diff --git a/pipeline/mmocr/.coveragerc b/pipeline/mmocr/.coveragerc
deleted file mode 100644
index a7ee638287be67483ce907295325c53264af4c8c..0000000000000000000000000000000000000000
--- a/pipeline/mmocr/.coveragerc
+++ /dev/null
@@ -1,3 +0,0 @@
-[run]
-omit =
- */__init__.py
diff --git a/pipeline/mmocr/.dev_scripts/benchmark_full_models.txt b/pipeline/mmocr/.dev_scripts/benchmark_full_models.txt
deleted file mode 100644
index 5d7d7bf4e36369bdd34ad1c73e99104a37191ab8..0000000000000000000000000000000000000000
--- a/pipeline/mmocr/.dev_scripts/benchmark_full_models.txt
+++ /dev/null
@@ -1,18 +0,0 @@
-textdet/dbnet/dbnet_resnet18_fpnc_1200e_icdar2015.py
-textdet/dbnetpp/dbnetpp_resnet50-dcnv2_fpnc_1200e_icdar2015.py
-textdet/drrg/drrg_resnet50_fpn-unet_1200e_ctw1500.py
-textdet/fcenet/fcenet_resnet50_fpn_1500e_icdar2015.py
-textdet/maskrcnn/mask-rcnn_resnet50_fpn_160e_icdar2015.py
-textdet/panet/panet_resnet18_fpem-ffm_600e_icdar2015.py
-textdet/psenet/psenet_resnet50_fpnf_600e_icdar2015.py
-textdet/textsnake/textsnake_resnet50_fpn-unet_1200e_ctw1500.py
-textrecog/abinet/abinet-vision_20e_st-an_mj.py
-textrecog/crnn/crnn_mini-vgg_5e_mj.py
-textrecog/master/master_resnet31_12e_st_mj_sa.py
-textrecog/nrtr/nrtr_resnet31-1by16-1by8_6e_st_mj.py
-textrecog/robust_scanner/robustscanner_resnet31_5e_st-sub_mj-sub_sa_real.py
-textrecog/sar/sar_resnet31_parallel-decoder_5e_st-sub_mj-sub_sa_real.py
-textrecog/satrn/satrn_shallow-small_5e_st_mj.py
-textrecog/satrn/satrn_shallow-small_5e_st_mj.py
-textrecog/aster/aster_resnet45_6e_st_mj.py
-textrecog/svtr/svtr-small_20e_st_mj.py
diff --git a/pipeline/mmocr/.dev_scripts/benchmark_options.py b/pipeline/mmocr/.dev_scripts/benchmark_options.py
deleted file mode 100644
index e10c7adbccb430f513ae58517a47050444cdecc1..0000000000000000000000000000000000000000
--- a/pipeline/mmocr/.dev_scripts/benchmark_options.py
+++ /dev/null
@@ -1,7 +0,0 @@
-# Copyright (c) OpenMMLab. All rights reserved.
-
-third_part_libs = [
- 'pip install -r ../requirements/albu.txt',
-]
-
-default_floating_range = 0.5
diff --git a/pipeline/mmocr/.dev_scripts/benchmark_train_models.txt b/pipeline/mmocr/.dev_scripts/benchmark_train_models.txt
deleted file mode 100644
index 8cba62d0cae75b93cac6b004aff0c860abcfcaaa..0000000000000000000000000000000000000000
--- a/pipeline/mmocr/.dev_scripts/benchmark_train_models.txt
+++ /dev/null
@@ -1,9 +0,0 @@
-textdet/dbnetpp/dbnetpp_resnet50-dcnv2_fpnc_1200e_icdar2015.py
-textdet/fcenet/fcenet_resnet50_fpn_1500e_icdar2015.py
-textdet/maskrcnn/mask-rcnn_resnet50_fpn_160e_icdar2015.py
-textrecog/abinet/abinet-vision_20e_st-an_mj.py
-textrecog/crnn/crnn_mini-vgg_5e_mj.py
-textrecog/aster/aster_resnet45_6e_st_mj.py
-textrecog/nrtr/nrtr_resnet31-1by16-1by8_6e_st_mj.py
-textrecog/sar/sar_resnet31_parallel-decoder_5e_st-sub_mj-sub_sa_real.py
-textrecog/svtr/svtr-small_20e_st_mj.py
diff --git a/pipeline/mmocr/.dev_scripts/covignore.cfg b/pipeline/mmocr/.dev_scripts/covignore.cfg
deleted file mode 100644
index 00ec54b01a343447ab555390112b6d3e9676738c..0000000000000000000000000000000000000000
--- a/pipeline/mmocr/.dev_scripts/covignore.cfg
+++ /dev/null
@@ -1,18 +0,0 @@
-# Each line should be the relative path to the root directory
-# of this repo. Support regular expression as well.
-# For example:
-# mmocr/models/textdet/postprocess/utils.py
-# .*/utils.py
-.*/__init__.py
-
-# It will be removed after all models have been refactored
-mmocr/utils/bbox_utils.py
-
-# Major part is covered, however, it's hard to cover model's output.
-mmocr/models/textdet/detectors/mmdet_wrapper.py
-
-# It will be removed after KieVisualizer and TextSpotterVisualizer
-mmocr/visualization/visualize.py
-
-# Add tests for data preparers later
-mmocr/datasets/preparers
diff --git a/pipeline/mmocr/.dev_scripts/diff_coverage_test.sh b/pipeline/mmocr/.dev_scripts/diff_coverage_test.sh
deleted file mode 100644
index 588d6dbd4070e314f7fa4c5fda1311b3589221b2..0000000000000000000000000000000000000000
--- a/pipeline/mmocr/.dev_scripts/diff_coverage_test.sh
+++ /dev/null
@@ -1,43 +0,0 @@
-#!/bin/bash
-
-set -e
-
-readarray -t IGNORED_FILES < $( dirname "$0" )/covignore.cfg
-
-REUSE_COVERAGE_REPORT=${REUSE_COVERAGE_REPORT:-0}
-REPO=${1:-"origin"}
-BRANCH=${2:-"refactor_dev"}
-
-git fetch $REPO $BRANCH
-
-PY_FILES=""
-for FILE_NAME in $(git diff --name-only ${REPO}/${BRANCH}); do
- # Only test python files in mmocr/ existing in current branch, and not ignored in covignore.cfg
- if [ ${FILE_NAME: -3} == ".py" ] && [ ${FILE_NAME:0:6} == "mmocr/" ] && [ -f "$FILE_NAME" ]; then
- IGNORED=false
- for IGNORED_FILE_NAME in "${IGNORED_FILES[@]}"; do
- # Skip blank lines
- if [ -z "$IGNORED_FILE_NAME" ]; then
- continue
- fi
- if [ "${IGNORED_FILE_NAME::1}" != "#" ] && [[ "$FILE_NAME" =~ $IGNORED_FILE_NAME ]]; then
- echo "Ignoring $FILE_NAME"
- IGNORED=true
- break
- fi
- done
- if [ "$IGNORED" = false ]; then
- PY_FILES="$PY_FILES $FILE_NAME"
- fi
- fi
-done
-
-# Only test the coverage when PY_FILES are not empty, otherwise they will test the entire project
-if [ ! -z "${PY_FILES}" ]
-then
- if [ "$REUSE_COVERAGE_REPORT" == "0" ]; then
- coverage run --branch --source mmocr -m pytest tests/
- fi
- coverage report --fail-under 90 -m $PY_FILES
- interrogate -v --ignore-init-method --ignore-module --ignore-nested-functions --ignore-magic --ignore-regex "__repr__" --fail-under 95 $PY_FILES
-fi
diff --git a/pipeline/mmocr/.github/CODE_OF_CONDUCT.md b/pipeline/mmocr/.github/CODE_OF_CONDUCT.md
deleted file mode 100644
index 92afad1c5ab5d5781115dee45c131d3751d3cd31..0000000000000000000000000000000000000000
--- a/pipeline/mmocr/.github/CODE_OF_CONDUCT.md
+++ /dev/null
@@ -1,76 +0,0 @@
-# Contributor Covenant Code of Conduct
-
-## Our Pledge
-
-In the interest of fostering an open and welcoming environment, we as
-contributors and maintainers pledge to making participation in our project and
-our community a harassment-free experience for everyone, regardless of age, body
-size, disability, ethnicity, sex characteristics, gender identity and expression,
-level of experience, education, socio-economic status, nationality, personal
-appearance, race, religion, or sexual identity and orientation.
-
-## Our Standards
-
-Examples of behavior that contributes to creating a positive environment
-include:
-
-- Using welcoming and inclusive language
-- Being respectful of differing viewpoints and experiences
-- Gracefully accepting constructive criticism
-- Focusing on what is best for the community
-- Showing empathy towards other community members
-
-Examples of unacceptable behavior by participants include:
-
-- The use of sexualized language or imagery and unwelcome sexual attention or
- advances
-- Trolling, insulting/derogatory comments, and personal or political attacks
-- Public or private harassment
-- Publishing others' private information, such as a physical or electronic
- address, without explicit permission
-- Other conduct which could reasonably be considered inappropriate in a
- professional setting
-
-## Our Responsibilities
-
-Project maintainers are responsible for clarifying the standards of acceptable
-behavior and are expected to take appropriate and fair corrective action in
-response to any instances of unacceptable behavior.
-
-Project maintainers have the right and responsibility to remove, edit, or
-reject comments, commits, code, wiki edits, issues, and other contributions
-that are not aligned to this Code of Conduct, or to ban temporarily or
-permanently any contributor for other behaviors that they deem inappropriate,
-threatening, offensive, or harmful.
-
-## Scope
-
-This Code of Conduct applies both within project spaces and in public spaces
-when an individual is representing the project or its community. Examples of
-representing a project or community include using an official project e-mail
-address, posting via an official social media account, or acting as an appointed
-representative at an online or offline event. Representation of a project may be
-further defined and clarified by project maintainers.
-
-## Enforcement
-
-Instances of abusive, harassing, or otherwise unacceptable behavior may be
-reported by contacting the project team at chenkaidev@gmail.com. All
-complaints will be reviewed and investigated and will result in a response that
-is deemed necessary and appropriate to the circumstances. The project team is
-obligated to maintain confidentiality with regard to the reporter of an incident.
-Further details of specific enforcement policies may be posted separately.
-
-Project maintainers who do not follow or enforce the Code of Conduct in good
-faith may face temporary or permanent repercussions as determined by other
-members of the project's leadership.
-
-## Attribution
-
-This Code of Conduct is adapted from the [Contributor Covenant][homepage], version 1.4,
-available at https://www.contributor-covenant.org/version/1/4/code-of-conduct.html
-
-For answers to common questions about this code of conduct, see
-https://www.contributor-covenant.org/faq
-
-[homepage]: https://www.contributor-covenant.org
diff --git a/pipeline/mmocr/.github/CONTRIBUTING.md b/pipeline/mmocr/.github/CONTRIBUTING.md
deleted file mode 100644
index 7c7d23f22866eae2c12844e365c0f8a03c0e501b..0000000000000000000000000000000000000000
--- a/pipeline/mmocr/.github/CONTRIBUTING.md
+++ /dev/null
@@ -1 +0,0 @@
-We appreciate all contributions to improve MMOCR. Please read [Contribution Guide](/docs/en/notes/contribution_guide.md) for step-by-step instructions to make a contribution to MMOCR, and [CONTRIBUTING.md](https://github.com/open-mmlab/mmcv/blob/master/CONTRIBUTING.md) in MMCV for more details about the contributing guideline.
diff --git a/pipeline/mmocr/.github/ISSUE_TEMPLATE/1-bug-report.yml b/pipeline/mmocr/.github/ISSUE_TEMPLATE/1-bug-report.yml
deleted file mode 100644
index 6faa7b762abbec60f3444db838dcdfc072ba5f25..0000000000000000000000000000000000000000
--- a/pipeline/mmocr/.github/ISSUE_TEMPLATE/1-bug-report.yml
+++ /dev/null
@@ -1,121 +0,0 @@
-name: "๐ Bug report"
-description: "Create a report to help us reproduce and fix the bug"
-labels: kind/bug
-title: "[Bug] "
-
-body:
- - type: markdown
- attributes:
- value: |
- ## Note
- For general usage questions or idea discussions, please post it to our [**Forum**](https://github.com/open-mmlab/mmocr/discussions)
- If this issue is about installing MMCV, please file an issue at [MMCV](https://github.com/open-mmlab/mmcv/issues/new/choose).
- If it's anything about model deployment, please raise it to [MMDeploy](https://github.com/open-mmlab/mmdeploy)
-
- Please fill in as **much** of the following form as you're able to. **The clearer the description, the shorter it will take to solve it.**
-
- - type: checkboxes
- attributes:
- label: Prerequisite
- description: Please check the following items before creating a new issue.
- options:
- - label: I have searched [Issues](https://github.com/open-mmlab/mmocr/issues) and [Discussions](https://github.com/open-mmlab/mmocr/discussions) but cannot get the expected help.
- required: true
- # - label: I have read the [FAQ documentation](https://mmocr.readthedocs.io/en/1.x/notes/4_faq.html) but cannot get the expected help.
- # required: true
- - label: The bug has not been fixed in the [latest version (0.x)](https://github.com/open-mmlab/mmocr) or [latest version (1.x)](https://github.com/open-mmlab/mmocr/tree/dev-1.x).
- required: true
-
- - type: dropdown
- id: task
- attributes:
- label: Task
- description: The problem arises when
- options:
- - I'm using the official example scripts/configs for the officially supported tasks/models/datasets.
- - I have modified the scripts/configs, or I'm working on my own tasks/models/datasets.
- validations:
- required: true
-
- - type: dropdown
- id: branch
- attributes:
- label: Branch
- description: The problem arises when I'm working on
- options:
- - main branch https://github.com/open-mmlab/mmocr
- - 1.x branch https://github.com/open-mmlab/mmocr/tree/dev-1.x
- validations:
- required: true
-
- - type: textarea
- attributes:
- label: Environment
- description: |
- Please run `python mmocr/utils/collect_env.py` to collect necessary environment information and copy-paste it here.
- You may add additional information that may be helpful for locating the problem, such as
- - How you installed PyTorch \[e.g., pip, conda, source\]
- - Other environment variables that may be related (such as `$PATH`, `$LD_LIBRARY_PATH`, `$PYTHONPATH`, etc.)
- validations:
- required: true
-
- - type: textarea
- attributes:
- label: Reproduces the problem - code sample
- description: |
- Please provide a code sample that reproduces the problem you ran into. It can be a Colab link or just a code snippet.
- placeholder: |
- ```python
- # Sample code to reproduce the problem
- ```
- validations:
- required: true
-
- - type: textarea
- attributes:
- label: Reproduces the problem - command or script
- description: |
- What command or script did you run?
- placeholder: |
- ```shell
- The command or script you run.
- ```
- validations:
- required: true
-
- - type: textarea
- attributes:
- label: Reproduces the problem - error message
- description: |
- Please provide the error message or logs you got, with the full traceback.
-
- Tip: You can attach images or log files by dragging them into the text area..
- placeholder: |
- ```
- The error message or logs you got, with the full traceback.
- ```
- validations:
- required: true
-
- - type: textarea
- attributes:
- label: Additional information
- description: |
- Tell us anything else you think we should know.
-
- Tip: You can attach images or log files by dragging them into the text area.
- placeholder: |
- 1. What's your expected result?
- 2. What dataset did you use?
- 3. What do you think might be the reason?
-
- - type: markdown
- attributes:
- value: |
- ## Acknowledgement
- Thanks for taking the time to fill out this report.
-
- If you have already identified the reason, we strongly appreciate you creating a new PR to fix it [**Here**](https://github.com/open-mmlab/mmocr/pulls)!
- Please refer to [**Contribution Guide**](https://mmocr.readthedocs.io/en/dev-1.x/notes/contribution_guide.html) for contributing.
-
- Welcome to join our [**Community**](https://mmocr.readthedocs.io/en/latest/contact.html) to discuss together. ๐ฌ
diff --git a/pipeline/mmocr/.github/ISSUE_TEMPLATE/2-feature_request.yml b/pipeline/mmocr/.github/ISSUE_TEMPLATE/2-feature_request.yml
deleted file mode 100644
index 56dad87138c0285a155fb7366f27ab7da869ed9b..0000000000000000000000000000000000000000
--- a/pipeline/mmocr/.github/ISSUE_TEMPLATE/2-feature_request.yml
+++ /dev/null
@@ -1,39 +0,0 @@
-name: ๐ Feature request
-description: Suggest an idea for this project
-labels: [feature-request]
-title: "[Feature] "
-
-body:
- - type: markdown
- attributes:
- value: |
- ## Note
- For general usage questions or idea discussions, please post it to our [**Forum**](https://github.com/open-mmlab/mmocr/discussions)
-
- Please fill in as **much** of the following form as you're able to. **The clearer the description, the shorter it will take to solve it.**
-
- - type: textarea
- attributes:
- label: What is the feature?
- description: Tell us more about the feature and how this feature can help.
- placeholder: |
- E.g., It is inconvenient when \[....\].
- validations:
- required: true
-
- - type: textarea
- attributes:
- label: Any other context?
- description: |
- Have you considered any alternative solutions or features? If so, what are they? Also, feel free to add any other context or screenshots about the feature request here.
-
- - type: markdown
- attributes:
- value: |
- ## Acknowledgement
- Thanks for taking the time to fill out this report.
-
- We strongly appreciate you creating a new PR to implement it [**Here**](https://github.com/open-mmlab/mmocr/pulls)!
- Please refer to [**Contribution Guide**](https://mmocr.readthedocs.io/en/dev-1.x/notes/contribution_guide.html) for contributing.
-
- Welcome to join our [**Community**](https://mmocr.readthedocs.io/en/latest/contact.html) to discuss together. ๐ฌ
diff --git a/pipeline/mmocr/.github/ISSUE_TEMPLATE/3-new-model.yml b/pipeline/mmocr/.github/ISSUE_TEMPLATE/3-new-model.yml
deleted file mode 100644
index ea5491cca4ebb4392bb3413a547d43ea14c2f021..0000000000000000000000000000000000000000
--- a/pipeline/mmocr/.github/ISSUE_TEMPLATE/3-new-model.yml
+++ /dev/null
@@ -1,51 +0,0 @@
-name: "\U0001F31F New model/dataset/scheduler addition"
-description: Submit a proposal/request to implement a new model / dataset / scheduler
-labels: [ "feature-request" ]
-title: "[New Models] "
-
-
-body:
- - type: markdown
- attributes:
- value: |
- ## Note
- For general usage questions or idea discussions, please post it to our [**Forum**](https://github.com/open-mmlab/mmocr/discussions)
-
- Please fill in as **much** of the following form as you're able to. **The clearer the description, the shorter it will take to solve it.**
-
- - type: textarea
- id: description-request
- validations:
- required: true
- attributes:
- label: Model/Dataset/Scheduler description
- description: |
- Put any and all important information relative to the model/dataset/scheduler
-
- - type: checkboxes
- attributes:
- label: Open source status
- description: |
- Please provide the open-source status, which would be very helpful
- options:
- - label: "The model implementation is available"
- - label: "The model weights are available."
-
- - type: textarea
- id: additional-info
- attributes:
- label: Provide useful links for the implementation
- description: |
- Please provide information regarding the implementation, the weights, and the authors.
- Please mention the authors by @gh-username if you're aware of their usernames.
-
- - type: markdown
- attributes:
- value: |
- ## Acknowledgement
- Thanks for taking the time to fill out this report.
-
- We strongly appreciate you creating a new PR to implement it [**Here**](https://github.com/open-mmlab/mmocr/pulls)!
- Please refer to [**Contribution Guide**](https://mmocr.readthedocs.io/en/dev-1.x/notes/contribution_guide.html) for contributing.
-
- Welcome to join our [**Community**](https://mmocr.readthedocs.io/en/latest/contact.html) to discuss together. ๐ฌ
diff --git a/pipeline/mmocr/.github/ISSUE_TEMPLATE/4-documentation.yml b/pipeline/mmocr/.github/ISSUE_TEMPLATE/4-documentation.yml
deleted file mode 100644
index f19e070f56fda1fed144a1241ba3827c84bd4f18..0000000000000000000000000000000000000000
--- a/pipeline/mmocr/.github/ISSUE_TEMPLATE/4-documentation.yml
+++ /dev/null
@@ -1,48 +0,0 @@
-name: ๐ Documentation
-description: Report an issue related to the documentation.
-labels: "docs"
-title: "[Docs] "
-
-body:
- - type: markdown
- attributes:
- value: |
- ## Note
- For general usage questions or idea discussions, please post it to our [**Forum**](https://github.com/open-mmlab/mmocr/discussions)
- Please fill in as **much** of the following form as you're able to. **The clearer the description, the shorter it will take to solve it.**
-
- - type: dropdown
- id: branch
- attributes:
- label: Branch
- description: This issue is related to the
- options:
- - master branch https://mmocr.readthedocs.io/en/latest/
- - 1.x branch https://mmocr.readthedocs.io/en/dev-1.x/
- validations:
- required: true
-
- - type: textarea
- attributes:
- label: ๐ The doc issue
- description: >
- A clear and concise description the issue.
- validations:
- required: true
-
- - type: textarea
- attributes:
- label: Suggest a potential alternative/fix
- description: >
- Tell us how we could improve the documentation in this regard.
-
- - type: markdown
- attributes:
- value: |
- ## Acknowledgement
- Thanks for taking the time to fill out this report.
-
- If you have already identified the reason, we strongly appreciate you creating a new PR to fix it [**here**](https://github.com/open-mmlab/mmocr/pulls)!
- Please refer to [**Contribution Guide**](https://mmocr.readthedocs.io/en/dev-1.x/notes/contribution_guide.html) for contributing.
-
- Welcome to join our [**Community**](https://mmocr.readthedocs.io/en/latest/contact.html) to discuss together. ๐ฌ
diff --git a/pipeline/mmocr/.github/ISSUE_TEMPLATE/config.yml b/pipeline/mmocr/.github/ISSUE_TEMPLATE/config.yml
deleted file mode 100644
index fca6615a0531179134ef7bcd94a37a10fc0718c2..0000000000000000000000000000000000000000
--- a/pipeline/mmocr/.github/ISSUE_TEMPLATE/config.yml
+++ /dev/null
@@ -1,12 +0,0 @@
-blank_issues_enabled: false
-
-contact_links:
- - name: โ FAQ
- url: https://mmocr.readthedocs.io/en/dev-1.x/get_started/faq.html
- about: Is your question frequently asked?
- - name: ๐ฌ Forum
- url: https://github.com/open-mmlab/mmocr/discussions
- about: Ask general usage questions and discuss with other MMOCR community members
- - name: ๐ Explore OpenMMLab
- url: https://openmmlab.com/
- about: Get know more about OpenMMLab
diff --git a/pipeline/mmocr/.github/pull_request_template.md b/pipeline/mmocr/.github/pull_request_template.md
deleted file mode 100644
index e010f972caa5699d8cfcac64a859b6eb7d1d7749..0000000000000000000000000000000000000000
--- a/pipeline/mmocr/.github/pull_request_template.md
+++ /dev/null
@@ -1,33 +0,0 @@
-Thanks for your contribution and we appreciate it a lot. The following instructions would make your pull request more healthy and more easily get feedback. If you do not understand some items, don't worry, just make the pull request and seek help from maintainers.
-
-## Motivation
-
-Please describe the motivation of this PR and the goal you want to achieve through this PR.
-
-## Modification
-
-Please briefly describe what modification is made in this PR.
-
-## BC-breaking (Optional)
-
-Does the modification introduce changes that break the backward-compatibility of the downstream repositories?
-If so, please describe how it breaks the compatibility and how the downstream projects should modify their code to keep compatibility with this PR.
-
-## Use cases (Optional)
-
-If this PR introduces a new feature, it is better to list some use cases here, and update the documentation.
-
-## Checklist
-
-**Before PR**:
-
-- [ ] I have read and followed the workflow indicated in the [CONTRIBUTING.md](https://github.com/open-mmlab/mmocr/blob/main/.github/CONTRIBUTING.md) to create this PR.
-- [ ] Pre-commit or linting tools indicated in [CONTRIBUTING.md](https://github.com/open-mmlab/mmocr/blob/main/.github/CONTRIBUTING.md) are used to fix the potential lint issues.
-- [ ] Bug fixes are covered by unit tests, the case that causes the bug should be added in the unit tests.
-- [ ] New functionalities are covered by complete unit tests. If not, please add more unit test to ensure the correctness.
-- [ ] The documentation has been modified accordingly, including docstring or example tutorials.
-
-**After PR**:
-
-- [ ] If the modification has potential influence on downstream or other related projects, this PR should be tested with some of those projects.
-- [ ] CLA has been signed and all committers have signed the CLA in this PR.
diff --git a/pipeline/mmocr/.github/workflows/lint.yml b/pipeline/mmocr/.github/workflows/lint.yml
deleted file mode 100644
index e9cdba667ba986019473046d13315b2755bd5de6..0000000000000000000000000000000000000000
--- a/pipeline/mmocr/.github/workflows/lint.yml
+++ /dev/null
@@ -1,27 +0,0 @@
-name: lint
-
-on: [push, pull_request]
-
-concurrency:
- group: ${{ github.workflow }}-${{ github.ref }}
- cancel-in-progress: true
-
-jobs:
- lint:
- runs-on: ubuntu-latest
- steps:
- - uses: actions/checkout@v2
- - name: Set up Python 3.7
- uses: actions/setup-python@v2
- with:
- python-version: 3.7
- - name: Install pre-commit hook
- run: |
- pip install pre-commit
- pre-commit install
- - name: Linting
- run: pre-commit run --all-files
- - name: Check docstring coverage
- run: |
- pip install interrogate
- interrogate -v --ignore-init-method --ignore-module --ignore-nested-functions --ignore-regex "__repr__" --fail-under 90 mmocr
diff --git a/pipeline/mmocr/.github/workflows/merge_stage_test.yml b/pipeline/mmocr/.github/workflows/merge_stage_test.yml
deleted file mode 100644
index 856ede8335a691ccb5585ecae04728fcd1e958bb..0000000000000000000000000000000000000000
--- a/pipeline/mmocr/.github/workflows/merge_stage_test.yml
+++ /dev/null
@@ -1,160 +0,0 @@
-name: merge_stage_test
-
-on:
- push:
- paths-ignore:
- - 'README.md'
- - 'README_zh-CN.md'
- - 'docs/**'
- - 'demo/**'
- - '.dev_scripts/**'
- - '.circleci/**'
- - 'projects/**'
- branches:
- - dev-1.x
-
-concurrency:
- group: ${{ github.workflow }}-${{ github.ref }}
- cancel-in-progress: true
-
-jobs:
- build_cpu_py:
- runs-on: ubuntu-22.04
- strategy:
- matrix:
- python-version: [3.8, 3.9]
- torch: [1.8.1]
- include:
- - torch: 1.8.1
- torchvision: 0.9.1
- steps:
- - uses: actions/checkout@v3
- - name: Set up Python ${{ matrix.python-version }}
- uses: actions/setup-python@v4
- with:
- python-version: ${{ matrix.python-version }}
- - name: Upgrade pip
- run: pip install pip --upgrade
- - name: Install PyTorch
- run: pip install torch==${{matrix.torch}}+cpu torchvision==${{matrix.torchvision}}+cpu -f https://download.pytorch.org/whl/cpu/torch_stable.html
- - name: Install MMEngine
- run: pip install git+https://github.com/open-mmlab/mmengine.git@main
- - name: Install MMCV
- run: |
- pip install -U openmim
- mim install 'mmcv >= 2.0.0rc1'
- - name: Install MMDet
- run: pip install git+https://github.com/open-mmlab/mmdetection.git@dev-3.x
- - name: Install other dependencies
- run: pip install -r requirements/tests.txt
- - name: Build and install
- run: rm -rf .eggs && pip install -e .
- - name: Run unittests and generate coverage report
- run: |
- coverage run --branch --source mmocr -m pytest tests/
- coverage xml
- coverage report -m
-
- build_cpu_pt:
- runs-on: ubuntu-22.04
- strategy:
- matrix:
- python-version: [3.7]
- torch: [1.6.0, 1.7.1, 1.8.1, 1.9.1, 1.10.1, 1.11.0, 1.12.1, 1.13.0]
- include:
- - torch: 1.6.0
- torchvision: 0.7.0
- - torch: 1.7.1
- torchvision: 0.8.2
- - torch: 1.8.1
- torchvision: 0.9.1
- - torch: 1.9.1
- torchvision: 0.10.1
- - torch: 1.10.1
- torchvision: 0.11.2
- - torch: 1.11.0
- torchvision: 0.12.0
- - torch: 1.12.1
- torchvision: 0.13.1
- - torch: 1.13.0
- torchvision: 0.14.0
- - torch: 2.0.0
- torchvision: 0.15.1
- python-version: 3.8
- steps:
- - uses: actions/checkout@v3
- - name: Set up Python ${{ matrix.python-version }}
- uses: actions/setup-python@v4
- with:
- python-version: ${{ matrix.python-version }}
- - name: Upgrade pip
- run: pip install pip --upgrade
- - name: Install PyTorch
- run: pip install torch==${{matrix.torch}}+cpu torchvision==${{matrix.torchvision}}+cpu -f https://download.pytorch.org/whl/cpu/torch_stable.html
- - name: Install MMEngine
- run: pip install git+https://github.com/open-mmlab/mmengine.git@main
- - name: Install MMCV
- run: |
- pip install -U openmim
- mim install 'mmcv >= 2.0.0rc1'
- - name: Install MMDet
- run: pip install git+https://github.com/open-mmlab/mmdetection.git@dev-3.x
- - name: Install other dependencies
- run: pip install -r requirements/tests.txt
- - name: Build and install
- run: rm -rf .eggs && pip install -e .
- - name: Run unittests and generate coverage report
- run: |
- coverage run --branch --source mmocr -m pytest tests/
- coverage xml
- coverage report -m
- # Only upload coverage report for python3.7 && pytorch1.8.1 cpu
- - name: Upload coverage to Codecov
- if: ${{matrix.torch == '1.8.1' && matrix.python-version == '3.7'}}
- uses: codecov/codecov-action@v1.0.14
- with:
- file: ./coverage.xml
- flags: unittests
- env_vars: OS,PYTHON
- name: codecov-umbrella
- fail_ci_if_error: false
-
-
- build_windows:
- runs-on: windows-2022
- strategy:
- matrix:
- python: [3.7]
- platform: [cpu, cu111]
- torch: [1.8.1]
- torchvision: [0.9.1]
- include:
- - python-version: 3.8
- platform: cu117
- torch: 2.0.0
- torchvision: 0.15.1
- steps:
- - uses: actions/checkout@v2
- - name: Set up Python ${{ matrix.python }}
- uses: actions/setup-python@v2
- with:
- python-version: ${{ matrix.python }}
- - name: Upgrade pip
- run: python -m pip install --upgrade pip
- - name: Install lmdb
- run: pip install lmdb
- - name: Install PyTorch
- run: pip install torch==${{matrix.torch}}+${{matrix.platform}} torchvision==${{matrix.torchvision}}+${{matrix.platform}} -f https://download.pytorch.org/whl/${{matrix.platform}}/torch_stable.html
- - name: Install mmocr dependencies
- run: |
- pip install git+https://github.com/open-mmlab/mmengine.git@main
- pip install -U openmim
- mim install 'mmcv >= 2.0.0rc1'
- pip install git+https://github.com/open-mmlab/mmdetection.git@dev-3.x
- pip install -r requirements/tests.txt
- - name: Build and install
- run: |
- pip install -e .
- - name: Run unittests and generate coverage report
- run: |
- pytest tests/
diff --git a/pipeline/mmocr/.github/workflows/pr_stage_test.yml b/pipeline/mmocr/.github/workflows/pr_stage_test.yml
deleted file mode 100644
index e9344e5a056f58db0e3c5e0bfd588078f2817613..0000000000000000000000000000000000000000
--- a/pipeline/mmocr/.github/workflows/pr_stage_test.yml
+++ /dev/null
@@ -1,102 +0,0 @@
-name: pr_stage_test
-
-on:
- pull_request:
- paths-ignore:
- - 'README.md'
- - 'README_zh-CN.md'
- - 'docs/**'
- - 'demo/**'
- - '.dev_scripts/**'
- - '.circleci/**'
- - 'projects/**'
-
-concurrency:
- group: ${{ github.workflow }}-${{ github.ref }}
- cancel-in-progress: true
-
-jobs:
- build_cpu:
- runs-on: ubuntu-22.04
- strategy:
- matrix:
- python-version: [3.7]
- include:
- - torch: 1.8.1
- torchvision: 0.9.1
- steps:
- - uses: actions/checkout@v3
- - name: Set up Python ${{ matrix.python-version }}
- uses: actions/setup-python@v4
- with:
- python-version: ${{ matrix.python-version }}
- - name: Upgrade pip
- run: pip install pip --upgrade
- - name: Install PyTorch
- run: pip install torch==${{matrix.torch}}+cpu torchvision==${{matrix.torchvision}}+cpu -f https://download.pytorch.org/whl/cpu/torch_stable.html
- - name: Install MMEngine
- run: pip install git+https://github.com/open-mmlab/mmengine.git@main
- - name: Install MMCV
- run: |
- pip install -U openmim
- mim install 'mmcv >= 2.0.0rc1'
- - name: Install MMDet
- run: pip install git+https://github.com/open-mmlab/mmdetection.git@dev-3.x
- - name: Install other dependencies
- run: pip install -r requirements/tests.txt
- - name: Build and install
- run: rm -rf .eggs && pip install -e .
- - name: Run unittests and generate coverage report
- run: |
- coverage run --branch --source mmocr -m pytest tests/
- coverage xml
- coverage report -m
- # Upload coverage report for python3.7 && pytorch1.8.1 cpu
- - name: Upload coverage to Codecov
- uses: codecov/codecov-action@v1.0.14
- with:
- file: ./coverage.xml
- flags: unittests
- env_vars: OS,PYTHON
- name: codecov-umbrella
- fail_ci_if_error: false
-
-
- build_windows:
- runs-on: windows-2022
- strategy:
- matrix:
- python: [3.7]
- platform: [cpu, cu111]
- torch: [1.8.1]
- torchvision: [0.9.1]
- include:
- - python-version: 3.8
- platform: cu117
- torch: 2.0.0
- torchvision: 0.15.1
- steps:
- - uses: actions/checkout@v3
- - name: Set up Python ${{ matrix.python }}
- uses: actions/setup-python@v4
- with:
- python-version: ${{ matrix.python }}
- - name: Upgrade pip
- run: python -m pip install --upgrade pip
- - name: Install lmdb
- run: pip install lmdb
- - name: Install PyTorch
- run: pip install torch==${{matrix.torch}}+${{matrix.platform}} torchvision==${{matrix.torchvision}}+${{matrix.platform}} -f https://download.pytorch.org/whl/${{matrix.platform}}/torch_stable.html
- - name: Install mmocr dependencies
- run: |
- pip install git+https://github.com/open-mmlab/mmengine.git@main
- pip install -U openmim
- mim install 'mmcv >= 2.0.0rc1'
- pip install git+https://github.com/open-mmlab/mmdetection.git@dev-3.x
- pip install -r requirements/tests.txt
- - name: Build and install
- run: |
- pip install -e .
- - name: Run unittests and generate coverage report
- run: |
- pytest tests/
diff --git a/pipeline/mmocr/.github/workflows/publish-to-pypi.yml b/pipeline/mmocr/.github/workflows/publish-to-pypi.yml
deleted file mode 100644
index fc8e5f4fa230670134149e69cf301723a7176de9..0000000000000000000000000000000000000000
--- a/pipeline/mmocr/.github/workflows/publish-to-pypi.yml
+++ /dev/null
@@ -1,26 +0,0 @@
-name: deploy
-
-on: push
-
-concurrency:
- group: ${{ github.workflow }}-${{ github.ref }}
- cancel-in-progress: true
-
-jobs:
- build-n-publish:
- runs-on: ubuntu-latest
- if: startsWith(github.event.ref, 'refs/tags')
- steps:
- - uses: actions/checkout@v2
- - name: Set up Python 3.7
- uses: actions/setup-python@v1
- with:
- python-version: 3.7
- - name: Build MMOCR
- run: |
- pip install wheel
- python setup.py sdist bdist_wheel
- - name: Publish distribution to PyPI
- run: |
- pip install twine
- twine upload dist/* -u __token__ -p ${{ secrets.pypi_password }}
diff --git a/pipeline/mmocr/.github/workflows/test_mim.yml b/pipeline/mmocr/.github/workflows/test_mim.yml
deleted file mode 100644
index 2c1c170d6375a7bedc761404f228db80030cec44..0000000000000000000000000000000000000000
--- a/pipeline/mmocr/.github/workflows/test_mim.yml
+++ /dev/null
@@ -1,44 +0,0 @@
-name: test-mim
-
-on:
- push:
- paths:
- - 'model-index.yml'
- - 'configs/**'
-
- pull_request:
- paths:
- - 'model-index.yml'
- - 'configs/**'
-
-concurrency:
- group: ${{ github.workflow }}-${{ github.ref }}
- cancel-in-progress: true
-
-jobs:
- build_cpu:
- runs-on: ubuntu-18.04
- strategy:
- matrix:
- python-version: [3.7]
- torch: [1.8.0]
- include:
- - torch: 1.8.0
- torch_version: torch1.8
- torchvision: 0.9.0
- steps:
- - uses: actions/checkout@v2
- - name: Set up Python ${{ matrix.python-version }}
- uses: actions/setup-python@v2
- with:
- python-version: ${{ matrix.python-version }}
- - name: Upgrade pip
- run: pip install pip --upgrade
- - name: Install PyTorch
- run: pip install torch==${{matrix.torch}}+cpu torchvision==${{matrix.torchvision}}+cpu -f https://download.pytorch.org/whl/torch_stable.html
- - name: Install openmim
- run: pip install openmim
- - name: Build and install
- run: rm -rf .eggs && mim install -e .
- - name: test commands of mim
- run: mim search mmocr
diff --git a/pipeline/mmocr/.owners.yml b/pipeline/mmocr/.owners.yml
deleted file mode 100644
index c21ef0dc20db0d2994136a04637844f726a82162..0000000000000000000000000000000000000000
--- a/pipeline/mmocr/.owners.yml
+++ /dev/null
@@ -1,9 +0,0 @@
-assign:
- strategy:
- random
- # daily-shift-based
- scedule:
- '*/1 * * * *'
- assignees:
- - gaotongxiao
- - Harold-lkk
diff --git a/pipeline/mmocr/.pre-commit-config.yaml b/pipeline/mmocr/.pre-commit-config.yaml
deleted file mode 100644
index bf71f4f9b198b3d81c4bfe335de3fc3a7df35ca0..0000000000000000000000000000000000000000
--- a/pipeline/mmocr/.pre-commit-config.yaml
+++ /dev/null
@@ -1,63 +0,0 @@
-exclude: ^tests/data/
-repos:
- - repo: https://github.com/PyCQA/flake8
- rev: 5.0.4
- hooks:
- - id: flake8
- - repo: https://github.com/zhouzaida/isort
- rev: 5.12.1
- hooks:
- - id: isort
- - repo: https://github.com/pre-commit/mirrors-yapf
- rev: v0.32.0
- hooks:
- - id: yapf
- - repo: https://github.com/codespell-project/codespell
- rev: v2.2.1
- hooks:
- - id: codespell
- - repo: https://github.com/pre-commit/pre-commit-hooks
- rev: v4.3.0
- hooks:
- - id: trailing-whitespace
- exclude: |
- (?x)^(
- dicts/|
- projects/.*?/dicts/
- )
- - id: check-yaml
- - id: end-of-file-fixer
- exclude: |
- (?x)^(
- dicts/|
- projects/.*?/dicts/
- )
- - id: requirements-txt-fixer
- - id: double-quote-string-fixer
- - id: check-merge-conflict
- - id: fix-encoding-pragma
- args: ["--remove"]
- - id: mixed-line-ending
- args: ["--fix=lf"]
- - id: mixed-line-ending
- args: ["--fix=lf"]
- - repo: https://github.com/executablebooks/mdformat
- rev: 0.7.9
- hooks:
- - id: mdformat
- args: ["--number", "--table-width", "200"]
- additional_dependencies:
- - mdformat-openmmlab
- - mdformat_frontmatter
- - linkify-it-py
- - repo: https://github.com/myint/docformatter
- rev: v1.3.1
- hooks:
- - id: docformatter
- args: ["--in-place", "--wrap-descriptions", "79"]
- - repo: https://github.com/open-mmlab/pre-commit-hooks
- rev: v0.2.0 # Use the ref you want to point at
- hooks:
- - id: check-algo-readme
- - id: check-copyright
- args: ["mmocr", "tests", "tools"] # these directories will be checked
diff --git a/pipeline/mmocr/.pylintrc b/pipeline/mmocr/.pylintrc
deleted file mode 100644
index d7a39be85d13c69aea978374a5edf921a5d4cc39..0000000000000000000000000000000000000000
--- a/pipeline/mmocr/.pylintrc
+++ /dev/null
@@ -1,621 +0,0 @@
-[MASTER]
-
-# A comma-separated list of package or module names from where C extensions may
-# be loaded. Extensions are loading into the active Python interpreter and may
-# run arbitrary code.
-extension-pkg-whitelist=
-
-# Specify a score threshold to be exceeded before program exits with error.
-fail-under=10.0
-
-# Add files or directories to the blacklist. They should be base names, not
-# paths.
-ignore=CVS,configs
-
-# Add files or directories matching the regex patterns to the blacklist. The
-# regex matches against base names, not paths.
-ignore-patterns=
-
-# Python code to execute, usually for sys.path manipulation such as
-# pygtk.require().
-#init-hook=
-
-# Use multiple processes to speed up Pylint. Specifying 0 will auto-detect the
-# number of processors available to use.
-jobs=1
-
-# Control the amount of potential inferred values when inferring a single
-# object. This can help the performance when dealing with large functions or
-# complex, nested conditions.
-limit-inference-results=100
-
-# List of plugins (as comma separated values of python module names) to load,
-# usually to register additional checkers.
-load-plugins=
-
-# Pickle collected data for later comparisons.
-persistent=yes
-
-# When enabled, pylint would attempt to guess common misconfiguration and emit
-# user-friendly hints instead of false-positive error messages.
-suggestion-mode=yes
-
-# Allow loading of arbitrary C extensions. Extensions are imported into the
-# active Python interpreter and may run arbitrary code.
-unsafe-load-any-extension=no
-
-
-[MESSAGES CONTROL]
-
-# Only show warnings with the listed confidence levels. Leave empty to show
-# all. Valid levels: HIGH, INFERENCE, INFERENCE_FAILURE, UNDEFINED.
-confidence=
-
-# Disable the message, report, category or checker with the given id(s). You
-# can either give multiple identifiers separated by comma (,) or put this
-# option multiple times (only on the command line, not in the configuration
-# file where it should appear only once). You can also use "--disable=all" to
-# disable everything first and then reenable specific checks. For example, if
-# you want to run only the similarities checker, you can use "--disable=all
-# --enable=similarities". If you want to run only the classes checker, but have
-# no Warning level messages displayed, use "--disable=all --enable=classes
-# --disable=W".
-disable=print-statement,
- parameter-unpacking,
- unpacking-in-except,
- old-raise-syntax,
- backtick,
- long-suffix,
- old-ne-operator,
- old-octal-literal,
- import-star-module-level,
- non-ascii-bytes-literal,
- raw-checker-failed,
- bad-inline-option,
- locally-disabled,
- file-ignored,
- suppressed-message,
- useless-suppression,
- deprecated-pragma,
- use-symbolic-message-instead,
- apply-builtin,
- basestring-builtin,
- buffer-builtin,
- cmp-builtin,
- coerce-builtin,
- execfile-builtin,
- file-builtin,
- long-builtin,
- raw_input-builtin,
- reduce-builtin,
- standarderror-builtin,
- unicode-builtin,
- xrange-builtin,
- coerce-method,
- delslice-method,
- getslice-method,
- setslice-method,
- no-absolute-import,
- old-division,
- dict-iter-method,
- dict-view-method,
- next-method-called,
- metaclass-assignment,
- indexing-exception,
- raising-string,
- reload-builtin,
- oct-method,
- hex-method,
- nonzero-method,
- cmp-method,
- input-builtin,
- round-builtin,
- intern-builtin,
- unichr-builtin,
- map-builtin-not-iterating,
- zip-builtin-not-iterating,
- range-builtin-not-iterating,
- filter-builtin-not-iterating,
- using-cmp-argument,
- eq-without-hash,
- div-method,
- idiv-method,
- rdiv-method,
- exception-message-attribute,
- invalid-str-codec,
- sys-max-int,
- bad-python3-import,
- deprecated-string-function,
- deprecated-str-translate-call,
- deprecated-itertools-function,
- deprecated-types-field,
- next-method-defined,
- dict-items-not-iterating,
- dict-keys-not-iterating,
- dict-values-not-iterating,
- deprecated-operator-function,
- deprecated-urllib-function,
- xreadlines-attribute,
- deprecated-sys-function,
- exception-escape,
- comprehension-escape,
- no-member,
- invalid-name,
- too-many-branches,
- wrong-import-order,
- too-many-arguments,
- missing-function-docstring,
- missing-module-docstring,
- too-many-locals,
- too-few-public-methods,
- abstract-method,
- broad-except,
- too-many-nested-blocks,
- too-many-instance-attributes,
- missing-class-docstring,
- duplicate-code,
- not-callable,
- protected-access,
- dangerous-default-value,
- no-name-in-module,
- logging-fstring-interpolation,
- super-init-not-called,
- redefined-builtin,
- attribute-defined-outside-init,
- arguments-differ,
- cyclic-import,
- bad-super-call,
- too-many-statements
-
-# Enable the message, report, category or checker with the given id(s). You can
-# either give multiple identifier separated by comma (,) or put this option
-# multiple time (only on the command line, not in the configuration file where
-# it should appear only once). See also the "--disable" option for examples.
-enable=c-extension-no-member
-
-
-[REPORTS]
-
-# Python expression which should return a score less than or equal to 10. You
-# have access to the variables 'error', 'warning', 'refactor', and 'convention'
-# which contain the number of messages in each category, as well as 'statement'
-# which is the total number of statements analyzed. This score is used by the
-# global evaluation report (RP0004).
-evaluation=10.0 - ((float(5 * error + warning + refactor + convention) / statement) * 10)
-
-# Template used to display messages. This is a python new-style format string
-# used to format the message information. See doc for all details.
-#msg-template=
-
-# Set the output format. Available formats are text, parseable, colorized, json
-# and msvs (visual studio). You can also give a reporter class, e.g.
-# mypackage.mymodule.MyReporterClass.
-output-format=text
-
-# Tells whether to display a full report or only the messages.
-reports=no
-
-# Activate the evaluation score.
-score=yes
-
-
-[REFACTORING]
-
-# Maximum number of nested blocks for function / method body
-max-nested-blocks=5
-
-# Complete name of functions that never returns. When checking for
-# inconsistent-return-statements if a never returning function is called then
-# it will be considered as an explicit return statement and no message will be
-# printed.
-never-returning-functions=sys.exit
-
-
-[TYPECHECK]
-
-# List of decorators that produce context managers, such as
-# contextlib.contextmanager. Add to this list to register other decorators that
-# produce valid context managers.
-contextmanager-decorators=contextlib.contextmanager
-
-# List of members which are set dynamically and missed by pylint inference
-# system, and so shouldn't trigger E1101 when accessed. Python regular
-# expressions are accepted.
-generated-members=
-
-# Tells whether missing members accessed in mixin class should be ignored. A
-# mixin class is detected if its name ends with "mixin" (case insensitive).
-ignore-mixin-members=yes
-
-# Tells whether to warn about missing members when the owner of the attribute
-# is inferred to be None.
-ignore-none=yes
-
-# This flag controls whether pylint should warn about no-member and similar
-# checks whenever an opaque object is returned when inferring. The inference
-# can return multiple potential results while evaluating a Python object, but
-# some branches might not be evaluated, which results in partial inference. In
-# that case, it might be useful to still emit no-member and other checks for
-# the rest of the inferred objects.
-ignore-on-opaque-inference=yes
-
-# List of class names for which member attributes should not be checked (useful
-# for classes with dynamically set attributes). This supports the use of
-# qualified names.
-ignored-classes=optparse.Values,thread._local,_thread._local
-
-# List of module names for which member attributes should not be checked
-# (useful for modules/projects where namespaces are manipulated during runtime
-# and thus existing member attributes cannot be deduced by static analysis). It
-# supports qualified module names, as well as Unix pattern matching.
-ignored-modules=
-
-# Show a hint with possible names when a member name was not found. The aspect
-# of finding the hint is based on edit distance.
-missing-member-hint=yes
-
-# The minimum edit distance a name should have in order to be considered a
-# similar match for a missing member name.
-missing-member-hint-distance=1
-
-# The total number of similar names that should be taken in consideration when
-# showing a hint for a missing member.
-missing-member-max-choices=1
-
-# List of decorators that change the signature of a decorated function.
-signature-mutators=
-
-
-[SPELLING]
-
-# Limits count of emitted suggestions for spelling mistakes.
-max-spelling-suggestions=4
-
-# Spelling dictionary name. Available dictionaries: none. To make it work,
-# install the python-enchant package.
-spelling-dict=
-
-# List of comma separated words that should not be checked.
-spelling-ignore-words=
-
-# A path to a file that contains the private dictionary; one word per line.
-spelling-private-dict-file=
-
-# Tells whether to store unknown words to the private dictionary (see the
-# --spelling-private-dict-file option) instead of raising a message.
-spelling-store-unknown-words=no
-
-
-[LOGGING]
-
-# The type of string formatting that logging methods do. `old` means using %
-# formatting, `new` is for `{}` formatting.
-logging-format-style=old
-
-# Logging modules to check that the string format arguments are in logging
-# function parameter format.
-logging-modules=logging
-
-
-[VARIABLES]
-
-# List of additional names supposed to be defined in builtins. Remember that
-# you should avoid defining new builtins when possible.
-additional-builtins=
-
-# Tells whether unused global variables should be treated as a violation.
-allow-global-unused-variables=yes
-
-# List of strings which can identify a callback function by name. A callback
-# name must start or end with one of those strings.
-callbacks=cb_,
- _cb
-
-# A regular expression matching the name of dummy variables (i.e. expected to
-# not be used).
-dummy-variables-rgx=_+$|(_[a-zA-Z0-9_]*[a-zA-Z0-9]+?$)|dummy|^ignored_|^unused_
-
-# Argument names that match this expression will be ignored. Default to name
-# with leading underscore.
-ignored-argument-names=_.*|^ignored_|^unused_
-
-# Tells whether we should check for unused import in __init__ files.
-init-import=no
-
-# List of qualified module names which can have objects that can redefine
-# builtins.
-redefining-builtins-modules=six.moves,past.builtins,future.builtins,builtins,io
-
-
-[FORMAT]
-
-# Expected format of line ending, e.g. empty (any line ending), LF or CRLF.
-expected-line-ending-format=
-
-# Regexp for a line that is allowed to be longer than the limit.
-ignore-long-lines=^\s*(# )??$
-
-# Number of spaces of indent required inside a hanging or continued line.
-indent-after-paren=4
-
-# String used as indentation unit. This is usually " " (4 spaces) or "\t" (1
-# tab).
-indent-string=' '
-
-# Maximum number of characters on a single line.
-max-line-length=100
-
-# Maximum number of lines in a module.
-max-module-lines=1000
-
-# Allow the body of a class to be on the same line as the declaration if body
-# contains single statement.
-single-line-class-stmt=no
-
-# Allow the body of an if to be on the same line as the test if there is no
-# else.
-single-line-if-stmt=no
-
-
-[STRING]
-
-# This flag controls whether inconsistent-quotes generates a warning when the
-# character used as a quote delimiter is used inconsistently within a module.
-check-quote-consistency=no
-
-# This flag controls whether the implicit-str-concat should generate a warning
-# on implicit string concatenation in sequences defined over several lines.
-check-str-concat-over-line-jumps=no
-
-
-[SIMILARITIES]
-
-# Ignore comments when computing similarities.
-ignore-comments=yes
-
-# Ignore docstrings when computing similarities.
-ignore-docstrings=yes
-
-# Ignore imports when computing similarities.
-ignore-imports=no
-
-# Minimum lines number of a similarity.
-min-similarity-lines=4
-
-
-[MISCELLANEOUS]
-
-# List of note tags to take in consideration, separated by a comma.
-notes=FIXME,
- XXX,
- TODO
-
-# Regular expression of note tags to take in consideration.
-#notes-rgx=
-
-
-[BASIC]
-
-# Naming style matching correct argument names.
-argument-naming-style=snake_case
-
-# Regular expression matching correct argument names. Overrides argument-
-# naming-style.
-#argument-rgx=
-
-# Naming style matching correct attribute names.
-attr-naming-style=snake_case
-
-# Regular expression matching correct attribute names. Overrides attr-naming-
-# style.
-#attr-rgx=
-
-# Bad variable names which should always be refused, separated by a comma.
-bad-names=foo,
- bar,
- baz,
- toto,
- tutu,
- tata
-
-# Bad variable names regexes, separated by a comma. If names match any regex,
-# they will always be refused
-bad-names-rgxs=
-
-# Naming style matching correct class attribute names.
-class-attribute-naming-style=any
-
-# Regular expression matching correct class attribute names. Overrides class-
-# attribute-naming-style.
-#class-attribute-rgx=
-
-# Naming style matching correct class names.
-class-naming-style=PascalCase
-
-# Regular expression matching correct class names. Overrides class-naming-
-# style.
-#class-rgx=
-
-# Naming style matching correct constant names.
-const-naming-style=UPPER_CASE
-
-# Regular expression matching correct constant names. Overrides const-naming-
-# style.
-#const-rgx=
-
-# Minimum line length for functions/classes that require docstrings, shorter
-# ones are exempt.
-docstring-min-length=-1
-
-# Naming style matching correct function names.
-function-naming-style=snake_case
-
-# Regular expression matching correct function names. Overrides function-
-# naming-style.
-#function-rgx=
-
-# Good variable names which should always be accepted, separated by a comma.
-good-names=i,
- j,
- k,
- ex,
- Run,
- _,
- x,
- y,
- w,
- h,
- a,
- b
-
-# Good variable names regexes, separated by a comma. If names match any regex,
-# they will always be accepted
-good-names-rgxs=
-
-# Include a hint for the correct naming format with invalid-name.
-include-naming-hint=no
-
-# Naming style matching correct inline iteration names.
-inlinevar-naming-style=any
-
-# Regular expression matching correct inline iteration names. Overrides
-# inlinevar-naming-style.
-#inlinevar-rgx=
-
-# Naming style matching correct method names.
-method-naming-style=snake_case
-
-# Regular expression matching correct method names. Overrides method-naming-
-# style.
-#method-rgx=
-
-# Naming style matching correct module names.
-module-naming-style=snake_case
-
-# Regular expression matching correct module names. Overrides module-naming-
-# style.
-#module-rgx=
-
-# Colon-delimited sets of names that determine each other's naming style when
-# the name regexes allow several styles.
-name-group=
-
-# Regular expression which should only match function or class names that do
-# not require a docstring.
-no-docstring-rgx=^_
-
-# List of decorators that produce properties, such as abc.abstractproperty. Add
-# to this list to register other decorators that produce valid properties.
-# These decorators are taken in consideration only for invalid-name.
-property-classes=abc.abstractproperty
-
-# Naming style matching correct variable names.
-variable-naming-style=snake_case
-
-# Regular expression matching correct variable names. Overrides variable-
-# naming-style.
-#variable-rgx=
-
-
-[DESIGN]
-
-# Maximum number of arguments for function / method.
-max-args=5
-
-# Maximum number of attributes for a class (see R0902).
-max-attributes=7
-
-# Maximum number of boolean expressions in an if statement (see R0916).
-max-bool-expr=5
-
-# Maximum number of branch for function / method body.
-max-branches=12
-
-# Maximum number of locals for function / method body.
-max-locals=15
-
-# Maximum number of parents for a class (see R0901).
-max-parents=7
-
-# Maximum number of public methods for a class (see R0904).
-max-public-methods=20
-
-# Maximum number of return / yield for function / method body.
-max-returns=6
-
-# Maximum number of statements in function / method body.
-max-statements=50
-
-# Minimum number of public methods for a class (see R0903).
-min-public-methods=2
-
-
-[IMPORTS]
-
-# List of modules that can be imported at any level, not just the top level
-# one.
-allow-any-import-level=
-
-# Allow wildcard imports from modules that define __all__.
-allow-wildcard-with-all=no
-
-# Analyse import fallback blocks. This can be used to support both Python 2 and
-# 3 compatible code, which means that the block might have code that exists
-# only in one or another interpreter, leading to false positives when analysed.
-analyse-fallback-blocks=no
-
-# Deprecated modules which should not be used, separated by a comma.
-deprecated-modules=optparse,tkinter.tix
-
-# Create a graph of external dependencies in the given file (report RP0402 must
-# not be disabled).
-ext-import-graph=
-
-# Create a graph of every (i.e. internal and external) dependencies in the
-# given file (report RP0402 must not be disabled).
-import-graph=
-
-# Create a graph of internal dependencies in the given file (report RP0402 must
-# not be disabled).
-int-import-graph=
-
-# Force import order to recognize a module as part of the standard
-# compatibility libraries.
-known-standard-library=
-
-# Force import order to recognize a module as part of a third party library.
-known-third-party=enchant
-
-# Couples of modules and preferred modules, separated by a comma.
-preferred-modules=
-
-
-[CLASSES]
-
-# List of method names used to declare (i.e. assign) instance attributes.
-defining-attr-methods=__init__,
- __new__,
- setUp,
- __post_init__
-
-# List of member names, which should be excluded from the protected access
-# warning.
-exclude-protected=_asdict,
- _fields,
- _replace,
- _source,
- _make
-
-# List of valid names for the first argument in a class method.
-valid-classmethod-first-arg=cls
-
-# List of valid names for the first argument in a metaclass class method.
-valid-metaclass-classmethod-first-arg=cls
-
-
-[EXCEPTIONS]
-
-# Exceptions that will emit a warning when being caught. Defaults to
-# "BaseException, Exception".
-overgeneral-exceptions=BaseException,
- Exception
diff --git a/pipeline/mmocr/.readthedocs.yml b/pipeline/mmocr/.readthedocs.yml
deleted file mode 100644
index 5d508503d475b736ef08545e5c130cb8f373cd1a..0000000000000000000000000000000000000000
--- a/pipeline/mmocr/.readthedocs.yml
+++ /dev/null
@@ -1,9 +0,0 @@
-version: 2
-
-formats: all
-
-python:
- version: 3.7
- install:
- - requirements: requirements/docs.txt
- - requirements: requirements/readthedocs.txt
diff --git a/pipeline/mmocr/CITATION.cff b/pipeline/mmocr/CITATION.cff
deleted file mode 100644
index 7d1d93a7c68daf442bc6540b197b401e7a38b91c..0000000000000000000000000000000000000000
--- a/pipeline/mmocr/CITATION.cff
+++ /dev/null
@@ -1,9 +0,0 @@
-cff-version: 1.2.0
-message: "If you use this software, please cite it as below."
-title: "OpenMMLab Text Detection, Recognition and Understanding Toolbox"
-authors:
- - name: "MMOCR Contributors"
-version: 0.3.0
-date-released: 2020-08-15
-repository-code: "https://github.com/open-mmlab/mmocr"
-license: Apache-2.0
diff --git a/pipeline/mmocr/LICENSE b/pipeline/mmocr/LICENSE
deleted file mode 100644
index 3076a4378396deea4db311adbe1fbfd8b8b05920..0000000000000000000000000000000000000000
--- a/pipeline/mmocr/LICENSE
+++ /dev/null
@@ -1,203 +0,0 @@
-Copyright (c) MMOCR Authors. All rights reserved.
-
- Apache License
- Version 2.0, January 2004
- http://www.apache.org/licenses/
-
- TERMS AND CONDITIONS FOR USE, REPRODUCTION, AND DISTRIBUTION
-
- 1. Definitions.
-
- "License" shall mean the terms and conditions for use, reproduction,
- and distribution as defined by Sections 1 through 9 of this document.
-
- "Licensor" shall mean the copyright owner or entity authorized by
- the copyright owner that is granting the License.
-
- "Legal Entity" shall mean the union of the acting entity and all
- other entities that control, are controlled by, or are under common
- control with that entity. For the purposes of this definition,
- "control" means (i) the power, direct or indirect, to cause the
- direction or management of such entity, whether by contract or
- otherwise, or (ii) ownership of fifty percent (50%) or more of the
- outstanding shares, or (iii) beneficial ownership of such entity.
-
- "You" (or "Your") shall mean an individual or Legal Entity
- exercising permissions granted by this License.
-
- "Source" form shall mean the preferred form for making modifications,
- including but not limited to software source code, documentation
- source, and configuration files.
-
- "Object" form shall mean any form resulting from mechanical
- transformation or translation of a Source form, including but
- not limited to compiled object code, generated documentation,
- and conversions to other media types.
-
- "Work" shall mean the work of authorship, whether in Source or
- Object form, made available under the License, as indicated by a
- copyright notice that is included in or attached to the work
- (an example is provided in the Appendix below).
-
- "Derivative Works" shall mean any work, whether in Source or Object
- form, that is based on (or derived from) the Work and for which the
- editorial revisions, annotations, elaborations, or other modifications
- represent, as a whole, an original work of authorship. For the purposes
- of this License, Derivative Works shall not include works that remain
- separable from, or merely link (or bind by name) to the interfaces of,
- the Work and Derivative Works thereof.
-
- "Contribution" shall mean any work of authorship, including
- the original version of the Work and any modifications or additions
- to that Work or Derivative Works thereof, that is intentionally
- submitted to Licensor for inclusion in the Work by the copyright owner
- or by an individual or Legal Entity authorized to submit on behalf of
- the copyright owner. For the purposes of this definition, "submitted"
- means any form of electronic, verbal, or written communication sent
- to the Licensor or its representatives, including but not limited to
- communication on electronic mailing lists, source code control systems,
- and issue tracking systems that are managed by, or on behalf of, the
- Licensor for the purpose of discussing and improving the Work, but
- excluding communication that is conspicuously marked or otherwise
- designated in writing by the copyright owner as "Not a Contribution."
-
- "Contributor" shall mean Licensor and any individual or Legal Entity
- on behalf of whom a Contribution has been received by Licensor and
- subsequently incorporated within the Work.
-
- 2. Grant of Copyright License. Subject to the terms and conditions of
- this License, each Contributor hereby grants to You a perpetual,
- worldwide, non-exclusive, no-charge, royalty-free, irrevocable
- copyright license to reproduce, prepare Derivative Works of,
- publicly display, publicly perform, sublicense, and distribute the
- Work and such Derivative Works in Source or Object form.
-
- 3. Grant of Patent License. Subject to the terms and conditions of
- this License, each Contributor hereby grants to You a perpetual,
- worldwide, non-exclusive, no-charge, royalty-free, irrevocable
- (except as stated in this section) patent license to make, have made,
- use, offer to sell, sell, import, and otherwise transfer the Work,
- where such license applies only to those patent claims licensable
- by such Contributor that are necessarily infringed by their
- Contribution(s) alone or by combination of their Contribution(s)
- with the Work to which such Contribution(s) was submitted. If You
- institute patent litigation against any entity (including a
- cross-claim or counterclaim in a lawsuit) alleging that the Work
- or a Contribution incorporated within the Work constitutes direct
- or contributory patent infringement, then any patent licenses
- granted to You under this License for that Work shall terminate
- as of the date such litigation is filed.
-
- 4. Redistribution. You may reproduce and distribute copies of the
- Work or Derivative Works thereof in any medium, with or without
- modifications, and in Source or Object form, provided that You
- meet the following conditions:
-
- (a) You must give any other recipients of the Work or
- Derivative Works a copy of this License; and
-
- (b) You must cause any modified files to carry prominent notices
- stating that You changed the files; and
-
- (c) You must retain, in the Source form of any Derivative Works
- that You distribute, all copyright, patent, trademark, and
- attribution notices from the Source form of the Work,
- excluding those notices that do not pertain to any part of
- the Derivative Works; and
-
- (d) If the Work includes a "NOTICE" text file as part of its
- distribution, then any Derivative Works that You distribute must
- include a readable copy of the attribution notices contained
- within such NOTICE file, excluding those notices that do not
- pertain to any part of the Derivative Works, in at least one
- of the following places: within a NOTICE text file distributed
- as part of the Derivative Works; within the Source form or
- documentation, if provided along with the Derivative Works; or,
- within a display generated by the Derivative Works, if and
- wherever such third-party notices normally appear. The contents
- of the NOTICE file are for informational purposes only and
- do not modify the License. You may add Your own attribution
- notices within Derivative Works that You distribute, alongside
- or as an addendum to the NOTICE text from the Work, provided
- that such additional attribution notices cannot be construed
- as modifying the License.
-
- You may add Your own copyright statement to Your modifications and
- may provide additional or different license terms and conditions
- for use, reproduction, or distribution of Your modifications, or
- for any such Derivative Works as a whole, provided Your use,
- reproduction, and distribution of the Work otherwise complies with
- the conditions stated in this License.
-
- 5. Submission of Contributions. Unless You explicitly state otherwise,
- any Contribution intentionally submitted for inclusion in the Work
- by You to the Licensor shall be under the terms and conditions of
- this License, without any additional terms or conditions.
- Notwithstanding the above, nothing herein shall supersede or modify
- the terms of any separate license agreement you may have executed
- with Licensor regarding such Contributions.
-
- 6. Trademarks. This License does not grant permission to use the trade
- names, trademarks, service marks, or product names of the Licensor,
- except as required for reasonable and customary use in describing the
- origin of the Work and reproducing the content of the NOTICE file.
-
- 7. Disclaimer of Warranty. Unless required by applicable law or
- agreed to in writing, Licensor provides the Work (and each
- Contributor provides its Contributions) on an "AS IS" BASIS,
- WITHOUT WARRANTIES OR CONDITIONS OF ANY KIND, either express or
- implied, including, without limitation, any warranties or conditions
- of TITLE, NON-INFRINGEMENT, MERCHANTABILITY, or FITNESS FOR A
- PARTICULAR PURPOSE. You are solely responsible for determining the
- appropriateness of using or redistributing the Work and assume any
- risks associated with Your exercise of permissions under this License.
-
- 8. Limitation of Liability. In no event and under no legal theory,
- whether in tort (including negligence), contract, or otherwise,
- unless required by applicable law (such as deliberate and grossly
- negligent acts) or agreed to in writing, shall any Contributor be
- liable to You for damages, including any direct, indirect, special,
- incidental, or consequential damages of any character arising as a
- result of this License or out of the use or inability to use the
- Work (including but not limited to damages for loss of goodwill,
- work stoppage, computer failure or malfunction, or any and all
- other commercial damages or losses), even if such Contributor
- has been advised of the possibility of such damages.
-
- 9. Accepting Warranty or Additional Liability. While redistributing
- the Work or Derivative Works thereof, You may choose to offer,
- and charge a fee for, acceptance of support, warranty, indemnity,
- or other liability obligations and/or rights consistent with this
- License. However, in accepting such obligations, You may act only
- on Your own behalf and on Your sole responsibility, not on behalf
- of any other Contributor, and only if You agree to indemnify,
- defend, and hold each Contributor harmless for any liability
- incurred by, or claims asserted against, such Contributor by reason
- of your accepting any such warranty or additional liability.
-
- END OF TERMS AND CONDITIONS
-
- APPENDIX: How to apply the Apache License to your work.
-
- To apply the Apache License to your work, attach the following
- boilerplate notice, with the fields enclosed by brackets "[]"
- replaced with your own identifying information. (Don't include
- the brackets!) The text should be enclosed in the appropriate
- comment syntax for the file format. We also recommend that a
- file or class name and description of purpose be included on the
- same "printed page" as the copyright notice for easier
- identification within third-party archives.
-
- Copyright 2021 MMOCR Authors. All rights reserved.
-
- Licensed under the Apache License, Version 2.0 (the "License");
- you may not use this file except in compliance with the License.
- You may obtain a copy of the License at
-
- http://www.apache.org/licenses/LICENSE-2.0
-
- Unless required by applicable law or agreed to in writing, software
- distributed under the License is distributed on an "AS IS" BASIS,
- WITHOUT WARRANTIES OR CONDITIONS OF ANY KIND, either express or implied.
- See the License for the specific language governing permissions and
- limitations under the License.
diff --git a/pipeline/mmocr/MANIFEST.in b/pipeline/mmocr/MANIFEST.in
deleted file mode 100644
index 2ba112301a27e08af6939187dbd8e24cd85e852d..0000000000000000000000000000000000000000
--- a/pipeline/mmocr/MANIFEST.in
+++ /dev/null
@@ -1,5 +0,0 @@
-include requirements/*.txt
-include mmocr/.mim/model-index.yml
-include mmocr/.mim/dicts/*.txt
-recursive-include mmocr/.mim/configs *.py *.yml
-recursive-include mmocr/.mim/tools *.sh *.py
diff --git a/pipeline/mmocr/README_zh-CN.md b/pipeline/mmocr/README_zh-CN.md
deleted file mode 100644
index c38839637ec2e410e830cbcc8eb45160b178f8fd..0000000000000000000000000000000000000000
--- a/pipeline/mmocr/README_zh-CN.md
+++ /dev/null
@@ -1,250 +0,0 @@
-
-
-
-
-
-
-[](https://github.com/open-mmlab/mmocr/actions)
-[](https://mmocr.readthedocs.io/en/dev-1.x/?badge=dev-1.x)
-[](https://codecov.io/gh/open-mmlab/mmocr)
-[](https://github.com/open-mmlab/mmocr/blob/main/LICENSE)
-[](https://pypi.org/project/mmocr/)
-[](https://github.com/open-mmlab/mmocr/issues)
-[](https://github.com/open-mmlab/mmocr/issues)
-
-
-[๐ๆๆกฃ](https://mmocr.readthedocs.io/zh_CN/dev-1.x/) |
-[๐ ๏ธๅฎ่ฃ
](https://mmocr.readthedocs.io/zh_CN/dev-1.x/get_started/install.html) |
-[๐ๆจกๅๅบ](https://mmocr.readthedocs.io/zh_CN/dev-1.x/modelzoo.html) |
-[๐ๆดๆฐๆฅๅฟ](https://mmocr.readthedocs.io/en/dev-1.x/notes/changelog.html) |
-[๐คๆฅๅ้ฎ้ข](https://github.com/open-mmlab/mmocr/issues/new/choose)
-
-
-
-
-
-[English](/README.md) | ็ฎไฝไธญๆ
-
-
-
-
-
-
-
-
-
-
-
-
-
-
-
-
-
-
-
-
-
-
-
-## ่ฟๆๆดๆฐ
-
-**้ป่ฎคๅๆฏ็ฎๅไธบ `main`๏ผไธๅๆฏไธ็ไปฃ็ ๅทฒ็ปๅๆขๅฐ v1.0.0 ็ๆฌใๆง็ `main` ๅๆฏ๏ผv0.6.3๏ผ็ไปฃ็ ็ฐๅญๅจ `0.x` ๅๆฏไธใ** ๅฆๆๆจไธ็ดๅจไฝฟ็จ `main` ๅๆฏ๏ผๅนถ้ๅฐๅ็บง้ฎ้ข๏ผ่ฏท้
่ฏป [่ฟ็งปๆๅ](https://mmocr.readthedocs.io/zh_CN/dev-1.x/migration/overview.html) ๅ [ๅๆฏ่ฏดๆ](https://mmocr.readthedocs.io/zh_CN/dev-1.x/migration/branches.html) ใ
-
-ๆๆฐ็็ๆฌ v1.0.0 ไบ 2023-04-06 ๅๅธใๅ
ถ็ธๅฏนไบ 1.0.0rc6 ็ไธป่ฆๆดๆฐๅฆไธ๏ผ
-
-1. Dataset Preparer ไธญๆฏๆไบ SCUT-CTW1500, SynthText ๅ MJSynth ๆฐๆฎ้๏ผ
-2. ๆดๆฐไบๆๆกฃๅ FAQ๏ผ
-3. ๅ็บงๆไปถๅ็ซฏ๏ผไฝฟ็จไบ `backend_args` ๆฟๆข `file_client_args`;
-4. ๅขๅ ไบ MMOCR ๆ็จ notebookใ
-
-ๅฆๆ้่ฆไบ่งฃ MMOCR 1.0 ็ธๅฏนไบ 0.x ็ๅ็บงๅ
ๅฎน๏ผ่ฏท้
่ฏป [MMOCR 1.x ๆดๆฐๆฑๆป](https://mmocr.readthedocs.io/zh_CN/dev-1.x/migration/news.html)๏ผๆ่
้
่ฏป[ๆดๆฐๆฅๅฟ](https://mmocr.readthedocs.io/zh_CN/dev-1.x/notes/changelog.html)ไปฅ่ทๅๆดๅคไฟกๆฏใ
-
-## ็ฎไป
-
-MMOCR ๆฏๅบไบ PyTorch ๅ mmdetection ็ๅผๆบๅทฅๅ
ท็ฎฑ๏ผไธๆณจไบๆๆฌๆฃๆต๏ผๆๆฌ่ฏๅซไปฅๅ็ธๅบ็ไธๆธธไปปๅก๏ผๅฆๅ
ณ้ฎไฟกๆฏๆๅใ ๅฎๆฏ OpenMMLab ้กน็ฎ็ไธ้จๅใ
-
-ไธปๅๆฏ็ฎๅๆฏๆ **PyTorch 1.6 ไปฅไธ**็็ๆฌใ
-
-
-
-
-
-### ไธป่ฆ็นๆง
-
--**ๅ
จๆต็จ**
-
-่ฏฅๅทฅๅ
ท็ฎฑไธไป
ๆฏๆๆๆฌๆฃๆตๅๆๆฌ่ฏๅซ๏ผ่ฟๆฏๆๅ
ถไธๆธธไปปๅก๏ผไพๅฆๅ
ณ้ฎไฟกๆฏๆๅใ
-
--**ๅค็งๆจกๅ**
-
-่ฏฅๅทฅๅ
ท็ฎฑๆฏๆ็จไบๆๆฌๆฃๆต๏ผๆๆฌ่ฏๅซๅๅ
ณ้ฎไฟกๆฏๆๅ็ๅ็งๆๆฐๆจกๅใ
-
--**ๆจกๅๅ่ฎพ่ฎก**
-
-MMOCR ็ๆจกๅๅ่ฎพ่ฎกไฝฟ็จๆทๅฏไปฅๅฎไน่ชๅทฑ็ไผๅๅจ๏ผๆฐๆฎ้ขๅค็ๅจ๏ผๆจกๅ็ปไปถๅฆไธปๅนฒๆจกๅ๏ผ้ข้จๆจกๅๅๅคด้จๆจกๅ๏ผไปฅๅๆๅคฑๅฝๆฐใๆๅ
ณๅฆไฝๆๅปบ่ชๅฎไนๆจกๅ็ไฟกๆฏ๏ผ่ฏทๅ่[ๆฆ่ง](https://mmocr.readthedocs.io/zh_CN/dev-1.x/get_started/overview.html)ใ
-
--**ไผๅคๅฎ็จๅทฅๅ
ท**
-
-่ฏฅๅทฅๅ
ท็ฎฑๆไพไบไธๅฅๅ
จ้ข็ๅฎ็จ็จๅบ๏ผๅฏไปฅๅธฎๅฉ็จๆท่ฏไผฐๆจกๅ็ๆง่ฝใๅฎๅ
ๆฌๅฏๅฏนๅพๅ๏ผๆ ๆณจ็็ๅผไปฅๅ้ขๆต็ปๆ่ฟ่กๅฏ่งๅ็ๅฏ่งๅๅทฅๅ
ท๏ผไปฅๅ็จไบๅจ่ฎญ็ป่ฟ็จไธญ่ฏไผฐๆจกๅ็้ช่ฏๅทฅๅ
ทใๅฎ่ฟๅ
ๆฌๆฐๆฎ่ฝฌๆขๅจ๏ผๆผ็คบไบๅฆไฝๅฐ็จๆท่ชๅปบ็ๆ ๆณจๆฐๆฎ่ฝฌๆขไธบ MMOCR ๆฏๆ็ๆ ๆณจๆไปถใ
-
-## ๅฎ่ฃ
-
-MMOCR ไพ่ต [PyTorch](https://pytorch.org/), [MMEngine](https://github.com/open-mmlab/mmengine), [MMCV](https://github.com/open-mmlab/mmcv) ๅ [MMDetection](https://github.com/open-mmlab/mmdetection)๏ผไปฅไธๆฏๅฎ่ฃ
็็ฎ่ฆๆญฅ้ชคใ
-ๆด่ฏฆ็ป็ๅฎ่ฃ
ๆๅ่ฏทๅ่ [ๅฎ่ฃ
ๆๆกฃ](https://mmocr.readthedocs.io/zh_CN/dev-1.x/get_started/install.html)ใ
-
-```shell
-conda create -n open-mmlab python=3.8 pytorch=1.10 cudatoolkit=11.3 torchvision -c pytorch -y
-conda activate open-mmlab
-pip3 install openmim
-git clone https://github.com/open-mmlab/mmocr.git
-cd mmocr
-mim install -e .
