File size: 9,986 Bytes
a03b3ba |
1 2 3 4 5 6 7 8 9 10 11 12 13 14 15 16 17 18 19 20 21 22 23 24 25 26 27 28 29 30 31 32 33 34 35 36 37 38 39 40 41 42 43 44 45 46 47 48 49 50 51 52 53 54 55 56 57 58 59 60 61 62 63 64 65 66 67 68 69 70 71 72 73 74 75 76 77 78 79 80 81 82 83 84 85 86 87 88 89 90 91 92 93 94 95 96 97 98 99 100 101 102 103 104 105 106 107 108 109 110 111 112 113 114 115 116 117 118 119 120 121 122 123 124 125 126 127 128 129 130 131 132 133 134 135 136 137 138 139 140 141 142 143 144 145 146 147 148 149 150 151 152 153 154 155 156 157 158 159 160 161 162 163 164 165 166 167 168 169 170 171 172 173 174 175 176 177 178 179 180 181 182 183 184 185 186 187 188 189 190 191 192 193 194 195 196 197 198 199 200 201 202 203 204 205 206 207 208 209 210 211 212 213 214 215 216 217 218 219 220 221 222 223 224 225 226 227 228 229 230 231 232 233 234 235 236 237 238 239 240 241 242 243 244 245 246 247 248 249 250 251 252 253 254 255 256 257 258 259 |
# Getting Started with the Gradio Python client
Tags: CLIENT, API, SPACES
The Gradio Python client makes it very easy to use any Gradio app as an API. As an example, consider this [Hugging Face Space that transcribes audio files](https://huggingface.co/spaces/abidlabs/whisper) that are recorded from the microphone.
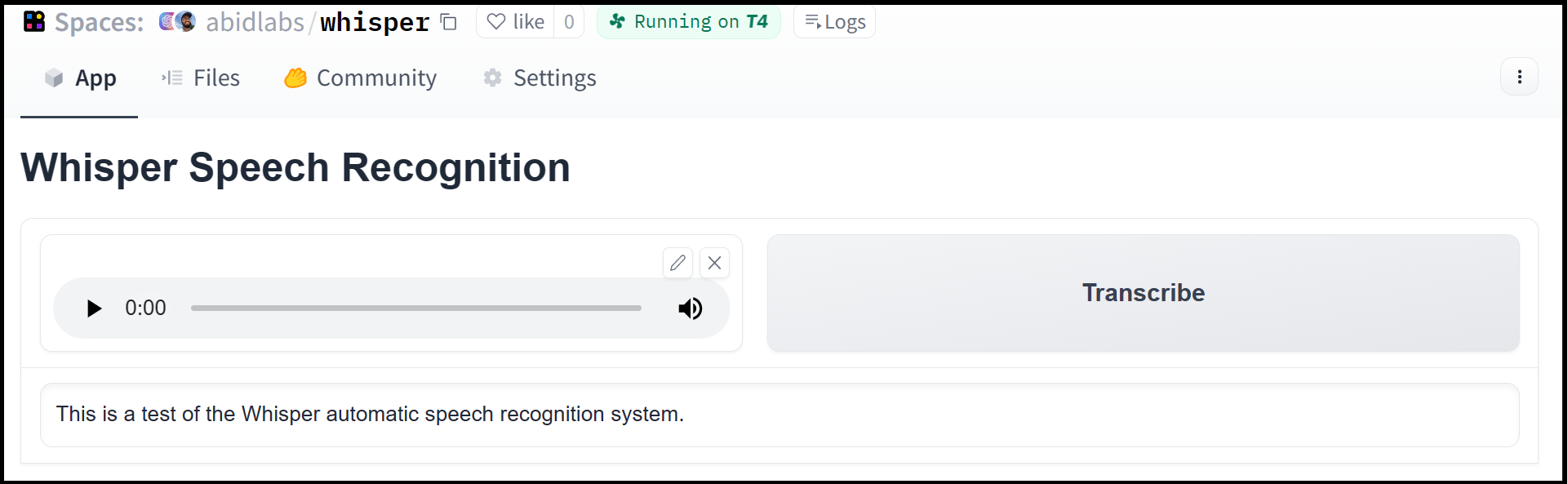
Using the `gradio_client` library, we can easily use the Gradio as an API to transcribe audio files programmatically.
