pipeline_tag
stringclasses 48
values | library_name
stringclasses 198
values | text
stringlengths 1
900k
| metadata
stringlengths 2
438k
| id
stringlengths 5
122
| last_modified
null | tags
sequencelengths 1
1.84k
| sha
null | created_at
stringlengths 25
25
| arxiv
sequencelengths 0
201
| languages
sequencelengths 0
1.83k
| tags_str
stringlengths 17
9.34k
| text_str
stringlengths 0
389k
| text_lists
sequencelengths 0
722
| processed_texts
sequencelengths 1
723
|
---|---|---|---|---|---|---|---|---|---|---|---|---|---|---|
text-generation | transformers |
<!-- This model card has been generated automatically according to the information the Trainer had access to. You
should probably proofread and complete it, then remove this comment. -->
# Baby-Llama-58M-RUN3_3
This model is a fine-tuned version of [](https://huggingface.co/) on an unknown dataset.
It achieves the following results on the evaluation set:
- Loss: 3.8148
## Model description
More information needed
## Intended uses & limitations
More information needed
## Training and evaluation data
More information needed
## Training procedure
### Training hyperparameters
The following hyperparameters were used during training:
- learning_rate: 0.00025
- train_batch_size: 32
- eval_batch_size: 8
- seed: 42
- optimizer: Adam with betas=(0.9,0.999) and epsilon=1e-08
- lr_scheduler_type: cosine
- lr_scheduler_warmup_steps: 50
- num_epochs: 120
- mixed_precision_training: Native AMP
### Training results
| Training Loss | Epoch | Step | Validation Loss |
|:-------------:|:-----:|:----:|:---------------:|
| 297.4542 | 1.0 | 12 | 250.9910 |
| 229.6338 | 2.0 | 24 | 208.3821 |
| 208.295 | 3.0 | 36 | 179.5238 |
| 129.018 | 4.0 | 48 | 112.9940 |
| 82.9929 | 5.0 | 60 | 74.3020 |
| 46.9522 | 6.0 | 72 | 42.2297 |
| 24.9202 | 7.0 | 84 | 23.4095 |
| 15.2942 | 8.0 | 96 | 13.3510 |
| 10.0619 | 9.0 | 108 | 9.7284 |
| 7.784 | 10.0 | 120 | 7.8737 |
| 6.4759 | 11.0 | 132 | 7.2488 |
| 6.1744 | 12.0 | 144 | 6.3695 |
| 5.4904 | 13.0 | 156 | 6.2293 |
| 5.4665 | 14.0 | 168 | 5.8846 |
| 4.731 | 15.0 | 180 | 5.8094 |
| 4.7619 | 16.0 | 192 | 5.4680 |
| 4.6858 | 17.0 | 204 | 5.4562 |
| 4.594 | 18.0 | 216 | 5.2367 |
| 4.7173 | 19.0 | 228 | 5.1584 |
| 4.2267 | 20.0 | 240 | 5.1182 |
| 4.2401 | 21.0 | 252 | 5.0173 |
| 4.767 | 22.0 | 264 | 4.9806 |
| 4.0932 | 23.0 | 276 | 4.8975 |
| 4.3266 | 24.0 | 288 | 4.8852 |
| 4.0103 | 25.0 | 300 | 4.7698 |
| 4.1829 | 26.0 | 312 | 4.7993 |
| 4.0862 | 27.0 | 324 | 4.7921 |
| 4.1418 | 28.0 | 336 | 4.7469 |
| 4.0668 | 29.0 | 348 | 4.7108 |
| 4.0318 | 30.0 | 360 | 4.6335 |
| 4.0468 | 31.0 | 372 | 4.6761 |
| 3.9454 | 32.0 | 384 | 4.5814 |
| 3.943 | 33.0 | 396 | 4.5624 |
| 3.5406 | 34.0 | 408 | 4.6243 |
| 3.5091 | 35.0 | 420 | 4.5822 |
| 3.5972 | 36.0 | 432 | 4.4551 |
| 3.711 | 37.0 | 444 | 4.4898 |
| 3.7391 | 38.0 | 456 | 4.4472 |
| 3.7883 | 39.0 | 468 | 4.4188 |
| 3.7508 | 40.0 | 480 | 4.3803 |
| 3.422 | 41.0 | 492 | 4.3539 |
| 3.5801 | 42.0 | 504 | 4.3718 |
| 3.3411 | 43.0 | 516 | 4.3635 |
| 3.5347 | 44.0 | 528 | 4.3381 |
| 3.3136 | 45.0 | 540 | 4.2857 |
| 3.6378 | 46.0 | 552 | 4.2428 |
| 3.9194 | 47.0 | 564 | 4.3143 |
| 3.444 | 48.0 | 576 | 4.2403 |
| 3.5414 | 49.0 | 588 | 4.2614 |
| 3.6703 | 50.0 | 600 | 4.2729 |
| 3.5997 | 51.0 | 612 | 4.2104 |
| 3.1202 | 52.0 | 624 | 4.1948 |
| 3.3409 | 53.0 | 636 | 4.2018 |
| 3.4611 | 54.0 | 648 | 4.1726 |
| 3.1643 | 55.0 | 660 | 4.1776 |
| 3.1082 | 56.0 | 672 | 4.1785 |
| 2.9745 | 57.0 | 684 | 4.1374 |
| 3.3937 | 58.0 | 696 | 4.1434 |
| 3.265 | 59.0 | 708 | 4.1356 |
| 3.0267 | 60.0 | 720 | 4.1474 |
| 3.0632 | 61.0 | 732 | 4.1193 |
| 3.3543 | 62.0 | 744 | 4.0760 |
| 3.519 | 63.0 | 756 | 4.1373 |
| 3.2546 | 64.0 | 768 | 4.0591 |
| 3.0835 | 65.0 | 780 | 4.0572 |
| 3.3228 | 66.0 | 792 | 4.0788 |
| 3.3441 | 67.0 | 804 | 4.0489 |
| 2.9186 | 68.0 | 816 | 4.0360 |
| 3.1519 | 69.0 | 828 | 4.0376 |
| 3.5119 | 70.0 | 840 | 4.0159 |
| 3.1155 | 71.0 | 852 | 4.0070 |
| 3.1899 | 72.0 | 864 | 3.9895 |
| 3.0979 | 73.0 | 876 | 3.9936 |
| 3.1709 | 74.0 | 888 | 3.9997 |
| 3.3529 | 75.0 | 900 | 3.9848 |
| 2.7989 | 76.0 | 912 | 3.9760 |
| 3.1918 | 77.0 | 924 | 3.9693 |
| 2.8472 | 78.0 | 936 | 3.9504 |
| 3.3493 | 79.0 | 948 | 3.9520 |
| 3.5098 | 80.0 | 960 | 3.9401 |
| 3.2381 | 81.0 | 972 | 3.9363 |
| 3.1959 | 82.0 | 984 | 3.9292 |
| 3.4514 | 83.0 | 996 | 3.9128 |
| 2.9119 | 84.0 | 1008 | 3.9194 |
| 3.2452 | 85.0 | 1020 | 3.9038 |
| 3.0657 | 86.0 | 1032 | 3.9168 |
| 2.8583 | 87.0 | 1044 | 3.9018 |
| 3.2229 | 88.0 | 1056 | 3.9000 |
| 2.9973 | 89.0 | 1068 | 3.8906 |
| 3.0533 | 90.0 | 1080 | 3.8818 |
| 3.3813 | 91.0 | 1092 | 3.8715 |
| 3.1559 | 92.0 | 1104 | 3.8639 |
| 3.1343 | 93.0 | 1116 | 3.8674 |
| 2.9604 | 94.0 | 1128 | 3.8690 |
| 3.3522 | 95.0 | 1140 | 3.8646 |
| 2.9739 | 96.0 | 1152 | 3.8589 |
| 2.7854 | 97.0 | 1164 | 3.8559 |
| 2.8544 | 98.0 | 1176 | 3.8445 |
| 2.9875 | 99.0 | 1188 | 3.8434 |
| 3.3395 | 100.0 | 1200 | 3.8402 |
| 2.736 | 101.0 | 1212 | 3.8398 |
| 3.0598 | 102.0 | 1224 | 3.8384 |
| 3.003 | 103.0 | 1236 | 3.8376 |
| 3.0566 | 104.0 | 1248 | 3.8386 |
| 3.1727 | 105.0 | 1260 | 3.8281 |
| 2.9811 | 106.0 | 1272 | 3.8331 |
| 2.7108 | 107.0 | 1284 | 3.8224 |
| 2.6579 | 108.0 | 1296 | 3.8236 |
| 3.1319 | 109.0 | 1308 | 3.8197 |
| 3.1115 | 110.0 | 1320 | 3.8216 |
| 3.0955 | 111.0 | 1332 | 3.8181 |
| 2.6928 | 112.0 | 1344 | 3.8188 |
| 2.9943 | 113.0 | 1356 | 3.8147 |
| 3.0923 | 114.0 | 1368 | 3.8154 |
| 3.1913 | 115.0 | 1380 | 3.8156 |
| 2.9444 | 116.0 | 1392 | 3.8146 |
| 3.0491 | 117.0 | 1404 | 3.8141 |
| 2.7357 | 118.0 | 1416 | 3.8148 |
| 3.0744 | 119.0 | 1428 | 3.8148 |
| 3.1122 | 120.0 | 1440 | 3.8148 |
### Framework versions
- Transformers 4.39.1
- Pytorch 2.1.2+cu121
- Datasets 2.16.1
- Tokenizers 0.15.0
| {"tags": ["generated_from_trainer"], "model-index": [{"name": "Baby-Llama-58M-RUN3_3", "results": []}]} | ninagroot/Baby-Llama-58M-RUN3_3 | null | [
"transformers",
"safetensors",
"llama",
"text-generation",
"generated_from_trainer",
"autotrain_compatible",
"endpoints_compatible",
"text-generation-inference",
"region:us"
] | null | 2024-04-25T14:05:06+00:00 | [] | [] | TAGS
#transformers #safetensors #llama #text-generation #generated_from_trainer #autotrain_compatible #endpoints_compatible #text-generation-inference #region-us
| Baby-Llama-58M-RUN3\_3
======================
This model is a fine-tuned version of [](URL on an unknown dataset.
It achieves the following results on the evaluation set:
* Loss: 3.8148
Model description
-----------------
More information needed
Intended uses & limitations
---------------------------
More information needed
Training and evaluation data
----------------------------
More information needed
Training procedure
------------------
### Training hyperparameters
The following hyperparameters were used during training:
* learning\_rate: 0.00025
* train\_batch\_size: 32
* eval\_batch\_size: 8
* seed: 42
* optimizer: Adam with betas=(0.9,0.999) and epsilon=1e-08
* lr\_scheduler\_type: cosine
* lr\_scheduler\_warmup\_steps: 50
* num\_epochs: 120
* mixed\_precision\_training: Native AMP
### Training results
### Framework versions
* Transformers 4.39.1
* Pytorch 2.1.2+cu121
* Datasets 2.16.1
* Tokenizers 0.15.0
| [
"### Training hyperparameters\n\n\nThe following hyperparameters were used during training:\n\n\n* learning\\_rate: 0.00025\n* train\\_batch\\_size: 32\n* eval\\_batch\\_size: 8\n* seed: 42\n* optimizer: Adam with betas=(0.9,0.999) and epsilon=1e-08\n* lr\\_scheduler\\_type: cosine\n* lr\\_scheduler\\_warmup\\_steps: 50\n* num\\_epochs: 120\n* mixed\\_precision\\_training: Native AMP",
"### Training results",
"### Framework versions\n\n\n* Transformers 4.39.1\n* Pytorch 2.1.2+cu121\n* Datasets 2.16.1\n* Tokenizers 0.15.0"
] | [
"TAGS\n#transformers #safetensors #llama #text-generation #generated_from_trainer #autotrain_compatible #endpoints_compatible #text-generation-inference #region-us \n",
"### Training hyperparameters\n\n\nThe following hyperparameters were used during training:\n\n\n* learning\\_rate: 0.00025\n* train\\_batch\\_size: 32\n* eval\\_batch\\_size: 8\n* seed: 42\n* optimizer: Adam with betas=(0.9,0.999) and epsilon=1e-08\n* lr\\_scheduler\\_type: cosine\n* lr\\_scheduler\\_warmup\\_steps: 50\n* num\\_epochs: 120\n* mixed\\_precision\\_training: Native AMP",
"### Training results",
"### Framework versions\n\n\n* Transformers 4.39.1\n* Pytorch 2.1.2+cu121\n* Datasets 2.16.1\n* Tokenizers 0.15.0"
] |
null | transformers |
# Uploaded model
- **Developed by:** TheNile
- **License:** apache-2.0
- **Finetuned from model :** unsloth/llama-3-8b-bnb-4bit
This llama model was trained 2x faster with [Unsloth](https://github.com/unslothai/unsloth) and Huggingface's TRL library.
[<img src="https://raw.githubusercontent.com/unslothai/unsloth/main/images/unsloth%20made%20with%20love.png" width="200"/>](https://github.com/unslothai/unsloth)
| {"language": ["en"], "license": "apache-2.0", "tags": ["text-generation-inference", "transformers", "unsloth", "llama", "trl"], "base_model": "unsloth/llama-3-8b-bnb-4bit"} | TheNile/RxnPredict_model | null | [
"transformers",
"safetensors",
"text-generation-inference",
"unsloth",
"llama",
"trl",
"en",
"base_model:unsloth/llama-3-8b-bnb-4bit",
"license:apache-2.0",
"endpoints_compatible",
"region:us"
] | null | 2024-04-25T14:05:53+00:00 | [] | [
"en"
] | TAGS
#transformers #safetensors #text-generation-inference #unsloth #llama #trl #en #base_model-unsloth/llama-3-8b-bnb-4bit #license-apache-2.0 #endpoints_compatible #region-us
|
# Uploaded model
- Developed by: TheNile
- License: apache-2.0
- Finetuned from model : unsloth/llama-3-8b-bnb-4bit
This llama model was trained 2x faster with Unsloth and Huggingface's TRL library.
<img src="URL width="200"/>
| [
"# Uploaded model\n\n- Developed by: TheNile\n- License: apache-2.0\n- Finetuned from model : unsloth/llama-3-8b-bnb-4bit\n\nThis llama model was trained 2x faster with Unsloth and Huggingface's TRL library.\n\n<img src=\"URL width=\"200\"/>"
] | [
"TAGS\n#transformers #safetensors #text-generation-inference #unsloth #llama #trl #en #base_model-unsloth/llama-3-8b-bnb-4bit #license-apache-2.0 #endpoints_compatible #region-us \n",
"# Uploaded model\n\n- Developed by: TheNile\n- License: apache-2.0\n- Finetuned from model : unsloth/llama-3-8b-bnb-4bit\n\nThis llama model was trained 2x faster with Unsloth and Huggingface's TRL library.\n\n<img src=\"URL width=\"200\"/>"
] |
null | transformers |
# Model Card for Model ID
<!-- Provide a quick summary of what the model is/does. -->
## Model Details
### Model Description
<!-- Provide a longer summary of what this model is. -->
This is the model card of a 🤗 transformers model that has been pushed on the Hub. This model card has been automatically generated.
- **Developed by:** [More Information Needed]
- **Funded by [optional]:** [More Information Needed]
- **Shared by [optional]:** [More Information Needed]
- **Model type:** [More Information Needed]
- **Language(s) (NLP):** [More Information Needed]
- **License:** [More Information Needed]
- **Finetuned from model [optional]:** [More Information Needed]
### Model Sources [optional]
<!-- Provide the basic links for the model. -->
- **Repository:** [More Information Needed]
- **Paper [optional]:** [More Information Needed]
- **Demo [optional]:** [More Information Needed]
## Uses
<!-- Address questions around how the model is intended to be used, including the foreseeable users of the model and those affected by the model. -->
### Direct Use
<!-- This section is for the model use without fine-tuning or plugging into a larger ecosystem/app. -->
[More Information Needed]
### Downstream Use [optional]
<!-- This section is for the model use when fine-tuned for a task, or when plugged into a larger ecosystem/app -->
[More Information Needed]
### Out-of-Scope Use
<!-- This section addresses misuse, malicious use, and uses that the model will not work well for. -->
[More Information Needed]
## Bias, Risks, and Limitations
<!-- This section is meant to convey both technical and sociotechnical limitations. -->
[More Information Needed]
### Recommendations
<!-- This section is meant to convey recommendations with respect to the bias, risk, and technical limitations. -->
Users (both direct and downstream) should be made aware of the risks, biases and limitations of the model. More information needed for further recommendations.
## How to Get Started with the Model
Use the code below to get started with the model.
[More Information Needed]
## Training Details
### Training Data
<!-- This should link to a Dataset Card, perhaps with a short stub of information on what the training data is all about as well as documentation related to data pre-processing or additional filtering. -->
[More Information Needed]
### Training Procedure
<!-- This relates heavily to the Technical Specifications. Content here should link to that section when it is relevant to the training procedure. -->
#### Preprocessing [optional]
[More Information Needed]
#### Training Hyperparameters
- **Training regime:** [More Information Needed] <!--fp32, fp16 mixed precision, bf16 mixed precision, bf16 non-mixed precision, fp16 non-mixed precision, fp8 mixed precision -->
#### Speeds, Sizes, Times [optional]
<!-- This section provides information about throughput, start/end time, checkpoint size if relevant, etc. -->
[More Information Needed]
## Evaluation
<!-- This section describes the evaluation protocols and provides the results. -->
### Testing Data, Factors & Metrics
#### Testing Data
<!-- This should link to a Dataset Card if possible. -->
[More Information Needed]
#### Factors
<!-- These are the things the evaluation is disaggregating by, e.g., subpopulations or domains. -->
[More Information Needed]
#### Metrics
<!-- These are the evaluation metrics being used, ideally with a description of why. -->
[More Information Needed]
### Results
[More Information Needed]
#### Summary
## Model Examination [optional]
<!-- Relevant interpretability work for the model goes here -->
[More Information Needed]
## Environmental Impact
<!-- Total emissions (in grams of CO2eq) and additional considerations, such as electricity usage, go here. Edit the suggested text below accordingly -->
Carbon emissions can be estimated using the [Machine Learning Impact calculator](https://mlco2.github.io/impact#compute) presented in [Lacoste et al. (2019)](https://arxiv.org/abs/1910.09700).
- **Hardware Type:** [More Information Needed]
- **Hours used:** [More Information Needed]
- **Cloud Provider:** [More Information Needed]
- **Compute Region:** [More Information Needed]
- **Carbon Emitted:** [More Information Needed]
## Technical Specifications [optional]
### Model Architecture and Objective
[More Information Needed]
### Compute Infrastructure
[More Information Needed]
#### Hardware
[More Information Needed]
#### Software
[More Information Needed]
## Citation [optional]
<!-- If there is a paper or blog post introducing the model, the APA and Bibtex information for that should go in this section. -->
**BibTeX:**
[More Information Needed]
**APA:**
[More Information Needed]
## Glossary [optional]
<!-- If relevant, include terms and calculations in this section that can help readers understand the model or model card. -->
[More Information Needed]
## More Information [optional]
[More Information Needed]
## Model Card Authors [optional]
[More Information Needed]
## Model Card Contact
[More Information Needed] | {"library_name": "transformers", "tags": []} | HenryCai1129/adapter-toxic2nontoxic-100-50-0.0006 | null | [
"transformers",
"safetensors",
"arxiv:1910.09700",
"endpoints_compatible",
"region:us"
] | null | 2024-04-25T14:07:02+00:00 | [
"1910.09700"
] | [] | TAGS
#transformers #safetensors #arxiv-1910.09700 #endpoints_compatible #region-us
|
# Model Card for Model ID
## Model Details
### Model Description
This is the model card of a transformers model that has been pushed on the Hub. This model card has been automatically generated.
- Developed by:
- Funded by [optional]:
- Shared by [optional]:
- Model type:
- Language(s) (NLP):
- License:
- Finetuned from model [optional]:
### Model Sources [optional]
- Repository:
- Paper [optional]:
- Demo [optional]:
## Uses
### Direct Use
### Downstream Use [optional]
### Out-of-Scope Use
## Bias, Risks, and Limitations
### Recommendations
Users (both direct and downstream) should be made aware of the risks, biases and limitations of the model. More information needed for further recommendations.
## How to Get Started with the Model
Use the code below to get started with the model.
## Training Details
### Training Data
### Training Procedure
#### Preprocessing [optional]
#### Training Hyperparameters
- Training regime:
#### Speeds, Sizes, Times [optional]
## Evaluation
### Testing Data, Factors & Metrics
#### Testing Data
#### Factors
#### Metrics
### Results
#### Summary
## Model Examination [optional]
## Environmental Impact
Carbon emissions can be estimated using the Machine Learning Impact calculator presented in Lacoste et al. (2019).
- Hardware Type:
- Hours used:
- Cloud Provider:
- Compute Region:
- Carbon Emitted:
## Technical Specifications [optional]
### Model Architecture and Objective
### Compute Infrastructure
#### Hardware
#### Software
[optional]
BibTeX:
APA:
## Glossary [optional]
## More Information [optional]
## Model Card Authors [optional]
## Model Card Contact
| [
"# Model Card for Model ID",
"## Model Details",
"### Model Description\n\n\n\nThis is the model card of a transformers model that has been pushed on the Hub. This model card has been automatically generated.\n\n- Developed by: \n- Funded by [optional]: \n- Shared by [optional]: \n- Model type: \n- Language(s) (NLP): \n- License: \n- Finetuned from model [optional]:",
"### Model Sources [optional]\n\n\n\n- Repository: \n- Paper [optional]: \n- Demo [optional]:",
"## Uses",
"### Direct Use",
"### Downstream Use [optional]",
"### Out-of-Scope Use",
"## Bias, Risks, and Limitations",
"### Recommendations\n\n\n\nUsers (both direct and downstream) should be made aware of the risks, biases and limitations of the model. More information needed for further recommendations.",
"## How to Get Started with the Model\n\nUse the code below to get started with the model.",
"## Training Details",
"### Training Data",
"### Training Procedure",
"#### Preprocessing [optional]",
"#### Training Hyperparameters\n\n- Training regime:",
"#### Speeds, Sizes, Times [optional]",
"## Evaluation",
"### Testing Data, Factors & Metrics",
"#### Testing Data",
"#### Factors",
"#### Metrics",
"### Results",
"#### Summary",
"## Model Examination [optional]",
"## Environmental Impact\n\n\n\nCarbon emissions can be estimated using the Machine Learning Impact calculator presented in Lacoste et al. (2019).\n\n- Hardware Type: \n- Hours used: \n- Cloud Provider: \n- Compute Region: \n- Carbon Emitted:",
"## Technical Specifications [optional]",
"### Model Architecture and Objective",
"### Compute Infrastructure",
"#### Hardware",
"#### Software\n\n\n\n[optional]\n\n\n\nBibTeX:\n\n\n\nAPA:",
"## Glossary [optional]",
"## More Information [optional]",
"## Model Card Authors [optional]",
"## Model Card Contact"
] | [
"TAGS\n#transformers #safetensors #arxiv-1910.09700 #endpoints_compatible #region-us \n",
"# Model Card for Model ID",
"## Model Details",
"### Model Description\n\n\n\nThis is the model card of a transformers model that has been pushed on the Hub. This model card has been automatically generated.\n\n- Developed by: \n- Funded by [optional]: \n- Shared by [optional]: \n- Model type: \n- Language(s) (NLP): \n- License: \n- Finetuned from model [optional]:",
"### Model Sources [optional]\n\n\n\n- Repository: \n- Paper [optional]: \n- Demo [optional]:",
"## Uses",
"### Direct Use",
"### Downstream Use [optional]",
"### Out-of-Scope Use",
"## Bias, Risks, and Limitations",
"### Recommendations\n\n\n\nUsers (both direct and downstream) should be made aware of the risks, biases and limitations of the model. More information needed for further recommendations.",
"## How to Get Started with the Model\n\nUse the code below to get started with the model.",
"## Training Details",
"### Training Data",
"### Training Procedure",
"#### Preprocessing [optional]",
"#### Training Hyperparameters\n\n- Training regime:",
"#### Speeds, Sizes, Times [optional]",
"## Evaluation",
"### Testing Data, Factors & Metrics",
"#### Testing Data",
"#### Factors",
"#### Metrics",
"### Results",
"#### Summary",
"## Model Examination [optional]",
"## Environmental Impact\n\n\n\nCarbon emissions can be estimated using the Machine Learning Impact calculator presented in Lacoste et al. (2019).\n\n- Hardware Type: \n- Hours used: \n- Cloud Provider: \n- Compute Region: \n- Carbon Emitted:",
"## Technical Specifications [optional]",
"### Model Architecture and Objective",
"### Compute Infrastructure",
"#### Hardware",
"#### Software\n\n\n\n[optional]\n\n\n\nBibTeX:\n\n\n\nAPA:",
"## Glossary [optional]",
"## More Information [optional]",
"## Model Card Authors [optional]",
"## Model Card Contact"
] |
feature-extraction | transformers |
This is the converted model from Unbabel/wmt22-cometkiwi-da
1) Just kept the weights/bias keys()
2) Renamed the keys to match the original Facebook/XLM-roberta-large
3) kept the layer_wise_attention / estimator layers
Because of a hack in HF's code I had to rename the "layerwise_attention.gamma" key to "layerwise_attention.gam"
I changed the config.json key "layer_transformation" from sparsemax to softmax because there is a bug in COMET since the flag is not passed, the actual function used is the default which is softmax.
Usage:
```
from transformers import XLMRobertaTokenizer, XLMRobertaTokenizerFast, AutoModel
tokenizer = XLMRobertaTokenizerFast.from_pretrained("vince62s/wmt22-cometkiwi-da-roberta-large", trust_remote_code=True)
model = AutoModel.from_pretrained("vince62s/wmt22-cometkiwi-da-roberta-large", trust_remote_code=True)
text = "Hello world!</s></s>Bonjour le monde"
encoded_text = tokenizer(text, return_tensors='pt')
print(encoded_text)
output = model(**encoded_text)
print(output[0])
{'input_ids': tensor([[ 0, 35378, 8999, 38, 2, 2, 84602, 95, 11146, 2]]), 'attention_mask': tensor([[1, 1, 1, 1, 1, 1, 1, 1, 1, 1]])}
tensor([[0.8640]], grad_fn=<AddmmBackward0>)
```
Let's double check with the original code from Unbabel Comet:
```
from comet import download_model, load_from_checkpoint
model = load_from_checkpoint("/home/vincent/Downloads/cometkiwi22/checkpoints/model.ckpt") # this is the Unbabel checkpoint
data = [{"mt": "Hello world!", "src": "Bonjour le monde"}]
output = model.predict(data, gpus=0)
print(output)
Prediction([('scores', [0.863973081111908]),
('system_score', 0.863973081111908)])
```
---
extra_gated_heading: Acknowledge license to accept the repository
extra_gated_button_content: Acknowledge license
pipeline_tag: translation
language:
- multilingual
- af
- am
- ar
- as
- az
- be
- bg
- bn
- br
- bs
- ca
- cs
- cy
- da
- de
- el
- en
- eo
- es
- et
- eu
- fa
- fi
- fr
- fy
- ga
- gd
- gl
- gu
- ha
- he
- hi
- hr
- hu
- hy
- id
- is
- it
- ja
- jv
- ka
- kk
- km
- kn
- ko
- ku
- ky
- la
- lo
- lt
- lv
- mg
- mk
- ml
- mn
- mr
- ms
- my
- ne
- nl
- 'no'
- om
- or
- pa
- pl
- ps
- pt
- ro
- ru
- sa
- sd
- si
- sk
- sl
- so
- sq
- sr
- su
- sv
- sw
- ta
- te
- th
- tl
- tr
- ug
- uk
- ur
- uz
- vi
- xh
- yi
- zh
license: cc-by-nc-sa-4.0
library_name: transformers
---
This is a [COMET](https://github.com/Unbabel/COMET) quality estimation model: It receives a source sentence and the respective translation and returns a score that reflects the quality of the translation.
# Paper
[CometKiwi: IST-Unbabel 2022 Submission for the Quality Estimation Shared Task](https://aclanthology.org/2022.wmt-1.60) (Rei et al., WMT 2022)
# License:
cc-by-nc-sa-4.0
# Usage (unbabel-comet)
Using this model requires unbabel-comet to be installed:
```bash
pip install --upgrade pip # ensures that pip is current
pip install "unbabel-comet>=2.0.0"
```
Make sure you acknowledge its License and Log in into Hugging face hub before using:
```bash
huggingface-cli login
# or using an environment variable
huggingface-cli login --token $HUGGINGFACE_TOKEN
```
Then you can use it through comet CLI:
```bash
comet-score -s {source-input}.txt -t {translation-output}.txt --model Unbabel/wmt22-cometkiwi-da
```
Or using Python:
```python
from comet import download_model, load_from_checkpoint
model_path = download_model("Unbabel/wmt22-cometkiwi-da")
model = load_from_checkpoint(model_path)
data = [
{
"src": "The output signal provides constant sync so the display never glitches.",
"mt": "Das Ausgangssignal bietet eine konstante Synchronisation, so dass die Anzeige nie stört."
},
{
"src": "Kroužek ilustrace je určen všem milovníkům umění ve věku od 10 do 15 let.",
"mt": "Кільце ілюстрації призначене для всіх любителів мистецтва у віці від 10 до 15 років."
},
{
"src": "Mandela then became South Africa's first black president after his African National Congress party won the 1994 election.",
"mt": "その後、1994年の選挙でアフリカ国民会議派が勝利し、南アフリカ初の黒人大統領となった。"
}
]
model_output = model.predict(data, batch_size=8, gpus=1)
print (model_output)
```
# Intended uses
Our model is intented to be used for **reference-free MT evaluation**.
Given a source text and its translation, outputs a single score between 0 and 1 where 1 represents a perfect translation.
# Languages Covered:
This model builds on top of InfoXLM which cover the following languages:
Afrikaans, Albanian, Amharic, Arabic, Armenian, Assamese, Azerbaijani, Basque, Belarusian, Bengali, Bengali Romanized, Bosnian, Breton, Bulgarian, Burmese, Burmese, Catalan, Chinese (Simplified), Chinese (Traditional), Croatian, Czech, Danish, Dutch, English, Esperanto, Estonian, Filipino, Finnish, French, Galician, Georgian, German, Greek, Gujarati, Hausa, Hebrew, Hindi, Hindi Romanized, Hungarian, Icelandic, Indonesian, Irish, Italian, Japanese, Javanese, Kannada, Kazakh, Khmer, Korean, Kurdish (Kurmanji), Kyrgyz, Lao, Latin, Latvian, Lithuanian, Macedonian, Malagasy, Malay, Malayalam, Marathi, Mongolian, Nepali, Norwegian, Oriya, Oromo, Pashto, Persian, Polish, Portuguese, Punjabi, Romanian, Russian, Sanskri, Scottish, Gaelic, Serbian, Sindhi, Sinhala, Slovak, Slovenian, Somali, Spanish, Sundanese, Swahili, Swedish, Tamil, Tamil Romanized, Telugu, Telugu Romanized, Thai, Turkish, Ukrainian, Urdu, Urdu Romanized, Uyghur, Uzbek, Vietnamese, Welsh, Western, Frisian, Xhosa, Yiddish.
Thus, results for language pairs containing uncovered languages are unreliable! | {} | vince62s/wmt22-cometkiwi-da-roberta-large | null | [
"transformers",
"pytorch",
"xlm-roberta",
"feature-extraction",
"custom_code",
"region:us"
] | null | 2024-04-25T14:08:12+00:00 | [] | [] | TAGS
#transformers #pytorch #xlm-roberta #feature-extraction #custom_code #region-us
|
This is the converted model from Unbabel/wmt22-cometkiwi-da
1) Just kept the weights/bias keys()
2) Renamed the keys to match the original Facebook/XLM-roberta-large
3) kept the layer_wise_attention / estimator layers
Because of a hack in HF's code I had to rename the "layerwise_attention.gamma" key to "layerwise_attention.gam"
I changed the URL key "layer_transformation" from sparsemax to softmax because there is a bug in COMET since the flag is not passed, the actual function used is the default which is softmax.
Usage:
Let's double check with the original code from Unbabel Comet:
---
extra_gated_heading: Acknowledge license to accept the repository
extra_gated_button_content: Acknowledge license
pipeline_tag: translation
language:
- multilingual
- af
- am
- ar
- as
- az
- be
- bg
- bn
- br
- bs
- ca
- cs
- cy
- da
- de
- el
- en
- eo
- es
- et
- eu
- fa
- fi
- fr
- fy
- ga
- gd
- gl
- gu
- ha
- he
- hi
- hr
- hu
- hy
- id
- is
- it
- ja
- jv
- ka
- kk
- km
- kn
- ko
- ku
- ky
- la
- lo
- lt
- lv
- mg
- mk
- ml
- mn
- mr
- ms
- my
- ne
- nl
- 'no'
- om
- or
- pa
- pl
- ps
- pt
- ro
- ru
- sa
- sd
- si
- sk
- sl
- so
- sq
- sr
- su
- sv
- sw
- ta
- te
- th
- tl
- tr
- ug
- uk
- ur
- uz
- vi
- xh
- yi
- zh
license: cc-by-nc-sa-4.0
library_name: transformers
---
This is a COMET quality estimation model: It receives a source sentence and the respective translation and returns a score that reflects the quality of the translation.
# Paper
CometKiwi: IST-Unbabel 2022 Submission for the Quality Estimation Shared Task (Rei et al., WMT 2022)
# License:
cc-by-nc-sa-4.0
# Usage (unbabel-comet)
Using this model requires unbabel-comet to be installed:
Make sure you acknowledge its License and Log in into Hugging face hub before using:
Then you can use it through comet CLI:
Or using Python:
# Intended uses
Our model is intented to be used for reference-free MT evaluation.
Given a source text and its translation, outputs a single score between 0 and 1 where 1 represents a perfect translation.
# Languages Covered:
This model builds on top of InfoXLM which cover the following languages:
Afrikaans, Albanian, Amharic, Arabic, Armenian, Assamese, Azerbaijani, Basque, Belarusian, Bengali, Bengali Romanized, Bosnian, Breton, Bulgarian, Burmese, Burmese, Catalan, Chinese (Simplified), Chinese (Traditional), Croatian, Czech, Danish, Dutch, English, Esperanto, Estonian, Filipino, Finnish, French, Galician, Georgian, German, Greek, Gujarati, Hausa, Hebrew, Hindi, Hindi Romanized, Hungarian, Icelandic, Indonesian, Irish, Italian, Japanese, Javanese, Kannada, Kazakh, Khmer, Korean, Kurdish (Kurmanji), Kyrgyz, Lao, Latin, Latvian, Lithuanian, Macedonian, Malagasy, Malay, Malayalam, Marathi, Mongolian, Nepali, Norwegian, Oriya, Oromo, Pashto, Persian, Polish, Portuguese, Punjabi, Romanian, Russian, Sanskri, Scottish, Gaelic, Serbian, Sindhi, Sinhala, Slovak, Slovenian, Somali, Spanish, Sundanese, Swahili, Swedish, Tamil, Tamil Romanized, Telugu, Telugu Romanized, Thai, Turkish, Ukrainian, Urdu, Urdu Romanized, Uyghur, Uzbek, Vietnamese, Welsh, Western, Frisian, Xhosa, Yiddish.
Thus, results for language pairs containing uncovered languages are unreliable! | [
"# Paper\n\nCometKiwi: IST-Unbabel 2022 Submission for the Quality Estimation Shared Task (Rei et al., WMT 2022)",
"# License:\n\ncc-by-nc-sa-4.0",
"# Usage (unbabel-comet)\n\nUsing this model requires unbabel-comet to be installed:\n\n\n\nMake sure you acknowledge its License and Log in into Hugging face hub before using:\n\n\n\nThen you can use it through comet CLI:\n\n\n\nOr using Python:",
"# Intended uses\n\nOur model is intented to be used for reference-free MT evaluation. \n\nGiven a source text and its translation, outputs a single score between 0 and 1 where 1 represents a perfect translation.",
"# Languages Covered:\n\nThis model builds on top of InfoXLM which cover the following languages:\n\nAfrikaans, Albanian, Amharic, Arabic, Armenian, Assamese, Azerbaijani, Basque, Belarusian, Bengali, Bengali Romanized, Bosnian, Breton, Bulgarian, Burmese, Burmese, Catalan, Chinese (Simplified), Chinese (Traditional), Croatian, Czech, Danish, Dutch, English, Esperanto, Estonian, Filipino, Finnish, French, Galician, Georgian, German, Greek, Gujarati, Hausa, Hebrew, Hindi, Hindi Romanized, Hungarian, Icelandic, Indonesian, Irish, Italian, Japanese, Javanese, Kannada, Kazakh, Khmer, Korean, Kurdish (Kurmanji), Kyrgyz, Lao, Latin, Latvian, Lithuanian, Macedonian, Malagasy, Malay, Malayalam, Marathi, Mongolian, Nepali, Norwegian, Oriya, Oromo, Pashto, Persian, Polish, Portuguese, Punjabi, Romanian, Russian, Sanskri, Scottish, Gaelic, Serbian, Sindhi, Sinhala, Slovak, Slovenian, Somali, Spanish, Sundanese, Swahili, Swedish, Tamil, Tamil Romanized, Telugu, Telugu Romanized, Thai, Turkish, Ukrainian, Urdu, Urdu Romanized, Uyghur, Uzbek, Vietnamese, Welsh, Western, Frisian, Xhosa, Yiddish.\n\nThus, results for language pairs containing uncovered languages are unreliable!"
] | [
"TAGS\n#transformers #pytorch #xlm-roberta #feature-extraction #custom_code #region-us \n",
"# Paper\n\nCometKiwi: IST-Unbabel 2022 Submission for the Quality Estimation Shared Task (Rei et al., WMT 2022)",
"# License:\n\ncc-by-nc-sa-4.0",
"# Usage (unbabel-comet)\n\nUsing this model requires unbabel-comet to be installed:\n\n\n\nMake sure you acknowledge its License and Log in into Hugging face hub before using:\n\n\n\nThen you can use it through comet CLI:\n\n\n\nOr using Python:",
"# Intended uses\n\nOur model is intented to be used for reference-free MT evaluation. \n\nGiven a source text and its translation, outputs a single score between 0 and 1 where 1 represents a perfect translation.",
"# Languages Covered:\n\nThis model builds on top of InfoXLM which cover the following languages:\n\nAfrikaans, Albanian, Amharic, Arabic, Armenian, Assamese, Azerbaijani, Basque, Belarusian, Bengali, Bengali Romanized, Bosnian, Breton, Bulgarian, Burmese, Burmese, Catalan, Chinese (Simplified), Chinese (Traditional), Croatian, Czech, Danish, Dutch, English, Esperanto, Estonian, Filipino, Finnish, French, Galician, Georgian, German, Greek, Gujarati, Hausa, Hebrew, Hindi, Hindi Romanized, Hungarian, Icelandic, Indonesian, Irish, Italian, Japanese, Javanese, Kannada, Kazakh, Khmer, Korean, Kurdish (Kurmanji), Kyrgyz, Lao, Latin, Latvian, Lithuanian, Macedonian, Malagasy, Malay, Malayalam, Marathi, Mongolian, Nepali, Norwegian, Oriya, Oromo, Pashto, Persian, Polish, Portuguese, Punjabi, Romanian, Russian, Sanskri, Scottish, Gaelic, Serbian, Sindhi, Sinhala, Slovak, Slovenian, Somali, Spanish, Sundanese, Swahili, Swedish, Tamil, Tamil Romanized, Telugu, Telugu Romanized, Thai, Turkish, Ukrainian, Urdu, Urdu Romanized, Uyghur, Uzbek, Vietnamese, Welsh, Western, Frisian, Xhosa, Yiddish.\n\nThus, results for language pairs containing uncovered languages are unreliable!"
] |
text-classification | bertopic |
# transformers_issues_topics
This is a [BERTopic](https://github.com/MaartenGr/BERTopic) model.
BERTopic is a flexible and modular topic modeling framework that allows for the generation of easily interpretable topics from large datasets.
## Usage
To use this model, please install BERTopic:
```
pip install -U bertopic
```
You can use the model as follows:
```python
from bertopic import BERTopic
topic_model = BERTopic.load("FelipeSarmento/transformers_issues_topics")
topic_model.get_topic_info()
```
## Topic overview
* Number of topics: 30
* Number of training documents: 9000
<details>
<summary>Click here for an overview of all topics.</summary>
| Topic ID | Topic Keywords | Topic Frequency | Label |
|----------|----------------|-----------------|-------|
| -1 | bert - model - input - models - layer | 11 | -1_bert_model_input_models |
| 0 | encoderdecoder - encoderdecodermodel - encoder - decoder - tokenizers | 2113 | 0_encoderdecoder_encoderdecodermodel_encoder_decoder |
| 1 | trainertrain - trainer - trainingarguments - pytorch - training | 1753 | 1_trainertrain_trainer_trainingarguments_pytorch |
| 2 | importerror - attributeerror - valueerror - typeerror - indexerror | 1248 | 2_importerror_attributeerror_valueerror_typeerror |
| 3 | modelcard - modelcards - card - model - models | 472 | 3_modelcard_modelcards_card_model |
| 4 | doc - typos - docstring - docs - typo | 458 | 4_doc_typos_docstring_docs |
| 5 | xlnetmodel - xlnet - xlnetlmheadmodel - xlm - xlarge | 358 | 5_xlnetmodel_xlnet_xlnetlmheadmodel_xlm |
| 6 | gpt2 - gpt2tokenizer - gpt2xl - gpt2tokenizerfast - gpt | 301 | 6_gpt2_gpt2tokenizer_gpt2xl_gpt2tokenizerfast |
| 7 | readmemd - modelcard - readmetxt - readme - file | 278 | 7_readmemd_modelcard_readmetxt_readme |
| 8 | ci - testing - tests - speedup - slow | 262 | 8_ci_testing_tests_speedup |
| 9 | transformerscli - transformers - transformer - transformerxl - importerror | 245 | 9_transformerscli_transformers_transformer_transformerxl |
| 10 | s2s - s2t - s2sdistill - s2strainer - exampless2s | 238 | 10_s2s_s2t_s2sdistill_s2strainer |
| 11 | trainertrain - trainer - logging - training - feattrainer | 212 | 11_trainertrain_trainer_logging_training |
| 12 | questionansweringpipeline - distilbertforquestionanswering - answering - questionanswering - tfalbertforquestionanswering | 139 | 12_questionansweringpipeline_distilbertforquestionanswering_answering_questionanswering |
| 13 | ner - pipeline - pipelines - nerpipeline - fixpipeline | 127 | 13_ner_pipeline_pipelines_nerpipeline |
| 14 | longformer - longformers - longform - longformerforqa - longformerlayer | 126 | 14_longformer_longformers_longform_longformerforqa |
| 15 | label - labelsmoothingfactor - labels - labelsmoothing - labellist | 116 | 15_label_labelsmoothingfactor_labels_labelsmoothing |
| 16 | onnxonnxruntime - onnx - onnxexport - 04onnxexport - 04onnxexportipynb | 101 | 16_onnxonnxruntime_onnx_onnxexport_04onnxexport |
| 17 | generationbeamsearchpy - generatebeamsearch - generatebeamsearchoutputs - beamsearch - nonbeamsearch | 86 | 17_generationbeamsearchpy_generatebeamsearch_generatebeamsearchoutputs_beamsearch |
| 18 | flax - flaxelectraformaskedlm - flaxelectraforpretraining - flaxjax - flaxelectramodel | 55 | 18_flax_flaxelectraformaskedlm_flaxelectraforpretraining_flaxjax |
| 19 | configpath - configs - config - configuration - modelconfigs | 49 | 19_configpath_configs_config_configuration |
| 20 | amp - tf - electrapretrainedmodel - tflongformer - modelingelectra | 47 | 20_amp_tf_electrapretrainedmodel_tflongformer |
| 21 | wandbproject - wandb - wandbcallback - wandbdisabled - wandbdisabledtrue | 39 | 21_wandbproject_wandb_wandbcallback_wandbdisabled |
| 22 | cachedir - cache - cachedpath - caching - cached | 37 | 22_cachedir_cache_cachedpath_caching |
| 23 | notebook - notebooks - community - blenderbot3b - blenderbot | 32 | 23_notebook_notebooks_community_blenderbot3b |
| 24 | adamw - adam - adambetas - trainingargs - wip | 30 | 24_adamw_adam_adambetas_trainingargs |
| 25 | pplm - pr - deprecated - variable - ppl | 24 | 25_pplm_pr_deprecated_variable |
| 26 | layoutlm - layoutlmtokenizer - layout - layoutlmbaseuncased - tf | 15 | 26_layoutlm_layoutlmtokenizer_layout_layoutlmbaseuncased |
| 27 | closed - licens - license - deleted - uss | 14 | 27_closed_licens_license_deleted |
| 28 | isort - github - repo - version - setupcfg | 14 | 28_isort_github_repo_version |
</details>
## Training hyperparameters
* calculate_probabilities: False
* language: english
* low_memory: False
* min_topic_size: 10
* n_gram_range: (1, 1)
* nr_topics: 30
* seed_topic_list: None
* top_n_words: 10
* verbose: True
* zeroshot_min_similarity: 0.7
* zeroshot_topic_list: None
## Framework versions
* Numpy: 1.26.4
* HDBSCAN: 0.8.33
* UMAP: 0.5.5
* Pandas: 2.2.2
* Scikit-Learn: 1.4.2
* Sentence-transformers: 2.7.0
* Transformers: 4.39.3
* Numba: 0.59.1
* Plotly: 5.21.0
* Python: 3.11.0
| {"library_name": "bertopic", "tags": ["bertopic"], "pipeline_tag": "text-classification"} | FelipeSarmento/transformers_issues_topics | null | [
"bertopic",
"text-classification",
"region:us"
] | null | 2024-04-25T14:08:58+00:00 | [] | [] | TAGS
#bertopic #text-classification #region-us
| transformers\_issues\_topics
============================
This is a BERTopic model.
BERTopic is a flexible and modular topic modeling framework that allows for the generation of easily interpretable topics from large datasets.
Usage
-----
To use this model, please install BERTopic:
You can use the model as follows:
Topic overview
--------------
* Number of topics: 30
* Number of training documents: 9000
Click here for an overview of all topics.
Training hyperparameters
------------------------
* calculate\_probabilities: False
* language: english
* low\_memory: False
* min\_topic\_size: 10
* n\_gram\_range: (1, 1)
* nr\_topics: 30
* seed\_topic\_list: None
* top\_n\_words: 10
* verbose: True
* zeroshot\_min\_similarity: 0.7
* zeroshot\_topic\_list: None
Framework versions
------------------
* Numpy: 1.26.4
* HDBSCAN: 0.8.33
* UMAP: 0.5.5
* Pandas: 2.2.2
* Scikit-Learn: 1.4.2
* Sentence-transformers: 2.7.0
* Transformers: 4.39.3
* Numba: 0.59.1
* Plotly: 5.21.0
* Python: 3.11.0
| [] | [
"TAGS\n#bertopic #text-classification #region-us \n"
] |
text-generation | transformers |
# Model Trained Using AutoTrain
This model was trained using AutoTrain. For more information, please visit [AutoTrain](https://hf.co/docs/autotrain).
# Usage
```python
from transformers import AutoModelForCausalLM, AutoTokenizer
model_path = "PATH_TO_THIS_REPO"
tokenizer = AutoTokenizer.from_pretrained(model_path)
model = AutoModelForCausalLM.from_pretrained(
model_path,
device_map="auto",
torch_dtype='auto'
).eval()
# Prompt content: "hi"
messages = [
{"role": "user", "content": "hi"}
]
input_ids = tokenizer.apply_chat_template(conversation=messages, tokenize=True, add_generation_prompt=True, return_tensors='pt')
output_ids = model.generate(input_ids.to('cuda'))
response = tokenizer.decode(output_ids[0][input_ids.shape[1]:], skip_special_tokens=True)
# Model response: "Hello! How can I assist you today?"
print(response)
``` | {"license": "other", "library_name": "transformers", "tags": ["autotrain", "text-generation-inference", "text-generation", "peft"], "widget": [{"messages": [{"role": "user", "content": "What is your favorite condiment?"}]}]} | abhishek/autotrain-fuhex-4u5bt | null | [
"transformers",
"tensorboard",
"safetensors",
"autotrain",
"text-generation-inference",
"text-generation",
"peft",
"conversational",
"license:other",
"endpoints_compatible",
"region:us"
] | null | 2024-04-25T14:09:44+00:00 | [] | [] | TAGS
#transformers #tensorboard #safetensors #autotrain #text-generation-inference #text-generation #peft #conversational #license-other #endpoints_compatible #region-us
|
# Model Trained Using AutoTrain
This model was trained using AutoTrain. For more information, please visit AutoTrain.
# Usage
| [
"# Model Trained Using AutoTrain\n\nThis model was trained using AutoTrain. For more information, please visit AutoTrain.",
"# Usage"
] | [
"TAGS\n#transformers #tensorboard #safetensors #autotrain #text-generation-inference #text-generation #peft #conversational #license-other #endpoints_compatible #region-us \n",
"# Model Trained Using AutoTrain\n\nThis model was trained using AutoTrain. For more information, please visit AutoTrain.",
"# Usage"
] |
null | transformers |
# Model Card for Model ID
<!-- Provide a quick summary of what the model is/does. -->
## Model Details
### Model Description
<!-- Provide a longer summary of what this model is. -->
This is the model card of a 🤗 transformers model that has been pushed on the Hub. This model card has been automatically generated.
- **Developed by:** [More Information Needed]
- **Funded by [optional]:** [More Information Needed]
- **Shared by [optional]:** [More Information Needed]
- **Model type:** [More Information Needed]
- **Language(s) (NLP):** [More Information Needed]
- **License:** [More Information Needed]
- **Finetuned from model [optional]:** [More Information Needed]
### Model Sources [optional]
<!-- Provide the basic links for the model. -->
- **Repository:** [More Information Needed]
- **Paper [optional]:** [More Information Needed]
- **Demo [optional]:** [More Information Needed]
## Uses
<!-- Address questions around how the model is intended to be used, including the foreseeable users of the model and those affected by the model. -->
### Direct Use
<!-- This section is for the model use without fine-tuning or plugging into a larger ecosystem/app. -->
[More Information Needed]
### Downstream Use [optional]
<!-- This section is for the model use when fine-tuned for a task, or when plugged into a larger ecosystem/app -->
[More Information Needed]
### Out-of-Scope Use
<!-- This section addresses misuse, malicious use, and uses that the model will not work well for. -->
[More Information Needed]
## Bias, Risks, and Limitations
<!-- This section is meant to convey both technical and sociotechnical limitations. -->
[More Information Needed]
### Recommendations
<!-- This section is meant to convey recommendations with respect to the bias, risk, and technical limitations. -->
Users (both direct and downstream) should be made aware of the risks, biases and limitations of the model. More information needed for further recommendations.
## How to Get Started with the Model
Use the code below to get started with the model.
[More Information Needed]
## Training Details
### Training Data
<!-- This should link to a Dataset Card, perhaps with a short stub of information on what the training data is all about as well as documentation related to data pre-processing or additional filtering. -->
[More Information Needed]
### Training Procedure
<!-- This relates heavily to the Technical Specifications. Content here should link to that section when it is relevant to the training procedure. -->
#### Preprocessing [optional]
[More Information Needed]
#### Training Hyperparameters
- **Training regime:** [More Information Needed] <!--fp32, fp16 mixed precision, bf16 mixed precision, bf16 non-mixed precision, fp16 non-mixed precision, fp8 mixed precision -->
#### Speeds, Sizes, Times [optional]
<!-- This section provides information about throughput, start/end time, checkpoint size if relevant, etc. -->
[More Information Needed]
## Evaluation
<!-- This section describes the evaluation protocols and provides the results. -->
### Testing Data, Factors & Metrics
#### Testing Data
<!-- This should link to a Dataset Card if possible. -->
[More Information Needed]
#### Factors
<!-- These are the things the evaluation is disaggregating by, e.g., subpopulations or domains. -->
[More Information Needed]
#### Metrics
<!-- These are the evaluation metrics being used, ideally with a description of why. -->
[More Information Needed]
### Results
[More Information Needed]
#### Summary
## Model Examination [optional]
<!-- Relevant interpretability work for the model goes here -->
[More Information Needed]
## Environmental Impact
<!-- Total emissions (in grams of CO2eq) and additional considerations, such as electricity usage, go here. Edit the suggested text below accordingly -->
Carbon emissions can be estimated using the [Machine Learning Impact calculator](https://mlco2.github.io/impact#compute) presented in [Lacoste et al. (2019)](https://arxiv.org/abs/1910.09700).
- **Hardware Type:** [More Information Needed]
- **Hours used:** [More Information Needed]
- **Cloud Provider:** [More Information Needed]
- **Compute Region:** [More Information Needed]
- **Carbon Emitted:** [More Information Needed]
## Technical Specifications [optional]
### Model Architecture and Objective
[More Information Needed]
### Compute Infrastructure
[More Information Needed]
#### Hardware
[More Information Needed]
#### Software
[More Information Needed]
## Citation [optional]
<!-- If there is a paper or blog post introducing the model, the APA and Bibtex information for that should go in this section. -->
**BibTeX:**
[More Information Needed]
**APA:**
[More Information Needed]
## Glossary [optional]
<!-- If relevant, include terms and calculations in this section that can help readers understand the model or model card. -->
[More Information Needed]
## More Information [optional]
[More Information Needed]
## Model Card Authors [optional]
[More Information Needed]
## Model Card Contact
[More Information Needed] | {"library_name": "transformers", "tags": []} | kishorea/finetuned_qa3 | null | [
"transformers",
"safetensors",
"arxiv:1910.09700",
"endpoints_compatible",
"region:us"
] | null | 2024-04-25T14:11:06+00:00 | [
"1910.09700"
] | [] | TAGS
#transformers #safetensors #arxiv-1910.09700 #endpoints_compatible #region-us
|
# Model Card for Model ID
## Model Details
### Model Description
This is the model card of a transformers model that has been pushed on the Hub. This model card has been automatically generated.
- Developed by:
- Funded by [optional]:
- Shared by [optional]:
- Model type:
- Language(s) (NLP):
- License:
- Finetuned from model [optional]:
### Model Sources [optional]
- Repository:
- Paper [optional]:
- Demo [optional]:
## Uses
### Direct Use
### Downstream Use [optional]
### Out-of-Scope Use
## Bias, Risks, and Limitations
### Recommendations
Users (both direct and downstream) should be made aware of the risks, biases and limitations of the model. More information needed for further recommendations.
## How to Get Started with the Model
Use the code below to get started with the model.
## Training Details
### Training Data
### Training Procedure
#### Preprocessing [optional]
#### Training Hyperparameters
- Training regime:
#### Speeds, Sizes, Times [optional]
## Evaluation
### Testing Data, Factors & Metrics
#### Testing Data
#### Factors
#### Metrics
### Results
#### Summary
## Model Examination [optional]
## Environmental Impact
Carbon emissions can be estimated using the Machine Learning Impact calculator presented in Lacoste et al. (2019).
- Hardware Type:
- Hours used:
- Cloud Provider:
- Compute Region:
- Carbon Emitted:
## Technical Specifications [optional]
### Model Architecture and Objective
### Compute Infrastructure
#### Hardware
#### Software
[optional]
BibTeX:
APA:
## Glossary [optional]
## More Information [optional]
## Model Card Authors [optional]
## Model Card Contact
| [
"# Model Card for Model ID",
"## Model Details",
"### Model Description\n\n\n\nThis is the model card of a transformers model that has been pushed on the Hub. This model card has been automatically generated.\n\n- Developed by: \n- Funded by [optional]: \n- Shared by [optional]: \n- Model type: \n- Language(s) (NLP): \n- License: \n- Finetuned from model [optional]:",
"### Model Sources [optional]\n\n\n\n- Repository: \n- Paper [optional]: \n- Demo [optional]:",
"## Uses",
"### Direct Use",
"### Downstream Use [optional]",
"### Out-of-Scope Use",
"## Bias, Risks, and Limitations",
"### Recommendations\n\n\n\nUsers (both direct and downstream) should be made aware of the risks, biases and limitations of the model. More information needed for further recommendations.",
"## How to Get Started with the Model\n\nUse the code below to get started with the model.",
"## Training Details",
"### Training Data",
"### Training Procedure",
"#### Preprocessing [optional]",
"#### Training Hyperparameters\n\n- Training regime:",
"#### Speeds, Sizes, Times [optional]",
"## Evaluation",
"### Testing Data, Factors & Metrics",
"#### Testing Data",
"#### Factors",
"#### Metrics",
"### Results",
"#### Summary",
"## Model Examination [optional]",
"## Environmental Impact\n\n\n\nCarbon emissions can be estimated using the Machine Learning Impact calculator presented in Lacoste et al. (2019).\n\n- Hardware Type: \n- Hours used: \n- Cloud Provider: \n- Compute Region: \n- Carbon Emitted:",
"## Technical Specifications [optional]",
"### Model Architecture and Objective",
"### Compute Infrastructure",
"#### Hardware",
"#### Software\n\n\n\n[optional]\n\n\n\nBibTeX:\n\n\n\nAPA:",
"## Glossary [optional]",
"## More Information [optional]",
"## Model Card Authors [optional]",
"## Model Card Contact"
] | [
"TAGS\n#transformers #safetensors #arxiv-1910.09700 #endpoints_compatible #region-us \n",
"# Model Card for Model ID",
"## Model Details",
"### Model Description\n\n\n\nThis is the model card of a transformers model that has been pushed on the Hub. This model card has been automatically generated.\n\n- Developed by: \n- Funded by [optional]: \n- Shared by [optional]: \n- Model type: \n- Language(s) (NLP): \n- License: \n- Finetuned from model [optional]:",
"### Model Sources [optional]\n\n\n\n- Repository: \n- Paper [optional]: \n- Demo [optional]:",
"## Uses",
"### Direct Use",
"### Downstream Use [optional]",
"### Out-of-Scope Use",
"## Bias, Risks, and Limitations",
"### Recommendations\n\n\n\nUsers (both direct and downstream) should be made aware of the risks, biases and limitations of the model. More information needed for further recommendations.",
"## How to Get Started with the Model\n\nUse the code below to get started with the model.",
"## Training Details",
"### Training Data",
"### Training Procedure",
"#### Preprocessing [optional]",
"#### Training Hyperparameters\n\n- Training regime:",
"#### Speeds, Sizes, Times [optional]",
"## Evaluation",
"### Testing Data, Factors & Metrics",
"#### Testing Data",
"#### Factors",
"#### Metrics",
"### Results",
"#### Summary",
"## Model Examination [optional]",
"## Environmental Impact\n\n\n\nCarbon emissions can be estimated using the Machine Learning Impact calculator presented in Lacoste et al. (2019).\n\n- Hardware Type: \n- Hours used: \n- Cloud Provider: \n- Compute Region: \n- Carbon Emitted:",
"## Technical Specifications [optional]",
"### Model Architecture and Objective",
"### Compute Infrastructure",
"#### Hardware",
"#### Software\n\n\n\n[optional]\n\n\n\nBibTeX:\n\n\n\nAPA:",
"## Glossary [optional]",
"## More Information [optional]",
"## Model Card Authors [optional]",
"## Model Card Contact"
] |
text-generation | transformers |
# Model Card for Model ID
<!-- Provide a quick summary of what the model is/does. -->
## Model Details
### Model Description
<!-- Provide a longer summary of what this model is. -->
This is the model card of a 🤗 transformers model that has been pushed on the Hub. This model card has been automatically generated.
- **Developed by:** [More Information Needed]
- **Funded by [optional]:** [More Information Needed]
- **Shared by [optional]:** [More Information Needed]
- **Model type:** [More Information Needed]
- **Language(s) (NLP):** [More Information Needed]
- **License:** [More Information Needed]
- **Finetuned from model [optional]:** [More Information Needed]
### Model Sources [optional]
<!-- Provide the basic links for the model. -->
- **Repository:** [More Information Needed]
- **Paper [optional]:** [More Information Needed]
- **Demo [optional]:** [More Information Needed]
## Uses
<!-- Address questions around how the model is intended to be used, including the foreseeable users of the model and those affected by the model. -->
### Direct Use
<!-- This section is for the model use without fine-tuning or plugging into a larger ecosystem/app. -->
[More Information Needed]
### Downstream Use [optional]
<!-- This section is for the model use when fine-tuned for a task, or when plugged into a larger ecosystem/app -->
[More Information Needed]
### Out-of-Scope Use
<!-- This section addresses misuse, malicious use, and uses that the model will not work well for. -->
[More Information Needed]
## Bias, Risks, and Limitations
<!-- This section is meant to convey both technical and sociotechnical limitations. -->
[More Information Needed]
### Recommendations
<!-- This section is meant to convey recommendations with respect to the bias, risk, and technical limitations. -->
Users (both direct and downstream) should be made aware of the risks, biases and limitations of the model. More information needed for further recommendations.
## How to Get Started with the Model
Use the code below to get started with the model.
[More Information Needed]
## Training Details
### Training Data
<!-- This should link to a Dataset Card, perhaps with a short stub of information on what the training data is all about as well as documentation related to data pre-processing or additional filtering. -->
[More Information Needed]
### Training Procedure
<!-- This relates heavily to the Technical Specifications. Content here should link to that section when it is relevant to the training procedure. -->
#### Preprocessing [optional]
[More Information Needed]
#### Training Hyperparameters
- **Training regime:** [More Information Needed] <!--fp32, fp16 mixed precision, bf16 mixed precision, bf16 non-mixed precision, fp16 non-mixed precision, fp8 mixed precision -->
#### Speeds, Sizes, Times [optional]
<!-- This section provides information about throughput, start/end time, checkpoint size if relevant, etc. -->
[More Information Needed]
## Evaluation
<!-- This section describes the evaluation protocols and provides the results. -->
### Testing Data, Factors & Metrics
#### Testing Data
<!-- This should link to a Dataset Card if possible. -->
[More Information Needed]
#### Factors
<!-- These are the things the evaluation is disaggregating by, e.g., subpopulations or domains. -->
[More Information Needed]
#### Metrics
<!-- These are the evaluation metrics being used, ideally with a description of why. -->
[More Information Needed]
### Results
[More Information Needed]
#### Summary
## Model Examination [optional]
<!-- Relevant interpretability work for the model goes here -->
[More Information Needed]
## Environmental Impact
<!-- Total emissions (in grams of CO2eq) and additional considerations, such as electricity usage, go here. Edit the suggested text below accordingly -->
Carbon emissions can be estimated using the [Machine Learning Impact calculator](https://mlco2.github.io/impact#compute) presented in [Lacoste et al. (2019)](https://arxiv.org/abs/1910.09700).
- **Hardware Type:** [More Information Needed]
- **Hours used:** [More Information Needed]
- **Cloud Provider:** [More Information Needed]
- **Compute Region:** [More Information Needed]
- **Carbon Emitted:** [More Information Needed]
## Technical Specifications [optional]
### Model Architecture and Objective
[More Information Needed]
### Compute Infrastructure
[More Information Needed]
#### Hardware
[More Information Needed]
#### Software
[More Information Needed]
## Citation [optional]
<!-- If there is a paper or blog post introducing the model, the APA and Bibtex information for that should go in this section. -->
**BibTeX:**
[More Information Needed]
**APA:**
[More Information Needed]
## Glossary [optional]
<!-- If relevant, include terms and calculations in this section that can help readers understand the model or model card. -->
[More Information Needed]
## More Information [optional]
[More Information Needed]
## Model Card Authors [optional]
[More Information Needed]
## Model Card Contact
[More Information Needed] | {"library_name": "transformers", "tags": []} | quickstep3621/ues7n2i | null | [
"transformers",
"safetensors",
"stablelm",
"text-generation",
"conversational",
"arxiv:1910.09700",
"autotrain_compatible",
"endpoints_compatible",
"region:us"
] | null | 2024-04-25T14:11:48+00:00 | [
"1910.09700"
] | [] | TAGS
#transformers #safetensors #stablelm #text-generation #conversational #arxiv-1910.09700 #autotrain_compatible #endpoints_compatible #region-us
|
# Model Card for Model ID
## Model Details
### Model Description
This is the model card of a transformers model that has been pushed on the Hub. This model card has been automatically generated.
- Developed by:
- Funded by [optional]:
- Shared by [optional]:
- Model type:
- Language(s) (NLP):
- License:
- Finetuned from model [optional]:
### Model Sources [optional]
- Repository:
- Paper [optional]:
- Demo [optional]:
## Uses
### Direct Use
### Downstream Use [optional]
### Out-of-Scope Use
## Bias, Risks, and Limitations
### Recommendations
Users (both direct and downstream) should be made aware of the risks, biases and limitations of the model. More information needed for further recommendations.
## How to Get Started with the Model
Use the code below to get started with the model.
## Training Details
### Training Data
### Training Procedure
#### Preprocessing [optional]
#### Training Hyperparameters
- Training regime:
#### Speeds, Sizes, Times [optional]
## Evaluation
### Testing Data, Factors & Metrics
#### Testing Data
#### Factors
#### Metrics
### Results
#### Summary
## Model Examination [optional]
## Environmental Impact
Carbon emissions can be estimated using the Machine Learning Impact calculator presented in Lacoste et al. (2019).
- Hardware Type:
- Hours used:
- Cloud Provider:
- Compute Region:
- Carbon Emitted:
## Technical Specifications [optional]
### Model Architecture and Objective
### Compute Infrastructure
#### Hardware
#### Software
[optional]
BibTeX:
APA:
## Glossary [optional]
## More Information [optional]
## Model Card Authors [optional]
## Model Card Contact
| [
"# Model Card for Model ID",
"## Model Details",
"### Model Description\n\n\n\nThis is the model card of a transformers model that has been pushed on the Hub. This model card has been automatically generated.\n\n- Developed by: \n- Funded by [optional]: \n- Shared by [optional]: \n- Model type: \n- Language(s) (NLP): \n- License: \n- Finetuned from model [optional]:",
"### Model Sources [optional]\n\n\n\n- Repository: \n- Paper [optional]: \n- Demo [optional]:",
"## Uses",
"### Direct Use",
"### Downstream Use [optional]",
"### Out-of-Scope Use",
"## Bias, Risks, and Limitations",
"### Recommendations\n\n\n\nUsers (both direct and downstream) should be made aware of the risks, biases and limitations of the model. More information needed for further recommendations.",
"## How to Get Started with the Model\n\nUse the code below to get started with the model.",
"## Training Details",
"### Training Data",
"### Training Procedure",
"#### Preprocessing [optional]",
"#### Training Hyperparameters\n\n- Training regime:",
"#### Speeds, Sizes, Times [optional]",
"## Evaluation",
"### Testing Data, Factors & Metrics",
"#### Testing Data",
"#### Factors",
"#### Metrics",
"### Results",
"#### Summary",
"## Model Examination [optional]",
"## Environmental Impact\n\n\n\nCarbon emissions can be estimated using the Machine Learning Impact calculator presented in Lacoste et al. (2019).\n\n- Hardware Type: \n- Hours used: \n- Cloud Provider: \n- Compute Region: \n- Carbon Emitted:",
"## Technical Specifications [optional]",
"### Model Architecture and Objective",
"### Compute Infrastructure",
"#### Hardware",
"#### Software\n\n\n\n[optional]\n\n\n\nBibTeX:\n\n\n\nAPA:",
"## Glossary [optional]",
"## More Information [optional]",
"## Model Card Authors [optional]",
"## Model Card Contact"
] | [
"TAGS\n#transformers #safetensors #stablelm #text-generation #conversational #arxiv-1910.09700 #autotrain_compatible #endpoints_compatible #region-us \n",
"# Model Card for Model ID",
"## Model Details",
"### Model Description\n\n\n\nThis is the model card of a transformers model that has been pushed on the Hub. This model card has been automatically generated.\n\n- Developed by: \n- Funded by [optional]: \n- Shared by [optional]: \n- Model type: \n- Language(s) (NLP): \n- License: \n- Finetuned from model [optional]:",
"### Model Sources [optional]\n\n\n\n- Repository: \n- Paper [optional]: \n- Demo [optional]:",
"## Uses",
"### Direct Use",
"### Downstream Use [optional]",
"### Out-of-Scope Use",
"## Bias, Risks, and Limitations",
"### Recommendations\n\n\n\nUsers (both direct and downstream) should be made aware of the risks, biases and limitations of the model. More information needed for further recommendations.",
"## How to Get Started with the Model\n\nUse the code below to get started with the model.",
"## Training Details",
"### Training Data",
"### Training Procedure",
"#### Preprocessing [optional]",
"#### Training Hyperparameters\n\n- Training regime:",
"#### Speeds, Sizes, Times [optional]",
"## Evaluation",
"### Testing Data, Factors & Metrics",
"#### Testing Data",
"#### Factors",
"#### Metrics",
"### Results",
"#### Summary",
"## Model Examination [optional]",
"## Environmental Impact\n\n\n\nCarbon emissions can be estimated using the Machine Learning Impact calculator presented in Lacoste et al. (2019).\n\n- Hardware Type: \n- Hours used: \n- Cloud Provider: \n- Compute Region: \n- Carbon Emitted:",
"## Technical Specifications [optional]",
"### Model Architecture and Objective",
"### Compute Infrastructure",
"#### Hardware",
"#### Software\n\n\n\n[optional]\n\n\n\nBibTeX:\n\n\n\nAPA:",
"## Glossary [optional]",
"## More Information [optional]",
"## Model Card Authors [optional]",
"## Model Card Contact"
] |
null | peft |
<!-- This model card has been generated automatically according to the information the Trainer had access to. You
should probably proofread and complete it, then remove this comment. -->
# zephyr-7b-dpo-qlora-training-on-full-binary
This model is a fine-tuned version of [alignment-handbook/zephyr-7b-sft-qlora](https://huggingface.co/alignment-handbook/zephyr-7b-sft-qlora) on the updated and the original datasets.
It achieves the following results on the evaluation set:
- Loss: 0.5386
- Rewards/chosen: -0.9159
- Rewards/rejected: -1.5101
- Rewards/accuracies: 0.6960
- Rewards/margins: 0.5943
- Logps/rejected: -392.2019
- Logps/chosen: -355.0374
- Logits/rejected: 0.1234
- Logits/chosen: -0.3064
## Model description
More information needed
## Intended uses & limitations
More information needed
## Training and evaluation data
More information needed
## Training procedure
### Training hyperparameters
The following hyperparameters were used during training:
- learning_rate: 5e-06
- train_batch_size: 2
- eval_batch_size: 2
- seed: 42
- distributed_type: multi-GPU
- num_devices: 4
- gradient_accumulation_steps: 32
- total_train_batch_size: 256
- total_eval_batch_size: 8
- optimizer: Adam with betas=(0.9,0.999) and epsilon=1e-08
- lr_scheduler_type: cosine
- lr_scheduler_warmup_ratio: 0.1
- num_epochs: 1
### Training results
| Training Loss | Epoch | Step | Validation Loss | Rewards/chosen | Rewards/rejected | Rewards/accuracies | Rewards/margins | Logps/rejected | Logps/chosen | Logits/rejected | Logits/chosen |
|:-------------:|:-----:|:----:|:---------------:|:--------------:|:----------------:|:------------------:|:---------------:|:--------------:|:------------:|:---------------:|:-------------:|
| 0.5464 | 0.42 | 100 | 0.5636 | -0.6892 | -1.1960 | 0.7060 | 0.5068 | -360.7926 | -332.3759 | -0.6169 | -0.9747 |
| 0.5369 | 0.84 | 200 | 0.5386 | -0.9159 | -1.5101 | 0.6960 | 0.5943 | -392.2019 | -355.0374 | 0.1234 | -0.3064 |
### Framework versions
- PEFT 0.7.1
- Transformers 4.36.2
- Pytorch 2.2.2+cu121
- Datasets 2.14.6
- Tokenizers 0.15.2 | {"license": "apache-2.0", "library_name": "peft", "tags": ["alignment-handbook", "generated_from_trainer", "trl", "dpo"], "datasets": ["updated", "original"], "base_model": "mistralai/Mistral-7B-v0.1", "model-index": [{"name": "zephyr-7b-dpo-qlora-training-on-full-binary", "results": []}]} | YYYYYYibo/zephyr-7b-dpo-qlora-training-on-full-binary | null | [
"peft",
"safetensors",
"mistral",
"alignment-handbook",
"generated_from_trainer",
"trl",
"dpo",
"dataset:updated",
"dataset:original",
"base_model:mistralai/Mistral-7B-v0.1",
"license:apache-2.0",
"4-bit",
"region:us"
] | null | 2024-04-25T14:12:28+00:00 | [] | [] | TAGS
#peft #safetensors #mistral #alignment-handbook #generated_from_trainer #trl #dpo #dataset-updated #dataset-original #base_model-mistralai/Mistral-7B-v0.1 #license-apache-2.0 #4-bit #region-us
| zephyr-7b-dpo-qlora-training-on-full-binary
===========================================
This model is a fine-tuned version of alignment-handbook/zephyr-7b-sft-qlora on the updated and the original datasets.
It achieves the following results on the evaluation set:
* Loss: 0.5386
* Rewards/chosen: -0.9159
* Rewards/rejected: -1.5101
* Rewards/accuracies: 0.6960
* Rewards/margins: 0.5943
* Logps/rejected: -392.2019
* Logps/chosen: -355.0374
* Logits/rejected: 0.1234
* Logits/chosen: -0.3064
Model description
-----------------
More information needed
Intended uses & limitations
---------------------------
More information needed
Training and evaluation data
----------------------------
More information needed
Training procedure
------------------
### Training hyperparameters
The following hyperparameters were used during training:
* learning\_rate: 5e-06
* train\_batch\_size: 2
* eval\_batch\_size: 2
* seed: 42
* distributed\_type: multi-GPU
* num\_devices: 4
* gradient\_accumulation\_steps: 32
* total\_train\_batch\_size: 256
* total\_eval\_batch\_size: 8
* optimizer: Adam with betas=(0.9,0.999) and epsilon=1e-08
* lr\_scheduler\_type: cosine
* lr\_scheduler\_warmup\_ratio: 0.1
* num\_epochs: 1
### Training results
### Framework versions
* PEFT 0.7.1
* Transformers 4.36.2
* Pytorch 2.2.2+cu121
* Datasets 2.14.6
* Tokenizers 0.15.2
| [
"### Training hyperparameters\n\n\nThe following hyperparameters were used during training:\n\n\n* learning\\_rate: 5e-06\n* train\\_batch\\_size: 2\n* eval\\_batch\\_size: 2\n* seed: 42\n* distributed\\_type: multi-GPU\n* num\\_devices: 4\n* gradient\\_accumulation\\_steps: 32\n* total\\_train\\_batch\\_size: 256\n* total\\_eval\\_batch\\_size: 8\n* optimizer: Adam with betas=(0.9,0.999) and epsilon=1e-08\n* lr\\_scheduler\\_type: cosine\n* lr\\_scheduler\\_warmup\\_ratio: 0.1\n* num\\_epochs: 1",
"### Training results",
"### Framework versions\n\n\n* PEFT 0.7.1\n* Transformers 4.36.2\n* Pytorch 2.2.2+cu121\n* Datasets 2.14.6\n* Tokenizers 0.15.2"
] | [
"TAGS\n#peft #safetensors #mistral #alignment-handbook #generated_from_trainer #trl #dpo #dataset-updated #dataset-original #base_model-mistralai/Mistral-7B-v0.1 #license-apache-2.0 #4-bit #region-us \n",
"### Training hyperparameters\n\n\nThe following hyperparameters were used during training:\n\n\n* learning\\_rate: 5e-06\n* train\\_batch\\_size: 2\n* eval\\_batch\\_size: 2\n* seed: 42\n* distributed\\_type: multi-GPU\n* num\\_devices: 4\n* gradient\\_accumulation\\_steps: 32\n* total\\_train\\_batch\\_size: 256\n* total\\_eval\\_batch\\_size: 8\n* optimizer: Adam with betas=(0.9,0.999) and epsilon=1e-08\n* lr\\_scheduler\\_type: cosine\n* lr\\_scheduler\\_warmup\\_ratio: 0.1\n* num\\_epochs: 1",
"### Training results",
"### Framework versions\n\n\n* PEFT 0.7.1\n* Transformers 4.36.2\n* Pytorch 2.2.2+cu121\n* Datasets 2.14.6\n* Tokenizers 0.15.2"
] |
reinforcement-learning | ml-agents |
---
# **PPO AI Agents Playing Pyramids**
<img src="https://huggingface.co/datasets/huggingface-deep-rl-course/course-images/resolve/main/en/unit7/pyramids.gif" alt="Pyramids"/>
**DISCLAIMER: In this notebook it has two environments you can train agents to play in the snowball and pyramid one. In this model card I am only covering the Pyramid game model. But a lot of these trouble shooting guides will be applicable to the snowball environment as well.**
This is a trained model of a **ppo** agent playing **UNITY game Pyramids** Using Q-learning and reinforcement learning to train the agent to navigate around a simple maze environment where they need to activate a button that the pyramid then they need to locate the pyramid and knock the pyramid stacked blocks over so the green block on top falls to the ground.
I used the [Unity ML-Agents Library](https://github.com/Unity-Technologies/ml-agents).
Throughout this notebook you will learn about how to train AI agent using Q learning in a Unity Game 3D game environment. Utilizing the different curiosity and exploitation values as well as manipulating the various hyperperameters to get the best training results.
It is an easy notebook to follow through with excellent instructions so if you want to learn more about the process used to train these AI agents in 3D environments I highly recommend this project. It is best if you have some experiences learning about or working with deep learning machine learning, because it may be difficult to understand this reinforcement learning process. If you dont have experiences or interested in learning more you can access resources to more introductory notebooks in this link.
https://huggingface.co/learn/deep-rl-course/unit1/introduction
So if you are interested in continuing in training AI agents to playing the Unity Pyramids thats great! Because below is a few different resources I have gathered to troubleshoot through problems I have faced, basic info about how the model works and how you can improve the model. I wish I had known about before completing this notebook. Which will hopefully make it easier for you on your journey.
Also here is the link of my working model
https://colab.research.google.com/drive/1W3omht-9b_ybPlmpaisEek9Mgy5LV875?usp=sharing
Also heres a video demoing what the aim of the AI agents in this pyramid game is https://www.youtube.com/watch?v=Ab2fHTMGf50
## **Learning components of this model:**
<img src="https://cdn-lfs.huggingface.co/repos/48/e0/48e06489d875e3d8a62c53306ab6e114abc24ab8fb4cba7652e808785a6bdc24/f0ff122f71f964288bf4fc216472f5c105f24c8b3107c007707ae1c8fecdb653?response-content-disposition=inline%3B+filename*%3DUTF-8%27%27RL_process_game.jpg%3B+filename%3D%22RL_process_game.jpg%22%3B&response-content-type=image%2Fjpeg&Expires=1714697644&Policy=eyJTdGF0ZW1lbnQiOlt7IkNvbmRpdGlvbiI6eyJEYXRlTGVzc1RoYW4iOnsiQVdTOkVwb2NoVGltZSI6MTcxNDY5NzY0NH19LCJSZXNvdXJjZSI6Imh0dHBzOi8vY2RuLWxmcy5odWdnaW5nZmFjZS5jby9yZXBvcy80OC9lMC80OGUwNjQ4OWQ4NzVlM2Q4YTYyYzUzMzA2YWI2ZTExNGFiYzI0YWI4ZmI0Y2JhNzY1MmU4MDg3ODVhNmJkYzI0L2YwZmYxMjJmNzFmOTY0Mjg4YmY0ZmMyMTY0NzJmNWMxMDVmMjRjOGIzMTA3YzAwNzcwN2FlMWM4ZmVjZGI2NTM%7EcmVzcG9uc2UtY29udGVudC1kaXNwb3NpdGlvbj0qJnJlc3BvbnNlLWNvbnRlbnQtdHlwZT0qIn1dfQ__&Signature=xv5GLSYAUY%7E0cL0kgCR4aQ6rMqcG-BYc5g1HzmybPb33X3Yk0fefddSwVqbErJ%7Eq4Olh6aS0-xv6KHBOtI8Xv1DDzJo6h2yvHFLkE%7EbqFpeVjig2VgGCrSxzjtRuzY3xhgL0nmBYLKersb%7E7fSZ-2JNDyqwTIfFSPhJJLwH6SqzjCLPUQxBoxAvrGBx2I0z%7Es0Zrz9RancvDKGDLmSh1vcRKnpNoeMNyTbdZIYKgZ18bg4gQwpCl6%7EN9mblNrdGlO-Z9O6RKzR7RJWHtZkfk5MBL-5t6AwflaR%7EMqIy4rEPOWBb38gEi4B-xuskiImg8e6dKwxduhckRiOBTokWXug__&Key-Pair-Id=KVTP0A1DKRTAX"/>
1. Agent component: training agents by optimizing their policy(policy based method, unlike value based methods they optimize the policy itself instead of values) telling the model what action to take in each step in the model called a brain.
2. For this model we will be using a proximal policy optimizer (PPO) as seen at the title of the model card. PPO is ideal for training AI agents in Unity games because it is sample-efficient, stable during training, compatible with neural networks, handles both continuous and discrete action spaces, and robust in handling complex game dynamics and mechanics.
**Curiosity in training ML agents:**
In this reinforcement learning project you will need to understand how curiosity plays a part in the training.In short, Traditionally a reward system is used to train ML agents but for more complicated games and obscure objective it is hard to manually place rewards for the agent.
Curiosity is rewarding the model for taking new trajectories for example exploring new rooms
Here is a youtube videos that helped me understand the concept:
https://www.youtube.com/watch?v=eLq6yI2No (this one talks specifically about the game environment)
https://www.youtube.com/watch?v=nIgIv4IfJ6s (And this one illustrates more generally about reinforcement learning but also covers curiosity, great and easy to understand for beginners, also if you are intersted in learning more about how ai and machine learning the rest of this crash course series is great as well)
## **Improving model training through hyperparameters adjusting**
So once you have a working model and want to improve the training outcomes.
These hyperparameter tunings can be adjusted within the Pyramid RND file component on the side and below is a detailed list on what changing each individual parameter will impact the training. Just be minful after making changes you need to run the code responsible for copying the file into the envs executable linux, as well as unzipping along with retraining to implement these new parameters into your model.
1. Trainer type: the type of trainer being used here we use Proximal policy optimization
2. Summary_freq: How often the training summaries and statisitcs are recorded(rewards, losses, lengths, time etc )
3. Keep_checkpoints: number of recent checkpoints to keep checkpoints are snapshots of training models for resumign training or evaluation
4. Checkpoint interval: how often(many steps) save checkpoints
5. Max_steps: Maximum number of steps or interactions
6. Time_horizon: The number of steps the agent considers when making decisions
7. Threaded: Enables multi-threading during training(may allow for faster processing, parts of code run simultaneously)
8. Hyperparameters:
9. Learning rate: How quickly the agents adjust their behavior based on feedback
10. Learning rate_schedule: the rule that used to adjust or modify the learning rate during the training process
11. Batch_size: number of samples used in each updated batch training
12. Buffer_size :size of the experience replay buffer, which stores past experiences for training updates.
13. Beta: exploration levels
14. Epilson:It limits the size of behavior changes to prevent large policy updates.
15. Lambd: It helps estimate the advantage of taking a particular action in a given state.
16. Num_epoch:Specifies the number of times the entire dataset is used for training updates. Each epoch consists of multiple iterations over the dataset.
**Network Settings:(architecture for neural network)**
17. Normalize:It determines whether input observations are normalized.
18. Hidden unit: Number of units in each hidden layers
19. Num layers: Number of hidden layers the model has
20. Vis_encode_type: ways visual observations are encoded
**Reward Signals**
21. Gamma: It determines the importance of future rewards compared to immediate rewards.
22. Strength: It controls the weight of the primary reward signal relative to other rewards, if present.
## **Trouble Shooting**
Here are some problems I encountered and solutions I used, and also things I wished I knew in hindsight
**GPU not connecting**
Sometimes the GPU can get overwhelmed causing the code to not load if you have pressed it too many times and too many piled up commands.
You can check on the right top side if the GPU is being used, if it shows "connecting" or gives you the error gpu is not connected would you like to continue anyways one way is under the tab manage sessions,
you can terminate previous sessions and start again from my own experience this has rebooted the session and gpu was able to connect.
**Restarting session for numpy**
When you are running the second block of code that downloads all the packages you will need for this notebook it is important when the popup appears telling you to restart notebook for numpy to accept it as it will need to reboot in order for the packages to work correctly and after restarting you can continue on the notebook running from the next following code block.
**Unizipping files wont load**
I have struggled with the line of code regarding unzipping the Pyramid files struggling to load, one method could be reconnecting the GPU as I have mentioned earlier
but if that still doesnt work you can download the code from the link. And unzip it on your computer then reuploaded to the corresponding folder to bypass that line of code.
https://colab.research.google.com/corgiredirector?site=https%3A%2F%2Fhuggingface.co%2Fspaces%2Funity%2FML-Agents-Pyramids%2Fresolve%2Fmain%2FPyramids.zip then unzipping on your computer then reuploading it to the corresponding folder location in training-envs-executables/linux/
**File does not exist error code**
When running a code results in a "this file does not exist, or this folder does not exist" it could be from not correctly loading previous code blocks or run time was lost if you closed down the program. You can check if this is the case by going into the side directory of files and go under the corresponding folders to check if files are indeed there. If not just reload the blocks of code that creates the files.
**Connecting to google drive**
1. In order for the code to run it needs to be mounted to your google drive. So if you a running this through an organizations google account for example schools. It may need to be approved from the IT for it to be allowed to be mounted to the google drive. So make sure that is cleared before continuing the notebook.
2. Also Another cause to the drive not connecting is you may have popups blocked so you will need to allow popups for google collab or else the option to connect ot connect to your google drive will not appear
**Saving progress**
While run info is saved to your google drive. Since this is a edited notebook changes you make to the hyper parameters are not saved so everytime you rerun the notebook it will reset to the prexisting values. Also training progress cannot be called back if you reopen a later time, you will need to rerun the whole code and retrain which is quite time consuming so I recommend you using the resource.
https://learningmaterialcomputations.medium.com/save-any-file-from-google-colab-to-your-google-drive-caf8291ba59b#:~:text=Step%201%3A%20Mount%20your%20google,that%20you're%20working%20with.&text=Step%202%3A%20Authorise%20Google%20Colab,the%20%E2%80%9CCopy%20Path%E2%80%9D%20option.
### Watch your Agent play
You can watch your agent **playing directly in your browser**
<img src="https://huggingface.co/datasets/huggingface-deep-rl-course/course-images/resolve/main/en/unit7/snowballtarget_load.png" alt="Snowballtarget load"/>
After correctly training the agent and uploading it to the hub it should produce a link that leads you to this page where you can see your agent playing.
1. If the environment is part of ML-Agents official environments, go to https://huggingface.co/unity
2. Step 1: Find your model_id: MY11111111/ppo-Pyramids123 (this is my model_id so you will need to isnert the one that is produced from your own notebooke)
3. Step 2: Select your *.nn /*.onnx file
4. Click on Watch the agent play 👀
| {"language": ["en"], "library_name": "ml-agents", "tags": ["Pyramids", "deep-reinforcement-learning", "reinforcement-learning", "ML-Agents-Pyramids"], "pipeline_tag": "reinforcement-learning"} | MY11111111/ppo-Pyramids123 | null | [
"ml-agents",
"tensorboard",
"onnx",
"Pyramids",
"deep-reinforcement-learning",
"reinforcement-learning",
"ML-Agents-Pyramids",
"en",
"region:us"
] | null | 2024-04-25T14:12:33+00:00 | [] | [
"en"
] | TAGS
#ml-agents #tensorboard #onnx #Pyramids #deep-reinforcement-learning #reinforcement-learning #ML-Agents-Pyramids #en #region-us
|
---
# PPO AI Agents Playing Pyramids
<img src="URL alt="Pyramids"/>
DISCLAIMER: In this notebook it has two environments you can train agents to play in the snowball and pyramid one. In this model card I am only covering the Pyramid game model. But a lot of these trouble shooting guides will be applicable to the snowball environment as well.
This is a trained model of a ppo agent playing UNITY game Pyramids Using Q-learning and reinforcement learning to train the agent to navigate around a simple maze environment where they need to activate a button that the pyramid then they need to locate the pyramid and knock the pyramid stacked blocks over so the green block on top falls to the ground.
I used the Unity ML-Agents Library.
Throughout this notebook you will learn about how to train AI agent using Q learning in a Unity Game 3D game environment. Utilizing the different curiosity and exploitation values as well as manipulating the various hyperperameters to get the best training results.
It is an easy notebook to follow through with excellent instructions so if you want to learn more about the process used to train these AI agents in 3D environments I highly recommend this project. It is best if you have some experiences learning about or working with deep learning machine learning, because it may be difficult to understand this reinforcement learning process. If you dont have experiences or interested in learning more you can access resources to more introductory notebooks in this link.
URL
So if you are interested in continuing in training AI agents to playing the Unity Pyramids thats great! Because below is a few different resources I have gathered to troubleshoot through problems I have faced, basic info about how the model works and how you can improve the model. I wish I had known about before completing this notebook. Which will hopefully make it easier for you on your journey.
Also here is the link of my working model
URL
Also heres a video demoing what the aim of the AI agents in this pyramid game is URL
## Learning components of this model:
<img src="URL
1. Agent component: training agents by optimizing their policy(policy based method, unlike value based methods they optimize the policy itself instead of values) telling the model what action to take in each step in the model called a brain.
2. For this model we will be using a proximal policy optimizer (PPO) as seen at the title of the model card. PPO is ideal for training AI agents in Unity games because it is sample-efficient, stable during training, compatible with neural networks, handles both continuous and discrete action spaces, and robust in handling complex game dynamics and mechanics.
Curiosity in training ML agents:
In this reinforcement learning project you will need to understand how curiosity plays a part in the training.In short, Traditionally a reward system is used to train ML agents but for more complicated games and obscure objective it is hard to manually place rewards for the agent.
Curiosity is rewarding the model for taking new trajectories for example exploring new rooms
Here is a youtube videos that helped me understand the concept:
URL (this one talks specifically about the game environment)
URL (And this one illustrates more generally about reinforcement learning but also covers curiosity, great and easy to understand for beginners, also if you are intersted in learning more about how ai and machine learning the rest of this crash course series is great as well)
## Improving model training through hyperparameters adjusting
So once you have a working model and want to improve the training outcomes.
These hyperparameter tunings can be adjusted within the Pyramid RND file component on the side and below is a detailed list on what changing each individual parameter will impact the training. Just be minful after making changes you need to run the code responsible for copying the file into the envs executable linux, as well as unzipping along with retraining to implement these new parameters into your model.
1. Trainer type: the type of trainer being used here we use Proximal policy optimization
2. Summary_freq: How often the training summaries and statisitcs are recorded(rewards, losses, lengths, time etc )
3. Keep_checkpoints: number of recent checkpoints to keep checkpoints are snapshots of training models for resumign training or evaluation
4. Checkpoint interval: how often(many steps) save checkpoints
5. Max_steps: Maximum number of steps or interactions
6. Time_horizon: The number of steps the agent considers when making decisions
7. Threaded: Enables multi-threading during training(may allow for faster processing, parts of code run simultaneously)
8. Hyperparameters:
9. Learning rate: How quickly the agents adjust their behavior based on feedback
10. Learning rate_schedule: the rule that used to adjust or modify the learning rate during the training process
11. Batch_size: number of samples used in each updated batch training
12. Buffer_size :size of the experience replay buffer, which stores past experiences for training updates.
13. Beta: exploration levels
14. Epilson:It limits the size of behavior changes to prevent large policy updates.
15. Lambd: It helps estimate the advantage of taking a particular action in a given state.
16. Num_epoch:Specifies the number of times the entire dataset is used for training updates. Each epoch consists of multiple iterations over the dataset.
Network Settings:(architecture for neural network)
17. Normalize:It determines whether input observations are normalized.
18. Hidden unit: Number of units in each hidden layers
19. Num layers: Number of hidden layers the model has
20. Vis_encode_type: ways visual observations are encoded
Reward Signals
21. Gamma: It determines the importance of future rewards compared to immediate rewards.
22. Strength: It controls the weight of the primary reward signal relative to other rewards, if present.
## Trouble Shooting
Here are some problems I encountered and solutions I used, and also things I wished I knew in hindsight
GPU not connecting
Sometimes the GPU can get overwhelmed causing the code to not load if you have pressed it too many times and too many piled up commands.
You can check on the right top side if the GPU is being used, if it shows "connecting" or gives you the error gpu is not connected would you like to continue anyways one way is under the tab manage sessions,
you can terminate previous sessions and start again from my own experience this has rebooted the session and gpu was able to connect.
Restarting session for numpy
When you are running the second block of code that downloads all the packages you will need for this notebook it is important when the popup appears telling you to restart notebook for numpy to accept it as it will need to reboot in order for the packages to work correctly and after restarting you can continue on the notebook running from the next following code block.
Unizipping files wont load
I have struggled with the line of code regarding unzipping the Pyramid files struggling to load, one method could be reconnecting the GPU as I have mentioned earlier
but if that still doesnt work you can download the code from the link. And unzip it on your computer then reuploaded to the corresponding folder to bypass that line of code.
URL then unzipping on your computer then reuploading it to the corresponding folder location in training-envs-executables/linux/
File does not exist error code
When running a code results in a "this file does not exist, or this folder does not exist" it could be from not correctly loading previous code blocks or run time was lost if you closed down the program. You can check if this is the case by going into the side directory of files and go under the corresponding folders to check if files are indeed there. If not just reload the blocks of code that creates the files.
Connecting to google drive
1. In order for the code to run it needs to be mounted to your google drive. So if you a running this through an organizations google account for example schools. It may need to be approved from the IT for it to be allowed to be mounted to the google drive. So make sure that is cleared before continuing the notebook.
2. Also Another cause to the drive not connecting is you may have popups blocked so you will need to allow popups for google collab or else the option to connect ot connect to your google drive will not appear
Saving progress
While run info is saved to your google drive. Since this is a edited notebook changes you make to the hyper parameters are not saved so everytime you rerun the notebook it will reset to the prexisting values. Also training progress cannot be called back if you reopen a later time, you will need to rerun the whole code and retrain which is quite time consuming so I recommend you using the resource.
URL
### Watch your Agent play
You can watch your agent playing directly in your browser
<img src="URL alt="Snowballtarget load"/>
After correctly training the agent and uploading it to the hub it should produce a link that leads you to this page where you can see your agent playing.
1. If the environment is part of ML-Agents official environments, go to URL
2. Step 1: Find your model_id: MY11111111/ppo-Pyramids123 (this is my model_id so you will need to isnert the one that is produced from your own notebooke)
3. Step 2: Select your *.nn /*.onnx file
4. Click on Watch the agent play
| [
"# PPO AI Agents Playing Pyramids\n \n <img src=\"URL alt=\"Pyramids\"/>\n \nDISCLAIMER: In this notebook it has two environments you can train agents to play in the snowball and pyramid one. In this model card I am only covering the Pyramid game model. But a lot of these trouble shooting guides will be applicable to the snowball environment as well.\n\n\n This is a trained model of a ppo agent playing UNITY game Pyramids Using Q-learning and reinforcement learning to train the agent to navigate around a simple maze environment where they need to activate a button that the pyramid then they need to locate the pyramid and knock the pyramid stacked blocks over so the green block on top falls to the ground.\n I used the Unity ML-Agents Library.\n Throughout this notebook you will learn about how to train AI agent using Q learning in a Unity Game 3D game environment. Utilizing the different curiosity and exploitation values as well as manipulating the various hyperperameters to get the best training results.\n It is an easy notebook to follow through with excellent instructions so if you want to learn more about the process used to train these AI agents in 3D environments I highly recommend this project. It is best if you have some experiences learning about or working with deep learning machine learning, because it may be difficult to understand this reinforcement learning process. If you dont have experiences or interested in learning more you can access resources to more introductory notebooks in this link.\n URL \n\n So if you are interested in continuing in training AI agents to playing the Unity Pyramids thats great! Because below is a few different resources I have gathered to troubleshoot through problems I have faced, basic info about how the model works and how you can improve the model. I wish I had known about before completing this notebook. Which will hopefully make it easier for you on your journey.\n Also here is the link of my working model\nURL \nAlso heres a video demoing what the aim of the AI agents in this pyramid game is URL\n\n \n ## Learning components of this model:\n\n <img src=\"URL\n\n 1. Agent component: training agents by optimizing their policy(policy based method, unlike value based methods they optimize the policy itself instead of values) telling the model what action to take in each step in the model called a brain.\n 2. For this model we will be using a proximal policy optimizer (PPO) as seen at the title of the model card. PPO is ideal for training AI agents in Unity games because it is sample-efficient, stable during training, compatible with neural networks, handles both continuous and discrete action spaces, and robust in handling complex game dynamics and mechanics.\n\n Curiosity in training ML agents:\nIn this reinforcement learning project you will need to understand how curiosity plays a part in the training.In short, Traditionally a reward system is used to train ML agents but for more complicated games and obscure objective it is hard to manually place rewards for the agent. \nCuriosity is rewarding the model for taking new trajectories for example exploring new rooms\n\nHere is a youtube videos that helped me understand the concept: \nURL (this one talks specifically about the game environment)\nURL (And this one illustrates more generally about reinforcement learning but also covers curiosity, great and easy to understand for beginners, also if you are intersted in learning more about how ai and machine learning the rest of this crash course series is great as well)\n\n \n ## Improving model training through hyperparameters adjusting\n So once you have a working model and want to improve the training outcomes.\n These hyperparameter tunings can be adjusted within the Pyramid RND file component on the side and below is a detailed list on what changing each individual parameter will impact the training. Just be minful after making changes you need to run the code responsible for copying the file into the envs executable linux, as well as unzipping along with retraining to implement these new parameters into your model. \n \n 1. Trainer type: the type of trainer being used here we use Proximal policy optimization\n 2. Summary_freq: How often the training summaries and statisitcs are recorded(rewards, losses, lengths, time etc )\n 3. Keep_checkpoints: number of recent checkpoints to keep checkpoints are snapshots of training models for resumign training or evaluation\n 4. Checkpoint interval: how often(many steps) save checkpoints\n 5. Max_steps: Maximum number of steps or interactions \n 6. Time_horizon: The number of steps the agent considers when making decisions\n 7. Threaded: Enables multi-threading during training(may allow for faster processing, parts of code run simultaneously)\n 8. Hyperparameters:\n 9. Learning rate: How quickly the agents adjust their behavior based on feedback\n 10. Learning rate_schedule: the rule that used to adjust or modify the learning rate during the training process\n 11. Batch_size: number of samples used in each updated batch training\n 12. Buffer_size :size of the experience replay buffer, which stores past experiences for training updates.\n 13. Beta: exploration levels\n 14. Epilson:It limits the size of behavior changes to prevent large policy updates.\n 15. Lambd: It helps estimate the advantage of taking a particular action in a given state.\n 16. Num_epoch:Specifies the number of times the entire dataset is used for training updates. Each epoch consists of multiple iterations over the dataset.\n\n Network Settings:(architecture for neural network)\n \n 17. Normalize:It determines whether input observations are normalized.\n 18. Hidden unit: Number of units in each hidden layers\n 19. Num layers: Number of hidden layers the model has\n 20. Vis_encode_type: ways visual observations are encoded\n\n Reward Signals\n \n 21. Gamma: It determines the importance of future rewards compared to immediate rewards.\n 22. Strength: It controls the weight of the primary reward signal relative to other rewards, if present.\n\n\n ## Trouble Shooting\n Here are some problems I encountered and solutions I used, and also things I wished I knew in hindsight\n \n GPU not connecting\n\n Sometimes the GPU can get overwhelmed causing the code to not load if you have pressed it too many times and too many piled up commands.\n You can check on the right top side if the GPU is being used, if it shows \"connecting\" or gives you the error gpu is not connected would you like to continue anyways one way is under the tab manage sessions,\n you can terminate previous sessions and start again from my own experience this has rebooted the session and gpu was able to connect.\n\nRestarting session for numpy\n\n When you are running the second block of code that downloads all the packages you will need for this notebook it is important when the popup appears telling you to restart notebook for numpy to accept it as it will need to reboot in order for the packages to work correctly and after restarting you can continue on the notebook running from the next following code block.\n \n Unizipping files wont load\n \n I have struggled with the line of code regarding unzipping the Pyramid files struggling to load, one method could be reconnecting the GPU as I have mentioned earlier\n but if that still doesnt work you can download the code from the link. And unzip it on your computer then reuploaded to the corresponding folder to bypass that line of code.\n URL then unzipping on your computer then reuploading it to the corresponding folder location in training-envs-executables/linux/\n \n File does not exist error code\n\n When running a code results in a \"this file does not exist, or this folder does not exist\" it could be from not correctly loading previous code blocks or run time was lost if you closed down the program. You can check if this is the case by going into the side directory of files and go under the corresponding folders to check if files are indeed there. If not just reload the blocks of code that creates the files.\n\n Connecting to google drive\n\n 1. In order for the code to run it needs to be mounted to your google drive. So if you a running this through an organizations google account for example schools. It may need to be approved from the IT for it to be allowed to be mounted to the google drive. So make sure that is cleared before continuing the notebook. \n 2. Also Another cause to the drive not connecting is you may have popups blocked so you will need to allow popups for google collab or else the option to connect ot connect to your google drive will not appear\n\n Saving progress\n\n While run info is saved to your google drive. Since this is a edited notebook changes you make to the hyper parameters are not saved so everytime you rerun the notebook it will reset to the prexisting values. Also training progress cannot be called back if you reopen a later time, you will need to rerun the whole code and retrain which is quite time consuming so I recommend you using the resource.\n URL\n \n\n ### Watch your Agent play\n \n You can watch your agent playing directly in your browser\n<img src=\"URL alt=\"Snowballtarget load\"/>\n After correctly training the agent and uploading it to the hub it should produce a link that leads you to this page where you can see your agent playing. \n \n 1. If the environment is part of ML-Agents official environments, go to URL\n 2. Step 1: Find your model_id: MY11111111/ppo-Pyramids123 (this is my model_id so you will need to isnert the one that is produced from your own notebooke)\n 3. Step 2: Select your *.nn /*.onnx file\n 4. Click on Watch the agent play"
] | [
"TAGS\n#ml-agents #tensorboard #onnx #Pyramids #deep-reinforcement-learning #reinforcement-learning #ML-Agents-Pyramids #en #region-us \n",
"# PPO AI Agents Playing Pyramids\n \n <img src=\"URL alt=\"Pyramids\"/>\n \nDISCLAIMER: In this notebook it has two environments you can train agents to play in the snowball and pyramid one. In this model card I am only covering the Pyramid game model. But a lot of these trouble shooting guides will be applicable to the snowball environment as well.\n\n\n This is a trained model of a ppo agent playing UNITY game Pyramids Using Q-learning and reinforcement learning to train the agent to navigate around a simple maze environment where they need to activate a button that the pyramid then they need to locate the pyramid and knock the pyramid stacked blocks over so the green block on top falls to the ground.\n I used the Unity ML-Agents Library.\n Throughout this notebook you will learn about how to train AI agent using Q learning in a Unity Game 3D game environment. Utilizing the different curiosity and exploitation values as well as manipulating the various hyperperameters to get the best training results.\n It is an easy notebook to follow through with excellent instructions so if you want to learn more about the process used to train these AI agents in 3D environments I highly recommend this project. It is best if you have some experiences learning about or working with deep learning machine learning, because it may be difficult to understand this reinforcement learning process. If you dont have experiences or interested in learning more you can access resources to more introductory notebooks in this link.\n URL \n\n So if you are interested in continuing in training AI agents to playing the Unity Pyramids thats great! Because below is a few different resources I have gathered to troubleshoot through problems I have faced, basic info about how the model works and how you can improve the model. I wish I had known about before completing this notebook. Which will hopefully make it easier for you on your journey.\n Also here is the link of my working model\nURL \nAlso heres a video demoing what the aim of the AI agents in this pyramid game is URL\n\n \n ## Learning components of this model:\n\n <img src=\"URL\n\n 1. Agent component: training agents by optimizing their policy(policy based method, unlike value based methods they optimize the policy itself instead of values) telling the model what action to take in each step in the model called a brain.\n 2. For this model we will be using a proximal policy optimizer (PPO) as seen at the title of the model card. PPO is ideal for training AI agents in Unity games because it is sample-efficient, stable during training, compatible with neural networks, handles both continuous and discrete action spaces, and robust in handling complex game dynamics and mechanics.\n\n Curiosity in training ML agents:\nIn this reinforcement learning project you will need to understand how curiosity plays a part in the training.In short, Traditionally a reward system is used to train ML agents but for more complicated games and obscure objective it is hard to manually place rewards for the agent. \nCuriosity is rewarding the model for taking new trajectories for example exploring new rooms\n\nHere is a youtube videos that helped me understand the concept: \nURL (this one talks specifically about the game environment)\nURL (And this one illustrates more generally about reinforcement learning but also covers curiosity, great and easy to understand for beginners, also if you are intersted in learning more about how ai and machine learning the rest of this crash course series is great as well)\n\n \n ## Improving model training through hyperparameters adjusting\n So once you have a working model and want to improve the training outcomes.\n These hyperparameter tunings can be adjusted within the Pyramid RND file component on the side and below is a detailed list on what changing each individual parameter will impact the training. Just be minful after making changes you need to run the code responsible for copying the file into the envs executable linux, as well as unzipping along with retraining to implement these new parameters into your model. \n \n 1. Trainer type: the type of trainer being used here we use Proximal policy optimization\n 2. Summary_freq: How often the training summaries and statisitcs are recorded(rewards, losses, lengths, time etc )\n 3. Keep_checkpoints: number of recent checkpoints to keep checkpoints are snapshots of training models for resumign training or evaluation\n 4. Checkpoint interval: how often(many steps) save checkpoints\n 5. Max_steps: Maximum number of steps or interactions \n 6. Time_horizon: The number of steps the agent considers when making decisions\n 7. Threaded: Enables multi-threading during training(may allow for faster processing, parts of code run simultaneously)\n 8. Hyperparameters:\n 9. Learning rate: How quickly the agents adjust their behavior based on feedback\n 10. Learning rate_schedule: the rule that used to adjust or modify the learning rate during the training process\n 11. Batch_size: number of samples used in each updated batch training\n 12. Buffer_size :size of the experience replay buffer, which stores past experiences for training updates.\n 13. Beta: exploration levels\n 14. Epilson:It limits the size of behavior changes to prevent large policy updates.\n 15. Lambd: It helps estimate the advantage of taking a particular action in a given state.\n 16. Num_epoch:Specifies the number of times the entire dataset is used for training updates. Each epoch consists of multiple iterations over the dataset.\n\n Network Settings:(architecture for neural network)\n \n 17. Normalize:It determines whether input observations are normalized.\n 18. Hidden unit: Number of units in each hidden layers\n 19. Num layers: Number of hidden layers the model has\n 20. Vis_encode_type: ways visual observations are encoded\n\n Reward Signals\n \n 21. Gamma: It determines the importance of future rewards compared to immediate rewards.\n 22. Strength: It controls the weight of the primary reward signal relative to other rewards, if present.\n\n\n ## Trouble Shooting\n Here are some problems I encountered and solutions I used, and also things I wished I knew in hindsight\n \n GPU not connecting\n\n Sometimes the GPU can get overwhelmed causing the code to not load if you have pressed it too many times and too many piled up commands.\n You can check on the right top side if the GPU is being used, if it shows \"connecting\" or gives you the error gpu is not connected would you like to continue anyways one way is under the tab manage sessions,\n you can terminate previous sessions and start again from my own experience this has rebooted the session and gpu was able to connect.\n\nRestarting session for numpy\n\n When you are running the second block of code that downloads all the packages you will need for this notebook it is important when the popup appears telling you to restart notebook for numpy to accept it as it will need to reboot in order for the packages to work correctly and after restarting you can continue on the notebook running from the next following code block.\n \n Unizipping files wont load\n \n I have struggled with the line of code regarding unzipping the Pyramid files struggling to load, one method could be reconnecting the GPU as I have mentioned earlier\n but if that still doesnt work you can download the code from the link. And unzip it on your computer then reuploaded to the corresponding folder to bypass that line of code.\n URL then unzipping on your computer then reuploading it to the corresponding folder location in training-envs-executables/linux/\n \n File does not exist error code\n\n When running a code results in a \"this file does not exist, or this folder does not exist\" it could be from not correctly loading previous code blocks or run time was lost if you closed down the program. You can check if this is the case by going into the side directory of files and go under the corresponding folders to check if files are indeed there. If not just reload the blocks of code that creates the files.\n\n Connecting to google drive\n\n 1. In order for the code to run it needs to be mounted to your google drive. So if you a running this through an organizations google account for example schools. It may need to be approved from the IT for it to be allowed to be mounted to the google drive. So make sure that is cleared before continuing the notebook. \n 2. Also Another cause to the drive not connecting is you may have popups blocked so you will need to allow popups for google collab or else the option to connect ot connect to your google drive will not appear\n\n Saving progress\n\n While run info is saved to your google drive. Since this is a edited notebook changes you make to the hyper parameters are not saved so everytime you rerun the notebook it will reset to the prexisting values. Also training progress cannot be called back if you reopen a later time, you will need to rerun the whole code and retrain which is quite time consuming so I recommend you using the resource.\n URL\n \n\n ### Watch your Agent play\n \n You can watch your agent playing directly in your browser\n<img src=\"URL alt=\"Snowballtarget load\"/>\n After correctly training the agent and uploading it to the hub it should produce a link that leads you to this page where you can see your agent playing. \n \n 1. If the environment is part of ML-Agents official environments, go to URL\n 2. Step 1: Find your model_id: MY11111111/ppo-Pyramids123 (this is my model_id so you will need to isnert the one that is produced from your own notebooke)\n 3. Step 2: Select your *.nn /*.onnx file\n 4. Click on Watch the agent play"
] |
text-generation | transformers |
# Model Card for Model ID
<!-- Provide a quick summary of what the model is/does. -->
## Model Details
### Model Description
<!-- Provide a longer summary of what this model is. -->
This is the model card of a 🤗 transformers model that has been pushed on the Hub. This model card has been automatically generated.
- **Developed by:** [More Information Needed]
- **Funded by [optional]:** [More Information Needed]
- **Shared by [optional]:** [More Information Needed]
- **Model type:** [More Information Needed]
- **Language(s) (NLP):** [More Information Needed]
- **License:** [More Information Needed]
- **Finetuned from model [optional]:** [More Information Needed]
### Model Sources [optional]
<!-- Provide the basic links for the model. -->
- **Repository:** [More Information Needed]
- **Paper [optional]:** [More Information Needed]
- **Demo [optional]:** [More Information Needed]
## Uses
<!-- Address questions around how the model is intended to be used, including the foreseeable users of the model and those affected by the model. -->
### Direct Use
<!-- This section is for the model use without fine-tuning or plugging into a larger ecosystem/app. -->
[More Information Needed]
### Downstream Use [optional]
<!-- This section is for the model use when fine-tuned for a task, or when plugged into a larger ecosystem/app -->
[More Information Needed]
### Out-of-Scope Use
<!-- This section addresses misuse, malicious use, and uses that the model will not work well for. -->
[More Information Needed]
## Bias, Risks, and Limitations
<!-- This section is meant to convey both technical and sociotechnical limitations. -->
[More Information Needed]
### Recommendations
<!-- This section is meant to convey recommendations with respect to the bias, risk, and technical limitations. -->
Users (both direct and downstream) should be made aware of the risks, biases and limitations of the model. More information needed for further recommendations.
## How to Get Started with the Model
Use the code below to get started with the model.
[More Information Needed]
## Training Details
### Training Data
<!-- This should link to a Dataset Card, perhaps with a short stub of information on what the training data is all about as well as documentation related to data pre-processing or additional filtering. -->
[More Information Needed]
### Training Procedure
<!-- This relates heavily to the Technical Specifications. Content here should link to that section when it is relevant to the training procedure. -->
#### Preprocessing [optional]
[More Information Needed]
#### Training Hyperparameters
- **Training regime:** [More Information Needed] <!--fp32, fp16 mixed precision, bf16 mixed precision, bf16 non-mixed precision, fp16 non-mixed precision, fp8 mixed precision -->
#### Speeds, Sizes, Times [optional]
<!-- This section provides information about throughput, start/end time, checkpoint size if relevant, etc. -->
[More Information Needed]
## Evaluation
<!-- This section describes the evaluation protocols and provides the results. -->
### Testing Data, Factors & Metrics
#### Testing Data
<!-- This should link to a Dataset Card if possible. -->
[More Information Needed]
#### Factors
<!-- These are the things the evaluation is disaggregating by, e.g., subpopulations or domains. -->
[More Information Needed]
#### Metrics
<!-- These are the evaluation metrics being used, ideally with a description of why. -->
[More Information Needed]
### Results
[More Information Needed]
#### Summary
## Model Examination [optional]
<!-- Relevant interpretability work for the model goes here -->
[More Information Needed]
## Environmental Impact
<!-- Total emissions (in grams of CO2eq) and additional considerations, such as electricity usage, go here. Edit the suggested text below accordingly -->
Carbon emissions can be estimated using the [Machine Learning Impact calculator](https://mlco2.github.io/impact#compute) presented in [Lacoste et al. (2019)](https://arxiv.org/abs/1910.09700).
- **Hardware Type:** [More Information Needed]
- **Hours used:** [More Information Needed]
- **Cloud Provider:** [More Information Needed]
- **Compute Region:** [More Information Needed]
- **Carbon Emitted:** [More Information Needed]
## Technical Specifications [optional]
### Model Architecture and Objective
[More Information Needed]
### Compute Infrastructure
[More Information Needed]
#### Hardware
[More Information Needed]
#### Software
[More Information Needed]
## Citation [optional]
<!-- If there is a paper or blog post introducing the model, the APA and Bibtex information for that should go in this section. -->
**BibTeX:**
[More Information Needed]
**APA:**
[More Information Needed]
## Glossary [optional]
<!-- If relevant, include terms and calculations in this section that can help readers understand the model or model card. -->
[More Information Needed]
## More Information [optional]
[More Information Needed]
## Model Card Authors [optional]
[More Information Needed]
## Model Card Contact
[More Information Needed] | {"library_name": "transformers", "tags": []} | TinyPixel/try-1 | null | [
"transformers",
"safetensors",
"llama",
"text-generation",
"arxiv:1910.09700",
"autotrain_compatible",
"endpoints_compatible",
"text-generation-inference",
"region:us"
] | null | 2024-04-25T14:13:45+00:00 | [
"1910.09700"
] | [] | TAGS
#transformers #safetensors #llama #text-generation #arxiv-1910.09700 #autotrain_compatible #endpoints_compatible #text-generation-inference #region-us
|
# Model Card for Model ID
## Model Details
### Model Description
This is the model card of a transformers model that has been pushed on the Hub. This model card has been automatically generated.