-```
-
-## ๅฟซ้ๅ
ฅ้จ
-
-่ฏทๅ่[ๅฟซ้ๅ
ฅ้จ](https://mmocr.readthedocs.io/zh_CN/dev-1.x/get_started/quick_run.html)ๆๆกฃๅญฆไน MMOCR ็ๅบๆฌไฝฟ็จใ
-
-## [ๆจกๅๅบ](https://mmocr.readthedocs.io/zh_CN/dev-1.x/modelzoo.html)
-
-ๆฏๆ็็ฎๆณ๏ผ
-
-
-้ชจๅนฒ็ฝ็ป
-
-- [x] [oCLIP](configs/backbone/oclip/README.md) (ECCV'2022)
-
-
-
-
-ๆๅญๆฃๆต
-
-- [x] [DBNet](configs/textdet/dbnet/README.md) (AAAI'2020) / [DBNet++](configs/textdet/dbnetpp/README.md) (TPAMI'2022)
-- [x] [Mask R-CNN](configs/textdet/maskrcnn/README.md) (ICCV'2017)
-- [x] [PANet](configs/textdet/panet/README.md) (ICCV'2019)
-- [x] [PSENet](configs/textdet/psenet/README.md) (CVPR'2019)
-- [x] [TextSnake](configs/textdet/textsnake/README.md) (ECCV'2018)
-- [x] [DRRG](configs/textdet/drrg/README.md) (CVPR'2020)
-- [x] [FCENet](configs/textdet/fcenet/README.md) (CVPR'2021)
-
-
-
-
-ๆๅญ่ฏๅซ
-
-- [x] [ABINet](configs/textrecog/abinet/README.md) (CVPR'2021)
-- [x] [ASTER](configs/textrecog/aster/README.md) (TPAMI'2018)
-- [x] [CRNN](configs/textrecog/crnn/README.md) (TPAMI'2016)
-- [x] [MASTER](configs/textrecog/master/README.md) (PR'2021)
-- [x] [NRTR](configs/textrecog/nrtr/README.md) (ICDAR'2019)
-- [x] [RobustScanner](configs/textrecog/robust_scanner/README.md) (ECCV'2020)
-- [x] [SAR](configs/textrecog/sar/README.md) (AAAI'2019)
-- [x] [SATRN](configs/textrecog/satrn/README.md) (CVPR'2020 Workshop on Text and Documents in the Deep Learning Era)
-- [x] [SVTR](configs/textrecog/svtr/README.md) (IJCAI'2022)
-
-
-
-
-ๅ
ณ้ฎไฟกๆฏๆๅ
-
-- [x] [SDMG-R](configs/kie/sdmgr/README.md) (ArXiv'2021)
-
-
-
-
-็ซฏๅฏน็ซฏ OCR
-
-- [x] [ABCNet](projects/ABCNet/README.md) (CVPR'2020)
-- [x] [ABCNetV2](projects/ABCNet/README_V2.md) (TPAMI'2021)
-- [x] [SPTS](projects/SPTS/README.md) (ACM MM'2022)
-
-
-
-่ฏท็นๅป[ๆจกๅๅบ](https://mmocr.readthedocs.io/zh_CN/dev-1.x/modelzoo.html)ๆฅ็ๆดๅคๅ
ณไบไธ่ฟฐ็ฎๆณ็่ฏฆ็ปไฟกๆฏใ
-
-## ็คพๅบ้กน็ฎ
-
-[่ฟ้](projects/README.md)ๆไธไบ็ฑ็คพๅบ็จๆทๆฏๆๅ็ปดๆค็ๅบไบ MMOCR ็ SOTA ๆจกๅๅ่งฃๅณๆนๆก็ๅฎ็ฐใ่ฟไบ้กน็ฎๅฑ็คบไบๅบไบ MMOCR ็็ ็ฉถๅไบงๅๅผๅ็ๆไฝณๅฎ่ทตใ
-ๆไปฌๆฌข่ฟๅนถๆ่ฐขๅฏน OpenMMLab ็ๆ็ณป็ป็ๆๆ่ดก็ฎใ
-
-## ่ดก็ฎๆๅ
-
-ๆไปฌๆ่ฐขๆๆ็่ดก็ฎ่
ไธบๆน่ฟๅๆๅ MMOCR ๆไฝๅบ็ๅชๅใ่ฏทๅ่[่ดก็ฎๆๅ](.github/CONTRIBUTING.md)ๆฅไบ่งฃๅไธ้กน็ฎ่ดก็ฎ็็ธๅ
ณๆๅผใ
-
-## ่ด่ฐข
-
-MMOCR ๆฏไธๆฌพ็ฑๆฅ่ชไธๅ้ซๆ กๅไผไธ็็ ๅไบบๅๅ
ฑๅๅไธ่ดก็ฎ็ๅผๆบ้กน็ฎใๆไปฌๆ่ฐขๆๆไธบ้กน็ฎๆไพ็ฎๆณๅค็ฐๅๆฐๅ่ฝๆฏๆ็่ดก็ฎ่
๏ผไปฅๅๆไพๅฎ่ดตๅ้ฆ็็จๆทใ ๆไปฌๅธๆๆญคๅทฅๅ
ท็ฎฑๅฏไปฅๅธฎๅฉๅคงๅฎถๆฅๅค็ฐๅทฒๆ็ๆนๆณๅๅผๅๆฐ็ๆนๆณ๏ผไป่ไธบ็ ็ฉถ็คพๅบ่ดก็ฎๅ้ใ
-
-## ๅผ็จ
-
-ๅฆๆๆจๅ็ฐๆญค้กน็ฎๅฏนๆจ็็ ็ฉถๆ็จ๏ผ่ฏท่่ๅผ็จ๏ผ
-
-```bibtex
-@article{mmocr2021,
- title={MMOCR: A Comprehensive Toolbox for Text Detection, Recognition and Understanding},
- author={Kuang, Zhanghui and Sun, Hongbin and Li, Zhizhong and Yue, Xiaoyu and Lin, Tsui Hin and Chen, Jianyong and Wei, Huaqiang and Zhu, Yiqin and Gao, Tong and Zhang, Wenwei and Chen, Kai and Zhang, Wayne and Lin, Dahua},
- journal= {arXiv preprint arXiv:2108.06543},
- year={2021}
-}
-```
-
-## ๅผๆบ่ฎธๅฏ่ฏ
-
-่ฏฅ้กน็ฎ้็จ [Apache 2.0 license](LICENSE) ๅผๆบ่ฎธๅฏ่ฏใ
-
-## OpenMMLab ็ๅ
ถไป้กน็ฎ
-
-- [MMEngine](https://github.com/open-mmlab/mmengine): OpenMMLab ๆทฑๅบฆๅญฆไน ๆจกๅ่ฎญ็ปๅบ็กๅบ
-- [MMCV](https://github.com/open-mmlab/mmcv): OpenMMLab ่ฎก็ฎๆบ่ง่งๅบ็กๅบ
-- [MIM](https://github.com/open-mmlab/mim): MIM ๆฏ OpenMMlab ้กน็ฎใ็ฎๆณใๆจกๅ็็ปไธๅ
ฅๅฃ
-- [MMClassification](https://github.com/open-mmlab/mmclassification): OpenMMLab ๅพๅๅ็ฑปๅทฅๅ
ท็ฎฑ
-- [MMDetection](https://github.com/open-mmlab/mmdetection): OpenMMLab ็ฎๆ ๆฃๆตๅทฅๅ
ท็ฎฑ
-- [MMDetection3D](https://github.com/open-mmlab/mmdetection3d): OpenMMLab ๆฐไธไปฃ้็จ 3D ็ฎๆ ๆฃๆตๅนณๅฐ
-- [MMRotate](https://github.com/open-mmlab/mmrotate): OpenMMLab ๆ่ฝฌๆกๆฃๆตๅทฅๅ
ท็ฎฑไธๆต่ฏๅบๅ
-- [MMSegmentation](https://github.com/open-mmlab/mmsegmentation): OpenMMLab ่ฏญไนๅๅฒๅทฅๅ
ท็ฎฑ
-- [MMOCR](https://github.com/open-mmlab/mmocr): OpenMMLab ๅ
จๆต็จๆๅญๆฃๆต่ฏๅซ็่งฃๅทฅๅ
ท็ฎฑ
-- [MMPose](https://github.com/open-mmlab/mmpose): OpenMMLab ๅงฟๆไผฐ่ฎกๅทฅๅ
ท็ฎฑ
-- [MMHuman3D](https://github.com/open-mmlab/mmhuman3d): OpenMMLab ไบบไฝๅๆฐๅๆจกๅๅทฅๅ
ท็ฎฑไธๆต่ฏๅบๅ
-- [MMSelfSup](https://github.com/open-mmlab/mmselfsup): OpenMMLab ่ช็็ฃๅญฆไน ๅทฅๅ
ท็ฎฑไธๆต่ฏๅบๅ
-- [MMRazor](https://github.com/open-mmlab/mmrazor): OpenMMLab ๆจกๅๅ็ผฉๅทฅๅ
ท็ฎฑไธๆต่ฏๅบๅ
-- [MMFewShot](https://github.com/open-mmlab/mmfewshot): OpenMMLab ๅฐๆ ทๆฌๅญฆไน ๅทฅๅ
ท็ฎฑไธๆต่ฏๅบๅ
-- [MMAction2](https://github.com/open-mmlab/mmaction2): OpenMMLab ๆฐไธไปฃ่ง้ข็่งฃๅทฅๅ
ท็ฎฑ
-- [MMTracking](https://github.com/open-mmlab/mmtracking): OpenMMLab ไธไฝๅ่ง้ข็ฎๆ ๆ็ฅๅนณๅฐ
-- [MMFlow](https://github.com/open-mmlab/mmflow): OpenMMLab ๅ
ๆตไผฐ่ฎกๅทฅๅ
ท็ฎฑไธๆต่ฏๅบๅ
-- [MMEditing](https://github.com/open-mmlab/mmediting): OpenMMLab ๅพๅ่ง้ข็ผ่พๅทฅๅ
ท็ฎฑ
-- [MMGeneration](https://github.com/open-mmlab/mmgeneration): OpenMMLab ๅพ็่ง้ข็ๆๆจกๅๅทฅๅ
ท็ฎฑ
-- [MMDeploy](https://github.com/open-mmlab/mmdeploy): OpenMMLab ๆจกๅ้จ็ฝฒๆกๆถ
-
-## ๆฌข่ฟๅ ๅ
ฅ OpenMMLab ็คพๅบ
-
-ๆซๆไธๆน็ไบ็ปด็ ๅฏๅ
ณๆณจ OpenMMLab ๅข้็ [็ฅไนๅฎๆน่ดฆๅท](https://www.zhihu.com/people/openmmlab)๏ผๅ ๅ
ฅ OpenMMLab ๅข้็ [ๅฎๆนไบคๆต QQ ็พค](https://r.vansin.top/?r=join-qq)๏ผๆ้่ฟๆทปๅ ๅพฎไฟกโOpenๅฐๅตLabโๅ ๅ
ฅๅฎๆนไบคๆตๅพฎไฟก็พคใ
-
-
-
-ๆไปฌไผๅจ OpenMMLab ็คพๅบไธบๅคงๅฎถ
-
-- ๐ข ๅไบซ AI ๆกๆถ็ๅๆฒฟๆ ธๅฟๆๆฏ
-- ๐ป ่งฃ่ฏป PyTorch ๅธธ็จๆจกๅๆบ็
-- ๐ฐ ๅๅธ OpenMMLab ็็ธๅ
ณๆฐ้ป
-- ๐ ไป็ป OpenMMLab ๅผๅ็ๅๆฒฟ็ฎๆณ
-- ๐ ่ทๅๆด้ซๆ็้ฎ้ข็ญ็ๅๆ่งๅ้ฆ
-- ๐ฅ ๆไพไธๅ่กๅไธๅผๅ่
ๅ
ๅไบคๆต็ๅนณๅฐ
-
-ๅนฒ่ดงๆปกๆปก ๐๏ผ็ญไฝ ๆฅๆฉ ๐๏ผOpenMMLab ็คพๅบๆๅพ
ๆจ็ๅ ๅ
ฅ ๐ฌ
diff --git a/pipeline/mmocr/__init__.py b/pipeline/mmocr/__init__.py
deleted file mode 100644
index f8b5d0dd213a040186f5431c3439072395abfe85..0000000000000000000000000000000000000000
--- a/pipeline/mmocr/__init__.py
+++ /dev/null
@@ -1 +0,0 @@
-from mmocr import *
\ No newline at end of file
diff --git a/pipeline/mmocr/__pycache__/__init__.cpython-39.pyc b/pipeline/mmocr/__pycache__/__init__.cpython-39.pyc
deleted file mode 100644
index 4e919aa89a3d0508ed8e311b4d4395831fbaee52..0000000000000000000000000000000000000000
Binary files a/pipeline/mmocr/__pycache__/__init__.cpython-39.pyc and /dev/null differ
diff --git a/pipeline/mmocr/dicts/chinese_english_digits.txt b/pipeline/mmocr/dicts/chinese_english_digits.txt
deleted file mode 100644
index 2fb5f7326d863a2eefb67777df6becfb15671275..0000000000000000000000000000000000000000
--- a/pipeline/mmocr/dicts/chinese_english_digits.txt
+++ /dev/null
@@ -1,11377 +0,0 @@
-!
-"
-#
-$
-%
-&
-'
-(
-)
-*
-+
-,
--
-.
-/
-0
-1
-2
-3
-4
-5
-6
-7
-8
-9
-:
-;
-<
-=
->
-?
-@
-A
-B
-C
-D
-E
-F
-G
-H
-I
-J
-K
-L
-M
-N
-O
-P
-Q
-R
-S
-T
-U
-V
-W
-X
-Y
-Z
-[
-\
-]
-^
-_
-`
-a
-b
-c
-d
-e
-f
-g
-h
-i
-j
-k
-l
-m
-n
-o
-p
-q
-r
-s
-t
-u
-v
-w
-x
-y
-z
-{
-|
-}
-~
-ยฎ
-ยฐ
-ยฑ
-ยณ
-ยด
-ยท
-ร
-ร
-ร
-ร
-ร
-ร
-ร
-ร
-ร
-ร
-ร
-รค
-รจ
-รฉ
-รฌ
-รฒ
-รณ
-รด
-รถ
-รท
-รผ
-ล
-ษ
-ห
-ฮฉ
-ฮฒ
-ฮด
-ฮฟ
-ะ
-ะท
-ู
-โ
-โ
-โ
-โ
-โ
-โ
-โ
-โ
-โข
-โฅ
-โฆ
-โฐ
-โฒ
-โป
-โฌ
-โ
-โข
-โ
-โ
ก
-โ
ข
-โ
ฃ
-โ
ค
-โ
ฅ
-โ
-โ
-โ
-โ
-โ
-โ
-โถ
-โ
-โค
-โ
-โ
-โ
-โ
-โ
-โก
-โช
-โฒ
-โณ
-โถ
-โธ
-โผ
-โฝ
-โ
-โ
-โ
-โ
-โฅ
-โ
-โ
-โ
-โ
-โฅ
-โช
-โญ
-โ
-โค
-ใ
-ใ
-ใ
-ใ
-ใ
-ใ
-ใ
-ใ
-ใ
-ใ
-ใ
-ใ
-ใ
-ใ
-ใ
-ใ
-ใ
-ใ
-ใ
-ใ
-ใ
-ใ
-ใ
-ใ
-ใ
-ใ
-ใ
-ใ
-ใ
-ใ
-ใ
-ใ
-ใ
-ใ
-ใ
-ใ
-ใ
-ใ
-ใ
-ใ
-ใ
-ใ
-ใ
-ใ
-ใ
-ใ
-ใ
-ใ
-ใ
-ใ
-ใก
-ใข
-ใฃ
-ใค
-ใฅ
-ใฆ
-ใง
-ใจ
-ใฉ
-ใช
-ใซ
-ใฌ
-ใญ
-ใฎ
-ใฏ
-ใฐ
-ใฑ
-ใฒ
-ใณ
-ใด
-ใต
-ใถ
-ใท
-ใธ
-ใน
-ใบ
-ใป
-ใผ
-ใฝ
-ใพ
-ใฟ
-ใ
-ใ
-ใ
-ใ
-ใ
-ใ
-ใ
-ใ
-ใ
-ใ
-ใ
-ใ
-ใ
-ใ
-ใ
-ใ
-ใ
-ใ
-ใ
-ใก
-ใข
-ใฃ
-ใค
-ใฅ
-ใฆ
-ใง
-ใจ
-ใฉ
-ใช
-ใซ
-ใฌ
-ใญ
-ใฎ
-ใฏ
-ใฐ
-ใฑ
-ใฒ
-ใณ
-ใด
-ใต
-ใถ
-ใท
-ใธ
-ใน
-ใบ
-ใป
-ใผ
-ใฝ
-ใพ
-ใฟ
-ใ
-ใ
-ใ
-ใ
-ใ
-ใ
-ใ
-ใ
-ใ
-ใ
-ใ
-ใ
-ใ
-ใ
-ใ
-ใ
-ใ
-ใ
-ใ
-ใ
-ใ
-ใ
-ใ
-ใ
-ใ
-ใ
-ใ
-ใ
-ใ
-ใ
-ใ
-ใ
-ใก
-ใข
-ใฃ
-ใค
-ใฅ
-ใฆ
-ใง
-ใจ
-ใฉ
-ใช
-ใซ
-ใฌ
-ใญ
-ใฏ
-ใฑ
-ใฒ
-ใณ
-ใด
-ใต
-ใถ
-ใผ
-ใ
-ใก
-ใฅ
-ใ
-ใ
-ใง
-ใถ
-ใบ
-ไ
-ไฒ
-ไท
-ไธ
-ไน
-ไฝ
-ไ
-ไ
-ไ
-ไฃ
-ไ
-ไ
-ไฏ
-ได
-ได
-ได
-ได
-ไธ
-ไธ
-ไธ
-ไธ
-ไธ
-ไธ
-ไธ
-ไธ
-ไธ
-ไธ
-ไธ
-ไธ
-ไธ
-ไธ
-ไธ
-ไธ
-ไธ
-ไธ
-ไธ
-ไธ
-ไธ
-ไธ
-ไธ
-ไธก
-ไธข
-ไธฃ
-ไธค
-ไธฅ
-ไธง
-ไธจ
-ไธช
-ไธซ
-ไธฌ
-ไธญ
-ไธฐ
-ไธฑ
-ไธฒ
-ไธด
-ไธถ
-ไธธ
-ไธน
-ไธบ
-ไธป
-ไธผ
-ไธฝ
-ไธพ
-ไธฟ
-ไน
-ไน
-ไน
-ไน
-ไน
-ไน
-ไน
-ไน
-ไน
-ไน
-ไน
-ไน
-ไน
-ไน
-ไน
-ไน
-ไน
-ไน
-ไน
-ไน
-ไน
-ไน
-ไน
-ไน
-ไนก
-ไนฆ
-ไนฉ
-ไนฐ
-ไนฑ
-ไนณ
-ไนธ
-ไนพ
-ไบ
-ไบ
-ไบ
-ไบ
-ไบ
-ไบ
-ไบ
-ไบ
-ไบ
-ไบ
-ไบ
-ไบ
-ไบ
-ไบ
-ไบ
-ไบ
-ไบ
-ไบ
-ไบ
-ไบ
-ไบ
-ไบก
-ไบข
-ไบค
-ไบฅ
-ไบฆ
-ไบง
-ไบจ
-ไบฉ
-ไบซ
-ไบฌ
-ไบญ
-ไบฎ
-ไบฒ
-ไบณ
-ไบต
-ไบถ
-ไบธ
-ไบน
-ไบบ
-ไบป
-ไบฟ
-ไป
-ไป
-ไป
-ไป
-ไป
-ไป
-ไป
-ไป
-ไป
-ไป
-ไป
-ไป
-ไป
-ไป
-ไป
-ไป
-ไป
-ไป
-ไป
-ไป
-ไป
-ไป
-ไป
-ไป
-ไป
-ไป
-ไปก
-ไปฃ
-ไปค
-ไปฅ
-ไปจ
-ไปช
-ไปซ
-ไปฌ
-ไปญ
-ไปฎ
-ไปฐ
-ไปฑ
-ไปฒ
-ไปณ
-ไปต
-ไปถ
-ไปท
-ไปป
-ไปฝ
-ไปฟ
-ไผ
-ไผ
-ไผ
-ไผ
-ไผ
-ไผ
-ไผ
-ไผ
-ไผ
-ไผ
-ไผ
-ไผ
-ไผ
-ไผ
-ไผ
-ไผ
-ไผ
-ไผ
-ไผ
-ไผ
-ไผ
-ไผ
-ไผข
-ไผฃ
-ไผค
-ไผฅ
-ไผฆ
-ไผง
-ไผฉ
-ไผช
-ไผซ
-ไผฌ
-ไผฏ
-ไผฐ
-ไผฑ
-ไผฒ
-ไผด
-ไผถ
-ไผท
-ไผธ
-ไผบ
-ไผป
-ไผผ
-ไผฝ
-ไผพ
-ไฝ
-ไฝ
-ไฝ
-ไฝ
-ไฝ
-ไฝ
-ไฝ
-ไฝ
-ไฝ
-ไฝ
-ไฝ
-ไฝ
-ไฝ
-ไฝ
-ไฝ
-ไฝ
-ไฝ
-ไฝ
-ไฝ
-ไฝ
-ไฝ
-ไฝ
-ไฝ
-ไฝข
-ไฝฃ
-ไฝค
-ไฝฅ
-ไฝง
-ไฝฉ
-ไฝฌ
-ไฝฎ
-ไฝฏ
-ไฝฐ
-ไฝณ
-ไฝด
-ไฝต
-ไฝถ
-ไฝท
-ไฝธ
-ไฝน
-ไฝบ
-ไฝป
-ไฝผ
-ไฝฝ
-ไฝพ
-ไฝฟ
-ไพ
-ไพ
-ไพ
-ไพ
-ไพ
-ไพ
-ไพ
-ไพ
-ไพ
-ไพ
-ไพ
-ไพ
-ไพ
-ไพ
-ไพ
-ไพ
-ไพ
-ไพ
-ไพก
-ไพฃ
-ไพฅ
-ไพฆ
-ไพง
-ไพจ
-ไพฉ
-ไพช
-ไพฌ
-ไพญ
-ไพฎ
-ไพฏ
-ไพฒ
-ไพต
-ไพน
-ไพผ
-ไพฟ
-ไฟ
-ไฟ
-ไฟ
-ไฟ
-ไฟ
-ไฟ
-ไฟ
-ไฟ
-ไฟ
-ไฟ
-ไฟ
-ไฟ
-ไฟ
-ไฟ
-ไฟ
-ไฟ
-ไฟ
-ไฟ
-ไฟ
-ไฟ
-ไฟ
-ไฟก
-ไฟฃ
-ไฟค
-ไฟฆ
-ไฟง
-ไฟจ
-ไฟฉ
-ไฟช
-ไฟซ
-ไฟฌ
-ไฟญ
-ไฟฎ
-ไฟฏ
-ไฟฑ
-ไฟณ
-ไฟด
-ไฟต
-ไฟถ
-ไฟท
-ไฟธ
-ไฟบ
-ไฟพ
-ๅ
-ๅ
-ๅ
-ๅ
-ๅ
-ๅ
-ๅ
-ๅ
-ๅ
-ๅ
-ๅ
-ๅ
-ๅ
-ๅ
-ๅ
-ๅ
-ๅ
-ๅ
-ๅ
-ๅ
-ๅก
-ๅข
-ๅค
-ๅฅ
-ๅฆ
-ๅจ
-ๅฉ
-ๅช
-ๅซ
-ๅฌ
-ๅญ
-ๅฎ
-ๅณ
-ๅด
-ๅถ
-ๅท
-ๅธ
-ๅน
-ๅบ
-ๅผ
-ๅพ
-ๅ
-ๅ
-ๅ
-ๅ
-ๅ
-ๅ
-ๅ
-ๅ
-ๅ
-ๅ
-ๅ
-ๅ
-ๅ
-ๅ
-ๅข
-ๅฅ
-ๅช
-ๅฌ
-ๅญ
-ๅฐ
-ๅฑ
-ๅฒ
-ๅด
-ๅถ
-ๅท
-ๅป
-ๅพ
-ๅฟ
-ๅ
-ๅ
-ๅ
-ๅ
-ๅ
-ๅ
-ๅ
-ๅ
-ๅ
-ๅ
-ๅ
-ๅฃ
-ๅฅ
-ๅฆ
-ๅง
-ๅจ
-ๅฉ
-ๅช
-ๅซ
-ๅฌ
-ๅฏ
-ๅฒ
-ๅณ
-ๅต
-ๅท
-ๅบ
-ๅป
-ๅพ
-ๅ
-ๅ
-ๅ
-ๅ
-ๅ
-ๅ
-ๅ
-ๅ
-ๅ
-ๅ
-ๅ
-ๅ
-ๅ
-ๅ
-ๅก
-ๅค
-ๅฆ
-ๅง
-ๅช
-ๅฌ
-ๅญ
-ๅฎ
-ๅฐ
-ๅฑ
-ๅณ
-ๅด
-ๅต
-ๅถ
-ๅธ
-ๅน
-ๅป
-ๅฝ
-ๅพ
-ๅฟ
-ๅ
-ๅ
-ๅ
-ๅ
-ๅ
-ๅ
-ๅ
-ๅ
-ๅ
-ๅ
-ๅ
-ๅ
-ๅ
-ๅก
-ๅฃ
-ๅฆ
-ๅฒ
-ๅด
-ๅต
-ๅฝ
-ๅพ
-ๅฟ
-ๅ
-ๅ
-ๅ
-ๅ
-ๅ
-ๅ
-ๅ
-ๅ
-ๅ
-ๅ
-ๅ
-ๅ
-ๅ
-ๅ
-ๅ
-ๅ
-ๅ
-ๅ
-ๅ
-ๅ
-ๅ
ข
-ๅ
ฅ
-ๅ
ง
-ๅ
จ
-ๅ
ฉ
-ๅ
ซ
-ๅ
ฌ
-ๅ
ญ
-ๅ
ฎ
-ๅ
ฐ
-ๅ
ฑ
-ๅ
ณ
-ๅ
ด
-ๅ
ต
-ๅ
ถ
-ๅ
ท
-ๅ
ธ
-ๅ
น
-ๅ
ป
-ๅ
ผ
-ๅ
ฝ
-ๅ
ฟ
-ๅ
-ๅ
-ๅ
-ๅ
-ๅ
-ๅ
-ๅ
-ๅ
-ๅ
-ๅ
-ๅ
-ๅ
-ๅ
-ๅ
-ๅ
-ๅ
-ๅ
-ๅ
-ๅ
-ๅ
-ๅ
-ๅ
-ๅ
-ๅข
-ๅค
-ๅฅ
-ๅง
-ๅจ
-ๅฌ
-ๅฏ
-ๅฐ
-ๅฑ
-ๅฒ
-ๅณ
-ๅด
-ๅต
-ๅถ
-ๅท
-ๅป
-ๅผ
-ๅฝ
-ๅฟ
-ๅ
-ๅ
-ๅ
-ๅ
-ๅ
-ๅ
-ๅ
-ๅ
-ๅ
-ๅ
-ๅ
-ๅ
-ๅ
-ๅ
-ๅ
-ๅ
-ๅก
-ๅค
-ๅฆ
-ๅง
-ๅช
-ๅซ
-ๅญ
-ๅฏ
-ๅฐ
-ๅฑ
-ๅณ
-ๅถ
-ๅธ
-ๅน
-ๅบ
-ๅป
-ๅผ
-ๅฝ
-ๅฟ
-ๅ
-ๅ
-ๅ
-ๅ
-ๅ
-ๅ
-ๅ
-ๅ
-ๅ
-ๅ
-ๅ
-ๅ
-ๅ
-ๅ
-ๅ
-ๅ
-ๅ
-ๅ
-ๅ
-ๅ
-ๅ
-ๅ
-ๅค
-ๅฅ
-ๅจ
-ๅฉ
-ๅซ
-ๅฌ
-ๅญ
-ๅฎ
-ๅฐ
-ๅฒ
-ๅณ
-ๅต
-ๅถ
-ๅท
-ๅธ
-ๅน
-ๅบ
-ๅป
-ๅฝ
-ๅฟ
-ๅ
-ๅ
-ๅ
-ๅ
-ๅ
-ๅ
-ๅ
-ๅ
-ๅ
-ๅ
-ๅ
-ๅ
-ๅ
-ๅ
-ๅ
-ๅ
-ๅ
-ๅ
-ๅ
-ๅก
-ๅฃ
-ๅค
-ๅฅ
-ๅง
-ๅจ
-ๅฉ
-ๅช
-ๅฏ
-ๅฐ
-ๅฒ
-ๅธ
-ๅบ
-ๅฝ
-ๅฟ
-ๅ
-ๅ
-ๅ
-ๅ
-ๅ
-ๅ
-ๅ
-ๅ
-ๅ
-ๅ
-ๅ
-ๅ
-ๅ
-ๅก
-ๅข
-ๅฃ
-ๅจ
-ๅฉ
-ๅช
-ๅซ
-ๅฌ
-ๅญ
-ๅฑ
-ๅฒ
-ๅณ
-ๅด
-ๅต
-ๅท
-ๅน
-ๅป
-ๅผ
-ๅพ
-ๅฟ
-ๅ
-ๅ
-ๅ
-ๅ
-ๅ
-ๅ
-ๅ
-ๅ
-ๅ
-ๅ
-ๅ
-ๅ
-ๅ
-ๅ
-ๅ
-ๅ
-ๅข
-ๅค
-ๅง
-ๅฉ
-ๅฎ
-ๅฐ
-ๅฒ
-ๅท
-ๅธ
-ๅบ
-ๅผ
-ๅพ
-ๅฟ
-ๅ
-ๅ
-ๅ
-ๅ
-ๅ
-ๅ
-ๅ
-ๅ
-ๅ
-ๅ
-ๅ
-ๅ
-ๅ
-ๅ
-ๅ
-ๅ
-ๅ
-ๅ
-ๅ
-ๅก
-ๅฃ
-ๅค
-ๅฆ
-ๅช
-ๅฎ
-ๅฏ
-ๅณ
-ๅน
-ๅบ
-ๅป
-ๅผ
-ๅฝ
-ๅพ
-ๅฟ
-ๅ
-ๅ
-ๅ
-ๅ
-ๅ
-ๅ
-ๅ
-ๅ
-ๅ
-ๅ
-ๅ
-ๅ
-ๅ
-ๅ
-ๅ
-ๅ
-ๅ
-ๅ
-ๅ
-ๅ
-ๅ
-ๅ
-ๅ
-ๅ
-ๅก
-ๅข
-ๅฃ
-ๅค
-ๅฆ
-ๅง
-ๅจ
-ๅซ
-ๅฌ
-ๅญ
-ๅฎ
-ๅฏ
-ๅฐ
-ๅฑ
-ๅฒ
-ๅณ
-ๅด
-ๅต
-ๅท
-ๅธ
-ๅบ
-ๅป
-ๅผ
-ๅฟ
-ๅ
-ๅ
-ๅ
-ๅ
-ๅ
-ๅ
-ๅ
-ๅ
-ๅ
-ๅ
-ๅ
-ๅ
-ๅ
-ๅ
-ๅ
-ๅ
-ๅ
-ๅ
-ๅข
-ๅฃ
-ๅฅ
-ๅฆ
-ๅจ
-ๅฉ
-ๅญ
-ๅฎ
-ๅฒ
-ๅณ
-ๅน
-ๅป
-ๅพ
-ๅฟ
-ๅ
-ๅ
-ๅ
-ๅ
-ๅ
-ๅ
-ๅ
-ๅ
-ๅ
-ๅ
-ๅ
-ๅ
-ๅ
-ๅ
-ๅ
-ๅ
-ๅ
-ๅ
-ๅ
-ๅ
-ๅ
-ๅ
-ๅก
-ๅฃ
-ๅค
-ๅฅ
-ๅฆ
-ๅจ
-ๅฉ
-ๅช
-ๅซ
-ๅฌ
-ๅญ
-ๅฎ
-ๅฏ
-ๅฐ
-ๅฑ
-ๅฒ
-ๅณ
-ๅต
-ๅถ
-ๅท
-ๅธ
-ๅน
-ๅป
-ๅผ
-ๅฝ
-ๅ
-ๅ
-ๅ
-ๅ
-ๅ
-ๅ
-ๅ
-ๅ
-ๅ
-ๅ
-ๅ
-ๅ
-ๅ
-ๅ
-ๅ
-ๅ
-ๅ
-ๅ
-ๅ
-ๅ
-ๅ
-ๅ
-ๅ
-ๅ
-ๅ
-ๅก
-ๅฆ
-ๅง
-ๅจ
-ๅฉ
-ๅช
-ๅซ
-ๅฌ
-ๅญ
-ๅฎ
-ๅฏ
-ๅฐ
-ๅฑ
-ๅฒ
-ๅณ
-ๅด
-ๅต
-ๅท
-ๅธ
-ๅน
-ๅบ
-ๅป
-ๅผ
-ๅฝ
-ๅพ
-ๅฟ
-ๅ
-ๅ
-ๅ
-ๅ
-ๅ
-ๅ
-ๅ
-ๅ
-ๅ
-ๅ
-ๅ
-ๅ
-ๅ
-ๅ
-ๅ
-ๅ
-ๅ
-ๅ
-ๅ
-ๅ
-ๅข
-ๅฃ
-ๅค
-ๅฅ
-ๅฆ
-ๅจ
-ๅช
-ๅซ
-ๅฐ
-ๅฑ
-ๅฒ
-ๅณ
-ๅด
-ๅต
-ๅถ
-ๅท
-ๅธ
-ๅบ
-ๅป
-ๅผ
-ๅฝ
-ๅฟ
-ๅ
-ๅ
-ๅ
-ๅ
-ๅ
-ๅ
-ๅ
-ๅ
-ๅ
-ๅ
-ๅ
-ๅ
-ๅ
-ๅ
-ๅ
-ๅ
-ๅ
-ๅ
-ๅ
-ๅ
-ๅ
-ๅ
-ๅ
-ๅ
-ๅ
-ๅข
-ๅฃ
-ๅค
-ๅฅ
-ๅฆ
-ๅง
-ๅจ
-ๅฉ
-ๅช
-ๅซ
-ๅฌ
-ๅญ
-ๅฎ
-ๅฏ
-ๅฑ
-ๅฒ
-ๅณ
-ๅถ
-ๅท
-ๅธ
-ๅบ
-ๅป
-ๅฝ
-ๅพ
-ๅฟ
-ๅ
-ๅ
-ๅ
-ๅ
-ๅ
-ๅ
-ๅ
-ๅ
-ๅ
-ๅ
-ๅ
-ๅ
-ๅ
-ๅ
-ๅ
-ๅ
-ๅ
-ๅ
-ๅ
-ๅ
-ๅ
-ๅ
-ๅ
-ๅ
-ๅ
-ๅ
-ๅ
-ๅก
-ๅข
-ๅฃ
-ๅค
-ๅฅ
-ๅฆ
-ๅง
-ๅจ
-ๅฉ
-ๅช
-ๅญ
-ๅฎ
-ๅฒ
-ๅณ
-ๅบ
-ๅผ
-ๅฝ
-ๅฟ
-ๅ
-ๅ
-ๅ
-ๅ
-ๅ
-ๅ
-ๅ
-ๅ
-ๅ
-ๅ
-ๅ
-ๅ
-ๅ
-ๅ
-ๅ
-ๅ
-ๅก
-ๅข
-ๅฃ
-ๅค
-ๅฅ
-ๅฆ
-ๅง
-ๅช
-ๅซ
-ๅฌ
-ๅญ
-ๅฎ
-ๅฏ
-ๅฐ
-ๅฑ
-ๅฒ
-ๅณ
-ๅต
-ๅถ
-ๅท
-ๅธ
-ๅป
-ๅผ
-ๅฝ
-ๅพ
-ๅฟ
-ๅ
-ๅ
-ๅ
-ๅ
-ๅ
-ๅ
-ๅ
-ๅ
-ๅ
-ๅ
-ๅ
-ๅ
-ๅ
-ๅ
-ๅ
-ๅ
-ๅ
-ๅ
-ๅ
-ๅ
-ๅ
-ๅ
-ๅก
-ๅค
-ๅฅ
-ๅฆ
-ๅง
-ๅฉ
-ๅช
-ๅซ
-ๅฌ
-ๅญ
-ๅฎ
-ๅฐ
-ๅฑ
-ๅฒ
-ๅณ
-ๅด
-ๅต
-ๅถ
-ๅท
-ๅธ
-ๅป
-ๅผ
-ๅพ
-ๅ
-ๅ
-ๅ
-ๅ
-ๅ
-ๅ
-ๅ
-ๅ
-ๅ
-ๅ
-ๅ
-ๅ
-ๅ
-ๅ
-ๅ
-ๅ
-ๅ
-ๅ
-ๅ
-ๅ
-ๅ
-ๅ
-ๅข
-ๅฃ
-ๅค
-ๅฆ
-ๅง
-ๅจ
-ๅช
-ๅซ
-ๅฌ
-ๅญ
-ๅฎ
-ๅฏ
-ๅฐ
-ๅฑ
-ๅณ
-ๅต
-ๅถ
-ๅท
-ๅน
-ๅบ
-ๅป
-ๅผ
-ๅฝ
-ๅพ
-ๅ
-ๅ
-ๅ
-ๅ
-ๅ
-ๅ
-ๅ
-ๅ
-ๅ
-ๅ
-ๅ
-ๅ
-ๅ
-ๅ
-ๅ
-ๅ
-ๅ
-ๅ
-ๅ
-ๅ
-ๅก
-ๅข
-ๅฃ
-ๅค
-ๅฅ
-ๅฆ
-ๅจ
-ๅช
-ๅซ
-ๅฌ
-ๅฎ
-ๅฏ
-ๅฐ
-ๅฑ
-ๅฒ
-ๅณ
-ๅต
-ๅท
-ๅป
-ๅฝ
-ๅพ
-ๅฟ
-ๅ
-ๅ
-ๅ
-ๅ
-ๅ
-ๅ
-ๅ
-ๅ
-ๅ
-ๅ
-ๅ
-ๅ
-ๅ
-ๅ
-ๅ
-ๅ
-ๅ
-ๅ
-ๅ
-ๅก
-ๅข
-ๅฃ
-ๅค
-ๅฅ
-ๅง
-ๅฌ
-ๅญ
-ๅฑ
-ๅฒ
-ๅด
-ๅถ
-ๅท
-ๅน
-ๅป
-ๅฟ
-ๅ
-ๅ
-ๅ
-ๅ
-ๅ
-ๅ
-ๅ
-ๅ
-ๅ
-ๅ
-ๅ
-ๅ
-ๅ
-ๅ
-ๅ
-ๅ
-ๅ
-ๅ
-ๅข
-ๅฃ
-ๅค
-ๅจ
-ๅฉ
-ๅช
-ๅซ
-ๅฌ
-ๅญ
-ๅฑ
-ๅณ
-ๅถ
-ๅท
-ๅป
-ๅผ
-ๅ
-ๅ
-ๅ
-ๅ
-ๅ
-ๅ
-ๅ
-ๅ
-ๅ
-ๅ
-ๅ
-ๅ
-ๅก
-ๅข
-ๅฃ
-ๅค
-ๅฅ
-ๅฌ
-ๅญ
-ๅฏ
-ๅฐ
-ๅฑ
-ๅด
-ๅต
-ๅท
-ๅบ
-ๅผ
-ๅฟ
-ๅ
-ๅ
-ๅ
-ๅ
-ๅ
-ๅ
-ๅ
-ๅ
-ๅ
-ๅ
-ๅ
-ๅ
-ๅ
-ๅ
-ๅ
-ๅ
-ๅก
-ๅข
-ๅฃ
-ๅค
-ๅฆ
-ๅง
-ๅจ
-ๅซ
-ๅญ
-ๅฎ
-ๅฏ
-ๅฐ
-ๅฑ
-ๅฒ
-ๅณ
-ๅด
-ๅต
-ๅท
-ๅน
-ๅบ
-ๅฝ
-ๅพ
-ๅฟ
-ๅ
-ๅ
-ๅ
-ๅ
-ๅ
-ๅ
-ๅ
-ๅ
-ๅ
-ๅ
-ๅ
-ๅ
-ๅ
-ๅ
-ๅ
-ๅ
-ๅ
-ๅ
-ๅ
-ๅ
-ๅ
-ๅฃ
-ๅฅ
-ๅง
-ๅจ
-ๅฉ
-ๅช
-ๅฌ
-ๅญ
-ๅฎ
-ๅฏ
-ๅฐ
-ๅณ
-ๅด
-ๅน
-ๅบ
-ๅป
-ๅพ
-ๅฟ
-ๅ
-ๅ
-ๅ
-ๅ
-ๅ
-ๅ
-ๅ
-ๅ
-ๅ
-ๅ
-ๅ
-ๅ
-ๅ
-ๅ
-ๅ
-ๅ
-ๅ
-ๅ
-ๅก
-ๅฃ
-ๅค
-ๅฆ
-ๅจ
-ๅฉ
-ๅช
-ๅซ
-ๅฌ
-ๅญ
-ๅฏ
-ๅฐ
-ๅฑ
-ๅณ
-ๅต
-ๅท
-ๅบ
-ๅป
-ๅผ
-ๅพ
-ๅ
-ๅ
-ๅ
-ๅ
-ๅ
-ๅ
-ๅ
-ๅ
-ๅ
-ๅ
-ๅ
-ๅ
-ๅ
-ๅ
-ๅ
-ๅ
-ๅ
-ๅ
-ๅ
-ๅ
-ๅ
-ๅ
-ๅก
-ๅข
-ๅฃ
-ๅค
-ๅฆ
-ๅง
-ๅจ
-ๅฉ
-ๅซ
-ๅญ
-ๅฎ
-ๅฏ
-ๅฑ
-ๅฒ
-ๅด
-ๅต
-ๅธ
-ๅบ
-ๅพ
-ๅฟ
-ๅ
-ๅ
-ๅ
-ๅ
-ๅ
-ๅ
-ๅ
-ๅ
-ๅ
-ๅ
-ๅ
-ๅ
-ๅ
-ๅ
-ๅ
-ๅ
-ๅ
-ๅ
-ๅ
-ๅ
-ๅ
-ๅค
-ๅฅ
-ๅง
-ๅจ
-ๅฉ
-ๅช
-ๅซ
-ๅญ
-ๅฎ
-ๅฏ
-ๅณ
-ๅด
-ๅต
-ๅถ
-ๅท
-ๅธ
-ๅน
-ๅบ
-ๅผ
-ๅฝ
-ๅฟ
-ๅ
-ๅ
-ๅ
-ๅ
-ๅ
-ๅ
-ๅ
-ๅ
-ๅ
-ๅ
-ๅ
-ๅ
-ๅ
-ๅ
-ๅ
-ๅ
-ๅ ก
-ๅ ฃ
-ๅ ค
-ๅ ฆ
-ๅ ง
-ๅ จ
-ๅ ช
-ๅ ญ
-ๅ ฎ
-ๅ ฐ
-ๅ ฑ
-ๅ ณ
-ๅ ด
-ๅ ต
-ๅ ถ
-ๅ บ
-ๅ ผ
-ๅ ฝ
-ๅก
-ๅก
-ๅก
-ๅก
-ๅก
-ๅก
-ๅก
-ๅก
-ๅก
-ๅก
-ๅก
-ๅก
-ๅก
-ๅก
-ๅก
-ๅก
-ๅก
-ๅก
-ๅกจ
-ๅกฉ
-ๅกซ
-ๅกฌ
-ๅกญ
-ๅกฏ
-ๅกฑ
-ๅกธ
-ๅกผ
-ๅกฝ
-ๅกพ
-ๅกฟ
-ๅข
-ๅข
-ๅข
-ๅข
-ๅข
-ๅข
-ๅข
-ๅข
-ๅข
-ๅข
-ๅข
-ๅข
-ๅข
-ๅข
-ๅข
-ๅข
-ๅข
-ๅข
-ๅข
-ๅข
-ๅขก
-ๅขฆ
-ๅขจ
-ๅขฉ
-ๅขน
-ๅขบ
-ๅฃ
-ๅฃ
-ๅฃ
-ๅฃ
-ๅฃ
-ๅฃ
-ๅฃ
-ๅฃ
-ๅฃ
-ๅฃ
-ๅฃ
-ๅฃ
-ๅฃ
-ๅฃ
-ๅฃก
-ๅฃค
-ๅฃฉ
-ๅฃซ
-ๅฃฌ
-ๅฃฎ
-ๅฃฐ
-ๅฃฑ
-ๅฃฒ
-ๅฃณ
-ๅฃถ
-ๅฃธ
-ๅฃน
-ๅฃบ
-ๅฃผ
-ๅฃฝ
-ๅค
-ๅค
-ๅค
-ๅค
-ๅค
-ๅค
-ๅค
-ๅค
-ๅค
-ๅค
-ๅค
-ๅค
-ๅค
-ๅค
-ๅค
-ๅค
-ๅคก
-ๅคข
-ๅคค
-ๅคฅ
-ๅคง
-ๅคฉ
-ๅคช
-ๅคซ
-ๅคฌ
-ๅคญ
-ๅคฎ
-ๅคฏ
-ๅคฐ
-ๅคฑ
-ๅคด
-ๅคท
-ๅคธ
-ๅคน
-ๅคบ
-ๅคผ
-ๅคพ
-ๅคฟ
-ๅฅ
-ๅฅ
-ๅฅ
-ๅฅ
-ๅฅ
-ๅฅ
-ๅฅ
-ๅฅ
-ๅฅ
-ๅฅ
-ๅฅ
-ๅฅ
-ๅฅ
-ๅฅ
-ๅฅ
-ๅฅ
-ๅฅ
-ๅฅ
-ๅฅ
-ๅฅ
-ๅฅ
-ๅฅก
-ๅฅข
-ๅฅฃ
-ๅฅค
-ๅฅฅ
-ๅฅง
-ๅฅจ
-ๅฅซ
-ๅฅญ
-ๅฅฐ
-ๅฅณ
-ๅฅด
-ๅฅถ
-ๅฅธ
-ๅฅน
-ๅฅฝ
-ๅฆ
-ๅฆ
-ๅฆ
-ๅฆ
-ๅฆ
-ๅฆ
-ๅฆ
-ๅฆ
-ๅฆ
-ๅฆ
-ๅฆ
-ๅฆ
-ๅฆ
-ๅฆ
-ๅฆ
-ๅฆ
-ๅฆ
-ๅฆฃ
-ๅฆค
-ๅฆฅ
-ๅฆจ
-ๅฆฉ
-ๅฆช
-ๅฆซ
-ๅฆฌ
-ๅฆฎ
-ๅฆฏ
-ๅฆฒ
-ๅฆณ
-ๅฆน
-ๅฆป
-ๅฆผ
-ๅฆพ
-ๅง
-ๅง
-ๅง
-ๅง
-ๅง
-ๅง
-ๅง
-ๅง
-ๅง
-ๅง
-ๅง
-ๅง
-ๅง
-ๅง
-ๅง
-ๅง
-ๅง
-ๅง
-ๅงฃ
-ๅงค
-ๅงฅ
-ๅงจ
-ๅงช
-ๅงซ
-ๅงฌ
-ๅงฎ
-ๅงฑ
-ๅงต
-ๅงน
-ๅงบ
-ๅงป
-ๅงฟ
-ๅจ
-ๅจ
-ๅจ
-ๅจ
-ๅจ
-ๅจ
-ๅจ
-ๅจ
-ๅจ
-ๅจ
-ๅจ
-ๅจ
-ๅจ
-ๅจ
-ๅจ
-ๅจ
-ๅจ
-ๅจ
-ๅจ
-ๅจ
-ๅจก
-ๅจฃ
-ๅจฅ
-ๅจฉ
-ๅจญ
-ๅจฏ
-ๅจฑ
-ๅจฒ
-ๅจด
-ๅจต
-ๅจถ
-ๅจผ
-ๅฉ
-ๅฉ
-ๅฉ
-ๅฉ
-ๅฉ
-ๅฉ
-ๅฉ
-ๅฉ
-ๅฉ
-ๅฉ
-ๅฉ
-ๅฉ
-ๅฉข
-ๅฉฃ
-ๅฉฅ
-ๅฉฆ
-ๅฉง
-ๅฉจ
-ๅฉช
-ๅฉฒ
-ๅฉด
-ๅฉต
-ๅฉถ
-ๅฉท
-ๅฉบ
-ๅฉผ
-ๅฉฟ
-ๅช
-ๅช
-ๅช
-ๅช
-ๅช
-ๅช
-ๅช
-ๅช
-ๅช
-ๅชข
-ๅชฅ
-ๅชช
-ๅชญ
-ๅชฎ
-ๅชฒ
-ๅชณ
-ๅชต
-ๅชธ
-ๅชป
-ๅชฝ
-ๅชพ
-ๅชฟ
-ๅซ
-ๅซ
-ๅซ
-ๅซ
-ๅซ
-ๅซ
-ๅซ
-ๅซ
-ๅซ
-ๅซ
-ๅซ
-ๅซ
-ๅซ
-ๅซ
-ๅซ
-ๅซ
-ๅซก
-ๅซฃ
-ๅซฆ
-ๅซง
-ๅซฉ
-ๅซช
-ๅซซ
-ๅซญ
-ๅซฎ
-ๅซฐ
-ๅซฑ
-ๅซฒ
-ๅซณ
-ๅซถ
-ๅซฝ
-ๅฌ
-ๅฌ
-ๅฌ
-ๅฌ
-ๅฌ
-ๅฌ
-ๅฌ
-ๅฌ
-ๅฌ
-ๅฌ
-ๅฌข
-ๅฌฌ
-ๅฌฒ
-ๅฌด
-ๅฌท
-ๅฌพ
-ๅฌฟ
-ๅญ
-ๅญ
-ๅญ
-ๅญ
-ๅญ
-ๅญ
-ๅญ
-ๅญ
-ๅญ
-ๅญ
-ๅญ
-ๅญ
-ๅญ
-ๅญ
-ๅญ
-ๅญ
-ๅญ
-ๅญข
-ๅญฃ
-ๅญค
-ๅญฅ
-ๅญฆ
-ๅญฉ
-ๅญช
-ๅญฌ
-ๅญญ
-ๅญฐ
-ๅญฑ
-ๅญฒ
-ๅญณ
-ๅญต
-ๅญธ
-ๅญบ
-ๅญป
-ๅญฝ
-ๅฎ
-ๅฎ
-ๅฎ
-ๅฎ
-ๅฎ
-ๅฎ
-ๅฎ
-ๅฎ
-ๅฎ
-ๅฎ
-ๅฎ
-ๅฎ
-ๅฎ
-ๅฎ
-ๅฎ
-ๅฎ
-ๅฎ
-ๅฎ
-ๅฎ
-ๅฎ
-ๅฎ
-ๅฎ
-ๅฎ
-ๅฎ
-ๅฎ
-ๅฎก
-ๅฎข
-ๅฎฃ
-ๅฎค
-ๅฎฅ
-ๅฎฆ
-ๅฎช
-ๅฎซ
-ๅฎฌ
-ๅฎฎ
-ๅฎฐ
-ๅฎณ
-ๅฎด
-ๅฎต
-ๅฎถ
-ๅฎธ
-ๅฎน
-ๅฎผ
-ๅฎฝ
-ๅฎพ
-ๅฎฟ
-ๅฏ
-ๅฏ
-ๅฏ
-ๅฏ
-ๅฏ
-ๅฏ
-ๅฏ
-ๅฏ
-ๅฏ
-ๅฏ
-ๅฏ
-ๅฏ
-ๅฏ
-ๅฏ
-ๅฏ
-ๅฏ
-ๅฏ
-ๅฏ
-ๅฏ
-ๅฏ
-ๅฏ
-ๅฏก
-ๅฏข
-ๅฏค
-ๅฏฅ
-ๅฏฆ
-ๅฏง
-ๅฏจ
-ๅฏฉ
-ๅฏฎ
-ๅฏฏ
-ๅฏฐ
-ๅฏถ
-ๅฏธ
-ๅฏน
-ๅฏบ
-ๅฏป
-ๅฏผ
-ๅฏพ
-ๅฏฟ
-ๅฐ
-ๅฐ
-ๅฐ
-ๅฐ
-ๅฐ
-ๅฐ
-ๅฐ
-ๅฐ
-ๅฐ
-ๅฐ
-ๅฐ
-ๅฐ
-ๅฐ
-ๅฐ
-ๅฐ
-ๅฐ
-ๅฐ
-ๅฐ
-ๅฐ
-ๅฐ
-ๅฐ
-ๅฐ
-ๅฐ
-ๅฐค
-ๅฐฅ
-ๅฐง
-ๅฐจ
-ๅฐช
-ๅฐซ
-ๅฐฌ
-ๅฐญ
-ๅฐฐ
-ๅฐฑ
-ๅฐด
-ๅฐธ
-ๅฐน
-ๅฐบ
-ๅฐป
-ๅฐผ
-ๅฐฝ
-ๅฐพ
-ๅฐฟ
-ๅฑ
-ๅฑ
-ๅฑ
-ๅฑ
-ๅฑ
-ๅฑ
-ๅฑ
-ๅฑ
-ๅฑ
-ๅฑ
-ๅฑ
-ๅฑ
-ๅฑ
-ๅฑ
-ๅฑ
-ๅฑ
-ๅฑ
-ๅฑ
-ๅฑ
-ๅฑ
-ๅฑ
-ๅฑ
-ๅฑ
-ๅฑก
-ๅฑฃ
-ๅฑฅ
-ๅฑฆ
-ๅฑง
-ๅฑฉ
-ๅฑญ
-ๅฑฎ
-ๅฑฏ
-ๅฑฑ
-ๅฑณ
-ๅฑด
-ๅฑน
-ๅฑบ
-ๅฑผ
-ๅฑพ
-ๅฑฟ
-ๅฒ
-ๅฒ
-ๅฒ
-ๅฒ
-ๅฒ
-ๅฒ
-ๅฒ
-ๅฒ
-ๅฒ
-ๅฒ
-ๅฒ
-ๅฒ
-ๅฒ
-ๅฒ
-ๅฒ
-ๅฒ
-ๅฒ
-ๅฒ
-ๅฒ
-ๅฒ
-ๅฒก
-ๅฒข
-ๅฒฃ
-ๅฒง
-ๅฒจ
-ๅฒฉ
-ๅฒซ
-ๅฒฌ
-ๅฒญ
-ๅฒฑ
-ๅฒณ
-ๅฒต
-ๅฒท
-ๅฒธ
-ๅฒฝ
-ๅฒฟ
-ๅณ
-ๅณ
-ๅณ
-ๅณ
-ๅณ
-ๅณ
-ๅณ
-ๅณ
-ๅณ
-ๅณ
-ๅณ
-ๅณก
-ๅณฃ
-ๅณค
-ๅณฅ
-ๅณฆ
-ๅณง
-ๅณจ
-ๅณฉ
-ๅณช
-ๅณญ
-ๅณฏ
-ๅณฐ
-ๅณป
-ๅณฟ
-ๅด
-ๅด
-ๅด
-ๅด
-ๅด
-ๅด
-ๅด
-ๅด
-ๅด
-ๅด
-ๅด
-ๅด
-ๅด
-ๅด
-ๅด
-ๅด
-ๅด
-ๅด
-ๅด
-ๅด
-ๅด
-ๅด
-ๅด
-ๅดฃ
-ๅดค
-ๅดฆ
-ๅดง
-ๅดฉ
-ๅดญ
-ๅดฎ
-ๅดฑ
-ๅดด
-ๅดถ
-ๅดท
-ๅดฝ
-ๅดพ
-ๅดฟ
-ๅต
-ๅต
-ๅต
-ๅต
-ๅต
-ๅต
-ๅต
-ๅต
-ๅต
-ๅต
-ๅต
-ๅต
-ๅต
-ๅต
-ๅต
-ๅต
-ๅต
-ๅตก
-ๅตฅ
-ๅตฆ
-ๅตฉ
-ๅตซ
-ๅตฌ
-ๅตฏ
-ๅตฐ
-ๅตฒ
-ๅตด
-ๅตท
-ๅตธ
-ๅตบ
-ๅตฝ
-ๅตพ
-ๅถ
-ๅถ
-ๅถ
-ๅถ
-ๅถ
-ๅถ
-ๅถ
-ๅถ
-ๅถ
-ๅถ
-ๅถ
-ๅถ
-ๅถ
-ๅถจ
-ๅถช
-ๅถญ
-ๅถฎ
-ๅถฐ
-ๅถฑ
-ๅถฒ
-ๅถถ
-ๅถท
-ๅถฝ
-ๅถพ
-ๅท
-ๅท
-ๅท
-ๅท
-ๅท
-ๅท
-ๅท
-ๅท
-ๅท
-ๅท
-ๅท
-ๅท
-ๅท
-ๅท
-ๅท
-ๅทก
-ๅทข
-ๅทฃ
-ๅทฅ
-ๅทฆ
-ๅทง
-ๅทจ
-ๅทฉ
-ๅทซ
-ๅทฎ
-ๅทฏ
-ๅทฑ
-ๅทฒ
-ๅทณ
-ๅทด
-ๅทต
-ๅทท
-ๅทป
-ๅทฝ
-ๅทพ
-ๅทฟ
-ๅธ
-ๅธ
-ๅธ
-ๅธ
-ๅธ
-ๅธ
-ๅธ
-ๅธ
-ๅธ
-ๅธ
-ๅธ
-ๅธ
-ๅธ
-ๅธ
-ๅธ
-ๅธ
-ๅธ
-ๅธ
-ๅธ
-ๅธ
-ๅธ
-ๅธ
-ๅธก
-ๅธข
-ๅธฃ
-ๅธฆ
-ๅธง
-ๅธจ
-ๅธซ
-ๅธญ
-ๅธฎ
-ๅธฏ
-ๅธฐ
-ๅธฑ
-ๅธณ
-ๅธถ
-ๅธท
-ๅธธ
-ๅธป
-ๅธผ
-ๅธฝ
-ๅธฟ
-ๅน
-ๅน
-ๅน
-ๅน
-ๅน
-ๅน
-ๅน
-ๅน
-ๅน
-ๅน
-ๅน
-ๅน
-ๅนก
-ๅนข
-ๅนฃ
-ๅนค
-ๅนฅ
-ๅนจ
-ๅนฉ
-ๅนช
-ๅนญ
-ๅนฎ
-ๅนฐ
-ๅนฒ
-ๅนณ
-ๅนด
-ๅนต
-ๅนถ
-ๅนท
-ๅนธ
-ๅนน
-ๅนบ
-ๅนป
-ๅนผ
-ๅนฝ
-ๅนพ
-ๅนฟ
-ๅบ
-ๅบ
-ๅบ
-ๅบ
-ๅบ
-ๅบ
-ๅบ
-ๅบ
-ๅบ
-ๅบ
-ๅบ
-ๅบ
-ๅบ
-ๅบ
-ๅบ
-ๅบ
-ๅบ
-ๅบ
-ๅบ
-ๅบ
-ๅบ
-ๅบ
-ๅบค
-ๅบฅ
-ๅบฆ
-ๅบง
-ๅบช
-ๅบญ
-ๅบณ
-ๅบต
-ๅบถ
-ๅบท
-ๅบธ
-ๅบน
-ๅบพ
-ๅป
-ๅป
-ๅป
-ๅป
-ๅป
-ๅป
-ๅป
-ๅป
-ๅป
-ๅป
-ๅป
-ๅปข
-ๅปฃ
-ๅปฅ
-ๅปฆ
-ๅปง
-ๅปจ
-ๅปช
-ๅปญ
-ๅปถ
-ๅปท
-ๅปธ
-ๅปบ
-ๅปป
-ๅปผ
-ๅปฟ
-ๅผ
-ๅผ
-ๅผ
-ๅผ
-ๅผ
-ๅผ
-ๅผ
-ๅผ
-ๅผ
-ๅผ
-ๅผ
-ๅผ
-ๅผ
-ๅผ
-ๅผ
-ๅผ
-ๅผ
-ๅผ
-ๅผ
-ๅผ
-ๅผ
-ๅผ
-ๅผ
-ๅผข
-ๅผฅ
-ๅผฆ
-ๅผง
-ๅผจ
-ๅผฉ
-ๅผช
-ๅผญ
-ๅผฎ
-ๅผฏ
-ๅผฐ
-ๅผฑ
-ๅผต
-ๅผถ
-ๅผท
-ๅผธ
-ๅผน
-ๅผบ
-ๅผผ
-ๅผพ
-ๅฝ
-ๅฝ
-ๅฝ
-ๅฝ
-ๅฝ
-ๅฝ
-ๅฝ
-ๅฝ
-ๅฝ
-ๅฝ
-ๅฝ
-ๅฝ
-ๅฝ
-ๅฝ
-ๅฝก
-ๅฝข
-ๅฝค
-ๅฝฆ
-ๅฝง
-ๅฝฉ
-ๅฝช
-ๅฝซ
-ๅฝฌ
-ๅฝญ
-ๅฝฏ
-ๅฝฐ
-ๅฝฑ
-ๅฝณ
-ๅฝด
-ๅฝท
-ๅฝน
-ๅฝป
-ๅฝผ
-ๅฝฟ
-ๅพ
-ๅพ
-ๅพ
-ๅพ
-ๅพ
-ๅพ
-ๅพ
-ๅพ
-ๅพ
-ๅพ
-ๅพ
-ๅพ
-ๅพ
-ๅพ
-ๅพ
-ๅพ
-ๅพ
-ๅพ
-ๅพ
-ๅพ
-ๅพ
-ๅพก
-ๅพง
-ๅพจ
-ๅพช
-ๅพฌ
-ๅพญ
-ๅพฎ
-ๅพฏ
-ๅพณ
-ๅพด
-ๅพต
-ๅพถ
-ๅพท
-ๅพน
-ๅพผ
-ๅพฝ
-ๅฟ
-ๅฟ
-ๅฟ
-ๅฟ
-ๅฟ
-ๅฟ
-ๅฟ
-ๅฟ
-ๅฟ
-ๅฟ
-ๅฟ
-ๅฟ
-ๅฟ
-ๅฟ
-ๅฟ
-ๅฟ
-ๅฟ
-ๅฟ
-ๅฟ
-ๅฟ
-ๅฟก
-ๅฟค
-ๅฟง
-ๅฟช
-ๅฟซ
-ๅฟญ
-ๅฟฎ
-ๅฟฑ
-ๅฟณ
-ๅฟต
-ๅฟธ
-ๅฟบ
-ๅฟป
-ๅฟผ
-ๅฟฝ
-ๅฟพ
-ๅฟฟ
-ๆ
-ๆ
-ๆ
-ๆ
-ๆ
-ๆ
-ๆ
-ๆ
-ๆ
-ๆ
-ๆ
-ๆ
-ๆ
-ๆ
-ๆ
-ๆ
-ๆ
-ๆ
-ๆ
-ๆ
-ๆ
-ๆ
-ๆ
-ๆ
-ๆ
-ๆก
-ๆฅ
-ๆฆ
-ๆง
-ๆจ
-ๆฉ
-ๆช
-ๆซ
-ๆฏ
-ๆฑ
-ๆฒ
-ๆณ
-ๆต
-ๆป
-ๆผ
-ๆฟ
-ๆ
-ๆ
-ๆ
-ๆ
-ๆ
-ๆ
-ๆ
-ๆ
-ๆ
-ๆ
-ๆ
-ๆ
-ๆ
-ๆ
-ๆ
-ๆ
-ๆ
-ๆ
-ๆ
-ๆ
-ๆข
-ๆฃ
-ๆค
-ๆง
-ๆจ
-ๆฉ
-ๆช
-ๆซ
-ๆฌ
-ๆญ
-ๆฏ
-ๆฐ
-ๆณ
-ๆต
-ๆถ
-ๆธ
-ๆน
-ๆบ
-ๆป
-ๆผ
-ๆฝ
-ๆฟ
-ๆ
-ๆ
-ๆ
-ๆ
-ๆ
-ๆ
-ๆ
-ๆ
-ๆ
-ๆ
-ๆ
-ๆ
-ๆ
-ๆ
-ๆ
-ๆ
-ๆ
-ๆ
-ๆข
-ๆฃ
-ๆฆ
-ๆจ
-ๆฉ
-ๆช
-ๆซ
-ๆฌ
-ๆญ
-ๆฎ
-ๆฏ
-ๆฐ
-ๆฑ
-ๆฒ
-ๆด
-ๆธ
-ๆป
-ๆผ
-ๆ
-ๆ
-ๆ
-ๆ
-ๆ
-ๆ
-ๆ
-ๆ
-ๆ
-ๆ
-ๆ
-ๆ
-ๆ
-ๆ
-ๆ
-ๆ
-ๆ
-ๆ
-ๆ
-ๆ
-ๆ
-ๆก
-ๆฆ
-ๆง
-ๆจ
-ๆฉ
-ๆซ
-ๆฌ
-ๆญ
-ๆฎ
-ๆฏ
-ๆฐ
-ๆณ
-ๆด
-ๆต
-ๆถ
-ๆธ
-ๆน
-ๆบ
-ๆ
-ๆ
-ๆ
-ๆ
-ๆ
-ๆ
-ๆ
-ๆ
-ๆ
-ๆ
-ๆ
-ๆ
-ๆ
-ๆ
-ๆ
-ๆ
-ๆ
-ๆ
-ๆฃ
-ๆค
-ๆฅ
-ๆฆ
-ๆง
-ๆซ
-ๆฌ
-ๆต
-ๆฟ
-ๆ
-ๆ
-ๆ
-ๆ
-ๆ
-ๆ
-ๆ
-ๆ
-ๆ
-ๆ
-ๆ
-ๆ
-ๆ
-ๆ
-ๆ
-ๆ
-ๆ
-ๆ
ข
-ๆ
ฅ
-ๆ
ง
-ๆ
จ
-ๆ
ฌ
-ๆ
ญ
-ๆ
ฐ
-ๆ
ฑ
-ๆ
ด
-ๆ
ต
-ๆ
ถ
-ๆ
ท
-ๆ
ฝ
-ๆ
พ
-ๆ
-ๆ
-ๆ
-ๆ
-ๆ
-ๆ
-ๆ
-ๆ
-ๆ
-ๆ
-ๆ
-ๆ
-ๆง
-ๆจ
-ๆฉ
-ๆฌ
-ๆญ
-ๆฏ
-ๆฐ
-ๆฒ
-ๆท
-ๆธ
-ๆน
-ๆบ
-ๆพ
-ๆ
-ๆ
-ๆ
-ๆ
-ๆ
-ๆ
-ๆ
-ๆ
-ๆ
-ๆ
-ๆ
-ๆ
-ๆ
-ๆ
-ๆ
-ๆ
-ๆ
-ๆก
-ๆฆ
-ๆญ
-ๆฐ
-ๆฒ
-ๆต
-ๆน
-ๆป
-ๆฝ
-ๆฟ
-ๆ
-ๆ
-ๆ
-ๆ
-ๆ
-ๆ
-ๆ
-ๆ
-ๆ
-ๆ
-ๆ
-ๆ
-ๆ
-ๆ
-ๆ
-ๆ
-ๆ
-ๆ
-ๆ
-ๆ
-ๆ
-ๆ
-ๆ
-ๆก
-ๆข
-ๆฅ
-ๆฆ
-ๆช
-ๆซ
-ๆฌ
-ๆญ
-ๆฎ
-ๆฒ
-ๆณ
-ๆด
-ๆถ
-ๆท
-ๆธ
-ๆบ
-ๆป
-ๆฝ
-ๆพ
-ๆฟ
-ๆ
-ๆ
-ๆ
-ๆ
-ๆ
-ๆ
-ๆ
-ๆ
-ๆ
-ๆ
-ๆ
-ๆ
-ๆ
-ๆ
-ๆ
-ๆ
-ๆ
-ๆ
-ๆ
-ๆ
-ๆ
-ๆ
-ๆ
-ๆ
-ๆ
-ๆ
-ๆ
-ๆก
-ๆข
-ๆฃ
-ๆค
-ๆฅ
-ๆฆ
-ๆง
-ๆฉ
-ๆช
-ๆซ
-ๆฌ
-ๆญ
-ๆฎ
-ๆฏ
-ๆฐ
-ๆฑ
-ๆณ
-ๆถ
-ๆน
-ๆผ
-ๆฝ
-ๆพ
-ๆฟ
-ๆ
-ๆ
-ๆ
-ๆ
-ๆ
-ๆ
-ๆ
-ๆ
-ๆ
-ๆ
-ๆ
-ๆ
-ๆ
-ๆ
-ๆ
-ๆ
-ๆ
-ๆ
-ๆ
-ๆ
-ๆ
-ๆ
-ๆ
-ๆก
-ๆข
-ๆค
-ๆฅ
-ๆจ
-ๆซ
-ๆฌ
-ๆฑ
-ๆต
-ๆถ
-ๆน
-ๆป
-ๆผ
-ๆฝ
-ๆฟ
-ๆ
-ๆ
-ๆ
-ๆ
-ๆ
-ๆ
-ๆ
-ๆ
-ๆ
-ๆ
-ๆ
-ๆ
-ๆ
-ๆ
-ๆ
-ๆ
-ๆ
-ๆ
-ๆ
-ๆ
-ๆ
-ๆ
-ๆ
-ๆ
-ๆ
-ๆ
-ๆ
-ๆ
-ๆ
-ๆก
-ๆข
-ๆฃ
-ๆฅ
-ๆฆ
-ๆง
-ๆจ
-ๆฉ
-ๆซ
-ๆฌ
-ๆญ
-ๆฎ
-ๆฏ
-ๆฑ
-ๆณ
-ๆด
-ๆถ
-ๆท
-ๆผ
-ๆฝ
-ๆพ
-ๆฟ
-ๆ
-ๆ
-ๆ
-ๆ
-ๆ
-ๆ
-ๆ
-ๆ
-ๆ
-ๆ
-ๆ
-ๆ
-ๆ
-ๆ
-ๆ
-ๆ
-ๆ
-ๆ
-ๆ
-ๆ
-ๆก
-ๆข
-ๆฃ
-ๆค
-ๆฅ
-ๆฆ
-ๆจ
-ๆช
-ๆซ
-ๆญ
-ๆฏ
-ๆฑ
-ๆฒ
-ๆต
-ๆน
-ๆบ
-ๆผ
-ๆฝ
-ๆฟ
-ๆ
-ๆ
-ๆ
-ๆ
-ๆ
-ๆ
-ๆ
-ๆ
-ๆ
-ๆ
-ๆ
-ๆ
-ๆ
-ๆ
-ๆ
-ๆ
-ๆ
-ๆ
-ๆก
-ๆข
-ๆฃ
-ๆง
-ๆจ
-ๆฉ
-ๆญ
-ๆฎ
-ๆฐ
-ๆฑ
-ๆฒ
-ๆด
-ๆถ
-ๆท
-ๆบ
-ๆป
-ๆฝ
-ๆ
-ๆ
-ๆ
-ๆ
-ๆ
-ๆ
-ๆ
-ๆ
-ๆ
-ๆ
-ๆ
-ๆ
-ๆ
-ๆ
-ๆ
-ๆ
-ๆ
-ๆ
-ๆ
-ๆก
-ๆข
-ๆฃ
-ๆค
-ๆฅ
-ๆง
-ๆจ
-ๆฉ
-ๆช
-ๆฌ
-ๆฎ
-ๆฏ
-ๆฐ
-ๆฑ
-ๆฒ
-ๆณ
-ๆด
-ๆท
-ๆธ
-ๆน
-ๆบ
-ๆป
-ๆผ
-ๆพ
-ๆฟ
-ๆ
-ๆ
-ๆ
-ๆ
-ๆ
-ๆ
-ๆ
-ๆ
-ๆ
-ๆ
-ๆ
-ๆ
-ๆ
-ๆ
-ๆ
-ๆ
-ๆ
-ๆ
-ๆ
-ๆก
-ๆฃ
-ๆฅ
-ๆฆ
-ๆฉ
-ๆช
-ๆซ
-ๆญ
-ๆฐ
-ๆฒ
-ๆด
-ๆต
-ๆถ
-ๆธ
-ๆบ
-ๆผ
-ๆฝ
-ๆพ
-ๆฟ
-ๆ
-ๆ
-ๆ
-ๆ
-ๆ
-ๆ
-ๆ
-ๆ
-ๆ
-ๆ
-ๆ
-ๆ
-ๆ
-ๆ
-ๆ
-ๆ
-ๆ
-ๆ
-ๆ
-ๆ
-ๆ
-ๆก
-ๆข
-ๆฃ
-ๆค
-ๆฅ
-ๆฆ
-ๆง
-ๆจ
-ๆช
-ๆฌ
-ๆญ
-ๆฏ
-ๆฐ
-ๆด
-ๆต
-ๆบ
-ๆฝ
-ๆพ
-ๆ
-ๆ
-ๆ
-ๆ
-ๆ
-ๆ
-ๆ
-ๆ
-ๆ
-ๆ
-ๆ
-ๆ
-ๆ
-ๆ
-ๆ
-ๆ
-ๆ
-ๆ
-ๆ
-ๆง
-ๆฉ
-ๆญ
-ๆด
-ๆต
-ๆธ
-ๆน
-ๆบ
-ๆฝ
-ๆ
-ๆ
-ๆ
-ๆ
-ๆ
-ๆ
-ๆ
-ๆ
-ๆ
-ๆ
-ๆ
-ๆ
-ๆ
-ๆ
-ๆค
-ๆฅ
-ๆฆ
-ๆง
-ๆฉ
-ๆฌ
-ๆญ
-ๆฎ
-ๆฐ
-ๆฑ
-ๆด
-ๆต
-ๆถ
-ๆท
-ๆธ
-ๆบ
-ๆผ
-ๆ
-ๆ
-ๆ
-ๆ
-ๆ
-ๆ
-ๆ
-ๆ
-ๆ
-ๆ
-ๆ
-ๆ
-ๆ
-ๆ
-ๆ
-ๆข
-ๆค
-ๆฆ
-ๆฉ
-ๆซ
-ๆท
-ๆธ
-ๆฟ
-ๆ
-ๆ
-ๆ
-ๆ
-ๆ
-ๆ
-ๆ
-ๆ
-ๆ
-ๆ
-ๆฅ
-ๆง
-ๆฉ
-ๆซ
-ๆฎ
-ๆฏ
-ๆฐ
-ๆฒ
-ๆถ
-ๆธ
-ๆน
-ๆป
-ๆฝ
-ๆพ
-ๆฟ
-ๆ
-ๆ
-ๆ
-ๆ
-ๆ
-ๆ
-ๆ
-ๆ
-ๆ
-ๆ
-ๆ
-ๆ
-ๆข
-ๆฃ
-ๆฆ
-ๆง
-ๆฉ
-ๆซ
-ๆฌ
-ๆฐ
-ๆฑ
-ๆฒ
-ๆด
-ๆท
-ๆธ
-ๆบ
-ๆป
-ๆ
-ๆ
-ๆ
-ๆ
-ๆ
-ๆ
-ๆ
-ๆ
-ๆ
-ๆ
-ๆ
-ๆ
-ๆ
-ๆ
-ๆ
-ๆ
-ๆ
-ๆ
-ๆ
-ๆก
-ๆค
-ๆฅ
-ๆง
-ๆจ
-ๆฉ
-ๆซ
-ๆญ
-ๆฎ
-ๆฏ
-ๆฐ
-ๆฒ
-ๆด
-ๆถ
-ๆธ
-ๆน
-ๆผ
-ๆฝ
-ๆฟ
-ๆ
-ๆ
-ๆ
-ๆ
-ๆ
-ๆ
-ๆ
-ๆ
-ๆ
-ๆ
-ๆ
-ๆ
-ๆ
-ๆ
-ๆ
-ๆ
-ๆ
-ๆ
-ๆ
-ๆ
-ๆ
-ๆก
-ๆข
-ๆฅ
-ๆฆ
-ๆง
-ๆจ
-ๆฉ
-ๆฌ
-ๆญ
-ๆฎ
-ๆฏ
-ๆฐ
-ๆฑ
-ๆณ
-ๆด
-ๆต
-ๆถ
-ๆท
-ๆธ
-ๆบ
-ๆป
-ๆผ
-ๆฟ
-ๆ
-ๆ
-ๆ
-ๆ
-ๆ
-ๆ
-ๆ
-ๆ
-ๆ
-ๆ
-ๆ
-ๆ
-ๆ
-ๆ
-ๆ
-ๆ
-ๆ
-ๆ
-ๆ
-ๆ
-ๆ
-ๆ
-ๆฃ
-ๆค
-ๆฅ
-ๆง
-ๆจ
-ๆช
-ๆซ
-ๆฌ
-ๆญ
-ๆฏ
-ๆฐ
-ๆฑ
-ๆณ
-ๆด
-ๆต
-ๆถ
-ๆบ
-ๆผ
-ๆฝ
-ๆพ
-ๆ
-ๆ
-ๆ
-ๆ
-ๆ
-ๆ
-ๆ
-ๆ
-ๆ
-ๆ
-ๆ
-ๆ
-ๆ
-ๆ
-ๆ
-ๆ
-ๆ
-ๆ
-ๆ
-ๆก
-ๆข
-ๆฃ
-ๆค
-ๆฅ
-ๆฆ
-ๆง
-ๆจ
-ๆฉ
-ๆซ
-ๆฌ
-ๆฎ
-ๆฏ
-ๆฐ
-ๆณ
-ๆด
-ๆถ
-ๆท
-ๆน
-ๆบ
-ๆป
-ๆผ
-ๆพ
-ๆ
-ๆ
-ๆ
-ๆ
-ๆ
-ๆ
-ๆ
-ๆ
-ๆ
-ๆ
-ๆ
-ๆ
-ๆ
-ๆ
-ๆ
-ๆฃ
-ๆฆ
-ๆง
-ๆจ
-ๆฌ
-ๆฎ
-ๆฑ
-ๆฒ
-ๆด
-ๆต
-ๆถ
-ๆน
-ๆป
-ๆพ
-ๆ
-ๆ
-ๆ
-ๆ
-ๆ
-ๆ
-ๆ
-ๆ
-ๆ
-ๆข
-ๆฆ
-ๆง
-ๆจ
-ๆฉ
-ๆช
-ๆญ
-ๆฐ
-ๆฑ
-ๆฒ
-ๆณ
-ๆด
-ๆต
-ๆท
-ๆธ
-ๆน
-ๆผ
-ๆฝ
-ๆพ
-ๆฟ
-ๆ
-ๆ
-ๆ
-ๆ
-ๆ
-ๆ
-ๆ
-ๆ
-ๆ
-ๆ
-ๆ
-ๆ
-ๆ
-ๆ
-ๆ
-ๆ
-ๆ
-ๆ
-ๆ
-ๆ
-ๆ
-ๆฃ
-ๆฆ
-ๆจ
-ๆช
-ๆซ
-ๆฌ
-ๆญ
-ๆฎ
-ๆฏ
-ๆฑ
-ๆณ
-ๆด
-ๆต
-ๆถ
-ๆบ
-ๆฝ
-ๆฟ
-ๆ
-ๆ
-ๆ
-ๆ
-ๆ
-ๆ
-ๆ
-ๆ
-ๆ
-ๆ
-ๆ
-ๆ
-ๆ
-ๆ
-ๆ
-ๆ
-ๆ
-ๆ
-ๆ
-ๆ
-ๆก
-ๆข
-ๆฅ
-ๆง
-ๆจ
-ๆฉ
-ๆช
-ๆฌ
-ๆญ
-ๆฏ
-ๆฐ
-ๆฑ
-ๆฒ
-ๆณ
-ๆต
-ๆท
-ๆป
-ๆผ
-ๆพ
-ๆฟ
-ๆ
-ๆ
-ๆ
-ๆ
-ๆ
-ๆ
-ๆ
-ๆ
-ๆ
-ๆ
-ๆ
-ๆ
-ๆ
-ๆ
-ๆ
-ๆ
-ๆ
-ๆ
-ๆ
-ๆ
-ๆ
-ๆก
-ๆข
-ๆฃ
-ๆฅ
-ๆฆ
-ๆง
-ๆจ
-ๆช
-ๆซ
-ๆฌ
-ๆญ
-ๆฎ
-ๆฏ
-ๆฐ
-ๆฒ
-ๆณ
-ๆต
-ๆถ
-ๆท
-ๆธ
-ๆน
-ๆป
-ๆฟ
-ๆ
-ๆ
-ๆ
-ๆ
-ๆ
-ๆ
-ๆ
-ๆ
-ๆ
-ๆ
-ๆ
-ๆ
-ๆ
-ๆ
-ๆ
-ๆ
-ๆ
-ๆ
-ๆ
-ๆ
-ๆ
-ๆ
-ๆ
-ๆข
-ๆฃ
-ๆค
-ๆฅ
-ๆฉ
-ๆฌ
-ๆฎ
-ๆฏ
-ๆฐ
-ๆฑ
-ๆณ
-ๆด
-ๆท
-ๆน
-ๆบ
-ๆป
-ๆฝ
-ๆฟ
-ๆ
-ๆ
-ๆ
-ๆ
-ๆ
-ๆ
-ๆ
-ๆ
-ๆ
-ๆ
-ๆ
-ๆ
-ๆ
-ๆ
-ๆ
-ๆ
-ๆ
-ๆ
-ๆ
-ๆ
-ๆ
-ๆ
-ๆ
-ๆ
-ๆ ก
-ๆ ข
-ๆ ฉ
-ๆ ช
-ๆ ฏ
-ๆ ฑ
-ๆ ฒ
-ๆ ณ
-ๆ ด
-ๆ ต
-ๆ ท
-ๆ ธ
-ๆ น
-ๆ ป
-ๆ ผ
-ๆ ฝ
-ๆ พ
-ๆ ฟ
-ๆก
-ๆก
-ๆก
-ๆก
-ๆก
-ๆก
-ๆก
-ๆก
-ๆก
-ๆก
-ๆก
-ๆก
-ๆก
-ๆก
-ๆก
-ๆก
-ๆก
-ๆก
-ๆก
-ๆก
-ๆก
-ๆก
-ๆก
-ๆกก
-ๆกข
-ๆกฃ
-ๆกค
-ๆกฅ
-ๆกฆ
-ๆกง
-ๆกจ
-ๆกฉ
-ๆกซ
-ๆกฎ
-ๆกฏ
-ๆกฒ
-ๆกด
-ๆกถ
-ๆกท
-ๆกน
-ๆกป
-ๆข
-ๆข
-ๆข
-ๆข
-ๆข
-ๆข
-ๆข
-ๆข
-ๆข
-ๆข
-ๆข
-ๆขก
-ๆขข
-ๆขฃ
-ๆขฆ
-ๆขง
-ๆขจ
-ๆขฉ
-ๆขช
-ๆขซ
-ๆขฌ
-ๆขญ
-ๆขฏ
-ๆขฐ
-ๆขฑ
-ๆขฒ
-ๆขณ
-ๆขด
-ๆขต
-ๆขถ
-ๆขผ
-ๆขฝ
-ๆขพ
-ๆขฟ
-ๆฃ
-ๆฃ
-ๆฃ
-ๆฃ
-ๆฃ
-ๆฃ
-ๆฃ
-ๆฃ
-ๆฃ
-ๆฃ
-ๆฃ
-ๆฃ
-ๆฃ
-ๆฃ
-ๆฃ
-ๆฃ
-ๆฃ
-ๆฃ
-ๆฃ
-ๆฃ
-ๆฃ
-ๆฃก
-ๆฃฃ
-ๆฃจ
-ๆฃช
-ๆฃซ
-ๆฃฌ
-ๆฃฎ
-ๆฃฏ
-ๆฃฐ
-ๆฃฑ
-ๆฃฒ
-ๆฃต
-ๆฃธ
-ๆฃน
-ๆฃบ
-ๆฃป
-ๆฃผ
-ๆฃฝ
-ๆค
-ๆค
-ๆค
-ๆค
-ๆค
-ๆค
-ๆค
-ๆค
-ๆค
-ๆค
-ๆค
-ๆค
-ๆค
-ๆค
-ๆค
-ๆค
-ๆค
-ๆคค
-ๆคฉ
-ๆคซ
-ๆคญ
-ๆคฎ
-ๆคฐ
-ๆคฒ
-ๆคด
-ๆคธ
-ๆคน
-ๆคฝ
-ๆคฟ
-ๆฅ
-ๆฅ
-ๆฅ
-ๆฅ
-ๆฅ
-ๆฅ
-ๆฅ
-ๆฅ
-ๆฅ
-ๆฅ
-ๆฅ
-ๆฅ
-ๆฅ
-ๆฅ
-ๆฅ
-ๆฅ
-ๆฅข
-ๆฅฃ
-ๆฅค
-ๆฅฅ
-ๆฅฆ
-ๆฅจ
-ๆฅฉ
-ๆฅช
-ๆฅซ
-ๆฅญ
-ๆฅฎ
-ๆฅฏ
-ๆฅฐ
-ๆฅฑ
-ๆฅต
-ๆฅถ
-ๆฅท
-ๆฅธ
-ๆฅน
-ๆฅบ
-ๆฅผ
-ๆฅฝ
-ๆฆ
-ๆฆ
-ๆฆ
-ๆฆ
-ๆฆ
-ๆฆ
-ๆฆ
-ๆฆ
-ๆฆ
-ๆฆ
-ๆฆ
-ๆฆ
-ๆฆ
-ๆฆ
-ๆฆ
-ๆฆ
-ๆฆ
-ๆฆ
-ๆฆ
-ๆฆฅ
-ๆฆง
-ๆฆจ
-ๆฆซ
-ๆฆญ
-ๆฆฐ
-ๆฆฑ
-ๆฆด
-ๆฆท
-ๆฆบ
-ๆฆป
-ๆฆผ
-ๆฆพ
-ๆง
-ๆง
-ๆง
-ๆง
-ๆง
-ๆง
-ๆง
-ๆง
-ๆง
-ๆง
-ๆง
-ๆง
-ๆง
-ๆง
-ๆง
-ๆง
-ๆง
-ๆง
-ๆง
-ๆงข
-ๆงฅ
-ๆงฉ
-ๆงฌ
-ๆงญ
-ๆงฑ
-ๆงฒ
-ๆงต
-ๆงป
-ๆงฝ
-ๆงพ
-ๆงฟ
-ๆจ
-ๆจ
-ๆจ
-ๆจ
-ๆจ
-ๆจ
-ๆจ
-ๆจ
-ๆจ
-ๆจ
-ๆจ
-ๆจ
-ๆจ
-ๆจก
-ๆจฃ
-ๆจจ
-ๆจฉ
-ๆจช
-ๆจซ
-ๆจญ
-ๆจฏ
-ๆจฑ
-ๆจต
-ๆจน
-ๆจฝ
-ๆจพ
-ๆฉ
-ๆฉ
-ๆฉ
-ๆฉ
-ๆฉ
-ๆฉ
-ๆฉ
-ๆฉ
-ๆฉ
-ๆฉ
-ๆฉ
-ๆฉ
-ๆฉ
-ๆฉ
-ๆฉก
-ๆฉฆ
-ๆฉช
-ๆฉฑ
-ๆฉน
-ๆฉบ
-ๆฉผ
-ๆฉฟ
-ๆช
-ๆช
-ๆช
-ๆช
-ๆช
-ๆช
-ๆช
-ๆช
-ๆช
-ๆช
-ๆช
-ๆช
-ๆช
-ๆช
-ๆช
-ๆช
-ๆช
-ๆชฅ
-ๆชง
-ๆชจ
-ๆชฉ
-ๆชซ
-ๆชฌ
-ๆชฐ
-ๆชต
-ๆชป
-ๆชฟ
-ๆซ
-ๆซ
-ๆซ
-ๆซ
-ๆซ
-ๆซ
-ๆซ
-ๆซ
-ๆซ
-ๆซก
-ๆซฐ
-ๆซป
-ๆซผ
-ๆฌ
-ๆฌ
-ๆฌ
-ๆฌ
-ๆฌ
-ๆฌ
-ๆฌ
-ๆฌ
-ๆฌ
-ๆฌก
-ๆฌข
-ๆฌฃ
-ๆฌค
-ๆฌฆ
-ๆฌง
-ๆฌฌ
-ๆฌฑ
-ๆฌฒ
-ๆฌท
-ๆฌธ
-ๆฌน
-ๆฌบ
-ๆฌป
-ๆฌพ
-ๆฌฟ
-ๆญ
-ๆญ
-ๆญ
-ๆญ
-ๆญ
-ๆญ
-ๆญ
-ๆญ
-ๆญ
-ๆญ
-ๆญ
-ๆญ
-ๆญ
-ๆญ
-ๆญ
-ๆญ
-ๆญข
-ๆญฃ
-ๆญค
-ๆญฅ
-ๆญฆ
-ๆญง
-ๆญฉ
-ๆญช
-ๆญฏ
-ๆญณ
-ๆญด
-ๆญธ
-ๆญน
-ๆญป
-ๆญผ
-ๆฎ
-ๆฎ
-ๆฎ
-ๆฎ
-ๆฎ
-ๆฎ
-ๆฎ
-ๆฎ
-ๆฎ
-ๆฎ
-ๆฎ
-ๆฎ
-ๆฎ
-ๆฎ
-ๆฎ
-ๆฎ
-ๆฎ
-ๆฎก
-ๆฎข
-ๆฎฃ
-ๆฎช
-ๆฎญ
-ๆฎณ
-ๆฎด
-ๆฎต
-ๆฎท
-ๆฎฝ
-ๆฎฟ
-ๆฏ
-ๆฏ
-ๆฏ
-ๆฏ
-ๆฏ
-ๆฏ
-ๆฏ
-ๆฏ
-ๆฏ
-ๆฏ
-ๆฏ
-ๆฏ
-ๆฏ
-ๆฏ
-ๆฏ
-ๆฏ
-ๆฏ
-ๆฏ
-ๆฏ
-ๆฏ
-ๆฏก
-ๆฏจ
-ๆฏซ
-ๆฏฌ
-ๆฏฏ
-ๆฏฐ
-ๆฏณ
-ๆฏต
-ๆฏถ
-ๆฏธ
-ๆฏน
-ๆฏป
-ๆฏฝ
-ๆฐ
-ๆฐ
-ๆฐ
-ๆฐ
-ๆฐ
-ๆฐ
-ๆฐ
-ๆฐ
-ๆฐ
-ๆฐ
-ๆฐ
-ๆฐ
-ๆฐ
-ๆฐ
-ๆฐ
-ๆฐ
-ๆฐ
-ๆฐ
-ๆฐ
-ๆฐก
-ๆฐข
-ๆฐฃ
-ๆฐค
-ๆฐฆ
-ๆฐง
-ๆฐจ
-ๆฐฉ
-ๆฐช
-ๆฐฎ
-ๆฐฏ
-ๆฐฐ
-ๆฐฒ
-ๆฐด
-ๆฐต
-ๆฐท
-ๆฐธ
-ๆฐน
-ๆฐฝ
-ๆฐพ
-ๆฐฟ
-ๆฑ
-ๆฑ
-ๆฑ
-ๆฑ
-ๆฑ
-ๆฑ
-ๆฑ
-ๆฑ
-ๆฑ
-ๆฑ
-ๆฑ
-ๆฑ
-ๆฑ
-ๆฑ
-ๆฑ
-ๆฑ
-ๆฑ
-ๆฑ
-ๆฑ
-ๆฑ
-ๆฑ
-ๆฑ
-ๆฑก
-ๆฑฃ
-ๆฑค
-ๆฑง
-ๆฑจ
-ๆฑฉ
-ๆฑช
-ๆฑซ
-ๆฑญ
-ๆฑฏ
-ๆฑฐ
-ๆฑฒ
-ๆฑด
-ๆฑถ
-ๆฑธ
-ๆฑน
-ๆฑบ
-ๆฑฝ
-ๆฑพ
-ๆฒ
-ๆฒ
-ๆฒ
-ๆฒ
-ๆฒ
-ๆฒ
-ๆฒ
-ๆฒ
-ๆฒ
-ๆฒ
-ๆฒ
-ๆฒ
-ๆฒ
-ๆฒ
-ๆฒ
-ๆฒ
-ๆฒ
-ๆฒ
-ๆฒ
-ๆฒ
-ๆฒ
-ๆฒ
-ๆฒ
-ๆฒก
-ๆฒข
-ๆฒฃ
-ๆฒค
-ๆฒฅ
-ๆฒฆ
-ๆฒง
-ๆฒจ
-ๆฒฉ
-ๆฒช
-ๆฒซ
-ๆฒฌ
-ๆฒญ
-ๆฒฎ
-ๆฒฑ
-ๆฒฒ
-ๆฒณ
-ๆฒด
-ๆฒต
-ๆฒถ
-ๆฒธ
-ๆฒน
-ๆฒป
-ๆฒผ
-ๆฒฝ
-ๆฒพ
-ๆฒฟ
-ๆณ
-ๆณ
-ๆณ
-ๆณ
-ๆณ
-ๆณ
-ๆณ
-ๆณ
-ๆณ
-ๆณ
-ๆณ
-ๆณ
-ๆณ
-ๆณ
-ๆณ
-ๆณ
-ๆณ
-ๆณ
-ๆณ
-ๆณ
-ๆณ
-ๆณ
-ๆณ
-ๆณก
-ๆณข
-ๆณฃ
-ๆณฅ
-ๆณจ
-ๆณช
-ๆณซ
-ๆณฌ
-ๆณฎ
-ๆณฏ
-ๆณฐ
-ๆณฑ
-ๆณฒ
-ๆณณ
-ๆณต
-ๆณท
-ๆณธ
-ๆณบ
-ๆณป
-ๆณผ
-ๆณฝ
-ๆณพ
-ๆด
-ๆด
-ๆด
-ๆด
-ๆด
-ๆด
-ๆด
-ๆด
-ๆด
-ๆด
-ๆด
-ๆด
-ๆด
-ๆด
-ๆด
-ๆด
-ๆด
-ๆดฃ
-ๆดค
-ๆดฅ
-ๆดง
-ๆดจ
-ๆดฉ
-ๆดช
-ๆดซ
-ๆดญ
-ๆดฎ
-ๆดฑ
-ๆดฒ
-ๆดณ
-ๆดด
-ๆดต
-ๆดธ
-ๆดน
-ๆดบ
-ๆดป
-ๆดผ
-ๆดฝ
-ๆดพ
-ๆดฟ
-ๆต
-ๆต
-ๆต
-ๆต
-ๆต
-ๆต
-ๆต
-ๆต
-ๆต
-ๆต
-ๆต
-ๆต
-ๆต
-ๆต
-ๆต
-ๆต
-ๆต
-ๆต
-ๆต
-ๆต
-ๆต
-ๆต
-ๆต
-ๆต
-ๆต
-ๆต
-ๆตก
-ๆตฃ
-ๆตค
-ๆตฅ
-ๆตฆ
-ๆตฉ
-ๆตช
-ๆตฌ
-ๆตฎ
-ๆตฏ
-ๆตฐ
-ๆตฑ
-ๆตฒ
-ๆตด
-ๆตท
-ๆตธ
-ๆตผ
-ๆตฟ
-ๆถ
-ๆถ
-ๆถ
-ๆถ
-ๆถ
-ๆถ
-ๆถ
-ๆถ
-ๆถ
-ๆถ
-ๆถ
-ๆถ
-ๆถ
-ๆถ
-ๆถ
-ๆถ
-ๆถ
-ๆถ
-ๆถ
-ๆถ
-ๆถ
-ๆถก
-ๆถข
-ๆถฃ
-ๆถค
-ๆถฅ
-ๆถฆ
-ๆถง
-ๆถจ
-ๆถฉ
-ๆถช
-ๆถซ
-ๆถฌ
-ๆถฎ
-ๆถฏ
-ๆถฒ
-ๆถด
-ๆถต
-ๆถท
-ๆถธ
-ๆถผ
-ๆถฟ
-ๆท
-ๆท
-ๆท
-ๆท
-ๆท
-ๆท
-ๆท
-ๆท
-ๆท
-ๆท
