi:\nOtomatik Vites\nMotor Hacmi:\n1300 cc ve altı\nMotor Gücü:\nBilmiyorum\nKimden:\nGaleriden\n***********************************************+1 8.araç verisi -------------------------------------------------\n450.000 TL\n8.706 TL den başlayan taksitlerle »\nARABA DEĞERİ HESAPLA\nİstanbul\nİlan No:\n#1788037\nİlan Tarihi:\n27/12/2020\nMarka:\nMercedes\nModel:\nE Serisi\nVaryant:\nE 250 CDI Elite\nModel Yılı:\n2014\nKilometre:\n195.315\nYakıt Türü:\nDizel\nVites Tipi:\nYarı Otomatik Vites\nMotor Hacmi:\n2001-2500 cc\nMotor Gücü:\nBilmiyorum\nKimden:\nGaleriden\n***********************************************+1 9.araç verisi -------------------------------------------------\nThe error has occured in this page\nThe error has occurred in this process\nThe error has occured in this page\nThe error has occurred in this process\nThe error has occured in this page\nThe error has occurred in this process\nThe error has occured in this page\nThe error has occurred in this process\nThe error has occured in this page\nThe error has occurred in this process\nThe error has occured in this page\nThe error has occurred in this process\nThe error has occured in this page\nThe error has occurred in this process\nThe error has occured in this page\nThe error has occurred in this process\nThe error has occured in this page\nThe error has occurred in this process\nThe error has occured in this page\nThe error has occurred in this process\nThe error has occured in this page\nThe error has occurred in this process\nThe error has occured in this page\nThe error has occurred in this process\nThe error has occured in this page\nThe error has occurred in this process\nThe error has occured in this page\nThe error has occurred in this process\nThe error has occured in this page\nThe error has occurred in this process\nThe error has occured in this page\nThe error has occurred in this process\nThe error has occured in this page\nThe error has occurred in this process\nThe error has occured in this page\nThe error has occurred in this process\nThe error has occured in this page\nThe error has occurred in this process\nThe error has occured in this page\nThe error has occurred in this process\nThe error has occured in this page\nThe error has occurred in this process\nThe error has occured in this page\nThe error has occurred in this process\nThe error has occured in this page\nThe error has occurred in this process\nThe error has occured in this page\nThe error has occurred in this process\nThe error has occured in this page\nThe error has occurred in this process\nThe error has occured in this page\nThe error has occurred in this process\nThe error has occured in this page\nThe error has occurred in this process\nThe error has occured in this page\n"
],
[
"from selenium import webdriver\nimport time\nimport pandas as pd\n\ndriver_path='C:\\Program Files (x86)\\Google\\Chrome\\Application\\chromedriver.exe'\nbrowser=webdriver.Chrome(driver_path)\nurl='https://www.araba.com/otomobil/mercedes-e-serisi'\nbrowser.get(url)\n\nempty_list=[]\ndf_simple = pd.DataFrame(empty_list,columns=['advertisement_number','brand','series','version','year','km', 'price','advertisement_date','gear','fuel','engine_capacity','engine_power','from','city']) \ndf_simple.to_csv(r\"istihza3.csv\",encoding=\"utf-8\",index=False,mode=\"a\")\n\nfor k in range(2,50):\n for i in range(2,23):\n if(i==6 or i==11):\n continue\n box_ = browser.find_element_by_xpath('//*[@id=\"park_or_compare\"]/table/tbody/tr['+str(i)+ ']')\n box_.click()\n\n content=browser.find_elements_by_xpath('/html/body/div[1]/div[7]/div[2]/div[3]/div[5]/div[1]/div[1]/div[2]')\n\n #content\n contlist=[]\n for i in content:\n a=i.text\n contlist=a.split(\"\\n\")\n for i in contlist:\n if ( i.startswith('/')):\n contlist.remove(str(i)) \n try:\n lst = [ [ contlist[5],contlist[9],contlist[11],contlist[13],contlist[15],contlist[17],contlist[0],contlist[7],contlist[0],contlist[8],contlist[21],contlist[19],contlist[23],contlist[3] ] ] \n except:\n print('The error has occurred in this process')\n df = pd.DataFrame(lst) \n df.to_csv(r\"istihza3.csv\",encoding=\"utf-8\",index=False,mode=\"a\")\n\n browser.execute_script(\"window.history.go(-1)\")\n for j in contlist:\n print(j)\n\n print('***********************************************+1 '+str(i)+ '.araç verisi -------------------------------------------------')\n contlist=[]\n print('*-*-*-*-*-*-*-*-*************Sayfa '+str(k) + ' Sonu*-*-*-*-*-*-*-*-*-*-***********')\n\n #tıkla = browser.find_element_by_xpath('/html/body/div[1]/div[7]/div[2]/div[3]/div[2]/div[4]/div[1]/div/a['+str(k)+ ']')\n #tıkla.click()\n url='https://www.araba.com/otomobil/mercedes-e-serisi?sayfa='+str(k)\n browser.get(url)\n",
"525.000 TL\n10.157 TL den başlayan taksitlerle »\nARABA DEĞERİ HESAPLA\nAnkara\nİlan No:\n#2798325\nİlan Tarihi:\n27/03/2021\nMarka:\nMercedes\nModel:\nE Serisi\nVaryant:\nE 250 BlueTEC\nModel Yılı:\n2015\nKilometre:\n150.000\nYakıt Türü:\nBenzin\nVites Tipi:\nOtomatik Vites\nMotor Hacmi:\n1801-2000 cc\nMotor Gücü:\n126-150 BG\nKimden:\nGaleriden\n***********************************************+1 Galeriden.araç verisi -------------------------------------------------\n217.900 TL\n4.215 TL den başlayan taksitlerle »\nARABA DEĞERİ HESAPLA\nİstanbul\nİlan No:\n#2921626\nİlan Tarihi:\n23/03/2021\nMarka:\nMercedes\nModel:\nE Serisi\nVaryant:\nE 200 Komp. Avantgarde\nModel Yılı:\n2004\nKilometre:\n215.000\nYakıt Türü:\nBenzin\nVites Tipi:\nYarı Otomatik Vites\nMotor Hacmi:\n1601-1800 cc\nMotor Gücü:\nBilmiyorum\nKimden:\nGaleriden\n***********************************************+1 Galeriden.araç verisi -------------------------------------------------\n389.000 TL\n7.526 TL den başlayan taksitlerle »\nARABA DEĞERİ HESAPLA\nAnkara\nİlan No:\n#4324911\nİlan Tarihi:\n21/03/2021\nMarka:\nMercedes\nModel:\nE Serisi\nVaryant:\nE 180 Elite\nModel Yılı:\n2013\nKilometre:\n80.200\nYakıt Türü:\nBenzin\nVites Tipi:\nYarı Otomatik Vites\nMotor Hacmi:\n1301-1600 cc\nMotor Gücü:\nBilmiyorum\nKimden:\nGaleriden\n***********************************************+1 Galeriden.araç verisi -------------------------------------------------\n310.000 TL\n5.997 TL den başlayan taksitlerle »\nARABA DEĞERİ HESAPLA\nKilis\nİlan No:\n#4590792\nİlan Tarihi:\n21/03/2021\nMarka:\nMercedes\nModel:\nE Serisi\nVaryant:\nE 250 CDI BlueEfficiency Avantgarde\nModel Yılı:\n2010\nKilometre:\n302.000\nYakıt Türü:\nDizel\nVites Tipi:\nYarı Otomatik Vites\nMotor Hacmi:\n2001-2500 cc\nMotor Gücü:\nBilmiyorum\nKimden:\nGaleriden\n***********************************************+1 Galeriden.araç verisi -------------------------------------------------\n215.000 TL\n4.159 TL den başlayan taksitlerle »\nARABA DEĞERİ HESAPLA\nKilis\nİlan No:\n#2743421\nİlan Tarihi:\n21/03/2021\nMarka:\nMercedes\nModel:\nE Serisi\nVaryant:\nE 220 CDI Elegance\nModel Yılı:\n2005\nKilometre:\n237.000\nYakıt Türü:\nDizel\nVites Tipi:\nYarı Otomatik Vites\nMotor Hacmi:\n2001-2500 cc\nMotor Gücü:\nBilmiyorum\nKimden:\nGaleriden\n***********************************************+1 Galeriden.araç verisi -------------------------------------------------\n695.000 TL\n13.445 TL den başlayan taksitlerle »\nARABA DEĞERİ HESAPLA\nİstanbul\nİlan No:\n#1184828\nİlan Tarihi:\n21/03/2021\nMarka:\nMercedes\nModel:\nE Serisi\nVaryant:\nE 180 Exclusive\nModel Yılı:\n2018\nKilometre:\n33.500\nYakıt Türü:\nBenzin\nVites Tipi:\nOtomatik Vites\nMotor Hacmi:\n1301-1600 cc\nMotor Gücü:\nBilmiyorum\nKimden:\nGaleriden\n***********************************************+1 Galeriden.araç verisi -------------------------------------------------\n555.000 TL\n10.737 TL den başlayan taksitlerle »\nARABA DEĞERİ HESAPLA\nAdana\nİlan No:\n#1371734\nİlan Tarihi:\n21/03/2021\nMarka:\nMercedes\nModel:\nE Serisi\nVaryant:\nE 250 CDI Edition\nModel Yılı:\n2015\nKilometre:\n184.000\nYakıt Türü:\nDizel\nVites Tipi:\nYarı Otomatik Vites\nMotor Hacmi:\n2001-2500 cc\nMotor Gücü:\nBilmiyorum\nKimden:\nGaleriden\n***********************************************+1 Galeriden.araç verisi -------------------------------------------------\n895.000 TL\n17.315 TL den başlayan taksitlerle »\nARABA DEĞERİ HESAPLA\nİstanbul\nİlan No:\n#4764206\nİlan Tarihi:\n21/03/2021\nMarka:\nMercedes\nModel:\nE Serisi\nVaryant:\nE 300 AMG\nModel Yılı:\n2017\nKilometre:\n104.000\nYakıt Türü:\nBenzin\nVites Tipi:\nOtomatik Vites\nMotor Hacmi:\n1801-2000 cc\nMotor Gücü:\nBilmiyorum\nKimden:\nGaleriden\n***********************************************+1 Galeriden.araç verisi -------------------------------------------------\n"
],
[
"/html/body/div[1]/div[7]/div[2]/div[3]/div[2]/div[4]/div[1]/div/a[2]\n\n/html/body/div[1]/div[7]/div[2]/div[3]/div[2]/div[4]/div[1]/div/a[3]",
"2\n3\n4\n5\n6\n7\n8\n9\n10\n11\n12\n13\n14\n"
],
[
"df_car=pd.read_csv('istihza3.csv')\n\ncars =[]\ni = 1\nwhile i <= 49:\n cars.append(df_car[i])\n i = i+2\ndf_cars=pd.DataFrame(cars)\ndf_cars",
"_____no_output_____"
],
[
"df_car=pd.read_csv('istihza3.csv')\ndf_car=df_car[1::2]\ndf_car.reset_index(inplace=True)\ndf_car.drop(\"index\", axis = 1, inplace = True)\ndf_car\ndf_car.to_csv(r'istihza4.csv', index = False)",
"_____no_output_____"
]
]
] | [
"code"
] | [
[
"code",
"code",
"code",
"code",
"code",
"code",
"code",
"code",
"code",
"code",
"code",
"code",
"code",
"code",
"code",
"code",
"code",
"code"
]
] |
e78666e82878193bb96a1d3b0aab7094e3bbc1bb | 25,775 | ipynb | Jupyter Notebook | AI-ML/Tensorflow fcc/Instructor notebooks/Neural Networks.ipynb | f-dufour/cheat-sheets-and-snippets | 8156089787ba58ba3a569464ee08cea9f90095a3 | [
"Unlicense"
] | null | null | null | AI-ML/Tensorflow fcc/Instructor notebooks/Neural Networks.ipynb | f-dufour/cheat-sheets-and-snippets | 8156089787ba58ba3a569464ee08cea9f90095a3 | [
"Unlicense"
] | null | null | null | AI-ML/Tensorflow fcc/Instructor notebooks/Neural Networks.ipynb | f-dufour/cheat-sheets-and-snippets | 8156089787ba58ba3a569464ee08cea9f90095a3 | [
"Unlicense"
] | null | null | null | 25,775 | 25,775 | 0.734549 | [
[
[
"#Introduction to Neural Networks\nIn this notebook you will learn how to create and use a neural network to classify articles of clothing. To achieve this, we will use a sub module of TensorFlow called *keras*.\n\n*This guide is based on the following TensorFlow documentation.*\n\nhttps://www.tensorflow.org/tutorials/keras/classification\n\n\n",
"_____no_output_____"
],
[
"##Keras\nBefore we dive in and start discussing neural networks, I'd like to give a breif introduction to keras.\n\nFrom the keras official documentation (https://keras.io/) keras is described as follows.\n\n\"Keras is a high-level neural networks API, written in Python and capable of running on top of TensorFlow, CNTK, or Theano. It was developed with a focus on enabling fast experimentation. \n\nUse Keras if you need a deep learning library that:\n\n- Allows for easy and fast prototyping (through user friendliness, modularity, and extensibility).\n- Supports both convolutional networks and recurrent networks, as well as combinations of the two.\n- Runs seamlessly on CPU and GPU.\"\n\nKeras is a very powerful module that allows us to avoid having to build neural networks from scratch. It also hides a lot of mathematical complexity (that otherwise we would have to implement) inside of helpful packages, modules and methods.\n\nIn this guide we will use keras to quickly develop neural networks.\n\n",
"_____no_output_____"
],
[
"##What is a Neural Network\nSo, what are these magical things that have been beating chess grandmasters, driving cars, detecting cancer cells and winning video games? \n\nA deep neural network is a layered representation of data. The term \"deep\" refers to the presence of multiple layers. Recall that in our core learning algorithms (like linear regression) data was not transformed or modified within the model, it simply existed in one layer. We passed some features to our model, some math was done, an answer was returned. The data was not changed or transformed throughout this process. A neural network processes our data differently. It attempts to represent our data in different ways and in different dimensions by applying specific operations to transform our data at each layer. Another way to express this is that at each layer our data is transformed in order to learn more about it. By performing these transformations, the model can better understand our data and therefore provide a better prediction. \n\n",
"_____no_output_____"
],
[
"##How it Works\nBefore going into too much detail I will provide a very surface level explination of how neural networks work on a mathematical level. All the terms and concepts I discuss will be defined and explained in more detail below.\n\nOn a lower level neural networks are simply a combination of elementry math operations and some more advanced linear algebra. Each neural network consists of a sequence of layers in which data passes through. These layers are made up on neurons and the neurons of one layer are connected to the next (see below). These connections are defined by what we call a weight (some numeric value). Each layer also has something called a bias, this is simply an extra neuron that has no connections and holds a single numeric value. Data starts at the input layer and is trasnformed as it passes through subsequent layers. The data at each subsequent neuron is defined as the following.\n\n> $Y =(\\sum_{i=0}^n w_i x_i) + b$\n\n> $w$ stands for the weight of each connection to the neuron\n\n> $x$ stands for the value of the connected neuron from the previous value\n\n> $b$ stands for the bias at each layer, this is a constant\n\n> $n$ is the number of connections\n\n> $Y$ is the output of the current neuron\n\n> $\\sum$ stands for sum\n\nThe equation you just read is called a weighed sum. We will take this weighted sum at each and every neuron as we pass information through the network. Then we will add what's called a bias to this sum. The bias allows us to shift the network up or down by a constant value. It is like the y-intercept of a line.\n\nBut that equation is the not complete one! We forgot a crucial part, **the activation function**. This is a function that we apply to the equation seen above to add complexity and dimensionality to our network. Our new equation with the addition of an activation function $F(x)$ is seen below.\n\n> $Y =F((\\sum_{i=0}^n w_i x_i) + b)$\n\nOur network will start with predefined activation functions (they may be different at each layer) but random weights and biases. As we train the network by feeding it data it will learn the correct weights and biases and adjust the network accordingly using a technqiue called **backpropagation** (explained below). Once the correct weights and biases have been learned our network will hopefully be able to give us meaningful predictions. We get these predictions by observing the values at our final layer, the output layer. \n\n\n",
"_____no_output_____"
],
[
"##Breaking Down The Neural Network!\n\nBefore we dive into any code lets break down how a neural network works and what it does.\n\n\n*Figure 1*\n\n\n",
"_____no_output_____"
],
[
"###Data\nThe type of data a neural network processes varies drastically based on the problem being solved. When we build a neural network, we define what shape and kind of data it can accept. It may sometimes be neccessary to modify our dataset so that it can be passed to our neural network. \n\nSome common types of data a neural network uses are listed below.\n- Vector Data (2D)\n- Timeseries or Sequence (3D)\n- Image Data (4D)\n- Video Data (5D)\n\nThere are of course many different types or data, but these are the main categories.\n\n",
"_____no_output_____"
],
[
"###Layers\nAs we mentioned earlier each neural network consists of multiple layers. At each layer a different transformation of data occurs. Our initial input data is fed through the layers and eventually arrives at the output layer where we will obtain the result.\n####Input Layer\nThe input layer is the layer that our initial data is passed to. It is the first layer in our neural network.\n####Output Layer\nThe output layer is the layer that we will retrive our results from. Once the data has passed through all other layers it will arrive here.\n####Hidden Layer(s)\nAll the other layers in our neural network are called \"hidden layers\". This is because they are hidden to us, we cannot observe them. Most neural networks consist of at least one hidden layer but can have an unlimited amount. Typically, the more complex the model the more hidden layers.\n####Neurons\nEach layer is made up of what are called neurons. Neurons have a few different properties that we will discuss later. The important aspect to understand now is that each neuron is responsible for generating/holding/passing ONE numeric value. \n\nThis means that in the case of our input layer it will have as many neurons as we have input information. For example, say we want to pass an image that is 28x28 pixels, thats 784 pixels. We would need 784 neurons in our input layer to capture each of these pixels. \n\nThis also means that our output layer will have as many neurons as we have output information. The output is a little more complicated to understand so I'll refrain from an example right now but hopefully you're getting the idea.\n\nBut what about our hidden layers? Well these have as many neurons as we decide. We'll discuss how we can pick these values later but understand a hidden layer can have any number of neurons.\n####Connected Layers\nSo how are all these layers connected? Well the neurons in one layer will be connected to neurons in the subsequent layer. However, the neurons can be connected in a variety of different ways. \n\nTake for example *Figure 1* (look above). Each neuron in one layer is connected to every neuron in the next layer. This is called a **dense** layer. There are many other ways of connecting layers but well discuss those as we see them. \n\n",
"_____no_output_____"
],
[
"###Weights\nWeights are associated with each connection in our neural network. Every pair of connected nodes will have one weight that denotes the strength of the connection between them. These are vital to the inner workings of a neural network and will be tweaked as the neural network is trained. The model will try to determine what these weights should be to achieve the best result. Weights start out at a constant or random value and will change as the network sees training data.",
"_____no_output_____"
],
[
"###Biases\nBiases are another important part of neural networks and will also be tweaked as the model is trained. A bias is simply a constant value associated with each layer. It can be thought of as an extra neuron that has no connections. The purpose of a bias is to shift an entire activation function by a constant value. This allows a lot more flexibllity when it comes to choosing an activation and training the network. There is one bias for each layer.",
"_____no_output_____"
],
[
"###Activation Function\nActivation functions are simply a function that is applied to the weighed sum of a neuron. They can be anything we want but are typically higher order/degree functions that aim to add a higher dimension to our data. We would want to do this to introduce more comolexity to our model. By transforming our data to a higher dimension, we can typically make better, more complex predictions.\n\nA list of some common activation functions and their graphs can be seen below.\n\n- Relu (Rectified Linear Unit)\n\n\n- Tanh (Hyperbolic Tangent)\n\n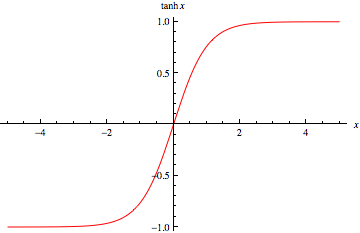\n- Sigmoid \n\n\n\n",
"_____no_output_____"
],
[
"###Backpropagation\nBackpropagation is the fundemental algorithm behind training neural networks. It is what changes the weights and biases of our network. To fully explain this process, we need to start by discussing something called a cost/loss function.\n\n####Loss/Cost Function\nAs we now know our neural network feeds information through the layers until it eventually reaches an output layer. This layer contains the results that we look at to determine the prediciton from our network. In the training phase it is likely that our network will make many mistakes and poor predicitions. In fact, at the start of training our network doesn't know anything (it has random weights and biases)! \n\nWe need some way of evaluating if the network is doing well and how well it is doing. For our training data we have the features (input) and the labels (expected output), because of this we can compare the output from our network to the expected output. Based on the difference between these values we can determine if our network has done a good job or poor job. If the network has done a good job, we'll make minor changes to the weights and biases. If it has done a poor job our changes may be more drastic.\n\nSo, this is where the cost/loss function comes in. This function is responsible for determining how well the network did. We pass it the output and the expected output, and it returns to us some value representing the cost/loss of the network. This effectively makes the networks job to optimize this cost function, trying to make it as low as possible. \n\nSome common loss/cost functions include.\n- Mean Squared Error\n- Mean Absolute Error\n- Hinge Loss\n\n####Gradient Descent\nGradient descent and backpropagation are closely related. Gradient descent is the algorithm used to find the optimal paramaters (weights and biases) for our network, while backpropagation is the process of calculating the gradient that is used in the gradient descent step. \n\nGradient descent requires some pretty advanced calculus and linear algebra to understand so we'll stay away from that for now. Let's just read the formal definition for now.\n\n\"Gradient descent is an optimization algorithm used to minimize some function by iteratively moving in the direction of steepest descent as defined by the negative of the gradient. In machine learning, we use gradient descent to update the parameters of our model.\" (https://ml-cheatsheet.readthedocs.io/en/latest/gradient_descent.html)\n\nAnd that's all we really need to know for now. I'll direct you to the video for a more in depth explination.\n\n\n\n",
"_____no_output_____"
],
[
"###Optimizer\nYou may sometimes see the term optimizer or optimization function. This is simply the function that implements the backpropagation algorithm described above. Here's a list of a few common ones.\n- Gradient Descent\n- Stochastic Gradient Descent\n- Mini-Batch Gradient Descent\n- Momentum\n- Nesterov Accelerated Gradient\n\n*This article explains them quite well is where I've pulled this list from.*\n\n(https://medium.com/@sdoshi579/optimizers-for-training-neural-network-59450d71caf6)",
"_____no_output_____"
],
[
"##Creating a Neural Network\nOkay now you have reached the exciting part of this tutorial! No more math and complex explinations. Time to get hands on and train a very basic neural network.\n\n*As stated earlier this guide is based off of the following TensorFlow tutorial.*\nhttps://www.tensorflow.org/tutorials/keras/classification\n",
"_____no_output_____"
],
[
"###Imports",
"_____no_output_____"
]
],
[
[
"%tensorflow_version 2.x # this line is not required unless you are in a notebook\n# TensorFlow and tf.keras\nimport tensorflow as tf\nfrom tensorflow import keras\n\n# Helper libraries\nimport numpy as np\nimport matplotlib.pyplot as plt",
"_____no_output_____"
]
],
[
[
"###Dataset\nFor this tutorial we will use the MNIST Fashion Dataset. This is a dataset that is included in keras.\n\nThis dataset includes 60,000 images for training and 10,000 images for validation/testing.",
"_____no_output_____"
]
],
[
[
"fashion_mnist = keras.datasets.fashion_mnist # load dataset\n\n(train_images, train_labels), (test_images, test_labels) = fashion_mnist.load_data() # split into tetsing and training",
"_____no_output_____"
]
],
[
[
"Let's have a look at this data to see what we are working with.",
"_____no_output_____"
]
],
[
[
"train_images.shape",
"_____no_output_____"
]
],
[
[
"So we've got 60,000 images that are made up of 28x28 pixels (784 in total).",
"_____no_output_____"
]
],
[
[
"train_images[0,23,23] # let's have a look at one pixel",
"_____no_output_____"
]
],
[
[
"Our pixel values are between 0 and 255, 0 being black and 255 being white. This means we have a grayscale image as there are no color channels.",
"_____no_output_____"
]
],
[
[
"train_labels[:10] # let's have a look at the first 10 training labels",
"_____no_output_____"
]
],
[
[
"Our labels are integers ranging from 0 - 9. Each integer represents a specific article of clothing. We'll create an array of label names to indicate which is which.",
"_____no_output_____"
]
],
[
[
"class_names = ['T-shirt/top', 'Trouser', 'Pullover', 'Dress', 'Coat',\n 'Sandal', 'Shirt', 'Sneaker', 'Bag', 'Ankle boot']",
"_____no_output_____"
]
],
[
[
"Fianlly let's look at what some of these images look like!",
"_____no_output_____"
]
],
[
[
"plt.figure()\nplt.imshow(train_images[1])\nplt.colorbar()\nplt.grid(False)\nplt.show()",
"_____no_output_____"
]
],
[
[
"##Data Preprocessing\nThe last step before creating our model is to *preprocess* our data. This simply means applying some prior transformations to our data before feeding it the model. In this case we will simply scale all our greyscale pixel values (0-255) to be between 0 and 1. We can do this by dividing each value in the training and testing sets by 255.0. We do this because smaller values will make it easier for the model to process our values. \n\n",
"_____no_output_____"
]
],
[
[
"train_images = train_images / 255.0\n\ntest_images = test_images / 255.0",
"_____no_output_____"
]
],
[
[
"##Building the Model\nNow it's time to build the model! We are going to use a keras *sequential* model with three different layers. This model represents a feed-forward neural network (one that passes values from left to right). We'll break down each layer and its architecture below.",
"_____no_output_____"
]
],
[
[
"model = keras.Sequential([\n keras.layers.Flatten(input_shape=(28, 28)), # input layer (1)\n keras.layers.Dense(128, activation='relu'), # hidden layer (2)\n keras.layers.Dense(10, activation='softmax') # output layer (3)\n])",
"_____no_output_____"
]
],
[
[
"**Layer 1:** This is our input layer and it will conist of 784 neurons. We use the flatten layer with an input shape of (28,28) to denote that our input should come in in that shape. The flatten means that our layer will reshape the shape (28,28) array into a vector of 784 neurons so that each pixel will be associated with one neuron.\n\n**Layer 2:** This is our first and only hidden layer. The *dense* denotes that this layer will be fully connected and each neuron from the previous layer connects to each neuron of this layer. It has 128 neurons and uses the rectify linear unit activation function.\n\n**Layer 3:** This is our output later and is also a dense layer. It has 10 neurons that we will look at to determine our models output. Each neuron represnts the probabillity of a given image being one of the 10 different classes. The activation function *softmax* is used on this layer to calculate a probabillity distribution for each class. This means the value of any neuron in this layer will be between 0 and 1, where 1 represents a high probabillity of the image being that class.",
"_____no_output_____"
],
[
"###Compile the Model\nThe last step in building the model is to define the loss function, optimizer and metrics we would like to track. I won't go into detail about why we chose each of these right now.",
"_____no_output_____"
]
],
[
[
"model.compile(optimizer='adam',\n loss='sparse_categorical_crossentropy',\n metrics=['accuracy'])",
"_____no_output_____"
]
],
[
[
"##Training the Model\nNow it's finally time to train the model. Since we've already done all the work on our data this step is as easy as calling a single method.",
"_____no_output_____"
]
],
[
[
"model.fit(train_images, train_labels, epochs=10) # we pass the data, labels and epochs and watch the magic!",
"_____no_output_____"
]
],
[
[
"##Evaluating the Model\nNow it's time to test/evaluate the model. We can do this quite easily using another builtin method from keras.\n\nThe *verbose* argument is defined from the keras documentation as:\n\"verbose: 0 or 1. Verbosity mode. 0 = silent, 1 = progress bar.\"\n(https://keras.io/models/sequential/)",
"_____no_output_____"
]
],
[
[
"test_loss, test_acc = model.evaluate(test_images, test_labels, verbose=1) \n\nprint('Test accuracy:', test_acc)",
"_____no_output_____"
]
],
[
[
"You'll likely notice that the accuracy here is lower than when training the model. This difference is reffered to as **overfitting**.\n\nAnd now we have a trained model that's ready to use to predict some values!",
"_____no_output_____"
],
[
"##Making Predictions\nTo make predictions we simply need to pass an array of data in the form we've specified in the input layer to ```.predict()``` method.",
"_____no_output_____"
]
],
[
[
"predictions = model.predict(test_images)",
"_____no_output_____"
]
],
[
[
"This method returns to us an array of predictions for each image we passed it. Let's have a look at the predictions for image 1.",
"_____no_output_____"
]
],
[
[
"predictions[0]",
"_____no_output_____"
]
],
[
[
"If we wan't to get the value with the highest score we can use a useful function from numpy called ```argmax()```. This simply returns the index of the maximium value from a numpy array. ",
"_____no_output_____"
]
],
[
[
"np.argmax(predictions[0])",
"_____no_output_____"
]
],
[
[
"And we can check if this is correct by looking at the value of the cooresponding test label.",
"_____no_output_____"
]
],
[
[
"test_labels[0]",
"_____no_output_____"
]
],
[
[
"##Verifying Predictions\nI've written a small function here to help us verify predictions with some simple visuals.",
"_____no_output_____"
]
],
[
[
"COLOR = 'white'\nplt.rcParams['text.color'] = COLOR\nplt.rcParams['axes.labelcolor'] = COLOR\n\ndef predict(model, image, correct_label):\n class_names = ['T-shirt/top', 'Trouser', 'Pullover', 'Dress', 'Coat',\n 'Sandal', 'Shirt', 'Sneaker', 'Bag', 'Ankle boot']\n prediction = model.predict(np.array([image]))\n predicted_class = class_names[np.argmax(prediction)]\n\n show_image(image, class_names[correct_label], predicted_class)\n\n\ndef show_image(img, label, guess):\n plt.figure()\n plt.imshow(img, cmap=plt.cm.binary)\n plt.title(\"Excpected: \" + label)\n plt.xlabel(\"Guess: \" + guess)\n plt.colorbar()\n plt.grid(False)\n plt.show()\n\n\ndef get_number():\n while True:\n num = input(\"Pick a number: \")\n if num.isdigit():\n num = int(num)\n if 0 <= num <= 1000:\n return int(num)\n else:\n print(\"Try again...\")\n\nnum = get_number()\nimage = test_images[num]\nlabel = test_labels[num]\npredict(model, image, label)\n",
"_____no_output_____"
]
],
[
[
"And that's pretty much it for an introduction to neural networks!",
"_____no_output_____"
],
[
"##Sources\n\n1. Doshi, Sanket. “Various Optimization Algorithms For Training Neural Network.” Medium, Medium, 10 Mar. 2019, www.medium.com/@sdoshi579/optimizers-for-training-neural-network-59450d71caf6.\n\n2. “Basic Classification: Classify Images of Clothing : TensorFlow Core.” TensorFlow, www.tensorflow.org/tutorials/keras/classification.\n\n3. “Gradient Descent¶.” Gradient Descent - ML Glossary Documentation, www.ml-cheatsheet.readthedocs.io/en/latest/gradient_descent.html.\n\n4. Chollet François. Deep Learning with Python. Manning Publications Co., 2018.\n\n5. “Keras: The Python Deep Learning Library.” Home - Keras Documentation, www.keras.io/.",
"_____no_output_____"
]
]
] | [
"markdown",
"code",
"markdown",
"code",
"markdown",
"code",
"markdown",
"code",
"markdown",
"code",
"markdown",
"code",
"markdown",
"code",
"markdown",
"code",
"markdown",
"code",
"markdown",
"code",
"markdown",
"code",
"markdown",
"code",
"markdown",
"code",
"markdown",
"code",
"markdown",
"code",
"markdown",
"code",
"markdown",
"code",
"markdown"
] | [
[
"markdown",
"markdown",
"markdown",
"markdown",
"markdown",
"markdown",
"markdown",
"markdown",
"markdown",
"markdown",
"markdown",
"markdown",
"markdown",
"markdown"
],
[
"code"
],
[
"markdown"
],
[
"code"
],
[
"markdown"
],
[
"code"
],
[
"markdown"
],
[
"code"
],
[
"markdown"
],
[
"code"
],
[
"markdown"
],
[
"code"
],
[
"markdown"
],
[
"code"
],
[
"markdown"
],
[
"code"
],
[
"markdown"
],
[
"code"
],
[
"markdown",
"markdown"
],
[
"code"
],
[
"markdown"
],
[
"code"
],
[
"markdown"
