Commit
•
9914952
1
Parent(s):
8184b34
Update README.md
Browse files
README.md
CHANGED
@@ -1,199 +1,190 @@
|
|
1 |
---
|
2 |
-
|
3 |
-
|
|
|
|
|
|
|
|
|
|
|
|
|
|
|
|
|
|
|
|
|
|
|
|
|
|
|
|
|
|
|
|
|
|
|
|
|
|
|
|
|
|
|
|
|
|
|
|
|
|
|
|
|
|
|
|
|
|
|
|
|
|
|
|
|
|
|
4 |
---
|
|
|
|
|
|
|
|
|
|
|
|
|
5 |
|
6 |
-
|
7 |
|
8 |
-
|
9 |
|
10 |
-
|
11 |
-
|
12 |
-
|
13 |
-
|
14 |
-
|
15 |
-
|
16 |
-
|
17 |
-
|
18 |
-
|
19 |
-
|
20 |
-
|
21 |
-
|
22 |
-
|
23 |
-
|
24 |
-
|
25 |
-
|
26 |
-
|
27 |
-
|
28 |
-
|
29 |
-
|
30 |
-
|
31 |
-
|
32 |
-
|
33 |
-
|
34 |
-
|
35 |
-
|
36 |
-
|
37 |
-
|
38 |
-
|
39 |
-
|
40 |
-
###
|
41 |
-
|
42 |
-
|
43 |
-
|
44 |
-
|
45 |
-
|
46 |
-
|
47 |
-
|
48 |
-
|
49 |
-
|
50 |
-
|
51 |
-
|
52 |
-
|
53 |
-
|
54 |
-
|
55 |
-
|
56 |
-
|
57 |
-
|
58 |
-
|
59 |
-
|
60 |
-
|
61 |
-
|
62 |
-
|
63 |
-
|
64 |
-
|
65 |
-
|
66 |
-
|
67 |
-
|
68 |
-
|
69 |
-
|
70 |
-
|
71 |
-
|
72 |
-
|
73 |
-
|
74 |
-
|
75 |
-
|
76 |
-
|
77 |
-
|
78 |
-
|
79 |
-
|
80 |
-
|
81 |
-
|
82 |
-
[
|
83 |
-
|
84 |
-
|
85 |
-
|
86 |
-
|
87 |
-
|
88 |
-
|
89 |
-
|
90 |
-
|
91 |
-
|
92 |
-
|
93 |
-
|
94 |
-
|
95 |
-
|
96 |
-
|
97 |
-
|
98 |
-
|
99 |
-
|
100 |
-
|
101 |
-
|
102 |
-
|
103 |
-
|
104 |
-
|
105 |
-
|
106 |
-
|
107 |
-
|
108 |
-
|
109 |
-
|
110 |
-
|
111 |
-
|
112 |
-
|
113 |
-
|
114 |
-
|
115 |
-
|
116 |
-
|
117 |
-
|
118 |
-
|
119 |
-
|
120 |
-
|
121 |
-
|
122 |
-
|
123 |
-
|
124 |
-
|
125 |
-
|
126 |
-
|
127 |
-
###
|
128 |
-
|
129 |
-
|
130 |
-
|
131 |
-
|
132 |
-
|
133 |
-
|
134 |
-
|
135 |
-
|
136 |
-
|
137 |
-
|
138 |
-
|
139 |
-
|
140 |
-
|
141 |
-
|
142 |
-
|
143 |
-
|
144 |
-
|
145 |
-
|
146 |
-
|
147 |
-
|
148 |
-
-
|
149 |
-
|
150 |
-
|
151 |
-
|
152 |
-
|
153 |
-
## Technical Specifications [optional]
|
154 |
-
|
155 |
-
### Model Architecture and Objective
|
156 |
-
|
157 |
-
[More Information Needed]
|
158 |
-
|
159 |
-
### Compute Infrastructure
|
160 |
-
|
161 |
-
[More Information Needed]
|
162 |
-
|
163 |
-
#### Hardware
|
164 |
-
|
165 |
-
[More Information Needed]
|
166 |
-
|
167 |
-
#### Software
|
168 |
-
|
169 |
-
[More Information Needed]
|
170 |
-
|
171 |
-
## Citation [optional]
|
172 |
-
|
173 |
-
<!-- If there is a paper or blog post introducing the model, the APA and Bibtex information for that should go in this section. -->
|
174 |
-
|
175 |
-
**BibTeX:**
|
176 |
-
|
177 |
-
[More Information Needed]
|
178 |
-
|
179 |
-
**APA:**
|
180 |
-
|
181 |
-
[More Information Needed]
|
182 |
-
|
183 |
-
## Glossary [optional]
|
184 |
-
|
185 |
-
<!-- If relevant, include terms and calculations in this section that can help readers understand the model or model card. -->
|
186 |
-
|
187 |
-
[More Information Needed]
|
188 |
-
|
189 |
-
## More Information [optional]
|
190 |
-
|
191 |
-
[More Information Needed]
|
192 |
-
|
193 |
-
## Model Card Authors [optional]
|
194 |
-
|
195 |
-
[More Information Needed]
|
196 |
-
|
197 |