-ๆท
-ๆท
-ๆท
-ๆท
-ๆท
-ๆท
-ๆท
-ๆท
-ๆท
-ๆท
-ๆท
-ๆทก
-ๆทข
-ๆทค
-ๆทฆ
-ๆทจ
-ๆทซ
-ๆทฌ
-ๆทฎ
-ๆทฏ
-ๆทฐ
-ๆทฑ
-ๆทณ
-ๆทท
-ๆทน
-ๆทป
-ๆทผ
-ๆธ
-ๆธ
-ๆธ
-ๆธ
-ๆธ
-ๆธ
-ๆธ
-ๆธ
-ๆธ
-ๆธ
-ๆธ
-ๆธ
-ๆธ
-ๆธ
-ๆธ
-ๆธ
-ๆธ
-ๆธ
-ๆธ
-ๆธ
-ๆธ
-ๆธ
-ๆธ
-ๆธ
-ๆธก
-ๆธข
-ๆธฃ
-ๆธค
-ๆธฅ
-ๆธง
-ๆธฉ
-ๆธซ
-ๆธฌ
-ๆธญ
-ๆธฏ
-ๆธฐ
-ๆธฑ
-ๆธฒ
-ๆธด
-ๆธถ
-ๆธธ
-ๆธบ
-ๆธป
-ๆธผ
-ๆน
-ๆน
-ๆน
-ๆน
-ๆน
-ๆน
-ๆน
-ๆน
-ๆน
-ๆน
-ๆน
-ๆน
-ๆน
-ๆน
-ๆน
-ๆน
-ๆน
-ๆนก
-ๆนข
-ๆนฃ
-ๆนฆ
-ๆนง
-ๆนฉ
-ๆนซ
-ๆนฎ
-ๆนฒ
-ๆนณ
-ๆนด
-ๆนผ
-ๆนพ
-ๆนฟ
-ๆบ
-ๆบ
-ๆบ
-ๆบ
-ๆบ
-ๆบ
-ๆบ
-ๆบ
-ๆบ
-ๆบ
-ๆบ
-ๆบ
-ๆบ
-ๆบ
-ๆบ
-ๆบ
-ๆบ
-ๆบ
-ๆบ
-ๆบ
-ๆบ
-ๆบ
-ๆบก
-ๆบข
-ๆบฅ
-ๆบฆ
-ๆบง
-ๆบช
-ๆบซ
-ๆบฏ
-ๆบฑ
-ๆบฒ
-ๆบณ
-ๆบด
-ๆบต
-ๆบถ
-ๆบท
-ๆบบ
-ๆบป
-ๆบฝ
-ๆป
-ๆป
-ๆป
-ๆป
-ๆป
-ๆป
-ๆป
-ๆป
-ๆป
-ๆป
-ๆป
-ๆป
-ๆป
-ๆป
-ๆป
-ๆป
-ๆป
-ๆป
-ๆป
-ๆป
-ๆป
-ๆป
-ๆป
-ๆปก
-ๆปข
-ๆปค
-ๆปฅ
-ๆปฆ
-ๆปง
-ๆปจ
-ๆปฉ
-ๆปช
-ๆปซ
-ๆปฎ
-ๆปด
-ๆปน
-ๆปบ
-ๆปฝ
-ๆผ
-ๆผ
-ๆผ
-ๆผ
-ๆผ
-ๆผ
-ๆผ
-ๆผ
-ๆผ
-ๆผ
-ๆผ
-ๆผ
-ๆผ
-ๆผ
-ๆผ
-ๆผข
-ๆผค
-ๆผฆ
-ๆผฉ
-ๆผช
-ๆผซ
-ๆผญ
-ๆผฏ
-ๆผฐ
-ๆผฑ
-ๆผณ
-ๆผด
-ๆผถ
-ๆผท
-ๆผน
-ๆผป
-ๆผผ
-ๆผพ
-ๆฝ
-ๆฝ
-ๆฝ
-ๆฝ
-ๆฝ
-ๆฝ
-ๆฝ
-ๆฝ
-ๆฝ
-ๆฝ
-ๆฝ
-ๆฝ
-ๆฝ
-ๆฝ
-ๆฝ
-ๆฝ
-ๆฝ
-ๆฝ
-ๆฝข
-ๆฝค
-ๆฝฆ
-ๆฝฉ
-ๆฝฌ
-ๆฝญ
-ๆฝฎ
-ๆฝฒ
-ๆฝด
-ๆฝต
-ๆฝธ
-ๆฝบ
-ๆฝผ
-ๆฝฝ
-ๆฝพ
-ๆพ
-ๆพ
-ๆพ
-ๆพ
-ๆพ
-ๆพ
-ๆพ
-ๆพ
-ๆพ
-ๆพ
-ๆพ
-ๆพ
-ๆพ
-ๆพ
-ๆพก
-ๆพฃ
-ๆพค
-ๆพฅ
-ๆพง
-ๆพจ
-ๆพช
-ๆพซ
-ๆพญ
-ๆพณ
-ๆพด
-ๆพถ
-ๆพน
-ๆพผ
-ๆพพ
-ๆฟ
-ๆฟ
-ๆฟ
-ๆฟ
-ๆฟ
-ๆฟ
-ๆฟ
-ๆฟ
-ๆฟ
-ๆฟ
-ๆฟ
-ๆฟ
-ๆฟ
-ๆฟ
-ๆฟ
-ๆฟ
-ๆฟก
-ๆฟฆ
-ๆฟฉ
-ๆฟซ
-ๆฟฌ
-ๆฟฎ
-ๆฟฏ
-ๆฟธ
-็
-็
-็
-็
-็
-็
-็
-็
-็
-็
-็
-็
-็ก
-็ฃ
-็ฉ
-็ฌ
-็ฐ
-็ฑ
-็ด
-็ต
-็น
-็บ
-็ผ
-็ฝ
-็
-็
-็
-็
-็
-็
-็
-็
-็
-็ฉ
-็ซ
-็ฌ
-็ญ
-็ฏ
-็ฐ
-็ต
-็ถ
-็ธ
-็บ
-็ผ
-็พ
-็ฟ
-็
-็
-็
-็
-็
-็
-็
-็
-็
-็
-็
-็
-็
-็
-็
-็
-็
-็ก
-็ค
-็ง
-็ซ
-็ฌ
-็ญ
-็ฎ
-็ฏ
-็ฐ
-็ฑ
-็ณ
-็ต
-็ท
-็ธ
-็น
-็บ
-็ผ
-็ฝ
-็ฟ
-็
-็
-็
-็
-็
-็
-็
-็
-็
-็
-็
-็
-็
-็
-็
-็
-็ค
-็ฆ
-็ง
-็จ
-็ฉ
-็ซ
-็ฌ
-็ญ
-็ฏ
-็ท
-็น
-็บ
-็ป
-็ฝ
-็
-็
-็
-็
-็
-็
-็
-็
-็
-็
-็
-็
-็
-็
-็
-็
-็
-็
-็ก
-็ฆ
-็ฉ
-็ซ
-็ฎ
-็ฏ
-็ฐ
-็ฑ
-็ฒ
-็ด
-็ถ
-็น
-็ป
-็ผ
-็
-็
-็
-็
-็
-็
-็
-็
-็
-็
-็
-็
-็
-็
-็
-็
-็
ค
-็
ฆ
-็
ง
-็
จ
-็
ฎ
-็
ฒ
-็
ณ
-็
ด
-็
ต
-็
ถ
-็
ธ
-็
น
-็
บ
-็
ฝ
-็
ฟ
-็
-็
-็
-็
-็
-็
-็
-็
-็
-็
-็
-็
-็
-็
-็
-็ค
-็จ
-็ฌ
-็ญ
-็ฏ
-็ฑ
-็ณ
-็ด
-็ต
-็ธ
-็น
-็ฟ
-็
-็
-็
-็
-็
-็
-็
-็
-็
-็
-็
-็
-็
-็
-็
-็
-็
-็
-็ข
-็ฅ
-็ง
-็ฌ
-็ฎ
-็น
-็ฟ
-็
-็
-็
-็
-็
-็
-็
-็
-็
-็
-็
-็ข
-็ง
-็จ
-็ช
-็ฌ
-็ญ
-็ฐ
-็ฑ
-็ต
-็ถ
-็ท
-็ธ
-็น
-็ป
-็ฝ
-็ฟ
-็
-็
-็
-็
-็
-็
-็
-็
-็
-็
-็
-็
-็
-็
-็ก
-็ข
-็ฃ
-็ค
-็ฅ
-็ฆ
-็ง
-็ฉ
-็ฎ
-็ฏ
-็ฒ
-็ด
-็ต
-็ท
-็ธ
-็น
-็บ
-็ป
-็พ
-็ฟ
-็
-็
-็
-็
-็
-็
-็
-็
-็
-็
-็
-็
-็
-็จ
-็ฌ
-็ญ
-็ฏ
-็ฐ
-็ด
-็ถ
-็ท
-็ธ
-็น
-็บ
-็ผ
-็ฝ
-็
-็
-็
-็
-็
-็
-็
-็
-็
-็
-็
-็
-็
-็
-็
-็
-็
-็
-็
-็ก
-็ข
-็ฅ
-็ง
-็จ
-็ฉ
-็ฌ
-็ญ
-็ฎ
-็ฏ
-็ฐ
-็ฑ
-็ฒ
-็ณ
-็ด
-็ถ
-็ท
-็ธ
-็บ
-็ป
-็ผ
-็
-็
-็
-็
-็
-็
-็
-็
-็
-็
-็
-็
-็
-็
-็
-็
-็ก
-็ข
-็ฅ
-็ง
-็ฉ
-็ช
-็ซ
-็ฌ
-็ฎ
-็ฐ
-็ฑ
-็ฒ
-็ณ
-็ด
-็ต
-็ถ
-็ท
-็ธ
-็พ
-็ฟ
-็
-็
-็
-็
-็
-็
-็
-็
-็
-็
-็
-็
-็ฃ
-็ฆ
-็จ
-็ฌ
-็ญ
-็ฎ
-็ฏ
-็ฒ
-็ด
-็ถ
-็ป
-็พ
-็
-็
-็
-็
-็
-็
-็
-็
-็
-็
-็
-็
-็
-็
-็
-็
-็
-็
-็
-็
-็
-็
-็ก
-็ข
-็ฅ
-็ฆ
-็ฉ
-็ซ
-็ญ
-็ฎ
-็ฏ
-็ฐ
-็ฑ
-็ฒ
-็ณ
-็ถ
-็ท
-็บ
-็ป
-็ผ
-็
-็
-็
-็
-็
-็
-็
-็
-็
-็
-็
-็
-็
-็
-็
-็
-็
-็
-็
-็ฃ
-็ฅ
-็ฆ
-็ง
-็ฉ
-็ช
-็ญ
-็ฎ
-็ฐ
-็ฒ
-็ต
-็ธ
-็น
-็บ
-็ฝ
-็พ
-็
-็
-็
-็
-็
-็
-็
-็
-็
-็
-็
-็
-็
-็
-็ข
-็ฃ
-็ค
-็ฅ
-็ฆ
-็จ
-็ช
-็ซ
-็ฌ
-็ญ
-็ฎ
-็ฏ
-็ฐ
-็ฑ
-็ฒ
-็ณ
-็ด
-็ต
-็ถ
-็ป
-็ผ
-็
-็
-็
-็
-็
-็
-็
-็
-็
-็
-็
-็
-็
-็
-็
-็
-็
-็
-็
-็
-็
-็ข
-็ง
-็จ
-็ช
-็ญ
-็ฎ
-็ฐ
-็ฑ
-็ณ
-็ด
-็ถ
-็ท
-็ธ
-็ฝ
-็พ
-็ฟ
-็
-็
-็
-็
-็
-็
-็
-็
-็
-็
-็
-็
-็
-็
-็
-็
-็
-็
-็
-็
-็
-็ค
-็ฅ
-็ง
-็จ
-็ฉ
-็ช
-็ฒ
-็ต
-็ท
-็ธ
-็น
-็บ
-็ฟ
-็
-็
-็
-็
-็
-็
-็
-็
-็
-็
-็
-็
-็
-็
-็ข
-็ฃ
-็ค
-็ฆ
-็จ
-็ฌ
-็ฎ
-็ฏ
-็ด
-็ถ
-็ท
-็น
-็ป
-็ฟ
-็
-็
-็
-็
-็
-็
-็
-็
-็
-็
-็
-็
-็
-็
-็
-็
-็
-็
-็
-็ก
-็ข
-็ฅ
-็ฆ
-็จ
-็ฉ
-็ช
-็ซ
-็ฌ
-็ญ
-็ฏ
-็ฐ
-็ฑ
-็ฒ
-็ณ
-็ด
-็ต
-็ท
-็ธ
-็บ
-็ป
-็ฝ
-็พ
-็ฟ
-็
-็
-็
-็
-็
-็
-็
-็
-็
-็
-็
-็
-็
-็
-็
-็
-็
-็
-็
-็ค
-็ฅ
-็ฆ
-็ง
-็ช
-็ซ
-็ฌ
-็ฏ
-็ฒ
-็ณ
-็ด
-็ถ
-็ท
-็ธ
-็น
-็ผ
-็ฝ
-็ฟ
-็
-็
-็
-็
-็
-็
-็
-็
-็
-็
-็
-็
-็
-็
-็
-็
-็
-็
-็ก
-็ข
-็ฃ
-็ค
-็ฅ
-็ง
-็ซ
-็ฌ
-็ญ
-็ฎ
-็ฏ
-็ฐ
-็ฑ
-็ฒ
-็ณ
-็ด
-็ต
-็ธ
-็น
-็ป
-็ผ
-็ฝ
-็พ
-็
-็
-็
-็
-็
-็
-็
-็
-็
-็
-็
-็
-็
-็
-็
-็
-็
-็
-็
-็
-็
-็
-็
-็ก
-็ข
-็ฃ
-็ค
-็ฆ
-็ง
-็จ
-็ฉ
-็ช
-็ซ
-็ฏ
-็ฐ
-็ฑ
-็ฒ
-็ด
-็น
-็บ
-็ป
-็ผ
-็พ
-็ฟ
-็
-็
-็
-็
-็
-็
-็
-็
-็
-็
-็
-็
-็
-็
-็
-็
-็
-็
-็
-็ข
-็ฃ
-็ค
-็ฅ
-็ฆ
-็จ
-็ฉ
-็ช
-็ซ
-็ญ
-็ฐ
-็ฑ
-็ณ
-็ด
-็ต
-็ธ
-็ผ
-็พ
-็ฟ
-็
-็
-็
-็
-็
-็
-็
-็
-็
-็
-็ข
-็ฃ
-็ฅ
-็ฆ
-็จ
-็ช
-็ซ
-็ฏ
-็ด
-็ถ
-็ธ
-็น
-็บ
-็ป
-็ผ
-็ฝ
-็พ
-็ฟ
-็
-็
-็
-็
-็
-็
-็
-็
-็
-็
-็
-็
-็
-็
-็
-็ค
-็ฆ
-็ช
-็ฎ
-็ฑ
-็ฒ
-็ด
-็ฟ
-็
-็
-็
-็
-็
-็
-็
-็
-็
-็
-็
-็
-็
-็
-็
-็
-็
-็
-็
-็ก
-็ข
-็ฃ
-็ฅ
-็ฉ
-็ฌ
-็ญ
-็ฎ
-็ฏ
-็ฑ
-็ฒ
-็ด
-็ต
-็ธ
-็น
-็ป
-็ผ
-็พ
-็
-็
-็
-็
-็
-็
-็
-็
-็
-็
-็
-็
-็
-็
-็
-็
-็
-็
-็ข
-็ฆ
-็จ
-็ฉ
-็ฌ
-็ญ
-็ฏ
-็ด
-็ต
-็ถ
-็ท
-็ธ
-็น
-็บ
-็ผ
-็ฝ
-็
-็
-็
-็
-็
-็
-็
-็
-็
-็
-็
-็
-็
-็
-็
-็
-็
-็ก
-็ข
-็ฃ
-็ฅ
-็ฆ
-็จ
-็ฉ
-็ช
-็ซ
-็ฌ
-็ญ
-็ฐ
-็ณ
-็ท
-็น
-็บ
-็ฝ
-็พ
-็ฟ
-็
-็
-็
-็
-็
-็
-็
-็
-็
-็
-็
-็
-็
-็
-็
-็ข
-็ค
-็ฅ
-็ง
-็ฉ
-็ช
-็ฌ
-็ญ
-็ฏ
-็ฐ
-็ณ
-็ต
-็ป
-็ฝ
-็พ
-็ฟ
-็
-็
-็
-็
-็
-็
-็
-็
-็
-็
-็
-็
-็ข
-็ฃ
-็ฅ
-็ง
-็จ
-็ฉ
-็ซ
-็ฌ
-็ญ
-็ฎ
-็ฐ
-็ฑ
-็ฒ
-็ณ
-็ถ
-็ธ
-็ป
-็ผ
-็ฝ
-็พ
-็ฟ
-็
-็
-็
-็
-็
-็
-็
-็
-็
-็
-็
-็
-็
-็
-็
-็
-็
-็
-็
-็
-็
-็ ข
-็ ฃ
-็ ฅ
-็ ฆ
-็ ง
-็ ฌ
-็ ญ
-็ ฎ
-็ ฏ
-็ ฐ
-็ ฒ
-็ ด
-็ ท
-็ ธ
-็ น
-็ บ
-็ ป
-็ ผ
-็ พ
-็ก
-็ก
-็ก
-็ก
-็ก
-็ก
-็ก
-็ก
-็ก
-็ก
-็ก
-็ก
-็ก
-็ก
-็ก
-็ก
-็ก
-็ก
-็กก
-็กช
-็กซ
-็กฌ
-็กฎ
-็กฐ
-็กต
-็กท
-็กผ
-็กพ
-็กฟ
-็ข
-็ข
-็ข
-็ข
-็ข
-็ข
-็ข
-็ข
-็ข
-็ข
-็ข
-็ข
-็ข
-็ข
-็ข
-็ข
-็ข
-็ข
-็ข
-็ข
-็ขก
-็ขฃ
-็ขฅ
-็ขง
-็ขจ
-็ขช
-็ขซ
-็ขฐ
-็ขฑ
-็ขฒ
-็ขณ
-็ขด
-็ขถ
-็ขบ
-็ขป
-็ขพ
-็ฃ
-็ฃ
-็ฃ
-็ฃ
-็ฃ
-็ฃ
-็ฃ
-็ฃ
-็ฃ
-็ฃ
-็ฃ
-็ฃ
-็ฃ
-็ฃ
-็ฃ
-็ฃ
-็ฃ
-็ฃ
-็ฃ
-็ฃก
-็ฃข
-็ฃจ
-็ฃฌ
-็ฃฒ
-็ฃณ
-็ฃด
-็ฃต
-็ฃท
-็ฃน
-็ฃบ
-็ฃป
-็ฃพ
-็ฃฟ
-็ค
-็ค
-็ค
-็ค
-็ค
-็ค
-็ค
-็ค
-็ค
-็คก
-็คค
-็คง
-็คจ
-็คฎ
-็คฒ
-็คด
-็คถ
-็คบ
-็คผ
-็คฝ
-็คพ
-็คฟ
-็ฅ
-็ฅ
-็ฅ
-็ฅ
-็ฅ
-็ฅ
-็ฅ
-็ฅ
-็ฅ
-็ฅ
-็ฅ
-็ฅ
-็ฅ
-็ฅ
-็ฅ
-็ฅ
-็ฅ
-็ฅ
-็ฅ
-็ฅ
-็ฅ
-็ฅ
-็ฅ
-็ฅ
-็ฅ
-็ฅ
-็ฅข
-็ฅค
-็ฅฅ
-็ฅง
-็ฅจ
-็ฅซ
-็ฅญ
-็ฅฏ
-็ฅฑ
-็ฅฒ
-็ฅด
-็ฅถ
-็ฅท
-็ฅธ
-็ฅบ
-็ฅผ
-็ฅพ
-็ฅฟ
-็ฆ
-็ฆ
-็ฆ
-็ฆ
-็ฆ
-็ฆ
-็ฆ
-็ฆ
-็ฆ
-็ฆ
-็ฆ
-็ฆ
-็ฆ
-็ฆ
-็ฆ
-็ฆ
-็ฆ
-็ฆ
-็ฆ
-็ฆค
-็ฆฅ
-็ฆง
-็ฆจ
-็ฆฉ
-็ฆซ
-็ฆฎ
-็ฆณ
-็ฆด
-็ฆต
-็ฆท
-็ฆน
-็ฆบ
-็ฆป
-็ฆฝ
-็ฆพ
-็ง
-็ง
-็ง
-็ง
-็ง
-็ง
-็ง
-็ง
-็ง
-็ง
-็ง
-็ง
-็ง
-็ง
-็ง
-็ง
-็ง
-็ง
-็ง
-็ง
-็ง
-็งฃ
-็งค
-็งฆ
-็งง
-็งฉ
-็งช
-็งซ
-็งฌ
-็งญ
-็งฏ
-็งฐ
-็งด
-็งธ
-็งป
-็งฝ
-็งพ
-็งฟ
-็จ
-็จ
-็จ
-็จ
-็จ
-็จ
-็จ
-็จ
-็จ
-็จ
-็จ
-็จ
-็จ
-็จ
-็จ
-็จ
-็จ
-็จ
-็จฃ
-็จญ
-็จฎ
-็จฑ
-็จฒ
-็จณ
-็จท
-็จน
-็จบ
-็จป
-็จผ
-็จฝ
-็จพ
-็จฟ
-็ฉ
-็ฉ
-็ฉ
-็ฉ
-็ฉ
-็ฉ
-็ฉ
-็ฉ
-็ฉ
-็ฉ
-็ฉ
-็ฉ
-็ฉ
-็ฉ
-็ฉ
-็ฉ
-็ฉก
-็ฉง
-็ฉจ
-็ฉฌ
-็ฉฐ
-็ฉด
-็ฉต
-็ฉถ
-็ฉท
-็ฉธ
-็ฉน
-็ฉบ
-็ฉผ
-็ฉฝ
-็ฉพ
-็ฉฟ
-็ช
-็ช
-็ช
-็ช
-็ช
-็ช
-็ช
-็ช
-็ช
-็ช
-็ช
-็ช
-็ช
-็ช
-็ช
-็ช
-็ช
-็ช
-็ช
-็ช
-็ช
-็ช
-็ช
-็ช
-็ช
-็ชก
-็ชฃ
-็ชฅ
-็ชฆ
-็ชจ
-็ชฉ
-็ชซ
-็ชฌ
-็ชญ
-็ชฐ
-็ชฑ
-็ชณ
-็ชด
-็ชธ
-็ชน
-็ชบ
-็ชป
-็ชฝ
-็ชพ
-็ชฟ
-็ซ
-็ซ
-็ซ
-็ซ
-็ซ
-็ซ
-็ซ
-็ซ
-็ซ
-็ซ
-็ซ
-็ซ
-็ซ
-็ซฃ
-็ซฅ
-็ซฆ
-็ซญ
-็ซฎ
-็ซฏ
-็ซน
-็ซบ
-็ซป
-็ซฝ
-็ซฟ
-็ฌ
-็ฌ
-็ฌ
-็ฌ
-็ฌ
-็ฌ
-็ฌ
-็ฌ
-็ฌ
-็ฌ
-็ฌ
-็ฌ
-็ฌ
-็ฌ
-็ฌ
-็ฌ
-็ฌก
-็ฌค
-็ฌฅ
-็ฌฆ
-็ฌจ
-็ฌช
-็ฌซ
-็ฌฌ
-็ฌญ
-็ฌฎ
-็ฌฏ
-็ฌฑ
-็ฌฒ
-็ฌณ
-็ฌด
-็ฌต
-็ฌธ
-็ฌน
-็ฌบ
-็ฌผ
-็ฌพ
-็ญ
-็ญ
-็ญ
-็ญ
-็ญ
-็ญ
-็ญ
-็ญ
-็ญ
-็ญ
-็ญ
-็ญ
-็ญ
-็ญ
-็ญ
-็ญ
-็ญ
-็ญข
-็ญค
-็ญฅ
-็ญฆ
-็ญฉ
-็ญญ
-็ญฎ
-็ญฏ
-็ญฐ
-็ญฑ
-็ญฒ
-็ญณ
-็ญด
-็ญต
-็ญท
-็ญน
-็ญป
-็ญผ
-็ญพ
-็ฎ
-็ฎ
-็ฎ
-็ฎ
-็ฎ
-็ฎ
-็ฎ
-็ฎ
-็ฎ
-็ฎ
-็ฎ
-็ฎ
-็ฎ
-็ฎ
-็ฎ
-็ฎ
-็ฎ
-็ฎ
-็ฎก
-็ฎข
-็ฎฃ
-็ฎฆ
-็ฎง
-็ฎจ
-็ฎฉ
-็ฎช
-็ฎซ
-็ฎฌ
-็ฎญ
-็ฎฏ
-็ฎฑ
-็ฎด
-็ฎต
-็ฎธ
-็ฎป
-็ฎผ
-็ฎพ
-็ฏ
-็ฏ
-็ฏ
-็ฏ
-็ฏ
-็ฏ
-็ฏ
-็ฏ
-็ฏ
-็ฏ
-็ฏ
-็ฏ
-็ฏก
-็ฏข
-็ฏฅ
-็ฏฆ
-็ฏจ
-็ฏช
-็ฏญ
-็ฏฎ
-็ฏฏ
-็ฏฑ
-็ฏฒ
-็ฏท
-็ฏธ
-็ฏน
-็ฏป
-็ฏผ
-็ฏพ
-็ฐ
-็ฐ
-็ฐ
-็ฐ
-็ฐ
-็ฐ
-็ฐ
-็ฐ
-็ฐ
-็ฐ
-็ฐ
-็ฐ
-็ฐ
-็ฐ
-็ฐก
-็ฐฆ
-็ฐง
-็ฐจ
-็ฐช
-็ฐฌ
-็ฐฐ
-็ฐณ
-็ฐด
-็ฐต
-็ฐธ
-็ฐฟ
-็ฑ
-็ฑ
-็ฑ
-็ฑ
-็ฑ
-็ฑ
-็ฑ
-็ฑ
-็ฑ
-็ฑ
-็ฑ
-็ฑ
-็ฑข
-็ฑฃ
-็ฑค
-็ฑฅ
-็ฑง
-็ฑฏ
-็ฑณ
-็ฑด
-็ฑน
-็ฑบ
-็ฑป
-็ฑผ
-็ฑฝ
-็ฑพ
-็ฒ
-็ฒ
-็ฒ
-็ฒ
-็ฒ
-็ฒ
-็ฒ
-็ฒ
-็ฒ
-็ฒ
-็ฒ
-็ฒ
-็ฒ
-็ฒ
-็ฒ
-็ฒ
-็ฒข
-็ฒค
-็ฒฅ
-็ฒง
-็ฒฉ
-็ฒช
-็ฒฎ
-็ฒฑ
-็ฒฒ
-็ฒณ
-็ฒถ
-็ฒน
-็ฒบ
-็ฒป
-็ฒผ
-็ฒฝ
-็ฒพ
-็ฒฟ
-็ณ
-็ณ
-็ณ
-็ณ
-็ณ
-็ณ
-็ณ
-็ณ
-็ณ
-็ณ
-็ณ
-็ณ
-็ณ
-็ณ
-็ณ
-็ณ
-็ณฆ
-็ณง
-็ณจ
-็ณฏ
-็ณต
-็ณธ
-็ณป
-็ด
-็ด
-็ด
-็ด
-็ด
-็ด
-็ด
-็ด
-็ด
-็ด
-็ด
-็ดข
-็ดง
-็ดซ
-็ดฏ
-็ดต
-็ดฝ
-็ดพ
-็ต
-็ต
-็ต
-็ต
-็ต
-็ต
-็ต
-็ต
-็ต
-็ต
-็ต
-็ต
-็ต
-็ตฃ
-็ตฆ
-็ตฉ
-็ตช
-็ตซ
-็ตฎ
-็ตต
-็ตท
-็ตบ
-็ตป
-็ตฟ
-็ถ
-็ถ
-็ถ
-็ถ
-็ถ
-็ถ
-็ถ
-็ถ
-็ถ
-็ถ
-็ถ
-็ถฆ
-็ถช
-็ถฎ
-็ถฏ
-็ถด
-็ถท
-็ท
-็ท
-็ท
-็ท
-็ท
-็ท
-็ท
-็ท
-็ทฃ
-็ทฉ
-็ทบ
-็ทผ
-็ธ
-็ธ
-็ธ
-็ธ
-็ธ
-็ธ
-็ธข
-็ธฆ
-็ธฉ
-็ธฎ
-็ธฏ
-็ธบ
-็ธป
-็ธฟ
-็น
-็น
-็น
-็น
-็น
-็น
-็น
-็น
-็น
-็น
-็น
-็น
-็น
-็นฃ
-็นณ
-็นธ
-็นป
-็บ
-็บ
-็บ
-็บ
-็บ
-็บ
-็บ
-็บ
-็บ
-็บ
-็บ
-็บก
-็บข
-็บฃ
-็บค
-็บฅ
-็บฆ
-็บง
-็บจ
-็บฉ
-็บช
-็บซ
-็บฌ
-็บญ
-็บฎ
-็บฏ
-็บฐ
-็บฑ
-็บฒ
-็บณ
-็บด
-็บต
-็บถ
-็บท
-็บธ
-็บน
-็บบ
-็บป
-็บผ
-็บฝ
-็บพ
-็บฟ
-็ป
-็ป
-็ป
-็ป
-็ป
-็ป
-็ป
-็ป
-็ป
-็ป
-็ป
-็ป
-็ป
-็ป
-็ป
-็ป
-็ป
-็ป
-็ป
-็ป
-็ป
-็ป
-็ป
-็ป
-็ป
-็ป
-็ป
-็ป
-็ป
-็ป
-็ป
-็ป
-็ป
-็ปก
-็ปข
-็ปฃ
-็ปค
-็ปฅ
-็ปฆ
-็ปง
-็ปจ
-็ปฉ
-็ปช
-็ปซ
-็ปญ
-็ปฎ
-็ปฏ
-็ปฐ
-็ปฑ
-็ปฒ
-็ปณ
-็ปด
-็ปต
-็ปถ
-็ปท
-็ปธ
-็ปน
-็ปบ
-็ปป
-็ปผ
-็ปฝ
-็ปพ
-็ปฟ
-็ผ
-็ผ
-็ผ
-็ผ
-็ผ
-็ผ
-็ผ
-็ผ
-็ผ
-็ผ
-็ผ
-็ผ
-็ผ
-็ผ
-็ผ
-็ผ
-็ผ
-็ผ
-็ผ
-็ผ
-็ผ
-็ผ
-็ผ
-็ผ
-็ผ
-็ผ
-็ผ
-็ผ
-็ผ
-็ผ
-็ผ
-็ผ
-็ผก
-็ผข
-็ผฃ
-็ผค
-็ผฅ
-็ผฆ
-็ผง
-็ผจ
-็ผฉ
-็ผช
-็ผซ
-็ผฌ
-็ผญ
-็ผฎ
-็ผฏ
-็ผฐ
-็ผฑ
-็ผฒ
-็ผณ
-็ผด
-็ผต
-็ผถ
-็ผธ
-็ผบ
-็ผผ
-็ผพ
-็ฝ
-็ฝ
-็ฝ
-็ฝ
-็ฝ
-็ฝ
-็ฝ
-็ฝ
-็ฝ
-็ฝ
-็ฝ
-็ฝ
-็ฝ
-็ฝ
-็ฝ
-็ฝ
-็ฝ
-็ฝ
-็ฝ
-็ฝก
-็ฝข
-็ฝฃ
-็ฝฅ
-็ฝฆ
-็ฝจ
-็ฝฉ
-็ฝช
-็ฝญ
-็ฝฎ
-็ฝฏ
-็ฝฒ
-็ฝณ
-็ฝด
-็ฝถ
-็ฝน
-็ฝป
-็ฝฝ
-็ฝพ
-็ฝฟ
-็พ
-็พ
-็พ
-็พ
-็พ
-็พ
-็พ
-็พ
-็พ
-็พ
-็พ
-็พ
-็พ
-็พ
-็พ
-็พ
-็พ
-็พ
-็พ
-็พ
-็พ
-็พ
-็พก
-็พฃ
-็พค
-็พง
-็พจ
-็พฉ
-็พซ
-็พญ
-็พฏ
-็พฐ
-็พฑ
-็พฒ
-็พด
-็พต
-็พถ
-็พธ
-็พน
-็พผ
-็พฝ
-็พพ
-็พฟ
-็ฟ
-็ฟ
-็ฟ
-็ฟ
-็ฟ
-็ฟ
-็ฟ
-็ฟ
-็ฟ
-็ฟ
-็ฟ
-็ฟ
-็ฟ
-็ฟ
-็ฟ
-็ฟ
-็ฟ
-็ฟ
-็ฟ
-็ฟ
-็ฟก
-็ฟฃ
-็ฟฅ
-็ฟฆ
-็ฟฉ
-็ฟซ
-็ฟฌ
-็ฟฎ
-็ฟฏ
-็ฟฐ
-็ฟฑ
-็ฟฒ
-็ฟณ
-็ฟถ
-็ฟป
-็ฟผ
-็ฟพ
-็ฟฟ
-่
-่
-่
-่
-่
-่
-่
-่
-่
-่
-่
-่
-่
-่
-่
-่
-่
-่
-่
-่
-่
-่ก
-่ข
-่ค
-่ฅ
-่ฆ
-่ง
-่จ
-่ฉ
-่ญ
-่ฐ
-่ฑ
-่ณ
-่ต
-่ถ
-่ท
-่ธ
-่ป
-่ฝ
-่ฟ
-่
-่
-่
-่
-่
-่
-่
-่
-่
-่
-่
-่
-่
-่ก
-่ฉ
-่ช
-่ฐ
-่ฑ
-่ฒ
-่ด
-่ป
-่ฝ
-่ฟ
-่
-่
-่
-่
-่
-่
-่
-่
-่
-่
-่
-่
-่
-่
-่
-่
-่
-่
-่
-่ก
-่ข
-่ฃ
-่ค
-่ฅ
-่ฆ
-่ฉ
-่ช
-่ซ
-่ฌ
-่ญ
-่ฎ
-่ฏ
-่ฑ
-่ฒ
-่ณ
-่ด
-่ถ
-่ธ
-่บ
-่ผ
-่ฝ
-่พ
-่ฟ
-่
-่
-่
-่
-่
-่
-่
-่
-่
-่
-่
-่
-่
-่
-่
-่
-่
-่
-่
-่
-่
-่
-่ก
-่ค
-่ฅ
-่ง
-่จ
-่ฉ
-่ช
-่ซ
-่ฌ
-่ญ
-่ฎ
-่ฏ
-่ฐ
-่ฑ
-่ฒ
-่ณ
-่ด
-่ถ
-่ธ
-่น
-่บ
-่ป
-่ผ
-่ฝ
-่พ
-่
-่
-่
-่
-่
-่
-่
-่
-่
-่
-่
-่
-่
-่
-่
-่
-่
-่
-่
-่
-่
-่
-่ก
-่ข
-่ฃ
-่ค
-่ฉ
-่ฌ
-่ฏ
-่ฐ
-่ฑ
-่ฒ
-่ณ
-่ถ
-่ท
-่ธ
-่น
-่ฝ
-่พ
-่ฟ
-่
-่
-่
-่
-่
-่
-่
-่
-่
-่
-่
-่
-่
-่
-่
-่
-่
-่
ฅ
-่
ง
-่
ฉ
-่
ฌ
-่
ญ
-่
ฎ
-่
ฏ
-่
ฐ
-่
ฑ
-่
ฒ
-่
ด
-่
ท
-่
น
-่
บ
-่
ป
-่
ผ
-่
ฝ
-่
พ
-่
ฟ
-่
-่
-่
-่
-่
-่
-่
-่
-่
-่
-่
-่
-่
-่
-่
-่ฃ
-่ฆ
-่จ
-่ช
-่ซ
-่ฐ
-่ณ
-่ด
-่ถ
-่บ
-่ป
-่
-่
-่
-่
-่
-่
-่
-่
-่
-่
-่
-่
-่
-่
-่
-่
-่
-่ก
-่ฃ
-่ง
-่จ
-่ฉ
-่ช
-่ฌ
-่ญ
-่ฏ
-่ฑ
-่ฒ
-่ณ
-่ด
-่บ
-่ป
-่ผ
-่พ
-่ฟ
-่
-่
-่
-่
-่
-่
-่
-่
-่
-่
-่
-่
-่
-่
-่
-่
-่
-่
-่
-่
-่
-่
-่
-่
-่
-่
-่ก
-่ข
-่ฃ
-่ฅ
-่ฆ
-่จ
-่ช
-่ซ
-่ฌ
-่ฐ
-่ฑ
-่ฒ
-่ณ
-่ด
-่ต
-่ถ
-่ท
-่ธ
-่น
-่บ
-่ป
-่ผ
-่
-่
-่
-่
-่
-่
-่
-่
-่
-่
-่
-่
-่
-่
-่จ
-่ฎ
-่ฏ
-่ฐ
-่ฒ
-่ณ
-่ด
-่น
-่บ
-่ป
-่ฝ
-่พ
-่ฟ
-่
-่
-่
-่
-่
-่
-่
-่
-่
-่
-่
-่
-่
-่
-่
-่
-่
-่
-่
-่
-่ก
-่ฃ
-่ค
-่ฅ
-่ฆ
-่ง
-่จ
-่ฉ
-่ช
-่ซ
-่ฌ
-่ญ
-่ฎ
-่ฏ
-่ฐ
-่ฑ
-่ณ
-่ด
-่ถ
-่ท
-่ธ
-่น
-่บ
-่ผ
-่ฝ
-่พ
-่
-่
-่
-่
-่
-่
-่
-่
-่
-่
-่
-่
-่
-่
-่
-่
-่
-่
-่
-่
-่
-่
-่
-่
-่
-่
-่ก
-่ฃ
-่ค
-่ฅ
-่ฆ
-่ง
-่จ
-่ซ
-่ฏ
-่ฑ
-่ณ
-่ด
-่ถ
-่ท
-่น
-่บ
-่ป
-่พ
-่
-่
-่
-่
-่
-่
-่
-่
-่
-่
-่
-่
-่
-่
-่
-่
-่
-่
-่
-่
-่
-่
-่
-่
-่
-่
-่ง
-่จ
-่ซ
-่ฌ
-่ญ
-่ฏ
-่ฑ
-่ฒ
-่ณ
-่ด
-่ต
-่ถ
-่ท
-่ธ
-่น
-่บ
-่ผ
-่
-่
-่
-่
-่
-่
-่
-่
-่
-่
-่
-่
-่
-่
-่
-่
-่
-่
-่
-่
-่
-่
-่
-่
-่
-่
-่ก
-่ฃ
-่ค
-่ฅ
-่ฆ
-่ง
-่จ
-่ฉ
-่ช
-่ซ
-่ฌ
-่ญ
-่ฎ
-่ฏ
-่ด
-่ต
-่ท
-่ธ
-่ป
-่ผ
-่ฝ
-่
-่
-่
-่
-่
-่
-่
-่
-่
-่
-่
-่
-่
-่
-่
-่
-่จ
-่ฉ
-่ช
-่ซ
-่ฐ
-่ฑ
-่ฒ
-่ณ
-่ด
-่ถ
-่ท
-่ธ
-่น
-่บ
-่ผ
-่ฝ
-่ฟ
-่
-่
-่
-่
-่
-่
-่
-่
-่
-่
-่
-่
-่
-่
-่
-่
-่
-่
-่
-่
-่
-่ก
-่ข
-่ฅ
-่ง
-่ฉ
-่ช
-่ซ
-่ญ
-่ฏ
-่ฐ
-่ฑ
-่ฒ
-่ด
-่ต
-่ถ
-่ท
-่ธ
-่น
-่บ
-่ผ
-่ฝ
-่พ
-่
-่
-่
-่
-่
-่
-่
-่
-่
-่
-่
-่
-่
-่
-่
-่ฃ
-่ค
-่ฅ
-่ฆ
-่ง
-่จ
-่ฉ
-่ฌ
-่ฎ
-่ฏ
-่ฑ
-่ถ
-่ท
-่ธ
-่น
-่ผ
-่ฝ
-่
-่
-่
-่
-่
-่
-่
-่
-่
-่
-่
-่
-่
-่
-่
-่
-่ก
-่ฃ
-่จ
-่ฉ
-่ซ
-่ฌ
-่ญ
-่ฐ
-่ฑ
-่ณ
-่ด
-่ต
-่ถ
-่ธ
-่น
-่บ
-่ฝ
-่
-่
-่
-่
-่
-่
-่
-่
-่
-่
-่
-่
-่
-่
-่
-่ก
-่ข
-่ง
-่จ
-่ฎ
-่ฏ
-่ฑ
-่ฒ
-่ด
-่ถ
-่ธ
-่น
-่บ
-่ป
-่ผ
-่ฝ
-่พ
-่ฟ
-่
-่
-่
-่
-่
-่
-่
-่
-่
-่
-่
-่
-่
-่
-่
-่
-่
-่ข
-่ฃ
-่ฅ
-่ฆ
-่ง
-่ช
-่ซ
-่ฌ
-่ฎ
-่ฐ
-่ฑ
-่ณ
-่ด
-่ต
-่ท
-่บ
-่ผ
-่ฟ
-่
-่
-่
-่
-่
-่
-่
-่
-่
-่
-่
-่
-่
-่
-่ก
-่ช
-่ซ
-่ฌ
-่ฏ
-่ต
-่ท
-่ธ
-่น
-่บ
-่ป
-่ผ
-่ฝ
-่
-่
-่
-่
-่
-่
-่
-่
-่
-่
-่
-่
-่ก
-่ฃ
-่ค
-่จ
-่ซ
-่ฐ
-่ฒ
-่ณ
-่ด
-่น
-่บ
-่ป
-่พ
-่
-่
-่
-่
-่
-่
-่
-่
-่
-่
-่
-่
-่
-่ก
-่ข
-่ค
-่ฆ
-่จ
-่ช
-่ซ
-่ฌ
-่ฎ
-่ฏ
-่ฐ
-่ถ
-่ท
-่ธ
-่น
-่ฟ
-่
-่
-่
-่
-่
-่
-่
-่
-่
-่
-่
-่
-่
-่
-่
-่
-่
-่
-่ข
-่ค
-่ฅ
-่ฆ
-่จ
-่ฉ
-่ซ
-่ญ
-่ณ
-่น
-่ป
-่พ
-่ฟ
-่
-่
-่
-่
-่
-่
-่
-่
-่
-่ก
-่ค
-่ง
-่ฉ
-่ญ
-่ต
-่ธ
-่ผ
-่ฝ
-่
-่
-่
-่
-่
-่
-่
-่
-่
-่
-่
-่
-่
-่
-่
-่
-่
-่ก
-่ข
-่ฅ
-่ง
-่ซ
-่ฌ
-่ฎ
-่ฑ
-่ต
-่น
-่บ
-่ป
-่ผ
-่ฝ
-่พ
-่ฟ
-่
-่
-่
-่
-่
-่
-่
-่
-่
-่
-่
-่
-่
-่
-่
-่
-่
-่ก
-่ข
-่ฃ
-่ค
-่ง
-่จ
-่ฉ
-่ช
-่ฌ
-่ฏ
-่ฐ
-่ฑ
-่ณ
-่ด
-่ต
-่ถ
-่ท
-่น
-่บ
-่ป
-่พ
-่ฟ
-่
-่
-่
-่
-่
-่
-่
-่
-่
-่
-่
-่
-่
-่
-่
-่
-่
-่
-่
-่
-่
-่ฃ
-่ค
-่ฆ
-่ฉ
-่ช
-่ซ
-่ญ
-่ฎ
-่ฐ
-่ฑ
-่ฒ
-่ณ
-่ด
-่ธ
-่น
-่พ
-่
-่
-่
-่
-่
-่
-่
-่
-่
-่
-่
-่
-่
-่
-่
-่
-่
-่
-่
-่
-่
-่
-่ก
-่ข
-่ฃ
-่ค
-่ฅ
-่จ
-่ฉ
-่ฎ
-่ฐ
-่ฑ
-่ด
-่ท
-่บ
-่ป
-่ผ
-่พ
-่ฟ
-่
-่
-่
-่
-่
-่
-่
-่
-่
-่
-่
-่
-่
-่
-่
-่
-่
-่
-่ก
-่ฃ
-่ค
-่ฅ
-่ฎ
-่ฏ
-่ฐ
-่ฑ
-่ฒ
-่ด
-่ถ
-่ป
-่ผ
-่ฝ
-่พ
-่
-่
-่
-่
-่
-่
-่
-่
-่
-่
-่
-่
-่
-่
-่
-่ฃ
-่จ
-่ฉ
-่ซ
-่ฌ
-่ญ
-่ฎ
-่ฏ
-่ณ
-่ต
-่น
-่บ
-่ฝ
-่พ
-่ฟ
-่
-่
-่
-่
-่
-่
-่
-่
-่
-่
-่
-่
-่
-่
-่
-่ข
-่ฅ
-่ฆ
-่ง
-่ฉ
-่ช
-่ซ
-่ญ
-่ฎ
-่ณ
-่น
-่บ
-่พ
-่
-่
-่
-่
-่
-่
-่
-่
-่
-่
-่
-่
-่
-่
-่ ก
-่ ข
-่ จ
-่ ช
-่ ญ
-่ ฒ
-่ น
-่ ป
-่ ผ
-่ก
-่ก
-่ก
-่ก
-่ก
-่ก
-่ก
-่ก
-่ก
-่ก
-่ก
-่ก
-่ก
-่ก
-่กก
-่กข
-่กฃ
-่กค
-่กฅ
-่กจ
-่กฉ
-่กซ
-่กฌ
-่กฎ
-่กฐ
-่กฑ
-่กฒ
-่กต
-่กท
-่กน
-่กฝ
-่กพ
-่กฟ
-่ข
-่ข
-่ข
-่ข
-่ข
-่ข
-่ข
-่ข
-่ข
-่ข
-่ข
-่ข
-่ข
-่ข
-่ข
-่ข
-่ข
-่ขข
-่ขค
-่ขจ
-่ขฉ
-่ขช
-่ขซ
-่ขญ
-่ขฏ
-่ขฑ
-่ขด
-่ขท
-่ขธ
-่ขบ
-่ขผ
-่ขฟ
-่ฃ
-่ฃ
-่ฃ
-่ฃ
-่ฃ
-่ฃ
-่ฃ
-่ฃ
-่ฃ
-่ฃ
-่ฃ
-่ฃ
-่ฃ
-่ฃ
-่ฃ
-่ฃ
-่ฃ
-่ฃ
-่ฃ
-่ฃ
-่ฃ
-่ฃข
-่ฃค
-่ฃฅ
-่ฃจ
-่ฃช
-่ฃฏ
-่ฃฐ
-่ฃฑ
-่ฃณ
-่ฃด
-่ฃธ
-่ฃน
-่ฃผ
-่ฃฝ
-่ฃพ
-่ค
-่ค
-่ค
-่ค
-่ค
-่ค
-่ค
-่ค
-่ค
-่ค
-่ค
-่ค
-่คก
-่คฃ
-่คฅ
-่คฆ
-่คง
-่คช
-่คซ
-่คญ
-่คฏ
-่คฐ
-่คฑ
-่คด
-่คต
-่คถ
-่คท
-่คพ
-่ฅ
-่ฅ
-่ฅ
-่ฅ
-่ฅ
-่ฅ
-่ฅ
-่ฅ
-่ฅ
-่ฅ
-่ฅ
-่ฅ
-่ฅฆ
-่ฅช
-่ฅซ
-่ฅญ
-่ฅฎ
-่ฅฐ
-่ฅต
-่ฅถ
-่ฅป
-่ฅผ
-่ฅฟ
-่ฆ
-่ฆ
-่ฆ
-่ฆ
-่ฆ
-่ฆ
-่ฆ
-่ฆ
-่ฆ
-่ฆ
-่ฆ
-่ฆ
-่ฆง
-่ฆช
-่ฆณ
-่ง
-่ง
-่ง
-่ง
-่ง
-่ง
-่ง
-่ง
-่ง
-่ง
-่ง
-่ง
-่ง
-่ง
-่ง
-่ง
-่ง
-่ง
-่ง
-่ง
-่ง
-่ง
-่งฃ
-่งฅ
-่งฆ
-่งฉ
-่งซ
-่งญ
-่งฏ
-่งฑ
-่งณ
-่งบ
-่งผ
-่งฝ
-่งฟ
-่จ
-่จ
-่จ
-่จ
-่จ
-่จ
-่จ
-่จ
-่จ
-่จ
-่จ
-่จฆ
-่จง
-่จช
-่จญ
-่จฑ
-่จณ
-่จด
-่จน
-่จป
-่จผ
-่จพ
-่จฟ
-่ฉ
-่ฉ
-่ฉ
-่ฉ
-่ฉ
-่ฉ
-่ฉข
-่ฉง
-่ฉฐ
-่ฉฑ
-่ฉน
-่ช
-่ช
-่ช
-่ช
-่ช
-่ช
-่ช
-่ชจ
-่ชญ
-่ชฐ
-่ชฒ
-่ชท
-่ชพ
-่ชฟ
-่ซ
-่ซ
-่ซ
-่ซ
-่ซ
-่ซ
-่ซก
-่ซฎ
-่ซฒ
-่ซด
-่ซต
-่ซพ
-่ฌ
-่ฌ
-่ฌ
-่ฌฅ
-่ฌฆ
-่ฌท
-่ญ
-่ญ
-่ญ
-่ญ
-่ญ
-่ญ
-่ญ
-่ญ
-่ญฆ
-่ญฉ
-่ญฌ
-่ญฐ
-่ญฒ
-่ญณ
-่ญถ
-่ญบ
-่ญพ
-่ฎ
-่ฎ
-่ฎ
-่ฎ
-่ฎ
-่ฎ
-่ฎก
-่ฎข
-่ฎฃ
-่ฎค
-่ฎฅ
-่ฎฆ
-่ฎง
-่ฎจ
-่ฎฉ
-่ฎช
-่ฎซ
-่ฎฌ
-่ฎญ
-่ฎฎ
-่ฎฏ
-่ฎฐ
-่ฎฑ
-่ฎฒ
-่ฎณ
-่ฎด
-่ฎต
-่ฎถ
-่ฎท
-่ฎธ
-่ฎน
-่ฎบ
-่ฎป
-่ฎผ
-่ฎฝ
-่ฎพ
-่ฎฟ
-่ฏ
-่ฏ
-่ฏ
-่ฏ
-่ฏ
-่ฏ
-่ฏ
-่ฏ
-่ฏ
-่ฏ
-่ฏ
-่ฏ
-่ฏ
-่ฏ
-่ฏ
-่ฏ
-่ฏ
-่ฏ
-่ฏ
-่ฏ
-่ฏ
-่ฏ
-่ฏ
-่ฏ
-่ฏ
-่ฏ
-่ฏ
-่ฏ
-่ฏ
-่ฏ
-่ฏ
-่ฏ
-่ฏ
-่ฏก
-่ฏข
-่ฏฃ
-่ฏค
-่ฏฅ
-่ฏฆ
-่ฏง
-่ฏจ
-่ฏฉ
-่ฏช
-่ฏซ
-่ฏฌ
-่ฏญ
-่ฏฎ
-่ฏฏ
-่ฏฐ
-่ฏฑ
-่ฏฒ
-่ฏณ
-่ฏด
-่ฏต
-่ฏถ
-่ฏท
-่ฏธ
-่ฏน
-่ฏบ
-่ฏป
-่ฏผ
-่ฏฝ
-่ฏพ
-่ฏฟ
-่ฐ
-่ฐ
-่ฐ
-่ฐ
-่ฐ
-่ฐ
-่ฐ
-่ฐ
-่ฐ
-่ฐ
-่ฐ
-่ฐ
-่ฐ
-่ฐ
-่ฐ
-่ฐ
-่ฐ
-่ฐ
-่ฐ
-่ฐ
-่ฐ
-่ฐ
-่ฐ
-่ฐ
-่ฐ
-่ฐ
-่ฐ
-่ฐ
-่ฐ
-่ฐ
-่ฐ
-่ฐ
-่ฐก
-่ฐข
-่ฐฃ
-่ฐค
-่ฐฅ
-่ฐฆ
-่ฐง
-่ฐจ
-่ฐฉ
-่ฐช
-่ฐซ
-่ฐฌ
-่ฐญ
-่ฐฎ
-่ฐฏ
-่ฐฐ
-่ฐฑ
-่ฐฒ
-่ฐณ
-่ฐด
-่ฐต
-่ฐถ
-่ฐท
-่ฐน
-่ฐบ
-่ฐผ
-่ฐฝ
-่ฐพ
-่ฐฟ
-่ฑ
-่ฑ
-่ฑ
-่ฑ
-่ฑ
-่ฑ
-่ฑ
-่ฑ
-่ฑ
-่ฑ
-่ฑ
-่ฑ
-่ฑ
-่ฑ
-่ฑ
-่ฑก
-่ฑข
-่ฑจ
-่ฑฉ
-่ฑช
-่ฑซ
-่ฑญ
-่ฑฏ
-่ฑฐ
-่ฑณ
-่ฑต
-่ฑธ
-่ฑน
-่ฑบ
-่ฑฟ
-่ฒ
-่ฒ
-่ฒ
-่ฒ
-่ฒ
-่ฒ
-่ฒ
-่ฒ
-่ฒ
-่ฒ
-่ฒ
-่ฒ
-่ฒ
-่ฒ
-่ฒ
-่ฒ
-่ฒ
-่ฒ
-่ฒก
-่ฒค
-่ฒจ
-่ฒฌ
-่ฒฎ
-่ฒณ
-่ฒด
-่ฒท
-่ฒธ
-่ฒป
-่ฒผ
-่ฒฟ
-่ณ
-่ณ
-่ณ
-่ณ
-่ณ
-่ณ
-่ณฃ
-่ณฆ
-่ณจ
-่ณธ
-่ณผ
-่ด
-่ด
-่ด
-่ด
-่ด
-่ด
-่ด
-่ด
-่ดก
-่ดข
-่ดฃ
-่ดค
-่ดฅ
-่ดฆ
-่ดง
-่ดจ
-่ดฉ
-่ดช
-่ดซ
-่ดฌ
-่ดญ
-่ดฎ
-่ดฏ
-่ดฐ
-่ดฑ
-่ดฒ
-่ดณ
-่ดด
-่ดต
-่ดถ
-่ดท
-่ดธ
-่ดน
-่ดบ
-่ดป
-่ดผ
-่ดฝ
-่ดพ
-่ดฟ
-่ต
-่ต
-่ต
-่ต
-่ต
-่ต
-่ต
-่ต
-่ต
-่ต
-่ต
-่ต
-่ต
-่ต
-่ต
-่ต
-่ต
-่ต
-่ต
-่ต
-่ต
-่ต
-่ต
-่ต
-่ต
-่ต
-่ต
-่ต
-่ต
-่ต
-่ต
-่ต
-่ตก
-่ตข
-่ตฃ
-่ตค
-่ตฅ
-่ตฆ
-่ตง
-่ตฉ
-่ตช
-่ตซ
-่ตญ
-่ตฐ
-่ตณ
-่ตด
-่ตต
-่ตถ
-่ตท
-่ตบ
-่ถ
-่ถ
-่ถ
-่ถ
-่ถ
-่ถ
-่ถ
-่ถ
-่ถ
-่ถ
-่ถ
-่ถ
-่ถ
-่ถข
-่ถฃ
-่ถจ
-่ถซ
-่ถฎ
-่ถฏ
-่ถฑ
-่ถณ
-่ถด
-่ถต
-่ถท
-่ถธ
-่ถน
-่ถบ
-่ถผ
-่ถพ
-่ถฟ
-่ท
-่ท
-่ท
-่ท
-่ท
-่ท
-่ท
-่ท
-่ท
-่ท
-่ท
-่ท
-่ท
-่ท
-่ท
-่ท
-่ท
-่ท
-่ท
-่ท
-่ท
-่ท
-่ทก
-่ทฃ
-่ทค
-่ทง
-่ทจ
-่ทฉ
-่ทช
-่ทซ
-่ทฌ
-่ทฏ
-่ทฑ
-่ทณ
-่ทต
-่ทถ
-่ทท
-่ทธ
-่ทน
-่ทบ
-่ทป
-่ทผ
-่ทฝ
-่ทฟ
-่ธ
-่ธ
-่ธ
-่ธ
-่ธ
-่ธ
-่ธ
-่ธ
-่ธ
-่ธ
-่ธ
-่ธ
-่ธ
-่ธ
-่ธ
-่ธ
-่ธ
-่ธก
-่ธข
-่ธฃ
-่ธฆ
-่ธง
-่ธฉ
-่ธช
-่ธฌ
-่ธญ
-่ธฎ
-่ธฏ
-่ธฐ
-่ธฑ
-่ธต
-่ธถ
-่ธธ
-่ธน
-่ธบ
-่ธผ
-่ธฝ
-่น
-่น
-่น
-่น
-่น
-่น
-่น
-่น
-่น
-่น
-่น
-่น
-่น
-่น
-่น
-่น
-่น
-่น
-่น
-่น
-่น
-่น
-่นก
-่นข
-่นฆ
-่นฉ
-่นฌ
-่นญ
-่นฎ
-่นฏ
-่นฐ
-่นฑ
-่นฒ
-่นณ
-่นด
-่นถ
-่นธ
-่นป
-่นผ
-่นฟ
-่บ
-่บ
-่บ
-่บ
-่บ
-่บ
-่บ
-่บ
-่บ
-่บ
-่บ
-่บ
-่บง
-่บจ
-่บฉ
-่บซ
-่บฌ
-่บญ
-่บฏ
-่บฒ
-่บด
-่บบ
-่บฟ
-่ป
-่ป
-่ป
-่ป
-่ป
-่ปข
-่ปฅ
-่ปง
-่ปจ
-่ปฑ
-่ปต
-่ปถ
-่ปท
-่ปฝ
-่ปฟ
-่ผ
-่ผ
-่ผ
-่ผ
-่ผ
-่ผฃ
-่ผค
-่ผง
-่ผฎ
-่ผด
-่ผต
-่ผถ
-่ผท
-่ผธ
-่ผผ
-่ฝ
-่ฝ
-่ฝ
-่ฝ
-่ฝ
-่ฝ
-่ฝ
-่ฝฃ
-่ฝฅ
-่ฝฆ
-่ฝง
-่ฝจ
-่ฝฉ
-่ฝช
-่ฝซ
-่ฝฌ
-่ฝญ
-่ฝฎ
-่ฝฏ
-่ฝฐ
-่ฝฑ
-่ฝฒ
-่ฝณ
-่ฝด
-่ฝต
-่ฝถ
-่ฝธ
-่ฝน
-่ฝบ
-่ฝป
-่ฝผ
-่ฝฝ
-่ฝพ
-่ฝฟ
-่พ
-่พ
-่พ
-่พ
-่พ
-่พ
-่พ
-่พ
-่พ
-่พ
-่พ
-่พ
-่พ
-่พ
-่พ
-่พ
-่พ
-่พ
-่พ
-่พ
-่พ
-่พ
-่พ
-่พ
-่พ
-่พ
-่พ
-่พ
-่พ
-่พฃ
-่พฆ
-่พจ
-่พฉ
-่พซ
-่พฐ
-่พฑ
-่พด
-่พถ
-่พท
-่พน
-่พบ
-่พป
-่พผ
-่พฝ
-่พพ
-่พฟ
-่ฟ
-่ฟ
-่ฟ
-่ฟ
-่ฟ
-่ฟ
-่ฟ
-่ฟ
-่ฟ
-่ฟ
-่ฟ
-่ฟ
-่ฟ
-่ฟ
-่ฟ
-่ฟ
-่ฟ
-่ฟ
-่ฟ
-่ฟ
-่ฟ
-่ฟ
-่ฟข
-่ฟค
-่ฟฅ
-่ฟฆ
-่ฟจ
-่ฟฉ
-่ฟช
-่ฟซ
-่ฟญ
-่ฟฎ
-่ฟฐ
-่ฟณ
-่ฟด
-่ฟต
-่ฟท
-่ฟธ
-่ฟน
-่ฟบ
-่ฟฝ
-่ฟพ
-้
-้
-้
-้
-้
-้
-้
-้
-้
-้
-้
-้
-้
-้
-้
-้
-้
-้
-้
-้
-้
-้
-้
-้
-้
-้
-้ก
-้ข
-้ฃ
-้ค
-้ฅ
-้ฆ
-้ญ
-้ฎ
-้ฏ
-้ฒ
-้ด
-้ต
-้ถ
-้ท
-้ธ
-้ป
-้ผ
-้พ
-้ฟ
-้
-้
-้
-้
-้
-้
-้
-้
-้
-้
-้
-้
-้
-้
-้
-้
-้
-้
-้
-้ก
-้ข
-้ฃ
-้ฅ
-้จ
-้ฉ
-้ซ
-้ญ
-้ฎ
-้ฏ
-้ฑ
-้ด
-้ต
-้ถ
-้น
-้บ
-้ฝ
-้ฟ
-้
-้
-้
-้
-้
-้
-้
-้
-้
-้
-้
-้
-้
-้
-้
-้
-้
-้
-้
-้ก
-้ข
-้ฃ
-้ค
-้ฅ
-้ฆ
-้จ
-้ช
-้ฌ
-้ฎ
-้ฏ
-้ฐ
-้ฑ
-้ฒ
-้ณ
-้ด
-้ต
-้ถ
-้ท
-้ธ
-้น
-้บ
-้ป
-้ฝ
-้พ
-้
-้
-้
-้
-้
-้
-้
-้
-้
-้
-้
-้
-้
-้
-้
-้
-้
-้
-้
-้
-้ก
-้ข
-้ค
-้ฆ
-้ง
-้จ
-้ฉ
-้ช
-้ซ
-้ญ
-้ฏ
-้ด
-้ท
-้ธ
-้ฝ
-้พ
-้ฟ
-้
-้
-้
-้
-้
-้
-้
-้
-้
-้
-้
-้
-้
-้
-้
-้ก
-้ข
-้ฃ
-้ค
-้ฆ
-้จ
-้ฉ
-้ช
-้ฌ
-้ฎ
-้ฏ
-้ฐ
-้ฑ
-้ณ
-้น
-้ผ
-้ฝ
-้
-้
-้
-้
-้
-้
-้
-้
-้
-้
-้
-้
-้
-้
-้
-้
-้
-้
-้
-้
-้
-้
-้
-้
-้
ก
-้
ข
-้
ฃ
-้
ค
-้
ฅ
-้
ฆ
-้
ง
-้
ฉ
-้
ช
-้
ฌ
-้
ญ
-้
ฎ
-้
ฏ
-้
ฐ
-้
ฑ
-้
ฒ
-้
ด
-้
ต
-้
ถ
-้
ท
-้
ธ
-้
น
-้
บ
-้
ฝ
-้
พ
-้
ฟ
-้
-้
-้
-้
-้
-้
-้
-้
-้
-้
-้
-้
-้
-้
-้
-้
-้
-้
-้
-้ก
-้ข
-้ค
-้ฅ
-้ฆ
-้จ
-้ช
-้ซ
-้ญ
-้ฎ
-้ฏ
-้ฒ
-้ณ
-้ด
-้ต
-้ธ
-้น
-้บ
-้ป
-้ฝ
-้พ
-้ฟ
-้
-้
-้
-้
-้
-้
-้
-้
-้
-้
-้
-้
-้
-้
-้ญ
-้ฑ
-้ด
-้
-้
-้
-้
-้
-้
-้
-้ก
-้ฃ
-้ด
-้ต
-้
-้
-้
-้
-้
-้
-้
-้
-้ข
-้ค
-้ฅ
-้ง
-้จ
-้ฑ
-้ฒ
-้ด
-้ผ
-้ฝ
-้
-้
-้
-้
-้
-้ญ
-้ฎ
-้ถ
-้บ
-้
-้
-้
-้
-้
-้
-้
-้
-้ฃ
-้ช
-้น
-้
-้
-้
-้ข
-้ค
-้ง
-้ซ
-้ฌ
-้ฏ
-้ฑ
-้บ
-้ป
-้ฝ
-้พ
-้
-้
-้
-้ง
-้ช
-้ญ
-้ฎ
-้ฑ
-้ผ
-้
-้
-้
-้
-้
-้
-้
-้
-้
-้
-้ฌ
-้ถ
-้ท
-้น
-้
-้
-้
-้ฃ
-้ฆ
-้ธ
-้
-้
-้
-้
-้
-้ป
-้
-้
-้
-้จ
-้ซ
-้ฏ
-้ด
-้
-้
-้
-้
-้
-้
-้
-้
-้
-้
-้
-้
-้
-้
-้
-้
-้
-้
-้
-้
-้
-้
-้
-้
-้
-้
-้
-้ก
-้ข
-้ฃ
-้ค
-้ฅ
-้ฆ
-้ง
-้จ
-้ฉ
-้ช
-้ซ
-้ฌ
-้ญ
-้ฎ
-้ฏ
-้ฐ
-้ฑ
-้ฒ
-้ณ
-้ด
-้ต
-้ธ
-้น
-้บ
-้ป
-้ผ
-้ฝ
-้พ
-้ฟ
-้
-้
-้
-้
-้
-้
-้
-้
-้
-้
-้
-้
-้
-้
-้
-้
-้
-้
-้
-้
-้
-้
-้
-้
-้
-้
-้
-้
-้
-้
-้
-้
-้ก
-้ข
-้ฃ
-้ค
-้ฅ
-้ฆ
-้ง
-้จ
-้ฉ
-้ช
-้ซ
-้ฌ
-้ญ
-้ฎ
-้ฏ
-้ฐ
-้ฑ
-้ฒ
-้ณ
-้ด
-้ต
-้ถ
-้ท
-้ธ
-้บ
-้ป
-้ผ
-้ฝ
-้พ
-้ฟ
-้
-้
-้
-้
-้
-้
-้
-้
-้
-้
-้
-้
-้
-้
-้
-้
-้
-้
-้
-้
-้
-้
-้
-้
-้
-้
-้
-้
-้
-้
-้
-้ก
-้ข
-้ฃ
-้ค
-้ฅ
-้ฆ
-้ง
-้จ
-้ช
-้ญ
-้ฎ
-้ฏ
-้ฐ
-้ฑ
-้ฒ
-้ด
-้ต
-้ถ
-้ท
-้ธ
-้น
-้บ
-้ป
-้ผ
-้ฝ
-้พ
-้
-้
-้
-้
-้
-้
-้
-้
-้
-้
-้
-้
-้
-้
-้
-้
-้
-้
-้
-้
-้
-้
-้
-้
-้
-้
-้
-้
-้
-้ก
-้ข
-้ฃ
-้ค
-้ฅ
-้ฆ
-้ง
-้จ
-้ฉ
-้ช
-้ซ
-้ฌ
-้ญ
-้ฎ
-้ฏ
-้ฐ
-้ฑ
-้ฒ
-้ณ
-้ด
-้ต
-้ถ
-้ท
-้ฟ
-้
-้
-้
-้
-้
-้
-้
-้
-้
-้
-้
-้ข
-้ค
-้ฆ
-้ง
-้ช
-้ด
-้
-้
-้
-้
-้
-้
-้
-้
-้
-้ข
-้จ
-้ฉ
-้ช
-้ซ
-้ฌ
-้ญ
-้ฎ
-้ฏ
-้ฐ
-้ฑ
-้ฒ
-้ณ
-้ด
-้ต
-้ท
-้ธ
-้น
-้บ
-้ป
-้ผ
-้ฝ
-้พ
-้ฟ
-้
-้
-้
-้
-้
-้
-้
-้
-้
-้
-้
-้
-้
-้
-้
-้
-้
-้
-้
-้
-้
-้
-้
-้
-้
-้
-้
-้
-้
-้
-้
-้
-้ก
-้จ
-้ช
-้ฌ
-้ฎ
-้ฏ
-้ฑ
-้ฒ
-้ณ
-้ด
-้ต
-้ถ
-้ป
-้ผ
-้ฝ
-้ฟ
-้
-้
-้
-้
-้
-้
-้
-้
-้
-้
-้
-้
-้
-้
-้
-้
-้
-้
-้
-้ก
-้ข
-้ค
-้ฅ
-้ง
-้จ
-้ฉ
-้ช
-้ฌ
-้ญ
-้ฒ
-้ด
-้ต
-้ถ
-้ท
-้บ
-้ป
-้ผ
-้ฝ
-้พ
-้
-้
-้
-้
-้
-้
-้
-้
-้
-้
-้
-้
-้
-้
-้
-้
-้
-้
-้
-้
-้ฃ
-้ค
-้ฅ
-้ฆ
-้ง
-้จ
-้ฉ
-้ช
-้ฎ
-้ฐ
-้ณ
-้ถ
-้ท
-้น
-้ป
-้ผ
-้ฝ
-้พ
-้
-้
-้
-้
-้
-้
-้
-้
-้
-้
-้
-้
-้
-้
-้
-้
-้
-้
-้
-้
-้ฃ
-้จ
-้ฉ
-้ช
-้ซ
-้ฏ
-้ฐ
-้ฑ
-้ฒ
-้ณ
-้ถ
-้ท
-้น
-้ป
-้พ
-้
-้
-้
-้
-้
-้
-้
-้
-้
-้
-้
-้
-้
-้
-้
-้
-้
-้
-้
-้
-้
-้
-้ก
-้ข
-้ฃ
-้ค
-้จ
-้ช
-้ญ
-้ฎ
-้ฐ
-้ฒ
-้ณ
-้ธ
-้น
-้พ
-้ฟ
-้
-้
-้
-้
-้
-้
-้
-้
-้
-้
-้ก
-้ข
-้ฅ
-้ฆ
-้ง
-้ฉ
-้ซ
-้ฎ
-้ฐ
-้ณ
-้ด
-้ถ
-้ท
-้ธ
-้บ
-้ผ
-้ฟ
-้
-้
-้
-้
-้
-้
-้
-้
-้
-้
-้
-้
-้
-้
-้
-้
-้
-้
-้
-้ฃ
-้จ
-้ซ
-้ฌ
-้ญ
-้ฎ
-้ฏ
-้ฒ
-้ณ
-้ด
-้ต
-้ถ
-้ธ
-้น
-้บ
-้พ
-้ฟ
-้
-้
-้
-้
-้
-้
-้
-้
-้
-้
-้ก
-้ฆ
-้ง
-้จ
-้ฉ
-้ช
-้ซ
-้ฌ
-้ญ
-้ฎ
-้ณ
-้ต
-้ถ
-้น
-้ป
-้
-้
-้
-้
-้
-้
-้
-้
-้
-้
-้
-้ ฃ
-้ ฉ
-้ ซ
-้ ฌ
-้ ญ
-้ ฏ
-้ ณ
-้ ต
-้ ผ
-้ก
-้ก
-้ก
-้ก
-้ก
-้ก
-้ก
-้ก
-้ก
-้กก
-้กฆ
-้กต
-้กถ
-้กท
-้กธ
-้กน
-้กบ
-้กป
-้กผ
-้กฝ
-้กพ
-้กฟ
-้ข
-้ข
-้ข
-้ข
-้ข
-้ข
-้ข
-้ข
-้ข
-้ข
-้ข
-้ข
-้ข
-้ข
-้ข
-้ข
-้ข
-้ข
-้ข
-้ข
-้ข
-้ข
-้ข
-้ข
-้ข
-้ข
-้ข
-้ข
-้ข
-้ข
-้ข
-้ข
-้ข
-้ขก
-้ขข
-้ขฃ
-้ขค
-้ขฅ
-้ขฆ
-้ขง
-้ขจ
-้ขพ
-้ขฟ
-้ฃ
-้ฃ
-้ฃ
-้ฃ
-้ฃ
-้ฃ
-้ฃ
-้ฃ
-้ฃ
-้ฃ
-้ฃ
-้ฃ
-้ฃ
-้ฃ
-้ฃ
-้ฃ
-้ฃ
-้ฃ
-้ฃ
-้ฃ
-้ฃก
-้ฃฃ
-้ฃง
-้ฃจ
-้ฃฏ
-้ฃฐ
-้ฃฑ
-้ฃถ
-้ฃฝ
-้ค
-้ค
-้ค
-้ค
-้ค
-้ค
-้ค
-้ค
-้คค
-้คง
-้คจ
-้คฌ
-้คฎ
-้คฒ
-้คด
-้คธ
-้คฝ
-้ฅ
-้ฅ
-้ฅ
-้ฅ
-้ฅ
-้ฅ
-้ฅ
-้ฅ
-้ฅ
-้ฅ
-้ฅ
-้ฅ
-้ฅ
-้ฅฃ
-้ฅค
-้ฅฅ
-้ฅฆ
-้ฅง
-้ฅจ
-้ฅฉ
-้ฅช
-้ฅซ
-้ฅฌ
-้ฅญ
-้ฅฎ
-้ฅฏ
-้ฅฐ
-้ฅฑ
-้ฅฒ
-้ฅด
-้ฅต
-้ฅถ
-้ฅท
-้ฅธ
-้ฅน
-้ฅบ
-้ฅผ
-้ฅฝ
-้ฅพ
-้ฅฟ
-้ฆ
-้ฆ
-้ฆ
-้ฆ
-้ฆ
-้ฆ
-้ฆ
-้ฆ
-้ฆ
-้ฆ
-้ฆ
-้ฆ
-้ฆ
-้ฆ
-้ฆ
-้ฆ
-้ฆ
-้ฆ
-้ฆ
-้ฆ
-้ฆ
-้ฆ
-้ฆ
-้ฆ
-้ฆ
-้ฆ
-้ฆ
-้ฆ
-้ฆก
-้ฆค
-้ฆฅ
-้ฆง
-้ฆจ
-้ฆฌ
-้ฆฏ
-้ฆน
-้ฆบ
-้ฆฝ
-้ง
-้ง
-้ง
-้ง
-้ง
-้ง
-้ง
-้ง
-้ง
-้ง
-้งช
-้งฌ
-้งฎ
-้งฐ
-้งฑ
-้งท
-้งธ
-้งน
-้งป
-้งผ
-้งฝ
-้งพ
-้งฟ
-้จ
-้จ
-้จ
-้จ
-้จ
-้จ
-้จ
-้จ
-้จ
-้จ
-้จ
-้จ
-้จ
-้จ
-้จก
-้จข
-้จฃ
-้จง
-้จช
-้จฐ
-้จฑ
-้จณ
-้จต
-้จน
-้จบ
-้ฉ
-้ฉ
-้ฉ
-้ฉ
-้ฉ
-้ฉ
-้ฉ
-้ฉฉ
-้ฉฌ
-้ฉญ
-้ฉฎ
-้ฉฏ
-้ฉฐ
-้ฉฑ
-้ฉฒ
-้ฉณ
-้ฉด
-้ฉต
-้ฉถ
-้ฉท
-้ฉธ
-้ฉน
-้ฉบ
-้ฉป
-้ฉผ
-้ฉฝ
-้ฉพ
-้ฉฟ
-้ช
-้ช
-้ช
-้ช
-้ช
-้ช
-้ช
-้ช
-้ช
-้ช
-้ช
-้ช
-้ช
-้ช
-้ช
-้ช
-้ช
-้ช
-้ช
-้ช
-้ช
-้ช
-้ช
-้ช
-้ช
-้ช
-้ช
-้ช
-้ช
-้ช
-้ช
-้ช
-้ชก
-้ชข
-้ชฃ
-้ชค
-้ชฅ
-้ชฆ
-้ชง
-้ชจ
-้ชซ
-้ชญ
-้ชฐ
-้ชฑ
-้ชถ
-้ชท
-้ชธ
-้ชน
-้ชบ
-้ชผ
-้ซ
-้ซ
-้ซ
-้ซ
-้ซ
-้ซ
-้ซ
-้ซ
-้ซ
-้ซ
-้ซ
-้ซ
-้ซ
-้ซ
-้ซ
-้ซ
-้ซ
-้ซ
-้ซก
-้ซข
-้ซค
-้ซฆ
-้ซง
-้ซช
-้ซซ
-้ซญ
-้ซฎ
-้ซฏ
-้ซฒ
-้ซณ
-้ซต
-้ซน
-้ซป
-้ซฝ
-้ซพ
-้ฌ
-้ฌ
-้ฌ
-้ฌ
-้ฌ
-้ฌ
-้ฌ
-้ฌ
-้ฌ
-้ฌ
-้ฌ
-้ฌ
-้ฌ
-้ฌ
-้ฌก
-้ฌฃ
-้ฌฌ
-้ฌฏ
-้ฌฑ
-้ฌฒ
-้ฌต
-้ฌท
-้ฌบ
-้ฌป
-้ฌผ
-้ฌฝ
-้ญ
-้ญ
-้ญ
-้ญ
-้ญ
-้ญ
-้ญ
-้ญ
-้ญ
-้ญ
-้ญ
-้ญ
-้ญ
-้ญ
-้ญ
-้ญ
-้ญ
-้ญ
-้ญ
-้ญฃ
-้ญญ
-้ญฎ
-้ญฏ
-้ญถ
-้ญผ
-้ญพ
-้ญฟ
-้ฎ
-้ฎ
-้ฎ
-้ฎ
-้ฎ
-้ฎ
-้ฎ
-้ฎ
-้ฎ
-้ฎ
-้ฎ
-้ฎก
-้ฎฃ
-้ฎง
-้ฎจ
-้ฎฎ
-้ฎฐ
-้ฎธ
-้ฎน
-้ฎป
-้ฎผ
-้ฏ
-้ฏ
-้ฏ
-้ฏ
-้ฏฅ
-้ฏฎ
-้ฏต
-้ฏถ
-้ฏบ
-้ฏป
-้ฏพ
-้ฐ
-้ฐ
-้ฐ
-้ฐ
-้ฐ
-้ฐ
-้ฐ
-้ฐค
-้ฐง
-้ฐฌ
-้ฐถ
-้ฐฝ
-้ฑ
-้ฑ
-้ฑ
-้ฑ
-้ฑ
-้ฑ
-้ฑ
-้ฑ
-้ฑ
-้ฑ
-้ฑฅ
-้ฑฎ
-้ฑฒ
-้ฑณ
-้ฑต
-้ฑป
-้ฑผ
-้ฑพ
-้ฑฟ
-้ฒ
-้ฒ
-้ฒ
-้ฒ
-้ฒ
-้ฒ
-้ฒ
-้ฒ
-้ฒ
-้ฒ
-้ฒ
-้ฒ
-้ฒ
-้ฒ
-้ฒ
-้ฒ
-้ฒ
-้ฒ
-้ฒ
-้ฒ
-้ฒ
-้ฒ
-้ฒ
-้ฒ
-้ฒ
-้ฒ
-้ฒ
-้ฒ
-้ฒ
-้ฒ
-้ฒก
-้ฒข
-้ฒฃ
-้ฒค
-้ฒฅ
-้ฒฆ
-้ฒง
-้ฒจ
-้ฒฉ
-้ฒช
-้ฒซ
-้ฒฌ
-้ฒญ
-้ฒฎ
-้ฒฏ
-้ฒฐ
-้ฒฑ
-้ฒฒ
-้ฒณ
-้ฒด
-้ฒต
-้ฒถ
-้ฒท
-้ฒธ
-้ฒน
-้ฒบ
-้ฒป
-้ฒผ
-้ฒฝ
-้ฒพ
-้ฒฟ
-้ณ
-้ณ
-้ณ
-้ณ
-้ณ
-้ณ
-้ณ
-้ณ
-้ณ
-้ณ
-้ณ
-้ณ
-้ณ
-้ณ
-้ณ
-้ณ
-้ณ
-้ณ
-้ณ
-้ณ
-้ณ
-้ณ
-้ณ
-้ณ
-้ณ
-้ณ
-้ณ
-้ณ
-้ณ
-้ณ
-้ณ
-้ณก
-้ณข
-้ณฃ
-้ณค
-้ณฅ
-้ณฆ
-้ณง
-้ณฉ
-้ณฒ
-้ณณ
-้ณด
-้ณถ
-้ณท
-้ณธ
-้ณบ
-้ณผ
-้ณฝ
-้ด
-้ด
-้ด
-้ด
-้ด
-้ด
-้ด
-้ด
-้ดฅ
-้ดณ
-้ดน
-้ดฝ
-้ต
-้ต
-้ต
-้ต
-้ต
-้ต
-้ต
-้ตฉ
-้ตฐ
-้ตฑ
-้ตป
-้ถ
-้ถ
-้ถ
-้ถ
-้ถ
-้ถ
-้ถ
-้ถ
-้ถก
-้ถข
-้ถค
-้ถฌ
-้ถฑ
-้ถต
-้ถน
-้ถบ
-้ท
-้ท
-้ท
-้ท
-้ท
-้ท
-้ท
-้ท
-้ท
-้ทข
-้ทฃ
-้ทค
-้ทฉ
-้ทซ
-้ทฎ
-้ทพ
-้ทฟ
-้ธ
-้ธ
-้ธ
-้ธ
-้ธ
-้ธ
-้ธ
-้ธ
-้ธ
-้ธ
-้ธ
-้ธ
-้ธ
-้ธก
-้ธข
-้ธฃ
-้ธค
-้ธฅ
-้ธฆ
-้ธง
-้ธจ
-้ธฉ
-้ธช
-้ธซ
-้ธฌ
-้ธญ
-้ธฎ
-้ธฏ
-้ธฐ
-้ธฑ
-้ธฒ
-้ธณ
-้ธด
-้ธต
-้ธถ
-้ธท
-้ธธ
-้ธน
-้ธบ
-้ธป
-้ธฝ
-้ธพ
-้ธฟ
-้น
-้น
-้น
-้น
-้น
-้น
-้น
-้น
-้น
-้น
-้น
-้น
-้น
-้น
-้น
-้น
-้น
-้น
-้น
-้น
-้น
-้น
-้น
-้น
-้น
-้น
-้น
-้น
-้น
-้น
-้น
-้น
-้น
-้นก
-้นข
-้นฃ
-้นค
-้นฅ
-้นฆ
-้นง
-้นจ
-้นฉ
-้นช
-้นซ
-้นฌ
-้นญ
-้นฎ
-้นฏ
-้นฐ
-้นฑ
-้นฒ
-้นณ
-้นด
-้นพ
-้นฟ
-้บ
-้บ
-้บ
-้บ
-้บ
-้บ
-้บ
-้บ
-้บ
-้บ
-้บ
-้บ
-้บ
-้บ
-้บ
-้บ
-้บ
-้บ
-้บ
-้บ
-้บค
-้บฆ
-้บง
-้บจ
-้บฏ
-้บฐ
-้บด
-้บธ
-้บน
-้บบ
-้บป
-้บผ
-้บฝ
-้บพ
-้บฟ
-้ป
-้ป
-้ป
-้ป
-้ป
-้ป
-้ป
-้ป
-้ป
-้ป
-้ป
-้ป
-้ป
-้ป
-้ป
-้ป
-้ป
-้ป
-้ป
-้ป
-้ป
-้ป
-้ป
-้ปก
-้ปข
-้ปค
-้ปฅ
-้ปฆ
-้ปง
-้ปจ
-้ปฉ
-้ปช
-้ปซ
-้ปฌ
-้ปฎ
-้ปฏ
-้ปณ
-้ปธ
-้ปป
-้ปผ
-้ปพ
-้ผ
-้ผ
-้ผ
-้ผ
-้ผ
-้ผ
-้ผ
-้ผ
-้ผ
-้ผ
-้ผ
-้ผ
-้ผข
-้ผฉ
-้ผช
-้ผซ
-้ผฌ
-้ผฎ
-้ผฏ
-้ผท
-้ผน
-้ผบ
-้ผป
-้ผฝ
-้ผพ
-้ผฟ
-้ฝ
-้ฝ
-้ฝ
-้ฝ
-้ฝ
-้ฝ
-้ฝ
-้ฝ
-้ฝ
-้ฝข
-้ฝง
-้ฝจ
-้ฝฎ
-้ฝฐ
-้ฝพ
-้ฝฟ
-้พ
-้พ
-้พ
-้พ
-้พ
-้พ
-้พ
-้พ
-้พ
-้พ
-้พ
-้พ
-้พ
-้พ
-้พ
-้พ
-้พ
-้พ
-้พ
-้พ
-้พ
-้พ
-๊ฑฐ
-๋
-๋ฌ
-๋ค
-๋
-๋
-๋ฆฌ
-๋งฅ
-๋ฒ
-์
-์
-ํ
-๎
-๎ข
-๏ฅน
-๏ธฐ
-๏น
-๏น
-๏น
-๏ผ
-๏ผ
-๏ผ
-๏ผ
-๏ผ
-๏ผ
-๏ผ
-๏ผ
-๏ผ
-๏ผ
-๏ผ
-๏ผ
-๏ผ
-๏ผ
-๏ผ
-๏ผฟ
-๏ฝ
-๏ฝ
-๏ฝ
-๏ฟฅ
-๐ก
-๐จฑ
\ No newline at end of file
diff --git a/pipeline/mmocr/dicts/english_digits_symbols.txt b/pipeline/mmocr/dicts/english_digits_symbols.txt
deleted file mode 100644
index a945ae9c526e4faa68852eb3fb47d078a2f3f6ce..0000000000000000000000000000000000000000
--- a/pipeline/mmocr/dicts/english_digits_symbols.txt
+++ /dev/null
@@ -1,90 +0,0 @@
-0
-1
-2
-3
-4
-5
-6
-7
-8
-9
-a
-b
-c
-d
-e
-f
-g
-h
-i
-j
-k
-l
-m
-n
-o
-p
-q
-r
-s
-t
-u
-v
-w
-x
-y
-z
-A
-B
-C
-D
-E
-F
-G
-H
-I
-J
-K
-L
-M
-N
-O
-P
-Q
-R
-S
-T
-U
-V
-W
-X
-Y
-Z
-!
