Spaces:
Sleeping
Sleeping
bracken576
commited on
Commit
·
ea5b7d5
1
Parent(s):
625ab44
updated streamlit and requirements
Browse files- API_Download_DS2_en_csv_v2_5657328.csv +0 -0
- Metadata_Country_API_Download_DS2_en_csv_v2_5657328.csv +0 -9
- Metadata_Indicator_API_Download_DS2_en_csv_v2_5657328.csv +0 -0
- challenge.md +0 -14
- dat_munged.csv +0 -3
- dat_vars.csv +0 -0
- remark_slides.md +0 -10
- requirements.txt +3 -4
- slides.html +0 -304
- streamlit.py +25 -86
API_Download_DS2_en_csv_v2_5657328.csv
DELETED
The diff for this file is too large to render.
See raw diff
|
|
Metadata_Country_API_Download_DS2_en_csv_v2_5657328.csv
DELETED
@@ -1,9 +0,0 @@
|
|
1 |
-
"Country Code","Region","IncomeGroup","SpecialNotes","TableName",
|
2 |
-
"COD","Sub-Saharan Africa","Low income","The World Bank systematically assesses the appropriateness of official exchange rates as conversion factors. In this country, multiple or dual exchange rate activity exists and must be accounted for appropriately in underlying statistics. An alternative estimate (“alternative conversion factor” - PA.NUS.ATLS) is thus calculated as a weighted average of the different exchange rates in use in the country. Doing so better reflects economic reality and leads to more accurate cross-country comparisons and country classifications by income level. For this country, this applies to the period 1999-2004. Alternative conversion factors are used in the Atlas methodology and elsewhere in World Development Indicators as single-year conversion factors.","Congo, Dem. Rep.",
|
3 |
-
"GHA","Sub-Saharan Africa","Lower middle income","The World Bank systematically assesses the appropriateness of official exchange rates as conversion factors. In this country, multiple or dual exchange rate activity exists and must be accounted for appropriately in underlying statistics. An alternative estimate (“alternative conversion factor” - PA.NUS.ATLS) is thus calculated as a weighted average of the different exchange rates in use in the country. Doing so better reflects economic reality and leads to more accurate cross-country comparisons and country classifications by income level. For this country, this applies to the period 1974-1987. Alternative conversion factors are used in the Atlas methodology and elsewhere in World Development Indicators as single-year conversion factors.","Ghana",
|
4 |
-
"KEN","Sub-Saharan Africa","Lower middle income","Fiscal year end: June 30; reporting period for national accounts data: CY.","Kenya",
|
5 |
-
"NGA","Sub-Saharan Africa","Lower middle income","The World Bank systematically assesses the appropriateness of official exchange rates as conversion factors. In this country, multiple or dual exchange rate activity exists and must be accounted for appropriately in underlying statistics. An alternative estimate (“alternative conversion factor” - PA.NUS.ATLS) is thus calculated as a weighted average of the different exchange rates in use in the country. Doing so better reflects economic reality and leads to more accurate cross-country comparisons and country classifications by income level. For this country, this applies to 1970-2020. Alternative conversion factors are used in the Atlas methodology and elsewhere in World Development Indicators as single-year conversion factors.","Nigeria",
|
6 |
-
"ZAF","Sub-Saharan Africa","Upper middle income","Fiscal year end: March 31; reporting period for national accounts data: CY.","South Africa",
|
7 |
-
"ZWE","Sub-Saharan Africa","Lower middle income","National Accounts data are reported in Zimbabwean Dollar (ZWL). Before 2017, one ZWL is set to be equal to one USD.
|
8 |
-
|
9 |
-
The World Bank systematically assesses the appropriateness of official exchange rates as conversion factors. In this country, multiple or dual exchange rate activity exists and must be accounted for appropriately in underlying statistics. An alternative estimate (“alternative conversion factor” - PA.NUS.ATLS) is thus calculated as a weighted average of the different exchange rates in use in the country. Doing so better reflects economic reality and leads to more accurate cross-country comparisons and country classifications by income level. For this country, this applies to the period 2017-2022. Alternative conversion factors are used in the Atlas methodology and elsewhere in World Development Indicators as single-year conversion factors.","Zimbabwe",
|
|
|
|
|
|
|
|
|
|
|
|
|
|
|
|
|
|
|
|
Metadata_Indicator_API_Download_DS2_en_csv_v2_5657328.csv
DELETED
The diff for this file is too large to render.