-
## Model Card Contact
|
198 |
-
|
199 |
-
[More Information Needed]
|
|
|
1 |
---
|
2 |
+
license: cc-by-nc-4.0
|
3 |
+
inference: false
|
4 |
+
base_model: naver-clova-ix/donut-base
|
5 |
+
tags:
|
6 |
+
- donut
|
7 |
+
- image-to-text
|
8 |
+
- vision
|
9 |
+
model-index:
|
10 |
+
- name: donut-receipts-extract
|
11 |
+
results:
|
12 |
+
- task:
|
13 |
+
type: image-to-text
|
14 |
+
name: Image to text
|
15 |
+
metrics:
|
16 |
+
- type: loss
|
17 |
+
value: 0.326069
|
18 |
+
- type: accuracy
|
19 |
+
value: 0.895219
|
20 |
+
name: Accuracy
|
21 |
+
- type: cer
|
22 |
+
value: 0.158358
|
23 |
+
name: CER
|
24 |
+
- type: wer
|
25 |
+
value: 1.673989
|
26 |
+
name: WER
|
27 |
+
- type: edit distance
|
28 |
+
value: 0.145293
|
29 |
+
name: Edit_distance
|
30 |
+
metrics:
|
31 |
+
- cer
|
32 |
+
- wer
|
33 |
+
- accuracy
|
34 |
+
datasets:
|
35 |
+
- AdamCodd/donut-receipts
|
36 |
+
pipeline_tag: image-to-text
|
37 |
---
|
38 |
+
# Note
|
39 |
+
This model was forked from [AdamCodd/donut-receipts-extract](https://huggingface.co/AdamCodd/donut-receipts-extract).
|
40 |
+
|
41 |
+
# Donut-receipts-extract
|
42 |
+
|
43 |
+
Donut model was introduced in the paper [OCR-free Document Understanding Transformer](https://arxiv.org/abs/2111.15664) by Geewok et al. and first released in [this repository](https://github.com/clovaai/donut).
|
44 |
|
45 |
+
## === V2 ===
|
46 |
|
47 |
+
This model has been retrained on an improved version of the [AdamCodd/donut-receipts](https://huggingface.co/datasets/AdamCodd/donut-receipts) dataset (deduplicated, manually corrected). The new license for the V2 model is **cc-by-nc-4.0**. For commercial use rights, please contact me ([email protected]). Meanwhile, the V1 model remains available under the MIT license (under v1 branch).
|
48 |
|
49 |
+
It achieves the following results on the evaluation set:
|
50 |
+
* Loss: 0.326069
|
51 |
+
* Edit distance: 0.145293
|
52 |
+
* CER: 0.158358
|
53 |
+
* WER: 1.673989
|
54 |
+
* Mean accuracy: 0.895219
|
55 |
+
* F1: 0.977897
|
56 |
+
|
57 |
+
The task_prompt has been changed to ``<s_receipt>`` for the V2 (previously ``<s_cord-v2>`` for V1). Two new keys ``<s_svc>`` and ``<s_discount>`` have been added, ``<s_telephone>`` has been renamed to ``<s_phone>``.
|
58 |
+
|
59 |
+
The V2 performs way better than the V1 as it has been trained on twice the resolution for the receipts, using a better dataset. Despite that, it's not perfect due to a lack of diverse receipts (the training dataset is still ~1100 receipts); for a future version, that will be the main focus.
|
60 |
+
|
61 |
+
## === V1 ====
|
62 |
+
|
63 |
+
This model is a finetune of the [donut base model](https://huggingface.co/naver-clova-ix/donut-base/) on the [AdamCodd/donut-receipts](https://huggingface.co/datasets/AdamCodd/donut-receipts) dataset. Its purpose is to efficiently extract text from receipts.