-"
-#
-$
-%
-&
-'
-(
-)
-*
-+
-,
--
-.
-/
-:
-;
-<
-=
->
-?
-@
-[
-\
-]
-_
-`
-~
\ No newline at end of file
diff --git a/pipeline/mmocr/dicts/english_digits_symbols_space.txt b/pipeline/mmocr/dicts/english_digits_symbols_space.txt
deleted file mode 100644
index 5bd66a185eeeb64e39b78f33ba96c8120ca28112..0000000000000000000000000000000000000000
--- a/pipeline/mmocr/dicts/english_digits_symbols_space.txt
+++ /dev/null
@@ -1,91 +0,0 @@
-0
-1
-2
-3
-4
-5
-6
-7
-8
-9
-a
-b
-c
-d
-e
-f
-g
-h
-i
-j
-k
-l
-m
-n
-o
-p
-q
-r
-s
-t
-u
-v
-w
-x
-y
-z
-A
-B
-C
-D
-E
-F
-G
-H
-I
-J
-K
-L
-M
-N
-O
-P
-Q
-R
-S
-T
-U
-V
-W
-X
-Y
-Z
-!
-"
-#
-$
-%
-&
-'
-(
-)
-*
-+
-,
--
-.
-/
-:
-;
-<
-=
->
-?
-@
-[
-\
-]
-_
-`
-~
-
\ No newline at end of file
diff --git a/pipeline/mmocr/dicts/korean_english_digits_symbols.txt b/pipeline/mmocr/dicts/korean_english_digits_symbols.txt
deleted file mode 100644
index 764080d5486ebd77cb7b5db26ac82022eb84cc4e..0000000000000000000000000000000000000000
--- a/pipeline/mmocr/dicts/korean_english_digits_symbols.txt
+++ /dev/null
@@ -1,1803 +0,0 @@
-0
-1
-2
-3
-4
-5
-6
-7
-8
-9
-!
-"
-#
-$
-%
-&
-\
-'
-(
-)
-*
-+
-,
--
-.
-/
-:
-;
-<
-=
->
-?
-@
-[
-]
-^
-_
-`
-{
-|
-}
-~
-
-a
-b
-c
-d
-e
-f
-g
-h
-i
-j
-k
-l
-m
-n
-o
-p
-q
-r
-s
-t
-u
-v
-w
-x
-y
-z
-A
-B
-C
-D
-E
-F
-G
-H
-I
-J
-K
-L
-M
-N
-O
-P
-Q
-R
-S
-T
-U
-V
-W
-X
-Y
-Z
-ใ
-๊ฐ
-๊ฐ
-๊ฐ
-๊ฐ
-๊ฐ
-๊ฐ
-๊ฐ
-๊ฐ
-๊ฐ
-๊ฐ
-๊ฐ
-๊ฐ
-๊ฐ
-๊ฐ
-๊ฐ
-๊ฐ
-๊ฐ
-๊ฐ
-๊ฐค
-๊ฐฌ
-๊ฐญ
-๊ฐฏ
-๊ฐฑ
-๊ฐธ
-๊ฑ
-๊ฑฐ
-๊ฑฑ
-๊ฑด
-๊ฑท
-๊ฑธ
-๊ฒ
-๊ฒ
-๊ฒ
-๊ฒ
-๊ฒ
-๊ฒ
-๊ฒ
-๊ฒ
-๊ฒ
-๊ฒ
-๊ฒจ
-๊ฒฉ
-๊ฒช
-๊ฒฌ
-๊ฒฐ
-๊ฒธ
-๊ฒน
-๊ฒผ
-๊ฒฝ
-๊ณ
-๊ณ
-๊ณ
-๊ณก
-๊ณค
-๊ณง
-๊ณจ
-๊ณฐ
-๊ณฑ
-๊ณณ
-๊ณต
-๊ณถ
-๊ณผ
-๊ณฝ
-๊ด
-๊ด
-๊ด
-๊ด
-๊ด
-๊ด
-๊ดญ
-๊ดด
-๊ดผ
-๊ต
-๊ต
-๊ต
-๊ตฌ
-๊ตญ
-๊ตฐ
-๊ตณ
-๊ตด
-๊ตต
-๊ตถ
-๊ตผ
-๊ตฝ
-๊ตฟ
-๊ถ
-๊ถ
-๊ถ
-๊ถ
-๊ถ
-๊ถค
-๊ท
-๊ท
-๊ท
-๊ท
-๊ท
-๊ทค
-๊ทธ
-๊ทน
-๊ทผ
-๊ธ
-๊ธ
-๊ธ
-๊ธ
-๊ธ
-๊ธ
-๊ธฐ
-๊ธด
-๊ธธ
-๊น
-๊น
-๊น
-๊น
-๊น
-๊น
-๊น
-๊น
-๊น
-๊น
-๊น
-๊น
-๊นก
-๊นฅ
-๊นจ
-๊นฌ
-๊นป
-๊นฝ
-๊บ
-๊บต
-๊บผ
-๊บฝ
-๊บพ
-๊ป
-๊ป
-๊ป
-๊ป
-๊ป
-๊ป
-๊ป
-๊ปด
-๊ผ
-๊ผฌ
-๊ผญ
-๊ผด
-๊ผผ
-๊ผฝ
-๊ฝ
-๊ฝ
-๊ฝ
-๊ฝ
-๊ฝ
-๊ฝ
-๊ฝ
-๊ฝค
-๊ฝฅ
-๊พ
-๊พธ
-๊พน
-๊พผ
-๊ฟ
-๊ฟ
-๊ฟ
-๊ฟ
-๊ฟ
-๊ฟ
-๊ฟจ
-๊ฟฉ
-๊ฟฐ
-๋
-๋
-๋
-๋
-๋
-๋
-๋
-๋
-๋
-๋
-๋
-๋
-๋ผ
-๋
-๋
-๋
-๋
-๋
-๋
-๋
-๋
-๋ก
-๋ข
-๋จ
-๋ฉ
-๋ซ
-๋ฌ
-๋ญ
-๋ฎ
-๋ฏ
-๋ฑ
-๋ณ
-๋ด
-๋ต
-๋ธ
-๋ผ
-๋
-๋
-๋
-๋
-๋
-๋
-๋
-๋
-๋ฅ
-๋
-๋
-๋
-๋
-๋
-๋
-๋
-๋
-๋
-๋ฃ
-๋ค
-๋ฅ
-๋จ
-๋ฌ
-๋ด
-๋ต
-๋ท
-๋
-๋
-๋
-๋
-๋
-๋
-๋
-๋
-๋
-๋
ธ
-๋
น
-๋
ผ
-๋
-๋
-๋
-๋
-๋
-๋
-๋
-๋
-๋จ
-๋
-๋จ
-๋ฐ
-๋ธ
-๋ฝ
-๋
-๋
-๋
-๋
-๋
-๋
-๋
-๋
-๋
-๋
-๋ด
-๋ผ
-๋
-๋
-๋
-๋
-๋
-๋
-๋
-๋ฅ
-๋ฆ
-๋ช
-๋ฌ
-๋
-๋
-๋
-๋
-๋
-๋
-๋
-๋
-๋ค
-๋ฅ
-๋ฆ
-๋จ
-๋ซ
-๋ฌ
-๋ญ
-๋ฎ
-๋ณ
-๋ด
-๋ต
-๋ท
-๋น
-๋ป
-๋ฟ
-๋
-๋
-๋
-๋
-๋
-๋
-๋
-๋
-๋
-๋
-๋
-๋
-๋
-๋
-๋ค
-๋ฅ
-๋ง
-๋ฉ
-๋ซ
-๋ฎ
-๋ฐ
-๋ฑ
-๋ด
-๋ธ
-๋
-๋
-๋
-๋ฌ
-๋
-๋
-๋
-๋
-๋
-๋
-๋
-๋
-๋
-๋
-๋ผ
-๋
-๋
-๋
-๋
-๋จ
-๋ฉ
-๋
-๋
-๋
-๋
-๋
-๋ก
-๋ฃ
-๋ฅ
-๋ฌ
-๋
-๋ค
-๋ท
-๋น
-๋
-๋
-๋
-๋
-๋
-๋
-๋ฃ
-๋ค
-๋ฆ
-๋ฌ
-๋ญ
-๋ฏ
-๋ฑ
-๋ธ
-๋
-๋
-๋
-๋
-๋
-๋
-๋
-๋ค
-๋ฅ
-๋ง
-๋ฉ
-๋ช
-๋ฐ
-๋ฑ
-๋ด
-๋ธ
-๋
-๋
-๋
-๋
-๋
-๋
-๋
-๋ก
-๋
-๋ก
-๋ค
-๋จ
-๋ด
-๋ป
-๋ผ
-๋ด
-๋
-๋
-๋
-๋
-๋ฃ
-๋ฅ
-๋
-๋
-๋ซ
-๋ฑ
-๋ฐ
-๋ด
-๋ธ
-๋
-๋จ
-๋ฉ
-๋ฌ
-๋ฏ
-๋ฐ
-๋ธ
-๋ป
-๋
-๋
-๋
-๋ค
-๋ต
-๋ผ
-๋ฝ
-๋
-๋
-๋
-๋
-๋
-๋
-๋
-๋
-๋
-๋
-๋
-๋
-๋จ
-๋ฉ
-๋ซ
-๋ฌ
-๋ญ
-๋ฒ
-๋ด
-๋ต
-๋ธ
-๋
-๋ฌ
-๋ญ
-๋ฐ
-๋ด
-๋ผ
-๋ฝ
-๋ฟ
-๋
-๋
-๋
-๋
-๋
-๋
-๋
-๋
-๋
-๋
-๋
-๋ ค
-๋ ฅ
-๋ จ
-๋ ฌ
-๋ ด
-๋ ต
-๋ ท
-๋ ธ
-๋ น
-๋ก
-๋ก
-๋ก
-๋ก
-๋ก
-๋กค
-๋กฌ
-๋กญ
-๋กฏ
-๋กฑ
-๋กธ
-๋ขฐ
-๋ฃ
-๋ฃ
-๋ฃก
-๋ฃจ
-๋ฃฉ
-๋ฃฌ
-๋ฃฐ
-๋ฃธ
-๋ฃน
-๋ฃป
-๋ฃฝ
-๋ค
-๋ค
-๋คผ
-๋คฝ
-๋ฅ
-๋ฅ
-๋ฅ
-๋ฅ
-๋ฅ
-๋ฅ
-๋ฅจ
-๋ฅซ
-๋ฅญ
-๋ฅด
-๋ฅต
-๋ฅธ
-๋ฅผ
-๋ฆ
-๋ฆ
-๋ฆ
-๋ฆ
-๋ฆ
-๋ฆฌ
-๋ฆญ
-๋ฆฐ
-๋ฆณ
-๋ฆด
-๋ฆผ
-๋ฆฝ
-๋ฆฟ
-๋ง
-๋ง
-๋ง
-๋ง
-๋ง
-๋ง
-๋ง
-๋ง
-๋ง
-๋ง
-๋ง
-๋ง
-๋ง
-๋งก
-๋งฃ
-๋งค
-๋งฅ
-๋งจ
-๋งฌ
-๋งด
-๋งต
-๋งท
-๋งน
-๋งบ
-๋จ
-๋จธ
-๋จน
-๋จผ
-๋ฉ
-๋ฉ
-๋ฉ
-๋ฉ
-๋ฉ
-๋ฉ
-๋ฉ
-๋ฉ
-๋ฉค
-๋ฉฅ
-๋ฉง
-๋ฉฉ
-๋ฉฐ
-๋ฉฑ
-๋ฉด
-๋ฉธ
-๋ช
-๋ช
-๋ช
-๋ชจ
-๋ชฉ
-๋ชซ
-๋ชฌ
-๋ชฐ
-๋ชธ
-๋ชน
-๋ชป
-๋ชฝ
-๋ซผ
-๋ฌ
-๋ฌ
-๋ฌด
-๋ฌต
-๋ฌถ
-๋ฌธ
-๋ฌป
-๋ฌผ
-๋ญ
-๋ญ
-๋ญ
-๋ญ
-๋ญ
-๋ญ
-๋ฎ
-๋ฎ
-๋ฎ
-๋ฎค
-๋ฎฌ
-๋ฏ
-๋ฏ
-๋ฏ
-๋ฏ
-๋ฏธ
-๋ฏน
-๋ฏผ
-๋ฏฟ
-๋ฐ
-๋ฐ
-๋ฐ
-๋ฐ
-๋ฐ
-๋ฐ
-๋ฐ
-๋ฐ
-๋ฐ
-๋ฐ
-๋ฐ
-๋ฐ
-๋ฐ
-๋ฐ
-๋ฐ
-๋ฐค
-๋ฐฅ
-๋ฐง
-๋ฐฉ
-๋ฐญ
-๋ฐฐ
-๋ฐฑ
-๋ฐด
-๋ฐธ
-๋ฑ
-๋ฑ
-๋ฑ
-๋ฑ
-๋ฑ
-๋ฒ
-๋ฒ
-๋ฒ
-๋ฒ
-๋ฒ
-๋ฒ
-๋ฒ
-๋ฒ
-๋ฒ
-๋ฒ
-๋ฒก
-๋ฒค
-๋ฒง
-๋ฒจ
-๋ฒฐ
-๋ฒณ
-๋ฒต
-๋ฒผ
-๋ฒฝ
-๋ณ
-๋ณ
-๋ณ
-๋ณ
-๋ณ
-๋ณด
-๋ณต
-๋ณถ
-๋ณธ
-๋ณผ
-๋ด
-๋ด
-๋ด
-๋ด
-๋ด
-๋ดค
-๋ต
-๋ต
-๋ตค
-๋ถ
-๋ถ
-๋ถ
-๋ถ
-๋ถ
-๋ถ
-๋ถ
-๋ถ
-๋ถ
-๋ถ
-๋ท
-๋ท
-๋ทฐ
-๋ธ
-๋ธ
-๋ธ
-๋ธ
-๋ธจ
-๋น
-๋น
-๋น
-๋น
-๋น
-๋น
-๋น
-๋น
-๋น
-๋น
-๋น
-๋นก
-๋นค
-๋นจ
-๋นต
-๋นป
-๋นผ
-๋นฝ
-๋บ
-๋บ
-๋บ
-๋บ
-๋บ
-๋บจ
-๋บด
-๋บต
-๋ป
-๋ป
-๋ป
-๋ป
-๋ป
-๋ปค
-๋ปฅ
-๋ปฌ
-๋ผ
-๋ผ
-๋ฝ
-๋ฝ
-๋ฝ
-๋ฝ
-๋ฝ
-๋ฝ
-๋พฐ
-๋ฟ
-๋ฟ
-๋ฟ
-๋ฟ
-๋ฟ
-๋ฟ
-์
-์
-์
-์จ
-์
-์
-์
-์ฌ
-์ญ
-์ฐ
-์ด
-์ถ
-์ผ
-์ฝ
-์ฟ
-์
-์
-์
-์
-์
-์
-์
-์
-์
-์ค
-์ฅ
-์จ
-์ฌ
-์ด
-์ต
-์ท
-์น
-์พ
-์
-์
-์
-์
-์
-์ฃ
-์ค
-์ฌ
-์ญ
-์ฏ
-์ฐ
-์ฑ
-์ธ
-์น
-์ผ
-์
-์
-์
-์
-์
-์
-์
-์
-์
ค
-์
ง
-์
จ
-์
ฐ
-์
ด
-์
ธ
-์
-์
-์
-์
-์
-์
-์
-์ก
-์ฅ
-์ฝ
-์
-์
-์ณ
-์ผ
-์
-์
-์
-์
-์
-์
-์
-์
-์
-์
-์
-์จ
-์ซ
-์ญ
-์ฎ
-์ฏ
-์ฑ
-์ฒ
-์ด
-์
-์
-์ฌ
-์ด
-์ผ
-์ฝ
-์ฟ
-์
-์
-์
-์
-์
-์ค
-์จ
-์ฌ
-์ญ
-์ด
-์ต
-์ท
-์น
-์
-์
-์
-์ฃ
-์ค
-์ซ
-์ฌ
-์ญ
-์ฏ
-์ฐ
-์ฑ
-์ถ
-์ธ
-์น
-์ผ
-์
-์
-์
-์
-์
-์
-์
-์ค
-์ฉ
-์
-์จ
-์ฉ
-์ฌ
-์ฐ
-์ธ
-์น
-์ผ
-์ฝ
-์
-์
-์
-์ด
-์
-์
-์
-์
-์
-์ฉ
-์ญ
-์ด
-์
-์
-์
-์
-์ค
-์ฅ
-์จ
-์ฌ
-์น
-์ฐ
-์
-์ฐ
-์ฑ
-์ด
-์ธ
-์
-์
-์
-์จ
-์ฉ
-์ฌ
-์ฐ
-์ท
-์น
-์ป
-์ฝ
-์
-์
-์
-์
-์
-์
-์
-์
-์
-์
-์
-์
-์
-์
-์ก
-์ค
-์จ
-์ฐ
-์ฑ
-์ณ
-์ด
-์ต
-์ผ
-์ฝ
-์
-์
-์
-์
-์
-์
-์
-์
-์
-์ด
-์ต
-์ธ
-์น
-์ป
-์ผ
-์ฝ
-์
-์
-์
-์
-์
-์
-์
-์
-์
-์
-์
-์
-์
-์ก
-์ฃ
-์ฅ
-์ฌ
-์ญ
-์ฎ
-์ฐ
-์ด
-์ท
-์ผ
-์ฝ
-์ฟ
-์
-์
-์
-์
-์
-์
-์
-์
-์
-์ค
-์ฅ
-์จ
-์ฌ
-์ญ
-์ฎ
-์ณ
-์ด
-์ต
-์ท
-์น
-์ป
-์
-์
-์
-์
-์
-์
-์
-์
-์
-์ธ
-์ผ
-์
-์
-์
-์
-์ค
-์ฉ
-์ฐ
-์ฑ
-์ด
-์ธ
-์
-์
-์
-์
-์
-์
-์
-์
-์
-์
-์จ
-์ฉ
-์ฌ
-์ฐ
-์ธ
-์น
-์
-์
-์
-์
-์
-์
-์
-์
-์ก
-์ค
-์จ
-์ฐ
-์ณ
-์ต
-์ผ
-์ฝ
-์
-์
-์
-์
-์
-์
-์
-์ด
-์ต
-์ธ
-์ผ
-์ฝ
-์
-์
-์
-์
-์
-์
-์
-์
-์
-์
-์
-์
-์
-์
-์
-์ก
-์ฃ
-์ฅ
-์ฆ
-์ฌ
-์ญ
-์ผ
-์ฟ
-์
-์
-์
-์
-์
-์
-์
-์
-์
-์
-์
-์
-์
-์
-์
-์
-์
-์
-์
-์ ค
-์ ฌ
-์ ญ
-์ ฏ
-์ ฑ
-์ ธ
-์ ผ
-์ก
-์กฐ
-์กฑ
-์กด
-์กธ
-์ข
-์ข
-์ข
-์ข
-์ข
-์ข
-์ขก
-์ฃ
-์ฃ
-์ฃค
-์ฃผ
-์ฃฝ
-์ค
-์ค
-์ค
-์ค
-์ค
-์ค
-์ค
-์คฌ
-์ฅ
-์ฅ
-์ฅ
-์ฅฌ
-์ฅฐ
-์ฅด
-์ฆ
-์ฆ
-์ฆ
-์ฆ
-์ฆ
-์ฆ
-์ฆ
-์ง
-์ง
-์ง
-์ง
-์ง
-์ง
-์ง
-์ง
-์ง
-์ง
-์ง
-์ง
-์ง
-์ง
-์ง
-์ง
-์งค
-์งง
-์งฌ
-์งญ
-์งฑ
-์งธ
-์จ
-์จ
-์จ
-์จฐ
-์ฉ
-์ฉ
-์ฉ
-์ฉ
-์ฉ
-์ฉก
-์ฉฐ
-์ช
-์ชผ
-์ชฝ
-์ซ
-์ซ
-์ซ
-์ซ
-์ฌ
-์ฌ
-์ญ
-์ญ
-์ญ
-์ญค
-์ฎธ
-์ฏ
-์ฏค
-์ฐ
-์ฐ
-์ฐ
-์ฐ
-์ฐ
-์ฐ
-์ฐก
-์ฐข
-์ฐง
-์ฐจ
-์ฐฉ
-์ฐฌ
-์ฐฎ
-์ฐฐ
-์ฐธ
-์ฐน
-์ฐป
-์ฐฝ
-์ฐพ
-์ฑ
-์ฑ
-์ฑ
-์ฑ
-์ฑ
-์ฑ
-์ฑ
-์ฑ
-์ฑ
-์ฑค
-์ฒ
-์ฒ
-์ฒ
-์ฒ
-์ฒจ
-์ฒฉ
-์ฒซ
-์ฒญ
-์ฒด
-์ฒต
-์ฒธ
-์ฒผ
-์ณ
-์ณ
-์ณค
-์ด
-์ด
-์ด
-์ด
-์ด
-์ด
-์ด
-์ดจ
-์ดฌ
-์ต
-์ตธ
-์ตน
-์ถ
-์ถ
-์ถ
-์ถ
-์ถค
-์ถฅ
-์ถง
-์ถฉ
-์ถฐ
-์ท
-์ท
-์ทจ
-์ธ
-์ธ
-์ธก
-์ธจ
-์ธฐ
-์ธณ
-์ธต
-์น
-์น
-์น
-์น
-์นก
-์นจ
-์นฉ
-์นซ
-์นญ
-์นฒ
-์นด
-์นต
-์นธ
-์นผ
-์บ
-์บ
-์บ
-์บ
-์บ
-์บ
-์บ
-์บ
-์บก
-์บฃ
-์บฅ
-์บฌ
-์ปค
-์ปฅ
-์ปจ
-์ปซ
-์ปฌ
-์ปด
-์ปต
-์ปท
-์ปธ
-์ปน
-์ผ
-์ผ
-์ผ
-์ผ
-์ผ
-์ผ
-์ผ
-์ผฐ
-์ฝ
-์ฝ
-์ฝ
-์ฝ
-์ฝค
-์ฝฅ
-์ฝง
-์ฝฉ
-์ฝฐ
-์ฝด
-์ฝธ
-์พ
-์พ
-์พจ
-์พฐ
-์ฟ
-์ฟ
-์ฟก
-์ฟค
-์ฟจ
-์ฟฐ
-์ฟต
-์ฟผ
-ํ
-ํ
-ํ
-ํด
-ํต
-ํธ
-ํผ
-ํ
-ํ
-ํ
-ํ
-ํฌ
-ํฐ
-ํด
-ํผ
-ํค
-ํฅ
-ํจ
-ํฌ
-ํด
-ํต
-ํท
-ํน
-ํ
-ํ
-ํ
-ํ
-ํ
-ํ
-ํ
-ํ
-ํ
-ํ
-ํ
-ํ
-ํ
-ํค
-ํฌ
-ํญ
-ํฑ
-ํธ
-ํฐ
-ํฑ
-ํด
-ํธ
-ํ
-ํ
-ํ
-ํ
-ํ
-ํ
-ํ
-ํ
-ํ
-ํ
-ํ
-ํ
ก
-ํ
จ
-ํ
-ํ
-ํก
-ํค
-ํจ
-ํฐ
-ํฑ
-ํณ
-ํต
-ํบ
-ํ
-ํด
-ํฌ
-ํญ
-ํฐ
-ํด
-ํผ
-ํ
-ํ
-ํ
-ํ
-ํ
-ํฌ
-ํธ
-ํน
-ํผ
-ํฟ
-ํ
-ํ
-ํ
-ํฐ
-ํฑ
-ํด
-ํธ
-ํ
-ํ
-ํ
-ํ
-ํ
-ํ
-ํ
-ํ
-ํ
-ํ
-ํ
-ํ
-ํก
-ํฅ
-ํจ
-ํฉ
-ํฌ
-ํฐ
-ํธ
-ํน
-ํป
-ํฝ
-ํผ
-ํฝ
-ํ
-ํ
-ํ
-ํ
-ํ
-ํ
-ํ
-ํ
-ํ
-ํ
-ํจ
-ํฉ
-ํซ
-ํญ
-ํด
-ํธ
-ํผ
-ํ
-ํ
-ํ
-ํ
-ํฌ
-ํญ
-ํฐ
-ํด
-ํผ
-ํ
-ํ
-ํ
-ํธ
-ํน
-ํผ
-ํ
-ํ
-ํ
-ํ
-ํ
-ํจ
-ํฐ
-ํธ
-ํ
-ํ
-ํ
-ํ
-ํผ
-ํฝ
-ํ
-ํ
-ํ
-ํ
-ํ
-ํ
-ํ
-ํ
-ํ
-ํ
-ํฅ
-ํจ
-ํฉ
-ํซ
-ํญ
-ํด
-ํต
-ํธ
-ํผ
-ํ
-ํ
-ํ
-ํ
-ํ
-ํ
-ํฅ
-ํ
-ํ
-ํ
-ํ
-ํ
-ํ
-ํ
-ํค
-ํฅ
-ํจ
-ํฌ
-ํด
-ํต
-ํธ
-ํน
-ํ
-ํ
-ํ
-ํ
-ํ
-ํ
-ํ
-ํ
-ํ
-ํ
-ํธ
-ํน
-ํผ
-ํ
-ํ
-ํ
-ํ
-ํ
-ํ
-ํ
-ํ
-ํ
-ํฉ
-ํฐ
-ํ
-ํ
-ํ
-ํ
-ํ
-ํก
-ํจ
-ํ
-ํ
-ํ
-ํ
-ํ
-ํ
-ํ
-ํ
-ํ
-ํค
-ํจ
-ํผ
-ํ
-ํ
-ํ
-ํ
-ํ
-ํฉ
-ํด
-ํ
-ํ
-ํ
-ํ
-ํ
-ํ
-ํ
-ํ
-ํก
-ํฅ
-ํฉ
-ํฌ
-ํฐ
-ํ
-ํ
-ํ
-ํ
-ํ
-ํ
-๊ณน
-๋
-์จ
-๋
-์ฑฑ
-์ฌฌ
-ํฌ
-์ดค
-ํค
-๊ฐ
-๋จ
-๋ฐ
-๊ฑ
-์ฅ
-๋ฝ
-๊ท
-๋ฝ
-์ด
-์ฟฑ
-์
-์ผ
-์ข
-๋ฝ
-์
-๋ฆ
-์ก
-์จ
-์ฝ
-๋ณฝ
-๋ข
-๊ผฐ
-๋ณ
-์ฐ
-๋ท
-์
-์ก
-์ธ
-์
-์ฅ
-์
-๋ค
-๋
-๋ผ
-์ผ
-๊ธฑ
-์ฃ
-์ฐ
-ํ
ฌ
-์ฐ
-๋ค
-๋คจ
-๋
-๋ทธ
-๋
-๋
-๋ฌ
-์
-์ฉจ
-๋นณ
-๊บณ
-์
-๋
-๊น
-๋ฅ
-์ฌ
-์ฉ
\ No newline at end of file
diff --git a/pipeline/mmocr/dicts/lower_english_digits.txt b/pipeline/mmocr/dicts/lower_english_digits.txt
deleted file mode 100644
index 474060366f8a2a00c108d5c743821c0a61867cd5..0000000000000000000000000000000000000000
--- a/pipeline/mmocr/dicts/lower_english_digits.txt
+++ /dev/null
@@ -1,36 +0,0 @@
-0
-1
-2
-3
-4
-5
-6
-7
-8
-9
-a
-b
-c
-d
-e
-f
-g
-h
-i
-j
-k
-l
-m
-n
-o
-p
-q
-r
-s
-t
-u
-v
-w
-x
-y
-z
\ No newline at end of file
diff --git a/pipeline/mmocr/dicts/lower_english_digits_space.txt b/pipeline/mmocr/dicts/lower_english_digits_space.txt
deleted file mode 100644
index a80a4a20b6f47e0338d5cccb9b71504eb38f62bb..0000000000000000000000000000000000000000
--- a/pipeline/mmocr/dicts/lower_english_digits_space.txt
+++ /dev/null
@@ -1,37 +0,0 @@
-0
-1
-2
-3
-4
-5
-6
-7
-8
-9
-a
-b
-c
-d
-e
-f
-g
-h
-i
-j
-k
-l
-m
-n
-o
-p
-q
-r
-s
-t
-u
-v
-w
-x
-y
-z
-
\ No newline at end of file
diff --git a/pipeline/mmocr/dicts/sdmgr_dict.txt b/pipeline/mmocr/dicts/sdmgr_dict.txt
deleted file mode 100644
index b68274119a13962dc989c7330edd371d5c43ced4..0000000000000000000000000000000000000000
--- a/pipeline/mmocr/dicts/sdmgr_dict.txt
+++ /dev/null
@@ -1,91 +0,0 @@
-/
-\
-.
-$
-ยฃ
-โฌ
-ยฅ
-:
--
-,
-*
-#
-(
-)
-%
-@
-!
-'
-&
-=
->
-+
-"
-ร
-?
-<
-[
-]
-_
-0
-1
-2
-3
-4
-5
-6
-7
-8
-9
-a
-b
-c
-d
-e
-f
-g
-h
-i
-j
-k
-l
-m
-n
-o
-p
-q
-r
-s
-t
-u
-v
-w
-x
-y
-z
-A
-B
-C
-D
-E
-F
-G
-H
-I
-J
-K
-L
-M
-N
-O
-P
-Q
-R
-S
-T
-U
-V
-W
-X
-Y
-Z
\ No newline at end of file
diff --git a/pipeline/mmocr/docker/Dockerfile b/pipeline/mmocr/docker/Dockerfile
deleted file mode 100644
index ba1f95416d2fb1278284c80e5a07a9dc3ebcf2f4..0000000000000000000000000000000000000000
--- a/pipeline/mmocr/docker/Dockerfile
+++ /dev/null
@@ -1,24 +0,0 @@
-ARG PYTORCH="1.9.0"
-ARG CUDA="11.1"
-ARG CUDNN="8"
-
-FROM pytorch/pytorch:${PYTORCH}-cuda${CUDA}-cudnn${CUDNN}-devel
-
-# To fix GPG key error when running apt-get update
-RUN apt-key adv --fetch-keys https://developer.download.nvidia.com/compute/cuda/repos/ubuntu1804/x86_64/3bf863cc.pub
-RUN apt-key adv --fetch-keys https://developer.download.nvidia.com/compute/machine-learning/repos/ubuntu1804/x86_64/7fa2af80.pub
-
-RUN apt-get update && apt-get install -y git ninja-build libglib2.0-0 libsm6 libxrender-dev libxext6 libgl1-mesa-glx \
- && apt-get clean \
- && rm -rf /var/lib/apt/lists/*
-
-RUN pip install -U openmim
-RUN mim install mmengine
-RUN mim install 'mmcv>=2.0.0rc1'
-RUN pip install 'mmdet>=3.0.0rc0'
-RUN git clone https://github.com/open-mmlab/mmocr.git /mmocr
-WORKDIR /mmocr
-ENV FORCE_CUDA="1"
-RUN pip install -r requirements.txt
-RUN pip install --no-cache-dir -e .
-RUN pip install -r requirements/albu.txt
diff --git a/pipeline/mmocr/docker/serve/Dockerfile b/pipeline/mmocr/docker/serve/Dockerfile
deleted file mode 100644
index 617112708f4b2336b0ceac9cd579b34cc6353d13..0000000000000000000000000000000000000000
--- a/pipeline/mmocr/docker/serve/Dockerfile
+++ /dev/null
@@ -1,54 +0,0 @@
-ARG PYTORCH="1.6.0"
-ARG CUDA="10.1"
-ARG CUDNN="7"
-FROM pytorch/pytorch:${PYTORCH}-cuda${CUDA}-cudnn${CUDNN}-devel
-RUN rm /etc/apt/sources.list.d/cuda.list
-RUN rm /etc/apt/sources.list.d/nvidia-ml.list
-RUN apt-key del 7fa2af80
-RUN apt-key adv --fetch-keys https://developer.download.nvidia.com/compute/cuda/repos/ubuntu1804/x86_64/3bf863cc.pub
-RUN apt-key adv --fetch-keys https://developer.download.nvidia.com/compute/machine-learning/repos/ubuntu1804/x86_64/7fa2af80.pub
-
-ARG MMCV="1.3.17"
-ARG MMDET="2.25.0"
-
-ENV PYTHONUNBUFFERED TRUE
-
-RUN apt-get update && \
- DEBIAN_FRONTEND=noninteractive apt-get install --no-install-recommends -y \
- ca-certificates \
- g++ \
- openjdk-11-jre-headless \
- # MMDet Requirements
- ffmpeg libsm6 libxext6 git ninja-build libglib2.0-0 libsm6 libxrender-dev libxext6 \
- && rm -rf /var/lib/apt/lists/*
-
-ENV PATH="/opt/conda/bin:$PATH"
-RUN export FORCE_CUDA=1
-
-# TORCHSEVER
-RUN pip install torchserve torch-model-archiver
-
-# MMLAB
-ARG PYTORCH
-ARG CUDA
-RUN ["/bin/bash", "-c", "pip install mmcv-full==${MMCV} -f https://download.openmmlab.com/mmcv/dist/cu${CUDA//./}/torch${PYTORCH}/index.html"]
-RUN pip install mmdet==${MMDET}
-RUN pip install mmocr
-RUN useradd -m model-server \
- && mkdir -p /home/model-server/tmp
-
-COPY entrypoint.sh /usr/local/bin/entrypoint.sh
-
-RUN chmod +x /usr/local/bin/entrypoint.sh \
- && chown -R model-server /home/model-server
-
-COPY config.properties /home/model-server/config.properties
-RUN mkdir /home/model-server/model-store && chown -R model-server /home/model-server/model-store
-
-EXPOSE 8080 8081 8082
-
-USER model-server
-WORKDIR /home/model-server
-ENV TEMP=/home/model-server/tmp
-ENTRYPOINT ["/usr/local/bin/entrypoint.sh"]
-CMD ["serve"]
diff --git a/pipeline/mmocr/docker/serve/config.properties b/pipeline/mmocr/docker/serve/config.properties
deleted file mode 100644
index efb9c47e40ab550bac765611e6c6c6f2a7152f11..0000000000000000000000000000000000000000
--- a/pipeline/mmocr/docker/serve/config.properties
+++ /dev/null
@@ -1,5 +0,0 @@
-inference_address=http://0.0.0.0:8080
-management_address=http://0.0.0.0:8081
-metrics_address=http://0.0.0.0:8082
-model_store=/home/model-server/model-store
-load_models=all
diff --git a/pipeline/mmocr/docker/serve/entrypoint.sh b/pipeline/mmocr/docker/serve/entrypoint.sh
deleted file mode 100644
index 41ba00b048aed84b45c5a8015a016ff148e97d86..0000000000000000000000000000000000000000
--- a/pipeline/mmocr/docker/serve/entrypoint.sh
+++ /dev/null
@@ -1,12 +0,0 @@
-#!/bin/bash
-set -e
-
-if [[ "$1" = "serve" ]]; then
- shift 1
- torchserve --start --ts-config /home/model-server/config.properties
-else
- eval "$@"
-fi
-
-# prevent docker exit
-tail -f /dev/null
diff --git a/pipeline/mmocr/docs/en/Makefile b/pipeline/mmocr/docs/en/Makefile
deleted file mode 100644
index d4bb2cbb9eddb1bb1b4f366623044af8e4830919..0000000000000000000000000000000000000000
--- a/pipeline/mmocr/docs/en/Makefile
+++ /dev/null
@@ -1,20 +0,0 @@
-# Minimal makefile for Sphinx documentation
-#
-
-# You can set these variables from the command line, and also
-# from the environment for the first two.
-SPHINXOPTS ?=
-SPHINXBUILD ?= sphinx-build
-SOURCEDIR = .
-BUILDDIR = _build
-
-# Put it first so that "make" without argument is like "make help".
-help:
- @$(SPHINXBUILD) -M help "$(SOURCEDIR)" "$(BUILDDIR)" $(SPHINXOPTS) $(O)
-
-.PHONY: help Makefile
-
-# Catch-all target: route all unknown targets to Sphinx using the new
-# "make mode" option. $(O) is meant as a shortcut for $(SPHINXOPTS).
-%: Makefile
- @$(SPHINXBUILD) -M $@ "$(SOURCEDIR)" "$(BUILDDIR)" $(SPHINXOPTS) $(O)
diff --git a/pipeline/mmocr/docs/en/_static/css/readthedocs.css b/pipeline/mmocr/docs/en/_static/css/readthedocs.css
deleted file mode 100644
index c4736f9dc728b2b0a49fd8e10d759c5d58e506d1..0000000000000000000000000000000000000000
--- a/pipeline/mmocr/docs/en/_static/css/readthedocs.css
+++ /dev/null
@@ -1,6 +0,0 @@
-.header-logo {
- background-image: url("../images/mmocr.png");
- background-size: 110px 40px;
- height: 40px;
- width: 110px;
-}
diff --git a/pipeline/mmocr/docs/en/_static/images/mmocr.png b/pipeline/mmocr/docs/en/_static/images/mmocr.png
deleted file mode 100644
index 363e34989e376b23b78ca4c31933542f15ec78ee..0000000000000000000000000000000000000000
Binary files a/pipeline/mmocr/docs/en/_static/images/mmocr.png and /dev/null differ
diff --git a/pipeline/mmocr/docs/en/_static/js/collapsed.js b/pipeline/mmocr/docs/en/_static/js/collapsed.js
deleted file mode 100644
index 3124c144dfdfc01a9510cfe39b1c8ff2240644ce..0000000000000000000000000000000000000000
--- a/pipeline/mmocr/docs/en/_static/js/collapsed.js
+++ /dev/null
@@ -1 +0,0 @@
-var collapsedSections = ['Migration Guides', 'API Reference']
diff --git a/pipeline/mmocr/docs/en/_static/js/table.js b/pipeline/mmocr/docs/en/_static/js/table.js
deleted file mode 100644
index 8dacf477f33e81bba3a0c0edc11b135f648b1f0a..0000000000000000000000000000000000000000
--- a/pipeline/mmocr/docs/en/_static/js/table.js
+++ /dev/null
@@ -1,31 +0,0 @@
-$(document).ready(function () {
- table = $('.model-summary').DataTable({
- "stateSave": false,
- "lengthChange": false,
- "pageLength": 10,
- "order": [],
- "scrollX": true,
- "columnDefs": [
- { "type": "summary", targets: '_all' },
- ]
- });
- // Override the default sorting for the summary columns, which
- // never takes the "-" character into account.
- jQuery.extend(jQuery.fn.dataTableExt.oSort, {
- "summary-asc": function (str1, str2) {
- if (str1 == "-
")
- return 1;
- if (str2 == "-
")
- return -1;
- return ((str1 < str2) ? -1 : ((str1 > str2) ? 1 : 0));
- },
-
- "summary-desc": function (str1, str2) {
- if (str1 == "-
")
- return 1;
- if (str2 == "-
")
- return -1;
- return ((str1 < str2) ? 1 : ((str1 > str2) ? -1 : 0));
- }
- });
-})
diff --git a/pipeline/mmocr/docs/en/_templates/classtemplate.rst b/pipeline/mmocr/docs/en/_templates/classtemplate.rst
deleted file mode 100644
index 4f74842394ec9807fb1ae2d8f05a8a57e9a2e24c..0000000000000000000000000000000000000000
--- a/pipeline/mmocr/docs/en/_templates/classtemplate.rst
+++ /dev/null
@@ -1,14 +0,0 @@
-.. role:: hidden
- :class: hidden-section
-.. currentmodule:: {{ module }}
-
-
-{{ name | underline}}
-
-.. autoclass:: {{ name }}
- :members:
-
-
-..
- autogenerated from source/_templates/classtemplate.rst
- note it does not have :inherited-members:
diff --git a/pipeline/mmocr/docs/en/api/apis.rst b/pipeline/mmocr/docs/en/api/apis.rst
deleted file mode 100644
index fcca6a24ea6147a55010e4f3d6a5141a36d2d295..0000000000000000000000000000000000000000
--- a/pipeline/mmocr/docs/en/api/apis.rst
+++ /dev/null
@@ -1,26 +0,0 @@
-.. role:: hidden
- :class: hidden-section
-
-mmocr.apis
-===================================
-
-.. contents:: mmocr.apis
- :depth: 2
- :local:
- :backlinks: top
-
-.. currentmodule:: mmocr.apis.inferencers
-
-Inferencers
----------------------------------------------
-
-.. autosummary::
- :toctree: generated
- :nosignatures:
- :template: classtemplate.rst
-
- MMOCRInferencer
- TextDetInferencer
- TextRecInferencer
- TextSpotInferencer
- KIEInferencer
diff --git a/pipeline/mmocr/docs/en/api/datasets.rst b/pipeline/mmocr/docs/en/api/datasets.rst
deleted file mode 100644
index 8b63debf9a88a68fe97930066c2e1b8090859854..0000000000000000000000000000000000000000
--- a/pipeline/mmocr/docs/en/api/datasets.rst
+++ /dev/null
@@ -1,57 +0,0 @@
-.. role:: hidden
- :class: hidden-section
-
-mmocr.datasets
-===================================
-
-.. contents:: mmocr.datasets
- :depth: 2
- :local:
- :backlinks: top
-
-.. currentmodule:: mmocr.datasets.samplers
-
-Samplers
----------------------------------------------
-
-.. autosummary::
- :toctree: generated
- :nosignatures:
- :template: classtemplate.rst
-
- BatchAugSampler
-
-.. currentmodule:: mmocr.datasets
-
-Datasets
----------------------------------------------
-
-.. autosummary::
- :toctree: generated
- :nosignatures:
- :template: classtemplate.rst
-
- OCRDataset
- WildReceiptDataset
-
-Compatible Datasets
----------------------------------------------
-
-.. autosummary::
- :toctree: generated
- :nosignatures:
- :template: classtemplate.rst
-
- IcdarDataset
- RecogLMDBDataset
- RecogTextDataset
-
-Dataset Wrapper
----------------------------------------------
-
-.. autosummary::
- :toctree: generated
- :nosignatures:
- :template: classtemplate.rst
-
- ConcatDataset
diff --git a/pipeline/mmocr/docs/en/api/engine.rst b/pipeline/mmocr/docs/en/api/engine.rst
deleted file mode 100644
index ecc8fec238acd8a4be2f2453502aac2b8e3cb7ff..0000000000000000000000000000000000000000
--- a/pipeline/mmocr/docs/en/api/engine.rst
+++ /dev/null
@@ -1,22 +0,0 @@
-.. role:: hidden
- :class: hidden-section
-
-mmocr.engine
-===================================
-
-.. contents:: mmocr.engine
- :depth: 2
- :local:
- :backlinks: top
-
-.. currentmodule:: mmocr.engine.hooks
-
-Hooks
----------------------------------------------
-
-.. autosummary::
- :toctree: generated
- :nosignatures:
- :template: classtemplate.rst
-
- VisualizationHook
diff --git a/pipeline/mmocr/docs/en/api/evaluation.rst b/pipeline/mmocr/docs/en/api/evaluation.rst
deleted file mode 100644
index d92d51a4ffbdeb6c7b13559e6a927682c1f246ef..0000000000000000000000000000000000000000
--- a/pipeline/mmocr/docs/en/api/evaluation.rst
+++ /dev/null
@@ -1,57 +0,0 @@
-.. role:: hidden
- :class: hidden-section
-
-mmocr.evaluation
-===================================
-
-.. contents:: mmocr.evaluation
- :depth: 2
- :local:
- :backlinks: top
-
-.. currentmodule:: mmocr.evaluation.evaluator
-
-Evaluator
----------------------------------------------
-
-.. autosummary::
- :toctree: generated
- :nosignatures:
- :template: classtemplate.rst
-
- MultiDatasetsEvaluator
-
-.. currentmodule:: mmocr.evaluation.metrics
-
-TextDet Metric
----------------------------------------------
-
-.. autosummary::
- :toctree: generated
- :nosignatures:
- :template: classtemplate.rst
-
- HmeanIOUMetric
-
-TextRecog Metric
----------------------------------------------
-
-.. autosummary::
- :toctree: generated
- :nosignatures:
- :template: classtemplate.rst
-
- WordMetric
- CharMetric
- OneMinusNEDMetric
-
-
-KIE Metric
----------------------------------------------
-
-.. autosummary::
- :toctree: generated
- :nosignatures:
- :template: classtemplate.rst
-
- F1Metric
diff --git a/pipeline/mmocr/docs/en/api/models.rst b/pipeline/mmocr/docs/en/api/models.rst
deleted file mode 100644
index 9ac53908debea467cc0a23ff6e6d71b670e2534e..0000000000000000000000000000000000000000
--- a/pipeline/mmocr/docs/en/api/models.rst
+++ /dev/null
@@ -1,423 +0,0 @@
-.. role:: hidden
- :class: hidden-section
-
-mmocr.models
-===================================
-
-- :mod:`~mmocr.models.common`
-
- - :ref:`commombackbones`
- - :ref:`commomdictionary`
- - :ref:`commomlayers`
- - :ref:`commomlosses`
- - :ref:`commommodules`
-
-- :mod:`~mmocr.models.textdet`
-
- - :ref:`detdetectors`
- - :ref:`detdatapreprocessors`
- - :ref:`detnecks`
- - :ref:`detheads`
- - :ref:`detmodulelosses`
- - :ref:`detpostprocessors`
-
-- :mod:`~mmocr.models.textrecog`
-
- - :ref:`recrecognizers`
- - :ref:`recdatapreprocessors`
- - :ref:`recpreprocessors`
- - :ref:`recencoders`
- - :ref:`recdecoders`
- - :ref:`recmodulelosses`
- - :ref:`recpostprocessors`
- - :ref:`reclayers`
-
-- :mod:`~mmocr.models.kie`
-
- - :ref:`kieextractors`
- - :ref:`kieheads`
- - :ref:`kiemodulelosses`
- - :ref:`kiepostprocessors`
-
-
-.. module:: mmocr.models.common
-models.common
----------------------------------------------
-.. currentmodule:: mmocr.models.common
-
-.. _commombackbones:
-
-BackBones
-^^^^^^^^^^^^^^^^^^^^^^^^^^^^^^^^^^^^^^^^^^^^^
-.. autosummary::
- :toctree: generated
- :nosignatures:
- :template: classtemplate.rst
-
- UNet
-
-.. _commomdictionary:
-
-Dictionary
-^^^^^^^^^^^^^^^^^^^^^^^^^^^^^^^^^^^^^^^^^^^^^
-.. autosummary::
- :toctree: generated
- :nosignatures:
- :template: classtemplate.rst
-
- Dictionary
-
-.. _commomlosses:
-
-Losses
-^^^^^^^^^^^^^^^^^^^^^^^^^^^^^^^^^^^^^^^^^^^^^
-.. autosummary::
- :toctree: generated
- :nosignatures:
- :template: classtemplate.rst
-
- MaskedBalancedBCEWithLogitsLoss
- MaskedDiceLoss
- MaskedSmoothL1Loss
- MaskedSquareDiceLoss
- MaskedBCEWithLogitsLoss
- SmoothL1Loss
- CrossEntropyLoss
- MaskedBalancedBCELoss
- MaskedBCELoss
-
-.. _commomlayers:
-
-Layers
-^^^^^^^^^^^^^^^^^^^^^^^^^^^^^^^^^^^^^^^^^^^^^
-.. autosummary::
- :toctree: generated
- :nosignatures:
- :template: classtemplate.rst
-
- TFEncoderLayer
- TFDecoderLayer
-
-.. _commommodules:
-
-Modules
-^^^^^^^^^^^^^^^^^^^^^^^^^^^^^^^^^^^^^^^^^^^^^
-.. autosummary::
- :toctree: generated
- :nosignatures:
- :template: classtemplate.rst
-
- ScaledDotProductAttention
- MultiHeadAttention
- PositionwiseFeedForward
- PositionalEncoding
-
-
-.. module:: mmocr.models.textdet
-models.textdet
----------------------------------------------
-.. currentmodule:: mmocr.models.textdet
-
-.. _detdetectors:
-
-Detectors
-^^^^^^^^^^^^^^^^^^^^^^^^^^^^^^^^^^^^^^^^^^^^^
-.. autosummary::
- :toctree: generated
- :nosignatures:
- :template: classtemplate.rst
-
- SingleStageTextDetector
- DBNet
- PANet
- PSENet
- TextSnake
- FCENet
- DRRG
- MMDetWrapper
-
-
-.. _detdatapreprocessors:
-
-Data Preprocessors
-^^^^^^^^^^^^^^^^^^^^^^^^^^^^^^^^^^^^^^^^^^^^^
-.. autosummary::
- :toctree: generated
- :nosignatures:
- :template: classtemplate.rst
-
- TextDetDataPreprocessor
-
-
-.. _detnecks:
-
-Necks
-^^^^^^^^^^^^^^^^^^^^^^^^^^^^^^^^^^^^^^^^^^^^^
-.. autosummary::
- :toctree: generated
- :nosignatures:
- :template: classtemplate.rst
-
- FPEM_FFM
- FPNF
- FPNC
- FPN_UNet
-
-
-.. _detheads:
-
-Heads
-^^^^^^^^^^^^^^^^^^^^^^^^^^^^^^^^^^^^^^^^^^^^^
-.. autosummary::
- :toctree: generated
- :nosignatures:
- :template: classtemplate.rst
-
- BaseTextDetHead
- PSEHead
- PANHead
- DBHead
- FCEHead
- TextSnakeHead
- DRRGHead
-
-
-.. _detmodulelosses:
-
-Module Losses
-^^^^^^^^^^^^^^^^^^^^^^^^^^^^^^^^^^^^^^^^^^^^^
-.. autosummary::
- :toctree: generated
- :nosignatures:
- :template: classtemplate.rst
-
- SegBasedModuleLoss
- PANModuleLoss
- PSEModuleLoss
- DBModuleLoss
- TextSnakeModuleLoss
- FCEModuleLoss
- DRRGModuleLoss
-
-
-.. _detpostprocessors:
-
-Postprocessors
-^^^^^^^^^^^^^^^^^^^^^^^^^^^^^^^^^^^^^^^^^^^^^
-.. autosummary::
- :toctree: generated
- :nosignatures:
- :template: classtemplate.rst
-
- BaseTextDetPostProcessor
- PSEPostprocessor
- PANPostprocessor
- DBPostprocessor
- DRRGPostprocessor
- FCEPostprocessor
- TextSnakePostprocessor
-
-
-
-.. module:: mmocr.models.textrecog
-models.textrecog
----------------------------------------------
-.. currentmodule:: mmocr.models.textrecog
-
-.. _recrecognizers:
-
-
-Recognizers
-^^^^^^^^^^^^^^^^^^^^^^^^^^^^^^^^^^^^^^^^^^^^^
-
-.. autosummary::
- :toctree: generated
- :nosignatures:
- :template: classtemplate.rst
-
- BaseRecognizer
- EncoderDecoderRecognizer
- CRNN
- SARNet
- NRTR
- RobustScanner
- SATRN
- ABINet
- MASTER
- ASTER
-
-.. _recdatapreprocessors:
-
-Data Preprocessors
-^^^^^^^^^^^^^^^^^^^^^^^^^^^^^^^^^^^^^^^^^^^^^
-.. autosummary::
- :toctree: generated
- :nosignatures:
- :template: classtemplate.rst
-
- TextRecogDataPreprocessor
-
-.. _recpreprocessors:
-
-Preprocessors
-^^^^^^^^^^^^^^^^^^^^^^^^^^^^^^^^^^^^^^^^^^^^^
-.. autosummary::
- :toctree: generated
- :nosignatures:
- :template: classtemplate.rst
-
- STN
-
-.. _recbackbones:
-
-BackBones
-^^^^^^^^^^^^^^^^^^^^^^^^^^^^^^^^^^^^^^^^^^^^^
-.. autosummary::
- :toctree: generated
- :nosignatures:
- :template: classtemplate.rst
-
- ResNet31OCR
- MiniVGG
- NRTRModalityTransform
- ShallowCNN
- ResNetABI
- ResNet
- MobileNetV2
-
-
-.. _recencoders:
-
-Encoders
-^^^^^^^^^^^^^^^^^^^^^^^^^^^^^^^^^^^^^^^^^^^^^
-.. autosummary::
- :toctree: generated
- :nosignatures:
- :template: classtemplate.rst
-
- SAREncoder
- NRTREncoder
- BaseEncoder
- ChannelReductionEncoder
- SATRNEncoder
- ABIEncoder
- ASTEREncoder
-
-.. _recdecoders:
-
-Decoders
-^^^^^^^^^^^^^^^^^^^^^^^^^^^^^^^^^^^^^^^^^^^^^
-.. autosummary::
- :toctree: generated
- :nosignatures:
- :template: classtemplate.rst
-
- BaseDecoder
- ABILanguageDecoder
- ABIVisionDecoder
- ABIFuser
- CRNNDecoder
- ParallelSARDecoder
- SequentialSARDecoder
- ParallelSARDecoderWithBS
- NRTRDecoder
- SequenceAttentionDecoder
- PositionAttentionDecoder
- RobustScannerFuser
- MasterDecoder
- ASTERDecoder
-
-.. _recmodulelosses:
-
-Module Losses
-^^^^^^^^^^^^^^^^^^^^^^^^^^^^^^^^^^^^^^^^^^^^^
-.. autosummary::
- :toctree: generated
- :nosignatures:
- :template: classtemplate.rst
-
- BaseTextRecogModuleLoss
- CEModuleLoss
- CTCModuleLoss
- ABIModuleLoss
-
-.. _recpostprocessors:
-
-Postprocessors
-^^^^^^^^^^^^^^^^^^^^^^^^^^^^^^^^^^^^^^^^^^^^^
-.. autosummary::
- :toctree: generated
- :nosignatures:
- :template: classtemplate.rst
-
- BaseTextRecogPostprocessor
- AttentionPostprocessor
- CTCPostProcessor
-
-.. _reclayers:
-
-Layers
-^^^^^^^^^^^^^^^^^^^^^^^^^^^^^^^^^^^^^^^^^^^^^
-.. autosummary::
- :toctree: generated
- :nosignatures:
- :template: classtemplate.rst
-
- BidirectionalLSTM
- Adaptive2DPositionalEncoding
- BasicBlock
- Bottleneck
- RobustScannerFusionLayer
- DotProductAttentionLayer
- PositionAwareLayer
- SATRNEncoderLayer
-
-
-.. module:: mmocr.models.kie
-models.kie
----------------------------------------------
-.. currentmodule:: mmocr.models.kie
-
-.. _kieextractors:
-
-Extractors
-^^^^^^^^^^^^^^^^^^^^^^^^^^^^^^^^^^^^^^^^^^^^^
-.. autosummary::
- :toctree: generated
- :nosignatures:
- :template: classtemplate.rst
-
- SDMGR
-
-.. _kieheads:
-
-Heads
-^^^^^^^^^^^^^^^^^^^^^^^^^^^^^^^^^^^^^^^^^^^^^
-.. autosummary::
- :toctree: generated
- :nosignatures:
- :template: classtemplate.rst
-
- SDMGRHead
-
-.. _kiemodulelosses:
-
-Module Losses
-^^^^^^^^^^^^^^^^^^^^^^^^^^^^^^^^^^^^^^^^^^^^^
-.. autosummary::
- :toctree: generated
- :nosignatures:
- :template: classtemplate.rst
-
- SDMGRModuleLoss
-
-.. _kiepostprocessors:
-
-Postprocessors
-^^^^^^^^^^^^^^^^^^^^^^^^^^^^^^^^^^^^^^^^^^^^^
-.. autosummary::
- :toctree: generated
- :nosignatures:
- :template: classtemplate.rst
-
- SDMGRPostProcessor
diff --git a/pipeline/mmocr/docs/en/api/structures.rst b/pipeline/mmocr/docs/en/api/structures.rst
deleted file mode 100644
index 920c4d440d65861b380427c8d077936b49515d00..0000000000000000000000000000000000000000
--- a/pipeline/mmocr/docs/en/api/structures.rst
+++ /dev/null
@@ -1,15 +0,0 @@
-.. role:: hidden
- :class: hidden-section
-
-mmocr.structures
-===================================
-
-.. currentmodule:: mmocr.structures
-.. autosummary::
- :toctree: generated
- :nosignatures:
- :template: classtemplate.rst
-
- TextDetDataSample
- TextRecogDataSample
- KIEDataSample
diff --git a/pipeline/mmocr/docs/en/api/transforms.rst b/pipeline/mmocr/docs/en/api/transforms.rst
deleted file mode 100644
index 08b7e854ee19c15100a9d4779862e7523f74cb03..0000000000000000000000000000000000000000
--- a/pipeline/mmocr/docs/en/api/transforms.rst
+++ /dev/null
@@ -1,111 +0,0 @@
-.. role:: hidden
- :class: hidden-section
-
-mmocr.datasets
-===================================
-
-.. contents:: mmocr.datasets.transforms
- :depth: 2
- :local:
- :backlinks: top
-
-.. currentmodule:: mmocr.datasets.transforms
-
-Loading
----------------------------------------------
-
-.. autosummary::
- :toctree: generated
- :nosignatures:
- :template: classtemplate.rst
-
- LoadImageFromFile
- LoadOCRAnnotations
- LoadKIEAnnotations
- InferencerLoader
-
-
-TextDet Transforms
----------------------------------------------
-
-.. autosummary::
- :toctree: generated
- :nosignatures:
- :template: classtemplate.rst
-
- BoundedScaleAspectJitter
- RandomFlip
- SourceImagePad
- ShortScaleAspectJitter
- TextDetRandomCrop
- TextDetRandomCropFlip
-
-
-TextRecog Transforms
----------------------------------------------
-
-.. autosummary::
- :toctree: generated
- :nosignatures:
- :template: classtemplate.rst
-
- TextRecogGeneralAug
- CropHeight
- ImageContentJitter
- ReversePixels
- PyramidRescale
- PadToWidth
- RescaleToHeight
-
-
-OCR Transforms
----------------------------------------------
-
-.. autosummary::
- :toctree: generated
- :nosignatures:
- :template: classtemplate.rst
-
- RandomCrop
- RandomRotate
- Resize
- FixInvalidPolygon
- RemoveIgnored
-
-
-
-Formatting
----------------------------------------------
-
-.. autosummary::
- :toctree: generated
- :nosignatures:
- :template: classtemplate.rst
-
- PackTextDetInputs
- PackTextRecogInputs
- PackKIEInputs
-
-
-Transform Wrapper
----------------------------------------------
-
-.. autosummary::
- :toctree: generated
- :nosignatures:
- :template: classtemplate.rst
-
- ImgAugWrapper
- TorchVisionWrapper
-
-
-Adapter
----------------------------------------------
-
-.. autosummary::
- :toctree: generated
- :nosignatures:
- :template: classtemplate.rst
-
- MMDet2MMOCR
- MMOCR2MMDet
diff --git a/pipeline/mmocr/docs/en/api/utils.rst b/pipeline/mmocr/docs/en/api/utils.rst
deleted file mode 100644
index 06cc5c64383e094d5ea9d5edbc955a4f4acbfc4d..0000000000000000000000000000000000000000
--- a/pipeline/mmocr/docs/en/api/utils.rst
+++ /dev/null
@@ -1,101 +0,0 @@
-.. role:: hidden
- :class: hidden-section
-
-mmocr.utils
-===================================
-
-.. contents:: mmocr.utils
- :depth: 2
- :local:
- :backlinks: top
-
-.. currentmodule:: mmocr.utils
-
-Image Utils
----------------------------------------------
-.. autosummary::
- :toctree: generated
- :nosignatures:
- crop_img
- warp_img
-
-
-Box Utils
----------------------------------------------
-.. autosummary::
- :toctree: generated
- :nosignatures:
-
- bbox2poly
- bbox_center_distance
- bbox_diag_distance
- bezier2polygon
- is_on_same_line
- rescale_bboxes
-
- stitch_boxes_into_lines
-
-
-Point Utils
----------------------------------------------
-.. autosummary::
- :toctree: generated
- :nosignatures:
-
- point_distance
- points_center
-
-Polygon Utils
----------------------------------------------
-.. autosummary::
- :toctree: generated
- :nosignatures:
-
- boundary_iou
- crop_polygon
- is_poly_inside_rect
- offset_polygon
- poly2bbox
- poly2shapely
- poly_intersection
- poly_iou
- poly_make_valid
- poly_union
- polys2shapely
- rescale_polygon
- rescale_polygons
- shapely2poly
- sort_points
- sort_vertex
- sort_vertex8
-
-
-Mask Utils
----------------------------------------------
-.. autosummary::
- :toctree: generated
- :nosignatures:
-
- fill_hole
-
-
-Misc Utils
----------------------------------------------
-.. autosummary::
- :toctree: generated
- :nosignatures:
-
- equal_len
- is_2dlist
- is_3dlist
- is_none_or_type
- is_type_list
-
-
-Setup Env
----------------------------------------------
-.. autosummary::
- :toctree: generated
- :nosignatures:
-
- register_all_modules
diff --git a/pipeline/mmocr/docs/en/api/visualization.rst b/pipeline/mmocr/docs/en/api/visualization.rst
deleted file mode 100644
index e48469226cd9dac973ea7d5739af78bbe98e1992..0000000000000000000000000000000000000000
--- a/pipeline/mmocr/docs/en/api/visualization.rst
+++ /dev/null
@@ -1,18 +0,0 @@
-.. role:: hidden
- :class: hidden-section
-
-mmocr.visualization
-===================================
-
-.. currentmodule:: mmocr.visualization
-
-.. autosummary::
- :toctree: generated
- :nosignatures:
- :template: classtemplate.rst
-
- BaseLocalVisualizer
- TextDetLocalVisualizer
- TextRecogLocalVisualizer
- TextSpottingLocalVisualizer
- KIELocalVisualizer
diff --git a/pipeline/mmocr/docs/en/basic_concepts/convention.md b/pipeline/mmocr/docs/en/basic_concepts/convention.md
deleted file mode 100644
index 4964cacbced155f72e8a6c114dbb1d21255ccd1d..0000000000000000000000000000000000000000
--- a/pipeline/mmocr/docs/en/basic_concepts/convention.md
+++ /dev/null
@@ -1,3 +0,0 @@
-# Convention\[coming soon\]
-
-Coming Soon!
diff --git a/pipeline/mmocr/docs/en/basic_concepts/data_flow.md b/pipeline/mmocr/docs/en/basic_concepts/data_flow.md
deleted file mode 100644
index 11957fa1fe3bb256db6d9a0ee553476d9bbb21b6..0000000000000000000000000000000000000000
--- a/pipeline/mmocr/docs/en/basic_concepts/data_flow.md
+++ /dev/null
@@ -1,3 +0,0 @@
-# Data Flow\[coming soon\]
-
-Coming Soon!
diff --git a/pipeline/mmocr/docs/en/basic_concepts/datasets.md b/pipeline/mmocr/docs/en/basic_concepts/datasets.md
deleted file mode 100644
index 7e121d2b36ce00360b62f97f574d07101c50a83e..0000000000000000000000000000000000000000
--- a/pipeline/mmocr/docs/en/basic_concepts/datasets.md
+++ /dev/null
@@ -1,491 +0,0 @@
-# Dataset
-
-## Overview
-
-In MMOCR, all the datasets are processed via different Dataset classes based on [mmengine.BaseDataset](mmengine.dataset.BaseDataset). Dataset classes are responsible for loading the data and performing initial parsing, then fed to [data pipeline](./transforms.md) for data preprocessing, augmentation, formatting, etc.
-
-
-
-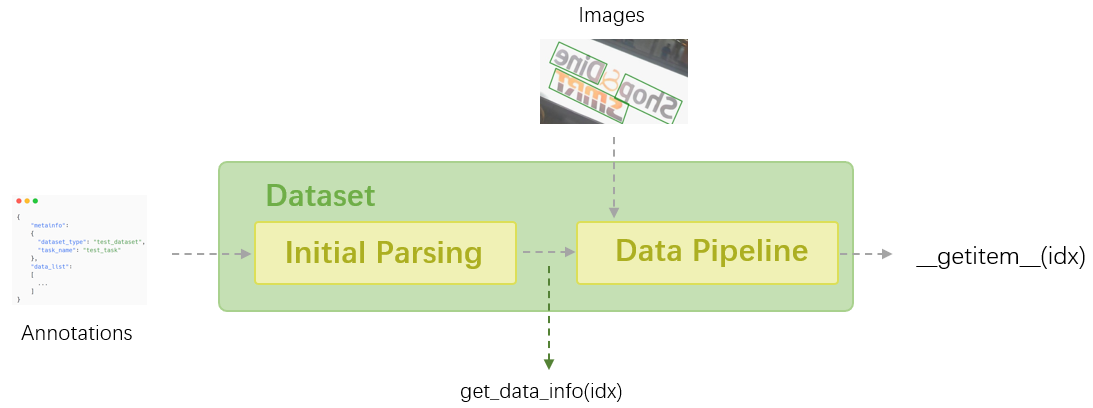
-
-
-
-In this tutorial, we will introduce some common interfaces of the Dataset class, and the usage of Dataset implementations in MMOCR as well as the annotation types they support.
-
-```{tip}
-Dataset class supports some advanced features, such as lazy initialization and data serialization, and takes advantage of various dataset wrappers to perform data concatenation, repeating, and category balancing. These content will not be covered in this tutorial, but you can read {external+mmengine:doc}`MMEngine: BaseDataset ` for more details.
-```
-
-## Common Interfaces
-
-Now, let's look at a concrete example and learn some typical interfaces of a Dataset class.
-`OCRDataset` is a widely used Dataset implementation in MMOCR, and is suggested as a default Dataset type in MMOCR as its associated annotation format is flexible enough to support *all* the OCR tasks ([more info](#ocrdataset)). Now we will instantiate an `OCRDataset` object wherein the toy dataset in `tests/data/det_toy_dataset` will be loaded.
-
-```python
-from mmocr.datasets import OCRDataset
-from mmengine.registry import init_default_scope
-init_default_scope('mmocr')
-
-train_pipeline = [
- dict(
- type='LoadImageFromFile'),
- dict(
- type='LoadOCRAnnotations',
- with_polygon=True,
- with_bbox=True,
- with_label=True,
- ),
- dict(type='RandomCrop', min_side_ratio=0.1),
- dict(type='Resize', scale=(640, 640), keep_ratio=True),
- dict(type='Pad', size=(640, 640)),
- dict(
- type='PackTextDetInputs',
- meta_keys=('img_path', 'ori_shape', 'img_shape'))
-]
-dataset = OCRDataset(
- data_root='tests/data/det_toy_dataset',
- ann_file='textdet_test.json',
- test_mode=False,
- pipeline=train_pipeline)
-
-```
-
-Let's peek the size of this dataset:
-
-```python
->>> print(len(dataset))
-
-10
-```
-
-Typically, a Dataset class loads and stores two types of information: (1) **meta information**: Some meta descriptors of the dataset's property, such as available object categories in this dataset. (2) **annotation**: The path to images, and their labels. We can access the meta information in `dataset.metainfo`:
-
-```python
->>> from pprint import pprint
->>> pprint(dataset.metainfo)
-
-{'category': [{'id': 0, 'name': 'text'}],
- 'dataset_type': 'TextDetDataset',
- 'task_name': 'textdet'}
-```
-
-As for the annotations, we can access them via `dataset.get_data_info(idx)`, which returns a dictionary containing the information of the `idx`-th sample in the dataset that is initially parsed, but not yet processed by [data pipeline](./transforms.md).
-
-```python
->>> from pprint import pprint
->>> pprint(dataset.get_data_info(0))
-
-{'height': 720,
- 'img_path': 'tests/data/det_toy_dataset/test/img_10.jpg',
- 'instances': [{'bbox': [260.0, 138.0, 284.0, 158.0],
- 'bbox_label': 0,
- 'ignore': True,
- 'polygon': [261, 138, 284, 140, 279, 158, 260, 158]},
- ...,
- {'bbox': [1011.0, 157.0, 1079.0, 173.0],
- 'bbox_label': 0,
- 'ignore': True,
- 'polygon': [1011, 157, 1079, 160, 1076, 173, 1011, 170]}],
- 'sample_idx': 0,
- 'seg_map': 'test/gt_img_10.txt',
- 'width': 1280}
-
-```
-
-On the other hand, we can get the sample fully processed by data pipeline via `dataset[idx]` or `dataset.__getitem__(idx)`, which is directly feedable to models and perform a full train/test cycle. It has two fields:
-
-- `inputs`: The image after data augmentation;
-- `data_samples`: The [DataSample](./structures.md) that contains the augmented annotations, and meta information appended by some data transforms to keep track of some key properties of this sample.