- Developed by:
- Funded by [optional]:
- Shared by [optional]:
- Model type:
- Language(s) (NLP):
- License:
- Finetuned from model [optional]:
### Model Sources [optional]
- Repository:
- Paper [optional]:
- Demo [optional]:
## Uses
### Direct Use
### Downstream Use [optional]
### Out-of-Scope Use
## Bias, Risks, and Limitations
### Recommendations
Users (both direct and downstream) should be made aware of the risks, biases and limitations of the model. More information needed for further recommendations.
## How to Get Started with the Model
Use the code below to get started with the model.
## Training Details
### Training Data
### Training Procedure
#### Preprocessing [optional]
#### Training Hyperparameters
- Training regime:
#### Speeds, Sizes, Times [optional]
## Evaluation
### Testing Data, Factors & Metrics
#### Testing Data
#### Factors
#### Metrics
### Results
#### Summary
## Model Examination [optional]
## Environmental Impact
Carbon emissions can be estimated using the Machine Learning Impact calculator presented in Lacoste et al. (2019).
- Hardware Type:
- Hours used:
- Cloud Provider:
- Compute Region:
- Carbon Emitted:
## Technical Specifications [optional]
### Model Architecture and Objective
### Compute Infrastructure
#### Hardware
#### Software
[optional]
BibTeX:
APA:
## Glossary [optional]
## More Information [optional]
## Model Card Authors [optional]
## Model Card Contact
| [
"# Model Card for Model ID",
"## Model Details",
"### Model Description\n\n\n\nThis is the model card of a transformers model that has been pushed on the Hub. This model card has been automatically generated.\n\n- Developed by: \n- Funded by [optional]: \n- Shared by [optional]: \n- Model type: \n- Language(s) (NLP): \n- License: \n- Finetuned from model [optional]:",
"### Model Sources [optional]\n\n\n\n- Repository: \n- Paper [optional]: \n- Demo [optional]:",
"## Uses",
"### Direct Use",
"### Downstream Use [optional]",
"### Out-of-Scope Use",
"## Bias, Risks, and Limitations",
"### Recommendations\n\n\n\nUsers (both direct and downstream) should be made aware of the risks, biases and limitations of the model. More information needed for further recommendations.",
"## How to Get Started with the Model\n\nUse the code below to get started with the model.",
"## Training Details",
"### Training Data",
"### Training Procedure",
"#### Preprocessing [optional]",
"#### Training Hyperparameters\n\n- Training regime:",
"#### Speeds, Sizes, Times [optional]",
"## Evaluation",
"### Testing Data, Factors & Metrics",
"#### Testing Data",
"#### Factors",
"#### Metrics",
"### Results",
"#### Summary",
"## Model Examination [optional]",
"## Environmental Impact\n\n\n\nCarbon emissions can be estimated using the Machine Learning Impact calculator presented in Lacoste et al. (2019).\n\n- Hardware Type: \n- Hours used: \n- Cloud Provider: \n- Compute Region: \n- Carbon Emitted:",
"## Technical Specifications [optional]",
"### Model Architecture and Objective",
"### Compute Infrastructure",
"#### Hardware",
"#### Software\n\n\n\n[optional]\n\n\n\nBibTeX:\n\n\n\nAPA:",
"## Glossary [optional]",
"## More Information [optional]",
"## Model Card Authors [optional]",
"## Model Card Contact"
] | [
"TAGS\n#transformers #safetensors #llama #text-generation #arxiv-1910.09700 #autotrain_compatible #endpoints_compatible #text-generation-inference #region-us \n",
"# Model Card for Model ID",
"## Model Details",
"### Model Description\n\n\n\nThis is the model card of a transformers model that has been pushed on the Hub. This model card has been automatically generated.\n\n- Developed by: \n- Funded by [optional]: \n- Shared by [optional]: \n- Model type: \n- Language(s) (NLP): \n- License: \n- Finetuned from model [optional]:",
"### Model Sources [optional]\n\n\n\n- Repository: \n- Paper [optional]: \n- Demo [optional]:",
"## Uses",
"### Direct Use",
"### Downstream Use [optional]",
"### Out-of-Scope Use",
"## Bias, Risks, and Limitations",
"### Recommendations\n\n\n\nUsers (both direct and downstream) should be made aware of the risks, biases and limitations of the model. More information needed for further recommendations.",
"## How to Get Started with the Model\n\nUse the code below to get started with the model.",
"## Training Details",
"### Training Data",
"### Training Procedure",
"#### Preprocessing [optional]",
"#### Training Hyperparameters\n\n- Training regime:",
"#### Speeds, Sizes, Times [optional]",
"## Evaluation",
"### Testing Data, Factors & Metrics",
"#### Testing Data",
"#### Factors",
"#### Metrics",
"### Results",
"#### Summary",
"## Model Examination [optional]",
"## Environmental Impact\n\n\n\nCarbon emissions can be estimated using the Machine Learning Impact calculator presented in Lacoste et al. (2019).\n\n- Hardware Type: \n- Hours used: \n- Cloud Provider: \n- Compute Region: \n- Carbon Emitted:",
"## Technical Specifications [optional]",
"### Model Architecture and Objective",
"### Compute Infrastructure",
"#### Hardware",
"#### Software\n\n\n\n[optional]\n\n\n\nBibTeX:\n\n\n\nAPA:",
"## Glossary [optional]",
"## More Information [optional]",
"## Model Card Authors [optional]",
"## Model Card Contact"
] |
text-to-image | diffusers | # Novakid_Pony-XL
<Gallery />
## Model description
**Please post your creations! I love seeing the fruits of my hard work enjoyed!**
words used while training...
Novakid,
1boy, 1girl,
solo,
faceless, no eyes, no mouth, no nose, no facial features, long fiery hair, body made of plasma, cowboy shot, cowboy hat, cowboy boots, cowboy western, jeans, black leather jacket, brown coat, shirt under vest,
**Face symbol prompts per line.**
heart symbol on face,
x-cross symbol on face,
circle symbol on face,
star symbol on face,
4-point-compass symbol on face,
omega symbol on face,
Open-Centre-Cross
6-pointed-star symbol on face,
triangle symbol on face,
**Body color prompts are per line.**
blue fiery hair, blue plasma, blue skin,
green fiery hair, green plasma, green skin,
red fiery hair, red plasma, red skin,
white fiery hair, white plasma, white skin,
yellow fiery hair, yellow plasma, yellow skin,
## Trigger words
You should use `Novakid` to trigger the image generation.
You should use `faceless` to trigger the image generation.
You should use `no eyes` to trigger the image generation.
You should use `no mouth` to trigger the image generation.
You should use `no nose` to trigger the image generation.
You should use `no facial features` to trigger the image generation.
You should use `long fiery hair` to trigger the image generation.
You should use `body made of plasma` to trigger the image generation.
## Download model
Weights for this model are available in Safetensors format.
[Download](/crystalkalem/Novakid_Pony-XL/tree/main) them in the Files & versions tab.
| {"license": "afl-3.0", "tags": ["text-to-image", "stable-diffusion", "lora", "diffusers", "template:sd-lora"], "widget": [{"text": "UNICODE\u0000\u0000N\u0000o\u0000v\u0000a\u0000k\u0000i\u0000d\u0000,\u0000 \u00001\u0000g\u0000i\u0000r\u0000l\u0000,\u0000 \u0000s\u0000o\u0000l\u0000o\u0000,\u0000 \u0000o\u0000m\u0000e\u0000g\u0000a\u0000 \u0000s\u0000y\u0000m\u0000b\u0000o\u0000l\u0000 \u0000o\u0000n\u0000 \u0000f\u0000a\u0000c\u0000e\u0000,\u0000 \u0000f\u0000a\u0000c\u0000e\u0000l\u0000e\u0000s\u0000s\u0000,\u0000 \u0000n\u0000o\u0000 \u0000e\u0000y\u0000e\u0000s\u0000,\u0000 \u0000n\u0000o\u0000 \u0000m\u0000o\u0000u\u0000t\u0000h\u0000,\u0000 \u0000n\u0000o\u0000 \u0000n\u0000o\u0000s\u0000e\u0000,\u0000 \u0000n\u0000o\u0000 \u0000f\u0000a\u0000c\u0000i\u0000a\u0000l\u0000 \u0000f\u0000e\u0000a\u0000t\u0000u\u0000r\u0000e\u0000s\u0000,\u0000 \u0000w\u0000h\u0000i\u0000t\u0000e\u0000 \u0000f\u0000i\u0000e\u0000r\u0000y\u0000 \u0000h\u0000a\u0000i\u0000r\u0000,\u0000 \u0000l\u0000o\u0000n\u0000g\u0000 \u0000f\u0000i\u0000e\u0000r\u0000y\u0000 \u0000h\u0000a\u0000i\u0000r\u0000,\u0000 \u0000b\u0000o\u0000d\u0000y\u0000 \u0000m\u0000a\u0000d\u0000e\u0000 \u0000o\u0000f\u0000 \u0000p\u0000l\u0000a\u0000s\u0000m\u0000a\u0000,\u0000 \u0000w\u0000h\u0000i\u0000t\u0000e\u0000 \u0000p\u0000l\u0000a\u0000s\u0000m\u0000a\u0000,\u0000 \u0000w\u0000h\u0000i\u0000t\u0000e\u0000 \u0000s\u0000k\u0000i\u0000n\u0000,\u0000 \u0000c\u0000o\u0000w\u0000b\u0000o\u0000y\u0000 \u0000h\u0000a\u0000t\u0000,\u0000 \u0000b\u0000l\u0000a\u0000c\u0000k\u0000 \u0000v\u0000e\u0000s\u0000t\u0000,\u0000 \u0000w\u0000h\u0000i\u0000t\u0000e\u0000 \u0000s\u0000h\u0000i\u0000r\u0000t\u0000,\u0000 \u0000j\u0000e\u0000a\u0000n\u0000s\u0000,\u0000 \u0000b\u0000r\u0000o\u0000w\u0000n\u0000 \u0000j\u0000a\u0000c\u0000k\u0000e\u0000t\u0000,\u0000 \u0000s\u0000t\u0000a\u0000n\u0000d\u0000i\u0000n\u0000g\u0000,\u0000 \u0000p\u0000o\u0000n\u0000y\u0000t\u0000a\u0000i\u0000l\u0000,\u0000 \u0000f\u0000a\u0000c\u0000i\u0000n\u0000g\u0000 \u0000v\u0000i\u0000e\u0000w\u0000e\u0000r\u0000,\u0000 \u0000f\u0000u\u0000l\u0000l\u0000 \u0000b\u0000o\u0000d\u0000y\u0000,\u0000 \u0000c\u0000o\u0000l\u0000l\u0000a\u0000r\u0000b\u0000o\u0000n\u0000e\u0000,\u0000 \u0000r\u0000e\u0000d\u0000 \u0000n\u0000e\u0000c\u0000k\u0000e\u0000r\u0000c\u0000h\u0000i\u0000e\u0000f\u0000,\u0000 \u0000b\u0000l\u0000a\u0000c\u0000k\u0000 \u0000b\u0000a\u0000c\u0000k\u0000g\u0000r\u0000o\u0000u\u0000n\u0000d\u0000,\u0000 \u0000s\u0000i\u0000m\u0000p\u0000l\u0000e\u0000 \u0000b\u0000a\u0000c\u0000k\u0000g\u0000r\u0000o\u0000u\u0000n\u0000d\u0000,\u0000,\u0000", "output": {"url": "images/DED619DB92F6A41F6EF6D105EB8C210DA8F7096618B8FBB05B0A46BB662C238C.jpeg"}}], "base_model": "stablediffusionapi/pony-diffusion-v6-xl", "instance_prompt": "Novakid, faceless, no eyes, no mouth, no nose, no facial features, long fiery hair, body made of plasma"} | crystalkalem/Novakid_Pony-XL | null | [
"diffusers",
"text-to-image",
"stable-diffusion",
"lora",
"template:sd-lora",
"base_model:stablediffusionapi/pony-diffusion-v6-xl",
"license:afl-3.0",
"region:us"
] | null | 2024-04-25T14:14:32+00:00 | [] | [] | TAGS
#diffusers #text-to-image #stable-diffusion #lora #template-sd-lora #base_model-stablediffusionapi/pony-diffusion-v6-xl #license-afl-3.0 #region-us
| # Novakid_Pony-XL
<Gallery />
## Model description
Please post your creations! I love seeing the fruits of my hard work enjoyed!
words used while training...
Novakid,
1boy, 1girl,
solo,
faceless, no eyes, no mouth, no nose, no facial features, long fiery hair, body made of plasma, cowboy shot, cowboy hat, cowboy boots, cowboy western, jeans, black leather jacket, brown coat, shirt under vest,
Face symbol prompts per line.
heart symbol on face,
x-cross symbol on face,
circle symbol on face,
star symbol on face,
4-point-compass symbol on face,
omega symbol on face,
Open-Centre-Cross
6-pointed-star symbol on face,
triangle symbol on face,
Body color prompts are per line.
blue fiery hair, blue plasma, blue skin,
green fiery hair, green plasma, green skin,
red fiery hair, red plasma, red skin,
white fiery hair, white plasma, white skin,
yellow fiery hair, yellow plasma, yellow skin,
## Trigger words
You should use 'Novakid' to trigger the image generation.
You should use 'faceless' to trigger the image generation.
You should use 'no eyes' to trigger the image generation.
You should use 'no mouth' to trigger the image generation.
You should use 'no nose' to trigger the image generation.
You should use 'no facial features' to trigger the image generation.
You should use 'long fiery hair' to trigger the image generation.
You should use 'body made of plasma' to trigger the image generation.
## Download model
Weights for this model are available in Safetensors format.
Download them in the Files & versions tab.
| [
"# Novakid_Pony-XL\n\n<Gallery />",
"## Model description \n\nPlease post your creations! I love seeing the fruits of my hard work enjoyed!\n\nwords used while training...\n\nNovakid,\n\n1boy, 1girl,\n\nsolo,\n\nfaceless, no eyes, no mouth, no nose, no facial features, long fiery hair, body made of plasma, cowboy shot, cowboy hat, cowboy boots, cowboy western, jeans, black leather jacket, brown coat, shirt under vest,\n\nFace symbol prompts per line.\n\nheart symbol on face,\n\nx-cross symbol on face,\n\ncircle symbol on face,\n\nstar symbol on face,\n\n4-point-compass symbol on face,\n\nomega symbol on face,\n\nOpen-Centre-Cross\n\n6-pointed-star symbol on face,\n\ntriangle symbol on face,\n\n\nBody color prompts are per line.\n\nblue fiery hair, blue plasma, blue skin,\n\ngreen fiery hair, green plasma, green skin,\n\nred fiery hair, red plasma, red skin,\n\nwhite fiery hair, white plasma, white skin,\n\nyellow fiery hair, yellow plasma, yellow skin,",
"## Trigger words\n\nYou should use 'Novakid' to trigger the image generation.\n\nYou should use 'faceless' to trigger the image generation.\n\nYou should use 'no eyes' to trigger the image generation.\n\nYou should use 'no mouth' to trigger the image generation.\n\nYou should use 'no nose' to trigger the image generation.\n\nYou should use 'no facial features' to trigger the image generation.\n\nYou should use 'long fiery hair' to trigger the image generation.\n\nYou should use 'body made of plasma' to trigger the image generation.",
"## Download model\n\nWeights for this model are available in Safetensors format.\n\nDownload them in the Files & versions tab."
] | [
"TAGS\n#diffusers #text-to-image #stable-diffusion #lora #template-sd-lora #base_model-stablediffusionapi/pony-diffusion-v6-xl #license-afl-3.0 #region-us \n",
"# Novakid_Pony-XL\n\n<Gallery />",
"## Model description \n\nPlease post your creations! I love seeing the fruits of my hard work enjoyed!\n\nwords used while training...\n\nNovakid,\n\n1boy, 1girl,\n\nsolo,\n\nfaceless, no eyes, no mouth, no nose, no facial features, long fiery hair, body made of plasma, cowboy shot, cowboy hat, cowboy boots, cowboy western, jeans, black leather jacket, brown coat, shirt under vest,\n\nFace symbol prompts per line.\n\nheart symbol on face,\n\nx-cross symbol on face,\n\ncircle symbol on face,\n\nstar symbol on face,\n\n4-point-compass symbol on face,\n\nomega symbol on face,\n\nOpen-Centre-Cross\n\n6-pointed-star symbol on face,\n\ntriangle symbol on face,\n\n\nBody color prompts are per line.\n\nblue fiery hair, blue plasma, blue skin,\n\ngreen fiery hair, green plasma, green skin,\n\nred fiery hair, red plasma, red skin,\n\nwhite fiery hair, white plasma, white skin,\n\nyellow fiery hair, yellow plasma, yellow skin,",
"## Trigger words\n\nYou should use 'Novakid' to trigger the image generation.\n\nYou should use 'faceless' to trigger the image generation.\n\nYou should use 'no eyes' to trigger the image generation.\n\nYou should use 'no mouth' to trigger the image generation.\n\nYou should use 'no nose' to trigger the image generation.\n\nYou should use 'no facial features' to trigger the image generation.\n\nYou should use 'long fiery hair' to trigger the image generation.\n\nYou should use 'body made of plasma' to trigger the image generation.",
"## Download model\n\nWeights for this model are available in Safetensors format.\n\nDownload them in the Files & versions tab."
] |
reinforcement-learning | stable-baselines3 |
# **PPO** Agent playing **LunarLander-v2**
This is a trained model of a **PPO** agent playing **LunarLander-v2**
using the [stable-baselines3 library](https://github.com/DLR-RM/stable-baselines3).
## Usage (with Stable-baselines3)
TODO: Add your code
```python
from stable_baselines3 import ...
from huggingface_sb3 import load_from_hub
...
```
| {"library_name": "stable-baselines3", "tags": ["LunarLander-v2", "deep-reinforcement-learning", "reinforcement-learning", "stable-baselines3"], "model-index": [{"name": "PPO", "results": [{"task": {"type": "reinforcement-learning", "name": "reinforcement-learning"}, "dataset": {"name": "LunarLander-v2", "type": "LunarLander-v2"}, "metrics": [{"type": "mean_reward", "value": "259.93 +/- 13.45", "name": "mean_reward", "verified": false}]}]}]} | raulgadea/ppo-LunarLander-v2 | null | [
"stable-baselines3",
"LunarLander-v2",
"deep-reinforcement-learning",
"reinforcement-learning",
"model-index",
"region:us"
] | null | 2024-04-25T14:14:34+00:00 | [] | [] | TAGS
#stable-baselines3 #LunarLander-v2 #deep-reinforcement-learning #reinforcement-learning #model-index #region-us
|
# PPO Agent playing LunarLander-v2
This is a trained model of a PPO agent playing LunarLander-v2
using the stable-baselines3 library.
## Usage (with Stable-baselines3)
TODO: Add your code
| [
"# PPO Agent playing LunarLander-v2\nThis is a trained model of a PPO agent playing LunarLander-v2\nusing the stable-baselines3 library.",
"## Usage (with Stable-baselines3)\nTODO: Add your code"
] | [
"TAGS\n#stable-baselines3 #LunarLander-v2 #deep-reinforcement-learning #reinforcement-learning #model-index #region-us \n",
"# PPO Agent playing LunarLander-v2\nThis is a trained model of a PPO agent playing LunarLander-v2\nusing the stable-baselines3 library.",
"## Usage (with Stable-baselines3)\nTODO: Add your code"
] |
null | null |
MambaBit. Bit-level cursed model with vocab size=2
* 4 layers, vocab size=2, embedded size = 4096 float32 parm per bit.
* Training was done on first 8030848 bits of tiny Shakespeare in 10 hours on laptop with 16GB VRAM on 9 batches of 128*8 bit each. Training code included in trainer.ipynb
* To run the model run `python mambabit.py "As sun raised over"`.
Expected output
```
As sun raised over me.
LEONTES:
Now means means me not so much as my father,
In the good many lord, and my father come.
KING RICHARD III:
What is my father come and my father,
In the good lord, and my father come and before his father.
GLOUCESTER:
Now the goes of men, a
```
* Bytes are encoded with most significant bit fed first. Eg '7' = [0, 0, 1, 1, 0, 1, 1, 1], so MSB 0 is being fed first
rather than last as if it was with [1, 1, 1, 0, 1, 1, 0, 0]. Intuition with that is that bits at the beginning change less frequent than in the end, so model will be like "I think I will produce a digit" then "I think I will produce 7" instead of "so I spat something. Should it be a number? a letter? dunno"
* I tried to use BF16 originally, but model went into nan (with default big LR) or gradients were so small weights didn't change(smaller LR). I switched back to F32, however some layers still initialize weight with factor x0.001 as I hoped it
would stop model from going to nan.
--------
Also next morning I realized that Mamba module doesn't come with built-in normalization and residual as I thought,
which explains why BF16 attempts failed. Oops.
| {"license": "apache-2.0"} | Maykeye/MambaBitPet-NoRes-NoNorm-TinyShakespeare | null | [
"license:apache-2.0",
"region:us"
] | null | 2024-04-25T14:16:12+00:00 | [] | [] | TAGS
#license-apache-2.0 #region-us
|
MambaBit. Bit-level cursed model with vocab size=2
* 4 layers, vocab size=2, embedded size = 4096 float32 parm per bit.
* Training was done on first 8030848 bits of tiny Shakespeare in 10 hours on laptop with 16GB VRAM on 9 batches of 128*8 bit each. Training code included in URL
* To run the model run 'python URL "As sun raised over"'.
Expected output
* Bytes are encoded with most significant bit fed first. Eg '7' = [0, 0, 1, 1, 0, 1, 1, 1], so MSB 0 is being fed first
rather than last as if it was with [1, 1, 1, 0, 1, 1, 0, 0]. Intuition with that is that bits at the beginning change less frequent than in the end, so model will be like "I think I will produce a digit" then "I think I will produce 7" instead of "so I spat something. Should it be a number? a letter? dunno"
* I tried to use BF16 originally, but model went into nan (with default big LR) or gradients were so small weights didn't change(smaller LR). I switched back to F32, however some layers still initialize weight with factor x0.001 as I hoped it
would stop model from going to nan.
--------
Also next morning I realized that Mamba module doesn't come with built-in normalization and residual as I thought,
which explains why BF16 attempts failed. Oops.
| [] | [
"TAGS\n#license-apache-2.0 #region-us \n"
] |
text-generation | transformers |
<!-- This model card has been generated automatically according to the information the Trainer had access to. You
should probably proofread and complete it, then remove this comment. -->
# ds-math-7rl-ft3
This model is a fine-tuned version of [deepseek-ai/deepseek-math-7b-rl](https://huggingface.co/deepseek-ai/deepseek-math-7b-rl) on an unknown dataset.
## Model description
More information needed
## Intended uses & limitations
More information needed
## Training and evaluation data
More information needed
## Training procedure
### Training hyperparameters
The following hyperparameters were used during training:
- learning_rate: 1e-06
- train_batch_size: 1
- eval_batch_size: 8
- seed: 42
- optimizer: Adam with betas=(0.9,0.999) and epsilon=1e-08
- lr_scheduler_type: linear
- lr_scheduler_warmup_steps: 100
- training_steps: 3000
### Training results
### Framework versions
- Transformers 4.40.1
- Pytorch 2.3.0+cu121
- Datasets 2.19.0
- Tokenizers 0.19.1
| {"license": "other", "tags": ["trl", "sft", "generated_from_trainer"], "base_model": "deepseek-ai/deepseek-math-7b-rl", "model-index": [{"name": "ds-math-7rl-ft3", "results": []}]} | ihork/ds-math-7rl-ft3 | null | [
"transformers",
"tensorboard",
"safetensors",
"llama",
"text-generation",
"trl",
"sft",
"generated_from_trainer",
"conversational",
"base_model:deepseek-ai/deepseek-math-7b-rl",
"license:other",
"autotrain_compatible",
"endpoints_compatible",
"text-generation-inference",
"region:us"
] | null | 2024-04-25T14:16:27+00:00 | [] | [] | TAGS
#transformers #tensorboard #safetensors #llama #text-generation #trl #sft #generated_from_trainer #conversational #base_model-deepseek-ai/deepseek-math-7b-rl #license-other #autotrain_compatible #endpoints_compatible #text-generation-inference #region-us
|
# ds-math-7rl-ft3
This model is a fine-tuned version of deepseek-ai/deepseek-math-7b-rl on an unknown dataset.
## Model description
More information needed
## Intended uses & limitations
More information needed
## Training and evaluation data
More information needed
## Training procedure
### Training hyperparameters
The following hyperparameters were used during training:
- learning_rate: 1e-06
- train_batch_size: 1
- eval_batch_size: 8
- seed: 42
- optimizer: Adam with betas=(0.9,0.999) and epsilon=1e-08
- lr_scheduler_type: linear
- lr_scheduler_warmup_steps: 100
- training_steps: 3000
### Training results
### Framework versions
- Transformers 4.40.1
- Pytorch 2.3.0+cu121
- Datasets 2.19.0
- Tokenizers 0.19.1
| [
"# ds-math-7rl-ft3\n\nThis model is a fine-tuned version of deepseek-ai/deepseek-math-7b-rl on an unknown dataset.",
"## Model description\n\nMore information needed",
"## Intended uses & limitations\n\nMore information needed",
"## Training and evaluation data\n\nMore information needed",
"## Training procedure",
"### Training hyperparameters\n\nThe following hyperparameters were used during training:\n- learning_rate: 1e-06\n- train_batch_size: 1\n- eval_batch_size: 8\n- seed: 42\n- optimizer: Adam with betas=(0.9,0.999) and epsilon=1e-08\n- lr_scheduler_type: linear\n- lr_scheduler_warmup_steps: 100\n- training_steps: 3000",
"### Training results",
"### Framework versions\n\n- Transformers 4.40.1\n- Pytorch 2.3.0+cu121\n- Datasets 2.19.0\n- Tokenizers 0.19.1"
] | [
"TAGS\n#transformers #tensorboard #safetensors #llama #text-generation #trl #sft #generated_from_trainer #conversational #base_model-deepseek-ai/deepseek-math-7b-rl #license-other #autotrain_compatible #endpoints_compatible #text-generation-inference #region-us \n",
"# ds-math-7rl-ft3\n\nThis model is a fine-tuned version of deepseek-ai/deepseek-math-7b-rl on an unknown dataset.",
"## Model description\n\nMore information needed",
"## Intended uses & limitations\n\nMore information needed",
"## Training and evaluation data\n\nMore information needed",
"## Training procedure",
"### Training hyperparameters\n\nThe following hyperparameters were used during training:\n- learning_rate: 1e-06\n- train_batch_size: 1\n- eval_batch_size: 8\n- seed: 42\n- optimizer: Adam with betas=(0.9,0.999) and epsilon=1e-08\n- lr_scheduler_type: linear\n- lr_scheduler_warmup_steps: 100\n- training_steps: 3000",
"### Training results",
"### Framework versions\n\n- Transformers 4.40.1\n- Pytorch 2.3.0+cu121\n- Datasets 2.19.0\n- Tokenizers 0.19.1"
] |
text-generation | transformers |
# Model Card for Model ID
<!-- Provide a quick summary of what the model is/does. -->
## Model Details
### Model Description
<!-- Provide a longer summary of what this model is. -->
This is the model card of a 🤗 transformers model that has been pushed on the Hub. This model card has been automatically generated.
- **Developed by:** [More Information Needed]
- **Funded by [optional]:** [More Information Needed]
- **Shared by [optional]:** [More Information Needed]
- **Model type:** [More Information Needed]
- **Language(s) (NLP):** [More Information Needed]
- **License:** [More Information Needed]
- **Finetuned from model [optional]:** [More Information Needed]
### Model Sources [optional]
<!-- Provide the basic links for the model. -->
- **Repository:** [More Information Needed]
- **Paper [optional]:** [More Information Needed]
- **Demo [optional]:** [More Information Needed]
## Uses
<!-- Address questions around how the model is intended to be used, including the foreseeable users of the model and those affected by the model. -->
### Direct Use
<!-- This section is for the model use without fine-tuning or plugging into a larger ecosystem/app. -->
[More Information Needed]
### Downstream Use [optional]
<!-- This section is for the model use when fine-tuned for a task, or when plugged into a larger ecosystem/app -->
[More Information Needed]
### Out-of-Scope Use
<!-- This section addresses misuse, malicious use, and uses that the model will not work well for. -->
[More Information Needed]
## Bias, Risks, and Limitations
<!-- This section is meant to convey both technical and sociotechnical limitations. -->
[More Information Needed]
### Recommendations
<!-- This section is meant to convey recommendations with respect to the bias, risk, and technical limitations. -->
Users (both direct and downstream) should be made aware of the risks, biases and limitations of the model. More information needed for further recommendations.
## How to Get Started with the Model
Use the code below to get started with the model.
[More Information Needed]
## Training Details
### Training Data
<!-- This should link to a Dataset Card, perhaps with a short stub of information on what the training data is all about as well as documentation related to data pre-processing or additional filtering. -->
[More Information Needed]
### Training Procedure
<!-- This relates heavily to the Technical Specifications. Content here should link to that section when it is relevant to the training procedure. -->
#### Preprocessing [optional]
[More Information Needed]
#### Training Hyperparameters
- **Training regime:** [More Information Needed] <!--fp32, fp16 mixed precision, bf16 mixed precision, bf16 non-mixed precision, fp16 non-mixed precision, fp8 mixed precision -->
#### Speeds, Sizes, Times [optional]
<!-- This section provides information about throughput, start/end time, checkpoint size if relevant, etc. -->
[More Information Needed]
## Evaluation
<!-- This section describes the evaluation protocols and provides the results. -->
### Testing Data, Factors & Metrics
#### Testing Data
<!-- This should link to a Dataset Card if possible. -->
[More Information Needed]
#### Factors
<!-- These are the things the evaluation is disaggregating by, e.g., subpopulations or domains. -->
[More Information Needed]
#### Metrics
<!-- These are the evaluation metrics being used, ideally with a description of why. -->
[More Information Needed]
### Results
[More Information Needed]
#### Summary
## Model Examination [optional]
<!-- Relevant interpretability work for the model goes here -->
[More Information Needed]
## Environmental Impact
<!-- Total emissions (in grams of CO2eq) and additional considerations, such as electricity usage, go here. Edit the suggested text below accordingly -->
Carbon emissions can be estimated using the [Machine Learning Impact calculator](https://mlco2.github.io/impact#compute) presented in [Lacoste et al. (2019)](https://arxiv.org/abs/1910.09700).
- **Hardware Type:** [More Information Needed]
- **Hours used:** [More Information Needed]
- **Cloud Provider:** [More Information Needed]
- **Compute Region:** [More Information Needed]
- **Carbon Emitted:** [More Information Needed]
## Technical Specifications [optional]
### Model Architecture and Objective
[More Information Needed]
### Compute Infrastructure
[More Information Needed]
#### Hardware
[More Information Needed]
#### Software
[More Information Needed]
## Citation [optional]
<!-- If there is a paper or blog post introducing the model, the APA and Bibtex information for that should go in this section. -->
**BibTeX:**
[More Information Needed]
**APA:**
[More Information Needed]
## Glossary [optional]
<!-- If relevant, include terms and calculations in this section that can help readers understand the model or model card. -->
[More Information Needed]
## More Information [optional]
[More Information Needed]
## Model Card Authors [optional]
[More Information Needed]
## Model Card Contact
[More Information Needed] | {"library_name": "transformers", "tags": []} | DBangshu/Gemma-2b | null | [
"transformers",
"safetensors",
"gemma",
"text-generation",
"arxiv:1910.09700",
"autotrain_compatible",
"endpoints_compatible",
"text-generation-inference",
"region:us"
] | null | 2024-04-25T14:16:49+00:00 | [
"1910.09700"
] | [] | TAGS
#transformers #safetensors #gemma #text-generation #arxiv-1910.09700 #autotrain_compatible #endpoints_compatible #text-generation-inference #region-us
|
# Model Card for Model ID
## Model Details
### Model Description
This is the model card of a transformers model that has been pushed on the Hub. This model card has been automatically generated.
- Developed by:
- Funded by [optional]:
- Shared by [optional]:
- Model type:
- Language(s) (NLP):
- License:
- Finetuned from model [optional]:
### Model Sources [optional]
- Repository:
- Paper [optional]:
- Demo [optional]:
## Uses
### Direct Use
### Downstream Use [optional]
### Out-of-Scope Use
## Bias, Risks, and Limitations
### Recommendations
Users (both direct and downstream) should be made aware of the risks, biases and limitations of the model. More information needed for further recommendations.
## How to Get Started with the Model
Use the code below to get started with the model.
## Training Details
### Training Data
### Training Procedure
#### Preprocessing [optional]
#### Training Hyperparameters
- Training regime:
#### Speeds, Sizes, Times [optional]
## Evaluation
### Testing Data, Factors & Metrics
#### Testing Data
#### Factors
#### Metrics
### Results
#### Summary
## Model Examination [optional]
## Environmental Impact
Carbon emissions can be estimated using the Machine Learning Impact calculator presented in Lacoste et al. (2019).
- Hardware Type:
- Hours used:
- Cloud Provider:
- Compute Region:
- Carbon Emitted:
## Technical Specifications [optional]
### Model Architecture and Objective
### Compute Infrastructure
#### Hardware
#### Software
[optional]
BibTeX:
APA:
## Glossary [optional]
## More Information [optional]
## Model Card Authors [optional]
## Model Card Contact
| [
"# Model Card for Model ID",
"## Model Details",
"### Model Description\n\n\n\nThis is the model card of a transformers model that has been pushed on the Hub. This model card has been automatically generated.\n\n- Developed by: \n- Funded by [optional]: \n- Shared by [optional]: \n- Model type: \n- Language(s) (NLP): \n- License: \n- Finetuned from model [optional]:",
"### Model Sources [optional]\n\n\n\n- Repository: \n- Paper [optional]: \n- Demo [optional]:",
"## Uses",
"### Direct Use",
"### Downstream Use [optional]",
"### Out-of-Scope Use",
"## Bias, Risks, and Limitations",
"### Recommendations\n\n\n\nUsers (both direct and downstream) should be made aware of the risks, biases and limitations of the model. More information needed for further recommendations.",
"## How to Get Started with the Model\n\nUse the code below to get started with the model.",
"## Training Details",
"### Training Data",
"### Training Procedure",
"#### Preprocessing [optional]",
"#### Training Hyperparameters\n\n- Training regime:",
"#### Speeds, Sizes, Times [optional]",
"## Evaluation",
"### Testing Data, Factors & Metrics",
"#### Testing Data",
"#### Factors",
"#### Metrics",
"### Results",
"#### Summary",
"## Model Examination [optional]",
"## Environmental Impact\n\n\n\nCarbon emissions can be estimated using the Machine Learning Impact calculator presented in Lacoste et al. (2019).\n\n- Hardware Type: \n- Hours used: \n- Cloud Provider: \n- Compute Region: \n- Carbon Emitted:",
"## Technical Specifications [optional]",
"### Model Architecture and Objective",
"### Compute Infrastructure",
"#### Hardware",
"#### Software\n\n\n\n[optional]\n\n\n\nBibTeX:\n\n\n\nAPA:",
"## Glossary [optional]",
"## More Information [optional]",
"## Model Card Authors [optional]",
"## Model Card Contact"
] | [
"TAGS\n#transformers #safetensors #gemma #text-generation #arxiv-1910.09700 #autotrain_compatible #endpoints_compatible #text-generation-inference #region-us \n",
"# Model Card for Model ID",
"## Model Details",
"### Model Description\n\n\n\nThis is the model card of a transformers model that has been pushed on the Hub. This model card has been automatically generated.\n\n- Developed by: \n- Funded by [optional]: \n- Shared by [optional]: \n- Model type: \n- Language(s) (NLP): \n- License: \n- Finetuned from model [optional]:",
"### Model Sources [optional]\n\n\n\n- Repository: \n- Paper [optional]: \n- Demo [optional]:",
"## Uses",
"### Direct Use",
"### Downstream Use [optional]",
"### Out-of-Scope Use",
"## Bias, Risks, and Limitations",
"### Recommendations\n\n\n\nUsers (both direct and downstream) should be made aware of the risks, biases and limitations of the model. More information needed for further recommendations.",
"## How to Get Started with the Model\n\nUse the code below to get started with the model.",
"## Training Details",
"### Training Data",
"### Training Procedure",
"#### Preprocessing [optional]",
"#### Training Hyperparameters\n\n- Training regime:",
"#### Speeds, Sizes, Times [optional]",
"## Evaluation",
"### Testing Data, Factors & Metrics",
"#### Testing Data",
"#### Factors",
"#### Metrics",
"### Results",
"#### Summary",
"## Model Examination [optional]",
"## Environmental Impact\n\n\n\nCarbon emissions can be estimated using the Machine Learning Impact calculator presented in Lacoste et al. (2019).\n\n- Hardware Type: \n- Hours used: \n- Cloud Provider: \n- Compute Region: \n- Carbon Emitted:",
"## Technical Specifications [optional]",
"### Model Architecture and Objective",
"### Compute Infrastructure",
"#### Hardware",
"#### Software\n\n\n\n[optional]\n\n\n\nBibTeX:\n\n\n\nAPA:",
"## Glossary [optional]",
"## More Information [optional]",
"## Model Card Authors [optional]",
"## Model Card Contact"
] |
text-generation | transformers |
# Model Card for Model ID
<!-- Provide a quick summary of what the model is/does. -->
## Model Details
### Model Description
<!-- Provide a longer summary of what this model is. -->
This is the model card of a 🤗 transformers model that has been pushed on the Hub. This model card has been automatically generated.
- **Developed by:** [More Information Needed]
- **Funded by [optional]:** [More Information Needed]
- **Shared by [optional]:** [More Information Needed]
- **Model type:** [More Information Needed]
- **Language(s) (NLP):** [More Information Needed]
- **License:** [More Information Needed]
- **Finetuned from model [optional]:** [More Information Needed]
### Model Sources [optional]
<!-- Provide the basic links for the model. -->
- **Repository:** [More Information Needed]
- **Paper [optional]:** [More Information Needed]
- **Demo [optional]:** [More Information Needed]
## Uses
<!-- Address questions around how the model is intended to be used, including the foreseeable users of the model and those affected by the model. -->
### Direct Use
<!-- This section is for the model use without fine-tuning or plugging into a larger ecosystem/app. -->
[More Information Needed]
### Downstream Use [optional]
<!-- This section is for the model use when fine-tuned for a task, or when plugged into a larger ecosystem/app -->
[More Information Needed]
### Out-of-Scope Use
<!-- This section addresses misuse, malicious use, and uses that the model will not work well for. -->
[More Information Needed]
## Bias, Risks, and Limitations
<!-- This section is meant to convey both technical and sociotechnical limitations. -->
[More Information Needed]
### Recommendations
<!-- This section is meant to convey recommendations with respect to the bias, risk, and technical limitations. -->
Users (both direct and downstream) should be made aware of the risks, biases and limitations of the model. More information needed for further recommendations.
## How to Get Started with the Model
Use the code below to get started with the model.
[More Information Needed]
## Training Details
### Training Data
<!-- This should link to a Dataset Card, perhaps with a short stub of information on what the training data is all about as well as documentation related to data pre-processing or additional filtering. -->
[More Information Needed]
### Training Procedure
<!-- This relates heavily to the Technical Specifications. Content here should link to that section when it is relevant to the training procedure. -->
#### Preprocessing [optional]
[More Information Needed]
#### Training Hyperparameters
- **Training regime:** [More Information Needed] <!--fp32, fp16 mixed precision, bf16 mixed precision, bf16 non-mixed precision, fp16 non-mixed precision, fp8 mixed precision -->
#### Speeds, Sizes, Times [optional]
<!-- This section provides information about throughput, start/end time, checkpoint size if relevant, etc. -->
[More Information Needed]
## Evaluation
<!-- This section describes the evaluation protocols and provides the results. -->
### Testing Data, Factors & Metrics
#### Testing Data
<!-- This should link to a Dataset Card if possible. -->
[More Information Needed]
#### Factors
<!-- These are the things the evaluation is disaggregating by, e.g., subpopulations or domains. -->
[More Information Needed]
#### Metrics
<!-- These are the evaluation metrics being used, ideally with a description of why. -->
[More Information Needed]
### Results
[More Information Needed]
#### Summary
## Model Examination [optional]
<!-- Relevant interpretability work for the model goes here -->
[More Information Needed]
## Environmental Impact
<!-- Total emissions (in grams of CO2eq) and additional considerations, such as electricity usage, go here. Edit the suggested text below accordingly -->
Carbon emissions can be estimated using the [Machine Learning Impact calculator](https://mlco2.github.io/impact#compute) presented in [Lacoste et al. (2019)](https://arxiv.org/abs/1910.09700).
- **Hardware Type:** [More Information Needed]
- **Hours used:** [More Information Needed]
- **Cloud Provider:** [More Information Needed]
- **Compute Region:** [More Information Needed]
- **Carbon Emitted:** [More Information Needed]
## Technical Specifications [optional]
### Model Architecture and Objective
[More Information Needed]
### Compute Infrastructure
[More Information Needed]
#### Hardware
[More Information Needed]
#### Software
[More Information Needed]
## Citation [optional]
<!-- If there is a paper or blog post introducing the model, the APA and Bibtex information for that should go in this section. -->
**BibTeX:**
[More Information Needed]
**APA:**
[More Information Needed]
## Glossary [optional]
<!-- If relevant, include terms and calculations in this section that can help readers understand the model or model card. -->
[More Information Needed]
## More Information [optional]
[More Information Needed]
## Model Card Authors [optional]
[More Information Needed]
## Model Card Contact
[More Information Needed] | {"library_name": "transformers", "tags": []} | happylayers/sc21 | null | [
"transformers",
"safetensors",
"stablelm",
"text-generation",
"conversational",
"arxiv:1910.09700",
"autotrain_compatible",
"endpoints_compatible",
"region:us"
] | null | 2024-04-25T14:17:07+00:00 | [
"1910.09700"
] | [] | TAGS
#transformers #safetensors #stablelm #text-generation #conversational #arxiv-1910.09700 #autotrain_compatible #endpoints_compatible #region-us
|
# Model Card for Model ID
## Model Details
### Model Description
This is the model card of a transformers model that has been pushed on the Hub. This model card has been automatically generated.
- Developed by:
- Funded by [optional]:
- Shared by [optional]:
- Model type:
- Language(s) (NLP):
- License:
- Finetuned from model [optional]:
### Model Sources [optional]
- Repository:
- Paper [optional]:
- Demo [optional]:
## Uses
### Direct Use
### Downstream Use [optional]
### Out-of-Scope Use
## Bias, Risks, and Limitations
### Recommendations
Users (both direct and downstream) should be made aware of the risks, biases and limitations of the model. More information needed for further recommendations.
## How to Get Started with the Model
Use the code below to get started with the model.
## Training Details
### Training Data
### Training Procedure
#### Preprocessing [optional]
#### Training Hyperparameters
- Training regime:
#### Speeds, Sizes, Times [optional]
## Evaluation
### Testing Data, Factors & Metrics
#### Testing Data
#### Factors
#### Metrics
### Results
#### Summary
## Model Examination [optional]
## Environmental Impact
Carbon emissions can be estimated using the Machine Learning Impact calculator presented in Lacoste et al. (2019).
- Hardware Type:
- Hours used:
- Cloud Provider:
- Compute Region:
- Carbon Emitted:
## Technical Specifications [optional]
### Model Architecture and Objective
### Compute Infrastructure
#### Hardware
#### Software
[optional]
BibTeX:
APA:
## Glossary [optional]
## More Information [optional]
## Model Card Authors [optional]
## Model Card Contact
| [
"# Model Card for Model ID",
"## Model Details",
"### Model Description\n\n\n\nThis is the model card of a transformers model that has been pushed on the Hub. This model card has been automatically generated.\n\n- Developed by: \n- Funded by [optional]: \n- Shared by [optional]: \n- Model type: \n- Language(s) (NLP): \n- License: \n- Finetuned from model [optional]:",
"### Model Sources [optional]\n\n\n\n- Repository: \n- Paper [optional]: \n- Demo [optional]:",
"## Uses",
"### Direct Use",
"### Downstream Use [optional]",
"### Out-of-Scope Use",
"## Bias, Risks, and Limitations",
"### Recommendations\n\n\n\nUsers (both direct and downstream) should be made aware of the risks, biases and limitations of the model. More information needed for further recommendations.",
"## How to Get Started with the Model\n\nUse the code below to get started with the model.",
"## Training Details",
"### Training Data",
"### Training Procedure",
"#### Preprocessing [optional]",
"#### Training Hyperparameters\n\n- Training regime:",
"#### Speeds, Sizes, Times [optional]",
"## Evaluation",
"### Testing Data, Factors & Metrics",
"#### Testing Data",
"#### Factors",
"#### Metrics",
"### Results",
"#### Summary",
"## Model Examination [optional]",
"## Environmental Impact\n\n\n\nCarbon emissions can be estimated using the Machine Learning Impact calculator presented in Lacoste et al. (2019).\n\n- Hardware Type: \n- Hours used: \n- Cloud Provider: \n- Compute Region: \n- Carbon Emitted:",
"## Technical Specifications [optional]",
"### Model Architecture and Objective",
"### Compute Infrastructure",
"#### Hardware",
"#### Software\n\n\n\n[optional]\n\n\n\nBibTeX:\n\n\n\nAPA:",
"## Glossary [optional]",
"## More Information [optional]",
"## Model Card Authors [optional]",
"## Model Card Contact"
] | [
"TAGS\n#transformers #safetensors #stablelm #text-generation #conversational #arxiv-1910.09700 #autotrain_compatible #endpoints_compatible #region-us \n",
"# Model Card for Model ID",
"## Model Details",
"### Model Description\n\n\n\nThis is the model card of a transformers model that has been pushed on the Hub. This model card has been automatically generated.\n\n- Developed by: \n- Funded by [optional]: \n- Shared by [optional]: \n- Model type: \n- Language(s) (NLP): \n- License: \n- Finetuned from model [optional]:",
"### Model Sources [optional]\n\n\n\n- Repository: \n- Paper [optional]: \n- Demo [optional]:",
"## Uses",
"### Direct Use",
"### Downstream Use [optional]",
"### Out-of-Scope Use",
"## Bias, Risks, and Limitations",
"### Recommendations\n\n\n\nUsers (both direct and downstream) should be made aware of the risks, biases and limitations of the model. More information needed for further recommendations.",
"## How to Get Started with the Model\n\nUse the code below to get started with the model.",
"## Training Details",
"### Training Data",
"### Training Procedure",
"#### Preprocessing [optional]",
"#### Training Hyperparameters\n\n- Training regime:",
"#### Speeds, Sizes, Times [optional]",
"## Evaluation",
"### Testing Data, Factors & Metrics",
"#### Testing Data",
"#### Factors",
"#### Metrics",
"### Results",
"#### Summary",
"## Model Examination [optional]",
"## Environmental Impact\n\n\n\nCarbon emissions can be estimated using the Machine Learning Impact calculator presented in Lacoste et al. (2019).\n\n- Hardware Type: \n- Hours used: \n- Cloud Provider: \n- Compute Region: \n- Carbon Emitted:",
"## Technical Specifications [optional]",
"### Model Architecture and Objective",
"### Compute Infrastructure",
"#### Hardware",
"#### Software\n\n\n\n[optional]\n\n\n\nBibTeX:\n\n\n\nAPA:",
"## Glossary [optional]",
"## More Information [optional]",
"## Model Card Authors [optional]",
"## Model Card Contact"
] |
text-generation | transformers |
## llama2.c-stories110M-pruned50
This repo contains model files for [llama2.c 110M tinystories](https://huggingface.co/Xenova/llama2.c-stories110M) optimized for [NM-vLLM](https://github.com/neuralmagic/nm-vllm), a high-throughput serving engine for compressed LLMs.
This model was pruned with [SparseGPT](https://arxiv.org/abs/2301.00774), using [SparseML](https://github.com/neuralmagic/sparseml).
The weights for this model were saved using [compressed-tensors](https://github.com/neuralmagic/compressed-tensors/pull/30) library. The chosen compression is format bitmask-compression.
## Inference
Install [NM-vLLM](https://github.com/neuralmagic/nm-vllm) for fast inference and low memory-usage:
```bash
pip install nm-vllm[sparse]
```
Run in a Python pipeline for local inference:
```python
from vllm import LLM, SamplingParams
model = LLM("nm-testing/llama2.c-stories110M-pruned50", sparsity="sparse_w16a16")
prompt = "Hello my name is"
sampling_params = SamplingParams(max_tokens=100, temperature=0)
outputs = model.generate(prompt, sampling_params=sampling_params)
print(outputs[0].outputs[0].text)
```
## Prompt template
N/A
## Sparsification
For details on how this model was sparsified, see the `recipe.yaml` in this repo and follow the instructions below.
Install [SparseML](https://github.com/neuralmagic/sparseml):
```bash
git clone https://github.com/neuralmagic/sparseml
pip install -e "sparseml[transformers]"
```
Replace the recipe as you like and run this one-shot compression script to apply SparseGPT:
```python
import sparseml.transformers
original_model_name = "Xenova/llama2.c-stories110M"
calibration_dataset = "open_platypus"
output_directory = "output/"
recipe = """
test_stage:
obcq_modifiers:
SparseGPTModifier:
sparsity: 0.5
sequential_update: true
targets: ['re:model.layers.\d*$']
"""
# Apply SparseGPT to the model
sparseml.transformers.oneshot(
model=original_model_name,
dataset=calibration_dataset,
recipe=recipe,
output_dir=output_directory,
)
```
## Slack
For further support, and discussions on these models and AI in general, join [Neural Magic's Slack Community](https://join.slack.com/t/discuss-neuralmagic/shared_invite/zt-q1a1cnvo-YBoICSIw3L1dmQpjBeDurQ) | {"tags": ["nm-vllm", "sparse"], "base_model": "Xenova/llama2.c-stories110M", "inference": true, "model_type": "llama", "quantized_by": "mgoin"} | nm-testing/llama2.c-stories110M-pruned50-compressed-tensors | null | [
"transformers",
"safetensors",
"llama",
"text-generation",
"nm-vllm",
"sparse",
"arxiv:2301.00774",
"base_model:Xenova/llama2.c-stories110M",
"autotrain_compatible",
"endpoints_compatible",
"text-generation-inference",
"region:us"
] | null | 2024-04-25T14:17:32+00:00 | [
"2301.00774"
] | [] | TAGS
#transformers #safetensors #llama #text-generation #nm-vllm #sparse #arxiv-2301.00774 #base_model-Xenova/llama2.c-stories110M #autotrain_compatible #endpoints_compatible #text-generation-inference #region-us
|
## llama2.c-stories110M-pruned50
This repo contains model files for llama2.c 110M tinystories optimized for NM-vLLM, a high-throughput serving engine for compressed LLMs.
This model was pruned with SparseGPT, using SparseML.
The weights for this model were saved using compressed-tensors library. The chosen compression is format bitmask-compression.
## Inference
Install NM-vLLM for fast inference and low memory-usage:
Run in a Python pipeline for local inference:
## Prompt template
N/A
## Sparsification
For details on how this model was sparsified, see the 'URL' in this repo and follow the instructions below.
Install SparseML:
Replace the recipe as you like and run this one-shot compression script to apply SparseGPT:
## Slack
For further support, and discussions on these models and AI in general, join Neural Magic's Slack Community | [
"## llama2.c-stories110M-pruned50\nThis repo contains model files for llama2.c 110M tinystories optimized for NM-vLLM, a high-throughput serving engine for compressed LLMs.\n\nThis model was pruned with SparseGPT, using SparseML.\nThe weights for this model were saved using compressed-tensors library. The chosen compression is format bitmask-compression.",
"## Inference\nInstall NM-vLLM for fast inference and low memory-usage: \n\nRun in a Python pipeline for local inference:",
"## Prompt template\n\nN/A",
"## Sparsification\nFor details on how this model was sparsified, see the 'URL' in this repo and follow the instructions below.\n\nInstall SparseML:\n\n\nReplace the recipe as you like and run this one-shot compression script to apply SparseGPT:",
"## Slack\n\nFor further support, and discussions on these models and AI in general, join Neural Magic's Slack Community"
] | [
"TAGS\n#transformers #safetensors #llama #text-generation #nm-vllm #sparse #arxiv-2301.00774 #base_model-Xenova/llama2.c-stories110M #autotrain_compatible #endpoints_compatible #text-generation-inference #region-us \n",
"## llama2.c-stories110M-pruned50\nThis repo contains model files for llama2.c 110M tinystories optimized for NM-vLLM, a high-throughput serving engine for compressed LLMs.\n\nThis model was pruned with SparseGPT, using SparseML.\nThe weights for this model were saved using compressed-tensors library. The chosen compression is format bitmask-compression.",
"## Inference\nInstall NM-vLLM for fast inference and low memory-usage: \n\nRun in a Python pipeline for local inference:",
"## Prompt template\n\nN/A",
"## Sparsification\nFor details on how this model was sparsified, see the 'URL' in this repo and follow the instructions below.\n\nInstall SparseML:\n\n\nReplace the recipe as you like and run this one-shot compression script to apply SparseGPT:",
"## Slack\n\nFor further support, and discussions on these models and AI in general, join Neural Magic's Slack Community"
] |
text-classification | transformers |
<!-- This model card has been generated automatically according to the information the Trainer had access to. You
should probably proofread and complete it, then remove this comment. -->
# mi-super-modelo
This model is a fine-tuned version of [bert-base-cased](https://huggingface.co/bert-base-cased) on an unknown dataset.
It achieves the following results on the evaluation set:
- Loss: 1.6244
- Accuracy: 0.15
## Model description
More information needed
## Intended uses & limitations
More information needed
## Training and evaluation data
More information needed
## Training procedure
### Training hyperparameters
The following hyperparameters were used during training:
- learning_rate: 5e-05
- train_batch_size: 8
- eval_batch_size: 8
- seed: 42
- optimizer: Adam with betas=(0.9,0.999) and epsilon=1e-08
- lr_scheduler_type: linear
- num_epochs: 1
### Training results
| Training Loss | Epoch | Step | Validation Loss | Accuracy |
|:-------------:|:-----:|:----:|:---------------:|:--------:|
| 1.6526 | 0.5 | 5 | 1.7443 | 0.125 |
| 1.6784 | 1.0 | 10 | 1.6244 | 0.15 |
### Framework versions
- Transformers 4.40.0
- Pytorch 2.2.1+cu121
- Datasets 2.19.0
- Tokenizers 0.19.1
| {"license": "apache-2.0", "tags": ["generated_from_trainer"], "metrics": ["accuracy"], "base_model": "bert-base-cased", "model-index": [{"name": "mi-super-modelo", "results": []}]} | cristiangalvis/mi-super-modelo | null | [
"transformers",
"tensorboard",
"safetensors",
"bert",
"text-classification",
"generated_from_trainer",
"base_model:bert-base-cased",
"license:apache-2.0",
"autotrain_compatible",
"endpoints_compatible",
"region:us"
] | null | 2024-04-25T14:17:41+00:00 | [] | [] | TAGS
#transformers #tensorboard #safetensors #bert #text-classification #generated_from_trainer #base_model-bert-base-cased #license-apache-2.0 #autotrain_compatible #endpoints_compatible #region-us
| mi-super-modelo
===============
This model is a fine-tuned version of bert-base-cased on an unknown dataset.
It achieves the following results on the evaluation set:
* Loss: 1.6244
* Accuracy: 0.15
Model description
-----------------
More information needed
Intended uses & limitations
---------------------------
More information needed
Training and evaluation data
----------------------------
More information needed
Training procedure
------------------
### Training hyperparameters
The following hyperparameters were used during training:
* learning\_rate: 5e-05
* train\_batch\_size: 8
* eval\_batch\_size: 8
* seed: 42
* optimizer: Adam with betas=(0.9,0.999) and epsilon=1e-08
* lr\_scheduler\_type: linear
* num\_epochs: 1
### Training results
### Framework versions
* Transformers 4.40.0
* Pytorch 2.2.1+cu121
* Datasets 2.19.0
* Tokenizers 0.19.1
| [
"### Training hyperparameters\n\n\nThe following hyperparameters were used during training:\n\n\n* learning\\_rate: 5e-05\n* train\\_batch\\_size: 8\n* eval\\_batch\\_size: 8\n* seed: 42\n* optimizer: Adam with betas=(0.9,0.999) and epsilon=1e-08\n* lr\\_scheduler\\_type: linear\n* num\\_epochs: 1",
"### Training results",
"### Framework versions\n\n\n* Transformers 4.40.0\n* Pytorch 2.2.1+cu121\n* Datasets 2.19.0\n* Tokenizers 0.19.1"
] | [
"TAGS\n#transformers #tensorboard #safetensors #bert #text-classification #generated_from_trainer #base_model-bert-base-cased #license-apache-2.0 #autotrain_compatible #endpoints_compatible #region-us \n",
"### Training hyperparameters\n\n\nThe following hyperparameters were used during training:\n\n\n* learning\\_rate: 5e-05\n* train\\_batch\\_size: 8\n* eval\\_batch\\_size: 8\n* seed: 42\n* optimizer: Adam with betas=(0.9,0.999) and epsilon=1e-08\n* lr\\_scheduler\\_type: linear\n* num\\_epochs: 1",
"### Training results",
"### Framework versions\n\n\n* Transformers 4.40.0\n* Pytorch 2.2.1+cu121\n* Datasets 2.19.0\n* Tokenizers 0.19.1"
] |
null | transformers |
# Uploaded model
- **Developed by:** Ketan3101
- **License:** apache-2.0
- **Finetuned from model :** unsloth/llama-3-8b-bnb-4bit
This llama model was trained 2x faster with [Unsloth](https://github.com/unslothai/unsloth) and Huggingface's TRL library.
[<img src="https://raw.githubusercontent.com/unslothai/unsloth/main/images/unsloth%20made%20with%20love.png" width="200"/>](https://github.com/unslothai/unsloth)
| {"language": ["en"], "license": "apache-2.0", "tags": ["text-generation-inference", "transformers", "unsloth", "llama", "trl"], "base_model": "unsloth/llama-3-8b-bnb-4bit"} | Ketan3101/llama-3_8b_lora_model | null | [
"transformers",
"safetensors",
"text-generation-inference",
"unsloth",
"llama",
"trl",
"en",
"base_model:unsloth/llama-3-8b-bnb-4bit",
"license:apache-2.0",
"endpoints_compatible",
"region:us"
] | null | 2024-04-25T14:18:58+00:00 | [] | [
"en"
] | TAGS
#transformers #safetensors #text-generation-inference #unsloth #llama #trl #en #base_model-unsloth/llama-3-8b-bnb-4bit #license-apache-2.0 #endpoints_compatible #region-us
|
# Uploaded model
- Developed by: Ketan3101
- License: apache-2.0
- Finetuned from model : unsloth/llama-3-8b-bnb-4bit
This llama model was trained 2x faster with Unsloth and Huggingface's TRL library.
<img src="URL width="200"/>
| [
"# Uploaded model\n\n- Developed by: Ketan3101\n- License: apache-2.0\n- Finetuned from model : unsloth/llama-3-8b-bnb-4bit\n\nThis llama model was trained 2x faster with Unsloth and Huggingface's TRL library.\n\n<img src=\"URL width=\"200\"/>"
] | [
"TAGS\n#transformers #safetensors #text-generation-inference #unsloth #llama #trl #en #base_model-unsloth/llama-3-8b-bnb-4bit #license-apache-2.0 #endpoints_compatible #region-us \n",
"# Uploaded model\n\n- Developed by: Ketan3101\n- License: apache-2.0\n- Finetuned from model : unsloth/llama-3-8b-bnb-4bit\n\nThis llama model was trained 2x faster with Unsloth and Huggingface's TRL library.\n\n<img src=\"URL width=\"200\"/>"
] |
multiple-choice | transformers |
<!-- This model card has been generated automatically according to the information the Trainer had access to. You
should probably proofread and complete it, then remove this comment. -->
# NLPGroupProject-Finetune-bio-mobilebert-AL-Promt
This model is a fine-tuned version of [nlpie/bio-mobilebert](https://huggingface.co/nlpie/bio-mobilebert) on an unknown dataset.
It achieves the following results on the evaluation set:
- Loss: 1.0324
- Accuracy: 0.742
## Model description
More information needed
## Intended uses & limitations
More information needed
## Training and evaluation data
More information needed
## Training procedure
### Training hyperparameters
The following hyperparameters were used during training:
- learning_rate: 5e-05
- train_batch_size: 4
- eval_batch_size: 4
- seed: 42
- optimizer: Adam with betas=(0.9,0.999) and epsilon=1e-08
- lr_scheduler_type: linear
- num_epochs: 3
### Training results
| Training Loss | Epoch | Step | Validation Loss | Accuracy |
|:-------------:|:------:|:----:|:---------------:|:--------:|
| No log | 0.3121 | 250 | 0.8727 | 0.727 |
| 35.354 | 0.6242 | 500 | 0.7830 | 0.738 |
| 35.354 | 0.9363 | 750 | 0.7660 | 0.745 |
| 0.8233 | 1.2484 | 1000 | 0.9794 | 0.744 |
| 0.8233 | 1.5605 | 1250 | 0.8635 | 0.746 |
| 0.7285 | 1.8727 | 1500 | 0.6671 | 0.747 |
| 0.7285 | 2.1848 | 1750 | 1.0348 | 0.758 |
| 0.5734 | 2.4969 | 2000 | 1.0761 | 0.747 |
| 0.5734 | 2.8090 | 2250 | 1.0324 | 0.742 |
### Framework versions
- Transformers 4.40.0
- Pytorch 2.2.2+cu118
- Datasets 2.19.0
- Tokenizers 0.19.1
| {"license": "mit", "tags": ["generated_from_trainer"], "metrics": ["accuracy"], "base_model": "nlpie/bio-mobilebert", "model-index": [{"name": "NLPGroupProject-Finetune-bio-mobilebert-AL-Promt", "results": []}]} | BenjaminTT/NLPGroupProject-Finetune-bio-mobilebert-AL-Promt | null | [
"transformers",
"safetensors",
"mobilebert",
"multiple-choice",
"generated_from_trainer",
"base_model:nlpie/bio-mobilebert",
"license:mit",
"endpoints_compatible",
"region:us"
] | null | 2024-04-25T14:24:15+00:00 | [] | [] | TAGS
#transformers #safetensors #mobilebert #multiple-choice #generated_from_trainer #base_model-nlpie/bio-mobilebert #license-mit #endpoints_compatible #region-us
| NLPGroupProject-Finetune-bio-mobilebert-AL-Promt
================================================
This model is a fine-tuned version of nlpie/bio-mobilebert on an unknown dataset.
It achieves the following results on the evaluation set:
* Loss: 1.0324
* Accuracy: 0.742
Model description
-----------------
More information needed
Intended uses & limitations
---------------------------
More information needed
Training and evaluation data
----------------------------
More information needed
Training procedure
------------------
### Training hyperparameters
The following hyperparameters were used during training:
* learning\_rate: 5e-05
* train\_batch\_size: 4
* eval\_batch\_size: 4
* seed: 42
* optimizer: Adam with betas=(0.9,0.999) and epsilon=1e-08
* lr\_scheduler\_type: linear
* num\_epochs: 3
### Training results
### Framework versions
* Transformers 4.40.0
* Pytorch 2.2.2+cu118
* Datasets 2.19.0
* Tokenizers 0.19.1
| [
"### Training hyperparameters\n\n\nThe following hyperparameters were used during training:\n\n\n* learning\\_rate: 5e-05\n* train\\_batch\\_size: 4\n* eval\\_batch\\_size: 4\n* seed: 42\n* optimizer: Adam with betas=(0.9,0.999) and epsilon=1e-08\n* lr\\_scheduler\\_type: linear\n* num\\_epochs: 3",
"### Training results",
"### Framework versions\n\n\n* Transformers 4.40.0\n* Pytorch 2.2.2+cu118\n* Datasets 2.19.0\n* Tokenizers 0.19.1"
] | [
"TAGS\n#transformers #safetensors #mobilebert #multiple-choice #generated_from_trainer #base_model-nlpie/bio-mobilebert #license-mit #endpoints_compatible #region-us \n",
"### Training hyperparameters\n\n\nThe following hyperparameters were used during training:\n\n\n* learning\\_rate: 5e-05\n* train\\_batch\\_size: 4\n* eval\\_batch\\_size: 4\n* seed: 42\n* optimizer: Adam with betas=(0.9,0.999) and epsilon=1e-08\n* lr\\_scheduler\\_type: linear\n* num\\_epochs: 3",
"### Training results",
"### Framework versions\n\n\n* Transformers 4.40.0\n* Pytorch 2.2.2+cu118\n* Datasets 2.19.0\n* Tokenizers 0.19.1"
] |
text-generation | transformers |
<!-- This model card has been generated automatically according to the information the Trainer had access to. You
should probably proofread and complete it, then remove this comment. -->
# 0.0_ablation_5iters_bs256_useresponse_iter_5
This model is a fine-tuned version of [ZhangShenao/0.0_ablation_5iters_bs256_useresponse_iter_4](https://huggingface.co/ZhangShenao/0.0_ablation_5iters_bs256_useresponse_iter_4) on the ZhangShenao/0.0_ablation_5iters_bs256_useresponse_dataset dataset.
## Model description
More information needed
## Intended uses & limitations
More information needed
## Training and evaluation data
More information needed
## Training procedure
### Training hyperparameters
The following hyperparameters were used during training:
- learning_rate: 5e-07
- train_batch_size: 8
- eval_batch_size: 8
- seed: 42
- distributed_type: multi-GPU
- num_devices: 8
- gradient_accumulation_steps: 4
- total_train_batch_size: 256
- total_eval_batch_size: 64
- optimizer: Adam with betas=(0.9,0.999) and epsilon=1e-08
- lr_scheduler_type: cosine
- lr_scheduler_warmup_ratio: 0.1
- num_epochs: 1
### Training results
### Framework versions
- Transformers 4.36.2
- Pytorch 2.1.2+cu121
- Datasets 2.14.6
- Tokenizers 0.15.2
| {"license": "mit", "tags": ["alignment-handbook", "generated_from_trainer", "trl", "dpo", "generated_from_trainer"], "datasets": ["ZhangShenao/0.0_ablation_5iters_bs256_useresponse_dataset"], "base_model": "ZhangShenao/0.0_ablation_5iters_bs256_useresponse_iter_4", "model-index": [{"name": "0.0_ablation_5iters_bs256_useresponse_iter_5", "results": []}]} | ZhangShenao/0.0_ablation_5iters_bs256_useresponse_iter_5 | null | [
"transformers",
"safetensors",
"mistral",
"text-generation",
"alignment-handbook",
"generated_from_trainer",
"trl",
"dpo",
"conversational",
"dataset:ZhangShenao/0.0_ablation_5iters_bs256_useresponse_dataset",
"base_model:ZhangShenao/0.0_ablation_5iters_bs256_useresponse_iter_4",
"license:mit",
"autotrain_compatible",
"endpoints_compatible",
"text-generation-inference",
"region:us"
] | null | 2024-04-25T14:25:39+00:00 | [] | [] | TAGS
#transformers #safetensors #mistral #text-generation #alignment-handbook #generated_from_trainer #trl #dpo #conversational #dataset-ZhangShenao/0.0_ablation_5iters_bs256_useresponse_dataset #base_model-ZhangShenao/0.0_ablation_5iters_bs256_useresponse_iter_4 #license-mit #autotrain_compatible #endpoints_compatible #text-generation-inference #region-us
|
# 0.0_ablation_5iters_bs256_useresponse_iter_5
This model is a fine-tuned version of ZhangShenao/0.0_ablation_5iters_bs256_useresponse_iter_4 on the ZhangShenao/0.0_ablation_5iters_bs256_useresponse_dataset dataset.
## Model description
More information needed
## Intended uses & limitations
More information needed
## Training and evaluation data
More information needed
## Training procedure
### Training hyperparameters
The following hyperparameters were used during training:
- learning_rate: 5e-07
- train_batch_size: 8
- eval_batch_size: 8
- seed: 42
- distributed_type: multi-GPU
- num_devices: 8
- gradient_accumulation_steps: 4
- total_train_batch_size: 256
- total_eval_batch_size: 64
- optimizer: Adam with betas=(0.9,0.999) and epsilon=1e-08
- lr_scheduler_type: cosine
- lr_scheduler_warmup_ratio: 0.1
- num_epochs: 1
### Training results
### Framework versions
- Transformers 4.36.2
- Pytorch 2.1.2+cu121
- Datasets 2.14.6
- Tokenizers 0.15.2
| [
"# 0.0_ablation_5iters_bs256_useresponse_iter_5\n\nThis model is a fine-tuned version of ZhangShenao/0.0_ablation_5iters_bs256_useresponse_iter_4 on the ZhangShenao/0.0_ablation_5iters_bs256_useresponse_dataset dataset.",
"## Model description\n\nMore information needed",
"## Intended uses & limitations\n\nMore information needed",
"## Training and evaluation data\n\nMore information needed",
"## Training procedure",
"### Training hyperparameters\n\nThe following hyperparameters were used during training:\n- learning_rate: 5e-07\n- train_batch_size: 8\n- eval_batch_size: 8\n- seed: 42\n- distributed_type: multi-GPU\n- num_devices: 8\n- gradient_accumulation_steps: 4\n- total_train_batch_size: 256\n- total_eval_batch_size: 64\n- optimizer: Adam with betas=(0.9,0.999) and epsilon=1e-08\n- lr_scheduler_type: cosine\n- lr_scheduler_warmup_ratio: 0.1\n- num_epochs: 1",
"### Training results",
"### Framework versions\n\n- Transformers 4.36.2\n- Pytorch 2.1.2+cu121\n- Datasets 2.14.6\n- Tokenizers 0.15.2"
] | [
"TAGS\n#transformers #safetensors #mistral #text-generation #alignment-handbook #generated_from_trainer #trl #dpo #conversational #dataset-ZhangShenao/0.0_ablation_5iters_bs256_useresponse_dataset #base_model-ZhangShenao/0.0_ablation_5iters_bs256_useresponse_iter_4 #license-mit #autotrain_compatible #endpoints_compatible #text-generation-inference #region-us \n",
"# 0.0_ablation_5iters_bs256_useresponse_iter_5\n\nThis model is a fine-tuned version of ZhangShenao/0.0_ablation_5iters_bs256_useresponse_iter_4 on the ZhangShenao/0.0_ablation_5iters_bs256_useresponse_dataset dataset.",
"## Model description\n\nMore information needed",
"## Intended uses & limitations\n\nMore information needed",
"## Training and evaluation data\n\nMore information needed",
"## Training procedure",
"### Training hyperparameters\n\nThe following hyperparameters were used during training:\n- learning_rate: 5e-07\n- train_batch_size: 8\n- eval_batch_size: 8\n- seed: 42\n- distributed_type: multi-GPU\n- num_devices: 8\n- gradient_accumulation_steps: 4\n- total_train_batch_size: 256\n- total_eval_batch_size: 64\n- optimizer: Adam with betas=(0.9,0.999) and epsilon=1e-08\n- lr_scheduler_type: cosine\n- lr_scheduler_warmup_ratio: 0.1\n- num_epochs: 1",
"### Training results",
"### Framework versions\n\n- Transformers 4.36.2\n- Pytorch 2.1.2+cu121\n- Datasets 2.14.6\n- Tokenizers 0.15.2"
] |
null | transformers | ## About
<!-- ### quantize_version: 1 -->
<!-- ### output_tensor_quantised: 1 -->
<!-- ### convert_type: -->
<!-- ### vocab_type: -->
static quants of https://huggingface.co/aidiary/Llama-3-Gozaru-8B-Instruct
<!-- provided-files -->
weighted/imatrix quants seem not to be available (by me) at this time. If they do not show up a week or so after the static ones, I have probably not planned for them. Feel free to request them by opening a Community Discussion.
## Usage
If you are unsure how to use GGUF files, refer to one of [TheBloke's
READMEs](https://huggingface.co/TheBloke/KafkaLM-70B-German-V0.1-GGUF) for
more details, including on how to concatenate multi-part files.
## Provided Quants
(sorted by size, not necessarily quality. IQ-quants are often preferable over similar sized non-IQ quants)
| Link | Type | Size/GB | Notes |
|:-----|:-----|--------:|:------|
| [GGUF](https://huggingface.co/mradermacher/Llama-3-Gozaru-8B-Instruct-GGUF/resolve/main/Llama-3-Gozaru-8B-Instruct.Q2_K.gguf) | Q2_K | 3.3 | |
| [GGUF](https://huggingface.co/mradermacher/Llama-3-Gozaru-8B-Instruct-GGUF/resolve/main/Llama-3-Gozaru-8B-Instruct.IQ3_XS.gguf) | IQ3_XS | 3.6 | |
| [GGUF](https://huggingface.co/mradermacher/Llama-3-Gozaru-8B-Instruct-GGUF/resolve/main/Llama-3-Gozaru-8B-Instruct.Q3_K_S.gguf) | Q3_K_S | 3.8 | |
| [GGUF](https://huggingface.co/mradermacher/Llama-3-Gozaru-8B-Instruct-GGUF/resolve/main/Llama-3-Gozaru-8B-Instruct.IQ3_S.gguf) | IQ3_S | 3.8 | beats Q3_K* |
| [GGUF](https://huggingface.co/mradermacher/Llama-3-Gozaru-8B-Instruct-GGUF/resolve/main/Llama-3-Gozaru-8B-Instruct.IQ3_M.gguf) | IQ3_M | 3.9 | |
| [GGUF](https://huggingface.co/mradermacher/Llama-3-Gozaru-8B-Instruct-GGUF/resolve/main/Llama-3-Gozaru-8B-Instruct.Q3_K_M.gguf) | Q3_K_M | 4.1 | lower quality |
| [GGUF](https://huggingface.co/mradermacher/Llama-3-Gozaru-8B-Instruct-GGUF/resolve/main/Llama-3-Gozaru-8B-Instruct.Q3_K_L.gguf) | Q3_K_L | 4.4 | |
| [GGUF](https://huggingface.co/mradermacher/Llama-3-Gozaru-8B-Instruct-GGUF/resolve/main/Llama-3-Gozaru-8B-Instruct.IQ4_XS.gguf) | IQ4_XS | 4.6 | |
| [GGUF](https://huggingface.co/mradermacher/Llama-3-Gozaru-8B-Instruct-GGUF/resolve/main/Llama-3-Gozaru-8B-Instruct.Q4_K_S.gguf) | Q4_K_S | 4.8 | fast, recommended |
| [GGUF](https://huggingface.co/mradermacher/Llama-3-Gozaru-8B-Instruct-GGUF/resolve/main/Llama-3-Gozaru-8B-Instruct.Q4_K_M.gguf) | Q4_K_M | 5.0 | fast, recommended |
| [GGUF](https://huggingface.co/mradermacher/Llama-3-Gozaru-8B-Instruct-GGUF/resolve/main/Llama-3-Gozaru-8B-Instruct.Q5_K_S.gguf) | Q5_K_S | 5.7 | |
| [GGUF](https://huggingface.co/mradermacher/Llama-3-Gozaru-8B-Instruct-GGUF/resolve/main/Llama-3-Gozaru-8B-Instruct.Q5_K_M.gguf) | Q5_K_M | 5.8 | |
| [GGUF](https://huggingface.co/mradermacher/Llama-3-Gozaru-8B-Instruct-GGUF/resolve/main/Llama-3-Gozaru-8B-Instruct.Q6_K.gguf) | Q6_K | 6.7 | very good quality |
| [GGUF](https://huggingface.co/mradermacher/Llama-3-Gozaru-8B-Instruct-GGUF/resolve/main/Llama-3-Gozaru-8B-Instruct.Q8_0.gguf) | Q8_0 | 8.6 | fast, best quality |
| [GGUF](https://huggingface.co/mradermacher/Llama-3-Gozaru-8B-Instruct-GGUF/resolve/main/Llama-3-Gozaru-8B-Instruct.f16.gguf) | f16 | 16.2 | 16 bpw, overkill |
Here is a handy graph by ikawrakow comparing some lower-quality quant
types (lower is better):

And here are Artefact2's thoughts on the matter:
https://gist.github.com/Artefact2/b5f810600771265fc1e39442288e8ec9
## FAQ / Model Request
See https://huggingface.co/mradermacher/model_requests for some answers to
questions you might have and/or if you want some other model quantized.
## Thanks
I thank my company, [nethype GmbH](https://www.nethype.de/), for letting
me use its servers and providing upgrades to my workstation to enable
this work in my free time.
<!-- end -->
| {"language": ["en"], "library_name": "transformers", "tags": [], "base_model": "aidiary/Llama-3-Gozaru-8B-Instruct", "quantized_by": "mradermacher"} | mradermacher/Llama-3-Gozaru-8B-Instruct-GGUF | null | [
"transformers",
"gguf",
"en",
"base_model:aidiary/Llama-3-Gozaru-8B-Instruct",
"endpoints_compatible",
"region:us"
] | null | 2024-04-25T14:26:28+00:00 | [] | [
"en"
] | TAGS
#transformers #gguf #en #base_model-aidiary/Llama-3-Gozaru-8B-Instruct #endpoints_compatible #region-us
| About
-----
static quants of URL
weighted/imatrix quants seem not to be available (by me) at this time. If they do not show up a week or so after the static ones, I have probably not planned for them. Feel free to request them by opening a Community Discussion.
Usage
-----
If you are unsure how to use GGUF files, refer to one of TheBloke's
READMEs for
more details, including on how to concatenate multi-part files.
Provided Quants
---------------
(sorted by size, not necessarily quality. IQ-quants are often preferable over similar sized non-IQ quants)
Here is a handy graph by ikawrakow comparing some lower-quality quant
types (lower is better):
!URL
And here are Artefact2's thoughts on the matter:
URL
FAQ / Model Request
-------------------
See URL for some answers to
questions you might have and/or if you want some other model quantized.
Thanks
------
I thank my company, nethype GmbH, for letting
me use its servers and providing upgrades to my workstation to enable
this work in my free time.
| [] | [
"TAGS\n#transformers #gguf #en #base_model-aidiary/Llama-3-Gozaru-8B-Instruct #endpoints_compatible #region-us \n"
] |
text-generation | transformers |
# Model Card for Model ID
<!-- Provide a quick summary of what the model is/does. -->
## Model Details
### Model Description
<!-- Provide a longer summary of what this model is. -->
This is the model card of a 🤗 transformers model that has been pushed on the Hub. This model card has been automatically generated.
- **Developed by:** [More Information Needed]
- **Funded by [optional]:** [More Information Needed]
- **Shared by [optional]:** [More Information Needed]
- **Model type:** [More Information Needed]
- **Language(s) (NLP):** [More Information Needed]
- **License:** [More Information Needed]
- **Finetuned from model [optional]:** [More Information Needed]
### Model Sources [optional]
<!-- Provide the basic links for the model. -->
- **Repository:** [More Information Needed]
- **Paper [optional]:** [More Information Needed]
- **Demo [optional]:** [More Information Needed]
## Uses
<!-- Address questions around how the model is intended to be used, including the foreseeable users of the model and those affected by the model. -->
### Direct Use
<!-- This section is for the model use without fine-tuning or plugging into a larger ecosystem/app. -->
[More Information Needed]
### Downstream Use [optional]
<!-- This section is for the model use when fine-tuned for a task, or when plugged into a larger ecosystem/app -->
[More Information Needed]
### Out-of-Scope Use
<!-- This section addresses misuse, malicious use, and uses that the model will not work well for. -->
[More Information Needed]
## Bias, Risks, and Limitations
<!-- This section is meant to convey both technical and sociotechnical limitations. -->
[More Information Needed]
### Recommendations
<!-- This section is meant to convey recommendations with respect to the bias, risk, and technical limitations. -->
Users (both direct and downstream) should be made aware of the risks, biases and limitations of the model. More information needed for further recommendations.
## How to Get Started with the Model
Use the code below to get started with the model.
[More Information Needed]
## Training Details
### Training Data
<!-- This should link to a Dataset Card, perhaps with a short stub of information on what the training data is all about as well as documentation related to data pre-processing or additional filtering. -->
[More Information Needed]
### Training Procedure
<!-- This relates heavily to the Technical Specifications. Content here should link to that section when it is relevant to the training procedure. -->
#### Preprocessing [optional]
[More Information Needed]
#### Training Hyperparameters
- **Training regime:** [More Information Needed] <!--fp32, fp16 mixed precision, bf16 mixed precision, bf16 non-mixed precision, fp16 non-mixed precision, fp8 mixed precision -->
#### Speeds, Sizes, Times [optional]
<!-- This section provides information about throughput, start/end time, checkpoint size if relevant, etc. -->
[More Information Needed]
## Evaluation
<!-- This section describes the evaluation protocols and provides the results. -->
### Testing Data, Factors & Metrics
#### Testing Data
<!-- This should link to a Dataset Card if possible. -->
[More Information Needed]
#### Factors
<!-- These are the things the evaluation is disaggregating by, e.g., subpopulations or domains. -->
[More Information Needed]
#### Metrics
<!-- These are the evaluation metrics being used, ideally with a description of why. -->
[More Information Needed]
### Results
[More Information Needed]
#### Summary
## Model Examination [optional]
<!-- Relevant interpretability work for the model goes here -->
[More Information Needed]
## Environmental Impact
<!-- Total emissions (in grams of CO2eq) and additional considerations, such as electricity usage, go here. Edit the suggested text below accordingly -->
Carbon emissions can be estimated using the [Machine Learning Impact calculator](https://mlco2.github.io/impact#compute) presented in [Lacoste et al. (2019)](https://arxiv.org/abs/1910.09700).
- **Hardware Type:** [More Information Needed]
- **Hours used:** [More Information Needed]
- **Cloud Provider:** [More Information Needed]
- **Compute Region:** [More Information Needed]
- **Carbon Emitted:** [More Information Needed]
## Technical Specifications [optional]
### Model Architecture and Objective
[More Information Needed]
### Compute Infrastructure
[More Information Needed]
#### Hardware
[More Information Needed]
#### Software
[More Information Needed]
## Citation [optional]
<!-- If there is a paper or blog post introducing the model, the APA and Bibtex information for that should go in this section. -->
**BibTeX:**
[More Information Needed]
**APA:**
[More Information Needed]
## Glossary [optional]
<!-- If relevant, include terms and calculations in this section that can help readers understand the model or model card. -->
[More Information Needed]
## More Information [optional]
[More Information Needed]
## Model Card Authors [optional]
[More Information Needed]
## Model Card Contact
[More Information Needed] | {"library_name": "transformers", "tags": []} | liquid9212/khurkx6 | null | [
"transformers",
"safetensors",
"stablelm",
"text-generation",
"conversational",
"arxiv:1910.09700",
"autotrain_compatible",
"endpoints_compatible",
"region:us"
] | null | 2024-04-25T14:26:53+00:00 | [
"1910.09700"
] | [] | TAGS
#transformers #safetensors #stablelm #text-generation #conversational #arxiv-1910.09700 #autotrain_compatible #endpoints_compatible #region-us
|
# Model Card for Model ID
## Model Details
### Model Description
This is the model card of a transformers model that has been pushed on the Hub. This model card has been automatically generated.
- Developed by:
- Funded by [optional]:
- Shared by [optional]:
- Model type:
- Language(s) (NLP):
- License:
- Finetuned from model [optional]:
### Model Sources [optional]
- Repository:
- Paper [optional]:
- Demo [optional]:
## Uses
### Direct Use
### Downstream Use [optional]
### Out-of-Scope Use
## Bias, Risks, and Limitations
### Recommendations
Users (both direct and downstream) should be made aware of the risks, biases and limitations of the model. More information needed for further recommendations.
## How to Get Started with the Model
Use the code below to get started with the model.
## Training Details
### Training Data
### Training Procedure
#### Preprocessing [optional]
#### Training Hyperparameters
- Training regime:
#### Speeds, Sizes, Times [optional]
## Evaluation
### Testing Data, Factors & Metrics
#### Testing Data
#### Factors
#### Metrics
### Results
#### Summary
## Model Examination [optional]
## Environmental Impact
Carbon emissions can be estimated using the Machine Learning Impact calculator presented in Lacoste et al. (2019).
- Hardware Type:
- Hours used:
- Cloud Provider:
- Compute Region:
- Carbon Emitted:
## Technical Specifications [optional]
### Model Architecture and Objective
### Compute Infrastructure
#### Hardware
#### Software
[optional]
BibTeX:
APA:
## Glossary [optional]
## More Information [optional]
## Model Card Authors [optional]
## Model Card Contact
| [
"# Model Card for Model ID",
"## Model Details",
"### Model Description\n\n\n\nThis is the model card of a transformers model that has been pushed on the Hub. This model card has been automatically generated.\n\n- Developed by: \n- Funded by [optional]: \n- Shared by [optional]: \n- Model type: \n- Language(s) (NLP): \n- License: \n- Finetuned from model [optional]:",
"### Model Sources [optional]\n\n\n\n- Repository: \n- Paper [optional]: \n- Demo [optional]:",
"## Uses",
"### Direct Use",
"### Downstream Use [optional]",
"### Out-of-Scope Use",
"## Bias, Risks, and Limitations",
"### Recommendations\n\n\n\nUsers (both direct and downstream) should be made aware of the risks, biases and limitations of the model. More information needed for further recommendations.",
"## How to Get Started with the Model\n\nUse the code below to get started with the model.",
"## Training Details",
"### Training Data",
"### Training Procedure",
"#### Preprocessing [optional]",
"#### Training Hyperparameters\n\n- Training regime:",
"#### Speeds, Sizes, Times [optional]",
"## Evaluation",
"### Testing Data, Factors & Metrics",
"#### Testing Data",
"#### Factors",
"#### Metrics",
"### Results",
"#### Summary",
"## Model Examination [optional]",
"## Environmental Impact\n\n\n\nCarbon emissions can be estimated using the Machine Learning Impact calculator presented in Lacoste et al. (2019).\n\n- Hardware Type: \n- Hours used: \n- Cloud Provider: \n- Compute Region: \n- Carbon Emitted:",
"## Technical Specifications [optional]",
"### Model Architecture and Objective",
"### Compute Infrastructure",
"#### Hardware",
"#### Software\n\n\n\n[optional]\n\n\n\nBibTeX:\n\n\n\nAPA:",
"## Glossary [optional]",
"## More Information [optional]",
"## Model Card Authors [optional]",
"## Model Card Contact"
] | [
"TAGS\n#transformers #safetensors #stablelm #text-generation #conversational #arxiv-1910.09700 #autotrain_compatible #endpoints_compatible #region-us \n",
"# Model Card for Model ID",
"## Model Details",
"### Model Description\n\n\n\nThis is the model card of a transformers model that has been pushed on the Hub. This model card has been automatically generated.\n\n- Developed by: \n- Funded by [optional]: \n- Shared by [optional]: \n- Model type: \n- Language(s) (NLP): \n- License: \n- Finetuned from model [optional]:",
"### Model Sources [optional]\n\n\n\n- Repository: \n- Paper [optional]: \n- Demo [optional]:",
"## Uses",
"### Direct Use",
"### Downstream Use [optional]",
"### Out-of-Scope Use",
"## Bias, Risks, and Limitations",
"### Recommendations\n\n\n\nUsers (both direct and downstream) should be made aware of the risks, biases and limitations of the model. More information needed for further recommendations.",
"## How to Get Started with the Model\n\nUse the code below to get started with the model.",
"## Training Details",
"### Training Data",
"### Training Procedure",
"#### Preprocessing [optional]",
"#### Training Hyperparameters\n\n- Training regime:",
"#### Speeds, Sizes, Times [optional]",
"## Evaluation",
"### Testing Data, Factors & Metrics",
"#### Testing Data",
"#### Factors",
"#### Metrics",
"### Results",
"#### Summary",
"## Model Examination [optional]",
"## Environmental Impact\n\n\n\nCarbon emissions can be estimated using the Machine Learning Impact calculator presented in Lacoste et al. (2019).\n\n- Hardware Type: \n- Hours used: \n- Cloud Provider: \n- Compute Region: \n- Carbon Emitted:",
"## Technical Specifications [optional]",
"### Model Architecture and Objective",
"### Compute Infrastructure",
"#### Hardware",
"#### Software\n\n\n\n[optional]\n\n\n\nBibTeX:\n\n\n\nAPA:",
"## Glossary [optional]",
"## More Information [optional]",
"## Model Card Authors [optional]",
"## Model Card Contact"
] |
text-generation | null | # Meta-Llama-3-11.5B-Instruct
This is a merge of pre-trained language models created using [mergekit](https://github.com/cg123/mergekit).
I had this idea at night that it would make sense to make a frankenmerge of Llama 3.. since we didn't get a 13B or 34B versions this time..
Here's the same thing but for the base model: [mpasila/Meta-Llama-3-11.5B](https://huggingface.co/mpasila/Meta-Llama-3-11.5B/)
## Merge Details
### Merge Method
This model was merged using the passthrough merge method.
### Models Merged
The following models were included in the merge:
* [Undi95/Meta-Llama-3-8B-Instruct-hf](https://huggingface.co/Undi95/Meta-Llama-3-8B-Instruct-hf)
### Configuration
The following YAML configuration was used to produce this model:
```yaml
slices:
- sources:
- model: Undi95/Meta-Llama-3-8B-Instruct-hf
layer_range: [0, 24]
- sources:
- model: Undi95/Meta-Llama-3-8B-Instruct-hf
layer_range: [8, 32]
merge_method: passthrough
dtype: bfloat16
``` | {"language": ["en"], "license": "other", "tags": ["mergekit", "merge", "facebook", "meta", "pytorch", "llama", "llama-3"], "base_model": ["Undi95/Meta-Llama-3-8B-Instruct-hf"], "pipeline_tag": "text-generation", "license_name": "llama3", "license_link": "LICENSE", "extra_gated_prompt": "### META LLAMA 3 COMMUNITY LICENSE AGREEMENT Meta Llama 3 Version Release Date: April 18, 2024\n\"Agreement\" means the terms and conditions for use, reproduction, distribution and modification of the Llama Materials set forth herein.\n\"Documentation\" means the specifications, manuals and documentation accompanying Meta Llama 3 distributed by Meta at https://llama.meta.com/get-started/.\n\"Licensee\" or \"you\" means you, or your employer or any other person or entity (if you are entering into this Agreement on such person or entity\u2019s behalf), of the age required under applicable laws, rules or regulations to provide legal consent and that has legal authority to bind your employer or such other person or entity if you are entering in this Agreement on their behalf.\n\"Meta Llama 3\" means the foundational large language models and software and algorithms, including machine-learning model code, trained model weights, inference-enabling code, training-enabling code, fine-tuning enabling code and other elements of the foregoing distributed by Meta at https://llama.meta.com/llama-downloads.\n\"Llama Materials\" means, collectively, Meta\u2019s proprietary Meta Llama 3 and Documentation (and any portion thereof) made available under this Agreement.\n\"Meta\" or \"we\" means Meta Platforms Ireland Limited (if you are located in or, if you are an entity, your principal place of business is in the EEA or Switzerland) and Meta Platforms, Inc. (if you are located outside of the EEA or Switzerland).\n \n1. License Rights and Redistribution.\na. Grant of Rights. You are granted a non-exclusive, worldwide, non-transferable and royalty-free limited license under Meta\u2019s intellectual property or other rights owned by Meta embodied in the Llama Materials to use, reproduce, distribute, copy, create derivative works of, and make modifications to the Llama Materials.\nb. Redistribution and Use.\ni. If you distribute or make available the Llama Materials (or any derivative works thereof), or a product or service that uses any of them, including another AI model, you shall (A) provide a copy of this Agreement with any such Llama Materials; and (B) prominently display \u201cBuilt with Meta Llama 3\u201d on a related website, user interface, blogpost, about page, or product documentation. If you use the Llama Materials to create, train, fine tune, or otherwise improve an AI model, which is distributed or made available, you shall also include \u201cLlama 3\u201d at the beginning of any such AI model name.\nii. If you receive Llama Materials, or any derivative works thereof, from a Licensee as part of an integrated end user product, then Section 2 of this Agreement will not apply to you.\niii. You must retain in all copies of the Llama Materials that you distribute the following attribution notice within a \u201cNotice\u201d text file distributed as a part of such copies: \u201cMeta Llama 3 is licensed under the Meta Llama 3 Community License, Copyright \u00a9 Meta Platforms, Inc. All Rights Reserved.\u201d\niv. Your use of the Llama Materials must comply with applicable laws and regulations (including trade compliance laws and regulations) and adhere to the Acceptable Use Policy for the Llama Materials (available at https://llama.meta.com/llama3/use-policy), which is hereby incorporated by reference into this Agreement.\nv. You will not use the Llama Materials or any output or results of the Llama Materials to improve any other large language model (excluding Meta Llama 3 or derivative works thereof).\n2. Additional Commercial Terms. If, on the Meta Llama 3 version release date, the monthly active users of the products or services made available by or for Licensee, or Licensee\u2019s affiliates, is greater than 700 million monthly active users in the preceding calendar month, you must request a license from Meta, which Meta may grant to you in its sole discretion, and you are not authorized to exercise any of the rights under this Agreement unless or until Meta otherwise expressly grants you such rights.\n3. Disclaimer of Warranty. UNLESS REQUIRED BY APPLICABLE LAW, THE LLAMA MATERIALS AND ANY OUTPUT AND RESULTS THEREFROM ARE PROVIDED ON AN \u201cAS IS\u201d BASIS, WITHOUT WARRANTIES OF ANY KIND, AND META DISCLAIMS ALL WARRANTIES OF ANY KIND, BOTH EXPRESS AND IMPLIED, INCLUDING, WITHOUT LIMITATION, ANY WARRANTIES OF TITLE, NON-INFRINGEMENT, MERCHANTABILITY, OR FITNESS FOR A PARTICULAR PURPOSE. YOU ARE SOLELY RESPONSIBLE FOR DETERMINING THE APPROPRIATENESS OF USING OR REDISTRIBUTING THE LLAMA MATERIALS AND ASSUME ANY RISKS ASSOCIATED WITH YOUR USE OF THE LLAMA MATERIALS AND ANY OUTPUT AND RESULTS.\n4. Limitation of Liability. IN NO EVENT WILL META OR ITS AFFILIATES BE LIABLE UNDER ANY THEORY OF LIABILITY, WHETHER IN CONTRACT, TORT, NEGLIGENCE, PRODUCTS LIABILITY, OR OTHERWISE, ARISING OUT OF THIS AGREEMENT, FOR ANY LOST PROFITS OR ANY INDIRECT, SPECIAL, CONSEQUENTIAL, INCIDENTAL, EXEMPLARY OR PUNITIVE DAMAGES, EVEN IF META OR ITS AFFILIATES HAVE BEEN ADVISED OF THE POSSIBILITY OF ANY OF THE FOREGOING.\n5. Intellectual Property.\na. No trademark licenses are granted under this Agreement, and in connection with the Llama Materials, neither Meta nor Licensee may use any name or mark owned by or associated with the other or any of its affiliates, except as required for reasonable and customary use in describing and redistributing the Llama Materials or as set forth in this Section 5(a). Meta hereby grants you a license to use \u201cLlama 3\u201d (the \u201cMark\u201d) solely as required to comply with the last sentence of Section 1.b.i. You will comply with Meta\u2019s brand guidelines (currently accessible at https://about.meta.com/brand/resources/meta/company-brand/ ). All goodwill arising out of your use of the Mark will inure to the benefit of Meta.\nb. Subject to Meta\u2019s ownership of Llama Materials and derivatives made by or for Meta, with respect to any derivative works and modifications of the Llama Materials that are made by you, as between you and Meta, you are and will be the owner of such derivative works and modifications.\nc. If you institute litigation or other proceedings against Meta or any entity (including a cross-claim or counterclaim in a lawsuit) alleging that the Llama Materials or Meta Llama 3 outputs or results, or any portion of any of the foregoing, constitutes infringement of intellectual property or other rights owned or licensable by you, then any licenses granted to you under this Agreement shall terminate as of the date such litigation or claim is filed or instituted. You will indemnify and hold harmless Meta from and against any claim by any third party arising out of or related to your use or distribution of the Llama Materials.\n6. Term and Termination. The term of this Agreement will commence upon your acceptance of this Agreement or access to the Llama Materials and will continue in full force and effect until terminated in accordance with the terms and conditions herein. Meta may terminate this Agreement if you are in breach of any term or condition of this Agreement. Upon termination of this Agreement, you shall delete and cease use of the Llama Materials. Sections 3, 4 and 7 shall survive the termination of this Agreement.\n7. Governing Law and Jurisdiction. This Agreement will be governed and construed under the laws of the State of California without regard to choice of law principles, and the UN Convention on Contracts for the International Sale of Goods does not apply to this Agreement. The courts of California shall have exclusive jurisdiction of any dispute arising out of this Agreement.\n### Meta Llama 3 Acceptable Use Policy\nMeta is committed to promoting safe and fair use of its tools and features, including Meta Llama 3. If you access or use Meta Llama 3, you agree to this Acceptable Use Policy (\u201cPolicy\u201d). The most recent copy of this policy can be found at [https://llama.meta.com/llama3/use-policy](https://llama.meta.com/llama3/use-policy)\n#### Prohibited Uses\nWe want everyone to use Meta Llama 3 safely and responsibly. You agree you will not use, or allow others to use, Meta Llama 3 to: 1. Violate the law or others\u2019 rights, including to:\n 1. Engage in, promote, generate, contribute to, encourage, plan, incite, or further illegal or unlawful activity or content, such as:\n 1. Violence or terrorism\n 2. Exploitation or harm to children, including the solicitation, creation, acquisition, or dissemination of child exploitative content or failure to report Child Sexual Abuse Material\n 3. Human trafficking, exploitation, and sexual violence\n 4. The illegal distribution of information or materials to minors, including obscene materials, or failure to employ legally required age-gating in connection with such information or materials.\n 5. Sexual solicitation\n 6. Any other criminal activity\n 2. Engage in, promote, incite, or facilitate the harassment, abuse, threatening, or bullying of individuals or groups of individuals\n 3. Engage in, promote, incite, or facilitate discrimination or other unlawful or harmful conduct in the provision of employment, employment benefits, credit, housing, other economic benefits, or other essential goods and services\n 4. Engage in the unauthorized or unlicensed practice of any profession including, but not limited to, financial, legal, medical/health, or related professional practices\n 5. Collect, process, disclose, generate, or infer health, demographic, or other sensitive personal or private information about individuals without rights and consents required by applicable laws\n 6. Engage in or facilitate any action or generate any content that infringes, misappropriates, or otherwise violates any third-party rights, including the outputs or results of any products or services using the Llama Materials\n 7. Create, generate, or facilitate the creation of malicious code, malware, computer viruses or do anything else that could disable, overburden, interfere with or impair the proper working, integrity, operation or appearance of a website or computer system\n2. Engage in, promote, incite, facilitate, or assist in the planning or development of activities that present a risk of death or bodily harm to individuals, including use of Meta Llama 3 related to the following:\n 1. Military, warfare, nuclear industries or applications, espionage, use for materials or activities that are subject to the International Traffic Arms Regulations (ITAR) maintained by the United States Department of State\n 2. Guns and illegal weapons (including weapon development)\n 3. Illegal drugs and regulated/controlled substances\n 4. Operation of critical infrastructure, transportation technologies, or heavy machinery\n 5. Self-harm or harm to others, including suicide, cutting, and eating disorders\n 6. Any content intended to incite or promote violence, abuse, or any infliction of bodily harm to an individual\n3. Intentionally deceive or mislead others, including use of Meta Llama 3 related to the following:\n 1. Generating, promoting, or furthering fraud or the creation or promotion of disinformation\n 2. Generating, promoting, or furthering defamatory content, including the creation of defamatory statements, images, or other content\n 3. Generating, promoting, or further distributing spam\n 4. Impersonating another individual without consent, authorization, or legal right\n 5. Representing that the use of Meta Llama 3 or outputs are human-generated\n 6. Generating or facilitating false online engagement, including fake reviews and other means of fake online engagement\n4. Fail to appropriately disclose to end users any known dangers of your AI system\nPlease report any violation of this Policy, software \u201cbug,\u201d or other problems that could lead to a violation of this Policy through one of the following means:\n * Reporting issues with the model: [https://github.com/meta-llama/llama3](https://github.com/meta-llama/llama3)\n * Reporting risky content generated by the model:\n developers.facebook.com/llama_output_feedback\n * Reporting bugs and security concerns: facebook.com/whitehat/info\n * Reporting violations of the Acceptable Use Policy or unlicensed uses of Meta Llama 3: [email protected]", "extra_gated_fields": {"First Name": "text", "Last Name": "text", "Date of birth": "date_picker", "Country": "country", "Affiliation": "text", "geo": "ip_location", "By clicking Submit below I accept the terms of the license and acknowledge that the information I provide will be collected stored processed and shared in accordance with the Meta Privacy Policy": "checkbox"}, "extra_gated_description": "The information you provide will be collected, stored, processed and shared in accordance with the [Meta Privacy Policy](https://www.facebook.com/privacy/policy/).", "extra_gated_button_content": "Submit"} | asiansoul/Meta-Llama-3-11.5B-Instruct-GGUF | null | [
"gguf",
"mergekit",
"merge",
"facebook",
"meta",
"pytorch",
"llama",
"llama-3",
"text-generation",
"en",
"base_model:Undi95/Meta-Llama-3-8B-Instruct-hf",
"license:other",
"region:us"
] | null | 2024-04-25T14:27:13+00:00 | [] | [
"en"
] | TAGS
#gguf #mergekit #merge #facebook #meta #pytorch #llama #llama-3 #text-generation #en #base_model-Undi95/Meta-Llama-3-8B-Instruct-hf #license-other #region-us
| # Meta-Llama-3-11.5B-Instruct
This is a merge of pre-trained language models created using mergekit.
I had this idea at night that it would make sense to make a frankenmerge of Llama 3.. since we didn't get a 13B or 34B versions this time..
Here's the same thing but for the base model: mpasila/Meta-Llama-3-11.5B
## Merge Details
### Merge Method
This model was merged using the passthrough merge method.
### Models Merged
The following models were included in the merge:
* Undi95/Meta-Llama-3-8B-Instruct-hf
### Configuration
The following YAML configuration was used to produce this model:
| [
"# Meta-Llama-3-11.5B-Instruct\n\nThis is a merge of pre-trained language models created using mergekit.\n\nI had this idea at night that it would make sense to make a frankenmerge of Llama 3.. since we didn't get a 13B or 34B versions this time..\n\nHere's the same thing but for the base model: mpasila/Meta-Llama-3-11.5B",
"## Merge Details",
"### Merge Method\n\nThis model was merged using the passthrough merge method.",
"### Models Merged\n\nThe following models were included in the merge:\n* Undi95/Meta-Llama-3-8B-Instruct-hf",
"### Configuration\n\nThe following YAML configuration was used to produce this model:"
] | [
"TAGS\n#gguf #mergekit #merge #facebook #meta #pytorch #llama #llama-3 #text-generation #en #base_model-Undi95/Meta-Llama-3-8B-Instruct-hf #license-other #region-us \n",
"# Meta-Llama-3-11.5B-Instruct\n\nThis is a merge of pre-trained language models created using mergekit.\n\nI had this idea at night that it would make sense to make a frankenmerge of Llama 3.. since we didn't get a 13B or 34B versions this time..\n\nHere's the same thing but for the base model: mpasila/Meta-Llama-3-11.5B",
"## Merge Details",
"### Merge Method\n\nThis model was merged using the passthrough merge method.",
"### Models Merged\n\nThe following models were included in the merge:\n* Undi95/Meta-Llama-3-8B-Instruct-hf",
"### Configuration\n\nThe following YAML configuration was used to produce this model:"
] |
text-generation | transformers |
# Model Card for Model ID
<!-- Provide a quick summary of what the model is/does. -->
## Model Details
### Model Description
<!-- Provide a longer summary of what this model is. -->
This is the model card of a 🤗 transformers model that has been pushed on the Hub. This model card has been automatically generated.
- **Developed by:** [More Information Needed]
- **Funded by [optional]:** [More Information Needed]
- **Shared by [optional]:** [More Information Needed]
- **Model type:** [More Information Needed]
- **Language(s) (NLP):** [More Information Needed]
- **License:** [More Information Needed]
- **Finetuned from model [optional]:** [More Information Needed]
### Model Sources [optional]
<!-- Provide the basic links for the model. -->
- **Repository:** [More Information Needed]
- **Paper [optional]:** [More Information Needed]
- **Demo [optional]:** [More Information Needed]
## Uses
<!-- Address questions around how the model is intended to be used, including the foreseeable users of the model and those affected by the model. -->
### Direct Use
<!-- This section is for the model use without fine-tuning or plugging into a larger ecosystem/app. -->
[More Information Needed]
### Downstream Use [optional]
<!-- This section is for the model use when fine-tuned for a task, or when plugged into a larger ecosystem/app -->
[More Information Needed]
### Out-of-Scope Use
<!-- This section addresses misuse, malicious use, and uses that the model will not work well for. -->
[More Information Needed]
## Bias, Risks, and Limitations
<!-- This section is meant to convey both technical and sociotechnical limitations. -->
[More Information Needed]
### Recommendations
<!-- This section is meant to convey recommendations with respect to the bias, risk, and technical limitations. -->
Users (both direct and downstream) should be made aware of the risks, biases and limitations of the model. More information needed for further recommendations.
## How to Get Started with the Model
Use the code below to get started with the model.
[More Information Needed]
## Training Details
### Training Data
<!-- This should link to a Dataset Card, perhaps with a short stub of information on what the training data is all about as well as documentation related to data pre-processing or additional filtering. -->
[More Information Needed]
### Training Procedure
<!-- This relates heavily to the Technical Specifications. Content here should link to that section when it is relevant to the training procedure. -->
#### Preprocessing [optional]
[More Information Needed]
#### Training Hyperparameters
- **Training regime:** [More Information Needed] <!--fp32, fp16 mixed precision, bf16 mixed precision, bf16 non-mixed precision, fp16 non-mixed precision, fp8 mixed precision -->
#### Speeds, Sizes, Times [optional]
<!-- This section provides information about throughput, start/end time, checkpoint size if relevant, etc. -->
[More Information Needed]
## Evaluation
<!-- This section describes the evaluation protocols and provides the results. -->
### Testing Data, Factors & Metrics
#### Testing Data
<!-- This should link to a Dataset Card if possible. -->
[More Information Needed]
#### Factors
<!-- These are the things the evaluation is disaggregating by, e.g., subpopulations or domains. -->
[More Information Needed]
#### Metrics
<!-- These are the evaluation metrics being used, ideally with a description of why. -->
[More Information Needed]
### Results
[More Information Needed]
#### Summary
## Model Examination [optional]
<!-- Relevant interpretability work for the model goes here -->
[More Information Needed]
## Environmental Impact
<!-- Total emissions (in grams of CO2eq) and additional considerations, such as electricity usage, go here. Edit the suggested text below accordingly -->
Carbon emissions can be estimated using the [Machine Learning Impact calculator](https://mlco2.github.io/impact#compute) presented in [Lacoste et al. (2019)](https://arxiv.org/abs/1910.09700).
- **Hardware Type:** [More Information Needed]
- **Hours used:** [More Information Needed]
- **Cloud Provider:** [More Information Needed]
- **Compute Region:** [More Information Needed]
- **Carbon Emitted:** [More Information Needed]
## Technical Specifications [optional]
### Model Architecture and Objective
[More Information Needed]
### Compute Infrastructure
[More Information Needed]
#### Hardware
[More Information Needed]
#### Software
[More Information Needed]
## Citation [optional]
<!-- If there is a paper or blog post introducing the model, the APA and Bibtex information for that should go in this section. -->
**BibTeX:**
[More Information Needed]
**APA:**
[More Information Needed]
## Glossary [optional]
<!-- If relevant, include terms and calculations in this section that can help readers understand the model or model card. -->
[More Information Needed]
## More Information [optional]
[More Information Needed]
## Model Card Authors [optional]
[More Information Needed]
## Model Card Contact
[More Information Needed] | {"library_name": "transformers", "tags": []} | quickstep3621/sb8jwe1 | null | [
"transformers",
"safetensors",
"stablelm",
"text-generation",
"conversational",
"arxiv:1910.09700",
"autotrain_compatible",
"endpoints_compatible",
"region:us"
] | null | 2024-04-25T14:27:23+00:00 | [
"1910.09700"
] | [] | TAGS
#transformers #safetensors #stablelm #text-generation #conversational #arxiv-1910.09700 #autotrain_compatible #endpoints_compatible #region-us
|
# Model Card for Model ID
## Model Details
### Model Description
This is the model card of a transformers model that has been pushed on the Hub. This model card has been automatically generated.
- Developed by:
- Funded by [optional]:
- Shared by [optional]:
- Model type:
- Language(s) (NLP):
- License:
- Finetuned from model [optional]:
### Model Sources [optional]
- Repository:
- Paper [optional]:
- Demo [optional]:
## Uses
### Direct Use
### Downstream Use [optional]
### Out-of-Scope Use
## Bias, Risks, and Limitations
### Recommendations
Users (both direct and downstream) should be made aware of the risks, biases and limitations of the model. More information needed for further recommendations.
## How to Get Started with the Model
Use the code below to get started with the model.
## Training Details
### Training Data
### Training Procedure
#### Preprocessing [optional]
#### Training Hyperparameters
- Training regime:
#### Speeds, Sizes, Times [optional]
## Evaluation
### Testing Data, Factors & Metrics
#### Testing Data
#### Factors
#### Metrics
### Results
#### Summary
## Model Examination [optional]
## Environmental Impact
Carbon emissions can be estimated using the Machine Learning Impact calculator presented in Lacoste et al. (2019).
- Hardware Type:
- Hours used:
- Cloud Provider:
- Compute Region:
- Carbon Emitted:
## Technical Specifications [optional]
### Model Architecture and Objective
### Compute Infrastructure
#### Hardware
#### Software
[optional]
BibTeX:
APA:
## Glossary [optional]
## More Information [optional]
## Model Card Authors [optional]
## Model Card Contact
| [
"# Model Card for Model ID",
"## Model Details",
"### Model Description\n\n\n\nThis is the model card of a transformers model that has been pushed on the Hub. This model card has been automatically generated.\n\n- Developed by: \n- Funded by [optional]: \n- Shared by [optional]: \n- Model type: \n- Language(s) (NLP): \n- License: \n- Finetuned from model [optional]:",
"### Model Sources [optional]\n\n\n\n- Repository: \n- Paper [optional]: \n- Demo [optional]:",
"## Uses",
"### Direct Use",
"### Downstream Use [optional]",
"### Out-of-Scope Use",
"## Bias, Risks, and Limitations",
"### Recommendations\n\n\n\nUsers (both direct and downstream) should be made aware of the risks, biases and limitations of the model. More information needed for further recommendations.",
"## How to Get Started with the Model\n\nUse the code below to get started with the model.",
"## Training Details",
"### Training Data",
"### Training Procedure",
"#### Preprocessing [optional]",
"#### Training Hyperparameters\n\n- Training regime:",
"#### Speeds, Sizes, Times [optional]",
"## Evaluation",
"### Testing Data, Factors & Metrics",
"#### Testing Data",
"#### Factors",
"#### Metrics",
"### Results",
"#### Summary",
"## Model Examination [optional]",
"## Environmental Impact\n\n\n\nCarbon emissions can be estimated using the Machine Learning Impact calculator presented in Lacoste et al. (2019).\n\n- Hardware Type: \n- Hours used: \n- Cloud Provider: \n- Compute Region: \n- Carbon Emitted:",
"## Technical Specifications [optional]",
"### Model Architecture and Objective",
"### Compute Infrastructure",
"#### Hardware",
"#### Software\n\n\n\n[optional]\n\n\n\nBibTeX:\n\n\n\nAPA:",
"## Glossary [optional]",
"## More Information [optional]",
"## Model Card Authors [optional]",
"## Model Card Contact"
] | [
"TAGS\n#transformers #safetensors #stablelm #text-generation #conversational #arxiv-1910.09700 #autotrain_compatible #endpoints_compatible #region-us \n",
"# Model Card for Model ID",
"## Model Details",
"### Model Description\n\n\n\nThis is the model card of a transformers model that has been pushed on the Hub. This model card has been automatically generated.\n\n- Developed by: \n- Funded by [optional]: \n- Shared by [optional]: \n- Model type: \n- Language(s) (NLP): \n- License: \n- Finetuned from model [optional]:",
"### Model Sources [optional]\n\n\n\n- Repository: \n- Paper [optional]: \n- Demo [optional]:",
"## Uses",
"### Direct Use",
"### Downstream Use [optional]",
"### Out-of-Scope Use",
"## Bias, Risks, and Limitations",
"### Recommendations\n\n\n\nUsers (both direct and downstream) should be made aware of the risks, biases and limitations of the model. More information needed for further recommendations.",
"## How to Get Started with the Model\n\nUse the code below to get started with the model.",
"## Training Details",
"### Training Data",
"### Training Procedure",
"#### Preprocessing [optional]",
"#### Training Hyperparameters\n\n- Training regime:",
"#### Speeds, Sizes, Times [optional]",
"## Evaluation",
"### Testing Data, Factors & Metrics",
"#### Testing Data",
"#### Factors",
"#### Metrics",
"### Results",
"#### Summary",
"## Model Examination [optional]",
"## Environmental Impact\n\n\n\nCarbon emissions can be estimated using the Machine Learning Impact calculator presented in Lacoste et al. (2019).\n\n- Hardware Type: \n- Hours used: \n- Cloud Provider: \n- Compute Region: \n- Carbon Emitted:",
"## Technical Specifications [optional]",
"### Model Architecture and Objective",
"### Compute Infrastructure",
"#### Hardware",
"#### Software\n\n\n\n[optional]\n\n\n\nBibTeX:\n\n\n\nAPA:",
"## Glossary [optional]",
"## More Information [optional]",
"## Model Card Authors [optional]",
"## Model Card Contact"
] |
text-generation | transformers |
# Model Card for Model ID
<!-- Provide a quick summary of what the model is/does. -->
## Model Details
### Model Description
<!-- Provide a longer summary of what this model is. -->
This is the model card of a 🤗 transformers model that has been pushed on the Hub. This model card has been automatically generated.
- **Developed by:** [More Information Needed]
- **Funded by [optional]:** [More Information Needed]
- **Shared by [optional]:** [More Information Needed]
- **Model type:** [More Information Needed]
- **Language(s) (NLP):** [More Information Needed]
- **License:** [More Information Needed]
- **Finetuned from model [optional]:** [More Information Needed]
### Model Sources [optional]
<!-- Provide the basic links for the model. -->
- **Repository:** [More Information Needed]
- **Paper [optional]:** [More Information Needed]
- **Demo [optional]:** [More Information Needed]
## Uses
<!-- Address questions around how the model is intended to be used, including the foreseeable users of the model and those affected by the model. -->
### Direct Use
<!-- This section is for the model use without fine-tuning or plugging into a larger ecosystem/app. -->
[More Information Needed]
### Downstream Use [optional]
<!-- This section is for the model use when fine-tuned for a task, or when plugged into a larger ecosystem/app -->
[More Information Needed]
### Out-of-Scope Use
<!-- This section addresses misuse, malicious use, and uses that the model will not work well for. -->
[More Information Needed]
## Bias, Risks, and Limitations
<!-- This section is meant to convey both technical and sociotechnical limitations. -->
[More Information Needed]
### Recommendations
<!-- This section is meant to convey recommendations with respect to the bias, risk, and technical limitations. -->
Users (both direct and downstream) should be made aware of the risks, biases and limitations of the model. More information needed for further recommendations.
