Spaces:
Running
Running
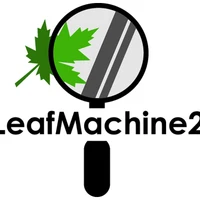
Major update. Support for 15 LLMs, World Flora Online taxonomy validation, geolocation, 2 OCR methods, significant UI changes, stability improvements, consistent JSON parsing
c824976
import os, yaml, datetime, argparse, re, cv2, random, shutil, tiktoken, json, csv | |
from collections import Counter | |
import pandas as pd | |
from pathlib import Path | |
from dataclasses import dataclass | |
from tqdm import tqdm | |
import numpy as np | |
import concurrent.futures | |
from time import perf_counter | |
import torch | |
from vouchervision.model_maps import ModelMaps | |
''' | |
TIFF --> DNG | |
Install | |
https://helpx.adobe.com/camera-raw/using/adobe-dng-converter.html | |
Read | |
https://helpx.adobe.com/content/dam/help/en/photoshop/pdf/dng_commandline.pdf | |
''' | |
# https://stackoverflow.com/questions/287871/how-do-i-print-colored-text-to-the-terminal | |
def validate_dir(dir): | |
if not os.path.exists(dir): | |
os.makedirs(dir, exist_ok=True) | |
def get_cfg_from_full_path(path_cfg): | |
with open(path_cfg, "r") as ymlfile: | |
cfg = yaml.full_load(ymlfile) | |
return cfg | |
def num_tokens_from_string(string, encoding_name): | |
try: | |
# Ensure the encoding is obtained correctly. | |
encoding = tiktoken.get_encoding(encoding_name) | |
# Convert dictionary to string if it is not already a string | |
if isinstance(string, dict): | |
string = json.dumps(string, ensure_ascii=False) | |
# Encode the string and return the number of tokens. | |
num_tokens = len(encoding.encode(string)) | |
except Exception as e: | |
# If there's any error, log it and return 0. | |
print(f"An error occurred: {e}") | |
num_tokens = 0 | |
return num_tokens | |
def add_to_expense_report(dir_home, data): | |
path_expense_report = os.path.join(dir_home, 'expense_report','expense_report.csv') | |
# Check if the file exists | |
file_exists = os.path.isfile(path_expense_report) | |
# Open the file in append mode if it exists, or write mode if it doesn't | |
mode = 'a' if file_exists else 'w' | |
with open(path_expense_report, mode=mode, newline='') as file: | |
writer = csv.writer(file) | |
# If the file does not exist, write the header first | |
if not file_exists: | |
writer.writerow(['run','date','api_version','total_cost', 'n_images', 'tokens_in', 'tokens_out', 'rate_in', 'rate_out', 'cost_in', 'cost_out',]) | |
# Write the data row | |
writer.writerow(data) | |
def save_token_info_as_csv(Dirs, LLM_version0, path_api_cost, total_tokens_in, total_tokens_out, n_images, dir_home, logger): | |
if path_api_cost: | |
LLM_version = ModelMaps.get_version_mapping_cost(LLM_version0) | |
# Define the CSV file path | |
csv_file_path = os.path.join(Dirs.path_cost, Dirs.run_name + '.csv') | |
cost_in, cost_out, total_cost, rate_in, rate_out = calculate_cost(LLM_version, path_api_cost, total_tokens_in, total_tokens_out) | |
# The data to be written to the CSV file | |
data = [Dirs.run_name, get_datetime(),LLM_version, total_cost, n_images, total_tokens_in, total_tokens_out, rate_in, rate_out, cost_in, cost_out,] | |
# Open the file in write mode | |
with open(csv_file_path, mode='w', newline='') as file: | |
writer = csv.writer(file) | |
# Write the header | |
writer.writerow(['run','date','api_version','total_cost', 'n_images', 'tokens_in', 'tokens_out', 'rate_in', 'rate_out', 'cost_in', 'cost_out',]) | |
# Write the data | |
writer.writerow(data) | |
# Create a summary string | |
cost_summary = (f"Cost Summary for {Dirs.run_name}:\n" | |
f" API Cost In: ${rate_in} per 1000 Tokens\n" | |
f" API Cost Out: ${rate_out} per 1000 Tokens\n" | |
f" Tokens In: {total_tokens_in} - Cost: ${cost_in:.4f}\n" | |
f" Tokens Out: {total_tokens_out} - Cost: ${cost_out:.4f}\n" | |
f" Images Processed: {n_images}\n" | |
f" Total Cost: ${total_cost:.4f}") | |
add_to_expense_report(dir_home, data) | |
logger.info(cost_summary) | |
return total_cost | |
else: | |
return None #TODO add config tests to expense_report | |
def summarize_expense_report(path_expense_report): | |
# Initialize counters and sums | |
run_count = 0 | |
total_cost_sum = 0 | |
tokens_in_sum = 0 | |
tokens_out_sum = 0 | |
rate_in_sum = 0 | |
rate_out_sum = 0 | |
cost_in_sum = 0 | |
cost_out_sum = 0 | |
n_images_sum = 0 | |
api_version_counts = Counter() | |
# Try to read the CSV file into a DataFrame | |
try: | |
df = pd.read_csv(path_expense_report) | |
# Process each row in the DataFrame | |
for index, row in df.iterrows(): | |
run_count += 1 | |
total_cost_sum += row['total_cost'] | |
tokens_in_sum += row['tokens_in'] | |
tokens_out_sum += row['tokens_out'] | |
rate_in_sum += row['rate_in'] | |
rate_out_sum += row['rate_out'] | |
cost_in_sum += row['cost_in'] | |
cost_out_sum += row['cost_out'] | |
n_images_sum += row['n_images'] | |
api_version_counts[row['api_version']] += 1 | |
except FileNotFoundError: | |
print(f"The file {path_expense_report} does not exist.") | |
return None | |
# Calculate API version percentages | |
api_version_percentages = {version: (count / run_count) * 100 for version, count in api_version_counts.items()} | |
# Calculate cost per image for each API version | |
cost_per_image_dict = {} | |
for version, count in api_version_counts.items(): | |
total_cost = df[df['api_version'] == version]['total_cost'].sum() | |
n_images = df[df['api_version'] == version]['n_images'].sum() | |
cost_per_image = total_cost / n_images if n_images > 0 else 0 | |
cost_per_image_dict[version] = cost_per_image | |
# Return the DataFrame and all summaries | |
return { | |
'run_count': run_count, | |
'total_cost_sum': total_cost_sum, | |
'tokens_in_sum': tokens_in_sum, | |
'tokens_out_sum': tokens_out_sum, | |
'rate_in_sum': rate_in_sum, | |
'rate_out_sum': rate_out_sum, | |
'cost_in_sum': cost_in_sum, | |
'cost_out_sum': cost_out_sum, | |
'n_images_sum':n_images_sum, | |
'api_version_percentages': api_version_percentages, | |
'cost_per_image': cost_per_image_dict | |
}, df | |
def calculate_cost(LLM_version, path_api_cost, total_tokens_in, total_tokens_out): | |
# Load the rates from the YAML file | |
with open(path_api_cost, 'r') as file: | |
cost_data = yaml.safe_load(file) | |
# Get the rates for the specified LLM version | |
if LLM_version in cost_data: | |
rates = cost_data[LLM_version] | |
cost_in = rates['in'] * (total_tokens_in/1000) | |
cost_out = rates['out'] * (total_tokens_out/1000) | |
total_cost = cost_in + cost_out | |
else: | |
raise ValueError(f"LLM version {LLM_version} not found in the cost data") | |
return cost_in, cost_out, total_cost, rates['in'], rates['out'] | |
