diff --git a/.env.example b/.env.example
index a790e320464ebc778ca07f5bcd826a9c8412ed0e..5da97050ea5f276f8cf7973dc6358c8489febc7e 100644
--- a/.env.example
+++ b/.env.example
@@ -1,6 +1,6 @@
-# example of file for storing private and user specific environment variables, like keys or system paths
-# rename it to ".env" (excluded from version control by default)
-# .env is loaded by train.py automatically
-# hydra allows you to reference variables in .yaml configs with special syntax: ${oc.env:MY_VAR}
-
-MY_VAR="/home/user/my/system/path"
+# example of file for storing private and user specific environment variables, like keys or system paths
+# rename it to ".env" (excluded from version control by default)
+# .env is loaded by train.py automatically
+# hydra allows you to reference variables in .yaml configs with special syntax: ${oc.env:MY_VAR}
+
+MY_VAR="/home/user/my/system/path"
diff --git a/.gitattributes b/.gitattributes
index a6344aac8c09253b3b630fb776ae94478aa0275b..5daa0eb51dcfaa3a464bfd1d06123192f9cc908f 100644
--- a/.gitattributes
+++ b/.gitattributes
@@ -1,35 +1,42 @@
-*.7z filter=lfs diff=lfs merge=lfs -text
-*.arrow filter=lfs diff=lfs merge=lfs -text
-*.bin filter=lfs diff=lfs merge=lfs -text
-*.bz2 filter=lfs diff=lfs merge=lfs -text
-*.ckpt filter=lfs diff=lfs merge=lfs -text
-*.ftz filter=lfs diff=lfs merge=lfs -text
-*.gz filter=lfs diff=lfs merge=lfs -text
-*.h5 filter=lfs diff=lfs merge=lfs -text
-*.joblib filter=lfs diff=lfs merge=lfs -text
-*.lfs.* filter=lfs diff=lfs merge=lfs -text
-*.mlmodel filter=lfs diff=lfs merge=lfs -text
-*.model filter=lfs diff=lfs merge=lfs -text
-*.msgpack filter=lfs diff=lfs merge=lfs -text
-*.npy filter=lfs diff=lfs merge=lfs -text
-*.npz filter=lfs diff=lfs merge=lfs -text
-*.onnx filter=lfs diff=lfs merge=lfs -text
-*.ot filter=lfs diff=lfs merge=lfs -text
-*.parquet filter=lfs diff=lfs merge=lfs -text
-*.pb filter=lfs diff=lfs merge=lfs -text
-*.pickle filter=lfs diff=lfs merge=lfs -text
-*.pkl filter=lfs diff=lfs merge=lfs -text
-*.pt filter=lfs diff=lfs merge=lfs -text
-*.pth filter=lfs diff=lfs merge=lfs -text
-*.rar filter=lfs diff=lfs merge=lfs -text
-*.safetensors filter=lfs diff=lfs merge=lfs -text
-saved_model/**/* filter=lfs diff=lfs merge=lfs -text
-*.tar.* filter=lfs diff=lfs merge=lfs -text
-*.tar filter=lfs diff=lfs merge=lfs -text
-*.tflite filter=lfs diff=lfs merge=lfs -text
-*.tgz filter=lfs diff=lfs merge=lfs -text
-*.wasm filter=lfs diff=lfs merge=lfs -text
-*.xz filter=lfs diff=lfs merge=lfs -text
-*.zip filter=lfs diff=lfs merge=lfs -text
-*.zst filter=lfs diff=lfs merge=lfs -text
-*tfevents* filter=lfs diff=lfs merge=lfs -text
+*.7z filter=lfs diff=lfs merge=lfs -text
+*.arrow filter=lfs diff=lfs merge=lfs -text
+*.bin filter=lfs diff=lfs merge=lfs -text
+*.bz2 filter=lfs diff=lfs merge=lfs -text
+*.ckpt filter=lfs diff=lfs merge=lfs -text
+*.ftz filter=lfs diff=lfs merge=lfs -text
+*.gz filter=lfs diff=lfs merge=lfs -text
+*.h5 filter=lfs diff=lfs merge=lfs -text
+*.joblib filter=lfs diff=lfs merge=lfs -text
+*.lfs.* filter=lfs diff=lfs merge=lfs -text
+*.mlmodel filter=lfs diff=lfs merge=lfs -text
+*.model filter=lfs diff=lfs merge=lfs -text
+*.msgpack filter=lfs diff=lfs merge=lfs -text
+*.npy filter=lfs diff=lfs merge=lfs -text
+*.npz filter=lfs diff=lfs merge=lfs -text
+*.onnx filter=lfs diff=lfs merge=lfs -text
+*.ot filter=lfs diff=lfs merge=lfs -text
+*.parquet filter=lfs diff=lfs merge=lfs -text
+*.pb filter=lfs diff=lfs merge=lfs -text
+*.pickle filter=lfs diff=lfs merge=lfs -text
+*.pkl filter=lfs diff=lfs merge=lfs -text
+*.pt filter=lfs diff=lfs merge=lfs -text
+*.pth filter=lfs diff=lfs merge=lfs -text
+*.rar filter=lfs diff=lfs merge=lfs -text
+*.safetensors filter=lfs diff=lfs merge=lfs -text
+saved_model/**/* filter=lfs diff=lfs merge=lfs -text
+*.tar.* filter=lfs diff=lfs merge=lfs -text
+*.tar filter=lfs diff=lfs merge=lfs -text
+*.tflite filter=lfs diff=lfs merge=lfs -text
+*.tgz filter=lfs diff=lfs merge=lfs -text
+*.wasm filter=lfs diff=lfs merge=lfs -text
+*.xz filter=lfs diff=lfs merge=lfs -text
+*.zip filter=lfs diff=lfs merge=lfs -text
+*.zst filter=lfs diff=lfs merge=lfs -text
+*tfevents* filter=lfs diff=lfs merge=lfs -text
+references/\[2017[[:space:]]RSE\][[:space:]]L8_Biome.pdf filter=lfs diff=lfs merge=lfs -text
+references/\[2019[[:space:]]ISPRS\][[:space:]]HRC_WHU.pdf filter=lfs diff=lfs merge=lfs -text
+references/\[2019[[:space:]]TGRS\][[:space:]]CDnet.pdf filter=lfs diff=lfs merge=lfs -text
+references/\[2021[[:space:]]TGRS\][[:space:]]CDnetV2.pdf filter=lfs diff=lfs merge=lfs -text
+references/\[2022[[:space:]]TGRS\][[:space:]]DBNet.pdf filter=lfs diff=lfs merge=lfs -text
+references/\[2024[[:space:]]ISPRS\][[:space:]]SCNN.pdf filter=lfs diff=lfs merge=lfs -text
+references/\[2024[[:space:]]TGRS\][[:space:]]GaoFen12.pdf filter=lfs diff=lfs merge=lfs -text
diff --git a/.gitignore b/.gitignore
index 04a06484441a3d09afd793ef8a7107931de8e06f..6b6decba74116c6191adeab79f9302b170235a1e 100644
--- a/.gitignore
+++ b/.gitignore
@@ -1,154 +1,153 @@
-# Byte-compiled / optimized / DLL files
-__pycache__/
-*.py[cod]
-*$py.class
-
-# C extensions
-*.so
-
-# Distribution / packaging
-.Python
-build/
-develop-eggs/
-dist/
-downloads/
-eggs/
-.eggs/
-lib/
-lib64/
-parts/
-sdist/
-var/
-wheels/
-pip-wheel-metadata/
-share/python-wheels/
-*.egg-info/
-.installed.cfg
-*.egg
-MANIFEST
-
-# PyInstaller
-# Usually these files are written by a python script from a template
-# before PyInstaller builds the exe, so as to inject date/other infos into it.
-*.manifest
-*.spec
-
-# Installer logs
-pip-log.txt
-pip-delete-this-directory.txt
-
-# Unit test / coverage reports
-htmlcov/
-.tox/
-.nox/
-.coverage
-.coverage.*
-.cache
-nosetests.xml
-coverage.xml
-*.cover
-*.py,cover
-.hypothesis/
-.pytest_cache/
-
-# Translations
-*.mo
-*.pot
-
-# Django stuff:
-*.log
-local_settings.py
-db.sqlite3
-db.sqlite3-journal
-
-# Flask stuff:
-instance/
-.webassets-cache
-
-# Scrapy stuff:
-.scrapy
-
-# Sphinx documentation
-docs/_build/
-
-# PyBuilder
-target/
-
-# Jupyter Notebook
-.ipynb_checkpoints
-
-# IPython
-profile_default/
-ipython_config.py
-
-# pyenv
-.python-version
-
-# pipenv
-# According to pypa/pipenv#598, it is recommended to include Pipfile.lock in version control.
-# However, in case of collaboration, if having platform-specific dependencies or dependencies
-# having no cross-platform support, pipenv may install dependencies that don't work, or not
-# install all needed dependencies.
-#Pipfile.lock
-
-# PEP 582; used by e.g. github.com/David-OConnor/pyflow
-__pypackages__/
-
-# Celery stuff
-celerybeat-schedule
-celerybeat.pid
-
-# SageMath parsed files
-*.sage.py
-
-# Environments
-.venv
-env/
-venv/
-ENV/
-env.bak/
-venv.bak/
-
-# Spyder project settings
-.spyderproject
-.spyproject
-
-# Rope project settings
-.ropeproject
-
-# mkdocs documentation
-/site
-
-# mypy
-.mypy_cache/
-.dmypy.json
-dmypy.json
-
-# Pyre type checker
-.pyre/
-
-### VisualStudioCode
-.vscode/*
-!.vscode/settings.json
-!.vscode/tasks.json
-!.vscode/launch.json
-!.vscode/extensions.json
-*.code-workspace
-**/.vscode
-
-# JetBrains
-.idea/
-
-# Data & Models
-*.h5
-*.tar
-*.tar.gz
-
-# Lightning-Hydra-Template
-configs/local/default.yaml
-/data/
-/logs/
-.env
-
-# Aim logging
-.aim
+# Byte-compiled / optimized / DLL files
+__pycache__/
+*.py[cod]
+*$py.class
+
+# C extensions
+*.so
+
+# Distribution / packaging
+.Python
+build/
+develop-eggs/
+dist/
+downloads/
+eggs/
+.eggs/
+lib/
+lib64/
+parts/
+sdist/
+var/
+wheels/
+pip-wheel-metadata/
+share/python-wheels/
+*.egg-info/
+.installed.cfg
+*.egg
+MANIFEST
+
+# PyInstaller
+# Usually these files are written by a python script from a template
+# before PyInstaller builds the exe, so as to inject date/other infos into it.
+*.manifest
+*.spec
+
+# Installer logs
+pip-log.txt
+pip-delete-this-directory.txt
+
+# Unit test / coverage reports
+htmlcov/
+.tox/
+.nox/
+.coverage
+.coverage.*
+.cache
+nosetests.xml
+coverage.xml
+*.cover
+*.py,cover
+.hypothesis/
+.pytest_cache/
+
+# Translations
+*.mo
+*.pot
+
+# Django stuff:
+
+local_settings.py
+db.sqlite3
+db.sqlite3-journal
+
+# Flask stuff:
+instance/
+.webassets-cache
+
+# Scrapy stuff:
+.scrapy
+
+# Sphinx documentation
+docs/_build/
+
+# PyBuilder
+target/
+
+# Jupyter Notebook
+.ipynb_checkpoints
+
+# IPython
+profile_default/
+ipython_config.py
+
+# pyenv
+.python-version
+
+# pipenv
+# According to pypa/pipenv#598, it is recommended to include Pipfile.lock in version control.
+# However, in case of collaboration, if having platform-specific dependencies or dependencies
+# having no cross-platform support, pipenv may install dependencies that don't work, or not
+# install all needed dependencies.
+#Pipfile.lock
+
+# PEP 582; used by e.g. github.com/David-OConnor/pyflow
+__pypackages__/
+
+# Celery stuff
+celerybeat-schedule
+celerybeat.pid
+
+# SageMath parsed files
+*.sage.py
+
+# Environments
+.venv
+env/
+venv/
+ENV/
+env.bak/
+venv.bak/
+
+# Spyder project settings
+.spyderproject
+.spyproject
+
+# Rope project settings
+.ropeproject
+
+# mkdocs documentation
+/site
+
+# mypy
+.mypy_cache/
+.dmypy.json
+dmypy.json
+
+# Pyre type checker
+.pyre/
+
+### VisualStudioCode
+.vscode/*
+!.vscode/settings.json
+!.vscode/tasks.json
+!.vscode/launch.json
+!.vscode/extensions.json
+*.code-workspace
+**/.vscode
+
+# JetBrains
+.idea/
+
+# Data & Models
+*.h5
+*.tar
+*.tar.gz
+
+# Lightning-Hydra-Template
+configs/local/default.yaml
+/data/
+.env
+
+# Aim logging
+.aim
diff --git a/.pre-commit-config.yaml b/.pre-commit-config.yaml
index ee45ce1946f075adb092b2d574abcbdb96169984..bebafad8fdad1205ec2987fdd9cfb984320a9172 100644
--- a/.pre-commit-config.yaml
+++ b/.pre-commit-config.yaml
@@ -1,147 +1,147 @@
-default_language_version:
- python: python3
-
-repos:
- - repo: https://github.com/pre-commit/pre-commit-hooks
- rev: v4.4.0
- hooks:
- # list of supported hooks: https://pre-commit.com/hooks.html
- - id: trailing-whitespace
- - id: end-of-file-fixer
- - id: check-docstring-first
- - id: check-yaml
- - id: debug-statements
- - id: detect-private-key
- - id: check-executables-have-shebangs
- - id: check-toml
- - id: check-case-conflict
- - id: check-added-large-files
-
- # python code formatting
- - repo: https://github.com/psf/black
- rev: 23.1.0
- hooks:
- - id: black
- args: [--line-length, "99"]
-
- # python import sorting
- - repo: https://github.com/PyCQA/isort
- rev: 5.12.0
- hooks:
- - id: isort
- args: ["--profile", "black", "--filter-files"]
-
- # python upgrading syntax to newer version
- - repo: https://github.com/asottile/pyupgrade
- rev: v3.3.1
- hooks:
- - id: pyupgrade
- args: [--py38-plus]
-
- # python docstring formatting
- - repo: https://github.com/myint/docformatter
- rev: v1.7.4
- hooks:
- - id: docformatter
- args:
- [
- --in-place,
- --wrap-summaries=99,
- --wrap-descriptions=99,
- --style=sphinx,
- --black,
- ]
-
- # python docstring coverage checking
- - repo: https://github.com/econchick/interrogate
- rev: 1.5.0 # or master if you're bold
- hooks:
- - id: interrogate
- args:
- [
- --verbose,
- --fail-under=80,
- --ignore-init-module,
- --ignore-init-method,
- --ignore-module,
- --ignore-nested-functions,
- -vv,
- ]
-
- # python check (PEP8), programming errors and code complexity
- - repo: https://github.com/PyCQA/flake8
- rev: 6.0.0
- hooks:
- - id: flake8
- args:
- [
- "--extend-ignore",
- "E203,E402,E501,F401,F841,RST2,RST301",
- "--exclude",
- "logs/*,data/*",
- ]
- additional_dependencies: [flake8-rst-docstrings==0.3.0]
-
- # python security linter
- - repo: https://github.com/PyCQA/bandit
- rev: "1.7.5"
- hooks:
- - id: bandit
- args: ["-s", "B101"]
-
- # yaml formatting
- - repo: https://github.com/pre-commit/mirrors-prettier
- rev: v3.0.0-alpha.6
- hooks:
- - id: prettier
- types: [yaml]
- exclude: "environment.yaml"
-
- # shell scripts linter
- - repo: https://github.com/shellcheck-py/shellcheck-py
- rev: v0.9.0.2
- hooks:
- - id: shellcheck
-
- # md formatting
- - repo: https://github.com/executablebooks/mdformat
- rev: 0.7.16
- hooks:
- - id: mdformat
- args: ["--number"]
- additional_dependencies:
- - mdformat-gfm
- - mdformat-tables
- - mdformat_frontmatter
- # - mdformat-toc
- # - mdformat-black
-
- # word spelling linter
- - repo: https://github.com/codespell-project/codespell
- rev: v2.2.4
- hooks:
- - id: codespell
- args:
- - --skip=logs/**,data/**,*.ipynb
- # - --ignore-words-list=abc,def
-
- # jupyter notebook cell output clearing
- - repo: https://github.com/kynan/nbstripout
- rev: 0.6.1
- hooks:
- - id: nbstripout
-
- # jupyter notebook linting
- - repo: https://github.com/nbQA-dev/nbQA
- rev: 1.6.3
- hooks:
- - id: nbqa-black
- args: ["--line-length=99"]
- - id: nbqa-isort
- args: ["--profile=black"]
- - id: nbqa-flake8
- args:
- [
- "--extend-ignore=E203,E402,E501,F401,F841",
- "--exclude=logs/*,data/*",
- ]
+default_language_version:
+ python: python3
+
+repos:
+ - repo: https://github.com/pre-commit/pre-commit-hooks
+ rev: v4.4.0
+ hooks:
+ # list of supported hooks: https://pre-commit.com/hooks.html
+ - id: trailing-whitespace
+ - id: end-of-file-fixer
+ - id: check-docstring-first
+ - id: check-yaml
+ - id: debug-statements
+ - id: detect-private-key
+ - id: check-executables-have-shebangs
+ - id: check-toml
+ - id: check-case-conflict
+ - id: check-added-large-files
+
+ # python code formatting
+ - repo: https://github.com/psf/black
+ rev: 23.1.0
+ hooks:
+ - id: black
+ args: [--line-length, "99"]
+
+ # python import sorting
+ - repo: https://github.com/PyCQA/isort
+ rev: 5.12.0
+ hooks:
+ - id: isort
+ args: ["--profile", "black", "--filter-files"]
+
+ # python upgrading syntax to newer version
+ - repo: https://github.com/asottile/pyupgrade
+ rev: v3.3.1
+ hooks:
+ - id: pyupgrade
+ args: [--py38-plus]
+
+ # python docstring formatting
+ - repo: https://github.com/myint/docformatter
+ rev: v1.7.4
+ hooks:
+ - id: docformatter
+ args:
+ [
+ --in-place,
+ --wrap-summaries=99,
+ --wrap-descriptions=99,
+ --style=sphinx,
+ --black,
+ ]
+
+ # python docstring coverage checking
+ - repo: https://github.com/econchick/interrogate
+ rev: 1.5.0 # or master if you're bold
+ hooks:
+ - id: interrogate
+ args:
+ [
+ --verbose,
+ --fail-under=80,
+ --ignore-init-module,
+ --ignore-init-method,
+ --ignore-module,
+ --ignore-nested-functions,
+ -vv,
+ ]
+
+ # python check (PEP8), programming errors and code complexity
+ - repo: https://github.com/PyCQA/flake8
+ rev: 6.0.0
+ hooks:
+ - id: flake8
+ args:
+ [
+ "--extend-ignore",
+ "E203,E402,E501,F401,F841,RST2,RST301",
+ "--exclude",
+ "logs/*,data/*",
+ ]
+ additional_dependencies: [flake8-rst-docstrings==0.3.0]
+
+ # python security linter
+ - repo: https://github.com/PyCQA/bandit
+ rev: "1.7.5"
+ hooks:
+ - id: bandit
+ args: ["-s", "B101"]
+
+ # yaml formatting
+ - repo: https://github.com/pre-commit/mirrors-prettier
+ rev: v3.0.0-alpha.6
+ hooks:
+ - id: prettier
+ types: [yaml]
+ exclude: "environment.yaml"
+
+ # shell scripts linter
+ - repo: https://github.com/shellcheck-py/shellcheck-py
+ rev: v0.9.0.2
+ hooks:
+ - id: shellcheck
+
+ # md formatting
+ - repo: https://github.com/executablebooks/mdformat
+ rev: 0.7.16
+ hooks:
+ - id: mdformat
+ args: ["--number"]
+ additional_dependencies:
+ - mdformat-gfm
+ - mdformat-tables
+ - mdformat_frontmatter
+ # - mdformat-toc
+ # - mdformat-black
+
+ # word spelling linter
+ - repo: https://github.com/codespell-project/codespell
+ rev: v2.2.4
+ hooks:
+ - id: codespell
+ args:
+ - --skip=logs/**,data/**,*.ipynb
+ # - --ignore-words-list=abc,def
+
+ # jupyter notebook cell output clearing
+ - repo: https://github.com/kynan/nbstripout
+ rev: 0.6.1
+ hooks:
+ - id: nbstripout
+
+ # jupyter notebook linting
+ - repo: https://github.com/nbQA-dev/nbQA
+ rev: 1.6.3
+ hooks:
+ - id: nbqa-black
+ args: ["--line-length=99"]
+ - id: nbqa-isort
+ args: ["--profile=black"]
+ - id: nbqa-flake8
+ args:
+ [
+ "--extend-ignore=E203,E402,E501,F401,F841",
+ "--exclude=logs/*,data/*",
+ ]
diff --git a/.project-root b/.project-root
index 63eab774b9e36aa1a46cbd31b59cbd373bc5477f..93eddd6ac93054524ecfc0654be88006cbd8b15b 100644
--- a/.project-root
+++ b/.project-root
@@ -1,2 +1,2 @@
-# this file is required for inferring the project root directory
-# do not delete
+# this file is required for inferring the project root directory
+# do not delete
diff --git a/Makefile b/Makefile
index 38184df93ea2c09f6d527abbb7f7c804b014284c..400a4e175b311b95a89c26ead521600c3fef18ef 100644
--- a/Makefile
+++ b/Makefile
@@ -1,30 +1,30 @@
-
-help: ## Show help
- @grep -E '^[.a-zA-Z_-]+:.*?## .*$$' $(MAKEFILE_LIST) | awk 'BEGIN {FS = ":.*?## "}; {printf "\033[36m%-30s\033[0m %s\n", $$1, $$2}'
-
-clean: ## Clean autogenerated files
- rm -rf dist
- find . -type f -name "*.DS_Store" -ls -delete
- find . | grep -E "(__pycache__|\.pyc|\.pyo)" | xargs rm -rf
- find . | grep -E ".pytest_cache" | xargs rm -rf
- find . | grep -E ".ipynb_checkpoints" | xargs rm -rf
- rm -f .coverage
-
-clean-logs: ## Clean logs
- rm -rf logs/**
-
-format: ## Run pre-commit hooks
- pre-commit run -a
-
-sync: ## Merge changes from main branch to your current branch
- git pull
- git pull origin main
-
-test: ## Run not slow tests
- pytest -k "not slow"
-
-test-full: ## Run all tests
- pytest
-
-train: ## Train the model
- python src/train.py
+
+help: ## Show help
+ @grep -E '^[.a-zA-Z_-]+:.*?## .*$$' $(MAKEFILE_LIST) | awk 'BEGIN {FS = ":.*?## "}; {printf "\033[36m%-30s\033[0m %s\n", $$1, $$2}'
+
+clean: ## Clean autogenerated files
+ rm -rf dist
+ find . -type f -name "*.DS_Store" -ls -delete
+ find . | grep -E "(__pycache__|\.pyc|\.pyo)" | xargs rm -rf
+ find . | grep -E ".pytest_cache" | xargs rm -rf
+ find . | grep -E ".ipynb_checkpoints" | xargs rm -rf
+ rm -f .coverage
+
+clean-logs: ## Clean logs
+ rm -rf logs/**
+
+format: ## Run pre-commit hooks
+ pre-commit run -a
+
+sync: ## Merge changes from main branch to your current branch
+ git pull
+ git pull origin main
+
+test: ## Run not slow tests
+ pytest -k "not slow"
+
+test-full: ## Run all tests
+ pytest
+
+train: ## Train the model
+ python src/train.py
diff --git a/README.md b/README.md
index 9b293b532fb6d73ee7a2468a4f4ad3da93159382..597fe0da1f7cfe665a293a50859a1d4170e8645a 100644
--- a/README.md
+++ b/README.md
@@ -1,104 +1,104 @@
----
-title: Cloudseg
-emoji: 📚
-colorFrom: blue
-colorTo: red
-sdk: gradio
-sdk_version: 4.40.0
-app_file: app.py
-pinned: false
-license: apache-2.0
----
-# Cloud Segmentation
-
-[](https://huggingface.co/spaces/caixiaoshun/cloudseg)
-[](https://github.com/pre-commit/pre-commit)
-[](https://pytorch.org/get-started/locally/)
-[](https://pytorchlightning.ai/)
-[](https://hydra.cc/)
-[](https://github.com/XavierJiezou/cloudseg#license)
-[](https://github.com/XavierJiezou/cloudseg/graphs/contributors)
-[](https://github.com/ashleve/lightning-hydra-template)
-[](https://www.nature.com/articles/nature14539)
-[](https://papers.nips.cc/paper/2020)
-
-## Datasets
-
-```bash
-cloudseg
-├── src
-├── configs
-├── data
-│ ├── hrcwhu
-│ │ ├── train.txt
-│ │ ├── test.txt
-│ │ ├── img_dir
-│ │ │ ├── train
-│ │ │ ├── test
-│ │ ├── ann_dir
-│ │ │ ├── train
-│ │ │ ├── test
-```
-
-## Supported Methods
-
-- [UNet (MICCAI 2016)](configs/model/unet)
-- [CDNetv1 (TGRS 2019)](configs/model/cdnetv1)
-- [CDNetv2 (TGRS 2021)](configs/model/cdnetv2)
-- [DBNet (TGRS 2022)](configs/model/dbnet)
-- [HrCloudNet (JEI 2024)](configs/model/hrcloudnet)
-- [McdNet (International Journal of Applied Earth Observation and Geoinformation 2024)](configs/model/mcdnet)
-- [Scnn (ISPRS 2024)](configs/model/scnn)
-
-## Installation
-
-```bash
-git clone https://github.com/XavierJiezou/cloudseg.git
-cd cloudseg
-conda env create -f environment.yaml
-conda activate cloudseg
-```
-
-## Usage
-
-**Train model with default configuration**
-
-```bash
-# train on CPU
-python src/train.py trainer=cpu
-
-# train on GPU
-python src/train.py trainer=gpu
-```
-
-**Train model with chosen experiment configuration from [configs/experiment/](configs/experiment/)**
-
-```bash
-python src/train.py experiment=experiment_name.yaml
-```
-
-**Tranin Example**
-
-```bash
-python src/train.py experiment=hrcwhu_cdnetv1.yaml
-```
-
-**You can override any parameter from command line like this**
-
-```bash
-python src/train.py trainer.max_epochs=20 data.batch_size=64
-```
-
-**Visualization in wandb**
-
-```bash
-python wand_vis.py --model-name model_name
-```
-
-**Example**
-
-```bash
-python wand_vis.py --model-name cdnetv1
-```
-
-
+---
+title: Cloudseg
+emoji: 📚
+colorFrom: blue
+colorTo: red
+sdk: gradio
+sdk_version: 4.40.0
+app_file: app.py
+pinned: false
+license: apache-2.0
+---
+# Cloud Segmentation
+
+[](https://huggingface.co/spaces/caixiaoshun/cloudseg)
+[](https://github.com/pre-commit/pre-commit)
+[](https://pytorch.org/get-started/locally/)
+[](https://pytorchlightning.ai/)
+[](https://hydra.cc/)
+[](https://github.com/XavierJiezou/cloudseg#license)
+[](https://github.com/XavierJiezou/cloudseg/graphs/contributors)
+[](https://github.com/ashleve/lightning-hydra-template)
+[](https://www.nature.com/articles/nature14539)
+[](https://papers.nips.cc/paper/2020)
+
+## Datasets
+
+```bash
+cloudseg
+├── src
+├── configs
+├── data
+│ ├── hrcwhu
+│ │ ├── train.txt
+│ │ ├── test.txt
+│ │ ├── img_dir
+│ │ │ ├── train
+│ │ │ ├── test
+│ │ ├── ann_dir
+│ │ │ ├── train
+│ │ │ ├── test
+```
+
+## Supported Methods
+
+- [UNet (MICCAI 2016)](configs/model/unet)
+- [CDNetv1 (TGRS 2019)](configs/model/cdnetv1)
+- [CDNetv2 (TGRS 2021)](configs/model/cdnetv2)
+- [DBNet (TGRS 2022)](configs/model/dbnet)
+- [HrCloudNet (JEI 2024)](configs/model/hrcloudnet)
+- [McdNet (International Journal of Applied Earth Observation and Geoinformation 2024)](configs/model/mcdnet)
+- [Scnn (ISPRS 2024)](configs/model/scnn)
+
+## Installation
+
+```bash
+git clone https://github.com/XavierJiezou/cloudseg.git
+cd cloudseg
+conda env create -f environment.yaml
+conda activate cloudseg
+```
+
+## Usage
+
+**Train model with default configuration**
+
+```bash
+# train on CPU
+python src/train.py trainer=cpu
+
+# train on GPU
+python src/train.py trainer=gpu
+```
+
+**Train model with chosen experiment configuration from [configs/experiment/](configs/experiment/)**
+
+```bash
+python src/train.py experiment=experiment_name.yaml
+```
+
+**Tranin Example**
+
+```bash
+python src/train.py experiment=hrcwhu_cdnetv1.yaml
+```
+
+**You can override any parameter from command line like this**
+
+```bash
+python src/train.py trainer.max_epochs=20 data.batch_size=64
+```
+
+**Visualization in wandb**
+
+```bash
+python wand_vis.py --model-name model_name
+```
+
+**Example**
+
+```bash
+python wand_vis.py --model-name cdnetv1
+```
+
+
diff --git a/app.py b/app.py
index bf716a2f7485ab8544195ff5c40df7baca596c77..68032bee96284a475f2a41d052ed1f634ae523dc 100644
--- a/app.py
+++ b/app.py
@@ -1,140 +1,142 @@
-# -*- coding: utf-8 -*-
-# @Time : 2024/8/4 下午2:38
-# @Author : xiaoshun
-# @Email : 3038523973@qq.com
-# @File : app.py
-# @Software: PyCharm
-
-from glob import glob
-
-import albumentations as albu
-import gradio as gr
-import numpy as np
-import torch
-from PIL import Image
-from albumentations.pytorch.transforms import ToTensorV2
-
-from src.models.components.cdnetv1 import CDnetV1
-from src.models.components.cdnetv2 import CDnetV2
-from src.models.components.dbnet import DBNet
-from src.models.components.hrcloudnet import HRCloudNet
-from src.models.components.mcdnet import MCDNet
-from src.models.components.scnn import SCNN
-
-
-class Application:
- def __init__(self):
- self.device = "cuda" if torch.cuda.is_available() else "cpu"
- self.models = {
- "cdnetv1": CDnetV1(num_classes=2).to(self.device),
- "cdnetv2": CDnetV2(num_classes=2).to(self.device),
- "hrcloudnet": HRCloudNet(num_classes=2).to(self.device),
- "mcdnet": MCDNet(in_channels=3, num_classes=2).to(self.device),
- "scnn": SCNN(num_classes=2).to(self.device),
- "dbnet": DBNet(img_size=256, in_channels=3, num_classes=2).to(
- self.device
- ),
- }
- self.__load_weight()
- self.transform = albu.Compose(
- [
- albu.Resize(256, 256, always_apply=True),
- ToTensorV2(),
- ]
- )
-
- def __load_weight(self):
- """
- 将模型权重加载进来
- """
- for model_name, model in self.models.items():
- weight_path = glob(
- f"logs/train/runs/*{model_name}*/*/checkpoints/*epoch*.ckpt"
- )[0]
- weight = torch.load(weight_path, map_location=self.device)
- state_dict = {}
- for key, value in weight["state_dict"].items():
- new_key = key[4:]
- state_dict[new_key] = value
- model.load_state_dict(state_dict)
- model.eval()
- print(f"{model_name} weight loaded!")
-
- @torch.no_grad
- def inference(self, image: torch.Tensor, model_name: str):
- x = image.float()
- x = x.unsqueeze(0)
- x = x.to(self.device)
- logits = self.models[model_name](x)
- if isinstance(logits, tuple):
- logits = logits[0]
- fake_mask = torch.argmax(logits, 1).detach().cpu().squeeze(0).numpy()
- return fake_mask
-
- def give_colors_to_mask(self, mask: np.ndarray):
- """
- 赋予mask颜色
- """
- assert len(mask.shape) == 2, "Value Error,mask的形状为(height,width)"
- colors_mask = np.zeros((mask.shape[0], mask.shape[1], 3)).astype(np.float32)
- colors = ((255, 255, 255), (128, 192, 128))
- for color in range(2):
- segc = mask == color
- colors_mask[:, :, 0] += segc * (colors[color][0])
- colors_mask[:, :, 1] += segc * (colors[color][1])
- colors_mask[:, :, 2] += segc * (colors[color][2])
- return colors_mask
-
- def to_pil(self, image: np.ndarray, width=None, height=None):
- colors_np = self.give_colors_to_mask(image)
- pil_np = Image.fromarray(np.uint8(colors_np))
- if width and height:
- pil_np = pil_np.resize((width, height))
- return pil_np
-
- def flip(self, image_pil: Image.Image, model_name: str):
- if image_pil is None:
- return Image.fromarray(np.uint8(np.random.random((32,32,3)) * 255)), "请上传一张图片"
- if model_name is None:
- return Image.fromarray(np.uint8(np.random.random((32,32,3)) * 255)), "请选择模型名称"
- image = np.array(image_pil)
- raw_height, raw_width = image.shape[0], image.shape[1]
- transform = self.transform(image=image)
- image = transform["image"]
- image = image / 255.0
- fake_image = self.inference(image, model_name)
- fake_image = self.to_pil(fake_image, raw_width, raw_height)
- return fake_image,"success"
-
- def tiff_to_png(image: Image.Image):
- if image.format == "TIFF":
- image = image.convert("RGB")
- return np.array(image)
-
- def run(self):
- app = gr.Interface(
- self.flip,
- [
- gr.Image(sources=["clipboard", "upload"], type="pil"),
- gr.Radio(
- ["cdnetv1", "cdnetv2", "hrcloud", "mcdnet", "scnn", "dbnet"],
- label="model_name",
- info="选择使用的模型",
- ),
- ],
- [gr.Image(), gr.Textbox(label="提示信息")],
- examples=[
- ["examples_png/barren_11.png", "dbnet"],
- ["examples_png/snow_10.png", "scnn"],
- ["examples_png/vegetation_21.png", "cdnetv2"],
- ["examples_png/water_22.png", "hrcloud"],
- ],
- title="云检测模型在线演示",
- submit_btn=gr.Button("Submit", variant="primary")
- )
- app.launch(share=True)
-
-
-if __name__ == "__main__":
- app = Application()
- app.run()
+# -*- coding: utf-8 -*-
+# @Time : 2024/8/4 下午2:38
+# @Author : xiaoshun
+# @Email : 3038523973@qq.com
+# @File : app.py
+# @Software: PyCharm
+
+from glob import glob
+
+import albumentations as albu
+import gradio as gr
+import numpy as np
+import torch
+from PIL import Image
+from albumentations.pytorch.transforms import ToTensorV2
+
+from src.models.components.cdnetv1 import CDnetV1
+from src.models.components.cdnetv2 import CDnetV2
+from src.models.components.dbnet import DBNet
+from src.models.components.hrcloudnet import HRCloudNet
+from src.models.components.mcdnet import MCDNet
+from src.models.components.scnn import SCNN
+from src.models.components.unetmobv2 import UNetMobV2
+
+
+class Application:
+ def __init__(self):
+ self.device = "cuda" if torch.cuda.is_available() else "cpu"
+ self.models = {
+ "cdnetv1": CDnetV1(num_classes=2).to(self.device),
+ "cdnetv2": CDnetV2(num_classes=2).to(self.device),
+ "hrcloudnet": HRCloudNet(num_classes=2).to(self.device),
+ "mcdnet": MCDNet(in_channels=3, num_classes=2).to(self.device),
+ "scnn": SCNN(num_classes=2).to(self.device),
+ "dbnet": DBNet(img_size=256, in_channels=3, num_classes=2).to(
+ self.device
+ ),
+ "unetmobv2": UNetMobV2(num_classes=2).to(self.device),
+ }
+ self.__load_weight()
+ self.transform = albu.Compose(
+ [
+ albu.Resize(256, 256, always_apply=True),
+ ToTensorV2(),
+ ]
+ )
+
+ def __load_weight(self):
+ """
+ 将模型权重加载进来
+ """
+ for model_name, model in self.models.items():
+ weight_path = glob(
+ f"logs/train/runs/*{model_name}*/*/checkpoints/*epoch*.ckpt"
+ )[0]
+ weight = torch.load(weight_path, map_location=self.device)
+ state_dict = {}
+ for key, value in weight["state_dict"].items():
+ new_key = key[4:]
+ state_dict[new_key] = value
+ model.load_state_dict(state_dict)
+ model.eval()
+ print(f"{model_name} weight loaded!")
+
+ @torch.no_grad
+ def inference(self, image: torch.Tensor, model_name: str):
+ x = image.float()
+ x = x.unsqueeze(0)
+ x = x.to(self.device)
+ logits = self.models[model_name](x)
+ if isinstance(logits, tuple):
+ logits = logits[0]
+ fake_mask = torch.argmax(logits, 1).detach().cpu().squeeze(0).numpy()
+ return fake_mask
+
+ def give_colors_to_mask(self, mask: np.ndarray):
+ """
+ 赋予mask颜色
+ """
+ assert len(mask.shape) == 2, "Value Error,mask的形状为(height,width)"
+ colors_mask = np.zeros((mask.shape[0], mask.shape[1], 3)).astype(np.float32)
+ colors = ((255, 255, 255), (128, 192, 128))
+ for color in range(2):
+ segc = mask == color
+ colors_mask[:, :, 0] += segc * (colors[color][0])
+ colors_mask[:, :, 1] += segc * (colors[color][1])
+ colors_mask[:, :, 2] += segc * (colors[color][2])
+ return colors_mask
+
+ def to_pil(self, image: np.ndarray, width=None, height=None):
+ colors_np = self.give_colors_to_mask(image)
+ pil_np = Image.fromarray(np.uint8(colors_np))
+ if width and height:
+ pil_np = pil_np.resize((width, height))
+ return pil_np
+
+ def flip(self, image_pil: Image.Image, model_name: str):
+ if image_pil is None:
+ return Image.fromarray(np.uint8(np.random.random((32, 32, 3)) * 255)), "请上传一张图片"
+ if model_name is None:
+ return Image.fromarray(np.uint8(np.random.random((32, 32, 3)) * 255)), "请选择模型名称"
+ image = np.array(image_pil)
+ raw_height, raw_width = image.shape[0], image.shape[1]
+ transform = self.transform(image=image)
+ image = transform["image"]
+ image = image / 255.0
+ fake_image = self.inference(image, model_name)
+ fake_image = self.to_pil(fake_image, raw_width, raw_height)
+ return fake_image, "success"
+
+ def tiff_to_png(image: Image.Image):
+ if image.format == "TIFF":
+ image = image.convert("RGB")
+ return np.array(image)
+
+ def run(self):
+ app = gr.Interface(
+ self.flip,
+ [
+ gr.Image(sources=["clipboard", "upload"], type="pil"),
+ gr.Radio(
+ ["cdnetv1", "cdnetv2", "hrcloud", "mcdnet", "scnn", "dbnet", "unetmobv2"],
+ label="model_name",
+ info="选择使用的模型",
+ ),
+ ],
+ [gr.Image(), gr.Textbox(label="提示信息")],
+ examples=[
+ ["examples_png/barren_11.png", "dbnet"],
+ ["examples_png/snow_10.png", "scnn"],
+ ["examples_png/vegetation_21.png", "cdnetv2"],
+ ["examples_png/water_22.png", "hrcloud"],
+ ],
+ title="云检测模型在线演示",
+ submit_btn=gr.Button("Submit", variant="primary")
+ )
+ app.launch(share=True)
+
+
+if __name__ == "__main__":
+ app = Application()
+ app.run()
diff --git a/configs/__init__.py b/configs/__init__.py
index 56bf7f4aa4906bc0f997132708cc0826c198e4aa..3d3602a030a6ad96964fc76f1b38934ff36640cb 100644
--- a/configs/__init__.py
+++ b/configs/__init__.py
@@ -1 +1 @@
-# this file is needed here to include configs when building project as a package
+# this file is needed here to include configs when building project as a package
diff --git a/configs/callbacks/default.yaml b/configs/callbacks/default.yaml
index c9bf2fb8e6846c55916653a7b520e2cd624eef35..8c75adb00fcffb430b64dcaf6a94f1c7c707eb93 100644
--- a/configs/callbacks/default.yaml
+++ b/configs/callbacks/default.yaml
@@ -1,22 +1,22 @@
-defaults:
- - model_checkpoint
- - early_stopping
- - model_summary
- - rich_progress_bar
- - _self_
-
-model_checkpoint:
- dirpath: ${paths.output_dir}/checkpoints
- filename: "epoch_{epoch:03d}"
- monitor: "val/acc"
- mode: "max"
- save_last: True
- auto_insert_metric_name: False
-
-early_stopping:
- monitor: "val/acc"
- patience: 100
- mode: "max"
-
-model_summary:
- max_depth: -1
+defaults:
+ - model_checkpoint
+ - early_stopping
+ - model_summary
+ - rich_progress_bar
+ - _self_
+
+model_checkpoint:
+ dirpath: ${paths.output_dir}/checkpoints
+ filename: "epoch_{epoch:03d}"
+ monitor: "val/acc"
+ mode: "max"
+ save_last: True
+ auto_insert_metric_name: False
+
+early_stopping:
+ monitor: "val/acc"
+ patience: 100
+ mode: "max"
+
+model_summary:
+ max_depth: -1
diff --git a/configs/callbacks/early_stopping.yaml b/configs/callbacks/early_stopping.yaml
index c826c8d58651a5e2c7cca0e99948a9b6ccabccf3..2c19a5f7f49c801cbbccc0c8358f0cefa9cb2aaf 100644
--- a/configs/callbacks/early_stopping.yaml
+++ b/configs/callbacks/early_stopping.yaml
@@ -1,15 +1,15 @@
-# https://lightning.ai/docs/pytorch/stable/api/lightning.pytorch.callbacks.EarlyStopping.html
-
-early_stopping:
- _target_: lightning.pytorch.callbacks.EarlyStopping
- monitor: ??? # quantity to be monitored, must be specified !!!
- min_delta: 0. # minimum change in the monitored quantity to qualify as an improvement
- patience: 3 # number of checks with no improvement after which training will be stopped
- verbose: False # verbosity mode
- mode: "min" # "max" means higher metric value is better, can be also "min"
- strict: True # whether to crash the training if monitor is not found in the validation metrics
- check_finite: True # when set True, stops training when the monitor becomes NaN or infinite
- stopping_threshold: null # stop training immediately once the monitored quantity reaches this threshold
- divergence_threshold: null # stop training as soon as the monitored quantity becomes worse than this threshold
- check_on_train_epoch_end: null # whether to run early stopping at the end of the training epoch
- # log_rank_zero_only: False # this keyword argument isn't available in stable version
+# https://lightning.ai/docs/pytorch/stable/api/lightning.pytorch.callbacks.EarlyStopping.html
+
+early_stopping:
+ _target_: lightning.pytorch.callbacks.EarlyStopping
+ monitor: ??? # quantity to be monitored, must be specified !!!
+ min_delta: 0. # minimum change in the monitored quantity to qualify as an improvement
+ patience: 3 # number of checks with no improvement after which training will be stopped
+ verbose: False # verbosity mode
+ mode: "min" # "max" means higher metric value is better, can be also "min"
+ strict: True # whether to crash the training if monitor is not found in the validation metrics
+ check_finite: True # when set True, stops training when the monitor becomes NaN or infinite
+ stopping_threshold: null # stop training immediately once the monitored quantity reaches this threshold
+ divergence_threshold: null # stop training as soon as the monitored quantity becomes worse than this threshold
+ check_on_train_epoch_end: null # whether to run early stopping at the end of the training epoch
+ # log_rank_zero_only: False # this keyword argument isn't available in stable version
diff --git a/configs/callbacks/model_checkpoint.yaml b/configs/callbacks/model_checkpoint.yaml
index bf946e88b1ecfaf96efa91428e4f38e17267b25f..114140863bd717654090d69f17fe69eaf228e5a6 100644
--- a/configs/callbacks/model_checkpoint.yaml
+++ b/configs/callbacks/model_checkpoint.yaml
@@ -1,17 +1,17 @@
-# https://lightning.ai/docs/pytorch/stable/api/lightning.pytorch.callbacks.ModelCheckpoint.html
-
-model_checkpoint:
- _target_: lightning.pytorch.callbacks.ModelCheckpoint
- dirpath: null # directory to save the model file
- filename: null # checkpoint filename
- monitor: null # name of the logged metric which determines when model is improving
- verbose: False # verbosity mode
- save_last: null # additionally always save an exact copy of the last checkpoint to a file last.ckpt
- save_top_k: 1 # save k best models (determined by above metric)
- mode: "min" # "max" means higher metric value is better, can be also "min"
- auto_insert_metric_name: True # when True, the checkpoints filenames will contain the metric name
- save_weights_only: False # if True, then only the model’s weights will be saved
- every_n_train_steps: null # number of training steps between checkpoints
- train_time_interval: null # checkpoints are monitored at the specified time interval
- every_n_epochs: null # number of epochs between checkpoints
- save_on_train_epoch_end: null # whether to run checkpointing at the end of the training epoch or the end of validation
+# https://lightning.ai/docs/pytorch/stable/api/lightning.pytorch.callbacks.ModelCheckpoint.html
+
+model_checkpoint:
+ _target_: lightning.pytorch.callbacks.ModelCheckpoint
+ dirpath: null # directory to save the model file
+ filename: null # checkpoint filename
+ monitor: null # name of the logged metric which determines when model is improving
+ verbose: False # verbosity mode
+ save_last: null # additionally always save an exact copy of the last checkpoint to a file last.ckpt
+ save_top_k: 1 # save k best models (determined by above metric)
+ mode: "min" # "max" means higher metric value is better, can be also "min"
+ auto_insert_metric_name: True # when True, the checkpoints filenames will contain the metric name
+ save_weights_only: False # if True, then only the model’s weights will be saved
+ every_n_train_steps: null # number of training steps between checkpoints
+ train_time_interval: null # checkpoints are monitored at the specified time interval
+ every_n_epochs: null # number of epochs between checkpoints
+ save_on_train_epoch_end: null # whether to run checkpointing at the end of the training epoch or the end of validation
diff --git a/configs/callbacks/model_summary.yaml b/configs/callbacks/model_summary.yaml
index b75981d8cd5d73f61088d80495dc540274bca3d1..65534b948e02850fe384dd2e4dc0c6b4732220ae 100644
--- a/configs/callbacks/model_summary.yaml
+++ b/configs/callbacks/model_summary.yaml
@@ -1,5 +1,5 @@
-# https://lightning.ai/docs/pytorch/stable/api/lightning.pytorch.callbacks.RichModelSummary.html
-
-model_summary:
- _target_: lightning.pytorch.callbacks.RichModelSummary
- max_depth: 1 # the maximum depth of layer nesting that the summary will include
+# https://lightning.ai/docs/pytorch/stable/api/lightning.pytorch.callbacks.RichModelSummary.html
+
+model_summary:
+ _target_: lightning.pytorch.callbacks.RichModelSummary
+ max_depth: 1 # the maximum depth of layer nesting that the summary will include
diff --git a/configs/callbacks/rich_progress_bar.yaml b/configs/callbacks/rich_progress_bar.yaml
index de6f1ccb11205a4db93645fb6f297e50205de172..e07975864ea35ca3f8984f4cf94dab9aa979e534 100644
--- a/configs/callbacks/rich_progress_bar.yaml
+++ b/configs/callbacks/rich_progress_bar.yaml
@@ -1,4 +1,4 @@
-# https://lightning.ai/docs/pytorch/latest/api/lightning.pytorch.callbacks.RichProgressBar.html
-
-rich_progress_bar:
- _target_: lightning.pytorch.callbacks.RichProgressBar
+# https://lightning.ai/docs/pytorch/latest/api/lightning.pytorch.callbacks.RichProgressBar.html
+
+rich_progress_bar:
+ _target_: lightning.pytorch.callbacks.RichProgressBar
diff --git a/configs/data/CloudSEN12/README.md b/configs/data/CloudSEN12/README.md
index 738132e3f672c3222181b8834f4f6e4d8efd398f..239eaae623996d0e3bfdcabb17c42f81984c0b5c 100644
--- a/configs/data/CloudSEN12/README.md
+++ b/configs/data/CloudSEN12/README.md
@@ -1,52 +1,52 @@
-# CloudSEN12
-
-> [CloudSEN12, a global dataset for semantic understanding of cloud and cloud shadow in Sentinel-2](https://www.nature.com/articles/s41597-022-01878-2)
-
-## Introduction
-
-- [Official Site](https://cloudsen12.github.io/download.html)
-- [Paper Download](https://www.nature.com/articles/s41597-022-01878-2.pdf)
-- Data Download: [Hugging Face](https://huggingface.co/datasets/csaybar/CloudSEN12-high)
-
-## Abstract
-
-Accurately characterizing clouds and their shadows is a long-standing problem in the Earth Observation community. Recent works showcase the necessity to improve cloud detection methods for imagery acquired by the Sentinel-2 satellites. However, the lack of consensus and transparency in existing reference datasets hampers the benchmarking of current cloud detection methods. Exploiting the analysis-ready data offered by the Copernicus program, we created CloudSEN12, a new multi-temporal global dataset to foster research in cloud and cloud shadow detection. CloudSEN12 has 49,400 image patches, including Sentinel-2 level-1C and level-2A multi-spectral data, Sentinel-1 synthetic aperture radar data, auxiliary remote sensing products, different hand-crafted annotations to label the presence of thick and thin clouds and cloud shadows, and the results from eight state-of-the-art cloud detection algorithms. At present, CloudSEN12 exceeds all previous efforts in terms of annotation richness, scene variability, geographic distribution, metadata complexity, quality control, and number of samples.
-
-## Dataset
-
-CloudSEN12 is a LARGE dataset (~1 TB) for cloud semantic understanding that consists of 49,400 image patches (IP) that are evenly spread throughout all continents except Antarctica. Each IP covers 5090 x 5090 meters and contains data from Sentinel-2 levels 1C and 2A, hand-crafted annotations of thick and thin clouds and cloud shadows, Sentinel-1 Synthetic Aperture Radar (SAR), digital elevation model, surface water occurrence, land cover classes, and cloud mask results from six cutting-edge cloud detection algorithms.
-
-
-
-```
-name: CloudSEN12
-source: Sentinel-1,2
-band: 12
-resolution: 10m
-pixel: 512x512
-train: 8490
-val: 535
-test: 975
-disk: (~1 TB)
-annotation:
- - 0: Clear
- - 1: Thick cloud
- - 2: Thin cloud
- - 3: Cloud shadow
-scene: -
-```
-
-## Citation
-
-```
-@article{cloudsen12,
- title={CloudSEN12, a global dataset for semantic understanding of cloud and cloud shadow in Sentinel-2},
- author={Aybar, Cesar and Ysuhuaylas, Luis and Loja, Jhomira and Gonzales, Karen and Herrera, Fernando and Bautista, Lesly and Yali, Roy and Flores, Angie and Diaz, Lissette and Cuenca, Nicole and others},
- journal={Scientific data},
- volume={9},
- number={1},
- pages={782},
- year={2022},
- publisher={Nature Publishing Group UK London}
-}
-```
+# CloudSEN12
+
+> [CloudSEN12, a global dataset for semantic understanding of cloud and cloud shadow in Sentinel-2](https://www.nature.com/articles/s41597-022-01878-2)
+
+## Introduction
+
+- [Official Site](https://cloudsen12.github.io/download.html)
+- [Paper Download](https://www.nature.com/articles/s41597-022-01878-2.pdf)
+- Data Download: [Hugging Face](https://huggingface.co/datasets/csaybar/CloudSEN12-high)
+
+## Abstract
+
+Accurately characterizing clouds and their shadows is a long-standing problem in the Earth Observation community. Recent works showcase the necessity to improve cloud detection methods for imagery acquired by the Sentinel-2 satellites. However, the lack of consensus and transparency in existing reference datasets hampers the benchmarking of current cloud detection methods. Exploiting the analysis-ready data offered by the Copernicus program, we created CloudSEN12, a new multi-temporal global dataset to foster research in cloud and cloud shadow detection. CloudSEN12 has 49,400 image patches, including Sentinel-2 level-1C and level-2A multi-spectral data, Sentinel-1 synthetic aperture radar data, auxiliary remote sensing products, different hand-crafted annotations to label the presence of thick and thin clouds and cloud shadows, and the results from eight state-of-the-art cloud detection algorithms. At present, CloudSEN12 exceeds all previous efforts in terms of annotation richness, scene variability, geographic distribution, metadata complexity, quality control, and number of samples.
+
+## Dataset
+
+CloudSEN12 is a LARGE dataset (~1 TB) for cloud semantic understanding that consists of 49,400 image patches (IP) that are evenly spread throughout all continents except Antarctica. Each IP covers 5090 x 5090 meters and contains data from Sentinel-2 levels 1C and 2A, hand-crafted annotations of thick and thin clouds and cloud shadows, Sentinel-1 Synthetic Aperture Radar (SAR), digital elevation model, surface water occurrence, land cover classes, and cloud mask results from six cutting-edge cloud detection algorithms.
+
+
+
+```
+name: CloudSEN12
+source: Sentinel-1,2
+band: 12
+resolution: 10m
+pixel: 512x512
+train: 8490
+val: 535
+test: 975
+disk: (~1 TB)
+annotation:
+ - 0: Clear
+ - 1: Thick cloud
+ - 2: Thin cloud
+ - 3: Cloud shadow
+scene: -
+```
+
+## Citation
+
+```
+@article{cloudsen12,
+ title={CloudSEN12, a global dataset for semantic understanding of cloud and cloud shadow in Sentinel-2},
+ author={Aybar, Cesar and Ysuhuaylas, Luis and Loja, Jhomira and Gonzales, Karen and Herrera, Fernando and Bautista, Lesly and Yali, Roy and Flores, Angie and Diaz, Lissette and Cuenca, Nicole and others},
+ journal={Scientific data},
+ volume={9},
+ number={1},
+ pages={782},
+ year={2022},
+ publisher={Nature Publishing Group UK London}
+}
+```
diff --git a/configs/data/GF12-MS-WHU/README.md b/configs/data/GF12-MS-WHU/README.md
index b9489e4d9195cd9c627d063ce0be1616a2914de2..865265f3dbd9288f124146b2566ec2da282aa71c 100644
--- a/configs/data/GF12-MS-WHU/README.md
+++ b/configs/data/GF12-MS-WHU/README.md
@@ -1,72 +1,72 @@
-# GaoFen12
-
-> [Transferring Deep Models for Cloud Detection in Multisensor Images via Weakly Supervised Learning](https://ieeexplore.ieee.org/document/10436637)
-
-## Introduction
-
-- [Official Site](https://github.com/whu-ZSC/GF1-GF2MS-WHU)
-- [Paper Download](https://zhiweili.net/assets/pdf/2024.2_TGRS_Transferring%20Deep%20Models%20for%20Cloud%20Detection%20in%20Multisensor%20Images%20via%20Weakly%20Supervised%20Learning.pdf)
-- Data Download: [Baidu Disk](https://pan.baidu.com/s/1kBpym0mW_TS9YL1GQ9t8Hw) (password: 9zuf)
-
-## Abstract
-
-Recently, deep learning has been widely used for cloud detection in satellite images; however, due to radiometric and spatial resolution differences in images from different sensors and time-consuming process of manually labeling cloud detection datasets, it is difficult to effectively generalize deep learning models for cloud detection in multisensor images. This article propose a weakly supervised learning method for transferring deep models for cloud detection in multisensor images (TransMCD), which leverages the generalization of deep models and the spectral features of clouds to construct pseudo-label dataset to improve the generalization of models. A deep model is first pretrained using a well-annotated cloud detection dataset, which is used to obtain a rough cloud mask of unlabeled target image. The rough mask can be used to determine the spectral threshold adaptively for cloud segmentation of target image. Block-level pseudo labels with high confidence in target image are selected using the rough mask and spectral mask. Unsupervised segmentation technique is used to construct a high-quality pixel-level pseudo-label dataset. Finally, the pseudo-label dataset is used as supervised information for transferring the pretrained model to target image. The TransMCD method was validated by transferring model trained on 16-m Gaofen-1 wide field of view(WFV)images to 8-m Gaofen-1, 4-m Gaofen-2, and 10-m Sentinel-2 images. The F1-score of the transferred models on target images achieves improvements of 1.23%–9.63% over the pretrained models, which is comparable to the fully-supervised models trained with well-annotated target images, suggesting the efficiency of the TransMCD method for cloud detection in multisensor images.
-
-## Dataset
-
-### GF1MS-WHU Dataset
-
-> The two GF-1 PMS sensors have four MS bands with an 8-m spatial resolution and a panchromatic (PAN) band with a higher spatial resolution of 2 m. The spectral range of the MS bands is identical to that of the WFV sensors. In this study, 141 unlabeled images collected from various regions in China were used as the training data for the proposed method. In addition, 33 labeled images were used as the training data for the fully supervised methods, as well as the validation data for the different methods. The acquisition of the images spanned from June 2014 to December 2020 and encompassed four MS bands in both PMS sensors. Note that Fig. 7 only presents the distribution regions of the labeled images.
-
-```yaml
-name: GF1MS-WHU
-source: GaoFen-1
-band: 4 (MS)
-resolution: 8m (MS), 2m (PAN)
-pixel: 250x250
-train: 6343
-val: -
-test: 4085
-disk: 10.8GB
-annotation:
- - 0: clear sky
- - 1: cloud
-scene: [Forest,Urban,Barren,Water,Farmland,Grass,Wetland]
-```
-
-### GF2MS-WHU Dataset
-
-> The GF-2 satellite is configured with two PMS sensors. Each sensor has four MS bands with a 4-m spatial resolution and a PAN band with a 1-m spatial resolution. The GF-2 PMS sensors have the same bandwidth as the GF-1 WFV sensors. In this study, 163 unlabeled images obtained from Hubei, Jilin, and Hainan provinces were used as the training data for the proposed method, and 29 labeled images were used as the training data for the fully supervised methods, as well as the validation data for the different methods. The images were acquired from June 2014 to October 2020 and included four MS bands in both PMS sensors.
-
-```yaml
-name: GF2MS-WHU
-source: GaoFen-2
-band: 4 (MS)
-resolution: 4m (MS), 1m (PAN)
-pixel: 250x250
-train: 14357
-val: -
-test: 7560
-disk: 26.7GB
-annotation:
- - 0: clear sky
- - 1: cloud
-scene: [Forest,Urban,Barren,Water,Farmland,Grass,Wetland]
-```
-
-
-## Citation
-
-```bibtex
-@ARTICLE{gaofen12,
- author={Zhu, Shaocong and Li, Zhiwei and Shen, Huanfeng},
- journal={IEEE Transactions on Geoscience and Remote Sensing},
- title={Transferring Deep Models for Cloud Detection in Multisensor Images via Weakly Supervised Learning},
- year={2024},
- volume={62},
- number={},
- pages={1-18},
- keywords={Cloud computing;Clouds;Sensors;Predictive models;Supervised learning;Image segmentation;Deep learning;Cloud detection;deep learning;multisensor images;weakly supervised learning},
- doi={10.1109/TGRS.2024.3358824}
-}
-```
+# GaoFen12
+
+> [Transferring Deep Models for Cloud Detection in Multisensor Images via Weakly Supervised Learning](https://ieeexplore.ieee.org/document/10436637)
+
+## Introduction
+
+- [Official Site](https://github.com/whu-ZSC/GF1-GF2MS-WHU)
+- [Paper Download](https://zhiweili.net/assets/pdf/2024.2_TGRS_Transferring%20Deep%20Models%20for%20Cloud%20Detection%20in%20Multisensor%20Images%20via%20Weakly%20Supervised%20Learning.pdf)
+- Data Download: [Baidu Disk](https://pan.baidu.com/s/1kBpym0mW_TS9YL1GQ9t8Hw) (password: 9zuf)
+
+## Abstract
+
+Recently, deep learning has been widely used for cloud detection in satellite images; however, due to radiometric and spatial resolution differences in images from different sensors and time-consuming process of manually labeling cloud detection datasets, it is difficult to effectively generalize deep learning models for cloud detection in multisensor images. This article propose a weakly supervised learning method for transferring deep models for cloud detection in multisensor images (TransMCD), which leverages the generalization of deep models and the spectral features of clouds to construct pseudo-label dataset to improve the generalization of models. A deep model is first pretrained using a well-annotated cloud detection dataset, which is used to obtain a rough cloud mask of unlabeled target image. The rough mask can be used to determine the spectral threshold adaptively for cloud segmentation of target image. Block-level pseudo labels with high confidence in target image are selected using the rough mask and spectral mask. Unsupervised segmentation technique is used to construct a high-quality pixel-level pseudo-label dataset. Finally, the pseudo-label dataset is used as supervised information for transferring the pretrained model to target image. The TransMCD method was validated by transferring model trained on 16-m Gaofen-1 wide field of view(WFV)images to 8-m Gaofen-1, 4-m Gaofen-2, and 10-m Sentinel-2 images. The F1-score of the transferred models on target images achieves improvements of 1.23%–9.63% over the pretrained models, which is comparable to the fully-supervised models trained with well-annotated target images, suggesting the efficiency of the TransMCD method for cloud detection in multisensor images.
+
+## Dataset
+
+### GF1MS-WHU Dataset
+
+> The two GF-1 PMS sensors have four MS bands with an 8-m spatial resolution and a panchromatic (PAN) band with a higher spatial resolution of 2 m. The spectral range of the MS bands is identical to that of the WFV sensors. In this study, 141 unlabeled images collected from various regions in China were used as the training data for the proposed method. In addition, 33 labeled images were used as the training data for the fully supervised methods, as well as the validation data for the different methods. The acquisition of the images spanned from June 2014 to December 2020 and encompassed four MS bands in both PMS sensors. Note that Fig. 7 only presents the distribution regions of the labeled images.
+
+```yaml
+name: GF1MS-WHU
+source: GaoFen-1
+band: 4 (MS)
+resolution: 8m (MS), 2m (PAN)
+pixel: 250x250
+train: 6343
+val: -
+test: 4085
+disk: 10.8GB
+annotation:
+ - 0: clear sky
+ - 1: cloud
+scene: [Forest,Urban,Barren,Water,Farmland,Grass,Wetland]
+```
+
+### GF2MS-WHU Dataset
+
+> The GF-2 satellite is configured with two PMS sensors. Each sensor has four MS bands with a 4-m spatial resolution and a PAN band with a 1-m spatial resolution. The GF-2 PMS sensors have the same bandwidth as the GF-1 WFV sensors. In this study, 163 unlabeled images obtained from Hubei, Jilin, and Hainan provinces were used as the training data for the proposed method, and 29 labeled images were used as the training data for the fully supervised methods, as well as the validation data for the different methods. The images were acquired from June 2014 to October 2020 and included four MS bands in both PMS sensors.
+
+```yaml
+name: GF2MS-WHU
+source: GaoFen-2
+band: 4 (MS)
+resolution: 4m (MS), 1m (PAN)
+pixel: 250x250
+train: 14357
+val: -
+test: 7560
+disk: 26.7GB
+annotation:
+ - 0: clear sky
+ - 1: cloud
+scene: [Forest,Urban,Barren,Water,Farmland,Grass,Wetland]
+```
+
+
+## Citation
+
+```bibtex
+@ARTICLE{gaofen12,
+ author={Zhu, Shaocong and Li, Zhiwei and Shen, Huanfeng},
+ journal={IEEE Transactions on Geoscience and Remote Sensing},
+ title={Transferring Deep Models for Cloud Detection in Multisensor Images via Weakly Supervised Learning},
+ year={2024},
+ volume={62},
+ number={},
+ pages={1-18},
+ keywords={Cloud computing;Clouds;Sensors;Predictive models;Supervised learning;Image segmentation;Deep learning;Cloud detection;deep learning;multisensor images;weakly supervised learning},
+ doi={10.1109/TGRS.2024.3358824}
+}
+```
diff --git a/configs/data/L8-Biome/README.md b/configs/data/L8-Biome/README.md
index ba834dc4a86721a313f18746482a0d2b30a04364..71e2b5ca8ee104785507e8e75d158b584c1d0c2b 100644
--- a/configs/data/L8-Biome/README.md
+++ b/configs/data/L8-Biome/README.md
@@ -1,56 +1,56 @@
-# L8-Biome
-
-> [Cloud detection algorithm comparison and validation for operational Landsat data products](https://www.sciencedirect.com/science/article/abs/pii/S0034425717301293)
-
-## Introduction
-
-- [Official Site](https://landsat.usgs.gov/landsat-8-cloud-cover-assessment-validation-data)
-- [Paper Download](https://gerslab.cahnr.uconn.edu/wp-content/uploads/sites/2514/2021/06/1-s2.0-S0034425717301293-Steve_Foga_cloud_detection_2017.pdf)
-- Data Download: [USGS](https://landsat.usgs.gov/landsat-8-cloud-cover-assessment-validation-data)
-
-## Abstract
-
-Clouds are a pervasive and unavoidable issue in satellite-borne optical imagery. Accurate, well-documented, and automated cloud detection algorithms are necessary to effectively leverage large collections of remotely sensed data. The Landsat project is uniquely suited for comparative validation of cloud assessment algorithms because the modular architecture of the Landsat ground system allows for quick evaluation of new code, and because Landsat has the most comprehensive manual truth masks of any current satellite data archive. Currently, the Landsat Level-1 Product Generation System (LPGS) uses separate algorithms for determining clouds, cirrus clouds, and snow and/or ice probability on a per-pixel basis. With more bands onboard the Landsat 8 Operational Land Imager (OLI)/Thermal Infrared Sensor (TIRS) satellite, and a greater number of cloud masking algorithms, the U.S. Geological Survey (USGS) is replacing the current cloud masking workflow with a more robust algorithm that is capable of working across multiple Landsat sensors with minimal modification. Because of the inherent error from stray light and intermittent data availability of TIRS, these algorithms need to operate both with and without thermal data. In this study, we created a workflow to evaluate cloud and cloud shadow masking algorithms using cloud validation masks manually derived from both Landsat 7 Enhanced Thematic Mapper Plus (ETM+) and Landsat 8 OLI/TIRS data. We created a new validation dataset consisting of 96 Landsat 8 scenes, representing different biomes and proportions of cloud cover. We evaluated algorithm performance by overall accuracy, omission error, and commission error for both cloud and cloud shadow. We found that CFMask, C code based on the Function of Mask (Fmask) algorithm, and its confidence bands have the best overall accuracy among the many algorithms tested using our validation data. The Artificial Thermal-Automated Cloud Cover Algorithm (AT-ACCA) is the most accurate nonthermal-based algorithm. We give preference to CFMask for operational cloud and cloud shadow detection, as it is derived from a priori knowledge of physical phenomena and is operable without geographic restriction, making it useful for current and future land imaging missions without having to be retrained in a machine-learning environment.
-
-## Dataset
-
-This collection contains 96 Pre-Collection Landsat 8 Operational Land Imager (OLI) Thermal Infrared Sensor (TIRS) terrain-corrected (Level-1T) scenes, displayed in the biomes listed below. Manually generated cloud masks are used to validate cloud cover assessment algorithms, which in turn are intended to compute the percentage of cloud cover in each scene.
-
-
-
-
-```yaml
-name: hrc_whu
-source: Landsat-8 OLI/TIRS
-band: 9
-resolution: 30m
-pixel: ∼7000 × 6000
-train: -
-val: -
-test: -
-disk: 88GB
-annotation:
- - 0: Fill
- - 64: Cloud Shadow
- - 128: Clear
- - 192: Thin Cloud
- - 255: Cloud
-scene: [Barren,Forest,Grass/Crops,Shrubland,Snow/Ice,Urban,Water,Wetlands]
-```
-
-## Citation
-
-```bibtex
-@article{l8biome,
- title = {Cloud detection algorithm comparison and validation for operational Landsat data products},
- journal = {Remote Sensing of Environment},
- volume = {194},
- pages = {379-390},
- year = {2017},
- issn = {0034-4257},
- doi = {https://doi.org/10.1016/j.rse.2017.03.026},
- url = {https://www.sciencedirect.com/science/article/pii/S0034425717301293},
- author = {Steve Foga and Pat L. Scaramuzza and Song Guo and Zhe Zhu and Ronald D. Dilley and Tim Beckmann and Gail L. Schmidt and John L. Dwyer and M. {Joseph Hughes} and Brady Laue},
- keywords = {Landsat, CFMask, Cloud detection, Cloud validation masks, Biome sampling, Data products},
-}
-```
+# L8-Biome
+
+> [Cloud detection algorithm comparison and validation for operational Landsat data products](https://www.sciencedirect.com/science/article/abs/pii/S0034425717301293)
+
+## Introduction
+
+- [Official Site](https://landsat.usgs.gov/landsat-8-cloud-cover-assessment-validation-data)
+- [Paper Download](https://gerslab.cahnr.uconn.edu/wp-content/uploads/sites/2514/2021/06/1-s2.0-S0034425717301293-Steve_Foga_cloud_detection_2017.pdf)
+- Data Download: [USGS](https://landsat.usgs.gov/landsat-8-cloud-cover-assessment-validation-data)
+
+## Abstract
+
+Clouds are a pervasive and unavoidable issue in satellite-borne optical imagery. Accurate, well-documented, and automated cloud detection algorithms are necessary to effectively leverage large collections of remotely sensed data. The Landsat project is uniquely suited for comparative validation of cloud assessment algorithms because the modular architecture of the Landsat ground system allows for quick evaluation of new code, and because Landsat has the most comprehensive manual truth masks of any current satellite data archive. Currently, the Landsat Level-1 Product Generation System (LPGS) uses separate algorithms for determining clouds, cirrus clouds, and snow and/or ice probability on a per-pixel basis. With more bands onboard the Landsat 8 Operational Land Imager (OLI)/Thermal Infrared Sensor (TIRS) satellite, and a greater number of cloud masking algorithms, the U.S. Geological Survey (USGS) is replacing the current cloud masking workflow with a more robust algorithm that is capable of working across multiple Landsat sensors with minimal modification. Because of the inherent error from stray light and intermittent data availability of TIRS, these algorithms need to operate both with and without thermal data. In this study, we created a workflow to evaluate cloud and cloud shadow masking algorithms using cloud validation masks manually derived from both Landsat 7 Enhanced Thematic Mapper Plus (ETM+) and Landsat 8 OLI/TIRS data. We created a new validation dataset consisting of 96 Landsat 8 scenes, representing different biomes and proportions of cloud cover. We evaluated algorithm performance by overall accuracy, omission error, and commission error for both cloud and cloud shadow. We found that CFMask, C code based on the Function of Mask (Fmask) algorithm, and its confidence bands have the best overall accuracy among the many algorithms tested using our validation data. The Artificial Thermal-Automated Cloud Cover Algorithm (AT-ACCA) is the most accurate nonthermal-based algorithm. We give preference to CFMask for operational cloud and cloud shadow detection, as it is derived from a priori knowledge of physical phenomena and is operable without geographic restriction, making it useful for current and future land imaging missions without having to be retrained in a machine-learning environment.
+
+## Dataset
+
+This collection contains 96 Pre-Collection Landsat 8 Operational Land Imager (OLI) Thermal Infrared Sensor (TIRS) terrain-corrected (Level-1T) scenes, displayed in the biomes listed below. Manually generated cloud masks are used to validate cloud cover assessment algorithms, which in turn are intended to compute the percentage of cloud cover in each scene.
+
+
+
+
+```yaml
+name: hrc_whu
+source: Landsat-8 OLI/TIRS
+band: 9
+resolution: 30m
+pixel: ∼7000 × 6000
+train: -
+val: -
+test: -
+disk: 88GB
+annotation:
+ - 0: Fill
+ - 64: Cloud Shadow
+ - 128: Clear
+ - 192: Thin Cloud
+ - 255: Cloud
+scene: [Barren,Forest,Grass/Crops,Shrubland,Snow/Ice,Urban,Water,Wetlands]
+```
+
+## Citation
+
+```bibtex
+@article{l8biome,
+ title = {Cloud detection algorithm comparison and validation for operational Landsat data products},
+ journal = {Remote Sensing of Environment},
+ volume = {194},
+ pages = {379-390},
+ year = {2017},
+ issn = {0034-4257},
+ doi = {https://doi.org/10.1016/j.rse.2017.03.026},
+ url = {https://www.sciencedirect.com/science/article/pii/S0034425717301293},
+ author = {Steve Foga and Pat L. Scaramuzza and Song Guo and Zhe Zhu and Ronald D. Dilley and Tim Beckmann and Gail L. Schmidt and John L. Dwyer and M. {Joseph Hughes} and Brady Laue},
+ keywords = {Landsat, CFMask, Cloud detection, Cloud validation masks, Biome sampling, Data products},
+}
+```
diff --git a/configs/data/celeba.yaml b/configs/data/celeba.yaml
index 25890188131c0231ff3f155a6a6713082f235777..b9bb14edf44d0bc45eec5d926f911c1ecf3be153 100644
--- a/configs/data/celeba.yaml
+++ b/configs/data/celeba.yaml
@@ -1,8 +1,8 @@
-_target_: src.data.celeba_datamodule.CelebADataModule
-size: 512 # image size
-test_dataset_size: 3000
-conditions: [] # [] for image-only, ['seg_mask', 'text'] for multi-modal conditions, ['seg_mask'] for segmentation mask only, ['text'] for text only
-batch_size: 2
-num_workers: 8
-pin_memory: False
+_target_: src.data.celeba_datamodule.CelebADataModule
+size: 512 # image size
+test_dataset_size: 3000
+conditions: [] # [] for image-only, ['seg_mask', 'text'] for multi-modal conditions, ['seg_mask'] for segmentation mask only, ['text'] for text only
+batch_size: 2
+num_workers: 8
+pin_memory: False
persistent_workers: False
\ No newline at end of file
diff --git a/configs/data/hrcwhu/README.md b/configs/data/hrcwhu/README.md
index e51ea6785e39c9a6c8fbfaa3b14d38273822e1bd..727a5d16710a7f5590aaf98acdd6073ab522bcb0 100644
--- a/configs/data/hrcwhu/README.md
+++ b/configs/data/hrcwhu/README.md
@@ -1,56 +1,56 @@
-# HRC_WHU
-
-> [Deep learning based cloud detection for medium and high resolution remote sensing images of different sensors](https://www.sciencedirect.com/science/article/pii/S0924271619300565)
-
-## Introduction
-
-- [Official Site](http://sendimage.whu.edu.cn/en/hrc_whu/)
-- [Paper Download](http://sendimage.whu.edu.cn/en/wp-content/uploads/2019/03/2019_PHOTO_Zhiwei-Li_Deep-learning-based-cloud-detection-for-medium-and-high-resolution-remote-sensing-images-of-different-sensors.pdf)
-- Data Download: [Baidu Disk](https://pan.baidu.com/s/1thOTKVO2iTAalFAjFI2_ZQ) (password: ihfb) or [Google Drive](https://drive.google.com/file/d/1qqikjaX7tkfOONsF5EtR4vl6J7sToA6p/view?usp=sharing)
-
-## Abstract
-
-Cloud detection is an important preprocessing step for the precise application of optical satellite imagery. In this paper, we propose a deep learning based cloud detection method named multi-scale convolutional feature fusion (MSCFF) for remote sensing images of different sensors. In the network architecture of MSCFF, the symmetric encoder-decoder module, which provides both local and global context by densifying feature maps with trainable convolutional filter banks, is utilized to extract multi-scale and high-level spatial features. The feature maps of multiple scales are then up-sampled and concatenated, and a novel multi-scale feature fusion module is designed to fuse the features of different scales for the output. The two output feature maps of the network are cloud and cloud shadow maps, which are in turn fed to binary classifiers outside the model to obtain the final cloud and cloud shadow mask. The MSCFF method was validated on hundreds of globally distributed optical satellite images, with spatial resolutions ranging from 0.5 to 50 m, including Landsat-5/7/8, Gaofen-1/2/4, Sentinel-2, Ziyuan-3, CBERS-04, Huanjing-1, and collected high-resolution images exported from Google Earth. The experimental results show that MSCFF achieves a higher accuracy than the traditional rule-based cloud detection methods and the state-of-the-art deep learning models, especially in bright surface covered areas. The effectiveness of MSCFF means that it has great promise for the practical application of cloud detection for multiple types of medium and high-resolution remote sensing images. Our established global high-resolution cloud detection validation dataset has been made available online (http://sendimage.whu.edu.cn/en/mscff/).
-
-## Dataset
-
-The high-resolution cloud cover validation dataset was created by the SENDIMAGE Lab in Wuhan University, and has been termed HRC_WHU. The HRC_WHU data comprise 150 high-resolution images acquired with three RGB channels and a resolution varying from 0.5 to 15 m in different global regions. As shown in Fig. 1, the images were collected from Google Earth (Google Inc.) in five main land-cover types, i.e., water, vegetation, urban, snow/ice, and barren. The associated reference cloud masks were digitized by experts in the field of remote sensing image interpretation. The established high-resolution cloud cover validation dataset has been made available online.
-
-
-
-```yaml
-name: hrc_whu
-source: google earth
-band: 3 (rgb)
-resolution: 0.5m-15m
-pixel: 1280x720
-train: 120
-val: null
-test: 30
-disk: 168mb
-annotation:
- - 0: clear sky
- - 1: cloud
-scene: [water, vegetation, urban, snow/ice, barren]
-```
-
-## Annotation
-
-In the procedure of delineating the cloud mask for high-resolution imagery, we first stretched the cloudy image to the appropriate contrast in Adobe Photoshop. The lasso tool and magic wand tool were then alternately used to mark the locations of the clouds in the image. The manually labeled reference mask was finally created by assigning the pixel values of cloud and clear sky to 255 and 0, respectively. Note that a tolerance of 5–30 was set when using the magic wand tool, and the lasso tool was used to modify the areas that could not be correctly selected by the magic wand tool. As we did in a previous study (Li et al., 2017), the thin clouds were labeled as cloud if they were visually identifiable and the underlying surface could not be seen clearly. Considering that cloud shadows in high-resolution images are rare and hard to accurately select, only clouds were labeled in the reference masks.
-
-## Citation
-
-```bibtex
-@article{hrc_whu,
- title = {Deep learning based cloud detection for medium and high resolution remote sensing images of different sensors},
- journal = {ISPRS Journal of Photogrammetry and Remote Sensing},
- volume = {150},
- pages = {197-212},
- year = {2019},
- issn = {0924-2716},
- doi = {https://doi.org/10.1016/j.isprsjprs.2019.02.017},
- url = {https://www.sciencedirect.com/science/article/pii/S0924271619300565},
- author = {Zhiwei Li and Huanfeng Shen and Qing Cheng and Yuhao Liu and Shucheng You and Zongyi He},
- keywords = {Cloud detection, Cloud shadow, Convolutional neural network, Multi-scale, Convolutional feature fusion, MSCFF}
-}
-```
+# HRC_WHU
+
+> [Deep learning based cloud detection for medium and high resolution remote sensing images of different sensors](https://www.sciencedirect.com/science/article/pii/S0924271619300565)
+
+## Introduction
+
+- [Official Site](http://sendimage.whu.edu.cn/en/hrc_whu/)
+- [Paper Download](http://sendimage.whu.edu.cn/en/wp-content/uploads/2019/03/2019_PHOTO_Zhiwei-Li_Deep-learning-based-cloud-detection-for-medium-and-high-resolution-remote-sensing-images-of-different-sensors.pdf)
+- Data Download: [Baidu Disk](https://pan.baidu.com/s/1thOTKVO2iTAalFAjFI2_ZQ) (password: ihfb) or [Google Drive](https://drive.google.com/file/d/1qqikjaX7tkfOONsF5EtR4vl6J7sToA6p/view?usp=sharing)
+
+## Abstract
+
+Cloud detection is an important preprocessing step for the precise application of optical satellite imagery. In this paper, we propose a deep learning based cloud detection method named multi-scale convolutional feature fusion (MSCFF) for remote sensing images of different sensors. In the network architecture of MSCFF, the symmetric encoder-decoder module, which provides both local and global context by densifying feature maps with trainable convolutional filter banks, is utilized to extract multi-scale and high-level spatial features. The feature maps of multiple scales are then up-sampled and concatenated, and a novel multi-scale feature fusion module is designed to fuse the features of different scales for the output. The two output feature maps of the network are cloud and cloud shadow maps, which are in turn fed to binary classifiers outside the model to obtain the final cloud and cloud shadow mask. The MSCFF method was validated on hundreds of globally distributed optical satellite images, with spatial resolutions ranging from 0.5 to 50 m, including Landsat-5/7/8, Gaofen-1/2/4, Sentinel-2, Ziyuan-3, CBERS-04, Huanjing-1, and collected high-resolution images exported from Google Earth. The experimental results show that MSCFF achieves a higher accuracy than the traditional rule-based cloud detection methods and the state-of-the-art deep learning models, especially in bright surface covered areas. The effectiveness of MSCFF means that it has great promise for the practical application of cloud detection for multiple types of medium and high-resolution remote sensing images. Our established global high-resolution cloud detection validation dataset has been made available online (http://sendimage.whu.edu.cn/en/mscff/).
+
+## Dataset
+
+The high-resolution cloud cover validation dataset was created by the SENDIMAGE Lab in Wuhan University, and has been termed HRC_WHU. The HRC_WHU data comprise 150 high-resolution images acquired with three RGB channels and a resolution varying from 0.5 to 15 m in different global regions. As shown in Fig. 1, the images were collected from Google Earth (Google Inc.) in five main land-cover types, i.e., water, vegetation, urban, snow/ice, and barren. The associated reference cloud masks were digitized by experts in the field of remote sensing image interpretation. The established high-resolution cloud cover validation dataset has been made available online.
+
+
+
+```yaml
+name: hrc_whu
+source: google earth
+band: 3 (rgb)
+resolution: 0.5m-15m
+pixel: 1280x720
+train: 120
+val: null
+test: 30
+disk: 168mb
+annotation:
+ - 0: clear sky
+ - 1: cloud
+scene: [water, vegetation, urban, snow/ice, barren]
+```
+
+## Annotation
+
+In the procedure of delineating the cloud mask for high-resolution imagery, we first stretched the cloudy image to the appropriate contrast in Adobe Photoshop. The lasso tool and magic wand tool were then alternately used to mark the locations of the clouds in the image. The manually labeled reference mask was finally created by assigning the pixel values of cloud and clear sky to 255 and 0, respectively. Note that a tolerance of 5–30 was set when using the magic wand tool, and the lasso tool was used to modify the areas that could not be correctly selected by the magic wand tool. As we did in a previous study (Li et al., 2017), the thin clouds were labeled as cloud if they were visually identifiable and the underlying surface could not be seen clearly. Considering that cloud shadows in high-resolution images are rare and hard to accurately select, only clouds were labeled in the reference masks.
+
+## Citation
+
+```bibtex
+@article{hrc_whu,
+ title = {Deep learning based cloud detection for medium and high resolution remote sensing images of different sensors},
+ journal = {ISPRS Journal of Photogrammetry and Remote Sensing},
+ volume = {150},
+ pages = {197-212},
+ year = {2019},
+ issn = {0924-2716},
+ doi = {https://doi.org/10.1016/j.isprsjprs.2019.02.017},
+ url = {https://www.sciencedirect.com/science/article/pii/S0924271619300565},
+ author = {Zhiwei Li and Huanfeng Shen and Qing Cheng and Yuhao Liu and Shucheng You and Zongyi He},
+ keywords = {Cloud detection, Cloud shadow, Convolutional neural network, Multi-scale, Convolutional feature fusion, MSCFF}
+}
+```
diff --git a/configs/data/hrcwhu/hrcwhu.yaml b/configs/data/hrcwhu/hrcwhu.yaml
index 0d8d8fb405c3b22400a55a74f60a29d78ee42354..81ffdeeeefb451068aa8d04f84b8358636865271 100644
--- a/configs/data/hrcwhu/hrcwhu.yaml
+++ b/configs/data/hrcwhu/hrcwhu.yaml
@@ -1,89 +1,89 @@
-_target_: src.data.hrcwhu_datamodule.HRCWHUDataModule
-root: data/hrcwhu
-train_pipeline:
- all_transform:
- _target_: albumentations.Compose
- transforms:
- - _target_: albumentations.HorizontalFlip
- p: 0.5
- - _target_: albumentations.ShiftScaleRotate
- p: 1
- - _target_: albumentations.RandomCrop
- height: 256
- width: 256
- always_apply: true
- - _target_: albumentations.GaussNoise
- p: 0.2
- - _target_: albumentations.Perspective
- p: 0.5
- - _target_: albumentations.OneOf
- transforms:
- - _target_: albumentations.CLAHE
- p: 1
- - _target_: albumentations.RandomGamma
- p: 1
- p: 0.9
-
- - _target_: albumentations.OneOf
- transforms:
- - _target_: albumentations.Sharpen
- p: 1
- - _target_: albumentations.Blur
- p: 1
- - _target_: albumentations.MotionBlur
- p: 1
- p: 0.9
-
- - _target_: albumentations.OneOf
- transforms:
- - _target_: albumentations.RandomBrightnessContrast
- p: 1
- - _target_: albumentations.HueSaturationValue
- p: 1
- p: 0.9
-
- img_transform:
- _target_: albumentations.Compose
- transforms:
- - _target_: albumentations.ToFloat
- max_value: 255.0
- - _target_: albumentations.pytorch.transforms.ToTensorV2
-
- ann_transform: null
-val_pipeline:
- all_transform:
- _target_: albumentations.Compose
- transforms:
- - _target_: albumentations.Resize
- height: 256
- width: 256
-
- img_transform:
- _target_: albumentations.Compose
- transforms:
- - _target_: albumentations.ToFloat
- max_value: 255.0
- - _target_: albumentations.pytorch.transforms.ToTensorV2
- ann_transform: null
-
-test_pipeline:
- all_transform:
- _target_: albumentations.Compose
- transforms:
- - _target_: albumentations.Resize
- height: 256
- width: 256
-
- img_transform:
- _target_: albumentations.Compose
- transforms:
- - _target_: albumentations.ToFloat
- max_value: 255.0
- - _target_: albumentations.pytorch.transforms.ToTensorV2
- ann_transform: null
-
-seed: 42
-batch_size: 8
-num_workers: 8
-pin_memory: True
+_target_: src.data.hrcwhu_datamodule.HRCWHUDataModule
+root: data/hrcwhu
+train_pipeline:
+ all_transform:
+ _target_: albumentations.Compose
+ transforms:
+ - _target_: albumentations.HorizontalFlip
+ p: 0.5
+ - _target_: albumentations.ShiftScaleRotate
+ p: 1
+ - _target_: albumentations.RandomCrop
+ height: 256
+ width: 256
+ always_apply: true
+ - _target_: albumentations.GaussNoise
+ p: 0.2
+ - _target_: albumentations.Perspective
+ p: 0.5
+ - _target_: albumentations.OneOf
+ transforms:
+ - _target_: albumentations.CLAHE
+ p: 1
+ - _target_: albumentations.RandomGamma
+ p: 1
+ p: 0.9
+
+ - _target_: albumentations.OneOf
+ transforms:
+ - _target_: albumentations.Sharpen
+ p: 1
+ - _target_: albumentations.Blur
+ p: 1
+ - _target_: albumentations.MotionBlur
+ p: 1
+ p: 0.9
+
+ - _target_: albumentations.OneOf
+ transforms:
+ - _target_: albumentations.RandomBrightnessContrast
+ p: 1
+ - _target_: albumentations.HueSaturationValue
+ p: 1
+ p: 0.9
+
+ img_transform:
+ _target_: albumentations.Compose
+ transforms:
+ - _target_: albumentations.ToFloat
+ max_value: 255.0
+ - _target_: albumentations.pytorch.transforms.ToTensorV2
+
+ ann_transform: null
+val_pipeline:
+ all_transform:
+ _target_: albumentations.Compose
+ transforms:
+ - _target_: albumentations.Resize
+ height: 256
+ width: 256
+
+ img_transform:
+ _target_: albumentations.Compose
+ transforms:
+ - _target_: albumentations.ToFloat
+ max_value: 255.0
+ - _target_: albumentations.pytorch.transforms.ToTensorV2
+ ann_transform: null
+
+test_pipeline:
+ all_transform:
+ _target_: albumentations.Compose
+ transforms:
+ - _target_: albumentations.Resize
+ height: 256
+ width: 256
+
+ img_transform:
+ _target_: albumentations.Compose
+ transforms:
+ - _target_: albumentations.ToFloat
+ max_value: 255.0
+ - _target_: albumentations.pytorch.transforms.ToTensorV2
+ ann_transform: null
+
+seed: 42
+batch_size: 8
+num_workers: 8
+pin_memory: True
persistent_workers: True
\ No newline at end of file
diff --git a/configs/data/mnist.yaml b/configs/data/mnist.yaml
index 51bfaff092a1e3fe2551c89dafa7c7b90ebffe40..085f177e9006bd9d4306e2219776edb79488c5e7 100644
--- a/configs/data/mnist.yaml
+++ b/configs/data/mnist.yaml
@@ -1,6 +1,6 @@
-_target_: src.data.mnist_datamodule.MNISTDataModule
-data_dir: ${paths.data_dir}
-batch_size: 128 # Needs to be divisible by the number of devices (e.g., if in a distributed setup)
-train_val_test_split: [55_000, 5_000, 10_000]
-num_workers: 0
-pin_memory: False
+_target_: src.data.mnist_datamodule.MNISTDataModule
+data_dir: ${paths.data_dir}
+batch_size: 128 # Needs to be divisible by the number of devices (e.g., if in a distributed setup)
+train_val_test_split: [55_000, 5_000, 10_000]
+num_workers: 0
+pin_memory: False
diff --git a/configs/debug/default.yaml b/configs/debug/default.yaml
index 1886902b39f1be560e314bce7b3778f95b44754c..45ae915b81c319b264f27e8f5507a38c17f61cfc 100644
--- a/configs/debug/default.yaml
+++ b/configs/debug/default.yaml
@@ -1,35 +1,35 @@
-# @package _global_
-
-# default debugging setup, runs 1 full epoch
-# other debugging configs can inherit from this one
-
-# overwrite task name so debugging logs are stored in separate folder
-task_name: "debug"
-
-# disable callbacks and loggers during debugging
-callbacks: null
-logger: null
-
-extras:
- ignore_warnings: False
- enforce_tags: False
-
-# sets level of all command line loggers to 'DEBUG'
-# https://hydra.cc/docs/tutorials/basic/running_your_app/logging/
-hydra:
- job_logging:
- root:
- level: DEBUG
-
- # use this to also set hydra loggers to 'DEBUG'
- # verbose: True
-
-trainer:
- max_epochs: 1
- accelerator: cpu # debuggers don't like gpus
- devices: 1 # debuggers don't like multiprocessing
- detect_anomaly: true # raise exception if NaN or +/-inf is detected in any tensor
-
-data:
- num_workers: 0 # debuggers don't like multiprocessing
- pin_memory: False # disable gpu memory pin
+# @package _global_
+
+# default debugging setup, runs 1 full epoch
+# other debugging configs can inherit from this one
+
+# overwrite task name so debugging logs are stored in separate folder
+task_name: "debug"
+
+# disable callbacks and loggers during debugging
+callbacks: null
+logger: null
+
+extras:
+ ignore_warnings: False
+ enforce_tags: False
+
+# sets level of all command line loggers to 'DEBUG'
+# https://hydra.cc/docs/tutorials/basic/running_your_app/logging/
+hydra:
+ job_logging:
+ root:
+ level: DEBUG
+
+ # use this to also set hydra loggers to 'DEBUG'
+ # verbose: True
+
+trainer:
+ max_epochs: 1
+ accelerator: cpu # debuggers don't like gpus
+ devices: 1 # debuggers don't like multiprocessing
+ detect_anomaly: true # raise exception if NaN or +/-inf is detected in any tensor
+
+data:
+ num_workers: 0 # debuggers don't like multiprocessing
+ pin_memory: False # disable gpu memory pin
diff --git a/configs/debug/fdr.yaml b/configs/debug/fdr.yaml
index 7f2d34fa37c31017e749d5a4fc5ae6763e688b46..08964d8c300e9b1cbf67cfed480d0789e519ada5 100644
--- a/configs/debug/fdr.yaml
+++ b/configs/debug/fdr.yaml
@@ -1,9 +1,9 @@
-# @package _global_
-
-# runs 1 train, 1 validation and 1 test step
-
-defaults:
- - default
-
-trainer:
- fast_dev_run: true
+# @package _global_
+
+# runs 1 train, 1 validation and 1 test step
+
+defaults:
+ - default
+
+trainer:
+ fast_dev_run: true
diff --git a/configs/debug/limit.yaml b/configs/debug/limit.yaml
index 514d77fbd1475b03fff0372e3da3c2fa7ea7d190..dcaa1a744d04ad81a6dace82864d4c941e56195c 100644
--- a/configs/debug/limit.yaml
+++ b/configs/debug/limit.yaml
@@ -1,12 +1,12 @@
-# @package _global_
-
-# uses only 1% of the training data and 5% of validation/test data
-
-defaults:
- - default
-
-trainer:
- max_epochs: 3
- limit_train_batches: 0.01
- limit_val_batches: 0.05
- limit_test_batches: 0.05
+# @package _global_
+
+# uses only 1% of the training data and 5% of validation/test data
+
+defaults:
+ - default
+
+trainer:
+ max_epochs: 3
+ limit_train_batches: 0.01
+ limit_val_batches: 0.05
+ limit_test_batches: 0.05
diff --git a/configs/debug/overfit.yaml b/configs/debug/overfit.yaml
index 9906586a67a12aa81ff69138f589a366dbe2222f..0bdf4ea56c0d5962062360c272136d3798fc866f 100644
--- a/configs/debug/overfit.yaml
+++ b/configs/debug/overfit.yaml
@@ -1,13 +1,13 @@
-# @package _global_
-
-# overfits to 3 batches
-
-defaults:
- - default
-
-trainer:
- max_epochs: 20
- overfit_batches: 3
-
-# model ckpt and early stopping need to be disabled during overfitting
-callbacks: null
+# @package _global_
+
+# overfits to 3 batches
+
+defaults:
+ - default
+
+trainer:
+ max_epochs: 20
+ overfit_batches: 3
+
+# model ckpt and early stopping need to be disabled during overfitting
+callbacks: null
diff --git a/configs/debug/profiler.yaml b/configs/debug/profiler.yaml
index 2bd7da87ae23ed425ace99b09250a76a5634a3fb..bbb5c8b2826b7011b60095184f522b680e520f44 100644
--- a/configs/debug/profiler.yaml
+++ b/configs/debug/profiler.yaml
@@ -1,12 +1,12 @@
-# @package _global_
-
-# runs with execution time profiling
-
-defaults:
- - default
-
-trainer:
- max_epochs: 1
- profiler: "simple"
- # profiler: "advanced"
- # profiler: "pytorch"
+# @package _global_
+
+# runs with execution time profiling
+
+defaults:
+ - default
+
+trainer:
+ max_epochs: 1
+ profiler: "simple"
+ # profiler: "advanced"
+ # profiler: "pytorch"
diff --git a/configs/eval.yaml b/configs/eval.yaml
index be312992b2a486b04d83a54dbd8f670d94979709..d94707c0bc5b939cd3ad4baab2927e730d26e919 100644
--- a/configs/eval.yaml
+++ b/configs/eval.yaml
@@ -1,18 +1,18 @@
-# @package _global_
-
-defaults:
- - _self_
- - data: mnist # choose datamodule with `test_dataloader()` for evaluation
- - model: mnist
- - logger: null
- - trainer: default
- - paths: default
- - extras: default
- - hydra: default
-
-task_name: "eval"
-
-tags: ["dev"]
-
-# passing checkpoint path is necessary for evaluation
-ckpt_path: ???
+# @package _global_
+
+defaults:
+ - _self_
+ - data: mnist # choose datamodule with `test_dataloader()` for evaluation
+ - model: mnist
+ - logger: null
+ - trainer: default
+ - paths: default
+ - extras: default
+ - hydra: default
+
+task_name: "eval"
+
+tags: ["dev"]
+
+# passing checkpoint path is necessary for evaluation
+ckpt_path: ???
diff --git a/configs/experiment/hrcwhu_cdnetv1.yaml b/configs/experiment/hrcwhu_cdnetv1.yaml
index bce0bdcb8b33c209a21287bc1cbbb89f83f7c7db..545b3e3f7b82080722b75b8246c0cc7216d95075 100644
--- a/configs/experiment/hrcwhu_cdnetv1.yaml
+++ b/configs/experiment/hrcwhu_cdnetv1.yaml
@@ -1,47 +1,47 @@
-# @package _global_
-
-# to execute this experiment run:
-# python train.py experiment=example
-
-defaults:
- - override /trainer: gpu
- - override /data: hrcwhu/hrcwhu
- - override /model: cdnetv1/cdnetv1
- - override /logger: wandb
- - override /callbacks: default
-
-# all parameters below will be merged with parameters from default configurations set above
-# this allows you to overwrite only specified parameters
-
-tags: ["hrcWhu", "cdnetv1"]
-
-seed: 42
-
-
- # scheduler:
- # _target_: torch.optim.lr_scheduler.ReduceLROnPlateau
- # _partial_: true
- # mode: min
- # factor: 0.1
- # patience: 10
-
-logger:
- wandb:
- project: "hrcWhu"
- name: "cdnetv1"
- aim:
- experiment: "hrcwhu_cdnetv1"
-
-callbacks:
- model_checkpoint:
- dirpath: ${paths.output_dir}/checkpoints
- filename: "epoch_{epoch:03d}"
- monitor: "val/loss"
- mode: "min"
- save_last: True
- auto_insert_metric_name: False
-
- early_stopping:
- monitor: "val/loss"
- patience: 10
+# @package _global_
+
+# to execute this experiment run:
+# python train.py experiment=example
+
+defaults:
+ - override /trainer: gpu
+ - override /data: hrcwhu/hrcwhu
+ - override /model: cdnetv1/cdnetv1
+ - override /logger: wandb
+ - override /callbacks: default
+
+# all parameters below will be merged with parameters from default configurations set above
+# this allows you to overwrite only specified parameters
+
+tags: ["hrcWhu", "cdnetv1"]
+
+seed: 42
+
+
+ # scheduler:
+ # _target_: torch.optim.lr_scheduler.ReduceLROnPlateau
+ # _partial_: true
+ # mode: min
+ # factor: 0.1
+ # patience: 10
+
+logger:
+ wandb:
+ project: "hrcWhu"
+ name: "cdnetv1"
+ aim:
+ experiment: "hrcwhu_cdnetv1"
+
+callbacks:
+ model_checkpoint:
+ dirpath: ${paths.output_dir}/checkpoints
+ filename: "epoch_{epoch:03d}"
+ monitor: "val/loss"
+ mode: "min"
+ save_last: True
+ auto_insert_metric_name: False
+
+ early_stopping:
+ monitor: "val/loss"
+ patience: 10
mode: "min"
\ No newline at end of file
diff --git a/configs/experiment/hrcwhu_cdnetv2.yaml b/configs/experiment/hrcwhu_cdnetv2.yaml
index 2b21dc6d872411bdf06db64300725ace1fa4f0a0..d30c95e5aa5e9cf7ab7759a048c2449fd92fee7d 100644
--- a/configs/experiment/hrcwhu_cdnetv2.yaml
+++ b/configs/experiment/hrcwhu_cdnetv2.yaml
@@ -1,47 +1,47 @@
-# @package _global_
-
-# to execute this experiment run:
-# python train.py experiment=example
-
-defaults:
- - override /trainer: gpu
- - override /data: hrcwhu/hrcwhu
- - override /model: cdnetv2/cdnetv2
- - override /logger: wandb
- - override /callbacks: default
-
-# all parameters below will be merged with parameters from default configurations set above
-# this allows you to overwrite only specified parameters
-
-tags: ["hrcWhu", "cdnetv2"]
-
-seed: 42
-
-
- # scheduler:
- # _target_: torch.optim.lr_scheduler.ReduceLROnPlateau
- # _partial_: true
- # mode: min
- # factor: 0.1
- # patience: 10
-
-logger:
- wandb:
- project: "hrcWhu"
- name: "cdnetv2"
- aim:
- experiment: "hrcwhu_cdnetv2"
-
-callbacks:
- model_checkpoint:
- dirpath: ${paths.output_dir}/checkpoints
- filename: "epoch_{epoch:03d}"
- monitor: "val/loss"
- mode: "min"
- save_last: True
- auto_insert_metric_name: False
-
- early_stopping:
- monitor: "val/loss"
- patience: 10
+# @package _global_
+
+# to execute this experiment run:
+# python train.py experiment=example
+
+defaults:
+ - override /trainer: gpu
+ - override /data: hrcwhu/hrcwhu
+ - override /model: cdnetv2/cdnetv2
+ - override /logger: wandb
+ - override /callbacks: default
+
+# all parameters below will be merged with parameters from default configurations set above
+# this allows you to overwrite only specified parameters
+
+tags: ["hrcWhu", "cdnetv2"]
+
+seed: 42
+
+
+ # scheduler:
+ # _target_: torch.optim.lr_scheduler.ReduceLROnPlateau
+ # _partial_: true
+ # mode: min
+ # factor: 0.1
+ # patience: 10
+
+logger:
+ wandb:
+ project: "hrcWhu"
+ name: "cdnetv2"
+ aim:
+ experiment: "hrcwhu_cdnetv2"
+
+callbacks:
+ model_checkpoint:
+ dirpath: ${paths.output_dir}/checkpoints
+ filename: "epoch_{epoch:03d}"
+ monitor: "val/loss"
+ mode: "min"
+ save_last: True
+ auto_insert_metric_name: False
+
+ early_stopping:
+ monitor: "val/loss"
+ patience: 10
mode: "min"
\ No newline at end of file
diff --git a/configs/experiment/hrcwhu_dbnet.yaml b/configs/experiment/hrcwhu_dbnet.yaml
index 388af5a19489a1e09bce6f6c397d8d8525ff634c..5bc8a198eefb8703bcfb0e4d28f32a295640079b 100644
--- a/configs/experiment/hrcwhu_dbnet.yaml
+++ b/configs/experiment/hrcwhu_dbnet.yaml
@@ -1,48 +1,48 @@
-# @package _global_
-
-# to execute this experiment run:
-# python train.py experiment=example
-
-defaults:
- - override /trainer: gpu
- - override /data: hrcwhu/hrcwhu
- - override /model: dbnet/dbnet
- - override /logger: wandb
- - override /callbacks: default
-
-# all parameters below will be merged with parameters from default configurations set above
-# this allows you to overwrite only specified parameters
-
-tags: ["hrcWhu", "dbnet"]
-
-seed: 42
-
-
-
- # scheduler:
- # _target_: torch.optim.lr_scheduler.ReduceLROnPlateau
- # _partial_: true
- # mode: min
- # factor: 0.1
- # patience: 10
-
-logger:
- wandb:
- project: "hrcWhu"
- name: "dbnet"
- aim:
- experiment: "hrcwhu_dbnet"
-
-callbacks:
- model_checkpoint:
- dirpath: ${paths.output_dir}/checkpoints
- filename: "epoch_{epoch:03d}"
- monitor: "val/loss"
- mode: "min"
- save_last: True
- auto_insert_metric_name: False
-
- early_stopping:
- monitor: "val/loss"
- patience: 10
+# @package _global_
+
+# to execute this experiment run:
+# python train.py experiment=example
+
+defaults:
+ - override /trainer: gpu
+ - override /data: hrcwhu/hrcwhu
+ - override /model: dbnet/dbnet
+ - override /logger: wandb
+ - override /callbacks: default
+
+# all parameters below will be merged with parameters from default configurations set above
+# this allows you to overwrite only specified parameters
+
+tags: ["hrcWhu", "dbnet"]
+
+seed: 42
+
+
+
+ # scheduler:
+ # _target_: torch.optim.lr_scheduler.ReduceLROnPlateau
+ # _partial_: true
+ # mode: min
+ # factor: 0.1
+ # patience: 10
+
+logger:
+ wandb:
+ project: "hrcWhu"
+ name: "dbnet"
+ aim:
+ experiment: "hrcwhu_dbnet"
+
+callbacks:
+ model_checkpoint:
+ dirpath: ${paths.output_dir}/checkpoints
+ filename: "epoch_{epoch:03d}"
+ monitor: "val/loss"
+ mode: "min"
+ save_last: True
+ auto_insert_metric_name: False
+
+ early_stopping:
+ monitor: "val/loss"
+ patience: 10
mode: "min"
\ No newline at end of file
diff --git a/configs/experiment/hrcwhu_hrcloudnet.yaml b/configs/experiment/hrcwhu_hrcloudnet.yaml
new file mode 100644
index 0000000000000000000000000000000000000000..9f2080ff8b84921b24d3f00610e76cc80e1d9d54
--- /dev/null
+++ b/configs/experiment/hrcwhu_hrcloudnet.yaml
@@ -0,0 +1,47 @@
+# @package _global_
+
+# to execute this experiment run:
+# python train.py experiment=example
+
+defaults:
+ - override /trainer: gpu
+ - override /data: hrcwhu/hrcwhu
+ - override /model: hrcloudnet/hrcloudnet
+ - override /logger: wandb
+ - override /callbacks: default
+
+# all parameters below will be merged with parameters from default configurations set above
+# this allows you to overwrite only specified parameters
+
+tags: ["hrcWhu", "hrcloudnet"]
+
+seed: 42
+
+
+ # scheduler:
+ # _target_: torch.optim.lr_scheduler.ReduceLROnPlateau
+ # _partial_: true
+ # mode: min
+ # factor: 0.1
+ # patience: 10
+
+logger:
+ wandb:
+ project: "hrcWhu"
+ name: "hrcloudnet"
+ aim:
+ experiment: "hrcwhu_hrcloudnet"
+
+callbacks:
+ model_checkpoint:
+ dirpath: ${paths.output_dir}/checkpoints
+ filename: "epoch_{epoch:03d}"
+ monitor: "val/loss"
+ mode: "min"
+ save_last: True
+ auto_insert_metric_name: False
+
+ early_stopping:
+ monitor: "val/loss"
+ patience: 10
+ mode: "min"
diff --git a/configs/experiment/hrcwhu_mcdnet.yaml b/configs/experiment/hrcwhu_mcdnet.yaml
index cf8c4cb41259143277f6d40b3d7ce46071ebc96f..1b6e826a728cdee9ced48e6d6b1c01b190ff9482 100644
--- a/configs/experiment/hrcwhu_mcdnet.yaml
+++ b/configs/experiment/hrcwhu_mcdnet.yaml
@@ -1,47 +1,47 @@
-# @package _global_
-
-# to execute this experiment run:
-# python train.py experiment=example
-
-defaults:
- - override /trainer: gpu
- - override /data: hrcwhu/hrcwhu
- - override /model: mcdnet/mcdnet
- - override /logger: wandb
- - override /callbacks: default
-
-# all parameters below will be merged with parameters from default configurations set above
-# this allows you to overwrite only specified parameters
-
-tags: ["hrcWhu", "mcdnet"]
-
-seed: 42
-
-
-# scheduler:
-# _target_: torch.optim.lr_scheduler.ReduceLROnPlateau
-# _partial_: true
-# mode: min
-# factor: 0.1
-# patience: 10
-
-logger:
- wandb:
- project: "hrcWhu"
- name: "mcdnet"
- aim:
- experiment: "hrcwhu_mcdnet"
-
-callbacks:
- model_checkpoint:
- dirpath: ${paths.output_dir}/checkpoints
- filename: "epoch_{epoch:03d}"
- monitor: "val/loss"
- mode: "min"
- save_last: True
- auto_insert_metric_name: False
-
- early_stopping:
- monitor: "val/loss"
- patience: 10
+# @package _global_
+
+# to execute this experiment run:
+# python train.py experiment=example
+
+defaults:
+ - override /trainer: gpu
+ - override /data: hrcwhu/hrcwhu
+ - override /model: mcdnet/mcdnet
+ - override /logger: wandb
+ - override /callbacks: default
+
+# all parameters below will be merged with parameters from default configurations set above
+# this allows you to overwrite only specified parameters
+
+tags: ["hrcWhu", "mcdnet"]
+
+seed: 42
+
+
+# scheduler:
+# _target_: torch.optim.lr_scheduler.ReduceLROnPlateau
+# _partial_: true
+# mode: min
+# factor: 0.1
+# patience: 10
+
+logger:
+ wandb:
+ project: "hrcWhu"
+ name: "mcdnet"
+ aim:
+ experiment: "hrcwhu_mcdnet"
+
+callbacks:
+ model_checkpoint:
+ dirpath: ${paths.output_dir}/checkpoints
+ filename: "epoch_{epoch:03d}"
+ monitor: "val/loss"
+ mode: "min"
+ save_last: True
+ auto_insert_metric_name: False
+
+ early_stopping:
+ monitor: "val/loss"
+ patience: 10
mode: "min"
\ No newline at end of file
diff --git a/configs/experiment/hrcwhu_scnn.yaml b/configs/experiment/hrcwhu_scnn.yaml
index 89145349426347d5c22762778d06fbacde21ce74..99302b88ba401712d2a63df7f1b738b19965e508 100644
--- a/configs/experiment/hrcwhu_scnn.yaml
+++ b/configs/experiment/hrcwhu_scnn.yaml
@@ -1,47 +1,47 @@
-# @package _global_
-
-# to execute this experiment run:
-# python train.py experiment=example
-
-defaults:
- - override /trainer: gpu
- - override /data: hrcwhu/hrcwhu
- - override /model: scnn/scnn
- - override /logger: wandb
- - override /callbacks: default
-
-# all parameters below will be merged with parameters from default configurations set above
-# this allows you to overwrite only specified parameters
-
-tags: ["hrcWhu", "scnn"]
-
-seed: 42
-
-
- # scheduler:
- # _target_: torch.optim.lr_scheduler.ReduceLROnPlateau
- # _partial_: true
- # mode: min
- # factor: 0.1
- # patience: 10
-
-logger:
- wandb:
- project: "hrcWhu"
- name: "scnn"
- aim:
- experiment: "hrcwhu_scnn"
-
-callbacks:
- model_checkpoint:
- dirpath: ${paths.output_dir}/checkpoints
- filename: "epoch_{epoch:03d}"
- monitor: "val/loss"
- mode: "min"
- save_last: True
- auto_insert_metric_name: False
-
- early_stopping:
- monitor: "val/loss"
- patience: 10
+# @package _global_
+
+# to execute this experiment run:
+# python train.py experiment=example
+
+defaults:
+ - override /trainer: gpu
+ - override /data: hrcwhu/hrcwhu
+ - override /model: scnn/scnn
+ - override /logger: wandb
+ - override /callbacks: default
+
+# all parameters below will be merged with parameters from default configurations set above
+# this allows you to overwrite only specified parameters
+
+tags: ["hrcWhu", "scnn"]
+
+seed: 42
+
+
+ # scheduler:
+ # _target_: torch.optim.lr_scheduler.ReduceLROnPlateau
+ # _partial_: true
+ # mode: min
+ # factor: 0.1
+ # patience: 10
+
+logger:
+ wandb:
+ project: "hrcWhu"
+ name: "scnn"
+ aim:
+ experiment: "hrcwhu_scnn"
+
+callbacks:
+ model_checkpoint:
+ dirpath: ${paths.output_dir}/checkpoints
+ filename: "epoch_{epoch:03d}"
+ monitor: "val/loss"
+ mode: "min"
+ save_last: True
+ auto_insert_metric_name: False
+
+ early_stopping:
+ monitor: "val/loss"
+ patience: 10
mode: "min"
\ No newline at end of file
diff --git a/configs/experiment/hrcwhu_unet.yaml b/configs/experiment/hrcwhu_unet.yaml
index 5247691cc1b5d258a7aa9e335c19f021670215b5..cd97a7a3f93688d56e41ac7d67ed874f8bc4e847 100644
--- a/configs/experiment/hrcwhu_unet.yaml
+++ b/configs/experiment/hrcwhu_unet.yaml
@@ -1,68 +1,68 @@
-# @package _global_
-
-# to execute this experiment run:
-# python train.py experiment=example
-
-defaults:
- - override /trainer: gpu
- - override /data: hrcwhu/hrcwhu
- - override /model: null
- - override /logger: wandb
- - override /callbacks: default
-
-# all parameters below will be merged with parameters from default configurations set above
-# this allows you to overwrite only specified parameters
-
-tags: ["hrcWhu", "unet"]
-
-seed: 42
-
-
-
-model:
- _target_: src.models.base_module.BaseLitModule
-
- net:
- _target_: src.models.components.unet.UNet
- in_channels: 3
- out_channels: 2
-
- num_classes: 2
-
- criterion:
- _target_: torch.nn.CrossEntropyLoss
-
- optimizer:
- _target_: torch.optim.SGD
- _partial_: true
- lr: 0.1
-
- scheduler: null
-
- # scheduler:
- # _target_: torch.optim.lr_scheduler.ReduceLROnPlateau
- # _partial_: true
- # mode: min
- # factor: 0.1
- # patience: 10
-
-logger:
- wandb:
- project: "hrcWhu"
- name: "unet"
- aim:
- experiment: "hrcwhu_unet"
-
-callbacks:
- model_checkpoint:
- dirpath: ${paths.output_dir}/checkpoints
- filename: "epoch_{epoch:03d}"
- monitor: "val/loss"
- mode: "min"
- save_last: True
- auto_insert_metric_name: False
-
- early_stopping:
- monitor: "val/loss"
- patience: 10
+# @package _global_
+
+# to execute this experiment run:
+# python train.py experiment=example
+
+defaults:
+ - override /trainer: gpu
+ - override /data: hrcwhu/hrcwhu
+ - override /model: null
+ - override /logger: wandb
+ - override /callbacks: default
+
+# all parameters below will be merged with parameters from default configurations set above
+# this allows you to overwrite only specified parameters
+
+tags: ["hrcWhu", "unet"]
+
+seed: 42
+
+
+
+model:
+ _target_: src.models.base_module.BaseLitModule
+
+ net:
+ _target_: src.models.components.unet.UNet
+ in_channels: 3
+ out_channels: 2
+
+ num_classes: 2
+
+ criterion:
+ _target_: torch.nn.CrossEntropyLoss
+
+ optimizer:
+ _target_: torch.optim.SGD
+ _partial_: true
+ lr: 0.1
+
+ scheduler: null
+
+ # scheduler:
+ # _target_: torch.optim.lr_scheduler.ReduceLROnPlateau
+ # _partial_: true
+ # mode: min
+ # factor: 0.1
+ # patience: 10
+
+logger:
+ wandb:
+ project: "hrcWhu"
+ name: "unet"
+ aim:
+ experiment: "hrcwhu_unet"
+
+callbacks:
+ model_checkpoint:
+ dirpath: ${paths.output_dir}/checkpoints
+ filename: "epoch_{epoch:03d}"
+ monitor: "val/loss"
+ mode: "min"
+ save_last: True
+ auto_insert_metric_name: False
+
+ early_stopping:
+ monitor: "val/loss"
+ patience: 10
mode: "min"
\ No newline at end of file
diff --git a/configs/experiment/hrcwhu_unetmobv2.yaml b/configs/experiment/hrcwhu_unetmobv2.yaml
new file mode 100644
index 0000000000000000000000000000000000000000..138c913b2c931f02dd45c2f39b8adcc9f586f4ee
--- /dev/null
+++ b/configs/experiment/hrcwhu_unetmobv2.yaml
@@ -0,0 +1,47 @@
+# @package _global_
+
+# to execute this experiment run:
+# python train.py experiment=example
+
+defaults:
+ - override /trainer: gpu
+ - override /data: hrcwhu/hrcwhu
+ - override /model: unetmobv2/unetmobv2
+ - override /logger: wandb
+ - override /callbacks: default
+
+# all parameters below will be merged with parameters from default configurations set above
+# this allows you to overwrite only specified parameters
+
+tags: ["hrcWhu", "unetmobv2"]
+
+seed: 42
+
+
+ # scheduler:
+ # _target_: torch.optim.lr_scheduler.ReduceLROnPlateau
+ # _partial_: true
+ # mode: min
+ # factor: 0.1
+ # patience: 10
+
+logger:
+ wandb:
+ project: "hrcWhu"
+ name: "unetmobv2"
+ aim:
+ experiment: "hrcwhu_unetmobv2"
+
+callbacks:
+ model_checkpoint:
+ dirpath: ${paths.output_dir}/checkpoints
+ filename: "epoch_{epoch:03d}"
+ monitor: "val/loss"
+ mode: "min"
+ save_last: True
+ auto_insert_metric_name: False
+
+ early_stopping:
+ monitor: "val/loss"
+ patience: 10
+ mode: "min"
\ No newline at end of file
diff --git a/configs/extras/default.yaml b/configs/extras/default.yaml
index b9c6b622283a647fbc513166fc14f016cc3ed8a0..cbb617cbbcd68fba87e84126e9f59fef8acc6e41 100644
--- a/configs/extras/default.yaml
+++ b/configs/extras/default.yaml
@@ -1,8 +1,8 @@
-# disable python warnings if they annoy you
-ignore_warnings: False
-
-# ask user for tags if none are provided in the config
-enforce_tags: True
-
-# pretty print config tree at the start of the run using Rich library
-print_config: True
+# disable python warnings if they annoy you
+ignore_warnings: False
+
+# ask user for tags if none are provided in the config
+enforce_tags: True
+
+# pretty print config tree at the start of the run using Rich library
+print_config: True
diff --git a/configs/hparams_search/mnist_optuna.yaml b/configs/hparams_search/mnist_optuna.yaml
index 1391183ebcdec3d8f5eb61374e0719d13c7545da..3ca6a40aacae8a9dc1985a5909b9a05a931b584a 100644
--- a/configs/hparams_search/mnist_optuna.yaml
+++ b/configs/hparams_search/mnist_optuna.yaml
@@ -1,52 +1,52 @@
-# @package _global_
-
-# example hyperparameter optimization of some experiment with Optuna:
-# python train.py -m hparams_search=mnist_optuna experiment=example
-
-defaults:
- - override /hydra/sweeper: optuna
-
-# choose metric which will be optimized by Optuna
-# make sure this is the correct name of some metric logged in lightning module!