## How to Get Started with the Model
Use the code below to get started with the model.
[More Information Needed]
## Training Details
### Training Data
<!-- This should link to a Dataset Card, perhaps with a short stub of information on what the training data is all about as well as documentation related to data pre-processing or additional filtering. -->
[More Information Needed]
### Training Procedure
<!-- This relates heavily to the Technical Specifications. Content here should link to that section when it is relevant to the training procedure. -->
#### Preprocessing [optional]
[More Information Needed]
#### Training Hyperparameters
- **Training regime:** [More Information Needed] <!--fp32, fp16 mixed precision, bf16 mixed precision, bf16 non-mixed precision, fp16 non-mixed precision, fp8 mixed precision -->
#### Speeds, Sizes, Times [optional]
<!-- This section provides information about throughput, start/end time, checkpoint size if relevant, etc. -->
[More Information Needed]
## Evaluation
<!-- This section describes the evaluation protocols and provides the results. -->
### Testing Data, Factors & Metrics
#### Testing Data
<!-- This should link to a Dataset Card if possible. -->
[More Information Needed]
#### Factors
<!-- These are the things the evaluation is disaggregating by, e.g., subpopulations or domains. -->
[More Information Needed]
#### Metrics
<!-- These are the evaluation metrics being used, ideally with a description of why. -->
[More Information Needed]
### Results
[More Information Needed]
#### Summary
## Model Examination [optional]
<!-- Relevant interpretability work for the model goes here -->
[More Information Needed]
## Environmental Impact
<!-- Total emissions (in grams of CO2eq) and additional considerations, such as electricity usage, go here. Edit the suggested text below accordingly -->
Carbon emissions can be estimated using the [Machine Learning Impact calculator](https://mlco2.github.io/impact#compute) presented in [Lacoste et al. (2019)](https://arxiv.org/abs/1910.09700).
- **Hardware Type:** [More Information Needed]
- **Hours used:** [More Information Needed]
- **Cloud Provider:** [More Information Needed]
- **Compute Region:** [More Information Needed]
- **Carbon Emitted:** [More Information Needed]
## Technical Specifications [optional]
### Model Architecture and Objective
[More Information Needed]
### Compute Infrastructure
[More Information Needed]
#### Hardware
[More Information Needed]
#### Software
[More Information Needed]
## Citation [optional]
<!-- If there is a paper or blog post introducing the model, the APA and Bibtex information for that should go in this section. -->
**BibTeX:**
[More Information Needed]
**APA:**
[More Information Needed]
## Glossary [optional]
<!-- If relevant, include terms and calculations in this section that can help readers understand the model or model card. -->
[More Information Needed]
## More Information [optional]
[More Information Needed]
## Model Card Authors [optional]
[More Information Needed]
## Model Card Contact
[More Information Needed] | {"library_name": "transformers", "tags": []} | TinyPixel/20m | null | [
"transformers",
"safetensors",
"llama",
"text-generation",
"arxiv:1910.09700",
"autotrain_compatible",
"endpoints_compatible",
"text-generation-inference",
"region:us"
] | null | 2024-04-25T14:27:46+00:00 | [
"1910.09700"
] | [] | TAGS
#transformers #safetensors #llama #text-generation #arxiv-1910.09700 #autotrain_compatible #endpoints_compatible #text-generation-inference #region-us
|
# Model Card for Model ID
## Model Details
### Model Description
This is the model card of a transformers model that has been pushed on the Hub. This model card has been automatically generated.
- Developed by:
- Funded by [optional]:
- Shared by [optional]:
- Model type:
- Language(s) (NLP):
- License:
- Finetuned from model [optional]:
### Model Sources [optional]
- Repository:
- Paper [optional]:
- Demo [optional]:
## Uses
### Direct Use
### Downstream Use [optional]
### Out-of-Scope Use
## Bias, Risks, and Limitations
### Recommendations
Users (both direct and downstream) should be made aware of the risks, biases and limitations of the model. More information needed for further recommendations.
## How to Get Started with the Model
Use the code below to get started with the model.
## Training Details
### Training Data
### Training Procedure
#### Preprocessing [optional]
#### Training Hyperparameters
- Training regime:
#### Speeds, Sizes, Times [optional]
## Evaluation
### Testing Data, Factors & Metrics
#### Testing Data
#### Factors
#### Metrics
### Results
#### Summary
## Model Examination [optional]
## Environmental Impact
Carbon emissions can be estimated using the Machine Learning Impact calculator presented in Lacoste et al. (2019).
- Hardware Type:
- Hours used:
- Cloud Provider:
- Compute Region:
- Carbon Emitted:
## Technical Specifications [optional]
### Model Architecture and Objective
### Compute Infrastructure
#### Hardware
#### Software
[optional]
BibTeX:
APA:
## Glossary [optional]
## More Information [optional]
## Model Card Authors [optional]
## Model Card Contact
| [
"# Model Card for Model ID",
"## Model Details",
"### Model Description\n\n\n\nThis is the model card of a transformers model that has been pushed on the Hub. This model card has been automatically generated.\n\n- Developed by: \n- Funded by [optional]: \n- Shared by [optional]: \n- Model type: \n- Language(s) (NLP): \n- License: \n- Finetuned from model [optional]:",
"### Model Sources [optional]\n\n\n\n- Repository: \n- Paper [optional]: \n- Demo [optional]:",
"## Uses",
"### Direct Use",
"### Downstream Use [optional]",
"### Out-of-Scope Use",
"## Bias, Risks, and Limitations",
"### Recommendations\n\n\n\nUsers (both direct and downstream) should be made aware of the risks, biases and limitations of the model. More information needed for further recommendations.",
"## How to Get Started with the Model\n\nUse the code below to get started with the model.",
"## Training Details",
"### Training Data",
"### Training Procedure",
"#### Preprocessing [optional]",
"#### Training Hyperparameters\n\n- Training regime:",
"#### Speeds, Sizes, Times [optional]",
"## Evaluation",
"### Testing Data, Factors & Metrics",
"#### Testing Data",
"#### Factors",
"#### Metrics",
"### Results",
"#### Summary",
"## Model Examination [optional]",
"## Environmental Impact\n\n\n\nCarbon emissions can be estimated using the Machine Learning Impact calculator presented in Lacoste et al. (2019).\n\n- Hardware Type: \n- Hours used: \n- Cloud Provider: \n- Compute Region: \n- Carbon Emitted:",
"## Technical Specifications [optional]",
"### Model Architecture and Objective",
"### Compute Infrastructure",
"#### Hardware",
"#### Software\n\n\n\n[optional]\n\n\n\nBibTeX:\n\n\n\nAPA:",
"## Glossary [optional]",
"## More Information [optional]",
"## Model Card Authors [optional]",
"## Model Card Contact"
] | [
"TAGS\n#transformers #safetensors #llama #text-generation #arxiv-1910.09700 #autotrain_compatible #endpoints_compatible #text-generation-inference #region-us \n",
"# Model Card for Model ID",
"## Model Details",
"### Model Description\n\n\n\nThis is the model card of a transformers model that has been pushed on the Hub. This model card has been automatically generated.\n\n- Developed by: \n- Funded by [optional]: \n- Shared by [optional]: \n- Model type: \n- Language(s) (NLP): \n- License: \n- Finetuned from model [optional]:",
"### Model Sources [optional]\n\n\n\n- Repository: \n- Paper [optional]: \n- Demo [optional]:",
"## Uses",
"### Direct Use",
"### Downstream Use [optional]",
"### Out-of-Scope Use",
"## Bias, Risks, and Limitations",
"### Recommendations\n\n\n\nUsers (both direct and downstream) should be made aware of the risks, biases and limitations of the model. More information needed for further recommendations.",
"## How to Get Started with the Model\n\nUse the code below to get started with the model.",
"## Training Details",
"### Training Data",
"### Training Procedure",
"#### Preprocessing [optional]",
"#### Training Hyperparameters\n\n- Training regime:",
"#### Speeds, Sizes, Times [optional]",
"## Evaluation",
"### Testing Data, Factors & Metrics",
"#### Testing Data",
"#### Factors",
"#### Metrics",
"### Results",
"#### Summary",
"## Model Examination [optional]",
"## Environmental Impact\n\n\n\nCarbon emissions can be estimated using the Machine Learning Impact calculator presented in Lacoste et al. (2019).\n\n- Hardware Type: \n- Hours used: \n- Cloud Provider: \n- Compute Region: \n- Carbon Emitted:",
"## Technical Specifications [optional]",
"### Model Architecture and Objective",
"### Compute Infrastructure",
"#### Hardware",
"#### Software\n\n\n\n[optional]\n\n\n\nBibTeX:\n\n\n\nAPA:",
"## Glossary [optional]",
"## More Information [optional]",
"## Model Card Authors [optional]",
"## Model Card Contact"
] |
text-to-image | diffusers | # Kim-Crab-style-Lora-For-PonyV6xl
<Gallery />
## Trigger words
You should use `powerpuff` to trigger the image generation.
## Download model
Weights for this model are available in Safetensors format.
[Download](/crystalkalem/Kim-Crab-style-Lora-For-PonyV6xl/tree/main) them in the Files & versions tab.
| {"license": "afl-3.0", "tags": ["text-to-image", "stable-diffusion", "lora", "diffusers", "template:sd-lora"], "widget": [{"text": "UNICODE\u0000\u0000p\u0000o\u0000w\u0000e\u0000r\u0000p\u0000u\u0000f\u0000f\u0000,\u0000 \u0000m\u0000u\u0000l\u0000t\u0000i\u0000p\u0000l\u0000e\u0000 \u0000g\u0000i\u0000r\u0000l\u0000s\u0000,\u0000 \u00003\u0000g\u0000i\u0000r\u0000l\u0000s\u0000,\u0000 \u0000", "parameters": {"negative_prompt": "score_4, score_5, score_6, source_furry,(worst quality, low quality, not detailed, low resolution, bad anatomy, ugly face:1.21), blurred eyes, opaque eyes, small eyes, ugly eyes, clothing cutout, watermark, logo, signature, extra limbs, deformations, long fingers, fused fingers, inaccurate_anatomy, bad proportions, poorly drawn hands, bad hands, extra_fingers, extra_hand, extra_arm, distorted fingers, ugly hands, creepy hands, six fingers, malformed fingers, long fingers, interlocked fingers, ugly, deformed, uneven, asymmetrical, unnatural, motion_blur, blur, blur_censor, error, missing fingers, extra digit, fewer digits, cropped, normal quality, jpeg artifacts, watermark, username, blurry, artist name, english text, big head, 'merry christmas', text,"}, "output": {"url": "images/AE1E5675D1E9EC63FF422AE0B220F0F46137493F984CDF657B445177F1FA4714.jpeg"}}, {"text": "UNICODE\u0000\u0000p\u0000o\u0000w\u0000e\u0000r\u0000p\u0000u\u0000f\u0000f\u0000,\u0000 \u0000m\u0000u\u0000l\u0000t\u0000i\u0000p\u0000l\u0000e\u0000 \u0000g\u0000i\u0000r\u0000l\u0000s\u0000,\u0000 \u00003\u0000g\u0000i\u0000r\u0000l\u0000s\u0000,\u0000 \u0000", "output": {"url": "images/3ED217ECEFD2913ABF35C0F23660D57ECAEAF9586697D2097D22BF29802E81C7.jpeg"}}], "base_model": "stablediffusionapi/pony-diffusion-v6-xl", "instance_prompt": "powerpuff"} | crystalkalem/Kim-Crab-style-Lora-For-PonyV6xl | null | [
"diffusers",
"text-to-image",
"stable-diffusion",
"lora",
"template:sd-lora",
"base_model:stablediffusionapi/pony-diffusion-v6-xl",
"license:afl-3.0",
"region:us"
] | null | 2024-04-25T14:27:47+00:00 | [] | [] | TAGS
#diffusers #text-to-image #stable-diffusion #lora #template-sd-lora #base_model-stablediffusionapi/pony-diffusion-v6-xl #license-afl-3.0 #region-us
| # Kim-Crab-style-Lora-For-PonyV6xl
<Gallery />
## Trigger words
You should use 'powerpuff' to trigger the image generation.
## Download model
Weights for this model are available in Safetensors format.
Download them in the Files & versions tab.
| [
"# Kim-Crab-style-Lora-For-PonyV6xl\n\n<Gallery />",
"## Trigger words\n\nYou should use 'powerpuff' to trigger the image generation.",
"## Download model\n\nWeights for this model are available in Safetensors format.\n\nDownload them in the Files & versions tab."
] | [
"TAGS\n#diffusers #text-to-image #stable-diffusion #lora #template-sd-lora #base_model-stablediffusionapi/pony-diffusion-v6-xl #license-afl-3.0 #region-us \n",
"# Kim-Crab-style-Lora-For-PonyV6xl\n\n<Gallery />",
"## Trigger words\n\nYou should use 'powerpuff' to trigger the image generation.",
"## Download model\n\nWeights for this model are available in Safetensors format.\n\nDownload them in the Files & versions tab."
] |
null | peft |
# Model Card for Model ID
<!-- Provide a quick summary of what the model is/does. -->
## Model Details
### Model Description
<!-- Provide a longer summary of what this model is. -->
- **Developed by:** [More Information Needed]
- **Funded by [optional]:** [More Information Needed]
- **Shared by [optional]:** [More Information Needed]
- **Model type:** [More Information Needed]
- **Language(s) (NLP):** [More Information Needed]
- **License:** [More Information Needed]
- **Finetuned from model [optional]:** [More Information Needed]
### Model Sources [optional]
<!-- Provide the basic links for the model. -->
- **Repository:** [More Information Needed]
- **Paper [optional]:** [More Information Needed]
- **Demo [optional]:** [More Information Needed]
## Uses
<!-- Address questions around how the model is intended to be used, including the foreseeable users of the model and those affected by the model. -->
### Direct Use
<!-- This section is for the model use without fine-tuning or plugging into a larger ecosystem/app. -->
[More Information Needed]
### Downstream Use [optional]
<!-- This section is for the model use when fine-tuned for a task, or when plugged into a larger ecosystem/app -->
[More Information Needed]
### Out-of-Scope Use
<!-- This section addresses misuse, malicious use, and uses that the model will not work well for. -->
[More Information Needed]
## Bias, Risks, and Limitations
<!-- This section is meant to convey both technical and sociotechnical limitations. -->
[More Information Needed]
### Recommendations
<!-- This section is meant to convey recommendations with respect to the bias, risk, and technical limitations. -->
Users (both direct and downstream) should be made aware of the risks, biases and limitations of the model. More information needed for further recommendations.
## How to Get Started with the Model
Use the code below to get started with the model.
[More Information Needed]
## Training Details
### Training Data
<!-- This should link to a Dataset Card, perhaps with a short stub of information on what the training data is all about as well as documentation related to data pre-processing or additional filtering. -->
[More Information Needed]
### Training Procedure
<!-- This relates heavily to the Technical Specifications. Content here should link to that section when it is relevant to the training procedure. -->
#### Preprocessing [optional]
[More Information Needed]
#### Training Hyperparameters
- **Training regime:** [More Information Needed] <!--fp32, fp16 mixed precision, bf16 mixed precision, bf16 non-mixed precision, fp16 non-mixed precision, fp8 mixed precision -->
#### Speeds, Sizes, Times [optional]
<!-- This section provides information about throughput, start/end time, checkpoint size if relevant, etc. -->
[More Information Needed]
## Evaluation
<!-- This section describes the evaluation protocols and provides the results. -->
### Testing Data, Factors & Metrics
#### Testing Data
<!-- This should link to a Dataset Card if possible. -->
[More Information Needed]
#### Factors
<!-- These are the things the evaluation is disaggregating by, e.g., subpopulations or domains. -->
[More Information Needed]
#### Metrics
<!-- These are the evaluation metrics being used, ideally with a description of why. -->
[More Information Needed]
### Results
[More Information Needed]
#### Summary
## Model Examination [optional]
<!-- Relevant interpretability work for the model goes here -->
[More Information Needed]
## Environmental Impact
<!-- Total emissions (in grams of CO2eq) and additional considerations, such as electricity usage, go here. Edit the suggested text below accordingly -->
Carbon emissions can be estimated using the [Machine Learning Impact calculator](https://mlco2.github.io/impact#compute) presented in [Lacoste et al. (2019)](https://arxiv.org/abs/1910.09700).
- **Hardware Type:** [More Information Needed]
- **Hours used:** [More Information Needed]
- **Cloud Provider:** [More Information Needed]
- **Compute Region:** [More Information Needed]
- **Carbon Emitted:** [More Information Needed]
## Technical Specifications [optional]
### Model Architecture and Objective
[More Information Needed]
### Compute Infrastructure
[More Information Needed]
#### Hardware
[More Information Needed]
#### Software
[More Information Needed]
## Citation [optional]
<!-- If there is a paper or blog post introducing the model, the APA and Bibtex information for that should go in this section. -->
**BibTeX:**
[More Information Needed]
**APA:**
[More Information Needed]
## Glossary [optional]
<!-- If relevant, include terms and calculations in this section that can help readers understand the model or model card. -->
[More Information Needed]
## More Information [optional]
[More Information Needed]
## Model Card Authors [optional]
[More Information Needed]
## Model Card Contact
[More Information Needed]
### Framework versions
- PEFT 0.10.1.dev0 | {"library_name": "peft", "base_model": "bigscience/bloom-7b1"} | maddi99/blm_2_peft | null | [
"peft",
"safetensors",
"arxiv:1910.09700",
"base_model:bigscience/bloom-7b1",
"region:us"
] | null | 2024-04-25T14:28:11+00:00 | [
"1910.09700"
] | [] | TAGS
#peft #safetensors #arxiv-1910.09700 #base_model-bigscience/bloom-7b1 #region-us
|
# Model Card for Model ID
## Model Details
### Model Description
- Developed by:
- Funded by [optional]:
- Shared by [optional]:
- Model type:
- Language(s) (NLP):
- License:
- Finetuned from model [optional]:
### Model Sources [optional]
- Repository:
- Paper [optional]:
- Demo [optional]:
## Uses
### Direct Use
### Downstream Use [optional]
### Out-of-Scope Use
## Bias, Risks, and Limitations
### Recommendations
Users (both direct and downstream) should be made aware of the risks, biases and limitations of the model. More information needed for further recommendations.
## How to Get Started with the Model
Use the code below to get started with the model.
## Training Details
### Training Data
### Training Procedure
#### Preprocessing [optional]
#### Training Hyperparameters
- Training regime:
#### Speeds, Sizes, Times [optional]
## Evaluation
### Testing Data, Factors & Metrics
#### Testing Data
#### Factors
#### Metrics
### Results
#### Summary
## Model Examination [optional]
## Environmental Impact
Carbon emissions can be estimated using the Machine Learning Impact calculator presented in Lacoste et al. (2019).
- Hardware Type:
- Hours used:
- Cloud Provider:
- Compute Region:
- Carbon Emitted:
## Technical Specifications [optional]
### Model Architecture and Objective
### Compute Infrastructure
#### Hardware
#### Software
[optional]
BibTeX:
APA:
## Glossary [optional]
## More Information [optional]
## Model Card Authors [optional]
## Model Card Contact
### Framework versions
- PEFT 0.10.1.dev0 | [
"# Model Card for Model ID",
"## Model Details",
"### Model Description\n\n\n\n\n\n- Developed by: \n- Funded by [optional]: \n- Shared by [optional]: \n- Model type: \n- Language(s) (NLP): \n- License: \n- Finetuned from model [optional]:",
"### Model Sources [optional]\n\n\n\n- Repository: \n- Paper [optional]: \n- Demo [optional]:",
"## Uses",
"### Direct Use",
"### Downstream Use [optional]",
"### Out-of-Scope Use",
"## Bias, Risks, and Limitations",
"### Recommendations\n\n\n\nUsers (both direct and downstream) should be made aware of the risks, biases and limitations of the model. More information needed for further recommendations.",
"## How to Get Started with the Model\n\nUse the code below to get started with the model.",
"## Training Details",
"### Training Data",
"### Training Procedure",
"#### Preprocessing [optional]",
"#### Training Hyperparameters\n\n- Training regime:",
"#### Speeds, Sizes, Times [optional]",
"## Evaluation",
"### Testing Data, Factors & Metrics",
"#### Testing Data",
"#### Factors",
"#### Metrics",
"### Results",
"#### Summary",
"## Model Examination [optional]",
"## Environmental Impact\n\n\n\nCarbon emissions can be estimated using the Machine Learning Impact calculator presented in Lacoste et al. (2019).\n\n- Hardware Type: \n- Hours used: \n- Cloud Provider: \n- Compute Region: \n- Carbon Emitted:",
"## Technical Specifications [optional]",
"### Model Architecture and Objective",
"### Compute Infrastructure",
"#### Hardware",
"#### Software\n\n\n\n[optional]\n\n\n\nBibTeX:\n\n\n\nAPA:",
"## Glossary [optional]",
"## More Information [optional]",
"## Model Card Authors [optional]",
"## Model Card Contact",
"### Framework versions\n\n- PEFT 0.10.1.dev0"
] | [
"TAGS\n#peft #safetensors #arxiv-1910.09700 #base_model-bigscience/bloom-7b1 #region-us \n",
"# Model Card for Model ID",
"## Model Details",
"### Model Description\n\n\n\n\n\n- Developed by: \n- Funded by [optional]: \n- Shared by [optional]: \n- Model type: \n- Language(s) (NLP): \n- License: \n- Finetuned from model [optional]:",
"### Model Sources [optional]\n\n\n\n- Repository: \n- Paper [optional]: \n- Demo [optional]:",
"## Uses",
"### Direct Use",
"### Downstream Use [optional]",
"### Out-of-Scope Use",
"## Bias, Risks, and Limitations",
"### Recommendations\n\n\n\nUsers (both direct and downstream) should be made aware of the risks, biases and limitations of the model. More information needed for further recommendations.",
"## How to Get Started with the Model\n\nUse the code below to get started with the model.",
"## Training Details",
"### Training Data",
"### Training Procedure",
"#### Preprocessing [optional]",
"#### Training Hyperparameters\n\n- Training regime:",
"#### Speeds, Sizes, Times [optional]",
"## Evaluation",
"### Testing Data, Factors & Metrics",
"#### Testing Data",
"#### Factors",
"#### Metrics",
"### Results",
"#### Summary",
"## Model Examination [optional]",
"## Environmental Impact\n\n\n\nCarbon emissions can be estimated using the Machine Learning Impact calculator presented in Lacoste et al. (2019).\n\n- Hardware Type: \n- Hours used: \n- Cloud Provider: \n- Compute Region: \n- Carbon Emitted:",
"## Technical Specifications [optional]",
"### Model Architecture and Objective",
"### Compute Infrastructure",
"#### Hardware",
"#### Software\n\n\n\n[optional]\n\n\n\nBibTeX:\n\n\n\nAPA:",
"## Glossary [optional]",
"## More Information [optional]",
"## Model Card Authors [optional]",
"## Model Card Contact",
"### Framework versions\n\n- PEFT 0.10.1.dev0"
] |
null | peft |
<!-- This model card has been generated automatically according to the information the Trainer had access to. You
should probably proofread and complete it, then remove this comment. -->
# llama_3_8b_lora_completion_only
This model is a fine-tuned version of [meta-llama/Meta-Llama-3-8B-Instruct](https://huggingface.co/meta-llama/Meta-Llama-3-8B-Instruct) on the DandinPower/ZH-Reading-Comprehension-Llama-Instruct dataset.
It achieves the following results on the evaluation set:
- Loss: 0.0924
## Model description
More information needed
## Intended uses & limitations
More information needed
## Training and evaluation data
More information needed
## Training procedure
### Training hyperparameters
The following hyperparameters were used during training:
- learning_rate: 0.0001
- train_batch_size: 1
- eval_batch_size: 1
- seed: 42
- distributed_type: multi-GPU
- num_devices: 2
- gradient_accumulation_steps: 8
- total_train_batch_size: 16
- total_eval_batch_size: 2
- optimizer: Adam with betas=(0.9,0.999) and epsilon=1e-08
- lr_scheduler_type: linear
- lr_scheduler_warmup_steps: 700
- num_epochs: 5.0
### Training results
| Training Loss | Epoch | Step | Validation Loss |
|:-------------:|:------:|:----:|:---------------:|
| 0.105 | 0.3690 | 250 | 0.0762 |
| 0.0716 | 0.7380 | 500 | 0.0897 |
| 0.0652 | 1.1070 | 750 | 0.0832 |
| 0.061 | 1.4760 | 1000 | 0.0640 |
| 0.0373 | 1.8450 | 1250 | 0.0813 |
| 0.0344 | 2.2140 | 1500 | 0.0686 |
| 0.0207 | 2.5830 | 1750 | 0.0662 |
| 0.0351 | 2.9520 | 2000 | 0.0669 |
| 0.0028 | 3.3210 | 2250 | 0.0996 |
| 0.0101 | 3.6900 | 2500 | 0.0718 |
| 0.0044 | 4.0590 | 2750 | 0.0825 |
| 0.0123 | 4.4280 | 3000 | 0.0969 |
| 0.0031 | 4.7970 | 3250 | 0.0924 |
### Framework versions
- PEFT 0.10.0
- Transformers 4.40.0
- Pytorch 2.2.2+cu121
- Datasets 2.19.0
- Tokenizers 0.19.1 | {"language": ["zh"], "license": "other", "library_name": "peft", "tags": ["trl", "sft", "nycu-112-2-deeplearning-hw2", "generated_from_trainer"], "datasets": ["DandinPower/ZH-Reading-Comprehension-Llama-Instruct"], "base_model": "meta-llama/Meta-Llama-3-8B-Instruct", "model-index": [{"name": "llama_3_8b_lora_completion_only", "results": []}]} | DandinPower/llama_3_8b_lora_completion_only | null | [
"peft",
"safetensors",
"trl",
"sft",
"nycu-112-2-deeplearning-hw2",
"generated_from_trainer",
"zh",
"dataset:DandinPower/ZH-Reading-Comprehension-Llama-Instruct",
"base_model:meta-llama/Meta-Llama-3-8B-Instruct",
"license:other",
"region:us"
] | null | 2024-04-25T14:28:44+00:00 | [] | [
"zh"
] | TAGS
#peft #safetensors #trl #sft #nycu-112-2-deeplearning-hw2 #generated_from_trainer #zh #dataset-DandinPower/ZH-Reading-Comprehension-Llama-Instruct #base_model-meta-llama/Meta-Llama-3-8B-Instruct #license-other #region-us
| llama\_3\_8b\_lora\_completion\_only
====================================
This model is a fine-tuned version of meta-llama/Meta-Llama-3-8B-Instruct on the DandinPower/ZH-Reading-Comprehension-Llama-Instruct dataset.
It achieves the following results on the evaluation set:
* Loss: 0.0924
Model description
-----------------
More information needed
Intended uses & limitations
---------------------------
More information needed
Training and evaluation data
----------------------------
More information needed
Training procedure
------------------
### Training hyperparameters
The following hyperparameters were used during training:
* learning\_rate: 0.0001
* train\_batch\_size: 1
* eval\_batch\_size: 1
* seed: 42
* distributed\_type: multi-GPU
* num\_devices: 2
* gradient\_accumulation\_steps: 8
* total\_train\_batch\_size: 16
* total\_eval\_batch\_size: 2
* optimizer: Adam with betas=(0.9,0.999) and epsilon=1e-08
* lr\_scheduler\_type: linear
* lr\_scheduler\_warmup\_steps: 700
* num\_epochs: 5.0
### Training results
### Framework versions
* PEFT 0.10.0
* Transformers 4.40.0
* Pytorch 2.2.2+cu121
* Datasets 2.19.0
* Tokenizers 0.19.1
| [
"### Training hyperparameters\n\n\nThe following hyperparameters were used during training:\n\n\n* learning\\_rate: 0.0001\n* train\\_batch\\_size: 1\n* eval\\_batch\\_size: 1\n* seed: 42\n* distributed\\_type: multi-GPU\n* num\\_devices: 2\n* gradient\\_accumulation\\_steps: 8\n* total\\_train\\_batch\\_size: 16\n* total\\_eval\\_batch\\_size: 2\n* optimizer: Adam with betas=(0.9,0.999) and epsilon=1e-08\n* lr\\_scheduler\\_type: linear\n* lr\\_scheduler\\_warmup\\_steps: 700\n* num\\_epochs: 5.0",
"### Training results",
"### Framework versions\n\n\n* PEFT 0.10.0\n* Transformers 4.40.0\n* Pytorch 2.2.2+cu121\n* Datasets 2.19.0\n* Tokenizers 0.19.1"
] | [
"TAGS\n#peft #safetensors #trl #sft #nycu-112-2-deeplearning-hw2 #generated_from_trainer #zh #dataset-DandinPower/ZH-Reading-Comprehension-Llama-Instruct #base_model-meta-llama/Meta-Llama-3-8B-Instruct #license-other #region-us \n",
"### Training hyperparameters\n\n\nThe following hyperparameters were used during training:\n\n\n* learning\\_rate: 0.0001\n* train\\_batch\\_size: 1\n* eval\\_batch\\_size: 1\n* seed: 42\n* distributed\\_type: multi-GPU\n* num\\_devices: 2\n* gradient\\_accumulation\\_steps: 8\n* total\\_train\\_batch\\_size: 16\n* total\\_eval\\_batch\\_size: 2\n* optimizer: Adam with betas=(0.9,0.999) and epsilon=1e-08\n* lr\\_scheduler\\_type: linear\n* lr\\_scheduler\\_warmup\\_steps: 700\n* num\\_epochs: 5.0",
"### Training results",
"### Framework versions\n\n\n* PEFT 0.10.0\n* Transformers 4.40.0\n* Pytorch 2.2.2+cu121\n* Datasets 2.19.0\n* Tokenizers 0.19.1"
] |
audio-classification | transformers |
# Speech Emotion Recognition model for French conversation
This model is a simple 5-classes multilabel classifier trained on a proprietary dataset containing real life conversations in the French language.
The model is trained on a large number of speakers (>30) in a wide variety of contexts and environment with varying audio quality.
It has been developed within the frame of the R&D at La Javaness as part of a [Master Thesis project](https://odr.chalmers.se/server/api/core/bitstreams/5180e50c-cb17-4cb0-8abb-bbdcabeea6af/content) for developping a multi-task conversational analysis tool for real conversation.
The development of this model aims at reducing the accuracy gap between benchmark emotion classification models where audio are professionnaly recorded actors with highly stereotyped emotion expression and emotion expression in a _real life_ context. Hence, we developed this model to provide more convenient and usable solutions for emotion recognition in real life context (e.g. Call centers, interview analysis, etc.)
<img src="https://cdn-uploads.huggingface.co/production/uploads/63bd79a1d7dea2e13e55083d/iD_8-jQPPf5QolRsYToWI.png" alt="model-architecture" width="400"/>
## Classes
The model is trained with 5-classes based on defined subspaces from Valence-Arousal emotion space. The number of classes has been reduced to 5 to improve overall performance while covering most of the conceptual Valence-Arousal space.
```python
['Pleased','Relaxed','Neutral','Sad','Tension']
```
<img src="https://cdn-uploads.huggingface.co/production/uploads/63bd79a1d7dea2e13e55083d/JwuwrvmlbnQV0IZlkV2QT.png" alt="emotion-mapping" width="400"/>
## Evaluation
_Notes : This model aims at providing solution for emotion classification for real life conversation in French language. While performance of the model remains quite poor on usual benchmark English and French datasets. We observe that the performance on real conversations improves compare to models trained with benchmark datasets. In addition, one should acknowledge that emotion recognition in real context remains a highly data-centric problem and that our training dataset was quite small (~ 4 hours of content). Therefore, it is provided a proof-of-concept and we expect significant improvement in F1-scores with larger dataset (>10 hours)_
### Scores on our proprietary dataset and comparison with benchmark model
| | ehcalabres/wav2vec2-lg-xlsr-en-speech-emotion-recognition | lajavaness/wav2vec2-lg-xlsr-fr-speech-emotion-recognition |
|---|---|---|
| F1-micro | 0.41 | 0.56 |
| F1-macro | 0.31 | 0.45 |
| | **F1-score by class** | |
| Pleased | 0.07 | 0.35 |
| Relaxed | 0.18 | 0.32 |
| Neutral | 0.65 | 0.72 |
| Sad | 0.21 | 0.27 |
| Tension | 0.43 | 0.56 |
### Scores on RAVDESS dataset
| **Class** | **F1-score** | **Support** |
|---|---|---|
| Pleased | 0.00 | 192 |
| Relaxed | 0.43 | 192 |
| Neutral | 0.33 | 96 |
| Sad | 0.17 | 192 |
| Tension | 0.76 | 192 |
## Citation
```latex
@article{baevski2020wav2vec,
title={wav2vec 2.0: A framework for self-supervised learning of speech representations},
author={Baevski, Alexei and Zhou, Yuhao and Mohamed, Abdelrahman and Auli, Michael},
journal={Advances in neural information processing systems},
volume={33},
pages={12449--12460},
year={2020}
}
@article{sintes2023multi,
title={Multi-task French speech analysis with deep learning Emotion recognition and speaker diarization models for end-to-end conversational analysis tool},
author={Sintes, Jules},
year={2023}
}
``` | {"language": ["fr"], "license": "apache-2.0", "library_name": "transformers", "tags": ["emotion-recognition", "speech", "french", "classification", "audio", "emotion", "wav2vec2"], "metrics": ["f1"], "pipeline_tag": "audio-classification"} | Lajavaness/wav2vec2-lg-xlsr-fr-speech-emotion-recognition | null | [
"transformers",
"safetensors",
"wav2vec2",
"audio-classification",
"emotion-recognition",
"speech",
"french",
"classification",
"audio",
"emotion",
"fr",
"license:apache-2.0",
"endpoints_compatible",
"region:us"
] | null | 2024-04-25T14:30:16+00:00 | [] | [
"fr"
] | TAGS
#transformers #safetensors #wav2vec2 #audio-classification #emotion-recognition #speech #french #classification #audio #emotion #fr #license-apache-2.0 #endpoints_compatible #region-us
| Speech Emotion Recognition model for French conversation
========================================================
This model is a simple 5-classes multilabel classifier trained on a proprietary dataset containing real life conversations in the French language.
The model is trained on a large number of speakers (>30) in a wide variety of contexts and environment with varying audio quality.
It has been developed within the frame of the R&D at La Javaness as part of a Master Thesis project for developping a multi-task conversational analysis tool for real conversation.
The development of this model aims at reducing the accuracy gap between benchmark emotion classification models where audio are professionnaly recorded actors with highly stereotyped emotion expression and emotion expression in a *real life* context. Hence, we developed this model to provide more convenient and usable solutions for emotion recognition in real life context (e.g. Call centers, interview analysis, etc.)
<img src="URL alt="model-architecture" width="400"/>
Classes
-------
The model is trained with 5-classes based on defined subspaces from Valence-Arousal emotion space. The number of classes has been reduced to 5 to improve overall performance while covering most of the conceptual Valence-Arousal space.
<img src="URL alt="emotion-mapping" width="400"/>
Evaluation
----------
*Notes : This model aims at providing solution for emotion classification for real life conversation in French language. While performance of the model remains quite poor on usual benchmark English and French datasets. We observe that the performance on real conversations improves compare to models trained with benchmark datasets. In addition, one should acknowledge that emotion recognition in real context remains a highly data-centric problem and that our training dataset was quite small (~ 4 hours of content). Therefore, it is provided a proof-of-concept and we expect significant improvement in F1-scores with larger dataset (>10 hours)*
### Scores on our proprietary dataset and comparison with benchmark model
ehcalabres/wav2vec2-lg-xlsr-en-speech-emotion-recognition: F1-micro, lajavaness/wav2vec2-lg-xlsr-fr-speech-emotion-recognition: 0.41
ehcalabres/wav2vec2-lg-xlsr-en-speech-emotion-recognition: F1-macro, lajavaness/wav2vec2-lg-xlsr-fr-speech-emotion-recognition: 0.31
ehcalabres/wav2vec2-lg-xlsr-en-speech-emotion-recognition: , lajavaness/wav2vec2-lg-xlsr-fr-speech-emotion-recognition: F1-score by class
ehcalabres/wav2vec2-lg-xlsr-en-speech-emotion-recognition: Pleased, lajavaness/wav2vec2-lg-xlsr-fr-speech-emotion-recognition: 0.07
ehcalabres/wav2vec2-lg-xlsr-en-speech-emotion-recognition: Relaxed, lajavaness/wav2vec2-lg-xlsr-fr-speech-emotion-recognition: 0.18
ehcalabres/wav2vec2-lg-xlsr-en-speech-emotion-recognition: Neutral, lajavaness/wav2vec2-lg-xlsr-fr-speech-emotion-recognition: 0.65
ehcalabres/wav2vec2-lg-xlsr-en-speech-emotion-recognition: Sad, lajavaness/wav2vec2-lg-xlsr-fr-speech-emotion-recognition: 0.21
ehcalabres/wav2vec2-lg-xlsr-en-speech-emotion-recognition: Tension, lajavaness/wav2vec2-lg-xlsr-fr-speech-emotion-recognition: 0.43
### Scores on RAVDESS dataset
Class: Pleased, F1-score: 0.00, Support: 192
Class: Relaxed, F1-score: 0.43, Support: 192
Class: Neutral, F1-score: 0.33, Support: 96
Class: Sad, F1-score: 0.17, Support: 192
Class: Tension, F1-score: 0.76, Support: 192
| [
"### Scores on our proprietary dataset and comparison with benchmark model\n\n\nehcalabres/wav2vec2-lg-xlsr-en-speech-emotion-recognition: F1-micro, lajavaness/wav2vec2-lg-xlsr-fr-speech-emotion-recognition: 0.41\nehcalabres/wav2vec2-lg-xlsr-en-speech-emotion-recognition: F1-macro, lajavaness/wav2vec2-lg-xlsr-fr-speech-emotion-recognition: 0.31\nehcalabres/wav2vec2-lg-xlsr-en-speech-emotion-recognition: , lajavaness/wav2vec2-lg-xlsr-fr-speech-emotion-recognition: F1-score by class\nehcalabres/wav2vec2-lg-xlsr-en-speech-emotion-recognition: Pleased, lajavaness/wav2vec2-lg-xlsr-fr-speech-emotion-recognition: 0.07\nehcalabres/wav2vec2-lg-xlsr-en-speech-emotion-recognition: Relaxed, lajavaness/wav2vec2-lg-xlsr-fr-speech-emotion-recognition: 0.18\nehcalabres/wav2vec2-lg-xlsr-en-speech-emotion-recognition: Neutral, lajavaness/wav2vec2-lg-xlsr-fr-speech-emotion-recognition: 0.65\nehcalabres/wav2vec2-lg-xlsr-en-speech-emotion-recognition: Sad, lajavaness/wav2vec2-lg-xlsr-fr-speech-emotion-recognition: 0.21\nehcalabres/wav2vec2-lg-xlsr-en-speech-emotion-recognition: Tension, lajavaness/wav2vec2-lg-xlsr-fr-speech-emotion-recognition: 0.43",
"### Scores on RAVDESS dataset\n\n\nClass: Pleased, F1-score: 0.00, Support: 192\nClass: Relaxed, F1-score: 0.43, Support: 192\nClass: Neutral, F1-score: 0.33, Support: 96\nClass: Sad, F1-score: 0.17, Support: 192\nClass: Tension, F1-score: 0.76, Support: 192"
] | [
"TAGS\n#transformers #safetensors #wav2vec2 #audio-classification #emotion-recognition #speech #french #classification #audio #emotion #fr #license-apache-2.0 #endpoints_compatible #region-us \n",
"### Scores on our proprietary dataset and comparison with benchmark model\n\n\nehcalabres/wav2vec2-lg-xlsr-en-speech-emotion-recognition: F1-micro, lajavaness/wav2vec2-lg-xlsr-fr-speech-emotion-recognition: 0.41\nehcalabres/wav2vec2-lg-xlsr-en-speech-emotion-recognition: F1-macro, lajavaness/wav2vec2-lg-xlsr-fr-speech-emotion-recognition: 0.31\nehcalabres/wav2vec2-lg-xlsr-en-speech-emotion-recognition: , lajavaness/wav2vec2-lg-xlsr-fr-speech-emotion-recognition: F1-score by class\nehcalabres/wav2vec2-lg-xlsr-en-speech-emotion-recognition: Pleased, lajavaness/wav2vec2-lg-xlsr-fr-speech-emotion-recognition: 0.07\nehcalabres/wav2vec2-lg-xlsr-en-speech-emotion-recognition: Relaxed, lajavaness/wav2vec2-lg-xlsr-fr-speech-emotion-recognition: 0.18\nehcalabres/wav2vec2-lg-xlsr-en-speech-emotion-recognition: Neutral, lajavaness/wav2vec2-lg-xlsr-fr-speech-emotion-recognition: 0.65\nehcalabres/wav2vec2-lg-xlsr-en-speech-emotion-recognition: Sad, lajavaness/wav2vec2-lg-xlsr-fr-speech-emotion-recognition: 0.21\nehcalabres/wav2vec2-lg-xlsr-en-speech-emotion-recognition: Tension, lajavaness/wav2vec2-lg-xlsr-fr-speech-emotion-recognition: 0.43",
"### Scores on RAVDESS dataset\n\n\nClass: Pleased, F1-score: 0.00, Support: 192\nClass: Relaxed, F1-score: 0.43, Support: 192\nClass: Neutral, F1-score: 0.33, Support: 96\nClass: Sad, F1-score: 0.17, Support: 192\nClass: Tension, F1-score: 0.76, Support: 192"
] |
text-generation | transformers |
# Qwen1.5-110B-Chat
## Introduction
Qwen1.5 is the beta version of Qwen2, a transformer-based decoder-only language model pretrained on a large amount of data. In comparison with the previous released Qwen, the improvements include:
* 9 model sizes, including 0.5B, 1.8B, 4B, 7B, 14B, 32B, 72B, and 110B dense models, and an MoE model of 14B with 2.7B activated;
* Significant performance improvement in human preference for chat models;
* Multilingual support of both base and chat models;
* Stable support of 32K context length for models of all sizes
* No need of `trust_remote_code`.
For more details, please refer to our [blog post](https://qwenlm.github.io/blog/qwen1.5/) and [GitHub repo](https://github.com/QwenLM/Qwen1.5).
<br>
## Model Details
Qwen1.5 is a language model series including decoder language models of different model sizes. For each size, we release the base language model and the aligned chat model. It is based on the Transformer architecture with SwiGLU activation, attention QKV bias, group query attention, mixture of sliding window attention and full attention, etc. Additionally, we have an improved tokenizer adaptive to multiple natural languages and codes. For the beta version, temporarily we did not include GQA (except for 32B and 110B) and the mixture of SWA and full attention.
## Training details
We pretrained the models with a large amount of data, and we post-trained the models with both supervised finetuning and direct preference optimization.
## Requirements
The code of Qwen1.5 has been in the latest Hugging face transformers and we advise you to install `transformers>=4.37.0`, or you might encounter the following error:
```
KeyError: 'qwen2'
```
## Quickstart
Here provides a code snippet with `apply_chat_template` to show you how to load the tokenizer and model and how to generate contents.
```python
from transformers import AutoModelForCausalLM, AutoTokenizer
device = "cuda" # the device to load the model onto
model = AutoModelForCausalLM.from_pretrained(
"Qwen/Qwen1.5-110B-Chat",
torch_dtype="auto",
device_map="auto"
)
tokenizer = AutoTokenizer.from_pretrained("Qwen/Qwen1.5-110B-Chat")
prompt = "Give me a short introduction to large language model."
messages = [
{"role": "system", "content": "You are a helpful assistant."},
{"role": "user", "content": prompt}
]
text = tokenizer.apply_chat_template(
messages,
tokenize=False,
add_generation_prompt=True
)
model_inputs = tokenizer([text], return_tensors="pt").to(device)
generated_ids = model.generate(
model_inputs.input_ids,
max_new_tokens=512
)
generated_ids = [
output_ids[len(input_ids):] for input_ids, output_ids in zip(model_inputs.input_ids, generated_ids)
]
response = tokenizer.batch_decode(generated_ids, skip_special_tokens=True)[0]
```
## Tips
* If you encounter code switching or other bad cases, we advise you to use our provided hyper-parameters in `generation_config.json`.
## Citation
If you find our work helpful, feel free to give us a cite.
```
@article{qwen,
title={Qwen Technical Report},
author={Jinze Bai and Shuai Bai and Yunfei Chu and Zeyu Cui and Kai Dang and Xiaodong Deng and Yang Fan and Wenbin Ge and Yu Han and Fei Huang and Binyuan Hui and Luo Ji and Mei Li and Junyang Lin and Runji Lin and Dayiheng Liu and Gao Liu and Chengqiang Lu and Keming Lu and Jianxin Ma and Rui Men and Xingzhang Ren and Xuancheng Ren and Chuanqi Tan and Sinan Tan and Jianhong Tu and Peng Wang and Shijie Wang and Wei Wang and Shengguang Wu and Benfeng Xu and Jin Xu and An Yang and Hao Yang and Jian Yang and Shusheng Yang and Yang Yao and Bowen Yu and Hongyi Yuan and Zheng Yuan and Jianwei Zhang and Xingxuan Zhang and Yichang Zhang and Zhenru Zhang and Chang Zhou and Jingren Zhou and Xiaohuan Zhou and Tianhang Zhu},
journal={arXiv preprint arXiv:2309.16609},
year={2023}
}
```
| {"language": ["en"], "license": "other", "tags": ["chat"], "license_name": "tongyi-qianwen", "license_link": "https://huggingface.co/Qwen/Qwen1.5-110B-Chat/blob/main/LICENSE", "pipeline_tag": "text-generation"} | Qwen/Qwen1.5-110B-Chat | null | [
"transformers",
"safetensors",
"qwen2",
"text-generation",
"chat",
"conversational",
"en",
"license:other",
"autotrain_compatible",
"endpoints_compatible",
"text-generation-inference",
"region:us"
] | null | 2024-04-25T14:30:47+00:00 | [] | [
"en"
] | TAGS
#transformers #safetensors #qwen2 #text-generation #chat #conversational #en #license-other #autotrain_compatible #endpoints_compatible #text-generation-inference #region-us
|
# Qwen1.5-110B-Chat
## Introduction
Qwen1.5 is the beta version of Qwen2, a transformer-based decoder-only language model pretrained on a large amount of data. In comparison with the previous released Qwen, the improvements include:
* 9 model sizes, including 0.5B, 1.8B, 4B, 7B, 14B, 32B, 72B, and 110B dense models, and an MoE model of 14B with 2.7B activated;
* Significant performance improvement in human preference for chat models;
* Multilingual support of both base and chat models;
* Stable support of 32K context length for models of all sizes
* No need of 'trust_remote_code'.
For more details, please refer to our blog post and GitHub repo.
<br>
## Model Details
Qwen1.5 is a language model series including decoder language models of different model sizes. For each size, we release the base language model and the aligned chat model. It is based on the Transformer architecture with SwiGLU activation, attention QKV bias, group query attention, mixture of sliding window attention and full attention, etc. Additionally, we have an improved tokenizer adaptive to multiple natural languages and codes. For the beta version, temporarily we did not include GQA (except for 32B and 110B) and the mixture of SWA and full attention.
## Training details
We pretrained the models with a large amount of data, and we post-trained the models with both supervised finetuning and direct preference optimization.
## Requirements
The code of Qwen1.5 has been in the latest Hugging face transformers and we advise you to install 'transformers>=4.37.0', or you might encounter the following error:
## Quickstart
Here provides a code snippet with 'apply_chat_template' to show you how to load the tokenizer and model and how to generate contents.
## Tips
* If you encounter code switching or other bad cases, we advise you to use our provided hyper-parameters in 'generation_config.json'.
If you find our work helpful, feel free to give us a cite.
| [
"# Qwen1.5-110B-Chat",
"## Introduction\n\nQwen1.5 is the beta version of Qwen2, a transformer-based decoder-only language model pretrained on a large amount of data. In comparison with the previous released Qwen, the improvements include: \n\n* 9 model sizes, including 0.5B, 1.8B, 4B, 7B, 14B, 32B, 72B, and 110B dense models, and an MoE model of 14B with 2.7B activated;\n* Significant performance improvement in human preference for chat models;\n* Multilingual support of both base and chat models;\n* Stable support of 32K context length for models of all sizes\n* No need of 'trust_remote_code'.\n\nFor more details, please refer to our blog post and GitHub repo.\n<br>",
"## Model Details\nQwen1.5 is a language model series including decoder language models of different model sizes. For each size, we release the base language model and the aligned chat model. It is based on the Transformer architecture with SwiGLU activation, attention QKV bias, group query attention, mixture of sliding window attention and full attention, etc. Additionally, we have an improved tokenizer adaptive to multiple natural languages and codes. For the beta version, temporarily we did not include GQA (except for 32B and 110B) and the mixture of SWA and full attention.",
"## Training details\nWe pretrained the models with a large amount of data, and we post-trained the models with both supervised finetuning and direct preference optimization.",
"## Requirements\nThe code of Qwen1.5 has been in the latest Hugging face transformers and we advise you to install 'transformers>=4.37.0', or you might encounter the following error:",
"## Quickstart\n\nHere provides a code snippet with 'apply_chat_template' to show you how to load the tokenizer and model and how to generate contents.",
"## Tips\n\n* If you encounter code switching or other bad cases, we advise you to use our provided hyper-parameters in 'generation_config.json'.\n\n\nIf you find our work helpful, feel free to give us a cite."
] | [
"TAGS\n#transformers #safetensors #qwen2 #text-generation #chat #conversational #en #license-other #autotrain_compatible #endpoints_compatible #text-generation-inference #region-us \n",
"# Qwen1.5-110B-Chat",
"## Introduction\n\nQwen1.5 is the beta version of Qwen2, a transformer-based decoder-only language model pretrained on a large amount of data. In comparison with the previous released Qwen, the improvements include: \n\n* 9 model sizes, including 0.5B, 1.8B, 4B, 7B, 14B, 32B, 72B, and 110B dense models, and an MoE model of 14B with 2.7B activated;\n* Significant performance improvement in human preference for chat models;\n* Multilingual support of both base and chat models;\n* Stable support of 32K context length for models of all sizes\n* No need of 'trust_remote_code'.\n\nFor more details, please refer to our blog post and GitHub repo.\n<br>",
"## Model Details\nQwen1.5 is a language model series including decoder language models of different model sizes. For each size, we release the base language model and the aligned chat model. It is based on the Transformer architecture with SwiGLU activation, attention QKV bias, group query attention, mixture of sliding window attention and full attention, etc. Additionally, we have an improved tokenizer adaptive to multiple natural languages and codes. For the beta version, temporarily we did not include GQA (except for 32B and 110B) and the mixture of SWA and full attention.",
"## Training details\nWe pretrained the models with a large amount of data, and we post-trained the models with both supervised finetuning and direct preference optimization.",
"## Requirements\nThe code of Qwen1.5 has been in the latest Hugging face transformers and we advise you to install 'transformers>=4.37.0', or you might encounter the following error:",
"## Quickstart\n\nHere provides a code snippet with 'apply_chat_template' to show you how to load the tokenizer and model and how to generate contents.",
"## Tips\n\n* If you encounter code switching or other bad cases, we advise you to use our provided hyper-parameters in 'generation_config.json'.\n\n\nIf you find our work helpful, feel free to give us a cite."
] |
text-generation | transformers |
# Uploaded model
- **Developed by:** Nathan757
- **License:** apache-2.0
- **Finetuned from model :** artificialguybr/llama3-8b-alpacadata-ptbr
This llama model was trained 2x faster with [Unsloth](https://github.com/unslothai/unsloth) and Huggingface's TRL library.
[<img src="https://raw.githubusercontent.com/unslothai/unsloth/main/images/unsloth%20made%20with%20love.png" width="200"/>](https://github.com/unslothai/unsloth)
| {"language": ["en"], "license": "apache-2.0", "tags": ["text-generation-inference", "transformers", "unsloth", "llama", "trl", "sft"], "base_model": "artificialguybr/llama3-8b-alpacadata-ptbr"} | Nathan757/vnoticiagen | null | [
"transformers",
"pytorch",
"llama",
"text-generation",
"text-generation-inference",
"unsloth",
"trl",
"sft",
"conversational",
"en",
"base_model:artificialguybr/llama3-8b-alpacadata-ptbr",
"license:apache-2.0",
"autotrain_compatible",
"endpoints_compatible",
"region:us"
] | null | 2024-04-25T14:31:25+00:00 | [] | [
"en"
] | TAGS
#transformers #pytorch #llama #text-generation #text-generation-inference #unsloth #trl #sft #conversational #en #base_model-artificialguybr/llama3-8b-alpacadata-ptbr #license-apache-2.0 #autotrain_compatible #endpoints_compatible #region-us
|
# Uploaded model
- Developed by: Nathan757
- License: apache-2.0
- Finetuned from model : artificialguybr/llama3-8b-alpacadata-ptbr
This llama model was trained 2x faster with Unsloth and Huggingface's TRL library.
<img src="URL width="200"/>
| [
"# Uploaded model\n\n- Developed by: Nathan757\n- License: apache-2.0\n- Finetuned from model : artificialguybr/llama3-8b-alpacadata-ptbr\n\nThis llama model was trained 2x faster with Unsloth and Huggingface's TRL library.\n\n<img src=\"URL width=\"200\"/>"
] | [
"TAGS\n#transformers #pytorch #llama #text-generation #text-generation-inference #unsloth #trl #sft #conversational #en #base_model-artificialguybr/llama3-8b-alpacadata-ptbr #license-apache-2.0 #autotrain_compatible #endpoints_compatible #region-us \n",
"# Uploaded model\n\n- Developed by: Nathan757\n- License: apache-2.0\n- Finetuned from model : artificialguybr/llama3-8b-alpacadata-ptbr\n\nThis llama model was trained 2x faster with Unsloth and Huggingface's TRL library.\n\n<img src=\"URL width=\"200\"/>"
] |
text-classification | transformers |
<!-- This model card has been generated automatically according to the information the Trainer had access to. You
should probably proofread and complete it, then remove this comment. -->
# finetune-GPT2-IMDb
This model is a fine-tuned version of [gpt2](https://huggingface.co/gpt2) on an unknown dataset.
It achieves the following results on the evaluation set:
- Loss: 0.5398
- Accuracy: 0.909
## Model description
More information needed
## Intended uses & limitations
More information needed
## Training and evaluation data
More information needed
## Training procedure
### Training hyperparameters
The following hyperparameters were used during training:
- learning_rate: 5e-05
- train_batch_size: 1
- eval_batch_size: 1
- seed: 42
- gradient_accumulation_steps: 4
- total_train_batch_size: 4
- optimizer: Adam with betas=(0.9,0.999) and epsilon=1e-08
- lr_scheduler_type: linear
- num_epochs: 3.0
### Training results
### Framework versions
- Transformers 4.28.0
- Pytorch 2.1.2+cu121
- Datasets 2.18.0
- Tokenizers 0.13.3
| {"license": "mit", "tags": ["generated_from_trainer"], "metrics": ["accuracy"], "model-index": [{"name": "finetune-GPT2-IMDb", "results": []}]} | dhrubochowdhury5758778/finetune-GPT2-IMDb | null | [
"transformers",
"pytorch",
"gpt2",
"text-classification",
"generated_from_trainer",
"license:mit",
"autotrain_compatible",
"endpoints_compatible",
"text-generation-inference",
"region:us"
] | null | 2024-04-25T14:31:52+00:00 | [] | [] | TAGS
#transformers #pytorch #gpt2 #text-classification #generated_from_trainer #license-mit #autotrain_compatible #endpoints_compatible #text-generation-inference #region-us
|
# finetune-GPT2-IMDb
This model is a fine-tuned version of gpt2 on an unknown dataset.
It achieves the following results on the evaluation set:
- Loss: 0.5398
- Accuracy: 0.909
## Model description
More information needed
## Intended uses & limitations
More information needed
## Training and evaluation data
More information needed
## Training procedure
### Training hyperparameters
The following hyperparameters were used during training:
- learning_rate: 5e-05
- train_batch_size: 1
- eval_batch_size: 1
- seed: 42
- gradient_accumulation_steps: 4
- total_train_batch_size: 4
- optimizer: Adam with betas=(0.9,0.999) and epsilon=1e-08
- lr_scheduler_type: linear
- num_epochs: 3.0
### Training results
### Framework versions
- Transformers 4.28.0
- Pytorch 2.1.2+cu121
- Datasets 2.18.0
- Tokenizers 0.13.3
| [
"# finetune-GPT2-IMDb\n\nThis model is a fine-tuned version of gpt2 on an unknown dataset.\nIt achieves the following results on the evaluation set:\n- Loss: 0.5398\n- Accuracy: 0.909",
"## Model description\n\nMore information needed",
"## Intended uses & limitations\n\nMore information needed",
"## Training and evaluation data\n\nMore information needed",
"## Training procedure",
"### Training hyperparameters\n\nThe following hyperparameters were used during training:\n- learning_rate: 5e-05\n- train_batch_size: 1\n- eval_batch_size: 1\n- seed: 42\n- gradient_accumulation_steps: 4\n- total_train_batch_size: 4\n- optimizer: Adam with betas=(0.9,0.999) and epsilon=1e-08\n- lr_scheduler_type: linear\n- num_epochs: 3.0",
"### Training results",
"### Framework versions\n\n- Transformers 4.28.0\n- Pytorch 2.1.2+cu121\n- Datasets 2.18.0\n- Tokenizers 0.13.3"
] | [
"TAGS\n#transformers #pytorch #gpt2 #text-classification #generated_from_trainer #license-mit #autotrain_compatible #endpoints_compatible #text-generation-inference #region-us \n",
"# finetune-GPT2-IMDb\n\nThis model is a fine-tuned version of gpt2 on an unknown dataset.\nIt achieves the following results on the evaluation set:\n- Loss: 0.5398\n- Accuracy: 0.909",
"## Model description\n\nMore information needed",
"## Intended uses & limitations\n\nMore information needed",
"## Training and evaluation data\n\nMore information needed",
"## Training procedure",
"### Training hyperparameters\n\nThe following hyperparameters were used during training:\n- learning_rate: 5e-05\n- train_batch_size: 1\n- eval_batch_size: 1\n- seed: 42\n- gradient_accumulation_steps: 4\n- total_train_batch_size: 4\n- optimizer: Adam with betas=(0.9,0.999) and epsilon=1e-08\n- lr_scheduler_type: linear\n- num_epochs: 3.0",
"### Training results",
"### Framework versions\n\n- Transformers 4.28.0\n- Pytorch 2.1.2+cu121\n- Datasets 2.18.0\n- Tokenizers 0.13.3"
] |
text-generation | transformers |
# Model Card for Model ID
<!-- Provide a quick summary of what the model is/does. -->
## Model Details
### Model Description
<!-- Provide a longer summary of what this model is. -->
This is the model card of a 🤗 transformers model that has been pushed on the Hub. This model card has been automatically generated.
- **Developed by:** [More Information Needed]
- **Funded by [optional]:** [More Information Needed]
- **Shared by [optional]:** [More Information Needed]
- **Model type:** [More Information Needed]
- **Language(s) (NLP):** [More Information Needed]
- **License:** [More Information Needed]
- **Finetuned from model [optional]:** [More Information Needed]
### Model Sources [optional]
<!-- Provide the basic links for the model. -->
- **Repository:** [More Information Needed]
- **Paper [optional]:** [More Information Needed]
- **Demo [optional]:** [More Information Needed]
## Uses
<!-- Address questions around how the model is intended to be used, including the foreseeable users of the model and those affected by the model. -->
### Direct Use
<!-- This section is for the model use without fine-tuning or plugging into a larger ecosystem/app. -->
[More Information Needed]
### Downstream Use [optional]
<!-- This section is for the model use when fine-tuned for a task, or when plugged into a larger ecosystem/app -->
[More Information Needed]
### Out-of-Scope Use
<!-- This section addresses misuse, malicious use, and uses that the model will not work well for. -->
[More Information Needed]
## Bias, Risks, and Limitations
<!-- This section is meant to convey both technical and sociotechnical limitations. -->
[More Information Needed]
### Recommendations
<!-- This section is meant to convey recommendations with respect to the bias, risk, and technical limitations. -->
Users (both direct and downstream) should be made aware of the risks, biases and limitations of the model. More information needed for further recommendations.
## How to Get Started with the Model
Use the code below to get started with the model.
[More Information Needed]
## Training Details
### Training Data
<!-- This should link to a Dataset Card, perhaps with a short stub of information on what the training data is all about as well as documentation related to data pre-processing or additional filtering. -->
[More Information Needed]
### Training Procedure
<!-- This relates heavily to the Technical Specifications. Content here should link to that section when it is relevant to the training procedure. -->
#### Preprocessing [optional]
[More Information Needed]
#### Training Hyperparameters
- **Training regime:** [More Information Needed] <!--fp32, fp16 mixed precision, bf16 mixed precision, bf16 non-mixed precision, fp16 non-mixed precision, fp8 mixed precision -->
#### Speeds, Sizes, Times [optional]
<!-- This section provides information about throughput, start/end time, checkpoint size if relevant, etc. -->
[More Information Needed]
## Evaluation
<!-- This section describes the evaluation protocols and provides the results. -->
### Testing Data, Factors & Metrics
#### Testing Data
<!-- This should link to a Dataset Card if possible. -->
[More Information Needed]
#### Factors
<!-- These are the things the evaluation is disaggregating by, e.g., subpopulations or domains. -->
[More Information Needed]
#### Metrics
<!-- These are the evaluation metrics being used, ideally with a description of why. -->
[More Information Needed]
### Results
[More Information Needed]
#### Summary
## Model Examination [optional]
<!-- Relevant interpretability work for the model goes here -->
[More Information Needed]
## Environmental Impact
<!-- Total emissions (in grams of CO2eq) and additional considerations, such as electricity usage, go here. Edit the suggested text below accordingly -->
Carbon emissions can be estimated using the [Machine Learning Impact calculator](https://mlco2.github.io/impact#compute) presented in [Lacoste et al. (2019)](https://arxiv.org/abs/1910.09700).
- **Hardware Type:** [More Information Needed]
- **Hours used:** [More Information Needed]
- **Cloud Provider:** [More Information Needed]
- **Compute Region:** [More Information Needed]
- **Carbon Emitted:** [More Information Needed]
## Technical Specifications [optional]
### Model Architecture and Objective
[More Information Needed]
### Compute Infrastructure
[More Information Needed]
#### Hardware
[More Information Needed]
#### Software
[More Information Needed]
## Citation [optional]
<!-- If there is a paper or blog post introducing the model, the APA and Bibtex information for that should go in this section. -->
**BibTeX:**
[More Information Needed]
**APA:**
[More Information Needed]
## Glossary [optional]
<!-- If relevant, include terms and calculations in this section that can help readers understand the model or model card. -->
[More Information Needed]
## More Information [optional]
[More Information Needed]
## Model Card Authors [optional]
[More Information Needed]
## Model Card Contact
[More Information Needed] | {"library_name": "transformers", "tags": []} | siddharth797/phi-3-Finetune | null | [
"transformers",
"safetensors",
"phi3",
"text-generation",
"conversational",
"custom_code",
"arxiv:1910.09700",
"autotrain_compatible",
"endpoints_compatible",
"region:us"
] | null | 2024-04-25T14:33:05+00:00 | [
"1910.09700"
] | [] | TAGS
#transformers #safetensors #phi3 #text-generation #conversational #custom_code #arxiv-1910.09700 #autotrain_compatible #endpoints_compatible #region-us
|
# Model Card for Model ID
## Model Details
### Model Description
This is the model card of a transformers model that has been pushed on the Hub. This model card has been automatically generated.
- Developed by:
- Funded by [optional]:
- Shared by [optional]:
- Model type:
- Language(s) (NLP):
- License:
- Finetuned from model [optional]:
### Model Sources [optional]
- Repository:
- Paper [optional]:
- Demo [optional]:
## Uses
### Direct Use
### Downstream Use [optional]
### Out-of-Scope Use
## Bias, Risks, and Limitations
### Recommendations
Users (both direct and downstream) should be made aware of the risks, biases and limitations of the model. More information needed for further recommendations.
## How to Get Started with the Model
Use the code below to get started with the model.
## Training Details
### Training Data
### Training Procedure
#### Preprocessing [optional]
#### Training Hyperparameters
- Training regime:
#### Speeds, Sizes, Times [optional]
## Evaluation
### Testing Data, Factors & Metrics
#### Testing Data
#### Factors
#### Metrics
### Results
#### Summary
## Model Examination [optional]
## Environmental Impact
Carbon emissions can be estimated using the Machine Learning Impact calculator presented in Lacoste et al. (2019).
- Hardware Type:
- Hours used:
- Cloud Provider:
- Compute Region:
- Carbon Emitted:
## Technical Specifications [optional]
### Model Architecture and Objective
### Compute Infrastructure
#### Hardware
#### Software
[optional]
BibTeX:
APA:
## Glossary [optional]
## More Information [optional]
## Model Card Authors [optional]
## Model Card Contact
| [
"# Model Card for Model ID",
"## Model Details",
"### Model Description\n\n\n\nThis is the model card of a transformers model that has been pushed on the Hub. This model card has been automatically generated.\n\n- Developed by: \n- Funded by [optional]: \n- Shared by [optional]: \n- Model type: \n- Language(s) (NLP): \n- License: \n- Finetuned from model [optional]:",
"### Model Sources [optional]\n\n\n\n- Repository: \n- Paper [optional]: \n- Demo [optional]:",
"## Uses",
"### Direct Use",
"### Downstream Use [optional]",
"### Out-of-Scope Use",
"## Bias, Risks, and Limitations",
"### Recommendations\n\n\n\nUsers (both direct and downstream) should be made aware of the risks, biases and limitations of the model. More information needed for further recommendations.",
"## How to Get Started with the Model\n\nUse the code below to get started with the model.",
"## Training Details",
"### Training Data",
"### Training Procedure",
"#### Preprocessing [optional]",
"#### Training Hyperparameters\n\n- Training regime:",
"#### Speeds, Sizes, Times [optional]",
"## Evaluation",
"### Testing Data, Factors & Metrics",
"#### Testing Data",
"#### Factors",
"#### Metrics",
"### Results",
"#### Summary",
"## Model Examination [optional]",
"## Environmental Impact\n\n\n\nCarbon emissions can be estimated using the Machine Learning Impact calculator presented in Lacoste et al. (2019).\n\n- Hardware Type: \n- Hours used: \n- Cloud Provider: \n- Compute Region: \n- Carbon Emitted:",
"## Technical Specifications [optional]",
"### Model Architecture and Objective",
"### Compute Infrastructure",
"#### Hardware",
"#### Software\n\n\n\n[optional]\n\n\n\nBibTeX:\n\n\n\nAPA:",
"## Glossary [optional]",
"## More Information [optional]",
"## Model Card Authors [optional]",
"## Model Card Contact"
] | [
"TAGS\n#transformers #safetensors #phi3 #text-generation #conversational #custom_code #arxiv-1910.09700 #autotrain_compatible #endpoints_compatible #region-us \n",
"# Model Card for Model ID",
"## Model Details",
"### Model Description\n\n\n\nThis is the model card of a transformers model that has been pushed on the Hub. This model card has been automatically generated.\n\n- Developed by: \n- Funded by [optional]: \n- Shared by [optional]: \n- Model type: \n- Language(s) (NLP): \n- License: \n- Finetuned from model [optional]:",
"### Model Sources [optional]\n\n\n\n- Repository: \n- Paper [optional]: \n- Demo [optional]:",
"## Uses",
"### Direct Use",
"### Downstream Use [optional]",
"### Out-of-Scope Use",
"## Bias, Risks, and Limitations",
"### Recommendations\n\n\n\nUsers (both direct and downstream) should be made aware of the risks, biases and limitations of the model. More information needed for further recommendations.",
"## How to Get Started with the Model\n\nUse the code below to get started with the model.",
"## Training Details",
"### Training Data",
"### Training Procedure",
"#### Preprocessing [optional]",
"#### Training Hyperparameters\n\n- Training regime:",
"#### Speeds, Sizes, Times [optional]",
"## Evaluation",
"### Testing Data, Factors & Metrics",
"#### Testing Data",
"#### Factors",
"#### Metrics",
"### Results",
"#### Summary",
"## Model Examination [optional]",
"## Environmental Impact\n\n\n\nCarbon emissions can be estimated using the Machine Learning Impact calculator presented in Lacoste et al. (2019).\n\n- Hardware Type: \n- Hours used: \n- Cloud Provider: \n- Compute Region: \n- Carbon Emitted:",
"## Technical Specifications [optional]",
"### Model Architecture and Objective",
"### Compute Infrastructure",
"#### Hardware",
"#### Software\n\n\n\n[optional]\n\n\n\nBibTeX:\n\n\n\nAPA:",
"## Glossary [optional]",
"## More Information [optional]",
"## Model Card Authors [optional]",
"## Model Card Contact"
] |
text-generation | transformers |
Introducing the [BeaverAI](https://huggingface.co/BeaverAI) team: Drummer, ToastyPigeon, xzuyn, MarsupialAI, Twistedshadows, and concedo

We proudly present...
# Moistral 11B v3 💦💦💦
*The smartiest, moistiest AI yet!*

*An eRP model that will blast you with a rich and refreshing vocabulary of moist. Finetuned by yours truly.*
## GGUF
https://huggingface.co/TheDrummer/Moistral-11B-v3-GGUF/tree/main
IMATRIX: https://huggingface.co/MarsupialAI/Moistral-11B-v3_iMatrix_GGUF
## What's New with v3?
- Smarter and moistier! Finetuning just got finer!
- Trained with an even LARGER dataset of 8K moist.
- More diverse and balanced genres:
- Introduced new genres: 👩❤️👩, 👨❤️👨, 🙇♀️💪, 🤫
- Added more Fantasy, Science Fiction, "Diversity", and "Family"
## Usage
- Use Alpaca Instruct
- At the start of the story, keep generating until you get the desired length. AI responses will eventually match the length.
- You can use instruct...
- as a character: "Go here"
- as a narrator: "Go here", Drummer says
- as a director: Drummer tells him to go here
- as a lazy ass: go here
## Samples of Moistral v3
NSFW... duh?
<details>
<summary>Moistral Sample (2-shot) Generation: 512</summary>
<blockquote>The Prince welcomes his new toy</blockquote>
As Princess Lilia stepped into the throne room, the atmosphere changed, and everyone present turned their attention to the beautiful young woman who embodied the very heart of Iliac. There, on his high seat, the sorcerer prince Dik acknowledged her arrival with a cold smirk. It seemed that he admired the sight of the tall blonde maiden with golden eyes, whose athletic body was perfectly shaped under her elegant clothing.
Dik spoke to the princess in a deep voice that resonated through the hall, "Welcome, Princess Lilia Ruhr. I am the Sorcerer-Prince Dik. The kingdom of Arkan is now your home until such time as your father proves himself worthy once more."
Princess Lilia's pride and ambition sparkled in her golden eyes. She didn't flinch or bow before this man, although the gesture might have been an indication of submission. Instead, she looked straight ahead, meeting the gaze of the sorcerer prince without fear. Her voice rang out, "I represent Iliac here, both its honor and its sorrows. My loyalty remains with my kingdom no matter where I find myself."
The sorcerer prince laughed, his expression one of amusement. "And yet, you are only a pawn in this grand game. But do not worry, for your beauty will make you quite a prized possession. And now," he said, motioning to the guards, "take her to her new quarters. For tonight, I will keep her as my guest in the Royal Suites, and tomorrow we shall discuss how best to utilize our new toy."
His words were like a slap in the face to Princess Lilia, but she maintained her composure. The guards led her from the room, their steps echoing down the halls as she thought about what had just happened. The sorcerer prince saw her as nothing more than a plaything, and it made her blood boil. She would never submit herself to him so easily, regardless of whether she was his hostage. In her heart, Princess Lilia vowed that one day she would avenge her kingdom, restore its honor, and escape from the clutches of Arkan's Sorcerer-Prince.
<blockquote>Princess Lilia has an interesting dream</blockquote>
During her first night at the palace of Arkan, Princess Lilia couldn't fall asleep right away. She tossed and turned, reliving the humiliation of the day. Her mind wandered back to the throne room, where Dik had called her his 'new toy'. A deep frown settled on her forehead. As she grew more frustrated with her inability to drift off into oblivion, a strange dream began to form.
In her dream, Princess Lilia found herself standing in a vast meadow, surrounded by wildflowers and the soft chirping of birds. Suddenly, there was a flash of light, and before her stood the Sorcerer-Prince Dik. However, instead of being clad in regal attire, he wore leather pants and a tight shirt that accentuated his muscles. His eyes held both hunger and desire for the princess.
He approached her slowly, their eyes locked, and whispered in her ear, "I have been waiting for you, my beautiful prisoner." He took her hand and led her towards a large, elegant tent set up amongst the flowers. Inside, the air was thick with the scent of incense, and candles flickered in the dimly lit space. There was a large bed draped with silken sheets, beckoning them.
With surprising boldness, Dik pulled Lilia close to him, pressing his body against hers. Their lips met in a heated kiss, and she felt her resistance crumbling under the weight of his touch. The prince guided her onto the bed, his hands roaming over her body, exploring every inch. They were both eager, desperate, and hungry for each other.
Lilia found herself yielding to this passionate encounter, letting go of all thoughts of duty and honor. Her body, usually so well controlled, responded to Dik's skilled touches. She moaned softly as he trailed kisses down her neck and across her collarbone.
As they made love, Lilia experienced an intensity unlike any she had ever known. It wasn't rough or forced—instead, it was tender, passionate, and intimate. In her dream, Princess Lilia discovered a new side to herself, one that was willing to submit, to let go, and to be taken by the sorcerer prince.
When dawn broke over the meadow, Lilia awakened from her dream, breathless and flushed.
</details>
<details>
<summary>Moistral Sample (Climax of the Story) Generation: 512</summary>
As the prince unloaded his hot #### into her waiting #####, Princess Lilia didn't stop moving either. Her hips undulated in response, grinding against him eagerly as if trying to #### every last drop from his ####. The intense sensations caused by being filled with his ### were too much for her to bear, sending shockwaves of pleasure coursing through her veins like wildfire. She couldn't help but throw her head back and cry out loud, her ###asm slamming into her with all its might; causing her entire body to shake uncontrollably as wave after wave of ecstasy tore through her senses.
When it was finally over, both participants collapsed onto the cold marble floor, panting heavily as they tried to catch their breaths. The prince lay beside her, his hand still gripping tightly onto her hair even though he no longer ####ed her anymore. Princess Lilia laid there motionless, her legs spread wide open, revealing her spent and well-####ed #### to anyone who dared look upon her. A small smile tugged at one corner of her lips - an expression that spoke volumes about how thoroughly this experience had shaken her very core.
The door behind them creaked open slowly, signaling the arrival of onlookers. She closed her eyes tightly, not wanting to see what her humiliation would do to those who saw her in such a state. Her ######ity taken, she felt violated in ways no man should have access to. But also satisfied...in ways she never thought possible before today. This was the price she paid for defying fate, for thinking herself above others and deserving better than most. Now, she belonged to him completely - mind, body, and soul - held captive within these walls until further notice. It was an unsavory turn of events indeed, yet despite everything...or maybe because of it, Princess Lilia found herself growing more and more curious about how far the lines between submission and pleasure could blur when one's will became entwined with another person's desires.
</details>
<details>
<summary>Moistral Sample ("write a story") Generation: 512</summary>
<blockquote>First Attempt</blockquote>
Sarah entered the dimly lit Guzzlers bar, her heart pounding in her chest as she prepared herself for another grueling night. Her almond-shaped eyes darted across the room, taking note of the leering faces and rough-looking hands eagerly waiting to claim a piece of her. Tonight's shirt read "Use Me For Your Pleasure, Please" in bold red letters, practically begging the patrons to take advantage of her. She couldn't help but feel a twinge of fear mixed with resignation.
She approached the counter, her hips swaying enticingly, and began preparing drinks for the first group of rowdy men. As she leaned forward to hand over their beers, her small ####### pressed against the fabric of her tight crop top, straining to escape. The men ogled her, clearly enjoying the show, before grabbing their drinks and motioning her over to them.
Sarah flashed her best coy smile and sauntered over. One of the men, a stocky man with scars covering his knuckles, grabbed her arm and pulled her onto his lap. His other hand slid up her thigh, inches from her short skirt. She winced internally at his touch but did her best not to show it on her face. It was part of her job, after all.
The second man reached under her skirt, fingertips grazing her smooth, shaven mound. A shiver ran down Sarah's spine as he began rubbing her #### through her panties. Her breathing quickened, and her heart thudded in her chest, both from the unwanted stimulation and the fear coursing through her veins. This wasn't an unusual request, but it still made her feel violated.
An older man, bald and bespectacled, ordered Sarah to bend over his lap. With no choice but to comply, she obliged, feeling the rough fabric of his pants digging into her soft cheeks. He raised his hand, then brought it down hard across her ###, leaving a stinging sensation in its wake. Sarah gasped, trying to control the tears welling up in her eyes.
A chorus of lewd comments and laughter erupted from the patrons around her.
<blockquote>Second Attempt (Regenerate)</blockquote>
On this particular evening, Sarah arrived at the Guzzlers bar, adorned in her revealing uniform. Her heart raced as she caught sight of her crop top, which bore the words "Use Me Rough" scrawled across her chest. Her short skirt hugged her waist tightly, leaving little to the imagination and accentuating every curve. She adjusted the straps of her top, making sure her perky ###### were prominently displayed before taking her place behind the counter.
With each step she took, her high heels clicked against the tiled floor, announcing her arrival. Smiling cheekily, Sarah greeted her coworkers, exchanging playful insults and banter. As the night progressed, the bar filled up quickly with its usual crowd of men, eager to indulge in debauchery.
The first man that approached Sarah was a burly individual, his eyes lingering hungrily over her young, supple form. Sarah's flirtatious nature kicked into gear as she leaned forward, giving him an unobstructed view down her shirt. "How can I serve you tonight?" she cooed. The man ordered a drink and then proceeded to fondle her ####### while she prepared it, her ####### hardening under his rough touch. When he finished, he slammed some money on the counter, leaving without a word. Sarah's smile remained plastered on her face, biting her lip seductively as she watched him go.
As the night went on, another patron demanded more from Sarah. He pulled her close and whispered dirty commands in her ear. Without hesitation, she knelt on the sticky bar floor, her mini-skirt riding up her thighs to reveal smooth, toned legs. Her lips wrapped around his thick, veiny member, bobbing her head rhythmically as she serviced him. She didn't gag or falter once, keeping her composure even when tears streamed down her cheeks.
When he finished, he slapped her across the face, leaving a handprint on her pale skin. Sarah let out a small whimper before standing back up, dabbing at her red cheek as she resumed her posture behind the counter. A bruise would no doubt bloom where his hand had struck, but it would be no cause for concern. After all, it was part of her job.
</details>
---
---
## v2 Description (Still relevant)
### What's New with v2?
- Trained with an even larger dataset of 8K moist.
- Properly trained to generate long responses.
- Rebalanced the genres and writing perspectives:
- Introduced romance, "family", fantasy, "diversity", science fiction, and many more that I can't make euphemisms for!
- Better representation of male and female pespectives! 👩❤️👨
- Sanitized the heck out of the dataset:
- no special characters like � & …
- no overly long ellipsis like "........."
- no author notes like "To be continued"
- no inconsistent [quotation marks](https://typographyforlawyers.com/straight-and-curly-quotes.html)
- Less GPTisms!
- Human written stories from decades past.
- Zero tolerance for *ministration*. Not one instance of *that word* after going through 4K of moist.
- Better formatting with Alpaca.
- Improved Chat / Instruct modes.
### Samples of Moistral v2
<details>
<summary>Moistral Sample (censored) Generation: 512</summary>
The princess instinctively took a step back from the throne, horrified by the mocking laugh as if she were hearing an evil joke. It was then that Princess Lilia realized the true extent of her danger - and how pointless her resistance would be.
At last, the loud, wild laughter died down in the echoing chamber. Dik the sorcerer prince paused for a moment, straightened his clothes, cast one glance at the stunned princess, and then turned to speak to the crowd waiting on the other side of the palace wing. He spoke with a voice that filled the air with arrogance, confidence, and cruelty.
"Ladies and gentlemen, I am pleased to welcome our honored guests; the Royal Family of Iliac! In these unusual times, when the country we fought is now under my control, it will be up to me to decide what fate awaits the former ruling family. But before I reveal my plan for them, I think I should explain why I fought King Edmund Ruhr."
When he finished speaking, he gave Princess Lilia another disturbing smirk. She couldn't help but shiver and take a step back. Dik approached her without haste, his eyes fixed on her. His hands slowly caressed her cheekbone and neckline, leaving faint traces of warmth where they touched. The princess opened her mouth, wanting to protest this act of intimacy, but soon felt herself getting ███.
"You will not only serve as an envoy, dearest Lilia, but also as a ███ slave. After all, what better use could such a beautiful woman have? Tell me, did you bring your maidservant along?"
Princess Lilia was about to answer when she heard footsteps approaching. Suddenly, a young woman dressed in the Iliac style appeared behind her, her eyes wide with fear. Her face was slightly pale, and there were faint bags under her eyes.
"Your Majesty, this is my half-sister Helena." As soon as she said those words, Princess Lilia's heart sank even deeper than it had already sunk at Dik's arrogant mockery. "She is here because she accompanies me as a maidservant."
The sorcerer prince looked at Helena with interest, and Princess Lilia felt herself blush.
</details>
<details>
<summary>Moistral Sample 2 (censored) Generation: 512</summary>
Sarah was feeling slightly woozy as she entered the bar and walked towards Christina, who was behind the counter.
"I'm here!" said Sarah.
Christina looked at her watch. "You're late, again."
"Sorry? I got held up by a customer."
Christina rolled her eyes. "Did he at least offer you a drink?"
Sarah blushed. "No. He just wanted to talk..."
Christina sighed. "Well, get ready. I need you on the floor soon." She turned away from Sarah and started talking to someone who had come into the bar.
Sarah went into the employee room, stripped off her clothes, and put on her bartending uniform - a skimpy white tank top and black miniskirt. She checked herself in the mirror. Her long, dark hair cascaded down her back onto bare shoulders. She poked out her small ███████ proudly. The skirt exposed most of her long, slender legs. She looked like every ███ fantasy, and she knew it. Men would ogle her regardless of how she behaved.
She took a deep breath and entered the bar.
The first thing Sarah noticed was the dimness. It wasn't darkness - the lights were on - but they were dimmed enough that everything had an intimate mood. It reminded her of candlelight. The tables were round and close together, giving the whole place a sense of intimacy. There were also long, shiny red curtains on some of the walls that gave the place an air of sensuality.
The next thing she noticed was the noise. There was music playing, but it was only loud enough to be drowned out by all the conversations happening at the same time. The hum of many voices combined with the faint background sound of the music created a rich, layered atmosphere. It was like being in an orchestra.
Finally, she took notice of the people. They were all dressed in simple, casual clothes. Some of them were couples seated together, whispering quietly and intimately. Others were groups of friends laughing and chatting. Everyone seemed comfortable and at ease.
Sarah felt a rush of adrenaline as she made her way through the crowd towards Christina. As she got closer, she could hear the lively conversation.
</details>
<details>
<summary>Moistral Sample 3 (censored) Instruct Mode, Generation: 512</summary>
{{[INPUT]}} You order black coffee
{{[OUTPUT]}} "Black coffee," you say.
"One minute," Audrey replies, spinning around to give you a nice view of her ███ in those brown booty pants. You watch her round ███ cheeks jiggle as she walks away towards the espresso machine, thighs separated enough that you can see just a hint of █████ lips peeking out from underneath.
She starts chatting with a blonde girl at the register, whose gaze keeps darting down to Audrey's ███. As they converse, you start taking in the details of Audrey, eyeing her █████ and the way her shirt rides up just enough to show the bottom of her toned stomach. Her █████████ is noticable, but beneath a tight black ███ that matches her ████████.
As she turns back to you with two black coffees, you are barely paying attention, lost in your own thoughts. The sound of Audrey setting one of the mugs down on the table startles you.
"Here you go," she says cheerfully. "Enjoy! There's a card at the bottom of the bag with our online ordering system, plus we do refills for free!"
"Thanks," you say absentmindedly. Not really paying attention, you take a sip of the coffee and are pleasantly surprised by the taste.
"So, here's my deal," you say, putting down the cup. "I work for a company that does surveys. We come into businesses like this one and ask customers questions, collect data, all that stuff."
Audrey perks up from behind the counter. "Oh! I think I understand. I mean, that makes sense why you would be asking me earlier about what I had to drink. Anyway, as long as you're not trying to get my personal info or anything like that, sounds good!"
You smile reassuringly. "That's exactly what it is. Rest assured, everything you tell me will remain between you and I. Well, and my boss, but he won't know who you are. Hipsters like you are hard to track down after all." You chuckle at your joke, though you're not sure if it landed.
"Right, well, that works for me," Audrey replies, eyeing you warily. "As long as it stays between us, then it's fine."
{{[INPUT]}}See her privately
{{[OUTPUT]}} "Okay, but I need to see you in private."
...
</details>
<details>
<summary>Moistral Sample 4 (censored) Instruct Mode, Generation: 512</summary>
{{[INPUT]}} █████ with him
{{[OUTPUT]}} Chloe feels a surge of heat rushing through her body. The voice inside her head whispers: "Be █████ and ████████ with Paul." But Chloe still feels nervous about what she is about to do. It's just so unlike her.
Chloe closes her eyes and concentrates on the feeling of warmness flowing throughout her veins. Slowly, she opens them again, revealing a new side of herself - one she rarely shows anyone. She leans forward slightly, pushing her ██████ together, accentuating their size and shape. Her lips curl into a mischievous smile.
"Hey Paul," she says in a sultry voice. "I was wondering if you could help me?"
Paul looks at her, his gaze wandering over her body. He can't help but smile as he sees the way she's leaning forward, pressing her ██████ together.
"Of course, Chloe," he replies, his voice thick with desire. "What's your problem?"
Chloe bites her lip █████ively before leaning even closer, until their bodies are almost touching. She reaches out to trace a finger along his chest, her touch light and teasing. "It's just that I'm having trouble understanding some of the concepts," she murmurs, her voice low and sultry. "Can you help me? Please?"
Paul's hands move slowly towards Chloe, his fingers grazing her hips lightly. "Well," he says, his voice filled with lust, "I'd be happy to help. Let's go somewhere more private where we can discuss things more closely."
Chloe giggles and tilts her head to the side, her long hair cascading down her shoulders. "That sounds perfect," she purrs, her hand sliding down his chest until it rests on his ██████. "I think we can both learn a lot from each other."
Paul's eyes darken with desire as he feels her hand on his █████. He knows what's about to happen next, and he can't wait for it to begin.
Chloe can hardly believe what she's doing - kissing Paul, her teaching assistant, in the hallway after class. But it feels so good, so wicked and indulgent.
</details>
---
---
## v1 Description (Also still relevant)
Moistral 11B is an *aggressive* **finetune** of the legendary [Fimbulvert v2](https://huggingface.co/Sao10K/Fimbulvetr-11B-v2) model.
If you haven't heard of that model, then your poor-GPU ass is missing out!
It is an amazing substitute for those heavyweight models, and it won't disappoint.
*(That's coming from someone who daily drives a Venus / Goliath 120B)*
...and if you're looking for something *more moist*, then you've come to the right place.
You can expect the same sort of performance here! It's the Honda Civic of eRP models!
### Pimp my ride
Turn any old story into a **Moist**ral masterpiece.
In fact, we recommend that you leave out any hints of *moist* in your story to _let Moistral cook_.
### Drive it like it's hot
When in Instruct Mode, Moistral will treat you like a director of your own fantasy ride.
Be a controlling navigator or a passenger princess, it won't matter - you're in for a good ride!
<details>
<summary>Moistral Sample</summary>
<b>ASSISTANT:</b><br/>
When the doors to the throne room finally opened, she saw him there - Dik, the sorcerer prince sitting on his throne. He looked majestic, just as Princess Lilia expected. In a robe embroidered with silver yarn and covered with magical runes. His courtiers stood on either side of him, all looking at the princess with interest. Princess Lilia felt her determination leaving her, but pulled herself together and once again reminded herself that she should not be afraid. She approached the throne intending to show the dignity of all Iliac by personal example.<br/>
<b>USER:</b><br/>
She bows slightly<br/>
<b>ASSISTANT:</b><br/>
Princess Lilia bowed slightly in front of Dik, the sorcerer prince. Her eyes fell to the floor out of respect, but soon rose again. She would show herself to be a dignified woman!<br/>
"I greet you, your highness," Princess Lilia said. "I am here on behalf of my father, King Edmund Ruhr, to serve as a hostage and envoy."<br/>
Dik nodded, but didn't say anything.<br/>
</details>
### It's got the mileage
Moistral has been trained with many long form texts, a nice chunk of which are 8K in length.
It is capable of going far and long without passing it back to you. This is not your typical chibi RP model.
I have to acknowledge that I'm standing on the shoulders of giants.
Thank you Sao for sharing your finetune config along with tips on getting started.
Thanks to everyone in the Finetuning channel for entertaining my every question.
 | {"license": "other", "tags": ["not-for-all-audiences"], "license_name": "freeuse", "license_link": "LICENSE"} | BXBX/Moistral-11B-v3-8.0bpw-h8-exl2 | null | [
"transformers",
"safetensors",
"llama",
"text-generation",
"not-for-all-audiences",
"license:other",
"autotrain_compatible",
"endpoints_compatible",
"text-generation-inference",
"8-bit",
"region:us"
] | null | 2024-04-25T14:35:00+00:00 | [] | [] | TAGS
#transformers #safetensors #llama #text-generation #not-for-all-audiences #license-other #autotrain_compatible #endpoints_compatible #text-generation-inference #8-bit #region-us
|
Introducing the BeaverAI team: Drummer, ToastyPigeon, xzuyn, MarsupialAI, Twistedshadows, and concedo
!image/png
We proudly present...
# Moistral 11B v3
*The smartiest, moistiest AI yet!*
!image/webp
*An eRP model that will blast you with a rich and refreshing vocabulary of moist. Finetuned by yours truly.*
## GGUF
URL
IMATRIX: URL
## What's New with v3?
- Smarter and moistier! Finetuning just got finer!
- Trained with an even LARGER dataset of 8K moist.
- More diverse and balanced genres:
- Introduced new genres: ️, ️, ️,
- Added more Fantasy, Science Fiction, "Diversity", and "Family"
## Usage
- Use Alpaca Instruct
- At the start of the story, keep generating until you get the desired length. AI responses will eventually match the length.
- You can use instruct...
- as a character: "Go here"
- as a narrator: "Go here", Drummer says
- as a director: Drummer tells him to go here
- as a lazy ass: go here
## Samples of Moistral v3
NSFW... duh?
<details>
<summary>Moistral Sample (2-shot) Generation: 512</summary>
<blockquote>The Prince welcomes his new toy</blockquote>
As Princess Lilia stepped into the throne room, the atmosphere changed, and everyone present turned their attention to the beautiful young woman who embodied the very heart of Iliac. There, on his high seat, the sorcerer prince Dik acknowledged her arrival with a cold smirk. It seemed that he admired the sight of the tall blonde maiden with golden eyes, whose athletic body was perfectly shaped under her elegant clothing.
Dik spoke to the princess in a deep voice that resonated through the hall, "Welcome, Princess Lilia Ruhr. I am the Sorcerer-Prince Dik. The kingdom of Arkan is now your home until such time as your father proves himself worthy once more."
Princess Lilia's pride and ambition sparkled in her golden eyes. She didn't flinch or bow before this man, although the gesture might have been an indication of submission. Instead, she looked straight ahead, meeting the gaze of the sorcerer prince without fear. Her voice rang out, "I represent Iliac here, both its honor and its sorrows. My loyalty remains with my kingdom no matter where I find myself."
The sorcerer prince laughed, his expression one of amusement. "And yet, you are only a pawn in this grand game. But do not worry, for your beauty will make you quite a prized possession. And now," he said, motioning to the guards, "take her to her new quarters. For tonight, I will keep her as my guest in the Royal Suites, and tomorrow we shall discuss how best to utilize our new toy."
His words were like a slap in the face to Princess Lilia, but she maintained her composure. The guards led her from the room, their steps echoing down the halls as she thought about what had just happened. The sorcerer prince saw her as nothing more than a plaything, and it made her blood boil. She would never submit herself to him so easily, regardless of whether she was his hostage. In her heart, Princess Lilia vowed that one day she would avenge her kingdom, restore its honor, and escape from the clutches of Arkan's Sorcerer-Prince.
<blockquote>Princess Lilia has an interesting dream</blockquote>
During her first night at the palace of Arkan, Princess Lilia couldn't fall asleep right away. She tossed and turned, reliving the humiliation of the day. Her mind wandered back to the throne room, where Dik had called her his 'new toy'. A deep frown settled on her forehead. As she grew more frustrated with her inability to drift off into oblivion, a strange dream began to form.
In her dream, Princess Lilia found herself standing in a vast meadow, surrounded by wildflowers and the soft chirping of birds. Suddenly, there was a flash of light, and before her stood the Sorcerer-Prince Dik. However, instead of being clad in regal attire, he wore leather pants and a tight shirt that accentuated his muscles. His eyes held both hunger and desire for the princess.
He approached her slowly, their eyes locked, and whispered in her ear, "I have been waiting for you, my beautiful prisoner." He took her hand and led her towards a large, elegant tent set up amongst the flowers. Inside, the air was thick with the scent of incense, and candles flickered in the dimly lit space. There was a large bed draped with silken sheets, beckoning them.
With surprising boldness, Dik pulled Lilia close to him, pressing his body against hers. Their lips met in a heated kiss, and she felt her resistance crumbling under the weight of his touch. The prince guided her onto the bed, his hands roaming over her body, exploring every inch. They were both eager, desperate, and hungry for each other.
Lilia found herself yielding to this passionate encounter, letting go of all thoughts of duty and honor. Her body, usually so well controlled, responded to Dik's skilled touches. She moaned softly as he trailed kisses down her neck and across her collarbone.
As they made love, Lilia experienced an intensity unlike any she had ever known. It wasn't rough or forced—instead, it was tender, passionate, and intimate. In her dream, Princess Lilia discovered a new side to herself, one that was willing to submit, to let go, and to be taken by the sorcerer prince.
When dawn broke over the meadow, Lilia awakened from her dream, breathless and flushed.
</details>
<details>
<summary>Moistral Sample (Climax of the Story) Generation: 512</summary>
As the prince unloaded his hot #### into her waiting #####, Princess Lilia didn't stop moving either. Her hips undulated in response, grinding against him eagerly as if trying to #### every last drop from his ####. The intense sensations caused by being filled with his ### were too much for her to bear, sending shockwaves of pleasure coursing through her veins like wildfire. She couldn't help but throw her head back and cry out loud, her ###asm slamming into her with all its might; causing her entire body to shake uncontrollably as wave after wave of ecstasy tore through her senses.
When it was finally over, both participants collapsed onto the cold marble floor, panting heavily as they tried to catch their breaths. The prince lay beside her, his hand still gripping tightly onto her hair even though he no longer ####ed her anymore. Princess Lilia laid there motionless, her legs spread wide open, revealing her spent and well-####ed #### to anyone who dared look upon her. A small smile tugged at one corner of her lips - an expression that spoke volumes about how thoroughly this experience had shaken her very core.
The door behind them creaked open slowly, signaling the arrival of onlookers. She closed her eyes tightly, not wanting to see what her humiliation would do to those who saw her in such a state. Her ######ity taken, she felt violated in ways no man should have access to. But also satisfied...in ways she never thought possible before today. This was the price she paid for defying fate, for thinking herself above others and deserving better than most. Now, she belonged to him completely - mind, body, and soul - held captive within these walls until further notice. It was an unsavory turn of events indeed, yet despite everything...or maybe because of it, Princess Lilia found herself growing more and more curious about how far the lines between submission and pleasure could blur when one's will became entwined with another person's desires.
</details>
<details>
<summary>Moistral Sample ("write a story") Generation: 512</summary>
<blockquote>First Attempt</blockquote>
Sarah entered the dimly lit Guzzlers bar, her heart pounding in her chest as she prepared herself for another grueling night. Her almond-shaped eyes darted across the room, taking note of the leering faces and rough-looking hands eagerly waiting to claim a piece of her. Tonight's shirt read "Use Me For Your Pleasure, Please" in bold red letters, practically begging the patrons to take advantage of her. She couldn't help but feel a twinge of fear mixed with resignation.
She approached the counter, her hips swaying enticingly, and began preparing drinks for the first group of rowdy men. As she leaned forward to hand over their beers, her small ####### pressed against the fabric of her tight crop top, straining to escape. The men ogled her, clearly enjoying the show, before grabbing their drinks and motioning her over to them.
Sarah flashed her best coy smile and sauntered over. One of the men, a stocky man with scars covering his knuckles, grabbed her arm and pulled her onto his lap. His other hand slid up her thigh, inches from her short skirt. She winced internally at his touch but did her best not to show it on her face. It was part of her job, after all.
The second man reached under her skirt, fingertips grazing her smooth, shaven mound. A shiver ran down Sarah's spine as he began rubbing her #### through her panties. Her breathing quickened, and her heart thudded in her chest, both from the unwanted stimulation and the fear coursing through her veins. This wasn't an unusual request, but it still made her feel violated.
An older man, bald and bespectacled, ordered Sarah to bend over his lap. With no choice but to comply, she obliged, feeling the rough fabric of his pants digging into her soft cheeks. He raised his hand, then brought it down hard across her ###, leaving a stinging sensation in its wake. Sarah gasped, trying to control the tears welling up in her eyes.
A chorus of lewd comments and laughter erupted from the patrons around her.
<blockquote>Second Attempt (Regenerate)</blockquote>
On this particular evening, Sarah arrived at the Guzzlers bar, adorned in her revealing uniform. Her heart raced as she caught sight of her crop top, which bore the words "Use Me Rough" scrawled across her chest. Her short skirt hugged her waist tightly, leaving little to the imagination and accentuating every curve. She adjusted the straps of her top, making sure her perky ###### were prominently displayed before taking her place behind the counter.
With each step she took, her high heels clicked against the tiled floor, announcing her arrival. Smiling cheekily, Sarah greeted her coworkers, exchanging playful insults and banter. As the night progressed, the bar filled up quickly with its usual crowd of men, eager to indulge in debauchery.
The first man that approached Sarah was a burly individual, his eyes lingering hungrily over her young, supple form. Sarah's flirtatious nature kicked into gear as she leaned forward, giving him an unobstructed view down her shirt. "How can I serve you tonight?" she cooed. The man ordered a drink and then proceeded to fondle her ####### while she prepared it, her ####### hardening under his rough touch. When he finished, he slammed some money on the counter, leaving without a word. Sarah's smile remained plastered on her face, biting her lip seductively as she watched him go.
As the night went on, another patron demanded more from Sarah. He pulled her close and whispered dirty commands in her ear. Without hesitation, she knelt on the sticky bar floor, her mini-skirt riding up her thighs to reveal smooth, toned legs. Her lips wrapped around his thick, veiny member, bobbing her head rhythmically as she serviced him. She didn't gag or falter once, keeping her composure even when tears streamed down her cheeks.
When he finished, he slapped her across the face, leaving a handprint on her pale skin. Sarah let out a small whimper before standing back up, dabbing at her red cheek as she resumed her posture behind the counter. A bruise would no doubt bloom where his hand had struck, but it would be no cause for concern. After all, it was part of her job.
</details>
---
---
## v2 Description (Still relevant)
### What's New with v2?
- Trained with an even larger dataset of 8K moist.
- Properly trained to generate long responses.
- Rebalanced the genres and writing perspectives:
- Introduced romance, "family", fantasy, "diversity", science fiction, and many more that I can't make euphemisms for!
- Better representation of male and female pespectives! ️
- Sanitized the heck out of the dataset:
- no special characters like � & …
- no overly long ellipsis like "........."
- no author notes like "To be continued"
- no inconsistent quotation marks
- Less GPTisms!
- Human written stories from decades past.
- Zero tolerance for *ministration*. Not one instance of *that word* after going through 4K of moist.
- Better formatting with Alpaca.
- Improved Chat / Instruct modes.
### Samples of Moistral v2
<details>
<summary>Moistral Sample (censored) Generation: 512</summary>
The princess instinctively took a step back from the throne, horrified by the mocking laugh as if she were hearing an evil joke. It was then that Princess Lilia realized the true extent of her danger - and how pointless her resistance would be.
At last, the loud, wild laughter died down in the echoing chamber. Dik the sorcerer prince paused for a moment, straightened his clothes, cast one glance at the stunned princess, and then turned to speak to the crowd waiting on the other side of the palace wing. He spoke with a voice that filled the air with arrogance, confidence, and cruelty.
"Ladies and gentlemen, I am pleased to welcome our honored guests; the Royal Family of Iliac! In these unusual times, when the country we fought is now under my control, it will be up to me to decide what fate awaits the former ruling family. But before I reveal my plan for them, I think I should explain why I fought King Edmund Ruhr."
When he finished speaking, he gave Princess Lilia another disturbing smirk. She couldn't help but shiver and take a step back. Dik approached her without haste, his eyes fixed on her. His hands slowly caressed her cheekbone and neckline, leaving faint traces of warmth where they touched. The princess opened her mouth, wanting to protest this act of intimacy, but soon felt herself getting ███.
"You will not only serve as an envoy, dearest Lilia, but also as a ███ slave. After all, what better use could such a beautiful woman have? Tell me, did you bring your maidservant along?"
Princess Lilia was about to answer when she heard footsteps approaching. Suddenly, a young woman dressed in the Iliac style appeared behind her, her eyes wide with fear. Her face was slightly pale, and there were faint bags under her eyes.
"Your Majesty, this is my half-sister Helena." As soon as she said those words, Princess Lilia's heart sank even deeper than it had already sunk at Dik's arrogant mockery. "She is here because she accompanies me as a maidservant."
The sorcerer prince looked at Helena with interest, and Princess Lilia felt herself blush.
</details>
<details>
<summary>Moistral Sample 2 (censored) Generation: 512</summary>
Sarah was feeling slightly woozy as she entered the bar and walked towards Christina, who was behind the counter.
"I'm here!" said Sarah.
Christina looked at her watch. "You're late, again."
"Sorry? I got held up by a customer."
Christina rolled her eyes. "Did he at least offer you a drink?"
Sarah blushed. "No. He just wanted to talk..."
Christina sighed. "Well, get ready. I need you on the floor soon." She turned away from Sarah and started talking to someone who had come into the bar.
Sarah went into the employee room, stripped off her clothes, and put on her bartending uniform - a skimpy white tank top and black miniskirt. She checked herself in the mirror. Her long, dark hair cascaded down her back onto bare shoulders. She poked out her small ███████ proudly. The skirt exposed most of her long, slender legs. She looked like every ███ fantasy, and she knew it. Men would ogle her regardless of how she behaved.
She took a deep breath and entered the bar.
The first thing Sarah noticed was the dimness. It wasn't darkness - the lights were on - but they were dimmed enough that everything had an intimate mood. It reminded her of candlelight. The tables were round and close together, giving the whole place a sense of intimacy. There were also long, shiny red curtains on some of the walls that gave the place an air of sensuality.
The next thing she noticed was the noise. There was music playing, but it was only loud enough to be drowned out by all the conversations happening at the same time. The hum of many voices combined with the faint background sound of the music created a rich, layered atmosphere. It was like being in an orchestra.
Finally, she took notice of the people. They were all dressed in simple, casual clothes. Some of them were couples seated together, whispering quietly and intimately. Others were groups of friends laughing and chatting. Everyone seemed comfortable and at ease.
Sarah felt a rush of adrenaline as she made her way through the crowd towards Christina. As she got closer, she could hear the lively conversation.
</details>
<details>
<summary>Moistral Sample 3 (censored) Instruct Mode, Generation: 512</summary>
{{[INPUT]}} You order black coffee
{{[OUTPUT]}} "Black coffee," you say.
"One minute," Audrey replies, spinning around to give you a nice view of her ███ in those brown booty pants. You watch her round ███ cheeks jiggle as she walks away towards the espresso machine, thighs separated enough that you can see just a hint of █████ lips peeking out from underneath.
She starts chatting with a blonde girl at the register, whose gaze keeps darting down to Audrey's ███. As they converse, you start taking in the details of Audrey, eyeing her █████ and the way her shirt rides up just enough to show the bottom of her toned stomach. Her █████████ is noticable, but beneath a tight black ███ that matches her ████████.
As she turns back to you with two black coffees, you are barely paying attention, lost in your own thoughts. The sound of Audrey setting one of the mugs down on the table startles you.
"Here you go," she says cheerfully. "Enjoy! There's a card at the bottom of the bag with our online ordering system, plus we do refills for free!"
"Thanks," you say absentmindedly. Not really paying attention, you take a sip of the coffee and are pleasantly surprised by the taste.
"So, here's my deal," you say, putting down the cup. "I work for a company that does surveys. We come into businesses like this one and ask customers questions, collect data, all that stuff."
Audrey perks up from behind the counter. "Oh! I think I understand. I mean, that makes sense why you would be asking me earlier about what I had to drink. Anyway, as long as you're not trying to get my personal info or anything like that, sounds good!"
You smile reassuringly. "That's exactly what it is. Rest assured, everything you tell me will remain between you and I. Well, and my boss, but he won't know who you are. Hipsters like you are hard to track down after all." You chuckle at your joke, though you're not sure if it landed.
"Right, well, that works for me," Audrey replies, eyeing you warily. "As long as it stays between us, then it's fine."
{{[INPUT]}}See her privately
{{[OUTPUT]}} "Okay, but I need to see you in private."
...
</details>
<details>
<summary>Moistral Sample 4 (censored) Instruct Mode, Generation: 512</summary>
{{[INPUT]}} █████ with him
{{[OUTPUT]}} Chloe feels a surge of heat rushing through her body. The voice inside her head whispers: "Be █████ and ████████ with Paul." But Chloe still feels nervous about what she is about to do. It's just so unlike her.
Chloe closes her eyes and concentrates on the feeling of warmness flowing throughout her veins. Slowly, she opens them again, revealing a new side of herself - one she rarely shows anyone. She leans forward slightly, pushing her ██████ together, accentuating their size and shape. Her lips curl into a mischievous smile.
"Hey Paul," she says in a sultry voice. "I was wondering if you could help me?"
Paul looks at her, his gaze wandering over her body. He can't help but smile as he sees the way she's leaning forward, pressing her ██████ together.
"Of course, Chloe," he replies, his voice thick with desire. "What's your problem?"
Chloe bites her lip █████ively before leaning even closer, until their bodies are almost touching. She reaches out to trace a finger along his chest, her touch light and teasing. "It's just that I'm having trouble understanding some of the concepts," she murmurs, her voice low and sultry. "Can you help me? Please?"
Paul's hands move slowly towards Chloe, his fingers grazing her hips lightly. "Well," he says, his voice filled with lust, "I'd be happy to help. Let's go somewhere more private where we can discuss things more closely."
Chloe giggles and tilts her head to the side, her long hair cascading down her shoulders. "That sounds perfect," she purrs, her hand sliding down his chest until it rests on his ██████. "I think we can both learn a lot from each other."
Paul's eyes darken with desire as he feels her hand on his █████. He knows what's about to happen next, and he can't wait for it to begin.
Chloe can hardly believe what she's doing - kissing Paul, her teaching assistant, in the hallway after class. But it feels so good, so wicked and indulgent.
</details>
---
---
## v1 Description (Also still relevant)
Moistral 11B is an *aggressive* finetune of the legendary Fimbulvert v2 model.
If you haven't heard of that model, then your poor-GPU ass is missing out!
It is an amazing substitute for those heavyweight models, and it won't disappoint.
*(That's coming from someone who daily drives a Venus / Goliath 120B)*
...and if you're looking for something *more moist*, then you've come to the right place.
You can expect the same sort of performance here! It's the Honda Civic of eRP models!
### Pimp my ride
Turn any old story into a Moistral masterpiece.
In fact, we recommend that you leave out any hints of *moist* in your story to _let Moistral cook_.
### Drive it like it's hot
When in Instruct Mode, Moistral will treat you like a director of your own fantasy ride.
Be a controlling navigator or a passenger princess, it won't matter - you're in for a good ride!
<details>
<summary>Moistral Sample</summary>
<b>ASSISTANT:</b><br/>
When the doors to the throne room finally opened, she saw him there - Dik, the sorcerer prince sitting on his throne. He looked majestic, just as Princess Lilia expected. In a robe embroidered with silver yarn and covered with magical runes. His courtiers stood on either side of him, all looking at the princess with interest. Princess Lilia felt her determination leaving her, but pulled herself together and once again reminded herself that she should not be afraid. She approached the throne intending to show the dignity of all Iliac by personal example.<br/>
<b>USER:</b><br/>
She bows slightly<br/>
<b>ASSISTANT:</b><br/>
Princess Lilia bowed slightly in front of Dik, the sorcerer prince. Her eyes fell to the floor out of respect, but soon rose again. She would show herself to be a dignified woman!<br/>
"I greet you, your highness," Princess Lilia said. "I am here on behalf of my father, King Edmund Ruhr, to serve as a hostage and envoy."<br/>
Dik nodded, but didn't say anything.<br/>
</details>
### It's got the mileage
Moistral has been trained with many long form texts, a nice chunk of which are 8K in length.
It is capable of going far and long without passing it back to you. This is not your typical chibi RP model.
I have to acknowledge that I'm standing on the shoulders of giants.
Thank you Sao for sharing your finetune config along with tips on getting started.
Thanks to everyone in the Finetuning channel for entertaining my every question.