def create_google_ocr_yaml_config(output_file, dir_images_local, dir_output): | |
# Define the configuration dictionary | |
config = { | |
'leafmachine': { | |
'LLM_version': 'PaLM 2', | |
'archival_component_detector': { | |
'detector_iteration': 'PREP_final', | |
'detector_type': 'Archival_Detector', | |
'detector_version': 'PREP_final', | |
'detector_weights': 'best.pt', | |
'do_save_prediction_overlay_images': True, | |
'ignore_objects_for_overlay': [], | |
'minimum_confidence_threshold': 0.5 | |
}, | |
'cropped_components': { | |
'binarize_labels': False, | |
'binarize_labels_skeletonize': False, | |
'do_save_cropped_annotations': True, | |
'save_cropped_annotations': ['label', 'barcode'], | |
'save_per_annotation_class': True, | |
'save_per_image': False | |
}, | |
'data': { | |
'do_apply_conversion_factor': False, | |
'include_darwin_core_data_from_combined_file': False, | |
'save_individual_csv_files_landmarks': False, | |
'save_individual_csv_files_measurements': False, | |
'save_individual_csv_files_rulers': False, | |
'save_individual_efd_files': False, | |
'save_json_measurements': False, | |
'save_json_rulers': False | |
}, | |
'do': { | |
'check_for_corrupt_images_make_vertical': True, | |
'check_for_illegal_filenames': False | |
}, | |
'logging': { | |
'log_level': None | |
}, | |
'modules': { | |
'specimen_crop': True | |
}, | |
'overlay': { | |
'alpha_transparency_archival': 0.3, | |
'alpha_transparency_plant': 0, | |
'alpha_transparency_seg_partial_leaf': 0.3, | |
'alpha_transparency_seg_whole_leaf': 0.4, | |
'ignore_archival_detections_classes': [], | |
'ignore_landmark_classes': [], | |
'ignore_plant_detections_classes': ['leaf_whole', 'specimen'], | |
'line_width_archival': 12, | |
'line_width_efd': 12, | |
'line_width_plant': 12, | |
'line_width_seg': 12, | |
'overlay_background_color': 'black', | |
'overlay_dpi': 300, | |
'save_overlay_to_jpgs': True, | |
'save_overlay_to_pdf': False, | |
'show_archival_detections': True, | |
'show_landmarks': True, | |
'show_plant_detections': True, | |
'show_segmentations': True | |
}, | |
'print': { | |
'optional_warnings': True, | |
'verbose': True | |
}, | |
'project': { | |
'batch_size': 500, | |
'build_new_embeddings_database': False, | |
'catalog_numerical_only': False, | |
'continue_run_from_partial_xlsx': '', | |
'delete_all_temps': False, | |
'delete_temps_keep_VVE': False, | |
'dir_images_local': dir_images_local, | |
'dir_output': dir_output, | |
'embeddings_database_name': 'SLTP_UM_AllAsiaMinimalInRegion', | |
'image_location': 'local', | |
'num_workers': 1, | |
'path_to_domain_knowledge_xlsx': '', | |
'prefix_removal': '', | |
'prompt_version': 'Version 2 PaLM 2', | |
'run_name': 'google_vision_ocr_test', | |
'suffix_removal': '', | |
'use_domain_knowledge': False | |
}, | |
'use_RGB_label_images': False | |
} | |
} | |
# Generate the YAML string from the data structure | |
validate_dir(os.path.dirname(output_file)) | |
yaml_str = yaml.dump(config, sort_keys=False) | |
# Write the YAML string to a file | |
with open(output_file, 'w') as file: | |
file.write(yaml_str) | |
def test_GPU(): | |
info = [] | |
success = False | |
if torch.cuda.is_available(): | |
num_gpus = torch.cuda.device_count() | |
info.append(f"Number of GPUs: {num_gpus}") | |
for i in range(num_gpus): | |
gpu = torch.cuda.get_device_properties(i) | |
info.append(f"GPU {i}: {gpu.name}") | |
success = True | |
else: | |
info.append("No GPU found!") | |
info.append("LeafMachine2 collages will run slowly, trOCR may not be available.") | |
return success, info | |
# def load_cfg(pathToCfg): | |
# try: | |
# with open(os.path.join(pathToCfg,"LeafMachine2.yaml"), "r") as ymlfile: | |
# cfg = yaml.full_load(ymlfile) | |
# except: | |
# with open(os.path.join(os.path.dirname(os.path.dirname(pathToCfg)),"LeafMachine2.yaml"), "r") as ymlfile: | |
# cfg = yaml.full_load(ymlfile) | |
# return cfg | |
# def load_cfg_VV(pathToCfg): | |
# try: | |
# with open(os.path.join(pathToCfg,"VoucherVision.yaml"), "r") as ymlfile: | |
# cfg = yaml.full_load(ymlfile) | |
# except: | |
# with open(os.path.join(os.path.dirname(os.path.dirname(pathToCfg)),"VoucherVision.yaml"), "r") as ymlfile: | |
# cfg = yaml.full_load(ymlfile) | |
# return cfg | |
def load_cfg(pathToCfg, system='LeafMachine2'): | |
if system not in ['LeafMachine2', 'VoucherVision', 'SpecimenCrop']: | |
raise ValueError("Invalid system. Expected 'LeafMachine2', 'VoucherVision' or 'SpecimenCrop'.") | |
try: | |
with open(os.path.join(pathToCfg, f"{system}.yaml"), "r") as ymlfile: | |
cfg = yaml.full_load(ymlfile) | |
except: | |
with open(os.path.join(os.path.dirname(os.path.dirname(pathToCfg)), f"{system}.yaml"), "r") as ymlfile: | |
cfg = yaml.full_load(ymlfile) | |
return cfg | |
def import_csv(full_path): | |
csv_data = pd.read_csv(full_path,sep=',',header=0, low_memory=False, dtype=str) | |
return csv_data | |
def import_tsv(full_path): | |
csv_data = pd.read_csv(full_path,sep='\t',header=0, low_memory=False, dtype=str) | |
return csv_data | |
def parse_cfg(): | |
parser = argparse.ArgumentParser( | |
description='Parse inputs to read config file', | |
formatter_class=argparse.ArgumentDefaultsHelpFormatter) | |
optional_args = parser._action_groups.pop() | |
required_args = parser.add_argument_group('MANDATORY arguments') | |
required_args.add_argument('--path-to-cfg', | |
type=str, | |
required=True, | |
help='Path to config file - LeafMachine.yaml. Do not include the file name, just the parent dir.') | |
parser._action_groups.append(optional_args) | |
args = parser.parse_args() | |
return args | |
def check_for_subdirs(cfg): | |
original_in = cfg['leafmachine']['project']['dir_images_local'] | |
dirs_list = [] | |
run_name = [] | |
has_subdirs = False | |
if os.path.isdir(original_in): | |
# list contents of the directory | |
contents = os.listdir(original_in) | |
# check if any of the contents is a directory | |
subdirs = [f for f in contents if os.path.isdir(os.path.join(original_in, f))] | |
if len(subdirs) > 0: | |
print("The directory contains subdirectories:") | |
for subdir in subdirs: | |
has_subdirs = True | |
print(os.path.join(original_in, subdir)) | |
dirs_list.append(os.path.join(original_in, subdir)) | |
run_name.append(subdir) | |
else: | |
print("The directory does not contain any subdirectories.") | |
dirs_list.append(original_in) | |
run_name.append(cfg['leafmachine']['project']['run_name']) | |
else: | |
print("The specified path is not a directory.") | |
return run_name, dirs_list, has_subdirs | |
def check_for_subdirs_VV(cfg): | |
original_in = cfg['leafmachine']['project']['dir_images_local'] | |