-optimized_metric: "val/acc_best"
-
-# here we define Optuna hyperparameter search
-# it optimizes for value returned from function with @hydra.main decorator
-# docs: https://hydra.cc/docs/next/plugins/optuna_sweeper
-hydra:
- mode: "MULTIRUN" # set hydra to multirun by default if this config is attached
-
- sweeper:
- _target_: hydra_plugins.hydra_optuna_sweeper.optuna_sweeper.OptunaSweeper
-
- # storage URL to persist optimization results
- # for example, you can use SQLite if you set 'sqlite:///example.db'
- storage: null
-
- # name of the study to persist optimization results
- study_name: null
-
- # number of parallel workers
- n_jobs: 1
-
- # 'minimize' or 'maximize' the objective
- direction: maximize
-
- # total number of runs that will be executed
- n_trials: 20
-
- # choose Optuna hyperparameter sampler
- # you can choose bayesian sampler (tpe), random search (without optimization), grid sampler, and others
- # docs: https://optuna.readthedocs.io/en/stable/reference/samplers.html
- sampler:
- _target_: optuna.samplers.TPESampler
- seed: 1234
- n_startup_trials: 10 # number of random sampling runs before optimization starts
-
- # define hyperparameter search space
- params:
- model.optimizer.lr: interval(0.0001, 0.1)
- data.batch_size: choice(32, 64, 128, 256)
- model.net.lin1_size: choice(64, 128, 256)
- model.net.lin2_size: choice(64, 128, 256)
- model.net.lin3_size: choice(32, 64, 128, 256)
+# @package _global_
+
+# example hyperparameter optimization of some experiment with Optuna:
+# python train.py -m hparams_search=mnist_optuna experiment=example
+
+defaults:
+ - override /hydra/sweeper: optuna
+
+# choose metric which will be optimized by Optuna
+# make sure this is the correct name of some metric logged in lightning module!
+optimized_metric: "val/acc_best"
+
+# here we define Optuna hyperparameter search
+# it optimizes for value returned from function with @hydra.main decorator
+# docs: https://hydra.cc/docs/next/plugins/optuna_sweeper
+hydra:
+ mode: "MULTIRUN" # set hydra to multirun by default if this config is attached
+
+ sweeper:
+ _target_: hydra_plugins.hydra_optuna_sweeper.optuna_sweeper.OptunaSweeper
+
+ # storage URL to persist optimization results
+ # for example, you can use SQLite if you set 'sqlite:///example.db'
+ storage: null
+
+ # name of the study to persist optimization results
+ study_name: null
+
+ # number of parallel workers
+ n_jobs: 1
+
+ # 'minimize' or 'maximize' the objective
+ direction: maximize
+
+ # total number of runs that will be executed
+ n_trials: 20
+
+ # choose Optuna hyperparameter sampler
+ # you can choose bayesian sampler (tpe), random search (without optimization), grid sampler, and others
+ # docs: https://optuna.readthedocs.io/en/stable/reference/samplers.html
+ sampler:
+ _target_: optuna.samplers.TPESampler
+ seed: 1234
+ n_startup_trials: 10 # number of random sampling runs before optimization starts
+
+ # define hyperparameter search space
+ params:
+ model.optimizer.lr: interval(0.0001, 0.1)
+ data.batch_size: choice(32, 64, 128, 256)
+ model.net.lin1_size: choice(64, 128, 256)
+ model.net.lin2_size: choice(64, 128, 256)
+ model.net.lin3_size: choice(32, 64, 128, 256)
diff --git a/configs/hydra/default.yaml b/configs/hydra/default.yaml
index aace38d320b808a7e53ea4ee230992e5abe804e9..b976c54c28e5f1a79f0609423c1f732bb848dfa0 100644
--- a/configs/hydra/default.yaml
+++ b/configs/hydra/default.yaml
@@ -1,19 +1,19 @@
-# https://hydra.cc/docs/configure_hydra/intro/
-
-# enable color logging
-defaults:
- - override hydra_logging: colorlog
- - override job_logging: colorlog
-
-# output directory, generated dynamically on each run
-run:
- dir: ${paths.log_dir}/${task_name}/runs/${logger.aim.experiment}/${now:%Y-%m-%d}_${now:%H-%M-%S}
-sweep:
- dir: ${paths.log_dir}/${task_name}/multiruns/${logger.aim.experiment}/${now:%Y-%m-%d}_${now:%H-%M-%S}
- subdir: ${hydra.job.num}
-
-job_logging:
- handlers:
- file:
- # Incorporates fix from https://github.com/facebookresearch/hydra/pull/2242
- filename: ${hydra.runtime.output_dir}/${task_name}.log
+# https://hydra.cc/docs/configure_hydra/intro/
+
+# enable color logging
+defaults:
+ - override hydra_logging: colorlog
+ - override job_logging: colorlog
+
+# output directory, generated dynamically on each run
+run:
+ dir: ${paths.log_dir}/${task_name}/runs/${logger.aim.experiment}/${now:%Y-%m-%d}_${now:%H-%M-%S}
+sweep:
+ dir: ${paths.log_dir}/${task_name}/multiruns/${logger.aim.experiment}/${now:%Y-%m-%d}_${now:%H-%M-%S}
+ subdir: ${hydra.job.num}
+
+job_logging:
+ handlers:
+ file:
+ # Incorporates fix from https://github.com/facebookresearch/hydra/pull/2242
+ filename: ${hydra.runtime.output_dir}/${task_name}.log
diff --git a/configs/logger/aim.yaml b/configs/logger/aim.yaml
index 8f9f6adad7feb2780c2efd5ddb0ed053621e05f8..b1cc6bc7364b69086a947e772f73752d3e576316 100644
--- a/configs/logger/aim.yaml
+++ b/configs/logger/aim.yaml
@@ -1,28 +1,28 @@
-# https://aimstack.io/
-
-# example usage in lightning module:
-# https://github.com/aimhubio/aim/blob/main/examples/pytorch_lightning_track.py
-
-# open the Aim UI with the following command (run in the folder containing the `.aim` folder):
-# `aim up`
-
-aim:
- _target_: aim.pytorch_lightning.AimLogger
- repo: ${paths.root_dir} # .aim folder will be created here
- # repo: "aim://ip_address:port" # can instead provide IP address pointing to Aim remote tracking server which manages the repo, see https://aimstack.readthedocs.io/en/latest/using/remote_tracking.html#
-
- # aim allows to group runs under experiment name
- experiment: null # any string, set to "default" if not specified
-
- train_metric_prefix: "train/"
- val_metric_prefix: "val/"
- test_metric_prefix: "test/"
-
- # sets the tracking interval in seconds for system usage metrics (CPU, GPU, memory, etc.)
- system_tracking_interval: 10 # set to null to disable system metrics tracking
-
- # enable/disable logging of system params such as installed packages, git info, env vars, etc.
- log_system_params: true
-
- # enable/disable tracking console logs (default value is true)
- capture_terminal_logs: false # set to false to avoid infinite console log loop issue https://github.com/aimhubio/aim/issues/2550
+# https://aimstack.io/
+
+# example usage in lightning module:
+# https://github.com/aimhubio/aim/blob/main/examples/pytorch_lightning_track.py
+
+# open the Aim UI with the following command (run in the folder containing the `.aim` folder):
+# `aim up`
+
+aim:
+ _target_: aim.pytorch_lightning.AimLogger
+ repo: ${paths.root_dir} # .aim folder will be created here
+ # repo: "aim://ip_address:port" # can instead provide IP address pointing to Aim remote tracking server which manages the repo, see https://aimstack.readthedocs.io/en/latest/using/remote_tracking.html#
+
+ # aim allows to group runs under experiment name
+ experiment: null # any string, set to "default" if not specified
+
+ train_metric_prefix: "train/"
+ val_metric_prefix: "val/"
+ test_metric_prefix: "test/"
+
+ # sets the tracking interval in seconds for system usage metrics (CPU, GPU, memory, etc.)
+ system_tracking_interval: 10 # set to null to disable system metrics tracking
+
+ # enable/disable logging of system params such as installed packages, git info, env vars, etc.
+ log_system_params: true
+
+ # enable/disable tracking console logs (default value is true)
+ capture_terminal_logs: false # set to false to avoid infinite console log loop issue https://github.com/aimhubio/aim/issues/2550
diff --git a/configs/logger/comet.yaml b/configs/logger/comet.yaml
index e0789274e2137ee6c97ca37a5d56c2b8abaf0aaa..dc2d533da7308bdf65318218676ddd3e67dffa9b 100644
--- a/configs/logger/comet.yaml
+++ b/configs/logger/comet.yaml
@@ -1,12 +1,12 @@
-# https://www.comet.ml
-
-comet:
- _target_: lightning.pytorch.loggers.comet.CometLogger
- api_key: ${oc.env:COMET_API_TOKEN} # api key is loaded from environment variable
- save_dir: "${paths.output_dir}"
- project_name: "lightning-hydra-template"
- rest_api_key: null
- # experiment_name: ""
- experiment_key: null # set to resume experiment
- offline: False
- prefix: ""
+# https://www.comet.ml
+
+comet:
+ _target_: lightning.pytorch.loggers.comet.CometLogger
+ api_key: ${oc.env:COMET_API_TOKEN} # api key is loaded from environment variable
+ save_dir: "${paths.output_dir}"
+ project_name: "lightning-hydra-template"
+ rest_api_key: null
+ # experiment_name: ""
+ experiment_key: null # set to resume experiment
+ offline: False
+ prefix: ""
diff --git a/configs/logger/csv.yaml b/configs/logger/csv.yaml
index fa028e9c146430c319101ffdfce466514338591c..72252ca86a7adfa465cce8ae526239bf94ca3ad9 100644
--- a/configs/logger/csv.yaml
+++ b/configs/logger/csv.yaml
@@ -1,7 +1,7 @@
-# csv logger built in lightning
-
-csv:
- _target_: lightning.pytorch.loggers.csv_logs.CSVLogger
- save_dir: "${paths.output_dir}"
- name: "csv/"
- prefix: ""
+# csv logger built in lightning
+
+csv:
+ _target_: lightning.pytorch.loggers.csv_logs.CSVLogger
+ save_dir: "${paths.output_dir}"
+ name: "csv/"
+ prefix: ""
diff --git a/configs/logger/many_loggers.yaml b/configs/logger/many_loggers.yaml
index dd586800bdccb4e8f4b0236a181b7ddd756ba9ab..590d207c895bf8eeeb269a1f44acbe04c45533fa 100644
--- a/configs/logger/many_loggers.yaml
+++ b/configs/logger/many_loggers.yaml
@@ -1,9 +1,9 @@
-# train with many loggers at once
-
-defaults:
- # - comet
- - csv
- # - mlflow
- # - neptune
- - tensorboard
- - wandb
+# train with many loggers at once
+
+defaults:
+ # - comet
+ - csv
+ # - mlflow
+ # - neptune
+ - tensorboard
+ - wandb
diff --git a/configs/logger/mlflow.yaml b/configs/logger/mlflow.yaml
index f8fb7e685fa27fc8141387a421b90a0b9b492d9e..72e807a5a21ae9bbd9760b8c97de0eccb8547cd9 100644
--- a/configs/logger/mlflow.yaml
+++ b/configs/logger/mlflow.yaml
@@ -1,12 +1,12 @@
-# https://mlflow.org
-
-mlflow:
- _target_: lightning.pytorch.loggers.mlflow.MLFlowLogger
- # experiment_name: ""
- # run_name: ""
- tracking_uri: ${paths.log_dir}/mlflow/mlruns # run `mlflow ui` command inside the `logs/mlflow/` dir to open the UI
- tags: null
- # save_dir: "./mlruns"
- prefix: ""
- artifact_location: null
- # run_id: ""
+# https://mlflow.org
+
+mlflow:
+ _target_: lightning.pytorch.loggers.mlflow.MLFlowLogger
+ # experiment_name: ""
+ # run_name: ""
+ tracking_uri: ${paths.log_dir}/mlflow/mlruns # run `mlflow ui` command inside the `logs/mlflow/` dir to open the UI
+ tags: null
+ # save_dir: "./mlruns"
+ prefix: ""
+ artifact_location: null
+ # run_id: ""
diff --git a/configs/logger/neptune.yaml b/configs/logger/neptune.yaml
index 8233c140018ecce6ab62971beed269991d31c89b..e3eeea4d28628c1a208a47ec8861776badd48458 100644
--- a/configs/logger/neptune.yaml
+++ b/configs/logger/neptune.yaml
@@ -1,9 +1,9 @@
-# https://neptune.ai
-
-neptune:
- _target_: lightning.pytorch.loggers.neptune.NeptuneLogger
- api_key: ${oc.env:NEPTUNE_API_TOKEN} # api key is loaded from environment variable
- project: username/lightning-hydra-template
- # name: ""
- log_model_checkpoints: True
- prefix: ""
+# https://neptune.ai
+
+neptune:
+ _target_: lightning.pytorch.loggers.neptune.NeptuneLogger
+ api_key: ${oc.env:NEPTUNE_API_TOKEN} # api key is loaded from environment variable
+ project: username/lightning-hydra-template
+ # name: ""
+ log_model_checkpoints: True
+ prefix: ""
diff --git a/configs/logger/tensorboard.yaml b/configs/logger/tensorboard.yaml
index 2bd31f6d8ba68d1f5c36a804885d5b9f9c1a9302..e47ca0acfd61e6c92837a715ea06137821354ab2 100644
--- a/configs/logger/tensorboard.yaml
+++ b/configs/logger/tensorboard.yaml
@@ -1,10 +1,10 @@
-# https://www.tensorflow.org/tensorboard/
-
-tensorboard:
- _target_: lightning.pytorch.loggers.tensorboard.TensorBoardLogger
- save_dir: "${paths.output_dir}/tensorboard/"
- name: null
- log_graph: False
- default_hp_metric: True
- prefix: ""
- # version: ""
+# https://www.tensorflow.org/tensorboard/
+
+tensorboard:
+ _target_: lightning.pytorch.loggers.tensorboard.TensorBoardLogger
+ save_dir: "${paths.output_dir}/tensorboard/"
+ name: null
+ log_graph: False
+ default_hp_metric: True
+ prefix: ""
+ # version: ""
diff --git a/configs/logger/wandb.yaml b/configs/logger/wandb.yaml
index ece165889b3d0d9dc750a8f3c7454188cfdf12b7..8c77afd3257eebffa10c7d39fdf056242bdfa5eb 100644
--- a/configs/logger/wandb.yaml
+++ b/configs/logger/wandb.yaml
@@ -1,16 +1,16 @@
-# https://wandb.ai
-
-wandb:
- _target_: lightning.pytorch.loggers.wandb.WandbLogger
- # name: "" # name of the run (normally generated by wandb)
- save_dir: "${paths.output_dir}"
- offline: False
- id: null # pass correct id to resume experiment!
- anonymous: null # enable anonymous logging
- project: "lightning-hydra-template"
- log_model: False # upload lightning ckpts
- prefix: "" # a string to put at the beginning of metric keys
- # entity: "" # set to name of your wandb team
- group: ""
- tags: []
- job_type: ""
+# https://wandb.ai
+
+wandb:
+ _target_: lightning.pytorch.loggers.wandb.WandbLogger
+ # name: "" # name of the run (normally generated by wandb)
+ save_dir: "${paths.output_dir}"
+ offline: False
+ id: null # pass correct id to resume experiment!
+ anonymous: null # enable anonymous logging
+ project: "lightning-hydra-template"
+ log_model: False # upload lightning ckpts
+ prefix: "" # a string to put at the beginning of metric keys
+ # entity: "" # set to name of your wandb team
+ group: ""
+ tags: []
+ job_type: ""
diff --git a/configs/model/cdnetv1/README.md b/configs/model/cdnetv1/README.md
index 05bf8cdddde31a57408ac919b3de6fdd09bdd832..56c951b831a8842dd498d76ad16bac84540248c3 100644
--- a/configs/model/cdnetv1/README.md
+++ b/configs/model/cdnetv1/README.md
@@ -1,117 +1,117 @@
-# CDnet: CNN-Based Cloud Detection for Remote Sensing Imagery
-
-> [CDnet: CNN-Based Cloud Detection for Remote Sensing Imagery](https://ieeexplore.ieee.org/document/8681238)
-
-## Introduction
-
-
-
-Official Repo
-
-Code Snippet
-
-## Abstract
-
-
-
-Cloud detection is one of the important tasks for remote sensing image (RSI) preprocessing. In this paper, we utilize the thumbnail (i.e., preview image) of RSI, which contains the information of original multispectral or panchromatic imagery, to extract cloud mask efficiently. Compared with detection cloud mask from original RSI, it is more challenging to detect cloud mask using thumbnails due to the loss of resolution and spectrum information. To tackle this problem, we propose a cloud detection neural network (CDnet) with an encoder–decoder structure, a feature pyramid module (FPM), and a boundary refinement (BR) block. The FPM extracts the multiscale contextual information without the loss of resolution and coverage; the BR block refines object boundaries; and the encoder–decoder structure gradually recovers segmentation results with the same size as input image. Experimental results on the ZY-3 satellite thumbnails cloud cover validation data set and two other validation data sets (GF-1 WFV Cloud and Cloud Shadow Cover Validation Data and Landsat-8 Cloud Cover Assessment Validation Data) demonstrate that the proposed method achieves accurate detection accuracy and outperforms several state-of-the-art methods.
-
-
-
-
-
-

-
-
-## Results and models
-
-### CLOUD EXTRACTION ACCURACY (%)
-
-
-| Method | OA | MIoU | Kappa | PA | UA |
-|----------|-------|-------|-------|-------|-------|
-| CDnet(ASPP+GAP) | 95.41 | 89.38 | 82.05 | 87.82 | 89.85 |
-| CDnet(FPM) | 96.47 | 91.70 | 85.06 | 89.75 | 90.41 |
-
-
-### CLOUD EXTRACTION ACCURACY (%) FOR MODULES AND VARIANTS OF THE CDNET
-
-
-
-| Method | OA | MIoU | Kappa | PA | UA |
-|----------|-------|-------|-------|-------|-------|
-| ResNet50 | 91.13 | 82.83 | 73.38 | 81.99 | 80.34 |
-| MRN* | 93.03 | 85.24 | 77.51 | 82.59 | 82.82 |
-| MRN+FPM | 93.89 | 88.50 | 81.82 | 87.10 | 85.51 |
-| MRN+FPM+BR| 94.31 | 88.97 | 82.59 | 87.12 | 87.04 |
-| CDnet-FPM | 93.14 | 88.14 | 80.44 | 87.64 | 84.46 |
-| CDnet-BR | 95.04 | 89.63 | 83.78 | 87.36 | 88.67 |
-| CDnet-FPM-BR| 93.10 | 87.91 | 80.01 | 87.01 | 83.84 |
-| CDnet-A | 94.84 | 89.41 | 82.91 | 87.32 | 88.07 |
-| CDnet-B | 95.27 | 90.51 | 84.01 | 88.97 | 89.71 |
-| CDnet-C | 96.09 | 90.73 | 84.27 | 88.74 | 90.28 |
-| CDnet | 96.47 | 91.70 | 85.06 | 89.75 | 90.41 |
-
-MRN stands for the modified ResNet-50.
-
-
-
-### CLOUD EXTRACTION ACCURACY (%)
-
-
-| Method | OA | MIoU | Kappa | PA | UA |
-|----------|-------|-------|-------|-------|-------|
-| Maxlike | 77.73 | 66.16 | 53.55 | 91.30 | 54.98 |
-| SVM | 78.21 | 66.79 | 54.87 | 91.77 | 56.37 |
-| L-unet | 86.51 | 73.67 | 63.79 | 83.15 | 64.79 |
-| FCN-8 | 90.53 | 81.08 | 68.08 | 82.91 | 78.87 |
-| MVGG-16 | 92.73 | 86.65 | 78.94 | 88.12 | 81.84 |
-| DPN | 93.11 | 86.73 | 79.05 | 87.68 | 83.96 |
-| DeeplabV2 | 93.36 | 87.56 | 79.12 | 87.50 | 84.65 |
-| PSPnet | 94.24 | 88.37 | 81.41 | 86.67 | 89.17 |
-| DeeplabV3 | 95.03 | 88.74 | 81.53 | 87.63 | 89.72 |
-| DeeplabV3+| 96.01 | 90.45 | 83.92 | 88.47 | 90.03 |
-| CDnet | 96.47 | 91.70 | 85.06 | 89.75 | 90.41 |
-
-
-### Cloud Extraction Accuracy (%) of GF-1 Satellite Imagery
-
-| Method | OA | MIoU | Kappa | PA | UA |
-|----------|-------|-------|-------|-------|-------|
-| MFC | 92.36 | 80.32 | 74.64 | 83.58 | 75.32 |
-| L-unet | 92.44 | 82.39 | 76.26 | 87.61 | 74.98 |
-| FCN-8 | 92.61 | 82.71 | 76.45 | 87.45 | 75.61 |
-| MVGG-16 | 93.07 | 86.17 | 77.13 | 87.68 | 79.50 |
-| DPN | 93.19 | 86.32 | 77.25 | 86.85 | 80.93 |
-| DeeplabV2 | 95.07 | 87.00 | 80.07 | 86.60 | 82.18 |
-| PSPnet | 95.30 | 87.45 | 80.74 | 85.87 | 83.27 |
-| DeeplabV3 | 95.95 | 88.13 | 81.05 | 86.36 | 88.72 |
-| DeeplabV3+| 96.18 | 89.11 | 82.31 | 87.37 | 89.05 |
-| CDnet | 96.73 | 89.83 | 83.23 | 87.94 | 89.60 |
-
-### Cloud Extraction Accuracy (%) of Landsat-8 Satellite Imagery
-
-| Method | OA | MIoU | Kappa | PA | UA |
-|----------|-------|-------|-------|-------|-------|
-| Fmask | 85.21 | 71.52 | 63.01 | 86.24 | 70.38 |
-| L-unet | 90.56 | 77.95 | 68.79 | 79.32 | 78.94 |
-| FCN-8 | 90.88 | 78.84 | 71.32 | 76.28 | 82.31 |
-| MVGG-16 | 93.31 | 81.59 | 77.08 | 77.29 | 83.00 |
-| DPN | 93.40 | 86.34 | 81.52 | 84.61 | 89.93 |
-| DeeplabV2 | 94.11 | 86.90 | 81.63 | 84.93 | 89.87 |
-| PSPnet | 95.43 | 88.29 | 83.12 | 86.98 | 90.59 |
-| DeeplabV3 | 96.38 | 90.32 | 84.31 | 89.52 | 91.92 |
-| CDnet | 97.16 | 90.84 | 84.91 | 90.15 | 92.08 |
-
-## Citation
-
-```bibtex
-@ARTICLE{8681238,
-author={J. {Yang} and J. {Guo} and H. {Yue} and Z. {Liu} and H. {Hu} and K. {Li}},
-journal={IEEE Transactions on Geoscience and Remote Sensing},
-title={CDnet: CNN-Based Cloud Detection for Remote Sensing Imagery},
-year={2019},
-volume={57},
-number={8},
-pages={6195-6211}, doi={10.1109/TGRS.2019.2904868} }
-```
+# CDnet: CNN-Based Cloud Detection for Remote Sensing Imagery
+
+> [CDnet: CNN-Based Cloud Detection for Remote Sensing Imagery](https://ieeexplore.ieee.org/document/8681238)
+
+## Introduction
+
+
+
+Official Repo
+
+Code Snippet
+
+## Abstract
+
+
+
+Cloud detection is one of the important tasks for remote sensing image (RSI) preprocessing. In this paper, we utilize the thumbnail (i.e., preview image) of RSI, which contains the information of original multispectral or panchromatic imagery, to extract cloud mask efficiently. Compared with detection cloud mask from original RSI, it is more challenging to detect cloud mask using thumbnails due to the loss of resolution and spectrum information. To tackle this problem, we propose a cloud detection neural network (CDnet) with an encoder–decoder structure, a feature pyramid module (FPM), and a boundary refinement (BR) block. The FPM extracts the multiscale contextual information without the loss of resolution and coverage; the BR block refines object boundaries; and the encoder–decoder structure gradually recovers segmentation results with the same size as input image. Experimental results on the ZY-3 satellite thumbnails cloud cover validation data set and two other validation data sets (GF-1 WFV Cloud and Cloud Shadow Cover Validation Data and Landsat-8 Cloud Cover Assessment Validation Data) demonstrate that the proposed method achieves accurate detection accuracy and outperforms several state-of-the-art methods.
+
+
+
+
+
+

+
+
+## Results and models
+
+### CLOUD EXTRACTION ACCURACY (%)
+
+
+| Method | OA | MIoU | Kappa | PA | UA |
+|----------|-------|-------|-------|-------|-------|
+| CDnet(ASPP+GAP) | 95.41 | 89.38 | 82.05 | 87.82 | 89.85 |
+| CDnet(FPM) | 96.47 | 91.70 | 85.06 | 89.75 | 90.41 |
+
+
+### CLOUD EXTRACTION ACCURACY (%) FOR MODULES AND VARIANTS OF THE CDNET
+
+
+
+| Method | OA | MIoU | Kappa | PA | UA |
+|----------|-------|-------|-------|-------|-------|
+| ResNet50 | 91.13 | 82.83 | 73.38 | 81.99 | 80.34 |
+| MRN* | 93.03 | 85.24 | 77.51 | 82.59 | 82.82 |
+| MRN+FPM | 93.89 | 88.50 | 81.82 | 87.10 | 85.51 |
+| MRN+FPM+BR| 94.31 | 88.97 | 82.59 | 87.12 | 87.04 |
+| CDnet-FPM | 93.14 | 88.14 | 80.44 | 87.64 | 84.46 |
+| CDnet-BR | 95.04 | 89.63 | 83.78 | 87.36 | 88.67 |
+| CDnet-FPM-BR| 93.10 | 87.91 | 80.01 | 87.01 | 83.84 |
+| CDnet-A | 94.84 | 89.41 | 82.91 | 87.32 | 88.07 |
+| CDnet-B | 95.27 | 90.51 | 84.01 | 88.97 | 89.71 |
+| CDnet-C | 96.09 | 90.73 | 84.27 | 88.74 | 90.28 |
+| CDnet | 96.47 | 91.70 | 85.06 | 89.75 | 90.41 |
+
+MRN stands for the modified ResNet-50.
+
+
+
+### CLOUD EXTRACTION ACCURACY (%)
+
+
+| Method | OA | MIoU | Kappa | PA | UA |
+|----------|-------|-------|-------|-------|-------|
+| Maxlike | 77.73 | 66.16 | 53.55 | 91.30 | 54.98 |
+| SVM | 78.21 | 66.79 | 54.87 | 91.77 | 56.37 |
+| L-unet | 86.51 | 73.67 | 63.79 | 83.15 | 64.79 |
+| FCN-8 | 90.53 | 81.08 | 68.08 | 82.91 | 78.87 |
+| MVGG-16 | 92.73 | 86.65 | 78.94 | 88.12 | 81.84 |
+| DPN | 93.11 | 86.73 | 79.05 | 87.68 | 83.96 |
+| DeeplabV2 | 93.36 | 87.56 | 79.12 | 87.50 | 84.65 |
+| PSPnet | 94.24 | 88.37 | 81.41 | 86.67 | 89.17 |
+| DeeplabV3 | 95.03 | 88.74 | 81.53 | 87.63 | 89.72 |
+| DeeplabV3+| 96.01 | 90.45 | 83.92 | 88.47 | 90.03 |
+| CDnet | 96.47 | 91.70 | 85.06 | 89.75 | 90.41 |
+
+
+### Cloud Extraction Accuracy (%) of GF-1 Satellite Imagery
+
+| Method | OA | MIoU | Kappa | PA | UA |
+|----------|-------|-------|-------|-------|-------|
+| MFC | 92.36 | 80.32 | 74.64 | 83.58 | 75.32 |
+| L-unet | 92.44 | 82.39 | 76.26 | 87.61 | 74.98 |
+| FCN-8 | 92.61 | 82.71 | 76.45 | 87.45 | 75.61 |
+| MVGG-16 | 93.07 | 86.17 | 77.13 | 87.68 | 79.50 |
+| DPN | 93.19 | 86.32 | 77.25 | 86.85 | 80.93 |
+| DeeplabV2 | 95.07 | 87.00 | 80.07 | 86.60 | 82.18 |
+| PSPnet | 95.30 | 87.45 | 80.74 | 85.87 | 83.27 |
+| DeeplabV3 | 95.95 | 88.13 | 81.05 | 86.36 | 88.72 |
+| DeeplabV3+| 96.18 | 89.11 | 82.31 | 87.37 | 89.05 |
+| CDnet | 96.73 | 89.83 | 83.23 | 87.94 | 89.60 |
+
+### Cloud Extraction Accuracy (%) of Landsat-8 Satellite Imagery
+
+| Method | OA | MIoU | Kappa | PA | UA |
+|----------|-------|-------|-------|-------|-------|
+| Fmask | 85.21 | 71.52 | 63.01 | 86.24 | 70.38 |
+| L-unet | 90.56 | 77.95 | 68.79 | 79.32 | 78.94 |
+| FCN-8 | 90.88 | 78.84 | 71.32 | 76.28 | 82.31 |
+| MVGG-16 | 93.31 | 81.59 | 77.08 | 77.29 | 83.00 |
+| DPN | 93.40 | 86.34 | 81.52 | 84.61 | 89.93 |
+| DeeplabV2 | 94.11 | 86.90 | 81.63 | 84.93 | 89.87 |
+| PSPnet | 95.43 | 88.29 | 83.12 | 86.98 | 90.59 |
+| DeeplabV3 | 96.38 | 90.32 | 84.31 | 89.52 | 91.92 |
+| CDnet | 97.16 | 90.84 | 84.91 | 90.15 | 92.08 |
+
+## Citation
+
+```bibtex
+@ARTICLE{8681238,
+author={J. {Yang} and J. {Guo} and H. {Yue} and Z. {Liu} and H. {Hu} and K. {Li}},
+journal={IEEE Transactions on Geoscience and Remote Sensing},
+title={CDnet: CNN-Based Cloud Detection for Remote Sensing Imagery},
+year={2019},
+volume={57},
+number={8},
+pages={6195-6211}, doi={10.1109/TGRS.2019.2904868} }
+```
diff --git a/configs/model/cdnetv1/cdnetv1.yaml b/configs/model/cdnetv1/cdnetv1.yaml
index f6d6b0e08e806029f3e50d0fb4fe3cc6915814c5..d0cc5c77ccb4d3c9854c9ca55ae2ed594ebc4feb 100644
--- a/configs/model/cdnetv1/cdnetv1.yaml
+++ b/configs/model/cdnetv1/cdnetv1.yaml
@@ -1,19 +1,19 @@
-_target_: src.models.base_module.BaseLitModule
-num_classes: 2
-
-net:
- _target_: src.models.components.cdnetv1.CDnetV1
- num_classes: 2
-
-criterion:
- _target_: torch.nn.CrossEntropyLoss
-
-optimizer:
- _target_: torch.optim.SGD
- _partial_: true
- lr: 0.0001
-
-scheduler: null
-
-# compile model for faster training with pytorch 2.0
-compile: false
+_target_: src.models.base_module.BaseLitModule
+num_classes: 2
+
+net:
+ _target_: src.models.components.cdnetv1.CDnetV1
+ num_classes: 2
+
+criterion:
+ _target_: torch.nn.CrossEntropyLoss
+
+optimizer:
+ _target_: torch.optim.SGD
+ _partial_: true
+ lr: 0.0001
+
+scheduler: null
+
+# compile model for faster training with pytorch 2.0
+compile: false
diff --git a/configs/model/cdnetv2/README.md b/configs/model/cdnetv2/README.md
index 45b3b2fc058643144a9f420a9a22157e763fa48d..8567bcb037297883fd48943142ec116136ac5b2c 100644
--- a/configs/model/cdnetv2/README.md
+++ b/configs/model/cdnetv2/README.md
@@ -1,90 +1,90 @@
-# CDnetV2: CNN-Based Cloud Detection for Remote Sensing Imagery With Cloud-Snow Coexistence
-
-> [CDnetV2: CNN-Based Cloud Detection for Remote Sensing Imagery With Cloud-Snow Coexistence](https://ieeexplore.ieee.org/document/9094671)
-
-## Introduction
-
-
-
-Official Repo
-
-Code Snippet
-
-## Abstract
-
-
-
-Cloud detection is a crucial preprocessing step for optical satellite remote sensing (RS) images. This article focuses on the cloud detection for RS imagery with cloud-snow coexistence and the utilization of the satellite thumbnails that lose considerable amount of high resolution and spectrum information of original RS images to extract cloud mask efficiently. To tackle this problem, we propose a novel cloud detection neural network with an encoder-decoder structure, named CDnetV2, as a series work on cloud detection. Compared with our previous CDnetV1, CDnetV2 contains two novel modules, that is, adaptive feature fusing model (AFFM) and high-level semantic information guidance flows (HSIGFs). AFFM is used to fuse multilevel feature maps by three submodules: channel attention fusion model (CAFM), spatial attention fusion model (SAFM), and channel attention refinement model (CARM). HSIGFs are designed to make feature layers at decoder of CDnetV2 be aware of the locations of the cloud objects. The high-level semantic information of HSIGFs is extracted by a proposed high-level feature fusing model (HFFM). By being equipped with these two proposed key modules, AFFM and HSIGFs, CDnetV2 is able to fully utilize features extracted from encoder layers and yield accurate cloud detection results. Experimental results on the ZY-3 satellite thumbnail data set demonstrate that the proposed CDnetV2 achieves accurate detection accuracy and outperforms several state-of-the-art methods.
-
-
-
-
-

-
-
-## Results and models
-
-### CLOUD EXTRACTION ACCURACY (%) OF DIFFERENT CNN-BASED METHODS ON ZY-3 SATELLITE THUMBNAILS
-
-
-| Method | OA | MIoU | Kappa | PA | UA |
-|-------|-------|-------|-------|-------|-------|
-| MSegNet | 90.86 | 81.20 | 75.57 | 73.78 | 86.13 |
-| MUnet | 91.62 | 82.51 | 76.70 | 74.44 | 87.39 |
-| PSPnet | 90.58 | 81.63 | 75.36 | 76.02 | 87.52 |
-| DeeplabV3+ | 91.80 | 82.62 | 77.65 | 75.30 | 87.76 |
-| CDnetV1 | 93.15 | 82.80 | 79.21 | 82.37 | 86.72 |
-| CDnetV2 | 95.76 | 86.62 | 82.51 | 87.75 | 88.58 |
-
-
-
-### STATISTICAL RESULTS OF CLOUDAGE ESTIMATION ERROR IN TERMS OF THE MAD AND ITS VARIANCE
-
-
-| Methods | Mean value ($\mu$) | Standard Deviation ($\sigma^2$)) |
-|------------|--------------------|----------------------------------|
-| CDnetV2 | 0.0241 | 0.0220 |
-| CDnetV1 | 0.0357 | 0.0288 |
-| DeeplabV3+ | 0.0456 | 0.0301 |
-| PSPnet | 0.0487 | 0.0380 |
-| MUnet | 0.0544 | 0.0583 |
-| MSegNet | 0.0572 | 0.0591 |
-
-
-
-
-### COMPUTATIONAL COMPLEXITY ANALYS IS OF DIFFERENT CNN-BASED METHODS
-
-| Methods | GFLOPs(224×224) | Trainable params | Running time (s)(1k×1k) |
-|------------|-----------------|------------------|-------------------------|
-| CDnetV2 | 31.5 | 65.9 M | 1.31 |
-| CDnetV1 | 48.5 | 64.8 M | 1.26 |
-| DeeplabV3+ | 31.8 | 40.3 M | 1.14 |
-| PSPnet | 19.3 | 46.6 M | 1.05 |
-| MUnet | 25.2 | 8.6 M | 1.09 |
-| MSegNet | 90.2 | 29.7 M | 1.28 |
-
-
-
-
-## Citation
-
-```bibtex
-@ARTICLE{8681238,
-author={J. {Yang} and J. {Guo} and H. {Yue} and Z. {Liu} and H. {Hu} and K. {Li}},
-journal={IEEE Transactions on Geoscience and Remote Sensing},
-title={CDnet: CNN-Based Cloud Detection for Remote Sensing Imagery},
-year={2019}, volume={57},
-number={8}, pages={6195-6211},
-doi={10.1109/TGRS.2019.2904868} }
-
-@ARTICLE{9094671,
-author={J. {Guo} and J. {Yang} and H. {Yue} and H. {Tan} and C. {Hou} and K. {Li}},
-journal={IEEE Transactions on Geoscience and Remote Sensing},
-title={CDnetV2: CNN-Based Cloud Detection for Remote Sensing Imagery With Cloud-Snow Coexistence},
-year={2021},
-volume={59},
-number={1},
-pages={700-713},
-doi={10.1109/TGRS.2020.2991398} }
-```
+# CDnetV2: CNN-Based Cloud Detection for Remote Sensing Imagery With Cloud-Snow Coexistence
+
+> [CDnetV2: CNN-Based Cloud Detection for Remote Sensing Imagery With Cloud-Snow Coexistence](https://ieeexplore.ieee.org/document/9094671)
+
+## Introduction
+
+
+
+Official Repo
+
+Code Snippet
+
+## Abstract
+
+
+
+Cloud detection is a crucial preprocessing step for optical satellite remote sensing (RS) images. This article focuses on the cloud detection for RS imagery with cloud-snow coexistence and the utilization of the satellite thumbnails that lose considerable amount of high resolution and spectrum information of original RS images to extract cloud mask efficiently. To tackle this problem, we propose a novel cloud detection neural network with an encoder-decoder structure, named CDnetV2, as a series work on cloud detection. Compared with our previous CDnetV1, CDnetV2 contains two novel modules, that is, adaptive feature fusing model (AFFM) and high-level semantic information guidance flows (HSIGFs). AFFM is used to fuse multilevel feature maps by three submodules: channel attention fusion model (CAFM), spatial attention fusion model (SAFM), and channel attention refinement model (CARM). HSIGFs are designed to make feature layers at decoder of CDnetV2 be aware of the locations of the cloud objects. The high-level semantic information of HSIGFs is extracted by a proposed high-level feature fusing model (HFFM). By being equipped with these two proposed key modules, AFFM and HSIGFs, CDnetV2 is able to fully utilize features extracted from encoder layers and yield accurate cloud detection results. Experimental results on the ZY-3 satellite thumbnail data set demonstrate that the proposed CDnetV2 achieves accurate detection accuracy and outperforms several state-of-the-art methods.
+
+
+
+
+

+
+
+## Results and models
+
+### CLOUD EXTRACTION ACCURACY (%) OF DIFFERENT CNN-BASED METHODS ON ZY-3 SATELLITE THUMBNAILS
+
+
+| Method | OA | MIoU | Kappa | PA | UA |
+|-------|-------|-------|-------|-------|-------|
+| MSegNet | 90.86 | 81.20 | 75.57 | 73.78 | 86.13 |
+| MUnet | 91.62 | 82.51 | 76.70 | 74.44 | 87.39 |
+| PSPnet | 90.58 | 81.63 | 75.36 | 76.02 | 87.52 |
+| DeeplabV3+ | 91.80 | 82.62 | 77.65 | 75.30 | 87.76 |
+| CDnetV1 | 93.15 | 82.80 | 79.21 | 82.37 | 86.72 |
+| CDnetV2 | 95.76 | 86.62 | 82.51 | 87.75 | 88.58 |
+
+
+
+### STATISTICAL RESULTS OF CLOUDAGE ESTIMATION ERROR IN TERMS OF THE MAD AND ITS VARIANCE
+
+
+| Methods | Mean value ($\mu$) | Standard Deviation ($\sigma^2$)) |
+|------------|--------------------|----------------------------------|
+| CDnetV2 | 0.0241 | 0.0220 |
+| CDnetV1 | 0.0357 | 0.0288 |
+| DeeplabV3+ | 0.0456 | 0.0301 |
+| PSPnet | 0.0487 | 0.0380 |
+| MUnet | 0.0544 | 0.0583 |
+| MSegNet | 0.0572 | 0.0591 |
+
+
+
+
+### COMPUTATIONAL COMPLEXITY ANALYS IS OF DIFFERENT CNN-BASED METHODS
+
+| Methods | GFLOPs(224×224) | Trainable params | Running time (s)(1k×1k) |
+|------------|-----------------|------------------|-------------------------|
+| CDnetV2 | 31.5 | 65.9 M | 1.31 |
+| CDnetV1 | 48.5 | 64.8 M | 1.26 |
+| DeeplabV3+ | 31.8 | 40.3 M | 1.14 |
+| PSPnet | 19.3 | 46.6 M | 1.05 |
+| MUnet | 25.2 | 8.6 M | 1.09 |
+| MSegNet | 90.2 | 29.7 M | 1.28 |
+
+
+
+
+## Citation
+
+```bibtex
+@ARTICLE{8681238,
+author={J. {Yang} and J. {Guo} and H. {Yue} and Z. {Liu} and H. {Hu} and K. {Li}},
+journal={IEEE Transactions on Geoscience and Remote Sensing},
+title={CDnet: CNN-Based Cloud Detection for Remote Sensing Imagery},
+year={2019}, volume={57},
+number={8}, pages={6195-6211},
+doi={10.1109/TGRS.2019.2904868} }
+
+@ARTICLE{9094671,
+author={J. {Guo} and J. {Yang} and H. {Yue} and H. {Tan} and C. {Hou} and K. {Li}},
+journal={IEEE Transactions on Geoscience and Remote Sensing},
+title={CDnetV2: CNN-Based Cloud Detection for Remote Sensing Imagery With Cloud-Snow Coexistence},
+year={2021},
+volume={59},
+number={1},
+pages={700-713},
+doi={10.1109/TGRS.2020.2991398} }
+```
diff --git a/configs/model/cdnetv2/cdnetv2.yaml b/configs/model/cdnetv2/cdnetv2.yaml
index 24fa0179f836ac8419181a78527a27ccf626c4d0..be0d092f2d0bded127714e71e35f12a70bebb23f 100644
--- a/configs/model/cdnetv2/cdnetv2.yaml
+++ b/configs/model/cdnetv2/cdnetv2.yaml
@@ -1,19 +1,19 @@
-_target_: src.models.cdnetv2_module.CDNetv2LitModule
-
-net:
- _target_: src.models.components.cdnetv2.CDnetV2
- num_classes: 2
-
-num_classes: 2
-
-criterion:
- _target_: src.loss.cdnetv2_loss.CDnetv2Loss
- loss_fn:
- _target_: torch.nn.CrossEntropyLoss
-
-optimizer:
- _target_: torch.optim.SGD
- _partial_: true
- lr: 0.0001
-
+_target_: src.models.cdnetv2_module.CDNetv2LitModule
+
+net:
+ _target_: src.models.components.cdnetv2.CDnetV2
+ num_classes: 2
+
+num_classes: 2
+
+criterion:
+ _target_: src.loss.cdnetv2_loss.CDnetv2Loss
+ loss_fn:
+ _target_: torch.nn.CrossEntropyLoss
+
+optimizer:
+ _target_: torch.optim.SGD
+ _partial_: true
+ lr: 0.0001
+
scheduler: null
\ No newline at end of file
diff --git a/configs/model/cnn.yaml b/configs/model/cnn.yaml
index add5938cc4c4740056b8dbbf6edf32415dcf4ccc..4bd0feed03354eabb744179e117bebef500c1591 100644
--- a/configs/model/cnn.yaml
+++ b/configs/model/cnn.yaml
@@ -1,21 +1,21 @@
-_target_: src.models.mnist_module.MNISTLitModule
-
-optimizer:
- _target_: torch.optim.Adam
- _partial_: true
- lr: 0.001
- weight_decay: 0.0
-
-scheduler:
- _target_: torch.optim.lr_scheduler.ReduceLROnPlateau
- _partial_: true
- mode: min
- factor: 0.1
- patience: 10
-
-net:
- _target_: src.models.components.cnn.CNN
- dim: 32
-
-# compile model for faster training with pytorch 2.0
-compile: false
+_target_: src.models.mnist_module.MNISTLitModule
+
+optimizer:
+ _target_: torch.optim.Adam
+ _partial_: true
+ lr: 0.001
+ weight_decay: 0.0
+
+scheduler:
+ _target_: torch.optim.lr_scheduler.ReduceLROnPlateau
+ _partial_: true
+ mode: min
+ factor: 0.1
+ patience: 10
+
+net:
+ _target_: src.models.components.cnn.CNN
+ dim: 32
+
+# compile model for faster training with pytorch 2.0
+compile: false
diff --git a/configs/model/dbnet/README.md b/configs/model/dbnet/README.md
index 3a7dc71d248fd114b0801957965f2f08c86243b4..2a607909c49c54ae8e09a1f55e89b2504c442f65 100644
--- a/configs/model/dbnet/README.md
+++ b/configs/model/dbnet/README.md
@@ -1,107 +1,107 @@
-# Dual-Branch Network for Cloud and Cloud Shadow Segmentation
-
-
-> [Dual-Branch Network for Cloud and Cloud Shadow Segmentation](https://ieeexplore.ieee.org/document/9775689)
-
-## Introduction
-
-
-
-Official Repo
-
-Code Snippet
-
-## Abstract
-
-
-
-— Cloud and cloud shadow segmentation is one of the
-most important issues in remote sensing image processing. Most
-of the remote sensing images are very complicated. In this work,
-a dual-branch model composed of transformer and convolution
-network is proposed to extract semantic and spatial detail information of the image, respectively, to solve the problems of false
-detection and missed detection. To improve the model’s feature
-extraction, a mutual guidance module (MGM) is introduced,
-so that the transformer branch and the convolution branch can
-guide each other for feature mining. Finally, in view of the problem of rough segmentation boundary, this work uses different
-features extracted by the transformer branch and the convolution
-branch for decoding and repairs the rough segmentation boundary in the decoding part to make the segmentation boundary
-clearer. Experimental results on the Landsat-8, Sentinel-2 data,
-the public dataset high-resolution cloud cover validation dataset
-created by researchers at Wuhan University (HRC_WHU), and
-the public dataset Spatial Procedures for Automated Removal
-of Cloud and Shadow (SPARCS) demonstrate the effectiveness
-of our method and its superiority to the existing state-of-the-art
-cloud and cloud shadow segmentation approaches.
-
-
-
-
-

-
-
-## Results and models
-
-### COMPARISON OF EVALUATION METRICS OF DIFFERENT MODELS ON CLOUD AND CLOUD SHADOW DATASET
-
-
-| **Method** | **Cloud** | | | | **Cloud Shadow** | | | | | | |
-|--------------------|-----------|----------|----------|-----------|------------------|----------|----------|-----------|-----------|------------|-------------|
-| | **OA(%)** | **P(%)** | **R(%)** | **F₁(%)** | **OA(%)** | **P(%)** | **R(%)** | **F₁(%)** | **PA(%)** | **MPA(%)** | **MIoU(%)** |
-| FCN-8S [7] | 95.87 | 88.47 | 92.8 | 90.63 | 97.19 | 86.87 | 88.72 | 87.79 | 93.40 | 90.52 | 84.01 |
-| PAN [38] | 98.25 | 96.29 | 95.49 | 95.89 | 98.31 | 92.71 | 92.46 | 92.59 | 96.73 | 95.52 | 91.26 |
-| BiseNet V2 [35] | 98.27 | 96.57 | 95.28 | 95.92 | 98.34 | 94.18 | 90.99 | 92.59 | 96.68 | 95.96 | 91.20 |
-| PSPNet [11] | 98.35 | 96.13 | 96.13 | 96.13 | 98.40 | 92.70 | 93.27 | 92.99 | 96.87 | 95.55 | 91.69 |
-| DeepLab V3Plus [9] | 98.65 | 97.70 | 95.94 | 96.82 | 98.66 | 93.88 | 94.32 | 94.10 | 97.37 | 96.48 | 92.99 |
-| LinkNet [39] | 98.61 | 96.59 | 96.91 | 96.75 | 98.54 | 94.19 | 92.91 | 93.55 | 97.23 | 96.24 | 92.55 |
-| ExtremeC3Net [40] | 98.64 | 97.32 | 96.28 | 96.80 | 98.60 | 94.68 | 92.95 | 93.82 | 97.30 | 96.57 | 92.76 |
-| DANet [41] | 96.45 | 91.68 | 91.72 | 91.71 | 97.29 | 88.40 | 87.68 | 88.04 | 94.03 | 91.93 | 85.07 |
-| CGNet [42] | 98.37 | 95.93 | 96.48 | 96.20 | 98.27 | 93.33 | 91.34 | 92.34 | 96.73 | 95.60 | 91.27 |
-| PVT [23] | 98.57 | 97.45 | 95.84 | 96.65 | 98.55 | 93.28 | 94.08 | 93.68 | 97.21 | 96.18 | 92.55 |
-| CvT [24] | 98.44 | 95.89 | 96.88 | 96.38 | 98.32 | 92.90 | 92.24 | 92.57 | 96.85 | 95.54 | 91.57 |
-| modified VGG [12] | 98.40 | 98.13 | 94.30 | 96.22 | 98.57 | 94.41 | 92.88 | 93.64 | 97.04 | 96.56 | 92.17 |
-| CloudNet [13] | 98.70 | 97.22 | 96.68 | 96.95 | 98.40 | 92.05 | 94.05 | 93.05 | 97.17 | 95.77 | 92.36 |
-| GAFFNet [43] | 98.53 | 96.49 | 96.63 | 96.56 | 98.41 | 92.71 | 93.40 | 93.05 | 97.06 | 95.73 | 92.08 |
-| Our | 98.76 | 97.95 | 96.22 | 97.08 | 98.73 | 94.39 | 94.39 | 94.39 | 97.56 | 96.77 | 93.42 |
-
-### COMPARISON OF EVALUATION METRICS OF DIFFERENT MODELS ON THE SPARCS DATASET
-
-
-| Method | Class Pixel Accuracy | | | | | Overall Results | | | | |
-|---|---|---|---|---|---|---|---|---|---|---|
-| | Cloud(%) | Cloud Shadow(%) | Snow/Ice(%) | Water(%) | Land(%) | PA(%) | Recall(%) | Precision(%) | F₁(%) | MIoU(%) |
-| PAN [38] | 89.10 | 75.27 | 86.60 | 79.96 | 95.64 | 91.20 | 87.34 | 85.32 | 81.53 | 76.57 |
-| BiSeNet V2 [35] | 85.87 | 64.75 | 93.84 | 81.44 | 97.17 | 91.31 | 89.77 | 84.61 | 83.09 | 77.79 |
-| PSPNet [11] | 90.79 | 63.75 | 94.22 | 77.73 | 96.84 | 91.78 | 90.29 | 84.67 | 83.48 | 78.20 |
-| DeepLab V3Plus [9] | 87.81 | 72.12 | 85.17 | 81.27 | 97.84 | 91.99 | 90.75 | 84.85 | 84.01 | 78.44 |
-| LinkNet [39] | 85.35 | 74.38 | 91.92 | 80.30 | 96.44 | 91.31 | 88.66 | 85.68 | 82.81 | 77.87 |
-| ExtremeC3Net [40] | 91.09 | 75.47 | 95.43 | 83.62 | 96.13 | 92.77 | 90.32 | 88.35 | 85.46 | 81.29 |
-| DANet [41] | 82.06 | 42.25 | 91.28 | 73.65 | 95.03 | 86.92 | 83.86 | 76.85 | 74.64 | 68.33 |
-| CGNet [42] | 90.63 | 72.78 | 95.37 | 83.30 | 96.51 | 93.22 | 91.00 | 88.95 | 86.30 | 82.28 |
-| PVT [23] | 88.22 | 75.77 | 92.00 | 86.27 | 95.92 | 92.02 | 89.76 | 87.64 | 84.66 | 80.24 |
-| CvT [24] | 88.24 | 71.63 | 95.41 | 87.71 | 96.14 | 92.17 | 89.83 | 87.83 | 84.80 | 80.55 |
-| modified VGG [12] | 85.55 | 58.53 | 94.87 | 79.35 | 95.98 | 89.99 | 86.38 | 82.85 | 79.36 | 74.00 |
-| CloudNet [13] | 85.99 | 74.58 | 91.78 | 80.34 | 96.52 | 91.50 | 88.49 | 85.84 | 82.79 | 77.95 |
-| GAFFNet [43] | 86.97 | 59.00 | 85.21 | 78.06 | 94.47 | 88.62 | 86.70 | 80.74 | 78.56 | 72.32 |
-| our | 91.12 | 78.38 | 96.59 | 89.99 | 97.52 | 94.31 | 92.90 | 90.72 | 88.83 | 85.26 |
-
-
-
-
-
-
-## Citation
-
-```bibtex
-@ARTICLE{9775689,
- author={Lu, Chen and Xia, Min and Qian, Ming and Chen, Binyu},
- journal={IEEE Transactions on Geoscience and Remote Sensing},
- title={Dual-Branch Network for Cloud and Cloud Shadow Segmentation},
- year={2022},
- volume={60},
- number={},
- pages={1-12},
- keywords={Feature extraction;Transformers;Convolution;Clouds;Image segmentation;Decoding;Task analysis;Deep learning;dual branch;remote sensing image;segmentation},
- doi={10.1109/TGRS.2022.3175613}}
-
-```
+# Dual-Branch Network for Cloud and Cloud Shadow Segmentation
+
+
+> [Dual-Branch Network for Cloud and Cloud Shadow Segmentation](https://ieeexplore.ieee.org/document/9775689)
+
+## Introduction
+
+
+
+Official Repo
+
+Code Snippet
+
+## Abstract
+
+
+
+— Cloud and cloud shadow segmentation is one of the
+most important issues in remote sensing image processing. Most
+of the remote sensing images are very complicated. In this work,
+a dual-branch model composed of transformer and convolution
+network is proposed to extract semantic and spatial detail information of the image, respectively, to solve the problems of false
+detection and missed detection. To improve the model’s feature
+extraction, a mutual guidance module (MGM) is introduced,
+so that the transformer branch and the convolution branch can
+guide each other for feature mining. Finally, in view of the problem of rough segmentation boundary, this work uses different
+features extracted by the transformer branch and the convolution
+branch for decoding and repairs the rough segmentation boundary in the decoding part to make the segmentation boundary
+clearer. Experimental results on the Landsat-8, Sentinel-2 data,
+the public dataset high-resolution cloud cover validation dataset
+created by researchers at Wuhan University (HRC_WHU), and
+the public dataset Spatial Procedures for Automated Removal
+of Cloud and Shadow (SPARCS) demonstrate the effectiveness
+of our method and its superiority to the existing state-of-the-art
+cloud and cloud shadow segmentation approaches.
+
+
+
+
+

+
+
+## Results and models
+
+### COMPARISON OF EVALUATION METRICS OF DIFFERENT MODELS ON CLOUD AND CLOUD SHADOW DATASET
+
+
+| **Method** | **Cloud** | | | | **Cloud Shadow** | | | | | | |
+|--------------------|-----------|----------|----------|-----------|------------------|----------|----------|-----------|-----------|------------|-------------|
+| | **OA(%)** | **P(%)** | **R(%)** | **F₁(%)** | **OA(%)** | **P(%)** | **R(%)** | **F₁(%)** | **PA(%)** | **MPA(%)** | **MIoU(%)** |
+| FCN-8S [7] | 95.87 | 88.47 | 92.8 | 90.63 | 97.19 | 86.87 | 88.72 | 87.79 | 93.40 | 90.52 | 84.01 |
+| PAN [38] | 98.25 | 96.29 | 95.49 | 95.89 | 98.31 | 92.71 | 92.46 | 92.59 | 96.73 | 95.52 | 91.26 |
+| BiseNet V2 [35] | 98.27 | 96.57 | 95.28 | 95.92 | 98.34 | 94.18 | 90.99 | 92.59 | 96.68 | 95.96 | 91.20 |
+| PSPNet [11] | 98.35 | 96.13 | 96.13 | 96.13 | 98.40 | 92.70 | 93.27 | 92.99 | 96.87 | 95.55 | 91.69 |
+| DeepLab V3Plus [9] | 98.65 | 97.70 | 95.94 | 96.82 | 98.66 | 93.88 | 94.32 | 94.10 | 97.37 | 96.48 | 92.99 |
+| LinkNet [39] | 98.61 | 96.59 | 96.91 | 96.75 | 98.54 | 94.19 | 92.91 | 93.55 | 97.23 | 96.24 | 92.55 |
+| ExtremeC3Net [40] | 98.64 | 97.32 | 96.28 | 96.80 | 98.60 | 94.68 | 92.95 | 93.82 | 97.30 | 96.57 | 92.76 |
+| DANet [41] | 96.45 | 91.68 | 91.72 | 91.71 | 97.29 | 88.40 | 87.68 | 88.04 | 94.03 | 91.93 | 85.07 |
+| CGNet [42] | 98.37 | 95.93 | 96.48 | 96.20 | 98.27 | 93.33 | 91.34 | 92.34 | 96.73 | 95.60 | 91.27 |
+| PVT [23] | 98.57 | 97.45 | 95.84 | 96.65 | 98.55 | 93.28 | 94.08 | 93.68 | 97.21 | 96.18 | 92.55 |
+| CvT [24] | 98.44 | 95.89 | 96.88 | 96.38 | 98.32 | 92.90 | 92.24 | 92.57 | 96.85 | 95.54 | 91.57 |
+| modified VGG [12] | 98.40 | 98.13 | 94.30 | 96.22 | 98.57 | 94.41 | 92.88 | 93.64 | 97.04 | 96.56 | 92.17 |
+| CloudNet [13] | 98.70 | 97.22 | 96.68 | 96.95 | 98.40 | 92.05 | 94.05 | 93.05 | 97.17 | 95.77 | 92.36 |
+| GAFFNet [43] | 98.53 | 96.49 | 96.63 | 96.56 | 98.41 | 92.71 | 93.40 | 93.05 | 97.06 | 95.73 | 92.08 |
+| Our | 98.76 | 97.95 | 96.22 | 97.08 | 98.73 | 94.39 | 94.39 | 94.39 | 97.56 | 96.77 | 93.42 |
+
+### COMPARISON OF EVALUATION METRICS OF DIFFERENT MODELS ON THE SPARCS DATASET
+
+
+| Method | Class Pixel Accuracy | | | | | Overall Results | | | | |
+|---|---|---|---|---|---|---|---|---|---|---|
+| | Cloud(%) | Cloud Shadow(%) | Snow/Ice(%) | Water(%) | Land(%) | PA(%) | Recall(%) | Precision(%) | F₁(%) | MIoU(%) |
+| PAN [38] | 89.10 | 75.27 | 86.60 | 79.96 | 95.64 | 91.20 | 87.34 | 85.32 | 81.53 | 76.57 |
+| BiSeNet V2 [35] | 85.87 | 64.75 | 93.84 | 81.44 | 97.17 | 91.31 | 89.77 | 84.61 | 83.09 | 77.79 |
+| PSPNet [11] | 90.79 | 63.75 | 94.22 | 77.73 | 96.84 | 91.78 | 90.29 | 84.67 | 83.48 | 78.20 |
+| DeepLab V3Plus [9] | 87.81 | 72.12 | 85.17 | 81.27 | 97.84 | 91.99 | 90.75 | 84.85 | 84.01 | 78.44 |
+| LinkNet [39] | 85.35 | 74.38 | 91.92 | 80.30 | 96.44 | 91.31 | 88.66 | 85.68 | 82.81 | 77.87 |
+| ExtremeC3Net [40] | 91.09 | 75.47 | 95.43 | 83.62 | 96.13 | 92.77 | 90.32 | 88.35 | 85.46 | 81.29 |
+| DANet [41] | 82.06 | 42.25 | 91.28 | 73.65 | 95.03 | 86.92 | 83.86 | 76.85 | 74.64 | 68.33 |
+| CGNet [42] | 90.63 | 72.78 | 95.37 | 83.30 | 96.51 | 93.22 | 91.00 | 88.95 | 86.30 | 82.28 |
+| PVT [23] | 88.22 | 75.77 | 92.00 | 86.27 | 95.92 | 92.02 | 89.76 | 87.64 | 84.66 | 80.24 |
+| CvT [24] | 88.24 | 71.63 | 95.41 | 87.71 | 96.14 | 92.17 | 89.83 | 87.83 | 84.80 | 80.55 |
+| modified VGG [12] | 85.55 | 58.53 | 94.87 | 79.35 | 95.98 | 89.99 | 86.38 | 82.85 | 79.36 | 74.00 |
+| CloudNet [13] | 85.99 | 74.58 | 91.78 | 80.34 | 96.52 | 91.50 | 88.49 | 85.84 | 82.79 | 77.95 |
+| GAFFNet [43] | 86.97 | 59.00 | 85.21 | 78.06 | 94.47 | 88.62 | 86.70 | 80.74 | 78.56 | 72.32 |
+| our | 91.12 | 78.38 | 96.59 | 89.99 | 97.52 | 94.31 | 92.90 | 90.72 | 88.83 | 85.26 |
+
+
+
+
+
+
+## Citation
+
+```bibtex
+@ARTICLE{9775689,
+ author={Lu, Chen and Xia, Min and Qian, Ming and Chen, Binyu},
+ journal={IEEE Transactions on Geoscience and Remote Sensing},
+ title={Dual-Branch Network for Cloud and Cloud Shadow Segmentation},
+ year={2022},
+ volume={60},
+ number={},
+ pages={1-12},
+ keywords={Feature extraction;Transformers;Convolution;Clouds;Image segmentation;Decoding;Task analysis;Deep learning;dual branch;remote sensing image;segmentation},
+ doi={10.1109/TGRS.2022.3175613}}
+
+```
diff --git a/configs/model/dbnet/dbnet.yaml b/configs/model/dbnet/dbnet.yaml
index f91ea3aea3a70e46dd69c02582312258164f33cd..0d071ef61b6bab1cd6f38ed2862d3c648db3dbc2 100644
--- a/configs/model/dbnet/dbnet.yaml
+++ b/configs/model/dbnet/dbnet.yaml
@@ -1,23 +1,23 @@
-_target_: src.models.base_module.BaseLitModule
-
-net:
- _target_: src.models.components.dbnet.DBNet
- img_size: 256
- in_channels: 3
- num_classes: 2
-
-num_classes: 2
-
-criterion:
- _target_: torch.nn.CrossEntropyLoss
-
-optimizer:
- _target_: torch.optim.Adam
- _partial_: true
- weight_decay: 0.0001
- lr: 0.0001
-
-scheduler:
- _target_: torch.optim.lr_scheduler.StepLR
- _partial_: true
+_target_: src.models.base_module.BaseLitModule
+
+net:
+ _target_: src.models.components.dbnet.DBNet
+ img_size: 256
+ in_channels: 3
+ num_classes: 2
+
+num_classes: 2
+
+criterion:
+ _target_: torch.nn.CrossEntropyLoss
+
+optimizer:
+ _target_: torch.optim.Adam
+ _partial_: true
+ weight_decay: 0.0001
+ lr: 0.0001
+
+scheduler:
+ _target_: torch.optim.lr_scheduler.StepLR
+ _partial_: true
step_size: 3
\ No newline at end of file
diff --git a/configs/model/hrcloudnet/README.md b/configs/model/hrcloudnet/README.md
index 5fbadccde7b98620e39ea3afdf7b25e3e05eabdb..aecbc66cdf300dce23c6f8c12c1d1c6d8109abfe 100644
--- a/configs/model/hrcloudnet/README.md
+++ b/configs/model/hrcloudnet/README.md
@@ -1,102 +1,102 @@
-# High-Resolution Cloud Detection Network
-
-> [High-Resolution Cloud Detection Network](https://arxiv.org/abs/2407.07365)
-
-## Introduction
-
-
-
-Official Repo
-
-Code Snippet
-
-## Abstract
-
-
-
-The complexity of clouds, particularly in terms of texture
-detail at high resolutions, has not been well explored by most
-existing cloud detection networks. This paper introduces
-the High-Resolution Cloud Detection Network (HR-cloudNet), which utilizes a hierarchical high-resolution integration approach. HR-cloud-Net integrates a high-resolution
-representation module, layer-wise cascaded feature fusion
-module, and multi-resolution pyramid pooling module to
-effectively capture complex cloud features. This architecture
-preserves detailed cloud texture information while facilitating feature exchange across different resolutions, thereby
-enhancing overall performance in cloud detection. Additionally, a novel approach is introduced wherein a student
-view, trained on noisy augmented images, is supervised by a
-teacher view processing normal images. This setup enables
-the student to learn from cleaner supervisions provided by
-the teacher, leading to improved performance. Extensive
-evaluations on three optical satellite image cloud detection
-datasets validate the superior performance of HR-cloud-Net
-compared to existing methods.
-
-
-
-
-
-

-
-
-## Results and models
-
-### CHLandSat-8 dataset
-
-
-| method | mae | weight-F-measure | structure-measure |
-|--------------|------------|------------------|-------------------|
-| U-Net | 0.1130 | 0.7448 | 0.7228 |
-| PSPNet | 0.0969 | 0.7989 | 0.7672 |
-| SegNet | 0.1023 | 0.7780 | 0.7540 |
-| Cloud-Net | 0.1012 | 0.7641 | 0.7368 |
-| CDNet | 0.1286 | 0.7222 | 0.7087 |
-| CDNet-v2 | 0.1254 | 0.7350 | 0.7141 |
-| HRNet | **0.0737** | 0.8279 | **0.8141** |
-| GANet | 0.0751 | **0.8396** | 0.8106 |
-| HR-cloud-Net | **0.0628** | **0.8503** | **0.8337** |
-
-### 38-cloud dataset
-
-
-| method | mae | weight-F-measure | structure-measure |
-|--------------|------------|------------------|-------------------|
-| U-Net | 0.0638 | 0.7966 | 0.7845 |
-| PSPNet | 0.0653 | 0.7592 | 0.7766 |
-| SegNet | 0.0556 | 0.8002 | 0.8059 |
-| Cloud-Net | 0.0556 | 0.7615 | 0.7987 |
-| CDNet | 0.1057 | 0.7378 | 0.7270 |
-| CDNet-v2 | 0.1084 | 0.7183 | 0.7213 |
-| HRNet | 0.0538 | 0.8086 | 0.8183 |
-| GANet | **0.0410** | **0.8159** | **0.8342** |
-| HR-cloud-Net | **0.0395** | **0.8673** | **0.8479** |
-
-
-### SPARCS dataset
-
-
-| method | mae | weight-F-measure | structure-measure |
-|--------------|------------|------------------|-------------------|
-| U-Net | 0.1314 | 0.3651 | 0.5416 |
-| PSPNet | 0.1263 | 0.3758 | 0.5414 |
-| SegNet | 0.1100 | 0.4697 | 0.5918 |
-| Cloud-Net | 0.1213 | 0.3804 | 0.5536 |
-| CDNet | 0.1157 | 0.4585 | 0.5919 |
-| CDNet-v2 | 0.1219 | 0.4247 | 0.5704 |
-| HRNet | 0.1008 | 0.3742 | 0.5777 |
-| GANet | **0.0987** | **0.5134** | **0.6210** |
-| HR-cloud-Net | **0.0833** | **0.5202** | **0.6327** |
-
-
-
-
-## Citation
-
-```bibtex
-@InProceedings{LiJEI2024,
- author = {Jingsheng Li and Tianxiang Xue and Jiayi Zhao and
- Jingmin Ge and Yufang Min and Wei Su and Kun Zhan},
- title = {High-Resolution Cloud Detection Network},
- booktitle = {Journal of Electronic Imaging},
- year = {2024},
-}
+# High-Resolution Cloud Detection Network
+
+> [High-Resolution Cloud Detection Network](https://arxiv.org/abs/2407.07365)
+
+## Introduction
+
+
+
+Official Repo
+
+Code Snippet
+
+## Abstract
+
+
+
+The complexity of clouds, particularly in terms of texture
+detail at high resolutions, has not been well explored by most
+existing cloud detection networks. This paper introduces
+the High-Resolution Cloud Detection Network (HR-cloudNet), which utilizes a hierarchical high-resolution integration approach. HR-cloud-Net integrates a high-resolution
+representation module, layer-wise cascaded feature fusion
+module, and multi-resolution pyramid pooling module to
+effectively capture complex cloud features. This architecture
+preserves detailed cloud texture information while facilitating feature exchange across different resolutions, thereby
+enhancing overall performance in cloud detection. Additionally, a novel approach is introduced wherein a student
+view, trained on noisy augmented images, is supervised by a
+teacher view processing normal images. This setup enables
+the student to learn from cleaner supervisions provided by
+the teacher, leading to improved performance. Extensive
+evaluations on three optical satellite image cloud detection
+datasets validate the superior performance of HR-cloud-Net
+compared to existing methods.
+
+
+
+
+
+

+
+
+## Results and models
+
+### CHLandSat-8 dataset
+
+
+| method | mae | weight-F-measure | structure-measure |
+|--------------|------------|------------------|-------------------|
+| U-Net | 0.1130 | 0.7448 | 0.7228 |
+| PSPNet | 0.0969 | 0.7989 | 0.7672 |
+| SegNet | 0.1023 | 0.7780 | 0.7540 |
+| Cloud-Net | 0.1012 | 0.7641 | 0.7368 |
+| CDNet | 0.1286 | 0.7222 | 0.7087 |
+| CDNet-v2 | 0.1254 | 0.7350 | 0.7141 |
+| HRNet | **0.0737** | 0.8279 | **0.8141** |
+| GANet | 0.0751 | **0.8396** | 0.8106 |
+| HR-cloud-Net | **0.0628** | **0.8503** | **0.8337** |
+
+### 38-cloud dataset
+
+
+| method | mae | weight-F-measure | structure-measure |
+|--------------|------------|------------------|-------------------|
+| U-Net | 0.0638 | 0.7966 | 0.7845 |
+| PSPNet | 0.0653 | 0.7592 | 0.7766 |
+| SegNet | 0.0556 | 0.8002 | 0.8059 |
+| Cloud-Net | 0.0556 | 0.7615 | 0.7987 |
+| CDNet | 0.1057 | 0.7378 | 0.7270 |
+| CDNet-v2 | 0.1084 | 0.7183 | 0.7213 |
+| HRNet | 0.0538 | 0.8086 | 0.8183 |
+| GANet | **0.0410** | **0.8159** | **0.8342** |
+| HR-cloud-Net | **0.0395** | **0.8673** | **0.8479** |
+
+
+### SPARCS dataset
+
+
+| method | mae | weight-F-measure | structure-measure |
+|--------------|------------|------------------|-------------------|
+| U-Net | 0.1314 | 0.3651 | 0.5416 |
+| PSPNet | 0.1263 | 0.3758 | 0.5414 |
+| SegNet | 0.1100 | 0.4697 | 0.5918 |
+| Cloud-Net | 0.1213 | 0.3804 | 0.5536 |
+| CDNet | 0.1157 | 0.4585 | 0.5919 |
+| CDNet-v2 | 0.1219 | 0.4247 | 0.5704 |
+| HRNet | 0.1008 | 0.3742 | 0.5777 |
+| GANet | **0.0987** | **0.5134** | **0.6210** |
+| HR-cloud-Net | **0.0833** | **0.5202** | **0.6327** |
+
+
+
+
+## Citation
+
+```bibtex
+@InProceedings{LiJEI2024,
+ author = {Jingsheng Li and Tianxiang Xue and Jiayi Zhao and
+ Jingmin Ge and Yufang Min and Wei Su and Kun Zhan},
+ title = {High-Resolution Cloud Detection Network},
+ booktitle = {Journal of Electronic Imaging},
+ year = {2024},
+}
```
\ No newline at end of file
diff --git a/configs/model/hrcloudnet/hrcloudnet.yaml b/configs/model/hrcloudnet/hrcloudnet.yaml
index 848b9833e9ddba1293effebd73ed1b3adf9faa04..5044d2ad6330de7e42e84accc56517f2b8bab338 100644
--- a/configs/model/hrcloudnet/hrcloudnet.yaml
+++ b/configs/model/hrcloudnet/hrcloudnet.yaml
@@ -1,18 +1,18 @@
-_target_: src.models.base_module.BaseLitModule
-
-net:
- _target_: src.models.components.hrcloudnet.HRCloudNet
- num_classes: 2
-
-num_classes: 2
-
-criterion:
- _target_: torch.nn.CrossEntropyLoss
-
-optimizer:
- _target_: torch.optim.Adam
- _partial_: true
- lr: 0.00005
- weight_decay: 0.0005
-
+_target_: src.models.base_module.BaseLitModule
+
+net:
+ _target_: src.models.components.hrcloudnet.HRCloudNet
+ num_classes: 2
+
+num_classes: 2
+
+criterion:
+ _target_: torch.nn.CrossEntropyLoss
+
+optimizer:
+ _target_: torch.optim.Adam
+ _partial_: true
+ lr: 0.00005
+ weight_decay: 0.0005
+
scheduler: null
\ No newline at end of file
diff --git a/configs/model/lnn.yaml b/configs/model/lnn.yaml
index d02662f8cdd442ee987695d83b33fb2ed4178142..53c93c2e72eaf768531e9303a2bbbbd423330398 100644
--- a/configs/model/lnn.yaml
+++ b/configs/model/lnn.yaml
@@ -1,21 +1,21 @@
-_target_: src.models.mnist_module.MNISTLitModule
-
-optimizer:
- _target_: torch.optim.Adam
- _partial_: true
- lr: 0.001
- weight_decay: 0.0
-
-scheduler:
- _target_: torch.optim.lr_scheduler.ReduceLROnPlateau
- _partial_: true
- mode: min
- factor: 0.1
- patience: 10
-
-net:
- _target_: src.models.components.lnn.LNN
- dim: 32
-
-# compile model for faster training with pytorch 2.0
-compile: false
+_target_: src.models.mnist_module.MNISTLitModule
+
+optimizer:
+ _target_: torch.optim.Adam
+ _partial_: true
+ lr: 0.001
+ weight_decay: 0.0
+
+scheduler:
+ _target_: torch.optim.lr_scheduler.ReduceLROnPlateau
+ _partial_: true
+ mode: min
+ factor: 0.1
+ patience: 10
+
+net:
+ _target_: src.models.components.lnn.LNN
+ dim: 32
+
+# compile model for faster training with pytorch 2.0
+compile: false
diff --git a/configs/model/mcdnet/README.md b/configs/model/mcdnet/README.md
index ea6597541e4afa90a2621807c61164b6bfc86b94..87f9c2d060f7107dedb3c87d3aaaa582a85108db 100644
--- a/configs/model/mcdnet/README.md
+++ b/configs/model/mcdnet/README.md
@@ -1,115 +1,115 @@
-# MCDNet: Multilevel cloud detection network for remote sensing images based on dual-perspective change-guided and multi-scale feature fusion
-
-> [MCDNet: Multilevel cloud detection network for remote sensing images based on dual-perspective change-guided and multi-scale feature fusion](https://www.sciencedirect.com/science/article/pii/S1569843224001742?via%3Dihub)
-
-## Introduction
-
-
-
-Official Repo
-
-Code Snippet
-
-## Abstract
-
-
-Cloud detection plays a crucial role in the preprocessing of optical remote sensing images. While extensive deep learning-based methods have shown strong performance in detecting thick clouds, their ability to identify thin and broken clouds is often inadequate due to their sparse distribution, semi-transparency, and similarity to background regions. To address this limitation, we introduce a multilevel cloud detection network (MCDNet) capable of simultaneously detecting thick and thin clouds. This network effectively enhances the accuracy of identifying thin and broken clouds by integrating a dual-perspective change-guided mechanism (DPCG) and a multi-scale feature fusion module (MSFF). The DPCG creates a dual-input stream by combining the original image with the thin cloud removal image, and then utilizes a dual-perspective feature fusion module (DPFF) to perform feature fusion and extract change features, thereby improving the model's ability to perceive thin cloud regions and mitigate inter-class similarity in multilevel cloud detection. The MSFF enhances the model's sensitivity to broken clouds by utilizing multiple non-adjacent low-level features to remedy the missing spatial information in the high-level features during multiple downsampling. Experimental results on the L8-Biome and WHUS2-CD datasets demonstrate that MCDNet significantly enhances the detection performance of both thin and broken clouds, and outperforms state-of-the-art methods in accuracy and efficiency.
-
-
-
-
-

-
-
-
-## Results and models
-
-### Quantitative performance of MCDNet with different thin cloud removal methods on the L8-Biome dataset.
-
-
-| Thin cloud removal method | OA | IoU | Specificity | Kappa |
-|--------------------------|------|------|-------------|--------|
-| DCP | 94.18| 89.55| 97.09 | 70.42 |
-| HF | 94.22| 89.69| 97.11 | 70.59 |
-| BCCR | 94.25| 89.75| 97.13 | 71.01 |
-
-### Quantitative performance of MCDNet with different components using BCCR thin cloud removal method on the L8-Biome dataset
-
-
-| Baseline | √ | √ | √ | √ |
-|----------------|-------|-------|-------|-------|
-| MSFF | × | √ | × | √ |
-| DPFF | × | × | √ | √ |
-| OA | 92.91 | 93.25 | 93.81 | 94.25 |
-| IoU | 87.58 | 88.13 | 88.99 | 89.75 |
-| Specificity | 96.46 | 96.62 | 96.90 | 97.13 |
-| Kappa | 67.22 | 68.88 | 69.94 | 71.01 |
-| Parameters (M) | 8.89 | 10.67 | 11.34 | 13.11 |
-
-
-
-### Quantitative comparisons of different methods on the L8-Biome dataset.
-
-
-| Method | OA | IoU | Specificity | Kappa |
-|----------|-------|-------|-------------|-------|
-| UNet | 90.78 | 84.44 | 95.39 | 63.22 |
-| SegNet | 91.44 | 85.33 | 95.72 | 63.98 |
-| HRNet | 91.17 | 84.87 | 95.59 | 62.32 |
-| SwinUnet | 91.73 | 85.74 | 95.86 | 64.36 |
-| MFCNN | 92.39 | 86.76 | 96.20 | 66.72 |
-| MSCFF | 92.49 | 86.99 | 96.25 | 65.82 |
-| CDNetV2 | 91.55 | 85.51 | 95.77 | 64.91 |
-| CloudNet | 92.19 | 86.55 | 96.09 | 65.99 |
-| MCDNet | 94.25 | 89.75 | 97.13 | 71.01 |
-
-
-### Quantitative comparisons of different methods on the WHUS2-CD dataset.
-
-| Method | OA | IoU | Specificity | Kappa |
-|----------|-------|-------|-------------|-------|
-| UNet | 98.24 | 63.72 | 99.51 | 63.45 |
-| SegNet | 98.09 | 62.53 | 99.15 | 61.71 |
-| HRNet | 97.91 | 61.73 | 99.41 | 59.29 |
-| SwinUnet | 98.69 | 61.93 | 99.48 | 67.81 |
-| MFCNN | 98.56 | 64.79 | 98.92 | 65.52 |
-| MSCFF | 98.84 | 66.21 | 99.59 | 68.73 |
-| CDNetV2 | 98.68 | 65.69 | 99.54 | 67.21 |
-| CloudNet | 98.58 | 65.82 | 99.55 | 68.57 |
-| MCDNet | 98.97 | 66.45 | 99.58 | 69.42 |
-
-
-### Quantitative performance of different fusion schemes for extracting change features on L8-Biome dataset.
-
-| Methods | Fusion schemes | OA | IoU | Specificity | Kappa | Params (M) |
-|---------|----------------|-------|-------|-------------|-------|------------|
-| (a) | no fusion | 93.25 | 88.13 | 96.62 | 68.88 | 10.67 |
-| (b) | Concatenation | 94.14 | 89.62 | 97.07 | 66.49 | 11.36 |
-| (c) | Subtraction | 91.05 | 84.85 | 95.52 | 64.32 | 10.67 |
-| (d) | CDM | 93.77 | 89.02 | 96.88 | 65.46 | 12.07 |
-| (e) | DPFF | 94.25 | 89.75 | 97.13 | 71.01 | 13.11 |
-
-
-### Quantitative performance of different multi-scale feature fusion schemes on L8-Biome dataset.
-
-| Methods | Fusion schemes | OA | IoU | Specificity | Kappa | Params (M) |
-|-----------|----------------|------|------|-------------|--------|------------|
-| (a) | no fusion | 93.81| 88.99| 96.90 | 69.94 | 11.34 |
-| (b) | HRNet | 94.03| 89.47| 97.02 | 69.59 | 12.58 |
-| (c) | MSCFF | 93.45| 88.45| 96.72 | 68.87 | 14.86 |
-| (d) | CloudNet | 93.92| 89.29| 96.96 | 66.97 | 11.34 |
-| (e) | MSFF | 94.25| 89.75| 97.13 | 71.01 | 13.11 |
-
-## Citation
-
-```bibtex
-@article{MCDNet,
-title = {MCDNet: Multilevel cloud detection network for remote sensing images based on dual-perspective change-guided and multi-scale feature fusion},
-journal = {International Journal of Applied Earth Observation and Geoinformation},
-volume = {129},
-pages = {103820},
-year = {2024},
-issn = {1569-8432},
-doi = {10.1016/j.jag.2024.103820}
-}
-```
+# MCDNet: Multilevel cloud detection network for remote sensing images based on dual-perspective change-guided and multi-scale feature fusion
+
+> [MCDNet: Multilevel cloud detection network for remote sensing images based on dual-perspective change-guided and multi-scale feature fusion](https://www.sciencedirect.com/science/article/pii/S1569843224001742?via%3Dihub)
+
+## Introduction
+
+
+
+Official Repo
+
+Code Snippet
+
+## Abstract
+
+
+Cloud detection plays a crucial role in the preprocessing of optical remote sensing images. While extensive deep learning-based methods have shown strong performance in detecting thick clouds, their ability to identify thin and broken clouds is often inadequate due to their sparse distribution, semi-transparency, and similarity to background regions. To address this limitation, we introduce a multilevel cloud detection network (MCDNet) capable of simultaneously detecting thick and thin clouds. This network effectively enhances the accuracy of identifying thin and broken clouds by integrating a dual-perspective change-guided mechanism (DPCG) and a multi-scale feature fusion module (MSFF). The DPCG creates a dual-input stream by combining the original image with the thin cloud removal image, and then utilizes a dual-perspective feature fusion module (DPFF) to perform feature fusion and extract change features, thereby improving the model's ability to perceive thin cloud regions and mitigate inter-class similarity in multilevel cloud detection. The MSFF enhances the model's sensitivity to broken clouds by utilizing multiple non-adjacent low-level features to remedy the missing spatial information in the high-level features during multiple downsampling. Experimental results on the L8-Biome and WHUS2-CD datasets demonstrate that MCDNet significantly enhances the detection performance of both thin and broken clouds, and outperforms state-of-the-art methods in accuracy and efficiency.
+
+
+
+
+

+
+
+
+## Results and models
+
+### Quantitative performance of MCDNet with different thin cloud removal methods on the L8-Biome dataset.
+
+
+| Thin cloud removal method | OA | IoU | Specificity | Kappa |
+|--------------------------|------|------|-------------|--------|
+| DCP | 94.18| 89.55| 97.09 | 70.42 |
+| HF | 94.22| 89.69| 97.11 | 70.59 |
+| BCCR | 94.25| 89.75| 97.13 | 71.01 |
+
+### Quantitative performance of MCDNet with different components using BCCR thin cloud removal method on the L8-Biome dataset
+
+
+| Baseline | √ | √ | √ | √ |
+|----------------|-------|-------|-------|-------|
+| MSFF | × | √ | × | √ |
+| DPFF | × | × | √ | √ |
+| OA | 92.91 | 93.25 | 93.81 | 94.25 |
+| IoU | 87.58 | 88.13 | 88.99 | 89.75 |
+| Specificity | 96.46 | 96.62 | 96.90 | 97.13 |
+| Kappa | 67.22 | 68.88 | 69.94 | 71.01 |
+| Parameters (M) | 8.89 | 10.67 | 11.34 | 13.11 |
+
+
+
+### Quantitative comparisons of different methods on the L8-Biome dataset.
+
+
+| Method | OA | IoU | Specificity | Kappa |
+|----------|-------|-------|-------------|-------|
+| UNet | 90.78 | 84.44 | 95.39 | 63.22 |
+| SegNet | 91.44 | 85.33 | 95.72 | 63.98 |
+| HRNet | 91.17 | 84.87 | 95.59 | 62.32 |
+| SwinUnet | 91.73 | 85.74 | 95.86 | 64.36 |
+| MFCNN | 92.39 | 86.76 | 96.20 | 66.72 |
+| MSCFF | 92.49 | 86.99 | 96.25 | 65.82 |
+| CDNetV2 | 91.55 | 85.51 | 95.77 | 64.91 |
+| CloudNet | 92.19 | 86.55 | 96.09 | 65.99 |
+| MCDNet | 94.25 | 89.75 | 97.13 | 71.01 |
+
+
+### Quantitative comparisons of different methods on the WHUS2-CD dataset.
+
+| Method | OA | IoU | Specificity | Kappa |
+|----------|-------|-------|-------------|-------|
+| UNet | 98.24 | 63.72 | 99.51 | 63.45 |
+| SegNet | 98.09 | 62.53 | 99.15 | 61.71 |
+| HRNet | 97.91 | 61.73 | 99.41 | 59.29 |
+| SwinUnet | 98.69 | 61.93 | 99.48 | 67.81 |
+| MFCNN | 98.56 | 64.79 | 98.92 | 65.52 |
+| MSCFF | 98.84 | 66.21 | 99.59 | 68.73 |
+| CDNetV2 | 98.68 | 65.69 | 99.54 | 67.21 |
+| CloudNet | 98.58 | 65.82 | 99.55 | 68.57 |
+| MCDNet | 98.97 | 66.45 | 99.58 | 69.42 |
+
+
+### Quantitative performance of different fusion schemes for extracting change features on L8-Biome dataset.
+
+| Methods | Fusion schemes | OA | IoU | Specificity | Kappa | Params (M) |
+|---------|----------------|-------|-------|-------------|-------|------------|
+| (a) | no fusion | 93.25 | 88.13 | 96.62 | 68.88 | 10.67 |
+| (b) | Concatenation | 94.14 | 89.62 | 97.07 | 66.49 | 11.36 |
+| (c) | Subtraction | 91.05 | 84.85 | 95.52 | 64.32 | 10.67 |
+| (d) | CDM | 93.77 | 89.02 | 96.88 | 65.46 | 12.07 |
+| (e) | DPFF | 94.25 | 89.75 | 97.13 | 71.01 | 13.11 |
+
+
+### Quantitative performance of different multi-scale feature fusion schemes on L8-Biome dataset.
+
+| Methods | Fusion schemes | OA | IoU | Specificity | Kappa | Params (M) |
+|-----------|----------------|------|------|-------------|--------|------------|
+| (a) | no fusion | 93.81| 88.99| 96.90 | 69.94 | 11.34 |
+| (b) | HRNet | 94.03| 89.47| 97.02 | 69.59 | 12.58 |
+| (c) | MSCFF | 93.45| 88.45| 96.72 | 68.87 | 14.86 |
+| (d) | CloudNet | 93.92| 89.29| 96.96 | 66.97 | 11.34 |
+| (e) | MSFF | 94.25| 89.75| 97.13 | 71.01 | 13.11 |
+
+## Citation
+
+```bibtex
+@article{MCDNet,
+title = {MCDNet: Multilevel cloud detection network for remote sensing images based on dual-perspective change-guided and multi-scale feature fusion},
+journal = {International Journal of Applied Earth Observation and Geoinformation},
+volume = {129},
+pages = {103820},
+year = {2024},
+issn = {1569-8432},
+doi = {10.1016/j.jag.2024.103820}
+}
+```
diff --git a/configs/model/mcdnet/mcdnet.yaml b/configs/model/mcdnet/mcdnet.yaml
index fe0faa2092a56e0abea70b49cef73921c92d286f..b5663edc3996520075fa49f86a9e917c080579f5 100644
--- a/configs/model/mcdnet/mcdnet.yaml
+++ b/configs/model/mcdnet/mcdnet.yaml
@@ -1,22 +1,22 @@
-_target_: src.models.base_module.BaseLitModule
-
-net:
- _target_: src.models.components.mcdnet.MCDNet
- in_channels: 3
- num_classes: 2
-
-num_classes: 2
-
-criterion:
- _target_: torch.nn.CrossEntropyLoss
-
-optimizer:
- _target_: torch.optim.SGD
- _partial_: true
- lr: 0.0001
-scheduler: null
-
-#scheduler:
-# _target_: torch.optim.lr_scheduler.LambdaLR
-# _partial_: true
+_target_: src.models.base_module.BaseLitModule
+
+net:
+ _target_: src.models.components.mcdnet.MCDNet
+ in_channels: 3
+ num_classes: 2
+
+num_classes: 2
+
+criterion:
+ _target_: torch.nn.CrossEntropyLoss
+
+optimizer:
+ _target_: torch.optim.SGD
+ _partial_: true
+ lr: 0.0001
+scheduler: null
+
+#scheduler:
+# _target_: torch.optim.lr_scheduler.LambdaLR
+# _partial_: true
# lr_lambda: src.models.components.mcdnet.lr_lambda
\ No newline at end of file
diff --git a/configs/model/scnn/README.md b/configs/model/scnn/README.md
index ef8f2f66e2b956f985ae132fb238be15f789d36b..eca95b934024e62a29dfdd8252f759f03829849e 100644
--- a/configs/model/scnn/README.md
+++ b/configs/model/scnn/README.md
@@ -1,109 +1,109 @@
-# Remote sensing image cloud detection using a shallow convolutional neural network
-
-> [Remote sensing image cloud detection using a shallow convolutional neural network](https://www.sciencedirect.com/science/article/abs/pii/S0924271624000352?via%3Dihub#fn1)
-
-## Introduction
-
-
-
-Official Repo
-
-Code Snippet
-
-## Abstract
-
-
-
-The state-of-the-art methods for cloud detection are dominated by deep convolutional neural networks (DCNNs). However, it is very expensive to train DCNNs for cloud detection and the trained DCNNs do not always perform well as expected. This paper proposes a shallow CNN (SCNN) by removing pooling/unpooling layers and normalization layers in DCNNs, retaining only three convolutional layers, and equipping them with 3 filters of
-1
-×
-1
-,
-1
-×
-1
-,
-3
-×
-3
- in spatial dimensions. It demonstrates that the three convolutional layers are sufficient for cloud detection. Since the label output by the SCNN for a pixel depends on a 3 × 3 patch around this pixel, the SCNN can be trained using some thousands 3 × 3 patches together with ground truth of their center pixels. It is very cheap to train a SCNN using some thousands 3 × 3 patches and to provide ground truth of their center pixels. Despite of its low cost, SCNN training is stabler than DCNN training, and the trained SCNN outperforms the representative state-of-the-art DCNNs for cloud detection. The same resolution of original image, feature maps and final label map assures that details are not lost as by pooling/unpooling in DCNNs. The border artifacts suffering from deep convolutional and pooling/unpooling layers are minimized by 3 convolutional layers with
-1
-×
-1
-,
-1
-×
-1
-,
-3
-×
-3
- filters. Incoherent patches suffering from patch-by-patch segmentation and batch normalization are eliminated by SCNN without normalization layers. Extensive experiments based on the L7 Irish, L8 Biome and GF1 WHU datasets are carried out to evaluate the proposed method and compare with state-of-the-art methods. The proposed SCNN promises to deal with images from any other sensors.
-
-
-## Results and models
-
-###
-
-| Dataset | Zone/Biome | Clear | | | | Cloud | | |
-|----------|------------|-------|-------|-------|-------|-------|-------|-------|
-| | | A^O | A^P | A^U | F_1 | A^P | A^U | F_1 |
-| L7 Irish | Austral | 88.87 | 86.94 | 76.29 | 81.27 | 89.61 | 94.69 | 92.08 |
-| | Boreal | 97.06 | 98.78 | 96.53 | 97.64 | 94.31 | 97.96 | 96.10 |
-| | Mid.N. | 93.90 | 97.04 | 89.02 | 92.86 | 91.74 | 97.82 | 94.68 |
-| | Mid.S. | 97.19 | 98.96 | 95.94 | 97.43 | 95.13 | 98.75 | 96.91 |
-| | Polar.N. | 83.55 | 81.99 | 84.55 | 83.25 | 85.11 | 82.61 | 83.84 |
-| | SubT.N. | 98.16 | 99.44 | 98.65 | 99.04 | 69.09 | 84.38 | 75.97 |
-| | SubT.S. | 95.35 | 99.02 | 95.10 | 97.02 | 83.51 | 96.34 | 89.47 |
-| | Tropical | 89.72 | 86.48 | 42.76 | 57.23 | 90.00 | 98.72 | 94.16 |
-| | Mean | 94.13 | 97.17 | 93.73 | 95.42 | 88.97 | 94.88 | 91.83 |
-| L8 Biome | Barren | 93.46 | 90.81 | 97.89 | 94.22 | 97.23 | 88.19 | 92.49 |
-| | Forest | 96.65 | 90.65 | 88.18 | 89.40 | 97.76 | 98.26 | 98.01 |
-| | Grass | 94.40 | 93.75 | 99.51 | 96.54 | 97.67 | 75.56 | 85.20 |
-| | Shrubland | 99.24 | 98.69 | 99.83 | 99.26 | 99.83 | 98.64 | 99.23 |
-| | Snow | 86.50 | 78.41 | 91.65 | 84.51 | 93.67 | 83.04 | 88.03 |
-| | Urban | 95.29 | 88.87 | 98.29 | 93.34 | 99.08 | 93.76 | 96.35 |
-| | Wetlands | 93.72 | 93.90 | 98.36 | 96.07 | 92.92 | 77.14 | 84.30 |
-| | Water | 97.52 | 96.65 | 99.29 | 97.95 | 98.90 | 94.92 | 96.87 |
-| | Mean | 94.35 | 91.75 | 97.75 | 94.65 | 97.47 | 90.79 | 94.01 |
-| GF1 WHU | Mean | 94.46 | 92.07 | 97.62 | 94.76 | 97.31 | 91.11 | 94.11 |
-
-
-### Quantitative evaluation for SCNN on L7 Irish, L8 Biome and GF1 WHU datasets
-
-| Dataset | Zone/Biome | Clear | | | | Cloud | | |
-|----------|------------|-------|-------|-------|-------|-------|-------|-------|
-| | | A^O | A^P | A^U | F_1 | A^P | A^U | F_1 |
-| L7 Irish | Austral | 88.87 | 86.94 | 76.29 | 81.27 | 89.61 | 94.69 | 92.08 |
-| | Boreal | 97.06 | 98.78 | 96.53 | 97.64 | 94.31 | 97.96 | 96.10 |
-| | Mid.N. | 93.90 | 97.04 | 89.02 | 92.86 | 91.74 | 97.82 | 94.68 |
-| | Mid.S. | 97.19 | 98.96 | 95.94 | 97.43 | 95.13 | 98.75 | 96.91 |
-| | Polar.N. | 83.55 | 81.99 | 84.55 | 83.25 | 85.11 | 82.61 | 83.84 |
-| | SubT.N. | 98.16 | 99.44 | 98.65 | 99.04 | 69.09 | 84.38 | 75.97 |
-| | SubT.S. | 95.35 | 99.02 | 95.10 | 97.02 | 83.51 | 96.34 | 89.47 |
-| | Tropical | 89.72 | 86.48 | 42.76 | 57.23 | 90.00 | 98.72 | 94.16 |
-| | Mean | 94.13 | 97.17 | 93.73 | 95.42 | 88.97 | 94.88 | 91.83 |
-| L8 Biome | Barren | 93.46 | 90.81 | 97.89 | 94.22 | 97.23 | 88.19 | 92.49 |
-| | Forest | 96.65 | 90.65 | 88.18 | 89.40 | 97.76 | 98.26 | 98.01 |
-| | Grass | 94.40 | 93.75 | 99.51 | 96.54 | 97.67 | 75.56 | 85.20 |
-| | Shrubland | 99.24 | 98.69 | 99.83 | 99.26 | 99.83 | 98.64 | 99.23 |
-| | Snow | 86.50 | 78.41 | 91.65 | 84.51 | 93.67 | 83.04 | 88.03 |
-| | Urban | 95.29 | 88.87 | 98.29 | 93.34 | 99.08 | 93.76 | 96.35 |
-| | Wetlands | 93.72 | 93.90 | 98.36 | 96.07 | 92.92 | 77.14 | 84.30 |
-| | Water | 97.52 | 96.65 | 99.29 | 97.95 | 98.90 | 94.92 | 96.87 |
-| | Mean | 94.35 | 91.75 | 97.75 | 94.65 | 97.47 | 90.79 | 94.01 |
-| GF1 WHU | Mean | 94.46 | 92.07 | 97.62 | 94.76 | 97.31 | 91.11 | 94.11 |
-
-
-
-## Citation
-
-```bibtex
-@InProceedings{LiJEI2024,
- author = {Dengfeng Chai and Jingfeng Huang and Minghui Wu and
- Xiaoping Yang and Ruisheng Wang},
- title = {Remote sensing image cloud detection using a shallow convolutional neural network},
- booktitle = {ISPRS Journal of Photogrammetry and Remote Sensing},
- year = {2024},
-}
+# Remote sensing image cloud detection using a shallow convolutional neural network
+
+> [Remote sensing image cloud detection using a shallow convolutional neural network](https://www.sciencedirect.com/science/article/abs/pii/S0924271624000352?via%3Dihub#fn1)
+
+## Introduction
+
+
+
+Official Repo
+
+Code Snippet
+
+## Abstract
+
+
+
+The state-of-the-art methods for cloud detection are dominated by deep convolutional neural networks (DCNNs). However, it is very expensive to train DCNNs for cloud detection and the trained DCNNs do not always perform well as expected. This paper proposes a shallow CNN (SCNN) by removing pooling/unpooling layers and normalization layers in DCNNs, retaining only three convolutional layers, and equipping them with 3 filters of
+1
+×
+1
+,
+1
+×
+1
+,
+3
+×
+3
+ in spatial dimensions. It demonstrates that the three convolutional layers are sufficient for cloud detection. Since the label output by the SCNN for a pixel depends on a 3 × 3 patch around this pixel, the SCNN can be trained using some thousands 3 × 3 patches together with ground truth of their center pixels. It is very cheap to train a SCNN using some thousands 3 × 3 patches and to provide ground truth of their center pixels. Despite of its low cost, SCNN training is stabler than DCNN training, and the trained SCNN outperforms the representative state-of-the-art DCNNs for cloud detection. The same resolution of original image, feature maps and final label map assures that details are not lost as by pooling/unpooling in DCNNs. The border artifacts suffering from deep convolutional and pooling/unpooling layers are minimized by 3 convolutional layers with
+1
+×
+1
+,
+1
+×
+1
+,
+3
+×
+3
+ filters. Incoherent patches suffering from patch-by-patch segmentation and batch normalization are eliminated by SCNN without normalization layers. Extensive experiments based on the L7 Irish, L8 Biome and GF1 WHU datasets are carried out to evaluate the proposed method and compare with state-of-the-art methods. The proposed SCNN promises to deal with images from any other sensors.
+
+
+## Results and models
+
+###
+
+| Dataset | Zone/Biome | Clear | | | | Cloud | | |
+|----------|------------|-------|-------|-------|-------|-------|-------|-------|
+| | | A^O | A^P | A^U | F_1 | A^P | A^U | F_1 |
+| L7 Irish | Austral | 88.87 | 86.94 | 76.29 | 81.27 | 89.61 | 94.69 | 92.08 |
+| | Boreal | 97.06 | 98.78 | 96.53 | 97.64 | 94.31 | 97.96 | 96.10 |
+| | Mid.N. | 93.90 | 97.04 | 89.02 | 92.86 | 91.74 | 97.82 | 94.68 |
+| | Mid.S. | 97.19 | 98.96 | 95.94 | 97.43 | 95.13 | 98.75 | 96.91 |
+| | Polar.N. | 83.55 | 81.99 | 84.55 | 83.25 | 85.11 | 82.61 | 83.84 |
+| | SubT.N. | 98.16 | 99.44 | 98.65 | 99.04 | 69.09 | 84.38 | 75.97 |
+| | SubT.S. | 95.35 | 99.02 | 95.10 | 97.02 | 83.51 | 96.34 | 89.47 |
+| | Tropical | 89.72 | 86.48 | 42.76 | 57.23 | 90.00 | 98.72 | 94.16 |
+| | Mean | 94.13 | 97.17 | 93.73 | 95.42 | 88.97 | 94.88 | 91.83 |
+| L8 Biome | Barren | 93.46 | 90.81 | 97.89 | 94.22 | 97.23 | 88.19 | 92.49 |
+| | Forest | 96.65 | 90.65 | 88.18 | 89.40 | 97.76 | 98.26 | 98.01 |
+| | Grass | 94.40 | 93.75 | 99.51 | 96.54 | 97.67 | 75.56 | 85.20 |
+| | Shrubland | 99.24 | 98.69 | 99.83 | 99.26 | 99.83 | 98.64 | 99.23 |
+| | Snow | 86.50 | 78.41 | 91.65 | 84.51 | 93.67 | 83.04 | 88.03 |
+| | Urban | 95.29 | 88.87 | 98.29 | 93.34 | 99.08 | 93.76 | 96.35 |
+| | Wetlands | 93.72 | 93.90 | 98.36 | 96.07 | 92.92 | 77.14 | 84.30 |
+| | Water | 97.52 | 96.65 | 99.29 | 97.95 | 98.90 | 94.92 | 96.87 |
+| | Mean | 94.35 | 91.75 | 97.75 | 94.65 | 97.47 | 90.79 | 94.01 |
+| GF1 WHU | Mean | 94.46 | 92.07 | 97.62 | 94.76 | 97.31 | 91.11 | 94.11 |
+
+
+### Quantitative evaluation for SCNN on L7 Irish, L8 Biome and GF1 WHU datasets
+
+| Dataset | Zone/Biome | Clear | | | | Cloud | | |
+|----------|------------|-------|-------|-------|-------|-------|-------|-------|
+| | | A^O | A^P | A^U | F_1 | A^P | A^U | F_1 |
+| L7 Irish | Austral | 88.87 | 86.94 | 76.29 | 81.27 | 89.61 | 94.69 | 92.08 |
+| | Boreal | 97.06 | 98.78 | 96.53 | 97.64 | 94.31 | 97.96 | 96.10 |
+| | Mid.N. | 93.90 | 97.04 | 89.02 | 92.86 | 91.74 | 97.82 | 94.68 |
+| | Mid.S. | 97.19 | 98.96 | 95.94 | 97.43 | 95.13 | 98.75 | 96.91 |
+| | Polar.N. | 83.55 | 81.99 | 84.55 | 83.25 | 85.11 | 82.61 | 83.84 |
+| | SubT.N. | 98.16 | 99.44 | 98.65 | 99.04 | 69.09 | 84.38 | 75.97 |
+| | SubT.S. | 95.35 | 99.02 | 95.10 | 97.02 | 83.51 | 96.34 | 89.47 |
+| | Tropical | 89.72 | 86.48 | 42.76 | 57.23 | 90.00 | 98.72 | 94.16 |
+| | Mean | 94.13 | 97.17 | 93.73 | 95.42 | 88.97 | 94.88 | 91.83 |
+| L8 Biome | Barren | 93.46 | 90.81 | 97.89 | 94.22 | 97.23 | 88.19 | 92.49 |
+| | Forest | 96.65 | 90.65 | 88.18 | 89.40 | 97.76 | 98.26 | 98.01 |
+| | Grass | 94.40 | 93.75 | 99.51 | 96.54 | 97.67 | 75.56 | 85.20 |
+| | Shrubland | 99.24 | 98.69 | 99.83 | 99.26 | 99.83 | 98.64 | 99.23 |
+| | Snow | 86.50 | 78.41 | 91.65 | 84.51 | 93.67 | 83.04 | 88.03 |
+| | Urban | 95.29 | 88.87 | 98.29 | 93.34 | 99.08 | 93.76 | 96.35 |
+| | Wetlands | 93.72 | 93.90 | 98.36 | 96.07 | 92.92 | 77.14 | 84.30 |
+| | Water | 97.52 | 96.65 | 99.29 | 97.95 | 98.90 | 94.92 | 96.87 |
+| | Mean | 94.35 | 91.75 | 97.75 | 94.65 | 97.47 | 90.79 | 94.01 |
+| GF1 WHU | Mean | 94.46 | 92.07 | 97.62 | 94.76 | 97.31 | 91.11 | 94.11 |
+
+
+
+## Citation
+
+```bibtex
+@InProceedings{LiJEI2024,
+ author = {Dengfeng Chai and Jingfeng Huang and Minghui Wu and
+ Xiaoping Yang and Ruisheng Wang},
+ title = {Remote sensing image cloud detection using a shallow convolutional neural network},
+ booktitle = {ISPRS Journal of Photogrammetry and Remote Sensing},
+ year = {2024},
+}
```
\ No newline at end of file
diff --git a/configs/model/scnn/scnn.yaml b/configs/model/scnn/scnn.yaml
index 4246f8fa792f88b9a20a02b8ae6b258419b13959..1343839006a88ca7adbb8ab5da38a9d1159bc86c 100644
--- a/configs/model/scnn/scnn.yaml
+++ b/configs/model/scnn/scnn.yaml
@@ -1,17 +1,17 @@
-_target_: src.models.base_module.BaseLitModule
-
-net:
- _target_: src.models.components.scnn.SCNN
- num_classes: 2
-
-num_classes: 2
-
-criterion:
- _target_: torch.nn.CrossEntropyLoss
-
-optimizer:
- _target_: torch.optim.RMSprop
- _partial_: true
- lr: 0.0001
-
+_target_: src.models.base_module.BaseLitModule
+
+net:
+ _target_: src.models.components.scnn.SCNN
+ num_classes: 2
+
+num_classes: 2
+
+criterion:
+ _target_: torch.nn.CrossEntropyLoss
+
+optimizer:
+ _target_: torch.optim.RMSprop
+ _partial_: true
+ lr: 0.0001
+
scheduler: null
\ No newline at end of file
diff --git a/configs/model/unet/README.md b/configs/model/unet/README.md
index ee75a24e7068dd6cfbf0fcb9c5d1b76edfb834bf..b741105baf35789adddfd565fd3ce92242aa576a 100644
--- a/configs/model/unet/README.md
+++ b/configs/model/unet/README.md
@@ -1,92 +1,92 @@
-# UNet
-
-> [U-Net: Convolutional Networks for Biomedical Image Segmentation](https://arxiv.org/abs/1505.04597)
-
-## Introduction
-
-
-
-Official Repo
-
-Code Snippet
-
-## Abstract
-
-
-
-There is large consent that successful training of deep networks requires many thousand annotated training samples. In this paper, we present a network and training strategy that relies on the strong use of data augmentation to use the available annotated samples more efficiently. The architecture consists of a contracting path to capture context and a symmetric expanding path that enables precise localization. We show that such a network can be trained end-to-end from very few images and outperforms the prior best method (a sliding-window convolutional network) on the ISBI challenge for segmentation of neuronal structures in electron microscopic stacks. Using the same network trained on transmitted light microscopy images (phase contrast and DIC) we won the ISBI cell tracking challenge 2015 in these categories by a large margin. Moreover, the network is fast. Segmentation of a 512x512 image takes less than a second on a recent GPU. The full implementation (based on Caffe) and the trained networks are available at [this http URL](https://lmb.informatik.uni-freiburg.de/people/ronneber/u-net/).