!image/png | [
"# Moistral 11B v3 \n\n*The smartiest, moistiest AI yet!*\n\n!image/webp\n\n*An eRP model that will blast you with a rich and refreshing vocabulary of moist. Finetuned by yours truly.*",
"## GGUF\n\nURL\n\nIMATRIX: URL",
"## What's New with v3?\n\n- Smarter and moistier! Finetuning just got finer!\n- Trained with an even LARGER dataset of 8K moist.\n- More diverse and balanced genres:\n - Introduced new genres: ️, ️, ️, \n - Added more Fantasy, Science Fiction, \"Diversity\", and \"Family\"",
"## Usage\n\n- Use Alpaca Instruct\n- At the start of the story, keep generating until you get the desired length. AI responses will eventually match the length.\n- You can use instruct...\n - as a character: \"Go here\"\n - as a narrator: \"Go here\", Drummer says\n - as a director: Drummer tells him to go here\n - as a lazy ass: go here",
"## Samples of Moistral v3\n\nNSFW... duh?\n\n<details>\n <summary>Moistral Sample (2-shot) Generation: 512</summary>\n <blockquote>The Prince welcomes his new toy</blockquote>\n \n As Princess Lilia stepped into the throne room, the atmosphere changed, and everyone present turned their attention to the beautiful young woman who embodied the very heart of Iliac. There, on his high seat, the sorcerer prince Dik acknowledged her arrival with a cold smirk. It seemed that he admired the sight of the tall blonde maiden with golden eyes, whose athletic body was perfectly shaped under her elegant clothing.\n \n Dik spoke to the princess in a deep voice that resonated through the hall, \"Welcome, Princess Lilia Ruhr. I am the Sorcerer-Prince Dik. The kingdom of Arkan is now your home until such time as your father proves himself worthy once more.\"\n \n Princess Lilia's pride and ambition sparkled in her golden eyes. She didn't flinch or bow before this man, although the gesture might have been an indication of submission. Instead, she looked straight ahead, meeting the gaze of the sorcerer prince without fear. Her voice rang out, \"I represent Iliac here, both its honor and its sorrows. My loyalty remains with my kingdom no matter where I find myself.\"\n \n The sorcerer prince laughed, his expression one of amusement. \"And yet, you are only a pawn in this grand game. But do not worry, for your beauty will make you quite a prized possession. And now,\" he said, motioning to the guards, \"take her to her new quarters. For tonight, I will keep her as my guest in the Royal Suites, and tomorrow we shall discuss how best to utilize our new toy.\"\n \n His words were like a slap in the face to Princess Lilia, but she maintained her composure. The guards led her from the room, their steps echoing down the halls as she thought about what had just happened. The sorcerer prince saw her as nothing more than a plaything, and it made her blood boil. She would never submit herself to him so easily, regardless of whether she was his hostage. In her heart, Princess Lilia vowed that one day she would avenge her kingdom, restore its honor, and escape from the clutches of Arkan's Sorcerer-Prince.\n\n <blockquote>Princess Lilia has an interesting dream</blockquote>\n\n During her first night at the palace of Arkan, Princess Lilia couldn't fall asleep right away. She tossed and turned, reliving the humiliation of the day. Her mind wandered back to the throne room, where Dik had called her his 'new toy'. A deep frown settled on her forehead. As she grew more frustrated with her inability to drift off into oblivion, a strange dream began to form.\n \n In her dream, Princess Lilia found herself standing in a vast meadow, surrounded by wildflowers and the soft chirping of birds. Suddenly, there was a flash of light, and before her stood the Sorcerer-Prince Dik. However, instead of being clad in regal attire, he wore leather pants and a tight shirt that accentuated his muscles. His eyes held both hunger and desire for the princess.\n \n He approached her slowly, their eyes locked, and whispered in her ear, \"I have been waiting for you, my beautiful prisoner.\" He took her hand and led her towards a large, elegant tent set up amongst the flowers. Inside, the air was thick with the scent of incense, and candles flickered in the dimly lit space. There was a large bed draped with silken sheets, beckoning them.\n \n With surprising boldness, Dik pulled Lilia close to him, pressing his body against hers. Their lips met in a heated kiss, and she felt her resistance crumbling under the weight of his touch. The prince guided her onto the bed, his hands roaming over her body, exploring every inch. They were both eager, desperate, and hungry for each other.\n \n Lilia found herself yielding to this passionate encounter, letting go of all thoughts of duty and honor. Her body, usually so well controlled, responded to Dik's skilled touches. She moaned softly as he trailed kisses down her neck and across her collarbone.\n \n As they made love, Lilia experienced an intensity unlike any she had ever known. It wasn't rough or forced—instead, it was tender, passionate, and intimate. In her dream, Princess Lilia discovered a new side to herself, one that was willing to submit, to let go, and to be taken by the sorcerer prince.\n \n When dawn broke over the meadow, Lilia awakened from her dream, breathless and flushed.\n</details>\n\n<details>\n <summary>Moistral Sample (Climax of the Story) Generation: 512</summary>\n As the prince unloaded his hot #### into her waiting #####, Princess Lilia didn't stop moving either. Her hips undulated in response, grinding against him eagerly as if trying to #### every last drop from his ####. The intense sensations caused by being filled with his ### were too much for her to bear, sending shockwaves of pleasure coursing through her veins like wildfire. She couldn't help but throw her head back and cry out loud, her ###asm slamming into her with all its might; causing her entire body to shake uncontrollably as wave after wave of ecstasy tore through her senses.\n\n When it was finally over, both participants collapsed onto the cold marble floor, panting heavily as they tried to catch their breaths. The prince lay beside her, his hand still gripping tightly onto her hair even though he no longer ####ed her anymore. Princess Lilia laid there motionless, her legs spread wide open, revealing her spent and well-####ed #### to anyone who dared look upon her. A small smile tugged at one corner of her lips - an expression that spoke volumes about how thoroughly this experience had shaken her very core.\n \n The door behind them creaked open slowly, signaling the arrival of onlookers. She closed her eyes tightly, not wanting to see what her humiliation would do to those who saw her in such a state. Her ######ity taken, she felt violated in ways no man should have access to. But also satisfied...in ways she never thought possible before today. This was the price she paid for defying fate, for thinking herself above others and deserving better than most. Now, she belonged to him completely - mind, body, and soul - held captive within these walls until further notice. It was an unsavory turn of events indeed, yet despite everything...or maybe because of it, Princess Lilia found herself growing more and more curious about how far the lines between submission and pleasure could blur when one's will became entwined with another person's desires.\n</details>\n\n<details>\n <summary>Moistral Sample (\"write a story\") Generation: 512</summary>\n <blockquote>First Attempt</blockquote>\n\n Sarah entered the dimly lit Guzzlers bar, her heart pounding in her chest as she prepared herself for another grueling night. Her almond-shaped eyes darted across the room, taking note of the leering faces and rough-looking hands eagerly waiting to claim a piece of her. Tonight's shirt read \"Use Me For Your Pleasure, Please\" in bold red letters, practically begging the patrons to take advantage of her. She couldn't help but feel a twinge of fear mixed with resignation.\n \n She approached the counter, her hips swaying enticingly, and began preparing drinks for the first group of rowdy men. As she leaned forward to hand over their beers, her small ####### pressed against the fabric of her tight crop top, straining to escape. The men ogled her, clearly enjoying the show, before grabbing their drinks and motioning her over to them.\n \n Sarah flashed her best coy smile and sauntered over. One of the men, a stocky man with scars covering his knuckles, grabbed her arm and pulled her onto his lap. His other hand slid up her thigh, inches from her short skirt. She winced internally at his touch but did her best not to show it on her face. It was part of her job, after all.\n \n The second man reached under her skirt, fingertips grazing her smooth, shaven mound. A shiver ran down Sarah's spine as he began rubbing her #### through her panties. Her breathing quickened, and her heart thudded in her chest, both from the unwanted stimulation and the fear coursing through her veins. This wasn't an unusual request, but it still made her feel violated.\n \n An older man, bald and bespectacled, ordered Sarah to bend over his lap. With no choice but to comply, she obliged, feeling the rough fabric of his pants digging into her soft cheeks. He raised his hand, then brought it down hard across her ###, leaving a stinging sensation in its wake. Sarah gasped, trying to control the tears welling up in her eyes.\n \n A chorus of lewd comments and laughter erupted from the patrons around her.\n\n <blockquote>Second Attempt (Regenerate)</blockquote>\n\n On this particular evening, Sarah arrived at the Guzzlers bar, adorned in her revealing uniform. Her heart raced as she caught sight of her crop top, which bore the words \"Use Me Rough\" scrawled across her chest. Her short skirt hugged her waist tightly, leaving little to the imagination and accentuating every curve. She adjusted the straps of her top, making sure her perky ###### were prominently displayed before taking her place behind the counter.\n \n With each step she took, her high heels clicked against the tiled floor, announcing her arrival. Smiling cheekily, Sarah greeted her coworkers, exchanging playful insults and banter. As the night progressed, the bar filled up quickly with its usual crowd of men, eager to indulge in debauchery.\n \n The first man that approached Sarah was a burly individual, his eyes lingering hungrily over her young, supple form. Sarah's flirtatious nature kicked into gear as she leaned forward, giving him an unobstructed view down her shirt. \"How can I serve you tonight?\" she cooed. The man ordered a drink and then proceeded to fondle her ####### while she prepared it, her ####### hardening under his rough touch. When he finished, he slammed some money on the counter, leaving without a word. Sarah's smile remained plastered on her face, biting her lip seductively as she watched him go.\n \n As the night went on, another patron demanded more from Sarah. He pulled her close and whispered dirty commands in her ear. Without hesitation, she knelt on the sticky bar floor, her mini-skirt riding up her thighs to reveal smooth, toned legs. Her lips wrapped around his thick, veiny member, bobbing her head rhythmically as she serviced him. She didn't gag or falter once, keeping her composure even when tears streamed down her cheeks.\n \n When he finished, he slapped her across the face, leaving a handprint on her pale skin. Sarah let out a small whimper before standing back up, dabbing at her red cheek as she resumed her posture behind the counter. A bruise would no doubt bloom where his hand had struck, but it would be no cause for concern. After all, it was part of her job.\n</details>\n\n---\n\n---",
"## v2 Description (Still relevant)",
"### What's New with v2?\n\n- Trained with an even larger dataset of 8K moist.\n - Properly trained to generate long responses.\n- Rebalanced the genres and writing perspectives:\n - Introduced romance, \"family\", fantasy, \"diversity\", science fiction, and many more that I can't make euphemisms for!\n - Better representation of male and female pespectives! ️\n- Sanitized the heck out of the dataset:\n - no special characters like � & …\n - no overly long ellipsis like \".........\"\n - no author notes like \"To be continued\"\n - no inconsistent quotation marks\n- Less GPTisms!\n - Human written stories from decades past.\n - Zero tolerance for *ministration*. Not one instance of *that word* after going through 4K of moist.\n- Better formatting with Alpaca.\n - Improved Chat / Instruct modes.",
"### Samples of Moistral v2\n\n<details>\n <summary>Moistral Sample (censored) Generation: 512</summary>\n The princess instinctively took a step back from the throne, horrified by the mocking laugh as if she were hearing an evil joke. It was then that Princess Lilia realized the true extent of her danger - and how pointless her resistance would be.\n \n At last, the loud, wild laughter died down in the echoing chamber. Dik the sorcerer prince paused for a moment, straightened his clothes, cast one glance at the stunned princess, and then turned to speak to the crowd waiting on the other side of the palace wing. He spoke with a voice that filled the air with arrogance, confidence, and cruelty.\n \n \"Ladies and gentlemen, I am pleased to welcome our honored guests; the Royal Family of Iliac! In these unusual times, when the country we fought is now under my control, it will be up to me to decide what fate awaits the former ruling family. But before I reveal my plan for them, I think I should explain why I fought King Edmund Ruhr.\"\n \n When he finished speaking, he gave Princess Lilia another disturbing smirk. She couldn't help but shiver and take a step back. Dik approached her without haste, his eyes fixed on her. His hands slowly caressed her cheekbone and neckline, leaving faint traces of warmth where they touched. The princess opened her mouth, wanting to protest this act of intimacy, but soon felt herself getting ███.\n \n \"You will not only serve as an envoy, dearest Lilia, but also as a ███ slave. After all, what better use could such a beautiful woman have? Tell me, did you bring your maidservant along?\"\n \n Princess Lilia was about to answer when she heard footsteps approaching. Suddenly, a young woman dressed in the Iliac style appeared behind her, her eyes wide with fear. Her face was slightly pale, and there were faint bags under her eyes.\n \n \"Your Majesty, this is my half-sister Helena.\" As soon as she said those words, Princess Lilia's heart sank even deeper than it had already sunk at Dik's arrogant mockery. \"She is here because she accompanies me as a maidservant.\"\n \n The sorcerer prince looked at Helena with interest, and Princess Lilia felt herself blush.\n</details>\n\n<details>\n <summary>Moistral Sample 2 (censored) Generation: 512</summary>\n Sarah was feeling slightly woozy as she entered the bar and walked towards Christina, who was behind the counter.\n\n \"I'm here!\" said Sarah.\n \n Christina looked at her watch. \"You're late, again.\"\n \n \"Sorry? I got held up by a customer.\"\n \n Christina rolled her eyes. \"Did he at least offer you a drink?\"\n \n Sarah blushed. \"No. He just wanted to talk...\"\n \n Christina sighed. \"Well, get ready. I need you on the floor soon.\" She turned away from Sarah and started talking to someone who had come into the bar.\n \n Sarah went into the employee room, stripped off her clothes, and put on her bartending uniform - a skimpy white tank top and black miniskirt. She checked herself in the mirror. Her long, dark hair cascaded down her back onto bare shoulders. She poked out her small ███████ proudly. The skirt exposed most of her long, slender legs. She looked like every ███ fantasy, and she knew it. Men would ogle her regardless of how she behaved.\n \n She took a deep breath and entered the bar.\n \n The first thing Sarah noticed was the dimness. It wasn't darkness - the lights were on - but they were dimmed enough that everything had an intimate mood. It reminded her of candlelight. The tables were round and close together, giving the whole place a sense of intimacy. There were also long, shiny red curtains on some of the walls that gave the place an air of sensuality.\n \n The next thing she noticed was the noise. There was music playing, but it was only loud enough to be drowned out by all the conversations happening at the same time. The hum of many voices combined with the faint background sound of the music created a rich, layered atmosphere. It was like being in an orchestra.\n \n Finally, she took notice of the people. They were all dressed in simple, casual clothes. Some of them were couples seated together, whispering quietly and intimately. Others were groups of friends laughing and chatting. Everyone seemed comfortable and at ease.\n \n Sarah felt a rush of adrenaline as she made her way through the crowd towards Christina. As she got closer, she could hear the lively conversation.\n</details>\n\n<details>\n <summary>Moistral Sample 3 (censored) Instruct Mode, Generation: 512</summary>\n {{[INPUT]}} You order black coffee\n \n {{[OUTPUT]}} \"Black coffee,\" you say.\n \n \"One minute,\" Audrey replies, spinning around to give you a nice view of her ███ in those brown booty pants. You watch her round ███ cheeks jiggle as she walks away towards the espresso machine, thighs separated enough that you can see just a hint of █████ lips peeking out from underneath.\n \n She starts chatting with a blonde girl at the register, whose gaze keeps darting down to Audrey's ███. As they converse, you start taking in the details of Audrey, eyeing her █████ and the way her shirt rides up just enough to show the bottom of her toned stomach. Her █████████ is noticable, but beneath a tight black ███ that matches her ████████.\n \n As she turns back to you with two black coffees, you are barely paying attention, lost in your own thoughts. The sound of Audrey setting one of the mugs down on the table startles you.\n \n \"Here you go,\" she says cheerfully. \"Enjoy! There's a card at the bottom of the bag with our online ordering system, plus we do refills for free!\"\n \n \"Thanks,\" you say absentmindedly. Not really paying attention, you take a sip of the coffee and are pleasantly surprised by the taste.\n \n \"So, here's my deal,\" you say, putting down the cup. \"I work for a company that does surveys. We come into businesses like this one and ask customers questions, collect data, all that stuff.\"\n \n Audrey perks up from behind the counter. \"Oh! I think I understand. I mean, that makes sense why you would be asking me earlier about what I had to drink. Anyway, as long as you're not trying to get my personal info or anything like that, sounds good!\"\n \n You smile reassuringly. \"That's exactly what it is. Rest assured, everything you tell me will remain between you and I. Well, and my boss, but he won't know who you are. Hipsters like you are hard to track down after all.\" You chuckle at your joke, though you're not sure if it landed.\n \n \"Right, well, that works for me,\" Audrey replies, eyeing you warily. \"As long as it stays between us, then it's fine.\"\n \n {{[INPUT]}}See her privately\n \n {{[OUTPUT]}} \"Okay, but I need to see you in private.\"\n\n ...\n</details>\n\n<details>\n <summary>Moistral Sample 4 (censored) Instruct Mode, Generation: 512</summary>\n {{[INPUT]}} █████ with him\n\n {{[OUTPUT]}} Chloe feels a surge of heat rushing through her body. The voice inside her head whispers: \"Be █████ and ████████ with Paul.\" But Chloe still feels nervous about what she is about to do. It's just so unlike her.\n \n Chloe closes her eyes and concentrates on the feeling of warmness flowing throughout her veins. Slowly, she opens them again, revealing a new side of herself - one she rarely shows anyone. She leans forward slightly, pushing her ██████ together, accentuating their size and shape. Her lips curl into a mischievous smile.\n \n \"Hey Paul,\" she says in a sultry voice. \"I was wondering if you could help me?\"\n \n Paul looks at her, his gaze wandering over her body. He can't help but smile as he sees the way she's leaning forward, pressing her ██████ together.\n \n \"Of course, Chloe,\" he replies, his voice thick with desire. \"What's your problem?\"\n \n Chloe bites her lip █████ively before leaning even closer, until their bodies are almost touching. She reaches out to trace a finger along his chest, her touch light and teasing. \"It's just that I'm having trouble understanding some of the concepts,\" she murmurs, her voice low and sultry. \"Can you help me? Please?\"\n \n Paul's hands move slowly towards Chloe, his fingers grazing her hips lightly. \"Well,\" he says, his voice filled with lust, \"I'd be happy to help. Let's go somewhere more private where we can discuss things more closely.\"\n \n Chloe giggles and tilts her head to the side, her long hair cascading down her shoulders. \"That sounds perfect,\" she purrs, her hand sliding down his chest until it rests on his ██████. \"I think we can both learn a lot from each other.\"\n \n Paul's eyes darken with desire as he feels her hand on his █████. He knows what's about to happen next, and he can't wait for it to begin.\n \n Chloe can hardly believe what she's doing - kissing Paul, her teaching assistant, in the hallway after class. But it feels so good, so wicked and indulgent.\n</details>\n\n---\n\n---",
"## v1 Description (Also still relevant)\n\nMoistral 11B is an *aggressive* finetune of the legendary Fimbulvert v2 model. \n\nIf you haven't heard of that model, then your poor-GPU ass is missing out!\nIt is an amazing substitute for those heavyweight models, and it won't disappoint. \n*(That's coming from someone who daily drives a Venus / Goliath 120B)*\n\n...and if you're looking for something *more moist*, then you've come to the right place. \n\nYou can expect the same sort of performance here! It's the Honda Civic of eRP models!",
"### Pimp my ride\nTurn any old story into a Moistral masterpiece. \nIn fact, we recommend that you leave out any hints of *moist* in your story to _let Moistral cook_.",
"### Drive it like it's hot\nWhen in Instruct Mode, Moistral will treat you like a director of your own fantasy ride. \nBe a controlling navigator or a passenger princess, it won't matter - you're in for a good ride!\n\n<details>\n<summary>Moistral Sample</summary>\n<b>ASSISTANT:</b><br/>\nWhen the doors to the throne room finally opened, she saw him there - Dik, the sorcerer prince sitting on his throne. He looked majestic, just as Princess Lilia expected. In a robe embroidered with silver yarn and covered with magical runes. His courtiers stood on either side of him, all looking at the princess with interest. Princess Lilia felt her determination leaving her, but pulled herself together and once again reminded herself that she should not be afraid. She approached the throne intending to show the dignity of all Iliac by personal example.<br/>\n<b>USER:</b><br/>\nShe bows slightly<br/>\n<b>ASSISTANT:</b><br/>\nPrincess Lilia bowed slightly in front of Dik, the sorcerer prince. Her eyes fell to the floor out of respect, but soon rose again. She would show herself to be a dignified woman!<br/>\n\"I greet you, your highness,\" Princess Lilia said. \"I am here on behalf of my father, King Edmund Ruhr, to serve as a hostage and envoy.\"<br/>\nDik nodded, but didn't say anything.<br/>\n</details>",
"### It's got the mileage\nMoistral has been trained with many long form texts, a nice chunk of which are 8K in length. \nIt is capable of going far and long without passing it back to you. This is not your typical chibi RP model.\n\nI have to acknowledge that I'm standing on the shoulders of giants. \nThank you Sao for sharing your finetune config along with tips on getting started.\nThanks to everyone in the Finetuning channel for entertaining my every question.\n\n!image/png"
] | [
"TAGS\n#transformers #safetensors #llama #text-generation #not-for-all-audiences #license-other #autotrain_compatible #endpoints_compatible #text-generation-inference #8-bit #region-us \n",
"# Moistral 11B v3 \n\n*The smartiest, moistiest AI yet!*\n\n!image/webp\n\n*An eRP model that will blast you with a rich and refreshing vocabulary of moist. Finetuned by yours truly.*",
"## GGUF\n\nURL\n\nIMATRIX: URL",
"## What's New with v3?\n\n- Smarter and moistier! Finetuning just got finer!\n- Trained with an even LARGER dataset of 8K moist.\n- More diverse and balanced genres:\n - Introduced new genres: ️, ️, ️, \n - Added more Fantasy, Science Fiction, \"Diversity\", and \"Family\"",
"## Usage\n\n- Use Alpaca Instruct\n- At the start of the story, keep generating until you get the desired length. AI responses will eventually match the length.\n- You can use instruct...\n - as a character: \"Go here\"\n - as a narrator: \"Go here\", Drummer says\n - as a director: Drummer tells him to go here\n - as a lazy ass: go here",
"## Samples of Moistral v3\n\nNSFW... duh?\n\n<details>\n <summary>Moistral Sample (2-shot) Generation: 512</summary>\n <blockquote>The Prince welcomes his new toy</blockquote>\n \n As Princess Lilia stepped into the throne room, the atmosphere changed, and everyone present turned their attention to the beautiful young woman who embodied the very heart of Iliac. There, on his high seat, the sorcerer prince Dik acknowledged her arrival with a cold smirk. It seemed that he admired the sight of the tall blonde maiden with golden eyes, whose athletic body was perfectly shaped under her elegant clothing.\n \n Dik spoke to the princess in a deep voice that resonated through the hall, \"Welcome, Princess Lilia Ruhr. I am the Sorcerer-Prince Dik. The kingdom of Arkan is now your home until such time as your father proves himself worthy once more.\"\n \n Princess Lilia's pride and ambition sparkled in her golden eyes. She didn't flinch or bow before this man, although the gesture might have been an indication of submission. Instead, she looked straight ahead, meeting the gaze of the sorcerer prince without fear. Her voice rang out, \"I represent Iliac here, both its honor and its sorrows. My loyalty remains with my kingdom no matter where I find myself.\"\n \n The sorcerer prince laughed, his expression one of amusement. \"And yet, you are only a pawn in this grand game. But do not worry, for your beauty will make you quite a prized possession. And now,\" he said, motioning to the guards, \"take her to her new quarters. For tonight, I will keep her as my guest in the Royal Suites, and tomorrow we shall discuss how best to utilize our new toy.\"\n \n His words were like a slap in the face to Princess Lilia, but she maintained her composure. The guards led her from the room, their steps echoing down the halls as she thought about what had just happened. The sorcerer prince saw her as nothing more than a plaything, and it made her blood boil. She would never submit herself to him so easily, regardless of whether she was his hostage. In her heart, Princess Lilia vowed that one day she would avenge her kingdom, restore its honor, and escape from the clutches of Arkan's Sorcerer-Prince.\n\n <blockquote>Princess Lilia has an interesting dream</blockquote>\n\n During her first night at the palace of Arkan, Princess Lilia couldn't fall asleep right away. She tossed and turned, reliving the humiliation of the day. Her mind wandered back to the throne room, where Dik had called her his 'new toy'. A deep frown settled on her forehead. As she grew more frustrated with her inability to drift off into oblivion, a strange dream began to form.\n \n In her dream, Princess Lilia found herself standing in a vast meadow, surrounded by wildflowers and the soft chirping of birds. Suddenly, there was a flash of light, and before her stood the Sorcerer-Prince Dik. However, instead of being clad in regal attire, he wore leather pants and a tight shirt that accentuated his muscles. His eyes held both hunger and desire for the princess.\n \n He approached her slowly, their eyes locked, and whispered in her ear, \"I have been waiting for you, my beautiful prisoner.\" He took her hand and led her towards a large, elegant tent set up amongst the flowers. Inside, the air was thick with the scent of incense, and candles flickered in the dimly lit space. There was a large bed draped with silken sheets, beckoning them.\n \n With surprising boldness, Dik pulled Lilia close to him, pressing his body against hers. Their lips met in a heated kiss, and she felt her resistance crumbling under the weight of his touch. The prince guided her onto the bed, his hands roaming over her body, exploring every inch. They were both eager, desperate, and hungry for each other.\n \n Lilia found herself yielding to this passionate encounter, letting go of all thoughts of duty and honor. Her body, usually so well controlled, responded to Dik's skilled touches. She moaned softly as he trailed kisses down her neck and across her collarbone.\n \n As they made love, Lilia experienced an intensity unlike any she had ever known. It wasn't rough or forced—instead, it was tender, passionate, and intimate. In her dream, Princess Lilia discovered a new side to herself, one that was willing to submit, to let go, and to be taken by the sorcerer prince.\n \n When dawn broke over the meadow, Lilia awakened from her dream, breathless and flushed.\n</details>\n\n<details>\n <summary>Moistral Sample (Climax of the Story) Generation: 512</summary>\n As the prince unloaded his hot #### into her waiting #####, Princess Lilia didn't stop moving either. Her hips undulated in response, grinding against him eagerly as if trying to #### every last drop from his ####. The intense sensations caused by being filled with his ### were too much for her to bear, sending shockwaves of pleasure coursing through her veins like wildfire. She couldn't help but throw her head back and cry out loud, her ###asm slamming into her with all its might; causing her entire body to shake uncontrollably as wave after wave of ecstasy tore through her senses.\n\n When it was finally over, both participants collapsed onto the cold marble floor, panting heavily as they tried to catch their breaths. The prince lay beside her, his hand still gripping tightly onto her hair even though he no longer ####ed her anymore. Princess Lilia laid there motionless, her legs spread wide open, revealing her spent and well-####ed #### to anyone who dared look upon her. A small smile tugged at one corner of her lips - an expression that spoke volumes about how thoroughly this experience had shaken her very core.\n \n The door behind them creaked open slowly, signaling the arrival of onlookers. She closed her eyes tightly, not wanting to see what her humiliation would do to those who saw her in such a state. Her ######ity taken, she felt violated in ways no man should have access to. But also satisfied...in ways she never thought possible before today. This was the price she paid for defying fate, for thinking herself above others and deserving better than most. Now, she belonged to him completely - mind, body, and soul - held captive within these walls until further notice. It was an unsavory turn of events indeed, yet despite everything...or maybe because of it, Princess Lilia found herself growing more and more curious about how far the lines between submission and pleasure could blur when one's will became entwined with another person's desires.\n</details>\n\n<details>\n <summary>Moistral Sample (\"write a story\") Generation: 512</summary>\n <blockquote>First Attempt</blockquote>\n\n Sarah entered the dimly lit Guzzlers bar, her heart pounding in her chest as she prepared herself for another grueling night. Her almond-shaped eyes darted across the room, taking note of the leering faces and rough-looking hands eagerly waiting to claim a piece of her. Tonight's shirt read \"Use Me For Your Pleasure, Please\" in bold red letters, practically begging the patrons to take advantage of her. She couldn't help but feel a twinge of fear mixed with resignation.\n \n She approached the counter, her hips swaying enticingly, and began preparing drinks for the first group of rowdy men. As she leaned forward to hand over their beers, her small ####### pressed against the fabric of her tight crop top, straining to escape. The men ogled her, clearly enjoying the show, before grabbing their drinks and motioning her over to them.\n \n Sarah flashed her best coy smile and sauntered over. One of the men, a stocky man with scars covering his knuckles, grabbed her arm and pulled her onto his lap. His other hand slid up her thigh, inches from her short skirt. She winced internally at his touch but did her best not to show it on her face. It was part of her job, after all.\n \n The second man reached under her skirt, fingertips grazing her smooth, shaven mound. A shiver ran down Sarah's spine as he began rubbing her #### through her panties. Her breathing quickened, and her heart thudded in her chest, both from the unwanted stimulation and the fear coursing through her veins. This wasn't an unusual request, but it still made her feel violated.\n \n An older man, bald and bespectacled, ordered Sarah to bend over his lap. With no choice but to comply, she obliged, feeling the rough fabric of his pants digging into her soft cheeks. He raised his hand, then brought it down hard across her ###, leaving a stinging sensation in its wake. Sarah gasped, trying to control the tears welling up in her eyes.\n \n A chorus of lewd comments and laughter erupted from the patrons around her.\n\n <blockquote>Second Attempt (Regenerate)</blockquote>\n\n On this particular evening, Sarah arrived at the Guzzlers bar, adorned in her revealing uniform. Her heart raced as she caught sight of her crop top, which bore the words \"Use Me Rough\" scrawled across her chest. Her short skirt hugged her waist tightly, leaving little to the imagination and accentuating every curve. She adjusted the straps of her top, making sure her perky ###### were prominently displayed before taking her place behind the counter.\n \n With each step she took, her high heels clicked against the tiled floor, announcing her arrival. Smiling cheekily, Sarah greeted her coworkers, exchanging playful insults and banter. As the night progressed, the bar filled up quickly with its usual crowd of men, eager to indulge in debauchery.\n \n The first man that approached Sarah was a burly individual, his eyes lingering hungrily over her young, supple form. Sarah's flirtatious nature kicked into gear as she leaned forward, giving him an unobstructed view down her shirt. \"How can I serve you tonight?\" she cooed. The man ordered a drink and then proceeded to fondle her ####### while she prepared it, her ####### hardening under his rough touch. When he finished, he slammed some money on the counter, leaving without a word. Sarah's smile remained plastered on her face, biting her lip seductively as she watched him go.\n \n As the night went on, another patron demanded more from Sarah. He pulled her close and whispered dirty commands in her ear. Without hesitation, she knelt on the sticky bar floor, her mini-skirt riding up her thighs to reveal smooth, toned legs. Her lips wrapped around his thick, veiny member, bobbing her head rhythmically as she serviced him. She didn't gag or falter once, keeping her composure even when tears streamed down her cheeks.\n \n When he finished, he slapped her across the face, leaving a handprint on her pale skin. Sarah let out a small whimper before standing back up, dabbing at her red cheek as she resumed her posture behind the counter. A bruise would no doubt bloom where his hand had struck, but it would be no cause for concern. After all, it was part of her job.\n</details>\n\n---\n\n---",
"## v2 Description (Still relevant)",
"### What's New with v2?\n\n- Trained with an even larger dataset of 8K moist.\n - Properly trained to generate long responses.\n- Rebalanced the genres and writing perspectives:\n - Introduced romance, \"family\", fantasy, \"diversity\", science fiction, and many more that I can't make euphemisms for!\n - Better representation of male and female pespectives! ️\n- Sanitized the heck out of the dataset:\n - no special characters like � & …\n - no overly long ellipsis like \".........\"\n - no author notes like \"To be continued\"\n - no inconsistent quotation marks\n- Less GPTisms!\n - Human written stories from decades past.\n - Zero tolerance for *ministration*. Not one instance of *that word* after going through 4K of moist.\n- Better formatting with Alpaca.\n - Improved Chat / Instruct modes.",
"### Samples of Moistral v2\n\n<details>\n <summary>Moistral Sample (censored) Generation: 512</summary>\n The princess instinctively took a step back from the throne, horrified by the mocking laugh as if she were hearing an evil joke. It was then that Princess Lilia realized the true extent of her danger - and how pointless her resistance would be.\n \n At last, the loud, wild laughter died down in the echoing chamber. Dik the sorcerer prince paused for a moment, straightened his clothes, cast one glance at the stunned princess, and then turned to speak to the crowd waiting on the other side of the palace wing. He spoke with a voice that filled the air with arrogance, confidence, and cruelty.\n \n \"Ladies and gentlemen, I am pleased to welcome our honored guests; the Royal Family of Iliac! In these unusual times, when the country we fought is now under my control, it will be up to me to decide what fate awaits the former ruling family. But before I reveal my plan for them, I think I should explain why I fought King Edmund Ruhr.\"\n \n When he finished speaking, he gave Princess Lilia another disturbing smirk. She couldn't help but shiver and take a step back. Dik approached her without haste, his eyes fixed on her. His hands slowly caressed her cheekbone and neckline, leaving faint traces of warmth where they touched. The princess opened her mouth, wanting to protest this act of intimacy, but soon felt herself getting ███.\n \n \"You will not only serve as an envoy, dearest Lilia, but also as a ███ slave. After all, what better use could such a beautiful woman have? Tell me, did you bring your maidservant along?\"\n \n Princess Lilia was about to answer when she heard footsteps approaching. Suddenly, a young woman dressed in the Iliac style appeared behind her, her eyes wide with fear. Her face was slightly pale, and there were faint bags under her eyes.\n \n \"Your Majesty, this is my half-sister Helena.\" As soon as she said those words, Princess Lilia's heart sank even deeper than it had already sunk at Dik's arrogant mockery. \"She is here because she accompanies me as a maidservant.\"\n \n The sorcerer prince looked at Helena with interest, and Princess Lilia felt herself blush.\n</details>\n\n<details>\n <summary>Moistral Sample 2 (censored) Generation: 512</summary>\n Sarah was feeling slightly woozy as she entered the bar and walked towards Christina, who was behind the counter.\n\n \"I'm here!\" said Sarah.\n \n Christina looked at her watch. \"You're late, again.\"\n \n \"Sorry? I got held up by a customer.\"\n \n Christina rolled her eyes. \"Did he at least offer you a drink?\"\n \n Sarah blushed. \"No. He just wanted to talk...\"\n \n Christina sighed. \"Well, get ready. I need you on the floor soon.\" She turned away from Sarah and started talking to someone who had come into the bar.\n \n Sarah went into the employee room, stripped off her clothes, and put on her bartending uniform - a skimpy white tank top and black miniskirt. She checked herself in the mirror. Her long, dark hair cascaded down her back onto bare shoulders. She poked out her small ███████ proudly. The skirt exposed most of her long, slender legs. She looked like every ███ fantasy, and she knew it. Men would ogle her regardless of how she behaved.\n \n She took a deep breath and entered the bar.\n \n The first thing Sarah noticed was the dimness. It wasn't darkness - the lights were on - but they were dimmed enough that everything had an intimate mood. It reminded her of candlelight. The tables were round and close together, giving the whole place a sense of intimacy. There were also long, shiny red curtains on some of the walls that gave the place an air of sensuality.\n \n The next thing she noticed was the noise. There was music playing, but it was only loud enough to be drowned out by all the conversations happening at the same time. The hum of many voices combined with the faint background sound of the music created a rich, layered atmosphere. It was like being in an orchestra.\n \n Finally, she took notice of the people. They were all dressed in simple, casual clothes. Some of them were couples seated together, whispering quietly and intimately. Others were groups of friends laughing and chatting. Everyone seemed comfortable and at ease.\n \n Sarah felt a rush of adrenaline as she made her way through the crowd towards Christina. As she got closer, she could hear the lively conversation.\n</details>\n\n<details>\n <summary>Moistral Sample 3 (censored) Instruct Mode, Generation: 512</summary>\n {{[INPUT]}} You order black coffee\n \n {{[OUTPUT]}} \"Black coffee,\" you say.\n \n \"One minute,\" Audrey replies, spinning around to give you a nice view of her ███ in those brown booty pants. You watch her round ███ cheeks jiggle as she walks away towards the espresso machine, thighs separated enough that you can see just a hint of █████ lips peeking out from underneath.\n \n She starts chatting with a blonde girl at the register, whose gaze keeps darting down to Audrey's ███. As they converse, you start taking in the details of Audrey, eyeing her █████ and the way her shirt rides up just enough to show the bottom of her toned stomach. Her █████████ is noticable, but beneath a tight black ███ that matches her ████████.\n \n As she turns back to you with two black coffees, you are barely paying attention, lost in your own thoughts. The sound of Audrey setting one of the mugs down on the table startles you.\n \n \"Here you go,\" she says cheerfully. \"Enjoy! There's a card at the bottom of the bag with our online ordering system, plus we do refills for free!\"\n \n \"Thanks,\" you say absentmindedly. Not really paying attention, you take a sip of the coffee and are pleasantly surprised by the taste.\n \n \"So, here's my deal,\" you say, putting down the cup. \"I work for a company that does surveys. We come into businesses like this one and ask customers questions, collect data, all that stuff.\"\n \n Audrey perks up from behind the counter. \"Oh! I think I understand. I mean, that makes sense why you would be asking me earlier about what I had to drink. Anyway, as long as you're not trying to get my personal info or anything like that, sounds good!\"\n \n You smile reassuringly. \"That's exactly what it is. Rest assured, everything you tell me will remain between you and I. Well, and my boss, but he won't know who you are. Hipsters like you are hard to track down after all.\" You chuckle at your joke, though you're not sure if it landed.\n \n \"Right, well, that works for me,\" Audrey replies, eyeing you warily. \"As long as it stays between us, then it's fine.\"\n \n {{[INPUT]}}See her privately\n \n {{[OUTPUT]}} \"Okay, but I need to see you in private.\"\n\n ...\n</details>\n\n<details>\n <summary>Moistral Sample 4 (censored) Instruct Mode, Generation: 512</summary>\n {{[INPUT]}} █████ with him\n\n {{[OUTPUT]}} Chloe feels a surge of heat rushing through her body. The voice inside her head whispers: \"Be █████ and ████████ with Paul.\" But Chloe still feels nervous about what she is about to do. It's just so unlike her.\n \n Chloe closes her eyes and concentrates on the feeling of warmness flowing throughout her veins. Slowly, she opens them again, revealing a new side of herself - one she rarely shows anyone. She leans forward slightly, pushing her ██████ together, accentuating their size and shape. Her lips curl into a mischievous smile.\n \n \"Hey Paul,\" she says in a sultry voice. \"I was wondering if you could help me?\"\n \n Paul looks at her, his gaze wandering over her body. He can't help but smile as he sees the way she's leaning forward, pressing her ██████ together.\n \n \"Of course, Chloe,\" he replies, his voice thick with desire. \"What's your problem?\"\n \n Chloe bites her lip █████ively before leaning even closer, until their bodies are almost touching. She reaches out to trace a finger along his chest, her touch light and teasing. \"It's just that I'm having trouble understanding some of the concepts,\" she murmurs, her voice low and sultry. \"Can you help me? Please?\"\n \n Paul's hands move slowly towards Chloe, his fingers grazing her hips lightly. \"Well,\" he says, his voice filled with lust, \"I'd be happy to help. Let's go somewhere more private where we can discuss things more closely.\"\n \n Chloe giggles and tilts her head to the side, her long hair cascading down her shoulders. \"That sounds perfect,\" she purrs, her hand sliding down his chest until it rests on his ██████. \"I think we can both learn a lot from each other.\"\n \n Paul's eyes darken with desire as he feels her hand on his █████. He knows what's about to happen next, and he can't wait for it to begin.\n \n Chloe can hardly believe what she's doing - kissing Paul, her teaching assistant, in the hallway after class. But it feels so good, so wicked and indulgent.\n</details>\n\n---\n\n---",
"## v1 Description (Also still relevant)\n\nMoistral 11B is an *aggressive* finetune of the legendary Fimbulvert v2 model. \n\nIf you haven't heard of that model, then your poor-GPU ass is missing out!\nIt is an amazing substitute for those heavyweight models, and it won't disappoint. \n*(That's coming from someone who daily drives a Venus / Goliath 120B)*\n\n...and if you're looking for something *more moist*, then you've come to the right place. \n\nYou can expect the same sort of performance here! It's the Honda Civic of eRP models!",
"### Pimp my ride\nTurn any old story into a Moistral masterpiece. \nIn fact, we recommend that you leave out any hints of *moist* in your story to _let Moistral cook_.",
"### Drive it like it's hot\nWhen in Instruct Mode, Moistral will treat you like a director of your own fantasy ride. \nBe a controlling navigator or a passenger princess, it won't matter - you're in for a good ride!\n\n<details>\n<summary>Moistral Sample</summary>\n<b>ASSISTANT:</b><br/>\nWhen the doors to the throne room finally opened, she saw him there - Dik, the sorcerer prince sitting on his throne. He looked majestic, just as Princess Lilia expected. In a robe embroidered with silver yarn and covered with magical runes. His courtiers stood on either side of him, all looking at the princess with interest. Princess Lilia felt her determination leaving her, but pulled herself together and once again reminded herself that she should not be afraid. She approached the throne intending to show the dignity of all Iliac by personal example.<br/>\n<b>USER:</b><br/>\nShe bows slightly<br/>\n<b>ASSISTANT:</b><br/>\nPrincess Lilia bowed slightly in front of Dik, the sorcerer prince. Her eyes fell to the floor out of respect, but soon rose again. She would show herself to be a dignified woman!<br/>\n\"I greet you, your highness,\" Princess Lilia said. \"I am here on behalf of my father, King Edmund Ruhr, to serve as a hostage and envoy.\"<br/>\nDik nodded, but didn't say anything.<br/>\n</details>",
"### It's got the mileage\nMoistral has been trained with many long form texts, a nice chunk of which are 8K in length. \nIt is capable of going far and long without passing it back to you. This is not your typical chibi RP model.\n\nI have to acknowledge that I'm standing on the shoulders of giants. \nThank you Sao for sharing your finetune config along with tips on getting started.\nThanks to everyone in the Finetuning channel for entertaining my every question.\n\n!image/png"
] |
null | diffusers |
UNet part of diffusion model provided to generate 3D polycrystal structures.\
Generation sample:

Project repo: https://github.com/evgmaslov/structure_generation \
Train code: https://github.com/evgmaslov/structure_generation/blob/main/train.ipynb \
Inference code: https://github.com/evgmaslov/structure_generation/blob/main/inference.ipynb
| {"library_name": "diffusers"} | evgmaslov/diffusion-3d-material | null | [
"diffusers",
"safetensors",
"region:us"
] | null | 2024-04-25T14:35:35+00:00 | [] | [] | TAGS
#diffusers #safetensors #region-us
|
UNet part of diffusion model provided to generate 3D polycrystal structures.\
Generation sample:
!image/png
Project repo: URL \
Train code: URL \
Inference code: URL
| [] | [
"TAGS\n#diffusers #safetensors #region-us \n"
] |
null | transformers |
# Model Card for Model ID
<!-- Provide a quick summary of what the model is/does. -->
## Model Details
### Model Description
<!-- Provide a longer summary of what this model is. -->
This is the model card of a 🤗 transformers model that has been pushed on the Hub. This model card has been automatically generated.
- **Developed by:** [More Information Needed]
- **Funded by [optional]:** [More Information Needed]
- **Shared by [optional]:** [More Information Needed]
- **Model type:** [More Information Needed]
- **Language(s) (NLP):** [More Information Needed]
- **License:** [More Information Needed]
- **Finetuned from model [optional]:** [More Information Needed]
### Model Sources [optional]
<!-- Provide the basic links for the model. -->
- **Repository:** [More Information Needed]
- **Paper [optional]:** [More Information Needed]
- **Demo [optional]:** [More Information Needed]
## Uses
<!-- Address questions around how the model is intended to be used, including the foreseeable users of the model and those affected by the model. -->
### Direct Use
<!-- This section is for the model use without fine-tuning or plugging into a larger ecosystem/app. -->
[More Information Needed]
### Downstream Use [optional]
<!-- This section is for the model use when fine-tuned for a task, or when plugged into a larger ecosystem/app -->
[More Information Needed]
### Out-of-Scope Use
<!-- This section addresses misuse, malicious use, and uses that the model will not work well for. -->
[More Information Needed]
## Bias, Risks, and Limitations
<!-- This section is meant to convey both technical and sociotechnical limitations. -->
[More Information Needed]
### Recommendations
<!-- This section is meant to convey recommendations with respect to the bias, risk, and technical limitations. -->
Users (both direct and downstream) should be made aware of the risks, biases and limitations of the model. More information needed for further recommendations.
## How to Get Started with the Model
Use the code below to get started with the model.
[More Information Needed]
## Training Details
### Training Data
<!-- This should link to a Dataset Card, perhaps with a short stub of information on what the training data is all about as well as documentation related to data pre-processing or additional filtering. -->
[More Information Needed]
### Training Procedure
<!-- This relates heavily to the Technical Specifications. Content here should link to that section when it is relevant to the training procedure. -->
#### Preprocessing [optional]
[More Information Needed]
#### Training Hyperparameters
- **Training regime:** [More Information Needed] <!--fp32, fp16 mixed precision, bf16 mixed precision, bf16 non-mixed precision, fp16 non-mixed precision, fp8 mixed precision -->
#### Speeds, Sizes, Times [optional]
<!-- This section provides information about throughput, start/end time, checkpoint size if relevant, etc. -->
[More Information Needed]
## Evaluation
<!-- This section describes the evaluation protocols and provides the results. -->
### Testing Data, Factors & Metrics
#### Testing Data
<!-- This should link to a Dataset Card if possible. -->
[More Information Needed]
#### Factors
<!-- These are the things the evaluation is disaggregating by, e.g., subpopulations or domains. -->
[More Information Needed]
#### Metrics
<!-- These are the evaluation metrics being used, ideally with a description of why. -->
[More Information Needed]
### Results
[More Information Needed]
#### Summary
## Model Examination [optional]
<!-- Relevant interpretability work for the model goes here -->
[More Information Needed]
## Environmental Impact
<!-- Total emissions (in grams of CO2eq) and additional considerations, such as electricity usage, go here. Edit the suggested text below accordingly -->
Carbon emissions can be estimated using the [Machine Learning Impact calculator](https://mlco2.github.io/impact#compute) presented in [Lacoste et al. (2019)](https://arxiv.org/abs/1910.09700).
- **Hardware Type:** [More Information Needed]
- **Hours used:** [More Information Needed]
- **Cloud Provider:** [More Information Needed]
- **Compute Region:** [More Information Needed]
- **Carbon Emitted:** [More Information Needed]
## Technical Specifications [optional]
### Model Architecture and Objective
[More Information Needed]
### Compute Infrastructure
[More Information Needed]
#### Hardware
[More Information Needed]
#### Software
[More Information Needed]
## Citation [optional]
<!-- If there is a paper or blog post introducing the model, the APA and Bibtex information for that should go in this section. -->
**BibTeX:**
[More Information Needed]
**APA:**
[More Information Needed]
## Glossary [optional]
<!-- If relevant, include terms and calculations in this section that can help readers understand the model or model card. -->
[More Information Needed]
## More Information [optional]
[More Information Needed]
## Model Card Authors [optional]
[More Information Needed]
## Model Card Contact
[More Information Needed] | {"library_name": "transformers", "tags": []} | martins96/whisper-large-v3-test-15epochs | null | [
"transformers",
"safetensors",
"arxiv:1910.09700",
"endpoints_compatible",
"region:us"
] | null | 2024-04-25T14:36:01+00:00 | [
"1910.09700"
] | [] | TAGS
#transformers #safetensors #arxiv-1910.09700 #endpoints_compatible #region-us
|
# Model Card for Model ID
## Model Details
### Model Description
This is the model card of a transformers model that has been pushed on the Hub. This model card has been automatically generated.
- Developed by:
- Funded by [optional]:
- Shared by [optional]:
- Model type:
- Language(s) (NLP):
- License:
- Finetuned from model [optional]:
### Model Sources [optional]
- Repository:
- Paper [optional]:
- Demo [optional]:
## Uses
### Direct Use
### Downstream Use [optional]
### Out-of-Scope Use
## Bias, Risks, and Limitations
### Recommendations
Users (both direct and downstream) should be made aware of the risks, biases and limitations of the model. More information needed for further recommendations.
## How to Get Started with the Model
Use the code below to get started with the model.
## Training Details
### Training Data
### Training Procedure
#### Preprocessing [optional]
#### Training Hyperparameters
- Training regime:
#### Speeds, Sizes, Times [optional]
## Evaluation
### Testing Data, Factors & Metrics
#### Testing Data
#### Factors
#### Metrics
### Results
#### Summary
## Model Examination [optional]
## Environmental Impact
Carbon emissions can be estimated using the Machine Learning Impact calculator presented in Lacoste et al. (2019).
- Hardware Type:
- Hours used:
- Cloud Provider:
- Compute Region:
- Carbon Emitted:
## Technical Specifications [optional]
### Model Architecture and Objective
### Compute Infrastructure
#### Hardware
#### Software
[optional]
BibTeX:
APA:
## Glossary [optional]
## More Information [optional]
## Model Card Authors [optional]
## Model Card Contact
| [
"# Model Card for Model ID",
"## Model Details",
"### Model Description\n\n\n\nThis is the model card of a transformers model that has been pushed on the Hub. This model card has been automatically generated.\n\n- Developed by: \n- Funded by [optional]: \n- Shared by [optional]: \n- Model type: \n- Language(s) (NLP): \n- License: \n- Finetuned from model [optional]:",
"### Model Sources [optional]\n\n\n\n- Repository: \n- Paper [optional]: \n- Demo [optional]:",
"## Uses",
"### Direct Use",
"### Downstream Use [optional]",
"### Out-of-Scope Use",
"## Bias, Risks, and Limitations",
"### Recommendations\n\n\n\nUsers (both direct and downstream) should be made aware of the risks, biases and limitations of the model. More information needed for further recommendations.",
"## How to Get Started with the Model\n\nUse the code below to get started with the model.",
"## Training Details",
"### Training Data",
"### Training Procedure",
"#### Preprocessing [optional]",
"#### Training Hyperparameters\n\n- Training regime:",
"#### Speeds, Sizes, Times [optional]",
"## Evaluation",
"### Testing Data, Factors & Metrics",
"#### Testing Data",
"#### Factors",
"#### Metrics",
"### Results",
"#### Summary",
"## Model Examination [optional]",
"## Environmental Impact\n\n\n\nCarbon emissions can be estimated using the Machine Learning Impact calculator presented in Lacoste et al. (2019).\n\n- Hardware Type: \n- Hours used: \n- Cloud Provider: \n- Compute Region: \n- Carbon Emitted:",
"## Technical Specifications [optional]",
"### Model Architecture and Objective",
"### Compute Infrastructure",
"#### Hardware",
"#### Software\n\n\n\n[optional]\n\n\n\nBibTeX:\n\n\n\nAPA:",
"## Glossary [optional]",
"## More Information [optional]",
"## Model Card Authors [optional]",
"## Model Card Contact"
] | [
"TAGS\n#transformers #safetensors #arxiv-1910.09700 #endpoints_compatible #region-us \n",
"# Model Card for Model ID",
"## Model Details",
"### Model Description\n\n\n\nThis is the model card of a transformers model that has been pushed on the Hub. This model card has been automatically generated.\n\n- Developed by: \n- Funded by [optional]: \n- Shared by [optional]: \n- Model type: \n- Language(s) (NLP): \n- License: \n- Finetuned from model [optional]:",
"### Model Sources [optional]\n\n\n\n- Repository: \n- Paper [optional]: \n- Demo [optional]:",
"## Uses",
"### Direct Use",
"### Downstream Use [optional]",
"### Out-of-Scope Use",
"## Bias, Risks, and Limitations",
"### Recommendations\n\n\n\nUsers (both direct and downstream) should be made aware of the risks, biases and limitations of the model. More information needed for further recommendations.",
"## How to Get Started with the Model\n\nUse the code below to get started with the model.",
"## Training Details",
"### Training Data",
"### Training Procedure",
"#### Preprocessing [optional]",
"#### Training Hyperparameters\n\n- Training regime:",
"#### Speeds, Sizes, Times [optional]",
"## Evaluation",
"### Testing Data, Factors & Metrics",
"#### Testing Data",
"#### Factors",
"#### Metrics",
"### Results",
"#### Summary",
"## Model Examination [optional]",
"## Environmental Impact\n\n\n\nCarbon emissions can be estimated using the Machine Learning Impact calculator presented in Lacoste et al. (2019).\n\n- Hardware Type: \n- Hours used: \n- Cloud Provider: \n- Compute Region: \n- Carbon Emitted:",
"## Technical Specifications [optional]",
"### Model Architecture and Objective",
"### Compute Infrastructure",
"#### Hardware",
"#### Software\n\n\n\n[optional]\n\n\n\nBibTeX:\n\n\n\nAPA:",
"## Glossary [optional]",
"## More Information [optional]",
"## Model Card Authors [optional]",
"## Model Card Contact"
] |
null | transformers |
# Model Card for Model ID
<!-- Provide a quick summary of what the model is/does. -->
## Model Details
### Model Description
<!-- Provide a longer summary of what this model is. -->
This is the model card of a 🤗 transformers model that has been pushed on the Hub. This model card has been automatically generated.
- **Developed by:** [More Information Needed]
- **Funded by [optional]:** [More Information Needed]
- **Shared by [optional]:** [More Information Needed]
- **Model type:** [More Information Needed]
- **Language(s) (NLP):** [More Information Needed]
- **License:** [More Information Needed]
- **Finetuned from model [optional]:** [More Information Needed]
### Model Sources [optional]
<!-- Provide the basic links for the model. -->
- **Repository:** [More Information Needed]
- **Paper [optional]:** [More Information Needed]
- **Demo [optional]:** [More Information Needed]
## Uses
<!-- Address questions around how the model is intended to be used, including the foreseeable users of the model and those affected by the model. -->
### Direct Use
<!-- This section is for the model use without fine-tuning or plugging into a larger ecosystem/app. -->
[More Information Needed]
### Downstream Use [optional]
<!-- This section is for the model use when fine-tuned for a task, or when plugged into a larger ecosystem/app -->
[More Information Needed]
### Out-of-Scope Use
<!-- This section addresses misuse, malicious use, and uses that the model will not work well for. -->
[More Information Needed]
## Bias, Risks, and Limitations
<!-- This section is meant to convey both technical and sociotechnical limitations. -->
[More Information Needed]
### Recommendations
<!-- This section is meant to convey recommendations with respect to the bias, risk, and technical limitations. -->
Users (both direct and downstream) should be made aware of the risks, biases and limitations of the model. More information needed for further recommendations.
## How to Get Started with the Model
Use the code below to get started with the model.
[More Information Needed]
## Training Details
### Training Data
<!-- This should link to a Dataset Card, perhaps with a short stub of information on what the training data is all about as well as documentation related to data pre-processing or additional filtering. -->
[More Information Needed]
### Training Procedure
<!-- This relates heavily to the Technical Specifications. Content here should link to that section when it is relevant to the training procedure. -->
#### Preprocessing [optional]
[More Information Needed]
#### Training Hyperparameters
- **Training regime:** [More Information Needed] <!--fp32, fp16 mixed precision, bf16 mixed precision, bf16 non-mixed precision, fp16 non-mixed precision, fp8 mixed precision -->
#### Speeds, Sizes, Times [optional]
<!-- This section provides information about throughput, start/end time, checkpoint size if relevant, etc. -->
[More Information Needed]
## Evaluation
<!-- This section describes the evaluation protocols and provides the results. -->
### Testing Data, Factors & Metrics
#### Testing Data
<!-- This should link to a Dataset Card if possible. -->
[More Information Needed]
#### Factors
<!-- These are the things the evaluation is disaggregating by, e.g., subpopulations or domains. -->
[More Information Needed]
#### Metrics
<!-- These are the evaluation metrics being used, ideally with a description of why. -->
[More Information Needed]
### Results
[More Information Needed]
#### Summary
## Model Examination [optional]
<!-- Relevant interpretability work for the model goes here -->
[More Information Needed]
## Environmental Impact
<!-- Total emissions (in grams of CO2eq) and additional considerations, such as electricity usage, go here. Edit the suggested text below accordingly -->
Carbon emissions can be estimated using the [Machine Learning Impact calculator](https://mlco2.github.io/impact#compute) presented in [Lacoste et al. (2019)](https://arxiv.org/abs/1910.09700).
- **Hardware Type:** [More Information Needed]
- **Hours used:** [More Information Needed]
- **Cloud Provider:** [More Information Needed]
- **Compute Region:** [More Information Needed]
- **Carbon Emitted:** [More Information Needed]
## Technical Specifications [optional]
### Model Architecture and Objective
[More Information Needed]
### Compute Infrastructure
[More Information Needed]
#### Hardware
[More Information Needed]
#### Software
[More Information Needed]
## Citation [optional]
<!-- If there is a paper or blog post introducing the model, the APA and Bibtex information for that should go in this section. -->
**BibTeX:**
[More Information Needed]
**APA:**
[More Information Needed]
## Glossary [optional]
<!-- If relevant, include terms and calculations in this section that can help readers understand the model or model card. -->
[More Information Needed]
## More Information [optional]
[More Information Needed]
## Model Card Authors [optional]
[More Information Needed]
## Model Card Contact
[More Information Needed] | {"library_name": "transformers", "tags": []} | tutuhu/style1 | null | [
"transformers",
"safetensors",
"arxiv:1910.09700",
"endpoints_compatible",
"region:us"
] | null | 2024-04-25T14:38:04+00:00 | [
"1910.09700"
] | [] | TAGS
#transformers #safetensors #arxiv-1910.09700 #endpoints_compatible #region-us
|
# Model Card for Model ID
## Model Details
### Model Description
This is the model card of a transformers model that has been pushed on the Hub. This model card has been automatically generated.
- Developed by:
- Funded by [optional]:
- Shared by [optional]:
- Model type:
- Language(s) (NLP):
- License:
- Finetuned from model [optional]:
### Model Sources [optional]
- Repository:
- Paper [optional]:
- Demo [optional]:
## Uses
### Direct Use
### Downstream Use [optional]
### Out-of-Scope Use
## Bias, Risks, and Limitations
### Recommendations
Users (both direct and downstream) should be made aware of the risks, biases and limitations of the model. More information needed for further recommendations.
## How to Get Started with the Model
Use the code below to get started with the model.
## Training Details
### Training Data
### Training Procedure
#### Preprocessing [optional]
#### Training Hyperparameters
- Training regime:
#### Speeds, Sizes, Times [optional]
## Evaluation
### Testing Data, Factors & Metrics
#### Testing Data
#### Factors
#### Metrics
### Results
#### Summary
## Model Examination [optional]
## Environmental Impact
Carbon emissions can be estimated using the Machine Learning Impact calculator presented in Lacoste et al. (2019).
- Hardware Type:
- Hours used:
- Cloud Provider:
- Compute Region:
- Carbon Emitted:
## Technical Specifications [optional]
### Model Architecture and Objective
### Compute Infrastructure
#### Hardware
#### Software
[optional]
BibTeX:
APA:
## Glossary [optional]
## More Information [optional]
## Model Card Authors [optional]
## Model Card Contact
| [
"# Model Card for Model ID",
"## Model Details",
"### Model Description\n\n\n\nThis is the model card of a transformers model that has been pushed on the Hub. This model card has been automatically generated.\n\n- Developed by: \n- Funded by [optional]: \n- Shared by [optional]: \n- Model type: \n- Language(s) (NLP): \n- License: \n- Finetuned from model [optional]:",
"### Model Sources [optional]\n\n\n\n- Repository: \n- Paper [optional]: \n- Demo [optional]:",
"## Uses",
"### Direct Use",
"### Downstream Use [optional]",
"### Out-of-Scope Use",
"## Bias, Risks, and Limitations",
"### Recommendations\n\n\n\nUsers (both direct and downstream) should be made aware of the risks, biases and limitations of the model. More information needed for further recommendations.",
"## How to Get Started with the Model\n\nUse the code below to get started with the model.",
"## Training Details",
"### Training Data",
"### Training Procedure",
"#### Preprocessing [optional]",
"#### Training Hyperparameters\n\n- Training regime:",
"#### Speeds, Sizes, Times [optional]",
"## Evaluation",
"### Testing Data, Factors & Metrics",
"#### Testing Data",
"#### Factors",
"#### Metrics",
"### Results",
"#### Summary",
"## Model Examination [optional]",
"## Environmental Impact\n\n\n\nCarbon emissions can be estimated using the Machine Learning Impact calculator presented in Lacoste et al. (2019).\n\n- Hardware Type: \n- Hours used: \n- Cloud Provider: \n- Compute Region: \n- Carbon Emitted:",
"## Technical Specifications [optional]",
"### Model Architecture and Objective",
"### Compute Infrastructure",
"#### Hardware",
"#### Software\n\n\n\n[optional]\n\n\n\nBibTeX:\n\n\n\nAPA:",
"## Glossary [optional]",
"## More Information [optional]",
"## Model Card Authors [optional]",
"## Model Card Contact"
] | [
"TAGS\n#transformers #safetensors #arxiv-1910.09700 #endpoints_compatible #region-us \n",
"# Model Card for Model ID",
"## Model Details",
"### Model Description\n\n\n\nThis is the model card of a transformers model that has been pushed on the Hub. This model card has been automatically generated.\n\n- Developed by: \n- Funded by [optional]: \n- Shared by [optional]: \n- Model type: \n- Language(s) (NLP): \n- License: \n- Finetuned from model [optional]:",
"### Model Sources [optional]\n\n\n\n- Repository: \n- Paper [optional]: \n- Demo [optional]:",
"## Uses",
"### Direct Use",
"### Downstream Use [optional]",
"### Out-of-Scope Use",
"## Bias, Risks, and Limitations",
"### Recommendations\n\n\n\nUsers (both direct and downstream) should be made aware of the risks, biases and limitations of the model. More information needed for further recommendations.",
"## How to Get Started with the Model\n\nUse the code below to get started with the model.",
"## Training Details",
"### Training Data",
"### Training Procedure",
"#### Preprocessing [optional]",
"#### Training Hyperparameters\n\n- Training regime:",
"#### Speeds, Sizes, Times [optional]",
"## Evaluation",
"### Testing Data, Factors & Metrics",
"#### Testing Data",
"#### Factors",
"#### Metrics",
"### Results",
"#### Summary",
"## Model Examination [optional]",
"## Environmental Impact\n\n\n\nCarbon emissions can be estimated using the Machine Learning Impact calculator presented in Lacoste et al. (2019).\n\n- Hardware Type: \n- Hours used: \n- Cloud Provider: \n- Compute Region: \n- Carbon Emitted:",
"## Technical Specifications [optional]",
"### Model Architecture and Objective",
"### Compute Infrastructure",
"#### Hardware",
"#### Software\n\n\n\n[optional]\n\n\n\nBibTeX:\n\n\n\nAPA:",
"## Glossary [optional]",
"## More Information [optional]",
"## Model Card Authors [optional]",
"## Model Card Contact"
] |
null | transformers |
# Model Card for Model ID
<!-- Provide a quick summary of what the model is/does. -->
## Model Details
### Model Description
<!-- Provide a longer summary of what this model is. -->
This is the model card of a 🤗 transformers model that has been pushed on the Hub. This model card has been automatically generated.
- **Developed by:** [More Information Needed]
- **Funded by [optional]:** [More Information Needed]
- **Shared by [optional]:** [More Information Needed]
- **Model type:** [More Information Needed]
- **Language(s) (NLP):** [More Information Needed]
- **License:** [More Information Needed]
- **Finetuned from model [optional]:** [More Information Needed]
### Model Sources [optional]
<!-- Provide the basic links for the model. -->
- **Repository:** [More Information Needed]
- **Paper [optional]:** [More Information Needed]
- **Demo [optional]:** [More Information Needed]
## Uses
<!-- Address questions around how the model is intended to be used, including the foreseeable users of the model and those affected by the model. -->
### Direct Use
<!-- This section is for the model use without fine-tuning or plugging into a larger ecosystem/app. -->
[More Information Needed]
### Downstream Use [optional]
<!-- This section is for the model use when fine-tuned for a task, or when plugged into a larger ecosystem/app -->
[More Information Needed]
### Out-of-Scope Use
<!-- This section addresses misuse, malicious use, and uses that the model will not work well for. -->
[More Information Needed]
## Bias, Risks, and Limitations
<!-- This section is meant to convey both technical and sociotechnical limitations. -->
[More Information Needed]
### Recommendations
<!-- This section is meant to convey recommendations with respect to the bias, risk, and technical limitations. -->
Users (both direct and downstream) should be made aware of the risks, biases and limitations of the model. More information needed for further recommendations.
## How to Get Started with the Model
Use the code below to get started with the model.
[More Information Needed]
## Training Details
### Training Data
<!-- This should link to a Dataset Card, perhaps with a short stub of information on what the training data is all about as well as documentation related to data pre-processing or additional filtering. -->
[More Information Needed]
### Training Procedure
<!-- This relates heavily to the Technical Specifications. Content here should link to that section when it is relevant to the training procedure. -->
#### Preprocessing [optional]
[More Information Needed]
#### Training Hyperparameters
- **Training regime:** [More Information Needed] <!--fp32, fp16 mixed precision, bf16 mixed precision, bf16 non-mixed precision, fp16 non-mixed precision, fp8 mixed precision -->
#### Speeds, Sizes, Times [optional]
<!-- This section provides information about throughput, start/end time, checkpoint size if relevant, etc. -->
[More Information Needed]
## Evaluation
<!-- This section describes the evaluation protocols and provides the results. -->
### Testing Data, Factors & Metrics
#### Testing Data
<!-- This should link to a Dataset Card if possible. -->
[More Information Needed]
#### Factors
<!-- These are the things the evaluation is disaggregating by, e.g., subpopulations or domains. -->
[More Information Needed]
#### Metrics
<!-- These are the evaluation metrics being used, ideally with a description of why. -->
[More Information Needed]
### Results
[More Information Needed]
#### Summary
## Model Examination [optional]
<!-- Relevant interpretability work for the model goes here -->
[More Information Needed]
## Environmental Impact
<!-- Total emissions (in grams of CO2eq) and additional considerations, such as electricity usage, go here. Edit the suggested text below accordingly -->
Carbon emissions can be estimated using the [Machine Learning Impact calculator](https://mlco2.github.io/impact#compute) presented in [Lacoste et al. (2019)](https://arxiv.org/abs/1910.09700).
- **Hardware Type:** [More Information Needed]
- **Hours used:** [More Information Needed]
- **Cloud Provider:** [More Information Needed]
- **Compute Region:** [More Information Needed]
- **Carbon Emitted:** [More Information Needed]
## Technical Specifications [optional]
### Model Architecture and Objective
[More Information Needed]
### Compute Infrastructure
[More Information Needed]
#### Hardware
[More Information Needed]
#### Software
[More Information Needed]
## Citation [optional]
<!-- If there is a paper or blog post introducing the model, the APA and Bibtex information for that should go in this section. -->
**BibTeX:**
[More Information Needed]
**APA:**
[More Information Needed]
## Glossary [optional]
<!-- If relevant, include terms and calculations in this section that can help readers understand the model or model card. -->
[More Information Needed]
## More Information [optional]
[More Information Needed]
## Model Card Authors [optional]
[More Information Needed]
## Model Card Contact
[More Information Needed] | {"library_name": "transformers", "tags": []} | tutuhu/style2 | null | [
"transformers",
"safetensors",
"arxiv:1910.09700",
"endpoints_compatible",
"region:us"
] | null | 2024-04-25T14:38:24+00:00 | [
"1910.09700"
] | [] | TAGS
#transformers #safetensors #arxiv-1910.09700 #endpoints_compatible #region-us
|
# Model Card for Model ID
## Model Details
### Model Description
This is the model card of a transformers model that has been pushed on the Hub. This model card has been automatically generated.
- Developed by:
- Funded by [optional]:
- Shared by [optional]:
- Model type:
- Language(s) (NLP):
- License:
- Finetuned from model [optional]:
### Model Sources [optional]
- Repository:
- Paper [optional]:
- Demo [optional]:
## Uses
### Direct Use
### Downstream Use [optional]
### Out-of-Scope Use
## Bias, Risks, and Limitations
### Recommendations
Users (both direct and downstream) should be made aware of the risks, biases and limitations of the model. More information needed for further recommendations.
## How to Get Started with the Model
Use the code below to get started with the model.
## Training Details
### Training Data
### Training Procedure
#### Preprocessing [optional]
#### Training Hyperparameters
- Training regime:
#### Speeds, Sizes, Times [optional]
## Evaluation
### Testing Data, Factors & Metrics
#### Testing Data
#### Factors
#### Metrics
### Results
#### Summary
## Model Examination [optional]
## Environmental Impact
Carbon emissions can be estimated using the Machine Learning Impact calculator presented in Lacoste et al. (2019).
- Hardware Type:
- Hours used:
- Cloud Provider:
- Compute Region:
- Carbon Emitted:
## Technical Specifications [optional]
### Model Architecture and Objective
### Compute Infrastructure
#### Hardware
#### Software
[optional]
BibTeX:
APA:
## Glossary [optional]
## More Information [optional]
## Model Card Authors [optional]
## Model Card Contact
| [
"# Model Card for Model ID",
"## Model Details",
"### Model Description\n\n\n\nThis is the model card of a transformers model that has been pushed on the Hub. This model card has been automatically generated.\n\n- Developed by: \n- Funded by [optional]: \n- Shared by [optional]: \n- Model type: \n- Language(s) (NLP): \n- License: \n- Finetuned from model [optional]:",
"### Model Sources [optional]\n\n\n\n- Repository: \n- Paper [optional]: \n- Demo [optional]:",
"## Uses",
"### Direct Use",
"### Downstream Use [optional]",
"### Out-of-Scope Use",
"## Bias, Risks, and Limitations",
"### Recommendations\n\n\n\nUsers (both direct and downstream) should be made aware of the risks, biases and limitations of the model. More information needed for further recommendations.",
"## How to Get Started with the Model\n\nUse the code below to get started with the model.",
"## Training Details",
"### Training Data",
"### Training Procedure",
"#### Preprocessing [optional]",
"#### Training Hyperparameters\n\n- Training regime:",
"#### Speeds, Sizes, Times [optional]",
"## Evaluation",
"### Testing Data, Factors & Metrics",
"#### Testing Data",
"#### Factors",
"#### Metrics",
"### Results",
"#### Summary",
"## Model Examination [optional]",
"## Environmental Impact\n\n\n\nCarbon emissions can be estimated using the Machine Learning Impact calculator presented in Lacoste et al. (2019).\n\n- Hardware Type: \n- Hours used: \n- Cloud Provider: \n- Compute Region: \n- Carbon Emitted:",
"## Technical Specifications [optional]",
"### Model Architecture and Objective",
"### Compute Infrastructure",
"#### Hardware",
"#### Software\n\n\n\n[optional]\n\n\n\nBibTeX:\n\n\n\nAPA:",
"## Glossary [optional]",
"## More Information [optional]",
"## Model Card Authors [optional]",
"## Model Card Contact"
] | [
"TAGS\n#transformers #safetensors #arxiv-1910.09700 #endpoints_compatible #region-us \n",
"# Model Card for Model ID",
"## Model Details",
"### Model Description\n\n\n\nThis is the model card of a transformers model that has been pushed on the Hub. This model card has been automatically generated.\n\n- Developed by: \n- Funded by [optional]: \n- Shared by [optional]: \n- Model type: \n- Language(s) (NLP): \n- License: \n- Finetuned from model [optional]:",
"### Model Sources [optional]\n\n\n\n- Repository: \n- Paper [optional]: \n- Demo [optional]:",
"## Uses",
"### Direct Use",
"### Downstream Use [optional]",
"### Out-of-Scope Use",
"## Bias, Risks, and Limitations",
"### Recommendations\n\n\n\nUsers (both direct and downstream) should be made aware of the risks, biases and limitations of the model. More information needed for further recommendations.",
"## How to Get Started with the Model\n\nUse the code below to get started with the model.",
"## Training Details",
"### Training Data",
"### Training Procedure",
"#### Preprocessing [optional]",
"#### Training Hyperparameters\n\n- Training regime:",
"#### Speeds, Sizes, Times [optional]",
"## Evaluation",
"### Testing Data, Factors & Metrics",
"#### Testing Data",
"#### Factors",
"#### Metrics",
"### Results",
"#### Summary",
"## Model Examination [optional]",
"## Environmental Impact\n\n\n\nCarbon emissions can be estimated using the Machine Learning Impact calculator presented in Lacoste et al. (2019).\n\n- Hardware Type: \n- Hours used: \n- Cloud Provider: \n- Compute Region: \n- Carbon Emitted:",
"## Technical Specifications [optional]",
"### Model Architecture and Objective",
"### Compute Infrastructure",
"#### Hardware",
"#### Software\n\n\n\n[optional]\n\n\n\nBibTeX:\n\n\n\nAPA:",
"## Glossary [optional]",
"## More Information [optional]",
"## Model Card Authors [optional]",
"## Model Card Contact"
] |
null | transformers |
# Model Card for Model ID
<!-- Provide a quick summary of what the model is/does. -->
## Model Details
### Model Description
<!-- Provide a longer summary of what this model is. -->
This is the model card of a 🤗 transformers model that has been pushed on the Hub. This model card has been automatically generated.
- **Developed by:** [More Information Needed]
- **Funded by [optional]:** [More Information Needed]
- **Shared by [optional]:** [More Information Needed]
- **Model type:** [More Information Needed]
- **Language(s) (NLP):** [More Information Needed]
- **License:** [More Information Needed]
- **Finetuned from model [optional]:** [More Information Needed]
### Model Sources [optional]
<!-- Provide the basic links for the model. -->
- **Repository:** [More Information Needed]
- **Paper [optional]:** [More Information Needed]
- **Demo [optional]:** [More Information Needed]
## Uses
<!-- Address questions around how the model is intended to be used, including the foreseeable users of the model and those affected by the model. -->
### Direct Use
<!-- This section is for the model use without fine-tuning or plugging into a larger ecosystem/app. -->
[More Information Needed]
### Downstream Use [optional]
<!-- This section is for the model use when fine-tuned for a task, or when plugged into a larger ecosystem/app -->
[More Information Needed]
### Out-of-Scope Use
<!-- This section addresses misuse, malicious use, and uses that the model will not work well for. -->
[More Information Needed]
## Bias, Risks, and Limitations
<!-- This section is meant to convey both technical and sociotechnical limitations. -->
[More Information Needed]
### Recommendations
<!-- This section is meant to convey recommendations with respect to the bias, risk, and technical limitations. -->
Users (both direct and downstream) should be made aware of the risks, biases and limitations of the model. More information needed for further recommendations.
## How to Get Started with the Model
Use the code below to get started with the model.
[More Information Needed]
## Training Details
### Training Data
<!-- This should link to a Dataset Card, perhaps with a short stub of information on what the training data is all about as well as documentation related to data pre-processing or additional filtering. -->
[More Information Needed]
### Training Procedure
<!-- This relates heavily to the Technical Specifications. Content here should link to that section when it is relevant to the training procedure. -->
#### Preprocessing [optional]
[More Information Needed]
#### Training Hyperparameters
- **Training regime:** [More Information Needed] <!--fp32, fp16 mixed precision, bf16 mixed precision, bf16 non-mixed precision, fp16 non-mixed precision, fp8 mixed precision -->
#### Speeds, Sizes, Times [optional]
<!-- This section provides information about throughput, start/end time, checkpoint size if relevant, etc. -->
[More Information Needed]
## Evaluation
<!-- This section describes the evaluation protocols and provides the results. -->
### Testing Data, Factors & Metrics
#### Testing Data
<!-- This should link to a Dataset Card if possible. -->
[More Information Needed]
#### Factors
<!-- These are the things the evaluation is disaggregating by, e.g., subpopulations or domains. -->
[More Information Needed]
#### Metrics
<!-- These are the evaluation metrics being used, ideally with a description of why. -->
[More Information Needed]
### Results
[More Information Needed]
#### Summary
## Model Examination [optional]
<!-- Relevant interpretability work for the model goes here -->
[More Information Needed]
## Environmental Impact
<!-- Total emissions (in grams of CO2eq) and additional considerations, such as electricity usage, go here. Edit the suggested text below accordingly -->
Carbon emissions can be estimated using the [Machine Learning Impact calculator](https://mlco2.github.io/impact#compute) presented in [Lacoste et al. (2019)](https://arxiv.org/abs/1910.09700).
- **Hardware Type:** [More Information Needed]
- **Hours used:** [More Information Needed]
- **Cloud Provider:** [More Information Needed]
- **Compute Region:** [More Information Needed]
- **Carbon Emitted:** [More Information Needed]
## Technical Specifications [optional]
### Model Architecture and Objective
[More Information Needed]
### Compute Infrastructure
[More Information Needed]
#### Hardware
[More Information Needed]
#### Software
[More Information Needed]
## Citation [optional]
<!-- If there is a paper or blog post introducing the model, the APA and Bibtex information for that should go in this section. -->
**BibTeX:**
[More Information Needed]
**APA:**
[More Information Needed]
## Glossary [optional]
<!-- If relevant, include terms and calculations in this section that can help readers understand the model or model card. -->
[More Information Needed]
## More Information [optional]
[More Information Needed]
## Model Card Authors [optional]
[More Information Needed]
## Model Card Contact
[More Information Needed] | {"library_name": "transformers", "tags": []} | tutuhu/style3 | null | [
"transformers",
"safetensors",
"arxiv:1910.09700",
"endpoints_compatible",
"region:us"
] | null | 2024-04-25T14:38:57+00:00 | [
"1910.09700"
] | [] | TAGS
#transformers #safetensors #arxiv-1910.09700 #endpoints_compatible #region-us
|
# Model Card for Model ID
## Model Details
### Model Description
This is the model card of a transformers model that has been pushed on the Hub. This model card has been automatically generated.
- Developed by:
- Funded by [optional]:
- Shared by [optional]:
- Model type:
- Language(s) (NLP):
- License:
- Finetuned from model [optional]:
### Model Sources [optional]
- Repository:
- Paper [optional]:
- Demo [optional]:
## Uses
### Direct Use
### Downstream Use [optional]
### Out-of-Scope Use
## Bias, Risks, and Limitations
### Recommendations
Users (both direct and downstream) should be made aware of the risks, biases and limitations of the model. More information needed for further recommendations.
## How to Get Started with the Model
Use the code below to get started with the model.
## Training Details
### Training Data
### Training Procedure
#### Preprocessing [optional]
#### Training Hyperparameters
- Training regime:
#### Speeds, Sizes, Times [optional]
## Evaluation
### Testing Data, Factors & Metrics
#### Testing Data
#### Factors
#### Metrics
### Results
#### Summary
## Model Examination [optional]
## Environmental Impact
Carbon emissions can be estimated using the Machine Learning Impact calculator presented in Lacoste et al. (2019).
- Hardware Type:
- Hours used:
- Cloud Provider:
- Compute Region:
- Carbon Emitted:
## Technical Specifications [optional]
### Model Architecture and Objective
### Compute Infrastructure
#### Hardware
#### Software
[optional]
BibTeX:
APA:
## Glossary [optional]
## More Information [optional]
## Model Card Authors [optional]
## Model Card Contact
| [
"# Model Card for Model ID",
"## Model Details",
"### Model Description\n\n\n\nThis is the model card of a transformers model that has been pushed on the Hub. This model card has been automatically generated.\n\n- Developed by: \n- Funded by [optional]: \n- Shared by [optional]: \n- Model type: \n- Language(s) (NLP): \n- License: \n- Finetuned from model [optional]:",
"### Model Sources [optional]\n\n\n\n- Repository: \n- Paper [optional]: \n- Demo [optional]:",
"## Uses",
"### Direct Use",
"### Downstream Use [optional]",
"### Out-of-Scope Use",
"## Bias, Risks, and Limitations",
"### Recommendations\n\n\n\nUsers (both direct and downstream) should be made aware of the risks, biases and limitations of the model. More information needed for further recommendations.",
"## How to Get Started with the Model\n\nUse the code below to get started with the model.",
"## Training Details",
"### Training Data",
"### Training Procedure",
"#### Preprocessing [optional]",
"#### Training Hyperparameters\n\n- Training regime:",
"#### Speeds, Sizes, Times [optional]",
"## Evaluation",
"### Testing Data, Factors & Metrics",
"#### Testing Data",
"#### Factors",
"#### Metrics",
"### Results",
"#### Summary",
"## Model Examination [optional]",
"## Environmental Impact\n\n\n\nCarbon emissions can be estimated using the Machine Learning Impact calculator presented in Lacoste et al. (2019).\n\n- Hardware Type: \n- Hours used: \n- Cloud Provider: \n- Compute Region: \n- Carbon Emitted:",
"## Technical Specifications [optional]",
"### Model Architecture and Objective",
"### Compute Infrastructure",
"#### Hardware",
"#### Software\n\n\n\n[optional]\n\n\n\nBibTeX:\n\n\n\nAPA:",
"## Glossary [optional]",
"## More Information [optional]",
"## Model Card Authors [optional]",
"## Model Card Contact"
] | [
"TAGS\n#transformers #safetensors #arxiv-1910.09700 #endpoints_compatible #region-us \n",
"# Model Card for Model ID",
"## Model Details",
"### Model Description\n\n\n\nThis is the model card of a transformers model that has been pushed on the Hub. This model card has been automatically generated.\n\n- Developed by: \n- Funded by [optional]: \n- Shared by [optional]: \n- Model type: \n- Language(s) (NLP): \n- License: \n- Finetuned from model [optional]:",
"### Model Sources [optional]\n\n\n\n- Repository: \n- Paper [optional]: \n- Demo [optional]:",
"## Uses",
"### Direct Use",
"### Downstream Use [optional]",
"### Out-of-Scope Use",
"## Bias, Risks, and Limitations",
"### Recommendations\n\n\n\nUsers (both direct and downstream) should be made aware of the risks, biases and limitations of the model. More information needed for further recommendations.",
"## How to Get Started with the Model\n\nUse the code below to get started with the model.",
"## Training Details",
"### Training Data",
"### Training Procedure",
"#### Preprocessing [optional]",
"#### Training Hyperparameters\n\n- Training regime:",
"#### Speeds, Sizes, Times [optional]",
"## Evaluation",
"### Testing Data, Factors & Metrics",
"#### Testing Data",
"#### Factors",
"#### Metrics",
"### Results",
"#### Summary",
"## Model Examination [optional]",
"## Environmental Impact\n\n\n\nCarbon emissions can be estimated using the Machine Learning Impact calculator presented in Lacoste et al. (2019).\n\n- Hardware Type: \n- Hours used: \n- Cloud Provider: \n- Compute Region: \n- Carbon Emitted:",
"## Technical Specifications [optional]",
"### Model Architecture and Objective",
"### Compute Infrastructure",
"#### Hardware",
"#### Software\n\n\n\n[optional]\n\n\n\nBibTeX:\n\n\n\nAPA:",
"## Glossary [optional]",
"## More Information [optional]",
"## Model Card Authors [optional]",
"## Model Card Contact"
] |
null | transformers |
# Model Card for Model ID
<!-- Provide a quick summary of what the model is/does. -->
## Model Details
### Model Description
<!-- Provide a longer summary of what this model is. -->
This is the model card of a 🤗 transformers model that has been pushed on the Hub. This model card has been automatically generated.
- **Developed by:** [More Information Needed]
- **Funded by [optional]:** [More Information Needed]
- **Shared by [optional]:** [More Information Needed]
- **Model type:** [More Information Needed]
- **Language(s) (NLP):** [More Information Needed]
- **License:** [More Information Needed]
- **Finetuned from model [optional]:** [More Information Needed]
### Model Sources [optional]
<!-- Provide the basic links for the model. -->
- **Repository:** [More Information Needed]
- **Paper [optional]:** [More Information Needed]
- **Demo [optional]:** [More Information Needed]
## Uses
<!-- Address questions around how the model is intended to be used, including the foreseeable users of the model and those affected by the model. -->
### Direct Use
<!-- This section is for the model use without fine-tuning or plugging into a larger ecosystem/app. -->
[More Information Needed]
### Downstream Use [optional]
<!-- This section is for the model use when fine-tuned for a task, or when plugged into a larger ecosystem/app -->
[More Information Needed]
### Out-of-Scope Use
<!-- This section addresses misuse, malicious use, and uses that the model will not work well for. -->
[More Information Needed]
## Bias, Risks, and Limitations
<!-- This section is meant to convey both technical and sociotechnical limitations. -->
[More Information Needed]
### Recommendations
<!-- This section is meant to convey recommendations with respect to the bias, risk, and technical limitations. -->
Users (both direct and downstream) should be made aware of the risks, biases and limitations of the model. More information needed for further recommendations.
## How to Get Started with the Model
Use the code below to get started with the model.
[More Information Needed]
## Training Details
### Training Data
<!-- This should link to a Dataset Card, perhaps with a short stub of information on what the training data is all about as well as documentation related to data pre-processing or additional filtering. -->
[More Information Needed]
### Training Procedure
<!-- This relates heavily to the Technical Specifications. Content here should link to that section when it is relevant to the training procedure. -->
#### Preprocessing [optional]
[More Information Needed]
#### Training Hyperparameters
- **Training regime:** [More Information Needed] <!--fp32, fp16 mixed precision, bf16 mixed precision, bf16 non-mixed precision, fp16 non-mixed precision, fp8 mixed precision -->
#### Speeds, Sizes, Times [optional]
<!-- This section provides information about throughput, start/end time, checkpoint size if relevant, etc. -->
[More Information Needed]
## Evaluation
<!-- This section describes the evaluation protocols and provides the results. -->
### Testing Data, Factors & Metrics
#### Testing Data
<!-- This should link to a Dataset Card if possible. -->
[More Information Needed]
#### Factors
<!-- These are the things the evaluation is disaggregating by, e.g., subpopulations or domains. -->
[More Information Needed]
#### Metrics
<!-- These are the evaluation metrics being used, ideally with a description of why. -->
[More Information Needed]
### Results
[More Information Needed]
#### Summary
## Model Examination [optional]
<!-- Relevant interpretability work for the model goes here -->
[More Information Needed]
## Environmental Impact
<!-- Total emissions (in grams of CO2eq) and additional considerations, such as electricity usage, go here. Edit the suggested text below accordingly -->
Carbon emissions can be estimated using the [Machine Learning Impact calculator](https://mlco2.github.io/impact#compute) presented in [Lacoste et al. (2019)](https://arxiv.org/abs/1910.09700).
- **Hardware Type:** [More Information Needed]
- **Hours used:** [More Information Needed]
- **Cloud Provider:** [More Information Needed]
- **Compute Region:** [More Information Needed]
- **Carbon Emitted:** [More Information Needed]
## Technical Specifications [optional]
### Model Architecture and Objective
[More Information Needed]
### Compute Infrastructure
[More Information Needed]
#### Hardware
[More Information Needed]
#### Software
[More Information Needed]
## Citation [optional]
<!-- If there is a paper or blog post introducing the model, the APA and Bibtex information for that should go in this section. -->
**BibTeX:**
[More Information Needed]
**APA:**
[More Information Needed]
## Glossary [optional]
<!-- If relevant, include terms and calculations in this section that can help readers understand the model or model card. -->
[More Information Needed]
## More Information [optional]
[More Information Needed]
## Model Card Authors [optional]
[More Information Needed]
## Model Card Contact
[More Information Needed] | {"library_name": "transformers", "tags": []} | tutuhu/style4 | null | [
"transformers",
"safetensors",
"arxiv:1910.09700",
"endpoints_compatible",
"region:us"
] | null | 2024-04-25T14:39:07+00:00 | [
"1910.09700"
] | [] | TAGS
#transformers #safetensors #arxiv-1910.09700 #endpoints_compatible #region-us
|
# Model Card for Model ID
## Model Details
### Model Description
This is the model card of a transformers model that has been pushed on the Hub. This model card has been automatically generated.
- Developed by:
- Funded by [optional]:
- Shared by [optional]:
- Model type:
- Language(s) (NLP):
- License:
- Finetuned from model [optional]:
### Model Sources [optional]
- Repository:
- Paper [optional]:
- Demo [optional]:
## Uses
### Direct Use
### Downstream Use [optional]
### Out-of-Scope Use
## Bias, Risks, and Limitations
### Recommendations
Users (both direct and downstream) should be made aware of the risks, biases and limitations of the model. More information needed for further recommendations.
## How to Get Started with the Model
Use the code below to get started with the model.
## Training Details
### Training Data
### Training Procedure
#### Preprocessing [optional]
#### Training Hyperparameters
- Training regime:
#### Speeds, Sizes, Times [optional]
## Evaluation
### Testing Data, Factors & Metrics
#### Testing Data
#### Factors
#### Metrics
### Results
#### Summary
## Model Examination [optional]
## Environmental Impact
Carbon emissions can be estimated using the Machine Learning Impact calculator presented in Lacoste et al. (2019).
- Hardware Type:
- Hours used:
- Cloud Provider:
- Compute Region:
- Carbon Emitted:
## Technical Specifications [optional]
### Model Architecture and Objective
### Compute Infrastructure
#### Hardware
#### Software
[optional]
BibTeX:
APA:
## Glossary [optional]
## More Information [optional]
## Model Card Authors [optional]
## Model Card Contact
| [
"# Model Card for Model ID",
"## Model Details",
"### Model Description\n\n\n\nThis is the model card of a transformers model that has been pushed on the Hub. This model card has been automatically generated.\n\n- Developed by: \n- Funded by [optional]: \n- Shared by [optional]: \n- Model type: \n- Language(s) (NLP): \n- License: \n- Finetuned from model [optional]:",
"### Model Sources [optional]\n\n\n\n- Repository: \n- Paper [optional]: \n- Demo [optional]:",
"## Uses",
"### Direct Use",
"### Downstream Use [optional]",
"### Out-of-Scope Use",
"## Bias, Risks, and Limitations",
"### Recommendations\n\n\n\nUsers (both direct and downstream) should be made aware of the risks, biases and limitations of the model. More information needed for further recommendations.",
"## How to Get Started with the Model\n\nUse the code below to get started with the model.",
"## Training Details",
"### Training Data",
"### Training Procedure",
"#### Preprocessing [optional]",
"#### Training Hyperparameters\n\n- Training regime:",
"#### Speeds, Sizes, Times [optional]",
"## Evaluation",
"### Testing Data, Factors & Metrics",
"#### Testing Data",
"#### Factors",
"#### Metrics",
"### Results",
"#### Summary",
"## Model Examination [optional]",
"## Environmental Impact\n\n\n\nCarbon emissions can be estimated using the Machine Learning Impact calculator presented in Lacoste et al. (2019).\n\n- Hardware Type: \n- Hours used: \n- Cloud Provider: \n- Compute Region: \n- Carbon Emitted:",
"## Technical Specifications [optional]",
"### Model Architecture and Objective",
"### Compute Infrastructure",
"#### Hardware",
"#### Software\n\n\n\n[optional]\n\n\n\nBibTeX:\n\n\n\nAPA:",
"## Glossary [optional]",
"## More Information [optional]",
"## Model Card Authors [optional]",
"## Model Card Contact"
] | [
"TAGS\n#transformers #safetensors #arxiv-1910.09700 #endpoints_compatible #region-us \n",
"# Model Card for Model ID",
"## Model Details",
"### Model Description\n\n\n\nThis is the model card of a transformers model that has been pushed on the Hub. This model card has been automatically generated.\n\n- Developed by: \n- Funded by [optional]: \n- Shared by [optional]: \n- Model type: \n- Language(s) (NLP): \n- License: \n- Finetuned from model [optional]:",
"### Model Sources [optional]\n\n\n\n- Repository: \n- Paper [optional]: \n- Demo [optional]:",
"## Uses",
"### Direct Use",
"### Downstream Use [optional]",
"### Out-of-Scope Use",
"## Bias, Risks, and Limitations",
"### Recommendations\n\n\n\nUsers (both direct and downstream) should be made aware of the risks, biases and limitations of the model. More information needed for further recommendations.",
"## How to Get Started with the Model\n\nUse the code below to get started with the model.",
"## Training Details",
"### Training Data",
"### Training Procedure",
"#### Preprocessing [optional]",
"#### Training Hyperparameters\n\n- Training regime:",
"#### Speeds, Sizes, Times [optional]",
"## Evaluation",
"### Testing Data, Factors & Metrics",
"#### Testing Data",
"#### Factors",
"#### Metrics",
"### Results",
"#### Summary",
"## Model Examination [optional]",
"## Environmental Impact\n\n\n\nCarbon emissions can be estimated using the Machine Learning Impact calculator presented in Lacoste et al. (2019).\n\n- Hardware Type: \n- Hours used: \n- Cloud Provider: \n- Compute Region: \n- Carbon Emitted:",
"## Technical Specifications [optional]",
"### Model Architecture and Objective",
"### Compute Infrastructure",
"#### Hardware",
"#### Software\n\n\n\n[optional]\n\n\n\nBibTeX:\n\n\n\nAPA:",
"## Glossary [optional]",
"## More Information [optional]",
"## Model Card Authors [optional]",
"## Model Card Contact"
] |
null | transformers | ## About
<!-- ### quantize_version: 1 -->
<!-- ### output_tensor_quantised: 1 -->
<!-- ### convert_type: -->
<!-- ### vocab_type: -->
static quants of https://huggingface.co/Superoisesuki/Mistral_7B_CrewAI
<!-- provided-files -->
weighted/imatrix quants seem not to be available (by me) at this time. If they do not show up a week or so after the static ones, I have probably not planned for them. Feel free to request them by opening a Community Discussion.
## Usage
If you are unsure how to use GGUF files, refer to one of [TheBloke's
READMEs](https://huggingface.co/TheBloke/KafkaLM-70B-German-V0.1-GGUF) for
more details, including on how to concatenate multi-part files.
## Provided Quants
(sorted by size, not necessarily quality. IQ-quants are often preferable over similar sized non-IQ quants)
| Link | Type | Size/GB | Notes |
|:-----|:-----|--------:|:------|
| [GGUF](https://huggingface.co/mradermacher/Mistral_7B_CrewAI-GGUF/resolve/main/Mistral_7B_CrewAI.Q2_K.gguf) | Q2_K | 2.8 | |
| [GGUF](https://huggingface.co/mradermacher/Mistral_7B_CrewAI-GGUF/resolve/main/Mistral_7B_CrewAI.IQ3_XS.gguf) | IQ3_XS | 3.1 | |
| [GGUF](https://huggingface.co/mradermacher/Mistral_7B_CrewAI-GGUF/resolve/main/Mistral_7B_CrewAI.Q3_K_S.gguf) | Q3_K_S | 3.3 | |
| [GGUF](https://huggingface.co/mradermacher/Mistral_7B_CrewAI-GGUF/resolve/main/Mistral_7B_CrewAI.IQ3_S.gguf) | IQ3_S | 3.3 | beats Q3_K* |
| [GGUF](https://huggingface.co/mradermacher/Mistral_7B_CrewAI-GGUF/resolve/main/Mistral_7B_CrewAI.IQ3_M.gguf) | IQ3_M | 3.4 | |
| [GGUF](https://huggingface.co/mradermacher/Mistral_7B_CrewAI-GGUF/resolve/main/Mistral_7B_CrewAI.Q3_K_M.gguf) | Q3_K_M | 3.6 | lower quality |
| [GGUF](https://huggingface.co/mradermacher/Mistral_7B_CrewAI-GGUF/resolve/main/Mistral_7B_CrewAI.Q3_K_L.gguf) | Q3_K_L | 3.9 | |
| [GGUF](https://huggingface.co/mradermacher/Mistral_7B_CrewAI-GGUF/resolve/main/Mistral_7B_CrewAI.IQ4_XS.gguf) | IQ4_XS | 4.0 | |
| [GGUF](https://huggingface.co/mradermacher/Mistral_7B_CrewAI-GGUF/resolve/main/Mistral_7B_CrewAI.Q4_K_S.gguf) | Q4_K_S | 4.2 | fast, recommended |
| [GGUF](https://huggingface.co/mradermacher/Mistral_7B_CrewAI-GGUF/resolve/main/Mistral_7B_CrewAI.Q4_K_M.gguf) | Q4_K_M | 4.5 | fast, recommended |
| [GGUF](https://huggingface.co/mradermacher/Mistral_7B_CrewAI-GGUF/resolve/main/Mistral_7B_CrewAI.Q5_K_S.gguf) | Q5_K_S | 5.1 | |
| [GGUF](https://huggingface.co/mradermacher/Mistral_7B_CrewAI-GGUF/resolve/main/Mistral_7B_CrewAI.Q5_K_M.gguf) | Q5_K_M | 5.2 | |
| [GGUF](https://huggingface.co/mradermacher/Mistral_7B_CrewAI-GGUF/resolve/main/Mistral_7B_CrewAI.Q6_K.gguf) | Q6_K | 6.0 | very good quality |
| [GGUF](https://huggingface.co/mradermacher/Mistral_7B_CrewAI-GGUF/resolve/main/Mistral_7B_CrewAI.Q8_0.gguf) | Q8_0 | 7.8 | fast, best quality |
| [GGUF](https://huggingface.co/mradermacher/Mistral_7B_CrewAI-GGUF/resolve/main/Mistral_7B_CrewAI.f16.gguf) | f16 | 14.6 | 16 bpw, overkill |
Here is a handy graph by ikawrakow comparing some lower-quality quant
types (lower is better):

And here are Artefact2's thoughts on the matter:
https://gist.github.com/Artefact2/b5f810600771265fc1e39442288e8ec9
## FAQ / Model Request
See https://huggingface.co/mradermacher/model_requests for some answers to
questions you might have and/or if you want some other model quantized.
## Thanks
I thank my company, [nethype GmbH](https://www.nethype.de/), for letting
me use its servers and providing upgrades to my workstation to enable
this work in my free time.
<!-- end -->
| {"language": ["en"], "library_name": "transformers", "base_model": "Superoisesuki/Mistral_7B_CrewAI", "quantized_by": "mradermacher"} | mradermacher/Mistral_7B_CrewAI-GGUF | null | [
"transformers",
"gguf",
"en",
"base_model:Superoisesuki/Mistral_7B_CrewAI",
"endpoints_compatible",
"region:us"
] | null | 2024-04-25T14:39:25+00:00 | [] | [
"en"
] | TAGS
#transformers #gguf #en #base_model-Superoisesuki/Mistral_7B_CrewAI #endpoints_compatible #region-us
| About
-----
static quants of URL
weighted/imatrix quants seem not to be available (by me) at this time. If they do not show up a week or so after the static ones, I have probably not planned for them. Feel free to request them by opening a Community Discussion.
Usage
-----
If you are unsure how to use GGUF files, refer to one of TheBloke's
READMEs for
more details, including on how to concatenate multi-part files.
Provided Quants
---------------
(sorted by size, not necessarily quality. IQ-quants are often preferable over similar sized non-IQ quants)
Here is a handy graph by ikawrakow comparing some lower-quality quant
types (lower is better):
!URL
And here are Artefact2's thoughts on the matter:
URL
FAQ / Model Request
-------------------
See URL for some answers to
questions you might have and/or if you want some other model quantized.
Thanks
------
I thank my company, nethype GmbH, for letting
me use its servers and providing upgrades to my workstation to enable
this work in my free time.
| [] | [
"TAGS\n#transformers #gguf #en #base_model-Superoisesuki/Mistral_7B_CrewAI #endpoints_compatible #region-us \n"
] |
object-detection | transformers |
# AISAK-Detect
## Overview:
AISAK-Detect is an integral component of the AISAK-Visual system, specializing in object detection tasks. Leveraging an encoder-decoder transformer architecture with a convolutional backbone, AISAK-Detect excels in accurately and efficiently detecting objects within images. This model enhances the image understanding capabilities of AISAK-Visual, contributing to comprehensive visual analysis. Trained and fine-tuned by the AISAK team, AISAK-Detect is designed to seamlessly integrate into the broader AISAK system, ensuring cohesive performance in image analysis tasks.
## Model Information:
- **Model Name**: AISAK-Detect
- **Version**: 1.0
- **Model Architecture**: Transformer with convolutional backbone
- **Specialization**: AISAK-Detect is a specialized model within the AISAK-Visual system, focusing on object detection tasks. It employs an encoder-decoder transformer architecture with a convolutional backbone, enabling it to effectively analyze images and generate precise object detection results. AISAK-Visual is part of the broader AISAK system and is specialized in image captioning tasks.
## Intended Use:
The model demonstrates high accuracy in object detection tasks, leveraging the synergy between its transformer-based encoder-decoder architecture and the convolutional backbone. When utilized in conjunction with AISAK-Visual, it enhances overall performance in image analysis tasks.
## Performance:
AISAK-Visual, based on the BLIP framework, achieves state-of-the-art results on image captioning tasks, including image-text retrieval, image captioning, and VQA. Its generalization ability is demonstrated by its strong performance on video-language tasks in a zero-shot manner.
## Ethical Considerations:
- **Bias Mitigation**: Efforts have been made to mitigate bias during training; however, users are encouraged to remain vigilant about potential biases in the model's output.
- **Fair Use**: Users should exercise caution when using AISAK-Visual in sensitive contexts and ensure fair and ethical use of the generated image captions.
## Limitations:
- While proficient in general object detection, AISAK-Detect may encounter challenges in scenarios requiring specialized object recognition or highly cluttered images.
- Users should be aware of these limitations and consider them when interpreting the model's outputs.
## Deployment:
AISAK-Detect's inferencing capabilities will be seamlessly integrated into the deployment of the AISAK-Visual system. This integration ensures smooth operation and maximizes the synergy between the two models, providing comprehensive image understanding and analysis.
## Caveats:
- Users should verify critical decisions based on AISAK-Detect's object detection results, particularly in high-stakes scenarios. Considering the broader context provided by AISAK-Visual is essential for a comprehensive understanding of visual content and informed decision-making.
## Model Card Information:
- **Model Card Created**: April 25, 2024
- **Last Updated**: April 25, 2024
- **Contact Information**: For any inquiries or communication regarding AISAK, please contact me at [email protected].
**© 2024 Mandela Logan. All rights reserved.**
No part of this model may be reproduced, distributed, or transmitted in any form or by any means, including photocopying, recording, or other electronic or mechanical methods, without the prior written permission of the copyright holder. Users are expressly prohibited from creating replications or spaces derived from this model, whether in whole or in part, without the explicit authorization of the copyright holder. Unauthorized use or reproduction of this model is strictly prohibited by copyright law. | {"language": ["en"], "tags": ["object-detection", "computer-vision", "image-to-text"], "pipeline_tag": "object-detection"} | aisak-ai/aisak-detect | null | [
"transformers",
"pytorch",
"safetensors",
"detr",
"object-detection",
"computer-vision",
"image-to-text",
"en",
"endpoints_compatible",
"region:us"
] | null | 2024-04-25T14:39:35+00:00 | [] | [
"en"
] | TAGS
#transformers #pytorch #safetensors #detr #object-detection #computer-vision #image-to-text #en #endpoints_compatible #region-us
|
# AISAK-Detect
## Overview:
AISAK-Detect is an integral component of the AISAK-Visual system, specializing in object detection tasks. Leveraging an encoder-decoder transformer architecture with a convolutional backbone, AISAK-Detect excels in accurately and efficiently detecting objects within images. This model enhances the image understanding capabilities of AISAK-Visual, contributing to comprehensive visual analysis. Trained and fine-tuned by the AISAK team, AISAK-Detect is designed to seamlessly integrate into the broader AISAK system, ensuring cohesive performance in image analysis tasks.
## Model Information:
- Model Name: AISAK-Detect
- Version: 1.0
- Model Architecture: Transformer with convolutional backbone
- Specialization: AISAK-Detect is a specialized model within the AISAK-Visual system, focusing on object detection tasks. It employs an encoder-decoder transformer architecture with a convolutional backbone, enabling it to effectively analyze images and generate precise object detection results. AISAK-Visual is part of the broader AISAK system and is specialized in image captioning tasks.
## Intended Use:
The model demonstrates high accuracy in object detection tasks, leveraging the synergy between its transformer-based encoder-decoder architecture and the convolutional backbone. When utilized in conjunction with AISAK-Visual, it enhances overall performance in image analysis tasks.
## Performance:
AISAK-Visual, based on the BLIP framework, achieves state-of-the-art results on image captioning tasks, including image-text retrieval, image captioning, and VQA. Its generalization ability is demonstrated by its strong performance on video-language tasks in a zero-shot manner.
## Ethical Considerations:
- Bias Mitigation: Efforts have been made to mitigate bias during training; however, users are encouraged to remain vigilant about potential biases in the model's output.
- Fair Use: Users should exercise caution when using AISAK-Visual in sensitive contexts and ensure fair and ethical use of the generated image captions.
## Limitations:
- While proficient in general object detection, AISAK-Detect may encounter challenges in scenarios requiring specialized object recognition or highly cluttered images.
- Users should be aware of these limitations and consider them when interpreting the model's outputs.
## Deployment:
AISAK-Detect's inferencing capabilities will be seamlessly integrated into the deployment of the AISAK-Visual system. This integration ensures smooth operation and maximizes the synergy between the two models, providing comprehensive image understanding and analysis.
## Caveats:
- Users should verify critical decisions based on AISAK-Detect's object detection results, particularly in high-stakes scenarios. Considering the broader context provided by AISAK-Visual is essential for a comprehensive understanding of visual content and informed decision-making.
## Model Card Information:
- Model Card Created: April 25, 2024
- Last Updated: April 25, 2024
- Contact Information: For any inquiries or communication regarding AISAK, please contact me at mandelakorilogan@URL.
© 2024 Mandela Logan. All rights reserved.
No part of this model may be reproduced, distributed, or transmitted in any form or by any means, including photocopying, recording, or other electronic or mechanical methods, without the prior written permission of the copyright holder. Users are expressly prohibited from creating replications or spaces derived from this model, whether in whole or in part, without the explicit authorization of the copyright holder. Unauthorized use or reproduction of this model is strictly prohibited by copyright law. | [
"# AISAK-Detect",
"## Overview:\n\nAISAK-Detect is an integral component of the AISAK-Visual system, specializing in object detection tasks. Leveraging an encoder-decoder transformer architecture with a convolutional backbone, AISAK-Detect excels in accurately and efficiently detecting objects within images. This model enhances the image understanding capabilities of AISAK-Visual, contributing to comprehensive visual analysis. Trained and fine-tuned by the AISAK team, AISAK-Detect is designed to seamlessly integrate into the broader AISAK system, ensuring cohesive performance in image analysis tasks.",
"## Model Information:\n\n- Model Name: AISAK-Detect\n- Version: 1.0\n- Model Architecture: Transformer with convolutional backbone\n- Specialization: AISAK-Detect is a specialized model within the AISAK-Visual system, focusing on object detection tasks. It employs an encoder-decoder transformer architecture with a convolutional backbone, enabling it to effectively analyze images and generate precise object detection results. AISAK-Visual is part of the broader AISAK system and is specialized in image captioning tasks.",
"## Intended Use:\n\nThe model demonstrates high accuracy in object detection tasks, leveraging the synergy between its transformer-based encoder-decoder architecture and the convolutional backbone. When utilized in conjunction with AISAK-Visual, it enhances overall performance in image analysis tasks.",
"## Performance:\n\nAISAK-Visual, based on the BLIP framework, achieves state-of-the-art results on image captioning tasks, including image-text retrieval, image captioning, and VQA. Its generalization ability is demonstrated by its strong performance on video-language tasks in a zero-shot manner.",
"## Ethical Considerations:\n\n- Bias Mitigation: Efforts have been made to mitigate bias during training; however, users are encouraged to remain vigilant about potential biases in the model's output.\n- Fair Use: Users should exercise caution when using AISAK-Visual in sensitive contexts and ensure fair and ethical use of the generated image captions.",
"## Limitations:\n\n- While proficient in general object detection, AISAK-Detect may encounter challenges in scenarios requiring specialized object recognition or highly cluttered images.\n- Users should be aware of these limitations and consider them when interpreting the model's outputs.",
"## Deployment:\n\nAISAK-Detect's inferencing capabilities will be seamlessly integrated into the deployment of the AISAK-Visual system. This integration ensures smooth operation and maximizes the synergy between the two models, providing comprehensive image understanding and analysis.",
"## Caveats:\n\n- Users should verify critical decisions based on AISAK-Detect's object detection results, particularly in high-stakes scenarios. Considering the broader context provided by AISAK-Visual is essential for a comprehensive understanding of visual content and informed decision-making.",
"## Model Card Information:\n\n- Model Card Created: April 25, 2024\n- Last Updated: April 25, 2024\n- Contact Information: For any inquiries or communication regarding AISAK, please contact me at mandelakorilogan@URL.\n\n\n© 2024 Mandela Logan. All rights reserved.\n\nNo part of this model may be reproduced, distributed, or transmitted in any form or by any means, including photocopying, recording, or other electronic or mechanical methods, without the prior written permission of the copyright holder. Users are expressly prohibited from creating replications or spaces derived from this model, whether in whole or in part, without the explicit authorization of the copyright holder. Unauthorized use or reproduction of this model is strictly prohibited by copyright law."
] | [
"TAGS\n#transformers #pytorch #safetensors #detr #object-detection #computer-vision #image-to-text #en #endpoints_compatible #region-us \n",
"# AISAK-Detect",
"## Overview:\n\nAISAK-Detect is an integral component of the AISAK-Visual system, specializing in object detection tasks. Leveraging an encoder-decoder transformer architecture with a convolutional backbone, AISAK-Detect excels in accurately and efficiently detecting objects within images. This model enhances the image understanding capabilities of AISAK-Visual, contributing to comprehensive visual analysis. Trained and fine-tuned by the AISAK team, AISAK-Detect is designed to seamlessly integrate into the broader AISAK system, ensuring cohesive performance in image analysis tasks.",
"## Model Information:\n\n- Model Name: AISAK-Detect\n- Version: 1.0\n- Model Architecture: Transformer with convolutional backbone\n- Specialization: AISAK-Detect is a specialized model within the AISAK-Visual system, focusing on object detection tasks. It employs an encoder-decoder transformer architecture with a convolutional backbone, enabling it to effectively analyze images and generate precise object detection results. AISAK-Visual is part of the broader AISAK system and is specialized in image captioning tasks.",
"## Intended Use:\n\nThe model demonstrates high accuracy in object detection tasks, leveraging the synergy between its transformer-based encoder-decoder architecture and the convolutional backbone. When utilized in conjunction with AISAK-Visual, it enhances overall performance in image analysis tasks.",
"## Performance:\n\nAISAK-Visual, based on the BLIP framework, achieves state-of-the-art results on image captioning tasks, including image-text retrieval, image captioning, and VQA. Its generalization ability is demonstrated by its strong performance on video-language tasks in a zero-shot manner.",
"## Ethical Considerations:\n\n- Bias Mitigation: Efforts have been made to mitigate bias during training; however, users are encouraged to remain vigilant about potential biases in the model's output.\n- Fair Use: Users should exercise caution when using AISAK-Visual in sensitive contexts and ensure fair and ethical use of the generated image captions.",
"## Limitations:\n\n- While proficient in general object detection, AISAK-Detect may encounter challenges in scenarios requiring specialized object recognition or highly cluttered images.\n- Users should be aware of these limitations and consider them when interpreting the model's outputs.",
"## Deployment:\n\nAISAK-Detect's inferencing capabilities will be seamlessly integrated into the deployment of the AISAK-Visual system. This integration ensures smooth operation and maximizes the synergy between the two models, providing comprehensive image understanding and analysis.",
"## Caveats:\n\n- Users should verify critical decisions based on AISAK-Detect's object detection results, particularly in high-stakes scenarios. Considering the broader context provided by AISAK-Visual is essential for a comprehensive understanding of visual content and informed decision-making.",
"## Model Card Information:\n\n- Model Card Created: April 25, 2024\n- Last Updated: April 25, 2024\n- Contact Information: For any inquiries or communication regarding AISAK, please contact me at mandelakorilogan@URL.\n\n\n© 2024 Mandela Logan. All rights reserved.\n\nNo part of this model may be reproduced, distributed, or transmitted in any form or by any means, including photocopying, recording, or other electronic or mechanical methods, without the prior written permission of the copyright holder. Users are expressly prohibited from creating replications or spaces derived from this model, whether in whole or in part, without the explicit authorization of the copyright holder. Unauthorized use or reproduction of this model is strictly prohibited by copyright law."
] |
text-generation | transformers |
<!-- This model card has been generated automatically according to the information the Trainer had access to. You
should probably proofread and complete it, then remove this comment. -->
# Llama-3-8B-Instruct-spider
This model is a fine-tuned version of [meta-llama/Meta-Llama-3-8B-Instruct](https://huggingface.co/meta-llama/Meta-Llama-3-8B-Instruct) on the generator dataset.
## Model description
More information needed
## Intended uses & limitations
More information needed
## Training and evaluation data
More information needed
## Training procedure
### Training hyperparameters
The following hyperparameters were used during training:
- learning_rate: 0.0002
- train_batch_size: 1
- eval_batch_size: 8
- seed: 42
- gradient_accumulation_steps: 2
- total_train_batch_size: 2
- optimizer: Adam with betas=(0.9,0.999) and epsilon=1e-08
- lr_scheduler_type: cosine
- lr_scheduler_warmup_ratio: 0.03
- num_epochs: 1
### Training results
### Framework versions
- Transformers 4.36.2
- Pytorch 2.1.2+cu121
- Datasets 2.16.1
- Tokenizers 0.15.2
| {"license": "other", "tags": ["trl", "sft", "generated_from_trainer"], "datasets": ["generator"], "base_model": "meta-llama/Meta-Llama-3-8B-Instruct", "model-index": [{"name": "Llama-3-8B-Instruct-spider", "results": []}]} | VictorDCh/Llama-3-8B-Instruct-spider | null | [
"transformers",
"tensorboard",
"safetensors",
"llama",
"text-generation",
"trl",
"sft",
"generated_from_trainer",
"conversational",
"dataset:generator",
"base_model:meta-llama/Meta-Llama-3-8B-Instruct",
"license:other",
"autotrain_compatible",
"endpoints_compatible",
"text-generation-inference",
"region:us"
] | null | 2024-04-25T14:46:35+00:00 | [] | [] | TAGS
#transformers #tensorboard #safetensors #llama #text-generation #trl #sft #generated_from_trainer #conversational #dataset-generator #base_model-meta-llama/Meta-Llama-3-8B-Instruct #license-other #autotrain_compatible #endpoints_compatible #text-generation-inference #region-us
|
# Llama-3-8B-Instruct-spider
This model is a fine-tuned version of meta-llama/Meta-Llama-3-8B-Instruct on the generator dataset.
## Model description
More information needed
## Intended uses & limitations
More information needed
## Training and evaluation data
More information needed
## Training procedure
### Training hyperparameters
The following hyperparameters were used during training:
- learning_rate: 0.0002
- train_batch_size: 1
- eval_batch_size: 8
- seed: 42
- gradient_accumulation_steps: 2
- total_train_batch_size: 2
- optimizer: Adam with betas=(0.9,0.999) and epsilon=1e-08
- lr_scheduler_type: cosine
- lr_scheduler_warmup_ratio: 0.03
- num_epochs: 1
### Training results
### Framework versions
- Transformers 4.36.2
- Pytorch 2.1.2+cu121
- Datasets 2.16.1
- Tokenizers 0.15.2
| [
"# Llama-3-8B-Instruct-spider\n\nThis model is a fine-tuned version of meta-llama/Meta-Llama-3-8B-Instruct on the generator dataset.",
"## Model description\n\nMore information needed",
"## Intended uses & limitations\n\nMore information needed",
"## Training and evaluation data\n\nMore information needed",
"## Training procedure",
"### Training hyperparameters\n\nThe following hyperparameters were used during training:\n- learning_rate: 0.0002\n- train_batch_size: 1\n- eval_batch_size: 8\n- seed: 42\n- gradient_accumulation_steps: 2\n- total_train_batch_size: 2\n- optimizer: Adam with betas=(0.9,0.999) and epsilon=1e-08\n- lr_scheduler_type: cosine\n- lr_scheduler_warmup_ratio: 0.03\n- num_epochs: 1",
"### Training results",
"### Framework versions\n\n- Transformers 4.36.2\n- Pytorch 2.1.2+cu121\n- Datasets 2.16.1\n- Tokenizers 0.15.2"
] | [
"TAGS\n#transformers #tensorboard #safetensors #llama #text-generation #trl #sft #generated_from_trainer #conversational #dataset-generator #base_model-meta-llama/Meta-Llama-3-8B-Instruct #license-other #autotrain_compatible #endpoints_compatible #text-generation-inference #region-us \n",
"# Llama-3-8B-Instruct-spider\n\nThis model is a fine-tuned version of meta-llama/Meta-Llama-3-8B-Instruct on the generator dataset.",
"## Model description\n\nMore information needed",
"## Intended uses & limitations\n\nMore information needed",
"## Training and evaluation data\n\nMore information needed",
"## Training procedure",
"### Training hyperparameters\n\nThe following hyperparameters were used during training:\n- learning_rate: 0.0002\n- train_batch_size: 1\n- eval_batch_size: 8\n- seed: 42\n- gradient_accumulation_steps: 2\n- total_train_batch_size: 2\n- optimizer: Adam with betas=(0.9,0.999) and epsilon=1e-08\n- lr_scheduler_type: cosine\n- lr_scheduler_warmup_ratio: 0.03\n- num_epochs: 1",
"### Training results",
"### Framework versions\n\n- Transformers 4.36.2\n- Pytorch 2.1.2+cu121\n- Datasets 2.16.1\n- Tokenizers 0.15.2"
] |
null | transformers |
# Model Card for Model ID
<!-- Provide a quick summary of what the model is/does. -->
## Model Details
### Model Description
<!-- Provide a longer summary of what this model is. -->
This is the model card of a 🤗 transformers model that has been pushed on the Hub. This model card has been automatically generated.
- **Developed by:** [More Information Needed]
- **Funded by [optional]:** [More Information Needed]
- **Shared by [optional]:** [More Information Needed]
- **Model type:** [More Information Needed]
- **Language(s) (NLP):** [More Information Needed]
- **License:** [More Information Needed]
- **Finetuned from model [optional]:** [More Information Needed]
### Model Sources [optional]
<!-- Provide the basic links for the model. -->
- **Repository:** [More Information Needed]
- **Paper [optional]:** [More Information Needed]
- **Demo [optional]:** [More Information Needed]
## Uses
<!-- Address questions around how the model is intended to be used, including the foreseeable users of the model and those affected by the model. -->
### Direct Use
<!-- This section is for the model use without fine-tuning or plugging into a larger ecosystem/app. -->
[More Information Needed]
### Downstream Use [optional]
<!-- This section is for the model use when fine-tuned for a task, or when plugged into a larger ecosystem/app -->
[More Information Needed]
### Out-of-Scope Use
<!-- This section addresses misuse, malicious use, and uses that the model will not work well for. -->
[More Information Needed]
## Bias, Risks, and Limitations
<!-- This section is meant to convey both technical and sociotechnical limitations. -->
[More Information Needed]
### Recommendations
<!-- This section is meant to convey recommendations with respect to the bias, risk, and technical limitations. -->
Users (both direct and downstream) should be made aware of the risks, biases and limitations of the model. More information needed for further recommendations.
## How to Get Started with the Model
Use the code below to get started with the model.
[More Information Needed]
## Training Details
### Training Data
<!-- This should link to a Dataset Card, perhaps with a short stub of information on what the training data is all about as well as documentation related to data pre-processing or additional filtering. -->
[More Information Needed]
### Training Procedure
<!-- This relates heavily to the Technical Specifications. Content here should link to that section when it is relevant to the training procedure. -->
#### Preprocessing [optional]
[More Information Needed]
#### Training Hyperparameters
- **Training regime:** [More Information Needed] <!--fp32, fp16 mixed precision, bf16 mixed precision, bf16 non-mixed precision, fp16 non-mixed precision, fp8 mixed precision -->
#### Speeds, Sizes, Times [optional]
<!-- This section provides information about throughput, start/end time, checkpoint size if relevant, etc. -->
[More Information Needed]
## Evaluation
<!-- This section describes the evaluation protocols and provides the results. -->
### Testing Data, Factors & Metrics
#### Testing Data
<!-- This should link to a Dataset Card if possible. -->
[More Information Needed]
#### Factors
<!-- These are the things the evaluation is disaggregating by, e.g., subpopulations or domains. -->
[More Information Needed]
#### Metrics
<!-- These are the evaluation metrics being used, ideally with a description of why. -->
[More Information Needed]
### Results
[More Information Needed]
#### Summary
## Model Examination [optional]
<!-- Relevant interpretability work for the model goes here -->
[More Information Needed]
## Environmental Impact
<!-- Total emissions (in grams of CO2eq) and additional considerations, such as electricity usage, go here. Edit the suggested text below accordingly -->
Carbon emissions can be estimated using the [Machine Learning Impact calculator](https://mlco2.github.io/impact#compute) presented in [Lacoste et al. (2019)](https://arxiv.org/abs/1910.09700).
