+ DAS3R: Dynamics-Aware Gaussian Splatting for Static Scene Reconstruction
+
+
+
+
+
+
+
+
+
+
+
+
+
+* Official demo of [DAS3R: Dynamics-Aware Gaussian Splatting for Static Scene Reconstruction](https://kai422.github.io/DAS3R/).
+* You can explore the sample results by clicking the sequence names at the bottom of the page.
+* Due to GPU memory and time constraints, the total processing frame number is constrained at 20 and the iterations for GS training is constrained at 2000. We apply uniform sampling when the total number of input frames exceeds 20.
+* This Gradio demo is built upon InstantSplat, which can be found at [https://huggingface.co/spaces/kairunwen/InstantSplat](https://huggingface.co/spaces/kairunwen/InstantSplat).
+
+'''
+
+block = gr.Blocks().queue()
+with block:
+ with gr.Row():
+ with gr.Column(scale=1):
+ # gr.Markdown('# ' + _TITLE)
+ gr.Markdown(_DESCRIPTION)
+
+ with gr.Row(variant='panel'):
+ with gr.Tab("Input"):
+ inputfiles = gr.File(file_count="multiple", label="images")
+ input_path = gr.Textbox(visible=False, label="example_path")
+ button_gen = gr.Button("RUN")
+
+ with gr.Row(variant='panel'):
+ with gr.Tab("Output"):
+ with gr.Column(scale=2):
+ with gr.Group():
+ output_model = gr.Model3D(
+ label="3D Dense Model under Gaussian Splats Formats, need more time to visualize",
+ interactive=False,
+ camera_position=[0.5, 0.5, 1], # 稍微偏移一点,以便更好地查看模型
+ )
+ gr.Markdown(
+ """
+
+ Use the left mouse button to rotate, the scroll wheel to zoom, and the right mouse button to move.
+
+ """
+ )
+ output_file = gr.File(label="ply")
+ with gr.Column(scale=1):
+ output_video = gr.Video(label="video")
+
+ button_gen.click(process, inputs=[inputfiles], outputs=[output_video, output_file, output_model])
+
+ gr.Examples(
+ examples=[
+ "davis-dog",
+ # "sintel-market_2",
+ ],
+ inputs=[input_path],
+ outputs=[output_video, output_file, output_model],
+ fn=lambda x: process(inputfiles=None, input_path=x),
+ cache_examples=True,
+ label='Sparse-view Examples'
+ )
+block.launch(server_name="0.0.0.0", share=False)
\ No newline at end of file
diff --git a/app_wrapper.py b/app_wrapper.py
new file mode 100644
index 0000000000000000000000000000000000000000..be1af7756d476cbd12c07cf0c901bb1efaab1dd7
--- /dev/null
+++ b/app_wrapper.py
@@ -0,0 +1,19 @@
+import gradio as gr
+import os
+# import spaces
+
+hf_token = os.getenv("instantsplat_token")
+
+# gr.load("kairunwen/tmp", hf_token=token, src="spaces").launch()
+
+
+
+import shlex
+import subprocess
+
+from huggingface_hub import HfApi
+
+api = HfApi()
+api.snapshot_download(repo_id="kairunwen/tmp", repo_type="space", local_dir=".", token=hf_token)
+subprocess.run(shlex.split("pip install -r requirements.txt"))
+subprocess.run(shlex.split("python app.py"))
diff --git a/arguments/__init__.py b/arguments/__init__.py
new file mode 100755
index 0000000000000000000000000000000000000000..9ee5acb1717345919959219c9fac89a31b5c8591
--- /dev/null
+++ b/arguments/__init__.py
@@ -0,0 +1,112 @@
+#
+# Copyright (C) 2023, Inria
+# GRAPHDECO research group, https://team.inria.fr/graphdeco
+# All rights reserved.
+#
+# This software is free for non-commercial, research and evaluation use
+# under the terms of the LICENSE.md file.
+#
+# For inquiries contact george.drettakis@inria.fr
+#
+
+from argparse import ArgumentParser, Namespace
+import sys
+import os
+
+class GroupParams:
+ pass
+
+class ParamGroup:
+ def __init__(self, parser: ArgumentParser, name : str, fill_none = False):
+ group = parser.add_argument_group(name)
+ for key, value in vars(self).items():
+ shorthand = False
+ if key.startswith("_"):
+ shorthand = True
+ key = key[1:]
+ t = type(value)
+ value = value if not fill_none else None
+ if shorthand:
+ if t == bool:
+ group.add_argument("--" + key, ("-" + key[0:1]), default=value, action="store_true")
+ else:
+ group.add_argument("--" + key, ("-" + key[0:1]), default=value, type=t)
+ else:
+ if t == bool:
+ group.add_argument("--" + key, default=value, action="store_true")
+ else:
+ group.add_argument("--" + key, default=value, type=t)
+
+ def extract(self, args):
+ group = GroupParams()
+ for arg in vars(args).items():
+ if arg[0] in vars(self) or ("_" + arg[0]) in vars(self):
+ setattr(group, arg[0], arg[1])
+ return group
+
+class ModelParams(ParamGroup):
+ def __init__(self, parser, sentinel=False):
+ self.sh_degree = 3
+ self._source_path = ""
+ self._model_path = ""
+ self._images = "images"
+ self._resolution = -1
+ self._white_background = False
+ self.data_device = "cuda"
+ self.eval = False
+ super().__init__(parser, "Loading Parameters", sentinel)
+
+ def extract(self, args):
+ g = super().extract(args)
+ g.source_path = os.path.abspath(g.source_path)
+ return g
+
+class PipelineParams(ParamGroup):
+ def __init__(self, parser):
+ self.convert_SHs_python = False
+ self.compute_cov3D_python = False
+ self.debug = False
+ super().__init__(parser, "Pipeline Parameters")
+
+class OptimizationParams(ParamGroup):
+ def __init__(self, parser):
+ self.iterations = 30_000
+ self.position_lr_init = 0.00016
+ self.position_lr_final = 0.0000016
+ self.position_lr_delay_mult = 0.01
+ self.position_lr_max_steps = 30_000
+ self.feature_lr = 0.0025
+ self.opacity_lr = 0.05
+ self.scaling_lr = 0.005
+ self.rotation_lr = 0.001
+ self.percent_dense = 0.01
+ self.lambda_dssim = 0.2
+ self.densification_interval = 100
+ self.opacity_reset_interval = 3000
+ self.densify_from_iter = 500
+ self.densify_until_iter = 15_000
+ self.densify_grad_threshold = 0.0002
+ self.random_background = False
+ super().__init__(parser, "Optimization Parameters")
+
+def get_combined_args(parser : ArgumentParser):
+ cmdlne_string = sys.argv[1:]
+ cfgfile_string = "Namespace()"
+ args_cmdline = parser.parse_args(cmdlne_string)
+
+ try:
+ cfgfilepath = os.path.join(args_cmdline.model_path, "cfg_args")
+ print("Looking for config file in", cfgfilepath)
+ with open(cfgfilepath) as cfg_file:
+ print("Config file found: {}".format(cfgfilepath))
+ cfgfile_string = cfg_file.read()
+ except TypeError:
+ print("Config file not found at")
+ pass
+ args_cfgfile = eval(cfgfile_string)
+
+ merged_dict = vars(args_cfgfile).copy()
+ for k,v in vars(args_cmdline).items():
+ if v != None:
+ merged_dict[k] = v
+ return Namespace(**merged_dict)
diff --git a/assets/example/davis-dog/00000.jpg b/assets/example/davis-dog/00000.jpg
new file mode 100644
index 0000000000000000000000000000000000000000..a80ffa8856d21e0e0ce248aaa28f1d0c0b34e552
Binary files /dev/null and b/assets/example/davis-dog/00000.jpg differ
diff --git a/assets/example/davis-dog/00001.jpg b/assets/example/davis-dog/00001.jpg
new file mode 100644
index 0000000000000000000000000000000000000000..b5043f31ee12e6d787058445ef84e610ac1c497f
Binary files /dev/null and b/assets/example/davis-dog/00001.jpg differ
diff --git a/assets/example/davis-dog/00002.jpg b/assets/example/davis-dog/00002.jpg
new file mode 100644
index 0000000000000000000000000000000000000000..d903ae0d308b92af6fbfae6e3bd4a60eb59094d2
Binary files /dev/null and b/assets/example/davis-dog/00002.jpg differ
diff --git a/assets/example/davis-dog/00003.jpg b/assets/example/davis-dog/00003.jpg
new file mode 100644
index 0000000000000000000000000000000000000000..3a03a653b661b3642cae3252d5cff8ce34d62424
Binary files /dev/null and b/assets/example/davis-dog/00003.jpg differ
diff --git a/assets/example/davis-dog/00004.jpg b/assets/example/davis-dog/00004.jpg
new file mode 100644
index 0000000000000000000000000000000000000000..e070d2fd3521bb9faefbb3224b009a69924c0c7f
Binary files /dev/null and b/assets/example/davis-dog/00004.jpg differ
diff --git a/assets/example/davis-dog/00005.jpg b/assets/example/davis-dog/00005.jpg
new file mode 100644
index 0000000000000000000000000000000000000000..1a3c068a167483ff572a9dfe594bec572f0716b9
Binary files /dev/null and b/assets/example/davis-dog/00005.jpg differ
diff --git a/assets/example/davis-dog/00006.jpg b/assets/example/davis-dog/00006.jpg
new file mode 100644
index 0000000000000000000000000000000000000000..45d4cee6499a17d42e2cb802a072f7dcf56484d3
Binary files /dev/null and b/assets/example/davis-dog/00006.jpg differ
diff --git a/assets/example/davis-dog/00007.jpg b/assets/example/davis-dog/00007.jpg
new file mode 100644
index 0000000000000000000000000000000000000000..2c55dcc000b853d186a6ee8248b0a209948b53b2
Binary files /dev/null and b/assets/example/davis-dog/00007.jpg differ
diff --git a/assets/example/davis-dog/00008.jpg b/assets/example/davis-dog/00008.jpg
new file mode 100644
index 0000000000000000000000000000000000000000..426b5b7e7ad81995ba4c2d6de0f8929047cafc38
Binary files /dev/null and b/assets/example/davis-dog/00008.jpg differ
diff --git a/assets/example/davis-dog/00009.jpg b/assets/example/davis-dog/00009.jpg
new file mode 100644
index 0000000000000000000000000000000000000000..8d104333700949e4158ca6214124b66a5294a3e3
Binary files /dev/null and b/assets/example/davis-dog/00009.jpg differ
diff --git a/assets/example/davis-dog/00010.jpg b/assets/example/davis-dog/00010.jpg
new file mode 100644
index 0000000000000000000000000000000000000000..28c61aeb441a87a6108037645f810aed1a8c3b7f
Binary files /dev/null and b/assets/example/davis-dog/00010.jpg differ
diff --git a/assets/example/davis-dog/00011.jpg b/assets/example/davis-dog/00011.jpg
new file mode 100644
index 0000000000000000000000000000000000000000..c1d9c6d9b4219c86576f685534108943b85e6c72
Binary files /dev/null and b/assets/example/davis-dog/00011.jpg differ
diff --git a/assets/example/davis-dog/00012.jpg b/assets/example/davis-dog/00012.jpg
new file mode 100644
index 0000000000000000000000000000000000000000..53a8905ae8238221eee9de488da84f85ae5e1149
Binary files /dev/null and b/assets/example/davis-dog/00012.jpg differ
diff --git a/assets/example/davis-dog/00013.jpg b/assets/example/davis-dog/00013.jpg
new file mode 100644
index 0000000000000000000000000000000000000000..69a7808771ad9852a4fd7c917d8ead20252e6aa7
Binary files /dev/null and b/assets/example/davis-dog/00013.jpg differ
diff --git a/assets/example/davis-dog/00014.jpg b/assets/example/davis-dog/00014.jpg
new file mode 100644
index 0000000000000000000000000000000000000000..faa0edeb2c7d8a48875679d94d8be54ff7cdf600
Binary files /dev/null and b/assets/example/davis-dog/00014.jpg differ
diff --git a/assets/example/davis-dog/00015.jpg b/assets/example/davis-dog/00015.jpg
new file mode 100644
index 0000000000000000000000000000000000000000..4a01fcfed9eaa6896fa256cbd8e0f9b7a130a3ab
Binary files /dev/null and b/assets/example/davis-dog/00015.jpg differ
diff --git a/assets/example/davis-dog/00016.jpg b/assets/example/davis-dog/00016.jpg
new file mode 100644
index 0000000000000000000000000000000000000000..95286db9843bd396b624fe03b5001d6a081fd4f4
Binary files /dev/null and b/assets/example/davis-dog/00016.jpg differ
diff --git a/assets/example/davis-dog/00017.jpg b/assets/example/davis-dog/00017.jpg
new file mode 100644
index 0000000000000000000000000000000000000000..370e3c646003af019aecbd9c0fdb1409e573f6cf
Binary files /dev/null and b/assets/example/davis-dog/00017.jpg differ
diff --git a/assets/example/davis-dog/00018.jpg b/assets/example/davis-dog/00018.jpg
new file mode 100644
index 0000000000000000000000000000000000000000..dc590cbba10f2351aad3ca13b749e632e258197e
Binary files /dev/null and b/assets/example/davis-dog/00018.jpg differ
diff --git a/assets/example/davis-dog/00019.jpg b/assets/example/davis-dog/00019.jpg
new file mode 100644
index 0000000000000000000000000000000000000000..e8eddf5cbfda3c67793d700255200a7bcc1f06df
Binary files /dev/null and b/assets/example/davis-dog/00019.jpg differ
diff --git a/assets/example/davis-dog/00020.jpg b/assets/example/davis-dog/00020.jpg
new file mode 100644
index 0000000000000000000000000000000000000000..fbc9a27dca64d96309a17b035e5ab1a9c02547b4
Binary files /dev/null and b/assets/example/davis-dog/00020.jpg differ
diff --git a/assets/example/davis-dog/00021.jpg b/assets/example/davis-dog/00021.jpg
new file mode 100644
index 0000000000000000000000000000000000000000..016945e123b82ae86187e1494dfa31e420ba90ec
Binary files /dev/null and b/assets/example/davis-dog/00021.jpg differ
diff --git a/assets/example/davis-dog/00022.jpg b/assets/example/davis-dog/00022.jpg
new file mode 100644
index 0000000000000000000000000000000000000000..def7fe8991fbcad609ba6b2efdd2aff5c40faa5c
Binary files /dev/null and b/assets/example/davis-dog/00022.jpg differ
diff --git a/assets/example/davis-dog/00023.jpg b/assets/example/davis-dog/00023.jpg
new file mode 100644
index 0000000000000000000000000000000000000000..8d2b892b347978cd66e231d0563a963858254b4b
Binary files /dev/null and b/assets/example/davis-dog/00023.jpg differ
diff --git a/assets/example/davis-dog/00024.jpg b/assets/example/davis-dog/00024.jpg
new file mode 100644
index 0000000000000000000000000000000000000000..890d85970686785162e4d34080ef1c9bb2fb2a9b
Binary files /dev/null and b/assets/example/davis-dog/00024.jpg differ
diff --git a/assets/example/davis-dog/00025.jpg b/assets/example/davis-dog/00025.jpg
new file mode 100644
index 0000000000000000000000000000000000000000..5f584e194a871a655b1c4f186cce3c9167d30caa
Binary files /dev/null and b/assets/example/davis-dog/00025.jpg differ
diff --git a/assets/example/davis-dog/00026.jpg b/assets/example/davis-dog/00026.jpg
new file mode 100644
index 0000000000000000000000000000000000000000..8d7767c35ed165fab73a6a0d3a94651f3135ef52
Binary files /dev/null and b/assets/example/davis-dog/00026.jpg differ
diff --git a/assets/example/davis-dog/00027.jpg b/assets/example/davis-dog/00027.jpg
new file mode 100644
index 0000000000000000000000000000000000000000..f379d96ad082123aa91298e352488419d7d733b2
Binary files /dev/null and b/assets/example/davis-dog/00027.jpg differ
diff --git a/assets/example/davis-dog/00028.jpg b/assets/example/davis-dog/00028.jpg
new file mode 100644
index 0000000000000000000000000000000000000000..4cbab43498888a3e7ec94dd47834ce667a0cf97b
Binary files /dev/null and b/assets/example/davis-dog/00028.jpg differ
diff --git a/assets/example/davis-dog/00029.jpg b/assets/example/davis-dog/00029.jpg
new file mode 100644
index 0000000000000000000000000000000000000000..b76893a6827834da544fd0e828c8a4dc7231e723
Binary files /dev/null and b/assets/example/davis-dog/00029.jpg differ
diff --git a/assets/example/davis-dog/00030.jpg b/assets/example/davis-dog/00030.jpg
new file mode 100644
index 0000000000000000000000000000000000000000..e15962042fc33ac86dc98876990ef9e0f9d7d969
Binary files /dev/null and b/assets/example/davis-dog/00030.jpg differ
diff --git a/assets/example/davis-dog/00031.jpg b/assets/example/davis-dog/00031.jpg
new file mode 100644
index 0000000000000000000000000000000000000000..e193d3944dd1450bee86661db921d1442b853ca9
Binary files /dev/null and b/assets/example/davis-dog/00031.jpg differ
diff --git a/assets/example/davis-dog/00032.jpg b/assets/example/davis-dog/00032.jpg
new file mode 100644
index 0000000000000000000000000000000000000000..9f796529e61eb479da9d93e0402394a890024556
Binary files /dev/null and b/assets/example/davis-dog/00032.jpg differ
diff --git a/assets/example/davis-dog/00033.jpg b/assets/example/davis-dog/00033.jpg
new file mode 100644
index 0000000000000000000000000000000000000000..9cab6d2f908ef604db3196fd4448857eae8e9688
Binary files /dev/null and b/assets/example/davis-dog/00033.jpg differ
diff --git a/assets/example/davis-dog/00034.jpg b/assets/example/davis-dog/00034.jpg
new file mode 100644
index 0000000000000000000000000000000000000000..61400eed0aea2429a7e4c60776d650836973c7a6
Binary files /dev/null and b/assets/example/davis-dog/00034.jpg differ
diff --git a/assets/example/davis-dog/00035.jpg b/assets/example/davis-dog/00035.jpg
new file mode 100644
index 0000000000000000000000000000000000000000..d41e97096523f8cc7602cf2386a162773082b32e
Binary files /dev/null and b/assets/example/davis-dog/00035.jpg differ
diff --git a/assets/example/davis-dog/00036.jpg b/assets/example/davis-dog/00036.jpg
new file mode 100644
index 0000000000000000000000000000000000000000..b9345f5dda0debc8405e30326c987451964fab3f
Binary files /dev/null and b/assets/example/davis-dog/00036.jpg differ
diff --git a/assets/example/davis-dog/00037.jpg b/assets/example/davis-dog/00037.jpg
new file mode 100644
index 0000000000000000000000000000000000000000..995c87a63915a1ca50143e07460faa29afe6f5ff
Binary files /dev/null and b/assets/example/davis-dog/00037.jpg differ
diff --git a/assets/example/davis-dog/00038.jpg b/assets/example/davis-dog/00038.jpg
new file mode 100644
index 0000000000000000000000000000000000000000..7701e865db962a9ec3ac4c2d60f536d88f15eea2
Binary files /dev/null and b/assets/example/davis-dog/00038.jpg differ
diff --git a/assets/example/davis-dog/00039.jpg b/assets/example/davis-dog/00039.jpg
new file mode 100644
index 0000000000000000000000000000000000000000..fb2e8d88fefa5a844b69e0591c8f6f9d61967eaf
Binary files /dev/null and b/assets/example/davis-dog/00039.jpg differ
diff --git a/assets/example/davis-dog/00040.jpg b/assets/example/davis-dog/00040.jpg
new file mode 100644
index 0000000000000000000000000000000000000000..887dda87b9613c14a1ccd47cfe7272baa44452b7
Binary files /dev/null and b/assets/example/davis-dog/00040.jpg differ
diff --git a/assets/example/davis-dog/00041.jpg b/assets/example/davis-dog/00041.jpg
new file mode 100644
index 0000000000000000000000000000000000000000..977cfe7ac19254429dac1748f6441eca5eb6266d
Binary files /dev/null and b/assets/example/davis-dog/00041.jpg differ
diff --git a/assets/example/davis-dog/00042.jpg b/assets/example/davis-dog/00042.jpg
new file mode 100644
index 0000000000000000000000000000000000000000..caff6b3feb8cba9a00e036a93b660bc6d28324c6
Binary files /dev/null and b/assets/example/davis-dog/00042.jpg differ
diff --git a/assets/example/davis-dog/00043.jpg b/assets/example/davis-dog/00043.jpg
new file mode 100644
index 0000000000000000000000000000000000000000..0fc4f42cd2f4b68cc3e8cf75976ebac28b5727ef
Binary files /dev/null and b/assets/example/davis-dog/00043.jpg differ
diff --git a/assets/example/davis-dog/00044.jpg b/assets/example/davis-dog/00044.jpg
new file mode 100644
index 0000000000000000000000000000000000000000..263d0053571571b501962f5cf62e61dbf9178a02
Binary files /dev/null and b/assets/example/davis-dog/00044.jpg differ
diff --git a/assets/example/davis-dog/00045.jpg b/assets/example/davis-dog/00045.jpg
new file mode 100644
index 0000000000000000000000000000000000000000..85ca5a410828b249a508417e3f8a0c1d59904078
Binary files /dev/null and b/assets/example/davis-dog/00045.jpg differ
diff --git a/assets/example/davis-dog/00046.jpg b/assets/example/davis-dog/00046.jpg
new file mode 100644
index 0000000000000000000000000000000000000000..40763b0aab3c771e858ec789d6e75057aebd4265
Binary files /dev/null and b/assets/example/davis-dog/00046.jpg differ
diff --git a/assets/example/davis-dog/00047.jpg b/assets/example/davis-dog/00047.jpg
new file mode 100644
index 0000000000000000000000000000000000000000..3a89b557776fa616c3cae224f21a518759bd9338
Binary files /dev/null and b/assets/example/davis-dog/00047.jpg differ
diff --git a/assets/example/davis-dog/00048.jpg b/assets/example/davis-dog/00048.jpg
new file mode 100644
index 0000000000000000000000000000000000000000..8dd6700e13d3cdbdd7947756dc43595ce1e99ac5
Binary files /dev/null and b/assets/example/davis-dog/00048.jpg differ
diff --git a/assets/example/davis-dog/00049.jpg b/assets/example/davis-dog/00049.jpg
new file mode 100644
index 0000000000000000000000000000000000000000..ed36dec3a55e73a5f1b5ccb2aa78ec690939c2a7
Binary files /dev/null and b/assets/example/davis-dog/00049.jpg differ
diff --git a/assets/example/davis-dog/00050.jpg b/assets/example/davis-dog/00050.jpg
new file mode 100644
index 0000000000000000000000000000000000000000..a14bec357e4aeccc245abed522320efa3e33bc1f
Binary files /dev/null and b/assets/example/davis-dog/00050.jpg differ
diff --git a/assets/example/davis-dog/00051.jpg b/assets/example/davis-dog/00051.jpg
new file mode 100644
index 0000000000000000000000000000000000000000..974ed8d6bad6a788578f370a1943a491c89c4f4b
Binary files /dev/null and b/assets/example/davis-dog/00051.jpg differ
diff --git a/assets/example/davis-dog/00052.jpg b/assets/example/davis-dog/00052.jpg
new file mode 100644
index 0000000000000000000000000000000000000000..394e7b0416a74a1ee004e58193043c4b71447415
Binary files /dev/null and b/assets/example/davis-dog/00052.jpg differ
diff --git a/assets/example/davis-dog/00053.jpg b/assets/example/davis-dog/00053.jpg
new file mode 100644
index 0000000000000000000000000000000000000000..7e2f0adc92b587a82cacd9d825ad992e204e9f95
Binary files /dev/null and b/assets/example/davis-dog/00053.jpg differ
diff --git a/assets/example/davis-dog/00054.jpg b/assets/example/davis-dog/00054.jpg
new file mode 100644
index 0000000000000000000000000000000000000000..e3d517eb86ca001ceee5e40a63c6ea242942800c
Binary files /dev/null and b/assets/example/davis-dog/00054.jpg differ
diff --git a/assets/example/davis-dog/00055.jpg b/assets/example/davis-dog/00055.jpg
new file mode 100644
index 0000000000000000000000000000000000000000..8c7ead10f769c6cc83cfc5ccc86dd1b11eac8e06
Binary files /dev/null and b/assets/example/davis-dog/00055.jpg differ
diff --git a/assets/example/davis-dog/00056.jpg b/assets/example/davis-dog/00056.jpg
new file mode 100644
index 0000000000000000000000000000000000000000..9fb21a9902b73e755e8f8f3efbc8dc8e4d4bb85c
Binary files /dev/null and b/assets/example/davis-dog/00056.jpg differ
diff --git a/assets/example/davis-dog/00057.jpg b/assets/example/davis-dog/00057.jpg
new file mode 100644
index 0000000000000000000000000000000000000000..89deb5393c73c54f05d5b5ae6a625f1cd4ad48d7
Binary files /dev/null and b/assets/example/davis-dog/00057.jpg differ
diff --git a/assets/example/davis-dog/00058.jpg b/assets/example/davis-dog/00058.jpg
new file mode 100644
index 0000000000000000000000000000000000000000..8f982159c7e295b3e2b4d1227036816efd53a125
Binary files /dev/null and b/assets/example/davis-dog/00058.jpg differ
diff --git a/assets/example/davis-dog/00059.jpg b/assets/example/davis-dog/00059.jpg
new file mode 100644
index 0000000000000000000000000000000000000000..59b038929f2dfe78b35cf9de4acc4695d076e41c
Binary files /dev/null and b/assets/example/davis-dog/00059.jpg differ
diff --git a/assets/example/sintel-market_2/frame_0001.png b/assets/example/sintel-market_2/frame_0001.png
new file mode 100644
index 0000000000000000000000000000000000000000..cc417d1e038ee6ede1f4149297eb9aab7dd75a7c
Binary files /dev/null and b/assets/example/sintel-market_2/frame_0001.png differ
diff --git a/assets/example/sintel-market_2/frame_0002.png b/assets/example/sintel-market_2/frame_0002.png
new file mode 100644
index 0000000000000000000000000000000000000000..9162dcb0d961b2d7e132f7389087bb6a82e4c83c
Binary files /dev/null and b/assets/example/sintel-market_2/frame_0002.png differ
diff --git a/assets/example/sintel-market_2/frame_0003.png b/assets/example/sintel-market_2/frame_0003.png
new file mode 100644
index 0000000000000000000000000000000000000000..0aad46e8214aeea908ac99bacee28769d3260b98
Binary files /dev/null and b/assets/example/sintel-market_2/frame_0003.png differ
diff --git a/assets/example/sintel-market_2/frame_0004.png b/assets/example/sintel-market_2/frame_0004.png
new file mode 100644
index 0000000000000000000000000000000000000000..dabef3ab83c3acc5304e0edcbbf5236d31cf1e44
Binary files /dev/null and b/assets/example/sintel-market_2/frame_0004.png differ
diff --git a/assets/example/sintel-market_2/frame_0005.png b/assets/example/sintel-market_2/frame_0005.png
new file mode 100644
index 0000000000000000000000000000000000000000..3158ce5d72f2446c0e55543d08b00f5ad91ad81f
Binary files /dev/null and b/assets/example/sintel-market_2/frame_0005.png differ
diff --git a/assets/example/sintel-market_2/frame_0006.png b/assets/example/sintel-market_2/frame_0006.png
new file mode 100644
index 0000000000000000000000000000000000000000..579d47d726bc6f7aad9954b852e725dab8ebd10a
Binary files /dev/null and b/assets/example/sintel-market_2/frame_0006.png differ
diff --git a/assets/example/sintel-market_2/frame_0007.png b/assets/example/sintel-market_2/frame_0007.png
new file mode 100644
index 0000000000000000000000000000000000000000..ff28354433478595e7cdae0c417a5be5ba5a35b8
Binary files /dev/null and b/assets/example/sintel-market_2/frame_0007.png differ
diff --git a/assets/example/sintel-market_2/frame_0008.png b/assets/example/sintel-market_2/frame_0008.png
new file mode 100644
index 0000000000000000000000000000000000000000..d4f45aac79f20b602219cf3cfb64e897cb6195c6
Binary files /dev/null and b/assets/example/sintel-market_2/frame_0008.png differ
diff --git a/assets/example/sintel-market_2/frame_0009.png b/assets/example/sintel-market_2/frame_0009.png
new file mode 100644
index 0000000000000000000000000000000000000000..1e0e2d5667e3a60ffa22fe585bea1b56b8375088
Binary files /dev/null and b/assets/example/sintel-market_2/frame_0009.png differ
diff --git a/assets/example/sintel-market_2/frame_0010.png b/assets/example/sintel-market_2/frame_0010.png
new file mode 100644
index 0000000000000000000000000000000000000000..bbdfb686ca6b19f4b1a0000b243948b78723c5d1
Binary files /dev/null and b/assets/example/sintel-market_2/frame_0010.png differ
diff --git a/assets/example/sintel-market_2/frame_0011.png b/assets/example/sintel-market_2/frame_0011.png
new file mode 100644
index 0000000000000000000000000000000000000000..b4765fcce889a97352ca0f636c07deb24fd90c26
Binary files /dev/null and b/assets/example/sintel-market_2/frame_0011.png differ
diff --git a/assets/example/sintel-market_2/frame_0012.png b/assets/example/sintel-market_2/frame_0012.png
new file mode 100644
index 0000000000000000000000000000000000000000..200c174326503bf86ccb1f5f2b8caa3b26917a71
Binary files /dev/null and b/assets/example/sintel-market_2/frame_0012.png differ
diff --git a/assets/example/sintel-market_2/frame_0013.png b/assets/example/sintel-market_2/frame_0013.png
new file mode 100644
index 0000000000000000000000000000000000000000..9b395e2fa3b0b0dd6a1c29c67167830d1998c397
Binary files /dev/null and b/assets/example/sintel-market_2/frame_0013.png differ
diff --git a/assets/example/sintel-market_2/frame_0014.png b/assets/example/sintel-market_2/frame_0014.png
new file mode 100644
index 0000000000000000000000000000000000000000..f3600170b066fce09ca497b5b962898255963c64
Binary files /dev/null and b/assets/example/sintel-market_2/frame_0014.png differ
diff --git a/assets/example/sintel-market_2/frame_0015.png b/assets/example/sintel-market_2/frame_0015.png
new file mode 100644
index 0000000000000000000000000000000000000000..3bd5ab51cecae5ddd96995f8d7b5a3e4ada98531
Binary files /dev/null and b/assets/example/sintel-market_2/frame_0015.png differ
diff --git a/assets/example/sintel-market_2/frame_0016.png b/assets/example/sintel-market_2/frame_0016.png
new file mode 100644
index 0000000000000000000000000000000000000000..99e56e262148a6c3c6f6808111d591a774171a82
Binary files /dev/null and b/assets/example/sintel-market_2/frame_0016.png differ
diff --git a/assets/example/sintel-market_2/frame_0017.png b/assets/example/sintel-market_2/frame_0017.png
new file mode 100644
index 0000000000000000000000000000000000000000..66e6b756183fbd05e1b9e0569f1e8a9eaf8a8b63
Binary files /dev/null and b/assets/example/sintel-market_2/frame_0017.png differ
diff --git a/assets/example/sintel-market_2/frame_0018.png b/assets/example/sintel-market_2/frame_0018.png
new file mode 100644
index 0000000000000000000000000000000000000000..bbb9598c578a34c51e8f53592769d343961e7592
Binary files /dev/null and b/assets/example/sintel-market_2/frame_0018.png differ
diff --git a/assets/example/sintel-market_2/frame_0019.png b/assets/example/sintel-market_2/frame_0019.png
new file mode 100644
index 0000000000000000000000000000000000000000..367c6ec52d040aefdd2a56b9a9cb1a038b581f02
Binary files /dev/null and b/assets/example/sintel-market_2/frame_0019.png differ
diff --git a/assets/example/sintel-market_2/frame_0020.png b/assets/example/sintel-market_2/frame_0020.png
new file mode 100644
index 0000000000000000000000000000000000000000..f9293f00a1f52bdc2eb5bd9c478137d1777bb00d
Binary files /dev/null and b/assets/example/sintel-market_2/frame_0020.png differ
diff --git a/assets/example/sintel-market_2/frame_0021.png b/assets/example/sintel-market_2/frame_0021.png
new file mode 100644
index 0000000000000000000000000000000000000000..8a470396bd7128b0962db1fe32ddf02ead3e3f9b
Binary files /dev/null and b/assets/example/sintel-market_2/frame_0021.png differ
diff --git a/assets/example/sintel-market_2/frame_0022.png b/assets/example/sintel-market_2/frame_0022.png
new file mode 100644
index 0000000000000000000000000000000000000000..99d7094b53c1189409ee0f0a0fb20d7c1ca31603
Binary files /dev/null and b/assets/example/sintel-market_2/frame_0022.png differ
diff --git a/assets/example/sintel-market_2/frame_0023.png b/assets/example/sintel-market_2/frame_0023.png
new file mode 100644
index 0000000000000000000000000000000000000000..a9d26b3c094a6d9176318d486e3e890449b48559
Binary files /dev/null and b/assets/example/sintel-market_2/frame_0023.png differ
diff --git a/assets/example/sintel-market_2/frame_0024.png b/assets/example/sintel-market_2/frame_0024.png
new file mode 100644
index 0000000000000000000000000000000000000000..6efb0879ac57cd2ef60d938928fd9a8db2ca884b
Binary files /dev/null and b/assets/example/sintel-market_2/frame_0024.png differ
diff --git a/assets/example/sintel-market_2/frame_0025.png b/assets/example/sintel-market_2/frame_0025.png
new file mode 100644
index 0000000000000000000000000000000000000000..29d00e8857e2367d971073c7a715ef71ac0c1057
Binary files /dev/null and b/assets/example/sintel-market_2/frame_0025.png differ
diff --git a/assets/example/sintel-market_2/frame_0026.png b/assets/example/sintel-market_2/frame_0026.png
new file mode 100644
index 0000000000000000000000000000000000000000..78715311e0cc632c609c86c39828dd56c78a79ba
Binary files /dev/null and b/assets/example/sintel-market_2/frame_0026.png differ
diff --git a/assets/example/sintel-market_2/frame_0027.png b/assets/example/sintel-market_2/frame_0027.png
new file mode 100644
index 0000000000000000000000000000000000000000..949c875a5135fb8296d115815cfbe74848b66b71
Binary files /dev/null and b/assets/example/sintel-market_2/frame_0027.png differ
diff --git a/assets/example/sintel-market_2/frame_0028.png b/assets/example/sintel-market_2/frame_0028.png
new file mode 100644
index 0000000000000000000000000000000000000000..f75fa75e984a56c2dfa432b612d973a51e0b1586
Binary files /dev/null and b/assets/example/sintel-market_2/frame_0028.png differ
diff --git a/assets/example/sintel-market_2/frame_0029.png b/assets/example/sintel-market_2/frame_0029.png
new file mode 100644
index 0000000000000000000000000000000000000000..0c4993499a2320336ff4ad7b2154043cc2d71b0f
Binary files /dev/null and b/assets/example/sintel-market_2/frame_0029.png differ
diff --git a/assets/example/sintel-market_2/frame_0030.png b/assets/example/sintel-market_2/frame_0030.png
new file mode 100644
index 0000000000000000000000000000000000000000..e859be76f5110adacba3c33b4f4b9e9f2cbb1072
Binary files /dev/null and b/assets/example/sintel-market_2/frame_0030.png differ
diff --git a/assets/example/sintel-market_2/frame_0031.png b/assets/example/sintel-market_2/frame_0031.png
new file mode 100644
index 0000000000000000000000000000000000000000..ce64437a76e4cb2e24c3b4c0a950a0034da483c0
Binary files /dev/null and b/assets/example/sintel-market_2/frame_0031.png differ
diff --git a/assets/example/sintel-market_2/frame_0032.png b/assets/example/sintel-market_2/frame_0032.png
new file mode 100644
index 0000000000000000000000000000000000000000..f3c7edcbadd550642a8280877354371e8e0da972
Binary files /dev/null and b/assets/example/sintel-market_2/frame_0032.png differ
diff --git a/assets/example/sintel-market_2/frame_0033.png b/assets/example/sintel-market_2/frame_0033.png
new file mode 100644
index 0000000000000000000000000000000000000000..76669846fbaf400e301550b5e679a3c656a52e14
Binary files /dev/null and b/assets/example/sintel-market_2/frame_0033.png differ
diff --git a/assets/example/sintel-market_2/frame_0034.png b/assets/example/sintel-market_2/frame_0034.png
new file mode 100644
index 0000000000000000000000000000000000000000..7a2de6b882e6885a2e5fb3e1f22a2e331939daf5
Binary files /dev/null and b/assets/example/sintel-market_2/frame_0034.png differ
diff --git a/assets/example/sintel-market_2/frame_0035.png b/assets/example/sintel-market_2/frame_0035.png
new file mode 100644
index 0000000000000000000000000000000000000000..247be1ae88d60ae251a32c914495e57bdb29040f
Binary files /dev/null and b/assets/example/sintel-market_2/frame_0035.png differ
diff --git a/assets/example/sintel-market_2/frame_0036.png b/assets/example/sintel-market_2/frame_0036.png
new file mode 100644
index 0000000000000000000000000000000000000000..357ea68119652e216ed8575e0b8c63a4d82ad9bc
Binary files /dev/null and b/assets/example/sintel-market_2/frame_0036.png differ
diff --git a/assets/example/sintel-market_2/frame_0037.png b/assets/example/sintel-market_2/frame_0037.png
new file mode 100644
index 0000000000000000000000000000000000000000..f6ce642a1fc5df57080a4ebd591cd2097694b74e
Binary files /dev/null and b/assets/example/sintel-market_2/frame_0037.png differ
diff --git a/assets/example/sintel-market_2/frame_0038.png b/assets/example/sintel-market_2/frame_0038.png
new file mode 100644
index 0000000000000000000000000000000000000000..0e3aca24bcc6b512aa8740e80e7f8a22ed3b16c0
Binary files /dev/null and b/assets/example/sintel-market_2/frame_0038.png differ
diff --git a/assets/example/sintel-market_2/frame_0039.png b/assets/example/sintel-market_2/frame_0039.png
new file mode 100644
index 0000000000000000000000000000000000000000..727e8202b105aec0c540f9b74d14394efc4b8ec0
Binary files /dev/null and b/assets/example/sintel-market_2/frame_0039.png differ
diff --git a/assets/example/sintel-market_2/frame_0040.png b/assets/example/sintel-market_2/frame_0040.png
new file mode 100644
index 0000000000000000000000000000000000000000..c221145d9a4859441872d6cd23eb0cc844178456
Binary files /dev/null and b/assets/example/sintel-market_2/frame_0040.png differ
diff --git a/assets/example/sintel-market_2/frame_0041.png b/assets/example/sintel-market_2/frame_0041.png
new file mode 100644
index 0000000000000000000000000000000000000000..3554e0fae2b5c41dd1455e2fe50565725519bcb8
Binary files /dev/null and b/assets/example/sintel-market_2/frame_0041.png differ
diff --git a/assets/example/sintel-market_2/frame_0042.png b/assets/example/sintel-market_2/frame_0042.png
new file mode 100644
index 0000000000000000000000000000000000000000..6f5e297ec9c84247818b0e1d94c075e2f5b0da76
Binary files /dev/null and b/assets/example/sintel-market_2/frame_0042.png differ
diff --git a/assets/example/sintel-market_2/frame_0043.png b/assets/example/sintel-market_2/frame_0043.png
new file mode 100644
index 0000000000000000000000000000000000000000..b69dee44da56b869ebc3465cf0f8a75b599475ac
Binary files /dev/null and b/assets/example/sintel-market_2/frame_0043.png differ
diff --git a/assets/example/sintel-market_2/frame_0044.png b/assets/example/sintel-market_2/frame_0044.png
new file mode 100644
index 0000000000000000000000000000000000000000..3721b598d49c8c7ed7b424ea94e3623bcbe04949
Binary files /dev/null and b/assets/example/sintel-market_2/frame_0044.png differ
diff --git a/assets/example/sintel-market_2/frame_0045.png b/assets/example/sintel-market_2/frame_0045.png
new file mode 100644
index 0000000000000000000000000000000000000000..344f23104fcdf9318d380f8d9e23c0d83108c3e9
Binary files /dev/null and b/assets/example/sintel-market_2/frame_0045.png differ
diff --git a/assets/example/sintel-market_2/frame_0046.png b/assets/example/sintel-market_2/frame_0046.png
new file mode 100644
index 0000000000000000000000000000000000000000..7b4265e2024d026d0f4ad1b006779d20b7759771
Binary files /dev/null and b/assets/example/sintel-market_2/frame_0046.png differ
diff --git a/assets/example/sintel-market_2/frame_0047.png b/assets/example/sintel-market_2/frame_0047.png
new file mode 100644
index 0000000000000000000000000000000000000000..f1562294a9ca4c1ce2c04121422e0e1649b7b9ff
Binary files /dev/null and b/assets/example/sintel-market_2/frame_0047.png differ
diff --git a/assets/example/sintel-market_2/frame_0048.png b/assets/example/sintel-market_2/frame_0048.png
new file mode 100644
index 0000000000000000000000000000000000000000..3d68f4f1076fb193ba6d7b1a5b7c5c4dbdb2a420
Binary files /dev/null and b/assets/example/sintel-market_2/frame_0048.png differ
diff --git a/assets/example/sintel-market_2/frame_0049.png b/assets/example/sintel-market_2/frame_0049.png
new file mode 100644
index 0000000000000000000000000000000000000000..07bb3a2ce25e25e7644f5425ad6ec852b6f18dfa
Binary files /dev/null and b/assets/example/sintel-market_2/frame_0049.png differ
diff --git a/assets/example/sintel-market_2/frame_0050.png b/assets/example/sintel-market_2/frame_0050.png
new file mode 100644
index 0000000000000000000000000000000000000000..a01c6a6f161f0eaf14a758c93ff2ede355a602ef
Binary files /dev/null and b/assets/example/sintel-market_2/frame_0050.png differ
diff --git a/datasets_preprocess/pointodyssey_rearrange.py b/datasets_preprocess/pointodyssey_rearrange.py
new file mode 100644
index 0000000000000000000000000000000000000000..5c309db58122c3554a3a210800bfe8a48bf3ba80
--- /dev/null
+++ b/datasets_preprocess/pointodyssey_rearrange.py
@@ -0,0 +1,64 @@
+import sys
+import torch
+sys.path.append('.')