-
-```python
->>> pprint(dataset[0])
-
-{'data_samples':
-) at 0x7f735a0508e0>,
- 'inputs': tensor([[[129, 111, 131, ..., 0, 0, 0], ...
- [ 19, 18, 15, ..., 0, 0, 0]]], dtype=torch.uint8)}
-```
-
-## Dataset Classes and Annotation Formats
-
-Each Dataset implementation can only load datasets in a specific annotation format. Here lists all supported Dataset classes and their compatible annotation formats, as well as an example config that showcases how to use them in practice.
-
-```{note}
-If you are not familiar with the config system, you may find [Dataset Configuration](../user_guides/dataset_prepare.md#dataset-configuration) helpful.
-```
-
-### OCRDataset
-
-Usually, there are many different types of annotations in OCR datasets, and the formats often vary between different subtasks, such as text detection and text recognition. These differences can result in the need for different data loading code when using different datasets, increasing the learning and maintenance costs for users.
-
-In MMOCR, we propose a unified dataset format that can adapt to all three subtasks of OCR: text detection, text recognition, and text spotting. This design maximizes the uniformity of the dataset, allows for the reuse of data annotations across different tasks, and makes dataset management more convenient. Considering that popular dataset formats are still inconsistent, MMOCR provides [Dataset Preparer](../user_guides/data_prepare/dataset_preparer.md) to help users convert their datasets to MMOCR format. We also strongly encourage researchers to develop their own datasets based on this data format.
-
-#### Annotation Format
-
-This annotation file is a `.json` file that stores a `dict`, containing both `metainfo` and `data_list`, where the former includes basic information about the dataset and the latter consists of the label item of each target instance. Here presents an extensive list of all the fields in the annotation file, but some fields are used in a subset of tasks and can be ignored in other tasks.
-
-```python
-{
- "metainfo":
- {
- "dataset_type": "TextDetDataset", # Options: TextDetDataset/TextRecogDataset/TextSpotterDataset
- "task_name": "textdet", # Options: textdet/textspotter/textrecog
- "category": [{"id": 0, "name": "text"}] # Used in textdet/textspotter
- },
- "data_list":
- [
- {
- "img_path": "test_img.jpg",
- "height": 604,
- "width": 640,
- "instances": # multiple instances in one image
- [
- {
- "bbox": [0, 0, 10, 20], # in textdet/textspotter, [x1, y1, x2, y2].
- "bbox_label": 0, # The object category, always 0 (text) in MMOCR
- "polygon": [0, 0, 0, 10, 10, 20, 20, 0], # in textdet/textspotter. [x1, y1, x2, y2, ....]
- "text": "mmocr", # in textspotter/textrecog
- "ignore": False # in textspotter/textdet. Whether to ignore this sample during training
- },
- #...
- ],
- }
- #... multiple images
- ]
-}
-```
-
-#### Example Config
-
-Here is a part of config example where we make `train_dataloader` use `OCRDataset` to load the ICDAR2015 dataset for a text detection model. Keep in mind that `OCRDataset` can load any OCR datasets prepared by Dataset Preparer regardless of its task. That is, you can use it for text recognition and text spotting, but you still have to modify the transform types in `pipeline` according to the needs of different tasks.
-
-```python
-pipeline = [
- dict(
- type='LoadImageFromFile'),
- dict(
- type='LoadOCRAnnotations',
- with_polygon=True,
- with_bbox=True,
- with_label=True,
- ),
- dict(
- type='PackTextDetInputs',
- meta_keys=('img_path', 'ori_shape', 'img_shape'))
-]
-
-icdar2015_textdet_train = dict(
- type='OCRDataset',
- data_root='data/icdar2015',
- ann_file='textdet_train.json',
- filter_cfg=dict(filter_empty_gt=True, min_size=32),
- pipeline=pipeline)
-
-train_dataloader = dict(
- batch_size=16,
- num_workers=8,
- persistent_workers=True,
- sampler=dict(type='DefaultSampler', shuffle=True),
- dataset=icdar2015_textdet_train)
-```
-
-### RecogLMDBDataset
-
-Reading images or labels from files can be slow when data are excessive, e.g. on a scale of millions. Besides, in academia, most of the scene text recognition datasets are stored in lmdb format, including images and labels. ([Example](https://github.com/clovaai/deep-text-recognition-benchmark))
-
-To get closer to the mainstream practice and enhance the data storage efficiency, MMOCR supports loading images and labels from lmdb datasets via `RecogLMDBDataset`.
-
-#### Annotation Format
-
-MMOCR requires the following keys for LMDB datasets:
-
-- `num_samples`: The parameter describing the data volume of the dataset.
-- The keys of images and labels are in the
- format of `image-000000001` and `label-000000001`, respectively. The index starts from 1.
-
-MMOCR has a toy LMDB dataset in `tests/data/rec_toy_dataset/imgs.lmdb`.
-You can get a sense of the format with the following code snippet.
-
-```python
->>> import lmdb
->>>
->>> env = lmdb.open('tests/data/rec_toy_dataset/imgs.lmdb')
->>> txn = env.begin()
->>> for k, v in txn.cursor():
->>> print(k, v)
-
-b'image-000000001' b'\xff...'
-b'image-000000002' b'\xff...'
-b'image-000000003' b'\xff...'
-b'image-000000004' b'\xff...'
-b'image-000000005' b'\xff...'
-b'image-000000006' b'\xff...'
-b'image-000000007' b'\xff...'
-b'image-000000008' b'\xff...'
-b'image-000000009' b'\xff...'
-b'image-000000010' b'\xff...'
-b'label-000000001' b'GRAND'
-b'label-000000002' b'HOTEL'
-b'label-000000003' b'HOTEL'
-b'label-000000004' b'PACIFIC'
-b'label-000000005' b'03/09/2009'
-b'label-000000006' b'ANING'
-b'label-000000007' b'Virgin'
-b'label-000000008' b'america'
-b'label-000000009' b'ATTACK'
-b'label-000000010' b'DAVIDSON'
-b'num-samples' b'10'
-```
-
-#### Example Config
-
-Here is a part of config example where we make `train_dataloader` use `RecogLMDBDataset` to load the toy dataset. Since `RecogLMDBDataset` loads images as numpy arrays, don't forget to use `LoadImageFromNDArray` instead of `LoadImageFromFile` in the pipeline for successful loading.
-
-```python
-pipeline = [
- dict(
- type='LoadImageFromNDArray'),
- dict(
- type='LoadOCRAnnotations',
- with_text=True,
- ),
- dict(
- type='PackTextRecogInputs',
- meta_keys=('img_path', 'ori_shape', 'img_shape'))
-]
-
-toy_textrecog_train = dict(
- type='RecogLMDBDataset',
- data_root='tests/data/rec_toy_dataset/',
- ann_file='imgs.lmdb',
- pipeline=pipeline)
-
-train_dataloader = dict(
- batch_size=16,
- num_workers=8,
- persistent_workers=True,
- sampler=dict(type='DefaultSampler', shuffle=True),
- dataset=toy_textrecog_train)
-```
-
-### RecogTextDataset
-
-Prior to MMOCR 1.0, MMOCR 0.x takes text files as input for text recognition. These formats has been deprecated in MMOCR 1.0, and this class could be removed anytime in the future. [More info](../migration/dataset.md)
-
-#### Annotation Format
-
-Text files can either be in `txt` format or `jsonl` format. The simple `.txt` annotations separate image name and word annotation by a blank space, which cannot handle the case when spaces are included in a text instance.
-
-```text
-img1.jpg OpenMMLab
-img2.jpg MMOCR
-```
-
-The JSON Line format uses a dictionary-like structure to represent the annotations, where the keys `filename` and `text` store the image name and word label, respectively.
-
-```json
-{"filename": "img1.jpg", "text": "OpenMMLab"}
-{"filename": "img2.jpg", "text": "MMOCR"}
-```
-
-#### Example Config
-
-Here is a part of config example where we use `RecogTextDataset` to load the old txt labels in training, and the old jsonl labels in testing.
-
-```python
-pipeline = [
- dict(
- type='LoadImageFromFile'),
- dict(
- type='LoadOCRAnnotations',
- with_polygon=True,
- with_bbox=True,
- with_label=True,
- ),
- dict(
- type='PackTextDetInputs',
- meta_keys=('img_path', 'ori_shape', 'img_shape'))
-]
-
- # loading 0.x txt format annos
- txt_dataset = dict(
- type='RecogTextDataset',
- data_root=data_root,
- ann_file='old_label.txt',
- data_prefix=dict(img_path='imgs'),
- parser_cfg=dict(
- type='LineStrParser',
- keys=['filename', 'text'],
- keys_idx=[0, 1]),
- pipeline=pipeline)
-
-
-train_dataloader = dict(
- batch_size=16,
- num_workers=8,
- persistent_workers=True,
- sampler=dict(type='DefaultSampler', shuffle=True),
- dataset=txt_dataset)
-
- # loading 0.x json line format annos
- jsonl_dataset = dict(
- type='RecogTextDataset',
- data_root=data_root,
- ann_file='old_label.jsonl',
- data_prefix=dict(img_path='imgs'),
- parser_cfg=dict(
- type='LineJsonParser',
- keys=['filename', 'text'],
- pipeline=pipeline))
-
-test_dataloader = dict(
- batch_size=16,
- num_workers=8,
- persistent_workers=True,
- sampler=dict(type='DefaultSampler', shuffle=False),
- dataset=jsonl_dataset)
-```
-
-### IcdarDataset
-
-Prior to MMOCR 1.0, MMOCR 0.x takes COCO-like format annotations as input for text detection. These formats has been deprecated in MMOCR 1.0, and this class could be removed anytime in the future. [More info](../migration/dataset.md)
-
-#### Annotation Format
-
-```json
-{
- "images": [
- {
- "id": 1,
- "width": 800,
- "height": 600,
- "file_name": "test.jpg"
- }
- ],
- "annotations": [
- {
- "id": 1,
- "image_id": 1,
- "category_id": 1,
- "bbox": [0,0,10,10],
- "segmentation": [
- [0,0,10,0,10,10,0,10]
- ],
- "area": 100,
- "iscrowd": 0
- }
- ]
-}
-```
-
-#### Example Config
-
-Here is a part of config example where we make `train_dataloader` use `IcdarDataset` to load the old labels.
-
-```python
-pipeline = [
- dict(
- type='LoadImageFromFile'),
- dict(
- type='LoadOCRAnnotations',
- with_polygon=True,
- with_bbox=True,
- with_label=True,
- ),
- dict(
- type='PackTextDetInputs',
- meta_keys=('img_path', 'ori_shape', 'img_shape'))
-]
-
-icdar2015_textdet_train = dict(
- type='IcdarDatasetDataset',
- data_root='data/det/icdar2015',
- ann_file='instances_training.json',
- filter_cfg=dict(filter_empty_gt=True, min_size=32),
- pipeline=pipeline)
-
-train_dataloader = dict(
- batch_size=16,
- num_workers=8,
- persistent_workers=True,
- sampler=dict(type='DefaultSampler', shuffle=True),
- dataset=icdar2015_textdet_train)
-```
-
-### WildReceiptDataset
-
-It's customized for [WildReceipt](https://mmocr.readthedocs.io/en/dev-1.x/user_guides/data_prepare/datasetzoo.html#wildreceipt) dataset only.
-
-#### Annotation Format
-
-```json
-// Close Set
-{
- "file_name": "image_files/Image_16/11/d5de7f2a20751e50b84c747c17a24cd98bed3554.jpeg",
- "height": 1200,
- "width": 1600,
- "annotations":
- [
- {
- "box": [550.0, 190.0, 937.0, 190.0, 937.0, 104.0, 550.0, 104.0],
- "text": "SAFEWAY",
- "label": 1
- },
- {
- "box": [1048.0, 211.0, 1074.0, 211.0, 1074.0, 196.0, 1048.0, 196.0],
- "text": "TM",
- "label": 25
- }
- ], //...
-}
-
-// Open Set
-{
- "file_name": "image_files/Image_12/10/845be0dd6f5b04866a2042abd28d558032ef2576.jpeg",
- "height": 348,
- "width": 348,
- "annotations":
- [
- {
- "box": [114.0, 19.0, 230.0, 19.0, 230.0, 1.0, 114.0, 1.0],
- "text": "CHOEUN",
- "label": 2,
- "edge": 1
- },
- {
- "box": [97.0, 35.0, 236.0, 35.0, 236.0, 19.0, 97.0, 19.0],
- "text": "KOREANRESTAURANT",
- "label": 2,
- "edge": 1
- }
- ]
-}
-```
-
-#### Example Config
-
-Please refer to [SDMGR's config](https://github.com/open-mmlab/mmocr/blob/f30c16ce96bd2393570c04eeb9cf48a7916315cc/configs/kie/sdmgr/sdmgr_novisual_60e_wildreceipt.py) for more details.
diff --git a/pipeline/mmocr/docs/en/basic_concepts/engine.md b/pipeline/mmocr/docs/en/basic_concepts/engine.md
deleted file mode 100644
index a113015ac6e77292e4e43779c2c498af12ea927c..0000000000000000000000000000000000000000
--- a/pipeline/mmocr/docs/en/basic_concepts/engine.md
+++ /dev/null
@@ -1,3 +0,0 @@
-# Engine\[coming soon\]
-
-Coming Soon!
diff --git a/pipeline/mmocr/docs/en/basic_concepts/evaluation.md b/pipeline/mmocr/docs/en/basic_concepts/evaluation.md
deleted file mode 100644
index ef477e967d646c6b000e44a75587440896d80490..0000000000000000000000000000000000000000
--- a/pipeline/mmocr/docs/en/basic_concepts/evaluation.md
+++ /dev/null
@@ -1,197 +0,0 @@
-# Evaluation
-
-```{note}
-Before reading this document, we recommend that you first read {external+mmengine:doc}`MMEngine: Model Accuracy Evaluation Basics `.
-```
-
-## Metrics
-
-MMOCR implements widely-used evaluation metrics for text detection, text recognition and key information extraction tasks based on the {external+mmengine:doc}`MMEngine: BaseMetric ` base class. Users can specify the metric used in the validation and test phases by modifying the `val_evaluator` and `test_evaluator` fields in the configuration file. For example, the following config shows how to use `HmeanIOUMetric` to evaluate the model performance in text detection task.
-
-```python
-val_evaluator = dict(type='HmeanIOUMetric')
-test_evaluator = val_evaluator
-
-# In addition, MMOCR also supports the combined evaluation of multiple metrics for the same task, such as using WordMetric and CharMetric at the same time
-val_evaluator = [
- dict(type='WordMetric', mode=['exact', 'ignore_case', 'ignore_case_symbol']),
- dict(type='CharMetric')
-]
-```
-
-```{tip}
-More evaluation related configurations can be found in the [evaluation configuration tutorial](../user_guides/config.md#evaluation-configuration).
-```
-
-As shown in the following table, MMOCR currently supports 5 evaluation metrics for text detection, text recognition, and key information extraction tasks, including `HmeanIOUMetric`, `WordMetric`, `CharMetric`, `OneMinusNEDMetric`, and `F1Metric`.
-
-| | | | |
-| --------------------------------------- | ------- | ------------------------------------------------- | --------------------------------------------------------------------- |
-| Metric | Task | Input Field | Output Field |
-| [HmeanIOUMetric](#hmeanioumetric) | TextDet | `pred_polygons` `pred_scores` `gt_polygons` | `recall` `precision` `hmean` |
-| [WordMetric](#wordmetric) | TextRec | `pred_text` `gt_text` | `word_acc` `word_acc_ignore_case` `word_acc_ignore_case_symbol` |
-| [CharMetric](#charmetric) | TextRec | `pred_text` `gt_text` | `char_recall` `char_precision` |
-| [OneMinusNEDMetric](#oneminusnedmetric) | TextRec | `pred_text` `gt_text` | `1-N.E.D` |
-| [F1Metric](#f1metric) | KIE | `pred_labels` `gt_labels` | `macro_f1` `micro_f1` |
-
-In general, the evaluation metric used in each task is conventionally determined. Users usually do not need to understand or manually modify the internal implementation of the evaluation metric. However, to facilitate more customized requirements, this document will further introduce the specific implementation details and configurable parameters of the built-in metrics in MMOCR.
-
-### HmeanIOUMetric
-
-[HmeanIOUMetric](mmocr.evaluation.metrics.hmean_iou_metric.HmeanIOUMetric) is one of the most widely used evaluation metrics in text detection tasks, because it calculates the harmonic mean (H-mean) between the detection precision (P) and recall rate (R). The `HmeanIOUMetric` can be calculated by the following equation:
-
-```{math}
-H = \frac{2}{\frac{1}{P} + \frac{1}{R}} = \frac{2PR}{P+R}
-```
-
-In addition, since it is equivalent to the F-score (also known as F-measure or F-metric) when {math}`\beta = 1`, `HmeanIOUMetric` is sometimes written as `F1Metric` or `f1-score`:
-
-```{math}
-F_1=(1+\beta^2)\cdot\frac{PR}{\beta^2\cdot P+R} = \frac{2PR}{P+R}
-```
-
-In MMOCR, the calculation of `HmeanIOUMetric` can be summarized as the following steps:
-
-1. Filter out invalid predictions
-
- - Filter out predictions with a score is lower than `pred_score_thrs`
- - Filter out predictions overlapping with `ignored` ground truth boxes with an overlap ratio higher than `ignore_precision_thr`
-
- It is worth noting that `pred_score_thrs` will **automatically search** for the **best threshold** within a certain range by default, and users can also customize the search range by manually modifying the configuration file:
-
- ```python
- # By default, HmeanIOUMetric searches the best threshold within the range [0.3, 0.9] with a step size of 0.1
- val_evaluator = dict(type='HmeanIOUMetric', pred_score_thrs=dict(start=0.3, stop=0.9, step=0.1))
- ```
-
-2. Calculate the IoU matrix
-
- - At the data processing stage, `HmeanIOUMetric` will calculate and maintain an {math}`M \times N` IoU matrix `iou_metric` for the convenience of the subsequent bounding box pairing step. Here, M and N represent the number of label bounding boxes and filtered prediction bounding boxes, respectively. Therefore, each element of this matrix stores the IoU between the m-th label bounding box and the n-th prediction bounding box.
-
-3. Compute the number of GT samples that can be accurately matched based on the corresponding pairing strategy
-
- Although `HmeanIOUMetric` can be calculated by a fixed formula, there may still be some subtle differences in the specific implementations. These differences mainly reflect the use of different strategies to match gt and predicted bounding boxes, which leads to the difference in final scores. Currently, MMOCR supports two matching strategies, namely `vanilla` and `max_matching`, for the `HmeanIOUMetric`. As shown below, users can specify the matching strategies in the config.
-
- - `vanilla` matching strategy
-
- By default, `HmeanIOUMetric` adopts the `vanilla` matching strategy, which is consistent with the `hmean-iou` implementation in MMOCR 0.x and the **official** text detection competition evaluation standard of ICDAR series. The matching strategy adopts the first-come-first-served matching method to pair the labels and predictions.
-
- ```python
- # By default, HmeanIOUMetric adopts 'vanilla' matching strategy
- val_evaluator = dict(type='HmeanIOUMetric')
- ```
-
- - `max_matching` matching strategy
-
- To address the shortcomings of the existing matching mechanism, MMOCR has implemented a more efficient matching strategy to maximize the number of matches.
-
- ```python
- # Specify to use 'max_matching' matching strategy
- val_evaluator = dict(type='HmeanIOUMetric', strategy='max_matching')
- ```
-
- ```{note}
- We recommend that research-oriented developers use the default `vanilla` matching strategy to ensure consistency with other papers. For industry-oriented developers, you can use the `max_matching` matching strategy to achieve optimized performance.
- ```
-
-4. Compute the final evaluation score according to the aforementioned matching strategy
-
-### WordMetric
-
-[WordMetric](mmocr.evaluation.metrics.recog_metric.WordMetric) implements **word-level** text recognition evaluation metrics and includes three text matching modes, namely `exact`, `ignore_case`, and `ignore_case_symbol`. Users can freely combine the output of one or more text matching modes in the configuration file by modifying the `mode` field.
-
-```python
-# Use WordMetric for text recognition task
-val_evaluator = [
- dict(type='WordMetric', mode=['exact', 'ignore_case', 'ignore_case_symbol'])
-]
-```
-
-- `exact`๏ผFull matching mode, i.e., only when the predicted text and the ground truth text are exactly the same, the predicted text is considered to be correct.
-- `ignore_case`๏ผThe mode ignores the case of the predicted text and the ground truth text.
-- `ignore_case_symbol`๏ผThe mode ignores the case and symbols of the predicted text and the ground truth text. This is also the text recognition accuracy reported by most academic papers. The performance reported by MMOCR uses the `ignore_case_symbol` mode by default.
-
-Assume that the real label is `MMOCR!` and the model output is `mmocr`. The `WordMetric` scores under the three matching modes are: `{'exact': 0, 'ignore_case': 0, 'ignore_case_symbol': 1}`.
-
-### CharMetric
-
-[CharMetric](mmocr.evaluation.metrics.recog_metric.CharMetric) implements **character-level** text recognition evaluation metrics that are **case-insensitive**.
-
-```python
-# Use CharMetric for text recognition task
-val_evaluator = [dict(type='CharMetric')]
-```
-
-Specifically, `CharMetric` will output two evaluation metrics, namely `char_precision` and `char_recall`. Let the number of correctly predicted characters (True Positive) be {math}`\sigma_{tp}`, then the precision *P* and recall *R* can be calculated by the following equation:
-
-```{math}
-P=\frac{\sigma_{tp}}{\sigma_{pred}}, R = \frac{\sigma_{tp}}{\sigma_{gt}}
-```
-
-where {math}`\sigma_{gt}` and {math}`\sigma_{pred}` represent the total number of characters in the label text and the predicted text, respectively.
-
-For example, assume that the label text is "MM**O**CR" and the predicted text is "mm**0**cR**1**". The score of the `CharMetric` is:
-
-```{math}
-P=\frac{4}{6}, R=\frac{4}{5}
-```
-
-### OneMinusNEDMetric
-
-[OneMinusNEDMetric(1-N.E.D)](mmocr.evaluation.metrics.recog_metric.OneMinusNEDMetric) is commonly used for text recognition evaluation of Chinese or English **text line-level** annotations. Unlike the full matching metric that requires the prediction and the gt text to be exactly the same, `1-N.E.D` uses the normalized [edit distance](https://en.wikipedia.org/wiki/Edit_distance) (also known as Levenshtein Distance) to measure the difference between the predicted and the gt text, so that the performance difference of the model can be better distinguished when evaluating long texts. Assume that the real and predicted texts are {math}`s_i` and {math}`\hat{s_i}`, respectively, and their lengths are {math}`l_{i}` and {math}`\hat{l_i}`, respectively. The `OneMinusNEDMetric` score can be calculated by the following formula:
-
-```{math}
-score = 1 - \frac{1}{N}\sum_{i=1}^{N}\frac{D(s_i, \hat{s_{i}})}{max(l_{i},\hat{l_{i}})}
-```
-
-where *N* is the total number of samples, and {math}`D(s_1, s_2)` is the edit distance between two strings.
-
-For example, assume that the real label is "OpenMMLabMMOCR", the prediction of model A is "0penMMLabMMOCR", and the prediction of model B is "uvwxyz". The results of the full matching and `OneMinusNEDMetric` evaluation metrics are as follows:
-
-| | | |
-| ------- | ---------- | ---------- |
-| | Full-match | 1 - N.E.D. |
-| Model A | 0 | 0.92857 |
-| Model B | 0 | 0 |
-
-As shown in the table above, although the model A only predicted one letter incorrectly, both models got 0 in when using full-match strategy. However, the `OneMinusNEDMetric` evaluation metric can better distinguish the performance of the two models on **long texts**.
-
-### F1Metric
-
-[F1Metric](mmocr.evaluation.metrics.f_metric.F1Metric) implements the F1-Metric evaluation metric for KIE tasks and provides two modes, namely `micro` and `macro`.
-
-```python
-val_evaluator = [
- dict(type='F1Metric', mode=['micro', 'macro'],
-]
-```
-
-- `micro` mode: Calculate the global F1-Metric score based on the total number of True Positive, False Negative, and False Positive.
-
-- `macro` mode๏ผCalculate the F1-Metric score for each class and then take the average.
-
-### Customized Metric
-
-MMOCR supports the implementation of customized evaluation metrics for users who pursue higher customization. In general, users only need to create a customized evaluation metric class `CustomizedMetric` and inherit {external+mmengine:doc}`MMEngine: BaseMetric `. Then, the data format processing method `process` and the metric calculation method `compute_metrics` need to be overwritten respectively. Finally, add it to the `METRICS` registry to implement any customized evaluation metric.
-
-```python
-from mmengine.evaluator import BaseMetric
-from mmocr.registry import METRICS
-
-@METRICS.register_module()
-class CustomizedMetric(BaseMetric):
-
- def process(self, data_batch: Sequence[Dict], predictions: Sequence[Dict]):
- """ process receives two parameters, data_batch stores the gt label information, and predictions stores the predicted results.
- """
- pass
-
- def compute_metrics(self, results: List):
- """ compute_metric receives the results of the process method as input and returns the evaluation results.
- """
- pass
-```
-
-```{note}
-More details can be found in {external+mmengine:doc}`MMEngine Documentation: BaseMetric `.
-```
diff --git a/pipeline/mmocr/docs/en/basic_concepts/models.md b/pipeline/mmocr/docs/en/basic_concepts/models.md
deleted file mode 100644
index 7eab561e7276af01c63ca7ae8c1452c5c6317c25..0000000000000000000000000000000000000000
--- a/pipeline/mmocr/docs/en/basic_concepts/models.md
+++ /dev/null
@@ -1,3 +0,0 @@
-# Models\[coming soon\]
-
-Coming Soon!
diff --git a/pipeline/mmocr/docs/en/basic_concepts/overview.md b/pipeline/mmocr/docs/en/basic_concepts/overview.md
deleted file mode 100644
index 9e31fefa5fc8cc9e7f86be30b18f0b62aa1c85d5..0000000000000000000000000000000000000000
--- a/pipeline/mmocr/docs/en/basic_concepts/overview.md
+++ /dev/null
@@ -1,3 +0,0 @@
-# Overview & Features\[coming soon\]
-
-Coming Soon!
diff --git a/pipeline/mmocr/docs/en/basic_concepts/structures.md b/pipeline/mmocr/docs/en/basic_concepts/structures.md
deleted file mode 100644
index 0f73a77286457a4f1360fcb8cd83353202ea786d..0000000000000000000000000000000000000000
--- a/pipeline/mmocr/docs/en/basic_concepts/structures.md
+++ /dev/null
@@ -1,219 +0,0 @@
-# Data Structures and Elements
-
-MMOCR uses {external+mmengine:doc}`MMEngine: Abstract Data Element ` to encapsulate the data required for each task into `data_sample`. The base class has implemented basic add/delete/update/check functions and supports data migration between different devices, as well as dictionary-like and tensor-like operations, which also allows the interfaces of different algorithms to be unified.
-
-Thanks to the unified data structures, the data flow between each module in the algorithm libraries, such as [`visualizer`](./visualizers.md), [`evaluator`](./evaluation.md), [`dataset`](./datasets.md), is greatly simplified. In MMOCR, we have the following conventions for different data types.
-
-- **xxxData**: Single granularity data annotation or model output. Currently MMEngine has three built-in granularities of {external+mmengine:doc}`data elements `, including instance-level data (`InstanceData`), pixel-level data (`PixelData`) and image-level label data (`LabelData`). Among the tasks currently supported by MMOCR, text detection and key information extraction tasks use `InstanceData` to encapsulate the bounding boxes and the corresponding box label, while the text recognition task uses `LabelData` to encapsulate the text content.
-- **xxxDataSample**: inherited from {external+mmengine:doc}`MMEngine: Base Data Element `, used to hold **all** annotation and prediction information that required by a single task. For example, [`TextDetDataSample`](mmocr.structures.textdet_data_sample.TextDetDataSample) for the text detection, [`TextRecogDataSample`](mmocr.structures.textrecog_data_sample.TextRecogDataSample) for text recognition, and [`KIEDataSample`](mmocr.structures.kie_data_sample.KIEDataSample) for the key information extraction task.
-
-In the following, we will introduce the practical application of data elements **xxxData** and data samples **xxxDataSample** in MMOCR, respectively.
-
-## Data Elements - xxxData
-
-`InstanceData` and `LabelData` are the `BaseDataElement` defined in `MMEngine` to encapsulate different granularity of annotation data or model output. In MMOCR, we have used `InstanceData` and `LabelData` for encapsulating the data types actually used in OCR-related tasks.
-
-### InstanceData
-
-In the **text detection** task, the detector concentrate on instance-level text samples, so we use `InstanceData` to encapsulate the data needed for this task. Typically, its required training annotation and prediction output contain rectangular or polygonal bounding boxes, as well as bounding box labels. Since the text detection task has only one positive sample class, "text", in MMOCR we use `0` to number this class by default. The following code example shows how to use the `InstanceData` to encapsulate the data used in the text detection task.
-
-```python
-import torch
-from mmengine.structures import InstanceData
-
-# defining gt_instance for encapsulating the ground truth data
-gt_instance = InstanceData()
-gt_instance.bbox = torch.Tensor([[0, 0, 10, 10], [10, 10, 20, 20]])
-gt_instance.polygons = torch.Tensor([[[0, 0], [10, 0], [10, 10], [0, 10]],
- [[10, 10], [20, 10], [20, 20], [10, 20]]])
-gt_instance.label = torch.Tensor([0, 0])
-
-# defining pred_instance for encapsulating the prediction data
-pred_instances = InstanceData()
-pred_polygons, scores = model(input)
-pred_instances.polygons = pred_polygons
-pred_instances.scores = scores
-```
-
-The conventions for the fields in `InstanceData` in MMOCR are shown in the table below. It is important to note that the length of each field in `InstanceData` must be equal to the number of instances `N` in the sample.
-
-| | | |
-| ----------- | ---------------------------------- | ----------------------------------------------------------------------------------------------------------------------------------------------------------- |
-| Field | Type | Description |
-| bboxes | `torch.FloatTensor` | Bounding boxes `[x1, y1, x2, y2]` with the shape `(N, 4)`. |
-| labels | `torch.LongTensor` | Instance label with the shape `(N, )`. By default, MMOCR uses `0` to represent the "text" class. |
-| polygons | `list[np.array(dtype=np.float32)]` | Polygonal bounding boxes with the shape `(N, )`. |
-| scores | `torch.Tensor` | Confidence scores of the predictions of bounding boxes. `(N, )`. |
-| ignored | `torch.BoolTensor` | Whether to ignore the current sample with the shape `(N, )`. |
-| texts | `list[str]` | The text content of each instance with the shape `(N, )`๏ผused for e2e text spotting or KIE task. |
-| text_scores | `torch.FloatTensor` | Confidence score of the predictions of text contents with the shape `(N, )`๏ผused for e2e text spotting task. |
-| edge_labels | `torch.IntTensor` | The node adjacency matrix with the shape `(N, N)`. In KIE, the optional values for the state between nodes are `-1` (ignored, not involved in loss calculation)๏ผ`0` (disconnected) and `1`(connected). |
-| edge_scores | `torch.FloatTensor` | The prediction confidence of each edge in the KIE task, with the shape `(N, N)`. |
-
-### LabelData
-
-For **text recognition** tasks, both labeled content and predicted content are wrapped using `LabelData`.
-
-```python
-import torch
-from mmengine.data import LabelData
-
-# defining gt_text for encapsulating the ground truth data
-gt_text = LabelData()
-gt_text.item = 'MMOCR'
-
-# defining pred_text for encapsulating the prediction data
-pred_text = LabelData()
-index, score = model(input)
-text = dictionary.idx2str(index)
-pred_text.score = score
-pred_text.item = text
-```
-
-The conventions for the `LabelData` fields in MMOCR are shown in the following table.
-
-| | | |
-| -------------- | ------------------ | ------------------------------------------------------------------------------------------------------------------------------------------------------------------------ |
-| Field | Type | Description |
-| item | `str` | Text content. |
-| score | `list[float]` | Confidence socre of the predicted text. |
-| indexes | `torch.LongTensor` | A sequence of text characters encoded by [dictionary](../basic_concepts/models.md#dictionary) and containing all special characters except ``. |
-| padded_indexes | `torch.LongTensor` | If the length of indexes is less than the maximum sequence length and `pad_idx` exists, this field holds the encoded text sequence padded to the maximum sequence length of `max_seq_len`. |
-
-## DataSample xxxDataSample
-
-By defining a uniform data structure, we can easily encapsulate the annotation data and prediction results in a unified way, making data transfer between different modules of the code base easier. In MMOCR, we have designed three data structures based on the data needed in three tasks: [`TextDetDataSample`](mmocr.structures.textdet_data_sample.TextDetDataSample), [`TextRecogDataSample`](mmocr.structures.textrecog_data_sample.TextRecogDataSample), and [`KIEDataSample`](mmocr.structures.kie_data_sample.KIEDataSample). These data structures all inherit from {external+mmengine:doc}`MMEngine: Base Data Element `, which is used to hold all annotation and prediction information required by each task.
-
-### Text Detection - TextDetDataSample
-
-[TextDetDataSample](mmocr.structures.textdet_data_sample.TextDetDataSample) is used to encapsulate the data needed for the text detection task. It contains two main fields `gt_instances` and `pred_instances`, which are used to store the annotation information and prediction results respectively.
-
-| | | |
-| -------------- | ------------------------------- | ----------------------- |
-| Field | Type | Description |
-| gt_instances | [`InstanceData`](#instancedata) | Annotation information. |
-| pred_instances | [`InstanceData`](#instancedata) | Prediction results. |
-
-The fields of [`InstanceData`](#instancedata) that will be used are:
-
-| | | |
-| -------- | ---------------------------------- | ------------------------------------------------------------------------------------------------ |
-| Field | Type | Description |
-| bboxes | `torch.FloatTensor` | Bounding boxes `[x1, y1, x2, y2]` with the shape `(N, 4)`. |
-| labels | `torch.LongTensor` | Instance label with the shape `(N, )`. By default, MMOCR uses `0` to represent the "text" class. |
-| polygons | `list[np.array(dtype=np.float32)]` | Polygonal bounding boxes with the shape `(N, )`. |
-| scores | `torch.Tensor` | Confidence scores of the predictions of bounding boxes. `(N, )`. |
-| ignored | `torch.BoolTensor` | Boolean flags with the shape `(N, )`, indicating whether to ignore the current sample. |
-
-Since text detection models usually only output one of the bboxes/polygons, we only need to make sure that one of these two is assigned a value.
-
-The following sample code demonstrates the use of `TextDetDataSample`.
-
-```python
-import torch
-from mmengine.data import TextDetDataSample
-
-data_sample = TextDetDataSample()
-# Define the ground truth data
-img_meta = dict(img_shape=(800, 1196, 3), pad_shape=(800, 1216, 3))
-gt_instances = InstanceData(metainfo=img_meta)
-gt_instances.bboxes = torch.rand((5, 4))
-gt_instances.labels = torch.zeros((5,), dtype=torch.long)
-data_sample.gt_instances = gt_instances
-
-# Define the prediction data
-pred_instances = InstanceData()
-pred_instances.bboxes = torch.rand((5, 4))
-pred_instances.labels = torch.zeros((5,), dtype=torch.long)
-data_sample.pred_instances = pred_instances
-```
-
-### Text Recognition - TextRecogDataSample
-
-[`TextRecogDataSample`](mmocr.structures.textrecog_data_sample.TextRecogDataSample) is used to encapsulate the data for the text recognition task. It has two fields, `gt_text` and `pred_text` , which are used to store annotation information and prediction results, respectively.
-
-| | | |
-| --------- | ------------------------------------------ | ------------------- |
-| Field | Type | Description |
-| gt_text | [`LabelData`](#text-recognition-labeldata) | Label information. |
-| pred_text | [`LabelData`](#text-recognition-labeldata) | Prediction results. |
-
-The following sample code demonstrates the use of [`TextRecogDataSample`](mmocr.structures.textrecog_data_sample.TextRecogDataSample).
-
-```python
-import torch
-from mmengine.data import TextRecogDataSample
-
-data_sample = TextRecogDataSample()
-# Define the ground truth data
-img_meta = dict(img_shape=(800, 1196, 3), pad_shape=(800, 1216, 3))
-gt_text = LabelData(metainfo=img_meta)
-gt_text.item = 'mmocr'
-data_sample.gt_text = gt_text
-
-# Define the prediction data
-pred_text = LabelData(metainfo=img_meta)
-pred_text.item = 'mmocr'
-data_sample.pred_text = pred_text
-```
-
-The fields of `LabelData` that will be used are:
-
-| | | |
-| -------------- | ------------------- | ----------------------------------------------------------------------------------------------------------------------------------------------------------------------- |
-| Field | Type | Description |
-| item | `list[str]` | The text corresponding to the instance, of length (N, ), for end-to-end OCR tasks and KIE |
-| score | `torch.FloatTensor` | Confidence of the text prediction, of length (N, ), for the end-to-end OCR task |
-| indexes | `torch.LongTensor` | A sequence of text characters encoded by [dictionary](../basic_concepts/models.md#dictionary) and containing all special characters except ``. |
-| padded_indexes | `torch.LongTensor` | If the length of indexes is less than the maximum sequence length and `pad_idx` exists, this field holds the encoded text sequence padded to the maximum sequence length of `max_seq_len`. |
-
-### Key Information Extraction - KIEDataSample
-
-[`KIEDataSample`](mmocr.structures.kie_data_sample.KIEDataSample) is used to encapsulate the data needed for the KIE task. It also contains two fields, `gt_instances` and `pred_instances`, which are used to store annotation information and prediction results respectively.
-
-| | | |
-| -------------- | ---------------------------------------------- | ----------------------- |
-| Field | Type | Description |
-| gt_instances | [`InstanceData`](#text-detection-instancedata) | Annotation information. |
-| pred_instances | [`InstanceData`](#text-detection-instancedata) | Prediction results. |
-
-The [`InstanceData`](#text-detection-instancedata) fields that will be used by this task are shown in the following table.
-
-| | | |
-| ----------- | ------------------- | -------------------------------------------------------------------------------------------------------------------------------------------------------------------------- |
-| Field | Type | Description |
-| bboxes | `torch.FloatTensor` | Bounding boxes `[x1, y1, x2, y2]` with the shape `(N, 4)`. |
-| labels | `torch.LongTensor` | Instance label with the shape `(N, )`. |
-| texts | `list[str]` | The text content of each instance with the shape `(N, )`๏ผused for e2e text spotting or KIE task. |
-| edge_labels | `torch.IntTensor` | The node adjacency matrix with the shape `(N, N)`. In the KIE task, the optional values for the state between nodes are `-1` (ignored, not involved in loss calculation)๏ผ`0` (disconnected) and `1`(connected). |
-| edge_scores | `torch.FloatTensor` | The prediction confidence of each edge in the KIE task, with the shape `(N, N)`. |
-| scores | `torch.FloatTensor` | The confidence scores for node label predictions, with the shape `(N,)`. |
-
-```{warning}
-Since there is no unified standard for model implementation of KIE tasks, the design currently considers only [SDMGR](../../../configs/kie/sdmgr/README.md) model usage scenarios. Therefore, the design is subject to change as we support more KIE models.
-```
-
-The following sample code shows the use of [`KIEDataSample`](mmocr.structures.kie_data_sample.KIEDataSample).
-
-```python
-import torch
-from mmengine.data import KIEDataSample
-
-data_sample = KIEDataSample()
-# Define the ground truth data
-img_meta = dict(img_shape=(800, 1196, 3),pad_shape=(800, 1216, 3))
-gt_instances = InstanceData(metainfo=img_meta)
-gt_instances.bboxes = torch.rand((5, 4))
-gt_instances.labels = torch.zeros((5,), dtype=torch.long)
-gt_instances.texts = ['text1', 'text2', 'text3', 'text4', 'text5']
-gt_instances.edge_lebels = torch.randint(-1, 2, (5, 5))
-data_sample.gt_instances = gt_instances
-
-# Define the prediction data
-pred_instances = InstanceData()
-pred_instances.bboxes = torch.rand((5, 4))
-pred_instances.labels = torch.rand((5,))
-pred_instances.edge_labels = torch.randint(-1, 2, (10, 10))
-pred_instances.edge_scores = torch.rand((10, 10))
-data_sample.pred_instances = pred_instances
-```
diff --git a/pipeline/mmocr/docs/en/basic_concepts/transforms.md b/pipeline/mmocr/docs/en/basic_concepts/transforms.md
deleted file mode 100644
index 0a19208156b4b6b0e7ad3b7eaf9014e09e586d00..0000000000000000000000000000000000000000
--- a/pipeline/mmocr/docs/en/basic_concepts/transforms.md
+++ /dev/null
@@ -1,226 +0,0 @@
-# Data Transforms and Pipeline
-
-In the design of MMOCR, dataset construction and preparation are decoupled. That is, dataset construction classes such as [`OCRDataset`](mmocr.datasets.ocr_dataset.OCRDataset) are responsible for loading and parsing annotation files; while data transforms further apply data preprocessing, augmentation, formatting, and other related functions. Currently, there are five types of data transforms implemented in MMOCR, as shown in the following table.
-
-| | | |
-| -------------------------------- | --------------------------------------------------------------------- | --------------------------------------------------------------------------------------------------- |
-| Transforms Type | File | Description |
-| Data Loading | loading.py | Implemented the data loading functions. |
-| Data Formatting | formatting.py | Formatting the data required by different tasks. |
-| Cross Project Data Adapter | adapters.py | Converting the data format between other OpenMMLab projects and MMOCR. |
-| Data Augmentation Functions | ocr_transforms.py textdet_transforms.py textrecog_transforms.py | Various built-in data augmentation methods designed for different tasks. |
-| Wrappers of Third Party Packages | wrappers.py | Wrapping the transforms implemented in popular third party packages such as [ImgAug](https://github.com/aleju/imgaug), and adapting them to MMOCR format. |
-
-Since each data transform class is independent of each other, we can easily combine any data transforms to build a data pipeline after we have defined the data fields. As shown in the following figure, in MMOCR, a typical training data pipeline consists of three stages: **data loading**, **data augmentation**, and **data formatting**. Users only need to define the data pipeline list in the configuration file and specify the specific data transform class and its parameters:
-
-
-
-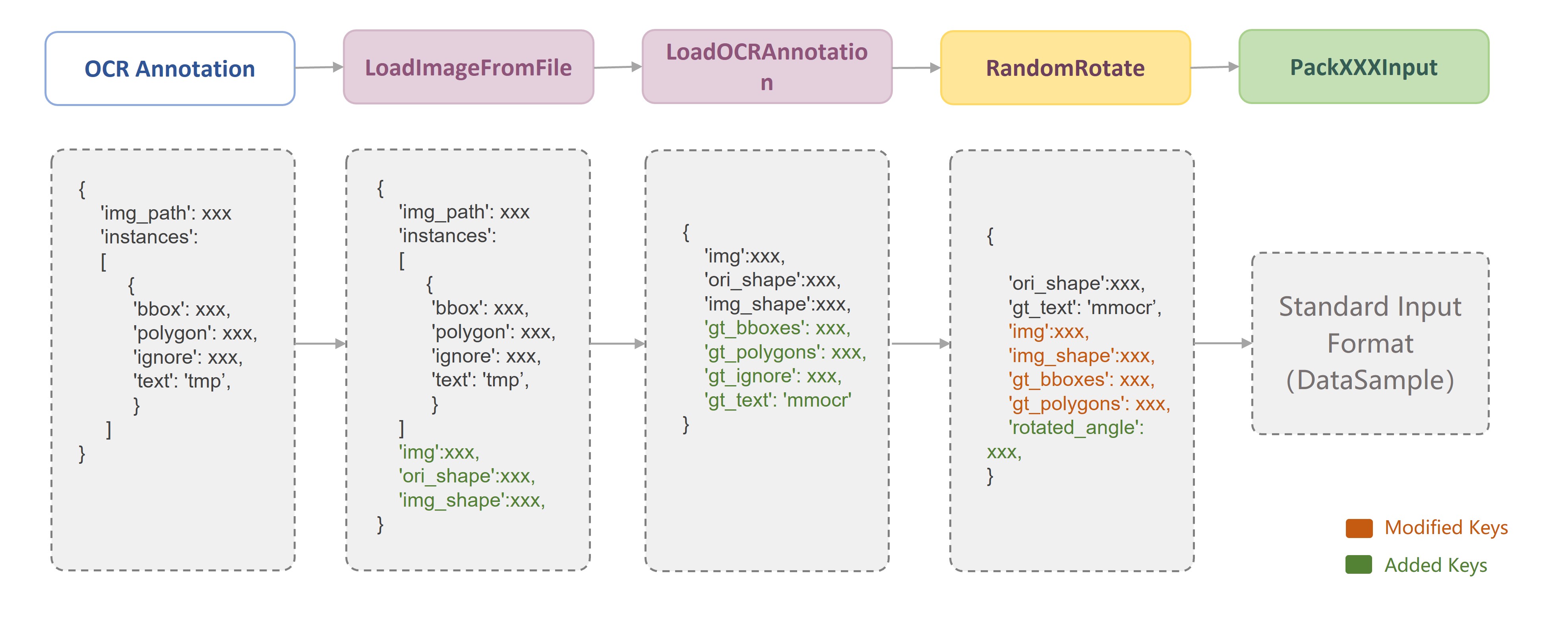
-
-
-
-```python
-train_pipeline_r18 = [
- # Loading images
- dict(
- type='LoadImageFromFile',
- color_type='color_ignore_orientation'),
- # Loading annotations
- dict(
- type='LoadOCRAnnotations',
- with_polygon=True,
- with_bbox=True,
- with_label=True,
- ),
- # Data augmentation
- dict(
- type='ImgAugWrapper',
- args=[['Fliplr', 0.5],
- dict(cls='Affine', rotate=[-10, 10]), ['Resize', [0.5, 3.0]]]),
- dict(type='RandomCrop', min_side_ratio=0.1),
- dict(type='Resize', scale=(640, 640), keep_ratio=True),
- dict(type='Pad', size=(640, 640)),
- # Data formatting
- dict(
- type='PackTextDetInputs',
- meta_keys=('img_path', 'ori_shape', 'img_shape'))
-]
-```
-
-```{tip}
-More tutorials about data pipeline configuration can be found in the [Config Doc](../user_guides/config.md#data-pipeline-configuration). Next, we will briefly introduce the data transforms supported in MMOCR according to their categories.
-```
-
-For each data transform, MMOCR provides a detailed docstring. For example, in the header of each data transform class, we annotate `Required Keys`, `Modified Keys` and `Added Keys`. The `Required Keys` represent the mandatory fields that should be included in the input required by the data transform, while the `Modified Keys` and `Added Keys` indicate that the transform may modify or add the fields into the original data. For example, `LoadImageFromFile` implements the image loading function, whose `Required Keys` is the image path `img_path`, and the `Modified Keys` includes the loaded image `img`, the current size of the image `img_shape`, the original size of the image `ori_shape`, and other image attributes.
-
-```python
-@TRANSFORMS.register_module()
-class LoadImageFromFile(MMCV_LoadImageFromFile):
- # We provide detailed docstring for each data transform.
- """Load an image from file.
-
- Required Keys:
-
- - img_path
-
- Modified Keys:
-
- - img
- - img_shape
- - ori_shape
- """
-```
-
-```{note}
-In the data pipeline of MMOCR, the image and label information are saved in a dictionary. By using the unified fields, the data can be freely transferred between different data transforms. Therefore, it is very important to understand the conventional fields used in MMOCR.
-```
-
-For your convenience, the following table lists the conventional keys used in MMOCR data transforms.
-
-| | | |
-| ---------------- | --------------------------------- | ------------------------------------------------------------------------------------------------------------------------------------------------------- |
-| Key | Type | Description |
-| img | `np.array(dtype=np.uint8)` | Image array, shape of `(h, w, c)`. |
-| img_shape | `tuple(int, int)` | Current image size `(h, w)`. |
-| ori_shape | `tuple(int, int)` | Original image size `(h, w)`. |
-| scale | `tuple(int, int)` | Stores the target image size `(h, w)` specified by the user in the `Resize` data transform series. Note: This value may not correspond to the actual image size after the transformation. |
-| scale_factor | `tuple(float, float)` | Stores the target image scale factor `(w_scale, h_scale)` specified by the user in the `Resize` data transform series. Note: This value may not correspond to the actual image size after the transformation. |
-| keep_ratio | `bool` | Boolean flag determines whether to keep the aspect ratio while scaling images. |
-| flip | `bool` | Boolean flags to indicate whether the image has been flipped. |
-| flip_direction | `str` | Flipping direction, options are `horizontal`, `vertical`, `diagonal`. |
-| gt_bboxes | `np.array(dtype=np.float32)` | Ground-truth bounding boxes. |
-| gt_polygons | `list[np.array(dtype=np.float32)` | Ground-truth polygons. |
-| gt_bboxes_labels | `np.array(dtype=np.int64)` | Category label of bounding boxes. By default, MMOCR uses `0` to represent "text" instances. |
-| gt_texts | `list[str]` | Ground-truth text content of the instance. |
-| gt_ignored | `np.array(dtype=np.bool_)` | Boolean flag indicating whether ignoring the instance (used in text detection). |
-
-## Data Loading
-
-Data loading transforms mainly implement the functions of loading data from different formats and backends. Currently, the following data loading transforms are implemented in MMOCR:
-
-| | | | |
-| ------------------ | --------------------------------------------------------- | -------------------------------------------------------------- | --------------------------------------------------------------- |
-| Transforms Name | Required Keys | Modified/Added Keys | Description |
-| LoadImageFromFile | `img_path` | `img` `img_shape` `ori_shape` | Load image from the specified path๏ผsupporting different file storage backends (e.g. `disk`, `http`, `petrel`) and decoding backends (e.g. `cv2`, `turbojpeg`, `pillow`, `tifffile`). |
-| LoadOCRAnnotations | `bbox` `bbox_label` `polygon` `ignore` `text` | `gt_bboxes` `gt_bboxes_labels` `gt_polygons` `gt_ignored` `gt_texts` | Parse the annotation required by OCR task. |
-| LoadKIEAnnotations | `bboxes` `bbox_labels` `edge_labels` `texts` | `gt_bboxes` `gt_bboxes_labels` `gt_edge_labels` `gt_texts` `ori_shape` | Parse the annotation required by KIE task. |
-
-## Data Augmentation
-
-Data augmentation is an indispensable process in text detection and recognition tasks. Currently, MMOCR has implemented dozens of data augmentation modules commonly used in OCR fields, which are classified into [ocr_transforms.py](/mmocr/datasets/transforms/ocr_transforms.py), [textdet_transforms.py](/mmocr/datasets/transforms/textdet_transforms.py), and [textrecog_transforms.py](/mmocr/datasets/transforms/textrecog_transforms.py).
-
-Specifically, `ocr_transforms.py` implements generic OCR data augmentation modules such as `RandomCrop` and `RandomRotate`:
-
-| | | | |
-| --------------- | ------------------------------------------------------------- | -------------------------------------------------------------- | -------------------------------------------------------------- |
-| Transforms Name | Required Keys | Modified/Added Keys | Description |
-| RandomCrop | `img` `gt_bboxes` `gt_bboxes_labels` `gt_polygons` `gt_ignored` `gt_texts` (optional) | `img` `img_shape` `gt_bboxes` `gt_bboxes_labels` `gt_polygons` `gt_ignored` `gt_texts` (optional) | Randomly crop the image and make sure the cropped image contains at least one text instance. The optional parameter is `min_side_ratio`, which controls the ratio of the short side of the cropped image to the original image, the default value is `0.4`. |
-| RandomRotate | `img` `img_shape` `gt_bboxes` (optional) `gt_polygons` (optional) | `img` `img_shape` `gt_bboxes` (optional) `gt_polygons` (optional) `rotated_angle` | Randomly rotate the image and optionally fill the blank areas of the rotated image. |
-| | | | |
-
-`textdet_transforms.py` implements text detection related data augmentation modules:
-
-| | | | |
-| ----------------- | ------------------------------------- | ------------------------------------------------------------------- | ------------------------------------------------------------------------------- |
-| Transforms Name | Required Keys | Modified/Added Keys | Description |
-| RandomFlip | `img` `gt_bboxes` `gt_polygons` | `img` `gt_bboxes` `gt_polygons` `flip` `flip_direction` | Random flip, support `horizontal`, `vertical` and `diagonal` modes. Defaults to `horizontal`. |
-| FixInvalidPolygon | `gt_polygons` `gt_ignored` | `gt_polygons` `gt_ignored` | Automatically fixing the invalid polygons included in the annotations. |
-
-`textrecog_transforms.py` implements text recognition related data augmentation modules:
-
-| | | | |
-| --------------- | ------------- | ----------------------------------------------------------------- | ----------------------------------------------------------------------------------------------------------- |
-| Transforms Name | Required Keys | Modified/Added Keys | Description |
-| RescaleToHeight | `img` | `img` `img_shape` `scale` `scale_factor` `keep_ratio` | Scales the image to the specified height while keeping the aspect ratio. When `min_width` and `max_width` are specified, the aspect ratio may be changed. |
-| | | | |
-
-```{warning}
-The above table only briefly introduces some selected data augmentation methods, for more information please refer to the [API documentation](../api.rst) or the code docstrings.
-```
-
-## Data Formatting
-
-Data formatting transforms are responsible for packaging images, ground truth labels, and other information into a dictionary. Different tasks usually rely on different formatting transforms. For example:
-
-| | | | |
-| ------------------- | ------------- | ------------------- | --------------------------------------------- |
-| Transforms Name | Required Keys | Modified/Added Keys | Description |
-| PackTextDetInputs | - | - | Pack the inputs required by text detection. |
-| PackTextRecogInputs | - | - | Pack the inputs required by text recognition. |
-| PackKIEInputs | - | - | Pack the inputs required by KIE. |
-
-## Cross Project Data Adapters
-
-The cross-project data adapters bridge the data formats between MMOCR and other OpenMMLab libraries such as [MMDetection](https://github.com/open-mmlab/mmdetection), making it possible to call models implemented in other OpenMMLab projects. Currently, MMOCR has implemented [`MMDet2MMOCR`](mmocr.datasets.transforms.MMDet2MMOCR) and [`MMOCR2MMDet`](mmocr.datasets.transforms.MMOCR2MMDet), allowing data to be converted between MMDetection and MMOCR formats; with these adapters, users can easily train any detectors supported by MMDetection in MMOCR. For example, we provide a [tutorial](#todo) to show how to train Mask R-CNN as a text detector in MMOCR.
-
-| | | | |
-| --------------- | -------------------------------------------- | ----------------------------- | ------------------------------------------ |
-| Transforms Name | Required Keys | Modified/Added Keys | Description |
-| MMDet2MMOCR | `gt_masks` `gt_ignore_flags` | `gt_polygons` `gt_ignored` | Convert the fields used in MMDet to MMOCR. |
-| MMOCR2MMDet | `img_shape` `gt_polygons` `gt_ignored` | `gt_masks` `gt_ignore_flags` | Convert the fields used in MMOCR to MMDet. |
-
-## Wrappers
-
-To facilitate the use of popular third-party CV libraries in MMOCR, we provide wrappers in `wrappers.py` to unify the data format between MMOCR and other third-party libraries. Users can directly configure the data transforms provided by these libraries in the configuration file of MMOCR. The supported wrappers are as follows:
-
-| | | | |
-| ------------------ | ------------------------------------------------------------ | ------------------------------------------------------------- | ------------------------------------------------------------- |
-| Transforms Name | Required Keys | Modified/Added Keys | Description |
-| ImgAugWrapper | `img` `gt_polygons` (optional for text recognition) `gt_bboxes` (optional for text recognition) `gt_bboxes_labels` (optional for text recognition) `gt_ignored` (optional for text recognition) `gt_texts` (optional) | `img` `gt_polygons` (optional for text recognition) `gt_bboxes` (optional for text recognition) `gt_bboxes_labels` (optional for text recognition) `gt_ignored` (optional for text recognition) `img_shape` (optional) `gt_texts` (optional) | [ImgAug](https://github.com/aleju/imgaug) wrapper, which bridges the data format and configuration between ImgAug and MMOCR, allowing users to config the data augmentation methods supported by ImgAug in MMOCR. |
-| TorchVisionWrapper | `img` | `img` `img_shape` | [TorchVision](https://github.com/pytorch/vision) wrapper, which bridges the data format and configuration between TorchVision and MMOCR, allowing users to config the data transforms supported by `torchvision.transforms` in MMOCR. |
-
-### `ImgAugWrapper` Example
-
-For example, in the original ImgAug, we can define a `Sequential` type data augmentation pipeline as follows to perform random flipping, random rotation and random scaling on the image:
-
-```python
-import imgaug.augmenters as iaa
-
-aug = iaa.Sequential(
- iaa.Fliplr(0.5), # horizontally flip 50% of all images
- iaa.Affine(rotate=(-10, 10)), # rotate by -10 to +10 degrees
- iaa.Resize((0.5, 3.0)) # scale images to 50-300% of their size
-)
-```
-
-In MMOCR, we can directly configure the above data augmentation pipeline in `train_pipeline` as follows:
-
-```python
-dict(
- type='ImgAugWrapper',
- args=[
- ['Fliplr', 0.5],
- dict(cls='Affine', rotate=[-10, 10]),
- ['Resize', [0.5, 3.0]],
- ]
-)
-```
-
-Specifically, the `args` parameter accepts a list, and each element in the list can be a list or a dictionary. If it is a list, the first element of the list is the class name in `imgaug.augmenters`, and the following elements are the initialization parameters of the class; if it is a dictionary, the `cls` key corresponds to the class name in `imgaug.augmenters`, and the other key-value pairs correspond to the initialization parameters of the class.
-
-### `TorchVisionWrapper` Example
-
-For example, in the original TorchVision, we can define a `Compose` type data transformation pipeline as follows to perform color jittering on the image:
-
-```python
-import torchvision.transforms as transforms
-
-aug = transforms.Compose([
- transforms.ColorJitter(
- brightness=32.0 / 255, # brightness jittering range
- saturation=0.5) # saturation jittering range
-])
-```
-
-In MMOCR, we can directly configure the above data transformation pipeline in `train_pipeline` as follows:
-
-```python
-dict(
- type='TorchVisionWrapper',
- op='ColorJitter',
- brightness=32.0 / 255,
- saturation=0.5
-)
-```
-
-Specifically, the `op` parameter is the class name in `torchvision.transforms`, and the following parameters correspond to the initialization parameters of the class.
diff --git a/pipeline/mmocr/docs/en/basic_concepts/visualizers.md b/pipeline/mmocr/docs/en/basic_concepts/visualizers.md
deleted file mode 100644
index bf620e1b7f531a8638242bbea7879f0d4430536f..0000000000000000000000000000000000000000
--- a/pipeline/mmocr/docs/en/basic_concepts/visualizers.md
+++ /dev/null
@@ -1,3 +0,0 @@
-# Visualizers\[coming soon\]
-
-Coming Soon!
diff --git a/pipeline/mmocr/docs/en/conf.py b/pipeline/mmocr/docs/en/conf.py
deleted file mode 100644
index b406fa6debf1ab5e0a98d0f0d51eef1a8461830e..0000000000000000000000000000000000000000
--- a/pipeline/mmocr/docs/en/conf.py
+++ /dev/null
@@ -1,176 +0,0 @@
-# Copyright (c) OpenMMLab. All rights reserved.
-# Configuration file for the Sphinx documentation builder.
-#
-# This file only contains a selection of the most common options. For a full
-# list see the documentation:
-# https://www.sphinx-doc.org/en/master/usage/configuration.html
-
-# -- Path setup --------------------------------------------------------------
-
-# If extensions (or modules to document with autodoc) are in another directory,
-# add these directories to sys.path here. If the directory is relative to the
-# documentation root, use os.path.abspath to make it absolute, like shown here.
-
-import os
-import subprocess
-import sys
-
-import pytorch_sphinx_theme
-
-sys.path.insert(0, os.path.abspath('../../'))
-
-# -- Project information -----------------------------------------------------
-
-project = 'MMOCR'
-copyright = '2020-2030, OpenMMLab'
-author = 'OpenMMLab'
-
-# The full version, including alpha/beta/rc tags
-version_file = '../../mmocr/version.py'
-with open(version_file) as f:
- exec(compile(f.read(), version_file, 'exec'))
-__version__ = locals()['__version__']
-release = __version__
-
-# -- General configuration ---------------------------------------------------
-
-# Add any Sphinx extension module names here, as strings. They can be
-# extensions coming with Sphinx (named 'sphinx.ext.*') or your custom
-# ones.
-extensions = [
- 'sphinx.ext.autodoc',
- 'sphinx.ext.napoleon',
- 'sphinx.ext.viewcode',
- 'sphinx_markdown_tables',
- 'sphinx_copybutton',
- 'myst_parser',
- 'sphinx.ext.intersphinx',
- 'sphinx.ext.autodoc.typehints',
- 'sphinx.ext.autosummary',
- 'sphinx.ext.autosectionlabel',
- 'sphinx_tabs.tabs',
-]
-autodoc_typehints = 'description'
-autodoc_mock_imports = ['mmcv._ext']
-autosummary_generate = True # Turn on sphinx.ext.autosummary
-
-# Ignore >>> when copying code
-copybutton_prompt_text = r'>>> |\.\.\. '
-copybutton_prompt_is_regexp = True
-
-myst_enable_extensions = ['colon_fence']
-
-# Add any paths that contain templates here, relative to this directory.
-templates_path = ['_templates']
-
-# The suffix(es) of source filenames.
-# You can specify multiple suffix as a list of string:
-#
-source_suffix = {
- '.rst': 'restructuredtext',
- '.md': 'markdown',
-}
-
-# The master toctree document.
-master_doc = 'index'
-
-# List of patterns, relative to source directory, that match files and
-# directories to ignore when looking for source files.
-# This pattern also affects html_static_path and html_extra_path.
-exclude_patterns = ['_build', 'Thumbs.db', '.DS_Store']
-
-# -- Options for HTML output -------------------------------------------------
-
-# The theme to use for HTML and HTML Help pages. See the documentation for
-# a list of builtin themes.
-#
-# html_theme = 'sphinx_rtd_theme'
-html_theme = 'pytorch_sphinx_theme'
-html_theme_path = [pytorch_sphinx_theme.get_html_theme_path()]
-html_theme_options = {
- 'logo_url':
- 'https://mmocr.readthedocs.io/en/dev-1.x/',
- 'menu': [
- {
- 'name':
- 'Tutorial',
- 'url':
- 'https://colab.research.google.com/github/open-mmlab/mmocr/blob/'
- 'dev-1.x/demo/tutorial.ipynb'
- },
- {
- 'name': 'GitHub',
- 'url': 'https://github.com/open-mmlab/mmocr'
- },
- {
- 'name':
- 'Upstream',
- 'children': [
- {
- 'name':
- 'MMEngine',
- 'url':
- 'https://github.com/open-mmlab/mmengine',
- 'description':
- 'Foundational library for training deep '
- 'learning models'
- },
- {
- 'name': 'MMCV',
- 'url': 'https://github.com/open-mmlab/mmcv',
- 'description': 'Foundational library for computer vision'
- },
- {
- 'name': 'MMDetection',
- 'url': 'https://github.com/open-mmlab/mmdetection',
- 'description': 'Object detection toolbox and benchmark'
- },
- ]
- },
- ],
- # Specify the language of shared menu
- 'menu_lang':
- 'en'
-}
-
-language = 'en'
-
-master_doc = 'index'
-
-# Add any paths that contain custom static files (such as style sheets) here,
-# relative to this directory. They are copied after the builtin static files,
-# so a file named "default.css" will overwrite the builtin "default.css".
-html_static_path = ['_static']
-
-html_css_files = [
- 'https://cdn.datatables.net/1.13.2/css/dataTables.bootstrap5.min.css',
- 'css/readthedocs.css'
-]
-html_js_files = [
- 'https://cdn.datatables.net/1.13.2/js/jquery.dataTables.min.js',
- 'https://cdn.datatables.net/1.13.2/js/dataTables.bootstrap5.min.js',
- 'js/collapsed.js',
- 'js/table.js',
-]
-
-myst_heading_anchors = 4
-
-intersphinx_mapping = {
- 'python': ('https://docs.python.org/3', None),
- 'numpy': ('https://numpy.org/doc/stable', None),
- 'torch': ('https://pytorch.org/docs/stable/', None),
- 'mmcv': ('https://mmcv.readthedocs.io/en/2.x/', None),
- 'mmengine': ('https://mmengine.readthedocs.io/en/latest/', None),
- 'mmdetection': ('https://mmdetection.readthedocs.io/en/dev-3.x/', None),
-}
-
-
-def builder_inited_handler(app):
- subprocess.run(['./merge_docs.sh'])
- subprocess.run(['./stats.py'])
- subprocess.run(['./dataset_zoo.py'])
- subprocess.run(['./project_zoo.py'])
-
-
-def setup(app):
- app.connect('builder-inited', builder_inited_handler)
diff --git a/pipeline/mmocr/docs/en/contact.md b/pipeline/mmocr/docs/en/contact.md
deleted file mode 100644
index c8a4321e3b1dd21457c82f8823a0a5c5d71e256e..0000000000000000000000000000000000000000
--- a/pipeline/mmocr/docs/en/contact.md
+++ /dev/null
@@ -1,18 +0,0 @@
-## Welcome to the OpenMMLab community
-
-Scan the QR code below to follow the OpenMMLab team's [**Zhihu Official Account**](https://www.zhihu.com/people/openmmlab) and join the OpenMMLab team's [**QQ Group**](https://jq.qq.com/?_wv=1027&k=aCvMxdr3), or join the official communication WeChat group by adding the WeChat, or join our [**Slack**](https://join.slack.com/t/mmocrworkspace/shared_invite/zt-1ifqhfla8-yKnLO_aKhVA2h71OrK8GZw)
-
-
-
-We will provide you with the OpenMMLab community
-
-- ๐ข share the latest core technologies of AI frameworks
-- ๐ป Explaining PyTorch common module source Code
-- ๐ฐ News related to the release of OpenMMLab
-- ๐ Introduction of cutting-edge algorithms developed by OpenMMLab
- ๐ Get the more efficient answer and feedback
-- ๐ฅ Provide a platform for communication with developers from all walks of life
-
-The OpenMMLab community looks forward to your participation! ๐ฌ
diff --git a/pipeline/mmocr/docs/en/dataset_zoo.py b/pipeline/mmocr/docs/en/dataset_zoo.py
deleted file mode 100644
index 733dc5cdaff09922f6a52c3405602dff8e28d011..0000000000000000000000000000000000000000
--- a/pipeline/mmocr/docs/en/dataset_zoo.py
+++ /dev/null
@@ -1,69 +0,0 @@
-#!/usr/bin/env python
-import os
-import os.path as osp
-import re
-
-import yaml
-
-dataset_zoo_path = '../../dataset_zoo'
-datasets = os.listdir(dataset_zoo_path)
-datasets.sort()
-
-table = '# Overview\n'
-table += '## Supported Datasets\n'
-table += '| Dataset Name | Text Detection | Text Recognition | Text Spotting | KIE |\n' \
- '|--------------|----------------|------------------|---------------|-----|\n' # noqa: E501
-details = '## Dataset Details\n'
-
-for dataset in datasets:
- meta = yaml.safe_load(
- open(osp.join(dataset_zoo_path, dataset, 'metafile.yml')))
- dataset_name = meta['Name']
- detail_link = re.sub('[^A-Za-z0-9- ]', '',
- dataset_name).replace(' ', '-').lower()
- paper = meta['Paper']
- data = meta['Data']
-
- table += '| [{}](#{}) | {} | {} | {} | {} |\n'.format(
- dataset,
- detail_link,
- 'โ' if 'textdet' in data['Tasks'] else '',
- 'โ' if 'textrecog' in data['Tasks'] else '',
- 'โ' if 'textspotting' in data['Tasks'] else '',
- 'โ' if 'kie' in data['Tasks'] else '',
- )
-
- details += '### {}\n'.format(dataset_name)
- details += "> \"{}\", *{}*, {}. [PDF]({})\n\n".format(
- paper['Title'], paper['Venue'], paper['Year'], paper['URL'])
-
- # Basic Info
- details += 'A. Basic Info\n'
- details += ' - Official Website: [{}]({})\n'.format(
- dataset, data['Website'])
- details += ' - Year: {}\n'.format(paper['Year'])
- details += ' - Language: {}\n'.format(data['Language'])
- details += ' - Scene: {}\n'.format(data['Scene'])
- details += ' - Annotation Granularity: {}\n'.format(data['Granularity'])
- details += ' - Supported Tasks: {}\n'.format(data['Tasks'])
- details += ' - License: [{}]({})\n'.format(data['License']['Type'],
- data['License']['Link'])
-
- # Format
- details += ' B. Annotation Format \n\n'
- sample_path = osp.join(dataset_zoo_path, dataset, 'sample_anno.md')
- if osp.exists(sample_path):
- with open(sample_path, 'r') as f:
- samples = f.readlines()
- samples = ''.join(samples)
- details += samples
- details += ' \n\n'
-
- # Reference
- details += 'C. Reference\n'
- details += '```bibtex\n{}\n```\n'.format(paper['BibTeX'])
-
-datasetzoo = table + details
-
-with open('user_guides/data_prepare/datasetzoo.md', 'w') as f:
- f.write(datasetzoo)
diff --git a/pipeline/mmocr/docs/en/docutils.conf b/pipeline/mmocr/docs/en/docutils.conf
deleted file mode 100644
index 0c00c84688701117f231fd0c8ec295fb747b7d8f..0000000000000000000000000000000000000000
--- a/pipeline/mmocr/docs/en/docutils.conf
+++ /dev/null
@@ -1,2 +0,0 @@
-[html writers]
-table_style: colwidths-auto
diff --git a/pipeline/mmocr/docs/en/get_started/faq.md b/pipeline/mmocr/docs/en/get_started/faq.md
deleted file mode 100644
index 0c72f3238db0a079edb0236ec54267e759d8da6a..0000000000000000000000000000000000000000
--- a/pipeline/mmocr/docs/en/get_started/faq.md
+++ /dev/null
@@ -1,83 +0,0 @@
-# FAQ
-
-## General
-
-**Q1** I'm getting the warning like `unexpected key in source state_dict: fc.weight, fc.bias`, is there something wrong?
-
-**A** It's not an error. It occurs because the backbone network is pretrained on image classification tasks, where the last fc layer is required to generate the classification output. However, the fc layer is no longer needed when the backbone network is used to extract features in downstream tasks, and therefore these weights can be safely skipped when loading the checkpoint.
-
-**Q2** MMOCR terminates with an error: `shapely.errors.TopologicalError: The operation 'GEOSIntersection_r' could not be performed. Likely cause is invalidity of the geometry`. How could I fix it?
-
-**A** This error occurs because of some invalid polygons (e.g., polygons with self-intersections) existing in the dataset or generated by some non-rigorous data transforms. These polygons can be fixed by adding `FixInvalidPolygon` transform after the transform likely to introduce invalid polygons. For example, a common practice is to append it after `LoadOCRAnnotations` in both train and test pipeline. The resulting pipeline should look like:
-
-```python
-train_pipeline = [
- ...
- dict(
- type='LoadOCRAnnotations',
- with_polygon=True,
- with_bbox=True,
- with_label=True,
- ),
- dict(type='FixInvalidPolygon', min_poly_points=4),
- ...
-]
-```
-
-In practice, we find that Totaltext contains some invalid polygons and using `FixInvalidPolygon` is a must. [Here](https://github.com/open-mmlab/mmocr/blob/27b6a68586b9a040678fe083bcf60662ae1b9261/configs/textdet/dbnet/dbnet_resnet18_fpnc_1200e_totaltext.py) is an example config.
-
-**Q3** Getting `libpng warning: iCCP: known incorrect sRGB profile` when loading images with `cv2` backend.
-
-**A** This is a warning from `libpng` and it is safe to ignore. It is caused by the `icc` profile in the image. You can use `pillow` backend to avoid this warning:
-
-```python
-train_pipeline = [
- dict(
- type='LoadImageFromFile',
- imdecode_backend='pillow'),
- ...
-]
-```
-
-## Text Recognition
-
-**Q1** What are the steps to train text recognition models with my own dictionary?
-
-**A** In MMOCR 1.0, you only need to modify the config and point `Dictionary` to your custom dict file. For example, if you want to train SAR model (https://github.com/open-mmlab/mmocr/blob/75c06d34bbc01d3d11dfd7afc098b6cdeee82579/configs/textrecog/sar/sar_resnet31_parallel-decoder_5e_st-sub_mj-sub_sa_real.py) with your own dictionary placed at `/my/dict.txt`, you can modify `dictionary.dict_file` term in [base config](https://github.com/open-mmlab/mmocr/blob/75c06d34bbc01d3d11dfd7afc098b6cdeee82579/configs/textrecog/sar/_base_sar_resnet31_parallel-decoder.py#L1) to:
-
-```python
-dictionary = dict(
- type='Dictionary',
- dict_file='/my/dict.txt',
- with_start=True,
- with_end=True,
- same_start_end=True,
- with_padding=True,
- with_unknown=True)
-```
-
-Now you are good to go. You can also find more information in [Dictionary API](https://mmocr.readthedocs.io/en/dev-1.x/api/generated/mmocr.models.common.Dictionary.html#mmocr.models.common.Dictionary).
-
-**Q2** How to properly visualize non-English characters?
-
-**A** You can customize `font_families` or `font_properties` in visualizer. For example, to visualize Korean:
-
-`configs/textrecog/_base_/default_runtime.py`:
-
-```python
-visualizer = dict(
- type='TextRecogLocalVisualizer',
- name='visualizer',
- font_families='NanumGothic', # new feature
- vis_backends=vis_backends)
-```
-
-It's also fine to pass the font path to visualizer:
-
-```python
-visualizer = dict(
- type='TextRecogLocalVisualizer',
- name='visualizer',
- font_properties='path/to/font_file',
- vis_backends=vis_backends)
-```
diff --git a/pipeline/mmocr/docs/en/get_started/install.md b/pipeline/mmocr/docs/en/get_started/install.md
deleted file mode 100644
index e892ba37272903e853a60cf7d7a2f0c8ee0cdc05..0000000000000000000000000000000000000000
--- a/pipeline/mmocr/docs/en/get_started/install.md
+++ /dev/null
@@ -1,244 +0,0 @@
-# Installation
-
-## Prerequisites
-
-- Linux | Windows | macOS
-- Python 3.7
-- PyTorch 1.6 or higher
-- torchvision 0.7.0
-- CUDA 10.1
-- NCCL 2
-- GCC 5.4.0 or higher
-
-## Environment Setup
-
-```{note}
-If you are experienced with PyTorch and have already installed it, just skip this part and jump to the [next section](#installation-steps). Otherwise, you can follow these steps for the preparation.
-```
-
-**Step 0.** Download and install Miniconda from the [official website](https://docs.conda.io/en/latest/miniconda.html).
-
-**Step 1.** Create a conda environment and activate it.
-
-```shell
-conda create --name openmmlab python=3.8 -y
-conda activate openmmlab
-```
-
-**Step 2.** Install PyTorch following [official instructions](https://pytorch.org/get-started/locally/), e.g.
-
-````{tabs}
-
-```{code-tab} shell GPU Platform
-conda install pytorch torchvision -c pytorch
-```
-
-```{code-tab} shell CPU Platform
-conda install pytorch torchvision cpuonly -c pytorch
-```
-
-````
-
-## Installation Steps
-
-We recommend that users follow our best practices to install MMOCR. However, the whole process is highly customizable. See [Customize Installation](#customize-installation) section for more information.
-
-### Best Practices
-
-**Step 0.** Install [MMEngine](https://github.com/open-mmlab/mmengine), [MMCV](https://github.com/open-mmlab/mmcv) and [MMDetection](https://github.com/open-mmlab/mmdetection) using [MIM](https://github.com/open-mmlab/mim).
-
-```shell
-pip install -U openmim
-mim install mmengine
-mim install mmcv
-mim install mmdet
-```
-
-**Step 1.** Install MMOCR.
-
-If you wish to run and develop MMOCR directly, install it from **source** (recommended).
-
-If you use MMOCR as a dependency or third-party package, install it via **MIM**.
-
-`````{tabs}
-
-````{group-tab} Install from Source
-
-```shell
-
-git clone https://github.com/open-mmlab/mmocr.git
-cd mmocr
-pip install -v -e .
-# "-v" increases pip's verbosity.
-# "-e" means installing the project in editable mode,
-# That is, any local modifications on the code will take effect immediately.
-
-```
-
-````
-
-````{group-tab} Install via MIM
-
-```shell
-
-mim install mmocr
-
-```
-
-````
-
-`````
-
-**Step 2. (Optional)** If you wish to use any transform involving `albumentations` (For example, `Albu` in ABINet's pipeline), or any dependency for building documentation or running unit tests, please install the dependency using the following command:
-
-`````{tabs}
-
-````{group-tab} Install from Source
-
-```shell
-# install albu
-pip install -r requirements/albu.txt
-# install the dependencies for building documentation and running unit tests
-pip install -r requirements.txt
-```
-
-````
-
-````{group-tab} Install via MIM
-
-```shell
-pip install albumentations>=1.1.0 --no-binary qudida,albumentations
-```
-
-````
-
-`````
-
-```{note}
-
-We recommend checking the environment after installing `albumentations` to
-ensure that `opencv-python` and `opencv-python-headless` are not installed together, otherwise it might cause unexpected issues. If that's unfortunately the case, please uninstall `opencv-python-headless` to make sure MMOCR's visualization utilities can work.
-
-Refer
-to [albumentations's official documentation](https://albumentations.ai/docs/getting_started/installation/#note-on-opencv-dependencies) for more details.
-
-```
-
-### Verify the installation
-
-You may verify the installation via this inference demo.
-
-`````{tabs}
-
-````{tab} Python
-
-Run the following code in a Python interpreter:
-
-```python
->>> from mmocr.apis import MMOCRInferencer
->>> ocr = MMOCRInferencer(det='DBNet', rec='CRNN')
->>> ocr('demo/demo_text_ocr.jpg', show=True, print_result=True)
-```
-````
-
-````{tab} Shell
-
-If you installed MMOCR from source, you can run the following in MMOCR's root directory:
-
-```shell
-python tools/infer.py demo/demo_text_ocr.jpg --det DBNet --rec CRNN --show --print-result
-```
-````
-
-`````
-
-You should be able to see a pop-up image and the inference result printed out in the console upon successful verification.
-
-
-
-
-
-
-```bash
-# Inference result
-{'predictions': [{'rec_texts': ['cbanks', 'docecea', 'grouf', 'pwate', 'chobnsonsg', 'soxee', 'oeioh', 'c', 'sones', 'lbrandec', 'sretalg', '11', 'to8', 'round', 'sale', 'year',
-'ally', 'sie', 'sall'], 'rec_scores': [...], 'det_polygons': [...], 'det_scores':
-[...]}]}
-```
-
-```{note}
-If you are running MMOCR on a server without GUI or via SSH tunnel with X11 forwarding disabled, you may not see the pop-up window.
-```
-
-## Customize Installation
-
-### CUDA versions
-
-When installing PyTorch, you need to specify the version of CUDA. If you are not clear on which to choose, follow our recommendations:
-
-- For Ampere-based NVIDIA GPUs, such as GeForce 30 series and NVIDIA A100, CUDA 11 is a must.
-- For older NVIDIA GPUs, CUDA 11 is backward compatible, but CUDA 10.2 offers better compatibility and is more lightweight.
-
-Please make sure the GPU driver satisfies the minimum version requirements. See [this table](https://docs.nvidia.com/cuda/cuda-toolkit-release-notes/index.html#cuda-major-component-versions__table-cuda-toolkit-driver-versions) for more information.
-
-```{note}
-Installing CUDA runtime libraries is enough if you follow our best practices, because no CUDA code will be compiled locally. However if you hope to compile MMCV from source or develop other CUDA operators, you need to install the complete CUDA toolkit from NVIDIA's [website](https://developer.nvidia.com/cuda-downloads), and its version should match the CUDA version of PyTorch. i.e., the specified version of cudatoolkit in `conda install` command.
-```
-
-### Install MMCV without MIM
-
-MMCV contains C++ and CUDA extensions, thus depending on PyTorch in a complex way. MIM solves such dependencies automatically and makes the installation easier. However, it is not a must.
-
-To install MMCV with pip instead of MIM, please follow [MMCV installation guides](https://mmcv.readthedocs.io/en/latest/get_started/installation.html). This requires manually specifying a find-url based on PyTorch version and its CUDA version.
-
-For example, the following command install mmcv-full built for PyTorch 1.10.x and CUDA 11.3.
-
-```shell
-pip install `mmcv>=2.0.0rc1` -f https://download.openmmlab.com/mmcv/dist/cu113/torch1.10/index.html
-```
-
-### Install on CPU-only platforms
-
-MMOCR can be built for CPU-only environment. In CPU mode you can train (requires MMCV version >= 1.4.4), test or inference a model.
-
-However, some functionalities are gone in this mode:
-
-- Deformable Convolution
-- Modulated Deformable Convolution
-- ROI pooling
-- SyncBatchNorm
-
-If you try to train/test/inference a model containing above ops, an error will be raised.
-The following table lists affected algorithms.