- **Hardware Type:** [More Information Needed]
- **Hours used:** [More Information Needed]
- **Cloud Provider:** [More Information Needed]
- **Compute Region:** [More Information Needed]
- **Carbon Emitted:** [More Information Needed]
## Technical Specifications [optional]
### Model Architecture and Objective
[More Information Needed]
### Compute Infrastructure
[More Information Needed]
#### Hardware
[More Information Needed]
#### Software
[More Information Needed]
## Citation [optional]
<!-- If there is a paper or blog post introducing the model, the APA and Bibtex information for that should go in this section. -->
**BibTeX:**
[More Information Needed]
**APA:**
[More Information Needed]
## Glossary [optional]
<!-- If relevant, include terms and calculations in this section that can help readers understand the model or model card. -->
[More Information Needed]
## More Information [optional]
[More Information Needed]
## Model Card Authors [optional]
[More Information Needed]
## Model Card Contact
[More Information Needed] | {"library_name": "transformers", "tags": []} | kishorea/finetuned_qa4 | null | [
"transformers",
"safetensors",
"arxiv:1910.09700",
"endpoints_compatible",
"region:us"
] | null | 2024-04-25T14:46:48+00:00 | [
"1910.09700"
] | [] | TAGS
#transformers #safetensors #arxiv-1910.09700 #endpoints_compatible #region-us
|
# Model Card for Model ID
## Model Details
### Model Description
This is the model card of a transformers model that has been pushed on the Hub. This model card has been automatically generated.
- Developed by:
- Funded by [optional]:
- Shared by [optional]:
- Model type:
- Language(s) (NLP):
- License:
- Finetuned from model [optional]:
### Model Sources [optional]
- Repository:
- Paper [optional]:
- Demo [optional]:
## Uses
### Direct Use
### Downstream Use [optional]
### Out-of-Scope Use
## Bias, Risks, and Limitations
### Recommendations
Users (both direct and downstream) should be made aware of the risks, biases and limitations of the model. More information needed for further recommendations.
## How to Get Started with the Model
Use the code below to get started with the model.
## Training Details
### Training Data
### Training Procedure
#### Preprocessing [optional]
#### Training Hyperparameters
- Training regime:
#### Speeds, Sizes, Times [optional]
## Evaluation
### Testing Data, Factors & Metrics
#### Testing Data
#### Factors
#### Metrics
### Results
#### Summary
## Model Examination [optional]
## Environmental Impact
Carbon emissions can be estimated using the Machine Learning Impact calculator presented in Lacoste et al. (2019).
- Hardware Type:
- Hours used:
- Cloud Provider:
- Compute Region:
- Carbon Emitted:
## Technical Specifications [optional]
### Model Architecture and Objective
### Compute Infrastructure
#### Hardware
#### Software
[optional]
BibTeX:
APA:
## Glossary [optional]
## More Information [optional]
## Model Card Authors [optional]
## Model Card Contact
| [
"# Model Card for Model ID",
"## Model Details",
"### Model Description\n\n\n\nThis is the model card of a transformers model that has been pushed on the Hub. This model card has been automatically generated.\n\n- Developed by: \n- Funded by [optional]: \n- Shared by [optional]: \n- Model type: \n- Language(s) (NLP): \n- License: \n- Finetuned from model [optional]:",
"### Model Sources [optional]\n\n\n\n- Repository: \n- Paper [optional]: \n- Demo [optional]:",
"## Uses",
"### Direct Use",
"### Downstream Use [optional]",
"### Out-of-Scope Use",
"## Bias, Risks, and Limitations",
"### Recommendations\n\n\n\nUsers (both direct and downstream) should be made aware of the risks, biases and limitations of the model. More information needed for further recommendations.",
"## How to Get Started with the Model\n\nUse the code below to get started with the model.",
"## Training Details",
"### Training Data",
"### Training Procedure",
"#### Preprocessing [optional]",
"#### Training Hyperparameters\n\n- Training regime:",
"#### Speeds, Sizes, Times [optional]",
"## Evaluation",
"### Testing Data, Factors & Metrics",
"#### Testing Data",
"#### Factors",
"#### Metrics",
"### Results",
"#### Summary",
"## Model Examination [optional]",
"## Environmental Impact\n\n\n\nCarbon emissions can be estimated using the Machine Learning Impact calculator presented in Lacoste et al. (2019).\n\n- Hardware Type: \n- Hours used: \n- Cloud Provider: \n- Compute Region: \n- Carbon Emitted:",
"## Technical Specifications [optional]",
"### Model Architecture and Objective",
"### Compute Infrastructure",
"#### Hardware",
"#### Software\n\n\n\n[optional]\n\n\n\nBibTeX:\n\n\n\nAPA:",
"## Glossary [optional]",
"## More Information [optional]",
"## Model Card Authors [optional]",
"## Model Card Contact"
] | [
"TAGS\n#transformers #safetensors #arxiv-1910.09700 #endpoints_compatible #region-us \n",
"# Model Card for Model ID",
"## Model Details",
"### Model Description\n\n\n\nThis is the model card of a transformers model that has been pushed on the Hub. This model card has been automatically generated.\n\n- Developed by: \n- Funded by [optional]: \n- Shared by [optional]: \n- Model type: \n- Language(s) (NLP): \n- License: \n- Finetuned from model [optional]:",
"### Model Sources [optional]\n\n\n\n- Repository: \n- Paper [optional]: \n- Demo [optional]:",
"## Uses",
"### Direct Use",
"### Downstream Use [optional]",
"### Out-of-Scope Use",
"## Bias, Risks, and Limitations",
"### Recommendations\n\n\n\nUsers (both direct and downstream) should be made aware of the risks, biases and limitations of the model. More information needed for further recommendations.",
"## How to Get Started with the Model\n\nUse the code below to get started with the model.",
"## Training Details",
"### Training Data",
"### Training Procedure",
"#### Preprocessing [optional]",
"#### Training Hyperparameters\n\n- Training regime:",
"#### Speeds, Sizes, Times [optional]",
"## Evaluation",
"### Testing Data, Factors & Metrics",
"#### Testing Data",
"#### Factors",
"#### Metrics",
"### Results",
"#### Summary",
"## Model Examination [optional]",
"## Environmental Impact\n\n\n\nCarbon emissions can be estimated using the Machine Learning Impact calculator presented in Lacoste et al. (2019).\n\n- Hardware Type: \n- Hours used: \n- Cloud Provider: \n- Compute Region: \n- Carbon Emitted:",
"## Technical Specifications [optional]",
"### Model Architecture and Objective",
"### Compute Infrastructure",
"#### Hardware",
"#### Software\n\n\n\n[optional]\n\n\n\nBibTeX:\n\n\n\nAPA:",
"## Glossary [optional]",
"## More Information [optional]",
"## Model Card Authors [optional]",
"## Model Card Contact"
] |
text-generation | transformers | # Model Card for Model ID
<!-- Provide a quick summary of what the model is/does. -->
This modelcard aims to be a base template for new models. It has been generated using [this raw template](https://github.com/huggingface/huggingface_hub/blob/main/src/huggingface_hub/templates/modelcard_template.md?plain=1).
## Model Details
### Model Description
<!-- Provide a longer summary of what this model is. -->
- **Developed by:** [More Information Needed]
- **Funded by [optional]:** [More Information Needed]
- **Shared by [optional]:** [More Information Needed]
- **Model type:** [More Information Needed]
- **Language(s) (NLP):** [More Information Needed]
- **License:** [More Information Needed]
- **Finetuned from model [optional]:** [More Information Needed]
### Model Sources [optional]
<!-- Provide the basic links for the model. -->
- **Repository:** [More Information Needed]
- **Paper [optional]:** [More Information Needed]
- **Demo [optional]:** [More Information Needed]
## Uses
<!-- Address questions around how the model is intended to be used, including the foreseeable users of the model and those affected by the model. -->
### Direct Use
<!-- This section is for the model use without fine-tuning or plugging into a larger ecosystem/app. -->
[More Information Needed]
### Downstream Use [optional]
<!-- This section is for the model use when fine-tuned for a task, or when plugged into a larger ecosystem/app -->
[More Information Needed]
### Out-of-Scope Use
<!-- This section addresses misuse, malicious use, and uses that the model will not work well for. -->
[More Information Needed]
## Bias, Risks, and Limitations
<!-- This section is meant to convey both technical and sociotechnical limitations. -->
[More Information Needed]
### Recommendations
<!-- This section is meant to convey recommendations with respect to the bias, risk, and technical limitations. -->
Users (both direct and downstream) should be made aware of the risks, biases and limitations of the model. More information needed for further recommendations.
## How to Get Started with the Model
Use the code below to get started with the model.
[More Information Needed]
## Training Details
### Training Data
<!-- This should link to a Dataset Card, perhaps with a short stub of information on what the training data is all about as well as documentation related to data pre-processing or additional filtering. -->
[More Information Needed]
### Training Procedure
<!-- This relates heavily to the Technical Specifications. Content here should link to that section when it is relevant to the training procedure. -->
#### Preprocessing [optional]
[More Information Needed]
#### Training Hyperparameters
- **Training regime:** [More Information Needed] <!--fp32, fp16 mixed precision, bf16 mixed precision, bf16 non-mixed precision, fp16 non-mixed precision, fp8 mixed precision -->
#### Speeds, Sizes, Times [optional]
<!-- This section provides information about throughput, start/end time, checkpoint size if relevant, etc. -->
[More Information Needed]
## Evaluation
<!-- This section describes the evaluation protocols and provides the results. -->
### Testing Data, Factors & Metrics
#### Testing Data
<!-- This should link to a Dataset Card if possible. -->
[More Information Needed]
#### Factors
<!-- These are the things the evaluation is disaggregating by, e.g., subpopulations or domains. -->
[More Information Needed]
#### Metrics
<!-- These are the evaluation metrics being used, ideally with a description of why. -->
[More Information Needed]
### Results
[More Information Needed]
#### Summary
## Model Examination [optional]
<!-- Relevant interpretability work for the model goes here -->
[More Information Needed]
## Environmental Impact
<!-- Total emissions (in grams of CO2eq) and additional considerations, such as electricity usage, go here. Edit the suggested text below accordingly -->
Carbon emissions can be estimated using the [Machine Learning Impact calculator](https://mlco2.github.io/impact#compute) presented in [Lacoste et al. (2019)](https://arxiv.org/abs/1910.09700).
- **Hardware Type:** [More Information Needed]
- **Hours used:** [More Information Needed]
- **Cloud Provider:** [More Information Needed]
- **Compute Region:** [More Information Needed]
- **Carbon Emitted:** [More Information Needed]
## Technical Specifications [optional]
### Model Architecture and Objective
[More Information Needed]
### Compute Infrastructure
[More Information Needed]
#### Hardware
[More Information Needed]
#### Software
[More Information Needed]
## Citation [optional]
<!-- If there is a paper or blog post introducing the model, the APA and Bibtex information for that should go in this section. -->
**BibTeX:**
[More Information Needed]
**APA:**
[More Information Needed]
## Glossary [optional]
<!-- If relevant, include terms and calculations in this section that can help readers understand the model or model card. -->
[More Information Needed]
## More Information [optional]
[More Information Needed]
## Model Card Authors [optional]
[More Information Needed]
## Model Card Contact
[More Information Needed] | {"language": ["en"], "library_name": "transformers", "datasets": ["sohamslc5/curr1"], "metrics": ["accuracy"], "pipeline_tag": "text-generation", "base_model": "microsoft/Phi-3-mini-4k-instruct"} | sohamslc5/PHI3 | null | [
"transformers",
"text-generation",
"en",
"dataset:sohamslc5/curr1",
"arxiv:1910.09700",
"base_model:microsoft/Phi-3-mini-4k-instruct",
"endpoints_compatible",
"region:us"
] | null | 2024-04-25T14:47:00+00:00 | [
"1910.09700"
] | [
"en"
] | TAGS
#transformers #text-generation #en #dataset-sohamslc5/curr1 #arxiv-1910.09700 #base_model-microsoft/Phi-3-mini-4k-instruct #endpoints_compatible #region-us
| # Model Card for Model ID
This modelcard aims to be a base template for new models. It has been generated using this raw template.
## Model Details
### Model Description
- Developed by:
- Funded by [optional]:
- Shared by [optional]:
- Model type:
- Language(s) (NLP):
- License:
- Finetuned from model [optional]:
### Model Sources [optional]
- Repository:
- Paper [optional]:
- Demo [optional]:
## Uses
### Direct Use
### Downstream Use [optional]
### Out-of-Scope Use
## Bias, Risks, and Limitations
### Recommendations
Users (both direct and downstream) should be made aware of the risks, biases and limitations of the model. More information needed for further recommendations.
## How to Get Started with the Model
Use the code below to get started with the model.
## Training Details
### Training Data
### Training Procedure
#### Preprocessing [optional]
#### Training Hyperparameters
- Training regime:
#### Speeds, Sizes, Times [optional]
## Evaluation
### Testing Data, Factors & Metrics
#### Testing Data
#### Factors
#### Metrics
### Results
#### Summary
## Model Examination [optional]
## Environmental Impact
Carbon emissions can be estimated using the Machine Learning Impact calculator presented in Lacoste et al. (2019).
- Hardware Type:
- Hours used:
- Cloud Provider:
- Compute Region:
- Carbon Emitted:
## Technical Specifications [optional]
### Model Architecture and Objective
### Compute Infrastructure
#### Hardware
#### Software
[optional]
BibTeX:
APA:
## Glossary [optional]
## More Information [optional]
## Model Card Authors [optional]
## Model Card Contact
| [
"# Model Card for Model ID\n\n\n\nThis modelcard aims to be a base template for new models. It has been generated using this raw template.",
"## Model Details",
"### Model Description\n\n\n\n\n\n- Developed by: \n- Funded by [optional]: \n- Shared by [optional]: \n- Model type: \n- Language(s) (NLP): \n- License: \n- Finetuned from model [optional]:",
"### Model Sources [optional]\n\n\n\n- Repository: \n- Paper [optional]: \n- Demo [optional]:",
"## Uses",
"### Direct Use",
"### Downstream Use [optional]",
"### Out-of-Scope Use",
"## Bias, Risks, and Limitations",
"### Recommendations\n\n\n\nUsers (both direct and downstream) should be made aware of the risks, biases and limitations of the model. More information needed for further recommendations.",
"## How to Get Started with the Model\n\nUse the code below to get started with the model.",
"## Training Details",
"### Training Data",
"### Training Procedure",
"#### Preprocessing [optional]",
"#### Training Hyperparameters\n\n- Training regime:",
"#### Speeds, Sizes, Times [optional]",
"## Evaluation",
"### Testing Data, Factors & Metrics",
"#### Testing Data",
"#### Factors",
"#### Metrics",
"### Results",
"#### Summary",
"## Model Examination [optional]",
"## Environmental Impact\n\n\n\nCarbon emissions can be estimated using the Machine Learning Impact calculator presented in Lacoste et al. (2019).\n\n- Hardware Type: \n- Hours used: \n- Cloud Provider: \n- Compute Region: \n- Carbon Emitted:",
"## Technical Specifications [optional]",
"### Model Architecture and Objective",
"### Compute Infrastructure",
"#### Hardware",
"#### Software\n\n\n\n[optional]\n\n\n\nBibTeX:\n\n\n\nAPA:",
"## Glossary [optional]",
"## More Information [optional]",
"## Model Card Authors [optional]",
"## Model Card Contact"
] | [
"TAGS\n#transformers #text-generation #en #dataset-sohamslc5/curr1 #arxiv-1910.09700 #base_model-microsoft/Phi-3-mini-4k-instruct #endpoints_compatible #region-us \n",
"# Model Card for Model ID\n\n\n\nThis modelcard aims to be a base template for new models. It has been generated using this raw template.",
"## Model Details",
"### Model Description\n\n\n\n\n\n- Developed by: \n- Funded by [optional]: \n- Shared by [optional]: \n- Model type: \n- Language(s) (NLP): \n- License: \n- Finetuned from model [optional]:",
"### Model Sources [optional]\n\n\n\n- Repository: \n- Paper [optional]: \n- Demo [optional]:",
"## Uses",
"### Direct Use",
"### Downstream Use [optional]",
"### Out-of-Scope Use",
"## Bias, Risks, and Limitations",
"### Recommendations\n\n\n\nUsers (both direct and downstream) should be made aware of the risks, biases and limitations of the model. More information needed for further recommendations.",
"## How to Get Started with the Model\n\nUse the code below to get started with the model.",
"## Training Details",
"### Training Data",
"### Training Procedure",
"#### Preprocessing [optional]",
"#### Training Hyperparameters\n\n- Training regime:",
"#### Speeds, Sizes, Times [optional]",
"## Evaluation",
"### Testing Data, Factors & Metrics",
"#### Testing Data",
"#### Factors",
"#### Metrics",
"### Results",
"#### Summary",
"## Model Examination [optional]",
"## Environmental Impact\n\n\n\nCarbon emissions can be estimated using the Machine Learning Impact calculator presented in Lacoste et al. (2019).\n\n- Hardware Type: \n- Hours used: \n- Cloud Provider: \n- Compute Region: \n- Carbon Emitted:",
"## Technical Specifications [optional]",
"### Model Architecture and Objective",
"### Compute Infrastructure",
"#### Hardware",
"#### Software\n\n\n\n[optional]\n\n\n\nBibTeX:\n\n\n\nAPA:",
"## Glossary [optional]",
"## More Information [optional]",
"## Model Card Authors [optional]",
"## Model Card Contact"
] |
null | transformers |
# Model Card for Model ID
<!-- Provide a quick summary of what the model is/does. -->
## Model Details
### Model Description
<!-- Provide a longer summary of what this model is. -->
This is the model card of a 🤗 transformers model that has been pushed on the Hub. This model card has been automatically generated.
- **Developed by:** [More Information Needed]
- **Funded by [optional]:** [More Information Needed]
- **Shared by [optional]:** [More Information Needed]
- **Model type:** [More Information Needed]
- **Language(s) (NLP):** [More Information Needed]
- **License:** [More Information Needed]
- **Finetuned from model [optional]:** [More Information Needed]
### Model Sources [optional]
<!-- Provide the basic links for the model. -->
- **Repository:** [More Information Needed]
- **Paper [optional]:** [More Information Needed]
- **Demo [optional]:** [More Information Needed]
## Uses
<!-- Address questions around how the model is intended to be used, including the foreseeable users of the model and those affected by the model. -->
### Direct Use
<!-- This section is for the model use without fine-tuning or plugging into a larger ecosystem/app. -->
[More Information Needed]
### Downstream Use [optional]
<!-- This section is for the model use when fine-tuned for a task, or when plugged into a larger ecosystem/app -->
[More Information Needed]
### Out-of-Scope Use
<!-- This section addresses misuse, malicious use, and uses that the model will not work well for. -->
[More Information Needed]
## Bias, Risks, and Limitations
<!-- This section is meant to convey both technical and sociotechnical limitations. -->
[More Information Needed]
### Recommendations
<!-- This section is meant to convey recommendations with respect to the bias, risk, and technical limitations. -->
Users (both direct and downstream) should be made aware of the risks, biases and limitations of the model. More information needed for further recommendations.
## How to Get Started with the Model
Use the code below to get started with the model.
[More Information Needed]
## Training Details
### Training Data
<!-- This should link to a Dataset Card, perhaps with a short stub of information on what the training data is all about as well as documentation related to data pre-processing or additional filtering. -->
[More Information Needed]
### Training Procedure
<!-- This relates heavily to the Technical Specifications. Content here should link to that section when it is relevant to the training procedure. -->
#### Preprocessing [optional]
[More Information Needed]
#### Training Hyperparameters
- **Training regime:** [More Information Needed] <!--fp32, fp16 mixed precision, bf16 mixed precision, bf16 non-mixed precision, fp16 non-mixed precision, fp8 mixed precision -->
#### Speeds, Sizes, Times [optional]
<!-- This section provides information about throughput, start/end time, checkpoint size if relevant, etc. -->
[More Information Needed]
## Evaluation
<!-- This section describes the evaluation protocols and provides the results. -->
### Testing Data, Factors & Metrics
#### Testing Data
<!-- This should link to a Dataset Card if possible. -->
[More Information Needed]
#### Factors
<!-- These are the things the evaluation is disaggregating by, e.g., subpopulations or domains. -->
[More Information Needed]
#### Metrics
<!-- These are the evaluation metrics being used, ideally with a description of why. -->
[More Information Needed]
### Results
[More Information Needed]
#### Summary
## Model Examination [optional]
<!-- Relevant interpretability work for the model goes here -->
[More Information Needed]
## Environmental Impact
<!-- Total emissions (in grams of CO2eq) and additional considerations, such as electricity usage, go here. Edit the suggested text below accordingly -->
Carbon emissions can be estimated using the [Machine Learning Impact calculator](https://mlco2.github.io/impact#compute) presented in [Lacoste et al. (2019)](https://arxiv.org/abs/1910.09700).
- **Hardware Type:** [More Information Needed]
- **Hours used:** [More Information Needed]
- **Cloud Provider:** [More Information Needed]
- **Compute Region:** [More Information Needed]
- **Carbon Emitted:** [More Information Needed]
## Technical Specifications [optional]
### Model Architecture and Objective
[More Information Needed]
### Compute Infrastructure
[More Information Needed]
#### Hardware
[More Information Needed]
#### Software
[More Information Needed]
## Citation [optional]
<!-- If there is a paper or blog post introducing the model, the APA and Bibtex information for that should go in this section. -->
**BibTeX:**
[More Information Needed]
**APA:**
[More Information Needed]
## Glossary [optional]
<!-- If relevant, include terms and calculations in this section that can help readers understand the model or model card. -->
[More Information Needed]
## More Information [optional]
[More Information Needed]
## Model Card Authors [optional]
[More Information Needed]
## Model Card Contact
[More Information Needed] | {"library_name": "transformers", "tags": []} | quickstep3621/kw25bda | null | [
"transformers",
"arxiv:1910.09700",
"endpoints_compatible",
"region:us"
] | null | 2024-04-25T14:49:03+00:00 | [
"1910.09700"
] | [] | TAGS
#transformers #arxiv-1910.09700 #endpoints_compatible #region-us
|
# Model Card for Model ID
## Model Details
### Model Description
This is the model card of a transformers model that has been pushed on the Hub. This model card has been automatically generated.
- Developed by:
- Funded by [optional]:
- Shared by [optional]:
- Model type:
- Language(s) (NLP):
- License:
- Finetuned from model [optional]:
### Model Sources [optional]
- Repository:
- Paper [optional]:
- Demo [optional]:
## Uses
### Direct Use
### Downstream Use [optional]
### Out-of-Scope Use
## Bias, Risks, and Limitations
### Recommendations
Users (both direct and downstream) should be made aware of the risks, biases and limitations of the model. More information needed for further recommendations.
## How to Get Started with the Model
Use the code below to get started with the model.
## Training Details
### Training Data
### Training Procedure
#### Preprocessing [optional]
#### Training Hyperparameters
- Training regime:
#### Speeds, Sizes, Times [optional]
## Evaluation
### Testing Data, Factors & Metrics
#### Testing Data
#### Factors
#### Metrics
### Results
#### Summary
## Model Examination [optional]
## Environmental Impact
Carbon emissions can be estimated using the Machine Learning Impact calculator presented in Lacoste et al. (2019).
- Hardware Type:
- Hours used:
- Cloud Provider:
- Compute Region:
- Carbon Emitted:
## Technical Specifications [optional]
### Model Architecture and Objective
### Compute Infrastructure
#### Hardware
#### Software
[optional]
BibTeX:
APA:
## Glossary [optional]
## More Information [optional]
## Model Card Authors [optional]
## Model Card Contact
| [
"# Model Card for Model ID",
"## Model Details",
"### Model Description\n\n\n\nThis is the model card of a transformers model that has been pushed on the Hub. This model card has been automatically generated.\n\n- Developed by: \n- Funded by [optional]: \n- Shared by [optional]: \n- Model type: \n- Language(s) (NLP): \n- License: \n- Finetuned from model [optional]:",
"### Model Sources [optional]\n\n\n\n- Repository: \n- Paper [optional]: \n- Demo [optional]:",
"## Uses",
"### Direct Use",
"### Downstream Use [optional]",
"### Out-of-Scope Use",
"## Bias, Risks, and Limitations",
"### Recommendations\n\n\n\nUsers (both direct and downstream) should be made aware of the risks, biases and limitations of the model. More information needed for further recommendations.",
"## How to Get Started with the Model\n\nUse the code below to get started with the model.",
"## Training Details",
"### Training Data",
"### Training Procedure",
"#### Preprocessing [optional]",
"#### Training Hyperparameters\n\n- Training regime:",
"#### Speeds, Sizes, Times [optional]",
"## Evaluation",
"### Testing Data, Factors & Metrics",
"#### Testing Data",
"#### Factors",
"#### Metrics",
"### Results",
"#### Summary",
"## Model Examination [optional]",
"## Environmental Impact\n\n\n\nCarbon emissions can be estimated using the Machine Learning Impact calculator presented in Lacoste et al. (2019).\n\n- Hardware Type: \n- Hours used: \n- Cloud Provider: \n- Compute Region: \n- Carbon Emitted:",
"## Technical Specifications [optional]",
"### Model Architecture and Objective",
"### Compute Infrastructure",
"#### Hardware",
"#### Software\n\n\n\n[optional]\n\n\n\nBibTeX:\n\n\n\nAPA:",
"## Glossary [optional]",
"## More Information [optional]",
"## Model Card Authors [optional]",
"## Model Card Contact"
] | [
"TAGS\n#transformers #arxiv-1910.09700 #endpoints_compatible #region-us \n",
"# Model Card for Model ID",
"## Model Details",
"### Model Description\n\n\n\nThis is the model card of a transformers model that has been pushed on the Hub. This model card has been automatically generated.\n\n- Developed by: \n- Funded by [optional]: \n- Shared by [optional]: \n- Model type: \n- Language(s) (NLP): \n- License: \n- Finetuned from model [optional]:",
"### Model Sources [optional]\n\n\n\n- Repository: \n- Paper [optional]: \n- Demo [optional]:",
"## Uses",
"### Direct Use",
"### Downstream Use [optional]",
"### Out-of-Scope Use",
"## Bias, Risks, and Limitations",
"### Recommendations\n\n\n\nUsers (both direct and downstream) should be made aware of the risks, biases and limitations of the model. More information needed for further recommendations.",
"## How to Get Started with the Model\n\nUse the code below to get started with the model.",
"## Training Details",
"### Training Data",
"### Training Procedure",
"#### Preprocessing [optional]",
"#### Training Hyperparameters\n\n- Training regime:",
"#### Speeds, Sizes, Times [optional]",
"## Evaluation",
"### Testing Data, Factors & Metrics",
"#### Testing Data",
"#### Factors",
"#### Metrics",
"### Results",
"#### Summary",
"## Model Examination [optional]",
"## Environmental Impact\n\n\n\nCarbon emissions can be estimated using the Machine Learning Impact calculator presented in Lacoste et al. (2019).\n\n- Hardware Type: \n- Hours used: \n- Cloud Provider: \n- Compute Region: \n- Carbon Emitted:",
"## Technical Specifications [optional]",
"### Model Architecture and Objective",
"### Compute Infrastructure",
"#### Hardware",
"#### Software\n\n\n\n[optional]\n\n\n\nBibTeX:\n\n\n\nAPA:",
"## Glossary [optional]",
"## More Information [optional]",
"## Model Card Authors [optional]",
"## Model Card Contact"
] |
null | transformers |
# Model Card for Model ID
<!-- Provide a quick summary of what the model is/does. -->
## Model Details
### Model Description
<!-- Provide a longer summary of what this model is. -->
This is the model card of a 🤗 transformers model that has been pushed on the Hub. This model card has been automatically generated.
- **Developed by:** [More Information Needed]
- **Funded by [optional]:** [More Information Needed]
- **Shared by [optional]:** [More Information Needed]
- **Model type:** [More Information Needed]
- **Language(s) (NLP):** [More Information Needed]
- **License:** [More Information Needed]
- **Finetuned from model [optional]:** [More Information Needed]
### Model Sources [optional]
<!-- Provide the basic links for the model. -->
- **Repository:** [More Information Needed]
- **Paper [optional]:** [More Information Needed]
- **Demo [optional]:** [More Information Needed]
## Uses
<!-- Address questions around how the model is intended to be used, including the foreseeable users of the model and those affected by the model. -->
### Direct Use
<!-- This section is for the model use without fine-tuning or plugging into a larger ecosystem/app. -->
[More Information Needed]
### Downstream Use [optional]
<!-- This section is for the model use when fine-tuned for a task, or when plugged into a larger ecosystem/app -->
[More Information Needed]
### Out-of-Scope Use
<!-- This section addresses misuse, malicious use, and uses that the model will not work well for. -->
[More Information Needed]
## Bias, Risks, and Limitations
<!-- This section is meant to convey both technical and sociotechnical limitations. -->
[More Information Needed]
### Recommendations
<!-- This section is meant to convey recommendations with respect to the bias, risk, and technical limitations. -->
Users (both direct and downstream) should be made aware of the risks, biases and limitations of the model. More information needed for further recommendations.
## How to Get Started with the Model
Use the code below to get started with the model.
[More Information Needed]
## Training Details
### Training Data
<!-- This should link to a Dataset Card, perhaps with a short stub of information on what the training data is all about as well as documentation related to data pre-processing or additional filtering. -->
[More Information Needed]
### Training Procedure
<!-- This relates heavily to the Technical Specifications. Content here should link to that section when it is relevant to the training procedure. -->
#### Preprocessing [optional]
[More Information Needed]
#### Training Hyperparameters
- **Training regime:** [More Information Needed] <!--fp32, fp16 mixed precision, bf16 mixed precision, bf16 non-mixed precision, fp16 non-mixed precision, fp8 mixed precision -->
#### Speeds, Sizes, Times [optional]
<!-- This section provides information about throughput, start/end time, checkpoint size if relevant, etc. -->
[More Information Needed]
## Evaluation
<!-- This section describes the evaluation protocols and provides the results. -->
### Testing Data, Factors & Metrics
#### Testing Data
<!-- This should link to a Dataset Card if possible. -->
[More Information Needed]
#### Factors
<!-- These are the things the evaluation is disaggregating by, e.g., subpopulations or domains. -->
[More Information Needed]
#### Metrics
<!-- These are the evaluation metrics being used, ideally with a description of why. -->
[More Information Needed]
### Results
[More Information Needed]
#### Summary
## Model Examination [optional]
<!-- Relevant interpretability work for the model goes here -->
[More Information Needed]
## Environmental Impact
<!-- Total emissions (in grams of CO2eq) and additional considerations, such as electricity usage, go here. Edit the suggested text below accordingly -->
Carbon emissions can be estimated using the [Machine Learning Impact calculator](https://mlco2.github.io/impact#compute) presented in [Lacoste et al. (2019)](https://arxiv.org/abs/1910.09700).
- **Hardware Type:** [More Information Needed]
- **Hours used:** [More Information Needed]
- **Cloud Provider:** [More Information Needed]
- **Compute Region:** [More Information Needed]
- **Carbon Emitted:** [More Information Needed]
## Technical Specifications [optional]
### Model Architecture and Objective
[More Information Needed]
### Compute Infrastructure
[More Information Needed]
#### Hardware
[More Information Needed]
#### Software
[More Information Needed]
## Citation [optional]
<!-- If there is a paper or blog post introducing the model, the APA and Bibtex information for that should go in this section. -->
**BibTeX:**
[More Information Needed]
**APA:**
[More Information Needed]
## Glossary [optional]
<!-- If relevant, include terms and calculations in this section that can help readers understand the model or model card. -->
[More Information Needed]
## More Information [optional]
[More Information Needed]
## Model Card Authors [optional]
[More Information Needed]
## Model Card Contact
[More Information Needed] | {"library_name": "transformers", "tags": []} | liquid9212/y4jvxqj | null | [
"transformers",
"arxiv:1910.09700",
"endpoints_compatible",
"region:us"
] | null | 2024-04-25T14:49:40+00:00 | [
"1910.09700"
] | [] | TAGS
#transformers #arxiv-1910.09700 #endpoints_compatible #region-us
|
# Model Card for Model ID
## Model Details
### Model Description
This is the model card of a transformers model that has been pushed on the Hub. This model card has been automatically generated.
- Developed by:
- Funded by [optional]:
- Shared by [optional]:
- Model type:
- Language(s) (NLP):
- License:
- Finetuned from model [optional]:
### Model Sources [optional]
- Repository:
- Paper [optional]:
- Demo [optional]:
## Uses
### Direct Use
### Downstream Use [optional]
### Out-of-Scope Use
## Bias, Risks, and Limitations
### Recommendations
Users (both direct and downstream) should be made aware of the risks, biases and limitations of the model. More information needed for further recommendations.
## How to Get Started with the Model
Use the code below to get started with the model.
## Training Details
### Training Data
### Training Procedure
#### Preprocessing [optional]
#### Training Hyperparameters
- Training regime:
#### Speeds, Sizes, Times [optional]
## Evaluation
### Testing Data, Factors & Metrics
#### Testing Data
#### Factors
#### Metrics
### Results
#### Summary
## Model Examination [optional]
## Environmental Impact
Carbon emissions can be estimated using the Machine Learning Impact calculator presented in Lacoste et al. (2019).
- Hardware Type:
- Hours used:
- Cloud Provider:
- Compute Region:
- Carbon Emitted:
## Technical Specifications [optional]
### Model Architecture and Objective
### Compute Infrastructure
#### Hardware
#### Software
[optional]
BibTeX:
APA:
## Glossary [optional]
## More Information [optional]
## Model Card Authors [optional]
## Model Card Contact
| [
"# Model Card for Model ID",
"## Model Details",
"### Model Description\n\n\n\nThis is the model card of a transformers model that has been pushed on the Hub. This model card has been automatically generated.\n\n- Developed by: \n- Funded by [optional]: \n- Shared by [optional]: \n- Model type: \n- Language(s) (NLP): \n- License: \n- Finetuned from model [optional]:",
"### Model Sources [optional]\n\n\n\n- Repository: \n- Paper [optional]: \n- Demo [optional]:",
"## Uses",
"### Direct Use",
"### Downstream Use [optional]",
"### Out-of-Scope Use",
"## Bias, Risks, and Limitations",
"### Recommendations\n\n\n\nUsers (both direct and downstream) should be made aware of the risks, biases and limitations of the model. More information needed for further recommendations.",
"## How to Get Started with the Model\n\nUse the code below to get started with the model.",
"## Training Details",
"### Training Data",
"### Training Procedure",
"#### Preprocessing [optional]",
"#### Training Hyperparameters\n\n- Training regime:",
"#### Speeds, Sizes, Times [optional]",
"## Evaluation",
"### Testing Data, Factors & Metrics",
"#### Testing Data",
"#### Factors",
"#### Metrics",
"### Results",
"#### Summary",
"## Model Examination [optional]",
"## Environmental Impact\n\n\n\nCarbon emissions can be estimated using the Machine Learning Impact calculator presented in Lacoste et al. (2019).\n\n- Hardware Type: \n- Hours used: \n- Cloud Provider: \n- Compute Region: \n- Carbon Emitted:",
"## Technical Specifications [optional]",
"### Model Architecture and Objective",
"### Compute Infrastructure",
"#### Hardware",
"#### Software\n\n\n\n[optional]\n\n\n\nBibTeX:\n\n\n\nAPA:",
"## Glossary [optional]",
"## More Information [optional]",
"## Model Card Authors [optional]",
"## Model Card Contact"
] | [
"TAGS\n#transformers #arxiv-1910.09700 #endpoints_compatible #region-us \n",
"# Model Card for Model ID",
"## Model Details",
"### Model Description\n\n\n\nThis is the model card of a transformers model that has been pushed on the Hub. This model card has been automatically generated.\n\n- Developed by: \n- Funded by [optional]: \n- Shared by [optional]: \n- Model type: \n- Language(s) (NLP): \n- License: \n- Finetuned from model [optional]:",
"### Model Sources [optional]\n\n\n\n- Repository: \n- Paper [optional]: \n- Demo [optional]:",
"## Uses",
"### Direct Use",
"### Downstream Use [optional]",
"### Out-of-Scope Use",
"## Bias, Risks, and Limitations",
"### Recommendations\n\n\n\nUsers (both direct and downstream) should be made aware of the risks, biases and limitations of the model. More information needed for further recommendations.",
"## How to Get Started with the Model\n\nUse the code below to get started with the model.",
"## Training Details",
"### Training Data",
"### Training Procedure",
"#### Preprocessing [optional]",
"#### Training Hyperparameters\n\n- Training regime:",
"#### Speeds, Sizes, Times [optional]",
"## Evaluation",
"### Testing Data, Factors & Metrics",
"#### Testing Data",
"#### Factors",
"#### Metrics",
"### Results",
"#### Summary",
"## Model Examination [optional]",
"## Environmental Impact\n\n\n\nCarbon emissions can be estimated using the Machine Learning Impact calculator presented in Lacoste et al. (2019).\n\n- Hardware Type: \n- Hours used: \n- Cloud Provider: \n- Compute Region: \n- Carbon Emitted:",
"## Technical Specifications [optional]",
"### Model Architecture and Objective",
"### Compute Infrastructure",
"#### Hardware",
"#### Software\n\n\n\n[optional]\n\n\n\nBibTeX:\n\n\n\nAPA:",
"## Glossary [optional]",
"## More Information [optional]",
"## Model Card Authors [optional]",
"## Model Card Contact"
] |
text-generation | transformers |
- Original model is [yanolja/EEVE-Korean-Instruct-10.8B-v1.0](https://huggingface.co/yanolja/EEVE-Korean-Instruct-10.8B-v1.0)
- quantized using [llama.cpp](https://github.com/ggerganov/llama.cpp)
## Ollama
Modelfile
```
FROM EEVE-Korean-Instruct-10.8B-v1.0-Q8_0.gguf
TEMPLATE """{{- if .System }}
<s>{{ .System }}</s>
{{- end }}
<s>Human:
{{ .Prompt }}</s>
<s>Assistant:
"""
SYSTEM """A chat between a curious user and an artificial intelligence assistant. The assistant gives helpful, detailed, and polite answers to the user's questions."""
PARAMETER temperature 0
PARAMETER num_predict 3000
PARAMETER num_ctx 4096
PARAMETER stop <s>
PARAMETER stop </s>
```
### Training Data
- Korean-translated version of [Open-Orca/SlimOrca-Dedup](https://huggingface.co/datasets/Open-Orca/SlimOrca-Dedup)
- Korean-translated version of [argilla/ultrafeedback-binarized-preferences-cleaned](https://huggingface.co/datasets/argilla/ultrafeedback-binarized-preferences-cleaned)
- No other dataset was used
## Citation
```
@misc{kim2024efficient,
title={Efficient and Effective Vocabulary Expansion Towards Multilingual Large Language Models},
author={Seungduk Kim and Seungtaek Choi and Myeongho Jeong},
year={2024},
eprint={2402.14714},
archivePrefix={arXiv},
primaryClass={cs.CL}
}
```
```
@misc{cui2023ultrafeedback,
title={UltraFeedback: Boosting Language Models with High-quality Feedback},
author={Ganqu Cui and Lifan Yuan and Ning Ding and Guanming Yao and Wei Zhu and Yuan Ni and Guotong Xie and Zhiyuan Liu and Maosong Sun},
year={2023},
eprint={2310.01377},
archivePrefix={arXiv},
primaryClass={cs.CL}
}
```
```
@misc{SlimOrcaDedup,
title = {SlimOrca Dedup: A Deduplicated Subset of SlimOrca},
author = {Wing Lian and Guan Wang and Bleys Goodson and Eugene Pentland and Austin Cook and Chanvichet Vong and "Teknium" and Nathan Hoos},
year = {2023},
publisher = {HuggingFace},
url = {https://huggingface.co/datasets/Open-Orca/SlimOrca-Dedup/}
}
```
```
@misc{mukherjee2023orca,
title={Orca: Progressive Learning from Complex Explanation Traces of GPT-4},
author={Subhabrata Mukherjee and Arindam Mitra and Ganesh Jawahar and Sahaj Agarwal and Hamid Palangi and Ahmed Awadallah},
year={2023},
eprint={2306.02707},
archivePrefix={arXiv},
primaryClass={cs.CL}
}
```
| {"license": "apache-2.0", "tags": ["generated_from_trainer"], "base_model": "yanolja/EEVE-Korean-10.8B-v1.0", "model-index": [{"name": "yanolja/EEVE-Korean-Instruct-10.8B-v1.0", "results": []}]} | teddylee777/EEVE-Korean-Instruct-10.8B-v1.0-gguf | null | [
"transformers",
"gguf",
"llama",
"text-generation",
"generated_from_trainer",
"conversational",
"arxiv:2402.14714",
"arxiv:2310.01377",
"arxiv:2306.02707",
"base_model:yanolja/EEVE-Korean-10.8B-v1.0",
"license:apache-2.0",
"autotrain_compatible",
"endpoints_compatible",
"text-generation-inference",
"region:us"
] | null | 2024-04-25T14:51:55+00:00 | [
"2402.14714",
"2310.01377",
"2306.02707"
] | [] | TAGS
#transformers #gguf #llama #text-generation #generated_from_trainer #conversational #arxiv-2402.14714 #arxiv-2310.01377 #arxiv-2306.02707 #base_model-yanolja/EEVE-Korean-10.8B-v1.0 #license-apache-2.0 #autotrain_compatible #endpoints_compatible #text-generation-inference #region-us
|
- Original model is yanolja/EEVE-Korean-Instruct-10.8B-v1.0
- quantized using URL
## Ollama
Modelfile
### Training Data
- Korean-translated version of Open-Orca/SlimOrca-Dedup
- Korean-translated version of argilla/ultrafeedback-binarized-preferences-cleaned
- No other dataset was used
| [
"## Ollama\n\nModelfile",
"### Training Data\n - Korean-translated version of Open-Orca/SlimOrca-Dedup\n - Korean-translated version of argilla/ultrafeedback-binarized-preferences-cleaned\n - No other dataset was used"
] | [
"TAGS\n#transformers #gguf #llama #text-generation #generated_from_trainer #conversational #arxiv-2402.14714 #arxiv-2310.01377 #arxiv-2306.02707 #base_model-yanolja/EEVE-Korean-10.8B-v1.0 #license-apache-2.0 #autotrain_compatible #endpoints_compatible #text-generation-inference #region-us \n",
"## Ollama\n\nModelfile",
"### Training Data\n - Korean-translated version of Open-Orca/SlimOrca-Dedup\n - Korean-translated version of argilla/ultrafeedback-binarized-preferences-cleaned\n - No other dataset was used"
] |
fill-mask | transformers |
# Model Card for Model ID
<!-- Provide a quick summary of what the model is/does. -->
## Model Details
### Model Description
<!-- Provide a longer summary of what this model is. -->
This is the model card of a 🤗 transformers model that has been pushed on the Hub. This model card has been automatically generated.
- **Developed by:** [More Information Needed]
- **Funded by [optional]:** [More Information Needed]
- **Shared by [optional]:** [More Information Needed]
- **Model type:** [More Information Needed]
- **Language(s) (NLP):** [More Information Needed]
- **License:** [More Information Needed]
- **Finetuned from model [optional]:** [More Information Needed]
### Model Sources [optional]
<!-- Provide the basic links for the model. -->
- **Repository:** [More Information Needed]
- **Paper [optional]:** [More Information Needed]
- **Demo [optional]:** [More Information Needed]
## Uses
<!-- Address questions around how the model is intended to be used, including the foreseeable users of the model and those affected by the model. -->
### Direct Use
<!-- This section is for the model use without fine-tuning or plugging into a larger ecosystem/app. -->
[More Information Needed]
### Downstream Use [optional]
<!-- This section is for the model use when fine-tuned for a task, or when plugged into a larger ecosystem/app -->
[More Information Needed]
### Out-of-Scope Use
<!-- This section addresses misuse, malicious use, and uses that the model will not work well for. -->
[More Information Needed]
## Bias, Risks, and Limitations
<!-- This section is meant to convey both technical and sociotechnical limitations. -->
[More Information Needed]
### Recommendations
<!-- This section is meant to convey recommendations with respect to the bias, risk, and technical limitations. -->
Users (both direct and downstream) should be made aware of the risks, biases and limitations of the model. More information needed for further recommendations.
## How to Get Started with the Model
Use the code below to get started with the model.
[More Information Needed]
## Training Details
### Training Data
<!-- This should link to a Dataset Card, perhaps with a short stub of information on what the training data is all about as well as documentation related to data pre-processing or additional filtering. -->
[More Information Needed]
### Training Procedure
<!-- This relates heavily to the Technical Specifications. Content here should link to that section when it is relevant to the training procedure. -->
#### Preprocessing [optional]
[More Information Needed]
#### Training Hyperparameters
- **Training regime:** [More Information Needed] <!--fp32, fp16 mixed precision, bf16 mixed precision, bf16 non-mixed precision, fp16 non-mixed precision, fp8 mixed precision -->
#### Speeds, Sizes, Times [optional]
<!-- This section provides information about throughput, start/end time, checkpoint size if relevant, etc. -->
[More Information Needed]
## Evaluation
<!-- This section describes the evaluation protocols and provides the results. -->
### Testing Data, Factors & Metrics
#### Testing Data
<!-- This should link to a Dataset Card if possible. -->
[More Information Needed]
#### Factors
<!-- These are the things the evaluation is disaggregating by, e.g., subpopulations or domains. -->
[More Information Needed]
#### Metrics
<!-- These are the evaluation metrics being used, ideally with a description of why. -->
[More Information Needed]
### Results
[More Information Needed]
#### Summary
## Model Examination [optional]
<!-- Relevant interpretability work for the model goes here -->
[More Information Needed]
## Environmental Impact
<!-- Total emissions (in grams of CO2eq) and additional considerations, such as electricity usage, go here. Edit the suggested text below accordingly -->
Carbon emissions can be estimated using the [Machine Learning Impact calculator](https://mlco2.github.io/impact#compute) presented in [Lacoste et al. (2019)](https://arxiv.org/abs/1910.09700).
- **Hardware Type:** [More Information Needed]
- **Hours used:** [More Information Needed]
- **Cloud Provider:** [More Information Needed]
- **Compute Region:** [More Information Needed]
- **Carbon Emitted:** [More Information Needed]
## Technical Specifications [optional]
### Model Architecture and Objective
[More Information Needed]
### Compute Infrastructure
[More Information Needed]
#### Hardware
[More Information Needed]
#### Software
[More Information Needed]
## Citation [optional]
<!-- If there is a paper or blog post introducing the model, the APA and Bibtex information for that should go in this section. -->
**BibTeX:**
[More Information Needed]
**APA:**
[More Information Needed]
## Glossary [optional]
<!-- If relevant, include terms and calculations in this section that can help readers understand the model or model card. -->
[More Information Needed]
## More Information [optional]
[More Information Needed]
## Model Card Authors [optional]
[More Information Needed]
## Model Card Contact
[More Information Needed] | {"library_name": "transformers", "tags": []} | Lancelot53/rna_tokenizer_v4_4096 | null | [
"transformers",
"bert",
"fill-mask",
"custom_code",
"arxiv:1910.09700",
"autotrain_compatible",
"endpoints_compatible",
"region:us"
] | null | 2024-04-25T14:54:51+00:00 | [
"1910.09700"
] | [] | TAGS
#transformers #bert #fill-mask #custom_code #arxiv-1910.09700 #autotrain_compatible #endpoints_compatible #region-us
|
# Model Card for Model ID
## Model Details
### Model Description
This is the model card of a transformers model that has been pushed on the Hub. This model card has been automatically generated.
- Developed by:
- Funded by [optional]:
- Shared by [optional]:
- Model type:
- Language(s) (NLP):
- License:
- Finetuned from model [optional]:
### Model Sources [optional]
- Repository:
- Paper [optional]:
- Demo [optional]:
## Uses
### Direct Use
### Downstream Use [optional]
### Out-of-Scope Use
## Bias, Risks, and Limitations
### Recommendations
Users (both direct and downstream) should be made aware of the risks, biases and limitations of the model. More information needed for further recommendations.
## How to Get Started with the Model
Use the code below to get started with the model.
## Training Details
### Training Data
### Training Procedure
#### Preprocessing [optional]
#### Training Hyperparameters
- Training regime:
#### Speeds, Sizes, Times [optional]
## Evaluation
### Testing Data, Factors & Metrics
#### Testing Data
#### Factors
#### Metrics
### Results
#### Summary
## Model Examination [optional]
## Environmental Impact
Carbon emissions can be estimated using the Machine Learning Impact calculator presented in Lacoste et al. (2019).
- Hardware Type:
- Hours used:
- Cloud Provider:
- Compute Region:
- Carbon Emitted:
## Technical Specifications [optional]
### Model Architecture and Objective
### Compute Infrastructure
#### Hardware
#### Software
[optional]
BibTeX:
APA:
## Glossary [optional]
## More Information [optional]
## Model Card Authors [optional]
## Model Card Contact
| [
"# Model Card for Model ID",
"## Model Details",
"### Model Description\n\n\n\nThis is the model card of a transformers model that has been pushed on the Hub. This model card has been automatically generated.\n\n- Developed by: \n- Funded by [optional]: \n- Shared by [optional]: \n- Model type: \n- Language(s) (NLP): \n- License: \n- Finetuned from model [optional]:",
"### Model Sources [optional]\n\n\n\n- Repository: \n- Paper [optional]: \n- Demo [optional]:",
"## Uses",
"### Direct Use",
"### Downstream Use [optional]",
"### Out-of-Scope Use",
"## Bias, Risks, and Limitations",
"### Recommendations\n\n\n\nUsers (both direct and downstream) should be made aware of the risks, biases and limitations of the model. More information needed for further recommendations.",
"## How to Get Started with the Model\n\nUse the code below to get started with the model.",
"## Training Details",
"### Training Data",
"### Training Procedure",
"#### Preprocessing [optional]",
"#### Training Hyperparameters\n\n- Training regime:",
"#### Speeds, Sizes, Times [optional]",
"## Evaluation",
"### Testing Data, Factors & Metrics",
"#### Testing Data",
"#### Factors",
"#### Metrics",
"### Results",
"#### Summary",
"## Model Examination [optional]",
"## Environmental Impact\n\n\n\nCarbon emissions can be estimated using the Machine Learning Impact calculator presented in Lacoste et al. (2019).\n\n- Hardware Type: \n- Hours used: \n- Cloud Provider: \n- Compute Region: \n- Carbon Emitted:",
"## Technical Specifications [optional]",
"### Model Architecture and Objective",
"### Compute Infrastructure",
"#### Hardware",
"#### Software\n\n\n\n[optional]\n\n\n\nBibTeX:\n\n\n\nAPA:",
"## Glossary [optional]",
"## More Information [optional]",
"## Model Card Authors [optional]",
"## Model Card Contact"
] | [
"TAGS\n#transformers #bert #fill-mask #custom_code #arxiv-1910.09700 #autotrain_compatible #endpoints_compatible #region-us \n",
"# Model Card for Model ID",
"## Model Details",
"### Model Description\n\n\n\nThis is the model card of a transformers model that has been pushed on the Hub. This model card has been automatically generated.\n\n- Developed by: \n- Funded by [optional]: \n- Shared by [optional]: \n- Model type: \n- Language(s) (NLP): \n- License: \n- Finetuned from model [optional]:",
"### Model Sources [optional]\n\n\n\n- Repository: \n- Paper [optional]: \n- Demo [optional]:",
"## Uses",
"### Direct Use",
"### Downstream Use [optional]",
"### Out-of-Scope Use",
"## Bias, Risks, and Limitations",
"### Recommendations\n\n\n\nUsers (both direct and downstream) should be made aware of the risks, biases and limitations of the model. More information needed for further recommendations.",
"## How to Get Started with the Model\n\nUse the code below to get started with the model.",
"## Training Details",
"### Training Data",
"### Training Procedure",
"#### Preprocessing [optional]",
"#### Training Hyperparameters\n\n- Training regime:",
"#### Speeds, Sizes, Times [optional]",
"## Evaluation",
"### Testing Data, Factors & Metrics",
"#### Testing Data",
"#### Factors",
"#### Metrics",
"### Results",
"#### Summary",
"## Model Examination [optional]",
"## Environmental Impact\n\n\n\nCarbon emissions can be estimated using the Machine Learning Impact calculator presented in Lacoste et al. (2019).\n\n- Hardware Type: \n- Hours used: \n- Cloud Provider: \n- Compute Region: \n- Carbon Emitted:",
"## Technical Specifications [optional]",
"### Model Architecture and Objective",
"### Compute Infrastructure",
"#### Hardware",
"#### Software\n\n\n\n[optional]\n\n\n\nBibTeX:\n\n\n\nAPA:",
"## Glossary [optional]",
"## More Information [optional]",
"## Model Card Authors [optional]",
"## Model Card Contact"
] |
text-generation | transformers |
# Model Card for Model ID
<!-- Provide a quick summary of what the model is/does. -->
## Model Details
### Model Description
<!-- Provide a longer summary of what this model is. -->
This is the model card of a 🤗 transformers model that has been pushed on the Hub. This model card has been automatically generated.
- **Developed by:** [More Information Needed]
- **Funded by [optional]:** [More Information Needed]
- **Shared by [optional]:** [More Information Needed]
- **Model type:** [More Information Needed]
- **Language(s) (NLP):** [More Information Needed]
- **License:** [More Information Needed]
- **Finetuned from model [optional]:** [More Information Needed]
### Model Sources [optional]
<!-- Provide the basic links for the model. -->
- **Repository:** [More Information Needed]
- **Paper [optional]:** [More Information Needed]
- **Demo [optional]:** [More Information Needed]
## Uses
<!-- Address questions around how the model is intended to be used, including the foreseeable users of the model and those affected by the model. -->
### Direct Use
<!-- This section is for the model use without fine-tuning or plugging into a larger ecosystem/app. -->
[More Information Needed]
### Downstream Use [optional]
<!-- This section is for the model use when fine-tuned for a task, or when plugged into a larger ecosystem/app -->
[More Information Needed]
### Out-of-Scope Use
<!-- This section addresses misuse, malicious use, and uses that the model will not work well for. -->
[More Information Needed]
## Bias, Risks, and Limitations
<!-- This section is meant to convey both technical and sociotechnical limitations. -->
[More Information Needed]
### Recommendations
<!-- This section is meant to convey recommendations with respect to the bias, risk, and technical limitations. -->
Users (both direct and downstream) should be made aware of the risks, biases and limitations of the model. More information needed for further recommendations.
## How to Get Started with the Model
Use the code below to get started with the model.
[More Information Needed]
## Training Details
### Training Data
<!-- This should link to a Dataset Card, perhaps with a short stub of information on what the training data is all about as well as documentation related to data pre-processing or additional filtering. -->
[More Information Needed]
### Training Procedure
<!-- This relates heavily to the Technical Specifications. Content here should link to that section when it is relevant to the training procedure. -->
#### Preprocessing [optional]
[More Information Needed]
#### Training Hyperparameters
- **Training regime:** [More Information Needed] <!--fp32, fp16 mixed precision, bf16 mixed precision, bf16 non-mixed precision, fp16 non-mixed precision, fp8 mixed precision -->
#### Speeds, Sizes, Times [optional]
<!-- This section provides information about throughput, start/end time, checkpoint size if relevant, etc. -->
[More Information Needed]
## Evaluation
<!-- This section describes the evaluation protocols and provides the results. -->
### Testing Data, Factors & Metrics
#### Testing Data
<!-- This should link to a Dataset Card if possible. -->
[More Information Needed]
#### Factors
<!-- These are the things the evaluation is disaggregating by, e.g., subpopulations or domains. -->
[More Information Needed]
#### Metrics
<!-- These are the evaluation metrics being used, ideally with a description of why. -->
[More Information Needed]
### Results
[More Information Needed]
#### Summary
## Model Examination [optional]
<!-- Relevant interpretability work for the model goes here -->
[More Information Needed]
## Environmental Impact
<!-- Total emissions (in grams of CO2eq) and additional considerations, such as electricity usage, go here. Edit the suggested text below accordingly -->
Carbon emissions can be estimated using the [Machine Learning Impact calculator](https://mlco2.github.io/impact#compute) presented in [Lacoste et al. (2019)](https://arxiv.org/abs/1910.09700).
- **Hardware Type:** [More Information Needed]
- **Hours used:** [More Information Needed]
- **Cloud Provider:** [More Information Needed]
- **Compute Region:** [More Information Needed]
- **Carbon Emitted:** [More Information Needed]
## Technical Specifications [optional]
### Model Architecture and Objective
[More Information Needed]
### Compute Infrastructure
[More Information Needed]
#### Hardware
[More Information Needed]
#### Software
[More Information Needed]
## Citation [optional]
<!-- If there is a paper or blog post introducing the model, the APA and Bibtex information for that should go in this section. -->
**BibTeX:**
[More Information Needed]
**APA:**
[More Information Needed]
## Glossary [optional]
<!-- If relevant, include terms and calculations in this section that can help readers understand the model or model card. -->
[More Information Needed]
## More Information [optional]
[More Information Needed]
## Model Card Authors [optional]
[More Information Needed]
## Model Card Contact
[More Information Needed] | {"library_name": "transformers", "tags": []} | liquid9212/m4dn6bf | null | [
"transformers",
"safetensors",
"stablelm",
"text-generation",
"conversational",
"arxiv:1910.09700",
"autotrain_compatible",
"endpoints_compatible",
"region:us"
] | null | 2024-04-25T14:55:25+00:00 | [
"1910.09700"
] | [] | TAGS
#transformers #safetensors #stablelm #text-generation #conversational #arxiv-1910.09700 #autotrain_compatible #endpoints_compatible #region-us
|
# Model Card for Model ID
## Model Details
### Model Description
This is the model card of a transformers model that has been pushed on the Hub. This model card has been automatically generated.
- Developed by:
- Funded by [optional]:
- Shared by [optional]:
- Model type:
- Language(s) (NLP):
- License:
- Finetuned from model [optional]:
### Model Sources [optional]
- Repository:
- Paper [optional]:
- Demo [optional]:
## Uses
### Direct Use
### Downstream Use [optional]
### Out-of-Scope Use
## Bias, Risks, and Limitations
### Recommendations
Users (both direct and downstream) should be made aware of the risks, biases and limitations of the model. More information needed for further recommendations.
## How to Get Started with the Model
Use the code below to get started with the model.
## Training Details
### Training Data
### Training Procedure
#### Preprocessing [optional]
#### Training Hyperparameters
- Training regime:
#### Speeds, Sizes, Times [optional]
## Evaluation
### Testing Data, Factors & Metrics
#### Testing Data
#### Factors
#### Metrics
### Results
#### Summary
## Model Examination [optional]
## Environmental Impact
Carbon emissions can be estimated using the Machine Learning Impact calculator presented in Lacoste et al. (2019).
- Hardware Type:
- Hours used:
- Cloud Provider:
- Compute Region:
- Carbon Emitted:
## Technical Specifications [optional]
### Model Architecture and Objective
### Compute Infrastructure
#### Hardware
#### Software
[optional]
BibTeX:
APA:
## Glossary [optional]
## More Information [optional]
## Model Card Authors [optional]
## Model Card Contact
| [
"# Model Card for Model ID",
"## Model Details",
"### Model Description\n\n\n\nThis is the model card of a transformers model that has been pushed on the Hub. This model card has been automatically generated.\n\n- Developed by: \n- Funded by [optional]: \n- Shared by [optional]: \n- Model type: \n- Language(s) (NLP): \n- License: \n- Finetuned from model [optional]:",
"### Model Sources [optional]\n\n\n\n- Repository: \n- Paper [optional]: \n- Demo [optional]:",
"## Uses",
"### Direct Use",
"### Downstream Use [optional]",
"### Out-of-Scope Use",
"## Bias, Risks, and Limitations",
"### Recommendations\n\n\n\nUsers (both direct and downstream) should be made aware of the risks, biases and limitations of the model. More information needed for further recommendations.",
"## How to Get Started with the Model\n\nUse the code below to get started with the model.",
"## Training Details",
"### Training Data",
"### Training Procedure",
"#### Preprocessing [optional]",
"#### Training Hyperparameters\n\n- Training regime:",
"#### Speeds, Sizes, Times [optional]",
"## Evaluation",
"### Testing Data, Factors & Metrics",
"#### Testing Data",
"#### Factors",
"#### Metrics",
"### Results",
"#### Summary",
"## Model Examination [optional]",
"## Environmental Impact\n\n\n\nCarbon emissions can be estimated using the Machine Learning Impact calculator presented in Lacoste et al. (2019).\n\n- Hardware Type: \n- Hours used: \n- Cloud Provider: \n- Compute Region: \n- Carbon Emitted:",
"## Technical Specifications [optional]",
"### Model Architecture and Objective",
"### Compute Infrastructure",
"#### Hardware",
"#### Software\n\n\n\n[optional]\n\n\n\nBibTeX:\n\n\n\nAPA:",
"## Glossary [optional]",
"## More Information [optional]",
"## Model Card Authors [optional]",
"## Model Card Contact"
] | [
"TAGS\n#transformers #safetensors #stablelm #text-generation #conversational #arxiv-1910.09700 #autotrain_compatible #endpoints_compatible #region-us \n",
"# Model Card for Model ID",
"## Model Details",
"### Model Description\n\n\n\nThis is the model card of a transformers model that has been pushed on the Hub. This model card has been automatically generated.\n\n- Developed by: \n- Funded by [optional]: \n- Shared by [optional]: \n- Model type: \n- Language(s) (NLP): \n- License: \n- Finetuned from model [optional]:",
"### Model Sources [optional]\n\n\n\n- Repository: \n- Paper [optional]: \n- Demo [optional]:",
"## Uses",
"### Direct Use",
"### Downstream Use [optional]",
"### Out-of-Scope Use",
"## Bias, Risks, and Limitations",
"### Recommendations\n\n\n\nUsers (both direct and downstream) should be made aware of the risks, biases and limitations of the model. More information needed for further recommendations.",
"## How to Get Started with the Model\n\nUse the code below to get started with the model.",
"## Training Details",
"### Training Data",
"### Training Procedure",
"#### Preprocessing [optional]",
"#### Training Hyperparameters\n\n- Training regime:",
"#### Speeds, Sizes, Times [optional]",
"## Evaluation",
"### Testing Data, Factors & Metrics",
"#### Testing Data",
"#### Factors",
"#### Metrics",
"### Results",
"#### Summary",
"## Model Examination [optional]",
"## Environmental Impact\n\n\n\nCarbon emissions can be estimated using the Machine Learning Impact calculator presented in Lacoste et al. (2019).\n\n- Hardware Type: \n- Hours used: \n- Cloud Provider: \n- Compute Region: \n- Carbon Emitted:",
"## Technical Specifications [optional]",
"### Model Architecture and Objective",
"### Compute Infrastructure",
"#### Hardware",
"#### Software\n\n\n\n[optional]\n\n\n\nBibTeX:\n\n\n\nAPA:",
"## Glossary [optional]",
"## More Information [optional]",
"## Model Card Authors [optional]",
"## Model Card Contact"
] |
text-generation | transformers |
# Model Card for Model ID
<!-- Provide a quick summary of what the model is/does. -->
## Model Details
### Model Description
<!-- Provide a longer summary of what this model is. -->
This is the model card of a 🤗 transformers model that has been pushed on the Hub. This model card has been automatically generated.
- **Developed by:** [More Information Needed]
- **Funded by [optional]:** [More Information Needed]
- **Shared by [optional]:** [More Information Needed]
- **Model type:** [More Information Needed]
- **Language(s) (NLP):** [More Information Needed]
- **License:** [More Information Needed]
- **Finetuned from model [optional]:** [More Information Needed]
### Model Sources [optional]
<!-- Provide the basic links for the model. -->
- **Repository:** [More Information Needed]
- **Paper [optional]:** [More Information Needed]
- **Demo [optional]:** [More Information Needed]
## Uses
<!-- Address questions around how the model is intended to be used, including the foreseeable users of the model and those affected by the model. -->
### Direct Use
<!-- This section is for the model use without fine-tuning or plugging into a larger ecosystem/app. -->
[More Information Needed]
### Downstream Use [optional]
<!-- This section is for the model use when fine-tuned for a task, or when plugged into a larger ecosystem/app -->
[More Information Needed]
### Out-of-Scope Use
<!-- This section addresses misuse, malicious use, and uses that the model will not work well for. -->
[More Information Needed]
## Bias, Risks, and Limitations
<!-- This section is meant to convey both technical and sociotechnical limitations. -->
[More Information Needed]
### Recommendations
<!-- This section is meant to convey recommendations with respect to the bias, risk, and technical limitations. -->
Users (both direct and downstream) should be made aware of the risks, biases and limitations of the model. More information needed for further recommendations.
## How to Get Started with the Model
Use the code below to get started with the model.
[More Information Needed]
## Training Details
### Training Data
<!-- This should link to a Dataset Card, perhaps with a short stub of information on what the training data is all about as well as documentation related to data pre-processing or additional filtering. -->
[More Information Needed]
### Training Procedure
<!-- This relates heavily to the Technical Specifications. Content here should link to that section when it is relevant to the training procedure. -->
#### Preprocessing [optional]
[More Information Needed]
#### Training Hyperparameters
- **Training regime:** [More Information Needed] <!--fp32, fp16 mixed precision, bf16 mixed precision, bf16 non-mixed precision, fp16 non-mixed precision, fp8 mixed precision -->
#### Speeds, Sizes, Times [optional]
<!-- This section provides information about throughput, start/end time, checkpoint size if relevant, etc. -->
[More Information Needed]
## Evaluation
<!-- This section describes the evaluation protocols and provides the results. -->
### Testing Data, Factors & Metrics
#### Testing Data
<!-- This should link to a Dataset Card if possible. -->
[More Information Needed]
#### Factors
<!-- These are the things the evaluation is disaggregating by, e.g., subpopulations or domains. -->
[More Information Needed]
#### Metrics
<!-- These are the evaluation metrics being used, ideally with a description of why. -->
[More Information Needed]
### Results
[More Information Needed]
#### Summary
## Model Examination [optional]
<!-- Relevant interpretability work for the model goes here -->
[More Information Needed]
## Environmental Impact
<!-- Total emissions (in grams of CO2eq) and additional considerations, such as electricity usage, go here. Edit the suggested text below accordingly -->
Carbon emissions can be estimated using the [Machine Learning Impact calculator](https://mlco2.github.io/impact#compute) presented in [Lacoste et al. (2019)](https://arxiv.org/abs/1910.09700).
- **Hardware Type:** [More Information Needed]
- **Hours used:** [More Information Needed]
- **Cloud Provider:** [More Information Needed]
- **Compute Region:** [More Information Needed]
- **Carbon Emitted:** [More Information Needed]
## Technical Specifications [optional]
### Model Architecture and Objective
[More Information Needed]
### Compute Infrastructure
[More Information Needed]
#### Hardware
[More Information Needed]
#### Software
[More Information Needed]
## Citation [optional]
<!-- If there is a paper or blog post introducing the model, the APA and Bibtex information for that should go in this section. -->
**BibTeX:**
[More Information Needed]
**APA:**
[More Information Needed]
## Glossary [optional]
<!-- If relevant, include terms and calculations in this section that can help readers understand the model or model card. -->
[More Information Needed]
## More Information [optional]
[More Information Needed]
## Model Card Authors [optional]
[More Information Needed]
## Model Card Contact
[More Information Needed] | {"library_name": "transformers", "tags": []} | quickstep3621/aqnb2s0 | null | [
"transformers",
"safetensors",
"stablelm",
"text-generation",
"conversational",
"arxiv:1910.09700",
"autotrain_compatible",
"endpoints_compatible",
"region:us"
] | null | 2024-04-25T14:55:56+00:00 | [
"1910.09700"
] | [] | TAGS
#transformers #safetensors #stablelm #text-generation #conversational #arxiv-1910.09700 #autotrain_compatible #endpoints_compatible #region-us
|
# Model Card for Model ID
## Model Details
### Model Description
This is the model card of a transformers model that has been pushed on the Hub. This model card has been automatically generated.
- Developed by:
- Funded by [optional]:
- Shared by [optional]:
- Model type:
- Language(s) (NLP):
- License:
- Finetuned from model [optional]:
### Model Sources [optional]
- Repository:
- Paper [optional]:
- Demo [optional]:
## Uses
### Direct Use
### Downstream Use [optional]
### Out-of-Scope Use
## Bias, Risks, and Limitations
### Recommendations
Users (both direct and downstream) should be made aware of the risks, biases and limitations of the model. More information needed for further recommendations.
## How to Get Started with the Model
Use the code below to get started with the model.
## Training Details
### Training Data
### Training Procedure
#### Preprocessing [optional]
#### Training Hyperparameters
- Training regime:
#### Speeds, Sizes, Times [optional]
## Evaluation
### Testing Data, Factors & Metrics
#### Testing Data
#### Factors
#### Metrics
### Results
#### Summary
## Model Examination [optional]
## Environmental Impact
Carbon emissions can be estimated using the Machine Learning Impact calculator presented in Lacoste et al. (2019).
- Hardware Type:
- Hours used:
- Cloud Provider:
- Compute Region:
- Carbon Emitted:
## Technical Specifications [optional]
### Model Architecture and Objective
### Compute Infrastructure
#### Hardware
#### Software
[optional]
BibTeX:
APA:
## Glossary [optional]
## More Information [optional]
## Model Card Authors [optional]
## Model Card Contact
| [
"# Model Card for Model ID",
"## Model Details",
"### Model Description\n\n\n\nThis is the model card of a transformers model that has been pushed on the Hub. This model card has been automatically generated.\n\n- Developed by: \n- Funded by [optional]: \n- Shared by [optional]: \n- Model type: \n- Language(s) (NLP): \n- License: \n- Finetuned from model [optional]:",
"### Model Sources [optional]\n\n\n\n- Repository: \n- Paper [optional]: \n- Demo [optional]:",
"## Uses",
"### Direct Use",
"### Downstream Use [optional]",
"### Out-of-Scope Use",
"## Bias, Risks, and Limitations",
"### Recommendations\n\n\n\nUsers (both direct and downstream) should be made aware of the risks, biases and limitations of the model. More information needed for further recommendations.",
"## How to Get Started with the Model\n\nUse the code below to get started with the model.",
"## Training Details",
"### Training Data",
"### Training Procedure",
"#### Preprocessing [optional]",
"#### Training Hyperparameters\n\n- Training regime:",
"#### Speeds, Sizes, Times [optional]",
"## Evaluation",
"### Testing Data, Factors & Metrics",
"#### Testing Data",
"#### Factors",
"#### Metrics",
"### Results",
"#### Summary",
"## Model Examination [optional]",
"## Environmental Impact\n\n\n\nCarbon emissions can be estimated using the Machine Learning Impact calculator presented in Lacoste et al. (2019).\n\n- Hardware Type: \n- Hours used: \n- Cloud Provider: \n- Compute Region: \n- Carbon Emitted:",
"## Technical Specifications [optional]",
"### Model Architecture and Objective",
"### Compute Infrastructure",
"#### Hardware",
"#### Software\n\n\n\n[optional]\n\n\n\nBibTeX:\n\n\n\nAPA:",
"## Glossary [optional]",
"## More Information [optional]",
"## Model Card Authors [optional]",
"## Model Card Contact"
] | [
"TAGS\n#transformers #safetensors #stablelm #text-generation #conversational #arxiv-1910.09700 #autotrain_compatible #endpoints_compatible #region-us \n",
"# Model Card for Model ID",
"## Model Details",
"### Model Description\n\n\n\nThis is the model card of a transformers model that has been pushed on the Hub. This model card has been automatically generated.\n\n- Developed by: \n- Funded by [optional]: \n- Shared by [optional]: \n- Model type: \n- Language(s) (NLP): \n- License: \n- Finetuned from model [optional]:",
"### Model Sources [optional]\n\n\n\n- Repository: \n- Paper [optional]: \n- Demo [optional]:",
"## Uses",
"### Direct Use",
"### Downstream Use [optional]",
"### Out-of-Scope Use",
"## Bias, Risks, and Limitations",
"### Recommendations\n\n\n\nUsers (both direct and downstream) should be made aware of the risks, biases and limitations of the model. More information needed for further recommendations.",
"## How to Get Started with the Model\n\nUse the code below to get started with the model.",
"## Training Details",
"### Training Data",
"### Training Procedure",
"#### Preprocessing [optional]",
"#### Training Hyperparameters\n\n- Training regime:",
"#### Speeds, Sizes, Times [optional]",
"## Evaluation",
"### Testing Data, Factors & Metrics",
"#### Testing Data",
"#### Factors",
"#### Metrics",
"### Results",
"#### Summary",
"## Model Examination [optional]",
"## Environmental Impact\n\n\n\nCarbon emissions can be estimated using the Machine Learning Impact calculator presented in Lacoste et al. (2019).\n\n- Hardware Type: \n- Hours used: \n- Cloud Provider: \n- Compute Region: \n- Carbon Emitted:",
"## Technical Specifications [optional]",
"### Model Architecture and Objective",
"### Compute Infrastructure",
"#### Hardware",
"#### Software\n\n\n\n[optional]\n\n\n\nBibTeX:\n\n\n\nAPA:",
"## Glossary [optional]",
"## More Information [optional]",
"## Model Card Authors [optional]",
"## Model Card Contact"
] |
text-generation | null |
## Exllama v2 Quantizations of L3-TheSpice-8b-v0.8.3
Using <a href="https://github.com/turboderp/exllamav2/releases/tag/v0.0.19">turboderp's ExLlamaV2 v0.0.19</a> for quantization.
<b>The "main" branch only contains the measurement.json, download one of the other branches for the model (see below)</b>
Each branch contains an individual bits per weight, with the main one containing only the meaurement.json for further conversions.
Original model: https://huggingface.co/cgato/L3-TheSpice-8b-v0.8.3
## Prompt format
```
{System Prompt}
Username: {Input}
BotName: {Response}
Username: {Input}
BotName: {Response}
```
## Available sizes
| Branch | Bits | lm_head bits | VRAM (4k) | VRAM (8K) | VRAM (16k) | VRAM (32k) | Description |
| ----- | ---- | ------- | ------ | ------ | ------ | ------ | ------------ |
| [8_0](https://huggingface.co/bartowski/L3-TheSpice-8b-v0.8.3-exl2/tree/8_0) | 8.0 | 8.0 | 10.1 GB | 10.5 GB | 11.5 GB | 13.6 GB | Maximum quality that ExLlamaV2 can produce, near unquantized performance. |
| [6_5](https://huggingface.co/bartowski/L3-TheSpice-8b-v0.8.3-exl2/tree/6_5) | 6.5 | 8.0 | 8.9 GB | 9.3 GB | 10.3 GB | 12.4 GB | Very similar to 8.0, good tradeoff of size vs performance, **recommended**. |
| [5_0](https://huggingface.co/bartowski/L3-TheSpice-8b-v0.8.3-exl2/tree/5_0) | 5.0 | 6.0 | 7.7 GB | 8.1 GB | 9.1 GB | 11.2 GB | Slightly lower quality vs 6.5, but usable on 8GB cards. |
| [4_25](https://huggingface.co/bartowski/L3-TheSpice-8b-v0.8.3-exl2/tree/4_25) | 4.25 | 6.0 | 7.0 GB | 7.4 GB | 8.4 GB | 10.5 GB | GPTQ equivalent bits per weight, slightly higher quality. |
| [3_5](https://huggingface.co/bartowski/L3-TheSpice-8b-v0.8.3-exl2/tree/3_5) | 3.5 | 6.0 | 6.4 GB | 6.8 GB | 7.8 GB | 9.9 GB | Lower quality, only use if you have to. |
## Download instructions
With git:
```shell
git clone --single-branch --branch 6_5 https://huggingface.co/bartowski/L3-TheSpice-8b-v0.8.3-exl2 L3-TheSpice-8b-v0.8.3-exl2-6_5
```
With huggingface hub (credit to TheBloke for instructions):
```shell
pip3 install huggingface-hub
```
To download a specific branch, use the `--revision` parameter. For example, to download the 6.5 bpw branch:
Linux:
```shell
huggingface-cli download bartowski/L3-TheSpice-8b-v0.8.3-exl2 --revision 6_5 --local-dir L3-TheSpice-8b-v0.8.3-exl2-6_5 --local-dir-use-symlinks False
```
Windows (which apparently doesn't like _ in folders sometimes?):
```shell
huggingface-cli download bartowski/L3-TheSpice-8b-v0.8.3-exl2 --revision 6_5 --local-dir L3-TheSpice-8b-v0.8.3-exl2-6.5 --local-dir-use-symlinks False
```
Want to support my work? Visit my ko-fi page here: https://ko-fi.com/bartowski
| {"license": "cc-by-nc-4.0", "quantized_by": "bartowski", "pipeline_tag": "text-generation"} | bartowski/L3-TheSpice-8b-v0.8.3-exl2 | null | [
"text-generation",
"license:cc-by-nc-4.0",
"region:us"
] | null | 2024-04-25T14:56:56+00:00 | [] | [] | TAGS
#text-generation #license-cc-by-nc-4.0 #region-us
| Exllama v2 Quantizations of L3-TheSpice-8b-v0.8.3
-------------------------------------------------
Using <a href="URL ExLlamaV2 v0.0.19 for quantization.
**The "main" branch only contains the URL, download one of the other branches for the model (see below)**
Each branch contains an individual bits per weight, with the main one containing only the URL for further conversions.
Original model: URL
Prompt format
-------------
Available sizes
---------------
Download instructions
---------------------
With git:
With huggingface hub (credit to TheBloke for instructions):
To download a specific branch, use the '--revision' parameter. For example, to download the 6.5 bpw branch:
Linux:
Windows (which apparently doesn't like \_ in folders sometimes?):
Want to support my work? Visit my ko-fi page here: URL
| [] | [
"TAGS\n#text-generation #license-cc-by-nc-4.0 #region-us \n"
] |
text-to-image | diffusers |
# API Inference
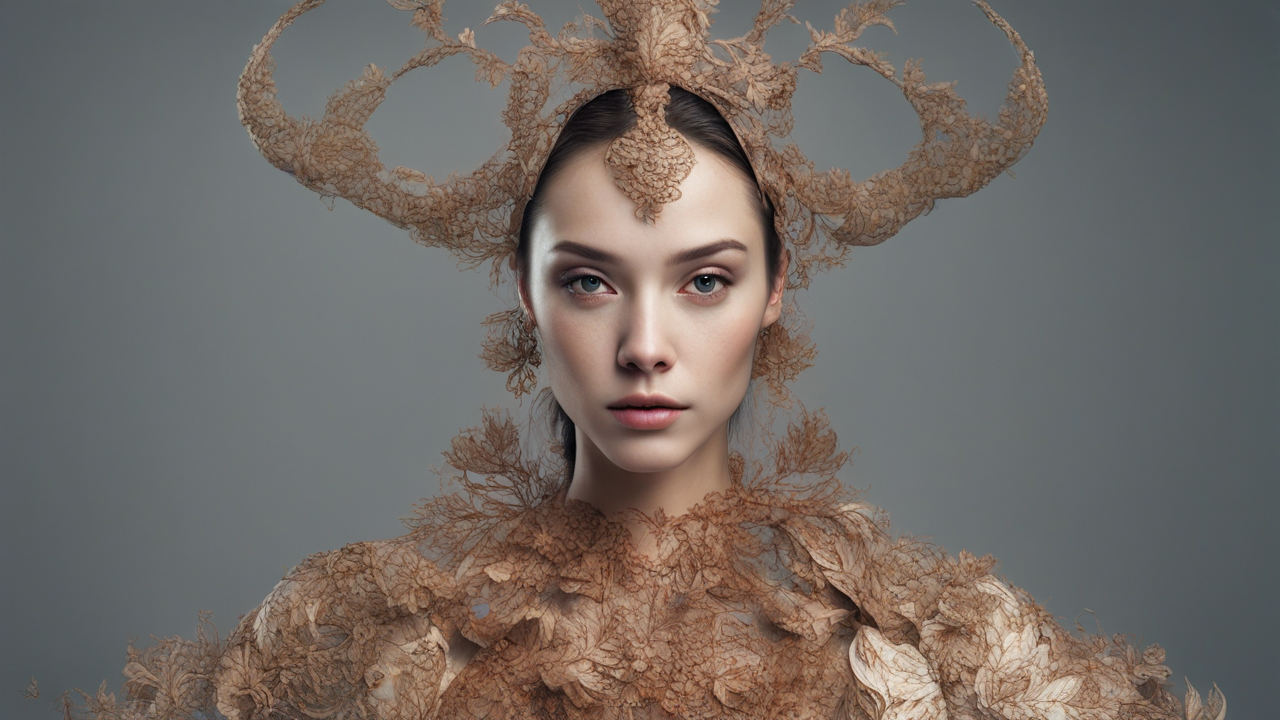
## Get API Key
Get API key from [ModelsLab API](http://modelslab.com), No Payment needed.
Replace Key in below code, change **model_id** to "realspice3"
Coding in PHP/Node/Java etc? Have a look at docs for more code examples: [View docs](https://modelslab.com/docs)
Try model for free: [Generate Images](https://modelslab.com/models/realspice3)
Model link: [View model](https://modelslab.com/models/realspice3)
View all models: [View Models](https://modelslab.com/models)
import requests
import json
url = "https://modelslab.com/api/v6/images/text2img"
payload = json.dumps({
"key": "your_api_key",
"model_id": "realspice3",
"prompt": "ultra realistic close up portrait ((beautiful pale cyberpunk female with heavy black eyeliner)), blue eyes, shaved side haircut, hyper detail, cinematic lighting, magic neon, dark red city, Canon EOS R3, nikon, f/1.4, ISO 200, 1/160s, 8K, RAW, unedited, symmetrical balance, in-frame, 8K",
"negative_prompt": "painting, extra fingers, mutated hands, poorly drawn hands, poorly drawn face, deformed, ugly, blurry, bad anatomy, bad proportions, extra limbs, cloned face, skinny, glitchy, double torso, extra arms, extra hands, mangled fingers, missing lips, ugly face, distorted face, extra legs, anime",
"width": "512",
"height": "512",
"samples": "1",
"num_inference_steps": "30",
"safety_checker": "no",
"enhance_prompt": "yes",
"seed": None,
"guidance_scale": 7.5,
"multi_lingual": "no",
"panorama": "no",
"self_attention": "no",
"upscale": "no",
"embeddings": "embeddings_model_id",
"lora": "lora_model_id",
"webhook": None,
"track_id": None
})
headers = {
'Content-Type': 'application/json'
}
response = requests.request("POST", url, headers=headers, data=payload)
print(response.text)
> Use this coupon code to get 25% off **DMGG0RBN** | {"license": "creativeml-openrail-m", "tags": ["modelslab.com", "stable-diffusion-api", "text-to-image", "ultra-realistic"], "pinned": true} | stablediffusionapi/realspice3 | null | [
"diffusers",
"modelslab.com",
"stable-diffusion-api",
"text-to-image",
"ultra-realistic",
"license:creativeml-openrail-m",
"endpoints_compatible",
"diffusers:StableDiffusionPipeline",
"region:us"
] | null | 2024-04-25T14:56:58+00:00 | [] | [] | TAGS
#diffusers #modelslab.com #stable-diffusion-api #text-to-image #ultra-realistic #license-creativeml-openrail-m #endpoints_compatible #diffusers-StableDiffusionPipeline #region-us
|
# API Inference
!generated from URL
## Get API Key
Get API key from ModelsLab API, No Payment needed.
Replace Key in below code, change model_id to "realspice3"
Coding in PHP/Node/Java etc? Have a look at docs for more code examples: View docs
Try model for free: Generate Images
Model link: View model
View all models: View Models
import requests
import json
url = "URL
payload = URL({
"key": "your_api_key",
"model_id": "realspice3",
"prompt": "ultra realistic close up portrait ((beautiful pale cyberpunk female with heavy black eyeliner)), blue eyes, shaved side haircut, hyper detail, cinematic lighting, magic neon, dark red city, Canon EOS R3, nikon, f/1.4, ISO 200, 1/160s, 8K, RAW, unedited, symmetrical balance, in-frame, 8K",
"negative_prompt": "painting, extra fingers, mutated hands, poorly drawn hands, poorly drawn face, deformed, ugly, blurry, bad anatomy, bad proportions, extra limbs, cloned face, skinny, glitchy, double torso, extra arms, extra hands, mangled fingers, missing lips, ugly face, distorted face, extra legs, anime",
"width": "512",
"height": "512",
"samples": "1",
"num_inference_steps": "30",
"safety_checker": "no",
"enhance_prompt": "yes",
"seed": None,
"guidance_scale": 7.5,
"multi_lingual": "no",
"panorama": "no",
"self_attention": "no",
"upscale": "no",
"embeddings": "embeddings_model_id",
"lora": "lora_model_id",
"webhook": None,
"track_id": None
})
headers = {
'Content-Type': 'application/json'
}
response = requests.request("POST", url, headers=headers, data=payload)
print(URL)
> Use this coupon code to get 25% off DMGG0RBN | [
"# API Inference\n\n!generated from URL",
"## Get API Key\n\nGet API key from ModelsLab API, No Payment needed. \n\nReplace Key in below code, change model_id to \"realspice3\"\n\nCoding in PHP/Node/Java etc? Have a look at docs for more code examples: View docs\n\nTry model for free: Generate Images\n\nModel link: View model\n\nView all models: View Models\n\n import requests \n import json \n \n url = \"URL \n \n payload = URL({ \n \"key\": \"your_api_key\", \n \"model_id\": \"realspice3\", \n \"prompt\": \"ultra realistic close up portrait ((beautiful pale cyberpunk female with heavy black eyeliner)), blue eyes, shaved side haircut, hyper detail, cinematic lighting, magic neon, dark red city, Canon EOS R3, nikon, f/1.4, ISO 200, 1/160s, 8K, RAW, unedited, symmetrical balance, in-frame, 8K\", \n \"negative_prompt\": \"painting, extra fingers, mutated hands, poorly drawn hands, poorly drawn face, deformed, ugly, blurry, bad anatomy, bad proportions, extra limbs, cloned face, skinny, glitchy, double torso, extra arms, extra hands, mangled fingers, missing lips, ugly face, distorted face, extra legs, anime\", \n \"width\": \"512\", \n \"height\": \"512\", \n \"samples\": \"1\", \n \"num_inference_steps\": \"30\", \n \"safety_checker\": \"no\", \n \"enhance_prompt\": \"yes\", \n \"seed\": None, \n \"guidance_scale\": 7.5, \n \"multi_lingual\": \"no\", \n \"panorama\": \"no\", \n \"self_attention\": \"no\", \n \"upscale\": \"no\", \n \"embeddings\": \"embeddings_model_id\", \n \"lora\": \"lora_model_id\", \n \"webhook\": None, \n \"track_id\": None \n }) \n \n headers = { \n 'Content-Type': 'application/json' \n } \n \n response = requests.request(\"POST\", url, headers=headers, data=payload) \n \n print(URL)\n\n> Use this coupon code to get 25% off DMGG0RBN"
] | [
"TAGS\n#diffusers #modelslab.com #stable-diffusion-api #text-to-image #ultra-realistic #license-creativeml-openrail-m #endpoints_compatible #diffusers-StableDiffusionPipeline #region-us \n",
"# API Inference\n\n!generated from URL",
"## Get API Key\n\nGet API key from ModelsLab API, No Payment needed. \n\nReplace Key in below code, change model_id to \"realspice3\"\n\nCoding in PHP/Node/Java etc? Have a look at docs for more code examples: View docs\n\nTry model for free: Generate Images\n\nModel link: View model\n\nView all models: View Models\n\n import requests \n import json \n \n url = \"URL \n \n payload = URL({ \n \"key\": \"your_api_key\", \n \"model_id\": \"realspice3\", \n \"prompt\": \"ultra realistic close up portrait ((beautiful pale cyberpunk female with heavy black eyeliner)), blue eyes, shaved side haircut, hyper detail, cinematic lighting, magic neon, dark red city, Canon EOS R3, nikon, f/1.4, ISO 200, 1/160s, 8K, RAW, unedited, symmetrical balance, in-frame, 8K\", \n \"negative_prompt\": \"painting, extra fingers, mutated hands, poorly drawn hands, poorly drawn face, deformed, ugly, blurry, bad anatomy, bad proportions, extra limbs, cloned face, skinny, glitchy, double torso, extra arms, extra hands, mangled fingers, missing lips, ugly face, distorted face, extra legs, anime\", \n \"width\": \"512\", \n \"height\": \"512\", \n \"samples\": \"1\", \n \"num_inference_steps\": \"30\", \n \"safety_checker\": \"no\", \n \"enhance_prompt\": \"yes\", \n \"seed\": None, \n \"guidance_scale\": 7.5, \n \"multi_lingual\": \"no\", \n \"panorama\": \"no\", \n \"self_attention\": \"no\", \n \"upscale\": \"no\", \n \"embeddings\": \"embeddings_model_id\", \n \"lora\": \"lora_model_id\", \n \"webhook\": None, \n \"track_id\": None \n }) \n \n headers = { \n 'Content-Type': 'application/json' \n } \n \n response = requests.request(\"POST\", url, headers=headers, data=payload) \n \n print(URL)\n\n> Use this coupon code to get 25% off DMGG0RBN"
] |
reinforcement-learning | stable-baselines3 |
# **A2C** Agent playing **PandaReachDense-v3**
This is a trained model of a **A2C** agent playing **PandaReachDense-v3**
using the [stable-baselines3 library](https://github.com/DLR-RM/stable-baselines3).
## Usage (with Stable-baselines3)
TODO: Add your code
```python
from stable_baselines3 import ...
from huggingface_sb3 import load_from_hub
...
```
| {"library_name": "stable-baselines3", "tags": ["PandaReachDense-v3", "deep-reinforcement-learning", "reinforcement-learning", "stable-baselines3"], "model-index": [{"name": "A2C", "results": [{"task": {"type": "reinforcement-learning", "name": "reinforcement-learning"}, "dataset": {"name": "PandaReachDense-v3", "type": "PandaReachDense-v3"}, "metrics": [{"type": "mean_reward", "value": "-0.36 +/- 0.17", "name": "mean_reward", "verified": false}]}]}]} | i-pj/a2c-PandaReachDense-v3 | null | [
"stable-baselines3",
"PandaReachDense-v3",
"deep-reinforcement-learning",
"reinforcement-learning",
"model-index",
"region:us"
] | null | 2024-04-25T14:59:37+00:00 | [] | [] | TAGS
#stable-baselines3 #PandaReachDense-v3 #deep-reinforcement-learning #reinforcement-learning #model-index #region-us
|
# A2C Agent playing PandaReachDense-v3
This is a trained model of a A2C agent playing PandaReachDense-v3
using the stable-baselines3 library.
## Usage (with Stable-baselines3)
TODO: Add your code
| [
"# A2C Agent playing PandaReachDense-v3\nThis is a trained model of a A2C agent playing PandaReachDense-v3\nusing the stable-baselines3 library.",
"## Usage (with Stable-baselines3)\nTODO: Add your code"
] | [
"TAGS\n#stable-baselines3 #PandaReachDense-v3 #deep-reinforcement-learning #reinforcement-learning #model-index #region-us \n",
"# A2C Agent playing PandaReachDense-v3\nThis is a trained model of a A2C agent playing PandaReachDense-v3\nusing the stable-baselines3 library.",
"## Usage (with Stable-baselines3)\nTODO: Add your code"
] |
text-generation | transformers | You can deploy it using vllm. And this is the script for deploying.
``` bash
python -O -u -m vllm.entrypoints.openai.api_server \
--host=127.0.0.1 \
--port=8090 \
--model=Melon/Meta-Llama-3-70B-Instruct-AutoAWQ-4bit \
--tokenizer=meta-llama/Meta-Llama-3-70B-Instruct \
--tensor-parallel-size=1 \
--quantization awq \
--dtype half
``` | {} | Melon/Meta-Llama-3-70B-Instruct-AutoAWQ-4bit | null | [
"transformers",
"safetensors",
"llama",
"text-generation",
"conversational",
"autotrain_compatible",
"endpoints_compatible",
"text-generation-inference",
"4-bit",
"region:us"
] | null | 2024-04-25T15:01:06+00:00 | [] | [] | TAGS
#transformers #safetensors #llama #text-generation #conversational #autotrain_compatible #endpoints_compatible #text-generation-inference #4-bit #region-us
| You can deploy it using vllm. And this is the script for deploying.
| [] | [
"TAGS\n#transformers #safetensors #llama #text-generation #conversational #autotrain_compatible #endpoints_compatible #text-generation-inference #4-bit #region-us \n"
] |
automatic-speech-recognition | transformers |
<!-- This model card has been generated automatically according to the information the Trainer had access to. You
should probably proofread and complete it, then remove this comment. -->
# wav2vec2_base_1.5.3
This model was trained from scratch on an unknown dataset.
It achieves the following results on the evaluation set:
- Loss: 0.2868
- Wer: 0.2168
- Cer: 0.0758
## Model description
More information needed
## Intended uses & limitations
More information needed
## Training and evaluation data
More information needed
## Training procedure
### Training hyperparameters
The following hyperparameters were used during training:
- learning_rate: 0.0003
- train_batch_size: 16
- eval_batch_size: 8
- seed: 42
- optimizer: Adam with betas=(0.9,0.999) and epsilon=1e-08
- lr_scheduler_type: linear
- lr_scheduler_warmup_steps: 500
- num_epochs: 10
### Training results
| Training Loss | Epoch | Step | Validation Loss | Wer | Cer |
|:-------------:|:-----:|:----:|:---------------:|:------:|:------:|
| 0.7186 | 0.89 | 500 | 0.6028 | 0.4056 | 0.1610 |
| 0.9547 | 1.78 | 1000 | 0.6175 | 0.4180 | 0.1697 |
| 0.8084 | 2.66 | 1500 | 0.4960 | 0.3489 | 0.1377 |
| 0.6525 | 3.55 | 2000 | 0.5084 | 0.3329 | 0.1323 |
| 0.548 | 4.44 | 2500 | 0.4705 | 0.2990 | 0.1166 |
| 0.4519 | 5.33 | 3000 | 0.4278 | 0.2805 | 0.1084 |
| 0.3772 | 6.22 | 3500 | 0.3823 | 0.2698 | 0.1019 |
| 0.3045 | 7.1 | 4000 | 0.3604 | 0.2528 | 0.0910 |
| 0.243 | 7.99 | 4500 | 0.3134 | 0.2345 | 0.0829 |
| 0.1969 | 8.88 | 5000 | 0.2982 | 0.2252 | 0.0808 |
| 0.1612 | 9.77 | 5500 | 0.2868 | 0.2168 | 0.0758 |
### Framework versions
- Transformers 4.39.3
- Pytorch 2.1.2
- Datasets 2.18.0
- Tokenizers 0.15.2
| {"tags": ["generated_from_trainer"], "metrics": ["wer"], "model-index": [{"name": "wav2vec2_base_1.5.3", "results": []}]} | Myriam123/wav2vec2_base_1.5.3 | null | [
"transformers",
"tensorboard",
"safetensors",
"wav2vec2",
"automatic-speech-recognition",
"generated_from_trainer",
"endpoints_compatible",
"region:us"
] | null | 2024-04-25T15:01:18+00:00 | [] | [] | TAGS
#transformers #tensorboard #safetensors #wav2vec2 #automatic-speech-recognition #generated_from_trainer #endpoints_compatible #region-us
| wav2vec2\_base\_1.5.3
=====================
This model was trained from scratch on an unknown dataset.
It achieves the following results on the evaluation set:
* Loss: 0.2868
* Wer: 0.2168
* Cer: 0.0758
Model description
-----------------
More information needed
Intended uses & limitations
---------------------------
More information needed
Training and evaluation data
----------------------------
More information needed
Training procedure
------------------
### Training hyperparameters
The following hyperparameters were used during training:
* learning\_rate: 0.0003
* train\_batch\_size: 16
* eval\_batch\_size: 8
* seed: 42
* optimizer: Adam with betas=(0.9,0.999) and epsilon=1e-08
* lr\_scheduler\_type: linear
* lr\_scheduler\_warmup\_steps: 500
* num\_epochs: 10
### Training results
### Framework versions
* Transformers 4.39.3
* Pytorch 2.1.2
* Datasets 2.18.0
* Tokenizers 0.15.2
| [
"### Training hyperparameters\n\n\nThe following hyperparameters were used during training:\n\n\n* learning\\_rate: 0.0003\n* train\\_batch\\_size: 16\n* eval\\_batch\\_size: 8\n* seed: 42\n* optimizer: Adam with betas=(0.9,0.999) and epsilon=1e-08\n* lr\\_scheduler\\_type: linear\n* lr\\_scheduler\\_warmup\\_steps: 500\n* num\\_epochs: 10",
"### Training results",
"### Framework versions\n\n\n* Transformers 4.39.3\n* Pytorch 2.1.2\n* Datasets 2.18.0\n* Tokenizers 0.15.2"
] | [
"TAGS\n#transformers #tensorboard #safetensors #wav2vec2 #automatic-speech-recognition #generated_from_trainer #endpoints_compatible #region-us \n",
"### Training hyperparameters\n\n\nThe following hyperparameters were used during training:\n\n\n* learning\\_rate: 0.0003\n* train\\_batch\\_size: 16\n* eval\\_batch\\_size: 8\n* seed: 42\n* optimizer: Adam with betas=(0.9,0.999) and epsilon=1e-08\n* lr\\_scheduler\\_type: linear\n* lr\\_scheduler\\_warmup\\_steps: 500\n* num\\_epochs: 10",
"### Training results",
"### Framework versions\n\n\n* Transformers 4.39.3\n* Pytorch 2.1.2\n* Datasets 2.18.0\n* Tokenizers 0.15.2"
] |
text-generation | transformers |
<!-- This model card has been generated automatically according to the information the Trainer had access to. You
should probably proofread and complete it, then remove this comment. -->
# codeparrot-ds
This model is a fine-tuned version of [gpt2](https://huggingface.co/gpt2) on an unknown dataset.
## Model description
More information needed
## Intended uses & limitations
More information needed
## Training and evaluation data
More information needed
## Training procedure
### Training hyperparameters
The following hyperparameters were used during training:
- learning_rate: 0.0005
- train_batch_size: 16
- eval_batch_size: 16
- seed: 42
- gradient_accumulation_steps: 8
- total_train_batch_size: 128
- optimizer: Adam with betas=(0.9,0.999) and epsilon=1e-08
- lr_scheduler_type: cosine
- lr_scheduler_warmup_steps: 1000
- num_epochs: 2
- mixed_precision_training: Native AMP
### Training results
### Framework versions
- Transformers 4.38.2
- Pytorch 2.2.1+cu121
- Datasets 2.18.0
- Tokenizers 0.15.2
| {"license": "mit", "tags": ["generated_from_trainer"], "base_model": "gpt2", "model-index": [{"name": "codeparrot-ds", "results": []}]} | cj94/codeparrot-ds | null | [
"transformers",
"tensorboard",
"safetensors",
"gpt2",
"text-generation",
"generated_from_trainer",
"base_model:gpt2",
"license:mit",
"autotrain_compatible",
"endpoints_compatible",
"text-generation-inference",
"region:us"
] | null | 2024-04-25T15:01:32+00:00 | [] | [] | TAGS
#transformers #tensorboard #safetensors #gpt2 #text-generation #generated_from_trainer #base_model-gpt2 #license-mit #autotrain_compatible #endpoints_compatible #text-generation-inference #region-us
|
# codeparrot-ds
This model is a fine-tuned version of gpt2 on an unknown dataset.
## Model description
More information needed
## Intended uses & limitations
More information needed
## Training and evaluation data
More information needed
## Training procedure
### Training hyperparameters
The following hyperparameters were used during training:
- learning_rate: 0.0005
- train_batch_size: 16
- eval_batch_size: 16
- seed: 42
- gradient_accumulation_steps: 8
- total_train_batch_size: 128
- optimizer: Adam with betas=(0.9,0.999) and epsilon=1e-08
- lr_scheduler_type: cosine
- lr_scheduler_warmup_steps: 1000
- num_epochs: 2
- mixed_precision_training: Native AMP
### Training results
### Framework versions
- Transformers 4.38.2
- Pytorch 2.2.1+cu121
- Datasets 2.18.0
- Tokenizers 0.15.2
| [
"# codeparrot-ds\n\nThis model is a fine-tuned version of gpt2 on an unknown dataset.",
"## Model description\n\nMore information needed",
"## Intended uses & limitations\n\nMore information needed",
"## Training and evaluation data\n\nMore information needed",
"## Training procedure",
"### Training hyperparameters\n\nThe following hyperparameters were used during training:\n- learning_rate: 0.0005\n- train_batch_size: 16\n- eval_batch_size: 16\n- seed: 42\n- gradient_accumulation_steps: 8\n- total_train_batch_size: 128\n- optimizer: Adam with betas=(0.9,0.999) and epsilon=1e-08\n- lr_scheduler_type: cosine\n- lr_scheduler_warmup_steps: 1000\n- num_epochs: 2\n- mixed_precision_training: Native AMP",
"### Training results",
"### Framework versions\n\n- Transformers 4.38.2\n- Pytorch 2.2.1+cu121\n- Datasets 2.18.0\n- Tokenizers 0.15.2"
] | [
"TAGS\n#transformers #tensorboard #safetensors #gpt2 #text-generation #generated_from_trainer #base_model-gpt2 #license-mit #autotrain_compatible #endpoints_compatible #text-generation-inference #region-us \n",
"# codeparrot-ds\n\nThis model is a fine-tuned version of gpt2 on an unknown dataset.",
"## Model description\n\nMore information needed",
"## Intended uses & limitations\n\nMore information needed",
"## Training and evaluation data\n\nMore information needed",
"## Training procedure",
"### Training hyperparameters\n\nThe following hyperparameters were used during training:\n- learning_rate: 0.0005\n- train_batch_size: 16\n- eval_batch_size: 16\n- seed: 42\n- gradient_accumulation_steps: 8\n- total_train_batch_size: 128\n- optimizer: Adam with betas=(0.9,0.999) and epsilon=1e-08\n- lr_scheduler_type: cosine\n- lr_scheduler_warmup_steps: 1000\n- num_epochs: 2\n- mixed_precision_training: Native AMP",
"### Training results",
"### Framework versions\n\n- Transformers 4.38.2\n- Pytorch 2.2.1+cu121\n- Datasets 2.18.0\n- Tokenizers 0.15.2"
] |
sentence-similarity | sentence-transformers |
# SentenceTransformer based on distilbert/distilroberta-base
This is a [sentence-transformers](https://www.SBERT.net) model finetuned from [distilbert/distilroberta-base](https://huggingface.co/distilbert/distilroberta-base) on the [sentence-transformers/all-nli](https://huggingface.co/datasets/sentence-transformers/all-nli) dataset. It maps sentences & paragraphs to a 768-dimensional dense vector space and can be used for semantic textual similarity, semantic search, paraphrase mining, text classification, clustering, and more.
## Model Details
### Model Description
- **Model Type:** Sentence Transformer
- **Base model:** [distilbert/distilroberta-base](https://huggingface.co/distilbert/distilroberta-base) <!-- at revision fb53ab8802853c8e4fbdbcd0529f21fc6f459b2b -->
- **Maximum Sequence Length:** 512 tokens
- **Output Dimensionality:** 768 tokens
- **Similarity Function:** Cosine Similarity
- **Training Dataset:**
- [sentence-transformers/all-nli](https://huggingface.co/datasets/sentence-transformers/all-nli)
- **Language:** en
<!-- - **License:** Unknown -->
### Model Sources
- **Documentation:** [Sentence Transformers Documentation](https://sbert.net)
- **Repository:** [Sentence Transformers on GitHub](https://github.com/UKPLab/sentence-transformers)
- **Hugging Face:** [Sentence Transformers on Hugging Face](https://huggingface.co/models?library=sentence-transformers)
### Full Model Architecture
```
SentenceTransformer(
(0): Transformer({'max_seq_length': 512, 'do_lower_case': False}) with Transformer model: RobertaModel
(1): Pooling({'word_embedding_dimension': 768, 'pooling_mode_cls_token': False, 'pooling_mode_mean_tokens': True, 'pooling_mode_max_tokens': False, 'pooling_mode_mean_sqrt_len_tokens': False, 'pooling_mode_weightedmean_tokens': False, 'pooling_mode_lasttoken': False, 'include_prompt': True})
)
```
## Usage
### Direct Usage (Sentence Transformers)
First install the Sentence Transformers library:
```bash
pip install -U sentence-transformers
```
Then you can load this model and run inference.
```python
from sentence_transformers import SentenceTransformer
# Download from the 🤗 Hub
model = SentenceTransformer("tomaarsen/distilroberta-base-nli-adaptive-layer")
# Run inference
sentences = [
'Introduction',
'Analytical Perspectives.',
'A man reads the paper.',
]
embeddings = model.encode(sentences)
print(embeddings.shape)
# [3, 768]
# Get the similarity scores for the embeddings
similarities = model.similarity(embeddings)
print(similarities.shape)
# [3, 3]
```
<!--
### Direct Usage (Transformers)
<details><summary>Click to see the direct usage in Transformers</summary>
</details>
-->
<!--
### Downstream Usage (Sentence Transformers)
You can finetune this model on your own dataset.
<details><summary>Click to expand</summary>
</details>
-->
<!--
### Out-of-Scope Use
*List how the model may foreseeably be misused and address what users ought not to do with the model.*
-->
## Evaluation
### Metrics
#### Semantic Similarity
* Dataset: `sts-dev`
* Evaluated with [<code>EmbeddingSimilarityEvaluator</code>](https://sbert.net/docs/package_reference/evaluation.html#sentence_transformers.evaluation.EmbeddingSimilarityEvaluator)
| Metric | Value |
|:--------------------|:-----------|
| pearson_cosine | 0.8456 |
| **spearman_cosine** | **0.8486** |
| pearson_manhattan | 0.8475 |
| spearman_manhattan | 0.8506 |
| pearson_euclidean | 0.8495 |
| spearman_euclidean | 0.8527 |
| pearson_dot | 0.7867 |
| spearman_dot | 0.7816 |
| pearson_max | 0.8495 |
| spearman_max | 0.8527 |
#### Semantic Similarity
* Dataset: `sts-test`
* Evaluated with [<code>EmbeddingSimilarityEvaluator</code>](https://sbert.net/docs/package_reference/evaluation.html#sentence_transformers.evaluation.EmbeddingSimilarityEvaluator)
| Metric | Value |
|:--------------------|:-----------|
| pearson_cosine | 0.8183 |
| **spearman_cosine** | **0.8148** |
| pearson_manhattan | 0.8132 |
| spearman_manhattan | 0.8088 |
| pearson_euclidean | 0.8148 |
| spearman_euclidean | 0.8105 |
| pearson_dot | 0.75 |
| spearman_dot | 0.735 |
| pearson_max | 0.8183 |
| spearman_max | 0.8148 |
<!--
## Bias, Risks and Limitations
*What are the known or foreseeable issues stemming from this model? You could also flag here known failure cases or weaknesses of the model.*
-->
<!--
### Recommendations
*What are recommendations with respect to the foreseeable issues? For example, filtering explicit content.*
-->
## Training Details
### Training Dataset
#### sentence-transformers/all-nli
* Dataset: [sentence-transformers/all-nli](https://huggingface.co/datasets/sentence-transformers/all-nli) at [e587f0c](https://huggingface.co/datasets/sentence-transformers/all-nli/tree/e587f0c494c20fb9a1853cdfb43d42576d60a7e5)
* Size: 557,850 training samples
* Columns: <code>anchor</code>, <code>positive</code>, and <code>negative</code>
* Approximate statistics based on the first 1000 samples:
| | anchor | positive | negative |
|:--------|:----------------------------------------------------------------------------------|:---------------------------------------------------------------------------------|:---------------------------------------------------------------------------------|
| type | string | string | string |
| details | <ul><li>min: 7 tokens</li><li>mean: 10.38 tokens</li><li>max: 45 tokens</li></ul> | <ul><li>min: 6 tokens</li><li>mean: 12.8 tokens</li><li>max: 39 tokens</li></ul> | <ul><li>min: 6 tokens</li><li>mean: 13.4 tokens</li><li>max: 50 tokens</li></ul> |
* Samples:
| anchor | positive | negative |
|:---------------------------------------------------------------------------|:-------------------------------------------------|:-----------------------------------------------------------|
| <code>A person on a horse jumps over a broken down airplane.</code> | <code>A person is outdoors, on a horse.</code> | <code>A person is at a diner, ordering an omelette.</code> |
| <code>Children smiling and waving at camera</code> | <code>There are children present</code> | <code>The kids are frowning</code> |
| <code>A boy is jumping on skateboard in the middle of a red bridge.</code> | <code>The boy does a skateboarding trick.</code> | <code>The boy skates down the sidewalk.</code> |
* Loss: [<code>AdaptiveLayerLoss</code>](https://sbert.net/docs/package_reference/losses.html#adaptivelayerloss) with these parameters:
```json
{
"loss": "MultipleNegativesRankingLoss",
"n_layers_per_step": 1,
"last_layer_weight": 1.0,
"prior_layers_weight": 1.0,
"kl_div_weight": 1.0,
"kl_temperature": 0.3
}
```
### Evaluation Dataset
#### sentence-transformers/all-nli
* Dataset: [sentence-transformers/all-nli](https://huggingface.co/datasets/sentence-transformers/all-nli) at [e587f0c](https://huggingface.co/datasets/sentence-transformers/all-nli/tree/e587f0c494c20fb9a1853cdfb43d42576d60a7e5)
* Size: 6,584 evaluation samples
* Columns: <code>anchor</code>, <code>positive</code>, and <code>negative</code>
* Approximate statistics based on the first 1000 samples:
| | anchor | positive | negative |
|:--------|:----------------------------------------------------------------------------------|:---------------------------------------------------------------------------------|:----------------------------------------------------------------------------------|
| type | string | string | string |
| details | <ul><li>min: 6 tokens</li><li>mean: 18.02 tokens</li><li>max: 66 tokens</li></ul> | <ul><li>min: 5 tokens</li><li>mean: 9.81 tokens</li><li>max: 29 tokens</li></ul> | <ul><li>min: 5 tokens</li><li>mean: 10.37 tokens</li><li>max: 29 tokens</li></ul> |
* Samples:
| anchor | positive | negative |
|:-------------------------------------------------------------------------------------------------------------------------------------------------------------------------------|:------------------------------------------------------------|:--------------------------------------------------------|
| <code>Two women are embracing while holding to go packages.</code> | <code>Two woman are holding packages.</code> | <code>The men are fighting outside a deli.</code> |
| <code>Two young children in blue jerseys, one with the number 9 and one with the number 2 are standing on wooden steps in a bathroom and washing their hands in a sink.</code> | <code>Two kids in numbered jerseys wash their hands.</code> | <code>Two kids in jackets walk to school.</code> |
| <code>A man selling donuts to a customer during a world exhibition event held in the city of Angeles</code> | <code>A man selling donuts to a customer.</code> | <code>A woman drinks her coffee in a small cafe.</code> |
* Loss: [<code>AdaptiveLayerLoss</code>](https://sbert.net/docs/package_reference/losses.html#adaptivelayerloss) with these parameters:
```json
{
"loss": "MultipleNegativesRankingLoss",
"n_layers_per_step": 1,
"last_layer_weight": 1.0,
"prior_layers_weight": 1.0,
"kl_div_weight": 1.0,
"kl_temperature": 0.3
}
```
### Training Hyperparameters
#### Non-Default Hyperparameters
- `eval_strategy`: steps
- `per_device_train_batch_size`: 128
- `per_device_eval_batch_size`: 128
- `num_train_epochs`: 1
- `warmup_ratio`: 0.1
- `fp16`: True
- `batch_sampler`: no_duplicates
#### All Hyperparameters
<details><summary>Click to expand</summary>
- `overwrite_output_dir`: False
- `do_predict`: False
- `eval_strategy`: steps
- `prediction_loss_only`: False
- `per_device_train_batch_size`: 128
- `per_device_eval_batch_size`: 128
- `per_gpu_train_batch_size`: None
- `per_gpu_eval_batch_size`: None
- `gradient_accumulation_steps`: 1
- `eval_accumulation_steps`: None
- `learning_rate`: 5e-05
- `weight_decay`: 0.0
- `adam_beta1`: 0.9
- `adam_beta2`: 0.999
- `adam_epsilon`: 1e-08
- `max_grad_norm`: 1.0
- `num_train_epochs`: 1
- `max_steps`: -1
- `lr_scheduler_type`: linear
- `lr_scheduler_kwargs`: {}
- `warmup_ratio`: 0.1
- `warmup_steps`: 0
- `log_level`: passive
- `log_level_replica`: warning
- `log_on_each_node`: True
- `logging_nan_inf_filter`: True
- `save_safetensors`: True
- `save_on_each_node`: False
- `save_only_model`: False
- `no_cuda`: False
- `use_cpu`: False
- `use_mps_device`: False
- `seed`: 42
- `data_seed`: None
- `jit_mode_eval`: False
- `use_ipex`: False
- `bf16`: False
- `fp16`: True
- `fp16_opt_level`: O1
- `half_precision_backend`: auto
- `bf16_full_eval`: False
- `fp16_full_eval`: False
- `tf32`: None
- `local_rank`: 0
- `ddp_backend`: None
- `tpu_num_cores`: None
- `tpu_metrics_debug`: False
- `debug`: []
- `dataloader_drop_last`: False
- `dataloader_num_workers`: 0
- `dataloader_prefetch_factor`: None
- `past_index`: -1
- `disable_tqdm`: False
- `remove_unused_columns`: True
- `label_names`: None
- `load_best_model_at_end`: False
- `ignore_data_skip`: False
- `fsdp`: []
- `fsdp_min_num_params`: 0
- `fsdp_config`: {'min_num_params': 0, 'xla': False, 'xla_fsdp_v2': False, 'xla_fsdp_grad_ckpt': False}
- `fsdp_transformer_layer_cls_to_wrap`: None
- `accelerator_config`: {'split_batches': False, 'dispatch_batches': None, 'even_batches': True, 'use_seedable_sampler': True, 'non_blocking': False, 'gradient_accumulation_kwargs': None}
- `deepspeed`: None
- `label_smoothing_factor`: 0.0
- `optim`: adamw_torch
- `optim_args`: None
- `adafactor`: False
- `group_by_length`: False
- `length_column_name`: length
- `ddp_find_unused_parameters`: None
- `ddp_bucket_cap_mb`: None
- `ddp_broadcast_buffers`: None
- `dataloader_pin_memory`: True
- `dataloader_persistent_workers`: False
- `skip_memory_metrics`: True
- `use_legacy_prediction_loop`: False
- `push_to_hub`: False
- `resume_from_checkpoint`: None
- `hub_model_id`: None
- `hub_strategy`: every_save
- `hub_private_repo`: False
- `hub_always_push`: False
- `gradient_checkpointing`: False
- `gradient_checkpointing_kwargs`: None
- `include_inputs_for_metrics`: False
- `eval_do_concat_batches`: True
- `fp16_backend`: auto
- `push_to_hub_model_id`: None
- `push_to_hub_organization`: None
- `mp_parameters`:
- `auto_find_batch_size`: False
- `full_determinism`: False
- `torchdynamo`: None
- `ray_scope`: last
- `ddp_timeout`: 1800
- `torch_compile`: False
- `torch_compile_backend`: None
- `torch_compile_mode`: None
- `dispatch_batches`: None
- `split_batches`: None
- `include_tokens_per_second`: False
- `include_num_input_tokens_seen`: False
- `neftune_noise_alpha`: None
- `optim_target_modules`: None
- `batch_sampler`: no_duplicates
- `multi_dataset_batch_sampler`: proportional
</details>
### Training Logs
| Epoch | Step | Training Loss | loss | sts-dev_spearman_cosine | sts-test_spearman_cosine |
|:------:|:----:|:-------------:|:------:|:-----------------------:|:------------------------:|
| 0.0229 | 100 | 7.0517 | 3.9378 | 0.7889 | - |
| 0.0459 | 200 | 4.4877 | 3.8105 | 0.7906 | - |
| 0.0688 | 300 | 4.0315 | 3.6401 | 0.7966 | - |
| 0.0918 | 400 | 3.822 | 3.3537 | 0.7883 | - |
| 0.1147 | 500 | 3.0608 | 2.5975 | 0.7973 | - |
| 0.1376 | 600 | 2.6304 | 2.3956 | 0.7943 | - |
| 0.1606 | 700 | 2.7723 | 2.0379 | 0.8009 | - |
| 0.1835 | 800 | 2.3556 | 1.9645 | 0.7984 | - |
| 0.2065 | 900 | 2.4998 | 1.9086 | 0.8017 | - |
| 0.2294 | 1000 | 2.1834 | 1.8400 | 0.7973 | - |
| 0.2524 | 1100 | 2.2793 | 1.5831 | 0.8102 | - |
| 0.2753 | 1200 | 2.1042 | 1.6485 | 0.8004 | - |
| 0.2982 | 1300 | 2.1365 | 1.7084 | 0.8013 | - |
| 0.3212 | 1400 | 2.0096 | 1.5520 | 0.8064 | - |
| 0.3441 | 1500 | 2.0492 | 1.4917 | 0.8084 | - |
| 0.3671 | 1600 | 1.8764 | 1.5447 | 0.8018 | - |
| 0.3900 | 1700 | 1.8611 | 1.5480 | 0.8046 | - |
| 0.4129 | 1800 | 1.972 | 1.5353 | 0.8075 | - |
| 0.4359 | 1900 | 1.8062 | 1.4633 | 0.8039 | - |
| 0.4588 | 2000 | 1.8565 | 1.4213 | 0.8027 | - |
| 0.4818 | 2100 | 1.8852 | 1.3860 | 0.8002 | - |
| 0.5047 | 2200 | 1.7939 | 1.5468 | 0.7910 | - |
| 0.5276 | 2300 | 1.7398 | 1.6041 | 0.7888 | - |
| 0.5506 | 2400 | 1.8535 | 1.5791 | 0.7949 | - |
| 0.5735 | 2500 | 1.8486 | 1.4871 | 0.7951 | - |
| 0.5965 | 2600 | 1.7379 | 1.5427 | 0.8019 | - |
| 0.6194 | 2700 | 1.7325 | 1.4585 | 0.8087 | - |
| 0.6423 | 2800 | 1.7664 | 1.5264 | 0.7965 | - |
| 0.6653 | 2900 | 1.7517 | 1.6344 | 0.7930 | - |
| 0.6882 | 3000 | 1.8329 | 1.4947 | 0.8008 | - |
| 0.7112 | 3100 | 1.7206 | 1.4917 | 0.8089 | - |
| 0.7341 | 3200 | 1.7138 | 1.4185 | 0.8065 | - |
| 0.7571 | 3300 | 1.3705 | 1.2040 | 0.8446 | - |
| 0.7800 | 3400 | 1.1289 | 1.1363 | 0.8447 | - |
| 0.8029 | 3500 | 1.0174 | 1.1049 | 0.8464 | - |
| 0.8259 | 3600 | 1.0188 | 1.0362 | 0.8466 | - |
| 0.8488 | 3700 | 0.9841 | 1.1391 | 0.8470 | - |
| 0.8718 | 3800 | 0.8466 | 1.0116 | 0.8485 | - |
| 0.8947 | 3900 | 0.9268 | 1.1323 | 0.8488 | - |
| 0.9176 | 4000 | 0.8686 | 1.0296 | 0.8495 | - |
| 0.9406 | 4100 | 0.9255 | 1.1737 | 0.8484 | - |
| 0.9635 | 4200 | 0.7991 | 1.0609 | 0.8486 | - |
| 0.9865 | 4300 | 0.8431 | 0.9976 | 0.8486 | - |
| 1.0 | 4359 | - | - | - | 0.8148 |
### Environmental Impact
Carbon emissions were measured using [CodeCarbon](https://github.com/mlco2/codecarbon).
