Update README.md
Browse files
README.md
CHANGED
@@ -6,7 +6,7 @@ license: apache-2.0
|
|
6 |
|
7 |
<p>
|
8 |
<img src="https://s3.amazonaws.com/moonup/production/uploads/62441d1d9fdefb55a0b7d12c/F1LWM9MXjHJsiAtgBFpDP.png" alt="Model architecture">
|
9 |
-
<em>Detailed architecture of Segment Anything Model (SAM).</em>
|
10 |
</p>
|
11 |
|
12 |
|
@@ -35,12 +35,75 @@ The abstract of the paper states:
|
|
35 |
|
36 |
# Model Details
|
37 |
|
|
|
|
|
|
|
|
|
|
|
|
|
38 |
|
39 |
|
40 |
-
|
41 |
|
42 |
-
|
|
|
|
|
|
|
|
|
|
|
|
|
|
|
|
|
|
|
|
|
|
|
|
|
|
|
|
|
|
|
|
|
|
|
|
|
43 |
Among other arguments to generate masks, you can pass 2D locations on the approximate position of your object of interest, a bounding box wrapping the object of interest (the format should be x, y coordinate of the top right and bottom left point of the bounding box), a segmentation mask. At this time of writing, passing a text as input is not supported by the official model according to [the official repository](https://github.com/facebookresearch/segment-anything/issues/4#issuecomment-1497626844).
|
|
|
|
|
|
|
|
|
|
|
|
|
|
|
|
|
|
|
|
|
|
|
|
|
|
|
|
|
|
|
|
|
|
|
|
|
|
|
|
|
|
|
|
|
|
|
|
|
|
|
|
|
|
|
|
|
|
|
|
|
|
|
|
|
|
|
|
|
|
|
|
|
|
|
|
|
|
|
44 |
|
45 |
|
46 |
# Citation
|
|
|
6 |
|
7 |
<p>
|
8 |
<img src="https://s3.amazonaws.com/moonup/production/uploads/62441d1d9fdefb55a0b7d12c/F1LWM9MXjHJsiAtgBFpDP.png" alt="Model architecture">
|
9 |
+
<em> Detailed architecture of Segment Anything Model (SAM).</em>
|
10 |
</p>
|
11 |
|
12 |
|
|
|
35 |
|
36 |
# Model Details
|
37 |
|
38 |
+
The SAM model is made up of 3 modules:
|
39 |
+
- The `VisionEncoder`: a VIT based image encoder. It computes the image embeddings using attention on patches of the image. Relative Positional Embedding is used.
|
40 |
+
- The `PromptEncoder`: generates embeddings for points and bounding boxes
|
41 |
+
- The `MaskDecoder`: a two-ways transformer which performs cross attention between the image embedding and the point embeddings (->) and between the point embeddings and the image embeddings. The outputs are fed
|
42 |
+
- The `Neck`: predicts the output masks based on the contextualized masks produced by the `MaskDecoder`.
|
43 |
+
# Usage
|
44 |
|
45 |
|
46 |
+
## Prompted-Mask-Generation
|
47 |
|
48 |
+
```python
|
49 |
+
>>> from PIL import Image
|
50 |
+
>>> import requests
|
51 |
+
>>> from transformers import SamModelForMaskedGeneration, SamProcessor
|
52 |
+
>>> model = SamModelForMaskedGeneration.from_pretrained("facebook/sam-vit-huge")
|
53 |
+
>>> processsor = SamProcessor.from_pretrained("facebook/sam-vit-huge")
|
54 |
+
|
55 |
+
>>> img_url = "https://huggingface.co/ybelkada/segment-anything/resolve/main/assets/car.png"
|
56 |
+
>>> raw_image = Image.open(requests.get(img_url, stream=True).raw).convert("RGB")
|
57 |
+
>>> input_points = [[[450, 600]]]
|
58 |
+
```
|
59 |
+
|
60 |
+
|
61 |
+
```python
|
62 |
+
>>> inputs = processor(raw_image, input_points=input_points, return_tensors="pt").to(device)
|
63 |
+
>>> outputs = model(**inputs)
|
64 |
+
>>> masks = processor.image_processor.post_process_masks(outputs.pred_masks.cpu(), inputs["original_sizes"].cpu(), inputs["reshaped_input_sizes"].cpu())
|
65 |
+
>>> scores = outputs.iou_scores
|
66 |
+
```
|
67 |
Among other arguments to generate masks, you can pass 2D locations on the approximate position of your object of interest, a bounding box wrapping the object of interest (the format should be x, y coordinate of the top right and bottom left point of the bounding box), a segmentation mask. At this time of writing, passing a text as input is not supported by the official model according to [the official repository](https://github.com/facebookresearch/segment-anything/issues/4#issuecomment-1497626844).
