Spaces:
Running
Running
File size: 29,356 Bytes
c8cb798 |
1 2 3 4 5 6 7 8 9 10 11 12 13 14 15 16 17 18 19 20 21 22 23 24 25 26 27 28 29 30 31 32 33 34 35 36 37 38 39 40 41 42 43 44 45 46 47 48 49 50 51 52 53 54 55 56 57 58 59 60 61 62 63 64 65 66 67 68 69 70 71 72 73 74 75 76 77 78 79 80 81 82 83 84 85 86 87 88 89 90 91 92 93 94 95 96 97 98 99 100 101 102 103 104 105 106 107 108 109 110 111 112 113 114 115 116 117 118 119 120 121 122 123 124 125 126 127 128 129 130 131 132 133 134 135 136 137 138 139 140 141 142 143 144 145 146 147 148 149 150 151 152 153 154 155 156 157 158 159 160 161 162 163 164 165 166 167 168 169 170 171 172 173 174 175 176 177 178 179 180 181 182 183 184 185 186 187 188 189 190 191 192 193 194 195 196 197 198 199 200 201 202 203 204 205 206 207 208 209 210 211 212 213 214 215 216 217 218 219 220 221 222 223 224 225 226 227 228 229 230 231 232 233 234 235 236 237 238 239 240 241 242 243 244 245 246 247 248 249 250 251 252 253 254 255 256 257 258 259 260 261 262 263 264 265 266 267 268 269 270 271 272 273 274 275 276 277 278 279 280 281 282 283 284 285 286 287 288 289 290 291 292 293 294 295 296 297 298 299 300 301 302 303 304 305 306 307 308 309 310 311 312 313 314 315 316 317 318 319 320 321 322 323 324 325 326 327 328 329 330 331 332 333 334 335 336 337 338 339 340 341 342 343 344 345 346 347 348 349 350 351 352 353 354 355 356 357 358 359 360 361 362 363 364 365 366 367 368 369 370 371 372 373 374 375 376 377 378 379 380 381 382 383 384 385 386 387 388 389 390 391 392 393 394 395 396 397 398 399 400 401 402 403 404 405 406 407 408 409 410 411 412 413 414 415 416 417 418 419 420 421 422 423 424 425 426 427 428 429 430 431 432 433 434 435 436 437 438 439 440 441 442 443 444 445 446 447 448 449 450 451 452 453 454 455 456 457 458 459 460 461 462 463 464 465 466 467 468 469 470 471 472 473 474 475 476 477 478 479 480 481 482 483 484 485 486 487 488 489 490 491 492 493 494 495 496 497 498 499 500 501 502 503 504 505 506 507 508 509 510 511 512 513 514 515 516 517 518 519 520 521 522 523 524 525 526 527 528 |
[](#load-pipelines)Load pipelines
=================================


Diffusion systems consist of multiple components like parameterized models and schedulers that interact in complex ways. That is why we designed the [DiffusionPipeline](/docs/diffusers/main/en/api/pipelines/overview#diffusers.DiffusionPipeline) to wrap the complexity of the entire diffusion system into an easy-to-use API. At the same time, the [DiffusionPipeline](/docs/diffusers/main/en/api/pipelines/overview#diffusers.DiffusionPipeline) is entirely customizable so you can modify each component to build a diffusion system for your use case.
This guide will show you how to load:
* pipelines from the Hub and locally
* different components into a pipeline
* multiple pipelines without increasing memory usage
* checkpoint variants such as different floating point types or non-exponential mean averaged (EMA) weights
[](#load-a-pipeline)Load a pipeline
-----------------------------------
Skip to the [DiffusionPipeline explained](#diffusionpipeline-explained) section if you’re interested in an explanation about how the [DiffusionPipeline](/docs/diffusers/main/en/api/pipelines/overview#diffusers.DiffusionPipeline) class works.
There are two ways to load a pipeline for a task:
1. Load the generic [DiffusionPipeline](/docs/diffusers/main/en/api/pipelines/overview#diffusers.DiffusionPipeline) class and allow it to automatically detect the correct pipeline class from the checkpoint.
2. Load a specific pipeline class for a specific task.
generic pipeline
specific pipeline
The [DiffusionPipeline](/docs/diffusers/main/en/api/pipelines/overview#diffusers.DiffusionPipeline) class is a simple and generic way to load the latest trending diffusion model from the [Hub](https://huggingface.co/models?library=diffusers&sort=trending). It uses the [from\_pretrained()](/docs/diffusers/main/en/api/pipelines/overview#diffusers.DiffusionPipeline.from_pretrained) method to automatically detect the correct pipeline class for a task from the checkpoint, downloads and caches all the required configuration and weight files, and returns a pipeline ready for inference.