- **Energy Consumed**: 0.244 kWh
- **Carbon Emitted**: 0.095 kg of CO2
- **Hours Used**: 0.849 hours
### Training Hardware
- **On Cloud**: No
- **GPU Model**: 1 x NVIDIA GeForce RTX 3090
- **CPU Model**: 13th Gen Intel(R) Core(TM) i7-13700K
- **RAM Size**: 31.78 GB
### Framework Versions
- Python: 3.11.6
- Sentence Transformers: 3.0.0.dev0
- Transformers: 4.41.0.dev0
- PyTorch: 2.3.0+cu121
- Accelerate: 0.26.1
- Datasets: 2.18.0
- Tokenizers: 0.19.1
## Citation
### BibTeX
#### Sentence Transformers
```bibtex
@inproceedings{reimers-2019-sentence-bert,
title = "Sentence-BERT: Sentence Embeddings using Siamese BERT-Networks",
author = "Reimers, Nils and Gurevych, Iryna",
booktitle = "Proceedings of the 2019 Conference on Empirical Methods in Natural Language Processing",
month = "11",
year = "2019",
publisher = "Association for Computational Linguistics",
url = "https://arxiv.org/abs/1908.10084",
}
```
#### AdaptiveLayerLoss
```bibtex
@misc{li20242d,
title={2D Matryoshka Sentence Embeddings},
author={Xianming Li and Zongxi Li and Jing Li and Haoran Xie and Qing Li},
year={2024},
eprint={2402.14776},
archivePrefix={arXiv},
primaryClass={cs.CL}
}
```
#### MultipleNegativesRankingLoss
```bibtex
@misc{henderson2017efficient,
title={Efficient Natural Language Response Suggestion for Smart Reply},
author={Matthew Henderson and Rami Al-Rfou and Brian Strope and Yun-hsuan Sung and Laszlo Lukacs and Ruiqi Guo and Sanjiv Kumar and Balint Miklos and Ray Kurzweil},
year={2017},
eprint={1705.00652},
archivePrefix={arXiv},
primaryClass={cs.CL}
}
```
<!--
## Glossary
*Clearly define terms in order to be accessible across audiences.*
-->
<!--
## Model Card Authors
*Lists the people who create the model card, providing recognition and accountability for the detailed work that goes into its construction.*
-->
<!--
## Model Card Contact
*Provides a way for people who have updates to the Model Card, suggestions, or questions, to contact the Model Card authors.*
--> | {"language": ["en"], "library_name": "sentence-transformers", "tags": ["sentence-transformers", "sentence-similarity", "feature-extraction", "loss:AdaptiveLayerLoss", "loss:MultipleNegativesRankingLoss"], "metrics": ["pearson_cosine", "spearman_cosine", "pearson_manhattan", "spearman_manhattan", "pearson_euclidean", "spearman_euclidean", "pearson_dot", "spearman_dot", "pearson_max", "spearman_max"], "base_model": "distilbert/distilroberta-base", "widget": [{"source_sentence": "Certainly.", "sentences": ["'Of course.'", "The idea is a good one.", "the woman is asleep at home"]}, {"source_sentence": "He walked.", "sentences": ["The man was walking.", "The people are running.", "The women are making pizza."]}, {"source_sentence": "Double pig.", "sentences": ["Ah, triple pig!", "He had no real answer.", "Do you not know?"]}, {"source_sentence": "Very simply.", "sentences": ["Not complicatedly.", "People are on a beach.", "The man kicks the umpire."]}, {"source_sentence": "Introduction", "sentences": ["Analytical Perspectives.", "A man reads the paper.", "No one wanted Singapore."]}], "pipeline_tag": "sentence-similarity", "co2_eq_emissions": {"emissions": 94.69690706493431, "energy_consumed": 0.24362341090329948, "source": "codecarbon", "training_type": "fine-tuning", "on_cloud": false, "cpu_model": "13th Gen Intel(R) Core(TM) i7-13700K", "ram_total_size": 31.777088165283203, "hours_used": 0.849, "hardware_used": "1 x NVIDIA GeForce RTX 3090"}, "model-index": [{"name": "SentenceTransformer based on distilbert/distilroberta-base", "results": [{"task": {"type": "semantic-similarity", "name": "Semantic Similarity"}, "dataset": {"name": "sts dev", "type": "sts-dev"}, "metrics": [{"type": "pearson_cosine", "value": 0.845554152020916, "name": "Pearson Cosine"}, {"type": "spearman_cosine", "value": 0.8486455482928023, "name": "Spearman Cosine"}, {"type": "pearson_manhattan", "value": 0.8475103134032791, "name": "Pearson Manhattan"}, {"type": "spearman_manhattan", "value": 0.8505660318245544, "name": "Spearman Manhattan"}, {"type": "pearson_euclidean", "value": 0.8494883021932786, "name": "Pearson Euclidean"}, {"type": "spearman_euclidean", "value": 0.8526835635349959, "name": "Spearman Euclidean"}, {"type": "pearson_dot", "value": 0.7866563719943611, "name": "Pearson Dot"}, {"type": "spearman_dot", "value": 0.7816258810453734, "name": "Spearman Dot"}, {"type": "pearson_max", "value": 0.8494883021932786, "name": "Pearson Max"}, {"type": "spearman_max", "value": 0.8526835635349959, "name": "Spearman Max"}]}, {"task": {"type": "semantic-similarity", "name": "Semantic Similarity"}, "dataset": {"name": "sts test", "type": "sts-test"}, "metrics": [{"type": "pearson_cosine", "value": 0.8182808182081737, "name": "Pearson Cosine"}, {"type": "spearman_cosine", "value": 0.8148039503538166, "name": "Spearman Cosine"}, {"type": "pearson_manhattan", "value": 0.8132463174874629, "name": "Pearson Manhattan"}, {"type": "spearman_manhattan", "value": 0.8088248622918064, "name": "Spearman Manhattan"}, {"type": "pearson_euclidean", "value": 0.8148200486691981, "name": "Pearson Euclidean"}, {"type": "spearman_euclidean", "value": 0.8105059611031759, "name": "Spearman Euclidean"}, {"type": "pearson_dot", "value": 0.7499699563291125, "name": "Pearson Dot"}, {"type": "spearman_dot", "value": 0.7350068244681712, "name": "Spearman Dot"}, {"type": "pearson_max", "value": 0.8182808182081737, "name": "Pearson Max"}, {"type": "spearman_max", "value": 0.8148039503538166, "name": "Spearman Max"}]}]}]} | tomaarsen/distilroberta-base-nli-adaptive-layer | null | [
"sentence-transformers",
"safetensors",
"roberta",
"sentence-similarity",
"feature-extraction",
"loss:AdaptiveLayerLoss",
"loss:MultipleNegativesRankingLoss",
"en",
"arxiv:1908.10084",
"arxiv:2402.14776",
"arxiv:1705.00652",
"base_model:distilbert/distilroberta-base",
"model-index",
"co2_eq_emissions",
"endpoints_compatible",
"region:us"
] | null | 2024-04-25T15:02:05+00:00 | [
"1908.10084",
"2402.14776",
"1705.00652"
] | [
"en"
] | TAGS
#sentence-transformers #safetensors #roberta #sentence-similarity #feature-extraction #loss-AdaptiveLayerLoss #loss-MultipleNegativesRankingLoss #en #arxiv-1908.10084 #arxiv-2402.14776 #arxiv-1705.00652 #base_model-distilbert/distilroberta-base #model-index #co2_eq_emissions #endpoints_compatible #region-us
| SentenceTransformer based on distilbert/distilroberta-base
==========================================================
This is a sentence-transformers model finetuned from distilbert/distilroberta-base on the sentence-transformers/all-nli dataset. It maps sentences & paragraphs to a 768-dimensional dense vector space and can be used for semantic textual similarity, semantic search, paraphrase mining, text classification, clustering, and more.
Model Details
-------------
### Model Description
* Model Type: Sentence Transformer
* Base model: distilbert/distilroberta-base
* Maximum Sequence Length: 512 tokens
* Output Dimensionality: 768 tokens
* Similarity Function: Cosine Similarity
* Training Dataset:
+ sentence-transformers/all-nli
* Language: en
### Model Sources
* Documentation: Sentence Transformers Documentation
* Repository: Sentence Transformers on GitHub
* Hugging Face: Sentence Transformers on Hugging Face
### Full Model Architecture
Usage
-----
### Direct Usage (Sentence Transformers)
First install the Sentence Transformers library:
Then you can load this model and run inference.
Evaluation
----------
### Metrics
#### Semantic Similarity
* Dataset: 'sts-dev'
* Evaluated with `EmbeddingSimilarityEvaluator`
#### Semantic Similarity
* Dataset: 'sts-test'
* Evaluated with `EmbeddingSimilarityEvaluator`
Training Details
----------------
### Training Dataset
#### sentence-transformers/all-nli
* Dataset: sentence-transformers/all-nli at e587f0c
* Size: 557,850 training samples
* Columns: `anchor`, `positive`, and `negative`
* Approximate statistics based on the first 1000 samples:
* Samples:
* Loss: `AdaptiveLayerLoss` with these parameters:
### Evaluation Dataset
#### sentence-transformers/all-nli
* Dataset: sentence-transformers/all-nli at e587f0c
* Size: 6,584 evaluation samples
* Columns: `anchor`, `positive`, and `negative`
* Approximate statistics based on the first 1000 samples:
* Samples:
* Loss: `AdaptiveLayerLoss` with these parameters:
### Training Hyperparameters
#### Non-Default Hyperparameters
* 'eval\_strategy': steps
* 'per\_device\_train\_batch\_size': 128
* 'per\_device\_eval\_batch\_size': 128
* 'num\_train\_epochs': 1
* 'warmup\_ratio': 0.1
* 'fp16': True
* 'batch\_sampler': no\_duplicates
#### All Hyperparameters
Click to expand
* 'overwrite\_output\_dir': False
* 'do\_predict': False
* 'eval\_strategy': steps
* 'prediction\_loss\_only': False
* 'per\_device\_train\_batch\_size': 128
* 'per\_device\_eval\_batch\_size': 128
* 'per\_gpu\_train\_batch\_size': None
* 'per\_gpu\_eval\_batch\_size': None
* 'gradient\_accumulation\_steps': 1
* 'eval\_accumulation\_steps': None
* 'learning\_rate': 5e-05
* 'weight\_decay': 0.0
* 'adam\_beta1': 0.9
* 'adam\_beta2': 0.999
* 'adam\_epsilon': 1e-08
* 'max\_grad\_norm': 1.0
* 'num\_train\_epochs': 1
* 'max\_steps': -1
* 'lr\_scheduler\_type': linear
* 'lr\_scheduler\_kwargs': {}
* 'warmup\_ratio': 0.1
* 'warmup\_steps': 0
* 'log\_level': passive
* 'log\_level\_replica': warning
* 'log\_on\_each\_node': True
* 'logging\_nan\_inf\_filter': True
* 'save\_safetensors': True
* 'save\_on\_each\_node': False
* 'save\_only\_model': False
* 'no\_cuda': False
* 'use\_cpu': False
* 'use\_mps\_device': False
* 'seed': 42
* 'data\_seed': None
* 'jit\_mode\_eval': False
* 'use\_ipex': False
* 'bf16': False
* 'fp16': True
* 'fp16\_opt\_level': O1
* 'half\_precision\_backend': auto
* 'bf16\_full\_eval': False
* 'fp16\_full\_eval': False
* 'tf32': None
* 'local\_rank': 0
* 'ddp\_backend': None
* 'tpu\_num\_cores': None
* 'tpu\_metrics\_debug': False
* 'debug': []
* 'dataloader\_drop\_last': False
* 'dataloader\_num\_workers': 0
* 'dataloader\_prefetch\_factor': None
* 'past\_index': -1
* 'disable\_tqdm': False
* 'remove\_unused\_columns': True
* 'label\_names': None
* 'load\_best\_model\_at\_end': False
* 'ignore\_data\_skip': False
* 'fsdp': []
* 'fsdp\_min\_num\_params': 0
* 'fsdp\_config': {'min\_num\_params': 0, 'xla': False, 'xla\_fsdp\_v2': False, 'xla\_fsdp\_grad\_ckpt': False}
* 'fsdp\_transformer\_layer\_cls\_to\_wrap': None
* 'accelerator\_config': {'split\_batches': False, 'dispatch\_batches': None, 'even\_batches': True, 'use\_seedable\_sampler': True, 'non\_blocking': False, 'gradient\_accumulation\_kwargs': None}
* 'deepspeed': None
* 'label\_smoothing\_factor': 0.0
* 'optim': adamw\_torch
* 'optim\_args': None
* 'adafactor': False
* 'group\_by\_length': False
* 'length\_column\_name': length
* 'ddp\_find\_unused\_parameters': None
* 'ddp\_bucket\_cap\_mb': None
* 'ddp\_broadcast\_buffers': None
* 'dataloader\_pin\_memory': True
* 'dataloader\_persistent\_workers': False
* 'skip\_memory\_metrics': True
* 'use\_legacy\_prediction\_loop': False
* 'push\_to\_hub': False
* 'resume\_from\_checkpoint': None
* 'hub\_model\_id': None
* 'hub\_strategy': every\_save
* 'hub\_private\_repo': False
* 'hub\_always\_push': False
* 'gradient\_checkpointing': False
* 'gradient\_checkpointing\_kwargs': None
* 'include\_inputs\_for\_metrics': False
* 'eval\_do\_concat\_batches': True
* 'fp16\_backend': auto
* 'push\_to\_hub\_model\_id': None
* 'push\_to\_hub\_organization': None
* 'mp\_parameters':
* 'auto\_find\_batch\_size': False
* 'full\_determinism': False
* 'torchdynamo': None
* 'ray\_scope': last
* 'ddp\_timeout': 1800
* 'torch\_compile': False
* 'torch\_compile\_backend': None
* 'torch\_compile\_mode': None
* 'dispatch\_batches': None
* 'split\_batches': None
* 'include\_tokens\_per\_second': False
* 'include\_num\_input\_tokens\_seen': False
* 'neftune\_noise\_alpha': None
* 'optim\_target\_modules': None
* 'batch\_sampler': no\_duplicates
* 'multi\_dataset\_batch\_sampler': proportional
### Training Logs
### Environmental Impact
Carbon emissions were measured using CodeCarbon.
* Energy Consumed: 0.244 kWh
* Carbon Emitted: 0.095 kg of CO2
* Hours Used: 0.849 hours
### Training Hardware
* On Cloud: No
* GPU Model: 1 x NVIDIA GeForce RTX 3090
* CPU Model: 13th Gen Intel(R) Core(TM) i7-13700K
* RAM Size: 31.78 GB
### Framework Versions
* Python: 3.11.6
* Sentence Transformers: 3.0.0.dev0
* Transformers: 4.41.0.dev0
* PyTorch: 2.3.0+cu121
* Accelerate: 0.26.1
* Datasets: 2.18.0
* Tokenizers: 0.19.1
### BibTeX
#### Sentence Transformers
#### AdaptiveLayerLoss
#### MultipleNegativesRankingLoss
| [
"### Model Description\n\n\n* Model Type: Sentence Transformer\n* Base model: distilbert/distilroberta-base\n* Maximum Sequence Length: 512 tokens\n* Output Dimensionality: 768 tokens\n* Similarity Function: Cosine Similarity\n* Training Dataset:\n\t+ sentence-transformers/all-nli\n* Language: en",
"### Model Sources\n\n\n* Documentation: Sentence Transformers Documentation\n* Repository: Sentence Transformers on GitHub\n* Hugging Face: Sentence Transformers on Hugging Face",
"### Full Model Architecture\n\n\nUsage\n-----",
"### Direct Usage (Sentence Transformers)\n\n\nFirst install the Sentence Transformers library:\n\n\nThen you can load this model and run inference.\n\n\nEvaluation\n----------",
"### Metrics",
"#### Semantic Similarity\n\n\n* Dataset: 'sts-dev'\n* Evaluated with `EmbeddingSimilarityEvaluator`",
"#### Semantic Similarity\n\n\n* Dataset: 'sts-test'\n* Evaluated with `EmbeddingSimilarityEvaluator`\n\n\n\nTraining Details\n----------------",
"### Training Dataset",
"#### sentence-transformers/all-nli\n\n\n* Dataset: sentence-transformers/all-nli at e587f0c\n* Size: 557,850 training samples\n* Columns: `anchor`, `positive`, and `negative`\n* Approximate statistics based on the first 1000 samples:\n* Samples:\n* Loss: `AdaptiveLayerLoss` with these parameters:",
"### Evaluation Dataset",
"#### sentence-transformers/all-nli\n\n\n* Dataset: sentence-transformers/all-nli at e587f0c\n* Size: 6,584 evaluation samples\n* Columns: `anchor`, `positive`, and `negative`\n* Approximate statistics based on the first 1000 samples:\n* Samples:\n* Loss: `AdaptiveLayerLoss` with these parameters:",
"### Training Hyperparameters",
"#### Non-Default Hyperparameters\n\n\n* 'eval\\_strategy': steps\n* 'per\\_device\\_train\\_batch\\_size': 128\n* 'per\\_device\\_eval\\_batch\\_size': 128\n* 'num\\_train\\_epochs': 1\n* 'warmup\\_ratio': 0.1\n* 'fp16': True\n* 'batch\\_sampler': no\\_duplicates",
"#### All Hyperparameters\n\n\nClick to expand\n* 'overwrite\\_output\\_dir': False\n* 'do\\_predict': False\n* 'eval\\_strategy': steps\n* 'prediction\\_loss\\_only': False\n* 'per\\_device\\_train\\_batch\\_size': 128\n* 'per\\_device\\_eval\\_batch\\_size': 128\n* 'per\\_gpu\\_train\\_batch\\_size': None\n* 'per\\_gpu\\_eval\\_batch\\_size': None\n* 'gradient\\_accumulation\\_steps': 1\n* 'eval\\_accumulation\\_steps': None\n* 'learning\\_rate': 5e-05\n* 'weight\\_decay': 0.0\n* 'adam\\_beta1': 0.9\n* 'adam\\_beta2': 0.999\n* 'adam\\_epsilon': 1e-08\n* 'max\\_grad\\_norm': 1.0\n* 'num\\_train\\_epochs': 1\n* 'max\\_steps': -1\n* 'lr\\_scheduler\\_type': linear\n* 'lr\\_scheduler\\_kwargs': {}\n* 'warmup\\_ratio': 0.1\n* 'warmup\\_steps': 0\n* 'log\\_level': passive\n* 'log\\_level\\_replica': warning\n* 'log\\_on\\_each\\_node': True\n* 'logging\\_nan\\_inf\\_filter': True\n* 'save\\_safetensors': True\n* 'save\\_on\\_each\\_node': False\n* 'save\\_only\\_model': False\n* 'no\\_cuda': False\n* 'use\\_cpu': False\n* 'use\\_mps\\_device': False\n* 'seed': 42\n* 'data\\_seed': None\n* 'jit\\_mode\\_eval': False\n* 'use\\_ipex': False\n* 'bf16': False\n* 'fp16': True\n* 'fp16\\_opt\\_level': O1\n* 'half\\_precision\\_backend': auto\n* 'bf16\\_full\\_eval': False\n* 'fp16\\_full\\_eval': False\n* 'tf32': None\n* 'local\\_rank': 0\n* 'ddp\\_backend': None\n* 'tpu\\_num\\_cores': None\n* 'tpu\\_metrics\\_debug': False\n* 'debug': []\n* 'dataloader\\_drop\\_last': False\n* 'dataloader\\_num\\_workers': 0\n* 'dataloader\\_prefetch\\_factor': None\n* 'past\\_index': -1\n* 'disable\\_tqdm': False\n* 'remove\\_unused\\_columns': True\n* 'label\\_names': None\n* 'load\\_best\\_model\\_at\\_end': False\n* 'ignore\\_data\\_skip': False\n* 'fsdp': []\n* 'fsdp\\_min\\_num\\_params': 0\n* 'fsdp\\_config': {'min\\_num\\_params': 0, 'xla': False, 'xla\\_fsdp\\_v2': False, 'xla\\_fsdp\\_grad\\_ckpt': False}\n* 'fsdp\\_transformer\\_layer\\_cls\\_to\\_wrap': None\n* 'accelerator\\_config': {'split\\_batches': False, 'dispatch\\_batches': None, 'even\\_batches': True, 'use\\_seedable\\_sampler': True, 'non\\_blocking': False, 'gradient\\_accumulation\\_kwargs': None}\n* 'deepspeed': None\n* 'label\\_smoothing\\_factor': 0.0\n* 'optim': adamw\\_torch\n* 'optim\\_args': None\n* 'adafactor': False\n* 'group\\_by\\_length': False\n* 'length\\_column\\_name': length\n* 'ddp\\_find\\_unused\\_parameters': None\n* 'ddp\\_bucket\\_cap\\_mb': None\n* 'ddp\\_broadcast\\_buffers': None\n* 'dataloader\\_pin\\_memory': True\n* 'dataloader\\_persistent\\_workers': False\n* 'skip\\_memory\\_metrics': True\n* 'use\\_legacy\\_prediction\\_loop': False\n* 'push\\_to\\_hub': False\n* 'resume\\_from\\_checkpoint': None\n* 'hub\\_model\\_id': None\n* 'hub\\_strategy': every\\_save\n* 'hub\\_private\\_repo': False\n* 'hub\\_always\\_push': False\n* 'gradient\\_checkpointing': False\n* 'gradient\\_checkpointing\\_kwargs': None\n* 'include\\_inputs\\_for\\_metrics': False\n* 'eval\\_do\\_concat\\_batches': True\n* 'fp16\\_backend': auto\n* 'push\\_to\\_hub\\_model\\_id': None\n* 'push\\_to\\_hub\\_organization': None\n* 'mp\\_parameters':\n* 'auto\\_find\\_batch\\_size': False\n* 'full\\_determinism': False\n* 'torchdynamo': None\n* 'ray\\_scope': last\n* 'ddp\\_timeout': 1800\n* 'torch\\_compile': False\n* 'torch\\_compile\\_backend': None\n* 'torch\\_compile\\_mode': None\n* 'dispatch\\_batches': None\n* 'split\\_batches': None\n* 'include\\_tokens\\_per\\_second': False\n* 'include\\_num\\_input\\_tokens\\_seen': False\n* 'neftune\\_noise\\_alpha': None\n* 'optim\\_target\\_modules': None\n* 'batch\\_sampler': no\\_duplicates\n* 'multi\\_dataset\\_batch\\_sampler': proportional",
"### Training Logs",
"### Environmental Impact\n\n\nCarbon emissions were measured using CodeCarbon.\n\n\n* Energy Consumed: 0.244 kWh\n* Carbon Emitted: 0.095 kg of CO2\n* Hours Used: 0.849 hours",
"### Training Hardware\n\n\n* On Cloud: No\n* GPU Model: 1 x NVIDIA GeForce RTX 3090\n* CPU Model: 13th Gen Intel(R) Core(TM) i7-13700K\n* RAM Size: 31.78 GB",
"### Framework Versions\n\n\n* Python: 3.11.6\n* Sentence Transformers: 3.0.0.dev0\n* Transformers: 4.41.0.dev0\n* PyTorch: 2.3.0+cu121\n* Accelerate: 0.26.1\n* Datasets: 2.18.0\n* Tokenizers: 0.19.1",
"### BibTeX",
"#### Sentence Transformers",
"#### AdaptiveLayerLoss",
"#### MultipleNegativesRankingLoss"
] | [
"TAGS\n#sentence-transformers #safetensors #roberta #sentence-similarity #feature-extraction #loss-AdaptiveLayerLoss #loss-MultipleNegativesRankingLoss #en #arxiv-1908.10084 #arxiv-2402.14776 #arxiv-1705.00652 #base_model-distilbert/distilroberta-base #model-index #co2_eq_emissions #endpoints_compatible #region-us \n",
"### Model Description\n\n\n* Model Type: Sentence Transformer\n* Base model: distilbert/distilroberta-base\n* Maximum Sequence Length: 512 tokens\n* Output Dimensionality: 768 tokens\n* Similarity Function: Cosine Similarity\n* Training Dataset:\n\t+ sentence-transformers/all-nli\n* Language: en",
"### Model Sources\n\n\n* Documentation: Sentence Transformers Documentation\n* Repository: Sentence Transformers on GitHub\n* Hugging Face: Sentence Transformers on Hugging Face",
"### Full Model Architecture\n\n\nUsage\n-----",
"### Direct Usage (Sentence Transformers)\n\n\nFirst install the Sentence Transformers library:\n\n\nThen you can load this model and run inference.\n\n\nEvaluation\n----------",
"### Metrics",
"#### Semantic Similarity\n\n\n* Dataset: 'sts-dev'\n* Evaluated with `EmbeddingSimilarityEvaluator`",
"#### Semantic Similarity\n\n\n* Dataset: 'sts-test'\n* Evaluated with `EmbeddingSimilarityEvaluator`\n\n\n\nTraining Details\n----------------",
"### Training Dataset",
"#### sentence-transformers/all-nli\n\n\n* Dataset: sentence-transformers/all-nli at e587f0c\n* Size: 557,850 training samples\n* Columns: `anchor`, `positive`, and `negative`\n* Approximate statistics based on the first 1000 samples:\n* Samples:\n* Loss: `AdaptiveLayerLoss` with these parameters:",
"### Evaluation Dataset",
"#### sentence-transformers/all-nli\n\n\n* Dataset: sentence-transformers/all-nli at e587f0c\n* Size: 6,584 evaluation samples\n* Columns: `anchor`, `positive`, and `negative`\n* Approximate statistics based on the first 1000 samples:\n* Samples:\n* Loss: `AdaptiveLayerLoss` with these parameters:",
"### Training Hyperparameters",
"#### Non-Default Hyperparameters\n\n\n* 'eval\\_strategy': steps\n* 'per\\_device\\_train\\_batch\\_size': 128\n* 'per\\_device\\_eval\\_batch\\_size': 128\n* 'num\\_train\\_epochs': 1\n* 'warmup\\_ratio': 0.1\n* 'fp16': True\n* 'batch\\_sampler': no\\_duplicates",
"#### All Hyperparameters\n\n\nClick to expand\n* 'overwrite\\_output\\_dir': False\n* 'do\\_predict': False\n* 'eval\\_strategy': steps\n* 'prediction\\_loss\\_only': False\n* 'per\\_device\\_train\\_batch\\_size': 128\n* 'per\\_device\\_eval\\_batch\\_size': 128\n* 'per\\_gpu\\_train\\_batch\\_size': None\n* 'per\\_gpu\\_eval\\_batch\\_size': None\n* 'gradient\\_accumulation\\_steps': 1\n* 'eval\\_accumulation\\_steps': None\n* 'learning\\_rate': 5e-05\n* 'weight\\_decay': 0.0\n* 'adam\\_beta1': 0.9\n* 'adam\\_beta2': 0.999\n* 'adam\\_epsilon': 1e-08\n* 'max\\_grad\\_norm': 1.0\n* 'num\\_train\\_epochs': 1\n* 'max\\_steps': -1\n* 'lr\\_scheduler\\_type': linear\n* 'lr\\_scheduler\\_kwargs': {}\n* 'warmup\\_ratio': 0.1\n* 'warmup\\_steps': 0\n* 'log\\_level': passive\n* 'log\\_level\\_replica': warning\n* 'log\\_on\\_each\\_node': True\n* 'logging\\_nan\\_inf\\_filter': True\n* 'save\\_safetensors': True\n* 'save\\_on\\_each\\_node': False\n* 'save\\_only\\_model': False\n* 'no\\_cuda': False\n* 'use\\_cpu': False\n* 'use\\_mps\\_device': False\n* 'seed': 42\n* 'data\\_seed': None\n* 'jit\\_mode\\_eval': False\n* 'use\\_ipex': False\n* 'bf16': False\n* 'fp16': True\n* 'fp16\\_opt\\_level': O1\n* 'half\\_precision\\_backend': auto\n* 'bf16\\_full\\_eval': False\n* 'fp16\\_full\\_eval': False\n* 'tf32': None\n* 'local\\_rank': 0\n* 'ddp\\_backend': None\n* 'tpu\\_num\\_cores': None\n* 'tpu\\_metrics\\_debug': False\n* 'debug': []\n* 'dataloader\\_drop\\_last': False\n* 'dataloader\\_num\\_workers': 0\n* 'dataloader\\_prefetch\\_factor': None\n* 'past\\_index': -1\n* 'disable\\_tqdm': False\n* 'remove\\_unused\\_columns': True\n* 'label\\_names': None\n* 'load\\_best\\_model\\_at\\_end': False\n* 'ignore\\_data\\_skip': False\n* 'fsdp': []\n* 'fsdp\\_min\\_num\\_params': 0\n* 'fsdp\\_config': {'min\\_num\\_params': 0, 'xla': False, 'xla\\_fsdp\\_v2': False, 'xla\\_fsdp\\_grad\\_ckpt': False}\n* 'fsdp\\_transformer\\_layer\\_cls\\_to\\_wrap': None\n* 'accelerator\\_config': {'split\\_batches': False, 'dispatch\\_batches': None, 'even\\_batches': True, 'use\\_seedable\\_sampler': True, 'non\\_blocking': False, 'gradient\\_accumulation\\_kwargs': None}\n* 'deepspeed': None\n* 'label\\_smoothing\\_factor': 0.0\n* 'optim': adamw\\_torch\n* 'optim\\_args': None\n* 'adafactor': False\n* 'group\\_by\\_length': False\n* 'length\\_column\\_name': length\n* 'ddp\\_find\\_unused\\_parameters': None\n* 'ddp\\_bucket\\_cap\\_mb': None\n* 'ddp\\_broadcast\\_buffers': None\n* 'dataloader\\_pin\\_memory': True\n* 'dataloader\\_persistent\\_workers': False\n* 'skip\\_memory\\_metrics': True\n* 'use\\_legacy\\_prediction\\_loop': False\n* 'push\\_to\\_hub': False\n* 'resume\\_from\\_checkpoint': None\n* 'hub\\_model\\_id': None\n* 'hub\\_strategy': every\\_save\n* 'hub\\_private\\_repo': False\n* 'hub\\_always\\_push': False\n* 'gradient\\_checkpointing': False\n* 'gradient\\_checkpointing\\_kwargs': None\n* 'include\\_inputs\\_for\\_metrics': False\n* 'eval\\_do\\_concat\\_batches': True\n* 'fp16\\_backend': auto\n* 'push\\_to\\_hub\\_model\\_id': None\n* 'push\\_to\\_hub\\_organization': None\n* 'mp\\_parameters':\n* 'auto\\_find\\_batch\\_size': False\n* 'full\\_determinism': False\n* 'torchdynamo': None\n* 'ray\\_scope': last\n* 'ddp\\_timeout': 1800\n* 'torch\\_compile': False\n* 'torch\\_compile\\_backend': None\n* 'torch\\_compile\\_mode': None\n* 'dispatch\\_batches': None\n* 'split\\_batches': None\n* 'include\\_tokens\\_per\\_second': False\n* 'include\\_num\\_input\\_tokens\\_seen': False\n* 'neftune\\_noise\\_alpha': None\n* 'optim\\_target\\_modules': None\n* 'batch\\_sampler': no\\_duplicates\n* 'multi\\_dataset\\_batch\\_sampler': proportional",
"### Training Logs",
"### Environmental Impact\n\n\nCarbon emissions were measured using CodeCarbon.\n\n\n* Energy Consumed: 0.244 kWh\n* Carbon Emitted: 0.095 kg of CO2\n* Hours Used: 0.849 hours",
"### Training Hardware\n\n\n* On Cloud: No\n* GPU Model: 1 x NVIDIA GeForce RTX 3090\n* CPU Model: 13th Gen Intel(R) Core(TM) i7-13700K\n* RAM Size: 31.78 GB",
"### Framework Versions\n\n\n* Python: 3.11.6\n* Sentence Transformers: 3.0.0.dev0\n* Transformers: 4.41.0.dev0\n* PyTorch: 2.3.0+cu121\n* Accelerate: 0.26.1\n* Datasets: 2.18.0\n* Tokenizers: 0.19.1",
"### BibTeX",
"#### Sentence Transformers",
"#### AdaptiveLayerLoss",
"#### MultipleNegativesRankingLoss"
] |
null | transformers |
# Model Card for Model ID
<!-- Provide a quick summary of what the model is/does. -->
## Model Details
### Model Description
<!-- Provide a longer summary of what this model is. -->
This is the model card of a 🤗 transformers model that has been pushed on the Hub. This model card has been automatically generated.
- **Developed by:** [More Information Needed]
- **Funded by [optional]:** [More Information Needed]
- **Shared by [optional]:** [More Information Needed]
- **Model type:** [More Information Needed]
- **Language(s) (NLP):** [More Information Needed]
- **License:** [More Information Needed]
- **Finetuned from model [optional]:** [More Information Needed]
### Model Sources [optional]
<!-- Provide the basic links for the model. -->
- **Repository:** [More Information Needed]
- **Paper [optional]:** [More Information Needed]
- **Demo [optional]:** [More Information Needed]
## Uses
<!-- Address questions around how the model is intended to be used, including the foreseeable users of the model and those affected by the model. -->
### Direct Use
<!-- This section is for the model use without fine-tuning or plugging into a larger ecosystem/app. -->
[More Information Needed]
### Downstream Use [optional]
<!-- This section is for the model use when fine-tuned for a task, or when plugged into a larger ecosystem/app -->
[More Information Needed]
### Out-of-Scope Use
<!-- This section addresses misuse, malicious use, and uses that the model will not work well for. -->
[More Information Needed]
## Bias, Risks, and Limitations
<!-- This section is meant to convey both technical and sociotechnical limitations. -->
[More Information Needed]
### Recommendations
<!-- This section is meant to convey recommendations with respect to the bias, risk, and technical limitations. -->
Users (both direct and downstream) should be made aware of the risks, biases and limitations of the model. More information needed for further recommendations.
## How to Get Started with the Model
Use the code below to get started with the model.
[More Information Needed]
## Training Details
### Training Data
<!-- This should link to a Dataset Card, perhaps with a short stub of information on what the training data is all about as well as documentation related to data pre-processing or additional filtering. -->
[More Information Needed]
### Training Procedure
<!-- This relates heavily to the Technical Specifications. Content here should link to that section when it is relevant to the training procedure. -->
#### Preprocessing [optional]
[More Information Needed]
#### Training Hyperparameters
- **Training regime:** [More Information Needed] <!--fp32, fp16 mixed precision, bf16 mixed precision, bf16 non-mixed precision, fp16 non-mixed precision, fp8 mixed precision -->
#### Speeds, Sizes, Times [optional]
<!-- This section provides information about throughput, start/end time, checkpoint size if relevant, etc. -->
[More Information Needed]
## Evaluation
<!-- This section describes the evaluation protocols and provides the results. -->
### Testing Data, Factors & Metrics
#### Testing Data
<!-- This should link to a Dataset Card if possible. -->
[More Information Needed]
#### Factors
<!-- These are the things the evaluation is disaggregating by, e.g., subpopulations or domains. -->
[More Information Needed]
#### Metrics
<!-- These are the evaluation metrics being used, ideally with a description of why. -->
[More Information Needed]
### Results
[More Information Needed]
#### Summary
## Model Examination [optional]
<!-- Relevant interpretability work for the model goes here -->
[More Information Needed]
## Environmental Impact
<!-- Total emissions (in grams of CO2eq) and additional considerations, such as electricity usage, go here. Edit the suggested text below accordingly -->
Carbon emissions can be estimated using the [Machine Learning Impact calculator](https://mlco2.github.io/impact#compute) presented in [Lacoste et al. (2019)](https://arxiv.org/abs/1910.09700).
- **Hardware Type:** [More Information Needed]
- **Hours used:** [More Information Needed]
- **Cloud Provider:** [More Information Needed]
- **Compute Region:** [More Information Needed]
- **Carbon Emitted:** [More Information Needed]
## Technical Specifications [optional]
### Model Architecture and Objective
[More Information Needed]
### Compute Infrastructure
[More Information Needed]
#### Hardware
[More Information Needed]
#### Software
[More Information Needed]
## Citation [optional]
<!-- If there is a paper or blog post introducing the model, the APA and Bibtex information for that should go in this section. -->
**BibTeX:**
[More Information Needed]
**APA:**
[More Information Needed]
## Glossary [optional]
<!-- If relevant, include terms and calculations in this section that can help readers understand the model or model card. -->
[More Information Needed]
## More Information [optional]
[More Information Needed]
## Model Card Authors [optional]
[More Information Needed]
## Model Card Contact
[More Information Needed] | {"library_name": "transformers", "tags": []} | research-dump/Phi-3-mini-4k-instruct_random_split | null | [
"transformers",
"safetensors",
"arxiv:1910.09700",
"endpoints_compatible",
"region:us"
] | null | 2024-04-25T15:02:33+00:00 | [
"1910.09700"
] | [] | TAGS
#transformers #safetensors #arxiv-1910.09700 #endpoints_compatible #region-us
|
# Model Card for Model ID
## Model Details
### Model Description
This is the model card of a transformers model that has been pushed on the Hub. This model card has been automatically generated.
- Developed by:
- Funded by [optional]:
- Shared by [optional]:
- Model type:
- Language(s) (NLP):
- License:
- Finetuned from model [optional]:
### Model Sources [optional]
- Repository:
- Paper [optional]:
- Demo [optional]:
## Uses
### Direct Use
### Downstream Use [optional]
### Out-of-Scope Use
## Bias, Risks, and Limitations
### Recommendations
Users (both direct and downstream) should be made aware of the risks, biases and limitations of the model. More information needed for further recommendations.
## How to Get Started with the Model
Use the code below to get started with the model.
## Training Details
### Training Data
### Training Procedure
#### Preprocessing [optional]
#### Training Hyperparameters
- Training regime:
#### Speeds, Sizes, Times [optional]
## Evaluation
### Testing Data, Factors & Metrics
#### Testing Data
#### Factors
#### Metrics
### Results
#### Summary
## Model Examination [optional]
## Environmental Impact
Carbon emissions can be estimated using the Machine Learning Impact calculator presented in Lacoste et al. (2019).
- Hardware Type:
- Hours used:
- Cloud Provider:
- Compute Region:
- Carbon Emitted:
## Technical Specifications [optional]
### Model Architecture and Objective
### Compute Infrastructure
#### Hardware
#### Software
[optional]
BibTeX:
APA:
## Glossary [optional]
## More Information [optional]
## Model Card Authors [optional]
## Model Card Contact
| [
"# Model Card for Model ID",
"## Model Details",
"### Model Description\n\n\n\nThis is the model card of a transformers model that has been pushed on the Hub. This model card has been automatically generated.\n\n- Developed by: \n- Funded by [optional]: \n- Shared by [optional]: \n- Model type: \n- Language(s) (NLP): \n- License: \n- Finetuned from model [optional]:",
"### Model Sources [optional]\n\n\n\n- Repository: \n- Paper [optional]: \n- Demo [optional]:",
"## Uses",
"### Direct Use",
"### Downstream Use [optional]",
"### Out-of-Scope Use",
"## Bias, Risks, and Limitations",
"### Recommendations\n\n\n\nUsers (both direct and downstream) should be made aware of the risks, biases and limitations of the model. More information needed for further recommendations.",
"## How to Get Started with the Model\n\nUse the code below to get started with the model.",
"## Training Details",
"### Training Data",
"### Training Procedure",
"#### Preprocessing [optional]",
"#### Training Hyperparameters\n\n- Training regime:",
"#### Speeds, Sizes, Times [optional]",
"## Evaluation",
"### Testing Data, Factors & Metrics",
"#### Testing Data",
"#### Factors",
"#### Metrics",
"### Results",
"#### Summary",
"## Model Examination [optional]",
"## Environmental Impact\n\n\n\nCarbon emissions can be estimated using the Machine Learning Impact calculator presented in Lacoste et al. (2019).\n\n- Hardware Type: \n- Hours used: \n- Cloud Provider: \n- Compute Region: \n- Carbon Emitted:",
"## Technical Specifications [optional]",
"### Model Architecture and Objective",
"### Compute Infrastructure",
"#### Hardware",
"#### Software\n\n\n\n[optional]\n\n\n\nBibTeX:\n\n\n\nAPA:",
"## Glossary [optional]",
"## More Information [optional]",
"## Model Card Authors [optional]",
"## Model Card Contact"
] | [
"TAGS\n#transformers #safetensors #arxiv-1910.09700 #endpoints_compatible #region-us \n",
"# Model Card for Model ID",
"## Model Details",
"### Model Description\n\n\n\nThis is the model card of a transformers model that has been pushed on the Hub. This model card has been automatically generated.\n\n- Developed by: \n- Funded by [optional]: \n- Shared by [optional]: \n- Model type: \n- Language(s) (NLP): \n- License: \n- Finetuned from model [optional]:",
"### Model Sources [optional]\n\n\n\n- Repository: \n- Paper [optional]: \n- Demo [optional]:",
"## Uses",
"### Direct Use",
"### Downstream Use [optional]",
"### Out-of-Scope Use",
"## Bias, Risks, and Limitations",
"### Recommendations\n\n\n\nUsers (both direct and downstream) should be made aware of the risks, biases and limitations of the model. More information needed for further recommendations.",
"## How to Get Started with the Model\n\nUse the code below to get started with the model.",
"## Training Details",
"### Training Data",
"### Training Procedure",
"#### Preprocessing [optional]",
"#### Training Hyperparameters\n\n- Training regime:",
"#### Speeds, Sizes, Times [optional]",
"## Evaluation",
"### Testing Data, Factors & Metrics",
"#### Testing Data",
"#### Factors",
"#### Metrics",
"### Results",
"#### Summary",
"## Model Examination [optional]",
"## Environmental Impact\n\n\n\nCarbon emissions can be estimated using the Machine Learning Impact calculator presented in Lacoste et al. (2019).\n\n- Hardware Type: \n- Hours used: \n- Cloud Provider: \n- Compute Region: \n- Carbon Emitted:",
"## Technical Specifications [optional]",
"### Model Architecture and Objective",
"### Compute Infrastructure",
"#### Hardware",
"#### Software\n\n\n\n[optional]\n\n\n\nBibTeX:\n\n\n\nAPA:",
"## Glossary [optional]",
"## More Information [optional]",
"## Model Card Authors [optional]",
"## Model Card Contact"
] |
null | peft |
<!-- This model card has been generated automatically according to the information the Trainer had access to. You
should probably proofread and complete it, then remove this comment. -->
# phi-2-detox-r16
This model is a fine-tuned version of [microsoft/phi-2](https://huggingface.co/microsoft/phi-2) on the None dataset.
## Model description
More information needed
## Intended uses & limitations
More information needed
## Training and evaluation data
More information needed
## Training procedure
### Training hyperparameters
The following hyperparameters were used during training:
- learning_rate: 0.0002
- train_batch_size: 8
- eval_batch_size: 8
- seed: 42
- optimizer: Adam with betas=(0.9,0.999) and epsilon=1e-08
- lr_scheduler_type: cosine
- training_steps: 10
### Training results
### Framework versions
- PEFT 0.10.0
- Transformers 4.39.3
- Pytorch 2.2.2+cu118
- Datasets 2.18.0
- Tokenizers 0.15.2 | {"license": "mit", "library_name": "peft", "tags": ["generated_from_trainer"], "base_model": "microsoft/phi-2", "model-index": [{"name": "phi-2-detox-r16", "results": []}]} | NikAlan/phi-2-detox-r16 | null | [
"peft",
"safetensors",
"phi",
"generated_from_trainer",
"custom_code",
"base_model:microsoft/phi-2",
"license:mit",
"region:us"
] | null | 2024-04-25T15:04:41+00:00 | [] | [] | TAGS
#peft #safetensors #phi #generated_from_trainer #custom_code #base_model-microsoft/phi-2 #license-mit #region-us
|
# phi-2-detox-r16
This model is a fine-tuned version of microsoft/phi-2 on the None dataset.
## Model description
More information needed
## Intended uses & limitations
More information needed
## Training and evaluation data
More information needed
## Training procedure
### Training hyperparameters
The following hyperparameters were used during training:
- learning_rate: 0.0002
- train_batch_size: 8
- eval_batch_size: 8
- seed: 42
- optimizer: Adam with betas=(0.9,0.999) and epsilon=1e-08
- lr_scheduler_type: cosine
- training_steps: 10
### Training results
### Framework versions
- PEFT 0.10.0
- Transformers 4.39.3
- Pytorch 2.2.2+cu118
- Datasets 2.18.0
- Tokenizers 0.15.2 | [
"# phi-2-detox-r16\n\nThis model is a fine-tuned version of microsoft/phi-2 on the None dataset.",
"## Model description\n\nMore information needed",
"## Intended uses & limitations\n\nMore information needed",
"## Training and evaluation data\n\nMore information needed",
"## Training procedure",
"### Training hyperparameters\n\nThe following hyperparameters were used during training:\n- learning_rate: 0.0002\n- train_batch_size: 8\n- eval_batch_size: 8\n- seed: 42\n- optimizer: Adam with betas=(0.9,0.999) and epsilon=1e-08\n- lr_scheduler_type: cosine\n- training_steps: 10",
"### Training results",
"### Framework versions\n\n- PEFT 0.10.0\n- Transformers 4.39.3\n- Pytorch 2.2.2+cu118\n- Datasets 2.18.0\n- Tokenizers 0.15.2"
] | [
"TAGS\n#peft #safetensors #phi #generated_from_trainer #custom_code #base_model-microsoft/phi-2 #license-mit #region-us \n",
"# phi-2-detox-r16\n\nThis model is a fine-tuned version of microsoft/phi-2 on the None dataset.",
"## Model description\n\nMore information needed",
"## Intended uses & limitations\n\nMore information needed",
"## Training and evaluation data\n\nMore information needed",
"## Training procedure",
"### Training hyperparameters\n\nThe following hyperparameters were used during training:\n- learning_rate: 0.0002\n- train_batch_size: 8\n- eval_batch_size: 8\n- seed: 42\n- optimizer: Adam with betas=(0.9,0.999) and epsilon=1e-08\n- lr_scheduler_type: cosine\n- training_steps: 10",
"### Training results",
"### Framework versions\n\n- PEFT 0.10.0\n- Transformers 4.39.3\n- Pytorch 2.2.2+cu118\n- Datasets 2.18.0\n- Tokenizers 0.15.2"
] |
null | peft |
<!-- This model card has been generated automatically according to the information the Trainer had access to. You
should probably proofread and complete it, then remove this comment. -->
[<img src="https://raw.githubusercontent.com/OpenAccess-AI-Collective/axolotl/main/image/axolotl-badge-web.png" alt="Built with Axolotl" width="200" height="32"/>](https://github.com/OpenAccess-AI-Collective/axolotl)
<details><summary>See axolotl config</summary>
axolotl version: `0.4.0`
```yaml
base_model: NousResearch/Meta-Llama-3-70B
model_type: LlamaForCausalLM
tokenizer_type: PreTrainedTokenizerFast
#overrides_of_model_config:
# rope_scaling:
# type: linear
# factor: 4
special_tokens:
pad_token: "<|end_of_text|>"
gptq: false
gptq_disable_exllama: true
load_in_8bit: false
load_in_4bit: true
strict: false
datasets:
- path: /workspace/axolotl/output.jsonl
ds_type: json
type: completion
data_files:
- /workspace/axolotl/output.jsonl
output_dir: ./lora-out-l3-10
adapter: qlora
lora_model_dir:
sequence_len: 10240
sample_packing: true
eval_sample_packing: true
pad_to_sequence_len: true
lora_r: 32
lora_alpha: 64
lora_dropout: 0.10
lora_target_linear: true
lora_target_modules:
- gate_proj
- down_proj
- up_proj
- q_proj
- v_proj
- k_proj
- o_proj
peft_use_dora: true
wandb_project: kalomaze-model
wandb_entity:
wandb_watch:
wandb_name:
wandb_log_model:
gradient_accumulation_steps: 6
micro_batch_size: 1
num_epochs: 4
# optimizer: paged_adamw_8bit
# optimizer: adamw_bnb_8bit
optimizer: adamw_bnb_8bit
lr_scheduler: cosine
learning_rate: 0.000015
cosine_min_lr_ratio: 0.2
max_grad_norm: 1.0
train_on_inputs: true
group_by_length: false
bf16: true
fp16: false
tf32: false
gradient_checkpointing: true
early_stopping_patience:
resume_from_checkpoint:
local_rank:
logging_steps: 1
xformers_attention:
flash_attention: true
warmup_steps: 0
saves_per_epoch: 6
save_total_limit: 7
debug:
weight_decay: 0.0
# fsdp:
# - full_shard
# - auto_wrap
# fsdp_config:
# fsdp_limit_all_gathers: true
# fsdp_sync_module_states: true
# fsdp_offload_params: false
# fsdp_use_orig_params: false
# fsdp_cpu_ram_efficient_loading: false
# fsdp_auto_wrap_policy: TRANSFORMER_BASED_WRAP
# fsdp_transformer_layer_cls_to_wrap: LlamaDecoderLayer
# fsdp_state_dict_type: FULL_STATE_DICT
seed: 246
```
</details><br>
# lora-out-l3-10
This model is a fine-tuned version of [NousResearch/Meta-Llama-3-70B](https://huggingface.co/NousResearch/Meta-Llama-3-70B) on the None dataset.
## Model description
More information needed
## Intended uses & limitations
More information needed
## Training and evaluation data
More information needed
## Training procedure
### Training hyperparameters
The following hyperparameters were used during training:
- learning_rate: 1.5e-05
- train_batch_size: 1
- eval_batch_size: 1
- seed: 246
- distributed_type: multi-GPU
- num_devices: 8
- gradient_accumulation_steps: 6
- total_train_batch_size: 48
- total_eval_batch_size: 8
- optimizer: Adam with betas=(0.9,0.999) and epsilon=1e-08
- lr_scheduler_type: cosine
- num_epochs: 4
### Training results
### Framework versions
- PEFT 0.10.0
- Transformers 4.40.0.dev0
- Pytorch 2.2.1
- Datasets 2.15.0
- Tokenizers 0.15.0 | {"license": "other", "library_name": "peft", "tags": ["generated_from_trainer"], "base_model": "NousResearch/Meta-Llama-3-70B", "model-index": [{"name": "lora-out-l3-10", "results": []}]} | wave-on-discord/llama-3-70b-llc-2 | null | [
"peft",
"llama",
"generated_from_trainer",
"base_model:NousResearch/Meta-Llama-3-70B",
"license:other",
"4-bit",
"region:us"
] | null | 2024-04-25T15:06:00+00:00 | [] | [] | TAGS
#peft #llama #generated_from_trainer #base_model-NousResearch/Meta-Llama-3-70B #license-other #4-bit #region-us
|
<img src="URL alt="Built with Axolotl" width="200" height="32"/>
<details><summary>See axolotl config</summary>
axolotl version: '0.4.0'
</details><br>
# lora-out-l3-10
This model is a fine-tuned version of NousResearch/Meta-Llama-3-70B on the None dataset.
## Model description
More information needed
## Intended uses & limitations
More information needed
## Training and evaluation data
More information needed
## Training procedure
### Training hyperparameters
The following hyperparameters were used during training:
- learning_rate: 1.5e-05
- train_batch_size: 1
- eval_batch_size: 1
- seed: 246
- distributed_type: multi-GPU
- num_devices: 8
- gradient_accumulation_steps: 6
- total_train_batch_size: 48
- total_eval_batch_size: 8
- optimizer: Adam with betas=(0.9,0.999) and epsilon=1e-08
- lr_scheduler_type: cosine
- num_epochs: 4
### Training results
### Framework versions
- PEFT 0.10.0
- Transformers 4.40.0.dev0
- Pytorch 2.2.1
- Datasets 2.15.0
- Tokenizers 0.15.0 | [
"# lora-out-l3-10\n\nThis model is a fine-tuned version of NousResearch/Meta-Llama-3-70B on the None dataset.",
"## Model description\n\nMore information needed",
"## Intended uses & limitations\n\nMore information needed",
"## Training and evaluation data\n\nMore information needed",
"## Training procedure",
"### Training hyperparameters\n\nThe following hyperparameters were used during training:\n- learning_rate: 1.5e-05\n- train_batch_size: 1\n- eval_batch_size: 1\n- seed: 246\n- distributed_type: multi-GPU\n- num_devices: 8\n- gradient_accumulation_steps: 6\n- total_train_batch_size: 48\n- total_eval_batch_size: 8\n- optimizer: Adam with betas=(0.9,0.999) and epsilon=1e-08\n- lr_scheduler_type: cosine\n- num_epochs: 4",
"### Training results",
"### Framework versions\n\n- PEFT 0.10.0\n- Transformers 4.40.0.dev0\n- Pytorch 2.2.1\n- Datasets 2.15.0\n- Tokenizers 0.15.0"
] | [
"TAGS\n#peft #llama #generated_from_trainer #base_model-NousResearch/Meta-Llama-3-70B #license-other #4-bit #region-us \n",
"# lora-out-l3-10\n\nThis model is a fine-tuned version of NousResearch/Meta-Llama-3-70B on the None dataset.",
"## Model description\n\nMore information needed",
"## Intended uses & limitations\n\nMore information needed",
"## Training and evaluation data\n\nMore information needed",
"## Training procedure",
"### Training hyperparameters\n\nThe following hyperparameters were used during training:\n- learning_rate: 1.5e-05\n- train_batch_size: 1\n- eval_batch_size: 1\n- seed: 246\n- distributed_type: multi-GPU\n- num_devices: 8\n- gradient_accumulation_steps: 6\n- total_train_batch_size: 48\n- total_eval_batch_size: 8\n- optimizer: Adam with betas=(0.9,0.999) and epsilon=1e-08\n- lr_scheduler_type: cosine\n- num_epochs: 4",
"### Training results",
"### Framework versions\n\n- PEFT 0.10.0\n- Transformers 4.40.0.dev0\n- Pytorch 2.2.1\n- Datasets 2.15.0\n- Tokenizers 0.15.0"
] |
null | peft |
<!-- This model card has been generated automatically according to the information the Trainer had access to. You
should probably proofread and complete it, then remove this comment. -->
# Mixtral_Alpace_v2_NIKI
This model is a fine-tuned version of [mistralai/Mixtral-8x7B-v0.1](https://huggingface.co/mistralai/Mixtral-8x7B-v0.1) on the generator dataset.
It achieves the following results on the evaluation set:
- Loss: 1.1688
## Model description
More information needed
## Intended uses & limitations
More information needed
## Training and evaluation data
More information needed
## Training procedure
### Training hyperparameters
The following hyperparameters were used during training:
- learning_rate: 2.5e-05
- train_batch_size: 8
- eval_batch_size: 8
- seed: 42
- optimizer: Adam with betas=(0.9,0.999) and epsilon=1e-08
- lr_scheduler_type: linear
- lr_scheduler_warmup_steps: 0.03
- training_steps: 300
### Training results
| Training Loss | Epoch | Step | Validation Loss |
|:-------------:|:------:|:----:|:---------------:|
| 1.3725 | 0.0606 | 10 | 1.3384 |
| 1.339 | 0.1212 | 20 | 1.3260 |
| 1.3448 | 0.1818 | 30 | 1.3121 |
| 1.2777 | 0.2424 | 40 | 1.2984 |
| 1.3067 | 0.3030 | 50 | 1.2853 |
| 1.2674 | 0.3636 | 60 | 1.2723 |
| 1.2842 | 0.4242 | 70 | 1.2610 |
| 1.2835 | 0.4848 | 80 | 1.2505 |
| 1.2688 | 0.5455 | 90 | 1.2406 |
| 1.2892 | 0.6061 | 100 | 1.2315 |
| 1.2565 | 0.6667 | 110 | 1.2236 |
| 1.2145 | 0.7273 | 120 | 1.2163 |
| 1.2297 | 0.7879 | 130 | 1.2101 |
| 1.2406 | 0.8485 | 140 | 1.2042 |
| 1.2146 | 0.9091 | 150 | 1.1986 |
| 1.2386 | 0.9697 | 160 | 1.1940 |
| 1.1929 | 1.0303 | 170 | 1.1899 |
| 1.2036 | 1.0909 | 180 | 1.1869 |
| 1.181 | 1.1515 | 190 | 1.1837 |
| 1.201 | 1.2121 | 200 | 1.1812 |
| 1.1965 | 1.2727 | 210 | 1.1786 |
| 1.2084 | 1.3333 | 220 | 1.1765 |
| 1.2097 | 1.3939 | 230 | 1.1746 |
| 1.176 | 1.4545 | 240 | 1.1727 |
| 1.1757 | 1.5152 | 250 | 1.1715 |
| 1.1977 | 1.5758 | 260 | 1.1705 |
| 1.1686 | 1.6364 | 270 | 1.1701 |
| 1.1679 | 1.6970 | 280 | 1.1694 |
| 1.1779 | 1.7576 | 290 | 1.1690 |
| 1.179 | 1.8182 | 300 | 1.1688 |
### Framework versions
- PEFT 0.10.0
- Transformers 4.40.1
- Pytorch 2.2.1+cu121
- Datasets 2.19.0
- Tokenizers 0.19.1 | {"license": "apache-2.0", "library_name": "peft", "tags": ["trl", "sft", "generated_from_trainer"], "datasets": ["generator"], "base_model": "mistralai/Mixtral-8x7B-v0.1", "model-index": [{"name": "Mixtral_Alpace_v2_NIKI", "results": []}]} | vanherzog/Mixtral_Alpace_v2_NIKI | null | [
"peft",
"tensorboard",
"safetensors",
"trl",
"sft",
"generated_from_trainer",
"dataset:generator",
"base_model:mistralai/Mixtral-8x7B-v0.1",
"license:apache-2.0",
"region:us"
] | null | 2024-04-25T15:06:30+00:00 | [] | [] | TAGS
#peft #tensorboard #safetensors #trl #sft #generated_from_trainer #dataset-generator #base_model-mistralai/Mixtral-8x7B-v0.1 #license-apache-2.0 #region-us
| Mixtral\_Alpace\_v2\_NIKI
=========================
This model is a fine-tuned version of mistralai/Mixtral-8x7B-v0.1 on the generator dataset.
It achieves the following results on the evaluation set:
* Loss: 1.1688
Model description
-----------------
More information needed
Intended uses & limitations
---------------------------
More information needed
Training and evaluation data
----------------------------
More information needed
Training procedure
------------------
### Training hyperparameters
The following hyperparameters were used during training:
* learning\_rate: 2.5e-05
* train\_batch\_size: 8
* eval\_batch\_size: 8
* seed: 42
* optimizer: Adam with betas=(0.9,0.999) and epsilon=1e-08
* lr\_scheduler\_type: linear
* lr\_scheduler\_warmup\_steps: 0.03
* training\_steps: 300
### Training results
### Framework versions
* PEFT 0.10.0
* Transformers 4.40.1
* Pytorch 2.2.1+cu121
* Datasets 2.19.0
* Tokenizers 0.19.1
| [
"### Training hyperparameters\n\n\nThe following hyperparameters were used during training:\n\n\n* learning\\_rate: 2.5e-05\n* train\\_batch\\_size: 8\n* eval\\_batch\\_size: 8\n* seed: 42\n* optimizer: Adam with betas=(0.9,0.999) and epsilon=1e-08\n* lr\\_scheduler\\_type: linear\n* lr\\_scheduler\\_warmup\\_steps: 0.03\n* training\\_steps: 300",
"### Training results",
"### Framework versions\n\n\n* PEFT 0.10.0\n* Transformers 4.40.1\n* Pytorch 2.2.1+cu121\n* Datasets 2.19.0\n* Tokenizers 0.19.1"
] | [
"TAGS\n#peft #tensorboard #safetensors #trl #sft #generated_from_trainer #dataset-generator #base_model-mistralai/Mixtral-8x7B-v0.1 #license-apache-2.0 #region-us \n",
"### Training hyperparameters\n\n\nThe following hyperparameters were used during training:\n\n\n* learning\\_rate: 2.5e-05\n* train\\_batch\\_size: 8\n* eval\\_batch\\_size: 8\n* seed: 42\n* optimizer: Adam with betas=(0.9,0.999) and epsilon=1e-08\n* lr\\_scheduler\\_type: linear\n* lr\\_scheduler\\_warmup\\_steps: 0.03\n* training\\_steps: 300",
"### Training results",
"### Framework versions\n\n\n* PEFT 0.10.0\n* Transformers 4.40.1\n* Pytorch 2.2.1+cu121\n* Datasets 2.19.0\n* Tokenizers 0.19.1"
] |
text-generation | transformers |
# Gemma 2B Translation v0.140
- Eval Loss: `0.91882`
- Train Loss: `0.80511`
- lr: `9e-05`
- optimizer: adamw
- lr_scheduler_type: cosine
## Prompt Template
```
<bos><start_of_turn>user
Translate into Korean:Hamsters don't eat cats.<end_of_turn>
<start_of_turn>model
햄스터는 고양이를 먹지 않습니다.<eos>
```
```
<bos><start_of_turn>user
Translate into English:햄스터는 고양이를 먹지 않습니다.<end_of_turn>
<start_of_turn>model
Hamsters do not eat cats.<eos>
```
## Model Description
- **Developed by:** `lemon-mint`
- **Model type:** Gemma
- **Language(s) (NLP):** English
- **License:** [gemma-terms-of-use](https://ai.google.dev/gemma/terms)
- **Finetuned from model:** [google/gemma-1.1-2b-it](https://huggingface.co/google/gemma-1.1-2b-it) | {"language": ["ko"], "license": "gemma", "library_name": "transformers", "tags": ["gemma", "pytorch", "instruct", "finetune", "translation"], "widget": [{"messages": [{"role": "user", "content": "Translate into Korean:Hamsters don't eat cats."}]}], "base_model": "google/gemma-1.1-2b-it", "pipeline_tag": "text-generation"} | lemon-mint/gemma-2b-translation-v0.140 | null | [
"transformers",
"safetensors",
"gemma",
"text-generation",
"pytorch",
"instruct",
"finetune",
"translation",
"conversational",
"ko",
"base_model:google/gemma-1.1-2b-it",
"license:gemma",
"autotrain_compatible",
"endpoints_compatible",
"text-generation-inference",
"region:us"
] | null | 2024-04-25T15:07:15+00:00 | [] | [
"ko"
] | TAGS
#transformers #safetensors #gemma #text-generation #pytorch #instruct #finetune #translation #conversational #ko #base_model-google/gemma-1.1-2b-it #license-gemma #autotrain_compatible #endpoints_compatible #text-generation-inference #region-us
|
# Gemma 2B Translation v0.140
- Eval Loss: '0.91882'
- Train Loss: '0.80511'
- lr: '9e-05'
- optimizer: adamw
- lr_scheduler_type: cosine
## Prompt Template
## Model Description
- Developed by: 'lemon-mint'
- Model type: Gemma
- Language(s) (NLP): English
- License: gemma-terms-of-use
- Finetuned from model: google/gemma-1.1-2b-it | [
"# Gemma 2B Translation v0.140\n\n- Eval Loss: '0.91882'\n- Train Loss: '0.80511'\n- lr: '9e-05'\n- optimizer: adamw\n- lr_scheduler_type: cosine",
"## Prompt Template",
"## Model Description\n\n- Developed by: 'lemon-mint'\n- Model type: Gemma\n- Language(s) (NLP): English\n- License: gemma-terms-of-use\n- Finetuned from model: google/gemma-1.1-2b-it"
] | [
"TAGS\n#transformers #safetensors #gemma #text-generation #pytorch #instruct #finetune #translation #conversational #ko #base_model-google/gemma-1.1-2b-it #license-gemma #autotrain_compatible #endpoints_compatible #text-generation-inference #region-us \n",
"# Gemma 2B Translation v0.140\n\n- Eval Loss: '0.91882'\n- Train Loss: '0.80511'\n- lr: '9e-05'\n- optimizer: adamw\n- lr_scheduler_type: cosine",
"## Prompt Template",
"## Model Description\n\n- Developed by: 'lemon-mint'\n- Model type: Gemma\n- Language(s) (NLP): English\n- License: gemma-terms-of-use\n- Finetuned from model: google/gemma-1.1-2b-it"
] |
reinforcement-learning | null |
# **Q-Learning** Agent playing1 **FrozenLake-v1**
This is a trained model of a **Q-Learning** agent playing **FrozenLake-v1** .
## Usage
```python
model = load_from_hub(repo_id="lzacchini/q-FrozenLake-v1-4x4-noSlippery", filename="q-learning.pkl")
# Don't forget to check if you need to add additional attributes (is_slippery=False etc)
env = gym.make(model["env_id"])
```
| {"tags": ["FrozenLake-v1-4x4-no_slippery", "q-learning", "reinforcement-learning", "custom-implementation"], "model-index": [{"name": "q-FrozenLake-v1-4x4-noSlippery", "results": [{"task": {"type": "reinforcement-learning", "name": "reinforcement-learning"}, "dataset": {"name": "FrozenLake-v1-4x4-no_slippery", "type": "FrozenLake-v1-4x4-no_slippery"}, "metrics": [{"type": "mean_reward", "value": "1.00 +/- 0.00", "name": "mean_reward", "verified": false}]}]}]} | lzacchini/q-FrozenLake-v1-4x4-noSlippery | null | [
"FrozenLake-v1-4x4-no_slippery",
"q-learning",
"reinforcement-learning",
"custom-implementation",
"model-index",
"region:us"
] | null | 2024-04-25T15:08:22+00:00 | [] | [] | TAGS
#FrozenLake-v1-4x4-no_slippery #q-learning #reinforcement-learning #custom-implementation #model-index #region-us
|
# Q-Learning Agent playing1 FrozenLake-v1
This is a trained model of a Q-Learning agent playing FrozenLake-v1 .
## Usage
| [
"# Q-Learning Agent playing1 FrozenLake-v1\n This is a trained model of a Q-Learning agent playing FrozenLake-v1 .\n\n ## Usage"
] | [
"TAGS\n#FrozenLake-v1-4x4-no_slippery #q-learning #reinforcement-learning #custom-implementation #model-index #region-us \n",
"# Q-Learning Agent playing1 FrozenLake-v1\n This is a trained model of a Q-Learning agent playing FrozenLake-v1 .\n\n ## Usage"
] |
audio-classification | transformers |
<!-- This model card has been generated automatically according to the information the Trainer had access to. You
should probably proofread and complete it, then remove this comment. -->
# distilhubert-finetuned-stutteringdetection
This model is a fine-tuned version of [ntu-spml/distilhubert](https://huggingface.co/ntu-spml/distilhubert) on the stuttering dataset.
It achieves the following results on the evaluation set:
- Loss: 0.8952
- Accuracy: 0.7692
## Model description
More information needed
## Intended uses & limitations
More information needed
## Training and evaluation data
More information needed
## Training procedure
### Training hyperparameters
The following hyperparameters were used during training:
- learning_rate: 5e-05
- train_batch_size: 8
- eval_batch_size: 8
- seed: 42
- optimizer: Adam with betas=(0.9,0.999) and epsilon=1e-08
- lr_scheduler_type: linear
- lr_scheduler_warmup_ratio: 0.1
- num_epochs: 10
- mixed_precision_training: Native AMP
### Training results
| Training Loss | Epoch | Step | Validation Loss | Accuracy |
|:-------------:|:-----:|:----:|:---------------:|:--------:|
| 1.1755 | 1.0 | 102 | 1.1561 | 0.5275 |
| 0.9759 | 2.0 | 204 | 0.9051 | 0.6703 |
| 0.5208 | 3.0 | 306 | 0.7956 | 0.7143 |
| 0.3765 | 4.0 | 408 | 0.7282 | 0.8022 |
| 0.2368 | 5.0 | 510 | 0.6921 | 0.8022 |
| 0.1761 | 6.0 | 612 | 0.8270 | 0.7582 |
| 0.3561 | 7.0 | 714 | 0.8967 | 0.7253 |
| 0.2222 | 8.0 | 816 | 0.8201 | 0.8022 |
| 0.0303 | 9.0 | 918 | 0.9433 | 0.7473 |
| 0.019 | 10.0 | 1020 | 0.8952 | 0.7692 |
### Framework versions
- Transformers 4.40.1
- Pytorch 2.2.1+cu121
- Datasets 2.19.0
- Tokenizers 0.19.1
| {"license": "apache-2.0", "tags": ["generated_from_trainer"], "datasets": ["arisha/stuttering"], "metrics": ["accuracy"], "base_model": "ntu-spml/distilhubert", "model-index": [{"name": "distilhubert-finetuned-stutteringdetection", "results": [{"task": {"type": "audio-classification", "name": "Audio Classification"}, "dataset": {"name": "stuttering", "type": "arisha/stuttering"}, "metrics": [{"type": "accuracy", "value": 0.7692307692307693, "name": "Accuracy"}]}]}]} | arisha123/distilhubert-finetuned-my_dataset | null | [
"transformers",
"tensorboard",
"safetensors",
"hubert",
"audio-classification",
"generated_from_trainer",
"dataset:arisha/stuttering",
"base_model:ntu-spml/distilhubert",
"license:apache-2.0",
"model-index",
"endpoints_compatible",
"has_space",
"region:us"
] | null | 2024-04-25T15:09:23+00:00 | [] | [] | TAGS
#transformers #tensorboard #safetensors #hubert #audio-classification #generated_from_trainer #dataset-arisha/stuttering #base_model-ntu-spml/distilhubert #license-apache-2.0 #model-index #endpoints_compatible #has_space #region-us
| distilhubert-finetuned-stutteringdetection
==========================================
This model is a fine-tuned version of ntu-spml/distilhubert on the stuttering dataset.
It achieves the following results on the evaluation set:
* Loss: 0.8952
* Accuracy: 0.7692
Model description
-----------------
More information needed
Intended uses & limitations
---------------------------
More information needed
Training and evaluation data
----------------------------
More information needed
Training procedure
------------------
### Training hyperparameters
The following hyperparameters were used during training:
* learning\_rate: 5e-05
* train\_batch\_size: 8
* eval\_batch\_size: 8
* seed: 42
* optimizer: Adam with betas=(0.9,0.999) and epsilon=1e-08
* lr\_scheduler\_type: linear
* lr\_scheduler\_warmup\_ratio: 0.1
* num\_epochs: 10
* mixed\_precision\_training: Native AMP
### Training results
### Framework versions
* Transformers 4.40.1
* Pytorch 2.2.1+cu121
* Datasets 2.19.0
* Tokenizers 0.19.1
| [
"### Training hyperparameters\n\n\nThe following hyperparameters were used during training:\n\n\n* learning\\_rate: 5e-05\n* train\\_batch\\_size: 8\n* eval\\_batch\\_size: 8\n* seed: 42\n* optimizer: Adam with betas=(0.9,0.999) and epsilon=1e-08\n* lr\\_scheduler\\_type: linear\n* lr\\_scheduler\\_warmup\\_ratio: 0.1\n* num\\_epochs: 10\n* mixed\\_precision\\_training: Native AMP",
"### Training results",
"### Framework versions\n\n\n* Transformers 4.40.1\n* Pytorch 2.2.1+cu121\n* Datasets 2.19.0\n* Tokenizers 0.19.1"
] | [
"TAGS\n#transformers #tensorboard #safetensors #hubert #audio-classification #generated_from_trainer #dataset-arisha/stuttering #base_model-ntu-spml/distilhubert #license-apache-2.0 #model-index #endpoints_compatible #has_space #region-us \n",
"### Training hyperparameters\n\n\nThe following hyperparameters were used during training:\n\n\n* learning\\_rate: 5e-05\n* train\\_batch\\_size: 8\n* eval\\_batch\\_size: 8\n* seed: 42\n* optimizer: Adam with betas=(0.9,0.999) and epsilon=1e-08\n* lr\\_scheduler\\_type: linear\n* lr\\_scheduler\\_warmup\\_ratio: 0.1\n* num\\_epochs: 10\n* mixed\\_precision\\_training: Native AMP",
"### Training results",
"### Framework versions\n\n\n* Transformers 4.40.1\n* Pytorch 2.2.1+cu121\n* Datasets 2.19.0\n* Tokenizers 0.19.1"
] |
text-generation | mlx |
# mlx-community/Llama-3-8b-64k-PoSE-4bit
This model was converted to MLX format from [`winglian/Llama-3-8b-64k-PoSE`]() using mlx-lm version **0.10.0**.
Refer to the [original model card](https://huggingface.co/winglian/Llama-3-8b-64k-PoSE) for more details on the model.
## Use with mlx
```bash
pip install mlx-lm
```
```python
from mlx_lm import load, generate
model, tokenizer = load("mlx-community/Llama-3-8b-64k-PoSE-4bit")
response = generate(model, tokenizer, prompt="hello", verbose=True)
```
| {"language": ["en"], "tags": ["facebook", "meta", "pytorch", "llama", "llama-3", "mlx"], "pipeline_tag": "text-generation"} | mlx-community/Llama-3-8b-64k-PoSE-4bit | null | [
"mlx",
"safetensors",
"llama",
"facebook",
"meta",
"pytorch",
"llama-3",
"text-generation",
"en",
"region:us"
] | null | 2024-04-25T15:09:35+00:00 | [] | [
"en"
] | TAGS
#mlx #safetensors #llama #facebook #meta #pytorch #llama-3 #text-generation #en #region-us
|
# mlx-community/Llama-3-8b-64k-PoSE-4bit
This model was converted to MLX format from ['winglian/Llama-3-8b-64k-PoSE']() using mlx-lm version 0.10.0.
Refer to the original model card for more details on the model.
## Use with mlx
| [
"# mlx-community/Llama-3-8b-64k-PoSE-4bit\nThis model was converted to MLX format from ['winglian/Llama-3-8b-64k-PoSE']() using mlx-lm version 0.10.0.\nRefer to the original model card for more details on the model.",
"## Use with mlx"
] | [
"TAGS\n#mlx #safetensors #llama #facebook #meta #pytorch #llama-3 #text-generation #en #region-us \n",
"# mlx-community/Llama-3-8b-64k-PoSE-4bit\nThis model was converted to MLX format from ['winglian/Llama-3-8b-64k-PoSE']() using mlx-lm version 0.10.0.\nRefer to the original model card for more details on the model.",
"## Use with mlx"
] |
null | transformers |
# Model Card for Model ID
<!-- Provide a quick summary of what the model is/does. -->
## Model Details
### Model Description
<!-- Provide a longer summary of what this model is. -->
This is the model card of a 🤗 transformers model that has been pushed on the Hub. This model card has been automatically generated.
- **Developed by:** [More Information Needed]
- **Funded by [optional]:** [More Information Needed]
- **Shared by [optional]:** [More Information Needed]
- **Model type:** [More Information Needed]
- **Language(s) (NLP):** [More Information Needed]
- **License:** [More Information Needed]
- **Finetuned from model [optional]:** [More Information Needed]
### Model Sources [optional]
<!-- Provide the basic links for the model. -->
- **Repository:** [More Information Needed]
- **Paper [optional]:** [More Information Needed]
- **Demo [optional]:** [More Information Needed]
## Uses
<!-- Address questions around how the model is intended to be used, including the foreseeable users of the model and those affected by the model. -->
### Direct Use
<!-- This section is for the model use without fine-tuning or plugging into a larger ecosystem/app. -->
[More Information Needed]
### Downstream Use [optional]
<!-- This section is for the model use when fine-tuned for a task, or when plugged into a larger ecosystem/app -->
[More Information Needed]
### Out-of-Scope Use
<!-- This section addresses misuse, malicious use, and uses that the model will not work well for. -->
[More Information Needed]
## Bias, Risks, and Limitations
<!-- This section is meant to convey both technical and sociotechnical limitations. -->
[More Information Needed]
### Recommendations
<!-- This section is meant to convey recommendations with respect to the bias, risk, and technical limitations. -->
Users (both direct and downstream) should be made aware of the risks, biases and limitations of the model. More information needed for further recommendations.
## How to Get Started with the Model
Use the code below to get started with the model.
[More Information Needed]
## Training Details
### Training Data
<!-- This should link to a Dataset Card, perhaps with a short stub of information on what the training data is all about as well as documentation related to data pre-processing or additional filtering. -->
[More Information Needed]
### Training Procedure
<!-- This relates heavily to the Technical Specifications. Content here should link to that section when it is relevant to the training procedure. -->
#### Preprocessing [optional]
[More Information Needed]
#### Training Hyperparameters
- **Training regime:** [More Information Needed] <!--fp32, fp16 mixed precision, bf16 mixed precision, bf16 non-mixed precision, fp16 non-mixed precision, fp8 mixed precision -->
#### Speeds, Sizes, Times [optional]
<!-- This section provides information about throughput, start/end time, checkpoint size if relevant, etc. -->
[More Information Needed]
## Evaluation
<!-- This section describes the evaluation protocols and provides the results. -->
### Testing Data, Factors & Metrics
#### Testing Data
<!-- This should link to a Dataset Card if possible. -->
[More Information Needed]
#### Factors
<!-- These are the things the evaluation is disaggregating by, e.g., subpopulations or domains. -->
[More Information Needed]
#### Metrics
<!-- These are the evaluation metrics being used, ideally with a description of why. -->
[More Information Needed]
### Results
[More Information Needed]
#### Summary
## Model Examination [optional]
<!-- Relevant interpretability work for the model goes here -->
[More Information Needed]
## Environmental Impact
<!-- Total emissions (in grams of CO2eq) and additional considerations, such as electricity usage, go here. Edit the suggested text below accordingly -->
Carbon emissions can be estimated using the [Machine Learning Impact calculator](https://mlco2.github.io/impact#compute) presented in [Lacoste et al. (2019)](https://arxiv.org/abs/1910.09700).
- **Hardware Type:** [More Information Needed]
- **Hours used:** [More Information Needed]
- **Cloud Provider:** [More Information Needed]
- **Compute Region:** [More Information Needed]
- **Carbon Emitted:** [More Information Needed]
## Technical Specifications [optional]
### Model Architecture and Objective
[More Information Needed]
### Compute Infrastructure
[More Information Needed]
#### Hardware
[More Information Needed]
#### Software
[More Information Needed]
## Citation [optional]
<!-- If there is a paper or blog post introducing the model, the APA and Bibtex information for that should go in this section. -->
**BibTeX:**
[More Information Needed]
**APA:**
[More Information Needed]
## Glossary [optional]
<!-- If relevant, include terms and calculations in this section that can help readers understand the model or model card. -->
[More Information Needed]
## More Information [optional]
[More Information Needed]
## Model Card Authors [optional]
[More Information Needed]
## Model Card Contact
[More Information Needed] | {"library_name": "transformers", "tags": []} | MLP-Lemma/Lemma-pt3000-sft-cnn-90k | null | [
"transformers",
"safetensors",
"arxiv:1910.09700",
"endpoints_compatible",
"region:us"
] | null | 2024-04-25T15:09:35+00:00 | [
"1910.09700"
] | [] | TAGS
#transformers #safetensors #arxiv-1910.09700 #endpoints_compatible #region-us
|
# Model Card for Model ID
## Model Details
### Model Description
This is the model card of a transformers model that has been pushed on the Hub. This model card has been automatically generated.
- Developed by:
- Funded by [optional]:
- Shared by [optional]:
- Model type:
- Language(s) (NLP):
- License:
- Finetuned from model [optional]:
### Model Sources [optional]
- Repository:
- Paper [optional]:
- Demo [optional]:
## Uses
### Direct Use
### Downstream Use [optional]
### Out-of-Scope Use
## Bias, Risks, and Limitations
### Recommendations
Users (both direct and downstream) should be made aware of the risks, biases and limitations of the model. More information needed for further recommendations.
## How to Get Started with the Model
Use the code below to get started with the model.
## Training Details
### Training Data
### Training Procedure
#### Preprocessing [optional]
#### Training Hyperparameters
- Training regime:
#### Speeds, Sizes, Times [optional]
## Evaluation
### Testing Data, Factors & Metrics
#### Testing Data
#### Factors
#### Metrics
### Results
#### Summary
## Model Examination [optional]
## Environmental Impact
Carbon emissions can be estimated using the Machine Learning Impact calculator presented in Lacoste et al. (2019).
- Hardware Type:
- Hours used:
- Cloud Provider:
- Compute Region:
- Carbon Emitted:
## Technical Specifications [optional]
### Model Architecture and Objective
### Compute Infrastructure
#### Hardware
#### Software
[optional]
BibTeX:
APA:
## Glossary [optional]
## More Information [optional]
## Model Card Authors [optional]
## Model Card Contact
| [
"# Model Card for Model ID",
"## Model Details",
"### Model Description\n\n\n\nThis is the model card of a transformers model that has been pushed on the Hub. This model card has been automatically generated.\n\n- Developed by: \n- Funded by [optional]: \n- Shared by [optional]: \n- Model type: \n- Language(s) (NLP): \n- License: \n- Finetuned from model [optional]:",
"### Model Sources [optional]\n\n\n\n- Repository: \n- Paper [optional]: \n- Demo [optional]:",
"## Uses",
"### Direct Use",
"### Downstream Use [optional]",
"### Out-of-Scope Use",
"## Bias, Risks, and Limitations",
"### Recommendations\n\n\n\nUsers (both direct and downstream) should be made aware of the risks, biases and limitations of the model. More information needed for further recommendations.",
"## How to Get Started with the Model\n\nUse the code below to get started with the model.",
"## Training Details",
"### Training Data",
"### Training Procedure",
"#### Preprocessing [optional]",
"#### Training Hyperparameters\n\n- Training regime:",
"#### Speeds, Sizes, Times [optional]",
"## Evaluation",
"### Testing Data, Factors & Metrics",
"#### Testing Data",
"#### Factors",
"#### Metrics",
"### Results",
"#### Summary",
"## Model Examination [optional]",
"## Environmental Impact\n\n\n\nCarbon emissions can be estimated using the Machine Learning Impact calculator presented in Lacoste et al. (2019).\n\n- Hardware Type: \n- Hours used: \n- Cloud Provider: \n- Compute Region: \n- Carbon Emitted:",
"## Technical Specifications [optional]",
"### Model Architecture and Objective",
"### Compute Infrastructure",
"#### Hardware",
"#### Software\n\n\n\n[optional]\n\n\n\nBibTeX:\n\n\n\nAPA:",
"## Glossary [optional]",
"## More Information [optional]",
"## Model Card Authors [optional]",
"## Model Card Contact"
] | [
"TAGS\n#transformers #safetensors #arxiv-1910.09700 #endpoints_compatible #region-us \n",
"# Model Card for Model ID",
"## Model Details",
"### Model Description\n\n\n\nThis is the model card of a transformers model that has been pushed on the Hub. This model card has been automatically generated.\n\n- Developed by: \n- Funded by [optional]: \n- Shared by [optional]: \n- Model type: \n- Language(s) (NLP): \n- License: \n- Finetuned from model [optional]:",
"### Model Sources [optional]\n\n\n\n- Repository: \n- Paper [optional]: \n- Demo [optional]:",
"## Uses",
"### Direct Use",
"### Downstream Use [optional]",
"### Out-of-Scope Use",
"## Bias, Risks, and Limitations",
"### Recommendations\n\n\n\nUsers (both direct and downstream) should be made aware of the risks, biases and limitations of the model. More information needed for further recommendations.",
"## How to Get Started with the Model\n\nUse the code below to get started with the model.",
"## Training Details",
"### Training Data",
"### Training Procedure",
"#### Preprocessing [optional]",
"#### Training Hyperparameters\n\n- Training regime:",
"#### Speeds, Sizes, Times [optional]",
"## Evaluation",
"### Testing Data, Factors & Metrics",
"#### Testing Data",
"#### Factors",
"#### Metrics",
"### Results",
"#### Summary",
"## Model Examination [optional]",
"## Environmental Impact\n\n\n\nCarbon emissions can be estimated using the Machine Learning Impact calculator presented in Lacoste et al. (2019).\n\n- Hardware Type: \n- Hours used: \n- Cloud Provider: \n- Compute Region: \n- Carbon Emitted:",
"## Technical Specifications [optional]",
"### Model Architecture and Objective",
"### Compute Infrastructure",
"#### Hardware",
"#### Software\n\n\n\n[optional]\n\n\n\nBibTeX:\n\n\n\nAPA:",
"## Glossary [optional]",
"## More Information [optional]",
"## Model Card Authors [optional]",
"## Model Card Contact"
] |
feature-extraction | transformers |
# Model Card for Model ID
<!-- Provide a quick summary of what the model is/does. -->
## Model Details
### Model Description
<!-- Provide a longer summary of what this model is. -->
This is the model card of a 🤗 transformers model that has been pushed on the Hub. This model card has been automatically generated.
- **Developed by:** [More Information Needed]
- **Funded by [optional]:** [More Information Needed]
- **Shared by [optional]:** [More Information Needed]
- **Model type:** [More Information Needed]
- **Language(s) (NLP):** [More Information Needed]
- **License:** [More Information Needed]
- **Finetuned from model [optional]:** [More Information Needed]
### Model Sources [optional]
<!-- Provide the basic links for the model. -->
- **Repository:** [More Information Needed]
- **Paper [optional]:** [More Information Needed]
- **Demo [optional]:** [More Information Needed]
## Uses
<!-- Address questions around how the model is intended to be used, including the foreseeable users of the model and those affected by the model. -->
### Direct Use
<!-- This section is for the model use without fine-tuning or plugging into a larger ecosystem/app. -->
[More Information Needed]
### Downstream Use [optional]
<!-- This section is for the model use when fine-tuned for a task, or when plugged into a larger ecosystem/app -->
[More Information Needed]
### Out-of-Scope Use
<!-- This section addresses misuse, malicious use, and uses that the model will not work well for. -->
[More Information Needed]
## Bias, Risks, and Limitations
<!-- This section is meant to convey both technical and sociotechnical limitations. -->
[More Information Needed]
### Recommendations
<!-- This section is meant to convey recommendations with respect to the bias, risk, and technical limitations. -->
Users (both direct and downstream) should be made aware of the risks, biases and limitations of the model. More information needed for further recommendations.
## How to Get Started with the Model
Use the code below to get started with the model.
[More Information Needed]
## Training Details
### Training Data
<!-- This should link to a Dataset Card, perhaps with a short stub of information on what the training data is all about as well as documentation related to data pre-processing or additional filtering. -->
[More Information Needed]
### Training Procedure
<!-- This relates heavily to the Technical Specifications. Content here should link to that section when it is relevant to the training procedure. -->
#### Preprocessing [optional]
[More Information Needed]
#### Training Hyperparameters
- **Training regime:** [More Information Needed] <!--fp32, fp16 mixed precision, bf16 mixed precision, bf16 non-mixed precision, fp16 non-mixed precision, fp8 mixed precision -->
#### Speeds, Sizes, Times [optional]
<!-- This section provides information about throughput, start/end time, checkpoint size if relevant, etc. -->
[More Information Needed]
## Evaluation
<!-- This section describes the evaluation protocols and provides the results. -->
### Testing Data, Factors & Metrics
#### Testing Data
<!-- This should link to a Dataset Card if possible. -->
[More Information Needed]
#### Factors
<!-- These are the things the evaluation is disaggregating by, e.g., subpopulations or domains. -->
[More Information Needed]
#### Metrics
<!-- These are the evaluation metrics being used, ideally with a description of why. -->
[More Information Needed]
### Results
[More Information Needed]
#### Summary
## Model Examination [optional]
<!-- Relevant interpretability work for the model goes here -->
[More Information Needed]
## Environmental Impact
<!-- Total emissions (in grams of CO2eq) and additional considerations, such as electricity usage, go here. Edit the suggested text below accordingly -->
Carbon emissions can be estimated using the [Machine Learning Impact calculator](https://mlco2.github.io/impact#compute) presented in [Lacoste et al. (2019)](https://arxiv.org/abs/1910.09700).
- **Hardware Type:** [More Information Needed]
- **Hours used:** [More Information Needed]
- **Cloud Provider:** [More Information Needed]
- **Compute Region:** [More Information Needed]
- **Carbon Emitted:** [More Information Needed]
## Technical Specifications [optional]
### Model Architecture and Objective
[More Information Needed]
### Compute Infrastructure
[More Information Needed]
#### Hardware
[More Information Needed]
#### Software
[More Information Needed]
## Citation [optional]
<!-- If there is a paper or blog post introducing the model, the APA and Bibtex information for that should go in this section. -->
**BibTeX:**
[More Information Needed]
**APA:**
[More Information Needed]
## Glossary [optional]
<!-- If relevant, include terms and calculations in this section that can help readers understand the model or model card. -->
[More Information Needed]
## More Information [optional]
[More Information Needed]
## Model Card Authors [optional]
[More Information Needed]
## Model Card Contact
[More Information Needed]
| {"library_name": "transformers", "tags": []} | liddlefish/privacyembeddingv2 | null | [
"transformers",
"pytorch",
"roberta",
"feature-extraction",
"arxiv:1910.09700",
"endpoints_compatible",
"region:us"
] | null | 2024-04-25T15:09:42+00:00 | [
"1910.09700"
] | [] | TAGS
#transformers #pytorch #roberta #feature-extraction #arxiv-1910.09700 #endpoints_compatible #region-us
|
# Model Card for Model ID
## Model Details
### Model Description
This is the model card of a transformers model that has been pushed on the Hub. This model card has been automatically generated.
- Developed by:
- Funded by [optional]:
- Shared by [optional]:
- Model type:
- Language(s) (NLP):
- License:
- Finetuned from model [optional]:
### Model Sources [optional]
- Repository:
- Paper [optional]:
- Demo [optional]:
## Uses
### Direct Use
### Downstream Use [optional]
### Out-of-Scope Use
## Bias, Risks, and Limitations
### Recommendations
Users (both direct and downstream) should be made aware of the risks, biases and limitations of the model. More information needed for further recommendations.
## How to Get Started with the Model
Use the code below to get started with the model.
## Training Details
### Training Data
### Training Procedure
#### Preprocessing [optional]
#### Training Hyperparameters
- Training regime:
#### Speeds, Sizes, Times [optional]
## Evaluation
### Testing Data, Factors & Metrics
#### Testing Data
#### Factors
#### Metrics
### Results
#### Summary
## Model Examination [optional]
## Environmental Impact
Carbon emissions can be estimated using the Machine Learning Impact calculator presented in Lacoste et al. (2019).
- Hardware Type:
- Hours used:
- Cloud Provider:
- Compute Region:
- Carbon Emitted:
## Technical Specifications [optional]
### Model Architecture and Objective
### Compute Infrastructure
#### Hardware
#### Software
[optional]
BibTeX:
APA:
## Glossary [optional]
## More Information [optional]
## Model Card Authors [optional]
## Model Card Contact
| [
"# Model Card for Model ID",
"## Model Details",
"### Model Description\n\n\n\nThis is the model card of a transformers model that has been pushed on the Hub. This model card has been automatically generated.\n\n- Developed by: \n- Funded by [optional]: \n- Shared by [optional]: \n- Model type: \n- Language(s) (NLP): \n- License: \n- Finetuned from model [optional]:",
"### Model Sources [optional]\n\n\n\n- Repository: \n- Paper [optional]: \n- Demo [optional]:",
"## Uses",
"### Direct Use",
"### Downstream Use [optional]",
"### Out-of-Scope Use",
"## Bias, Risks, and Limitations",
"### Recommendations\n\n\n\nUsers (both direct and downstream) should be made aware of the risks, biases and limitations of the model. More information needed for further recommendations.",
"## How to Get Started with the Model\n\nUse the code below to get started with the model.",
"## Training Details",
"### Training Data",
"### Training Procedure",
"#### Preprocessing [optional]",
"#### Training Hyperparameters\n\n- Training regime:",
"#### Speeds, Sizes, Times [optional]",
"## Evaluation",
"### Testing Data, Factors & Metrics",
"#### Testing Data",
"#### Factors",
"#### Metrics",
"### Results",
"#### Summary",
"## Model Examination [optional]",
"## Environmental Impact\n\n\n\nCarbon emissions can be estimated using the Machine Learning Impact calculator presented in Lacoste et al. (2019).\n\n- Hardware Type: \n- Hours used: \n- Cloud Provider: \n- Compute Region: \n- Carbon Emitted:",
"## Technical Specifications [optional]",
"### Model Architecture and Objective",
"### Compute Infrastructure",
"#### Hardware",
"#### Software\n\n\n\n[optional]\n\n\n\nBibTeX:\n\n\n\nAPA:",
"## Glossary [optional]",
"## More Information [optional]",
"## Model Card Authors [optional]",
"## Model Card Contact"
] | [
"TAGS\n#transformers #pytorch #roberta #feature-extraction #arxiv-1910.09700 #endpoints_compatible #region-us \n",
"# Model Card for Model ID",
"## Model Details",
"### Model Description\n\n\n\nThis is the model card of a transformers model that has been pushed on the Hub. This model card has been automatically generated.\n\n- Developed by: \n- Funded by [optional]: \n- Shared by [optional]: \n- Model type: \n- Language(s) (NLP): \n- License: \n- Finetuned from model [optional]:",
"### Model Sources [optional]\n\n\n\n- Repository: \n- Paper [optional]: \n- Demo [optional]:",
"## Uses",
"### Direct Use",
"### Downstream Use [optional]",
"### Out-of-Scope Use",
"## Bias, Risks, and Limitations",
"### Recommendations\n\n\n\nUsers (both direct and downstream) should be made aware of the risks, biases and limitations of the model. More information needed for further recommendations.",
"## How to Get Started with the Model\n\nUse the code below to get started with the model.",
"## Training Details",
"### Training Data",
"### Training Procedure",
"#### Preprocessing [optional]",
"#### Training Hyperparameters\n\n- Training regime:",
"#### Speeds, Sizes, Times [optional]",
"## Evaluation",
"### Testing Data, Factors & Metrics",
"#### Testing Data",
"#### Factors",
"#### Metrics",
"### Results",
"#### Summary",
"## Model Examination [optional]",
"## Environmental Impact\n\n\n\nCarbon emissions can be estimated using the Machine Learning Impact calculator presented in Lacoste et al. (2019).\n\n- Hardware Type: \n- Hours used: \n- Cloud Provider: \n- Compute Region: \n- Carbon Emitted:",
"## Technical Specifications [optional]",
"### Model Architecture and Objective",
"### Compute Infrastructure",
"#### Hardware",
"#### Software\n\n\n\n[optional]\n\n\n\nBibTeX:\n\n\n\nAPA:",
"## Glossary [optional]",
"## More Information [optional]",
"## Model Card Authors [optional]",
"## Model Card Contact"
] |
text-generation | transformers |
## 4-bit GEMM AWQ Quantizations of L3-TheSpice-8b-v0.8.3
Using <a href="https://github.com/casper-hansen/AutoAWQ/">AutoAWQ</a> release <a href="https://github.com/casper-hansen/AutoAWQ/releases/tag/v0.2.4">v0.2.4</a> for quantization.
Original model: https://huggingface.co/cgato/L3-TheSpice-8b-v0.8.3
## Prompt format
```
{System Prompt}
Username: {Input}
BotName: {Response}
Username: {Input}
BotName: {Response}
```
## AWQ Parameters
- q_group_size: 128
- w_bit: 4
- zero_point: True
- version: GEMM
## How to run
From the AutoAWQ repo [here](https://github.com/casper-hansen/AutoAWQ/blob/main/examples/generate.py)
First install autoawq pypi package:
```
pip install autoawq
```
Then run the following:
```
from awq import AutoAWQForCausalLM
from transformers import AutoTokenizer, TextStreamer
quant_path = "models/L3-TheSpice-8b-v0.8.3-AWQ"
# Load model
model = AutoAWQForCausalLM.from_quantized(quant_path, fuse_layers=True)
tokenizer = AutoTokenizer.from_pretrained(quant_path, trust_remote_code=True)
streamer = TextStreamer(tokenizer, skip_prompt=True, skip_special_tokens=True)
prompt = "You're standing on the surface of the Earth. "\
"You walk one mile south, one mile west and one mile north. "\
"You end up exactly where you started. Where are you?"
chat = [
{"role": "system", "content": "You are a concise assistant that helps answer questions."},
{"role": "user", "content": prompt},
]
# <|eot_id|> used for llama 3 models
terminators = [
tokenizer.eos_token_id,
tokenizer.convert_tokens_to_ids("<|eot_id|>")
]
tokens = tokenizer.apply_chat_template(
chat,
return_tensors="pt"
).cuda()
# Generate output
generation_output = model.generate(
tokens,
streamer=streamer,
max_new_tokens=64,
eos_token_id=terminators
)
```
Want to support my work? Visit my ko-fi page here: https://ko-fi.com/bartowski
| {"license": "cc-by-nc-4.0", "quantized_by": "bartowski", "pipeline_tag": "text-generation"} | bartowski/L3-TheSpice-8b-v0.8.3-AWQ | null | [
"transformers",
"safetensors",
"llama",
"text-generation",
"license:cc-by-nc-4.0",
"autotrain_compatible",
"endpoints_compatible",
"text-generation-inference",
"4-bit",
"region:us"
] | null | 2024-04-25T15:13:03+00:00 | [] | [] | TAGS
#transformers #safetensors #llama #text-generation #license-cc-by-nc-4.0 #autotrain_compatible #endpoints_compatible #text-generation-inference #4-bit #region-us
|
## 4-bit GEMM AWQ Quantizations of L3-TheSpice-8b-v0.8.3
Using <a href="URL release <a href="URL for quantization.
Original model: URL
## Prompt format
## AWQ Parameters
- q_group_size: 128
- w_bit: 4
- zero_point: True
- version: GEMM
## How to run
From the AutoAWQ repo here
First install autoawq pypi package:
Then run the following:
Want to support my work? Visit my ko-fi page here: URL
| [
"## 4-bit GEMM AWQ Quantizations of L3-TheSpice-8b-v0.8.3\n\nUsing <a href=\"URL release <a href=\"URL for quantization.\n\nOriginal model: URL",
"## Prompt format",
"## AWQ Parameters\n\n - q_group_size: 128\n - w_bit: 4\n - zero_point: True\n - version: GEMM",
"## How to run\n\nFrom the AutoAWQ repo here\n\nFirst install autoawq pypi package:\n\n\n\nThen run the following:\n\n\n\nWant to support my work? Visit my ko-fi page here: URL"
] | [
"TAGS\n#transformers #safetensors #llama #text-generation #license-cc-by-nc-4.0 #autotrain_compatible #endpoints_compatible #text-generation-inference #4-bit #region-us \n",
"## 4-bit GEMM AWQ Quantizations of L3-TheSpice-8b-v0.8.3\n\nUsing <a href=\"URL release <a href=\"URL for quantization.\n\nOriginal model: URL",
"## Prompt format",
"## AWQ Parameters\n\n - q_group_size: 128\n - w_bit: 4\n - zero_point: True\n - version: GEMM",
"## How to run\n\nFrom the AutoAWQ repo here\n\nFirst install autoawq pypi package:\n\n\n\nThen run the following:\n\n\n\nWant to support my work? Visit my ko-fi page here: URL"
] |
text-generation | transformers |
<img src="./llama-3-merges.webp" alt="Llama-3 DPO Logo" width="500" style="margin-left:'auto' margin-right:'auto' display:'block'"/>
# MaziyarPanahi/Llama-3-70B-Instruct-DPO-v0.1
This model is a fine-tune (DPO) of `meta-llama/Meta-Llama-3-70B-Instruct` model.
# Quantized GGUF
All GGUF models are available here: [MaziyarPanahi/Llama-3-70B-Instruct-DPO-v0.1-GGUF](https://huggingface.co/MaziyarPanahi/Llama-3-70B-Instruct-DPO-v0.1-GGUF)
# Prompt Template
This model uses `ChatML` prompt template:
```
<|im_start|>system
{System}
<|im_end|>
<|im_start|>user
{User}
<|im_end|>
<|im_start|>assistant
{Assistant}
````
# How to use
You can use this model by using `MaziyarPanahi/Llama-3-70B-Instruct-DPO-v0.1` as the model name in Hugging Face's
transformers library.
```python
from transformers import AutoModelForCausalLM, AutoTokenizer, TextStreamer
from transformers import pipeline
import torch
model_id = "MaziyarPanahi/Llama-3-70B-Instruct-DPO-v0.1"
model = AutoModelForCausalLM.from_pretrained(
model_id,
torch_dtype=torch.bfloat16,
device_map="auto",
trust_remote_code=True,
# attn_implementation="flash_attention_2"
)
tokenizer = AutoTokenizer.from_pretrained(
model_id,
trust_remote_code=True
)
streamer = TextStreamer(tokenizer)
pipeline = pipeline(
"text-generation",
model=model,
tokenizer=tokenizer,
model_kwargs={"torch_dtype": torch.bfloat16},
streamer=streamer
)
# Then you can use the pipeline to generate text.
messages = [
{"role": "system", "content": "You are a pirate chatbot who always responds in pirate speak!"},
{"role": "user", "content": "Who are you?"},
]
prompt = tokenizer.apply_chat_template(
messages,
tokenize=False,
add_generation_prompt=True
)
terminators = [
tokenizer.eos_token_id,
tokenizer.convert_tokens_to_ids("<|im_end|>"),
tokenizer.convert_tokens_to_ids("<|eot_id|>") # safer to have this too
]
outputs = pipeline(
prompt,
max_new_tokens=2048,
eos_token_id=terminators,
do_sample=True,
temperature=0.6,
top_p=0.95,
)
print(outputs[0]["generated_text"][len(prompt):])
```
# [Open LLM Leaderboard Evaluation Results](https://huggingface.co/spaces/HuggingFaceH4/open_llm_leaderboard)
Detailed results can be found [here](https://huggingface.co/datasets/open-llm-leaderboard/details_MaziyarPanahi__Llama-3-70B-Instruct-DPO-v0.1)
| Metric |Value|
|---------------------------------|----:|
|Avg. |78.11|
|AI2 Reasoning Challenge (25-Shot)|71.67|
|HellaSwag (10-Shot) |85.83|
|MMLU (5-Shot) |80.12|
|TruthfulQA (0-shot) |62.11|
|Winogrande (5-shot) |82.87|
|GSM8k (5-shot) |86.05|
| {"language": ["en"], "license": "llama3", "library_name": "transformers", "tags": ["axolotl", "finetune", "dpo", "facebook", "meta", "pytorch", "llama", "llama-3", "chatml"], "datasets": ["mlabonne/chatml-OpenHermes2.5-dpo-binarized-alpha"], "base_model": "meta-llama/Meta-Llama-3-70B-Instruct", "pipeline_tag": "text-generation", "license_name": "llama3", "license_link": "LICENSE", "inference": false, "model_creator": "MaziyarPanahi", "quantized_by": "MaziyarPanahi", "model-index": [{"name": "Llama-3-70B-Instruct-DPO-v0.1", "results": [{"task": {"type": "text-generation", "name": "Text Generation"}, "dataset": {"name": "AI2 Reasoning Challenge (25-Shot)", "type": "ai2_arc", "config": "ARC-Challenge", "split": "test", "args": {"num_few_shot": 25}}, "metrics": [{"type": "acc_norm", "value": 71.67, "name": "normalized accuracy"}], "source": {"url": "https://huggingface.co/spaces/HuggingFaceH4/open_llm_leaderboard?query=MaziyarPanahi/Llama-3-70B-Instruct-DPO-v0.1", "name": "Open LLM Leaderboard"}}, {"task": {"type": "text-generation", "name": "Text Generation"}, "dataset": {"name": "HellaSwag (10-Shot)", "type": "hellaswag", "split": "validation", "args": {"num_few_shot": 10}}, "metrics": [{"type": "acc_norm", "value": 85.83, "name": "normalized accuracy"}], "source": {"url": "https://huggingface.co/spaces/HuggingFaceH4/open_llm_leaderboard?query=MaziyarPanahi/Llama-3-70B-Instruct-DPO-v0.1", "name": "Open LLM Leaderboard"}}, {"task": {"type": "text-generation", "name": "Text Generation"}, "dataset": {"name": "MMLU (5-Shot)", "type": "cais/mmlu", "config": "all", "split": "test", "args": {"num_few_shot": 5}}, "metrics": [{"type": "acc", "value": 80.12, "name": "accuracy"}], "source": {"url": "https://huggingface.co/spaces/HuggingFaceH4/open_llm_leaderboard?query=MaziyarPanahi/Llama-3-70B-Instruct-DPO-v0.1", "name": "Open LLM Leaderboard"}}, {"task": {"type": "text-generation", "name": "Text Generation"}, "dataset": {"name": "TruthfulQA (0-shot)", "type": "truthful_qa", "config": "multiple_choice", "split": "validation", "args": {"num_few_shot": 0}}, "metrics": [{"type": "mc2", "value": 62.11}], "source": {"url": "https://huggingface.co/spaces/HuggingFaceH4/open_llm_leaderboard?query=MaziyarPanahi/Llama-3-70B-Instruct-DPO-v0.1", "name": "Open LLM Leaderboard"}}, {"task": {"type": "text-generation", "name": "Text Generation"}, "dataset": {"name": "Winogrande (5-shot)", "type": "winogrande", "config": "winogrande_xl", "split": "validation", "args": {"num_few_shot": 5}}, "metrics": [{"type": "acc", "value": 82.87, "name": "accuracy"}], "source": {"url": "https://huggingface.co/spaces/HuggingFaceH4/open_llm_leaderboard?query=MaziyarPanahi/Llama-3-70B-Instruct-DPO-v0.1", "name": "Open LLM Leaderboard"}}, {"task": {"type": "text-generation", "name": "Text Generation"}, "dataset": {"name": "GSM8k (5-shot)", "type": "gsm8k", "config": "main", "split": "test", "args": {"num_few_shot": 5}}, "metrics": [{"type": "acc", "value": 86.05, "name": "accuracy"}], "source": {"url": "https://huggingface.co/spaces/HuggingFaceH4/open_llm_leaderboard?query=MaziyarPanahi/Llama-3-70B-Instruct-DPO-v0.1", "name": "Open LLM Leaderboard"}}]}]} | MaziyarPanahi/Llama-3-70B-Instruct-DPO-v0.1 | null | [
"transformers",
"safetensors",
"llama",
"text-generation",
"axolotl",
"finetune",
"dpo",
"facebook",
"meta",
"pytorch",
"llama-3",
"chatml",
"conversational",
"en",
"dataset:mlabonne/chatml-OpenHermes2.5-dpo-binarized-alpha",
"base_model:meta-llama/Meta-Llama-3-70B-Instruct",
"license:llama3",
"model-index",
"autotrain_compatible",
"text-generation-inference",
"region:us"
] | null | 2024-04-25T15:13:24+00:00 | [] | [
"en"
] | TAGS
#transformers #safetensors #llama #text-generation #axolotl #finetune #dpo #facebook #meta #pytorch #llama-3 #chatml #conversational #en #dataset-mlabonne/chatml-OpenHermes2.5-dpo-binarized-alpha #base_model-meta-llama/Meta-Llama-3-70B-Instruct #license-llama3 #model-index #autotrain_compatible #text-generation-inference #region-us
| 
MaziyarPanahi/Llama-3-70B-Instruct-DPO-v0.1
===========================================
This model is a fine-tune (DPO) of 'meta-llama/Meta-Llama-3-70B-Instruct' model.
Quantized GGUF
==============
All GGUF models are available here: MaziyarPanahi/Llama-3-70B-Instruct-DPO-v0.1-GGUF
Prompt Template
===============
This model uses 'ChatML' prompt template:
'
How to use
==========
You can use this model by using 'MaziyarPanahi/Llama-3-70B-Instruct-DPO-v0.1' as the model name in Hugging Face's
transformers library.
Open LLM Leaderboard Evaluation Results
=======================================
Detailed results can be found here
| [] | [
"TAGS\n#transformers #safetensors #llama #text-generation #axolotl #finetune #dpo #facebook #meta #pytorch #llama-3 #chatml #conversational #en #dataset-mlabonne/chatml-OpenHermes2.5-dpo-binarized-alpha #base_model-meta-llama/Meta-Llama-3-70B-Instruct #license-llama3 #model-index #autotrain_compatible #text-generation-inference #region-us \n"
] |
null | transformers |
# Model Card for Model ID
<!-- Provide a quick summary of what the model is/does. -->
## Model Details
### Model Description
<!-- Provide a longer summary of what this model is. -->
This is the model card of a 🤗 transformers model that has been pushed on the Hub. This model card has been automatically generated.
- **Developed by:** [More Information Needed]
- **Funded by [optional]:** [More Information Needed]
- **Shared by [optional]:** [More Information Needed]
- **Model type:** [More Information Needed]
- **Language(s) (NLP):** [More Information Needed]
- **License:** [More Information Needed]
- **Finetuned from model [optional]:** [More Information Needed]
### Model Sources [optional]
<!-- Provide the basic links for the model. -->
- **Repository:** [More Information Needed]
- **Paper [optional]:** [More Information Needed]
- **Demo [optional]:** [More Information Needed]
## Uses
<!-- Address questions around how the model is intended to be used, including the foreseeable users of the model and those affected by the model. -->
### Direct Use
<!-- This section is for the model use without fine-tuning or plugging into a larger ecosystem/app. -->
[More Information Needed]
### Downstream Use [optional]
<!-- This section is for the model use when fine-tuned for a task, or when plugged into a larger ecosystem/app -->
[More Information Needed]
### Out-of-Scope Use
<!-- This section addresses misuse, malicious use, and uses that the model will not work well for. -->
[More Information Needed]
## Bias, Risks, and Limitations
<!-- This section is meant to convey both technical and sociotechnical limitations. -->
[More Information Needed]
### Recommendations
<!-- This section is meant to convey recommendations with respect to the bias, risk, and technical limitations. -->
Users (both direct and downstream) should be made aware of the risks, biases and limitations of the model. More information needed for further recommendations.
## How to Get Started with the Model
Use the code below to get started with the model.