-
-| Operator | Model |
-| :-----------------------------------------------------: | :-----------------------------------------------------: |
-| Deformable Convolution/Modulated Deformable Convolution | DBNet (r50dcnv2), DBNet++ (r50dcnv2), FCENet (r50dcnv2) |
-| SyncBatchNorm | PANet, PSENet |
-
-### Using MMOCR with Docker
-
-We provide a [Dockerfile](https://github.com/open-mmlab/mmocr/blob/master/docker/Dockerfile) to build an image.
-
-```shell
-# build an image with PyTorch 1.6, CUDA 10.1
-docker build -t mmocr docker/
-```
-
-Run it with
-
-```shell
-docker run --gpus all --shm-size=8g -it -v {DATA_DIR}:/mmocr/data mmocr
-```
-
-## Dependency on MMEngine, MMCV & MMDetection
-
-MMOCR has different version requirements on MMEngine, MMCV and MMDetection at each release to guarantee the implementation correctness. Please refer to the table below and ensure the package versions fit the requirement.
-
-| MMOCR | MMEngine | MMCV | MMDetection |
-| -------------- | --------------------------- | -------------------------- | --------------------------- |
-| dev-1.x | 0.7.1 \<= mmengine \< 1.0.0 | 2.0.0rc4 \<= mmcv \< 2.1.0 | 3.0.0rc5 \<= mmdet \< 3.1.0 |
-| 1.0.0 | 0.7.1 \<= mmengine \< 1.0.0 | 2.0.0rc4 \<= mmcv \< 2.1.0 | 3.0.0rc5 \<= mmdet \< 3.1.0 |
-| 1.0.0rc6 | 0.6.0 \<= mmengine \< 1.0.0 | 2.0.0rc4 \<= mmcv \< 2.1.0 | 3.0.0rc5 \<= mmdet \< 3.1.0 |
-| 1.0.0rc\[4-5\] | 0.1.0 \<= mmengine \< 1.0.0 | 2.0.0rc1 \<= mmcv \< 2.1.0 | 3.0.0rc0 \<= mmdet \< 3.1.0 |
-| 1.0.0rc\[0-3\] | 0.0.0 \<= mmengine \< 0.2.0 | 2.0.0rc1 \<= mmcv \< 2.1.0 | 3.0.0rc0 \<= mmdet \< 3.1.0 |
diff --git a/pipeline/mmocr/docs/en/get_started/overview.md b/pipeline/mmocr/docs/en/get_started/overview.md
deleted file mode 100644
index 7bbb67b142750fb1d148b44c0f9d79d605a061ad..0000000000000000000000000000000000000000
--- a/pipeline/mmocr/docs/en/get_started/overview.md
+++ /dev/null
@@ -1,20 +0,0 @@
-# Overview
-
-MMOCR is an open source toolkit based on [PyTorch](https://pytorch.org/) and [MMDetection](https://github.com/open-mmlab/mmdetection), supporting numerous OCR-related models, including text detection, text recognition, and key information extraction. In addition, it supports widely-used academic datasets and provides many useful tools, assisting users in exploring various aspects of models and datasets and implementing high-quality algorithms. Generally, it has the following features.
-
-- **One-stop, Multi-model**: MMOCR supports various OCR-related tasks and implements the latest models for text detection, recognition, and key information extraction.
-- **Modular Design**: MMOCR's modular design allows users to define and reuse modules in the model on demand.
-- **Various Useful Tools**: MMOCR provides a number of analysis tools, including visualizers, validation scripts, evaluators, etc., to help users troubleshoot, finetune or compare models.
-- **Powered by [OpenMMLab](https://openmmlab.com/)**: Like other algorithm libraries in OpenMMLab family, MMOCR follows OpenMMLab's rigorous development guidelines and interface conventions, significantly reducing the learning cost of users familiar with other projects in OpenMMLab family. In addition, benefiting from the unified interfaces among OpenMMLab, you can easily call the models implemented in other OpenMMLab projects (e.g. MMDetection) in MMOCR, facilitating cross-domain research and real-world applications.
-
-Together with the release of OpenMMLab 2.0, MMOCR now also comes to its 1.0.0 version, which has made significant BC-breaking changes, resulting in less code redundancy, higher code efficiency and an overall more systematic and consistent design.
-
-Considering that there are some backward incompatible changes in this version compared to 0.x, we have prepared a detailed [migration guide](../migration/overview.md). It lists all the changes made in the new version and the steps required to migrate. We hope this guide can help users familiar with the old framework to complete the upgrade as quickly as possible. Though this may take some time, we believe that the new features brought by MMOCR and the OpenMMLab ecosystem will make it all worthwhile. ๐
-
-Next, please read the section according to your actual needs.
-
-- We recommend that beginners go through [Quick Run](quick_run.md) to get familiar with MMOCR and master the usage of MMOCR by reading the examples in **User Guides**.
-- Intermediate and advanced developers are suggested to learn the background, conventions, and recommended implementations of each component from **Basic Concepts**.
-- Read our [FAQ](faq.md) to find answers to frequently asked questions.
-- If you can't find the answers you need in the documentation, feel free to raise an [issue](https://github.com/open-mmlab/mmocr/issues).
-- Everyone is welcome to be a contributor! Read the [contribution guide](../notes/contribution_guide.md) to learn how to contribute to MMOCR!
diff --git a/pipeline/mmocr/docs/en/get_started/quick_run.md b/pipeline/mmocr/docs/en/get_started/quick_run.md
deleted file mode 100644
index 5c5f01a4491fbbc64e2c4bbc63bf69b1d7f949d4..0000000000000000000000000000000000000000
--- a/pipeline/mmocr/docs/en/get_started/quick_run.md
+++ /dev/null
@@ -1,203 +0,0 @@
-# Quick Run
-
-This chapter will take you through the basic functions of MMOCR. And we assume you [installed MMOCR from source](install.md#best-practices). You may check out the [tutorial notebook](https://colab.research.google.com/github/open-mmlab/mmocr/blob/dev-1.x/demo/tutorial.ipynb) for how to perform inference, training and testing interactively.
-
-## Inference
-
-Run the following in MMOCR's root directory:
-
-```shell
-python tools/infer.py demo/demo_text_ocr.jpg --det DBNet --rec CRNN --show --print-result
-```
-
-You should be able to see a pop-up image and the inference result printed out in the console.
-
-
-
-
-
-
-```bash
-# Inference result
-{'predictions': [{'rec_texts': ['cbanks', 'docecea', 'grouf', 'pwate', 'chobnsonsg', 'soxee', 'oeioh', 'c', 'sones', 'lbrandec', 'sretalg', '11', 'to8', 'round', 'sale', 'year',
-'ally', 'sie', 'sall'], 'rec_scores': [...], 'det_polygons': [...], 'det_scores':
-[...]}]}
-```
-
-```{note}
-If you are running MMOCR on a server without GUI or via SSH tunnel with X11 forwarding disabled, you may not see the pop-up window.
-```
-
-A detailed description of MMOCR's inference interface can be found [here](../user_guides/inference.md)
-
-In addition to using our well-provided pre-trained models, you can also train models on your own datasets. In the next section, we will take you through the basic functions of MMOCR by training DBNet on the mini [ICDAR 2015](https://rrc.cvc.uab.es/?ch=4&com=downloads) dataset as an example.
-
-## Prepare a Dataset
-
-Since the variety of OCR dataset formats are not conducive to either switching or joint training of multiple datasets, MMOCR proposes a uniform [data format](../user_guides/dataset_prepare.md), and provides [dataset preparer](../user_guides/data_prepare/dataset_preparer.md) for commonly used OCR datasets. Usually, to use those datasets in MMOCR, you just need to follow the steps to get them ready for use.
-
-```{note}
-But here, efficiency means everything.
-```
-
-Here, we have prepared a lite version of ICDAR 2015 dataset for demonstration purposes. Download our pre-prepared [zip](https://download.openmmlab.com/mmocr/data/icdar2015/mini_icdar2015.tar.gz) and extract it to the `data/` directory under mmocr to get our prepared image and annotation file.
-
-```Bash
-wget https://download.openmmlab.com/mmocr/data/icdar2015/mini_icdar2015.tar.gz
-mkdir -p data/
-tar xzvf mini_icdar2015.tar.gz -C data/
-```
-
-## Modify the Config
-
-Once the dataset is prepared, we will then specify the location of the training set and the training parameters by modifying the config file.
-
-In this example, we will train a DBNet using resnet18 as its backbone. Since MMOCR already has a config file for the full ICDAR 2015 dataset (`configs/textdet/dbnet/dbnet_resnet18_fpnc_1200e_icdar2015.py`), we just need to make some modifications on top of it.
-
-We first need to modify the path to the dataset. In this config, most of the key config files are imported in `_base_`, such as the database configuration from `configs/textdet/_base_/datasets/icdar2015.py`. Open that file and replace the path pointed to by `icdar2015_textdet_data_root` in the first line with:
-
-```Python
-icdar2015_textdet_data_root = 'data/mini_icdar2015'
-```
-
-Also, because of the reduced dataset size, we have to reduce the number of training epochs to 400 accordingly, shorten the validation interval as well as the weight storage interval to 10 rounds, and drop the learning rate decay strategy. The following lines of configuration can be directly put into `configs/textdet/dbnet/dbnet_resnet18_fpnc_1200e_icdar2015.py` to take effect.
-
-```Python
-# Save checkpoints every 10 epochs, and only keep the latest checkpoint
-default_hooks = dict(
- checkpoint=dict(
- type='CheckpointHook',
- interval=10,
- max_keep_ckpts=1,
- ))
-# Set the maximum number of epochs to 400, and validate the model every 10 epochs
-train_cfg = dict(type='EpochBasedTrainLoop', max_epochs=400, val_interval=10)
-# Fix learning rate as a constant
-param_scheduler = [
- dict(type='ConstantLR', factor=1.0),
-]
-```
-
-Here, we have rewritten the corresponding parameters in the base configuration directly through the inheritance ({external+mmengine:doc}`MMEngine: Config `) mechanism of the config. The original fields are distributed in `configs/textdet/_base_/schedules/schedule_sgd_1200e.py` and `configs/textdet/_base_/default_runtime.py`.
-
-```{note}
-For a more detailed description of config, please refer to [here](../user_guides/config.md).
-```
-
-## Browse the Dataset
-
-Before we start the training, we can also visualize the image processed by training-time [data transforms](../basic_concepts/transforms.md). It's quite simple: pass the config file we need to visualize into the [browse_dataset.py](/tools/analysis_tools/browse_dataset.py) script.
-
-```Bash
-python tools/analysis_tools/browse_dataset.py configs/textdet/dbnet/dbnet_resnet18_fpnc_1200e_icdar2015.py
-```
-
-The transformed images and annotations will be displayed one by one in a pop-up window.
-
-
-
-
-
-```{note}
-For details on the parameters and usage of this script, please refer to [here](../user_guides/useful_tools.md).
-```
-
-```{tip}
-In addition to satisfying our curiosity, visualization can also help us check the parts that may affect the model's performance before training, such as problems in configs, datasets and data transforms.
-```
-
-## Training
-
-Start the training by running the following command:
-
-```Bash
-python tools/train.py configs/textdet/dbnet/dbnet_resnet18_fpnc_1200e_icdar2015.py
-```
-
-Depending on the system environment, MMOCR will automatically use the best device for training. If a GPU is available, a single GPU training will be started by default. When you start to see the output of the losses, you have successfully started the training.
-
-```Bash
-2022/08/22 18:42:22 - mmengine - INFO - Epoch(train) [1][5/7] lr: 7.0000e-03 memory: 7730 data_time: 0.4496 loss_prob: 14.6061 loss_thr: 2.2904 loss_db: 0.9879 loss: 17.8843 time: 1.8666
-2022/08/22 18:42:24 - mmengine - INFO - Exp name: dbnet_resnet18_fpnc_1200e_icdar2015
-2022/08/22 18:42:28 - mmengine - INFO - Epoch(train) [2][5/7] lr: 7.0000e-03 memory: 6695 data_time: 0.2052 loss_prob: 6.7840 loss_thr: 1.4114 loss_db: 0.9855 loss: 9.1809 time: 0.7506
-2022/08/22 18:42:29 - mmengine - INFO - Exp name: dbnet_resnet18_fpnc_1200e_icdar2015
-2022/08/22 18:42:33 - mmengine - INFO - Epoch(train) [3][5/7] lr: 7.0000e-03 memory: 6690 data_time: 0.2101 loss_prob: 3.0700 loss_thr: 1.1800 loss_db: 0.9967 loss: 5.2468 time: 0.6244
-2022/08/22 18:42:33 - mmengine - INFO - Exp name: dbnet_resnet18_fpnc_1200e_icdar2015
-```
-
-Without extra configurations, model weights will be saved to `work_dirs/dbnet_resnet18_fpnc_1200e_icdar2015/`, while the logs will be stored in `work_dirs/dbnet_resnet18_fpnc_1200e_icdar2015/TIMESTAMP/`. Next, we just need to wait with some patience for training to finish.
-
-```{note}
-For advanced usage of training, such as CPU training, multi-GPU training, and cluster training, please refer to [Training and Testing](../user_guides/train_test.md).
-```
-
-## Testing
-
-After 400 epochs, we observe that DBNet performs best in the last epoch, with `hmean` reaching 60.86 (You may see a different result):
-
-```Bash
-08/22 19:24:52 - mmengine - INFO - Epoch(val) [400][100/100] icdar/precision: 0.7285 icdar/recall: 0.5226 icdar/hmean: 0.6086
-```
-
-```{note}
-It may not have been trained to be optimal, but it is sufficient for a demo.
-```
-
-However, this value only reflects the performance of DBNet on the mini ICDAR 2015 dataset. For a comprehensive evaluation, we also need to see how it performs on out-of-distribution datasets. For example, `tests/data/det_toy_dataset` is a very small real dataset that we can use to verify the actual performance of DBNet.
-
-Before testing, we also need to make some changes to the location of the dataset. Open `configs/textdet/_base_/datasets/icdar2015.py` and change `data_root` of `icdar2015_textdet_test` to `tests/data/det_toy_dataset`:
-
-```Python
-# ...
-icdar2015_textdet_test = dict(
- type='OCRDataset',
- data_root='tests/data/det_toy_dataset',
- # ...
- )
-```
-
-Start testing:
-
-```Bash
-python tools/test.py configs/textdet/dbnet/dbnet_resnet18_fpnc_1200e_icdar2015.py work_dirs/dbnet_resnet18_fpnc_1200e_icdar2015/epoch_400.pth
-```
-
-And get the outputs like:
-
-```Bash
-08/21 21:45:59 - mmengine - INFO - Epoch(test) [5/10] memory: 8562
-08/21 21:45:59 - mmengine - INFO - Epoch(test) [10/10] eta: 0:00:00 time: 0.4893 data_time: 0.0191 memory: 283
-08/21 21:45:59 - mmengine - INFO - Evaluating hmean-iou...
-08/21 21:45:59 - mmengine - INFO - prediction score threshold: 0.30, recall: 0.6190, precision: 0.4815, hmean: 0.5417
-08/21 21:45:59 - mmengine - INFO - prediction score threshold: 0.40, recall: 0.6190, precision: 0.5909, hmean: 0.6047
-08/21 21:45:59 - mmengine - INFO - prediction score threshold: 0.50, recall: 0.6190, precision: 0.6842, hmean: 0.6500
-08/21 21:45:59 - mmengine - INFO - prediction score threshold: 0.60, recall: 0.6190, precision: 0.7222, hmean: 0.6667
-08/21 21:45:59 - mmengine - INFO - prediction score threshold: 0.70, recall: 0.3810, precision: 0.8889, hmean: 0.5333
-08/21 21:45:59 - mmengine - INFO - prediction score threshold: 0.80, recall: 0.0000, precision: 0.0000, hmean: 0.0000
-08/21 21:45:59 - mmengine - INFO - prediction score threshold: 0.90, recall: 0.0000, precision: 0.0000, hmean: 0.0000
-08/21 21:45:59 - mmengine - INFO - Epoch(test) [10/10] icdar/precision: 0.7222 icdar/recall: 0.6190 icdar/hmean: 0.6667
-```
-
-The model achieves an hmean of 0.6667 on this dataset.
-
-```{note}
-For advanced usage of testing, such as CPU testing, multi-GPU testing, and cluster testing, please refer to [Training and Testing](../user_guides/train_test.md).
-```
-
-## Visualize the Outputs
-
-We can also visualize its prediction output in `test.py`. You can open a pop-up visualization window with the `show` parameter; and can also specify the directory where the prediction result images are exported with the `show-dir` parameter.
-
-```Bash
-python tools/test.py configs/textdet/dbnet/dbnet_resnet18_fpnc_1200e_icdar2015.py work_dirs/dbnet_resnet18_fpnc_1200e_icdar2015/epoch_400.pth --show-dir imgs/
-```
-
-The true labels and predicted values are displayed in a tiled fashion in the visualization results. The green boxes in the left panel indicate the true labels and the red boxes in the right panel indicate the predicted values.
-
-
-
-
-
-```{note}
-For a description of more visualization features, see [here](../user_guides/visualization.md).
-```
diff --git a/pipeline/mmocr/docs/en/index.rst b/pipeline/mmocr/docs/en/index.rst
deleted file mode 100644
index 123b9b933e987b201f9265baac0f1a0225e38f8f..0000000000000000000000000000000000000000
--- a/pipeline/mmocr/docs/en/index.rst
+++ /dev/null
@@ -1,113 +0,0 @@
-Welcome to MMOCR's documentation!
-=======================================
-
-You can switch between English and Chinese in the lower-left corner of the layout.
-
-.. toctree::
- :maxdepth: 2
- :caption: Get Started
-
- get_started/overview.md
- get_started/install.md
- get_started/quick_run.md
- get_started/faq.md
-
-.. toctree::
- :maxdepth: 2
- :caption: User Guides
-
- user_guides/inference.md
- user_guides/config.md
- user_guides/dataset_prepare.md
- user_guides/train_test.md
- user_guides/visualization.md
- user_guides/useful_tools.md
-
-.. toctree::
- :maxdepth: 2
- :caption: Basic Concepts
-
- basic_concepts/structures.md
- basic_concepts/transforms.md
- basic_concepts/evaluation.md
- basic_concepts/datasets.md
- basic_concepts/overview.md
- basic_concepts/data_flow.md
- basic_concepts/models.md
- basic_concepts/visualizers.md
- basic_concepts/convention.md
- basic_concepts/engine.md
-
-
-.. toctree::
- :maxdepth: 2
- :caption: Dataset Zoo
-
- user_guides/data_prepare/datasetzoo.md
- user_guides/data_prepare/dataset_preparer.md
- user_guides/data_prepare/det.md
- user_guides/data_prepare/recog.md
- user_guides/data_prepare/kie.md
-
-.. toctree::
- :maxdepth: 2
- :caption: Model Zoo
-
- modelzoo.md
- projectzoo.md
- backbones.md
- textdet_models.md
- textrecog_models.md
- kie_models.md
-
-.. toctree::
- :maxdepth: 2
- :caption: Notes
-
- notes/branches.md
- notes/contribution_guide.md
- notes/changelog.md
-
-.. toctree::
- :maxdepth: 2
- :caption: Migrating from MMOCR 0.x
-
- migration/overview.md
- migration/news.md
- migration/branches.md
- migration/code.md
- migration/dataset.md
- migration/model.md
- migration/transforms.md
-
-.. toctree::
- :maxdepth: 1
- :caption: API Reference
-
- mmocr.apis
- mmocr.structures
- mmocr.datasets
- mmocr.transforms
- mmocr.models
- mmocr.evaluation
- mmocr.visualization
- mmocr.engine
- mmocr.utils
-
-.. toctree::
- :maxdepth: 2
- :caption: Contact US
-
- contact.md
-
-.. toctree::
- :caption: Switch Language
-
- switch_language.md
-
-
-Indices and tables
-==================
-
-* :ref:`genindex`
-* :ref:`search`
diff --git a/pipeline/mmocr/docs/en/make.bat b/pipeline/mmocr/docs/en/make.bat
deleted file mode 100644
index 8a3a0e25b49a52ade52c4f69ddeb0bc3d12527ff..0000000000000000000000000000000000000000
--- a/pipeline/mmocr/docs/en/make.bat
+++ /dev/null
@@ -1,36 +0,0 @@
-@ECHO OFF
-
-pushd %~dp0
-
-REM Command file for Sphinx documentation
-
-if "%SPHINXBUILD%" == "" (
- set SPHINXBUILD=sphinx-build
-)
-set SOURCEDIR=.
-set BUILDDIR=_build
-
-if "%1" == "" goto help
-
-%SPHINXBUILD% >NUL 2>NUL
-if errorlevel 9009 (
- echo.
- echo.The 'sphinx-build' command was not found. Make sure you have Sphinx
- echo.installed, then set the SPHINXBUILD environment variable to point
- echo.to the full path of the 'sphinx-build' executable. Alternatively you
- echo.may add the Sphinx directory to PATH.
- echo.
- echo.If you don't have Sphinx installed, grab it from
- echo.http://sphinx-doc.org/
- exit /b 1
-)
-
-
-%SPHINXBUILD% -M %1 %SOURCEDIR% %BUILDDIR% %SPHINXOPTS% %O%
-goto end
-
-:help
-%SPHINXBUILD% -M help %SOURCEDIR% %BUILDDIR% %SPHINXOPTS% %O%
-
-:end
-popd
diff --git a/pipeline/mmocr/docs/en/merge_docs.sh b/pipeline/mmocr/docs/en/merge_docs.sh
deleted file mode 100644
index 9835eab21fc3dc8a2de63332148dcd0b4f2fa6a2..0000000000000000000000000000000000000000
--- a/pipeline/mmocr/docs/en/merge_docs.sh
+++ /dev/null
@@ -1,7 +0,0 @@
-#!/usr/bin/env bash
-
-# gather models
-sed -e '$a\\n' -s ../../configs/kie/*/*.md | sed "s/md###t/html#t/g" | sed "s/#/#&/" | sed '1i\# Key Information Extraction Models' | sed 's/](\/docs\//](/g' | sed 's=](/=](https://github.com/open-mmlab/mmocr/tree/master/=g' >kie_models.md
-sed -e '$a\\n' -s ../../configs/textdet/*/*.md | sed "s/md###t/html#t/g" | sed "s/#/#&/" | sed '1i\# Text Detection Models' | sed 's/](\/docs\//](/g' | sed 's=](/=](https://github.com/open-mmlab/mmocr/tree/master/=g' >textdet_models.md
-sed -e '$a\\n' -s ../../configs/textrecog/*/*.md | sed "s/md###t/html#t/g" | sed "s/#/#&/" | sed '1i\# Text Recognition Models' | sed 's/](\/docs\//](/g' | sed 's=](/=](https://github.com/open-mmlab/mmocr/tree/master/=g' >textrecog_models.md
-sed -e '$a\\n' -s ../../configs/backbone/*/*.md | sed "s/md###t/html#t/g" | sed "s/#/#&/" | sed '1i\# BackBones' | sed 's/](\/docs\//](/g' | sed 's=](/=](https://github.com/open-mmlab/mmocr/tree/master/=g' >backbones.md
diff --git a/pipeline/mmocr/docs/en/migration/branches.md b/pipeline/mmocr/docs/en/migration/branches.md
deleted file mode 100644
index d5b02ae2461f2e743d5323d9f1978c622740ef86..0000000000000000000000000000000000000000
--- a/pipeline/mmocr/docs/en/migration/branches.md
+++ /dev/null
@@ -1,38 +0,0 @@
-# Branch Migration
-
-At an earlier stage, MMOCR had three branches: `main`, `1.x`, and `dev-1.x`. Some of these branches have been renamed together with the official MMOCR 1.0.0 release, and here is the changelog.
-
-- `main` branch housed the code for MMOCR 0.x (e.g., v0.6.3). Now it has been renamed to `0.x`.
-- `1.x` contained the code for MMOCR 1.x (e.g., 1.0.0rc6). Now it is an alias of `main`, and will be removed in mid 2023.
-- `dev-1.x` was the development branch for MMOCR 1.x. Now it remains unchanged.
-
-For more information about the branches, check out [branches](../notes/branches.md).
-
-## Resolving Conflicts When Upgrading the `main` branch
-
-For users who wish to upgrade from the old `main` branch that has the code for MMOCR 0.x, the non-fast-forwarded-able nature of the upgrade may cause conflicts. To resolve these conflicts, follow the steps below:
-
-1. Commit all the changes you have on `main` if you have any. Backup your current `main` branch by creating a copy.
-
- ```bash
- git checkout main
- git add --all
- git commit -m 'backup'
- git checkout -b main_backup
- ```
-
-2. Fetch the latest changes from the remote repository.
-
- ```bash
- git remote add openmmlab git@github.com:open-mmlab/mmocr.git
- git fetch openmmlab
- ```
-
-3. Reset the `main` branch to the latest `main` branch on the remote repository by running `git reset --hard openmmlab/main`.
-
- ```bash
- git checkout main
- git reset --hard openmmlab/main
- ```
-
-By following these steps, you can successfully upgrade your `main` branch.
diff --git a/pipeline/mmocr/docs/en/migration/code.md b/pipeline/mmocr/docs/en/migration/code.md
deleted file mode 100644
index 31b84b4985bd6690a7b79a0fce4463f0c7d26f73..0000000000000000000000000000000000000000
--- a/pipeline/mmocr/docs/en/migration/code.md
+++ /dev/null
@@ -1,151 +0,0 @@
-# Code Migration
-
-MMOCR has been designed in a way that there are a lot of shortcomings in the initial version in order to balance the tasks of text detection, recognition and key information extraction. In this 1.0 release, MMOCR synchronizes its new model architecture to align as much as possible with the overall OpenMMLab design and to achieve structural uniformity within the algorithm library. Although this upgrade is not fully backward compatible, we summarize the changes that may be of interest to developers for those who need them.
-
-## Fundamental Changes
-
-Functional boundaries of modules has not been clearly defined in MMOCR 0.x. In MMOCR 1.0, we address this issue by refactoring the design of model modules. Here are some major changes in 1.0:
-
-- MMOCR 1.0 no longer supports named entity recognition tasks since it's not in the scope of OCR.
-
-- The module that computes the loss in a model is named as *Module Loss*, which is also responsible for the conversion of gold annotations into loss targets. Another module, *Postprocessor*, is responsible for decoding the model raw output into `DataSample` for the corresponding task at prediction time.
-
-- The inputs of all models are now organized as a dictionary that consists of two keys: `inputs`, containing the original features of the images, and `List[DataSample]`, containing the meta-information of the images. At training time, the output format of a model is standardized to a dictionary containing the loss tensors. Similarly, a model generates a sequence of `DataSample`s containing the prediction outputs in testing.
-
-- In MMOCR 0.x, the majority of classes named `XXLoss` have the implementations closely bound to the corresponding model, while their names made users hard to tell them apart from other generic losses like `DiceLoss`. In 1.0, they are renamed to the form `XXModuleLoss`. (e.g. `DBLoss` was renamed to `DBModuleLoss`). The key to their configurations in config files is also changed from `loss` to `module_loss`.
-
-- The names of generic loss classes that are not related to the model implementation are kept as `XXLoss`. (e.g. [`MaskedBCELoss`](mmocr.models.common.losses.MaskedBCELoss)) They are all placed under `mmocr/models/common/losses`.
-
-- Changes under `mmocr/models/common/losses`: `DiceLoss` is renamed to [`MaskedDiceLoss`](mmocr.models.common.losses.MaskedDiceLoss). `FocalLoss` has been removed.
-
-- MMOCR 1.0 adds a *Dictionary* module which originates from *label converter*. It is used in text recognition and key information extraction tasks.
-
-## Text Detection Models
-
-### Key Changes (TL;DR)
-
-- The model weights from MMOCR 0.x still works in the 1.0, but the fields starting with `bbox_head` in the state dict `state_dict` need to be renamed to `det_head`.
-
-- `XXTargets` transforms, which were responsible for genearting detection targets, have been merged into `XXModuleLoss`.
-
-### SingleStageTextDetector
-
-- The original inheritance chain was `mmdet.BaseDetector->SingleStageDetector->SingleStageTextDetector`. Now `SingleStageTextDetector` is directly inherited from `BaseDetector` without extra dependency on MMDetection, and `SingleStageDetector` is deleted.
-
-- `bbox_head` is renamed to `det_head`.
-
-- `train_cfg`, `test_cfg` and `pretrained` fields are removed.
-
-- `forward_train()` and `simple_test()` are refactored to `loss()` and `predict()`. The part of `simple_test()` that was responsible for splitting the raw output of the model and feeding it into `head.get_bounary()` is integrated into `BaseTextDetPostProcessor`.
-
-- `TextDetectorMixin` has been removed since its implementation overlaps with `TextDetLocalVisualizer`.
-
-### Head
-
-- `HeadMixin`, the base class that `XXXHead` had to inherit from in version 0.x, has been replaced by `BaseTextDetHead`. `get_boundary()` and `resize_boundary()` are now rewritten as `__call__()` and `rescale()` in `BaseTextDetPostProcessor`.
-
-### ModuleLoss
-
-- Data transforms `XXXTargets` in text detection tasks are all moved to `XXXModuleLoss._get_target_single()`. Target-related configurations are no longer specified in the data pipeline but in `XXXLoss` instead.
-
-### Postprocessor
-
-- The logic in the original `XXXPostprocessor.__call__()` are transferred to the refactored `XXXPostprocessor.get_text_instances()`.
-
-- `BasePostprocessor` is refactored to `BaseTextDetPostProcessor`. This base class splits and processes the model output predictions one by one and supports automatic scaling of the output polygon or bounding box based on `scale_factor`.
-
-## Text Recognition
-
-### Key Changes (TL;DR)
-
-- Due to the change of the character order and some bugs in the model architecture being fixed, the recognition model weights in 0.x can no longer be directly used in 1.0. We will provide a migration script and tutorial for those who need it.
-
-- The support of SegOCR has been removed. TPS-CRNN will still be supported in a later version.
-
-- Test time augmentation will be supported in the upcoming release.
-
-- *Label converter* module has been removed and its functions have been split into *Dictionary*, *ModuleLoss* and *Postprocessor*.
-
-- The definition of `max_seq_len` has been unified and now it represents the original output length of the model.
-
-### Label Converter
-
-- The original label converters had spelling errors (written as label convertors). We fixed them by removing label converters from this project.
-
-- The part responsible for converting characters/strings to and from numeric indexes was extracted to *Dictionary*.
-
-- In older versions, different label converters would have different special character sets and character order. In version 0.x, the character order was as follows.
-
-| Converter | Character order |
-| ------------------------------- | ----------------------------------------- |
-| `AttnConvertor`, `ABIConvertor` | ``, ``, ``, characters |
-| `CTCConvertor` | ``, ``, characters |
-
-In 1.0, instead of designing different dictionaries and character orders for different tasks, we have a unified *Dictionary* implementation with the character order always as characters, \, \, \. \ in `CTCConvertor` has been equivalently replaced by \.
-
-- *Label convertor* originally supported three ways to initialize dictionaries: `dict_type`, `dict_file` and `dict_list`, which are now reduced to `dict_file` only in `Dictionary`. Also, we have put those pre-defined character sets originally supported in `dict_type` into `dicts/` directory now. The corresponding mapping is as follows:
-
- | MMOCR 0.x: `dict_type` | MMOCR 1.0: Dict path |
- | ---------------------- | -------------------------------------- |
- | DICT90 | dicts/english_digits_symbols.txt |
- | DICT91 | dicts/english_digits_symbols_space.txt |
- | DICT36 | dicts/lower_english_digits.txt |
- | DICT37 | dicts/lower_english_digits_space.txt |
-
-- The implementation of `str2tensor()` in *label converter* has been moved to `ModuleLoss.get_targets()`. The following table shows the correspondence between the old and new method implementations. Note that the old and new implementations are not identical.
-
- | MMOCR 0.x | MMOCR 1.0 | Note |
- | --------------------------------------------------------- | --------------------------------------- | -------------------------------------------------------------------------------------------------------- |
- | `ABIConvertor.str2tensor()`, `AttnConvertor.str2tensor()` | `BaseTextRecogModuleLoss.get_targets()` | The different implementations between `ABIConvertor.str2tensor()` and `AttnConvertor.str2tensor()` have been unified in the new version. |
- | `CTCConvertor.str2tensor()` | `CTCModuleLoss.get_targets()` | |
-
-- The implementation of `tensor2idx()` in *label converter* has been moved to `Postprocessor.get_single_prediction()`. The following table shows the correspondence between the old and new method implementations. Note that the old and new implementations are not identical.
-
- | MMOCR 0.x | MMOCR 1.0 |
- | --------------------------------------------------------- | ------------------------------------------------ |
- | `ABIConvertor.tensor2idx()`, `AttnConvertor.tensor2idx()` | `AttentionPostprocessor.get_single_prediction()` |
- | `CTCConvertor.tensor2idx()` | `CTCPostProcessor.get_single_prediction()` |
-
-## Key Information Extraction
-
-### Key Changes (TL;DR)
-
-- Due to changes in the inputs to the model, the model weights obtained in 0.x can no longer be directly used in 1.0.
-
-### KIEDataset & OpensetKIEDataset
-
-- The part that reads data is kept in `WildReceiptDataset`.
-
-- The part that additionally processes the nodes and edges is moved to `LoadKIEAnnotation`.
-
-- The part that uses dictionaries to transform text is moved to `SDMGRHead.convert_text()`, with the help of *Dictionary*.
-
-- The part of `compute_relation()` that computes the relationships between text boxes is moved to `SDMGRHead.compute_relations()`. It's now done inside the model.
-
-- The part that evaluates the model performance is done in [`F1Metric`](mmocr.evaluation.metric.F1Metric).
-
-- The part of `OpensetKIEDataset` that processes model's edge outputs is moved to `SDMGRPostProcessor`.
-
-### SDMGR
-
-- `show_result()` is integrated into `KIEVisualizer`.
-
-- The part of `forward_test()` that post-processes the output is organized in `SDMGRPostProcessor`.
-
-## Utils Migration
-
-Utility functions are now grouped together under `mmocr/utils/`. Here are the scopes of the files in this directory:
-
-- bbox_utils.py: bounding box related functions.
-- check_argument.py: used to check argument type.
-- collect_env.py: used to collect running environment.
-- data_converter_utils.py: used for data format conversion.
-- fileio.py: file input and output related functions.
-- img_utils.py: image processing related functions.
-- mask_utils.py: mask related functions.
-- ocr.py: used for MMOCR inference.
-- parsers.py: used for parsing datasets.
-- polygon_utils.py: polygon related functions.
-- setup_env.py: used for initialize MMOCR.
-- string_utils.py: string related functions.
-- typing.py: defines the abbreviation of types used in MMOCR.
diff --git a/pipeline/mmocr/docs/en/migration/dataset.md b/pipeline/mmocr/docs/en/migration/dataset.md
deleted file mode 100644
index 6238c344fee15183e43bb67d67ea573b669f586d..0000000000000000000000000000000000000000
--- a/pipeline/mmocr/docs/en/migration/dataset.md
+++ /dev/null
@@ -1,257 +0,0 @@
-# Dataset Migration
-
-Based on the new design of [BaseDataset](mmengine.dataset.BaseDataset) in [MMEngine](https://github.com/open-mmlab/mmengine), we have refactored the base OCR dataset class [`OCRDataset`](mmocr.datasets.OCRDataset) in MMOCR 1.0. The following document describes the differences between the old and new dataset formats in MMOCR, and how to migrate from the deprecated version to the latest. For users who do not want to migrate datasets at this time, we also provide a temporary solution in [Section Compatibility](#compatibility).
-
-```{note}
-The Key Information Extraction task still uses the original WildReceipt dataset annotation format.
-```
-
-## Review of Old Dataset Formats
-
-MMOCR version 0.x implements a number of dataset classes, such as `IcdarDataset`, `TextDetDataset` for text detection tasks, and `OCRDataset`, `OCRSegDataset` for text recognition tasks. At the same time, the annotations may vary in different formats, such as `.txt`, `.json`, `.jsonl`. Users have to manually configure the `Loader` and the `Parser` while customizing the datasets.
-
-### Text Detection
-
-For the text detection task, `IcdarDataset` uses a COCO-like annotation format.
-
-```json
-{
- "images": [
- {
- "id": 1,
- "width": 800,
- "height": 600,
- "file_name": "test.jpg"
- }
- ],
- "annotations": [
- {
- "id": 1,
- "image_id": 1,
- "category_id": 1,
- "bbox": [0,0,10,10],
- "segmentation": [
- [0,0,10,0,10,10,0,10]
- ],
- "area": 100,
- "iscrowd": 0
- }
- ]
-}
-```
-
-The `TextDetDataset` uses the JSON Line storage format, converting COCO-like labels to strings and saves them in `.txt` or `.jsonl` format files.
-
-```text
-{"file_name": "test/img_2.jpg", "height": 720, "width": 1280, "annotations": [{"iscrowd": 0, "category_id": 1, "bbox": [602.0, 173.0, 33.0, 24.0], "segmentation": [[602, 173, 635, 175, 634, 197, 602, 196]]}, {"iscrowd": 0, "category_id": 1, "bbox": [734.0, 310.0, 58.0, 54.0], "segmentation": [[734, 310, 792, 320, 792, 364, 738, 361]]}]}
-{"file_name": "test/img_5.jpg", "height": 720, "width": 1280, "annotations": [{"iscrowd": 1, "category_id": 1, "bbox": [405.0, 409.0, 32.0, 52.0], "segmentation": [[408, 409, 437, 436, 434, 461, 405, 433]]}, {"iscrowd": 1, "category_id": 1, "bbox": [435.0, 434.0, 8.0, 33.0], "segmentation": [[437, 434, 443, 440, 441, 467, 435, 462]]}]}
-```
-
-### Text Recognition
-
-For text recognition tasks, there are two annotation formats in MMOCR version 0.x. The simple `.txt` annotations separate image name and word annotation by a blank space, which cannot handle the case when spaces are included in a text instance.
-
-```text
-img1.jpg OpenMMLab
-img2.jpg MMOCR
-```
-
-The JSON Line format uses a dictionary-like structure to represent the annotations, where the keys `filename` and `text` store the image name and word label, respectively.
-
-```json
-{"filename": "img1.jpg", "text": "OpenMMLab"}
-{"filename": "img2.jpg", "text": "MMOCR"}
-```
-
-## New Dataset Format
-
-To solve the dataset issues, MMOCR 1.x adopts a unified dataset design introduced in MMEngine. Each annotation file is a `.json` file that stores a `dict`, containing both `metainfo` and `data_list`, where the former includes basic information about the dataset and the latter consists of the label item of each target instance.
-
-```json
-{
- "metainfo":
- {
- "classes": ("cat", "dog"),
- // ...
- },
- "data_list":
- [
- {
- "img_path": "xxx/xxx_0.jpg",
- "img_label": 0,
- // ...
- },
- // ...
- ]
-}
-```
-
-Based on the above structure, we introduced `TextDetDataset`, `TextRecogDataset` for MMOCR-specific tasks.
-
-### Text Detection
-
-#### Introduction of the New Format
-
-The `TextDetDataset` holds the information required by the text detection task, such as bounding boxes and labels. We refer users to `tests/data/det_toy_dataset/instances_test.json` which is an example annotation for `TextDetDataset`.
-
-```json
-{
- "metainfo":
- {
- "dataset_type": "TextDetDataset",
- "task_name": "textdet",
- "category": [{"id": 0, "name": "text"}]
- },
- "data_list":
- [
- {
- "img_path": "test_img.jpg",
- "height": 640,
- "width": 640,
- "instances":
- [
- {
- "polygon": [0, 0, 0, 10, 10, 20, 20, 0],
- "bbox": [0, 0, 10, 20],
- "bbox_label": 0,
- "ignore": False
- }๏ผ
- // ...
- ]
- }
- ]
-}
-```
-
-The bounding box format is as follows: `[min_x, min_y, max_x, max_y]`
-
-#### Migration Script
-
-We provide a migration script to help users migrate old annotation files to the new format.
-
-```bash
-python tools/dataset_converters/textdet/data_migrator.py ${IN_PATH} ${OUT_PATH}
-```
-
-| ARGS | Type | Description |
-| -------- | -------------------------------- | ---------------------------------------------------------------------------------------------------------------------------------------------------------------- |
-| in_path | str | ๏ผRequired๏ผPath to the old annotation file. |
-| out_path | str | ๏ผRequired๏ผPath to the new annotation file. |
-| --task | 'auto', 'textdet', 'textspotter' | Specifies the compatible task for the output dataset annotation. If 'textdet' is specified, the text field in coco format will not be dumped. The default is 'auto', which automatically determines the output format based on the the old annotation files. |
-
-### Text Recognition
-
-#### Introduction of the New Format
-
-The `TextRecogDataset` holds the information required by the text detection task, such as text and image path. We refer users to `tests/data/rec_toy_dataset/labels.json` which is an example annotation for `TextRecogDataset`.
-
-```json
-{
- "metainfo":
- {
- "dataset_type": "TextRecogDataset",
- "task_name": "textrecog",
- },
- "data_list":
- [
- {
- "img_path": "test_img.jpg",
- "instances":
- [
- {
- "text": "GRAND"
- }
- ]
- }
- ]
-}
-```
-
-#### Migration Script
-
-We provide a migration script to help users migrate old annotation files to the new format.
-
-```bash
-python tools/dataset_converters/textrecog/data_migrator.py ${IN_PATH} ${OUT_PATH} --format ${txt, jsonl, lmdb}
-```
-
-| ARGS | Type | Description |
-| -------- | ---------------------- | ------------------------------------------------- |
-| in_path | str | ๏ผRequired๏ผPath to the old annotation file. |
-| out_path | str | ๏ผRequired๏ผPath to the new annotation file. |
-| --format | 'txt', 'jsonl', 'lmdb' | Specify the format of the old dataset annotation. |
-
-## Compatibility
-
-In consideration of the cost to users for data migration, we have temporarily made MMOCR version 1.x compatible with the old MMOCR 0.x format.
-
-```{note}
-The code and components used for compatibility with the old data format may be completely removed in a future release. Therefore, we strongly recommend that users migrate their datasets to the new data format.
-```
-
-Specifically, we provide three dataset classes [IcdarDataset](mmocr.datasets.IcdarDataset), [RecogTextDataset](mmocr.datasets.RecogTextDataset), [RecogLMDBDataset](mmocr.datasets.RecogLMDBDataset) to support the old formats.
-
-1. [IcdarDataset](mmocr.datasets.IcdarDataset) supports COCO-like format annotations for text detection. You just need to add a new dataset config to `configs/textdet/_base_/datasets` and specify its dataset type as `IcdarDataset`.
-
- ```python
- data_root = 'data/det/icdar2015'
- train_anno_path = 'instances_training.json'
-
- train_dataset = dict(
- type='IcdarDataset',
- data_root=data_root,
- ann_file=train_anno_path,
- data_prefix=dict(img_path='imgs/'),
- filter_cfg=dict(filter_empty_gt=True, min_size=32),
- pipeline=None)
- ```
-
-2. [RecogTextDataset](mmocr.datasets.RecogTextDataset) supports `.txt` and `.jsonl` format annotations for text recognition. You just need to add a new dataset config to `configs/textrecog/_base_/datasets` and specify its dataset type as `RecogTextDataset`. For example, the following example shows how to configure and load the 0.x format labels `old_label.txt` and `old_label.jsonl` from the toy dataset.
-
- ```python
- data_root = 'tests/data/rec_toy_dataset/'
-
- # loading 0.x txt format annos
- txt_dataset = dict(
- type='RecogTextDataset',
- data_root=data_root,
- ann_file='old_label.txt',
- data_prefix=dict(img_path='imgs'),
- parser_cfg=dict(
- type='LineStrParser',
- keys=['filename', 'text'],
- keys_idx=[0, 1]),
- pipeline=[])
-
- # loading 0.x json line format annos
- jsonl_dataset = dict(
- type='RecogTextDataset',
- data_root=data_root,
- ann_file='old_label.jsonl',
- data_prefix=dict(img_path='imgs'),
- parser_cfg=dict(
- type='LineJsonParser',
- keys=['filename', 'text'],
- pipeline=[]))
- ```
-
-3. [RecogLMDBDataset](mmocr.datasets.RecogLMDBDataset) supports LMDB format dataset (img+labels) for text recognition. You just need to add a new dataset config to `configs/textrecog/_base_/datasets` and specify its dataset type as `RecogLMDBDataset`. For example, the following example shows how to configure and load the **both labels and images** `imgs.lmdb` from the toy dataset.
-
-- set the dataset type to `RecogLMDBDataset`
-
-```python
-# Specify the dataset type as RecogLMDBDataset
- data_root = 'tests/data/rec_toy_dataset/'
-
- lmdb_dataset = dict(
- type='RecogLMDBDataset',
- data_root=data_root,
- ann_file='imgs.lmdb',
- pipeline=None)
-```
-
-- replace the [`LoadImageFromFile`](mmocr.datasets.transforms.LoadImageFromFile) with [`LoadImageFromNDArray`](mmocr.datasets.transforms.LoadImageFromNDArray) in the data pipelines in `train_pipeline` and `test_pipeline`., for example๏ผ
-
-```python
- train_pipeline = [dict(type='LoadImageFromNDArray')]
-```
diff --git a/pipeline/mmocr/docs/en/migration/model.md b/pipeline/mmocr/docs/en/migration/model.md
deleted file mode 100644
index 2ab507470ed9892b12f420c40eb81bbecd0bfd29..0000000000000000000000000000000000000000
--- a/pipeline/mmocr/docs/en/migration/model.md
+++ /dev/null
@@ -1,5 +0,0 @@
-# Pretrained Model Migration
-
-Due to the extensive refactoring and fixing of the model structure in the new version, MMOCR 1.x does not support load weights trained by the old version. We have updated the pre-training weights and logs of all models on our website.
-
-In addition, we are working on the development of a weight migration tool for text detection tasks and plan to release it in the near future. Since the text recognition and key information extraction models are too much modified and the migration is lossy, we do not plan to support them accordingly for the time being. If you have specific requirements, please feel free to raise an [Issue](https://github.com/open-mmlab/mmocr/issues).
diff --git a/pipeline/mmocr/docs/en/migration/news.md b/pipeline/mmocr/docs/en/migration/news.md
deleted file mode 100644
index 1dc991186953916a4a6d4a47d4a1c30c7d8ada7d..0000000000000000000000000000000000000000
--- a/pipeline/mmocr/docs/en/migration/news.md
+++ /dev/null
@@ -1,19 +0,0 @@
-# What's New in MMOCR 1.x
-
-Here are some highlights of MMOCR 1.x compared to 0.x.
-
-1. **New engines**. MMOCR 1.x is based on [MMEngine](https://github.com/open-mmlab/mmengine), which provides a general and powerful runner that allows more flexible customizations and significantly simplifies the entrypoints of high-level interfaces.
-
-2. **Unified interfaces**. As a part of the OpenMMLab 2.0 projects, MMOCR 1.x unifies and refactors the interfaces and internal logics of train, testing, datasets, models, evaluation, and visualization. All the OpenMMLab 2.0 projects share the same design in those interfaces and logics to allow the emergence of multi-task/modality algorithms.
-
-3. **Cross project calling**. Benefiting from the unified design, you can use the models implemented in other OpenMMLab projects, such as MMDet. We provide an example of how to use MMDetection's Mask R-CNN through `MMDetWrapper`. Check our documents for more details. More wrappers will be released in the future.
-
-4. **Stronger visualization**. We provide a series of useful tools which are mostly based on brand-new visualizers. As a result, it is more convenient for the users to explore the models and datasets now.
-
-5. **More documentation and tutorials**. We add a bunch of documentation and tutorials to help users get started more smoothly.
-
-6. **One-stop Dataset Preparaion**. Multiple datasets are instantly ready with only one line of command, via our [Dataset Preparer](https://mmocr.readthedocs.io/en/dev-1.x/user_guides/data_prepare/dataset_preparer.html).
-
-7. **Embracing more `projects/`**: We now introduce `projects/` folder, where some experimental features, frameworks and models can be placed, only needed to satisfy the minimum requirement on the code quality. Everyone is welcome to post their implementation of any great ideas in this folder! Learn more from our [example project](https://github.com/open-mmlab/mmocr/blob/dev-1.x/projects/example_project/).
-
-8. **More models**. MMOCR 1.0 supports more tasks and more state-of-the-art models!
diff --git a/pipeline/mmocr/docs/en/migration/overview.md b/pipeline/mmocr/docs/en/migration/overview.md
deleted file mode 100644
index e389781dc5279340ef43301e373e0e5fa10d63cc..0000000000000000000000000000000000000000
--- a/pipeline/mmocr/docs/en/migration/overview.md
+++ /dev/null
@@ -1,18 +0,0 @@
-# Overview
-
-Along with the release of OpenMMLab 2.0, MMOCR 1.0 made many significant changes, resulting in less redundant, more efficient code and a more consistent overall design. However, these changes break backward compatibility. We understand that with such huge changes, it is not easy for users familiar with the old version to adapt to the new version. Therefore, we prepared a detailed migration guide to make the transition as smooth as possible so that all users can enjoy the productivity benefits of the new MMOCR and the entire OpenMMLab 2.0 ecosystem.
-
-```{warning}
-MMOCR 1.0 depends on the new foundational library for training deep learning models [MMEngine](https://github.com/open-mmlab/mmengine), and therefore has an entirely different dependency chain compared with MMOCR 0.x. Even if you have a well-rounded MMOCR 0.x environment before, you still need to create a new python environment for MMOCR 1.0. We provide a detailed [installation guide](../get_started/install.md) for reference.
-```
-
-Next, please read the sections according to your requirements.
-
-- Read [What's new in MMOCR 1.x](./news.md) to learn about the new features and changes in MMOCR 1.x.
-- If you want to migrate a model trained in version 0.x to use it directly in version 1.0, please read [Pretrained Model Migration](./model.md).
-- If you want to train the model, please read [Dataset Migration](./dataset.md) and [Data Transform Migration](./transforms.md).
-- If you want to develop on MMOCR, please read [Code Migration](code.md), [Branch Migration](branches.md) and [Upstream Library Changes](https://github.com/open-mmlab/mmengine/tree/main/docs/en/migration).
-
-As shown in the following figure, the maintenance plan of MMOCR 1.x version is mainly divided into three stages, namely "RC Period", "Compatibility Period" and "Maintenance Period". For old versions, we will no longer add major new features. Therefore, we strongly recommend users to migrate to MMOCR 1.x version as soon as possible.
-
-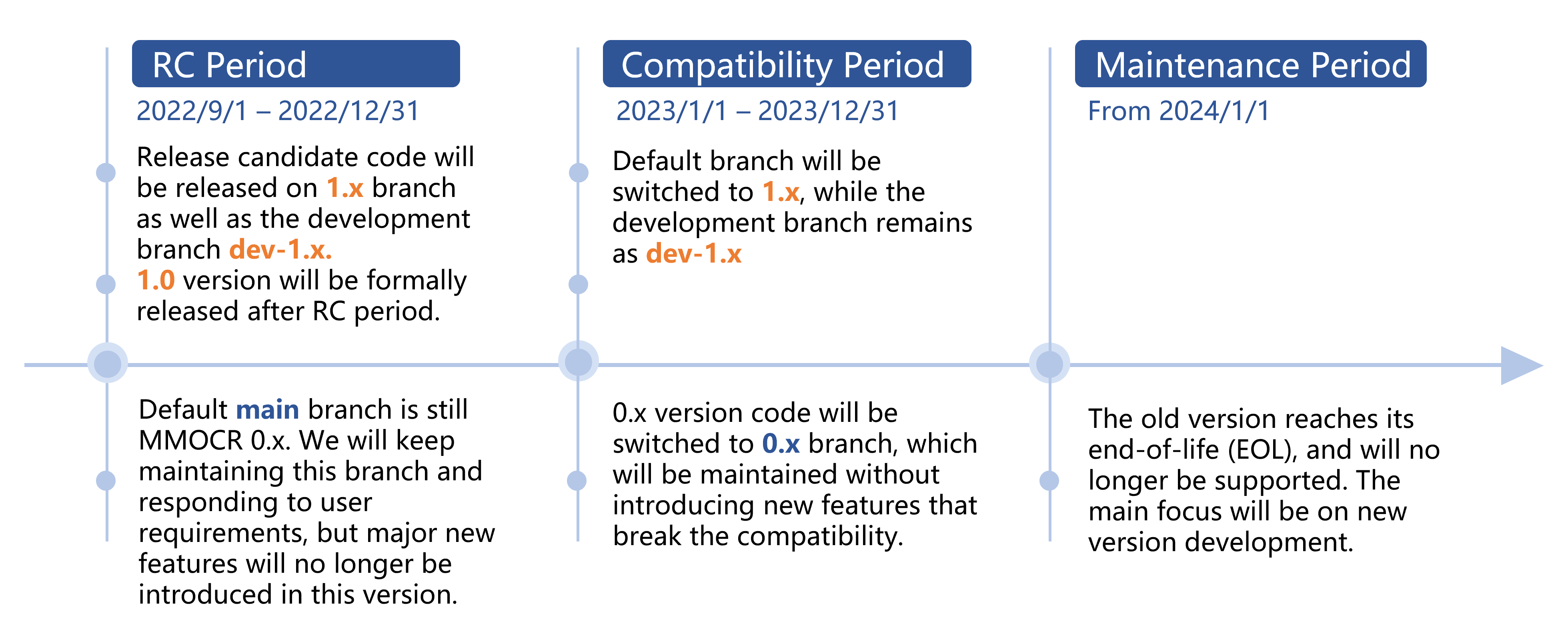
diff --git a/pipeline/mmocr/docs/en/migration/transforms.md b/pipeline/mmocr/docs/en/migration/transforms.md
deleted file mode 100644
index 33661313d5abae59b49e59e6fcf53591c40b3959..0000000000000000000000000000000000000000
--- a/pipeline/mmocr/docs/en/migration/transforms.md
+++ /dev/null
@@ -1,583 +0,0 @@
-# Data Transform Migration
-
-## Introduction
-
-In MMOCR version 0.x, we implemented a series of **Data Transform** methods in `mmocr/datasets/pipelines/xxx_transforms.py`. However, these modules are scattered all over the place and lack a standardized design. Therefore, we refactored all the data transform modules in MMOCR version 1.x. According to the task type, they are now defined in `ocr_transforms.py`, `textdet_transforms.py`, and `textrecog_transforms.py`, respectively, under `mmocr/datasets/transforms`. Specifically, `ocr_transforms.py` implements the data augmentation methods for OCR-related tasks in general, while `textdet_transforms.py` and `textrecog_transforms.py` implement data augmentation transforms related to text detection and text recognition tasks, respectively.
-
-Since some of the modules were renamed, merged or separated during the refactoring process, the new interface and default parameters may be inconsistent with the old version. Therefore, this migration guide will introduce how to configure the new data transforms to achieve the identical behavior as the old version.
-
-## Configuration Migration Guide
-
-### Data Formatting Related Data Transforms
-
-1. `Collect` + `CustomFormatBundle` -> [`PackTextDetInputs`](mmocr.datasets.transforms.formatting.PackTextDetInputs)/[`PackTextRecogInputs`](mmocr.datasets.transforms.formatting.PackTextRecogInputs)
-
-`PackxxxInputs` implements both `Collect` and `CustomFormatBundle` functions, and no longer has `key` parameters, the generation of training targets is moved to be done in `loss` modules.
-
-
-
-
- MMOCR 0.x Configuration
- MMOCR 1.x Configuration
-
-
-
-
-```python
-dict(
- type='CustomFormatBundle',
- keys=['gt_shrink', 'gt_shrink_mask', 'gt_thr', 'gt_thr_mask'],
- meta_keys=['img_path', 'ori_shape', 'img_shape'],
- visualize=dict(flag=False, boundary_key='gt_shrink')),
-dict(
- type='Collect',
- keys=['img', 'gt_shrink', 'gt_shrink_mask', 'gt_thr', 'gt_thr_mask'])
-```
-
-
-
-```python
-dict(
- type='PackTextDetInputs',
- meta_keys=('img_path', 'ori_shape', 'img_shape'))
-```
-
-
-
-
-
-### Data Augmentation Related Data Transforms
-
-1. `ResizeOCR` -> [`Resize`](mmocr.datasets.transforms.Resize), [`RescaleToHeight`](mmocr.datasets.transforms.RescaleToHeight), [`PadToWidth`](mmocr.datasets.transforms.PadToWidth)
-
- The original `ResizeOCR` is now split into three data augmentation modules.
-
- When `keep_aspect_ratio=False`, it is equivalent to `Resize` in version 1.x. Its configuration can be modified as follows.
-
-
-
-
- MMOCR 0.x Configuration
- MMOCR 1.x Configuration
-
-
-
-
-```python
-dict(
- type='ResizeOCR',
- height=32,
- min_width=100,
- max_width=100,
- keep_aspect_ratio=False)
-```
-
-
-
-```python
-dict(
- type='Resize',
- scale=(100, 32),
- keep_ratio=False)
-```
-
-
-
-
-
-When `keep_aspect_ratio=True` and `max_width=None`. The image will be rescaled to a fixed size alongside the height while keeping the aspect ratio the same as the origin.
-
-
-
-
- MMOCR 0.x Configuration
- MMOCR 1.x Configuration
-
-
-
-
-```python
-dict(
- type='ResizeOCR',
- height=32,
- min_width=32,
- max_width=None,
- width_downsample_ratio = 1.0 / 16
- keep_aspect_ratio=True)
-```
-
-
-
-```python
-dict(
- type='RescaleToHeight',
- height=32,
- min_width=32,
- max_width=None,
- width_divisor=16),
-```
-
-
-
-
-
-When `keep_aspect_ratio=True` and `max_width` is a fixed value. The image will be rescaled to a fixed size alongside the height while keeping the aspect ratio the same as the origin. Then, the width will be padded or cropped to `max_width`. That is to say, the shape of the output image is always `(height, max_width)`.
-
-
-
-
- MMOCR 0.x Configuration
- MMOCR 1.x Configuration
-
-
-
-
-```python
-dict(
- type='ResizeOCR',
- height=32,
- min_width=32,
- max_width=100,
- width_downsample_ratio = 1.0 / 16,
- keep_aspect_ratio=True)
-```
-
-
-
-```python
-dict(
- type='RescaleToHeight',
- height=32,
- min_width=32,
- max_width=100,
- width_divisor=16),
-dict(
- type='PadToWidth',
- width=100)
-```
-
-
-
-
-
-2. `RandomRotateTextDet` & `RandomRotatePolyInstances` -> [`RandomRotate`](mmocr.datasets.transforms.RandomRotate)
-
- We implemented all random rotation-related data augmentation in `RandomRotate` in version 1.x. Its default behavior is identical to the `RandomRotateTextDet` in version 0.x.
-
-```{note}
- The default value of "max_angle" might be different from the old version, so the users are suggested to manually set the number.
-```
-
-
-
-
- MMOCR 0.x Configuration
- MMOCR 1.x Configuration
-
-
-
-
-```python
-dict(type='RandomRotateTextDet')
-```
-
-
-
-```python
-dict(type='RandomRotate', max_angle=10)
-```
-
-
-
-
-
-For `RandomRotatePolyInstances`๏ผit is supposed to set `use_canvas=True`ใ
-
-
-
-
- MMOCR 0.x Configuration
- MMOCR 1.x Configuration
-
-
-
-
-```python
-dict(
- type='RandomRotatePolyInstances',
- rotate_ratio=0.5, # Specify the execution probability
- max_angle=60,
- pad_with_fixed_color=False)
-```
-
-
-
-```python
-# Wrap the data transforms with RandomApply and specify the execution probability
-dict(
- type='RandomApply',
- transforms=[
- dict(type='RandomRotate',
- max_angle=60,
- pad_with_fixed_color=False,
- use_canvas=True)],
- prob=0.5) # Specify the execution probability
-```
-
-
-
-
-
-```{note}
-In version 0.x, some data augmentation methods specified execution probability by defining an internal variable "xxx_ratio", such as "rotate_ratio", "crop_ratio", etc. In version 1.x, these parameters have been removed. Now we can use "RandomApply" to wrap different data transforms and specify their execution probabilities.
-```
-
-3. `RandomCropFlip` -> [`TextDetRandomCropFlip`](mmocr.datasets.transforms.TextDetRandomCropFlip)
-
- Currently, only the method name has been changed, and other parameters remain the same.
-
-4. `RandomCropPolyInstances` -> [`RandomCrop`](mmocr.datasets.transforms.RandomCrop)
-
- In MMOCR version 1.x, `crop_ratio` and `instance_key` are removed. The `gt_polygons` is now used as the target for cropping.
-
-
-
-
- MMOCR 0.x Configuration
- MMOCR 1.x Configuration
-
-
-
-
-```python
-dict(
- type='RandomCropPolyInstances',
- instance_key='gt_masks',
- crop_ratio=0.8, # Specify the execution probability
- min_side_ratio=0.3)
-```
-
-
-
-```python
-# Wrap the data transforms with RandomApply and specify the execution probability
-dict(
- type='RandomApply',
- transforms=[dict(type='RandomCrop', min_side_ratio=0.3)],
- prob=0.8) # Specify the execution probability
-```
-
-
-
-
-
-5. `RandomCropInstances` -> [`TextDetRandomCrop`](mmocr.datasets.transforms.TextDetRandomCrop)
-
- In MMOCR version 1.x, `crop_ratio` and `instance_key` are removed. The `gt_polygons` is now used as the target for cropping.
-
-
-
-
- MMOCR 0.x Configuration
- MMOCR 1.x Configuration
-
-
-
-
-```python
-dict(
- type='RandomCropInstances',
- target_size=(800๏ผ800),
- instance_key='gt_kernels')
-```
-
-
-
-```python
-dict(
- type='TextDetRandomCrop',
- target_size=(800๏ผ800))
-```
-
-
-
-
-
-6. `EastRandomCrop` -> [`RandomCrop`](mmocr.datasets.transforms.RandomCrop) + [`Resize`](mmocr.datasets.transforms.Resize) + [`mmengine.Pad`](mmcv.transforms.Pad)
-
- `EastRandomCrop` was implemented by applying cropping, scaling and padding to the input image. Now, the same effect can be achieved by combining three data transforms.
-
-
-
-
- MMOCR 0.x Configuration
- MMOCR 1.x Configuration
-
-
-
-
-```python
-dict(
- type='EastRandomCrop',
- max_tries=10,
- min_crop_side_ratio=0.1,
- target_size=(640, 640))
-```
-
-
-
-```python
-dict(type='RandomCrop', min_side_ratio=0.1),
-dict(type='Resize', scale=(640,640), keep_ratio=True),
-dict(type='Pad', size=(640,640))
-```
-
-
-
-
-
-7. `RandomScaling` -> [`mmengine.RandomResize`](mmcv.transforms.RandomResize)
-
- The `RandomScaling` is now replaced with [`mmengine.RandomResize`](mmcv.transforms.RandomResize).
-
-
-
-
- MMOCR 0.x Configuration
- MMOCR 1.x Configuration
-
-
-
-
-```python
- dict(
- type='RandomScaling',
- size=800,
- scale=(0.75, 2.5))
-```
-
-
-
-```python
-dict(
- type='RandomResize',
- scale=(800, 800),
- ratio_range=(0.75, 2.5),
- keep_ratio=True)
-```
-
-
-
-
-
-```{note}
-By default, the data pipeline will search for the corresponding data transforms from the register of the current *scope*, and if that data transform does not exist, it will continue to search in the upstream library, such as MMCV and MMEngine. For example, the `RandomResize` transform is not implemented in MMOCR, but it can be directly called in the configuration, as the program will automatically search for it from MMCV. In addition, you can also specify *scope* by adding a prefix. For example, `mmengine.RandomResize` will force it to use `RandomResize` implemented in MMEngine, which is useful when a method of the same name exists in both upstream and downstream libraries. It is noteworthy that all of the data transforms implemented in MMCV are registered to MMEngine, that is why we use `mmengine.RandomResize` but not `mmcv.RandomResize`.
-```
-
-8. `SquareResizePad` -> [`Resize`](mmocr.datasets.transforms.Resize) + [`SourceImagePad`](mmocr.datasets.transforms.SourceImagePad)
-
- `SquareResizePad` implements two branches and uses one of them randomly based on the `pad_ratio`. Specifically, one branch first resizes the image and then pads it to a certain size; while the other branch only resizes the image. To enhance the reusability of the different modules, we split this data transform into a combination of `Resize` + `SourceImagePad` in version 1.x, and control the branches via `RandomChoice`.
-
-
-
-
- MMOCR 0.x Configuration
- MMOCR 1.x Configuration
-
-
-
-
-```python
-dict(
- type='SquareResizePad',
- target_size=800,
- pad_ratio=0.6)
-```
-
-
-
-```python
-dict(
- type='RandomChoice',
- transforms=[
- [
- dict(
- type='Resize',
- scale=800,
- keep_ratio=True),
- dict(
- type='SourceImagePad',
- target_scale=800)
- ],
- [
- dict(
- type='Resize',
- scale=800,
- keep_ratio=False)
- ]
- ],
- prob=[0.4, 0.6]), # Probability of selection of two combinations
-```
-
-
-
-
-
-```{note}
-In version 1.x, the random choice wrapper "RandomChoice" replaces "OneOfWrapper", allowing random selection of data transform combinations.
-```
-
-9. `RandomWrapper` -> [`mmengine.RandomApply`](mmcv.transforms.RandomApply)
-
- In version 1.x, the `RandomWrapper` wrapper has been replaced with `RandomApply` in MMEngine, which is used to specify the probability of performing a data transform. And the probability `p` is now named `prob`.
-
-
-
-
- MMOCR 0.x Configuration
- MMOCR 1.x Configuration
-
-
-
-
-```python
- dict(
- type='RandomWrapper',
- p=0.25,
- transforms=[
- dict(type='PyramidRescale'),
- ])
-```
-
-
-
-```python
-dict(
- type='RandomApply',
- prob=0.25,
- transforms=[
- dict(type='PyramidRescale'),
- ])
-```
-
-
-
-
-
-10. `OneOfWrapper` -> [`mmengine.RandomChoice`](mmcv.transforms.RandomChoice)
-
- The random choice wrapper is now renamed to `RandomChoice` and is used in exactly the same way as before.
-
-11. `ScaleAspectJitter` -> [`ShortScaleAspectJitter`](mmocr.datasets.transforms.ShortScaleAspectJitter), [`BoundedScaleAspectJitter`](mmocr.datasets.transforms.BoundedScaleAspectJitter)
-
- The `ScaleAspectJitter` implemented several different image size jittering strategies, which has now been split into several independent data transforms.
-
- When `resize_type='indep_sample_in_range'`, it is equivalent to `RandomResize`.
-
-
-
-
- MMOCR 0.x Configuration
- MMOCR 1.x Configuration
-
-
-
-
-```python
-dict(
- type='ScaleAspectJitter',
- img_scale=None,
- keep_ratio=False,
- resize_type='indep_sample_in_range',
- scale_range=(640, 2560))
-```
-
-
-
-```python
- dict(
- type='RandomResize',
- scale=(640, 640),
- ratio_range=(1.0, 4.125),
- resize_type='Resize',
- keep_ratio=True))
-```
-
-
-
-
-
-When `resize_type='long_short_bound'`, we implemented `BoundedScaleAspectJitter`, which randomly rescales the image so that the long and short sides of the image are around the bound; then jitters the aspect ratio.
-
-
-
-
- MMOCR 0.x Configuration
- MMOCR 1.x Configuration
-
-
-
-
-```python
-dict(
- type='ScaleAspectJitter',
- img_scale=[(3000, 736)], # Unused
- ratio_range=(0.7, 1.3),
- aspect_ratio_range=(0.9, 1.1),
- multiscale_mode='value',
- long_size_bound=800,
- short_size_bound=480,
- resize_type='long_short_bound',
- keep_ratio=False)
-```
-
-
-
-```python
-dict(
- type='BoundedScaleAspectJitter',
- long_size_bound=800,
- short_size_bound=480,
- ratio_range=(0.7, 1.3),
- aspect_ratio_range=(0.9, 1.1))
-```
-
-
-
-
-
-When `resize_type='round_min_img_scale'`, we implemented `ShortScaleAspectJitter`, which rescales the image for its shorter side to reach the `short_size` and then jitters its aspect ratio, finally rescales the shape guaranteed to be divided by scale_divisor.
-
-
-
-
- MMOCR 0.x Configuration
- MMOCR 1.x Configuration
-
-
-
-
-```python
-dict(
- type='ScaleAspectJitter',
- img_scale=[(3000, 640)],
- ratio_range=(0.7, 1.3),
- aspect_ratio_range=(0.9, 1.1),
- multiscale_mode='value',
- keep_ratio=False)
-```
-
-
-
-```python
-dict(
- type='ShortScaleAspectJitter',
- short_size=640,
- ratio_range=(0.7, 1.3),
- aspect_ratio_range=(0.9, 1.1),
- scale_divisor=32),
-```
-
-
-
-
diff --git a/pipeline/mmocr/docs/en/notes/branches.md b/pipeline/mmocr/docs/en/notes/branches.md
deleted file mode 100644
index 9b799946895de88065724684dd28939d635cfc83..0000000000000000000000000000000000000000
--- a/pipeline/mmocr/docs/en/notes/branches.md
+++ /dev/null
@@ -1,25 +0,0 @@
-# Branches
-
-This documentation aims to provide a comprehensive understanding of the purpose and features of each branch in MMOCR.
-
-## Branch Overview
-
-### 1. `main`
-
-The `main` branch serves as the default branch for the MMOCR project. It contains the latest stable version of MMOCR, currently housing the code for MMOCR 1.x (e.g. v1.0.0). The `main` branch ensures users have access to the most recent and reliable version of the software.
-
-### 2. `dev-1.x`
-
-The `dev-1.x` branch is dedicated to the development of the next major version of MMOCR. This branch will routinely undergo reliance tests, and the passing commits will be squashed in a release and published to the `main` branch. By having a separate development branch, the project can continue to evolve without impacting the stability of the `main` branch. **All the PRs should be merged into the `dev-1.x` branch.**
-
-### 3. `0.x`
-
-The `0.x` branch serves as an archive for MMOCR 0.x (e.g. v0.6.3). This branch will no longer actively receive updates or improvements, but it remains accessible for historical reference or for users who have not yet upgraded to MMOCR 1.x.
-
-### 3. `1.x`
-
-It's an alias of `main` branch, which is intended for a smooth transition from the compatibility period. It will be removed in mid 2023.
-
-```{note}
-The branches mapping has been changed in 2023.04.06. For the legacy branches mapping and the guide for migration, please refer to the [branch migration guide](../migration/branches.md).
-```
diff --git a/pipeline/mmocr/docs/en/notes/changelog.md b/pipeline/mmocr/docs/en/notes/changelog.md
deleted file mode 100644
index b04e69531cb606f4f7e2e9d326d9955c6deab5f2..0000000000000000000000000000000000000000
--- a/pipeline/mmocr/docs/en/notes/changelog.md
+++ /dev/null
@@ -1,458 +0,0 @@
-# Changelog of v1.x
-
-## v1.0.0 (04/06/2023)
-
-We are excited to announce the first official release of MMOCR 1.0, with numerous enhancements, bug fixes, and the introduction of new dataset support!
-
-### ๐ Highlights
-
-- Support for SCUT-CTW1500, SynthText, and MJSynth datasets
-- Updated FAQ and documentation
-- Deprecation of file_client_args in favor of backend_args
-- Added a new MMOCR tutorial notebook
-
-### ๐ New Features & Enhancement
-
-- Add SCUT-CTW1500 by @Mountchicken in https://github.com/open-mmlab/mmocr/pull/1677
-- Cherry Pick #1205 by @gaotongxiao in https://github.com/open-mmlab/mmocr/pull/1774
-- Make lanms-neo optional by @gaotongxiao in https://github.com/open-mmlab/mmocr/pull/1772
-- SynthText by @gaotongxiao in https://github.com/open-mmlab/mmocr/pull/1779
-- Deprecate file_client_args and use backend_args instead by @gaotongxiao in https://github.com/open-mmlab/mmocr/pull/1765
-- MJSynth by @gaotongxiao in https://github.com/open-mmlab/mmocr/pull/1791
-- Add MMOCR tutorial notebook by @gaotongxiao in https://github.com/open-mmlab/mmocr/pull/1771
-- decouple batch_size to det_batch_size, rec_batch_size and kie_batch_size in MMOCRInferencer by @hugotong6425 in https://github.com/open-mmlab/mmocr/pull/1801
-- Accepts local-rank in train.py and test.py by @gaotongxiao in https://github.com/open-mmlab/mmocr/pull/1806
-- update stitch_boxes_into_lines by @cherryjm in https://github.com/open-mmlab/mmocr/pull/1824
-- Add tests for pytorch 2.0 by @gaotongxiao in https://github.com/open-mmlab/mmocr/pull/1836
-
-### ๐ Docs
-
-- FAQ by @gaotongxiao in https://github.com/open-mmlab/mmocr/pull/1773
-- Remove LoadImageFromLMDB from docs by @gaotongxiao in https://github.com/open-mmlab/mmocr/pull/1767
-- Mark projects in docs by @gaotongxiao in https://github.com/open-mmlab/mmocr/pull/1766
-- add opendatalab download link by @jorie-peng in https://github.com/open-mmlab/mmocr/pull/1753
-- Fix some deadlinks in the docs by @gaotongxiao in https://github.com/open-mmlab/mmocr/pull/1469
-- Fix quick run by @gaotongxiao in https://github.com/open-mmlab/mmocr/pull/1775
-- Dataset by @gaotongxiao in https://github.com/open-mmlab/mmocr/pull/1782
-- Update faq by @gaotongxiao in https://github.com/open-mmlab/mmocr/pull/1817
-- more social network links by @fengshiwest in https://github.com/open-mmlab/mmocr/pull/1818
-- Update docs after branch switching by @gaotongxiao in https://github.com/open-mmlab/mmocr/pull/1834
-
-### ๐ ๏ธ Bug Fixes:
-
-- Place dicts to .mim by @gaotongxiao in https://github.com/open-mmlab/mmocr/pull/1781
-- Test svtr_small instead of svtr_tiny by @gaotongxiao in https://github.com/open-mmlab/mmocr/pull/1786
-- Add pse weight to metafile by @gaotongxiao in https://github.com/open-mmlab/mmocr/pull/1787
-- Synthtext metafile by @gaotongxiao in https://github.com/open-mmlab/mmocr/pull/1788
-- Clear up some unused scripts by @gaotongxiao in https://github.com/open-mmlab/mmocr/pull/1798
-- if dst not exists, when move a single file may raise a file not exists error. by @KevinNuNu in https://github.com/open-mmlab/mmocr/pull/1803
-- CTW1500 by @gaotongxiao in https://github.com/open-mmlab/mmocr/pull/1814
-- MJSynth & SynthText Dataset Preparer config by @gaotongxiao in https://github.com/open-mmlab/mmocr/pull/1805
-- Use poly_intersection instead of poly.intersection to avoid supโฆ by @gaotongxiao in https://github.com/open-mmlab/mmocr/pull/1811
-- Abinet: fix ValueError: Blur limit must be odd when centered=True. Got: (3, 6) by @hugotong6425 in https://github.com/open-mmlab/mmocr/pull/1821
-- Bug generated during kie inference visualization by @Yangget in https://github.com/open-mmlab/mmocr/pull/1830
-- Revert sync bn in inferencer by @gaotongxiao in https://github.com/open-mmlab/mmocr/pull/1832
-- Fix mmdet digit version by @gaotongxiao in https://github.com/open-mmlab/mmocr/pull/1840
-
-### ๐ New Contributors
-
-- @jorie-peng made their first contribution in https://github.com/open-mmlab/mmocr/pull/1753
-- @hugotong6425 made their first contribution in https://github.com/open-mmlab/mmocr/pull/1801
-- @fengshiwest made their first contribution in https://github.com/open-mmlab/mmocr/pull/1818
-- @cherryjm made their first contribution in https://github.com/open-mmlab/mmocr/pull/1824
-- @Yangget made their first contribution in https://github.com/open-mmlab/mmocr/pull/1830
-
-Thank you to all the contributors for making this release possible! We're excited about the new features and enhancements in this version, and we're looking forward to your feedback and continued support. Happy coding! ๐
-
-**Full Changelog**: https://github.com/open-mmlab/mmocr/compare/v1.0.0rc6...v1.0.0
-
-### Highlights
-
-## v1.0.0rc6 (03/07/2023)
-
-### Highlights
-
-1. Two new models, ABCNet v2 (inference only) and SPTS are added to `projects/` folder.
-2. Announcing `Inferencer`, a unified inference interface in OpenMMLab for everyone's easy access and quick inference with all the pre-trained weights. [Docs](https://mmocr.readthedocs.io/en/dev-1.x/user_guides/inference.html)
-3. Users can use test-time augmentation for text recognition tasks. [Docs](https://mmocr.readthedocs.io/en/dev-1.x/user_guides/train_test.html#test-time-augmentation)
-4. Support [batch augmentation](https://openaccess.thecvf.com/content_CVPR_2020/papers/Hoffer_Augment_Your_Batch_Improving_Generalization_Through_Instance_Repetition_CVPR_2020_paper.pdf) through [`BatchAugSampler`](https://github.com/open-mmlab/mmocr/pull/1757), which is a technique used in SPTS.
-5. Dataset Preparer has been refactored to allow more flexible configurations. Besides, users are now able to prepare text recognition datasets in LMDB formats. [Docs](https://mmocr.readthedocs.io/en/dev-1.x/user_guides/data_prepare/dataset_preparer.html#lmdb-format)
-6. Some textspotting datasets have been revised to enhance the correctness and consistency with the common practice.
-7. Potential spurious warnings from `shapely` have been eliminated.
-
-### Dependency
-
-This version requires MMEngine >= 0.6.0, MMCV >= 2.0.0rc4 and MMDet >= 3.0.0rc5.
-
-### New Features & Enhancements
-
-- Discard deprecated lmdb dataset format and only support img+label now by @gaotongxiao in https://github.com/open-mmlab/mmocr/pull/1681
-- abcnetv2 inference by @Harold-lkk in https://github.com/open-mmlab/mmocr/pull/1657
-- Add RepeatAugSampler by @gaotongxiao in https://github.com/open-mmlab/mmocr/pull/1678
-- SPTS by @gaotongxiao in https://github.com/open-mmlab/mmocr/pull/1696
-- Refactor Inferencers by @gaotongxiao in https://github.com/open-mmlab/mmocr/pull/1608
-- Dynamic return type for rescale_polygons by @gaotongxiao in https://github.com/open-mmlab/mmocr/pull/1702
-- Revise upstream version limit by @gaotongxiao in https://github.com/open-mmlab/mmocr/pull/1703
-- TextRecogCropConverter add crop with opencv warpPersepective function by @KevinNuNu in https://github.com/open-mmlab/mmocr/pull/1667
-- change cudnn benchmark to false by @Harold-lkk in https://github.com/open-mmlab/mmocr/pull/1705
-- Add ST-pretrained DB-series models and logs by @gaotongxiao in https://github.com/open-mmlab/mmocr/pull/1635
-- Only keep meta and state_dict when publish model by @Harold-lkk in https://github.com/open-mmlab/mmocr/pull/1729
-- Rec TTA by @Harold-lkk in https://github.com/open-mmlab/mmocr/pull/1401
-- Speedup formatting by replacing np.transpose with torchโฆ by @gaotongxiao in https://github.com/open-mmlab/mmocr/pull/1719
-- Support auto import modules from registry. by @Harold-lkk in https://github.com/open-mmlab/mmocr/pull/1731
-- Support batch visualization & dumping in Inferencer by @gaotongxiao in https://github.com/open-mmlab/mmocr/pull/1722
-- add a new argument font_properties to set a specific font file in order to draw Chinese characters properly by @KevinNuNu in https://github.com/open-mmlab/mmocr/pull/1709
-- Refactor data converter and gather by @Harold-lkk in https://github.com/open-mmlab/mmocr/pull/1707
-- Support batch augmentation through BatchAugSampler by @gaotongxiao in https://github.com/open-mmlab/mmocr/pull/1757
-- Put all registry into registry.py by @Harold-lkk in https://github.com/open-mmlab/mmocr/pull/1760
-- train by @gaotongxiao in https://github.com/open-mmlab/mmocr/pull/1756
-- configs for regression benchmark by @gaotongxiao in https://github.com/open-mmlab/mmocr/pull/1755
-- Support lmdb format in Dataset Preparer by @gaotongxiao in https://github.com/open-mmlab/mmocr/pull/1762
-
-### Docs
-
-- update the link of DBNet by @AllentDan in https://github.com/open-mmlab/mmocr/pull/1672
-- Add notice for default branch switching by @gaotongxiao in https://github.com/open-mmlab/mmocr/pull/1693
-- docs: Add twitter discord medium youtube link by @vansin in https://github.com/open-mmlab/mmocr/pull/1724
-- Remove unsupported datasets in docs by @gaotongxiao in https://github.com/open-mmlab/mmocr/pull/1670
-
-### Bug Fixes
-
-- Update dockerfile by @gaotongxiao in https://github.com/open-mmlab/mmocr/pull/1671
-- Explicitly create np object array for compatibility by @gaotongxiao in https://github.com/open-mmlab/mmocr/pull/1691
-- Fix a minor error in docstring by @Mountchicken in https://github.com/open-mmlab/mmocr/pull/1685
-- Fix lint by @triple-Mu in https://github.com/open-mmlab/mmocr/pull/1694
-- Fix LoadOCRAnnotation ut by @Harold-lkk in https://github.com/open-mmlab/mmocr/pull/1695
-- Fix isort pre-commit error by @KevinNuNu in https://github.com/open-mmlab/mmocr/pull/1697
-- Update owners by @xinke-wang in https://github.com/open-mmlab/mmocr/pull/1699
-- Detect intersection before using shapley.intersection to eliminate spurious warnings by @gaotongxiao in https://github.com/open-mmlab/mmocr/pull/1710
-- Fix some inferencer bugs by @gaotongxiao in https://github.com/open-mmlab/mmocr/pull/1706
-- Fix textocr ignore flag by @xinke-wang in https://github.com/open-mmlab/mmocr/pull/1712
-- Add missing softmax in ASTER forward_test by @Mountchicken in https://github.com/open-mmlab/mmocr/pull/1718
-- Fix head in readme by @vansin in https://github.com/open-mmlab/mmocr/pull/1727
-- Fix some browse dataset script bugs and draw textdet gt instance with ignore flags by @KevinNuNu in https://github.com/open-mmlab/mmocr/pull/1701
-- icdar textrecog ann parser skip data with ignore flag by @KevinNuNu in https://github.com/open-mmlab/mmocr/pull/1708
-- bezier_to_polygon -> bezier2polygon by @double22a in https://github.com/open-mmlab/mmocr/pull/1739
-- Fix docs recog CharMetric P/R error definition by @KevinNuNu in https://github.com/open-mmlab/mmocr/pull/1740
-- Remove outdated resources in demo/ by @gaotongxiao in https://github.com/open-mmlab/mmocr/pull/1747
-- Fix wrong ic13 textspotting split data; add lexicons to ic13, ic15 and totaltext by @gaotongxiao in https://github.com/open-mmlab/mmocr/pull/1758
-- SPTS readme by @gaotongxiao in https://github.com/open-mmlab/mmocr/pull/1761
-
-### New Contributors
-
-- @triple-Mu made their first contribution in https://github.com/open-mmlab/mmocr/pull/1694
-- @double22a made their first contribution in https://github.com/open-mmlab/mmocr/pull/1739
-
-**Full Changelog**: https://github.com/open-mmlab/mmocr/compare/v1.0.0rc5...v1.0.0rc6
-
-## v1.0.0rc5 (01/06/2023)
-
-### Highlights
-
-1. Two models, Aster and SVTR, are added to our model zoo. The full implementation of ABCNet is also available now.