+import os
+import numpy as np
+import glob
+from tqdm import tqdm
+
+dataset_location = '../data/point_odyssey'
+# print(dataset_location)
+for dset in ["train", "test", "sample"]:
+ sequences = []
+ subdir = os.path.join(dataset_location, dset)
+ for seq in glob.glob(os.path.join(subdir, "*/")):
+ sequences.append(seq)
+ # sequences = sorted(sequences)
+ squences = sorted(sequences)
+
+ print('found %d unique videos in %s (dset=%s)' % (len(sequences), dataset_location, dset))
+
+ ## load trajectories
+ print('loading trajectories...')
+
+ for seq in sequences:
+ # print('seq', seq)
+ # if os.path.exists(os.path.join(seq, 'trajs_2d')):
+ # print('skipping', seq)
+ # continue
+ info_path = os.path.join(seq, 'info.npz')
+ info = np.load(info_path, allow_pickle=True)
+ trajs_3d_shape = info['trajs_3d'].astype(np.float32)
+
+ if len(trajs_3d_shape):
+ print('processing', seq)
+ rgb_path = os.path.join(seq, 'rgbs')
+ info_path = os.path.join(seq, 'info.npz')
+ annotations_path = os.path.join(seq, 'anno.npz')
+
+ trajs_3d_path = os.path.join(seq, 'trajs_3d')
+ trajs_2d_path = os.path.join(seq, 'trajs_2d')
+ os.makedirs(trajs_3d_path, exist_ok=True)
+ os.makedirs(trajs_2d_path, exist_ok=True)
+
+
+ info = np.load(info_path, allow_pickle=True)
+ trajs_3d_shape = info['trajs_3d']
+ anno = np.load(annotations_path, allow_pickle=True)
+ keys = {'trajs_2d': 'traj_2d', 'trajs_3d': 'traj_3d', 'valids': 'valid', 'visibs': 'visib', 'intrinsics': 'intrinsic', 'extrinsics': 'extrinsic'}
+ if len(trajs_3d_shape) == 0:
+ print(anno['trajs_3d'])
+ print('skipping', seq)
+ continue
+ tensors = {key: torch.tensor(anno[key]).cuda() for key in keys}
+
+ for t in tqdm(range(trajs_3d_shape[0])):
+ for key, item_key in keys.items():
+ path = os.path.join(seq, key)
+ os.makedirs(path, exist_ok=True)
+ filename = os.path.join(path, f'{item_key}_{t:05d}.npy')
+ np.save(filename, tensors[key][t].cpu().numpy())
+
+
+
+
diff --git a/datasets_preprocess/sintel_get_dynamics.py b/datasets_preprocess/sintel_get_dynamics.py
new file mode 100644
index 0000000000000000000000000000000000000000..e116380fafcbf1dbd9d633412045b7b84ca34b10
--- /dev/null
+++ b/datasets_preprocess/sintel_get_dynamics.py
@@ -0,0 +1,170 @@
+import numpy as np
+import cv2
+import os
+from tqdm import tqdm
+import argparse
+import torch
+
+TAG_FLOAT = 202021.25
+def flow_read(filename):
+ """ Read optical flow from file, return (U,V) tuple.
+
+ Original code by Deqing Sun, adapted from Daniel Scharstein.
+ """
+ f = open(filename,'rb')
+ check = np.fromfile(f,dtype=np.float32,count=1)[0]
+ assert check == TAG_FLOAT, 'flow_read:: Wrong tag in flow file (should be: {0}, is: {1}). Big-endian machine?'.format(TAG_FLOAT,check)
+ width = np.fromfile(f,dtype=np.int32,count=1)[0]
+ height = np.fromfile(f,dtype=np.int32,count=1)[0]
+ size = width*height
+ assert width > 0 and height > 0 and size > 1 and size < 100000000, 'flow_read:: Invalid input size (width = {0}, height = {1}).'.format(width,height)
+ tmp = np.fromfile(f,dtype=np.float32,count=-1).reshape((height,width*2))
+ u = tmp[:,np.arange(width)*2]
+ v = tmp[:,np.arange(width)*2 + 1]
+ return u,v
+
+def cam_read(filename):
+ """ Read camera data, return (M,N) tuple.
+
+ M is the intrinsic matrix, N is the extrinsic matrix, so that
+
+ x = M*N*X,
+ where x is a point in homogeneous image pixel coordinates, and X is a
+ point in homogeneous world coordinates.
+ """
+ f = open(filename,'rb')
+ check = np.fromfile(f,dtype=np.float32,count=1)[0]
+ assert check == TAG_FLOAT, 'cam_read:: Wrong tag in cam file (should be: {0}, is: {1}). Big-endian machine?'.format(TAG_FLOAT,check)
+ M = np.fromfile(f,dtype='float64',count=9).reshape((3,3))
+ N = np.fromfile(f,dtype='float64',count=12).reshape((3,4))
+ return M,N
+
+def depth_read(filename):
+ """ Read depth data from file, return as numpy array. """
+ f = open(filename,'rb')
+ check = np.fromfile(f,dtype=np.float32,count=1)[0]
+ assert check == TAG_FLOAT, 'depth_read:: Wrong tag in depth file (should be: {0}, is: {1}). Big-endian machine?'.format(TAG_FLOAT,check)
+ width = np.fromfile(f,dtype=np.int32,count=1)[0]
+ height = np.fromfile(f,dtype=np.int32,count=1)[0]
+ size = width*height
+ assert width > 0 and height > 0 and size > 1 and size < 100000000, 'depth_read:: Invalid input size (width = {0}, height = {1}).'.format(width,height)
+ depth = np.fromfile(f,dtype=np.float32,count=-1).reshape((height,width))
+ return depth
+
+def RT_to_extrinsic_matrix(R, T):
+ extrinsic_matrix = np.concatenate([R, T], axis=-1)
+ extrinsic_matrix = np.concatenate([extrinsic_matrix, np.array([[0, 0, 0, 1]])], axis=0)
+ return np.linalg.inv(extrinsic_matrix)
+
+def depth_to_3d(depth_map, intrinsic_matrix):
+ height, width = depth_map.shape
+ i, j = np.meshgrid(np.arange(width), np.arange(height))
+
+ # Convert pixel coordinates and depth values to 3D points
+ x = (i - intrinsic_matrix[0, 2]) * depth_map / intrinsic_matrix[0, 0]
+ y = (j - intrinsic_matrix[1, 2]) * depth_map / intrinsic_matrix[1, 1]
+ z = depth_map
+
+ points_3d = np.stack([x, y, z], axis=-1)
+ return points_3d
+
+def project_3d_to_2d(points_3d, intrinsic_matrix):
+ # Convert 3D points to homogeneous coordinates
+ projected_2d_hom = intrinsic_matrix @ points_3d.T
+ # Convert from homogeneous coordinates to 2D image coordinates
+ projected_2d = projected_2d_hom[:2, :] / projected_2d_hom[2, :]
+ return projected_2d.T
+
+def compute_optical_flow(depth1, depth2, pose1, pose2, intrinsic_matrix1, intrinsic_matrix2):
+ # Input: All inputs as numpy arrays; convert torch tensors to numpy arrays if needed
+ if isinstance(depth1, torch.Tensor):
+ depth1 = depth1.cpu().numpy()
+ if isinstance(depth2, torch.Tensor):
+ depth2 = depth2.cpu().numpy()
+ if isinstance(pose1, torch.Tensor):
+ pose1 = pose1.cpu().numpy()
+ if isinstance(pose2, torch.Tensor):
+ pose2 = pose2.cpu().numpy()
+ if isinstance(intrinsic_matrix1, torch.Tensor):
+ intrinsic_matrix1 = intrinsic_matrix1.cpu().numpy()
+ if isinstance(intrinsic_matrix2, torch.Tensor):
+ intrinsic_matrix2 = intrinsic_matrix2.cpu().numpy()
+
+ points_3d_frame1 = depth_to_3d(depth1, intrinsic_matrix1).reshape(-1, 3)
+ points_3d_frame1_hom = np.concatenate([points_3d_frame1, np.ones((points_3d_frame1.shape[0], 1))], axis=1).T
+
+ # Calculate the transformation matrix from frame 1 to frame 2
+ transformation_matrix = (pose2) @ np.linalg.inv(pose1)
+ points_3d_frame2_hom = transformation_matrix @ points_3d_frame1_hom
+ points_3d_frame2 = (points_3d_frame2_hom[:3, :]).T
+
+ points_2d_frame1 = project_3d_to_2d(points_3d_frame1, intrinsic_matrix1)
+ points_2d_frame2 = project_3d_to_2d(points_3d_frame2, intrinsic_matrix2)
+
+ # Compute optical flow vectors
+ optical_flow = points_2d_frame2 - points_2d_frame1
+ return optical_flow
+
+def get_dynamic_label(base_dir, seq, continuous=False, threshold=13.75, save_dir='dynamic_label'):
+ depth_dir = os.path.join(base_dir, 'depth', seq)
+ cam_dir = os.path.join(base_dir, 'camdata_left', seq)
+ flow_dir = os.path.join(base_dir, 'flow', seq)
+ dynamic_label_dir = os.path.join(base_dir, save_dir, seq)
+ os.makedirs(dynamic_label_dir, exist_ok=True)
+
+ frames = sorted([f for f in os.listdir(depth_dir) if f.endswith('.dpt')])
+ for i, frame1 in enumerate(frames):
+ if i == len(frames) - 1:
+ continue
+ frame2 = frames[i + 1]
+
+ frame1_id = frame1.split('.')[0]
+ frame2_id = frame2.split('.')[0]
+
+ # Load depth maps
+ depth_map_frame1 = depth_read(os.path.join(depth_dir, frame1))
+ depth_map_frame2 = depth_read(os.path.join(depth_dir, frame2))
+
+ # Load camera intrinsics and poses
+ intrinsic_matrix1, pose_frame1 = cam_read(os.path.join(cam_dir, f'{frame1_id}.cam'))
+ intrinsic_matrix2, pose_frame2 = cam_read(os.path.join(cam_dir, f'{frame2_id}.cam'))
+
+ # Pad pose with [0,0,0,1]
+ pose_frame1 = np.concatenate([pose_frame1, np.array([[0, 0, 0, 1]])], axis=0)
+ pose_frame2 = np.concatenate([pose_frame2, np.array([[0, 0, 0, 1]])], axis=0)
+
+ # Compute optical flow
+ optical_flow = compute_optical_flow(depth_map_frame1, depth_map_frame2, pose_frame1, pose_frame2, intrinsic_matrix1, intrinsic_matrix2)
+
+ # Reshape the optical flow to the image dimensions
+ height, width = depth_map_frame1.shape
+ optical_flow_image = optical_flow.reshape(height, width, 2)
+
+ # Load ground truth optical flow
+ u, v = flow_read(os.path.join(flow_dir, f'{frame1_id}.flo'))
+ gt_flow = np.stack([u, v], axis=-1)
+
+ # Compute the error map
+ error_map = np.linalg.norm(gt_flow - optical_flow_image, axis=-1)
+ if not continuous:
+ binary_error_map = error_map > threshold
+
+ # Save the binary error map
+ cv2.imwrite(os.path.join(dynamic_label_dir, f'{frame1_id}.png'), binary_error_map.astype(np.uint8) * 255)
+ else:
+ # Normalize the error map
+ error_map = error_map / error_map.max()
+ cv2.imwrite(os.path.join(dynamic_label_dir, f'{frame1_id}.png'), (error_map * 255).astype(np.uint8))
+
+if __name__ == '__main__':
+ # Process all sequences
+ sequences = sorted(os.listdir('data/sintel/training/depth'))
+ base_dir = 'data/sintel/training'
+ parser = argparse.ArgumentParser()
+ parser.add_argument('--continuous', action='store_true')
+ parser.add_argument('--threshold', type=float, default=13.75)
+ parser.add_argument('--save_dir', type=str, default='dynamic_label')
+ args = parser.parse_args()
+ for seq in tqdm(sequences):
+ get_dynamic_label(base_dir, seq, continuous=args.continuous, threshold=args.threshold, save_dir=args.save_dir)
+ print(f'Finished processing {seq}')
diff --git a/dynamic_predictor/DAS3R_b32_g4.sh b/dynamic_predictor/DAS3R_b32_g4.sh
new file mode 100644
index 0000000000000000000000000000000000000000..58f65de331aea15f65d44e9de288d99cec3753eb
--- /dev/null
+++ b/dynamic_predictor/DAS3R_b32_g4.sh
@@ -0,0 +1,19 @@
+# Set environment variables
+export CUDA_VISIBLE_DEVICES="0,1,2,3"
+
+# Run the Python script using torchrun (adjust if using distributed training)
+torchrun --nproc_per_node=1 --master_port=27777 launch.py --mode=train \
+ --model="AsymmetricCroCo3DStereo(pos_embed='RoPE100', patch_embed_cls='ManyAR_PatchEmbed', \
+ img_size=(512, 512), head_type='dpt', output_mode='pts3d', depth_mode=('exp', -inf, inf), conf_mode=('exp', 1, inf), \
+ enc_embed_dim=1024, enc_depth=24, enc_num_heads=16, dec_embed_dim=768, dec_depth=12, dec_num_heads=12, freeze='encoder_and_3d_predictor')" \
+ --train_dataset="10_000 @ PointOdysseyDUSt3R(dset='train', z_far=80, dataset_location='../data/point_odyssey', S=2, aug_crop=16, resolution=[(512, 288), (512, 384), (512, 336)], transform=ColorJitter, strides=[1,2,3,4,5,6,7,8,9], dist_type='linear_1_2', aug_focal=0.9)" \
+ --test_dataset="1 * PointOdysseyDUSt3R(dset='test', z_far=80, dataset_location='../data/point_odyssey', S=2, strides=[1,2,3,4,5,6,7,8,9], resolution=[(512, 288)], seed=777) + 1 * SintelDUSt3R(dset='final', z_far=80, S=2, strides=[1,2,3,4,5,6,7,8,9], resolution=[(512, 224)], seed=777)" \
+ --train_criterion="ConfLoss(Regr3D_MMask(L21, norm_mode='avg_dis'), alpha=0.2)" \
+ --test_criterion="Regr3D_ScaleShiftInv_MMask(L21, gt_scale=True)" \
+ --pretrained="Junyi42/MonST3R_PO-TA-S-W_ViTLarge_BaseDecoder_512_dpt" \
+ --lr=0.00005 --min_lr=1e-06 --warmup_epochs=3 --epochs=50 --batch_size=8 --accum_iter=1 \
+ --test_batch_size=8 \
+ --save_freq=3 --keep_freq=5 --eval_freq=50 \
+ --output_dir=results/MSeg_from_monst3r_b32_g4 \
+ --num_workers=16 --wandb \
+
diff --git a/dynamic_predictor/croco/.gitignore b/dynamic_predictor/croco/.gitignore
new file mode 100644
index 0000000000000000000000000000000000000000..b6e47617de110dea7ca47e087ff1347cc2646eda
--- /dev/null
+++ b/dynamic_predictor/croco/.gitignore
@@ -0,0 +1,129 @@
+# Byte-compiled / optimized / DLL files
+__pycache__/
+*.py[cod]
+*$py.class
+
+# C extensions
+*.so
+
+# Distribution / packaging
+.Python
+build/
+develop-eggs/
+dist/
+downloads/
+eggs/
+.eggs/
+lib/
+lib64/
+parts/
+sdist/
+var/
+wheels/
+pip-wheel-metadata/
+share/python-wheels/
+*.egg-info/
+.installed.cfg
+*.egg
+MANIFEST
+
+# PyInstaller
+# Usually these files are written by a python script from a template
+# before PyInstaller builds the exe, so as to inject date/other infos into it.
+*.manifest
+*.spec
+
+# Installer logs
+pip-log.txt
+pip-delete-this-directory.txt
+
+# Unit test / coverage reports
+htmlcov/
+.tox/
+.nox/
+.coverage
+.coverage.*
+.cache
+nosetests.xml
+coverage.xml
+*.cover
+*.py,cover
+.hypothesis/
+.pytest_cache/
+
+# Translations
+*.mo
+*.pot
+
+# Django stuff:
+*.log
+local_settings.py
+db.sqlite3
+db.sqlite3-journal
+
+# Flask stuff:
+instance/
+.webassets-cache
+
+# Scrapy stuff:
+.scrapy
+
+# Sphinx documentation
+docs/_build/
+
+# PyBuilder
+target/
+
+# Jupyter Notebook
+.ipynb_checkpoints
+
+# IPython
+profile_default/
+ipython_config.py
+
+# pyenv
+.python-version
+
+# pipenv
+# According to pypa/pipenv#598, it is recommended to include Pipfile.lock in version control.
+# However, in case of collaboration, if having platform-specific dependencies or dependencies
+# having no cross-platform support, pipenv may install dependencies that don't work, or not
+# install all needed dependencies.
+#Pipfile.lock
+
+# PEP 582; used by e.g. github.com/David-OConnor/pyflow
+__pypackages__/
+
+# Celery stuff
+celerybeat-schedule
+celerybeat.pid
+
+# SageMath parsed files
+*.sage.py
+
+# Environments
+.env
+.venv
+env/
+venv/
+ENV/
+env.bak/
+venv.bak/
+
+# Spyder project settings
+.spyderproject
+.spyproject
+
+# Rope project settings
+.ropeproject
+
+# mkdocs documentation
+/site
+
+# mypy
+.mypy_cache/
+.dmypy.json
+dmypy.json
+
+# Pyre type checker
+.pyre/
diff --git a/dynamic_predictor/croco/LICENSE b/dynamic_predictor/croco/LICENSE
new file mode 100644
index 0000000000000000000000000000000000000000..d9b84b1a65f9db6d8920a9048d162f52ba3ea56d
--- /dev/null
+++ b/dynamic_predictor/croco/LICENSE
@@ -0,0 +1,52 @@
+CroCo, Copyright (c) 2022-present Naver Corporation, is licensed under the Creative Commons Attribution-NonCommercial-ShareAlike 4.0 license.
+
+A summary of the CC BY-NC-SA 4.0 license is located here:
+ https://creativecommons.org/licenses/by-nc-sa/4.0/
+
+The CC BY-NC-SA 4.0 license is located here:
+ https://creativecommons.org/licenses/by-nc-sa/4.0/legalcode
+
+
+SEE NOTICE BELOW WITH RESPECT TO THE FILE: models/pos_embed.py, models/blocks.py
+
+***************************
+
+NOTICE WITH RESPECT TO THE FILE: models/pos_embed.py
+
+This software is being redistributed in a modifiled form. The original form is available here:
+
+https://github.com/facebookresearch/mae/blob/main/util/pos_embed.py
+
+This software in this file incorporates parts of the following software available here:
+
+Transformer: https://github.com/tensorflow/models/blob/master/official/legacy/transformer/model_utils.py
+available under the following license: https://github.com/tensorflow/models/blob/master/LICENSE
+
+MoCo v3: https://github.com/facebookresearch/moco-v3
+available under the following license: https://github.com/facebookresearch/moco-v3/blob/main/LICENSE
+
+DeiT: https://github.com/facebookresearch/deit
+available under the following license: https://github.com/facebookresearch/deit/blob/main/LICENSE
+
+
+ORIGINAL COPYRIGHT NOTICE AND PERMISSION NOTICE AVAILABLE HERE IS REPRODUCE BELOW:
+
+https://github.com/facebookresearch/mae/blob/main/LICENSE
+
+Attribution-NonCommercial 4.0 International
+
+***************************
+
+NOTICE WITH RESPECT TO THE FILE: models/blocks.py
+
+This software is being redistributed in a modifiled form. The original form is available here:
+
+https://github.com/rwightman/pytorch-image-models
+
+ORIGINAL COPYRIGHT NOTICE AND PERMISSION NOTICE AVAILABLE HERE IS REPRODUCE BELOW:
+
+https://github.com/rwightman/pytorch-image-models/blob/master/LICENSE
+
+Apache License
+Version 2.0, January 2004
+http://www.apache.org/licenses/
\ No newline at end of file
diff --git a/dynamic_predictor/croco/NOTICE b/dynamic_predictor/croco/NOTICE
new file mode 100644
index 0000000000000000000000000000000000000000..d51bb365036c12d428d6e3a4fd00885756d5261c
--- /dev/null
+++ b/dynamic_predictor/croco/NOTICE
@@ -0,0 +1,21 @@
+CroCo
+Copyright 2022-present NAVER Corp.
+
+This project contains subcomponents with separate copyright notices and license terms.
+Your use of the source code for these subcomponents is subject to the terms and conditions of the following licenses.
+
+====
+
+facebookresearch/mae
+https://github.com/facebookresearch/mae
+
+Attribution-NonCommercial 4.0 International
+
+====
+
+rwightman/pytorch-image-models
+https://github.com/rwightman/pytorch-image-models
+
+Apache License
+Version 2.0, January 2004
+http://www.apache.org/licenses/
\ No newline at end of file
diff --git a/dynamic_predictor/croco/README.MD b/dynamic_predictor/croco/README.MD
new file mode 100644
index 0000000000000000000000000000000000000000..38e33b001a60bd16749317fb297acd60f28a6f1b
--- /dev/null
+++ b/dynamic_predictor/croco/README.MD
@@ -0,0 +1,124 @@
+# CroCo + CroCo v2 / CroCo-Stereo / CroCo-Flow
+
+[[`CroCo arXiv`](https://arxiv.org/abs/2210.10716)] [[`CroCo v2 arXiv`](https://arxiv.org/abs/2211.10408)] [[`project page and demo`](https://croco.europe.naverlabs.com/)]
+
+This repository contains the code for our CroCo model presented in our NeurIPS'22 paper [CroCo: Self-Supervised Pre-training for 3D Vision Tasks by Cross-View Completion](https://openreview.net/pdf?id=wZEfHUM5ri) and its follow-up extension published at ICCV'23 [Improved Cross-view Completion Pre-training for Stereo Matching and Optical Flow](https://openaccess.thecvf.com/content/ICCV2023/html/Weinzaepfel_CroCo_v2_Improved_Cross-view_Completion_Pre-training_for_Stereo_Matching_and_ICCV_2023_paper.html), refered to as CroCo v2:
+
+
+
+```bibtex
+@inproceedings{croco,
+ title={{CroCo: Self-Supervised Pre-training for 3D Vision Tasks by Cross-View Completion}},
+ author={{Weinzaepfel, Philippe and Leroy, Vincent and Lucas, Thomas and Br\'egier, Romain and Cabon, Yohann and Arora, Vaibhav and Antsfeld, Leonid and Chidlovskii, Boris and Csurka, Gabriela and Revaud J\'er\^ome}},
+ booktitle={{NeurIPS}},
+ year={2022}
+}
+
+@inproceedings{croco_v2,
+ title={{CroCo v2: Improved Cross-view Completion Pre-training for Stereo Matching and Optical Flow}},
+ author={Weinzaepfel, Philippe and Lucas, Thomas and Leroy, Vincent and Cabon, Yohann and Arora, Vaibhav and Br{\'e}gier, Romain and Csurka, Gabriela and Antsfeld, Leonid and Chidlovskii, Boris and Revaud, J{\'e}r{\^o}me},
+ booktitle={ICCV},
+ year={2023}
+}
+```
+
+## License
+
+The code is distributed under the CC BY-NC-SA 4.0 License. See [LICENSE](LICENSE) for more information.
+Some components are based on code from [MAE](https://github.com/facebookresearch/mae) released under the CC BY-NC-SA 4.0 License and [timm](https://github.com/rwightman/pytorch-image-models) released under the Apache 2.0 License.
+Some components for stereo matching and optical flow are based on code from [unimatch](https://github.com/autonomousvision/unimatch) released under the MIT license.
+
+## Preparation
+
+1. Install dependencies on a machine with a NVidia GPU using e.g. conda. Note that `habitat-sim` is required only for the interactive demo and the synthetic pre-training data generation. If you don't plan to use it, you can ignore the line installing it and use a more recent python version.
+
+```bash
+conda create -n croco python=3.7 cmake=3.14.0
+conda activate croco
+conda install habitat-sim headless -c conda-forge -c aihabitat
+conda install pytorch torchvision -c pytorch
+conda install notebook ipykernel matplotlib
+conda install ipywidgets widgetsnbextension
+conda install scikit-learn tqdm quaternion opencv # only for pretraining / habitat data generation
+
+```
+
+2. Compile cuda kernels for RoPE
+
+CroCo v2 relies on RoPE positional embeddings for which you need to compile some cuda kernels.
+```bash
+cd models/curope/
+python setup.py build_ext --inplace
+cd ../../
+```
+
+This can be a bit long as we compile for all cuda architectures, feel free to update L9 of `models/curope/setup.py` to compile for specific architectures only.
+You might also need to set the environment `CUDA_HOME` in case you use a custom cuda installation.
+
+In case you cannot provide, we also provide a slow pytorch version, which will be automatically loaded.
+
+3. Download pre-trained model
+
+We provide several pre-trained models:
+
+| modelname | pre-training data | pos. embed. | Encoder | Decoder |
+|------------------------------------------------------------------------------------------------------------------------------------|-------------------|-------------|---------|---------|
+| [`CroCo.pth`](https://download.europe.naverlabs.com/ComputerVision/CroCo/CroCo.pth) | Habitat | cosine | ViT-B | Small |
+| [`CroCo_V2_ViTBase_SmallDecoder.pth`](https://download.europe.naverlabs.com/ComputerVision/CroCo/CroCo_V2_ViTBase_SmallDecoder.pth) | Habitat + real | RoPE | ViT-B | Small |
+| [`CroCo_V2_ViTBase_BaseDecoder.pth`](https://download.europe.naverlabs.com/ComputerVision/CroCo/CroCo_V2_ViTBase_BaseDecoder.pth) | Habitat + real | RoPE | ViT-B | Base |
+| [`CroCo_V2_ViTLarge_BaseDecoder.pth`](https://download.europe.naverlabs.com/ComputerVision/CroCo/CroCo_V2_ViTLarge_BaseDecoder.pth) | Habitat + real | RoPE | ViT-L | Base |
+
+To download a specific model, i.e., the first one (`CroCo.pth`)
+```bash
+mkdir -p pretrained_models/
+wget https://download.europe.naverlabs.com/ComputerVision/CroCo/CroCo.pth -P pretrained_models/
+```
+
+## Reconstruction example
+
+Simply run after downloading the `CroCo_V2_ViTLarge_BaseDecoder` pretrained model (or update the corresponding line in `demo.py`)
+```bash
+python demo.py
+```
+
+## Interactive demonstration of cross-view completion reconstruction on the Habitat simulator
+
+First download the test scene from Habitat:
+```bash
+python -m habitat_sim.utils.datasets_download --uids habitat_test_scenes --data-path habitat-sim-data/
+```
+
+Then, run the Notebook demo `interactive_demo.ipynb`.
+
+In this demo, you should be able to sample a random reference viewpoint from an [Habitat](https://github.com/facebookresearch/habitat-sim) test scene. Use the sliders to change viewpoint and select a masked target view to reconstruct using CroCo.
+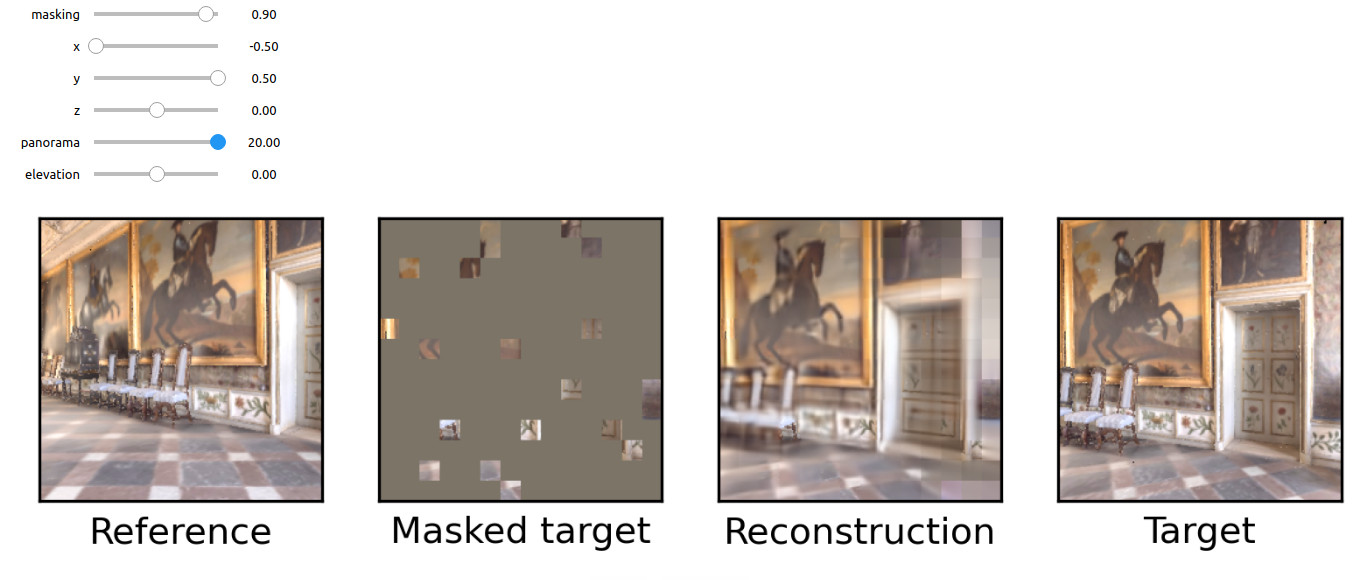
+
+## Pre-training
+
+### CroCo
+
+To pre-train CroCo, please first generate the pre-training data from the Habitat simulator, following the instructions in [datasets/habitat_sim/README.MD](datasets/habitat_sim/README.MD) and then run the following command:
+```
+torchrun --nproc_per_node=4 pretrain.py --output_dir ./output/pretraining/
+```
+
+Our CroCo pre-training was launched on a single server with 4 GPUs.