dirs_list = [] | |
run_name = [] | |
has_subdirs = False | |
if os.path.isdir(original_in): | |
dirs_list.append(original_in) | |
run_name.append(os.path.basename(os.path.normpath(original_in))) | |
# list contents of the directory | |
contents = os.listdir(original_in) | |
# check if any of the contents is a directory | |
subdirs = [f for f in contents if os.path.isdir(os.path.join(original_in, f))] | |
if len(subdirs) > 0: | |
print("The directory contains subdirectories:") | |
for subdir in subdirs: | |
has_subdirs = True | |
print(os.path.join(original_in, subdir)) | |
dirs_list.append(os.path.join(original_in, subdir)) | |
run_name.append(subdir) | |
else: | |
print("The directory does not contain any subdirectories.") | |
dirs_list.append(original_in) | |
run_name.append(cfg['leafmachine']['project']['run_name']) | |
else: | |
print("The specified path is not a directory.") | |
return run_name, dirs_list, has_subdirs | |
def get_datetime(): | |
day = "_".join([str(datetime.datetime.now().strftime("%Y")),str(datetime.datetime.now().strftime("%m")),str(datetime.datetime.now().strftime("%d"))]) | |
time = "-".join([str(datetime.datetime.now().strftime("%H")),str(datetime.datetime.now().strftime("%M")),str(datetime.datetime.now().strftime("%S"))]) | |
new_time = "__".join([day,time]) | |
return new_time | |
def save_config_file(cfg, logger, Dirs): | |
logger.info("Save config file") | |
name_yaml = ''.join([Dirs.run_name,'.yaml']) | |
write_yaml(cfg, os.path.join(Dirs.path_config_file, name_yaml)) | |
def write_yaml(cfg, path_cfg): | |
with open(path_cfg, 'w') as file: | |
yaml.dump(cfg, file, sort_keys=False) | |
def split_into_batches(Project, logger, cfg): | |
logger.name = 'Creating Batches' | |
n_batches, n_images = Project.process_in_batches(cfg) | |
m = f'Created {n_batches} Batches to Process {n_images} Images' | |
logger.info(m) | |
return Project, n_batches, m | |
def make_images_in_dir_vertical(dir_images_unprocessed, cfg): | |
skip_vertical = cfg['leafmachine']['do']['skip_vertical'] | |
if cfg['leafmachine']['do']['check_for_corrupt_images_make_vertical']: | |
n_rotate = 0 | |
n_corrupt = 0 | |
n_total = len(os.listdir(dir_images_unprocessed)) | |
for image_name_jpg in tqdm(os.listdir(dir_images_unprocessed), desc=f'{bcolors.BOLD} Checking Image Dimensions{bcolors.ENDC}',colour="cyan",position=0,total = n_total): | |
if image_name_jpg.endswith((".jpg",".JPG",".jpeg",".JPEG")): | |
try: | |
image = cv2.imread(os.path.join(dir_images_unprocessed, image_name_jpg)) | |
if not skip_vertical: | |
h, w, img_c = image.shape | |
image, img_h, img_w, did_rotate = make_image_vertical(image, h, w, do_rotate_180=False) | |
if did_rotate: | |
n_rotate += 1 | |
cv2.imwrite(os.path.join(dir_images_unprocessed,image_name_jpg), image) | |
except: | |
n_corrupt +=1 | |
os.remove(os.path.join(dir_images_unprocessed, image_name_jpg)) | |
# TODO check that below works as intended | |
elif image_name_jpg.endswith((".tiff",".tif",".png",".PNG",".TIFF",".TIF",".jp2",".JP2",".bmp",".BMP",".dib",".DIB")): | |
try: | |
image = cv2.imread(os.path.join(dir_images_unprocessed, image_name_jpg)) | |
if not skip_vertical: | |
h, w, img_c = image.shape | |
image, img_h, img_w, did_rotate = make_image_vertical(image, h, w, do_rotate_180=False) | |
if did_rotate: | |
n_rotate += 1 | |
image_name_jpg = '.'.join([image_name_jpg.split('.')[0], 'jpg']) | |
cv2.imwrite(os.path.join(dir_images_unprocessed,image_name_jpg), image) | |
except: | |
n_corrupt +=1 | |
os.remove(os.path.join(dir_images_unprocessed, image_name_jpg)) | |
m = ''.join(['Number of Images Rotated: ', str(n_rotate)]) | |
Print_Verbose(cfg, 2, m).bold() | |
m2 = ''.join(['Number of Images Corrupted: ', str(n_corrupt)]) | |
if n_corrupt > 0: | |
Print_Verbose(cfg, 2, m2).warning | |
else: | |
Print_Verbose(cfg, 2, m2).bold | |
def make_image_vertical(image, h, w, do_rotate_180): | |
did_rotate = False | |
if do_rotate_180: | |
# try: | |
image = cv2.rotate(image, cv2.ROTATE_180) | |
img_h, img_w, img_c = image.shape | |
did_rotate = True | |
# print(" Rotated 180") | |
else: | |
if h < w: | |
# try: | |
image = cv2.rotate(image, cv2.ROTATE_90_CLOCKWISE) | |
img_h, img_w, img_c = image.shape | |
did_rotate = True | |
# print(" Rotated 90 CW") | |
elif h >= w: | |
image = image | |
img_h = h | |
img_w = w | |
# print(" Not Rotated") | |
return image, img_h, img_w, did_rotate | |
def make_image_horizontal(image, h, w, do_rotate_180): | |
if h > w: | |
if do_rotate_180: | |
image = cv2.rotate(image, cv2.ROTATE_180) | |
return cv2.rotate(image, cv2.ROTATE_90_COUNTERCLOCKWISE), w, h, True | |
return image, w, h, False | |
def make_images_in_dir_horizontal(dir_images_unprocessed, cfg): | |
# if cfg['leafmachine']['do']['check_for_corrupt_images_make_horizontal']: | |
n_rotate = 0 | |
n_corrupt = 0 | |
n_total = len(os.listdir(dir_images_unprocessed)) | |
for image_name_jpg in tqdm(os.listdir(dir_images_unprocessed), desc=f'{bcolors.BOLD} Checking Image Dimensions{bcolors.ENDC}', colour="cyan", position=0, total=n_total): | |
if image_name_jpg.endswith((".jpg",".JPG",".jpeg",".JPEG")): | |
try: | |
image = cv2.imread(os.path.join(dir_images_unprocessed, image_name_jpg)) | |
h, w, img_c = image.shape | |
image, img_h, img_w, did_rotate = make_image_horizontal(image, h, w, do_rotate_180=False) | |
if did_rotate: | |
n_rotate += 1 | |
cv2.imwrite(os.path.join(dir_images_unprocessed,image_name_jpg), image) | |
except: | |
n_corrupt +=1 | |
os.remove(os.path.join(dir_images_unprocessed, image_name_jpg)) | |
# TODO check that below works as intended | |
elif image_name_jpg.endswith((".tiff",".tif",".png",".PNG",".TIFF",".TIF",".jp2",".JP2",".bmp",".BMP",".dib",".DIB")): | |
try: | |
image = cv2.imread(os.path.join(dir_images_unprocessed, image_name_jpg)) | |
h, w, img_c = image.shape | |
image, img_h, img_w, did_rotate = make_image_horizontal(image, h, w, do_rotate_180=False) | |
if did_rotate: | |
n_rotate += 1 | |
image_name_jpg = '.'.join([image_name_jpg.split('.')[0], 'jpg']) | |
cv2.imwrite(os.path.join(dir_images_unprocessed,image_name_jpg), image) | |
except: | |
n_corrupt +=1 | |
os.remove(os.path.join(dir_images_unprocessed, image_name_jpg)) | |
m = ''.join(['Number of Images Rotated: ', str(n_rotate)]) | |
print(m) | |
# Print_Verbose(cfg, 2, m).bold() | |
m2 = ''.join(['Number of Images Corrupted: ', str(n_corrupt)]) | |
print(m2) | |
class Print_Verbose_Error(): | |
cfg: str = '' | |
indent_level: int = 0 | |
message: str = '' | |
error: str = '' | |
def __init__(self, cfg,indent_level,message,error) -> None: | |
self.cfg = cfg | |
self.indent_level = indent_level | |