-
-
-
-
-
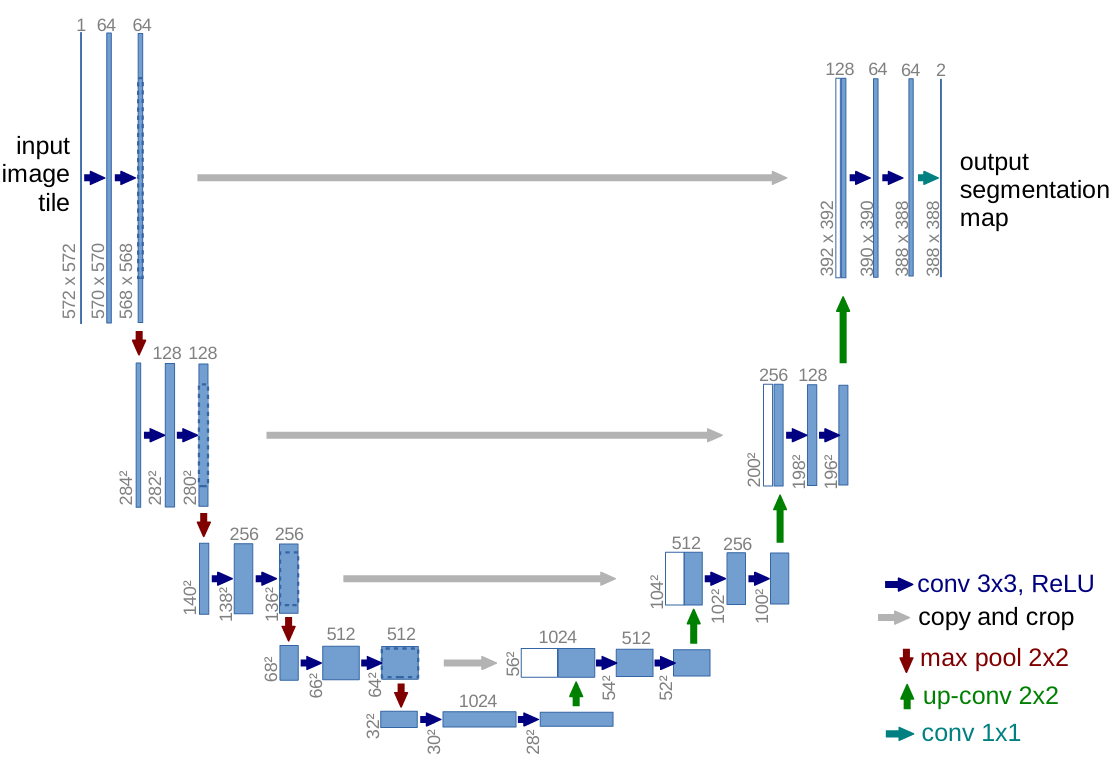
-
-
-## Results and models
-
-### Cityscapes
-
-| Method | Backbone | Loss | Crop Size | Lr schd | Mem (GB) | Inf time (fps) | Device | mIoU | mIoU(ms+flip) | config | download |
-| ---------- | ----------- | ------------- | --------- | ------: | -------- | -------------- | ------ | ----: | ------------: | ------------------------------------------------------------------------------------------------------------------------------ | ---------------------------------------------------------------------------------------------------------------------------------------------------------------------------------------------------------------------------------------------------------------------------------------------------------------------------------------------------------------------------- |
-| UNet + FCN | UNet-S5-D16 | Cross Entropy | 512x1024 | 160000 | 17.91 | 3.05 | V100 | 69.10 | 71.05 | [config](https://github.com/open-mmlab/mmsegmentation/blob/main/configs/unet/unet-s5-d16_fcn_4xb4-160k_cityscapes-512x1024.py) | [model](https://download.openmmlab.com/mmsegmentation/v0.5/unet/fcn_unet_s5-d16_4x4_512x1024_160k_cityscapes/fcn_unet_s5-d16_4x4_512x1024_160k_cityscapes_20211210_145204-6860854e.pth) \| [log](https://download.openmmlab.com/mmsegmentation/v0.5/unet/fcn_unet_s5-d16_4x4_512x1024_160k_cityscapes/fcn_unet_s5-d16_4x4_512x1024_160k_cityscapes_20211210_145204.log.json) |
-
-### DRIVE
-
-| Method | Backbone | Loss | Image Size | Crop Size | Stride | Lr schd | Mem (GB) | Inf time (fps) | Device | mDice | Dice | config | download |
-| ---------------- | ----------- | -------------------- | ---------- | --------- | -----: | ------- | -------- | -------------: | ------ | ----: | ----: | ------------------------------------------------------------------------------------------------------------------------------------------- | ---------------------------------------------------------------------------------------------------------------------------------------------------------------------------------------------------------------------------------------------------------------------------------------------------------------------------------------------------------------------------------------------------------------- |
-| UNet + FCN | UNet-S5-D16 | Cross Entropy | 584x565 | 64x64 | 42x42 | 40000 | 0.680 | - | V100 | 88.38 | 78.67 | [config](https://github.com/open-mmlab/mmsegmentation/blob/main/configs/unet/unet-s5-d16_fcn_4xb4-40k_drive-64x64.py) | [model](https://download.openmmlab.com/mmsegmentation/v0.5/unet/fcn_unet_s5-d16_64x64_40k_drive/fcn_unet_s5-d16_64x64_40k_drive_20201223_191051-5daf6d3b.pth) \| [log](https://download.openmmlab.com/mmsegmentation/v0.5/unet/unet_s5-d16_64x64_40k_drive/unet_s5-d16_64x64_40k_drive-20201223_191051.log.json) |
-| UNet + FCN | UNet-S5-D16 | Cross Entropy + Dice | 584x565 | 64x64 | 42x42 | 40000 | 0.582 | - | V100 | 88.71 | 79.32 | [config](https://github.com/open-mmlab/mmsegmentation/blob/main/configs/unet/unet-s5-d16_fcn_4xb4-ce-1.0-dice-3.0-40k_drive-64x64.py) | [model](https://download.openmmlab.com/mmsegmentation/v0.5/unet/fcn_unet_s5-d16_ce-1.0-dice-3.0_64x64_40k_drive/fcn_unet_s5-d16_ce-1.0-dice-3.0_64x64_40k_drive_20211210_201820-785de5c2.pth) \| [log](https://download.openmmlab.com/mmsegmentation/v0.5/unet/fcn_unet_s5-d16_ce-1.0-dice-3.0_64x64_40k_drive/fcn_unet_s5-d16_ce-1.0-dice-3.0_64x64_40k_drive_20211210_201820.log.json) |
-| UNet + PSPNet | UNet-S5-D16 | Cross Entropy | 584x565 | 64x64 | 42x42 | 40000 | 0.599 | - | V100 | 88.35 | 78.62 | [config](https://github.com/open-mmlab/mmsegmentation/blob/main/configs/unet/unet-s5-d16_pspnet_4xb4-40k_drive-64x64.py) | [model](https://download.openmmlab.com/mmsegmentation/v0.5/unet/pspnet_unet_s5-d16_64x64_40k_drive/pspnet_unet_s5-d16_64x64_40k_drive_20201227_181818-aac73387.pth) \| [log](https://download.openmmlab.com/mmsegmentation/v0.5/unet/pspnet_unet_s5-d16_64x64_40k_drive/pspnet_unet_s5-d16_64x64_40k_drive-20201227_181818.log.json) |
-| UNet + PSPNet | UNet-S5-D16 | Cross Entropy + Dice | 584x565 | 64x64 | 42x42 | 40000 | 0.585 | - | V100 | 88.76 | 79.42 | [config](https://github.com/open-mmlab/mmsegmentation/blob/main/configs/unet/unet-s5-d16_pspnet_4xb4-ce-1.0-dice-3.0-40k_drive-64x64.py) | [model](https://download.openmmlab.com/mmsegmentation/v0.5/unet/pspnet_unet_s5-d16_ce-1.0-dice-3.0_64x64_40k_drive/pspnet_unet_s5-d16_ce-1.0-dice-3.0_64x64_40k_drive_20211210_201821-22b3e3ba.pth) \| [log](https://download.openmmlab.com/mmsegmentation/v0.5/unet/pspnet_unet_s5-d16_ce-1.0-dice-3.0_64x64_40k_drive/pspnet_unet_s5-d16_ce-1.0-dice-3.0_64x64_40k_drive_20211210_201821.log.json) |
-| UNet + DeepLabV3 | UNet-S5-D16 | Cross Entropy | 584x565 | 64x64 | 42x42 | 40000 | 0.596 | - | V100 | 88.38 | 78.69 | [config](https://github.com/open-mmlab/mmsegmentation/blob/main/configs/unet/unet-s5-d16_deeplabv3_4xb4-40k_drive-64x64.py) | [model](https://download.openmmlab.com/mmsegmentation/v0.5/unet/deeplabv3_unet_s5-d16_64x64_40k_drive/deeplabv3_unet_s5-d16_64x64_40k_drive_20201226_094047-0671ff20.pth) \| [log](https://download.openmmlab.com/mmsegmentation/v0.5/unet/deeplabv3_unet_s5-d16_64x64_40k_drive/deeplabv3_unet_s5-d16_64x64_40k_drive-20201226_094047.log.json) |
-| UNet + DeepLabV3 | UNet-S5-D16 | Cross Entropy + Dice | 584x565 | 64x64 | 42x42 | 40000 | 0.582 | - | V100 | 88.84 | 79.56 | [config](https://github.com/open-mmlab/mmsegmentation/blob/main/configs/unet/unet-s5-d16_deeplabv3_4xb4-ce-1.0-dice-3.0-40k_drive-64x64.py) | [model](https://download.openmmlab.com/mmsegmentation/v0.5/unet/deeplabv3_unet_s5-d16_ce-1.0-dice-3.0_64x64_40k_drive/deeplabv3_unet_s5-d16_ce-1.0-dice-3.0_64x64_40k_drive_20211210_201825-6bf0efd7.pth) \| [log](https://download.openmmlab.com/mmsegmentation/v0.5/unet/deeplabv3_unet_s5-d16_ce-1.0-dice-3.0_64x64_40k_drive/deeplabv3_unet_s5-d16_ce-1.0-dice-3.0_64x64_40k_drive_20211210_201825.log.json) |
-
-### STARE
-
-| Method | Backbone | Loss | Image Size | Crop Size | Stride | Lr schd | Mem (GB) | Inf time (fps) | Device | mDice | Dice | config | download |
-| ---------------- | ----------- | -------------------- | ---------- | --------- | -----: | ------- | -------- | -------------: | ------ | ----: | ----: | --------------------------------------------------------------------------------------------------------------------------------------------- | ------------------------------------------------------------------------------------------------------------------------------------------------------------------------------------------------------------------------------------------------------------------------------------------------------------------------------------------------------------------------------------------------------------------------ |
-| UNet + FCN | UNet-S5-D16 | Cross Entropy | 605x700 | 128x128 | 85x85 | 40000 | 0.968 | - | V100 | 89.78 | 81.02 | [config](https://github.com/open-mmlab/mmsegmentation/blob/main/configs/unet/unet-s5-d16_fcn_4xb4-40k_stare-128x128.py) | [model](https://download.openmmlab.com/mmsegmentation/v0.5/unet/fcn_unet_s5-d16_128x128_40k_stare/fcn_unet_s5-d16_128x128_40k_stare_20201223_191051-7d77e78b.pth) \| [log](https://download.openmmlab.com/mmsegmentation/v0.5/unet/unet_s5-d16_128x128_40k_stare/unet_s5-d16_128x128_40k_stare-20201223_191051.log.json) |
-| UNet + FCN | UNet-S5-D16 | Cross Entropy + Dice | 605x700 | 128x128 | 85x85 | 40000 | 0.986 | - | V100 | 90.65 | 82.70 | [config](https://github.com/open-mmlab/mmsegmentation/blob/main/configs/unet/unet-s5-d16_fcn_4xb4-ce-1.0-dice-3.0-40k_stare-128x128.py) | [model](https://download.openmmlab.com/mmsegmentation/v0.5/unet/fcn_unet_s5-d16_ce-1.0-dice-3.0_128x128_40k_stare/fcn_unet_s5-d16_ce-1.0-dice-3.0_128x128_40k_stare_20211210_201821-f75705a9.pth) \| [log](https://download.openmmlab.com/mmsegmentation/v0.5/unet/fcn_unet_s5-d16_ce-1.0-dice-3.0_128x128_40k_stare/fcn_unet_s5-d16_ce-1.0-dice-3.0_128x128_40k_stare_20211210_201821.log.json) |
-| UNet + PSPNet | UNet-S5-D16 | Cross Entropy | 605x700 | 128x128 | 85x85 | 40000 | 0.982 | - | V100 | 89.89 | 81.22 | [config](https://github.com/open-mmlab/mmsegmentation/blob/main/configs/unet/unet-s5-d16_pspnet_4xb4-40k_stare-128x128.py) | [model](https://download.openmmlab.com/mmsegmentation/v0.5/unet/pspnet_unet_s5-d16_128x128_40k_stare/pspnet_unet_s5-d16_128x128_40k_stare_20201227_181818-3c2923c4.pth) \| [log](https://download.openmmlab.com/mmsegmentation/v0.5/unet/pspnet_unet_s5-d16_128x128_40k_stare/pspnet_unet_s5-d16_128x128_40k_stare-20201227_181818.log.json) |
-| UNet + PSPNet | UNet-S5-D16 | Cross Entropy + Dice | 605x700 | 128x128 | 85x85 | 40000 | 1.028 | - | V100 | 90.72 | 82.84 | [config](https://github.com/open-mmlab/mmsegmentation/blob/main/configs/unet/unet-s5-d16_pspnet_4xb4-ce-1.0-dice-3.0-40k_stare-128x128.py) | [model](https://download.openmmlab.com/mmsegmentation/v0.5/unet/pspnet_unet_s5-d16_ce-1.0-dice-3.0_128x128_40k_stare/pspnet_unet_s5-d16_ce-1.0-dice-3.0_128x128_40k_stare_20211210_201823-f1063ef7.pth) \| [log](https://download.openmmlab.com/mmsegmentation/v0.5/unet/pspnet_unet_s5-d16_ce-1.0-dice-3.0_128x128_40k_stare/pspnet_unet_s5-d16_ce-1.0-dice-3.0_128x128_40k_stare_20211210_201823.log.json) |
-| UNet + DeepLabV3 | UNet-S5-D16 | Cross Entropy | 605x700 | 128x128 | 85x85 | 40000 | 0.999 | - | V100 | 89.73 | 80.93 | [config](https://github.com/open-mmlab/mmsegmentation/blob/main/configs/unet/unet-s5-d16_deeplabv3_4xb4-40k_stare-128x128.py) | [model](https://download.openmmlab.com/mmsegmentation/v0.5/unet/deeplabv3_unet_s5-d16_128x128_40k_stare/deeplabv3_unet_s5-d16_128x128_40k_stare_20201226_094047-93dcb93c.pth) \| [log](https://download.openmmlab.com/mmsegmentation/v0.5/unet/deeplabv3_unet_s5-d16_128x128_40k_stare/deeplabv3_unet_s5-d16_128x128_40k_stare-20201226_094047.log.json) |
-| UNet + DeepLabV3 | UNet-S5-D16 | Cross Entropy + Dice | 605x700 | 128x128 | 85x85 | 40000 | 1.010 | - | V100 | 90.65 | 82.71 | [config](https://github.com/open-mmlab/mmsegmentation/blob/main/configs/unet/unet-s5-d16_deeplabv3_4xb4-ce-1.0-dice-3.0-40k_stare-128x128.py) | [model](https://download.openmmlab.com/mmsegmentation/v0.5/unet/deeplabv3_unet_s5-d16_ce-1.0-dice-3.0_128x128_40k_stare/deeplabv3_unet_s5-d16_ce-1.0-dice-3.0_128x128_40k_stare_20211210_201825-21db614c.pth) \| [log](https://download.openmmlab.com/mmsegmentation/v0.5/unet/deeplabv3_unet_s5-d16_ce-1.0-dice-3.0_128x128_40k_stare/deeplabv3_unet_s5-d16_ce-1.0-dice-3.0_128x128_40k_stare_20211210_201825.log.json) |
-
-### CHASE_DB1
-
-| Method | Backbone | Loss | Image Size | Crop Size | Stride | Lr schd | Mem (GB) | Inf time (fps) | Device | mDice | Dice | config | download |
-| ---------------- | ----------- | -------------------- | ---------- | --------- | -----: | ------- | -------- | -------------: | ------ | ----: | ----: | ------------------------------------------------------------------------------------------------------------------------------------------------- | ---------------------------------------------------------------------------------------------------------------------------------------------------------------------------------------------------------------------------------------------------------------------------------------------------------------------------------------------------------------------------------------------------------------------------------------- |
-| UNet + FCN | UNet-S5-D16 | Cross Entropy | 960x999 | 128x128 | 85x85 | 40000 | 0.968 | - | V100 | 89.46 | 80.24 | [config](https://github.com/open-mmlab/mmsegmentation/blob/main/configs/unet/unet-s5-d16_fcn_4xb4-40k_chase-db1-128x128.py) | [model](https://download.openmmlab.com/mmsegmentation/v0.5/unet/fcn_unet_s5-d16_128x128_40k_chase_db1/fcn_unet_s5-d16_128x128_40k_chase_db1_20201223_191051-11543527.pth) \| [log](https://download.openmmlab.com/mmsegmentation/v0.5/unet/unet_s5-d16_128x128_40k_chase_db1/unet_s5-d16_128x128_40k_chase_db1-20201223_191051.log.json) |
-| UNet + FCN | UNet-S5-D16 | Cross Entropy + Dice | 960x999 | 128x128 | 85x85 | 40000 | 0.986 | - | V100 | 89.52 | 80.40 | [config](https://github.com/open-mmlab/mmsegmentation/blob/main/configs/unet/unet-s5-d16_fcn_4xb4-ce-1.0-dice-3.0-40k_chase-db1-128x128.py) | [model](https://download.openmmlab.com/mmsegmentation/v0.5/unet/fcn_unet_s5-d16_ce-1.0-dice-3.0_128x128_40k_chase-db1/fcn_unet_s5-d16_ce-1.0-dice-3.0_128x128_40k_chase-db1_20211210_201821-1c4eb7cf.pth) \| [log](https://download.openmmlab.com/mmsegmentation/v0.5/unet/fcn_unet_s5-d16_ce-1.0-dice-3.0_128x128_40k_chase-db1/fcn_unet_s5-d16_ce-1.0-dice-3.0_128x128_40k_chase-db1_20211210_201821.log.json) |
-| UNet + PSPNet | UNet-S5-D16 | Cross Entropy | 960x999 | 128x128 | 85x85 | 40000 | 0.982 | - | V100 | 89.52 | 80.36 | [config](https://github.com/open-mmlab/mmsegmentation/blob/main/configs/unet/unet-s5-d16_pspnet_4xb4-40k_chase-db1-128x128.py) | [model](https://download.openmmlab.com/mmsegmentation/v0.5/unet/pspnet_unet_s5-d16_128x128_40k_chase_db1/pspnet_unet_s5-d16_128x128_40k_chase_db1_20201227_181818-68d4e609.pth) \| [log](https://download.openmmlab.com/mmsegmentation/v0.5/unet/pspnet_unet_s5-d16_128x128_40k_chase_db1/pspnet_unet_s5-d16_128x128_40k_chase_db1-20201227_181818.log.json) |
-| UNet + PSPNet | UNet-S5-D16 | Cross Entropy + Dice | 960x999 | 128x128 | 85x85 | 40000 | 1.028 | - | V100 | 89.45 | 80.28 | [config](https://github.com/open-mmlab/mmsegmentation/blob/main/configs/unet/unet-s5-d16_pspnet_4xb4-ce-1.0-dice-3.0-40k_chase-db1-128x128.py) | [model](https://download.openmmlab.com/mmsegmentation/v0.5/unet/pspnet_unet_s5-d16_ce-1.0-dice-3.0_128x128_40k_chase-db1/pspnet_unet_s5-d16_ce-1.0-dice-3.0_128x128_40k_chase-db1_20211210_201823-c0802c4d.pth) \| [log](https://download.openmmlab.com/mmsegmentation/v0.5/unet/pspnet_unet_s5-d16_ce-1.0-dice-3.0_128x128_40k_chase-db1/pspnet_unet_s5-d16_ce-1.0-dice-3.0_128x128_40k_chase-db1_20211210_201823.log.json) |
-| UNet + DeepLabV3 | UNet-S5-D16 | Cross Entropy | 960x999 | 128x128 | 85x85 | 40000 | 0.999 | - | V100 | 89.57 | 80.47 | [config](https://github.com/open-mmlab/mmsegmentation/blob/main/configs/unet/unet_s5-d16_deeplabv3_4xb4-40k_chase-db1-128x128.py) | [model](https://download.openmmlab.com/mmsegmentation/v0.5/unet/deeplabv3_unet_s5-d16_128x128_40k_chase_db1/deeplabv3_unet_s5-d16_128x128_40k_chase_db1_20201226_094047-4c5aefa3.pth) \| [log](https://download.openmmlab.com/mmsegmentation/v0.5/unet/deeplabv3_unet_s5-d16_128x128_40k_chase_db1/deeplabv3_unet_s5-d16_128x128_40k_chase_db1-20201226_094047.log.json) |
-| UNet + DeepLabV3 | UNet-S5-D16 | Cross Entropy + Dice | 960x999 | 128x128 | 85x85 | 40000 | 1.010 | - | V100 | 89.49 | 80.37 | [config](https://github.com/open-mmlab/mmsegmentation/blob/main/configs/unet/unet-s5-d16_deeplabv3_4xb4-ce-1.0-dice-3.0-40k_chase-db1-128x128.py) | [model](https://download.openmmlab.com/mmsegmentation/v0.5/unet/deeplabv3_unet_s5-d16_ce-1.0-dice-3.0_128x128_40k_chase-db1/deeplabv3_unet_s5-d16_ce-1.0-dice-3.0_128x128_40k_chase-db1_20211210_201825-4ef29df5.pth) \| [log](https://download.openmmlab.com/mmsegmentation/v0.5/unet/deeplabv3_unet_s5-d16_ce-1.0-dice-3.0_128x128_40k_chase-db1/deeplabv3_unet_s5-d16_ce-1.0-dice-3.0_128x128_40k_chase-db1_20211210_201825.log.json) |
-
-### HRF
-
-| Method | Backbone | Loss | Image Size | Crop Size | Stride | Lr schd | Mem (GB) | Inf time (fps) | Device | mDice | Dice | config | download |
-| ---------------- | ----------- | -------------------- | ---------- | --------- | ------: | ------- | -------- | -------------: | ------ | ----: | ----: | ------------------------------------------------------------------------------------------------------------------------------------------- | ---------------------------------------------------------------------------------------------------------------------------------------------------------------------------------------------------------------------------------------------------------------------------------------------------------------------------------------------------------------------------------------------------------------- |
-| UNet + FCN | UNet-S5-D16 | Cross Entropy | 2336x3504 | 256x256 | 170x170 | 40000 | 2.525 | - | V100 | 88.92 | 79.45 | [config](https://github.com/open-mmlab/mmsegmentation/blob/main/configs/unet/unet-s5-d16_fcn_4xb4-40k_hrf-256x256.py) | [model](https://download.openmmlab.com/mmsegmentation/v0.5/unet/fcn_unet_s5-d16_256x256_40k_hrf/fcn_unet_s5-d16_256x256_40k_hrf_20201223_173724-d89cf1ed.pth) \| [log](https://download.openmmlab.com/mmsegmentation/v0.5/unet/unet_s5-d16_256x256_40k_hrf/unet_s5-d16_256x256_40k_hrf-20201223_173724.log.json) |
-| UNet + FCN | UNet-S5-D16 | Cross Entropy + Dice | 2336x3504 | 256x256 | 170x170 | 40000 | 2.623 | - | V100 | 89.64 | 80.87 | [config](https://github.com/open-mmlab/mmsegmentation/blob/main/configs/unet/unet-s5-d16_fcn_4xb4-ce-1.0-dice-3.0-40k_hrf-256x256.py) | [model](https://download.openmmlab.com/mmsegmentation/v0.5/unet/fcn_unet_s5-d16_ce-1.0-dice-3.0_256x256_40k_hrf/fcn_unet_s5-d16_ce-1.0-dice-3.0_256x256_40k_hrf_20211210_201821-c314da8a.pth) \| [log](https://download.openmmlab.com/mmsegmentation/v0.5/unet/fcn_unet_s5-d16_ce-1.0-dice-3.0_256x256_40k_hrf/fcn_unet_s5-d16_ce-1.0-dice-3.0_256x256_40k_hrf_20211210_201821.log.json) |
-| UNet + PSPNet | UNet-S5-D16 | Cross Entropy | 2336x3504 | 256x256 | 170x170 | 40000 | 2.588 | - | V100 | 89.24 | 80.07 | [config](https://github.com/open-mmlab/mmsegmentation/blob/main/configs/unet/unet-s5-d16_pspnet_4xb4-40k_hrf-256x256.py) | [model](https://download.openmmlab.com/mmsegmentation/v0.5/unet/pspnet_unet_s5-d16_256x256_40k_hrf/pspnet_unet_s5-d16_256x256_40k_hrf_20201227_181818-fdb7e29b.pth) \| [log](https://download.openmmlab.com/mmsegmentation/v0.5/unet/pspnet_unet_s5-d16_256x256_40k_hrf/pspnet_unet_s5-d16_256x256_40k_hrf-20201227_181818.log.json) |
-| UNet + PSPNet | UNet-S5-D16 | Cross Entropy + Dice | 2336x3504 | 256x256 | 170x170 | 40000 | 2.798 | - | V100 | 89.69 | 80.96 | [config](https://github.com/open-mmlab/mmsegmentation/blob/main/configs/unet/unet-s5-d16_pspnet_4xb4-ce-1.0-dice-3.0-40k_hrf-256x256.py) | [model](https://download.openmmlab.com/mmsegmentation/v0.5/unet/pspnet_unet_s5-d16_ce-1.0-dice-3.0_256x256_40k_hrf/pspnet_unet_s5-d16_ce-1.0-dice-3.0_256x256_40k_hrf_20211210_201823-53d492fa.pth) \| [log](https://download.openmmlab.com/mmsegmentation/v0.5/unet/pspnet_unet_s5-d16_ce-1.0-dice-3.0_256x256_40k_hrf/pspnet_unet_s5-d16_ce-1.0-dice-3.0_256x256_40k_hrf_20211210_201823.log.json) |
-| UNet + DeepLabV3 | UNet-S5-D16 | Cross Entropy | 2336x3504 | 256x256 | 170x170 | 40000 | 2.604 | - | V100 | 89.32 | 80.21 | [config](https://github.com/open-mmlab/mmsegmentation/blob/main/configs/unet/unet-s5-d16_deeplabv3_4xb4-40k_hrf-256x256.py) | [model](https://download.openmmlab.com/mmsegmentation/v0.5/unet/deeplabv3_unet_s5-d16_256x256_40k_hrf/deeplabv3_unet_s5-d16_256x256_40k_hrf_20201226_094047-3a1fdf85.pth) \| [log](https://download.openmmlab.com/mmsegmentation/v0.5/unet/deeplabv3_unet_s5-d16_256x256_40k_hrf/deeplabv3_unet_s5-d16_256x256_40k_hrf-20201226_094047.log.json) |
-| UNet + DeepLabV3 | UNet-S5-D16 | Cross Entropy + Dice | 2336x3504 | 256x256 | 170x170 | 40000 | 2.607 | - | V100 | 89.56 | 80.71 | [config](https://github.com/open-mmlab/mmsegmentation/blob/main/configs/unet/unet-s5-d16_deeplabv3_4xb4-ce-1.0-dice-3.0-40k_hrf-256x256.py) | [model](https://download.openmmlab.com/mmsegmentation/v0.5/unet/deeplabv3_unet_s5-d16_ce-1.0-dice-3.0_256x256_40k_hrf/deeplabv3_unet_s5-d16_ce-1.0-dice-3.0_256x256_40k_hrf_20211210_202032-59daf7a4.pth) \| [log](https://download.openmmlab.com/mmsegmentation/v0.5/unet/deeplabv3_unet_s5-d16_ce-1.0-dice-3.0_256x256_40k_hrf/deeplabv3_unet_s5-d16_ce-1.0-dice-3.0_256x256_40k_hrf_20211210_202032.log.json) |
-
-Note:
-
-- In `DRIVE`, `STARE`, `CHASE_DB1`, and `HRF` dataset, `mDice` is mean dice of background and vessel, while `Dice` is dice metric of vessel(foreground) only.
-
-## Citation
-
-```bibtex
-@inproceedings{ronneberger2015u,
- title={U-net: Convolutional networks for biomedical image segmentation},
- author={Ronneberger, Olaf and Fischer, Philipp and Brox, Thomas},
- booktitle={International Conference on Medical image computing and computer-assisted intervention},
- pages={234--241},
- year={2015},
- organization={Springer}
-}
+# UNet
+
+> [U-Net: Convolutional Networks for Biomedical Image Segmentation](https://arxiv.org/abs/1505.04597)
+
+## Introduction
+
+
+
+Official Repo
+
+Code Snippet
+
+## Abstract
+
+
+
+There is large consent that successful training of deep networks requires many thousand annotated training samples. In this paper, we present a network and training strategy that relies on the strong use of data augmentation to use the available annotated samples more efficiently. The architecture consists of a contracting path to capture context and a symmetric expanding path that enables precise localization. We show that such a network can be trained end-to-end from very few images and outperforms the prior best method (a sliding-window convolutional network) on the ISBI challenge for segmentation of neuronal structures in electron microscopic stacks. Using the same network trained on transmitted light microscopy images (phase contrast and DIC) we won the ISBI cell tracking challenge 2015 in these categories by a large margin. Moreover, the network is fast. Segmentation of a 512x512 image takes less than a second on a recent GPU. The full implementation (based on Caffe) and the trained networks are available at [this http URL](https://lmb.informatik.uni-freiburg.de/people/ronneber/u-net/).
+
+
+
+
+
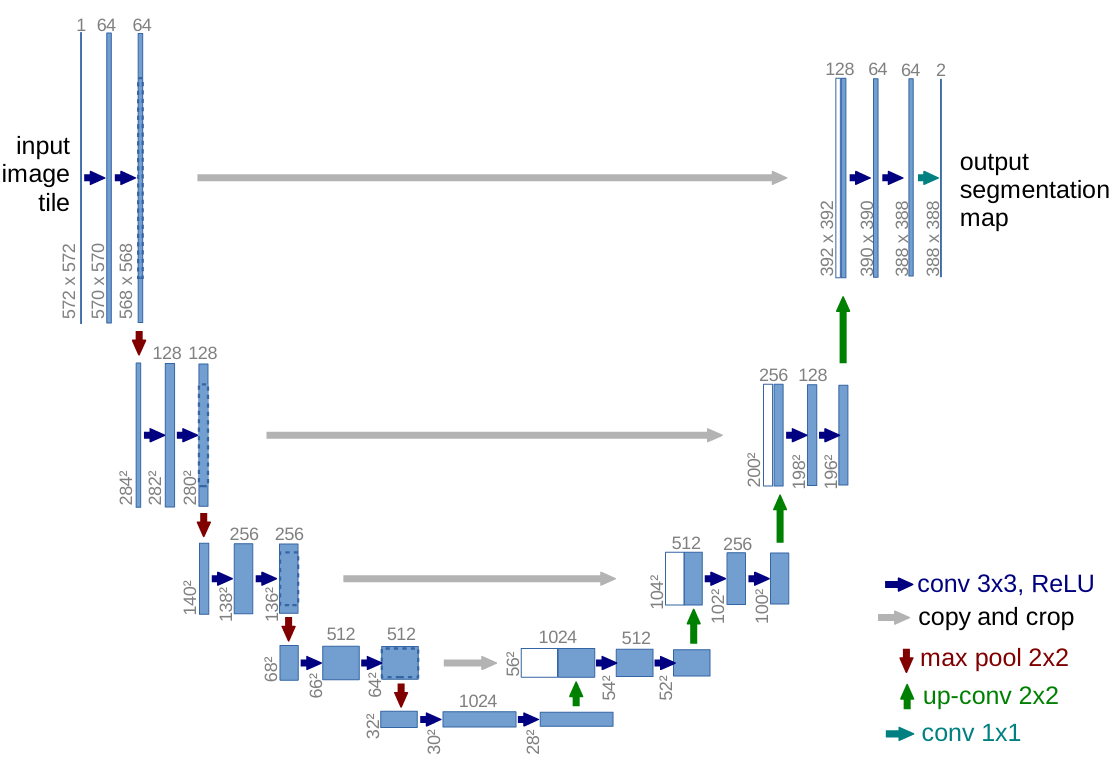
+
+
+## Results and models
+
+### Cityscapes
+
+| Method | Backbone | Loss | Crop Size | Lr schd | Mem (GB) | Inf time (fps) | Device | mIoU | mIoU(ms+flip) | config | download |
+| ---------- | ----------- | ------------- | --------- | ------: | -------- | -------------- | ------ | ----: | ------------: | ------------------------------------------------------------------------------------------------------------------------------ | ---------------------------------------------------------------------------------------------------------------------------------------------------------------------------------------------------------------------------------------------------------------------------------------------------------------------------------------------------------------------------- |
+| UNet + FCN | UNet-S5-D16 | Cross Entropy | 512x1024 | 160000 | 17.91 | 3.05 | V100 | 69.10 | 71.05 | [config](https://github.com/open-mmlab/mmsegmentation/blob/main/configs/unet/unet-s5-d16_fcn_4xb4-160k_cityscapes-512x1024.py) | [model](https://download.openmmlab.com/mmsegmentation/v0.5/unet/fcn_unet_s5-d16_4x4_512x1024_160k_cityscapes/fcn_unet_s5-d16_4x4_512x1024_160k_cityscapes_20211210_145204-6860854e.pth) \| [log](https://download.openmmlab.com/mmsegmentation/v0.5/unet/fcn_unet_s5-d16_4x4_512x1024_160k_cityscapes/fcn_unet_s5-d16_4x4_512x1024_160k_cityscapes_20211210_145204.log.json) |
+
+### DRIVE
+
+| Method | Backbone | Loss | Image Size | Crop Size | Stride | Lr schd | Mem (GB) | Inf time (fps) | Device | mDice | Dice | config | download |
+| ---------------- | ----------- | -------------------- | ---------- | --------- | -----: | ------- | -------- | -------------: | ------ | ----: | ----: | ------------------------------------------------------------------------------------------------------------------------------------------- | ---------------------------------------------------------------------------------------------------------------------------------------------------------------------------------------------------------------------------------------------------------------------------------------------------------------------------------------------------------------------------------------------------------------- |
+| UNet + FCN | UNet-S5-D16 | Cross Entropy | 584x565 | 64x64 | 42x42 | 40000 | 0.680 | - | V100 | 88.38 | 78.67 | [config](https://github.com/open-mmlab/mmsegmentation/blob/main/configs/unet/unet-s5-d16_fcn_4xb4-40k_drive-64x64.py) | [model](https://download.openmmlab.com/mmsegmentation/v0.5/unet/fcn_unet_s5-d16_64x64_40k_drive/fcn_unet_s5-d16_64x64_40k_drive_20201223_191051-5daf6d3b.pth) \| [log](https://download.openmmlab.com/mmsegmentation/v0.5/unet/unet_s5-d16_64x64_40k_drive/unet_s5-d16_64x64_40k_drive-20201223_191051.log.json) |
+| UNet + FCN | UNet-S5-D16 | Cross Entropy + Dice | 584x565 | 64x64 | 42x42 | 40000 | 0.582 | - | V100 | 88.71 | 79.32 | [config](https://github.com/open-mmlab/mmsegmentation/blob/main/configs/unet/unet-s5-d16_fcn_4xb4-ce-1.0-dice-3.0-40k_drive-64x64.py) | [model](https://download.openmmlab.com/mmsegmentation/v0.5/unet/fcn_unet_s5-d16_ce-1.0-dice-3.0_64x64_40k_drive/fcn_unet_s5-d16_ce-1.0-dice-3.0_64x64_40k_drive_20211210_201820-785de5c2.pth) \| [log](https://download.openmmlab.com/mmsegmentation/v0.5/unet/fcn_unet_s5-d16_ce-1.0-dice-3.0_64x64_40k_drive/fcn_unet_s5-d16_ce-1.0-dice-3.0_64x64_40k_drive_20211210_201820.log.json) |
+| UNet + PSPNet | UNet-S5-D16 | Cross Entropy | 584x565 | 64x64 | 42x42 | 40000 | 0.599 | - | V100 | 88.35 | 78.62 | [config](https://github.com/open-mmlab/mmsegmentation/blob/main/configs/unet/unet-s5-d16_pspnet_4xb4-40k_drive-64x64.py) | [model](https://download.openmmlab.com/mmsegmentation/v0.5/unet/pspnet_unet_s5-d16_64x64_40k_drive/pspnet_unet_s5-d16_64x64_40k_drive_20201227_181818-aac73387.pth) \| [log](https://download.openmmlab.com/mmsegmentation/v0.5/unet/pspnet_unet_s5-d16_64x64_40k_drive/pspnet_unet_s5-d16_64x64_40k_drive-20201227_181818.log.json) |
+| UNet + PSPNet | UNet-S5-D16 | Cross Entropy + Dice | 584x565 | 64x64 | 42x42 | 40000 | 0.585 | - | V100 | 88.76 | 79.42 | [config](https://github.com/open-mmlab/mmsegmentation/blob/main/configs/unet/unet-s5-d16_pspnet_4xb4-ce-1.0-dice-3.0-40k_drive-64x64.py) | [model](https://download.openmmlab.com/mmsegmentation/v0.5/unet/pspnet_unet_s5-d16_ce-1.0-dice-3.0_64x64_40k_drive/pspnet_unet_s5-d16_ce-1.0-dice-3.0_64x64_40k_drive_20211210_201821-22b3e3ba.pth) \| [log](https://download.openmmlab.com/mmsegmentation/v0.5/unet/pspnet_unet_s5-d16_ce-1.0-dice-3.0_64x64_40k_drive/pspnet_unet_s5-d16_ce-1.0-dice-3.0_64x64_40k_drive_20211210_201821.log.json) |
+| UNet + DeepLabV3 | UNet-S5-D16 | Cross Entropy | 584x565 | 64x64 | 42x42 | 40000 | 0.596 | - | V100 | 88.38 | 78.69 | [config](https://github.com/open-mmlab/mmsegmentation/blob/main/configs/unet/unet-s5-d16_deeplabv3_4xb4-40k_drive-64x64.py) | [model](https://download.openmmlab.com/mmsegmentation/v0.5/unet/deeplabv3_unet_s5-d16_64x64_40k_drive/deeplabv3_unet_s5-d16_64x64_40k_drive_20201226_094047-0671ff20.pth) \| [log](https://download.openmmlab.com/mmsegmentation/v0.5/unet/deeplabv3_unet_s5-d16_64x64_40k_drive/deeplabv3_unet_s5-d16_64x64_40k_drive-20201226_094047.log.json) |
+| UNet + DeepLabV3 | UNet-S5-D16 | Cross Entropy + Dice | 584x565 | 64x64 | 42x42 | 40000 | 0.582 | - | V100 | 88.84 | 79.56 | [config](https://github.com/open-mmlab/mmsegmentation/blob/main/configs/unet/unet-s5-d16_deeplabv3_4xb4-ce-1.0-dice-3.0-40k_drive-64x64.py) | [model](https://download.openmmlab.com/mmsegmentation/v0.5/unet/deeplabv3_unet_s5-d16_ce-1.0-dice-3.0_64x64_40k_drive/deeplabv3_unet_s5-d16_ce-1.0-dice-3.0_64x64_40k_drive_20211210_201825-6bf0efd7.pth) \| [log](https://download.openmmlab.com/mmsegmentation/v0.5/unet/deeplabv3_unet_s5-d16_ce-1.0-dice-3.0_64x64_40k_drive/deeplabv3_unet_s5-d16_ce-1.0-dice-3.0_64x64_40k_drive_20211210_201825.log.json) |
+
+### STARE
+
+| Method | Backbone | Loss | Image Size | Crop Size | Stride | Lr schd | Mem (GB) | Inf time (fps) | Device | mDice | Dice | config | download |
+| ---------------- | ----------- | -------------------- | ---------- | --------- | -----: | ------- | -------- | -------------: | ------ | ----: | ----: | --------------------------------------------------------------------------------------------------------------------------------------------- | ------------------------------------------------------------------------------------------------------------------------------------------------------------------------------------------------------------------------------------------------------------------------------------------------------------------------------------------------------------------------------------------------------------------------ |
+| UNet + FCN | UNet-S5-D16 | Cross Entropy | 605x700 | 128x128 | 85x85 | 40000 | 0.968 | - | V100 | 89.78 | 81.02 | [config](https://github.com/open-mmlab/mmsegmentation/blob/main/configs/unet/unet-s5-d16_fcn_4xb4-40k_stare-128x128.py) | [model](https://download.openmmlab.com/mmsegmentation/v0.5/unet/fcn_unet_s5-d16_128x128_40k_stare/fcn_unet_s5-d16_128x128_40k_stare_20201223_191051-7d77e78b.pth) \| [log](https://download.openmmlab.com/mmsegmentation/v0.5/unet/unet_s5-d16_128x128_40k_stare/unet_s5-d16_128x128_40k_stare-20201223_191051.log.json) |
+| UNet + FCN | UNet-S5-D16 | Cross Entropy + Dice | 605x700 | 128x128 | 85x85 | 40000 | 0.986 | - | V100 | 90.65 | 82.70 | [config](https://github.com/open-mmlab/mmsegmentation/blob/main/configs/unet/unet-s5-d16_fcn_4xb4-ce-1.0-dice-3.0-40k_stare-128x128.py) | [model](https://download.openmmlab.com/mmsegmentation/v0.5/unet/fcn_unet_s5-d16_ce-1.0-dice-3.0_128x128_40k_stare/fcn_unet_s5-d16_ce-1.0-dice-3.0_128x128_40k_stare_20211210_201821-f75705a9.pth) \| [log](https://download.openmmlab.com/mmsegmentation/v0.5/unet/fcn_unet_s5-d16_ce-1.0-dice-3.0_128x128_40k_stare/fcn_unet_s5-d16_ce-1.0-dice-3.0_128x128_40k_stare_20211210_201821.log.json) |
+| UNet + PSPNet | UNet-S5-D16 | Cross Entropy | 605x700 | 128x128 | 85x85 | 40000 | 0.982 | - | V100 | 89.89 | 81.22 | [config](https://github.com/open-mmlab/mmsegmentation/blob/main/configs/unet/unet-s5-d16_pspnet_4xb4-40k_stare-128x128.py) | [model](https://download.openmmlab.com/mmsegmentation/v0.5/unet/pspnet_unet_s5-d16_128x128_40k_stare/pspnet_unet_s5-d16_128x128_40k_stare_20201227_181818-3c2923c4.pth) \| [log](https://download.openmmlab.com/mmsegmentation/v0.5/unet/pspnet_unet_s5-d16_128x128_40k_stare/pspnet_unet_s5-d16_128x128_40k_stare-20201227_181818.log.json) |
+| UNet + PSPNet | UNet-S5-D16 | Cross Entropy + Dice | 605x700 | 128x128 | 85x85 | 40000 | 1.028 | - | V100 | 90.72 | 82.84 | [config](https://github.com/open-mmlab/mmsegmentation/blob/main/configs/unet/unet-s5-d16_pspnet_4xb4-ce-1.0-dice-3.0-40k_stare-128x128.py) | [model](https://download.openmmlab.com/mmsegmentation/v0.5/unet/pspnet_unet_s5-d16_ce-1.0-dice-3.0_128x128_40k_stare/pspnet_unet_s5-d16_ce-1.0-dice-3.0_128x128_40k_stare_20211210_201823-f1063ef7.pth) \| [log](https://download.openmmlab.com/mmsegmentation/v0.5/unet/pspnet_unet_s5-d16_ce-1.0-dice-3.0_128x128_40k_stare/pspnet_unet_s5-d16_ce-1.0-dice-3.0_128x128_40k_stare_20211210_201823.log.json) |
+| UNet + DeepLabV3 | UNet-S5-D16 | Cross Entropy | 605x700 | 128x128 | 85x85 | 40000 | 0.999 | - | V100 | 89.73 | 80.93 | [config](https://github.com/open-mmlab/mmsegmentation/blob/main/configs/unet/unet-s5-d16_deeplabv3_4xb4-40k_stare-128x128.py) | [model](https://download.openmmlab.com/mmsegmentation/v0.5/unet/deeplabv3_unet_s5-d16_128x128_40k_stare/deeplabv3_unet_s5-d16_128x128_40k_stare_20201226_094047-93dcb93c.pth) \| [log](https://download.openmmlab.com/mmsegmentation/v0.5/unet/deeplabv3_unet_s5-d16_128x128_40k_stare/deeplabv3_unet_s5-d16_128x128_40k_stare-20201226_094047.log.json) |
+| UNet + DeepLabV3 | UNet-S5-D16 | Cross Entropy + Dice | 605x700 | 128x128 | 85x85 | 40000 | 1.010 | - | V100 | 90.65 | 82.71 | [config](https://github.com/open-mmlab/mmsegmentation/blob/main/configs/unet/unet-s5-d16_deeplabv3_4xb4-ce-1.0-dice-3.0-40k_stare-128x128.py) | [model](https://download.openmmlab.com/mmsegmentation/v0.5/unet/deeplabv3_unet_s5-d16_ce-1.0-dice-3.0_128x128_40k_stare/deeplabv3_unet_s5-d16_ce-1.0-dice-3.0_128x128_40k_stare_20211210_201825-21db614c.pth) \| [log](https://download.openmmlab.com/mmsegmentation/v0.5/unet/deeplabv3_unet_s5-d16_ce-1.0-dice-3.0_128x128_40k_stare/deeplabv3_unet_s5-d16_ce-1.0-dice-3.0_128x128_40k_stare_20211210_201825.log.json) |
+
+### CHASE_DB1
+
+| Method | Backbone | Loss | Image Size | Crop Size | Stride | Lr schd | Mem (GB) | Inf time (fps) | Device | mDice | Dice | config | download |
+| ---------------- | ----------- | -------------------- | ---------- | --------- | -----: | ------- | -------- | -------------: | ------ | ----: | ----: | ------------------------------------------------------------------------------------------------------------------------------------------------- | ---------------------------------------------------------------------------------------------------------------------------------------------------------------------------------------------------------------------------------------------------------------------------------------------------------------------------------------------------------------------------------------------------------------------------------------- |
+| UNet + FCN | UNet-S5-D16 | Cross Entropy | 960x999 | 128x128 | 85x85 | 40000 | 0.968 | - | V100 | 89.46 | 80.24 | [config](https://github.com/open-mmlab/mmsegmentation/blob/main/configs/unet/unet-s5-d16_fcn_4xb4-40k_chase-db1-128x128.py) | [model](https://download.openmmlab.com/mmsegmentation/v0.5/unet/fcn_unet_s5-d16_128x128_40k_chase_db1/fcn_unet_s5-d16_128x128_40k_chase_db1_20201223_191051-11543527.pth) \| [log](https://download.openmmlab.com/mmsegmentation/v0.5/unet/unet_s5-d16_128x128_40k_chase_db1/unet_s5-d16_128x128_40k_chase_db1-20201223_191051.log.json) |
+| UNet + FCN | UNet-S5-D16 | Cross Entropy + Dice | 960x999 | 128x128 | 85x85 | 40000 | 0.986 | - | V100 | 89.52 | 80.40 | [config](https://github.com/open-mmlab/mmsegmentation/blob/main/configs/unet/unet-s5-d16_fcn_4xb4-ce-1.0-dice-3.0-40k_chase-db1-128x128.py) | [model](https://download.openmmlab.com/mmsegmentation/v0.5/unet/fcn_unet_s5-d16_ce-1.0-dice-3.0_128x128_40k_chase-db1/fcn_unet_s5-d16_ce-1.0-dice-3.0_128x128_40k_chase-db1_20211210_201821-1c4eb7cf.pth) \| [log](https://download.openmmlab.com/mmsegmentation/v0.5/unet/fcn_unet_s5-d16_ce-1.0-dice-3.0_128x128_40k_chase-db1/fcn_unet_s5-d16_ce-1.0-dice-3.0_128x128_40k_chase-db1_20211210_201821.log.json) |
+| UNet + PSPNet | UNet-S5-D16 | Cross Entropy | 960x999 | 128x128 | 85x85 | 40000 | 0.982 | - | V100 | 89.52 | 80.36 | [config](https://github.com/open-mmlab/mmsegmentation/blob/main/configs/unet/unet-s5-d16_pspnet_4xb4-40k_chase-db1-128x128.py) | [model](https://download.openmmlab.com/mmsegmentation/v0.5/unet/pspnet_unet_s5-d16_128x128_40k_chase_db1/pspnet_unet_s5-d16_128x128_40k_chase_db1_20201227_181818-68d4e609.pth) \| [log](https://download.openmmlab.com/mmsegmentation/v0.5/unet/pspnet_unet_s5-d16_128x128_40k_chase_db1/pspnet_unet_s5-d16_128x128_40k_chase_db1-20201227_181818.log.json) |
+| UNet + PSPNet | UNet-S5-D16 | Cross Entropy + Dice | 960x999 | 128x128 | 85x85 | 40000 | 1.028 | - | V100 | 89.45 | 80.28 | [config](https://github.com/open-mmlab/mmsegmentation/blob/main/configs/unet/unet-s5-d16_pspnet_4xb4-ce-1.0-dice-3.0-40k_chase-db1-128x128.py) | [model](https://download.openmmlab.com/mmsegmentation/v0.5/unet/pspnet_unet_s5-d16_ce-1.0-dice-3.0_128x128_40k_chase-db1/pspnet_unet_s5-d16_ce-1.0-dice-3.0_128x128_40k_chase-db1_20211210_201823-c0802c4d.pth) \| [log](https://download.openmmlab.com/mmsegmentation/v0.5/unet/pspnet_unet_s5-d16_ce-1.0-dice-3.0_128x128_40k_chase-db1/pspnet_unet_s5-d16_ce-1.0-dice-3.0_128x128_40k_chase-db1_20211210_201823.log.json) |
+| UNet + DeepLabV3 | UNet-S5-D16 | Cross Entropy | 960x999 | 128x128 | 85x85 | 40000 | 0.999 | - | V100 | 89.57 | 80.47 | [config](https://github.com/open-mmlab/mmsegmentation/blob/main/configs/unet/unet_s5-d16_deeplabv3_4xb4-40k_chase-db1-128x128.py) | [model](https://download.openmmlab.com/mmsegmentation/v0.5/unet/deeplabv3_unet_s5-d16_128x128_40k_chase_db1/deeplabv3_unet_s5-d16_128x128_40k_chase_db1_20201226_094047-4c5aefa3.pth) \| [log](https://download.openmmlab.com/mmsegmentation/v0.5/unet/deeplabv3_unet_s5-d16_128x128_40k_chase_db1/deeplabv3_unet_s5-d16_128x128_40k_chase_db1-20201226_094047.log.json) |
+| UNet + DeepLabV3 | UNet-S5-D16 | Cross Entropy + Dice | 960x999 | 128x128 | 85x85 | 40000 | 1.010 | - | V100 | 89.49 | 80.37 | [config](https://github.com/open-mmlab/mmsegmentation/blob/main/configs/unet/unet-s5-d16_deeplabv3_4xb4-ce-1.0-dice-3.0-40k_chase-db1-128x128.py) | [model](https://download.openmmlab.com/mmsegmentation/v0.5/unet/deeplabv3_unet_s5-d16_ce-1.0-dice-3.0_128x128_40k_chase-db1/deeplabv3_unet_s5-d16_ce-1.0-dice-3.0_128x128_40k_chase-db1_20211210_201825-4ef29df5.pth) \| [log](https://download.openmmlab.com/mmsegmentation/v0.5/unet/deeplabv3_unet_s5-d16_ce-1.0-dice-3.0_128x128_40k_chase-db1/deeplabv3_unet_s5-d16_ce-1.0-dice-3.0_128x128_40k_chase-db1_20211210_201825.log.json) |
+
+### HRF
+
+| Method | Backbone | Loss | Image Size | Crop Size | Stride | Lr schd | Mem (GB) | Inf time (fps) | Device | mDice | Dice | config | download |
+| ---------------- | ----------- | -------------------- | ---------- | --------- | ------: | ------- | -------- | -------------: | ------ | ----: | ----: | ------------------------------------------------------------------------------------------------------------------------------------------- | ---------------------------------------------------------------------------------------------------------------------------------------------------------------------------------------------------------------------------------------------------------------------------------------------------------------------------------------------------------------------------------------------------------------- |
+| UNet + FCN | UNet-S5-D16 | Cross Entropy | 2336x3504 | 256x256 | 170x170 | 40000 | 2.525 | - | V100 | 88.92 | 79.45 | [config](https://github.com/open-mmlab/mmsegmentation/blob/main/configs/unet/unet-s5-d16_fcn_4xb4-40k_hrf-256x256.py) | [model](https://download.openmmlab.com/mmsegmentation/v0.5/unet/fcn_unet_s5-d16_256x256_40k_hrf/fcn_unet_s5-d16_256x256_40k_hrf_20201223_173724-d89cf1ed.pth) \| [log](https://download.openmmlab.com/mmsegmentation/v0.5/unet/unet_s5-d16_256x256_40k_hrf/unet_s5-d16_256x256_40k_hrf-20201223_173724.log.json) |
+| UNet + FCN | UNet-S5-D16 | Cross Entropy + Dice | 2336x3504 | 256x256 | 170x170 | 40000 | 2.623 | - | V100 | 89.64 | 80.87 | [config](https://github.com/open-mmlab/mmsegmentation/blob/main/configs/unet/unet-s5-d16_fcn_4xb4-ce-1.0-dice-3.0-40k_hrf-256x256.py) | [model](https://download.openmmlab.com/mmsegmentation/v0.5/unet/fcn_unet_s5-d16_ce-1.0-dice-3.0_256x256_40k_hrf/fcn_unet_s5-d16_ce-1.0-dice-3.0_256x256_40k_hrf_20211210_201821-c314da8a.pth) \| [log](https://download.openmmlab.com/mmsegmentation/v0.5/unet/fcn_unet_s5-d16_ce-1.0-dice-3.0_256x256_40k_hrf/fcn_unet_s5-d16_ce-1.0-dice-3.0_256x256_40k_hrf_20211210_201821.log.json) |
+| UNet + PSPNet | UNet-S5-D16 | Cross Entropy | 2336x3504 | 256x256 | 170x170 | 40000 | 2.588 | - | V100 | 89.24 | 80.07 | [config](https://github.com/open-mmlab/mmsegmentation/blob/main/configs/unet/unet-s5-d16_pspnet_4xb4-40k_hrf-256x256.py) | [model](https://download.openmmlab.com/mmsegmentation/v0.5/unet/pspnet_unet_s5-d16_256x256_40k_hrf/pspnet_unet_s5-d16_256x256_40k_hrf_20201227_181818-fdb7e29b.pth) \| [log](https://download.openmmlab.com/mmsegmentation/v0.5/unet/pspnet_unet_s5-d16_256x256_40k_hrf/pspnet_unet_s5-d16_256x256_40k_hrf-20201227_181818.log.json) |
+| UNet + PSPNet | UNet-S5-D16 | Cross Entropy + Dice | 2336x3504 | 256x256 | 170x170 | 40000 | 2.798 | - | V100 | 89.69 | 80.96 | [config](https://github.com/open-mmlab/mmsegmentation/blob/main/configs/unet/unet-s5-d16_pspnet_4xb4-ce-1.0-dice-3.0-40k_hrf-256x256.py) | [model](https://download.openmmlab.com/mmsegmentation/v0.5/unet/pspnet_unet_s5-d16_ce-1.0-dice-3.0_256x256_40k_hrf/pspnet_unet_s5-d16_ce-1.0-dice-3.0_256x256_40k_hrf_20211210_201823-53d492fa.pth) \| [log](https://download.openmmlab.com/mmsegmentation/v0.5/unet/pspnet_unet_s5-d16_ce-1.0-dice-3.0_256x256_40k_hrf/pspnet_unet_s5-d16_ce-1.0-dice-3.0_256x256_40k_hrf_20211210_201823.log.json) |
+| UNet + DeepLabV3 | UNet-S5-D16 | Cross Entropy | 2336x3504 | 256x256 | 170x170 | 40000 | 2.604 | - | V100 | 89.32 | 80.21 | [config](https://github.com/open-mmlab/mmsegmentation/blob/main/configs/unet/unet-s5-d16_deeplabv3_4xb4-40k_hrf-256x256.py) | [model](https://download.openmmlab.com/mmsegmentation/v0.5/unet/deeplabv3_unet_s5-d16_256x256_40k_hrf/deeplabv3_unet_s5-d16_256x256_40k_hrf_20201226_094047-3a1fdf85.pth) \| [log](https://download.openmmlab.com/mmsegmentation/v0.5/unet/deeplabv3_unet_s5-d16_256x256_40k_hrf/deeplabv3_unet_s5-d16_256x256_40k_hrf-20201226_094047.log.json) |
+| UNet + DeepLabV3 | UNet-S5-D16 | Cross Entropy + Dice | 2336x3504 | 256x256 | 170x170 | 40000 | 2.607 | - | V100 | 89.56 | 80.71 | [config](https://github.com/open-mmlab/mmsegmentation/blob/main/configs/unet/unet-s5-d16_deeplabv3_4xb4-ce-1.0-dice-3.0-40k_hrf-256x256.py) | [model](https://download.openmmlab.com/mmsegmentation/v0.5/unet/deeplabv3_unet_s5-d16_ce-1.0-dice-3.0_256x256_40k_hrf/deeplabv3_unet_s5-d16_ce-1.0-dice-3.0_256x256_40k_hrf_20211210_202032-59daf7a4.pth) \| [log](https://download.openmmlab.com/mmsegmentation/v0.5/unet/deeplabv3_unet_s5-d16_ce-1.0-dice-3.0_256x256_40k_hrf/deeplabv3_unet_s5-d16_ce-1.0-dice-3.0_256x256_40k_hrf_20211210_202032.log.json) |
+
+Note:
+
+- In `DRIVE`, `STARE`, `CHASE_DB1`, and `HRF` dataset, `mDice` is mean dice of background and vessel, while `Dice` is dice metric of vessel(foreground) only.
+
+## Citation
+
+```bibtex
+@inproceedings{ronneberger2015u,
+ title={U-net: Convolutional networks for biomedical image segmentation},
+ author={Ronneberger, Olaf and Fischer, Philipp and Brox, Thomas},
+ booktitle={International Conference on Medical image computing and computer-assisted intervention},
+ pages={234--241},
+ year={2015},
+ organization={Springer}
+}
```
\ No newline at end of file
diff --git a/configs/model/unet/unet.yaml b/configs/model/unet/unet.yaml
index 91e70a9bdfad1102b183d69b0fb4b7b2e9960d3b..438d096c8050a7ffe17702de28ee8a3b3cfdc4b0 100644
--- a/configs/model/unet/unet.yaml
+++ b/configs/model/unet/unet.yaml
@@ -1,57 +1,57 @@
-# @package _global_
-
-# to execute this experiment run:
-# python train.py experiment=example
-
-defaults:
- - override /trainer: gpu
- - override /data: hrcWhu
- - override /model: unet
- - override /logger: wandb
- - override /callbacks: default
-
-# all parameters below will be merged with parameters from default configurations set above
-# this allows you to overwrite only specified parameters
-
-tags: ["hrcWhu", "unet"]
-
-seed: 42
-
-trainer:
- min_epochs: 10
- max_epochs: 10
- gradient_clip_val: 0.5
- devices: 1
-
-data:
- batch_size: 128
- train_val_test_split: [55_000, 5_000, 10_000]
- num_workers: 31
- pin_memory: False
- persistent_workers: False
-
-model:
- in_channels: 3
- out_channels: 7
-
-
-logger:
- wandb:
- project: "hrcWhu"
- name: "unet"
- aim:
- experiment: "unet"
-
-callbacks:
- model_checkpoint:
- dirpath: ${paths.output_dir}/checkpoints
- filename: "epoch_{epoch:03d}"
- monitor: "val/loss"
- mode: "min"
- save_last: True
- auto_insert_metric_name: False
-
- early_stopping:
- monitor: "val/loss"
- patience: 100
+# @package _global_
+
+# to execute this experiment run:
+# python train.py experiment=example
+
+defaults:
+ - override /trainer: gpu
+ - override /data: hrcWhu
+ - override /model: unet
+ - override /logger: wandb
+ - override /callbacks: default
+
+# all parameters below will be merged with parameters from default configurations set above
+# this allows you to overwrite only specified parameters
+
+tags: ["hrcWhu", "unet"]
+
+seed: 42
+
+trainer:
+ min_epochs: 10
+ max_epochs: 10
+ gradient_clip_val: 0.5
+ devices: 1
+
+data:
+ batch_size: 128
+ train_val_test_split: [55_000, 5_000, 10_000]
+ num_workers: 31
+ pin_memory: False
+ persistent_workers: False
+
+model:
+ in_channels: 3
+ out_channels: 7
+
+
+logger:
+ wandb:
+ project: "hrcWhu"
+ name: "unet"
+ aim:
+ experiment: "unet"
+
+callbacks:
+ model_checkpoint:
+ dirpath: ${paths.output_dir}/checkpoints
+ filename: "epoch_{epoch:03d}"
+ monitor: "val/loss"
+ mode: "min"
+ save_last: True
+ auto_insert_metric_name: False
+
+ early_stopping:
+ monitor: "val/loss"
+ patience: 100
mode: "min"
\ No newline at end of file
diff --git a/configs/model/unetmobv2/unetmobv2.yaml b/configs/model/unetmobv2/unetmobv2.yaml
new file mode 100644
index 0000000000000000000000000000000000000000..8f2aa6a9acb3f0fa021f1c18a9446b4960afb94d
--- /dev/null
+++ b/configs/model/unetmobv2/unetmobv2.yaml
@@ -0,0 +1,27 @@
+_target_: src.models.base_module.BaseLitModule
+
+net:
+ _target_: src.models.components.unetmobv2.UNetMobV2
+ num_classes: 2
+
+num_classes: 2
+
+criterion:
+ _target_: torch.nn.CrossEntropyLoss
+
+optimizer:
+ _target_: torch.optim.AdamW
+ _partial_: true
+ lr: 0.001
+
+scheduler:
+ _target_: torch.optim.lr_scheduler.ReduceLROnPlateau
+ _partial_: true
+ mode: min
+ factor: 0.1
+ patience: 4
+
+#scheduler:
+# _target_: torch.optim.lr_scheduler.LambdaLR
+# _partial_: true
+# lr_lambda: src.models.components.mcdnet.lr_lambda
\ No newline at end of file
diff --git a/configs/paths/default.yaml b/configs/paths/default.yaml
index ec81db2d34712909a79be3e42e65efe08c35ecee..c0e945f51f1bbba5aa48f01ddf15094cee26a476 100644
--- a/configs/paths/default.yaml
+++ b/configs/paths/default.yaml
@@ -1,18 +1,18 @@
-# path to root directory
-# this requires PROJECT_ROOT environment variable to exist
-# you can replace it with "." if you want the root to be the current working directory
-root_dir: ${oc.env:PROJECT_ROOT}
-
-# path to data directory
-data_dir: ${paths.root_dir}/data/
-
-# path to logging directory
-log_dir: ${paths.root_dir}/logs/
-
-# path to output directory, created dynamically by hydra
-# path generation pattern is specified in `configs/hydra/default.yaml`
-# use it to store all files generated during the run, like ckpts and metrics
-output_dir: ${hydra:runtime.output_dir}
-
-# path to working directory
-work_dir: ${hydra:runtime.cwd}
+# path to root directory
+# this requires PROJECT_ROOT environment variable to exist
+# you can replace it with "." if you want the root to be the current working directory
+root_dir: ${oc.env:PROJECT_ROOT}
+
+# path to data directory
+data_dir: ${paths.root_dir}/data/
+
+# path to logging directory
+log_dir: ${paths.root_dir}/logs/
+
+# path to output directory, created dynamically by hydra
+# path generation pattern is specified in `configs/hydra/default.yaml`
+# use it to store all files generated during the run, like ckpts and metrics
+output_dir: ${hydra:runtime.output_dir}
+
+# path to working directory
+work_dir: ${hydra:runtime.cwd}
diff --git a/configs/train.yaml b/configs/train.yaml
index 8e8d3e515725d75347d8b2f6aa4e0b80fa68fd82..526f5876ed828191793da5ba784455e5a4c54d7b 100644
--- a/configs/train.yaml
+++ b/configs/train.yaml
@@ -1,49 +1,49 @@
-# @package _global_
-
-# specify here default configuration
-# order of defaults determines the order in which configs override each other
-defaults:
- - _self_
- - data: null
- - model: null
- - callbacks: default
- - logger: null # set logger here or use command line (e.g. `python train.py logger=tensorboard`)
- - trainer: default
- - paths: default
- - extras: default
- - hydra: default
-
- # experiment configs allow for version control of specific hyperparameters
- # e.g. best hyperparameters for given model and datamodule
- - experiment: null
-
- # config for hyperparameter optimization
- - hparams_search: null
-
- # optional local config for machine/user specific settings
- # it's optional since it doesn't need to exist and is excluded from version control
- - optional local: default
-
- # debugging config (enable through command line, e.g. `python train.py debug=default)
- - debug: null
-
-# task name, determines output directory path
-task_name: "train"
-
-# tags to help you identify your experiments
-# you can overwrite this in experiment configs
-# overwrite from command line with `python train.py tags="[first_tag, second_tag]"`
-tags: ["dev"]
-
-# set False to skip model training
-train: True
-
-# evaluate on test set, using best model weights achieved during training
-# lightning chooses best weights based on the metric specified in checkpoint callback
-test: True
-
-# simply provide checkpoint path to resume training
-ckpt_path: null
-
-# seed for random number generators in pytorch, numpy and python.random
-seed: null
+# @package _global_
+
+# specify here default configuration
+# order of defaults determines the order in which configs override each other
+defaults:
+ - _self_
+ - data: null
+ - model: null
+ - callbacks: default
+ - logger: null # set logger here or use command line (e.g. `python train.py logger=tensorboard`)
+ - trainer: default
+ - paths: default
+ - extras: default
+ - hydra: default
+
+ # experiment configs allow for version control of specific hyperparameters
+ # e.g. best hyperparameters for given model and datamodule
+ - experiment: null
+
+ # config for hyperparameter optimization
+ - hparams_search: null
+
+ # optional local config for machine/user specific settings
+ # it's optional since it doesn't need to exist and is excluded from version control
+ - optional local: default
+
+ # debugging config (enable through command line, e.g. `python train.py debug=default)
+ - debug: null
+
+# task name, determines output directory path
+task_name: "train"
+
+# tags to help you identify your experiments
+# you can overwrite this in experiment configs
+# overwrite from command line with `python train.py tags="[first_tag, second_tag]"`
+tags: ["dev"]
+
+# set False to skip model training
+train: True
+
+# evaluate on test set, using best model weights achieved during training
+# lightning chooses best weights based on the metric specified in checkpoint callback
+test: True
+
+# simply provide checkpoint path to resume training
+ckpt_path: null
+
+# seed for random number generators in pytorch, numpy and python.random
+seed: null
diff --git a/configs/trainer/cpu.yaml b/configs/trainer/cpu.yaml
index b7d6767e60c956567555980654f15e7bb673a41f..570307e434697e7eaff51be2d7dd2a262c19a26f 100644
--- a/configs/trainer/cpu.yaml
+++ b/configs/trainer/cpu.yaml
@@ -1,5 +1,5 @@
-defaults:
- - default
-
-accelerator: cpu
-devices: 1
+defaults:
+ - default
+
+accelerator: cpu
+devices: 1
diff --git a/configs/trainer/ddp.yaml b/configs/trainer/ddp.yaml
index ab8f89004c399a33440f014fa27e040d4e952bc2..eacb9e5194e1ff9faf87b979e0383e2623f95b7e 100644
--- a/configs/trainer/ddp.yaml
+++ b/configs/trainer/ddp.yaml
@@ -1,9 +1,9 @@
-defaults:
- - default
-
-strategy: ddp
-
-accelerator: gpu
-devices: 4
-num_nodes: 1
-sync_batchnorm: True
+defaults:
+ - default
+
+strategy: ddp
+
+accelerator: gpu
+devices: 4
+num_nodes: 1
+sync_batchnorm: True
diff --git a/configs/trainer/ddp_sim.yaml b/configs/trainer/ddp_sim.yaml
index 8404419e5c295654967d0dfb73a7366e75be2f1f..7a24bba5cdc2759ceec606d0267e5b8f66a480c7 100644
--- a/configs/trainer/ddp_sim.yaml
+++ b/configs/trainer/ddp_sim.yaml
@@ -1,7 +1,7 @@
-defaults:
- - default
-
-# simulate DDP on CPU, useful for debugging
-accelerator: cpu
-devices: 2
-strategy: ddp_spawn
+defaults:
+ - default
+
+# simulate DDP on CPU, useful for debugging
+accelerator: cpu
+devices: 2
+strategy: ddp_spawn
diff --git a/configs/trainer/default.yaml b/configs/trainer/default.yaml
index 50905e7fdf158999e7c726edfff1a4dc16d548da..f880aab1e76b322b742e41a812fbc272ef86d889 100644
--- a/configs/trainer/default.yaml
+++ b/configs/trainer/default.yaml
@@ -1,19 +1,19 @@
-_target_: lightning.pytorch.trainer.Trainer
-
-default_root_dir: ${paths.output_dir}
-
-min_epochs: 1 # prevents early stopping
-max_epochs: 10
-
-accelerator: cpu
-devices: 1
-
-# mixed precision for extra speed-up
-# precision: 16
-
-# perform a validation loop every N training epochs
-check_val_every_n_epoch: 1
-
-# set True to to ensure deterministic results
-# makes training slower but gives more reproducibility than just setting seeds
-deterministic: False
+_target_: lightning.pytorch.trainer.Trainer
+
+default_root_dir: ${paths.output_dir}
+
+min_epochs: 1 # prevents early stopping
+max_epochs: 10
+
+accelerator: cpu
+devices: 1
+
+# mixed precision for extra speed-up
+# precision: 16
+
+# perform a validation loop every N training epochs
+check_val_every_n_epoch: 1
+
+# set True to to ensure deterministic results
+# makes training slower but gives more reproducibility than just setting seeds
+deterministic: False
diff --git a/configs/trainer/gpu.yaml b/configs/trainer/gpu.yaml
index 54d2c957bd106cf39352440c185ff4976b16e899..23f2c6e1906efa46b57f9bfeb501cae5a832d2c7 100644
--- a/configs/trainer/gpu.yaml
+++ b/configs/trainer/gpu.yaml
@@ -1,7 +1,7 @@
-defaults:
- - default
-
-accelerator: gpu
-devices: 1
-min_epochs: 10
-max_epochs: 10000
+defaults:
+ - default
+
+accelerator: gpu
+devices: 1
+min_epochs: 10
+max_epochs: 10000
diff --git a/configs/trainer/mps.yaml b/configs/trainer/mps.yaml
index 1ecf6d5cc3a34ca127c5510f4a18e989561e38e4..9706d4f2c23dde09527baceb63b85365321eca01 100644
--- a/configs/trainer/mps.yaml
+++ b/configs/trainer/mps.yaml
@@ -1,5 +1,5 @@
-defaults:
- - default
-
-accelerator: mps
-devices: 1
+defaults:
+ - default
+
+accelerator: mps
+devices: 1
diff --git a/environment.yaml b/environment.yaml
index 76cbd9be60c9cb15921bd94d0dae0c8573c4c507..d5c45514e88d0629223952f28f33708e0d639348 100644
--- a/environment.yaml
+++ b/environment.yaml
@@ -1,27 +1,27 @@
-name: cloudseg
-
-channels:
- - pytorch
- - conda-forge
- - defaults
-
-dependencies:
- - python=3.8.0
- - pytorch=2.0.0
- - torchvision=0.15.0
- - lightning==2.0.0
- - pip=24.2
-
- - pip:
- - torchmetrics
- - hydra-core
- - hydra-optuna-sweeper
- - hydra-colorlog
- - rich
- - pytest
- - rootutils
- - wandb
- - aim
- - gradio
- - image-dehazer
+name: cloudseg
+
+channels:
+ - pytorch
+ - conda-forge
+ - defaults
+
+dependencies:
+ - python=3.8.0
+ - pytorch=2.0.0
+ - torchvision=0.15.0
+ - lightning==2.0.0
+ - pip=24.2
+
+ - pip:
+ - torchmetrics
+ - hydra-core
+ - hydra-optuna-sweeper
+ - hydra-colorlog
+ - rich
+ - pytest
+ - rootutils
+ - wandb
+ - aim
+ - gradio
+ - image-dehazer
- thop
\ No newline at end of file
diff --git a/logs/train/runs/hrcwhu_hrcloudnet/2024-08-03_18-55-52/checkpoints/epoch_024.ckpt b/logs/train/runs/hrcwhu_hrcloudnet/2024-08-03_18-55-52/checkpoints/epoch_024.ckpt
new file mode 100644
index 0000000000000000000000000000000000000000..c165011a4a45d48f526f7d267d8d7fd08d5f9ef6
--- /dev/null
+++ b/logs/train/runs/hrcwhu_hrcloudnet/2024-08-03_18-55-52/checkpoints/epoch_024.ckpt
@@ -0,0 +1,3 @@
+version https://git-lfs.github.com/spec/v1
+oid sha256:3554e396cddb6e56b89062d84c1a6538d980cdc3adb9e27e53bc0d241a8cffbf
+size 893819887
diff --git a/logs/train/runs/hrcwhu_hrcloudnet/2024-08-03_18-55-52/checkpoints/last.ckpt b/logs/train/runs/hrcwhu_hrcloudnet/2024-08-03_18-55-52/checkpoints/last.ckpt
new file mode 100644
index 0000000000000000000000000000000000000000..e7b0c0386511ce94301c16745477fd2c24090a2f
--- /dev/null
+++ b/logs/train/runs/hrcwhu_hrcloudnet/2024-08-03_18-55-52/checkpoints/last.ckpt
@@ -0,0 +1,3 @@
+version https://git-lfs.github.com/spec/v1
+oid sha256:ef0c18c6eef14e194ec38fd287f07a8532f753130289a729c9101ad28f76d8e9
+size 893819887
diff --git a/logs/train/runs/hrcwhu_unetmobv2/2024-08-06_16-29-28/checkpoints/epoch_018.ckpt b/logs/train/runs/hrcwhu_unetmobv2/2024-08-06_16-29-28/checkpoints/epoch_018.ckpt
new file mode 100644
index 0000000000000000000000000000000000000000..04326b1469719f35a8ae82e8a05606e95f71a179
--- /dev/null
+++ b/logs/train/runs/hrcwhu_unetmobv2/2024-08-06_16-29-28/checkpoints/epoch_018.ckpt
@@ -0,0 +1,3 @@
+version https://git-lfs.github.com/spec/v1
+oid sha256:8d0d03fc29ae011b292e452ed6e6d094afafefa4dd1519804f9b374bdd994421
+size 80017178
diff --git a/logs/train/runs/hrcwhu_unetmobv2/2024-08-06_16-29-28/checkpoints/last.ckpt b/logs/train/runs/hrcwhu_unetmobv2/2024-08-06_16-29-28/checkpoints/last.ckpt
new file mode 100644
index 0000000000000000000000000000000000000000..d8644bcdfa30da1e782243398c90acb08b789dde
--- /dev/null
+++ b/logs/train/runs/hrcwhu_unetmobv2/2024-08-06_16-29-28/checkpoints/last.ckpt
@@ -0,0 +1,3 @@
+version https://git-lfs.github.com/spec/v1
+oid sha256:c446e9b789f27e25640984d8ea8c2489c50b8241132b8eee7825de0e1ce198e2
+size 80017178
diff --git a/pyproject.toml b/pyproject.toml
index 300ebf04f0594f1c517e7017d3697490009b203f..c5b7c6a5e3ff6faa26babae102852c08d1db1f88 100644
--- a/pyproject.toml
+++ b/pyproject.toml
@@ -1,25 +1,25 @@
-[tool.pytest.ini_options]
-addopts = [
- "--color=yes",
- "--durations=0",
- "--strict-markers",
- "--doctest-modules",
-]
-filterwarnings = [
- "ignore::DeprecationWarning",
- "ignore::UserWarning",
-]
-log_cli = "True"
-markers = [
- "slow: slow tests",
-]
-minversion = "6.0"
-testpaths = "tests/"
-
-[tool.coverage.report]
-exclude_lines = [
- "pragma: nocover",
- "raise NotImplementedError",
- "raise NotImplementedError()",
- "if __name__ == .__main__.:",
-]
+[tool.pytest.ini_options]
+addopts = [
+ "--color=yes",
+ "--durations=0",
+ "--strict-markers",
+ "--doctest-modules",
+]
+filterwarnings = [
+ "ignore::DeprecationWarning",
+ "ignore::UserWarning",
+]
+log_cli = "True"
+markers = [
+ "slow: slow tests",
+]
+minversion = "6.0"
+testpaths = "tests/"
+
+[tool.coverage.report]
+exclude_lines = [
+ "pragma: nocover",
+ "raise NotImplementedError",
+ "raise NotImplementedError()",
+ "if __name__ == .__main__.:",
+]
diff --git a/references/README.md b/references/README.md
new file mode 100644
index 0000000000000000000000000000000000000000..0293a5939d3277222929872c781285a5ed7875a5
--- /dev/null
+++ b/references/README.md
@@ -0,0 +1,6 @@
+# References
+
+## Datasets
+
+
+## Methods
\ No newline at end of file
diff --git a/references/[2017 RSE] L8_Biome.pdf b/references/[2017 RSE] L8_Biome.pdf
new file mode 100644
index 0000000000000000000000000000000000000000..527f048f9628a6bd77ec1206104299b8c0ead549
--- /dev/null
+++ b/references/[2017 RSE] L8_Biome.pdf
@@ -0,0 +1,3 @@
+version https://git-lfs.github.com/spec/v1
+oid sha256:7e1f66474ef5ae91107b843af4ab4496dfbbccd143f399c1e2b99c45afd78301
+size 1528606
diff --git a/references/[2019 ISPRS] HRC_WHU.pdf b/references/[2019 ISPRS] HRC_WHU.pdf
new file mode 100644
index 0000000000000000000000000000000000000000..bebc152c094262cede13c5694f9ba0c83eb147ce
--- /dev/null
+++ b/references/[2019 ISPRS] HRC_WHU.pdf
@@ -0,0 +1,3 @@
+version https://git-lfs.github.com/spec/v1
+oid sha256:e435482264330f748e3a8bd989736c6057f673af281d78837f74d67e1b161a76
+size 22667428
diff --git a/references/[2019 TGRS] CDnet.pdf b/references/[2019 TGRS] CDnet.pdf
new file mode 100644
index 0000000000000000000000000000000000000000..9edd74d4f3957fd78edb5373460f414687fba7da
--- /dev/null
+++ b/references/[2019 TGRS] CDnet.pdf
@@ -0,0 +1,3 @@
+version https://git-lfs.github.com/spec/v1
+oid sha256:305fb03f8f0a1faacb3c7d7d5d7dacd2abf9c3f37751a4cfd4e69b0e5b41d4eb
+size 24399164
diff --git a/references/[2021 TGRS] CDnetV2.pdf b/references/[2021 TGRS] CDnetV2.pdf
new file mode 100644
index 0000000000000000000000000000000000000000..3823b654e886b8b949d3ab9a5b294d46c60a0689
--- /dev/null
+++ b/references/[2021 TGRS] CDnetV2.pdf
@@ -0,0 +1,3 @@
+version https://git-lfs.github.com/spec/v1
+oid sha256:307a02864ac0be1a57fa041400bc0a6a273d3a4dd7848f640292fcce37f952ad
+size 22659838
diff --git a/references/[2022 TGRS] DBNet.pdf b/references/[2022 TGRS] DBNet.pdf
new file mode 100644
index 0000000000000000000000000000000000000000..adee5c78a4bc0386cce49775ed1cf67a7982bfbc
--- /dev/null
+++ b/references/[2022 TGRS] DBNet.pdf
@@ -0,0 +1,3 @@
+version https://git-lfs.github.com/spec/v1
+oid sha256:e1e09c33e1774f569901a685dc4e73c59308f51bc18dc9ef5e209dc8b51561b3
+size 5127467
diff --git a/references/[2024 ISPRS] SCNN.pdf b/references/[2024 ISPRS] SCNN.pdf
new file mode 100644
index 0000000000000000000000000000000000000000..b444accd2d231d358e8de3223566ae95ad96a897
--- /dev/null
+++ b/references/[2024 ISPRS] SCNN.pdf
@@ -0,0 +1,3 @@
+version https://git-lfs.github.com/spec/v1
+oid sha256:c0b23ea58709272022a4ccae02cda66c4e3e02549da3d96c1846aad3ac40a8be
+size 8572879
diff --git a/references/[2024 TGRS] GaoFen12.pdf b/references/[2024 TGRS] GaoFen12.pdf
new file mode 100644
index 0000000000000000000000000000000000000000..e586144ced9a79c40f94561650c850f2f64fea85
--- /dev/null
+++ b/references/[2024 TGRS] GaoFen12.pdf
@@ -0,0 +1,3 @@
+version https://git-lfs.github.com/spec/v1
+oid sha256:72caec256dc71d0c55b41b55a53516f0d865d37f6fd6b6565787615558b6327c
+size 4881310
diff --git a/requirements.txt b/requirements.txt
index c4602e18a0619a4af5be84bcf9600169acf1c7ae..de8e3cb173f8f092afc55f53727e39613510874d 100644
--- a/requirements.txt
+++ b/requirements.txt
@@ -1,11 +1,12 @@
-gradio==4.40.0
-opencv-python==4.10.0.84
-pillow==10.4.0
-albumentations==1.4.12
-image_dehazer==0.0.9
-einops==0.8.0
-yacs==0.1.8
-omegaconf==2.3.0
-torch
-torchvision
-torchaudio
\ No newline at end of file
+gradio==4.40.0
+opencv-python==4.10.0.84
+pillow==10.4.0
+albumentations==1.4.12
+image_dehazer==0.0.9
+einops==0.8.0
+yacs==0.1.8
+omegaconf==2.3.0
+torch
+torchvision
+torchaudio
+segmentation_models_pytorch
\ No newline at end of file
diff --git a/scripts/schedule.sh b/scripts/schedule.sh
index 44b3da1116ef4d54e9acffee7d639d549e136d45..ea7e6626181ada3736a9883f32402df56d4668ae 100644
--- a/scripts/schedule.sh
+++ b/scripts/schedule.sh
@@ -1,7 +1,7 @@
-#!/bin/bash
-# Schedule execution of many runs
-# Run from root folder with: bash scripts/schedule.sh
-
-python src/train.py trainer.max_epochs=5 logger=csv
-
-python src/train.py trainer.max_epochs=10 logger=csv
+#!/bin/bash
+# Schedule execution of many runs
+# Run from root folder with: bash scripts/schedule.sh
+
+python src/train.py trainer.max_epochs=5 logger=csv
+
+python src/train.py trainer.max_epochs=10 logger=csv
diff --git a/setup.py b/setup.py
index 40ad3e19f3be584f5b79248f5185634deb15dc4b..f0b07b84c993f41f58364da8535432ffcc991575 100644
--- a/setup.py
+++ b/setup.py
@@ -1,21 +1,21 @@
-#!/usr/bin/env python
-
-from setuptools import find_packages, setup
-
-setup(
- name="src",
- version="0.0.1",
- description="Describe Your Cool Project",
- author="",
- author_email="",
- url="https://github.com/XavierJiezou/cloudseg",
- install_requires=["lightning", "hydra-core"],
- packages=find_packages(),
- # use this to customize global commands available in the terminal after installing the package
- entry_points={
- "console_scripts": [
- "train_command = src.train:main",
- "eval_command = src.eval:main",
- ]
- },
-)
+#!/usr/bin/env python
+
+from setuptools import find_packages, setup
+
+setup(
+ name="src",
+ version="0.0.1",
+ description="Describe Your Cool Project",
+ author="",
+ author_email="",
+ url="https://github.com/XavierJiezou/cloudseg",
+ install_requires=["lightning", "hydra-core"],
+ packages=find_packages(),
+ # use this to customize global commands available in the terminal after installing the package
+ entry_points={
+ "console_scripts": [
+ "train_command = src.train:main",
+ "eval_command = src.eval:main",
+ ]
+ },
+)
diff --git a/src/data/components/celeba.py b/src/data/components/celeba.py
index 826a1a45ecd6eceaa0e8ce5ec9336d564eb2e85b..e98fe055b8f5e4ac775c4e662809e4755be024e5 100644
--- a/src/data/components/celeba.py
+++ b/src/data/components/celeba.py
@@ -1,234 +1,234 @@
-import json
-import os
-import random
-
-import albumentations
-import matplotlib.pyplot as plt
-import numpy as np
-import torch
-from PIL import Image
-from torch.utils.data import Dataset
-
-
-class DalleTransformerPreprocessor(object):
- def __init__(self,
- size=256,
- phase='train',
- additional_targets=None):
-
- self.size = size
- self.phase = phase
- # ddc: following dalle to use randomcrop
- self.train_preprocessor = albumentations.Compose([albumentations.RandomCrop(height=size, width=size)],
- additional_targets=additional_targets)
- self.val_preprocessor = albumentations.Compose([albumentations.CenterCrop(height=size, width=size)],
- additional_targets=additional_targets)
-
-
- def __call__(self, image, **kargs):
- """
- image: PIL.Image
- """
- if isinstance(image, np.ndarray):
- image = Image.fromarray(image.astype(np.uint8))
-
- w, h = image.size
- s_min = min(h, w)
-
- if self.phase == 'train':
- off_h = int(random.uniform(3*(h-s_min)//8, max(3*(h-s_min)//8+1, 5*(h-s_min)//8)))
- off_w = int(random.uniform(3*(w-s_min)//8, max(3*(w-s_min)//8+1, 5*(w-s_min)//8)))
-
- image = image.crop((off_w, off_h, off_w + s_min, off_h + s_min))
-
- # resize image
- t_max = min(s_min, round(9/8*self.size))
- t_max = max(t_max, self.size)
- t = int(random.uniform(self.size, t_max+1))
- image = image.resize((t, t))
- image = np.array(image).astype(np.uint8)
- image = self.train_preprocessor(image=image)
- else:
- if w < h:
- w_ = self.size
- h_ = int(h * w_/w)
- else:
- h_ = self.size
- w_ = int(w * h_/h)
- image = image.resize((w_, h_))
- image = np.array(image).astype(np.uint8)
- image = self.val_preprocessor(image=image)
- return image
-
-
-class CelebA(Dataset):
-
- """
- This Dataset can be used for:
- - image-only: setting 'conditions' = []
- - image and multi-modal 'conditions': setting conditions as the list of modalities you need
-
- To toggle between 256 and 512 image resolution, simply change the 'image_folder'
- """
-
- def __init__(
- self,
- phase='train',
- size=512,
- test_dataset_size=3000,
- conditions=['seg_mask', 'text', 'sketch'],
- image_folder='data/celeba/image/image_512_downsampled_from_hq_1024',
- text_file='data/celeba/text/captions_hq_beard_and_age_2022-08-19.json',
- mask_folder='data/celeba/mask/CelebAMask-HQ-mask-color-palette_32_nearest_downsampled_from_hq_512_one_hot_2d_tensor',
- sketch_folder='data/celeba/sketch/sketch_1x1024_tensor',
- ):
- self.transform = DalleTransformerPreprocessor(size=size, phase=phase)
- self.conditions = conditions
-
- self.image_folder = image_folder
-
- # conditions directory
- self.text_file = text_file
- with open(self.text_file, 'r') as f:
- self.text_file_content = json.load(f)
- if 'seg_mask' in self.conditions:
- self.mask_folder = mask_folder
- if 'sketch' in self.conditions:
- self.sketch_folder = sketch_folder
-
- # list of valid image names & train test split
- self.image_name_list = list(self.text_file_content.keys())
-
- # train test split
- if phase == 'train':
- self.image_name_list = self.image_name_list[:-test_dataset_size]
- elif phase == 'test':
- self.image_name_list = self.image_name_list[-test_dataset_size:]
- else:
- raise NotImplementedError
- self.num = len(self.image_name_list)
-
- def __len__(self):
- return self.num
-
- def __getitem__(self, index):
-
- # ---------- (1) get image ----------
- image_name = self.image_name_list[index]
- image_path = os.path.join(self.image_folder, image_name)
- image = Image.open(image_path).convert('RGB')
- image = np.array(image).astype(np.uint8)
- image = self.transform(image=image)['image']
- image = image.astype(np.float32)/127.5 - 1.0
-
- # record into data entry
- if len(self.conditions) == 1:
- data = {
- 'image': image,
- }
- else:
- data = {
- 'image': image,
- 'conditions': {}
- }
-
- # ---------- (2) get text ----------
- if 'text' in self.conditions:
- text = self.text_file_content[image_name]["Beard_and_Age"].lower()
- # record into data entry
- if len(self.conditions) == 1:
- data['caption'] = text
- else:
- data['conditions']['text'] = text
-
- # ---------- (3) get mask ----------
- if 'seg_mask' in self.conditions:
- mask_idx = image_name.split('.')[0]
- mask_name = f'{mask_idx}.pt'
- mask_path = os.path.join(self.mask_folder, mask_name)
- mask_one_hot_tensor = torch.load(mask_path)
-
- # record into data entry
- if len(self.conditions) == 1:
- data['seg_mask'] = mask_one_hot_tensor
- else:
- data['conditions']['seg_mask'] = mask_one_hot_tensor
-
- # ---------- (4) get sketch ----------
- if 'sketch' in self.conditions:
- sketch_idx = image_name.split('.')[0]
- sketch_name = f'{sketch_idx}.pt'
- sketch_path = os.path.join(self.sketch_folder, sketch_name)
- sketch_one_hot_tensor = torch.load(sketch_path)
-
- # record into data entry
- if len(self.conditions) == 1:
- data['sketch'] = sketch_one_hot_tensor
- else:
- data['conditions']['sketch'] = sketch_one_hot_tensor
- data["image_name"] = image_name.split('.')[0]
- return data
-
-
-if __name__ == '__main__':
- # The caption file only has 29999 captions: https://github.com/ziqihuangg/CelebA-Dialog/issues/1
-
- # Testing for `phase`
- train_dataset = CelebA(phase="train")
- test_dataset = CelebA(phase="test")
- assert len(train_dataset)==26999
- assert len(test_dataset)==3000
-
- # Testing for `size`
- size_512 = CelebA(size=512)
- assert size_512[0]['image'].shape == (512, 512, 3)
- assert size_512[0]["conditions"]['seg_mask'].shape == (19, 1024)
- assert size_512[0]["conditions"]['sketch'].shape == (1, 1024)
- size_512 = CelebA(size=256)
- assert size_512[0]['image'].shape == (256, 256, 3)
- assert size_512[0]["conditions"]['seg_mask'].shape == (19, 1024)
- assert size_512[0]["conditions"]['sketch'].shape == (1, 1024)
-
- # Testing for `conditions`
- dataset = CelebA(conditions = ['seg_mask', 'text', 'sketch'])
- image = dataset[0]["image"]
- seg_mask= dataset[0]["conditions"]['seg_mask']
- sketch = dataset[0]["conditions"]['sketch']
- text = dataset[0]["conditions"]['text']
- # show image, seg_mask, sketch in 3x3 grid, and text in title
- fig, ax = plt.subplots(1, 3, figsize=(12, 4))
-
- # Show image
- ax[0].imshow((image + 1) / 2)
- ax[0].set_title('Image')
- ax[0].axis('off')
-
- # # Show segmentation mask
- seg_mask = torch.argmax(seg_mask, dim=0).reshape(32, 32).numpy().astype(np.uint8)
- # resize to 512x512 using nearest neighbor interpolation
- seg_mask = Image.fromarray(seg_mask).resize((512, 512), Image.NEAREST)
- seg_mask = np.array(seg_mask)
- ax[1].imshow(seg_mask, cmap='tab20')
- ax[1].set_title('Segmentation Mask')
- ax[1].axis('off')
-
- # # # Show sketch
- sketch = sketch.reshape(32, 32).numpy().astype(np.uint8)
- # resize to 512x512 using nearest neighbor interpolation
- sketch = Image.fromarray(sketch).resize((512, 512), Image.NEAREST)
- sketch = np.array(sketch)
- ax[2].imshow(sketch, cmap='gray')
- ax[2].set_title('Sketch')
- ax[2].axis('off')
-
- # Add title with text
- fig.suptitle(text, fontsize=16)
- plt.tight_layout()
- plt.savefig('celeba_sample.png')
-
- # save seg_mask with name such as "27000.png, 270001.png, ..., 279999.png" of test dataset to "/mnt/chongqinggeminiceph1fs/geminicephfs/wx-mm-spr-xxxx/zouxuechao/Collaborative-Diffusion/evaluation/CollDiff/real_mask"
- from tqdm import tqdm
- for data in tqdm(test_dataset):
- mask = torch.argmax(data["conditions"]['seg_mask'], dim=0).reshape(32, 32).numpy().astype(np.uint8)
- mask = Image.fromarray(mask).resize((512, 512), Image.NEAREST)
+import json
+import os
+import random
+
+import albumentations
+import matplotlib.pyplot as plt
+import numpy as np
+import torch
+from PIL import Image
+from torch.utils.data import Dataset
+
+
+class DalleTransformerPreprocessor(object):
+ def __init__(self,
+ size=256,
+ phase='train',
+ additional_targets=None):
+
+ self.size = size
+ self.phase = phase
+ # ddc: following dalle to use randomcrop
+ self.train_preprocessor = albumentations.Compose([albumentations.RandomCrop(height=size, width=size)],
+ additional_targets=additional_targets)
+ self.val_preprocessor = albumentations.Compose([albumentations.CenterCrop(height=size, width=size)],
+ additional_targets=additional_targets)
+
+
+ def __call__(self, image, **kargs):
+ """
+ image: PIL.Image
+ """
+ if isinstance(image, np.ndarray):
+ image = Image.fromarray(image.astype(np.uint8))
+
+ w, h = image.size
+ s_min = min(h, w)
+
+ if self.phase == 'train':
+ off_h = int(random.uniform(3*(h-s_min)//8, max(3*(h-s_min)//8+1, 5*(h-s_min)//8)))
+ off_w = int(random.uniform(3*(w-s_min)//8, max(3*(w-s_min)//8+1, 5*(w-s_min)//8)))
+
+ image = image.crop((off_w, off_h, off_w + s_min, off_h + s_min))
+
+ # resize image
+ t_max = min(s_min, round(9/8*self.size))
+ t_max = max(t_max, self.size)
+ t = int(random.uniform(self.size, t_max+1))
+ image = image.resize((t, t))
+ image = np.array(image).astype(np.uint8)
+ image = self.train_preprocessor(image=image)
+ else:
+ if w < h:
+ w_ = self.size
+ h_ = int(h * w_/w)
+ else:
+ h_ = self.size
+ w_ = int(w * h_/h)
+ image = image.resize((w_, h_))
+ image = np.array(image).astype(np.uint8)
+ image = self.val_preprocessor(image=image)
+ return image
+
+
+class CelebA(Dataset):
+
+ """
+ This Dataset can be used for:
+ - image-only: setting 'conditions' = []
+ - image and multi-modal 'conditions': setting conditions as the list of modalities you need
+
+ To toggle between 256 and 512 image resolution, simply change the 'image_folder'
+ """
+
+ def __init__(
+ self,
+ phase='train',
+ size=512,
+ test_dataset_size=3000,
+ conditions=['seg_mask', 'text', 'sketch'],
+ image_folder='data/celeba/image/image_512_downsampled_from_hq_1024',
+ text_file='data/celeba/text/captions_hq_beard_and_age_2022-08-19.json',
+ mask_folder='data/celeba/mask/CelebAMask-HQ-mask-color-palette_32_nearest_downsampled_from_hq_512_one_hot_2d_tensor',
+ sketch_folder='data/celeba/sketch/sketch_1x1024_tensor',
+ ):
+ self.transform = DalleTransformerPreprocessor(size=size, phase=phase)
+ self.conditions = conditions
+
+ self.image_folder = image_folder
+
+ # conditions directory
+ self.text_file = text_file
+ with open(self.text_file, 'r') as f:
+ self.text_file_content = json.load(f)
+ if 'seg_mask' in self.conditions:
+ self.mask_folder = mask_folder
+ if 'sketch' in self.conditions:
+ self.sketch_folder = sketch_folder
+
+ # list of valid image names & train test split
+ self.image_name_list = list(self.text_file_content.keys())
+
+ # train test split
+ if phase == 'train':
+ self.image_name_list = self.image_name_list[:-test_dataset_size]
+ elif phase == 'test':
+ self.image_name_list = self.image_name_list[-test_dataset_size:]
+ else:
+ raise NotImplementedError
+ self.num = len(self.image_name_list)
+
+ def __len__(self):
+ return self.num
+
+ def __getitem__(self, index):
+
+ # ---------- (1) get image ----------
+ image_name = self.image_name_list[index]
+ image_path = os.path.join(self.image_folder, image_name)
+ image = Image.open(image_path).convert('RGB')
+ image = np.array(image).astype(np.uint8)
+ image = self.transform(image=image)['image']
+ image = image.astype(np.float32)/127.5 - 1.0
+
+ # record into data entry
+ if len(self.conditions) == 1:
+ data = {
+ 'image': image,
+ }
+ else:
+ data = {
+ 'image': image,
+ 'conditions': {}
+ }
+
+ # ---------- (2) get text ----------
+ if 'text' in self.conditions:
+ text = self.text_file_content[image_name]["Beard_and_Age"].lower()
+ # record into data entry
+ if len(self.conditions) == 1:
+ data['caption'] = text
+ else:
+ data['conditions']['text'] = text
+
+ # ---------- (3) get mask ----------
+ if 'seg_mask' in self.conditions:
+ mask_idx = image_name.split('.')[0]
+ mask_name = f'{mask_idx}.pt'
+ mask_path = os.path.join(self.mask_folder, mask_name)
+ mask_one_hot_tensor = torch.load(mask_path)
+
+ # record into data entry
+ if len(self.conditions) == 1:
+ data['seg_mask'] = mask_one_hot_tensor
+ else:
+ data['conditions']['seg_mask'] = mask_one_hot_tensor
+
+ # ---------- (4) get sketch ----------
+ if 'sketch' in self.conditions:
+ sketch_idx = image_name.split('.')[0]
+ sketch_name = f'{sketch_idx}.pt'
+ sketch_path = os.path.join(self.sketch_folder, sketch_name)
+ sketch_one_hot_tensor = torch.load(sketch_path)
+
+ # record into data entry
+ if len(self.conditions) == 1:
+ data['sketch'] = sketch_one_hot_tensor
+ else:
+ data['conditions']['sketch'] = sketch_one_hot_tensor
+ data["image_name"] = image_name.split('.')[0]
+ return data
+
+
+if __name__ == '__main__':
+ # The caption file only has 29999 captions: https://github.com/ziqihuangg/CelebA-Dialog/issues/1
+
+ # Testing for `phase`
+ train_dataset = CelebA(phase="train")
+ test_dataset = CelebA(phase="test")
+ assert len(train_dataset)==26999
+ assert len(test_dataset)==3000
+
+ # Testing for `size`
+ size_512 = CelebA(size=512)
+ assert size_512[0]['image'].shape == (512, 512, 3)
+ assert size_512[0]["conditions"]['seg_mask'].shape == (19, 1024)
+ assert size_512[0]["conditions"]['sketch'].shape == (1, 1024)
+ size_512 = CelebA(size=256)
+ assert size_512[0]['image'].shape == (256, 256, 3)
+ assert size_512[0]["conditions"]['seg_mask'].shape == (19, 1024)
+ assert size_512[0]["conditions"]['sketch'].shape == (1, 1024)
+
+ # Testing for `conditions`
+ dataset = CelebA(conditions = ['seg_mask', 'text', 'sketch'])
+ image = dataset[0]["image"]
+ seg_mask= dataset[0]["conditions"]['seg_mask']
+ sketch = dataset[0]["conditions"]['sketch']
+ text = dataset[0]["conditions"]['text']
+ # show image, seg_mask, sketch in 3x3 grid, and text in title
+ fig, ax = plt.subplots(1, 3, figsize=(12, 4))
+
+ # Show image
+ ax[0].imshow((image + 1) / 2)
+ ax[0].set_title('Image')
+ ax[0].axis('off')
+
+ # # Show segmentation mask
+ seg_mask = torch.argmax(seg_mask, dim=0).reshape(32, 32).numpy().astype(np.uint8)
+ # resize to 512x512 using nearest neighbor interpolation
+ seg_mask = Image.fromarray(seg_mask).resize((512, 512), Image.NEAREST)
+ seg_mask = np.array(seg_mask)
+ ax[1].imshow(seg_mask, cmap='tab20')
+ ax[1].set_title('Segmentation Mask')
+ ax[1].axis('off')
+
+ # # # Show sketch
+ sketch = sketch.reshape(32, 32).numpy().astype(np.uint8)
+ # resize to 512x512 using nearest neighbor interpolation
+ sketch = Image.fromarray(sketch).resize((512, 512), Image.NEAREST)
+ sketch = np.array(sketch)
+ ax[2].imshow(sketch, cmap='gray')
+ ax[2].set_title('Sketch')
+ ax[2].axis('off')
+
+ # Add title with text
+ fig.suptitle(text, fontsize=16)
+ plt.tight_layout()
+ plt.savefig('celeba_sample.png')
+
+ # save seg_mask with name such as "27000.png, 270001.png, ..., 279999.png" of test dataset to "/mnt/chongqinggeminiceph1fs/geminicephfs/wx-mm-spr-xxxx/zouxuechao/Collaborative-Diffusion/evaluation/CollDiff/real_mask"
+ from tqdm import tqdm
+ for data in tqdm(test_dataset):
+ mask = torch.argmax(data["conditions"]['seg_mask'], dim=0).reshape(32, 32).numpy().astype(np.uint8)
+ mask = Image.fromarray(mask).resize((512, 512), Image.NEAREST)
mask.save(f"/mnt/chongqinggeminiceph1fs/geminicephfs/wx-mm-spr-xxxx/zouxuechao/Collaborative-Diffusion/evaluation/CollDiff/real_mask/{data['image_name']}.png")
\ No newline at end of file
diff --git a/src/data/components/hrcwhu.py b/src/data/components/hrcwhu.py
index f37d6bb085701221178907e84bec684177ea1fac..ca0b16a84487a2514565bf527a5efd4bfa3b2c7d 100644
--- a/src/data/components/hrcwhu.py
+++ b/src/data/components/hrcwhu.py
@@ -1,137 +1,137 @@
-import os
-
-import albumentations
-import numpy as np
-from PIL import Image
-from torch.utils.data import Dataset
-
-
-class HRCWHU(Dataset):
- METAINFO = dict(
- classes=('clear sky', 'cloud'),
- palette=((128, 192, 128), (255, 255, 255)),
- img_size=(3, 256, 256), # C, H, W
- ann_size=(256, 256), # C, H, W
- train_size=120,
- test_size=30,
- )
-
- def __init__(self, root, phase, all_transform: albumentations.Compose = None,
- img_transform: albumentations.Compose = None,
- ann_transform: albumentations.Compose = None, seed: int = 42):
- self.root = root
- self.phase = phase
- self.all_transform = all_transform
- self.img_transform = img_transform
- self.ann_transform = ann_transform
- self.seed = seed
- self.data = self.load_data()
-
-
- def load_data(self):
- data_list = []
- split = 'train' if self.phase == 'train' else 'test'
- split_file = os.path.join(self.root, f'{split}.txt')
- with open(split_file, 'r') as f:
- for line in f:
- image_file = line.strip()
- img_path = os.path.join(self.root, 'img_dir', split, image_file)
- ann_path = os.path.join(self.root, 'ann_dir', split, image_file)
- lac_type = image_file.split('_')[0]
- data_list.append((img_path, ann_path, lac_type))
- return data_list
-
- def __len__(self):
- return len(self.data)
-
- def __getitem__(self, idx):
- img_path, ann_path, lac_type = self.data[idx]
- img = Image.open(img_path)
- ann = Image.open(ann_path)
-
- img = np.array(img)
- ann = np.array(ann)
-
- if self.all_transform:
- albumention = self.all_transform(image=img, mask=ann)
- img = albumention['image']
- ann = albumention['mask']
-
- if self.img_transform:
- img = self.img_transform(image=img)['image']
-
- if self.ann_transform:
- ann = self.ann_transform(image=img)['image']
-
- # if self.img_transform is not None:
- # img = self.img_transform(img)
- # if self.ann_transform is not None:
- # ann = self.ann_transform(ann)
- # if self.all_transform is not None:
- # # 对img和ann实现相同的随机变换操作
- # # seed_everything(self.seed, workers=True)
- # # random.seed(self.seed)
- # # img= self.all_transform(img)
- # # seed_everything(self.seed, workers=True)
- # # random.seed(self.seed)
- # # ann= self.all_transform(ann)
- # merge = torch.cat((img, ann), dim=0)
- # merge = self.all_transform(merge)
- # img = merge[:-1]
- # ann = merge[-1]
-
- return {
- 'img': img,
- 'ann': np.int64(ann),
- 'img_path': img_path,
- 'ann_path': ann_path,
- 'lac_type': lac_type,
- }
-
-
-if __name__ == '__main__':
- import torchvision.transforms as transforms
- import torch
-
- # all_transform = transforms.Compose([
- # transforms.RandomCrop((256, 256)),
- # ])
- all_transform = transforms.RandomCrop((256, 256))
-
- # img_transform = transforms.Compose([
- # transforms.ToTensor(),
- # ])
-
- img_transform = transforms.ToTensor()
-
- # ann_transform = transforms.Compose([
- # transforms.PILToTensor(),
- # ])
- ann_transform = transforms.PILToTensor()
-
- train_dataset = HRCWHU(root='data/hrcwhu', phase='train', all_transform=all_transform, img_transform=img_transform,
- ann_transform=ann_transform)
- test_dataset = HRCWHU(root='data/hrcwhu', phase='test', all_transform=all_transform, img_transform=img_transform,
- ann_transform=ann_transform)
-
- assert len(train_dataset) == train_dataset.METAINFO['train_size']
- assert len(test_dataset) == test_dataset.METAINFO['test_size']
-
- train_sample = train_dataset[0]
- test_sample = test_dataset[0]
-
- assert train_sample['img'].shape == test_sample['img'].shape == train_dataset.METAINFO['img_size']
- assert train_sample['ann'].shape == test_sample['ann'].shape == train_dataset.METAINFO['ann_size']
-
- import matplotlib.pyplot as plt
-
- fig, axs = plt.subplots(1, 2, figsize=(10, 5))
- for train_sample in train_dataset:
- axs[0].imshow(train_sample['img'].permute(1, 2, 0))
- axs[0].set_title('Image')
- axs[1].imshow(torch.tensor(train_dataset.METAINFO['palette'])[train_sample['ann']])
- axs[1].set_title('Annotation')
- plt.suptitle(f'Land Cover Type: {train_sample["lac_type"].capitalize()}', y=0.8)
- plt.tight_layout()
- plt.savefig('HRCWHU_sample.png', bbox_inches="tight")
- # break
+import os
+
+import albumentations
+import numpy as np
+from PIL import Image
+from torch.utils.data import Dataset
+
+
+class HRCWHU(Dataset):
+ METAINFO = dict(
+ classes=('clear sky', 'cloud'),
+ palette=((128, 192, 128), (255, 255, 255)),
+ img_size=(3, 256, 256), # C, H, W
+ ann_size=(256, 256), # C, H, W
+ train_size=120,
+ test_size=30,
+ )
+
+ def __init__(self, root, phase, all_transform: albumentations.Compose = None,
+ img_transform: albumentations.Compose = None,
+ ann_transform: albumentations.Compose = None, seed: int = 42):
+ self.root = root
+ self.phase = phase
+ self.all_transform = all_transform
+ self.img_transform = img_transform
+ self.ann_transform = ann_transform
+ self.seed = seed
+ self.data = self.load_data()
+
+
+ def load_data(self):
+ data_list = []
+ split = 'train' if self.phase == 'train' else 'test'
+ split_file = os.path.join(self.root, f'{split}.txt')
+ with open(split_file, 'r') as f:
+ for line in f:
+ image_file = line.strip()
+ img_path = os.path.join(self.root, 'img_dir', split, image_file)
+ ann_path = os.path.join(self.root, 'ann_dir', split, image_file)
+ lac_type = image_file.split('_')[0]
+ data_list.append((img_path, ann_path, lac_type))
+ return data_list
+
+ def __len__(self):
+ return len(self.data)
+
+ def __getitem__(self, idx):
+ img_path, ann_path, lac_type = self.data[idx]
+ img = Image.open(img_path)
+ ann = Image.open(ann_path)
+
+ img = np.array(img)
+ ann = np.array(ann)
+
+ if self.all_transform:
+ albumention = self.all_transform(image=img, mask=ann)
+ img = albumention['image']
+ ann = albumention['mask']
+
+ if self.img_transform:
+ img = self.img_transform(image=img)['image']
+
+ if self.ann_transform:
+ ann = self.ann_transform(image=img)['image']
+
+ # if self.img_transform is not None:
+ # img = self.img_transform(img)
+ # if self.ann_transform is not None:
+ # ann = self.ann_transform(ann)
+ # if self.all_transform is not None:
+ # # 对img和ann实现相同的随机变换操作
+ # # seed_everything(self.seed, workers=True)
+ # # random.seed(self.seed)
+ # # img= self.all_transform(img)
+ # # seed_everything(self.seed, workers=True)
+ # # random.seed(self.seed)
+ # # ann= self.all_transform(ann)
+ # merge = torch.cat((img, ann), dim=0)
+ # merge = self.all_transform(merge)
+ # img = merge[:-1]
+ # ann = merge[-1]
+
+ return {
+ 'img': img,
+ 'ann': np.int64(ann),
+ 'img_path': img_path,
+ 'ann_path': ann_path,
+ 'lac_type': lac_type,
+ }
+
+
+if __name__ == '__main__':
+ import torchvision.transforms as transforms
+ import torch
+
+ # all_transform = transforms.Compose([
+ # transforms.RandomCrop((256, 256)),
+ # ])
+ all_transform = transforms.RandomCrop((256, 256))
+
+ # img_transform = transforms.Compose([
+ # transforms.ToTensor(),
+ # ])
+
+ img_transform = transforms.ToTensor()
+
+ # ann_transform = transforms.Compose([
+ # transforms.PILToTensor(),
+ # ])
+ ann_transform = transforms.PILToTensor()
+
+ train_dataset = HRCWHU(root='data/hrcwhu', phase='train', all_transform=all_transform, img_transform=img_transform,
+ ann_transform=ann_transform)
+ test_dataset = HRCWHU(root='data/hrcwhu', phase='test', all_transform=all_transform, img_transform=img_transform,
+ ann_transform=ann_transform)
+
+ assert len(train_dataset) == train_dataset.METAINFO['train_size']
+ assert len(test_dataset) == test_dataset.METAINFO['test_size']
+
+ train_sample = train_dataset[0]
+ test_sample = test_dataset[0]
+
+ assert train_sample['img'].shape == test_sample['img'].shape == train_dataset.METAINFO['img_size']
+ assert train_sample['ann'].shape == test_sample['ann'].shape == train_dataset.METAINFO['ann_size']
+
+ import matplotlib.pyplot as plt
+
+ fig, axs = plt.subplots(1, 2, figsize=(10, 5))
+ for train_sample in train_dataset:
+ axs[0].imshow(train_sample['img'].permute(1, 2, 0))
+ axs[0].set_title('Image')
+ axs[1].imshow(torch.tensor(train_dataset.METAINFO['palette'])[train_sample['ann']])
+ axs[1].set_title('Annotation')
+ plt.suptitle(f'Land Cover Type: {train_sample["lac_type"].capitalize()}', y=0.8)
+ plt.tight_layout()
+ plt.savefig('HRCWHU_sample.png', bbox_inches="tight")
+ # break
diff --git a/src/data/components/mnist.py b/src/data/components/mnist.py
index f6a1ad6ceb4ea1a5a73060bab69ba2a2752cac4a..2742cda984ca5bed389af0f842c04aa32fc6316c 100644
--- a/src/data/components/mnist.py
+++ b/src/data/components/mnist.py
@@ -1,51 +1,51 @@
-import h5py
-import matplotlib.pyplot as plt
-import numpy as np
-from torch.utils.data import Dataset
-from torchvision.transforms import ToTensor
-
-
-class MNIST(Dataset):
- def __init__(self, h5_file, transform=ToTensor()):
- self.h5_file = h5_file
- self.transform = transform
- # 读取HDF5文件
- with h5py.File(self.h5_file, 'r') as file:
- self.data = []
- self.labels = []
- for i in range(10):
- images = file[str(i)][()]
- for img in images:
- self.data.append(img)
- self.labels.append(i)
- self.data = np.array(self.data)
- self.labels = np.array(self.labels)
-
- def __len__(self):
- return len(self.data)
-
- def __getitem__(self, idx):
- image = self.data[idx]
- label = self.labels[idx]
-
- if self.transform:
- image = self.transform(image)
-
- return image, label
-
-
-if __name__ == '__main__':
- mnist_h5_dataset = MNIST('data/mnist.h5')
-
- assert len(mnist_h5_dataset) == 70000
-
- # Display the first 10 images of each digit, along with their labels, in a 10x10 grid
- fig, axs = plt.subplots(10, 10, figsize=(10, 10))
- for i in range(10):
- images = mnist_h5_dataset.data[mnist_h5_dataset.labels == i]
- for j in range(10):
- axs[i, j].imshow(images[j], cmap='gray')
- axs[i, j].axis('off')
- axs[i, j].set_title(i)
- plt.tight_layout()
- plt.savefig("mnist_h5_dataset.png")
+import h5py
+import matplotlib.pyplot as plt
+import numpy as np
+from torch.utils.data import Dataset
+from torchvision.transforms import ToTensor
+
+
+class MNIST(Dataset):
+ def __init__(self, h5_file, transform=ToTensor()):
+ self.h5_file = h5_file
+ self.transform = transform
+ # 读取HDF5文件
+ with h5py.File(self.h5_file, 'r') as file:
+ self.data = []
+ self.labels = []
+ for i in range(10):
+ images = file[str(i)][()]
+ for img in images:
+ self.data.append(img)
+ self.labels.append(i)
+ self.data = np.array(self.data)
+ self.labels = np.array(self.labels)
+
+ def __len__(self):
+ return len(self.data)
+
+ def __getitem__(self, idx):
+ image = self.data[idx]
+ label = self.labels[idx]
+
+ if self.transform:
+ image = self.transform(image)
+
+ return image, label
+
+
+if __name__ == '__main__':
+ mnist_h5_dataset = MNIST('data/mnist.h5')
+
+ assert len(mnist_h5_dataset) == 70000
+
+ # Display the first 10 images of each digit, along with their labels, in a 10x10 grid
+ fig, axs = plt.subplots(10, 10, figsize=(10, 10))
+ for i in range(10):
+ images = mnist_h5_dataset.data[mnist_h5_dataset.labels == i]
+ for j in range(10):
+ axs[i, j].imshow(images[j], cmap='gray')
+ axs[i, j].axis('off')
+ axs[i, j].set_title(i)
+ plt.tight_layout()
+ plt.savefig("mnist_h5_dataset.png")
diff --git a/src/data/hrcwhu_datamodule.py b/src/data/hrcwhu_datamodule.py
index 9626f557e55cb719d8fbc4c3733b9edffa8bcf1f..d4d24d7a05409b3e94243f4a693e6d3bd579b264 100644
--- a/src/data/hrcwhu_datamodule.py
+++ b/src/data/hrcwhu_datamodule.py
@@ -1,164 +1,164 @@
-from typing import Any, Dict, Optional
-
-from lightning import LightningDataModule
-from torch.utils.data import DataLoader, Dataset
-
-from src.data.components.hrcwhu import HRCWHU
-
-
-class HRCWHUDataModule(LightningDataModule):
- def __init__(
- self,
- root: str,
- train_pipeline: None,
- val_pipeline: None,
- test_pipeline: None,
- seed: int=42,
- batch_size: int = 1,
- num_workers: int = 0,
- pin_memory: bool = False,
- persistent_workers: bool = False,
- ) -> None:
- super().__init__()
-
- # this line allows to access init params with 'self.hparams' attribute
- # also ensures init params will be stored in ckpt
- self.save_hyperparameters(logger=False)
-
- self.train_dataset: Optional[Dataset] = None
- self.val_dataset: Optional[Dataset] = None
- self.test_dataset: Optional[Dataset] = None
-
- self.batch_size_per_device = batch_size
-
- @property
- def num_classes(self) -> int:
- return len(HRCWHU.METAINFO["classes"])
-
- def prepare_data(self) -> None:
- """Download data if needed. Lightning ensures that `self.prepare_data()` is called only
- within a single process on CPU, so you can safely add your downloading logic within. In
- case of multi-node training, the execution of this hook depends upon
- `self.prepare_data_per_node()`.