],
[
"code"
],
[
"markdown",
"markdown"
],
[
"code"
],
[
"markdown"
],
[
"code"
],
[
"markdown"
],
[
"code"
],
[
"markdown"
],
[
"code"
],
[
"markdown"
],
[
"code"
],
[
"markdown",
"markdown"
]
] |
e7867ca7277c7688b988dd08daf047dce3f1ee65 | 70,054 | ipynb | Jupyter Notebook | python/moved-from-mxnet/tutorial.ipynb | marktab/mxnet-notebooks | 5872e3d8d590e4472375f36380d9cb140a926e91 | [
"Apache-2.0"
] | 731 | 2016-08-15T06:41:53.000Z | 2022-03-09T06:06:07.000Z | python/moved-from-mxnet/tutorial.ipynb | marktab/mxnet-notebooks | 5872e3d8d590e4472375f36380d9cb140a926e91 | [
"Apache-2.0"
] | 50 | 2016-08-21T01:41:06.000Z | 2020-10-16T07:15:51.000Z | python/moved-from-mxnet/tutorial.ipynb | marktab/mxnet-notebooks | 5872e3d8d590e4472375f36380d9cb140a926e91 | [
"Apache-2.0"
] | 370 | 2016-08-15T03:57:18.000Z | 2022-01-27T10:50:45.000Z | 78.185268 | 13,746 | 0.726026 | [
[
[
"# MXNet Tutorial and Hand Written Digit Recognition\n\nIn this tutorial we will go through the basic use case of MXNet and also touch on some advanced usages. This example is based on the MNIST dataset, which contains 70,000 images of hand written characters with 28-by-28 pixel size.\n\nThis tutorial covers the following topics:\n- network definition.\n- Variable naming.\n- Basic data loading and training with feed-forward deep neural networks.\n- Monitoring intermediate outputs for debuging.\n- Custom training loop for advanced models.",
"_____no_output_____"
],
[
"First let's import the modules and setup logging:",
"_____no_output_____"
]
],
[
[
"%matplotlib inline\nimport mxnet as mx\nimport numpy as np\nimport cv2\nimport matplotlib.pyplot as plt\nimport logging\n\nlogger = logging.getLogger()\nlogger.setLevel(logging.DEBUG)",
"_____no_output_____"
]
],
[
[
"## Network Definition\nNow we can start constructing our network:",
"_____no_output_____"
]
],
[
[
"# Variables are place holders for input arrays. We give each variable a unique name.\ndata = mx.symbol.Variable('data')\n\n# The input is fed to a fully connected layer that computes Y=WX+b.\n# This is the main computation module in the network.\n# Each layer also needs an unique name. We'll talk more about naming in the next section.\nfc1 = mx.symbol.FullyConnected(data = data, name='fc1', num_hidden=128)\n# Activation layers apply a non-linear function on the previous layer's output.\n# Here we use Rectified Linear Unit (ReLU) that computes Y = max(X, 0).\nact1 = mx.symbol.Activation(data = fc1, name='relu1', act_type=\"relu\")\n\nfc2 = mx.symbol.FullyConnected(data = act1, name = 'fc2', num_hidden = 64)\nact2 = mx.symbol.Activation(data = fc2, name='relu2', act_type=\"relu\")\n\nfc3 = mx.symbol.FullyConnected(data = act2, name='fc3', num_hidden=10)\n# Finally we have a loss layer that compares the network's output with label and generates gradient signals.\nmlp = mx.symbol.SoftmaxOutput(data = fc3, name = 'softmax')",
"_____no_output_____"
]
],
[
[
"We can visualize the network we just defined with MXNet's visualization module:",
"_____no_output_____"
]
],
[
[
"mx.viz.plot_network(mlp)",
"_____no_output_____"
]
],
[
[
"## Variable Naming\n\nMXNet requires variable names to follow certain conventions:\n- All input arrays have a name. This includes inputs (data & label) and model parameters (weight, bias, etc).\n- Arrays can be renamed by creating named variable. Otherwise, a default name is given as 'SymbolName_ArrayName'. For example, FullyConnected symbol fc1's weight array is named as 'fc1_weight'.\n- Although you can also rename weight arrays with variables, weight array's name should always end with '_weight' and bias array '_bias'. MXNet relies on the suffixes of array names to correctly initialize & update them.\n\nCall list_arguments method on a symbol to get the names of all its inputs:",
"_____no_output_____"
]
],
[
[
"mlp.list_arguments()",
"_____no_output_____"
]
],
[
[
"## Data Loading\n\nWe fetch and load the MNIST dataset and partition it into two sets: 60000 examples for training and 10000 examples for testing. We also visualize a few examples to get an idea of what the dataset looks like.",
"_____no_output_____"
]
],
[
[
"from sklearn.datasets import fetch_mldata\nmnist = fetch_mldata('MNIST original')\nnp.random.seed(1234) # set seed for deterministic ordering\np = np.random.permutation(mnist.data.shape[0])\nX = mnist.data[p]\nY = mnist.target[p]\n\nfor i in range(10):\n plt.subplot(1,10,i+1)\n plt.imshow(X[i].reshape((28,28)), cmap='Greys_r')\n plt.axis('off')\nplt.show()\n\nX = X.astype(np.float32)/255\nX_train = X[:60000]\nX_test = X[60000:]\nY_train = Y[:60000]\nY_test = Y[60000:]",
"_____no_output_____"
]
],
[
[
"Now we can create data iterators from our MNIST data. A data iterator returns a batch of data examples each time for the network to process. MXNet provide a suite of basic DataIters for parsing different data format. Here we use NDArrayIter, which wraps around a numpy array and each time slice a chunk from it along the first dimension.",
"_____no_output_____"
]
],
[
[
"batch_size = 100\ntrain_iter = mx.io.NDArrayIter(X_train, Y_train, batch_size=batch_size)\ntest_iter = mx.io.NDArrayIter(X_test, Y_test, batch_size=batch_size)",
"_____no_output_____"
]
],
[
[
"## Training\n\nWith the network and data source defined, we can finally start to train our model. We do this with MXNet's convenience wrapper for feed forward neural networks (it can also be made to handle RNNs with explicit unrolling). ",
"_____no_output_____"
]
],
[
[
"model = mx.model.FeedForward(\n ctx = mx.gpu(0), # Run on GPU 0\n symbol = mlp, # Use the network we just defined\n num_epoch = 10, # Train for 10 epochs\n learning_rate = 0.1, # Learning rate\n momentum = 0.9, # Momentum for SGD with momentum\n wd = 0.00001) # Weight decay for regularization\nmodel.fit(\n X=train_iter, # Training data set\n eval_data=test_iter, # Testing data set. MXNet computes scores on test set every epoch\n batch_end_callback = mx.callback.Speedometer(batch_size, 200)) # Logging module to print out progress",
"INFO:root:Start training with [gpu(0)]\nINFO:root:Epoch[0] Batch [200]\tSpeed: 70941.64 samples/sec\tTrain-accuracy=0.389050\nINFO:root:Epoch[0] Batch [400]\tSpeed: 97857.94 samples/sec\tTrain-accuracy=0.646450\nINFO:root:Epoch[0] Batch [600]\tSpeed: 70507.97 samples/sec\tTrain-accuracy=0.743333\nINFO:root:Epoch[0] Resetting Data Iterator\nINFO:root:Epoch[0] Train-accuracy=0.743333\nINFO:root:Epoch[0] Time cost=1.069\nINFO:root:Epoch[0] Validation-accuracy=0.950800\nINFO:root:Epoch[1] Batch [200]\tSpeed: 79912.66 samples/sec\tTrain-accuracy=0.947300\nINFO:root:Epoch[1] Batch [400]\tSpeed: 58822.31 samples/sec\tTrain-accuracy=0.954425\nINFO:root:Epoch[1] Batch [600]\tSpeed: 67124.87 samples/sec\tTrain-accuracy=0.957733\nINFO:root:Epoch[1] Resetting Data Iterator\nINFO:root:Epoch[1] Train-accuracy=0.957733\nINFO:root:Epoch[1] Time cost=0.893\nINFO:root:Epoch[1] Validation-accuracy=0.959400\nINFO:root:Epoch[2] Batch [200]\tSpeed: 87015.23 samples/sec\tTrain-accuracy=0.964450\nINFO:root:Epoch[2] Batch [400]\tSpeed: 91101.30 samples/sec\tTrain-accuracy=0.968875\nINFO:root:Epoch[2] Batch [600]\tSpeed: 88963.21 samples/sec\tTrain-accuracy=0.970017\nINFO:root:Epoch[2] Resetting Data Iterator\nINFO:root:Epoch[2] Train-accuracy=0.970017\nINFO:root:Epoch[2] Time cost=0.678\nINFO:root:Epoch[2] Validation-accuracy=0.963000\nINFO:root:Epoch[3] Batch [200]\tSpeed: 66986.68 samples/sec\tTrain-accuracy=0.973750\nINFO:root:Epoch[3] Batch [400]\tSpeed: 65680.34 samples/sec\tTrain-accuracy=0.976575\nINFO:root:Epoch[3] Batch [600]\tSpeed: 91931.16 samples/sec\tTrain-accuracy=0.977050\nINFO:root:Epoch[3] Resetting Data Iterator\nINFO:root:Epoch[3] Train-accuracy=0.977050\nINFO:root:Epoch[3] Time cost=0.825\nINFO:root:Epoch[3] Validation-accuracy=0.968000\nINFO:root:Epoch[4] Batch [200]\tSpeed: 73709.59 samples/sec\tTrain-accuracy=0.978950\nINFO:root:Epoch[4] Batch [400]\tSpeed: 85750.82 samples/sec\tTrain-accuracy=0.980425\nINFO:root:Epoch[4] Batch [600]\tSpeed: 87061.38 samples/sec\tTrain-accuracy=0.981183\nINFO:root:Epoch[4] Resetting Data Iterator\nINFO:root:Epoch[4] Train-accuracy=0.981183\nINFO:root:Epoch[4] Time cost=0.739\nINFO:root:Epoch[4] Validation-accuracy=0.967600\nINFO:root:Epoch[5] Batch [200]\tSpeed: 85031.11 samples/sec\tTrain-accuracy=0.981950\nINFO:root:Epoch[5] Batch [400]\tSpeed: 94063.25 samples/sec\tTrain-accuracy=0.983475\nINFO:root:Epoch[5] Batch [600]\tSpeed: 97417.46 samples/sec\tTrain-accuracy=0.984183\nINFO:root:Epoch[5] Resetting Data Iterator\nINFO:root:Epoch[5] Train-accuracy=0.984183\nINFO:root:Epoch[5] Time cost=0.657\nINFO:root:Epoch[5] Validation-accuracy=0.972000\nINFO:root:Epoch[6] Batch [200]\tSpeed: 96185.84 samples/sec\tTrain-accuracy=0.984650\nINFO:root:Epoch[6] Batch [400]\tSpeed: 95023.61 samples/sec\tTrain-accuracy=0.985850\nINFO:root:Epoch[6] Batch [600]\tSpeed: 97022.32 samples/sec\tTrain-accuracy=0.986683\nINFO:root:Epoch[6] Resetting Data Iterator\nINFO:root:Epoch[6] Train-accuracy=0.986683\nINFO:root:Epoch[6] Time cost=0.628\nINFO:root:Epoch[6] Validation-accuracy=0.971900\nINFO:root:Epoch[7] Batch [200]\tSpeed: 84764.84 samples/sec\tTrain-accuracy=0.986350\nINFO:root:Epoch[7] Batch [400]\tSpeed: 87358.40 samples/sec\tTrain-accuracy=0.986425\nINFO:root:Epoch[7] Batch [600]\tSpeed: 74520.63 samples/sec\tTrain-accuracy=0.986517\nINFO:root:Epoch[7] Resetting Data Iterator\nINFO:root:Epoch[7] Train-accuracy=0.986517\nINFO:root:Epoch[7] Time cost=0.737\nINFO:root:Epoch[7] Validation-accuracy=0.973700\nINFO:root:Epoch[8] Batch [200]\tSpeed: 91634.21 samples/sec\tTrain-accuracy=0.987450\nINFO:root:Epoch[8] Batch [400]\tSpeed: 94328.96 samples/sec\tTrain-accuracy=0.987250\nINFO:root:Epoch[8] Batch [600]\tSpeed: 91991.24 samples/sec\tTrain-accuracy=0.987850\nINFO:root:Epoch[8] Resetting Data Iterator\nINFO:root:Epoch[8] Train-accuracy=0.987850\nINFO:root:Epoch[8] Time cost=0.652\nINFO:root:Epoch[8] Validation-accuracy=0.976800\nINFO:root:Epoch[9] Batch [200]\tSpeed: 66583.86 samples/sec\tTrain-accuracy=0.986800\nINFO:root:Epoch[9] Batch [400]\tSpeed: 67393.86 samples/sec\tTrain-accuracy=0.987500\nINFO:root:Epoch[9] Batch [600]\tSpeed: 65748.40 samples/sec\tTrain-accuracy=0.987900\nINFO:root:Epoch[9] Resetting Data Iterator\nINFO:root:Epoch[9] Train-accuracy=0.987900\nINFO:root:Epoch[9] Time cost=0.906\nINFO:root:Epoch[9] Validation-accuracy=0.973800\n"
]
],
[
[
"## Evaluation\n\nAfter the model is trained, we can evaluate it on a held out test set.\nFirst, lets classity a sample image:",
"_____no_output_____"
]
],
[
[
"plt.imshow((X_test[0].reshape((28,28))*255).astype(np.uint8), cmap='Greys_r')\nplt.show()\nprint 'Result:', model.predict(X_test[0:1])[0].argmax()",
"_____no_output_____"
]
],
[
[
"We can also evaluate the model's accuracy on the entire test set:",
"_____no_output_____"
]
],
[
[
"print 'Accuracy:', model.score(test_iter)*100, '%'",
"Accuracy: 97.38 %\n"
]
],
[
[
"Now, try if your model recognizes your own hand writing.\n\nWrite a digit from 0 to 9 in the box below. Try to put your digit in the middle of the box.",
"_____no_output_____"
]
],
[
[
"# run hand drawing test\nfrom IPython.display import HTML\n\ndef classify(img):\n img = img[len('data:image/png;base64,'):].decode('base64')\n img = cv2.imdecode(np.fromstring(img, np.uint8), -1)\n img = cv2.resize(img[:,:,3], (28,28))\n img = img.astype(np.float32).reshape((1, 784))/255.0\n return model.predict(img)[0].argmax()\n\nhtml = \"\"\"<style type=\"text/css\">canvas { border: 1px solid black; }</style><div id=\"board\"><canvas id=\"myCanvas\" width=\"100px\" height=\"100px\">Sorry, your browser doesn't support canvas technology.</canvas><p><button id=\"classify\" onclick=\"classify()\">Classify</button><button id=\"clear\" onclick=\"myClear()\">Clear</button>Result: <input type=\"text\" id=\"result_output\" size=\"5\" value=\"\"></p></div>\"\"\"\nscript = \"\"\"<script type=\"text/JavaScript\" src=\"https://ajax.googleapis.com/ajax/libs/jquery/1.4.2/jquery.min.js?ver=1.4.2\"></script><script type=\"text/javascript\">function init() {var myCanvas = document.getElementById(\"myCanvas\");var curColor = $('#selectColor option:selected').val();if(myCanvas){var isDown = false;var ctx = myCanvas.getContext(\"2d\");var canvasX, canvasY;ctx.lineWidth = 8;$(myCanvas).mousedown(function(e){isDown = true;ctx.beginPath();var parentOffset = $(this).parent().offset(); canvasX = e.pageX - parentOffset.left;canvasY = e.pageY - parentOffset.top;ctx.moveTo(canvasX, canvasY);}).mousemove(function(e){if(isDown != false) {var parentOffset = $(this).parent().offset(); canvasX = e.pageX - parentOffset.left;canvasY = e.pageY - parentOffset.top;ctx.lineTo(canvasX, canvasY);ctx.strokeStyle = curColor;ctx.stroke();}}).mouseup(function(e){isDown = false;ctx.closePath();});}$('#selectColor').change(function () {curColor = $('#selectColor option:selected').val();});}init();function handle_output(out) {document.getElementById(\"result_output\").value = out.content.data[\"text/plain\"];}function classify() {var kernel = IPython.notebook.kernel;var myCanvas = document.getElementById(\"myCanvas\");data = myCanvas.toDataURL('image/png');document.getElementById(\"result_output\").value = \"\";kernel.execute(\"classify('\" + data +\"')\", { 'iopub' : {'output' : handle_output}}, {silent:false});}function myClear() {var myCanvas = document.getElementById(\"myCanvas\");myCanvas.getContext(\"2d\").clearRect(0, 0, myCanvas.width, myCanvas.height);}</script>\"\"\"\nHTML(html+script)",
"_____no_output_____"
]
],
[
[
"## Debugging\n\nDNNs can perform poorly for a lot of reasons, like learning rate too big/small, initialization too big/small, network structure not reasonable, etc. When this happens it's often helpful to print out the weights and intermediate outputs to understand what's going on. MXNet provides a monitor utility that does this:",
"_____no_output_____"
]
],
[
[
"def norm_stat(d):\n \"\"\"The statistics you want to see.\n We compute the L2 norm here but you can change it to anything you like.\"\"\"\n return mx.nd.norm(d)/np.sqrt(d.size)\nmon = mx.mon.Monitor(\n 100, # Print every 100 batches\n norm_stat, # The statistics function defined above\n pattern='.*weight', # A regular expression. Only arrays with name matching this pattern will be included.\n sort=True) # Sort output by name\nmodel = mx.model.FeedForward(ctx = mx.gpu(0), symbol = mlp, num_epoch = 1,\n learning_rate = 0.1, momentum = 0.9, wd = 0.00001)\nmodel.fit(X=train_iter, eval_data=test_iter, monitor=mon, # Set the monitor here\n batch_end_callback = mx.callback.Speedometer(100, 100))",
"INFO:root:Start training with [gpu(0)]\nINFO:root:Batch: 1 fc1_backward_weight 0.000519617\t\nINFO:root:Batch: 1 fc1_weight 0.00577777\t\nINFO:root:Batch: 1 fc2_backward_weight 0.00164324\t\nINFO:root:Batch: 1 fc2_weight 0.00577121\t\nINFO:root:Batch: 1 fc3_backward_weight 0.00490826\t\nINFO:root:Batch: 1 fc3_weight 0.00581168\t\nINFO:root:Epoch[0] Batch [100]\tSpeed: 56125.81 samples/sec\tTrain-accuracy=0.141400\nINFO:root:Batch: 101 fc1_backward_weight 0.170696\t\nINFO:root:Batch: 101 fc1_weight 0.0077417\t\nINFO:root:Batch: 101 fc2_backward_weight 0.300237\t\nINFO:root:Batch: 101 fc2_weight 0.0188219\t\nINFO:root:Batch: 101 fc3_backward_weight 1.26234\t\nINFO:root:Batch: 101 fc3_weight 0.0678799\t\nINFO:root:Epoch[0] Batch [200]\tSpeed: 76573.19 samples/sec\tTrain-accuracy=0.419000\nINFO:root:Batch: 201 fc1_backward_weight 0.224993\t\nINFO:root:Batch: 201 fc1_weight 0.0224456\t\nINFO:root:Batch: 201 fc2_backward_weight 0.574649\t\nINFO:root:Batch: 201 fc2_weight 0.0481841\t\nINFO:root:Batch: 201 fc3_backward_weight 1.50356\t\nINFO:root:Batch: 201 fc3_weight 0.223626\t\nINFO:root:Epoch[0] Batch [300]\tSpeed: 82821.98 samples/sec\tTrain-accuracy=0.574900\nINFO:root:Batch: 301 fc1_backward_weight 0.128922\t\nINFO:root:Batch: 301 fc1_weight 0.0297723\t\nINFO:root:Batch: 301 fc2_backward_weight 0.25938\t\nINFO:root:Batch: 301 fc2_weight 0.0623646\t\nINFO:root:Batch: 301 fc3_backward_weight 0.623773\t\nINFO:root:Batch: 301 fc3_weight 0.243092\t\nINFO:root:Epoch[0] Batch [400]\tSpeed: 81133.86 samples/sec\tTrain-accuracy=0.662375\nINFO:root:Batch: 401 fc1_backward_weight 0.244692\t\nINFO:root:Batch: 401 fc1_weight 0.0343876\t\nINFO:root:Batch: 401 fc2_backward_weight 0.42573\t\nINFO:root:Batch: 401 fc2_weight 0.0708167\t\nINFO:root:Batch: 401 fc3_backward_weight 0.813565\t\nINFO:root:Batch: 401 fc3_weight 0.252606\t\nINFO:root:Epoch[0] Batch [500]\tSpeed: 79695.23 samples/sec\tTrain-accuracy=0.716540\nINFO:root:Batch: 501 fc1_backward_weight 0.208892\t\nINFO:root:Batch: 501 fc1_weight 0.0385131\t\nINFO:root:Batch: 501 fc2_backward_weight 0.475372\t\nINFO:root:Batch: 501 fc2_weight 0.0783694\t\nINFO:root:Batch: 501 fc3_backward_weight 0.984594\t\nINFO:root:Batch: 501 fc3_weight 0.2605\t\nINFO:root:Epoch[0] Batch [600]\tSpeed: 78154.25 samples/sec\tTrain-accuracy=0.754600\nINFO:root:Epoch[0] Resetting Data Iterator\nINFO:root:Epoch[0] Train-accuracy=0.754600\nINFO:root:Epoch[0] Time cost=0.831\nINFO:root:Epoch[0] Validation-accuracy=0.953200\n"
]
],
[
[
"## Under the hood: Custom Training Loop\n\n`mx.model.FeedForward` is a convenience wrapper for training standard feed forward networks. What if the model you are working with is more complicated? With MXNet, you can easily control every aspect of training by writing your own training loop.\n\nNeural network training typically has 3 steps: forward, backward (gradient), and update. With custom training loop, you can control the details in each step as while as insert complicated computations in between. You can also connect multiple networks together.",
"_____no_output_____"
]
],
[
[
"# ==================Binding=====================\n# The symbol we created is only a graph description.\n# To run it, we first need to allocate memory and create an executor by 'binding' it.\n# In order to bind a symbol, we need at least two pieces of information: context and input shapes.\n# Context specifies which device the executor runs on, e.g. cpu, GPU0, GPU1, etc.\n# Input shapes define the executor's input array dimensions.\n# MXNet then run automatic shape inference to determine the dimensions of intermediate and output arrays.\n\n# data iterators defines shapes of its output with provide_data and provide_label property.\ninput_shapes = dict(train_iter.provide_data+train_iter.provide_label)\nprint 'input_shapes', input_shapes\n# We use simple_bind to let MXNet allocate memory for us.\n# You can also allocate memory youself and use bind to pass it to MXNet.\nexe = mlp.simple_bind(ctx=mx.gpu(0), **input_shapes)\n\n# ===============Initialization=================\n# First we get handle to input arrays\narg_arrays = dict(zip(mlp.list_arguments(), exe.arg_arrays))\ndata = arg_arrays[train_iter.provide_data[0][0]]\nlabel = arg_arrays[train_iter.provide_label[0][0]]\n\n# We initialize the weights with uniform distribution on (-0.01, 0.01).\ninit = mx.init.Uniform(scale=0.01)\nfor name, arr in arg_arrays.items():\n if name not in input_shapes:\n init(name, arr)\n \n# We also need to create an optimizer for updating weights\nopt = mx.optimizer.SGD(\n learning_rate=0.1,\n momentum=0.9,\n wd=0.00001,\n rescale_grad=1.0/train_iter.batch_size)\nupdater = mx.optimizer.get_updater(opt)\n\n# Finally we need a metric to print out training progress\nmetric = mx.metric.Accuracy()\n\n# Training loop begines\nfor epoch in range(10):\n train_iter.reset()\n metric.reset()\n t = 0\n for batch in train_iter:\n # Copy data to executor input. Note the [:].\n data[:] = batch.data[0]\n label[:] = batch.label[0]\n \n # Forward\n exe.forward(is_train=True)\n \n # You perform operations on exe.outputs here if you need to.\n # For example, you can stack a CRF on top of a neural network.\n \n # Backward\n exe.backward()\n \n # Update\n for i, pair in enumerate(zip(exe.arg_arrays, exe.grad_arrays)):\n weight, grad = pair\n updater(i, grad, weight)\n metric.update(batch.label, exe.outputs)\n t += 1\n if t % 100 == 0:\n print 'epoch:', epoch, 'iter:', t, 'metric:', metric.get()\n",
"input_shapes {'softmax_label': (100,), 'data': (100, 784)}\nepoch: 0 iter: 100 metric: ('accuracy', 0.1427)\nepoch: 0 iter: 200 metric: ('accuracy', 0.42695)\nepoch: 0 iter: 300 metric: ('accuracy', 0.5826333333333333)\nepoch: 0 iter: 400 metric: ('accuracy', 0.66875)\nepoch: 0 iter: 500 metric: ('accuracy', 0.72238)\nepoch: 0 iter: 600 metric: ('accuracy', 0.7602166666666667)\nepoch: 1 iter: 100 metric: ('accuracy', 0.9504)\nepoch: 1 iter: 200 metric: ('accuracy', 0.9515)\nepoch: 1 iter: 300 metric: ('accuracy', 0.9547666666666667)\nepoch: 1 iter: 400 metric: ('accuracy', 0.95665)\nepoch: 1 iter: 500 metric: ('accuracy', 0.95794)\nepoch: 1 iter: 600 metric: ('accuracy', 0.95935)\nepoch: 2 iter: 100 metric: ('accuracy', 0.9657)\nepoch: 2 iter: 200 metric: ('accuracy', 0.96715)\nepoch: 2 iter: 300 metric: ('accuracy', 0.9698)\nepoch: 2 iter: 400 metric: ('accuracy', 0.9702)\nepoch: 2 iter: 500 metric: ('accuracy', 0.97104)\nepoch: 2 iter: 600 metric: ('accuracy', 0.9717)\nepoch: 3 iter: 100 metric: ('accuracy', 0.976)\nepoch: 3 iter: 200 metric: ('accuracy', 0.97575)\nepoch: 3 iter: 300 metric: ('accuracy', 0.9772666666666666)\nepoch: 3 iter: 400 metric: ('accuracy', 0.9771)\nepoch: 3 iter: 500 metric: ('accuracy', 0.9771)\nepoch: 3 iter: 600 metric: ('accuracy', 0.97755)\nepoch: 4 iter: 100 metric: ('accuracy', 0.9805)\nepoch: 4 iter: 200 metric: ('accuracy', 0.9803)\nepoch: 4 iter: 300 metric: ('accuracy', 0.9814666666666667)\nepoch: 4 iter: 400 metric: ('accuracy', 0.981175)\nepoch: 4 iter: 500 metric: ('accuracy', 0.98132)\nepoch: 4 iter: 600 metric: ('accuracy', 0.98145)\nepoch: 5 iter: 100 metric: ('accuracy', 0.9837)\nepoch: 5 iter: 200 metric: ('accuracy', 0.98365)\nepoch: 5 iter: 300 metric: ('accuracy', 0.9835333333333334)\nepoch: 5 iter: 400 metric: ('accuracy', 0.98395)\nepoch: 5 iter: 500 metric: ('accuracy', 0.984)\nepoch: 5 iter: 600 metric: ('accuracy', 0.9842166666666666)\nepoch: 6 iter: 100 metric: ('accuracy', 0.9848)\nepoch: 6 iter: 200 metric: ('accuracy', 0.98475)\nepoch: 6 iter: 300 metric: ('accuracy', 0.9858333333333333)\nepoch: 6 iter: 400 metric: ('accuracy', 0.98555)\nepoch: 6 iter: 500 metric: ('accuracy', 0.9855)\nepoch: 6 iter: 600 metric: ('accuracy', 0.9856333333333334)\nepoch: 7 iter: 100 metric: ('accuracy', 0.9842)\nepoch: 7 iter: 200 metric: ('accuracy', 0.98625)\nepoch: 7 iter: 300 metric: ('accuracy', 0.9869)\nepoch: 7 iter: 400 metric: ('accuracy', 0.9877)\nepoch: 7 iter: 500 metric: ('accuracy', 0.98774)\nepoch: 7 iter: 600 metric: ('accuracy', 0.9875333333333334)\nepoch: 8 iter: 100 metric: ('accuracy', 0.9864)\nepoch: 8 iter: 200 metric: ('accuracy', 0.9878)\nepoch: 8 iter: 300 metric: ('accuracy', 0.9886666666666667)\nepoch: 8 iter: 400 metric: ('accuracy', 0.98885)\nepoch: 8 iter: 500 metric: ('accuracy', 0.98918)\nepoch: 8 iter: 600 metric: ('accuracy', 0.9894666666666667)\nepoch: 9 iter: 100 metric: ('accuracy', 0.9884)\nepoch: 9 iter: 200 metric: ('accuracy', 0.98855)\nepoch: 9 iter: 300 metric: ('accuracy', 0.9894666666666667)\nepoch: 9 iter: 400 metric: ('accuracy', 0.98945)\nepoch: 9 iter: 500 metric: ('accuracy', 0.98972)\nepoch: 9 iter: 600 metric: ('accuracy', 0.9899333333333333)\n"
]
],
[
[
"## New Operators\n\nMXNet provides a repository of common operators (or layers). However, new models often require new layers. There are several ways to [create new operators](https://mxnet.readthedocs.org/en/latest/tutorial/new_op_howto.html) with MXNet. Here we talk about the easiest way: pure python. ",
"_____no_output_____"
]
],
[
[
"# Define custom softmax operator\nclass NumpySoftmax(mx.operator.NumpyOp):\n def __init__(self):\n # Call the parent class constructor. \n # Because NumpySoftmax is a loss layer, it doesn't need gradient input from layers above.\n super(NumpySoftmax, self).__init__(need_top_grad=False)\n \n def list_arguments(self):\n # Define the input to NumpySoftmax.\n return ['data', 'label']\n\n def list_outputs(self):\n # Define the output.\n return ['output']\n\n def infer_shape(self, in_shape):\n # Calculate the dimensions of the output (and missing inputs) from (some) input shapes.\n data_shape = in_shape[0] # shape of first argument 'data'\n label_shape = (in_shape[0][0],) # 'label' should be one dimensional and has batch_size instances.\n output_shape = in_shape[0] # 'output' dimension is the same as the input.\n return [data_shape, label_shape], [output_shape]\n\n def forward(self, in_data, out_data):\n x = in_data[0] # 'data'\n y = out_data[0] # 'output'\n \n # Compute softmax\n y[:] = np.exp(x - x.max(axis=1).reshape((x.shape[0], 1)))\n y /= y.sum(axis=1).reshape((x.shape[0], 1))\n\n def backward(self, out_grad, in_data, out_data, in_grad):\n l = in_data[1] # 'label'\n l = l.reshape((l.size,)).astype(np.int) # cast to int\n y = out_data[0] # 'output'\n dx = in_grad[0] # gradient for 'data'\n \n # Compute gradient\n dx[:] = y\n dx[np.arange(l.shape[0]), l] -= 1.0\n\nnumpy_softmax = NumpySoftmax()\n\ndata = mx.symbol.Variable('data')\nfc1 = mx.symbol.FullyConnected(data = data, name='fc1', num_hidden=128)\nact1 = mx.symbol.Activation(data = fc1, name='relu1', act_type=\"relu\")\nfc2 = mx.symbol.FullyConnected(data = act1, name = 'fc2', num_hidden = 64)\nact2 = mx.symbol.Activation(data = fc2, name='relu2', act_type=\"relu\")\nfc3 = mx.symbol.FullyConnected(data = act2, name='fc3', num_hidden=10)\n# Use the new operator we just defined instead of the standard softmax operator.\nmlp = numpy_softmax(data=fc3, name = 'softmax')\n\nmodel = mx.model.FeedForward(ctx = mx.gpu(0), symbol = mlp, num_epoch = 2,\n learning_rate = 0.1, momentum = 0.9, wd = 0.00001)\nmodel.fit(X=train_iter, eval_data=test_iter,\n batch_end_callback = mx.callback.Speedometer(100, 100))",
"INFO:root:Start training with [gpu(0)]\nINFO:root:Epoch[0] Batch [100]\tSpeed: 53975.81 samples/sec\tTrain-accuracy=0.167800\nINFO:root:Epoch[0] Batch [200]\tSpeed: 75720.80 samples/sec\tTrain-accuracy=0.455800\nINFO:root:Epoch[0] Batch [300]\tSpeed: 73701.82 samples/sec\tTrain-accuracy=0.602833\nINFO:root:Epoch[0] Batch [400]\tSpeed: 65162.74 samples/sec\tTrain-accuracy=0.684375\nINFO:root:Epoch[0] Batch [500]\tSpeed: 65920.09 samples/sec\tTrain-accuracy=0.735120\nINFO:root:Epoch[0] Batch [600]\tSpeed: 67870.31 samples/sec\tTrain-accuracy=0.770333\nINFO:root:Epoch[0] Resetting Data Iterator\nINFO:root:Epoch[0] Train-accuracy=0.770333\nINFO:root:Epoch[0] Time cost=0.923\nINFO:root:Epoch[0] Validation-accuracy=0.950400\nINFO:root:Epoch[1] Batch [100]\tSpeed: 54063.96 samples/sec\tTrain-accuracy=0.946700\nINFO:root:Epoch[1] Batch [200]\tSpeed: 74701.53 samples/sec\tTrain-accuracy=0.949700\nINFO:root:Epoch[1] Batch [300]\tSpeed: 69534.33 samples/sec\tTrain-accuracy=0.953400\nINFO:root:Epoch[1] Batch [400]\tSpeed: 76418.05 samples/sec\tTrain-accuracy=0.954875\nINFO:root:Epoch[1] Batch [500]\tSpeed: 68825.54 samples/sec\tTrain-accuracy=0.956340\nINFO:root:Epoch[1] Batch [600]\tSpeed: 74324.13 samples/sec\tTrain-accuracy=0.958083\nINFO:root:Epoch[1] Resetting Data Iterator\nINFO:root:Epoch[1] Train-accuracy=0.958083\nINFO:root:Epoch[1] Time cost=0.879\nINFO:root:Epoch[1] Validation-accuracy=0.957200\n"
]
]
] | [
"markdown",
"code",
"markdown",
"code",
"markdown",
"code",
"markdown",
"code",
"markdown",
"code",
"markdown",
"code",
"markdown",
"code",
"markdown",
"code",
"markdown",
"code",
"markdown",
"code",
"markdown",
"code",
"markdown",
"code",
"markdown",
"code"
] | [
[
"markdown",
"markdown"
],
[
"code"
],
[
"markdown"
],
[
"code"
],
[
"markdown"
],
[
"code"
],
[
"markdown"
],
[
"code"
],
[
"markdown"
],
[
"code"
],
[
"markdown"
],
[
"code"
],
[
"markdown"
],
[
"code"
],
[
"markdown"
],
[
"code"
],
[
"markdown"
],
[
"code"
],
[
"markdown"
],
[
"code"
],
[
"markdown"
],
[
"code"
],
[
"markdown"
],
[
"code"
],
[
"markdown"
],
[
"code"
]
] |
e78680b14a30c358caa148e883d3b177f8b4d0a0 | 206,305 | ipynb | Jupyter Notebook | final.ipynb | invokred/ride-sharing-optimizer | e8b3f6c6caa5110a79678b458c520e4cef68ea46 | [
"MIT"
] | null | null | null | final.ipynb | invokred/ride-sharing-optimizer | e8b3f6c6caa5110a79678b458c520e4cef68ea46 | [
"MIT"
] | null | null | null | final.ipynb | invokred/ride-sharing-optimizer | e8b3f6c6caa5110a79678b458c520e4cef68ea46 | [
"MIT"
] | null | null | null | 320.349379 | 51,692 | 0.882087 | [
[
[
"empty"
]
]
] | [
"empty"
] | [
[
"empty"
]
] |
e7868f85ef3a3bc9268f4ecc4ce829764635e2c1 | 14,110 | ipynb | Jupyter Notebook | challenges/026-Giant_Squid/026-Day04_Giant_Squid.ipynb | jfdaniel77/interview-challenge | 9af3a675c8ca38ae282c38d1be4fb9d5dca13fce | [
"MIT"
] | null | null | null | challenges/026-Giant_Squid/026-Day04_Giant_Squid.ipynb | jfdaniel77/interview-challenge | 9af3a675c8ca38ae282c38d1be4fb9d5dca13fce | [
"MIT"
] | null | null | null | challenges/026-Giant_Squid/026-Day04_Giant_Squid.ipynb | jfdaniel77/interview-challenge | 9af3a675c8ca38ae282c38d1be4fb9d5dca13fce | [
"MIT"
] | null | null | null | 39.085873 | 327 | 0.488802 | [
[
[
"# Challenge 026 - Giant Squid!\n\nThis challenge is taken from Advent of Code 2021 - Day 4: Giant Squid (https://adventofcode.com/2021/day/4).\n\n## Problem - Part 1\n\nYou're already almost 1.5km (almost a mile) below the surface of the ocean, already so deep that you can't see any sunlight. What you can see, however, is a giant squid that has attached itself to the outside of your submarine.\n\nMaybe it wants to play bingo?\n\nBingo is played on a set of boards each consisting of a 5x5 grid of numbers. Numbers are chosen at random, and the chosen number is marked on all boards on which it appears. (Numbers may not appear on all boards.) If all numbers in any row or any column of a board are marked, that board wins. (Diagonals don't count.)\n\nThe submarine has a bingo subsystem to help passengers (currently, you and the giant squid) pass the time. It automatically generates a random order in which to draw numbers and a random set of boards (your puzzle input). For example:\n```\n7,4,9,5,11,17,23,2,0,14,21,24,10,16,13,6,15,25,12,22,18,20,8,19,3,26,1\n\n22 13 17 11 0\n 8 2 23 4 24\n21 9 14 16 7\n 6 10 3 18 5\n 1 12 20 15 19\n\n 3 15 0 2 22\n 9 18 13 17 5\n19 8 7 25 23\n20 11 10 24 4\n14 21 16 12 6\n\n14 21 17 24 4\n10 16 15 9 19\n18 8 23 26 20\n22 11 13 6 5\n 2 0 12 3 7\n```\nAfter the first five numbers are drawn (7, 4, 9, 5, and 11), there are no winners, but the boards are marked as follows (shown here adjacent to each other to save space):\n```\n22 13 17 11 0 3 15 0 2 22 14 21 17 24 4\n 8 2 23 4 24 9 18 13 17 5 10 16 15 9 19\n21 9 14 16 7 19 8 7 25 23 18 8 23 26 20\n 6 10 3 18 5 20 11 10 24 4 22 11 13 6 5\n 1 12 20 15 19 14 21 16 12 6 2 0 12 3 7\n```\n\nAfter the next six numbers are drawn (17, 23, 2, 0, 14, and 21), there are still no winners:\n```\n22 13 17 11 0 3 15 0 2 22 14 21 17 24 4\n 8 2 23 4 24 9 18 13 17 5 10 16 15 9 19\n21 9 14 16 7 19 8 7 25 23 18 8 23 26 20\n 6 10 3 18 5 20 11 10 24 4 22 11 13 6 5\n 1 12 20 15 19 14 21 16 12 6 2 0 12 3 7\n```\n\nFinally, 24 is drawn:\n```\n22 13 17 11 0 3 15 0 2 22 14 21 17 24 4\n 8 2 23 4 24 9 18 13 17 5 10 16 15 9 19\n21 9 14 16 7 19 8 7 25 23 18 8 23 26 20\n 6 10 3 18 5 20 11 10 24 4 22 11 13 6 5\n 1 12 20 15 19 14 21 16 12 6 2 0 12 3 7\n``` \nAt this point, the third board wins because it has at least one complete row or column of marked numbers (in this case, the entire top row is marked: 14 21 17 24 4).\n\nThe score of the winning board can now be calculated. Start by finding the sum of all unmarked numbers on that board; in this case, the sum is 188. Then, multiply that sum by the number that was just called when the board won, 24, to get the final score, 188 * 24 = 4512.\n\nTo guarantee victory against the giant squid, figure out which board will win first. What will your final score be if you choose that board?\n\n## Solution - Part 1\n```\nTo run this script, you need to save input-day-04-numbers.txt and input-day-boards.txt and run it in your local machine.\n```",
"_____no_output_____"
]
],
[
[