self.message = message | |
self.error = error | |
def print_error_to_console(self): | |
white_space = " " * 5 * self.indent_level | |
if self.cfg['leafmachine']['print']['optional_warnings']: | |
print(f"{bcolors.FAIL}{white_space}{self.message} ERROR: {self.error}{bcolors.ENDC}") | |
def print_warning_to_console(self): | |
white_space = " " * 5 * self.indent_level | |
if self.cfg['leafmachine']['print']['optional_warnings']: | |
print(f"{bcolors.WARNING}{white_space}{self.message} ERROR: {self.error}{bcolors.ENDC}") | |
class Print_Verbose(): | |
cfg: str = '' | |
indent_level: int = 0 | |
message: str = '' | |
def __init__(self, cfg, indent_level, message) -> None: | |
self.cfg = cfg | |
self.indent_level = indent_level | |
self.message = message | |
def bold(self): | |
white_space = " " * 5 * self.indent_level | |
if self.cfg['leafmachine']['print']['verbose']: | |
print(f"{bcolors.BOLD}{white_space}{self.message}{bcolors.ENDC}") | |
def green(self): | |
white_space = " " * 5 * self.indent_level | |
if self.cfg['leafmachine']['print']['verbose']: | |
print(f"{bcolors.OKGREEN}{white_space}{self.message}{bcolors.ENDC}") | |
def cyan(self): | |
white_space = " " * 5 * self.indent_level | |
if self.cfg['leafmachine']['print']['verbose']: | |
print(f"{bcolors.OKCYAN}{white_space}{self.message}{bcolors.ENDC}") | |
def blue(self): | |
white_space = " " * 5 * self.indent_level | |
if self.cfg['leafmachine']['print']['verbose']: | |
print(f"{bcolors.OKBLUE}{white_space}{self.message}{bcolors.ENDC}") | |
def warning(self): | |
white_space = " " * 5 * self.indent_level | |
if self.cfg['leafmachine']['print']['verbose']: | |
print(f"{bcolors.WARNING}{white_space}{self.message}{bcolors.ENDC}") | |
def plain(self): | |
white_space = " " * 5 * self.indent_level | |
if self.cfg['leafmachine']['print']['verbose']: | |
print(f"{white_space}{self.message}") | |
def print_main_start(message): | |
indent_level = 1 | |
white_space = " " * 5 * indent_level | |
end = " " * int(80 - len(message) - len(white_space)) | |
# end_white_space = " " * end | |
blank = " " * 80 | |
print(f"{bcolors.CBLUEBG2}{blank}{bcolors.ENDC}") | |
print(f"{bcolors.CBLUEBG2}{white_space}{message}{end}{bcolors.ENDC}") | |
print(f"{bcolors.CBLUEBG2}{blank}{bcolors.ENDC}") | |
def print_main_success(message): | |
indent_level = 1 | |
white_space = " " * 5 * indent_level | |
end = " " * int(80 - len(message) - len(white_space)) | |
blank = " " * 80 | |
# end_white_space = " " * end | |
print(f"{bcolors.CGREENBG2}{blank}{bcolors.ENDC}") | |
print(f"{bcolors.CGREENBG2}{white_space}{message}{end}{bcolors.ENDC}") | |
print(f"{bcolors.CGREENBG2}{blank}{bcolors.ENDC}") | |
def print_main_warn(message): | |
indent_level = 1 | |
white_space = " " * 5 * indent_level | |
end = " " * int(80 - len(message) - len(white_space)) | |
# end_white_space = " " * end | |
blank = " " * 80 | |
print(f"{bcolors.CYELLOWBG2}{blank}{bcolors.ENDC}") | |
print(f"{bcolors.CYELLOWBG2}{white_space}{message}{end}{bcolors.ENDC}") | |
print(f"{bcolors.CYELLOWBG2}{blank}{bcolors.ENDC}") | |
def print_main_fail(message): | |
indent_level = 1 | |
white_space = " " * 5 * indent_level | |
end = " " * int(80 - len(message) - len(white_space)) | |
# end_white_space = " " * end | |
blank = " " * 80 | |
print(f"{bcolors.CREDBG2}{blank}{bcolors.ENDC}") | |
print(f"{bcolors.CREDBG2}{white_space}{message}{end}{bcolors.ENDC}") | |
print(f"{bcolors.CREDBG2}{blank}{bcolors.ENDC}") | |
def print_main_info(message): | |
indent_level = 2 | |
white_space = " " * 5 * indent_level | |
end = " " * int(80 - len(message) - len(white_space)) | |
# end_white_space = " " * end | |
print(f"{bcolors.CGREYBG}{white_space}{message}{end}{bcolors.ENDC}") | |
# def report_config(dir_home, cfg_file_path): | |
# print_main_start("Loading Configuration File") | |
# if cfg_file_path == None: | |
# print_main_info(''.join([os.path.join(dir_home, 'LeafMachine2.yaml')])) | |
# elif cfg_file_path == 'test_installation': | |
# print_main_info(''.join([os.path.join(dir_home, 'demo','LeafMachine2_demo.yaml')])) | |
# else: | |
# print_main_info(cfg_file_path) | |
# def report_config_VV(dir_home, cfg_file_path): | |
# print_main_start("Loading Configuration File") | |
# if cfg_file_path == None: | |
# print_main_info(''.join([os.path.join(dir_home, 'VoucherVision.yaml')])) | |
# elif cfg_file_path == 'test_installation': | |
# print_main_info(''.join([os.path.join(dir_home, 'demo','VoucherVision_demo.yaml')])) | |
# else: | |
# print_main_info(cfg_file_path) | |
def report_config(dir_home, cfg_file_path, system='VoucherVision'): | |
print_main_start("Loading Configuration File") | |
if system not in ['LeafMachine2', 'VoucherVision', 'SpecimenCrop']: | |
raise ValueError("Invalid system. Expected 'LeafMachine2' or 'VoucherVision' or 'SpecimenCrop'.") | |
if cfg_file_path == None: | |
print_main_info(''.join([os.path.join(dir_home, f'{system}.yaml')])) | |
elif cfg_file_path == 'test_installation': | |
print_main_info(''.join([os.path.join(dir_home, 'demo', f'{system}_demo.yaml')])) | |
else: | |
print_main_info(cfg_file_path) | |
def make_file_names_valid(dir, cfg): | |
if cfg['leafmachine']['do']['check_for_illegal_filenames']: | |
n_total = len(os.listdir(dir)) | |
for file in tqdm(os.listdir(dir), desc=f'{bcolors.HEADER} Removing illegal characters from file names{bcolors.ENDC}',colour="cyan",position=0,total = n_total): | |
name = Path(file).stem | |
ext = Path(file).suffix | |
name_cleaned = re.sub(r"[^a-zA-Z0-9_-]","-",name) | |
name_new = ''.join([name_cleaned,ext]) | |
i = 0 | |
try: | |
os.rename(os.path.join(dir,file), os.path.join(dir,name_new)) | |
except: | |
while os.path.exists(os.path.join(dir,name_new)): | |
i += 1 | |
name_new = '_'.join([name_cleaned, str(i), ext]) | |
os.rename(os.path.join(dir,file), os.path.join(dir,name_new)) | |
# def load_config_file(dir_home, cfg_file_path): | |
# if cfg_file_path == None: # Default path | |
# return load_cfg(dir_home) | |
# else: | |
# if cfg_file_path == 'test_installation': | |
# path_cfg = os.path.join(dir_home,'demo','LeafMachine2_demo.yaml') | |
# return get_cfg_from_full_path(path_cfg) | |
# else: # Custom path | |
# return get_cfg_from_full_path(cfg_file_path) | |
# def load_config_file_VV(dir_home, cfg_file_path): | |
# if cfg_file_path == None: # Default path | |
# return load_cfg_VV(dir_home) | |
# else: | |
# if cfg_file_path == 'test_installation': | |
# path_cfg = os.path.join(dir_home,'demo','VoucherVision_demo.yaml') | |
# return get_cfg_from_full_path(path_cfg) | |
# else: # Custom path | |
# return get_cfg_from_full_path(cfg_file_path) | |
def load_config_file(dir_home, cfg_file_path, system='LeafMachine2'): | |