See raw diff
|
|
challenge.md
DELETED
@@ -1,14 +0,0 @@
|
|
1 |
-
# Learning Challenge
|
2 |
-
|
3 |
-
- Add the ability to filter the chart to a specified year range with [st.date_input()](https://docs.streamlit.io/develop/api-reference/widgets/st.date_input)
|
4 |
-
- Add [Dataframes - st.data_editor()](https://docs.streamlit.io/develop/concepts/design/dataframes) to allow the user to pick which variables are displayed in the drop down.
|
5 |
-
- Add a few metrics to your dashboard using [st.metric()](https://docs.streamlit.io/develop/api-reference/data/st.metric)
|
6 |
-
- Report the year range of data available for the variable selected over all countries
|
7 |
-
- Add the percent growth from 2000 to the latest available year
|
8 |
-
- Add the country with the highest value in the latest year.
|
9 |
-
- Give the user of your app the ability to take a picture using [st.camera_input()](https://docs.streamlit.io/develop/api-reference/widgets/st.camera_input).
|
10 |
-
- Try to use a third party extension to allow the user to draw on the camera picture taken using [streamlit-drawable-canvas](https://github.com/andfanilo/streamlit-drawable-canvas?tab=readme-ov-file).
|
11 |
-
- Now organize your application using
|
12 |
-
- [st.set_page_config()](https://docs.streamlit.io/develop/api-reference/configuration/st.set_page_config)
|
13 |
-
- [st.columns()](https://docs.streamlit.io/develop/api-reference/layout/st.columns)
|
14 |
-
|
|
|
|
|
|
|
|
|
|
|
|
|
|
|
|
|
|
|
|
|
|
|
|
|
|
|
|
|
|
dat_munged.csv
DELETED
@@ -1,3 +0,0 @@
|
|
1 |
-
version https://git-lfs.github.com/spec/v1
|
2 |
-
oid sha256:afc45ef4e1dd519b46ef23416f0f656148b469e96b744cb8ebe88add1f3a5980
|
3 |
-
size 27673782
|
|
|
|
|
|
|
|
dat_vars.csv
DELETED
The diff for this file is too large to render.
See raw diff
|
|
remark_slides.md
DELETED
@@ -1,10 +0,0 @@
|
|
1 |
-
# GitHub Pages Slideshow with [Remark](https://github.com/gnab/remark)
|
2 |
-
|
3 |
-
This template is made from [Remark](https://github.com/gnab/remark), an open source tool to help create and display slideshows from markdown. For questions, see [Remark's documentation](https://github.com/gnab/remark). I have added a Github action to convert the slides to a pdf in the `slides` branch.
|
4 |
-
|
5 |
-
The most important things to know are:
|
6 |
-
- Edit the `slides.html` file to edit the slides
|
7 |
-
- Slides are separated by `----`
|
8 |
-
- Presenter notes after `???` within one slide
|
9 |
-
- Toggle presenter notes during presentation with `P`
|
10 |
-
- Read the full guide to [remark markdown](https://github.com/gnab/remark/wiki)
|
|
|
|
|
|
|
|
|
|
|
|
|
|
|
|
|
|
|
|
|
|
requirements.txt
CHANGED
@@ -1,7 +1,6 @@
|
|
1 |
-
altair
|
2 |
-
polars
|
3 |
pandas
|
4 |
streamlit
|
5 |
-
scikit-learn
|
6 |
numpy
|
7 |
-
plotly
|
|
|
|
|
|
|
|
|
|
1 |
pandas
|
2 |
streamlit
|
|
|
3 |
numpy
|
4 |
+
plotly
|
5 |
+
tensorflow
|
6 |
+
keras
|
slides.html
DELETED
@@ -1,304 +0,0 @@
|
|
1 |
-
<!DOCTYPE html>
|
2 |
-
<html>
|
3 |
-
<head>
|
4 |
-
<title>Introduction to Data Science Programming in Python</title>
|
5 |
-
<meta http-equiv="Content-Type" content="text/html; charset=UTF-8"/>
|
6 |
-
<style type="text/css">
|
7 |
-
@import url(https://fonts.googleapis.com/css?family=Yanone+Kaffeesatz);
|
8 |
-
@import url(https://fonts.googleapis.com/css?family=Droid+Serif:400,700,400italic);
|
9 |
-
@import url(https://fonts.googleapis.com/css?family=Ubuntu+Mono:400,700,400italic);
|
10 |
-
|
11 |
-
body { font-family: 'Droid Serif'; }
|
12 |
-
h1 {
|
13 |
-
font-family: 'Yanone Kaffeesatz';
|
14 |
-
font-weight: normal;
|
15 |
-
color:darkslategrey;
|
16 |
-
}
|
17 |
-
h2, h3 {
|
18 |
-
font-family: 'Yanone Kaffeesatz';
|
19 |
-
font-weight: normal;
|
