Commit
•
53c3ce9
1
Parent(s):
37de0cb
Update README.md
Browse files
README.md
CHANGED
@@ -28,4 +28,40 @@ language:
|
|
28 |
metrics:
|
29 |
- rouge
|
30 |
pipeline_tag: summarization
|
31 |
-
---
|
|
|
|
|
|
|
|
|
|
|
|
|
|
|
|
|
|
|
|
|
|
|
|
|
|
|
|
|
|
|
|
|
|
|
|
|
|
|
|
|
|
|
|
|
|
|
|
|
|
|
|
|
|
|
|
|
|
|
|
|
|
|
|
|
|
|
|
|
|
|
|
|
|
28 |
metrics:
|
29 |
- rouge
|
30 |
pipeline_tag: summarization
|
31 |
+
---
|
32 |
+
|
33 |
+
# mT5-small based Azerbaijani Summarization
|
34 |
+
|
35 |
+
In this project, [Google's Multilingual T5-small](https://github.com/google-research/multilingual-t5) is fine-tuned on [Azerbaijani News Summary Dataset](https://huggingface.co/datasets/nijatzeynalov/azerbaijani-multi-news) for **Summarization** downstream task. The model is trained with 3 epochs, 64 batch size and 10e-4 learning rate. It took almost 12 hours on GPU instance with Ubuntu Server 20.04 LTS image in Microsoft Azure. The max news length is kept as 2048 and max summary length is determined as 128.
|
36 |
+
|
37 |
+
|
38 |
+
mT5 is a multilingual variant of __T5__ and only pre-trained on [mC4](https://www.tensorflow.org/datasets/catalog/c4#c4multilingual)
|
39 |
+
excluding any supervised training. Therefore, the mT5 model has to be fine-tuned before it is useable on a downstream task.
|
40 |
+
|
41 |
+
### Text-to-Text Transfer Transformer (T5)
|
42 |
+
|
43 |
+
The paper [“Exploring the Limits of Transfer Learning with a Unified Text-to-Text Transformer”](https://arxiv.org/pdf/1910.10683.pdf) presents a large-scale empirical survey to determine which transfer learning techniques work best and apply these insights at scale to create a new model called the Text-To-Text Transfer Transformer.
|
44 |
+
|
45 |
+
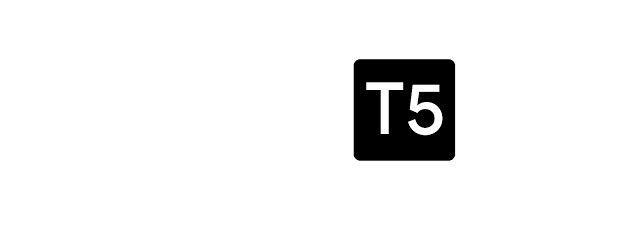
|
46 |
+
|
47 |
+
|
48 |
+
|
49 |
+
T5, or Text-to-Text Transfer Transformer, is a Transformer based architecture that uses a text-to-text approach. Every task – including translation, question answering, and classification – is cast as feeding the model text as input and training it to generate some target text. This allows for the use of the same model, loss function, hyperparameters, etc. across our diverse set of tasks.
|
50 |
+
|
51 |
+
The changes compared to BERT include:
|
52 |
+
|
53 |
+
- adding a causal decoder to the bidirectional architecture.
|
54 |
+
- replacing the fill-in-the-blank cloze task with a mix of alternative pre-training tasks.
|
55 |
+
|
56 |
+
The model was trained on a cleaned version of Common Crawl that is two orders of magnitude larger than Wikipedia.
|
57 |
+
|
58 |
+
The T5 model, pre-trained on C4, achieves state-of-the-art results on many NLP benchmarks while being flexible enough to be fine-tuned to several downstream tasks. The pre-trained T5 in Hugging Face is also trained on the mixture of unsupervised training (which is trained by reconstructing the masked sentence) and task-specific training.
|
59 |
+
|
60 |
+
### Multilingual t5
|
61 |
+
|
62 |
+
["mt5"](https://arxiv.org/pdf/2010.11934v3.pdf) is a multilingual variant of T5 that was pre-trained on a new Common Crawl-based dataset covering
|
63 |
+
101 languages.
|
64 |
+
|
65 |
+
mT5 is pre-trained only by unsupervised manner with multiple languages, and it’s not trained for specific downstream tasks. To dare say, this pre-trained model has ability to build correct text in Azerbaijani, but it doesn’t have any ability for specific tasks, such as, summarization, correction, machine translation, etc.
|
66 |
+
|
67 |
+
Therefore I trained (fine-tune) this model for summarization in Azerbaijani using [Azerbaijani News Summary Dataset](https://huggingface.co/datasets/nijatzeynalov/azerbaijani-multi-news).
|