+It should take around 10 days with A100 or 15 days with V100 to do the 400 pre-training epochs, but decent performances are obtained earlier in training.
+Note that, while the code contains the same scaling rule of the learning rate as MAE when changing the effective batch size, we did not experimented if it is valid in our case.
+The first run can take a few minutes to start, to parse all available pre-training pairs.
+
+### CroCo v2
+
+For CroCo v2 pre-training, in addition to the generation of the pre-training data from the Habitat simulator above, please pre-extract the crops from the real datasets following the instructions in [datasets/crops/README.MD](datasets/crops/README.MD).
+Then, run the following command for the largest model (ViT-L encoder, Base decoder):
+```
+torchrun --nproc_per_node=8 pretrain.py --model "CroCoNet(enc_embed_dim=1024, enc_depth=24, enc_num_heads=16, dec_embed_dim=768, dec_num_heads=12, dec_depth=12, pos_embed='RoPE100')" --dataset "habitat_release+ARKitScenes+MegaDepth+3DStreetView+IndoorVL" --warmup_epochs 12 --max_epoch 125 --epochs 250 --amp 0 --keep_freq 5 --output_dir ./output/pretraining_crocov2/
+```
+
+Our CroCo v2 pre-training was launched on a single server with 8 GPUs for the largest model, and on a single server with 4 GPUs for the smaller ones, keeping a batch size of 64 per gpu in all cases.
+The largest model should take around 12 days on A100.
+Note that, while the code contains the same scaling rule of the learning rate as MAE when changing the effective batch size, we did not experimented if it is valid in our case.
+
+## Stereo matching and Optical flow downstream tasks
+
+For CroCo-Stereo and CroCo-Flow, please refer to [stereoflow/README.MD](stereoflow/README.MD).
diff --git a/dynamic_predictor/croco/assets/Chateau1.png b/dynamic_predictor/croco/assets/Chateau1.png
new file mode 100644
index 0000000000000000000000000000000000000000..d282fc6a51c00b8dd8267d5d507220ae253c2d65
Binary files /dev/null and b/dynamic_predictor/croco/assets/Chateau1.png differ
diff --git a/dynamic_predictor/croco/assets/Chateau2.png b/dynamic_predictor/croco/assets/Chateau2.png
new file mode 100644
index 0000000000000000000000000000000000000000..722b2fc553ec089346722efb9445526ddfa8e7bd
Binary files /dev/null and b/dynamic_predictor/croco/assets/Chateau2.png differ
diff --git a/dynamic_predictor/croco/assets/arch.jpg b/dynamic_predictor/croco/assets/arch.jpg
new file mode 100644
index 0000000000000000000000000000000000000000..3f5b032729ddc58c06d890a0ebda1749276070c4
Binary files /dev/null and b/dynamic_predictor/croco/assets/arch.jpg differ
diff --git a/dynamic_predictor/croco/croco-stereo-flow-demo.ipynb b/dynamic_predictor/croco/croco-stereo-flow-demo.ipynb
new file mode 100644
index 0000000000000000000000000000000000000000..2b00a7607ab5f82d1857041969bfec977e56b3e0
--- /dev/null
+++ b/dynamic_predictor/croco/croco-stereo-flow-demo.ipynb
@@ -0,0 +1,191 @@
+{
+ "cells": [
+ {
+ "cell_type": "markdown",
+ "id": "9bca0f41",
+ "metadata": {},
+ "source": [
+ "# Simple inference example with CroCo-Stereo or CroCo-Flow"
+ ]
+ },
+ {
+ "cell_type": "code",
+ "execution_count": null,
+ "id": "80653ef7",
+ "metadata": {},
+ "outputs": [],
+ "source": [
+ "# Copyright (C) 2022-present Naver Corporation. All rights reserved.\n",
+ "# Licensed under CC BY-NC-SA 4.0 (non-commercial use only)."
+ ]
+ },
+ {
+ "cell_type": "markdown",
+ "id": "4f033862",
+ "metadata": {},
+ "source": [
+ "First download the model(s) of your choice by running\n",
+ "```\n",
+ "bash stereoflow/download_model.sh crocostereo.pth\n",
+ "bash stereoflow/download_model.sh crocoflow.pth\n",
+ "```"
+ ]
+ },
+ {
+ "cell_type": "code",
+ "execution_count": null,
+ "id": "1fb2e392",
+ "metadata": {},
+ "outputs": [],
+ "source": [
+ "import torch\n",
+ "use_gpu = torch.cuda.is_available() and torch.cuda.device_count()>0\n",
+ "device = torch.device('cuda:0' if use_gpu else 'cpu')\n",
+ "import matplotlib.pylab as plt"
+ ]
+ },
+ {
+ "cell_type": "code",
+ "execution_count": null,
+ "id": "e0e25d77",
+ "metadata": {},
+ "outputs": [],
+ "source": [
+ "from stereoflow.test import _load_model_and_criterion\n",
+ "from stereoflow.engine import tiled_pred\n",
+ "from stereoflow.datasets_stereo import img_to_tensor, vis_disparity\n",
+ "from stereoflow.datasets_flow import flowToColor\n",
+ "tile_overlap=0.7 # recommended value, higher value can be slightly better but slower"
+ ]
+ },
+ {
+ "cell_type": "markdown",
+ "id": "86a921f5",
+ "metadata": {},
+ "source": [
+ "### CroCo-Stereo example"
+ ]
+ },
+ {
+ "cell_type": "code",
+ "execution_count": null,
+ "id": "64e483cb",
+ "metadata": {},
+ "outputs": [],
+ "source": [
+ "image1 = np.asarray(Image.open(''))\n",
+ "image2 = np.asarray(Image.open(''))"
+ ]
+ },
+ {
+ "cell_type": "code",
+ "execution_count": null,
+ "id": "f0d04303",
+ "metadata": {},
+ "outputs": [],
+ "source": [
+ "model, _, cropsize, with_conf, task, tile_conf_mode = _load_model_and_criterion('stereoflow_models/crocostereo.pth', None, device)\n"
+ ]
+ },
+ {
+ "cell_type": "code",
+ "execution_count": null,
+ "id": "47dc14b5",
+ "metadata": {},
+ "outputs": [],
+ "source": [
+ "im1 = img_to_tensor(image1).to(device).unsqueeze(0)\n",
+ "im2 = img_to_tensor(image2).to(device).unsqueeze(0)\n",
+ "with torch.inference_mode():\n",
+ " pred, _, _ = tiled_pred(model, None, im1, im2, None, conf_mode=tile_conf_mode, overlap=tile_overlap, crop=cropsize, with_conf=with_conf, return_time=False)\n",
+ "pred = pred.squeeze(0).squeeze(0).cpu().numpy()"
+ ]
+ },
+ {
+ "cell_type": "code",
+ "execution_count": null,
+ "id": "583b9f16",
+ "metadata": {},
+ "outputs": [],
+ "source": [
+ "plt.imshow(vis_disparity(pred))\n",
+ "plt.axis('off')"
+ ]
+ },
+ {
+ "cell_type": "markdown",
+ "id": "d2df5d70",
+ "metadata": {},
+ "source": [
+ "### CroCo-Flow example"
+ ]
+ },
+ {
+ "cell_type": "code",
+ "execution_count": null,
+ "id": "9ee257a7",
+ "metadata": {},
+ "outputs": [],
+ "source": [
+ "image1 = np.asarray(Image.open(''))\n",
+ "image2 = np.asarray(Image.open(''))"
+ ]
+ },
+ {
+ "cell_type": "code",
+ "execution_count": null,
+ "id": "d5edccf0",
+ "metadata": {},
+ "outputs": [],
+ "source": [
+ "model, _, cropsize, with_conf, task, tile_conf_mode = _load_model_and_criterion('stereoflow_models/crocoflow.pth', None, device)\n"
+ ]
+ },
+ {
+ "cell_type": "code",
+ "execution_count": null,
+ "id": "b19692c3",
+ "metadata": {},
+ "outputs": [],
+ "source": [
+ "im1 = img_to_tensor(image1).to(device).unsqueeze(0)\n",
+ "im2 = img_to_tensor(image2).to(device).unsqueeze(0)\n",
+ "with torch.inference_mode():\n",
+ " pred, _, _ = tiled_pred(model, None, im1, im2, None, conf_mode=tile_conf_mode, overlap=tile_overlap, crop=cropsize, with_conf=with_conf, return_time=False)\n",
+ "pred = pred.squeeze(0).permute(1,2,0).cpu().numpy()"
+ ]
+ },
+ {
+ "cell_type": "code",
+ "execution_count": null,
+ "id": "26f79db3",
+ "metadata": {},
+ "outputs": [],
+ "source": [
+ "plt.imshow(flowToColor(pred))\n",
+ "plt.axis('off')"
+ ]
+ }
+ ],
+ "metadata": {
+ "kernelspec": {
+ "display_name": "Python 3 (ipykernel)",
+ "language": "python",
+ "name": "python3"
+ },
+ "language_info": {
+ "codemirror_mode": {
+ "name": "ipython",
+ "version": 3
+ },
+ "file_extension": ".py",
+ "mimetype": "text/x-python",
+ "name": "python",
+ "nbconvert_exporter": "python",
+ "pygments_lexer": "ipython3",
+ "version": "3.9.7"
+ }
+ },
+ "nbformat": 4,
+ "nbformat_minor": 5
+}
diff --git a/dynamic_predictor/croco/datasets/__init__.py b/dynamic_predictor/croco/datasets/__init__.py
new file mode 100644
index 0000000000000000000000000000000000000000..e69de29bb2d1d6434b8b29ae775ad8c2e48c5391
diff --git a/dynamic_predictor/croco/datasets/crops/README.MD b/dynamic_predictor/croco/datasets/crops/README.MD
new file mode 100644
index 0000000000000000000000000000000000000000..47ddabebb177644694ee247ae878173a3a16644f
--- /dev/null
+++ b/dynamic_predictor/croco/datasets/crops/README.MD
@@ -0,0 +1,104 @@
+## Generation of crops from the real datasets
+
+The instructions below allow to generate the crops used for pre-training CroCo v2 from the following real-world datasets: ARKitScenes, MegaDepth, 3DStreetView and IndoorVL.
+
+### Download the metadata of the crops to generate
+
+First, download the metadata and put them in `./data/`:
+```
+mkdir -p data
+cd data/
+wget https://download.europe.naverlabs.com/ComputerVision/CroCo/data/crop_metadata.zip
+unzip crop_metadata.zip
+rm crop_metadata.zip
+cd ..
+```
+
+### Prepare the original datasets
+
+Second, download the original datasets in `./data/original_datasets/`.
+```
+mkdir -p data/original_datasets
+```
+
+##### ARKitScenes
+
+Download the `raw` dataset from https://github.com/apple/ARKitScenes/blob/main/DATA.md and put it in `./data/original_datasets/ARKitScenes/`.
+The resulting file structure should be like:
+```
+./data/original_datasets/ARKitScenes/
+└───Training
+ └───40753679
+ │ │ ultrawide
+ │ │ ...
+ └───40753686
+ │
+ ...
+```
+
+##### MegaDepth
+
+Download `MegaDepth v1 Dataset` from https://www.cs.cornell.edu/projects/megadepth/ and put it in `./data/original_datasets/MegaDepth/`.
+The resulting file structure should be like:
+
+```
+./data/original_datasets/MegaDepth/
+└───0000
+│ └───images
+│ │ │ 1000557903_87fa96b8a4_o.jpg
+│ │ └ ...
+│ └─── ...
+└───0001
+│ │
+│ └ ...
+└─── ...
+```
+
+##### 3DStreetView
+
+Download `3D_Street_View` dataset from https://github.com/amir32002/3D_Street_View and put it in `./data/original_datasets/3DStreetView/`.
+The resulting file structure should be like:
+
+```
+./data/original_datasets/3DStreetView/
+└───dataset_aligned
+│ └───0002
+│ │ │ 0000002_0000001_0000002_0000001.jpg
+│ │ └ ...
+│ └─── ...
+└───dataset_unaligned
+│ └───0003
+│ │ │ 0000003_0000001_0000002_0000001.jpg
+│ │ └ ...
+│ └─── ...
+```
+
+##### IndoorVL
+
+Download the `IndoorVL` datasets using [Kapture](https://github.com/naver/kapture).
+
+```
+pip install kapture
+mkdir -p ./data/original_datasets/IndoorVL
+cd ./data/original_datasets/IndoorVL
+kapture_download_dataset.py update
+kapture_download_dataset.py install "HyundaiDepartmentStore_*"
+kapture_download_dataset.py install "GangnamStation_*"
+cd -
+```
+
+### Extract the crops
+
+Now, extract the crops for each of the dataset:
+```
+for dataset in ARKitScenes MegaDepth 3DStreetView IndoorVL;
+do
+ python3 datasets/crops/extract_crops_from_images.py --crops ./data/crop_metadata/${dataset}/crops_release.txt --root-dir ./data/original_datasets/${dataset}/ --output-dir ./data/${dataset}_crops/ --imsize 256 --nthread 8 --max-subdir-levels 5 --ideal-number-pairs-in-dir 500;
+done
+```
+
+##### Note for IndoorVL
+
+Due to some legal issues, we can only release 144,228 pairs out of the 1,593,689 pairs used in the paper.
+To account for it in terms of number of pre-training iterations, the pre-training command in this repository uses 125 training epochs including 12 warm-up epochs and learning rate cosine schedule of 250, instead of 100, 10 and 200 respectively.
+The impact on the performance is negligible.
diff --git a/dynamic_predictor/croco/datasets/crops/extract_crops_from_images.py b/dynamic_predictor/croco/datasets/crops/extract_crops_from_images.py
new file mode 100644
index 0000000000000000000000000000000000000000..eb66a0474ce44b54c44c08887cbafdb045b11ff3
--- /dev/null
+++ b/dynamic_predictor/croco/datasets/crops/extract_crops_from_images.py
@@ -0,0 +1,159 @@
+# Copyright (C) 2022-present Naver Corporation. All rights reserved.
+# Licensed under CC BY-NC-SA 4.0 (non-commercial use only).
+#
+# --------------------------------------------------------
+# Extracting crops for pre-training
+# --------------------------------------------------------
+
+import os
+import argparse
+from tqdm import tqdm
+from PIL import Image
+import functools
+from multiprocessing import Pool
+import math
+
+
+def arg_parser():
+ parser = argparse.ArgumentParser('Generate cropped image pairs from image crop list')
+
+ parser.add_argument('--crops', type=str, required=True, help='crop file')
+ parser.add_argument('--root-dir', type=str, required=True, help='root directory')
+ parser.add_argument('--output-dir', type=str, required=True, help='output directory')
+ parser.add_argument('--imsize', type=int, default=256, help='size of the crops')
+ parser.add_argument('--nthread', type=int, required=True, help='number of simultaneous threads')
+ parser.add_argument('--max-subdir-levels', type=int, default=5, help='maximum number of subdirectories')
+ parser.add_argument('--ideal-number-pairs-in-dir', type=int, default=500, help='number of pairs stored in a dir')
+ return parser
+
+
+def main(args):
+ listing_path = os.path.join(args.output_dir, 'listing.txt')
+
+ print(f'Loading list of crops ... ({args.nthread} threads)')
+ crops, num_crops_to_generate = load_crop_file(args.crops)
+
+ print(f'Preparing jobs ({len(crops)} candidate image pairs)...')
+ num_levels = min(math.ceil(math.log(num_crops_to_generate, args.ideal_number_pairs_in_dir)), args.max_subdir_levels)
+ num_pairs_in_dir = math.ceil(num_crops_to_generate ** (1/num_levels))
+
+ jobs = prepare_jobs(crops, num_levels, num_pairs_in_dir)
+ del crops
+
+ os.makedirs(args.output_dir, exist_ok=True)
+ mmap = Pool(args.nthread).imap_unordered if args.nthread > 1 else map
+ call = functools.partial(save_image_crops, args)
+
+ print(f"Generating cropped images to {args.output_dir} ...")
+ with open(listing_path, 'w') as listing:
+ listing.write('# pair_path\n')
+ for results in tqdm(mmap(call, jobs), total=len(jobs)):
+ for path in results:
+ listing.write(f'{path}\n')
+ print('Finished writing listing to', listing_path)
+
+
+def load_crop_file(path):
+ data = open(path).read().splitlines()
+ pairs = []
+ num_crops_to_generate = 0
+ for line in tqdm(data):
+ if line.startswith('#'):
+ continue
+ line = line.split(', ')
+ if len(line) < 8:
+ img1, img2, rotation = line
+ pairs.append((img1, img2, int(rotation), []))
+ else:
+ l1, r1, t1, b1, l2, r2, t2, b2 = map(int, line)
+ rect1, rect2 = (l1, t1, r1, b1), (l2, t2, r2, b2)
+ pairs[-1][-1].append((rect1, rect2))
+ num_crops_to_generate += 1
+ return pairs, num_crops_to_generate
+
+
+def prepare_jobs(pairs, num_levels, num_pairs_in_dir):
+ jobs = []
+ powers = [num_pairs_in_dir**level for level in reversed(range(num_levels))]
+
+ def get_path(idx):
+ idx_array = []
+ d = idx
+ for level in range(num_levels - 1):
+ idx_array.append(idx // powers[level])
+ idx = idx % powers[level]
+ idx_array.append(d)
+ return '/'.join(map(lambda x: hex(x)[2:], idx_array))
+
+ idx = 0
+ for pair_data in tqdm(pairs):
+ img1, img2, rotation, crops = pair_data
+ if -60 <= rotation and rotation <= 60:
+ rotation = 0 # most likely not a true rotation
+ paths = [get_path(idx + k) for k in range(len(crops))]
+ idx += len(crops)
+ jobs.append(((img1, img2), rotation, crops, paths))
+ return jobs
+
+
+def load_image(path):
+ try:
+ return Image.open(path).convert('RGB')
+ except Exception as e:
+ print('skipping', path, e)
+ raise OSError()
+
+
+def save_image_crops(args, data):
+ # load images
+ img_pair, rot, crops, paths = data
+ try:
+ img1, img2 = [load_image(os.path.join(args.root_dir, impath)) for impath in img_pair]
+ except OSError as e:
+ return []
+
+ def area(sz):
+ return sz[0] * sz[1]
+
+ tgt_size = (args.imsize, args.imsize)
+
+ def prepare_crop(img, rect, rot=0):
+ # actual crop
+ img = img.crop(rect)
+
+ # resize to desired size
+ interp = Image.Resampling.LANCZOS if area(img.size) > 4*area(tgt_size) else Image.Resampling.BICUBIC
+ img = img.resize(tgt_size, resample=interp)
+
+ # rotate the image
+ rot90 = (round(rot/90) % 4) * 90
+ if rot90 == 90:
+ img = img.transpose(Image.Transpose.ROTATE_90)
+ elif rot90 == 180:
+ img = img.transpose(Image.Transpose.ROTATE_180)
+ elif rot90 == 270:
+ img = img.transpose(Image.Transpose.ROTATE_270)
+ return img
+
+ results = []
+ for (rect1, rect2), path in zip(crops, paths):
+ crop1 = prepare_crop(img1, rect1)
+ crop2 = prepare_crop(img2, rect2, rot)
+
+ fullpath1 = os.path.join(args.output_dir, path+'_1.jpg')
+ fullpath2 = os.path.join(args.output_dir, path+'_2.jpg')
+ os.makedirs(os.path.dirname(fullpath1), exist_ok=True)
+
+ assert not os.path.isfile(fullpath1), fullpath1
+ assert not os.path.isfile(fullpath2), fullpath2
+ crop1.save(fullpath1)
+ crop2.save(fullpath2)
+ results.append(path)
+
+ return results
+
+
+if __name__ == '__main__':
+ args = arg_parser().parse_args()
+ main(args)
+
diff --git a/dynamic_predictor/croco/datasets/habitat_sim/README.MD b/dynamic_predictor/croco/datasets/habitat_sim/README.MD
new file mode 100644
index 0000000000000000000000000000000000000000..a505781ff9eb91bce7f1d189e848f8ba1c560940
--- /dev/null
+++ b/dynamic_predictor/croco/datasets/habitat_sim/README.MD
@@ -0,0 +1,76 @@
+## Generation of synthetic image pairs using Habitat-Sim
+
+These instructions allow to generate pre-training pairs from the Habitat simulator.
+As we did not save metadata of the pairs used in the original paper, they are not strictly the same, but these data use the same setting and are equivalent.
+
+### Download Habitat-Sim scenes
+Download Habitat-Sim scenes:
+- Download links can be found here: https://github.com/facebookresearch/habitat-sim/blob/main/DATASETS.md
+- We used scenes from the HM3D, habitat-test-scenes, Replica, ReplicaCad and ScanNet datasets.
+- Please put the scenes under `./data/habitat-sim-data/scene_datasets/` following the structure below, or update manually paths in `paths.py`.
+```
+./data/
+└──habitat-sim-data/
+ └──scene_datasets/
+ ├──hm3d/
+ ├──gibson/
+ ├──habitat-test-scenes/
+ ├──replica_cad_baked_lighting/
+ ├──replica_cad/
+ ├──ReplicaDataset/
+ └──scannet/
+```
+
+### Image pairs generation
+We provide metadata to generate reproducible images pairs for pretraining and validation.
+Experiments described in the paper used similar data, but whose generation was not reproducible at the time.
+
+Specifications:
+- 256x256 resolution images, with 60 degrees field of view .
+- Up to 1000 image pairs per scene.
+- Number of scenes considered/number of images pairs per dataset:
+ - Scannet: 1097 scenes / 985 209 pairs
+ - HM3D:
+ - hm3d/train: 800 / 800k pairs
+ - hm3d/val: 100 scenes / 100k pairs
+ - hm3d/minival: 10 scenes / 10k pairs
+ - habitat-test-scenes: 3 scenes / 3k pairs
+ - replica_cad_baked_lighting: 13 scenes / 13k pairs
+
+- Scenes from hm3d/val and hm3d/minival pairs were not used for the pre-training but kept for validation purposes.
+
+Download metadata and extract it:
+```bash
+mkdir -p data/habitat_release_metadata/
+cd data/habitat_release_metadata/
+wget https://download.europe.naverlabs.com/ComputerVision/CroCo/data/habitat_release_metadata/multiview_habitat_metadata.tar.gz
+tar -xvf multiview_habitat_metadata.tar.gz
+cd ../..
+# Location of the metadata
+METADATA_DIR="./data/habitat_release_metadata/multiview_habitat_metadata"
+```
+
+Generate image pairs from metadata:
+- The following command will print a list of commandlines to generate image pairs for each scene:
+```bash
+# Target output directory
+PAIRS_DATASET_DIR="./data/habitat_release/"
+python datasets/habitat_sim/generate_from_metadata_files.py --input_dir=$METADATA_DIR --output_dir=$PAIRS_DATASET_DIR
+```
+- One can launch multiple of such commands in parallel e.g. using GNU Parallel:
+```bash
+python datasets/habitat_sim/generate_from_metadata_files.py --input_dir=$METADATA_DIR --output_dir=$PAIRS_DATASET_DIR | parallel -j 16
+```
+
+## Metadata generation
+
+Image pairs were randomly sampled using the following commands, whose outputs contain randomness and are thus not exactly reproducible:
+```bash
+# Print commandlines to generate image pairs from the different scenes available.
+PAIRS_DATASET_DIR=MY_CUSTOM_PATH
+python datasets/habitat_sim/generate_multiview_images.py --list_commands --output_dir=$PAIRS_DATASET_DIR
+
+# Once a dataset is generated, pack metadata files for reproducibility.
+METADATA_DIR=MY_CUSTON_PATH
+python datasets/habitat_sim/pack_metadata_files.py $PAIRS_DATASET_DIR $METADATA_DIR
+```
diff --git a/dynamic_predictor/croco/datasets/habitat_sim/__init__.py b/dynamic_predictor/croco/datasets/habitat_sim/__init__.py
new file mode 100644
index 0000000000000000000000000000000000000000..e69de29bb2d1d6434b8b29ae775ad8c2e48c5391
diff --git a/dynamic_predictor/croco/datasets/habitat_sim/generate_from_metadata.py b/dynamic_predictor/croco/datasets/habitat_sim/generate_from_metadata.py
new file mode 100644
index 0000000000000000000000000000000000000000..fbe0d399084359495250dc8184671ff498adfbf2
--- /dev/null
+++ b/dynamic_predictor/croco/datasets/habitat_sim/generate_from_metadata.py
@@ -0,0 +1,92 @@
+# Copyright (C) 2022-present Naver Corporation. All rights reserved.
+# Licensed under CC BY-NC-SA 4.0 (non-commercial use only).
+
+"""
+Script to generate image pairs for a given scene reproducing poses provided in a metadata file.
+"""
+import os
+from datasets.habitat_sim.multiview_habitat_sim_generator import MultiviewHabitatSimGenerator
+from datasets.habitat_sim.paths import SCENES_DATASET
+import argparse
+import quaternion
+import PIL.Image
+import cv2
+import json
+from tqdm import tqdm
+
+def generate_multiview_images_from_metadata(metadata_filename,
+ output_dir,
+ overload_params = dict(),
+ scene_datasets_paths=None,
+ exist_ok=False):
+ """
+ Generate images from a metadata file for reproducibility purposes.
+ """
+ # Reorder paths by decreasing label length, to avoid collisions when testing if a string by such label
+ if scene_datasets_paths is not None:
+ scene_datasets_paths = dict(sorted(scene_datasets_paths.items(), key= lambda x: len(x[0]), reverse=True))
+
+ with open(metadata_filename, 'r') as f:
+ input_metadata = json.load(f)
+ metadata = dict()
+ for key, value in input_metadata.items():
+ # Optionally replace some paths
+ if key in ("scene_dataset_config_file", "scene", "navmesh") and value != "":
+ if scene_datasets_paths is not None:
+ for dataset_label, dataset_path in scene_datasets_paths.items():
+ if value.startswith(dataset_label):
+ value = os.path.normpath(os.path.join(dataset_path, os.path.relpath(value, dataset_label)))
+ break
+ metadata[key] = value
+
+ # Overload some parameters
+ for key, value in overload_params.items():
+ metadata[key] = value
+
+ generation_entries = dict([(key, value) for key, value in metadata.items() if not (key in ('multiviews', 'output_dir', 'generate_depth'))])
+ generate_depth = metadata["generate_depth"]
+
+ os.makedirs(output_dir, exist_ok=exist_ok)
+
+ generator = MultiviewHabitatSimGenerator(**generation_entries)
+
+ # Generate views
+ for idx_label, data in tqdm(metadata['multiviews'].items()):
+ positions = data["positions"]
+ orientations = data["orientations"]
+ n = len(positions)
+ for oidx in range(n):
+ observation = generator.render_viewpoint(positions[oidx], quaternion.from_float_array(orientations[oidx]))
+ observation_label = f"{oidx + 1}" # Leonid is indexing starting from 1
+ # Color image saved using PIL
+ img = PIL.Image.fromarray(observation['color'][:,:,:3])
+ filename = os.path.join(output_dir, f"{idx_label}_{observation_label}.jpeg")
+ img.save(filename)
+ if generate_depth:
+ # Depth image as EXR file
+ filename = os.path.join(output_dir, f"{idx_label}_{observation_label}_depth.exr")
+ cv2.imwrite(filename, observation['depth'], [cv2.IMWRITE_EXR_TYPE, cv2.IMWRITE_EXR_TYPE_HALF])
+ # Camera parameters
+ camera_params = dict([(key, observation[key].tolist()) for key in ("camera_intrinsics", "R_cam2world", "t_cam2world")])
+ filename = os.path.join(output_dir, f"{idx_label}_{observation_label}_camera_params.json")
+ with open(filename, "w") as f:
+ json.dump(camera_params, f)
+ # Save metadata
+ with open(os.path.join(output_dir, "metadata.json"), "w") as f:
+ json.dump(metadata, f)
+
+ generator.close()
+
+if __name__ == "__main__":
+ parser = argparse.ArgumentParser()
+ parser.add_argument("--metadata_filename", required=True)
+ parser.add_argument("--output_dir", required=True)
+ args = parser.parse_args()
+
+ generate_multiview_images_from_metadata(metadata_filename=args.metadata_filename,
+ output_dir=args.output_dir,
+ scene_datasets_paths=SCENES_DATASET,
+ overload_params=dict(),
+ exist_ok=True)
+
+
\ No newline at end of file
diff --git a/dynamic_predictor/croco/datasets/habitat_sim/generate_from_metadata_files.py b/dynamic_predictor/croco/datasets/habitat_sim/generate_from_metadata_files.py
new file mode 100644
index 0000000000000000000000000000000000000000..962ef849d8c31397b8622df4f2d9140175d78873
--- /dev/null
+++ b/dynamic_predictor/croco/datasets/habitat_sim/generate_from_metadata_files.py
@@ -0,0 +1,27 @@
+# Copyright (C) 2022-present Naver Corporation. All rights reserved.
+# Licensed under CC BY-NC-SA 4.0 (non-commercial use only).
+
+"""
+Script generating commandlines to generate image pairs from metadata files.
+"""
+import os
+import glob
+from tqdm import tqdm
+import argparse
+
+if __name__ == "__main__":
+ parser = argparse.ArgumentParser()
+ parser.add_argument("--input_dir", required=True)
+ parser.add_argument("--output_dir", required=True)
+ parser.add_argument("--prefix", default="", help="Commanline prefix, useful e.g. to setup environment.")
+ args = parser.parse_args()
+
+ input_metadata_filenames = glob.iglob(f"{args.input_dir}/**/metadata.json", recursive=True)
+
+ for metadata_filename in tqdm(input_metadata_filenames):
+ output_dir = os.path.join(args.output_dir, os.path.relpath(os.path.dirname(metadata_filename), args.input_dir))
+ # Do not process the scene if the metadata file already exists
+ if os.path.exists(os.path.join(output_dir, "metadata.json")):
+ continue
+ commandline = f"{args.prefix}python datasets/habitat_sim/generate_from_metadata.py --metadata_filename={metadata_filename} --output_dir={output_dir}"
+ print(commandline)
diff --git a/dynamic_predictor/croco/datasets/habitat_sim/generate_multiview_images.py b/dynamic_predictor/croco/datasets/habitat_sim/generate_multiview_images.py
new file mode 100644
index 0000000000000000000000000000000000000000..421d49a1696474415940493296b3f2d982398850
--- /dev/null
+++ b/dynamic_predictor/croco/datasets/habitat_sim/generate_multiview_images.py
@@ -0,0 +1,177 @@
+# Copyright (C) 2022-present Naver Corporation. All rights reserved.
+# Licensed under CC BY-NC-SA 4.0 (non-commercial use only).
+
+import os
+from tqdm import tqdm
+import argparse
+import PIL.Image
+import numpy as np
+import json
+from datasets.habitat_sim.multiview_habitat_sim_generator import MultiviewHabitatSimGenerator, NoNaviguableSpaceError
+from datasets.habitat_sim.paths import list_scenes_available
+import cv2
+import quaternion
+import shutil
+
+def generate_multiview_images_for_scene(scene_dataset_config_file,
+ scene,
+ navmesh,
+ output_dir,
+ views_count,
+ size,
+ exist_ok=False,
+ generate_depth=False,
+ **kwargs):
+ """
+ Generate tuples of overlapping views for a given scene.
+ generate_depth: generate depth images and camera parameters.
+ """
+ if os.path.exists(output_dir) and not exist_ok:
+ print(f"Scene {scene}: data already generated. Ignoring generation.")
+ return
+ try:
+ print(f"Scene {scene}: {size} multiview acquisitions to generate...")
+ os.makedirs(output_dir, exist_ok=exist_ok)
+
+ metadata_filename = os.path.join(output_dir, "metadata.json")
+
+ metadata_template = dict(scene_dataset_config_file=scene_dataset_config_file,
+ scene=scene,
+ navmesh=navmesh,
+ views_count=views_count,
+ size=size,
+ generate_depth=generate_depth,
+ **kwargs)
+ metadata_template["multiviews"] = dict()
+
+ if os.path.exists(metadata_filename):
+ print("Metadata file already exists:", metadata_filename)
+ print("Loading already generated metadata file...")
+ with open(metadata_filename, "r") as f:
+ metadata = json.load(f)
+
+ for key in metadata_template.keys():
+ if key != "multiviews":
+ assert metadata_template[key] == metadata[key], f"existing file is inconsistent with the input parameters:\nKey: {key}\nmetadata: {metadata[key]}\ntemplate: {metadata_template[key]}."
+ else:
+ print("No temporary file found. Starting generation from scratch...")
+ metadata = metadata_template
+
+ starting_id = len(metadata["multiviews"])
+ print(f"Starting generation from index {starting_id}/{size}...")
+ if starting_id >= size:
+ print("Generation already done.")
+ return
+
+ generator = MultiviewHabitatSimGenerator(scene_dataset_config_file=scene_dataset_config_file,
+ scene=scene,
+ navmesh=navmesh,
+ views_count = views_count,
+ size = size,
+ **kwargs)
+
+ for idx in tqdm(range(starting_id, size)):
+ # Generate / re-generate the observations
+ try:
+ data = generator[idx]
+ observations = data["observations"]
+ positions = data["positions"]
+ orientations = data["orientations"]
+
+ idx_label = f"{idx:08}"
+ for oidx, observation in enumerate(observations):
+ observation_label = f"{oidx + 1}" # Leonid is indexing starting from 1
+ # Color image saved using PIL
+ img = PIL.Image.fromarray(observation['color'][:,:,:3])
+ filename = os.path.join(output_dir, f"{idx_label}_{observation_label}.jpeg")
+ img.save(filename)
+ if generate_depth:
+ # Depth image as EXR file
+ filename = os.path.join(output_dir, f"{idx_label}_{observation_label}_depth.exr")
+ cv2.imwrite(filename, observation['depth'], [cv2.IMWRITE_EXR_TYPE, cv2.IMWRITE_EXR_TYPE_HALF])
+ # Camera parameters
+ camera_params = dict([(key, observation[key].tolist()) for key in ("camera_intrinsics", "R_cam2world", "t_cam2world")])
+ filename = os.path.join(output_dir, f"{idx_label}_{observation_label}_camera_params.json")
+ with open(filename, "w") as f:
+ json.dump(camera_params, f)
+ metadata["multiviews"][idx_label] = {"positions": positions.tolist(),
+ "orientations": orientations.tolist(),
+ "covisibility_ratios": data["covisibility_ratios"].tolist(),
+ "valid_fractions": data["valid_fractions"].tolist(),
+ "pairwise_visibility_ratios": data["pairwise_visibility_ratios"].tolist()}
+ except RecursionError:
+ print("Recursion error: unable to sample observations for this scene. We will stop there.")
+ break
+
+ # Regularly save a temporary metadata file, in case we need to restart the generation
+ if idx % 10 == 0:
+ with open(metadata_filename, "w") as f:
+ json.dump(metadata, f)
+
+ # Save metadata
+ with open(metadata_filename, "w") as f:
+ json.dump(metadata, f)
+
+ generator.close()
+ except NoNaviguableSpaceError:
+ pass
+
+def create_commandline(scene_data, generate_depth, exist_ok=False):
+ """
+ Create a commandline string to generate a scene.