-
- Do not use it to assign state (self.x = y).
- """
- # train
- HRCWHU(
- root=self.hparams.root,
- phase="train",
- **self.hparams.train_pipeline,
- seed=self.hparams.seed,
- )
-
- # val or test
- HRCWHU(
- root=self.hparams.root,
- phase="test",
- **self.hparams.test_pipeline,
- seed=self.hparams.seed,
- )
-
- def setup(self, stage: Optional[str] = None) -> None:
- """Load data. Set variables: `self.data_train`, `self.data_val`, `self.data_test`.
-
- This method is called by Lightning before `trainer.fit()`, `trainer.validate()`, `trainer.test()`, and
- `trainer.predict()`, so be careful not to execute things like random split twice! Also, it is called after
- `self.prepare_data()` and there is a barrier in between which ensures that all the processes proceed to
- `self.setup()` once the data is prepared and available for use.
-
- :param stage: The stage to setup. Either `"fit"`, `"validate"`, `"test"`, or `"predict"`. Defaults to ``None``.
- """
- # Divide batch size by the number of devices.
- if self.trainer is not None:
- if self.hparams.batch_size % self.trainer.world_size != 0:
- raise RuntimeError(
- f"Batch size ({self.hparams.batch_size}) is not divisible by the number of devices ({self.trainer.world_size})."
- )
- self.batch_size_per_device = self.hparams.batch_size // self.trainer.world_size
-
- # load and split datasets only if not loaded already
- if not self.train_dataset and not self.val_dataset and not self.test_dataset:
- self.train_dataset = HRCWHU(
- root=self.hparams.root,
- phase="train",
- **self.hparams.train_pipeline,
- seed=self.hparams.seed,
- )
-
- self.val_dataset = self.test_dataset = HRCWHU(
- root=self.hparams.root,
- phase="test",
- **self.hparams.test_pipeline,
- seed=self.hparams.seed,
- )
-
- def train_dataloader(self) -> DataLoader[Any]:
- """Create and return the train dataloader.
-
- :return: The train dataloader.
- """
- return DataLoader(
- dataset=self.train_dataset,
- batch_size=self.batch_size_per_device,
- num_workers=self.hparams.num_workers,
- pin_memory=self.hparams.pin_memory,
- persistent_workers=self.hparams.persistent_workers,
- shuffle=True,
- )
-
- def val_dataloader(self) -> DataLoader[Any]:
- """Create and return the validation dataloader.
-
- :return: The validation dataloader.
- """
- return DataLoader(
- dataset=self.val_dataset,
- batch_size=self.batch_size_per_device,
- num_workers=self.hparams.num_workers,
- pin_memory=self.hparams.pin_memory,
- persistent_workers=self.hparams.persistent_workers,
- shuffle=False,
- )
-
- def test_dataloader(self) -> DataLoader[Any]:
- """Create and return the test dataloader.
-
- :return: The test dataloader.
- """
- return DataLoader(
- dataset=self.test_dataset,
- batch_size=self.batch_size_per_device,
- num_workers=self.hparams.num_workers,
- pin_memory=self.hparams.pin_memory,
- persistent_workers=self.hparams.persistent_workers,
- shuffle=False,
- )
-
- def teardown(self, stage: Optional[str] = None) -> None:
- """Lightning hook for cleaning up after `trainer.fit()`, `trainer.validate()`,
- `trainer.test()`, and `trainer.predict()`.
-
- :param stage: The stage being torn down. Either `"fit"`, `"validate"`, `"test"`, or `"predict"`.
- Defaults to ``None``.
- """
- pass
-
- def state_dict(self) -> Dict[Any, Any]:
- """Called when saving a checkpoint. Implement to generate and save the datamodule state.
-
- :return: A dictionary containing the datamodule state that you want to save.
- """
- return {}
-
- def load_state_dict(self, state_dict: Dict[str, Any]) -> None:
- """Called when loading a checkpoint. Implement to reload datamodule state given datamodule
- `state_dict()`.
-
- :param state_dict: The datamodule state returned by `self.state_dict()`.
- """
- pass
-
-
-if __name__ == "__main__":
- _ = HRCWHUDataModule()
+from typing import Any, Dict, Optional
+
+from lightning import LightningDataModule
+from torch.utils.data import DataLoader, Dataset
+
+from src.data.components.hrcwhu import HRCWHU
+
+
+class HRCWHUDataModule(LightningDataModule):
+ def __init__(
+ self,
+ root: str,
+ train_pipeline: None,
+ val_pipeline: None,
+ test_pipeline: None,
+ seed: int=42,
+ batch_size: int = 1,
+ num_workers: int = 0,
+ pin_memory: bool = False,
+ persistent_workers: bool = False,
+ ) -> None:
+ super().__init__()
+
+ # this line allows to access init params with 'self.hparams' attribute
+ # also ensures init params will be stored in ckpt
+ self.save_hyperparameters(logger=False)
+
+ self.train_dataset: Optional[Dataset] = None
+ self.val_dataset: Optional[Dataset] = None
+ self.test_dataset: Optional[Dataset] = None
+
+ self.batch_size_per_device = batch_size
+
+ @property
+ def num_classes(self) -> int:
+ return len(HRCWHU.METAINFO["classes"])
+
+ def prepare_data(self) -> None:
+ """Download data if needed. Lightning ensures that `self.prepare_data()` is called only
+ within a single process on CPU, so you can safely add your downloading logic within. In
+ case of multi-node training, the execution of this hook depends upon
+ `self.prepare_data_per_node()`.
+
+ Do not use it to assign state (self.x = y).
+ """
+ # train
+ HRCWHU(
+ root=self.hparams.root,
+ phase="train",
+ **self.hparams.train_pipeline,
+ seed=self.hparams.seed,
+ )
+
+ # val or test
+ HRCWHU(
+ root=self.hparams.root,
+ phase="test",
+ **self.hparams.test_pipeline,
+ seed=self.hparams.seed,
+ )
+
+ def setup(self, stage: Optional[str] = None) -> None:
+ """Load data. Set variables: `self.data_train`, `self.data_val`, `self.data_test`.
+
+ This method is called by Lightning before `trainer.fit()`, `trainer.validate()`, `trainer.test()`, and
+ `trainer.predict()`, so be careful not to execute things like random split twice! Also, it is called after
+ `self.prepare_data()` and there is a barrier in between which ensures that all the processes proceed to
+ `self.setup()` once the data is prepared and available for use.
+
+ :param stage: The stage to setup. Either `"fit"`, `"validate"`, `"test"`, or `"predict"`. Defaults to ``None``.
+ """
+ # Divide batch size by the number of devices.
+ if self.trainer is not None:
+ if self.hparams.batch_size % self.trainer.world_size != 0:
+ raise RuntimeError(
+ f"Batch size ({self.hparams.batch_size}) is not divisible by the number of devices ({self.trainer.world_size})."
+ )
+ self.batch_size_per_device = self.hparams.batch_size // self.trainer.world_size
+
+ # load and split datasets only if not loaded already
+ if not self.train_dataset and not self.val_dataset and not self.test_dataset:
+ self.train_dataset = HRCWHU(
+ root=self.hparams.root,
+ phase="train",
+ **self.hparams.train_pipeline,
+ seed=self.hparams.seed,
+ )
+
+ self.val_dataset = self.test_dataset = HRCWHU(
+ root=self.hparams.root,
+ phase="test",
+ **self.hparams.test_pipeline,
+ seed=self.hparams.seed,
+ )
+
+ def train_dataloader(self) -> DataLoader[Any]:
+ """Create and return the train dataloader.
+
+ :return: The train dataloader.
+ """
+ return DataLoader(
+ dataset=self.train_dataset,
+ batch_size=self.batch_size_per_device,
+ num_workers=self.hparams.num_workers,
+ pin_memory=self.hparams.pin_memory,
+ persistent_workers=self.hparams.persistent_workers,
+ shuffle=True,
+ )
+
+ def val_dataloader(self) -> DataLoader[Any]:
+ """Create and return the validation dataloader.
+
+ :return: The validation dataloader.
+ """
+ return DataLoader(
+ dataset=self.val_dataset,
+ batch_size=self.batch_size_per_device,
+ num_workers=self.hparams.num_workers,
+ pin_memory=self.hparams.pin_memory,
+ persistent_workers=self.hparams.persistent_workers,
+ shuffle=False,
+ )
+
+ def test_dataloader(self) -> DataLoader[Any]:
+ """Create and return the test dataloader.
+
+ :return: The test dataloader.
+ """
+ return DataLoader(
+ dataset=self.test_dataset,
+ batch_size=self.batch_size_per_device,
+ num_workers=self.hparams.num_workers,
+ pin_memory=self.hparams.pin_memory,
+ persistent_workers=self.hparams.persistent_workers,
+ shuffle=False,
+ )
+
+ def teardown(self, stage: Optional[str] = None) -> None:
+ """Lightning hook for cleaning up after `trainer.fit()`, `trainer.validate()`,
+ `trainer.test()`, and `trainer.predict()`.
+
+ :param stage: The stage being torn down. Either `"fit"`, `"validate"`, `"test"`, or `"predict"`.
+ Defaults to ``None``.
+ """
+ pass
+
+ def state_dict(self) -> Dict[Any, Any]:
+ """Called when saving a checkpoint. Implement to generate and save the datamodule state.
+
+ :return: A dictionary containing the datamodule state that you want to save.
+ """
+ return {}
+
+ def load_state_dict(self, state_dict: Dict[str, Any]) -> None:
+ """Called when loading a checkpoint. Implement to reload datamodule state given datamodule
+ `state_dict()`.
+
+ :param state_dict: The datamodule state returned by `self.state_dict()`.
+ """
+ pass
+
+
+if __name__ == "__main__":
+ _ = HRCWHUDataModule()
diff --git a/src/data/mnist_datamodule.py b/src/data/mnist_datamodule.py
index 312dc0178322ed8908f9420396aae7e5e8333a2c..087de63d01c4aa2fdaab3eca9feb428befa2955b 100644
--- a/src/data/mnist_datamodule.py
+++ b/src/data/mnist_datamodule.py
@@ -1,210 +1,210 @@
-from typing import Any, Dict, Optional, Tuple
-
-import torch
-from lightning import LightningDataModule
-from torch.utils.data import DataLoader, Dataset, random_split
-from torchvision.transforms import transforms
-
-from src.data.components.mnist import MNIST
-
-
-class MNISTDataModule(LightningDataModule):
- """`LightningDataModule` for the MNIST dataset.
-
- The MNIST database of handwritten digits has a training set of 60,000 examples, and a test set of 10,000 examples.
- It is a subset of a larger set available from NIST. The digits have been size-normalized and centered in a
- fixed-size image. The original black and white images from NIST were size normalized to fit in a 20x20 pixel box
- while preserving their aspect ratio. The resulting images contain grey levels as a result of the anti-aliasing
- technique used by the normalization algorithm. the images were centered in a 28x28 image by computing the center of
- mass of the pixels, and translating the image so as to position this point at the center of the 28x28 field.
-
- A `LightningDataModule` implements 7 key methods:
-
- ```python
- def prepare_data(self):
- # Things to do on 1 GPU/TPU (not on every GPU/TPU in DDP).
- # Download data, pre-process, split, save to disk, etc...
-
- def setup(self, stage):
- # Things to do on every process in DDP.
- # Load data, set variables, etc...
-
- def train_dataloader(self):
- # return train dataloader
-
- def val_dataloader(self):
- # return validation dataloader
-
- def test_dataloader(self):
- # return test dataloader
-
- def predict_dataloader(self):
- # return predict dataloader
-
- def teardown(self, stage):
- # Called on every process in DDP.
- # Clean up after fit or test.
- ```
-
- This allows you to share a full dataset without explaining how to download,
- split, transform and process the data.
-
- Read the docs:
- https://lightning.ai/docs/pytorch/latest/data/datamodule.html
- """
-
- def __init__(
- self,
- data_dir: str = "data/",
- train_val_test_split: Tuple[int, int, int] = (55_000, 5_000, 10_000),
- batch_size: int = 64,
- num_workers: int = 0,
- pin_memory: bool = False,
- persistent_workers: bool = False,
- ) -> None:
- """Initialize a `MNISTDataModule`.
-
- :param data_dir: The data directory. Defaults to `"data/"`.
- :param train_val_test_split: The train, validation and test split. Defaults to `(55_000, 5_000, 10_000)`.
- :param batch_size: The batch size. Defaults to `64`.
- :param num_workers: The number of workers. Defaults to `0`.
- :param pin_memory: Whether to pin memory. Defaults to `False`.
- :param persistent_workers: Whether to keep workers alive between data loading. Defaults to `False`.
- """
- super().__init__()
-
- # this line allows to access init params with 'self.hparams' attribute
- # also ensures init params will be stored in ckpt
- self.save_hyperparameters(logger=False)
-
- # data transformations
- self.transforms = transforms.Compose(
- [transforms.ToTensor(), transforms.Normalize((0.1307,), (0.3081,))]
- )
-
- self.data_train: Optional[Dataset] = None
- self.data_val: Optional[Dataset] = None
- self.data_test: Optional[Dataset] = None
-
- self.batch_size_per_device = batch_size
-
- @property
- def num_classes(self) -> int:
- """Get the number of classes.
-
- :return: The number of MNIST classes (10).
- """
- return 10
-
- def prepare_data(self) -> None:
- """Download data if needed. Lightning ensures that `self.prepare_data()` is called only
- within a single process on CPU, so you can safely add your downloading logic within. In
- case of multi-node training, the execution of this hook depends upon
- `self.prepare_data_per_node()`.
-
- Do not use it to assign state (self.x = y).
- """
- MNIST(
- h5_file=f"{self.hparams.data_dir}/mnist.h5",
- transform=self.transforms,
- )
-
- def setup(self, stage: Optional[str] = None) -> None:
- """Load data. Set variables: `self.data_train`, `self.data_val`, `self.data_test`.
-
- This method is called by Lightning before `trainer.fit()`, `trainer.validate()`, `trainer.test()`, and
- `trainer.predict()`, so be careful not to execute things like random split twice! Also, it is called after
- `self.prepare_data()` and there is a barrier in between which ensures that all the processes proceed to
- `self.setup()` once the data is prepared and available for use.
-
- :param stage: The stage to setup. Either `"fit"`, `"validate"`, `"test"`, or `"predict"`. Defaults to ``None``.
- """
- # Divide batch size by the number of devices.
- if self.trainer is not None:
- if self.hparams.batch_size % self.trainer.world_size != 0:
- raise RuntimeError(
- f"Batch size ({self.hparams.batch_size}) is not divisible by the number of devices ({self.trainer.world_size})."
- )
- self.batch_size_per_device = self.hparams.batch_size // self.trainer.world_size
-
- # load and split datasets only if not loaded already
- if not self.data_train and not self.data_val and not self.data_test:
- dataset = MNIST(
- h5_file=f"{self.hparams.data_dir}/mnist.h5",
- transform=self.transforms,
- )
- self.data_train, self.data_val, self.data_test = random_split(
- dataset=dataset,
- lengths=self.hparams.train_val_test_split,
- generator=torch.Generator().manual_seed(42),
- )
-
- def train_dataloader(self) -> DataLoader[Any]:
- """Create and return the train dataloader.
-
- :return: The train dataloader.
- """
- return DataLoader(
- dataset=self.data_train,
- batch_size=self.batch_size_per_device,
- num_workers=self.hparams.num_workers,
- pin_memory=self.hparams.pin_memory,
- persistent_workers=self.hparams.persistent_workers,
- shuffle=True,
- )
-
- def val_dataloader(self) -> DataLoader[Any]:
- """Create and return the validation dataloader.
-
- :return: The validation dataloader.
- """
- return DataLoader(
- dataset=self.data_val,
- batch_size=self.batch_size_per_device,
- num_workers=self.hparams.num_workers,
- pin_memory=self.hparams.pin_memory,
- persistent_workers=self.hparams.persistent_workers,
- shuffle=False,
- )
-
- def test_dataloader(self) -> DataLoader[Any]:
- """Create and return the test dataloader.
-
- :return: The test dataloader.
- """
- return DataLoader(
- dataset=self.data_test,
- batch_size=self.batch_size_per_device,
- num_workers=self.hparams.num_workers,
- pin_memory=self.hparams.pin_memory,
- persistent_workers=self.hparams.persistent_workers,
- shuffle=False,
- )
-
- def teardown(self, stage: Optional[str] = None) -> None:
- """Lightning hook for cleaning up after `trainer.fit()`, `trainer.validate()`,
- `trainer.test()`, and `trainer.predict()`.
-
- :param stage: The stage being torn down. Either `"fit"`, `"validate"`, `"test"`, or `"predict"`.
- Defaults to ``None``.
- """
- pass
-
- def state_dict(self) -> Dict[Any, Any]:
- """Called when saving a checkpoint. Implement to generate and save the datamodule state.
-
- :return: A dictionary containing the datamodule state that you want to save.
- """
- return {}
-
- def load_state_dict(self, state_dict: Dict[str, Any]) -> None:
- """Called when loading a checkpoint. Implement to reload datamodule state given datamodule
- `state_dict()`.
-
- :param state_dict: The datamodule state returned by `self.state_dict()`.
- """
- pass
-
-
-if __name__ == "__main__":
- _ = MNISTDataModule()
+from typing import Any, Dict, Optional, Tuple
+
+import torch
+from lightning import LightningDataModule
+from torch.utils.data import DataLoader, Dataset, random_split
+from torchvision.transforms import transforms
+
+from src.data.components.mnist import MNIST
+
+
+class MNISTDataModule(LightningDataModule):
+ """`LightningDataModule` for the MNIST dataset.
+
+ The MNIST database of handwritten digits has a training set of 60,000 examples, and a test set of 10,000 examples.
+ It is a subset of a larger set available from NIST. The digits have been size-normalized and centered in a
+ fixed-size image. The original black and white images from NIST were size normalized to fit in a 20x20 pixel box
+ while preserving their aspect ratio. The resulting images contain grey levels as a result of the anti-aliasing
+ technique used by the normalization algorithm. the images were centered in a 28x28 image by computing the center of
+ mass of the pixels, and translating the image so as to position this point at the center of the 28x28 field.
+
+ A `LightningDataModule` implements 7 key methods:
+
+ ```python
+ def prepare_data(self):
+ # Things to do on 1 GPU/TPU (not on every GPU/TPU in DDP).
+ # Download data, pre-process, split, save to disk, etc...
+
+ def setup(self, stage):
+ # Things to do on every process in DDP.
+ # Load data, set variables, etc...
+
+ def train_dataloader(self):
+ # return train dataloader
+
+ def val_dataloader(self):
+ # return validation dataloader
+
+ def test_dataloader(self):
+ # return test dataloader
+
+ def predict_dataloader(self):
+ # return predict dataloader
+
+ def teardown(self, stage):
+ # Called on every process in DDP.
+ # Clean up after fit or test.
+ ```
+
+ This allows you to share a full dataset without explaining how to download,
+ split, transform and process the data.
+
+ Read the docs:
+ https://lightning.ai/docs/pytorch/latest/data/datamodule.html
+ """
+
+ def __init__(
+ self,
+ data_dir: str = "data/",
+ train_val_test_split: Tuple[int, int, int] = (55_000, 5_000, 10_000),
+ batch_size: int = 64,
+ num_workers: int = 0,
+ pin_memory: bool = False,
+ persistent_workers: bool = False,
+ ) -> None:
+ """Initialize a `MNISTDataModule`.
+
+ :param data_dir: The data directory. Defaults to `"data/"`.
+ :param train_val_test_split: The train, validation and test split. Defaults to `(55_000, 5_000, 10_000)`.
+ :param batch_size: The batch size. Defaults to `64`.
+ :param num_workers: The number of workers. Defaults to `0`.
+ :param pin_memory: Whether to pin memory. Defaults to `False`.
+ :param persistent_workers: Whether to keep workers alive between data loading. Defaults to `False`.
+ """
+ super().__init__()
+
+ # this line allows to access init params with 'self.hparams' attribute
+ # also ensures init params will be stored in ckpt
+ self.save_hyperparameters(logger=False)
+
+ # data transformations
+ self.transforms = transforms.Compose(
+ [transforms.ToTensor(), transforms.Normalize((0.1307,), (0.3081,))]
+ )
+
+ self.data_train: Optional[Dataset] = None
+ self.data_val: Optional[Dataset] = None
+ self.data_test: Optional[Dataset] = None
+
+ self.batch_size_per_device = batch_size
+
+ @property
+ def num_classes(self) -> int:
+ """Get the number of classes.
+
+ :return: The number of MNIST classes (10).
+ """
+ return 10
+
+ def prepare_data(self) -> None:
+ """Download data if needed. Lightning ensures that `self.prepare_data()` is called only
+ within a single process on CPU, so you can safely add your downloading logic within. In
+ case of multi-node training, the execution of this hook depends upon
+ `self.prepare_data_per_node()`.
+
+ Do not use it to assign state (self.x = y).
+ """
+ MNIST(
+ h5_file=f"{self.hparams.data_dir}/mnist.h5",
+ transform=self.transforms,
+ )
+
+ def setup(self, stage: Optional[str] = None) -> None:
+ """Load data. Set variables: `self.data_train`, `self.data_val`, `self.data_test`.
+
+ This method is called by Lightning before `trainer.fit()`, `trainer.validate()`, `trainer.test()`, and
+ `trainer.predict()`, so be careful not to execute things like random split twice! Also, it is called after
+ `self.prepare_data()` and there is a barrier in between which ensures that all the processes proceed to
+ `self.setup()` once the data is prepared and available for use.
+
+ :param stage: The stage to setup. Either `"fit"`, `"validate"`, `"test"`, or `"predict"`. Defaults to ``None``.
+ """
+ # Divide batch size by the number of devices.
+ if self.trainer is not None:
+ if self.hparams.batch_size % self.trainer.world_size != 0:
+ raise RuntimeError(
+ f"Batch size ({self.hparams.batch_size}) is not divisible by the number of devices ({self.trainer.world_size})."
+ )
+ self.batch_size_per_device = self.hparams.batch_size // self.trainer.world_size
+
+ # load and split datasets only if not loaded already
+ if not self.data_train and not self.data_val and not self.data_test:
+ dataset = MNIST(
+ h5_file=f"{self.hparams.data_dir}/mnist.h5",
+ transform=self.transforms,
+ )
+ self.data_train, self.data_val, self.data_test = random_split(
+ dataset=dataset,
+ lengths=self.hparams.train_val_test_split,
+ generator=torch.Generator().manual_seed(42),
+ )
+
+ def train_dataloader(self) -> DataLoader[Any]:
+ """Create and return the train dataloader.
+
+ :return: The train dataloader.
+ """
+ return DataLoader(
+ dataset=self.data_train,
+ batch_size=self.batch_size_per_device,
+ num_workers=self.hparams.num_workers,
+ pin_memory=self.hparams.pin_memory,
+ persistent_workers=self.hparams.persistent_workers,
+ shuffle=True,
+ )
+
+ def val_dataloader(self) -> DataLoader[Any]:
+ """Create and return the validation dataloader.
+
+ :return: The validation dataloader.
+ """
+ return DataLoader(
+ dataset=self.data_val,
+ batch_size=self.batch_size_per_device,
+ num_workers=self.hparams.num_workers,
+ pin_memory=self.hparams.pin_memory,
+ persistent_workers=self.hparams.persistent_workers,
+ shuffle=False,
+ )
+
+ def test_dataloader(self) -> DataLoader[Any]:
+ """Create and return the test dataloader.
+
+ :return: The test dataloader.
+ """
+ return DataLoader(
+ dataset=self.data_test,
+ batch_size=self.batch_size_per_device,
+ num_workers=self.hparams.num_workers,
+ pin_memory=self.hparams.pin_memory,
+ persistent_workers=self.hparams.persistent_workers,
+ shuffle=False,
+ )
+
+ def teardown(self, stage: Optional[str] = None) -> None:
+ """Lightning hook for cleaning up after `trainer.fit()`, `trainer.validate()`,
+ `trainer.test()`, and `trainer.predict()`.
+
+ :param stage: The stage being torn down. Either `"fit"`, `"validate"`, `"test"`, or `"predict"`.
+ Defaults to ``None``.
+ """
+ pass
+
+ def state_dict(self) -> Dict[Any, Any]:
+ """Called when saving a checkpoint. Implement to generate and save the datamodule state.
+
+ :return: A dictionary containing the datamodule state that you want to save.
+ """
+ return {}
+
+ def load_state_dict(self, state_dict: Dict[str, Any]) -> None:
+ """Called when loading a checkpoint. Implement to reload datamodule state given datamodule
+ `state_dict()`.
+
+ :param state_dict: The datamodule state returned by `self.state_dict()`.
+ """
+ pass
+
+
+if __name__ == "__main__":
+ _ = MNISTDataModule()
diff --git a/src/eval.py b/src/eval.py
index b70faae8b59c2d508a070cef7fa85ed39be0a3c1..72ce0f447902234474c346e48fc7761df4591479 100644
--- a/src/eval.py
+++ b/src/eval.py
@@ -1,99 +1,99 @@
-from typing import Any, Dict, List, Tuple
-
-import hydra
-import rootutils
-from lightning import LightningDataModule, LightningModule, Trainer
-from lightning.pytorch.loggers import Logger
-from omegaconf import DictConfig
-
-rootutils.setup_root(__file__, indicator=".project-root", pythonpath=True)
-# ------------------------------------------------------------------------------------ #
-# the setup_root above is equivalent to:
-# - adding project root dir to PYTHONPATH
-# (so you don't need to force user to install project as a package)
-# (necessary before importing any local modules e.g. `from src import utils`)
-# - setting up PROJECT_ROOT environment variable
-# (which is used as a base for paths in "configs/paths/default.yaml")
-# (this way all filepaths are the same no matter where you run the code)
-# - loading environment variables from ".env" in root dir
-#
-# you can remove it if you:
-# 1. either install project as a package or move entry files to project root dir
-# 2. set `root_dir` to "." in "configs/paths/default.yaml"
-#
-# more info: https://github.com/ashleve/rootutils
-# ------------------------------------------------------------------------------------ #
-
-from src.utils import (
- RankedLogger,
- extras,
- instantiate_loggers,
- log_hyperparameters,
- task_wrapper,
-)
-
-log = RankedLogger(__name__, rank_zero_only=True)
-
-
-@task_wrapper
-def evaluate(cfg: DictConfig) -> Tuple[Dict[str, Any], Dict[str, Any]]:
- """Evaluates given checkpoint on a datamodule testset.
-
- This method is wrapped in optional @task_wrapper decorator, that controls the behavior during
- failure. Useful for multiruns, saving info about the crash, etc.
-
- :param cfg: DictConfig configuration composed by Hydra.
- :return: Tuple[dict, dict] with metrics and dict with all instantiated objects.
- """
- assert cfg.ckpt_path
-
- log.info(f"Instantiating datamodule <{cfg.data._target_}>")
- datamodule: LightningDataModule = hydra.utils.instantiate(cfg.data)
-
- log.info(f"Instantiating model <{cfg.model._target_}>")
- model: LightningModule = hydra.utils.instantiate(cfg.model)
-
- log.info("Instantiating loggers...")
- logger: List[Logger] = instantiate_loggers(cfg.get("logger"))
-
- log.info(f"Instantiating trainer <{cfg.trainer._target_}>")
- trainer: Trainer = hydra.utils.instantiate(cfg.trainer, logger=logger)
-
- object_dict = {
- "cfg": cfg,
- "datamodule": datamodule,
- "model": model,
- "logger": logger,
- "trainer": trainer,
- }
-
- if logger:
- log.info("Logging hyperparameters!")
- log_hyperparameters(object_dict)
-
- log.info("Starting testing!")
- trainer.test(model=model, datamodule=datamodule, ckpt_path=cfg.ckpt_path)
-
- # for predictions use trainer.predict(...)
- # predictions = trainer.predict(model=model, dataloaders=dataloaders, ckpt_path=cfg.ckpt_path)
-
- metric_dict = trainer.callback_metrics
-
- return metric_dict, object_dict
-
-
-@hydra.main(version_base="1.3", config_path="../configs", config_name="eval.yaml")
-def main(cfg: DictConfig) -> None:
- """Main entry point for evaluation.
-
- :param cfg: DictConfig configuration composed by Hydra.
- """
- # apply extra utilities
- # (e.g. ask for tags if none are provided in cfg, print cfg tree, etc.)
- extras(cfg)
-
- evaluate(cfg)
-
-
-if __name__ == "__main__":
- main()
+from typing import Any, Dict, List, Tuple
+
+import hydra
+import rootutils
+from lightning import LightningDataModule, LightningModule, Trainer
+from lightning.pytorch.loggers import Logger
+from omegaconf import DictConfig
+
+rootutils.setup_root(__file__, indicator=".project-root", pythonpath=True)
+# ------------------------------------------------------------------------------------ #
+# the setup_root above is equivalent to:
+# - adding project root dir to PYTHONPATH
+# (so you don't need to force user to install project as a package)
+# (necessary before importing any local modules e.g. `from src import utils`)
+# - setting up PROJECT_ROOT environment variable
+# (which is used as a base for paths in "configs/paths/default.yaml")
+# (this way all filepaths are the same no matter where you run the code)
+# - loading environment variables from ".env" in root dir
+#
+# you can remove it if you:
+# 1. either install project as a package or move entry files to project root dir
+# 2. set `root_dir` to "." in "configs/paths/default.yaml"
+#
+# more info: https://github.com/ashleve/rootutils
+# ------------------------------------------------------------------------------------ #
+
+from src.utils import (
+ RankedLogger,
+ extras,
+ instantiate_loggers,
+ log_hyperparameters,
+ task_wrapper,
+)
+
+log = RankedLogger(__name__, rank_zero_only=True)
+
+
+@task_wrapper
+def evaluate(cfg: DictConfig) -> Tuple[Dict[str, Any], Dict[str, Any]]:
+ """Evaluates given checkpoint on a datamodule testset.
+
+ This method is wrapped in optional @task_wrapper decorator, that controls the behavior during
+ failure. Useful for multiruns, saving info about the crash, etc.
+
+ :param cfg: DictConfig configuration composed by Hydra.
+ :return: Tuple[dict, dict] with metrics and dict with all instantiated objects.
+ """
+ assert cfg.ckpt_path
+
+ log.info(f"Instantiating datamodule <{cfg.data._target_}>")
+ datamodule: LightningDataModule = hydra.utils.instantiate(cfg.data)
+
+ log.info(f"Instantiating model <{cfg.model._target_}>")
+ model: LightningModule = hydra.utils.instantiate(cfg.model)
+
+ log.info("Instantiating loggers...")
+ logger: List[Logger] = instantiate_loggers(cfg.get("logger"))
+
+ log.info(f"Instantiating trainer <{cfg.trainer._target_}>")
+ trainer: Trainer = hydra.utils.instantiate(cfg.trainer, logger=logger)
+
+ object_dict = {
+ "cfg": cfg,
+ "datamodule": datamodule,
+ "model": model,
+ "logger": logger,
+ "trainer": trainer,
+ }
+
+ if logger:
+ log.info("Logging hyperparameters!")
+ log_hyperparameters(object_dict)
+
+ log.info("Starting testing!")
+ trainer.test(model=model, datamodule=datamodule, ckpt_path=cfg.ckpt_path)
+
+ # for predictions use trainer.predict(...)
+ # predictions = trainer.predict(model=model, dataloaders=dataloaders, ckpt_path=cfg.ckpt_path)
+
+ metric_dict = trainer.callback_metrics
+
+ return metric_dict, object_dict
+
+
+@hydra.main(version_base="1.3", config_path="../configs", config_name="eval.yaml")
+def main(cfg: DictConfig) -> None:
+ """Main entry point for evaluation.
+
+ :param cfg: DictConfig configuration composed by Hydra.
+ """
+ # apply extra utilities
+ # (e.g. ask for tags if none are provided in cfg, print cfg tree, etc.)
+ extras(cfg)
+
+ evaluate(cfg)
+
+
+if __name__ == "__main__":
+ main()
diff --git a/src/loss/__init__.py b/src/loss/__init__.py
index 0f1931cf7e39e6633c3415f02d0ba91f397d92cc..01ea204192e948adf5cf435c66b4cbfc9ab13488 100644
--- a/src/loss/__init__.py
+++ b/src/loss/__init__.py
@@ -1,6 +1,6 @@
-# -*- coding: utf-8 -*-
-# @Time : 2024/8/1 下午2:45
-# @Author : xiaoshun
-# @Email : 3038523973@qq.com
-# @File : __init__.py.py
-# @Software: PyCharm
+# -*- coding: utf-8 -*-
+# @Time : 2024/8/1 下午2:45
+# @Author : xiaoshun
+# @Email : 3038523973@qq.com
+# @File : __init__.py.py
+# @Software: PyCharm
diff --git a/src/loss/cdnetv2_loss.py b/src/loss/cdnetv2_loss.py
index c2fe74978b17c5b767257e485410f884b436c316..9d747fdc6104488045d31e578841e3ee782ce77a 100644
--- a/src/loss/cdnetv2_loss.py
+++ b/src/loss/cdnetv2_loss.py
@@ -1,20 +1,20 @@
-# -*- coding: utf-8 -*-
-# @Time : 2024/8/1 下午2:45
-# @Author : xiaoshun
-# @Email : 3038523973@qq.com
-# @File : cdnetv2_loss.py
-# @Software: PyCharm
-import torch
-import torch.nn as nn
-
-
-class CDnetv2Loss(nn.Module):
- def __init__(self, loss_fn: nn.Module) -> None:
- super().__init__()
- self.loss_fn = loss_fn
-
- def forward(self, logits: torch.Tensor, logits_aux,target: torch.Tensor) -> torch.Tensor:
- loss = self.loss_fn(logits, target)
- loss_aux = self.loss_fn(logits_aux, target)
- total_loss = loss + loss_aux
- return total_loss
+# -*- coding: utf-8 -*-
+# @Time : 2024/8/1 下午2:45
+# @Author : xiaoshun
+# @Email : 3038523973@qq.com
+# @File : cdnetv2_loss.py
+# @Software: PyCharm
+import torch
+import torch.nn as nn
+
+
+class CDnetv2Loss(nn.Module):
+ def __init__(self, loss_fn: nn.Module) -> None:
+ super().__init__()
+ self.loss_fn = loss_fn
+
+ def forward(self, logits: torch.Tensor, logits_aux,target: torch.Tensor) -> torch.Tensor:
+ loss = self.loss_fn(logits, target)
+ loss_aux = self.loss_fn(logits_aux, target)
+ total_loss = loss + loss_aux
+ return total_loss
diff --git a/src/models/base_module.py b/src/models/base_module.py
index 2049662e86ba75db570fa2fca937d0f5da098968..6e2ccb56c77375404bd211035146cf5074f6531e 100644
--- a/src/models/base_module.py
+++ b/src/models/base_module.py
@@ -1,280 +1,280 @@
-from typing import Any, Dict, Tuple
-
-import torch
-from lightning import LightningModule
-from torchmetrics import MaxMetric, MeanMetric
-from torchmetrics.classification import Accuracy, F1Score, Precision, Recall
-from torchmetrics.segmentation import MeanIoU, GeneralizedDiceScore
-
-
-class BaseLitModule(LightningModule):
- """Example of a `LightningModule` for MNIST classification.
-
- A `LightningModule` implements 8 key methods:
-
- ```python
- def __init__(self):
- # Define initialization code here.
-
- def setup(self, stage):
- # Things to setup before each stage, 'fit', 'validate', 'test', 'predict'.
- # This hook is called on every process when using DDP.
-
- def training_step(self, batch, batch_idx):
- # The complete training step.
-
- def validation_step(self, batch, batch_idx):
- # The complete validation step.
-
- def test_step(self, batch, batch_idx):
- # The complete test step.
-
- def predict_step(self, batch, batch_idx):
- # The complete predict step.
-
- def configure_optimizers(self):
- # Define and configure optimizers and LR schedulers.
- ```
-
- Docs:
- https://lightning.ai/docs/pytorch/latest/common/lightning_module.html
- """
-
- def __init__(
- self,
- net: torch.nn.Module,
- num_classes: int,
- criterion: torch.nn.Module,
- optimizer: torch.optim.Optimizer,
- scheduler: torch.optim.lr_scheduler,
- compile: bool = False,
- ) -> None:
- super().__init__()
-
- # this line allows to access init params with 'self.hparams' attribute
- # also ensures init params will be stored in ckpt
- self.save_hyperparameters(logger=False, ignore=['net'])
-
- self.net = net
-
- # metric objects for calculating and averaging accuracy across batches
- task = "binary" if self.hparams.num_classes==2 else "multiclass"
-
- self.train_accuracy = Accuracy(task=task, num_classes=num_classes)
- self.train_precision = Precision(task=task, num_classes=num_classes)
- self.train_recall = Recall(task=task, num_classes=num_classes)
- self.train_f1score = F1Score(task=task, num_classes=num_classes)
- self.train_miou = MeanIoU(num_classes=num_classes)
- self.train_dice = GeneralizedDiceScore(num_classes=num_classes)
-
- self.val_accuracy = Accuracy(task=task, num_classes=num_classes)
- self.val_precision = Precision(task=task, num_classes=num_classes)
- self.val_recall = Recall(task=task, num_classes=num_classes)
- self.val_f1score = F1Score(task=task, num_classes=num_classes)
- self.val_miou = MeanIoU(num_classes=num_classes)
- self.val_dice = GeneralizedDiceScore(num_classes=num_classes)
-
- self.test_accuracy = Accuracy(task=task, num_classes=num_classes)
- self.test_precision = Precision(task=task, num_classes=num_classes)
- self.test_recall = Recall(task=task, num_classes=num_classes)
- self.test_f1score = F1Score(task=task, num_classes=num_classes)
- self.test_miou = MeanIoU(num_classes=num_classes)
- self.test_dice = GeneralizedDiceScore(num_classes=num_classes)
-
- # for averaging loss across batches
- self.train_loss = MeanMetric()
- self.val_loss = MeanMetric()
- self.test_loss = MeanMetric()
-
- # for tracking best so far validation accuracy
- self.val_miou_best = MaxMetric()
-
- def forward(self, x: torch.Tensor) -> torch.Tensor:
- """Perform a forward pass through the model `self.net`.
-
- :param x: A tensor of images.
- :return: A tensor of logits.
- """
- return self.net(x)
-
- def on_train_start(self) -> None:
- """Lightning hook that is called when training begins."""
- # by default lightning executes validation step sanity checks before training starts,
- # so it's worth to make sure validation metrics don't store results from these checks
- self.val_loss.reset()
-
- self.val_accuracy.reset()
- self.val_precision.reset()
- self.val_recall.reset()
- self.val_f1score.reset()
- self.val_miou.reset()
- self.val_dice.reset()
-
- self.val_miou_best.reset()
-
- def model_step(
- self, batch: Tuple[torch.Tensor, torch.Tensor]
- ) -> Tuple[torch.Tensor, torch.Tensor, torch.Tensor]:
- """Perform a single model step on a batch of data.
-
- :param batch: A batch of data (a tuple) containing the input tensor of images and target labels.
-
- :return: A tuple containing (in order):
- - A tensor of losses.
- - A tensor of predictions.
- - A tensor of target labels.
- """
- x, y = batch["img"], batch["ann"]
- logits = self.forward(x)
- loss = self.hparams.criterion(logits, y)
- preds = torch.argmax(logits, dim=1)
- return loss, preds, y
-
- def training_step(
- self, batch: Tuple[torch.Tensor, torch.Tensor], batch_idx: int
- ) -> torch.Tensor:
- """Perform a single training step on a batch of data from the training set.
-
- :param batch: A batch of data (a tuple) containing the input tensor of images and target
- labels.
- :param batch_idx: The index of the current batch.
- :return: A tensor of losses between model predictions and targets.
- """
- loss, preds, targets = self.model_step(batch)
-
- # print(preds.shape) # (8, 256, 256)
- # print(targets.shape) # (8, 256, 256)
-
- # update and log metrics
- self.train_loss(loss)
-
- self.train_accuracy(preds, targets)
- self.train_precision(preds, targets)
- self.train_recall(preds, targets)
- self.train_f1score(preds, targets)
- self.train_miou(preds, targets)
- self.train_dice(preds, targets)
-
- self.log("train/loss", self.train_loss, on_step=False, on_epoch=True, prog_bar=True)
-
- self.log("train/accuracy", self.train_accuracy, on_step=False, on_epoch=True, prog_bar=True)
- self.log("train/precision", self.train_precision, on_step=False, on_epoch=True, prog_bar=True)
- self.log("train/recall", self.train_recall, on_step=False, on_epoch=True, prog_bar=True)
- self.log("train/f1score", self.train_f1score, on_step=False, on_epoch=True, prog_bar=True)
- self.log("train/miou", self.train_miou, on_step=False, on_epoch=True, prog_bar=True)
- self.log("train/dice", self.train_dice, on_step=False, on_epoch=True, prog_bar=True)
-
- # return loss or backpropagation will fail
- return loss
-
- def on_train_epoch_end(self) -> None:
- "Lightning hook that is called when a training epoch ends."
- pass
-
- def validation_step(self, batch: Tuple[torch.Tensor, torch.Tensor], batch_idx: int) -> None:
- """Perform a single validation step on a batch of data from the validation set.
-
- :param batch: A batch of data (a tuple) containing the input tensor of images and target
- labels.
- :param batch_idx: The index of the current batch.
- """
- loss, preds, targets = self.model_step(batch)
-
- # update and log metrics
- self.val_loss(loss)
-
- self.val_accuracy(preds, targets)
- self.val_precision(preds, targets)
- self.val_recall(preds, targets)
- self.val_f1score(preds, targets)
- self.val_miou(preds, targets)
- self.val_dice(preds, targets)
-
- self.log("val/loss", self.val_loss, on_step=False, on_epoch=True, prog_bar=True)
-
- self.log("val/accuracy", self.val_accuracy, on_step=False, on_epoch=True, prog_bar=True)
- self.log("val/precision", self.val_precision, on_step=False, on_epoch=True, prog_bar=True)
- self.log("val/recall", self.val_recall, on_step=False, on_epoch=True, prog_bar=True)
- self.log("val/f1score", self.val_f1score, on_step=False, on_epoch=True, prog_bar=True)
- self.log("val/miou", self.val_miou, on_step=False, on_epoch=True, prog_bar=True)
- self.log("val/dice", self.val_dice, on_step=False, on_epoch=True, prog_bar=True)
-
- def on_validation_epoch_end(self) -> None:
- "Lightning hook that is called when a validation epoch ends."
- miou = self.val_miou.compute() # get current val acc
- self.val_miou_best(miou) # update best so far val acc
- # log `val_acc_best` as a value through `.compute()` method, instead of as a metric object
- # otherwise metric would be reset by lightning after each epoch
- self.log("val/miou_best", self.val_miou_best.compute(), sync_dist=True, prog_bar=True)
-
- def test_step(self, batch: Tuple[torch.Tensor, torch.Tensor], batch_idx: int) -> None:
- """Perform a single test step on a batch of data from the test set.
-
- :param batch: A batch of data (a tuple) containing the input tensor of images and target
- labels.
- :param batch_idx: The index of the current batch.
- """
- loss, preds, targets = self.model_step(batch)
-
- # update and log metrics
- self.test_loss(loss)
-
- # update and log metrics
- self.test_accuracy(preds, targets)
- self.test_precision(preds, targets)
- self.test_recall(preds, targets)
- self.test_f1score(preds, targets)
- self.test_miou(preds, targets)
- self.test_dice(preds, targets)
-
- self.log("test/loss", self.test_loss, on_step=False, on_epoch=True, prog_bar=True)
-
- self.log("test/accuracy", self.test_accuracy, on_step=False, on_epoch=True, prog_bar=True)
- self.log("test/precision", self.test_precision, on_step=False, on_epoch=True, prog_bar=True)
- self.log("test/recall", self.test_recall, on_step=False, on_epoch=True, prog_bar=True)
- self.log("test/f1score", self.test_f1score, on_step=False, on_epoch=True, prog_bar=True)
- self.log("test/miou", self.test_miou, on_step=False, on_epoch=True, prog_bar=True)
- self.log("test/dice", self.test_dice, on_step=False, on_epoch=True, prog_bar=True)
-
- def on_test_epoch_end(self) -> None:
- """Lightning hook that is called when a test epoch ends."""
- pass
-
- def setup(self, stage: str) -> None:
- """Lightning hook that is called at the beginning of fit (train + validate), validate,
- test, or predict.
-
- This is a good hook when you need to build models dynamically or adjust something about
- them. This hook is called on every process when using DDP.
-
- :param stage: Either `"fit"`, `"validate"`, `"test"`, or `"predict"`.
- """
- if self.hparams.compile and stage == "fit":
- self.net = torch.compile(self.net)
-
- def configure_optimizers(self) -> Dict[str, Any]:
- """Choose what optimizers and learning-rate schedulers to use in your optimization.
- Normally you'd need one. But in the case of GANs or similar you might have multiple.
-
- Examples:
- https://lightning.ai/docs/pytorch/latest/common/lightning_module.html#configure-optimizers
-
- :return: A dict containing the configured optimizers and learning-rate schedulers to be used for training.
- """
- optimizer = self.hparams.optimizer(params=self.trainer.model.parameters())
- if self.hparams.scheduler is not None:
- scheduler = self.hparams.scheduler(optimizer=optimizer)
- return {
- "optimizer": optimizer,
- "lr_scheduler": {
- "scheduler": scheduler,
- "monitor": "val/loss",
- "interval": "epoch",
- "frequency": 1,
- },
- }
- return {"optimizer": optimizer}
-
-
-if __name__ == "__main__":
- _ = BaseLitModule(None, None, None, None)
+from typing import Any, Dict, Tuple
+
+import torch
+from lightning import LightningModule
+from torchmetrics import MaxMetric, MeanMetric
+from torchmetrics.classification import Accuracy, F1Score, Precision, Recall
+from torchmetrics.segmentation import MeanIoU, GeneralizedDiceScore
+
+
+class BaseLitModule(LightningModule):
+ """Example of a `LightningModule` for MNIST classification.
+
+ A `LightningModule` implements 8 key methods:
+
+ ```python
+ def __init__(self):
+ # Define initialization code here.
+
+ def setup(self, stage):
+ # Things to setup before each stage, 'fit', 'validate', 'test', 'predict'.
+ # This hook is called on every process when using DDP.
+
+ def training_step(self, batch, batch_idx):
+ # The complete training step.
+
+ def validation_step(self, batch, batch_idx):
+ # The complete validation step.
+
+ def test_step(self, batch, batch_idx):
+ # The complete test step.
+
+ def predict_step(self, batch, batch_idx):
+ # The complete predict step.
+
+ def configure_optimizers(self):
+ # Define and configure optimizers and LR schedulers.
+ ```
+
+ Docs:
+ https://lightning.ai/docs/pytorch/latest/common/lightning_module.html
+ """
+
+ def __init__(
+ self,
+ net: torch.nn.Module,
+ num_classes: int,
+ criterion: torch.nn.Module,
+ optimizer: torch.optim.Optimizer,
+ scheduler: torch.optim.lr_scheduler,
+ compile: bool = False,
+ ) -> None:
+ super().__init__()
+
+ # this line allows to access init params with 'self.hparams' attribute
+ # also ensures init params will be stored in ckpt
+ self.save_hyperparameters(logger=False, ignore=['net'])
+
+ self.net = net
+
+ # metric objects for calculating and averaging accuracy across batches
+ task = "binary" if self.hparams.num_classes==2 else "multiclass"
+
+ self.train_accuracy = Accuracy(task=task, num_classes=num_classes)
+ self.train_precision = Precision(task=task, num_classes=num_classes)
+ self.train_recall = Recall(task=task, num_classes=num_classes)
+ self.train_f1score = F1Score(task=task, num_classes=num_classes)
+ self.train_miou = MeanIoU(num_classes=num_classes)
+ self.train_dice = GeneralizedDiceScore(num_classes=num_classes)
+
+ self.val_accuracy = Accuracy(task=task, num_classes=num_classes)
+ self.val_precision = Precision(task=task, num_classes=num_classes)
+ self.val_recall = Recall(task=task, num_classes=num_classes)
+ self.val_f1score = F1Score(task=task, num_classes=num_classes)
+ self.val_miou = MeanIoU(num_classes=num_classes)
+ self.val_dice = GeneralizedDiceScore(num_classes=num_classes)
+
+ self.test_accuracy = Accuracy(task=task, num_classes=num_classes)
+ self.test_precision = Precision(task=task, num_classes=num_classes)
+ self.test_recall = Recall(task=task, num_classes=num_classes)
+ self.test_f1score = F1Score(task=task, num_classes=num_classes)
+ self.test_miou = MeanIoU(num_classes=num_classes)
+ self.test_dice = GeneralizedDiceScore(num_classes=num_classes)
+
+ # for averaging loss across batches
+ self.train_loss = MeanMetric()
+ self.val_loss = MeanMetric()
+ self.test_loss = MeanMetric()
+
+ # for tracking best so far validation accuracy
+ self.val_miou_best = MaxMetric()
+
+ def forward(self, x: torch.Tensor) -> torch.Tensor:
+ """Perform a forward pass through the model `self.net`.
+
+ :param x: A tensor of images.
+ :return: A tensor of logits.
+ """
+ return self.net(x)
+
+ def on_train_start(self) -> None:
+ """Lightning hook that is called when training begins."""
+ # by default lightning executes validation step sanity checks before training starts,
+ # so it's worth to make sure validation metrics don't store results from these checks
+ self.val_loss.reset()
+
+ self.val_accuracy.reset()
+ self.val_precision.reset()
+ self.val_recall.reset()
+ self.val_f1score.reset()
+ self.val_miou.reset()
+ self.val_dice.reset()
+
+ self.val_miou_best.reset()
+
+ def model_step(
+ self, batch: Tuple[torch.Tensor, torch.Tensor]
+ ) -> Tuple[torch.Tensor, torch.Tensor, torch.Tensor]:
+ """Perform a single model step on a batch of data.
+
+ :param batch: A batch of data (a tuple) containing the input tensor of images and target labels.
+
+ :return: A tuple containing (in order):
+ - A tensor of losses.
+ - A tensor of predictions.
+ - A tensor of target labels.
+ """
+ x, y = batch["img"], batch["ann"]
+ logits = self.forward(x)
+ loss = self.hparams.criterion(logits, y)
+ preds = torch.argmax(logits, dim=1)
+ return loss, preds, y
+
+ def training_step(
+ self, batch: Tuple[torch.Tensor, torch.Tensor], batch_idx: int
+ ) -> torch.Tensor:
+ """Perform a single training step on a batch of data from the training set.
+
+ :param batch: A batch of data (a tuple) containing the input tensor of images and target
+ labels.
+ :param batch_idx: The index of the current batch.
+ :return: A tensor of losses between model predictions and targets.
+ """
+ loss, preds, targets = self.model_step(batch)
+
+ # print(preds.shape) # (8, 256, 256)
+ # print(targets.shape) # (8, 256, 256)
+
+ # update and log metrics
+ self.train_loss(loss)
+
+ self.train_accuracy(preds, targets)
+ self.train_precision(preds, targets)
+ self.train_recall(preds, targets)
+ self.train_f1score(preds, targets)
+ self.train_miou(preds, targets)
+ self.train_dice(preds, targets)
+
+ self.log("train/loss", self.train_loss, on_step=False, on_epoch=True, prog_bar=True)
+
+ self.log("train/accuracy", self.train_accuracy, on_step=False, on_epoch=True, prog_bar=True)
+ self.log("train/precision", self.train_precision, on_step=False, on_epoch=True, prog_bar=True)
+ self.log("train/recall", self.train_recall, on_step=False, on_epoch=True, prog_bar=True)
+ self.log("train/f1score", self.train_f1score, on_step=False, on_epoch=True, prog_bar=True)
+ self.log("train/miou", self.train_miou, on_step=False, on_epoch=True, prog_bar=True)
+ self.log("train/dice", self.train_dice, on_step=False, on_epoch=True, prog_bar=True)
+
+ # return loss or backpropagation will fail
+ return loss
+
+ def on_train_epoch_end(self) -> None:
+ "Lightning hook that is called when a training epoch ends."
+ pass
+
+ def validation_step(self, batch: Tuple[torch.Tensor, torch.Tensor], batch_idx: int) -> None:
+ """Perform a single validation step on a batch of data from the validation set.
+
+ :param batch: A batch of data (a tuple) containing the input tensor of images and target
+ labels.
+ :param batch_idx: The index of the current batch.
+ """
+ loss, preds, targets = self.model_step(batch)
+
+ # update and log metrics
+ self.val_loss(loss)
+
+ self.val_accuracy(preds, targets)
+ self.val_precision(preds, targets)
+ self.val_recall(preds, targets)
+ self.val_f1score(preds, targets)
+ self.val_miou(preds, targets)
+ self.val_dice(preds, targets)
+
+ self.log("val/loss", self.val_loss, on_step=False, on_epoch=True, prog_bar=True)
+
+ self.log("val/accuracy", self.val_accuracy, on_step=False, on_epoch=True, prog_bar=True)
+ self.log("val/precision", self.val_precision, on_step=False, on_epoch=True, prog_bar=True)
+ self.log("val/recall", self.val_recall, on_step=False, on_epoch=True, prog_bar=True)
+ self.log("val/f1score", self.val_f1score, on_step=False, on_epoch=True, prog_bar=True)
+ self.log("val/miou", self.val_miou, on_step=False, on_epoch=True, prog_bar=True)
+ self.log("val/dice", self.val_dice, on_step=False, on_epoch=True, prog_bar=True)
+
+ def on_validation_epoch_end(self) -> None:
+ "Lightning hook that is called when a validation epoch ends."
+ miou = self.val_miou.compute() # get current val acc
+ self.val_miou_best(miou) # update best so far val acc
+ # log `val_acc_best` as a value through `.compute()` method, instead of as a metric object
+ # otherwise metric would be reset by lightning after each epoch
+ self.log("val/miou_best", self.val_miou_best.compute(), sync_dist=True, prog_bar=True)
+
+ def test_step(self, batch: Tuple[torch.Tensor, torch.Tensor], batch_idx: int) -> None:
+ """Perform a single test step on a batch of data from the test set.
+
+ :param batch: A batch of data (a tuple) containing the input tensor of images and target
+ labels.
+ :param batch_idx: The index of the current batch.
+ """
+ loss, preds, targets = self.model_step(batch)
+
+ # update and log metrics
+ self.test_loss(loss)
+
+ # update and log metrics
+ self.test_accuracy(preds, targets)
+ self.test_precision(preds, targets)
+ self.test_recall(preds, targets)
+ self.test_f1score(preds, targets)
+ self.test_miou(preds, targets)
+ self.test_dice(preds, targets)
+
+ self.log("test/loss", self.test_loss, on_step=False, on_epoch=True, prog_bar=True)
+
+ self.log("test/accuracy", self.test_accuracy, on_step=False, on_epoch=True, prog_bar=True)
+ self.log("test/precision", self.test_precision, on_step=False, on_epoch=True, prog_bar=True)
+ self.log("test/recall", self.test_recall, on_step=False, on_epoch=True, prog_bar=True)
+ self.log("test/f1score", self.test_f1score, on_step=False, on_epoch=True, prog_bar=True)
+ self.log("test/miou", self.test_miou, on_step=False, on_epoch=True, prog_bar=True)
+ self.log("test/dice", self.test_dice, on_step=False, on_epoch=True, prog_bar=True)
+
+ def on_test_epoch_end(self) -> None:
+ """Lightning hook that is called when a test epoch ends."""
+ pass
+
+ def setup(self, stage: str) -> None:
+ """Lightning hook that is called at the beginning of fit (train + validate), validate,
+ test, or predict.
+
+ This is a good hook when you need to build models dynamically or adjust something about
+ them. This hook is called on every process when using DDP.
+
+ :param stage: Either `"fit"`, `"validate"`, `"test"`, or `"predict"`.
+ """
+ if self.hparams.compile and stage == "fit":
+ self.net = torch.compile(self.net)
+
+ def configure_optimizers(self) -> Dict[str, Any]:
+ """Choose what optimizers and learning-rate schedulers to use in your optimization.
+ Normally you'd need one. But in the case of GANs or similar you might have multiple.
+
+ Examples:
+ https://lightning.ai/docs/pytorch/latest/common/lightning_module.html#configure-optimizers
+
+ :return: A dict containing the configured optimizers and learning-rate schedulers to be used for training.
+ """
+ optimizer = self.hparams.optimizer(params=self.trainer.model.parameters())
+ if self.hparams.scheduler is not None:
+ scheduler = self.hparams.scheduler(optimizer=optimizer)
+ return {
+ "optimizer": optimizer,
+ "lr_scheduler": {
+ "scheduler": scheduler,
+ "monitor": "val/loss",
+ "interval": "epoch",
+ "frequency": 1,
+ },
+ }
+ return {"optimizer": optimizer}
+
+
+if __name__ == "__main__":
+ _ = BaseLitModule(None, None, None, None)
diff --git a/src/models/cdnetv2_module.py b/src/models/cdnetv2_module.py
index f4e690d6fe25c403f23a6f9740292351cbe3f136..dd1bc23d12dcac9321be6435116a7c06fb7b88f1 100644
--- a/src/models/cdnetv2_module.py
+++ b/src/models/cdnetv2_module.py
@@ -1,34 +1,34 @@
-# -*- coding: utf-8 -*-
-# @Time : 2024/8/1 下午2:47
-# @Author : xiaoshun
-# @Email : 3038523973@qq.com
-# @File : cdnetv2_module.py
-# @Software: PyCharm
-from typing import Tuple
-
-import torch
-
-import src.models.base_module
-
-
-class CDNetv2LitModule(src.models.base_module.BaseLitModule):
- def __init__(self,**kwargs):
- super().__init__(**kwargs)
-
- def model_step(
- self, batch: Tuple[torch.Tensor, torch.Tensor]
- ) -> Tuple[torch.Tensor, torch.Tensor, torch.Tensor]:
- """Perform a single model step on a batch of data.
-
- :param batch: A batch of data (a tuple) containing the input tensor of images and target labels.
-
- :return: A tuple containing (in order):
- - A tensor of losses.
- - A tensor of predictions.
- - A tensor of target labels.
- """
- x, y = batch["img"], batch["ann"]
- logits ,logits_aux = self.forward(x)
- loss = self.hparams.criterion(logits ,logits_aux, y)
- preds = torch.argmax(logits, dim=1)
- return loss, preds, y
+# -*- coding: utf-8 -*-
+# @Time : 2024/8/1 下午2:47
+# @Author : xiaoshun
+# @Email : 3038523973@qq.com
+# @File : cdnetv2_module.py
+# @Software: PyCharm
+from typing import Tuple
+
+import torch
+
+import src.models.base_module
+
+
+class CDNetv2LitModule(src.models.base_module.BaseLitModule):
+ def __init__(self,**kwargs):
+ super().__init__(**kwargs)
+
+ def model_step(
+ self, batch: Tuple[torch.Tensor, torch.Tensor]
+ ) -> Tuple[torch.Tensor, torch.Tensor, torch.Tensor]:
+ """Perform a single model step on a batch of data.
+
+ :param batch: A batch of data (a tuple) containing the input tensor of images and target labels.
+
+ :return: A tuple containing (in order):
+ - A tensor of losses.
+ - A tensor of predictions.
+ - A tensor of target labels.
+ """
+ x, y = batch["img"], batch["ann"]
+ logits ,logits_aux = self.forward(x)
+ loss = self.hparams.criterion(logits ,logits_aux, y)
+ preds = torch.argmax(logits, dim=1)
+ return loss, preds, y
diff --git a/src/models/components/cdnetv1.py b/src/models/components/cdnetv1.py
index d0f733cfa80bf51db36c05481929d134aad4ffe9..e158194796c822e85dd6c78fe2211bc9d925ee11 100644
--- a/src/models/components/cdnetv1.py
+++ b/src/models/components/cdnetv1.py
@@ -1,389 +1,389 @@
-# -*- coding: utf-8 -*-
-# @Time : 2024/7/24 上午11:36
-# @Author : xiaoshun
-# @Email : 3038523973@qq.com
-# @File : cdnetv1.py
-# @Software: PyCharm
-
-"""Cloud detection Network"""
-
-"""Cloud detection Network"""
-
-"""
-This is the implementation of CDnetV1 without multi-scale inputs. This implementation uses ResNet by default.
-"""
-
-import torch
-import torch.nn as nn
-import torch.nn.functional as F
-
-affine_par = True
-
-
-def conv3x3(in_planes, out_planes, stride=1):
- "3x3 convolution with padding"
- return nn.Conv2d(in_planes, out_planes, kernel_size=3, stride=stride,
- padding=1, bias=False)
-
-
-class BasicBlock(nn.Module):
- expansion = 1
-
- def __init__(self, inplanes, planes, stride=1, downsample=None):
- super(BasicBlock, self).__init__()
- self.conv1 = conv3x3(inplanes, planes, stride)
- self.bn1 = nn.BatchNorm2d(planes, affine=affine_par)
- self.relu = nn.ReLU(inplace=True)
- self.conv2 = conv3x3(planes, planes)
- self.bn2 = nn.BatchNorm2d(planes, affine=affine_par)
- self.downsample = downsample
- self.stride = stride
-
- def forward(self, x):
- residual = x
-
- out = self.conv1(x)
- out = self.bn1(out)
- out = self.relu(out)
-
- out = self.conv2(out)
- out = self.bn2(out)
-
- if self.downsample is not None:
- residual = self.downsample(x)
-
- out += residual
- out = self.relu(out)
-
- return out
-
-
-class Bottleneck(nn.Module):
- expansion = 4
-
- def __init__(self, inplanes, planes, stride=1, dilation=1, downsample=None):
- super(Bottleneck, self).__init__()
- self.conv1 = nn.Conv2d(inplanes, planes, kernel_size=1, stride=stride, bias=False) # change
- self.bn1 = nn.BatchNorm2d(planes, affine=affine_par)
- for i in self.bn1.parameters():
- i.requires_grad = False
-
- padding = dilation
- self.conv2 = nn.Conv2d(planes, planes, kernel_size=3, stride=1, # change
- padding=padding, bias=False, dilation=dilation)
- self.bn2 = nn.BatchNorm2d(planes, affine=affine_par)
- for i in self.bn2.parameters():
- i.requires_grad = False
- self.conv3 = nn.Conv2d(planes, planes * 4, kernel_size=1, bias=False)
- self.bn3 = nn.BatchNorm2d(planes * 4, affine=affine_par)
- for i in self.bn3.parameters():
- i.requires_grad = False
- self.relu = nn.ReLU(inplace=True)
- self.downsample = downsample
- self.stride = stride
-
- def forward(self, x):
- residual = x
-
- out = self.conv1(x)
- out = self.bn1(out)
- out = self.relu(out)
-
- out = self.conv2(out)
- out = self.bn2(out)
- out = self.relu(out)
-
- out = self.conv3(out)
- out = self.bn3(out)
-
- if self.downsample is not None:
- residual = self.downsample(x)
-
- out += residual
- out = self.relu(out)
-
- return out
-
-
-class Classifier_Module(nn.Module):
-
- def __init__(self, dilation_series, padding_series, num_classes):
- super(Classifier_Module, self).__init__()
- self.conv2d_list = nn.ModuleList()
- for dilation, padding in zip(dilation_series, padding_series):
- self.conv2d_list.append(
- nn.Conv2d(2048, num_classes, kernel_size=3, stride=1, padding=padding, dilation=dilation, bias=True))
-
- for m in self.conv2d_list:
- m.weight.data.normal_(0, 0.01)
-
- def forward(self, x):
- out = self.conv2d_list[0](x)
- for i in range(len(self.conv2d_list) - 1):
- out += self.conv2d_list[i + 1](x)
- return out
-
-
-class _ConvBNReLU(nn.Module):
- def __init__(self, in_channels, out_channels, kernel_size, stride=1, padding=0,
- dilation=1, groups=1, norm_layer=nn.BatchNorm2d):
- super(_ConvBNReLU, self).__init__()
- self.conv = nn.Conv2d(in_channels, out_channels, kernel_size, stride, padding, dilation, groups, bias=False)
- self.bn = norm_layer(out_channels)
- self.relu = nn.ReLU(True)
-
- def forward(self, x):
- x = self.conv(x)
- x = self.bn(x)
- x = self.relu(x)
- return x
-
-
-class _ASPPConv(nn.Module):
- def __init__(self, in_channels, out_channels, atrous_rate, norm_layer):
- super(_ASPPConv, self).__init__()
- self.block = nn.Sequential(
- nn.Conv2d(in_channels, out_channels, 3, padding=atrous_rate, dilation=atrous_rate, bias=False),
- norm_layer(out_channels),
- nn.ReLU(True)
- )
-
- def forward(self, x):
- return self.block(x)
-
-
-class _AsppPooling(nn.Module):
- def __init__(self, in_channels, out_channels, norm_layer):
- super(_AsppPooling, self).__init__()
- self.gap = nn.Sequential(
- nn.AdaptiveAvgPool2d(1),
- nn.Conv2d(in_channels, out_channels, 1, bias=False),
- norm_layer(out_channels),
- nn.ReLU(True)
- )
-
- def forward(self, x):
- size = x.size()[2:]
- pool = self.gap(x)
- out = F.interpolate(pool, size, mode='bilinear', align_corners=True)
- return out
-
-
-class _ASPP(nn.Module):
- def __init__(self, in_channels, atrous_rates, norm_layer):
- super(_ASPP, self).__init__()
- out_channels = 512 # changed from 256
- self.b0 = nn.Sequential(
- nn.Conv2d(in_channels, out_channels, 1, bias=False),
- norm_layer(out_channels),
- nn.ReLU(True)
- )
-
- rate1, rate2, rate3 = tuple(atrous_rates)
- self.b1 = _ASPPConv(in_channels, out_channels, rate1, norm_layer)
- self.b2 = _ASPPConv(in_channels, out_channels, rate2, norm_layer)
- self.b3 = _ASPPConv(in_channels, out_channels, rate3, norm_layer)
- self.b4 = _AsppPooling(in_channels, out_channels, norm_layer=norm_layer)
-
- # self.project = nn.Sequential(
- # nn.Conv2d(5 * out_channels, out_channels, 1, bias=False),
- # norm_layer(out_channels),
- # nn.ReLU(True),
- # nn.Dropout(0.5))
- self.dropout2d = nn.Dropout2d(0.3)
-
- def forward(self, x):
- feat1 = self.dropout2d(self.b0(x))
- feat2 = self.dropout2d(self.b1(x))
- feat3 = self.dropout2d(self.b2(x))
- feat4 = self.dropout2d(self.b3(x))
- feat5 = self.dropout2d(self.b4(x))
- x = torch.cat((feat1, feat2, feat3, feat4, feat5), dim=1)
- # x = self.project(x)
- return x
-
-
-class _FPM(nn.Module):
- def __init__(self, in_channels, num_classes, norm_layer=nn.BatchNorm2d):
- super(_FPM, self).__init__()
- self.aspp = _ASPP(in_channels, [6, 12, 18], norm_layer=norm_layer)
- # self.dropout2d = nn.Dropout2d(0.5)
-
- def forward(self, x):
- x = torch.cat((x, self.aspp(x)), dim=1)
- # x = self.dropout2d(x) # added
- return x
-
-
-class BR(nn.Module):
- def __init__(self, num_classes, stride=1, downsample=None):
- super(BR, self).__init__()
- self.conv1 = conv3x3(num_classes, num_classes * 16, stride)
- self.relu = nn.ReLU(inplace=True)
- self.conv2 = conv3x3(num_classes * 16, num_classes)
- self.stride = stride
-
- def forward(self, x):
- residual = x
-
- out = self.conv1(x)
- out = self.relu(out)
-
- out = self.conv2(out)
- out += residual
-
- return out
-
-
-class CDnetV1(nn.Module):
- def __init__(self, block=Bottleneck, layers=[3, 4, 6, 3], num_classes=21, aux=True):
- self.inplanes = 64
- self.aux = aux
- super().__init__()
- # self.conv1 = nn.Conv2d(3, 64, kernel_size=7, stride=2, padding=3, bias=False)
- # self.bn1 = nn.BatchNorm2d(64, affine = affine_par)
-
- self.conv1 = nn.Conv2d(3, 64, kernel_size=3, stride=2, padding=1, bias=False)
- self.bn1 = nn.BatchNorm2d(64, affine=affine_par)
- self.conv2 = nn.Conv2d(64, 64, kernel_size=3, stride=1, padding=1, bias=False)
- self.bn2 = nn.BatchNorm2d(64, affine=affine_par)
- self.conv3 = nn.Conv2d(64, 64, kernel_size=3, stride=1, padding=1, bias=False)
- self.bn3 = nn.BatchNorm2d(64, affine=affine_par)
-
- for i in self.bn1.parameters():
- i.requires_grad = False
- self.relu = nn.ReLU(inplace=True)
- self.maxpool = nn.MaxPool2d(kernel_size=3, stride=2, padding=1, ceil_mode=True) # change
- self.layer1 = self._make_layer(block, 64, layers[0])
- self.layer2 = self._make_layer(block, 128, layers[1], stride=2)
- self.layer3 = self._make_layer(block, 256, layers[2], stride=1, dilation=2)
- self.layer4 = self._make_layer(block, 512, layers[3], stride=1, dilation=4)
- # self.layer5 = self._make_pred_layer(Classifier_Module, [6,12,18,24],[6,12,18,24],num_classes)
-
- self.res5_con1x1 = nn.Sequential(
- nn.Conv2d(1024 + 2048, 512, kernel_size=1, stride=1, padding=0),
- nn.BatchNorm2d(512),
- nn.ReLU(True)
- )
-
- self.fpm1 = _FPM(512, num_classes)
- self.fpm2 = _FPM(512, num_classes)
- self.fpm3 = _FPM(256, num_classes)
-
- self.br1 = BR(num_classes)
- self.br2 = BR(num_classes)
- self.br3 = BR(num_classes)
- self.br4 = BR(num_classes)
- self.br5 = BR(num_classes)
- self.br6 = BR(num_classes)
- self.br7 = BR(num_classes)
-
- self.predict1 = self._predict_layer(512 * 6, num_classes)
- self.predict2 = self._predict_layer(512 * 6, num_classes)
- self.predict3 = self._predict_layer(512 * 5 + 256, num_classes)
-
- for m in self.modules():
- if isinstance(m, nn.Conv2d):
- n = m.kernel_size[0] * m.kernel_size[1] * m.out_channels
- m.weight.data.normal_(0, 0.01)
- elif isinstance(m, nn.BatchNorm2d):
- m.weight.data.fill_(1)
- m.bias.data.zero_()
- # for i in m.parameters():
- # i.requires_grad = False
-
- def _predict_layer(self, in_channels, num_classes):
- return nn.Sequential(nn.Conv2d(in_channels, 256, kernel_size=1, stride=1, padding=0),
- nn.BatchNorm2d(256),
- nn.ReLU(True),
- nn.Dropout2d(0.1),
- nn.Conv2d(256, num_classes, kernel_size=3, stride=1, padding=1, bias=True))
-
- def _make_layer(self, block, planes, blocks, stride=1, dilation=1):
- downsample = None
- if stride != 1 or self.inplanes != planes * block.expansion or dilation == 2 or dilation == 4:
- downsample = nn.Sequential(
- nn.Conv2d(self.inplanes, planes * block.expansion,
- kernel_size=1, stride=stride, bias=False),
- nn.BatchNorm2d(planes * block.expansion, affine=affine_par))
- for i in downsample._modules['1'].parameters():
- i.requires_grad = False
- layers = []
- layers.append(block(self.inplanes, planes, stride, dilation=dilation, downsample=downsample))
- self.inplanes = planes * block.expansion
- for i in range(1, blocks):
- layers.append(block(self.inplanes, planes, dilation=dilation))
-
- return nn.Sequential(*layers)
-
- # def _make_pred_layer(self,block, dilation_series, padding_series,num_classes):
- # return block(dilation_series,padding_series,num_classes)
-
- def base_forward(self, x):
- x = self.relu(self.bn1(self.conv1(x)))
- size_conv1 = x.size()[2:]
- x = self.relu(self.bn2(self.conv2(x)))
- x = self.relu(self.bn3(self.conv3(x)))
- x = self.maxpool(x)
- x = self.layer1(x)
- res2 = x
- x = self.layer2(x)
- res3 = x
- x = self.layer3(x)
- res4 = x
- x = self.layer4(x)
- x = self.res5_con1x1(torch.cat([x, res4], dim=1))
-
- return x, res3, res2, size_conv1
-
- def forward(self, x):
- size = x.size()[2:]
- score1, score2, score3, size_conv1 = self.base_forward(x)
- # outputs = list()
- score1 = self.fpm1(score1)
- score1 = self.predict1(score1) # 1/8
- predict1 = score1
- score1 = self.br1(score1)
-
- score2 = self.fpm2(score2)
- score2 = self.predict2(score2) # 1/8
- predict2 = score2
-
- # first fusion
- score2 = self.br2(score2) + score1
- score2 = self.br3(score2)
-
- score3 = self.fpm3(score3)
- score3 = self.predict3(score3) # 1/4
- predict3 = score3
- score3 = self.br4(score3)
-
- # second fusion
- size_score3 = score3.size()[2:]
- score3 = score3 + F.interpolate(score2, size_score3, mode='bilinear', align_corners=True)
- score3 = self.br5(score3)
-
- # upsampling + BR
- score3 = F.interpolate(score3, size_conv1, mode='bilinear', align_corners=True)
- score3 = self.br6(score3)
- score3 = F.interpolate(score3, size, mode='bilinear', align_corners=True)
- score3 = self.br7(score3)
-
- # if self.aux:
- # auxout = self.dsn(mid)
- # auxout = F.interpolate(auxout, size, mode='bilinear', align_corners=True)
- # #outputs.append(auxout)
- return score3
- # return score3, predict1, predict2, predict3
-
-
-if __name__ == '__main__':
- model = CDnetV1(num_classes=21)
- fake_image = torch.randn(2, 3, 224, 224)
- outputs = model(fake_image)
- for out in outputs:
- print(out.shape)
- # torch.Size([2, 21, 224, 224])
- # torch.Size([2, 21, 29, 29])
- # torch.Size([2, 21, 29, 29])
- # torch.Size([2, 21, 57, 57])
+# -*- coding: utf-8 -*-
+# @Time : 2024/7/24 上午11:36
+# @Author : xiaoshun
+# @Email : 3038523973@qq.com
+# @File : cdnetv1.py
+# @Software: PyCharm
+
+"""Cloud detection Network"""
+
+"""Cloud detection Network"""
+
+"""
+This is the implementation of CDnetV1 without multi-scale inputs. This implementation uses ResNet by default.
+"""
+
+import torch
+import torch.nn as nn
+import torch.nn.functional as F
+
+affine_par = True
+
+
+def conv3x3(in_planes, out_planes, stride=1):
+ "3x3 convolution with padding"
+ return nn.Conv2d(in_planes, out_planes, kernel_size=3, stride=stride,
+ padding=1, bias=False)
+
+
+class BasicBlock(nn.Module):
+ expansion = 1
+
+ def __init__(self, inplanes, planes, stride=1, downsample=None):
+ super(BasicBlock, self).__init__()
+ self.conv1 = conv3x3(inplanes, planes, stride)
+ self.bn1 = nn.BatchNorm2d(planes, affine=affine_par)
+ self.relu = nn.ReLU(inplace=True)
+ self.conv2 = conv3x3(planes, planes)
+ self.bn2 = nn.BatchNorm2d(planes, affine=affine_par)
+ self.downsample = downsample
+ self.stride = stride
+
+ def forward(self, x):
+ residual = x
+
+ out = self.conv1(x)
+ out = self.bn1(out)
+ out = self.relu(out)
+
+ out = self.conv2(out)
+ out = self.bn2(out)
+
+ if self.downsample is not None:
+ residual = self.downsample(x)
+
+ out += residual
+ out = self.relu(out)
+
+ return out
+
+
+class Bottleneck(nn.Module):
+ expansion = 4
+
+ def __init__(self, inplanes, planes, stride=1, dilation=1, downsample=None):
+ super(Bottleneck, self).__init__()
+ self.conv1 = nn.Conv2d(inplanes, planes, kernel_size=1, stride=stride, bias=False) # change
+ self.bn1 = nn.BatchNorm2d(planes, affine=affine_par)
+ for i in self.bn1.parameters():
+ i.requires_grad = False
+
+ padding = dilation
+ self.conv2 = nn.Conv2d(planes, planes, kernel_size=3, stride=1, # change
+ padding=padding, bias=False, dilation=dilation)
+ self.bn2 = nn.BatchNorm2d(planes, affine=affine_par)
+ for i in self.bn2.parameters():
+ i.requires_grad = False
+ self.conv3 = nn.Conv2d(planes, planes * 4, kernel_size=1, bias=False)
+ self.bn3 = nn.BatchNorm2d(planes * 4, affine=affine_par)
+ for i in self.bn3.parameters():
+ i.requires_grad = False
+ self.relu = nn.ReLU(inplace=True)
+ self.downsample = downsample
+ self.stride = stride
+
+ def forward(self, x):
+ residual = x
+
+ out = self.conv1(x)
+ out = self.bn1(out)
+ out = self.relu(out)
+
+ out = self.conv2(out)
+ out = self.bn2(out)
+ out = self.relu(out)
+
+ out = self.conv3(out)
+ out = self.bn3(out)
+
+ if self.downsample is not None:
+ residual = self.downsample(x)
+
+ out += residual
+ out = self.relu(out)
+
+ return out
+
+
+class Classifier_Module(nn.Module):
+
+ def __init__(self, dilation_series, padding_series, num_classes):
+ super(Classifier_Module, self).__init__()
+ self.conv2d_list = nn.ModuleList()
+ for dilation, padding in zip(dilation_series, padding_series):
+ self.conv2d_list.append(
+ nn.Conv2d(2048, num_classes, kernel_size=3, stride=1, padding=padding, dilation=dilation, bias=True))
+
+ for m in self.conv2d_list:
+ m.weight.data.normal_(0, 0.01)
+
+ def forward(self, x):
+ out = self.conv2d_list[0](x)
+ for i in range(len(self.conv2d_list) - 1):
+ out += self.conv2d_list[i + 1](x)
+ return out
+
+
+class _ConvBNReLU(nn.Module):
+ def __init__(self, in_channels, out_channels, kernel_size, stride=1, padding=0,
+ dilation=1, groups=1, norm_layer=nn.BatchNorm2d):
+ super(_ConvBNReLU, self).__init__()
+ self.conv = nn.Conv2d(in_channels, out_channels, kernel_size, stride, padding, dilation, groups, bias=False)
+ self.bn = norm_layer(out_channels)
+ self.relu = nn.ReLU(True)
+
+ def forward(self, x):
+ x = self.conv(x)
+ x = self.bn(x)
+ x = self.relu(x)
+ return x
+
+
+class _ASPPConv(nn.Module):
+ def __init__(self, in_channels, out_channels, atrous_rate, norm_layer):
+ super(_ASPPConv, self).__init__()
+ self.block = nn.Sequential(
+ nn.Conv2d(in_channels, out_channels, 3, padding=atrous_rate, dilation=atrous_rate, bias=False),
+ norm_layer(out_channels),
+ nn.ReLU(True)
+ )
+
+ def forward(self, x):
+ return self.block(x)
+
+
+class _AsppPooling(nn.Module):
+ def __init__(self, in_channels, out_channels, norm_layer):
+ super(_AsppPooling, self).__init__()
+ self.gap = nn.Sequential(
+ nn.AdaptiveAvgPool2d(1),
+ nn.Conv2d(in_channels, out_channels, 1, bias=False),
+ norm_layer(out_channels),
+ nn.ReLU(True)
+ )
+
+ def forward(self, x):
+ size = x.size()[2:]
+ pool = self.gap(x)
+ out = F.interpolate(pool, size, mode='bilinear', align_corners=True)
+ return out
+
+
+class _ASPP(nn.Module):
+ def __init__(self, in_channels, atrous_rates, norm_layer):
+ super(_ASPP, self).__init__()
+ out_channels = 512 # changed from 256
+ self.b0 = nn.Sequential(
+ nn.Conv2d(in_channels, out_channels, 1, bias=False),
+ norm_layer(out_channels),
+ nn.ReLU(True)
+ )
+
+ rate1, rate2, rate3 = tuple(atrous_rates)
+ self.b1 = _ASPPConv(in_channels, out_channels, rate1, norm_layer)
+ self.b2 = _ASPPConv(in_channels, out_channels, rate2, norm_layer)
+ self.b3 = _ASPPConv(in_channels, out_channels, rate3, norm_layer)
+ self.b4 = _AsppPooling(in_channels, out_channels, norm_layer=norm_layer)
+
+ # self.project = nn.Sequential(
+ # nn.Conv2d(5 * out_channels, out_channels, 1, bias=False),
+ # norm_layer(out_channels),
+ # nn.ReLU(True),
+ # nn.Dropout(0.5))
+ self.dropout2d = nn.Dropout2d(0.3)
+
+ def forward(self, x):
+ feat1 = self.dropout2d(self.b0(x))
+ feat2 = self.dropout2d(self.b1(x))
+ feat3 = self.dropout2d(self.b2(x))
+ feat4 = self.dropout2d(self.b3(x))
+ feat5 = self.dropout2d(self.b4(x))
+ x = torch.cat((feat1, feat2, feat3, feat4, feat5), dim=1)
+ # x = self.project(x)
+ return x
+
+
+class _FPM(nn.Module):
+ def __init__(self, in_channels, num_classes, norm_layer=nn.BatchNorm2d):
+ super(_FPM, self).__init__()
+ self.aspp = _ASPP(in_channels, [6, 12, 18], norm_layer=norm_layer)
+ # self.dropout2d = nn.Dropout2d(0.5)
+
+ def forward(self, x):
+ x = torch.cat((x, self.aspp(x)), dim=1)
+ # x = self.dropout2d(x) # added
+ return x
+
+
+class BR(nn.Module):
+ def __init__(self, num_classes, stride=1, downsample=None):
+ super(BR, self).__init__()
+ self.conv1 = conv3x3(num_classes, num_classes * 16, stride)
+ self.relu = nn.ReLU(inplace=True)
+ self.conv2 = conv3x3(num_classes * 16, num_classes)
+ self.stride = stride
+
+ def forward(self, x):
+ residual = x
+
+ out = self.conv1(x)
+ out = self.relu(out)
+
+ out = self.conv2(out)
+ out += residual
+
+ return out
+
+
+class CDnetV1(nn.Module):
+ def __init__(self, block=Bottleneck, layers=[3, 4, 6, 3], num_classes=21, aux=True):
+ self.inplanes = 64
+ self.aux = aux
+ super().__init__()
+ # self.conv1 = nn.Conv2d(3, 64, kernel_size=7, stride=2, padding=3, bias=False)
+ # self.bn1 = nn.BatchNorm2d(64, affine = affine_par)
+
+ self.conv1 = nn.Conv2d(3, 64, kernel_size=3, stride=2, padding=1, bias=False)
+ self.bn1 = nn.BatchNorm2d(64, affine=affine_par)
+ self.conv2 = nn.Conv2d(64, 64, kernel_size=3, stride=1, padding=1, bias=False)
+ self.bn2 = nn.BatchNorm2d(64, affine=affine_par)
+ self.conv3 = nn.Conv2d(64, 64, kernel_size=3, stride=1, padding=1, bias=False)
+ self.bn3 = nn.BatchNorm2d(64, affine=affine_par)
+
+ for i in self.bn1.parameters():
+ i.requires_grad = False
+ self.relu = nn.ReLU(inplace=True)
+ self.maxpool = nn.MaxPool2d(kernel_size=3, stride=2, padding=1, ceil_mode=True) # change
+ self.layer1 = self._make_layer(block, 64, layers[0])
+ self.layer2 = self._make_layer(block, 128, layers[1], stride=2)
+ self.layer3 = self._make_layer(block, 256, layers[2], stride=1, dilation=2)
+ self.layer4 = self._make_layer(block, 512, layers[3], stride=1, dilation=4)
+ # self.layer5 = self._make_pred_layer(Classifier_Module, [6,12,18,24],[6,12,18,24],num_classes)
+
+ self.res5_con1x1 = nn.Sequential(
+ nn.Conv2d(1024 + 2048, 512, kernel_size=1, stride=1, padding=0),
+ nn.BatchNorm2d(512),
+ nn.ReLU(True)
+ )
+
+ self.fpm1 = _FPM(512, num_classes)
+ self.fpm2 = _FPM(512, num_classes)
+ self.fpm3 = _FPM(256, num_classes)
+
+ self.br1 = BR(num_classes)
+ self.br2 = BR(num_classes)
+ self.br3 = BR(num_classes)
+ self.br4 = BR(num_classes)
+ self.br5 = BR(num_classes)
+ self.br6 = BR(num_classes)
+ self.br7 = BR(num_classes)
+
+ self.predict1 = self._predict_layer(512 * 6, num_classes)
+ self.predict2 = self._predict_layer(512 * 6, num_classes)
+ self.predict3 = self._predict_layer(512 * 5 + 256, num_classes)
+
+ for m in self.modules():
+ if isinstance(m, nn.Conv2d):
+ n = m.kernel_size[0] * m.kernel_size[1] * m.out_channels
+ m.weight.data.normal_(0, 0.01)
+ elif isinstance(m, nn.BatchNorm2d):
+ m.weight.data.fill_(1)
+ m.bias.data.zero_()
+ # for i in m.parameters():
+ # i.requires_grad = False
+
+ def _predict_layer(self, in_channels, num_classes):
+ return nn.Sequential(nn.Conv2d(in_channels, 256, kernel_size=1, stride=1, padding=0),
+ nn.BatchNorm2d(256),
+ nn.ReLU(True),
+ nn.Dropout2d(0.1),
+ nn.Conv2d(256, num_classes, kernel_size=3, stride=1, padding=1, bias=True))
+
+ def _make_layer(self, block, planes, blocks, stride=1, dilation=1):
+ downsample = None
+ if stride != 1 or self.inplanes != planes * block.expansion or dilation == 2 or dilation == 4:
+ downsample = nn.Sequential(
+ nn.Conv2d(self.inplanes, planes * block.expansion,
+ kernel_size=1, stride=stride, bias=False),
+ nn.BatchNorm2d(planes * block.expansion, affine=affine_par))
+ for i in downsample._modules['1'].parameters():
+ i.requires_grad = False
+ layers = []
+ layers.append(block(self.inplanes, planes, stride, dilation=dilation, downsample=downsample))
+ self.inplanes = planes * block.expansion
+ for i in range(1, blocks):
+ layers.append(block(self.inplanes, planes, dilation=dilation))
+
+ return nn.Sequential(*layers)
+
+ # def _make_pred_layer(self,block, dilation_series, padding_series,num_classes):
+ # return block(dilation_series,padding_series,num_classes)
+
+ def base_forward(self, x):
+ x = self.relu(self.bn1(self.conv1(x)))
+ size_conv1 = x.size()[2:]
+ x = self.relu(self.bn2(self.conv2(x)))
+ x = self.relu(self.bn3(self.conv3(x)))
+ x = self.maxpool(x)
+ x = self.layer1(x)
+ res2 = x
+ x = self.layer2(x)
+ res3 = x
+ x = self.layer3(x)
+ res4 = x
+ x = self.layer4(x)
+ x = self.res5_con1x1(torch.cat([x, res4], dim=1))
+
+ return x, res3, res2, size_conv1
+
+ def forward(self, x):
+ size = x.size()[2:]
+ score1, score2, score3, size_conv1 = self.base_forward(x)
+ # outputs = list()
+ score1 = self.fpm1(score1)
+ score1 = self.predict1(score1) # 1/8
+ predict1 = score1
+ score1 = self.br1(score1)
+
+ score2 = self.fpm2(score2)
+ score2 = self.predict2(score2) # 1/8
+ predict2 = score2
+
+ # first fusion
+ score2 = self.br2(score2) + score1
+ score2 = self.br3(score2)
+
+ score3 = self.fpm3(score3)
+ score3 = self.predict3(score3) # 1/4
+ predict3 = score3
+ score3 = self.br4(score3)
+
+ # second fusion
+ size_score3 = score3.size()[2:]
+ score3 = score3 + F.interpolate(score2, size_score3, mode='bilinear', align_corners=True)
+ score3 = self.br5(score3)
+
+ # upsampling + BR
+ score3 = F.interpolate(score3, size_conv1, mode='bilinear', align_corners=True)
+ score3 = self.br6(score3)
+ score3 = F.interpolate(score3, size, mode='bilinear', align_corners=True)
+ score3 = self.br7(score3)
+
+ # if self.aux:
+ # auxout = self.dsn(mid)
+ # auxout = F.interpolate(auxout, size, mode='bilinear', align_corners=True)
+ # #outputs.append(auxout)
+ return score3
+ # return score3, predict1, predict2, predict3
+
+
+if __name__ == '__main__':
+ model = CDnetV1(num_classes=21)
+ fake_image = torch.randn(2, 3, 224, 224)
+ outputs = model(fake_image)
+ for out in outputs:
+ print(out.shape)
+ # torch.Size([2, 21, 224, 224])
+ # torch.Size([2, 21, 29, 29])
+ # torch.Size([2, 21, 29, 29])
+ # torch.Size([2, 21, 57, 57])
diff --git a/src/models/components/cdnetv2.py b/src/models/components/cdnetv2.py
index 0f24da3526c26fcea20d05e441df31098d767ec4..04699413cf288211e7c4e0cdd22e195f1a47fcb5 100644
--- a/src/models/components/cdnetv2.py
+++ b/src/models/components/cdnetv2.py
@@ -1,692 +1,692 @@
-# -*- coding: utf-8 -*-
-# @Time : 2024/7/24 下午3:41
-# @Author : xiaoshun
-# @Email : 3038523973@qq.com
-# @File : cdnetv2.py
-# @Software: PyCharm
-
-"""Cloud detection Network"""
-
-"""
-This is the implementation of CDnetV2 without multi-scale inputs. This implementation uses ResNet by default.
-"""
-# nn.GroupNorm
-
-import torch
-# import torch.nn as nn
-import torch.nn.functional as F
-from torch import nn
-
-affine_par = True
-
-
-def conv3x3(in_planes, out_planes, stride=1):
- "3x3 convolution with padding"
- return nn.Conv2d(in_planes, out_planes, kernel_size=3, stride=stride,
- padding=1, bias=False)
-
-
-class BasicBlock(nn.Module):
- expansion = 1
-
- def __init__(self, inplanes, planes, stride=1, downsample=None):
- super(BasicBlock, self).__init__()
- self.conv1 = conv3x3(inplanes, planes, stride)
- self.bn1 = nn.BatchNorm2d(planes, affine=affine_par)
- self.relu = nn.ReLU(inplace=True)
- self.conv2 = conv3x3(planes, planes)
- self.bn2 = nn.BatchNorm2d(planes, affine=affine_par)
- self.downsample = downsample
- self.stride = stride
-
- def forward(self, x):
- residual = x
-
- out = self.conv1(x)
- out = self.bn1(out)
- out = self.relu(out)
-
- out = self.conv2(out)
- out = self.bn2(out)
-
- if self.downsample is not None:
- residual = self.downsample(x)
-
- out += residual
- out = self.relu(out)
-
- return out
-
-
-class Bottleneck(nn.Module):
- expansion = 4
-
- def __init__(self, inplanes, planes, stride=1, dilation=1, downsample=None):
- super(Bottleneck, self).__init__()
- self.conv1 = nn.Conv2d(inplanes, planes, kernel_size=1, stride=stride, bias=False) # change
- self.bn1 = nn.BatchNorm2d(planes, affine=affine_par)
- for i in self.bn1.parameters():
- i.requires_grad = False
-
- padding = dilation
- self.conv2 = nn.Conv2d(planes, planes, kernel_size=3, stride=1, # change
- padding=padding, bias=False, dilation=dilation)
- self.bn2 = nn.BatchNorm2d(planes, affine=affine_par)
- for i in self.bn2.parameters():
- i.requires_grad = False
- self.conv3 = nn.Conv2d(planes, planes * 4, kernel_size=1, bias=False)
- self.bn3 = nn.BatchNorm2d(planes * 4, affine=affine_par)
- for i in self.bn3.parameters():
- i.requires_grad = False
- self.relu = nn.ReLU(inplace=True)
- self.downsample = downsample
- self.stride = stride
-
- def forward(self, x):
- residual = x
-
- out = self.conv1(x)
- out = self.bn1(out)
- out = self.relu(out)
-
- out = self.conv2(out)
- out = self.bn2(out)
- out = self.relu(out)
-
- out = self.conv3(out)
- out = self.bn3(out)
-
- if self.downsample is not None:
- residual = self.downsample(x)
-
- out += residual
- out = self.relu(out)
-
- return out
-
- # self.layerx_1 = Bottleneck_nosample(64, 64, stride=1, dilation=1)
- # self.layerx_2 = Bottleneck(256, 64, stride=1, dilation=1, downsample=None)
- # self.layerx_3 = Bottleneck_downsample(256, 64, stride=2, dilation=1)
-
-
-class Res_block_1(nn.Module):
- expansion = 4
-
- def __init__(self, inplanes=64, planes=64, stride=1, dilation=1):
- super(Res_block_1, self).__init__()
-
- self.conv1 = nn.Sequential(
- nn.Conv2d(inplanes, planes, kernel_size=1, stride=1, bias=False),
- nn.GroupNorm(8, planes),
- nn.ReLU(inplace=True))
-
- self.conv2 = nn.Sequential(
- nn.Conv2d(planes, planes, kernel_size=3, stride=1,
- padding=1, bias=False, dilation=1),
- nn.GroupNorm(8, planes),
- nn.ReLU(inplace=True))
-
- self.conv3 = nn.Sequential(
- nn.Conv2d(planes, planes * 4, kernel_size=1, bias=False),
- nn.GroupNorm(8, planes * 4))
-
- self.relu = nn.ReLU(inplace=True)
-
- self.down_sample = nn.Sequential(
- nn.Conv2d(inplanes, planes * 4,
- kernel_size=1, stride=1, bias=False),
- nn.GroupNorm(8, planes * 4))
-
- def forward(self, x):
- # residual = x
-
- out = self.conv1(x)
- out = self.conv2(out)
- out = self.conv3(out)
- residual = self.down_sample(x)
- out += residual
- out = self.relu(out)
-
- return out
-
-
-class Res_block_2(nn.Module):
- expansion = 4
-
- def __init__(self, inplanes=256, planes=64, stride=1, dilation=1):
- super(Res_block_2, self).__init__()
-
- self.conv1 = nn.Sequential(
- nn.Conv2d(inplanes, planes, kernel_size=1, stride=1, bias=False),
- nn.GroupNorm(8, planes),
- nn.ReLU(inplace=True))
-
- self.conv2 = nn.Sequential(
- nn.Conv2d(planes, planes, kernel_size=3, stride=1,
- padding=1, bias=False, dilation=1),
- nn.GroupNorm(8, planes),
- nn.ReLU(inplace=True))
-
- self.conv3 = nn.Sequential(
- nn.Conv2d(planes, planes * 4, kernel_size=1, bias=False),
- nn.GroupNorm(8, planes * 4))
-
- self.relu = nn.ReLU(inplace=True)
-
- def forward(self, x):
- residual = x
-
- out = self.conv1(x)
- out = self.conv2(out)
- out = self.conv3(out)
-
- out += residual
- out = self.relu(out)
-
- return out
-
-
-class Res_block_3(nn.Module):
- expansion = 4
-
- def __init__(self, inplanes=256, planes=64, stride=1, dilation=1):
- super(Res_block_3, self).__init__()
-
- self.conv1 = nn.Sequential(
- nn.Conv2d(inplanes, planes, kernel_size=1, stride=stride, bias=False),
- nn.GroupNorm(8, planes),
- nn.ReLU(inplace=True))
-
- self.conv2 = nn.Sequential(
- nn.Conv2d(planes, planes, kernel_size=3, stride=1,
- padding=1, bias=False, dilation=1),
- nn.GroupNorm(8, planes),
- nn.ReLU(inplace=True))
-
- self.conv3 = nn.Sequential(
- nn.Conv2d(planes, planes * 4, kernel_size=1, bias=False),
- nn.GroupNorm(8, planes * 4))
-
- self.relu = nn.ReLU(inplace=True)
-
- self.downsample = nn.Sequential(
- nn.Conv2d(inplanes, planes * 4,
- kernel_size=1, stride=stride, bias=False),
- nn.GroupNorm(8, planes * 4))
-
- def forward(self, x):
- # residual = x
-
- out = self.conv1(x)
- out = self.conv2(out)
- out = self.conv3(out)
- # residual = self.downsample(x)
- out += self.downsample(x)
- out = self.relu(out)
-
- return out
-
-
-class Classifier_Module(nn.Module):
-
- def __init__(self, dilation_series, padding_series, num_classes):
- super(Classifier_Module, self).__init__()
- self.conv2d_list = nn.ModuleList()
- for dilation, padding in zip(dilation_series, padding_series):
- self.conv2d_list.append(
- nn.Conv2d(2048, num_classes, kernel_size=3, stride=1, padding=padding, dilation=dilation, bias=True))
-
- for m in self.conv2d_list:
- m.weight.data.normal_(0, 0.01)
-
- def forward(self, x):
- out = self.conv2d_list[0](x)
- for i in range(len(self.conv2d_list) - 1):
- out += self.conv2d_list[i + 1](x)
- return out
-
-
-class _ConvBNReLU(nn.Module):
- def __init__(self, in_channels, out_channels, kernel_size, stride=1, padding=0,
- dilation=1, groups=1, relu6=False, norm_layer=nn.BatchNorm2d):
- super(_ConvBNReLU, self).__init__()
- self.conv = nn.Conv2d(in_channels, out_channels, kernel_size, stride, padding, dilation, groups, bias=False)
- self.bn = norm_layer(out_channels)
- self.relu = nn.ReLU6(True) if relu6 else nn.ReLU(True)
-
- def forward(self, x):
- x = self.conv(x)
- x = self.bn(x)
- x = self.relu(x)
- return x
-
-
-class _ASPPConv(nn.Module):
- def __init__(self, in_channels, out_channels, atrous_rate, norm_layer):
- super(_ASPPConv, self).__init__()
- self.block = nn.Sequential(
- nn.Conv2d(in_channels, out_channels, 3, padding=atrous_rate, dilation=atrous_rate, bias=False),
- norm_layer(out_channels),
- nn.ReLU(True)
- )
-
- def forward(self, x):
- return self.block(x)
-
-
-class _AsppPooling(nn.Module):
- def __init__(self, in_channels, out_channels, norm_layer):
- super(_AsppPooling, self).__init__()
- self.gap = nn.Sequential(
- nn.AdaptiveAvgPool2d(1),
- nn.Conv2d(in_channels, out_channels, 1, bias=False),
- norm_layer(out_channels),
- nn.ReLU(True)
- )
-
- def forward(self, x):
- size = x.size()[2:]
- pool = self.gap(x)
- out = F.interpolate(pool, size, mode='bilinear', align_corners=True)
- return out
-
-
-class _ASPP(nn.Module):
- def __init__(self, in_channels, atrous_rates, norm_layer):
- super(_ASPP, self).__init__()
- out_channels = 256
- self.b0 = nn.Sequential(
- nn.Conv2d(in_channels, out_channels, 1, bias=False),
- norm_layer(out_channels),
- nn.ReLU(True)
- )
-
- rate1, rate2, rate3 = tuple(atrous_rates)
- self.b1 = _ASPPConv(in_channels, out_channels, rate1, norm_layer)
- self.b2 = _ASPPConv(in_channels, out_channels, rate2, norm_layer)
- self.b3 = _ASPPConv(in_channels, out_channels, rate3, norm_layer)
- self.b4 = _AsppPooling(in_channels, out_channels, norm_layer=norm_layer)
-
- self.project = nn.Sequential(
- nn.Conv2d(5 * out_channels, out_channels, 1, bias=False),
- norm_layer(out_channels),
- nn.ReLU(True),
- nn.Dropout(0.5)
- )
-
- def forward(self, x):
- feat1 = self.b0(x)
- feat2 = self.b1(x)
- feat3 = self.b2(x)
- feat4 = self.b3(x)
- feat5 = self.b4(x)
- x = torch.cat((feat1, feat2, feat3, feat4, feat5), dim=1)
- x = self.project(x)
- return x
-
-
-class _DeepLabHead(nn.Module):
- def __init__(self, num_classes, c1_channels=256, norm_layer=nn.BatchNorm2d):
- super(_DeepLabHead, self).__init__()
- self.aspp = _ASPP(2048, [12, 24, 36], norm_layer=norm_layer)
- self.c1_block = _ConvBNReLU(c1_channels, 48, 3, padding=1, norm_layer=norm_layer)
- self.block = nn.Sequential(
- _ConvBNReLU(304, 256, 3, padding=1, norm_layer=norm_layer),
- nn.Dropout(0.5),
- _ConvBNReLU(256, 256, 3, padding=1, norm_layer=norm_layer),
- nn.Dropout(0.1),
- nn.Conv2d(256, num_classes, 1))
-
- def forward(self, x, c1):
- size = c1.size()[2:]
- c1 = self.c1_block(c1)
- x = self.aspp(x)
- x = F.interpolate(x, size, mode='bilinear', align_corners=True)
- return self.block(torch.cat([x, c1], dim=1))
-
-
-class _CARM(nn.Module):
- def __init__(self, in_planes, ratio=8):
- super(_CARM, self).__init__()
- self.avg_pool = nn.AdaptiveAvgPool2d(1)
- self.max_pool = nn.AdaptiveMaxPool2d(1)
-
- self.fc1_1 = nn.Linear(in_planes, in_planes // ratio)
- self.fc1_2 = nn.Linear(in_planes // ratio, in_planes)
-
- self.fc2_1 = nn.Linear(in_planes, in_planes // ratio)
- self.fc2_2 = nn.Linear(in_planes // ratio, in_planes)
- self.relu = nn.ReLU(True)
-
- self.sigmoid = nn.Sigmoid()
-
- def forward(self, x):
- avg_out = self.avg_pool(x)
- avg_out = avg_out.view(avg_out.size(0), -1)
- avg_out = self.fc1_2(self.relu(self.fc1_1(avg_out)))
-
- max_out = self.max_pool(x)
- max_out = max_out.view(max_out.size(0), -1)
- max_out = self.fc2_2(self.relu(self.fc2_1(max_out)))
-
- max_out_size = max_out.size()[1]
- avg_out = torch.reshape(avg_out, (-1, max_out_size, 1, 1))
- max_out = torch.reshape(max_out, (-1, max_out_size, 1, 1))
-
- out = self.sigmoid(avg_out + max_out)
-
- x = out * x
- return x
-
-
-class FSFB_CH(nn.Module):
- def __init__(self, in_planes, num, ratio=8):
- super(FSFB_CH, self).__init__()
- self.avg_pool = nn.AdaptiveAvgPool2d(1)
- self.max_pool = nn.AdaptiveMaxPool2d(1)
-
- self.fc1_1 = nn.Linear(in_planes, in_planes // ratio)
- self.fc1_2 = nn.Linear(in_planes // ratio, num * in_planes)
-
- self.fc2_1 = nn.Linear(in_planes, in_planes // ratio)
- self.fc2_2 = nn.Linear(in_planes // ratio, num * in_planes)
- self.relu = nn.ReLU(True)
-
- self.fc3 = nn.Linear(num * in_planes, 2 * num * in_planes)
- self.fc4 = nn.Linear(2 * num * in_planes, 2 * num * in_planes)
- self.fc5 = nn.Linear(2 * num * in_planes, num * in_planes)
-
- self.softmax = nn.Softmax(dim=3)
-
- def forward(self, x, num):
- avg_out = self.avg_pool(x)
- avg_out = avg_out.view(avg_out.size(0), -1)
- avg_out = self.fc1_2(self.relu(self.fc1_1(avg_out)))
-
- max_out = self.max_pool(x)
- max_out = max_out.view(max_out.size(0), -1)
- max_out = self.fc2_2(self.relu(self.fc2_1(max_out)))
-
- out = avg_out + max_out
- out = self.relu(self.fc3(out))
- out = self.relu(self.fc4(out))
- out = self.relu(self.fc5(out)) # (N, num*in_planes)
-
- out_size = out.size()[1]
- out = torch.reshape(out, (-1, out_size // num, 1, num)) # (N, in_planes, 1, num )
- out = self.softmax(out)
-
- channel_scale = torch.chunk(out, num, dim=3) # (N, in_planes, 1, 1 )
-
- return channel_scale
-
-
-class FSFB_SP(nn.Module):
- def __init__(self, num, norm_layer=nn.BatchNorm2d):
- super(FSFB_SP, self).__init__()
- self.conv = nn.Sequential(
- nn.Conv2d(2, 2 * num, kernel_size=3, padding=1, bias=False),
- norm_layer(2 * num),
- nn.ReLU(True),
- nn.Conv2d(2 * num, 4 * num, kernel_size=3, padding=1, bias=False),
- norm_layer(4 * num),
- nn.ReLU(True),
- nn.Conv2d(4 * num, 4 * num, kernel_size=3, padding=1, bias=False),
- norm_layer(4 * num),
- nn.ReLU(True),
- nn.Conv2d(4 * num, 2 * num, kernel_size=3, padding=1, bias=False),
- norm_layer(2 * num),
- nn.ReLU(True),
- nn.Conv2d(2 * num, num, kernel_size=3, padding=1, bias=False)
- )
- self.softmax = nn.Softmax(dim=1)
-
- def forward(self, x, num):
- avg_out = torch.mean(x, dim=1, keepdim=True)
- max_out, _ = torch.max(x, dim=1, keepdim=True)
- x = torch.cat([avg_out, max_out], dim=1)
- x = self.conv(x)
- x = self.softmax(x)
- spatial_scale = torch.chunk(x, num, dim=1)
- return spatial_scale
-
-
-##################################################################################################################
-
-
-class _HFFM(nn.Module):
- def __init__(self, in_channels, atrous_rates, norm_layer=nn.BatchNorm2d):
- super(_HFFM, self).__init__()
- out_channels = 256
- self.b0 = nn.Sequential(
- nn.Conv2d(in_channels, out_channels, 1, bias=False),
- norm_layer(out_channels),
- nn.ReLU(True)
- )
-
- rate1, rate2, rate3 = tuple(atrous_rates)
- self.b1 = _ASPPConv(in_channels, out_channels, rate1, norm_layer)
- self.b2 = _ASPPConv(in_channels, out_channels, rate2, norm_layer)
- self.b3 = _ASPPConv(in_channels, out_channels, rate3, norm_layer)
- self.b4 = _AsppPooling(in_channels, out_channels, norm_layer=norm_layer)
- self.carm = _CARM(in_channels)
- self.sa = FSFB_SP(4, norm_layer)
- self.ca = FSFB_CH(out_channels, 4, 8)
-
- def forward(self, x, num):
- x = self.carm(x)
- # feat1 = self.b0(x)
- feat1 = self.b1(x)
- feat2 = self.b2(x)
- feat3 = self.b3(x)
- feat4 = self.b4(x)
- feat = feat1 + feat2 + feat3 + feat4
- spatial_atten = self.sa(feat, num)
- channel_atten = self.ca(feat, num)
-
- feat_ca = channel_atten[0] * feat1 + channel_atten[1] * feat2 + channel_atten[2] * feat3 + channel_atten[
- 3] * feat4
- feat_sa = spatial_atten[0] * feat1 + spatial_atten[1] * feat2 + spatial_atten[2] * feat3 + spatial_atten[
- 3] * feat4
- feat_sa = feat_sa + feat_ca
-
- return feat_sa
-
-
-class _AFFM(nn.Module):
- def __init__(self, in_channels=256, norm_layer=nn.BatchNorm2d):
- super(_AFFM, self).__init__()
-
- self.sa = FSFB_SP(2, norm_layer)
- self.ca = FSFB_CH(in_channels, 2, 8)
- self.carm = _CARM(in_channels)
-
- def forward(self, feat1, feat2, hffm, num):
- feat = feat1 + feat2
- spatial_atten = self.sa(feat, num)
- channel_atten = self.ca(feat, num)
-
- feat_ca = channel_atten[0] * feat1 + channel_atten[1] * feat2
- feat_sa = spatial_atten[0] * feat1 + spatial_atten[1] * feat2
- output = self.carm(feat_sa + feat_ca + hffm)
- # output = self.carm (feat_sa + hffm)
-
- return output, channel_atten, spatial_atten
-
-
-class block_Conv3x3(nn.Module):
- def __init__(self, in_channels):
- super(block_Conv3x3, self).__init__()
- self.block = nn.Sequential(
- nn.Conv2d(in_channels, 256, kernel_size=3, stride=1, padding=1, bias=False),
- nn.BatchNorm2d(256),
- nn.ReLU(True)
- )
-
- def forward(self, x):
- return self.block(x)
-
-
-class CDnetV2(nn.Module):
- def __init__(self, block=Bottleneck, layers=[3, 4, 6, 3], num_classes=21, aux=True):
- self.inplanes = 256 # change
- self.aux = aux
- super().__init__()
- # self.conv1 = nn.Conv2d(3, 64, kernel_size=7, stride=2, padding=3, bias=False)
- # self.bn1 = nn.BatchNorm2d(64, affine = affine_par)
-
- self.conv1 = nn.Conv2d(3, 64, kernel_size=3, stride=2, padding=1, bias=False)
- self.bn1 = nn.BatchNorm2d(64, affine=affine_par)
-
- self.conv2 = nn.Conv2d(64, 64, kernel_size=3, stride=1, padding=1, bias=False)
- self.bn2 = nn.BatchNorm2d(64, affine=affine_par)
-
- self.conv3 = nn.Conv2d(64, 64, kernel_size=3, stride=1, padding=1, bias=False)
- self.bn3 = nn.BatchNorm2d(64, affine=affine_par)
-
- self.relu = nn.ReLU(inplace=True)
-
- self.dropout = nn.Dropout(0.3)
- for i in self.bn1.parameters():
- i.requires_grad = False
-
- self.maxpool = nn.MaxPool2d(kernel_size=3, stride=2, padding=1, ceil_mode=True) # change
-
- # self.layer1 = self._make_layer(block, 64, layers[0])
-
- self.layerx_1 = Res_block_1(64, 64, stride=1, dilation=1)
- self.layerx_2 = Res_block_2(256, 64, stride=1, dilation=1)
- self.layerx_3 = Res_block_3(256, 64, stride=2, dilation=1)
-
- self.layer2 = self._make_layer(block, 128, layers[1], stride=2)
- self.layer3 = self._make_layer(block, 256, layers[2], stride=1, dilation=2)
- self.layer4 = self._make_layer(block, 512, layers[3], stride=1, dilation=4)
- # self.layer5 = self._make_pred_layer(Classifier_Module, [6,12,18,24],[6,12,18,24],num_classes)
-
- self.hffm = _HFFM(2048, [6, 12, 18])
- self.affm_1 = _AFFM()
- self.affm_2 = _AFFM()
- self.affm_3 = _AFFM()
- self.affm_4 = _AFFM()
- self.carm = _CARM(256)
-
- self.con_layer1_1 = block_Conv3x3(256)
- self.con_res2 = block_Conv3x3(256)
- self.con_res3 = block_Conv3x3(512)
- self.con_res4 = block_Conv3x3(1024)
- self.con_res5 = block_Conv3x3(2048)
-
- self.dsn1 = nn.Sequential(
- nn.Conv2d(256, num_classes, kernel_size=1, stride=1, padding=0)
- )
-
- self.dsn2 = nn.Sequential(
- nn.Conv2d(256, num_classes, kernel_size=1, stride=1, padding=0)
- )
-
- for m in self.modules():
- if isinstance(m, nn.Conv2d):
- n = m.kernel_size[0] * m.kernel_size[1] * m.out_channels
- m.weight.data.normal_(0, 0.01)
- elif isinstance(m, nn.BatchNorm2d):
- m.weight.data.fill_(1)
- m.bias.data.zero_()
- # for i in m.parameters():
- # i.requires_grad = False
-
- # self.inplanes = 256 # change
-
- def _make_layer(self, block, planes, blocks, stride=1, dilation=1):
- downsample = None
- if stride != 1 or self.inplanes != planes * block.expansion or dilation == 2 or dilation == 4:
- downsample = nn.Sequential(
- nn.Conv2d(self.inplanes, planes * block.expansion,
- kernel_size=1, stride=stride, bias=False),
- nn.BatchNorm2d(planes * block.expansion, affine=affine_par))
- for i in downsample._modules['1'].parameters():
- i.requires_grad = False
- layers = []
- layers.append(block(self.inplanes, planes, stride, dilation=dilation, downsample=downsample))
- self.inplanes = planes * block.expansion
- for i in range(1, blocks):
- layers.append(block(self.inplanes, planes, dilation=dilation))
-
- return nn.Sequential(*layers)
-
- # def _make_pred_layer(self,block, dilation_series, padding_series,num_classes):
- # return block(dilation_series,padding_series,num_classes)
-
- def base_forward(self, x):
- x = self.relu(self.bn1(self.conv1(x))) # 1/2
- x = self.relu(self.bn2(self.conv2(x)))
- x = self.relu(self.bn3(self.conv3(x)))
- x = self.maxpool(x) # 1/4
-
- # x = self.layer1(x) # 1/8
-
- # layer1
- x = self.layerx_1(x) # 1/4
- layer1_0 = x
-
- x = self.layerx_2(x) # 1/4
- layer1_0 = self.con_layer1_1(x + layer1_0) # 256
- size_layer1_0 = layer1_0.size()[2:]
-
- x = self.layerx_3(x) # 1/8
- res2 = self.con_res2(x) # 256
- size_res2 = res2.size()[2:]
-
- # layer2-4
- x = self.layer2(x) # 1/16
- res3 = self.con_res3(x) # 256
- x = self.layer3(x) # 1/16
-
- res4 = self.con_res4(x) # 256
- x = self.layer4(x) # 1/16
- res5 = self.con_res5(x) # 256
-
- # x = self.res5_con1x1(torch.cat([x, res4], dim=1))
- return layer1_0, res2, res3, res4, res5, x, size_layer1_0, size_res2
-
- # return res2, res3, res4, res5, x, layer_1024, size_res2
-
- def forward(self, x):
- # size = x.size()[2:]
- layer1_0, res2, res3, res4, res5, layer4, size_layer1_0, size_res2 = self.base_forward(x)
-
- hffm = self.hffm(layer4, 4) # 256 HFFM
- res5 = res5 + hffm
- aux_feature = res5 # loss_aux
- # res5 = self.carm(res5)
- res5, _, _ = self.affm_1(res4, res5, hffm, 2) # 1/16
- # aux_feature = res5
- res5, _, _ = self.affm_2(res3, res5, hffm, 2) # 1/16
-
- res5 = F.interpolate(res5, size_res2, mode='bilinear', align_corners=True)
- res5, _, _ = self.affm_3(res2, res5, F.interpolate(hffm, size_res2, mode='bilinear', align_corners=True), 2)
-
- res5 = F.interpolate(res5, size_layer1_0, mode='bilinear', align_corners=True)
- res5, _, _ = self.affm_4(layer1_0, res5,
- F.interpolate(hffm, size_layer1_0, mode='bilinear', align_corners=True), 2)
-
- output = self.dsn1(res5)
-
- if self.aux:
- auxout = self.dsn2(aux_feature)
- # auxout = F.interpolate(auxout, size, mode='bilinear', align_corners=True)
- # outputs.append(auxout)
- size = x.size()[2:]
- pred, pred_aux = output, auxout
- pred = F.interpolate(pred, size, mode='bilinear', align_corners=True)
- pred_aux = F.interpolate(pred_aux, size, mode='bilinear', align_corners=True)
- return pred, pred_aux
-
-
-if __name__ == '__main__':
- model = CDnetV2(num_classes=3)
- fake_image = torch.rand(2, 3, 256, 256)
- output = model(fake_image)
- for out in output:
- print(out.shape)
- # torch.Size([2, 3, 256, 256])
- # torch.Size([2, 3, 256, 256])
+# -*- coding: utf-8 -*-
+# @Time : 2024/7/24 下午3:41
+# @Author : xiaoshun
+# @Email : 3038523973@qq.com
+# @File : cdnetv2.py
+# @Software: PyCharm
+
+"""Cloud detection Network"""
+
+"""
+This is the implementation of CDnetV2 without multi-scale inputs. This implementation uses ResNet by default.