"class Board:\n def __init__(self):\n self.position = {}\n self.bingo= {\n \"column\": [0,0,0,0,0],\n \"row\": [0,0,0,0,0]\n }\n self.playBoard = [\n [0,0,0,0,0],\n [0,0,0,0,0],\n [0,0,0,0,0],\n [0,0,0,0,0],\n [0,0,0,0,0],\n ]\n self.selected_number = []\n \n def populate_board(self, playBoard):\n self.playBoard = playBoard\n \n def checkBingo(self):\n return 5 in self.bingo[\"row\"] or 5 in self.bingo[\"column\"]\n \n def update_board(self, value):\n for idx, item in enumerate(self.playBoard):\n if value not in item:\n continue\n \n self.bingo[\"row\"][idx] = self.bingo[\"row\"][idx] + 1\n self.bingo[\"column\"][item.index(value)] = self.bingo[\"column\"][item.index(value)] + 1\n self.selected_number.append(value)\n \n def calculate_final_score(self):\n final_score = 0\n for item in self.playBoard:\n for number in item:\n if number in self.selected_number:\n continue\n final_score = final_score + number\n \n return final_score * self.selected_number.pop()\n \n def __str__(self):\n for item in self.playBoard:\n print(item)\n return \"\"\n\n\n# Get sequence of bingo number\ndef import_input_number():\n with open (\"input-day-04-numbers.txt\", \"r\") as file:\n input_data = file.read()\n return input_data\n\n# Populate Bingo playboard\ndef import_input_board():\n with open (\"input-day-04-boards.txt\", \"r\") as file:\n input_data = file.read().splitlines()\n\n board = []\n temp_board = []\n item_board = []\n for row, val in enumerate(input_data):\n if row == len(input_data) - 1:\n item_board.append(val)\n temp_board.append(item_board)\n elif val == '':\n temp_board.append(item_board)\n item_board = []\n else:\n item_board.append(val)\n \n for item in temp_board:\n temp_playboard = []\n for row in item:\n value = [ int(number) for number in row.split(\" \") if number != \"\"]\n temp_playboard.append(value)\n \n playBoard = Board()\n playBoard.populate_board(temp_playboard)\n board.append(playBoard)\n \n return board\n\nlist_number = list(map(int, import_input_number().split(',')))\nlist_board = import_input_board()\n\nfor number in list_number:\n is_bingo = False\n for idx, board in enumerate(list_board):\n board.update_board(number)\n is_bingo = board.checkBingo()\n \n if is_bingo:\n print(\"Board {} BINGO! Final score: {}\".format(idx, board.calculate_final_score()))\n break\n\n if is_bingo:\n break",
"_____no_output_____"
]
],
[
[
"## Problem - Part 2\n\nOn the other hand, it might be wise to try a different strategy: let the giant squid win.\n\nYou aren't sure how many bingo boards a giant squid could play at once, so rather than waste time counting its arms, the safe thing to do is to figure out which board will win last and choose that one. That way, no matter which boards it picks, it will win for sure.\n\nIn the above example, the second board is the last to win, which happens after 13 is eventually called and its middle column is completely marked. If you were to keep playing until this point, the second board would have a sum of unmarked numbers equal to 148 for a final score of 148 * 13 = 1924.\n\nFigure out which board will win last. Once it wins, what would its final score be?\n\n## Solution - Part 2\n\n```\nTo run this script, you need to save input-day-04-numbers.txt and input-day-boards.txt and run it in your local machine.\n```",
"_____no_output_____"
]
],
[
[
"class Board:\n def __init__(self):\n self.position = {}\n self.bingo= {\n \"column\": [0,0,0,0,0],\n \"row\": [0,0,0,0,0]\n }\n self.playBoard = [\n [0,0,0,0,0],\n [0,0,0,0,0],\n [0,0,0,0,0],\n [0,0,0,0,0],\n [0,0,0,0,0],\n ]\n self.selected_number = []\n self.id = 0\n \n def populate_board(self, idx, playBoard):\n self.playBoard = playBoard\n self.id = idx\n \n def checkBingo(self):\n return 5 in self.bingo[\"row\"] or 5 in self.bingo[\"column\"]\n \n def update_board(self, value):\n for idx, item in enumerate(self.playBoard):\n \n if value not in item:\n continue\n \n self.bingo[\"row\"][idx] = self.bingo[\"row\"][idx] + 1\n self.bingo[\"column\"][item.index(value)] = self.bingo[\"column\"][item.index(value)] + 1\n self.selected_number.append(value)\n \n \n def calculate_final_score(self):\n final_score = 0\n for item in self.playBoard:\n for number in item:\n if number in self.selected_number:\n continue\n final_score = final_score + number\n \n return final_score * self.selected_number.pop()\n \n def __str__(self):\n for item in self.playBoard:\n print(item)\n return \"\"\n\n\n# Get sequence of bingo number\ndef import_input_number():\n with open (\"input-day-04-numbers.txt\", \"r\") as file:\n input_data = file.read()\n return input_data\n\n# Populate Bingo playboard\ndef import_input_board():\n with open (\"input-day-04-boards.txt\", \"r\") as file:\n input_data = file.read().splitlines()\n\n board = []\n temp_board = []\n item_board = []\n for row, val in enumerate(input_data):\n if row == len(input_data) - 1:\n item_board.append(val)\n temp_board.append(item_board)\n elif val == '':\n temp_board.append(item_board)\n item_board = []\n else:\n item_board.append(val)\n \n for idx, item in enumerate(temp_board):\n temp_playboard = []\n for row in item:\n value = [ int(number) for number in row.split(\" \") if number != \"\"]\n temp_playboard.append(value)\n \n playBoard = Board()\n playBoard.populate_board(idx, temp_playboard)\n board.append(playBoard)\n \n return board\n\nlist_number = list(map(int, import_input_number().split(',')))\nlist_board = import_input_board()\n\nlast_board = 0\nbingo_board = []\nlast_number = 0\ncount_bingo = 0\nfor number in list_number:\n is_stop = False\n for idx, board in enumerate(list_board):\n \n if idx in bingo_board:\n continue\n \n board.update_board(number)\n is_bingo = board.checkBingo()\n \n if is_bingo:\n bingo_board.append(idx)\n count_bingo = count_bingo + 1\n \n if count_bingo == len(list_board):\n last_board = idx\n is_stop = True\n \n\n if is_stop:\n last_number = number\n break\n\nprint(\"Board {} BINGO! Final score: {}\".format(last_board, list_board[last_board].calculate_final_score()))",
"_____no_output_____"
]
]
] | [
"markdown",
"code",
"markdown",
"code"
] | [
[
"markdown"
],
[
"code"
],
[
"markdown"
],
[
"code"
]
] |
e7868fe39d6b9c7f26c72296ae1e79351e05da3b | 8,768 | ipynb | Jupyter Notebook | ml/Table_of_Contents.ipynb | Db2-DTE-POC/modernization | f6d52918b72850a2e3407c0beaef20a1b909a897 | [
"Apache-2.0"
] | null | null | null | ml/Table_of_Contents.ipynb | Db2-DTE-POC/modernization | f6d52918b72850a2e3407c0beaef20a1b909a897 | [
"Apache-2.0"
] | null | null | null | ml/Table_of_Contents.ipynb | Db2-DTE-POC/modernization | f6d52918b72850a2e3407c0beaef20a1b909a897 | [
"Apache-2.0"
] | 2 | 2021-11-17T08:17:36.000Z | 2021-11-23T09:26:42.000Z | 53.463415 | 435 | 0.538321 | [
[
[
"<img align=\"left\" src=\"media/Assets&ArchHeader.jpeg\">",
"_____no_output_____"
],
[
"# Modern Db2 Applications \nWelcome to this Db2 lab that highlights some of the new ways to access Db2 through REST services and build Machine Learning models for and with Db2. This lab uses Jupyter notebooks to demonstrate these features. If you are not familiar with the use of Jupyter notebooks or Python, the following notebooks will guide you through their usage. You can find a copy of these notebooks at https://github.com/Db2-DTE-POC/modernization. ",
"_____no_output_____"
],
[
"<div style=\"font-family: 'IBM Plex Sans';\"> \n<table style=\"float:left; width: 620px; height: 235px; border-spacing: 10px; border-collapse: separate; table-layout: fixed\"> \n <td style=\"padding: 15px; text-align:left; vertical-align: text-top; background-color:#F7F7F7; width: 300px; height: 250px;\"> \n <div style=\"height: 75px\"><p style=\"font-size: 24px\">An Introduction to Jupyter Notebooks</div> \n <div style=\"height: 125px\"><p style=\"font-size: 14px\"> \n If you are not familiar with the use of Jupyter notebooks or Python, the following notebook \n will guide you through their usage. \n </div> \n <div style=\"height: 25px\"><p style=\"font-size: 12px; text-align: right\"> \n <img style=\"display: inline-block;\"src=\"./media/clock.png\"> 10min \n </div> \n <div style=\"height: 10px\"><p style=\"font-size: 12px; text-align: right\"> \n <a href=\"./An_Introduction_to_Jupyter_Notebooks.ipynb\"> \n <img style=\"display: inline-block;\"src=\"./media/arrowblue.png\"></a> \n </div> \n </td> \n <td style=\"padding: 15px; text-align:left; vertical-align: text-top; background-color:#F7F7F7; width: 300px; height:250px\"> \n <div style=\"height: 75px\"><p style=\"font-size: 24px\">Db2 Magic Commands</div> \n <div style=\"height: 125px\"><p style=\"font-size: 14px\"> \n Db2 Magic commands are used in all of the notebooks used in this lab. \n The following notebook provides a tutorial on basics of using the Db2 magic commands. \n </div> \n <div style=\"height: 25px\"><p style=\"font-size: 12px; text-align: right\"> \n <img style=\"display: inline-block;\"src=\"./media/clock.png\"> 10min \n </div> \n <div style=\"height: 10px\"><p style=\"font-size: 12px; text-align: right\"> \n <a href=\"./Db2_Jupyter_Extensions_Tutorial.ipynb\"> \n <img style=\"display: inline-block;\"src=\"./media/arrowblue.png\"></a> \n </div> \n </td> \n <td style=\"padding: 15px; text-align:left; vertical-align: text-top; background-color:#F7F7F7; width: 300px; height:250px\"> \n <div style=\"height: 75px\"><p style=\"font-size: 24px\">Db2 Data Management Console</div> \n <div style=\"height: 125px\"><p style=\"font-size: 14px\"> \n The IBM Db2 Data Management Console builds on the best of Data Server Manager to help you manage one to hundreds of databases across the Db2 family from ground to cloud. \n </div> \n <div style=\"height: 25px\"><p style=\"font-size: 12px; text-align: right\"> \n <img style=\"display: inline-block;\"src=\"./media/clock.png\"> 10min \n </div> \n <div style=\"height: 10px\"><p style=\"font-size: 12px; text-align: right\"> \n <a href=\"./Db2_Data_Management_Console_Introduction.ipynb\"> \n <img style=\"display: inline-block;\"src=\"./media/arrowblue.png\"></a> \n </div> \n </td> \n </table> \n</div>",
"_____no_output_____"
],
[
"<!-- Row 1 -->\n<div style=\"font-family: 'IBM Plex Sans';\">\n<table style=\"float:left; width: 300px; height: 235px; border-spacing: 10px; border-collapse: separate; table-layout: fixed\">\n <td style=\"padding: 15px; text-align:left; vertical-align: text-top; background-color:#F7F7F7; width: 300px; height: 250px;\">\n <div style=\"height: 75px\"><p style=\"font-size: 24px\">\n<!-- Title -->\nBuild and Deploy an in-Db2 ML Model\n </div>\n <div style=\"height: 125px\"><p style=\"font-size: 14px\">\n<!-- Description -->\nThis lab contains an example of how to develop a customer segmentation machine learning model with Db2's native machine learning functionality.\n </div>\n <div style=\"height: 25px\"><p style=\"font-size: 12px; text-align: right\">\n <img style=\"display: inline-block;\"src=\"./media/clock.png\"> \n<!-- Duration -->\n90 min\n </div>\n <div style=\"height: 10px\"><p style=\"font-size: 12px; text-align: right\">\n<!-- URL --> \n<a href=\"./Db2MLTutorial1.ipynb\">\n <img style=\"display: inline-block;\"src=\"./media/arrowblue.png\"></a> \n </div> \n </td> \n <td style=\"padding: 15px; text-align:left; vertical-align: text-top; background-color:#F7F7F7; width: 300px; height: 250px;\">\n <div style=\"height: 75px\"><p style=\"font-size: 24px\">\n<!-- Title -->\nOperationalize a Scikit-learn Model with Db2\n </div>\n <div style=\"height: 125px\"><p style=\"font-size: 14px\">\n<!-- Description -->\nThis lab contains an example of how to develop a machine learning pipeline externally in a Jupyter notebook and deploy the pipeline to Db2 for in-database scoring with Python UDFs.\n </div>\n <div style=\"height: 25px\"><p style=\"font-size: 12px; text-align: right\">\n <img style=\"display: inline-block;\"src=\"./media/clock.png\"> \n<!-- Duration -->\n90 min\n </div>\n <div style=\"height: 10px\"><p style=\"font-size: 12px; text-align: right\">\n<!-- URL --> \n<a href=\"./Db2MLTutorial2.ipynb\">\n <img style=\"display: inline-block;\"src=\"./media/arrowblue.png\"></a> \n </div> \n </td> \n <td style=\"padding: 15px; text-align:left; vertical-align: text-top; background-color:#F7F7F7; width: 300px; height:250px\">\n <div style=\"height: 75px\"><p style=\"font-size: 24px\">Db2 RESTful Endpoint Service</div>\n <div style=\"height: 125px\"><p style=\"font-size: 14px\">\n You can extend your Db2 system so that application programmers can create Representational State Transfer (REST) endpoints \n to interact with your Db2 database\n </div>\n <div style=\"height: 25px\"><p style=\"font-size: 12px; text-align: right\">\n <img style=\"display: inline-block;\"src=\"./media/clock.png\"> 20min\n </div> \n <div style=\"height: 10px\"><p style=\"font-size: 12px; text-align: right\">\n <a href=\"./Db2 RESTful Example.ipynb\">\n <img style=\"display: inline-block;\"src=\"./media/arrowblue.png\"></a> \n </div> \n </td> \n</table>\n</div>",
"_____no_output_____"
],
[
"#### Questions and Comments: Peter Kohlmann [[email protected]], George Baklarz [[email protected]]",
"_____no_output_____"
]
]
] | [
"markdown"
] | [
[
"markdown",
"markdown",
"markdown",
"markdown",
"markdown"
]
] |
e7869cc394f975760af90284ca8d778529e3e6f4 | 11,600 | ipynb | Jupyter Notebook | new_vars.ipynb | brianbarbieri/Perimeter-of-an-Ellipse | 14fb667d8474068a68174ce7c31a258b288661ce | [
"Apache-2.0"
] | null | null | null | new_vars.ipynb | brianbarbieri/Perimeter-of-an-Ellipse | 14fb667d8474068a68174ce7c31a258b288661ce | [
"Apache-2.0"
] | null | null | null | new_vars.ipynb | brianbarbieri/Perimeter-of-an-Ellipse | 14fb667d8474068a68174ce7c31a258b288661ce | [
"Apache-2.0"
] | null | null | null | 57.711443 | 4,773 | 0.466293 | [
[
[
"import numpy as np\nimport pandas as pd\nimport itertools\nfrom scipy.special import ellipe",
"_____no_output_____"
],
[
"f = np.math.factorial\nratios = np.linspace(1.0, 10.0, num=21)\nb = np.linspace(1.0, 10.0, num=10)\ndata = np.array(list(itertools.product(b, ratios)))\ndf = pd.DataFrame(data, columns=[\"b\", \"ratios\"])\ndf[\"a\"] = df.b * df.ratios\ndf[\"h\"] = np.power((df.a-df.b), 2) / np.power((df.a+df.b), 2)\ndf[\"e\"] = np.sqrt(np.power(df.a, 2) - np.power(df.b, 2)) / df.a",
"_____no_output_____"
],
[
"class Ellipse:\n\n def __init__(self, a, b):\n self.a = a\n self.b = b\n self.h = self.calc_h()\n self.e = self.calc_e()\n\n def calc_h(self):\n return np.power((self.a-self.b), 2) / np.power((self.a+self.b), 2)\n\n def calc_e(self):\n return np.sqrt(np.power(self.a, 2) - np.power(self.b, 2)) / self.a\n\ndef euler_approx(x):\n return np.pi * np.sqrt(2*(np.power(x.a, 2) + np.power(x.b, 2)))\n\ndef ramanujan_I_approx(x):\n return np.pi * (3*(x.a+x.b) - np.sqrt((3*x.a + x.b) * (x.a + 3*x.b)))\n\ndef ramanujan_II_approx(x):\n return np.pi * (x.a+x.b) * (1 + ((3*x.h) / (10 + np.sqrt(4 - (3*x.h)))))\n\ndef inf_sum(x, n):\n start = 2 * x.a * np.pi\n summation = 0\n for i in range(1, n+1):\n f1 = f(2*i) ** 2 / ((2 ** i) * f(i)) ** 4\n f2 = (x.e ** (2*i)) / ((2*i) - 1)\n summation += f1 * f2\n return start * (1 - summation)\n\ndef error(exact, pred):\n '''Calculates the percentage error'''\n return np.abs(exact - pred) / exact",
"_____no_output_____"
],
[
"df[\"exact_inf_sum\"] = df.pipe(inf_sum, n=100)\ndf[\"exact_scipy\"] = 4 * df.a * ellipe(df.e)\ndf[\"euler\"] = df.pipe(euler_approx)\ndf[\"ramanujan_I\"] = df.pipe(ramanujan_I_approx)\ndf[\"ramanujan_II\"] = df.pipe(ramanujan_II_approx)",
"_____no_output_____"
],
[
"df[\"euler_error\"] = error(df.exact_inf_sum, df.euler)\ndf[\"ramanujan_I_error\"] = error(df.exact_inf_sum, df.ramanujan_I)\ndf[\"ramanujan_II_error\"] = error(df.exact_inf_sum, df.ramanujan_II)",
"_____no_output_____"
],
[
"df.to_csv(\"answer.csv\", index=False)",
"_____no_output_____"
],
[
"df",
"_____no_output_____"
]
]
] | [
"code"
] | [
[
"code",
"code",
"code",
"code",
"code",
"code",
"code"
]
] |
e786a8a306e49c99a8dc834490c8f5b06f23c126 | 341,091 | ipynb | Jupyter Notebook | notebooks/2_dask_colab.ipynb | oceanhackweek/Oceans19-data-science-tutorial | e97313c356be5cc0059c4b427ce109d087449330 | [
"CC-BY-4.0"
] | 6 | 2019-10-31T15:08:15.000Z | 2020-07-10T06:51:29.000Z | notebooks/2_dask_colab.ipynb | oceanhackweek/Oceans19-data-science-tutorial | e97313c356be5cc0059c4b427ce109d087449330 | [
"CC-BY-4.0"
] | null | null | null | notebooks/2_dask_colab.ipynb | oceanhackweek/Oceans19-data-science-tutorial | e97313c356be5cc0059c4b427ce109d087449330 | [
"CC-BY-4.0"
] | 1 | 2019-10-30T10:47:19.000Z | 2019-10-30T10:47:19.000Z | 259.779893 | 190,242 | 0.900786 | [
[
[
"<a href=\"https://colab.research.google.com/github/oceanhackweek/Oceans19-data-science-tutorial/blob/master/notebooks/2_dask_colab.ipynb\" target=\"_parent\"><img src=\"https://colab.research.google.com/assets/colab-badge.svg\" alt=\"Open In Colab\"/></a>",
"_____no_output_____"
],
[
"Parallel Processing with Dask\n====\n\n<img src=\"http://dask.readthedocs.io/en/latest/_images/dask_horizontal.svg\" \n width=\"30%\" \n align=right\n alt=\"Dask logo\">\n\n## Learning Objectives\n* get acquanted with the Python Dask Library\n* learn how to execute basic operations on large arrays which cannot fit in RAM\n* learn about the concepts of lazy evaluation and task scheduling graphs\n* learn how to work with Dask Arrays\n* learn how to work with Dask Delayed\n\n## Motivation\nResearchers are overloaded with data which their traditional processing workflows are incapable to handle. Usually they are faced with two possible options:\n\n* move the processing to large machines/clusters\n* modify their methods to access the data only pieces at a time.\n\nThey also like to test out things on their laptops, and later move to clusters, without having to modify their code a lot.\n\n\n[Dask](https://dask.org/) is a Python Library which makes this possible:\n\n* can perform computations on data which cannot fit into RAM\n* has interface similar to `numpy` and `scipy`, and lives under the hood of `xarray`\n* the same code used on your laptop can be run on a distributed cluster\n\n \n\n_Note: Pieces of this notebook comes from the following sources:_\n\n- https://github.com/rabernat/research_computing\n- https://github.com/dask/dask-examples",
"_____no_output_____"
],
[
"## Start a Dask distributed cluster and a Client for Dashboard\n\nWe can imitate starting a cluster on a local machine. Thus we can use the same code on a cluster.\n",
"_____no_output_____"
]
],
[
[
"",
"_____no_output_____"
],
[
"from dask.distributed import Client\nclient = Client(processes=False)\nclient",
"/usr/local/lib/python3.6/dist-packages/distributed/bokeh/core.py:57: UserWarning: \nFailed to start diagnostics server on port 8787. [Errno 99] Cannot assign requested address\n warnings.warn('\\n' + msg)\n/usr/local/lib/python3.6/dist-packages/distributed/deploy/local.py:197: UserWarning: \nCould not launch service 'bokeh' on port 8787. Got the following message:\n\n[Errno 99] Cannot assign requested address\n self.scheduler.start(scheduler_address)\n"
],
[
"",
"_____no_output_____"
]
],
[
[
"The distributed scheduler provides nice diagnostic tools which are useful to gain insight on the computation. They can reveal processing bottlenecks and are useful when running a scalable cluster (like kubernetes) and monitoring nodes.\n\n",
"_____no_output_____"
]
],
[
[
"# If we have set up a Kubernetes cluster we can start it in the following way:\n\n#from dask_kubernetes import KubeCluster\n#cluster = KubeCluster()\n#cluster.scale(4)\n#cluster",
"_____no_output_____"
]
],
[
[
"# Dask Arrays\n\nA dask array looks and feels a lot like a numpy array.\nHowever, a dask array doesn't directly hold any data.\nInstead, it symbolically represents the computations needed to generate the data.\nNothing is actually computed until the actual numerical values are needed.\nThis mode of operation is called \"lazy\"; it allows one to build up complex, large calculations symbolically before turning them over the scheduler for execution.\n\nIf we want to create a numpy array of all ones, we do it like this:",
"_____no_output_____"
]
],
[
[
"import numpy as np\nshape = (1000, 4000)\nones_np = np.ones(shape)\nones_np",
"_____no_output_____"
]
],
[
[
"This size of the array is:",
"_____no_output_____"
]
],
[
[
"print('%.1f MB' % (ones_np.nbytes / 1e6))",
"32.0 MB\n"
]
],
[
[
"Now let's create the same array using dask's array interface.",
"_____no_output_____"
]
],
[
[
"import dask.array as da\nones = da.ones(shape)\nones",
"_____no_output_____"
]
],
[
[
"This works, but we didn't tell dask how to split up the array, so it is not optimized for distributed computation.\n\nA crucal difference with dask is that we must specify the `chunks` argument. \"Chunks\" describes how the array is split up over many sub-arrays.\n\n\n_source: [Dask Array Documentation](http://dask.pydata.org/en/latest/array-overview.html)_\n\nThere are [several ways to specify chunks](http://dask.pydata.org/en/latest/array-creation.html#chunks).\nIn this lecture, we will use a block shape.",
"_____no_output_____"
]
],
[
[
"chunk_shape = (1000, 1000)\nones = da.ones(shape, chunks=chunk_shape)\nones",
"_____no_output_____"
]
],
[
[
"Notice that we just see a symbolic represetnation of the array, including its shape, dtype, and chunksize.\nNo data has been generated yet.\nWhen we call `.compute()` on a dask array, the computation is trigger and the dask array becomes a numpy array.",
"_____no_output_____"
]
],
[
[
"ones.compute() ",
"_____no_output_____"
],
[
"",
"_____no_output_____"
]
],
[
[
"## Task Graphs",
"_____no_output_____"
],
[
"In order to understand what happened when we called `.compute()`, we can visualize the dask _graph_, the symbolic operations that make up the array",
"_____no_output_____"
]
],
[
[
"ones.visualize()",
"_____no_output_____"
]
],
[
[
"Our array has four chunks. To generate it, dask calls `np.ones` four times and then concatenates this together into one array.\n\nRather than immediately loading a dask array (which puts all the data into RAM), it is more common to reduce the data somehow. For example:",
"_____no_output_____"
]
],
[
[
"sum_of_ones = ones.sum()\nsum_of_ones.visualize()",
"_____no_output_____"
]
],
[
[
"Here we see dask's strategy for finding the sum. This simple example illustrates the beauty of dask: it automatically designs an algorithm appropriate for custom operations with big data. \n\nIf we make our operation more complex, the graph gets more complex.",
"_____no_output_____"
]
],
[
[
"fancy_calculation = (ones * ones[::-1, ::-1]).mean()\nfancy_calculation.visualize()",
"_____no_output_____"
]
],
[
[
"### A Bigger Calculation\n\nThe examples above were toy examples; the data (32 MB) is nowhere nearly big enough to warrant the use of dask.\n\nWe can make it a lot bigger!",
"_____no_output_____"
]
],
[
[
"bigshape = (100000, 4000)\nbig_ones = da.ones(bigshape, chunks=chunk_shape)\nbig_ones",
"_____no_output_____"
],
[
"print('%.1f MB' % (big_ones.nbytes / 1e6))",
"3200.0 MB\n"
]
],
[
[
"This dataset is 6.4 GB, rather than 32 MB! This is probably close to or greater than the amount of available RAM than you have in your computer. Nevertheless, dask has no problem working on it.\n\n_Do not try to `.visualize()` this array!_\n\nWhen doing a big calculation, dask also has some tools to help us understand what is happening under the hood. Let's watch the dashboard again as we do a bigger computation.",
"_____no_output_____"
]
],
[
[
"pip install bokeh",
"Requirement already satisfied: bokeh in /usr/local/lib/python3.6/dist-packages (1.0.4)\nRequirement already satisfied: PyYAML>=3.10 in /usr/local/lib/python3.6/dist-packages (from bokeh) (3.13)\nRequirement already satisfied: python-dateutil>=2.1 in /usr/local/lib/python3.6/dist-packages (from bokeh) (2.6.1)\nRequirement already satisfied: numpy>=1.7.1 in /usr/local/lib/python3.6/dist-packages (from bokeh) (1.17.3)\nRequirement already satisfied: pillow>=4.0 in /usr/local/lib/python3.6/dist-packages (from bokeh) (4.3.0)\nRequirement already satisfied: six>=1.5.2 in /usr/local/lib/python3.6/dist-packages (from bokeh) (1.12.0)\nRequirement already satisfied: Jinja2>=2.7 in /usr/local/lib/python3.6/dist-packages (from bokeh) (2.10.3)\nRequirement already satisfied: packaging>=16.8 in /usr/local/lib/python3.6/dist-packages (from bokeh) (19.2)\nRequirement already satisfied: tornado>=4.3 in /usr/local/lib/python3.6/dist-packages (from bokeh) (4.5.3)\nRequirement already satisfied: olefile in /usr/local/lib/python3.6/dist-packages (from pillow>=4.0->bokeh) (0.46)\nRequirement already satisfied: MarkupSafe>=0.23 in /usr/local/lib/python3.6/dist-packages (from Jinja2>=2.7->bokeh) (1.1.1)\nRequirement already satisfied: pyparsing>=2.0.2 in /usr/local/lib/python3.6/dist-packages (from packaging>=16.8->bokeh) (2.4.2)\n"
],
[
"!jupyter nbextension enable --py widgetsnbextension",
"Enabling notebook extension jupyter-js-widgets/extension...\n - Validating: \u001b[32mOK\u001b[0m\n"
],
[
"big_calc = (big_ones * big_ones[::-1, ::-1]).mean()",
"_____no_output_____"
],
[
"from dask.distributed import get_task_stream\n\nwith get_task_stream(filename=\"task-stream.html\",plot=True) as ts:\n big_calc.compute()\n\n#client.profile(filename=\"dask-profile.html\")",
"_____no_output_____"
],
[
"from bokeh.plotting import output_notebook\noutput_notebook()",
"_____no_output_____"
],
[
"from bokeh.plotting import show\nts.figure.plot_height=400\nshow(ts.figure)",
"_____no_output_____"
],
[
"#import IPython\n#IPython.display.HTML(filename='task-stream.html')\n#IPython.display.HTML(filename='dask-profile.html')",
"_____no_output_____"
]
],
[
[
"### Reduction \n\nAll the usual numpy methods work on dask arrays.\nYou can also apply numpy function directly to a dask array, and it will stay lazy.",
"_____no_output_____"
]
],
[
[
"big_ones_reduce = (np.cos(big_ones)**2).mean(axis=1)\nbig_ones_reduce",
"_____no_output_____"
],
[
"from matplotlib import pyplot as plt\n%matplotlib inline\nplt.rcParams['figure.figsize'] = (12,8)",
"_____no_output_____"
]
],
[
[
"Plotting also triggers computation, since we need the actual values",
"_____no_output_____"
]
],
[
[
"plt.plot(big_ones_reduce)",
"_____no_output_____"
],
[
"",
"_____no_output_____"
]
],
[
[
"# Dask Delayed\n\nDask.delayed is a simple and powerful way to parallelize existing code. It allows users to delay function calls into a task graph with dependencies. Dask.delayed doesn't provide any fancy parallel algorithms like Dask.dataframe, but it does give the user complete control over what they want to build.\n\nSystems like Dask.dataframe are built with Dask.delayed. If you have a problem that is paralellizable, but isn't as simple as just a big array or a big dataframe, then dask.delayed may be the right choice for you.\n\n## Create simple functions\n\nThese functions do simple operations like add two numbers together, but they sleep for a random amount of time to simulate real work.",
"_____no_output_____"
]
],
[
[
"import time\n\ndef inc(x):\n time.sleep(0.1)\n return x + 1\n\ndef dec(x):\n time.sleep(0.1)\n return x - 1\n \ndef add(x, y):\n time.sleep(0.2)\n return x + y ",
"_____no_output_____"
]
],
[
[
"We can run them like normal Python functions below",
"_____no_output_____"
]
],
[
[
"%%time\nx = inc(1)\ny = dec(2)\nz = add(x, y)\nz",
"CPU times: user 64.8 ms, sys: 11.4 ms, total: 76.2 ms\nWall time: 401 ms\n"
]
],
[
[
"These ran one after the other, in sequence. Note though that the first two lines `inc(1)` and `dec(2)` don't depend on each other, we *could* have called them in parallel had we been clever.\n\n## Annotate functions with Dask Delayed to make them lazy\n\nWe can call `dask.delayed` on our funtions to make them lazy. Rather than compute their results immediately, they record what we want to compute as a task into a graph that we'll run later on parallel hardware.",
"_____no_output_____"
]
],
[
[
"import dask\ninc = dask.delayed(inc)\ndec = dask.delayed(dec)\nadd = dask.delayed(add)",
"_____no_output_____"
]
],
[
[
"Calling these lazy functions is now almost free. We're just constructing a graph",
"_____no_output_____"
]
],
[
[
"%%time\nx = inc(1)\ny = dec(2)\nz = add(x, y)\nz",
"CPU times: user 367 µs, sys: 0 ns, total: 367 µs\nWall time: 335 µs\n"
]
],
[
[
"## Visualize computation",
"_____no_output_____"
]
],
[
[
"z.visualize(rankdir='LR')",
"_____no_output_____"
]
],
[
[
"## Run in parallel\n\nCall `.compute()` when you want your result as a normal Python object\n\nIf you started `Client()` above then you may want to watch the status page during computation.",
"_____no_output_____"
]
],
[
[
"%%time\nz.compute()",
"CPU times: user 74.6 ms, sys: 7.71 ms, total: 82.3 ms\nWall time: 323 ms\n"
]
],
[
[
"## Parallelize Normal Python code\n\nNow we use Dask in normal for-loopy Python code. This generates graphs instead of doing computations directly, but still looks like the code we had before. Dask is a convenient way to add parallelism to existing workflows.",
"_____no_output_____"
]
],
[
[
"%%time\nzs = []\nfor i in range(256):\n x = inc(i)\n y = dec(x)\n z = add(x, y)\n zs.append(z)\n \nzs = dask.persist(*zs) # trigger computation in the background",
"CPU times: user 147 ms, sys: 16.9 ms, total: 164 ms\nWall time: 167 ms\n"
]
],
[
[
"In general `dask.delayed` is useful when the output of the individual parallel tasks are in a dask format (like dask.array) and are intended to be concatenated in one big dask object.",
"_____no_output_____"
],
[
"## Dask Schedulers\n\nThe Dask *Schedulers* orchestrate the tasks in the Task Graphs so that they can be run in parallel. *How* they run in parallel, though, is determined by which *Scheduler* you choose.\n\nThere are 3 *local* schedulers:\n\n- **Single-Thread Local:** For debugging, profiling, and diagnosing issues\n- **Multi-threaded:** Using the Python built-in `threading` package (the default for all Dask operations except `Bags`)\n- **Multi-process:** Using the Python built-in `multiprocessing` package (the default for Dask `Bags`)\n\nand 1 *distributed* scheduler, which we will talk about later:\n\n- **Distributed:** Using the `dask.distributed` module (which uses `tornado` for TCP communication). The distributed scheduler uses a `Cluster` to manage communication between the scheduler and the \"workers\". This is described in the next section.",
"_____no_output_____"
],
[
"## Distributed Clusters (http://distributed.dask.org/)\n\nDask can be deployed on distributed infrastructure, such as a an HPC system or a cloud computing system.\n\n- `LocalCluster` - Creates a `Cluster` that can be executed locally. Each `Cluster` includes a `Scheduler` and `Worker`s. \n- `Client` - Connects to and drives computation on a distributed `Cluster`\n\n### Dask Jobqueue (http://jobqueue.dask.org/)\n\n- `PBSCluster`\n- `SlurmCluster`\n- `LSFCluster`\n- etc.\n\n### Dask Kubernetes (http://kubernetes.dask.org/)\n\n- `KubeCluster`\n",
"_____no_output_____"
]
],
[
[
"",
"_____no_output_____"
]
]
] | [
"markdown",
"code",
"markdown",
"code",
"markdown",
"code",
"markdown",
"code",
"markdown",
"code",
"markdown",
"code",
"markdown",
"code",
"markdown",
"code",
"markdown",
"code",
"markdown",
"code",
"markdown",
"code",
"markdown",
"code",
"markdown",
"code",
"markdown",
"code",
"markdown",
"code",
"markdown",
"code",
"markdown",
"code",
"markdown",
"code",
"markdown",
"code",
"markdown",
"code",
"markdown",
"code",
"markdown",
"code"
] | [
[
"markdown",
"markdown",
"markdown"
],
[
"code",
"code",
"code"
],
[
"markdown"
],
[
"code"
],
[
"markdown"
],
[
"code"
],
[
"markdown"
],
[
"code"
],
[
"markdown"
],
[
"code"
],
[
"markdown"
],
[
"code"
],
[
"markdown"
],
[
"code",
"code"
],
[
"markdown",
"markdown"
],
[
"code"
],
[
"markdown"
],
[
"code"
],
[
"markdown"
],
[
"code"
],
[
"markdown"
],
[
"code",
"code"
],
[
"markdown"
],
[
"code",
"code",
"code",
"code",
"code",
"code",
"code"
],
[
"markdown"
],
[
"code",
"code"
],
[
"markdown"
],
[
"code",
"code"
],
[
"markdown"
],
[
"code"
],
[
"markdown"
],
[
"code"
],
[
"markdown"
],
[
"code"
],
[
"markdown"
],
[
"code"
],
[
"markdown"
],
[
"code"
],
[
"markdown"
],
[
"code"
],
[
"markdown"
],
[
"code"
],
[
"markdown",
"markdown",
"markdown"
],
[
"code"
]
] |
e786ab36aedabd9393579dd158c350f80da3ce21 | 3,536 | ipynb | Jupyter Notebook | chapter_supervised-learning/mlp-gluon.ipynb | kyoyo/gluon_tutorials_zh_git | 79579c35131ce59423e07565903f68ae92eebf4d | [
"Apache-2.0"
] | 1 | 2017-12-18T05:30:11.000Z | 2017-12-18T05:30:11.000Z | chapter_supervised-learning/mlp-gluon.ipynb | kyoyo/gluon_tutorials_zh_git | 79579c35131ce59423e07565903f68ae92eebf4d | [
"Apache-2.0"
] | null | null | null | chapter_supervised-learning/mlp-gluon.ipynb | kyoyo/gluon_tutorials_zh_git | 79579c35131ce59423e07565903f68ae92eebf4d | [
"Apache-2.0"
] | null | null | null | 23.891892 | 141 | 0.507919 | [
[
[
"# 多层感知机 --- 使用Gluon\n\n我们只需要稍微改动[多类Logistic回归](../chapter_crashcourse/softmax-regression-gluon.md)来实现多层感知机。\n\n## 定义模型\n\n唯一的区别在这里,我们加了一行进来。",
"_____no_output_____"
]
],
[
[
"from mxnet import gluon\n\nnet = gluon.nn.Sequential()\nwith net.name_scope():\n net.add(gluon.nn.Flatten())\n net.add(gluon.nn.Dense(256, activation=\"relu\"))\n net.add(gluon.nn.Dense(10))\nnet.initialize()",
"_____no_output_____"
]
],
[
[
"## 读取数据并训练",