+ """
+ def my_formatting(val):
+ if val is None or val == "":
+ return '""'
+ else:
+ return val
+ commandline = f"""python {__file__} --scene {my_formatting(scene_data.scene)}
+ --scene_dataset_config_file {my_formatting(scene_data.scene_dataset_config_file)}
+ --navmesh {my_formatting(scene_data.navmesh)}
+ --output_dir {my_formatting(scene_data.output_dir)}
+ --generate_depth {int(generate_depth)}
+ --exist_ok {int(exist_ok)}
+ """
+ commandline = " ".join(commandline.split())
+ return commandline
+
+if __name__ == "__main__":
+ os.umask(2)
+
+ parser = argparse.ArgumentParser(description="""Example of use -- listing commands to generate data for scenes available:
+ > python datasets/habitat_sim/generate_multiview_habitat_images.py --list_commands
+ """)
+
+ parser.add_argument("--output_dir", type=str, required=True)
+ parser.add_argument("--list_commands", action='store_true', help="list commandlines to run if true")
+ parser.add_argument("--scene", type=str, default="")
+ parser.add_argument("--scene_dataset_config_file", type=str, default="")
+ parser.add_argument("--navmesh", type=str, default="")
+
+ parser.add_argument("--generate_depth", type=int, default=1)
+ parser.add_argument("--exist_ok", type=int, default=0)
+
+ kwargs = dict(resolution=(256,256), hfov=60, views_count = 2, size=1000)
+
+ args = parser.parse_args()
+ generate_depth=bool(args.generate_depth)
+ exist_ok = bool(args.exist_ok)
+
+ if args.list_commands:
+ # Listing scenes available...
+ scenes_data = list_scenes_available(base_output_dir=args.output_dir)
+
+ for scene_data in scenes_data:
+ print(create_commandline(scene_data, generate_depth=generate_depth, exist_ok=exist_ok))
+ else:
+ if args.scene == "" or args.output_dir == "":
+ print("Missing scene or output dir argument!")
+ print(parser.format_help())
+ else:
+ generate_multiview_images_for_scene(scene=args.scene,
+ scene_dataset_config_file = args.scene_dataset_config_file,
+ navmesh = args.navmesh,
+ output_dir = args.output_dir,
+ exist_ok=exist_ok,
+ generate_depth=generate_depth,
+ **kwargs)
\ No newline at end of file
diff --git a/dynamic_predictor/croco/datasets/habitat_sim/multiview_habitat_sim_generator.py b/dynamic_predictor/croco/datasets/habitat_sim/multiview_habitat_sim_generator.py
new file mode 100644
index 0000000000000000000000000000000000000000..91e5f923b836a645caf5d8e4aacc425047e3c144
--- /dev/null
+++ b/dynamic_predictor/croco/datasets/habitat_sim/multiview_habitat_sim_generator.py
@@ -0,0 +1,390 @@
+# Copyright (C) 2022-present Naver Corporation. All rights reserved.
+# Licensed under CC BY-NC-SA 4.0 (non-commercial use only).
+
+import os
+import numpy as np
+import quaternion
+import habitat_sim
+import json
+from sklearn.neighbors import NearestNeighbors
+import cv2
+
+# OpenCV to habitat camera convention transformation
+R_OPENCV2HABITAT = np.stack((habitat_sim.geo.RIGHT, -habitat_sim.geo.UP, habitat_sim.geo.FRONT), axis=0)
+R_HABITAT2OPENCV = R_OPENCV2HABITAT.T
+DEG2RAD = np.pi / 180
+
+def compute_camera_intrinsics(height, width, hfov):
+ f = width/2 / np.tan(hfov/2 * np.pi/180)
+ cu, cv = width/2, height/2
+ return f, cu, cv
+
+def compute_camera_pose_opencv_convention(camera_position, camera_orientation):
+ R_cam2world = quaternion.as_rotation_matrix(camera_orientation) @ R_OPENCV2HABITAT
+ t_cam2world = np.asarray(camera_position)
+ return R_cam2world, t_cam2world
+
+def compute_pointmap(depthmap, hfov):
+ """ Compute a HxWx3 pointmap in camera frame from a HxW depth map."""
+ height, width = depthmap.shape
+ f, cu, cv = compute_camera_intrinsics(height, width, hfov)
+ # Cast depth map to point
+ z_cam = depthmap
+ u, v = np.meshgrid(range(width), range(height))
+ x_cam = (u - cu) / f * z_cam
+ y_cam = (v - cv) / f * z_cam
+ X_cam = np.stack((x_cam, y_cam, z_cam), axis=-1)
+ return X_cam
+
+def compute_pointcloud(depthmap, hfov, camera_position, camera_rotation):
+ """Return a 3D point cloud corresponding to valid pixels of the depth map"""
+ R_cam2world, t_cam2world = compute_camera_pose_opencv_convention(camera_position, camera_rotation)
+
+ X_cam = compute_pointmap(depthmap=depthmap, hfov=hfov)
+ valid_mask = (X_cam[:,:,2] != 0.0)
+
+ X_cam = X_cam.reshape(-1, 3)[valid_mask.flatten()]
+ X_world = X_cam @ R_cam2world.T + t_cam2world.reshape(1, 3)
+ return X_world
+
+def compute_pointcloud_overlaps_scikit(pointcloud1, pointcloud2, distance_threshold, compute_symmetric=False):
+ """
+ Compute 'overlapping' metrics based on a distance threshold between two point clouds.
+ """
+ nbrs = NearestNeighbors(n_neighbors=1, algorithm = 'kd_tree').fit(pointcloud2)
+ distances, indices = nbrs.kneighbors(pointcloud1)
+ intersection1 = np.count_nonzero(distances.flatten() < distance_threshold)
+
+ data = {"intersection1": intersection1,
+ "size1": len(pointcloud1)}
+ if compute_symmetric:
+ nbrs = NearestNeighbors(n_neighbors=1, algorithm = 'kd_tree').fit(pointcloud1)
+ distances, indices = nbrs.kneighbors(pointcloud2)
+ intersection2 = np.count_nonzero(distances.flatten() < distance_threshold)
+ data["intersection2"] = intersection2
+ data["size2"] = len(pointcloud2)
+
+ return data
+
+def _append_camera_parameters(observation, hfov, camera_location, camera_rotation):
+ """
+ Add camera parameters to the observation dictionnary produced by Habitat-Sim
+ In-place modifications.
+ """
+ R_cam2world, t_cam2world = compute_camera_pose_opencv_convention(camera_location, camera_rotation)
+ height, width = observation['depth'].shape
+ f, cu, cv = compute_camera_intrinsics(height, width, hfov)
+ K = np.asarray([[f, 0, cu],
+ [0, f, cv],
+ [0, 0, 1.0]])
+ observation["camera_intrinsics"] = K
+ observation["t_cam2world"] = t_cam2world
+ observation["R_cam2world"] = R_cam2world
+
+def look_at(eye, center, up, return_cam2world=True):
+ """
+ Return camera pose looking at a given center point.
+ Analogous of gluLookAt function, using OpenCV camera convention.
+ """
+ z = center - eye
+ z /= np.linalg.norm(z, axis=-1, keepdims=True)
+ y = -up
+ y = y - np.sum(y * z, axis=-1, keepdims=True) * z
+ y /= np.linalg.norm(y, axis=-1, keepdims=True)
+ x = np.cross(y, z, axis=-1)
+
+ if return_cam2world:
+ R = np.stack((x, y, z), axis=-1)
+ t = eye
+ else:
+ # World to camera transformation
+ # Transposed matrix
+ R = np.stack((x, y, z), axis=-2)
+ t = - np.einsum('...ij, ...j', R, eye)
+ return R, t
+
+def look_at_for_habitat(eye, center, up, return_cam2world=True):
+ R, t = look_at(eye, center, up)
+ orientation = quaternion.from_rotation_matrix(R @ R_OPENCV2HABITAT.T)
+ return orientation, t
+
+def generate_orientation_noise(pan_range, tilt_range, roll_range):
+ return (quaternion.from_rotation_vector(np.random.uniform(*pan_range) * DEG2RAD * habitat_sim.geo.UP)
+ * quaternion.from_rotation_vector(np.random.uniform(*tilt_range) * DEG2RAD * habitat_sim.geo.RIGHT)
+ * quaternion.from_rotation_vector(np.random.uniform(*roll_range) * DEG2RAD * habitat_sim.geo.FRONT))
+
+
+class NoNaviguableSpaceError(RuntimeError):
+ def __init__(self, *args):
+ super().__init__(*args)
+
+class MultiviewHabitatSimGenerator:
+ def __init__(self,
+ scene,
+ navmesh,
+ scene_dataset_config_file,
+ resolution = (240, 320),
+ views_count=2,
+ hfov = 60,
+ gpu_id = 0,
+ size = 10000,
+ minimum_covisibility = 0.5,
+ transform = None):
+ self.scene = scene
+ self.navmesh = navmesh
+ self.scene_dataset_config_file = scene_dataset_config_file
+ self.resolution = resolution
+ self.views_count = views_count
+ assert(self.views_count >= 1)
+ self.hfov = hfov
+ self.gpu_id = gpu_id
+ self.size = size
+ self.transform = transform
+
+ # Noise added to camera orientation
+ self.pan_range = (-3, 3)
+ self.tilt_range = (-10, 10)
+ self.roll_range = (-5, 5)
+
+ # Height range to sample cameras
+ self.height_range = (1.2, 1.8)
+
+ # Random steps between the camera views
+ self.random_steps_count = 5
+ self.random_step_variance = 2.0
+
+ # Minimum fraction of the scene which should be valid (well defined depth)
+ self.minimum_valid_fraction = 0.7
+
+ # Distance threshold to see to select pairs
+ self.distance_threshold = 0.05
+ # Minimum IoU of a view point cloud with respect to the reference view to be kept.
+ self.minimum_covisibility = minimum_covisibility
+
+ # Maximum number of retries.
+ self.max_attempts_count = 100
+
+ self.seed = None
+ self._lazy_initialization()
+
+ def _lazy_initialization(self):
+ # Lazy random seeding and instantiation of the simulator to deal with multiprocessing properly
+ if self.seed == None:
+ # Re-seed numpy generator
+ np.random.seed()
+ self.seed = np.random.randint(2**32-1)
+ sim_cfg = habitat_sim.SimulatorConfiguration()
+ sim_cfg.scene_id = self.scene
+ if self.scene_dataset_config_file is not None and self.scene_dataset_config_file != "":
+ sim_cfg.scene_dataset_config_file = self.scene_dataset_config_file
+ sim_cfg.random_seed = self.seed
+ sim_cfg.load_semantic_mesh = False
+ sim_cfg.gpu_device_id = self.gpu_id
+
+ depth_sensor_spec = habitat_sim.CameraSensorSpec()
+ depth_sensor_spec.uuid = "depth"
+ depth_sensor_spec.sensor_type = habitat_sim.SensorType.DEPTH
+ depth_sensor_spec.resolution = self.resolution
+ depth_sensor_spec.hfov = self.hfov
+ depth_sensor_spec.position = [0.0, 0.0, 0]
+ depth_sensor_spec.orientation
+
+ rgb_sensor_spec = habitat_sim.CameraSensorSpec()
+ rgb_sensor_spec.uuid = "color"
+ rgb_sensor_spec.sensor_type = habitat_sim.SensorType.COLOR
+ rgb_sensor_spec.resolution = self.resolution
+ rgb_sensor_spec.hfov = self.hfov
+ rgb_sensor_spec.position = [0.0, 0.0, 0]
+ agent_cfg = habitat_sim.agent.AgentConfiguration(sensor_specifications=[rgb_sensor_spec, depth_sensor_spec])
+
+ cfg = habitat_sim.Configuration(sim_cfg, [agent_cfg])
+ self.sim = habitat_sim.Simulator(cfg)
+ if self.navmesh is not None and self.navmesh != "":
+ # Use pre-computed navmesh when available (usually better than those generated automatically)
+ self.sim.pathfinder.load_nav_mesh(self.navmesh)
+
+ if not self.sim.pathfinder.is_loaded:
+ # Try to compute a navmesh
+ navmesh_settings = habitat_sim.NavMeshSettings()
+ navmesh_settings.set_defaults()
+ self.sim.recompute_navmesh(self.sim.pathfinder, navmesh_settings, True)
+
+ # Ensure that the navmesh is not empty
+ if not self.sim.pathfinder.is_loaded:
+ raise NoNaviguableSpaceError(f"No naviguable location (scene: {self.scene} -- navmesh: {self.navmesh})")
+
+ self.agent = self.sim.initialize_agent(agent_id=0)
+
+ def close(self):
+ self.sim.close()
+
+ def __del__(self):
+ self.sim.close()
+
+ def __len__(self):
+ return self.size
+
+ def sample_random_viewpoint(self):
+ """ Sample a random viewpoint using the navmesh """
+ nav_point = self.sim.pathfinder.get_random_navigable_point()
+
+ # Sample a random viewpoint height
+ viewpoint_height = np.random.uniform(*self.height_range)
+ viewpoint_position = nav_point + viewpoint_height * habitat_sim.geo.UP
+ viewpoint_orientation = quaternion.from_rotation_vector(np.random.uniform(0, 2 * np.pi) * habitat_sim.geo.UP) * generate_orientation_noise(self.pan_range, self.tilt_range, self.roll_range)
+ return viewpoint_position, viewpoint_orientation, nav_point
+
+ def sample_other_random_viewpoint(self, observed_point, nav_point):
+ """ Sample a random viewpoint close to an existing one, using the navmesh and a reference observed point."""
+ other_nav_point = nav_point
+
+ walk_directions = self.random_step_variance * np.asarray([1,0,1])
+ for i in range(self.random_steps_count):
+ temp = self.sim.pathfinder.snap_point(other_nav_point + walk_directions * np.random.normal(size=3))
+ # Snapping may return nan when it fails
+ if not np.isnan(temp[0]):
+ other_nav_point = temp
+
+ other_viewpoint_height = np.random.uniform(*self.height_range)
+ other_viewpoint_position = other_nav_point + other_viewpoint_height * habitat_sim.geo.UP
+
+ # Set viewing direction towards the central point
+ rotation, position = look_at_for_habitat(eye=other_viewpoint_position, center=observed_point, up=habitat_sim.geo.UP, return_cam2world=True)
+ rotation = rotation * generate_orientation_noise(self.pan_range, self.tilt_range, self.roll_range)
+ return position, rotation, other_nav_point
+
+ def is_other_pointcloud_overlapping(self, ref_pointcloud, other_pointcloud):
+ """ Check if a viewpoint is valid and overlaps significantly with a reference one. """
+ # Observation
+ pixels_count = self.resolution[0] * self.resolution[1]
+ valid_fraction = len(other_pointcloud) / pixels_count
+ assert valid_fraction <= 1.0 and valid_fraction >= 0.0
+ overlap = compute_pointcloud_overlaps_scikit(ref_pointcloud, other_pointcloud, self.distance_threshold, compute_symmetric=True)
+ covisibility = min(overlap["intersection1"] / pixels_count, overlap["intersection2"] / pixels_count)
+ is_valid = (valid_fraction >= self.minimum_valid_fraction) and (covisibility >= self.minimum_covisibility)
+ return is_valid, valid_fraction, covisibility
+
+ def is_other_viewpoint_overlapping(self, ref_pointcloud, observation, position, rotation):
+ """ Check if a viewpoint is valid and overlaps significantly with a reference one. """
+ # Observation
+ other_pointcloud = compute_pointcloud(observation['depth'], self.hfov, position, rotation)
+ return self.is_other_pointcloud_overlapping(ref_pointcloud, other_pointcloud)
+
+ def render_viewpoint(self, viewpoint_position, viewpoint_orientation):
+ agent_state = habitat_sim.AgentState()
+ agent_state.position = viewpoint_position
+ agent_state.rotation = viewpoint_orientation
+ self.agent.set_state(agent_state)
+ viewpoint_observations = self.sim.get_sensor_observations(agent_ids=0)
+ _append_camera_parameters(viewpoint_observations, self.hfov, viewpoint_position, viewpoint_orientation)
+ return viewpoint_observations
+
+ def __getitem__(self, useless_idx):
+ ref_position, ref_orientation, nav_point = self.sample_random_viewpoint()
+ ref_observations = self.render_viewpoint(ref_position, ref_orientation)
+ # Extract point cloud
+ ref_pointcloud = compute_pointcloud(depthmap=ref_observations['depth'], hfov=self.hfov,
+ camera_position=ref_position, camera_rotation=ref_orientation)
+
+ pixels_count = self.resolution[0] * self.resolution[1]
+ ref_valid_fraction = len(ref_pointcloud) / pixels_count
+ assert ref_valid_fraction <= 1.0 and ref_valid_fraction >= 0.0
+ if ref_valid_fraction < self.minimum_valid_fraction:
+ # This should produce a recursion error at some point when something is very wrong.
+ return self[0]
+ # Pick an reference observed point in the point cloud
+ observed_point = np.mean(ref_pointcloud, axis=0)
+
+ # Add the first image as reference
+ viewpoints_observations = [ref_observations]
+ viewpoints_covisibility = [ref_valid_fraction]
+ viewpoints_positions = [ref_position]
+ viewpoints_orientations = [quaternion.as_float_array(ref_orientation)]
+ viewpoints_clouds = [ref_pointcloud]
+ viewpoints_valid_fractions = [ref_valid_fraction]
+
+ for _ in range(self.views_count - 1):
+ # Generate an other viewpoint using some dummy random walk
+ successful_sampling = False
+ for sampling_attempt in range(self.max_attempts_count):
+ position, rotation, _ = self.sample_other_random_viewpoint(observed_point, nav_point)
+ # Observation
+ other_viewpoint_observations = self.render_viewpoint(position, rotation)
+ other_pointcloud = compute_pointcloud(other_viewpoint_observations['depth'], self.hfov, position, rotation)
+
+ is_valid, valid_fraction, covisibility = self.is_other_pointcloud_overlapping(ref_pointcloud, other_pointcloud)
+ if is_valid:
+ successful_sampling = True
+ break
+ if not successful_sampling:
+ print("WARNING: Maximum number of attempts reached.")
+ # Dirty hack, try using a novel original viewpoint
+ return self[0]
+ viewpoints_observations.append(other_viewpoint_observations)
+ viewpoints_covisibility.append(covisibility)
+ viewpoints_positions.append(position)
+ viewpoints_orientations.append(quaternion.as_float_array(rotation)) # WXYZ convention for the quaternion encoding.
+ viewpoints_clouds.append(other_pointcloud)
+ viewpoints_valid_fractions.append(valid_fraction)
+
+ # Estimate relations between all pairs of images
+ pairwise_visibility_ratios = np.ones((len(viewpoints_observations), len(viewpoints_observations)))
+ for i in range(len(viewpoints_observations)):
+ pairwise_visibility_ratios[i,i] = viewpoints_valid_fractions[i]
+ for j in range(i+1, len(viewpoints_observations)):
+ overlap = compute_pointcloud_overlaps_scikit(viewpoints_clouds[i], viewpoints_clouds[j], self.distance_threshold, compute_symmetric=True)
+ pairwise_visibility_ratios[i,j] = overlap['intersection1'] / pixels_count
+ pairwise_visibility_ratios[j,i] = overlap['intersection2'] / pixels_count
+
+ # IoU is relative to the image 0
+ data = {"observations": viewpoints_observations,
+ "positions": np.asarray(viewpoints_positions),
+ "orientations": np.asarray(viewpoints_orientations),
+ "covisibility_ratios": np.asarray(viewpoints_covisibility),
+ "valid_fractions": np.asarray(viewpoints_valid_fractions, dtype=float),
+ "pairwise_visibility_ratios": np.asarray(pairwise_visibility_ratios, dtype=float),
+ }
+
+ if self.transform is not None:
+ data = self.transform(data)
+ return data
+
+ def generate_random_spiral_trajectory(self, images_count = 100, max_radius=0.5, half_turns=5, use_constant_orientation=False):
+ """
+ Return a list of images corresponding to a spiral trajectory from a random starting point.
+ Useful to generate nice visualisations.
+ Use an even number of half turns to get a nice "C1-continuous" loop effect
+ """
+ ref_position, ref_orientation, navpoint = self.sample_random_viewpoint()
+ ref_observations = self.render_viewpoint(ref_position, ref_orientation)
+ ref_pointcloud = compute_pointcloud(depthmap=ref_observations['depth'], hfov=self.hfov,
+ camera_position=ref_position, camera_rotation=ref_orientation)
+ pixels_count = self.resolution[0] * self.resolution[1]
+ if len(ref_pointcloud) / pixels_count < self.minimum_valid_fraction:
+ # Dirty hack: ensure that the valid part of the image is significant
+ return self.generate_random_spiral_trajectory(images_count, max_radius, half_turns, use_constant_orientation)
+
+ # Pick an observed point in the point cloud
+ observed_point = np.mean(ref_pointcloud, axis=0)
+ ref_R, ref_t = compute_camera_pose_opencv_convention(ref_position, ref_orientation)
+
+ images = []
+ is_valid = []
+ # Spiral trajectory, use_constant orientation
+ for i, alpha in enumerate(np.linspace(0, 1, images_count)):
+ r = max_radius * np.abs(np.sin(alpha * np.pi)) # Increase then decrease the radius
+ theta = alpha * half_turns * np.pi
+ x = r * np.cos(theta)
+ y = r * np.sin(theta)
+ z = 0.0
+ position = ref_position + (ref_R @ np.asarray([x, y, z]).reshape(3,1)).flatten()
+ if use_constant_orientation:
+ orientation = ref_orientation
+ else:
+ # trajectory looking at a mean point in front of the ref observation
+ orientation, position = look_at_for_habitat(eye=position, center=observed_point, up=habitat_sim.geo.UP)
+ observations = self.render_viewpoint(position, orientation)
+ images.append(observations['color'][...,:3])
+ _is_valid, valid_fraction, iou = self.is_other_viewpoint_overlapping(ref_pointcloud, observations, position, orientation)
+ is_valid.append(_is_valid)
+ return images, np.all(is_valid)
\ No newline at end of file
diff --git a/dynamic_predictor/croco/datasets/habitat_sim/pack_metadata_files.py b/dynamic_predictor/croco/datasets/habitat_sim/pack_metadata_files.py
new file mode 100644
index 0000000000000000000000000000000000000000..10672a01f7dd615d3b4df37781f7f6f97e753ba6
--- /dev/null
+++ b/dynamic_predictor/croco/datasets/habitat_sim/pack_metadata_files.py
@@ -0,0 +1,69 @@
+# Copyright (C) 2022-present Naver Corporation. All rights reserved.
+# Licensed under CC BY-NC-SA 4.0 (non-commercial use only).
+"""
+Utility script to pack metadata files of the dataset in order to be able to re-generate it elsewhere.
+"""
+import os
+import glob
+from tqdm import tqdm
+import shutil
+import json
+from datasets.habitat_sim.paths import *
+import argparse
+import collections
+
+if __name__ == "__main__":
+ parser = argparse.ArgumentParser()
+ parser.add_argument("input_dir")
+ parser.add_argument("output_dir")
+ args = parser.parse_args()
+
+ input_dirname = args.input_dir
+ output_dirname = args.output_dir
+
+ input_metadata_filenames = glob.iglob(f"{input_dirname}/**/metadata.json", recursive=True)
+
+ images_count = collections.defaultdict(lambda : 0)
+
+ os.makedirs(output_dirname)
+ for input_filename in tqdm(input_metadata_filenames):
+ # Ignore empty files
+ with open(input_filename, "r") as f:
+ original_metadata = json.load(f)
+ if "multiviews" not in original_metadata or len(original_metadata["multiviews"]) == 0:
+ print("No views in", input_filename)
+ continue
+
+ relpath = os.path.relpath(input_filename, input_dirname)
+ print(relpath)
+
+ # Copy metadata, while replacing scene paths by generic keys depending on the dataset, for portability.
+ # Data paths are sorted by decreasing length to avoid potential bugs due to paths starting by the same string pattern.
+ scenes_dataset_paths = dict(sorted(SCENES_DATASET.items(), key=lambda x: len(x[1]), reverse=True))
+ metadata = dict()
+ for key, value in original_metadata.items():
+ if key in ("scene_dataset_config_file", "scene", "navmesh") and value != "":
+ known_path = False
+ for dataset, dataset_path in scenes_dataset_paths.items():
+ if value.startswith(dataset_path):
+ value = os.path.join(dataset, os.path.relpath(value, dataset_path))
+ known_path = True
+ break
+ if not known_path:
+ raise KeyError("Unknown path:" + value)
+ metadata[key] = value
+
+ # Compile some general statistics while packing data
+ scene_split = metadata["scene"].split("/")
+ upper_level = "/".join(scene_split[:2]) if scene_split[0] == "hm3d" else scene_split[0]
+ images_count[upper_level] += len(metadata["multiviews"])
+
+ output_filename = os.path.join(output_dirname, relpath)
+ os.makedirs(os.path.dirname(output_filename), exist_ok=True)
+ with open(output_filename, "w") as f:
+ json.dump(metadata, f)
+
+ # Print statistics
+ print("Images count:")
+ for upper_level, count in images_count.items():
+ print(f"- {upper_level}: {count}")
\ No newline at end of file
diff --git a/dynamic_predictor/croco/datasets/habitat_sim/paths.py b/dynamic_predictor/croco/datasets/habitat_sim/paths.py
new file mode 100644
index 0000000000000000000000000000000000000000..4d63b5fa29c274ddfeae084734a35ba66d7edee8
--- /dev/null
+++ b/dynamic_predictor/croco/datasets/habitat_sim/paths.py
@@ -0,0 +1,129 @@
+# Copyright (C) 2022-present Naver Corporation. All rights reserved.
+# Licensed under CC BY-NC-SA 4.0 (non-commercial use only).
+
+"""
+Paths to Habitat-Sim scenes
+"""
+
+import os
+import json
+import collections
+from tqdm import tqdm
+
+
+# Hardcoded path to the different scene datasets
+SCENES_DATASET = {
+ "hm3d": "./data/habitat-sim-data/scene_datasets/hm3d/",
+ "gibson": "./data/habitat-sim-data/scene_datasets/gibson/",
+ "habitat-test-scenes": "./data/habitat-sim/scene_datasets/habitat-test-scenes/",
+ "replica_cad_baked_lighting": "./data/habitat-sim/scene_datasets/replica_cad_baked_lighting/",
+ "replica_cad": "./data/habitat-sim/scene_datasets/replica_cad/",
+ "replica": "./data/habitat-sim/scene_datasets/ReplicaDataset/",
+ "scannet": "./data/habitat-sim/scene_datasets/scannet/"
+}
+
+SceneData = collections.namedtuple("SceneData", ["scene_dataset_config_file", "scene", "navmesh", "output_dir"])
+
+def list_replicacad_scenes(base_output_dir, base_path=SCENES_DATASET["replica_cad"]):
+ scene_dataset_config_file = os.path.join(base_path, "replicaCAD.scene_dataset_config.json")
+ scenes = [f"apt_{i}" for i in range(6)] + ["empty_stage"]
+ navmeshes = [f"navmeshes/apt_{i}_static_furniture.navmesh" for i in range(6)] + ["empty_stage.navmesh"]
+ scenes_data = []
+ for idx in range(len(scenes)):
+ output_dir = os.path.join(base_output_dir, "ReplicaCAD", scenes[idx])
+ # Add scene
+ data = SceneData(scene_dataset_config_file=scene_dataset_config_file,
+ scene = scenes[idx] + ".scene_instance.json",
+ navmesh = os.path.join(base_path, navmeshes[idx]),
+ output_dir = output_dir)
+ scenes_data.append(data)
+ return scenes_data
+
+def list_replica_cad_baked_lighting_scenes(base_output_dir, base_path=SCENES_DATASET["replica_cad_baked_lighting"]):
+ scene_dataset_config_file = os.path.join(base_path, "replicaCAD_baked.scene_dataset_config.json")
+ scenes = sum([[f"Baked_sc{i}_staging_{j:02}" for i in range(5)] for j in range(21)], [])
+ navmeshes = ""#[f"navmeshes/apt_{i}_static_furniture.navmesh" for i in range(6)] + ["empty_stage.navmesh"]
+ scenes_data = []
+ for idx in range(len(scenes)):
+ output_dir = os.path.join(base_output_dir, "replica_cad_baked_lighting", scenes[idx])
+ data = SceneData(scene_dataset_config_file=scene_dataset_config_file,
+ scene = scenes[idx],
+ navmesh = "",
+ output_dir = output_dir)
+ scenes_data.append(data)
+ return scenes_data
+
+def list_replica_scenes(base_output_dir, base_path):
+ scenes_data = []
+ for scene_id in os.listdir(base_path):
+ scene = os.path.join(base_path, scene_id, "mesh.ply")
+ navmesh = os.path.join(base_path, scene_id, "habitat/mesh_preseg_semantic.navmesh") # Not sure if I should use it
+ scene_dataset_config_file = ""
+ output_dir = os.path.join(base_output_dir, scene_id)
+ # Add scene only if it does not exist already, or if exist_ok
+ data = SceneData(scene_dataset_config_file = scene_dataset_config_file,
+ scene = scene,
+ navmesh = navmesh,
+ output_dir = output_dir)
+ scenes_data.append(data)
+ return scenes_data
+
+
+def list_scenes(base_output_dir, base_path):
+ """
+ Generic method iterating through a base_path folder to find scenes.
+ """
+ scenes_data = []
+ for root, dirs, files in os.walk(base_path, followlinks=True):
+ folder_scenes_data = []
+ for file in files:
+ name, ext = os.path.splitext(file)
+ if ext == ".glb":
+ scene = os.path.join(root, name + ".glb")
+ navmesh = os.path.join(root, name + ".navmesh")
+ if not os.path.exists(navmesh):
+ navmesh = ""
+ relpath = os.path.relpath(root, base_path)
+ output_dir = os.path.abspath(os.path.join(base_output_dir, relpath, name))
+ data = SceneData(scene_dataset_config_file="",
+ scene = scene,
+ navmesh = navmesh,
+ output_dir = output_dir)
+ folder_scenes_data.append(data)
+
+ # Specific check for HM3D:
+ # When two meshesxxxx.basis.glb and xxxx.glb are present, use the 'basis' version.
+ basis_scenes = [data.scene[:-len(".basis.glb")] for data in folder_scenes_data if data.scene.endswith(".basis.glb")]
+ if len(basis_scenes) != 0:
+ folder_scenes_data = [data for data in folder_scenes_data if not (data.scene[:-len(".glb")] in basis_scenes)]
+
+ scenes_data.extend(folder_scenes_data)
+ return scenes_data
+
+def list_scenes_available(base_output_dir, scenes_dataset_paths=SCENES_DATASET):
+ scenes_data = []
+
+ # HM3D
+ for split in ("minival", "train", "val", "examples"):
+ scenes_data += list_scenes(base_output_dir=os.path.join(base_output_dir, f"hm3d/{split}/"),
+ base_path=f"{scenes_dataset_paths['hm3d']}/{split}")
+
+ # Gibson
+ scenes_data += list_scenes(base_output_dir=os.path.join(base_output_dir, "gibson"),
+ base_path=scenes_dataset_paths["gibson"])
+
+ # Habitat test scenes (just a few)
+ scenes_data += list_scenes(base_output_dir=os.path.join(base_output_dir, "habitat-test-scenes"),
+ base_path=scenes_dataset_paths["habitat-test-scenes"])
+
+ # ReplicaCAD (baked lightning)
+ scenes_data += list_replica_cad_baked_lighting_scenes(base_output_dir=base_output_dir)
+
+ # ScanNet
+ scenes_data += list_scenes(base_output_dir=os.path.join(base_output_dir, "scannet"),
+ base_path=scenes_dataset_paths["scannet"])
+
+ # Replica
+ list_replica_scenes(base_output_dir=os.path.join(base_output_dir, "replica"),
+ base_path=scenes_dataset_paths["replica"])
+ return scenes_data
diff --git a/dynamic_predictor/croco/datasets/pairs_dataset.py b/dynamic_predictor/croco/datasets/pairs_dataset.py
new file mode 100644
index 0000000000000000000000000000000000000000..9f107526b34e154d9013a9a7a0bde3d5ff6f581c
--- /dev/null
+++ b/dynamic_predictor/croco/datasets/pairs_dataset.py
@@ -0,0 +1,109 @@
+# Copyright (C) 2022-present Naver Corporation. All rights reserved.
+# Licensed under CC BY-NC-SA 4.0 (non-commercial use only).
+
+import os
+from torch.utils.data import Dataset
+from PIL import Image
+
+from datasets.transforms import get_pair_transforms
+
+def load_image(impath):
+ return Image.open(impath)
+
+def load_pairs_from_cache_file(fname, root=''):
+ assert os.path.isfile(fname), "cannot parse pairs from {:s}, file does not exist".format(fname)
+ with open(fname, 'r') as fid:
+ lines = fid.read().strip().splitlines()
+ pairs = [ (os.path.join(root,l.split()[0]), os.path.join(root,l.split()[1])) for l in lines]
+ return pairs
+
+def load_pairs_from_list_file(fname, root=''):
+ assert os.path.isfile(fname), "cannot parse pairs from {:s}, file does not exist".format(fname)
+ with open(fname, 'r') as fid:
+ lines = fid.read().strip().splitlines()
+ pairs = [ (os.path.join(root,l+'_1.jpg'), os.path.join(root,l+'_2.jpg')) for l in lines if not l.startswith('#')]
+ return pairs
+
+
+def write_cache_file(fname, pairs, root=''):
+ if len(root)>0:
+ if not root.endswith('/'): root+='/'
+ assert os.path.isdir(root)
+ s = ''
+ for im1, im2 in pairs:
+ if len(root)>0:
+ assert im1.startswith(root), im1
+ assert im2.startswith(root), im2
+ s += '{:s} {:s}\n'.format(im1[len(root):], im2[len(root):])
+ with open(fname, 'w') as fid:
+ fid.write(s[:-1])
+
+def parse_and_cache_all_pairs(dname, data_dir='./data/'):
+ if dname=='habitat_release':
+ dirname = os.path.join(data_dir, 'habitat_release')
+ assert os.path.isdir(dirname), "cannot find folder for habitat_release pairs: "+dirname
+ cache_file = os.path.join(dirname, 'pairs.txt')
+ assert not os.path.isfile(cache_file), "cache file already exists: "+cache_file
+
+ print('Parsing pairs for dataset: '+dname)
+ pairs = []
+ for root, dirs, files in os.walk(dirname):
+ if 'val' in root: continue
+ dirs.sort()
+ pairs += [ (os.path.join(root,f), os.path.join(root,f[:-len('_1.jpeg')]+'_2.jpeg')) for f in sorted(files) if f.endswith('_1.jpeg')]
+ print('Found {:,} pairs'.format(len(pairs)))
+ print('Writing cache to: '+cache_file)
+ write_cache_file(cache_file, pairs, root=dirname)
+
+ else:
+ raise NotImplementedError('Unknown dataset: '+dname)
+
+def dnames_to_image_pairs(dnames, data_dir='./data/'):
+ """
+ dnames: list of datasets with image pairs, separated by +
+ """
+ all_pairs = []
+ for dname in dnames.split('+'):
+ if dname=='habitat_release':
+ dirname = os.path.join(data_dir, 'habitat_release')
+ assert os.path.isdir(dirname), "cannot find folder for habitat_release pairs: "+dirname
+ cache_file = os.path.join(dirname, 'pairs.txt')
+ assert os.path.isfile(cache_file), "cannot find cache file for habitat_release pairs, please first create the cache file, see instructions. "+cache_file
+ pairs = load_pairs_from_cache_file(cache_file, root=dirname)
+ elif dname in ['ARKitScenes', 'MegaDepth', '3DStreetView', 'IndoorVL']:
+ dirname = os.path.join(data_dir, dname+'_crops')
+ assert os.path.isdir(dirname), "cannot find folder for {:s} pairs: {:s}".format(dname, dirname)
+ list_file = os.path.join(dirname, 'listing.txt')
+ assert os.path.isfile(list_file), "cannot find list file for {:s} pairs, see instructions. {:s}".format(dname, list_file)
+ pairs = load_pairs_from_list_file(list_file, root=dirname)
+ print(' {:s}: {:,} pairs'.format(dname, len(pairs)))
+ all_pairs += pairs
+ if '+' in dnames: print(' Total: {:,} pairs'.format(len(all_pairs)))
+ return all_pairs
+
+
+class PairsDataset(Dataset):
+
+ def __init__(self, dnames, trfs='', totensor=True, normalize=True, data_dir='./data/'):
+ super().__init__()
+ self.image_pairs = dnames_to_image_pairs(dnames, data_dir=data_dir)
+ self.transforms = get_pair_transforms(transform_str=trfs, totensor=totensor, normalize=normalize)
+
+ def __len__(self):
+ return len(self.image_pairs)
+
+ def __getitem__(self, index):
+ im1path, im2path = self.image_pairs[index]
+ im1 = load_image(im1path)
+ im2 = load_image(im2path)
+ if self.transforms is not None: im1, im2 = self.transforms(im1, im2)
+ return im1, im2
+
+
+if __name__=="__main__":
+ import argparse
+ parser = argparse.ArgumentParser(prog="Computing and caching list of pairs for a given dataset")
+ parser.add_argument('--data_dir', default='./data/', type=str, help="path where data are stored")
+ parser.add_argument('--dataset', default='habitat_release', type=str, help="name of the dataset")
+ args = parser.parse_args()
+ parse_and_cache_all_pairs(dname=args.dataset, data_dir=args.data_dir)
diff --git a/dynamic_predictor/croco/datasets/transforms.py b/dynamic_predictor/croco/datasets/transforms.py
new file mode 100644
index 0000000000000000000000000000000000000000..216bac61f8254fd50e7f269ee80301f250a2d11e
--- /dev/null
+++ b/dynamic_predictor/croco/datasets/transforms.py
@@ -0,0 +1,95 @@
+# Copyright (C) 2022-present Naver Corporation. All rights reserved.