Copied
from diffusers import DiffusionPipeline
pipeline = DiffusionPipeline.from\_pretrained("stable-diffusion-v1-5/stable-diffusion-v1-5", use\_safetensors=True)
This same checkpoint can also be used for an image-to-image task. The [DiffusionPipeline](/docs/diffusers/main/en/api/pipelines/overview#diffusers.DiffusionPipeline) class can handle any task as long as you provide the appropriate inputs. For example, for an image-to-image task, you need to pass an initial image to the pipeline.
Copied
from diffusers import DiffusionPipeline
pipeline = DiffusionPipeline.from\_pretrained("stable-diffusion-v1-5/stable-diffusion-v1-5", use\_safetensors=True)
init\_image = load\_image("https://huggingface.co/datasets/huggingface/documentation-images/resolve/main/diffusers/img2img-init.png")
prompt = "Astronaut in a jungle, cold color palette, muted colors, detailed, 8k"
image = pipeline("Astronaut in a jungle, cold color palette, muted colors, detailed, 8k", image=init\_image).images\[0\]
Use the Space below to gauge a pipeline’s memory requirements before you download and load it to see if it runs on your hardware.
### [](#local-pipeline)Local pipeline
To load a pipeline locally, use [git-lfs](https://git-lfs.github.com/) to manually download a checkpoint to your local disk.
Copied
git-lfs install
git clone https://huggingface.co/stable-diffusion-v1-5/stable-diffusion-v1-5
This creates a local folder, ./stable-diffusion-v1-5, on your disk and you should pass its path to [from\_pretrained()](/docs/diffusers/main/en/api/pipelines/overview#diffusers.DiffusionPipeline.from_pretrained).
Copied
from diffusers import DiffusionPipeline
stable\_diffusion = DiffusionPipeline.from\_pretrained("./stable-diffusion-v1-5", use\_safetensors=True)
The [from\_pretrained()](/docs/diffusers/main/en/api/pipelines/overview#diffusers.DiffusionPipeline.from_pretrained) method won’t download files from the Hub when it detects a local path, but this also means it won’t download and cache the latest changes to a checkpoint.
[](#customize-a-pipeline)Customize a pipeline
---------------------------------------------
You can customize a pipeline by loading different components into it. This is important because you can:
* change to a scheduler with faster generation speed or higher generation quality depending on your needs (call the `scheduler.compatibles` method on your pipeline to see compatible schedulers)
* change a default pipeline component to a newer and better performing one
For example, let’s customize the default [stabilityai/stable-diffusion-xl-base-1.0](https://hf.co/stabilityai/stable-diffusion-xl-base-1.0) checkpoint with:
* The [HeunDiscreteScheduler](/docs/diffusers/main/en/api/schedulers/heun#diffusers.HeunDiscreteScheduler) to generate higher quality images at the expense of slower generation speed. You must pass the `subfolder="scheduler"` parameter in [from\_pretrained()](/docs/diffusers/main/en/api/schedulers/overview#diffusers.SchedulerMixin.from_pretrained) to load the scheduler configuration into the correct [subfolder](https://hf.co/stabilityai/stable-diffusion-xl-base-1.0/tree/main/scheduler) of the pipeline repository.
* A more stable VAE that runs in fp16.
Copied
from diffusers import StableDiffusionXLPipeline, HeunDiscreteScheduler, AutoencoderKL
import torch
scheduler = HeunDiscreteScheduler.from\_pretrained("stabilityai/stable-diffusion-xl-base-1.0", subfolder="scheduler")
vae = AutoencoderKL.from\_pretrained("madebyollin/sdxl-vae-fp16-fix", torch\_dtype=torch.float16, use\_safetensors=True)
Now pass the new scheduler and VAE to the [StableDiffusionXLPipeline](/docs/diffusers/main/en/api/pipelines/stable_diffusion/stable_diffusion_xl#diffusers.StableDiffusionXLPipeline).