+"""
+# nn.GroupNorm
+
+import torch
+# import torch.nn as nn
+import torch.nn.functional as F
+from torch import nn
+
+affine_par = True
+
+
+def conv3x3(in_planes, out_planes, stride=1):
+ "3x3 convolution with padding"
+ return nn.Conv2d(in_planes, out_planes, kernel_size=3, stride=stride,
+ padding=1, bias=False)
+
+
+class BasicBlock(nn.Module):
+ expansion = 1
+
+ def __init__(self, inplanes, planes, stride=1, downsample=None):
+ super(BasicBlock, self).__init__()
+ self.conv1 = conv3x3(inplanes, planes, stride)
+ self.bn1 = nn.BatchNorm2d(planes, affine=affine_par)
+ self.relu = nn.ReLU(inplace=True)
+ self.conv2 = conv3x3(planes, planes)
+ self.bn2 = nn.BatchNorm2d(planes, affine=affine_par)
+ self.downsample = downsample
+ self.stride = stride
+
+ def forward(self, x):
+ residual = x
+
+ out = self.conv1(x)
+ out = self.bn1(out)
+ out = self.relu(out)
+
+ out = self.conv2(out)
+ out = self.bn2(out)
+
+ if self.downsample is not None:
+ residual = self.downsample(x)
+
+ out += residual
+ out = self.relu(out)
+
+ return out
+
+
+class Bottleneck(nn.Module):
+ expansion = 4
+
+ def __init__(self, inplanes, planes, stride=1, dilation=1, downsample=None):
+ super(Bottleneck, self).__init__()
+ self.conv1 = nn.Conv2d(inplanes, planes, kernel_size=1, stride=stride, bias=False) # change
+ self.bn1 = nn.BatchNorm2d(planes, affine=affine_par)
+ for i in self.bn1.parameters():
+ i.requires_grad = False
+
+ padding = dilation
+ self.conv2 = nn.Conv2d(planes, planes, kernel_size=3, stride=1, # change
+ padding=padding, bias=False, dilation=dilation)
+ self.bn2 = nn.BatchNorm2d(planes, affine=affine_par)
+ for i in self.bn2.parameters():
+ i.requires_grad = False
+ self.conv3 = nn.Conv2d(planes, planes * 4, kernel_size=1, bias=False)
+ self.bn3 = nn.BatchNorm2d(planes * 4, affine=affine_par)
+ for i in self.bn3.parameters():
+ i.requires_grad = False
+ self.relu = nn.ReLU(inplace=True)
+ self.downsample = downsample
+ self.stride = stride
+
+ def forward(self, x):
+ residual = x
+
+ out = self.conv1(x)
+ out = self.bn1(out)
+ out = self.relu(out)
+
+ out = self.conv2(out)
+ out = self.bn2(out)
+ out = self.relu(out)
+
+ out = self.conv3(out)
+ out = self.bn3(out)
+
+ if self.downsample is not None:
+ residual = self.downsample(x)
+
+ out += residual
+ out = self.relu(out)
+
+ return out
+
+ # self.layerx_1 = Bottleneck_nosample(64, 64, stride=1, dilation=1)
+ # self.layerx_2 = Bottleneck(256, 64, stride=1, dilation=1, downsample=None)
+ # self.layerx_3 = Bottleneck_downsample(256, 64, stride=2, dilation=1)
+
+
+class Res_block_1(nn.Module):
+ expansion = 4
+
+ def __init__(self, inplanes=64, planes=64, stride=1, dilation=1):
+ super(Res_block_1, self).__init__()
+
+ self.conv1 = nn.Sequential(
+ nn.Conv2d(inplanes, planes, kernel_size=1, stride=1, bias=False),
+ nn.GroupNorm(8, planes),
+ nn.ReLU(inplace=True))
+
+ self.conv2 = nn.Sequential(
+ nn.Conv2d(planes, planes, kernel_size=3, stride=1,
+ padding=1, bias=False, dilation=1),
+ nn.GroupNorm(8, planes),
+ nn.ReLU(inplace=True))
+
+ self.conv3 = nn.Sequential(
+ nn.Conv2d(planes, planes * 4, kernel_size=1, bias=False),
+ nn.GroupNorm(8, planes * 4))
+
+ self.relu = nn.ReLU(inplace=True)
+
+ self.down_sample = nn.Sequential(
+ nn.Conv2d(inplanes, planes * 4,
+ kernel_size=1, stride=1, bias=False),
+ nn.GroupNorm(8, planes * 4))
+
+ def forward(self, x):
+ # residual = x
+
+ out = self.conv1(x)
+ out = self.conv2(out)
+ out = self.conv3(out)
+ residual = self.down_sample(x)
+ out += residual
+ out = self.relu(out)
+
+ return out
+
+
+class Res_block_2(nn.Module):
+ expansion = 4
+
+ def __init__(self, inplanes=256, planes=64, stride=1, dilation=1):
+ super(Res_block_2, self).__init__()
+
+ self.conv1 = nn.Sequential(
+ nn.Conv2d(inplanes, planes, kernel_size=1, stride=1, bias=False),
+ nn.GroupNorm(8, planes),
+ nn.ReLU(inplace=True))
+
+ self.conv2 = nn.Sequential(
+ nn.Conv2d(planes, planes, kernel_size=3, stride=1,
+ padding=1, bias=False, dilation=1),
+ nn.GroupNorm(8, planes),
+ nn.ReLU(inplace=True))
+
+ self.conv3 = nn.Sequential(
+ nn.Conv2d(planes, planes * 4, kernel_size=1, bias=False),
+ nn.GroupNorm(8, planes * 4))
+
+ self.relu = nn.ReLU(inplace=True)
+
+ def forward(self, x):
+ residual = x
+
+ out = self.conv1(x)
+ out = self.conv2(out)
+ out = self.conv3(out)
+
+ out += residual
+ out = self.relu(out)
+
+ return out
+
+
+class Res_block_3(nn.Module):
+ expansion = 4
+
+ def __init__(self, inplanes=256, planes=64, stride=1, dilation=1):
+ super(Res_block_3, self).__init__()
+
+ self.conv1 = nn.Sequential(
+ nn.Conv2d(inplanes, planes, kernel_size=1, stride=stride, bias=False),
+ nn.GroupNorm(8, planes),
+ nn.ReLU(inplace=True))
+
+ self.conv2 = nn.Sequential(
+ nn.Conv2d(planes, planes, kernel_size=3, stride=1,
+ padding=1, bias=False, dilation=1),
+ nn.GroupNorm(8, planes),
+ nn.ReLU(inplace=True))
+
+ self.conv3 = nn.Sequential(
+ nn.Conv2d(planes, planes * 4, kernel_size=1, bias=False),
+ nn.GroupNorm(8, planes * 4))
+
+ self.relu = nn.ReLU(inplace=True)
+
+ self.downsample = nn.Sequential(
+ nn.Conv2d(inplanes, planes * 4,
+ kernel_size=1, stride=stride, bias=False),
+ nn.GroupNorm(8, planes * 4))
+
+ def forward(self, x):
+ # residual = x
+
+ out = self.conv1(x)
+ out = self.conv2(out)
+ out = self.conv3(out)
+ # residual = self.downsample(x)
+ out += self.downsample(x)
+ out = self.relu(out)
+
+ return out
+
+
+class Classifier_Module(nn.Module):
+
+ def __init__(self, dilation_series, padding_series, num_classes):
+ super(Classifier_Module, self).__init__()
+ self.conv2d_list = nn.ModuleList()
+ for dilation, padding in zip(dilation_series, padding_series):
+ self.conv2d_list.append(
+ nn.Conv2d(2048, num_classes, kernel_size=3, stride=1, padding=padding, dilation=dilation, bias=True))
+
+ for m in self.conv2d_list:
+ m.weight.data.normal_(0, 0.01)
+
+ def forward(self, x):
+ out = self.conv2d_list[0](x)
+ for i in range(len(self.conv2d_list) - 1):
+ out += self.conv2d_list[i + 1](x)
+ return out
+
+
+class _ConvBNReLU(nn.Module):
+ def __init__(self, in_channels, out_channels, kernel_size, stride=1, padding=0,
+ dilation=1, groups=1, relu6=False, norm_layer=nn.BatchNorm2d):
+ super(_ConvBNReLU, self).__init__()
+ self.conv = nn.Conv2d(in_channels, out_channels, kernel_size, stride, padding, dilation, groups, bias=False)
+ self.bn = norm_layer(out_channels)
+ self.relu = nn.ReLU6(True) if relu6 else nn.ReLU(True)
+
+ def forward(self, x):
+ x = self.conv(x)
+ x = self.bn(x)
+ x = self.relu(x)
+ return x
+
+
+class _ASPPConv(nn.Module):
+ def __init__(self, in_channels, out_channels, atrous_rate, norm_layer):
+ super(_ASPPConv, self).__init__()
+ self.block = nn.Sequential(
+ nn.Conv2d(in_channels, out_channels, 3, padding=atrous_rate, dilation=atrous_rate, bias=False),
+ norm_layer(out_channels),
+ nn.ReLU(True)
+ )
+
+ def forward(self, x):
+ return self.block(x)
+
+
+class _AsppPooling(nn.Module):
+ def __init__(self, in_channels, out_channels, norm_layer):
+ super(_AsppPooling, self).__init__()
+ self.gap = nn.Sequential(
+ nn.AdaptiveAvgPool2d(1),
+ nn.Conv2d(in_channels, out_channels, 1, bias=False),
+ norm_layer(out_channels),
+ nn.ReLU(True)
+ )
+
+ def forward(self, x):
+ size = x.size()[2:]
+ pool = self.gap(x)
+ out = F.interpolate(pool, size, mode='bilinear', align_corners=True)
+ return out
+
+
+class _ASPP(nn.Module):
+ def __init__(self, in_channels, atrous_rates, norm_layer):
+ super(_ASPP, self).__init__()
+ out_channels = 256
+ self.b0 = nn.Sequential(
+ nn.Conv2d(in_channels, out_channels, 1, bias=False),
+ norm_layer(out_channels),
+ nn.ReLU(True)
+ )
+
+ rate1, rate2, rate3 = tuple(atrous_rates)
+ self.b1 = _ASPPConv(in_channels, out_channels, rate1, norm_layer)
+ self.b2 = _ASPPConv(in_channels, out_channels, rate2, norm_layer)
+ self.b3 = _ASPPConv(in_channels, out_channels, rate3, norm_layer)
+ self.b4 = _AsppPooling(in_channels, out_channels, norm_layer=norm_layer)
+
+ self.project = nn.Sequential(
+ nn.Conv2d(5 * out_channels, out_channels, 1, bias=False),
+ norm_layer(out_channels),
+ nn.ReLU(True),
+ nn.Dropout(0.5)
+ )
+
+ def forward(self, x):
+ feat1 = self.b0(x)
+ feat2 = self.b1(x)
+ feat3 = self.b2(x)
+ feat4 = self.b3(x)
+ feat5 = self.b4(x)
+ x = torch.cat((feat1, feat2, feat3, feat4, feat5), dim=1)
+ x = self.project(x)
+ return x
+
+
+class _DeepLabHead(nn.Module):
+ def __init__(self, num_classes, c1_channels=256, norm_layer=nn.BatchNorm2d):
+ super(_DeepLabHead, self).__init__()
+ self.aspp = _ASPP(2048, [12, 24, 36], norm_layer=norm_layer)
+ self.c1_block = _ConvBNReLU(c1_channels, 48, 3, padding=1, norm_layer=norm_layer)
+ self.block = nn.Sequential(
+ _ConvBNReLU(304, 256, 3, padding=1, norm_layer=norm_layer),
+ nn.Dropout(0.5),
+ _ConvBNReLU(256, 256, 3, padding=1, norm_layer=norm_layer),
+ nn.Dropout(0.1),
+ nn.Conv2d(256, num_classes, 1))
+
+ def forward(self, x, c1):
+ size = c1.size()[2:]
+ c1 = self.c1_block(c1)
+ x = self.aspp(x)
+ x = F.interpolate(x, size, mode='bilinear', align_corners=True)
+ return self.block(torch.cat([x, c1], dim=1))
+
+
+class _CARM(nn.Module):
+ def __init__(self, in_planes, ratio=8):
+ super(_CARM, self).__init__()
+ self.avg_pool = nn.AdaptiveAvgPool2d(1)
+ self.max_pool = nn.AdaptiveMaxPool2d(1)
+
+ self.fc1_1 = nn.Linear(in_planes, in_planes // ratio)
+ self.fc1_2 = nn.Linear(in_planes // ratio, in_planes)
+
+ self.fc2_1 = nn.Linear(in_planes, in_planes // ratio)
+ self.fc2_2 = nn.Linear(in_planes // ratio, in_planes)
+ self.relu = nn.ReLU(True)
+
+ self.sigmoid = nn.Sigmoid()
+
+ def forward(self, x):
+ avg_out = self.avg_pool(x)
+ avg_out = avg_out.view(avg_out.size(0), -1)
+ avg_out = self.fc1_2(self.relu(self.fc1_1(avg_out)))
+
+ max_out = self.max_pool(x)
+ max_out = max_out.view(max_out.size(0), -1)
+ max_out = self.fc2_2(self.relu(self.fc2_1(max_out)))
+
+ max_out_size = max_out.size()[1]
+ avg_out = torch.reshape(avg_out, (-1, max_out_size, 1, 1))
+ max_out = torch.reshape(max_out, (-1, max_out_size, 1, 1))
+
+ out = self.sigmoid(avg_out + max_out)
+
+ x = out * x
+ return x
+
+
+class FSFB_CH(nn.Module):
+ def __init__(self, in_planes, num, ratio=8):
+ super(FSFB_CH, self).__init__()
+ self.avg_pool = nn.AdaptiveAvgPool2d(1)
+ self.max_pool = nn.AdaptiveMaxPool2d(1)
+
+ self.fc1_1 = nn.Linear(in_planes, in_planes // ratio)
+ self.fc1_2 = nn.Linear(in_planes // ratio, num * in_planes)
+
+ self.fc2_1 = nn.Linear(in_planes, in_planes // ratio)
+ self.fc2_2 = nn.Linear(in_planes // ratio, num * in_planes)
+ self.relu = nn.ReLU(True)
+
+ self.fc3 = nn.Linear(num * in_planes, 2 * num * in_planes)
+ self.fc4 = nn.Linear(2 * num * in_planes, 2 * num * in_planes)
+ self.fc5 = nn.Linear(2 * num * in_planes, num * in_planes)
+
+ self.softmax = nn.Softmax(dim=3)
+
+ def forward(self, x, num):
+ avg_out = self.avg_pool(x)
+ avg_out = avg_out.view(avg_out.size(0), -1)
+ avg_out = self.fc1_2(self.relu(self.fc1_1(avg_out)))
+
+ max_out = self.max_pool(x)
+ max_out = max_out.view(max_out.size(0), -1)
+ max_out = self.fc2_2(self.relu(self.fc2_1(max_out)))
+
+ out = avg_out + max_out
+ out = self.relu(self.fc3(out))
+ out = self.relu(self.fc4(out))
+ out = self.relu(self.fc5(out)) # (N, num*in_planes)
+
+ out_size = out.size()[1]
+ out = torch.reshape(out, (-1, out_size // num, 1, num)) # (N, in_planes, 1, num )
+ out = self.softmax(out)
+
+ channel_scale = torch.chunk(out, num, dim=3) # (N, in_planes, 1, 1 )
+
+ return channel_scale
+
+
+class FSFB_SP(nn.Module):
+ def __init__(self, num, norm_layer=nn.BatchNorm2d):
+ super(FSFB_SP, self).__init__()
+ self.conv = nn.Sequential(
+ nn.Conv2d(2, 2 * num, kernel_size=3, padding=1, bias=False),
+ norm_layer(2 * num),
+ nn.ReLU(True),
+ nn.Conv2d(2 * num, 4 * num, kernel_size=3, padding=1, bias=False),
+ norm_layer(4 * num),
+ nn.ReLU(True),
+ nn.Conv2d(4 * num, 4 * num, kernel_size=3, padding=1, bias=False),
+ norm_layer(4 * num),
+ nn.ReLU(True),
+ nn.Conv2d(4 * num, 2 * num, kernel_size=3, padding=1, bias=False),
+ norm_layer(2 * num),
+ nn.ReLU(True),
+ nn.Conv2d(2 * num, num, kernel_size=3, padding=1, bias=False)
+ )
+ self.softmax = nn.Softmax(dim=1)
+
+ def forward(self, x, num):
+ avg_out = torch.mean(x, dim=1, keepdim=True)
+ max_out, _ = torch.max(x, dim=1, keepdim=True)
+ x = torch.cat([avg_out, max_out], dim=1)
+ x = self.conv(x)
+ x = self.softmax(x)
+ spatial_scale = torch.chunk(x, num, dim=1)
+ return spatial_scale
+
+
+##################################################################################################################
+
+
+class _HFFM(nn.Module):
+ def __init__(self, in_channels, atrous_rates, norm_layer=nn.BatchNorm2d):
+ super(_HFFM, self).__init__()
+ out_channels = 256
+ self.b0 = nn.Sequential(
+ nn.Conv2d(in_channels, out_channels, 1, bias=False),
+ norm_layer(out_channels),
+ nn.ReLU(True)
+ )
+
+ rate1, rate2, rate3 = tuple(atrous_rates)
+ self.b1 = _ASPPConv(in_channels, out_channels, rate1, norm_layer)
+ self.b2 = _ASPPConv(in_channels, out_channels, rate2, norm_layer)
+ self.b3 = _ASPPConv(in_channels, out_channels, rate3, norm_layer)
+ self.b4 = _AsppPooling(in_channels, out_channels, norm_layer=norm_layer)
+ self.carm = _CARM(in_channels)
+ self.sa = FSFB_SP(4, norm_layer)
+ self.ca = FSFB_CH(out_channels, 4, 8)
+
+ def forward(self, x, num):
+ x = self.carm(x)
+ # feat1 = self.b0(x)
+ feat1 = self.b1(x)
+ feat2 = self.b2(x)
+ feat3 = self.b3(x)
+ feat4 = self.b4(x)
+ feat = feat1 + feat2 + feat3 + feat4
+ spatial_atten = self.sa(feat, num)
+ channel_atten = self.ca(feat, num)
+
+ feat_ca = channel_atten[0] * feat1 + channel_atten[1] * feat2 + channel_atten[2] * feat3 + channel_atten[
+ 3] * feat4
+ feat_sa = spatial_atten[0] * feat1 + spatial_atten[1] * feat2 + spatial_atten[2] * feat3 + spatial_atten[
+ 3] * feat4
+ feat_sa = feat_sa + feat_ca
+
+ return feat_sa
+
+
+class _AFFM(nn.Module):
+ def __init__(self, in_channels=256, norm_layer=nn.BatchNorm2d):
+ super(_AFFM, self).__init__()
+
+ self.sa = FSFB_SP(2, norm_layer)
+ self.ca = FSFB_CH(in_channels, 2, 8)
+ self.carm = _CARM(in_channels)
+
+ def forward(self, feat1, feat2, hffm, num):
+ feat = feat1 + feat2
+ spatial_atten = self.sa(feat, num)
+ channel_atten = self.ca(feat, num)
+
+ feat_ca = channel_atten[0] * feat1 + channel_atten[1] * feat2
+ feat_sa = spatial_atten[0] * feat1 + spatial_atten[1] * feat2
+ output = self.carm(feat_sa + feat_ca + hffm)
+ # output = self.carm (feat_sa + hffm)
+
+ return output, channel_atten, spatial_atten
+
+
+class block_Conv3x3(nn.Module):
+ def __init__(self, in_channels):
+ super(block_Conv3x3, self).__init__()
+ self.block = nn.Sequential(
+ nn.Conv2d(in_channels, 256, kernel_size=3, stride=1, padding=1, bias=False),
+ nn.BatchNorm2d(256),
+ nn.ReLU(True)
+ )
+
+ def forward(self, x):
+ return self.block(x)
+
+
+class CDnetV2(nn.Module):
+ def __init__(self, block=Bottleneck, layers=[3, 4, 6, 3], num_classes=21, aux=True):
+ self.inplanes = 256 # change
+ self.aux = aux
+ super().__init__()
+ # self.conv1 = nn.Conv2d(3, 64, kernel_size=7, stride=2, padding=3, bias=False)
+ # self.bn1 = nn.BatchNorm2d(64, affine = affine_par)
+
+ self.conv1 = nn.Conv2d(3, 64, kernel_size=3, stride=2, padding=1, bias=False)
+ self.bn1 = nn.BatchNorm2d(64, affine=affine_par)
+
+ self.conv2 = nn.Conv2d(64, 64, kernel_size=3, stride=1, padding=1, bias=False)
+ self.bn2 = nn.BatchNorm2d(64, affine=affine_par)
+
+ self.conv3 = nn.Conv2d(64, 64, kernel_size=3, stride=1, padding=1, bias=False)
+ self.bn3 = nn.BatchNorm2d(64, affine=affine_par)
+
+ self.relu = nn.ReLU(inplace=True)
+
+ self.dropout = nn.Dropout(0.3)
+ for i in self.bn1.parameters():
+ i.requires_grad = False
+
+ self.maxpool = nn.MaxPool2d(kernel_size=3, stride=2, padding=1, ceil_mode=True) # change
+
+ # self.layer1 = self._make_layer(block, 64, layers[0])
+
+ self.layerx_1 = Res_block_1(64, 64, stride=1, dilation=1)
+ self.layerx_2 = Res_block_2(256, 64, stride=1, dilation=1)
+ self.layerx_3 = Res_block_3(256, 64, stride=2, dilation=1)
+
+ self.layer2 = self._make_layer(block, 128, layers[1], stride=2)
+ self.layer3 = self._make_layer(block, 256, layers[2], stride=1, dilation=2)
+ self.layer4 = self._make_layer(block, 512, layers[3], stride=1, dilation=4)
+ # self.layer5 = self._make_pred_layer(Classifier_Module, [6,12,18,24],[6,12,18,24],num_classes)
+
+ self.hffm = _HFFM(2048, [6, 12, 18])
+ self.affm_1 = _AFFM()
+ self.affm_2 = _AFFM()
+ self.affm_3 = _AFFM()
+ self.affm_4 = _AFFM()
+ self.carm = _CARM(256)
+
+ self.con_layer1_1 = block_Conv3x3(256)
+ self.con_res2 = block_Conv3x3(256)
+ self.con_res3 = block_Conv3x3(512)
+ self.con_res4 = block_Conv3x3(1024)
+ self.con_res5 = block_Conv3x3(2048)
+
+ self.dsn1 = nn.Sequential(
+ nn.Conv2d(256, num_classes, kernel_size=1, stride=1, padding=0)
+ )
+
+ self.dsn2 = nn.Sequential(
+ nn.Conv2d(256, num_classes, kernel_size=1, stride=1, padding=0)
+ )
+
+ for m in self.modules():
+ if isinstance(m, nn.Conv2d):
+ n = m.kernel_size[0] * m.kernel_size[1] * m.out_channels
+ m.weight.data.normal_(0, 0.01)
+ elif isinstance(m, nn.BatchNorm2d):
+ m.weight.data.fill_(1)
+ m.bias.data.zero_()
+ # for i in m.parameters():
+ # i.requires_grad = False
+
+ # self.inplanes = 256 # change
+
+ def _make_layer(self, block, planes, blocks, stride=1, dilation=1):
+ downsample = None
+ if stride != 1 or self.inplanes != planes * block.expansion or dilation == 2 or dilation == 4:
+ downsample = nn.Sequential(
+ nn.Conv2d(self.inplanes, planes * block.expansion,
+ kernel_size=1, stride=stride, bias=False),
+ nn.BatchNorm2d(planes * block.expansion, affine=affine_par))
+ for i in downsample._modules['1'].parameters():
+ i.requires_grad = False
+ layers = []
+ layers.append(block(self.inplanes, planes, stride, dilation=dilation, downsample=downsample))
+ self.inplanes = planes * block.expansion
+ for i in range(1, blocks):
+ layers.append(block(self.inplanes, planes, dilation=dilation))
+
+ return nn.Sequential(*layers)
+
+ # def _make_pred_layer(self,block, dilation_series, padding_series,num_classes):
+ # return block(dilation_series,padding_series,num_classes)
+
+ def base_forward(self, x):
+ x = self.relu(self.bn1(self.conv1(x))) # 1/2
+ x = self.relu(self.bn2(self.conv2(x)))
+ x = self.relu(self.bn3(self.conv3(x)))
+ x = self.maxpool(x) # 1/4
+
+ # x = self.layer1(x) # 1/8
+
+ # layer1
+ x = self.layerx_1(x) # 1/4
+ layer1_0 = x
+
+ x = self.layerx_2(x) # 1/4
+ layer1_0 = self.con_layer1_1(x + layer1_0) # 256
+ size_layer1_0 = layer1_0.size()[2:]
+
+ x = self.layerx_3(x) # 1/8
+ res2 = self.con_res2(x) # 256
+ size_res2 = res2.size()[2:]
+
+ # layer2-4
+ x = self.layer2(x) # 1/16
+ res3 = self.con_res3(x) # 256
+ x = self.layer3(x) # 1/16
+
+ res4 = self.con_res4(x) # 256
+ x = self.layer4(x) # 1/16
+ res5 = self.con_res5(x) # 256
+
+ # x = self.res5_con1x1(torch.cat([x, res4], dim=1))
+ return layer1_0, res2, res3, res4, res5, x, size_layer1_0, size_res2
+
+ # return res2, res3, res4, res5, x, layer_1024, size_res2
+
+ def forward(self, x):
+ # size = x.size()[2:]
+ layer1_0, res2, res3, res4, res5, layer4, size_layer1_0, size_res2 = self.base_forward(x)
+
+ hffm = self.hffm(layer4, 4) # 256 HFFM
+ res5 = res5 + hffm
+ aux_feature = res5 # loss_aux
+ # res5 = self.carm(res5)
+ res5, _, _ = self.affm_1(res4, res5, hffm, 2) # 1/16
+ # aux_feature = res5
+ res5, _, _ = self.affm_2(res3, res5, hffm, 2) # 1/16
+
+ res5 = F.interpolate(res5, size_res2, mode='bilinear', align_corners=True)
+ res5, _, _ = self.affm_3(res2, res5, F.interpolate(hffm, size_res2, mode='bilinear', align_corners=True), 2)
+
+ res5 = F.interpolate(res5, size_layer1_0, mode='bilinear', align_corners=True)
+ res5, _, _ = self.affm_4(layer1_0, res5,
+ F.interpolate(hffm, size_layer1_0, mode='bilinear', align_corners=True), 2)
+
+ output = self.dsn1(res5)
+
+ if self.aux:
+ auxout = self.dsn2(aux_feature)
+ # auxout = F.interpolate(auxout, size, mode='bilinear', align_corners=True)
+ # outputs.append(auxout)
+ size = x.size()[2:]
+ pred, pred_aux = output, auxout
+ pred = F.interpolate(pred, size, mode='bilinear', align_corners=True)
+ pred_aux = F.interpolate(pred_aux, size, mode='bilinear', align_corners=True)
+ return pred, pred_aux
+
+
+if __name__ == '__main__':
+ model = CDnetV2(num_classes=3)
+ fake_image = torch.rand(2, 3, 256, 256)
+ output = model(fake_image)
+ for out in output:
+ print(out.shape)
+ # torch.Size([2, 3, 256, 256])
+ # torch.Size([2, 3, 256, 256])
diff --git a/src/models/components/cnn.py b/src/models/components/cnn.py
index 73c103d61656f384b500ef5c8e6b603914210403..26ed9cf291c1573923cb8903966a590b7966f97e 100644
--- a/src/models/components/cnn.py
+++ b/src/models/components/cnn.py
@@ -1,26 +1,26 @@
-import torch
-from torch import nn
-
-
-class CNN(nn.Module):
- def __init__(self, dim=32):
- super(CNN, self).__init__()
- self.conv1 = nn.Conv2d(1, dim, 5)
- self.conv2 = nn.Conv2d(dim, dim * 2, 5)
- self.fc1 = nn.Linear(dim * 2 * 4 * 4, 10)
-
- def forward(self, x):
- x = torch.relu(self.conv1(x))
- x = torch.max_pool2d(x, 2)
- x = torch.relu(self.conv2(x))
- x = torch.max_pool2d(x, 2)
- x = x.view(-1, x.shape[1] * x.shape[2] * x.shape[3])
- x = self.fc1(x)
- return x
-
-
-if __name__ == "__main__":
- input = torch.randn(2, 1, 28, 28)
- model = CNN()
- output = model(input)
+import torch
+from torch import nn
+
+
+class CNN(nn.Module):
+ def __init__(self, dim=32):
+ super(CNN, self).__init__()
+ self.conv1 = nn.Conv2d(1, dim, 5)
+ self.conv2 = nn.Conv2d(dim, dim * 2, 5)
+ self.fc1 = nn.Linear(dim * 2 * 4 * 4, 10)
+
+ def forward(self, x):
+ x = torch.relu(self.conv1(x))
+ x = torch.max_pool2d(x, 2)
+ x = torch.relu(self.conv2(x))
+ x = torch.max_pool2d(x, 2)
+ x = x.view(-1, x.shape[1] * x.shape[2] * x.shape[3])
+ x = self.fc1(x)
+ return x
+
+
+if __name__ == "__main__":
+ input = torch.randn(2, 1, 28, 28)
+ model = CNN()
+ output = model(input)
assert output.shape == (2, 10)
\ No newline at end of file
diff --git a/src/models/components/dbnet.py b/src/models/components/dbnet.py
index 3d55855f7d0353bccc537fa5c7c13f44dc2929b4..27d8b5d910155faf7bc7c14c1555c23c2f2b5fa9 100644
--- a/src/models/components/dbnet.py
+++ b/src/models/components/dbnet.py
@@ -1,680 +1,680 @@
-# -*- coding: utf-8 -*-
-# @Time : 2024/7/26 上午11:19
-# @Author : xiaoshun
-# @Email : 3038523973@qq.com
-# @File : dbnet.py
-# @Software: PyCharm
-
-import torch
-import torch.nn as nn
-import torch.nn.functional as F
-from einops import rearrange
-
-
-# from models.Transformer.ViT import truncated_normal_
-
-# Decoder细化卷积模块
-class SBR(nn.Module):
- def __init__(self, in_ch):
- super(SBR, self).__init__()
- self.conv1x3 = nn.Sequential(
- nn.Conv2d(in_ch, in_ch, kernel_size=(1, 3), stride=1, padding=(0, 1)),
- nn.BatchNorm2d(in_ch),
- nn.ReLU(True)
- )
- self.conv3x1 = nn.Sequential(
- nn.Conv2d(in_ch, in_ch, kernel_size=(3, 1), stride=1, padding=(1, 0)),
- nn.BatchNorm2d(in_ch),
- nn.ReLU(True)
- )
-
- def forward(self, x):
- out = self.conv3x1(self.conv1x3(x)) # 先进行1x3的卷积,得到结果并将结果再进行3x1的卷积
- return out + x
-
-
-# 下采样卷积模块 stage 1,2,3
-class c_stage123(nn.Module):
- def __init__(self, in_chans, out_chans):
- super().__init__()
- self.stage123 = nn.Sequential(
- nn.Conv2d(in_channels=in_chans, out_channels=out_chans, kernel_size=3, stride=2, padding=1),
- nn.BatchNorm2d(out_chans),
- nn.ReLU(),
- nn.Conv2d(in_channels=out_chans, out_channels=out_chans, kernel_size=3, stride=1, padding=1),
- nn.BatchNorm2d(out_chans),
- nn.ReLU(),
- )
- self.conv1x1_123 = nn.Conv2d(in_channels=in_chans, out_channels=out_chans, kernel_size=1)
- self.maxpool = nn.MaxPool2d(kernel_size=3, stride=2, padding=1)
-
- def forward(self, x):
- stage123 = self.stage123(x) # 3*3卷积,两倍下采样 3*224*224-->64*112*112
- max = self.maxpool(x) # 最大值池化,两倍下采样 3*224*224-->3*112*112
- max = self.conv1x1_123(max) # 1*1卷积 3*112*112-->64*112*112
- stage123 = stage123 + max # 残差结构,广播机制
- return stage123
-
-
-# 下采样卷积模块 stage4,5
-class c_stage45(nn.Module):
- def __init__(self, in_chans, out_chans):
- super().__init__()
- self.stage45 = nn.Sequential(
- nn.Conv2d(in_channels=in_chans, out_channels=out_chans, kernel_size=3, stride=2, padding=1),
- nn.BatchNorm2d(out_chans),
- nn.ReLU(),
- nn.Conv2d(in_channels=out_chans, out_channels=out_chans, kernel_size=3, stride=1, padding=1),
- nn.BatchNorm2d(out_chans),
- nn.ReLU(),
- nn.Conv2d(in_channels=out_chans, out_channels=out_chans, kernel_size=3, stride=1, padding=1),
- nn.BatchNorm2d(out_chans),
- nn.ReLU(),
- )
- self.conv1x1_45 = nn.Conv2d(in_channels=in_chans, out_channels=out_chans, kernel_size=1)
- self.maxpool = nn.MaxPool2d(kernel_size=3, stride=2, padding=1)
-
- def forward(self, x):
- stage45 = self.stage45(x) # 3*3卷积模块 2倍下采样
- max = self.maxpool(x) # 最大值池化,两倍下采样
- max = self.conv1x1_45(max) # 1*1卷积模块 调整通道数
- stage45 = stage45 + max # 残差结构
- return stage45
-
-
-class Identity(nn.Module): # 恒等映射
- def __init__(self):
- super().__init__()
-
- def forward(self, x):
- return x
-
-
-# 轻量卷积模块
-class DepthwiseConv2d(nn.Module): # 用于自注意力机制
- def __init__(self, in_chans, out_chans, kernel_size=1, stride=1, padding=0, dilation=1):
- super().__init__()
- # depthwise conv
- self.depthwise = nn.Conv2d(
- in_channels=in_chans,
- out_channels=in_chans,
- kernel_size=kernel_size,
- stride=stride,
- padding=padding,
- dilation=dilation, # 深层卷积的膨胀率
- groups=in_chans # 指定分组卷积的组数
- )
- # batch norm
- self.bn = nn.BatchNorm2d(num_features=in_chans)
-
- # pointwise conv 逐点卷积
- self.pointwise = nn.Conv2d(
- in_channels=in_chans,
- out_channels=out_chans,
- kernel_size=1
- )
-
- def forward(self, x):
- x = self.depthwise(x)
- x = self.bn(x)
- x = self.pointwise(x)
- return x
-
-
-# residual skip connection 残差跳跃连接
-class Residual(nn.Module):
- def __init__(self, fn):
- super().__init__()
- self.fn = fn
-
- def forward(self, input, **kwargs):
- x = self.fn(input, **kwargs)
- return (x + input)
-
-
-# layer norm plus 层归一化
-class PreNorm(nn.Module): # 代表神经网络层
- def __init__(self, dim, fn):
- super().__init__()
- self.norm = nn.LayerNorm(dim)
- self.fn = fn
-
- def forward(self, input, **kwargs):
- return self.fn(self.norm(input), **kwargs)
-
-
-# FeedForward层使得representation的表达能力更强
-class FeedForward(nn.Module):
- def __init__(self, dim, hidden_dim, dropout=0.):
- super().__init__()
- self.net = nn.Sequential(
- nn.Linear(in_features=dim, out_features=hidden_dim),
- nn.GELU(),
- nn.Dropout(dropout),
- nn.Linear(in_features=hidden_dim, out_features=dim),
- nn.Dropout(dropout)
- )
-
- def forward(self, input):
- return self.net(input)
-
-
-class ConvAttnetion(nn.Module):
- '''
- using the Depth_Separable_Wise Conv2d to produce the q, k, v instead of using Linear Project in ViT
- '''
-
- def __init__(self, dim, img_size, heads=8, dim_head=64, kernel_size=3, q_stride=1, k_stride=1, v_stride=1,
- dropout=0., last_stage=False):
- super().__init__()
- self.last_stage = last_stage
- self.img_size = img_size
- inner_dim = dim_head * heads # 512
- project_out = not (heads == 1 and dim_head == dim)
-
- self.heads = heads
- self.scale = dim_head ** (-0.5)
-
- pad = (kernel_size - q_stride) // 2
-
- self.to_q = DepthwiseConv2d(in_chans=dim, out_chans=inner_dim, kernel_size=kernel_size, stride=q_stride,
- padding=pad) # 自注意力机制
- self.to_k = DepthwiseConv2d(in_chans=dim, out_chans=inner_dim, kernel_size=kernel_size, stride=k_stride,
- padding=pad)
- self.to_v = DepthwiseConv2d(in_chans=dim, out_chans=inner_dim, kernel_size=kernel_size, stride=v_stride,
- padding=pad)
-
- self.to_out = nn.Sequential(
- nn.Linear(
- in_features=inner_dim,
- out_features=dim
- ),
- nn.Dropout(dropout)
- ) if project_out else Identity()
-
- def forward(self, x):
- b, n, c, h = *x.shape, self.heads # * 星号的作用大概是去掉 tuple 属性吧
-
- # print(x.shape)
- # print('+++++++++++++++++++++++++++++++++')
-
- # if语句内容没有使用
- if self.last_stage:
- cls_token = x[:, 0]
- # print(cls_token.shape)
- # print('+++++++++++++++++++++++++++++++++')
- x = x[:, 1:] # 去掉每个数组的第一个元素
-
- cls_token = rearrange(torch.unsqueeze(cls_token, dim=1), 'b n (h d) -> b h n d', h=h)
-
- # rearrange:用于对张量的维度进行重新变换排序,可用于替换pytorch中的reshape,view,transpose和permute等操作
- x = rearrange(x, 'b (l w) n -> b n l w', l=self.img_size, w=self.img_size) # [1, 3136, 64]-->1*64*56*56
- # batch_size,N(通道数),h,w
-
- q = self.to_q(x) # 1*64*56*56-->1*64*56*56
- # print(q.shape)
- # print('++++++++++++++')
- q = rearrange(q, 'b (h d) l w -> b h (l w) d', h=h) # 1*64*56*56-->1*1*3136*64
- # print(q.shape)
- # print('=====================')
- # batch_size,head,h*w,dim_head
-
- k = self.to_k(x) # 操作和q一样
- k = rearrange(k, 'b (h d) l w -> b h (l w) d', h=h)
- # batch_size,head,h*w,dim_head
-
- v = self.to_v(x) ##操作和q一样
- # print(v.shape)
- # print('[[[[[[[[[[[[[[[[[[[[[[[[[[[[')
- v = rearrange(v, 'b (h d) l w -> b h (l w) d', h=h)
- # print(v.shape)
- # print(']]]]]]]]]]]]]]]]]]]]]]]]]]]')
- # batch_size,head,h*w,dim_head
-
- if self.last_stage:
- # print(q.shape)
- # print('================')
- q = torch.cat([cls_token, q], dim=2)
- # print(q.shape)
- # print('++++++++++++++++++')
- v = torch.cat([cls_token, v], dim=2)
- k = torch.cat([cls_token, k], dim=2)
-
- # calculate attention by matmul + scale
- # permute:(batch_size,head,dim_head,h*w
- # print(k.shape)
- # print('++++++++++++++++++++')
- k = k.permute(0, 1, 3, 2) # 1*1*3136*64-->1*1*64*3136
- # print(k.shape)
- # print('====================')
- attention = (q.matmul(k)) # 1*1*3136*3136
- # print(attention.shape)
- # print('--------------------')
- attention = attention * self.scale # 可以得到一个logit的向量,避免出现梯度下降和梯度爆炸
- # print(attention.shape)
- # print('####################')
- # pass a softmax
- attention = F.softmax(attention, dim=-1)
- # print(attention.shape)
- # print('********************')
-
- # matmul v
- # attention.matmul(v):(batch_size,head,h*w,dim_head)
- # permute:(batch_size,h*w,head,dim_head)
- out = (attention.matmul(v)).permute(0, 2, 1, 3).reshape(b, n,
- c) # 1*3136*64 这些操作的目的是将注意力权重和值向量相乘后得到的结果进行重塑,得到一个形状为 (batch size, 序列长度, 值向量或矩阵的维度) 的张量
-
- # linear project
- out = self.to_out(out)
- return out
-
-
-# Reshape Layers
-class Rearrange(nn.Module):
- def __init__(self, string, h, w):
- super().__init__()
- self.string = string
- self.h = h
- self.w = w
-
- def forward(self, input):
-
- if self.string == 'b c h w -> b (h w) c':
- N, C, H, W = input.shape
- # print(input.shape)
- x = torch.reshape(input, shape=(N, -1, self.h * self.w)).permute(0, 2, 1)
- # print(x.shape)
- # print('+++++++++++++++++++')
- if self.string == 'b (h w) c -> b c h w':
- N, _, C = input.shape
- # print(input.shape)
- x = torch.reshape(input, shape=(N, self.h, self.w, -1)).permute(0, 3, 1, 2)
- # print(x.shape)
- # print('=====================')
- return x
-
-
-# Transformer layers
-class Transformer(nn.Module):
- def __init__(self, dim, img_size, depth, heads, dim_head, mlp_dim, dropout=0., last_stage=False):
- super().__init__()
- self.layers = nn.ModuleList([ # 管理子模块,参数注册
- nn.ModuleList([
- PreNorm(dim=dim, fn=ConvAttnetion(dim, img_size, heads=heads, dim_head=dim_head, dropout=dropout,
- last_stage=last_stage)), # 归一化,重参数化
- PreNorm(dim=dim, fn=FeedForward(dim=dim, hidden_dim=mlp_dim, dropout=dropout))
- ]) for _ in range(depth)
- ])
-
- def forward(self, x):
- for attn, ff in self.layers:
- x = x + attn(x)
- x = x + ff(x)
- return x
-
-
-class DBNet(nn.Module): # 最主要的大函数
- def __init__(self, img_size, in_channels, num_classes, dim=64, kernels=[7, 3, 3, 3], strides=[4, 2, 2, 2],
- heads=[1, 3, 6, 6],
- depth=[1, 2, 10, 10], pool='cls', dropout=0., emb_dropout=0., scale_dim=4, ):
- super().__init__()
-
- assert pool in ['cls', 'mean'], f'pool type must be either cls or mean pooling'
- self.pool = pool
- self.dim = dim
-
- # stage1
- # k:7 s:4 in: 1, 64, 56, 56 out: 1, 3136, 64
- self.stage1_conv_embed = nn.Sequential(
- nn.Conv2d( # 1*3*224*224-->[1, 64, 56, 56]
- in_channels=in_channels,
- out_channels=dim,
- kernel_size=kernels[0],
- stride=strides[0],
- padding=2
- ),
- Rearrange('b c h w -> b (h w) c', h=img_size // 4, w=img_size // 4), # [1, 64, 56, 56]-->[1, 3136, 64]
- nn.LayerNorm(dim) # 对每个batch归一化
- )
-
- self.stage1_transformer = nn.Sequential(
- Transformer( #
- dim=dim,
- img_size=img_size // 4,
- depth=depth[0], # Transformer层中的编码器和解码器层数。
- heads=heads[0],
- dim_head=self.dim, # 它是每个注意力头的维度大小,通常是嵌入维度除以头数。
- mlp_dim=dim * scale_dim, # mlp_dim:它是Transformer中前馈神经网络的隐藏层维度大小,通常是嵌入维度乘以一个缩放因子。
- dropout=dropout,
- # last_stage=last_stage #它是一个标志位,用于表示该Transformer层是否是最后一层。
- ),
- Rearrange('b (h w) c -> b c h w', h=img_size // 4, w=img_size // 4)
- )
-
- # stage2
- # k:3 s:2 in: 1, 192, 28, 28 out: 1, 784, 192
- in_channels = dim
- scale = heads[1] // heads[0]
- dim = scale * dim
-
- self.stage2_conv_embed = nn.Sequential(
- nn.Conv2d(
- in_channels=in_channels,
- out_channels=dim,
- kernel_size=kernels[1],
- stride=strides[1],
- padding=1
- ),
- Rearrange('b c h w -> b (h w) c', h=img_size // 8, w=img_size // 8),
- nn.LayerNorm(dim)
- )
-
- self.stage2_transformer = nn.Sequential(
- Transformer(
- dim=dim,
- img_size=img_size // 8,
- depth=depth[1],
- heads=heads[1],
- dim_head=self.dim,
- mlp_dim=dim * scale_dim,
- dropout=dropout
- ),
- Rearrange('b (h w) c -> b c h w', h=img_size // 8, w=img_size // 8)
- )
-
- # stage3
- in_channels = dim
- scale = heads[2] // heads[1]
- dim = scale * dim
-
- self.stage3_conv_embed = nn.Sequential(
- nn.Conv2d(
- in_channels=in_channels,
- out_channels=dim,
- kernel_size=kernels[2],
- stride=strides[2],
- padding=1
- ),
- Rearrange('b c h w -> b (h w) c', h=img_size // 16, w=img_size // 16),
- nn.LayerNorm(dim)
- )
-
- self.stage3_transformer = nn.Sequential(
- Transformer(
- dim=dim,
- img_size=img_size // 16,
- depth=depth[2],
- heads=heads[2],
- dim_head=self.dim,
- mlp_dim=dim * scale_dim,
- dropout=dropout
- ),
- Rearrange('b (h w) c -> b c h w', h=img_size // 16, w=img_size // 16)
- )
-
- # stage4
- in_channels = dim
- scale = heads[3] // heads[2]
- dim = scale * dim
-
- self.stage4_conv_embed = nn.Sequential(
- nn.Conv2d(
- in_channels=in_channels,
- out_channels=dim,
- kernel_size=kernels[3],
- stride=strides[3],
- padding=1
- ),
- Rearrange('b c h w -> b (h w) c', h=img_size // 32, w=img_size // 32),
- nn.LayerNorm(dim)
- )
-
- self.stage4_transformer = nn.Sequential(
- Transformer(
- dim=dim, img_size=img_size // 32,
- depth=depth[3],
- heads=heads[3],
- dim_head=self.dim,
- mlp_dim=dim * scale_dim,
- dropout=dropout,
- ),
- Rearrange('b (h w) c -> b c h w', h=img_size // 32, w=img_size // 32)
- )
-
- ### CNN Branch ###
- self.c_stage1 = c_stage123(in_chans=3, out_chans=64)
- self.c_stage2 = c_stage123(in_chans=64, out_chans=128)
- self.c_stage3 = c_stage123(in_chans=128, out_chans=384)
- self.c_stage4 = c_stage45(in_chans=384, out_chans=512)
- self.c_stage5 = c_stage45(in_chans=512, out_chans=1024)
- self.c_max = nn.MaxPool2d(kernel_size=3, stride=2, padding=1)
- self.up_conv1 = nn.Conv2d(in_channels=192, out_channels=128, kernel_size=1)
- self.up_conv2 = nn.Conv2d(in_channels=384, out_channels=512, kernel_size=1)
-
- ### CTmerge ###
- self.CTmerge1 = nn.Sequential(
- nn.Conv2d(in_channels=128, out_channels=64, kernel_size=3, stride=1, padding=1),
- nn.BatchNorm2d(64),
- nn.ReLU(),
- nn.Conv2d(in_channels=64, out_channels=64, kernel_size=3, stride=1, padding=1),
- nn.BatchNorm2d(64),
- nn.ReLU(),
- )
- self.CTmerge2 = nn.Sequential(
- nn.Conv2d(in_channels=320, out_channels=128, kernel_size=3, stride=1, padding=1),
- nn.BatchNorm2d(128),
- nn.ReLU(),
- nn.Conv2d(in_channels=128, out_channels=128, kernel_size=3, stride=1, padding=1),
- nn.BatchNorm2d(128),
- nn.ReLU(),
- )
- self.CTmerge3 = nn.Sequential(
- nn.Conv2d(in_channels=768, out_channels=512, kernel_size=3, stride=1, padding=1),
- nn.BatchNorm2d(512),
- nn.ReLU(),
- nn.Conv2d(in_channels=512, out_channels=384, kernel_size=3, stride=1, padding=1),
- nn.BatchNorm2d(384),
- nn.ReLU(),
- nn.Conv2d(in_channels=384, out_channels=384, kernel_size=3, stride=1, padding=1),
- nn.BatchNorm2d(384),
- nn.ReLU(),
- )
-
- self.CTmerge4 = nn.Sequential(
- nn.Conv2d(in_channels=896, out_channels=640, kernel_size=3, stride=1, padding=1),
- nn.BatchNorm2d(640),
- nn.ReLU(),
- nn.Conv2d(in_channels=640, out_channels=512, kernel_size=3, stride=1, padding=1),
- nn.BatchNorm2d(512),
- nn.ReLU(),
- nn.Conv2d(in_channels=512, out_channels=512, kernel_size=3, stride=1, padding=1),
- nn.BatchNorm2d(512),
- nn.ReLU(),
- )
-
- # decoder
- self.decoder4 = nn.Sequential(
- DepthwiseConv2d(
- in_chans=1408,
- out_chans=1024,
- kernel_size=3,
- stride=1,
- padding=1
- ),
- DepthwiseConv2d(
- in_chans=1024,
- out_chans=512,
- kernel_size=3,
- stride=1,
- padding=1
- ),
- nn.GELU()
- )
- self.decoder3 = nn.Sequential(
- DepthwiseConv2d(
- in_chans=896,
- out_chans=512,
- kernel_size=3,
- stride=1,
- padding=1
- ),
- DepthwiseConv2d(
- in_chans=512,
- out_chans=384,
- kernel_size=3,
- stride=1,
- padding=1
- ),
- nn.GELU()
- )
-
- self.decoder2 = nn.Sequential(
- DepthwiseConv2d(
- in_chans=576,
- out_chans=256,
- kernel_size=3,
- stride=1,
- padding=1
- ),
- DepthwiseConv2d(
- in_chans=256,
- out_chans=192,
- kernel_size=3,
- stride=1,
- padding=1
- ),
- nn.GELU()
- )
-
- self.decoder1 = nn.Sequential(
- DepthwiseConv2d(
- in_chans=256,
- out_chans=64,
- kernel_size=3,
- stride=1,
- padding=1
- ),
- DepthwiseConv2d(
- in_chans=64,
- out_chans=16,
- kernel_size=3,
- stride=1,
- padding=1
- ),
- nn.GELU()
- )
- self.sbr4 = SBR(512)
- self.sbr3 = SBR(384)
- self.sbr2 = SBR(192)
- self.sbr1 = SBR(16)
-
- self.head = nn.Conv2d(in_channels=16, out_channels=num_classes, kernel_size=1)
-
- def forward(self, input):
- ### encoder ###
- # stage1 = ts1 cat cs1
- # t_s1 = self.t_stage1(input)
- # print(input.shape)
- # print('++++++++++++++++++++++')
-
- t_s1 = self.stage1_conv_embed(input) # 1*3*224*224-->1*3136*64
-
- # print(t_s1.shape)
- # print('======================')
-
- t_s1 = self.stage1_transformer(t_s1) # 1*3136*64-->1*64*56*56
-
- # print(t_s1.shape)
- # print('----------------------')
-
- c_s1 = self.c_stage1(input) # 1*3*224*224-->1*64*112*112
-
- # print(c_s1.shape)
- # print('!!!!!!!!!!!!!!!!!!!!!!!')
-
- stage1 = self.CTmerge1(torch.cat([t_s1, self.c_max(c_s1)], dim=1)) # 1*64*56*56 # 拼接两条分支
-
- # print(stage1.shape)
- # print('[[[[[[[[[[[[[[[[[[[[[[[')
-
- # stage2 = ts2 up cs2
- # t_s2 = self.t_stage2(stage1)
- t_s2 = self.stage2_conv_embed(stage1) # 1*64*56*56-->1*784*192 # stage2_conv_embed是转化为序列操作
-
- # print(t_s2.shape)
- # print('[[[[[[[[[[[[[[[[[[[[[[[')
- t_s2 = self.stage2_transformer(t_s2) # 1*784*192-->1*192*28*28
- # print(t_s2.shape)
- # print('+++++++++++++++++++++++++')
-
- c_s2 = self.c_stage2(c_s1) # 1*64*112*112-->1*128*56*56
- stage2 = self.CTmerge2(
- torch.cat([c_s2, F.interpolate(t_s2, size=c_s2.size()[2:], mode='bilinear', align_corners=True)],
- dim=1)) # mode='bilinear'表示使用双线性插值 1*128*56*56
-
- # stage3 = ts3 cat cs3
- # t_s3 = self.t_stage3(t_s2)
- t_s3 = self.stage3_conv_embed(t_s2) # 1*192*28*28-->1*196*384
- # print(t_s3.shape)
- # print('///////////////////////')
- t_s3 = self.stage3_transformer(t_s3) # 1*196*384-->1*384*14*14
- # print(t_s3.shape)
- # print('....................')
- c_s3 = self.c_stage3(stage2) # 1*128*56*56-->1*384*28*28
- stage3 = self.CTmerge3(torch.cat([t_s3, self.c_max(c_s3)], dim=1)) # 1*384*14*14
-
- # stage4 = ts4 up cs4
- # t_s4 = self.t_stage4(stage3)
- t_s4 = self.stage4_conv_embed(stage3) # 1*384*14*14-->1*49*384
- # print(t_s4.shape)
- # print(';;;;;;;;;;;;;;;;;;;;;;;')
- t_s4 = self.stage4_transformer(t_s4) # 1*49*384-->1*384*7*7
- # print(t_s4.shape)
- # print('::::::::::::::::::::')
-
- c_s4 = self.c_stage4(c_s3) # 1*384*28*28-->1*512*14*14
- stage4 = self.CTmerge4(
- torch.cat([c_s4, F.interpolate(t_s4, size=c_s4.size()[2:], mode='bilinear', align_corners=True)],
- dim=1)) # 1*512*14*14
-
- # cs5
- c_s5 = self.c_stage5(stage4) # 1*512*14*14-->1*1024*7*7
-
- ### decoder ###
- decoder4 = torch.cat([c_s5, t_s4], dim=1) # 1*1408*7*7
- decoder4 = self.decoder4(decoder4) # 1*1408*7*7-->1*512*7*7
- decoder4 = F.interpolate(decoder4, size=c_s3.size()[2:], mode='bilinear',
- align_corners=True) # 1*512*7*7-->1*512*28*28
- decoder4 = self.sbr4(decoder4) # 1*512*28*28
- # print(decoder4.shape)
-
- decoder3 = torch.cat([decoder4, c_s3], dim=1) # 1*896*28*28
- decoder3 = self.decoder3(decoder3) # 1*384*28*28
- decoder3 = F.interpolate(decoder3, size=t_s2.size()[2:], mode='bilinear', align_corners=True) # 1*384*28*28
- decoder3 = self.sbr3(decoder3) # 1*384*28*28
- # print(decoder3.shape)
-
- decoder2 = torch.cat([decoder3, t_s2], dim=1) # 1*576*28*28
- decoder2 = self.decoder2(decoder2) # 1*192*28*28
- decoder2 = F.interpolate(decoder2, size=c_s1.size()[2:], mode='bilinear', align_corners=True) # 1*192*112*112
- decoder2 = self.sbr2(decoder2) # 1*192*112*112
- # print(decoder2.shape)
-
- decoder1 = torch.cat([decoder2, c_s1], dim=1) # 1*256*112*112
- decoder1 = self.decoder1(decoder1) # 1*16*112*112
- # print(decoder1.shape)
- final = F.interpolate(decoder1, size=input.size()[2:], mode='bilinear', align_corners=True) # 1*16*224*224
- # print(final.shape)
- # final = self.sbr1(decoder1)
- # print(final.shape)
- final = self.head(final) # 1*3*224*224
-
- return final
-
-
-if __name__ == '__main__':
- x = torch.rand(1, 3, 224, 224).cuda()
- model = DBNet(img_size=224, in_channels=3, num_classes=7).cuda()
- y = model(x)
- print(y.shape)
- # torch.Size([1, 7, 224, 224])
+# -*- coding: utf-8 -*-
+# @Time : 2024/7/26 上午11:19
+# @Author : xiaoshun
+# @Email : 3038523973@qq.com
+# @File : dbnet.py
+# @Software: PyCharm
+
+import torch
+import torch.nn as nn
+import torch.nn.functional as F
+from einops import rearrange
+
+
+# from models.Transformer.ViT import truncated_normal_
+
+# Decoder细化卷积模块
+class SBR(nn.Module):
+ def __init__(self, in_ch):
+ super(SBR, self).__init__()
+ self.conv1x3 = nn.Sequential(
+ nn.Conv2d(in_ch, in_ch, kernel_size=(1, 3), stride=1, padding=(0, 1)),
+ nn.BatchNorm2d(in_ch),
+ nn.ReLU(True)
+ )
+ self.conv3x1 = nn.Sequential(
+ nn.Conv2d(in_ch, in_ch, kernel_size=(3, 1), stride=1, padding=(1, 0)),
+ nn.BatchNorm2d(in_ch),
+ nn.ReLU(True)
+ )
+
+ def forward(self, x):
+ out = self.conv3x1(self.conv1x3(x)) # 先进行1x3的卷积,得到结果并将结果再进行3x1的卷积
+ return out + x
+
+
+# 下采样卷积模块 stage 1,2,3
+class c_stage123(nn.Module):
+ def __init__(self, in_chans, out_chans):
+ super().__init__()
+ self.stage123 = nn.Sequential(
+ nn.Conv2d(in_channels=in_chans, out_channels=out_chans, kernel_size=3, stride=2, padding=1),
+ nn.BatchNorm2d(out_chans),
+ nn.ReLU(),
+ nn.Conv2d(in_channels=out_chans, out_channels=out_chans, kernel_size=3, stride=1, padding=1),
+ nn.BatchNorm2d(out_chans),
+ nn.ReLU(),
+ )
+ self.conv1x1_123 = nn.Conv2d(in_channels=in_chans, out_channels=out_chans, kernel_size=1)
+ self.maxpool = nn.MaxPool2d(kernel_size=3, stride=2, padding=1)
+
+ def forward(self, x):
+ stage123 = self.stage123(x) # 3*3卷积,两倍下采样 3*224*224-->64*112*112
+ max = self.maxpool(x) # 最大值池化,两倍下采样 3*224*224-->3*112*112
+ max = self.conv1x1_123(max) # 1*1卷积 3*112*112-->64*112*112
+ stage123 = stage123 + max # 残差结构,广播机制
+ return stage123
+
+
+# 下采样卷积模块 stage4,5
+class c_stage45(nn.Module):
+ def __init__(self, in_chans, out_chans):
+ super().__init__()
+ self.stage45 = nn.Sequential(
+ nn.Conv2d(in_channels=in_chans, out_channels=out_chans, kernel_size=3, stride=2, padding=1),
+ nn.BatchNorm2d(out_chans),
+ nn.ReLU(),
+ nn.Conv2d(in_channels=out_chans, out_channels=out_chans, kernel_size=3, stride=1, padding=1),
+ nn.BatchNorm2d(out_chans),
+ nn.ReLU(),
+ nn.Conv2d(in_channels=out_chans, out_channels=out_chans, kernel_size=3, stride=1, padding=1),
+ nn.BatchNorm2d(out_chans),
+ nn.ReLU(),
+ )
+ self.conv1x1_45 = nn.Conv2d(in_channels=in_chans, out_channels=out_chans, kernel_size=1)
+ self.maxpool = nn.MaxPool2d(kernel_size=3, stride=2, padding=1)
+
+ def forward(self, x):
+ stage45 = self.stage45(x) # 3*3卷积模块 2倍下采样
+ max = self.maxpool(x) # 最大值池化,两倍下采样
+ max = self.conv1x1_45(max) # 1*1卷积模块 调整通道数
+ stage45 = stage45 + max # 残差结构
+ return stage45
+
+
+class Identity(nn.Module): # 恒等映射
+ def __init__(self):
+ super().__init__()
+
+ def forward(self, x):
+ return x
+
+
+# 轻量卷积模块
+class DepthwiseConv2d(nn.Module): # 用于自注意力机制
+ def __init__(self, in_chans, out_chans, kernel_size=1, stride=1, padding=0, dilation=1):
+ super().__init__()
+ # depthwise conv
+ self.depthwise = nn.Conv2d(
+ in_channels=in_chans,
+ out_channels=in_chans,
+ kernel_size=kernel_size,
+ stride=stride,
+ padding=padding,
+ dilation=dilation, # 深层卷积的膨胀率
+ groups=in_chans # 指定分组卷积的组数
+ )
+ # batch norm
+ self.bn = nn.BatchNorm2d(num_features=in_chans)
+
+ # pointwise conv 逐点卷积
+ self.pointwise = nn.Conv2d(
+ in_channels=in_chans,
+ out_channels=out_chans,
+ kernel_size=1
+ )
+
+ def forward(self, x):
+ x = self.depthwise(x)
+ x = self.bn(x)
+ x = self.pointwise(x)
+ return x
+
+
+# residual skip connection 残差跳跃连接
+class Residual(nn.Module):
+ def __init__(self, fn):
+ super().__init__()
+ self.fn = fn
+
+ def forward(self, input, **kwargs):
+ x = self.fn(input, **kwargs)
+ return (x + input)
+
+
+# layer norm plus 层归一化
+class PreNorm(nn.Module): # 代表神经网络层
+ def __init__(self, dim, fn):
+ super().__init__()
+ self.norm = nn.LayerNorm(dim)
+ self.fn = fn
+
+ def forward(self, input, **kwargs):
+ return self.fn(self.norm(input), **kwargs)
+
+
+# FeedForward层使得representation的表达能力更强
+class FeedForward(nn.Module):
+ def __init__(self, dim, hidden_dim, dropout=0.):
+ super().__init__()
+ self.net = nn.Sequential(
+ nn.Linear(in_features=dim, out_features=hidden_dim),
+ nn.GELU(),
+ nn.Dropout(dropout),
+ nn.Linear(in_features=hidden_dim, out_features=dim),
+ nn.Dropout(dropout)
+ )
+
+ def forward(self, input):
+ return self.net(input)
+
+
+class ConvAttnetion(nn.Module):
+ '''
+ using the Depth_Separable_Wise Conv2d to produce the q, k, v instead of using Linear Project in ViT
+ '''
+
+ def __init__(self, dim, img_size, heads=8, dim_head=64, kernel_size=3, q_stride=1, k_stride=1, v_stride=1,
+ dropout=0., last_stage=False):
+ super().__init__()
+ self.last_stage = last_stage
+ self.img_size = img_size
+ inner_dim = dim_head * heads # 512
+ project_out = not (heads == 1 and dim_head == dim)
+
+ self.heads = heads
+ self.scale = dim_head ** (-0.5)
+
+ pad = (kernel_size - q_stride) // 2
+
+ self.to_q = DepthwiseConv2d(in_chans=dim, out_chans=inner_dim, kernel_size=kernel_size, stride=q_stride,
+ padding=pad) # 自注意力机制
+ self.to_k = DepthwiseConv2d(in_chans=dim, out_chans=inner_dim, kernel_size=kernel_size, stride=k_stride,
+ padding=pad)
+ self.to_v = DepthwiseConv2d(in_chans=dim, out_chans=inner_dim, kernel_size=kernel_size, stride=v_stride,
+ padding=pad)
+
+ self.to_out = nn.Sequential(
+ nn.Linear(
+ in_features=inner_dim,
+ out_features=dim
+ ),
+ nn.Dropout(dropout)
+ ) if project_out else Identity()
+
+ def forward(self, x):
+ b, n, c, h = *x.shape, self.heads # * 星号的作用大概是去掉 tuple 属性吧
+
+ # print(x.shape)
+ # print('+++++++++++++++++++++++++++++++++')
+
+ # if语句内容没有使用
+ if self.last_stage:
+ cls_token = x[:, 0]
+ # print(cls_token.shape)
+ # print('+++++++++++++++++++++++++++++++++')
+ x = x[:, 1:] # 去掉每个数组的第一个元素
+
+ cls_token = rearrange(torch.unsqueeze(cls_token, dim=1), 'b n (h d) -> b h n d', h=h)
+
+ # rearrange:用于对张量的维度进行重新变换排序,可用于替换pytorch中的reshape,view,transpose和permute等操作
+ x = rearrange(x, 'b (l w) n -> b n l w', l=self.img_size, w=self.img_size) # [1, 3136, 64]-->1*64*56*56
+ # batch_size,N(通道数),h,w
+
+ q = self.to_q(x) # 1*64*56*56-->1*64*56*56
+ # print(q.shape)
+ # print('++++++++++++++')
+ q = rearrange(q, 'b (h d) l w -> b h (l w) d', h=h) # 1*64*56*56-->1*1*3136*64
+ # print(q.shape)
+ # print('=====================')
+ # batch_size,head,h*w,dim_head
+
+ k = self.to_k(x) # 操作和q一样
+ k = rearrange(k, 'b (h d) l w -> b h (l w) d', h=h)
+ # batch_size,head,h*w,dim_head
+
+ v = self.to_v(x) ##操作和q一样
+ # print(v.shape)
+ # print('[[[[[[[[[[[[[[[[[[[[[[[[[[[[')
+ v = rearrange(v, 'b (h d) l w -> b h (l w) d', h=h)
+ # print(v.shape)
+ # print(']]]]]]]]]]]]]]]]]]]]]]]]]]]')
+ # batch_size,head,h*w,dim_head
+
+ if self.last_stage:
+ # print(q.shape)
+ # print('================')
+ q = torch.cat([cls_token, q], dim=2)
+ # print(q.shape)
+ # print('++++++++++++++++++')
+ v = torch.cat([cls_token, v], dim=2)
+ k = torch.cat([cls_token, k], dim=2)
+
+ # calculate attention by matmul + scale
+ # permute:(batch_size,head,dim_head,h*w
+ # print(k.shape)
+ # print('++++++++++++++++++++')
+ k = k.permute(0, 1, 3, 2) # 1*1*3136*64-->1*1*64*3136
+ # print(k.shape)
+ # print('====================')
+ attention = (q.matmul(k)) # 1*1*3136*3136
+ # print(attention.shape)
+ # print('--------------------')
+ attention = attention * self.scale # 可以得到一个logit的向量,避免出现梯度下降和梯度爆炸
+ # print(attention.shape)
+ # print('####################')
+ # pass a softmax
+ attention = F.softmax(attention, dim=-1)
+ # print(attention.shape)
+ # print('********************')
+
+ # matmul v
+ # attention.matmul(v):(batch_size,head,h*w,dim_head)
+ # permute:(batch_size,h*w,head,dim_head)
+ out = (attention.matmul(v)).permute(0, 2, 1, 3).reshape(b, n,
+ c) # 1*3136*64 这些操作的目的是将注意力权重和值向量相乘后得到的结果进行重塑,得到一个形状为 (batch size, 序列长度, 值向量或矩阵的维度) 的张量
+
+ # linear project
+ out = self.to_out(out)
+ return out
+
+
+# Reshape Layers
+class Rearrange(nn.Module):
+ def __init__(self, string, h, w):
+ super().__init__()
+ self.string = string
+ self.h = h
+ self.w = w
+
+ def forward(self, input):
+
+ if self.string == 'b c h w -> b (h w) c':
+ N, C, H, W = input.shape
+ # print(input.shape)
+ x = torch.reshape(input, shape=(N, -1, self.h * self.w)).permute(0, 2, 1)
+ # print(x.shape)
+ # print('+++++++++++++++++++')
+ if self.string == 'b (h w) c -> b c h w':
+ N, _, C = input.shape
+ # print(input.shape)
+ x = torch.reshape(input, shape=(N, self.h, self.w, -1)).permute(0, 3, 1, 2)
+ # print(x.shape)
+ # print('=====================')
+ return x
+
+
+# Transformer layers
+class Transformer(nn.Module):
+ def __init__(self, dim, img_size, depth, heads, dim_head, mlp_dim, dropout=0., last_stage=False):
+ super().__init__()
+ self.layers = nn.ModuleList([ # 管理子模块,参数注册
+ nn.ModuleList([
+ PreNorm(dim=dim, fn=ConvAttnetion(dim, img_size, heads=heads, dim_head=dim_head, dropout=dropout,
+ last_stage=last_stage)), # 归一化,重参数化
+ PreNorm(dim=dim, fn=FeedForward(dim=dim, hidden_dim=mlp_dim, dropout=dropout))
+ ]) for _ in range(depth)
+ ])
+
+ def forward(self, x):
+ for attn, ff in self.layers:
+ x = x + attn(x)
+ x = x + ff(x)
+ return x
+
+
+class DBNet(nn.Module): # 最主要的大函数
+ def __init__(self, img_size, in_channels, num_classes, dim=64, kernels=[7, 3, 3, 3], strides=[4, 2, 2, 2],
+ heads=[1, 3, 6, 6],
+ depth=[1, 2, 10, 10], pool='cls', dropout=0., emb_dropout=0., scale_dim=4, ):
+ super().__init__()
+
+ assert pool in ['cls', 'mean'], f'pool type must be either cls or mean pooling'
+ self.pool = pool
+ self.dim = dim
+
+ # stage1
+ # k:7 s:4 in: 1, 64, 56, 56 out: 1, 3136, 64
+ self.stage1_conv_embed = nn.Sequential(
+ nn.Conv2d( # 1*3*224*224-->[1, 64, 56, 56]
+ in_channels=in_channels,
+ out_channels=dim,
+ kernel_size=kernels[0],
+ stride=strides[0],
+ padding=2
+ ),
+ Rearrange('b c h w -> b (h w) c', h=img_size // 4, w=img_size // 4), # [1, 64, 56, 56]-->[1, 3136, 64]
+ nn.LayerNorm(dim) # 对每个batch归一化
+ )
+
+ self.stage1_transformer = nn.Sequential(
+ Transformer( #
+ dim=dim,
+ img_size=img_size // 4,
+ depth=depth[0], # Transformer层中的编码器和解码器层数。
+ heads=heads[0],
+ dim_head=self.dim, # 它是每个注意力头的维度大小,通常是嵌入维度除以头数。
+ mlp_dim=dim * scale_dim, # mlp_dim:它是Transformer中前馈神经网络的隐藏层维度大小,通常是嵌入维度乘以一个缩放因子。
+ dropout=dropout,
+ # last_stage=last_stage #它是一个标志位,用于表示该Transformer层是否是最后一层。
+ ),
+ Rearrange('b (h w) c -> b c h w', h=img_size // 4, w=img_size // 4)
+ )
+
+ # stage2
+ # k:3 s:2 in: 1, 192, 28, 28 out: 1, 784, 192
+ in_channels = dim
+ scale = heads[1] // heads[0]
+ dim = scale * dim
+
+ self.stage2_conv_embed = nn.Sequential(
+ nn.Conv2d(
+ in_channels=in_channels,
+ out_channels=dim,
+ kernel_size=kernels[1],
+ stride=strides[1],
+ padding=1
+ ),
+ Rearrange('b c h w -> b (h w) c', h=img_size // 8, w=img_size // 8),
+ nn.LayerNorm(dim)
+ )
+
+ self.stage2_transformer = nn.Sequential(
+ Transformer(
+ dim=dim,
+ img_size=img_size // 8,
+ depth=depth[1],
+ heads=heads[1],
+ dim_head=self.dim,
+ mlp_dim=dim * scale_dim,
+ dropout=dropout
+ ),
+ Rearrange('b (h w) c -> b c h w', h=img_size // 8, w=img_size // 8)
+ )
+
+ # stage3
+ in_channels = dim
+ scale = heads[2] // heads[1]
+ dim = scale * dim
+
+ self.stage3_conv_embed = nn.Sequential(
+ nn.Conv2d(
+ in_channels=in_channels,
+ out_channels=dim,
+ kernel_size=kernels[2],
+ stride=strides[2],
+ padding=1
+ ),
+ Rearrange('b c h w -> b (h w) c', h=img_size // 16, w=img_size // 16),
+ nn.LayerNorm(dim)
+ )
+
+ self.stage3_transformer = nn.Sequential(
+ Transformer(
+ dim=dim,
+ img_size=img_size // 16,
+ depth=depth[2],
+ heads=heads[2],
+ dim_head=self.dim,
+ mlp_dim=dim * scale_dim,
+ dropout=dropout
+ ),
+ Rearrange('b (h w) c -> b c h w', h=img_size // 16, w=img_size // 16)
+ )
+
+ # stage4
+ in_channels = dim
+ scale = heads[3] // heads[2]
+ dim = scale * dim
+
+ self.stage4_conv_embed = nn.Sequential(
+ nn.Conv2d(
+ in_channels=in_channels,
+ out_channels=dim,
+ kernel_size=kernels[3],
+ stride=strides[3],
+ padding=1
+ ),
+ Rearrange('b c h w -> b (h w) c', h=img_size // 32, w=img_size // 32),
+ nn.LayerNorm(dim)
+ )
+
+ self.stage4_transformer = nn.Sequential(
+ Transformer(
+ dim=dim, img_size=img_size // 32,
+ depth=depth[3],
+ heads=heads[3],
+ dim_head=self.dim,
+ mlp_dim=dim * scale_dim,
+ dropout=dropout,
+ ),
+ Rearrange('b (h w) c -> b c h w', h=img_size // 32, w=img_size // 32)
+ )
+
+ ### CNN Branch ###
+ self.c_stage1 = c_stage123(in_chans=3, out_chans=64)
+ self.c_stage2 = c_stage123(in_chans=64, out_chans=128)
+ self.c_stage3 = c_stage123(in_chans=128, out_chans=384)
+ self.c_stage4 = c_stage45(in_chans=384, out_chans=512)
+ self.c_stage5 = c_stage45(in_chans=512, out_chans=1024)
+ self.c_max = nn.MaxPool2d(kernel_size=3, stride=2, padding=1)
+ self.up_conv1 = nn.Conv2d(in_channels=192, out_channels=128, kernel_size=1)
+ self.up_conv2 = nn.Conv2d(in_channels=384, out_channels=512, kernel_size=1)
+
+ ### CTmerge ###
+ self.CTmerge1 = nn.Sequential(
+ nn.Conv2d(in_channels=128, out_channels=64, kernel_size=3, stride=1, padding=1),
+ nn.BatchNorm2d(64),
+ nn.ReLU(),
+ nn.Conv2d(in_channels=64, out_channels=64, kernel_size=3, stride=1, padding=1),
+ nn.BatchNorm2d(64),
+ nn.ReLU(),
+ )
+ self.CTmerge2 = nn.Sequential(
+ nn.Conv2d(in_channels=320, out_channels=128, kernel_size=3, stride=1, padding=1),
+ nn.BatchNorm2d(128),
+ nn.ReLU(),
+ nn.Conv2d(in_channels=128, out_channels=128, kernel_size=3, stride=1, padding=1),
+ nn.BatchNorm2d(128),
+ nn.ReLU(),
+ )
+ self.CTmerge3 = nn.Sequential(
+ nn.Conv2d(in_channels=768, out_channels=512, kernel_size=3, stride=1, padding=1),
+ nn.BatchNorm2d(512),
+ nn.ReLU(),
+ nn.Conv2d(in_channels=512, out_channels=384, kernel_size=3, stride=1, padding=1),
+ nn.BatchNorm2d(384),
+ nn.ReLU(),
+ nn.Conv2d(in_channels=384, out_channels=384, kernel_size=3, stride=1, padding=1),
+ nn.BatchNorm2d(384),
+ nn.ReLU(),
+ )
+
+ self.CTmerge4 = nn.Sequential(
+ nn.Conv2d(in_channels=896, out_channels=640, kernel_size=3, stride=1, padding=1),
+ nn.BatchNorm2d(640),
+ nn.ReLU(),
+ nn.Conv2d(in_channels=640, out_channels=512, kernel_size=3, stride=1, padding=1),
+ nn.BatchNorm2d(512),
+ nn.ReLU(),
+ nn.Conv2d(in_channels=512, out_channels=512, kernel_size=3, stride=1, padding=1),
+ nn.BatchNorm2d(512),
+ nn.ReLU(),
+ )
+
+ # decoder
+ self.decoder4 = nn.Sequential(
+ DepthwiseConv2d(
+ in_chans=1408,
+ out_chans=1024,
+ kernel_size=3,
+ stride=1,
+ padding=1
+ ),
+ DepthwiseConv2d(
+ in_chans=1024,
+ out_chans=512,
+ kernel_size=3,
+ stride=1,
+ padding=1
+ ),
+ nn.GELU()
+ )
+ self.decoder3 = nn.Sequential(
+ DepthwiseConv2d(
+ in_chans=896,
+ out_chans=512,
+ kernel_size=3,
+ stride=1,
+ padding=1
+ ),
+ DepthwiseConv2d(
+ in_chans=512,
+ out_chans=384,
+ kernel_size=3,
+ stride=1,
+ padding=1
+ ),
+ nn.GELU()
+ )
+
+ self.decoder2 = nn.Sequential(
+ DepthwiseConv2d(
+ in_chans=576,
+ out_chans=256,
+ kernel_size=3,
+ stride=1,
+ padding=1
+ ),
+ DepthwiseConv2d(
+ in_chans=256,
+ out_chans=192,
+ kernel_size=3,
+ stride=1,
+ padding=1
+ ),
+ nn.GELU()
+ )
+
+ self.decoder1 = nn.Sequential(
+ DepthwiseConv2d(
+ in_chans=256,
+ out_chans=64,
+ kernel_size=3,
+ stride=1,
+ padding=1
+ ),
+ DepthwiseConv2d(
+ in_chans=64,
+ out_chans=16,
+ kernel_size=3,
+ stride=1,
+ padding=1
+ ),
+ nn.GELU()
+ )
+ self.sbr4 = SBR(512)
+ self.sbr3 = SBR(384)
+ self.sbr2 = SBR(192)
+ self.sbr1 = SBR(16)
+
+ self.head = nn.Conv2d(in_channels=16, out_channels=num_classes, kernel_size=1)
+
+ def forward(self, input):
+ ### encoder ###
+ # stage1 = ts1 cat cs1
+ # t_s1 = self.t_stage1(input)
+ # print(input.shape)
+ # print('++++++++++++++++++++++')
+
+ t_s1 = self.stage1_conv_embed(input) # 1*3*224*224-->1*3136*64
+
+ # print(t_s1.shape)
+ # print('======================')
+
+ t_s1 = self.stage1_transformer(t_s1) # 1*3136*64-->1*64*56*56
+
+ # print(t_s1.shape)
+ # print('----------------------')
+
+ c_s1 = self.c_stage1(input) # 1*3*224*224-->1*64*112*112
+
+ # print(c_s1.shape)
+ # print('!!!!!!!!!!!!!!!!!!!!!!!')
+
+ stage1 = self.CTmerge1(torch.cat([t_s1, self.c_max(c_s1)], dim=1)) # 1*64*56*56 # 拼接两条分支
+
+ # print(stage1.shape)
+ # print('[[[[[[[[[[[[[[[[[[[[[[[')
+
+ # stage2 = ts2 up cs2
+ # t_s2 = self.t_stage2(stage1)
+ t_s2 = self.stage2_conv_embed(stage1) # 1*64*56*56-->1*784*192 # stage2_conv_embed是转化为序列操作
+
+ # print(t_s2.shape)
+ # print('[[[[[[[[[[[[[[[[[[[[[[[')
+ t_s2 = self.stage2_transformer(t_s2) # 1*784*192-->1*192*28*28
+ # print(t_s2.shape)
+ # print('+++++++++++++++++++++++++')
+
+ c_s2 = self.c_stage2(c_s1) # 1*64*112*112-->1*128*56*56
+ stage2 = self.CTmerge2(
+ torch.cat([c_s2, F.interpolate(t_s2, size=c_s2.size()[2:], mode='bilinear', align_corners=True)],
+ dim=1)) # mode='bilinear'表示使用双线性插值 1*128*56*56
+
+ # stage3 = ts3 cat cs3
+ # t_s3 = self.t_stage3(t_s2)
+ t_s3 = self.stage3_conv_embed(t_s2) # 1*192*28*28-->1*196*384
+ # print(t_s3.shape)
+ # print('///////////////////////')
+ t_s3 = self.stage3_transformer(t_s3) # 1*196*384-->1*384*14*14
+ # print(t_s3.shape)
+ # print('....................')
+ c_s3 = self.c_stage3(stage2) # 1*128*56*56-->1*384*28*28
+ stage3 = self.CTmerge3(torch.cat([t_s3, self.c_max(c_s3)], dim=1)) # 1*384*14*14
+
+ # stage4 = ts4 up cs4
+ # t_s4 = self.t_stage4(stage3)
+ t_s4 = self.stage4_conv_embed(stage3) # 1*384*14*14-->1*49*384
+ # print(t_s4.shape)
+ # print(';;;;;;;;;;;;;;;;;;;;;;;')
+ t_s4 = self.stage4_transformer(t_s4) # 1*49*384-->1*384*7*7
+ # print(t_s4.shape)
+ # print('::::::::::::::::::::')
+
+ c_s4 = self.c_stage4(c_s3) # 1*384*28*28-->1*512*14*14
+ stage4 = self.CTmerge4(
+ torch.cat([c_s4, F.interpolate(t_s4, size=c_s4.size()[2:], mode='bilinear', align_corners=True)],
+ dim=1)) # 1*512*14*14
+
+ # cs5
+ c_s5 = self.c_stage5(stage4) # 1*512*14*14-->1*1024*7*7
+
+ ### decoder ###
+ decoder4 = torch.cat([c_s5, t_s4], dim=1) # 1*1408*7*7
+ decoder4 = self.decoder4(decoder4) # 1*1408*7*7-->1*512*7*7
+ decoder4 = F.interpolate(decoder4, size=c_s3.size()[2:], mode='bilinear',
+ align_corners=True) # 1*512*7*7-->1*512*28*28
+ decoder4 = self.sbr4(decoder4) # 1*512*28*28
+ # print(decoder4.shape)
+
+ decoder3 = torch.cat([decoder4, c_s3], dim=1) # 1*896*28*28
+ decoder3 = self.decoder3(decoder3) # 1*384*28*28
+ decoder3 = F.interpolate(decoder3, size=t_s2.size()[2:], mode='bilinear', align_corners=True) # 1*384*28*28
+ decoder3 = self.sbr3(decoder3) # 1*384*28*28
+ # print(decoder3.shape)
+
+ decoder2 = torch.cat([decoder3, t_s2], dim=1) # 1*576*28*28
+ decoder2 = self.decoder2(decoder2) # 1*192*28*28
+ decoder2 = F.interpolate(decoder2, size=c_s1.size()[2:], mode='bilinear', align_corners=True) # 1*192*112*112
+ decoder2 = self.sbr2(decoder2) # 1*192*112*112
+ # print(decoder2.shape)
+
+ decoder1 = torch.cat([decoder2, c_s1], dim=1) # 1*256*112*112
+ decoder1 = self.decoder1(decoder1) # 1*16*112*112
+ # print(decoder1.shape)
+ final = F.interpolate(decoder1, size=input.size()[2:], mode='bilinear', align_corners=True) # 1*16*224*224
+ # print(final.shape)
+ # final = self.sbr1(decoder1)
+ # print(final.shape)
+ final = self.head(final) # 1*3*224*224
+
+ return final
+
+
+if __name__ == '__main__':
+ x = torch.rand(1, 3, 224, 224).cuda()
+ model = DBNet(img_size=224, in_channels=3, num_classes=7).cuda()
+ y = model(x)
+ print(y.shape)
+ # torch.Size([1, 7, 224, 224])
diff --git a/src/models/components/dual_branch.py b/src/models/components/dual_branch.py
index f291bfdf9f0ed39808ff4c82a986b91407fafb7e..b1c2d9f296278eef49cf0bf7e3d3eeb4626c1fa7 100644
--- a/src/models/components/dual_branch.py
+++ b/src/models/components/dual_branch.py
@@ -1,680 +1,680 @@
-# -*- coding: utf-8 -*-
-# @Time : 2024/7/26 上午11:19
-# @Author : xiaoshun
-# @Email : 3038523973@qq.com
-# @File : dual_branch.py
-# @Software: PyCharm
-
-from einops import rearrange
-import torch
-import torch.nn as nn
-import torch.nn.functional as F
-
-
-# from models.Transformer.ViT import truncated_normal_
-
-# Decoder细化卷积模块
-class SBR(nn.Module):
- def __init__(self, in_ch):
- super(SBR, self).__init__()
- self.conv1x3 = nn.Sequential(
- nn.Conv2d(in_ch, in_ch, kernel_size=(1, 3), stride=1, padding=(0, 1)),
- nn.BatchNorm2d(in_ch),
- nn.ReLU(True)
- )
- self.conv3x1 = nn.Sequential(
- nn.Conv2d(in_ch, in_ch, kernel_size=(3, 1), stride=1, padding=(1, 0)),
- nn.BatchNorm2d(in_ch),
- nn.ReLU(True)
- )
-
- def forward(self, x):
- out = self.conv3x1(self.conv1x3(x)) # 先进行1x3的卷积,得到结果并将结果再进行3x1的卷积
- return out + x
-
-
-# 下采样卷积模块 stage 1,2,3
-class c_stage123(nn.Module):
- def __init__(self, in_chans, out_chans):
- super().__init__()
- self.stage123 = nn.Sequential(
- nn.Conv2d(in_channels=in_chans, out_channels=out_chans, kernel_size=3, stride=2, padding=1),
- nn.BatchNorm2d(out_chans),
- nn.ReLU(),
- nn.Conv2d(in_channels=out_chans, out_channels=out_chans, kernel_size=3, stride=1, padding=1),
- nn.BatchNorm2d(out_chans),
- nn.ReLU(),
- )
- self.conv1x1_123 = nn.Conv2d(in_channels=in_chans, out_channels=out_chans, kernel_size=1)
- self.maxpool = nn.MaxPool2d(kernel_size=3, stride=2, padding=1)
-
- def forward(self, x):
- stage123 = self.stage123(x) # 3*3卷积,两倍下采样 3*224*224-->64*112*112
- max = self.maxpool(x) # 最大值池化,两倍下采样 3*224*224-->3*112*112
- max = self.conv1x1_123(max) # 1*1卷积 3*112*112-->64*112*112
- stage123 = stage123 + max # 残差结构,广播机制
- return stage123
-
-
-# 下采样卷积模块 stage4,5
-class c_stage45(nn.Module):
- def __init__(self, in_chans, out_chans):
- super().__init__()
- self.stage45 = nn.Sequential(
- nn.Conv2d(in_channels=in_chans, out_channels=out_chans, kernel_size=3, stride=2, padding=1),
- nn.BatchNorm2d(out_chans),
- nn.ReLU(),
- nn.Conv2d(in_channels=out_chans, out_channels=out_chans, kernel_size=3, stride=1, padding=1),
- nn.BatchNorm2d(out_chans),
- nn.ReLU(),
- nn.Conv2d(in_channels=out_chans, out_channels=out_chans, kernel_size=3, stride=1, padding=1),
- nn.BatchNorm2d(out_chans),
- nn.ReLU(),
- )
- self.conv1x1_45 = nn.Conv2d(in_channels=in_chans, out_channels=out_chans, kernel_size=1)
- self.maxpool = nn.MaxPool2d(kernel_size=3, stride=2, padding=1)
-
- def forward(self, x):
- stage45 = self.stage45(x) # 3*3卷积模块 2倍下采样
- max = self.maxpool(x) # 最大值池化,两倍下采样
- max = self.conv1x1_45(max) # 1*1卷积模块 调整通道数
- stage45 = stage45 + max # 残差结构
- return stage45
-
-
-class Identity(nn.Module): # 恒等映射
- def __init__(self):
- super().__init__()
-
- def forward(self, x):
- return x
-
-
-# 轻量卷积模块
-class DepthwiseConv2d(nn.Module): # 用于自注意力机制
- def __init__(self, in_chans, out_chans, kernel_size=1, stride=1, padding=0, dilation=1):
- super().__init__()
- # depthwise conv
- self.depthwise = nn.Conv2d(
- in_channels=in_chans,
- out_channels=in_chans,
- kernel_size=kernel_size,
- stride=stride,
- padding=padding,
- dilation=dilation, # 深层卷积的膨胀率
- groups=in_chans # 指定分组卷积的组数
- )
- # batch norm
- self.bn = nn.BatchNorm2d(num_features=in_chans)
-
- # pointwise conv 逐点卷积
- self.pointwise = nn.Conv2d(
- in_channels=in_chans,
- out_channels=out_chans,
- kernel_size=1
- )
-
- def forward(self, x):
- x = self.depthwise(x)
- x = self.bn(x)
- x = self.pointwise(x)
- return x
-
-
-# residual skip connection 残差跳跃连接
-class Residual(nn.Module):
- def __init__(self, fn):
- super().__init__()
- self.fn = fn
-
- def forward(self, input, **kwargs):
- x = self.fn(input, **kwargs)
- return (x + input)
-
-
-# layer norm plus 层归一化
-class PreNorm(nn.Module): # 代表神经网络层
- def __init__(self, dim, fn):
- super().__init__()
- self.norm = nn.LayerNorm(dim)
- self.fn = fn
-
- def forward(self, input, **kwargs):
- return self.fn(self.norm(input), **kwargs)
-
-
-# FeedForward层使得representation的表达能力更强
-class FeedForward(nn.Module):
- def __init__(self, dim, hidden_dim, dropout=0.):
- super().__init__()
- self.net = nn.Sequential(
- nn.Linear(in_features=dim, out_features=hidden_dim),
- nn.GELU(),
- nn.Dropout(dropout),
- nn.Linear(in_features=hidden_dim, out_features=dim),
- nn.Dropout(dropout)
- )
-
- def forward(self, input):
- return self.net(input)
-
-
-class ConvAttnetion(nn.Module):
- '''
- using the Depth_Separable_Wise Conv2d to produce the q, k, v instead of using Linear Project in ViT
- '''
-
- def __init__(self, dim, img_size, heads=8, dim_head=64, kernel_size=3, q_stride=1, k_stride=1, v_stride=1,
- dropout=0., last_stage=False):
- super().__init__()
- self.last_stage = last_stage
- self.img_size = img_size
- inner_dim = dim_head * heads # 512
- project_out = not (heads == 1 and dim_head == dim)
-
- self.heads = heads
- self.scale = dim_head ** (-0.5)
-
- pad = (kernel_size - q_stride) // 2
-
- self.to_q = DepthwiseConv2d(in_chans=dim, out_chans=inner_dim, kernel_size=kernel_size, stride=q_stride,
- padding=pad) # 自注意力机制
- self.to_k = DepthwiseConv2d(in_chans=dim, out_chans=inner_dim, kernel_size=kernel_size, stride=k_stride,
- padding=pad)
- self.to_v = DepthwiseConv2d(in_chans=dim, out_chans=inner_dim, kernel_size=kernel_size, stride=v_stride,
- padding=pad)
-
- self.to_out = nn.Sequential(
- nn.Linear(
- in_features=inner_dim,
- out_features=dim
- ),
- nn.Dropout(dropout)
- ) if project_out else Identity()
-
- def forward(self, x):
- b, n, c, h = *x.shape, self.heads # * 星号的作用大概是去掉 tuple 属性吧
-
- # print(x.shape)
- # print('+++++++++++++++++++++++++++++++++')
-
- # if语句内容没有使用
- if self.last_stage:
- cls_token = x[:, 0]
- # print(cls_token.shape)
- # print('+++++++++++++++++++++++++++++++++')
- x = x[:, 1:] # 去掉每个数组的第一个元素
-
- cls_token = rearrange(torch.unsqueeze(cls_token, dim=1), 'b n (h d) -> b h n d', h=h)
-
- # rearrange:用于对张量的维度进行重新变换排序,可用于替换pytorch中的reshape,view,transpose和permute等操作
- x = rearrange(x, 'b (l w) n -> b n l w', l=self.img_size, w=self.img_size) # [1, 3136, 64]-->1*64*56*56
- # batch_size,N(通道数),h,w
-
- q = self.to_q(x) # 1*64*56*56-->1*64*56*56
- # print(q.shape)
- # print('++++++++++++++')
- q = rearrange(q, 'b (h d) l w -> b h (l w) d', h=h) # 1*64*56*56-->1*1*3136*64
- # print(q.shape)
- # print('=====================')
- # batch_size,head,h*w,dim_head
-
- k = self.to_k(x) # 操作和q一样
- k = rearrange(k, 'b (h d) l w -> b h (l w) d', h=h)
- # batch_size,head,h*w,dim_head
-
- v = self.to_v(x) ##操作和q一样
- # print(v.shape)
- # print('[[[[[[[[[[[[[[[[[[[[[[[[[[[[')
- v = rearrange(v, 'b (h d) l w -> b h (l w) d', h=h)
- # print(v.shape)
- # print(']]]]]]]]]]]]]]]]]]]]]]]]]]]')
- # batch_size,head,h*w,dim_head
-
- if self.last_stage:
- # print(q.shape)
- # print('================')
- q = torch.cat([cls_token, q], dim=2)
- # print(q.shape)
- # print('++++++++++++++++++')
- v = torch.cat([cls_token, v], dim=2)
- k = torch.cat([cls_token, k], dim=2)
-
- # calculate attention by matmul + scale
- # permute:(batch_size,head,dim_head,h*w
- # print(k.shape)
- # print('++++++++++++++++++++')
- k = k.permute(0, 1, 3, 2) # 1*1*3136*64-->1*1*64*3136
- # print(k.shape)
- # print('====================')
- attention = (q.matmul(k)) # 1*1*3136*3136
- # print(attention.shape)
- # print('--------------------')
- attention = attention * self.scale # 可以得到一个logit的向量,避免出现梯度下降和梯度爆炸
- # print(attention.shape)
- # print('####################')
- # pass a softmax
- attention = F.softmax(attention, dim=-1)
- # print(attention.shape)
- # print('********************')
-
- # matmul v
- # attention.matmul(v):(batch_size,head,h*w,dim_head)
- # permute:(batch_size,h*w,head,dim_head)
- out = (attention.matmul(v)).permute(0, 2, 1, 3).reshape(b, n,
- c) # 1*3136*64 这些操作的目的是将注意力权重和值向量相乘后得到的结果进行重塑,得到一个形状为 (batch size, 序列长度, 值向量或矩阵的维度) 的张量
-
- # linear project
- out = self.to_out(out)
- return out
-
-
-# Reshape Layers
-class Rearrange(nn.Module):
- def __init__(self, string, h, w):
- super().__init__()
- self.string = string
- self.h = h
- self.w = w
-
- def forward(self, input):
-
- if self.string == 'b c h w -> b (h w) c':
- N, C, H, W = input.shape
- # print(input.shape)
- x = torch.reshape(input, shape=(N, -1, self.h * self.w)).permute(0, 2, 1)
- # print(x.shape)
- # print('+++++++++++++++++++')
- if self.string == 'b (h w) c -> b c h w':
- N, _, C = input.shape
- # print(input.shape)
- x = torch.reshape(input, shape=(N, self.h, self.w, -1)).permute(0, 3, 1, 2)
- # print(x.shape)
- # print('=====================')
- return x
-
-
-# Transformer layers
-class Transformer(nn.Module):
- def __init__(self, dim, img_size, depth, heads, dim_head, mlp_dim, dropout=0., last_stage=False):
- super().__init__()
- self.layers = nn.ModuleList([ # 管理子模块,参数注册
- nn.ModuleList([
- PreNorm(dim=dim, fn=ConvAttnetion(dim, img_size, heads=heads, dim_head=dim_head, dropout=dropout,
- last_stage=last_stage)), # 归一化,重参数化
- PreNorm(dim=dim, fn=FeedForward(dim=dim, hidden_dim=mlp_dim, dropout=dropout))
- ]) for _ in range(depth)
- ])
-
- def forward(self, x):
- for attn, ff in self.layers:
- x = x + attn(x)
- x = x + ff(x)
- return x
-
-
-class Dual_Branch(nn.Module): # 最主要的大函数
- def __init__(self, img_size, in_channels, num_classes, dim=64, kernels=[7, 3, 3, 3], strides=[4, 2, 2, 2],
- heads=[1, 3, 6, 6],
- depth=[1, 2, 10, 10], pool='cls', dropout=0., emb_dropout=0., scale_dim=4, ):
- super().__init__()
-
- assert pool in ['cls', 'mean'], f'pool type must be either cls or mean pooling'
- self.pool = pool
- self.dim = dim
-
- # stage1
- # k:7 s:4 in: 1, 64, 56, 56 out: 1, 3136, 64
- self.stage1_conv_embed = nn.Sequential(
- nn.Conv2d( # 1*3*224*224-->[1, 64, 56, 56]
- in_channels=in_channels,
- out_channels=dim,
- kernel_size=kernels[0],
- stride=strides[0],
- padding=2
- ),
- Rearrange('b c h w -> b (h w) c', h=img_size // 4, w=img_size // 4), # [1, 64, 56, 56]-->[1, 3136, 64]
- nn.LayerNorm(dim) # 对每个batch归一化
- )
-
- self.stage1_transformer = nn.Sequential(
- Transformer( #
- dim=dim,
- img_size=img_size // 4,
- depth=depth[0], # Transformer层中的编码器和解码器层数。
- heads=heads[0],
- dim_head=self.dim, # 它是每个注意力头的维度大小,通常是嵌入维度除以头数。
- mlp_dim=dim * scale_dim, # mlp_dim:它是Transformer中前馈神经网络的隐藏层维度大小,通常是嵌入维度乘以一个缩放因子。
- dropout=dropout,
- # last_stage=last_stage #它是一个标志位,用于表示该Transformer层是否是最后一层。
- ),
- Rearrange('b (h w) c -> b c h w', h=img_size // 4, w=img_size // 4)
- )
-
- # stage2
- # k:3 s:2 in: 1, 192, 28, 28 out: 1, 784, 192
- in_channels = dim
- scale = heads[1] // heads[0]
- dim = scale * dim
-
- self.stage2_conv_embed = nn.Sequential(
- nn.Conv2d(
- in_channels=in_channels,
- out_channels=dim,
- kernel_size=kernels[1],
- stride=strides[1],
- padding=1
- ),
- Rearrange('b c h w -> b (h w) c', h=img_size // 8, w=img_size // 8),
- nn.LayerNorm(dim)
- )
-
- self.stage2_transformer = nn.Sequential(
- Transformer(
- dim=dim,
- img_size=img_size // 8,
- depth=depth[1],
- heads=heads[1],
- dim_head=self.dim,
- mlp_dim=dim * scale_dim,
- dropout=dropout
- ),
- Rearrange('b (h w) c -> b c h w', h=img_size // 8, w=img_size // 8)
- )
-
- # stage3
- in_channels = dim
- scale = heads[2] // heads[1]
- dim = scale * dim
-
- self.stage3_conv_embed = nn.Sequential(
- nn.Conv2d(
- in_channels=in_channels,
- out_channels=dim,
- kernel_size=kernels[2],
- stride=strides[2],
- padding=1
- ),
- Rearrange('b c h w -> b (h w) c', h=img_size // 16, w=img_size // 16),
- nn.LayerNorm(dim)
- )
-
- self.stage3_transformer = nn.Sequential(
- Transformer(
- dim=dim,
- img_size=img_size // 16,
- depth=depth[2],
- heads=heads[2],
- dim_head=self.dim,
- mlp_dim=dim * scale_dim,
- dropout=dropout
- ),
- Rearrange('b (h w) c -> b c h w', h=img_size // 16, w=img_size // 16)
- )
-
- # stage4
- in_channels = dim
- scale = heads[3] // heads[2]
- dim = scale * dim
-
- self.stage4_conv_embed = nn.Sequential(
- nn.Conv2d(
- in_channels=in_channels,
- out_channels=dim,
- kernel_size=kernels[3],
- stride=strides[3],
- padding=1
- ),
- Rearrange('b c h w -> b (h w) c', h=img_size // 32, w=img_size // 32),
- nn.LayerNorm(dim)
- )
-
- self.stage4_transformer = nn.Sequential(
- Transformer(
- dim=dim, img_size=img_size // 32,
- depth=depth[3],
- heads=heads[3],
- dim_head=self.dim,
- mlp_dim=dim * scale_dim,
- dropout=dropout,
- ),
- Rearrange('b (h w) c -> b c h w', h=img_size // 32, w=img_size // 32)
- )
-
- ### CNN Branch ###
- self.c_stage1 = c_stage123(in_chans=3, out_chans=64)
- self.c_stage2 = c_stage123(in_chans=64, out_chans=128)
- self.c_stage3 = c_stage123(in_chans=128, out_chans=384)
- self.c_stage4 = c_stage45(in_chans=384, out_chans=512)
- self.c_stage5 = c_stage45(in_chans=512, out_chans=1024)
- self.c_max = nn.MaxPool2d(kernel_size=3, stride=2, padding=1)
- self.up_conv1 = nn.Conv2d(in_channels=192, out_channels=128, kernel_size=1)
- self.up_conv2 = nn.Conv2d(in_channels=384, out_channels=512, kernel_size=1)
-
- ### CTmerge ###
- self.CTmerge1 = nn.Sequential(
- nn.Conv2d(in_channels=128, out_channels=64, kernel_size=3, stride=1, padding=1),
- nn.BatchNorm2d(64),
- nn.ReLU(),
- nn.Conv2d(in_channels=64, out_channels=64, kernel_size=3, stride=1, padding=1),
- nn.BatchNorm2d(64),
- nn.ReLU(),
- )
- self.CTmerge2 = nn.Sequential(
- nn.Conv2d(in_channels=320, out_channels=128, kernel_size=3, stride=1, padding=1),
- nn.BatchNorm2d(128),
- nn.ReLU(),
- nn.Conv2d(in_channels=128, out_channels=128, kernel_size=3, stride=1, padding=1),
- nn.BatchNorm2d(128),
- nn.ReLU(),
- )
- self.CTmerge3 = nn.Sequential(
- nn.Conv2d(in_channels=768, out_channels=512, kernel_size=3, stride=1, padding=1),
- nn.BatchNorm2d(512),
- nn.ReLU(),
- nn.Conv2d(in_channels=512, out_channels=384, kernel_size=3, stride=1, padding=1),
- nn.BatchNorm2d(384),
- nn.ReLU(),
- nn.Conv2d(in_channels=384, out_channels=384, kernel_size=3, stride=1, padding=1),
- nn.BatchNorm2d(384),
- nn.ReLU(),
- )
-
- self.CTmerge4 = nn.Sequential(
- nn.Conv2d(in_channels=896, out_channels=640, kernel_size=3, stride=1, padding=1),
- nn.BatchNorm2d(640),
- nn.ReLU(),
- nn.Conv2d(in_channels=640, out_channels=512, kernel_size=3, stride=1, padding=1),
- nn.BatchNorm2d(512),
- nn.ReLU(),
- nn.Conv2d(in_channels=512, out_channels=512, kernel_size=3, stride=1, padding=1),
- nn.BatchNorm2d(512),
- nn.ReLU(),
- )
-
- # decoder
- self.decoder4 = nn.Sequential(
- DepthwiseConv2d(
- in_chans=1408,
- out_chans=1024,
- kernel_size=3,
- stride=1,
- padding=1
- ),
- DepthwiseConv2d(
- in_chans=1024,
- out_chans=512,
- kernel_size=3,
- stride=1,
- padding=1
- ),
- nn.GELU()
- )
- self.decoder3 = nn.Sequential(
- DepthwiseConv2d(
- in_chans=896,
- out_chans=512,
- kernel_size=3,
- stride=1,
- padding=1
- ),
- DepthwiseConv2d(
- in_chans=512,
- out_chans=384,
- kernel_size=3,
- stride=1,
- padding=1
- ),
- nn.GELU()
- )
-
- self.decoder2 = nn.Sequential(
- DepthwiseConv2d(
- in_chans=576,
- out_chans=256,
- kernel_size=3,
- stride=1,
- padding=1
- ),
- DepthwiseConv2d(
- in_chans=256,
- out_chans=192,
- kernel_size=3,
- stride=1,
- padding=1
- ),
- nn.GELU()
- )
-
- self.decoder1 = nn.Sequential(
- DepthwiseConv2d(
- in_chans=256,
- out_chans=64,
- kernel_size=3,
- stride=1,
- padding=1
- ),
- DepthwiseConv2d(
- in_chans=64,
- out_chans=16,
- kernel_size=3,
- stride=1,
- padding=1
- ),
- nn.GELU()
- )
- self.sbr4 = SBR(512)
- self.sbr3 = SBR(384)
- self.sbr2 = SBR(192)
- self.sbr1 = SBR(16)
-
- self.head = nn.Conv2d(in_channels=16, out_channels=num_classes, kernel_size=1)
-
- def forward(self, input):
- ### encoder ###
- # stage1 = ts1 cat cs1
- # t_s1 = self.t_stage1(input)
- # print(input.shape)
- # print('++++++++++++++++++++++')
-
- t_s1 = self.stage1_conv_embed(input) # 1*3*224*224-->1*3136*64
-
- # print(t_s1.shape)
- # print('======================')
-
- t_s1 = self.stage1_transformer(t_s1) # 1*3136*64-->1*64*56*56
-
- # print(t_s1.shape)
- # print('----------------------')
-
- c_s1 = self.c_stage1(input) # 1*3*224*224-->1*64*112*112
-
- # print(c_s1.shape)
- # print('!!!!!!!!!!!!!!!!!!!!!!!')