20 |
-
}
|
21 |
-
.font40 {
|
22 |
-
font-size: 40px;
|
23 |
-
}
|
24 |
-
.font30 {
|
25 |
-
font-size: 30px;
|
26 |
-
}
|
27 |
-
.font20 {
|
28 |
-
font-size: 20px;
|
29 |
-
}
|
30 |
-
.remark-code, .remark-inline-code {
|
31 |
-
font-family: 'Ubuntu Mono';
|
32 |
-
font-size: 20px;
|
33 |
-
}
|
34 |
-
/* Two-column layout */
|
35 |
-
.left-column {
|
36 |
-
color: #777;
|
37 |
-
width: 50%;
|
38 |
-
float: left;
|
39 |
-
}
|
40 |
-
.left-column h2:last-of-type, .left-column h3:last-child {
|
41 |
-
color: #000;
|
42 |
-
}
|
43 |
-
.right-column {
|
44 |
-
width: 50%;
|
45 |
-
float: right;
|
46 |
-
padding-top: 1em;
|
47 |
-
}
|
48 |
-
.right-column h2:last-of-type, .right-column h3:last-child {
|
49 |
-
color: #000;
|
50 |
-
}
|
51 |
-
.inverse {
|
52 |
-
background: #272822;
|
53 |
-
color: #e4e4e1;
|
54 |
-
text-shadow: 0 0 20px #333;
|
55 |
-
}
|
56 |
-
.inverse h1, .inverse h2, .inverse h3 {
|
57 |
-
color: #f3f3f3;
|
58 |
-
line-height: 0.8em;
|
59 |
-
}
|
60 |
-
.lightfont {color:rgb(129, 126, 126);
|
61 |
-
</style>
|
62 |
-
</head>
|
63 |
-
<body>
|
64 |
-
<textarea id="source">
|
65 |
-
|
66 |
-
class: center, middle, font30
|
67 |
-
|
68 |
-
# Introduction to Streamlit Apps
|
69 |
-
|
70 |
-
J. Hathaway - Data Science Program Chair (BYU-I)
|
71 |
-
|
72 |
-
---
|
73 |
-
|
74 |
-
class: font30
|
75 |
-
|
76 |
-
# Disclaimers
|
77 |
-
|
78 |
-
## Dashboarding is easy to start with modern tools like Streamlit.
|
79 |
-
|
80 |
-
### It is much harder to implement as [Full-Stack Developer](https://aws.amazon.com/what-is/full-stack-development) has it's own schooling and employment. Enjoy using these tools. However, know their purpose and use them accordingly.
|
81 |
-
|
82 |
-
|
83 |
-
---
|
84 |
-
class: font20
|
85 |
-
# Agenda
|
86 |
-
|
87 |
-
Exemplify the data science process - Extract, Transform, Load, Analyze
|
88 |
-
|
89 |
-
1. Checking installations (1 minute)
|
90 |
-
2. Creating an account and navigating Hugging Face (10 minutes)
|
91 |
-
3. Docker for dashboard development using Streamlit (10 minutes)
|
92 |
-
4. Polars for data munging (5 minutes). _Don't munge data in your app (unless you have to)!_
|
93 |
-
5. What are dashboards? (5 minutes)
|
94 |
-
6. Why Streamlit for dashboards? (10 minutes)
|
95 |
-
7. Visualization in dashboards (5 minutes)
|
96 |
-
8. Tables in dashboards (5 minutes)
|
97 |
-
9. Key Performance Indicators [KPIs] in dashboards (5 minutes)
|
98 |
-
10. Challenge yourself to some dasbhoard edits (20 minutes)
|
99 |
-
|
100 |
-
|
101 |
-
---
|
102 |
-
class: font40
|
103 |
-
# Checking our installation
|
104 |
-
|
105 |
-
1. [Python Installed](https://www.python.org/downloads/)
|
106 |
-
2. [VS Code Installed](https://code.visualstudio.com/download)
|
107 |
-
3. [Python VS Code Extension Installed](https://marketplace.visualstudio.com/items?itemName=ms-python.python)
|
108 |
-
4. [Docker Installed](https://www.docker.com/)
|
109 |
-
4. Python packages installed.
|
110 |
-
```python
|
111 |
-
pip install polars plotly streamlit
|
112 |
-
```
|
113 |
-
|
114 |
-
---
|
115 |
-
class: font20
|
116 |
-
# Hugging Face Accounts and Navigation
|
117 |
-
|
118 |
-
## [Create your Hugging Face](https://huggingface.co/join) account.
|
119 |
-
|
120 |
-
> The platform where the machine learning community collaborates on models, datasets, and applications.
|
121 |
-
|
122 |
-
- [Hugging Face Docs](https://huggingface.co/docs)
|
123 |
-
- [Hugging Face Spaces](https://huggingface.co/docs/hub/spaces) ([Youtube Intro](https://www.youtube.com/watch?v=3bSVKNKb_PY))
|
124 |
-
- [Hugging Face Repositories](https://huggingface.co/docs/hub/repositories)
|
125 |
-
- [Hugging Face Organizations](https://huggingface.co/docs/hub/organizations)
