File size: 3,803 Bytes
3d747d0 d37e08d c385977 89140e0 d37e08d 76ba888 c385977 d37e08d 76ba888 95f03df 76ba888 c385977 76ba888 c385977 76ba888 c385977 76ba888 d37e08d 6863ccc ac9635b 6863ccc d37e08d c385977 76ba888 d37e08d 18ab89f c385977 18ab89f 76ba888 |
1 2 3 4 5 6 7 8 9 10 11 12 13 14 15 16 17 18 19 20 21 22 23 24 25 26 27 28 29 30 31 32 33 34 35 36 37 38 39 40 41 42 43 44 45 46 47 48 49 50 51 52 53 54 55 56 57 58 59 60 61 62 63 64 65 66 67 68 69 70 71 72 |
---
license: apache-2.0
---
# Positive Transfer Of The Whisper Speech Transformer To Human And Animal Voice Activity Detection
We proposed **WhisperSeg**, utilizing the Whisper Transformer pre-trained for Automatic Speech Recognition (ASR) for both human and animal Voice Activity Detection (VAD). For more details, please refer to our paper:
> [**Positive Transfer of the Whisper Speech Transformer to Human and Animal Voice Activity Detection**](https://doi.org/10.1101/2023.09.30.560270)
>
> Nianlong Gu, Kanghwi Lee, Maris Basha, Sumit Kumar Ram, Guanghao You, Richard H. R. Hahnloser <br>
> University of Zurich and ETH Zurich
*Accepted to the 2024 IEEE International Conference on Acoustics, Speech and Signal Processing (ICASSP 2024)*
The model "nccratliri/whisperseg-large-ms" is the checkpoint of the multi-species WhisperSeg-large that was finetuned on the vocal segmentation datasets of five species.
## Usage
### Clone the GitHub repo and install dependencies
```bash
git clone https://github.com/nianlonggu/WhisperSeg.git
cd WhisperSeg; pip install -r requirements.txt
```
Then in the folder "WhisperSeg", run the following python script:
```python
from model import WhisperSegmenter
import librosa
import json
segmenter = WhisperSegmenter( "nccratliri/whisperseg-large-ms", device="cuda" )
sr = 32000
spec_time_step = 0.0025
audio, _ = librosa.load( "data/example_subset/Zebra_finch/test_adults/zebra_finch_g17y2U-f00007.wav",
sr = sr )
## Note if spec_time_step is not provided, a default value will be used by the model.
prediction = segmenter.segment( audio, sr = sr, spec_time_step = spec_time_step )
print(prediction)
```
{'onset': [0.01, 0.38, 0.603, 0.758, 0.912, 1.813, 1.967, 2.073, 2.838, 2.982, 3.112, 3.668, 3.828, 3.953, 5.158, 5.323, 5.467], 'offset': [0.073, 0.447, 0.673, 0.83, 1.483, 1.882, 2.037, 2.643, 2.893, 3.063, 3.283, 3.742, 3.898, 4.523, 5.223, 5.393, 6.043], 'cluster': ['zebra_finch_0', 'zebra_finch_0', 'zebra_finch_0', 'zebra_finch_0', 'zebra_finch_0', 'zebra_finch_0', 'zebra_finch_0', 'zebra_finch_0', 'zebra_finch_0', 'zebra_finch_0', 'zebra_finch_0', 'zebra_finch_0', 'zebra_finch_0', 'zebra_finch_0', 'zebra_finch_0', 'zebra_finch_0', 'zebra_finch_0']}
Visualize the results of WhisperSeg:
```python
from audio_utils import SpecViewer
spec_viewer = SpecViewer()
spec_viewer.visualize( audio = audio, sr = sr, min_frequency= 0, prediction = prediction,
window_size=8, precision_bits=1
)
```
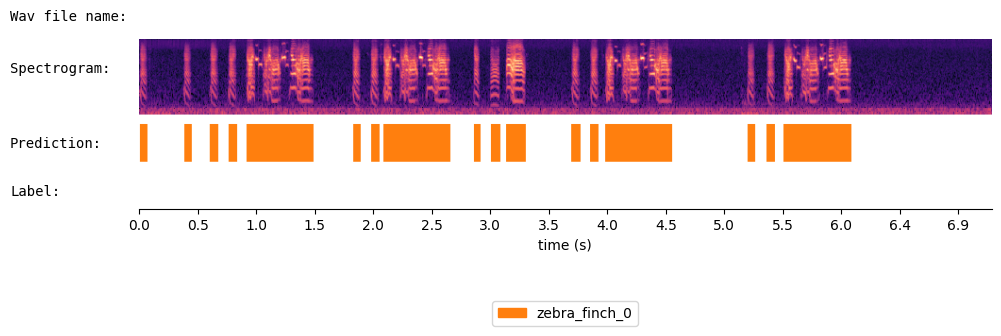
Run it in Google Colab:
<a href="https://colab.research.google.com/github/nianlonggu/WhisperSeg/blob/master/docs/WhisperSeg_Voice_Activity_Detection_Demo.ipynb" target="_parent"><img src="https://colab.research.google.com/assets/colab-badge.svg" alt="Open In Colab"/></a>
For more details, please refer to the GitHub repository: https://github.com/nianlonggu/WhisperSeg
## Citation
When using our code or models for your work, please cite the following paper:
```
@INPROCEEDINGS{10447620,
author={Gu, Nianlong and Lee, Kanghwi and Basha, Maris and Kumar Ram, Sumit and You, Guanghao and Hahnloser, Richard H. R.},
booktitle={ICASSP 2024 - 2024 IEEE International Conference on Acoustics, Speech and Signal Processing (ICASSP)},
title={Positive Transfer of the Whisper Speech Transformer to Human and Animal Voice Activity Detection},
year={2024},
volume={},
number={},
pages={7505-7509},
keywords={Voice activity detection;Adaptation models;Animals;Transformers;Acoustics;Human voice;Spectrogram;Voice activity detection;audio segmentation;Transformer;Whisper},
doi={10.1109/ICASSP48485.2024.10447620}}
```
## Contact
[email protected]
|