Copied
pipeline = StableDiffusionXLPipeline.from\_pretrained(
"stabilityai/stable-diffusion-xl-base-1.0",
scheduler=scheduler,
vae=vae,
torch\_dtype=torch.float16,
variant="fp16",
use\_safetensors=True
).to("cuda")
[](#reuse-a-pipeline)Reuse a pipeline
-------------------------------------
When you load multiple pipelines that share the same model components, it makes sense to reuse the shared components instead of reloading everything into memory again, especially if your hardware is memory-constrained. For example:
1. You generated an image with the [StableDiffusionPipeline](/docs/diffusers/main/en/api/pipelines/stable_diffusion/text2img#diffusers.StableDiffusionPipeline) but you want to improve its quality with the [StableDiffusionSAGPipeline](/docs/diffusers/main/en/api/pipelines/self_attention_guidance#diffusers.StableDiffusionSAGPipeline). Both of these pipelines share the same pretrained model, so it’d be a waste of memory to load the same model twice.
2. You want to add a model component, like a [`MotionAdapter`](../api/pipelines/animatediff#animatediffpipeline), to [AnimateDiffPipeline](/docs/diffusers/main/en/api/pipelines/animatediff#diffusers.AnimateDiffPipeline) which was instantiated from an existing [StableDiffusionPipeline](/docs/diffusers/main/en/api/pipelines/stable_diffusion/text2img#diffusers.StableDiffusionPipeline). Again, both pipelines share the same pretrained model, so it’d be a waste of memory to load an entirely new pipeline again.
With the [DiffusionPipeline.from\_pipe()](/docs/diffusers/main/en/api/pipelines/overview#diffusers.DiffusionPipeline.from_pipe) API, you can switch between multiple pipelines to take advantage of their different features without increasing memory-usage. It is similar to turning on and off a feature in your pipeline.
To switch between tasks (rather than features), use the [from\_pipe()](/docs/diffusers/main/en/api/pipelines/overview#diffusers.DiffusionPipeline.from_pipe) method with the [AutoPipeline](../api/pipelines/auto_pipeline) class, which automatically identifies the pipeline class based on the task (learn more in the [AutoPipeline](../tutorials/autopipeline) tutorial).
Let’s start with a [StableDiffusionPipeline](/docs/diffusers/main/en/api/pipelines/stable_diffusion/text2img#diffusers.StableDiffusionPipeline) and then reuse the loaded model components to create a [StableDiffusionSAGPipeline](/docs/diffusers/main/en/api/pipelines/self_attention_guidance#diffusers.StableDiffusionSAGPipeline) to increase generation quality. You’ll use the [StableDiffusionPipeline](/docs/diffusers/main/en/api/pipelines/stable_diffusion/text2img#diffusers.StableDiffusionPipeline) with an [IP-Adapter](./ip_adapter) to generate a bear eating pizza.
Copied
from diffusers import DiffusionPipeline, StableDiffusionSAGPipeline
import torch
import gc
from diffusers.utils import load\_image
from accelerate.utils import compute\_module\_sizes
image = load\_image("https://huggingface.co/datasets/huggingface/documentation-images/resolve/main/diffusers/load\_neg\_embed.png")
pipe\_sd = DiffusionPipeline.from\_pretrained("SG161222/Realistic\_Vision\_V6.0\_B1\_noVAE", torch\_dtype=torch.float16)
pipe\_sd.load\_ip\_adapter("h94/IP-Adapter", subfolder="models", weight\_name="ip-adapter\_sd15.bin")
pipe\_sd.set\_ip\_adapter\_scale(0.6)
pipe\_sd.to("cuda")
generator = torch.Generator(device="cpu").manual\_seed(33)
out\_sd = pipe\_sd(
prompt="bear eats pizza",
negative\_prompt="wrong white balance, dark, sketches,worst quality,low quality",
ip\_adapter\_image=image,
num\_inference\_steps=50,
generator=generator,
).images\[0\]
out\_sd
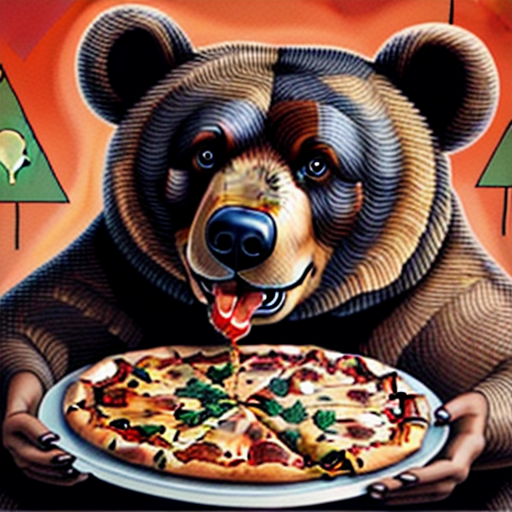
For reference, you can check how much memory this process consumed.