[More Information Needed]
## Training Details
### Training Data
<!-- This should link to a Dataset Card, perhaps with a short stub of information on what the training data is all about as well as documentation related to data pre-processing or additional filtering. -->
[More Information Needed]
### Training Procedure
<!-- This relates heavily to the Technical Specifications. Content here should link to that section when it is relevant to the training procedure. -->
#### Preprocessing [optional]
[More Information Needed]
#### Training Hyperparameters
- **Training regime:** [More Information Needed] <!--fp32, fp16 mixed precision, bf16 mixed precision, bf16 non-mixed precision, fp16 non-mixed precision, fp8 mixed precision -->
#### Speeds, Sizes, Times [optional]
<!-- This section provides information about throughput, start/end time, checkpoint size if relevant, etc. -->
[More Information Needed]
## Evaluation
<!-- This section describes the evaluation protocols and provides the results. -->
### Testing Data, Factors & Metrics
#### Testing Data
<!-- This should link to a Dataset Card if possible. -->
[More Information Needed]
#### Factors
<!-- These are the things the evaluation is disaggregating by, e.g., subpopulations or domains. -->
[More Information Needed]
#### Metrics
<!-- These are the evaluation metrics being used, ideally with a description of why. -->
[More Information Needed]
### Results
[More Information Needed]
#### Summary
## Model Examination [optional]
<!-- Relevant interpretability work for the model goes here -->
[More Information Needed]
## Environmental Impact
<!-- Total emissions (in grams of CO2eq) and additional considerations, such as electricity usage, go here. Edit the suggested text below accordingly -->
Carbon emissions can be estimated using the [Machine Learning Impact calculator](https://mlco2.github.io/impact#compute) presented in [Lacoste et al. (2019)](https://arxiv.org/abs/1910.09700).
- **Hardware Type:** [More Information Needed]
- **Hours used:** [More Information Needed]
- **Cloud Provider:** [More Information Needed]
- **Compute Region:** [More Information Needed]
- **Carbon Emitted:** [More Information Needed]
## Technical Specifications [optional]
### Model Architecture and Objective
[More Information Needed]
### Compute Infrastructure
[More Information Needed]
#### Hardware
[More Information Needed]
#### Software
[More Information Needed]
## Citation [optional]
<!-- If there is a paper or blog post introducing the model, the APA and Bibtex information for that should go in this section. -->
**BibTeX:**
[More Information Needed]
**APA:**
[More Information Needed]
## Glossary [optional]
<!-- If relevant, include terms and calculations in this section that can help readers understand the model or model card. -->
[More Information Needed]
## More Information [optional]
[More Information Needed]
## Model Card Authors [optional]
[More Information Needed]
## Model Card Contact
[More Information Needed] | {"library_name": "transformers", "tags": []} | MLP-Lemma/Lemma-pt3000-sft-xsum-90k | null | [
"transformers",
"safetensors",
"arxiv:1910.09700",
"endpoints_compatible",
"region:us"
] | null | 2024-04-25T15:13:42+00:00 | [
"1910.09700"
] | [] | TAGS
#transformers #safetensors #arxiv-1910.09700 #endpoints_compatible #region-us
|
# Model Card for Model ID
## Model Details
### Model Description
This is the model card of a transformers model that has been pushed on the Hub. This model card has been automatically generated.
- Developed by:
- Funded by [optional]:
- Shared by [optional]:
- Model type:
- Language(s) (NLP):
- License:
- Finetuned from model [optional]:
### Model Sources [optional]
- Repository:
- Paper [optional]:
- Demo [optional]:
## Uses
### Direct Use
### Downstream Use [optional]
### Out-of-Scope Use
## Bias, Risks, and Limitations
### Recommendations
Users (both direct and downstream) should be made aware of the risks, biases and limitations of the model. More information needed for further recommendations.
## How to Get Started with the Model
Use the code below to get started with the model.
## Training Details
### Training Data
### Training Procedure
#### Preprocessing [optional]
#### Training Hyperparameters
- Training regime:
#### Speeds, Sizes, Times [optional]
## Evaluation
### Testing Data, Factors & Metrics
#### Testing Data
#### Factors
#### Metrics
### Results
#### Summary
## Model Examination [optional]
## Environmental Impact
Carbon emissions can be estimated using the Machine Learning Impact calculator presented in Lacoste et al. (2019).
- Hardware Type:
- Hours used:
- Cloud Provider:
- Compute Region:
- Carbon Emitted:
## Technical Specifications [optional]
### Model Architecture and Objective
### Compute Infrastructure
#### Hardware
#### Software
[optional]
BibTeX:
APA:
## Glossary [optional]
## More Information [optional]
## Model Card Authors [optional]
## Model Card Contact
| [
"# Model Card for Model ID",
"## Model Details",
"### Model Description\n\n\n\nThis is the model card of a transformers model that has been pushed on the Hub. This model card has been automatically generated.\n\n- Developed by: \n- Funded by [optional]: \n- Shared by [optional]: \n- Model type: \n- Language(s) (NLP): \n- License: \n- Finetuned from model [optional]:",
"### Model Sources [optional]\n\n\n\n- Repository: \n- Paper [optional]: \n- Demo [optional]:",
"## Uses",
"### Direct Use",
"### Downstream Use [optional]",
"### Out-of-Scope Use",
"## Bias, Risks, and Limitations",
"### Recommendations\n\n\n\nUsers (both direct and downstream) should be made aware of the risks, biases and limitations of the model. More information needed for further recommendations.",
"## How to Get Started with the Model\n\nUse the code below to get started with the model.",
"## Training Details",
"### Training Data",
"### Training Procedure",
"#### Preprocessing [optional]",
"#### Training Hyperparameters\n\n- Training regime:",
"#### Speeds, Sizes, Times [optional]",
"## Evaluation",
"### Testing Data, Factors & Metrics",
"#### Testing Data",
"#### Factors",
"#### Metrics",
"### Results",
"#### Summary",
"## Model Examination [optional]",
"## Environmental Impact\n\n\n\nCarbon emissions can be estimated using the Machine Learning Impact calculator presented in Lacoste et al. (2019).\n\n- Hardware Type: \n- Hours used: \n- Cloud Provider: \n- Compute Region: \n- Carbon Emitted:",
"## Technical Specifications [optional]",
"### Model Architecture and Objective",
"### Compute Infrastructure",
"#### Hardware",
"#### Software\n\n\n\n[optional]\n\n\n\nBibTeX:\n\n\n\nAPA:",
"## Glossary [optional]",
"## More Information [optional]",
"## Model Card Authors [optional]",
"## Model Card Contact"
] | [
"TAGS\n#transformers #safetensors #arxiv-1910.09700 #endpoints_compatible #region-us \n",
"# Model Card for Model ID",
"## Model Details",
"### Model Description\n\n\n\nThis is the model card of a transformers model that has been pushed on the Hub. This model card has been automatically generated.\n\n- Developed by: \n- Funded by [optional]: \n- Shared by [optional]: \n- Model type: \n- Language(s) (NLP): \n- License: \n- Finetuned from model [optional]:",
"### Model Sources [optional]\n\n\n\n- Repository: \n- Paper [optional]: \n- Demo [optional]:",
"## Uses",
"### Direct Use",
"### Downstream Use [optional]",
"### Out-of-Scope Use",
"## Bias, Risks, and Limitations",
"### Recommendations\n\n\n\nUsers (both direct and downstream) should be made aware of the risks, biases and limitations of the model. More information needed for further recommendations.",
"## How to Get Started with the Model\n\nUse the code below to get started with the model.",
"## Training Details",
"### Training Data",
"### Training Procedure",
"#### Preprocessing [optional]",
"#### Training Hyperparameters\n\n- Training regime:",
"#### Speeds, Sizes, Times [optional]",
"## Evaluation",
"### Testing Data, Factors & Metrics",
"#### Testing Data",
"#### Factors",
"#### Metrics",
"### Results",
"#### Summary",
"## Model Examination [optional]",
"## Environmental Impact\n\n\n\nCarbon emissions can be estimated using the Machine Learning Impact calculator presented in Lacoste et al. (2019).\n\n- Hardware Type: \n- Hours used: \n- Cloud Provider: \n- Compute Region: \n- Carbon Emitted:",
"## Technical Specifications [optional]",
"### Model Architecture and Objective",
"### Compute Infrastructure",
"#### Hardware",
"#### Software\n\n\n\n[optional]\n\n\n\nBibTeX:\n\n\n\nAPA:",
"## Glossary [optional]",
"## More Information [optional]",
"## Model Card Authors [optional]",
"## Model Card Contact"
] |
reinforcement-learning | null |
# **Q-Learning** Agent playing1 **Taxi-v3**
This is a trained model of a **Q-Learning** agent playing **Taxi-v3** .
## Usage
```python
model = load_from_hub(repo_id="lzacchini/q-Taxi-v3", filename="q-learning.pkl")
# Don't forget to check if you need to add additional attributes (is_slippery=False etc)
env = gym.make(model["env_id"])
```
| {"tags": ["Taxi-v3", "q-learning", "reinforcement-learning", "custom-implementation"], "model-index": [{"name": "q-Taxi-v3", "results": [{"task": {"type": "reinforcement-learning", "name": "reinforcement-learning"}, "dataset": {"name": "Taxi-v3", "type": "Taxi-v3"}, "metrics": [{"type": "mean_reward", "value": "7.54 +/- 2.74", "name": "mean_reward", "verified": false}]}]}]} | lzacchini/q-Taxi-v3 | null | [
"Taxi-v3",
"q-learning",
"reinforcement-learning",
"custom-implementation",
"model-index",
"region:us"
] | null | 2024-04-25T15:14:50+00:00 | [] | [] | TAGS
#Taxi-v3 #q-learning #reinforcement-learning #custom-implementation #model-index #region-us
|
# Q-Learning Agent playing1 Taxi-v3
This is a trained model of a Q-Learning agent playing Taxi-v3 .
## Usage
| [
"# Q-Learning Agent playing1 Taxi-v3\n This is a trained model of a Q-Learning agent playing Taxi-v3 .\n\n ## Usage"
] | [
"TAGS\n#Taxi-v3 #q-learning #reinforcement-learning #custom-implementation #model-index #region-us \n",
"# Q-Learning Agent playing1 Taxi-v3\n This is a trained model of a Q-Learning agent playing Taxi-v3 .\n\n ## Usage"
] |
null | peft |
<!-- This model card has been generated automatically according to the information the Trainer had access to. You
should probably proofread and complete it, then remove this comment. -->
# mistral-7b-instruct-v0.2-advisegpt-v0.1
This model is a fine-tuned version of [mistralai/Mistral-7B-Instruct-v0.2](https://huggingface.co/mistralai/Mistral-7B-Instruct-v0.2) on the generator dataset.
It achieves the following results on the evaluation set:
- Loss: 0.5297
## Model description
More information needed
## Intended uses & limitations
More information needed
## Training and evaluation data
More information needed
## Training procedure
### Training hyperparameters
The following hyperparameters were used during training:
- learning_rate: 2e-05
- train_batch_size: 6
- eval_batch_size: 6
- seed: 42
- gradient_accumulation_steps: 15
- total_train_batch_size: 90
- optimizer: Adam with betas=(0.9,0.999) and epsilon=1e-08
- lr_scheduler_type: cosine
- num_epochs: 5
- mixed_precision_training: Native AMP
### Training results
| Training Loss | Epoch | Step | Validation Loss |
|:-------------:|:------:|:----:|:---------------:|
| 0.2215 | 0.9819 | 47 | 0.6548 |
| 0.1586 | 1.9847 | 95 | 0.5741 |
| 0.1421 | 2.9875 | 143 | 0.5422 |
| 0.1334 | 3.9903 | 191 | 0.5308 |
| 0.1298 | 4.9095 | 235 | 0.5297 |
### Framework versions
- PEFT 0.10.1.dev0
- Transformers 4.40.0
- Pytorch 2.2.2
- Datasets 2.19.0
- Tokenizers 0.19.1 | {"license": "apache-2.0", "library_name": "peft", "tags": ["trl", "sft", "generated_from_trainer"], "datasets": ["generator", "ninyx/data_advise-gpt-extended"], "base_model": "mistralai/Mistral-7B-Instruct-v0.2", "model-index": [{"name": "mistral-7b-instruct-v0.2-advisegpt-v0.1", "results": []}]} | ninyx/mistral-7b-instruct-v0.2-advisegpt-v0.1 | null | [
"peft",
"safetensors",
"trl",
"sft",
"generated_from_trainer",
"dataset:generator",
"dataset:ninyx/data_advise-gpt-extended",
"base_model:mistralai/Mistral-7B-Instruct-v0.2",
"license:apache-2.0",
"region:us"
] | null | 2024-04-25T15:15:18+00:00 | [] | [] | TAGS
#peft #safetensors #trl #sft #generated_from_trainer #dataset-generator #dataset-ninyx/data_advise-gpt-extended #base_model-mistralai/Mistral-7B-Instruct-v0.2 #license-apache-2.0 #region-us
| mistral-7b-instruct-v0.2-advisegpt-v0.1
=======================================
This model is a fine-tuned version of mistralai/Mistral-7B-Instruct-v0.2 on the generator dataset.
It achieves the following results on the evaluation set:
* Loss: 0.5297
Model description
-----------------
More information needed
Intended uses & limitations
---------------------------
More information needed
Training and evaluation data
----------------------------
More information needed
Training procedure
------------------
### Training hyperparameters
The following hyperparameters were used during training:
* learning\_rate: 2e-05
* train\_batch\_size: 6
* eval\_batch\_size: 6
* seed: 42
* gradient\_accumulation\_steps: 15
* total\_train\_batch\_size: 90
* optimizer: Adam with betas=(0.9,0.999) and epsilon=1e-08
* lr\_scheduler\_type: cosine
* num\_epochs: 5
* mixed\_precision\_training: Native AMP
### Training results
### Framework versions
* PEFT 0.10.1.dev0
* Transformers 4.40.0
* Pytorch 2.2.2
* Datasets 2.19.0
* Tokenizers 0.19.1
| [
"### Training hyperparameters\n\n\nThe following hyperparameters were used during training:\n\n\n* learning\\_rate: 2e-05\n* train\\_batch\\_size: 6\n* eval\\_batch\\_size: 6\n* seed: 42\n* gradient\\_accumulation\\_steps: 15\n* total\\_train\\_batch\\_size: 90\n* optimizer: Adam with betas=(0.9,0.999) and epsilon=1e-08\n* lr\\_scheduler\\_type: cosine\n* num\\_epochs: 5\n* mixed\\_precision\\_training: Native AMP",
"### Training results",
"### Framework versions\n\n\n* PEFT 0.10.1.dev0\n* Transformers 4.40.0\n* Pytorch 2.2.2\n* Datasets 2.19.0\n* Tokenizers 0.19.1"
] | [
"TAGS\n#peft #safetensors #trl #sft #generated_from_trainer #dataset-generator #dataset-ninyx/data_advise-gpt-extended #base_model-mistralai/Mistral-7B-Instruct-v0.2 #license-apache-2.0 #region-us \n",
"### Training hyperparameters\n\n\nThe following hyperparameters were used during training:\n\n\n* learning\\_rate: 2e-05\n* train\\_batch\\_size: 6\n* eval\\_batch\\_size: 6\n* seed: 42\n* gradient\\_accumulation\\_steps: 15\n* total\\_train\\_batch\\_size: 90\n* optimizer: Adam with betas=(0.9,0.999) and epsilon=1e-08\n* lr\\_scheduler\\_type: cosine\n* num\\_epochs: 5\n* mixed\\_precision\\_training: Native AMP",
"### Training results",
"### Framework versions\n\n\n* PEFT 0.10.1.dev0\n* Transformers 4.40.0\n* Pytorch 2.2.2\n* Datasets 2.19.0\n* Tokenizers 0.19.1"
] |
null | transformers |
# Model Card for Model ID
<!-- Provide a quick summary of what the model is/does. -->
## Model Details
### Model Description
<!-- Provide a longer summary of what this model is. -->
This is the model card of a 🤗 transformers model that has been pushed on the Hub. This model card has been automatically generated.
- **Developed by:** [More Information Needed]
- **Funded by [optional]:** [More Information Needed]
- **Shared by [optional]:** [More Information Needed]
- **Model type:** [More Information Needed]
- **Language(s) (NLP):** [More Information Needed]
- **License:** [More Information Needed]
- **Finetuned from model [optional]:** [More Information Needed]
### Model Sources [optional]
<!-- Provide the basic links for the model. -->
- **Repository:** [More Information Needed]
- **Paper [optional]:** [More Information Needed]
- **Demo [optional]:** [More Information Needed]
## Uses
<!-- Address questions around how the model is intended to be used, including the foreseeable users of the model and those affected by the model. -->
### Direct Use
<!-- This section is for the model use without fine-tuning or plugging into a larger ecosystem/app. -->
[More Information Needed]
### Downstream Use [optional]
<!-- This section is for the model use when fine-tuned for a task, or when plugged into a larger ecosystem/app -->
[More Information Needed]
### Out-of-Scope Use
<!-- This section addresses misuse, malicious use, and uses that the model will not work well for. -->
[More Information Needed]
## Bias, Risks, and Limitations
<!-- This section is meant to convey both technical and sociotechnical limitations. -->
[More Information Needed]
### Recommendations
<!-- This section is meant to convey recommendations with respect to the bias, risk, and technical limitations. -->
Users (both direct and downstream) should be made aware of the risks, biases and limitations of the model. More information needed for further recommendations.
## How to Get Started with the Model
Use the code below to get started with the model.
[More Information Needed]
## Training Details
### Training Data
<!-- This should link to a Dataset Card, perhaps with a short stub of information on what the training data is all about as well as documentation related to data pre-processing or additional filtering. -->
[More Information Needed]
### Training Procedure
<!-- This relates heavily to the Technical Specifications. Content here should link to that section when it is relevant to the training procedure. -->
#### Preprocessing [optional]
[More Information Needed]
#### Training Hyperparameters
- **Training regime:** [More Information Needed] <!--fp32, fp16 mixed precision, bf16 mixed precision, bf16 non-mixed precision, fp16 non-mixed precision, fp8 mixed precision -->
#### Speeds, Sizes, Times [optional]
<!-- This section provides information about throughput, start/end time, checkpoint size if relevant, etc. -->
[More Information Needed]
## Evaluation
<!-- This section describes the evaluation protocols and provides the results. -->
### Testing Data, Factors & Metrics
#### Testing Data
<!-- This should link to a Dataset Card if possible. -->
[More Information Needed]
#### Factors
<!-- These are the things the evaluation is disaggregating by, e.g., subpopulations or domains. -->
[More Information Needed]
#### Metrics
<!-- These are the evaluation metrics being used, ideally with a description of why. -->
[More Information Needed]
### Results
[More Information Needed]
#### Summary
## Model Examination [optional]
<!-- Relevant interpretability work for the model goes here -->
[More Information Needed]
## Environmental Impact
<!-- Total emissions (in grams of CO2eq) and additional considerations, such as electricity usage, go here. Edit the suggested text below accordingly -->
Carbon emissions can be estimated using the [Machine Learning Impact calculator](https://mlco2.github.io/impact#compute) presented in [Lacoste et al. (2019)](https://arxiv.org/abs/1910.09700).
- **Hardware Type:** [More Information Needed]
- **Hours used:** [More Information Needed]
- **Cloud Provider:** [More Information Needed]
- **Compute Region:** [More Information Needed]
- **Carbon Emitted:** [More Information Needed]
## Technical Specifications [optional]
### Model Architecture and Objective
[More Information Needed]
### Compute Infrastructure
[More Information Needed]
#### Hardware
[More Information Needed]
#### Software
[More Information Needed]
## Citation [optional]
<!-- If there is a paper or blog post introducing the model, the APA and Bibtex information for that should go in this section. -->
**BibTeX:**
[More Information Needed]
**APA:**
[More Information Needed]
## Glossary [optional]
<!-- If relevant, include terms and calculations in this section that can help readers understand the model or model card. -->
[More Information Needed]
## More Information [optional]
[More Information Needed]
## Model Card Authors [optional]
[More Information Needed]
## Model Card Contact
[More Information Needed] | {"license": "mit", "library_name": "transformers"} | kishorea/finetuned_qa5 | null | [
"transformers",
"safetensors",
"arxiv:1910.09700",
"license:mit",
"endpoints_compatible",
"region:us"
] | null | 2024-04-25T15:16:30+00:00 | [
"1910.09700"
] | [] | TAGS
#transformers #safetensors #arxiv-1910.09700 #license-mit #endpoints_compatible #region-us
|
# Model Card for Model ID
## Model Details
### Model Description
This is the model card of a transformers model that has been pushed on the Hub. This model card has been automatically generated.
- Developed by:
- Funded by [optional]:
- Shared by [optional]:
- Model type:
- Language(s) (NLP):
- License:
- Finetuned from model [optional]:
### Model Sources [optional]
- Repository:
- Paper [optional]:
- Demo [optional]:
## Uses
### Direct Use
### Downstream Use [optional]
### Out-of-Scope Use
## Bias, Risks, and Limitations
### Recommendations
Users (both direct and downstream) should be made aware of the risks, biases and limitations of the model. More information needed for further recommendations.
## How to Get Started with the Model
Use the code below to get started with the model.
## Training Details
### Training Data
### Training Procedure
#### Preprocessing [optional]
#### Training Hyperparameters
- Training regime:
#### Speeds, Sizes, Times [optional]
## Evaluation
### Testing Data, Factors & Metrics
#### Testing Data
#### Factors
#### Metrics
### Results
#### Summary
## Model Examination [optional]
## Environmental Impact
Carbon emissions can be estimated using the Machine Learning Impact calculator presented in Lacoste et al. (2019).
- Hardware Type:
- Hours used:
- Cloud Provider:
- Compute Region:
- Carbon Emitted:
## Technical Specifications [optional]
### Model Architecture and Objective
### Compute Infrastructure
#### Hardware
#### Software
[optional]
BibTeX:
APA:
## Glossary [optional]
## More Information [optional]
## Model Card Authors [optional]
## Model Card Contact
| [
"# Model Card for Model ID",
"## Model Details",
"### Model Description\n\n\n\nThis is the model card of a transformers model that has been pushed on the Hub. This model card has been automatically generated.\n\n- Developed by: \n- Funded by [optional]: \n- Shared by [optional]: \n- Model type: \n- Language(s) (NLP): \n- License: \n- Finetuned from model [optional]:",
"### Model Sources [optional]\n\n\n\n- Repository: \n- Paper [optional]: \n- Demo [optional]:",
"## Uses",
"### Direct Use",
"### Downstream Use [optional]",
"### Out-of-Scope Use",
"## Bias, Risks, and Limitations",
"### Recommendations\n\n\n\nUsers (both direct and downstream) should be made aware of the risks, biases and limitations of the model. More information needed for further recommendations.",
"## How to Get Started with the Model\n\nUse the code below to get started with the model.",
"## Training Details",
"### Training Data",
"### Training Procedure",
"#### Preprocessing [optional]",
"#### Training Hyperparameters\n\n- Training regime:",
"#### Speeds, Sizes, Times [optional]",
"## Evaluation",
"### Testing Data, Factors & Metrics",
"#### Testing Data",
"#### Factors",
"#### Metrics",
"### Results",
"#### Summary",
"## Model Examination [optional]",
"## Environmental Impact\n\n\n\nCarbon emissions can be estimated using the Machine Learning Impact calculator presented in Lacoste et al. (2019).\n\n- Hardware Type: \n- Hours used: \n- Cloud Provider: \n- Compute Region: \n- Carbon Emitted:",
"## Technical Specifications [optional]",
"### Model Architecture and Objective",
"### Compute Infrastructure",
"#### Hardware",
"#### Software\n\n\n\n[optional]\n\n\n\nBibTeX:\n\n\n\nAPA:",
"## Glossary [optional]",
"## More Information [optional]",
"## Model Card Authors [optional]",
"## Model Card Contact"
] | [
"TAGS\n#transformers #safetensors #arxiv-1910.09700 #license-mit #endpoints_compatible #region-us \n",
"# Model Card for Model ID",
"## Model Details",
"### Model Description\n\n\n\nThis is the model card of a transformers model that has been pushed on the Hub. This model card has been automatically generated.\n\n- Developed by: \n- Funded by [optional]: \n- Shared by [optional]: \n- Model type: \n- Language(s) (NLP): \n- License: \n- Finetuned from model [optional]:",
"### Model Sources [optional]\n\n\n\n- Repository: \n- Paper [optional]: \n- Demo [optional]:",
"## Uses",
"### Direct Use",
"### Downstream Use [optional]",
"### Out-of-Scope Use",
"## Bias, Risks, and Limitations",
"### Recommendations\n\n\n\nUsers (both direct and downstream) should be made aware of the risks, biases and limitations of the model. More information needed for further recommendations.",
"## How to Get Started with the Model\n\nUse the code below to get started with the model.",
"## Training Details",
"### Training Data",
"### Training Procedure",
"#### Preprocessing [optional]",
"#### Training Hyperparameters\n\n- Training regime:",
"#### Speeds, Sizes, Times [optional]",
"## Evaluation",
"### Testing Data, Factors & Metrics",
"#### Testing Data",
"#### Factors",
"#### Metrics",
"### Results",
"#### Summary",
"## Model Examination [optional]",
"## Environmental Impact\n\n\n\nCarbon emissions can be estimated using the Machine Learning Impact calculator presented in Lacoste et al. (2019).\n\n- Hardware Type: \n- Hours used: \n- Cloud Provider: \n- Compute Region: \n- Carbon Emitted:",
"## Technical Specifications [optional]",
"### Model Architecture and Objective",
"### Compute Infrastructure",
"#### Hardware",
"#### Software\n\n\n\n[optional]\n\n\n\nBibTeX:\n\n\n\nAPA:",
"## Glossary [optional]",
"## More Information [optional]",
"## Model Card Authors [optional]",
"## Model Card Contact"
] |
text-generation | transformers |
# Model Card for Model ID
<!-- Provide a quick summary of what the model is/does. -->
## Model Details
### Model Description
<!-- Provide a longer summary of what this model is. -->
This is the model card of a 🤗 transformers model that has been pushed on the Hub. This model card has been automatically generated.
- **Developed by:** [More Information Needed]
- **Funded by [optional]:** [More Information Needed]
- **Shared by [optional]:** [More Information Needed]
- **Model type:** [More Information Needed]
- **Language(s) (NLP):** [More Information Needed]
- **License:** [More Information Needed]
- **Finetuned from model [optional]:** [More Information Needed]
### Model Sources [optional]
<!-- Provide the basic links for the model. -->
- **Repository:** [More Information Needed]
- **Paper [optional]:** [More Information Needed]
- **Demo [optional]:** [More Information Needed]
## Uses
<!-- Address questions around how the model is intended to be used, including the foreseeable users of the model and those affected by the model. -->
### Direct Use
<!-- This section is for the model use without fine-tuning or plugging into a larger ecosystem/app. -->
[More Information Needed]
### Downstream Use [optional]
<!-- This section is for the model use when fine-tuned for a task, or when plugged into a larger ecosystem/app -->
[More Information Needed]
### Out-of-Scope Use
<!-- This section addresses misuse, malicious use, and uses that the model will not work well for. -->
[More Information Needed]
## Bias, Risks, and Limitations
<!-- This section is meant to convey both technical and sociotechnical limitations. -->
[More Information Needed]
### Recommendations
<!-- This section is meant to convey recommendations with respect to the bias, risk, and technical limitations. -->
Users (both direct and downstream) should be made aware of the risks, biases and limitations of the model. More information needed for further recommendations.
## How to Get Started with the Model
Use the code below to get started with the model.
[More Information Needed]
## Training Details
### Training Data
<!-- This should link to a Dataset Card, perhaps with a short stub of information on what the training data is all about as well as documentation related to data pre-processing or additional filtering. -->
[More Information Needed]
### Training Procedure
<!-- This relates heavily to the Technical Specifications. Content here should link to that section when it is relevant to the training procedure. -->
#### Preprocessing [optional]
[More Information Needed]
#### Training Hyperparameters
- **Training regime:** [More Information Needed] <!--fp32, fp16 mixed precision, bf16 mixed precision, bf16 non-mixed precision, fp16 non-mixed precision, fp8 mixed precision -->
#### Speeds, Sizes, Times [optional]
<!-- This section provides information about throughput, start/end time, checkpoint size if relevant, etc. -->
[More Information Needed]
## Evaluation
<!-- This section describes the evaluation protocols and provides the results. -->
### Testing Data, Factors & Metrics
#### Testing Data
<!-- This should link to a Dataset Card if possible. -->
[More Information Needed]
#### Factors
<!-- These are the things the evaluation is disaggregating by, e.g., subpopulations or domains. -->
[More Information Needed]
#### Metrics
<!-- These are the evaluation metrics being used, ideally with a description of why. -->
[More Information Needed]
### Results
[More Information Needed]
#### Summary
## Model Examination [optional]
<!-- Relevant interpretability work for the model goes here -->
[More Information Needed]
## Environmental Impact
<!-- Total emissions (in grams of CO2eq) and additional considerations, such as electricity usage, go here. Edit the suggested text below accordingly -->
Carbon emissions can be estimated using the [Machine Learning Impact calculator](https://mlco2.github.io/impact#compute) presented in [Lacoste et al. (2019)](https://arxiv.org/abs/1910.09700).
- **Hardware Type:** [More Information Needed]
- **Hours used:** [More Information Needed]
- **Cloud Provider:** [More Information Needed]
- **Compute Region:** [More Information Needed]
- **Carbon Emitted:** [More Information Needed]
## Technical Specifications [optional]
### Model Architecture and Objective
[More Information Needed]
### Compute Infrastructure
[More Information Needed]
#### Hardware
[More Information Needed]
#### Software
[More Information Needed]
## Citation [optional]
<!-- If there is a paper or blog post introducing the model, the APA and Bibtex information for that should go in this section. -->
**BibTeX:**
[More Information Needed]
**APA:**
[More Information Needed]
## Glossary [optional]
<!-- If relevant, include terms and calculations in this section that can help readers understand the model or model card. -->
[More Information Needed]
## More Information [optional]
[More Information Needed]
## Model Card Authors [optional]
[More Information Needed]
## Model Card Contact
[More Information Needed] | {"library_name": "transformers", "tags": []} | terry69/zephyr-7b-sft-qlora-10p-full | null | [
"transformers",
"safetensors",
"mistral",
"text-generation",
"arxiv:1910.09700",
"autotrain_compatible",
"endpoints_compatible",
"text-generation-inference",
"region:us"
] | null | 2024-04-25T15:16:48+00:00 | [
"1910.09700"
] | [] | TAGS
#transformers #safetensors #mistral #text-generation #arxiv-1910.09700 #autotrain_compatible #endpoints_compatible #text-generation-inference #region-us
|
# Model Card for Model ID
## Model Details
### Model Description
This is the model card of a transformers model that has been pushed on the Hub. This model card has been automatically generated.
- Developed by:
- Funded by [optional]:
- Shared by [optional]:
- Model type:
- Language(s) (NLP):
- License:
- Finetuned from model [optional]:
### Model Sources [optional]
- Repository:
- Paper [optional]:
- Demo [optional]:
## Uses
### Direct Use
### Downstream Use [optional]
### Out-of-Scope Use
## Bias, Risks, and Limitations
### Recommendations
Users (both direct and downstream) should be made aware of the risks, biases and limitations of the model. More information needed for further recommendations.
## How to Get Started with the Model
Use the code below to get started with the model.
## Training Details
### Training Data
### Training Procedure
#### Preprocessing [optional]
#### Training Hyperparameters
- Training regime:
#### Speeds, Sizes, Times [optional]
## Evaluation
### Testing Data, Factors & Metrics
#### Testing Data
#### Factors
#### Metrics
### Results
#### Summary
## Model Examination [optional]
## Environmental Impact
Carbon emissions can be estimated using the Machine Learning Impact calculator presented in Lacoste et al. (2019).
- Hardware Type:
- Hours used:
- Cloud Provider:
- Compute Region:
- Carbon Emitted:
## Technical Specifications [optional]
### Model Architecture and Objective
### Compute Infrastructure
#### Hardware
#### Software
[optional]
BibTeX:
APA:
## Glossary [optional]
## More Information [optional]
## Model Card Authors [optional]
## Model Card Contact
| [
"# Model Card for Model ID",
"## Model Details",
"### Model Description\n\n\n\nThis is the model card of a transformers model that has been pushed on the Hub. This model card has been automatically generated.\n\n- Developed by: \n- Funded by [optional]: \n- Shared by [optional]: \n- Model type: \n- Language(s) (NLP): \n- License: \n- Finetuned from model [optional]:",
"### Model Sources [optional]\n\n\n\n- Repository: \n- Paper [optional]: \n- Demo [optional]:",
"## Uses",
"### Direct Use",
"### Downstream Use [optional]",
"### Out-of-Scope Use",
"## Bias, Risks, and Limitations",
"### Recommendations\n\n\n\nUsers (both direct and downstream) should be made aware of the risks, biases and limitations of the model. More information needed for further recommendations.",
"## How to Get Started with the Model\n\nUse the code below to get started with the model.",
"## Training Details",
"### Training Data",
"### Training Procedure",
"#### Preprocessing [optional]",
"#### Training Hyperparameters\n\n- Training regime:",
"#### Speeds, Sizes, Times [optional]",
"## Evaluation",
"### Testing Data, Factors & Metrics",
"#### Testing Data",
"#### Factors",
"#### Metrics",
"### Results",
"#### Summary",
"## Model Examination [optional]",
"## Environmental Impact\n\n\n\nCarbon emissions can be estimated using the Machine Learning Impact calculator presented in Lacoste et al. (2019).\n\n- Hardware Type: \n- Hours used: \n- Cloud Provider: \n- Compute Region: \n- Carbon Emitted:",
"## Technical Specifications [optional]",
"### Model Architecture and Objective",
"### Compute Infrastructure",
"#### Hardware",
"#### Software\n\n\n\n[optional]\n\n\n\nBibTeX:\n\n\n\nAPA:",
"## Glossary [optional]",
"## More Information [optional]",
"## Model Card Authors [optional]",
"## Model Card Contact"
] | [
"TAGS\n#transformers #safetensors #mistral #text-generation #arxiv-1910.09700 #autotrain_compatible #endpoints_compatible #text-generation-inference #region-us \n",
"# Model Card for Model ID",
"## Model Details",
"### Model Description\n\n\n\nThis is the model card of a transformers model that has been pushed on the Hub. This model card has been automatically generated.\n\n- Developed by: \n- Funded by [optional]: \n- Shared by [optional]: \n- Model type: \n- Language(s) (NLP): \n- License: \n- Finetuned from model [optional]:",
"### Model Sources [optional]\n\n\n\n- Repository: \n- Paper [optional]: \n- Demo [optional]:",
"## Uses",
"### Direct Use",
"### Downstream Use [optional]",
"### Out-of-Scope Use",
"## Bias, Risks, and Limitations",
"### Recommendations\n\n\n\nUsers (both direct and downstream) should be made aware of the risks, biases and limitations of the model. More information needed for further recommendations.",
"## How to Get Started with the Model\n\nUse the code below to get started with the model.",
"## Training Details",
"### Training Data",
"### Training Procedure",
"#### Preprocessing [optional]",
"#### Training Hyperparameters\n\n- Training regime:",
"#### Speeds, Sizes, Times [optional]",
"## Evaluation",
"### Testing Data, Factors & Metrics",
"#### Testing Data",
"#### Factors",
"#### Metrics",
"### Results",
"#### Summary",
"## Model Examination [optional]",
"## Environmental Impact\n\n\n\nCarbon emissions can be estimated using the Machine Learning Impact calculator presented in Lacoste et al. (2019).\n\n- Hardware Type: \n- Hours used: \n- Cloud Provider: \n- Compute Region: \n- Carbon Emitted:",
"## Technical Specifications [optional]",
"### Model Architecture and Objective",
"### Compute Infrastructure",
"#### Hardware",
"#### Software\n\n\n\n[optional]\n\n\n\nBibTeX:\n\n\n\nAPA:",
"## Glossary [optional]",
"## More Information [optional]",
"## Model Card Authors [optional]",
"## Model Card Contact"
] |
image-classification | transformers |
<!-- This model card has been generated automatically according to the information the Trainer had access to. You
should probably proofread and complete it, then remove this comment. -->
# Boya1_RMSProp_1-e5_20Epoch_swin-base-window7-224-in22k_fold2
This model is a fine-tuned version of [microsoft/swin-base-patch4-window7-224-in22k](https://huggingface.co/microsoft/swin-base-patch4-window7-224-in22k) on the imagefolder dataset.
It achieves the following results on the evaluation set:
- Loss: 2.4218
- Accuracy: 0.6454
## Model description
More information needed
## Intended uses & limitations
More information needed
## Training and evaluation data
More information needed
## Training procedure
### Training hyperparameters
The following hyperparameters were used during training:
- learning_rate: 1e-05
- train_batch_size: 16
- eval_batch_size: 16
- seed: 42
- optimizer: Adam with betas=(0.9,0.999) and epsilon=1e-08
- lr_scheduler_type: linear
- lr_scheduler_warmup_ratio: 0.1
- num_epochs: 20
### Training results
| Training Loss | Epoch | Step | Validation Loss | Accuracy |
|:-------------:|:-----:|:-----:|:---------------:|:--------:|
| 1.1618 | 1.0 | 923 | 1.1839 | 0.5970 |
| 0.8976 | 2.0 | 1846 | 1.0699 | 0.6378 |
| 0.7736 | 3.0 | 2769 | 0.9583 | 0.6708 |
| 0.6954 | 4.0 | 3692 | 0.9868 | 0.6651 |
| 0.6308 | 5.0 | 4615 | 1.0373 | 0.6632 |
| 0.4596 | 6.0 | 5538 | 1.1537 | 0.6511 |
| 0.4024 | 7.0 | 6461 | 1.1814 | 0.6554 |
| 0.2437 | 8.0 | 7384 | 1.2764 | 0.65 |
| 0.2069 | 9.0 | 8307 | 1.4493 | 0.6457 |
| 0.1113 | 10.0 | 9230 | 1.5231 | 0.6497 |
| 0.1803 | 11.0 | 10153 | 1.6738 | 0.6414 |
| 0.1099 | 12.0 | 11076 | 1.7749 | 0.6473 |
| 0.1161 | 13.0 | 11999 | 1.9080 | 0.6473 |
| 0.1045 | 14.0 | 12922 | 2.0173 | 0.6505 |
| 0.085 | 15.0 | 13845 | 2.1608 | 0.6470 |
| 0.0137 | 16.0 | 14768 | 2.2375 | 0.6408 |
| 0.0385 | 17.0 | 15691 | 2.3465 | 0.6430 |
| 0.0121 | 18.0 | 16614 | 2.3696 | 0.6476 |
| 0.0316 | 19.0 | 17537 | 2.4233 | 0.6446 |
| 0.0511 | 20.0 | 18460 | 2.4218 | 0.6454 |
### Framework versions
- Transformers 4.35.0
- Pytorch 2.1.0
- Datasets 2.14.6
- Tokenizers 0.14.1
| {"license": "apache-2.0", "tags": ["generated_from_trainer"], "datasets": ["imagefolder"], "metrics": ["accuracy"], "base_model": "microsoft/swin-base-patch4-window7-224-in22k", "model-index": [{"name": "Boya1_RMSProp_1-e5_20Epoch_swin-base-window7-224-in22k_fold2", "results": [{"task": {"type": "image-classification", "name": "Image Classification"}, "dataset": {"name": "imagefolder", "type": "imagefolder", "config": "default", "split": "test", "args": "default"}, "metrics": [{"type": "accuracy", "value": 0.6454054054054054, "name": "Accuracy"}]}]}]} | onizukal/Boya1_RMSProp_1-e5_20Epoch_swin-base-window7-224-in22k_fold2 | null | [
"transformers",
"safetensors",
"swin",
"image-classification",
"generated_from_trainer",
"dataset:imagefolder",
"base_model:microsoft/swin-base-patch4-window7-224-in22k",
"license:apache-2.0",
"model-index",
"autotrain_compatible",
"endpoints_compatible",
"region:us"
] | null | 2024-04-25T15:17:22+00:00 | [] | [] | TAGS
#transformers #safetensors #swin #image-classification #generated_from_trainer #dataset-imagefolder #base_model-microsoft/swin-base-patch4-window7-224-in22k #license-apache-2.0 #model-index #autotrain_compatible #endpoints_compatible #region-us
| Boya1\_RMSProp\_1-e5\_20Epoch\_swin-base-window7-224-in22k\_fold2
=================================================================
This model is a fine-tuned version of microsoft/swin-base-patch4-window7-224-in22k on the imagefolder dataset.
It achieves the following results on the evaluation set:
* Loss: 2.4218
* Accuracy: 0.6454
Model description
-----------------
More information needed
Intended uses & limitations
---------------------------
More information needed
Training and evaluation data
----------------------------
More information needed
Training procedure
------------------
### Training hyperparameters
The following hyperparameters were used during training:
* learning\_rate: 1e-05
* train\_batch\_size: 16
* eval\_batch\_size: 16
* seed: 42
* optimizer: Adam with betas=(0.9,0.999) and epsilon=1e-08
* lr\_scheduler\_type: linear
* lr\_scheduler\_warmup\_ratio: 0.1
* num\_epochs: 20
### Training results
### Framework versions
* Transformers 4.35.0
* Pytorch 2.1.0
* Datasets 2.14.6
* Tokenizers 0.14.1
| [
"### Training hyperparameters\n\n\nThe following hyperparameters were used during training:\n\n\n* learning\\_rate: 1e-05\n* train\\_batch\\_size: 16\n* eval\\_batch\\_size: 16\n* seed: 42\n* optimizer: Adam with betas=(0.9,0.999) and epsilon=1e-08\n* lr\\_scheduler\\_type: linear\n* lr\\_scheduler\\_warmup\\_ratio: 0.1\n* num\\_epochs: 20",
"### Training results",
"### Framework versions\n\n\n* Transformers 4.35.0\n* Pytorch 2.1.0\n* Datasets 2.14.6\n* Tokenizers 0.14.1"
] | [
"TAGS\n#transformers #safetensors #swin #image-classification #generated_from_trainer #dataset-imagefolder #base_model-microsoft/swin-base-patch4-window7-224-in22k #license-apache-2.0 #model-index #autotrain_compatible #endpoints_compatible #region-us \n",
"### Training hyperparameters\n\n\nThe following hyperparameters were used during training:\n\n\n* learning\\_rate: 1e-05\n* train\\_batch\\_size: 16\n* eval\\_batch\\_size: 16\n* seed: 42\n* optimizer: Adam with betas=(0.9,0.999) and epsilon=1e-08\n* lr\\_scheduler\\_type: linear\n* lr\\_scheduler\\_warmup\\_ratio: 0.1\n* num\\_epochs: 20",
"### Training results",
"### Framework versions\n\n\n* Transformers 4.35.0\n* Pytorch 2.1.0\n* Datasets 2.14.6\n* Tokenizers 0.14.1"
] |
text2text-generation | transformers | ## Model description
This model is a sequence-to-sequence question generator that takes an answer and context as an input and generates a question as an output. It is based on a pre-trained mt5-base by [Google](https://github.com/google-research/multilingual-t5) model.
## Training data
The model was fine-tuned on [XQuAD](https://github.com/deepmind/xquad)
## Example usage
```python
from transformers import MT5ForConditionalGeneration, AutoTokenizer
import torch
model = MT5ForConditionalGeneration.from_pretrained("nluai/question-generation-vietnamese")
tokenizer = AutoTokenizer.from_pretrained("nluai/question-generation-vietnamese")
device = torch.device("cuda" if torch.cuda.is_available() else "cpu")
model = model.to(device)
# Content used to create a set of questions
context = '''Thành phố Hồ Chí Minh (còn gọi là Sài Gòn) tên gọi cũ trước 1975 là Sài Gòn hay Sài Gòn-Gia Định là thành phố lớn nhất ở Việt Nam về dân số và quy mô đô thị hóa. Đây còn là trung tâm kinh tế, chính trị, văn hóa và giáo dục tại Việt Nam. Thành phố Hồ Chí Minh là thành phố trực thuộc trung ương thuộc loại đô thị đặc biệt của Việt Nam cùng với thủ đô Hà Nội.Nằm trong vùng chuyển tiếp giữa Đông Nam Bộ và Tây Nam Bộ, thành phố này hiện có 16 quận, 1 thành phố và 5 huyện, tổng diện tích 2.061 km². Theo kết quả điều tra dân số chính thức vào thời điểm ngày một tháng 4 năm 2009 thì dân số thành phố là 7.162.864 người (chiếm 8,34% dân số Việt Nam), mật độ dân số trung bình 3.419 người/km². Đến năm 2019, dân số thành phố tăng lên 8.993.082 người và cũng là nơi có mật độ dân số cao nhất Việt Nam. Tuy nhiên, nếu tính những người cư trú không đăng ký hộ khẩu thì dân số thực tế của thành phố này năm 2018 là gần 14 triệu người.'''
encoding = tokenizer.encode_plus(context, return_tensors="pt")
input_ids, attention_masks = encoding["input_ids"].to(device), encoding["attention_mask"].to(device)
output = model.generate(input_ids=input_ids, attention_mask=attention_masks, max_length=256)
question = tokenizer.decode(output[0], skip_special_tokens=True,clean_up_tokenization_spaces=True)
question
#question: Thành phố hồ chí minh có bao nhiêu quận?
```
| {} | nluai/question-generation-vietnamese | null | [
"transformers",
"pytorch",
"mt5",
"text2text-generation",
"autotrain_compatible",
"endpoints_compatible",
"text-generation-inference",
"region:us"
] | null | 2024-04-25T15:18:33+00:00 | [] | [] | TAGS
#transformers #pytorch #mt5 #text2text-generation #autotrain_compatible #endpoints_compatible #text-generation-inference #region-us
| ## Model description
This model is a sequence-to-sequence question generator that takes an answer and context as an input and generates a question as an output. It is based on a pre-trained mt5-base by Google model.
## Training data
The model was fine-tuned on XQuAD
## Example usage
| [
"## Model description\nThis model is a sequence-to-sequence question generator that takes an answer and context as an input and generates a question as an output. It is based on a pre-trained mt5-base by Google model.",
"## Training data\nThe model was fine-tuned on XQuAD",
"## Example usage"
] | [
"TAGS\n#transformers #pytorch #mt5 #text2text-generation #autotrain_compatible #endpoints_compatible #text-generation-inference #region-us \n",
"## Model description\nThis model is a sequence-to-sequence question generator that takes an answer and context as an input and generates a question as an output. It is based on a pre-trained mt5-base by Google model.",
"## Training data\nThe model was fine-tuned on XQuAD",
"## Example usage"
] |
null | null | HyperSD 1-step LoRA baked-in model converted to OpenVINO(int8) and weights compressed with NNCF(10GB to 4.4GB).
Original Model : [Hyper-SD](https://huggingface.co/ByteDance/Hyper-SD)
You can use this model with [FastSD CPU](https://github.com/rupeshs/fastsdcpu).

To run the model yourself, you can leverage the 🧨 Diffusers library:
1. Install the dependencies:
```
pip install optimum-intel openvino diffusers onnx
```
2. Run the model:
```py
from optimum.intel.openvino.modeling_diffusion import OVStableDiffusionXLPipeline
pipeline = OVStableDiffusionXLPipeline.from_pretrained(
"rupeshs/hyper-sd-sdxl-1-step-openvino-int8",
ov_config={"CACHE_DIR": ""},
)
prompt = "a cute cat,flowers"
images = pipeline(
prompt=prompt,
width=768,
height=768,
num_inference_steps=1,
guidance_scale=1.0,
).images
images[0].save("out_image.png")
```
| {"language": ["en"], "license": "openrail++", "tags": ["stablediffusion", "openvino"]} | rupeshs/hyper-sd-sdxl-1-step-openvino-int8 | null | [
"stablediffusion",
"openvino",
"en",
"license:openrail++",
"region:us"
] | null | 2024-04-25T15:19:20+00:00 | [] | [
"en"
] | TAGS
#stablediffusion #openvino #en #license-openrail++ #region-us
| HyperSD 1-step LoRA baked-in model converted to OpenVINO(int8) and weights compressed with NNCF(10GB to 4.4GB).
Original Model : Hyper-SD
You can use this model with FastSD CPU.
!Sample
To run the model yourself, you can leverage the Diffusers library:
1. Install the dependencies:
2. Run the model:
| [] | [
"TAGS\n#stablediffusion #openvino #en #license-openrail++ #region-us \n"
] |
text-generation | transformers |
# Model Card for Model ID
<!-- Provide a quick summary of what the model is/does. -->
## Model Details
### Model Description
<!-- Provide a longer summary of what this model is. -->
This is the model card of a 🤗 transformers model that has been pushed on the Hub. This model card has been automatically generated.
- **Developed by:** [More Information Needed]
- **Funded by [optional]:** [More Information Needed]
- **Shared by [optional]:** [More Information Needed]
- **Model type:** [More Information Needed]
- **Language(s) (NLP):** [More Information Needed]
- **License:** [More Information Needed]
- **Finetuned from model [optional]:** [More Information Needed]
### Model Sources [optional]
<!-- Provide the basic links for the model. -->
- **Repository:** [More Information Needed]
- **Paper [optional]:** [More Information Needed]
- **Demo [optional]:** [More Information Needed]
## Uses
<!-- Address questions around how the model is intended to be used, including the foreseeable users of the model and those affected by the model. -->
### Direct Use
<!-- This section is for the model use without fine-tuning or plugging into a larger ecosystem/app. -->
[More Information Needed]
### Downstream Use [optional]
<!-- This section is for the model use when fine-tuned for a task, or when plugged into a larger ecosystem/app -->
[More Information Needed]
### Out-of-Scope Use
<!-- This section addresses misuse, malicious use, and uses that the model will not work well for. -->
[More Information Needed]
## Bias, Risks, and Limitations
<!-- This section is meant to convey both technical and sociotechnical limitations. -->
[More Information Needed]
### Recommendations
<!-- This section is meant to convey recommendations with respect to the bias, risk, and technical limitations. -->
Users (both direct and downstream) should be made aware of the risks, biases and limitations of the model. More information needed for further recommendations.
## How to Get Started with the Model
Use the code below to get started with the model.
[More Information Needed]
## Training Details
### Training Data
<!-- This should link to a Dataset Card, perhaps with a short stub of information on what the training data is all about as well as documentation related to data pre-processing or additional filtering. -->
[More Information Needed]
### Training Procedure
<!-- This relates heavily to the Technical Specifications. Content here should link to that section when it is relevant to the training procedure. -->
#### Preprocessing [optional]
[More Information Needed]
#### Training Hyperparameters
- **Training regime:** [More Information Needed] <!--fp32, fp16 mixed precision, bf16 mixed precision, bf16 non-mixed precision, fp16 non-mixed precision, fp8 mixed precision -->
#### Speeds, Sizes, Times [optional]
<!-- This section provides information about throughput, start/end time, checkpoint size if relevant, etc. -->
[More Information Needed]
## Evaluation
<!-- This section describes the evaluation protocols and provides the results. -->
### Testing Data, Factors & Metrics
#### Testing Data
<!-- This should link to a Dataset Card if possible. -->
[More Information Needed]
#### Factors
<!-- These are the things the evaluation is disaggregating by, e.g., subpopulations or domains. -->
[More Information Needed]
#### Metrics
<!-- These are the evaluation metrics being used, ideally with a description of why. -->
[More Information Needed]
### Results
[More Information Needed]
#### Summary
## Model Examination [optional]
<!-- Relevant interpretability work for the model goes here -->
[More Information Needed]
## Environmental Impact
<!-- Total emissions (in grams of CO2eq) and additional considerations, such as electricity usage, go here. Edit the suggested text below accordingly -->
Carbon emissions can be estimated using the [Machine Learning Impact calculator](https://mlco2.github.io/impact#compute) presented in [Lacoste et al. (2019)](https://arxiv.org/abs/1910.09700).
- **Hardware Type:** [More Information Needed]
- **Hours used:** [More Information Needed]
- **Cloud Provider:** [More Information Needed]
- **Compute Region:** [More Information Needed]
- **Carbon Emitted:** [More Information Needed]
## Technical Specifications [optional]
### Model Architecture and Objective
[More Information Needed]
### Compute Infrastructure
[More Information Needed]
#### Hardware
[More Information Needed]
#### Software
[More Information Needed]
## Citation [optional]
<!-- If there is a paper or blog post introducing the model, the APA and Bibtex information for that should go in this section. -->
**BibTeX:**
[More Information Needed]
**APA:**
[More Information Needed]
## Glossary [optional]
<!-- If relevant, include terms and calculations in this section that can help readers understand the model or model card. -->
[More Information Needed]
## More Information [optional]
[More Information Needed]
## Model Card Authors [optional]
[More Information Needed]
## Model Card Contact
[More Information Needed] | {"library_name": "transformers", "tags": []} | liquid9212/woh0lgj | null | [
"transformers",
"safetensors",
"stablelm",
"text-generation",
"conversational",
"arxiv:1910.09700",
"autotrain_compatible",
"endpoints_compatible",
"region:us"
] | null | 2024-04-25T15:20:27+00:00 | [
"1910.09700"
] | [] | TAGS
#transformers #safetensors #stablelm #text-generation #conversational #arxiv-1910.09700 #autotrain_compatible #endpoints_compatible #region-us
|
# Model Card for Model ID
## Model Details
### Model Description
This is the model card of a transformers model that has been pushed on the Hub. This model card has been automatically generated.
- Developed by:
- Funded by [optional]:
- Shared by [optional]:
- Model type:
- Language(s) (NLP):
- License:
- Finetuned from model [optional]:
### Model Sources [optional]
- Repository:
- Paper [optional]:
- Demo [optional]:
## Uses
### Direct Use
### Downstream Use [optional]
### Out-of-Scope Use
## Bias, Risks, and Limitations
### Recommendations
Users (both direct and downstream) should be made aware of the risks, biases and limitations of the model. More information needed for further recommendations.
## How to Get Started with the Model
Use the code below to get started with the model.
## Training Details
### Training Data
### Training Procedure
#### Preprocessing [optional]
#### Training Hyperparameters
- Training regime:
#### Speeds, Sizes, Times [optional]
## Evaluation
### Testing Data, Factors & Metrics
#### Testing Data
#### Factors
#### Metrics
### Results
#### Summary
## Model Examination [optional]
## Environmental Impact
Carbon emissions can be estimated using the Machine Learning Impact calculator presented in Lacoste et al. (2019).
- Hardware Type:
- Hours used:
- Cloud Provider:
- Compute Region:
- Carbon Emitted:
## Technical Specifications [optional]
### Model Architecture and Objective
### Compute Infrastructure
#### Hardware
#### Software
[optional]
BibTeX:
APA:
## Glossary [optional]
## More Information [optional]
## Model Card Authors [optional]
## Model Card Contact
| [
"# Model Card for Model ID",
"## Model Details",
"### Model Description\n\n\n\nThis is the model card of a transformers model that has been pushed on the Hub. This model card has been automatically generated.\n\n- Developed by: \n- Funded by [optional]: \n- Shared by [optional]: \n- Model type: \n- Language(s) (NLP): \n- License: \n- Finetuned from model [optional]:",
"### Model Sources [optional]\n\n\n\n- Repository: \n- Paper [optional]: \n- Demo [optional]:",
"## Uses",
"### Direct Use",
"### Downstream Use [optional]",
"### Out-of-Scope Use",
"## Bias, Risks, and Limitations",
"### Recommendations\n\n\n\nUsers (both direct and downstream) should be made aware of the risks, biases and limitations of the model. More information needed for further recommendations.",
"## How to Get Started with the Model\n\nUse the code below to get started with the model.",
"## Training Details",
"### Training Data",
"### Training Procedure",
"#### Preprocessing [optional]",
"#### Training Hyperparameters\n\n- Training regime:",
"#### Speeds, Sizes, Times [optional]",
"## Evaluation",
"### Testing Data, Factors & Metrics",
"#### Testing Data",
"#### Factors",
"#### Metrics",
"### Results",
"#### Summary",
"## Model Examination [optional]",
"## Environmental Impact\n\n\n\nCarbon emissions can be estimated using the Machine Learning Impact calculator presented in Lacoste et al. (2019).\n\n- Hardware Type: \n- Hours used: \n- Cloud Provider: \n- Compute Region: \n- Carbon Emitted:",
"## Technical Specifications [optional]",
"### Model Architecture and Objective",
"### Compute Infrastructure",
"#### Hardware",
"#### Software\n\n\n\n[optional]\n\n\n\nBibTeX:\n\n\n\nAPA:",
"## Glossary [optional]",
"## More Information [optional]",
"## Model Card Authors [optional]",
"## Model Card Contact"
] | [
"TAGS\n#transformers #safetensors #stablelm #text-generation #conversational #arxiv-1910.09700 #autotrain_compatible #endpoints_compatible #region-us \n",
"# Model Card for Model ID",
"## Model Details",
"### Model Description\n\n\n\nThis is the model card of a transformers model that has been pushed on the Hub. This model card has been automatically generated.\n\n- Developed by: \n- Funded by [optional]: \n- Shared by [optional]: \n- Model type: \n- Language(s) (NLP): \n- License: \n- Finetuned from model [optional]:",
"### Model Sources [optional]\n\n\n\n- Repository: \n- Paper [optional]: \n- Demo [optional]:",
"## Uses",
"### Direct Use",
"### Downstream Use [optional]",
"### Out-of-Scope Use",
"## Bias, Risks, and Limitations",
"### Recommendations\n\n\n\nUsers (both direct and downstream) should be made aware of the risks, biases and limitations of the model. More information needed for further recommendations.",
"## How to Get Started with the Model\n\nUse the code below to get started with the model.",
"## Training Details",
"### Training Data",
"### Training Procedure",
"#### Preprocessing [optional]",
"#### Training Hyperparameters\n\n- Training regime:",
"#### Speeds, Sizes, Times [optional]",
"## Evaluation",
"### Testing Data, Factors & Metrics",
"#### Testing Data",
"#### Factors",
"#### Metrics",
"### Results",
"#### Summary",
"## Model Examination [optional]",
"## Environmental Impact\n\n\n\nCarbon emissions can be estimated using the Machine Learning Impact calculator presented in Lacoste et al. (2019).\n\n- Hardware Type: \n- Hours used: \n- Cloud Provider: \n- Compute Region: \n- Carbon Emitted:",
"## Technical Specifications [optional]",
"### Model Architecture and Objective",
"### Compute Infrastructure",
"#### Hardware",
"#### Software\n\n\n\n[optional]\n\n\n\nBibTeX:\n\n\n\nAPA:",
"## Glossary [optional]",
"## More Information [optional]",
"## Model Card Authors [optional]",
"## Model Card Contact"
] |
text-generation | transformers |
# Model Card for Model ID
<!-- Provide a quick summary of what the model is/does. -->
## Model Details
### Model Description
<!-- Provide a longer summary of what this model is. -->
This is the model card of a 🤗 transformers model that has been pushed on the Hub. This model card has been automatically generated.
- **Developed by:** [More Information Needed]
- **Funded by [optional]:** [More Information Needed]
- **Shared by [optional]:** [More Information Needed]
- **Model type:** [More Information Needed]
- **Language(s) (NLP):** [More Information Needed]
- **License:** [More Information Needed]
- **Finetuned from model [optional]:** [More Information Needed]
### Model Sources [optional]
<!-- Provide the basic links for the model. -->
- **Repository:** [More Information Needed]
- **Paper [optional]:** [More Information Needed]
- **Demo [optional]:** [More Information Needed]
## Uses
<!-- Address questions around how the model is intended to be used, including the foreseeable users of the model and those affected by the model. -->
### Direct Use
<!-- This section is for the model use without fine-tuning or plugging into a larger ecosystem/app. -->
[More Information Needed]
### Downstream Use [optional]
<!-- This section is for the model use when fine-tuned for a task, or when plugged into a larger ecosystem/app -->
[More Information Needed]
### Out-of-Scope Use
<!-- This section addresses misuse, malicious use, and uses that the model will not work well for. -->
[More Information Needed]
## Bias, Risks, and Limitations
<!-- This section is meant to convey both technical and sociotechnical limitations. -->
[More Information Needed]
### Recommendations
<!-- This section is meant to convey recommendations with respect to the bias, risk, and technical limitations. -->
Users (both direct and downstream) should be made aware of the risks, biases and limitations of the model. More information needed for further recommendations.
## How to Get Started with the Model
Use the code below to get started with the model.
[More Information Needed]
## Training Details
### Training Data
<!-- This should link to a Dataset Card, perhaps with a short stub of information on what the training data is all about as well as documentation related to data pre-processing or additional filtering. -->
[More Information Needed]
### Training Procedure
<!-- This relates heavily to the Technical Specifications. Content here should link to that section when it is relevant to the training procedure. -->
#### Preprocessing [optional]
[More Information Needed]
#### Training Hyperparameters
- **Training regime:** [More Information Needed] <!--fp32, fp16 mixed precision, bf16 mixed precision, bf16 non-mixed precision, fp16 non-mixed precision, fp8 mixed precision -->
#### Speeds, Sizes, Times [optional]
<!-- This section provides information about throughput, start/end time, checkpoint size if relevant, etc. -->
[More Information Needed]
## Evaluation
<!-- This section describes the evaluation protocols and provides the results. -->
### Testing Data, Factors & Metrics
#### Testing Data
<!-- This should link to a Dataset Card if possible. -->
[More Information Needed]
#### Factors
<!-- These are the things the evaluation is disaggregating by, e.g., subpopulations or domains. -->
[More Information Needed]
#### Metrics
<!-- These are the evaluation metrics being used, ideally with a description of why. -->
[More Information Needed]
### Results
[More Information Needed]
#### Summary
## Model Examination [optional]
<!-- Relevant interpretability work for the model goes here -->
[More Information Needed]
## Environmental Impact
<!-- Total emissions (in grams of CO2eq) and additional considerations, such as electricity usage, go here. Edit the suggested text below accordingly -->
Carbon emissions can be estimated using the [Machine Learning Impact calculator](https://mlco2.github.io/impact#compute) presented in [Lacoste et al. (2019)](https://arxiv.org/abs/1910.09700).
- **Hardware Type:** [More Information Needed]
- **Hours used:** [More Information Needed]
- **Cloud Provider:** [More Information Needed]
- **Compute Region:** [More Information Needed]
- **Carbon Emitted:** [More Information Needed]
## Technical Specifications [optional]
### Model Architecture and Objective
[More Information Needed]
### Compute Infrastructure
[More Information Needed]
#### Hardware
[More Information Needed]
#### Software
[More Information Needed]
## Citation [optional]
<!-- If there is a paper or blog post introducing the model, the APA and Bibtex information for that should go in this section. -->
**BibTeX:**
[More Information Needed]
**APA:**
[More Information Needed]
## Glossary [optional]
<!-- If relevant, include terms and calculations in this section that can help readers understand the model or model card. -->
[More Information Needed]
## More Information [optional]
[More Information Needed]
## Model Card Authors [optional]
[More Information Needed]
## Model Card Contact
[More Information Needed] | {"library_name": "transformers", "tags": []} | quickstep3621/t7403i6 | null | [
"transformers",
"safetensors",
"stablelm",
"text-generation",
"conversational",
"arxiv:1910.09700",
"autotrain_compatible",
"endpoints_compatible",
"region:us"
] | null | 2024-04-25T15:20:59+00:00 | [
"1910.09700"
] | [] | TAGS
#transformers #safetensors #stablelm #text-generation #conversational #arxiv-1910.09700 #autotrain_compatible #endpoints_compatible #region-us
|
# Model Card for Model ID
## Model Details
### Model Description
This is the model card of a transformers model that has been pushed on the Hub. This model card has been automatically generated.
- Developed by:
- Funded by [optional]:
- Shared by [optional]:
- Model type:
- Language(s) (NLP):
- License:
- Finetuned from model [optional]:
### Model Sources [optional]
- Repository:
- Paper [optional]:
- Demo [optional]:
## Uses
### Direct Use
### Downstream Use [optional]
### Out-of-Scope Use
## Bias, Risks, and Limitations
### Recommendations
Users (both direct and downstream) should be made aware of the risks, biases and limitations of the model. More information needed for further recommendations.
## How to Get Started with the Model
Use the code below to get started with the model.
## Training Details
### Training Data
### Training Procedure
#### Preprocessing [optional]
#### Training Hyperparameters
- Training regime:
#### Speeds, Sizes, Times [optional]
## Evaluation
### Testing Data, Factors & Metrics
#### Testing Data
#### Factors
#### Metrics
### Results
#### Summary
## Model Examination [optional]
## Environmental Impact
Carbon emissions can be estimated using the Machine Learning Impact calculator presented in Lacoste et al. (2019).
- Hardware Type:
- Hours used:
- Cloud Provider:
- Compute Region:
- Carbon Emitted:
## Technical Specifications [optional]
### Model Architecture and Objective
### Compute Infrastructure
#### Hardware
#### Software
[optional]
BibTeX:
APA:
## Glossary [optional]
## More Information [optional]
## Model Card Authors [optional]
## Model Card Contact
| [
"# Model Card for Model ID",
"## Model Details",
"### Model Description\n\n\n\nThis is the model card of a transformers model that has been pushed on the Hub. This model card has been automatically generated.\n\n- Developed by: \n- Funded by [optional]: \n- Shared by [optional]: \n- Model type: \n- Language(s) (NLP): \n- License: \n- Finetuned from model [optional]:",
"### Model Sources [optional]\n\n\n\n- Repository: \n- Paper [optional]: \n- Demo [optional]:",
"## Uses",
"### Direct Use",
"### Downstream Use [optional]",
"### Out-of-Scope Use",
"## Bias, Risks, and Limitations",
"### Recommendations\n\n\n\nUsers (both direct and downstream) should be made aware of the risks, biases and limitations of the model. More information needed for further recommendations.",
"## How to Get Started with the Model\n\nUse the code below to get started with the model.",
"## Training Details",
"### Training Data",
"### Training Procedure",
"#### Preprocessing [optional]",
"#### Training Hyperparameters\n\n- Training regime:",
"#### Speeds, Sizes, Times [optional]",
"## Evaluation",
"### Testing Data, Factors & Metrics",
"#### Testing Data",
"#### Factors",
"#### Metrics",
"### Results",
"#### Summary",
"## Model Examination [optional]",
"## Environmental Impact\n\n\n\nCarbon emissions can be estimated using the Machine Learning Impact calculator presented in Lacoste et al. (2019).\n\n- Hardware Type: \n- Hours used: \n- Cloud Provider: \n- Compute Region: \n- Carbon Emitted:",
"## Technical Specifications [optional]",
"### Model Architecture and Objective",
"### Compute Infrastructure",
"#### Hardware",
"#### Software\n\n\n\n[optional]\n\n\n\nBibTeX:\n\n\n\nAPA:",
"## Glossary [optional]",
"## More Information [optional]",
"## Model Card Authors [optional]",
"## Model Card Contact"
] | [
"TAGS\n#transformers #safetensors #stablelm #text-generation #conversational #arxiv-1910.09700 #autotrain_compatible #endpoints_compatible #region-us \n",
"# Model Card for Model ID",
"## Model Details",
"### Model Description\n\n\n\nThis is the model card of a transformers model that has been pushed on the Hub. This model card has been automatically generated.\n\n- Developed by: \n- Funded by [optional]: \n- Shared by [optional]: \n- Model type: \n- Language(s) (NLP): \n- License: \n- Finetuned from model [optional]:",
"### Model Sources [optional]\n\n\n\n- Repository: \n- Paper [optional]: \n- Demo [optional]:",
"## Uses",
"### Direct Use",
"### Downstream Use [optional]",
"### Out-of-Scope Use",
"## Bias, Risks, and Limitations",
"### Recommendations\n\n\n\nUsers (both direct and downstream) should be made aware of the risks, biases and limitations of the model. More information needed for further recommendations.",
"## How to Get Started with the Model\n\nUse the code below to get started with the model.",
"## Training Details",
"### Training Data",
"### Training Procedure",
"#### Preprocessing [optional]",
"#### Training Hyperparameters\n\n- Training regime:",
"#### Speeds, Sizes, Times [optional]",
"## Evaluation",
"### Testing Data, Factors & Metrics",
"#### Testing Data",
"#### Factors",
"#### Metrics",
"### Results",
"#### Summary",
"## Model Examination [optional]",
"## Environmental Impact\n\n\n\nCarbon emissions can be estimated using the Machine Learning Impact calculator presented in Lacoste et al. (2019).\n\n- Hardware Type: \n- Hours used: \n- Cloud Provider: \n- Compute Region: \n- Carbon Emitted:",
"## Technical Specifications [optional]",
"### Model Architecture and Objective",
"### Compute Infrastructure",
"#### Hardware",
"#### Software\n\n\n\n[optional]\n\n\n\nBibTeX:\n\n\n\nAPA:",
"## Glossary [optional]",
"## More Information [optional]",
"## Model Card Authors [optional]",
"## Model Card Contact"
] |
text-generation | transformers |
# Model Card for Model ID
<!-- Provide a quick summary of what the model is/does. -->
## Model Details
### Model Description
<!-- Provide a longer summary of what this model is. -->
This is the model card of a 🤗 transformers model that has been pushed on the Hub. This model card has been automatically generated.
- **Developed by:** [More Information Needed]
- **Funded by [optional]:** [More Information Needed]
- **Shared by [optional]:** [More Information Needed]
- **Model type:** [More Information Needed]
- **Language(s) (NLP):** [More Information Needed]
- **License:** [More Information Needed]
- **Finetuned from model [optional]:** [More Information Needed]
### Model Sources [optional]
<!-- Provide the basic links for the model. -->
- **Repository:** [More Information Needed]
- **Paper [optional]:** [More Information Needed]
- **Demo [optional]:** [More Information Needed]
## Uses
<!-- Address questions around how the model is intended to be used, including the foreseeable users of the model and those affected by the model. -->
### Direct Use
<!-- This section is for the model use without fine-tuning or plugging into a larger ecosystem/app. -->
[More Information Needed]
### Downstream Use [optional]
<!-- This section is for the model use when fine-tuned for a task, or when plugged into a larger ecosystem/app -->
[More Information Needed]
### Out-of-Scope Use
<!-- This section addresses misuse, malicious use, and uses that the model will not work well for. -->
[More Information Needed]
## Bias, Risks, and Limitations
<!-- This section is meant to convey both technical and sociotechnical limitations. -->
[More Information Needed]
### Recommendations
<!-- This section is meant to convey recommendations with respect to the bias, risk, and technical limitations. -->
Users (both direct and downstream) should be made aware of the risks, biases and limitations of the model. More information needed for further recommendations.
## How to Get Started with the Model
Use the code below to get started with the model.
[More Information Needed]
## Training Details
### Training Data
<!-- This should link to a Dataset Card, perhaps with a short stub of information on what the training data is all about as well as documentation related to data pre-processing or additional filtering. -->
[More Information Needed]
### Training Procedure
<!-- This relates heavily to the Technical Specifications. Content here should link to that section when it is relevant to the training procedure. -->
#### Preprocessing [optional]
[More Information Needed]
#### Training Hyperparameters
- **Training regime:** [More Information Needed] <!--fp32, fp16 mixed precision, bf16 mixed precision, bf16 non-mixed precision, fp16 non-mixed precision, fp8 mixed precision -->
#### Speeds, Sizes, Times [optional]
<!-- This section provides information about throughput, start/end time, checkpoint size if relevant, etc. -->
[More Information Needed]
## Evaluation
<!-- This section describes the evaluation protocols and provides the results. -->
### Testing Data, Factors & Metrics
#### Testing Data
<!-- This should link to a Dataset Card if possible. -->
[More Information Needed]
#### Factors
<!-- These are the things the evaluation is disaggregating by, e.g., subpopulations or domains. -->
[More Information Needed]
#### Metrics
<!-- These are the evaluation metrics being used, ideally with a description of why. -->
[More Information Needed]
### Results
[More Information Needed]
#### Summary
## Model Examination [optional]
<!-- Relevant interpretability work for the model goes here -->
[More Information Needed]
## Environmental Impact
<!-- Total emissions (in grams of CO2eq) and additional considerations, such as electricity usage, go here. Edit the suggested text below accordingly -->
Carbon emissions can be estimated using the [Machine Learning Impact calculator](https://mlco2.github.io/impact#compute) presented in [Lacoste et al. (2019)](https://arxiv.org/abs/1910.09700).
- **Hardware Type:** [More Information Needed]
- **Hours used:** [More Information Needed]
- **Cloud Provider:** [More Information Needed]
- **Compute Region:** [More Information Needed]
- **Carbon Emitted:** [More Information Needed]
## Technical Specifications [optional]
### Model Architecture and Objective
[More Information Needed]
### Compute Infrastructure
[More Information Needed]
#### Hardware
[More Information Needed]
#### Software
[More Information Needed]
## Citation [optional]
<!-- If there is a paper or blog post introducing the model, the APA and Bibtex information for that should go in this section. -->
**BibTeX:**
[More Information Needed]
**APA:**
[More Information Needed]
## Glossary [optional]
<!-- If relevant, include terms and calculations in this section that can help readers understand the model or model card. -->
[More Information Needed]
## More Information [optional]
[More Information Needed]
## Model Card Authors [optional]
[More Information Needed]
## Model Card Contact
[More Information Needed] | {"library_name": "transformers", "tags": ["unsloth", "trl", "sft"]} | kiko2001/llama-3-coding-mkd | null | [
"transformers",
"pytorch",
"llama",
"text-generation",
"unsloth",
"trl",
"sft",
"arxiv:1910.09700",
"autotrain_compatible",
"endpoints_compatible",
"text-generation-inference",
"region:us"
] | null | 2024-04-25T15:21:23+00:00 | [
"1910.09700"
] | [] | TAGS
#transformers #pytorch #llama #text-generation #unsloth #trl #sft #arxiv-1910.09700 #autotrain_compatible #endpoints_compatible #text-generation-inference #region-us
|
# Model Card for Model ID
## Model Details
### Model Description
This is the model card of a transformers model that has been pushed on the Hub. This model card has been automatically generated.
- Developed by:
- Funded by [optional]:
- Shared by [optional]:
- Model type:
- Language(s) (NLP):
- License:
- Finetuned from model [optional]:
### Model Sources [optional]
- Repository:
- Paper [optional]:
- Demo [optional]:
## Uses
### Direct Use
### Downstream Use [optional]
### Out-of-Scope Use
## Bias, Risks, and Limitations
### Recommendations
Users (both direct and downstream) should be made aware of the risks, biases and limitations of the model. More information needed for further recommendations.
## How to Get Started with the Model
Use the code below to get started with the model.
## Training Details
### Training Data
### Training Procedure
#### Preprocessing [optional]
#### Training Hyperparameters
- Training regime:
#### Speeds, Sizes, Times [optional]
## Evaluation
### Testing Data, Factors & Metrics
#### Testing Data
#### Factors
#### Metrics
### Results
#### Summary
## Model Examination [optional]
## Environmental Impact
Carbon emissions can be estimated using the Machine Learning Impact calculator presented in Lacoste et al. (2019).
- Hardware Type:
- Hours used:
- Cloud Provider:
- Compute Region:
- Carbon Emitted:
## Technical Specifications [optional]
### Model Architecture and Objective
### Compute Infrastructure
#### Hardware
#### Software
[optional]
BibTeX:
APA:
## Glossary [optional]
## More Information [optional]
## Model Card Authors [optional]
## Model Card Contact
| [
"# Model Card for Model ID",
"## Model Details",
"### Model Description\n\n\n\nThis is the model card of a transformers model that has been pushed on the Hub. This model card has been automatically generated.\n\n- Developed by: \n- Funded by [optional]: \n- Shared by [optional]: \n- Model type: \n- Language(s) (NLP): \n- License: \n- Finetuned from model [optional]:",
"### Model Sources [optional]\n\n\n\n- Repository: \n- Paper [optional]: \n- Demo [optional]:",
"## Uses",
"### Direct Use",
"### Downstream Use [optional]",
"### Out-of-Scope Use",
"## Bias, Risks, and Limitations",
"### Recommendations\n\n\n\nUsers (both direct and downstream) should be made aware of the risks, biases and limitations of the model. More information needed for further recommendations.",
"## How to Get Started with the Model\n\nUse the code below to get started with the model.",
"## Training Details",
"### Training Data",
"### Training Procedure",
"#### Preprocessing [optional]",
"#### Training Hyperparameters\n\n- Training regime:",
"#### Speeds, Sizes, Times [optional]",
"## Evaluation",
"### Testing Data, Factors & Metrics",
"#### Testing Data",
"#### Factors",
"#### Metrics",
"### Results",
"#### Summary",
"## Model Examination [optional]",
"## Environmental Impact\n\n\n\nCarbon emissions can be estimated using the Machine Learning Impact calculator presented in Lacoste et al. (2019).\n\n- Hardware Type: \n- Hours used: \n- Cloud Provider: \n- Compute Region: \n- Carbon Emitted:",
"## Technical Specifications [optional]",
"### Model Architecture and Objective",
"### Compute Infrastructure",
"#### Hardware",
"#### Software\n\n\n\n[optional]\n\n\n\nBibTeX:\n\n\n\nAPA:",
"## Glossary [optional]",
"## More Information [optional]",
"## Model Card Authors [optional]",
"## Model Card Contact"
] | [
"TAGS\n#transformers #pytorch #llama #text-generation #unsloth #trl #sft #arxiv-1910.09700 #autotrain_compatible #endpoints_compatible #text-generation-inference #region-us \n",
"# Model Card for Model ID",
"## Model Details",
"### Model Description\n\n\n\nThis is the model card of a transformers model that has been pushed on the Hub. This model card has been automatically generated.\n\n- Developed by: \n- Funded by [optional]: \n- Shared by [optional]: \n- Model type: \n- Language(s) (NLP): \n- License: \n- Finetuned from model [optional]:",
"### Model Sources [optional]\n\n\n\n- Repository: \n- Paper [optional]: \n- Demo [optional]:",
"## Uses",
"### Direct Use",
"### Downstream Use [optional]",
"### Out-of-Scope Use",
"## Bias, Risks, and Limitations",
"### Recommendations\n\n\n\nUsers (both direct and downstream) should be made aware of the risks, biases and limitations of the model. More information needed for further recommendations.",
"## How to Get Started with the Model\n\nUse the code below to get started with the model.",
"## Training Details",
"### Training Data",
"### Training Procedure",
"#### Preprocessing [optional]",
"#### Training Hyperparameters\n\n- Training regime:",
"#### Speeds, Sizes, Times [optional]",
"## Evaluation",
"### Testing Data, Factors & Metrics",
"#### Testing Data",
"#### Factors",
"#### Metrics",
"### Results",
"#### Summary",
"## Model Examination [optional]",
"## Environmental Impact\n\n\n\nCarbon emissions can be estimated using the Machine Learning Impact calculator presented in Lacoste et al. (2019).\n\n- Hardware Type: \n- Hours used: \n- Cloud Provider: \n- Compute Region: \n- Carbon Emitted:",
"## Technical Specifications [optional]",
"### Model Architecture and Objective",
"### Compute Infrastructure",
"#### Hardware",
"#### Software\n\n\n\n[optional]\n\n\n\nBibTeX:\n\n\n\nAPA:",
"## Glossary [optional]",
"## More Information [optional]",
"## Model Card Authors [optional]",
"## Model Card Contact"
] |
image-classification | transformers |
<!-- This model card has been generated automatically according to the information the Trainer had access to. You
should probably proofread and complete it, then remove this comment. -->
# dinov2-base-finetuned-oxford
This model is a fine-tuned version of [facebook/dinov2-base](https://huggingface.co/facebook/dinov2-base) on an unknown dataset.
It achieves the following results on the evaluation set:
- Loss: 0.2296
- Accuracy: 0.9319
## Model description
More information needed
## Intended uses & limitations
More information needed
## Training and evaluation data
More information needed
## Training procedure
### Training hyperparameters
The following hyperparameters were used during training:
- learning_rate: 5e-05
- train_batch_size: 4
- eval_batch_size: 4
- seed: 42
- gradient_accumulation_steps: 4
- total_train_batch_size: 16
- optimizer: Adam with betas=(0.9,0.999) and epsilon=1e-08
- lr_scheduler_type: linear
- lr_scheduler_warmup_ratio: 0.1
- num_epochs: 3
### Training results
| Training Loss | Epoch | Step | Validation Loss | Accuracy |
|:-------------:|:-----:|:----:|:---------------:|:--------:|
| 1.0593 | 1.0 | 230 | 0.6444 | 0.7855 |
| 0.4115 | 2.0 | 460 | 0.4093 | 0.8705 |
| 0.0495 | 3.0 | 690 | 0.2296 | 0.9319 |
### Framework versions
- Transformers 4.40.0
- Pytorch 2.2.1+cu121
- Datasets 2.19.0
- Tokenizers 0.19.1
| {"license": "apache-2.0", "tags": ["generated_from_trainer"], "metrics": ["accuracy"], "base_model": "facebook/dinov2-base", "model-index": [{"name": "dinov2-base-finetuned-oxford", "results": []}]} | levent1/dinov2-base-finetuned-oxford | null | [
"transformers",
"tensorboard",
"safetensors",
"dinov2",
"image-classification",
"generated_from_trainer",
"base_model:facebook/dinov2-base",
"license:apache-2.0",
"autotrain_compatible",
"endpoints_compatible",
"region:us"
] | null | 2024-04-25T15:21:30+00:00 | [] | [] | TAGS
#transformers #tensorboard #safetensors #dinov2 #image-classification #generated_from_trainer #base_model-facebook/dinov2-base #license-apache-2.0 #autotrain_compatible #endpoints_compatible #region-us
| dinov2-base-finetuned-oxford
============================
This model is a fine-tuned version of facebook/dinov2-base on an unknown dataset.
It achieves the following results on the evaluation set:
* Loss: 0.2296
* Accuracy: 0.9319
Model description
-----------------
More information needed
Intended uses & limitations
---------------------------
More information needed
Training and evaluation data
----------------------------
More information needed
Training procedure
------------------
### Training hyperparameters
The following hyperparameters were used during training:
* learning\_rate: 5e-05
* train\_batch\_size: 4
* eval\_batch\_size: 4
* seed: 42
* gradient\_accumulation\_steps: 4
* total\_train\_batch\_size: 16
* optimizer: Adam with betas=(0.9,0.999) and epsilon=1e-08
* lr\_scheduler\_type: linear
* lr\_scheduler\_warmup\_ratio: 0.1
* num\_epochs: 3
### Training results
### Framework versions
* Transformers 4.40.0
* Pytorch 2.2.1+cu121
* Datasets 2.19.0
* Tokenizers 0.19.1
| [
"### Training hyperparameters\n\n\nThe following hyperparameters were used during training:\n\n\n* learning\\_rate: 5e-05\n* train\\_batch\\_size: 4\n* eval\\_batch\\_size: 4\n* seed: 42\n* gradient\\_accumulation\\_steps: 4\n* total\\_train\\_batch\\_size: 16\n* optimizer: Adam with betas=(0.9,0.999) and epsilon=1e-08\n* lr\\_scheduler\\_type: linear\n* lr\\_scheduler\\_warmup\\_ratio: 0.1\n* num\\_epochs: 3",
"### Training results",
"### Framework versions\n\n\n* Transformers 4.40.0\n* Pytorch 2.2.1+cu121\n* Datasets 2.19.0\n* Tokenizers 0.19.1"
] | [
"TAGS\n#transformers #tensorboard #safetensors #dinov2 #image-classification #generated_from_trainer #base_model-facebook/dinov2-base #license-apache-2.0 #autotrain_compatible #endpoints_compatible #region-us \n",
"### Training hyperparameters\n\n\nThe following hyperparameters were used during training:\n\n\n* learning\\_rate: 5e-05\n* train\\_batch\\_size: 4\n* eval\\_batch\\_size: 4\n* seed: 42\n* gradient\\_accumulation\\_steps: 4\n* total\\_train\\_batch\\_size: 16\n* optimizer: Adam with betas=(0.9,0.999) and epsilon=1e-08\n* lr\\_scheduler\\_type: linear\n* lr\\_scheduler\\_warmup\\_ratio: 0.1\n* num\\_epochs: 3",
"### Training results",
"### Framework versions\n\n\n* Transformers 4.40.0\n* Pytorch 2.2.1+cu121\n* Datasets 2.19.0\n* Tokenizers 0.19.1"
] |
null | transformers |
# Model Card for Model ID
<!-- Provide a quick summary of what the model is/does. -->
## Model Details
### Model Description
<!-- Provide a longer summary of what this model is. -->
This is the model card of a 🤗 transformers model that has been pushed on the Hub. This model card has been automatically generated.
- **Developed by:** [More Information Needed]
- **Funded by [optional]:** [More Information Needed]
- **Shared by [optional]:** [More Information Needed]
- **Model type:** [More Information Needed]
- **Language(s) (NLP):** [More Information Needed]
- **License:** [More Information Needed]
- **Finetuned from model [optional]:** [More Information Needed]
### Model Sources [optional]
<!-- Provide the basic links for the model. -->
- **Repository:** [More Information Needed]
- **Paper [optional]:** [More Information Needed]
- **Demo [optional]:** [More Information Needed]
## Uses
<!-- Address questions around how the model is intended to be used, including the foreseeable users of the model and those affected by the model. -->
### Direct Use
<!-- This section is for the model use without fine-tuning or plugging into a larger ecosystem/app. -->
[More Information Needed]
### Downstream Use [optional]
<!-- This section is for the model use when fine-tuned for a task, or when plugged into a larger ecosystem/app -->
[More Information Needed]
### Out-of-Scope Use
<!-- This section addresses misuse, malicious use, and uses that the model will not work well for. -->
[More Information Needed]
## Bias, Risks, and Limitations
<!-- This section is meant to convey both technical and sociotechnical limitations. -->
[More Information Needed]
### Recommendations
<!-- This section is meant to convey recommendations with respect to the bias, risk, and technical limitations. -->
Users (both direct and downstream) should be made aware of the risks, biases and limitations of the model. More information needed for further recommendations.
## How to Get Started with the Model
Use the code below to get started with the model.
[More Information Needed]
## Training Details
### Training Data
<!-- This should link to a Dataset Card, perhaps with a short stub of information on what the training data is all about as well as documentation related to data pre-processing or additional filtering. -->
[More Information Needed]
### Training Procedure
<!-- This relates heavily to the Technical Specifications. Content here should link to that section when it is relevant to the training procedure. -->
#### Preprocessing [optional]
[More Information Needed]
#### Training Hyperparameters
- **Training regime:** [More Information Needed] <!--fp32, fp16 mixed precision, bf16 mixed precision, bf16 non-mixed precision, fp16 non-mixed precision, fp8 mixed precision -->
#### Speeds, Sizes, Times [optional]
<!-- This section provides information about throughput, start/end time, checkpoint size if relevant, etc. -->
[More Information Needed]
## Evaluation
<!-- This section describes the evaluation protocols and provides the results. -->
### Testing Data, Factors & Metrics
#### Testing Data
<!-- This should link to a Dataset Card if possible. -->
[More Information Needed]
#### Factors
<!-- These are the things the evaluation is disaggregating by, e.g., subpopulations or domains. -->
[More Information Needed]
#### Metrics
<!-- These are the evaluation metrics being used, ideally with a description of why. -->
[More Information Needed]
### Results
[More Information Needed]
#### Summary
## Model Examination [optional]
<!-- Relevant interpretability work for the model goes here -->
[More Information Needed]
## Environmental Impact
<!-- Total emissions (in grams of CO2eq) and additional considerations, such as electricity usage, go here. Edit the suggested text below accordingly -->
Carbon emissions can be estimated using the [Machine Learning Impact calculator](https://mlco2.github.io/impact#compute) presented in [Lacoste et al. (2019)](https://arxiv.org/abs/1910.09700).
- **Hardware Type:** [More Information Needed]
- **Hours used:** [More Information Needed]
- **Cloud Provider:** [More Information Needed]
- **Compute Region:** [More Information Needed]
- **Carbon Emitted:** [More Information Needed]
## Technical Specifications [optional]
### Model Architecture and Objective
[More Information Needed]
### Compute Infrastructure
[More Information Needed]
#### Hardware
[More Information Needed]
#### Software
[More Information Needed]
## Citation [optional]
<!-- If there is a paper or blog post introducing the model, the APA and Bibtex information for that should go in this section. -->
**BibTeX:**
[More Information Needed]
**APA:**
[More Information Needed]
## Glossary [optional]
<!-- If relevant, include terms and calculations in this section that can help readers understand the model or model card. -->
[More Information Needed]
## More Information [optional]
[More Information Needed]
## Model Card Authors [optional]
[More Information Needed]
## Model Card Contact
[More Information Needed] | {"library_name": "transformers", "tags": []} | Ornelas7/code-search-net-tokenizer | null | [
"transformers",
"arxiv:1910.09700",
"endpoints_compatible",
"region:us"
] | null | 2024-04-25T15:22:23+00:00 | [
"1910.09700"
] | [] | TAGS
#transformers #arxiv-1910.09700 #endpoints_compatible #region-us
|
# Model Card for Model ID
## Model Details
### Model Description
This is the model card of a transformers model that has been pushed on the Hub. This model card has been automatically generated.
- Developed by:
- Funded by [optional]:
- Shared by [optional]:
- Model type:
- Language(s) (NLP):
- License:
- Finetuned from model [optional]:
### Model Sources [optional]
- Repository:
- Paper [optional]:
- Demo [optional]:
## Uses
### Direct Use
### Downstream Use [optional]
### Out-of-Scope Use
## Bias, Risks, and Limitations
### Recommendations
Users (both direct and downstream) should be made aware of the risks, biases and limitations of the model. More information needed for further recommendations.
## How to Get Started with the Model
Use the code below to get started with the model.
## Training Details
### Training Data
### Training Procedure
#### Preprocessing [optional]
#### Training Hyperparameters
- Training regime:
#### Speeds, Sizes, Times [optional]
## Evaluation
### Testing Data, Factors & Metrics
#### Testing Data
#### Factors
#### Metrics
### Results
#### Summary
## Model Examination [optional]
## Environmental Impact
Carbon emissions can be estimated using the Machine Learning Impact calculator presented in Lacoste et al. (2019).
- Hardware Type:
- Hours used:
- Cloud Provider:
- Compute Region:
- Carbon Emitted:
## Technical Specifications [optional]
### Model Architecture and Objective
### Compute Infrastructure
#### Hardware
#### Software
[optional]
BibTeX:
APA:
## Glossary [optional]
## More Information [optional]
## Model Card Authors [optional]
## Model Card Contact
| [
"# Model Card for Model ID",
"## Model Details",
"### Model Description\n\n\n\nThis is the model card of a transformers model that has been pushed on the Hub. This model card has been automatically generated.\n\n- Developed by: \n- Funded by [optional]: \n- Shared by [optional]: \n- Model type: \n- Language(s) (NLP): \n- License: \n- Finetuned from model [optional]:",
"### Model Sources [optional]\n\n\n\n- Repository: \n- Paper [optional]: \n- Demo [optional]:",
"## Uses",
"### Direct Use",
"### Downstream Use [optional]",
"### Out-of-Scope Use",
"## Bias, Risks, and Limitations",
"### Recommendations\n\n\n\nUsers (both direct and downstream) should be made aware of the risks, biases and limitations of the model. More information needed for further recommendations.",
"## How to Get Started with the Model\n\nUse the code below to get started with the model.",
"## Training Details",
"### Training Data",
"### Training Procedure",
"#### Preprocessing [optional]",
"#### Training Hyperparameters\n\n- Training regime:",
"#### Speeds, Sizes, Times [optional]",
"## Evaluation",
"### Testing Data, Factors & Metrics",
"#### Testing Data",
"#### Factors",
"#### Metrics",
"### Results",
"#### Summary",
"## Model Examination [optional]",
"## Environmental Impact\n\n\n\nCarbon emissions can be estimated using the Machine Learning Impact calculator presented in Lacoste et al. (2019).\n\n- Hardware Type: \n- Hours used: \n- Cloud Provider: \n- Compute Region: \n- Carbon Emitted:",
"## Technical Specifications [optional]",
"### Model Architecture and Objective",
"### Compute Infrastructure",
"#### Hardware",
"#### Software\n\n\n\n[optional]\n\n\n\nBibTeX:\n\n\n\nAPA:",
"## Glossary [optional]",
"## More Information [optional]",
"## Model Card Authors [optional]",
"## Model Card Contact"
] | [
"TAGS\n#transformers #arxiv-1910.09700 #endpoints_compatible #region-us \n",
"# Model Card for Model ID",
"## Model Details",
"### Model Description\n\n\n\nThis is the model card of a transformers model that has been pushed on the Hub. This model card has been automatically generated.\n\n- Developed by: \n- Funded by [optional]: \n- Shared by [optional]: \n- Model type: \n- Language(s) (NLP): \n- License: \n- Finetuned from model [optional]:",
"### Model Sources [optional]\n\n\n\n- Repository: \n- Paper [optional]: \n- Demo [optional]:",
"## Uses",
"### Direct Use",
"### Downstream Use [optional]",
"### Out-of-Scope Use",
"## Bias, Risks, and Limitations",
"### Recommendations\n\n\n\nUsers (both direct and downstream) should be made aware of the risks, biases and limitations of the model. More information needed for further recommendations.",
"## How to Get Started with the Model\n\nUse the code below to get started with the model.",
"## Training Details",
"### Training Data",
"### Training Procedure",
"#### Preprocessing [optional]",
"#### Training Hyperparameters\n\n- Training regime:",
"#### Speeds, Sizes, Times [optional]",
"## Evaluation",
"### Testing Data, Factors & Metrics",
"#### Testing Data",
"#### Factors",
"#### Metrics",
"### Results",
"#### Summary",
"## Model Examination [optional]",
"## Environmental Impact\n\n\n\nCarbon emissions can be estimated using the Machine Learning Impact calculator presented in Lacoste et al. (2019).\n\n- Hardware Type: \n- Hours used: \n- Cloud Provider: \n- Compute Region: \n- Carbon Emitted:",
"## Technical Specifications [optional]",
"### Model Architecture and Objective",
"### Compute Infrastructure",
"#### Hardware",
"#### Software\n\n\n\n[optional]\n\n\n\nBibTeX:\n\n\n\nAPA:",
"## Glossary [optional]",
"## More Information [optional]",
"## Model Card Authors [optional]",
"## Model Card Contact"
] |
null | mlx |
# GreenBitAI/Phi-3-mini-4k-instruct-layer-mix-bpw-2.2-mlx
This quantized low-bit model was converted to MLX format from [`GreenBitAI/Phi-3-mini-4k-instruct-layer-mix-bpw-2.2`]().
Refer to the [original model card](https://huggingface.co/GreenBitAI/Phi-3-mini-4k-instruct-layer-mix-bpw-2.2) for more details on the model.
## Use with mlx
```bash
pip install gbx-lm
```
```python
from gbx_lm import load, generate
model, tokenizer = load("GreenBitAI/Phi-3-mini-4k-instruct-layer-mix-bpw-2.2-mlx")
response = generate(model, tokenizer, prompt="hello", verbose=True)
```
| {"license": "apache-2.0", "tags": ["mlx"]} | GreenBitAI/Phi-3-mini-4k-instruct-layer-mix-bpw-2.2-mlx | null | [
"mlx",
"safetensors",
"phi3",
"custom_code",
"license:apache-2.0",
"region:us"
] | null | 2024-04-25T15:22:29+00:00 | [] | [] | TAGS
#mlx #safetensors #phi3 #custom_code #license-apache-2.0 #region-us
|
# GreenBitAI/Phi-3-mini-4k-instruct-layer-mix-bpw-2.2-mlx
This quantized low-bit model was converted to MLX format from ['GreenBitAI/Phi-3-mini-4k-instruct-layer-mix-bpw-2.2']().
Refer to the original model card for more details on the model.
## Use with mlx
| [
"# GreenBitAI/Phi-3-mini-4k-instruct-layer-mix-bpw-2.2-mlx\nThis quantized low-bit model was converted to MLX format from ['GreenBitAI/Phi-3-mini-4k-instruct-layer-mix-bpw-2.2']().\nRefer to the original model card for more details on the model.",
"## Use with mlx"
] | [
"TAGS\n#mlx #safetensors #phi3 #custom_code #license-apache-2.0 #region-us \n",
"# GreenBitAI/Phi-3-mini-4k-instruct-layer-mix-bpw-2.2-mlx\nThis quantized low-bit model was converted to MLX format from ['GreenBitAI/Phi-3-mini-4k-instruct-layer-mix-bpw-2.2']().\nRefer to the original model card for more details on the model.",
"## Use with mlx"
] |
text-generation | transformers |
# Model Card for Model ID
<!-- Provide a quick summary of what the model is/does. -->
## Model Details
### Model Description
<!-- Provide a longer summary of what this model is. -->
This is the model card of a 🤗 transformers model that has been pushed on the Hub. This model card has been automatically generated.
- **Developed by:** [More Information Needed]
- **Funded by [optional]:** [More Information Needed]
- **Shared by [optional]:** [More Information Needed]
- **Model type:** [More Information Needed]
- **Language(s) (NLP):** [More Information Needed]
- **License:** [More Information Needed]
- **Finetuned from model [optional]:** [More Information Needed]
### Model Sources [optional]
<!-- Provide the basic links for the model. -->
- **Repository:** [More Information Needed]
- **Paper [optional]:** [More Information Needed]
- **Demo [optional]:** [More Information Needed]
## Uses
<!-- Address questions around how the model is intended to be used, including the foreseeable users of the model and those affected by the model. -->
### Direct Use
<!-- This section is for the model use without fine-tuning or plugging into a larger ecosystem/app. -->
[More Information Needed]
### Downstream Use [optional]
<!-- This section is for the model use when fine-tuned for a task, or when plugged into a larger ecosystem/app -->
[More Information Needed]
### Out-of-Scope Use
<!-- This section addresses misuse, malicious use, and uses that the model will not work well for. -->
[More Information Needed]
## Bias, Risks, and Limitations
<!-- This section is meant to convey both technical and sociotechnical limitations. -->
[More Information Needed]
### Recommendations
<!-- This section is meant to convey recommendations with respect to the bias, risk, and technical limitations. -->
Users (both direct and downstream) should be made aware of the risks, biases and limitations of the model. More information needed for further recommendations.
## How to Get Started with the Model
Use the code below to get started with the model.
[More Information Needed]
## Training Details
### Training Data
<!-- This should link to a Dataset Card, perhaps with a short stub of information on what the training data is all about as well as documentation related to data pre-processing or additional filtering. -->
[More Information Needed]
### Training Procedure
<!-- This relates heavily to the Technical Specifications. Content here should link to that section when it is relevant to the training procedure. -->
#### Preprocessing [optional]
[More Information Needed]
#### Training Hyperparameters
- **Training regime:** [More Information Needed] <!--fp32, fp16 mixed precision, bf16 mixed precision, bf16 non-mixed precision, fp16 non-mixed precision, fp8 mixed precision -->
#### Speeds, Sizes, Times [optional]
<!-- This section provides information about throughput, start/end time, checkpoint size if relevant, etc. -->
[More Information Needed]
## Evaluation
<!-- This section describes the evaluation protocols and provides the results. -->
### Testing Data, Factors & Metrics
#### Testing Data
<!-- This should link to a Dataset Card if possible. -->
[More Information Needed]
#### Factors
<!-- These are the things the evaluation is disaggregating by, e.g., subpopulations or domains. -->
[More Information Needed]
#### Metrics
<!-- These are the evaluation metrics being used, ideally with a description of why. -->
[More Information Needed]
### Results
[More Information Needed]
#### Summary
## Model Examination [optional]
<!-- Relevant interpretability work for the model goes here -->
[More Information Needed]
## Environmental Impact
<!-- Total emissions (in grams of CO2eq) and additional considerations, such as electricity usage, go here. Edit the suggested text below accordingly -->
Carbon emissions can be estimated using the [Machine Learning Impact calculator](https://mlco2.github.io/impact#compute) presented in [Lacoste et al. (2019)](https://arxiv.org/abs/1910.09700).
- **Hardware Type:** [More Information Needed]
- **Hours used:** [More Information Needed]
- **Cloud Provider:** [More Information Needed]
- **Compute Region:** [More Information Needed]
- **Carbon Emitted:** [More Information Needed]
## Technical Specifications [optional]
### Model Architecture and Objective
[More Information Needed]
### Compute Infrastructure
[More Information Needed]
#### Hardware
[More Information Needed]
#### Software
[More Information Needed]
## Citation [optional]
<!-- If there is a paper or blog post introducing the model, the APA and Bibtex information for that should go in this section. -->
**BibTeX:**
[More Information Needed]
**APA:**
[More Information Needed]
## Glossary [optional]
<!-- If relevant, include terms and calculations in this section that can help readers understand the model or model card. -->
[More Information Needed]
## More Information [optional]
[More Information Needed]
## Model Card Authors [optional]
[More Information Needed]
## Model Card Contact
[More Information Needed] | {"library_name": "transformers", "tags": []} | yamaguchi-kota/gemma-medical_qa-Finetune | null | [
"transformers",
"safetensors",
"gemma",
"text-generation",
"conversational",
"arxiv:1910.09700",
"autotrain_compatible",
"endpoints_compatible",
"text-generation-inference",
"region:us"
] | null | 2024-04-25T15:23:27+00:00 | [
"1910.09700"
] | [] | TAGS
#transformers #safetensors #gemma #text-generation #conversational #arxiv-1910.09700 #autotrain_compatible #endpoints_compatible #text-generation-inference #region-us
|
# Model Card for Model ID
## Model Details
### Model Description
This is the model card of a transformers model that has been pushed on the Hub. This model card has been automatically generated.
- Developed by:
- Funded by [optional]:
- Shared by [optional]:
- Model type:
- Language(s) (NLP):
- License:
- Finetuned from model [optional]:
### Model Sources [optional]
- Repository:
- Paper [optional]:
- Demo [optional]:
## Uses
### Direct Use
### Downstream Use [optional]
### Out-of-Scope Use
## Bias, Risks, and Limitations
### Recommendations
Users (both direct and downstream) should be made aware of the risks, biases and limitations of the model. More information needed for further recommendations.
## How to Get Started with the Model
Use the code below to get started with the model.
## Training Details
### Training Data
### Training Procedure
#### Preprocessing [optional]
#### Training Hyperparameters
- Training regime:
#### Speeds, Sizes, Times [optional]
## Evaluation
### Testing Data, Factors & Metrics
#### Testing Data
#### Factors
#### Metrics
### Results
#### Summary
## Model Examination [optional]
## Environmental Impact
Carbon emissions can be estimated using the Machine Learning Impact calculator presented in Lacoste et al. (2019).
- Hardware Type:
- Hours used:
- Cloud Provider:
- Compute Region:
- Carbon Emitted:
## Technical Specifications [optional]
### Model Architecture and Objective
### Compute Infrastructure
#### Hardware
#### Software
[optional]
BibTeX:
APA:
## Glossary [optional]
## More Information [optional]
## Model Card Authors [optional]
## Model Card Contact
| [
"# Model Card for Model ID",
"## Model Details",
"### Model Description\n\n\n\nThis is the model card of a transformers model that has been pushed on the Hub. This model card has been automatically generated.\n\n- Developed by: \n- Funded by [optional]: \n- Shared by [optional]: \n- Model type: \n- Language(s) (NLP): \n- License: \n- Finetuned from model [optional]:",
"### Model Sources [optional]\n\n\n\n- Repository: \n- Paper [optional]: \n- Demo [optional]:",
"## Uses",
"### Direct Use",
"### Downstream Use [optional]",
"### Out-of-Scope Use",
"## Bias, Risks, and Limitations",
"### Recommendations\n\n\n\nUsers (both direct and downstream) should be made aware of the risks, biases and limitations of the model. More information needed for further recommendations.",
"## How to Get Started with the Model\n\nUse the code below to get started with the model.",
"## Training Details",
"### Training Data",
"### Training Procedure",
"#### Preprocessing [optional]",
"#### Training Hyperparameters\n\n- Training regime:",
"#### Speeds, Sizes, Times [optional]",
"## Evaluation",
"### Testing Data, Factors & Metrics",
"#### Testing Data",
"#### Factors",
"#### Metrics",
"### Results",
"#### Summary",
"## Model Examination [optional]",
"## Environmental Impact\n\n\n\nCarbon emissions can be estimated using the Machine Learning Impact calculator presented in Lacoste et al. (2019).\n\n- Hardware Type: \n- Hours used: \n- Cloud Provider: \n- Compute Region: \n- Carbon Emitted:",
"## Technical Specifications [optional]",
"### Model Architecture and Objective",
"### Compute Infrastructure",
"#### Hardware",
"#### Software\n\n\n\n[optional]\n\n\n\nBibTeX:\n\n\n\nAPA:",
"## Glossary [optional]",
"## More Information [optional]",
"## Model Card Authors [optional]",
"## Model Card Contact"
] | [
"TAGS\n#transformers #safetensors #gemma #text-generation #conversational #arxiv-1910.09700 #autotrain_compatible #endpoints_compatible #text-generation-inference #region-us \n",
"# Model Card for Model ID",
"## Model Details",
"### Model Description\n\n\n\nThis is the model card of a transformers model that has been pushed on the Hub. This model card has been automatically generated.\n\n- Developed by: \n- Funded by [optional]: \n- Shared by [optional]: \n- Model type: \n- Language(s) (NLP): \n- License: \n- Finetuned from model [optional]:",
"### Model Sources [optional]\n\n\n\n- Repository: \n- Paper [optional]: \n- Demo [optional]:",
"## Uses",
"### Direct Use",
"### Downstream Use [optional]",
"### Out-of-Scope Use",
"## Bias, Risks, and Limitations",
"### Recommendations\n\n\n\nUsers (both direct and downstream) should be made aware of the risks, biases and limitations of the model. More information needed for further recommendations.",
"## How to Get Started with the Model\n\nUse the code below to get started with the model.",
"## Training Details",
"### Training Data",
"### Training Procedure",
"#### Preprocessing [optional]",
"#### Training Hyperparameters\n\n- Training regime:",
"#### Speeds, Sizes, Times [optional]",
"## Evaluation",
"### Testing Data, Factors & Metrics",
"#### Testing Data",
"#### Factors",
"#### Metrics",
"### Results",
"#### Summary",
"## Model Examination [optional]",
"## Environmental Impact\n\n\n\nCarbon emissions can be estimated using the Machine Learning Impact calculator presented in Lacoste et al. (2019).\n\n- Hardware Type: \n- Hours used: \n- Cloud Provider: \n- Compute Region: \n- Carbon Emitted:",
"## Technical Specifications [optional]",
"### Model Architecture and Objective",
"### Compute Infrastructure",
"#### Hardware",
"#### Software\n\n\n\n[optional]\n\n\n\nBibTeX:\n\n\n\nAPA:",
"## Glossary [optional]",
"## More Information [optional]",
"## Model Card Authors [optional]",
"## Model Card Contact"
] |
null | diffusers |
# Model Card for Model ID
<!-- Provide a quick summary of what the model is/does. -->
## Model Details
### Model Description
<!-- Provide a longer summary of what this model is. -->
This is the model card of a 🧨 diffusers model that has been pushed on the Hub. This model card has been automatically generated.
- **Developed by:** [More Information Needed]
- **Funded by [optional]:** [More Information Needed]
- **Shared by [optional]:** [More Information Needed]
- **Model type:** [More Information Needed]
- **Language(s) (NLP):** [More Information Needed]
- **License:** [More Information Needed]
- **Finetuned from model [optional]:** [More Information Needed]
### Model Sources [optional]
<!-- Provide the basic links for the model. -->
- **Repository:** [More Information Needed]
- **Paper [optional]:** [More Information Needed]
- **Demo [optional]:** [More Information Needed]
## Uses
<!-- Address questions around how the model is intended to be used, including the foreseeable users of the model and those affected by the model. -->
### Direct Use
<!-- This section is for the model use without fine-tuning or plugging into a larger ecosystem/app. -->
[More Information Needed]
### Downstream Use [optional]
<!-- This section is for the model use when fine-tuned for a task, or when plugged into a larger ecosystem/app -->
[More Information Needed]
### Out-of-Scope Use
<!-- This section addresses misuse, malicious use, and uses that the model will not work well for. -->
[More Information Needed]
## Bias, Risks, and Limitations
<!-- This section is meant to convey both technical and sociotechnical limitations. -->
[More Information Needed]
### Recommendations
<!-- This section is meant to convey recommendations with respect to the bias, risk, and technical limitations. -->
Users (both direct and downstream) should be made aware of the risks, biases and limitations of the model. More information needed for further recommendations.
## How to Get Started with the Model
Use the code below to get started with the model.
[More Information Needed]
## Training Details
### Training Data
<!-- This should link to a Dataset Card, perhaps with a short stub of information on what the training data is all about as well as documentation related to data pre-processing or additional filtering. -->
[More Information Needed]
### Training Procedure
<!-- This relates heavily to the Technical Specifications. Content here should link to that section when it is relevant to the training procedure. -->
#### Preprocessing [optional]
[More Information Needed]
#### Training Hyperparameters
- **Training regime:** [More Information Needed] <!--fp32, fp16 mixed precision, bf16 mixed precision, bf16 non-mixed precision, fp16 non-mixed precision, fp8 mixed precision -->
#### Speeds, Sizes, Times [optional]
<!-- This section provides information about throughput, start/end time, checkpoint size if relevant, etc. -->
[More Information Needed]
## Evaluation
<!-- This section describes the evaluation protocols and provides the results. -->
### Testing Data, Factors & Metrics
#### Testing Data
<!-- This should link to a Dataset Card if possible. -->
[More Information Needed]
#### Factors
<!-- These are the things the evaluation is disaggregating by, e.g., subpopulations or domains. -->
[More Information Needed]
#### Metrics
<!-- These are the evaluation metrics being used, ideally with a description of why. -->
[More Information Needed]
### Results
[More Information Needed]
#### Summary
## Model Examination [optional]
<!-- Relevant interpretability work for the model goes here -->
[More Information Needed]
## Environmental Impact
<!-- Total emissions (in grams of CO2eq) and additional considerations, such as electricity usage, go here. Edit the suggested text below accordingly -->
Carbon emissions can be estimated using the [Machine Learning Impact calculator](https://mlco2.github.io/impact#compute) presented in [Lacoste et al. (2019)](https://arxiv.org/abs/1910.09700).
- **Hardware Type:** [More Information Needed]
- **Hours used:** [More Information Needed]
- **Cloud Provider:** [More Information Needed]
- **Compute Region:** [More Information Needed]
- **Carbon Emitted:** [More Information Needed]
## Technical Specifications [optional]
### Model Architecture and Objective
[More Information Needed]
### Compute Infrastructure
[More Information Needed]
#### Hardware
[More Information Needed]
#### Software
[More Information Needed]
## Citation [optional]
<!-- If there is a paper or blog post introducing the model, the APA and Bibtex information for that should go in this section. -->
**BibTeX:**
[More Information Needed]
**APA:**
[More Information Needed]
## Glossary [optional]
<!-- If relevant, include terms and calculations in this section that can help readers understand the model or model card. -->
[More Information Needed]
## More Information [optional]
[More Information Needed]
## Model Card Authors [optional]
[More Information Needed]
## Model Card Contact
[More Information Needed] | {"library_name": "diffusers"} | smacky42/sn17-6-2 | null | [
"diffusers",
"safetensors",
"arxiv:1910.09700",
"region:us"
] | null | 2024-04-25T15:23:30+00:00 | [
"1910.09700"
] | [] | TAGS
#diffusers #safetensors #arxiv-1910.09700 #region-us
|
# Model Card for Model ID
## Model Details
### Model Description
This is the model card of a diffusers model that has been pushed on the Hub. This model card has been automatically generated.
- Developed by:
- Funded by [optional]:
- Shared by [optional]:
- Model type:
- Language(s) (NLP):
- License:
- Finetuned from model [optional]:
### Model Sources [optional]
- Repository:
- Paper [optional]:
- Demo [optional]:
## Uses
### Direct Use
### Downstream Use [optional]
### Out-of-Scope Use
## Bias, Risks, and Limitations
### Recommendations
Users (both direct and downstream) should be made aware of the risks, biases and limitations of the model. More information needed for further recommendations.
## How to Get Started with the Model
Use the code below to get started with the model.
## Training Details
### Training Data
### Training Procedure
#### Preprocessing [optional]
#### Training Hyperparameters
- Training regime:
#### Speeds, Sizes, Times [optional]
## Evaluation
### Testing Data, Factors & Metrics
#### Testing Data
#### Factors
#### Metrics
### Results
#### Summary
## Model Examination [optional]
## Environmental Impact
Carbon emissions can be estimated using the Machine Learning Impact calculator presented in Lacoste et al. (2019).
- Hardware Type:
- Hours used:
- Cloud Provider:
- Compute Region:
- Carbon Emitted:
## Technical Specifications [optional]
### Model Architecture and Objective
### Compute Infrastructure
#### Hardware
#### Software
[optional]
BibTeX:
APA:
## Glossary [optional]
## More Information [optional]
## Model Card Authors [optional]
## Model Card Contact
| [
"# Model Card for Model ID",
"## Model Details",
"### Model Description\n\n\n\nThis is the model card of a diffusers model that has been pushed on the Hub. This model card has been automatically generated.\n\n- Developed by: \n- Funded by [optional]: \n- Shared by [optional]: \n- Model type: \n- Language(s) (NLP): \n- License: \n- Finetuned from model [optional]:",
"### Model Sources [optional]\n\n\n\n- Repository: \n- Paper [optional]: \n- Demo [optional]:",
"## Uses",
"### Direct Use",
"### Downstream Use [optional]",
"### Out-of-Scope Use",
"## Bias, Risks, and Limitations",
"### Recommendations\n\n\n\nUsers (both direct and downstream) should be made aware of the risks, biases and limitations of the model. More information needed for further recommendations.",
"## How to Get Started with the Model\n\nUse the code below to get started with the model.",
"## Training Details",
"### Training Data",
"### Training Procedure",
"#### Preprocessing [optional]",
"#### Training Hyperparameters\n\n- Training regime:",
"#### Speeds, Sizes, Times [optional]",
"## Evaluation",
"### Testing Data, Factors & Metrics",
"#### Testing Data",
"#### Factors",
"#### Metrics",
"### Results",
"#### Summary",
"## Model Examination [optional]",
"## Environmental Impact\n\n\n\nCarbon emissions can be estimated using the Machine Learning Impact calculator presented in Lacoste et al. (2019).\n\n- Hardware Type: \n- Hours used: \n- Cloud Provider: \n- Compute Region: \n- Carbon Emitted:",
"## Technical Specifications [optional]",
"### Model Architecture and Objective",
"### Compute Infrastructure",
"#### Hardware",
"#### Software\n\n\n\n[optional]\n\n\n\nBibTeX:\n\n\n\nAPA:",
"## Glossary [optional]",
"## More Information [optional]",
"## Model Card Authors [optional]",
"## Model Card Contact"
] | [
"TAGS\n#diffusers #safetensors #arxiv-1910.09700 #region-us \n",
"# Model Card for Model ID",
"## Model Details",
"### Model Description\n\n\n\nThis is the model card of a diffusers model that has been pushed on the Hub. This model card has been automatically generated.\n\n- Developed by: \n- Funded by [optional]: \n- Shared by [optional]: \n- Model type: \n- Language(s) (NLP): \n- License: \n- Finetuned from model [optional]:",
"### Model Sources [optional]\n\n\n\n- Repository: \n- Paper [optional]: \n- Demo [optional]:",
"## Uses",
"### Direct Use",
"### Downstream Use [optional]",
"### Out-of-Scope Use",
"## Bias, Risks, and Limitations",
"### Recommendations\n\n\n\nUsers (both direct and downstream) should be made aware of the risks, biases and limitations of the model. More information needed for further recommendations.",
"## How to Get Started with the Model\n\nUse the code below to get started with the model.",
"## Training Details",
"### Training Data",
"### Training Procedure",
"#### Preprocessing [optional]",
"#### Training Hyperparameters\n\n- Training regime:",
"#### Speeds, Sizes, Times [optional]",
"## Evaluation",
"### Testing Data, Factors & Metrics",
"#### Testing Data",
"#### Factors",
"#### Metrics",
"### Results",
"#### Summary",
"## Model Examination [optional]",
"## Environmental Impact\n\n\n\nCarbon emissions can be estimated using the Machine Learning Impact calculator presented in Lacoste et al. (2019).\n\n- Hardware Type: \n- Hours used: \n- Cloud Provider: \n- Compute Region: \n- Carbon Emitted:",
"## Technical Specifications [optional]",
"### Model Architecture and Objective",
"### Compute Infrastructure",
"#### Hardware",
"#### Software\n\n\n\n[optional]\n\n\n\nBibTeX:\n\n\n\nAPA:",
"## Glossary [optional]",
"## More Information [optional]",
"## Model Card Authors [optional]",
"## Model Card Contact"
] |
text-classification | transformers |
<!-- This model card has been generated automatically according to the information the Trainer had access to. You
should probably proofread and complete it, then remove this comment. -->
# NDD-pagekit_test-content_tags
This model is a fine-tuned version of [distilbert-base-uncased](https://huggingface.co/distilbert-base-uncased) on an unknown dataset.