Here's the entire code to do it:
```python
from gradio_client import Client
client = Client("abidlabs/whisper")
client.predict("audio_sample.wav")
>> "This is a test of the whisper speech recognition model."
```
The Gradio client works with any hosted Gradio app, whether it be an image generator, a text summarizer, a stateful chatbot, a tax calculator, or anything else! The Gradio Client is mostly used with apps hosted on [Hugging Face Spaces](https://hf.space), but your app can be hosted anywhere, such as your own server.
**Prerequisites**: To use the Gradio client, you do _not_ need to know the `gradio` library in great detail. However, it is helpful to have general familiarity with Gradio's concepts of input and output components.
## Installation
If you already have a recent version of `gradio`, then the `gradio_client` is included as a dependency.
Otherwise, the lightweight `gradio_client` package can be installed from pip (or pip3) and is tested to work with Python versions 3.9 or higher:
```bash
$ pip install gradio_client
```
## Connecting to a running Gradio App
Start by connecting instantiating a `Client` object and connecting it to a Gradio app that is running on Hugging Face Spaces or generally anywhere on the web.
## Connecting to a Hugging Face Space
```python
from gradio_client import Client
client = Client("abidlabs/en2fr") # a Space that translates from English to French
```
You can also connect to private Spaces by passing in your HF token with the `hf_token` parameter. You can get your HF token here: https://huggingface.co/settings/tokens
```python
from gradio_client import Client
client = Client("abidlabs/my-private-space", hf_token="...")
```
## Duplicating a Space for private use
While you can use any public Space as an API, you may get rate limited by Hugging Face if you make too many requests. For unlimited usage of a Space, simply duplicate the Space to create a private Space,
and then use it to make as many requests as you'd like!
The `gradio_client` includes a class method: `Client.duplicate()` to make this process simple (you'll need to pass in your [Hugging Face token](https://huggingface.co/settings/tokens) or be logged in using the Hugging Face CLI):
```python
import os
from gradio_client import Client
HF_TOKEN = os.environ.get("HF_TOKEN")
client = Client.duplicate("abidlabs/whisper", hf_token=HF_TOKEN)
client.predict("audio_sample.wav")
>> "This is a test of the whisper speech recognition model."
```
If you have previously duplicated a Space, re-running `duplicate()` will _not_ create a new Space. Instead, the Client will attach to the previously-created Space. So it is safe to re-run the `Client.duplicate()` method multiple times.
**Note:** if the original Space uses GPUs, your private Space will as well, and your Hugging Face account will get billed based on the price of the GPU. To minimize charges, your Space will automatically go to sleep after 1 hour of inactivity. You can also set the hardware using the `hardware` parameter of `duplicate()`.
## Connecting a general Gradio app
If your app is running somewhere else, just provide the full URL instead, including the "http://" or "https://". Here's an example of making predictions to a Gradio app that is running on a share URL:
```python
from gradio_client import Client
client = Client("https://bec81a83-5b5c-471e.gradio.live")
```
## Inspecting the API endpoints
Once you have connected to a Gradio app, you can view the APIs that are available to you by calling the `Client.view_api()` method. For the Whisper Space, we see the following:
```bash
Client.predict() Usage Info
---------------------------
Named API endpoints: 1
- predict(input_audio, api_name="/predict") -> value_0
Parameters:
- [Audio] input_audio: str (filepath or URL)
Returns:
- [Textbox] value_0: str (value)
```
This shows us that we have 1 API endpoint in this space, and shows us how to use the API endpoint to make a prediction: we should call the `.predict()` method (which we will explore below), providing a parameter `input_audio` of type `str`, which is a `filepath or URL`.
We should also provide the `api_name='/predict'` argument to the `predict()` method. Although this isn't necessary if a Gradio app has only 1 named endpoint, it does allow us to call different endpoints in a single app if they are available. If an app has unnamed API endpoints, these can also be displayed by running `.view_api(all_endpoints=True)`.