Copied
def bytes\_to\_giga\_bytes(bytes):
return bytes / 1024 / 1024 / 1024
print(f"Max memory allocated: {bytes\_to\_giga\_bytes(torch.cuda.max\_memory\_allocated())} GB")
"Max memory allocated: 4.406213283538818 GB"
Now, reuse the same pipeline components from [StableDiffusionPipeline](/docs/diffusers/main/en/api/pipelines/stable_diffusion/text2img#diffusers.StableDiffusionPipeline) in [StableDiffusionSAGPipeline](/docs/diffusers/main/en/api/pipelines/self_attention_guidance#diffusers.StableDiffusionSAGPipeline) with the [from\_pipe()](/docs/diffusers/main/en/api/pipelines/overview#diffusers.DiffusionPipeline.from_pipe) method.
Some pipeline methods may not function properly on new pipelines created with [from\_pipe()](/docs/diffusers/main/en/api/pipelines/overview#diffusers.DiffusionPipeline.from_pipe). For instance, the [enable\_model\_cpu\_offload()](/docs/diffusers/main/en/api/pipelines/overview#diffusers.DiffusionPipeline.enable_model_cpu_offload) method installs hooks on the model components based on a unique offloading sequence for each pipeline. If the models are executed in a different order in the new pipeline, the CPU offloading may not work correctly.
To ensure everything works as expected, we recommend re-applying a pipeline method on a new pipeline created with [from\_pipe()](/docs/diffusers/main/en/api/pipelines/overview#diffusers.DiffusionPipeline.from_pipe).
Copied
pipe\_sag = StableDiffusionSAGPipeline.from\_pipe(
pipe\_sd
)
generator = torch.Generator(device="cpu").manual\_seed(33)
out\_sag = pipe\_sag(
prompt="bear eats pizza",
negative\_prompt="wrong white balance, dark, sketches,worst quality,low quality",
ip\_adapter\_image=image,
num\_inference\_steps=50,
generator=generator,
guidance\_scale=1.0,
sag\_scale=0.75
).images\[0\]
out\_sag
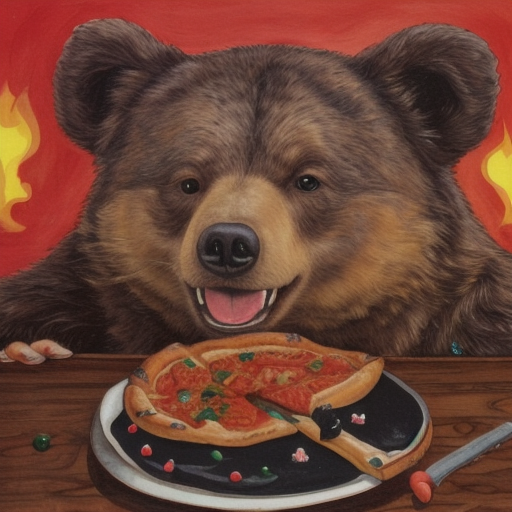
If you check the memory usage, you’ll see it remains the same as before because [StableDiffusionPipeline](/docs/diffusers/main/en/api/pipelines/stable_diffusion/text2img#diffusers.StableDiffusionPipeline) and [StableDiffusionSAGPipeline](/docs/diffusers/main/en/api/pipelines/self_attention_guidance#diffusers.StableDiffusionSAGPipeline) are sharing the same pipeline components. This allows you to use them interchangeably without any additional memory overhead.
Copied
print(f"Max memory allocated: {bytes\_to\_giga\_bytes(torch.cuda.max\_memory\_allocated())} GB")
"Max memory allocated: 4.406213283538818 GB"
Let’s animate the image with the [AnimateDiffPipeline](/docs/diffusers/main/en/api/pipelines/animatediff#diffusers.AnimateDiffPipeline) and also add a `MotionAdapter` module to the pipeline. For the [AnimateDiffPipeline](/docs/diffusers/main/en/api/pipelines/animatediff#diffusers.AnimateDiffPipeline), you need to unload the IP-Adapter first and reload it _after_ you’ve created your new pipeline (this only applies to the [AnimateDiffPipeline](/docs/diffusers/main/en/api/pipelines/animatediff#diffusers.AnimateDiffPipeline)).