"_____no_output_____"
]
],
[
[
"import sys\nsys.path.append('..')\nfrom mxnet import ndarray as nd\nfrom mxnet import autograd\nimport utils\n\n\nbatch_size = 256\ntrain_data, test_data = utils.load_data_fashion_mnist(batch_size)\n\nsoftmax_cross_entropy = gluon.loss.SoftmaxCrossEntropyLoss()\ntrainer = gluon.Trainer(net.collect_params(), 'sgd', {'learning_rate': 0.5})\n\nfor epoch in range(5):\n train_loss = 0.\n train_acc = 0.\n for data, label in train_data:\n with autograd.record():\n output = net(data)\n loss = softmax_cross_entropy(output, label)\n loss.backward()\n trainer.step(batch_size)\n\n train_loss += nd.mean(loss).asscalar()\n train_acc += utils.accuracy(output, label)\n\n test_acc = utils.evaluate_accuracy(test_data, net)\n print(\"Epoch %d. Loss: %f, Train acc %f, Test acc %f\" % (\n epoch, train_loss/len(train_data), train_acc/len(train_data), test_acc))",
"Epoch 0. Loss: 0.694022, Train acc 0.745893, Test acc 0.817508\n"
]
],
[
[
"## 结论\n\n通过Gluon我们可以更方便地构造多层神经网络。\n\n## 练习\n\n- 尝试多加入几个隐含层,对比从0开始的实现。\n- 尝试使用一个另外的激活函数,可以使用`help(nd.Activation)`或者[线上文档](https://mxnet.apache.org/api/python/ndarray.html#mxnet.ndarray.Activation)查看提供的选项。\n\n**吐槽和讨论欢迎点**[这里](https://discuss.gluon.ai/t/topic/738)",
"_____no_output_____"
]
]
] | [
"markdown",
"code",
"markdown",
"code",
"markdown"
] | [
[
"markdown"
],
[
"code"
],
[
"markdown"
],
[
"code"
],
[
"markdown"
]
] |
e786d85266cbc8d985ac2b4d06b8505f065eaf9b | 288,593 | ipynb | Jupyter Notebook | MovieLens_Recommendation_Notebook-Copy1.ipynb | nikita9604/Movie-Recommendation-Website-based-on-Genre | 23d699248db8f681a2d82d25798ceeb2d3de7ade | [
"MIT"
] | null | null | null | MovieLens_Recommendation_Notebook-Copy1.ipynb | nikita9604/Movie-Recommendation-Website-based-on-Genre | 23d699248db8f681a2d82d25798ceeb2d3de7ade | [
"MIT"
] | null | null | null | MovieLens_Recommendation_Notebook-Copy1.ipynb | nikita9604/Movie-Recommendation-Website-based-on-Genre | 23d699248db8f681a2d82d25798ceeb2d3de7ade | [
"MIT"
] | null | null | null | 34.08042 | 5,520 | 0.388901 | [
[
[
"#importing the required libraries\nimport numpy as np\nimport pandas as pd\nimport pickle\nimport matrix_factorization_utilities\nimport scipy.sparse as sp\nfrom scipy.sparse.linalg import svds",
"_____no_output_____"
]
],
[
[
"### Direct Links\n\n1. [To get top 10 by genre](#best_genre)\n2. [To get top 10 similar users](#top_ten)",
"_____no_output_____"
]
],
[
[
"# Reading the ratings data\nratings = pd.read_csv('Dataset/ratings.csv')",
"_____no_output_____"
],
[
"len(ratings)",
"_____no_output_____"
],
[
"#Just taking the required columns\nratings = ratings[['userId', 'movieId','rating']]",
"_____no_output_____"
],
[
"# Checking if the user has rated the same movie twice, in that case we just take max of them\nratings_df = ratings.groupby(['userId','movieId']).aggregate(np.max)",
"_____no_output_____"
],
[
"# In this case there are no such cases where the user has rated the same movie twice.\nlen(ratings_df)",
"_____no_output_____"
],
[
"# Inspecting the data\nratings.head()",
"_____no_output_____"
],
[
"ratings_df.head()",
"_____no_output_____"
],
[
"# Counting no of unique users\nlen(ratings['userId'].unique())",
"_____no_output_____"
],
[
"#Getting the percentage count of each rating value \ncount_ratings = ratings.groupby('rating').count()\ncount_ratings['perc_total']=round(count_ratings['userId']*100/count_ratings['userId'].sum(),1)",
"_____no_output_____"
],
[
"count_ratings",
"_____no_output_____"
],
[
"#Visualising the percentage total for each rating\ncount_ratings['perc_total'].plot.bar()",
"_____no_output_____"
],
[
"#reading the movies dataset\nmovie_list = pd.read_csv('Dataset/movies.csv')",
"_____no_output_____"
],
[
"len(movie_list)",
"_____no_output_____"
],
[
"# insepcting the movie list dataframe\nmovie_list.head()",
"_____no_output_____"
],
[
"# reading the tags datast\ntags = pd.read_csv('Dataset/tags.csv')",
"_____no_output_____"
],
[
"# inspecting the tags data frame\ntags.head()",
"_____no_output_____"
],
[
"# inspecting various genres\ngenres = movie_list['genres']",
"_____no_output_____"
],
[
"genres.head()",
"_____no_output_____"
],
[
"genre_list = \"\"\nfor index,row in movie_list.iterrows():\n genre_list += row.genres + \"|\"\n#split the string into a list of values\ngenre_list_split = genre_list.split('|')\n#de-duplicate values\nnew_list = list(set(genre_list_split))\n#remove the value that is blank\nnew_list.remove('')\n#inspect list of genres\nnew_list",
"_____no_output_____"
],
[
"#Enriching the movies dataset by adding the various genres columns.\nmovies_with_genres = movie_list.copy()\n\nfor genre in new_list :\n movies_with_genres[genre] = movies_with_genres.apply(lambda _:int(genre in _.genres), axis = 1)",
"_____no_output_____"
],
[
"movies_with_genres.head()",
"_____no_output_____"
],
[
"#Calculating the sparsity\nno_of_users = len(ratings['userId'].unique())\nno_of_movies = len(ratings['movieId'].unique())\n\nsparsity = round(1.0 - len(ratings)/(1.0*(no_of_movies*no_of_users)),3)\nprint(sparsity)",
"0.99\n"
],
[
"# Counting the number of unique movies in the dataset.\nlen(ratings['movieId'].unique())",
"_____no_output_____"
],
[
"# Finding the average rating for movie and the number of ratings for each movie\navg_movie_rating = pd.DataFrame(ratings.groupby('movieId')['rating'].agg(['mean','count']))\n#avg_movie_rating['movieId']= avg_movie_rating.index",
"_____no_output_____"
],
[
"# inspecting the average movie rating data frame\navg_movie_rating.head()",
"_____no_output_____"
],
[
"len(avg_movie_rating)",
"_____no_output_____"
],
[
"#calculate the percentile count. It gives the no of ratings at least 70% of the movies have\nnp.percentile(avg_movie_rating['count'],70)",
"_____no_output_____"
],
[
"#Get the average movie rating across all movies \navg_rating_all=ratings['rating'].mean()\navg_rating_all\n#set a minimum threshold for number of reviews that the movie has to have\nmin_reviews=30\nmin_reviews\nmovie_score = avg_movie_rating.loc[avg_movie_rating['count']>min_reviews]\nmovie_score.head()",
"_____no_output_____"
],
[
"len(movie_score)",
"_____no_output_____"
],
[
"#create a function for weighted rating score based off count of reviews\ndef weighted_rating(x, m=min_reviews, C=avg_rating_all):\n v = x['count']\n R = x['mean']\n # Calculation based on the IMDB formula\n return (v/(v+m) * R) + (m/(m+v) * C)",
"_____no_output_____"
],
[
"#Calculating the weighted score for each movie\nmovie_score['weighted_score'] = movie_score.apply(weighted_rating, axis=1)\nmovie_score.head()",
"<ipython-input-107-f069e78bacf9>:2: SettingWithCopyWarning: \nA value is trying to be set on a copy of a slice from a DataFrame.\nTry using .loc[row_indexer,col_indexer] = value instead\n\nSee the caveats in the documentation: https://pandas.pydata.org/pandas-docs/stable/user_guide/indexing.html#returning-a-view-versus-a-copy\n movie_score['weighted_score'] = movie_score.apply(weighted_rating, axis=1)\n"
],
[
"#join movie details to movie ratings\nmovies_with_genres.index.name = None\nmovies_with_genres = movies_with_genres.rename_axis(None) \nmovie_score = pd.merge(movie_score,movies_with_genres,on='movieId')\nmovie_score.head()",
"_____no_output_____"
],
[
"#list top scored movies over the whole range of movies\npd.DataFrame(movie_score.sort_values(['weighted_score'],ascending=False)[['title','count','mean','weighted_score','genres']][:10])",
"_____no_output_____"
]
],
[
[
"### Reading movie_score.csv directly\n<a id = \"best_genre\"></a>",
"_____no_output_____"
]
],
[
[
"#movie_score.to_csv('movie_score.csv', index = False)\n\nmovie_score = pd.read_csv('movie_score.csv')\nmovie_score.head()",
"_____no_output_____"
],
[
"# Gives the best movies according to genre based on weighted score which is calculated using IMDB formula\ndef best_movies_by_genre(genre,top_n):\n return pd.DataFrame(movie_score.loc[(movie_score[genre]==1)].sort_values(['weighted_score'],ascending=False)[['title','count','mean','weighted_score']][:top_n])",
"_____no_output_____"
],
[
"#run function to return top recommended movies by genre\nbest_movies_by_genre('Musical',10) ",
"_____no_output_____"
],
[
"#run function to return top recommended movies by genre\nbest_movies_by_genre('Action',10) ",
"_____no_output_____"
],
[
"#run function to return top recommended movies by genre\nbest_movies_by_genre('Children',10) ",
"_____no_output_____"
],
[
"#run function to return top recommended movies by genre\nbest_movies_by_genre('Drama',10) ",
"_____no_output_____"
],
[
"# Creating a data frame that has user ratings accross all movies in form of matrix used in matrix factorisation\nratings_df = pd.pivot_table(ratings, index='userId', columns='movieId', aggfunc=np.max)",
"_____no_output_____"
],
[
"ratings_df.head()",
"_____no_output_____"
],
[
"# Apply low rank matrix factorization to find the latent features\n# U, M = matrix_factorization_utilities.low_rank_matrix_factorization(ratings_df.to_numpy(),\n# num_features=5,\n# regularization_amount=1.0)",
"_____no_output_____"
],
[
"ratings_df",
"_____no_output_____"
],
[
"#merging ratings and movies dataframes\nratings_movies = pd.merge(ratings,movie_list, on = 'movieId')",
"_____no_output_____"
],
[
"ratings_movies.head()",
"_____no_output_____"
],
[
"ratings_movies",
"_____no_output_____"
]
],
[
[
"### Gets the other top 10 movies which are watched by the people who saw this particular movie\n<a id = \"top_ten\"></a>",
"_____no_output_____"
]
],
[
[
"#ratings_movies.to_csv('ratings_movies.csv', index = False)\n\nratings_movies = pd.read_csv('ratings_movies.csv')\nratings_movies.head()",
"_____no_output_____"
],
[
"#Gets the other top 10 movies which are watched by the people who saw this particular movie\ndef get_other_movies(movie_name):\n #get all users who watched a specific movie\n df_movie_users_series = ratings_movies.loc[ratings_movies['title']==movie_name]['userId']\n #convert to a data frame\n df_movie_users = pd.DataFrame(df_movie_users_series,columns=['userId'])\n #get a list of all other movies watched by these users\n other_movies = pd.merge(df_movie_users,ratings_movies,on='userId')\n #get a list of the most commonly watched movies by these other user\n other_users_watched = pd.DataFrame(other_movies.groupby('title')['userId'].count()).sort_values('userId',ascending=False)\n other_users_watched['perc_who_watched'] = round(other_users_watched['userId']*100/other_users_watched['userId'][0],1)\n return other_users_watched[1:11]",
"_____no_output_____"
],
[
"# Getting other top 10 movies which are watched by the people who saw 'Gone Girl'\nget_other_movies('Gone Girl (2014)')",
"_____no_output_____"
],
[
"from sklearn.neighbors import NearestNeighbors\n",
"_____no_output_____"
],
[
"avg_movie_rating.head()",
"_____no_output_____"
],
[
"#only include movies with more than 10 ratings\nmovie_plus_10_ratings = avg_movie_rating.loc[avg_movie_rating['count']>=10]\nprint(len(movie_plus_10_ratings))",
"6870\n"
],
[
"movie_plus_10_ratings",
"_____no_output_____"
],
[
"filtered_ratings = pd.merge(movie_plus_10_ratings, ratings, on=\"movieId\")\nlen(filtered_ratings)",
"_____no_output_____"
],
[
"filtered_ratings.head()",
"_____no_output_____"
],
[
"#create a matrix table with movieIds on the rows and userIds in the columns.\n#replace NAN values with 0\nmovie_wide = filtered_ratings.pivot(index = 'movieId', columns = 'userId', values = 'rating').fillna(0)\nmovie_wide.head()",
"_____no_output_____"
],
[
"#specify model parameters\nmodel_knn = NearestNeighbors(metric='cosine',algorithm='brute')\n#fit model to the data set\nmodel_knn.fit(movie_wide)",
"_____no_output_____"
],
[
"#Gets the top 10 nearest neighbours got the movie\ndef print_similar_movies(movie_name) :\n #get the list of user ratings for a specific userId\n query_index = movie_list.loc[movie_list['title']==movie_name]['movieId'].dropna().values[0]\n query_index_movie_ratings = movie_wide.loc[query_index,:].values.reshape(1,-1)\n #get the closest 10 movies and their distances from the movie specified\n distances,indices = model_knn.kneighbors(query_index_movie_ratings,n_neighbors = 11) \n #write a loop that prints the similar movies for a specified movie.\n for i in range(0,len(distances.flatten())):\n #get the title of the random movie that was chosen\n get_movie = movie_list.loc[movie_list['movieId']==query_index]['title']\n #for the first movie in the list i.e closest print the title\n if i==0:\n print('Recommendations for {0}:\\n'.format(get_movie))\n else :\n #get the indiciees for the closest movies\n indices_flat = indices.flatten()[i]\n #get the title of the movie\n get_movie = movie_list.loc[movie_list['movieId']==movie_wide.iloc[indices_flat,:].name]['title']\n #print the movie\n print('{0}: {1}, with distance of {2}:'.format(i,get_movie,distances.flatten()[i]))",
"_____no_output_____"
],
[
"print_similar_movies('Godfather, The (1972)')",
"Recommendations for 843 Godfather, The (1972)\nName: title, dtype: object:\n\n1: 1195 Godfather: Part II, The (1974)\nName: title, dtype: object, with distance of 0.23770491131534777:\n2: 1187 Goodfellas (1990)\nName: title, dtype: object, with distance of 0.4077577043082983:\n3: 1173 Raiders of the Lost Ark (Indiana Jones and the...\nName: title, dtype: object, with distance of 0.41957422766432895:\n4: 257 Star Wars: Episode IV - A New Hope (1977)\nName: title, dtype: object, with distance of 0.4220206766704162:\n5: 1171 Star Wars: Episode V - The Empire Strikes Back...\nName: title, dtype: object, with distance of 0.4373053916055094:\n6: 1169 One Flew Over the Cuckoo's Nest (1975)\nName: title, dtype: object, with distance of 0.44116502131188295:\n7: 602 Fargo (1996)\nName: title, dtype: object, with distance of 0.462544964302234:\n8: 1182 Apocalypse Now (1979)\nName: title, dtype: object, with distance of 0.4630624594884504:\n9: 1212 Terminator, The (1984)\nName: title, dtype: object, with distance of 0.46605747831264477:\n10: 293 Pulp Fiction (1994)\nName: title, dtype: object, with distance of 0.47000891643182396:\n"
],
[
"print_similar_movies('Toy Story (1995)')",
"Recommendations for 0 Toy Story (1995)\nName: title, dtype: object:\n\n1: 257 Star Wars: Episode IV - A New Hope (1977)\nName: title, dtype: object, with distance of 0.4203702182940916:\n2: 767 Independence Day (a.k.a. ID4) (1996)\nName: title, dtype: object, with distance of 0.4264775268701718:\n3: 1184 Star Wars: Episode VI - Return of the Jedi (1983)\nName: title, dtype: object, with distance of 0.44730365269962324:\n4: 352 Forrest Gump (1994)\nName: title, dtype: object, with distance of 0.45689872537724374:\n5: 640 Mission: Impossible (1996)\nName: title, dtype: object, with distance of 0.457736564895886:\n6: 3027 Toy Story 2 (1999)\nName: title, dtype: object, with distance of 0.4603017587650964:\n7: 1242 Back to the Future (1985)\nName: title, dtype: object, with distance of 0.46115899489577405:\n8: 582 Aladdin (1992)\nName: title, dtype: object, with distance of 0.46720198545017855:\n9: 476 Jurassic Park (1993)\nName: title, dtype: object, with distance of 0.4680072843818418:\n10: 1052 Willy Wonka & the Chocolate Factory (1971)\nName: title, dtype: object, with distance of 0.46967932613810226:\n"
],
[
"print_similar_movies('Skyfall (2012)')",
"Recommendations for 19338 Skyfall (2012)\nName: title, dtype: object:\n\n1: 18312 Dark Knight Rises, The (2012)\nName: title, dtype: object, with distance of 0.38339118524349736:\n2: 19473 Looper (2012)\nName: title, dtype: object, with distance of 0.4655465783348146:\n3: 20046 Hobbit: An Unexpected Journey, The (2012)\nName: title, dtype: object, with distance of 0.4791141110494047:\n4: 20906 Iron Man 3 (2013)\nName: title, dtype: object, with distance of 0.4900484774282041:\n5: 20994 Star Trek Into Darkness (2013)\nName: title, dtype: object, with distance of 0.4909417544965664:\n6: 20124 Django Unchained (2012)\nName: title, dtype: object, with distance of 0.495007359587433:\n7: 18318 Sherlock Holmes: A Game of Shadows (2011)\nName: title, dtype: object, with distance of 0.5006787501261142:\n8: 18304 Hunger Games, The (2012)\nName: title, dtype: object, with distance of 0.500874607555973:\n9: 18349 Mission: Impossible - Ghost Protocol (2011)\nName: title, dtype: object, with distance of 0.5080619200160614:\n10: 17874 Avengers, The (2012)\nName: title, dtype: object, with distance of 0.5138452712015772:\n"
],
[
"movies_with_genres.head()",
"_____no_output_____"
],
[
"#Getting the movies list with only genres like Musical and other such columns\nmovie_content_df_temp = movies_with_genres.copy()\nmovie_content_df_temp.set_index('movieId')\nmovie_content_df = movie_content_df_temp.drop(columns = ['movieId','title','genres'])\nmovie_content_df = movie_content_df.to_numpy()\nmovie_content_df",
"_____no_output_____"
],
[
"# Import linear_kernel\nfrom sklearn.metrics.pairwise import linear_kernel\n\n# Compute the cosine similarity matrix\ncosine_sim = linear_kernel(movie_content_df,movie_content_df)",
"_____no_output_____"
],
[
"# Similarity of the movies based on the content\ncosine_sim",
"_____no_output_____"
],
[
"cosine_sim.shape",
"_____no_output_____"
],
[
"# from numpy import savez_compressed\n\n# savez_compressed('cosine.npz', cosine_sim)\n#savetxt('cosine.csv', cosine_sim, delimiter=',')",
"_____no_output_____"
],
[
"#create a series of the movie id and title\nindicies = pd.Series(movie_content_df_temp.index, movie_content_df_temp['title'])\nindicies ",
"_____no_output_____"
],
[
"indcs = indicies.to_dict()\nrev_ind = {}\nfor key,val in indcs.items():\n rev_ind[val] = key\nrev_ind[19338]",
"_____no_output_____"
],
[
"# import pickle\n\n# a_file = open(\"indicies.pkl\", \"wb\")\n# pickle.dump(indcs, a_file)\n# a_file.close()",
"_____no_output_____"
],
[
"indcs['Skyfall (2012)']",
"_____no_output_____"
],
[
"# movie_content_df_temp.head()",
"_____no_output_____"
],
[
"# movie_content_df_temp.to_csv('mv_cnt_tmp.csv', index = False)",
"_____no_output_____"
]
],
[
[
"### Directly getting top 10 movies based on content similarity",
"_____no_output_____"
]
],
[
[
"movie_content_df_temp = pd.read_csv('mv_cnt_tmp.csv')\n\nmovie_content_df_temp.head()",
"_____no_output_____"
],
[
"a_file = open(\"indicies.pkl\", \"rb\")\ninds = pickle.load(a_file)\na_file.close()\ninds['Skyfall (2012)']",
"_____no_output_____"
],
[
"from numpy import load\n\ndata_dict = load('cosine.npz')\ncosine_sim = data_dict['arr_0']\ncosine_sim",
"_____no_output_____"
],
[
"cosine_sim.shape",
"_____no_output_____"
],
[
"#Gets the top 10 similar movies based on the content\ndef get_similar_movies_based_on_content(movie_name) :\n movie_index = inds[movie_name]\n sim_scores = list(enumerate(cosine_sim[movie_index]))\n # Sort the movies based on the similarity scores\n sim_scores = sorted(sim_scores, key=lambda x: x[1], reverse=True)\n \n # Get the scores of the 10 most similar movies\n sim_scores = sim_scores[0:11]\n print(sim_scores)\n # Get the movie indices\n movie_indices = [i[0] for i in sim_scores]\n if(movie_index in movie_indices):\n movie_indices.remove(movie_index)\n print(movie_indices)\n similar_movies = pd.DataFrame(movie_content_df_temp[['title','genres']].iloc[movie_indices])\n return similar_movies[:10]",
"_____no_output_____"
],
[
"#indicies[\"Skyfall (2012)\"]",
"_____no_output_____"
],
[
"get_similar_movies_based_on_content('Skyfall (2012)')",
"[(6260, 4.0), (6822, 4.0), (11018, 4.0), (11826, 4.0), (15203, 4.0), (16733, 4.0), (18315, 4.0), (18349, 4.0), (19338, 4.0), (20615, 4.0), (9, 3.0)]\n[6260, 6822, 11018, 11826, 15203, 16733, 18315, 18349, 20615, 9]\n"
],
[
"get_similar_movies_based_on_content('Jumanji (1995)')",
"[(0, 3.0), (1, 3.0), (55, 3.0), (59, 3.0), (124, 3.0), (255, 3.0), (542, 3.0), (553, 3.0), (624, 3.0), (653, 3.0), (664, 3.0)]\n[0, 55, 59, 124, 255, 542, 553, 624, 653, 664]\n"
],
[
"#get ordered list of movieIds\nitem_indices = pd.DataFrame(sorted(list(set(ratings['movieId']))),columns=['movieId'])\n#add in data frame index value to data frame\nitem_indices['movie_index']=item_indices.index\n#inspect data frame\nitem_indices.head()",
"_____no_output_____"
],
[
"#get ordered list of userIds\nuser_indices = pd.DataFrame(sorted(list(set(ratings['userId']))),columns=['userId'])\n#add in data frame index value to data frame\nuser_indices['user_index']=user_indices.index\n#inspect data frame\nuser_indices.head()",
"_____no_output_____"
],
[
"ratings.head()",
"_____no_output_____"
],
[
"#join the movie indices\ndf_with_index = pd.merge(ratings,item_indices,on='movieId')\n#join the user indices\ndf_with_index=pd.merge(df_with_index,user_indices,on='userId')\n#inspec the data frame\ndf_with_index.head()",
"_____no_output_____"
],
[
"#import train_test_split module\nfrom sklearn.model_selection import train_test_split\n#take 80% as the training set and 20% as the test set\ndf_train, df_test= train_test_split(df_with_index,test_size=0.2)\nprint(len(df_train))\nprint(len(df_test))",
"838860\n209715\n"
],
[
"df_train.head()",
"_____no_output_____"
],
[
"df_test.head()",
"_____no_output_____"
],
[
"n_users = ratings.userId.unique().shape[0]\nn_items = ratings.movieId.unique().shape[0]\nprint(n_users)\nprint(n_items)",
"7120\n14026\n"
]
],
[
[
"#### User_index is row and Movie_index is column and value is rating",
"_____no_output_____"
]
],
[
[
"#Create two user-item matrices, one for training and another for testing\ntrain_data_matrix = np.zeros((n_users, n_items))\n #for every line in the data\nfor line in df_train.itertuples():\n #set the value in the column and row to \n #line[1] is userId, line[2] is movieId and line[3] is rating, line[4] is movie_index and line[5] is user_index\n train_data_matrix[line[5], line[4]] = line[3]\ntrain_data_matrix.shape",
"_____no_output_____"
],
[
"#Create two user-item matrices, one for training and another for testing\ntest_data_matrix = np.zeros((n_users, n_items))\n #for every line in the data\nfor line in df_test[:1].itertuples():\n #set the value in the column and row to \n #line[1] is userId, line[2] is movieId and line[3] is rating, line[4] is movie_index and line[5] is user_index\n #print(line[2])\n test_data_matrix[line[5], line[4]] = line[3]\n #train_data_matrix[line['movieId'], line['userId']] = line['rating']\ntest_data_matrix.shape",
"_____no_output_____"
],
[
"pd.DataFrame(train_data_matrix).head()",
"_____no_output_____"
],
[
"df_train['rating'].max()",
"_____no_output_____"
],
[
"from sklearn.metrics import mean_squared_error\nfrom math import sqrt\ndef rmse(prediction, ground_truth):\n #select prediction values that are non-zero and flatten into 1 array\n prediction = prediction[ground_truth.nonzero()].flatten() \n #select test values that are non-zero and flatten into 1 array\n ground_truth = ground_truth[ground_truth.nonzero()].flatten()\n #return RMSE between values\n return sqrt(mean_squared_error(prediction, ground_truth))",
"_____no_output_____"
],
[
"#Calculate the rmse sscore of SVD using different values of k (latent features)\nfrom scipy.sparse.linalg import svds\n\nrmse_list = []\nfor i in [1,2,5,20,40,60,100,200]:\n #apply svd to the test data\n u,s,vt = svds(train_data_matrix,k=i)\n #get diagonal matrix\n s_diag_matrix=np.diag(s)\n #predict x with dot product of u s_diag and vt\n X_pred = np.dot(np.dot(u,s_diag_matrix),vt)\n #calculate rmse score of matrix factorisation predictions\n rmse_score = rmse(X_pred,test_data_matrix)\n rmse_list.append(rmse_score)\n print(\"Matrix Factorisation with \" + str(i) +\" latent features has a RMSE of \" + str(rmse_score))",
"Matrix Factorisation with 1 latent features has a RMSE of 3.3815340046294016\nMatrix Factorisation with 2 latent features has a RMSE of 2.8318466180645654\nMatrix Factorisation with 5 latent features has a RMSE of 2.270509008845397\nMatrix Factorisation with 20 latent features has a RMSE of 0.12056335049977118\nMatrix Factorisation with 40 latent features has a RMSE of 0.2529999325728296\nMatrix Factorisation with 60 latent features has a RMSE of 0.4145888651453946\nMatrix Factorisation with 100 latent features has a RMSE of 0.25500306534137174\nMatrix Factorisation with 200 latent features has a RMSE of 0.41988162205674007\n"
],
[
"#Convert predictions to a DataFrame\nmf_pred = pd.DataFrame(X_pred)\nmf_pred.head()",
"_____no_output_____"
],
[
"df_names = pd.merge(ratings,movie_list,on='movieId')\ndf_names.head()",
"_____no_output_____"
],
[
"#choose a user ID\nuser_id = 1\n#get movies rated by this user id\nusers_movies = df_names.loc[df_names[\"userId\"]==user_id]\n#print how many ratings user has made \nprint(\"User ID : \" + str(user_id) + \" has already rated \" + str(len(users_movies)) + \" movies\")\n#list movies that have been rated\nusers_movies",
"User ID : 1 has already rated 175 movies\n"
],
[
"user_index = df_train.loc[df_train[\"userId\"]==user_id]['user_index'][:1].values[0]\n#get movie ratings predicted for this user and sort by highest rating prediction\nsorted_user_predictions = pd.DataFrame(mf_pred.iloc[user_index].sort_values(ascending=False))\n#rename the columns\nsorted_user_predictions.columns=['ratings']\n#save the index values as movie id\nsorted_user_predictions['movieId']=sorted_user_predictions.index\nprint(\"Top 10 predictions for User \" + str(user_id))\n#display the top 10 predictions for this user\npd.merge(sorted_user_predictions,movie_list, on = 'movieId')[:10]",
"Top 10 predictions for User 1\n"
],
[
"#count number of unique users\nnumUsers = df_train.userId.unique().shape[0]\n#count number of unitque movies\nnumMovies = df_train.movieId.unique().shape[0]\nprint(len(df_train))\nprint(numUsers) \nprint(numMovies) ",
"838860\n7120\n13465\n"
],
[
"#Separate out the values of the df_train data set into separate variables\nUsers = df_train['userId'].values\nMovies = df_train['movieId'].values\nRatings = df_train['rating'].values\nprint(Users),print(len(Users))\nprint(Movies),print(len(Movies))\nprint(Ratings),print(len(Ratings))",
"[5832 531 3797 ... 3879 2397 238]\n838860\n[ 3618 2338 3224 ... 69757 51540 1196]\n838860\n[3. 1.5 2. ... 3. 4. 4.5]\n838860\n"
],
[
"#import libraries\nimport tensorflow as tf\nfrom tensorflow import keras\nfrom keras.layers import Embedding, Reshape \nfrom keras.models import Sequential\nfrom keras.optimizers import Adam\nfrom keras.callbacks import EarlyStopping, ModelCheckpoint",
"_____no_output_____"
],
[
"from keras.utils import plot_model",
"_____no_output_____"
],
[
"# Couting no of unique users and movies\nlen(ratings.userId.unique()), len(ratings.movieId.unique())",
"_____no_output_____"
],
[
"# Assigning a unique value to each user and movie in range 0,no_of_users and 0,no_of_movies respectively.\nratings.userId = ratings.userId.astype('category').cat.codes.values\nratings.movieId = ratings.movieId.astype('category').cat.codes.values",
"_____no_output_____"
],
[
"# Splitting the data into train and test.\ntrain, test = train_test_split(ratings, test_size=0.2)",
"_____no_output_____"
],
[
"train.head()",
"_____no_output_____"
],
[
"test.head()",
"_____no_output_____"
],
[
"n_users, n_movies = len(ratings.userId.unique()), len(ratings.movieId.unique())",
"_____no_output_____"
],
[
"# Returns a neural network model which performs matrix factorisation\ndef matrix_factorisation_model_with_n_latent_factors(n_latent_factors) :\n movie_input = keras.layers.Input(shape=[1],name='Item')\n movie_embedding = keras.layers.Embedding(n_movies + 1, n_latent_factors, name='Movie-Embedding')(movie_input)\n movie_vec = keras.layers.Flatten(name='FlattenMovies')(movie_embedding)\n\n user_input = keras.layers.Input(shape=[1],name='User')\n user_vec = keras.layers.Flatten(name='FlattenUsers')(keras.layers.Embedding(n_users + 1, n_latent_factors,name='User-Embedding')(user_input))\n prod = keras.layers.dot([movie_vec, user_vec], axes=1)\n \n model = keras.Model([user_input, movie_input], prod)\n model.compile('adam', 'mean_squared_error')\n \n return model",
"_____no_output_____"
],
[
"model = matrix_factorisation_model_with_n_latent_factors(20)",
"_____no_output_____"
],
[
"model.summary()",
"Model: \"functional_1\"\n__________________________________________________________________________________________________\nLayer (type) Output Shape Param # Connected to \n==================================================================================================\nItem (InputLayer) [(None, 1)] 0 \n__________________________________________________________________________________________________\nUser (InputLayer) [(None, 1)] 0 \n__________________________________________________________________________________________________\nMovie-Embedding (Embedding) (None, 1, 20) 280540 Item[0][0] \n__________________________________________________________________________________________________\nUser-Embedding (Embedding) (None, 1, 20) 142420 User[0][0] \n__________________________________________________________________________________________________\nFlattenMovies (Flatten) (None, 20) 0 Movie-Embedding[0][0] \n__________________________________________________________________________________________________\nFlattenUsers (Flatten) (None, 20) 0 User-Embedding[0][0] \n__________________________________________________________________________________________________\ndot (Dot) (None, 1) 0 FlattenMovies[0][0] \n FlattenUsers[0][0] \n==================================================================================================\nTotal params: 422,960\nTrainable params: 422,960\nNon-trainable params: 0\n__________________________________________________________________________________________________\n"
],
[
"#Training the model\nhistory = model.fit([train.userId, train.movieId], train.rating, epochs=10, verbose=1)",
"Epoch 1/10\n26215/26215 [==============================] - 238s 9ms/step - loss: 0.4897\nEpoch 2/10\n 3658/26215 [===>..........................] - ETA: 3:25 - loss: 0.4492"
],
[
"y_hat = np.round(model.predict([test.userId, test.movieId]),0)\ny_true = test.rating",
"_____no_output_____"
],
[
"from sklearn.metrics import mean_absolute_error\nmean_absolute_error(y_true, y_hat)",
"_____no_output_____"
],
[
"#Getting summary of movie embeddings\nmovie_embedding_learnt = model.get_layer(name='Movie-Embedding').get_weights()[0]\npd.DataFrame(movie_embedding_learnt).describe()",
"_____no_output_____"
],
[
"# Getting summary of user embeddings from the model\nuser_embedding_learnt = model.get_layer(name='User-Embedding').get_weights()[0]\npd.DataFrame(user_embedding_learnt).describe()",
"_____no_output_____"
],
[
"from keras.constraints import non_neg",
"_____no_output_____"
],
[
"# Returns a neural network model which performs matrix factorisation with additional constraint on embeddings(that they can't be negative)\ndef matrix_factorisation_model_with_n_latent_factors_and_non_negative_embedding(n_latent_factors) :\n movie_input = keras.layers.Input(shape=[1],name='Item')\n movie_embedding = keras.layers.Embedding(n_movies + 1, n_latent_factors, name='Non-Negative-Movie-Embedding',embeddings_constraint=non_neg())(movie_input)\n movie_vec = keras.layers.Flatten(name='FlattenMovies')(movie_embedding)\n\n user_input = keras.layers.Input(shape=[1],name='User')\n user_vec = keras.layers.Flatten(name='FlattenUsers')(keras.layers.Embedding(n_users + 1, n_latent_factors,name='Non-Negative-User-Embedding',embeddings_constraint=non_neg())(user_input))\n prod = keras.layers.merge([movie_vec, user_vec], mode='dot',name='DotProduct')\n \n model = keras.Model([user_input, movie_input], prod)\n model.compile('adam', 'mean_squared_error')\n \n return model",
"_____no_output_____"
],
[
"model2 = matrix_factorisation_model_with_n_latent_factors_and_non_negative_embedding(5)",
"C:\\Users\\gureddy\\AppData\\Local\\Continuum\\anaconda3\\lib\\site-packages\\ipykernel_launcher.py:8: UserWarning: The `merge` function is deprecated and will be removed after 08/2017. Use instead layers from `keras.layers.merge`, e.g. `add`, `concatenate`, etc.\n \nC:\\Users\\gureddy\\AppData\\Local\\Continuum\\anaconda3\\lib\\site-packages\\keras\\legacy\\layers.py:465: UserWarning: The `Merge` layer is deprecated and will be removed after 08/2017. Use instead layers from `keras.layers.merge`, e.g. `add`, `concatenate`, etc.\n name=name)\n"
],
[
"model2.summary()",