-
- stage1 = self.CTmerge1(torch.cat([t_s1, self.c_max(c_s1)], dim=1)) # 1*64*56*56 # 拼接两条分支
-
- # print(stage1.shape)
- # print('[[[[[[[[[[[[[[[[[[[[[[[')
-
- # stage2 = ts2 up cs2
- # t_s2 = self.t_stage2(stage1)
- t_s2 = self.stage2_conv_embed(stage1) # 1*64*56*56-->1*784*192 # stage2_conv_embed是转化为序列操作
-
- # print(t_s2.shape)
- # print('[[[[[[[[[[[[[[[[[[[[[[[')
- t_s2 = self.stage2_transformer(t_s2) # 1*784*192-->1*192*28*28
- # print(t_s2.shape)
- # print('+++++++++++++++++++++++++')
-
- c_s2 = self.c_stage2(c_s1) # 1*64*112*112-->1*128*56*56
- stage2 = self.CTmerge2(
- torch.cat([c_s2, F.interpolate(t_s2, size=c_s2.size()[2:], mode='bilinear', align_corners=True)],
- dim=1)) # mode='bilinear'表示使用双线性插值 1*128*56*56
-
- # stage3 = ts3 cat cs3
- # t_s3 = self.t_stage3(t_s2)
- t_s3 = self.stage3_conv_embed(t_s2) # 1*192*28*28-->1*196*384
- # print(t_s3.shape)
- # print('///////////////////////')
- t_s3 = self.stage3_transformer(t_s3) # 1*196*384-->1*384*14*14
- # print(t_s3.shape)
- # print('....................')
- c_s3 = self.c_stage3(stage2) # 1*128*56*56-->1*384*28*28
- stage3 = self.CTmerge3(torch.cat([t_s3, self.c_max(c_s3)], dim=1)) # 1*384*14*14
-
- # stage4 = ts4 up cs4
- # t_s4 = self.t_stage4(stage3)
- t_s4 = self.stage4_conv_embed(stage3) # 1*384*14*14-->1*49*384
- # print(t_s4.shape)
- # print(';;;;;;;;;;;;;;;;;;;;;;;')
- t_s4 = self.stage4_transformer(t_s4) # 1*49*384-->1*384*7*7
- # print(t_s4.shape)
- # print('::::::::::::::::::::')
-
- c_s4 = self.c_stage4(c_s3) # 1*384*28*28-->1*512*14*14
- stage4 = self.CTmerge4(
- torch.cat([c_s4, F.interpolate(t_s4, size=c_s4.size()[2:], mode='bilinear', align_corners=True)],
- dim=1)) # 1*512*14*14
-
- # cs5
- c_s5 = self.c_stage5(stage4) # 1*512*14*14-->1*1024*7*7
-
- ### decoder ###
- decoder4 = torch.cat([c_s5, t_s4], dim=1) # 1*1408*7*7
- decoder4 = self.decoder4(decoder4) # 1*1408*7*7-->1*512*7*7
- decoder4 = F.interpolate(decoder4, size=c_s3.size()[2:], mode='bilinear',
- align_corners=True) # 1*512*7*7-->1*512*28*28
- decoder4 = self.sbr4(decoder4) # 1*512*28*28
- # print(decoder4.shape)
-
- decoder3 = torch.cat([decoder4, c_s3], dim=1) # 1*896*28*28
- decoder3 = self.decoder3(decoder3) # 1*384*28*28
- decoder3 = F.interpolate(decoder3, size=t_s2.size()[2:], mode='bilinear', align_corners=True) # 1*384*28*28
- decoder3 = self.sbr3(decoder3) # 1*384*28*28
- # print(decoder3.shape)
-
- decoder2 = torch.cat([decoder3, t_s2], dim=1) # 1*576*28*28
- decoder2 = self.decoder2(decoder2) # 1*192*28*28
- decoder2 = F.interpolate(decoder2, size=c_s1.size()[2:], mode='bilinear', align_corners=True) # 1*192*112*112
- decoder2 = self.sbr2(decoder2) # 1*192*112*112
- # print(decoder2.shape)
-
- decoder1 = torch.cat([decoder2, c_s1], dim=1) # 1*256*112*112
- decoder1 = self.decoder1(decoder1) # 1*16*112*112
- # print(decoder1.shape)
- final = F.interpolate(decoder1, size=input.size()[2:], mode='bilinear', align_corners=True) # 1*16*224*224
- # print(final.shape)
- # final = self.sbr1(decoder1)
- # print(final.shape)
- final = self.head(final) # 1*3*224*224
-
- return final
-
-
-if __name__ == '__main__':
- x = torch.rand(1, 3, 224, 224).cuda()
- model = Dual_Branch(img_size=224, in_channels=3, num_classes=7).cuda()
- y = model(x)
- print(y.shape)
- # torch.Size([1, 7, 224, 224])
+# -*- coding: utf-8 -*-
+# @Time : 2024/7/26 上午11:19
+# @Author : xiaoshun
+# @Email : 3038523973@qq.com
+# @File : dual_branch.py
+# @Software: PyCharm
+
+from einops import rearrange
+import torch
+import torch.nn as nn
+import torch.nn.functional as F
+
+
+# from models.Transformer.ViT import truncated_normal_
+
+# Decoder细化卷积模块
+class SBR(nn.Module):
+ def __init__(self, in_ch):
+ super(SBR, self).__init__()
+ self.conv1x3 = nn.Sequential(
+ nn.Conv2d(in_ch, in_ch, kernel_size=(1, 3), stride=1, padding=(0, 1)),
+ nn.BatchNorm2d(in_ch),
+ nn.ReLU(True)
+ )
+ self.conv3x1 = nn.Sequential(
+ nn.Conv2d(in_ch, in_ch, kernel_size=(3, 1), stride=1, padding=(1, 0)),
+ nn.BatchNorm2d(in_ch),
+ nn.ReLU(True)
+ )
+
+ def forward(self, x):
+ out = self.conv3x1(self.conv1x3(x)) # 先进行1x3的卷积,得到结果并将结果再进行3x1的卷积
+ return out + x
+
+
+# 下采样卷积模块 stage 1,2,3
+class c_stage123(nn.Module):
+ def __init__(self, in_chans, out_chans):
+ super().__init__()
+ self.stage123 = nn.Sequential(
+ nn.Conv2d(in_channels=in_chans, out_channels=out_chans, kernel_size=3, stride=2, padding=1),
+ nn.BatchNorm2d(out_chans),
+ nn.ReLU(),
+ nn.Conv2d(in_channels=out_chans, out_channels=out_chans, kernel_size=3, stride=1, padding=1),
+ nn.BatchNorm2d(out_chans),
+ nn.ReLU(),
+ )
+ self.conv1x1_123 = nn.Conv2d(in_channels=in_chans, out_channels=out_chans, kernel_size=1)
+ self.maxpool = nn.MaxPool2d(kernel_size=3, stride=2, padding=1)
+
+ def forward(self, x):
+ stage123 = self.stage123(x) # 3*3卷积,两倍下采样 3*224*224-->64*112*112
+ max = self.maxpool(x) # 最大值池化,两倍下采样 3*224*224-->3*112*112
+ max = self.conv1x1_123(max) # 1*1卷积 3*112*112-->64*112*112
+ stage123 = stage123 + max # 残差结构,广播机制
+ return stage123
+
+
+# 下采样卷积模块 stage4,5
+class c_stage45(nn.Module):
+ def __init__(self, in_chans, out_chans):
+ super().__init__()
+ self.stage45 = nn.Sequential(
+ nn.Conv2d(in_channels=in_chans, out_channels=out_chans, kernel_size=3, stride=2, padding=1),
+ nn.BatchNorm2d(out_chans),
+ nn.ReLU(),
+ nn.Conv2d(in_channels=out_chans, out_channels=out_chans, kernel_size=3, stride=1, padding=1),
+ nn.BatchNorm2d(out_chans),
+ nn.ReLU(),
+ nn.Conv2d(in_channels=out_chans, out_channels=out_chans, kernel_size=3, stride=1, padding=1),
+ nn.BatchNorm2d(out_chans),
+ nn.ReLU(),
+ )
+ self.conv1x1_45 = nn.Conv2d(in_channels=in_chans, out_channels=out_chans, kernel_size=1)
+ self.maxpool = nn.MaxPool2d(kernel_size=3, stride=2, padding=1)
+
+ def forward(self, x):
+ stage45 = self.stage45(x) # 3*3卷积模块 2倍下采样
+ max = self.maxpool(x) # 最大值池化,两倍下采样
+ max = self.conv1x1_45(max) # 1*1卷积模块 调整通道数
+ stage45 = stage45 + max # 残差结构
+ return stage45
+
+
+class Identity(nn.Module): # 恒等映射
+ def __init__(self):
+ super().__init__()
+
+ def forward(self, x):
+ return x
+
+
+# 轻量卷积模块
+class DepthwiseConv2d(nn.Module): # 用于自注意力机制
+ def __init__(self, in_chans, out_chans, kernel_size=1, stride=1, padding=0, dilation=1):
+ super().__init__()
+ # depthwise conv
+ self.depthwise = nn.Conv2d(
+ in_channels=in_chans,
+ out_channels=in_chans,
+ kernel_size=kernel_size,
+ stride=stride,
+ padding=padding,
+ dilation=dilation, # 深层卷积的膨胀率
+ groups=in_chans # 指定分组卷积的组数
+ )
+ # batch norm
+ self.bn = nn.BatchNorm2d(num_features=in_chans)
+
+ # pointwise conv 逐点卷积
+ self.pointwise = nn.Conv2d(
+ in_channels=in_chans,
+ out_channels=out_chans,
+ kernel_size=1
+ )
+
+ def forward(self, x):
+ x = self.depthwise(x)
+ x = self.bn(x)
+ x = self.pointwise(x)
+ return x
+
+
+# residual skip connection 残差跳跃连接
+class Residual(nn.Module):
+ def __init__(self, fn):
+ super().__init__()
+ self.fn = fn
+
+ def forward(self, input, **kwargs):
+ x = self.fn(input, **kwargs)
+ return (x + input)
+
+
+# layer norm plus 层归一化
+class PreNorm(nn.Module): # 代表神经网络层
+ def __init__(self, dim, fn):
+ super().__init__()
+ self.norm = nn.LayerNorm(dim)
+ self.fn = fn
+
+ def forward(self, input, **kwargs):
+ return self.fn(self.norm(input), **kwargs)
+
+
+# FeedForward层使得representation的表达能力更强
+class FeedForward(nn.Module):
+ def __init__(self, dim, hidden_dim, dropout=0.):
+ super().__init__()
+ self.net = nn.Sequential(
+ nn.Linear(in_features=dim, out_features=hidden_dim),
+ nn.GELU(),
+ nn.Dropout(dropout),
+ nn.Linear(in_features=hidden_dim, out_features=dim),
+ nn.Dropout(dropout)
+ )
+
+ def forward(self, input):
+ return self.net(input)
+
+
+class ConvAttnetion(nn.Module):
+ '''
+ using the Depth_Separable_Wise Conv2d to produce the q, k, v instead of using Linear Project in ViT
+ '''
+
+ def __init__(self, dim, img_size, heads=8, dim_head=64, kernel_size=3, q_stride=1, k_stride=1, v_stride=1,
+ dropout=0., last_stage=False):
+ super().__init__()
+ self.last_stage = last_stage
+ self.img_size = img_size
+ inner_dim = dim_head * heads # 512
+ project_out = not (heads == 1 and dim_head == dim)
+
+ self.heads = heads
+ self.scale = dim_head ** (-0.5)
+
+ pad = (kernel_size - q_stride) // 2
+
+ self.to_q = DepthwiseConv2d(in_chans=dim, out_chans=inner_dim, kernel_size=kernel_size, stride=q_stride,
+ padding=pad) # 自注意力机制
+ self.to_k = DepthwiseConv2d(in_chans=dim, out_chans=inner_dim, kernel_size=kernel_size, stride=k_stride,
+ padding=pad)
+ self.to_v = DepthwiseConv2d(in_chans=dim, out_chans=inner_dim, kernel_size=kernel_size, stride=v_stride,
+ padding=pad)
+
+ self.to_out = nn.Sequential(
+ nn.Linear(
+ in_features=inner_dim,
+ out_features=dim
+ ),
+ nn.Dropout(dropout)
+ ) if project_out else Identity()
+
+ def forward(self, x):
+ b, n, c, h = *x.shape, self.heads # * 星号的作用大概是去掉 tuple 属性吧
+
+ # print(x.shape)
+ # print('+++++++++++++++++++++++++++++++++')
+
+ # if语句内容没有使用
+ if self.last_stage:
+ cls_token = x[:, 0]
+ # print(cls_token.shape)
+ # print('+++++++++++++++++++++++++++++++++')
+ x = x[:, 1:] # 去掉每个数组的第一个元素
+
+ cls_token = rearrange(torch.unsqueeze(cls_token, dim=1), 'b n (h d) -> b h n d', h=h)
+
+ # rearrange:用于对张量的维度进行重新变换排序,可用于替换pytorch中的reshape,view,transpose和permute等操作
+ x = rearrange(x, 'b (l w) n -> b n l w', l=self.img_size, w=self.img_size) # [1, 3136, 64]-->1*64*56*56
+ # batch_size,N(通道数),h,w
+
+ q = self.to_q(x) # 1*64*56*56-->1*64*56*56
+ # print(q.shape)
+ # print('++++++++++++++')
+ q = rearrange(q, 'b (h d) l w -> b h (l w) d', h=h) # 1*64*56*56-->1*1*3136*64
+ # print(q.shape)
+ # print('=====================')
+ # batch_size,head,h*w,dim_head
+
+ k = self.to_k(x) # 操作和q一样
+ k = rearrange(k, 'b (h d) l w -> b h (l w) d', h=h)
+ # batch_size,head,h*w,dim_head
+
+ v = self.to_v(x) ##操作和q一样
+ # print(v.shape)
+ # print('[[[[[[[[[[[[[[[[[[[[[[[[[[[[')
+ v = rearrange(v, 'b (h d) l w -> b h (l w) d', h=h)
+ # print(v.shape)
+ # print(']]]]]]]]]]]]]]]]]]]]]]]]]]]')
+ # batch_size,head,h*w,dim_head
+
+ if self.last_stage:
+ # print(q.shape)
+ # print('================')
+ q = torch.cat([cls_token, q], dim=2)
+ # print(q.shape)
+ # print('++++++++++++++++++')
+ v = torch.cat([cls_token, v], dim=2)
+ k = torch.cat([cls_token, k], dim=2)
+
+ # calculate attention by matmul + scale
+ # permute:(batch_size,head,dim_head,h*w
+ # print(k.shape)
+ # print('++++++++++++++++++++')
+ k = k.permute(0, 1, 3, 2) # 1*1*3136*64-->1*1*64*3136
+ # print(k.shape)
+ # print('====================')
+ attention = (q.matmul(k)) # 1*1*3136*3136
+ # print(attention.shape)
+ # print('--------------------')
+ attention = attention * self.scale # 可以得到一个logit的向量,避免出现梯度下降和梯度爆炸
+ # print(attention.shape)
+ # print('####################')
+ # pass a softmax
+ attention = F.softmax(attention, dim=-1)
+ # print(attention.shape)
+ # print('********************')
+
+ # matmul v
+ # attention.matmul(v):(batch_size,head,h*w,dim_head)
+ # permute:(batch_size,h*w,head,dim_head)
+ out = (attention.matmul(v)).permute(0, 2, 1, 3).reshape(b, n,
+ c) # 1*3136*64 这些操作的目的是将注意力权重和值向量相乘后得到的结果进行重塑,得到一个形状为 (batch size, 序列长度, 值向量或矩阵的维度) 的张量
+
+ # linear project
+ out = self.to_out(out)
+ return out
+
+
+# Reshape Layers
+class Rearrange(nn.Module):
+ def __init__(self, string, h, w):
+ super().__init__()
+ self.string = string
+ self.h = h
+ self.w = w
+
+ def forward(self, input):
+
+ if self.string == 'b c h w -> b (h w) c':
+ N, C, H, W = input.shape
+ # print(input.shape)
+ x = torch.reshape(input, shape=(N, -1, self.h * self.w)).permute(0, 2, 1)
+ # print(x.shape)
+ # print('+++++++++++++++++++')
+ if self.string == 'b (h w) c -> b c h w':
+ N, _, C = input.shape
+ # print(input.shape)
+ x = torch.reshape(input, shape=(N, self.h, self.w, -1)).permute(0, 3, 1, 2)
+ # print(x.shape)
+ # print('=====================')
+ return x
+
+
+# Transformer layers
+class Transformer(nn.Module):
+ def __init__(self, dim, img_size, depth, heads, dim_head, mlp_dim, dropout=0., last_stage=False):
+ super().__init__()
+ self.layers = nn.ModuleList([ # 管理子模块,参数注册
+ nn.ModuleList([
+ PreNorm(dim=dim, fn=ConvAttnetion(dim, img_size, heads=heads, dim_head=dim_head, dropout=dropout,
+ last_stage=last_stage)), # 归一化,重参数化
+ PreNorm(dim=dim, fn=FeedForward(dim=dim, hidden_dim=mlp_dim, dropout=dropout))
+ ]) for _ in range(depth)
+ ])
+
+ def forward(self, x):
+ for attn, ff in self.layers:
+ x = x + attn(x)
+ x = x + ff(x)
+ return x
+
+
+class Dual_Branch(nn.Module): # 最主要的大函数
+ def __init__(self, img_size, in_channels, num_classes, dim=64, kernels=[7, 3, 3, 3], strides=[4, 2, 2, 2],
+ heads=[1, 3, 6, 6],
+ depth=[1, 2, 10, 10], pool='cls', dropout=0., emb_dropout=0., scale_dim=4, ):
+ super().__init__()
+
+ assert pool in ['cls', 'mean'], f'pool type must be either cls or mean pooling'
+ self.pool = pool
+ self.dim = dim
+
+ # stage1
+ # k:7 s:4 in: 1, 64, 56, 56 out: 1, 3136, 64
+ self.stage1_conv_embed = nn.Sequential(
+ nn.Conv2d( # 1*3*224*224-->[1, 64, 56, 56]
+ in_channels=in_channels,
+ out_channels=dim,
+ kernel_size=kernels[0],
+ stride=strides[0],
+ padding=2
+ ),
+ Rearrange('b c h w -> b (h w) c', h=img_size // 4, w=img_size // 4), # [1, 64, 56, 56]-->[1, 3136, 64]
+ nn.LayerNorm(dim) # 对每个batch归一化
+ )
+
+ self.stage1_transformer = nn.Sequential(
+ Transformer( #
+ dim=dim,
+ img_size=img_size // 4,
+ depth=depth[0], # Transformer层中的编码器和解码器层数。
+ heads=heads[0],
+ dim_head=self.dim, # 它是每个注意力头的维度大小,通常是嵌入维度除以头数。
+ mlp_dim=dim * scale_dim, # mlp_dim:它是Transformer中前馈神经网络的隐藏层维度大小,通常是嵌入维度乘以一个缩放因子。
+ dropout=dropout,
+ # last_stage=last_stage #它是一个标志位,用于表示该Transformer层是否是最后一层。
+ ),
+ Rearrange('b (h w) c -> b c h w', h=img_size // 4, w=img_size // 4)
+ )
+
+ # stage2
+ # k:3 s:2 in: 1, 192, 28, 28 out: 1, 784, 192
+ in_channels = dim
+ scale = heads[1] // heads[0]
+ dim = scale * dim
+
+ self.stage2_conv_embed = nn.Sequential(
+ nn.Conv2d(
+ in_channels=in_channels,
+ out_channels=dim,
+ kernel_size=kernels[1],
+ stride=strides[1],
+ padding=1
+ ),
+ Rearrange('b c h w -> b (h w) c', h=img_size // 8, w=img_size // 8),
+ nn.LayerNorm(dim)
+ )
+
+ self.stage2_transformer = nn.Sequential(
+ Transformer(
+ dim=dim,
+ img_size=img_size // 8,
+ depth=depth[1],
+ heads=heads[1],
+ dim_head=self.dim,
+ mlp_dim=dim * scale_dim,
+ dropout=dropout
+ ),
+ Rearrange('b (h w) c -> b c h w', h=img_size // 8, w=img_size // 8)
+ )
+
+ # stage3
+ in_channels = dim
+ scale = heads[2] // heads[1]
+ dim = scale * dim
+
+ self.stage3_conv_embed = nn.Sequential(
+ nn.Conv2d(
+ in_channels=in_channels,
+ out_channels=dim,
+ kernel_size=kernels[2],
+ stride=strides[2],
+ padding=1
+ ),
+ Rearrange('b c h w -> b (h w) c', h=img_size // 16, w=img_size // 16),
+ nn.LayerNorm(dim)
+ )
+
+ self.stage3_transformer = nn.Sequential(
+ Transformer(
+ dim=dim,
+ img_size=img_size // 16,
+ depth=depth[2],
+ heads=heads[2],
+ dim_head=self.dim,
+ mlp_dim=dim * scale_dim,
+ dropout=dropout
+ ),
+ Rearrange('b (h w) c -> b c h w', h=img_size // 16, w=img_size // 16)
+ )
+
+ # stage4
+ in_channels = dim
+ scale = heads[3] // heads[2]
+ dim = scale * dim
+
+ self.stage4_conv_embed = nn.Sequential(
+ nn.Conv2d(
+ in_channels=in_channels,
+ out_channels=dim,
+ kernel_size=kernels[3],
+ stride=strides[3],
+ padding=1
+ ),
+ Rearrange('b c h w -> b (h w) c', h=img_size // 32, w=img_size // 32),
+ nn.LayerNorm(dim)
+ )
+
+ self.stage4_transformer = nn.Sequential(
+ Transformer(
+ dim=dim, img_size=img_size // 32,
+ depth=depth[3],
+ heads=heads[3],
+ dim_head=self.dim,
+ mlp_dim=dim * scale_dim,
+ dropout=dropout,
+ ),
+ Rearrange('b (h w) c -> b c h w', h=img_size // 32, w=img_size // 32)
+ )
+
+ ### CNN Branch ###
+ self.c_stage1 = c_stage123(in_chans=3, out_chans=64)
+ self.c_stage2 = c_stage123(in_chans=64, out_chans=128)
+ self.c_stage3 = c_stage123(in_chans=128, out_chans=384)
+ self.c_stage4 = c_stage45(in_chans=384, out_chans=512)
+ self.c_stage5 = c_stage45(in_chans=512, out_chans=1024)
+ self.c_max = nn.MaxPool2d(kernel_size=3, stride=2, padding=1)
+ self.up_conv1 = nn.Conv2d(in_channels=192, out_channels=128, kernel_size=1)
+ self.up_conv2 = nn.Conv2d(in_channels=384, out_channels=512, kernel_size=1)
+
+ ### CTmerge ###
+ self.CTmerge1 = nn.Sequential(
+ nn.Conv2d(in_channels=128, out_channels=64, kernel_size=3, stride=1, padding=1),
+ nn.BatchNorm2d(64),
+ nn.ReLU(),
+ nn.Conv2d(in_channels=64, out_channels=64, kernel_size=3, stride=1, padding=1),
+ nn.BatchNorm2d(64),
+ nn.ReLU(),
+ )
+ self.CTmerge2 = nn.Sequential(
+ nn.Conv2d(in_channels=320, out_channels=128, kernel_size=3, stride=1, padding=1),
+ nn.BatchNorm2d(128),
+ nn.ReLU(),
+ nn.Conv2d(in_channels=128, out_channels=128, kernel_size=3, stride=1, padding=1),
+ nn.BatchNorm2d(128),
+ nn.ReLU(),
+ )
+ self.CTmerge3 = nn.Sequential(
+ nn.Conv2d(in_channels=768, out_channels=512, kernel_size=3, stride=1, padding=1),
+ nn.BatchNorm2d(512),
+ nn.ReLU(),
+ nn.Conv2d(in_channels=512, out_channels=384, kernel_size=3, stride=1, padding=1),
+ nn.BatchNorm2d(384),
+ nn.ReLU(),
+ nn.Conv2d(in_channels=384, out_channels=384, kernel_size=3, stride=1, padding=1),
+ nn.BatchNorm2d(384),
+ nn.ReLU(),
+ )
+
+ self.CTmerge4 = nn.Sequential(
+ nn.Conv2d(in_channels=896, out_channels=640, kernel_size=3, stride=1, padding=1),
+ nn.BatchNorm2d(640),
+ nn.ReLU(),
+ nn.Conv2d(in_channels=640, out_channels=512, kernel_size=3, stride=1, padding=1),
+ nn.BatchNorm2d(512),
+ nn.ReLU(),
+ nn.Conv2d(in_channels=512, out_channels=512, kernel_size=3, stride=1, padding=1),
+ nn.BatchNorm2d(512),
+ nn.ReLU(),
+ )
+
+ # decoder
+ self.decoder4 = nn.Sequential(
+ DepthwiseConv2d(
+ in_chans=1408,
+ out_chans=1024,
+ kernel_size=3,
+ stride=1,
+ padding=1
+ ),
+ DepthwiseConv2d(
+ in_chans=1024,
+ out_chans=512,
+ kernel_size=3,
+ stride=1,
+ padding=1
+ ),
+ nn.GELU()
+ )
+ self.decoder3 = nn.Sequential(
+ DepthwiseConv2d(
+ in_chans=896,
+ out_chans=512,
+ kernel_size=3,
+ stride=1,
+ padding=1
+ ),
+ DepthwiseConv2d(
+ in_chans=512,
+ out_chans=384,
+ kernel_size=3,
+ stride=1,
+ padding=1
+ ),
+ nn.GELU()
+ )
+
+ self.decoder2 = nn.Sequential(
+ DepthwiseConv2d(
+ in_chans=576,
+ out_chans=256,
+ kernel_size=3,
+ stride=1,
+ padding=1
+ ),
+ DepthwiseConv2d(
+ in_chans=256,
+ out_chans=192,
+ kernel_size=3,
+ stride=1,
+ padding=1
+ ),
+ nn.GELU()
+ )
+
+ self.decoder1 = nn.Sequential(
+ DepthwiseConv2d(
+ in_chans=256,
+ out_chans=64,
+ kernel_size=3,
+ stride=1,
+ padding=1
+ ),
+ DepthwiseConv2d(
+ in_chans=64,
+ out_chans=16,
+ kernel_size=3,
+ stride=1,
+ padding=1
+ ),
+ nn.GELU()
+ )
+ self.sbr4 = SBR(512)
+ self.sbr3 = SBR(384)
+ self.sbr2 = SBR(192)
+ self.sbr1 = SBR(16)
+
+ self.head = nn.Conv2d(in_channels=16, out_channels=num_classes, kernel_size=1)
+
+ def forward(self, input):
+ ### encoder ###
+ # stage1 = ts1 cat cs1
+ # t_s1 = self.t_stage1(input)
+ # print(input.shape)
+ # print('++++++++++++++++++++++')
+
+ t_s1 = self.stage1_conv_embed(input) # 1*3*224*224-->1*3136*64
+
+ # print(t_s1.shape)
+ # print('======================')
+
+ t_s1 = self.stage1_transformer(t_s1) # 1*3136*64-->1*64*56*56
+
+ # print(t_s1.shape)
+ # print('----------------------')
+
+ c_s1 = self.c_stage1(input) # 1*3*224*224-->1*64*112*112
+
+ # print(c_s1.shape)
+ # print('!!!!!!!!!!!!!!!!!!!!!!!')
+
+ stage1 = self.CTmerge1(torch.cat([t_s1, self.c_max(c_s1)], dim=1)) # 1*64*56*56 # 拼接两条分支
+
+ # print(stage1.shape)
+ # print('[[[[[[[[[[[[[[[[[[[[[[[')
+
+ # stage2 = ts2 up cs2
+ # t_s2 = self.t_stage2(stage1)
+ t_s2 = self.stage2_conv_embed(stage1) # 1*64*56*56-->1*784*192 # stage2_conv_embed是转化为序列操作
+
+ # print(t_s2.shape)
+ # print('[[[[[[[[[[[[[[[[[[[[[[[')
+ t_s2 = self.stage2_transformer(t_s2) # 1*784*192-->1*192*28*28
+ # print(t_s2.shape)
+ # print('+++++++++++++++++++++++++')
+
+ c_s2 = self.c_stage2(c_s1) # 1*64*112*112-->1*128*56*56
+ stage2 = self.CTmerge2(
+ torch.cat([c_s2, F.interpolate(t_s2, size=c_s2.size()[2:], mode='bilinear', align_corners=True)],
+ dim=1)) # mode='bilinear'表示使用双线性插值 1*128*56*56
+
+ # stage3 = ts3 cat cs3
+ # t_s3 = self.t_stage3(t_s2)
+ t_s3 = self.stage3_conv_embed(t_s2) # 1*192*28*28-->1*196*384
+ # print(t_s3.shape)
+ # print('///////////////////////')
+ t_s3 = self.stage3_transformer(t_s3) # 1*196*384-->1*384*14*14
+ # print(t_s3.shape)
+ # print('....................')
+ c_s3 = self.c_stage3(stage2) # 1*128*56*56-->1*384*28*28
+ stage3 = self.CTmerge3(torch.cat([t_s3, self.c_max(c_s3)], dim=1)) # 1*384*14*14
+
+ # stage4 = ts4 up cs4
+ # t_s4 = self.t_stage4(stage3)
+ t_s4 = self.stage4_conv_embed(stage3) # 1*384*14*14-->1*49*384
+ # print(t_s4.shape)
+ # print(';;;;;;;;;;;;;;;;;;;;;;;')
+ t_s4 = self.stage4_transformer(t_s4) # 1*49*384-->1*384*7*7
+ # print(t_s4.shape)
+ # print('::::::::::::::::::::')
+
+ c_s4 = self.c_stage4(c_s3) # 1*384*28*28-->1*512*14*14
+ stage4 = self.CTmerge4(
+ torch.cat([c_s4, F.interpolate(t_s4, size=c_s4.size()[2:], mode='bilinear', align_corners=True)],
+ dim=1)) # 1*512*14*14
+
+ # cs5
+ c_s5 = self.c_stage5(stage4) # 1*512*14*14-->1*1024*7*7
+
+ ### decoder ###
+ decoder4 = torch.cat([c_s5, t_s4], dim=1) # 1*1408*7*7
+ decoder4 = self.decoder4(decoder4) # 1*1408*7*7-->1*512*7*7
+ decoder4 = F.interpolate(decoder4, size=c_s3.size()[2:], mode='bilinear',
+ align_corners=True) # 1*512*7*7-->1*512*28*28
+ decoder4 = self.sbr4(decoder4) # 1*512*28*28
+ # print(decoder4.shape)
+
+ decoder3 = torch.cat([decoder4, c_s3], dim=1) # 1*896*28*28
+ decoder3 = self.decoder3(decoder3) # 1*384*28*28
+ decoder3 = F.interpolate(decoder3, size=t_s2.size()[2:], mode='bilinear', align_corners=True) # 1*384*28*28
+ decoder3 = self.sbr3(decoder3) # 1*384*28*28
+ # print(decoder3.shape)
+
+ decoder2 = torch.cat([decoder3, t_s2], dim=1) # 1*576*28*28
+ decoder2 = self.decoder2(decoder2) # 1*192*28*28
+ decoder2 = F.interpolate(decoder2, size=c_s1.size()[2:], mode='bilinear', align_corners=True) # 1*192*112*112
+ decoder2 = self.sbr2(decoder2) # 1*192*112*112
+ # print(decoder2.shape)
+
+ decoder1 = torch.cat([decoder2, c_s1], dim=1) # 1*256*112*112
+ decoder1 = self.decoder1(decoder1) # 1*16*112*112
+ # print(decoder1.shape)
+ final = F.interpolate(decoder1, size=input.size()[2:], mode='bilinear', align_corners=True) # 1*16*224*224
+ # print(final.shape)
+ # final = self.sbr1(decoder1)
+ # print(final.shape)
+ final = self.head(final) # 1*3*224*224
+
+ return final
+
+
+if __name__ == '__main__':
+ x = torch.rand(1, 3, 224, 224).cuda()
+ model = Dual_Branch(img_size=224, in_channels=3, num_classes=7).cuda()
+ y = model(x)
+ print(y.shape)
+ # torch.Size([1, 7, 224, 224])
diff --git a/src/models/components/hrcloud.py b/src/models/components/hrcloud.py
index 5272936195ba96fbd6695e9983ae47a8040eaee0..94fe7b7d0471d36ac5465f128376d72369155d77 100644
--- a/src/models/components/hrcloud.py
+++ b/src/models/components/hrcloud.py
@@ -1,751 +1,751 @@
-# 论文地址:https://arxiv.org/abs/2407.07365
-#
-from __future__ import absolute_import
-from __future__ import division
-from __future__ import print_function
-
-import logging
-import os
-
-import numpy as np
-import torch
-import torch._utils
-import torch.nn as nn
-import torch.nn.functional as F
-
-BatchNorm2d = nn.BatchNorm2d
-# BN_MOMENTUM = 0.01
-relu_inplace = True
-BN_MOMENTUM = 0.1
-ALIGN_CORNERS = True
-
-logger = logging.getLogger(__name__)
-
-
-def conv3x3(in_planes, out_planes, stride=1):
- """3x3 convolution with padding"""
- return nn.Conv2d(in_planes, out_planes, kernel_size=3, stride=stride,
- padding=1, bias=False)
-
-
-from yacs.config import CfgNode as CN
-import math
-from einops import rearrange
-
-# configs for HRNet48
-HRNET_48 = CN()
-HRNET_48.FINAL_CONV_KERNEL = 1
-
-HRNET_48.STAGE1 = CN()
-HRNET_48.STAGE1.NUM_MODULES = 1
-HRNET_48.STAGE1.NUM_BRANCHES = 1
-HRNET_48.STAGE1.NUM_BLOCKS = [4]
-HRNET_48.STAGE1.NUM_CHANNELS = [64]
-HRNET_48.STAGE1.BLOCK = 'BOTTLENECK'
-HRNET_48.STAGE1.FUSE_METHOD = 'SUM'
-
-HRNET_48.STAGE2 = CN()
-HRNET_48.STAGE2.NUM_MODULES = 1
-HRNET_48.STAGE2.NUM_BRANCHES = 2
-HRNET_48.STAGE2.NUM_BLOCKS = [4, 4]
-HRNET_48.STAGE2.NUM_CHANNELS = [48, 96]
-HRNET_48.STAGE2.BLOCK = 'BASIC'
-HRNET_48.STAGE2.FUSE_METHOD = 'SUM'
-
-HRNET_48.STAGE3 = CN()
-HRNET_48.STAGE3.NUM_MODULES = 4
-HRNET_48.STAGE3.NUM_BRANCHES = 3
-HRNET_48.STAGE3.NUM_BLOCKS = [4, 4, 4]
-HRNET_48.STAGE3.NUM_CHANNELS = [48, 96, 192]
-HRNET_48.STAGE3.BLOCK = 'BASIC'
-HRNET_48.STAGE3.FUSE_METHOD = 'SUM'
-
-HRNET_48.STAGE4 = CN()
-HRNET_48.STAGE4.NUM_MODULES = 3
-HRNET_48.STAGE4.NUM_BRANCHES = 4
-HRNET_48.STAGE4.NUM_BLOCKS = [4, 4, 4, 4]
-HRNET_48.STAGE4.NUM_CHANNELS = [48, 96, 192, 384]
-HRNET_48.STAGE4.BLOCK = 'BASIC'
-HRNET_48.STAGE4.FUSE_METHOD = 'SUM'
-
-HRNET_32 = CN()
-HRNET_32.FINAL_CONV_KERNEL = 1
-
-HRNET_32.STAGE1 = CN()
-HRNET_32.STAGE1.NUM_MODULES = 1
-HRNET_32.STAGE1.NUM_BRANCHES = 1
-HRNET_32.STAGE1.NUM_BLOCKS = [4]
-HRNET_32.STAGE1.NUM_CHANNELS = [64]
-HRNET_32.STAGE1.BLOCK = 'BOTTLENECK'
-HRNET_32.STAGE1.FUSE_METHOD = 'SUM'
-
-HRNET_32.STAGE2 = CN()
-HRNET_32.STAGE2.NUM_MODULES = 1
-HRNET_32.STAGE2.NUM_BRANCHES = 2
-HRNET_32.STAGE2.NUM_BLOCKS = [4, 4]
-HRNET_32.STAGE2.NUM_CHANNELS = [32, 64]
-HRNET_32.STAGE2.BLOCK = 'BASIC'
-HRNET_32.STAGE2.FUSE_METHOD = 'SUM'
-
-HRNET_32.STAGE3 = CN()
-HRNET_32.STAGE3.NUM_MODULES = 4
-HRNET_32.STAGE3.NUM_BRANCHES = 3
-HRNET_32.STAGE3.NUM_BLOCKS = [4, 4, 4]
-HRNET_32.STAGE3.NUM_CHANNELS = [32, 64, 128]
-HRNET_32.STAGE3.BLOCK = 'BASIC'
-HRNET_32.STAGE3.FUSE_METHOD = 'SUM'
-
-HRNET_32.STAGE4 = CN()
-HRNET_32.STAGE4.NUM_MODULES = 3
-HRNET_32.STAGE4.NUM_BRANCHES = 4
-HRNET_32.STAGE4.NUM_BLOCKS = [4, 4, 4, 4]
-HRNET_32.STAGE4.NUM_CHANNELS = [32, 64, 128, 256]
-HRNET_32.STAGE4.BLOCK = 'BASIC'
-HRNET_32.STAGE4.FUSE_METHOD = 'SUM'
-
-HRNET_18 = CN()
-HRNET_18.FINAL_CONV_KERNEL = 1
-
-HRNET_18.STAGE1 = CN()
-HRNET_18.STAGE1.NUM_MODULES = 1
-HRNET_18.STAGE1.NUM_BRANCHES = 1
-HRNET_18.STAGE1.NUM_BLOCKS = [4]
-HRNET_18.STAGE1.NUM_CHANNELS = [64]
-HRNET_18.STAGE1.BLOCK = 'BOTTLENECK'
-HRNET_18.STAGE1.FUSE_METHOD = 'SUM'
-
-HRNET_18.STAGE2 = CN()
-HRNET_18.STAGE2.NUM_MODULES = 1
-HRNET_18.STAGE2.NUM_BRANCHES = 2
-HRNET_18.STAGE2.NUM_BLOCKS = [4, 4]
-HRNET_18.STAGE2.NUM_CHANNELS = [18, 36]
-HRNET_18.STAGE2.BLOCK = 'BASIC'
-HRNET_18.STAGE2.FUSE_METHOD = 'SUM'
-
-HRNET_18.STAGE3 = CN()
-HRNET_18.STAGE3.NUM_MODULES = 4
-HRNET_18.STAGE3.NUM_BRANCHES = 3
-HRNET_18.STAGE3.NUM_BLOCKS = [4, 4, 4]
-HRNET_18.STAGE3.NUM_CHANNELS = [18, 36, 72]
-HRNET_18.STAGE3.BLOCK = 'BASIC'
-HRNET_18.STAGE3.FUSE_METHOD = 'SUM'
-
-HRNET_18.STAGE4 = CN()
-HRNET_18.STAGE4.NUM_MODULES = 3
-HRNET_18.STAGE4.NUM_BRANCHES = 4
-HRNET_18.STAGE4.NUM_BLOCKS = [4, 4, 4, 4]
-HRNET_18.STAGE4.NUM_CHANNELS = [18, 36, 72, 144]
-HRNET_18.STAGE4.BLOCK = 'BASIC'
-HRNET_18.STAGE4.FUSE_METHOD = 'SUM'
-
-
-class PPM(nn.Module):
- def __init__(self, in_dim, reduction_dim, bins):
- super(PPM, self).__init__()
- self.features = []
- for bin in bins:
- self.features.append(nn.Sequential(
- nn.AdaptiveAvgPool2d(bin),
- nn.Conv2d(in_dim, reduction_dim, kernel_size=1, bias=False),
- nn.BatchNorm2d(reduction_dim),
- nn.ReLU(inplace=True)
- ))
- self.features = nn.ModuleList(self.features)
-
- def forward(self, x):
- x_size = x.size()
- out = [x]
- for f in self.features:
- out.append(F.interpolate(f(x), x_size[2:], mode='bilinear', align_corners=True))
- return torch.cat(out, 1)
-
-
-class BasicBlock(nn.Module):
- expansion = 1
-
- def __init__(self, inplanes, planes, stride=1, downsample=None):
- super(BasicBlock, self).__init__()
- self.conv1 = conv3x3(inplanes, planes, stride)
- self.bn1 = BatchNorm2d(planes, momentum=BN_MOMENTUM)
- self.relu = nn.ReLU(inplace=relu_inplace)
- self.conv2 = conv3x3(planes, planes)
- self.bn2 = BatchNorm2d(planes, momentum=BN_MOMENTUM)
- self.downsample = downsample
- self.stride = stride
-
- def forward(self, x):
- residual = x
-
- out = self.conv1(x)
- out = self.bn1(out)
- out = self.relu(out)
-
- out = self.conv2(out)
- out = self.bn2(out)
-
- if self.downsample is not None:
- residual = self.downsample(x)
- out = out + residual
- out = self.relu(out)
-
- return out
-
-
-class Bottleneck(nn.Module):
- expansion = 4
-
- def __init__(self, inplanes, planes, stride=1, downsample=None):
- super(Bottleneck, self).__init__()
- self.conv1 = nn.Conv2d(inplanes, planes, kernel_size=1, bias=False)
- self.bn1 = BatchNorm2d(planes, momentum=BN_MOMENTUM)
- self.conv2 = nn.Conv2d(planes, planes, kernel_size=3, stride=stride,
- padding=1, bias=False)
- self.bn2 = BatchNorm2d(planes, momentum=BN_MOMENTUM)
- self.conv3 = nn.Conv2d(planes, planes * self.expansion, kernel_size=1,
- bias=False)
- self.bn3 = BatchNorm2d(planes * self.expansion,
- momentum=BN_MOMENTUM)
- self.relu = nn.ReLU(inplace=relu_inplace)
- self.downsample = downsample
- self.stride = stride
-
- def forward(self, x):
- residual = x
-
- out = self.conv1(x)
- out = self.bn1(out)
- out = self.relu(out)
-
- out = self.conv2(out)
- out = self.bn2(out)
- out = self.relu(out)
-
- out = self.conv3(out)
- out = self.bn3(out)
-
- if self.downsample is not None:
- residual = self.downsample(x)
- # att = self.downsample(att)
- out = out + residual
- out = self.relu(out)
-
- return out
-
-
-class HighResolutionModule(nn.Module):
- def __init__(self, num_branches, blocks, num_blocks, num_inchannels,
- num_channels, fuse_method, multi_scale_output=True):
- super(HighResolutionModule, self).__init__()
- self._check_branches(
- num_branches, blocks, num_blocks, num_inchannels, num_channels)
-
- self.num_inchannels = num_inchannels
- self.fuse_method = fuse_method
- self.num_branches = num_branches
-
- self.multi_scale_output = multi_scale_output
-
- self.branches = self._make_branches(
- num_branches, blocks, num_blocks, num_channels)
- self.fuse_layers = self._make_fuse_layers()
- self.relu = nn.ReLU(inplace=relu_inplace)
-
- def _check_branches(self, num_branches, blocks, num_blocks,
- num_inchannels, num_channels):
- if num_branches != len(num_blocks):
- error_msg = 'NUM_BRANCHES({}) <> NUM_BLOCKS({})'.format(
- num_branches, len(num_blocks))
- logger.error(error_msg)
- raise ValueError(error_msg)
-
- if num_branches != len(num_channels):
- error_msg = 'NUM_BRANCHES({}) <> NUM_CHANNELS({})'.format(
- num_branches, len(num_channels))
- logger.error(error_msg)
- raise ValueError(error_msg)
-
- if num_branches != len(num_inchannels):
- error_msg = 'NUM_BRANCHES({}) <> NUM_INCHANNELS({})'.format(
- num_branches, len(num_inchannels))
- logger.error(error_msg)
- raise ValueError(error_msg)
-
- def _make_one_branch(self, branch_index, block, num_blocks, num_channels,
- stride=1):
- downsample = None
- if stride != 1 or \
- self.num_inchannels[branch_index] != num_channels[branch_index] * block.expansion:
- downsample = nn.Sequential(
- nn.Conv2d(self.num_inchannels[branch_index],
- num_channels[branch_index] * block.expansion,
- kernel_size=1, stride=stride, bias=False),
- BatchNorm2d(num_channels[branch_index] * block.expansion,
- momentum=BN_MOMENTUM),
- )
-
- layers = []
- layers.append(block(self.num_inchannels[branch_index],
- num_channels[branch_index], stride, downsample))
- self.num_inchannels[branch_index] = \
- num_channels[branch_index] * block.expansion
- for i in range(1, num_blocks[branch_index]):
- layers.append(block(self.num_inchannels[branch_index],
- num_channels[branch_index]))
-
- return nn.Sequential(*layers)
-
- # 创建平行层
- def _make_branches(self, num_branches, block, num_blocks, num_channels):
- branches = []
-
- for i in range(num_branches):
- branches.append(
- self._make_one_branch(i, block, num_blocks, num_channels))
-
- return nn.ModuleList(branches)
-
- def _make_fuse_layers(self):
- if self.num_branches == 1:
- return None
- num_branches = self.num_branches # 3
- num_inchannels = self.num_inchannels # [48, 96, 192]
- fuse_layers = []
- for i in range(num_branches if self.multi_scale_output else 1):
- fuse_layer = []
- for j in range(num_branches):
- if j > i:
- fuse_layer.append(nn.Sequential(
- nn.Conv2d(num_inchannels[j],
- num_inchannels[i],
- 1,
- 1,
- 0,
- bias=False),
- BatchNorm2d(num_inchannels[i], momentum=BN_MOMENTUM)))
- elif j == i:
- fuse_layer.append(None)
- else:
- conv3x3s = []
- for k in range(i - j):
- if k == i - j - 1:
- num_outchannels_conv3x3 = num_inchannels[i]
- conv3x3s.append(nn.Sequential(
- nn.Conv2d(num_inchannels[j],
- num_outchannels_conv3x3,
- 3, 2, 1, bias=False),
- BatchNorm2d(num_outchannels_conv3x3,
- momentum=BN_MOMENTUM)))
- else:
- num_outchannels_conv3x3 = num_inchannels[j]
- conv3x3s.append(nn.Sequential(
- nn.Conv2d(num_inchannels[j],
- num_outchannels_conv3x3,
- 3, 2, 1, bias=False),
- BatchNorm2d(num_outchannels_conv3x3,
- momentum=BN_MOMENTUM),
- nn.ReLU(inplace=relu_inplace)))
- fuse_layer.append(nn.Sequential(*conv3x3s))
- fuse_layers.append(nn.ModuleList(fuse_layer))
-
- return nn.ModuleList(fuse_layers)
-
- def get_num_inchannels(self):
- return self.num_inchannels
-
- def forward(self, x):
- if self.num_branches == 1:
- return [self.branches[0](x[0])]
-
- for i in range(self.num_branches):
- x[i] = self.branches[i](x[i])
-
- x_fuse = []
- for i in range(len(self.fuse_layers)):
- y = x[0] if i == 0 else self.fuse_layers[i][0](x[0])
- for j in range(1, self.num_branches):
- if i == j:
- y = y + x[j]
- elif j > i:
- width_output = x[i].shape[-1]
- height_output = x[i].shape[-2]
- y = y + F.interpolate(
- self.fuse_layers[i][j](x[j]),
- size=[height_output, width_output],
- mode='bilinear', align_corners=ALIGN_CORNERS)
- else:
- y = y + self.fuse_layers[i][j](x[j])
- x_fuse.append(self.relu(y))
-
- return x_fuse
-
-
-blocks_dict = {
- 'BASIC': BasicBlock,
- 'BOTTLENECK': Bottleneck
-}
-
-
-class HRcloudNet(nn.Module):
-
- def __init__(self, num_classes=2, base_c=48, **kwargs):
- global ALIGN_CORNERS
- extra = HRNET_48
- super(HRcloudNet, self).__init__()
- ALIGN_CORNERS = True
- # ALIGN_CORNERS = config.MODEL.ALIGN_CORNERS
- self.num_classes = num_classes
- # stem net
- self.conv1 = nn.Conv2d(3, 64, kernel_size=3, stride=2, padding=1,
- bias=False)
- self.bn1 = BatchNorm2d(64, momentum=BN_MOMENTUM)
- self.conv2 = nn.Conv2d(64, 64, kernel_size=3, stride=2, padding=1,
- bias=False)
- self.bn2 = BatchNorm2d(64, momentum=BN_MOMENTUM)
- self.relu = nn.ReLU(inplace=relu_inplace)
-
- self.stage1_cfg = extra['STAGE1']
- num_channels = self.stage1_cfg['NUM_CHANNELS'][0]
- block = blocks_dict[self.stage1_cfg['BLOCK']]
- num_blocks = self.stage1_cfg['NUM_BLOCKS'][0]
- self.layer1 = self._make_layer(block, 64, num_channels, num_blocks)
- stage1_out_channel = block.expansion * num_channels
-
- self.stage2_cfg = extra['STAGE2']
- num_channels = self.stage2_cfg['NUM_CHANNELS']
- block = blocks_dict[self.stage2_cfg['BLOCK']]
- num_channels = [
- num_channels[i] * block.expansion for i in range(len(num_channels))]
- self.transition1 = self._make_transition_layer(
- [stage1_out_channel], num_channels)
- self.stage2, pre_stage_channels = self._make_stage(
- self.stage2_cfg, num_channels)
-
- self.stage3_cfg = extra['STAGE3']
- num_channels = self.stage3_cfg['NUM_CHANNELS']
- block = blocks_dict[self.stage3_cfg['BLOCK']]
- num_channels = [
- num_channels[i] * block.expansion for i in range(len(num_channels))]
- self.transition2 = self._make_transition_layer(
- pre_stage_channels, num_channels) # 只在pre[-1]与cur[-1]之间下采样?
- self.stage3, pre_stage_channels = self._make_stage(
- self.stage3_cfg, num_channels)
-
- self.stage4_cfg = extra['STAGE4']
- num_channels = self.stage4_cfg['NUM_CHANNELS']
- block = blocks_dict[self.stage4_cfg['BLOCK']]
- num_channels = [
- num_channels[i] * block.expansion for i in range(len(num_channels))]
- self.transition3 = self._make_transition_layer(
- pre_stage_channels, num_channels)
- self.stage4, pre_stage_channels = self._make_stage(
- self.stage4_cfg, num_channels, multi_scale_output=True)
- self.out_conv = OutConv(base_c, num_classes)
- last_inp_channels = int(np.sum(pre_stage_channels))
-
- self.corr = Corr(nclass=2)
- self.proj = nn.Sequential(
- # 512 32
- nn.Conv2d(720, 48, kernel_size=3, stride=1, padding=1, bias=True),
- nn.BatchNorm2d(48),
- nn.ReLU(inplace=True),
- nn.Dropout2d(0.1),
- )
- # self.up1 = Up(base_c * 16, base_c * 8 // factor, bilinear)
- self.up2 = Up(base_c * 8, base_c * 4, True)
- self.up3 = Up(base_c * 4, base_c * 2, True)
- self.up4 = Up(base_c * 2, base_c, True)
- fea_dim = 720
- bins = (1, 2, 3, 6)
- self.ppm = PPM(fea_dim, int(fea_dim / len(bins)), bins)
- fea_dim *= 2
- self.cls = nn.Sequential(
- nn.Conv2d(fea_dim, 512, kernel_size=3, padding=1, bias=False),
- nn.BatchNorm2d(512),
- nn.ReLU(inplace=True),
- nn.Dropout2d(p=0.1),
- nn.Conv2d(512, 2, kernel_size=1)
- )
-
- '''
- 转换层的作用有两种情况:
-
- 当前分支数小于之前分支数时,仅对前几个分支进行通道数调整。
- 当前分支数大于之前分支数时,新建一些转换层,对多余的分支进行下采样,改变通道数以适应后续的连接。
- 最终,这些转换层会被组合成一个 nn.ModuleList 对象,并在网络的构建过程中使用。
- 这有助于确保每个分支的通道数在不同阶段之间能够正确匹配,以便进行特征的融合和连接
- '''
-
- def _make_transition_layer(
- self, num_channels_pre_layer, num_channels_cur_layer):
- # 现在的分支数
- num_branches_cur = len(num_channels_cur_layer) # 3
- # 处理前的分支数
- num_branches_pre = len(num_channels_pre_layer) # 2
-
- transition_layers = []
- for i in range(num_branches_cur):
- # 如果当前分支数小于之前分支数,仅针对第一到第二阶段
- if i < num_branches_pre:
- # 如果对应层的通道数不一致,则进行转化(
- if num_channels_cur_layer[i] != num_channels_pre_layer[i]:
- transition_layers.append(nn.Sequential(
-
- nn.Conv2d(num_channels_pre_layer[i],
- num_channels_cur_layer[i],
- 3,
- 1,
- 1,
- bias=False),
- BatchNorm2d(
- num_channels_cur_layer[i], momentum=BN_MOMENTUM),
- nn.ReLU(inplace=relu_inplace)))
- else:
- transition_layers.append(None)
- else: # 在新建层下采样改变通道数
- conv3x3s = []
- for j in range(i + 1 - num_branches_pre): # 3
- inchannels = num_channels_pre_layer[-1]
- outchannels = num_channels_cur_layer[i] \
- if j == i - num_branches_pre else inchannels
- conv3x3s.append(nn.Sequential(
- nn.Conv2d(
- inchannels, outchannels, 3, 2, 1, bias=False),
- BatchNorm2d(outchannels, momentum=BN_MOMENTUM),
- nn.ReLU(inplace=relu_inplace)))
- transition_layers.append(nn.Sequential(*conv3x3s))
-
- return nn.ModuleList(transition_layers)
-
- '''
- _make_layer 函数的主要作用是创建一个由多个相同类型的残差块(Residual Block)组成的层。
- '''
-
- def _make_layer(self, block, inplanes, planes, blocks, stride=1):
- downsample = None
- if stride != 1 or inplanes != planes * block.expansion:
- downsample = nn.Sequential(
- nn.Conv2d(inplanes, planes * block.expansion,
- kernel_size=1, stride=stride, bias=False),
- BatchNorm2d(planes * block.expansion, momentum=BN_MOMENTUM),
- )
-
- layers = []
- layers.append(block(inplanes, planes, stride, downsample))
- inplanes = planes * block.expansion
- for i in range(1, blocks):
- layers.append(block(inplanes, planes))
-
- return nn.Sequential(*layers)
-
- # 多尺度融合
- def _make_stage(self, layer_config, num_inchannels,
- multi_scale_output=True):
- num_modules = layer_config['NUM_MODULES']
- num_branches = layer_config['NUM_BRANCHES']
- num_blocks = layer_config['NUM_BLOCKS']
- num_channels = layer_config['NUM_CHANNELS']
- block = blocks_dict[layer_config['BLOCK']]
- fuse_method = layer_config['FUSE_METHOD']
-
- modules = []
- for i in range(num_modules): # 重复4次
- # multi_scale_output is only used last module
- if not multi_scale_output and i == num_modules - 1:
- reset_multi_scale_output = False
- else:
- reset_multi_scale_output = True
- modules.append(
- HighResolutionModule(num_branches,
- block,
- num_blocks,
- num_inchannels,
- num_channels,
- fuse_method,
- reset_multi_scale_output)
- )
- num_inchannels = modules[-1].get_num_inchannels()
-
- return nn.Sequential(*modules), num_inchannels
-
- def forward(self, input, need_fp=True, use_corr=True):
- # from ipdb import set_trace
- # set_trace()
- x = self.conv1(input)
- x = self.bn1(x)
- x = self.relu(x)
- # x_176 = x
- x = self.conv2(x)
- x = self.bn2(x)
- x = self.relu(x)
- x = self.layer1(x)
-
- x_list = []
- for i in range(self.stage2_cfg['NUM_BRANCHES']): # 2
- if self.transition1[i] is not None:
- x_list.append(self.transition1[i](x))
- else:
- x_list.append(x)
- y_list = self.stage2(x_list)
- # Y1
- x_list = []
- for i in range(self.stage3_cfg['NUM_BRANCHES']):
- if self.transition2[i] is not None:
- if i < self.stage2_cfg['NUM_BRANCHES']:
- x_list.append(self.transition2[i](y_list[i]))
- else:
- x_list.append(self.transition2[i](y_list[-1]))
- else:
- x_list.append(y_list[i])
- y_list = self.stage3(x_list)
-
- x_list = []
- for i in range(self.stage4_cfg['NUM_BRANCHES']):
- if self.transition3[i] is not None:
- if i < self.stage3_cfg['NUM_BRANCHES']:
- x_list.append(self.transition3[i](y_list[i]))
- else:
- x_list.append(self.transition3[i](y_list[-1]))
- else:
- x_list.append(y_list[i])
- x = self.stage4(x_list)
- dict_return = {}
- # Upsampling
- x0_h, x0_w = x[0].size(2), x[0].size(3)
-
- x3 = F.interpolate(x[3], size=(x0_h, x0_w), mode='bilinear', align_corners=ALIGN_CORNERS)
- # x = self.stage3_(x)
- x[2] = self.up2(x[3], x[2])
- x2 = F.interpolate(x[2], size=(x0_h, x0_w), mode='bilinear', align_corners=ALIGN_CORNERS)
- # x = self.stage2_(x)
- x[1] = self.up3(x[2], x[1])
- x1 = F.interpolate(x[1], size=(x0_h, x0_w), mode='bilinear', align_corners=ALIGN_CORNERS)
- x[0] = self.up4(x[1], x[0])
- xk = torch.cat([x[0], x1, x2, x3], 1)
- # PPM
- feat = self.ppm(xk)
- x = self.cls(feat)
- # fp分支
- if need_fp:
- logits = F.interpolate(x, size=input.size()[2:], mode='bilinear', align_corners=True)
- # logits = self.out_conv(torch.cat((x, nn.Dropout2d(0.5)(x))))
- out = logits
- out_fp = logits
- if use_corr:
- proj_feats = self.proj(xk)
- corr_out = self.corr(proj_feats, out)
- corr_out = F.interpolate(corr_out, size=(352, 352), mode="bilinear", align_corners=True)
- dict_return['corr_out'] = corr_out
- dict_return['out'] = out
- dict_return['out_fp'] = out_fp
-
- return dict_return['out']
-
- out = F.interpolate(x, size=input.size()[2:], mode='bilinear', align_corners=True)
- if use_corr: # True
- proj_feats = self.proj(xk)
- # 计算
- corr_out = self.corr(proj_feats, out)
- corr_out = F.interpolate(corr_out, size=(352, 352), mode="bilinear", align_corners=True)
- dict_return['corr_out'] = corr_out
- dict_return['out'] = out
- return dict_return['out']
- # return x
-
- def init_weights(self, pretrained='', ):
- logger.info('=> init weights from normal distribution')
- for m in self.modules():
- if isinstance(m, nn.Conv2d):
- nn.init.normal_(m.weight, std=0.001)
- elif isinstance(m, nn.BatchNorm2d):
- nn.init.constant_(m.weight, 1)
- nn.init.constant_(m.bias, 0)
- if os.path.isfile(pretrained):
- pretrained_dict = torch.load(pretrained)
- logger.info('=> loading pretrained model {}'.format(pretrained))
- model_dict = self.state_dict()
- pretrained_dict = {k: v for k, v in pretrained_dict.items()
- if k in model_dict.keys()}
- for k, _ in pretrained_dict.items():
- logger.info(
- '=> loading {} pretrained model {}'.format(k, pretrained))
- model_dict.update(pretrained_dict)
- self.load_state_dict(model_dict)
-
-
-class OutConv(nn.Sequential):
- def __init__(self, in_channels, num_classes):
- super(OutConv, self).__init__(
- nn.Conv2d(720, num_classes, kernel_size=1)
- )
-
-
-class DoubleConv(nn.Sequential):
- def __init__(self, in_channels, out_channels, mid_channels=None):
- if mid_channels is None:
- mid_channels = out_channels
- super(DoubleConv, self).__init__(
- nn.Conv2d(in_channels + out_channels, mid_channels, kernel_size=3, padding=1, bias=False),
- nn.BatchNorm2d(mid_channels),
- nn.ReLU(inplace=True),
- nn.Conv2d(mid_channels, out_channels, kernel_size=3, padding=1, bias=False),
- nn.BatchNorm2d(out_channels),
- nn.ReLU(inplace=True)
- )
-
-
-class Up(nn.Module):
- def __init__(self, in_channels, out_channels, bilinear=True):
- super(Up, self).__init__()
- if bilinear:
- self.up = nn.Upsample(scale_factor=2, mode='bilinear', align_corners=True)
- self.conv = DoubleConv(in_channels, out_channels, in_channels // 2)
- else:
- self.up = nn.ConvTranspose2d(in_channels, in_channels // 2, kernel_size=2, stride=2)
- self.conv = DoubleConv(in_channels, out_channels)
-
- def forward(self, x1: torch.Tensor, x2: torch.Tensor) -> torch.Tensor:
- x1 = self.up(x1)
- # [N, C, H, W]
- diff_y = x2.size()[2] - x1.size()[2]
- diff_x = x2.size()[3] - x1.size()[3]
-
- # padding_left, padding_right, padding_top, padding_bottom
- x1 = F.pad(x1, [diff_x // 2, diff_x - diff_x // 2,
- diff_y // 2, diff_y - diff_y // 2])
-
- x = torch.cat([x2, x1], dim=1)
- x = self.conv(x)
- return x
-
-
-class Corr(nn.Module):
- def __init__(self, nclass=2):
- super(Corr, self).__init__()
- self.nclass = nclass
- self.conv1 = nn.Conv2d(48, self.nclass, kernel_size=1, stride=1, padding=0, bias=True)
- self.conv2 = nn.Conv2d(48, self.nclass, kernel_size=1, stride=1, padding=0, bias=True)
-
- def forward(self, feature_in, out):
- # in torch.Size([4, 32, 22, 22])
- # out = [4 2 352 352]
- h_in, w_in = math.ceil(feature_in.shape[2] / (1)), math.ceil(feature_in.shape[3] / (1))
- out = F.interpolate(out.detach(), (h_in, w_in), mode='bilinear', align_corners=True)
- feature = F.interpolate(feature_in, (h_in, w_in), mode='bilinear', align_corners=True)
- f1 = rearrange(self.conv1(feature), 'n c h w -> n c (h w)')
- f2 = rearrange(self.conv2(feature), 'n c h w -> n c (h w)')
- out_temp = rearrange(out, 'n c h w -> n c (h w)')
- corr_map = torch.matmul(f1.transpose(1, 2), f2) / torch.sqrt(torch.tensor(f1.shape[1]).float())
- corr_map = F.softmax(corr_map, dim=-1)
- # out_temp 2 2 484
- # corr_map 4 484 484
- out = rearrange(torch.matmul(out_temp, corr_map), 'n c (h w) -> n c h w', h=h_in, w=w_in)
- # out torch.Size([4, 2, 22, 22])
- return out
-
-
-if __name__ == '__main__':
- input = torch.randn(4, 3, 352, 352)
- cloud = HRcloudNet(num_classes=2)
- output = cloud(input)
- print(output.shape)
+# 论文地址:https://arxiv.org/abs/2407.07365
+#
+from __future__ import absolute_import
+from __future__ import division
+from __future__ import print_function
+
+import logging
+import os
+
+import numpy as np
+import torch
+import torch._utils
+import torch.nn as nn
+import torch.nn.functional as F
+
+BatchNorm2d = nn.BatchNorm2d
+# BN_MOMENTUM = 0.01
+relu_inplace = True
+BN_MOMENTUM = 0.1
+ALIGN_CORNERS = True
+
+logger = logging.getLogger(__name__)
+
+
+def conv3x3(in_planes, out_planes, stride=1):
+ """3x3 convolution with padding"""
+ return nn.Conv2d(in_planes, out_planes, kernel_size=3, stride=stride,
+ padding=1, bias=False)
+
+
+from yacs.config import CfgNode as CN
+import math
+from einops import rearrange
+
+# configs for HRNet48
+HRNET_48 = CN()
+HRNET_48.FINAL_CONV_KERNEL = 1
+
+HRNET_48.STAGE1 = CN()
+HRNET_48.STAGE1.NUM_MODULES = 1
+HRNET_48.STAGE1.NUM_BRANCHES = 1
+HRNET_48.STAGE1.NUM_BLOCKS = [4]
+HRNET_48.STAGE1.NUM_CHANNELS = [64]
+HRNET_48.STAGE1.BLOCK = 'BOTTLENECK'
+HRNET_48.STAGE1.FUSE_METHOD = 'SUM'
+
+HRNET_48.STAGE2 = CN()
+HRNET_48.STAGE2.NUM_MODULES = 1
+HRNET_48.STAGE2.NUM_BRANCHES = 2
+HRNET_48.STAGE2.NUM_BLOCKS = [4, 4]
+HRNET_48.STAGE2.NUM_CHANNELS = [48, 96]
+HRNET_48.STAGE2.BLOCK = 'BASIC'
+HRNET_48.STAGE2.FUSE_METHOD = 'SUM'
+
+HRNET_48.STAGE3 = CN()
+HRNET_48.STAGE3.NUM_MODULES = 4
+HRNET_48.STAGE3.NUM_BRANCHES = 3
+HRNET_48.STAGE3.NUM_BLOCKS = [4, 4, 4]
+HRNET_48.STAGE3.NUM_CHANNELS = [48, 96, 192]
+HRNET_48.STAGE3.BLOCK = 'BASIC'
+HRNET_48.STAGE3.FUSE_METHOD = 'SUM'
+
+HRNET_48.STAGE4 = CN()
+HRNET_48.STAGE4.NUM_MODULES = 3
+HRNET_48.STAGE4.NUM_BRANCHES = 4
+HRNET_48.STAGE4.NUM_BLOCKS = [4, 4, 4, 4]
+HRNET_48.STAGE4.NUM_CHANNELS = [48, 96, 192, 384]
+HRNET_48.STAGE4.BLOCK = 'BASIC'
+HRNET_48.STAGE4.FUSE_METHOD = 'SUM'
+
+HRNET_32 = CN()
+HRNET_32.FINAL_CONV_KERNEL = 1
+
+HRNET_32.STAGE1 = CN()
+HRNET_32.STAGE1.NUM_MODULES = 1
+HRNET_32.STAGE1.NUM_BRANCHES = 1
+HRNET_32.STAGE1.NUM_BLOCKS = [4]
+HRNET_32.STAGE1.NUM_CHANNELS = [64]
+HRNET_32.STAGE1.BLOCK = 'BOTTLENECK'
+HRNET_32.STAGE1.FUSE_METHOD = 'SUM'
+
+HRNET_32.STAGE2 = CN()
+HRNET_32.STAGE2.NUM_MODULES = 1
+HRNET_32.STAGE2.NUM_BRANCHES = 2
+HRNET_32.STAGE2.NUM_BLOCKS = [4, 4]
+HRNET_32.STAGE2.NUM_CHANNELS = [32, 64]
+HRNET_32.STAGE2.BLOCK = 'BASIC'
+HRNET_32.STAGE2.FUSE_METHOD = 'SUM'
+
+HRNET_32.STAGE3 = CN()
+HRNET_32.STAGE3.NUM_MODULES = 4
+HRNET_32.STAGE3.NUM_BRANCHES = 3
+HRNET_32.STAGE3.NUM_BLOCKS = [4, 4, 4]
+HRNET_32.STAGE3.NUM_CHANNELS = [32, 64, 128]
+HRNET_32.STAGE3.BLOCK = 'BASIC'
+HRNET_32.STAGE3.FUSE_METHOD = 'SUM'
+
+HRNET_32.STAGE4 = CN()
+HRNET_32.STAGE4.NUM_MODULES = 3
+HRNET_32.STAGE4.NUM_BRANCHES = 4
+HRNET_32.STAGE4.NUM_BLOCKS = [4, 4, 4, 4]
+HRNET_32.STAGE4.NUM_CHANNELS = [32, 64, 128, 256]
+HRNET_32.STAGE4.BLOCK = 'BASIC'
+HRNET_32.STAGE4.FUSE_METHOD = 'SUM'
+
+HRNET_18 = CN()
+HRNET_18.FINAL_CONV_KERNEL = 1
+
+HRNET_18.STAGE1 = CN()
+HRNET_18.STAGE1.NUM_MODULES = 1
+HRNET_18.STAGE1.NUM_BRANCHES = 1
+HRNET_18.STAGE1.NUM_BLOCKS = [4]
+HRNET_18.STAGE1.NUM_CHANNELS = [64]
+HRNET_18.STAGE1.BLOCK = 'BOTTLENECK'
+HRNET_18.STAGE1.FUSE_METHOD = 'SUM'
+
+HRNET_18.STAGE2 = CN()
+HRNET_18.STAGE2.NUM_MODULES = 1
+HRNET_18.STAGE2.NUM_BRANCHES = 2
+HRNET_18.STAGE2.NUM_BLOCKS = [4, 4]
+HRNET_18.STAGE2.NUM_CHANNELS = [18, 36]
+HRNET_18.STAGE2.BLOCK = 'BASIC'
+HRNET_18.STAGE2.FUSE_METHOD = 'SUM'
+
+HRNET_18.STAGE3 = CN()
+HRNET_18.STAGE3.NUM_MODULES = 4
+HRNET_18.STAGE3.NUM_BRANCHES = 3
+HRNET_18.STAGE3.NUM_BLOCKS = [4, 4, 4]
+HRNET_18.STAGE3.NUM_CHANNELS = [18, 36, 72]
+HRNET_18.STAGE3.BLOCK = 'BASIC'
+HRNET_18.STAGE3.FUSE_METHOD = 'SUM'
+
+HRNET_18.STAGE4 = CN()
+HRNET_18.STAGE4.NUM_MODULES = 3
+HRNET_18.STAGE4.NUM_BRANCHES = 4
+HRNET_18.STAGE4.NUM_BLOCKS = [4, 4, 4, 4]
+HRNET_18.STAGE4.NUM_CHANNELS = [18, 36, 72, 144]
+HRNET_18.STAGE4.BLOCK = 'BASIC'
+HRNET_18.STAGE4.FUSE_METHOD = 'SUM'
+
+
+class PPM(nn.Module):
+ def __init__(self, in_dim, reduction_dim, bins):
+ super(PPM, self).__init__()
+ self.features = []
+ for bin in bins:
+ self.features.append(nn.Sequential(
+ nn.AdaptiveAvgPool2d(bin),
+ nn.Conv2d(in_dim, reduction_dim, kernel_size=1, bias=False),
+ nn.BatchNorm2d(reduction_dim),
+ nn.ReLU(inplace=True)
+ ))
+ self.features = nn.ModuleList(self.features)
+
+ def forward(self, x):
+ x_size = x.size()
+ out = [x]
+ for f in self.features:
+ out.append(F.interpolate(f(x), x_size[2:], mode='bilinear', align_corners=True))
+ return torch.cat(out, 1)
+
+
+class BasicBlock(nn.Module):
+ expansion = 1
+
+ def __init__(self, inplanes, planes, stride=1, downsample=None):
+ super(BasicBlock, self).__init__()
+ self.conv1 = conv3x3(inplanes, planes, stride)
+ self.bn1 = BatchNorm2d(planes, momentum=BN_MOMENTUM)
+ self.relu = nn.ReLU(inplace=relu_inplace)
+ self.conv2 = conv3x3(planes, planes)
+ self.bn2 = BatchNorm2d(planes, momentum=BN_MOMENTUM)
+ self.downsample = downsample
+ self.stride = stride
+
+ def forward(self, x):
+ residual = x
+
+ out = self.conv1(x)
+ out = self.bn1(out)
+ out = self.relu(out)
+
+ out = self.conv2(out)
+ out = self.bn2(out)
+
+ if self.downsample is not None:
+ residual = self.downsample(x)
+ out = out + residual
+ out = self.relu(out)
+
+ return out
+
+
+class Bottleneck(nn.Module):
+ expansion = 4
+
+ def __init__(self, inplanes, planes, stride=1, downsample=None):
+ super(Bottleneck, self).__init__()
+ self.conv1 = nn.Conv2d(inplanes, planes, kernel_size=1, bias=False)
+ self.bn1 = BatchNorm2d(planes, momentum=BN_MOMENTUM)
+ self.conv2 = nn.Conv2d(planes, planes, kernel_size=3, stride=stride,
+ padding=1, bias=False)
+ self.bn2 = BatchNorm2d(planes, momentum=BN_MOMENTUM)
+ self.conv3 = nn.Conv2d(planes, planes * self.expansion, kernel_size=1,
+ bias=False)
+ self.bn3 = BatchNorm2d(planes * self.expansion,
+ momentum=BN_MOMENTUM)
+ self.relu = nn.ReLU(inplace=relu_inplace)
+ self.downsample = downsample
+ self.stride = stride
+
+ def forward(self, x):
+ residual = x
+
+ out = self.conv1(x)
+ out = self.bn1(out)
+ out = self.relu(out)
+
+ out = self.conv2(out)
+ out = self.bn2(out)
+ out = self.relu(out)
+
+ out = self.conv3(out)
+ out = self.bn3(out)
+
+ if self.downsample is not None:
+ residual = self.downsample(x)
+ # att = self.downsample(att)
+ out = out + residual
+ out = self.relu(out)
+
+ return out
+
+
+class HighResolutionModule(nn.Module):
+ def __init__(self, num_branches, blocks, num_blocks, num_inchannels,
+ num_channels, fuse_method, multi_scale_output=True):
+ super(HighResolutionModule, self).__init__()
+ self._check_branches(
+ num_branches, blocks, num_blocks, num_inchannels, num_channels)
+
+ self.num_inchannels = num_inchannels
+ self.fuse_method = fuse_method
+ self.num_branches = num_branches
+
+ self.multi_scale_output = multi_scale_output
+
+ self.branches = self._make_branches(
+ num_branches, blocks, num_blocks, num_channels)
+ self.fuse_layers = self._make_fuse_layers()
+ self.relu = nn.ReLU(inplace=relu_inplace)
+
+ def _check_branches(self, num_branches, blocks, num_blocks,
+ num_inchannels, num_channels):
+ if num_branches != len(num_blocks):
+ error_msg = 'NUM_BRANCHES({}) <> NUM_BLOCKS({})'.format(
+ num_branches, len(num_blocks))
+ logger.error(error_msg)
+ raise ValueError(error_msg)
+
+ if num_branches != len(num_channels):
+ error_msg = 'NUM_BRANCHES({}) <> NUM_CHANNELS({})'.format(
+ num_branches, len(num_channels))
+ logger.error(error_msg)
+ raise ValueError(error_msg)
+
+ if num_branches != len(num_inchannels):
+ error_msg = 'NUM_BRANCHES({}) <> NUM_INCHANNELS({})'.format(
+ num_branches, len(num_inchannels))
+ logger.error(error_msg)
+ raise ValueError(error_msg)
+
+ def _make_one_branch(self, branch_index, block, num_blocks, num_channels,
+ stride=1):
+ downsample = None
+ if stride != 1 or \
+ self.num_inchannels[branch_index] != num_channels[branch_index] * block.expansion:
+ downsample = nn.Sequential(
+ nn.Conv2d(self.num_inchannels[branch_index],
+ num_channels[branch_index] * block.expansion,
+ kernel_size=1, stride=stride, bias=False),
+ BatchNorm2d(num_channels[branch_index] * block.expansion,
+ momentum=BN_MOMENTUM),
+ )
+
+ layers = []
+ layers.append(block(self.num_inchannels[branch_index],
+ num_channels[branch_index], stride, downsample))
+ self.num_inchannels[branch_index] = \
+ num_channels[branch_index] * block.expansion
+ for i in range(1, num_blocks[branch_index]):
+ layers.append(block(self.num_inchannels[branch_index],
+ num_channels[branch_index]))
+
+ return nn.Sequential(*layers)
+
+ # 创建平行层
+ def _make_branches(self, num_branches, block, num_blocks, num_channels):
+ branches = []
+
+ for i in range(num_branches):
+ branches.append(
+ self._make_one_branch(i, block, num_blocks, num_channels))
+
+ return nn.ModuleList(branches)
+
+ def _make_fuse_layers(self):
+ if self.num_branches == 1:
+ return None
+ num_branches = self.num_branches # 3
+ num_inchannels = self.num_inchannels # [48, 96, 192]
+ fuse_layers = []
+ for i in range(num_branches if self.multi_scale_output else 1):
+ fuse_layer = []
+ for j in range(num_branches):
+ if j > i:
+ fuse_layer.append(nn.Sequential(
+ nn.Conv2d(num_inchannels[j],
+ num_inchannels[i],
+ 1,
+ 1,
+ 0,
+ bias=False),
+ BatchNorm2d(num_inchannels[i], momentum=BN_MOMENTUM)))
+ elif j == i:
+ fuse_layer.append(None)
+ else:
+ conv3x3s = []
+ for k in range(i - j):
+ if k == i - j - 1:
+ num_outchannels_conv3x3 = num_inchannels[i]
+ conv3x3s.append(nn.Sequential(
+ nn.Conv2d(num_inchannels[j],
+ num_outchannels_conv3x3,
+ 3, 2, 1, bias=False),
+ BatchNorm2d(num_outchannels_conv3x3,
+ momentum=BN_MOMENTUM)))
+ else:
+ num_outchannels_conv3x3 = num_inchannels[j]
+ conv3x3s.append(nn.Sequential(
+ nn.Conv2d(num_inchannels[j],
+ num_outchannels_conv3x3,
+ 3, 2, 1, bias=False),
+ BatchNorm2d(num_outchannels_conv3x3,
+ momentum=BN_MOMENTUM),
+ nn.ReLU(inplace=relu_inplace)))
+ fuse_layer.append(nn.Sequential(*conv3x3s))
+ fuse_layers.append(nn.ModuleList(fuse_layer))
+
+ return nn.ModuleList(fuse_layers)
+
+ def get_num_inchannels(self):
+ return self.num_inchannels
+
+ def forward(self, x):
+ if self.num_branches == 1:
+ return [self.branches[0](x[0])]
+
+ for i in range(self.num_branches):
+ x[i] = self.branches[i](x[i])
+
+ x_fuse = []
+ for i in range(len(self.fuse_layers)):
+ y = x[0] if i == 0 else self.fuse_layers[i][0](x[0])
+ for j in range(1, self.num_branches):
+ if i == j:
+ y = y + x[j]
+ elif j > i:
+ width_output = x[i].shape[-1]
+ height_output = x[i].shape[-2]
+ y = y + F.interpolate(
+ self.fuse_layers[i][j](x[j]),
+ size=[height_output, width_output],
+ mode='bilinear', align_corners=ALIGN_CORNERS)
+ else:
+ y = y + self.fuse_layers[i][j](x[j])
+ x_fuse.append(self.relu(y))
+
+ return x_fuse
+
+
+blocks_dict = {
+ 'BASIC': BasicBlock,
+ 'BOTTLENECK': Bottleneck
+}
+
+
+class HRcloudNet(nn.Module):
+
+ def __init__(self, num_classes=2, base_c=48, **kwargs):
+ global ALIGN_CORNERS
+ extra = HRNET_48
+ super(HRcloudNet, self).__init__()
+ ALIGN_CORNERS = True
+ # ALIGN_CORNERS = config.MODEL.ALIGN_CORNERS
+ self.num_classes = num_classes
+ # stem net
+ self.conv1 = nn.Conv2d(3, 64, kernel_size=3, stride=2, padding=1,
+ bias=False)
+ self.bn1 = BatchNorm2d(64, momentum=BN_MOMENTUM)
+ self.conv2 = nn.Conv2d(64, 64, kernel_size=3, stride=2, padding=1,
+ bias=False)
+ self.bn2 = BatchNorm2d(64, momentum=BN_MOMENTUM)
+ self.relu = nn.ReLU(inplace=relu_inplace)
+
+ self.stage1_cfg = extra['STAGE1']
+ num_channels = self.stage1_cfg['NUM_CHANNELS'][0]
+ block = blocks_dict[self.stage1_cfg['BLOCK']]
+ num_blocks = self.stage1_cfg['NUM_BLOCKS'][0]
+ self.layer1 = self._make_layer(block, 64, num_channels, num_blocks)
+ stage1_out_channel = block.expansion * num_channels
+
+ self.stage2_cfg = extra['STAGE2']
+ num_channels = self.stage2_cfg['NUM_CHANNELS']
+ block = blocks_dict[self.stage2_cfg['BLOCK']]
+ num_channels = [
+ num_channels[i] * block.expansion for i in range(len(num_channels))]
+ self.transition1 = self._make_transition_layer(
+ [stage1_out_channel], num_channels)
+ self.stage2, pre_stage_channels = self._make_stage(
+ self.stage2_cfg, num_channels)
+
+ self.stage3_cfg = extra['STAGE3']
+ num_channels = self.stage3_cfg['NUM_CHANNELS']
+ block = blocks_dict[self.stage3_cfg['BLOCK']]
+ num_channels = [
+ num_channels[i] * block.expansion for i in range(len(num_channels))]
+ self.transition2 = self._make_transition_layer(
+ pre_stage_channels, num_channels) # 只在pre[-1]与cur[-1]之间下采样?
+ self.stage3, pre_stage_channels = self._make_stage(
+ self.stage3_cfg, num_channels)
+
+ self.stage4_cfg = extra['STAGE4']
+ num_channels = self.stage4_cfg['NUM_CHANNELS']
+ block = blocks_dict[self.stage4_cfg['BLOCK']]
+ num_channels = [
+ num_channels[i] * block.expansion for i in range(len(num_channels))]
+ self.transition3 = self._make_transition_layer(
+ pre_stage_channels, num_channels)
+ self.stage4, pre_stage_channels = self._make_stage(
+ self.stage4_cfg, num_channels, multi_scale_output=True)
+ self.out_conv = OutConv(base_c, num_classes)
+ last_inp_channels = int(np.sum(pre_stage_channels))
+
+ self.corr = Corr(nclass=2)
+ self.proj = nn.Sequential(
+ # 512 32
+ nn.Conv2d(720, 48, kernel_size=3, stride=1, padding=1, bias=True),
+ nn.BatchNorm2d(48),
+ nn.ReLU(inplace=True),
+ nn.Dropout2d(0.1),
+ )
+ # self.up1 = Up(base_c * 16, base_c * 8 // factor, bilinear)
+ self.up2 = Up(base_c * 8, base_c * 4, True)
+ self.up3 = Up(base_c * 4, base_c * 2, True)
+ self.up4 = Up(base_c * 2, base_c, True)
+ fea_dim = 720
+ bins = (1, 2, 3, 6)
+ self.ppm = PPM(fea_dim, int(fea_dim / len(bins)), bins)
+ fea_dim *= 2
+ self.cls = nn.Sequential(
+ nn.Conv2d(fea_dim, 512, kernel_size=3, padding=1, bias=False),
+ nn.BatchNorm2d(512),
+ nn.ReLU(inplace=True),
+ nn.Dropout2d(p=0.1),
+ nn.Conv2d(512, 2, kernel_size=1)
+ )
+
+ '''
+ 转换层的作用有两种情况:
+
+ 当前分支数小于之前分支数时,仅对前几个分支进行通道数调整。
+ 当前分支数大于之前分支数时,新建一些转换层,对多余的分支进行下采样,改变通道数以适应后续的连接。
+ 最终,这些转换层会被组合成一个 nn.ModuleList 对象,并在网络的构建过程中使用。
+ 这有助于确保每个分支的通道数在不同阶段之间能够正确匹配,以便进行特征的融合和连接
+ '''
+
+ def _make_transition_layer(
+ self, num_channels_pre_layer, num_channels_cur_layer):
+ # 现在的分支数
+ num_branches_cur = len(num_channels_cur_layer) # 3
+ # 处理前的分支数
+ num_branches_pre = len(num_channels_pre_layer) # 2
+
+ transition_layers = []
+ for i in range(num_branches_cur):
+ # 如果当前分支数小于之前分支数,仅针对第一到第二阶段
+ if i < num_branches_pre:
+ # 如果对应层的通道数不一致,则进行转化(
+ if num_channels_cur_layer[i] != num_channels_pre_layer[i]:
+ transition_layers.append(nn.Sequential(
+
+ nn.Conv2d(num_channels_pre_layer[i],
+ num_channels_cur_layer[i],
+ 3,
+ 1,
+ 1,
+ bias=False),
+ BatchNorm2d(
+ num_channels_cur_layer[i], momentum=BN_MOMENTUM),
+ nn.ReLU(inplace=relu_inplace)))
+ else:
+ transition_layers.append(None)
+ else: # 在新建层下采样改变通道数
+ conv3x3s = []
+ for j in range(i + 1 - num_branches_pre): # 3
+ inchannels = num_channels_pre_layer[-1]
+ outchannels = num_channels_cur_layer[i] \
+ if j == i - num_branches_pre else inchannels
+ conv3x3s.append(nn.Sequential(
+ nn.Conv2d(
+ inchannels, outchannels, 3, 2, 1, bias=False),
+ BatchNorm2d(outchannels, momentum=BN_MOMENTUM),
+ nn.ReLU(inplace=relu_inplace)))
+ transition_layers.append(nn.Sequential(*conv3x3s))
+
+ return nn.ModuleList(transition_layers)
+
+ '''
+ _make_layer 函数的主要作用是创建一个由多个相同类型的残差块(Residual Block)组成的层。
+ '''
+
+ def _make_layer(self, block, inplanes, planes, blocks, stride=1):
+ downsample = None
+ if stride != 1 or inplanes != planes * block.expansion:
+ downsample = nn.Sequential(
+ nn.Conv2d(inplanes, planes * block.expansion,
+ kernel_size=1, stride=stride, bias=False),
+ BatchNorm2d(planes * block.expansion, momentum=BN_MOMENTUM),
+ )
+
+ layers = []
+ layers.append(block(inplanes, planes, stride, downsample))
+ inplanes = planes * block.expansion
+ for i in range(1, blocks):
+ layers.append(block(inplanes, planes))
+
+ return nn.Sequential(*layers)
+
+ # 多尺度融合
+ def _make_stage(self, layer_config, num_inchannels,
+ multi_scale_output=True):
+ num_modules = layer_config['NUM_MODULES']
+ num_branches = layer_config['NUM_BRANCHES']
+ num_blocks = layer_config['NUM_BLOCKS']
+ num_channels = layer_config['NUM_CHANNELS']
+ block = blocks_dict[layer_config['BLOCK']]
+ fuse_method = layer_config['FUSE_METHOD']
+
+ modules = []
+ for i in range(num_modules): # 重复4次
+ # multi_scale_output is only used last module
+ if not multi_scale_output and i == num_modules - 1:
+ reset_multi_scale_output = False
+ else:
+ reset_multi_scale_output = True
+ modules.append(
+ HighResolutionModule(num_branches,
+ block,
+ num_blocks,
+ num_inchannels,
+ num_channels,
+ fuse_method,
+ reset_multi_scale_output)
+ )
+ num_inchannels = modules[-1].get_num_inchannels()
+
+ return nn.Sequential(*modules), num_inchannels
+
+ def forward(self, input, need_fp=True, use_corr=True):
+ # from ipdb import set_trace
+ # set_trace()
+ x = self.conv1(input)
+ x = self.bn1(x)
+ x = self.relu(x)
+ # x_176 = x
+ x = self.conv2(x)
+ x = self.bn2(x)
+ x = self.relu(x)
+ x = self.layer1(x)
+
+ x_list = []
+ for i in range(self.stage2_cfg['NUM_BRANCHES']): # 2
+ if self.transition1[i] is not None:
+ x_list.append(self.transition1[i](x))
+ else:
+ x_list.append(x)
+ y_list = self.stage2(x_list)
+ # Y1
+ x_list = []
+ for i in range(self.stage3_cfg['NUM_BRANCHES']):
+ if self.transition2[i] is not None:
+ if i < self.stage2_cfg['NUM_BRANCHES']:
+ x_list.append(self.transition2[i](y_list[i]))
+ else:
+ x_list.append(self.transition2[i](y_list[-1]))
+ else:
+ x_list.append(y_list[i])
+ y_list = self.stage3(x_list)
+
+ x_list = []
+ for i in range(self.stage4_cfg['NUM_BRANCHES']):
+ if self.transition3[i] is not None:
+ if i < self.stage3_cfg['NUM_BRANCHES']:
+ x_list.append(self.transition3[i](y_list[i]))
+ else:
+ x_list.append(self.transition3[i](y_list[-1]))
+ else:
+ x_list.append(y_list[i])
+ x = self.stage4(x_list)
+ dict_return = {}
+ # Upsampling
+ x0_h, x0_w = x[0].size(2), x[0].size(3)
+
+ x3 = F.interpolate(x[3], size=(x0_h, x0_w), mode='bilinear', align_corners=ALIGN_CORNERS)
+ # x = self.stage3_(x)
+ x[2] = self.up2(x[3], x[2])
+ x2 = F.interpolate(x[2], size=(x0_h, x0_w), mode='bilinear', align_corners=ALIGN_CORNERS)
+ # x = self.stage2_(x)
+ x[1] = self.up3(x[2], x[1])
+ x1 = F.interpolate(x[1], size=(x0_h, x0_w), mode='bilinear', align_corners=ALIGN_CORNERS)
+ x[0] = self.up4(x[1], x[0])
+ xk = torch.cat([x[0], x1, x2, x3], 1)
+ # PPM
+ feat = self.ppm(xk)
+ x = self.cls(feat)
+ # fp分支
+ if need_fp:
+ logits = F.interpolate(x, size=input.size()[2:], mode='bilinear', align_corners=True)
+ # logits = self.out_conv(torch.cat((x, nn.Dropout2d(0.5)(x))))
+ out = logits
+ out_fp = logits
+ if use_corr:
+ proj_feats = self.proj(xk)
+ corr_out = self.corr(proj_feats, out)
+ corr_out = F.interpolate(corr_out, size=(352, 352), mode="bilinear", align_corners=True)
+ dict_return['corr_out'] = corr_out
+ dict_return['out'] = out
+ dict_return['out_fp'] = out_fp
+
+ return dict_return['out']
+
+ out = F.interpolate(x, size=input.size()[2:], mode='bilinear', align_corners=True)
+ if use_corr: # True
+ proj_feats = self.proj(xk)
+ # 计算
+ corr_out = self.corr(proj_feats, out)
+ corr_out = F.interpolate(corr_out, size=(352, 352), mode="bilinear", align_corners=True)
+ dict_return['corr_out'] = corr_out
+ dict_return['out'] = out
+ return dict_return['out']
+ # return x
+
+ def init_weights(self, pretrained='', ):
+ logger.info('=> init weights from normal distribution')
+ for m in self.modules():
+ if isinstance(m, nn.Conv2d):
+ nn.init.normal_(m.weight, std=0.001)
+ elif isinstance(m, nn.BatchNorm2d):
+ nn.init.constant_(m.weight, 1)
+ nn.init.constant_(m.bias, 0)
+ if os.path.isfile(pretrained):
+ pretrained_dict = torch.load(pretrained)
+ logger.info('=> loading pretrained model {}'.format(pretrained))
+ model_dict = self.state_dict()
+ pretrained_dict = {k: v for k, v in pretrained_dict.items()
+ if k in model_dict.keys()}
+ for k, _ in pretrained_dict.items():
+ logger.info(
+ '=> loading {} pretrained model {}'.format(k, pretrained))
+ model_dict.update(pretrained_dict)
+ self.load_state_dict(model_dict)
+
+
+class OutConv(nn.Sequential):
+ def __init__(self, in_channels, num_classes):
+ super(OutConv, self).__init__(
+ nn.Conv2d(720, num_classes, kernel_size=1)
+ )
+
+
+class DoubleConv(nn.Sequential):
+ def __init__(self, in_channels, out_channels, mid_channels=None):
+ if mid_channels is None:
+ mid_channels = out_channels
+ super(DoubleConv, self).__init__(
+ nn.Conv2d(in_channels + out_channels, mid_channels, kernel_size=3, padding=1, bias=False),
+ nn.BatchNorm2d(mid_channels),
+ nn.ReLU(inplace=True),
+ nn.Conv2d(mid_channels, out_channels, kernel_size=3, padding=1, bias=False),
+ nn.BatchNorm2d(out_channels),
+ nn.ReLU(inplace=True)
+ )
+
+
+class Up(nn.Module):
+ def __init__(self, in_channels, out_channels, bilinear=True):
+ super(Up, self).__init__()
+ if bilinear:
+ self.up = nn.Upsample(scale_factor=2, mode='bilinear', align_corners=True)
+ self.conv = DoubleConv(in_channels, out_channels, in_channels // 2)
+ else:
+ self.up = nn.ConvTranspose2d(in_channels, in_channels // 2, kernel_size=2, stride=2)
+ self.conv = DoubleConv(in_channels, out_channels)
+
+ def forward(self, x1: torch.Tensor, x2: torch.Tensor) -> torch.Tensor:
+ x1 = self.up(x1)
+ # [N, C, H, W]
+ diff_y = x2.size()[2] - x1.size()[2]
+ diff_x = x2.size()[3] - x1.size()[3]
+
+ # padding_left, padding_right, padding_top, padding_bottom
+ x1 = F.pad(x1, [diff_x // 2, diff_x - diff_x // 2,
+ diff_y // 2, diff_y - diff_y // 2])
+
+ x = torch.cat([x2, x1], dim=1)
+ x = self.conv(x)
+ return x
+
+
+class Corr(nn.Module):
+ def __init__(self, nclass=2):
+ super(Corr, self).__init__()
+ self.nclass = nclass
+ self.conv1 = nn.Conv2d(48, self.nclass, kernel_size=1, stride=1, padding=0, bias=True)
+ self.conv2 = nn.Conv2d(48, self.nclass, kernel_size=1, stride=1, padding=0, bias=True)
+
+ def forward(self, feature_in, out):
+ # in torch.Size([4, 32, 22, 22])
+ # out = [4 2 352 352]
+ h_in, w_in = math.ceil(feature_in.shape[2] / (1)), math.ceil(feature_in.shape[3] / (1))
+ out = F.interpolate(out.detach(), (h_in, w_in), mode='bilinear', align_corners=True)
+ feature = F.interpolate(feature_in, (h_in, w_in), mode='bilinear', align_corners=True)
+ f1 = rearrange(self.conv1(feature), 'n c h w -> n c (h w)')
+ f2 = rearrange(self.conv2(feature), 'n c h w -> n c (h w)')
+ out_temp = rearrange(out, 'n c h w -> n c (h w)')
+ corr_map = torch.matmul(f1.transpose(1, 2), f2) / torch.sqrt(torch.tensor(f1.shape[1]).float())
+ corr_map = F.softmax(corr_map, dim=-1)
+ # out_temp 2 2 484
+ # corr_map 4 484 484
+ out = rearrange(torch.matmul(out_temp, corr_map), 'n c (h w) -> n c h w', h=h_in, w=w_in)
+ # out torch.Size([4, 2, 22, 22])
+ return out
+
+
+if __name__ == '__main__':
+ input = torch.randn(4, 3, 352, 352)
+ cloud = HRcloudNet(num_classes=2)
+ output = cloud(input)
+ print(output.shape)
# torch.Size([4, 2, 352, 352]) torch.Size([4, 2, 352, 352]) torch.Size([4, 2, 352, 352])
\ No newline at end of file
diff --git a/src/models/components/hrcloudnet.py b/src/models/components/hrcloudnet.py
index 61682e4b41bf606348e5e12fc98c2bfdae020091..5c6b38638addca4d521f24bd44f7e353d72df994 100644
--- a/src/models/components/hrcloudnet.py
+++ b/src/models/components/hrcloudnet.py
@@ -1,751 +1,751 @@
-# 论文地址:https://arxiv.org/abs/2407.07365
-#
-from __future__ import absolute_import
-from __future__ import division
-from __future__ import print_function
-
-import logging
-import os
-
-import numpy as np
-import torch
-import torch._utils
-import torch.nn as nn
-import torch.nn.functional as F
-
-BatchNorm2d = nn.BatchNorm2d
-# BN_MOMENTUM = 0.01
-relu_inplace = True
-BN_MOMENTUM = 0.1
-ALIGN_CORNERS = True
-
-logger = logging.getLogger(__name__)
-
-
-def conv3x3(in_planes, out_planes, stride=1):
- """3x3 convolution with padding"""
- return nn.Conv2d(in_planes, out_planes, kernel_size=3, stride=stride,
- padding=1, bias=False)
-
-
-from yacs.config import CfgNode as CN
-import math
-from einops import rearrange
-
-# configs for HRNet48
-HRNET_48 = CN()
-HRNET_48.FINAL_CONV_KERNEL = 1
-
-HRNET_48.STAGE1 = CN()
-HRNET_48.STAGE1.NUM_MODULES = 1
-HRNET_48.STAGE1.NUM_BRANCHES = 1
-HRNET_48.STAGE1.NUM_BLOCKS = [4]
-HRNET_48.STAGE1.NUM_CHANNELS = [64]
-HRNET_48.STAGE1.BLOCK = 'BOTTLENECK'
-HRNET_48.STAGE1.FUSE_METHOD = 'SUM'
-
-HRNET_48.STAGE2 = CN()
-HRNET_48.STAGE2.NUM_MODULES = 1
-HRNET_48.STAGE2.NUM_BRANCHES = 2
-HRNET_48.STAGE2.NUM_BLOCKS = [4, 4]
-HRNET_48.STAGE2.NUM_CHANNELS = [48, 96]
-HRNET_48.STAGE2.BLOCK = 'BASIC'
-HRNET_48.STAGE2.FUSE_METHOD = 'SUM'
-
-HRNET_48.STAGE3 = CN()
-HRNET_48.STAGE3.NUM_MODULES = 4
-HRNET_48.STAGE3.NUM_BRANCHES = 3
-HRNET_48.STAGE3.NUM_BLOCKS = [4, 4, 4]
-HRNET_48.STAGE3.NUM_CHANNELS = [48, 96, 192]
-HRNET_48.STAGE3.BLOCK = 'BASIC'
-HRNET_48.STAGE3.FUSE_METHOD = 'SUM'
-
-HRNET_48.STAGE4 = CN()
-HRNET_48.STAGE4.NUM_MODULES = 3
-HRNET_48.STAGE4.NUM_BRANCHES = 4
-HRNET_48.STAGE4.NUM_BLOCKS = [4, 4, 4, 4]
-HRNET_48.STAGE4.NUM_CHANNELS = [48, 96, 192, 384]
-HRNET_48.STAGE4.BLOCK = 'BASIC'
-HRNET_48.STAGE4.FUSE_METHOD = 'SUM'
-
-HRNET_32 = CN()
-HRNET_32.FINAL_CONV_KERNEL = 1
-
-HRNET_32.STAGE1 = CN()
-HRNET_32.STAGE1.NUM_MODULES = 1
-HRNET_32.STAGE1.NUM_BRANCHES = 1
-HRNET_32.STAGE1.NUM_BLOCKS = [4]
-HRNET_32.STAGE1.NUM_CHANNELS = [64]
-HRNET_32.STAGE1.BLOCK = 'BOTTLENECK'
-HRNET_32.STAGE1.FUSE_METHOD = 'SUM'
-
-HRNET_32.STAGE2 = CN()
-HRNET_32.STAGE2.NUM_MODULES = 1
-HRNET_32.STAGE2.NUM_BRANCHES = 2
-HRNET_32.STAGE2.NUM_BLOCKS = [4, 4]
-HRNET_32.STAGE2.NUM_CHANNELS = [32, 64]
-HRNET_32.STAGE2.BLOCK = 'BASIC'
-HRNET_32.STAGE2.FUSE_METHOD = 'SUM'
-
-HRNET_32.STAGE3 = CN()
-HRNET_32.STAGE3.NUM_MODULES = 4
-HRNET_32.STAGE3.NUM_BRANCHES = 3
-HRNET_32.STAGE3.NUM_BLOCKS = [4, 4, 4]
-HRNET_32.STAGE3.NUM_CHANNELS = [32, 64, 128]
-HRNET_32.STAGE3.BLOCK = 'BASIC'
-HRNET_32.STAGE3.FUSE_METHOD = 'SUM'
-
-HRNET_32.STAGE4 = CN()
-HRNET_32.STAGE4.NUM_MODULES = 3
-HRNET_32.STAGE4.NUM_BRANCHES = 4
-HRNET_32.STAGE4.NUM_BLOCKS = [4, 4, 4, 4]
-HRNET_32.STAGE4.NUM_CHANNELS = [32, 64, 128, 256]
-HRNET_32.STAGE4.BLOCK = 'BASIC'
-HRNET_32.STAGE4.FUSE_METHOD = 'SUM'
-
-HRNET_18 = CN()
-HRNET_18.FINAL_CONV_KERNEL = 1
-
-HRNET_18.STAGE1 = CN()
-HRNET_18.STAGE1.NUM_MODULES = 1
-HRNET_18.STAGE1.NUM_BRANCHES = 1
-HRNET_18.STAGE1.NUM_BLOCKS = [4]
-HRNET_18.STAGE1.NUM_CHANNELS = [64]
-HRNET_18.STAGE1.BLOCK = 'BOTTLENECK'
-HRNET_18.STAGE1.FUSE_METHOD = 'SUM'
-
-HRNET_18.STAGE2 = CN()
-HRNET_18.STAGE2.NUM_MODULES = 1
-HRNET_18.STAGE2.NUM_BRANCHES = 2
-HRNET_18.STAGE2.NUM_BLOCKS = [4, 4]
-HRNET_18.STAGE2.NUM_CHANNELS = [18, 36]
-HRNET_18.STAGE2.BLOCK = 'BASIC'
-HRNET_18.STAGE2.FUSE_METHOD = 'SUM'
-
-HRNET_18.STAGE3 = CN()
-HRNET_18.STAGE3.NUM_MODULES = 4
-HRNET_18.STAGE3.NUM_BRANCHES = 3
-HRNET_18.STAGE3.NUM_BLOCKS = [4, 4, 4]
-HRNET_18.STAGE3.NUM_CHANNELS = [18, 36, 72]
-HRNET_18.STAGE3.BLOCK = 'BASIC'
-HRNET_18.STAGE3.FUSE_METHOD = 'SUM'
-
-HRNET_18.STAGE4 = CN()
-HRNET_18.STAGE4.NUM_MODULES = 3
-HRNET_18.STAGE4.NUM_BRANCHES = 4
-HRNET_18.STAGE4.NUM_BLOCKS = [4, 4, 4, 4]
-HRNET_18.STAGE4.NUM_CHANNELS = [18, 36, 72, 144]
-HRNET_18.STAGE4.BLOCK = 'BASIC'
-HRNET_18.STAGE4.FUSE_METHOD = 'SUM'
-
-
-class PPM(nn.Module):
- def __init__(self, in_dim, reduction_dim, bins):
- super(PPM, self).__init__()
- self.features = []
- for bin in bins:
- self.features.append(nn.Sequential(
- nn.AdaptiveAvgPool2d(bin),
- nn.Conv2d(in_dim, reduction_dim, kernel_size=1, bias=False),
- nn.BatchNorm2d(reduction_dim),
- nn.ReLU(inplace=True)
- ))
- self.features = nn.ModuleList(self.features)
-
- def forward(self, x):
- x_size = x.size()
- out = [x]
- for f in self.features:
- out.append(F.interpolate(f(x), x_size[2:], mode='bilinear', align_corners=True))
- return torch.cat(out, 1)
-
-
-class BasicBlock(nn.Module):
- expansion = 1
-
- def __init__(self, inplanes, planes, stride=1, downsample=None):
- super(BasicBlock, self).__init__()
- self.conv1 = conv3x3(inplanes, planes, stride)
- self.bn1 = BatchNorm2d(planes, momentum=BN_MOMENTUM)
- self.relu = nn.ReLU(inplace=relu_inplace)
- self.conv2 = conv3x3(planes, planes)
- self.bn2 = BatchNorm2d(planes, momentum=BN_MOMENTUM)
- self.downsample = downsample
- self.stride = stride
-
- def forward(self, x):
- residual = x
-
- out = self.conv1(x)
- out = self.bn1(out)
- out = self.relu(out)
-
- out = self.conv2(out)
- out = self.bn2(out)
-
- if self.downsample is not None:
- residual = self.downsample(x)
- out = out + residual
- out = self.relu(out)
-
- return out
-
-
-class Bottleneck(nn.Module):
- expansion = 4
-
- def __init__(self, inplanes, planes, stride=1, downsample=None):
- super(Bottleneck, self).__init__()
- self.conv1 = nn.Conv2d(inplanes, planes, kernel_size=1, bias=False)
- self.bn1 = BatchNorm2d(planes, momentum=BN_MOMENTUM)
- self.conv2 = nn.Conv2d(planes, planes, kernel_size=3, stride=stride,
- padding=1, bias=False)
- self.bn2 = BatchNorm2d(planes, momentum=BN_MOMENTUM)
- self.conv3 = nn.Conv2d(planes, planes * self.expansion, kernel_size=1,
- bias=False)
- self.bn3 = BatchNorm2d(planes * self.expansion,
- momentum=BN_MOMENTUM)
- self.relu = nn.ReLU(inplace=relu_inplace)
- self.downsample = downsample
- self.stride = stride
-
- def forward(self, x):
- residual = x
-
- out = self.conv1(x)
- out = self.bn1(out)
- out = self.relu(out)
-
- out = self.conv2(out)
- out = self.bn2(out)
- out = self.relu(out)
-
- out = self.conv3(out)
- out = self.bn3(out)
-
- if self.downsample is not None:
- residual = self.downsample(x)
- # att = self.downsample(att)
- out = out + residual
- out = self.relu(out)
-
- return out
-
-
-class HighResolutionModule(nn.Module):
- def __init__(self, num_branches, blocks, num_blocks, num_inchannels,
- num_channels, fuse_method, multi_scale_output=True):
- super(HighResolutionModule, self).__init__()
- self._check_branches(
- num_branches, blocks, num_blocks, num_inchannels, num_channels)
-
- self.num_inchannels = num_inchannels
- self.fuse_method = fuse_method
- self.num_branches = num_branches
-
- self.multi_scale_output = multi_scale_output
-
- self.branches = self._make_branches(
- num_branches, blocks, num_blocks, num_channels)
- self.fuse_layers = self._make_fuse_layers()
- self.relu = nn.ReLU(inplace=relu_inplace)
-
- def _check_branches(self, num_branches, blocks, num_blocks,
- num_inchannels, num_channels):
- if num_branches != len(num_blocks):
- error_msg = 'NUM_BRANCHES({}) <> NUM_BLOCKS({})'.format(
- num_branches, len(num_blocks))
- logger.error(error_msg)
- raise ValueError(error_msg)
-
- if num_branches != len(num_channels):
- error_msg = 'NUM_BRANCHES({}) <> NUM_CHANNELS({})'.format(
- num_branches, len(num_channels))
- logger.error(error_msg)
- raise ValueError(error_msg)
-
- if num_branches != len(num_inchannels):
- error_msg = 'NUM_BRANCHES({}) <> NUM_INCHANNELS({})'.format(
- num_branches, len(num_inchannels))
- logger.error(error_msg)
- raise ValueError(error_msg)
-
- def _make_one_branch(self, branch_index, block, num_blocks, num_channels,
- stride=1):
- downsample = None
- if stride != 1 or \
- self.num_inchannels[branch_index] != num_channels[branch_index] * block.expansion:
- downsample = nn.Sequential(
- nn.Conv2d(self.num_inchannels[branch_index],
- num_channels[branch_index] * block.expansion,
- kernel_size=1, stride=stride, bias=False),
- BatchNorm2d(num_channels[branch_index] * block.expansion,
- momentum=BN_MOMENTUM),
- )
-
- layers = []
- layers.append(block(self.num_inchannels[branch_index],
- num_channels[branch_index], stride, downsample))
- self.num_inchannels[branch_index] = \
- num_channels[branch_index] * block.expansion
- for i in range(1, num_blocks[branch_index]):
- layers.append(block(self.num_inchannels[branch_index],
- num_channels[branch_index]))
-
- return nn.Sequential(*layers)
-
- # 创建平行层
- def _make_branches(self, num_branches, block, num_blocks, num_channels):
- branches = []
-
- for i in range(num_branches):
- branches.append(
- self._make_one_branch(i, block, num_blocks, num_channels))
-
- return nn.ModuleList(branches)
-
- def _make_fuse_layers(self):
- if self.num_branches == 1:
- return None
- num_branches = self.num_branches # 3
- num_inchannels = self.num_inchannels # [48, 96, 192]
- fuse_layers = []
- for i in range(num_branches if self.multi_scale_output else 1):
- fuse_layer = []
- for j in range(num_branches):
- if j > i:
- fuse_layer.append(nn.Sequential(
- nn.Conv2d(num_inchannels[j],
- num_inchannels[i],
- 1,
- 1,
- 0,
- bias=False),
- BatchNorm2d(num_inchannels[i], momentum=BN_MOMENTUM)))
- elif j == i:
- fuse_layer.append(None)
- else:
- conv3x3s = []
- for k in range(i - j):
- if k == i - j - 1:
- num_outchannels_conv3x3 = num_inchannels[i]
- conv3x3s.append(nn.Sequential(
- nn.Conv2d(num_inchannels[j],
- num_outchannels_conv3x3,
- 3, 2, 1, bias=False),
- BatchNorm2d(num_outchannels_conv3x3,
- momentum=BN_MOMENTUM)))
- else:
- num_outchannels_conv3x3 = num_inchannels[j]
- conv3x3s.append(nn.Sequential(
- nn.Conv2d(num_inchannels[j],
- num_outchannels_conv3x3,
- 3, 2, 1, bias=False),
- BatchNorm2d(num_outchannels_conv3x3,
- momentum=BN_MOMENTUM),
- nn.ReLU(inplace=relu_inplace)))
- fuse_layer.append(nn.Sequential(*conv3x3s))
- fuse_layers.append(nn.ModuleList(fuse_layer))
-
- return nn.ModuleList(fuse_layers)
-
- def get_num_inchannels(self):
- return self.num_inchannels
-
- def forward(self, x):
- if self.num_branches == 1:
- return [self.branches[0](x[0])]
-
- for i in range(self.num_branches):
- x[i] = self.branches[i](x[i])
-
- x_fuse = []
- for i in range(len(self.fuse_layers)):
- y = x[0] if i == 0 else self.fuse_layers[i][0](x[0])
- for j in range(1, self.num_branches):
- if i == j:
- y = y + x[j]
- elif j > i:
- width_output = x[i].shape[-1]
- height_output = x[i].shape[-2]
- y = y + F.interpolate(
- self.fuse_layers[i][j](x[j]),
- size=[height_output, width_output],
- mode='bilinear', align_corners=ALIGN_CORNERS)
- else:
- y = y + self.fuse_layers[i][j](x[j])
- x_fuse.append(self.relu(y))
-
- return x_fuse
-
-
-blocks_dict = {
- 'BASIC': BasicBlock,
- 'BOTTLENECK': Bottleneck
-}
-
-
-class HRCloudNet(nn.Module):
-
- def __init__(self, num_classes=2, base_c=48, **kwargs):
- global ALIGN_CORNERS
- extra = HRNET_48
- super(HRCloudNet, self).__init__()
- ALIGN_CORNERS = True
- # ALIGN_CORNERS = config.MODEL.ALIGN_CORNERS
- self.num_classes = num_classes
- # stem net
- self.conv1 = nn.Conv2d(3, 64, kernel_size=3, stride=2, padding=1,
- bias=False)
- self.bn1 = BatchNorm2d(64, momentum=BN_MOMENTUM)
- self.conv2 = nn.Conv2d(64, 64, kernel_size=3, stride=2, padding=1,
- bias=False)
- self.bn2 = BatchNorm2d(64, momentum=BN_MOMENTUM)
- self.relu = nn.ReLU(inplace=relu_inplace)
-
- self.stage1_cfg = extra['STAGE1']
- num_channels = self.stage1_cfg['NUM_CHANNELS'][0]
- block = blocks_dict[self.stage1_cfg['BLOCK']]
- num_blocks = self.stage1_cfg['NUM_BLOCKS'][0]
- self.layer1 = self._make_layer(block, 64, num_channels, num_blocks)
- stage1_out_channel = block.expansion * num_channels
-
- self.stage2_cfg = extra['STAGE2']
- num_channels = self.stage2_cfg['NUM_CHANNELS']
- block = blocks_dict[self.stage2_cfg['BLOCK']]
- num_channels = [
- num_channels[i] * block.expansion for i in range(len(num_channels))]
- self.transition1 = self._make_transition_layer(
- [stage1_out_channel], num_channels)
- self.stage2, pre_stage_channels = self._make_stage(
- self.stage2_cfg, num_channels)
-
- self.stage3_cfg = extra['STAGE3']
- num_channels = self.stage3_cfg['NUM_CHANNELS']
- block = blocks_dict[self.stage3_cfg['BLOCK']]
- num_channels = [
- num_channels[i] * block.expansion for i in range(len(num_channels))]
- self.transition2 = self._make_transition_layer(
- pre_stage_channels, num_channels) # 只在pre[-1]与cur[-1]之间下采样?