Copied
from diffusers import AnimateDiffPipeline, MotionAdapter, DDIMScheduler
from diffusers.utils import export\_to\_gif
pipe\_sag.unload\_ip\_adapter()
adapter = MotionAdapter.from\_pretrained("guoyww/animatediff-motion-adapter-v1-5-2", torch\_dtype=torch.float16)
pipe\_animate = AnimateDiffPipeline.from\_pipe(pipe\_sd, motion\_adapter=adapter)
pipe\_animate.scheduler = DDIMScheduler.from\_config(pipe\_animate.scheduler.config, beta\_schedule="linear")
\# load IP-Adapter and LoRA weights again
pipe\_animate.load\_ip\_adapter("h94/IP-Adapter", subfolder="models", weight\_name="ip-adapter\_sd15.bin")
pipe\_animate.load\_lora\_weights("guoyww/animatediff-motion-lora-zoom-out", adapter\_name="zoom-out")
pipe\_animate.to("cuda")
generator = torch.Generator(device="cpu").manual\_seed(33)
pipe\_animate.set\_adapters("zoom-out", adapter\_weights=0.75)
out = pipe\_animate(
prompt="bear eats pizza",
num\_frames=16,
num\_inference\_steps=50,
ip\_adapter\_image=image,
generator=generator,
).frames\[0\]
export\_to\_gif(out, "out\_animate.gif")
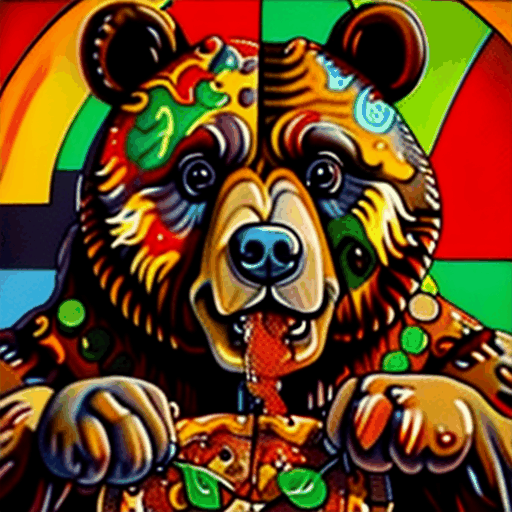
The [AnimateDiffPipeline](/docs/diffusers/main/en/api/pipelines/animatediff#diffusers.AnimateDiffPipeline) is more memory-intensive and consumes 15GB of memory (see the [Memory-usage of from\_pipe](#memory-usage-of-from_pipe) section to learn what this means for your memory-usage).
Copied
print(f"Max memory allocated: {bytes\_to\_giga\_bytes(torch.cuda.max\_memory\_allocated())} GB")
"Max memory allocated: 15.178664207458496 GB"
### [](#modify-frompipe-components)Modify from\_pipe components
Pipelines loaded with [from\_pipe()](/docs/diffusers/main/en/api/pipelines/overview#diffusers.DiffusionPipeline.from_pipe) can be customized with different model components or methods. However, whenever you modify the _state_ of the model components, it affects all the other pipelines that share the same components. For example, if you call [unload\_ip\_adapter()](/docs/diffusers/main/en/api/loaders/ip_adapter#diffusers.loaders.IPAdapterMixin.unload_ip_adapter) on the [StableDiffusionSAGPipeline](/docs/diffusers/main/en/api/pipelines/self_attention_guidance#diffusers.StableDiffusionSAGPipeline), you won’t be able to use IP-Adapter with the [StableDiffusionPipeline](/docs/diffusers/main/en/api/pipelines/stable_diffusion/text2img#diffusers.StableDiffusionPipeline) because it’s been removed from their shared components.
Copied
pipe.sag\_unload\_ip\_adapter()
generator = torch.Generator(device="cpu").manual\_seed(33)
out\_sd = pipe\_sd(
prompt="bear eats pizza",
negative\_prompt="wrong white balance, dark, sketches,worst quality,low quality",
ip\_adapter\_image=image,
num\_inference\_steps=50,
generator=generator,
).images\[0\]
"AttributeError: 'NoneType' object has no attribute 'image\_projection\_layers'"
### [](#memory-usage-of-frompipe)Memory usage of from\_pipe
The memory requirement of loading multiple pipelines with [from\_pipe()](/docs/diffusers/main/en/api/pipelines/overview#diffusers.DiffusionPipeline.from_pipe) is determined by the pipeline with the highest memory-usage regardless of the number of pipelines you create.