-2. Dataset Preparer supports 5 more datasets: CocoTextV2, FUNSD, TextOCR, NAF, SROIE.
-3. We have 4 more text recognition transforms, and two helper transforms. See https://github.com/open-mmlab/mmocr/pull/1646 https://github.com/open-mmlab/mmocr/pull/1632 https://github.com/open-mmlab/mmocr/pull/1645 for details.
-4. The transform, `FixInvalidPolygon`, is getting smarter at dealing with invalid polygons, and now capable of handling more weird annotations. As a result, a complete training cycle on TotalText dataset can be performed bug-free. The weights of DBNet and FCENet pretrained on TotalText are also released.
-
-### New Features & Enhancements
-
-- Update ic15 det config according to DataPrepare by @Harold-lkk in https://github.com/open-mmlab/mmocr/pull/1617
-- Refactor icdardataset metainfo to lowercase. by @Harold-lkk in https://github.com/open-mmlab/mmocr/pull/1620
-- Add ASTER Encoder by @Mountchicken in https://github.com/open-mmlab/mmocr/pull/1239
-- Add ASTER decoder by @Mountchicken in https://github.com/open-mmlab/mmocr/pull/1625
-- Add ASTER config by @Mountchicken in https://github.com/open-mmlab/mmocr/pull/1238
-- Update ASTER config by @Mountchicken in https://github.com/open-mmlab/mmocr/pull/1629
-- Support browse_dataset.py to visualize original dataset by @xinke-wang in https://github.com/open-mmlab/mmocr/pull/1503
-- Add CocoTextv2 to dataset preparer by @xinke-wang in https://github.com/open-mmlab/mmocr/pull/1514
-- Add Funsd to dataset preparer by @xinke-wang in https://github.com/open-mmlab/mmocr/pull/1550
-- Add TextOCR to Dataset Preparer by @xinke-wang in https://github.com/open-mmlab/mmocr/pull/1543
-- Refine example projects and readme by @gaotongxiao in https://github.com/open-mmlab/mmocr/pull/1628
-- Enhance FixInvalidPolygon, add RemoveIgnored transform by @gaotongxiao in https://github.com/open-mmlab/mmocr/pull/1632
-- ConditionApply by @Harold-lkk in https://github.com/open-mmlab/mmocr/pull/1646
-- Add NAF to dataset preparer by @Mountchicken in https://github.com/open-mmlab/mmocr/pull/1609
-- Add SROIE to dataset preparer by @FerryHuang in https://github.com/open-mmlab/mmocr/pull/1639
-- Add svtr decoder by @willpat1213 in https://github.com/open-mmlab/mmocr/pull/1448
-- Add missing unit tests by @Mountchicken in https://github.com/open-mmlab/mmocr/pull/1651
-- Add svtr encoder by @willpat1213 in https://github.com/open-mmlab/mmocr/pull/1483
-- ABCNet train by @Harold-lkk in https://github.com/open-mmlab/mmocr/pull/1610
-- Totaltext cfgs for DB and FCE by @gaotongxiao in https://github.com/open-mmlab/mmocr/pull/1633
-- Add Aliases to models by @gaotongxiao in https://github.com/open-mmlab/mmocr/pull/1611
-- SVTR transforms by @gaotongxiao in https://github.com/open-mmlab/mmocr/pull/1645
-- Add SVTR framework and configs by @gaotongxiao in https://github.com/open-mmlab/mmocr/pull/1621
-- Issue Template by @Harold-lkk in https://github.com/open-mmlab/mmocr/pull/1663
-
-### Docs
-
-- Add Chinese translation for browse_dataset.py by @xinke-wang in https://github.com/open-mmlab/mmocr/pull/1647
-- updata abcnet doc by @Harold-lkk in https://github.com/open-mmlab/mmocr/pull/1658
-- update the dbnetpp\`s readme file by @zhuyue66 in https://github.com/open-mmlab/mmocr/pull/1626
-- Inferencer docs by @gaotongxiao in https://github.com/open-mmlab/mmocr/pull/1744
-
-### Bug Fixes
-
-- nn.SmoothL1Loss beta can not be zero in PyTorch 1.13 version by @Harold-lkk in https://github.com/open-mmlab/mmocr/pull/1616
-- ctc loss bug if target is empty by @Harold-lkk in https://github.com/open-mmlab/mmocr/pull/1618
-- Add torch 1.13 by @gaotongxiao in https://github.com/open-mmlab/mmocr/pull/1619
-- Remove outdated tutorial link by @gaotongxiao in https://github.com/open-mmlab/mmocr/pull/1627
-- Dev 1.x some doc mistakes by @KevinNuNu in https://github.com/open-mmlab/mmocr/pull/1630
-- Support custom font to visualize some languages (e.g. Korean) by @ProtossDragoon in https://github.com/open-mmlab/mmocr/pull/1567
-- db_module_loss๏ผnegative number encountered in sqrt by @KevinNuNu in https://github.com/open-mmlab/mmocr/pull/1640
-- Use int instead of np.int by @gaotongxiao in https://github.com/open-mmlab/mmocr/pull/1636
-- Remove support for py3.6 by @gaotongxiao in https://github.com/open-mmlab/mmocr/pull/1660
-
-### New Contributors
-
-- @zhuyue66 made their first contribution in https://github.com/open-mmlab/mmocr/pull/1626
-- @KevinNuNu made their first contribution in https://github.com/open-mmlab/mmocr/pull/1630
-- @FerryHuang made their first contribution in https://github.com/open-mmlab/mmocr/pull/1639
-- @willpat1213 made their first contribution in https://github.com/open-mmlab/mmocr/pull/1448
-
-**Full Changelog**: https://github.com/open-mmlab/mmocr/compare/v1.0.0rc4...v1.0.0rc5
-
-## v1.0.0rc4 (12/06/2022)
-
-### Highlights
-
-1. Dataset Preparer can automatically generate base dataset configs at the end of the preparation process, and supports 6 more datasets: IIIT5k, CUTE80, ICDAR2013, ICDAR2015, SVT, SVTP.
-2. Introducing our `projects/` folder - implementing new models and features into OpenMMLab's algorithm libraries has long been complained to be troublesome due to the rigorous requirements on code quality, which could hinder the fast iteration of SOTA models and might discourage community members from sharing their latest outcome here. We now introduce `projects/` folder, where some experimental features, frameworks and models can be placed, only needed to satisfy the minimum requirement on the code quality. Everyone is welcome to post their implementation of any great ideas in this folder! We also add the first [example project](https://github.com/open-mmlab/mmocr/tree/dev-1.x/projects/example_project) to illustrate what we expect a good project to have (check out the raw content of README.md for more info!).
-3. Inside the `projects/` folder, we are releasing the preview version of ABCNet, which is the first implementation of text spotting models in MMOCR. It's inference-only now, but the full implementation will be available very soon.
-
-### New Features & Enhancements
-
-- Add SVT to dataset preparer by @xinke-wang in https://github.com/open-mmlab/mmocr/pull/1521
-- Polish bbox2poly by @gaotongxiao in https://github.com/open-mmlab/mmocr/pull/1532
-- Add SVTP to dataset preparer by @xinke-wang in https://github.com/open-mmlab/mmocr/pull/1523
-- Iiit5k converter by @Harold-lkk in https://github.com/open-mmlab/mmocr/pull/1530
-- Add cute80 to dataset preparer by @xinke-wang in https://github.com/open-mmlab/mmocr/pull/1522
-- Add IC13 preparer by @xinke-wang in https://github.com/open-mmlab/mmocr/pull/1531
-- Add 'Projects/' folder, and the first example project by @gaotongxiao in https://github.com/open-mmlab/mmocr/pull/1524
-- Rename to {dataset-name}\_task_train/test by @Harold-lkk in https://github.com/open-mmlab/mmocr/pull/1541
-- Add print_config.py to the tools by @IncludeMathH in https://github.com/open-mmlab/mmocr/pull/1547
-- Add get_md5 by @gaotongxiao in https://github.com/open-mmlab/mmocr/pull/1553
-- Add config generator by @gaotongxiao in https://github.com/open-mmlab/mmocr/pull/1552
-- Support IC15_1811 by @gaotongxiao in https://github.com/open-mmlab/mmocr/pull/1556
-- Update CT80 config by @gaotongxiao in https://github.com/open-mmlab/mmocr/pull/1555
-- Add config generators to all textdet and textrecog configs by @gaotongxiao in https://github.com/open-mmlab/mmocr/pull/1560
-- Refactor TPS by @Mountchicken in https://github.com/open-mmlab/mmocr/pull/1240
-- Add TextSpottingConfigGenerator by @gaotongxiao in https://github.com/open-mmlab/mmocr/pull/1561
-- Add common typing by @Harold-lkk in https://github.com/open-mmlab/mmocr/pull/1596
-- Update textrecog config and readme by @gaotongxiao in https://github.com/open-mmlab/mmocr/pull/1597
-- Support head loss or postprocessor is None for only infer by @Harold-lkk in https://github.com/open-mmlab/mmocr/pull/1594
-- Textspotting datasample by @Harold-lkk in https://github.com/open-mmlab/mmocr/pull/1593
-- Simplify mono_gather by @gaotongxiao in https://github.com/open-mmlab/mmocr/pull/1588
-- ABCNet v1 infer by @Harold-lkk in https://github.com/open-mmlab/mmocr/pull/1598
-
-### Docs
-
-- Add Chinese Guidance on How to Add New Datasets to Dataset Preparer by @xinke-wang in https://github.com/open-mmlab/mmocr/pull/1506
-- Update the qq group link by @vansin in https://github.com/open-mmlab/mmocr/pull/1569
-- Collapse some sections; update logo url by @gaotongxiao in https://github.com/open-mmlab/mmocr/pull/1571
-- Update dataset preparer (CN) by @gaotongxiao in https://github.com/open-mmlab/mmocr/pull/1591
-
-### Bug Fixes
-
-- Fix two bugs in dataset preparer by @xinke-wang in https://github.com/open-mmlab/mmocr/pull/1513
-- Register bug of CLIPResNet by @jyshee in https://github.com/open-mmlab/mmocr/pull/1517
-- Being more conservative on Dataset Preparer by @gaotongxiao in https://github.com/open-mmlab/mmocr/pull/1520
-- python -m pip upgrade in windows by @Harold-lkk in https://github.com/open-mmlab/mmocr/pull/1525
-- Fix wildreceipt metafile by @xinke-wang in https://github.com/open-mmlab/mmocr/pull/1528
-- Fix Dataset Preparer Extract by @xinke-wang in https://github.com/open-mmlab/mmocr/pull/1527
-- Fix ICDARTxtParser by @xinke-wang in https://github.com/open-mmlab/mmocr/pull/1529
-- Fix Dataset Zoo Script by @xinke-wang in https://github.com/open-mmlab/mmocr/pull/1533
-- Fix crop without padding and recog metainfo delete unuse info by @Harold-lkk in https://github.com/open-mmlab/mmocr/pull/1526
-- Automatically create nonexistent directory for base configs by @gaotongxiao in https://github.com/open-mmlab/mmocr/pull/1535
-- Change mmcv.dump to mmengine.dump by @ProtossDragoon in https://github.com/open-mmlab/mmocr/pull/1540
-- mmocr.utils.typing -> mmocr.utils.typing_utils by @gaotongxiao in https://github.com/open-mmlab/mmocr/pull/1538
-- Wildreceipt tests by @gaotongxiao in https://github.com/open-mmlab/mmocr/pull/1546
-- Fix judge exist dir by @Harold-lkk in https://github.com/open-mmlab/mmocr/pull/1542
-- Fix IC13 textdet config by @xinke-wang in https://github.com/open-mmlab/mmocr/pull/1563
-- Fix IC13 textrecog annotations by @gaotongxiao in https://github.com/open-mmlab/mmocr/pull/1568
-- Auto scale lr by @gaotongxiao in https://github.com/open-mmlab/mmocr/pull/1584
-- Fix icdar data parse for text containing separator by @Harold-lkk in https://github.com/open-mmlab/mmocr/pull/1587
-- Fix textspotting ut by @Harold-lkk in https://github.com/open-mmlab/mmocr/pull/1599
-- Fix TextSpottingConfigGenerator and TextSpottingDataConverter by @gaotongxiao in https://github.com/open-mmlab/mmocr/pull/1604
-- Keep E2E Inferencer output simple by @gaotongxiao in https://github.com/open-mmlab/mmocr/pull/1559
-
-### New Contributors
-
-- @jyshee made their first contribution in https://github.com/open-mmlab/mmocr/pull/1517
-- @ProtossDragoon made their first contribution in https://github.com/open-mmlab/mmocr/pull/1540
-- @IncludeMathH made their first contribution in https://github.com/open-mmlab/mmocr/pull/1547
-
-**Full Changelog**: https://github.com/open-mmlab/mmocr/compare/v1.0.0rc3...v1.0.0rc4
-
-## v1.0.0rc3 (11/03/2022)
-
-### Highlights
-
-1. We release several pretrained models using [oCLIP-ResNet](https://github.com/open-mmlab/mmocr/blob/1.x/configs/backbone/oclip/README.md) as the backbone, which is a ResNet variant trained with [oCLIP](https://www.ecva.net/papers/eccv_2022/papers_ECCV/papers/136880282.pdf) and can significantly boost the performance of text detection models.
-
-2. Preparing datasets is troublesome and tedious, especially in OCR domain where multiple datasets are usually required. In order to free our users from laborious work, we designed a [Dataset Preparer](https://mmocr.readthedocs.io/en/dev-1.x/user_guides/data_prepare/dataset_preparer.html) to help you get a bunch of datasets ready for use, with only **one line of command**! Dataset Preparer is also crafted to consist of a series of reusable modules, each responsible for handling one of the standardized phases throughout the preparation process, shortening the development cycle on supporting new datasets.
-
-### New Features & Enhancements
-
-- Add Dataset Preparer by @xinke-wang in https://github.com/open-mmlab/mmocr/pull/1484
-
-* support modified resnet structure used in oCLIP by @HannibalAPE in https://github.com/open-mmlab/mmocr/pull/1458
-* Add oCLIP configs by @gaotongxiao in https://github.com/open-mmlab/mmocr/pull/1509
-
-### Docs
-
-- Update install.md by @rogachevai in https://github.com/open-mmlab/mmocr/pull/1494
-- Refine some docs by @gaotongxiao in https://github.com/open-mmlab/mmocr/pull/1455
-- Update some dataset preparer related docs by @xinke-wang in https://github.com/open-mmlab/mmocr/pull/1502
-- oclip readme by @Harold-lkk in https://github.com/open-mmlab/mmocr/pull/1505
-
-### Bug Fixes
-
-- Fix offline_eval error caused by new data flow by @gaotongxiao in https://github.com/open-mmlab/mmocr/pull/1500
-
-### New Contributors
-
-- @rogachevai made their first contribution in https://github.com/open-mmlab/mmocr/pull/1494
-- @HannibalAPE made their first contribution in https://github.com/open-mmlab/mmocr/pull/1458
-
-**Full Changelog**: https://github.com/open-mmlab/mmocr/compare/v1.0.0rc2...v1.0.0rc3
-
-## v1.0.0rc2 (10/14/2022)
-
-This release relaxes the version requirement of `MMEngine` to `>=0.1.0, < 1.0.0`.
-
-## v1.0.0rc1 (10/09/2022)
-
-### Highlights
-
-This release fixes a severe bug leading to inaccurate metric report in multi-GPU training.
-We release the weights for all the text recognition models in MMOCR 1.0 architecture. The inference shorthand for them are also added back to `ocr.py`. Besides, more documentation chapters are available now.
-
-### New Features & Enhancements
-
-- Simplify the Mask R-CNN config by @xinke-wang in https://github.com/open-mmlab/mmocr/pull/1391
-- auto scale lr by @Harold-lkk in https://github.com/open-mmlab/mmocr/pull/1326
-- Update paths to pretrain weights by @gaotongxiao in https://github.com/open-mmlab/mmocr/pull/1416
-- Streamline duplicated split_result in pan_postprocessor by @gaotongxiao in https://github.com/open-mmlab/mmocr/pull/1418
-- Update model links in ocr.py and inference.md by @gaotongxiao in https://github.com/open-mmlab/mmocr/pull/1431
-- Update rec configs by @gaotongxiao in https://github.com/open-mmlab/mmocr/pull/1417
-- Visualizer refine by @Harold-lkk in https://github.com/open-mmlab/mmocr/pull/1411
-- Support get flops and parameters in dev-1.x by @vansin in https://github.com/open-mmlab/mmocr/pull/1414
-
-### Docs
-
-- intersphinx and api by @Harold-lkk in https://github.com/open-mmlab/mmocr/pull/1367
-- Fix quickrun by @gaotongxiao in https://github.com/open-mmlab/mmocr/pull/1374
-- Fix some docs issues by @gaotongxiao in https://github.com/open-mmlab/mmocr/pull/1385
-- Add Documents for DataElements by @xinke-wang in https://github.com/open-mmlab/mmocr/pull/1381
-- config english by @Harold-lkk in https://github.com/open-mmlab/mmocr/pull/1372
-- Metrics by @xinke-wang in https://github.com/open-mmlab/mmocr/pull/1399
-- Add version switcher to menu by @gaotongxiao in https://github.com/open-mmlab/mmocr/pull/1407
-- Data Transforms by @xinke-wang in https://github.com/open-mmlab/mmocr/pull/1392
-- Fix inference docs by @gaotongxiao in https://github.com/open-mmlab/mmocr/pull/1415
-- Fix some docs by @xinke-wang in https://github.com/open-mmlab/mmocr/pull/1410
-- Add maintenance plan to migration guide by @xinke-wang in https://github.com/open-mmlab/mmocr/pull/1413
-- Update Recog Models by @xinke-wang in https://github.com/open-mmlab/mmocr/pull/1402
-
-### Bug Fixes
-
-- clear metric.results only done in main process by @Harold-lkk in https://github.com/open-mmlab/mmocr/pull/1379
-- Fix a bug in MMDetWrapper by @xinke-wang in https://github.com/open-mmlab/mmocr/pull/1393
-- Fix browse_dataset.py by @Mountchicken in https://github.com/open-mmlab/mmocr/pull/1398
-- ImgAugWrapper: Do not cilp polygons if not applicable by @gaotongxiao in https://github.com/open-mmlab/mmocr/pull/1231
-- Fix CI by @gaotongxiao in https://github.com/open-mmlab/mmocr/pull/1365
-- Fix merge stage test by @gaotongxiao in https://github.com/open-mmlab/mmocr/pull/1370
-- Del CI support for torch 1.5.1 by @gaotongxiao in https://github.com/open-mmlab/mmocr/pull/1371
-- Test windows cu111 by @gaotongxiao in https://github.com/open-mmlab/mmocr/pull/1373
-- Fix windows CI by @gaotongxiao in https://github.com/open-mmlab/mmocr/pull/1387
-- Upgrade pre commit hooks by @Harold-lkk in https://github.com/open-mmlab/mmocr/pull/1429
-- Skip invalid augmented polygons in ImgAugWrapper by @gaotongxiao in https://github.com/open-mmlab/mmocr/pull/1434
-
-### New Contributors
-
-- @vansin made their first contribution in https://github.com/open-mmlab/mmocr/pull/1414
-
-**Full Changelog**: https://github.com/open-mmlab/mmocr/compare/v1.0.0rc0...v1.0.0rc1
-
-## v1.0.0rc0 (09/01/2022)
-
-We are excited to announce the release of MMOCR 1.0.0rc0.
-MMOCR 1.0.0rc0 is the first version of MMOCR 1.x, a part of the OpenMMLab 2.0 projects.
-Built upon the new [training engine](https://github.com/open-mmlab/mmengine),
-MMOCR 1.x unifies the interfaces of dataset, models, evaluation, and visualization with faster training and testing speed.
-
-### Highlights
-
-1. **New engines**. MMOCR 1.x is based on [MMEngine](https://github.com/open-mmlab/mmengine), which provides a general and powerful runner that allows more flexible customizations and significantly simplifies the entrypoints of high-level interfaces.
-
-2. **Unified interfaces**. As a part of the OpenMMLab 2.0 projects, MMOCR 1.x unifies and refactors the interfaces and internal logics of train, testing, datasets, models, evaluation, and visualization. All the OpenMMLab 2.0 projects share the same design in those interfaces and logics to allow the emergence of multi-task/modality algorithms.
-
-3. **Cross project calling**. Benefiting from the unified design, you can use the models implemented in other OpenMMLab projects, such as MMDet. We provide an example of how to use MMDetection's Mask R-CNN through `MMDetWrapper`. Check our documents for more details. More wrappers will be released in the future.
-
-4. **Stronger visualization**. We provide a series of useful tools which are mostly based on brand-new visualizers. As a result, it is more convenient for the users to explore the models and datasets now.
-
-5. **More documentation and tutorials**. We add a bunch of documentation and tutorials to help users get started more smoothly. Read it [here](https://mmocr.readthedocs.io/en/dev-1.x/).
-
-### Breaking Changes
-
-We briefly list the major breaking changes here.
-We will update the [migration guide](../migration.md) to provide complete details and migration instructions.
-
-#### Dependencies
-
-- MMOCR 1.x relies on MMEngine to run. MMEngine is a new foundational library for training deep learning models in OpenMMLab 2.0 models. The dependencies of file IO and training are migrated from MMCV 1.x to MMEngine.
-- MMOCR 1.x relies on MMCV>=2.0.0rc0. Although MMCV no longer maintains the training functionalities since 2.0.0rc0, MMOCR 1.x relies on the data transforms, CUDA operators, and image processing interfaces in MMCV. Note that the package `mmcv` is the version that provide pre-built CUDA operators and `mmcv-lite` does not since MMCV 2.0.0rc0, while `mmcv-full` has been deprecated.
-
-#### Training and testing
-
-- MMOCR 1.x uses Runner in [MMEngine](https://github.com/open-mmlab/mmengine) rather than that in MMCV. The new Runner implements and unifies the building logic of dataset, model, evaluation, and visualizer. Therefore, MMOCR 1.x no longer maintains the building logics of those modules in `mmocr.train.apis` and `tools/train.py`. Those code have been migrated into [MMEngine](https://github.com/open-mmlab/mmengine/blob/main/mmengine/runner/runner.py). Please refer to the [migration guide of Runner in MMEngine](https://mmengine.readthedocs.io/en/latest/migration/runner.html) for more details.
-- The Runner in MMEngine also supports testing and validation. The testing scripts are also simplified, which has similar logic as that in training scripts to build the runner.
-- The execution points of hooks in the new Runner have been enriched to allow more flexible customization. Please refer to the [migration guide of Hook in MMEngine](https://mmengine.readthedocs.io/en/latest/migration/hook.html) for more details.
-- Learning rate and momentum scheduling has been migrated from `Hook` to `Parameter Scheduler` in MMEngine. Please refer to the [migration guide of Parameter Scheduler in MMEngine](https://mmengine.readthedocs.io/en/latest/migration/param_scheduler.html) for more details.
-
-#### Configs
-
-- The [Runner in MMEngine](https://github.com/open-mmlab/mmengine/blob/main/mmengine/runner/runner.py) uses a different config structures to ease the understanding of the components in runner. Users can read the [config example of MMOCR](../user_guides/config.md) or refer to the [migration guide in MMEngine](https://mmengine.readthedocs.io/en/latest/migration/runner.html) for migration details.
-- The file names of configs and models are also refactored to follow the new rules unified across OpenMMLab 2.0 projects. Please refer to the [user guides of config](../user_guides/config.md) for more details.
-
-#### Dataset
-
-The Dataset classes implemented in MMOCR 1.x all inherits from the `BaseDetDataset`, which inherits from the [BaseDataset in MMEngine](https://mmengine.readthedocs.io/en/latest/advanced_tutorials/basedataset.html). There are several changes of Dataset in MMOCR 1.x.
-
-- All the datasets support to serialize the data list to reduce the memory when multiple workers are built to accelerate data loading.
-- The interfaces are changed accordingly.
-
-#### Data Transforms
-
-The data transforms in MMOCR 1.x all inherits from those in MMCV>=2.0.0rc0, which follows a new convention in OpenMMLab 2.0 projects.
-The changes are listed as below:
-
-- The interfaces are also changed. Please refer to the [API Reference](https://mmocr.readthedocs.io/en/dev-1.x/)
-- The functionality of some data transforms (e.g., `Resize`) are decomposed into several transforms.
-- The same data transforms in different OpenMMLab 2.0 libraries have the same augmentation implementation and the logic of the same arguments, i.e., `Resize` in MMDet 3.x and MMOCR 1.x will resize the image in the exact same manner given the same arguments.
-
-#### Model
-
-The models in MMOCR 1.x all inherits from `BaseModel` in MMEngine, which defines a new convention of models in OpenMMLab 2.0 projects. Users can refer to the [tutorial of model](https://mmengine.readthedocs.io/en/latest/tutorials/model.html) in MMengine for more details. Accordingly, there are several changes as the following:
-
-- The model interfaces, including the input and output formats, are significantly simplified and unified following the new convention in MMOCR 1.x. Specifically, all the input data in training and testing are packed into `inputs` and `data_samples`, where `inputs` contains model inputs like a list of image tensors, and `data_samples` contains other information of the current data sample such as ground truths and model predictions. In this way, different tasks in MMOCR 1.x can share the same input arguments, which makes the models more general and suitable for multi-task learning.
-- The model has a data preprocessor module, which is used to pre-process the input data of model. In MMOCR 1.x, the data preprocessor usually does necessary steps to form the input images into a batch, such as padding. It can also serve as a place for some special data augmentations or more efficient data transformations like normalization.
-- The internal logic of model have been changed. In MMOCR 0.x, model used `forward_train` and `simple_test` to deal with different model forward logics. In MMOCR 1.x and OpenMMLab 2.0, the forward function has three modes: `loss`, `predict`, and `tensor` for training, inference, and tracing or other purposes, respectively. The forward function calls `self.loss()`, `self.predict()`, and `self._forward()` given the modes `loss`, `predict`, and `tensor`, respectively.
-
-#### Evaluation
-
-MMOCR 1.x mainly implements corresponding metrics for each task, which are manipulated by [Evaluator](https://mmengine.readthedocs.io/en/latest/design/evaluator.html) to complete the evaluation.
-In addition, users can build evaluator in MMOCR 1.x to conduct offline evaluation, i.e., evaluate predictions that may not produced by MMOCR, prediction follows our dataset conventions. More details can be find in the [Evaluation Tutorial](https://mmengine.readthedocs.io/en/latest/tutorials/evaluation.html) in MMEngine.
-
-#### Visualization
-
-The functions of visualization in MMOCR 1.x are removed. Instead, in OpenMMLab 2.0 projects, we use [Visualizer](https://mmengine.readthedocs.io/en/latest/design/visualization.html) to visualize data. MMOCR 1.x implements `TextDetLocalVisualizer`, `TextRecogLocalVisualizer`, and `KIELocalVisualizer` to allow visualization of ground truths, model predictions, and feature maps, etc., at any place, for the three tasks supported in MMOCR. It also supports to dump the visualization data to any external visualization backends such as Tensorboard and Wandb. Check our [Visualization Document](https://mmocr.readthedocs.io/en/dev-1.x/user_guides/visualization.html) for more details.
-
-### Improvements
-
-- Most models enjoy a performance improvement from the new framework and refactor of data transforms. For example, in MMOCR 1.x, DBNet-R50 achieves **0.854** hmean score on ICDAR 2015, while the counterpart can only get **0.840** hmean score in MMOCR 0.x.
-- Support mixed precision training of most of the models. However, the [rest models](https://mmocr.readthedocs.io/en/dev-1.x/user_guides/train_test.html#mixed-precision-training) are not supported yet because the operators they used might not be representable in fp16. We will update the documentation and list the results of mixed precision training.
-
-### Ongoing changes
-
-1. Test-time augmentation: which was supported in MMOCR 0.x, is not implemented yet in this version due to limited time slot. We will support it in the following releases with a new and simplified design.
-2. Inference interfaces: a unified inference interfaces will be supported in the future to ease the use of released models.
-3. Interfaces of useful tools that can be used in notebook: more useful tools that implemented in the `tools/` directory will have their python interfaces so that they can be used through notebook and in downstream libraries.
-4. Documentation: we will add more design docs, tutorials, and migration guidance so that the community can deep dive into our new design, participate the future development, and smoothly migrate downstream libraries to MMOCR 1.x.
diff --git a/pipeline/mmocr/docs/en/notes/changelog_v0.x.md b/pipeline/mmocr/docs/en/notes/changelog_v0.x.md
deleted file mode 100644
index 6b087b1d55cc73b5051fcd4272d97afa0bcbf753..0000000000000000000000000000000000000000
--- a/pipeline/mmocr/docs/en/notes/changelog_v0.x.md
+++ /dev/null
@@ -1,904 +0,0 @@
-# Changelog
-
-## 0.6.0 (05/05/2022)
-
-### Highlights
-
-1. A new recognition algorithm [MASTER](https://arxiv.org/abs/1910.02562) has been added into MMOCR, which was the championship solution for the "ICDAR 2021 Competition on Scientific Table Image Recognition to Latex"! The model pre-trained on SynthText and MJSynth is available for testing! Credit to @JiaquanYe
-2. [DBNet++](https://arxiv.org/abs/2202.10304) has been released now! A new Adaptive Scale Fusion module has been equipped for feature enhancement. Benefiting from this, the new model achieved 2% better h-mean score than its predecessor on the ICDAR2015 dataset.
-3. Three more dataset converters are added: LSVT, RCTW and HierText. Check the dataset zoo ([Det](https://mmocr.readthedocs.io/en/latest/datasets/det.html#) & [Recog](https://mmocr.readthedocs.io/en/latest/datasets/recog.html) ) to explore further information.
-4. To enhance the data storage efficiency, MMOCR now supports loading both images and labels from .lmdb format annotations for the text recognition task. To enable such a feature, the new lmdb_converter.py is ready for use to pack your cropped images and labels into an lmdb file. For a detailed tutorial, please refer to the following sections and the [doc](https://mmocr.readthedocs.io/en/latest/tools.html#convert-text-recognition-dataset-to-lmdb-format).
-5. Testing models on multiple datasets is a widely used evaluation strategy. MMOCR now supports automatically reporting mean scores when there is more than one dataset to evaluate, which enables a more convenient comparison between checkpoints. [Doc](https://mmocr.readthedocs.io/en/latest/tutorials/dataset_types.html#getting-mean-evaluation-scores)
-6. Evaluation is more flexible and customizable now. For text detection tasks, you can set the score threshold range where the best results might come out. ([Doc](https://mmocr.readthedocs.io/en/latest/tutorials/dataset_types.html#evaluation)) If too many results are flooding your text recognition train log, you can trim it by specifying a subset of metrics in evaluation config. Check out the [Evaluation](https://mmocr.readthedocs.io/en/latest/tutorials/dataset_types.html#ocrdataset) section for details.
-7. MMOCR provides a script to convert the .json labels obtained by the popular annotation toolkit **Labelme** to MMOCR-supported data format. @Y-M-Y contributed a log analysis tool that helps users gain a better understanding of the entire training process. Read [tutorial docs](https://mmocr.readthedocs.io/en/latest/tools.html) to get started.
-
-### Lmdb Dataset
-
-Reading images or labels from files can be slow when data are excessive, e.g. on a scale of millions. Besides, in academia, most of the scene text recognition datasets are stored in lmdb format, including images and labels. To get closer to the mainstream practice and enhance the data storage efficiency, MMOCR now officially supports loading images and labels from lmdb datasets via a new pipeline [LoadImageFromLMDB](https://github.com/open-mmlab/mmocr/blob/878383b9de8d0e598f31fbb844ffcb0c305deb8b/mmocr/datasets/pipelines/loading.py#L140).
-This section is intended to serve as a quick walkthrough for you to master this update and apply it to facilitate your research.
-
-#### Specifications
-
-To better align with the academic community, MMOCR now requires the following specifications for lmdb datasets:
-
-- The parameter describing the data volume of the dataset is `num-samples` instead of `total_number` (deprecated).
-- Images and labels are stored with keys in the form of `image-000000001` and `label-000000001`, respectively.
-
-#### Usage
-
-1. Use existing academic lmdb datasets if they meet the specifications; or the tool provided by MMOCR to pack images & annotations into a lmdb dataset.
-
-- Previously, MMOCR had a function `txt2lmdb` (deprecated) that only supported converting labels to lmdb format. However, it is quite different from academic lmdb datasets, which usually contain both images and labels. Now MMOCR provides a new utility [lmdb_converter](https://github.com/open-mmlab/mmocr/blob/main/tools/data/utils/lmdb_converter.py) to convert recognition datasets with both images and labels to lmdb format.
-
-- Say that your recognition data in MMOCR's format are organized as follows. (See an example in [ocr_toy_dataset](https://github.com/open-mmlab/mmocr/tree/main/tests/data/ocr_toy_dataset)).
-
- ```text
- # Directory structure
-
- โโโimg_path
- | |โโ img1.jpg
- | |โโ img2.jpg
- | |โโ ...
- |โโlabel.txt (or label.jsonl)
-
- # Annotation format
-
- label.txt: img1.jpg HELLO
- img2.jpg WORLD
- ...
-
- label.jsonl: {'filename':'img1.jpg', 'text':'HELLO'}
- {'filename':'img2.jpg', 'text':'WORLD'}
- ...
- ```
-
-- Then pack these files up:
-
- ```bash
- python tools/data/utils/lmdb_converter.py {PATH_TO_LABEL} {OUTPUT_PATH} --i {PATH_TO_IMAGES}
- ```
-
-- Check out [tools.md](https://github.com/open-mmlab/mmocr/blob/main/docs/en/tools.md) for more details.
-
-2. The second step is to modify the configuration files. For example, to train CRNN on MJ and ST datasets:
-
-- Set parser as `LineJsonParser` and `file_format` as 'lmdb' in [dataset config](https://github.com/open-mmlab/mmocr/blob/main/configs/_base_/recog_datasets/ST_MJ_train.py#L9)
-
- ```python
- # configs/_base_/recog_datasets/ST_MJ_train.py
- train1 = dict(
- type='OCRDataset',
- img_prefix=train_img_prefix1,
- ann_file=train_ann_file1,
- loader=dict(
- type='AnnFileLoader',
- repeat=1,
- file_format='lmdb',
- parser=dict(
- type='LineJsonParser',
- keys=['filename', 'text'],
- )),
- pipeline=None,
- test_mode=False)
- ```
-
-- Use `LoadImageFromLMDB` in [pipeline](https://github.com/open-mmlab/mmocr/blob/main/configs/_base_/recog_pipelines/crnn_pipeline.py#L4):
-
- ```python
- # configs/_base_/recog_pipelines/crnn_pipeline.py
- train_pipeline = [
- dict(type='LoadImageFromLMDB', color_type='grayscale'),
- ...
- ```
-
-3. You are good to go! Start training and MMOCR will load data from your lmdb dataset.
-
-### New Features & Enhancements
-
-- Add analyze_logs in tools and its description in docs by @Y-M-Y in https://github.com/open-mmlab/mmocr/pull/899
-- Add LSVT Data Converter by @xinke-wang in https://github.com/open-mmlab/mmocr/pull/896
-- Add RCTW dataset converter by @xinke-wang in https://github.com/open-mmlab/mmocr/pull/914
-- Support computing mean scores in UniformConcatDataset by @gaotongxiao in https://github.com/open-mmlab/mmocr/pull/981
-- Support loading images and labels from lmdb file by @Mountchicken in https://github.com/open-mmlab/mmocr/pull/982
-- Add recog2lmdb and new toy dataset files by @Mountchicken in https://github.com/open-mmlab/mmocr/pull/979
-- Add labelme converter for textdet and textrecog by @cuhk-hbsun in https://github.com/open-mmlab/mmocr/pull/972
-- Update CircleCI configs by @xinke-wang in https://github.com/open-mmlab/mmocr/pull/918
-- Update Git Action by @xinke-wang in https://github.com/open-mmlab/mmocr/pull/930
-- More customizable fields in dataloaders by @gaotongxiao in https://github.com/open-mmlab/mmocr/pull/933
-- Skip CIs when docs are modified by @gaotongxiao in https://github.com/open-mmlab/mmocr/pull/941
-- Rename Github tests, fix ignored paths by @gaotongxiao in https://github.com/open-mmlab/mmocr/pull/946
-- Support latest MMCV by @gaotongxiao in https://github.com/open-mmlab/mmocr/pull/959
-- Support dynamic threshold range in eval_hmean by @gaotongxiao in https://github.com/open-mmlab/mmocr/pull/962
-- Update the version requirement of mmdet in docker by @Mountchicken in https://github.com/open-mmlab/mmocr/pull/966
-- Replace `opencv-python-headless` with `open-python` by @gaotongxiao in https://github.com/open-mmlab/mmocr/pull/970
-- Update Dataset Configs by @xinke-wang in https://github.com/open-mmlab/mmocr/pull/980
-- Add SynthText dataset config by @xinke-wang in https://github.com/open-mmlab/mmocr/pull/983
-- Automatically report mean scores when applicable by @gaotongxiao in https://github.com/open-mmlab/mmocr/pull/995
-- Add DBNet++ by @xinke-wang in https://github.com/open-mmlab/mmocr/pull/973
-- Add MASTER by @JiaquanYe in https://github.com/open-mmlab/mmocr/pull/807
-- Allow choosing metrics to report in text recognition tasks by @gaotongxiao in https://github.com/open-mmlab/mmocr/pull/989
-- Add HierText converter by @Mountchicken in https://github.com/open-mmlab/mmocr/pull/948
-- Fix lint_only in CircleCI by @gaotongxiao in https://github.com/open-mmlab/mmocr/pull/998
-
-### Bug Fixes
-
-- Fix CircleCi Main Branch Accidentally Run PR Stage Test by @xinke-wang in https://github.com/open-mmlab/mmocr/pull/927
-- Fix a deprecate warning about mmdet.datasets.pipelines.formating by @Mountchicken in https://github.com/open-mmlab/mmocr/pull/944
-- Fix a Bug in ResNet plugin by @Mountchicken in https://github.com/open-mmlab/mmocr/pull/967
-- revert a wrong setting in db_r18 cfg by @gaotongxiao in https://github.com/open-mmlab/mmocr/pull/978
-- Fix TotalText Anno version issue by @xinke-wang in https://github.com/open-mmlab/mmocr/pull/945
-- Update installation step of `albumentations` by @gaotongxiao in https://github.com/open-mmlab/mmocr/pull/984
-- Fix ImgAug transform by @gaotongxiao in https://github.com/open-mmlab/mmocr/pull/949
-- Fix GPG key error in CI and docker by @gaotongxiao in https://github.com/open-mmlab/mmocr/pull/988
-- update label.lmdb by @Mountchicken in https://github.com/open-mmlab/mmocr/pull/991
-- correct meta key by @garvan2021 in https://github.com/open-mmlab/mmocr/pull/926
-- Use new image by @gaotongxiao in https://github.com/open-mmlab/mmocr/pull/976
-- Fix Data Converter Issues by @xinke-wang in https://github.com/open-mmlab/mmocr/pull/955
-
-### Docs
-
-- Update CONTRIBUTING.md by @gaotongxiao in https://github.com/open-mmlab/mmocr/pull/905
-- Fix the misleading description in test.py by @gaotongxiao in https://github.com/open-mmlab/mmocr/pull/908
-- Update recog.md for lmdb Generation by @xinke-wang in https://github.com/open-mmlab/mmocr/pull/934
-- Add MMCV by @gaotongxiao in https://github.com/open-mmlab/mmocr/pull/954
-- Add wechat QR code to CN readme by @gaotongxiao in https://github.com/open-mmlab/mmocr/pull/960
-- Update CONTRIBUTING.md by @gaotongxiao in https://github.com/open-mmlab/mmocr/pull/947
-- Use QR codes from MMCV by @gaotongxiao in https://github.com/open-mmlab/mmocr/pull/971
-- Renew dataset_types.md by @gaotongxiao in https://github.com/open-mmlab/mmocr/pull/997
-
-### New Contributors
-
-- @Y-M-Y made their first contribution in https://github.com/open-mmlab/mmocr/pull/899
-
-**Full Changelog**: https://github.com/open-mmlab/mmocr/compare/v0.5.0...v0.6.0
-
-## 0.5.0 (31/03/2022)
-
-### Highlights
-
-1. MMOCR now supports SPACE recognition! (What a prominent feature!) Users only need to convert the recognition annotations that contain spaces from a plain `.txt` file to JSON line format `.jsonl`, and then revise a few configurations to enable the `LineJsonParser`. For more information, please read our step-by-step [tutorial](https://mmocr.readthedocs.io/en/latest/tutorials/blank_recog.html).
-2. [Tesseract](https://github.com/tesseract-ocr/tesseract) is now available in MMOCR! While MMOCR is more flexible to support various downstream tasks, users might sometimes not be satisfied with DL models and would like to turn to effective legacy solutions. Therefore, we offer this option in `mmocr.utils.ocr` by wrapping Tesseract as a detector and/or recognizer. Users can easily create an MMOCR object by `MMOCR(det=โTesseractโ, recog=โTesseractโ)`. Credit to @garvan2021
-3. We release data converters for **16** widely used OCR datasets, including multiple scenarios such as document, handwritten, and scene text. Now it is more convenient to generate annotation files for these datasets. Check the dataset zoo ( [Det](https://mmocr.readthedocs.io/en/latest/datasets/det.html#) & [Recog](https://mmocr.readthedocs.io/en/latest/datasets/recog.html) ) to explore further information.
-4. Special thanks to @EighteenSprings @BeyondYourself @yangrisheng, who had actively participated in documentation translation!
-
-### Migration Guide - ResNet
-
-Some refactoring processes are still going on. For text recognition models, we unified the [`ResNet-like` architectures](https://github.com/open-mmlab/mmocr/blob/72f945457324e700f0d14796dd10a51535c01a57/mmocr/models/textrecog/backbones/resnet.py) which are used as backbones. By introducing stage-wise and block-wise plugins, the refactored ResNet is highly flexible to support existing models, like ResNet31 and ResNet45, and other future designs of ResNet variants.
-
-#### Plugin
-
-- `Plugin` is a module category inherited from MMCV's implementation of `PLUGIN_LAYERS`, which can be inserted between each stage of ResNet or into a basicblock. You can find a simple implementation of plugin at [mmocr/models/textrecog/plugins/common.py](https://github.com/open-mmlab/mmocr/blob/72f945457324e700f0d14796dd10a51535c01a57/mmocr/models/textrecog/plugins/common.py), or click the button below.
-
-
- Plugin Example
-
- ```python
- @PLUGIN_LAYERS.register_module()
- class Maxpool2d(nn.Module):
- """A wrapper around nn.Maxpool2d().
-
- Args:
- kernel_size (int or tuple(int)): Kernel size for max pooling layer
- stride (int or tuple(int)): Stride for max pooling layer
- padding (int or tuple(int)): Padding for pooling layer
- """
-
- def __init__(self, kernel_size, stride, padding=0, **kwargs):
- super(Maxpool2d, self).__init__()
- self.model = nn.MaxPool2d(kernel_size, stride, padding)
-
- def forward(self, x):
- """
- Args:
- x (Tensor): Input feature map
-
- Returns:
- Tensor: The tensor after Maxpooling layer.
- """
- return self.model(x)
- ```
-
-
-
-#### Stage-wise Plugins
-
-- ResNet is composed of stages, and each stage is composed of blocks. E.g., ResNet18 is composed of 4 stages, and each stage is composed of basicblocks. For each stage, we provide two ports to insert stage-wise plugins by giving `plugins` parameters in ResNet.
-
- ```text
- [port1: before stage] ---> [stage] ---> [port2: after stage]
- ```
-
-- E.g. Using a ResNet with four stages as example. Suppose we want to insert an additional convolution layer before each stage, and an additional convolution layer at stage 1, 2, 4. Then you can define the special ResNet18 like this
-
- ```python
- resnet18_speical = ResNet(
- # for simplicity, some required
- # parameters are omitted
- plugins=[
- dict(
- cfg=dict(
- type='ConvModule',
- kernel_size=3,
- stride=1,
- padding=1,
- norm_cfg=dict(type='BN'),
- act_cfg=dict(type='ReLU')),
- stages=(True, True, True, True),
- position='before_stage')
- dict(
- cfg=dict(
- type='ConvModule',
- kernel_size=3,
- stride=1,
- padding=1,
- norm_cfg=dict(type='BN'),
- act_cfg=dict(type='ReLU')),
- stages=(True, True, False, True),
- position='after_stage')
- ])
- ```
-
-- You can also insert more than one plugin in each port and those plugins will be executed in order. Let's take ResNet in [MASTER](https://arxiv.org/abs/1910.02562) as an example:
-
-
- Multiple Plugins Example
-
- - ResNet in Master is based on ResNet31. And after each stage, a module named `GCAModule` will be used. The `GCAModule` is inserted before the stage-wise convolution layer in ResNet31. In conlusion, there will be two plugins at `after_stage` port in the same time.
-
- ```python
- resnet_master = ResNet(
- # for simplicity, some required
- # parameters are omitted
- plugins=[
- dict(
- cfg=dict(type='Maxpool2d', kernel_size=2, stride=(2, 2)),
- stages=(True, True, False, False),
- position='before_stage'),
- dict(
- cfg=dict(type='Maxpool2d', kernel_size=(2, 1), stride=(2, 1)),
- stages=(False, False, True, False),
- position='before_stage'),
- dict(
- cfg=dict(type='GCAModule', kernel_size=3, stride=1, padding=1),
- stages=[True, True, True, True],
- position='after_stage'),
- dict(
- cfg=dict(
- type='ConvModule',
- kernel_size=3,
- stride=1,
- padding=1,
- norm_cfg=dict(type='BN'),
- act_cfg=dict(type='ReLU')),
- stages=(True, True, True, True),
- position='after_stage')
- ])
-
- ```
-
-
-
- - In each plugin, we will pass two parameters (`in_channels`, `out_channels`) to support operations that need the information of current channels.
-
-#### Block-wise Plugin (Experimental)
-
-- We also refactored the `BasicBlock` used in ResNet. Now it can be customized with block-wise plugins. Check [here](https://github.com/open-mmlab/mmocr/blob/72f945457324e700f0d14796dd10a51535c01a57/mmocr/models/textrecog/layers/conv_layer.py) for more details.
-
-- BasicBlock is composed of two convolution layer in the main branch and a shortcut branch. We provide four ports to insert plugins.
-
- ```text
- [port1: before_conv1] ---> [conv1] --->
- [port2: after_conv1] ---> [conv2] --->
- [port3: after_conv2] ---> +(shortcut) ---> [port4: after_shortcut]
- ```
-
-- In each plugin, we will pass a parameter `in_channels` to support operations that need the information of current channels.
-
-- E.g. Build a ResNet with customized BasicBlock with an additional convolution layer before conv1:
-
-
- Block-wise Plugin Example
-
- ```python
- resnet_31 = ResNet(
- in_channels=3,
- stem_channels=[64, 128],
- block_cfgs=dict(type='BasicBlock'),
- arch_layers=[1, 2, 5, 3],
- arch_channels=[256, 256, 512, 512],
- strides=[1, 1, 1, 1],
- plugins=[
- dict(
- cfg=dict(type='Maxpool2d',
- kernel_size=2,
- stride=(2, 2)),
- stages=(True, True, False, False),
- position='before_stage'),
- dict(
- cfg=dict(type='Maxpool2d',
- kernel_size=(2, 1),
- stride=(2, 1)),
- stages=(False, False, True, False),
- position='before_stage'),
- dict(
- cfg=dict(
- type='ConvModule',
- kernel_size=3,
- stride=1,
- padding=1,
- norm_cfg=dict(type='BN'),
- act_cfg=dict(type='ReLU')),
- stages=(True, True, True, True),
- position='after_stage')
- ])
- ```
-
-
-
-#### Full Examples
-
-
-ResNet without plugins
-
-- ResNet45 is used in ASTER and ABINet without any plugins.
-
- ```python
- resnet45_aster = ResNet(
- in_channels=3,
- stem_channels=[64, 128],
- block_cfgs=dict(type='BasicBlock', use_conv1x1='True'),
- arch_layers=[3, 4, 6, 6, 3],
- arch_channels=[32, 64, 128, 256, 512],
- strides=[(2, 2), (2, 2), (2, 1), (2, 1), (2, 1)])
-
- resnet45_abi = ResNet(
- in_channels=3,
- stem_channels=32,
- block_cfgs=dict(type='BasicBlock', use_conv1x1='True'),
- arch_layers=[3, 4, 6, 6, 3],
- arch_channels=[32, 64, 128, 256, 512],
- strides=[2, 1, 2, 1, 1])
- ```
-
-
-
-ResNet with plugins
-
-- ResNet31 is a typical architecture to use stage-wise plugins. Before the first three stages, Maxpooling layer is used. After each stage, a convolution layer with BN and ReLU is used.
-
- ```python
- resnet_31 = ResNet(
- in_channels=3,
- stem_channels=[64, 128],
- block_cfgs=dict(type='BasicBlock'),
- arch_layers=[1, 2, 5, 3],
- arch_channels=[256, 256, 512, 512],
- strides=[1, 1, 1, 1],
- plugins=[
- dict(
- cfg=dict(type='Maxpool2d',
- kernel_size=2,
- stride=(2, 2)),
- stages=(True, True, False, False),
- position='before_stage'),
- dict(
- cfg=dict(type='Maxpool2d',
- kernel_size=(2, 1),
- stride=(2, 1)),
- stages=(False, False, True, False),
- position='before_stage'),
- dict(
- cfg=dict(
- type='ConvModule',
- kernel_size=3,
- stride=1,
- padding=1,
- norm_cfg=dict(type='BN'),
- act_cfg=dict(type='ReLU')),
- stages=(True, True, True, True),
- position='after_stage')
- ])
- ```
-
-
-
-### Migration Guide - Dataset Annotation Loader
-
-The annotation loaders, `LmdbLoader` and `HardDiskLoader`, are unified into `AnnFileLoader` for a more consistent design and wider support on different file formats and storage backends. `AnnFileLoader` can load the annotations from `disk`(default), `http` and `petrel` backend, and parse the annotation in `txt` or `lmdb` format. `LmdbLoader` and `HardDiskLoader` are deprecated, and users are recommended to modify their configs to use the new `AnnFileLoader`. Users can migrate their legacy loader `HardDiskLoader` referring to the following example:
-
-```python
-# Legacy config
-train = dict(
- type='OCRDataset',
- ...
- loader=dict(
- type='HardDiskLoader',
- ...))
-
-# Suggested config
-train = dict(
- type='OCRDataset',
- ...
- loader=dict(
- type='AnnFileLoader',
- file_storage_backend='disk',
- file_format='txt',
- ...))
-```
-
-Similarly, using `AnnFileLoader` with `file_format='lmdb'` instead of `LmdbLoader` is strongly recommended.
-
-### New Features & Enhancements
-
-- Update mmcv install by @Harold-lkk in https://github.com/open-mmlab/mmocr/pull/775
-- Upgrade isort by @gaotongxiao in https://github.com/open-mmlab/mmocr/pull/771
-- Automatically infer device for inference if not speicifed by @gaotongxiao in https://github.com/open-mmlab/mmocr/pull/781
-- Add open-mmlab precommit hooks by @gaotongxiao in https://github.com/open-mmlab/mmocr/pull/787
-- Add windows CI by @gaotongxiao in https://github.com/open-mmlab/mmocr/pull/790
-- Add CurvedSyntext150k Converter by @gaotongxiao in https://github.com/open-mmlab/mmocr/pull/719
-- Add FUNSD Converter by @xinke-wang in https://github.com/open-mmlab/mmocr/pull/808
-- Support loading annotation file with petrel/http backend by @cuhk-hbsun in https://github.com/open-mmlab/mmocr/pull/793
-- Support different seeds on different ranks by @gaotongxiao in https://github.com/open-mmlab/mmocr/pull/820
-- Support json in recognition converter by @Mountchicken in https://github.com/open-mmlab/mmocr/pull/844
-- Add args and docs for multi-machine training/testing by @gaotongxiao in https://github.com/open-mmlab/mmocr/pull/849
-- Add warning info for LineStrParser by @xinke-wang in https://github.com/open-mmlab/mmocr/pull/850
-- Deploy openmmlab-bot by @gaotongxiao in https://github.com/open-mmlab/mmocr/pull/876
-- Add Tesserocr Inference by @garvan2021 in https://github.com/open-mmlab/mmocr/pull/814
-- Add LV Dataset Converter by @xinke-wang in https://github.com/open-mmlab/mmocr/pull/871
-- Add SROIE Converter by @xinke-wang in https://github.com/open-mmlab/mmocr/pull/810
-- Add NAF Converter by @xinke-wang in https://github.com/open-mmlab/mmocr/pull/815
-- Add DeText Converter by @xinke-wang in https://github.com/open-mmlab/mmocr/pull/818
-- Add IMGUR Converter by @xinke-wang in https://github.com/open-mmlab/mmocr/pull/825
-- Add ILST Converter by @Mountchicken in https://github.com/open-mmlab/mmocr/pull/833
-- Add KAIST Converter by @xinke-wang in https://github.com/open-mmlab/mmocr/pull/835
-- Add IC11 (Born-digital Images) Data Converter by @xinke-wang in https://github.com/open-mmlab/mmocr/pull/857
-- Add IC13 (Focused Scene Text) Data Converter by @xinke-wang in https://github.com/open-mmlab/mmocr/pull/861
-- Add BID Converter by @Mountchicken in https://github.com/open-mmlab/mmocr/pull/862
-- Add Vintext Converter by @Mountchicken in https://github.com/open-mmlab/mmocr/pull/864
-- Add MTWI Data Converter by @xinke-wang in https://github.com/open-mmlab/mmocr/pull/867
-- Add COCO Text v2 Data Converter by @xinke-wang in https://github.com/open-mmlab/mmocr/pull/872
-- Add ReCTS Data Converter by @xinke-wang in https://github.com/open-mmlab/mmocr/pull/892
-- Refactor ResNets by @Mountchicken in https://github.com/open-mmlab/mmocr/pull/809
-
-### Bug Fixes
-
-- Bump mmdet version to 2.20.0 in Dockerfile by @GPhilo in https://github.com/open-mmlab/mmocr/pull/763
-- Update mmdet version limit by @cuhk-hbsun in https://github.com/open-mmlab/mmocr/pull/773
-- Minimum version requirement of albumentations by @gaotongxiao in https://github.com/open-mmlab/mmocr/pull/769
-- Disable worker in the dataloader of gpu unit test by @gaotongxiao in https://github.com/open-mmlab/mmocr/pull/780
-- Standardize the type of torch.device in ocr.py by @gaotongxiao in https://github.com/open-mmlab/mmocr/pull/800
-- Use RECOGNIZER instead of DETECTORS by @cuhk-hbsun in https://github.com/open-mmlab/mmocr/pull/685
-- Add num_classes to configs of ABINet by @gaotongxiao in https://github.com/open-mmlab/mmocr/pull/805
-- Support loading space character from dict file by @gaotongxiao in https://github.com/open-mmlab/mmocr/pull/854
-- Description in tools/data/utils/txt2lmdb.py by @Mountchicken in https://github.com/open-mmlab/mmocr/pull/870
-- ignore_index in SARLoss by @Mountchicken in https://github.com/open-mmlab/mmocr/pull/869
-- Fix a bug that may cause inplace operation error by @Mountchicken in https://github.com/open-mmlab/mmocr/pull/884
-- Use hyphen instead of underscores in script args by @gaotongxiao in https://github.com/open-mmlab/mmocr/pull/890
-
-### Docs
-
-- Add deprecation message for deploy tools by @xinke-wang in https://github.com/open-mmlab/mmocr/pull/801
-- Reorganizing OpenMMLab projects in readme by @xinke-wang in https://github.com/open-mmlab/mmocr/pull/806
-- Add demo/README_zh.md by @EighteenSprings in https://github.com/open-mmlab/mmocr/pull/802
-- Add detailed version requirement table by @gaotongxiao in https://github.com/open-mmlab/mmocr/pull/778
-- Correct misleading section title in training.md by @gaotongxiao in https://github.com/open-mmlab/mmocr/pull/819
-- Update README_zh-CN document URL by @BeyondYourself in https://github.com/open-mmlab/mmocr/pull/823
-- translate testing.md. by @yangrisheng in https://github.com/open-mmlab/mmocr/pull/822
-- Fix confused description for load-from and resume-from by @xinke-wang in https://github.com/open-mmlab/mmocr/pull/842
-- Add documents getting_started in docs/zh by @BeyondYourself in https://github.com/open-mmlab/mmocr/pull/841
-- Add the model serving translation document by @BeyondYourself in https://github.com/open-mmlab/mmocr/pull/845
-- Update docs about installation on Windows by @Mountchicken in https://github.com/open-mmlab/mmocr/pull/852
-- Update tutorial notebook by @gaotongxiao in https://github.com/open-mmlab/mmocr/pull/853
-- Update Instructions for New Data Converters by @xinke-wang in https://github.com/open-mmlab/mmocr/pull/900
-- Brief installation instruction in README by @Harold-lkk in https://github.com/open-mmlab/mmocr/pull/897
-- update doc for ILST, VinText, BID by @Mountchicken in https://github.com/open-mmlab/mmocr/pull/902
-- Fix typos in readme by @gaotongxiao in https://github.com/open-mmlab/mmocr/pull/903
-- Recog dataset doc by @Harold-lkk in https://github.com/open-mmlab/mmocr/pull/893
-- Reorganize the directory structure section in det.md by @gaotongxiao in https://github.com/open-mmlab/mmocr/pull/894
-
-### New Contributors
-
-- @GPhilo made their first contribution in https://github.com/open-mmlab/mmocr/pull/763
-- @xinke-wang made their first contribution in https://github.com/open-mmlab/mmocr/pull/801
-- @EighteenSprings made their first contribution in https://github.com/open-mmlab/mmocr/pull/802
-- @BeyondYourself made their first contribution in https://github.com/open-mmlab/mmocr/pull/823
-- @yangrisheng made their first contribution in https://github.com/open-mmlab/mmocr/pull/822
-- @Mountchicken made their first contribution in https://github.com/open-mmlab/mmocr/pull/844
-- @garvan2021 made their first contribution in https://github.com/open-mmlab/mmocr/pull/814
-
-**Full Changelog**: https://github.com/open-mmlab/mmocr/compare/v0.4.1...v0.5.0
-
-## v0.4.1 (27/01/2022)
-
-### Highlights
-
-1. Visualizing edge weights in OpenSet KIE is now supported! https://github.com/open-mmlab/mmocr/pull/677
-2. Some configurations have been optimized to significantly speed up the training and testing processes! Don't worry - you can still tune these parameters in case these modifications do not work. https://github.com/open-mmlab/mmocr/pull/757
-3. Now you can use CPU to train/debug your model! https://github.com/open-mmlab/mmocr/pull/752
-4. We have fixed a severe bug that causes users unable to call `mmocr.apis.test` with our pre-built wheels. https://github.com/open-mmlab/mmocr/pull/667
-
-### New Features & Enhancements
-
-- Show edge score for openset kie by @cuhk-hbsun in https://github.com/open-mmlab/mmocr/pull/677
-- Download flake8 from github as pre-commit hooks by @gaotongxiao in https://github.com/open-mmlab/mmocr/pull/695
-- Deprecate the support for 'python setup.py test' by @Harold-lkk in https://github.com/open-mmlab/mmocr/pull/722
-- Disable multi-processing feature of cv2 to speed up data loading by @gaotongxiao in https://github.com/open-mmlab/mmocr/pull/721
-- Extend ctw1500 converter to support text fields by @Harold-lkk in https://github.com/open-mmlab/mmocr/pull/729
-- Extend totaltext converter to support text fields by @Harold-lkk in https://github.com/open-mmlab/mmocr/pull/728
-- Speed up training by @gaotongxiao in https://github.com/open-mmlab/mmocr/pull/739
-- Add setup multi-processing both in train and test.py by @Harold-lkk in https://github.com/open-mmlab/mmocr/pull/757
-- Support CPU training/testing by @gaotongxiao in https://github.com/open-mmlab/mmocr/pull/752
-- Support specify gpu for testing and training with gpu-id instead of gpu-ids and gpus by @Harold-lkk in https://github.com/open-mmlab/mmocr/pull/756
-- Remove unnecessary custom_import from test.py by @Harold-lkk in https://github.com/open-mmlab/mmocr/pull/758
-
-### Bug Fixes
-
-- Fix satrn onnxruntime test by @AllentDan in https://github.com/open-mmlab/mmocr/pull/679
-- Support both ConcatDataset and UniformConcatDataset by @cuhk-hbsun in https://github.com/open-mmlab/mmocr/pull/675
-- Fix bugs of show_results in single_gpu_test by @cuhk-hbsun in https://github.com/open-mmlab/mmocr/pull/667
-- Fix a bug for sar decoder when bi-rnn is used by @MhLiao in https://github.com/open-mmlab/mmocr/pull/690
-- Fix opencv version to avoid some bugs by @gaotongxiao in https://github.com/open-mmlab/mmocr/pull/694
-- Fix py39 ci error by @Harold-lkk in https://github.com/open-mmlab/mmocr/pull/707
-- Update visualize.py by @TommyZihao in https://github.com/open-mmlab/mmocr/pull/715
-- Fix link of config by @cuhk-hbsun in https://github.com/open-mmlab/mmocr/pull/726
-- Use yaml.safe_load instead of load by @gaotongxiao in https://github.com/open-mmlab/mmocr/pull/753
-- Add necessary keys to test_pipelines to enable test-time visualization by @gaotongxiao in https://github.com/open-mmlab/mmocr/pull/754
-
-### Docs
-
-- Fix recog.md by @gaotongxiao in https://github.com/open-mmlab/mmocr/pull/674
-- Add config tutorial by @gaotongxiao in https://github.com/open-mmlab/mmocr/pull/683
-- Add MMSelfSup/MMRazor/MMDeploy in readme by @cuhk-hbsun in https://github.com/open-mmlab/mmocr/pull/692
-- Add recog & det model summary by @gaotongxiao in https://github.com/open-mmlab/mmocr/pull/693
-- Update docs link by @gaotongxiao in https://github.com/open-mmlab/mmocr/pull/710
-- add pull request template.md by @Harold-lkk in https://github.com/open-mmlab/mmocr/pull/711
-- Add website links to readme by @gaotongxiao in https://github.com/open-mmlab/mmocr/pull/731
-- update readme according to standard by @Harold-lkk in https://github.com/open-mmlab/mmocr/pull/742
-
-### New Contributors
-
-- @MhLiao made their first contribution in https://github.com/open-mmlab/mmocr/pull/690
-- @TommyZihao made their first contribution in https://github.com/open-mmlab/mmocr/pull/715
-
-**Full Changelog**: https://github.com/open-mmlab/mmocr/compare/v0.4.0...v0.4.1
-
-## v0.4.0 (15/12/2021)
-
-### Highlights
-
-1. We release a new text recognition model - [ABINet](https://arxiv.org/pdf/2103.06495.pdf) (CVPR 2021, Oral). With it dedicated model design and useful data augmentation transforms, ABINet can achieve the best performance on irregular text recognition tasks. [Check it out!](https://mmocr.readthedocs.io/en/latest/textrecog_models.html#read-like-humans-autonomous-bidirectional-and-iterative-language-modeling-for-scene-text-recognition)
-2. We are also working hard to fulfill the requests from our community.
- [OpenSet KIE](https://mmocr.readthedocs.io/en/latest/kie_models.html#wildreceiptopenset) is one of the achievement, which extends the application of SDMGR from text node classification to node-pair relation extraction. We also provide
- a demo script to convert WildReceipt to open set domain, though it cannot
- take the full advantage of OpenSet format. For more information, please read our
- [tutorial](https://mmocr.readthedocs.io/en/latest/tutorials/kie_closeset_openset.html).
-3. APIs of models can be exposed through TorchServe. [Docs](https://mmocr.readthedocs.io/en/latest/model_serving.html)
-
-### Breaking Changes & Migration Guide
-
-#### Postprocessor
-
-Some refactoring processes are still going on. For all text detection models, we unified their `decode` implementations into a new module category, `POSTPROCESSOR`, which is responsible for decoding different raw outputs into boundary instances. In all text detection configs, the `text_repr_type` argument in `bbox_head` is deprecated and will be removed in the future release.
-
-**Migration Guide**: Find a similar line from detection model's config:
-
-```
-text_repr_type=xxx,
-```
-
-And replace it with
-
-```
-postprocessor=dict(type='{MODEL_NAME}Postprocessor', text_repr_type=xxx)),
-```
-
-Take a snippet of PANet's config as an example. Before the change, its config for `bbox_head` looks like:
-
-```
- bbox_head=dict(
- type='PANHead',
- text_repr_type='poly',
- in_channels=[128, 128, 128, 128],
- out_channels=6,
- module_loss=dict(type='PANModuleLoss')),
-```
-
-Afterwards:
-
-```
- bbox_head=dict(
- type='PANHead',
- in_channels=[128, 128, 128, 128],
- out_channels=6,
- module_loss=dict(type='PANModuleLoss'),
- postprocessor=dict(type='PANPostprocessor', text_repr_type='poly')),
-```
-
-There are other postprocessors and each takes different arguments. Interested users can find their interfaces or implementations in `mmocr/models/textdet/postprocess` or through our [api docs](https://mmocr.readthedocs.io/en/latest/api.html#textdet-postprocess).
-
-#### New Config Structure
-
-We reorganized the `configs/` directory by extracting reusable sections into `configs/_base_`. Now the directory tree of `configs/_base_` is organized as follows:
-
-```
-_base_
-โโโ det_datasets
-โโโ det_models
-โโโ det_pipelines
-โโโ recog_datasets
-โโโ recog_models
-โโโ recog_pipelines
-โโโ schedules
-```
-
-Most of model configs are making full use of base configs now, which makes the overall structural clearer and facilitates fair
-comparison across models. Despite the seemingly significant hierarchical difference, **these changes would not break the backward compatibility** as the names of model configs remain the same.
-
-### New Features
-
-- Support openset kie by @cuhk-hbsun in https://github.com/open-mmlab/mmocr/pull/498
-- Add converter for the Open Images v5 text annotations by Krylov et al. by @baudm in https://github.com/open-mmlab/mmocr/pull/497
-- Support Chinese for kie show result by @cuhk-hbsun in https://github.com/open-mmlab/mmocr/pull/464
-- Add TorchServe support for text detection and recognition by @Harold-lkk in https://github.com/open-mmlab/mmocr/pull/522
-- Save filename in text detection test results by @cuhk-hbsun in https://github.com/open-mmlab/mmocr/pull/570
-- Add codespell pre-commit hook and fix typos by @gaotongxiao in https://github.com/open-mmlab/mmocr/pull/520
-- Avoid duplicate placeholder docs in CN by @gaotongxiao in https://github.com/open-mmlab/mmocr/pull/582
-- Save results to json file for kie. by @cuhk-hbsun in https://github.com/open-mmlab/mmocr/pull/589
-- Add SAR_CN to ocr.py by @gaotongxiao in https://github.com/open-mmlab/mmocr/pull/579
-- mim extension for windows by @gaotongxiao in https://github.com/open-mmlab/mmocr/pull/641
-- Support muitiple pipelines for different datasets by @cuhk-hbsun in https://github.com/open-mmlab/mmocr/pull/657
-- ABINet Framework by @gaotongxiao in https://github.com/open-mmlab/mmocr/pull/651
-
-### Refactoring
-
-- Refactor textrecog config structure by @cuhk-hbsun in https://github.com/open-mmlab/mmocr/pull/617
-- Refactor text detection config by @cuhk-hbsun in https://github.com/open-mmlab/mmocr/pull/626
-- refactor transformer modules by @cuhk-hbsun in https://github.com/open-mmlab/mmocr/pull/618
-- refactor textdet postprocess by @cuhk-hbsun in https://github.com/open-mmlab/mmocr/pull/640
-
-### Docs
-
-- C++ example section by @apiaccess21 in https://github.com/open-mmlab/mmocr/pull/593
-- install.md Chinese section by @A465539338 in https://github.com/open-mmlab/mmocr/pull/364
-- Add Chinese Translation of deployment.md. by @fatfishZhao in https://github.com/open-mmlab/mmocr/pull/506
-- Fix a model link and add the metafile for SATRN by @gaotongxiao in https://github.com/open-mmlab/mmocr/pull/473
-- Improve docs style by @gaotongxiao in https://github.com/open-mmlab/mmocr/pull/474
-- Enhancement & sync Chinese docs by @gaotongxiao in https://github.com/open-mmlab/mmocr/pull/492
-- TorchServe docs by @gaotongxiao in https://github.com/open-mmlab/mmocr/pull/539
-- Update docs menu by @gaotongxiao in https://github.com/open-mmlab/mmocr/pull/564
-- Docs for KIE CloseSet & OpenSet by @gaotongxiao in https://github.com/open-mmlab/mmocr/pull/573
-- Fix broken links by @gaotongxiao in https://github.com/open-mmlab/mmocr/pull/576
-- Docstring for text recognition models by @gaotongxiao in https://github.com/open-mmlab/mmocr/pull/562
-- Add MMFlow & MIM by @gaotongxiao in https://github.com/open-mmlab/mmocr/pull/597
-- Add MMFewShot by @gaotongxiao in https://github.com/open-mmlab/mmocr/pull/621
-- Update model readme by @gaotongxiao in https://github.com/open-mmlab/mmocr/pull/604
-- Add input size check to model_inference by @mpena-vina in https://github.com/open-mmlab/mmocr/pull/633
-- Docstring for textdet models by @gaotongxiao in https://github.com/open-mmlab/mmocr/pull/561
-- Add MMHuman3D in readme by @gaotongxiao in https://github.com/open-mmlab/mmocr/pull/644
-- Use shared menu from theme instead by @gaotongxiao in https://github.com/open-mmlab/mmocr/pull/655
-- Refactor docs structure by @gaotongxiao in https://github.com/open-mmlab/mmocr/pull/662
-- Docs fix by @gaotongxiao in https://github.com/open-mmlab/mmocr/pull/664
-
-### Enhancements
-
-- Use bounding box around polygon instead of within polygon by @alexander-soare in https://github.com/open-mmlab/mmocr/pull/469
-- Add CITATION.cff by @gaotongxiao in https://github.com/open-mmlab/mmocr/pull/476
-- Add py3.9 CI by @gaotongxiao in https://github.com/open-mmlab/mmocr/pull/475
-- update model-index.yml by @gaotongxiao in https://github.com/open-mmlab/mmocr/pull/484
-- Use container in CI by @gaotongxiao in https://github.com/open-mmlab/mmocr/pull/502
-- CircleCI Setup by @gaotongxiao in https://github.com/open-mmlab/mmocr/pull/611
-- Remove unnecessary custom_import from train.py by @gaotongxiao in https://github.com/open-mmlab/mmocr/pull/603
-- Change the upper version of mmcv to 1.5.0 by @zhouzaida in https://github.com/open-mmlab/mmocr/pull/628
-- Update CircleCI by @gaotongxiao in https://github.com/open-mmlab/mmocr/pull/631
-- Pass custom_hooks to MMCV by @gaotongxiao in https://github.com/open-mmlab/mmocr/pull/609
-- Skip CI when some specific files were changed by @gaotongxiao in https://github.com/open-mmlab/mmocr/pull/642
-- Add markdown linter in pre-commit hook by @gaotongxiao in https://github.com/open-mmlab/mmocr/pull/643
-- Use shape from loaded image by @cuhk-hbsun in https://github.com/open-mmlab/mmocr/pull/652
-- Cancel previous runs that are not completed by @Harold-lkk in https://github.com/open-mmlab/mmocr/pull/666
-
-### Bug Fixes
-
-- Modify algorithm "sar" weights path in metafile by @ShoupingShan in https://github.com/open-mmlab/mmocr/pull/581
-- Fix Cuda CI by @gaotongxiao in https://github.com/open-mmlab/mmocr/pull/472
-- Fix image export in test.py for KIE models by @gaotongxiao in https://github.com/open-mmlab/mmocr/pull/486
-- Allow invalid polygons in intersection and union by default by @gaotongxiao in https://github.com/open-mmlab/mmocr/pull/471
-- Update checkpoints' links for SATRN by @gaotongxiao in https://github.com/open-mmlab/mmocr/pull/518
-- Fix converting to onnx bug because of changing key from img_shape to resize_shape by @Harold-lkk in https://github.com/open-mmlab/mmocr/pull/523
-- Fix PyTorch 1.6 incompatible checkpoints by @gaotongxiao in https://github.com/open-mmlab/mmocr/pull/540
-- Fix paper field in metafiles by @gaotongxiao in https://github.com/open-mmlab/mmocr/pull/550
-- Unify recognition task names in metafiles by @gaotongxiao in https://github.com/open-mmlab/mmocr/pull/548
-- Fix py3.9 CI by @gaotongxiao in https://github.com/open-mmlab/mmocr/pull/563
-- Always map location to cpu when loading checkpoint by @gaotongxiao in https://github.com/open-mmlab/mmocr/pull/567
-- Fix wrong model builder in recog_test_imgs by @gaotongxiao in https://github.com/open-mmlab/mmocr/pull/574
-- Improve dbnet r50 by fixing img std by @gaotongxiao in https://github.com/open-mmlab/mmocr/pull/578
-- Fix resource warning: unclosed file by @cuhk-hbsun in https://github.com/open-mmlab/mmocr/pull/577
-- Fix bug that same start_point for different texts in draw_texts_by_pil by @cuhk-hbsun in https://github.com/open-mmlab/mmocr/pull/587
-- Keep original texts for kie by @cuhk-hbsun in https://github.com/open-mmlab/mmocr/pull/588
-- Fix random seed by @gaotongxiao in https://github.com/open-mmlab/mmocr/pull/600
-- Fix DBNet_r50 config by @gaotongxiao in https://github.com/open-mmlab/mmocr/pull/625
-- Change SBC case to DBC case by @cuhk-hbsun in https://github.com/open-mmlab/mmocr/pull/632
-- Fix kie demo by @innerlee in https://github.com/open-mmlab/mmocr/pull/610
-- fix type check by @cuhk-hbsun in https://github.com/open-mmlab/mmocr/pull/650
-- Remove depreciated image validator in totaltext converter by @gaotongxiao in https://github.com/open-mmlab/mmocr/pull/661
-- Fix change locals() dict by @Fei-Wang in https://github.com/open-mmlab/mmocr/pull/663
-- fix #614: textsnake targets by @HolyCrap96 in https://github.com/open-mmlab/mmocr/pull/660
-
-### New Contributors
-
-- @alexander-soare made their first contribution in https://github.com/open-mmlab/mmocr/pull/469
-- @A465539338 made their first contribution in https://github.com/open-mmlab/mmocr/pull/364
-- @fatfishZhao made their first contribution in https://github.com/open-mmlab/mmocr/pull/506
-- @baudm made their first contribution in https://github.com/open-mmlab/mmocr/pull/497
-- @ShoupingShan made their first contribution in https://github.com/open-mmlab/mmocr/pull/581
-- @apiaccess21 made their first contribution in https://github.com/open-mmlab/mmocr/pull/593
-- @zhouzaida made their first contribution in https://github.com/open-mmlab/mmocr/pull/628
-- @mpena-vina made their first contribution in https://github.com/open-mmlab/mmocr/pull/633
-- @Fei-Wang made their first contribution in https://github.com/open-mmlab/mmocr/pull/663
-
-**Full Changelog**: https://github.com/open-mmlab/mmocr/compare/v0.3.0...0.4.0
-
-## v0.3.0 (25/8/2021)
-
-### Highlights
-
-1. We add a new text recognition model -- SATRN! Its pretrained checkpoint achieves the best performance over other provided text recognition models. A lighter version of SATRN is also released which can obtain ~98% of the performance of the original model with only 45 MB in size. ([@2793145003](https://github.com/2793145003)) [#405](https://github.com/open-mmlab/mmocr/pull/405)
-2. Improve the demo script, `ocr.py`, which supports applying end-to-end text detection, text recognition and key information extraction models on images with easy-to-use commands. Users can find its full documentation in the demo section. ([@samayala22](https://github.com/samayala22), [@manjrekarom](https://github.com/manjrekarom)) [#371](https://github.com/open-mmlab/mmocr/pull/371), [#386](https://github.com/open-mmlab/mmocr/pull/386), [#400](https://github.com/open-mmlab/mmocr/pull/400), [#374](https://github.com/open-mmlab/mmocr/pull/374), [#428](https://github.com/open-mmlab/mmocr/pull/428)
-3. Our documentation is reorganized into a clearer structure. More useful contents are on the way! [#409](https://github.com/open-mmlab/mmocr/pull/409), [#454](https://github.com/open-mmlab/mmocr/pull/454)
-4. The requirement of `Polygon3` is removed since this project is no longer maintained or distributed. We unified all its references to equivalent substitutions in `shapely` instead. [#448](https://github.com/open-mmlab/mmocr/pull/448)
-
-### Breaking Changes & Migration Guide
-
-1. Upgrade version requirement of MMDetection to 2.14.0 to avoid bugs [#382](https://github.com/open-mmlab/mmocr/pull/382)
-2. MMOCR now has its own model and layer registries inherited from MMDetection's or MMCV's counterparts. ([#436](https://github.com/open-mmlab/mmocr/pull/436)) The modified hierarchical structure of the model registries are now organized as follows.
-
-```text
-mmcv.MODELS -> mmdet.BACKBONES -> BACKBONES
-mmcv.MODELS -> mmdet.NECKS -> NECKS
-mmcv.MODELS -> mmdet.ROI_EXTRACTORS -> ROI_EXTRACTORS
-mmcv.MODELS -> mmdet.HEADS -> HEADS
-mmcv.MODELS -> mmdet.LOSSES -> LOSSES
-mmcv.MODELS -> mmdet.DETECTORS -> DETECTORS
-mmcv.ACTIVATION_LAYERS -> ACTIVATION_LAYERS
-mmcv.UPSAMPLE_LAYERS -> UPSAMPLE_LAYERS
-```
-
-To migrate your old implementation to our new backend, you need to change the import path of any registries and their corresponding builder functions (including `build_detectors`) from `mmdet.models.builder` to `mmocr.models.builder`. If you have referred to any model or layer of MMDetection or MMCV in your model config, you need to add `mmdet.` or `mmcv.` prefix to its name to inform the model builder of the right namespace to work on.
-
-Interested users may check out [MMCV's tutorial on Registry](https://mmcv.readthedocs.io/en/latest/understand_mmcv/registry.html) for in-depth explanations on its mechanism.
-
-### New Features
-
-- Automatically replace SyncBN with BN for inference [#420](https://github.com/open-mmlab/mmocr/pull/420), [#453](https://github.com/open-mmlab/mmocr/pull/453)
-- Support batch inference for CRNN and SegOCR [#407](https://github.com/open-mmlab/mmocr/pull/407)
-- Support exporting documentation in pdf or epub format [#406](https://github.com/open-mmlab/mmocr/pull/406)
-- Support `persistent_workers` option in data loader [#459](https://github.com/open-mmlab/mmocr/pull/459)
-
-### Bug Fixes
-
-- Remove depreciated key in kie_test_imgs.py [#381](https://github.com/open-mmlab/mmocr/pull/381)
-- Fix dimension mismatch in batch testing/inference of DBNet [#383](https://github.com/open-mmlab/mmocr/pull/383)
-- Fix the problem of dice loss which stays at 1 with an empty target given [#408](https://github.com/open-mmlab/mmocr/pull/408)
-- Fix a wrong link in ocr.py ([@naarkhoo](https://github.com/naarkhoo)) [#417](https://github.com/open-mmlab/mmocr/pull/417)
-- Fix undesired assignment to "pretrained" in test.py [#418](https://github.com/open-mmlab/mmocr/pull/418)
-- Fix a problem in polygon generation of DBNet [#421](https://github.com/open-mmlab/mmocr/pull/421), [#443](https://github.com/open-mmlab/mmocr/pull/443)
-- Skip invalid annotations in totaltext_converter [#438](https://github.com/open-mmlab/mmocr/pull/438)
-- Add zero division handler in poly utils, remove Polygon3 [#448](https://github.com/open-mmlab/mmocr/pull/448)
-
-### Improvements
-
-- Replace lanms-proper with lanms-neo to support installation on Windows (with special thanks to [@gen-ko](https://github.com/gen-ko) who has re-distributed this package!)