"__________________________________________________________________________________________________\nLayer (type) Output Shape Param # Connected to \n==================================================================================================\nItem (InputLayer) (None, 1) 0 \n__________________________________________________________________________________________________\nUser (InputLayer) (None, 1) 0 \n__________________________________________________________________________________________________\nNon-Negative-Movie-Embedding (E (None, 1, 5) 70135 Item[0][0] \n__________________________________________________________________________________________________\nNon-Negative-User-Embedding (Em (None, 1, 5) 35605 User[0][0] \n__________________________________________________________________________________________________\nFlattenMovies (Flatten) (None, 5) 0 Non-Negative-Movie-Embedding[0][0\n__________________________________________________________________________________________________\nFlattenUsers (Flatten) (None, 5) 0 Non-Negative-User-Embedding[0][0]\n__________________________________________________________________________________________________\nDotProduct (Merge) (None, 1) 0 FlattenMovies[0][0] \n FlattenUsers[0][0] \n==================================================================================================\nTotal params: 105,740\nTrainable params: 105,740\nNon-trainable params: 0\n__________________________________________________________________________________________________\n"
],
[
"history_nonneg = model2.fit([train.userId, train.movieId], train.rating, epochs=50, verbose=0)",
"_____no_output_____"
],
[
"movie_embedding_learnt = model2.get_layer(name='Non-Negative-Movie-Embedding').get_weights()[0]\npd.DataFrame(movie_embedding_learnt).describe()",
"_____no_output_____"
],
[
"y_hat = np.round(model2.predict([test.userId, test.movieId]),0)\ny_true = test.rating",
"_____no_output_____"
],
[
"mean_absolute_error(y_true, y_hat)",
"_____no_output_____"
],
[
"# Returns a neural network model which does recommendation\ndef neural_network_model(n_latent_factors_user, n_latent_factors_movie):\n \n movie_input = keras.layers.Input(shape=[1],name='Item')\n movie_embedding = keras.layers.Embedding(n_movies + 1, n_latent_factors_movie, name='Movie-Embedding')(movie_input)\n movie_vec = keras.layers.Flatten(name='FlattenMovies')(movie_embedding)\n movie_vec = keras.layers.Dropout(0.2)(movie_vec)\n\n\n user_input = keras.layers.Input(shape=[1],name='User')\n user_vec = keras.layers.Flatten(name='FlattenUsers')(keras.layers.Embedding(n_users + 1, n_latent_factors_user,name='User-Embedding')(user_input))\n user_vec = keras.layers.Dropout(0.2)(user_vec)\n\n\n concat = keras.layers.merge([movie_vec, user_vec], mode='concat',name='Concat')\n concat_dropout = keras.layers.Dropout(0.2)(concat)\n dense = keras.layers.Dense(100,name='FullyConnected')(concat)\n dropout_1 = keras.layers.Dropout(0.2,name='Dropout')(dense)\n dense_2 = keras.layers.Dense(50,name='FullyConnected-1')(concat)\n dropout_2 = keras.layers.Dropout(0.2,name='Dropout')(dense_2)\n dense_3 = keras.layers.Dense(20,name='FullyConnected-2')(dense_2)\n dropout_3 = keras.layers.Dropout(0.2,name='Dropout')(dense_3)\n dense_4 = keras.layers.Dense(10,name='FullyConnected-3', activation='relu')(dense_3)\n\n\n result = keras.layers.Dense(1, activation='relu',name='Activation')(dense_4)\n adam = Adam(lr=0.005)\n model = keras.Model([user_input, movie_input], result)\n model.compile(optimizer=adam,loss= 'mean_absolute_error')\n return model",
"_____no_output_____"
],
[
"model3 = neural_network_model(10,13)",
"C:\\Users\\gureddy\\AppData\\Local\\Continuum\\anaconda3\\lib\\site-packages\\ipykernel_launcher.py:14: UserWarning: The `merge` function is deprecated and will be removed after 08/2017. Use instead layers from `keras.layers.merge`, e.g. `add`, `concatenate`, etc.\n \nC:\\Users\\gureddy\\AppData\\Local\\Continuum\\anaconda3\\lib\\site-packages\\keras\\legacy\\layers.py:465: UserWarning: The `Merge` layer is deprecated and will be removed after 08/2017. Use instead layers from `keras.layers.merge`, e.g. `add`, `concatenate`, etc.\n name=name)\n"
],
[
"history_neural_network = model3.fit([train.userId, train.movieId], train.rating, epochs=50, verbose=0)",
"_____no_output_____"
],
[
"model3.summary()",
"__________________________________________________________________________________________________\nLayer (type) Output Shape Param # Connected to \n==================================================================================================\nItem (InputLayer) (None, 1) 0 \n__________________________________________________________________________________________________\nUser (InputLayer) (None, 1) 0 \n__________________________________________________________________________________________________\nMovie-Embedding (Embedding) (None, 1, 13) 182351 Item[0][0] \n__________________________________________________________________________________________________\nUser-Embedding (Embedding) (None, 1, 10) 71210 User[0][0] \n__________________________________________________________________________________________________\nFlattenMovies (Flatten) (None, 13) 0 Movie-Embedding[0][0] \n__________________________________________________________________________________________________\nFlattenUsers (Flatten) (None, 10) 0 User-Embedding[0][0] \n__________________________________________________________________________________________________\ndropout_7 (Dropout) (None, 13) 0 FlattenMovies[0][0] \n__________________________________________________________________________________________________\ndropout_8 (Dropout) (None, 10) 0 FlattenUsers[0][0] \n__________________________________________________________________________________________________\nConcat (Merge) (None, 23) 0 dropout_7[0][0] \n dropout_8[0][0] \n__________________________________________________________________________________________________\nFullyConnected-1 (Dense) (None, 50) 1200 Concat[0][0] \n__________________________________________________________________________________________________\nFullyConnected-2 (Dense) (None, 20) 1020 FullyConnected-1[0][0] \n__________________________________________________________________________________________________\nFullyConnected-3 (Dense) (None, 10) 210 FullyConnected-2[0][0] \n__________________________________________________________________________________________________\nActivation (Dense) (None, 1) 11 FullyConnected-3[0][0] \n==================================================================================================\nTotal params: 256,002\nTrainable params: 256,002\nNon-trainable params: 0\n__________________________________________________________________________________________________\n"
],
[
"y_hat = np.round(model3.predict([test.userId, test.movieId]),0)\ny_true = test.rating",
"_____no_output_____"
],
[
"mean_absolute_error(y_true, y_hat)",
"_____no_output_____"
]
]
] | [
"code",
"markdown",
"code",
"markdown",
"code",
"markdown",
"code",
"markdown",
"code",
"markdown",
"code"
] | [
[
"code"
],
[
"markdown"
],
[
"code",
"code",
"code",
"code",
"code",
"code",
"code",
"code",
"code",
"code",
"code",
"code",
"code",
"code",
"code",
"code",
"code",
"code",
"code",
"code",
"code",
"code",
"code",
"code",
"code",
"code",
"code",
"code",
"code",
"code",
"code",
"code",
"code"
],
[
"markdown"
],
[
"code",
"code",
"code",
"code",
"code",
"code",
"code",
"code",
"code",
"code",
"code",
"code",
"code"
],
[
"markdown"
],
[
"code",
"code",
"code",
"code",
"code",
"code",
"code",
"code",
"code",
"code",
"code",
"code",
"code",
"code",
"code",
"code",
"code",
"code",
"code",
"code",
"code",
"code",
"code",
"code",
"code",
"code",
"code"
],
[
"markdown"
],
[
"code",
"code",
"code",
"code",
"code",
"code",
"code",
"code",
"code",
"code",
"code",
"code",
"code",
"code",
"code",
"code"
],
[
"markdown"
],
[
"code",
"code",
"code",
"code",
"code",
"code",
"code",
"code",
"code",
"code",
"code",
"code",
"code",
"code",
"code",
"code",
"code",
"code",
"code",
"code",
"code",
"code",
"code",
"code",
"code",
"code",
"code",
"code",
"code",
"code",
"code",
"code",
"code",
"code",
"code",
"code",
"code",
"code",
"code",
"code",
"code",
"code"
]
] |
e786f7f2ce7a97b58b9499a88f8db12833aa4c60 | 238,884 | ipynb | Jupyter Notebook | _notebooks/2021-06-23-collaborative-filtering-movielens-latest-small-01.ipynb | recohut/notebook | 610670666a1c3d8ef430d42f712ff72ecdbd8f86 | [
"Apache-2.0"
] | null | null | null | _notebooks/2021-06-23-collaborative-filtering-movielens-latest-small-01.ipynb | recohut/notebook | 610670666a1c3d8ef430d42f712ff72ecdbd8f86 | [
"Apache-2.0"
] | 1 | 2022-01-12T05:40:57.000Z | 2022-01-12T05:40:57.000Z | _notebooks/2021-06-23-collaborative-filtering-movielens-latest-small-01.ipynb | recohut/notebook | 610670666a1c3d8ef430d42f712ff72ecdbd8f86 | [
"Apache-2.0"
] | 1 | 2021-08-13T19:00:26.000Z | 2021-08-13T19:00:26.000Z | 396.159204 | 112,366 | 0.921297 | [
[
[
"# CF Part 1 - Data loading and EDA\n> Collaborative Filtering on MovieLens Latest-small Part 1 - Downloading movielens latest small dataset and exploratory data analysis\n\n- toc: false\n- badges: true\n- comments: true\n- categories: [movie, collaborative]\n- image:",
"_____no_output_____"
]
],
[
[
"import matplotlib.pyplot as plt\nimport pandas as pd\nimport numpy as np\nimport sys\nimport os\n\nfrom scipy.sparse import csr_matrix\nfrom sklearn.preprocessing import LabelEncoder",
"_____no_output_____"
],
[
"!wget http://files.grouplens.org/datasets/movielens/ml-latest-small.zip\n!unzip ml-latest-small.zip",
"--2021-06-23 06:11:54-- http://files.grouplens.org/datasets/movielens/ml-latest-small.zip\nResolving files.grouplens.org (files.grouplens.org)... 128.101.65.152\nConnecting to files.grouplens.org (files.grouplens.org)|128.101.65.152|:80... connected.\nHTTP request sent, awaiting response... 200 OK\nLength: 978202 (955K) [application/zip]\nSaving to: ‘ml-latest-small.zip’\n\nml-latest-small.zip 100%[===================>] 955.28K 3.95MB/s in 0.2s \n\n2021-06-23 06:11:54 (3.95 MB/s) - ‘ml-latest-small.zip’ saved [978202/978202]\n\nArchive: ml-latest-small.zip\n creating: ml-latest-small/\n inflating: ml-latest-small/links.csv \n inflating: ml-latest-small/tags.csv \n inflating: ml-latest-small/ratings.csv \n inflating: ml-latest-small/README.txt \n inflating: ml-latest-small/movies.csv \n"
],
[
"DOWNLOAD_DESTINATION_DIR = \"/content/ml-latest-small\"\n\nratings_path = os.path.join(DOWNLOAD_DESTINATION_DIR, 'ratings.csv')\nratings = pd.read_csv(\n ratings_path,\n sep=',',\n names=[\"userid\", \"itemid\", \"rating\", \"timestamp\"],\n skiprows=1\n)\n\nmovies_path = os.path.join(DOWNLOAD_DESTINATION_DIR, 'movies.csv')\nmovies = pd.read_csv(\n movies_path,\n sep=',',\n names=[\"itemid\", \"title\", \"genres\"],\n encoding='latin-1',\n skiprows=1\n)",
"_____no_output_____"
],
[
"ratings.head()",
"_____no_output_____"
],
[
"movies.head()",
"_____no_output_____"
],
[
"print(\"There are {} users and {} movies in this dataset.\"\\\n .format(ratings.userid.nunique(),\n ratings.itemid.nunique()))",
"There are 610 users and 9724 movies in this dataset.\n"
],
[
"# histogram of ratings\nratings.groupby('rating').size().plot(kind='bar');",
"_____no_output_____"
]
],
[
[
"Ratings range from $0.5$ to $5.0$, with a step of $0.5$. The above histogram presents the repartition of ratings in the dataset. the two most commun ratings are $4.0$ and $3.0$ and the less common ratings are $0.5$ and $1.5$",
"_____no_output_____"
]
],
[
[
"# average rating of movies\nmovie_means = ratings.join(movies['title'], on='itemid').groupby('title').rating.mean()\nmovie_means[:50].plot(kind='bar', grid=True, figsize=(16,6), title=\"mean ratings of 50 movies\");",
"_____no_output_____"
],
[
"# 30 most rated movies vs. 30 less rated movies\nfig, (ax1, ax2) = plt.subplots(ncols=2, figsize=(16,4), sharey=True)\nmovie_means.nlargest(30).plot(kind='bar', ax=ax1, title=\"Top 30 movies in data set\");\nmovie_means.nsmallest(30).plot(kind='bar', ax=ax2, title=\"Bottom 30 movies in data set\");",
"_____no_output_____"
],
[
"def ids_encoder(ratings):\n users = sorted(ratings['userid'].unique())\n items = sorted(ratings['itemid'].unique())\n\n # create users and items encoders\n uencoder = LabelEncoder()\n iencoder = LabelEncoder()\n\n # fit users and items ids to the corresponding encoder\n uencoder.fit(users)\n iencoder.fit(items)\n\n # encode userids and itemids\n ratings.userid = uencoder.transform(ratings.userid.tolist())\n ratings.itemid = iencoder.transform(ratings.itemid.tolist())\n\n return ratings, uencoder, iencoder",
"_____no_output_____"
],
[
"# userids and itemids encoding\nratings, uencoder, iencoder = ids_encoder(ratings)",
"_____no_output_____"
],
[
"# transform rating dataframe to matrix\ndef ratings_matrix(ratings): \n return csr_matrix(pd.crosstab(ratings.userid, ratings.itemid, ratings.rating, aggfunc=sum).fillna(0).values) \n\nR = ratings_matrix(ratings)",
"_____no_output_____"
],
[
"R[:10,:10].todense()",
"_____no_output_____"
],
[
"plt.figure(figsize=(20,10))\nplt.imshow(csr_matrix(R).todense(), cmap='hot', interpolation='nearest')\nplt.show()",
"_____no_output_____"
],
[
"plt.figure(figsize=(5,5))\nplt.imshow(csr_matrix(R[:100,:100]).todense(), cmap='hot', interpolation='nearest')\nplt.show()",
"_____no_output_____"
]
]
] | [
"markdown",
"code",
"markdown",
"code"
] | [
[
"markdown"
],
[
"code",
"code",
"code",
"code",
"code",
"code",
"code"
],
[
"markdown"
],
[
"code",
"code",
"code",
"code",
"code",
"code",
"code",
"code"
]
] |
e7871251e327a0ed60e2bb55e5749ba5d24b049f | 6,378 | ipynb | Jupyter Notebook | code/multi_variable_linear_regression_01_start.ipynb | zeran4/justdoit | 00ba46262f1fd636d9fde15812e341d73ced41ae | [
"MIT"
] | null | null | null | code/multi_variable_linear_regression_01_start.ipynb | zeran4/justdoit | 00ba46262f1fd636d9fde15812e341d73ced41ae | [
"MIT"
] | null | null | null | code/multi_variable_linear_regression_01_start.ipynb | zeran4/justdoit | 00ba46262f1fd636d9fde15812e341d73ced41ae | [
"MIT"
] | null | null | null | 31.264706 | 124 | 0.554406 | [
[
[
"### multi variable에 대한 linear regression의 코드를 리뷰해보자",
"_____no_output_____"
]
],
[
[
"import tensorflow as tf\nimport numpy as np\n\ntf.set_random_seed(777) # for reproducibility",
"_____no_output_____"
],
[
"x1_data = [73., 93., 89., 96., 73.]\nx2_data = [80., 88., 91., 98., 66.]\nx3_data = [75., 93., 90., 100., 70.]\n# Hypothesis / y hat\ny_data = [152., 185., 180., 196., 142.]",
"_____no_output_____"
],
[
"x1 = tf.placeholder(tf.float32)\nx2 = tf.placeholder(tf.float32)\nx3 = tf.placeholder(tf.float32)\n\ny = tf.placeholder(tf.float32)",
"_____no_output_____"
],
[
"w1 = tf.Variable(tf.random_normal([1]),name='weight_1')\nw2 = tf.Variable(tf.random_normal([1]), name='weight_2')\nw3 = tf.Variable(tf.random_normal([1]), name='weight_3')\nb = tf.Variable(tf.random_normal([1]), name='bias')\n\ny_hat = x1 * w1 + x2 * w2 + x3 * w3 + b",
"_____no_output_____"
],
[
"# cost/loss function\nloss = tf.reduce_mean(tf.square(y_hat - y)) # sum of the squares\n# optimizer\noptimizer = tf.train.GradientDescentOptimizer(learning_rate=1e-5)\ntrain = optimizer.minimize(loss)",
"_____no_output_____"
],
[
"init = tf.global_variables_initializer()\nsess = tf.Session()\nsess.run(init)",
"_____no_output_____"
],
[
"for step in range(2001):\n loss_value, y_hat_value, _ = sess.run([loss, y_hat, train], {x1: x1_data, x2: x2_data, x3: x3_data, y: y_data})\n\n if step % 100 == 0:\n print(step, \"loss: \", loss_value, \"\\nPrediction: \", y_hat_value)",
"0 loss: 62547.3 \nPrediction: [-75.96344757 -78.27629089 -83.83014679 -90.80435944 -56.97648239]\n100 loss: 13.2472 \nPrediction: [ 146.06376648 188.31446838 178.98654175 195.37893677 146.31259155]\n200 loss: 12.5621 \nPrediction: [ 146.20278931 188.21931458 179.02929688 195.40849304 146.18890381]\n300 loss: 11.9133 \nPrediction: [ 146.33810425 188.12670898 179.07095337 195.43722534 146.068573 ]\n400 loss: 11.2986 \nPrediction: [ 146.4697876 188.0365448 179.11146545 195.46513367 145.95143127]\n500 loss: 10.7164 \nPrediction: [ 146.59799194 187.94880676 179.15092468 195.49229431 145.83746338]\n600 loss: 10.1648 \nPrediction: [ 146.72277832 187.86340332 179.18934631 195.51869202 145.7265625 ]\n700 loss: 9.64238 \nPrediction: [ 146.84425354 187.7802887 179.22674561 195.54437256 145.61865234]\n800 loss: 9.14744 \nPrediction: [ 146.96246338 187.69935608 179.26315308 195.56930542 145.51361084]\n900 loss: 8.6786 \nPrediction: [ 147.07757568 187.62060547 179.29858398 195.59356689 145.41145325]\n1000 loss: 8.23449 \nPrediction: [ 147.18959045 187.54394531 179.33308411 195.61715698 145.31201172]\n1100 loss: 7.81377 \nPrediction: [ 147.29864502 187.46932983 179.3666687 195.64007568 145.21525574]\n1200 loss: 7.41522 \nPrediction: [ 147.40480042 187.396698 179.39938354 195.66235352 145.12110901]\n1300 loss: 7.0377 \nPrediction: [ 147.50811768 187.32600403 179.43119812 195.684021 145.02949524]\n1400 loss: 6.68003 \nPrediction: [ 147.60870361 187.25717163 179.46218872 195.70504761 144.94033813]\n1500 loss: 6.34126 \nPrediction: [ 147.706604 187.19020081 179.49237061 195.72554016 144.85360718]\n1600 loss: 6.02031 \nPrediction: [ 147.8019104 187.125 179.52174377 195.74542236 144.76919556]\n1700 loss: 5.71629 \nPrediction: [ 147.89468384 187.06155396 179.55033875 195.76475525 144.6870575 ]\n1800 loss: 5.4283 \nPrediction: [ 147.98498535 186.99978638 179.57817078 195.78353882 144.60714722]\n1900 loss: 5.15548 \nPrediction: [ 148.07289124 186.93965149 179.6053009 195.80180359 144.52940369]\n2000 loss: 4.89701 \nPrediction: [ 148.15844727 186.88110352 179.63166809 195.8195343 144.45372009]\n"
],
[
"# 대충 x1, x2, x3 값을 넣고 y 값을 prediction 해보자\nx1_test = [87.]\nx2_test = [82.]\nx3_test = [91.]\ny_hat_value = sess.run([y_hat], {x1: x1_test, x2: x2_test, x3: x3_test})\nprint(\"x1 : \", x1_test, \", x2 : \", x2_test, \", x3 : \", x3_test, \"\\nPrediction: \", y_hat_value[0])\n",
"x1 : [87.0] , x2 : [82.0] , x3 : [91.0] \nPrediction: [ 177.55586243]\n"
]
]
] | [
"markdown",
"code"
] | [
[
"markdown"
],
[
"code",
"code",
"code",
"code",
"code",
"code",
"code",
"code"
]
] |
e78716568bfb3746824115db109e66b507397b7b | 839,634 | ipynb | Jupyter Notebook | braiding/plotting.ipynb | afeinstein20/flares_soc | b120b6174c6a5b305823dc5bef668fe17ea2e9c0 | [
"MIT"
] | null | null | null | braiding/plotting.ipynb | afeinstein20/flares_soc | b120b6174c6a5b305823dc5bef668fe17ea2e9c0 | [
"MIT"
] | null | null | null | braiding/plotting.ipynb | afeinstein20/flares_soc | b120b6174c6a5b305823dc5bef668fe17ea2e9c0 | [
"MIT"
] | null | null | null | 634.643991 | 486,900 | 0.940893 | [
[
[
"import sys, os\nimport numpy as np\nimport matplotlib.pyplot as plt\nfrom astropy.table import Table,Column\nfrom tqdm import notebook\nfrom astropy import units\nimport emcee\nfrom mpmath import expint, mp\nfrom scipy.optimize import minimize\nfrom lightkurve.search import search_lightcurve\n\n%load_ext autoreload\n%autoreload 2\nfrom astroquery.mast import Catalogs\nfrom astroquery.gaia import Gaia\nfrom astropy.coordinates import SkyCoord\n\nimport stella\n\nsys.path.append('/Users/arcticfox/Documents/GitHub/flares_soc/scripts')\nfrom tools import *\n\nCOLOR = 'k'#'#FFFAF1'\nplt.rcParams['font.size'] = 20\nplt.rcParams['text.color'] = COLOR\nplt.rcParams['axes.labelcolor'] = COLOR\nplt.rcParams['xtick.color'] = COLOR\nplt.rcParams['ytick.color'] = COLOR\n\nplt.rcParams['xtick.major.width'] = 3\nplt.rcParams['ytick.major.width'] = 3\nplt.rcParams['xtick.major.size'] = 14 #12\nplt.rcParams['ytick.major.size'] = 14#12\n\nplt.rcParams['xtick.minor.width'] = 1\nplt.rcParams['ytick.minor.width'] = 1\nplt.rcParams['xtick.minor.size'] = 8\nplt.rcParams['ytick.minor.size'] = 8\n\nplt.rcParams['axes.linewidth'] = 3\nlw = 5\n\nplt.rcParams['text.color'] = COLOR\nplt.rcParams['xtick.color'] = COLOR\nplt.rcParams['ytick.color'] = COLOR\nplt.rcParams['axes.labelcolor'] = COLOR\n#plt.rcParams['axes.spines.top'] = False\n#plt.rcParams['axes.spines.right'] = False\nplt.rcParams['axes.labelcolor'] = COLOR\nplt.rcParams['axes.edgecolor'] = COLOR\nplt.rcParams['figure.facecolor'] = 'none'\nplt.rcParams['legend.facecolor'] = 'none'\nparula = np.load('/Users/arcticfox/parula_colors.npy')\n\nfrom matplotlib.colors import LinearSegmentedColormap\n\nparula_colors = np.load('/Users/arcticfox/parula_colors.npy')\nparula_map = LinearSegmentedColormap.from_list('parula', parula_colors[:220])\n\nsamples_short = np.load('/Users/arcticfox/Downloads/short_period.npy')\nsamples_long = np.load('/Users/arcticfox/Downloads/long_period.npy')",
"The autoreload extension is already loaded. To reload it, use:\n %reload_ext autoreload\n"
],
[
"parula = np.load('/Users/arcticfox/parula_colors.npy')\n\nmgun = Table.read('/Users/arcticfox/Documents/flares/criticality/all_star_param_catalog.tab',\n format='ascii')\n\nallflares = Table.read('/Users/arcticfox/Documents/flares/criticality/all_flares_param_catalog.tab', \n format='csv')\n\nfilters = [i for i in allflares.colnames if 'passed_' in i]\nallflares = allflares[(allflares[filters[0]]=='True')&\n (allflares[filters[1]]=='True')&\n (allflares[filters[2]]=='True')&\n (allflares[filters[3]]=='True')]",
"_____no_output_____"
],
[
"fakenews = 231799463\nbadinds = np.where(allflares['TIC_ID']==fakenews)[0]\nbadinds2= np.where(mgun['TIC_ID']==fakenews)[0]8\nallflares.remove_rows(badinds)\nmgun.remove_row(badinds2[0])",
"_____no_output_____"
],
[
"f20 = Table.read('feinstein2020.txt', format='ascii')\nh20 = Table.read('howard20.txt', format='csv')\n\ntics = np.append(f20['TIC'],h20['tic_id'])\nrots = np.append(f20['period_days'], h20['p_rot'])\n\n_,args=np.unique(tics,return_index=True)\ntics=tics[args]\nrots=rots[args]",
"_____no_output_____"
],
[
"mgun_rots = np.zeros(len(mgun))\nmissing=np.array([],dtype=int)\n\nfor i in range(len(tics)):\n ind = np.where(mgun['TIC_ID']==tics[i])[0]\n if len(ind)>0:\n mgun_rots[i] = rots[i]\n else:\n missing=np.append(missing,tics[i])",
"_____no_output_____"
]
],
[
[
"### Find the missing targets",
"_____no_output_____"
]
],
[
[
"ndat = np.load('more_targets.npy', allow_pickle=True)\nflare_table = Table.read('new_flares.tab', format='ascii')",
"_____no_output_____"
],
[
"lks = []\n\nfor tic in np.unique(flare_table['Target_ID']):\n s = search_lightcurve('TIC {}'.format(int(tic)), author='SPOC',\n exptime=120, mission='TESS')\n d = s[s.year<2020][0].download_all()\n lks.append(d)",
"Warning: 30% (5871/19412) of the cadences will be ignored due to the quality mask (quality_bitmask=175).\nWarning: 30% (5871/19412) of the cadences will be ignored due to the quality mask (quality_bitmask=175).\nWarning: 30% (5871/19412) of the cadences will be ignored due to the quality mask (quality_bitmask=175).\nWarning: 30% (5871/19412) of the cadences will be ignored due to the quality mask (quality_bitmask=175).\n"
],
[
"tcolnames = ['ID','ra', 'dec', 'Teff', 'Tmag', 'd', 'Hmag','Jmag', 'Kmag']\ngcolnames = ['teff_val', 'teff_percentile_lower', 'phot_rp_mean_mag', 'phot_bp_mean_mag',\n 'phot_g_mean_mag', 'bp_rp','lum_val','radius_val']",
"_____no_output_____"
],
[
"for i in range(len(lks)):\n row_mgun = np.zeros(len(mgun.colnames))\n row_allflares = np.zeros(len(allflares.colnames))\n \n output_t = Catalogs.query_region(SkyCoord(lks[i][0].meta['RA_OBJ'],\n lks[i][0].meta['DEC_OBJ'], unit=units.deg),\n radius=0.1,\n catalog='TIC')[0]\n \n for j,k in enumerate([0,2,3,4,5,6,-3,-2,-1]):\n row_mgun[k] = output_t[tcolnames[j]]\n \n row_mgun=row_mgun.tolist()\n row_mgun[1]=''\n row_mgun[7]=''\n mgun.add_row(row_mgun)\n \n output_g = Catalogs.query_region(SkyCoord(lks[i][0].meta['RA_OBJ'],\n lks[i][0].meta['DEC_OBJ'], unit=units.deg),\n radius=0.1,\n catalog='Gaia', version=2)[0]\n \n for j,k in enumerate([0,2,3,4,5,6,-8,-7,-6]):\n row_allflares[k] = output_t[tcolnames[j]]\n for j,k in enumerate([19,20,21,22,31,32,24,23]):\n row_allflares[k] = output_g[gcolnames[j]]\n \n for ind in np.where(flare_table['Target_ID']==int(output_t[0]))[0]:\n for j,k in enumerate(np.arange(7,13,1)):\n row_allflares[k]=flare_table[ind][j]\n try:\n row_allflares=row_allflares.tolist()\n except:\n pass\n row_allflares[1]=''\n row_allflares[7]=''\n for n in range(14,18):\n row_allflares[n]='True'\n allflares.add_row(row_allflares)",
"_____no_output_____"
],
[
"xmatch = np.zeros(len(mgun))\nfor i in range(len(tics)):\n i1 = np.where(tics==tics[i])[0]\n i2 = np.where(mgun['TIC_ID']==tics[i])[0]\n xmatch[i2] = rots[i1]\nmgun.add_column(Column(xmatch,'period_days'))",
"_____no_output_____"
],
[
"#mgun.add_column(Column(mgun_rots,'period_days'))\nsub_mgun = mgun[(mgun['period_days']>0) & (mgun['N_flares']>0)]\nlen(sub_mgun),len(mgun)",
"_____no_output_____"
],
[
"xmatch = np.zeros(len(allflares),dtype=int)\nxmatch_rots = np.zeros(len(allflares))\nxmatch_time = np.zeros(len(allflares))\nxmatch_lum = np.zeros(len(allflares))\nxmatch_rate = np.zeros(len(allflares))\n\nfor i in range(len(sub_mgun['TIC_ID'])):\n ind = np.where(allflares['TIC_ID']==sub_mgun['TIC_ID'][i])[0]\n\n xmatch[ind] = 1\n xmatch_rots[ind]=sub_mgun['period_days'][i]\n xmatch_time[ind]=sub_mgun['Total_obs_time'][i]\n xmatch_lum[ind]=sub_mgun['lum'][i]\n xmatch_rate[ind]=sub_mgun['N_flares_per_day'][i]\n\ntry:\n allflares.add_column(Column(xmatch_rots, 'Prot'))\n allflares.add_column(Column(xmatch_time, 'Total_obs_time'))\n allflares.add_column(Column(xmatch_rate, 'N_flares_per_day'))\nexcept:\n allflares.replace_column('Prot',xmatch_rots)\n allflares.replace_column('Total_obs_time',xmatch_time)\n allflares.replace_column('N_flares_per_day',xmatch_rate)",
"_____no_output_____"
],
[
"subflares = allflares[xmatch==1]\nlowlim = subflares[subflares['prob']>=0.99]\nmedlim = subflares[(subflares['prob']>=0.9)]\nupplim = subflares[(subflares['prob']>=0.5)]",
"_____no_output_____"
],
[
"mark_tab = Table()\nmark_tab.add_column(Column(upplim['TIC_ID'], 'TargetID'))\nmark_tab.add_column(Column(upplim['amp'], 'amp'))\nmark_tab.add_column(Column(upplim['flare_energy_erg'], 'flare_energy_erg'))\nmark_tab.add_column(Column(upplim['Prot'], 'Prot'))\nmark_tab.add_column(Column(upplim['prob'], 'flare_probability'))\nmark_tab.add_column(Column(upplim['N_flares_per_day'], 'N_flares_per_day'))",
"_____no_output_____"
],
[
"mark_tab.write('flare_outputs.csv', format='csv')",
"WARNING: AstropyDeprecationWarning: flare_outputs.csv already exists. Automatically overwriting ASCII files is deprecated. Use the argument 'overwrite=True' in the future. [astropy.io.ascii.ui]\n"
],
[
"medlim.write('medlim.csv',format='csv')\nlowlim.write('lowlim.csv',format='csv')\nupplim.write('upplim.csv',format='csv')",
"WARNING: AstropyDeprecationWarning: medlim.csv already exists. Automatically overwriting ASCII files is deprecated. Use the argument 'overwrite=True' in the future. [astropy.io.ascii.ui]\nWARNING: AstropyDeprecationWarning: lowlim.csv already exists. Automatically overwriting ASCII files is deprecated. Use the argument 'overwrite=True' in the future. [astropy.io.ascii.ui]\nWARNING: AstropyDeprecationWarning: upplim.csv already exists. Automatically overwriting ASCII files is deprecated. Use the argument 'overwrite=True' in the future. [astropy.io.ascii.ui]\n"
]
],
[
[
"# Load Tables",
"_____no_output_____"
]
],
[
[
"medlim = Table.read('medlim.csv',format='csv')\nlowlim = Table.read('lowlim.csv',format='csv')\nupplim = Table.read('upplim.csv',format='csv')",
"_____no_output_____"
]
],
[
[
"## Plot the light curves",
"_____no_output_____"
]
],
[
[
"outliers = np.unique(medlim[(medlim['amp']>=1) & (medlim['Prot']<3)]['TIC_ID'])\n\nlk = []\ntic_tracker=[]\nfor tic in outliers:\n print(tic)\n d = search_lightcurve('TIC {}'.format(tic), mission='TESS', \n author='SPOC').download_all().stitch()\n lk.append(d)\n tic_tracker.append(d.meta['TICID'])",
"31281820\n32068898\n"
],
[
"megaflares = medlim[(medlim['amp']>2) & (medlim['Prot']<3)]\nmegaflares.sort('amp')\nmegaflares.reverse()",
"_____no_output_____"
],
[
"plt.rcParams['font.size'] = 22",
"_____no_output_____"
],
[
"megaflares",
"_____no_output_____"
],
[
"fig, axes = plt.subplots(figsize=(16,25), nrows=5)\nfig.set_facecolor('w')\nax = axes.reshape(-1)\ninds = [0,1,2,5,6]#,5]\n\nfor x,i in enumerate(inds):\n ind = np.where(megaflares['TIC_ID'][i]==tic_tracker)[0][0]\n print(ind)\n\n for n in [megaflares['tpeak'][i]-2457000]:\n \n left, bottom, width, height = [0.08, 0.45, 0.55, 0.5]\n ax2 = ax[x].inset_axes([left, bottom, width, height])\n ax2.plot(lk[ind].time.value, lk[ind].flux.value,'k.',ms=1)\n ax2.vlines(n+0.01,0,100,lw=10,color=parula[100],alpha=0.4)\n ax2.set_ylim(np.nanmin(lk[ind].flux.value),1.08)\n \n if i == 0:\n ax2.set_xlim(1438.2,1450.)\n ax2.set_xticks(np.round(np.linspace(1438.2,1450.,4),1))\n ax2.set_ylim(0.97,1.03)\n ax2.set_yticks(np.round(np.linspace(0.98,1.02,3),2))\n elif i == 1:\n ax2.set_xlim(1328,1338)\n ax2.set_xticks(np.round(np.linspace(1328,1338,4),1))\n ax2.set_ylim(0.3,1.7)\n elif i == 2:\n ax2.set_xlim(1339.8,1353)\n ax2.set_xticks(np.round(np.linspace(1339.8,1353,4),1))\n elif i == 5:\n ax2.set_xlim(1630.5,1639.)\n ax2.set_xticks(np.round(np.linspace(1630.5,1639.,4),1))\n ax2.set_ylim(0.97,1.03)\n ax2.set_yticks(np.round(np.linspace(0.98,1.02,3),2))\n elif i == 6:\n ax2.set_xlim(1371,1381.5)\n ax2.set_xticks(np.round(np.linspace(1371,1381.5,4),1))\n \n q = ((lk[ind].time.value>=n-0.8) & (lk[ind].time.value<=n+0.2))\n ax[x].plot(lk[ind].time.value[q], lk[ind].flux.value[q],c='k',lw=3)\n ax[x].set_xlim(n-0.8,n+0.2)\n ax[x].set_xticks(np.round(np.arange(n-0.7,n+0.3,0.2),2))\n ax[x].set_xticklabels([str(e) for e in np.round(np.arange(n-0.7,n+0.3,0.2),2)])\n ax[x].vlines(n+0.01,0,100,lw=60,color=parula[100],alpha=0.4)\n ax[x].set_ylim(np.nanmin(lk[ind].flux.value[q])-0.08, \n np.nanmax(lk[ind].flux.value[q])+0.08)\n ax[x].set_rasterized(True)\n \n sec = ax[x].secondary_yaxis('right')\n sec.set_yticks([])\n sec.set_ylabel('\\nTIC {}'.format(megaflares['TIC_ID'][i]) + \n '\\n$P_{rot}$ = ' + str(np.round(megaflares['Prot'][i],2)) + ' days')\n \nax[-3].set_ylabel('Normalized Flux', fontsize=30)\nax[-1].set_xlabel('Time [BJD - 2457000]', fontsize=30)\nplt.savefig('/Users/arcticfox/Desktop/lightcurves.pdf',dpi=300,rasterize=True,\n bbox_inches='tight')",
"2\n4\n10\n14\n9\n"
]
],
[
[
"## Rotation period plot",
"_____no_output_____"
]
],
[
[
"fig = plt.figure(figsize=(8,6))\nfig.set_facecolor('w')\nplt.scatter(mgun['bp']-mgun['rp'], \n mgun['period_days'], \n c=mgun['flare_rates'], \n vmin=0, vmax=0.5,\n cmap=parula_map)\nplt.yscale('log')\nplt.ylabel('Rotation Period [days]')\nplt.xlabel('$B_p - R_p$')\nplt.xlim(-1,5)\nplt.colorbar(label='Flare Rate [days$^{-1}$]')\n#plt.savefig('/Users/arcticfox/Desktop/rots.pdf',dpi=250,rasterize=True,\n# bbox_inches='tight')",
"_____no_output_____"
],
[
"np.nanmin(mgun['bp']-mgun['rp']), np.nanmax(mgun['bp']-mgun['rp'])",
"_____no_output_____"
]
],
[
[
"## Fitting the Flare Frequency Distributions",
"_____no_output_____"
]
],
[
[
"def slope_fit(x, n, i=0, j=1, plot=False, init=[-1.5,-2], \n bounds=((-10.0, 10.0), (-1000, 1000))):\n \n logx = np.log10(x)\n logn = np.log10(n)\n q = ((np.isnan(logn) == False) & (np.isfinite(logn)==True))\n \n if plot:\n plt.plot(logx[i:j], np.log10(n[i:j]), '.', c='k')\n plt.plot(logx[i:j], linear(init, logx[i:j]), '--', c='g', linewidth=3)\n\n try:\n results = minimize(linear_fit, x0=init,\n args=(logx[q][i:j-1]-np.diff(logx[q][i:j])/2.,\n logn[q][i:j-1], \n np.sqrt(logn[q][i:j-1]) ),\n bounds=bounds,\n method='L-BFGS-B', tol=1e-8)\n results.x[1] = 10**results.x[1]\n\n results2 = leastsq(power_law_resid, results.x,\n args=(x[q][i:j-1]-np.diff(x[q][i:j])/2.,\n n[q][i:j-1],\n np.sqrt(n[q][i:j-1]) ),\n full_output=True)\n except:\n print(len(np.diff(logx[q][i:j])), len(logx[q][i:j-1]))\n results = minimize(linear_fit, x0=init,\n args=(logx[q][i+1:j-1]-np.diff(logx[q][i:j])/2.,\n logn[q][i+1:j-1], \n np.sqrt(logn[q][i+1:j-1]) ),\n bounds=bounds,\n method='L-BFGS-B', tol=1e-8)\n\n results.x[1] = 10**results.x[1]\n\n results2 = leastsq(power_law_resid, results.x,\n args=(x[q][i+1:j-1]-np.diff(x[q][i:j])/2.,\n n[q][i+1:j-1],\n np.sqrt(n[q][i+1:j-1]) ),\n full_output=True)\n \n fit_params = results2[0]\n \n slope_err = np.sqrt(results2[1][0][0])\n\n\n model = linear([fit_params[0], np.log10(fit_params[1])], logx)\n \n if plot:\n plt.plot(logx, model, c='r')\n plt.title('{} $\\pm$ {}'.format(np.round(fit_params[0],2),\n np.round(slope_err,2)))\n plt.show()\n \n return fit_params[0], slope_err, n, results.x[1], x, 10**model, np.log10(fit_params[1])",
"_____no_output_____"
]
],
[
[
"### Amplitude Binning ",
"_____no_output_____"
]
],
[
[
"bins = np.logspace(np.log10(1), np.log10(500),20)\ncut = 3",
"_____no_output_____"
],
[
"outslow = []\noutfast = []\n\nfor t in [medlim, upplim, lowlim]:\n\n os = plt.hist(t[t['Prot']>=cut]['amp']*100, \n bins=bins, \n weights=np.full(len(t[t['Prot']>=cut]['amp']),\n 1.0/np.nansum(t[t['Prot']>=cut]['Total_obs_time']*\n t[t['Prot']>=cut]['prob'])))\n outslow.append(os)\n\n of = plt.hist(t[t['Prot']<cut]['amp']*100, \n bins=bins, color=parula[100],\n weights=np.full(len(t[t['Prot']<cut]['amp']),\n 1.0/np.nansum(t[t['Prot']<cut]['Total_obs_time']*\n t[t['Prot']<cut]['prob'])))\n outfast.append(of)\n \nplt.close()",