|
64 |
+
|
65 |
+
It achieves the following results on the evaluation set:
|
66 |
+
* Loss: 0.498843
|
67 |
+
* Edit distance: 0.198315
|
68 |
+
* CER: 0.213929
|
69 |
+
* WER: 7.634032
|
70 |
+
* Mean accuracy: 0.843472
|
71 |
+
|
72 |
+
## Model description
|
73 |
+
|
74 |
+
Donut consists of a vision encoder (Swin Transformer) and a text decoder (BART). Given an image, the encoder first encodes the image into a tensor of embeddings (of shape batch_size, seq_len, hidden_size), after which the decoder autoregressively generates text, conditioned on the encoding of the encoder.
|
75 |
+
|
76 |
+
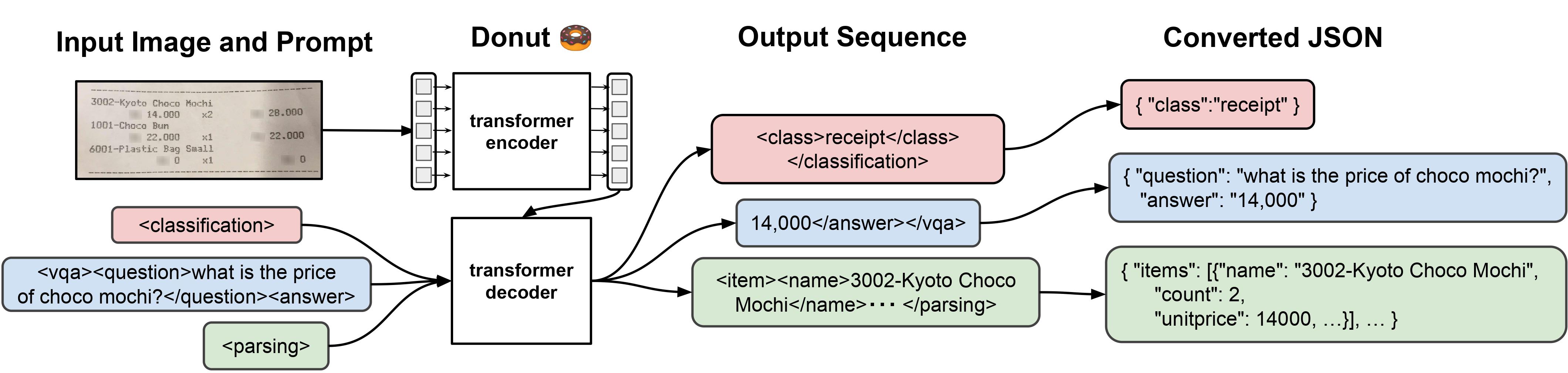
|
77 |
+
|
78 |
+
|
79 |
+
### How to use
|
80 |
+
|
81 |
+
```python
|
82 |
+
import torch
|
83 |
+
import re
|
84 |
+
from PIL import Image
|
85 |
+
from transformers import DonutProcessor, VisionEncoderDecoderModel
|
86 |
+
|
87 |
+
device = torch.device('cuda:0' if torch.cuda.is_available() else 'cpu')
|
88 |
+
processor = DonutProcessor.from_pretrained("AdamCodd/donut-receipts-extract")
|
89 |
+
model = VisionEncoderDecoderModel.from_pretrained("AdamCodd/donut-receipts-extract")
|
90 |
+
model.to(device)
|
91 |
+
|
92 |
+
def load_and_preprocess_image(image_path: str, processor):
|
93 |
+
"""
|
94 |
+
Load an image and preprocess it for the model.
|
95 |
+
"""
|
96 |
+
image = Image.open(image_path).convert("RGB")
|
97 |
+
pixel_values = processor(image, return_tensors="pt").pixel_values
|
98 |
+
return pixel_values
|
99 |
+
|
100 |
+
def generate_text_from_image(model, image_path: str, processor, device):
|
101 |
+
"""
|
102 |
+
Generate text from an image using the trained model.