|
68 |
+
For more details, refer to this notebook, which shows a walk throught of how to use the model, with a visual example!
|
69 |
+
|
70 |
+
## Automatic-Mask-Generation
|
71 |
+
|
72 |
+
The model can be used for generating segmentation masks in a "zero-shot" fashion, given an input image. The model is automatically prompt with a grid of `1024` points
|
73 |
+
which are all fed to the model.
|
74 |
+
|
75 |
+
The pipeline is made for automatic mask generation. The following snippet demonstrates how easy you can run it (on any device! Simply feed the appropriate `points_per_batch` argument)
|
76 |
+
```python
|
77 |
+
from transformers import pipeline
|
78 |
+
generator = pipeline("automatic-mask-generation", device = 0, points_per_batch = 256)
|
79 |
+
image_url = "https://huggingface.co/ybelkada/segment-anything/resolve/main/assets/car.png"
|
80 |
+
outputs = generator(image_url, points_per_batch = 256)
|
81 |
+
```
|
82 |
+
Now to display the image:
|
83 |
+
```python
|
84 |
+
import matplotlib.pyplot as plt
|
85 |
+
from PIL import Image
|
86 |
+
import numpy as np
|
87 |
+
|
88 |
+
def show_mask(mask, ax, random_color=False):
|
89 |
+
if random_color:
|
90 |
+
color = np.concatenate([np.random.random(3), np.array([0.6])], axis=0)
|
91 |
+
else:
|
92 |
+
color = np.array([30 / 255, 144 / 255, 255 / 255, 0.6])
|
93 |
+
h, w = mask.shape[-2:]
|
94 |
+
mask_image = mask.reshape(h, w, 1) * color.reshape(1, 1, -1)
|
95 |
+
ax.imshow(mask_image)
|
96 |
+
|
97 |
+
|
98 |
+
plt.imshow(np.array(raw_image))
|
99 |
+
ax = plt.gca()
|
100 |
+
for mask in outputs["masks"]:
|
101 |
+
show_mask(mask, ax=ax, random_color=True)
|
102 |
+
plt.axis("off")
|
103 |
+
plt.show()
|
104 |
+
```
|
105 |
+
This should give you the following 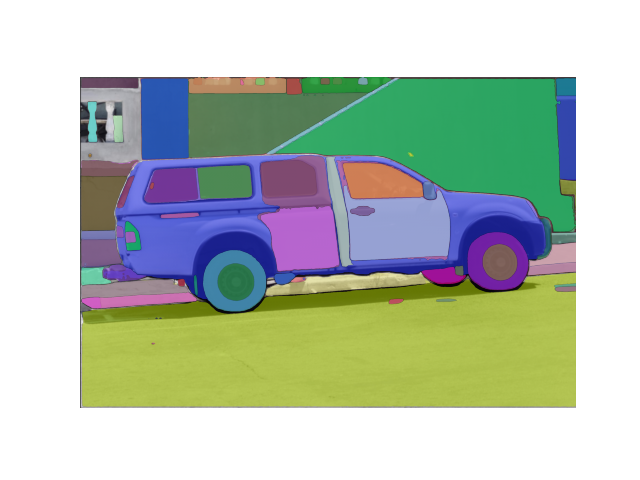
|
106 |
+
|
107 |
|
108 |
|
109 |
# Citation
|