|
126 |
-
|
127 |
-
|
128 |
-
---
|
129 |
-
class: font20
|
130 |
-
# Docker for Dashboard Development
|
131 |
-
|
132 |
-
.left-column[
|
133 |
-
1. Clone our Hugging Face repository
|
134 |
-
2. Explore the `DockerFile` and `docker-compose.yml` files.
|
135 |
-
3. Running `Docker compose up`
|
136 |
-
4. Editing our App
|
137 |
-
5. Pushing our changes
|
138 |
-
]
|
139 |
-
.right-column[
|
140 |
-

|
141 |
-
]
|
142 |
-
---
|
143 |
-
class: font20
|
144 |
-
# Polars for data munging
|
145 |
-
|
146 |
-
> Polars is a lightning fast DataFrame library/in-memory query engine. Its embarrassingly parallel execution, cache efficient algorithms and expressive API makes it perfect for efficient data wrangling, data pipelines, snappy APIs and so much more. Polars is about as fast as it gets, see the results in the [H2O.ai benchmark](https://h2oai.github.io/db-benchmark/).
|
147 |
-
> </br>
|
148 |
-
> [Polars Website](https://www.pola.rs/)
|
149 |
-
|
150 |
-

|
151 |
-
|
152 |
-
|
153 |
-
---
|
154 |
-
|
155 |
-
class: font20
|
156 |
-
# Introduction to Dashboarding (Structured design)
|
157 |
-
|
158 |
-
> A dashboard is a way of displaying various types of visual data in one place that let's the user focus on one general topic but explore questions within that topic.
|
159 |
-
|
160 |
-
|
161 |
-

|
162 |
-
|
163 |
-
---
|
164 |
-
class: font20
|
165 |
-
# Introduction to Dashboarding (Audience)
|
166 |
-
|
167 |
-
> A dashboard is a way of displaying various types of visual data in one place that let's the user focus on one general topic but explore questions within that topic.
|
168 |
-
|
169 |
-
> A poorly-designed dashboard doesn’t respect the reader’s time. The whole point of a dashboard is to create a product that will save the user’s time by including everything they need to know in one place. If they can’t go through the dashboard in a couple of minutes and get on with their job, the design needs to be changed.
|
170 |
-
|
171 |
-
|
172 |
-

|
173 |
-
|
174 |
-
[Reference 1](https://www.vmwareopsguide.com/dashboards/chapter-1-design-considerations/3.1.2-the-art-of-dashboard/) and [Reference 2](https://databox.com/bad-dashboard-examples)
|
175 |
-
|
176 |
-
---
|
177 |
-
|
178 |
-
class: font20
|
179 |
-
# Why Streamlit for dashboards?
|
180 |
-
|
181 |
-
|
182 |
-
Streamlit turns data scripts into shareable web apps in minutes in pure Python. A faster way to build and share data apps with no front‑end experience required.
|
183 |
-
|
184 |
-
|
185 |
-

|
186 |
-
|
187 |
-
---
|
188 |
-
class: font30
|
189 |
-
# Streamlit programming
|
190 |
-
|
191 |
-
Now let's practice using Streamlit with our installation of Python
|
192 |
-
|
193 |
-
__Streamlit practice (streamlit_try.py)__
|
194 |
-
|
195 |
-
_After deleting your Docker Image and Container, edit your `DockerFile` to build from a new `streamlit_try.py` script that you create in the folder. Use the code below for the app._
|
196 |
-
|
197 |
-
|
198 |
-
```python
|
199 |
-
import streamlit as st
|
200 |
-
import polars as pl
|
201 |
-
|
202 |
-
st.write("Here's our first attempt at using data to create a table:")
|
203 |
-
st.write(pl.DataFrame({
|
204 |
-
'first column': [1, 2, 3, 4],
|
205 |
-
'second column': [10, 20, 30, 40]
|
206 |
-
}))
|
207 |
-
```
|
208 |
-
|
209 |
-
---
|
210 |
-
class: font20
|
211 |
-
# Introduction to Data Visualization
|
212 |
-
|
213 |
-
Our eyes are drawn to [colors and patterns](https://www.tableau.com/learn/whitepapers/tableau-visual-guidebook). We can quickly identify red from blue, and squares from circles. Our culture is visual, including everything from art and advertisements to TV and movies. Data visualization is another form of visual art that grabs our interest and keeps our eyes on the message.
|
214 |
-
|
215 |
-
.left-column[
|
216 |
-
### Advantages of data visualization:
|
217 |
-
|
218 |
-
- Easily sharing information.
|
219 |
-
- Interactively explore opportunities.
|
220 |
-
- Visualize patterns and relationships.
|
221 |
-
]
|
222 |
-
.right-column[
|
223 |
-
### Disadvantages:
|
224 |
-
|
225 |
-
- Biased or inaccurate information.
|
226 |
-
- Correlation doesn’t always mean causation.
|
227 |
-
- Core messages can get lost in translation.