if system not in ['LeafMachine2', 'VoucherVision', 'SpecimenCrop']: | |
raise ValueError("Invalid system. Expected 'LeafMachine2' or 'VoucherVision' or 'SpecimenCrop'.") | |
if cfg_file_path is None: # Default path | |
if system == 'LeafMachine2': | |
return load_cfg(dir_home, system='LeafMachine2') # For LeafMachine2 | |
elif system == 'VoucherVision': # VoucherVision | |
return load_cfg(dir_home, system='VoucherVision') # For VoucherVision | |
elif system == 'SpecimenCrop': # SpecimenCrop | |
return load_cfg(dir_home, system='SpecimenCrop') # For SpecimenCrop | |
else: | |
if cfg_file_path == 'test_installation': | |
path_cfg = os.path.join(dir_home, 'demo', f'{system}_demo.yaml') | |
return get_cfg_from_full_path(path_cfg) | |
else: # Custom path | |
return get_cfg_from_full_path(cfg_file_path) | |
def load_config_file_testing(dir_home, cfg_file_path): | |
if cfg_file_path == None: # Default path | |
return load_cfg(dir_home) | |
else: | |
if cfg_file_path == 'test_installation': | |
path_cfg = os.path.join(dir_home,'demo','demo.yaml') | |
return get_cfg_from_full_path(path_cfg) | |
else: # Custom path | |
return get_cfg_from_full_path(cfg_file_path) | |
def subset_dir_images(cfg, Project, Dirs): | |
if cfg['leafmachine']['project']['process_subset_of_images']: | |
dir_images_subset = cfg['leafmachine']['project']['dir_images_subset'] | |
num_images_per_species = cfg['leafmachine']['project']['n_images_per_species'] | |
if cfg['leafmachine']['project']['species_list'] is not None: | |
species_list = import_csv(cfg['leafmachine']['project']['species_list']) | |
species_list = species_list.iloc[:, 0].tolist() | |
else: | |
species_list = None | |
validate_dir(dir_images_subset) | |
species_counts = {} | |
filenames = os.listdir(Project.dir_images) | |
random.shuffle(filenames) | |
for filename in filenames: | |
species_name = filename.split('.')[0] | |
species_name = species_name.split('_')[2:] | |
species_name = '_'.join([species_name[0], species_name[1], species_name[2]]) | |
if (species_list is None) or ((species_name in species_list) and (species_list is not None)): | |
if species_name not in species_counts: | |
species_counts[species_name] = 0 | |
if species_counts[species_name] < num_images_per_species: | |
species_counts[species_name] += 1 | |
src_path = os.path.join(Project.dir_images, filename) | |
dest_path = os.path.join(dir_images_subset, filename) | |
shutil.copy(src_path, dest_path) | |
Project.dir_images = dir_images_subset | |
subset_csv_name = os.path.join(Dirs.dir_images_subset, '.'.join([Dirs.run_name, 'csv'])) | |
df = pd.DataFrame({'species_name': list(species_counts.keys()), 'count': list(species_counts.values())}) | |
df.to_csv(subset_csv_name, index=False) | |
return Project | |
else: | |
return Project | |
'''# Define function to be executed by each worker | |
def worker_crop(rank, cfg, dir_home, Project, Dirs): | |
# Set worker seed based on rank | |
np.random.seed(rank) | |
# Call function for this worker | |
crop_detections_from_images(cfg, dir_home, Project, Dirs) | |
def crop_detections_from_images(cfg, dir_home, Project, Dirs): | |
num_workers = 6 | |
# Initialize and start worker processes | |
processes = [] | |
for rank in range(num_workers): | |
p = mp.Process(target=worker_crop, args=(rank, cfg, dir_home, Project, Dirs)) | |
p.start() | |
processes.append(p) | |
# Wait for all worker processes to finish | |
for p in processes: | |
p.join()''' | |
def crop_detections_from_images_worker_VV(filename, analysis, Project, Dirs, save_per_image, save_per_class, save_list, binarize_labels): | |
try: | |
full_image = cv2.imread(os.path.join(Project.dir_images, '.'.join([filename, 'jpg']))) | |
except: | |
full_image = cv2.imread(os.path.join(Project.dir_images, '.'.join([filename, 'jpeg']))) | |
try: | |
archival = analysis['Detections_Archival_Components'] | |
has_archival = True | |
except: | |
has_archival = False | |
try: | |
plant = analysis['Detections_Plant_Components'] | |
has_plant = True | |
except: | |
has_plant = False | |
if has_archival and (save_per_image or save_per_class): | |
crop_component_from_yolo_coords_VV('ARCHIVAL', Dirs, analysis, archival, full_image, filename, save_per_image, save_per_class, save_list) | |
def crop_detections_from_images_worker(filename, analysis, Project, Dirs, save_per_image, save_per_class, save_list, binarize_labels): | |
try: | |
full_image = cv2.imread(os.path.join(Project.dir_images, '.'.join([filename, 'jpg']))) | |
except: | |
full_image = cv2.imread(os.path.join(Project.dir_images, '.'.join([filename, 'jpeg']))) | |
try: | |
archival = analysis['Detections_Archival_Components'] | |
has_archival = True | |
except: | |
has_archival = False | |
try: | |
plant = analysis['Detections_Plant_Components'] | |
has_plant = True | |
except: | |
has_plant = False | |
if has_archival and (save_per_image or save_per_class): | |
crop_component_from_yolo_coords('ARCHIVAL', Dirs, analysis, archival, full_image, filename, save_per_image, save_per_class, save_list) | |
if has_plant and (save_per_image or save_per_class): | |
crop_component_from_yolo_coords('PLANT', Dirs, analysis, plant, full_image, filename, save_per_image, save_per_class, save_list) | |
def crop_detections_from_images(cfg, logger, dir_home, Project, Dirs, batch_size=50): | |
t2_start = perf_counter() | |
logger.name = 'Crop Components' | |
if cfg['leafmachine']['cropped_components']['do_save_cropped_annotations']: | |
detections = cfg['leafmachine']['cropped_components']['save_cropped_annotations'] | |
logger.info(f"Cropping {detections} components from images") | |
save_per_image = cfg['leafmachine']['cropped_components']['save_per_image'] | |
save_per_class = cfg['leafmachine']['cropped_components']['save_per_annotation_class'] | |
save_list = cfg['leafmachine']['cropped_components']['save_cropped_annotations'] | |
try: | |
binarize_labels = cfg['leafmachine']['cropped_components']['binarize_labels'] | |
except: | |
binarize_labels = False | |
if cfg['leafmachine']['project']['batch_size'] is None: | |
batch_size = 50 | |
else: | |
batch_size = int(cfg['leafmachine']['project']['batch_size']) | |
if cfg['leafmachine']['project']['num_workers'] is None: | |
num_workers = 4 | |
else: | |
num_workers = int(cfg['leafmachine']['project']['num_workers']) | |
if binarize_labels: | |
save_per_class = True | |
with concurrent.futures.ThreadPoolExecutor(max_workers=num_workers) as executor: | |
futures = [] | |
for i in range(0, len(Project.project_data), batch_size): | |