+# Licensed under CC BY-NC-SA 4.0 (non-commercial use only).
+
+import torch
+import torchvision.transforms
+import torchvision.transforms.functional as F
+
+# "Pair": apply a transform on a pair
+# "Both": apply the exact same transform to both images
+
+class ComposePair(torchvision.transforms.Compose):
+ def __call__(self, img1, img2):
+ for t in self.transforms:
+ img1, img2 = t(img1, img2)
+ return img1, img2
+
+class NormalizeBoth(torchvision.transforms.Normalize):
+ def forward(self, img1, img2):
+ img1 = super().forward(img1)
+ img2 = super().forward(img2)
+ return img1, img2
+
+class ToTensorBoth(torchvision.transforms.ToTensor):
+ def __call__(self, img1, img2):
+ img1 = super().__call__(img1)
+ img2 = super().__call__(img2)
+ return img1, img2
+
+class RandomCropPair(torchvision.transforms.RandomCrop):
+ # the crop will be intentionally different for the two images with this class
+ def forward(self, img1, img2):
+ img1 = super().forward(img1)
+ img2 = super().forward(img2)
+ return img1, img2
+
+class ColorJitterPair(torchvision.transforms.ColorJitter):
+ # can be symmetric (same for both images) or assymetric (different jitter params for each image) depending on assymetric_prob
+ def __init__(self, assymetric_prob, **kwargs):
+ super().__init__(**kwargs)
+ self.assymetric_prob = assymetric_prob
+ def jitter_one(self, img, fn_idx, brightness_factor, contrast_factor, saturation_factor, hue_factor):
+ for fn_id in fn_idx:
+ if fn_id == 0 and brightness_factor is not None:
+ img = F.adjust_brightness(img, brightness_factor)
+ elif fn_id == 1 and contrast_factor is not None:
+ img = F.adjust_contrast(img, contrast_factor)
+ elif fn_id == 2 and saturation_factor is not None:
+ img = F.adjust_saturation(img, saturation_factor)
+ elif fn_id == 3 and hue_factor is not None:
+ img = F.adjust_hue(img, hue_factor)
+ return img
+
+ def forward(self, img1, img2):
+
+ fn_idx, brightness_factor, contrast_factor, saturation_factor, hue_factor = self.get_params(
+ self.brightness, self.contrast, self.saturation, self.hue
+ )
+ img1 = self.jitter_one(img1, fn_idx, brightness_factor, contrast_factor, saturation_factor, hue_factor)
+ if torch.rand(1) < self.assymetric_prob: # assymetric:
+ fn_idx, brightness_factor, contrast_factor, saturation_factor, hue_factor = self.get_params(
+ self.brightness, self.contrast, self.saturation, self.hue
+ )
+ img2 = self.jitter_one(img2, fn_idx, brightness_factor, contrast_factor, saturation_factor, hue_factor)
+ return img1, img2
+
+def get_pair_transforms(transform_str, totensor=True, normalize=True):
+ # transform_str is eg crop224+color
+ trfs = []
+ for s in transform_str.split('+'):
+ if s.startswith('crop'):
+ size = int(s[len('crop'):])
+ trfs.append(RandomCropPair(size))
+ elif s=='acolor':
+ trfs.append(ColorJitterPair(assymetric_prob=1.0, brightness=(0.6, 1.4), contrast=(0.6, 1.4), saturation=(0.6, 1.4), hue=0.0))
+ elif s=='': # if transform_str was ""
+ pass
+ else:
+ raise NotImplementedError('Unknown augmentation: '+s)
+
+ if totensor:
+ trfs.append( ToTensorBoth() )
+ if normalize:
+ trfs.append( NormalizeBoth(mean=[0.485, 0.456, 0.406], std=[0.229, 0.224, 0.225]) )
+
+ if len(trfs)==0:
+ return None
+ elif len(trfs)==1:
+ return trfs
+ else:
+ return ComposePair(trfs)
+
+
+
+
+
diff --git a/dynamic_predictor/croco/demo.py b/dynamic_predictor/croco/demo.py
new file mode 100644
index 0000000000000000000000000000000000000000..91b80ccc5c98c18e20d1ce782511aa824ef28f77
--- /dev/null
+++ b/dynamic_predictor/croco/demo.py
@@ -0,0 +1,55 @@
+# Copyright (C) 2022-present Naver Corporation. All rights reserved.
+# Licensed under CC BY-NC-SA 4.0 (non-commercial use only).
+
+import torch
+from models.croco import CroCoNet
+from PIL import Image
+import torchvision.transforms
+from torchvision.transforms import ToTensor, Normalize, Compose
+
+def main():
+ device = torch.device('cuda:0' if torch.cuda.is_available() and torch.cuda.device_count()>0 else 'cpu')
+
+ # load 224x224 images and transform them to tensor
+ imagenet_mean = [0.485, 0.456, 0.406]
+ imagenet_mean_tensor = torch.tensor(imagenet_mean).view(1,3,1,1).to(device, non_blocking=True)
+ imagenet_std = [0.229, 0.224, 0.225]
+ imagenet_std_tensor = torch.tensor(imagenet_std).view(1,3,1,1).to(device, non_blocking=True)
+ trfs = Compose([ToTensor(), Normalize(mean=imagenet_mean, std=imagenet_std)])
+ image1 = trfs(Image.open('assets/Chateau1.png').convert('RGB')).to(device, non_blocking=True).unsqueeze(0)
+ image2 = trfs(Image.open('assets/Chateau2.png').convert('RGB')).to(device, non_blocking=True).unsqueeze(0)
+
+ # load model
+ ckpt = torch.load('pretrained_models/CroCo_V2_ViTLarge_BaseDecoder.pth', 'cpu')
+ model = CroCoNet( **ckpt.get('croco_kwargs',{})).to(device)
+ model.eval()
+ msg = model.load_state_dict(ckpt['model'], strict=True)
+
+ # forward
+ with torch.inference_mode():
+ out, mask, target = model(image1, image2)
+
+ # the output is normalized, thus use the mean/std of the actual image to go back to RGB space
+ patchified = model.patchify(image1)
+ mean = patchified.mean(dim=-1, keepdim=True)
+ var = patchified.var(dim=-1, keepdim=True)
+ decoded_image = model.unpatchify(out * (var + 1.e-6)**.5 + mean)
+ # undo imagenet normalization, prepare masked image
+ decoded_image = decoded_image * imagenet_std_tensor + imagenet_mean_tensor
+ input_image = image1 * imagenet_std_tensor + imagenet_mean_tensor
+ ref_image = image2 * imagenet_std_tensor + imagenet_mean_tensor
+ image_masks = model.unpatchify(model.patchify(torch.ones_like(ref_image)) * mask[:,:,None])
+ masked_input_image = ((1 - image_masks) * input_image)
+
+ # make visualization
+ visualization = torch.cat((ref_image, masked_input_image, decoded_image, input_image), dim=3) # 4*(B, 3, H, W) -> B, 3, H, W*4
+ B, C, H, W = visualization.shape
+ visualization = visualization.permute(1, 0, 2, 3).reshape(C, B*H, W)
+ visualization = torchvision.transforms.functional.to_pil_image(torch.clamp(visualization, 0, 1))
+ fname = "demo_output.png"
+ visualization.save(fname)
+ print('Visualization save in '+fname)
+
+
+if __name__=="__main__":
+ main()
diff --git a/dynamic_predictor/croco/interactive_demo.ipynb b/dynamic_predictor/croco/interactive_demo.ipynb
new file mode 100644
index 0000000000000000000000000000000000000000..6cfc960af5baac9a69029c29a16eea4e24123a71
--- /dev/null
+++ b/dynamic_predictor/croco/interactive_demo.ipynb
@@ -0,0 +1,271 @@
+{
+ "cells": [
+ {
+ "cell_type": "markdown",
+ "metadata": {},
+ "source": [
+ "# Interactive demo of Cross-view Completion."
+ ]
+ },
+ {
+ "cell_type": "code",
+ "execution_count": null,
+ "metadata": {},
+ "outputs": [],
+ "source": [
+ "# Copyright (C) 2022-present Naver Corporation. All rights reserved.\n",
+ "# Licensed under CC BY-NC-SA 4.0 (non-commercial use only)."
+ ]
+ },
+ {
+ "cell_type": "code",
+ "execution_count": null,
+ "metadata": {},
+ "outputs": [],
+ "source": [
+ "import torch\n",
+ "import numpy as np\n",
+ "from models.croco import CroCoNet\n",
+ "from ipywidgets import interact, interactive, fixed, interact_manual\n",
+ "import ipywidgets as widgets\n",
+ "import matplotlib.pyplot as plt\n",
+ "import quaternion\n",
+ "import models.masking"
+ ]
+ },
+ {
+ "cell_type": "markdown",
+ "metadata": {},
+ "source": [
+ "### Load CroCo model"
+ ]
+ },
+ {
+ "cell_type": "code",
+ "execution_count": null,
+ "metadata": {},
+ "outputs": [],
+ "source": [
+ "ckpt = torch.load('pretrained_models/CroCo_V2_ViTLarge_BaseDecoder.pth', 'cpu')\n",
+ "model = CroCoNet( **ckpt.get('croco_kwargs',{}))\n",
+ "msg = model.load_state_dict(ckpt['model'], strict=True)\n",
+ "use_gpu = torch.cuda.is_available() and torch.cuda.device_count()>0\n",
+ "device = torch.device('cuda:0' if use_gpu else 'cpu')\n",
+ "model = model.eval()\n",
+ "model = model.to(device=device)\n",
+ "print(msg)\n",
+ "\n",
+ "def process_images(ref_image, target_image, masking_ratio, reconstruct_unmasked_patches=False):\n",
+ " \"\"\"\n",
+ " Perform Cross-View completion using two input images, specified using Numpy arrays.\n",
+ " \"\"\"\n",
+ " # Replace the mask generator\n",
+ " model.mask_generator = models.masking.RandomMask(model.patch_embed.num_patches, masking_ratio)\n",
+ "\n",
+ " # ImageNet-1k color normalization\n",
+ " imagenet_mean = torch.as_tensor([0.485, 0.456, 0.406]).reshape(1,3,1,1).to(device)\n",
+ " imagenet_std = torch.as_tensor([0.229, 0.224, 0.225]).reshape(1,3,1,1).to(device)\n",
+ "\n",
+ " normalize_input_colors = True\n",
+ " is_output_normalized = True\n",
+ " with torch.no_grad():\n",
+ " # Cast data to torch\n",
+ " target_image = (torch.as_tensor(target_image, dtype=torch.float, device=device).permute(2,0,1) / 255)[None]\n",
+ " ref_image = (torch.as_tensor(ref_image, dtype=torch.float, device=device).permute(2,0,1) / 255)[None]\n",
+ "\n",
+ " if normalize_input_colors:\n",
+ " ref_image = (ref_image - imagenet_mean) / imagenet_std\n",
+ " target_image = (target_image - imagenet_mean) / imagenet_std\n",
+ "\n",
+ " out, mask, _ = model(target_image, ref_image)\n",
+ " # # get target\n",
+ " if not is_output_normalized:\n",
+ " predicted_image = model.unpatchify(out)\n",
+ " else:\n",
+ " # The output only contains higher order information,\n",
+ " # we retrieve mean and standard deviation from the actual target image\n",
+ " patchified = model.patchify(target_image)\n",
+ " mean = patchified.mean(dim=-1, keepdim=True)\n",
+ " var = patchified.var(dim=-1, keepdim=True)\n",
+ " pred_renorm = out * (var + 1.e-6)**.5 + mean\n",
+ " predicted_image = model.unpatchify(pred_renorm)\n",
+ "\n",
+ " image_masks = model.unpatchify(model.patchify(torch.ones_like(ref_image)) * mask[:,:,None])\n",
+ " masked_target_image = (1 - image_masks) * target_image\n",
+ " \n",
+ " if not reconstruct_unmasked_patches:\n",
+ " # Replace unmasked patches by their actual values\n",
+ " predicted_image = predicted_image * image_masks + masked_target_image\n",
+ "\n",
+ " # Unapply color normalization\n",
+ " if normalize_input_colors:\n",
+ " predicted_image = predicted_image * imagenet_std + imagenet_mean\n",
+ " masked_target_image = masked_target_image * imagenet_std + imagenet_mean\n",
+ " \n",
+ " # Cast to Numpy\n",
+ " masked_target_image = np.asarray(torch.clamp(masked_target_image.squeeze(0).permute(1,2,0) * 255, 0, 255).cpu().numpy(), dtype=np.uint8)\n",
+ " predicted_image = np.asarray(torch.clamp(predicted_image.squeeze(0).permute(1,2,0) * 255, 0, 255).cpu().numpy(), dtype=np.uint8)\n",
+ " return masked_target_image, predicted_image"
+ ]
+ },
+ {
+ "cell_type": "markdown",
+ "metadata": {},
+ "source": [
+ "### Use the Habitat simulator to render images from arbitrary viewpoints (requires habitat_sim to be installed)"
+ ]
+ },
+ {
+ "cell_type": "code",
+ "execution_count": null,
+ "metadata": {},
+ "outputs": [],
+ "source": [
+ "import os\n",
+ "os.environ[\"MAGNUM_LOG\"]=\"quiet\"\n",
+ "os.environ[\"HABITAT_SIM_LOG\"]=\"quiet\"\n",
+ "import habitat_sim\n",
+ "\n",
+ "scene = \"habitat-sim-data/scene_datasets/habitat-test-scenes/skokloster-castle.glb\"\n",
+ "navmesh = \"habitat-sim-data/scene_datasets/habitat-test-scenes/skokloster-castle.navmesh\"\n",
+ "\n",
+ "sim_cfg = habitat_sim.SimulatorConfiguration()\n",
+ "if use_gpu: sim_cfg.gpu_device_id = 0\n",
+ "sim_cfg.scene_id = scene\n",
+ "sim_cfg.load_semantic_mesh = False\n",
+ "rgb_sensor_spec = habitat_sim.CameraSensorSpec()\n",
+ "rgb_sensor_spec.uuid = \"color\"\n",
+ "rgb_sensor_spec.sensor_type = habitat_sim.SensorType.COLOR\n",
+ "rgb_sensor_spec.resolution = (224,224)\n",
+ "rgb_sensor_spec.hfov = 56.56\n",
+ "rgb_sensor_spec.position = [0.0, 0.0, 0.0]\n",
+ "rgb_sensor_spec.orientation = [0, 0, 0]\n",
+ "agent_cfg = habitat_sim.agent.AgentConfiguration(sensor_specifications=[rgb_sensor_spec])\n",
+ "\n",
+ "\n",
+ "cfg = habitat_sim.Configuration(sim_cfg, [agent_cfg])\n",
+ "sim = habitat_sim.Simulator(cfg)\n",
+ "if navmesh is not None:\n",
+ " sim.pathfinder.load_nav_mesh(navmesh)\n",
+ "agent = sim.initialize_agent(agent_id=0)\n",
+ "\n",
+ "def sample_random_viewpoint():\n",
+ " \"\"\" Sample a random viewpoint using the navmesh \"\"\"\n",
+ " nav_point = sim.pathfinder.get_random_navigable_point()\n",
+ " # Sample a random viewpoint height\n",
+ " viewpoint_height = np.random.uniform(1.0, 1.6)\n",
+ " viewpoint_position = nav_point + viewpoint_height * habitat_sim.geo.UP\n",
+ " viewpoint_orientation = quaternion.from_rotation_vector(np.random.uniform(-np.pi, np.pi) * habitat_sim.geo.UP)\n",
+ " return viewpoint_position, viewpoint_orientation\n",
+ "\n",
+ "def render_viewpoint(position, orientation):\n",
+ " agent_state = habitat_sim.AgentState()\n",
+ " agent_state.position = position\n",
+ " agent_state.rotation = orientation\n",
+ " agent.set_state(agent_state)\n",
+ " viewpoint_observations = sim.get_sensor_observations(agent_ids=0)\n",
+ " image = viewpoint_observations['color'][:,:,:3]\n",
+ " image = np.asarray(np.clip(1.5 * np.asarray(image, dtype=float), 0, 255), dtype=np.uint8)\n",
+ " return image"
+ ]
+ },
+ {
+ "cell_type": "markdown",
+ "metadata": {},
+ "source": [
+ "### Sample a random reference view"
+ ]
+ },
+ {
+ "cell_type": "code",
+ "execution_count": null,
+ "metadata": {},
+ "outputs": [],
+ "source": [
+ "ref_position, ref_orientation = sample_random_viewpoint()\n",
+ "ref_image = render_viewpoint(ref_position, ref_orientation)\n",
+ "plt.clf()\n",
+ "fig, axes = plt.subplots(1,1, squeeze=False, num=1)\n",
+ "axes[0,0].imshow(ref_image)\n",
+ "for ax in axes.flatten():\n",
+ " ax.set_xticks([])\n",
+ " ax.set_yticks([])"
+ ]
+ },
+ {
+ "cell_type": "markdown",
+ "metadata": {},
+ "source": [
+ "### Interactive cross-view completion using CroCo"
+ ]
+ },
+ {
+ "cell_type": "code",
+ "execution_count": null,
+ "metadata": {},
+ "outputs": [],
+ "source": [
+ "reconstruct_unmasked_patches = False\n",
+ "\n",
+ "def show_demo(masking_ratio, x, y, z, panorama, elevation):\n",
+ " R = quaternion.as_rotation_matrix(ref_orientation)\n",
+ " target_position = ref_position + x * R[:,0] + y * R[:,1] + z * R[:,2]\n",
+ " target_orientation = (ref_orientation\n",
+ " * quaternion.from_rotation_vector(-elevation * np.pi/180 * habitat_sim.geo.LEFT) \n",
+ " * quaternion.from_rotation_vector(-panorama * np.pi/180 * habitat_sim.geo.UP))\n",
+ " \n",
+ " ref_image = render_viewpoint(ref_position, ref_orientation)\n",
+ " target_image = render_viewpoint(target_position, target_orientation)\n",
+ "\n",
+ " masked_target_image, predicted_image = process_images(ref_image, target_image, masking_ratio, reconstruct_unmasked_patches)\n",
+ "\n",
+ " fig, axes = plt.subplots(1,4, squeeze=True, dpi=300)\n",
+ " axes[0].imshow(ref_image)\n",
+ " axes[0].set_xlabel(\"Reference\")\n",
+ " axes[1].imshow(masked_target_image)\n",
+ " axes[1].set_xlabel(\"Masked target\")\n",
+ " axes[2].imshow(predicted_image)\n",
+ " axes[2].set_xlabel(\"Reconstruction\") \n",
+ " axes[3].imshow(target_image)\n",
+ " axes[3].set_xlabel(\"Target\")\n",
+ " for ax in axes.flatten():\n",
+ " ax.set_xticks([])\n",
+ " ax.set_yticks([])\n",
+ "\n",
+ "interact(show_demo,\n",
+ " masking_ratio=widgets.FloatSlider(description='masking', value=0.9, min=0.0, max=1.0),\n",
+ " x=widgets.FloatSlider(value=0.0, min=-0.5, max=0.5, step=0.05),\n",
+ " y=widgets.FloatSlider(value=0.0, min=-0.5, max=0.5, step=0.05),\n",
+ " z=widgets.FloatSlider(value=0.0, min=-0.5, max=0.5, step=0.05),\n",
+ " panorama=widgets.FloatSlider(value=0.0, min=-20, max=20, step=0.5),\n",
+ " elevation=widgets.FloatSlider(value=0.0, min=-20, max=20, step=0.5));"
+ ]
+ }
+ ],
+ "metadata": {
+ "kernelspec": {
+ "display_name": "Python 3 (ipykernel)",
+ "language": "python",
+ "name": "python3"
+ },
+ "language_info": {
+ "codemirror_mode": {
+ "name": "ipython",
+ "version": 3
+ },
+ "file_extension": ".py",
+ "mimetype": "text/x-python",
+ "name": "python",
+ "nbconvert_exporter": "python",
+ "pygments_lexer": "ipython3",
+ "version": "3.7.13"
+ },
+ "vscode": {
+ "interpreter": {
+ "hash": "f9237820cd248d7e07cb4fb9f0e4508a85d642f19d831560c0a4b61f3e907e67"
+ }
+ }
+ },
+ "nbformat": 4,
+ "nbformat_minor": 2
+}
diff --git a/dynamic_predictor/croco/models/blocks.py b/dynamic_predictor/croco/models/blocks.py
new file mode 100644
index 0000000000000000000000000000000000000000..9fc2d82c43dbbfa7990aa55a418c74c8e011bb15
--- /dev/null
+++ b/dynamic_predictor/croco/models/blocks.py
@@ -0,0 +1,252 @@
+# Copyright (C) 2022-present Naver Corporation. All rights reserved.
+# Licensed under CC BY-NC-SA 4.0 (non-commercial use only).
+
+
+# --------------------------------------------------------
+# Main encoder/decoder blocks
+# --------------------------------------------------------
+# References:
+# timm
+# https://github.com/rwightman/pytorch-image-models/blob/master/timm/models/vision_transformer.py
+# https://github.com/rwightman/pytorch-image-models/blob/master/timm/models/layers/helpers.py
+# https://github.com/rwightman/pytorch-image-models/blob/master/timm/models/layers/drop.py
+# https://github.com/rwightman/pytorch-image-models/blob/master/timm/models/layers/mlp.py
+# https://github.com/rwightman/pytorch-image-models/blob/master/timm/models/layers/patch_embed.py
+
+
+import torch
+import torch.nn as nn
+
+from itertools import repeat
+import collections.abc
+
+
+def _ntuple(n):
+ def parse(x):
+ if isinstance(x, collections.abc.Iterable) and not isinstance(x, str):
+ return x
+ return tuple(repeat(x, n))
+ return parse
+to_2tuple = _ntuple(2)
+
+def drop_path(x, drop_prob: float = 0., training: bool = False, scale_by_keep: bool = True):
+ """Drop paths (Stochastic Depth) per sample (when applied in main path of residual blocks).
+ """
+ if drop_prob == 0. or not training:
+ return x
+ keep_prob = 1 - drop_prob
+ shape = (x.shape[0],) + (1,) * (x.ndim - 1) # work with diff dim tensors, not just 2D ConvNets
+ random_tensor = x.new_empty(shape).bernoulli_(keep_prob)
+ if keep_prob > 0.0 and scale_by_keep:
+ random_tensor.div_(keep_prob)
+ return x * random_tensor
+
+class DropPath(nn.Module):
+ """Drop paths (Stochastic Depth) per sample (when applied in main path of residual blocks).
+ """
+ def __init__(self, drop_prob: float = 0., scale_by_keep: bool = True):
+ super(DropPath, self).__init__()
+ self.drop_prob = drop_prob
+ self.scale_by_keep = scale_by_keep
+
+ def forward(self, x):
+ return drop_path(x, self.drop_prob, self.training, self.scale_by_keep)
+
+ def extra_repr(self):
+ return f'drop_prob={round(self.drop_prob,3):0.3f}'
+
+class Mlp(nn.Module):
+ """ MLP as used in Vision Transformer, MLP-Mixer and related networks"""
+ def __init__(self, in_features, hidden_features=None, out_features=None, act_layer=nn.GELU, bias=True, drop=0.):
+ super().__init__()
+ out_features = out_features or in_features
+ hidden_features = hidden_features or in_features
+ bias = to_2tuple(bias)
+ drop_probs = to_2tuple(drop)
+
+ self.fc1 = nn.Linear(in_features, hidden_features, bias=bias[0])
+ self.act = act_layer()
+ self.drop1 = nn.Dropout(drop_probs[0])
+ self.fc2 = nn.Linear(hidden_features, out_features, bias=bias[1])
+ self.drop2 = nn.Dropout(drop_probs[1])
+
+ def forward(self, x):
+ x = self.fc1(x)
+ x = self.act(x)
+ x = self.drop1(x)
+ x = self.fc2(x)
+ x = self.drop2(x)
+ return x
+
+class Attention(nn.Module):
+
+ def __init__(self, dim, rope=None, num_heads=8, qkv_bias=False, attn_drop=0., proj_drop=0.):
+ super().__init__()
+ self.num_heads = num_heads
+ head_dim = dim // num_heads
+ self.scale = head_dim ** -0.5
+ self.qkv = nn.Linear(dim, dim * 3, bias=qkv_bias)
+ self.attn_drop = nn.Dropout(attn_drop)
+ self.proj = nn.Linear(dim, dim)
+ self.proj_drop = nn.Dropout(proj_drop)
+ self.rope = rope
+
+ def forward(self, x, xpos):
+ B, N, C = x.shape
+
+ qkv = self.qkv(x).reshape(B, N, 3, self.num_heads, C // self.num_heads).transpose(1,3)
+ q, k, v = [qkv[:,:,i] for i in range(3)]
+ # q,k,v = qkv.unbind(2) # make torchscript happy (cannot use tensor as tuple)
+
+ if self.rope is not None:
+ q = self.rope(q, xpos)
+ k = self.rope(k, xpos)
+
+ attn = (q @ k.transpose(-2, -1)) * self.scale
+ attn = attn.softmax(dim=-1)
+ attn = self.attn_drop(attn)
+
+ x = (attn @ v).transpose(1, 2).reshape(B, N, C)
+ x = self.proj(x)
+ x = self.proj_drop(x)
+ return x
+
+class Block(nn.Module):
+
+ def __init__(self, dim, num_heads, mlp_ratio=4., qkv_bias=False, drop=0., attn_drop=0.,
+ drop_path=0., act_layer=nn.GELU, norm_layer=nn.LayerNorm, rope=None):
+ super().__init__()
+ self.norm1 = norm_layer(dim)
+ self.attn = Attention(dim, rope=rope, num_heads=num_heads, qkv_bias=qkv_bias, attn_drop=attn_drop, proj_drop=drop)
+ # NOTE: drop path for stochastic depth, we shall see if this is better than dropout here
+ self.drop_path = DropPath(drop_path) if drop_path > 0. else nn.Identity()
+ self.norm2 = norm_layer(dim)
+ mlp_hidden_dim = int(dim * mlp_ratio)
+ self.mlp = Mlp(in_features=dim, hidden_features=mlp_hidden_dim, act_layer=act_layer, drop=drop)
+
+ def forward(self, x, xpos):
+ x = x + self.drop_path(self.attn(self.norm1(x), xpos))
+ x = x + self.drop_path(self.mlp(self.norm2(x)))
+ return x
+
+class CrossAttention(nn.Module):
+
+ def __init__(self, dim, rope=None, num_heads=8, qkv_bias=False, attn_drop=0., proj_drop=0.):
+ super().__init__()
+ self.num_heads = num_heads
+ head_dim = dim // num_heads
+ self.scale = head_dim ** -0.5
+
+ self.projq = nn.Linear(dim, dim, bias=qkv_bias)
+ self.projk = nn.Linear(dim, dim, bias=qkv_bias)
+ self.projv = nn.Linear(dim, dim, bias=qkv_bias)
+ self.attn_drop = nn.Dropout(attn_drop)
+ self.proj = nn.Linear(dim, dim)
+ self.proj_drop = nn.Dropout(proj_drop)
+
+ self.rope = rope
+
+ def forward(self, query, key, value, qpos, kpos):
+ B, Nq, C = query.shape
+ Nk = key.shape[1]
+ Nv = value.shape[1]
+
+ q = self.projq(query).reshape(B,Nq,self.num_heads, C// self.num_heads).permute(0, 2, 1, 3)
+ k = self.projk(key).reshape(B,Nk,self.num_heads, C// self.num_heads).permute(0, 2, 1, 3)
+ v = self.projv(value).reshape(B,Nv,self.num_heads, C// self.num_heads).permute(0, 2, 1, 3)
+
+ if self.rope is not None:
+ q = self.rope(q, qpos)
+ k = self.rope(k, kpos)
+
+ attn = (q @ k.transpose(-2, -1)) * self.scale
+ attn = attn.softmax(dim=-1)
+ attn = self.attn_drop(attn)
+
+ x = (attn @ v).transpose(1, 2).reshape(B, Nq, C)
+ x = self.proj(x)
+ x = self.proj_drop(x)
+ return x
+
+class DecoderBlock(nn.Module):
+
+ def __init__(self, dim, num_heads, mlp_ratio=4., qkv_bias=False, drop=0., attn_drop=0.,
+ drop_path=0., act_layer=nn.GELU, norm_layer=nn.LayerNorm, norm_mem=True, rope=None):
+ super().__init__()
+ self.norm1 = norm_layer(dim)
+ self.attn = Attention(dim, rope=rope, num_heads=num_heads, qkv_bias=qkv_bias, attn_drop=attn_drop, proj_drop=drop)
+ self.cross_attn = CrossAttention(dim, rope=rope, num_heads=num_heads, qkv_bias=qkv_bias, attn_drop=attn_drop, proj_drop=drop)
+ self.drop_path = DropPath(drop_path) if drop_path > 0. else nn.Identity()
+ self.norm2 = norm_layer(dim)
+ self.norm3 = norm_layer(dim)
+ mlp_hidden_dim = int(dim * mlp_ratio)
+ self.mlp = Mlp(in_features=dim, hidden_features=mlp_hidden_dim, act_layer=act_layer, drop=drop)
+ self.norm_y = norm_layer(dim) if norm_mem else nn.Identity()
+
+ def forward(self, x, y, xpos, ypos):
+ x = x + self.drop_path(self.attn(self.norm1(x), xpos))
+ y_ = self.norm_y(y)
+ x = x + self.drop_path(self.cross_attn(self.norm2(x), y_, y_, xpos, ypos))
+ x = x + self.drop_path(self.mlp(self.norm3(x)))
+ return x, y
+
+
+# patch embedding
+class PositionGetter(object):
+ """ return positions of patches """
+
+ def __init__(self):
+ self.cache_positions = {}
+
+ def __call__(self, b, h, w, device):
+ if not (h,w) in self.cache_positions:
+ x = torch.arange(w, device=device)
+ y = torch.arange(h, device=device)
+ self.cache_positions[h,w] = torch.cartesian_prod(y, x) # (h, w, 2)
+ pos = self.cache_positions[h,w].view(1, h*w, 2).expand(b, -1, 2).clone()
+ return pos
+
+class PatchEmbed(nn.Module):
+ """ just adding _init_weights + position getter compared to timm.models.layers.patch_embed.PatchEmbed"""
+
+ def __init__(self, img_size=224, patch_size=16, in_chans=3, embed_dim=768, norm_layer=None, flatten=True, init='xavier'):
+ super().__init__()
+ img_size = to_2tuple(img_size)
+ patch_size = to_2tuple(patch_size)
+ self.img_size = img_size
+ self.patch_size = patch_size
+ self.grid_size = (img_size[0] // patch_size[0], img_size[1] // patch_size[1])
+ self.num_patches = self.grid_size[0] * self.grid_size[1]
+ self.flatten = flatten
+
+ self.proj = nn.Conv2d(in_chans, embed_dim, kernel_size=patch_size, stride=patch_size)
+ self.norm = norm_layer(embed_dim) if norm_layer else nn.Identity()
+
+ self.position_getter = PositionGetter()
+ self.init_type = init
+
+ def forward(self, x):
+ B, C, H, W = x.shape
+ torch._assert(H == self.img_size[0], f"Input image height ({H}) doesn't match model ({self.img_size[0]}).")
+ torch._assert(W == self.img_size[1], f"Input image width ({W}) doesn't match model ({self.img_size[1]}).")
+ x = self.proj(x)
+ pos = self.position_getter(B, x.size(2), x.size(3), x.device)
+ if self.flatten:
+ x = x.flatten(2).transpose(1, 2) # BCHW -> BNC
+ x = self.norm(x)
+ return x, pos
+
+ def _init_weights(self):
+ w = self.proj.weight.data
+ if self.init_type == 'xavier':
+ torch.nn.init.xavier_uniform_(w.view([w.shape[0], -1]))
+ elif self.init_type == 'kaiming':
+ torch.nn.init.kaiming_uniform_(w.view([w.shape[0], -1]))
+ elif self.init_type == 'zero':
+ torch.nn.init.zeros_(w)
+ bias = getattr(self.proj, 'bias', None)
+ if bias is not None:
+ torch.nn.init.zeros_(bias)
+ else:
+ raise ValueError(f"Unknown init type {self.init_type}")
+
diff --git a/dynamic_predictor/croco/models/criterion.py b/dynamic_predictor/croco/models/criterion.py
new file mode 100644
index 0000000000000000000000000000000000000000..11696c40865344490f23796ea45e8fbd5e654731
--- /dev/null
+++ b/dynamic_predictor/croco/models/criterion.py
@@ -0,0 +1,37 @@
+# Copyright (C) 2022-present Naver Corporation. All rights reserved.
+# Licensed under CC BY-NC-SA 4.0 (non-commercial use only).
+#
+# --------------------------------------------------------
+# Criterion to train CroCo
+# --------------------------------------------------------
+# References:
+# MAE: https://github.com/facebookresearch/mae
+# --------------------------------------------------------
+
+import torch
+
+class MaskedMSE(torch.nn.Module):
+
+ def __init__(self, norm_pix_loss=False, masked=True):
+ """
+ norm_pix_loss: normalize each patch by their pixel mean and variance
+ masked: compute loss over the masked patches only
+ """
+ super().__init__()
+ self.norm_pix_loss = norm_pix_loss
+ self.masked = masked
+
+ def forward(self, pred, mask, target):
+
+ if self.norm_pix_loss:
+ mean = target.mean(dim=-1, keepdim=True)
+ var = target.var(dim=-1, keepdim=True)
+ target = (target - mean) / (var + 1.e-6)**.5
+
+ loss = (pred - target) ** 2
+ loss = loss.mean(dim=-1) # [N, L], mean loss per patch
+ if self.masked:
+ loss = (loss * mask).sum() / mask.sum() # mean loss on masked patches
+ else:
+ loss = loss.mean() # mean loss
+ return loss
diff --git a/dynamic_predictor/croco/models/croco.py b/dynamic_predictor/croco/models/croco.py
new file mode 100644
index 0000000000000000000000000000000000000000..14c68634152d75555b4c35c25af268394c5821fe
--- /dev/null
+++ b/dynamic_predictor/croco/models/croco.py
@@ -0,0 +1,249 @@
+# Copyright (C) 2022-present Naver Corporation. All rights reserved.
+# Licensed under CC BY-NC-SA 4.0 (non-commercial use only).