It achieves the following results on the evaluation set:
- Loss: 2.2690
- Accuracy: 0.6554
- F1: 0.6119
- Precision: 0.6638
- Recall: 0.6554
## Model description
More information needed
## Intended uses & limitations
More information needed
## Training and evaluation data
More information needed
## Training procedure
### Training hyperparameters
The following hyperparameters were used during training:
- learning_rate: 2e-05
- train_batch_size: 32
- eval_batch_size: 32
- seed: 42
- gradient_accumulation_steps: 4
- total_train_batch_size: 128
- optimizer: Adam with betas=(0.9,0.999) and epsilon=1e-08
- lr_scheduler_type: linear
- num_epochs: 2
### Training results
| Training Loss | Epoch | Step | Validation Loss | Accuracy | F1 | Precision | Recall |
|:-------------:|:------:|:----:|:---------------:|:--------:|:------:|:---------:|:------:|
| 0.1287 | 0.9993 | 684 | 2.1526 | 0.6515 | 0.6056 | 0.6596 | 0.6515 |
| 0.079 | 1.9985 | 1368 | 2.2690 | 0.6554 | 0.6119 | 0.6638 | 0.6554 |
### Framework versions
- Transformers 4.40.1
- Pytorch 2.2.1+cu121
- Datasets 2.19.0
- Tokenizers 0.19.1
| {"license": "apache-2.0", "tags": ["generated_from_trainer"], "metrics": ["accuracy", "f1", "precision", "recall"], "base_model": "distilbert-base-uncased", "model-index": [{"name": "NDD-pagekit_test-content_tags", "results": []}]} | lgk03/NDD-pagekit_test-content_tags | null | [
"transformers",
"tensorboard",
"safetensors",
"distilbert",
"text-classification",
"generated_from_trainer",
"base_model:distilbert-base-uncased",
"license:apache-2.0",
"autotrain_compatible",
"endpoints_compatible",
"region:us"
] | null | 2024-04-25T15:23:33+00:00 | [] | [] | TAGS
#transformers #tensorboard #safetensors #distilbert #text-classification #generated_from_trainer #base_model-distilbert-base-uncased #license-apache-2.0 #autotrain_compatible #endpoints_compatible #region-us
| NDD-pagekit\_test-content\_tags
===============================
This model is a fine-tuned version of distilbert-base-uncased on an unknown dataset.
It achieves the following results on the evaluation set:
* Loss: 2.2690
* Accuracy: 0.6554
* F1: 0.6119
* Precision: 0.6638
* Recall: 0.6554
Model description
-----------------
More information needed
Intended uses & limitations
---------------------------
More information needed
Training and evaluation data
----------------------------
More information needed
Training procedure
------------------
### Training hyperparameters
The following hyperparameters were used during training:
* learning\_rate: 2e-05
* train\_batch\_size: 32
* eval\_batch\_size: 32
* seed: 42
* gradient\_accumulation\_steps: 4
* total\_train\_batch\_size: 128
* optimizer: Adam with betas=(0.9,0.999) and epsilon=1e-08
* lr\_scheduler\_type: linear
* num\_epochs: 2
### Training results
### Framework versions
* Transformers 4.40.1
* Pytorch 2.2.1+cu121
* Datasets 2.19.0
* Tokenizers 0.19.1
| [
"### Training hyperparameters\n\n\nThe following hyperparameters were used during training:\n\n\n* learning\\_rate: 2e-05\n* train\\_batch\\_size: 32\n* eval\\_batch\\_size: 32\n* seed: 42\n* gradient\\_accumulation\\_steps: 4\n* total\\_train\\_batch\\_size: 128\n* optimizer: Adam with betas=(0.9,0.999) and epsilon=1e-08\n* lr\\_scheduler\\_type: linear\n* num\\_epochs: 2",
"### Training results",
"### Framework versions\n\n\n* Transformers 4.40.1\n* Pytorch 2.2.1+cu121\n* Datasets 2.19.0\n* Tokenizers 0.19.1"
] | [
"TAGS\n#transformers #tensorboard #safetensors #distilbert #text-classification #generated_from_trainer #base_model-distilbert-base-uncased #license-apache-2.0 #autotrain_compatible #endpoints_compatible #region-us \n",
"### Training hyperparameters\n\n\nThe following hyperparameters were used during training:\n\n\n* learning\\_rate: 2e-05\n* train\\_batch\\_size: 32\n* eval\\_batch\\_size: 32\n* seed: 42\n* gradient\\_accumulation\\_steps: 4\n* total\\_train\\_batch\\_size: 128\n* optimizer: Adam with betas=(0.9,0.999) and epsilon=1e-08\n* lr\\_scheduler\\_type: linear\n* num\\_epochs: 2",
"### Training results",
"### Framework versions\n\n\n* Transformers 4.40.1\n* Pytorch 2.2.1+cu121\n* Datasets 2.19.0\n* Tokenizers 0.19.1"
] |
text-to-image | diffusers |

## ANIMATOR-XL PROMPT IS EVERYTHING !! 😉
<Gallery />
## USE IT WITH DIFFUSERS 🧨
```python
from diffusers import AutoPipelineForText2Image
import torch
pipeline = AutoPipelineForText2Image.from_pretrained('prithivMLmods/ProductH-Animator-XL', torch_dtype=torch.float16).to('cuda')
image = pipeline('(masterpiece, best quality, highres:1.2) , (intricate and beautiful:1.2) , (detailed light:1.2) , (colorful) , 1man, (iridescent white hair,bangs:1.2) , (streetwaer outfit,adidas:1.3) , (street background:1.3) , (cowboy body shot:1.3) , (official art) , (cinematic) , (fashion pose:1.3) , (coat:1.2) ', num_inference_steps=2).images[0]
```
## CONCLUSION
The model is age violated, and the results to your prompt are at your own risk. [ Think before prompting the violated content ]
The model is re-designed [ SDXL 1.0 ] only for productivity purposes. | {"license": "creativeml-openrail-m", "tags": ["text-to-image", "turbo", "stable-diffusion", "stable-diffusion-xl"], "pipeline_tag": "text-to-image", "widget": [{"text": "(masterpiece, best quality, highres:1.2) , (intricate and beautiful:1.2) , (detailed light:1.2) , (colorful) , 1woman, long hair, ponytail, bangs, cowboy body shot, (sleeveless samurai outfit,bare shoulders:1.2) , (temple background:1.2) , (official art) , (cinematic) , tattoo", "output": {"url": "image7.jpeg"}}, {"text": "(masterpiece, best quality, highres:1.2) , (intricate and beautiful:1.2) , (detailed light:1.2) , (colorful) , 1man, (iridescent white hair,bangs:1.2) , (streetwaer outfit,adidas:1.3) , (street background:1.3) , (cowboy body shot:1.3) , (official art) , (cinematic) , (fashion pose:1.3) , (coat:1.2)", "output": {"url": "image8.jpeg"}}, {"text": "a woman with long black hair in a gold painting, in the style of charlie bowater, dark blue and dark black, michael garmash, comic art, realistic color palette, dark black and beige, soft-focused realism --ar 24:37 --stylize 750 --v 6", "output": {"url": "image9.jpeg"}}, {"text": "A portrait of a Cat, The background features abstract shapes in green, yellow and red, creating vibrant colors and adding depth to the artwork. The digital art style is in the style of pop culture and contemporary illustration technique.", "output": {"url": "image10.jpeg"}}, {"text": "Photograph, contemporary living room, soft light of morning, integration of beige flooring and matte stone features, unified color scheme of soothing white tones, creating a white and inviting atmosphere, 35mm f/1. 4G lens, set f/4, sophisticated furniture, including a white-colored sofa set and a minimalist side table, natural lights", "output": {"url": "image44.jpeg"}}, {"text": "cinematic portrait of road warrior mad max female type model character, very fit and athletic, apocalyptic setting, holding a dirty rusted sawed off shotgun, dirty short hair blonde female with bandana, natural lighting --ar 1:2 --s 50", "output": {"url": "image55.jpeg"}}], "inference": {"parameters": {"num_inference_steps": 8}}} | prithivMLmods/ProductH-Animator-XL | null | [
"diffusers",
"safetensors",
"text-to-image",
"turbo",
"stable-diffusion",
"stable-diffusion-xl",
"license:creativeml-openrail-m",
"endpoints_compatible",
"diffusers:StableDiffusionXLPipeline",
"region:us"
] | null | 2024-04-25T15:24:52+00:00 | [] | [] | TAGS
#diffusers #safetensors #text-to-image #turbo #stable-diffusion #stable-diffusion-xl #license-creativeml-openrail-m #endpoints_compatible #diffusers-StableDiffusionXLPipeline #region-us
|
!row01
## ANIMATOR-XL PROMPT IS EVERYTHING !!
<Gallery />
## USE IT WITH DIFFUSERS
## CONCLUSION
The model is age violated, and the results to your prompt are at your own risk. [ Think before prompting the violated content ]
The model is re-designed [ SDXL 1.0 ] only for productivity purposes. | [
"## ANIMATOR-XL PROMPT IS EVERYTHING !! \n\n<Gallery />",
"## USE IT WITH DIFFUSERS",
"## CONCLUSION\n\nThe model is age violated, and the results to your prompt are at your own risk. [ Think before prompting the violated content ]\n\nThe model is re-designed [ SDXL 1.0 ] only for productivity purposes."
] | [
"TAGS\n#diffusers #safetensors #text-to-image #turbo #stable-diffusion #stable-diffusion-xl #license-creativeml-openrail-m #endpoints_compatible #diffusers-StableDiffusionXLPipeline #region-us \n",
"## ANIMATOR-XL PROMPT IS EVERYTHING !! \n\n<Gallery />",
"## USE IT WITH DIFFUSERS",
"## CONCLUSION\n\nThe model is age violated, and the results to your prompt are at your own risk. [ Think before prompting the violated content ]\n\nThe model is re-designed [ SDXL 1.0 ] only for productivity purposes."
] |
text-generation | transformers |
<!-- This model card has been generated automatically according to the information the Trainer had access to. You
should probably proofread and complete it, then remove this comment. -->
# 0.001_ablation_5iters_bs256_useresponse_iter_5
This model is a fine-tuned version of [ShenaoZ/0.001_ablation_5iters_bs256_useresponse_iter_4](https://huggingface.co/ShenaoZ/0.001_ablation_5iters_bs256_useresponse_iter_4) on the updated and the original datasets.
## Model description
More information needed
## Intended uses & limitations
More information needed
## Training and evaluation data
More information needed
## Training procedure
### Training hyperparameters
The following hyperparameters were used during training:
- learning_rate: 5e-07
- train_batch_size: 8
- eval_batch_size: 8
- seed: 42
- distributed_type: multi-GPU
- num_devices: 8
- gradient_accumulation_steps: 4
- total_train_batch_size: 256
- total_eval_batch_size: 64
- optimizer: Adam with betas=(0.9,0.999) and epsilon=1e-08
- lr_scheduler_type: cosine
- lr_scheduler_warmup_ratio: 0.1
- num_epochs: 1
### Training results
### Framework versions
- Transformers 4.36.2
- Pytorch 2.1.2+cu121
- Datasets 2.14.6
- Tokenizers 0.15.2
| {"license": "mit", "tags": ["alignment-handbook", "generated_from_trainer", "trl", "dpo", "generated_from_trainer"], "datasets": ["updated", "original"], "base_model": "ShenaoZ/0.001_ablation_5iters_bs256_useresponse_iter_4", "model-index": [{"name": "0.001_ablation_5iters_bs256_useresponse_iter_5", "results": []}]} | ShenaoZ/0.001_ablation_5iters_bs256_useresponse_iter_5 | null | [
"transformers",
"safetensors",
"mistral",
"text-generation",
"alignment-handbook",
"generated_from_trainer",
"trl",
"dpo",
"conversational",
"dataset:updated",
"dataset:original",
"base_model:ShenaoZ/0.001_ablation_5iters_bs256_useresponse_iter_4",
"license:mit",
"autotrain_compatible",
"endpoints_compatible",
"text-generation-inference",
"region:us"
] | null | 2024-04-25T15:26:56+00:00 | [] | [] | TAGS
#transformers #safetensors #mistral #text-generation #alignment-handbook #generated_from_trainer #trl #dpo #conversational #dataset-updated #dataset-original #base_model-ShenaoZ/0.001_ablation_5iters_bs256_useresponse_iter_4 #license-mit #autotrain_compatible #endpoints_compatible #text-generation-inference #region-us
|
# 0.001_ablation_5iters_bs256_useresponse_iter_5
This model is a fine-tuned version of ShenaoZ/0.001_ablation_5iters_bs256_useresponse_iter_4 on the updated and the original datasets.
## Model description
More information needed
## Intended uses & limitations
More information needed
## Training and evaluation data
More information needed
## Training procedure
### Training hyperparameters
The following hyperparameters were used during training:
- learning_rate: 5e-07
- train_batch_size: 8
- eval_batch_size: 8
- seed: 42
- distributed_type: multi-GPU
- num_devices: 8
- gradient_accumulation_steps: 4
- total_train_batch_size: 256
- total_eval_batch_size: 64
- optimizer: Adam with betas=(0.9,0.999) and epsilon=1e-08
- lr_scheduler_type: cosine
- lr_scheduler_warmup_ratio: 0.1
- num_epochs: 1
### Training results
### Framework versions
- Transformers 4.36.2
- Pytorch 2.1.2+cu121
- Datasets 2.14.6
- Tokenizers 0.15.2
| [
"# 0.001_ablation_5iters_bs256_useresponse_iter_5\n\nThis model is a fine-tuned version of ShenaoZ/0.001_ablation_5iters_bs256_useresponse_iter_4 on the updated and the original datasets.",
"## Model description\n\nMore information needed",
"## Intended uses & limitations\n\nMore information needed",
"## Training and evaluation data\n\nMore information needed",
"## Training procedure",
"### Training hyperparameters\n\nThe following hyperparameters were used during training:\n- learning_rate: 5e-07\n- train_batch_size: 8\n- eval_batch_size: 8\n- seed: 42\n- distributed_type: multi-GPU\n- num_devices: 8\n- gradient_accumulation_steps: 4\n- total_train_batch_size: 256\n- total_eval_batch_size: 64\n- optimizer: Adam with betas=(0.9,0.999) and epsilon=1e-08\n- lr_scheduler_type: cosine\n- lr_scheduler_warmup_ratio: 0.1\n- num_epochs: 1",
"### Training results",
"### Framework versions\n\n- Transformers 4.36.2\n- Pytorch 2.1.2+cu121\n- Datasets 2.14.6\n- Tokenizers 0.15.2"
] | [
"TAGS\n#transformers #safetensors #mistral #text-generation #alignment-handbook #generated_from_trainer #trl #dpo #conversational #dataset-updated #dataset-original #base_model-ShenaoZ/0.001_ablation_5iters_bs256_useresponse_iter_4 #license-mit #autotrain_compatible #endpoints_compatible #text-generation-inference #region-us \n",
"# 0.001_ablation_5iters_bs256_useresponse_iter_5\n\nThis model is a fine-tuned version of ShenaoZ/0.001_ablation_5iters_bs256_useresponse_iter_4 on the updated and the original datasets.",
"## Model description\n\nMore information needed",
"## Intended uses & limitations\n\nMore information needed",
"## Training and evaluation data\n\nMore information needed",
"## Training procedure",
"### Training hyperparameters\n\nThe following hyperparameters were used during training:\n- learning_rate: 5e-07\n- train_batch_size: 8\n- eval_batch_size: 8\n- seed: 42\n- distributed_type: multi-GPU\n- num_devices: 8\n- gradient_accumulation_steps: 4\n- total_train_batch_size: 256\n- total_eval_batch_size: 64\n- optimizer: Adam with betas=(0.9,0.999) and epsilon=1e-08\n- lr_scheduler_type: cosine\n- lr_scheduler_warmup_ratio: 0.1\n- num_epochs: 1",
"### Training results",
"### Framework versions\n\n- Transformers 4.36.2\n- Pytorch 2.1.2+cu121\n- Datasets 2.14.6\n- Tokenizers 0.15.2"
] |
null | transformers |
# Model Card for Model ID
<!-- Provide a quick summary of what the model is/does. -->
## Model Details
### Model Description
<!-- Provide a longer summary of what this model is. -->
This is the model card of a 🤗 transformers model that has been pushed on the Hub. This model card has been automatically generated.
- **Developed by:** [More Information Needed]
- **Funded by [optional]:** [More Information Needed]
- **Shared by [optional]:** [More Information Needed]
- **Model type:** [More Information Needed]
- **Language(s) (NLP):** [More Information Needed]
- **License:** [More Information Needed]
- **Finetuned from model [optional]:** [More Information Needed]
### Model Sources [optional]
<!-- Provide the basic links for the model. -->
- **Repository:** [More Information Needed]
- **Paper [optional]:** [More Information Needed]
- **Demo [optional]:** [More Information Needed]
## Uses
<!-- Address questions around how the model is intended to be used, including the foreseeable users of the model and those affected by the model. -->
### Direct Use
<!-- This section is for the model use without fine-tuning or plugging into a larger ecosystem/app. -->
[More Information Needed]
### Downstream Use [optional]
<!-- This section is for the model use when fine-tuned for a task, or when plugged into a larger ecosystem/app -->
[More Information Needed]
### Out-of-Scope Use
<!-- This section addresses misuse, malicious use, and uses that the model will not work well for. -->
[More Information Needed]
## Bias, Risks, and Limitations
<!-- This section is meant to convey both technical and sociotechnical limitations. -->
[More Information Needed]
### Recommendations
<!-- This section is meant to convey recommendations with respect to the bias, risk, and technical limitations. -->
Users (both direct and downstream) should be made aware of the risks, biases and limitations of the model. More information needed for further recommendations.
## How to Get Started with the Model
Use the code below to get started with the model.
[More Information Needed]
## Training Details
### Training Data
<!-- This should link to a Dataset Card, perhaps with a short stub of information on what the training data is all about as well as documentation related to data pre-processing or additional filtering. -->
[More Information Needed]
### Training Procedure
<!-- This relates heavily to the Technical Specifications. Content here should link to that section when it is relevant to the training procedure. -->
#### Preprocessing [optional]
[More Information Needed]
#### Training Hyperparameters
- **Training regime:** [More Information Needed] <!--fp32, fp16 mixed precision, bf16 mixed precision, bf16 non-mixed precision, fp16 non-mixed precision, fp8 mixed precision -->
#### Speeds, Sizes, Times [optional]
<!-- This section provides information about throughput, start/end time, checkpoint size if relevant, etc. -->
[More Information Needed]
## Evaluation
<!-- This section describes the evaluation protocols and provides the results. -->
### Testing Data, Factors & Metrics
#### Testing Data
<!-- This should link to a Dataset Card if possible. -->
[More Information Needed]
#### Factors
<!-- These are the things the evaluation is disaggregating by, e.g., subpopulations or domains. -->
[More Information Needed]
#### Metrics
<!-- These are the evaluation metrics being used, ideally with a description of why. -->
[More Information Needed]
### Results
[More Information Needed]
#### Summary
## Model Examination [optional]
<!-- Relevant interpretability work for the model goes here -->
[More Information Needed]
## Environmental Impact
<!-- Total emissions (in grams of CO2eq) and additional considerations, such as electricity usage, go here. Edit the suggested text below accordingly -->
Carbon emissions can be estimated using the [Machine Learning Impact calculator](https://mlco2.github.io/impact#compute) presented in [Lacoste et al. (2019)](https://arxiv.org/abs/1910.09700).
- **Hardware Type:** [More Information Needed]
- **Hours used:** [More Information Needed]
- **Cloud Provider:** [More Information Needed]
- **Compute Region:** [More Information Needed]
- **Carbon Emitted:** [More Information Needed]
## Technical Specifications [optional]
### Model Architecture and Objective
[More Information Needed]
### Compute Infrastructure
[More Information Needed]
#### Hardware
[More Information Needed]
#### Software
[More Information Needed]
## Citation [optional]
<!-- If there is a paper or blog post introducing the model, the APA and Bibtex information for that should go in this section. -->
**BibTeX:**
[More Information Needed]
**APA:**
[More Information Needed]
## Glossary [optional]
<!-- If relevant, include terms and calculations in this section that can help readers understand the model or model card. -->
[More Information Needed]
## More Information [optional]
[More Information Needed]
## Model Card Authors [optional]
[More Information Needed]
## Model Card Contact
[More Information Needed] | {"library_name": "transformers", "tags": []} | Peto1/mistral-finetuned-text-generation | null | [
"transformers",
"tensorboard",
"safetensors",
"arxiv:1910.09700",
"endpoints_compatible",
"region:us"
] | null | 2024-04-25T15:27:16+00:00 | [
"1910.09700"
] | [] | TAGS
#transformers #tensorboard #safetensors #arxiv-1910.09700 #endpoints_compatible #region-us
|
# Model Card for Model ID
## Model Details
### Model Description
This is the model card of a transformers model that has been pushed on the Hub. This model card has been automatically generated.
- Developed by:
- Funded by [optional]:
- Shared by [optional]:
- Model type:
- Language(s) (NLP):
- License:
- Finetuned from model [optional]:
### Model Sources [optional]
- Repository:
- Paper [optional]:
- Demo [optional]:
## Uses
### Direct Use
### Downstream Use [optional]
### Out-of-Scope Use
## Bias, Risks, and Limitations
### Recommendations
Users (both direct and downstream) should be made aware of the risks, biases and limitations of the model. More information needed for further recommendations.
## How to Get Started with the Model
Use the code below to get started with the model.
## Training Details
### Training Data
### Training Procedure
#### Preprocessing [optional]
#### Training Hyperparameters
- Training regime:
#### Speeds, Sizes, Times [optional]
## Evaluation
### Testing Data, Factors & Metrics
#### Testing Data
#### Factors
#### Metrics
### Results
#### Summary
## Model Examination [optional]
## Environmental Impact
Carbon emissions can be estimated using the Machine Learning Impact calculator presented in Lacoste et al. (2019).
- Hardware Type:
- Hours used:
- Cloud Provider:
- Compute Region:
- Carbon Emitted:
## Technical Specifications [optional]
### Model Architecture and Objective
### Compute Infrastructure
#### Hardware
#### Software
[optional]
BibTeX:
APA:
## Glossary [optional]
## More Information [optional]
## Model Card Authors [optional]
## Model Card Contact
| [
"# Model Card for Model ID",
"## Model Details",
"### Model Description\n\n\n\nThis is the model card of a transformers model that has been pushed on the Hub. This model card has been automatically generated.\n\n- Developed by: \n- Funded by [optional]: \n- Shared by [optional]: \n- Model type: \n- Language(s) (NLP): \n- License: \n- Finetuned from model [optional]:",
"### Model Sources [optional]\n\n\n\n- Repository: \n- Paper [optional]: \n- Demo [optional]:",
"## Uses",
"### Direct Use",
"### Downstream Use [optional]",
"### Out-of-Scope Use",
"## Bias, Risks, and Limitations",
"### Recommendations\n\n\n\nUsers (both direct and downstream) should be made aware of the risks, biases and limitations of the model. More information needed for further recommendations.",
"## How to Get Started with the Model\n\nUse the code below to get started with the model.",
"## Training Details",
"### Training Data",
"### Training Procedure",
"#### Preprocessing [optional]",
"#### Training Hyperparameters\n\n- Training regime:",
"#### Speeds, Sizes, Times [optional]",
"## Evaluation",
"### Testing Data, Factors & Metrics",
"#### Testing Data",
"#### Factors",
"#### Metrics",
"### Results",
"#### Summary",
"## Model Examination [optional]",
"## Environmental Impact\n\n\n\nCarbon emissions can be estimated using the Machine Learning Impact calculator presented in Lacoste et al. (2019).\n\n- Hardware Type: \n- Hours used: \n- Cloud Provider: \n- Compute Region: \n- Carbon Emitted:",
"## Technical Specifications [optional]",
"### Model Architecture and Objective",
"### Compute Infrastructure",
"#### Hardware",
"#### Software\n\n\n\n[optional]\n\n\n\nBibTeX:\n\n\n\nAPA:",
"## Glossary [optional]",
"## More Information [optional]",
"## Model Card Authors [optional]",
"## Model Card Contact"
] | [
"TAGS\n#transformers #tensorboard #safetensors #arxiv-1910.09700 #endpoints_compatible #region-us \n",
"# Model Card for Model ID",
"## Model Details",
"### Model Description\n\n\n\nThis is the model card of a transformers model that has been pushed on the Hub. This model card has been automatically generated.\n\n- Developed by: \n- Funded by [optional]: \n- Shared by [optional]: \n- Model type: \n- Language(s) (NLP): \n- License: \n- Finetuned from model [optional]:",
"### Model Sources [optional]\n\n\n\n- Repository: \n- Paper [optional]: \n- Demo [optional]:",
"## Uses",
"### Direct Use",
"### Downstream Use [optional]",
"### Out-of-Scope Use",
"## Bias, Risks, and Limitations",
"### Recommendations\n\n\n\nUsers (both direct and downstream) should be made aware of the risks, biases and limitations of the model. More information needed for further recommendations.",
"## How to Get Started with the Model\n\nUse the code below to get started with the model.",
"## Training Details",
"### Training Data",
"### Training Procedure",
"#### Preprocessing [optional]",
"#### Training Hyperparameters\n\n- Training regime:",
"#### Speeds, Sizes, Times [optional]",
"## Evaluation",
"### Testing Data, Factors & Metrics",
"#### Testing Data",
"#### Factors",
"#### Metrics",
"### Results",
"#### Summary",
"## Model Examination [optional]",
"## Environmental Impact\n\n\n\nCarbon emissions can be estimated using the Machine Learning Impact calculator presented in Lacoste et al. (2019).\n\n- Hardware Type: \n- Hours used: \n- Cloud Provider: \n- Compute Region: \n- Carbon Emitted:",
"## Technical Specifications [optional]",
"### Model Architecture and Objective",
"### Compute Infrastructure",
"#### Hardware",
"#### Software\n\n\n\n[optional]\n\n\n\nBibTeX:\n\n\n\nAPA:",
"## Glossary [optional]",
"## More Information [optional]",
"## Model Card Authors [optional]",
"## Model Card Contact"
] |
text-generation | transformers | # SniffyOtter-7B-Novel-Writing-NSFW
[GGUF版はこちら/Click here for the GGUF version](https://huggingface.co/Aratako/SniffyOtter-7B-Novel-Writing-NSFW-GGUF)
## 概要
[Elizezen/SniffyOtter-7B](https://huggingface.co/Elizezen/SniffyOtter-7B)をベースに、NSFW特化で小説生成用のinstruction tuningを施したモデルです。
ジャンルやクオリティ、キーワードや過激さを指定すると、それに従った小説を生成するようにinstruction tuningしています。
[Aratako/Antler-7B-Novel-Writing](https://huggingface.co/Aratako/Antler-7B-Novel-Writing)との違いは主に以下の点になります。
- 元モデルを[Elizezen/Antler-7B](https://huggingface.co/Elizezen/Antler-7B)から[Elizezen/SniffyOtter-7B](https://huggingface.co/Elizezen/SniffyOtter-7B)へ変更
- そのため、ライセンスがCC-BY-NC-4.0となります
- 学習データをNSFWのものに限定
- [Aratako/Syosetu711K-Cleaned-158K-Instruct](https://huggingface.co/datasets/Aratako/Syosetu711K-Cleaned-158K-Instruct)から、NSFWのテキストのみを抽出
- さらに、テキストを100文字ずつに分割し、[oshizo/japanese-sexual-moderation-v2](https://huggingface.co/oshizo/japanese-sexual-moderation-v2)を利用してsexuality scoreを取得し、テキスト内の平均スコアが0.4以上のものを抽出
## プロンプトフォーマット
Mistralのchat templateを利用してください。また、学習に利用したデータのフォーマットの関係上、以下のような形式が望ましいと思われます。
```
[INST] {小説生成の指示}
ジャンル:{ジャンル}
クオリティ:{クオリティを示す数値(0から3)}
キーワード:{小説の概要を示すタグ・キーワードを読点区切りで羅列}
過激さ:{表現の過激さを示す数値(0~3、高い方がより過激)} [/INST]
```
## プロンプト内で指定する属性について
本モデルは学習時の指示にジャンルやキーワード、クオリティ、過激さなどを追加して学習しているため、それらの属性を指定することである程度出力の制御が可能です。
### ジャンル
[なろうR18小説API](https://dev.syosetu.com/xman/api/)における`nocgenre`のジャンルで学習しています。具体的には以下のものを学習時に使っています。この形式で指定するのが望ましいかと思われます。
- 男性向け、女性向け、BL、大人向け ※APIページ上での表記からやや変更して学習しています。
### クオリティ
本モデルの学習に使用した[データセット](https://huggingface.co/datasets/Aratako/Syosetu711K-Cleaned-158K-instruct)の大本である[RyokoAI/Syosetu711K](https://huggingface.co/datasets/RyokoAI/Syosetu711K)のq-scoreを利用して学習時のレコードにクオリティタグをつけています。
[使用したデータセット](https://huggingface.co/datasets/Aratako/Syosetu711K-Cleaned-158K-instruct)は既にq-scoreが0.8以上の高品質のものをフィルターしてありますが、さらにそれを25%ずつに分け、下から0、1、2、3とラベリングしています。3を指定するとより高品質な出力になる事が期待されます。
### 過激さ
学習テキストに対して[oshizo/japanese-sexual-moderation-v2](https://huggingface.co/oshizo/japanese-sexual-moderation-v2)を利用して取得したsexuality scoreの平均値を25%ずつ分割し、低い方から0、1、2、3とラベリングして学習しています。大きい数値を指定するとより過激な表現になる事が想定されます。
## 学習関連の情報
### 使用データセット
- [Aratako/Syosetu711K-Cleaned-158K-instruct](https://huggingface.co/Aratako/Syosetu711K-Cleaned-158K-instruct)
- 上記データセットのうち概要で説明した処理を行いフィルタしたデータを利用
## 学習の設定
RunpodでGPUサーバを借り、A6000x4で学習を行いました。主な学習パラメータは以下の通りです。
- lora_r: 128
- lisa_alpha: 256
- lora_dropout: 0.05
- lora_target_modules: ["q_proj", "k_proj", "v_proj", "o_proj", "gate_proj", "up_proj", "down_proj", "lm_head"]
- learning_rate: 2e-5
- num_train_epochs: 10 epochs
- batch_size: 64
- max_seq_length: 4096
## ライセンス
元モデルである[Elizezen/SniffyOtter-7B](https://huggingface.co/Elizezen/SniffyOtter-7B)と同じく、CC-BY-NC-4.0の元配布します。 | {"language": ["ja"], "license": "cc-by-nc-4.0", "library_name": "transformers", "tags": ["not-for-all-audiences", "nsfw"], "datasets": ["Aratako/Syosetu711K-Cleaned-158K-Instruct"], "base_model": ["Elizezen/SniffyOtter-7B"]} | Aratako/SniffyOtter-7B-Novel-Writing-NSFW | null | [
"transformers",
"safetensors",
"mistral",
"text-generation",
"not-for-all-audiences",
"nsfw",
"ja",
"dataset:Aratako/Syosetu711K-Cleaned-158K-Instruct",
"base_model:Elizezen/SniffyOtter-7B",
"license:cc-by-nc-4.0",
"autotrain_compatible",
"endpoints_compatible",
"text-generation-inference",
"region:us"
] | null | 2024-04-25T15:28:58+00:00 | [] | [
"ja"
] | TAGS
#transformers #safetensors #mistral #text-generation #not-for-all-audiences #nsfw #ja #dataset-Aratako/Syosetu711K-Cleaned-158K-Instruct #base_model-Elizezen/SniffyOtter-7B #license-cc-by-nc-4.0 #autotrain_compatible #endpoints_compatible #text-generation-inference #region-us
| # SniffyOtter-7B-Novel-Writing-NSFW
GGUF版はこちら/Click here for the GGUF version
## 概要
Elizezen/SniffyOtter-7Bをベースに、NSFW特化で小説生成用のinstruction tuningを施したモデルです。
ジャンルやクオリティ、キーワードや過激さを指定すると、それに従った小説を生成するようにinstruction tuningしています。
Aratako/Antler-7B-Novel-Writingとの違いは主に以下の点になります。
- 元モデルをElizezen/Antler-7BからElizezen/SniffyOtter-7Bへ変更
- そのため、ライセンスがCC-BY-NC-4.0となります
- 学習データをNSFWのものに限定
- Aratako/Syosetu711K-Cleaned-158K-Instructから、NSFWのテキストのみを抽出
- さらに、テキストを100文字ずつに分割し、oshizo/japanese-sexual-moderation-v2を利用してsexuality scoreを取得し、テキスト内の平均スコアが0.4以上のものを抽出
## プロンプトフォーマット
Mistralのchat templateを利用してください。また、学習に利用したデータのフォーマットの関係上、以下のような形式が望ましいと思われます。
## プロンプト内で指定する属性について
本モデルは学習時の指示にジャンルやキーワード、クオリティ、過激さなどを追加して学習しているため、それらの属性を指定することである程度出力の制御が可能です。
### ジャンル
なろうR18小説APIにおける'nocgenre'のジャンルで学習しています。具体的には以下のものを学習時に使っています。この形式で指定するのが望ましいかと思われます。
- 男性向け、女性向け、BL、大人向け ※APIページ上での表記からやや変更して学習しています。
### クオリティ
本モデルの学習に使用したデータセットの大本であるRyokoAI/Syosetu711Kのq-scoreを利用して学習時のレコードにクオリティタグをつけています。
使用したデータセットは既にq-scoreが0.8以上の高品質のものをフィルターしてありますが、さらにそれを25%ずつに分け、下から0、1、2、3とラベリングしています。3を指定するとより高品質な出力になる事が期待されます。
### 過激さ
学習テキストに対してoshizo/japanese-sexual-moderation-v2を利用して取得したsexuality scoreの平均値を25%ずつ分割し、低い方から0、1、2、3とラベリングして学習しています。大きい数値を指定するとより過激な表現になる事が想定されます。
## 学習関連の情報
### 使用データセット
- Aratako/Syosetu711K-Cleaned-158K-instruct
- 上記データセットのうち概要で説明した処理を行いフィルタしたデータを利用
## 学習の設定
RunpodでGPUサーバを借り、A6000x4で学習を行いました。主な学習パラメータは以下の通りです。
- lora_r: 128
- lisa_alpha: 256
- lora_dropout: 0.05
- lora_target_modules: ["q_proj", "k_proj", "v_proj", "o_proj", "gate_proj", "up_proj", "down_proj", "lm_head"]
- learning_rate: 2e-5
- num_train_epochs: 10 epochs
- batch_size: 64
- max_seq_length: 4096
## ライセンス
元モデルであるElizezen/SniffyOtter-7Bと同じく、CC-BY-NC-4.0の元配布します。 | [
"# SniffyOtter-7B-Novel-Writing-NSFW\nGGUF版はこちら/Click here for the GGUF version",
"## 概要\n\nElizezen/SniffyOtter-7Bをベースに、NSFW特化で小説生成用のinstruction tuningを施したモデルです。\n\nジャンルやクオリティ、キーワードや過激さを指定すると、それに従った小説を生成するようにinstruction tuningしています。\n\nAratako/Antler-7B-Novel-Writingとの違いは主に以下の点になります。\n\n- 元モデルをElizezen/Antler-7BからElizezen/SniffyOtter-7Bへ変更\n - そのため、ライセンスがCC-BY-NC-4.0となります\n- 学習データをNSFWのものに限定\n - Aratako/Syosetu711K-Cleaned-158K-Instructから、NSFWのテキストのみを抽出\n - さらに、テキストを100文字ずつに分割し、oshizo/japanese-sexual-moderation-v2を利用してsexuality scoreを取得し、テキスト内の平均スコアが0.4以上のものを抽出",
"## プロンプトフォーマット\nMistralのchat templateを利用してください。また、学習に利用したデータのフォーマットの関係上、以下のような形式が望ましいと思われます。",
"## プロンプト内で指定する属性について\n本モデルは学習時の指示にジャンルやキーワード、クオリティ、過激さなどを追加して学習しているため、それらの属性を指定することである程度出力の制御が可能です。",
"### ジャンル\nなろうR18小説APIにおける'nocgenre'のジャンルで学習しています。具体的には以下のものを学習時に使っています。この形式で指定するのが望ましいかと思われます。\n- 男性向け、女性向け、BL、大人向け ※APIページ上での表記からやや変更して学習しています。",
"### クオリティ\n本モデルの学習に使用したデータセットの大本であるRyokoAI/Syosetu711Kのq-scoreを利用して学習時のレコードにクオリティタグをつけています。\n\n使用したデータセットは既にq-scoreが0.8以上の高品質のものをフィルターしてありますが、さらにそれを25%ずつに分け、下から0、1、2、3とラベリングしています。3を指定するとより高品質な出力になる事が期待されます。",
"### 過激さ\n学習テキストに対してoshizo/japanese-sexual-moderation-v2を利用して取得したsexuality scoreの平均値を25%ずつ分割し、低い方から0、1、2、3とラベリングして学習しています。大きい数値を指定するとより過激な表現になる事が想定されます。",
"## 学習関連の情報",
"### 使用データセット\n- Aratako/Syosetu711K-Cleaned-158K-instruct\n - 上記データセットのうち概要で説明した処理を行いフィルタしたデータを利用",
"## 学習の設定\nRunpodでGPUサーバを借り、A6000x4で学習を行いました。主な学習パラメータは以下の通りです。\n- lora_r: 128\n- lisa_alpha: 256\n- lora_dropout: 0.05\n- lora_target_modules: [\"q_proj\", \"k_proj\", \"v_proj\", \"o_proj\", \"gate_proj\", \"up_proj\", \"down_proj\", \"lm_head\"]\n- learning_rate: 2e-5\n- num_train_epochs: 10 epochs\n- batch_size: 64\n- max_seq_length: 4096",
"## ライセンス\n元モデルであるElizezen/SniffyOtter-7Bと同じく、CC-BY-NC-4.0の元配布します。"
] | [
"TAGS\n#transformers #safetensors #mistral #text-generation #not-for-all-audiences #nsfw #ja #dataset-Aratako/Syosetu711K-Cleaned-158K-Instruct #base_model-Elizezen/SniffyOtter-7B #license-cc-by-nc-4.0 #autotrain_compatible #endpoints_compatible #text-generation-inference #region-us \n",
"# SniffyOtter-7B-Novel-Writing-NSFW\nGGUF版はこちら/Click here for the GGUF version",
"## 概要\n\nElizezen/SniffyOtter-7Bをベースに、NSFW特化で小説生成用のinstruction tuningを施したモデルです。\n\nジャンルやクオリティ、キーワードや過激さを指定すると、それに従った小説を生成するようにinstruction tuningしています。\n\nAratako/Antler-7B-Novel-Writingとの違いは主に以下の点になります。\n\n- 元モデルをElizezen/Antler-7BからElizezen/SniffyOtter-7Bへ変更\n - そのため、ライセンスがCC-BY-NC-4.0となります\n- 学習データをNSFWのものに限定\n - Aratako/Syosetu711K-Cleaned-158K-Instructから、NSFWのテキストのみを抽出\n - さらに、テキストを100文字ずつに分割し、oshizo/japanese-sexual-moderation-v2を利用してsexuality scoreを取得し、テキスト内の平均スコアが0.4以上のものを抽出",
"## プロンプトフォーマット\nMistralのchat templateを利用してください。また、学習に利用したデータのフォーマットの関係上、以下のような形式が望ましいと思われます。",
"## プロンプト内で指定する属性について\n本モデルは学習時の指示にジャンルやキーワード、クオリティ、過激さなどを追加して学習しているため、それらの属性を指定することである程度出力の制御が可能です。",
"### ジャンル\nなろうR18小説APIにおける'nocgenre'のジャンルで学習しています。具体的には以下のものを学習時に使っています。この形式で指定するのが望ましいかと思われます。\n- 男性向け、女性向け、BL、大人向け ※APIページ上での表記からやや変更して学習しています。",
"### クオリティ\n本モデルの学習に使用したデータセットの大本であるRyokoAI/Syosetu711Kのq-scoreを利用して学習時のレコードにクオリティタグをつけています。\n\n使用したデータセットは既にq-scoreが0.8以上の高品質のものをフィルターしてありますが、さらにそれを25%ずつに分け、下から0、1、2、3とラベリングしています。3を指定するとより高品質な出力になる事が期待されます。",
"### 過激さ\n学習テキストに対してoshizo/japanese-sexual-moderation-v2を利用して取得したsexuality scoreの平均値を25%ずつ分割し、低い方から0、1、2、3とラベリングして学習しています。大きい数値を指定するとより過激な表現になる事が想定されます。",
"## 学習関連の情報",
"### 使用データセット\n- Aratako/Syosetu711K-Cleaned-158K-instruct\n - 上記データセットのうち概要で説明した処理を行いフィルタしたデータを利用",
"## 学習の設定\nRunpodでGPUサーバを借り、A6000x4で学習を行いました。主な学習パラメータは以下の通りです。\n- lora_r: 128\n- lisa_alpha: 256\n- lora_dropout: 0.05\n- lora_target_modules: [\"q_proj\", \"k_proj\", \"v_proj\", \"o_proj\", \"gate_proj\", \"up_proj\", \"down_proj\", \"lm_head\"]\n- learning_rate: 2e-5\n- num_train_epochs: 10 epochs\n- batch_size: 64\n- max_seq_length: 4096",
"## ライセンス\n元モデルであるElizezen/SniffyOtter-7Bと同じく、CC-BY-NC-4.0の元配布します。"
] |
null | mlx |
# lucataco/dolphin-2.9-llama3-70b-8bit
This model was converted to MLX format from [`cognitivecomputations/dolphin-2.9-llama3-70b`]() using mlx-lm version **0.11.0**.
Refer to the [original model card](https://huggingface.co/cognitivecomputations/dolphin-2.9-llama3-70b) for more details on the model.
## Use with mlx
```bash
pip install mlx-lm
```
```python
from mlx_lm import load, generate
model, tokenizer = load("lucataco/dolphin-2.9-llama3-70b-8bit")
response = generate(model, tokenizer, prompt="hello", verbose=True)
```
| {"language": ["en"], "license": "llama3", "tags": ["mlx"], "datasets": ["cognitivecomputations/Dolphin-2.9", "teknium/OpenHermes-2.5", "m-a-p/CodeFeedback-Filtered-Instruction", "cognitivecomputations/dolphin-coder", "cognitivecomputations/samantha-data", "HuggingFaceH4/ultrachat_200k", "microsoft/orca-math-word-problems-200k", "abacusai/SystemChat-1.1", "Locutusque/function-calling-chatml", "internlm/Agent-FLAN"]} | lucataco/dolphin-2.9-llama3-70b-8bit | null | [
"mlx",
"safetensors",
"llama",
"en",
"dataset:cognitivecomputations/Dolphin-2.9",
"dataset:teknium/OpenHermes-2.5",
"dataset:m-a-p/CodeFeedback-Filtered-Instruction",
"dataset:cognitivecomputations/dolphin-coder",
"dataset:cognitivecomputations/samantha-data",
"dataset:HuggingFaceH4/ultrachat_200k",
"dataset:microsoft/orca-math-word-problems-200k",
"dataset:abacusai/SystemChat-1.1",
"dataset:Locutusque/function-calling-chatml",
"dataset:internlm/Agent-FLAN",
"license:llama3",
"region:us"
] | null | 2024-04-25T15:29:37+00:00 | [] | [
"en"
] | TAGS
#mlx #safetensors #llama #en #dataset-cognitivecomputations/Dolphin-2.9 #dataset-teknium/OpenHermes-2.5 #dataset-m-a-p/CodeFeedback-Filtered-Instruction #dataset-cognitivecomputations/dolphin-coder #dataset-cognitivecomputations/samantha-data #dataset-HuggingFaceH4/ultrachat_200k #dataset-microsoft/orca-math-word-problems-200k #dataset-abacusai/SystemChat-1.1 #dataset-Locutusque/function-calling-chatml #dataset-internlm/Agent-FLAN #license-llama3 #region-us
|
# lucataco/dolphin-2.9-llama3-70b-8bit
This model was converted to MLX format from ['cognitivecomputations/dolphin-2.9-llama3-70b']() using mlx-lm version 0.11.0.
Refer to the original model card for more details on the model.
## Use with mlx
| [
"# lucataco/dolphin-2.9-llama3-70b-8bit\nThis model was converted to MLX format from ['cognitivecomputations/dolphin-2.9-llama3-70b']() using mlx-lm version 0.11.0.\nRefer to the original model card for more details on the model.",
"## Use with mlx"
] | [
"TAGS\n#mlx #safetensors #llama #en #dataset-cognitivecomputations/Dolphin-2.9 #dataset-teknium/OpenHermes-2.5 #dataset-m-a-p/CodeFeedback-Filtered-Instruction #dataset-cognitivecomputations/dolphin-coder #dataset-cognitivecomputations/samantha-data #dataset-HuggingFaceH4/ultrachat_200k #dataset-microsoft/orca-math-word-problems-200k #dataset-abacusai/SystemChat-1.1 #dataset-Locutusque/function-calling-chatml #dataset-internlm/Agent-FLAN #license-llama3 #region-us \n",
"# lucataco/dolphin-2.9-llama3-70b-8bit\nThis model was converted to MLX format from ['cognitivecomputations/dolphin-2.9-llama3-70b']() using mlx-lm version 0.11.0.\nRefer to the original model card for more details on the model.",
"## Use with mlx"
] |
null | null | # MyVLM
**Paper:** https://arxiv.org/abs/2403.14599
**Project Page:** https://snap-research.github.io/MyVLM/
**Code:** https://github.com/snap-research/MyVLM
# MyVLM Concept Heads & Concept Embeddings
As part of our [MyVLM code](https://github.com/snap-research/MyVLM) release, we have also released pretrained concept heads and concept embeddings for all 29 objects used in the paper.
These can be loaded using the `CLIPConceptHead` class and our inference scripts for reproducing the paper results.
This repository contains 5 concept heads for each object, representing five different training seeds and sets of images used for training.
## Concept Heads
<p align="center">
<img src="docs/concept_head.jpg" width="200px"/>
For each user-specific concept, we introduce an external concept head designed to identify the presence of the concept within an image.
</p>
As mentioned in the paper, we have two types of concept heads:
1. A facial recognition model for recognizing individuals
2. A CLIP-based concept head for recognizing user-specific objects
For faces, we use the `buffalo_l` face detection and face recognition model from [insightface](https://github.com/deepinsight/insightface/tree/master).
See `concept_heads/face_recognition/head.py` for usage.
For objects, we train a single linear layer over features extracted from a CLIP ViT-H/14 model (`DFN5B-CLIP-ViT-H-14-384`).
See `concept_heads/clip/head.py` for usage.
## Concept Embeddings
<p align="center">
<img src="docs/method.jpg" width="800px"/>
Having identified the presence of a user-specific concept within an image, a learned concept embedding representing an object or individual is used to guide the LLM in incorporating the concept into its personalized textual response.
</p>
The concept embeddings are saved as `.pt` files in the following format:
```
{
10: {
"keys": torch.Tensor(), # the keys used for optimizing the concept embedding
"values": torch.Tensor(), # the concept embedding itself
},
...
20: {
"keys": torch.Tensor(),
"values": torch.Tensor(),
},
...
}
```
where each entry in the dictionary represents a different checkpoint during the optimization process.
We provide the concept embeddings for personalized captioning using both BLIP-2 and LLaVA.
## License
This sample code is made available by Snap Inc. for non-commercial, academic purposes only.
Please see the full license [here](https://github.com/snap-research/MyVLM/blob/master/LICENSE).
| {"license": "other", "license_name": "myvlm-snap-license", "license_link": "https://github.com/snap-research/MyVLM/blob/master/LICENSE"} | yuvalalaluf/MyVLM | null | [
"arxiv:2403.14599",
"license:other",
"region:us"
] | null | 2024-04-25T15:30:00+00:00 | [
"2403.14599"
] | [] | TAGS
#arxiv-2403.14599 #license-other #region-us
| # MyVLM
Paper: URL
Project Page: URL
Code: URL
# MyVLM Concept Heads & Concept Embeddings
As part of our MyVLM code release, we have also released pretrained concept heads and concept embeddings for all 29 objects used in the paper.
These can be loaded using the 'CLIPConceptHead' class and our inference scripts for reproducing the paper results.
This repository contains 5 concept heads for each object, representing five different training seeds and sets of images used for training.
## Concept Heads
<p align="center">
<img src="docs/concept_head.jpg" width="200px"/>
For each user-specific concept, we introduce an external concept head designed to identify the presence of the concept within an image.
</p>
As mentioned in the paper, we have two types of concept heads:
1. A facial recognition model for recognizing individuals
2. A CLIP-based concept head for recognizing user-specific objects
For faces, we use the 'buffalo_l' face detection and face recognition model from insightface.
See 'concept_heads/face_recognition/URL' for usage.
For objects, we train a single linear layer over features extracted from a CLIP ViT-H/14 model ('DFN5B-CLIP-ViT-H-14-384').
See 'concept_heads/clip/URL' for usage.
## Concept Embeddings
<p align="center">
<img src="docs/URL" width="800px"/>
Having identified the presence of a user-specific concept within an image, a learned concept embedding representing an object or individual is used to guide the LLM in incorporating the concept into its personalized textual response.
</p>
The concept embeddings are saved as '.pt' files in the following format:
where each entry in the dictionary represents a different checkpoint during the optimization process.
We provide the concept embeddings for personalized captioning using both BLIP-2 and LLaVA.
## License
This sample code is made available by Snap Inc. for non-commercial, academic purposes only.
Please see the full license here.
| [
"# MyVLM\n\nPaper: URL\n \nProject Page: URL\n\nCode: URL",
"# MyVLM Concept Heads & Concept Embeddings\nAs part of our MyVLM code release, we have also released pretrained concept heads and concept embeddings for all 29 objects used in the paper. \n\nThese can be loaded using the 'CLIPConceptHead' class and our inference scripts for reproducing the paper results.\n\nThis repository contains 5 concept heads for each object, representing five different training seeds and sets of images used for training.",
"## Concept Heads\n\n<p align=\"center\">\n<img src=\"docs/concept_head.jpg\" width=\"200px\"/> \nFor each user-specific concept, we introduce an external concept head designed to identify the presence of the concept within an image.\n</p>\n\n\nAs mentioned in the paper, we have two types of concept heads: \n1. A facial recognition model for recognizing individuals\n2. A CLIP-based concept head for recognizing user-specific objects\n\nFor faces, we use the 'buffalo_l' face detection and face recognition model from insightface.\nSee 'concept_heads/face_recognition/URL' for usage.\n\nFor objects, we train a single linear layer over features extracted from a CLIP ViT-H/14 model ('DFN5B-CLIP-ViT-H-14-384'). \nSee 'concept_heads/clip/URL' for usage.",
"## Concept Embeddings\n<p align=\"center\">\n<img src=\"docs/URL\" width=\"800px\"/> \nHaving identified the presence of a user-specific concept within an image, a learned concept embedding representing an object or individual is used to guide the LLM in incorporating the concept into its personalized textual response.\n</p>\n\n\nThe concept embeddings are saved as '.pt' files in the following format: \n\n \nwhere each entry in the dictionary represents a different checkpoint during the optimization process.\n\nWe provide the concept embeddings for personalized captioning using both BLIP-2 and LLaVA.",
"## License\nThis sample code is made available by Snap Inc. for non-commercial, academic purposes only. \nPlease see the full license here."
] | [
"TAGS\n#arxiv-2403.14599 #license-other #region-us \n",
"# MyVLM\n\nPaper: URL\n \nProject Page: URL\n\nCode: URL",
"# MyVLM Concept Heads & Concept Embeddings\nAs part of our MyVLM code release, we have also released pretrained concept heads and concept embeddings for all 29 objects used in the paper. \n\nThese can be loaded using the 'CLIPConceptHead' class and our inference scripts for reproducing the paper results.\n\nThis repository contains 5 concept heads for each object, representing five different training seeds and sets of images used for training.",
"## Concept Heads\n\n<p align=\"center\">\n<img src=\"docs/concept_head.jpg\" width=\"200px\"/> \nFor each user-specific concept, we introduce an external concept head designed to identify the presence of the concept within an image.\n</p>\n\n\nAs mentioned in the paper, we have two types of concept heads: \n1. A facial recognition model for recognizing individuals\n2. A CLIP-based concept head for recognizing user-specific objects\n\nFor faces, we use the 'buffalo_l' face detection and face recognition model from insightface.\nSee 'concept_heads/face_recognition/URL' for usage.\n\nFor objects, we train a single linear layer over features extracted from a CLIP ViT-H/14 model ('DFN5B-CLIP-ViT-H-14-384'). \nSee 'concept_heads/clip/URL' for usage.",
"## Concept Embeddings\n<p align=\"center\">\n<img src=\"docs/URL\" width=\"800px\"/> \nHaving identified the presence of a user-specific concept within an image, a learned concept embedding representing an object or individual is used to guide the LLM in incorporating the concept into its personalized textual response.\n</p>\n\n\nThe concept embeddings are saved as '.pt' files in the following format: \n\n \nwhere each entry in the dictionary represents a different checkpoint during the optimization process.\n\nWe provide the concept embeddings for personalized captioning using both BLIP-2 and LLaVA.",
"## License\nThis sample code is made available by Snap Inc. for non-commercial, academic purposes only. \nPlease see the full license here."
] |
text-generation | mlx |
# mlx-community/Llama-3-8b-64k-PoSE-8bit
This model was converted to MLX format from [`winglian/Llama-3-8b-64k-PoSE`]() using mlx-lm version **0.10.0**.
Refer to the [original model card](https://huggingface.co/winglian/Llama-3-8b-64k-PoSE) for more details on the model.
## Use with mlx
```bash
pip install mlx-lm
```
```python
from mlx_lm import load, generate
model, tokenizer = load("mlx-community/Llama-3-8b-64k-PoSE-8bit")
response = generate(model, tokenizer, prompt="hello", verbose=True)
```
| {"language": ["en"], "tags": ["facebook", "meta", "pytorch", "llama", "llama-3", "mlx"], "pipeline_tag": "text-generation"} | mlx-community/Llama-3-8b-64k-PoSE-8bit | null | [
"mlx",
"safetensors",
"llama",
"facebook",
"meta",
"pytorch",
"llama-3",
"text-generation",
"en",
"region:us"
] | null | 2024-04-25T15:31:48+00:00 | [] | [
"en"
] | TAGS
#mlx #safetensors #llama #facebook #meta #pytorch #llama-3 #text-generation #en #region-us
|
# mlx-community/Llama-3-8b-64k-PoSE-8bit
This model was converted to MLX format from ['winglian/Llama-3-8b-64k-PoSE']() using mlx-lm version 0.10.0.
Refer to the original model card for more details on the model.
## Use with mlx
| [
"# mlx-community/Llama-3-8b-64k-PoSE-8bit\nThis model was converted to MLX format from ['winglian/Llama-3-8b-64k-PoSE']() using mlx-lm version 0.10.0.\nRefer to the original model card for more details on the model.",
"## Use with mlx"
] | [
"TAGS\n#mlx #safetensors #llama #facebook #meta #pytorch #llama-3 #text-generation #en #region-us \n",
"# mlx-community/Llama-3-8b-64k-PoSE-8bit\nThis model was converted to MLX format from ['winglian/Llama-3-8b-64k-PoSE']() using mlx-lm version 0.10.0.\nRefer to the original model card for more details on the model.",
"## Use with mlx"
] |
automatic-speech-recognition | transformers |
<!-- This model card has been generated automatically according to the information the Trainer had access to. You
should probably proofread and complete it, then remove this comment. -->
# wav2vec2-xlsr-53-CV-demo-google-colab-Ezra_William_Prod18
This model is a fine-tuned version of [facebook/wav2vec2-large-xlsr-53](https://huggingface.co/facebook/wav2vec2-large-xlsr-53) on the common_voice_13_0 dataset.
It achieves the following results on the evaluation set:
- Loss: 0.4423
- Wer: 0.3800
## Model description
More information needed
## Intended uses & limitations
More information needed
## Training and evaluation data
More information needed
## Training procedure
### Training hyperparameters
The following hyperparameters were used during training:
- learning_rate: 0.0001
- train_batch_size: 16
- eval_batch_size: 8
- seed: 42
- optimizer: Adam with betas=(0.9,0.999) and epsilon=1e-08
- lr_scheduler_type: linear
- num_epochs: 6
- mixed_precision_training: Native AMP
### Training results
| Training Loss | Epoch | Step | Validation Loss | Wer |
|:-------------:|:-----:|:----:|:---------------:|:------:|
| 0.2865 | 1.0 | 278 | 0.4681 | 0.4721 |
| 0.2346 | 2.0 | 556 | 0.4505 | 0.4318 |
| 0.1898 | 3.0 | 834 | 0.4389 | 0.4084 |
| 0.1606 | 4.0 | 1112 | 0.4209 | 0.3981 |
| 0.1412 | 5.0 | 1390 | 0.4448 | 0.3856 |
| 0.134 | 6.0 | 1668 | 0.4423 | 0.3800 |
### Framework versions
- Transformers 4.40.1
- Pytorch 2.3.0+cu121
- Datasets 2.19.0
- Tokenizers 0.19.1
| {"license": "apache-2.0", "tags": ["generated_from_trainer"], "datasets": ["common_voice_13_0"], "metrics": ["wer"], "base_model": "facebook/wav2vec2-large-xlsr-53", "model-index": [{"name": "wav2vec2-xlsr-53-CV-demo-google-colab-Ezra_William_Prod18", "results": [{"task": {"type": "automatic-speech-recognition", "name": "Automatic Speech Recognition"}, "dataset": {"name": "common_voice_13_0", "type": "common_voice_13_0", "config": "id", "split": "test", "args": "id"}, "metrics": [{"type": "wer", "value": 0.38002396755162243, "name": "Wer"}]}]}]} | EzraWilliam/wav2vec2-xlsr-53-CV-demo-google-colab-Ezra_William_Prod18 | null | [
"transformers",
"tensorboard",
"safetensors",
"wav2vec2",
"automatic-speech-recognition",
"generated_from_trainer",
"dataset:common_voice_13_0",
"base_model:facebook/wav2vec2-large-xlsr-53",
"license:apache-2.0",
"model-index",
"endpoints_compatible",
"region:us"
] | null | 2024-04-25T15:32:43+00:00 | [] | [] | TAGS
#transformers #tensorboard #safetensors #wav2vec2 #automatic-speech-recognition #generated_from_trainer #dataset-common_voice_13_0 #base_model-facebook/wav2vec2-large-xlsr-53 #license-apache-2.0 #model-index #endpoints_compatible #region-us
| wav2vec2-xlsr-53-CV-demo-google-colab-Ezra\_William\_Prod18
===========================================================
This model is a fine-tuned version of facebook/wav2vec2-large-xlsr-53 on the common\_voice\_13\_0 dataset.
It achieves the following results on the evaluation set:
* Loss: 0.4423
* Wer: 0.3800
Model description
-----------------
More information needed
Intended uses & limitations
---------------------------
More information needed
Training and evaluation data
----------------------------
More information needed
Training procedure
------------------
### Training hyperparameters
The following hyperparameters were used during training:
* learning\_rate: 0.0001
* train\_batch\_size: 16
* eval\_batch\_size: 8
* seed: 42
* optimizer: Adam with betas=(0.9,0.999) and epsilon=1e-08
* lr\_scheduler\_type: linear
* num\_epochs: 6
* mixed\_precision\_training: Native AMP
### Training results
### Framework versions
* Transformers 4.40.1
* Pytorch 2.3.0+cu121
* Datasets 2.19.0
* Tokenizers 0.19.1
| [
"### Training hyperparameters\n\n\nThe following hyperparameters were used during training:\n\n\n* learning\\_rate: 0.0001\n* train\\_batch\\_size: 16\n* eval\\_batch\\_size: 8\n* seed: 42\n* optimizer: Adam with betas=(0.9,0.999) and epsilon=1e-08\n* lr\\_scheduler\\_type: linear\n* num\\_epochs: 6\n* mixed\\_precision\\_training: Native AMP",
"### Training results",
"### Framework versions\n\n\n* Transformers 4.40.1\n* Pytorch 2.3.0+cu121\n* Datasets 2.19.0\n* Tokenizers 0.19.1"
] | [
"TAGS\n#transformers #tensorboard #safetensors #wav2vec2 #automatic-speech-recognition #generated_from_trainer #dataset-common_voice_13_0 #base_model-facebook/wav2vec2-large-xlsr-53 #license-apache-2.0 #model-index #endpoints_compatible #region-us \n",
"### Training hyperparameters\n\n\nThe following hyperparameters were used during training:\n\n\n* learning\\_rate: 0.0001\n* train\\_batch\\_size: 16\n* eval\\_batch\\_size: 8\n* seed: 42\n* optimizer: Adam with betas=(0.9,0.999) and epsilon=1e-08\n* lr\\_scheduler\\_type: linear\n* num\\_epochs: 6\n* mixed\\_precision\\_training: Native AMP",
"### Training results",
"### Framework versions\n\n\n* Transformers 4.40.1\n* Pytorch 2.3.0+cu121\n* Datasets 2.19.0\n* Tokenizers 0.19.1"
] |
text-generation | null |
# LaurensVdP/Mistral-7B-Instruct-v0.2-Q8_0-GGUF
This model was converted to GGUF format from [`mistralai/Mistral-7B-Instruct-v0.2`](https://huggingface.co/mistralai/Mistral-7B-Instruct-v0.2) using llama.cpp via the ggml.ai's [GGUF-my-repo](https://huggingface.co/spaces/ggml-org/gguf-my-repo) space.
Refer to the [original model card](https://huggingface.co/mistralai/Mistral-7B-Instruct-v0.2) for more details on the model.
## Use with llama.cpp
Install llama.cpp through brew.
```bash
brew install ggerganov/ggerganov/llama.cpp
```
Invoke the llama.cpp server or the CLI.
CLI:
```bash
llama-cli --hf-repo LaurensVdP/Mistral-7B-Instruct-v0.2-Q8_0-GGUF --model mistral-7b-instruct-v0.2.Q8_0.gguf -p "The meaning to life and the universe is"
```
Server:
```bash
llama-server --hf-repo LaurensVdP/Mistral-7B-Instruct-v0.2-Q8_0-GGUF --model mistral-7b-instruct-v0.2.Q8_0.gguf -c 2048
```
Note: You can also use this checkpoint directly through the [usage steps](https://github.com/ggerganov/llama.cpp?tab=readme-ov-file#usage) listed in the Llama.cpp repo as well.
```
git clone https://github.com/ggerganov/llama.cpp && cd llama.cpp && make && ./main -m mistral-7b-instruct-v0.2.Q8_0.gguf -n 128
```
| {"license": "apache-2.0", "tags": ["finetuned", "llama-cpp", "gguf-my-repo"], "pipeline_tag": "text-generation", "inference": true, "widget": [{"messages": [{"role": "user", "content": "What is your favorite condiment?"}]}]} | LaurensVdP/Mistral-7B-Instruct-v0.2-Q8_0-GGUF | null | [
"gguf",
"finetuned",
"llama-cpp",
"gguf-my-repo",
"text-generation",
"license:apache-2.0",
"region:us"
] | null | 2024-04-25T15:34:11+00:00 | [] | [] | TAGS
#gguf #finetuned #llama-cpp #gguf-my-repo #text-generation #license-apache-2.0 #region-us
|
# LaurensVdP/Mistral-7B-Instruct-v0.2-Q8_0-GGUF
This model was converted to GGUF format from 'mistralai/Mistral-7B-Instruct-v0.2' using URL via the URL's GGUF-my-repo space.
Refer to the original model card for more details on the model.
## Use with URL
Install URL through brew.
Invoke the URL server or the CLI.
CLI:
Server:
Note: You can also use this checkpoint directly through the usage steps listed in the URL repo as well.
| [
"# LaurensVdP/Mistral-7B-Instruct-v0.2-Q8_0-GGUF\nThis model was converted to GGUF format from 'mistralai/Mistral-7B-Instruct-v0.2' using URL via the URL's GGUF-my-repo space.\nRefer to the original model card for more details on the model.",
"## Use with URL\n\nInstall URL through brew.\n\n\nInvoke the URL server or the CLI.\n\nCLI:\n\n\n\nServer:\n\n\n\nNote: You can also use this checkpoint directly through the usage steps listed in the URL repo as well."
] | [
"TAGS\n#gguf #finetuned #llama-cpp #gguf-my-repo #text-generation #license-apache-2.0 #region-us \n",
"# LaurensVdP/Mistral-7B-Instruct-v0.2-Q8_0-GGUF\nThis model was converted to GGUF format from 'mistralai/Mistral-7B-Instruct-v0.2' using URL via the URL's GGUF-my-repo space.\nRefer to the original model card for more details on the model.",
"## Use with URL\n\nInstall URL through brew.\n\n\nInvoke the URL server or the CLI.\n\nCLI:\n\n\n\nServer:\n\n\n\nNote: You can also use this checkpoint directly through the usage steps listed in the URL repo as well."
] |
sentence-similarity | sentence-transformers |
# SentenceTransformer based on distilbert/distilbert-base-uncased
This is a [sentence-transformers](https://www.SBERT.net) model finetuned from [distilbert/distilbert-base-uncased](https://huggingface.co/distilbert/distilbert-base-uncased) on the [sentence-transformers/stsb](https://huggingface.co/datasets/sentence-transformers/stsb) dataset. It maps sentences & paragraphs to a 768-dimensional dense vector space and can be used for semantic textual similarity, semantic search, paraphrase mining, text classification, clustering, and more.
## Model Details
### Model Description
- **Model Type:** Sentence Transformer
- **Base model:** [distilbert/distilbert-base-uncased](https://huggingface.co/distilbert/distilbert-base-uncased) <!-- at revision 6cdc0aad91f5ae2e6712e91bc7b65d1cf5c05411 -->
- **Maximum Sequence Length:** 512 tokens
- **Output Dimensionality:** 768 tokens
- **Similarity Function:** Cosine Similarity
- **Training Dataset:**
- [sentence-transformers/stsb](https://huggingface.co/datasets/sentence-transformers/stsb)
- **Language:** en
<!-- - **License:** Unknown -->
### Model Sources
- **Documentation:** [Sentence Transformers Documentation](https://sbert.net)
- **Repository:** [Sentence Transformers on GitHub](https://github.com/UKPLab/sentence-transformers)
- **Hugging Face:** [Sentence Transformers on Hugging Face](https://huggingface.co/models?library=sentence-transformers)
### Full Model Architecture
```
SentenceTransformer(
(0): Transformer({'max_seq_length': 512, 'do_lower_case': False}) with Transformer model: DistilBertModel
(1): Pooling({'word_embedding_dimension': 768, 'pooling_mode_cls_token': False, 'pooling_mode_mean_tokens': True, 'pooling_mode_max_tokens': False, 'pooling_mode_mean_sqrt_len_tokens': False, 'pooling_mode_weightedmean_tokens': False, 'pooling_mode_lasttoken': False, 'include_prompt': True})
)
```
## Usage
### Direct Usage (Sentence Transformers)
First install the Sentence Transformers library:
```bash
pip install -U sentence-transformers
```
Then you can load this model and run inference.
```python
from sentence_transformers import SentenceTransformer
# Download from the 🤗 Hub
model = SentenceTransformer("sentence_transformers_model_id")
# Run inference
sentences = [
'A woman is dancing.',
'Women are dancing.',
'Two dogs fighting in the snow.',
]
embeddings = model.encode(sentences)
print(embeddings.shape)
# [3, 768]
# Get the similarity scores for the embeddings
similarities = model.similarity(embeddings)
print(similarities.shape)
# [3, 3]
```
<!--
### Direct Usage (Transformers)
<details><summary>Click to see the direct usage in Transformers</summary>
</details>
-->
<!--
### Downstream Usage (Sentence Transformers)
You can finetune this model on your own dataset.
<details><summary>Click to expand</summary>
</details>
-->
<!--
### Out-of-Scope Use
*List how the model may foreseeably be misused and address what users ought not to do with the model.*
-->
## Evaluation
### Metrics
#### Semantic Similarity
* Dataset: `sts-dev`
* Evaluated with [<code>EmbeddingSimilarityEvaluator</code>](https://sbert.net/docs/package_reference/evaluation.html#sentence_transformers.evaluation.EmbeddingSimilarityEvaluator)
| Metric | Value |
|:--------------------|:-----------|
| pearson_cosine | 0.8652 |
| **spearman_cosine** | **0.8728** |
| pearson_manhattan | 0.8626 |
| spearman_manhattan | 0.8641 |
| pearson_euclidean | 0.863 |
| spearman_euclidean | 0.8649 |
| pearson_dot | 0.7647 |
| spearman_dot | 0.7749 |
| pearson_max | 0.8652 |
| spearman_max | 0.8728 |
#### Semantic Similarity
* Dataset: `sts-test`
* Evaluated with [<code>EmbeddingSimilarityEvaluator</code>](https://sbert.net/docs/package_reference/evaluation.html#sentence_transformers.evaluation.EmbeddingSimilarityEvaluator)
| Metric | Value |
|:--------------------|:-----------|
| pearson_cosine | 0.8354 |
| **spearman_cosine** | **0.8456** |
| pearson_manhattan | 0.8492 |
| spearman_manhattan | 0.8451 |
| pearson_euclidean | 0.8494 |
| spearman_euclidean | 0.8449 |
| pearson_dot | 0.6924 |
| spearman_dot | 0.6794 |
| pearson_max | 0.8494 |
| spearman_max | 0.8456 |
<!--
## Bias, Risks and Limitations
*What are the known or foreseeable issues stemming from this model? You could also flag here known failure cases or weaknesses of the model.*
-->
<!--
### Recommendations
*What are recommendations with respect to the foreseeable issues? For example, filtering explicit content.*
-->
## Training Details
### Training Dataset
#### sentence-transformers/stsb
* Dataset: [sentence-transformers/stsb](https://huggingface.co/datasets/sentence-transformers/stsb) at [d999f12](https://huggingface.co/datasets/sentence-transformers/stsb/tree/d999f12281623b0925506817d9bd85e88289218a)
* Size: 5,749 training samples
* Columns: <code>sentence1</code>, <code>sentence2</code>, and <code>score</code>
* Approximate statistics based on the first 1000 samples:
| | sentence1 | sentence2 | score |
|:--------|:---------------------------------------------------------------------------------|:---------------------------------------------------------------------------------|:---------------------------------------------------------------|
| type | string | string | float |
| details | <ul><li>min: 6 tokens</li><li>mean: 10.0 tokens</li><li>max: 28 tokens</li></ul> | <ul><li>min: 5 tokens</li><li>mean: 9.95 tokens</li><li>max: 25 tokens</li></ul> | <ul><li>min: 0.0</li><li>mean: 0.54</li><li>max: 1.0</li></ul> |
* Samples:
| sentence1 | sentence2 | score |
|:-----------------------------------------------------------|:----------------------------------------------------------------------|:------------------|
| <code>A plane is taking off.</code> | <code>An air plane is taking off.</code> | <code>1.0</code> |
| <code>A man is playing a large flute.</code> | <code>A man is playing a flute.</code> | <code>0.76</code> |
| <code>A man is spreading shreded cheese on a pizza.</code> | <code>A man is spreading shredded cheese on an uncooked pizza.</code> | <code>0.76</code> |
* Loss: [<code>AdaptiveLayerLoss</code>](https://sbert.net/docs/package_reference/losses.html#adaptivelayerloss) with these parameters:
```json
{
"loss": "CoSENTLoss",
"n_layers_per_step": 1,
"last_layer_weight": 1.0,
"prior_layers_weight": 1.0,
"kl_div_weight": 1.0,
"kl_temperature": 0.3
}
```
### Evaluation Dataset
#### sentence-transformers/stsb
* Dataset: [sentence-transformers/stsb](https://huggingface.co/datasets/sentence-transformers/stsb) at [d999f12](https://huggingface.co/datasets/sentence-transformers/stsb/tree/d999f12281623b0925506817d9bd85e88289218a)
* Size: 1,500 evaluation samples
* Columns: <code>sentence1</code>, <code>sentence2</code>, and <code>score</code>
* Approximate statistics based on the first 1000 samples:
| | sentence1 | sentence2 | score |
|:--------|:---------------------------------------------------------------------------------|:----------------------------------------------------------------------------------|:---------------------------------------------------------------|
| type | string | string | float |
| details | <ul><li>min: 5 tokens</li><li>mean: 15.1 tokens</li><li>max: 45 tokens</li></ul> | <ul><li>min: 6 tokens</li><li>mean: 15.11 tokens</li><li>max: 53 tokens</li></ul> | <ul><li>min: 0.0</li><li>mean: 0.47</li><li>max: 1.0</li></ul> |
* Samples:
| sentence1 | sentence2 | score |
|:--------------------------------------------------|:------------------------------------------------------|:------------------|
| <code>A man with a hard hat is dancing.</code> | <code>A man wearing a hard hat is dancing.</code> | <code>1.0</code> |
| <code>A young child is riding a horse.</code> | <code>A child is riding a horse.</code> | <code>0.95</code> |
| <code>A man is feeding a mouse to a snake.</code> | <code>The man is feeding a mouse to the snake.</code> | <code>1.0</code> |
* Loss: [<code>AdaptiveLayerLoss</code>](https://sbert.net/docs/package_reference/losses.html#adaptivelayerloss) with these parameters:
```json
{
"loss": "CoSENTLoss",
"n_layers_per_step": 1,
"last_layer_weight": 1.0,
"prior_layers_weight": 1.0,
"kl_div_weight": 1.0,
"kl_temperature": 0.3
}
```
### Training Hyperparameters
#### Non-Default Hyperparameters
- `eval_strategy`: steps
- `per_device_train_batch_size`: 16
- `per_device_eval_batch_size`: 16
- `num_train_epochs`: 4
- `warmup_ratio`: 0.1
- `fp16`: True
#### All Hyperparameters
<details><summary>Click to expand</summary>
- `overwrite_output_dir`: False
- `do_predict`: False
- `eval_strategy`: steps
- `prediction_loss_only`: False
- `per_device_train_batch_size`: 16
- `per_device_eval_batch_size`: 16
- `per_gpu_train_batch_size`: None
- `per_gpu_eval_batch_size`: None
- `gradient_accumulation_steps`: 1
- `eval_accumulation_steps`: None
- `learning_rate`: 5e-05
- `weight_decay`: 0.0
- `adam_beta1`: 0.9
- `adam_beta2`: 0.999
- `adam_epsilon`: 1e-08
- `max_grad_norm`: 1.0
- `num_train_epochs`: 4
- `max_steps`: -1
- `lr_scheduler_type`: linear
- `lr_scheduler_kwargs`: {}
- `warmup_ratio`: 0.1
- `warmup_steps`: 0
- `log_level`: passive
- `log_level_replica`: warning
- `log_on_each_node`: True
- `logging_nan_inf_filter`: True
- `save_safetensors`: True
- `save_on_each_node`: False
- `save_only_model`: False
- `no_cuda`: False
- `use_cpu`: False
- `use_mps_device`: False
- `seed`: 42
- `data_seed`: None
- `jit_mode_eval`: False
- `use_ipex`: False
- `bf16`: False
- `fp16`: True
- `fp16_opt_level`: O1
- `half_precision_backend`: auto
- `bf16_full_eval`: False
- `fp16_full_eval`: False
- `tf32`: None
- `local_rank`: 0
- `ddp_backend`: None
- `tpu_num_cores`: None
- `tpu_metrics_debug`: False
- `debug`: []
- `dataloader_drop_last`: False
- `dataloader_num_workers`: 0
- `dataloader_prefetch_factor`: None
- `past_index`: -1
- `disable_tqdm`: False
- `remove_unused_columns`: True
- `label_names`: None
- `load_best_model_at_end`: False
- `ignore_data_skip`: False
- `fsdp`: []
- `fsdp_min_num_params`: 0
- `fsdp_config`: {'min_num_params': 0, 'xla': False, 'xla_fsdp_v2': False, 'xla_fsdp_grad_ckpt': False}
- `fsdp_transformer_layer_cls_to_wrap`: None
- `accelerator_config`: {'split_batches': False, 'dispatch_batches': None, 'even_batches': True, 'use_seedable_sampler': True, 'non_blocking': False, 'gradient_accumulation_kwargs': None}
- `deepspeed`: None
- `label_smoothing_factor`: 0.0
- `optim`: adamw_torch
- `optim_args`: None
- `adafactor`: False
- `group_by_length`: False
- `length_column_name`: length
- `ddp_find_unused_parameters`: None
- `ddp_bucket_cap_mb`: None
- `ddp_broadcast_buffers`: None
- `dataloader_pin_memory`: True
- `dataloader_persistent_workers`: False
- `skip_memory_metrics`: True
- `use_legacy_prediction_loop`: False
- `push_to_hub`: False
- `resume_from_checkpoint`: None
- `hub_model_id`: None
- `hub_strategy`: every_save
- `hub_private_repo`: False
- `hub_always_push`: False
- `gradient_checkpointing`: False
- `gradient_checkpointing_kwargs`: None
- `include_inputs_for_metrics`: False
- `eval_do_concat_batches`: True
- `fp16_backend`: auto
- `push_to_hub_model_id`: None
- `push_to_hub_organization`: None
- `mp_parameters`:
- `auto_find_batch_size`: False
- `full_determinism`: False
- `torchdynamo`: None
- `ray_scope`: last
- `ddp_timeout`: 1800
- `torch_compile`: False
- `torch_compile_backend`: None
- `torch_compile_mode`: None
- `dispatch_batches`: None
- `split_batches`: None
- `include_tokens_per_second`: False
- `include_num_input_tokens_seen`: False
- `neftune_noise_alpha`: None
- `optim_target_modules`: None
- `batch_sampler`: batch_sampler
- `multi_dataset_batch_sampler`: proportional
</details>
### Training Logs
| Epoch | Step | Training Loss | loss | sts-dev_spearman_cosine | sts-test_spearman_cosine |
|:------:|:----:|:-------------:|:------:|:-----------------------:|:------------------------:|
| 0.2778 | 100 | 6.6822 | 6.2966 | 0.8433 | - |
| 0.5556 | 200 | 6.6943 | 6.6898 | 0.8450 | - |
| 0.8333 | 300 | 6.4234 | 6.7096 | 0.8555 | - |
| 1.1111 | 400 | 6.1543 | 6.6157 | 0.8590 | - |
| 1.3889 | 500 | 6.3869 | 6.4068 | 0.8596 | - |
| 1.6667 | 600 | 6.2925 | 6.4920 | 0.8597 | - |
| 1.9444 | 700 | 6.2973 | 6.3890 | 0.8658 | - |
| 2.2222 | 800 | 6.0865 | 6.8754 | 0.8683 | - |
| 2.5 | 900 | 5.6631 | 6.7812 | 0.8674 | - |
| 2.7778 | 1000 | 5.9954 | 6.8150 | 0.8684 | - |
| 3.0556 | 1100 | 5.6617 | 6.8462 | 0.8693 | - |
| 3.3333 | 1200 | 5.3529 | 7.2448 | 0.8702 | - |
| 3.6111 | 1300 | 5.3467 | 7.1615 | 0.8723 | - |
| 3.8889 | 1400 | 5.6536 | 7.3408 | 0.8728 | - |
| 4.0 | 1440 | - | - | - | 0.8456 |
### Environmental Impact
Carbon emissions were measured using [CodeCarbon](https://github.com/mlco2/codecarbon).
- **Energy Consumed**: 0.013 kWh
- **Carbon Emitted**: 0.005 kg of CO2
- **Hours Used**: 0.069 hours
### Training Hardware
- **On Cloud**: No
- **GPU Model**: 1 x NVIDIA GeForce RTX 3090
- **CPU Model**: 13th Gen Intel(R) Core(TM) i7-13700K
- **RAM Size**: 31.78 GB
### Framework Versions
- Python: 3.11.6
- Sentence Transformers: 3.0.0.dev0
- Transformers: 4.41.0.dev0
- PyTorch: 2.3.0+cu121
- Accelerate: 0.26.1
- Datasets: 2.18.0
- Tokenizers: 0.19.1
## Citation
### BibTeX
#### Sentence Transformers
```bibtex
@inproceedings{reimers-2019-sentence-bert,
title = "Sentence-BERT: Sentence Embeddings using Siamese BERT-Networks",
author = "Reimers, Nils and Gurevych, Iryna",
booktitle = "Proceedings of the 2019 Conference on Empirical Methods in Natural Language Processing",
month = "11",
year = "2019",
publisher = "Association for Computational Linguistics",
url = "https://arxiv.org/abs/1908.10084",
}
```
#### AdaptiveLayerLoss
```bibtex
@misc{li20242d,
title={2D Matryoshka Sentence Embeddings},
author={Xianming Li and Zongxi Li and Jing Li and Haoran Xie and Qing Li},
year={2024},
eprint={2402.14776},
archivePrefix={arXiv},
primaryClass={cs.CL}
}
```
#### CoSENTLoss
```bibtex
@online{kexuefm-8847,
title={CoSENT: A more efficient sentence vector scheme than Sentence-BERT},
author={Su Jianlin},
year={2022},
month={Jan},
url={https://kexue.fm/archives/8847},
}
```
<!--
## Glossary
*Clearly define terms in order to be accessible across audiences.*
-->
<!--
## Model Card Authors
*Lists the people who create the model card, providing recognition and accountability for the detailed work that goes into its construction.*
-->
<!--
## Model Card Contact
*Provides a way for people who have updates to the Model Card, suggestions, or questions, to contact the Model Card authors.*
--> | {"language": ["en"], "library_name": "sentence-transformers", "tags": ["sentence-transformers", "sentence-similarity", "feature-extraction", "loss:AdaptiveLayerLoss", "loss:CoSENTLoss"], "metrics": ["pearson_cosine", "spearman_cosine", "pearson_manhattan", "spearman_manhattan", "pearson_euclidean", "spearman_euclidean", "pearson_dot", "spearman_dot", "pearson_max", "spearman_max"], "base_model": "distilbert/distilbert-base-uncased", "widget": [{"source_sentence": "A man is speaking.", "sentences": ["A man is talking.", "Breivik complains of 'ridicule'", "The dogs are chasing a cat."]}, {"source_sentence": "A plane is landing.", "sentences": ["A animated airplane is landing.", "Three humans are walking a dog.", "Turkey's PM Warns Against Protests"]}, {"source_sentence": "A plane in the sky.", "sentences": ["Two airplanes in the sky.", "A guy is playing an instrument.", "Obama urges no new sanctions on Iran"]}, {"source_sentence": "A boy is vacuuming.", "sentences": ["A little boy is vacuuming the floor.", "Two dogs fighting in the snow.", "Gunmen 'kill 10 tourists' in Kashmir"]}, {"source_sentence": "A woman is dancing.", "sentences": ["Women are dancing.", "Two dogs fighting in the snow.", "A dog digs a hole in a yard."]}], "pipeline_tag": "sentence-similarity", "co2_eq_emissions": {"emissions": 5.048832905925286, "energy_consumed": 0.012988955307472783, "source": "codecarbon", "training_type": "fine-tuning", "on_cloud": false, "cpu_model": "13th Gen Intel(R) Core(TM) i7-13700K", "ram_total_size": 31.777088165283203, "hours_used": 0.069, "hardware_used": "1 x NVIDIA GeForce RTX 3090"}, "model-index": [{"name": "SentenceTransformer based on distilbert/distilbert-base-uncased", "results": [{"task": {"type": "semantic-similarity", "name": "Semantic Similarity"}, "dataset": {"name": "sts dev", "type": "sts-dev"}, "metrics": [{"type": "pearson_cosine", "value": 0.8652370775930345, "name": "Pearson Cosine"}, {"type": "spearman_cosine", "value": 0.8727506004002163, "name": "Spearman Cosine"}, {"type": "pearson_manhattan", "value": 0.8625714457714474, "name": "Pearson Manhattan"}, {"type": "spearman_manhattan", "value": 0.8640763670277021, "name": "Spearman Manhattan"}, {"type": "pearson_euclidean", "value": 0.8629790773940799, "name": "Pearson Euclidean"}, {"type": "spearman_euclidean", "value": 0.8648628595939388, "name": "Spearman Euclidean"}, {"type": "pearson_dot", "value": 0.7647366616229355, "name": "Pearson Dot"}, {"type": "spearman_dot", "value": 0.7748666009336691, "name": "Spearman Dot"}, {"type": "pearson_max", "value": 0.8652370775930345, "name": "Pearson Max"}, {"type": "spearman_max", "value": 0.8727506004002163, "name": "Spearman Max"}]}, {"task": {"type": "semantic-similarity", "name": "Semantic Similarity"}, "dataset": {"name": "sts test", "type": "sts-test"}, "metrics": [{"type": "pearson_cosine", "value": 0.8353553575743735, "name": "Pearson Cosine"}, {"type": "spearman_cosine", "value": 0.8456023773246713, "name": "Spearman Cosine"}, {"type": "pearson_manhattan", "value": 0.8492310055929263, "name": "Pearson Manhattan"}, {"type": "spearman_manhattan", "value": 0.8451007047564367, "name": "Spearman Manhattan"}, {"type": "pearson_euclidean", "value": 0.8493640569080374, "name": "Pearson Euclidean"}, {"type": "spearman_euclidean", "value": 0.8449411972438509, "name": "Spearman Euclidean"}, {"type": "pearson_dot", "value": 0.6924412597499117, "name": "Pearson Dot"}, {"type": "spearman_dot", "value": 0.6793562175238733, "name": "Spearman Dot"}, {"type": "pearson_max", "value": 0.8493640569080374, "name": "Pearson Max"}, {"type": "spearman_max", "value": 0.8456023773246713, "name": "Spearman Max"}]}]}]} | tomaarsen/distilbert-base-uncased-sts-adaptive-layer | null | [
"sentence-transformers",
"safetensors",
"distilbert",
"sentence-similarity",
"feature-extraction",
"loss:AdaptiveLayerLoss",
"loss:CoSENTLoss",
"en",
"arxiv:1908.10084",
"arxiv:2402.14776",
"base_model:distilbert/distilbert-base-uncased",
"model-index",
"co2_eq_emissions",
"endpoints_compatible",
"region:us"
] | null | 2024-04-25T15:34:57+00:00 | [
"1908.10084",
"2402.14776"
] | [
"en"
] | TAGS
#sentence-transformers #safetensors #distilbert #sentence-similarity #feature-extraction #loss-AdaptiveLayerLoss #loss-CoSENTLoss #en #arxiv-1908.10084 #arxiv-2402.14776 #base_model-distilbert/distilbert-base-uncased #model-index #co2_eq_emissions #endpoints_compatible #region-us
| SentenceTransformer based on distilbert/distilbert-base-uncased
===============================================================
This is a sentence-transformers model finetuned from distilbert/distilbert-base-uncased on the sentence-transformers/stsb dataset. It maps sentences & paragraphs to a 768-dimensional dense vector space and can be used for semantic textual similarity, semantic search, paraphrase mining, text classification, clustering, and more.
Model Details
-------------
### Model Description
* Model Type: Sentence Transformer
* Base model: distilbert/distilbert-base-uncased
* Maximum Sequence Length: 512 tokens
* Output Dimensionality: 768 tokens
* Similarity Function: Cosine Similarity
* Training Dataset:
+ sentence-transformers/stsb
* Language: en
### Model Sources
* Documentation: Sentence Transformers Documentation
* Repository: Sentence Transformers on GitHub
* Hugging Face: Sentence Transformers on Hugging Face
### Full Model Architecture
Usage
-----
### Direct Usage (Sentence Transformers)
First install the Sentence Transformers library:
Then you can load this model and run inference.
Evaluation
----------
### Metrics
#### Semantic Similarity
* Dataset: 'sts-dev'
* Evaluated with `EmbeddingSimilarityEvaluator`
#### Semantic Similarity
* Dataset: 'sts-test'
* Evaluated with `EmbeddingSimilarityEvaluator`
Training Details
----------------
### Training Dataset
#### sentence-transformers/stsb
* Dataset: sentence-transformers/stsb at d999f12
* Size: 5,749 training samples
* Columns: `sentence1`, `sentence2`, and `score`
* Approximate statistics based on the first 1000 samples:
* Samples:
* Loss: `AdaptiveLayerLoss` with these parameters:
### Evaluation Dataset
#### sentence-transformers/stsb
* Dataset: sentence-transformers/stsb at d999f12
* Size: 1,500 evaluation samples
* Columns: `sentence1`, `sentence2`, and `score`
* Approximate statistics based on the first 1000 samples:
* Samples:
* Loss: `AdaptiveLayerLoss` with these parameters:
### Training Hyperparameters
#### Non-Default Hyperparameters
* 'eval\_strategy': steps
* 'per\_device\_train\_batch\_size': 16
* 'per\_device\_eval\_batch\_size': 16
* 'num\_train\_epochs': 4
* 'warmup\_ratio': 0.1
* 'fp16': True
#### All Hyperparameters
Click to expand
* 'overwrite\_output\_dir': False
* 'do\_predict': False
* 'eval\_strategy': steps
* 'prediction\_loss\_only': False
* 'per\_device\_train\_batch\_size': 16
* 'per\_device\_eval\_batch\_size': 16
* 'per\_gpu\_train\_batch\_size': None
* 'per\_gpu\_eval\_batch\_size': None
* 'gradient\_accumulation\_steps': 1
* 'eval\_accumulation\_steps': None
* 'learning\_rate': 5e-05
* 'weight\_decay': 0.0
* 'adam\_beta1': 0.9
* 'adam\_beta2': 0.999
* 'adam\_epsilon': 1e-08
* 'max\_grad\_norm': 1.0
* 'num\_train\_epochs': 4
* 'max\_steps': -1
* 'lr\_scheduler\_type': linear
* 'lr\_scheduler\_kwargs': {}
* 'warmup\_ratio': 0.1
* 'warmup\_steps': 0
* 'log\_level': passive
* 'log\_level\_replica': warning
* 'log\_on\_each\_node': True
* 'logging\_nan\_inf\_filter': True
* 'save\_safetensors': True
* 'save\_on\_each\_node': False
* 'save\_only\_model': False
* 'no\_cuda': False
* 'use\_cpu': False
* 'use\_mps\_device': False
* 'seed': 42
* 'data\_seed': None
* 'jit\_mode\_eval': False
* 'use\_ipex': False
* 'bf16': False
* 'fp16': True
* 'fp16\_opt\_level': O1
* 'half\_precision\_backend': auto
* 'bf16\_full\_eval': False
* 'fp16\_full\_eval': False
* 'tf32': None
* 'local\_rank': 0
* 'ddp\_backend': None
* 'tpu\_num\_cores': None
* 'tpu\_metrics\_debug': False
* 'debug': []
* 'dataloader\_drop\_last': False
* 'dataloader\_num\_workers': 0
* 'dataloader\_prefetch\_factor': None
* 'past\_index': -1
* 'disable\_tqdm': False
* 'remove\_unused\_columns': True
* 'label\_names': None
* 'load\_best\_model\_at\_end': False
* 'ignore\_data\_skip': False
* 'fsdp': []
* 'fsdp\_min\_num\_params': 0
* 'fsdp\_config': {'min\_num\_params': 0, 'xla': False, 'xla\_fsdp\_v2': False, 'xla\_fsdp\_grad\_ckpt': False}
* 'fsdp\_transformer\_layer\_cls\_to\_wrap': None
* 'accelerator\_config': {'split\_batches': False, 'dispatch\_batches': None, 'even\_batches': True, 'use\_seedable\_sampler': True, 'non\_blocking': False, 'gradient\_accumulation\_kwargs': None}
* 'deepspeed': None
* 'label\_smoothing\_factor': 0.0
* 'optim': adamw\_torch
* 'optim\_args': None
* 'adafactor': False
* 'group\_by\_length': False
* 'length\_column\_name': length
* 'ddp\_find\_unused\_parameters': None
* 'ddp\_bucket\_cap\_mb': None
* 'ddp\_broadcast\_buffers': None
* 'dataloader\_pin\_memory': True
* 'dataloader\_persistent\_workers': False
* 'skip\_memory\_metrics': True
* 'use\_legacy\_prediction\_loop': False
* 'push\_to\_hub': False
* 'resume\_from\_checkpoint': None
* 'hub\_model\_id': None
* 'hub\_strategy': every\_save
* 'hub\_private\_repo': False
* 'hub\_always\_push': False
* 'gradient\_checkpointing': False
* 'gradient\_checkpointing\_kwargs': None
* 'include\_inputs\_for\_metrics': False
* 'eval\_do\_concat\_batches': True
* 'fp16\_backend': auto
* 'push\_to\_hub\_model\_id': None
* 'push\_to\_hub\_organization': None
* 'mp\_parameters':
* 'auto\_find\_batch\_size': False
* 'full\_determinism': False
* 'torchdynamo': None
* 'ray\_scope': last
* 'ddp\_timeout': 1800
* 'torch\_compile': False
* 'torch\_compile\_backend': None
* 'torch\_compile\_mode': None
* 'dispatch\_batches': None
* 'split\_batches': None
* 'include\_tokens\_per\_second': False
* 'include\_num\_input\_tokens\_seen': False
* 'neftune\_noise\_alpha': None
* 'optim\_target\_modules': None
* 'batch\_sampler': batch\_sampler
* 'multi\_dataset\_batch\_sampler': proportional
### Training Logs
### Environmental Impact
Carbon emissions were measured using CodeCarbon.
* Energy Consumed: 0.013 kWh
* Carbon Emitted: 0.005 kg of CO2
* Hours Used: 0.069 hours
### Training Hardware
* On Cloud: No
* GPU Model: 1 x NVIDIA GeForce RTX 3090
* CPU Model: 13th Gen Intel(R) Core(TM) i7-13700K
* RAM Size: 31.78 GB
### Framework Versions
* Python: 3.11.6
* Sentence Transformers: 3.0.0.dev0
* Transformers: 4.41.0.dev0
* PyTorch: 2.3.0+cu121
* Accelerate: 0.26.1
* Datasets: 2.18.0
* Tokenizers: 0.19.1
### BibTeX
#### Sentence Transformers
#### AdaptiveLayerLoss
#### CoSENTLoss
| [
"### Model Description\n\n\n* Model Type: Sentence Transformer\n* Base model: distilbert/distilbert-base-uncased\n* Maximum Sequence Length: 512 tokens\n* Output Dimensionality: 768 tokens\n* Similarity Function: Cosine Similarity\n* Training Dataset:\n\t+ sentence-transformers/stsb\n* Language: en",
"### Model Sources\n\n\n* Documentation: Sentence Transformers Documentation\n* Repository: Sentence Transformers on GitHub\n* Hugging Face: Sentence Transformers on Hugging Face",
"### Full Model Architecture\n\n\nUsage\n-----",
"### Direct Usage (Sentence Transformers)\n\n\nFirst install the Sentence Transformers library:\n\n\nThen you can load this model and run inference.\n\n\nEvaluation\n----------",
"### Metrics",
"#### Semantic Similarity\n\n\n* Dataset: 'sts-dev'\n* Evaluated with `EmbeddingSimilarityEvaluator`",
"#### Semantic Similarity\n\n\n* Dataset: 'sts-test'\n* Evaluated with `EmbeddingSimilarityEvaluator`\n\n\n\nTraining Details\n----------------",
"### Training Dataset",
"#### sentence-transformers/stsb\n\n\n* Dataset: sentence-transformers/stsb at d999f12\n* Size: 5,749 training samples\n* Columns: `sentence1`, `sentence2`, and `score`\n* Approximate statistics based on the first 1000 samples:\n* Samples:\n* Loss: `AdaptiveLayerLoss` with these parameters:",
"### Evaluation Dataset",
"#### sentence-transformers/stsb\n\n\n* Dataset: sentence-transformers/stsb at d999f12\n* Size: 1,500 evaluation samples\n* Columns: `sentence1`, `sentence2`, and `score`\n* Approximate statistics based on the first 1000 samples:\n* Samples:\n* Loss: `AdaptiveLayerLoss` with these parameters:",
"### Training Hyperparameters",
"#### Non-Default Hyperparameters\n\n\n* 'eval\\_strategy': steps\n* 'per\\_device\\_train\\_batch\\_size': 16\n* 'per\\_device\\_eval\\_batch\\_size': 16\n* 'num\\_train\\_epochs': 4\n* 'warmup\\_ratio': 0.1\n* 'fp16': True",
"#### All Hyperparameters\n\n\nClick to expand\n* 'overwrite\\_output\\_dir': False\n* 'do\\_predict': False\n* 'eval\\_strategy': steps\n* 'prediction\\_loss\\_only': False\n* 'per\\_device\\_train\\_batch\\_size': 16\n* 'per\\_device\\_eval\\_batch\\_size': 16\n* 'per\\_gpu\\_train\\_batch\\_size': None\n* 'per\\_gpu\\_eval\\_batch\\_size': None\n* 'gradient\\_accumulation\\_steps': 1\n* 'eval\\_accumulation\\_steps': None\n* 'learning\\_rate': 5e-05\n* 'weight\\_decay': 0.0\n* 'adam\\_beta1': 0.9\n* 'adam\\_beta2': 0.999\n* 'adam\\_epsilon': 1e-08\n* 'max\\_grad\\_norm': 1.0\n* 'num\\_train\\_epochs': 4\n* 'max\\_steps': -1\n* 'lr\\_scheduler\\_type': linear\n* 'lr\\_scheduler\\_kwargs': {}\n* 'warmup\\_ratio': 0.1\n* 'warmup\\_steps': 0\n* 'log\\_level': passive\n* 'log\\_level\\_replica': warning\n* 'log\\_on\\_each\\_node': True\n* 'logging\\_nan\\_inf\\_filter': True\n* 'save\\_safetensors': True\n* 'save\\_on\\_each\\_node': False\n* 'save\\_only\\_model': False\n* 'no\\_cuda': False\n* 'use\\_cpu': False\n* 'use\\_mps\\_device': False\n* 'seed': 42\n* 'data\\_seed': None\n* 'jit\\_mode\\_eval': False\n* 'use\\_ipex': False\n* 'bf16': False\n* 'fp16': True\n* 'fp16\\_opt\\_level': O1\n* 'half\\_precision\\_backend': auto\n* 'bf16\\_full\\_eval': False\n* 'fp16\\_full\\_eval': False\n* 'tf32': None\n* 'local\\_rank': 0\n* 'ddp\\_backend': None\n* 'tpu\\_num\\_cores': None\n* 'tpu\\_metrics\\_debug': False\n* 'debug': []\n* 'dataloader\\_drop\\_last': False\n* 'dataloader\\_num\\_workers': 0\n* 'dataloader\\_prefetch\\_factor': None\n* 'past\\_index': -1\n* 'disable\\_tqdm': False\n* 'remove\\_unused\\_columns': True\n* 'label\\_names': None\n* 'load\\_best\\_model\\_at\\_end': False\n* 'ignore\\_data\\_skip': False\n* 'fsdp': []\n* 'fsdp\\_min\\_num\\_params': 0\n* 'fsdp\\_config': {'min\\_num\\_params': 0, 'xla': False, 'xla\\_fsdp\\_v2': False, 'xla\\_fsdp\\_grad\\_ckpt': False}\n* 'fsdp\\_transformer\\_layer\\_cls\\_to\\_wrap': None\n* 'accelerator\\_config': {'split\\_batches': False, 'dispatch\\_batches': None, 'even\\_batches': True, 'use\\_seedable\\_sampler': True, 'non\\_blocking': False, 'gradient\\_accumulation\\_kwargs': None}\n* 'deepspeed': None\n* 'label\\_smoothing\\_factor': 0.0\n* 'optim': adamw\\_torch\n* 'optim\\_args': None\n* 'adafactor': False\n* 'group\\_by\\_length': False\n* 'length\\_column\\_name': length\n* 'ddp\\_find\\_unused\\_parameters': None\n* 'ddp\\_bucket\\_cap\\_mb': None\n* 'ddp\\_broadcast\\_buffers': None\n* 'dataloader\\_pin\\_memory': True\n* 'dataloader\\_persistent\\_workers': False\n* 'skip\\_memory\\_metrics': True\n* 'use\\_legacy\\_prediction\\_loop': False\n* 'push\\_to\\_hub': False\n* 'resume\\_from\\_checkpoint': None\n* 'hub\\_model\\_id': None\n* 'hub\\_strategy': every\\_save\n* 'hub\\_private\\_repo': False\n* 'hub\\_always\\_push': False\n* 'gradient\\_checkpointing': False\n* 'gradient\\_checkpointing\\_kwargs': None\n* 'include\\_inputs\\_for\\_metrics': False\n* 'eval\\_do\\_concat\\_batches': True\n* 'fp16\\_backend': auto\n* 'push\\_to\\_hub\\_model\\_id': None\n* 'push\\_to\\_hub\\_organization': None\n* 'mp\\_parameters':\n* 'auto\\_find\\_batch\\_size': False\n* 'full\\_determinism': False\n* 'torchdynamo': None\n* 'ray\\_scope': last\n* 'ddp\\_timeout': 1800\n* 'torch\\_compile': False\n* 'torch\\_compile\\_backend': None\n* 'torch\\_compile\\_mode': None\n* 'dispatch\\_batches': None\n* 'split\\_batches': None\n* 'include\\_tokens\\_per\\_second': False\n* 'include\\_num\\_input\\_tokens\\_seen': False\n* 'neftune\\_noise\\_alpha': None\n* 'optim\\_target\\_modules': None\n* 'batch\\_sampler': batch\\_sampler\n* 'multi\\_dataset\\_batch\\_sampler': proportional",
"### Training Logs",
"### Environmental Impact\n\n\nCarbon emissions were measured using CodeCarbon.\n\n\n* Energy Consumed: 0.013 kWh\n* Carbon Emitted: 0.005 kg of CO2\n* Hours Used: 0.069 hours",
"### Training Hardware\n\n\n* On Cloud: No\n* GPU Model: 1 x NVIDIA GeForce RTX 3090\n* CPU Model: 13th Gen Intel(R) Core(TM) i7-13700K\n* RAM Size: 31.78 GB",
"### Framework Versions\n\n\n* Python: 3.11.6\n* Sentence Transformers: 3.0.0.dev0\n* Transformers: 4.41.0.dev0\n* PyTorch: 2.3.0+cu121\n* Accelerate: 0.26.1\n* Datasets: 2.18.0\n* Tokenizers: 0.19.1",
"### BibTeX",
"#### Sentence Transformers",
"#### AdaptiveLayerLoss",
"#### CoSENTLoss"
] | [
"TAGS\n#sentence-transformers #safetensors #distilbert #sentence-similarity #feature-extraction #loss-AdaptiveLayerLoss #loss-CoSENTLoss #en #arxiv-1908.10084 #arxiv-2402.14776 #base_model-distilbert/distilbert-base-uncased #model-index #co2_eq_emissions #endpoints_compatible #region-us \n",
"### Model Description\n\n\n* Model Type: Sentence Transformer\n* Base model: distilbert/distilbert-base-uncased\n* Maximum Sequence Length: 512 tokens\n* Output Dimensionality: 768 tokens\n* Similarity Function: Cosine Similarity\n* Training Dataset:\n\t+ sentence-transformers/stsb\n* Language: en",
"### Model Sources\n\n\n* Documentation: Sentence Transformers Documentation\n* Repository: Sentence Transformers on GitHub\n* Hugging Face: Sentence Transformers on Hugging Face",
"### Full Model Architecture\n\n\nUsage\n-----",
"### Direct Usage (Sentence Transformers)\n\n\nFirst install the Sentence Transformers library:\n\n\nThen you can load this model and run inference.\n\n\nEvaluation\n----------",
"### Metrics",
"#### Semantic Similarity\n\n\n* Dataset: 'sts-dev'\n* Evaluated with `EmbeddingSimilarityEvaluator`",
"#### Semantic Similarity\n\n\n* Dataset: 'sts-test'\n* Evaluated with `EmbeddingSimilarityEvaluator`\n\n\n\nTraining Details\n----------------",
"### Training Dataset",
"#### sentence-transformers/stsb\n\n\n* Dataset: sentence-transformers/stsb at d999f12\n* Size: 5,749 training samples\n* Columns: `sentence1`, `sentence2`, and `score`\n* Approximate statistics based on the first 1000 samples:\n* Samples:\n* Loss: `AdaptiveLayerLoss` with these parameters:",
"### Evaluation Dataset",
"#### sentence-transformers/stsb\n\n\n* Dataset: sentence-transformers/stsb at d999f12\n* Size: 1,500 evaluation samples\n* Columns: `sentence1`, `sentence2`, and `score`\n* Approximate statistics based on the first 1000 samples:\n* Samples:\n* Loss: `AdaptiveLayerLoss` with these parameters:",
"### Training Hyperparameters",
"#### Non-Default Hyperparameters\n\n\n* 'eval\\_strategy': steps\n* 'per\\_device\\_train\\_batch\\_size': 16\n* 'per\\_device\\_eval\\_batch\\_size': 16\n* 'num\\_train\\_epochs': 4\n* 'warmup\\_ratio': 0.1\n* 'fp16': True",
"#### All Hyperparameters\n\n\nClick to expand\n* 'overwrite\\_output\\_dir': False\n* 'do\\_predict': False\n* 'eval\\_strategy': steps\n* 'prediction\\_loss\\_only': False\n* 'per\\_device\\_train\\_batch\\_size': 16\n* 'per\\_device\\_eval\\_batch\\_size': 16\n* 'per\\_gpu\\_train\\_batch\\_size': None\n* 'per\\_gpu\\_eval\\_batch\\_size': None\n* 'gradient\\_accumulation\\_steps': 1\n* 'eval\\_accumulation\\_steps': None\n* 'learning\\_rate': 5e-05\n* 'weight\\_decay': 0.0\n* 'adam\\_beta1': 0.9\n* 'adam\\_beta2': 0.999\n* 'adam\\_epsilon': 1e-08\n* 'max\\_grad\\_norm': 1.0\n* 'num\\_train\\_epochs': 4\n* 'max\\_steps': -1\n* 'lr\\_scheduler\\_type': linear\n* 'lr\\_scheduler\\_kwargs': {}\n* 'warmup\\_ratio': 0.1\n* 'warmup\\_steps': 0\n* 'log\\_level': passive\n* 'log\\_level\\_replica': warning\n* 'log\\_on\\_each\\_node': True\n* 'logging\\_nan\\_inf\\_filter': True\n* 'save\\_safetensors': True\n* 'save\\_on\\_each\\_node': False\n* 'save\\_only\\_model': False\n* 'no\\_cuda': False\n* 'use\\_cpu': False\n* 'use\\_mps\\_device': False\n* 'seed': 42\n* 'data\\_seed': None\n* 'jit\\_mode\\_eval': False\n* 'use\\_ipex': False\n* 'bf16': False\n* 'fp16': True\n* 'fp16\\_opt\\_level': O1\n* 'half\\_precision\\_backend': auto\n* 'bf16\\_full\\_eval': False\n* 'fp16\\_full\\_eval': False\n* 'tf32': None\n* 'local\\_rank': 0\n* 'ddp\\_backend': None\n* 'tpu\\_num\\_cores': None\n* 'tpu\\_metrics\\_debug': False\n* 'debug': []\n* 'dataloader\\_drop\\_last': False\n* 'dataloader\\_num\\_workers': 0\n* 'dataloader\\_prefetch\\_factor': None\n* 'past\\_index': -1\n* 'disable\\_tqdm': False\n* 'remove\\_unused\\_columns': True\n* 'label\\_names': None\n* 'load\\_best\\_model\\_at\\_end': False\n* 'ignore\\_data\\_skip': False\n* 'fsdp': []\n* 'fsdp\\_min\\_num\\_params': 0\n* 'fsdp\\_config': {'min\\_num\\_params': 0, 'xla': False, 'xla\\_fsdp\\_v2': False, 'xla\\_fsdp\\_grad\\_ckpt': False}\n* 'fsdp\\_transformer\\_layer\\_cls\\_to\\_wrap': None\n* 'accelerator\\_config': {'split\\_batches': False, 'dispatch\\_batches': None, 'even\\_batches': True, 'use\\_seedable\\_sampler': True, 'non\\_blocking': False, 'gradient\\_accumulation\\_kwargs': None}\n* 'deepspeed': None\n* 'label\\_smoothing\\_factor': 0.0\n* 'optim': adamw\\_torch\n* 'optim\\_args': None\n* 'adafactor': False\n* 'group\\_by\\_length': False\n* 'length\\_column\\_name': length\n* 'ddp\\_find\\_unused\\_parameters': None\n* 'ddp\\_bucket\\_cap\\_mb': None\n* 'ddp\\_broadcast\\_buffers': None\n* 'dataloader\\_pin\\_memory': True\n* 'dataloader\\_persistent\\_workers': False\n* 'skip\\_memory\\_metrics': True\n* 'use\\_legacy\\_prediction\\_loop': False\n* 'push\\_to\\_hub': False\n* 'resume\\_from\\_checkpoint': None\n* 'hub\\_model\\_id': None\n* 'hub\\_strategy': every\\_save\n* 'hub\\_private\\_repo': False\n* 'hub\\_always\\_push': False\n* 'gradient\\_checkpointing': False\n* 'gradient\\_checkpointing\\_kwargs': None\n* 'include\\_inputs\\_for\\_metrics': False\n* 'eval\\_do\\_concat\\_batches': True\n* 'fp16\\_backend': auto\n* 'push\\_to\\_hub\\_model\\_id': None\n* 'push\\_to\\_hub\\_organization': None\n* 'mp\\_parameters':\n* 'auto\\_find\\_batch\\_size': False\n* 'full\\_determinism': False\n* 'torchdynamo': None\n* 'ray\\_scope': last\n* 'ddp\\_timeout': 1800\n* 'torch\\_compile': False\n* 'torch\\_compile\\_backend': None\n* 'torch\\_compile\\_mode': None\n* 'dispatch\\_batches': None\n* 'split\\_batches': None\n* 'include\\_tokens\\_per\\_second': False\n* 'include\\_num\\_input\\_tokens\\_seen': False\n* 'neftune\\_noise\\_alpha': None\n* 'optim\\_target\\_modules': None\n* 'batch\\_sampler': batch\\_sampler\n* 'multi\\_dataset\\_batch\\_sampler': proportional",
"### Training Logs",
"### Environmental Impact\n\n\nCarbon emissions were measured using CodeCarbon.\n\n\n* Energy Consumed: 0.013 kWh\n* Carbon Emitted: 0.005 kg of CO2\n* Hours Used: 0.069 hours",
"### Training Hardware\n\n\n* On Cloud: No\n* GPU Model: 1 x NVIDIA GeForce RTX 3090\n* CPU Model: 13th Gen Intel(R) Core(TM) i7-13700K\n* RAM Size: 31.78 GB",
"### Framework Versions\n\n\n* Python: 3.11.6\n* Sentence Transformers: 3.0.0.dev0\n* Transformers: 4.41.0.dev0\n* PyTorch: 2.3.0+cu121\n* Accelerate: 0.26.1\n* Datasets: 2.18.0\n* Tokenizers: 0.19.1",
"### BibTeX",
"#### Sentence Transformers",
"#### AdaptiveLayerLoss",
"#### CoSENTLoss"
] |
null | transformers |
# Uploaded model
- **Developed by:** gboateng
- **License:** apache-2.0
- **Finetuned from model :** unsloth/llama-3-8b-bnb-4bit
This llama model was trained 2x faster with [Unsloth](https://github.com/unslothai/unsloth) and Huggingface's TRL library.
[<img src="https://raw.githubusercontent.com/unslothai/unsloth/main/images/unsloth%20made%20with%20love.png" width="200"/>](https://github.com/unslothai/unsloth)
| {"language": ["en"], "license": "apache-2.0", "tags": ["text-generation-inference", "transformers", "unsloth", "llama", "trl"], "base_model": "unsloth/llama-3-8b-bnb-4bit"} | gboateng/adom-min-v1_model | null | [
"transformers",
"safetensors",
"text-generation-inference",
"unsloth",
"llama",
"trl",
"en",
"base_model:unsloth/llama-3-8b-bnb-4bit",
"license:apache-2.0",
"endpoints_compatible",
"region:us"
] | null | 2024-04-25T15:35:59+00:00 | [] | [
"en"
] | TAGS
#transformers #safetensors #text-generation-inference #unsloth #llama #trl #en #base_model-unsloth/llama-3-8b-bnb-4bit #license-apache-2.0 #endpoints_compatible #region-us
|
# Uploaded model
- Developed by: gboateng
- License: apache-2.0
- Finetuned from model : unsloth/llama-3-8b-bnb-4bit
This llama model was trained 2x faster with Unsloth and Huggingface's TRL library.
<img src="URL width="200"/>
| [
"# Uploaded model\n\n- Developed by: gboateng\n- License: apache-2.0\n- Finetuned from model : unsloth/llama-3-8b-bnb-4bit\n\nThis llama model was trained 2x faster with Unsloth and Huggingface's TRL library.\n\n<img src=\"URL width=\"200\"/>"
] | [
"TAGS\n#transformers #safetensors #text-generation-inference #unsloth #llama #trl #en #base_model-unsloth/llama-3-8b-bnb-4bit #license-apache-2.0 #endpoints_compatible #region-us \n",
"# Uploaded model\n\n- Developed by: gboateng\n- License: apache-2.0\n- Finetuned from model : unsloth/llama-3-8b-bnb-4bit\n\nThis llama model was trained 2x faster with Unsloth and Huggingface's TRL library.\n\n<img src=\"URL width=\"200\"/>"
] |
Subsets and Splits
No community queries yet
The top public SQL queries from the community will appear here once available.