Pipeline
Memory usage (GB)
StableDiffusionPipeline
4.400
StableDiffusionSAGPipeline
4.400
AnimateDiffPipeline
15.178
The [AnimateDiffPipeline](/docs/diffusers/main/en/api/pipelines/animatediff#diffusers.AnimateDiffPipeline) has the highest memory requirement, so the _total memory-usage_ is based only on the [AnimateDiffPipeline](/docs/diffusers/main/en/api/pipelines/animatediff#diffusers.AnimateDiffPipeline). Your memory-usage will not increase if you create additional pipelines as long as their memory requirements doesn’t exceed that of the [AnimateDiffPipeline](/docs/diffusers/main/en/api/pipelines/animatediff#diffusers.AnimateDiffPipeline). Each pipeline can be used interchangeably without any additional memory overhead.
[](#safety-checker)Safety checker
---------------------------------
Diffusers implements a [safety checker](https://github.com/huggingface/diffusers/blob/main/src/diffusers/pipelines/stable_diffusion/safety_checker.py) for Stable Diffusion models which can generate harmful content. The safety checker screens the generated output against known hardcoded not-safe-for-work (NSFW) content. If for whatever reason you’d like to disable the safety checker, pass `safety_checker=None` to the [from\_pretrained()](/docs/diffusers/main/en/api/pipelines/overview#diffusers.DiffusionPipeline.from_pretrained) method.
Copied
from diffusers import DiffusionPipeline
pipeline = DiffusionPipeline.from\_pretrained("stable-diffusion-v1-5/stable-diffusion-v1-5", safety\_checker=None, use\_safetensors=True)
"""
You have disabled the safety checker for <class 'diffusers.pipelines.stable\_diffusion.pipeline\_stable\_diffusion.StableDiffusionPipeline'> by passing \`safety\_checker=None\`. Ensure that you abide by the conditions of the Stable Diffusion license and do not expose unfiltered results in services or applications open to the public. Both the diffusers team and Hugging Face strongly recommend keeping the safety filter enabled in all public-facing circumstances, disabling it only for use cases that involve analyzing network behavior or auditing its results. For more information, please have a look at https://github.com/huggingface/diffusers/pull/254 .
"""
[](#checkpoint-variants)Checkpoint variants
-------------------------------------------
A checkpoint variant is usually a checkpoint whose weights are:
* Stored in a different floating point type, such as [torch.float16](https://pytorch.org/docs/stable/tensors.html#data-types), because it only requires half the bandwidth and storage to download. You can’t use this variant if you’re continuing training or using a CPU.
* Non-exponential mean averaged (EMA) weights which shouldn’t be used for inference. You should use this variant to continue finetuning a model.
When the checkpoints have identical model structures, but they were trained on different datasets and with a different training setup, they should be stored in separate repositories. For example, [stabilityai/stable-diffusion-2](https://hf.co/stabilityai/stable-diffusion-2) and [stabilityai/stable-diffusion-2-1](https://hf.co/stabilityai/stable-diffusion-2-1) are stored in separate repositories.
Otherwise, a variant is **identical** to the original checkpoint. They have exactly the same serialization format (like [safetensors](./using_safetensors)), model structure, and their weights have identical tensor shapes.
**checkpoint type**
**weight name**
**argument for loading weights**
original
diffusion\_pytorch\_model.safetensors
floating point
diffusion\_pytorch\_model.fp16.safetensors
`variant`, `torch_dtype`
non-EMA
diffusion\_pytorch\_model.non\_ema.safetensors
`variant`
There are two important arguments for loading variants:
* `torch_dtype` specifies the floating point precision of the loaded checkpoint. For example, if you want to save bandwidth by loading a fp16 variant, you should set `variant="fp16"` and `torch_dtype=torch.float16` to _convert the weights_ to fp16. Otherwise, the fp16 weights are converted to the default fp32 precision.
If you only set `torch_dtype=torch.float16`, the default fp32 weights are downloaded first and then converted to fp16.