+
+
+# --------------------------------------------------------
+# CroCo model during pretraining
+# --------------------------------------------------------
+
+
+
+import torch
+import torch.nn as nn
+torch.backends.cuda.matmul.allow_tf32 = True # for gpu >= Ampere and pytorch >= 1.12
+from functools import partial
+
+from models.blocks import Block, DecoderBlock, PatchEmbed
+from models.pos_embed import get_2d_sincos_pos_embed, RoPE2D
+from models.masking import RandomMask
+
+
+class CroCoNet(nn.Module):
+
+ def __init__(self,
+ img_size=224, # input image size
+ patch_size=16, # patch_size
+ mask_ratio=0.9, # ratios of masked tokens
+ enc_embed_dim=768, # encoder feature dimension
+ enc_depth=12, # encoder depth
+ enc_num_heads=12, # encoder number of heads in the transformer block
+ dec_embed_dim=512, # decoder feature dimension
+ dec_depth=8, # decoder depth
+ dec_num_heads=16, # decoder number of heads in the transformer block
+ mlp_ratio=4,
+ norm_layer=partial(nn.LayerNorm, eps=1e-6),
+ norm_im2_in_dec=True, # whether to apply normalization of the 'memory' = (second image) in the decoder
+ pos_embed='cosine', # positional embedding (either cosine or RoPE100)
+ ):
+
+ super(CroCoNet, self).__init__()
+
+ # patch embeddings (with initialization done as in MAE)
+ self._set_patch_embed(img_size, patch_size, enc_embed_dim)
+
+ # mask generations
+ self._set_mask_generator(self.patch_embed.num_patches, mask_ratio)
+
+ self.pos_embed = pos_embed
+ if pos_embed=='cosine':
+ # positional embedding of the encoder
+ enc_pos_embed = get_2d_sincos_pos_embed(enc_embed_dim, int(self.patch_embed.num_patches**.5), n_cls_token=0)
+ self.register_buffer('enc_pos_embed', torch.from_numpy(enc_pos_embed).float())
+ # positional embedding of the decoder
+ dec_pos_embed = get_2d_sincos_pos_embed(dec_embed_dim, int(self.patch_embed.num_patches**.5), n_cls_token=0)
+ self.register_buffer('dec_pos_embed', torch.from_numpy(dec_pos_embed).float())
+ # pos embedding in each block
+ self.rope = None # nothing for cosine
+ elif pos_embed.startswith('RoPE'): # eg RoPE100
+ self.enc_pos_embed = None # nothing to add in the encoder with RoPE
+ self.dec_pos_embed = None # nothing to add in the decoder with RoPE
+ if RoPE2D is None: raise ImportError("Cannot find cuRoPE2D, please install it following the README instructions")
+ freq = float(pos_embed[len('RoPE'):])
+ self.rope = RoPE2D(freq=freq)
+ else:
+ raise NotImplementedError('Unknown pos_embed '+pos_embed)
+
+ # transformer for the encoder
+ self.enc_depth = enc_depth
+ self.enc_embed_dim = enc_embed_dim
+ self.enc_blocks = nn.ModuleList([
+ Block(enc_embed_dim, enc_num_heads, mlp_ratio, qkv_bias=True, norm_layer=norm_layer, rope=self.rope)
+ for i in range(enc_depth)])
+ self.enc_norm = norm_layer(enc_embed_dim)
+
+ # masked tokens
+ self._set_mask_token(dec_embed_dim)
+
+ # decoder
+ self._set_decoder(enc_embed_dim, dec_embed_dim, dec_num_heads, dec_depth, mlp_ratio, norm_layer, norm_im2_in_dec)
+
+ # prediction head
+ self._set_prediction_head(dec_embed_dim, patch_size)
+
+ # initializer weights
+ self.initialize_weights()
+
+ def _set_patch_embed(self, img_size=224, patch_size=16, enc_embed_dim=768):
+ self.patch_embed = PatchEmbed(img_size, patch_size, 3, enc_embed_dim)
+
+ def _set_mask_generator(self, num_patches, mask_ratio):
+ self.mask_generator = RandomMask(num_patches, mask_ratio)
+
+ def _set_mask_token(self, dec_embed_dim):
+ self.mask_token = nn.Parameter(torch.zeros(1, 1, dec_embed_dim))
+
+ def _set_decoder(self, enc_embed_dim, dec_embed_dim, dec_num_heads, dec_depth, mlp_ratio, norm_layer, norm_im2_in_dec):
+ self.dec_depth = dec_depth
+ self.dec_embed_dim = dec_embed_dim
+ # transfer from encoder to decoder
+ self.decoder_embed = nn.Linear(enc_embed_dim, dec_embed_dim, bias=True)
+ # transformer for the decoder
+ self.dec_blocks = nn.ModuleList([
+ DecoderBlock(dec_embed_dim, dec_num_heads, mlp_ratio=mlp_ratio, qkv_bias=True, norm_layer=norm_layer, norm_mem=norm_im2_in_dec, rope=self.rope)
+ for i in range(dec_depth)])
+ # final norm layer
+ self.dec_norm = norm_layer(dec_embed_dim)
+
+ def _set_prediction_head(self, dec_embed_dim, patch_size):
+ self.prediction_head = nn.Linear(dec_embed_dim, patch_size**2 * 3, bias=True)
+
+
+ def initialize_weights(self):
+ # patch embed
+ self.patch_embed._init_weights()
+ # mask tokens
+ if self.mask_token is not None: torch.nn.init.normal_(self.mask_token, std=.02)
+ # linears and layer norms
+ self.apply(self._init_weights)
+
+ def _init_weights(self, m):
+ if isinstance(m, nn.Linear):
+ # we use xavier_uniform following official JAX ViT:
+ torch.nn.init.xavier_uniform_(m.weight)
+ if isinstance(m, nn.Linear) and m.bias is not None:
+ nn.init.constant_(m.bias, 0)
+ elif isinstance(m, nn.LayerNorm):
+ nn.init.constant_(m.bias, 0)
+ nn.init.constant_(m.weight, 1.0)
+
+ def _encode_image(self, image, do_mask=False, return_all_blocks=False):
+ """
+ image has B x 3 x img_size x img_size
+ do_mask: whether to perform masking or not
+ return_all_blocks: if True, return the features at the end of every block
+ instead of just the features from the last block (eg for some prediction heads)
+ """
+ # embed the image into patches (x has size B x Npatches x C)
+ # and get position if each return patch (pos has size B x Npatches x 2)
+ x, pos = self.patch_embed(image)
+ # add positional embedding without cls token
+ if self.enc_pos_embed is not None:
+ x = x + self.enc_pos_embed[None,...]
+ # apply masking
+ B,N,C = x.size()
+ if do_mask:
+ masks = self.mask_generator(x)
+ x = x[~masks].view(B, -1, C)
+ posvis = pos[~masks].view(B, -1, 2)
+ else:
+ B,N,C = x.size()
+ masks = torch.zeros((B,N), dtype=bool)
+ posvis = pos
+ # now apply the transformer encoder and normalization
+ if return_all_blocks:
+ out = []
+ for blk in self.enc_blocks:
+ x = blk(x, posvis)
+ out.append(x)
+ out[-1] = self.enc_norm(out[-1])
+ return out, pos, masks
+ else:
+ for blk in self.enc_blocks:
+ x = blk(x, posvis)
+ x = self.enc_norm(x)
+ return x, pos, masks
+
+ def _decoder(self, feat1, pos1, masks1, feat2, pos2, return_all_blocks=False):
+ """
+ return_all_blocks: if True, return the features at the end of every block
+ instead of just the features from the last block (eg for some prediction heads)
+
+ masks1 can be None => assume image1 fully visible
+ """
+ # encoder to decoder layer
+ visf1 = self.decoder_embed(feat1)
+ f2 = self.decoder_embed(feat2)
+ # append masked tokens to the sequence
+ B,Nenc,C = visf1.size()
+ if masks1 is None: # downstreams
+ f1_ = visf1
+ else: # pretraining
+ Ntotal = masks1.size(1)
+ f1_ = self.mask_token.repeat(B, Ntotal, 1).to(dtype=visf1.dtype)
+ f1_[~masks1] = visf1.view(B * Nenc, C)
+ # add positional embedding
+ if self.dec_pos_embed is not None:
+ f1_ = f1_ + self.dec_pos_embed
+ f2 = f2 + self.dec_pos_embed
+ # apply Transformer blocks
+ out = f1_
+ out2 = f2
+ if return_all_blocks:
+ _out, out = out, []
+ for blk in self.dec_blocks:
+ _out, out2 = blk(_out, out2, pos1, pos2)
+ out.append(_out)
+ out[-1] = self.dec_norm(out[-1])
+ else:
+ for blk in self.dec_blocks:
+ out, out2 = blk(out, out2, pos1, pos2)
+ out = self.dec_norm(out)
+ return out
+
+ def patchify(self, imgs):
+ """
+ imgs: (B, 3, H, W)
+ x: (B, L, patch_size**2 *3)
+ """
+ p = self.patch_embed.patch_size[0]
+ assert imgs.shape[2] == imgs.shape[3] and imgs.shape[2] % p == 0
+
+ h = w = imgs.shape[2] // p
+ x = imgs.reshape(shape=(imgs.shape[0], 3, h, p, w, p))
+ x = torch.einsum('nchpwq->nhwpqc', x)
+ x = x.reshape(shape=(imgs.shape[0], h * w, p**2 * 3))
+
+ return x
+
+ def unpatchify(self, x, channels=3):
+ """
+ x: (N, L, patch_size**2 *channels)
+ imgs: (N, 3, H, W)
+ """
+ patch_size = self.patch_embed.patch_size[0]
+ h = w = int(x.shape[1]**.5)
+ assert h * w == x.shape[1]
+ x = x.reshape(shape=(x.shape[0], h, w, patch_size, patch_size, channels))
+ x = torch.einsum('nhwpqc->nchpwq', x)
+ imgs = x.reshape(shape=(x.shape[0], channels, h * patch_size, h * patch_size))
+ return imgs
+
+ def forward(self, img1, img2):
+ """
+ img1: tensor of size B x 3 x img_size x img_size
+ img2: tensor of size B x 3 x img_size x img_size
+
+ out will be B x N x (3*patch_size*patch_size)
+ masks are also returned as B x N just in case
+ """
+ # encoder of the masked first image
+ feat1, pos1, mask1 = self._encode_image(img1, do_mask=True)
+ # encoder of the second image
+ feat2, pos2, _ = self._encode_image(img2, do_mask=False)
+ # decoder
+ decfeat = self._decoder(feat1, pos1, mask1, feat2, pos2)
+ # prediction head
+ out = self.prediction_head(decfeat)
+ # get target
+ target = self.patchify(img1)
+ return out, mask1, target
diff --git a/dynamic_predictor/croco/models/croco_downstream.py b/dynamic_predictor/croco/models/croco_downstream.py
new file mode 100644
index 0000000000000000000000000000000000000000..159dfff4d2c1461bc235e21441b57ce1e2088f76
--- /dev/null
+++ b/dynamic_predictor/croco/models/croco_downstream.py
@@ -0,0 +1,122 @@
+# Copyright (C) 2022-present Naver Corporation. All rights reserved.
+# Licensed under CC BY-NC-SA 4.0 (non-commercial use only).
+
+# --------------------------------------------------------
+# CroCo model for downstream tasks
+# --------------------------------------------------------
+
+import torch
+
+from .croco import CroCoNet
+
+
+def croco_args_from_ckpt(ckpt):
+ if 'croco_kwargs' in ckpt: # CroCo v2 released models
+ return ckpt['croco_kwargs']
+ elif 'args' in ckpt and hasattr(ckpt['args'], 'model'): # pretrained using the official code release
+ s = ckpt['args'].model # eg "CroCoNet(enc_embed_dim=1024, enc_num_heads=16, enc_depth=24)"
+ assert s.startswith('CroCoNet(')
+ return eval('dict'+s[len('CroCoNet'):]) # transform it into the string of a dictionary and evaluate it
+ else: # CroCo v1 released models
+ return dict()
+
+class CroCoDownstreamMonocularEncoder(CroCoNet):
+
+ def __init__(self,
+ head,
+ **kwargs):
+ """ Build network for monocular downstream task, only using the encoder.
+ It takes an extra argument head, that is called with the features
+ and a dictionary img_info containing 'width' and 'height' keys
+ The head is setup with the croconet arguments in this init function
+ NOTE: It works by *calling super().__init__() but with redefined setters
+
+ """
+ super(CroCoDownstreamMonocularEncoder, self).__init__(**kwargs)
+ head.setup(self)
+ self.head = head
+
+ def _set_mask_generator(self, *args, **kwargs):
+ """ No mask generator """
+ return
+
+ def _set_mask_token(self, *args, **kwargs):
+ """ No mask token """
+ self.mask_token = None
+ return
+
+ def _set_decoder(self, *args, **kwargs):
+ """ No decoder """
+ return
+
+ def _set_prediction_head(self, *args, **kwargs):
+ """ No 'prediction head' for downstream tasks."""
+ return
+
+ def forward(self, img):
+ """
+ img if of size batch_size x 3 x h x w
+ """
+ B, C, H, W = img.size()
+ img_info = {'height': H, 'width': W}
+ need_all_layers = hasattr(self.head, 'return_all_blocks') and self.head.return_all_blocks
+ out, _, _ = self._encode_image(img, do_mask=False, return_all_blocks=need_all_layers)
+ return self.head(out, img_info)
+
+
+class CroCoDownstreamBinocular(CroCoNet):
+
+ def __init__(self,
+ head,
+ **kwargs):
+ """ Build network for binocular downstream task
+ It takes an extra argument head, that is called with the features
+ and a dictionary img_info containing 'width' and 'height' keys
+ The head is setup with the croconet arguments in this init function
+ """
+ super(CroCoDownstreamBinocular, self).__init__(**kwargs)
+ head.setup(self)
+ self.head = head
+
+ def _set_mask_generator(self, *args, **kwargs):
+ """ No mask generator """
+ return
+
+ def _set_mask_token(self, *args, **kwargs):
+ """ No mask token """
+ self.mask_token = None
+ return
+
+ def _set_prediction_head(self, *args, **kwargs):
+ """ No prediction head for downstream tasks, define your own head """
+ return
+
+ def encode_image_pairs(self, img1, img2, return_all_blocks=False):
+ """ run encoder for a pair of images
+ it is actually ~5% faster to concatenate the images along the batch dimension
+ than to encode them separately
+ """
+ ## the two commented lines below is the naive version with separate encoding
+ #out, pos, _ = self._encode_image(img1, do_mask=False, return_all_blocks=return_all_blocks)
+ #out2, pos2, _ = self._encode_image(img2, do_mask=False, return_all_blocks=False)
+ ## and now the faster version
+ out, pos, _ = self._encode_image( torch.cat( (img1,img2), dim=0), do_mask=False, return_all_blocks=return_all_blocks )
+ if return_all_blocks:
+ out,out2 = list(map(list, zip(*[o.chunk(2, dim=0) for o in out])))
+ out2 = out2[-1]
+ else:
+ out,out2 = out.chunk(2, dim=0)
+ pos,pos2 = pos.chunk(2, dim=0)
+ return out, out2, pos, pos2
+
+ def forward(self, img1, img2):
+ B, C, H, W = img1.size()
+ img_info = {'height': H, 'width': W}
+ return_all_blocks = hasattr(self.head, 'return_all_blocks') and self.head.return_all_blocks
+ out, out2, pos, pos2 = self.encode_image_pairs(img1, img2, return_all_blocks=return_all_blocks)
+ if return_all_blocks:
+ decout = self._decoder(out[-1], pos, None, out2, pos2, return_all_blocks=return_all_blocks)
+ decout = out+decout
+ else:
+ decout = self._decoder(out, pos, None, out2, pos2, return_all_blocks=return_all_blocks)
+ return self.head(decout, img_info)
\ No newline at end of file
diff --git a/dynamic_predictor/croco/models/curope/__init__.py b/dynamic_predictor/croco/models/curope/__init__.py
new file mode 100644
index 0000000000000000000000000000000000000000..25e3d48a162760260826080f6366838e83e26878
--- /dev/null
+++ b/dynamic_predictor/croco/models/curope/__init__.py
@@ -0,0 +1,4 @@
+# Copyright (C) 2022-present Naver Corporation. All rights reserved.
+# Licensed under CC BY-NC-SA 4.0 (non-commercial use only).
+
+from .curope2d import cuRoPE2D
diff --git a/dynamic_predictor/croco/models/curope/curope.cpp b/dynamic_predictor/croco/models/curope/curope.cpp
new file mode 100644
index 0000000000000000000000000000000000000000..8fe9058e05aa1bf3f37b0d970edc7312bc68455b
--- /dev/null
+++ b/dynamic_predictor/croco/models/curope/curope.cpp
@@ -0,0 +1,69 @@
+/*
+ Copyright (C) 2022-present Naver Corporation. All rights reserved.
+ Licensed under CC BY-NC-SA 4.0 (non-commercial use only).
+*/
+
+#include
+
+// forward declaration
+void rope_2d_cuda( torch::Tensor tokens, const torch::Tensor pos, const float base, const float fwd );
+
+void rope_2d_cpu( torch::Tensor tokens, const torch::Tensor positions, const float base, const float fwd )
+{
+ const int B = tokens.size(0);
+ const int N = tokens.size(1);
+ const int H = tokens.size(2);
+ const int D = tokens.size(3) / 4;
+
+ auto tok = tokens.accessor();
+ auto pos = positions.accessor();
+
+ for (int b = 0; b < B; b++) {
+ for (int x = 0; x < 2; x++) { // y and then x (2d)
+ for (int n = 0; n < N; n++) {
+
+ // grab the token position
+ const int p = pos[b][n][x];
+
+ for (int h = 0; h < H; h++) {
+ for (int d = 0; d < D; d++) {
+ // grab the two values
+ float u = tok[b][n][h][d+0+x*2*D];
+ float v = tok[b][n][h][d+D+x*2*D];
+
+ // grab the cos,sin
+ const float inv_freq = fwd * p / powf(base, d/float(D));
+ float c = cosf(inv_freq);
+ float s = sinf(inv_freq);
+
+ // write the result
+ tok[b][n][h][d+0+x*2*D] = u*c - v*s;
+ tok[b][n][h][d+D+x*2*D] = v*c + u*s;
+ }
+ }
+ }
+ }
+ }
+}
+
+void rope_2d( torch::Tensor tokens, // B,N,H,D
+ const torch::Tensor positions, // B,N,2
+ const float base,
+ const float fwd )
+{
+ TORCH_CHECK(tokens.dim() == 4, "tokens must have 4 dimensions");
+ TORCH_CHECK(positions.dim() == 3, "positions must have 3 dimensions");
+ TORCH_CHECK(tokens.size(0) == positions.size(0), "batch size differs between tokens & positions");
+ TORCH_CHECK(tokens.size(1) == positions.size(1), "seq_length differs between tokens & positions");
+ TORCH_CHECK(positions.size(2) == 2, "positions.shape[2] must be equal to 2");
+ TORCH_CHECK(tokens.is_cuda() == positions.is_cuda(), "tokens and positions are not on the same device" );
+
+ if (tokens.is_cuda())
+ rope_2d_cuda( tokens, positions, base, fwd );
+ else
+ rope_2d_cpu( tokens, positions, base, fwd );
+}
+
+PYBIND11_MODULE(TORCH_EXTENSION_NAME, m) {
+ m.def("rope_2d", &rope_2d, "RoPE 2d forward/backward");
+}
diff --git a/dynamic_predictor/croco/models/curope/curope2d.py b/dynamic_predictor/croco/models/curope/curope2d.py
new file mode 100644
index 0000000000000000000000000000000000000000..a49c12f8c529e9a889b5ac20c5767158f238e17d
--- /dev/null
+++ b/dynamic_predictor/croco/models/curope/curope2d.py
@@ -0,0 +1,40 @@
+# Copyright (C) 2022-present Naver Corporation. All rights reserved.
+# Licensed under CC BY-NC-SA 4.0 (non-commercial use only).
+
+import torch
+
+try:
+ import curope as _kernels # run `python setup.py install`
+except ModuleNotFoundError:
+ from . import curope as _kernels # run `python setup.py build_ext --inplace`
+
+
+class cuRoPE2D_func (torch.autograd.Function):
+
+ @staticmethod
+ def forward(ctx, tokens, positions, base, F0=1):
+ ctx.save_for_backward(positions)
+ ctx.saved_base = base
+ ctx.saved_F0 = F0
+ # tokens = tokens.clone() # uncomment this if inplace doesn't work
+ _kernels.rope_2d( tokens, positions, base, F0 )
+ ctx.mark_dirty(tokens)
+ return tokens
+
+ @staticmethod
+ def backward(ctx, grad_res):
+ positions, base, F0 = ctx.saved_tensors[0], ctx.saved_base, ctx.saved_F0
+ _kernels.rope_2d( grad_res, positions, base, -F0 )
+ ctx.mark_dirty(grad_res)
+ return grad_res, None, None, None
+
+
+class cuRoPE2D(torch.nn.Module):
+ def __init__(self, freq=100.0, F0=1.0):
+ super().__init__()
+ self.base = freq
+ self.F0 = F0
+
+ def forward(self, tokens, positions):
+ cuRoPE2D_func.apply( tokens.transpose(1,2), positions, self.base, self.F0 )
+ return tokens
\ No newline at end of file
diff --git a/dynamic_predictor/croco/models/curope/kernels.cu b/dynamic_predictor/croco/models/curope/kernels.cu
new file mode 100644
index 0000000000000000000000000000000000000000..7156cd1bb935cb1f0be45e58add53f9c21505c20
--- /dev/null
+++ b/dynamic_predictor/croco/models/curope/kernels.cu
@@ -0,0 +1,108 @@
+/*
+ Copyright (C) 2022-present Naver Corporation. All rights reserved.
+ Licensed under CC BY-NC-SA 4.0 (non-commercial use only).
+*/
+
+#include
+#include
+#include
+#include
+
+#define CHECK_CUDA(tensor) {\
+ TORCH_CHECK((tensor).is_cuda(), #tensor " is not in cuda memory"); \
+ TORCH_CHECK((tensor).is_contiguous(), #tensor " is not contiguous"); }
+void CHECK_KERNEL() {auto error = cudaGetLastError(); TORCH_CHECK( error == cudaSuccess, cudaGetErrorString(error));}
+
+
+template < typename scalar_t >
+__global__ void rope_2d_cuda_kernel(
+ //scalar_t* __restrict__ tokens,
+ torch::PackedTensorAccessor32 tokens,
+ const int64_t* __restrict__ pos,
+ const float base,
+ const float fwd )
+ // const int N, const int H, const int D )
+{
+ // tokens shape = (B, N, H, D)
+ const int N = tokens.size(1);
+ const int H = tokens.size(2);
+ const int D = tokens.size(3);
+
+ // each block update a single token, for all heads
+ // each thread takes care of a single output
+ extern __shared__ float shared[];
+ float* shared_inv_freq = shared + D;
+
+ const int b = blockIdx.x / N;
+ const int n = blockIdx.x % N;
+
+ const int Q = D / 4;
+ // one token = [0..Q : Q..2Q : 2Q..3Q : 3Q..D]
+ // u_Y v_Y u_X v_X
+
+ // shared memory: first, compute inv_freq
+ if (threadIdx.x < Q)
+ shared_inv_freq[threadIdx.x] = fwd / powf(base, threadIdx.x/float(Q));
+ __syncthreads();
+
+ // start of X or Y part
+ const int X = threadIdx.x < D/2 ? 0 : 1;
+ const int m = (X*D/2) + (threadIdx.x % Q); // index of u_Y or u_X
+
+ // grab the cos,sin appropriate for me
+ const float freq = pos[blockIdx.x*2+X] * shared_inv_freq[threadIdx.x % Q];
+ const float cos = cosf(freq);
+ const float sin = sinf(freq);
+ /*
+ float* shared_cos_sin = shared + D + D/4;
+ if ((threadIdx.x % (D/2)) < Q)
+ shared_cos_sin[m+0] = cosf(freq);
+ else
+ shared_cos_sin[m+Q] = sinf(freq);
+ __syncthreads();
+ const float cos = shared_cos_sin[m+0];
+ const float sin = shared_cos_sin[m+Q];
+ */
+
+ for (int h = 0; h < H; h++)
+ {
+ // then, load all the token for this head in shared memory
+ shared[threadIdx.x] = tokens[b][n][h][threadIdx.x];
+ __syncthreads();
+
+ const float u = shared[m];
+ const float v = shared[m+Q];
+
+ // write output
+ if ((threadIdx.x % (D/2)) < Q)
+ tokens[b][n][h][threadIdx.x] = u*cos - v*sin;
+ else
+ tokens[b][n][h][threadIdx.x] = v*cos + u*sin;
+ }
+}
+
+void rope_2d_cuda( torch::Tensor tokens, const torch::Tensor pos, const float base, const float fwd )
+{
+ const int B = tokens.size(0); // batch size
+ const int N = tokens.size(1); // sequence length
+ const int H = tokens.size(2); // number of heads
+ const int D = tokens.size(3); // dimension per head
+
+ TORCH_CHECK(tokens.stride(3) == 1 && tokens.stride(2) == D, "tokens are not contiguous");
+ TORCH_CHECK(pos.is_contiguous(), "positions are not contiguous");
+ TORCH_CHECK(pos.size(0) == B && pos.size(1) == N && pos.size(2) == 2, "bad pos.shape");
+ TORCH_CHECK(D % 4 == 0, "token dim must be multiple of 4");
+
+ // one block for each layer, one thread per local-max
+ const int THREADS_PER_BLOCK = D;
+ const int N_BLOCKS = B * N; // each block takes care of H*D values
+ const int SHARED_MEM = sizeof(float) * (D + D/4);
+
+ AT_DISPATCH_FLOATING_TYPES_AND_HALF(tokens.type(), "rope_2d_cuda", ([&] {
+ rope_2d_cuda_kernel <<>> (
+ //tokens.data_ptr(),
+ tokens.packed_accessor32(),
+ pos.data_ptr(),
+ base, fwd); //, N, H, D );
+ }));
+}
diff --git a/dynamic_predictor/croco/models/curope/setup.py b/dynamic_predictor/croco/models/curope/setup.py
new file mode 100644
index 0000000000000000000000000000000000000000..230632ed05e309200e8f93a3a852072333975009
--- /dev/null
+++ b/dynamic_predictor/croco/models/curope/setup.py
@@ -0,0 +1,34 @@
+# Copyright (C) 2022-present Naver Corporation. All rights reserved.
+# Licensed under CC BY-NC-SA 4.0 (non-commercial use only).
+
+from setuptools import setup
+from torch import cuda
+from torch.utils.cpp_extension import BuildExtension, CUDAExtension
+
+# compile for all possible CUDA architectures
+all_cuda_archs = cuda.get_gencode_flags().replace('compute=','arch=').split()
+# alternatively, you can list cuda archs that you want, eg:
+# all_cuda_archs = [
+ # '-gencode', 'arch=compute_70,code=sm_70',
+ # '-gencode', 'arch=compute_75,code=sm_75',
+ # '-gencode', 'arch=compute_80,code=sm_80',
+ # '-gencode', 'arch=compute_86,code=sm_86'
+# ]
+
+setup(
+ name = 'curope',
+ ext_modules = [
+ CUDAExtension(
+ name='curope',
+ sources=[
+ "curope.cpp",
+ "kernels.cu",
+ ],
+ extra_compile_args = dict(
+ nvcc=['-O3','--ptxas-options=-v',"--use_fast_math"]+all_cuda_archs,
+ cxx=['-O3'])
+ )
+ ],
+ cmdclass = {
+ 'build_ext': BuildExtension
+ })
diff --git a/dynamic_predictor/croco/models/dpt_block.py b/dynamic_predictor/croco/models/dpt_block.py
new file mode 100644
index 0000000000000000000000000000000000000000..d4ddfb74e2769ceca88720d4c730e00afd71c763
--- /dev/null
+++ b/dynamic_predictor/croco/models/dpt_block.py
@@ -0,0 +1,450 @@
+# Copyright (C) 2022-present Naver Corporation. All rights reserved.
+# Licensed under CC BY-NC-SA 4.0 (non-commercial use only).
+
+# --------------------------------------------------------
+# DPT head for ViTs
+# --------------------------------------------------------
+# References:
+# https://github.com/isl-org/DPT
+# https://github.com/EPFL-VILAB/MultiMAE/blob/main/multimae/output_adapters.py
+
+import torch
+import torch.nn as nn
+import torch.nn.functional as F
+from einops import rearrange, repeat
+from typing import Union, Tuple, Iterable, List, Optional, Dict
+
+def pair(t):
+ return t if isinstance(t, tuple) else (t, t)
+
+def make_scratch(in_shape, out_shape, groups=1, expand=False):
+ scratch = nn.Module()
+
+ out_shape1 = out_shape
+ out_shape2 = out_shape
+ out_shape3 = out_shape
+ out_shape4 = out_shape
+ if expand == True:
+ out_shape1 = out_shape
+ out_shape2 = out_shape * 2
+ out_shape3 = out_shape * 4
+ out_shape4 = out_shape * 8
+
+ scratch.layer1_rn = nn.Conv2d(
+ in_shape[0],
+ out_shape1,
+ kernel_size=3,
+ stride=1,
+ padding=1,
+ bias=False,
+ groups=groups,
+ )
+ scratch.layer2_rn = nn.Conv2d(
+ in_shape[1],
+ out_shape2,
+ kernel_size=3,
+ stride=1,
+ padding=1,
+ bias=False,
+ groups=groups,
+ )
+ scratch.layer3_rn = nn.Conv2d(
+ in_shape[2],
+ out_shape3,
+ kernel_size=3,
+ stride=1,
+ padding=1,
+ bias=False,
+ groups=groups,
+ )
+ scratch.layer4_rn = nn.Conv2d(
+ in_shape[3],
+ out_shape4,
+ kernel_size=3,
+ stride=1,
+ padding=1,
+ bias=False,
+ groups=groups,
+ )
+
+ scratch.layer_rn = nn.ModuleList([
+ scratch.layer1_rn,
+ scratch.layer2_rn,
+ scratch.layer3_rn,
+ scratch.layer4_rn,
+ ])
+
+ return scratch
+
+class ResidualConvUnit_custom(nn.Module):
+ """Residual convolution module."""
+
+ def __init__(self, features, activation, bn):
+ """Init.
+ Args:
+ features (int): number of features
+ """
+ super().__init__()
+
+ self.bn = bn
+
+ self.groups = 1
+
+ self.conv1 = nn.Conv2d(
+ features,
+ features,
+ kernel_size=3,
+ stride=1,
+ padding=1,
+ bias=not self.bn,
+ groups=self.groups,
+ )
+
+ self.conv2 = nn.Conv2d(
+ features,
+ features,
+ kernel_size=3,
+ stride=1,
+ padding=1,
+ bias=not self.bn,
+ groups=self.groups,
+ )
+
+ if self.bn == True:
+ self.bn1 = nn.BatchNorm2d(features)
+ self.bn2 = nn.BatchNorm2d(features)
+
+ self.activation = activation
+
+ self.skip_add = nn.quantized.FloatFunctional()
+
+ def forward(self, x):
+ """Forward pass.
+ Args:
+ x (tensor): input
+ Returns:
+ tensor: output
+ """
+
+ out = self.activation(x)
+ out = self.conv1(out)
+ if self.bn == True:
+ out = self.bn1(out)
+
+ out = self.activation(out)
+ out = self.conv2(out)
+ if self.bn == True:
+ out = self.bn2(out)
+
+ if self.groups > 1:
+ out = self.conv_merge(out)
+
+ return self.skip_add.add(out, x)
+
+class FeatureFusionBlock_custom(nn.Module):
+ """Feature fusion block."""
+
+ def __init__(
+ self,
+ features,
+ activation,
+ deconv=False,
+ bn=False,
+ expand=False,
+ align_corners=True,
+ width_ratio=1,
+ ):
+ """Init.
+ Args:
+ features (int): number of features
+ """
+ super(FeatureFusionBlock_custom, self).__init__()
+ self.width_ratio = width_ratio
+
+ self.deconv = deconv
+ self.align_corners = align_corners
+
+ self.groups = 1
+
+ self.expand = expand
+ out_features = features
+ if self.expand == True:
+ out_features = features // 2
+
+ self.out_conv = nn.Conv2d(
+ features,
+ out_features,
+ kernel_size=1,
+ stride=1,
+ padding=0,
+ bias=True,
+ groups=1,
+ )
+
+ self.resConfUnit1 = ResidualConvUnit_custom(features, activation, bn)
+ self.resConfUnit2 = ResidualConvUnit_custom(features, activation, bn)
+
+ self.skip_add = nn.quantized.FloatFunctional()
+
+ def forward(self, *xs):
+ """Forward pass.
+ Returns:
+ tensor: output
+ """
+ output = xs[0]
+
+ if len(xs) == 2:
+ res = self.resConfUnit1(xs[1])
+ if self.width_ratio != 1:
+ res = F.interpolate(res, size=(output.shape[2], output.shape[3]), mode='bilinear')
+
+ output = self.skip_add.add(output, res)
+ # output += res
+
+ output = self.resConfUnit2(output)
+
+ if self.width_ratio != 1:
+ # and output.shape[3] < self.width_ratio * output.shape[2]
+ #size=(image.shape[])
+ if (output.shape[3] / output.shape[2]) < (2 / 3) * self.width_ratio:
+ shape = 3 * output.shape[3]
+ else:
+ shape = int(self.width_ratio * 2 * output.shape[2])
+ output = F.interpolate(output, size=(2* output.shape[2], shape), mode='bilinear')
+ else:
+ output = nn.functional.interpolate(output, scale_factor=2,
+ mode="bilinear", align_corners=self.align_corners)
+ output = self.out_conv(output)
+ return output
+
+def make_fusion_block(features, use_bn, width_ratio=1):
+ return FeatureFusionBlock_custom(
+ features,
+ nn.ReLU(False),
+ deconv=False,
+ bn=use_bn,
+ expand=False,
+ align_corners=True,
+ width_ratio=width_ratio,
+ )
+
+class Interpolate(nn.Module):
+ """Interpolation module."""
+
+ def __init__(self, scale_factor, mode, align_corners=False):
+ """Init.
+ Args:
+ scale_factor (float): scaling
+ mode (str): interpolation mode
+ """
+ super(Interpolate, self).__init__()
+
+ self.interp = nn.functional.interpolate
+ self.scale_factor = scale_factor
+ self.mode = mode
+ self.align_corners = align_corners
+
+ def forward(self, x):
+ """Forward pass.
+ Args:
+ x (tensor): input
+ Returns:
+ tensor: interpolated data
+ """
+
+ x = self.interp(
+ x,
+ scale_factor=self.scale_factor,
+ mode=self.mode,
+ align_corners=self.align_corners,
+ )
+
+ return x
+
+class DPTOutputAdapter(nn.Module):
+ """DPT output adapter.
+
+ :param num_cahnnels: Number of output channels
+ :param stride_level: tride level compared to the full-sized image.
+ E.g. 4 for 1/4th the size of the image.
+ :param patch_size_full: Int or tuple of the patch size over the full image size.
+ Patch size for smaller inputs will be computed accordingly.
+ :param hooks: Index of intermediate layers
+ :param layer_dims: Dimension of intermediate layers
+ :param feature_dim: Feature dimension
+ :param last_dim: out_channels/in_channels for the last two Conv2d when head_type == regression
+ :param use_bn: If set to True, activates batch norm
+ :param dim_tokens_enc: Dimension of tokens coming from encoder
+ """
+
+ def __init__(self,
+ num_channels: int = 1,
+ stride_level: int = 1,
+ patch_size: Union[int, Tuple[int, int]] = 16,
+ main_tasks: Iterable[str] = ('rgb',),
+ hooks: List[int] = [2, 5, 8, 11],
+ layer_dims: List[int] = [96, 192, 384, 768],
+ feature_dim: int = 256,
+ last_dim: int = 32,
+ use_bn: bool = False,
+ dim_tokens_enc: Optional[int] = None,
+ head_type: str = 'regression',
+ output_width_ratio=1,
+ **kwargs):
+ super().__init__()
+ self.num_channels = num_channels
+ self.stride_level = stride_level
+ self.patch_size = pair(patch_size)
+ self.main_tasks = main_tasks
+ self.hooks = hooks
+ self.layer_dims = layer_dims
+ self.feature_dim = feature_dim
+ self.dim_tokens_enc = dim_tokens_enc * len(self.main_tasks) if dim_tokens_enc is not None else None
+ self.head_type = head_type
+
+ # Actual patch height and width, taking into account stride of input
+ self.P_H = max(1, self.patch_size[0] // stride_level)
+ self.P_W = max(1, self.patch_size[1] // stride_level)
+
+ self.scratch = make_scratch(layer_dims, feature_dim, groups=1, expand=False)
+
+ self.scratch.refinenet1 = make_fusion_block(feature_dim, use_bn, output_width_ratio)
+ self.scratch.refinenet2 = make_fusion_block(feature_dim, use_bn, output_width_ratio)
+ self.scratch.refinenet3 = make_fusion_block(feature_dim, use_bn, output_width_ratio)
+ self.scratch.refinenet4 = make_fusion_block(feature_dim, use_bn, output_width_ratio)
+
+ if self.head_type == 'regression':
+ # The "DPTDepthModel" head
+ self.head = nn.Sequential(
+ nn.Conv2d(feature_dim, feature_dim // 2, kernel_size=3, stride=1, padding=1),
+ Interpolate(scale_factor=2, mode="bilinear", align_corners=True),
+ nn.Conv2d(feature_dim // 2, last_dim, kernel_size=3, stride=1, padding=1),
+ nn.ReLU(True),
+ nn.Conv2d(last_dim, self.num_channels, kernel_size=1, stride=1, padding=0)
+ )
+ elif self.head_type == 'semseg':
+ # The "DPTSegmentationModel" head
+ self.head = nn.Sequential(
+ nn.Conv2d(feature_dim, feature_dim, kernel_size=3, padding=1, bias=False),
+ nn.BatchNorm2d(feature_dim) if use_bn else nn.Identity(),
+ nn.ReLU(True),
+ nn.Dropout(0.1, False),
+ nn.Conv2d(feature_dim, self.num_channels, kernel_size=1),
+ Interpolate(scale_factor=2, mode="bilinear", align_corners=True),
+ )
+ else:
+ raise ValueError('DPT head_type must be "regression" or "semseg".')