## Making a prediction
The simplest way to make a prediction is simply to call the `.predict()` function with the appropriate arguments:
```python
from gradio_client import Client
client = Client("abidlabs/en2fr", api_name='/predict')
client.predict("Hello")
>> Bonjour
```
If there are multiple parameters, then you should pass them as separate arguments to `.predict()`, like this:
```python
from gradio_client import Client
client = Client("gradio/calculator")
client.predict(4, "add", 5)
>> 9.0
```
For certain inputs, such as images, you should pass in the filepath or URL to the file. Likewise, for the corresponding output types, you will get a filepath or URL returned.
```python
from gradio_client import Client
client = Client("abidlabs/whisper")
client.predict("https://audio-samples.github.io/samples/mp3/blizzard_unconditional/sample-0.mp3")
>> "My thought I have nobody by a beauty and will as you poured. Mr. Rochester is serve in that so don't find simpus, and devoted abode, to at might in a r—"
```
## Running jobs asynchronously
Oe should note that `.predict()` is a _blocking_ operation as it waits for the operation to complete before returning the prediction.
In many cases, you may be better off letting the job run in the background until you need the results of the prediction. You can do this by creating a `Job` instance using the `.submit()` method, and then later calling `.result()` on the job to get the result. For example:
```python
from gradio_client import Client
client = Client(space="abidlabs/en2fr")
job = client.submit("Hello", api_name="/predict") # This is not blocking
# Do something else
job.result() # This is blocking
>> Bonjour
```
## Adding callbacks
Alternatively, one can add one or more callbacks to perform actions after the job has completed running, like this:
```python
from gradio_client import Client
def print_result(x):
print("The translated result is: {x}")
client = Client(space="abidlabs/en2fr")
job = client.submit("Hello", api_name="/predict", result_callbacks=[print_result])
# Do something else
>> The translated result is: Bonjour
```
## Status
The `Job` object also allows you to get the status of the running job by calling the `.status()` method. This returns a `StatusUpdate` object with the following attributes: `code` (the status code, one of a set of defined strings representing the status. See the `utils.Status` class), `rank` (the current position of this job in the queue), `queue_size` (the total queue size), `eta` (estimated time this job will complete), `success` (a boolean representing whether the job completed successfully), and `time` (the time that the status was generated).
```py
from gradio_client import Client
client = Client(src="gradio/calculator")
job = client.submit(5, "add", 4, api_name="/predict")
job.status()
>> <Status.STARTING: 'STARTING'>
```
_Note_: The `Job` class also has a `.done()` instance method which returns a boolean indicating whether the job has completed.
## Cancelling Jobs
The `Job` class also has a `.cancel()` instance method that cancels jobs that have been queued but not started. For example, if you run:
```py
client = Client("abidlabs/whisper")
job1 = client.submit("audio_sample1.wav")
job2 = client.submit("audio_sample2.wav")
job1.cancel() # will return False, assuming the job has started
job2.cancel() # will return True, indicating that the job has been canceled
```
If the first job has started processing, then it will not be canceled. If the second job
has not yet started, it will be successfully canceled and removed from the queue.
## Generator Endpoints
Some Gradio API endpoints do not return a single value, rather they return a series of values. You can get the series of values that have been returned at any time from such a generator endpoint by running `job.outputs()`:
```py
from gradio_client import Client
client = Client(src="gradio/count_generator")
job = client.submit(3, api_name="/count")
while not job.done():
time.sleep(0.1)
job.outputs()
>> ['0', '1', '2']
```
Note that running `job.result()` on a generator endpoint only gives you the _first_ value returned by the endpoint.
The `Job` object is also iterable, which means you can use it to display the results of a generator function as they are returned from the endpoint. Here's the equivalent example using the `Job` as a generator:
```py
from gradio_client import Client
client = Client(src="gradio/count_generator")
job = client.submit(3, api_name="/count")
for o in job:
print(o)
>> 0
>> 1
>> 2
```
You can also cancel jobs that that have iterative outputs, in which case the job will finish as soon as the current iteration finishes running.
```py
from gradio_client import Client
import time
client = Client("abidlabs/test-yield")
job = client.submit("abcdef")
time.sleep(3)
job.cancel() # job cancels after 2 iterations
```
|