- self.stage3, pre_stage_channels = self._make_stage(
- self.stage3_cfg, num_channels)
-
- self.stage4_cfg = extra['STAGE4']
- num_channels = self.stage4_cfg['NUM_CHANNELS']
- block = blocks_dict[self.stage4_cfg['BLOCK']]
- num_channels = [
- num_channels[i] * block.expansion for i in range(len(num_channels))]
- self.transition3 = self._make_transition_layer(
- pre_stage_channels, num_channels)
- self.stage4, pre_stage_channels = self._make_stage(
- self.stage4_cfg, num_channels, multi_scale_output=True)
- self.out_conv = OutConv(base_c, num_classes)
- last_inp_channels = int(np.sum(pre_stage_channels))
-
- self.corr = Corr(nclass=2)
- self.proj = nn.Sequential(
- # 512 32
- nn.Conv2d(720, 48, kernel_size=3, stride=1, padding=1, bias=True),
- nn.BatchNorm2d(48),
- nn.ReLU(inplace=True),
- nn.Dropout2d(0.1),
- )
- # self.up1 = Up(base_c * 16, base_c * 8 // factor, bilinear)
- self.up2 = Up(base_c * 8, base_c * 4, True)
- self.up3 = Up(base_c * 4, base_c * 2, True)
- self.up4 = Up(base_c * 2, base_c, True)
- fea_dim = 720
- bins = (1, 2, 3, 6)
- self.ppm = PPM(fea_dim, int(fea_dim / len(bins)), bins)
- fea_dim *= 2
- self.cls = nn.Sequential(
- nn.Conv2d(fea_dim, 512, kernel_size=3, padding=1, bias=False),
- nn.BatchNorm2d(512),
- nn.ReLU(inplace=True),
- nn.Dropout2d(p=0.1),
- nn.Conv2d(512, 2, kernel_size=1)
- )
-
- '''
- 转换层的作用有两种情况:
-
- 当前分支数小于之前分支数时,仅对前几个分支进行通道数调整。
- 当前分支数大于之前分支数时,新建一些转换层,对多余的分支进行下采样,改变通道数以适应后续的连接。
- 最终,这些转换层会被组合成一个 nn.ModuleList 对象,并在网络的构建过程中使用。
- 这有助于确保每个分支的通道数在不同阶段之间能够正确匹配,以便进行特征的融合和连接
- '''
-
- def _make_transition_layer(
- self, num_channels_pre_layer, num_channels_cur_layer):
- # 现在的分支数
- num_branches_cur = len(num_channels_cur_layer) # 3
- # 处理前的分支数
- num_branches_pre = len(num_channels_pre_layer) # 2
-
- transition_layers = []
- for i in range(num_branches_cur):
- # 如果当前分支数小于之前分支数,仅针对第一到第二阶段
- if i < num_branches_pre:
- # 如果对应层的通道数不一致,则进行转化(
- if num_channels_cur_layer[i] != num_channels_pre_layer[i]:
- transition_layers.append(nn.Sequential(
-
- nn.Conv2d(num_channels_pre_layer[i],
- num_channels_cur_layer[i],
- 3,
- 1,
- 1,
- bias=False),
- BatchNorm2d(
- num_channels_cur_layer[i], momentum=BN_MOMENTUM),
- nn.ReLU(inplace=relu_inplace)))
- else:
- transition_layers.append(None)
- else: # 在新建层下采样改变通道数
- conv3x3s = []
- for j in range(i + 1 - num_branches_pre): # 3
- inchannels = num_channels_pre_layer[-1]
- outchannels = num_channels_cur_layer[i] \
- if j == i - num_branches_pre else inchannels
- conv3x3s.append(nn.Sequential(
- nn.Conv2d(
- inchannels, outchannels, 3, 2, 1, bias=False),
- BatchNorm2d(outchannels, momentum=BN_MOMENTUM),
- nn.ReLU(inplace=relu_inplace)))
- transition_layers.append(nn.Sequential(*conv3x3s))
-
- return nn.ModuleList(transition_layers)
-
- '''
- _make_layer 函数的主要作用是创建一个由多个相同类型的残差块(Residual Block)组成的层。
- '''
-
- def _make_layer(self, block, inplanes, planes, blocks, stride=1):
- downsample = None
- if stride != 1 or inplanes != planes * block.expansion:
- downsample = nn.Sequential(
- nn.Conv2d(inplanes, planes * block.expansion,
- kernel_size=1, stride=stride, bias=False),
- BatchNorm2d(planes * block.expansion, momentum=BN_MOMENTUM),
- )
-
- layers = []
- layers.append(block(inplanes, planes, stride, downsample))
- inplanes = planes * block.expansion
- for i in range(1, blocks):
- layers.append(block(inplanes, planes))
-
- return nn.Sequential(*layers)
-
- # 多尺度融合
- def _make_stage(self, layer_config, num_inchannels,
- multi_scale_output=True):
- num_modules = layer_config['NUM_MODULES']
- num_branches = layer_config['NUM_BRANCHES']
- num_blocks = layer_config['NUM_BLOCKS']
- num_channels = layer_config['NUM_CHANNELS']
- block = blocks_dict[layer_config['BLOCK']]
- fuse_method = layer_config['FUSE_METHOD']
-
- modules = []
- for i in range(num_modules): # 重复4次
- # multi_scale_output is only used last module
- if not multi_scale_output and i == num_modules - 1:
- reset_multi_scale_output = False
- else:
- reset_multi_scale_output = True
- modules.append(
- HighResolutionModule(num_branches,
- block,
- num_blocks,
- num_inchannels,
- num_channels,
- fuse_method,
- reset_multi_scale_output)
- )
- num_inchannels = modules[-1].get_num_inchannels()
-
- return nn.Sequential(*modules), num_inchannels
-
- def forward(self, input, need_fp=True, use_corr=True):
- # from ipdb import set_trace
- # set_trace()
- x = self.conv1(input)
- x = self.bn1(x)
- x = self.relu(x)
- # x_176 = x
- x = self.conv2(x)
- x = self.bn2(x)
- x = self.relu(x)
- x = self.layer1(x)
-
- x_list = []
- for i in range(self.stage2_cfg['NUM_BRANCHES']): # 2
- if self.transition1[i] is not None:
- x_list.append(self.transition1[i](x))
- else:
- x_list.append(x)
- y_list = self.stage2(x_list)
- # Y1
- x_list = []
- for i in range(self.stage3_cfg['NUM_BRANCHES']):
- if self.transition2[i] is not None:
- if i < self.stage2_cfg['NUM_BRANCHES']:
- x_list.append(self.transition2[i](y_list[i]))
- else:
- x_list.append(self.transition2[i](y_list[-1]))
- else:
- x_list.append(y_list[i])
- y_list = self.stage3(x_list)
-
- x_list = []
- for i in range(self.stage4_cfg['NUM_BRANCHES']):
- if self.transition3[i] is not None:
- if i < self.stage3_cfg['NUM_BRANCHES']:
- x_list.append(self.transition3[i](y_list[i]))
- else:
- x_list.append(self.transition3[i](y_list[-1]))
- else:
- x_list.append(y_list[i])
- x = self.stage4(x_list)
- dict_return = {}
- # Upsampling
- x0_h, x0_w = x[0].size(2), x[0].size(3)
-
- x3 = F.interpolate(x[3], size=(x0_h, x0_w), mode='bilinear', align_corners=ALIGN_CORNERS)
- # x = self.stage3_(x)
- x[2] = self.up2(x[3], x[2])
- x2 = F.interpolate(x[2], size=(x0_h, x0_w), mode='bilinear', align_corners=ALIGN_CORNERS)
- # x = self.stage2_(x)
- x[1] = self.up3(x[2], x[1])
- x1 = F.interpolate(x[1], size=(x0_h, x0_w), mode='bilinear', align_corners=ALIGN_CORNERS)
- x[0] = self.up4(x[1], x[0])
- xk = torch.cat([x[0], x1, x2, x3], 1)
- # PPM
- feat = self.ppm(xk)
- x = self.cls(feat)
- # fp分支
- if need_fp:
- logits = F.interpolate(x, size=input.size()[2:], mode='bilinear', align_corners=True)
- # logits = self.out_conv(torch.cat((x, nn.Dropout2d(0.5)(x))))
- out = logits
- out_fp = logits
- if use_corr:
- proj_feats = self.proj(xk)
- corr_out = self.corr(proj_feats, out)
- corr_out = F.interpolate(corr_out, size=(352, 352), mode="bilinear", align_corners=True)
- dict_return['corr_out'] = corr_out
- dict_return['out'] = out
- dict_return['out_fp'] = out_fp
-
- return dict_return['out']
-
- out = F.interpolate(x, size=input.size()[2:], mode='bilinear', align_corners=True)
- if use_corr: # True
- proj_feats = self.proj(xk)
- # 计算
- corr_out = self.corr(proj_feats, out)
- corr_out = F.interpolate(corr_out, size=(352, 352), mode="bilinear", align_corners=True)
- dict_return['corr_out'] = corr_out
- dict_return['out'] = out
- return dict_return['out']
- # return x
-
- def init_weights(self, pretrained='', ):
- logger.info('=> init weights from normal distribution')
- for m in self.modules():
- if isinstance(m, nn.Conv2d):
- nn.init.normal_(m.weight, std=0.001)
- elif isinstance(m, nn.BatchNorm2d):
- nn.init.constant_(m.weight, 1)
- nn.init.constant_(m.bias, 0)
- if os.path.isfile(pretrained):
- pretrained_dict = torch.load(pretrained)
- logger.info('=> loading pretrained model {}'.format(pretrained))
- model_dict = self.state_dict()
- pretrained_dict = {k: v for k, v in pretrained_dict.items()
- if k in model_dict.keys()}
- for k, _ in pretrained_dict.items():
- logger.info(
- '=> loading {} pretrained model {}'.format(k, pretrained))
- model_dict.update(pretrained_dict)
- self.load_state_dict(model_dict)
-
-
-class OutConv(nn.Sequential):
- def __init__(self, in_channels, num_classes):
- super(OutConv, self).__init__(
- nn.Conv2d(720, num_classes, kernel_size=1)
- )
-
-
-class DoubleConv(nn.Sequential):
- def __init__(self, in_channels, out_channels, mid_channels=None):
- if mid_channels is None:
- mid_channels = out_channels
- super(DoubleConv, self).__init__(
- nn.Conv2d(in_channels + out_channels, mid_channels, kernel_size=3, padding=1, bias=False),
- nn.BatchNorm2d(mid_channels),
- nn.ReLU(inplace=True),
- nn.Conv2d(mid_channels, out_channels, kernel_size=3, padding=1, bias=False),
- nn.BatchNorm2d(out_channels),
- nn.ReLU(inplace=True)
- )
-
-
-class Up(nn.Module):
- def __init__(self, in_channels, out_channels, bilinear=True):
- super(Up, self).__init__()
- if bilinear:
- self.up = nn.Upsample(scale_factor=2, mode='bilinear', align_corners=True)
- self.conv = DoubleConv(in_channels, out_channels, in_channels // 2)
- else:
- self.up = nn.ConvTranspose2d(in_channels, in_channels // 2, kernel_size=2, stride=2)
- self.conv = DoubleConv(in_channels, out_channels)
-
- def forward(self, x1: torch.Tensor, x2: torch.Tensor) -> torch.Tensor:
- x1 = self.up(x1)
- # [N, C, H, W]
- diff_y = x2.size()[2] - x1.size()[2]
- diff_x = x2.size()[3] - x1.size()[3]
-
- # padding_left, padding_right, padding_top, padding_bottom
- x1 = F.pad(x1, [diff_x // 2, diff_x - diff_x // 2,
- diff_y // 2, diff_y - diff_y // 2])
-
- x = torch.cat([x2, x1], dim=1)
- x = self.conv(x)
- return x
-
-
-class Corr(nn.Module):
- def __init__(self, nclass=2):
- super(Corr, self).__init__()
- self.nclass = nclass
- self.conv1 = nn.Conv2d(48, self.nclass, kernel_size=1, stride=1, padding=0, bias=True)
- self.conv2 = nn.Conv2d(48, self.nclass, kernel_size=1, stride=1, padding=0, bias=True)
-
- def forward(self, feature_in, out):
- # in torch.Size([4, 32, 22, 22])
- # out = [4 2 352 352]
- h_in, w_in = math.ceil(feature_in.shape[2] / (1)), math.ceil(feature_in.shape[3] / (1))
- out = F.interpolate(out.detach(), (h_in, w_in), mode='bilinear', align_corners=True)
- feature = F.interpolate(feature_in, (h_in, w_in), mode='bilinear', align_corners=True)
- f1 = rearrange(self.conv1(feature), 'n c h w -> n c (h w)')
- f2 = rearrange(self.conv2(feature), 'n c h w -> n c (h w)')
- out_temp = rearrange(out, 'n c h w -> n c (h w)')
- corr_map = torch.matmul(f1.transpose(1, 2), f2) / torch.sqrt(torch.tensor(f1.shape[1]).float())
- corr_map = F.softmax(corr_map, dim=-1)
- # out_temp 2 2 484
- # corr_map 4 484 484
- out = rearrange(torch.matmul(out_temp, corr_map), 'n c (h w) -> n c h w', h=h_in, w=w_in)
- # out torch.Size([4, 2, 22, 22])
- return out
-
-
-if __name__ == '__main__':
- input = torch.randn(4, 3, 352, 352)
- cloud = HRCloudNet(num_classes=2)
- output = cloud(input)
- print(output.shape)
+# 论文地址:https://arxiv.org/abs/2407.07365
+#
+from __future__ import absolute_import
+from __future__ import division
+from __future__ import print_function
+
+import logging
+import os
+
+import numpy as np
+import torch
+import torch._utils
+import torch.nn as nn
+import torch.nn.functional as F
+
+BatchNorm2d = nn.BatchNorm2d
+# BN_MOMENTUM = 0.01
+relu_inplace = True
+BN_MOMENTUM = 0.1
+ALIGN_CORNERS = True
+
+logger = logging.getLogger(__name__)
+
+
+def conv3x3(in_planes, out_planes, stride=1):
+ """3x3 convolution with padding"""
+ return nn.Conv2d(in_planes, out_planes, kernel_size=3, stride=stride,
+ padding=1, bias=False)
+
+
+from yacs.config import CfgNode as CN
+import math
+from einops import rearrange
+
+# configs for HRNet48
+HRNET_48 = CN()
+HRNET_48.FINAL_CONV_KERNEL = 1
+
+HRNET_48.STAGE1 = CN()
+HRNET_48.STAGE1.NUM_MODULES = 1
+HRNET_48.STAGE1.NUM_BRANCHES = 1
+HRNET_48.STAGE1.NUM_BLOCKS = [4]
+HRNET_48.STAGE1.NUM_CHANNELS = [64]
+HRNET_48.STAGE1.BLOCK = 'BOTTLENECK'
+HRNET_48.STAGE1.FUSE_METHOD = 'SUM'
+
+HRNET_48.STAGE2 = CN()
+HRNET_48.STAGE2.NUM_MODULES = 1
+HRNET_48.STAGE2.NUM_BRANCHES = 2
+HRNET_48.STAGE2.NUM_BLOCKS = [4, 4]
+HRNET_48.STAGE2.NUM_CHANNELS = [48, 96]
+HRNET_48.STAGE2.BLOCK = 'BASIC'
+HRNET_48.STAGE2.FUSE_METHOD = 'SUM'
+
+HRNET_48.STAGE3 = CN()
+HRNET_48.STAGE3.NUM_MODULES = 4
+HRNET_48.STAGE3.NUM_BRANCHES = 3
+HRNET_48.STAGE3.NUM_BLOCKS = [4, 4, 4]
+HRNET_48.STAGE3.NUM_CHANNELS = [48, 96, 192]
+HRNET_48.STAGE3.BLOCK = 'BASIC'
+HRNET_48.STAGE3.FUSE_METHOD = 'SUM'
+
+HRNET_48.STAGE4 = CN()
+HRNET_48.STAGE4.NUM_MODULES = 3
+HRNET_48.STAGE4.NUM_BRANCHES = 4
+HRNET_48.STAGE4.NUM_BLOCKS = [4, 4, 4, 4]
+HRNET_48.STAGE4.NUM_CHANNELS = [48, 96, 192, 384]
+HRNET_48.STAGE4.BLOCK = 'BASIC'
+HRNET_48.STAGE4.FUSE_METHOD = 'SUM'
+
+HRNET_32 = CN()
+HRNET_32.FINAL_CONV_KERNEL = 1
+
+HRNET_32.STAGE1 = CN()
+HRNET_32.STAGE1.NUM_MODULES = 1
+HRNET_32.STAGE1.NUM_BRANCHES = 1
+HRNET_32.STAGE1.NUM_BLOCKS = [4]
+HRNET_32.STAGE1.NUM_CHANNELS = [64]
+HRNET_32.STAGE1.BLOCK = 'BOTTLENECK'
+HRNET_32.STAGE1.FUSE_METHOD = 'SUM'
+
+HRNET_32.STAGE2 = CN()
+HRNET_32.STAGE2.NUM_MODULES = 1
+HRNET_32.STAGE2.NUM_BRANCHES = 2
+HRNET_32.STAGE2.NUM_BLOCKS = [4, 4]
+HRNET_32.STAGE2.NUM_CHANNELS = [32, 64]
+HRNET_32.STAGE2.BLOCK = 'BASIC'
+HRNET_32.STAGE2.FUSE_METHOD = 'SUM'
+
+HRNET_32.STAGE3 = CN()
+HRNET_32.STAGE3.NUM_MODULES = 4
+HRNET_32.STAGE3.NUM_BRANCHES = 3
+HRNET_32.STAGE3.NUM_BLOCKS = [4, 4, 4]
+HRNET_32.STAGE3.NUM_CHANNELS = [32, 64, 128]
+HRNET_32.STAGE3.BLOCK = 'BASIC'
+HRNET_32.STAGE3.FUSE_METHOD = 'SUM'
+
+HRNET_32.STAGE4 = CN()
+HRNET_32.STAGE4.NUM_MODULES = 3
+HRNET_32.STAGE4.NUM_BRANCHES = 4
+HRNET_32.STAGE4.NUM_BLOCKS = [4, 4, 4, 4]
+HRNET_32.STAGE4.NUM_CHANNELS = [32, 64, 128, 256]
+HRNET_32.STAGE4.BLOCK = 'BASIC'
+HRNET_32.STAGE4.FUSE_METHOD = 'SUM'
+
+HRNET_18 = CN()
+HRNET_18.FINAL_CONV_KERNEL = 1
+
+HRNET_18.STAGE1 = CN()
+HRNET_18.STAGE1.NUM_MODULES = 1
+HRNET_18.STAGE1.NUM_BRANCHES = 1
+HRNET_18.STAGE1.NUM_BLOCKS = [4]
+HRNET_18.STAGE1.NUM_CHANNELS = [64]
+HRNET_18.STAGE1.BLOCK = 'BOTTLENECK'
+HRNET_18.STAGE1.FUSE_METHOD = 'SUM'
+
+HRNET_18.STAGE2 = CN()
+HRNET_18.STAGE2.NUM_MODULES = 1
+HRNET_18.STAGE2.NUM_BRANCHES = 2
+HRNET_18.STAGE2.NUM_BLOCKS = [4, 4]
+HRNET_18.STAGE2.NUM_CHANNELS = [18, 36]
+HRNET_18.STAGE2.BLOCK = 'BASIC'
+HRNET_18.STAGE2.FUSE_METHOD = 'SUM'
+
+HRNET_18.STAGE3 = CN()
+HRNET_18.STAGE3.NUM_MODULES = 4
+HRNET_18.STAGE3.NUM_BRANCHES = 3
+HRNET_18.STAGE3.NUM_BLOCKS = [4, 4, 4]
+HRNET_18.STAGE3.NUM_CHANNELS = [18, 36, 72]
+HRNET_18.STAGE3.BLOCK = 'BASIC'
+HRNET_18.STAGE3.FUSE_METHOD = 'SUM'
+
+HRNET_18.STAGE4 = CN()
+HRNET_18.STAGE4.NUM_MODULES = 3
+HRNET_18.STAGE4.NUM_BRANCHES = 4
+HRNET_18.STAGE4.NUM_BLOCKS = [4, 4, 4, 4]
+HRNET_18.STAGE4.NUM_CHANNELS = [18, 36, 72, 144]
+HRNET_18.STAGE4.BLOCK = 'BASIC'
+HRNET_18.STAGE4.FUSE_METHOD = 'SUM'
+
+
+class PPM(nn.Module):
+ def __init__(self, in_dim, reduction_dim, bins):
+ super(PPM, self).__init__()
+ self.features = []
+ for bin in bins:
+ self.features.append(nn.Sequential(
+ nn.AdaptiveAvgPool2d(bin),
+ nn.Conv2d(in_dim, reduction_dim, kernel_size=1, bias=False),
+ nn.BatchNorm2d(reduction_dim),
+ nn.ReLU(inplace=True)
+ ))
+ self.features = nn.ModuleList(self.features)
+
+ def forward(self, x):
+ x_size = x.size()
+ out = [x]
+ for f in self.features:
+ out.append(F.interpolate(f(x), x_size[2:], mode='bilinear', align_corners=True))
+ return torch.cat(out, 1)
+
+
+class BasicBlock(nn.Module):
+ expansion = 1
+
+ def __init__(self, inplanes, planes, stride=1, downsample=None):
+ super(BasicBlock, self).__init__()
+ self.conv1 = conv3x3(inplanes, planes, stride)
+ self.bn1 = BatchNorm2d(planes, momentum=BN_MOMENTUM)
+ self.relu = nn.ReLU(inplace=relu_inplace)
+ self.conv2 = conv3x3(planes, planes)
+ self.bn2 = BatchNorm2d(planes, momentum=BN_MOMENTUM)
+ self.downsample = downsample
+ self.stride = stride
+
+ def forward(self, x):
+ residual = x
+
+ out = self.conv1(x)
+ out = self.bn1(out)
+ out = self.relu(out)
+
+ out = self.conv2(out)
+ out = self.bn2(out)
+
+ if self.downsample is not None:
+ residual = self.downsample(x)
+ out = out + residual
+ out = self.relu(out)
+
+ return out
+
+
+class Bottleneck(nn.Module):
+ expansion = 4
+
+ def __init__(self, inplanes, planes, stride=1, downsample=None):
+ super(Bottleneck, self).__init__()
+ self.conv1 = nn.Conv2d(inplanes, planes, kernel_size=1, bias=False)
+ self.bn1 = BatchNorm2d(planes, momentum=BN_MOMENTUM)
+ self.conv2 = nn.Conv2d(planes, planes, kernel_size=3, stride=stride,
+ padding=1, bias=False)
+ self.bn2 = BatchNorm2d(planes, momentum=BN_MOMENTUM)
+ self.conv3 = nn.Conv2d(planes, planes * self.expansion, kernel_size=1,
+ bias=False)
+ self.bn3 = BatchNorm2d(planes * self.expansion,
+ momentum=BN_MOMENTUM)
+ self.relu = nn.ReLU(inplace=relu_inplace)
+ self.downsample = downsample
+ self.stride = stride
+
+ def forward(self, x):
+ residual = x
+
+ out = self.conv1(x)
+ out = self.bn1(out)
+ out = self.relu(out)
+
+ out = self.conv2(out)
+ out = self.bn2(out)
+ out = self.relu(out)
+
+ out = self.conv3(out)
+ out = self.bn3(out)
+
+ if self.downsample is not None:
+ residual = self.downsample(x)
+ # att = self.downsample(att)
+ out = out + residual
+ out = self.relu(out)
+
+ return out
+
+
+class HighResolutionModule(nn.Module):
+ def __init__(self, num_branches, blocks, num_blocks, num_inchannels,
+ num_channels, fuse_method, multi_scale_output=True):
+ super(HighResolutionModule, self).__init__()
+ self._check_branches(
+ num_branches, blocks, num_blocks, num_inchannels, num_channels)
+
+ self.num_inchannels = num_inchannels
+ self.fuse_method = fuse_method
+ self.num_branches = num_branches
+
+ self.multi_scale_output = multi_scale_output
+
+ self.branches = self._make_branches(
+ num_branches, blocks, num_blocks, num_channels)
+ self.fuse_layers = self._make_fuse_layers()
+ self.relu = nn.ReLU(inplace=relu_inplace)
+
+ def _check_branches(self, num_branches, blocks, num_blocks,
+ num_inchannels, num_channels):
+ if num_branches != len(num_blocks):
+ error_msg = 'NUM_BRANCHES({}) <> NUM_BLOCKS({})'.format(
+ num_branches, len(num_blocks))
+ logger.error(error_msg)
+ raise ValueError(error_msg)
+
+ if num_branches != len(num_channels):
+ error_msg = 'NUM_BRANCHES({}) <> NUM_CHANNELS({})'.format(
+ num_branches, len(num_channels))
+ logger.error(error_msg)
+ raise ValueError(error_msg)
+
+ if num_branches != len(num_inchannels):
+ error_msg = 'NUM_BRANCHES({}) <> NUM_INCHANNELS({})'.format(
+ num_branches, len(num_inchannels))
+ logger.error(error_msg)
+ raise ValueError(error_msg)
+
+ def _make_one_branch(self, branch_index, block, num_blocks, num_channels,
+ stride=1):
+ downsample = None
+ if stride != 1 or \
+ self.num_inchannels[branch_index] != num_channels[branch_index] * block.expansion:
+ downsample = nn.Sequential(
+ nn.Conv2d(self.num_inchannels[branch_index],
+ num_channels[branch_index] * block.expansion,
+ kernel_size=1, stride=stride, bias=False),
+ BatchNorm2d(num_channels[branch_index] * block.expansion,
+ momentum=BN_MOMENTUM),
+ )
+
+ layers = []
+ layers.append(block(self.num_inchannels[branch_index],
+ num_channels[branch_index], stride, downsample))
+ self.num_inchannels[branch_index] = \
+ num_channels[branch_index] * block.expansion
+ for i in range(1, num_blocks[branch_index]):
+ layers.append(block(self.num_inchannels[branch_index],
+ num_channels[branch_index]))
+
+ return nn.Sequential(*layers)
+
+ # 创建平行层
+ def _make_branches(self, num_branches, block, num_blocks, num_channels):
+ branches = []
+
+ for i in range(num_branches):
+ branches.append(
+ self._make_one_branch(i, block, num_blocks, num_channels))
+
+ return nn.ModuleList(branches)
+
+ def _make_fuse_layers(self):
+ if self.num_branches == 1:
+ return None
+ num_branches = self.num_branches # 3
+ num_inchannels = self.num_inchannels # [48, 96, 192]
+ fuse_layers = []
+ for i in range(num_branches if self.multi_scale_output else 1):
+ fuse_layer = []
+ for j in range(num_branches):
+ if j > i:
+ fuse_layer.append(nn.Sequential(
+ nn.Conv2d(num_inchannels[j],
+ num_inchannels[i],
+ 1,
+ 1,
+ 0,
+ bias=False),
+ BatchNorm2d(num_inchannels[i], momentum=BN_MOMENTUM)))
+ elif j == i:
+ fuse_layer.append(None)
+ else:
+ conv3x3s = []
+ for k in range(i - j):
+ if k == i - j - 1:
+ num_outchannels_conv3x3 = num_inchannels[i]
+ conv3x3s.append(nn.Sequential(
+ nn.Conv2d(num_inchannels[j],
+ num_outchannels_conv3x3,
+ 3, 2, 1, bias=False),
+ BatchNorm2d(num_outchannels_conv3x3,
+ momentum=BN_MOMENTUM)))
+ else:
+ num_outchannels_conv3x3 = num_inchannels[j]
+ conv3x3s.append(nn.Sequential(
+ nn.Conv2d(num_inchannels[j],
+ num_outchannels_conv3x3,
+ 3, 2, 1, bias=False),
+ BatchNorm2d(num_outchannels_conv3x3,
+ momentum=BN_MOMENTUM),
+ nn.ReLU(inplace=relu_inplace)))
+ fuse_layer.append(nn.Sequential(*conv3x3s))
+ fuse_layers.append(nn.ModuleList(fuse_layer))
+
+ return nn.ModuleList(fuse_layers)
+
+ def get_num_inchannels(self):
+ return self.num_inchannels
+
+ def forward(self, x):
+ if self.num_branches == 1:
+ return [self.branches[0](x[0])]
+
+ for i in range(self.num_branches):
+ x[i] = self.branches[i](x[i])
+
+ x_fuse = []
+ for i in range(len(self.fuse_layers)):
+ y = x[0] if i == 0 else self.fuse_layers[i][0](x[0])
+ for j in range(1, self.num_branches):
+ if i == j:
+ y = y + x[j]
+ elif j > i:
+ width_output = x[i].shape[-1]
+ height_output = x[i].shape[-2]
+ y = y + F.interpolate(
+ self.fuse_layers[i][j](x[j]),
+ size=[height_output, width_output],
+ mode='bilinear', align_corners=ALIGN_CORNERS)
+ else:
+ y = y + self.fuse_layers[i][j](x[j])
+ x_fuse.append(self.relu(y))
+
+ return x_fuse
+
+
+blocks_dict = {
+ 'BASIC': BasicBlock,
+ 'BOTTLENECK': Bottleneck
+}
+
+
+class HRCloudNet(nn.Module):
+
+ def __init__(self, num_classes=2, base_c=48, **kwargs):
+ global ALIGN_CORNERS
+ extra = HRNET_48
+ super(HRCloudNet, self).__init__()
+ ALIGN_CORNERS = True
+ # ALIGN_CORNERS = config.MODEL.ALIGN_CORNERS
+ self.num_classes = num_classes
+ # stem net
+ self.conv1 = nn.Conv2d(3, 64, kernel_size=3, stride=2, padding=1,
+ bias=False)
+ self.bn1 = BatchNorm2d(64, momentum=BN_MOMENTUM)
+ self.conv2 = nn.Conv2d(64, 64, kernel_size=3, stride=2, padding=1,
+ bias=False)
+ self.bn2 = BatchNorm2d(64, momentum=BN_MOMENTUM)
+ self.relu = nn.ReLU(inplace=relu_inplace)
+
+ self.stage1_cfg = extra['STAGE1']
+ num_channels = self.stage1_cfg['NUM_CHANNELS'][0]
+ block = blocks_dict[self.stage1_cfg['BLOCK']]
+ num_blocks = self.stage1_cfg['NUM_BLOCKS'][0]
+ self.layer1 = self._make_layer(block, 64, num_channels, num_blocks)
+ stage1_out_channel = block.expansion * num_channels
+
+ self.stage2_cfg = extra['STAGE2']
+ num_channels = self.stage2_cfg['NUM_CHANNELS']
+ block = blocks_dict[self.stage2_cfg['BLOCK']]
+ num_channels = [
+ num_channels[i] * block.expansion for i in range(len(num_channels))]
+ self.transition1 = self._make_transition_layer(
+ [stage1_out_channel], num_channels)
+ self.stage2, pre_stage_channels = self._make_stage(
+ self.stage2_cfg, num_channels)
+
+ self.stage3_cfg = extra['STAGE3']
+ num_channels = self.stage3_cfg['NUM_CHANNELS']
+ block = blocks_dict[self.stage3_cfg['BLOCK']]
+ num_channels = [
+ num_channels[i] * block.expansion for i in range(len(num_channels))]
+ self.transition2 = self._make_transition_layer(
+ pre_stage_channels, num_channels) # 只在pre[-1]与cur[-1]之间下采样?
+ self.stage3, pre_stage_channels = self._make_stage(
+ self.stage3_cfg, num_channels)
+
+ self.stage4_cfg = extra['STAGE4']
+ num_channels = self.stage4_cfg['NUM_CHANNELS']
+ block = blocks_dict[self.stage4_cfg['BLOCK']]
+ num_channels = [
+ num_channels[i] * block.expansion for i in range(len(num_channels))]
+ self.transition3 = self._make_transition_layer(
+ pre_stage_channels, num_channels)
+ self.stage4, pre_stage_channels = self._make_stage(
+ self.stage4_cfg, num_channels, multi_scale_output=True)
+ self.out_conv = OutConv(base_c, num_classes)
+ last_inp_channels = int(np.sum(pre_stage_channels))
+
+ self.corr = Corr(nclass=2)
+ self.proj = nn.Sequential(
+ # 512 32
+ nn.Conv2d(720, 48, kernel_size=3, stride=1, padding=1, bias=True),
+ nn.BatchNorm2d(48),
+ nn.ReLU(inplace=True),
+ nn.Dropout2d(0.1),
+ )
+ # self.up1 = Up(base_c * 16, base_c * 8 // factor, bilinear)
+ self.up2 = Up(base_c * 8, base_c * 4, True)
+ self.up3 = Up(base_c * 4, base_c * 2, True)
+ self.up4 = Up(base_c * 2, base_c, True)
+ fea_dim = 720
+ bins = (1, 2, 3, 6)
+ self.ppm = PPM(fea_dim, int(fea_dim / len(bins)), bins)
+ fea_dim *= 2
+ self.cls = nn.Sequential(
+ nn.Conv2d(fea_dim, 512, kernel_size=3, padding=1, bias=False),
+ nn.BatchNorm2d(512),
+ nn.ReLU(inplace=True),
+ nn.Dropout2d(p=0.1),
+ nn.Conv2d(512, 2, kernel_size=1)
+ )
+
+ '''
+ 转换层的作用有两种情况:
+
+ 当前分支数小于之前分支数时,仅对前几个分支进行通道数调整。
+ 当前分支数大于之前分支数时,新建一些转换层,对多余的分支进行下采样,改变通道数以适应后续的连接。
+ 最终,这些转换层会被组合成一个 nn.ModuleList 对象,并在网络的构建过程中使用。
+ 这有助于确保每个分支的通道数在不同阶段之间能够正确匹配,以便进行特征的融合和连接
+ '''
+
+ def _make_transition_layer(
+ self, num_channels_pre_layer, num_channels_cur_layer):
+ # 现在的分支数
+ num_branches_cur = len(num_channels_cur_layer) # 3
+ # 处理前的分支数
+ num_branches_pre = len(num_channels_pre_layer) # 2
+
+ transition_layers = []
+ for i in range(num_branches_cur):
+ # 如果当前分支数小于之前分支数,仅针对第一到第二阶段
+ if i < num_branches_pre:
+ # 如果对应层的通道数不一致,则进行转化(
+ if num_channels_cur_layer[i] != num_channels_pre_layer[i]:
+ transition_layers.append(nn.Sequential(
+
+ nn.Conv2d(num_channels_pre_layer[i],
+ num_channels_cur_layer[i],
+ 3,
+ 1,
+ 1,
+ bias=False),
+ BatchNorm2d(
+ num_channels_cur_layer[i], momentum=BN_MOMENTUM),
+ nn.ReLU(inplace=relu_inplace)))
+ else:
+ transition_layers.append(None)
+ else: # 在新建层下采样改变通道数
+ conv3x3s = []
+ for j in range(i + 1 - num_branches_pre): # 3
+ inchannels = num_channels_pre_layer[-1]
+ outchannels = num_channels_cur_layer[i] \
+ if j == i - num_branches_pre else inchannels
+ conv3x3s.append(nn.Sequential(
+ nn.Conv2d(
+ inchannels, outchannels, 3, 2, 1, bias=False),
+ BatchNorm2d(outchannels, momentum=BN_MOMENTUM),
+ nn.ReLU(inplace=relu_inplace)))
+ transition_layers.append(nn.Sequential(*conv3x3s))
+
+ return nn.ModuleList(transition_layers)
+
+ '''
+ _make_layer 函数的主要作用是创建一个由多个相同类型的残差块(Residual Block)组成的层。
+ '''
+
+ def _make_layer(self, block, inplanes, planes, blocks, stride=1):
+ downsample = None
+ if stride != 1 or inplanes != planes * block.expansion:
+ downsample = nn.Sequential(
+ nn.Conv2d(inplanes, planes * block.expansion,
+ kernel_size=1, stride=stride, bias=False),
+ BatchNorm2d(planes * block.expansion, momentum=BN_MOMENTUM),
+ )
+
+ layers = []
+ layers.append(block(inplanes, planes, stride, downsample))
+ inplanes = planes * block.expansion
+ for i in range(1, blocks):
+ layers.append(block(inplanes, planes))
+
+ return nn.Sequential(*layers)
+
+ # 多尺度融合
+ def _make_stage(self, layer_config, num_inchannels,
+ multi_scale_output=True):
+ num_modules = layer_config['NUM_MODULES']
+ num_branches = layer_config['NUM_BRANCHES']
+ num_blocks = layer_config['NUM_BLOCKS']
+ num_channels = layer_config['NUM_CHANNELS']
+ block = blocks_dict[layer_config['BLOCK']]
+ fuse_method = layer_config['FUSE_METHOD']
+
+ modules = []
+ for i in range(num_modules): # 重复4次
+ # multi_scale_output is only used last module
+ if not multi_scale_output and i == num_modules - 1:
+ reset_multi_scale_output = False
+ else:
+ reset_multi_scale_output = True
+ modules.append(
+ HighResolutionModule(num_branches,
+ block,
+ num_blocks,
+ num_inchannels,
+ num_channels,
+ fuse_method,
+ reset_multi_scale_output)
+ )
+ num_inchannels = modules[-1].get_num_inchannels()
+
+ return nn.Sequential(*modules), num_inchannels
+
+ def forward(self, input, need_fp=True, use_corr=True):
+ # from ipdb import set_trace
+ # set_trace()
+ x = self.conv1(input)
+ x = self.bn1(x)
+ x = self.relu(x)
+ # x_176 = x
+ x = self.conv2(x)
+ x = self.bn2(x)
+ x = self.relu(x)
+ x = self.layer1(x)
+
+ x_list = []
+ for i in range(self.stage2_cfg['NUM_BRANCHES']): # 2
+ if self.transition1[i] is not None:
+ x_list.append(self.transition1[i](x))
+ else:
+ x_list.append(x)
+ y_list = self.stage2(x_list)
+ # Y1
+ x_list = []
+ for i in range(self.stage3_cfg['NUM_BRANCHES']):
+ if self.transition2[i] is not None:
+ if i < self.stage2_cfg['NUM_BRANCHES']:
+ x_list.append(self.transition2[i](y_list[i]))
+ else:
+ x_list.append(self.transition2[i](y_list[-1]))
+ else:
+ x_list.append(y_list[i])
+ y_list = self.stage3(x_list)
+
+ x_list = []
+ for i in range(self.stage4_cfg['NUM_BRANCHES']):
+ if self.transition3[i] is not None:
+ if i < self.stage3_cfg['NUM_BRANCHES']:
+ x_list.append(self.transition3[i](y_list[i]))
+ else:
+ x_list.append(self.transition3[i](y_list[-1]))
+ else:
+ x_list.append(y_list[i])
+ x = self.stage4(x_list)
+ dict_return = {}
+ # Upsampling
+ x0_h, x0_w = x[0].size(2), x[0].size(3)
+
+ x3 = F.interpolate(x[3], size=(x0_h, x0_w), mode='bilinear', align_corners=ALIGN_CORNERS)
+ # x = self.stage3_(x)
+ x[2] = self.up2(x[3], x[2])
+ x2 = F.interpolate(x[2], size=(x0_h, x0_w), mode='bilinear', align_corners=ALIGN_CORNERS)
+ # x = self.stage2_(x)
+ x[1] = self.up3(x[2], x[1])
+ x1 = F.interpolate(x[1], size=(x0_h, x0_w), mode='bilinear', align_corners=ALIGN_CORNERS)
+ x[0] = self.up4(x[1], x[0])
+ xk = torch.cat([x[0], x1, x2, x3], 1)
+ # PPM
+ feat = self.ppm(xk)
+ x = self.cls(feat)
+ # fp分支
+ if need_fp:
+ logits = F.interpolate(x, size=input.size()[2:], mode='bilinear', align_corners=True)
+ # logits = self.out_conv(torch.cat((x, nn.Dropout2d(0.5)(x))))
+ out = logits
+ out_fp = logits
+ if use_corr:
+ proj_feats = self.proj(xk)
+ corr_out = self.corr(proj_feats, out)
+ corr_out = F.interpolate(corr_out, size=(352, 352), mode="bilinear", align_corners=True)
+ dict_return['corr_out'] = corr_out
+ dict_return['out'] = out
+ dict_return['out_fp'] = out_fp
+
+ return dict_return['out']
+
+ out = F.interpolate(x, size=input.size()[2:], mode='bilinear', align_corners=True)
+ if use_corr: # True
+ proj_feats = self.proj(xk)
+ # 计算
+ corr_out = self.corr(proj_feats, out)
+ corr_out = F.interpolate(corr_out, size=(352, 352), mode="bilinear", align_corners=True)
+ dict_return['corr_out'] = corr_out
+ dict_return['out'] = out
+ return dict_return['out']
+ # return x
+
+ def init_weights(self, pretrained='', ):
+ logger.info('=> init weights from normal distribution')
+ for m in self.modules():
+ if isinstance(m, nn.Conv2d):
+ nn.init.normal_(m.weight, std=0.001)
+ elif isinstance(m, nn.BatchNorm2d):
+ nn.init.constant_(m.weight, 1)
+ nn.init.constant_(m.bias, 0)
+ if os.path.isfile(pretrained):
+ pretrained_dict = torch.load(pretrained)
+ logger.info('=> loading pretrained model {}'.format(pretrained))
+ model_dict = self.state_dict()
+ pretrained_dict = {k: v for k, v in pretrained_dict.items()
+ if k in model_dict.keys()}
+ for k, _ in pretrained_dict.items():
+ logger.info(
+ '=> loading {} pretrained model {}'.format(k, pretrained))
+ model_dict.update(pretrained_dict)
+ self.load_state_dict(model_dict)
+
+
+class OutConv(nn.Sequential):
+ def __init__(self, in_channels, num_classes):
+ super(OutConv, self).__init__(
+ nn.Conv2d(720, num_classes, kernel_size=1)
+ )
+
+
+class DoubleConv(nn.Sequential):
+ def __init__(self, in_channels, out_channels, mid_channels=None):
+ if mid_channels is None:
+ mid_channels = out_channels
+ super(DoubleConv, self).__init__(
+ nn.Conv2d(in_channels + out_channels, mid_channels, kernel_size=3, padding=1, bias=False),
+ nn.BatchNorm2d(mid_channels),
+ nn.ReLU(inplace=True),
+ nn.Conv2d(mid_channels, out_channels, kernel_size=3, padding=1, bias=False),
+ nn.BatchNorm2d(out_channels),
+ nn.ReLU(inplace=True)
+ )
+
+
+class Up(nn.Module):
+ def __init__(self, in_channels, out_channels, bilinear=True):
+ super(Up, self).__init__()
+ if bilinear:
+ self.up = nn.Upsample(scale_factor=2, mode='bilinear', align_corners=True)
+ self.conv = DoubleConv(in_channels, out_channels, in_channels // 2)
+ else:
+ self.up = nn.ConvTranspose2d(in_channels, in_channels // 2, kernel_size=2, stride=2)
+ self.conv = DoubleConv(in_channels, out_channels)
+
+ def forward(self, x1: torch.Tensor, x2: torch.Tensor) -> torch.Tensor:
+ x1 = self.up(x1)
+ # [N, C, H, W]
+ diff_y = x2.size()[2] - x1.size()[2]
+ diff_x = x2.size()[3] - x1.size()[3]
+
+ # padding_left, padding_right, padding_top, padding_bottom
+ x1 = F.pad(x1, [diff_x // 2, diff_x - diff_x // 2,
+ diff_y // 2, diff_y - diff_y // 2])
+
+ x = torch.cat([x2, x1], dim=1)
+ x = self.conv(x)
+ return x
+
+
+class Corr(nn.Module):
+ def __init__(self, nclass=2):
+ super(Corr, self).__init__()
+ self.nclass = nclass
+ self.conv1 = nn.Conv2d(48, self.nclass, kernel_size=1, stride=1, padding=0, bias=True)
+ self.conv2 = nn.Conv2d(48, self.nclass, kernel_size=1, stride=1, padding=0, bias=True)
+
+ def forward(self, feature_in, out):
+ # in torch.Size([4, 32, 22, 22])
+ # out = [4 2 352 352]
+ h_in, w_in = math.ceil(feature_in.shape[2] / (1)), math.ceil(feature_in.shape[3] / (1))
+ out = F.interpolate(out.detach(), (h_in, w_in), mode='bilinear', align_corners=True)
+ feature = F.interpolate(feature_in, (h_in, w_in), mode='bilinear', align_corners=True)
+ f1 = rearrange(self.conv1(feature), 'n c h w -> n c (h w)')
+ f2 = rearrange(self.conv2(feature), 'n c h w -> n c (h w)')
+ out_temp = rearrange(out, 'n c h w -> n c (h w)')
+ corr_map = torch.matmul(f1.transpose(1, 2), f2) / torch.sqrt(torch.tensor(f1.shape[1]).float())
+ corr_map = F.softmax(corr_map, dim=-1)
+ # out_temp 2 2 484
+ # corr_map 4 484 484
+ out = rearrange(torch.matmul(out_temp, corr_map), 'n c (h w) -> n c h w', h=h_in, w=w_in)
+ # out torch.Size([4, 2, 22, 22])
+ return out
+
+
+if __name__ == '__main__':
+ input = torch.randn(4, 3, 352, 352)
+ cloud = HRCloudNet(num_classes=2)
+ output = cloud(input)
+ print(output.shape)
# torch.Size([4, 2, 352, 352]) torch.Size([4, 2, 352, 352]) torch.Size([4, 2, 352, 352])
\ No newline at end of file
diff --git a/src/models/components/lnn.py b/src/models/components/lnn.py
index 751a68818abb03a4b27f49b154ac4d4229da6570..c2370649d5b8719e255ab59eb3803f0dfcd8962a 100644
--- a/src/models/components/lnn.py
+++ b/src/models/components/lnn.py
@@ -1,23 +1,23 @@
-import torch
-from torch import nn
-
-
-class LNN(nn.Module):
- # 创建一个全连接网络用于手写数字识别,并通过一个参数dim控制中间层的维度
- def __init__(self, dim=32):
- super(LNN, self).__init__()
- self.fc1 = nn.Linear(28 * 28, dim)
- self.fc2 = nn.Linear(dim, 10)
-
- def forward(self, x):
- x = x.view(-1, x.shape[1] * x.shape[2] * x.shape[3])
- x = torch.relu(self.fc1(x))
- x = self.fc2(x)
- return x
-
-
-if __name__ == "__main__":
- input = torch.randn(2, 1, 28, 28)
- model = LNN()
- output = model(input)
- assert output.shape == (2, 10)
+import torch
+from torch import nn
+
+
+class LNN(nn.Module):
+ # 创建一个全连接网络用于手写数字识别,并通过一个参数dim控制中间层的维度
+ def __init__(self, dim=32):
+ super(LNN, self).__init__()
+ self.fc1 = nn.Linear(28 * 28, dim)
+ self.fc2 = nn.Linear(dim, 10)
+
+ def forward(self, x):
+ x = x.view(-1, x.shape[1] * x.shape[2] * x.shape[3])
+ x = torch.relu(self.fc1(x))
+ x = self.fc2(x)
+ return x
+
+
+if __name__ == "__main__":
+ input = torch.randn(2, 1, 28, 28)
+ model = LNN()
+ output = model(input)
+ assert output.shape == (2, 10)
diff --git a/src/models/components/mcdnet.py b/src/models/components/mcdnet.py
index 054b6c15e52f3d3d4d3a4b4db644a8b3cc5746ae..23807def14bf8e91a3146d8d987f398f5fa41977 100644
--- a/src/models/components/mcdnet.py
+++ b/src/models/components/mcdnet.py
@@ -1,448 +1,448 @@
-# -*- coding: utf-8 -*-
-# @Time : 2024/7/21 下午3:51
-# @Author : xiaoshun
-# @Email : 3038523973@qq.com
-# @File : mcdnet.py
-# @Software: PyCharm
-import cv2
-import image_dehazer
-import numpy as np
-# 论文地址:https://www.sciencedirect.com/science/article/pii/S1569843224001742?via%3Dihub
-import torch
-import torch.nn as nn
-import torch.nn.functional as F
-
-
-class _DPFF(nn.Module):
- def __init__(self, in_channels) -> None:
- super(_DPFF, self).__init__()
- self.cbr1 = nn.Conv2d(in_channels * 2, in_channels, 1, 1, bias=False)
- self.cbr2 = nn.Conv2d(in_channels * 2, in_channels, 1, 1, bias=False)
- # self.sigmoid = nn.Sigmoid()
- self.cbr3 = nn.Conv2d(in_channels, in_channels, 1, 1, bias=False)
- self.cbr4 = nn.Conv2d(in_channels * 2, in_channels, 1, 1, bias=False)
-
- def forward(self, feature1, feature2):
- d1 = torch.abs(feature1 - feature2)
- d2 = self.cbr1(torch.cat([feature1, feature2], dim=1))
- d = torch.cat([d1, d2], dim=1)
- d = self.cbr2(d)
- # d = self.sigmoid(d)
-
- v1, v2 = self.cbr3(feature1), self.cbr3(feature2)
- v1, v2 = v1 * d, v2 * d
- features = torch.cat([v1, v2], dim=1)
- features = self.cbr4(features)
-
- return features
-
-
-class DPFF(nn.Module):
- def __init__(self, layer_channels) -> None:
- super(DPFF, self).__init__()
- self.cfes = nn.ModuleList()
- for layer_channel in layer_channels:
- self.cfes.append(_DPFF(layer_channel))
-
- def forward(self, features1, features2):
- outputs = []
- for feature1, feature2, cfe in zip(features1, features2, self.cfes):
- outputs.append(cfe(feature1, feature2))
- return outputs
-
-
-class DirectDPFF(nn.Module):
- def __init__(self, layer_channels) -> None:
- super(DirectDPFF, self).__init__()
- self.fusions = nn.ModuleList(
- [nn.Conv2d(layer_channel * 2, layer_channel, 1, 1) for layer_channel in layer_channels]
- )
-
- def forward(self, features1, features2):
- outputs = []
- for feature1, feature2, fusion in zip(features1, features2, self.fusions):
- feature = torch.cat([feature1, feature2], dim=1)
- outputs.append(fusion(feature))
- return outputs
-
-
-class ConvBlock(nn.Module):
- def __init__(self, input_size, output_size, kernel_size=4, stride=2, padding=1, bias=True,
- bn=False, activation=True, maxpool=True):
- super(ConvBlock, self).__init__()
- self.module = []
- if maxpool:
- down = nn.Sequential(
- *[
- nn.MaxPool2d(2),
- nn.Conv2d(input_size, output_size, 1, 1, 0, bias=bias)
- ]
- )
- else:
- down = nn.Conv2d(input_size, output_size, kernel_size, stride, padding, bias=bias)
- self.module.append(down)
- if bn:
- self.module.append(nn.BatchNorm2d(output_size))
- if activation:
- self.module.append(nn.PReLU())
- self.module = nn.Sequential(*self.module)
-
- def forward(self, x):
- out = self.module(x)
-
- return out
-
-
-class DeconvBlock(nn.Module):
- def __init__(self, input_size, output_size, kernel_size=4, stride=2, padding=1, bias=True,
- bn=False, activation=True, bilinear=True):
- super(DeconvBlock, self).__init__()
- self.module = []
- if bilinear:
- deconv = nn.Sequential(
- *[
- nn.Upsample(scale_factor=2, mode='bilinear', align_corners=True),
- nn.Conv2d(input_size, output_size, 1, 1, 0, bias=bias)
- ]
- )
- else:
- deconv = nn.ConvTranspose2d(input_size, output_size, kernel_size, stride, padding, bias=bias)
- self.module.append(deconv)
- if bn:
- self.module.append(nn.BatchNorm2d(output_size))
- if activation:
- self.module.append(nn.PReLU())
- self.module = nn.Sequential(*self.module)
-
- def forward(self, x):
- out = self.module(x)
-
- return out
-
-
-class FusionBlock(torch.nn.Module):
- def __init__(self, num_filter, num_ft, kernel_size=4, stride=2, padding=1, bias=True, maxpool=False,
- bilinear=False):
- super(FusionBlock, self).__init__()
- self.num_ft = num_ft
- self.up_convs = nn.ModuleList()
- self.down_convs = nn.ModuleList()
- for i in range(self.num_ft):
- self.up_convs.append(
- DeconvBlock(num_filter // (2 ** i), num_filter // (2 ** (i + 1)), kernel_size, stride, padding,
- bias=bias, bilinear=bilinear)
- )
- self.down_convs.append(
- ConvBlock(num_filter // (2 ** (i + 1)), num_filter // (2 ** i), kernel_size, stride, padding, bias=bias,
- maxpool=maxpool)
- )
-
- def forward(self, ft_l, ft_h_list):
- ft_fusion = ft_l
- for i in range(len(ft_h_list)):
- ft = ft_fusion
- for j in range(self.num_ft - i):
- ft = self.up_convs[j](ft)
- ft = ft - ft_h_list[i]
- for j in range(self.num_ft - i):
- ft = self.down_convs[self.num_ft - i - j - 1](ft)
- ft_fusion = ft_fusion + ft
-
- return ft_fusion
-
-
-class ConvLayer(nn.Module):
- def __init__(self, in_channels, out_channels, kernel_size, stride, bias=True):
- super(ConvLayer, self).__init__()
- reflection_padding = kernel_size // 2
- self.reflection_pad = nn.ReflectionPad2d(reflection_padding)
- self.conv2d = nn.Conv2d(in_channels, out_channels, kernel_size, stride, bias=bias)
-
- def forward(self, x):
- out = self.reflection_pad(x)
- out = self.conv2d(out)
- return out
-
-
-class UpsampleConvLayer(torch.nn.Module):
- def __init__(self, in_channels, out_channels, kernel_size, stride):
- super(UpsampleConvLayer, self).__init__()
- self.conv2d = nn.ConvTranspose2d(in_channels, out_channels, kernel_size, stride=stride)
-
- def forward(self, x):
- out = self.conv2d(x)
- return out
-
-
-class AddRelu(nn.Module):
- """It is for adding two feed forwards to the output of the two following conv layers in expanding path
- """
-
- def __init__(self) -> None:
- super(AddRelu, self).__init__()
- self.relu = nn.PReLU()
-
- def forward(self, input_tensor1, input_tensor2, input_tensor3):
- x = input_tensor1 + input_tensor2 + input_tensor3
- return self.relu(x)
-
-
-class BasicBlock(nn.Module):
- def __init__(self, in_channels, out_channels, mid_channels=None):
- super(BasicBlock, self).__init__()
- if not mid_channels:
- mid_channels = out_channels
- self.conv1 = ConvLayer(in_channels, mid_channels, kernel_size=3, stride=1)
- self.bn1 = nn.BatchNorm2d(mid_channels, momentum=0.1)
- self.relu = nn.PReLU()
-
- self.conv2 = ConvLayer(mid_channels, out_channels, kernel_size=3, stride=1)
- self.bn2 = nn.BatchNorm2d(out_channels, momentum=0.1)
-
- self.conv3 = ConvLayer(in_channels, out_channels, kernel_size=1, stride=1)
-
- def forward(self, x):
- out = self.conv1(x)
- out = self.bn1(out)
- out = self.relu(out)
-
- out = self.conv2(out)
- out = self.bn2(out)
-
- residual = self.conv3(x)
-
- out = out + residual
- out = self.relu(out)
-
- return out
-
-
-class Bottleneck(nn.Module):
- def __init__(self, in_channels, out_channels):
- super(Bottleneck, self).__init__()
- self.conv1 = ConvLayer(in_channels, out_channels, kernel_size=3, stride=1)
- self.bn1 = nn.BatchNorm2d(out_channels, momentum=0.1)
-
- self.conv2 = ConvLayer(out_channels, out_channels, kernel_size=3, stride=1)
- self.bn2 = nn.BatchNorm2d(out_channels, momentum=0.1)
-
- self.conv3 = ConvLayer(out_channels, out_channels, kernel_size=3, stride=1)
- self.bn3 = nn.BatchNorm2d(out_channels, momentum=0.1)
-
- self.conv4 = ConvLayer(in_channels, out_channels, kernel_size=1, stride=1)
-
- self.relu = nn.PReLU()
-
- def forward(self, x):
- out = self.conv1(x)
- out = self.bn1(out)
- out = self.relu(out)
-
- out = self.conv2(out)
- out = self.bn2(out)
- out = self.relu(out)
-
- out = self.conv3(out)
- out = self.bn3(out)
-
- residual = self.conv4(x)
-
- out = out + residual
- out = self.relu(out)
-
- return out
-
-
-class PPM(nn.Module):
- def __init__(self, in_channels, out_channels):
- super(PPM, self).__init__()
-
- self.pool_sizes = [1, 2, 3, 6] # subregion size in each level
- self.num_levels = len(self.pool_sizes) # number of pyramid levels
-
- self.conv_layers = nn.ModuleList()
- for i in range(self.num_levels):
- self.conv_layers.append(nn.Sequential(
- nn.AdaptiveAvgPool2d(output_size=self.pool_sizes[i]),
- nn.Conv2d(in_channels, in_channels // self.num_levels, kernel_size=1),
- nn.BatchNorm2d(in_channels // self.num_levels),
- nn.ReLU(inplace=True)
- ))
- self.out_conv = nn.Conv2d(in_channels * 2, out_channels, kernel_size=1, stride=1)
-
- def forward(self, x):
- input_size = x.size()[2:] # get input size
- output = [x]
-
- # pyramid pooling
- for i in range(self.num_levels):
- out = self.conv_layers[i](x)
- out = F.interpolate(out, size=input_size, mode='bilinear', align_corners=True)
- output.append(out)
-
- # concatenate features from different levels
- output = torch.cat(output, dim=1)
- output = self.out_conv(output)
-
- return output
-
-
-class MCDNet(nn.Module):
- def __init__(self, in_channels=4, num_classes=4, maxpool=False, bilinear=False) -> None:
- super(MCDNet, self).__init__()
- level = 1
- # encoder
- self.conv_input = ConvLayer(in_channels, 32 * level, kernel_size=3, stride=2)
-
- self.dense0 = BasicBlock(32 * level, 32 * level)
- self.conv2x = ConvLayer(32 * level, 64 * level, kernel_size=3, stride=2)
-
- self.dense1 = BasicBlock(64 * level, 64 * level)
- self.conv4x = ConvLayer(64 * level, 128 * level, kernel_size=3, stride=2)
-
- self.dense2 = BasicBlock(128 * level, 128 * level)
- self.conv8x = ConvLayer(128 * level, 256 * level, kernel_size=3, stride=2)
-
- self.dense3 = BasicBlock(256 * level, 256 * level)
- self.conv16x = ConvLayer(256 * level, 512 * level, kernel_size=3, stride=2)
-
- self.dense4 = PPM(512 * level, 512 * level)
-
- # dpff
- self.dpffm = DPFF([32, 64, 128, 256, 512])
-
- # decoder
- self.convd16x = UpsampleConvLayer(512 * level, 256 * level, kernel_size=3, stride=2)
- self.fusion4 = FusionBlock(256 * level, 3, maxpool=maxpool, bilinear=bilinear)
- self.dense_4 = Bottleneck(512 * level, 256 * level)
- self.add_block4 = AddRelu()
-
- self.convd8x = UpsampleConvLayer(256 * level, 128 * level, kernel_size=3, stride=2)
- self.fusion3 = FusionBlock(128 * level, 2, maxpool=maxpool, bilinear=bilinear)
- self.dense_3 = Bottleneck(256 * level, 128 * level)
- self.add_block3 = AddRelu()
-
- self.convd4x = UpsampleConvLayer(128 * level, 64 * level, kernel_size=3, stride=2)
- self.fusion2 = FusionBlock(64 * level, 1, maxpool=maxpool, bilinear=bilinear)
- self.dense_2 = Bottleneck(128 * level, 64 * level)
- self.add_block2 = AddRelu()
-
- self.convd2x = UpsampleConvLayer(64 * level, 32 * level, kernel_size=3, stride=2)
- self.dense_1 = Bottleneck(64 * level, 32 * level)
- self.add_block1 = AddRelu()
-
- self.head = UpsampleConvLayer(32 * level, num_classes, kernel_size=3, stride=2)
- self.apply(self._weights_init)
-
- def _weights_init(self, m):
- if isinstance(m, nn.Linear):
- nn.init.xavier_normal_(m.weight)
- nn.init.constant_(m.bias, 0)
- elif isinstance(m, nn.Conv2d):
- nn.init.kaiming_normal_(m.weight, mode='fan_out', nonlinearity='relu')
- elif isinstance(m, nn.BatchNorm2d):
- nn.init.constant_(m.weight, 1)
- nn.init.constant_(m.bias, 0)
-
- def get_lr_data(self, x: torch.Tensor) -> torch.Tensor:
- images = x.cpu().permute(0, 2, 3, 1).numpy()
- batch_size = images.shape[0]
- lr = []
- for i in range(batch_size):
- lr_image = cv2.cvtColor(images[i], cv2.COLOR_RGB2BGR)
- lr_image = image_dehazer.remove_haze(lr_image, showHazeTransmissionMap=False)[0]
- lr_image = cv2.cvtColor(lr_image, cv2.COLOR_BGR2RGB)
- max_pix = np.max(lr_image)
- min_pix = np.min(lr_image)
- lr_image = (lr_image - min_pix) / (max_pix - min_pix)
- lr_image = np.clip(lr_image, 0, 1)
- lr_tensor = torch.from_numpy(lr_image).permute(2, 0, 1).float()
- lr.append(lr_tensor)
- return torch.stack(lr, dim=0).to(x.device)
-
- def forward(self, x1):
- x2 = self.get_lr_data(x1)
- # encoder1
- res1x_1 = self.conv_input(x1)
- res1x_1 = self.dense0(res1x_1)
-
- res2x_1 = self.conv2x(res1x_1)
- res2x_1 = self.dense1(res2x_1)
-
- res4x_1 = self.conv4x(res2x_1)
- res4x_1 = self.dense2(res4x_1)
-
- res8x_1 = self.conv8x(res4x_1)
- res8x_1 = self.dense3(res8x_1)
-
- res16x_1 = self.conv16x(res8x_1)
- res16x_1 = self.dense4(res16x_1)
-
- # encoder2
- res1x_2 = self.conv_input(x2)
- res1x_2 = self.dense0(res1x_2)
-
- res2x_2 = self.conv2x(res1x_2)
- res2x_2 = self.dense1(res2x_2)
-
- res4x_2 = self.conv4x(res2x_2)
- res4x_2 = self.dense2(res4x_2)
-
- res8x_2 = self.conv8x(res4x_2)
- res8x_2 = self.dense3(res8x_2)
-
- res16x_2 = self.conv16x(res8x_2)
- res16x_2 = self.dense4(res16x_2)
-
- # dual-perspective feature fusion
- res1x, res2x, res4x, res8x, res16x = self.dpffm(
- [res1x_1, res2x_1, res4x_1, res8x_1, res16x_1],
- [res1x_2, res2x_2, res4x_2, res8x_2, res16x_2]
- )
-
- # decoder
- res8x1 = self.convd16x(res16x)
- res8x1 = F.interpolate(res8x1, res8x.size()[2:], mode='bilinear')
- res8x2 = self.fusion4(res8x, [res1x, res2x, res4x])
- res8x2 = torch.cat([res8x1, res8x2], dim=1)
- res8x2 = self.dense_4(res8x2)
- res8x2 = self.add_block4(res8x1, res8x, res8x2)
-
- res4x1 = self.convd8x(res8x2)
- res4x1 = F.interpolate(res4x1, res4x.size()[2:], mode='bilinear')
- res4x2 = self.fusion3(res4x, [res1x, res2x])
- res4x2 = torch.cat([res4x1, res4x2], dim=1)
- res4x2 = self.dense_3(res4x2)
- res4x2 = self.add_block3(res4x1, res4x, res4x2)
-
- res2x1 = self.convd4x(res4x2)
- res2x1 = F.interpolate(res2x1, res2x.size()[2:], mode='bilinear')
- res2x2 = self.fusion2(res2x, [res1x])
- res2x2 = torch.cat([res2x1, res2x2], dim=1)
- res2x2 = self.dense_2(res2x2)
- res2x2 = self.add_block2(res2x1, res2x, res2x2)
-
- res1x1 = self.convd2x(res2x2)
- res1x1 = F.interpolate(res1x1, res1x.size()[2:], mode='bilinear')
- res1x2 = torch.cat([res1x1, res1x], dim=1)
- res1x2 = self.dense_1(res1x2)
- res1x2 = self.add_block1(res1x1, res1x, res1x2)
-
- out = self.head(res1x2)
- out = F.interpolate(out, x1.size()[2:], mode='bilinear')
-
- return out
-
-
-def lr_lambda(epoch):
- return (1 - epoch / 50) ** 0.9
-
-
-if __name__ == "__main__":
- device = torch.device('cuda' if torch.cuda.is_available() else 'cpu')
- # device = 'cpu'
- model = MCDNet(in_channels=3, num_classes=7).to(device)
- fake_img = torch.randn(size=(2, 3, 256, 256)).to(device)
- out = model(fake_img).detach().cpu()
- print(out.shape)
-# torch.Size([2, 7, 256, 256])
+# -*- coding: utf-8 -*-
+# @Time : 2024/7/21 下午3:51
+# @Author : xiaoshun
+# @Email : 3038523973@qq.com
+# @File : mcdnet.py
+# @Software: PyCharm
+import cv2
+import image_dehazer
+import numpy as np
+# 论文地址:https://www.sciencedirect.com/science/article/pii/S1569843224001742?via%3Dihub
+import torch
+import torch.nn as nn
+import torch.nn.functional as F
+
+
+class _DPFF(nn.Module):
+ def __init__(self, in_channels) -> None:
+ super(_DPFF, self).__init__()
+ self.cbr1 = nn.Conv2d(in_channels * 2, in_channels, 1, 1, bias=False)
+ self.cbr2 = nn.Conv2d(in_channels * 2, in_channels, 1, 1, bias=False)
+ # self.sigmoid = nn.Sigmoid()
+ self.cbr3 = nn.Conv2d(in_channels, in_channels, 1, 1, bias=False)
+ self.cbr4 = nn.Conv2d(in_channels * 2, in_channels, 1, 1, bias=False)
+
+ def forward(self, feature1, feature2):
+ d1 = torch.abs(feature1 - feature2)
+ d2 = self.cbr1(torch.cat([feature1, feature2], dim=1))
+ d = torch.cat([d1, d2], dim=1)
+ d = self.cbr2(d)
+ # d = self.sigmoid(d)
+
+ v1, v2 = self.cbr3(feature1), self.cbr3(feature2)
+ v1, v2 = v1 * d, v2 * d
+ features = torch.cat([v1, v2], dim=1)
+ features = self.cbr4(features)
+
+ return features
+
+
+class DPFF(nn.Module):
+ def __init__(self, layer_channels) -> None:
+ super(DPFF, self).__init__()
+ self.cfes = nn.ModuleList()
+ for layer_channel in layer_channels:
+ self.cfes.append(_DPFF(layer_channel))
+
+ def forward(self, features1, features2):
+ outputs = []
+ for feature1, feature2, cfe in zip(features1, features2, self.cfes):
+ outputs.append(cfe(feature1, feature2))
+ return outputs
+
+
+class DirectDPFF(nn.Module):
+ def __init__(self, layer_channels) -> None:
+ super(DirectDPFF, self).__init__()
+ self.fusions = nn.ModuleList(
+ [nn.Conv2d(layer_channel * 2, layer_channel, 1, 1) for layer_channel in layer_channels]
+ )
+
+ def forward(self, features1, features2):
+ outputs = []
+ for feature1, feature2, fusion in zip(features1, features2, self.fusions):
+ feature = torch.cat([feature1, feature2], dim=1)
+ outputs.append(fusion(feature))
+ return outputs
+
+
+class ConvBlock(nn.Module):
+ def __init__(self, input_size, output_size, kernel_size=4, stride=2, padding=1, bias=True,
+ bn=False, activation=True, maxpool=True):
+ super(ConvBlock, self).__init__()
+ self.module = []
+ if maxpool:
+ down = nn.Sequential(
+ *[
+ nn.MaxPool2d(2),
+ nn.Conv2d(input_size, output_size, 1, 1, 0, bias=bias)
+ ]
+ )
+ else:
+ down = nn.Conv2d(input_size, output_size, kernel_size, stride, padding, bias=bias)
+ self.module.append(down)
+ if bn:
+ self.module.append(nn.BatchNorm2d(output_size))
+ if activation:
+ self.module.append(nn.PReLU())
+ self.module = nn.Sequential(*self.module)
+
+ def forward(self, x):
+ out = self.module(x)
+
+ return out
+
+
+class DeconvBlock(nn.Module):
+ def __init__(self, input_size, output_size, kernel_size=4, stride=2, padding=1, bias=True,
+ bn=False, activation=True, bilinear=True):
+ super(DeconvBlock, self).__init__()
+ self.module = []
+ if bilinear:
+ deconv = nn.Sequential(
+ *[
+ nn.Upsample(scale_factor=2, mode='bilinear', align_corners=True),
+ nn.Conv2d(input_size, output_size, 1, 1, 0, bias=bias)
+ ]
+ )
+ else:
+ deconv = nn.ConvTranspose2d(input_size, output_size, kernel_size, stride, padding, bias=bias)
+ self.module.append(deconv)
+ if bn:
+ self.module.append(nn.BatchNorm2d(output_size))
+ if activation:
+ self.module.append(nn.PReLU())
+ self.module = nn.Sequential(*self.module)
+
+ def forward(self, x):
+ out = self.module(x)
+
+ return out
+
+
+class FusionBlock(torch.nn.Module):
+ def __init__(self, num_filter, num_ft, kernel_size=4, stride=2, padding=1, bias=True, maxpool=False,
+ bilinear=False):
+ super(FusionBlock, self).__init__()
+ self.num_ft = num_ft
+ self.up_convs = nn.ModuleList()
+ self.down_convs = nn.ModuleList()
+ for i in range(self.num_ft):
+ self.up_convs.append(
+ DeconvBlock(num_filter // (2 ** i), num_filter // (2 ** (i + 1)), kernel_size, stride, padding,
+ bias=bias, bilinear=bilinear)
+ )
+ self.down_convs.append(
+ ConvBlock(num_filter // (2 ** (i + 1)), num_filter // (2 ** i), kernel_size, stride, padding, bias=bias,
+ maxpool=maxpool)
+ )
+
+ def forward(self, ft_l, ft_h_list):
+ ft_fusion = ft_l
+ for i in range(len(ft_h_list)):
+ ft = ft_fusion
+ for j in range(self.num_ft - i):
+ ft = self.up_convs[j](ft)
+ ft = ft - ft_h_list[i]
+ for j in range(self.num_ft - i):
+ ft = self.down_convs[self.num_ft - i - j - 1](ft)
+ ft_fusion = ft_fusion + ft
+
+ return ft_fusion
+
+
+class ConvLayer(nn.Module):
+ def __init__(self, in_channels, out_channels, kernel_size, stride, bias=True):
+ super(ConvLayer, self).__init__()
+ reflection_padding = kernel_size // 2
+ self.reflection_pad = nn.ReflectionPad2d(reflection_padding)
+ self.conv2d = nn.Conv2d(in_channels, out_channels, kernel_size, stride, bias=bias)
+
+ def forward(self, x):
+ out = self.reflection_pad(x)
+ out = self.conv2d(out)
+ return out
+
+
+class UpsampleConvLayer(torch.nn.Module):
+ def __init__(self, in_channels, out_channels, kernel_size, stride):
+ super(UpsampleConvLayer, self).__init__()
+ self.conv2d = nn.ConvTranspose2d(in_channels, out_channels, kernel_size, stride=stride)
+
+ def forward(self, x):
+ out = self.conv2d(x)
+ return out
+
+
+class AddRelu(nn.Module):
+ """It is for adding two feed forwards to the output of the two following conv layers in expanding path
+ """
+
+ def __init__(self) -> None:
+ super(AddRelu, self).__init__()
+ self.relu = nn.PReLU()
+
+ def forward(self, input_tensor1, input_tensor2, input_tensor3):
+ x = input_tensor1 + input_tensor2 + input_tensor3
+ return self.relu(x)
+
+
+class BasicBlock(nn.Module):
+ def __init__(self, in_channels, out_channels, mid_channels=None):
+ super(BasicBlock, self).__init__()
+ if not mid_channels:
+ mid_channels = out_channels
+ self.conv1 = ConvLayer(in_channels, mid_channels, kernel_size=3, stride=1)
+ self.bn1 = nn.BatchNorm2d(mid_channels, momentum=0.1)
+ self.relu = nn.PReLU()
+
+ self.conv2 = ConvLayer(mid_channels, out_channels, kernel_size=3, stride=1)
+ self.bn2 = nn.BatchNorm2d(out_channels, momentum=0.1)
+
+ self.conv3 = ConvLayer(in_channels, out_channels, kernel_size=1, stride=1)
+
+ def forward(self, x):
+ out = self.conv1(x)
+ out = self.bn1(out)
+ out = self.relu(out)
+
+ out = self.conv2(out)
+ out = self.bn2(out)
+
+ residual = self.conv3(x)
+
+ out = out + residual
+ out = self.relu(out)
+
+ return out
+
+
+class Bottleneck(nn.Module):
+ def __init__(self, in_channels, out_channels):
+ super(Bottleneck, self).__init__()
+ self.conv1 = ConvLayer(in_channels, out_channels, kernel_size=3, stride=1)
+ self.bn1 = nn.BatchNorm2d(out_channels, momentum=0.1)
+
+ self.conv2 = ConvLayer(out_channels, out_channels, kernel_size=3, stride=1)
+ self.bn2 = nn.BatchNorm2d(out_channels, momentum=0.1)
+
+ self.conv3 = ConvLayer(out_channels, out_channels, kernel_size=3, stride=1)
+ self.bn3 = nn.BatchNorm2d(out_channels, momentum=0.1)
+
+ self.conv4 = ConvLayer(in_channels, out_channels, kernel_size=1, stride=1)
+
+ self.relu = nn.PReLU()
+
+ def forward(self, x):
+ out = self.conv1(x)
+ out = self.bn1(out)
+ out = self.relu(out)
+
+ out = self.conv2(out)
+ out = self.bn2(out)
+ out = self.relu(out)
+
+ out = self.conv3(out)
+ out = self.bn3(out)
+
+ residual = self.conv4(x)
+
+ out = out + residual
+ out = self.relu(out)
+
+ return out
+
+
+class PPM(nn.Module):
+ def __init__(self, in_channels, out_channels):
+ super(PPM, self).__init__()
+
+ self.pool_sizes = [1, 2, 3, 6] # subregion size in each level
+ self.num_levels = len(self.pool_sizes) # number of pyramid levels
+
+ self.conv_layers = nn.ModuleList()
+ for i in range(self.num_levels):
+ self.conv_layers.append(nn.Sequential(
+ nn.AdaptiveAvgPool2d(output_size=self.pool_sizes[i]),
+ nn.Conv2d(in_channels, in_channels // self.num_levels, kernel_size=1),
+ nn.BatchNorm2d(in_channels // self.num_levels),
+ nn.ReLU(inplace=True)
+ ))
+ self.out_conv = nn.Conv2d(in_channels * 2, out_channels, kernel_size=1, stride=1)
+
+ def forward(self, x):
+ input_size = x.size()[2:] # get input size
+ output = [x]
+
+ # pyramid pooling
+ for i in range(self.num_levels):
+ out = self.conv_layers[i](x)
+ out = F.interpolate(out, size=input_size, mode='bilinear', align_corners=True)
+ output.append(out)
+
+ # concatenate features from different levels
+ output = torch.cat(output, dim=1)
+ output = self.out_conv(output)
+
+ return output
+
+
+class MCDNet(nn.Module):
+ def __init__(self, in_channels=4, num_classes=4, maxpool=False, bilinear=False) -> None:
+ super(MCDNet, self).__init__()
+ level = 1
+ # encoder
+ self.conv_input = ConvLayer(in_channels, 32 * level, kernel_size=3, stride=2)
+
+ self.dense0 = BasicBlock(32 * level, 32 * level)
+ self.conv2x = ConvLayer(32 * level, 64 * level, kernel_size=3, stride=2)
+
+ self.dense1 = BasicBlock(64 * level, 64 * level)
+ self.conv4x = ConvLayer(64 * level, 128 * level, kernel_size=3, stride=2)
+
+ self.dense2 = BasicBlock(128 * level, 128 * level)
+ self.conv8x = ConvLayer(128 * level, 256 * level, kernel_size=3, stride=2)
+
+ self.dense3 = BasicBlock(256 * level, 256 * level)
+ self.conv16x = ConvLayer(256 * level, 512 * level, kernel_size=3, stride=2)
+
+ self.dense4 = PPM(512 * level, 512 * level)
+
+ # dpff
+ self.dpffm = DPFF([32, 64, 128, 256, 512])
+
+ # decoder
+ self.convd16x = UpsampleConvLayer(512 * level, 256 * level, kernel_size=3, stride=2)
+ self.fusion4 = FusionBlock(256 * level, 3, maxpool=maxpool, bilinear=bilinear)
+ self.dense_4 = Bottleneck(512 * level, 256 * level)
+ self.add_block4 = AddRelu()
+
+ self.convd8x = UpsampleConvLayer(256 * level, 128 * level, kernel_size=3, stride=2)
+ self.fusion3 = FusionBlock(128 * level, 2, maxpool=maxpool, bilinear=bilinear)
+ self.dense_3 = Bottleneck(256 * level, 128 * level)
+ self.add_block3 = AddRelu()
+
+ self.convd4x = UpsampleConvLayer(128 * level, 64 * level, kernel_size=3, stride=2)
+ self.fusion2 = FusionBlock(64 * level, 1, maxpool=maxpool, bilinear=bilinear)
+ self.dense_2 = Bottleneck(128 * level, 64 * level)
+ self.add_block2 = AddRelu()
+
+ self.convd2x = UpsampleConvLayer(64 * level, 32 * level, kernel_size=3, stride=2)
+ self.dense_1 = Bottleneck(64 * level, 32 * level)
+ self.add_block1 = AddRelu()
+
+ self.head = UpsampleConvLayer(32 * level, num_classes, kernel_size=3, stride=2)
+ self.apply(self._weights_init)
+
+ def _weights_init(self, m):
+ if isinstance(m, nn.Linear):
+ nn.init.xavier_normal_(m.weight)
+ nn.init.constant_(m.bias, 0)
+ elif isinstance(m, nn.Conv2d):
+ nn.init.kaiming_normal_(m.weight, mode='fan_out', nonlinearity='relu')
+ elif isinstance(m, nn.BatchNorm2d):
+ nn.init.constant_(m.weight, 1)
+ nn.init.constant_(m.bias, 0)
+
+ def get_lr_data(self, x: torch.Tensor) -> torch.Tensor:
+ images = x.cpu().permute(0, 2, 3, 1).numpy()
+ batch_size = images.shape[0]
+ lr = []
+ for i in range(batch_size):
+ lr_image = cv2.cvtColor(images[i], cv2.COLOR_RGB2BGR)
+ lr_image = image_dehazer.remove_haze(lr_image, showHazeTransmissionMap=False)[0]
+ lr_image = cv2.cvtColor(lr_image, cv2.COLOR_BGR2RGB)
+ max_pix = np.max(lr_image)
+ min_pix = np.min(lr_image)
+ lr_image = (lr_image - min_pix) / (max_pix - min_pix)
+ lr_image = np.clip(lr_image, 0, 1)
+ lr_tensor = torch.from_numpy(lr_image).permute(2, 0, 1).float()
+ lr.append(lr_tensor)
+ return torch.stack(lr, dim=0).to(x.device)
+
+ def forward(self, x1):
+ x2 = self.get_lr_data(x1)
+ # encoder1
+ res1x_1 = self.conv_input(x1)
+ res1x_1 = self.dense0(res1x_1)
+
+ res2x_1 = self.conv2x(res1x_1)
+ res2x_1 = self.dense1(res2x_1)
+
+ res4x_1 = self.conv4x(res2x_1)
+ res4x_1 = self.dense2(res4x_1)
+
+ res8x_1 = self.conv8x(res4x_1)
+ res8x_1 = self.dense3(res8x_1)
+
+ res16x_1 = self.conv16x(res8x_1)
+ res16x_1 = self.dense4(res16x_1)
+
+ # encoder2
+ res1x_2 = self.conv_input(x2)
+ res1x_2 = self.dense0(res1x_2)
+
+ res2x_2 = self.conv2x(res1x_2)
+ res2x_2 = self.dense1(res2x_2)
+
+ res4x_2 = self.conv4x(res2x_2)
+ res4x_2 = self.dense2(res4x_2)
+
+ res8x_2 = self.conv8x(res4x_2)
+ res8x_2 = self.dense3(res8x_2)
+
+ res16x_2 = self.conv16x(res8x_2)
+ res16x_2 = self.dense4(res16x_2)
+
+ # dual-perspective feature fusion
+ res1x, res2x, res4x, res8x, res16x = self.dpffm(
+ [res1x_1, res2x_1, res4x_1, res8x_1, res16x_1],
+ [res1x_2, res2x_2, res4x_2, res8x_2, res16x_2]
+ )
+
+ # decoder
+ res8x1 = self.convd16x(res16x)
+ res8x1 = F.interpolate(res8x1, res8x.size()[2:], mode='bilinear')
+ res8x2 = self.fusion4(res8x, [res1x, res2x, res4x])
+ res8x2 = torch.cat([res8x1, res8x2], dim=1)
+ res8x2 = self.dense_4(res8x2)
+ res8x2 = self.add_block4(res8x1, res8x, res8x2)
+
+ res4x1 = self.convd8x(res8x2)
+ res4x1 = F.interpolate(res4x1, res4x.size()[2:], mode='bilinear')
+ res4x2 = self.fusion3(res4x, [res1x, res2x])
+ res4x2 = torch.cat([res4x1, res4x2], dim=1)
+ res4x2 = self.dense_3(res4x2)
+ res4x2 = self.add_block3(res4x1, res4x, res4x2)
+
+ res2x1 = self.convd4x(res4x2)
+ res2x1 = F.interpolate(res2x1, res2x.size()[2:], mode='bilinear')
+ res2x2 = self.fusion2(res2x, [res1x])
+ res2x2 = torch.cat([res2x1, res2x2], dim=1)
+ res2x2 = self.dense_2(res2x2)
+ res2x2 = self.add_block2(res2x1, res2x, res2x2)
+
+ res1x1 = self.convd2x(res2x2)
+ res1x1 = F.interpolate(res1x1, res1x.size()[2:], mode='bilinear')
+ res1x2 = torch.cat([res1x1, res1x], dim=1)
+ res1x2 = self.dense_1(res1x2)
+ res1x2 = self.add_block1(res1x1, res1x, res1x2)
+
+ out = self.head(res1x2)
+ out = F.interpolate(out, x1.size()[2:], mode='bilinear')
+
+ return out
+
+
+def lr_lambda(epoch):
+ return (1 - epoch / 50) ** 0.9
+
+
+if __name__ == "__main__":
+ device = torch.device('cuda' if torch.cuda.is_available() else 'cpu')
+ # device = 'cpu'
+ model = MCDNet(in_channels=3, num_classes=7).to(device)
+ fake_img = torch.randn(size=(2, 3, 256, 256)).to(device)
+ out = model(fake_img).detach().cpu()
+ print(out.shape)
+# torch.Size([2, 7, 256, 256])
diff --git a/src/models/components/scnn.py b/src/models/components/scnn.py
index 171722cfa7647ea8bc0de6de1484c656878aee1f..8f22f2e96f86bcd06388eacf99a58284eae053fb 100644
--- a/src/models/components/scnn.py
+++ b/src/models/components/scnn.py
@@ -1,36 +1,36 @@
-# -*- coding: utf-8 -*-
-# @Time : 2024/7/21 下午5:11
-# @Author : xiaoshun
-# @Email : 3038523973@qq.com
-# @File : scnn.py
-# @Software: PyCharm
-
-# 论文地址:https://www.sciencedirect.com/science/article/abs/pii/S0924271624000352?via%3Dihub#fn1
-
-import torch
-import torch.nn as nn
-import torch.nn.functional as F
-
-
-class SCNN(nn.Module):
- def __init__(self, in_channels=3, num_classes=2, dropout_p=0.5):
- super().__init__()
- self.conv1 = nn.Conv2d(in_channels, 64, kernel_size=1)
- self.conv2 = nn.Conv2d(64, num_classes, kernel_size=1)
- self.conv3 = nn.Conv2d(num_classes, num_classes, kernel_size=3, padding=1)
- self.dropout = nn.Dropout2d(p=dropout_p)
-
- def forward(self, x):
- x = F.relu(self.conv1(x))
- x = self.dropout(x)
- x = self.conv2(x)
- x = self.conv3(x)
- return x
-
-
-if __name__ == '__main__':
- model = SCNN(num_classes=7)
- fake_img = torch.randn((2, 3, 224, 224))
- out = model(fake_img)
- print(out.shape)
- # torch.Size([2, 7, 224, 224])
+# -*- coding: utf-8 -*-
+# @Time : 2024/7/21 下午5:11
+# @Author : xiaoshun
+# @Email : 3038523973@qq.com
+# @File : scnn.py
+# @Software: PyCharm
+
+# 论文地址:https://www.sciencedirect.com/science/article/abs/pii/S0924271624000352?via%3Dihub#fn1
+
+import torch
+import torch.nn as nn
+import torch.nn.functional as F
+
+
+class SCNN(nn.Module):
+ def __init__(self, in_channels=3, num_classes=2, dropout_p=0.5):
+ super().__init__()
+ self.conv1 = nn.Conv2d(in_channels, 64, kernel_size=1)
+ self.conv2 = nn.Conv2d(64, num_classes, kernel_size=1)
+ self.conv3 = nn.Conv2d(num_classes, num_classes, kernel_size=3, padding=1)
+ self.dropout = nn.Dropout2d(p=dropout_p)
+
+ def forward(self, x):
+ x = F.relu(self.conv1(x))
+ x = self.dropout(x)
+ x = self.conv2(x)
+ x = self.conv3(x)
+ return x
+
+
+if __name__ == '__main__':
+ model = SCNN(num_classes=7)
+ fake_img = torch.randn((2, 3, 224, 224))
+ out = model(fake_img)
+ print(out.shape)
+ # torch.Size([2, 7, 224, 224])
diff --git a/src/models/components/unet.py b/src/models/components/unet.py
index 5e1ed8561d4d02dbac4c9938260ff7878c23bda0..b166da4e76549299de61813369e9852a0efbc3b4 100644
--- a/src/models/components/unet.py
+++ b/src/models/components/unet.py
@@ -1,63 +1,63 @@
-import torch
-import torch.nn as nn
-import torch.nn.functional as F
-
-class UNet(nn.Module):
- def __init__(self, in_channels, out_channels):
- super(UNet, self).__init__()
-
- def conv_block(in_channels, out_channels):
- return nn.Sequential(
- nn.Conv2d(in_channels, out_channels, kernel_size=3, padding=1),
- nn.ReLU(inplace=True),
- nn.Conv2d(out_channels, out_channels, kernel_size=3, padding=1),
- nn.ReLU(inplace=True)
- )
-
- self.encoder1 = conv_block(in_channels, 64)
- self.encoder2 = conv_block(64, 128)
- self.encoder3 = conv_block(128, 256)
- self.encoder4 = conv_block(256, 512)
- self.bottleneck = conv_block(512, 1024)
-
- self.upconv4 = nn.ConvTranspose2d(1024, 512, kernel_size=2, stride=2)
- self.decoder4 = conv_block(1024, 512)
- self.upconv3 = nn.ConvTranspose2d(512, 256, kernel_size=2, stride=2)
- self.decoder3 = conv_block(512, 256)
- self.upconv2 = nn.ConvTranspose2d(256, 128, kernel_size=2, stride=2)
- self.decoder2 = conv_block(256, 128)
- self.upconv1 = nn.ConvTranspose2d(128, 64, kernel_size=2, stride=2)
- self.decoder1 = conv_block(128, 64)
-
- self.final = nn.Conv2d(64, out_channels, kernel_size=1)
-
- def forward(self, x):
- enc1 = self.encoder1(x)
- enc2 = self.encoder2(F.max_pool2d(enc1, kernel_size=2, stride=2))
- enc3 = self.encoder3(F.max_pool2d(enc2, kernel_size=2, stride=2))
- enc4 = self.encoder4(F.max_pool2d(enc3, kernel_size=2, stride=2))
- bottleneck = self.bottleneck(F.max_pool2d(enc4, kernel_size=2, stride=2))
-
- dec4 = self.upconv4(bottleneck)
- dec4 = torch.cat((dec4, enc4), dim=1)
- dec4 = self.decoder4(dec4)
- dec3 = self.upconv3(dec4)
- dec3 = torch.cat((dec3, enc3), dim=1)
- dec3 = self.decoder3(dec3)
- dec2 = self.upconv2(dec3)
- dec2 = torch.cat((dec2, enc2), dim=1)
- dec2 = self.decoder2(dec2)
- dec1 = self.upconv1(dec2)
- dec1 = torch.cat((dec1, enc1), dim=1)
- dec1 = self.decoder1(dec1)
-
- return self.final(dec1)
-
-if __name__ == "__main__":
- model = UNet(in_channels=3,out_channels=7)
- fake_img = torch.rand(size=(2,3,224,224))
- print(fake_img.shape)
- # torch.Size([2, 3, 224, 224])
- out = model(fake_img)
- print(out.shape)
+import torch
+import torch.nn as nn
+import torch.nn.functional as F
+
+class UNet(nn.Module):
+ def __init__(self, in_channels, out_channels):
+ super(UNet, self).__init__()
+
+ def conv_block(in_channels, out_channels):
+ return nn.Sequential(
+ nn.Conv2d(in_channels, out_channels, kernel_size=3, padding=1),
+ nn.ReLU(inplace=True),
+ nn.Conv2d(out_channels, out_channels, kernel_size=3, padding=1),
+ nn.ReLU(inplace=True)
+ )
+
+ self.encoder1 = conv_block(in_channels, 64)
+ self.encoder2 = conv_block(64, 128)
+ self.encoder3 = conv_block(128, 256)
+ self.encoder4 = conv_block(256, 512)
+ self.bottleneck = conv_block(512, 1024)
+
+ self.upconv4 = nn.ConvTranspose2d(1024, 512, kernel_size=2, stride=2)
+ self.decoder4 = conv_block(1024, 512)
+ self.upconv3 = nn.ConvTranspose2d(512, 256, kernel_size=2, stride=2)
+ self.decoder3 = conv_block(512, 256)
+ self.upconv2 = nn.ConvTranspose2d(256, 128, kernel_size=2, stride=2)
+ self.decoder2 = conv_block(256, 128)
+ self.upconv1 = nn.ConvTranspose2d(128, 64, kernel_size=2, stride=2)
+ self.decoder1 = conv_block(128, 64)
+
+ self.final = nn.Conv2d(64, out_channels, kernel_size=1)
+
+ def forward(self, x):
+ enc1 = self.encoder1(x)
+ enc2 = self.encoder2(F.max_pool2d(enc1, kernel_size=2, stride=2))
+ enc3 = self.encoder3(F.max_pool2d(enc2, kernel_size=2, stride=2))
+ enc4 = self.encoder4(F.max_pool2d(enc3, kernel_size=2, stride=2))
+ bottleneck = self.bottleneck(F.max_pool2d(enc4, kernel_size=2, stride=2))
+
+ dec4 = self.upconv4(bottleneck)
+ dec4 = torch.cat((dec4, enc4), dim=1)
+ dec4 = self.decoder4(dec4)
+ dec3 = self.upconv3(dec4)
+ dec3 = torch.cat((dec3, enc3), dim=1)
+ dec3 = self.decoder3(dec3)
+ dec2 = self.upconv2(dec3)
+ dec2 = torch.cat((dec2, enc2), dim=1)
+ dec2 = self.decoder2(dec2)
+ dec1 = self.upconv1(dec2)
+ dec1 = torch.cat((dec1, enc1), dim=1)
+ dec1 = self.decoder1(dec1)
+
+ return self.final(dec1)
+
+if __name__ == "__main__":
+ model = UNet(in_channels=3,out_channels=7)
+ fake_img = torch.rand(size=(2,3,224,224))
+ print(fake_img.shape)
+ # torch.Size([2, 3, 224, 224])
+ out = model(fake_img)
+ print(out.shape)
# torch.Size([2, 7, 224, 224])
\ No newline at end of file
diff --git a/src/models/components/unetmobv2.py b/src/models/components/unetmobv2.py
new file mode 100644
index 0000000000000000000000000000000000000000..acfdba24ef3574bd53f06fbbe5b7a3b447f0d1af
--- /dev/null
+++ b/src/models/components/unetmobv2.py
@@ -0,0 +1,31 @@
+# -*- coding: utf-8 -*-
+# @Time : 2024/8/6 下午3:44
+# @Author : xiaoshun
+# @Email : 3038523973@qq.com
+# @File : unetmobv2.py
+# @Software: PyCharm
+import segmentation_models_pytorch as smp
+import torch
+from torch import nn as nn
+
+
+class UNetMobV2(nn.Module):
+ def __init__(self,num_classes):
+ super().__init__()
+ self.backbone = smp.Unet(
+ encoder_name='mobilenet_v2',
+ encoder_weights='imagenet',
+ in_channels=3,
+ classes=num_classes,
+ )
+
+ def forward(self, x):
+ x = self.backbone(x)
+ return x
+
+
+if __name__ == '__main__':
+ fake_image = torch.rand(1, 3, 224, 224)
+ model = UNetMobV2(num_classes=2)
+ output = model(fake_image)
+ print(output.size())
diff --git a/src/models/components/vae.py b/src/models/components/vae.py
index 9bfaecea93df715d9e48a809fe38053f65361fe1..049a6fec6772f6366ab1bb2bf1817205fee32f97 100644
--- a/src/models/components/vae.py
+++ b/src/models/components/vae.py
@@ -1,144 +1,144 @@
-from typing import List
-
-import matplotlib.pyplot as plt
-import torch
-import torch.nn as nn
-from src.plugin.ldm.modules.diffusionmodules.model import Encoder, Decoder
-from src.plugin.ldm.modules.distributions.distributions import DiagonalGaussianDistribution
-
-
-class AutoencoderKL(nn.Module):
- def __init__(
- self,
- double_z: bool = True,
- z_channels: int = 3,
- resolution: int = 512,
- in_channels: int = 3,
- out_ch: int = 3,
- ch: int = 128,
- ch_mult: List = [1, 2, 4, 4],
- num_res_blocks: int = 2,
- attn_resolutions: List = [],
- dropout: float = 0.0,
- embed_dim: int = 3,
- ckpt_path: str = None,
- ignore_keys: List = [],
- ):
- super(AutoencoderKL, self).__init__()
- ddconfig = {
- "double_z": double_z,
- "z_channels": z_channels,
- "resolution": resolution,
- "in_channels": in_channels,
- "out_ch": out_ch,
- "ch": ch,
- "ch_mult": ch_mult,
- "num_res_blocks": num_res_blocks,
- "attn_resolutions": attn_resolutions,
- "dropout": dropout
- }
- self.encoder = Encoder(**ddconfig)
- self.decoder = Decoder(**ddconfig)
- assert ddconfig["double_z"]
- self.quant_conv = nn.Conv2d(
- 2 * ddconfig["z_channels"], 2 * embed_dim, 1)
- self.post_quant_conv = nn.Conv2d(embed_dim, ddconfig["z_channels"], 1)
- self.embed_dim = embed_dim
- if ckpt_path is not None:
- self.init_from_ckpt(ckpt_path, ignore_keys=ignore_keys)
-
- def init_from_ckpt(self, path, ignore_keys=list()):
- sd = torch.load(path, map_location="cpu")["state_dict"]
- keys = list(sd.keys())
- for k in keys:
- for ik in ignore_keys:
- if k.startswith(ik):
- print(f"Deleting key {k} from state_dict.")
- del sd[k]
- self.load_state_dict(sd, strict=False)
- print(f"Restored from {path}")
-
- def encode(self, x):
- h = self.encoder(x) # B, C, h, w
- moments = self.quant_conv(h) # B, 6, h, w
- posterior = DiagonalGaussianDistribution(moments)
- return posterior # 分布
-
- def decode(self, z):
- z = self.post_quant_conv(z)
- dec = self.decoder(z)
- return dec
-
- def forward(self, input, sample_posterior=True):
- posterior = self.encode(input) # 高斯分布
- if sample_posterior:
- z = posterior.sample() # 采样
- else:
- z = posterior.mode()
- dec = self.decode(z)
- last_layer_weight = self.decoder.conv_out.weight
- return dec, posterior, last_layer_weight
-
-
-if __name__ == '__main__':
- # Test the input and output shapes of the model
- model = AutoencoderKL()
- x = torch.randn(1, 3, 512, 512)
- dec, posterior, last_layer_weight = model(x)
-
- assert dec.shape == (1, 3, 512, 512)
- assert posterior.sample().shape == posterior.mode().shape == (1, 3, 64, 64)
- assert last_layer_weight.shape == (3, 128, 3, 3)
-
- # Plot the latent space and the reconstruction from the pretrained model
- model = AutoencoderKL(ckpt_path="/mnt/chongqinggeminiceph1fs/geminicephfs/wx-mm-spr-xxxx/zouxuechao/Collaborative-Diffusion/outputs/512_vae/2024-06-27T06-02-04_512_vae/checkpoints/epoch=000036.ckpt")
- model.eval()
- image_path = "data/celeba/image/image_512_downsampled_from_hq_1024/0.jpg"
-
- from PIL import Image
- import numpy as np
- from src.data.components.celeba import DalleTransformerPreprocessor
-
- image = Image.open(image_path).convert('RGB')
- image = np.array(image).astype(np.uint8)
- import copy
- original = copy.deepcopy(image)
- transform = DalleTransformerPreprocessor(size=512, phase='test')
- image = transform(image=image)['image']
- image = image.astype(np.float32)/127.5 - 1.0
- image = torch.from_numpy(image).permute(2, 0, 1).unsqueeze(0)
-
- dec, posterior, last_layer_weight = model(image)
-
- # original image
- plt.subplot(1, 3, 1)
- plt.imshow(original)
- plt.title("Original")
- plt.axis("off")
-
- # sampled image from the latent space
- plt.subplot(1, 3, 2)
- x = model.decode(posterior.sample())
- x = (x+1)/2
- x = x.squeeze(0).permute(1, 2, 0).cpu()
- x = x.detach().numpy()
- x = x.clip(0, 1)
- x = (x*255).astype(np.uint8)
- plt.imshow(x)
- plt.title("Sampled")
- plt.axis("off")
-
- # reconstructed image
- plt.subplot(1, 3, 3)
- x = dec
- x = (x+1)/2
- x = x.squeeze(0).permute(1, 2, 0).cpu()
- x = x.detach().numpy()
- x = x.clip(0, 1)
- x = (x*255).astype(np.uint8)
- plt.imshow(x)
- plt.title("Reconstructed")
- plt.axis("off")
-
- plt.tight_layout()
- plt.savefig("vae_reconstruction.png")
+from typing import List
+
+import matplotlib.pyplot as plt
+import torch
+import torch.nn as nn
+from src.plugin.ldm.modules.diffusionmodules.model import Encoder, Decoder
+from src.plugin.ldm.modules.distributions.distributions import DiagonalGaussianDistribution
+
+
+class AutoencoderKL(nn.Module):
+ def __init__(
+ self,
+ double_z: bool = True,
+ z_channels: int = 3,
+ resolution: int = 512,
+ in_channels: int = 3,
+ out_ch: int = 3,
+ ch: int = 128,
+ ch_mult: List = [1, 2, 4, 4],
+ num_res_blocks: int = 2,
+ attn_resolutions: List = [],
+ dropout: float = 0.0,
+ embed_dim: int = 3,
+ ckpt_path: str = None,
+ ignore_keys: List = [],
+ ):
+ super(AutoencoderKL, self).__init__()
+ ddconfig = {
+ "double_z": double_z,
+ "z_channels": z_channels,
+ "resolution": resolution,
+ "in_channels": in_channels,
+ "out_ch": out_ch,
+ "ch": ch,
+ "ch_mult": ch_mult,
+ "num_res_blocks": num_res_blocks,
+ "attn_resolutions": attn_resolutions,
+ "dropout": dropout
+ }
+ self.encoder = Encoder(**ddconfig)
+ self.decoder = Decoder(**ddconfig)
+ assert ddconfig["double_z"]
+ self.quant_conv = nn.Conv2d(
+ 2 * ddconfig["z_channels"], 2 * embed_dim, 1)
+ self.post_quant_conv = nn.Conv2d(embed_dim, ddconfig["z_channels"], 1)
+ self.embed_dim = embed_dim
+ if ckpt_path is not None:
+ self.init_from_ckpt(ckpt_path, ignore_keys=ignore_keys)
+
+ def init_from_ckpt(self, path, ignore_keys=list()):
+ sd = torch.load(path, map_location="cpu")["state_dict"]
+ keys = list(sd.keys())
+ for k in keys:
+ for ik in ignore_keys:
+ if k.startswith(ik):
+ print(f"Deleting key {k} from state_dict.")
+ del sd[k]
+ self.load_state_dict(sd, strict=False)
+ print(f"Restored from {path}")
+
+ def encode(self, x):
+ h = self.encoder(x) # B, C, h, w
+ moments = self.quant_conv(h) # B, 6, h, w
+ posterior = DiagonalGaussianDistribution(moments)
+ return posterior # 分布
+
+ def decode(self, z):
+ z = self.post_quant_conv(z)
+ dec = self.decoder(z)
+ return dec
+
+ def forward(self, input, sample_posterior=True):
+ posterior = self.encode(input) # 高斯分布
+ if sample_posterior:
+ z = posterior.sample() # 采样
+ else:
+ z = posterior.mode()
+ dec = self.decode(z)
+ last_layer_weight = self.decoder.conv_out.weight
+ return dec, posterior, last_layer_weight
+
+
+if __name__ == '__main__':
+ # Test the input and output shapes of the model
+ model = AutoencoderKL()
+ x = torch.randn(1, 3, 512, 512)
+ dec, posterior, last_layer_weight = model(x)
+
+ assert dec.shape == (1, 3, 512, 512)
+ assert posterior.sample().shape == posterior.mode().shape == (1, 3, 64, 64)
+ assert last_layer_weight.shape == (3, 128, 3, 3)
+
+ # Plot the latent space and the reconstruction from the pretrained model
+ model = AutoencoderKL(ckpt_path="/mnt/chongqinggeminiceph1fs/geminicephfs/wx-mm-spr-xxxx/zouxuechao/Collaborative-Diffusion/outputs/512_vae/2024-06-27T06-02-04_512_vae/checkpoints/epoch=000036.ckpt")
+ model.eval()
+ image_path = "data/celeba/image/image_512_downsampled_from_hq_1024/0.jpg"
+
+ from PIL import Image
+ import numpy as np
+ from src.data.components.celeba import DalleTransformerPreprocessor
+
+ image = Image.open(image_path).convert('RGB')
+ image = np.array(image).astype(np.uint8)
+ import copy
+ original = copy.deepcopy(image)
+ transform = DalleTransformerPreprocessor(size=512, phase='test')
+ image = transform(image=image)['image']
+ image = image.astype(np.float32)/127.5 - 1.0
+ image = torch.from_numpy(image).permute(2, 0, 1).unsqueeze(0)
+
+ dec, posterior, last_layer_weight = model(image)
+
+ # original image
+ plt.subplot(1, 3, 1)
+ plt.imshow(original)
+ plt.title("Original")
+ plt.axis("off")
+
+ # sampled image from the latent space
+ plt.subplot(1, 3, 2)
+ x = model.decode(posterior.sample())
+ x = (x+1)/2
+ x = x.squeeze(0).permute(1, 2, 0).cpu()
+ x = x.detach().numpy()
+ x = x.clip(0, 1)
+ x = (x*255).astype(np.uint8)
+ plt.imshow(x)
+ plt.title("Sampled")
+ plt.axis("off")
+
+ # reconstructed image
+ plt.subplot(1, 3, 3)
+ x = dec
+ x = (x+1)/2
+ x = x.squeeze(0).permute(1, 2, 0).cpu()
+ x = x.detach().numpy()
+ x = x.clip(0, 1)
+ x = (x*255).astype(np.uint8)
+ plt.imshow(x)
+ plt.title("Reconstructed")
+ plt.axis("off")
+
+ plt.tight_layout()
+ plt.savefig("vae_reconstruction.png")
diff --git a/src/models/mnist_module.py b/src/models/mnist_module.py
index fde934427b7ef0fbf6e4e5001b27bf52a48520a4..94de25be6ffd9141cf9ae013c6219f2e3bfb053b 100644
--- a/src/models/mnist_module.py
+++ b/src/models/mnist_module.py
@@ -1,217 +1,217 @@
-from typing import Any, Dict, Tuple
-
-import torch
-from lightning import LightningModule
-from torchmetrics import MaxMetric, MeanMetric
-from torchmetrics.classification.accuracy import Accuracy
-
-
-class MNISTLitModule(LightningModule):
- """Example of a `LightningModule` for MNIST classification.
-
- A `LightningModule` implements 8 key methods:
-
- ```python
- def __init__(self):
- # Define initialization code here.
-
- def setup(self, stage):
- # Things to setup before each stage, 'fit', 'validate', 'test', 'predict'.
- # This hook is called on every process when using DDP.
-
- def training_step(self, batch, batch_idx):
- # The complete training step.
-
- def validation_step(self, batch, batch_idx):
- # The complete validation step.
-
- def test_step(self, batch, batch_idx):
- # The complete test step.
-
- def predict_step(self, batch, batch_idx):
- # The complete predict step.
-
- def configure_optimizers(self):
- # Define and configure optimizers and LR schedulers.
- ```
-
- Docs:
- https://lightning.ai/docs/pytorch/latest/common/lightning_module.html
- """
-
- def __init__(
- self,
- net: torch.nn.Module,
- optimizer: torch.optim.Optimizer,
- scheduler: torch.optim.lr_scheduler,
- compile: bool,
- ) -> None:
- """Initialize a `MNISTLitModule`.
-
- :param net: The model to train.
- :param optimizer: The optimizer to use for training.
- :param scheduler: The learning rate scheduler to use for training.
- """
- super().__init__()
-
- # this line allows to access init params with 'self.hparams' attribute
- # also ensures init params will be stored in ckpt
- self.save_hyperparameters(logger=False, ignore=['net'])
-
- self.net = net
-
- # loss function
- self.criterion = torch.nn.CrossEntropyLoss()
-
- # metric objects for calculating and averaging accuracy across batches
- self.train_acc = Accuracy(task="multiclass", num_classes=10)
- self.val_acc = Accuracy(task="multiclass", num_classes=10)
- self.test_acc = Accuracy(task="multiclass", num_classes=10)
-
- # for averaging loss across batches
- self.train_loss = MeanMetric()
- self.val_loss = MeanMetric()
- self.test_loss = MeanMetric()
-
- # for tracking best so far validation accuracy
- self.val_acc_best = MaxMetric()
-
- def forward(self, x: torch.Tensor) -> torch.Tensor:
- """Perform a forward pass through the model `self.net`.
-
- :param x: A tensor of images.
- :return: A tensor of logits.
- """
- return self.net(x)
-
- def on_train_start(self) -> None:
- """Lightning hook that is called when training begins."""
- # by default lightning executes validation step sanity checks before training starts,
- # so it's worth to make sure validation metrics don't store results from these checks
- self.val_loss.reset()
- self.val_acc.reset()
- self.val_acc_best.reset()
-
- def model_step(
- self, batch: Tuple[torch.Tensor, torch.Tensor]
- ) -> Tuple[torch.Tensor, torch.Tensor, torch.Tensor]:
- """Perform a single model step on a batch of data.
-
- :param batch: A batch of data (a tuple) containing the input tensor of images and target labels.