* `variant` specifies which files should be loaded from the repository. For example, if you want to load a non-EMA variant of a UNet from [stable-diffusion-v1-5/stable-diffusion-v1-5](https://hf.co/stable-diffusion-v1-5/stable-diffusion-v1-5/tree/main/unet), set `variant="non_ema"` to download the `non_ema` file.
fp16
non-EMA
Copied
from diffusers import DiffusionPipeline
import torch
pipeline = DiffusionPipeline.from\_pretrained(
"stable-diffusion-v1-5/stable-diffusion-v1-5", variant="fp16", torch\_dtype=torch.float16, use\_safetensors=True
)
Use the `variant` parameter in the [DiffusionPipeline.save\_pretrained()](/docs/diffusers/main/en/api/pipelines/overview#diffusers.DiffusionPipeline.save_pretrained) method to save a checkpoint as a different floating point type or as a non-EMA variant. You should try save a variant to the same folder as the original checkpoint, so you have the option of loading both from the same folder.
fp16
non\_ema
Copied
from diffusers import DiffusionPipeline
pipeline.save\_pretrained("stable-diffusion-v1-5/stable-diffusion-v1-5", variant="fp16")
If you don’t save the variant to an existing folder, you must specify the `variant` argument otherwise it’ll throw an `Exception` because it can’t find the original checkpoint.
Copied
\# 👎 this won't work
pipeline = DiffusionPipeline.from\_pretrained(
"./stable-diffusion-v1-5", torch\_dtype=torch.float16, use\_safetensors=True
)
\# 👍 this works
pipeline = DiffusionPipeline.from\_pretrained(
"./stable-diffusion-v1-5", variant="fp16", torch\_dtype=torch.float16, use\_safetensors=True
)
[](#diffusionpipeline-explained)DiffusionPipeline explained
-----------------------------------------------------------
As a class method, [DiffusionPipeline.from\_pretrained()](/docs/diffusers/main/en/api/pipelines/overview#diffusers.DiffusionPipeline.from_pretrained) is responsible for two things:
* Download the latest version of the folder structure required for inference and cache it. If the latest folder structure is available in the local cache, [DiffusionPipeline.from\_pretrained()](/docs/diffusers/main/en/api/pipelines/overview#diffusers.DiffusionPipeline.from_pretrained) reuses the cache and won’t redownload the files.
* Load the cached weights into the correct pipeline [class](../api/pipelines/overview#diffusers-summary) - retrieved from the `model_index.json` file - and return an instance of it.
The pipelines’ underlying folder structure corresponds directly with their class instances. For example, the [StableDiffusionPipeline](/docs/diffusers/main/en/api/pipelines/stable_diffusion/text2img#diffusers.StableDiffusionPipeline) corresponds to the folder structure in [`stable-diffusion-v1-5/stable-diffusion-v1-5`](https://huggingface.co/stable-diffusion-v1-5/stable-diffusion-v1-5).
Copied
from diffusers import DiffusionPipeline
repo\_id = "stable-diffusion-v1-5/stable-diffusion-v1-5"
pipeline = DiffusionPipeline.from\_pretrained(repo\_id, use\_safetensors=True)
print(pipeline)
You’ll see pipeline is an instance of [StableDiffusionPipeline](/docs/diffusers/main/en/api/pipelines/stable_diffusion/text2img#diffusers.StableDiffusionPipeline), which consists of seven components:
* `"feature_extractor"`: a [CLIPImageProcessor](https://huggingface.co/docs/transformers/main/en/model_doc/clip#transformers.CLIPImageProcessor) from 🤗 Transformers.
* `"safety_checker"`: a [component](https://github.com/huggingface/diffusers/blob/e55687e1e15407f60f32242027b7bb8170e58266/src/diffusers/pipelines/stable_diffusion/safety_checker.py#L32) for screening against harmful content.
* `"scheduler"`: an instance of [PNDMScheduler](/docs/diffusers/main/en/api/schedulers/pndm#diffusers.PNDMScheduler).
* `"text_encoder"`: a [CLIPTextModel](https://huggingface.co/docs/transformers/main/en/model_doc/clip#transformers.CLIPTextModel) from 🤗 Transformers.
* `"tokenizer"`: a [CLIPTokenizer](https://huggingface.co/docs/transformers/main/en/model_doc/clip#transformers.CLIPTokenizer) from 🤗 Transformers.
* `"unet"`: an instance of [UNet2DConditionModel](/docs/diffusers/main/en/api/models/unet2d-cond#diffusers.UNet2DConditionModel).
* `"vae"`: an instance of [AutoencoderKL](/docs/diffusers/main/en/api/models/autoencoderkl#diffusers.AutoencoderKL).