batch = list(Project.project_data.items())[i:i+batch_size] | |
# print(f'Cropping Detections from Images {i} to {i+batch_size}') | |
logger.info(f'Cropping {detections} from images {i} to {i+batch_size} [{len(Project.project_data)}]') | |
for filename, analysis in batch: | |
if len(analysis) != 0: | |
futures.append(executor.submit(crop_detections_from_images_worker, filename, analysis, Project, Dirs, save_per_image, save_per_class, save_list, binarize_labels)) | |
for future in concurrent.futures.as_completed(futures): | |
pass | |
futures.clear() | |
t2_stop = perf_counter() | |
logger.info(f"Save cropped components --- elapsed time: {round(t2_stop - t2_start)} seconds") | |
def crop_detections_from_images_VV(cfg, logger, dir_home, Project, Dirs, batch_size=50): | |
t2_start = perf_counter() | |
logger.name = 'Crop Components' | |
if cfg['leafmachine']['cropped_components']['do_save_cropped_annotations']: | |
detections = cfg['leafmachine']['cropped_components']['save_cropped_annotations'] | |
logger.info(f"Cropping {detections} components from images") | |
save_per_image = cfg['leafmachine']['cropped_components']['save_per_image'] | |
save_per_class = cfg['leafmachine']['cropped_components']['save_per_annotation_class'] | |
save_list = cfg['leafmachine']['cropped_components']['save_cropped_annotations'] | |
binarize_labels = cfg['leafmachine']['cropped_components']['binarize_labels'] | |
if cfg['leafmachine']['project']['batch_size'] is None: | |
batch_size = 50 | |
else: | |
batch_size = int(cfg['leafmachine']['project']['batch_size']) | |
if cfg['leafmachine']['project']['num_workers'] is None: | |
num_workers = 4 | |
else: | |
num_workers = int(cfg['leafmachine']['project']['num_workers']) | |
if binarize_labels: | |
save_per_class = True | |
with concurrent.futures.ThreadPoolExecutor(max_workers=num_workers) as executor: | |
futures = [] | |
for i in range(0, len(Project.project_data), batch_size): | |
batch = list(Project.project_data.items())[i:i+batch_size] | |
# print(f'Cropping Detections from Images {i} to {i+batch_size}') | |
logger.info(f'Cropping {detections} from images {i} to {i+batch_size} [{len(Project.project_data)}]') | |
for filename, analysis in batch: | |
if len(analysis) != 0: | |
futures.append(executor.submit(crop_detections_from_images_worker_VV, filename, analysis, Project, Dirs, save_per_image, save_per_class, save_list, binarize_labels)) | |
for future in concurrent.futures.as_completed(futures): | |
pass | |
futures.clear() | |
t2_stop = perf_counter() | |
logger.info(f"Save cropped components --- elapsed time: {round(t2_stop - t2_start)} seconds") | |
# def crop_detections_from_images_VV(cfg, logger, dir_home, Project, Dirs, batch_size=50): | |
# t2_start = perf_counter() | |
# logger.name = 'Crop Components' | |
# if cfg['leafmachine']['cropped_components']['do_save_cropped_annotations']: | |
# detections = cfg['leafmachine']['cropped_components']['save_cropped_annotations'] | |
# logger.info(f"Cropping {detections} components from images") | |
# save_per_image = cfg['leafmachine']['cropped_components']['save_per_image'] | |
# save_per_class = cfg['leafmachine']['cropped_components']['save_per_annotation_class'] | |
# save_list = cfg['leafmachine']['cropped_components']['save_cropped_annotations'] | |
# binarize_labels = cfg['leafmachine']['cropped_components']['binarize_labels'] | |
# if cfg['leafmachine']['project']['batch_size'] is None: | |
# batch_size = 50 | |
# else: | |
# batch_size = int(cfg['leafmachine']['project']['batch_size']) | |
# if binarize_labels: | |
# save_per_class = True | |
# for i in range(0, len(Project.project_data), batch_size): | |
# batch = list(Project.project_data.items())[i:i+batch_size] | |
# logger.info(f"Cropping {detections} from images {i} to {i+batch_size} [{len(Project.project_data)}]") | |
# for filename, analysis in batch: | |
# if len(analysis) != 0: | |
# crop_detections_from_images_worker_VV(filename, analysis, Project, Dirs, save_per_image, save_per_class, save_list, binarize_labels) | |
# t2_stop = perf_counter() | |
# logger.info(f"Save cropped components --- elapsed time: {round(t2_stop - t2_start)} seconds") | |
# def crop_detections_from_images_SpecimenCrop(cfg, logger, dir_home, Project, Dirs, original_img_dir=None, batch_size=50): | |
# t2_start = perf_counter() | |
# logger.name = 'Crop Components --- Specimen Crop' | |
# if cfg['leafmachine']['modules']['specimen_crop']: | |
# # save_list = ['ruler', 'barcode', 'colorcard', 'label', 'map', 'envelope', 'photo', 'attached_item', 'weights', | |
# # 'leaf_whole', 'leaf_partial', 'leaflet', 'seed_fruit_one', 'seed_fruit_many', 'flower_one', 'flower_many', 'bud', 'specimen', 'roots', 'wood'] | |
# save_list = cfg['leafmachine']['cropped_components']['include_these_objects_in_specimen_crop'] | |
# logger.info(f"Cropping to include {save_list} components from images") | |
# if cfg['leafmachine']['project']['batch_size'] is None: | |
# batch_size = 50 | |
# else: | |
# batch_size = int(cfg['leafmachine']['project']['batch_size']) | |
# if cfg['leafmachine']['project']['num_workers'] is None: | |
# num_workers = 4 | |
# else: | |
# num_workers = int(cfg['leafmachine']['project']['num_workers']) | |
# with concurrent.futures.ThreadPoolExecutor(max_workers=num_workers) as executor: | |
# futures = [] | |
# for i in range(0, len(Project.project_data), batch_size): | |
# batch = list(Project.project_data.items())[i:i+batch_size] | |
# # print(f'Cropping Detections from Images {i} to {i+batch_size}') | |
# logger.info(f'Cropping {save_list} from images {i} to {i+batch_size} [{len(Project.project_data)}]') | |
# for filename, analysis in batch: | |
# if len(analysis) != 0: | |
# futures.append(executor.submit(crop_detections_from_images_worker_SpecimenCrop, filename, analysis, Project, Dirs, save_list, original_img_dir)) | |
# for future in concurrent.futures.as_completed(futures): | |
# pass | |
# futures.clear() | |
# t2_stop = perf_counter() | |
# logger.info(f"Save cropped components --- elapsed time: {round(t2_stop - t2_start)} seconds") | |
''' | |
# Single threaded | |
def crop_detections_from_images(cfg, dir_home, Project, Dirs): | |
if cfg['leafmachine']['cropped_components']['do_save_cropped_annotations']: | |
save_per_image = cfg['leafmachine']['cropped_components']['save_per_image'] | |
save_per_class = cfg['leafmachine']['cropped_components']['save_per_annotation_class'] | |
save_list = cfg['leafmachine']['cropped_components']['save_cropped_annotations'] | |
binarize_labels = cfg['leafmachine']['cropped_components']['binarize_labels'] | |
if binarize_labels: | |