|
228 |
-
]
|
229 |
-
|
230 |
-
[Tableau Reference](https://www.tableau.com/learn/articles/data-visualization)
|
231 |
-
|
232 |
-
---
|
233 |
-
class: font20
|
234 |
-
# Introduction to __Plotly__ for Data Visualization
|
235 |
-
|
236 |
-
The Plotly Python package leverages the plotly.js JavaScript library to enables Python users to create beautiful interactive web-based visualizations. Plotly.js is built on top of d3.js and stack.gl, Plotly.js is a high-level, declarative charting library. plotly.js ships with over 40 chart types, including 3D charts, statistical graphs, and SVG maps.
|
237 |
-
|
238 |
-
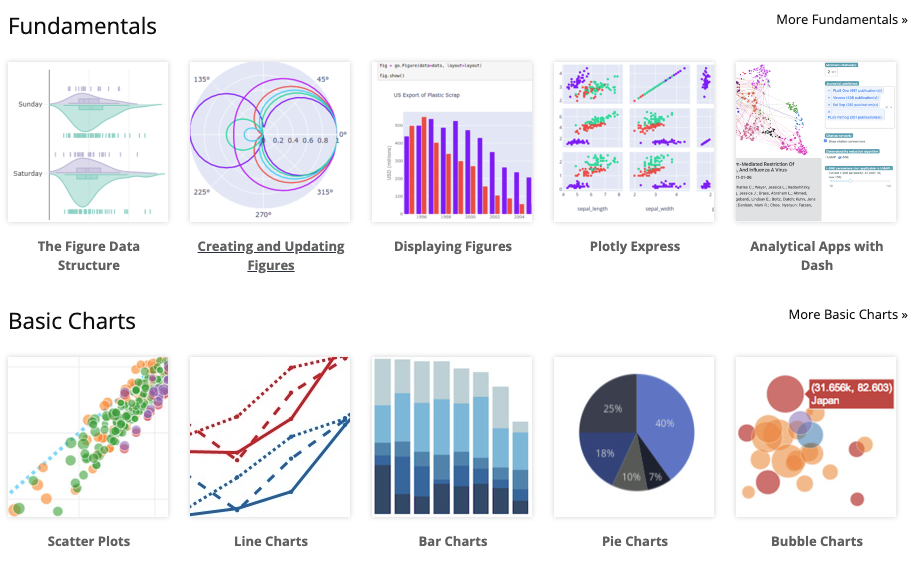
|
239 |
-
|
240 |
-
---
|
241 |
-
class: font20
|
242 |
-
# Tables in dashboards
|
243 |
-
|
244 |
-
> Complexity is the downfall of dashboards. Raw data is always complex.
|
245 |
-
|
246 |
-
- [How to Fit Big Tables on Small Screens](https://www.youtube.com/watch?v=s7nvF1PuAWY)
|
247 |
-
- [Examples of great tables](https://posit-dev.github.io/great-tables/examples/)
|
248 |
-
|
249 |
-
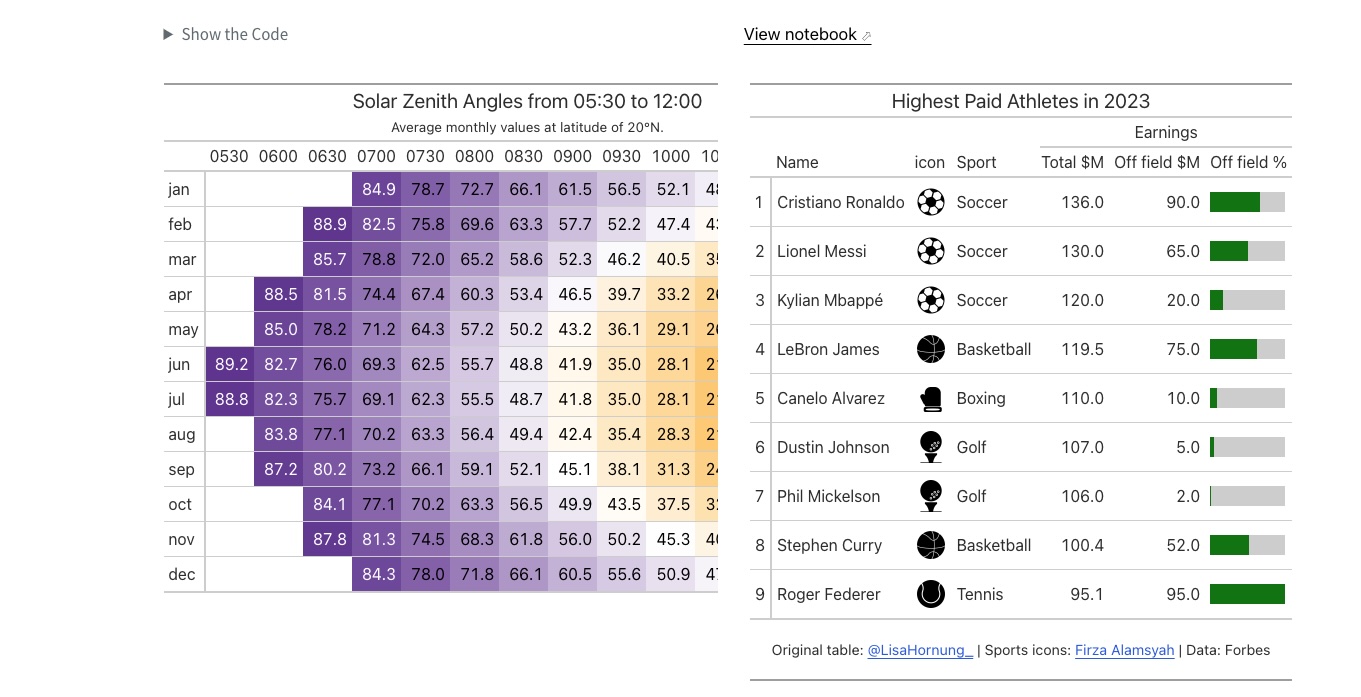
|
250 |
-
|
251 |
-
---
|
252 |
-
class: font40
|
253 |
-
# Key Performance Indicators (KPIs) in dashboards
|
254 |
-
|
255 |
-
> Too much summarization and too much dashboard real estate.