Copied
StableDiffusionPipeline {
"feature\_extractor": \[
"transformers",
"CLIPImageProcessor"
\],
"safety\_checker": \[
"stable\_diffusion",
"StableDiffusionSafetyChecker"
\],
"scheduler": \[
"diffusers",
"PNDMScheduler"
\],
"text\_encoder": \[
"transformers",
"CLIPTextModel"
\],
"tokenizer": \[
"transformers",
"CLIPTokenizer"
\],
"unet": \[
"diffusers",
"UNet2DConditionModel"
\],
"vae": \[
"diffusers",
"AutoencoderKL"
\]
}
Compare the components of the pipeline instance to the [`stable-diffusion-v1-5/stable-diffusion-v1-5`](https://huggingface.co/stable-diffusion-v1-5/stable-diffusion-v1-5/tree/main) folder structure, and you’ll see there is a separate folder for each of the components in the repository:
Copied
.
├── feature\_extractor
│ └── preprocessor\_config.json
├── model\_index.json
├── safety\_checker
│ ├── config.json
| ├── model.fp16.safetensors
│ ├── model.safetensors
│ ├── pytorch\_model.bin
| └── pytorch\_model.fp16.bin
├── scheduler
│ └── scheduler\_config.json
├── text\_encoder
│ ├── config.json
| ├── model.fp16.safetensors
│ ├── model.safetensors
│ |── pytorch\_model.bin
| └── pytorch\_model.fp16.bin
├── tokenizer
│ ├── merges.txt
│ ├── special\_tokens\_map.json
│ ├── tokenizer\_config.json
│ └── vocab.json
├── unet
│ ├── config.json
│ ├── diffusion\_pytorch\_model.bin
| |── diffusion\_pytorch\_model.fp16.bin
│ |── diffusion\_pytorch\_model.f16.safetensors
│ |── diffusion\_pytorch\_model.non\_ema.bin
│ |── diffusion\_pytorch\_model.non\_ema.safetensors
│ └── diffusion\_pytorch\_model.safetensors
|── vae
. ├── config.json
. ├── diffusion\_pytorch\_model.bin
├── diffusion\_pytorch\_model.fp16.bin
├── diffusion\_pytorch\_model.fp16.safetensors
└── diffusion\_pytorch\_model.safetensors
You can access each of the components of the pipeline as an attribute to view its configuration:
Copied
pipeline.tokenizer
CLIPTokenizer(
name\_or\_path="/root/.cache/huggingface/hub/models--runwayml--stable-diffusion-v1-5/snapshots/39593d5650112b4cc580433f6b0435385882d819/tokenizer",
vocab\_size=49408,
model\_max\_length=77,
is\_fast=False,
padding\_side="right",
truncation\_side="right",
special\_tokens={
"bos\_token": AddedToken("<|startoftext|>", rstrip=False, lstrip=False, single\_word=False, normalized=True),
"eos\_token": AddedToken("<|endoftext|>", rstrip=False, lstrip=False, single\_word=False, normalized=True),
"unk\_token": AddedToken("<|endoftext|>", rstrip=False, lstrip=False, single\_word=False, normalized=True),
"pad\_token": "<|endoftext|>",
},
clean\_up\_tokenization\_spaces=True
)
Every pipeline expects a [`model_index.json`](https://huggingface.co/stable-diffusion-v1-5/stable-diffusion-v1-5/blob/main/model_index.json) file that tells the [DiffusionPipeline](/docs/diffusers/main/en/api/pipelines/overview#diffusers.DiffusionPipeline):
* which pipeline class to load from `_class_name`
* which version of 🧨 Diffusers was used to create the model in `_diffusers_version`
* what components from which library are stored in the subfolders (`name` corresponds to the component and subfolder name, `library` corresponds to the name of the library to load the class from, and `class` corresponds to the class name)
Copied
{
"\_class\_name": "StableDiffusionPipeline",
"\_diffusers\_version": "0.6.0",
"feature\_extractor": \[
"transformers",
"CLIPImageProcessor"
\],
"safety\_checker": \[
"stable\_diffusion",
"StableDiffusionSafetyChecker"
\],
"scheduler": \[
"diffusers",
"PNDMScheduler"
\],
"text\_encoder": \[
"transformers",
"CLIPTextModel"
\],
"tokenizer": \[
"transformers",
"CLIPTokenizer"
\],
"unet": \[
"diffusers",
"UNet2DConditionModel"
\],
"vae": \[
"diffusers",
"AutoencoderKL"
\]
}
[< \> Update on GitHub](https://github.com/huggingface/diffusers/blob/main/docs/source/en/using-diffusers/loading.md)
[←Working with big models](/docs/diffusers/main/en/tutorials/inference_with_big_models) [Load community pipelines and components→](/docs/diffusers/main/en/using-diffusers/custom_pipeline_overview) |