save_per_class = True | |
for filename, analysis in tqdm(Project.project_data.items(), desc=f'{bcolors.BOLD} Cropping Detections from Images{bcolors.ENDC}',colour="cyan",position=0,total = len(Project.project_data.items())): | |
if len(analysis) != 0: | |
try: | |
full_image = cv2.imread(os.path.join(Project.dir_images, '.'.join([filename, 'jpg']))) | |
except: | |
full_image = cv2.imread(os.path.join(Project.dir_images, '.'.join([filename, 'jpeg']))) | |
try: | |
archival = analysis['Detections_Archival_Components'] | |
has_archival = True | |
except: | |
has_archival = False | |
try: | |
plant = analysis['Detections_Plant_Components'] | |
has_plant = True | |
except: | |
has_plant = False | |
if has_archival and (save_per_image or save_per_class): | |
crop_component_from_yolo_coords('ARCHIVAL', Dirs, analysis, archival, full_image, filename, save_per_image, save_per_class, save_list) | |
if has_plant and (save_per_image or save_per_class): | |
crop_component_from_yolo_coords('PLANT', Dirs, analysis, plant, full_image, filename, save_per_image, save_per_class, save_list) | |
''' | |
def process_detections(success, save_list, detections, detection_type, height, width, min_x, min_y, max_x, max_y): | |
for detection in detections: | |
detection_class = detection[0] | |
detection_class = set_index_for_annotation(detection_class, detection_type) | |
if (detection_class in save_list) or ('save_all' in save_list): | |
location = yolo_to_position_ruler(detection, height, width) | |
ruler_polygon = [ | |
(location[1], location[2]), | |
(location[3], location[2]), | |
(location[3], location[4]), | |
(location[1], location[4]) | |
] | |
x_coords = [x for x, y in ruler_polygon] | |
y_coords = [y for x, y in ruler_polygon] | |
min_x = min(min_x, *x_coords) | |
min_y = min(min_y, *y_coords) | |
max_x = max(max_x, *x_coords) | |
max_y = max(max_y, *y_coords) | |
success = True | |
return min_x, min_y, max_x, max_y, success | |
def crop_component_from_yolo_coords_VV(anno_type, Dirs, analysis, all_detections, full_image, filename, save_per_image, save_per_class, save_list): | |
height = analysis['height'] | |
width = analysis['width'] | |
# Initialize a list to hold all the cropped images | |
cropped_images = [] | |
if len(all_detections) < 1: | |
print(' MAKE THIS HAVE AN EMPTY PLACEHOLDER') # TODO ################################################################################### | |
else: | |
for detection in all_detections: | |
detection_class = detection[0] | |
detection_class = set_index_for_annotation(detection_class, anno_type) | |
if (detection_class in save_list) or ('save_all' in save_list): | |
location = yolo_to_position_ruler(detection, height, width) | |
ruler_polygon = [(location[1], location[2]), (location[3], location[2]), (location[3], location[4]), (location[1], location[4])] | |
x_coords = [x for x, y in ruler_polygon] | |
y_coords = [y for x, y in ruler_polygon] | |
min_x, min_y = min(x_coords), min(y_coords) | |
max_x, max_y = max(x_coords), max(y_coords) | |
detection_cropped = full_image[min_y:max_y, min_x:max_x] | |
cropped_images.append(detection_cropped) | |
loc = '-'.join([str(min_x), str(min_y), str(max_x), str(max_y)]) | |
detection_cropped_name = '.'.join(['__'.join([filename, detection_class, loc]), 'jpg']) | |
# detection_cropped_name = '.'.join([filename,'jpg']) | |
# save_per_image | |
if (detection_class in save_list) and save_per_image: | |
if detection_class == 'label': | |
detection_class2 = 'label_ind' | |
else: | |
detection_class2 = detection_class | |
dir_destination = os.path.join(Dirs.save_per_image, filename, detection_class2) | |
# print(os.path.join(dir_destination,detection_cropped_name)) | |
validate_dir(dir_destination) | |
# cv2.imwrite(os.path.join(dir_destination,detection_cropped_name), detection_cropped) | |
# save_per_class | |
if (detection_class in save_list) and save_per_class: | |
if detection_class == 'label': | |
detection_class2 = 'label_ind' | |
else: | |
detection_class2 = detection_class | |
dir_destination = os.path.join(Dirs.save_per_annotation_class, detection_class2) | |
# print(os.path.join(dir_destination,detection_cropped_name)) | |
validate_dir(dir_destination) | |
# cv2.imwrite(os.path.join(dir_destination,detection_cropped_name), detection_cropped) | |
else: | |
# print(f'detection_class: {detection_class} not in save_list: {save_list}') | |
pass | |
# Initialize a list to hold all the acceptable cropped images | |
acceptable_cropped_images = [] | |
for img in cropped_images: | |
# Calculate the aspect ratio of the image | |
aspect_ratio = min(img.shape[0], img.shape[1]) / max(img.shape[0], img.shape[1]) | |
# Only add the image to the acceptable list if the aspect ratio is more square than 1:8 | |
if aspect_ratio >= 1/8: | |
acceptable_cropped_images.append(img) | |
# Sort acceptable_cropped_images by area (largest first) | |
acceptable_cropped_images.sort(key=lambda img: img.shape[0] * img.shape[1], reverse=True) | |
# If there are no acceptable cropped images, set combined_image to None or to a placeholder image | |
if not acceptable_cropped_images: | |
combined_image = None # Or a placeholder image here | |
else: | |
# # Recalculate max_width and total_height for acceptable images | |
# max_width = max(img.shape[1] for img in acceptable_cropped_images) | |
# total_height = sum(img.shape[0] for img in acceptable_cropped_images) | |
# # Now, combine all the acceptable cropped images into a single image | |
# combined_image = np.zeros((total_height, max_width, 3), dtype=np.uint8) | |
# y_offset = 0 | |
# for img in acceptable_cropped_images: | |
# combined_image[y_offset:y_offset+img.shape[0], :img.shape[1]] = img | |
# y_offset += img.shape[0] | |
# Start with the first image | |
# Recalculate max_width and total_height for acceptable images | |
max_width = max(img.shape[1] for img in acceptable_cropped_images) | |
total_height = sum(img.shape[0] for img in acceptable_cropped_images) | |
combined_image = np.zeros((total_height, max_width, 3), dtype=np.uint8) | |
y_offset = 0 | |
y_offset_next_row = 0 | |
x_offset = 0 | |
# Start with the first image | |
combined_image[y_offset:y_offset+acceptable_cropped_images[0].shape[0], :acceptable_cropped_images[0].shape[1]] = acceptable_cropped_images[0] | |
y_offset_next_row += acceptable_cropped_images[0].shape[0] | |
# Add the second image below the first one | |
y_offset = y_offset_next_row | |
combined_image[y_offset:y_offset+acceptable_cropped_images[1].shape[0], :acceptable_cropped_images[1].shape[1]] = acceptable_cropped_images[1] | |
y_offset_next_row += acceptable_cropped_images[1].shape[0] | |