-
- :return: A tuple containing (in order):
- - A tensor of losses.
- - A tensor of predictions.
- - A tensor of target labels.
- """
- x, y = batch
- logits = self.forward(x)
- loss = self.criterion(logits, y)
- preds = torch.argmax(logits, dim=1)
- return loss, preds, y
-
- def training_step(
- self, batch: Tuple[torch.Tensor, torch.Tensor], batch_idx: int
- ) -> torch.Tensor:
- """Perform a single training step on a batch of data from the training set.
-
- :param batch: A batch of data (a tuple) containing the input tensor of images and target
- labels.
- :param batch_idx: The index of the current batch.
- :return: A tensor of losses between model predictions and targets.
- """
- loss, preds, targets = self.model_step(batch)
-
- # update and log metrics
- self.train_loss(loss)
- self.train_acc(preds, targets)
- self.log("train/loss", self.train_loss, on_step=False, on_epoch=True, prog_bar=True)
- self.log("train/acc", self.train_acc, on_step=False, on_epoch=True, prog_bar=True)
-
- # return loss or backpropagation will fail
- return loss
-
- def on_train_epoch_end(self) -> None:
- "Lightning hook that is called when a training epoch ends."
- pass
-
- def validation_step(self, batch: Tuple[torch.Tensor, torch.Tensor], batch_idx: int) -> None:
- """Perform a single validation step on a batch of data from the validation set.
-
- :param batch: A batch of data (a tuple) containing the input tensor of images and target
- labels.
- :param batch_idx: The index of the current batch.
- """
- loss, preds, targets = self.model_step(batch)
-
- # update and log metrics
- self.val_loss(loss)
- self.val_acc(preds, targets)
- self.log("val/loss", self.val_loss, on_step=False, on_epoch=True, prog_bar=True)
- self.log("val/acc", self.val_acc, on_step=False, on_epoch=True, prog_bar=True)
-
- def on_validation_epoch_end(self) -> None:
- "Lightning hook that is called when a validation epoch ends."
- acc = self.val_acc.compute() # get current val acc
- self.val_acc_best(acc) # update best so far val acc
- # log `val_acc_best` as a value through `.compute()` method, instead of as a metric object
- # otherwise metric would be reset by lightning after each epoch
- self.log("val/acc_best", self.val_acc_best.compute(), sync_dist=True, prog_bar=True)
-
- def test_step(self, batch: Tuple[torch.Tensor, torch.Tensor], batch_idx: int) -> None:
- """Perform a single test step on a batch of data from the test set.
-
- :param batch: A batch of data (a tuple) containing the input tensor of images and target
- labels.
- :param batch_idx: The index of the current batch.
- """
- loss, preds, targets = self.model_step(batch)
-
- # update and log metrics
- self.test_loss(loss)
- self.test_acc(preds, targets)
- self.log("test/loss", self.test_loss, on_step=False, on_epoch=True, prog_bar=True)
- self.log("test/acc", self.test_acc, on_step=False, on_epoch=True, prog_bar=True)
-
- def on_test_epoch_end(self) -> None:
- """Lightning hook that is called when a test epoch ends."""
- pass
-
- def setup(self, stage: str) -> None:
- """Lightning hook that is called at the beginning of fit (train + validate), validate,
- test, or predict.
-
- This is a good hook when you need to build models dynamically or adjust something about
- them. This hook is called on every process when using DDP.
-
- :param stage: Either `"fit"`, `"validate"`, `"test"`, or `"predict"`.
- """
- if self.hparams.compile and stage == "fit":
- self.net = torch.compile(self.net)
-
- def configure_optimizers(self) -> Dict[str, Any]:
- """Choose what optimizers and learning-rate schedulers to use in your optimization.
- Normally you'd need one. But in the case of GANs or similar you might have multiple.
-
- Examples:
- https://lightning.ai/docs/pytorch/latest/common/lightning_module.html#configure-optimizers
-
- :return: A dict containing the configured optimizers and learning-rate schedulers to be used for training.
- """
- optimizer = self.hparams.optimizer(params=self.trainer.model.parameters())
- if self.hparams.scheduler is not None:
- scheduler = self.hparams.scheduler(optimizer=optimizer)
- return {
- "optimizer": optimizer,
- "lr_scheduler": {
- "scheduler": scheduler,
- "monitor": "val/loss",
- "interval": "epoch",
- "frequency": 1,
- },
- }
- return {"optimizer": optimizer}
-
-
-if __name__ == "__main__":
- _ = MNISTLitModule(None, None, None, None)
+from typing import Any, Dict, Tuple
+
+import torch
+from lightning import LightningModule
+from torchmetrics import MaxMetric, MeanMetric
+from torchmetrics.classification.accuracy import Accuracy
+
+
+class MNISTLitModule(LightningModule):
+ """Example of a `LightningModule` for MNIST classification.
+
+ A `LightningModule` implements 8 key methods:
+
+ ```python
+ def __init__(self):
+ # Define initialization code here.
+
+ def setup(self, stage):
+ # Things to setup before each stage, 'fit', 'validate', 'test', 'predict'.
+ # This hook is called on every process when using DDP.
+
+ def training_step(self, batch, batch_idx):
+ # The complete training step.
+
+ def validation_step(self, batch, batch_idx):
+ # The complete validation step.
+
+ def test_step(self, batch, batch_idx):
+ # The complete test step.
+
+ def predict_step(self, batch, batch_idx):
+ # The complete predict step.
+
+ def configure_optimizers(self):
+ # Define and configure optimizers and LR schedulers.
+ ```
+
+ Docs:
+ https://lightning.ai/docs/pytorch/latest/common/lightning_module.html
+ """
+
+ def __init__(
+ self,
+ net: torch.nn.Module,
+ optimizer: torch.optim.Optimizer,
+ scheduler: torch.optim.lr_scheduler,
+ compile: bool,
+ ) -> None:
+ """Initialize a `MNISTLitModule`.
+
+ :param net: The model to train.
+ :param optimizer: The optimizer to use for training.
+ :param scheduler: The learning rate scheduler to use for training.
+ """
+ super().__init__()
+
+ # this line allows to access init params with 'self.hparams' attribute
+ # also ensures init params will be stored in ckpt
+ self.save_hyperparameters(logger=False, ignore=['net'])
+
+ self.net = net
+
+ # loss function
+ self.criterion = torch.nn.CrossEntropyLoss()
+
+ # metric objects for calculating and averaging accuracy across batches
+ self.train_acc = Accuracy(task="multiclass", num_classes=10)
+ self.val_acc = Accuracy(task="multiclass", num_classes=10)
+ self.test_acc = Accuracy(task="multiclass", num_classes=10)
+
+ # for averaging loss across batches
+ self.train_loss = MeanMetric()
+ self.val_loss = MeanMetric()
+ self.test_loss = MeanMetric()
+
+ # for tracking best so far validation accuracy
+ self.val_acc_best = MaxMetric()
+
+ def forward(self, x: torch.Tensor) -> torch.Tensor:
+ """Perform a forward pass through the model `self.net`.
+
+ :param x: A tensor of images.
+ :return: A tensor of logits.
+ """
+ return self.net(x)
+
+ def on_train_start(self) -> None:
+ """Lightning hook that is called when training begins."""
+ # by default lightning executes validation step sanity checks before training starts,
+ # so it's worth to make sure validation metrics don't store results from these checks
+ self.val_loss.reset()
+ self.val_acc.reset()
+ self.val_acc_best.reset()
+
+ def model_step(
+ self, batch: Tuple[torch.Tensor, torch.Tensor]
+ ) -> Tuple[torch.Tensor, torch.Tensor, torch.Tensor]:
+ """Perform a single model step on a batch of data.
+
+ :param batch: A batch of data (a tuple) containing the input tensor of images and target labels.
+
+ :return: A tuple containing (in order):
+ - A tensor of losses.
+ - A tensor of predictions.
+ - A tensor of target labels.
+ """
+ x, y = batch
+ logits = self.forward(x)
+ loss = self.criterion(logits, y)
+ preds = torch.argmax(logits, dim=1)
+ return loss, preds, y
+
+ def training_step(
+ self, batch: Tuple[torch.Tensor, torch.Tensor], batch_idx: int
+ ) -> torch.Tensor:
+ """Perform a single training step on a batch of data from the training set.
+
+ :param batch: A batch of data (a tuple) containing the input tensor of images and target
+ labels.
+ :param batch_idx: The index of the current batch.
+ :return: A tensor of losses between model predictions and targets.
+ """
+ loss, preds, targets = self.model_step(batch)
+
+ # update and log metrics
+ self.train_loss(loss)
+ self.train_acc(preds, targets)
+ self.log("train/loss", self.train_loss, on_step=False, on_epoch=True, prog_bar=True)
+ self.log("train/acc", self.train_acc, on_step=False, on_epoch=True, prog_bar=True)
+
+ # return loss or backpropagation will fail
+ return loss
+
+ def on_train_epoch_end(self) -> None:
+ "Lightning hook that is called when a training epoch ends."
+ pass
+
+ def validation_step(self, batch: Tuple[torch.Tensor, torch.Tensor], batch_idx: int) -> None:
+ """Perform a single validation step on a batch of data from the validation set.
+
+ :param batch: A batch of data (a tuple) containing the input tensor of images and target
+ labels.
+ :param batch_idx: The index of the current batch.
+ """
+ loss, preds, targets = self.model_step(batch)
+
+ # update and log metrics
+ self.val_loss(loss)
+ self.val_acc(preds, targets)
+ self.log("val/loss", self.val_loss, on_step=False, on_epoch=True, prog_bar=True)
+ self.log("val/acc", self.val_acc, on_step=False, on_epoch=True, prog_bar=True)
+
+ def on_validation_epoch_end(self) -> None:
+ "Lightning hook that is called when a validation epoch ends."
+ acc = self.val_acc.compute() # get current val acc
+ self.val_acc_best(acc) # update best so far val acc
+ # log `val_acc_best` as a value through `.compute()` method, instead of as a metric object
+ # otherwise metric would be reset by lightning after each epoch
+ self.log("val/acc_best", self.val_acc_best.compute(), sync_dist=True, prog_bar=True)
+
+ def test_step(self, batch: Tuple[torch.Tensor, torch.Tensor], batch_idx: int) -> None:
+ """Perform a single test step on a batch of data from the test set.
+
+ :param batch: A batch of data (a tuple) containing the input tensor of images and target
+ labels.
+ :param batch_idx: The index of the current batch.
+ """
+ loss, preds, targets = self.model_step(batch)
+
+ # update and log metrics
+ self.test_loss(loss)
+ self.test_acc(preds, targets)
+ self.log("test/loss", self.test_loss, on_step=False, on_epoch=True, prog_bar=True)
+ self.log("test/acc", self.test_acc, on_step=False, on_epoch=True, prog_bar=True)
+
+ def on_test_epoch_end(self) -> None:
+ """Lightning hook that is called when a test epoch ends."""
+ pass
+
+ def setup(self, stage: str) -> None:
+ """Lightning hook that is called at the beginning of fit (train + validate), validate,
+ test, or predict.
+
+ This is a good hook when you need to build models dynamically or adjust something about
+ them. This hook is called on every process when using DDP.
+
+ :param stage: Either `"fit"`, `"validate"`, `"test"`, or `"predict"`.
+ """
+ if self.hparams.compile and stage == "fit":
+ self.net = torch.compile(self.net)
+
+ def configure_optimizers(self) -> Dict[str, Any]:
+ """Choose what optimizers and learning-rate schedulers to use in your optimization.
+ Normally you'd need one. But in the case of GANs or similar you might have multiple.
+
+ Examples:
+ https://lightning.ai/docs/pytorch/latest/common/lightning_module.html#configure-optimizers
+
+ :return: A dict containing the configured optimizers and learning-rate schedulers to be used for training.
+ """
+ optimizer = self.hparams.optimizer(params=self.trainer.model.parameters())
+ if self.hparams.scheduler is not None:
+ scheduler = self.hparams.scheduler(optimizer=optimizer)
+ return {
+ "optimizer": optimizer,
+ "lr_scheduler": {
+ "scheduler": scheduler,
+ "monitor": "val/loss",
+ "interval": "epoch",
+ "frequency": 1,
+ },
+ }
+ return {"optimizer": optimizer}
+
+
+if __name__ == "__main__":
+ _ = MNISTLitModule(None, None, None, None)
diff --git a/src/train.py b/src/train.py
index c272227dbc704d1a9eeab67e7e5dcecb879e87ea..38289c0489288e8eaf37b7a958877823c9f0c981 100644
--- a/src/train.py
+++ b/src/train.py
@@ -1,133 +1,133 @@
-from typing import Any, Dict, List, Optional, Tuple
-
-import hydra
-import lightning as L
-import rootutils
-import torch
-from lightning import Callback, LightningDataModule, LightningModule, Trainer
-from lightning.pytorch.loggers import Logger
-from omegaconf import DictConfig
-
-rootutils.setup_root(__file__, indicator=".project-root", pythonpath=True)
-# ------------------------------------------------------------------------------------ #
-# the setup_root above is equivalent to:
-# - adding project root dir to PYTHONPATH
-# (so you don't need to force user to install project as a package)
-# (necessary before importing any local modules e.g. `from src import utils`)
-# - setting up PROJECT_ROOT environment variable
-# (which is used as a base for paths in "configs/paths/default.yaml")
-# (this way all filepaths are the same no matter where you run the code)
-# - loading environment variables from ".env" in root dir
-#
-# you can remove it if you:
-# 1. either install project as a package or move entry files to project root dir
-# 2. set `root_dir` to "." in "configs/paths/default.yaml"
-#
-# more info: https://github.com/ashleve/rootutils
-# ------------------------------------------------------------------------------------ #
-
-from src.utils import (
- RankedLogger,
- extras,
- get_metric_value,
- instantiate_callbacks,
- instantiate_loggers,
- log_hyperparameters,
- task_wrapper,
-)
-
-log = RankedLogger(__name__, rank_zero_only=True)
-
-
-@task_wrapper
-def train(cfg: DictConfig) -> Tuple[Dict[str, Any], Dict[str, Any]]:
- """Trains the model. Can additionally evaluate on a testset, using best weights obtained during
- training.
-
- This method is wrapped in optional @task_wrapper decorator, that controls the behavior during
- failure. Useful for multiruns, saving info about the crash, etc.
-
- :param cfg: A DictConfig configuration composed by Hydra.
- :return: A tuple with metrics and dict with all instantiated objects.
- """
- # set seed for random number generators in pytorch, numpy and python.random
- if cfg.get("seed"):
- L.seed_everything(cfg.seed, workers=True)
-
- log.info(f"Instantiating datamodule <{cfg.data._target_}>")
- datamodule: LightningDataModule = hydra.utils.instantiate(cfg.data)
-
- log.info(f"Instantiating model <{cfg.model._target_}>")
- model: LightningModule = hydra.utils.instantiate(cfg.model)
-
- log.info("Instantiating callbacks...")
- callbacks: List[Callback] = instantiate_callbacks(cfg.get("callbacks"))
-
- log.info("Instantiating loggers...")
- logger: List[Logger] = instantiate_loggers(cfg.get("logger"))
-
- log.info(f"Instantiating trainer <{cfg.trainer._target_}>")
- trainer: Trainer = hydra.utils.instantiate(cfg.trainer, callbacks=callbacks, logger=logger)
-
- object_dict = {
- "cfg": cfg,
- "datamodule": datamodule,
- "model": model,
- "callbacks": callbacks,
- "logger": logger,
- "trainer": trainer,
- }
-
- if logger:
- log.info("Logging hyperparameters!")
- log_hyperparameters(object_dict)
-
- if cfg.get("train"):
- log.info("Starting training!")
- trainer.fit(model=model, datamodule=datamodule, ckpt_path=cfg.get("ckpt_path"))
-
- train_metrics = trainer.callback_metrics
-
- if cfg.get("test"):
- log.info("Starting testing!")
- ckpt_path = trainer.checkpoint_callback.best_model_path
- if ckpt_path == "":
- log.warning("Best ckpt not found! Using current weights for testing...")
- ckpt_path = None
- trainer.test(model=model, datamodule=datamodule, ckpt_path=ckpt_path)
- log.info(f"Best ckpt path: {ckpt_path}")
-
- test_metrics = trainer.callback_metrics
-
- # merge train and test metrics
- metric_dict = {**train_metrics, **test_metrics}
-
- return metric_dict, object_dict
-
-
-@hydra.main(version_base="1.3", config_path="../configs", config_name="train.yaml")
-def main(cfg: DictConfig) -> Optional[float]:
- """Main entry point for training.
-
- :param cfg: DictConfig configuration composed by Hydra.
- :return: Optional[float] with optimized metric value.
- """
- # apply extra utilities
- # (e.g. ask for tags if none are provided in cfg, print cfg tree, etc.)
- extras(cfg)
-
- # train the model
- metric_dict, _ = train(cfg)
-
- # safely retrieve metric value for hydra-based hyperparameter optimization
- metric_value = get_metric_value(
- metric_dict=metric_dict, metric_name=cfg.get("optimized_metric")
- )
-
- # return optimized metric
- return metric_value
-
-
-if __name__ == "__main__":
- torch.set_float32_matmul_precision('high')
- main()
+from typing import Any, Dict, List, Optional, Tuple
+
+import hydra
+import lightning as L
+import rootutils
+import torch
+from lightning import Callback, LightningDataModule, LightningModule, Trainer
+from lightning.pytorch.loggers import Logger
+from omegaconf import DictConfig
+
+rootutils.setup_root(__file__, indicator=".project-root", pythonpath=True)
+# ------------------------------------------------------------------------------------ #
+# the setup_root above is equivalent to:
+# - adding project root dir to PYTHONPATH
+# (so you don't need to force user to install project as a package)
+# (necessary before importing any local modules e.g. `from src import utils`)
+# - setting up PROJECT_ROOT environment variable
+# (which is used as a base for paths in "configs/paths/default.yaml")
+# (this way all filepaths are the same no matter where you run the code)
+# - loading environment variables from ".env" in root dir
+#
+# you can remove it if you:
+# 1. either install project as a package or move entry files to project root dir
+# 2. set `root_dir` to "." in "configs/paths/default.yaml"
+#
+# more info: https://github.com/ashleve/rootutils
+# ------------------------------------------------------------------------------------ #
+
+from src.utils import (
+ RankedLogger,
+ extras,
+ get_metric_value,
+ instantiate_callbacks,
+ instantiate_loggers,
+ log_hyperparameters,
+ task_wrapper,
+)
+
+log = RankedLogger(__name__, rank_zero_only=True)
+
+
+@task_wrapper
+def train(cfg: DictConfig) -> Tuple[Dict[str, Any], Dict[str, Any]]:
+ """Trains the model. Can additionally evaluate on a testset, using best weights obtained during
+ training.
+
+ This method is wrapped in optional @task_wrapper decorator, that controls the behavior during
+ failure. Useful for multiruns, saving info about the crash, etc.
+
+ :param cfg: A DictConfig configuration composed by Hydra.
+ :return: A tuple with metrics and dict with all instantiated objects.
+ """
+ # set seed for random number generators in pytorch, numpy and python.random
+ if cfg.get("seed"):
+ L.seed_everything(cfg.seed, workers=True)
+
+ log.info(f"Instantiating datamodule <{cfg.data._target_}>")
+ datamodule: LightningDataModule = hydra.utils.instantiate(cfg.data)
+
+ log.info(f"Instantiating model <{cfg.model._target_}>")
+ model: LightningModule = hydra.utils.instantiate(cfg.model)
+
+ log.info("Instantiating callbacks...")
+ callbacks: List[Callback] = instantiate_callbacks(cfg.get("callbacks"))
+
+ log.info("Instantiating loggers...")
+ logger: List[Logger] = instantiate_loggers(cfg.get("logger"))
+
+ log.info(f"Instantiating trainer <{cfg.trainer._target_}>")
+ trainer: Trainer = hydra.utils.instantiate(cfg.trainer, callbacks=callbacks, logger=logger)
+
+ object_dict = {
+ "cfg": cfg,
+ "datamodule": datamodule,
+ "model": model,
+ "callbacks": callbacks,
+ "logger": logger,
+ "trainer": trainer,
+ }
+
+ if logger:
+ log.info("Logging hyperparameters!")
+ log_hyperparameters(object_dict)
+
+ if cfg.get("train"):
+ log.info("Starting training!")
+ trainer.fit(model=model, datamodule=datamodule, ckpt_path=cfg.get("ckpt_path"))
+
+ train_metrics = trainer.callback_metrics
+
+ if cfg.get("test"):
+ log.info("Starting testing!")
+ ckpt_path = trainer.checkpoint_callback.best_model_path
+ if ckpt_path == "":
+ log.warning("Best ckpt not found! Using current weights for testing...")
+ ckpt_path = None
+ trainer.test(model=model, datamodule=datamodule, ckpt_path=ckpt_path)
+ log.info(f"Best ckpt path: {ckpt_path}")
+
+ test_metrics = trainer.callback_metrics
+
+ # merge train and test metrics
+ metric_dict = {**train_metrics, **test_metrics}
+
+ return metric_dict, object_dict
+
+
+@hydra.main(version_base="1.3", config_path="../configs", config_name="train.yaml")
+def main(cfg: DictConfig) -> Optional[float]:
+ """Main entry point for training.
+
+ :param cfg: DictConfig configuration composed by Hydra.
+ :return: Optional[float] with optimized metric value.
+ """
+ # apply extra utilities
+ # (e.g. ask for tags if none are provided in cfg, print cfg tree, etc.)
+ extras(cfg)
+
+ # train the model
+ metric_dict, _ = train(cfg)
+
+ # safely retrieve metric value for hydra-based hyperparameter optimization
+ metric_value = get_metric_value(
+ metric_dict=metric_dict, metric_name=cfg.get("optimized_metric")
+ )
+
+ # return optimized metric
+ return metric_value
+
+
+if __name__ == "__main__":
+ torch.set_float32_matmul_precision('high')
+ main()
diff --git a/src/utils/__init__.py b/src/utils/__init__.py
index 5b0707ca57ec89fc5f5cb1a023135eeb756a8e1e..e67ddb8724eb81712d81ad550c2237e81e7512ea 100644
--- a/src/utils/__init__.py
+++ b/src/utils/__init__.py
@@ -1,5 +1,5 @@
-from src.utils.instantiators import instantiate_callbacks, instantiate_loggers
-from src.utils.logging_utils import log_hyperparameters
-from src.utils.pylogger import RankedLogger
-from src.utils.rich_utils import enforce_tags, print_config_tree
-from src.utils.utils import extras, get_metric_value, task_wrapper
+from src.utils.instantiators import instantiate_callbacks, instantiate_loggers
+from src.utils.logging_utils import log_hyperparameters
+from src.utils.pylogger import RankedLogger
+from src.utils.rich_utils import enforce_tags, print_config_tree
+from src.utils.utils import extras, get_metric_value, task_wrapper
diff --git a/src/utils/instantiators.py b/src/utils/instantiators.py
index 82b9278a465d39565942f862442ebe79549825d7..0b21fd211cf590a81f162335845978324f8223f0 100644
--- a/src/utils/instantiators.py
+++ b/src/utils/instantiators.py
@@ -1,56 +1,56 @@
-from typing import List
-
-import hydra
-from lightning import Callback
-from lightning.pytorch.loggers import Logger
-from omegaconf import DictConfig
-
-from src.utils import pylogger
-
-log = pylogger.RankedLogger(__name__, rank_zero_only=True)
-
-
-def instantiate_callbacks(callbacks_cfg: DictConfig) -> List[Callback]:
- """Instantiates callbacks from config.
-
- :param callbacks_cfg: A DictConfig object containing callback configurations.
- :return: A list of instantiated callbacks.
- """
- callbacks: List[Callback] = []
-
- if not callbacks_cfg:
- log.warning("No callback configs found! Skipping..")
- return callbacks
-
- if not isinstance(callbacks_cfg, DictConfig):
- raise TypeError("Callbacks config must be a DictConfig!")
-
- for _, cb_conf in callbacks_cfg.items():
- if isinstance(cb_conf, DictConfig) and "_target_" in cb_conf:
- log.info(f"Instantiating callback <{cb_conf._target_}>")
- callbacks.append(hydra.utils.instantiate(cb_conf))
-
- return callbacks
-
-
-def instantiate_loggers(logger_cfg: DictConfig) -> List[Logger]:
- """Instantiates loggers from config.
-
- :param logger_cfg: A DictConfig object containing logger configurations.
- :return: A list of instantiated loggers.
- """
- logger: List[Logger] = []
-
- if not logger_cfg:
- log.warning("No logger configs found! Skipping...")
- return logger
-
- if not isinstance(logger_cfg, DictConfig):
- raise TypeError("Logger config must be a DictConfig!")
-
- for _, lg_conf in logger_cfg.items():
- if isinstance(lg_conf, DictConfig) and "_target_" in lg_conf:
- log.info(f"Instantiating logger <{lg_conf._target_}>")
- logger.append(hydra.utils.instantiate(lg_conf))
-
- return logger
+from typing import List
+
+import hydra
+from lightning import Callback
+from lightning.pytorch.loggers import Logger
+from omegaconf import DictConfig
+
+from src.utils import pylogger
+
+log = pylogger.RankedLogger(__name__, rank_zero_only=True)
+
+
+def instantiate_callbacks(callbacks_cfg: DictConfig) -> List[Callback]:
+ """Instantiates callbacks from config.
+
+ :param callbacks_cfg: A DictConfig object containing callback configurations.
+ :return: A list of instantiated callbacks.
+ """
+ callbacks: List[Callback] = []
+
+ if not callbacks_cfg:
+ log.warning("No callback configs found! Skipping..")
+ return callbacks
+
+ if not isinstance(callbacks_cfg, DictConfig):
+ raise TypeError("Callbacks config must be a DictConfig!")
+
+ for _, cb_conf in callbacks_cfg.items():
+ if isinstance(cb_conf, DictConfig) and "_target_" in cb_conf:
+ log.info(f"Instantiating callback <{cb_conf._target_}>")
+ callbacks.append(hydra.utils.instantiate(cb_conf))
+
+ return callbacks
+
+
+def instantiate_loggers(logger_cfg: DictConfig) -> List[Logger]:
+ """Instantiates loggers from config.
+
+ :param logger_cfg: A DictConfig object containing logger configurations.
+ :return: A list of instantiated loggers.
+ """
+ logger: List[Logger] = []
+
+ if not logger_cfg:
+ log.warning("No logger configs found! Skipping...")
+ return logger
+
+ if not isinstance(logger_cfg, DictConfig):
+ raise TypeError("Logger config must be a DictConfig!")
+
+ for _, lg_conf in logger_cfg.items():
+ if isinstance(lg_conf, DictConfig) and "_target_" in lg_conf:
+ log.info(f"Instantiating logger <{lg_conf._target_}>")
+ logger.append(hydra.utils.instantiate(lg_conf))
+
+ return logger
diff --git a/src/utils/logging_utils.py b/src/utils/logging_utils.py
index 360abcdceec82e551995f756ce6ec3b2d06ae641..8b08e2613ddbd7b37040d3db2a8d4db6f90cd700 100644
--- a/src/utils/logging_utils.py
+++ b/src/utils/logging_utils.py
@@ -1,57 +1,57 @@
-from typing import Any, Dict
-
-from lightning_utilities.core.rank_zero import rank_zero_only
-from omegaconf import OmegaConf
-
-from src.utils import pylogger
-
-log = pylogger.RankedLogger(__name__, rank_zero_only=True)
-
-
-@rank_zero_only
-def log_hyperparameters(object_dict: Dict[str, Any]) -> None:
- """Controls which config parts are saved by Lightning loggers.
-
- Additionally saves:
- - Number of model parameters
-
- :param object_dict: A dictionary containing the following objects:
- - `"cfg"`: A DictConfig object containing the main config.
- - `"model"`: The Lightning model.
- - `"trainer"`: The Lightning trainer.
- """
- hparams = {}
-
- cfg = OmegaConf.to_container(object_dict["cfg"])
- model = object_dict["model"]
- trainer = object_dict["trainer"]
-
- if not trainer.logger:
- log.warning("Logger not found! Skipping hyperparameter logging...")
- return
-
- hparams["model"] = cfg["model"]
-
- # save number of model parameters
- hparams["model/params/total"] = sum(p.numel() for p in model.parameters())
- hparams["model/params/trainable"] = sum(
- p.numel() for p in model.parameters() if p.requires_grad
- )
- hparams["model/params/non_trainable"] = sum(
- p.numel() for p in model.parameters() if not p.requires_grad
- )
-
- hparams["data"] = cfg["data"]
- hparams["trainer"] = cfg["trainer"]
-
- hparams["callbacks"] = cfg.get("callbacks")
- hparams["extras"] = cfg.get("extras")
-
- hparams["task_name"] = cfg.get("task_name")
- hparams["tags"] = cfg.get("tags")
- hparams["ckpt_path"] = cfg.get("ckpt_path")
- hparams["seed"] = cfg.get("seed")
-
- # send hparams to all loggers
- for logger in trainer.loggers:
- logger.log_hyperparams(hparams)
+from typing import Any, Dict
+
+from lightning_utilities.core.rank_zero import rank_zero_only
+from omegaconf import OmegaConf
+
+from src.utils import pylogger
+
+log = pylogger.RankedLogger(__name__, rank_zero_only=True)
+
+
+@rank_zero_only
+def log_hyperparameters(object_dict: Dict[str, Any]) -> None:
+ """Controls which config parts are saved by Lightning loggers.
+
+ Additionally saves:
+ - Number of model parameters
+
+ :param object_dict: A dictionary containing the following objects:
+ - `"cfg"`: A DictConfig object containing the main config.
+ - `"model"`: The Lightning model.
+ - `"trainer"`: The Lightning trainer.
+ """
+ hparams = {}
+
+ cfg = OmegaConf.to_container(object_dict["cfg"])
+ model = object_dict["model"]
+ trainer = object_dict["trainer"]
+
+ if not trainer.logger:
+ log.warning("Logger not found! Skipping hyperparameter logging...")
+ return
+
+ hparams["model"] = cfg["model"]
+
+ # save number of model parameters
+ hparams["model/params/total"] = sum(p.numel() for p in model.parameters())
+ hparams["model/params/trainable"] = sum(
+ p.numel() for p in model.parameters() if p.requires_grad
+ )
+ hparams["model/params/non_trainable"] = sum(
+ p.numel() for p in model.parameters() if not p.requires_grad
+ )
+
+ hparams["data"] = cfg["data"]
+ hparams["trainer"] = cfg["trainer"]
+
+ hparams["callbacks"] = cfg.get("callbacks")
+ hparams["extras"] = cfg.get("extras")
+
+ hparams["task_name"] = cfg.get("task_name")
+ hparams["tags"] = cfg.get("tags")
+ hparams["ckpt_path"] = cfg.get("ckpt_path")
+ hparams["seed"] = cfg.get("seed")
+
+ # send hparams to all loggers
+ for logger in trainer.loggers:
+ logger.log_hyperparams(hparams)
diff --git a/src/utils/make_h5.py b/src/utils/make_h5.py
index 492c16d5f254e423615c8594b51fb8032dd65f12..00c8c358fc52b635d3db4c7ffa8fca4c7c8e7ab4 100644
--- a/src/utils/make_h5.py
+++ b/src/utils/make_h5.py
@@ -1,37 +1,37 @@
-import os
-
-import h5py
-import numpy as np
-from PIL import Image
-from tqdm import tqdm
-
-
-class MNISTH5Creator:
- def __init__(self, data_dir, h5_file):
- self.data_dir = data_dir
- self.h5_file = h5_file
-
- def create_h5_file(self):
- """创建HDF5文件,包含从0到9的子目录中的所有图像数据。"""
- with h5py.File(self.h5_file, 'w') as h5f:
- for i in range(10):
- images = self.load_images_for_digit(i)
- h5f.create_dataset(name=str(i), data=images)
- print("HDF5文件已创建.")
-
- def load_images_for_digit(self, digit):
- """为给定的数字加载所有图像,并将它们转换为numpy数组。"""
- digit_folder = os.path.join(self.data_dir, str(digit))
- images = []
- for img_name in tqdm(os.listdir(digit_folder), desc=f"Loading images for digit {digit}"):
- img_path = os.path.join(digit_folder, img_name)
- img = Image.open(img_path).convert('L')
- img_array = np.array(img)
- images.append(img_array)
- return images
-
-if __name__ == "__main__":
- data_dir = 'data/mnist'
- h5_file = 'data/mnist.h5'
- mnist_h5_creator = MNISTH5Creator(data_dir, h5_file)
- mnist_h5_creator.create_h5_file()
+import os
+
+import h5py
+import numpy as np
+from PIL import Image
+from tqdm import tqdm
+
+
+class MNISTH5Creator:
+ def __init__(self, data_dir, h5_file):
+ self.data_dir = data_dir
+ self.h5_file = h5_file
+
+ def create_h5_file(self):
+ """创建HDF5文件,包含从0到9的子目录中的所有图像数据。"""
+ with h5py.File(self.h5_file, 'w') as h5f:
+ for i in range(10):
+ images = self.load_images_for_digit(i)
+ h5f.create_dataset(name=str(i), data=images)
+ print("HDF5文件已创建.")
+
+ def load_images_for_digit(self, digit):
+ """为给定的数字加载所有图像,并将它们转换为numpy数组。"""
+ digit_folder = os.path.join(self.data_dir, str(digit))
+ images = []
+ for img_name in tqdm(os.listdir(digit_folder), desc=f"Loading images for digit {digit}"):
+ img_path = os.path.join(digit_folder, img_name)
+ img = Image.open(img_path).convert('L')
+ img_array = np.array(img)
+ images.append(img_array)
+ return images
+
+if __name__ == "__main__":
+ data_dir = 'data/mnist'
+ h5_file = 'data/mnist.h5'
+ mnist_h5_creator = MNISTH5Creator(data_dir, h5_file)
+ mnist_h5_creator.create_h5_file()
diff --git a/src/utils/pylogger.py b/src/utils/pylogger.py
index c4ee8675ebde11b2a43b0679a03cd88d9268bc71..08e329e08dc8758da6d58a488b96be4389a95e36 100644
--- a/src/utils/pylogger.py
+++ b/src/utils/pylogger.py
@@ -1,51 +1,51 @@
-import logging
-from typing import Mapping, Optional
-
-from lightning_utilities.core.rank_zero import rank_prefixed_message, rank_zero_only
-
-
-class RankedLogger(logging.LoggerAdapter):
- """A multi-GPU-friendly python command line logger."""
-
- def __init__(
- self,
- name: str = __name__,
- rank_zero_only: bool = False,
- extra: Optional[Mapping[str, object]] = None,
- ) -> None:
- """Initializes a multi-GPU-friendly python command line logger that logs on all processes
- with their rank prefixed in the log message.
-
- :param name: The name of the logger. Default is ``__name__``.
- :param rank_zero_only: Whether to force all logs to only occur on the rank zero process. Default is `False`.
- :param extra: (Optional) A dict-like object which provides contextual information. See `logging.LoggerAdapter`.
- """
- logger = logging.getLogger(name)
- super().__init__(logger=logger, extra=extra)
- self.rank_zero_only = rank_zero_only
-
- def log(self, level: int, msg: str, rank: Optional[int] = None, *args, **kwargs) -> None:
- """Delegate a log call to the underlying logger, after prefixing its message with the rank
- of the process it's being logged from. If `'rank'` is provided, then the log will only
- occur on that rank/process.
-
- :param level: The level to log at. Look at `logging.__init__.py` for more information.
- :param msg: The message to log.
- :param rank: The rank to log at.
- :param args: Additional args to pass to the underlying logging function.
- :param kwargs: Any additional keyword args to pass to the underlying logging function.
- """
- if self.isEnabledFor(level):
- msg, kwargs = self.process(msg, kwargs)
- current_rank = getattr(rank_zero_only, "rank", None)
- if current_rank is None:
- raise RuntimeError("The `rank_zero_only.rank` needs to be set before use")
- msg = rank_prefixed_message(msg, current_rank)
- if self.rank_zero_only:
- if current_rank == 0:
- self.logger.log(level, msg, *args, **kwargs)
- else:
- if rank is None:
- self.logger.log(level, msg, *args, **kwargs)
- elif current_rank == rank:
- self.logger.log(level, msg, *args, **kwargs)
+import logging
+from typing import Mapping, Optional
+
+from lightning_utilities.core.rank_zero import rank_prefixed_message, rank_zero_only
+
+
+class RankedLogger(logging.LoggerAdapter):
+ """A multi-GPU-friendly python command line logger."""
+
+ def __init__(
+ self,
+ name: str = __name__,
+ rank_zero_only: bool = False,
+ extra: Optional[Mapping[str, object]] = None,
+ ) -> None:
+ """Initializes a multi-GPU-friendly python command line logger that logs on all processes
+ with their rank prefixed in the log message.
+
+ :param name: The name of the logger. Default is ``__name__``.
+ :param rank_zero_only: Whether to force all logs to only occur on the rank zero process. Default is `False`.
+ :param extra: (Optional) A dict-like object which provides contextual information. See `logging.LoggerAdapter`.
+ """
+ logger = logging.getLogger(name)
+ super().__init__(logger=logger, extra=extra)
+ self.rank_zero_only = rank_zero_only
+
+ def log(self, level: int, msg: str, rank: Optional[int] = None, *args, **kwargs) -> None:
+ """Delegate a log call to the underlying logger, after prefixing its message with the rank
+ of the process it's being logged from. If `'rank'` is provided, then the log will only
+ occur on that rank/process.
+
+ :param level: The level to log at. Look at `logging.__init__.py` for more information.
+ :param msg: The message to log.
+ :param rank: The rank to log at.
+ :param args: Additional args to pass to the underlying logging function.
+ :param kwargs: Any additional keyword args to pass to the underlying logging function.
+ """
+ if self.isEnabledFor(level):
+ msg, kwargs = self.process(msg, kwargs)
+ current_rank = getattr(rank_zero_only, "rank", None)
+ if current_rank is None:
+ raise RuntimeError("The `rank_zero_only.rank` needs to be set before use")
+ msg = rank_prefixed_message(msg, current_rank)
+ if self.rank_zero_only:
+ if current_rank == 0:
+ self.logger.log(level, msg, *args, **kwargs)
+ else:
+ if rank is None:
+ self.logger.log(level, msg, *args, **kwargs)
+ elif current_rank == rank:
+ self.logger.log(level, msg, *args, **kwargs)
diff --git a/src/utils/rich_utils.py b/src/utils/rich_utils.py
index aeec6806bb1e4a15a04b91b710a546231590ab14..816e33a6cad1bd5fdcbf133364f95899efa59007 100644
--- a/src/utils/rich_utils.py
+++ b/src/utils/rich_utils.py
@@ -1,99 +1,99 @@
-from pathlib import Path
-from typing import Sequence
-
-import rich
-import rich.syntax
-import rich.tree
-from hydra.core.hydra_config import HydraConfig
-from lightning_utilities.core.rank_zero import rank_zero_only
-from omegaconf import DictConfig, OmegaConf, open_dict
-from rich.prompt import Prompt
-
-from src.utils import pylogger
-
-log = pylogger.RankedLogger(__name__, rank_zero_only=True)
-
-
-@rank_zero_only
-def print_config_tree(
- cfg: DictConfig,
- print_order: Sequence[str] = (
- "data",
- "model",
- "callbacks",
- "logger",
- "trainer",
- "paths",
- "extras",
- ),
- resolve: bool = False,
- save_to_file: bool = False,
-) -> None:
- """Prints the contents of a DictConfig as a tree structure using the Rich library.
-
- :param cfg: A DictConfig composed by Hydra.
- :param print_order: Determines in what order config components are printed. Default is ``("data", "model",
- "callbacks", "logger", "trainer", "paths", "extras")``.
- :param resolve: Whether to resolve reference fields of DictConfig. Default is ``False``.
- :param save_to_file: Whether to export config to the hydra output folder. Default is ``False``.
- """
- style = "dim"
- tree = rich.tree.Tree("CONFIG", style=style, guide_style=style)
-
- queue = []
-
- # add fields from `print_order` to queue
- for field in print_order:
- queue.append(field) if field in cfg else log.warning(
- f"Field '{field}' not found in config. Skipping '{field}' config printing..."
- )
-
- # add all the other fields to queue (not specified in `print_order`)
- for field in cfg:
- if field not in queue:
- queue.append(field)
-
- # generate config tree from queue
- for field in queue:
- branch = tree.add(field, style=style, guide_style=style)
-
- config_group = cfg[field]
- if isinstance(config_group, DictConfig):
- branch_content = OmegaConf.to_yaml(config_group, resolve=resolve)
- else:
- branch_content = str(config_group)
-
- branch.add(rich.syntax.Syntax(branch_content, "yaml"))
-
- # print config tree
- rich.print(tree)
-
- # save config tree to file
- if save_to_file:
- with open(Path(cfg.paths.output_dir, "config_tree.log"), "w") as file:
- rich.print(tree, file=file)
-
-
-@rank_zero_only
-def enforce_tags(cfg: DictConfig, save_to_file: bool = False) -> None:
- """Prompts user to input tags from command line if no tags are provided in config.
-
- :param cfg: A DictConfig composed by Hydra.
- :param save_to_file: Whether to export tags to the hydra output folder. Default is ``False``.
- """
- if not cfg.get("tags"):
- if "id" in HydraConfig().cfg.hydra.job:
- raise ValueError("Specify tags before launching a multirun!")
-
- log.warning("No tags provided in config. Prompting user to input tags...")
- tags = Prompt.ask("Enter a list of comma separated tags", default="dev")
- tags = [t.strip() for t in tags.split(",") if t != ""]
-
- with open_dict(cfg):
- cfg.tags = tags
-
- log.info(f"Tags: {cfg.tags}")
-
- if save_to_file:
- with open(Path(cfg.paths.output_dir, "tags.log"), "w") as file:
- rich.print(cfg.tags, file=file)
+from pathlib import Path
+from typing import Sequence
+
+import rich
+import rich.syntax
+import rich.tree
+from hydra.core.hydra_config import HydraConfig
+from lightning_utilities.core.rank_zero import rank_zero_only
+from omegaconf import DictConfig, OmegaConf, open_dict
+from rich.prompt import Prompt
+
+from src.utils import pylogger
+
+log = pylogger.RankedLogger(__name__, rank_zero_only=True)
+
+
+@rank_zero_only
+def print_config_tree(
+ cfg: DictConfig,
+ print_order: Sequence[str] = (
+ "data",
+ "model",
+ "callbacks",
+ "logger",
+ "trainer",
+ "paths",
+ "extras",
+ ),
+ resolve: bool = False,
+ save_to_file: bool = False,
+) -> None:
+ """Prints the contents of a DictConfig as a tree structure using the Rich library.
+
+ :param cfg: A DictConfig composed by Hydra.
+ :param print_order: Determines in what order config components are printed. Default is ``("data", "model",
+ "callbacks", "logger", "trainer", "paths", "extras")``.
+ :param resolve: Whether to resolve reference fields of DictConfig. Default is ``False``.
+ :param save_to_file: Whether to export config to the hydra output folder. Default is ``False``.
+ """
+ style = "dim"
+ tree = rich.tree.Tree("CONFIG", style=style, guide_style=style)
+
+ queue = []
+
+ # add fields from `print_order` to queue
+ for field in print_order:
+ queue.append(field) if field in cfg else log.warning(
+ f"Field '{field}' not found in config. Skipping '{field}' config printing..."
+ )
+
+ # add all the other fields to queue (not specified in `print_order`)
+ for field in cfg:
+ if field not in queue:
+ queue.append(field)
+
+ # generate config tree from queue
+ for field in queue:
+ branch = tree.add(field, style=style, guide_style=style)
+
+ config_group = cfg[field]
+ if isinstance(config_group, DictConfig):
+ branch_content = OmegaConf.to_yaml(config_group, resolve=resolve)
+ else:
+ branch_content = str(config_group)
+
+ branch.add(rich.syntax.Syntax(branch_content, "yaml"))
+
+ # print config tree
+ rich.print(tree)
+
+ # save config tree to file
+ if save_to_file:
+ with open(Path(cfg.paths.output_dir, "config_tree.log"), "w") as file:
+ rich.print(tree, file=file)
+
+
+@rank_zero_only
+def enforce_tags(cfg: DictConfig, save_to_file: bool = False) -> None:
+ """Prompts user to input tags from command line if no tags are provided in config.
+
+ :param cfg: A DictConfig composed by Hydra.
+ :param save_to_file: Whether to export tags to the hydra output folder. Default is ``False``.
+ """
+ if not cfg.get("tags"):
+ if "id" in HydraConfig().cfg.hydra.job:
+ raise ValueError("Specify tags before launching a multirun!")
+
+ log.warning("No tags provided in config. Prompting user to input tags...")
+ tags = Prompt.ask("Enter a list of comma separated tags", default="dev")
+ tags = [t.strip() for t in tags.split(",") if t != ""]
+
+ with open_dict(cfg):
+ cfg.tags = tags
+
+ log.info(f"Tags: {cfg.tags}")
+
+ if save_to_file:
+ with open(Path(cfg.paths.output_dir, "tags.log"), "w") as file:
+ rich.print(cfg.tags, file=file)
diff --git a/src/utils/utils.py b/src/utils/utils.py
index 02b55765ad3de9441bed931a577ebbb3b669fda4..a300cbd5906d4214863b3303abe0e4d3f2856e51 100644
--- a/src/utils/utils.py
+++ b/src/utils/utils.py
@@ -1,119 +1,119 @@
-import warnings
-from importlib.util import find_spec
-from typing import Any, Callable, Dict, Optional, Tuple
-
-from omegaconf import DictConfig
-
-from src.utils import pylogger, rich_utils
-
-log = pylogger.RankedLogger(__name__, rank_zero_only=True)
-
-
-def extras(cfg: DictConfig) -> None:
- """Applies optional utilities before the task is started.
-
- Utilities:
- - Ignoring python warnings
- - Setting tags from command line
- - Rich config printing
-
- :param cfg: A DictConfig object containing the config tree.
- """
- # return if no `extras` config
- if not cfg.get("extras"):
- log.warning("Extras config not found! ")
- return
-
- # disable python warnings
- if cfg.extras.get("ignore_warnings"):
- log.info("Disabling python warnings! ")
- warnings.filterwarnings("ignore")
-
- # prompt user to input tags from command line if none are provided in the config
- if cfg.extras.get("enforce_tags"):
- log.info("Enforcing tags! ")
- rich_utils.enforce_tags(cfg, save_to_file=True)
-
- # pretty print config tree using Rich library
- if cfg.extras.get("print_config"):
- log.info("Printing config tree with Rich! ")
- rich_utils.print_config_tree(cfg, resolve=True, save_to_file=True)
-
-
-def task_wrapper(task_func: Callable) -> Callable:
- """Optional decorator that controls the failure behavior when executing the task function.
-
- This wrapper can be used to:
- - make sure loggers are closed even if the task function raises an exception (prevents multirun failure)
- - save the exception to a `.log` file
- - mark the run as failed with a dedicated file in the `logs/` folder (so we can find and rerun it later)
- - etc. (adjust depending on your needs)
-
- Example:
- ```
- @utils.task_wrapper
- def train(cfg: DictConfig) -> Tuple[Dict[str, Any], Dict[str, Any]]:
- ...
- return metric_dict, object_dict
- ```
-
- :param task_func: The task function to be wrapped.
-
- :return: The wrapped task function.
- """
-
- def wrap(cfg: DictConfig) -> Tuple[Dict[str, Any], Dict[str, Any]]:
- # execute the task
- try:
- metric_dict, object_dict = task_func(cfg=cfg)
-
- # things to do if exception occurs
- except Exception as ex:
- # save exception to `.log` file
- log.exception("")
-
- # some hyperparameter combinations might be invalid or cause out-of-memory errors
- # so when using hparam search plugins like Optuna, you might want to disable
- # raising the below exception to avoid multirun failure
- raise ex
-
- # things to always do after either success or exception
- finally:
- # display output dir path in terminal
- log.info(f"Output dir: {cfg.paths.output_dir}")
-
- # always close wandb run (even if exception occurs so multirun won't fail)
- if find_spec("wandb"): # check if wandb is installed
- import wandb
-
- if wandb.run:
- log.info("Closing wandb!")
- wandb.finish()
-
- return metric_dict, object_dict
-
- return wrap
-
-
-def get_metric_value(metric_dict: Dict[str, Any], metric_name: Optional[str]) -> Optional[float]:
- """Safely retrieves value of the metric logged in LightningModule.
-
- :param metric_dict: A dict containing metric values.
- :param metric_name: If provided, the name of the metric to retrieve.
- :return: If a metric name was provided, the value of the metric.
- """
- if not metric_name:
- log.info("Metric name is None! Skipping metric value retrieval...")
- return None
-
- if metric_name not in metric_dict:
- raise Exception(
- f"Metric value not found! \n"
- "Make sure metric name logged in LightningModule is correct!\n"
- "Make sure `optimized_metric` name in `hparams_search` config is correct!"
- )
-
- metric_value = metric_dict[metric_name].item()
- log.info(f"Retrieved metric value! <{metric_name}={metric_value}>")
-
- return metric_value
+import warnings
+from importlib.util import find_spec
+from typing import Any, Callable, Dict, Optional, Tuple
+
+from omegaconf import DictConfig
+
+from src.utils import pylogger, rich_utils
+
+log = pylogger.RankedLogger(__name__, rank_zero_only=True)
+
+
+def extras(cfg: DictConfig) -> None:
+ """Applies optional utilities before the task is started.
+
+ Utilities:
+ - Ignoring python warnings
+ - Setting tags from command line
+ - Rich config printing
+
+ :param cfg: A DictConfig object containing the config tree.
+ """
+ # return if no `extras` config
+ if not cfg.get("extras"):
+ log.warning("Extras config not found! ")
+ return
+
+ # disable python warnings
+ if cfg.extras.get("ignore_warnings"):
+ log.info("Disabling python warnings! ")
+ warnings.filterwarnings("ignore")
+
+ # prompt user to input tags from command line if none are provided in the config
+ if cfg.extras.get("enforce_tags"):
+ log.info("Enforcing tags! ")
+ rich_utils.enforce_tags(cfg, save_to_file=True)
+
+ # pretty print config tree using Rich library
+ if cfg.extras.get("print_config"):
+ log.info("Printing config tree with Rich! ")
+ rich_utils.print_config_tree(cfg, resolve=True, save_to_file=True)
+
+
+def task_wrapper(task_func: Callable) -> Callable:
+ """Optional decorator that controls the failure behavior when executing the task function.
+
+ This wrapper can be used to:
+ - make sure loggers are closed even if the task function raises an exception (prevents multirun failure)
+ - save the exception to a `.log` file
+ - mark the run as failed with a dedicated file in the `logs/` folder (so we can find and rerun it later)
+ - etc. (adjust depending on your needs)
+
+ Example:
+ ```
+ @utils.task_wrapper
+ def train(cfg: DictConfig) -> Tuple[Dict[str, Any], Dict[str, Any]]:
+ ...
+ return metric_dict, object_dict
+ ```
+
+ :param task_func: The task function to be wrapped.
+
+ :return: The wrapped task function.
+ """
+
+ def wrap(cfg: DictConfig) -> Tuple[Dict[str, Any], Dict[str, Any]]:
+ # execute the task
+ try:
+ metric_dict, object_dict = task_func(cfg=cfg)
+
+ # things to do if exception occurs
+ except Exception as ex:
+ # save exception to `.log` file
+ log.exception("")
+
+ # some hyperparameter combinations might be invalid or cause out-of-memory errors
+ # so when using hparam search plugins like Optuna, you might want to disable
+ # raising the below exception to avoid multirun failure
+ raise ex
+
+ # things to always do after either success or exception
+ finally:
+ # display output dir path in terminal
+ log.info(f"Output dir: {cfg.paths.output_dir}")
+
+ # always close wandb run (even if exception occurs so multirun won't fail)
+ if find_spec("wandb"): # check if wandb is installed
+ import wandb
+
+ if wandb.run:
+ log.info("Closing wandb!")
+ wandb.finish()
+
+ return metric_dict, object_dict
+
+ return wrap
+
+
+def get_metric_value(metric_dict: Dict[str, Any], metric_name: Optional[str]) -> Optional[float]:
+ """Safely retrieves value of the metric logged in LightningModule.
+
+ :param metric_dict: A dict containing metric values.
+ :param metric_name: If provided, the name of the metric to retrieve.
+ :return: If a metric name was provided, the value of the metric.
+ """
+ if not metric_name:
+ log.info("Metric name is None! Skipping metric value retrieval...")
+ return None
+
+ if metric_name not in metric_dict:
+ raise Exception(
+ f"Metric value not found! \n"
+ "Make sure metric name logged in LightningModule is correct!\n"
+ "Make sure `optimized_metric` name in `hparams_search` config is correct!"
+ )
+
+ metric_value = metric_dict[metric_name].item()
+ log.info(f"Retrieved metric value! <{metric_name}={metric_value}>")
+
+ return metric_value
diff --git a/tests/conftest.py b/tests/conftest.py
index b5dea333ca4818bbb1a4fdd5e6e9a70a7ebad1b4..060be573d694e638da34c3e92d84f9f5e6f55b1e 100644
--- a/tests/conftest.py
+++ b/tests/conftest.py
@@ -1,107 +1,107 @@
-"""This file prepares config fixtures for other tests."""
-
-from pathlib import Path
-
-import pytest
-import rootutils
-from hydra import compose, initialize
-from hydra.core.global_hydra import GlobalHydra
-from omegaconf import DictConfig, open_dict
-
-
-@pytest.fixture(scope="package")
-def cfg_train_global() -> DictConfig:
- """A pytest fixture for setting up a default Hydra DictConfig for training.
-
- :return: A DictConfig object containing a default Hydra configuration for training.
- """
- with initialize(version_base="1.3", config_path="../configs"):
- cfg = compose(config_name="train.yaml", return_hydra_config=True, overrides=[])
-
- # set defaults for all tests
- with open_dict(cfg):
- cfg.paths.root_dir = str(rootutils.find_root(indicator=".project-root"))
- cfg.trainer.max_epochs = 1
- cfg.trainer.limit_train_batches = 0.01
- cfg.trainer.limit_val_batches = 0.1
- cfg.trainer.limit_test_batches = 0.1
- cfg.trainer.accelerator = "cpu"
- cfg.trainer.devices = 1
- cfg.data.num_workers = 0
- cfg.data.pin_memory = False
- cfg.extras.print_config = False
- cfg.extras.enforce_tags = False
- cfg.logger = None
-
- return cfg
-
-
-@pytest.fixture(scope="package")
-def cfg_eval_global() -> DictConfig:
- """A pytest fixture for setting up a default Hydra DictConfig for evaluation.
-
- :return: A DictConfig containing a default Hydra configuration for evaluation.
- """
- with initialize(version_base="1.3", config_path="../configs"):
- cfg = compose(config_name="eval.yaml", return_hydra_config=True, overrides=["ckpt_path=."])
-
- # set defaults for all tests
- with open_dict(cfg):
- cfg.paths.root_dir = str(rootutils.find_root(indicator=".project-root"))
- cfg.trainer.max_epochs = 1
- cfg.trainer.limit_test_batches = 0.1
- cfg.trainer.accelerator = "cpu"
- cfg.trainer.devices = 1
- cfg.data.num_workers = 0
- cfg.data.pin_memory = False
- cfg.extras.print_config = False
- cfg.extras.enforce_tags = False
- cfg.logger = None
-
- return cfg
-
-
-@pytest.fixture(scope="function")
-def cfg_train(cfg_train_global: DictConfig, tmp_path: Path) -> DictConfig:
- """A pytest fixture built on top of the `cfg_train_global()` fixture, which accepts a temporary
- logging path `tmp_path` for generating a temporary logging path.
-
- This is called by each test which uses the `cfg_train` arg. Each test generates its own temporary logging path.
-
- :param cfg_train_global: The input DictConfig object to be modified.
- :param tmp_path: The temporary logging path.
-
- :return: A DictConfig with updated output and log directories corresponding to `tmp_path`.
- """
- cfg = cfg_train_global.copy()
-
- with open_dict(cfg):
- cfg.paths.output_dir = str(tmp_path)
- cfg.paths.log_dir = str(tmp_path)
-
- yield cfg
-
- GlobalHydra.instance().clear()
-
-
-@pytest.fixture(scope="function")
-def cfg_eval(cfg_eval_global: DictConfig, tmp_path: Path) -> DictConfig:
- """A pytest fixture built on top of the `cfg_eval_global()` fixture, which accepts a temporary
- logging path `tmp_path` for generating a temporary logging path.
-
- This is called by each test which uses the `cfg_eval` arg. Each test generates its own temporary logging path.
-
- :param cfg_train_global: The input DictConfig object to be modified.
- :param tmp_path: The temporary logging path.
-
- :return: A DictConfig with updated output and log directories corresponding to `tmp_path`.
- """
- cfg = cfg_eval_global.copy()
-
- with open_dict(cfg):
- cfg.paths.output_dir = str(tmp_path)
- cfg.paths.log_dir = str(tmp_path)
-
- yield cfg
-
- GlobalHydra.instance().clear()
+"""This file prepares config fixtures for other tests."""
+
+from pathlib import Path
+
+import pytest
+import rootutils
+from hydra import compose, initialize
+from hydra.core.global_hydra import GlobalHydra
+from omegaconf import DictConfig, open_dict
+
+
+@pytest.fixture(scope="package")
+def cfg_train_global() -> DictConfig:
+ """A pytest fixture for setting up a default Hydra DictConfig for training.
+
+ :return: A DictConfig object containing a default Hydra configuration for training.
+ """
+ with initialize(version_base="1.3", config_path="../configs"):
+ cfg = compose(config_name="train.yaml", return_hydra_config=True, overrides=[])
+
+ # set defaults for all tests
+ with open_dict(cfg):
+ cfg.paths.root_dir = str(rootutils.find_root(indicator=".project-root"))
+ cfg.trainer.max_epochs = 1
+ cfg.trainer.limit_train_batches = 0.01
+ cfg.trainer.limit_val_batches = 0.1
+ cfg.trainer.limit_test_batches = 0.1
+ cfg.trainer.accelerator = "cpu"
+ cfg.trainer.devices = 1
+ cfg.data.num_workers = 0
+ cfg.data.pin_memory = False
+ cfg.extras.print_config = False
+ cfg.extras.enforce_tags = False
+ cfg.logger = None
+
+ return cfg
+
+
+@pytest.fixture(scope="package")
+def cfg_eval_global() -> DictConfig:
+ """A pytest fixture for setting up a default Hydra DictConfig for evaluation.
+
+ :return: A DictConfig containing a default Hydra configuration for evaluation.
+ """
+ with initialize(version_base="1.3", config_path="../configs"):
+ cfg = compose(config_name="eval.yaml", return_hydra_config=True, overrides=["ckpt_path=."])
+
+ # set defaults for all tests
+ with open_dict(cfg):
+ cfg.paths.root_dir = str(rootutils.find_root(indicator=".project-root"))
+ cfg.trainer.max_epochs = 1
+ cfg.trainer.limit_test_batches = 0.1
+ cfg.trainer.accelerator = "cpu"
+ cfg.trainer.devices = 1
+ cfg.data.num_workers = 0
+ cfg.data.pin_memory = False
+ cfg.extras.print_config = False
+ cfg.extras.enforce_tags = False
+ cfg.logger = None
+
+ return cfg
+
+
+@pytest.fixture(scope="function")
+def cfg_train(cfg_train_global: DictConfig, tmp_path: Path) -> DictConfig:
+ """A pytest fixture built on top of the `cfg_train_global()` fixture, which accepts a temporary
+ logging path `tmp_path` for generating a temporary logging path.
+
+ This is called by each test which uses the `cfg_train` arg. Each test generates its own temporary logging path.
+
+ :param cfg_train_global: The input DictConfig object to be modified.
+ :param tmp_path: The temporary logging path.
+
+ :return: A DictConfig with updated output and log directories corresponding to `tmp_path`.
+ """
+ cfg = cfg_train_global.copy()
+
+ with open_dict(cfg):
+ cfg.paths.output_dir = str(tmp_path)
+ cfg.paths.log_dir = str(tmp_path)
+
+ yield cfg
+
+ GlobalHydra.instance().clear()
+
+
+@pytest.fixture(scope="function")
+def cfg_eval(cfg_eval_global: DictConfig, tmp_path: Path) -> DictConfig:
+ """A pytest fixture built on top of the `cfg_eval_global()` fixture, which accepts a temporary
+ logging path `tmp_path` for generating a temporary logging path.
+
+ This is called by each test which uses the `cfg_eval` arg. Each test generates its own temporary logging path.
+
+ :param cfg_train_global: The input DictConfig object to be modified.
+ :param tmp_path: The temporary logging path.
+
+ :return: A DictConfig with updated output and log directories corresponding to `tmp_path`.
+ """
+ cfg = cfg_eval_global.copy()
+
+ with open_dict(cfg):
+ cfg.paths.output_dir = str(tmp_path)
+ cfg.paths.log_dir = str(tmp_path)
+
+ yield cfg
+
+ GlobalHydra.instance().clear()
diff --git a/tests/helpers/package_available.py b/tests/helpers/package_available.py
index 0afdba8dc1efd49f9d8c1a47ede62b7e206b99f3..57470436e40b9615e552abbac7f8e77dd7990603 100644
--- a/tests/helpers/package_available.py
+++ b/tests/helpers/package_available.py
@@ -1,32 +1,32 @@
-import platform
-
-import pkg_resources
-from lightning.fabric.accelerators import TPUAccelerator
-
-
-def _package_available(package_name: str) -> bool:
- """Check if a package is available in your environment.
-
- :param package_name: The name of the package to be checked.
-
- :return: `True` if the package is available. `False` otherwise.
- """
- try:
- return pkg_resources.require(package_name) is not None
- except pkg_resources.DistributionNotFound:
- return False
-
-
-_TPU_AVAILABLE = TPUAccelerator.is_available()
-
-_IS_WINDOWS = platform.system() == "Windows"
-
-_SH_AVAILABLE = not _IS_WINDOWS and _package_available("sh")
-
-_DEEPSPEED_AVAILABLE = not _IS_WINDOWS and _package_available("deepspeed")
-_FAIRSCALE_AVAILABLE = not _IS_WINDOWS and _package_available("fairscale")
-
-_WANDB_AVAILABLE = _package_available("wandb")
-_NEPTUNE_AVAILABLE = _package_available("neptune")
-_COMET_AVAILABLE = _package_available("comet_ml")
-_MLFLOW_AVAILABLE = _package_available("mlflow")
+import platform
+
+import pkg_resources
+from lightning.fabric.accelerators import TPUAccelerator
+
+
+def _package_available(package_name: str) -> bool:
+ """Check if a package is available in your environment.
+
+ :param package_name: The name of the package to be checked.
+
+ :return: `True` if the package is available. `False` otherwise.
+ """
+ try:
+ return pkg_resources.require(package_name) is not None
+ except pkg_resources.DistributionNotFound:
+ return False
+
+
+_TPU_AVAILABLE = TPUAccelerator.is_available()
+
+_IS_WINDOWS = platform.system() == "Windows"
+
+_SH_AVAILABLE = not _IS_WINDOWS and _package_available("sh")
+
+_DEEPSPEED_AVAILABLE = not _IS_WINDOWS and _package_available("deepspeed")
+_FAIRSCALE_AVAILABLE = not _IS_WINDOWS and _package_available("fairscale")
+
+_WANDB_AVAILABLE = _package_available("wandb")
+_NEPTUNE_AVAILABLE = _package_available("neptune")
+_COMET_AVAILABLE = _package_available("comet_ml")
+_MLFLOW_AVAILABLE = _package_available("mlflow")
diff --git a/tests/helpers/run_if.py b/tests/helpers/run_if.py
index 9703af425129d0225d0aeed20dedc3ed35bc7548..b3f1c673d9962110d58bba5b7b168a91e185a710 100644
--- a/tests/helpers/run_if.py
+++ b/tests/helpers/run_if.py
@@ -1,142 +1,142 @@
-"""Adapted from:
-
-https://github.com/PyTorchLightning/pytorch-lightning/blob/master/tests/helpers/runif.py
-"""
-
-import sys
-from typing import Any, Dict, Optional
-
-import pytest
-import torch
-from packaging.version import Version
-from pkg_resources import get_distribution
-from pytest import MarkDecorator
-
-from tests.helpers.package_available import (
- _COMET_AVAILABLE,
- _DEEPSPEED_AVAILABLE,
- _FAIRSCALE_AVAILABLE,
- _IS_WINDOWS,
- _MLFLOW_AVAILABLE,
- _NEPTUNE_AVAILABLE,
- _SH_AVAILABLE,
- _TPU_AVAILABLE,
- _WANDB_AVAILABLE,
-)
-
-
-class RunIf:
- """RunIf wrapper for conditional skipping of tests.
-
- Fully compatible with `@pytest.mark`.
-
- Example:
-
- ```python
- @RunIf(min_torch="1.8")
- @pytest.mark.parametrize("arg1", [1.0, 2.0])
- def test_wrapper(arg1):
- assert arg1 > 0
- ```
- """
-
- def __new__(
- cls,
- min_gpus: int = 0,
- min_torch: Optional[str] = None,
- max_torch: Optional[str] = None,
- min_python: Optional[str] = None,
- skip_windows: bool = False,
- sh: bool = False,
- tpu: bool = False,
- fairscale: bool = False,
- deepspeed: bool = False,
- wandb: bool = False,
- neptune: bool = False,
- comet: bool = False,
- mlflow: bool = False,
- **kwargs: Dict[Any, Any],
- ) -> MarkDecorator:
- """Creates a new `@RunIf` `MarkDecorator` decorator.
-
- :param min_gpus: Min number of GPUs required to run test.
- :param min_torch: Minimum pytorch version to run test.
- :param max_torch: Maximum pytorch version to run test.
- :param min_python: Minimum python version required to run test.
- :param skip_windows: Skip test for Windows platform.
- :param tpu: If TPU is available.
- :param sh: If `sh` module is required to run the test.
- :param fairscale: If `fairscale` module is required to run the test.
- :param deepspeed: If `deepspeed` module is required to run the test.
- :param wandb: If `wandb` module is required to run the test.
- :param neptune: If `neptune` module is required to run the test.
- :param comet: If `comet` module is required to run the test.
- :param mlflow: If `mlflow` module is required to run the test.
- :param kwargs: Native `pytest.mark.skipif` keyword arguments.
- """
- conditions = []
- reasons = []
-
- if min_gpus:
- conditions.append(torch.cuda.device_count() < min_gpus)
- reasons.append(f"GPUs>={min_gpus}")
-
- if min_torch:
- torch_version = get_distribution("torch").version
- conditions.append(Version(torch_version) < Version(min_torch))
- reasons.append(f"torch>={min_torch}")
-
- if max_torch:
- torch_version = get_distribution("torch").version
- conditions.append(Version(torch_version) >= Version(max_torch))
- reasons.append(f"torch<{max_torch}")
-
- if min_python:
- py_version = (
- f"{sys.version_info.major}.{sys.version_info.minor}.{sys.version_info.micro}"
- )
- conditions.append(Version(py_version) < Version(min_python))
- reasons.append(f"python>={min_python}")
-
- if skip_windows:
- conditions.append(_IS_WINDOWS)
- reasons.append("does not run on Windows")
-
- if tpu:
- conditions.append(not _TPU_AVAILABLE)
- reasons.append("TPU")
-
- if sh:
- conditions.append(not _SH_AVAILABLE)
- reasons.append("sh")
-
- if fairscale:
- conditions.append(not _FAIRSCALE_AVAILABLE)
- reasons.append("fairscale")
-
- if deepspeed:
- conditions.append(not _DEEPSPEED_AVAILABLE)
- reasons.append("deepspeed")
-
- if wandb:
- conditions.append(not _WANDB_AVAILABLE)
- reasons.append("wandb")
-
- if neptune:
- conditions.append(not _NEPTUNE_AVAILABLE)
- reasons.append("neptune")
-
- if comet:
- conditions.append(not _COMET_AVAILABLE)
- reasons.append("comet")
-
- if mlflow:
- conditions.append(not _MLFLOW_AVAILABLE)
- reasons.append("mlflow")
-
- reasons = [rs for cond, rs in zip(conditions, reasons) if cond]
- return pytest.mark.skipif(
- condition=any(conditions),
- reason=f"Requires: [{' + '.join(reasons)}]",
- **kwargs,
- )
+"""Adapted from:
+
+https://github.com/PyTorchLightning/pytorch-lightning/blob/master/tests/helpers/runif.py
+"""
+
+import sys
+from typing import Any, Dict, Optional
+
+import pytest
+import torch
+from packaging.version import Version
+from pkg_resources import get_distribution
+from pytest import MarkDecorator
+
+from tests.helpers.package_available import (
+ _COMET_AVAILABLE,
+ _DEEPSPEED_AVAILABLE,
+ _FAIRSCALE_AVAILABLE,
+ _IS_WINDOWS,
+ _MLFLOW_AVAILABLE,
+ _NEPTUNE_AVAILABLE,
+ _SH_AVAILABLE,
+ _TPU_AVAILABLE,
+ _WANDB_AVAILABLE,
+)
+
+
+class RunIf:
+ """RunIf wrapper for conditional skipping of tests.
+
+ Fully compatible with `@pytest.mark`.
+
+ Example:
+
+ ```python
+ @RunIf(min_torch="1.8")
+ @pytest.mark.parametrize("arg1", [1.0, 2.0])
+ def test_wrapper(arg1):
+ assert arg1 > 0
+ ```
+ """
+
+ def __new__(
+ cls,
+ min_gpus: int = 0,
+ min_torch: Optional[str] = None,
+ max_torch: Optional[str] = None,
+ min_python: Optional[str] = None,
+ skip_windows: bool = False,
+ sh: bool = False,
+ tpu: bool = False,
+ fairscale: bool = False,
+ deepspeed: bool = False,
+ wandb: bool = False,
+ neptune: bool = False,
+ comet: bool = False,
+ mlflow: bool = False,
+ **kwargs: Dict[Any, Any],
+ ) -> MarkDecorator:
+ """Creates a new `@RunIf` `MarkDecorator` decorator.
+
+ :param min_gpus: Min number of GPUs required to run test.
+ :param min_torch: Minimum pytorch version to run test.
+ :param max_torch: Maximum pytorch version to run test.
+ :param min_python: Minimum python version required to run test.
+ :param skip_windows: Skip test for Windows platform.
+ :param tpu: If TPU is available.
+ :param sh: If `sh` module is required to run the test.
+ :param fairscale: If `fairscale` module is required to run the test.
+ :param deepspeed: If `deepspeed` module is required to run the test.
+ :param wandb: If `wandb` module is required to run the test.
+ :param neptune: If `neptune` module is required to run the test.
+ :param comet: If `comet` module is required to run the test.
+ :param mlflow: If `mlflow` module is required to run the test.
+ :param kwargs: Native `pytest.mark.skipif` keyword arguments.
+ """
+ conditions = []
+ reasons = []
+
+ if min_gpus:
+ conditions.append(torch.cuda.device_count() < min_gpus)
+ reasons.append(f"GPUs>={min_gpus}")
+
+ if min_torch:
+ torch_version = get_distribution("torch").version
+ conditions.append(Version(torch_version) < Version(min_torch))
+ reasons.append(f"torch>={min_torch}")
+
+ if max_torch:
+ torch_version = get_distribution("torch").version
+ conditions.append(Version(torch_version) >= Version(max_torch))
+ reasons.append(f"torch<{max_torch}")
+
+ if min_python:
+ py_version = (
+ f"{sys.version_info.major}.{sys.version_info.minor}.{sys.version_info.micro}"
+ )
+ conditions.append(Version(py_version) < Version(min_python))
+ reasons.append(f"python>={min_python}")
+
+ if skip_windows:
+ conditions.append(_IS_WINDOWS)
+ reasons.append("does not run on Windows")
+
+ if tpu:
+ conditions.append(not _TPU_AVAILABLE)
+ reasons.append("TPU")
+
+ if sh:
+ conditions.append(not _SH_AVAILABLE)
+ reasons.append("sh")
+
+ if fairscale:
+ conditions.append(not _FAIRSCALE_AVAILABLE)
+ reasons.append("fairscale")
+
+ if deepspeed:
+ conditions.append(not _DEEPSPEED_AVAILABLE)
+ reasons.append("deepspeed")
+
+ if wandb:
+ conditions.append(not _WANDB_AVAILABLE)
+ reasons.append("wandb")
+
+ if neptune:
+ conditions.append(not _NEPTUNE_AVAILABLE)
+ reasons.append("neptune")
+
+ if comet:
+ conditions.append(not _COMET_AVAILABLE)
+ reasons.append("comet")
+
+ if mlflow:
+ conditions.append(not _MLFLOW_AVAILABLE)
+ reasons.append("mlflow")
+
+ reasons = [rs for cond, rs in zip(conditions, reasons) if cond]
+ return pytest.mark.skipif(
+ condition=any(conditions),
+ reason=f"Requires: [{' + '.join(reasons)}]",
+ **kwargs,
+ )
diff --git a/tests/helpers/run_sh_command.py b/tests/helpers/run_sh_command.py
index fdd2ed633f1185dd7936924616be6a6359a7bca7..a23bada8f5140c64e75bf9676969fa0d11bd5c70 100644
--- a/tests/helpers/run_sh_command.py
+++ b/tests/helpers/run_sh_command.py
@@ -1,22 +1,22 @@
-from typing import List
-
-import pytest
-
-from tests.helpers.package_available import _SH_AVAILABLE
-
-if _SH_AVAILABLE:
- import sh
-
-
-def run_sh_command(command: List[str]) -> None:
- """Default method for executing shell commands with `pytest` and `sh` package.
-
- :param command: A list of shell commands as strings.
- """
- msg = None
- try:
- sh.python(command)
- except sh.ErrorReturnCode as e:
- msg = e.stderr.decode()
- if msg:
- pytest.fail(msg=msg)
+from typing import List
+
+import pytest
+
+from tests.helpers.package_available import _SH_AVAILABLE
+
+if _SH_AVAILABLE:
+ import sh
+
+
+def run_sh_command(command: List[str]) -> None:
+ """Default method for executing shell commands with `pytest` and `sh` package.
+
+ :param command: A list of shell commands as strings.
+ """
+ msg = None
+ try:
+ sh.python(command)
+ except sh.ErrorReturnCode as e:
+ msg = e.stderr.decode()
+ if msg:
+ pytest.fail(msg=msg)
diff --git a/tests/test_configs.py b/tests/test_configs.py
index d7041dc78cc207489255d8618c4a2e75ba74464d..681330734437625d8be75685a16c5069bfa96610 100644
--- a/tests/test_configs.py
+++ b/tests/test_configs.py
@@ -1,37 +1,37 @@
-import hydra
-from hydra.core.hydra_config import HydraConfig
-from omegaconf import DictConfig
-
-
-def test_train_config(cfg_train: DictConfig) -> None:
- """Tests the training configuration provided by the `cfg_train` pytest fixture.
-
- :param cfg_train: A DictConfig containing a valid training configuration.
- """
- assert cfg_train
- assert cfg_train.data
- assert cfg_train.model
- assert cfg_train.trainer
-
- HydraConfig().set_config(cfg_train)
-
- hydra.utils.instantiate(cfg_train.data)
- hydra.utils.instantiate(cfg_train.model)
- hydra.utils.instantiate(cfg_train.trainer)
-
-
-def test_eval_config(cfg_eval: DictConfig) -> None:
- """Tests the evaluation configuration provided by the `cfg_eval` pytest fixture.
-
- :param cfg_train: A DictConfig containing a valid evaluation configuration.
- """
- assert cfg_eval
- assert cfg_eval.data
- assert cfg_eval.model
- assert cfg_eval.trainer
-
- HydraConfig().set_config(cfg_eval)
-
- hydra.utils.instantiate(cfg_eval.data)
- hydra.utils.instantiate(cfg_eval.model)
- hydra.utils.instantiate(cfg_eval.trainer)
+import hydra
+from hydra.core.hydra_config import HydraConfig
+from omegaconf import DictConfig
+
+
+def test_train_config(cfg_train: DictConfig) -> None:
+ """Tests the training configuration provided by the `cfg_train` pytest fixture.
+
+ :param cfg_train: A DictConfig containing a valid training configuration.
+ """
+ assert cfg_train
+ assert cfg_train.data
+ assert cfg_train.model
+ assert cfg_train.trainer
+
+ HydraConfig().set_config(cfg_train)
+
+ hydra.utils.instantiate(cfg_train.data)
+ hydra.utils.instantiate(cfg_train.model)
+ hydra.utils.instantiate(cfg_train.trainer)
+
+
+def test_eval_config(cfg_eval: DictConfig) -> None:
+ """Tests the evaluation configuration provided by the `cfg_eval` pytest fixture.
+
+ :param cfg_train: A DictConfig containing a valid evaluation configuration.
+ """
+ assert cfg_eval
+ assert cfg_eval.data
+ assert cfg_eval.model
+ assert cfg_eval.trainer
+
+ HydraConfig().set_config(cfg_eval)
+
+ hydra.utils.instantiate(cfg_eval.data)
+ hydra.utils.instantiate(cfg_eval.model)
+ hydra.utils.instantiate(cfg_eval.trainer)
diff --git a/tests/test_datamodules.py b/tests/test_datamodules.py
index bf909434415d50c5d181bf8cdc3261b024f52860..f173c7cc0a968273803adab4f63ad299553c0eb4 100644
--- a/tests/test_datamodules.py
+++ b/tests/test_datamodules.py
@@ -1,38 +1,38 @@
-from pathlib import Path
-
-import pytest
-import torch
-
-from src.data.celeba_datamodule import MNISTDataModule
-
-
-@pytest.mark.parametrize("batch_size", [32, 128])
-def test_mnist_datamodule(batch_size: int) -> None:
- """Tests `MNISTDataModule` to verify that it can be downloaded correctly, that the necessary
- attributes were created (e.g., the dataloader objects), and that dtypes and batch sizes
- correctly match.
-
- :param batch_size: Batch size of the data to be loaded by the dataloader.
- """
- data_dir = "data/"
-
- dm = MNISTDataModule(data_dir=data_dir, batch_size=batch_size)
- dm.prepare_data()
-
- assert not dm.data_train and not dm.data_val and not dm.data_test
- assert Path(data_dir, "MNIST").exists()
- assert Path(data_dir, "MNIST", "raw").exists()
-
- dm.setup()
- assert dm.data_train and dm.data_val and dm.data_test
- assert dm.train_dataloader() and dm.val_dataloader() and dm.test_dataloader()
-
- num_datapoints = len(dm.data_train) + len(dm.data_val) + len(dm.data_test)
- assert num_datapoints == 70_000
-
- batch = next(iter(dm.train_dataloader()))
- x, y = batch
- assert len(x) == batch_size
- assert len(y) == batch_size
- assert x.dtype == torch.float32
- assert y.dtype == torch.int64
+from pathlib import Path
+
+import pytest
+import torch
+
+from src.data.celeba_datamodule import MNISTDataModule
+
+
+@pytest.mark.parametrize("batch_size", [32, 128])
+def test_mnist_datamodule(batch_size: int) -> None:
+ """Tests `MNISTDataModule` to verify that it can be downloaded correctly, that the necessary
+ attributes were created (e.g., the dataloader objects), and that dtypes and batch sizes
+ correctly match.
+
+ :param batch_size: Batch size of the data to be loaded by the dataloader.
+ """
+ data_dir = "data/"
+
+ dm = MNISTDataModule(data_dir=data_dir, batch_size=batch_size)
+ dm.prepare_data()
+
+ assert not dm.data_train and not dm.data_val and not dm.data_test
+ assert Path(data_dir, "MNIST").exists()
+ assert Path(data_dir, "MNIST", "raw").exists()
+
+ dm.setup()
+ assert dm.data_train and dm.data_val and dm.data_test
+ assert dm.train_dataloader() and dm.val_dataloader() and dm.test_dataloader()
+
+ num_datapoints = len(dm.data_train) + len(dm.data_val) + len(dm.data_test)
+ assert num_datapoints == 70_000
+
+ batch = next(iter(dm.train_dataloader()))
+ x, y = batch
+ assert len(x) == batch_size
+ assert len(y) == batch_size
+ assert x.dtype == torch.float32
+ assert y.dtype == torch.int64
diff --git a/tests/test_eval.py b/tests/test_eval.py
index 423c9d295047ba3c2a8e9306a1b975a09c34de09..cca2df5e99ed8863f29b2bf61db134953e3a1ad6 100644
--- a/tests/test_eval.py
+++ b/tests/test_eval.py
@@ -1,39 +1,39 @@
-import os
-from pathlib import Path
-
-import pytest
-from hydra.core.hydra_config import HydraConfig
-from omegaconf import DictConfig, open_dict
-
-from src.eval import evaluate
-from src.train import train
-
-
-@pytest.mark.slow
-def test_train_eval(tmp_path: Path, cfg_train: DictConfig, cfg_eval: DictConfig) -> None:
- """Tests training and evaluation by training for 1 epoch with `train.py` then evaluating with
- `eval.py`.
-
- :param tmp_path: The temporary logging path.
- :param cfg_train: A DictConfig containing a valid training configuration.
- :param cfg_eval: A DictConfig containing a valid evaluation configuration.
- """
- assert str(tmp_path) == cfg_train.paths.output_dir == cfg_eval.paths.output_dir
-
- with open_dict(cfg_train):
- cfg_train.trainer.max_epochs = 1
- cfg_train.test = True
-
- HydraConfig().set_config(cfg_train)
- train_metric_dict, _ = train(cfg_train)
-
- assert "last.ckpt" in os.listdir(tmp_path / "checkpoints")
-
- with open_dict(cfg_eval):
- cfg_eval.ckpt_path = str(tmp_path / "checkpoints" / "last.ckpt")
-
- HydraConfig().set_config(cfg_eval)
- test_metric_dict, _ = evaluate(cfg_eval)
-
- assert test_metric_dict["test/acc"] > 0.0
- assert abs(train_metric_dict["test/acc"].item() - test_metric_dict["test/acc"].item()) < 0.001
+import os
+from pathlib import Path
+
+import pytest
+from hydra.core.hydra_config import HydraConfig
+from omegaconf import DictConfig, open_dict
+
+from src.eval import evaluate
+from src.train import train
+
+
+@pytest.mark.slow
+def test_train_eval(tmp_path: Path, cfg_train: DictConfig, cfg_eval: DictConfig) -> None:
+ """Tests training and evaluation by training for 1 epoch with `train.py` then evaluating with
+ `eval.py`.
+
+ :param tmp_path: The temporary logging path.
+ :param cfg_train: A DictConfig containing a valid training configuration.
+ :param cfg_eval: A DictConfig containing a valid evaluation configuration.
+ """
+ assert str(tmp_path) == cfg_train.paths.output_dir == cfg_eval.paths.output_dir
+
+ with open_dict(cfg_train):
+ cfg_train.trainer.max_epochs = 1
+ cfg_train.test = True
+
+ HydraConfig().set_config(cfg_train)
+ train_metric_dict, _ = train(cfg_train)
+
+ assert "last.ckpt" in os.listdir(tmp_path / "checkpoints")
+
+ with open_dict(cfg_eval):
+ cfg_eval.ckpt_path = str(tmp_path / "checkpoints" / "last.ckpt")
+
+ HydraConfig().set_config(cfg_eval)
+ test_metric_dict, _ = evaluate(cfg_eval)
+
+ assert test_metric_dict["test/acc"] > 0.0
+ assert abs(train_metric_dict["test/acc"].item() - test_metric_dict["test/acc"].item()) < 0.001
diff --git a/tests/test_sweeps.py b/tests/test_sweeps.py
index 7856b1551df4e3d4979110ede30076e6a703976f..40f2a55fc3b8aff68dda45cbe086527df67fa3eb 100644
--- a/tests/test_sweeps.py
+++ b/tests/test_sweeps.py
@@ -1,107 +1,107 @@
-from pathlib import Path
-
-import pytest
-
-from tests.helpers.run_if import RunIf
-from tests.helpers.run_sh_command import run_sh_command
-
-startfile = "src/train.py"
-overrides = ["logger=[]"]
-
-
-@RunIf(sh=True)
-@pytest.mark.slow
-def test_experiments(tmp_path: Path) -> None:
- """Test running all available experiment configs with `fast_dev_run=True.`
-
- :param tmp_path: The temporary logging path.
- """
- command = [
- startfile,
- "-m",
- "experiment=glob(*)",
- "hydra.sweep.dir=" + str(tmp_path),
- "++trainer.fast_dev_run=true",
- ] + overrides
- run_sh_command(command)
-
-
-@RunIf(sh=True)
-@pytest.mark.slow
-def test_hydra_sweep(tmp_path: Path) -> None:
- """Test default hydra sweep.
-
- :param tmp_path: The temporary logging path.
- """
- command = [
- startfile,
- "-m",
- "hydra.sweep.dir=" + str(tmp_path),
- "model.optimizer.lr=0.005,0.01",
- "++trainer.fast_dev_run=true",
- ] + overrides
-
- run_sh_command(command)
-
-
-@RunIf(sh=True)
-@pytest.mark.slow
-def test_hydra_sweep_ddp_sim(tmp_path: Path) -> None:
- """Test default hydra sweep with ddp sim.
-
- :param tmp_path: The temporary logging path.
- """
- command = [
- startfile,
- "-m",
- "hydra.sweep.dir=" + str(tmp_path),
- "trainer=ddp_sim",
- "trainer.max_epochs=3",
- "+trainer.limit_train_batches=0.01",
- "+trainer.limit_val_batches=0.1",
- "+trainer.limit_test_batches=0.1",
- "model.optimizer.lr=0.005,0.01,0.02",
- ] + overrides
- run_sh_command(command)
-
-
-@RunIf(sh=True)
-@pytest.mark.slow
-def test_optuna_sweep(tmp_path: Path) -> None:
- """Test Optuna hyperparam sweeping.
-
- :param tmp_path: The temporary logging path.
- """
- command = [
- startfile,
- "-m",
- "hparams_search=mnist_optuna",
- "hydra.sweep.dir=" + str(tmp_path),
- "hydra.sweeper.n_trials=10",
- "hydra.sweeper.sampler.n_startup_trials=5",
- "++trainer.fast_dev_run=true",
- ] + overrides
- run_sh_command(command)
-
-
-@RunIf(wandb=True, sh=True)
-@pytest.mark.slow
-def test_optuna_sweep_ddp_sim_wandb(tmp_path: Path) -> None:
- """Test Optuna sweep with wandb logging and ddp sim.
-
- :param tmp_path: The temporary logging path.
- """
- command = [
- startfile,
- "-m",
- "hparams_search=mnist_optuna",
- "hydra.sweep.dir=" + str(tmp_path),
- "hydra.sweeper.n_trials=5",
- "trainer=ddp_sim",
- "trainer.max_epochs=3",
- "+trainer.limit_train_batches=0.01",
- "+trainer.limit_val_batches=0.1",
- "+trainer.limit_test_batches=0.1",
- "logger=wandb",
- ]
- run_sh_command(command)
+from pathlib import Path
+
+import pytest
+
+from tests.helpers.run_if import RunIf
+from tests.helpers.run_sh_command import run_sh_command
+
+startfile = "src/train.py"
+overrides = ["logger=[]"]
+
+
+@RunIf(sh=True)
+@pytest.mark.slow
+def test_experiments(tmp_path: Path) -> None:
+ """Test running all available experiment configs with `fast_dev_run=True.`
+
+ :param tmp_path: The temporary logging path.
+ """
+ command = [
+ startfile,
+ "-m",
+ "experiment=glob(*)",
+ "hydra.sweep.dir=" + str(tmp_path),
+ "++trainer.fast_dev_run=true",
+ ] + overrides
+ run_sh_command(command)
+
+
+@RunIf(sh=True)
+@pytest.mark.slow
+def test_hydra_sweep(tmp_path: Path) -> None:
+ """Test default hydra sweep.
+
+ :param tmp_path: The temporary logging path.
+ """
+ command = [
+ startfile,
+ "-m",
+ "hydra.sweep.dir=" + str(tmp_path),
+ "model.optimizer.lr=0.005,0.01",
+ "++trainer.fast_dev_run=true",
+ ] + overrides
+
+ run_sh_command(command)
+
+
+@RunIf(sh=True)
+@pytest.mark.slow
+def test_hydra_sweep_ddp_sim(tmp_path: Path) -> None:
+ """Test default hydra sweep with ddp sim.
+
+ :param tmp_path: The temporary logging path.
+ """
+ command = [
+ startfile,
+ "-m",
+ "hydra.sweep.dir=" + str(tmp_path),
+ "trainer=ddp_sim",
+ "trainer.max_epochs=3",
+ "+trainer.limit_train_batches=0.01",
+ "+trainer.limit_val_batches=0.1",
+ "+trainer.limit_test_batches=0.1",
+ "model.optimizer.lr=0.005,0.01,0.02",
+ ] + overrides
+ run_sh_command(command)
+
+
+@RunIf(sh=True)
+@pytest.mark.slow
+def test_optuna_sweep(tmp_path: Path) -> None:
+ """Test Optuna hyperparam sweeping.
+
+ :param tmp_path: The temporary logging path.
+ """
+ command = [
+ startfile,
+ "-m",
+ "hparams_search=mnist_optuna",
+ "hydra.sweep.dir=" + str(tmp_path),
+ "hydra.sweeper.n_trials=10",
+ "hydra.sweeper.sampler.n_startup_trials=5",
+ "++trainer.fast_dev_run=true",
+ ] + overrides
+ run_sh_command(command)
+
+
+@RunIf(wandb=True, sh=True)
+@pytest.mark.slow
+def test_optuna_sweep_ddp_sim_wandb(tmp_path: Path) -> None:
+ """Test Optuna sweep with wandb logging and ddp sim.
+
+ :param tmp_path: The temporary logging path.
+ """
+ command = [
+ startfile,
+ "-m",
+ "hparams_search=mnist_optuna",
+ "hydra.sweep.dir=" + str(tmp_path),
+ "hydra.sweeper.n_trials=5",
+ "trainer=ddp_sim",
+ "trainer.max_epochs=3",
+ "+trainer.limit_train_batches=0.01",
+ "+trainer.limit_val_batches=0.1",
+ "+trainer.limit_test_batches=0.1",
+ "logger=wandb",
+ ]
+ run_sh_command(command)
diff --git a/tests/test_train.py b/tests/test_train.py
index c13ae02c8ae259553e0f0e8192cf054c228172dd..2e5415fa958c09a5929324d5919e751a38c435d0 100644
--- a/tests/test_train.py
+++ b/tests/test_train.py
@@ -1,108 +1,108 @@
-import os
-from pathlib import Path
-
-import pytest
-from hydra.core.hydra_config import HydraConfig
-from omegaconf import DictConfig, open_dict
-
-from src.train import train
-from tests.helpers.run_if import RunIf
-
-
-def test_train_fast_dev_run(cfg_train: DictConfig) -> None:
- """Run for 1 train, val and test step.
-
- :param cfg_train: A DictConfig containing a valid training configuration.
- """
- HydraConfig().set_config(cfg_train)
- with open_dict(cfg_train):
- cfg_train.trainer.fast_dev_run = True
- cfg_train.trainer.accelerator = "cpu"
- train(cfg_train)
-
-
-@RunIf(min_gpus=1)
-def test_train_fast_dev_run_gpu(cfg_train: DictConfig) -> None:
- """Run for 1 train, val and test step on GPU.
-
- :param cfg_train: A DictConfig containing a valid training configuration.
- """
- HydraConfig().set_config(cfg_train)
- with open_dict(cfg_train):
- cfg_train.trainer.fast_dev_run = True
- cfg_train.trainer.accelerator = "gpu"
- train(cfg_train)
-
-
-@RunIf(min_gpus=1)
-@pytest.mark.slow
-def test_train_epoch_gpu_amp(cfg_train: DictConfig) -> None:
- """Train 1 epoch on GPU with mixed-precision.
-
- :param cfg_train: A DictConfig containing a valid training configuration.
- """
- HydraConfig().set_config(cfg_train)
- with open_dict(cfg_train):
- cfg_train.trainer.max_epochs = 1
- cfg_train.trainer.accelerator = "gpu"
- cfg_train.trainer.precision = 16
- train(cfg_train)
-
-
-@pytest.mark.slow
-def test_train_epoch_double_val_loop(cfg_train: DictConfig) -> None:
- """Train 1 epoch with validation loop twice per epoch.
-
- :param cfg_train: A DictConfig containing a valid training configuration.
- """
- HydraConfig().set_config(cfg_train)
- with open_dict(cfg_train):
- cfg_train.trainer.max_epochs = 1
- cfg_train.trainer.val_check_interval = 0.5
- train(cfg_train)
-
-
-@pytest.mark.slow
-def test_train_ddp_sim(cfg_train: DictConfig) -> None:
- """Simulate DDP (Distributed Data Parallel) on 2 CPU processes.
-
- :param cfg_train: A DictConfig containing a valid training configuration.
- """
- HydraConfig().set_config(cfg_train)
- with open_dict(cfg_train):
- cfg_train.trainer.max_epochs = 2
- cfg_train.trainer.accelerator = "cpu"
- cfg_train.trainer.devices = 2
- cfg_train.trainer.strategy = "ddp_spawn"
- train(cfg_train)
-
-
-@pytest.mark.slow
-def test_train_resume(tmp_path: Path, cfg_train: DictConfig) -> None:
- """Run 1 epoch, finish, and resume for another epoch.
-
- :param tmp_path: The temporary logging path.
- :param cfg_train: A DictConfig containing a valid training configuration.
- """
- with open_dict(cfg_train):
- cfg_train.trainer.max_epochs = 1
-
- HydraConfig().set_config(cfg_train)
- metric_dict_1, _ = train(cfg_train)
-
- files = os.listdir(tmp_path / "checkpoints")
- assert "last.ckpt" in files
- assert "epoch_000.ckpt" in files
-
- with open_dict(cfg_train):
- cfg_train.ckpt_path = str(tmp_path / "checkpoints" / "last.ckpt")
- cfg_train.trainer.max_epochs = 2
-
- metric_dict_2, _ = train(cfg_train)
-
- files = os.listdir(tmp_path / "checkpoints")
- assert "epoch_001.ckpt" in files
- assert "epoch_002.ckpt" not in files
-
- assert metric_dict_1["train/acc"] < metric_dict_2["train/acc"]
- assert metric_dict_1["val/acc"] < metric_dict_2["val/acc"]
+import os
+from pathlib import Path
+
+import pytest
+from hydra.core.hydra_config import HydraConfig
+from omegaconf import DictConfig, open_dict
+
+from src.train import train
+from tests.helpers.run_if import RunIf
+
+
+def test_train_fast_dev_run(cfg_train: DictConfig) -> None:
+ """Run for 1 train, val and test step.
+
+ :param cfg_train: A DictConfig containing a valid training configuration.
+ """
+ HydraConfig().set_config(cfg_train)
+ with open_dict(cfg_train):
+ cfg_train.trainer.fast_dev_run = True
+ cfg_train.trainer.accelerator = "cpu"
+ train(cfg_train)
+
+
+@RunIf(min_gpus=1)
+def test_train_fast_dev_run_gpu(cfg_train: DictConfig) -> None:
+ """Run for 1 train, val and test step on GPU.
+
+ :param cfg_train: A DictConfig containing a valid training configuration.
+ """
+ HydraConfig().set_config(cfg_train)
+ with open_dict(cfg_train):
+ cfg_train.trainer.fast_dev_run = True
+ cfg_train.trainer.accelerator = "gpu"
+ train(cfg_train)
+
+
+@RunIf(min_gpus=1)
+@pytest.mark.slow
+def test_train_epoch_gpu_amp(cfg_train: DictConfig) -> None:
+ """Train 1 epoch on GPU with mixed-precision.
+
+ :param cfg_train: A DictConfig containing a valid training configuration.
+ """
+ HydraConfig().set_config(cfg_train)
+ with open_dict(cfg_train):
+ cfg_train.trainer.max_epochs = 1
+ cfg_train.trainer.accelerator = "gpu"
+ cfg_train.trainer.precision = 16
+ train(cfg_train)
+
+
+@pytest.mark.slow
+def test_train_epoch_double_val_loop(cfg_train: DictConfig) -> None:
+ """Train 1 epoch with validation loop twice per epoch.
+
+ :param cfg_train: A DictConfig containing a valid training configuration.
+ """
+ HydraConfig().set_config(cfg_train)
+ with open_dict(cfg_train):
+ cfg_train.trainer.max_epochs = 1
+ cfg_train.trainer.val_check_interval = 0.5
+ train(cfg_train)
+
+
+@pytest.mark.slow
+def test_train_ddp_sim(cfg_train: DictConfig) -> None:
+ """Simulate DDP (Distributed Data Parallel) on 2 CPU processes.
+
+ :param cfg_train: A DictConfig containing a valid training configuration.
+ """
+ HydraConfig().set_config(cfg_train)
+ with open_dict(cfg_train):
+ cfg_train.trainer.max_epochs = 2
+ cfg_train.trainer.accelerator = "cpu"
+ cfg_train.trainer.devices = 2
+ cfg_train.trainer.strategy = "ddp_spawn"
+ train(cfg_train)
+
+
+@pytest.mark.slow
+def test_train_resume(tmp_path: Path, cfg_train: DictConfig) -> None:
+ """Run 1 epoch, finish, and resume for another epoch.
+
+ :param tmp_path: The temporary logging path.
+ :param cfg_train: A DictConfig containing a valid training configuration.
+ """
+ with open_dict(cfg_train):
+ cfg_train.trainer.max_epochs = 1
+
+ HydraConfig().set_config(cfg_train)
+ metric_dict_1, _ = train(cfg_train)
+
+ files = os.listdir(tmp_path / "checkpoints")
+ assert "last.ckpt" in files
+ assert "epoch_000.ckpt" in files
+
+ with open_dict(cfg_train):
+ cfg_train.ckpt_path = str(tmp_path / "checkpoints" / "last.ckpt")
+ cfg_train.trainer.max_epochs = 2
+
+ metric_dict_2, _ = train(cfg_train)
+
+ files = os.listdir(tmp_path / "checkpoints")
+ assert "epoch_001.ckpt" in files
+ assert "epoch_002.ckpt" not in files
+
+ assert metric_dict_1["train/acc"] < metric_dict_2["train/acc"]
+ assert metric_dict_1["val/acc"] < metric_dict_2["val/acc"]
diff --git a/wandb_vis.py b/wandb_vis.py
index 97424a0fa466a8585b79be8543b8b2cacbd20364..dfa1bf881191268bc3f1ce09697fd1e4f5b8aef3 100644
--- a/wandb_vis.py
+++ b/wandb_vis.py
@@ -1,181 +1,185 @@
-# -*- coding: utf-8 -*-
-# @Time : 2024/8/3 上午10:46
-# @Author : xiaoshun
-# @Email : 3038523973@qq.com
-# @File : wandb_vis.py
-# @Software: PyCharm
-import argparse
-import os
-import shutil
-from glob import glob
-
-import albumentations as albu
-import numpy as np
-import torch
-import wandb
-from PIL import Image
-from albumentations.pytorch.transforms import ToTensorV2
-from matplotlib import pyplot as plt
-from rich.progress import track
-from thop import profile
-
-from src.data.components.hrcwhu import HRCWHU
-from src.data.hrcwhu_datamodule import HRCWHUDataModule
-from src.models.components.cdnetv1 import CDnetV1
-from src.models.components.cdnetv2 import CDnetV2
-from src.models.components.dbnet import DBNet
-from src.models.components.hrcloudnet import HRCloudNet
-from src.models.components.mcdnet import MCDNet
-from src.models.components.scnn import SCNN
-
-
-class WandbVis:
- def __init__(self, model_name):
- self.model_name = model_name
- self.device = "cuda:1" if torch.cuda.is_available() else "cpu"
- # self.device = "cpu"
- self.colors = ((255, 255, 255), (128, 192, 128))
- self.num_classes = 2
- self.model = self.load_model()
- self.dataloader = self.load_dataset()
- self.macs, self.params = None, None
- wandb.init(project='model_vis', name=self.model_name)
-
- def load_weight(self, weight_path: str):
- weight = torch.load(weight_path, map_location=self.device)
- state_dict = {}
- for key, value in weight["state_dict"].items():
- new_key = key[4:]
- state_dict[new_key] = value
- return state_dict
-
- def load_model_by_model_name(self):
- if self.model_name == 'dbnet':
- return DBNet(img_size=256, in_channels=3, num_classes=2).to(self.device)
- if self.model_name == "cdnetv1":
- return CDnetV1(num_classes=2).to(self.device)
- if self.model_name == "cdnetv2":
- return CDnetV2(num_classes=2).to(self.device)
-
- if self.model_name == "hrcloud":
- return HRCloudNet(num_classes=2).to(self.device)
- if self.model_name == "mcdnet":
- return MCDNet(in_channels=3, num_classes=2).to(self.device)
-
- if self.model_name == "scnn":
- return SCNN(num_classes=2).to(self.device)
-
- raise ValueError(f"{self.model_name}模型不存在")
-
- def load_model(self):
- weight_path = glob(f"logs/train/runs/hrcwhu_{self.model_name}/*/checkpoints/*.ckpt")[0]
- model = self.load_model_by_model_name()
- state_dict = self.load_weight(weight_path)
- model.load_state_dict(state_dict)
- model.eval()
- return model
-
- def load_dataset(self):
-
- all_transform = albu.Compose(
- [
- albu.Resize(
- height=HRCWHU.METAINFO["img_size"][1],
- width=HRCWHU.METAINFO["img_size"][2],
- always_apply=True
- )
- ]
- )
- img_transform = albu.Compose([
- albu.ToFloat(255.0),
- ToTensorV2()
- ])
- ann_transform = None
- val_pipeline = dict(
- all_transform=all_transform,
- img_transform=img_transform,
- ann_transform=ann_transform,
- )
- dataloader = HRCWHUDataModule(
- root="/home/liujie/liumin/cloudseg/data/hrcwhu",
- train_pipeline=val_pipeline,
- val_pipeline=val_pipeline,
- test_pipeline=val_pipeline,
- batch_size=1,
- )
- dataloader.setup()
- test_dataloader = dataloader.test_dataloader()
- return test_dataloader
- # for data in test_dataloader:
- # print(data['img'].shape,data['ann'].shape,data['img_path'],data['ann_path'],data['lac_type'])
- # break
- # torch.Size([1, 3, 256, 256])
- # torch.Size([1, 256, 256])
- # ['/home/liujie/liumin/cloudseg/data/hrcwhu/img_dir/test/barren_30.tif']
- # ['/home/liujie/liumin/cloudseg/data/hrcwhu/ann_dir/test/barren_30.tif']
- # ['barren']
-
- def give_colors_to_mask(self, mask: np.ndarray):
- """
- 赋予mask颜色
- """
- assert len(mask.shape) == 2, "Value Error,mask的形状为(height,width)"
- colors_mask = np.zeros((mask.shape[0], mask.shape[1], 3)).astype(np.float32)
- for color in range(2):
- segc = (mask == color)
- colors_mask[:, :, 0] += segc * (self.colors[color][0])
- colors_mask[:, :, 1] += segc * (self.colors[color][1])
- colors_mask[:, :, 2] += segc * (self.colors[color][2])
- return colors_mask
-
- @torch.no_grad
- def pred_mask(self, x: torch.Tensor):
- x = x.to(self.device)
- self.macs, self.params = profile(self.model, inputs=(x,),verbose=False)
- logits = self.model(x)
- if isinstance(logits, tuple):
- logits = logits[0]
- fake_mask = torch.argmax(logits, 1).detach().cpu().squeeze(0).numpy()
- return fake_mask
-
- def np_pil_np(self, image: np.ndarray, filename="colors_ann"):
- colors_np = self.give_colors_to_mask(image)
- pil_np = Image.fromarray(np.uint8(colors_np))
- return np.array(pil_np)
-
- def run(self, delete_wadb_log=True):
- # print(len(self.dataloader))
- # 30
- for data in track(self.dataloader):
- img = data["img"]
- ann = data["ann"].squeeze(0).numpy()
- img_path = data["img_path"]
- fake_mask = self.pred_mask(img)
-
- colors_ann = self.np_pil_np(ann)
- colors_fake = self.np_pil_np(fake_mask, "colors_fake")
- image_name = img_path[0].split(os.path.sep)[-1].split(".")[0]
-
- plt.subplot(1, 2, 1)
- plt.axis("off")
- plt.title("groud true")
- plt.imshow(colors_ann)
-
- plt.subplot(1, 2, 2)
- plt.axis("off")
- plt.title("predict mask")
- plt.imshow(colors_fake)
- wandb.log({image_name: wandb.Image(plt)})
- wandb.log({"macs":self.macs,"params":self.params})
- wandb.finish()
- if delete_wadb_log and os.path.exists("wandb"):
- shutil.rmtree("wandb")
-
-
-if __name__ == "__main__":
- parser = argparse.ArgumentParser()
- parser.add_argument("--model-name", type=str, default="dbnet")
- parser.add_argument("--delete-wadb-log", type=bool, default=True)
- args = parser.parse_args()
- vis = WandbVis(model_name=args.model_name)
- vis.run(delete_wadb_log=args.delete_wadb_log)
+# -*- coding: utf-8 -*-
+# @Time : 2024/8/3 上午10:46
+# @Author : xiaoshun
+# @Email : 3038523973@qq.com
+# @File : wandb_vis.py
+# @Software: PyCharm
+import argparse
+import os
+import shutil
+from glob import glob
+
+import albumentations as albu
+import numpy as np
+import torch
+import wandb
+from PIL import Image
+from albumentations.pytorch.transforms import ToTensorV2
+from matplotlib import pyplot as plt
+from rich.progress import track
+from thop import profile
+
+from src.data.components.hrcwhu import HRCWHU
+from src.data.hrcwhu_datamodule import HRCWHUDataModule
+from src.models.components.cdnetv1 import CDnetV1
+from src.models.components.cdnetv2 import CDnetV2
+from src.models.components.dbnet import DBNet
+from src.models.components.hrcloudnet import HRCloudNet
+from src.models.components.mcdnet import MCDNet
+from src.models.components.scnn import SCNN
+from src.models.components.unetmobv2 import UNetMobV2
+
+
+class WandbVis:
+ def __init__(self, model_name):
+ self.model_name = model_name
+ self.device = "cuda:1" if torch.cuda.is_available() else "cpu"
+ # self.device = "cpu"
+ self.colors = ((255, 255, 255), (128, 192, 128))
+ self.num_classes = 2
+ self.model = self.load_model()
+ self.dataloader = self.load_dataset()
+ self.macs, self.params = None, None
+ wandb.init(project='model_vis', name=self.model_name)
+
+ def load_weight(self, weight_path: str):
+ weight = torch.load(weight_path, map_location=self.device)
+ state_dict = {}
+ for key, value in weight["state_dict"].items():
+ new_key = key[4:]
+ state_dict[new_key] = value
+ return state_dict
+
+ def load_model_by_model_name(self):
+ if self.model_name == 'dbnet':
+ return DBNet(img_size=256, in_channels=3, num_classes=2).to(self.device)
+ if self.model_name == "cdnetv1":
+ return CDnetV1(num_classes=2).to(self.device)
+ if self.model_name == "cdnetv2":
+ return CDnetV2(num_classes=2).to(self.device)
+
+ if self.model_name == "hrcloud":
+ return HRCloudNet(num_classes=2).to(self.device)
+ if self.model_name == "mcdnet":
+ return MCDNet(in_channels=3, num_classes=2).to(self.device)
+
+ if self.model_name == "scnn":
+ return SCNN(num_classes=2).to(self.device)
+
+ if self.model_name == "unetmobv2":
+ return UNetMobV2(num_classes=2).to(self.device)
+
+ raise ValueError(f"{self.model_name}模型不存在")
+
+ def load_model(self):
+ weight_path = glob(f"logs/train/runs/hrcwhu_{self.model_name}/*/checkpoints/*.ckpt")[0]
+ model = self.load_model_by_model_name()
+ state_dict = self.load_weight(weight_path)
+ model.load_state_dict(state_dict)
+ model.eval()
+ return model
+
+ def load_dataset(self):
+
+ all_transform = albu.Compose(
+ [
+ albu.Resize(
+ height=HRCWHU.METAINFO["img_size"][1],
+ width=HRCWHU.METAINFO["img_size"][2],
+ always_apply=True
+ )
+ ]
+ )
+ img_transform = albu.Compose([
+ albu.ToFloat(255.0),
+ ToTensorV2()
+ ])
+ ann_transform = None
+ val_pipeline = dict(
+ all_transform=all_transform,
+ img_transform=img_transform,
+ ann_transform=ann_transform,
+ )
+ dataloader = HRCWHUDataModule(
+ root="/home/liujie/liumin/cloudseg/data/hrcwhu",
+ train_pipeline=val_pipeline,
+ val_pipeline=val_pipeline,
+ test_pipeline=val_pipeline,
+ batch_size=1,
+ )
+ dataloader.setup()
+ test_dataloader = dataloader.test_dataloader()
+ return test_dataloader
+ # for data in test_dataloader:
+ # print(data['img'].shape,data['ann'].shape,data['img_path'],data['ann_path'],data['lac_type'])
+ # break
+ # torch.Size([1, 3, 256, 256])
+ # torch.Size([1, 256, 256])
+ # ['/home/liujie/liumin/cloudseg/data/hrcwhu/img_dir/test/barren_30.tif']
+ # ['/home/liujie/liumin/cloudseg/data/hrcwhu/ann_dir/test/barren_30.tif']
+ # ['barren']
+
+ def give_colors_to_mask(self, mask: np.ndarray):
+ """
+ 赋予mask颜色
+ """
+ assert len(mask.shape) == 2, "Value Error,mask的形状为(height,width)"
+ colors_mask = np.zeros((mask.shape[0], mask.shape[1], 3)).astype(np.float32)
+ for color in range(2):
+ segc = (mask == color)
+ colors_mask[:, :, 0] += segc * (self.colors[color][0])
+ colors_mask[:, :, 1] += segc * (self.colors[color][1])
+ colors_mask[:, :, 2] += segc * (self.colors[color][2])
+ return colors_mask
+
+ @torch.no_grad
+ def pred_mask(self, x: torch.Tensor):
+ x = x.to(self.device)
+ self.macs, self.params = profile(self.model, inputs=(x,), verbose=False)
+ logits = self.model(x)
+ if isinstance(logits, tuple):
+ logits = logits[0]
+ fake_mask = torch.argmax(logits, 1).detach().cpu().squeeze(0).numpy()
+ return fake_mask
+
+ def np_pil_np(self, image: np.ndarray, filename="colors_ann"):
+ colors_np = self.give_colors_to_mask(image)
+ pil_np = Image.fromarray(np.uint8(colors_np))
+ return np.array(pil_np)
+
+ def run(self, delete_wadb_log=True):
+ # print(len(self.dataloader))
+ # 30
+ for data in track(self.dataloader):
+ img = data["img"]
+ ann = data["ann"].squeeze(0).numpy()
+ img_path = data["img_path"]
+ fake_mask = self.pred_mask(img)
+
+ colors_ann = self.np_pil_np(ann)
+ colors_fake = self.np_pil_np(fake_mask, "colors_fake")
+ image_name = img_path[0].split(os.path.sep)[-1].split(".")[0]
+
+ plt.subplot(1, 2, 1)
+ plt.axis("off")
+ plt.title("groud true")
+ plt.imshow(colors_ann)
+
+ plt.subplot(1, 2, 2)
+ plt.axis("off")
+ plt.title("predict mask")
+ plt.imshow(colors_fake)
+ wandb.log({image_name: wandb.Image(plt)})
+ wandb.log({"macs": self.macs, "params": self.params})
+ wandb.finish()
+ if delete_wadb_log and os.path.exists("wandb"):
+ shutil.rmtree("wandb")
+
+
+if __name__ == "__main__":
+ parser = argparse.ArgumentParser()
+ parser.add_argument("--model-name", type=str, default="dbnet")
+ parser.add_argument("--delete-wadb-log", type=bool, default=True)
+ args = parser.parse_args()
+ vis = WandbVis(model_name=args.model_name)
+ vis.run(delete_wadb_log=args.delete_wadb_log)