+
+ if self.dim_tokens_enc is not None:
+ self.init(dim_tokens_enc=dim_tokens_enc)
+
+ def init(self, dim_tokens_enc=768):
+ """
+ Initialize parts of decoder that are dependent on dimension of encoder tokens.
+ Should be called when setting up MultiMAE.
+
+ :param dim_tokens_enc: Dimension of tokens coming from encoder
+ """
+ #print(dim_tokens_enc)
+
+ # Set up activation postprocessing layers
+ if isinstance(dim_tokens_enc, int):
+ dim_tokens_enc = 4 * [dim_tokens_enc]
+
+ self.dim_tokens_enc = [dt * len(self.main_tasks) for dt in dim_tokens_enc]
+
+ self.act_1_postprocess = nn.Sequential(
+ nn.Conv2d(
+ in_channels=self.dim_tokens_enc[0],
+ out_channels=self.layer_dims[0],
+ kernel_size=1, stride=1, padding=0,
+ ),
+ nn.ConvTranspose2d(
+ in_channels=self.layer_dims[0],
+ out_channels=self.layer_dims[0],
+ kernel_size=4, stride=4, padding=0,
+ bias=True, dilation=1, groups=1,
+ )
+ )
+
+ self.act_2_postprocess = nn.Sequential(
+ nn.Conv2d(
+ in_channels=self.dim_tokens_enc[1],
+ out_channels=self.layer_dims[1],
+ kernel_size=1, stride=1, padding=0,
+ ),
+ nn.ConvTranspose2d(
+ in_channels=self.layer_dims[1],
+ out_channels=self.layer_dims[1],
+ kernel_size=2, stride=2, padding=0,
+ bias=True, dilation=1, groups=1,
+ )
+ )
+
+ self.act_3_postprocess = nn.Sequential(
+ nn.Conv2d(
+ in_channels=self.dim_tokens_enc[2],
+ out_channels=self.layer_dims[2],
+ kernel_size=1, stride=1, padding=0,
+ )
+ )
+
+ self.act_4_postprocess = nn.Sequential(
+ nn.Conv2d(
+ in_channels=self.dim_tokens_enc[3],
+ out_channels=self.layer_dims[3],
+ kernel_size=1, stride=1, padding=0,
+ ),
+ nn.Conv2d(
+ in_channels=self.layer_dims[3],
+ out_channels=self.layer_dims[3],
+ kernel_size=3, stride=2, padding=1,
+ )
+ )
+
+ self.act_postprocess = nn.ModuleList([
+ self.act_1_postprocess,
+ self.act_2_postprocess,
+ self.act_3_postprocess,
+ self.act_4_postprocess
+ ])
+
+ def adapt_tokens(self, encoder_tokens):
+ # Adapt tokens
+ x = []
+ x.append(encoder_tokens[:, :])
+ x = torch.cat(x, dim=-1)
+ return x
+
+ def forward(self, encoder_tokens: List[torch.Tensor], image_size):
+ #input_info: Dict):
+ assert self.dim_tokens_enc is not None, 'Need to call init(dim_tokens_enc) function first'
+ H, W = image_size
+
+ # Number of patches in height and width
+ N_H = H // (self.stride_level * self.P_H)
+ N_W = W // (self.stride_level * self.P_W)
+
+ # Hook decoder onto 4 layers from specified ViT layers
+ layers = [encoder_tokens[hook] for hook in self.hooks]
+
+ # Extract only task-relevant tokens and ignore global tokens.
+ layers = [self.adapt_tokens(l) for l in layers]
+
+ # Reshape tokens to spatial representation
+ layers = [rearrange(l, 'b (nh nw) c -> b c nh nw', nh=N_H, nw=N_W) for l in layers]
+
+ layers = [self.act_postprocess[idx](l) for idx, l in enumerate(layers)]
+ # Project layers to chosen feature dim
+ layers = [self.scratch.layer_rn[idx](l) for idx, l in enumerate(layers)]
+
+ # Fuse layers using refinement stages
+ path_4 = self.scratch.refinenet4(layers[3])
+ path_3 = self.scratch.refinenet3(path_4, layers[2])
+ path_2 = self.scratch.refinenet2(path_3, layers[1])
+ path_1 = self.scratch.refinenet1(path_2, layers[0])
+
+ # Output head
+ out = self.head(path_1)
+
+ return out
diff --git a/dynamic_predictor/croco/models/head_downstream.py b/dynamic_predictor/croco/models/head_downstream.py
new file mode 100644
index 0000000000000000000000000000000000000000..bd40c91ba244d6c3522c6efd4ed4d724b7bdc650
--- /dev/null
+++ b/dynamic_predictor/croco/models/head_downstream.py
@@ -0,0 +1,58 @@
+# Copyright (C) 2022-present Naver Corporation. All rights reserved.
+# Licensed under CC BY-NC-SA 4.0 (non-commercial use only).
+
+# --------------------------------------------------------
+# Heads for downstream tasks
+# --------------------------------------------------------
+
+"""
+A head is a module where the __init__ defines only the head hyperparameters.
+A method setup(croconet) takes a CroCoNet and set all layers according to the head and croconet attributes.
+The forward takes the features as well as a dictionary img_info containing the keys 'width' and 'height'
+"""
+
+import torch
+import torch.nn as nn
+from .dpt_block import DPTOutputAdapter
+
+
+class PixelwiseTaskWithDPT(nn.Module):
+ """ DPT module for CroCo.
+ by default, hooks_idx will be equal to:
+ * for encoder-only: 4 equally spread layers
+ * for encoder+decoder: last encoder + 3 equally spread layers of the decoder
+ """
+
+ def __init__(self, *, hooks_idx=None, layer_dims=[96,192,384,768],
+ output_width_ratio=1, num_channels=1, postprocess=None, **kwargs):
+ super(PixelwiseTaskWithDPT, self).__init__()
+ self.return_all_blocks = True # backbone needs to return all layers
+ self.postprocess = postprocess
+ self.output_width_ratio = output_width_ratio
+ self.num_channels = num_channels
+ self.hooks_idx = hooks_idx
+ self.layer_dims = layer_dims
+
+ def setup(self, croconet):
+ dpt_args = {'output_width_ratio': self.output_width_ratio, 'num_channels': self.num_channels}
+ if self.hooks_idx is None:
+ if hasattr(croconet, 'dec_blocks'): # encoder + decoder
+ step = {8: 3, 12: 4, 24: 8}[croconet.dec_depth]
+ hooks_idx = [croconet.dec_depth+croconet.enc_depth-1-i*step for i in range(3,-1,-1)]
+ else: # encoder only
+ step = croconet.enc_depth//4
+ hooks_idx = [croconet.enc_depth-1-i*step for i in range(3,-1,-1)]
+ self.hooks_idx = hooks_idx
+ print(f' PixelwiseTaskWithDPT: automatically setting hook_idxs={self.hooks_idx}')
+ dpt_args['hooks'] = self.hooks_idx
+ dpt_args['layer_dims'] = self.layer_dims
+ self.dpt = DPTOutputAdapter(**dpt_args)
+ dim_tokens = [croconet.enc_embed_dim if hook0:
+ pos_embed = np.concatenate([np.zeros([n_cls_token, embed_dim]), pos_embed], axis=0)
+ return pos_embed
+
+
+def get_2d_sincos_pos_embed_from_grid(embed_dim, grid):
+ assert embed_dim % 2 == 0
+
+ # use half of dimensions to encode grid_h
+ emb_h = get_1d_sincos_pos_embed_from_grid(embed_dim // 2, grid[0]) # (H*W, D/2)
+ emb_w = get_1d_sincos_pos_embed_from_grid(embed_dim // 2, grid[1]) # (H*W, D/2)
+
+ emb = np.concatenate([emb_h, emb_w], axis=1) # (H*W, D)
+ return emb
+
+
+def get_1d_sincos_pos_embed_from_grid(embed_dim, pos):
+ """
+ embed_dim: output dimension for each position
+ pos: a list of positions to be encoded: size (M,)
+ out: (M, D)
+ """
+ assert embed_dim % 2 == 0
+ omega = np.arange(embed_dim // 2, dtype=float)
+ omega /= embed_dim / 2.
+ omega = 1. / 10000**omega # (D/2,)
+
+ pos = pos.reshape(-1) # (M,)
+ out = np.einsum('m,d->md', pos, omega) # (M, D/2), outer product
+
+ emb_sin = np.sin(out) # (M, D/2)
+ emb_cos = np.cos(out) # (M, D/2)
+
+ emb = np.concatenate([emb_sin, emb_cos], axis=1) # (M, D)
+ return emb
+
+
+# --------------------------------------------------------
+# Interpolate position embeddings for high-resolution
+# References:
+# MAE: https://github.com/facebookresearch/mae/blob/main/util/pos_embed.py
+# DeiT: https://github.com/facebookresearch/deit
+# --------------------------------------------------------
+def interpolate_pos_embed(model, checkpoint_model):
+ if 'pos_embed' in checkpoint_model:
+ pos_embed_checkpoint = checkpoint_model['pos_embed']
+ embedding_size = pos_embed_checkpoint.shape[-1]
+ num_patches = model.patch_embed.num_patches
+ num_extra_tokens = model.pos_embed.shape[-2] - num_patches
+ # height (== width) for the checkpoint position embedding
+ orig_size = int((pos_embed_checkpoint.shape[-2] - num_extra_tokens) ** 0.5)
+ # height (== width) for the new position embedding
+ new_size = int(num_patches ** 0.5)
+ # class_token and dist_token are kept unchanged
+ if orig_size != new_size:
+ print("Position interpolate from %dx%d to %dx%d" % (orig_size, orig_size, new_size, new_size))
+ extra_tokens = pos_embed_checkpoint[:, :num_extra_tokens]
+ # only the position tokens are interpolated
+ pos_tokens = pos_embed_checkpoint[:, num_extra_tokens:]
+ pos_tokens = pos_tokens.reshape(-1, orig_size, orig_size, embedding_size).permute(0, 3, 1, 2)
+ pos_tokens = torch.nn.functional.interpolate(
+ pos_tokens, size=(new_size, new_size), mode='bicubic', align_corners=False)
+ pos_tokens = pos_tokens.permute(0, 2, 3, 1).flatten(1, 2)
+ new_pos_embed = torch.cat((extra_tokens, pos_tokens), dim=1)
+ checkpoint_model['pos_embed'] = new_pos_embed
+
+
+#----------------------------------------------------------
+# RoPE2D: RoPE implementation in 2D
+#----------------------------------------------------------
+
+try:
+ from models.curope import cuRoPE2D
+ RoPE2D = cuRoPE2D
+except ImportError:
+ print('Warning, cannot find cuda-compiled version of RoPE2D, using a slow pytorch version instead')
+
+ class RoPE2D(torch.nn.Module):
+
+ def __init__(self, freq=100.0, F0=1.0):
+ super().__init__()
+ self.base = freq
+ self.F0 = F0
+ self.cache = {}
+
+ def get_cos_sin(self, D, seq_len, device, dtype):
+ if (D,seq_len,device,dtype) not in self.cache:
+ inv_freq = 1.0 / (self.base ** (torch.arange(0, D, 2).float().to(device) / D))
+ t = torch.arange(seq_len, device=device, dtype=inv_freq.dtype)
+ freqs = torch.einsum("i,j->ij", t, inv_freq).to(dtype)
+ freqs = torch.cat((freqs, freqs), dim=-1)
+ cos = freqs.cos() # (Seq, Dim)
+ sin = freqs.sin()
+ self.cache[D,seq_len,device,dtype] = (cos,sin)
+ return self.cache[D,seq_len,device,dtype]
+
+ @staticmethod
+ def rotate_half(x):
+ x1, x2 = x[..., : x.shape[-1] // 2], x[..., x.shape[-1] // 2 :]
+ return torch.cat((-x2, x1), dim=-1)
+
+ def apply_rope1d(self, tokens, pos1d, cos, sin):
+ assert pos1d.ndim==2
+ cos = torch.nn.functional.embedding(pos1d, cos)[:, None, :, :]
+ sin = torch.nn.functional.embedding(pos1d, sin)[:, None, :, :]
+ return (tokens * cos) + (self.rotate_half(tokens) * sin)
+
+ def forward(self, tokens, positions):
+ """
+ input:
+ * tokens: batch_size x nheads x ntokens x dim
+ * positions: batch_size x ntokens x 2 (y and x position of each token)
+ output:
+ * tokens after appplying RoPE2D (batch_size x nheads x ntokens x dim)
+ """
+ assert tokens.size(3)%2==0, "number of dimensions should be a multiple of two"
+ D = tokens.size(3) // 2
+ assert positions.ndim==3 and positions.shape[-1] == 2 # Batch, Seq, 2
+ cos, sin = self.get_cos_sin(D, int(positions.max())+1, tokens.device, tokens.dtype)
+ # split features into two along the feature dimension, and apply rope1d on each half
+ y, x = tokens.chunk(2, dim=-1)
+ y = self.apply_rope1d(y, positions[:,:,0], cos, sin)
+ x = self.apply_rope1d(x, positions[:,:,1], cos, sin)
+ tokens = torch.cat((y, x), dim=-1)
+ return tokens
\ No newline at end of file
diff --git a/dynamic_predictor/croco/pretrain.py b/dynamic_predictor/croco/pretrain.py
new file mode 100644
index 0000000000000000000000000000000000000000..2c45e488015ef5380c71d0381ff453fdb860759e
--- /dev/null
+++ b/dynamic_predictor/croco/pretrain.py
@@ -0,0 +1,254 @@
+# Copyright (C) 2022-present Naver Corporation. All rights reserved.
+# Licensed under CC BY-NC-SA 4.0 (non-commercial use only).
+#
+# --------------------------------------------------------
+# Pre-training CroCo
+# --------------------------------------------------------
+# References:
+# MAE: https://github.com/facebookresearch/mae
+# DeiT: https://github.com/facebookresearch/deit
+# BEiT: https://github.com/microsoft/unilm/tree/master/beit
+# --------------------------------------------------------
+import argparse
+import datetime
+import json
+import numpy as np
+import os
+import sys
+import time
+import math
+from pathlib import Path
+from typing import Iterable
+
+import torch
+import torch.distributed as dist
+import torch.backends.cudnn as cudnn
+from torch.utils.tensorboard import SummaryWriter
+import torchvision.transforms as transforms
+import torchvision.datasets as datasets
+
+import utils.misc as misc
+from utils.misc import NativeScalerWithGradNormCount as NativeScaler
+from models.croco import CroCoNet
+from models.criterion import MaskedMSE
+from datasets.pairs_dataset import PairsDataset
+
+
+def get_args_parser():
+ parser = argparse.ArgumentParser('CroCo pre-training', add_help=False)
+ # model and criterion
+ parser.add_argument('--model', default='CroCoNet()', type=str, help="string containing the model to build")
+ parser.add_argument('--norm_pix_loss', default=1, choices=[0,1], help="apply per-patch mean/std normalization before applying the loss")
+ # dataset
+ parser.add_argument('--dataset', default='habitat_release', type=str, help="training set")
+ parser.add_argument('--transforms', default='crop224+acolor', type=str, help="transforms to apply") # in the paper, we also use some homography and rotation, but find later that they were not useful or even harmful
+ # training
+ parser.add_argument('--seed', default=0, type=int, help="Random seed")
+ parser.add_argument('--batch_size', default=64, type=int, help="Batch size per GPU (effective batch size is batch_size * accum_iter * # gpus")
+ parser.add_argument('--epochs', default=800, type=int, help="Maximum number of epochs for the scheduler")
+ parser.add_argument('--max_epoch', default=400, type=int, help="Stop training at this epoch")
+ parser.add_argument('--accum_iter', default=1, type=int, help="Accumulate gradient iterations (for increasing the effective batch size under memory constraints)")
+ parser.add_argument('--weight_decay', type=float, default=0.05, help="weight decay (default: 0.05)")
+ parser.add_argument('--lr', type=float, default=None, metavar='LR', help='learning rate (absolute lr)')
+ parser.add_argument('--blr', type=float, default=1.5e-4, metavar='LR', help='base learning rate: absolute_lr = base_lr * total_batch_size / 256')
+ parser.add_argument('--min_lr', type=float, default=0., metavar='LR', help='lower lr bound for cyclic schedulers that hit 0')
+ parser.add_argument('--warmup_epochs', type=int, default=40, metavar='N', help='epochs to warmup LR')
+ parser.add_argument('--amp', type=int, default=1, choices=[0,1], help="Use Automatic Mixed Precision for pretraining")
+ # others
+ parser.add_argument('--num_workers', default=8, type=int)
+ parser.add_argument('--world_size', default=1, type=int, help='number of distributed processes')
+ parser.add_argument('--local_rank', default=-1, type=int)
+ parser.add_argument('--dist_url', default='env://', help='url used to set up distributed training')
+ parser.add_argument('--save_freq', default=1, type=int, help='frequence (number of epochs) to save checkpoint in checkpoint-last.pth')
+ parser.add_argument('--keep_freq', default=20, type=int, help='frequence (number of epochs) to save checkpoint in checkpoint-%d.pth')
+ parser.add_argument('--print_freq', default=20, type=int, help='frequence (number of iterations) to print infos while training')
+ # paths
+ parser.add_argument('--output_dir', default='./output/', type=str, help="path where to save the output")
+ parser.add_argument('--data_dir', default='./data/', type=str, help="path where data are stored")
+ return parser
+
+
+
+
+def main(args):
+ misc.init_distributed_mode(args)
+ global_rank = misc.get_rank()
+ world_size = misc.get_world_size()
+
+ print("output_dir: "+args.output_dir)
+ if args.output_dir:
+ Path(args.output_dir).mkdir(parents=True, exist_ok=True)
+
+ # auto resume
+ last_ckpt_fname = os.path.join(args.output_dir, f'checkpoint-last.pth')
+ args.resume = last_ckpt_fname if os.path.isfile(last_ckpt_fname) else None
+
+ print('job dir: {}'.format(os.path.dirname(os.path.realpath(__file__))))
+ print("{}".format(args).replace(', ', ',\n'))
+
+ device = "cuda" if torch.cuda.is_available() else "cpu"
+ device = torch.device(device)
+
+ # fix the seed
+ seed = args.seed + misc.get_rank()
+ torch.manual_seed(seed)
+ np.random.seed(seed)
+
+ cudnn.benchmark = True
+
+ ## training dataset and loader
+ print('Building dataset for {:s} with transforms {:s}'.format(args.dataset, args.transforms))
+ dataset = PairsDataset(args.dataset, trfs=args.transforms, data_dir=args.data_dir)
+ if world_size>1:
+ sampler_train = torch.utils.data.DistributedSampler(
+ dataset, num_replicas=world_size, rank=global_rank, shuffle=True
+ )
+ print("Sampler_train = %s" % str(sampler_train))
+ else:
+ sampler_train = torch.utils.data.RandomSampler(dataset)
+ data_loader_train = torch.utils.data.DataLoader(
+ dataset, sampler=sampler_train,
+ batch_size=args.batch_size,
+ num_workers=args.num_workers,
+ pin_memory=True,
+ drop_last=True,
+ )
+
+ ## model
+ print('Loading model: {:s}'.format(args.model))
+ model = eval(args.model)
+ print('Loading criterion: MaskedMSE(norm_pix_loss={:s})'.format(str(bool(args.norm_pix_loss))))
+ criterion = MaskedMSE(norm_pix_loss=bool(args.norm_pix_loss))
+
+ model.to(device)
+ model_without_ddp = model
+ print("Model = %s" % str(model_without_ddp))
+
+ eff_batch_size = args.batch_size * args.accum_iter * misc.get_world_size()
+ if args.lr is None: # only base_lr is specified
+ args.lr = args.blr * eff_batch_size / 256
+ print("base lr: %.2e" % (args.lr * 256 / eff_batch_size))
+ print("actual lr: %.2e" % args.lr)
+ print("accumulate grad iterations: %d" % args.accum_iter)
+ print("effective batch size: %d" % eff_batch_size)
+
+ if args.distributed:
+ model = torch.nn.parallel.DistributedDataParallel(model, device_ids=[args.gpu], find_unused_parameters=True, static_graph=True)
+ model_without_ddp = model.module
+
+ param_groups = misc.get_parameter_groups(model_without_ddp, args.weight_decay) # following timm: set wd as 0 for bias and norm layers
+ optimizer = torch.optim.AdamW(param_groups, lr=args.lr, betas=(0.9, 0.95))
+ print(optimizer)
+ loss_scaler = NativeScaler()
+
+ misc.load_model(args=args, model_without_ddp=model_without_ddp, optimizer=optimizer, loss_scaler=loss_scaler)
+
+ if global_rank == 0 and args.output_dir is not None:
+ log_writer = SummaryWriter(log_dir=args.output_dir)
+ else:
+ log_writer = None
+
+ print(f"Start training until {args.max_epoch} epochs")
+ start_time = time.time()
+ for epoch in range(args.start_epoch, args.max_epoch):
+ if world_size>1:
+ data_loader_train.sampler.set_epoch(epoch)
+
+ train_stats = train_one_epoch(
+ model, criterion, data_loader_train,
+ optimizer, device, epoch, loss_scaler,
+ log_writer=log_writer,
+ args=args
+ )
+
+ if args.output_dir and epoch % args.save_freq == 0 :
+ misc.save_model(
+ args=args, model_without_ddp=model_without_ddp, optimizer=optimizer,
+ loss_scaler=loss_scaler, epoch=epoch, fname='last')
+
+ if args.output_dir and (epoch % args.keep_freq == 0 or epoch + 1 == args.max_epoch) and (epoch>0 or args.max_epoch==1):
+ misc.save_model(
+ args=args, model_without_ddp=model_without_ddp, optimizer=optimizer,
+ loss_scaler=loss_scaler, epoch=epoch)
+
+ log_stats = {**{f'train_{k}': v for k, v in train_stats.items()},
+ 'epoch': epoch,}
+
+ if args.output_dir and misc.is_main_process():
+ if log_writer is not None:
+ log_writer.flush()
+ with open(os.path.join(args.output_dir, "log.txt"), mode="a", encoding="utf-8") as f:
+ f.write(json.dumps(log_stats) + "\n")
+
+ total_time = time.time() - start_time
+ total_time_str = str(datetime.timedelta(seconds=int(total_time)))
+ print('Training time {}'.format(total_time_str))
+
+
+
+
+def train_one_epoch(model: torch.nn.Module, criterion: torch.nn.Module,
+ data_loader: Iterable, optimizer: torch.optim.Optimizer,
+ device: torch.device, epoch: int, loss_scaler,
+ log_writer=None,
+ args=None):
+ model.train(True)
+ metric_logger = misc.MetricLogger(delimiter=" ")
+ metric_logger.add_meter('lr', misc.SmoothedValue(window_size=1, fmt='{value:.6f}'))
+ header = 'Epoch: [{}]'.format(epoch)
+ accum_iter = args.accum_iter
+
+ optimizer.zero_grad()
+
+ if log_writer is not None:
+ print('log_dir: {}'.format(log_writer.log_dir))
+
+ for data_iter_step, (image1, image2) in enumerate(metric_logger.log_every(data_loader, args.print_freq, header)):
+
+ # we use a per iteration lr scheduler
+ if data_iter_step % accum_iter == 0:
+ misc.adjust_learning_rate(optimizer, data_iter_step / len(data_loader) + epoch, args)
+
+ image1 = image1.to(device, non_blocking=True)
+ image2 = image2.to(device, non_blocking=True)
+ with torch.cuda.amp.autocast(enabled=bool(args.amp)):
+ out, mask, target = model(image1, image2)
+ loss = criterion(out, mask, target)
+
+ loss_value = loss.item()
+
+ if not math.isfinite(loss_value):
+ print("Loss is {}, stopping training".format(loss_value))
+ sys.exit(1)
+
+ loss /= accum_iter
+ loss_scaler(loss, optimizer, parameters=model.parameters(),
+ update_grad=(data_iter_step + 1) % accum_iter == 0)
+ if (data_iter_step + 1) % accum_iter == 0:
+ optimizer.zero_grad()
+
+ torch.cuda.synchronize()
+
+ metric_logger.update(loss=loss_value)
+
+ lr = optimizer.param_groups[0]["lr"]
+ metric_logger.update(lr=lr)
+
+ loss_value_reduce = misc.all_reduce_mean(loss_value)
+ if log_writer is not None and ((data_iter_step + 1) % (accum_iter*args.print_freq)) == 0:
+ # x-axis is based on epoch_1000x in the tensorboard, calibrating differences curves when batch size changes
+ epoch_1000x = int((data_iter_step / len(data_loader) + epoch) * 1000)
+ log_writer.add_scalar('train_loss', loss_value_reduce, epoch_1000x)
+ log_writer.add_scalar('lr', lr, epoch_1000x)
+
+ # gather the stats from all processes
+ metric_logger.synchronize_between_processes()
+ print("Averaged stats:", metric_logger)
+ return {k: meter.global_avg for k, meter in metric_logger.meters.items()}
+
+
+
+if __name__ == '__main__':
+ args = get_args_parser()
+ args = args.parse_args()
+ main(args)
diff --git a/dynamic_predictor/croco/stereoflow/README.MD b/dynamic_predictor/croco/stereoflow/README.MD
new file mode 100644
index 0000000000000000000000000000000000000000..81595380fadd274b523e0cf77921b1b65cbedb34
--- /dev/null
+++ b/dynamic_predictor/croco/stereoflow/README.MD
@@ -0,0 +1,318 @@
+## CroCo-Stereo and CroCo-Flow
+
+This README explains how to use CroCo-Stereo and CroCo-Flow as well as how they were trained.
+All commands should be launched from the root directory.
+
+### Simple inference example
+
+We provide a simple inference exemple for CroCo-Stereo and CroCo-Flow in the Totebook `croco-stereo-flow-demo.ipynb`.
+Before running it, please download the trained models with:
+```
+bash stereoflow/download_model.sh crocostereo.pth
+bash stereoflow/download_model.sh crocoflow.pth
+```
+
+### Prepare data for training or evaluation
+
+Put the datasets used for training/evaluation in `./data/stereoflow` (or update the paths at the top of `stereoflow/datasets_stereo.py` and `stereoflow/datasets_flow.py`).
+Please find below on the file structure should look for each dataset:
+
+FlyingChairs
+
+```
+./data/stereoflow/FlyingChairs/
+└───chairs_split.txt
+└───data/
+ └─── ...
+```
+
+
+
+MPI-Sintel
+
+```
+./data/stereoflow/MPI-Sintel/
+└───training/
+│ └───clean/
+│ └───final/
+│ └───flow/
+└───test/
+ └───clean/
+ └───final/
+```
+
+
+
+SceneFlow (including FlyingThings)
+
+```
+./data/stereoflow/SceneFlow/
+└───Driving/
+│ └───disparity/
+│ └───frames_cleanpass/
+│ └───frames_finalpass/
+└───FlyingThings/
+│ └───disparity/
+│ └───frames_cleanpass/
+│ └───frames_finalpass/
+│ └───optical_flow/
+└───Monkaa/
+ └───disparity/
+ └───frames_cleanpass/
+ └───frames_finalpass/
+```
+
+
+
+TartanAir
+
+```
+./data/stereoflow/TartanAir/
+└───abandonedfactory/
+│ └───.../
+└───abandonedfactory_night/
+│ └───.../
+└───.../
+```
+
+
+
+Booster
+
+```
+./data/stereoflow/booster_gt/
+└───train/
+ └───balanced/
+ └───Bathroom/
+ └───Bedroom/
+ └───...
+```
+
+
+
+CREStereo
+
+```
+./data/stereoflow/crenet_stereo_trainset/
+└───stereo_trainset/
+ └───crestereo/
+ └───hole/
+ └───reflective/
+ └───shapenet/
+ └───tree/
+```
+
+
+
+ETH3D Two-view Low-res
+
+```
+./data/stereoflow/eth3d_lowres/
+└───test/
+│ └───lakeside_1l/
+│ └───...
+└───train/
+│ └───delivery_area_1l/
+│ └───...
+└───train_gt/
+ └───delivery_area_1l/
+ └───...
+```
+
+
+
+KITTI 2012
+
+```
+./data/stereoflow/kitti-stereo-2012/
+└───testing/
+│ └───colored_0/
+│ └───colored_1/
+└───training/
+ └───colored_0/
+ └───colored_1/
+ └───disp_occ/
+ └───flow_occ/
+```
+
+
+
+KITTI 2015
+
+```
+./data/stereoflow/kitti-stereo-2015/
+└───testing/
+│ └───image_2/
+│ └───image_3/
+└───training/
+ └───image_2/
+ └───image_3/
+ └───disp_occ_0/
+ └───flow_occ/
+```
+
+
+
+Middlebury
+
+```
+./data/stereoflow/middlebury
+└───2005/
+│ └───train/
+│ └───Art/
+│ └───...
+└───2006/
+│ └───Aloe/
+│ └───Baby1/
+│ └───...
+└───2014/
+│ └───Adirondack-imperfect/
+│ └───Adirondack-perfect/
+│ └───...
+└───2021/
+│ └───data/
+│ └───artroom1/
+│ └───artroom2/
+│ └───...
+└───MiddEval3_F/
+ └───test/
+ │ └───Australia/
+ │ └───...
+ └───train/
+ └───Adirondack/
+ └───...
+```
+
+
+
+Spring
+
+```
+./data/stereoflow/spring/
+└───test/
+│ └───0003/
+│ └───...
+└───train/
+ └───0001/
+ └───...
+```
+
+
+
+### CroCo-Stereo
+
+##### Main model
+
+The main training of CroCo-Stereo was performed on a series of datasets, and it was used as it for Middlebury v3 benchmark.
+
+```
+# Download the model
+bash stereoflow/download_model.sh crocostereo.pth
+# Middlebury v3 submission
+python stereoflow/test.py --model stereoflow_models/crocostereo.pth --dataset "MdEval3('all_full')" --save submission --tile_overlap 0.9
+# Training command that was used, using checkpoint-last.pth
+python -u stereoflow/train.py stereo --criterion "LaplacianLossBounded2()" --dataset "CREStereo('train')+SceneFlow('train_allpass')+30*ETH3DLowRes('train')+50*Md05('train')+50*Md06('train')+50*Md14('train')+50*Md21('train')+50*MdEval3('train_full')+Booster('train_balanced')" --val_dataset "SceneFlow('test1of100_finalpass')+SceneFlow('test1of100_cleanpass')+ETH3DLowRes('subval')+Md05('subval')+Md06('subval')+Md14('subval')+Md21('subval')+MdEval3('subval_full')+Booster('subval_balanced')" --lr 3e-5 --batch_size 6 --epochs 32 --pretrained pretrained_models/CroCo_V2_ViTLarge_BaseDecoder.pth --output_dir xps/crocostereo/main/
+# or it can be launched on multiple gpus (while maintaining the effective batch size), e.g. on 3 gpus:
+torchrun --nproc_per_node 3 stereoflow/train.py stereo --criterion "LaplacianLossBounded2()" --dataset "CREStereo('train')+SceneFlow('train_allpass')+30*ETH3DLowRes('train')+50*Md05('train')+50*Md06('train')+50*Md14('train')+50*Md21('train')+50*MdEval3('train_full')+Booster('train_balanced')" --val_dataset "SceneFlow('test1of100_finalpass')+SceneFlow('test1of100_cleanpass')+ETH3DLowRes('subval')+Md05('subval')+Md06('subval')+Md14('subval')+Md21('subval')+MdEval3('subval_full')+Booster('subval_balanced')" --lr 3e-5 --batch_size 2 --epochs 32 --pretrained pretrained_models/CroCo_V2_ViTLarge_BaseDecoder.pth --output_dir xps/crocostereo/main/
+```
+
+For evaluation of validation set, we also provide the model trained on the `subtrain` subset of the training sets.
+
+```
+# Download the model
+bash stereoflow/download_model.sh crocostereo_subtrain.pth
+# Evaluation on validation sets
+python stereoflow/test.py --model stereoflow_models/crocostereo_subtrain.pth --dataset "MdEval3('subval_full')+ETH3DLowRes('subval')+SceneFlow('test_finalpass')+SceneFlow('test_cleanpass')" --save metrics --tile_overlap 0.9
+# Training command that was used (same as above but on subtrain, using checkpoint-best.pth), can also be launched on multiple gpus
+python -u stereoflow/train.py stereo --criterion "LaplacianLossBounded2()" --dataset "CREStereo('train')+SceneFlow('train_allpass')+30*ETH3DLowRes('subtrain')+50*Md05('subtrain')+50*Md06('subtrain')+50*Md14('subtrain')+50*Md21('subtrain')+50*MdEval3('subtrain_full')+Booster('subtrain_balanced')" --val_dataset "SceneFlow('test1of100_finalpass')+SceneFlow('test1of100_cleanpass')+ETH3DLowRes('subval')+Md05('subval')+Md06('subval')+Md14('subval')+Md21('subval')+MdEval3('subval_full')+Booster('subval_balanced')" --lr 3e-5 --batch_size 6 --epochs 32 --pretrained pretrained_models/CroCo_V2_ViTLarge_BaseDecoder.pth --output_dir xps/crocostereo/main_subtrain/
+```
+
+##### Other models
+
+
+ Model for ETH3D
+ The model used for the submission on ETH3D is trained with the same command but using an unbounded Laplacian loss.