# Create a list to store the images that are too tall for the current row | |
too_tall_images = [] | |
# Now try to fill in to the right with the remaining images | |
current_width = acceptable_cropped_images[1].shape[1] | |
for img in acceptable_cropped_images[2:]: | |
if current_width + img.shape[1] > max_width: | |
# If this image doesn't fit, start a new row | |
y_offset = y_offset_next_row | |
combined_image[y_offset:y_offset+img.shape[0], :img.shape[1]] = img | |
current_width = img.shape[1] | |
y_offset_next_row = y_offset + img.shape[0] | |
else: | |
# If this image fits, add it to the right | |
max_height = y_offset_next_row - y_offset | |
if img.shape[0] > max_height: | |
too_tall_images.append(img) | |
else: | |
combined_image[y_offset:y_offset+img.shape[0], current_width:current_width+img.shape[1]] = img | |
current_width += img.shape[1] | |
# Process the images that were too tall for their rows | |
for img in too_tall_images: | |
y_offset = y_offset_next_row | |
combined_image[y_offset:y_offset+img.shape[0], :img.shape[1]] = img | |
y_offset_next_row += img.shape[0] | |
# Trim the combined_image to remove extra black space | |
combined_image = combined_image[:y_offset_next_row] | |
# save the combined image | |
# if (detection_class in save_list) and save_per_class: | |
dir_destination = os.path.join(Dirs.save_per_annotation_class, 'label') | |
validate_dir(dir_destination) | |
# combined_image_name = '__'.join([filename, detection_class]) + '.jpg' | |
combined_image_name = '.'.join([filename,'jpg']) | |
cv2.imwrite(os.path.join(dir_destination, combined_image_name), combined_image) | |
original_image_name = '.'.join([filename,'jpg']) | |
cv2.imwrite(os.path.join(Dirs.save_original, original_image_name), full_image) | |
def crop_component_from_yolo_coords(anno_type, Dirs, analysis, all_detections, full_image, filename, save_per_image, save_per_class, save_list): | |
height = analysis['height'] | |
width = analysis['width'] | |
if len(all_detections) < 1: | |
print(' MAKE THIS HAVE AN EMPTY PLACEHOLDER') # TODO ################################################################################### | |
else: | |
for detection in all_detections: | |
detection_class = detection[0] | |
detection_class = set_index_for_annotation(detection_class, anno_type) | |
if (detection_class in save_list) or ('save_all' in save_list): | |
location = yolo_to_position_ruler(detection, height, width) | |
ruler_polygon = [(location[1], location[2]), (location[3], location[2]), (location[3], location[4]), (location[1], location[4])] | |
x_coords = [x for x, y in ruler_polygon] | |
y_coords = [y for x, y in ruler_polygon] | |
min_x, min_y = min(x_coords), min(y_coords) | |
max_x, max_y = max(x_coords), max(y_coords) | |
detection_cropped = full_image[min_y:max_y, min_x:max_x] | |
loc = '-'.join([str(min_x), str(min_y), str(max_x), str(max_y)]) | |
detection_cropped_name = '.'.join(['__'.join([filename, detection_class, loc]), 'jpg']) | |
# save_per_image | |
if (detection_class in save_list) and save_per_image: | |
dir_destination = os.path.join(Dirs.save_per_image, filename, detection_class) | |
# print(os.path.join(dir_destination,detection_cropped_name)) | |
validate_dir(dir_destination) | |
cv2.imwrite(os.path.join(dir_destination,detection_cropped_name), detection_cropped) | |
# save_per_class | |
if (detection_class in save_list) and save_per_class: | |
dir_destination = os.path.join(Dirs.save_per_annotation_class, detection_class) | |
# print(os.path.join(dir_destination,detection_cropped_name)) | |
validate_dir(dir_destination) | |
cv2.imwrite(os.path.join(dir_destination,detection_cropped_name), detection_cropped) | |
else: | |
# print(f'detection_class: {detection_class} not in save_list: {save_list}') | |
pass | |
def yolo_to_position_ruler(annotation, height, width): | |
return ['ruler', | |
int((annotation[1] * width) - ((annotation[3] * width) / 2)), | |
int((annotation[2] * height) - ((annotation[4] * height) / 2)), | |
int(annotation[3] * width) + int((annotation[1] * width) - ((annotation[3] * width) / 2)), | |
int(annotation[4] * height) + int((annotation[2] * height) - ((annotation[4] * height) / 2))] | |
class bcolors: | |
HEADER = '\033[95m' | |
OKBLUE = '\033[94m' | |
OKCYAN = '\033[96m' | |
OKGREEN = '\033[92m' | |
WARNING = '\033[93m' | |
FAIL = '\033[91m' | |
ENDC = '\033[0m' | |
BOLD = '\033[1m' | |
UNDERLINE = '\033[4m' | |
CEND = '\33[0m' | |
CBOLD = '\33[1m' | |
CITALIC = '\33[3m' | |
CURL = '\33[4m' | |
CBLINK = '\33[5m' | |
CBLINK2 = '\33[6m' | |
CSELECTED = '\33[7m' | |
CBLACK = '\33[30m' | |
CRED = '\33[31m' | |
CGREEN = '\33[32m' | |
CYELLOW = '\33[33m' | |
CBLUE = '\33[34m' | |
CVIOLET = '\33[35m' | |
CBEIGE = '\33[36m' | |
CWHITE = '\33[37m' | |
CBLACKBG = '\33[40m' | |
CREDBG = '\33[41m' | |
CGREENBG = '\33[42m' | |
CYELLOWBG = '\33[43m' | |
CBLUEBG = '\33[44m' | |
CVIOLETBG = '\33[45m' | |
CBEIGEBG = '\33[46m' | |
CWHITEBG = '\33[47m' | |
CGREY = '\33[90m' | |
CRED2 = '\33[91m' | |
CGREEN2 = '\33[92m' | |
CYELLOW2 = '\33[93m' | |
CBLUE2 = '\33[94m' | |
CVIOLET2 = '\33[95m' | |
CBEIGE2 = '\33[96m' | |
CWHITE2 = '\33[97m' | |
CGREYBG = '\33[100m' | |
CREDBG2 = '\33[101m' | |
CGREENBG2 = '\33[102m' | |
CYELLOWBG2 = '\33[103m' | |
CBLUEBG2 = '\33[104m' | |
CVIOLETBG2 = '\33[105m' | |
CBEIGEBG2 = '\33[106m' | |
CWHITEBG2 = '\33[107m' | |
CBLUEBG3 = '\33[112m' | |
def set_index_for_annotation(cls,annoType): | |
if annoType == 'PLANT': | |
if cls == 0: | |
annoInd = 'Leaf_WHOLE' | |
elif cls == 1: | |
annoInd = 'Leaf_PARTIAL' | |
elif cls == 2: | |
annoInd = 'Leaflet' | |
elif cls == 3: | |
annoInd = 'Seed_Fruit_ONE' | |
elif cls == 4: | |
annoInd = 'Seed_Fruit_MANY' | |
elif cls == 5: | |
annoInd = 'Flower_ONE' | |
elif cls == 6: | |
annoInd = 'Flower_MANY' | |
elif cls == 7: | |
annoInd = 'Bud' | |
elif cls == 8: | |
annoInd = 'Specimen' | |
elif cls == 9: | |
annoInd = 'Roots' | |
elif cls == 10: | |
annoInd = 'Wood' | |
elif annoType == 'ARCHIVAL': | |
if cls == 0: | |
annoInd = 'Ruler' | |
elif cls == 1: | |
annoInd = 'Barcode' | |
elif cls == 2: | |
annoInd = 'Colorcard' | |
elif cls == 3: | |
annoInd = 'Label' | |
elif cls == 4: | |
annoInd = 'Map' | |
elif cls == 5: | |
annoInd = 'Envelope' | |
elif cls == 6: | |
annoInd = 'Photo' | |
elif cls == 7: | |
annoInd = 'Attached_item' | |
elif cls == 8: | |
annoInd = 'Weights' | |
return annoInd.lower() | |
# def set_yaml(path_to_yaml, value): | |
# with open('file_to_edit.yaml') as f: | |
# doc = yaml.load(f) | |
# doc['state'] = state | |
# with open('file_to_edit.yaml', 'w') as f: | |
# yaml.dump(doc, f) |