"_____no_output_____"
],
[
"plt.errorbar((outfast[0][1][1:]+outfast[0][1][:-1])/2,\n outfast[0][0],\n yerr=(outfast[1][0], outfast[2][0]), marker='o',\n linestyle='')\nplt.errorbar((outslow[0][1][1:]+outslow[0][1][:-1])/2,\n outslow[0][0],\n yerr=(outslow[1][0], outslow[2][0]), marker='o',\n linestyle='')\nplt.yscale('log')\nplt.xscale('log')",
"_____no_output_____"
],
[
"fitslow, fitfast = [], []\n\nfor i in range(len(outslow)):\n fl = slope_fit((outslow[i][1][1:]+outslow[i][1][:-1])/2,\n outslow[i][0],\n i=0,\n j=len(outslow[i][0]),\n plot=True, init=[0.988,10],\n bounds=((0.988-0.0076,0.988+0.0077), (-100, 100)))\n\n ff = slope_fit((outfast[i][1][1:]+outfast[i][1][:-1])/2,\n outfast[i][0],\n i=0,j=len(outfast[i][0]),\n plot=True, init=[0.961,-2],\n bounds=((0.961-0.0058,0.961+0.0060), (-100, 100)))\n fitslow.append(fl)\n fitfast.append(ff)",
"//anaconda3/lib/python3.7/site-packages/ipykernel_launcher.py:5: RuntimeWarning: divide by zero encountered in log10\n \"\"\"\n//anaconda3/lib/python3.7/site-packages/ipykernel_launcher.py:9: RuntimeWarning: divide by zero encountered in log10\n if __name__ == '__main__':\n//anaconda3/lib/python3.7/site-packages/ipykernel_launcher.py:31: RuntimeWarning: invalid value encountered in sqrt\n"
],
[
"samples_short = np.load('/Users/arcticfox/Downloads/short_period.npy')\nsamples_long = np.load('/Users/arcticfox/Downloads/long_period.npy')\n\nslow_dist = np.nanpercentile(samples_long[:,0], [5,50,95])\nslow_dist[0]=slow_dist[1]-slow_dist[0]\nslow_dist[2]=slow_dist[2]-slow_dist[1]\nfast_dist = np.nanpercentile(samples_short[:,0], [5,50,95])\nfast_dist[0]=fast_dist[1]-fast_dist[0]\nfast_dist[2]=fast_dist[2]-fast_dist[1]",
"_____no_output_____"
],
[
"def pdf(a, q, astar):\n norm = a0**(q-1) / mp.mpf(expint(q, a0/astar))\n return norm * a**-q * np.exp(-a/astar)\n\na0 = np.amin(mark_tab['amp'])\nasamp = np.logspace(np.log10(0.001), np.log10(1000),100)\nsize = 500\nshort_samp_pdf = np.zeros((size,len(asamp)))\nlong_samp_pdf = np.zeros((size,len(asamp)))\n\nschoices = np.random.choice(np.arange(0,len(samples_short),1,dtype=int),\n len(samples_short),replace=False)\nlchoices = np.random.choice(np.arange(0,len(samples_long),1,dtype=int),\n len(samples_long),replace=False)\n\nfor i in range(size):\n short_samp_pdf[i] = pdf(asamp, \n samples_short[schoices[i]][0], \n 10**samples_short[i][1]*100)\n long_samp_pdf[i] = pdf(asamp,\n samples_long[lchoices[i]][0],\n 10**samples_long[i][1]*100)",
"_____no_output_____"
],
[
"pdf_short = np.percentile(short_samp_pdf, [2.5,5,16,50,84,95,97.5], axis=0)\npdf_long = np.percentile(long_samp_pdf, [2.5,5,16,50,84,95,97.5], axis=0)",
"_____no_output_____"
],
[
"plt.plot(asamp, asamp*pdf_long[1],\n 'k', lw=3,zorder=4, label=label)\nplt.fill_between(asamp, \n y1=asamp*pdf_long[0], \n y2=asamp*pdf_long[-1],\n lw=0, color='k', alpha=0.3, zorder=4)\n\nplt.plot(asamp, asamp*pdf_short[1],\n 'r', lw=3,zorder=4, label=label)\nplt.fill_between(asamp, \n y1=asamp*pdf_short[0], \n y2=asamp*pdf_short[-1],\n lw=0, color='r', alpha=0.3, zorder=4)\n#plt.xlim([1e-2,3])\nplt.ylim([1e-6,100])\nplt.yscale('log')\nplt.xscale('log')",
"_____no_output_____"
],
[
"fig, (ax2,ax3)=plt.subplots(nrows=2,figsize=(8,10),sharex=True,sharey=True)\nfig.set_facecolor('w')\n\nc1=210\nc2=100\n\nax3.hist(medlim[medlim['Prot']>=cut]['amp']*100, \n bins=bins, color=parula[c1],\n weights=np.full(len(medlim[medlim['Prot']>=cut]['amp']),\n 1.0/np.nansum(medlim[medlim['Prot']>=cut]['Total_obs_time']*\n medlim[medlim['Prot']>=cut]['prob'])),\n alpha=0.6)\n\nax3.errorbar((outslow[0][1][1:]+outslow[0][1][:-1])/2,\n outslow[0][0],\n yerr=(outslow[1][0], outslow[2][0]), marker='',\n linestyle='', color=parula[c1], capsize=4, lw=3, \n capthick=3, zorder=3)\n\nlabel = str(np.round(slow_dist[0],4)) + '$_{-'+str(np.round(slow_dist[1],4))\nlabel += '}^{+'+str(np.round(slow_dist[2],3))+'}$'\nlabel = r\"$\\alpha$' = \" + label\nlabel = r\"$\\alpha$' = \" + str(np.round(slow_dist[1],3)) + '$\\pm$' + str(np.round(slow_dist[0],3))\n\nx = np.append(1,(outslow[0][1][1:]+outslow[0][1][:-1])/2)\nx = np.append(x,1000)\nN = 5\nax3.plot(asamp, pdf_long[3]/N,#*asamp,\n 'k', lw=3,zorder=4, label=label)\nax3.fill_between(asamp, \n y1=pdf_long[0]/N,#*asamp, \n y2=pdf_long[-1]/N,#*asamp,\n lw=0, color='k', alpha=0.3, zorder=4)\n\nax3.hist(medlim[medlim['Prot']>=cut]['amp']*100, \n bins=bins, color=parula[c1],\n weights=np.full(len(medlim[medlim['Prot']>=cut]['amp']),\n 1.0/np.nansum(medlim[medlim['Prot']>=cut]['Total_obs_time']*\n medlim[medlim['Prot']>=cut]['prob'])),\n histtype='bar', fill=None,edgecolor=parula[c1],lw=3)\nax3.set_title('P$_{rot} \\geq$'+str(cut)+ ' days')\n\nax2.hist(medlim[medlim['Prot']<cut]['amp']*100, \n bins=bins, color=parula[c2],\n weights=np.full(len(medlim[medlim['Prot']<cut]['amp']),\n 1.0/np.nansum(medlim[medlim['Prot']<cut]['Total_obs_time']*\n medlim[medlim['Prot']<cut]['prob'])),\n alpha=0.6)\nax2.hist(medlim[medlim['Prot']<cut]['amp']*100, \n bins=bins, color=parula[c2],\n weights=np.full(len(medlim[medlim['Prot']<cut]['amp']),\n 1.0/np.nansum(medlim[medlim['Prot']<cut]['Total_obs_time']*\n medlim[medlim['Prot']<cut]['prob'])),\n histtype='bar', fill=None, lw=3, edgecolor=parula[c2])\n\nax2.errorbar((outfast[0][1][1:]+outfast[0][1][:-1])/2,\n outfast[0][0],\n yerr=(outfast[1][0], outfast[2][0]), marker='',\n linestyle='', color=parula[c2], capsize=4, lw=3,\n capthick=3, zorder=3)\n\nlabel = str(np.round(fast_dist[0],4)) + '$_{-'+str(np.round(fast_dist[1],4))\nlabel += '}^{+'+str(np.round(fast_dist[2],4))+'}$'\nlabel = r\"$\\alpha$' = \" + label\n\nlabel = r\"$\\alpha$' = \" + str(np.round(fast_dist[1],3)) + '$\\pm$' + str(np.round(fast_dist[0],3))\n\nx = np.append(1,(outfast[0][1][1:]+outfast[0][1][:-1])/2)\nx = np.append(x,1000)\nN = 10\nax2.plot(asamp, pdf_short[3]/N,#*asamp,\n 'k', lw=3,zorder=4, label=label)\nax2.fill_between(asamp, \n y1=pdf_short[0]/N,#*asamp, \n y2=pdf_short[-1]/N,#*asamp,\n lw=0, color='k', alpha=0.3, zorder=4)\n\nax2.set_title('P$_{rot} <$'+str(cut)+ ' days')\n\nax2.set_xscale('log')\nax2.set_yscale('log')\nax3.set_xscale('log')\nax3.set_yscale('log')\nax2.set_ylabel('Flare Rate [day$^{-1}$]')\nax3.set_ylabel('Flare Rate [day$^{-1}$]')\nax3.set_xlabel('Flare Amplitude [%]')\nplt.subplots_adjust(hspace=0.3)\nplt.xlim(1,500)\nplt.ylim(1e-6,1e-2)\nax2.legend()\nax3.legend()\nplt.savefig('/Users/arcticfox/Desktop/hist_rots.pdf',\n dpi=250,rasterize=True,\n bbox_inches='tight')",
"//anaconda3/lib/python3.7/site-packages/ipykernel_launcher.py:94: MatplotlibDeprecationWarning: savefig() got unexpected keyword argument \"rasterize\" which is no longer supported as of 3.3 and will become an error in 3.6\n"
]
]
] | [
"code",
"markdown",
"code",
"markdown",
"code",
"markdown",
"code",
"markdown",
"code",
"markdown",
"code",
"markdown",
"code"
] | [
[
"code",
"code",
"code",
"code",
"code"
],
[
"markdown"
],
[
"code",
"code",
"code",
"code",
"code",
"code",
"code",
"code",
"code",
"code",
"code"
],
[
"markdown"
],
[
"code"
],
[
"markdown"
],
[
"code",
"code",
"code",
"code",
"code"
],
[
"markdown"
],
[
"code",
"code"
],
[
"markdown"
],
[
"code"
],
[
"markdown"
],
[
"code",
"code",
"code",
"code",
"code",
"code",
"code",
"code",
"code"
]
] |
e78730ff84ea82bff9194e70a7b4a00847222a5b | 29,819 | ipynb | Jupyter Notebook | 06_prepare/02_Prepare_Dataset_BERT_Scikit_ScriptMode_FeatureStore.ipynb | MarcusFra/workshop | 83f16d41f5e10f9c23242066f77a14bb61ac78d7 | [
"Apache-2.0"
] | 2,327 | 2020-03-01T09:47:34.000Z | 2021-11-25T12:38:42.000Z | 06_prepare/02_Prepare_Dataset_BERT_Scikit_ScriptMode_FeatureStore.ipynb | MarcusFra/workshop | 83f16d41f5e10f9c23242066f77a14bb61ac78d7 | [
"Apache-2.0"
] | 209 | 2020-03-01T17:14:12.000Z | 2021-11-08T20:35:42.000Z | 06_prepare/02_Prepare_Dataset_BERT_Scikit_ScriptMode_FeatureStore.ipynb | MarcusFra/workshop | 83f16d41f5e10f9c23242066f77a14bb61ac78d7 | [
"Apache-2.0"
] | 686 | 2020-03-03T17:24:51.000Z | 2021-11-25T23:39:12.000Z | 27.034451 | 478 | 0.571984 | [
[
[
"# Feature Transformation with Amazon a SageMaker Processing Job and Scikit-Learn\n\nIn this notebook, we convert raw text into BERT embeddings. This will allow us to perform natural language processing tasks such as text classification.\n\nTypically a machine learning (ML) process consists of few steps. First, gathering data with various ETL jobs, then pre-processing the data, featurizing the dataset by incorporating standard techniques or prior knowledge, and finally training an ML model using an algorithm.\n\nOften, distributed data processing frameworks such as Scikit-Learn are used to pre-process data sets in order to prepare them for training. In this notebook we'll use Amazon SageMaker Processing, and leverage the power of Scikit-Learn in a managed SageMaker environment to run our processing workload.",
"_____no_output_____"
],
[
"# NOTE: THIS NOTEBOOK WILL TAKE A 5-10 MINUTES TO COMPLETE.\n\n# PLEASE BE PATIENT.",
"_____no_output_____"
],
[
"\n\n\n",
"_____no_output_____"
],
[
"## Contents\n\n1. Setup Environment\n1. Setup Input Data\n1. Setup Output Data\n1. Build a Scikit-Learn container for running the processing job\n1. Run the Processing Job using Amazon SageMaker\n1. Inspect the Processed Output Data",
"_____no_output_____"
],
[
"# Setup Environment\n\nLet's start by specifying:\n* The S3 bucket and prefixes that you use for training and model data. Use the default bucket specified by the Amazon SageMaker session.\n* The IAM role ARN used to give processing and training access to the dataset.",
"_____no_output_____"
]
],
[
[
"import sagemaker\nimport boto3\n\nsess = sagemaker.Session()\nrole = sagemaker.get_execution_role()\nbucket = sess.default_bucket()\nregion = boto3.Session().region_name\n\nsm = boto3.Session().client(service_name=\"sagemaker\", region_name=region)\ns3 = boto3.Session().client(service_name=\"s3\", region_name=region)",
"_____no_output_____"
]
],
[
[
"# Setup Input Data",
"_____no_output_____"
]
],
[
[
"%store -r s3_public_path_tsv",
"_____no_output_____"
],
[
"try:\n s3_public_path_tsv\nexcept NameError:\n print(\"++++++++++++++++++++++++++++++++++++++++++++++++++++++++\")\n print(\"[ERROR] Please run the notebooks in the INGEST section before you continue.\")\n print(\"++++++++++++++++++++++++++++++++++++++++++++++++++++++++\")",
"_____no_output_____"
],
[
"print(s3_public_path_tsv)",
"_____no_output_____"
],
[
"%store -r s3_private_path_tsv",
"_____no_output_____"
],
[
"try:\n s3_private_path_tsv\nexcept NameError:\n print(\"++++++++++++++++++++++++++++++++++++++++++++++++++++++++\")\n print(\"[ERROR] Please run the notebooks in the INGEST section before you continue.\")\n print(\"++++++++++++++++++++++++++++++++++++++++++++++++++++++++\")",
"_____no_output_____"
],
[
"print(s3_private_path_tsv)",
"_____no_output_____"
],
[
"raw_input_data_s3_uri = \"s3://{}/amazon-reviews-pds/tsv/\".format(bucket)\nprint(raw_input_data_s3_uri)",
"_____no_output_____"
],
[
"!aws s3 ls $raw_input_data_s3_uri",
"_____no_output_____"
]
],
[
[
"# Run the Processing Job using Amazon SageMaker\n\nNext, use the Amazon SageMaker Python SDK to submit a processing job using our custom python script.",
"_____no_output_____"
],
[
"# Review the Processing Script",
"_____no_output_____"
]
],
[
[
"!pygmentize preprocess-scikit-text-to-bert-feature-store.py",
"_____no_output_____"
]
],
[
[
"Run this script as a processing job. You also need to specify one `ProcessingInput` with the `source` argument of the Amazon S3 bucket and `destination` is where the script reads this data from `/opt/ml/processing/input` (inside the Docker container.) All local paths inside the processing container must begin with `/opt/ml/processing/`.\n\nAlso give the `run()` method a `ProcessingOutput`, where the `source` is the path the script writes output data to. For outputs, the `destination` defaults to an S3 bucket that the Amazon SageMaker Python SDK creates for you, following the format `s3://sagemaker-<region>-<account_id>/<processing_job_name>/output/<output_name>/`. You also give the `ProcessingOutput` value for `output_name`, to make it easier to retrieve these output artifacts after the job is run.\n\nThe arguments parameter in the `run()` method are command-line arguments in our `preprocess-scikit-text-to-bert-feature-store.py` script.\n\nNote that we sharding the data using `ShardedByS3Key` to spread the transformations across all worker nodes in the cluster.",
"_____no_output_____"
],
[
"# Track the `Experiment`\nWe will track every step of this experiment throughout the `prepare`, `train`, `optimize`, and `deploy`.",
"_____no_output_____"
],
[
"# Concepts\n\n**Experiment**: A collection of related Trials. Add Trials to an Experiment that you wish to compare together.\n\n**Trial**: A description of a multi-step machine learning workflow. Each step in the workflow is described by a Trial Component. There is no relationship between Trial Components such as ordering.\n\n**Trial Component**: A description of a single step in a machine learning workflow. For example data cleaning, feature extraction, model training, model evaluation, etc.\n\n**Tracker**: A logger of information about a single TrialComponent.\n\n<img src=\"img/sagemaker-experiments.png\" width=\"90%\" align=\"left\">\n",
"_____no_output_____"
],
[
"# Create the `Experiment`",
"_____no_output_____"
]
],
[
[
"import time\nfrom smexperiments.experiment import Experiment\n\ntimestamp = int(time.time())\n\nexperiment = Experiment.create(\n experiment_name=\"Amazon-Customer-Reviews-BERT-Experiment-{}\".format(timestamp),\n description=\"Amazon Customer Reviews BERT Experiment\",\n sagemaker_boto_client=sm,\n)\n\nexperiment_name = experiment.experiment_name\nprint(\"Experiment name: {}\".format(experiment_name))",
"_____no_output_____"
]
],
[
[
"# Create the `Trial`",
"_____no_output_____"
]
],
[
[
"import time\nfrom smexperiments.trial import Trial\n\ntimestamp = int(time.time())\n\ntrial = Trial.create(\n trial_name=\"trial-{}\".format(timestamp), experiment_name=experiment_name, sagemaker_boto_client=sm\n)\n\ntrial_name = trial.trial_name\nprint(\"Trial name: {}\".format(trial_name))",
"_____no_output_____"
]
],
[
[
"# Create the `Experiment Config`",
"_____no_output_____"
]
],
[
[
"experiment_config = {\n \"ExperimentName\": experiment_name,\n \"TrialName\": trial_name,\n \"TrialComponentDisplayName\": \"prepare\",\n}",
"_____no_output_____"
],
[
"print(experiment_name)",
"_____no_output_____"
],
[
"%store experiment_name",
"_____no_output_____"
],
[
"print(trial_name)",
"_____no_output_____"
],
[
"%store trial_name",
"_____no_output_____"
]
],
[
[
"# Create Feature Store and Feature Group",
"_____no_output_____"
]
],
[
[
"featurestore_runtime = boto3.Session().client(service_name=\"sagemaker-featurestore-runtime\", region_name=region)",
"_____no_output_____"
],
[
"timestamp = int(time.time())\n\nfeature_store_offline_prefix = \"reviews-feature-store-\" + str(timestamp)\n\nprint(feature_store_offline_prefix)",
"_____no_output_____"
],
[
"feature_group_name = \"reviews-feature-group-\" + str(timestamp)\n\nprint(feature_group_name)",
"_____no_output_____"
],
[
"from sagemaker.feature_store.feature_definition import (\n FeatureDefinition,\n FeatureTypeEnum,\n)\n\nfeature_definitions = [\n FeatureDefinition(feature_name=\"input_ids\", feature_type=FeatureTypeEnum.STRING),\n FeatureDefinition(feature_name=\"input_mask\", feature_type=FeatureTypeEnum.STRING),\n FeatureDefinition(feature_name=\"segment_ids\", feature_type=FeatureTypeEnum.STRING),\n FeatureDefinition(feature_name=\"label_id\", feature_type=FeatureTypeEnum.INTEGRAL),\n FeatureDefinition(feature_name=\"review_id\", feature_type=FeatureTypeEnum.STRING),\n FeatureDefinition(feature_name=\"date\", feature_type=FeatureTypeEnum.STRING),\n FeatureDefinition(feature_name=\"label\", feature_type=FeatureTypeEnum.INTEGRAL),\n # FeatureDefinition(feature_name='review_body', feature_type=FeatureTypeEnum.STRING)\n]",
"_____no_output_____"
],
[
"from sagemaker.feature_store.feature_group import FeatureGroup\n\nfeature_group = FeatureGroup(name=feature_group_name, feature_definitions=feature_definitions, sagemaker_session=sess)\n\nprint(feature_group)",
"_____no_output_____"
]
],
[
[
"# Set the Processing Job Hyper-Parameters ",
"_____no_output_____"
]
],
[
[
"processing_instance_type = \"ml.c5.2xlarge\"\nprocessing_instance_count = 2\ntrain_split_percentage = 0.90\nvalidation_split_percentage = 0.05\ntest_split_percentage = 0.05\nbalance_dataset = True\nmax_seq_length = 64",
"_____no_output_____"
]
],
[
[
"# Choosing a `max_seq_length` for BERT\nSince a smaller `max_seq_length` leads to faster training and lower resource utilization, we want to find the smallest review length that captures `80%` of our reviews.\n\nRemember our distribution of review lengths from a previous section?\n\n```\nmean 51.683405\nstd 107.030844\nmin 1.000000\n10% 2.000000\n20% 7.000000\n30% 19.000000\n40% 22.000000\n50% 26.000000\n60% 32.000000\n70% 43.000000\n80% 63.000000\n90% 110.000000\n100% 5347.000000\nmax 5347.000000\n```\n\n\n\nReview length `63` represents the `80th` percentile for this dataset. However, it's best to stick with powers-of-2 when using BERT. So let's choose `64` as this is the smallest power-of-2 greater than `63`. Reviews with length > `64` will be truncated to `64`.",
"_____no_output_____"
]
],
[
[
"from sagemaker.sklearn.processing import SKLearnProcessor\n\nprocessor = SKLearnProcessor(\n framework_version=\"0.23-1\",\n role=role,\n instance_type=processing_instance_type,\n instance_count=processing_instance_count,\n env={\"AWS_DEFAULT_REGION\": region},\n max_runtime_in_seconds=7200,\n)",
"_____no_output_____"
],
[
"from sagemaker.processing import ProcessingInput, ProcessingOutput\n\nprocessor.run(\n code=\"preprocess-scikit-text-to-bert-feature-store.py\",\n inputs=[\n ProcessingInput(\n input_name=\"raw-input-data\",\n source=raw_input_data_s3_uri,\n destination=\"/opt/ml/processing/input/data/\",\n s3_data_distribution_type=\"ShardedByS3Key\",\n )\n ],\n outputs=[\n ProcessingOutput(\n output_name=\"bert-train\", s3_upload_mode=\"EndOfJob\", source=\"/opt/ml/processing/output/bert/train\"\n ),\n ProcessingOutput(\n output_name=\"bert-validation\",\n s3_upload_mode=\"EndOfJob\",\n source=\"/opt/ml/processing/output/bert/validation\",\n ),\n ProcessingOutput(\n output_name=\"bert-test\", s3_upload_mode=\"EndOfJob\", source=\"/opt/ml/processing/output/bert/test\"\n ),\n ],\n arguments=[\n \"--train-split-percentage\",\n str(train_split_percentage),\n \"--validation-split-percentage\",\n str(validation_split_percentage),\n \"--test-split-percentage\",\n str(test_split_percentage),\n \"--max-seq-length\",\n str(max_seq_length),\n \"--balance-dataset\",\n str(balance_dataset),\n \"--feature-store-offline-prefix\",\n str(feature_store_offline_prefix),\n \"--feature-group-name\",\n str(feature_group_name),\n ],\n experiment_config=experiment_config,\n logs=True,\n wait=False,\n)",
"_____no_output_____"
],
[
"scikit_processing_job_name = processor.jobs[-1].describe()[\"ProcessingJobName\"]\nprint(scikit_processing_job_name)",
"_____no_output_____"
],
[
"from IPython.core.display import display, HTML\n\ndisplay(\n HTML(\n '<b>Review <a target=\"blank\" href=\"https://console.aws.amazon.com/sagemaker/home?region={}#/processing-jobs/{}\">Processing Job</a></b>'.format(\n region, scikit_processing_job_name\n )\n )\n)",
"_____no_output_____"
],
[
"from IPython.core.display import display, HTML\n\ndisplay(\n HTML(\n '<b>Review <a target=\"blank\" href=\"https://console.aws.amazon.com/cloudwatch/home?region={}#logStream:group=/aws/sagemaker/ProcessingJobs;prefix={};streamFilter=typeLogStreamPrefix\">CloudWatch Logs</a> After About 5 Minutes</b>'.format(\n region, scikit_processing_job_name\n )\n )\n)",
"_____no_output_____"
],
[
"from IPython.core.display import display, HTML\n\ndisplay(\n HTML(\n '<b>Review <a target=\"blank\" href=\"https://s3.console.aws.amazon.com/s3/buckets/{}/{}/?region={}&tab=overview\">S3 Output Data</a> After The Processing Job Has Completed</b>'.format(\n bucket, scikit_processing_job_name, region\n )\n )\n)",
"_____no_output_____"
]
],
[
[
"# Monitor the Processing Job",
"_____no_output_____"
]
],
[
[
"running_processor = sagemaker.processing.ProcessingJob.from_processing_name(\n processing_job_name=scikit_processing_job_name, sagemaker_session=sess\n)\n\nprocessing_job_description = running_processor.describe()\n\nprint(processing_job_description)",
"_____no_output_____"
],
[
"running_processor.wait(logs=False)",
"_____no_output_____"
]
],
[
[
"# _Please Wait Until the ^^ Processing Job ^^ Completes Above._",
"_____no_output_____"
],
[
"# Inspect the Processed Output Data\n\nTake a look at a few rows of the transformed dataset to make sure the processing was successful.",
"_____no_output_____"
]
],
[
[
"processing_job_description = running_processor.describe()\n\noutput_config = processing_job_description[\"ProcessingOutputConfig\"]\nfor output in output_config[\"Outputs\"]:\n if output[\"OutputName\"] == \"bert-train\":\n processed_train_data_s3_uri = output[\"S3Output\"][\"S3Uri\"]\n if output[\"OutputName\"] == \"bert-validation\":\n processed_validation_data_s3_uri = output[\"S3Output\"][\"S3Uri\"]\n if output[\"OutputName\"] == \"bert-test\":\n processed_test_data_s3_uri = output[\"S3Output\"][\"S3Uri\"]\n\nprint(processed_train_data_s3_uri)\nprint(processed_validation_data_s3_uri)\nprint(processed_test_data_s3_uri)",
"_____no_output_____"
],
[
"!aws s3 ls $processed_train_data_s3_uri/",
"_____no_output_____"
],
[
"!aws s3 ls $processed_validation_data_s3_uri/",
"_____no_output_____"
],
[
"!aws s3 ls $processed_test_data_s3_uri/",
"_____no_output_____"
]
],
[
[
"# Pass Variables to the Next Notebook(s)",
"_____no_output_____"
]
],
[
[
"%store raw_input_data_s3_uri",
"_____no_output_____"
],
[
"%store max_seq_length",
"_____no_output_____"
],
[
"%store train_split_percentage",
"_____no_output_____"
],
[
"%store validation_split_percentage",
"_____no_output_____"
],
[
"%store test_split_percentage",
"_____no_output_____"
],
[
"%store balance_dataset",
"_____no_output_____"
],
[
"%store feature_store_offline_prefix",
"_____no_output_____"
],
[
"%store feature_group_name",
"_____no_output_____"
],
[
"%store processed_train_data_s3_uri",
"_____no_output_____"
],
[
"%store processed_validation_data_s3_uri",
"_____no_output_____"
],
[
"%store processed_test_data_s3_uri",
"_____no_output_____"
],
[
"%store",
"_____no_output_____"
]
],
[
[
"# Query The Feature Store",
"_____no_output_____"
]
],
[
[
"feature_store_query = feature_group.athena_query()",
"_____no_output_____"
],
[
"feature_store_table = feature_store_query.table_name",
"_____no_output_____"
],
[
"query_string = \"\"\"\nSELECT input_ids, input_mask, segment_ids, label_id, split_type FROM \"{}\" WHERE split_type='train' LIMIT 5\n\"\"\".format(\n feature_store_table\n)\n\nprint(\"Running \" + query_string)",
"_____no_output_____"
],
[
"feature_store_query.run(\n query_string=query_string,\n output_location=\"s3://\" + bucket + \"/\" + feature_store_offline_prefix + \"/query_results/\",\n)\n\nfeature_store_query.wait()",
"_____no_output_____"
],
[
"feature_store_query.as_dataframe()",
"_____no_output_____"
]
],
[
[
"# Show the Experiment Tracking Lineage",
"_____no_output_____"
]
],
[
[
"from sagemaker.analytics import ExperimentAnalytics\n\nimport pandas as pd\n\npd.set_option(\"max_colwidth\", 500)\n# pd.set_option(\"max_rows\", 100)\n\nexperiment_analytics = ExperimentAnalytics(\n sagemaker_session=sess, experiment_name=experiment_name, sort_by=\"CreationTime\", sort_order=\"Descending\"\n)\n\nexperiment_analytics_df = experiment_analytics.dataframe()\nexperiment_analytics_df",
"_____no_output_____"
],
[
"trial_component_name = experiment_analytics_df.TrialComponentName[0]\nprint(trial_component_name)",
"_____no_output_____"
],
[
"trial_component_description = sm.describe_trial_component(TrialComponentName=trial_component_name)\ntrial_component_description",
"_____no_output_____"
]
],
[
[
"# Show SageMaker ML Lineage Tracking \n\nAmazon SageMaker ML Lineage Tracking creates and stores information about the steps of a machine learning (ML) workflow from data preparation to model deployment. \n\nAmazon SageMaker Lineage enables events that happen within SageMaker to be traced via a graph structure. The data simplifies generating reports, making comparisons, or discovering relationships between events. For example easily trace both how a model was generated and where the model was deployed.\n\nThe lineage graph is created automatically by SageMaker and you can directly create or modify your own graphs.\n\n## Key Concepts\n\n* **Lineage Graph** - A connected graph tracing your machine learning workflow end to end.\n\n* **Artifacts** - Represents a URI addressable object or data. Artifacts are typically inputs or outputs to Actions.\n\n* **Actions** - Represents an action taken such as a computation, transformation, or job.\n\n* **Contexts** - Provides a method to logically group other entities.\n\n* **Associations** - A directed edge in the lineage graph that links two entities.\n\n* **Lineage Traversal** - Starting from an arbitrary point trace the lineage graph to discover and analyze relationships between steps in your workflow.\n\n* **Experiments** - Experiment entites (Experiments, Trials, and Trial Components) are also part of the lineage graph and can be associated wtih Artifacts, Actions, or Contexts.",
"_____no_output_____"
],
[
"## Show Lineage Artifacts For Our Processing Job",
"_____no_output_____"
]
],
[
[
"from sagemaker.lineage.visualizer import LineageTableVisualizer\n\nlineage_table_viz = LineageTableVisualizer(sess)\nlineage_table_viz_df = lineage_table_viz.show(processing_job_name=scikit_processing_job_name)\nlineage_table_viz_df",
"_____no_output_____"
]
],
[
[
"# Release Resources",
"_____no_output_____"
]
],
[
[
"%%html\n\n<p><b>Shutting down your kernel for this notebook to release resources.</b></p>\n<button class=\"sm-command-button\" data-commandlinker-command=\"kernelmenu:shutdown\" style=\"display:none;\">Shutdown Kernel</button>\n \n<script>\ntry {\n els = document.getElementsByClassName(\"sm-command-button\");\n els[0].click();\n}\ncatch(err) {\n // NoOp\n} \n</script>",
"_____no_output_____"
],
[
"%%javascript\n\ntry {\n Jupyter.notebook.save_checkpoint();\n Jupyter.notebook.session.delete();\n}\ncatch(err) {\n // NoOp\n}",
"_____no_output_____"
]
]
] | [
"markdown",
"code",
"markdown",
"code",
"markdown",
"code",
"markdown",
"code",
"markdown",
"code",
"markdown",
"code",
"markdown",
"code",
"markdown",
"code",
"markdown",
"code",
"markdown",
"code",
"markdown",
"code",
"markdown",
"code",
"markdown",
"code",
"markdown",
"code",
"markdown",
"code",
"markdown",
"code"
] | [
[
"markdown",
"markdown",
"markdown",
"markdown",
"markdown"
],
[
"code"
],
[
"markdown"
],
[
"code",
"code",
"code",
"code",
"code",
"code",
"code",
"code"
],
[
"markdown",
"markdown"
],
[
"code"
],
[
"markdown",
"markdown",
"markdown",
"markdown"
],
[
"code"
],
[
"markdown"
],
[
"code"
],
[
"markdown"
],
[
"code",
"code",
"code",
"code",
"code"
],
[
"markdown"
],
[
"code",
"code",
"code",
"code",
"code"
],
[
"markdown"
],
[
"code"
],
[
"markdown"
],
[
"code",
"code",
"code",
"code",
"code",
"code"
],
[
"markdown"
],
[
"code",
"code"
],
[
"markdown",
"markdown"
],
[
"code",
"code",
"code",
"code"
],
[
"markdown"
],
[
"code",
"code",
"code",
"code",
"code",
"code",
"code",
"code",
"code",
"code",
"code",
"code"
],
[
"markdown"
],
[
"code",
"code",
"code",
"code",
"code"
],
[
"markdown"
],
[
"code",
"code",
"code"
],
[
"markdown",
"markdown"
],
[
"code"
],
[
"markdown"
],
[
"code",
"code"
]
] |
e787400c8a10086472308a93f0a442398c525a90 | 693,157 | ipynb | Jupyter Notebook | Tea Sachets.ipynb | wordjelly/100-Scikit-Image-Notebooks | b849b37c08d39152ddba21ac40c24f52cb2a67dd | [
"Apache-2.0"
] | null | null | null | Tea Sachets.ipynb | wordjelly/100-Scikit-Image-Notebooks | b849b37c08d39152ddba21ac40c24f52cb2a67dd | [
"Apache-2.0"
] | null | null | null | Tea Sachets.ipynb | wordjelly/100-Scikit-Image-Notebooks | b849b37c08d39152ddba21ac40c24f52cb2a67dd | [
"Apache-2.0"
] | null | null | null | 2,014.991279 | 346,468 | 0.961887 | [
[
[
"## Imports\nimport numpy as np\nimport matplotlib.pyplot as plt\nimport skimage\nfrom skimage.filters import threshold_otsu\nfrom skimage import data\nfrom skimage.exposure import histogram\nfrom skimage.color import label2rgb\nimport matplotlib.patches as mpatches\nfrom skimage.measure import label, regionprops\nimport scipy.ndimage as ndimage\nimport scipy.ndimage.filters as filters\nfrom skimage.morphology import closing, erosion\nfrom skimage.io import imread, imsave, imshow, show, imread_collection, imshow_collection\nfrom skimage.exposure import histogram",
"_____no_output_____"
],
[
"## Viewing the image\nimage = imread(\"./images/tea_sachets.jpeg\")\nplt.figure(figsize=(10,5))\nplt.imshow(image)\nplt.axis('off')\nplt.title(\"Tea Sachets on The Floor\")\nplt.tight_layout();\nplt.show();",
"_____no_output_____"
],
[
"## Viewing the different channels\nimage_red = image[:,:,0]\nimage_green = image[:,:,1]\nimage_blue = image[:,:,2]\nfig,axes = plt.subplots(1,3,figsize=(10,5))\naxes[0].imshow(image_red);\naxes[0].set_title(\"red\");\naxes[1].imshow(image_green);\naxes[1].set_title(\"green\");\naxes[2].imshow(image_blue);\naxes[2].set_title(\"blue\");\nfor a in axes:\n a.axis('off')\nplt.tight_layout();\nplt.show();",
"_____no_output_____"
],
[
"## Viewing histograms of each channel\nred_histogram,red_centers = histogram(image_red)\ngreen_histogram,green_centers = histogram(image_green)\nblue_histogram,blue_centers = histogram(image_blue)\nfig,axes = plt.subplots(2,3,figsize=(10,5))\naxes[0,0].set_title(\"red image\");\naxes[0,0].imshow(image_red);\naxes[1,0].set_title(\"red histogram\");\naxes[1,0].plot(red_centers,red_histogram,lw=2);\n\naxes[0,1].set_title(\"green image\");\naxes[0,1].imshow(image_green);\naxes[1,1].set_title(\"green histogram\");\naxes[1,1].plot(green_centers,green_histogram,lw=2);\n\naxes[0,2].set_title(\"blue image\");\naxes[0,2].imshow(image_blue);\naxes[1,2].set_title(\"blue histogram\");\naxes[1,2].plot(blue_centers,blue_histogram,lw=2);\n\nplt.tight_layout();\nplt.show();",
"_____no_output_____"
],
[
"## Why the Blue Channel\n## consider all pixels in the blue channel with an intensity less than 10\nimage_blue = imread(\"./images/tea_sachets.jpeg\")[:,:,2]\ngray = skimage.color.rgb2gray(image_blue)\nthresh = threshold_otsu(gray)\nprint(thresh)\nthresh = 110\ngray[gray < thresh] = 1\ngray[gray >= thresh] = 0\nplt.figure(figsize=(10,5))\nplt.imshow(gray,cmap='gray')\nplt.show()",
"110\n"
],
[
"## Viewing Pixels above and below the threshold, and why the threshold matters.",
"_____no_output_____"
],
[
"## How closing works on a simple black and white image",
"_____no_output_____"
],
[
"## How erosion works on a simple black and white image\n## why did we set the sachets to white and the background to black ?\n## what would we do if it was the other way around?\n## ",
"_____no_output_____"
],
[
"## THE FINAL CODE\n## PROGRAM BEGINS HERE.\n## FOR A VIDEO EXPLANATION OF THIS NOTEBOOK, VISIT OUR YOUTUBE CHANNEL:\n## https://www.youtube.com/\n\n## START\n## -----\n\n## read the image\nimage = imread(\"./images/tea_sachets.jpeg\");\n\n## keep only the blue channel -> it has the maximum contrast, based on the color\n## of the items we want to detect\nimg = image[:,:,2]\n\n## convert the image to grayscale\nimg = skimage.color.rgb2gray(img);\n\n## compute a simple otsu threshold\nthresh = threshold_otsu(img);\n\nprint(\"otsu threshold:\" + str(thresh))\n## anything below the threshold - set to 1 (this sets all the packets, and the \n## the lines in the floor tiles to 1)\nimg[img < thresh] = 1\nimg[img >= thresh] = 0\n\n\n## perform a closing step.\nimg = closing(img,square(3))\n\n## perform 3 consecutive erosions, this helps to delineate the packets which are touching \n## the flooring lines.\ne1 = erosion(img)\ne2 = erosion(e1)\ne3 = erosion(e2)\n\nfig,axes = plt.subplots(1,3,figsize=(10,5))\naxes[0].imshow(e1,cmap=\"gray\");\naxes[0].set_title(\"first erosion - lots of lines\");\naxes[1].imshow(e2,cmap=\"gray\");\naxes[1].set_title(\"second erosion - some lines disappearing\");\naxes[2].imshow(e3,cmap=\"gray\");\naxes[2].set_title(\"third erosion - all lines gone\");\nfor a in axes:\n a.axis('off')\nplt.tight_layout();\nplt.show();\n\n\n## call label on the resulting image.\nlabelled = label(e3)\n\n## show the labels with rectangles, overlay on the original image, filtering rectangles that\n## are greater than 1000 pixels, this removes one or two noisy features.\nimage_label_overlay = label2rgb(labelled, image=image, bg_label=0)\nfig, ax = plt.subplots()\nax.imshow(image)\n\nfor region in regionprops(labelled):\n if region.area >= 1000:\n minr, minc, maxr, maxc = region.bbox\n rect = mpatches.Rectangle((minc, minr), maxc - minc, maxr - minr,\n fill=False, edgecolor='red', linewidth=2)\n ax.add_patch(rect)\n\nax.set_axis_off()\nplt.title(\"segmented\")\nplt.tight_layout()\nplt.show()",