+
+ # Download the model
+ bash stereoflow/download_model.sh crocostereo_eth3d.pth
+ # ETH3D submission
+ python stereoflow/test.py --model stereoflow_models/crocostereo_eth3d.pth --dataset "ETH3DLowRes('all')" --save submission --tile_overlap 0.9
+ # Training command that was used
+ python -u stereoflow/train.py stereo --criterion "LaplacianLoss()" --tile_conf_mode conf_expbeta3 --dataset "CREStereo('train')+SceneFlow('train_allpass')+30*ETH3DLowRes('train')+50*Md05('train')+50*Md06('train')+50*Md14('train')+50*Md21('train')+50*MdEval3('train_full')+Booster('train_balanced')" --val_dataset "SceneFlow('test1of100_finalpass')+SceneFlow('test1of100_cleanpass')+ETH3DLowRes('subval')+Md05('subval')+Md06('subval')+Md14('subval')+Md21('subval')+MdEval3('subval_full')+Booster('subval_balanced')" --lr 3e-5 --batch_size 6 --epochs 32 --pretrained pretrained_models/CroCo_V2_ViTLarge_BaseDecoder.pth --output_dir xps/crocostereo/main_eth3d/
+
+
+
+
+ Main model finetuned on Kitti
+
+ # Download the model
+ bash stereoflow/download_model.sh crocostereo_finetune_kitti.pth
+ # Kitti submission
+ python stereoflow/test.py --model stereoflow_models/crocostereo_finetune_kitti.pth --dataset "Kitti15('test')" --save submission --tile_overlap 0.9
+ # Training that was used
+ python -u stereoflow/train.py stereo --crop 352 1216 --criterion "LaplacianLossBounded2()" --dataset "Kitti12('train')+Kitti15('train')" --lr 3e-5 --batch_size 1 --accum_iter 6 --epochs 20 --pretrained pretrained_models/CroCo_V2_ViTLarge_BaseDecoder.pth --start_from stereoflow_models/crocostereo.pth --output_dir xps/crocostereo/finetune_kitti/ --save_every 5
+
+
+
+ Main model finetuned on Spring
+
+ # Download the model
+ bash stereoflow/download_model.sh crocostereo_finetune_spring.pth
+ # Spring submission
+ python stereoflow/test.py --model stereoflow_models/crocostereo_finetune_spring.pth --dataset "Spring('test')" --save submission --tile_overlap 0.9
+ # Training command that was used
+ python -u stereoflow/train.py stereo --criterion "LaplacianLossBounded2()" --dataset "Spring('train')" --lr 3e-5 --batch_size 6 --epochs 8 --pretrained pretrained_models/CroCo_V2_ViTLarge_BaseDecoder.pth --start_from stereoflow_models/crocostereo.pth --output_dir xps/crocostereo/finetune_spring/
+
+
+
+ Smaller models
+ To train CroCo-Stereo with smaller CroCo pretrained models, simply replace the --pretrained argument. To download the smaller CroCo-Stereo models based on CroCo v2 pretraining with ViT-Base encoder and Small encoder, use bash stereoflow/download_model.sh crocostereo_subtrain_vitb_smalldecoder.pth, and for the model with a ViT-Base encoder and a Base decoder, use bash stereoflow/download_model.sh crocostereo_subtrain_vitb_basedecoder.pth.
+
+
+
+### CroCo-Flow
+
+##### Main model
+
+The main training of CroCo-Flow was performed on the FlyingThings, FlyingChairs, MPI-Sintel and TartanAir datasets.
+It was used for our submission to the MPI-Sintel benchmark.
+
+```
+# Download the model
+bash stereoflow/download_model.sh crocoflow.pth
+# Evaluation
+python stereoflow/test.py --model stereoflow_models/crocoflow.pth --dataset "MPISintel('subval_cleanpass')+MPISintel('subval_finalpass')" --save metrics --tile_overlap 0.9
+# Sintel submission
+python stereoflow/test.py --model stereoflow_models/crocoflow.pth --dataset "MPISintel('test_allpass')" --save submission --tile_overlap 0.9
+# Training command that was used, with checkpoint-best.pth
+python -u stereoflow/train.py flow --criterion "LaplacianLossBounded()" --dataset "40*MPISintel('subtrain_cleanpass')+40*MPISintel('subtrain_finalpass')+4*FlyingThings('train_allpass')+4*FlyingChairs('train')+TartanAir('train')" --val_dataset "MPISintel('subval_cleanpass')+MPISintel('subval_finalpass')" --lr 2e-5 --batch_size 8 --epochs 240 --img_per_epoch 30000 --pretrained pretrained_models/CroCo_V2_ViTLarge_BaseDecoder.pth --output_dir xps/crocoflow/main/
+```
+
+##### Other models
+
+
+ Main model finetuned on Kitti
+
+ # Download the model
+ bash stereoflow/download_model.sh crocoflow_finetune_kitti.pth
+ # Kitti submission
+ python stereoflow/test.py --model stereoflow_models/crocoflow_finetune_kitti.pth --dataset "Kitti15('test')" --save submission --tile_overlap 0.99
+ # Training that was used, with checkpoint-last.pth
+ python -u stereoflow/train.py flow --crop 352 1216 --criterion "LaplacianLossBounded()" --dataset "Kitti15('train')+Kitti12('train')" --lr 2e-5 --batch_size 1 --accum_iter 8 --epochs 150 --save_every 5 --pretrained pretrained_models/CroCo_V2_ViTLarge_BaseDecoder.pth --start_from stereoflow_models/crocoflow.pth --output_dir xps/crocoflow/finetune_kitti/
+
+
+
+ Main model finetuned on Spring
+
+ # Download the model
+ bash stereoflow/download_model.sh crocoflow_finetune_spring.pth
+ # Spring submission
+ python stereoflow/test.py --model stereoflow_models/crocoflow_finetune_spring.pth --dataset "Spring('test')" --save submission --tile_overlap 0.9
+ # Training command that was used, with checkpoint-last.pth
+ python -u stereoflow/train.py flow --criterion "LaplacianLossBounded()" --dataset "Spring('train')" --lr 2e-5 --batch_size 8 --epochs 12 --pretrained pretrained_models/CroCo_V2_ViTLarge_BaseDecoder.pth --start_from stereoflow_models/crocoflow.pth --output_dir xps/crocoflow/finetune_spring/
+
+
+
+ Smaller models
+ To train CroCo-Flow with smaller CroCo pretrained models, simply replace the --pretrained argument. To download the smaller CroCo-Flow models based on CroCo v2 pretraining with ViT-Base encoder and Small encoder, use bash stereoflow/download_model.sh crocoflow_vitb_smalldecoder.pth, and for the model with a ViT-Base encoder and a Base decoder, use bash stereoflow/download_model.sh crocoflow_vitb_basedecoder.pth.
+
diff --git a/dynamic_predictor/croco/stereoflow/augmentor.py b/dynamic_predictor/croco/stereoflow/augmentor.py
new file mode 100644
index 0000000000000000000000000000000000000000..69e6117151988d94cbc4b385e0d88e982133bf10
--- /dev/null
+++ b/dynamic_predictor/croco/stereoflow/augmentor.py
@@ -0,0 +1,290 @@
+# Copyright (C) 2022-present Naver Corporation. All rights reserved.
+# Licensed under CC BY-NC-SA 4.0 (non-commercial use only).
+
+# --------------------------------------------------------
+# Data augmentation for training stereo and flow
+# --------------------------------------------------------
+
+# References
+# https://github.com/autonomousvision/unimatch/blob/master/dataloader/stereo/transforms.py
+# https://github.com/autonomousvision/unimatch/blob/master/dataloader/flow/transforms.py
+
+
+import numpy as np
+import random
+from PIL import Image
+
+import cv2
+cv2.setNumThreads(0)
+cv2.ocl.setUseOpenCL(False)
+
+import torch
+from torchvision.transforms import ColorJitter
+import torchvision.transforms.functional as FF
+
+class StereoAugmentor(object):
+
+ def __init__(self, crop_size, scale_prob=0.5, scale_xonly=True, lhth=800., lminscale=0.0, lmaxscale=1.0, hminscale=-0.2, hmaxscale=0.4, scale_interp_nearest=True, rightjitterprob=0.5, v_flip_prob=0.5, color_aug_asym=True, color_choice_prob=0.5):
+ self.crop_size = crop_size
+ self.scale_prob = scale_prob
+ self.scale_xonly = scale_xonly
+ self.lhth = lhth
+ self.lminscale = lminscale
+ self.lmaxscale = lmaxscale
+ self.hminscale = hminscale
+ self.hmaxscale = hmaxscale
+ self.scale_interp_nearest = scale_interp_nearest
+ self.rightjitterprob = rightjitterprob
+ self.v_flip_prob = v_flip_prob
+ self.color_aug_asym = color_aug_asym
+ self.color_choice_prob = color_choice_prob
+
+ def _random_scale(self, img1, img2, disp):
+ ch,cw = self.crop_size
+ h,w = img1.shape[:2]
+ if self.scale_prob>0. and np.random.rand()1.:
+ scale_x = clip_scale
+ scale_y = scale_x if not self.scale_xonly else 1.0
+ img1 = cv2.resize(img1, None, fx=scale_x, fy=scale_y, interpolation=cv2.INTER_LINEAR)
+ img2 = cv2.resize(img2, None, fx=scale_x, fy=scale_y, interpolation=cv2.INTER_LINEAR)
+ disp = cv2.resize(disp, None, fx=scale_x, fy=scale_y, interpolation=cv2.INTER_LINEAR if not self.scale_interp_nearest else cv2.INTER_NEAREST) * scale_x
+ return img1, img2, disp
+
+ def _random_crop(self, img1, img2, disp):
+ h,w = img1.shape[:2]
+ ch,cw = self.crop_size
+ assert ch<=h and cw<=w, (img1.shape, h,w,ch,cw)
+ offset_x = np.random.randint(w - cw + 1)
+ offset_y = np.random.randint(h - ch + 1)
+ img1 = img1[offset_y:offset_y+ch,offset_x:offset_x+cw]
+ img2 = img2[offset_y:offset_y+ch,offset_x:offset_x+cw]
+ disp = disp[offset_y:offset_y+ch,offset_x:offset_x+cw]
+ return img1, img2, disp
+
+ def _random_vflip(self, img1, img2, disp):
+ # vertical flip
+ if self.v_flip_prob>0 and np.random.rand() < self.v_flip_prob:
+ img1 = np.copy(np.flipud(img1))
+ img2 = np.copy(np.flipud(img2))
+ disp = np.copy(np.flipud(disp))
+ return img1, img2, disp
+
+ def _random_rotate_shift_right(self, img2):
+ if self.rightjitterprob>0. and np.random.rand() 0) & (xx < wd1) & (yy > 0) & (yy < ht1)
+ xx = xx[v]
+ yy = yy[v]
+ flow1 = flow1[v]
+
+ flow = np.inf * np.ones([ht1, wd1, 2], dtype=np.float32) # invalid value every where, before we fill it with the correct ones
+ flow[yy, xx] = flow1
+ return flow
+
+ def spatial_transform(self, img1, img2, flow, dname):
+
+ if np.random.rand() < self.spatial_aug_prob:
+ # randomly sample scale
+ ht, wd = img1.shape[:2]
+ clip_min_scale = np.maximum(
+ (self.crop_size[0] + 8) / float(ht),
+ (self.crop_size[1] + 8) / float(wd))
+ min_scale, max_scale = self.min_scale, self.max_scale
+ scale = 2 ** np.random.uniform(self.min_scale, self.max_scale)
+ scale_x = scale
+ scale_y = scale
+ if np.random.rand() < self.stretch_prob:
+ scale_x *= 2 ** np.random.uniform(-self.max_stretch, self.max_stretch)
+ scale_y *= 2 ** np.random.uniform(-self.max_stretch, self.max_stretch)
+ scale_x = np.clip(scale_x, clip_min_scale, None)
+ scale_y = np.clip(scale_y, clip_min_scale, None)
+ # rescale the images
+ img1 = cv2.resize(img1, None, fx=scale_x, fy=scale_y, interpolation=cv2.INTER_LINEAR)
+ img2 = cv2.resize(img2, None, fx=scale_x, fy=scale_y, interpolation=cv2.INTER_LINEAR)
+ flow = self._resize_flow(flow, scale_x, scale_y, factor=2.0 if dname=='Spring' else 1.0)
+ elif dname=="Spring":
+ flow = self._resize_flow(flow, 1.0, 1.0, factor=2.0)
+
+ if self.h_flip_prob>0. and np.random.rand() < self.h_flip_prob: # h-flip
+ img1 = img1[:, ::-1]
+ img2 = img2[:, ::-1]
+ flow = flow[:, ::-1] * [-1.0, 1.0]
+
+ if self.v_flip_prob>0. and np.random.rand() < self.v_flip_prob: # v-flip
+ img1 = img1[::-1, :]
+ img2 = img2[::-1, :]
+ flow = flow[::-1, :] * [1.0, -1.0]
+
+ # In case no cropping
+ if img1.shape[0] - self.crop_size[0] > 0:
+ y0 = np.random.randint(0, img1.shape[0] - self.crop_size[0])
+ else:
+ y0 = 0
+ if img1.shape[1] - self.crop_size[1] > 0:
+ x0 = np.random.randint(0, img1.shape[1] - self.crop_size[1])
+ else:
+ x0 = 0
+
+ img1 = img1[y0:y0 + self.crop_size[0], x0:x0 + self.crop_size[1]]
+ img2 = img2[y0:y0 + self.crop_size[0], x0:x0 + self.crop_size[1]]
+ flow = flow[y0:y0 + self.crop_size[0], x0:x0 + self.crop_size[1]]
+
+ return img1, img2, flow
+
+ def __call__(self, img1, img2, flow, dname):
+ img1, img2, flow = self.spatial_transform(img1, img2, flow, dname)
+ img1, img2 = self.color_transform(img1, img2)
+ img1 = np.ascontiguousarray(img1)
+ img2 = np.ascontiguousarray(img2)
+ flow = np.ascontiguousarray(flow)
+ return img1, img2, flow
\ No newline at end of file
diff --git a/dynamic_predictor/croco/stereoflow/criterion.py b/dynamic_predictor/croco/stereoflow/criterion.py
new file mode 100644
index 0000000000000000000000000000000000000000..57792ebeeee34827b317a4d32b7445837bb33f17
--- /dev/null
+++ b/dynamic_predictor/croco/stereoflow/criterion.py
@@ -0,0 +1,251 @@
+# Copyright (C) 2022-present Naver Corporation. All rights reserved.
+# Licensed under CC BY-NC-SA 4.0 (non-commercial use only).
+
+# --------------------------------------------------------
+# Losses, metrics per batch, metrics per dataset
+# --------------------------------------------------------
+
+import torch
+from torch import nn
+import torch.nn.functional as F
+
+def _get_gtnorm(gt):
+ if gt.size(1)==1: # stereo
+ return gt
+ # flow
+ return torch.sqrt(torch.sum(gt**2, dim=1, keepdims=True)) # Bx1xHxW
+
+############ losses without confidence
+
+class L1Loss(nn.Module):
+
+ def __init__(self, max_gtnorm=None):
+ super().__init__()
+ self.max_gtnorm = max_gtnorm
+ self.with_conf = False
+
+ def _error(self, gt, predictions):
+ return torch.abs(gt-predictions)
+
+ def forward(self, predictions, gt, inspect=False):
+ mask = torch.isfinite(gt)
+ if self.max_gtnorm is not None:
+ mask *= _get_gtnorm(gt).expand(-1,gt.size(1),-1,-1) which is a constant
+
+
+class LaplacianLossBounded(nn.Module): # used for CroCo-Flow ; in the equation of the paper, we have a=1/b
+ def __init__(self, max_gtnorm=10000., a=0.25, b=4.):
+ super().__init__()
+ self.max_gtnorm = max_gtnorm
+ self.with_conf = True
+ self.a, self.b = a, b
+
+ def forward(self, predictions, gt, conf):
+ mask = torch.isfinite(gt)
+ mask = mask[:,0,:,:]
+ if self.max_gtnorm is not None: mask *= _get_gtnorm(gt)[:,0,:,:] which is a constant
+
+class LaplacianLossBounded2(nn.Module): # used for CroCo-Stereo (except for ETH3D) ; in the equation of the paper, we have a=b
+ def __init__(self, max_gtnorm=None, a=3.0, b=3.0):
+ super().__init__()
+ self.max_gtnorm = max_gtnorm
+ self.with_conf = True
+ self.a, self.b = a, b
+
+ def forward(self, predictions, gt, conf):
+ mask = torch.isfinite(gt)
+ mask = mask[:,0,:,:]
+ if self.max_gtnorm is not None: mask *= _get_gtnorm(gt)[:,0,:,:] which is a constant
+
+############## metrics per batch
+
+class StereoMetrics(nn.Module):
+
+ def __init__(self, do_quantile=False):
+ super().__init__()
+ self.bad_ths = [0.5,1,2,3]
+ self.do_quantile = do_quantile
+
+ def forward(self, predictions, gt):
+ B = predictions.size(0)
+ metrics = {}
+ gtcopy = gt.clone()
+ mask = torch.isfinite(gtcopy)
+ gtcopy[~mask] = 999999.0 # we make a copy and put a non-infinite value, such that it does not become nan once multiplied by the mask value 0
+ Npx = mask.view(B,-1).sum(dim=1)
+ L1error = (torch.abs(gtcopy-predictions)*mask).view(B,-1)
+ L2error = (torch.square(gtcopy-predictions)*mask).view(B,-1)
+ # avgerr
+ metrics['avgerr'] = torch.mean(L1error.sum(dim=1)/Npx )
+ # rmse
+ metrics['rmse'] = torch.sqrt(L2error.sum(dim=1)/Npx).mean(dim=0)
+ # err > t for t in [0.5,1,2,3]
+ for ths in self.bad_ths:
+ metrics['bad@{:.1f}'.format(ths)] = (((L1error>ths)* mask.view(B,-1)).sum(dim=1)/Npx).mean(dim=0) * 100
+ return metrics
+
+class FlowMetrics(nn.Module):
+ def __init__(self):
+ super().__init__()
+ self.bad_ths = [1,3,5]
+
+ def forward(self, predictions, gt):
+ B = predictions.size(0)
+ metrics = {}
+ mask = torch.isfinite(gt[:,0,:,:]) # both x and y would be infinite
+ Npx = mask.view(B,-1).sum(dim=1)
+ gtcopy = gt.clone() # to compute L1/L2 error, we need to have non-infinite value, the error computed at this locations will be ignored
+ gtcopy[:,0,:,:][~mask] = 999999.0
+ gtcopy[:,1,:,:][~mask] = 999999.0
+ L1error = (torch.abs(gtcopy-predictions).sum(dim=1)*mask).view(B,-1)
+ L2error = (torch.sqrt(torch.sum(torch.square(gtcopy-predictions),dim=1))*mask).view(B,-1)
+ metrics['L1err'] = torch.mean(L1error.sum(dim=1)/Npx )
+ metrics['EPE'] = torch.mean(L2error.sum(dim=1)/Npx )
+ for ths in self.bad_ths:
+ metrics['bad@{:.1f}'.format(ths)] = (((L2error>ths)* mask.view(B,-1)).sum(dim=1)/Npx).mean(dim=0) * 100
+ return metrics
+
+############## metrics per dataset
+## we update the average and maintain the number of pixels while adding data batch per batch
+## at the beggining, call reset()
+## after each batch, call add_batch(...)
+## at the end: call get_results()
+
+class StereoDatasetMetrics(nn.Module):
+
+ def __init__(self):
+ super().__init__()
+ self.bad_ths = [0.5,1,2,3]
+
+ def reset(self):
+ self.agg_N = 0 # number of pixels so far
+ self.agg_L1err = torch.tensor(0.0) # L1 error so far
+ self.agg_Nbad = [0 for _ in self.bad_ths] # counter of bad pixels
+ self._metrics = None
+
+ def add_batch(self, predictions, gt):
+ assert predictions.size(1)==1, predictions.size()
+ assert gt.size(1)==1, gt.size()
+ if gt.size(2)==predictions.size(2)*2 and gt.size(3)==predictions.size(3)*2: # special case for Spring ...
+ L1err = torch.minimum( torch.minimum( torch.minimum(
+ torch.sum(torch.abs(gt[:,:,0::2,0::2]-predictions),dim=1),
+ torch.sum(torch.abs(gt[:,:,1::2,0::2]-predictions),dim=1)),
+ torch.sum(torch.abs(gt[:,:,0::2,1::2]-predictions),dim=1)),
+ torch.sum(torch.abs(gt[:,:,1::2,1::2]-predictions),dim=1))
+ valid = torch.isfinite(L1err)
+ else:
+ valid = torch.isfinite(gt[:,0,:,:]) # both x and y would be infinite
+ L1err = torch.sum(torch.abs(gt-predictions),dim=1)
+ N = valid.sum()
+ Nnew = self.agg_N + N
+ self.agg_L1err = float(self.agg_N)/Nnew * self.agg_L1err + L1err[valid].mean().cpu() * float(N)/Nnew
+ self.agg_N = Nnew
+ for i,th in enumerate(self.bad_ths):
+ self.agg_Nbad[i] += (L1err[valid]>th).sum().cpu()
+
+ def _compute_metrics(self):
+ if self._metrics is not None: return
+ out = {}
+ out['L1err'] = self.agg_L1err.item()
+ for i,th in enumerate(self.bad_ths):
+ out['bad@{:.1f}'.format(th)] = (float(self.agg_Nbad[i]) / self.agg_N).item() * 100.0
+ self._metrics = out
+
+ def get_results(self):
+ self._compute_metrics() # to avoid recompute them multiple times
+ return self._metrics
+
+class FlowDatasetMetrics(nn.Module):
+
+ def __init__(self):
+ super().__init__()
+ self.bad_ths = [0.5,1,3,5]
+ self.speed_ths = [(0,10),(10,40),(40,torch.inf)]
+
+ def reset(self):
+ self.agg_N = 0 # number of pixels so far
+ self.agg_L1err = torch.tensor(0.0) # L1 error so far
+ self.agg_L2err = torch.tensor(0.0) # L2 (=EPE) error so far
+ self.agg_Nbad = [0 for _ in self.bad_ths] # counter of bad pixels
+ self.agg_EPEspeed = [torch.tensor(0.0) for _ in self.speed_ths] # EPE per speed bin so far
+ self.agg_Nspeed = [0 for _ in self.speed_ths] # N pixels per speed bin so far
+ self._metrics = None
+ self.pairname_results = {}
+
+ def add_batch(self, predictions, gt):
+ assert predictions.size(1)==2, predictions.size()
+ assert gt.size(1)==2, gt.size()
+ if gt.size(2)==predictions.size(2)*2 and gt.size(3)==predictions.size(3)*2: # special case for Spring ...
+ L1err = torch.minimum( torch.minimum( torch.minimum(
+ torch.sum(torch.abs(gt[:,:,0::2,0::2]-predictions),dim=1),
+ torch.sum(torch.abs(gt[:,:,1::2,0::2]-predictions),dim=1)),
+ torch.sum(torch.abs(gt[:,:,0::2,1::2]-predictions),dim=1)),
+ torch.sum(torch.abs(gt[:,:,1::2,1::2]-predictions),dim=1))
+ L2err = torch.minimum( torch.minimum( torch.minimum(
+ torch.sqrt(torch.sum(torch.square(gt[:,:,0::2,0::2]-predictions),dim=1)),
+ torch.sqrt(torch.sum(torch.square(gt[:,:,1::2,0::2]-predictions),dim=1))),
+ torch.sqrt(torch.sum(torch.square(gt[:,:,0::2,1::2]-predictions),dim=1))),
+ torch.sqrt(torch.sum(torch.square(gt[:,:,1::2,1::2]-predictions),dim=1)))
+ valid = torch.isfinite(L1err)
+ gtspeed = (torch.sqrt(torch.sum(torch.square(gt[:,:,0::2,0::2]),dim=1)) + torch.sqrt(torch.sum(torch.square(gt[:,:,0::2,1::2]),dim=1)) +\
+ torch.sqrt(torch.sum(torch.square(gt[:,:,1::2,0::2]),dim=1)) + torch.sqrt(torch.sum(torch.square(gt[:,:,1::2,1::2]),dim=1)) ) / 4.0 # let's just average them
+ else:
+ valid = torch.isfinite(gt[:,0,:,:]) # both x and y would be infinite
+ L1err = torch.sum(torch.abs(gt-predictions),dim=1)
+ L2err = torch.sqrt(torch.sum(torch.square(gt-predictions),dim=1))
+ gtspeed = torch.sqrt(torch.sum(torch.square(gt),dim=1))
+ N = valid.sum()
+ Nnew = self.agg_N + N
+ self.agg_L1err = float(self.agg_N)/Nnew * self.agg_L1err + L1err[valid].mean().cpu() * float(N)/Nnew
+ self.agg_L2err = float(self.agg_N)/Nnew * self.agg_L2err + L2err[valid].mean().cpu() * float(N)/Nnew
+ self.agg_N = Nnew
+ for i,th in enumerate(self.bad_ths):
+ self.agg_Nbad[i] += (L2err[valid]>th).sum().cpu()
+ for i,(th1,th2) in enumerate(self.speed_ths):
+ vv = (gtspeed[valid]>=th1) * (gtspeed[valid] don't use batch_size>1 at test time)
+ self._prepare_data()
+ self._load_or_build_cache()
+
+ def prepare_data(self):
+ """
+ to be defined for each dataset
+ """
+ raise NotImplementedError
+
+ def __len__(self):
+ return len(self.pairnames) # each pairname is typically of the form (str, int1, int2)
+
+ def __getitem__(self, index):
+ pairname = self.pairnames[index]
+
+ # get filenames
+ img1name = self.pairname_to_img1name(pairname)
+ img2name = self.pairname_to_img2name(pairname)
+ flowname = self.pairname_to_flowname(pairname) if self.pairname_to_flowname is not None else None
+
+ # load images and disparities
+ img1 = _read_img(img1name)
+ img2 = _read_img(img2name)
+ flow = self.load_flow(flowname) if flowname is not None else None
+
+ # apply augmentations
+ if self.augmentor is not None:
+ img1, img2, flow = self.augmentor(img1, img2, flow, self.name)
+
+ if self.totensor:
+ img1 = img_to_tensor(img1)
+ img2 = img_to_tensor(img2)
+ if flow is not None:
+ flow = flow_to_tensor(flow)
+ else:
+ flow = torch.tensor([]) # to allow dataloader batching with default collate_gn
+ pairname = str(pairname) # transform potential tuple to str to be able to batch it
+
+ return img1, img2, flow, pairname
+
+ def __rmul__(self, v):
+ self.rmul *= v
+ self.pairnames = v * self.pairnames
+ return self
+
+ def __str__(self):
+ return f'{self.__class__.__name__}_{self.split}'
+
+ def __repr__(self):
+ s = f'{self.__class__.__name__}(split={self.split}, augmentor={self.augmentor_str}, crop_size={str(self.crop_size)}, totensor={self.totensor})'
+ if self.rmul==1:
+ s+=f'\n\tnum pairs: {len(self.pairnames)}'
+ else:
+ s+=f'\n\tnum pairs: {len(self.pairnames)} ({len(self.pairnames)//self.rmul}x{self.rmul})'
+ return s
+
+ def _set_root(self):
+ self.root = dataset_to_root[self.name]
+ assert os.path.isdir(self.root), f"could not find root directory for dataset {self.name}: {self.root}"
+
+ def _load_or_build_cache(self):
+ cache_file = osp.join(cache_dir, self.name+'.pkl')
+ if osp.isfile(cache_file):
+ with open(cache_file, 'rb') as fid:
+ self.pairnames = pickle.load(fid)[self.split]
+ else:
+ tosave = self._build_cache()
+ os.makedirs(cache_dir, exist_ok=True)
+ with open(cache_file, 'wb') as fid:
+ pickle.dump(tosave, fid)
+ self.pairnames = tosave[self.split]
+
+class TartanAirDataset(FlowDataset):
+
+ def _prepare_data(self):
+ self.name = "TartanAir"
+ self._set_root()
+ assert self.split in ['train']
+ self.pairname_to_img1name = lambda pairname: osp.join(self.root, pairname[0], 'image_left/{:06d}_left.png'.format(pairname[1]))
+ self.pairname_to_img2name = lambda pairname: osp.join(self.root, pairname[0], 'image_left/{:06d}_left.png'.format(pairname[2]))
+ self.pairname_to_flowname = lambda pairname: osp.join(self.root, pairname[0], 'flow/{:06d}_{:06d}_flow.npy'.format(pairname[1],pairname[2]))
+ self.pairname_to_str = lambda pairname: os.path.join(pairname[0][pairname[0].find('/')+1:], '{:06d}_{:06d}'.format(pairname[1], pairname[2]))
+ self.load_flow = _read_numpy_flow
+
+ def _build_cache(self):
+ seqs = sorted(os.listdir(self.root))
+ pairs = [(osp.join(s,s,difficulty,Pxxx),int(a[:6]),int(a[:6])+1) for s in seqs for difficulty in ['Easy','Hard'] for Pxxx in sorted(os.listdir(osp.join(self.root,s,s,difficulty))) for a in sorted(os.listdir(osp.join(self.root,s,s,difficulty,Pxxx,'image_left/')))[:-1]]
+ assert len(pairs)==306268, "incorrect parsing of pairs in TartanAir"
+ tosave = {'train': pairs}
+ return tosave
+
+class FlyingChairsDataset(FlowDataset):
+
+ def _prepare_data(self):
+ self.name = "FlyingChairs"
+ self._set_root()
+ assert self.split in ['train','val']
+ self.pairname_to_img1name = lambda pairname: osp.join(self.root, 'data', pairname+'_img1.ppm')
+ self.pairname_to_img2name = lambda pairname: osp.join(self.root, 'data', pairname+'_img2.ppm')
+ self.pairname_to_flowname = lambda pairname: osp.join(self.root, 'data', pairname+'_flow.flo')
+ self.pairname_to_str = lambda pairname: pairname
+ self.load_flow = _read_flo_file
+
+ def _build_cache(self):
+ split_file = osp.join(self.root, 'chairs_split.txt')
+ split_list = np.loadtxt(split_file, dtype=np.int32)
+ trainpairs = ['{:05d}'.format(i) for i in np.where(split_list==1)[0]+1]
+ valpairs = ['{:05d}'.format(i) for i in np.where(split_list==2)[0]+1]
+ assert len(trainpairs)==22232 and len(valpairs)==640, "incorrect parsing of pairs in MPI-Sintel"
+ tosave = {'train': trainpairs, 'val': valpairs}
+ return tosave
+
+class FlyingThingsDataset(FlowDataset):
+
+ def _prepare_data(self):
+ self.name = "FlyingThings"
+ self._set_root()
+ assert self.split in [f'{set_}_{pass_}pass{camstr}' for set_ in ['train','test','test1024'] for camstr in ['','_rightcam'] for pass_ in ['clean','final','all']]
+ self.pairname_to_img1name = lambda pairname: osp.join(self.root, f'frames_{pairname[3]}pass', pairname[0].replace('into_future','').replace('into_past',''), '{:04d}.png'.format(pairname[1]))
+ self.pairname_to_img2name = lambda pairname: osp.join(self.root, f'frames_{pairname[3]}pass', pairname[0].replace('into_future','').replace('into_past',''), '{:04d}.png'.format(pairname[2]))
+ self.pairname_to_flowname = lambda pairname: osp.join(self.root, 'optical_flow', pairname[0], 'OpticalFlowInto{f:s}_{i:04d}_{c:s}.pfm'.format(f='Future' if 'future' in pairname[0] else 'Past', i=pairname[1], c='L' if 'left' in pairname[0] else 'R' ))
+ self.pairname_to_str = lambda pairname: os.path.join(pairname[3]+'pass', pairname[0], 'Into{f:s}_{i:04d}_{c:s}'.format(f='Future' if 'future' in pairname[0] else 'Past', i=pairname[1], c='L' if 'left' in pairname[0] else 'R' ))
+ self.load_flow = _read_pfm_flow
+
+ def _build_cache(self):
+ tosave = {}
+ # train and test splits for the different passes
+ for set_ in ['train', 'test']:
+ sroot = osp.join(self.root, 'optical_flow', set_.upper())
+ fname_to_i = lambda f: int(f[len('OpticalFlowIntoFuture_'):-len('_L.pfm')])
+ pp = [(osp.join(set_.upper(), d, s, 'into_future/left'),fname_to_i(fname)) for d in sorted(os.listdir(sroot)) for s in sorted(os.listdir(osp.join(sroot,d))) for fname in sorted(os.listdir(osp.join(sroot,d, s, 'into_future/left')))[:-1]]
+ pairs = [(a,i,i+1) for a,i in pp]
+ pairs += [(a.replace('into_future','into_past'),i+1,i) for a,i in pp]
+ assert len(pairs)=={'train': 40302, 'test': 7866}[set_], "incorrect parsing of pairs Flying Things"
+ for cam in ['left','right']:
+ camstr = '' if cam=='left' else f'_{cam}cam'
+ for pass_ in ['final', 'clean']:
+ tosave[f'{set_}_{pass_}pass{camstr}'] = [(a.replace('left',cam),i,j,pass_) for a,i,j in pairs]
+ tosave[f'{set_}_allpass{camstr}'] = tosave[f'{set_}_cleanpass{camstr}'] + tosave[f'{set_}_finalpass{camstr}']
+ # test1024: this is the same split as unimatch 'validation' split
+ # see https://github.com/autonomousvision/unimatch/blob/master/dataloader/flow/datasets.py#L229
+ test1024_nsamples = 1024
+ alltest_nsamples = len(tosave['test_cleanpass']) # 7866
+ stride = alltest_nsamples // test1024_nsamples
+ remove = alltest_nsamples % test1024_nsamples
+ for cam in ['left','right']:
+ camstr = '' if cam=='left' else f'_{cam}cam'
+ for pass_ in ['final','clean']:
+ tosave[f'test1024_{pass_}pass{camstr}'] = sorted(tosave[f'test_{pass_}pass{camstr}'])[:-remove][::stride] # warning, it was not sorted before
+ assert len(tosave['test1024_cleanpass'])==1024, "incorrect parsing of pairs in Flying Things"
+ tosave[f'test1024_allpass{camstr}'] = tosave[f'test1024_cleanpass{camstr}'] + tosave[f'test1024_finalpass{camstr}']
+ return tosave
+
+
+class MPISintelDataset(FlowDataset):
+
+ def _prepare_data(self):
+ self.name = "MPISintel"
+ self._set_root()
+ assert self.split in [s+'_'+p for s in ['train','test','subval','subtrain'] for p in ['cleanpass','finalpass','allpass']]
+ self.pairname_to_img1name = lambda pairname: osp.join(self.root, pairname[0], 'frame_{:04d}.png'.format(pairname[1]))
+ self.pairname_to_img2name = lambda pairname: osp.join(self.root, pairname[0], 'frame_{:04d}.png'.format(pairname[1]+1))
+ self.pairname_to_flowname = lambda pairname: None if pairname[0].startswith('test/') else osp.join(self.root, pairname[0].replace('/clean/','/flow/').replace('/final/','/flow/'), 'frame_{:04d}.flo'.format(pairname[1]))
+ self.pairname_to_str = lambda pairname: osp.join(pairname[0], 'frame_{:04d}'.format(pairname[1]))
+ self.load_flow = _read_flo_file
+
+ def _build_cache(self):
+ trainseqs = sorted(os.listdir(self.root+'training/clean'))
+ trainpairs = [ (osp.join('training/clean', s),i) for s in trainseqs for i in range(1, len(os.listdir(self.root+'training/clean/'+s)))]
+ subvalseqs = ['temple_2','temple_3']
+ subtrainseqs = [s for s in trainseqs if s not in subvalseqs]
+ subvalpairs = [ (p,i) for p,i in trainpairs if any(s in p for s in subvalseqs)]
+ subtrainpairs = [ (p,i) for p,i in trainpairs if any(s in p for s in subtrainseqs)]
+ testseqs = sorted(os.listdir(self.root+'test/clean'))
+ testpairs = [ (osp.join('test/clean', s),i) for s in testseqs for i in range(1, len(os.listdir(self.root+'test/clean/'+s)))]
+ assert len(trainpairs)==1041 and len(testpairs)==552 and len(subvalpairs)==98 and len(subtrainpairs)==943, "incorrect parsing of pairs in MPI-Sintel"
+ tosave = {}
+ tosave['train_cleanpass'] = trainpairs
+ tosave['test_cleanpass'] = testpairs
+ tosave['subval_cleanpass'] = subvalpairs
+ tosave['subtrain_cleanpass'] = subtrainpairs
+ for t in ['train','test','subval','subtrain']:
+ tosave[t+'_finalpass'] = [(p.replace('/clean/','/final/'),i) for p,i in tosave[t+'_cleanpass']]
+ tosave[t+'_allpass'] = tosave[t+'_cleanpass'] + tosave[t+'_finalpass']
+ return tosave
+
+ def submission_save_pairname(self, pairname, prediction, outdir, _time):
+ assert prediction.shape[2]==2
+ outfile = os.path.join(outdir, 'submission', self.pairname_to_str(pairname)+'.flo')
+ os.makedirs( os.path.dirname(outfile), exist_ok=True)
+ writeFlowFile(prediction, outfile)
+
+ def finalize_submission(self, outdir):
+ assert self.split == 'test_allpass'
+ bundle_exe = "/nfs/data/ffs-3d/datasets/StereoFlow/MPI-Sintel/bundler/linux-x64/bundler" # eg