|
256 |
-
|
257 |
-
_[The Dark Side of KPIs: Uncovering the Limitations and Pitfalls](https://shahmm.medium.com/the-dark-side-of-kpis-uncovering-the-limitations-and-pitfalls-4139950e70ef)_
|
258 |
-
|
259 |
-

|
260 |
-
|
261 |
-
---
|
262 |
-
class: font20
|
263 |
-
# Streamlit Challenge Activity
|
264 |
-
|
265 |
-
- Add the ability to filter the chart to a specified year range with [st.date_input()](https://docs.streamlit.io/develop/api-reference/widgets/st.date_input)
|
266 |
-
- Add [Dataframes - st.data_editor()](https://docs.streamlit.io/develop/concepts/design/dataframes) to allow the user to pick which variables are displayed in the drop down.
|
267 |
-
- Add a few metrics to your dashboard using [st.metric()](https://docs.streamlit.io/develop/api-reference/data/st.metric)
|
268 |
-
- Report the year range of data available for the variable selected over all countries
|
269 |
-
- Add the percent growth from 2000 to the latest available year
|
270 |
-
- Add the country with the highest value in the latest year.
|
271 |
-
- Give the user of your app the ability to take a picture using [st.camera_input()](https://docs.streamlit.io/develop/api-reference/widgets/st.camera_input).
|
272 |
-
- Try to use a third party extension to allow the user to draw on the camera picture taken using [streamlit-drawable-canvas](https://github.com/andfanilo/streamlit-drawable-canvas?tab=readme-ov-file).
|
273 |
-
- Now organize your application using
|
274 |
-
- [st.set_page_config()](https://docs.streamlit.io/develop/api-reference/configuration/st.set_page_config)
|
275 |
-
- [st.columns()](https://docs.streamlit.io/develop/api-reference/layout/st.columns)
|
276 |
-
|
277 |
-
</textarea>
|
278 |
-
<script src="https://remarkjs.com/downloads/remark-latest.min.js" type="text/javascript">
|
279 |
-
</script>
|
280 |
-
<script type="text/javascript">
|
281 |
-
remark.macros.upper = function () {
|
282 |
-
// `this` is the value in the parenthesis, or undefined if left out
|
283 |
-
return this.toUpperCase();
|
284 |
-
};
|
285 |
-
|
286 |
-
remark.macros.random = function () {
|
287 |
-
// params are passed as function arguments: ["one", "of", "these", "words"]
|
288 |
-
var i = Math.floor(Math.random() * arguments.length);
|
289 |
-
return arguments[i];
|
290 |
-
};
|
291 |
-
|
292 |
-
remark.macros.scale = function (percentage) {
|
293 |
-
var url = this;
|
294 |
-
return '<img src="' + url + '" style="width: ' + percentage + '" />';