"otsu threshold:110\n"
]
]
] | [
"code"
] | [
[
"code",
"code",
"code",
"code",
"code",
"code",
"code",
"code",
"code"
]
] |
e78758a4b059abb1b37a85038b19ba64527cf77a | 5,023 | ipynb | Jupyter Notebook | Midterm_Exam.ipynb | JohnAngeloDazo/CPEN-21A-ECE-2-1 | 91d185b470e096a28ed312c3ba5435409ece0b0c | [
"Apache-2.0"
] | null | null | null | Midterm_Exam.ipynb | JohnAngeloDazo/CPEN-21A-ECE-2-1 | 91d185b470e096a28ed312c3ba5435409ece0b0c | [
"Apache-2.0"
] | null | null | null | Midterm_Exam.ipynb | JohnAngeloDazo/CPEN-21A-ECE-2-1 | 91d185b470e096a28ed312c3ba5435409ece0b0c | [
"Apache-2.0"
] | null | null | null | 25.115 | 240 | 0.406132 | [
[
[
"<a href=\"https://colab.research.google.com/github/JohnAngeloDazo/CPEN-21A-ECE-2-1/blob/main/Midterm_Exam.ipynb\" target=\"_parent\"><img src=\"https://colab.research.google.com/assets/colab-badge.svg\" alt=\"Open In Colab\"/></a>",
"_____no_output_____"
],
[
"#Midterm Exam",
"_____no_output_____"
],
[
"##PROBLEMSTATEMENT 1",
"_____no_output_____"
]
],
[
[
"a=\"John Angelo A. Dazo\"\nb=\"202012935\"\nc=\"19 years old\"\nd=\"June 09, 2002\"\ne=\"Blk 9 Lot 8 Persan Village, Sanja Mayor, Tanza, Cavite\"\nf=\"Programming Logic and Design\"\ng=\"1.33\"\n\nprint(\"Full Name: \"+a)\nprint(\"Student Number: \"+b)\nprint(\"Age: \"+c)\nprint(\"Birthday: \"+d)\nprint(\"Address: \"+e)\nprint(\"Course: \"+f)\nprint(\"Last Sem GWA: \"+g)",
"Full Name: John Angelo A. Dazo\nStudent Number: 202012935\nAge: 19 years old\nBirthday: June 09, 2002\nAddress: Blk 9 Lot 8 Persan Village, Sanja Mayor, Tanza, Cavite\nCourse: Programming Logic and Design\nLast Sem GWA: 1.33\n"
]
],
[
[
"##PROBLEMSTATEMENT 2",
"_____no_output_____"
]
],
[
[
"n=4 \nansw=\"Y\"\n\nprint(bool(2<n)and(n<6)) #a\nprint(bool(2<n)or(n==6)) #b\nprint(bool(not(2<n)or(n==6)))#c\nprint(bool(not(n<6))) #d\nprint(bool(answ==\"Y\")or(answ==\"y\")) #e\nprint(bool(answ==\"Y\")and(answ==\"y\")) #f\nprint(bool(not(answ==\"y\"))) #g\nprint(bool((2<n)and(n==5+1))or(answ==\"No\")) #h\nprint(bool((n==2)and(n==7))or(answ==\"Y\")) #i\nprint(bool(n==2)and((n==7)or(answ==\"Y\"))) #j",
"True\nTrue\nFalse\nFalse\nTrue\nFalse\nTrue\nFalse\nTrue\nFalse\n"
]
],
[
[
"##PROBLEMSTATEMENT 3",
"_____no_output_____"
]
],
[
[
"x=2\ny=-3\nw=7\nz=-10\n\nprint(x/y) #a\nprint(w/y/x) #b\nprint(z/y%x) #c\nprint(x%-y*w) #d\nprint(x%y) #e\nprint(z%w-y/x*5+5) #f\nprint(9-x%(2+y)) #g\nprint(z//w) #h\nprint((2+y)**2) #i\nprint(w/x*2) #j",
"-0.6666666666666666\n-1.1666666666666667\n1.3333333333333335\n14\n-1\n16.5\n9\n-2\n1\n7.0\n"
]
]
] | [
"markdown",
"code",
"markdown",
"code",
"markdown",
"code"
] | [
[
"markdown",
"markdown",
"markdown"
],
[
"code"
],
[
"markdown"
],
[
"code"
],
[
"markdown"
],
[
"code"
]
] |
e7876293ec2ca02ff809ab46dac24e81a9dd09fc | 301,916 | ipynb | Jupyter Notebook | examples/Pipeline.ipynb | Ricram2/Advanced-Lane-Lines-Detction-CarND- | fc4fe5de4a3693ef86e16d2893eab1c43736c307 | [
"MIT"
] | null | null | null | examples/Pipeline.ipynb | Ricram2/Advanced-Lane-Lines-Detction-CarND- | fc4fe5de4a3693ef86e16d2893eab1c43736c307 | [
"MIT"
] | null | null | null | examples/Pipeline.ipynb | Ricram2/Advanced-Lane-Lines-Detction-CarND- | fc4fe5de4a3693ef86e16d2893eab1c43736c307 | [
"MIT"
] | null | null | null | 535.312057 | 148,052 | 0.934399 | [
[
[
"import numpy as np\nimport cv2\nimport glob\nimport matplotlib.pyplot as plt\nimport matplotlib.image as mpimg\nfrom moviepy.editor import VideoFileClip\nfrom IPython.display import HTML\nimport os\nos.listdir(\"../test_images/\")\n%matplotlib inline",
"_____no_output_____"
],
[
"def calibrate(images):\n\n # prepare object points, like (0,0,0), (1,0,0), (2,0,0) ....,(6,5,0)\n objp = np.zeros((6*9,3), np.float32)\n objp[:,:2] = np.mgrid[0:9,0:6].T.reshape(-1,2)\n\n # Arrays to store object points and image points from all the images.\n objpoints = [] # 3d points in real world space\n imgpoints = [] # 2d points in image plane.\n\n # Make a list of calibration images\n images = glob.glob(images)\n\n # Step through the list and search for chessboard corners\n for fname in images:\n img = cv2.imread(fname)\n gray = cv2.cvtColor(img,cv2.COLOR_BGR2GRAY)\n # Find the chessboard corners\n ret, corners = cv2.findChessboardCorners(gray, (9,6),None)\n # If found, add object points, image points\n if ret == True:\n objpoints.append(objp)\n imgpoints.append(corners)\n img = cv2.drawChessboardCorners(img, (9,6), corners, ret)\n ret, mtx, dist, rvecs, tvecs = cv2.calibrateCamera(objpoints, imgpoints, gray.shape[::-1], None, None)\n #Extract Numbers to calibrate \n\n return ret, mtx, dist, rvecs, tvecs\n\ndef undistort(img, mtx, dist):\n \n dst = cv2.undistort(img, mtx, dist, None, mtx)\n img = cv2.cvtColor(img, cv2.COLOR_BGR2RGB)\n\n return dst\n\n\ndef wraping(img):\n \n img_size = (img.shape[1], img.shape[0])\n \n #Source coord\n src = np.float32(\n [[600,450],#Upper left\n [730,450],# Upper Right\n [200,720], #Bottom left\n [1240,720] # Bottom right \n ])\n \n #Desired coord\n \n dst = np.float32(\n [[460,0],#Upper left \n [990,0],#Upper Right\n [420,720],#Bottom left\n [900,720]# Bottom right \n ])\n \n M = cv2.getPerspectiveTransform(src, dst)\n warped = cv2.warpPerspective(img, M, img_size, flags=cv2.INTER_LINEAR)\n \n return warped\n\ndef detection(img, s_thresh=(90, 255), sx_thresh=(50, 100)):\n \n #Make img copy\n img = np.copy(img)\n \n #Gaussian to reduce noise\n img = cv2.GaussianBlur(img, (5, 5), 0)\n\n # Convert to HLS color space and separate the V channel\n hls = cv2.cvtColor(img, cv2.COLOR_RGB2HLS)\n l_channel = hls[:,:,1]\n s_channel = hls[:,:,2]\n \n # Sobel x\n sobelx = cv2.Sobel(l_channel, cv2.CV_64F, 1, 0) # Take the derivative in x\n abs_sobelx = np.absolute(sobelx) # Absolute x derivative to accentuate lines away from horizontal\n scaled_sobel = np.uint8(255*abs_sobelx/np.max(abs_sobelx))\n \n # Threshold x gradient\n sxbinary = np.zeros_like(scaled_sobel)\n sxbinary[(scaled_sobel >= sx_thresh[0]) & (scaled_sobel <= sx_thresh[1])] = 1\n \n # Threshold color channel\n s_binary = np.zeros_like(s_channel)\n s_binary[(s_channel >= s_thresh[0]) & (s_channel <= s_thresh[1])] = 1\n \n # Stack each channel\n color_binary = np.dstack(( np.zeros_like(sxbinary), sxbinary, s_binary))\n combined_binary = np.zeros_like(sxbinary)\n combined_binary[(s_binary == 1) | (sxbinary == 1)] = 1\n \n return combined_binary\n\ndef hist(img):\n \n # Grab only the bottom half of the image\n # Lane lines are likely to be mostly vertical nearest to the car\n bottom_half = img[img.shape[0]//2:,:]\n\n # Sum across image pixels vertically - make sure to set an `axis`\n # i.e. the highest areas of vertical lines should be larger values\n histogram = np.sum(bottom_half, axis=0)\n \n return histogram\n\ndef find_lane_pixels(binary_warped):\n \n # Take a histogram of the bottom half of the image\n histogram = np.sum(binary_warped[binary_warped.shape[0]//2:,:], axis=0)\n \n # Create an output image to draw on and visualize the result\n out_img = np.dstack((binary_warped, binary_warped, binary_warped))\n \n # Find the peak of the left and right halves of the histogram\n # These will be the starting point for the left and right lines\n midpoint = np.int(histogram.shape[0]//2)\n leftx_base = np.argmax(histogram[:midpoint])\n rightx_base = np.argmax(histogram[midpoint:]) + midpoint\n\n # HYPERPARAMETERS\n # Choose the number of sliding windows\n nwindows = 15\n # Set the width of the windows +/- margin\n margin = 90\n # Set minimum number of pixels found to recenter window\n minpix = 100\n\n # Set height of windows - based on nwindows above and image shape\n window_height = np.int(binary_warped.shape[0]//nwindows)\n \n # Identify the x and y positions of all nonzero pixels in the image\n nonzero = binary_warped.nonzero()\n nonzeroy = np.array(nonzero[0])\n nonzerox = np.array(nonzero[1])\n \n # Current positions to be updated later for each window in nwindows\n leftx_current = leftx_base\n rightx_current = rightx_base\n\n # Create empty lists to receive left and right lane pixel indices\n left_lane_inds = []\n right_lane_inds = []\n\n # Step through the windows one by one\n for window in range(nwindows):\n # Identify window boundaries in x and y (and right and left)\n win_y_low = binary_warped.shape[0] - (window+1)*window_height\n win_y_high = binary_warped.shape[0] - window*window_height\n win_xleft_low = leftx_current - margin\n win_xleft_high = leftx_current + margin\n win_xright_low = rightx_current - margin\n win_xright_high = rightx_current + margin\n \n # Draw the windows on the visualization image\n cv2.rectangle(out_img,(win_xleft_low,win_y_low),\n (win_xleft_high,win_y_high),(0,255,0), 2) \n cv2.rectangle(out_img,(win_xright_low,win_y_low),\n (win_xright_high,win_y_high),(0,255,0), 2) \n \n # Identify the nonzero pixels in x and y within the window #\n good_left_inds = ((nonzeroy >= win_y_low) & (nonzeroy < win_y_high) & \n (nonzerox >= win_xleft_low) & (nonzerox < win_xleft_high)).nonzero()[0]\n good_right_inds = ((nonzeroy >= win_y_low) & (nonzeroy < win_y_high) & \n (nonzerox >= win_xright_low) & (nonzerox < win_xright_high)).nonzero()[0]\n \n # Append these indices to the lists\n left_lane_inds.append(good_left_inds)\n right_lane_inds.append(good_right_inds)\n \n # If you found > minpix pixels, recenter next window on their mean position\n if len(good_left_inds) > minpix:\n leftx_current = np.int(np.mean(nonzerox[good_left_inds]))\n if len(good_right_inds) > minpix: \n rightx_current = np.int(np.mean(nonzerox[good_right_inds]))\n\n # Concatenate the arrays of indices (previously was a list of lists of pixels)\n try:\n left_lane_inds = np.concatenate(left_lane_inds)\n right_lane_inds = np.concatenate(right_lane_inds)\n except ValueError:\n # Avoids an error if the above is not implemented fully\n pass\n\n # Extract left and right line pixel positions\n leftx = nonzerox[left_lane_inds]\n lefty = nonzeroy[left_lane_inds] \n rightx = nonzerox[right_lane_inds]\n righty = nonzeroy[right_lane_inds]\n\n return leftx, lefty, rightx, righty, out_img\n\n\ndef fit_polynomial(binary_warped):\n # Find our lane pixels first\n leftx, lefty, rightx, righty, out_img = find_lane_pixels(binary_warped)\n\n # Fit a second order polynomial to each using `np.polyfit`\n left_fit = np.polyfit(lefty, leftx, 2)\n right_fit = np.polyfit(righty, rightx, 2)\n\n # Generate x and y values for plotting\n ploty = np.linspace(0, binary_warped.shape[0]-1, binary_warped.shape[0] )\n try:\n left_fitx = left_fit[0]*ploty**2 + left_fit[1]*ploty + left_fit[2]\n right_fitx = right_fit[0]*ploty**2 + right_fit[1]*ploty + right_fit[2]\n except TypeError:\n # Avoids an error if `left` and `right_fit` are still none or incorrect\n print('The function failed to fit a line!')\n left_fitx = 1*ploty**2 + 1*ploty\n right_fitx = 1*ploty**2 + 1*ploty\n\n ## Visualization ##\n # Colors in the left and right lane regions\n \n out_img[lefty, leftx] = [255, 0, 0]\n out_img[righty, rightx] = [0, 0, 255]\n\n return out_img\n\ndef fit_poly(img_shape, leftx, lefty, rightx, righty):\n \n # Fit a second order polynomial to each with np.polyfit() ###\n left_fit = np.polyfit(lefty, leftx, 2)\n right_fit = np.polyfit(righty, rightx, 2)\n \n # Generate x and y values for plotting\n ploty = np.linspace(0, img_shape[0]-1, img_shape[0])\n \n #Calc both polynomials using ploty, left_fit and right_fit ###\n left_fitx = left_fit[0]*ploty**2 + left_fit[1]*ploty + left_fit[2]\n right_fitx = right_fit[0]*ploty**2 + right_fit[1]*ploty + right_fit[2]\n\n return left_fitx, right_fitx, ploty\n\ndef search_around_poly(binary_warped):\n leftx, lefty, rightx, righty, out_img = find_lane_pixels(binary_warped)\n\n # Fit a second order polynomial to each using `np.polyfit`\n left_fit = np.polyfit(lefty, leftx, 2)\n right_fit = np.polyfit(righty, rightx, 2)\n \n \n # HYPERPARAMETER\n # Choose the width of the margin around the previous polynomial to search\n margin = 100\n\n # Grab activated pixels\n nonzero = binary_warped.nonzero()\n nonzeroy = np.array(nonzero[0])\n nonzerox = np.array(nonzero[1])\n \n ### TO-DO: Set the area of search based on activated x-values ###\n left_lane_inds = ((nonzerox > (left_fit[0]*(nonzeroy**2) + left_fit[1]*nonzeroy + \n left_fit[2] - margin)) & (nonzerox < (left_fit[0]*(nonzeroy**2) + \n left_fit[1]*nonzeroy + left_fit[2] + margin)))\n right_lane_inds = ((nonzerox > (right_fit[0]*(nonzeroy**2) + right_fit[1]*nonzeroy + \n right_fit[2] - margin)) & (nonzerox < (right_fit[0]*(nonzeroy**2) + \n right_fit[1]*nonzeroy + right_fit[2] + margin)))\n \n # Again, extract left and right line pixel positions\n leftx = nonzerox[left_lane_inds]\n lefty = nonzeroy[left_lane_inds] \n rightx = nonzerox[right_lane_inds]\n righty = nonzeroy[right_lane_inds]\n\n # Fit new polynomials\n left_fitx, right_fitx, ploty = fit_poly(binary_warped.shape, leftx, lefty, rightx, righty)\n \n ## Visualization ##\n # Create an image to draw on and an image to show the selection window\n out_img = np.dstack((binary_warped, binary_warped, binary_warped))*255\n window_img = np.zeros_like(out_img)\n \n # Color in left and right line pixels\n out_img[nonzeroy[left_lane_inds], nonzerox[left_lane_inds]] = [255, 0, 0]\n out_img[nonzeroy[right_lane_inds], nonzerox[right_lane_inds]] = [0, 0, 255]\n\n # Generate a polygon to illustrate the search window area\n # And recast the x and y points into usable format for cv2.fillPoly()\n left_line_window1 = np.array([np.transpose(np.vstack([left_fitx-margin, ploty]))])\n left_line_window2 = np.array([np.flipud(np.transpose(np.vstack([left_fitx+margin, \n ploty])))])\n left_line_pts = np.hstack((left_line_window1, left_line_window2))\n right_line_window1 = np.array([np.transpose(np.vstack([right_fitx-margin, ploty]))])\n right_line_window2 = np.array([np.flipud(np.transpose(np.vstack([right_fitx+margin, \n ploty])))])\n right_line_pts = np.hstack((right_line_window1, right_line_window2))\n\n # Draw the lane onto the warped blank image\n cv2.fillPoly(window_img, np.int_([left_line_pts]), (0,255, 0))\n cv2.fillPoly(window_img, np.int_([right_line_pts]), (0,255, 0))\n \n warp_zero = np.zeros_like(binary_warped).astype(np.uint8)\n color_warp = np.dstack((warp_zero, warp_zero, warp_zero))\n \n pts_left = np.array([np.transpose(np.vstack([left_fitx, ploty]))])\n pts_right = np.array([np.flipud(np.transpose(np.vstack([right_fitx, ploty])))])\n pts = np.hstack((pts_left, pts_right))\n \n lane = cv2.fillPoly(color_warp, np.int_([pts]), (0,255, 0))\n result = cv2.addWeighted(out_img, 1, lane, 0.3, 0)\n \n #Stablish meters per pixel\n ym_per_pix = 30/720 # meters per pixel in y dimension\n xm_per_pix = 3.7/520 # meters per pixel in x dimension\n \n #Extract line between bottom points of the lane's arrays\n center_lane = (left_fitx[-1] + right_fitx[-1]) /2\n offset = (out_img.shape[1]/2 - center_lane)* xm_per_pix\n y_eval = np.max(ploty)\n \n # Calculation of Radius_curves (radius of curvature)\n left_curverad = ((1 + (2*left_fit[0]*y_eval*ym_per_pix + left_fit[1])**2)**1.5) / np.absolute(2*left_fit[0])\n right_curverad = ((1 + (2*right_fit[0]*y_eval*ym_per_pix + right_fit[1])**2)**1.5) / np.absolute(2*right_fit[0])\n \n # Calculation of average radius\n average = (left_curverad+right_curverad)/2\n average_km = average/1000\n \n return result, left_curverad, right_curverad, average_km, offset\n \n\ndef unwraping(img):\n \n img_size = (img.shape[1], img.shape[0])\n #Source coord\n src = np.float32(\n [[600,450],#Upper left\n [730,450],# Upper Right\n [200,720], #Bottom left\n [1240,720] # Bottom right \n ])\n \n #Desired coord\n \n dst = np.float32(\n [[460,0], \n [990,0],\n [420,720],#punto ancla inferiror\n [900,720]#punto ancla inferiror \n ])\n \n M = cv2.getPerspectiveTransform(dst, src)\n unwarped = cv2.warpPerspective(img, M, img_size, flags=cv2.INTER_LINEAR)\n \n return unwarped ",
"_____no_output_____"
],
[
"#Calibrate camera.\nret, mtx, dist, rvecs, tvecs = calibrate('../camera_cal/calibration*.jpg')\n#Get Font to be rendered.\nfont = cv2.FONT_HERSHEY_SIMPLEX",
"_____no_output_____"
],
[
"def pipeline(image): \n\n \n dst = undistort(image, mtx, dist)\n binary_warped = wraping(dst)\n gobeled = detection(binary_warped)\n out_img = fit_polynomial(gobeled)\n result, left_curverad, right_curverad, average_km, offset = search_around_poly(gobeled)\n consolidated = cv2.addWeighted(unwraping(result), 0.8, dst, 1, 0) \n \n #Print text over image\n cv2.putText(consolidated,'{}{}'.format(\"%.4f\"%average_km, \" Km Curve R\"),(100,100), font, 1,(255,255,255),2,cv2.LINE_AA)\n cv2.putText(consolidated,'{}{}'.format(\"%.3f\"%offset,\" m distance to lane's center\"),(100,150), font, 0.5,(255,255,255),2,cv2.LINE_AA) \n plt.imshow(consolidated)\n \n return consolidated",
"_____no_output_____"
],
[
"def pipelineimg (path_in):\n image = mpimg.imread(path_in)\n dst = undistort(image, mtx, dist)\n binary_warped = wraping(dst)\n gobeled = detection(binary_warped)\n out_img = fit_polynomial(gobeled)\n result, left_curverad, right_curverad, average_km, offset = search_around_poly(gobeled)\n consolidatedimg = cv2.addWeighted(unwraping(result), 0.8, dst, 1, 0) \n \n #Print text over image\n cv2.putText(consolidatedimg,'{}{}'.format(\"%.4f\"%average_km, \" Km Curve R\"),(100,100), font, 1,(255,255,255),2,cv2.LINE_AA)\n cv2.putText(consolidatedimg,'{}{}'.format(\"%.3f\"%offset,\" m distance to lane's center\"),(100,150), font, 0.5,(255,255,255),2,cv2.LINE_AA) \n plt.imshow(consolidatedimg)\n return (consolidatedimg)\n\n##### load all images and sve the results.\n\n\nTEST_IMAGES = \"../test_images\"\nOUTPUT_FOLDER = \"../output_images\"\n\npath = os.scandir(TEST_IMAGES)\n\n\nif not os.path.exists(OUTPUT_FOLDER):\n os.makedirs(OUTPUT_FOLDER)\n \nwhile True:\n try:\n image_path = next(path)\n img_out = pipelineimg(image_path.path) # image_path is actually a DirFile Object\n mpimg.imsave(os.path.join(OUTPUT_FOLDER, image_path.name), img_out)\n except StopIteration:\n break # Iterator exhausted: stop the loop\n \nprint(\"finished\")",
"finished\n"
],
[
"white_output = '../output_images/output.mp4'\nclip1 = VideoFileClip(\"../challenge_video.mp4\")#.subclip(0,3)\nwhite_clip = clip1.fl_image(pipeline)\n%time white_clip.write_videofile(white_output, audio=False)",
"[MoviePy] >>>> Building video ../output_images/output.mp4\n[MoviePy] Writing video ../output_images/output.mp4\n"
]
]
] | [
"code"
] | [
[
"code",
"code",
"code",
"code",
"code",
"code"
]
] |
e787696641c32e2a00bab0d11e8f5421048dfe1b | 431,470 | ipynb | Jupyter Notebook | notebooks/gpu-scibert-uncased.ipynb | aadarshsingh191198/AAAI-21-SDU-shared-task-2-AD | 118270b8306583295e9210a015b03f1bcf2eb78d | [
"MIT"
] | 1 | 2021-08-08T19:53:21.000Z | 2021-08-08T19:53:21.000Z | notebooks/gpu-scibert-uncased.ipynb | aadarshsingh191198/AAAI-21-SDU-shared-task-2-AD | 118270b8306583295e9210a015b03f1bcf2eb78d | [
"MIT"
] | null | null | null | notebooks/gpu-scibert-uncased.ipynb | aadarshsingh191198/AAAI-21-SDU-shared-task-2-AD | 118270b8306583295e9210a015b03f1bcf2eb78d | [
"MIT"
] | 1 | 2021-10-30T14:35:17.000Z | 2021-10-30T14:35:17.000Z | 31.393335 | 134 | 0.561675 | [
[
[
"! pip install transformers -q\n! pip install tokenizers -q",
"_____no_output_____"
],
[
"import re\nimport os\nimport sys\nimport json\nimport ast\nimport pandas as pd\nfrom pathlib import Path\nimport matplotlib.cm as cm\nimport numpy as np\nimport pandas as pd\nfrom typing import *\nfrom tqdm.notebook import tqdm\nfrom sklearn.utils.extmath import softmax\nfrom sklearn import model_selection\nfrom sklearn.metrics import classification_report, f1_score",
"_____no_output_____"
],
[
"import torch\nimport torch.optim as optim\nimport torch.nn as nn\nimport transformers\nfrom transformers import AdamW\nimport tokenizers",
"_____no_output_____"
],
[
"def seed_all(seed = 42):\n \"\"\"\n Fix seed for reproducibility\n \"\"\"\n # python RNG\n import random\n random.seed(seed)\n\n # pytorch RNGs\n import torch\n torch.manual_seed(seed)\n torch.backends.cudnn.deterministic = True\n if torch.cuda.is_available(): torch.cuda.manual_seed_all(seed)\n\n # numpy RNG\n import numpy as np\n np.random.seed(seed)",
"_____no_output_____"
],
[
"class config:\n SEED = 42\n KFOLD = 5\n TRAIN_FILE = '../input/sdu-shared/train.csv'\n VAL_FILE = '../input/sdu-shared/dev.csv'\n SAVE_DIR = '.'\n MAX_LEN = 192\n MODEL = '../input/scibert-uncased'\n TOKENIZER = tokenizers.BertWordPieceTokenizer(f\"{MODEL}/vocab.txt\", lowercase=True)\n EPOCHS = 5\n TRAIN_BATCH_SIZE = 32\n VALID_BATCH_SIZE = 32\n DICTIONARY = json.load(open('../input/sdu-shared/diction.json'))\n \n A2ID = {}\n for k, v in DICTIONARY.items():\n for w in v:\n A2ID[w] = len(A2ID)\n",
"_____no_output_____"
],
[
"class AverageMeter:\n \"\"\"\n Computes and stores the average and current value\n Source : https://www.kaggle.com/abhishek/bert-base-uncased-using-pytorch/\n \"\"\"\n def __init__(self):\n self.reset()\n\n def reset(self):\n self.val = 0\n self.avg = 0\n self.sum = 0\n self.count = 0\n\n def update(self, val, n=1):\n self.val = val\n self.sum += val * n\n self.count += n\n self.avg = self.sum / self.count",
"_____no_output_____"
],
[
"class EarlyStopping:\n \"\"\"\n Early stopping utility\n Source : https://www.kaggle.com/abhishek/bert-base-uncased-using-pytorch/\n \"\"\"\n \n def __init__(self, patience=7, mode=\"max\", delta=0.001):\n self.patience = patience\n self.counter = 0\n self.mode = mode\n self.best_score = None\n self.early_stop = False\n self.delta = delta\n if self.mode == \"min\":\n self.val_score = np.Inf\n else:\n self.val_score = -np.Inf\n\n def __call__(self, epoch_score, model, model_path):\n if self.mode == \"min\":\n score = -1.0 * epoch_score\n else:\n score = np.copy(epoch_score)\n if self.best_score is None:\n self.best_score = score\n self.save_checkpoint(epoch_score, model, model_path)\n elif score < self.best_score + self.delta:\n self.counter += 1\n print('EarlyStopping counter: {} out of {}'.format(self.counter, self.patience))\n if self.counter >= self.patience:\n self.early_stop = True\n else:\n self.best_score = score\n self.save_checkpoint(epoch_score, model, model_path)\n self.counter = 0\n\n def save_checkpoint(self, epoch_score, model, model_path):\n if epoch_score not in [-np.inf, np.inf, -np.nan, np.nan]:\n print('Validation score improved ({} --> {}). Saving model!'.format(self.val_score, epoch_score))\n torch.save(model.state_dict(), model_path)\n self.val_score = epoch_score",
"_____no_output_____"
],
[
"def sample_text(text, acronym, max_len):\n text = text.split()\n idx = text.index(acronym)\n left_idx = max(0, idx - max_len//2)\n right_idx = min(len(text), idx + max_len//2)\n sampled_text = text[left_idx:right_idx]\n return ' '.join(sampled_text)",
"_____no_output_____"
],
[
"def process_data(text, acronym, expansion, tokenizer, max_len):\n\n text = str(text)\n expansion = str(expansion)\n acronym = str(acronym)\n\n n_tokens = len(text.split())\n if n_tokens>120:\n text = sample_text(text, acronym, 120)\n\n answers = acronym + ' ' + ' '.join(config.DICTIONARY[acronym])\n start = answers.find(expansion)\n end = start + len(expansion)\n\n char_mask = [0]*len(answers)\n for i in range(start, end):\n char_mask[i] = 1\n \n tok_answer = tokenizer.encode(answers)\n answer_ids = tok_answer.ids\n answer_offsets = tok_answer.offsets\n\n answer_ids = answer_ids[1:-1]\n answer_offsets = answer_offsets[1:-1]\n\n target_idx = []\n for i, (off1, off2) in enumerate(answer_offsets):\n if sum(char_mask[off1:off2])>0:\n target_idx.append(i)\n\n start = target_idx[0]\n end = target_idx[-1]\n\n \n text_ids = tokenizer.encode(text).ids[1:-1]\n\n token_ids = [101] + answer_ids + [102] + text_ids + [102]\n offsets = [(0,0)] + answer_offsets + [(0,0)]*(len(text_ids) + 2)\n mask = [1] * len(token_ids)\n token_type = [0]*(len(answer_ids) + 1) + [1]*(2+len(text_ids))\n\n text = answers + text\n start = start + 1\n end = end + 1\n\n padding = max_len - len(token_ids)\n \n\n if padding>=0:\n token_ids = token_ids + ([0] * padding)\n token_type = token_type + [1] * padding\n mask = mask + ([0] * padding)\n offsets = offsets + ([(0, 0)] * padding)\n else:\n token_ids = token_ids[0:max_len]\n token_type = token_type[0:max_len]\n mask = mask[0:max_len]\n offsets = offsets[0:max_len]\n \n\n assert len(token_ids)==max_len\n assert len(mask)==max_len\n assert len(offsets)==max_len\n assert len(token_type)==max_len\n\n return {\n 'ids': token_ids,\n 'mask': mask,\n 'token_type': token_type,\n 'offset': offsets,\n 'start': start,\n 'end': end, \n 'text': text,\n 'expansion': expansion,\n 'acronym': acronym,\n }",
"_____no_output_____"
],
[
"class Dataset:\n def __init__(self, text, acronym, expansion):\n self.text = text\n self.acronym = acronym\n self.expansion = expansion\n self.tokenizer = config.TOKENIZER\n self.max_len = config.MAX_LEN\n \n def __len__(self):\n return len(self.text)\n\n def __getitem__(self, item):\n data = process_data(\n self.text[item],\n self.acronym[item],\n self.expansion[item], \n self.tokenizer,\n self.max_len,\n \n )\n\n return {\n 'ids': torch.tensor(data['ids'], dtype=torch.long),\n 'mask': torch.tensor(data['mask'], dtype=torch.long),\n 'token_type': torch.tensor(data['token_type'], dtype=torch.long),\n 'offset': torch.tensor(data['offset'], dtype=torch.long),\n 'start': torch.tensor(data['start'], dtype=torch.long),\n 'end': torch.tensor(data['end'], dtype=torch.long),\n 'text': data['text'],\n 'expansion': data['expansion'],\n 'acronym': data['acronym'],\n }",
"_____no_output_____"
],
[
"def get_loss(start, start_logits, end, end_logits):\n loss_fn = nn.CrossEntropyLoss()\n start_loss = loss_fn(start_logits, start)\n end_loss = loss_fn(end_logits, end)\n loss = start_loss + end_loss\n return loss",
"_____no_output_____"
],
[
"class BertAD(nn.Module):\n def __init__(self):\n super(BertAD, self).__init__()\n self.bert = transformers.BertModel.from_pretrained(config.MODEL, output_hidden_states=True)\n self.layer = nn.Linear(768, 2)\n \n\n def forward(self, ids, mask, token_type, start=None, end=None):\n output = self.bert(input_ids = ids,\n attention_mask = mask,\n token_type_ids = token_type)\n \n logits = self.layer(output[0]) \n start_logits, end_logits = logits.split(1, dim=-1)\n \n start_logits = start_logits.squeeze(-1)\n end_logits = end_logits.squeeze(-1)\n\n loss = get_loss(start, start_logits, end, end_logits) \n\n return loss, start_logits, end_logits",
"_____no_output_____"
],
[
"def train_fn(data_loader, model, optimizer, device):\n model.train()\n losses = AverageMeter()\n tk0 = tqdm(data_loader, total=len(data_loader))\n \n for bi, d in enumerate(tk0):\n ids = d['ids']\n mask = d['mask']\n token_type = d['token_type']\n start = d['start']\n end = d['end']\n \n\n ids = ids.to(device, dtype=torch.long)\n token_type = token_type.to(device, dtype=torch.long)\n mask = mask.to(device, dtype=torch.long)\n start = start.to(device, dtype=torch.long)\n end = end.to(device, dtype=torch.long)\n \n\n model.zero_grad()\n loss, start_logits, end_logits = model(ids, mask, token_type, start, end)\n \n loss.backward()\n optimizer.step()\n # xm.optimizer_step(optimizer, barrier=True)\n \n losses.update(loss.item(), ids.size(0))\n tk0.set_postfix(loss=losses.avg)\n",
"_____no_output_____"
],
[
"def jaccard(str1, str2): \n a = set(str1.lower().split()) \n b = set(str2.lower().split())\n c = a.intersection(b)\n return float(len(c)) / (len(a) + len(b) - len(c))",
"_____no_output_____"
],
[
"def evaluate_jaccard(text, selected_text, acronym, offsets, idx_start, idx_end):\n filtered_output = \"\"\n for ix in range(idx_start, idx_end + 1):\n filtered_output += text[offsets[ix][0]: offsets[ix][1]]\n if (ix+1) < len(offsets) and offsets[ix][1] < offsets[ix+1][0]:\n filtered_output += \" \"\n\n candidates = config.DICTIONARY[acronym]\n candidate_jaccards = [jaccard(w.strip(), filtered_output.strip()) for w in candidates]\n idx = np.argmax(candidate_jaccards)\n\n return candidate_jaccards[idx], candidates[idx]",
"_____no_output_____"
],
[
"def eval_fn(data_loader, model, device):\n model.eval()\n losses = AverageMeter()\n jac = AverageMeter()\n\n tk0 = tqdm(data_loader, total=len(data_loader))\n\n pred_expansion_ = []\n true_expansion_ = []\n\n for bi, d in enumerate(tk0):\n ids = d['ids']\n mask = d['mask']\n token_type = d['token_type']\n start = d['start']\n end = d['end']\n \n text = d['text']\n expansion = d['expansion']\n offset = d['offset']\n acronym = d['acronym']\n\n\n ids = ids.to(device, dtype=torch.long)\n mask = mask.to(device, dtype=torch.long)\n token_type = token_type.to(device, dtype=torch.long)\n start = start.to(device, dtype=torch.long)\n end = end.to(device, dtype=torch.long)\n \n with torch.no_grad():\n loss, start_logits, end_logits = model(ids, mask, token_type, start, end)\n\n\n start_prob = torch.softmax(start_logits, dim=1).detach().cpu().numpy()\n end_prob = torch.softmax(end_logits, dim=1).detach().cpu().numpy()\n \n \n jac_= []\n \n for px, s in enumerate(text):\n start_idx = np.argmax(start_prob[px,:])\n end_idx = np.argmax(end_prob[px,:])\n\n js, exp = evaluate_jaccard(s, expansion[px], acronym[px], offset[px], start_idx, end_idx)\n jac_.append(js)\n pred_expansion_.append(exp)\n true_expansion_.append(expansion[px])\n\n \n jac.update(np.mean(jac_), len(jac_))\n losses.update(loss.item(), ids.size(0))\n\n tk0.set_postfix(loss=losses.avg, jaccard=jac.avg)\n\n\n pred_expansion_ = [config.A2ID[w] for w in pred_expansion_]\n true_expansion_ = [config.A2ID[w] for w in true_expansion_]\n \n f1 = f1_score(true_expansion_, pred_expansion_, average='macro')\n\n print('Average Jaccard : ', jac.avg)\n print('Macro F1 : ', f1)\n\n return f1 \n ",
"_____no_output_____"
],
[
"def run(df_train, df_val, fold):\n train_dataset = Dataset(\n text = df_train.text.values,\n acronym = df_train.acronym_.values,\n expansion = df_train.expansion.values\n )\n \n valid_dataset = Dataset(\n text = df_val.text.values,\n acronym = df_val.acronym_.values,\n expansion = df_val.expansion.values,\n )\n \n train_data_loader = torch.utils.data.DataLoader(\n train_dataset,\n batch_size=config.TRAIN_BATCH_SIZE,\n num_workers=4\n )\n\n valid_data_loader = torch.utils.data.DataLoader(\n valid_dataset,\n batch_size=config.VALID_BATCH_SIZE,\n num_workers=2\n )\n \n\n model = BertAD()\n device = torch.device('cuda:0' if torch.cuda.is_available else 'cpu')\n model.to(device)\n\n lr = 2e-5\n param_optimizer = list(model.named_parameters())\n no_decay = ['bias', 'gamma', 'beta']\n optimizer_grouped_parameters = [\n {'params': [p for n, p in param_optimizer if not any(nd in n for nd in no_decay)],\n 'weight_decay_rate': 0.01},\n {'params': [p for n, p in param_optimizer if any(nd in n for nd in no_decay)],\n 'weight_decay_rate': 0.0}\n ]\n optimizer = AdamW(optimizer_grouped_parameters, lr=lr)\n\n es = EarlyStopping(patience=2, mode=\"max\")\n\n print('Starting training....')\n for epoch in range(config.EPOCHS):\n train_fn(train_data_loader, model, optimizer, device)\n valid_loss = eval_fn(valid_data_loader, model, device)\n print(f'Fold {fold} | Epoch :{epoch + 1} | Validation Score :{valid_loss}')\n if fold is None:\n es(valid_loss, model, model_path=os.path.join(config.SAVE_DIR, \"model.bin\"))\n else:\n es(valid_loss, model, model_path=os.path.join(config.SAVE_DIR, f\"model_{fold}.bin\"))\n if es.early_stop:\n break\n\n return es.best_score",
"_____no_output_____"
],
[
"def run_k_fold(fold_id):\n '''\n Perform k-fold cross-validation\n '''\n seed_all()\n\n df_train = pd.read_csv(config.TRAIN_FILE)\n df_val = pd.read_csv(config.VAL_FILE)\n \n # concatenating train and validation set\n train = pd.concat([df_train, df_val]).reset_index()\n \n # train = df_train\n \n # dividing folds\n kf = model_selection.StratifiedKFold(n_splits=config.KFOLD, shuffle=False, random_state=config.SEED)\n for fold, (train_idx, val_idx) in enumerate(kf.split(X=train, y=train.acronym_.values)):\n train.loc[val_idx, 'kfold'] = fold\n\n print(f'################################################ Fold {fold_id} #################################################')\n df_train = train[train.kfold!=fold_id]\n df_val = train[train.kfold==fold_id]\n\n return run(df_train, df_val, fold_id)\n ",