|
103 |
+
"""
|
104 |
+
# Load and preprocess the image
|
105 |
+
pixel_values = load_and_preprocess_image(image_path, processor)
|
106 |
+
pixel_values = pixel_values.to(device)
|
107 |
+
|
108 |
+
# Generate output using model
|
109 |
+
model.eval()
|
110 |
+
with torch.no_grad():
|
111 |
+
task_prompt = "<s_receipt>" # <s_cord-v2> for v1
|
112 |
+
decoder_input_ids = processor.tokenizer(task_prompt, add_special_tokens=False, return_tensors="pt").input_ids
|
113 |
+
decoder_input_ids = decoder_input_ids.to(device)
|
114 |
+
generated_outputs = model.generate(
|
115 |
+
pixel_values,
|
116 |
+
decoder_input_ids=decoder_input_ids,
|
117 |
+
max_length=model.decoder.config.max_position_embeddings,
|
118 |
+
pad_token_id=processor.tokenizer.pad_token_id,
|
119 |
+
eos_token_id=processor.tokenizer.eos_token_id,
|
120 |
+
early_stopping=True,
|
121 |
+
bad_words_ids=[[processor.tokenizer.unk_token_id]],
|
122 |
+
return_dict_in_generate=True
|
123 |
+
)
|
124 |
+
|
125 |
+
# Decode generated output
|
126 |
+
decoded_text = processor.batch_decode(generated_outputs.sequences)[0]
|
127 |
+
decoded_text = decoded_text.replace(processor.tokenizer.eos_token, "").replace(processor.tokenizer.pad_token, "")
|
128 |
+
decoded_text = re.sub(r"<.*?>", "", decoded_text, count=1).strip() # remove first task start token
|
129 |
+
decoded_text = processor.token2json(decoded_text)
|
130 |
+
return decoded_text
|
131 |
+
|
132 |
+
# Example usage
|
133 |
+
image_path = "path_to_your_image" # Replace with your image path
|
134 |
+
extracted_text = generate_text_from_image(model, image_path, processor, device)
|
135 |
+
print("Extracted Text:", extracted_text)
|
136 |
+
```
|
137 |
+
|
138 |
+
Refer to the [documentation](https://huggingface.co/docs/transformers/main/en/model_doc/donut) for more code examples.
|
139 |
+
|
140 |
+
## Intended uses & limitations
|
141 |
+
|
142 |
+
This fine-tuned model is specifically designed for extracting text from receipts and may not perform optimally on other types of documents. The dataset used is still suboptimal (numerous errors are still there) so this model will need to be retrained at a later date to improve its performance.
|
143 |
+
|
144 |
+
### Training hyperparameters
|
145 |
+
|
146 |
+
The following hyperparameters were used during training:
|
147 |
+
- learning_rate: 3e-05
|
148 |
+
- train_batch_size: 2
|
149 |
+
- eval_batch_size: 4
|
150 |
+
- seed: 42
|
151 |
+
- optimizer: AdamW with betas=(0.9,0.999) and epsilon=1e-08
|
152 |
+
- lr_scheduler_type: linear
|
153 |
+
- lr_scheduler_warmup_steps: 300
|
154 |
+
- num_epochs: 35
|
155 |
+
- weight_decay: 0.01
|
156 |
+
|
157 |
+
### Framework versions
|
158 |
+
|
159 |
+
- Transformers 4.36.2
|
160 |
+
- Datasets 2.16.1
|
161 |
+
- Tokenizers 0.15.0
|
162 |
+
- Evaluate 0.4.1
|
163 |
+
|
164 |
+
If you want to support me, you can [here](https://ko-fi.com/adamcodd).
|
165 |
+
|
166 |
+
### BibTeX entry and citation info
|
167 |
+
|
168 |
+
```bibtex
|
169 |
+
@article{DBLP:journals/corr/abs-2111-15664,
|
170 |
+
author = {Geewook Kim and
|
171 |
+
Teakgyu Hong and
|
172 |
+
Moonbin Yim and
|
173 |
+
Jinyoung Park and
|
174 |
+
Jinyeong Yim and
|
175 |
+
Wonseok Hwang and
|
176 |
+
Sangdoo Yun and
|
177 |
+
Dongyoon Han and
|
178 |
+
Seunghyun Park},
|
179 |
+
title = {Donut: Document Understanding Transformer without {OCR}},
|
180 |
+
journal = {CoRR},
|
181 |
+
volume = {abs/2111.15664},
|
182 |
+
year = {2021},
|
183 |
+
url = {https://arxiv.org/abs/2111.15664},
|
184 |
+
eprinttype = {arXiv},
|
185 |
+
eprint = {2111.15664},
|
186 |
+
timestamp = {Thu, 02 Dec 2021 10:50:44 +0100},
|
187 |
+
biburl = {https://dblp.org/rec/journals/corr/abs-2111-15664.bib},
|
188 |
+
bibsource = {dblp computer science bibliography, https://dblp.org}
|
189 |
+
}
|
190 |
+
```
|
|
|
|
|
|
|
|
|
|
|
|
|
|
|
|
|
|
|
|
|
|
|
|
|
|
|
|
|
|
|
|
|
|
|
|
|
|
|
|
|
|
|
|
|
|
|
|
|
|
|
|
|
|
|
|
|
|
|
|
|
|
|
|
|
|
|
|
|
|
|
|
|
|
|
|
|
|
|
|
|
|
|
|
|
|
|
|
|
|
|
|
|
|
|
|