|
295 |
-
};
|
296 |
-
|
297 |
-
var slideshow = remark.create({
|
298 |
-
ratio: "16:9",
|
299 |
-
highlightLanguage: 'javascript',
|
300 |
-
highlightStyle: 'monokai'
|
301 |
-
});
|
302 |
-
</script>
|
303 |
-
</body>
|
304 |
-
</html>
|
|
|
|
|
|
|
|
|
|
|
|
|
|
|
|
|
|
|
|
|
|
|
|
|
|
|
|
|
|
|
|
|
|
|
|
|
|
|
|
|
|
|
|
|
|
|
|
|
|
|
|
|
|
|
|
|
|
|
|
|
|
|
|
|
|
|
|
|
|
|
|
|
|
|
|
|
|
|
|
|
|
|
|
|
|
|
|
|
|
|
|
|
|
|
|
|
|
|
|
|
|
|
|
|
|
|
|
|
|
|
|
|
|
|
|
|
|
|
|
|
|
|
|
|
|
|
|
|
|
|
|
|
|
|
|
|
|
|
|
|
|
|
|
|
|
|
|
|
|
|
|
|
|
|
|
|
|
|
|
|
|
|
|
|
|
|
|
|
|
|
|
|
|
|
|
|
|
|
|
|
|
|
|
|
|
|
|
|
|
|
|
|
|
|
|
|
|
|
|
|
|
|
|
|
|
|
|
|
|
|
|
|
|
|
|
|
|
|
|
|
|
|
|
|
|
|
|
|
|
|
|
|
|
|
|
|
|
|
|
|
|
|
|
|
|
|
|
|
|
|
|
|
|
|
|
|
|
|
|
|
|
|
|
|
|
|
|
|
|
|
|
|
|
|
|
|
|
|
|
|
|
|
|
|
|
|
|
|
|
|
|
|
|
|
|
|
|
|
|
|
|
|
|
|
|
|
|
|
|
|
|
|
|
|
|
|
|
|
|
|
|
|
|
|
|
|
|
|
|
|
|
|
|
|
|
|
|
|
|
|
|
|
|
|
|
|
|
|
|
|
|
|
|
|
|
|
|
|
|
|
|
|
|
|
|
|
|
|
|
|
|
|
|
|
|
|
|
|
|
|
|
|
|
|
|
|
|
|
|
|
|
|
|
|
|
|
|
|
|
|
|
|
|
|
|
|
|
|
|
|
|
|
|
|
|
|
|
|
|
|
|
|
|
|
|
|
|
|
|
|
|
|
|
|
|
|
|
|
|
|
|
|
|
|
|
|
|
|
|
|
|
|
|
|
|
|
|
|
|
|
|
|
|
|
|
|
|
|
|
|
|
|
|
|
|
|
|
|
|
|
|
|
|
|
|
|
|
|
|
|
|
|
|
|
|
|
|
|
|
|
|
|
|
|
|
|
|
|
|
|
|
|
|
|
|
|
|
|
|
|
|
|
|
|
|
|
|
|
|
|
|
|
|
|
|
|
|
|
|
|
|
|
|
|
|
|
|
|
|
|
|
|
|
|
|
|
|
|
|
|
|
|
|
|
|
|
|
|
|
|
|
|
|
|
|
|
|
|
|
|
|
|
|
|
|
|
|
|
|
|
|
|
|
|
|
|
|
|
|
|
|
|
|
|
|
|
|
|
|
streamlit.py
CHANGED
@@ -1,96 +1,35 @@
|
|
1 |
-
|
2 |
-
# packages
|
3 |
-
import streamlit as st
|
4 |
-
import polars as pl
|
5 |
import plotly.express as px
|
6 |
-
import
|
7 |
-
|
8 |
-
|
9 |
-
|
10 |
-
|
11 |
-
#
|
12 |
-
|
13 |
-
dat = pl.read_csv("dat_munged.csv")
|
14 |
-
info = pl.read_csv("Metadata_Indicator_API_Download_DS2_en_csv_v2_5657328.csv").rename({"INDICATOR_CODE":"Indicator Code", "INDICATOR_NAME":"Indicator Name"})
|
15 |
-
dat_vars = pl.read_csv("dat_vars.csv")
|
16 |
-
|
17 |
-
# %%
|
18 |
-
# Example Chart
|
19 |
-
# drop_country = ["ZAF"]
|
20 |
-
# indicator_code = "NY.GDP.PCAP.PP.KD"
|
21 |
-
list_name = dat_vars.select("Indicator Name").to_series().to_list()
|
22 |
-
list_code = dat_vars.select("Indicator Code").to_series().to_list()
|
23 |
-
list_country_code = ["ZAF", "ZWE", "KEN", "NGA", "GHA", "COD"]
|
24 |
-
list_country_name = ["South Africa", "Zimbabwe", "Kenya", "Nigeria", "Ghana", "Congo, Dem. Rep."]