"_____no_output_____"
],
[
"f0 = run_k_fold(0)",
"_____no_output_____"
],
[
"f1 = run_k_fold(1)",
"_____no_output_____"
],
[
"f2 = run_k_fold(2)",
"_____no_output_____"
],
[
"f3 = run_k_fold(3)",
"_____no_output_____"
],
[
"f4 = run_k_fold(4)",
"_____no_output_____"
],
[
"f = [f0, f1, f2, f3, f4]\nfor i, fs in enumerate(f):\n print(f'Fold {i} : {fs}')\nprint(f'Avg. {np.mean(f)}')",
"_____no_output_____"
]
]
] | [
"code"
] | [
[
"code",
"code",
"code",
"code",
"code",
"code",
"code",
"code",
"code",
"code",
"code",
"code",
"code",
"code",
"code",
"code",
"code",
"code",
"code",
"code",
"code",
"code",
"code",
"code"
]
] |
e7876fa8140d7215802e525c2afa84460aefae0c | 5,497 | ipynb | Jupyter Notebook | train_rs19.ipynb | Denbergvanthijs/railsem19_yolov5 | c5edd04f7586eb1d1af22bb19bb128c4ccd8ea2b | [
"Apache-2.0"
] | 15 | 2022-01-23T15:09:40.000Z | 2022-02-04T16:51:16.000Z | train_rs19.ipynb | Denbergvanthijs/railsem19_yolov5 | c5edd04f7586eb1d1af22bb19bb128c4ccd8ea2b | [
"Apache-2.0"
] | null | null | null | train_rs19.ipynb | Denbergvanthijs/railsem19_yolov5 | c5edd04f7586eb1d1af22bb19bb128c4ccd8ea2b | [
"Apache-2.0"
] | 1 | 2022-01-25T10:38:23.000Z | 2022-01-25T10:38:23.000Z | 2,748.5 | 5,496 | 0.727124 | [
[
[
"# Train YOLOv5 on RailSem19\n\nThis notebook is based on the [YOLOv5 tutorial](https://colab.research.google.com/github/ultralytics/yolov5/blob/master/tutorial.ipynb). This notebook is designed to run in Google Colab.\n\n---\n\n## Setup\n\nClone YOLOv5, install dependencies and check PyTorch and GPU:",
"_____no_output_____"
]
],
[
[
"!git clone https://github.com/ultralytics/yolov5 # clone\n%cd yolov5\n%pip install -qr requirements.txt # install\n\nfrom yolov5 import utils\ndisplay = utils.notebook_init() # checks",
"_____no_output_____"
],
[
"%cd ../\n!git clone https://github.com/Denbergvanthijs/railsem19_yolov5.git",
"_____no_output_____"
]
],
[
[
"Load Tensorboard and Weights & Biases (both optional):",
"_____no_output_____"
]
],
[
[
"# Tensorboard (optional)\n# %load_ext tensorboard\n# %tensorboard --logdir runs/train",
"_____no_output_____"
],
[
"# Weights & Biases (optional)\n%pip install -q wandb\nimport wandb\nwandb.login()",
"_____no_output_____"
]
],
[
[
"Mount personal Google Drive:",
"_____no_output_____"
]
],
[
[
"from google.colab import drive\ndrive.mount('/content/drive')",
"_____no_output_____"
]
],
[
[
"Copy training data from Google Drive to local disk:",
"_____no_output_____"
]
],
[
[
"!mkdir data\n!cp ./drive/MyDrive/rs19_person_semseg ./data/rs19_person_semseg -r",
"_____no_output_____"
]
],
[
[
"## Training\n\nTo train a model with the hyperparameters used in the research paper:",
"_____no_output_____"
]
],
[
[
"!python ./yolov5/train.py --batch-size 64 --epochs 50 --data ./railsem19_yolov5/data/rs19_person_semseg.yaml --weights yolov5s.pt --single-cls --cache --hyp ./railsem19_yolov5/data/hyp_evolve.yaml",
"_____no_output_____"
]
],
[
[
"## Hyperparameter optimalisation\n\nThe following will start the hyperparameter optimalisation:",
"_____no_output_____"
]
],
[
[
"!python ./yolov5/train.py --batch-size 64 --epochs 10 --data ./railsem19_yolov5/data/rs19_person_semseg.yaml --weights yolov5s.pt --single-cls --cache --evolve 40 --hyp \"./railsem19_yolov5/data/hyp_evolve.yaml\"",
"_____no_output_____"
]
]
] | [
"markdown",
"code",
"markdown",
"code",
"markdown",
"code",
"markdown",
"code",
"markdown",
"code",
"markdown",
"code"
] | [
[
"markdown"
],
[
"code",
"code"
],
[
"markdown"
],
[
"code",
"code"
],
[
"markdown"
],
[
"code"
],
[
"markdown"
],
[
"code"
],
[
"markdown"
],
[
"code"
],
[
"markdown"
],
[
"code"
]
] |
e787af49da17da7d898a1de9647acdea0723b436 | 263,027 | ipynb | Jupyter Notebook | jordan_analysis.ipynb | kdesai2018/DSL-final-project | 229de83b9f2f962332cf494dcb46f6d1aa863d81 | [
"MIT"
] | null | null | null | jordan_analysis.ipynb | kdesai2018/DSL-final-project | 229de83b9f2f962332cf494dcb46f6d1aa863d81 | [
"MIT"
] | null | null | null | jordan_analysis.ipynb | kdesai2018/DSL-final-project | 229de83b9f2f962332cf494dcb46f6d1aa863d81 | [
"MIT"
] | null | null | null | 75.474032 | 32,544 | 0.730092 | [
[
[
"# Imports\nimport matplotlib as mpl\nimport pandas as pd\nimport numpy as np\nimport matplotlib.pyplot as plt\nimport re",
"_____no_output_____"
]
],
[
[
"# Importing and Setuping Up Dataset",
"_____no_output_____"
]
],
[
[
"df = pd.read_csv('all_games.csv', low_memory=False)\ndf.head()",
"_____no_output_____"
],
[
"# Remove uncalibrated games\ndf = df[df['White Rating'] != '?']\ndf = df[df['Black Rating'] != '?']",
"_____no_output_____"
],
[
"df = df.astype({\n 'White Rating': int,\n 'Black Rating': int,\n})",
"_____no_output_____"
],
[
"df['Rating Diff'] = abs(df['White Rating'] - df['Black Rating'])\ndf['Avg Rating'] = (df['White Rating'] + df['Black Rating']) / 2",
"_____no_output_____"
]
],
[
[
"# Rating Analysis",
"_____no_output_____"
]
],
[
[
"Rating_Ranges = [1200, 1400, 1600, 1800, 2000, 2200]",
"_____no_output_____"
],
[
"rating = df['White Rating'].append(df['Black Rating'], ignore_index=True)\nbins = [rating.min()] + Rating_Ranges + [rating.max()]\ndisplay(rating.value_counts(bins=bins, sort=False))\nrating.hist(bins=bins)",
"_____no_output_____"
],
[
"display(df['Rating Diff'].value_counts(bins=20, sort=False))\ndf['Rating Diff'].hist(bins=20)",
"_____no_output_____"
],
[
"avg_bins = [df['Avg Rating'].min()] + Rating_Ranges + [df['Avg Rating'].max()]\ndisplay(df['Avg Rating'].value_counts(bins=avg_bins, sort=False))\ndf['Avg Rating'].hist(bins=avg_bins)",
"_____no_output_____"
],
[
"display(df['Avg Rating'].value_counts(bins=3, sort=False))",
"_____no_output_____"
],
[
"df['Event'].value_counts()",
"_____no_output_____"
],
[
"df['Event'].unique()",
"_____no_output_____"
]
],
[
[
"# Opening Analysis",
"_____no_output_____"
]
],
[
[
"def opening_prune(op):\n split = re.split('[:#,]', op)\n return split[0].rstrip()\ndf['Opening Short'] = df['Opening'].apply(opening_prune)",
"_____no_output_____"
],
[
"df['Opening Short'].value_counts(normalize=True)\ncounts = df['Opening Short'].value_counts()\npercentage = df['Opening Short'].value_counts(normalize=True).mul(100)\nopen_stats = pd.DataFrame({'counts': counts, 'percentage':percentage})\nprint('Overall Most Popular Openings')\nopen_stats.head(10)",
"Overall Most Popular Openings\n"
],
[
"df_q = df[df['Opening Short'] == \"Queen's Pawn Game\"]\ndf_q['Opening'].value_counts()",
"_____no_output_____"
],
[
"df_k = df[df['Opening Short'] == \"King's Pawn Game\"]\ndf_k['Opening'].value_counts()",
"_____no_output_____"
],
[
"for event in df['Event'].unique():\n df_event = df[df['Event'] == event]\n print(f'Popular Openings in {event}')\n counts = df_event['Opening Short'].value_counts()\n percentage = df_event['Opening Short'].value_counts(normalize=True).mul(100)\n open_stats = pd.DataFrame({'counts': counts, 'percentage':percentage})\n display(open_stats.head(10))",
"Popular Openings in Rated Blitz game\n"
]
],
[
[
"# Rating Breakdown",
"_____no_output_____"
]
],
[
[
"Rating_Ranges = [1500, 2200, 2200]",
"_____no_output_____"
],
[
"df_novice = df[df['Avg Rating'] < 1500]\ndf_n = pd.DataFrame(df_novice['Opening Short'].value_counts(normalize=True)).reset_index()\ndf_n[df_n['index'] == 'Scandinavian Defense']\n#df_n.loc('Scandinavian Defense')",
"_____no_output_____"
],
[
"df_master = df[df['Avg Rating'] > 2200]\ndf_m = pd.DataFrame(df_master['Opening Short'].value_counts(normalize=True)).reset_index()\ndf_m[df_m['index'] == 'Scandinavian Defense']",
"_____no_output_____"
],
[
"df_mid = df[(df['Avg Rating'] > 1500) & (df['Avg Rating'] < 2200)]",
"_____no_output_____"
],
[
"Rating_Ranges = [1200, 1400, 1600, 1800, 2000, 2200, 2500, 2500]\nfor i in range(len(Rating_Ranges)):\n if(i == 0):\n df_rate = df[(df['White Rating'] < Rating_Ranges[i]) & (df['Black Rating'] < Rating_Ranges[i])]\n print(f'Popular Openings in Rating Range: 0 - {Rating_Ranges[i]}')\n elif(i == len(Rating_Ranges) - 1):\n df_rate = df[(df['White Rating'] > Rating_Ranges[i]) & (df['Black Rating'] > Rating_Ranges[i])]\n print(f'Popular Openings in Rating Range: >{Rating_Ranges[i]}')\n else:\n df_rate = df[(df['White Rating'] > Rating_Ranges[i-1]) & (df['Black Rating'] > Rating_Ranges[i-1]) &\n (df['White Rating'] < Rating_Ranges[i]) & (df['Black Rating'] < Rating_Ranges[i])]\n print(f'Popular Openings in Rating Range: {Rating_Ranges[i-1]} - {Rating_Ranges[i]}') \n counts = df_rate['Opening Short'].value_counts()\n percentage = df_rate['Opening Short'].value_counts(normalize=True).mul(100)\n open_stats = pd.DataFrame({'counts': counts, 'percentage':percentage})\n open_stats.index.name='Opening'\n open_stats = open_stats.reset_index()\n display(open_stats.head(10)) \n # display(open_stats[open_stats['Opening'] == \"King's Pawn Game\"])",
"Popular Openings in Rating Range: 0 - 1200\n"
]
],
[
[
"# Effectiveness of Top Openings in Top Level Play",
"_____no_output_____"
]
],
[
[
"df_top = df[(df['Avg Rating'] > 2500)]\ncounts = df_top['Opening Short'].value_counts()\npercentage = df_top['Opening Short'].value_counts(normalize=True).mul(100)\ntop_stats = pd.DataFrame({'counts': counts, 'percentage':percentage})\ndisplay(top_stats.head(5))",
"_____no_output_____"
],
[
"openings = ['Sicilian Defense', 'French Defense', 'English Opening', 'Nimzo-Larsen Attack', 'Zukertort Opening']\nfor op in openings:\n df_op = df_top[df_top['Opening Short'] == op]\n df_results = pd.DataFrame(df_op['Result'].value_counts(normalize=True))\n print(f'>2500 ELO: Results for {op}')\n display(df_results)",
">2500 ELO: Results for Sicilian Defense\n"
],
[
"ratings.min()",
"_____no_output_____"
],
[
"prev_i = 750\ndata = []\nfor i in range(800, 2900, 50):\n df_op = df[(df['Opening Short'] == 'Sicilian Defense') & (df['Avg Rating'] > prev_i) & (df['Avg Rating'] < i)]\n if df_op.empty:\n prev_i = i\n continue\n df_results = pd.DataFrame(df_op['Result'].value_counts(normalize=True))\n try:\n data.append({'Rating': i, 'White': df_results.loc['1-0']['Result'], 'Black': df_results.loc['0-1']['Result'], 'Draw': df_results.loc['1/2-1/2']['Result']})\n except KeyError:\n continue\n prev_i = i\ndf_sicilian = pd.DataFrame(data)",
"_____no_output_____"
],
[
"df_sicilian = df_sicilian.set_index('Rating')",
"_____no_output_____"
],
[
"df_sicilian['Black/Draw'] = df_sicilian['Black'] + df_sicilian['Draw']",
"_____no_output_____"
],
[
"df_sicilian.plot.line()",
"_____no_output_____"
],
[
"prev_i = 750\ndata = []\nfor i in range(800, 2900, 50):\n df_op = df[(df['Opening Short'] == 'Nimzo-Larsen Attack') & (df['Avg Rating'] > prev_i) & (df['Avg Rating'] < i)]\n if df_op.empty:\n prev_i = i\n continue\n df_results = pd.DataFrame(df_op['Result'].value_counts(normalize=True))\n try:\n data.append({'Rating': i, 'White': df_results.loc['1-0']['Result'], 'Black': df_results.loc['0-1']['Result'], 'Draw': df_results.loc['1/2-1/2']['Result']})\n except KeyError:\n continue\n prev_i = i\ndf_lar = pd.DataFrame(data)\ndf_lar = df_lar.set_index('Rating')\ndf_lar.plot.line()",
"_____no_output_____"
],
[
"df_lar.plot.line()\nplt.suptitle(\"Nimzo-Larsen Attack: Rating vs Results\")",
"_____no_output_____"
],
[
"df_sicilian.plot.line()\nplt.suptitle(\"Sicilian Defense: Rating vs Results\")",
"_____no_output_____"
],
[
"df_sicilian['Black vs White'] = df_sicilian['Black'] - df_sicilian['White']",
"_____no_output_____"
],
[
"df['Opening Short'].value_counts(normalize=True)\ncounts = df['Opening Short'].value_counts()\npercentage = df['Opening Short'].value_counts(normalize=True).mul(100)\nopen_stats = pd.DataFrame({'counts': counts, 'percentage':percentage})\nopen_stats.index.name='Opening'\nopen_stats = open_stats.reset_index()\nprint('Overall Most Popular Openings')\nopen_stats.head(21)",
"Overall Most Popular Openings\n"
],
[
"open_stats[open_stats['Opening'] == 'Nimzo-Larsen Attack']",
"_____no_output_____"
]
]
] | [
"code",
"markdown",
"code",
"markdown",
"code",
"markdown",
"code",
"markdown",
"code",
"markdown",
"code"
] | [
[
"code"
],
[
"markdown"
],
[
"code",
"code",
"code",
"code"
],
[
"markdown"
],
[
"code",
"code",
"code",
"code",
"code",
"code",
"code"
],
[
"markdown"
],
[
"code",
"code",
"code",
"code",
"code"
],
[
"markdown"
],
[
"code",
"code",
"code",
"code",
"code"
],
[
"markdown"
],
[
"code",
"code",
"code",
"code",
"code",
"code",
"code",
"code",
"code",
"code",
"code",
"code",
"code"
]
] |
e787b1cf247de8ce35bec412c2179d7cb7d092a2 | 11,343 | ipynb | Jupyter Notebook | math/Math24_Dot_Product.ipynb | HasanIjaz-HB/Quantum-Computing | 53c2df99cd2efbfb827857125991342f336a3097 | [
"MIT"
] | null | null | null | math/Math24_Dot_Product.ipynb | HasanIjaz-HB/Quantum-Computing | 53c2df99cd2efbfb827857125991342f336a3097 | [
"MIT"
] | null | null | null | math/Math24_Dot_Product.ipynb | HasanIjaz-HB/Quantum-Computing | 53c2df99cd2efbfb827857125991342f336a3097 | [
"MIT"
] | null | null | null | 31.5961 | 309 | 0.498281 | [
[
[
"<table>\n <tr>\n <td style=\"background-color:#ffffff;\"><a href=\"https://qsoftware.lu.lv/index.php/qworld/\" target=\"_blank\"><img src=\"..\\images\\qworld.jpg\" width=\"70%\" align=\"left\"></a></td>\n <td style=\"background-color:#ffffff;\" width=\"*\"></td>\n <td style=\"background-color:#ffffff;vertical-align:text-top;\"><a href=\"https://qsoftware.lu.lv\" target=\"_blank\"><img src=\"..\\images\\logo.jpg\" width=\"25%\" align=\"right\"></a></td> \n </tr>\n <tr><td colspan=\"3\" align=\"right\" style=\"color:#777777;background-color:#ffffff;font-size:12px;\">\n prepared by <a href=\"http://abu.lu.lv\" target=\"_blank\">Abuzer Yakaryilmaz</a>\n </td></tr>\n <tr><td colspan=\"3\" align=\"right\" style=\"color:#bbbbbb;background-color:#ffffff;font-size:11px;font-style:italic;\">\n This cell contains some macros. If there is a problem with displaying mathematical formulas, please run this cell to load these macros.\n </td></tr>\n</table>\n$ \\newcommand{\\bra}[1]{\\langle #1|} $\n$ \\newcommand{\\ket}[1]{|#1\\rangle} $\n$ \\newcommand{\\braket}[2]{\\langle #1|#2\\rangle} $\n$ \\newcommand{\\dot}[2]{ #1 \\cdot #2} $\n$ \\newcommand{\\biginner}[2]{\\left\\langle #1,#2\\right\\rangle} $\n$ \\newcommand{\\mymatrix}[2]{\\left( \\begin{array}{#1} #2\\end{array} \\right)} $\n$ \\newcommand{\\myvector}[1]{\\mymatrix{c}{#1}} $\n$ \\newcommand{\\myrvector}[1]{\\mymatrix{r}{#1}} $\n$ \\newcommand{\\mypar}[1]{\\left( #1 \\right)} $\n$ \\newcommand{\\mybigpar}[1]{ \\Big( #1 \\Big)} $\n$ \\newcommand{\\sqrttwo}{\\frac{1}{\\sqrt{2}}} $\n$ \\newcommand{\\dsqrttwo}{\\dfrac{1}{\\sqrt{2}}} $\n$ \\newcommand{\\onehalf}{\\frac{1}{2}} $\n$ \\newcommand{\\donehalf}{\\dfrac{1}{2}} $\n$ \\newcommand{\\hadamard}{ \\mymatrix{rr}{ \\sqrttwo & \\sqrttwo \\\\ \\sqrttwo & -\\sqrttwo }} $\n$ \\newcommand{\\vzero}{\\myvector{1\\\\0}} $\n$ \\newcommand{\\vone}{\\myvector{0\\\\1}} $\n$ \\newcommand{\\vhadamardzero}{\\myvector{ \\sqrttwo \\\\ \\sqrttwo } } $\n$ \\newcommand{\\vhadamardone}{ \\myrvector{ \\sqrttwo \\\\ -\\sqrttwo } } $\n$ \\newcommand{\\myarray}[2]{ \\begin{array}{#1}#2\\end{array}} $\n$ \\newcommand{\\X}{ \\mymatrix{cc}{0 & 1 \\\\ 1 & 0} } $\n$ \\newcommand{\\Z}{ \\mymatrix{rr}{1 & 0 \\\\ 0 & -1} } $\n$ \\newcommand{\\Htwo}{ \\mymatrix{rrrr}{ \\frac{1}{2} & \\frac{1}{2} & \\frac{1}{2} & \\frac{1}{2} \\\\ \\frac{1}{2} & -\\frac{1}{2} & \\frac{1}{2} & -\\frac{1}{2} \\\\ \\frac{1}{2} & \\frac{1}{2} & -\\frac{1}{2} & -\\frac{1}{2} \\\\ \\frac{1}{2} & -\\frac{1}{2} & -\\frac{1}{2} & \\frac{1}{2} } } $\n$ \\newcommand{\\CNOT}{ \\mymatrix{cccc}{1 & 0 & 0 & 0 \\\\ 0 & 1 & 0 & 0 \\\\ 0 & 0 & 0 & 1 \\\\ 0 & 0 & 1 & 0} } $\n$ \\newcommand{\\norm}[1]{ \\left\\lVert #1 \\right\\rVert } $",
"_____no_output_____"
],
[
"<h2>Vectors: Dot (Scalar) Product</h2>\n\nTwo vectors can be multiplied with each other in different ways.\n\nOne of the very basic methods is <i>dot product</i>.\n\nIt is also called <i>scalar product</i>, because the result is a <i>scalar value</i>, e.g., a real number.\n\nConsider the following two vectors:\n$$\n u = \\myrvector{-3 \\\\ -2 \\\\ 0 \\\\ -1 \\\\ 4} \\mbox{ and } v = \\myrvector{-1\\\\ -1 \\\\2 \\\\ -3 \\\\ 5}.\n$$\n\nThe dot product of $ u $ and $ v $, denoted by $ \\dot{u}{v}$, can be defined algorithmically.\n\n<u>Pairwise multiplication</u>: the values in the same positions are multiplied with each other.\n\n<u>Summation of all pairwise multiplications</u>: Then we sum all the results obtained from the pairwise multiplications.\n\nWe write its Python code below.",
"_____no_output_____"
]
],
[
[
"# let's define both vectors\nu = [-3,-2,0,-1,4]\nv = [-1,-1,2,-3,5]\n\nuv = 0; # summation is initially zero\n\nfor i in range(len(u)): # iteratively access every pair with the same indices\n print(\"pairwise multiplication of the entries with index\",i,\"is\",u[i]*v[i])\n uv = uv + u[i]*v[i] # i-th entries are multiplied and then added to summation\n \nprint() # print an empty line \nprint(\"The dot product of\",u,'and',v,'is',uv)",
"_____no_output_____"
]
],
[
[
"The pairwise multiplications of entries are\n<ul>\n <li> $ (-3)\\cdot(-1) = 3 $, </li>\n <li> $ (-2)\\cdot(-1) = 2 $, </li>\n <li> $ 0\\cdot 2 = 0 $, </li>\n <li> $ (-1)\\cdot(-3) = 3 $, and, </li>\n <li> $ 4 \\cdot 5 = 20 $. </li>\n</ul>\n\nThus the summation of all pairwise multiplications of entries is $ 3+2+0+3+20 = 28 $.\n\n<b>Remark that the dimensions of the given vectors must be the same. Otherwise, the dot product is not defined.</b>",
"_____no_output_____"
],
[
"<h3> Task 1 </h3>\n\nFind the dot product of the following vectors in Python:\n\n$$\n v = \\myrvector{-3 \\\\ 4 \\\\ -5 \\\\ 6} ~~~~\\mbox{and}~~~~ u = \\myrvector{4 \\\\ 3 \\\\ 6 \\\\ 5}.\n$$\n\nYour outcome should be $0$.",
"_____no_output_____"
]
],
[
[
"#\n# your solution is here\n#\n",
"_____no_output_____"
]
],
[
[
"<a href=\"Math24_Dot_Product_Solutions.ipynb#task1\">click for our solution</a>",
"_____no_output_____"
],
[
"<h3> Task 2 </h3>\n\nLet $ u = \\myrvector{ -3 \\\\ -4 } $ be a 2 dimensional vector.\n\nFind $ \\dot{u}{u} $ in Python.",
"_____no_output_____"
]
],
[
[
"#\n# your solution is here\n#\n",
"_____no_output_____"
]
],
[
[
"<a href=\"Math24_Dot_Product_Solutions.ipynb#task2\">click for our solution</a>",
"_____no_output_____"
],
[
"<h3> Notes:</h3>\n\nAs may be observed from Task 2, the <b>length</b> of a vector can be calculated by using its <b>dot product</b> with itself.\n\n$$ \\norm{u} = \\sqrt{\\dot{u}{u}}. $$\n\n$ \\dot{u}{u} $ is $25$, and so $ \\norm{u} = \\sqrt{25} = 5 $. \n\n$ \\dot{u}{u} $ automatically accumulates the contribution of each entry to the length.",
"_____no_output_____"
],
[
"<h3> Orthogonal (perpendicular) vectors </h3>\n\nFor simplicity, we consider 2-dimensional vectors.\n\nThe following two vectors are perpendicular (orthogonal) to each other.\n\nThe angle between them is $ 90 $ degrees. \n\n<img src=\"../images/vector_-4_-5-small.jpg\" width=\"40%\">\n",
"_____no_output_____"
]
],
[
[
"# let's find the dot product of v and u\nv = [-4,0]\nu = [0,-5]\nresult = 0;\n\nfor i in range(2):\n result = result + v[i]*u[i]\n\nprint(\"the dot product of u and v is\",result)",
"_____no_output_____"
]
],
[
[
"Now, let's check the dot product of the following two vectors:\n\n<img src=\"../images/length_v_u.jpg\" width=\"40%\">",
"_____no_output_____"
]
],
[
[
"# we can use the same code\nv = [-4,3]\nu = [-3,-4]\nresult = 0;\n\nfor i in range(2):\n result = result + v[i]*u[i]\n\nprint(\"the dot product of u and v is\",result)",
"_____no_output_____"
]
],
[
[
"The dot product of new $ u $ and $ v $ is also $0$. \n\nThis is not surprising, because the vectors $u$ and $v$ (in both cases) are orthogonal to each other.\n\n<h3>Fact:</h3> \n<ul>\n <li>The dot product of two orthogonal (perpendicular) vectors is zero.</li>\n <li>If the dot product of two vectors is zero, then they are orthogonal to each other.</li>\n</ul>\n\n<i> This fact is important, because, as we will see later, orthogonal vectors (states) can be distinguished perfectly. </i>",
"_____no_output_____"
],
[
"<h3> Task 3 </h3>\n\nVerify that (i) $ u $ is orthogonal to $ -v $, (ii) $ -u $ is orthogonal to $ v $, and (iii) $ -u $ is orthogonal to $ -v $.\n\n<img src=\"../images/inner_v_u_-v_-u.jpg\" width=\"40%\">",
"_____no_output_____"
]
],
[
[
"# you may consider to write a function in Python for dot product\n\n#\n# your solution is here\n#\n",
"_____no_output_____"
]
],
[
[
"<a href=\"Math24_Dot_Product_Solutions.ipynb#task3\">click for our solution</a>",
"_____no_output_____"
],
[
"<h3> Task 4 </h3>\n\nFind the dot product of $ v $ and $ u $ in Python.\n\n$$\n v = \\myrvector{-1 \\\\ 2 \\\\ -3 \\\\ 4} ~~~~\\mbox{and}~~~~ u = \\myrvector{-2 \\\\ -1 \\\\ 5 \\\\ 2}.\n$$\n\nFind the dot product of $ -2v $ and $ 3u $ in Python.\n\nCompare both results.",
"_____no_output_____"
]
],
[
[
"#\n# your solution is here\n#\n",
"_____no_output_____"
]
],
[
[
"<a href=\"Math24_Dot_Product_Solutions.ipynb#task4\">click for our solution</a>",
"_____no_output_____"
]
]
] | [
"markdown",
"code",
"markdown",
"code",
"markdown",
"code",
"markdown",
"code",
"markdown",
"code",
"markdown",
"code",
"markdown",
"code",
"markdown"
] | [
[
"markdown",
"markdown"
],
[
"code"
],
[
"markdown",
"markdown"
],
[
"code"
],
[
"markdown",
"markdown"
],
[
"code"
],
[
"markdown",
"markdown",
"markdown"
],
[
"code"
],
[
"markdown"
],
[
"code"
],
[
"markdown",
"markdown"
],
[
"code"
],
[
"markdown",
"markdown"
],
[
"code"
],
[
"markdown"
]
] |
e787b2254948a5f541b607eaae2207bd211be5f8 | 421,417 | ipynb | Jupyter Notebook | 3_RNN_encoder_decoder.ipynb | YangLIN1997/DeepLearningForTimeSeriesForecasting | fc0b646e94009dc0e42d42b296dd0946e1692690 | [
"MIT"
] | 534 | 2019-06-06T18:32:22.000Z | 2022-03-15T13:05:02.000Z | 3_RNN_encoder_decoder.ipynb | YangLIN1997/DeepLearningForTimeSeriesForecasting | fc0b646e94009dc0e42d42b296dd0946e1692690 | [
"MIT"
] | 13 | 2019-06-10T16:16:11.000Z | 2020-11-13T18:01:30.000Z | 3_RNN_encoder_decoder.ipynb | YangLIN1997/DeepLearningForTimeSeriesForecasting | fc0b646e94009dc0e42d42b296dd0946e1692690 | [
"MIT"
] | 217 | 2019-06-17T09:51:20.000Z | 2022-03-01T16:39:46.000Z | 479.973804 | 262,180 | 0.932302 | [
[
[
"# Multi step model (simple encoder-decoder)\n\nIn this notebook, we demonstrate how to:\n- prepare time series data for training a RNN forecasting model\n- get data in the required shape for the keras API\n- implement a RNN model in keras to predict the next 3 steps ahead (time *t+1* to *t+3*) in the time series. This model uses a simple encoder decoder approach in which the final hidden state of the encoder is replicated across each time step of the decoder. \n- enable early stopping to reduce the likelihood of model overfitting\n- evaluate the model on a test dataset\n\nThe data in this example is taken from the GEFCom2014 forecasting competition<sup>1</sup>. It consists of 3 years of hourly electricity load and temperature values between 2012 and 2014. The task is to forecast future values of electricity load.\n\n<sup>1</sup>Tao Hong, Pierre Pinson, Shu Fan, Hamidreza Zareipour, Alberto Troccoli and Rob J. Hyndman, \"Probabilistic energy forecasting: Global Energy Forecasting Competition 2014 and beyond\", International Journal of Forecasting, vol.32, no.3, pp 896-913, July-September, 2016.",
"_____no_output_____"
]
],
[
[
"import os\nimport warnings\nimport matplotlib.pyplot as plt\nimport numpy as np\nimport pandas as pd\nimport datetime as dt\nfrom collections import UserDict\nfrom IPython.display import Image\n%matplotlib inline\n\nfrom common.utils import load_data, mape, TimeSeriesTensor, create_evaluation_df\n\npd.options.display.float_format = '{:,.2f}'.format\nnp.set_printoptions(precision=2)\nwarnings.filterwarnings(\"ignore\")",
"_____no_output_____"
]
],
[
[
"Load data into Pandas dataframe",
"_____no_output_____"
]
],
[
[
"energy = load_data('data/')\nenergy.head()",
"_____no_output_____"
],
[
"valid_start_dt = '2014-09-01 00:00:00'\ntest_start_dt = '2014-11-01 00:00:00'\n\nT = 6\nHORIZON = 3",
"_____no_output_____"
]
],
[
[
"Create training set containing only the model features",
"_____no_output_____"
]
],
[
[
"train = energy.copy()[energy.index < valid_start_dt][['load', 'temp']]",
"_____no_output_____"
]
],
[
[
"Scale data to be in range (0, 1). This transformation should be calibrated on the training set only. This is to prevent information from the validation or test sets leaking into the training data.",
"_____no_output_____"
]
],
[
[
"from sklearn.preprocessing import MinMaxScaler\n\ny_scaler = MinMaxScaler()\ny_scaler.fit(train[['load']])\n\nX_scaler = MinMaxScaler()\ntrain[['load', 'temp']] = X_scaler.fit_transform(train)",
"_____no_output_____"
]
],
[
[
"Use the TimeSeriesTensor convenience class to:\n1. Shift the values of the time series to create a Pandas dataframe containing all the data for a single training example\n2. Discard any samples with missing values\n3. Transform this Pandas dataframe into a numpy array of shape (samples, time steps, features) for input into Keras\n\nThe class takes the following parameters:\n\n- **dataset**: original time series\n- **H**: the forecast horizon\n- **tensor_structure**: a dictionary discribing the tensor structure in the form { 'tensor_name' : (range(max_backward_shift, max_forward_shift), [feature, feature, ...] ) }\n- **freq**: time series frequency\n- **drop_incomplete**: (Boolean) whether to drop incomplete samples",
"_____no_output_____"
]
],
[
[
"tensor_structure = {'X':(range(-T+1, 1), ['load', 'temp'])}\ntrain_inputs = TimeSeriesTensor(train, 'load', HORIZON, {'X':(range(-T+1, 1), ['load', 'temp'])})",
"_____no_output_____"
],
[
"train_inputs.dataframe.head()",
"_____no_output_____"
]
],
[
[
"Construct validation set (keeping T hours from the training set in order to construct initial features)",
"_____no_output_____"
]
],
[
[
"look_back_dt = dt.datetime.strptime(valid_start_dt, '%Y-%m-%d %H:%M:%S') - dt.timedelta(hours=T-1)\nvalid = energy.copy()[(energy.index >=look_back_dt) & (energy.index < test_start_dt)][['load', 'temp']]\nvalid[['load', 'temp']] = X_scaler.transform(valid)\nvalid_inputs = TimeSeriesTensor(valid, 'load', HORIZON, tensor_structure)",
"_____no_output_____"
]
],
[
[
"## Implement the RNN",
"_____no_output_____"
],
[
"We will implement a RNN forecasting model with the following structure:",
"_____no_output_____"
]
],
[
[
"Image('./images/simple_encoder_decoder.png')",
"_____no_output_____"
],
[
"from keras.models import Model, Sequential\nfrom keras.layers import GRU, Dense, RepeatVector, TimeDistributed, Flatten\nfrom keras.callbacks import EarlyStopping",
"Using TensorFlow backend.\n"
],
[
"LATENT_DIM = 5\nBATCH_SIZE = 32\nEPOCHS = 10",
"_____no_output_____"
],
[
"model = Sequential()\nmodel.add(GRU(LATENT_DIM, input_shape=(T, 2)))\nmodel.add(RepeatVector(HORIZON))\nmodel.add(GRU(LATENT_DIM, return_sequences=True))\nmodel.add(TimeDistributed(Dense(1)))\nmodel.add(Flatten())",
"_____no_output_____"
],
[
"model.compile(optimizer='RMSprop', loss='mse')",
"_____no_output_____"
],
[
"model.summary()",
"_________________________________________________________________\nLayer (type) Output Shape Param # \n=================================================================\ngru_1 (GRU) (None, 5) 120 \n_________________________________________________________________\nrepeat_vector_1 (RepeatVecto (None, 3, 5) 0 \n_________________________________________________________________\ngru_2 (GRU) (None, 3, 5) 165 \n_________________________________________________________________\ntime_distributed_1 (TimeDist (None, 3, 1) 6 \n_________________________________________________________________\nflatten_1 (Flatten) (None, 3) 0 \n=================================================================\nTotal params: 291\nTrainable params: 291\nNon-trainable params: 0\n_________________________________________________________________\n"
],
[
"earlystop = EarlyStopping(monitor='val_loss', min_delta=0, patience=5)",
"_____no_output_____"
],
[
"model.fit(train_inputs['X'],\n train_inputs['target'],\n batch_size=BATCH_SIZE,\n epochs=EPOCHS,\n validation_data=(valid_inputs['X'], valid_inputs['target']),\n callbacks=[earlystop],\n verbose=1)",
"Train on 23368 samples, validate on 1461 samples\nEpoch 1/50\n23368/23368 [==============================] - 4s 185us/step - loss: 0.0217 - val_loss: 0.0053\nEpoch 2/50\n23368/23368 [==============================] - 3s 129us/step - loss: 0.0050 - val_loss: 0.0042\nEpoch 3/50\n23368/23368 [==============================] - 3s 147us/step - loss: 0.0044 - val_loss: 0.0039\nEpoch 4/50\n23368/23368 [==============================] - 3s 129us/step - loss: 0.0041 - val_loss: 0.0035\nEpoch 5/50\n23368/23368 [==============================] - 3s 134us/step - loss: 0.0039 - val_loss: 0.0033\nEpoch 6/50\n23368/23368 [==============================] - 3s 138us/step - loss: 0.0037 - val_loss: 0.0032\nEpoch 7/50\n23368/23368 [==============================] - 3s 127us/step - loss: 0.0035 - val_loss: 0.0029\nEpoch 8/50\n23368/23368 [==============================] - 3s 130us/step - loss: 0.0034 - val_loss: 0.0029\nEpoch 9/50\n23368/23368 [==============================] - 4s 156us/step - loss: 0.0033 - val_loss: 0.0037\nEpoch 10/50\n23368/23368 [==============================] - 3s 123us/step - loss: 0.0033 - val_loss: 0.0029\nEpoch 11/50\n23368/23368 [==============================] - 4s 177us/step - loss: 0.0032 - val_loss: 0.0026\nEpoch 12/50\n23368/23368 [==============================] - 5s 195us/step - loss: 0.0032 - val_loss: 0.0032\nEpoch 13/50\n23368/23368 [==============================] - 4s 167us/step - loss: 0.0032 - val_loss: 0.0027\nEpoch 14/50\n23368/23368 [==============================] - 3s 135us/step - loss: 0.0032 - val_loss: 0.0028\nEpoch 15/50\n23368/23368 [==============================] - 4s 174us/step - loss: 0.0031 - val_loss: 0.0041\nEpoch 16/50\n23368/23368 [==============================] - 4s 172us/step - loss: 0.0031 - val_loss: 0.0034\n"
]
],
[
[
"## Evaluate the model",
"_____no_output_____"
]
],
[
[
"look_back_dt = dt.datetime.strptime(test_start_dt, '%Y-%m-%d %H:%M:%S') - dt.timedelta(hours=T-1)\ntest = energy.copy()[test_start_dt:][['load', 'temp']]\ntest[['load', 'temp']] = X_scaler.transform(test)\ntest_inputs = TimeSeriesTensor(test, 'load', HORIZON, tensor_structure)",
"_____no_output_____"
],
[
"predictions = model.predict(test_inputs['X'])",
"_____no_output_____"
],
[
"predictions",
"_____no_output_____"
],
[
"eval_df = create_evaluation_df(predictions, test_inputs, HORIZON, y_scaler)\neval_df.head()",
"_____no_output_____"
],
[
"eval_df['APE'] = (eval_df['prediction'] - eval_df['actual']).abs() / eval_df['actual']\neval_df.groupby('h')['APE'].mean()",
"_____no_output_____"
],
[
"mape(eval_df['prediction'], eval_df['actual'])",
"_____no_output_____"
]
],
[
[
"Plot actuals vs predictions at each horizon for first week of the test period. As is to be expected, predictions for one step ahead (*t+1*) are more accurate than those for 2 or 3 steps ahead",
"_____no_output_____"
]
],
[
[
"plot_df = eval_df[(eval_df.timestamp<'2014-11-08') & (eval_df.h=='t+1')][['timestamp', 'actual']]\nfor t in range(1, HORIZON+1):\n plot_df['t+'+str(t)] = eval_df[(eval_df.timestamp<'2014-11-08') & (eval_df.h=='t+'+str(t))]['prediction'].values\n\nfig = plt.figure(figsize=(15, 8))\nax = plt.plot(plot_df['timestamp'], plot_df['actual'], color='red', linewidth=4.0)\nax = fig.add_subplot(111)\nax.plot(plot_df['timestamp'], plot_df['t+1'], color='blue', linewidth=4.0, alpha=0.75)\nax.plot(plot_df['timestamp'], plot_df['t+2'], color='blue', linewidth=3.0, alpha=0.5)\nax.plot(plot_df['timestamp'], plot_df['t+3'], color='blue', linewidth=2.0, alpha=0.25)\nplt.xlabel('timestamp', fontsize=12)\nplt.ylabel('load', fontsize=12)\nax.legend(loc='best')\nplt.show()",
"_____no_output_____"
]
]
] | [
"markdown",
"code",
"markdown",
"code",
"markdown",
"code",
"markdown",
"code",
"markdown",
"code",
"markdown",
"code",
"markdown",
"code",
"markdown",
"code",
"markdown",
"code"
] | [
[
"markdown"
],
[
"code"
],
[
"markdown"
],
[
"code",
"code"
],
[
"markdown"
],
[
"code"
],
[
"markdown"
],
[
"code"
],
[
"markdown"
],
[
"code",
"code"
],
[
"markdown"
],
[
"code"
],
[
"markdown",
"markdown"
],
[
"code",
"code",
"code",
"code",
"code",
"code",
"code",
"code"
],
[
"markdown"
],
[
"code",
"code",
"code",
"code",
"code",
"code"
],
[
"markdown"
],
[
"code"
]
] |
Subsets and Splits
No saved queries yet
Save your SQL queries to embed, download, and access them later. Queries will appear here once saved.