|
25 |
-
|
26 |
-
|
27 |
-
drop_country = st.sidebar.multiselect("Remove Country (Country Code)", list_country_code)
|
28 |
-
|
29 |
-
checked_var = st.sidebar.checkbox("Use Variable Name")
|
30 |
-
|
31 |
-
|
32 |
-
if checked_var:
|
33 |
-
indicator_name = st.sidebar.selectbox("Select your variable", list_name)
|
34 |
-
indicator_code = dat_vars.filter(pl.col("Indicator Name") == indicator_name).select("Indicator Code").to_series()[0]
|
35 |
-
else:
|
36 |
-
indicator_code = st.sidebar.selectbox("Select your variable", list_code)
|
37 |
-
indicator_name = dat_vars.filter(pl.col("Indicator Code") == indicator_code).select("Indicator Name").to_series()[0]
|
38 |
-
|
39 |
-
title_text = indicator_name
|
40 |
-
subtitle_text = info.filter(pl.col("Indicator Code") == indicator_code).select("SOURCE_NOTE").to_series()[0]
|
41 |
-
|
42 |
-
y_axis_title = indicator_name[indicator_name.find("(")+1:indicator_name.find(")")]
|
43 |
-
|
44 |
-
use_dat = dat.filter((pl.col("Indicator Code").is_in([str(indicator_code)])) & (~pl.col("Country Code").is_in(drop_country)) & (pl.col("value").is_not_null()))
|
45 |
-
|
46 |
-
sp = px.line(use_dat.to_pandas(),
|
47 |
-
x="year", y="value", color="Country Name", markers=True,
|
48 |
-
labels = {"year":"Year", "value":y_axis_title},
|
49 |
-
title = title_text)
|
50 |
-
|
51 |
-
st.markdown("## Country performance over time")
|
52 |
-
|
53 |
-
st.markdown("_You can read about streamlit [here](slides.html)_")
|
54 |
-
|
55 |
-
st.markdown("__" + title_text + "__")
|
56 |
-
|
57 |
-
st.markdown(subtitle_text)
|
58 |
-
|
59 |
-
st.markdown("### Chart")
|
60 |
-
st.markdown("_Use the expand arrows visible when you hover over the upper right corner of the chart to see it in full screen._")
|
61 |
-
|
62 |
-
sp
|
63 |
-
|
64 |
-
st.markdown("### Table: " + title_text)
|
65 |
|
66 |
-
|
67 |
|
68 |
-
st.dataframe(
|
69 |
-
display_dat\
|
70 |
-
.pivot(index="year", on="Country Code", values="value", aggregate_function="first")\
|
71 |
-
.sort(pl.col("year"),descending=True), hide_index=True,
|
72 |
-
use_container_width=True,
|
73 |
-
column_config={
|
74 |
-
"value": y_axis_title,
|
75 |
-
"year": st.column_config.NumberColumn(
|
76 |
-
"Year",
|
77 |
-
help="Year of data",
|
78 |
-
format="%.0f"
|
79 |
-
)})
|
80 |
|
81 |
|
82 |
-
|
83 |
-
|
84 |
|
85 |
-
|
86 |
|
87 |
-
|
|
|
88 |
|
89 |
-
st.markdown("## My presentation")
|
90 |
|
91 |
-
|
92 |
-
|
93 |
-
|
|
|
|
|
|
|
|
|
94 |
|
95 |
-
|
96 |
-
|
|
|
|
|
|
1 |
+
#%%
|
|
|
|
|
|
|
2 |
import plotly.express as px
|
3 |
+
import numpy as np
|
4 |
+
import pandas as pd
|
5 |
+
import streamlit as st
|
6 |
+
import tensorflow as tf
|
7 |
+
from keras.preprocessing import image
|
8 |
+
#docker build -t streamlit
|
9 |
+
# docker compose up
|
|
|
|
|
|
|
|
|
|
|
|
|
|
|
|
|
|
|
|
|
|
|
|
|
|
|
|
|
|
|
|
|
|
|
|
|
|
|
|
|
|
|
|
|
|
|
|
|
|
|
|
|
|
|
|
|
|
|
|
|
|
|
|
|
|
|
|
|
|
|
|
|
|
|
|
|
|
|
|
|
|
|
|
|
|
|
|
|
|
|
|
|
|
|
|
|
|
|
|
|
|
|
|
|
10 |
|
11 |
+
st.markdown("## Bone Fracture Recognition with TensorFlow")
|
12 |
|
|
|
|
|
|
|
|
|
|
|
|
|
|
|
|
|
|
|
|
|
|
|
|
|
13 |
|
14 |
|
15 |
+
# actu_loc = [actu + f"_{loc}" for loc in locations]
|
16 |
+
# fore_loc = [fore + f"_{loc}" for loc in locations]
|
17 |
|
18 |
+
image_file = st.file_uploader("Upload X-Ray Image", type=['png', 'jpg'])
|
19 |
|
20 |
+
if image_file:
|
21 |
+
st.image(image_file, caption=None, width=None, use_column_width=None, clamp=False, channels="RGB", output_format="auto")
|
22 |
|
|
|
23 |
|
24 |
+
model = tf.keras.models.load_model("cnnBoneFracRec.h5")
|
25 |
+
target_names = ['Non-Fractured', 'Fractured']
|
26 |
+
temp_img = image.load_img(image_file, target_size=(100, 100))
|
27 |
+
x = image.img_to_array(temp_img)
|
28 |
+
x = np.expand_dims(x, axis=0)
|
29 |
+
images = np.vstack([x])
|
30 |
+
prediction = np.argmax(model.predict(images), axis=1)
|
31 |
|
32 |
+
prediction_str = target_names[prediction.item()]
|
33 |
+
7
|
34 |
+
if prediction_str:
|
35 |
+
st.markdown(f"##### Prediction : {prediction_str}")
|