-- Support MIM [#394](https://github.com/open-mmlab/mmocr/pull/394)
-- Add tests for PyTorch 1.9 in CI [#401](https://github.com/open-mmlab/mmocr/pull/401)
-- Enables fullscreen layout in readthedocs [#413](https://github.com/open-mmlab/mmocr/pull/413)
-- General documentation enhancement [#395](https://github.com/open-mmlab/mmocr/pull/395)
-- Update version checker [#427](https://github.com/open-mmlab/mmocr/pull/427)
-- Add copyright info [#439](https://github.com/open-mmlab/mmocr/pull/439)
-- Update citation information [#440](https://github.com/open-mmlab/mmocr/pull/440)
-
-### Contributors
-
-We thank [@2793145003](https://github.com/2793145003), [@samayala22](https://github.com/samayala22), [@manjrekarom](https://github.com/manjrekarom), [@naarkhoo](https://github.com/naarkhoo), [@gen-ko](https://github.com/gen-ko), [@duanjiaqi](https://github.com/duanjiaqi), [@gaotongxiao](https://github.com/gaotongxiao), [@cuhk-hbsun](https://github.com/cuhk-hbsun), [@innerlee](https://github.com/innerlee), [@wdsd641417025](https://github.com/wdsd641417025) for their contribution to this release!
-
-## v0.2.1 (20/7/2021)
-
-### Highlights
-
-1. Upgrade to use MMCV-full **>= 1.3.8** and MMDetection **>= 2.13.0** for latest features
-2. Add ONNX and TensorRT export tool, supporting the deployment of DBNet, PSENet, PANet and CRNN (experimental) [#278](https://github.com/open-mmlab/mmocr/pull/278), [#291](https://github.com/open-mmlab/mmocr/pull/291), [#300](https://github.com/open-mmlab/mmocr/pull/300), [#328](https://github.com/open-mmlab/mmocr/pull/328)
-3. Unified parameter initialization method which uses init_cfg in config files [#365](https://github.com/open-mmlab/mmocr/pull/365)
-
-### New Features
-
-- Support TextOCR dataset [#293](https://github.com/open-mmlab/mmocr/pull/293)
-- Support Total-Text dataset [#266](https://github.com/open-mmlab/mmocr/pull/266), [#273](https://github.com/open-mmlab/mmocr/pull/273), [#357](https://github.com/open-mmlab/mmocr/pull/357)
-- Support grouping text detection box into lines [#290](https://github.com/open-mmlab/mmocr/pull/290), [#304](https://github.com/open-mmlab/mmocr/pull/304)
-- Add benchmark_processing script that benchmarks data loading process [#261](https://github.com/open-mmlab/mmocr/pull/261)
-- Add SynthText preprocessor for text recognition models [#351](https://github.com/open-mmlab/mmocr/pull/351), [#361](https://github.com/open-mmlab/mmocr/pull/361)
-- Support batch inference during testing [#310](https://github.com/open-mmlab/mmocr/pull/310)
-- Add user-friendly OCR inference script [#366](https://github.com/open-mmlab/mmocr/pull/366)
-
-### Bug Fixes
-
-- Fix improper class ignorance in SDMGR Loss [#221](https://github.com/open-mmlab/mmocr/pull/221)
-- Fix potential numerical zero division error in DRRG [#224](https://github.com/open-mmlab/mmocr/pull/224)
-- Fix installing requirements with pip and mim [#242](https://github.com/open-mmlab/mmocr/pull/242)
-- Fix dynamic input error of DBNet [#269](https://github.com/open-mmlab/mmocr/pull/269)
-- Fix space parsing error in LineStrParser [#285](https://github.com/open-mmlab/mmocr/pull/285)
-- Fix textsnake decode error [#264](https://github.com/open-mmlab/mmocr/pull/264)
-- Correct isort setup [#288](https://github.com/open-mmlab/mmocr/pull/288)
-- Fix a bug in SDMGR config [#316](https://github.com/open-mmlab/mmocr/pull/316)
-- Fix kie_test_img for KIE nonvisual [#319](https://github.com/open-mmlab/mmocr/pull/319)
-- Fix metafiles [#342](https://github.com/open-mmlab/mmocr/pull/342)
-- Fix different device problem in FCENet [#334](https://github.com/open-mmlab/mmocr/pull/334)
-- Ignore improper tailing empty characters in annotation files [#358](https://github.com/open-mmlab/mmocr/pull/358)
-- Docs fixes [#247](https://github.com/open-mmlab/mmocr/pull/247), [#255](https://github.com/open-mmlab/mmocr/pull/255), [#265](https://github.com/open-mmlab/mmocr/pull/265), [#267](https://github.com/open-mmlab/mmocr/pull/267), [#268](https://github.com/open-mmlab/mmocr/pull/268), [#270](https://github.com/open-mmlab/mmocr/pull/270), [#276](https://github.com/open-mmlab/mmocr/pull/276), [#287](https://github.com/open-mmlab/mmocr/pull/287), [#330](https://github.com/open-mmlab/mmocr/pull/330), [#355](https://github.com/open-mmlab/mmocr/pull/355), [#367](https://github.com/open-mmlab/mmocr/pull/367)
-- Fix NRTR config [#356](https://github.com/open-mmlab/mmocr/pull/356), [#370](https://github.com/open-mmlab/mmocr/pull/370)
-
-### Improvements
-
-- Add backend for resizeocr [#244](https://github.com/open-mmlab/mmocr/pull/244)
-- Skip image processing pipelines in SDMGR novisual [#260](https://github.com/open-mmlab/mmocr/pull/260)
-- Speedup DBNet [#263](https://github.com/open-mmlab/mmocr/pull/263)
-- Update mmcv installation method in workflow [#323](https://github.com/open-mmlab/mmocr/pull/323)
-- Add part of Chinese documentations [#353](https://github.com/open-mmlab/mmocr/pull/353), [#362](https://github.com/open-mmlab/mmocr/pull/362)
-- Add support for ConcatDataset with two workflows [#348](https://github.com/open-mmlab/mmocr/pull/348)
-- Add list_from_file and list_to_file utils [#226](https://github.com/open-mmlab/mmocr/pull/226)
-- Speed up sort_vertex [#239](https://github.com/open-mmlab/mmocr/pull/239)
-- Support distributed evaluation of KIE [#234](https://github.com/open-mmlab/mmocr/pull/234)
-- Add pretrained FCENet on IC15 [#258](https://github.com/open-mmlab/mmocr/pull/258)
-- Support CPU for OCR demo [#227](https://github.com/open-mmlab/mmocr/pull/227)
-- Avoid extra image pre-processing steps [#375](https://github.com/open-mmlab/mmocr/pull/375)
-
-## v0.2.0 (18/5/2021)
-
-### Highlights
-
-1. Add the NER approach Bert-softmax (NAACL'2019)
-2. Add the text detection method DRRG (CVPR'2020)
-3. Add the text detection method FCENet (CVPR'2021)
-4. Increase the ease of use via adding text detection and recognition end-to-end demo, and colab online demo.
-5. Simplify the installation.
-
-### New Features
-
-- Add Bert-softmax for Ner task [#148](https://github.com/open-mmlab/mmocr/pull/148)
-- Add DRRG [#189](https://github.com/open-mmlab/mmocr/pull/189)
-- Add FCENet [#133](https://github.com/open-mmlab/mmocr/pull/133)
-- Add end-to-end demo [#105](https://github.com/open-mmlab/mmocr/pull/105)
-- Support batch inference [#86](https://github.com/open-mmlab/mmocr/pull/86) [#87](https://github.com/open-mmlab/mmocr/pull/87) [#178](https://github.com/open-mmlab/mmocr/pull/178)
-- Add TPS preprocessor for text recognition [#117](https://github.com/open-mmlab/mmocr/pull/117) [#135](https://github.com/open-mmlab/mmocr/pull/135)
-- Add demo documentation [#151](https://github.com/open-mmlab/mmocr/pull/151) [#166](https://github.com/open-mmlab/mmocr/pull/166) [#168](https://github.com/open-mmlab/mmocr/pull/168) [#170](https://github.com/open-mmlab/mmocr/pull/170) [#171](https://github.com/open-mmlab/mmocr/pull/171)
-- Add checkpoint for Chinese recognition [#156](https://github.com/open-mmlab/mmocr/pull/156)
-- Add metafile [#175](https://github.com/open-mmlab/mmocr/pull/175) [#176](https://github.com/open-mmlab/mmocr/pull/176) [#177](https://github.com/open-mmlab/mmocr/pull/177) [#182](https://github.com/open-mmlab/mmocr/pull/182) [#183](https://github.com/open-mmlab/mmocr/pull/183)
-- Add support for numpy array inference [#74](https://github.com/open-mmlab/mmocr/pull/74)
-
-### Bug Fixes
-
-- Fix the duplicated point bug due to transform for textsnake [#130](https://github.com/open-mmlab/mmocr/pull/130)
-- Fix CTC loss NaN [#159](https://github.com/open-mmlab/mmocr/pull/159)
-- Fix error raised if result is empty in demo [#144](https://github.com/open-mmlab/mmocr/pull/141)
-- Fix results missing if one image has a large number of boxes [#98](https://github.com/open-mmlab/mmocr/pull/98)
-- Fix package missing in dockerfile [#109](https://github.com/open-mmlab/mmocr/pull/109)
-
-### Improvements
-
-- Simplify installation procedure via removing compiling [#188](https://github.com/open-mmlab/mmocr/pull/188)
-- Speed up panet post processing so that it can detect dense texts [#188](https://github.com/open-mmlab/mmocr/pull/188)
-- Add zh-CN README [#70](https://github.com/open-mmlab/mmocr/pull/70) [#95](https://github.com/open-mmlab/mmocr/pull/95)
-- Support windows [#89](https://github.com/open-mmlab/mmocr/pull/89)
-- Add Colab [#147](https://github.com/open-mmlab/mmocr/pull/147) [#199](https://github.com/open-mmlab/mmocr/pull/199)
-- Add 1-step installation using conda environment [#193](https://github.com/open-mmlab/mmocr/pull/193) [#194](https://github.com/open-mmlab/mmocr/pull/194) [#195](https://github.com/open-mmlab/mmocr/pull/195)
-
-## v0.1.0 (7/4/2021)
-
-### Highlights
-
-- MMOCR is released.
-
-### Main Features
-
-- Support text detection, text recognition and the corresponding downstream tasks such as key information extraction.
-- For text detection, support both single-step (`PSENet`, `PANet`, `DBNet`, `TextSnake`) and two-step (`MaskRCNN`) methods.
-- For text recognition, support CTC-loss based method `CRNN`; Encoder-decoder (with attention) based methods `SAR`, `Robustscanner`; Segmentation based method `SegOCR`; Transformer based method `NRTR`.
-- For key information extraction, support GCN based method `SDMG-R`.
-- Provide checkpoints and log files for all of the methods above.
diff --git a/pipeline/mmocr/docs/en/notes/contribution_guide.md b/pipeline/mmocr/docs/en/notes/contribution_guide.md
deleted file mode 100644
index 94cf4ce165196baeaff18c6615f1f683dfaa70eb..0000000000000000000000000000000000000000
--- a/pipeline/mmocr/docs/en/notes/contribution_guide.md
+++ /dev/null
@@ -1,134 +0,0 @@
-# Contribution Guide
-
-OpenMMLab welcomes everyone who is interested in contributing to our projects and accepts contribution in the form of PR.
-
-## What is PR
-
-`PR` is the abbreviation of `Pull Request`. Here's the definition of `PR` in the [official document](https://docs.github.com/en/github/collaborating-with-pull-requests/proposing-changes-to-your-work-with-pull-requests/about-pull-requests) of Github.
-
-```
-Pull requests let you tell others about changes you have pushed to a branch in a repository on GitHub. Once a pull request is opened, you can discuss and review the potential changes with collaborators and add follow-up commits before your changes are merged into the base branch.
-```
-
-## Basic Workflow
-
-1. Get the most recent codebase
-2. Checkout a new branch from `dev-1.x` branch, depending on the version of the codebase you want to contribute to.
-3. Commit your changes ([Don't forget to use pre-commit hooks!](#3-commit-your-changes))
-4. Push your changes and create a PR
-5. Discuss and review your code
-6. Merge your branch to `dev-1.x` branch
-
-## Procedures in detail
-
-### 1. Get the most recent codebase
-
-- When you work on your first PR
-
- Fork the OpenMMLab repository: click the **fork** button at the top right corner of Github page
- 
-
- Clone forked repository to local
-
- ```bash
- git clone git@github.com:XXX/mmocr.git
- ```
-
- Add source repository to upstream
-
- ```bash
- git remote add upstream git@github.com:open-mmlab/mmocr
- ```
-
-- After your first PR
-
- Checkout the latest branch of the local repository and pull the latest branch of the source repository. Here we assume that you are working on the `dev-1.x` branch.
-
- ```bash
- git checkout dev-1.x
- git pull upstream dev-1.x
- ```
-
-### 2. Checkout a new branch from `dev-1.x` branch
-
-```bash
-git checkout -b branchname
-```
-
-```{tip}
-To make commit history clear, we strongly recommend you checkout the `dev-1.x` branch before creating a new branch.
-```
-
-### 3. Commit your changes
-
-- If you are a first-time contributor, please install and initialize pre-commit hooks from the repository root directory first.
-
- ```bash
- pip install -U pre-commit
- pre-commit install
- ```
-
-- Commit your changes as usual. Pre-commit hooks will be triggered to stylize your code before each commit.
-
- ```bash
- # coding
- git add [files]
- git commit -m 'messages'
- ```
-
- ```{note}
- Sometimes your code may be changed by pre-commit hooks. In this case, please remember to re-stage the modified files and commit again.
- ```
-
-### 4. Push your changes to the forked repository and create a PR
-
-- Push the branch to your forked remote repository
-
- ```bash
- git push origin branchname
- ```
-
-- Create a PR
- 
-
-- Revise PR message template to describe your motivation and modifications made in this PR. You can also link the related issue to the PR manually in the PR message (For more information, checkout the [official guidance](https://docs.github.com/en/issues/tracking-your-work-with-issues/linking-a-pull-request-to-an-issue)).
-
-- Specifically, if you are contributing to `dev-1.x`, you will have to change the base branch of the PR to `dev-1.x` in the PR page, since the default base branch is `main`.
-
- 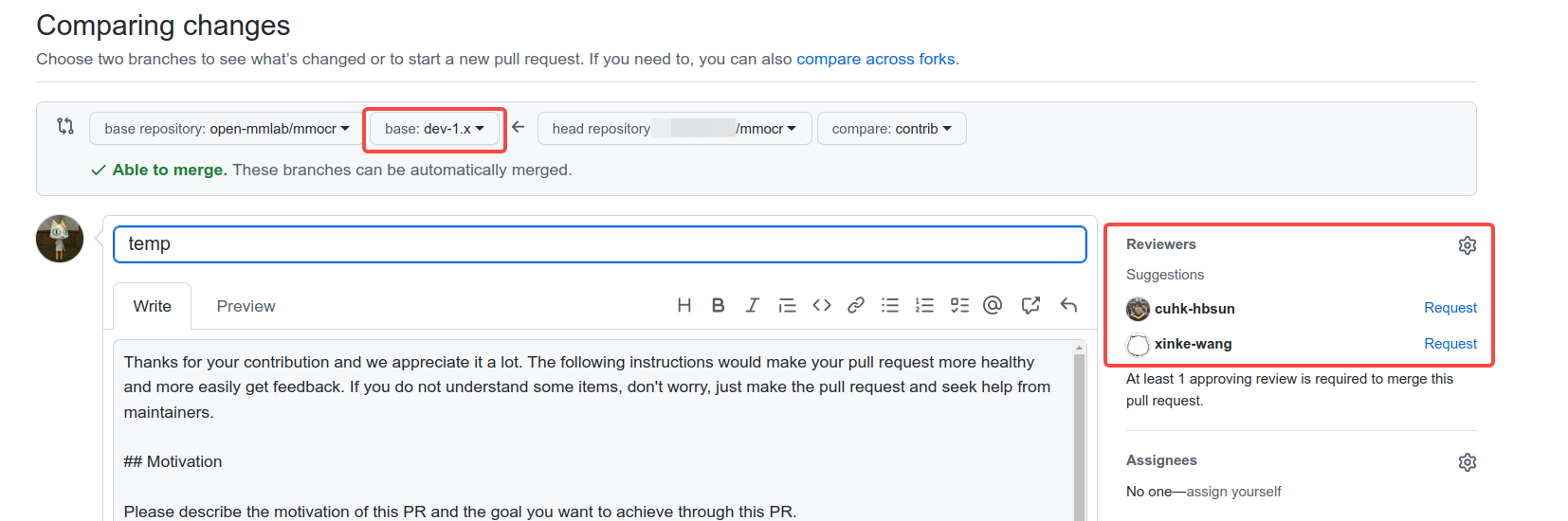
-
-- You can also ask a specific person to review the changes you've proposed.
-
-### 5. Discuss and review your code
-
-- Modify your codes according to reviewers' suggestions and then push your changes.
-
-### 6. Merge your branch to `dev-1.x` branch and delete the branch
-
-- After the PR is merged by the maintainer, you can delete the branch you created in your forked repository.
-
- ```bash
- git branch -d branchname # delete local branch
- git push origin --delete branchname # delete remote branch
- ```
-
-## PR Specs
-
-1. Use [pre-commit](https://pre-commit.com) hook to avoid issues of code style
-
-2. One short-time branch should be matched with only one PR
-
-3. Accomplish a detailed change in one PR. Avoid large PR
-
- - Bad: Support Faster R-CNN
- - Acceptable: Add a box head to Faster R-CNN
- - Good: Add a parameter to box head to support custom conv-layer number
-
-4. Provide clear and significant commit message
-
-5. Provide clear and meaningful PR description
-
- - Task name should be clarified in title. The general format is: \[Prefix\] Short description of the PR (Suffix)
- - Prefix: add new feature \[Feature\], fix bug \[Fix\], related to documents \[Docs\], in developing \[WIP\] (which will not be reviewed temporarily)
- - Introduce main changes, results and influences on other modules in short description
- - Associate related issues and pull requests with a milestone
diff --git a/pipeline/mmocr/docs/en/project_zoo.py b/pipeline/mmocr/docs/en/project_zoo.py
deleted file mode 100644
index ec5671793371fa22e754537b9fd12db22656ae42..0000000000000000000000000000000000000000
--- a/pipeline/mmocr/docs/en/project_zoo.py
+++ /dev/null
@@ -1,52 +0,0 @@
-#!/usr/bin/env python
-import os.path as osp
-import re
-
-# This script reads /projects/selected.txt and generate projectzoo.md
-
-files = []
-
-project_zoo = """
-# SOTA Models
-
-Here are some selected project implementations that are not yet included in
-MMOCR package, but are ready to use.
-
-"""
-
-files = open('../../projects/selected.txt').readlines()
-
-for file in files:
- file = file.strip()
- with open(osp.join('../../', file)) as f:
- content = f.read()
-
- # Extract title
- expr = '# (.*?)\n'
- title = re.search(expr, content).group(1)
- project_zoo += f'## {title}\n\n'
-
- # Locate the description
- expr = '## Description\n(.*?)##'
- description = re.search(expr, content, re.DOTALL).group(1)
- project_zoo += f'{description}\n'
-
- # check milestone 1
- expr = r'- \[(.?)\] Milestone 1'
- state = re.search(expr, content, re.DOTALL).group(1)
- infer_state = 'โ' if state == 'x' else 'โ'
-
- # check milestone 2
- expr = r'- \[(.?)\] Milestone 2'
- state = re.search(expr, content, re.DOTALL).group(1)
- training_state = 'โ' if state == 'x' else 'โ'
-
- # add table
- readme_link = f'https://github.com/open-mmlab/mmocr/blob/dev-1.x/{file}'
- project_zoo += '### Status \n'
- project_zoo += '| Inference | Train | README |\n'
- project_zoo += '| --------- | -------- | ------ |\n'
- project_zoo += f'|๏ธ{infer_state}|{training_state}|[link]({readme_link})|\n'
-
-with open('projectzoo.md', 'w') as f:
- f.write(project_zoo)
diff --git a/pipeline/mmocr/docs/en/requirements.txt b/pipeline/mmocr/docs/en/requirements.txt
deleted file mode 100644
index 89fbf86c01cb29f10f7e99c910248c4d5229da58..0000000000000000000000000000000000000000
--- a/pipeline/mmocr/docs/en/requirements.txt
+++ /dev/null
@@ -1,4 +0,0 @@
-recommonmark
-sphinx
-sphinx_markdown_tables
-sphinx_rtd_theme
diff --git a/pipeline/mmocr/docs/en/stats.py b/pipeline/mmocr/docs/en/stats.py
deleted file mode 100644
index 3238686937660a189b9db21b8653b519bb12627c..0000000000000000000000000000000000000000
--- a/pipeline/mmocr/docs/en/stats.py
+++ /dev/null
@@ -1,131 +0,0 @@
-#!/usr/bin/env python
-# Copyright (c) OpenMMLab. All rights reserved.
-import functools as func
-import re
-from os.path import basename, splitext
-
-import numpy as np
-import titlecase
-from weight_list import gen_weight_list
-
-
-def title2anchor(name):
- return re.sub(r'-+', '-', re.sub(r'[^a-zA-Z0-9]', '-',
- name.strip().lower())).strip('-')
-
-
-# Count algorithms
-
-files = [
- 'backbones.md', 'textdet_models.md', 'textrecog_models.md', 'kie_models.md'
-]
-
-stats = []
-
-for f in files:
- with open(f) as content_file:
- content = content_file.read()
-
- # Remove the blackquote notation from the paper link under the title
- # for better layout in readthedocs
- expr = r'(^## \s*?.*?\s+?)>\s*?(\[.*?\]\(.*?\))'
- content = re.sub(expr, r'\1\2', content, flags=re.MULTILINE)
- with open(f, 'w') as content_file:
- content_file.write(content)
-
- # title
- title = content.split('\n')[0].replace('#', '')
-
- # count papers
- exclude_papertype = ['ABSTRACT', 'IMAGE']
- exclude_expr = ''.join(f'(?!{s})' for s in exclude_papertype)
- expr = rf''\
- r'\s*\n.*?\btitle\s*=\s*{(.*?)}'
- papers = {(papertype, titlecase.titlecase(paper.lower().strip()))
- for (papertype, paper) in re.findall(expr, content, re.DOTALL)}
- print(papers)
- # paper links
- revcontent = '\n'.join(list(reversed(content.splitlines())))
- paperlinks = {}
- for _, p in papers:
- q = p.replace('\\', '\\\\').replace('?', '\\?')
- paper_link = title2anchor(
- re.search(
- rf'\btitle\s*=\s*{{\s*{q}\s*}}.*?\n## (.*?)\s*[,;]?\s*\n',
- revcontent, re.DOTALL | re.IGNORECASE).group(1))
- paperlinks[p] = f'[{p}]({splitext(basename(f))[0]}.md#{paper_link})'
- paperlist = '\n'.join(
- sorted(f' - [{t}] {paperlinks[x]}' for t, x in papers))
- # count configs
- configs = {
- x.lower().strip()
- for x in re.findall(r'https.*configs/.*\.py', content)
- }
-
- # count ckpts
- ckpts = {
- x.lower().strip()
- for x in re.findall(r'https://download.*\.pth', content)
- if 'mmocr' in x
- }
-
- statsmsg = f"""
-### [{title}]({f})
-
-* Number of checkpoints: {len(ckpts)}
-* Number of configs: {len(configs)}
-* Number of papers: {len(papers)}
-{paperlist}
-
- """
-
- stats.append((papers, configs, ckpts, statsmsg))
-
-allpapers = func.reduce(lambda a, b: a.union(b), [p for p, _, _, _ in stats])
-allconfigs = func.reduce(lambda a, b: a.union(b), [c for _, c, _, _ in stats])
-allckpts = func.reduce(lambda a, b: a.union(b), [c for _, _, c, _ in stats])
-msglist = '\n'.join(x for _, _, _, x in stats)
-
-papertypes, papercounts = np.unique([t for t, _ in allpapers],
- return_counts=True)
-countstr = '\n'.join(
- [f' - {t}: {c}' for t, c in zip(papertypes, papercounts)])
-
-# get model list
-weight_list = gen_weight_list()
-
-modelzoo = f"""
-# Overview
-
-## Weights
-
-Here are the list of weights available for
-[Inference](user_guides/inference.md).
-
-For the ease of reference, some weights may have shorter aliases, which will be
-separated by `/` in the table.
-For example, "`DB_r18 / dbnet_resnet18_fpnc_1200e_icdar2015`" means that you can
-use either `DB_r18` or `dbnet_resnet18_fpnc_1200e_icdar2015`
-to initialize the Inferencer:
-
-```python
->>> from mmocr.apis import TextDetInferencer
->>> inferencer = TextDetInferencer(model='DB_r18')
->>> # equivalent to
->>> inferencer = TextDetInferencer(model='dbnet_resnet18_fpnc_1200e_icdar2015')
-```
-
-{weight_list}
-
-## Statistics
-
-* Number of checkpoints: {len(allckpts)}
-* Number of configs: {len(allconfigs)}
-* Number of papers: {len(allpapers)}
-{countstr}
-
-{msglist}
-""" # noqa
-
-with open('modelzoo.md', 'w') as f:
- f.write(modelzoo)
diff --git a/pipeline/mmocr/docs/en/switch_language.md b/pipeline/mmocr/docs/en/switch_language.md
deleted file mode 100644
index 7baa29992eb3b36ab2804b577d3bb76db8cc4233..0000000000000000000000000000000000000000
--- a/pipeline/mmocr/docs/en/switch_language.md
+++ /dev/null
@@ -1,3 +0,0 @@
-## English
-
-## ็ฎไฝไธญๆ
diff --git a/pipeline/mmocr/docs/en/user_guides/config.md b/pipeline/mmocr/docs/en/user_guides/config.md
deleted file mode 100644
index c2573d8488c67173e23d0b50258bb970dbe48e22..0000000000000000000000000000000000000000
--- a/pipeline/mmocr/docs/en/user_guides/config.md
+++ /dev/null
@@ -1,707 +0,0 @@
-# Config
-
-MMOCR mainly uses Python files as configuration files. The design of its configuration file system integrates the ideas of modularity and inheritance to facilitate various experiments.
-
-## Common Usage
-
-```{note}
-This section is recommended to be read together with the primary usage in {external+mmengine:doc}`MMEngine: Config `.
-```
-
-There are three most common operations in MMOCR: inheritance of configuration files, reference to `_base_` variables, and modification of `_base_` variables. Config provides two syntaxes for inheriting and modifying `_base_`, one for Python, Json, and Yaml, and one for Python configuration files only. In MMOCR, we **prefer the Python-only syntax**, so this will be the basis for further description.
-
-The `configs/textdet/dbnet/dbnet_resnet18_fpnc_1200e_icdar2015.py` is used as an example to illustrate the three common uses.
-
-```Python
-_base_ = [
- '_base_dbnet_resnet18_fpnc.py',
- '../_base_/datasets/icdar2015.py',
- '../_base_/default_runtime.py',
- '../_base_/schedules/schedule_sgd_1200e.py',
-]
-
-# dataset settings
-icdar2015_textdet_train = _base_.icdar2015_textdet_train
-icdar2015_textdet_train.pipeline = _base_.train_pipeline
-icdar2015_textdet_test = _base_.icdar2015_textdet_test
-icdar2015_textdet_test.pipeline = _base_.test_pipeline
-
-train_dataloader = dict(
- batch_size=16,
- num_workers=8,
- persistent_workers=True,
- sampler=dict(type='DefaultSampler', shuffle=True),
- dataset=icdar2015_textdet_train)
-
-val_dataloader = dict(
- batch_size=1,
- num_workers=4,
- persistent_workers=True,
- sampler=dict(type='DefaultSampler', shuffle=False),
- dataset=icdar2015_textdet_test)
-```
-
-### Configuration Inheritance
-
-There is an inheritance mechanism for configuration files, i.e. one configuration file A can use another configuration file B as its base and inherit all the fields directly from it, thus avoiding a lot of copy-pasting.
-
-In `dbnet_resnet18_fpnc_1200e_icdar2015.py` you can see that
-
-```Python
-_base_ = [
- '_base_dbnet_resnet18_fpnc.py',
- '../_base_/datasets/icdar2015.py',
- '../_base_/default_runtime.py',
- '../_base_/schedules/schedule_sgd_1200e.py',
-]
-```
-
-The above statement reads all the base configuration files in the list, and all the fields in them are loaded into `dbnet_resnet18_fpnc_1200e_icdar2015.py`. We can see the structure of the configuration file after it has been parsed by running the following statement in a Python interpretation.
-
-```Python
-from mmengine import Config
-db_config = Config.fromfile('configs/textdet/dbnet/dbnet_resnet18_fpnc_1200e_icdar2015.py')
-print(db_config)
-```
-
-It can be found that the parsed configuration contains all the fields and information in the base configuration.
-
-```{note}
-Variables with the same name cannot exist in each `_base_` profile.
-```
-
-### `_base_` Variable References
-
-Sometimes we may need to reference some fields in the `_base_` configuration directly in order to avoid duplicate definitions. Suppose we want to get the variable `pseudo` in the `_base_` configuration, we can get the variable in the `_base_` configuration directly via `_base_.pseudo`.
-
-This syntax has been used extensively in the configuration of MMOCR, and the dataset and pipeline configurations for each model in MMOCR are referenced in the *_base_* configuration. For example,
-
-```Python
-icdar2015_textdet_train = _base_.icdar2015_textdet_train
-# ...
-train_dataloader = dict(
- # ...
- dataset=icdar2015_textdet_train)
-```
-
-
-
-### `_base_` Variable Modification
-
-In MMOCR, different algorithms usually have different pipelines in different datasets, so there are often scenarios to modify the `pipeline` in the dataset. There are also many scenarios where you need to modify variables in the `_base_` configuration, for example, modifying the training strategy of an algorithm, replacing some modules of an algorithm(backbone, etc.). Users can directly modify the referenced `_base_` variables using Python syntax. For dict, we also provide a method similar to class attribute modification to modify the contents of the dictionary directly.
-
-1. Dictionary
-
- Here is an example of modifying `pipeline` in a dataset.
-
- The dictionary can be modified using Python syntax:
-
- ```Python
- # Get the dataset in _base_
- icdar2015_textdet_train = _base_.icdar2015_textdet_train
- # You can modify the variables directly with Python's update
- icdar2015_textdet_train.update(pipeline=_base_.train_pipeline)
- ```
-
- It can also be modified in the same way as changing Python class attributes.
-
- ```Python
- # Get the dataset in _base_
- icdar2015_textdet_train = _base_.icdar2015_textdet_train
- # The class property method is modified
- icdar2015_textdet_train.pipeline = _base_.train_pipeline
- ```
-
-2. List
-
- Suppose the variable `pseudo = [1, 2, 3]` in the `_base_` configuration needs to be modified to `[1, 2, 4]`:
-
- ```Python
- # pseudo.py
- pseudo = [1, 2, 3]
- ```
-
- Can be rewritten directly as.
-
- ```Python
- _base_ = ['pseudo.py']
- pseudo = [1, 2, 4]
- ```
-
- Or modify the list using Python syntax:
-
- ```Python
- _base_ = ['pseudo.py']
- pseudo = _base_.pseudo
- pseudo[2] = 4
- ```
-
-### Command Line Modification
-
-Sometimes we only want to fix part of the configuration and do not want to modify the configuration file itself. For example, if you want to change the learning rate during an experiment but do not want to write a new configuration file, you can pass in parameters on the command line to override the relevant configuration.
-
-We can pass `--cfg-options` on the command line and modify the corresponding fields directly with the arguments after it. For example, we can run the following command to modify the learning rate temporarily for this training session.
-
-```Shell
-python tools/train.py example.py --cfg-options optim_wrapper.optimizer.lr=1
-```
-
-For more detailed usage, refer to {external+mmengine:doc}`MMEngine: Command Line Modification `.
-
-## Configuration Content
-
-With config files and Registry, MMOCR can modify the training parameters as well as the model configuration without invading the code. Specifically, users can customize the following modules in the configuration file: environment configuration, hook configuration, log configuration, training strategy configuration, data-related configuration, model-related configuration, evaluation configuration, and visualization configuration.
-
-This document will take the text detection algorithm `DBNet` and the text recognition algorithm `CRNN` as examples to introduce the contents of Config in detail.
-
-
-
-### Environment Configuration
-
-```Python
-default_scope = 'mmocr'
-env_cfg = dict(
- cudnn_benchmark=True,
- mp_cfg=dict(mp_start_method='fork', opencv_num_threads=0),
- dist_cfg=dict(backend='nccl'))
-randomness = dict(seed=None)
-```
-
-There are three main components:
-
-- Set the default `scope` of all registries to `mmocr`, ensuring that all modules are searched first from the `MMOCR` codebase. If the module does not exist, the search will continue from the upstream algorithm libraries `MMEngine` and `MMCV`, see {external+mmengine:doc}`MMEngine: Registry ` for more details.
-
-- `env_cfg` configures the distributed environment, see {external+mmengine:doc}`MMEngine: Runner ` for more details.
-
-- `randomness`: Some settings to make the experiment as reproducible
- as possible like seed and deterministic. See {external+mmengine:doc}`MMEngine: Runner ` for more details.
-
-
-
-### Hook Configuration
-
-Hooks are divided into two main parts, default hooks, which are required for all tasks to run, and custom hooks, which generally serve specific algorithms or specific tasks (there are no custom hooks in MMOCR so far).
-
-```Python
-default_hooks = dict(
- timer=dict(type='IterTimerHook'), # Time recording, including data time as well as model inference time
- logger=dict(type='LoggerHook', interval=1), # Collect logs from different components
- param_scheduler=dict(type='ParamSchedulerHook'), # Update some hyper-parameters in optimizer
- checkpoint=dict(type='CheckpointHook', interval=1),# Save checkpoint. `interval` control save interval
- sampler_seed=dict(type='DistSamplerSeedHook'), # Data-loading sampler for distributed training.
- sync_buffer=dict(type='SyncBuffersHook'), # Synchronize buffer in case of distributed training
- visualization=dict( # Visualize the results of val and test
- type='VisualizationHook',
- interval=1,
- enable=False,
- show=False,
- draw_gt=False,
- draw_pred=False))
- custom_hooks = []
-```
-
-Here is a brief description of a few hooks whose parameters may be changed frequently. For a general modification method, refer to Modify configuration .
-
-- `LoggerHook`: Used to configure the behavior of the logger. For example, by modifying `interval` you can control the interval of log printing, so that the log is printed once per `interval` iteration, for more settings refer to [LoggerHook API](mmengine.hooks.LoggerHook).
-
-- `CheckpointHook`: Used to configure checkpoint-related behavior, such as saving optimal and/or latest weights. You can also modify `interval` to control the checkpoint saving interval. More settings can be found in [CheckpointHook API](mmengine.hooks.CheckpointHook)
-
-- `VisualizationHook`: Used to configure visualization-related behavior, such as visualizing predicted results during validation or testing. **Default is off**. This Hook also depends on [Visualization Configuration](#Visualization-configuration). You can refer to [Visualizer](visualization.md) for more details. For more configuration, you can refer to [VisualizationHook API](mmocr.engine.hooks.VisualizationHook).
-
-If you want to learn more about the configuration of the default hooks and their functions, you can refer to {external+mmengine:doc}`MMEngine: Hooks `.
-
-
-
-### Log Configuration
-
-This section is mainly used to configure the log level and the log processor.
-
-```Python
-log_level = 'INFO' # Logging Level
-log_processor = dict(type='LogProcessor',
- window_size=10,
- by_epoch=True)
-```
-
-- The logging severity level is the same as that of {external+python:doc}`Python: logging `
-
-- The log processor is mainly used to control the format of the output, detailed functions can be found in {external+mmengine:doc}`MMEngine: logging `.
-
- - `by_epoch=True` indicates that the logs are output in accordance to "epoch", and the log format needs to be consistent with the `type='EpochBasedTrainLoop'` parameter in `train_cfg`. For example, if you want to output logs by iteration number, you need to set ` by_epoch=False` in `log_processor` and `type='IterBasedTrainLoop'` in `train_cfg`.
-
- - `window_size` indicates the smoothing window of the loss, i.e. the average value of the various losses for the last `window_size` iterations. the final loss value printed in logger is the average of all the losses.
-
-
-
-### Training Strategy Configuration
-
-This section mainly contains optimizer settings, learning rate schedules and `Loop` settings.
-
-Training strategies usually vary for different tasks (text detection, text recognition, key information extraction). Here we explain the example configuration in `CRNN`, which is a text recognition model.
-
-```Python
-# optimizer
-optim_wrapper = dict(
- type='OptimWrapper', optimizer=dict(type='Adadelta', lr=1.0))
-param_scheduler = [dict(type='ConstantLR', factor=1.0)]
-train_cfg = dict(type='EpochBasedTrainLoop',
- max_epochs=5, # train epochs
- val_interval=1) # val interval
-val_cfg = dict(type='ValLoop')
-test_cfg = dict(type='TestLoop')
-```
-
-- `optim_wrapper` : It contains two main parts, OptimWrapper and Optimizer. Detailed usage information can be found in {external+mmengine:doc}`MMEngine: Optimizer Wrapper `.
-
- - The Optimizer wrapper supports different training strategies, including mixed-accuracy training (AMP), gradient accumulation, and gradient truncation.
-
- - All PyTorch optimizers are supported in the optimizer settings. All supported optimizers are available in {external+torch:ref}`PyTorch Optimizer List `.
-
-- `param_scheduler` : learning rate tuning strategy, supports most of the learning rate schedulers in PyTorch, such as `ExponentialLR`, `LinearLR`, `StepLR`, `MultiStepLR`, etc., and is used in much the same way, see [scheduler interface](mmengine.optim.scheduler), and more features can be found in the {external+mmengine:doc}`MMEngine: Optimizer Parameter Tuning Strategy `.
-
-- `train/test/val_cfg` : the execution flow of the task, MMEngine provides four kinds of flow: `EpochBasedTrainLoop`, `IterBasedTrainLoop`, `ValLoop`, `TestLoop` More can be found in {external+mmengine:doc}`MMEngine: loop controller `.
-
-### Data-related Configuration
-
-
-
-#### Dataset Configuration
-
-It is mainly about two parts.
-
-- The location of the dataset(s), including images and annotation files.
-
-- Data augmentation related configurations. In the OCR domain, data augmentation is usually strongly associated with the model.
-
-More parameter configurations can be found in [Data Base Class](#TODO).
-
-The naming convention for dataset fields in MMOCR is
-
-```Python
-{dataset}_{task}_{train/val/test} = dict(...)
-```
-
-- dataset: See [dataset abbreviations](#TODO)
-
-- task: `det`(text detection), `rec`(text recognition), `kie`(key information extraction)
-
-- train/val/test: Dataset split.
-
-For example, for text recognition tasks, Syn90k is used as the training set, while icdar2013 and icdar2015 serve as the test sets. These are configured as follows.
-
-```Python
-# text recognition dataset configuration
-mjsynth_textrecog_train = dict(
- type='OCRDataset',
- data_root='data/rec/Syn90k/',
- data_prefix=dict(img_path='mnt/ramdisk/max/90kDICT32px'),
- ann_file='train_labels.json',
- test_mode=False,
- pipeline=None)
-
-icdar2013_textrecog_test = dict(
- type='OCRDataset',
- data_root='data/rec/icdar_2013/',
- data_prefix=dict(img_path='Challenge2_Test_Task3_Images/'),
- ann_file='test_labels.json',
- test_mode=True,
- pipeline=None)
-
-icdar2015_textrecog_test = dict(
- type='OCRDataset',
- data_root='data/rec/icdar_2015/',
- data_prefix=dict(img_path='ch4_test_word_images_gt/'),
- ann_file='test_labels.json',
- test_mode=True,
- pipeline=None)
-```
-
-
-
-#### Data Pipeline Configuration
-
-In MMOCR, dataset construction and data preparation are decoupled from each other. In other words, dataset classes such as `OCRDataset` are responsible for reading and parsing annotation files, while Data Transforms further implement data loading, data augmentation, data formatting and other related functions.
-
-In general, there are different augmentation strategies for training and testing, so there are usually `training_pipeline` and `testing_pipeline`. More information can be found in [Data Transforms](../basic_concepts/transforms.md)
-
-- The data augmentation process of the training pipeline is usually: data loading (LoadImageFromFile) -> annotation information loading (LoadXXXAnntation) -> data augmentation -> data formatting (PackXXXInputs).
-
-- The data augmentation flow of the test pipeline is usually: Data Loading (LoadImageFromFile) -> Data Augmentation -> Annotation Loading (LoadXXXAnntation) -> Data Formatting (PackXXXInputs).
-
-Due to the specificity of the OCR task, different models have different data augmentation techniques, and even the same model can have different data augmentation strategies for different datasets. Take `CRNN` as an example.
-
-```Python
-# Data Augmentation
-train_pipeline = [
- dict(
- type='LoadImageFromFile',
- color_type='grayscale',
- ignore_empty=True,
- min_size=5),
- dict(type='LoadOCRAnnotations', with_text=True),
- dict(type='Resize', scale=(100, 32), keep_ratio=False),
- dict(
- type='PackTextRecogInputs',
- meta_keys=('img_path', 'ori_shape', 'img_shape', 'valid_ratio'))
-]
-test_pipeline = [
- dict(
- type='LoadImageFromFile',
- color_type='grayscale'),
- dict(
- type='RescaleToHeight',
- height=32,
- min_width=32,
- max_width=None,
- width_divisor=16),
- dict(type='LoadOCRAnnotations', with_text=True),
- dict(
- type='PackTextRecogInputs',
- meta_keys=('img_path', 'ori_shape', 'img_shape', 'valid_ratio'))
-]
-```
-
-#### Dataloader Configuration
-
-The main configuration information needed to construct the dataset loader (dataloader), see {external+torch:doc}`PyTorch DataLoader
` for more tutorials.
-
-```Python
-# Dataloader
-train_dataloader = dict(
- batch_size=64,
- num_workers=8,
- persistent_workers=True,
- sampler=dict(type='DefaultSampler', shuffle=True),
- dataset=dict(
- type='ConcatDataset',
- datasets=[mjsynth_textrecog_train],
- pipeline=train_pipeline))
-val_dataloader = dict(
- batch_size=1,
- num_workers=4,
- persistent_workers=True,
- drop_last=False,
- sampler=dict(type='DefaultSampler', shuffle=False),
- dataset=dict(
- type='ConcatDataset',
- datasets=[icdar2013_textrecog_test, icdar2015_textrecog_test],
- pipeline=test_pipeline))
-test_dataloader = val_dataloader
-```
-
-### Model-related Configuration
-
-
-
-#### Network Configuration
-
-This section configures the network architecture. Different algorithmic tasks use different network architectures. Find more info about network architecture in [structures](../basic_concepts/structures.md)
-
-##### Text Detection
-
-Text detection consists of several parts:
-
-- `data_preprocessor`: [data_preprocessor](mmocr.models.textdet.data_preprocessors.TextDetDataPreprocessor)
-- `backbone`: backbone network configuration
-- `neck`: neck network configuration
-- `det_head`: detection head network configuration
- - `module_loss`: module loss configuration
- - `postprocessor`: postprocessor configuration
-
-We present the model configuration in text detection using DBNet as an example.
-
-```Python
-model = dict(
- type='DBNet',
- data_preprocessor=dict(
- type='TextDetDataPreprocessor',
- mean=[123.675, 116.28, 103.53],
- std=[58.395, 57.12, 57.375],
- bgr_to_rgb=True,
- pad_size_divisor=32)
- backbone=dict(
- type='mmdet.ResNet',
- depth=18,
- num_stages=4,
- out_indices=(0, 1, 2, 3),
- frozen_stages=-1,
- norm_cfg=dict(type='BN', requires_grad=True),
- init_cfg=dict(type='Pretrained', checkpoint='torchvision://resnet18'),
- norm_eval=False,
- style='caffe'),
- neck=dict(
- type='FPNC', in_channels=[64, 128, 256, 512], lateral_channels=256),
- det_head=dict(
- type='DBHead',
- in_channels=256,
- module_loss=dict(type='DBModuleLoss'),
- postprocessor=dict(type='DBPostprocessor', text_repr_type='quad')))
-```
-
-##### Text Recognition
-
-Text recognition mainly contains:
-
-- `data_processor`: [data preprocessor configuration](mmocr.models.textrecog.data_processors.TextRecDataPreprocessor)
-- `preprocessor`: network preprocessor configuration, e.g. TPS
-- `backbone`: backbone configuration
-- `encoder`: encoder configuration
-- `decoder`: decoder configuration
- - `module_loss`: decoder module loss configuration
- - `postprocessor`: decoder postprocessor configuration
- - `dictionary`: dictionary configuration
-
-Using CRNN as an example.
-
-```Python
-# model
-model = dict(
- type='CRNN',
- data_preprocessor=dict(
- type='TextRecogDataPreprocessor', mean=[127], std=[127])
- preprocessor=None,
- backbone=dict(type='VeryDeepVgg', leaky_relu=False, input_channels=1),
- encoder=None,
- decoder=dict(
- type='CRNNDecoder',
- in_channels=512,
- rnn_flag=True,
- module_loss=dict(type='CTCModuleLoss', letter_case='lower'),
- postprocessor=dict(type='CTCPostProcessor'),
- dictionary=dict(
- type='Dictionary',
- dict_file='dicts/lower_english_digits.txt',
- with_padding=True)))
-```
-
-
-
-#### Checkpoint Loading Configuration
-
-The model weights in the checkpoint file can be loaded via the `load_from` parameter, simply by setting the `load_from` parameter to the path of the checkpoint file.
-
-You can also resume training by setting `resume=True` to load the training status information in the checkpoint. When both `load_from` and `resume=True` are set, MMEngine will load the training state from the checkpoint file at the `load_from` path.
-
-If only `resume=True` is set, the executor will try to find and read the latest checkpoint file from the `work_dir` folder
-
-```Python
-load_from = None # Path to load checkpoint
-resume = False # whether resume
-```
-
-More can be found in {external+mmengine:doc}`MMEngine: Load Weights or Recover Training ` and [OCR Advanced Tips - Resume Training from Checkpoints](train_test.md#resume-training-from-a-checkpoint).
-
-
-
-### Evaluation Configuration
-
-In model validation and model testing, quantitative measurement of model accuracy is often required. MMOCR performs this function by means of `Metric` and `Evaluator`. For more information, please refer to {external+mmengine:doc}`MMEngine: Evaluation
` and [Evaluation](../basic_concepts/evaluation.md)
-
-#### Evaluator
-
-Evaluator is mainly used to manage multiple datasets and multiple `Metrics`. For single and multiple dataset cases, there are single and multiple dataset evaluators, both of which can manage multiple `Metrics`.
-
-The single-dataset evaluator is configured as follows.
-
-```Python
-# Single Dataset Single Metric
-val_evaluator = dict(
- type='Evaluator',
- metrics=dict())
-
-# Single Dataset Multiple Metric
-val_evaluator = dict(
- type='Evaluator',
- metrics=[...])
-```
-
-`MultiDatasetsEvaluator` differs from single-dataset evaluation in two aspects: `type` and `dataset_prefixes`. The evaluator type must be `MultiDatasetsEvaluator` and cannot be omitted. The `dataset_prefixes` is mainly used to distinguish the results of different datasets with the same evaluation metrics, see [MultiDatasetsEvaluation](../basic_concepts/evaluation.md).
-
-Assuming that we need to test accuracy on IC13 and IC15 datasets, the configuration is as follows.
-
-```Python
-# Multiple datasets, single Metric
-val_evaluator = dict(
- type='MultiDatasetsEvaluator',
- metrics=dict(),
- dataset_prefixes=['IC13', 'IC15'])
-
-# Multiple datasets, multiple Metrics
-val_evaluator = dict(
- type='MultiDatasetsEvaluator',
- metrics=[...],
- dataset_prefixes=['IC13', 'IC15'])
-```
-
-#### Metric
-
-A metric evaluates a model's performance from a specific perspective. While there is no such common metric that fits all the tasks, MMOCR provides enough flexibility such that multiple metrics serving the same task can be used simultaneously. Here we list task-specific metrics for reference.
-
-Text detection: [`HmeanIOUMetric`](mmocr.evaluation.metrics.HmeanIOUMetric)
-
-Text recognition: [`WordMetric`](mmocr.evaluation.metrics.WordMetric), [`CharMetric`](mmocr.evaluation.metrics.CharMetric), [`OneMinusNEDMetric`](mmocr.evaluation.metrics.OneMinusNEDMetric)
-
-Key information extraction: [`F1Metric`](mmocr.evaluation.metrics.F1Metric)
-
-Text detection as an example, using a single `Metric` in the case of single dataset evaluation.
-
-```Python
-val_evaluator = dict(type='HmeanIOUMetric')
-```
-
-Take text recognition as an example, multiple datasets (`IC13` and `IC15`) are evaluated using multiple `Metric`s (`WordMetric` and `CharMetric`).
-
-```Python
-val_evaluator = dict(
- type='MultiDatasetsEvaluator',
- metrics=[
- dict(
- type='WordMetric',
- mode=['exact', 'ignore_case', 'ignore_case_symbol']),
- dict(type='CharMetric')
- ],
- dataset_prefixes=['IC13', 'IC15'])
-test_evaluator = val_evaluator
-```
-
-
-
-### Visualization Configuration
-
-Each task is bound to a task-specific visualizer. The visualizer is mainly used for visualizing or storing intermediate results of user models and visualizing val and test prediction results. The visualization results can also be stored in different backends such as WandB, TensorBoard, etc. through the corresponding visualization backend. Commonly used modification operations can be found in [visualization](visualization.md).
-
-The default configuration of visualization for text detection is as follows.
-
-```Python
-vis_backends = [dict(type='LocalVisBackend')]
-visualizer = dict(
- type='TextDetLocalVisualizer', # Different visualizers for different tasks
- vis_backends=vis_backends,
- name='visualizer')
-```
-
-## Directory Structure
-
-All configuration files of `MMOCR` are placed under the `configs` folder. To avoid config files from being too long and improve their reusability and clarity, MMOCR takes advantage of the inheritance mechanism and split config files into eight sections. Since each section is closely related to the task type, MMOCR provides a task folder for each task in `configs/`, namely `textdet` (text detection task), `textrecog` (text recognition task), and `kie` (key information extraction). Each folder is further divided into two parts: `_base_` folder and algorithm configuration folders.
-
-1. the `_base_` folder stores some general config files unrelated to specific algorithms, and each section is divided into datasets, training strategies and runtime configurations by directory.
-
-2. The algorithm configuration folder stores config files that are strongly related to the algorithm. The algorithm configuration folder has two kinds of config files.
-
- 1. Config files starting with `_base_`: Configures the model and data pipeline of an algorithm. In OCR domain, data augmentation strategies are generally strongly related to the algorithm, so the model and data pipeline are usually placed in the same config file.
-
- 2. Other config files, i.e. the algorithm-specific configurations on the specific dataset(s): These are the full config files that further configure training and testing settings, aggregating `_base_` configurations that are scattered in different locations. Inside some modifications to the fields in `_base_` configs may be performed, such as data pipeline, training strategy, etc.
-
-All these config files are distributed in different folders according to their contents as follows:
-
-
-
-
-
-The final directory structure is as follows.
-
-```Python
-configs
-โโโ textdet
-โ โโโ _base_
-โ โ โโโ datasets
-โ โ โ โโโ icdar2015.py
-โ โ โ โโโ icdar2017.py
-โ โ โ โโโ totaltext.py
-โ โ โโโ schedules
-โ โ โ โโโ schedule_adam_600e.py
-โ โ โโโ default_runtime.py
-โ โโโ dbnet
-โ โโโ _base_dbnet_resnet18_fpnc.py
-โ โโโ dbnet_resnet18_fpnc_1200e_icdar2015.py
-โโโ textrecog
-โ โโโ _base_
-โ โ โโโ datasets
-โ โ โ โโโ icdar2015.py
-โ โ โ โโโ icdar2017.py
-โ โ โ โโโ totaltext.py
-โ โ โโโ schedules
-โ โ โ โโโ schedule_adam_base.py
-โ โ โโโ default_runtime.py
-โ โโโ crnn
-โ โโโ _base_crnn_mini-vgg.py
-โ โโโ crnn_mini-vgg_5e_mj.py
-โโโ kie
- โโโ _base_
- โ โโโdatasets
- โ โโโ default_runtime.py
- โโโ sgdmr
- โโโ sdmgr_novisual_60e_wildreceipt_openset.py
-```
-
-## Naming Conventions
-
-MMOCR has a convention to name config files, and contributors to the code base need to follow the same naming rules. The file names are divided into four sections: algorithm information, module information, training information, and data information. Words that logically belong to different sections are connected by an underscore `'_'`, and multiple words in the same section are connected by a hyphen `'-'`.
-
-```Python
-{{algorithm info}}_{{module info}}_{{training info}}_{{data info}}.py
-```
-
-- algorithm info: the name of the algorithm, such as dbnet, crnn, etc.
-
-- module info: list some intermediate modules in the order of data flow. Its content depends on the algorithm, and some modules strongly related to the model will be omitted to avoid an overly long name. For example:
-
- - For the text detection task and the key information extraction task :
-
- ```Python
- {{algorithm info}}_{{backbone}}_{{neck}}_{{head}}_{{training info}}_{{data info}}.py
- ```
-
- `{head}` is usually omitted since it's algorithm-specific.
-
- - For text recognition tasks.
-
- ```Python
- {{algorithm info}}_{{backbone}}_{{encoder}}_{{decoder}}_{{training info}}_{{data info}}.py
- ```
-
- Since encoder and decoder are generally bound to the algorithm, they are usually omitted.
-
-- training info: some settings of the training strategy, including batch size, schedule, etc.
-
-- data info: dataset name, modality, input size, etc., such as icdar2015 and synthtext.
diff --git a/pipeline/mmocr/docs/en/user_guides/data_prepare/dataset_preparer.md b/pipeline/mmocr/docs/en/user_guides/data_prepare/dataset_preparer.md
deleted file mode 100644
index 55174cc5894e54db0daf9706ef406fce6f8d14c6..0000000000000000000000000000000000000000
--- a/pipeline/mmocr/docs/en/user_guides/data_prepare/dataset_preparer.md
+++ /dev/null
@@ -1,776 +0,0 @@
-# Dataset Preparer (Beta)
-
-```{note}
-Dataset Preparer is still in beta version and might not be stable enough. You are welcome to try it out and report any issues to us.
-```
-
-## One-click data preparation script
-
-MMOCR provides a unified one-stop data preparation script `prepare_dataset.py`.
-
-Only one line of command is needed to complete the data download, decompression, format conversion, and basic configure generation.
-
-```bash
-python tools/dataset_converters/prepare_dataset.py [-h] [--nproc NPROC] [--task {textdet,textrecog,textspotting,kie}] [--splits SPLITS [SPLITS ...]] [--lmdb] [--overwrite-cfg] [--dataset-zoo-path DATASET_ZOO_PATH] datasets [datasets ...]
-```
-
-| ARGS | Type | Description |
-| ------------------ | ---- | ----------------------------------------------------------------------------------------------------------------------------------------- |
-| dataset_name | str | (required) dataset name. |
-| --nproc | int | Number of processes to be used. Defaults to 4. |
-| --task | str | Convert the dataset to the format of a specified task supported by MMOCR. options are: 'textdet', 'textrecog', 'textspotting', and 'kie'. |
-| --splits | str | Splits of the dataset to be prepared. Multiple splits can be accepted. Defaults to `train val test`. |
-| --lmdb | str | Store the data in LMDB format. Only valid when the task is `textrecog`. |
-| --overwrite-cfg | str | Whether to overwrite the dataset config file if it already exists in `configs/{task}/_base_/datasets`. |
-| --dataset-zoo-path | str | Path to the dataset config file. If not specified, the default path is `./dataset_zoo`. |
-
-For example, the following command shows how to use the script to prepare the ICDAR2015 dataset for text detection task.
-
-```bash
-python tools/dataset_converters/prepare_dataset.py icdar2015 --task textdet --overwrite-cfg
-```
-
-Also, the script supports preparing multiple datasets at the same time. For example, the following command shows how to prepare the ICDAR2015 and TotalText datasets for text recognition task.
-
-```bash
-python tools/dataset_converters/prepare_dataset.py icdar2015 totaltext --task textrecog --overwrite-cfg
-```
-
-To check the supported datasets of Dataset Preparer, please refer to [Dataset Zoo](./datasetzoo.md). Some of other datasets that need to be prepared manually are listed in [Text Detection](./det.md) and [Text Recognition](./recog.md).
-
-For users in China, more datasets can be downloaded from the opensource dataset platform: [OpenDataLab](https://opendatalab.com/). After downloading the data, you can place the files listed in `data_obtainer.save_name` in `data/cache` and rerun the script.
-
-## Advanced Usage
-
-### LMDB Format
-
-In text recognition tasks, we usually use LMDB format to store data to speed up data loading. When using the `prepare_dataset.py` script to prepare data, you can store data to the LMDB format by the `--lmdb` parameter. For example:
-
-```bash
-python tools/dataset_converters/prepare_dataset.py icdar2015 --task textrecog --lmdb
-```
-
-As soon as the dataset is prepared, Dataset Preparer will generate `icdar2015_lmdb.py` in the `configs/textrecog/_base_/datasets/` directory. You can inherit this file and point the `dataloader` to the LMDB dataset. Moreover, the LMDB dataset needs to be loaded by [`LoadImageFromNDArray`](mmocr.datasets.transforms.LoadImageFromNDArray), thus you also need to modify `pipeline`.
-
-For example, if we want to change the training set of `configs/textrecog/crnn/crnn_mini-vgg_5e_mj.py` to icdar2015 generated before, we need to perform the following modifications:
-
-1. Modify `configs/textrecog/crnn/crnn_mini-vgg_5e_mj.py`:
-
- ```python
- _base_ = [
- '../_base_/datasets/icdar2015_lmdb.py', # point to icdar2015 lmdb dataset
- ...
- ]
-
- train_list = [_base_.icdar2015_lmdb_textrecog_train]
- ...
- ```
-
-2. Modify `train_pipeline` in `configs/textrecog/crnn/_base_crnn_mini-vgg.py`, change `LoadImageFromFile` to `LoadImageFromNDArray`:
-
- ```python
- train_pipeline = [
- dict(
- type='LoadImageFromNDArray',
- color_type='grayscale',
- file_client_args=file_client_args,
- ignore_empty=True,
- min_size=2),
- ...
- ]
- ```
-
-## Design
-
-There are many OCR datasets with different languages, annotation formats, and scenarios. There are generally two ways to use these datasets: to quickly understand the relevant information about the dataset, or to use it to train models. To meet these two usage scenarios, MMOCR provides dataset automatic preparation scripts. The dataset automatic preparation script uses modular design, which greatly enhances scalability, and allows users to easily configure other public or private datasets. The configuration files for the dataset automatic preparation script are uniformly stored in the `dataset_zoo/` directory. Users can find all the configuration files for the dataset preparation scripts officially supported by MMOCR in this directory. The directory structure of this folder is as follows:
-
-```text
-dataset_zoo/
-โโโ icdar2015
-โ โโโ metafile.yml
-โ โโโ sample_anno.md
-โ โโโ textdet.py
-โ โโโ textrecog.py
-โ โโโ textspotting.py
-โโโ wildreceipt
- โโโ metafile.yml
- โโโ sample_anno.md
- โโโ kie.py
- โโโ textdet.py
- โโโ textrecog.py
- โโโ textspotting.py
-```
-
-### Dataset-related Information
-
-The relevant information of a dataset includes the annotation format, annotation examples, and basic statistical information of the dataset. Although this information can be found on the official website of each dataset, it is scattered across various websites, and users need to spend a lot of time to discover the basic information of the dataset. Therefore, MMOCR has designed some paradigms to help users quickly understand the basic information of the dataset. MMOCR divides the relevant information of the dataset into two parts. One part is the basic information of the dataset, including the year of publication, the authors of the paper, and copyright information, etc. The other part is the annotation information of the dataset, including the annotation format and annotation examples. MMOCR provides a paradigm for each part, and contributors can fill in the basic information of the dataset according to the paradigm. This way, users can quickly understand the basic information of the dataset. Based on the basic information of the dataset, MMOCR provides a `metafile.yml` file, which contains the basic information of the corresponding dataset, including the year of publication, the authors of the paper, and copyright information, etc. In this way, users can quickly understand the basic information of the dataset. This file is not mandatory during the dataset preparation process (so users can ignore it when adding their own private datasets), but to better understand the information of various public datasets, MMOCR recommends that users read the corresponding metafile information before using the dataset preparation script to understand whether the characteristics of the dataset meet the user's needs. MMOCR uses ICDAR2015 as an example, and its sample content is shown below:
-
-```yaml
-Name: 'Incidental Scene Text IC15'
-Paper:
- Title: ICDAR 2015 Competition on Robust Reading
- URL: https://rrc.cvc.uab.es/files/short_rrc_2015.pdf
- Venue: ICDAR
- Year: '2015'
- BibTeX: '@inproceedings{karatzas2015icdar,
- title={ICDAR 2015 competition on robust reading},
- author={Karatzas, Dimosthenis and Gomez-Bigorda, Lluis and Nicolaou, Anguelos and Ghosh, Suman and Bagdanov, Andrew and Iwamura, Masakazu and Matas, Jiri and Neumann, Lukas and Chandrasekhar, Vijay Ramaseshan and Lu, Shijian and others},
- booktitle={2015 13th international conference on document analysis and recognition (ICDAR)},
- pages={1156--1160},
- year={2015},
- organization={IEEE}}'
-Data:
- Website: https://rrc.cvc.uab.es/?ch=4
- Language:
- - English
- Scene:
- - Natural Scene
- Granularity:
- - Word
- Tasks:
- - textdet
- - textrecog
- - textspotting
- License:
- Type: CC BY 4.0
- Link: https://creativecommons.org/licenses/by/4.0/
-```
-
-Specifically, MMOCR lists the meaning of each field in the following table:
-
-| Field Name | Meaning |
-| :--------------- | :------------------------------------------------------------------------------------------------------- |
-| Name | The name of the dataset |
-| Paper.Title | The title of the paper for the dataset |
-| Paper.URL | The URL of the paper for the dataset |
-| Paper.Venue | The venue of the paper for the dataset |
-| Paper.Year | The year of publication for the paper |
-| Paper.BibTeX | The BibTeX citation of the paper for the dataset |
-| Data.Website | The official website of the dataset |
-| Data.Language | The supported languages of the dataset |
-| Data.Scene | The supported scenes of the dataset, such as `Natural Scene`, `Document`, `Handwritten`, etc. |
-| Data.Granularity | The supported granularities of the dataset, such as `Character`, `Word`, `Line`, etc. |
-| Data.Tasks | The supported tasks of the dataset, such as `textdet`, `textrecog`, `textspotting`, `kie`, etc. |
-| Data.License | License information for the dataset. Use `N/A` if no license exists. |
-| Data.Format | File format of the annotation files, such as `.txt`, `.xml`, `.json`, etc. |
-| Data.Keywords | Keywords describing the characteristics of the dataset, such as `Horizontal`, `Vertical`, `Curved`, etc. |
-
-For the annotation information of the dataset, MMOCR provides a `sample_anno.md` file, which users can use as a template to fill in the annotation information of the dataset, so that users can quickly understand the annotation information of the dataset. MMOCR uses ICDAR2015 as an example, and the sample content is as follows:
-
-````markdown
- **Text Detection**
-
- ```text
- # x1,y1,x2,y2,x3,y3,x4,y4,trans
-
- 377,117,463,117,465,130,378,130,Genaxis Theatre
- 493,115,519,115,519,131,493,131,[06]
- 374,155,409,155,409,170,374,170,###
-````
-
-`sample_anno.md` provides annotation information for different tasks of the dataset, including the format of the annotation files (text corresponds to `txt` files, and the format of the annotation files can also be found in `meta.yml`), and examples of the annotations.
-
-With the information in these two files, users can quickly understand the basic information of the dataset. Additionally, MMOCR has summarized the basic information of all datasets, and users can view the basic information of all datasets in the [Overview](.overview.md).
-
-### Dataset Usage
-
-After decades of development, the OCR field has seen a series of related datasets emerge, often providing text annotation files in various styles, making it necessary for users to perform format conversion when using these datasets. Therefore, to facilitate dataset preparation for users, we have designed the Dataset Preparer to help users quickly prepare datasets in the format supported by MMOCR. For details, please refer to the [Dataset Format](../../basic_concepts/datasets.md) document. The following figure shows a typical workflow for running the Dataset Preparer.
-
-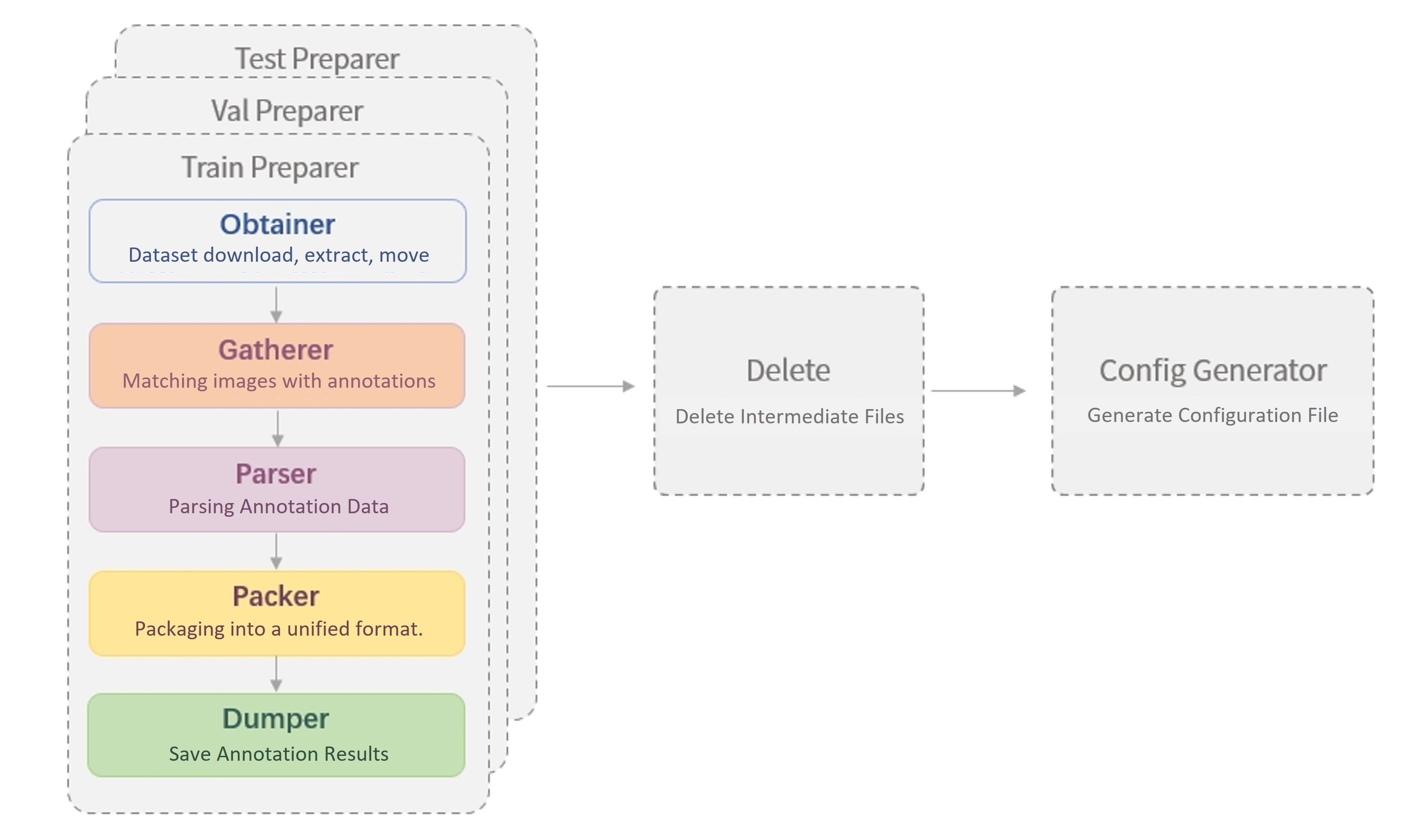
-
-The figure shows that when running the Dataset Preparer, the following operations will be performed in sequence:
-
-1. For the training set, validation set, and test set, the preparers will perform:
- 1. [Dataset download, extraction, and movement (Obtainer)](#Dataset-download-extraction-and-movement-obtainer)
- 2. [Matching annotations with images (Gatherer)](#dataset-collection-gatherer)
- 3. [Parsing original annotations (Parser)](#dataset-parsing-parser)
- 4. [Packing annotations into a unified format (Packer)](#dataset-conversion-packer)
- 5. [Saving annotations (Dumper)](#annotation-saving-dumper)
-2. Delete files (Delete)
-3. Generate the configuration file for the data set (Config Generator).
-
-To handle various types of datasets, MMOCR has designed each component as a plug-and-play module, and allows users to configure the dataset preparation process through configuration files located in `dataset_zoo/`. These configuration files are in Python format and can be used in the same way as other configuration files in MMOCR, as described in the [Configuration File documentation](../config.md).
-
-In `dataset_zoo/`, each dataset has its own folder, and the configuration files are named after the task to distinguish different configurations under different tasks. Taking the text detection part of ICDAR2015 as an example, the sample configuration file `dataset_zoo/icdar2015/textdet.py` is shown below:
-
-```python
-data_root = 'data/icdar2015'
-cache_path = 'data/cache'
-train_preparer = dict(
- obtainer=dict(
- type='NaiveDataObtainer',
- cache_path=cache_path,
- files=[
- dict(
- url='https://rrc.cvc.uab.es/downloads/ch4_training_images.zip',
- save_name='ic15_textdet_train_img.zip',
- md5='c51cbace155dcc4d98c8dd19d378f30d',
- content=['image'],
- mapping=[['ic15_textdet_train_img', 'textdet_imgs/train']]),
- dict(
- url='https://rrc.cvc.uab.es/downloads/'
- 'ch4_training_localization_transcription_gt.zip',
- save_name='ic15_textdet_train_gt.zip',
- md5='3bfaf1988960909014f7987d2343060b',
- content=['annotation'],
- mapping=[['ic15_textdet_train_gt', 'annotations/train']]),
- ]),
- gatherer=dict(
- type='PairGatherer',
- img_suffixes=['.jpg', '.JPG'],
- rule=[r'img_(\d+)\.([jJ][pP][gG])', r'gt_img_\1.txt']),
- parser=dict(type='ICDARTxtTextDetAnnParser', encoding='utf-8-sig'),
- packer=dict(type='TextDetPacker'),
- dumper=dict(type='JsonDumper'),
-)
-
-test_preparer = dict(
- obtainer=dict(
- type='NaiveDataObtainer',
- cache_path=cache_path,
- files=[
- dict(
- url='https://rrc.cvc.uab.es/downloads/ch4_test_images.zip',
- save_name='ic15_textdet_test_img.zip',
- md5='97e4c1ddcf074ffcc75feff2b63c35dd',
- content=['image'],
- mapping=[['ic15_textdet_test_img', 'textdet_imgs/test']]),
- dict(
- url='https://rrc.cvc.uab.es/downloads/'
- 'Challenge4_Test_Task4_GT.zip',
- save_name='ic15_textdet_test_gt.zip',
- md5='8bce173b06d164b98c357b0eb96ef430',
- content=['annotation'],
- mapping=[['ic15_textdet_test_gt', 'annotations/test']]),
- ]),
- gatherer=dict(
- type='PairGatherer',
- img_suffixes=['.jpg', '.JPG'],
- rule=[r'img_(\d+)\.([jJ][pP][gG])', r'gt_img_\1.txt']),
- parser=dict(type='ICDARTxtTextDetAnnParser', encoding='utf-8-sig'),
- packer=dict(type='TextDetPacker'),
- dumper=dict(type='JsonDumper'),
-)
-
-delete = ['annotations', 'ic15_textdet_test_img', 'ic15_textdet_train_img']
-config_generator = dict(type='TextDetConfigGenerator')
-```
-
-#### Dataset download extraction and movement (Obtainer)
-
-The `obtainer` module in Dataset Preparer is responsible for downloading, extracting, and moving the dataset. Currently, MMOCR only provides the `NaiveDataObtainer`. Generally speaking, the built-in `NaiveDataObtainer` is sufficient for downloading most datasets that can be accessed through direct links, and supports operations such as extraction, moving files, and renaming. However, MMOCR currently does not support automatically downloading datasets stored in resources that require login, such as Baidu or Google Drive. Here is a brief introduction to the `NaiveDataObtainer`.
-
-| Field Name | Meaning |
-| ---------- | -------------------------------------------------------------------------------------------- |
-| cache_path | Dataset cache path, used to store the compressed files downloaded during dataset preparation |
-| data_root | Root directory where the dataset is stored |
-| files | Dataset file list, used to describe the download information of the dataset |
-
-The `files` field is a list, and each element in the list is a dictionary used to describe the download information of a dataset file. The table below shows the meaning of each field:
-
-| Field Name | Meaning |
-| ------------------ | ------------------------------------------------------------------------------------------------------------------------------------------ |
-| url | Download link for the dataset file |
-| save_name | Name used to save the dataset file |
-| md5 (optional) | MD5 hash of the dataset file, used to check if the downloaded file is complete |
-| split (optional) | Dataset split the file belongs to, such as `train`, `test`, etc., this field can be omitted |
-| content (optional) | Content of the dataset file, such as `image`, `annotation`, etc., this field can be omitted |
-| mapping (optional) | Decompression mapping of the dataset file, used to specify the storage location of the file after decompression, this field can be omitted |
-
-The Dataset Preparer follows the following conventions:
-
-- Images of different types of datasets are moved to the corresponding category `{taskname}_imgs/{split}/` folder, such as `textdet_imgs/train/`.
-- For a annotation file containing annotation information for all images, the annotations are moved to `annotations/{split}.*` file, such as `annotations/train.json`.
-- For a annotation file containing annotation information for one image, all annotation files are moved to `annotations/{split}/` folder, such as `annotations/train/`.
-- For some other special cases, such as all training, testing, and validation images are in one folder, the images can be moved to a self-set folder, such as `{taskname}_imgs/imgs/`, and the image storage location should be specified in the subsequent `gatherer` module.
-
-An example configuration is as follows:
-
-```python
- obtainer=dict(
- type='NaiveDataObtainer',
- cache_path=cache_path,
- files=[
- dict(
- url='https://rrc.cvc.uab.es/downloads/ch4_training_images.zip',
- save_name='ic15_textdet_train_img.zip',
- md5='c51cbace155dcc4d98c8dd19d378f30d',
- content=['image'],
- mapping=[['ic15_textdet_train_img', 'textdet_imgs/train']]),
- dict(
- url='https://rrc.cvc.uab.es/downloads/'
- 'ch4_training_localization_transcription_gt.zip',
- save_name='ic15_textdet_train_gt.zip',
- md5='3bfaf1988960909014f7987d2343060b',
- content=['annotation'],
- mapping=[['ic15_textdet_train_gt', 'annotations/train']]),
- ]),
-```
-
-#### Dataset collection (Gatherer)
-
-The `gatherer` module traverses the files in the dataset directory, matches image files with their corresponding annotation files, and organizes a file list for the `parser` module to read. Therefore, it is necessary to know the matching rules between image files and annotation files in the current dataset. There are two commonly used annotation storage formats for OCR datasets: one is multiple annotation files corresponding to multiple images, and the other is a single annotation file corresponding to multiple images, for example:
-
-```text
-Many-to-Many
-โโโ {taskname}_imgs/{split}/img_img_1.jpg
-โโโ annotations/{split}/gt_img_1.txt
-โโโ {taskname}_imgs/{split}/img_2.jpg
-โโโ annotations/{split}/gt_img_2.txt
-โโโ {taskname}_imgs/{split}/img_3.JPG
-โโโ annotations/{split}/gt_img_3.txt
-
-One-to-Many
-โโโ {taskname}/{split}/img_1.jpg
-โโโ {taskname}/{split}/img_2.jpg
-โโโ {taskname}/{split}/img_3.JPG
-โโโ annotations/gt.txt
-```
-
-Specific design is as follows:
-
-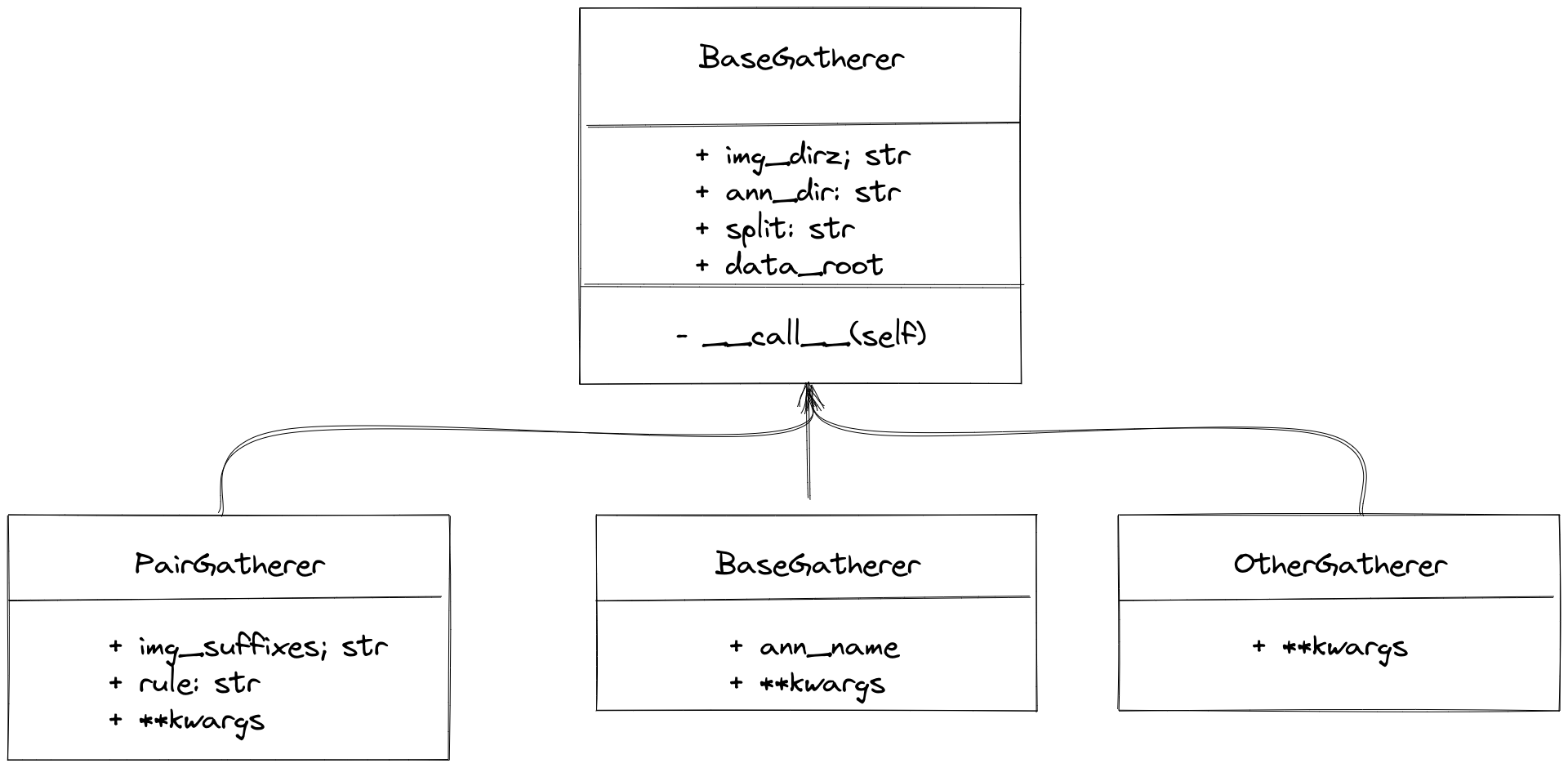
-
-MMOCR has built-in `PairGatherer` and `MonoGatherer` to handle the two common cases mentioned above. `PairGatherer` is used for many-to-many situations, while `MonoGatherer` is used for one-to-many situations.
-
-```{note}
-To simplify processing, the gatherer assumes that the dataset's images and annotations are stored separately in `{taskname}_imgs/{split}/` and `annotations/`, respectively. In particular, for many-to-many situations, the annotation file needs to be placed in `annotations/{split}`.
-```
-
-- In the many-to-many case, `PairGatherer` needs to find the image files and corresponding annotation files according to a certain naming convention. First, the suffix of the image needs to be specified by the `img_suffixes` parameter, as in the example above `img_suffixes=[.jpg,.JPG]`. In addition, a pair of [regular expressions](https://docs.python.org/3/library/re.html) `rule` is used to specify the correspondence between the image and annotation files. For example, `rule=[r'img_(\d+)\.([jJ][pP][gG])'๏ผr'gt_img_\1.txt']`. The first regular expression is used to match the image file name, `\d+` is used to match the image sequence number, and `([jJ][pP][gG])` is used to match the image suffix. The second regular expression is used to match the annotation file name, where `\1` associates the matched image sequence number with the annotation file sequence number. An example configuration is:
-
-```python
- gatherer=dict(
- type='PairGatherer',
- img_suffixes=['.jpg', '.JPG'],
- rule=[r'img_(\d+)\.([jJ][pP][gG])', r'gt_img_\1.txt']),
-```
-
-For the case of one-to-many, it is usually simple, and the user only needs to specify the annotation file name. For example, for the training set configuration:
-
-```python
- gatherer=dict(type='MonoGatherer', ann_name='train.txt'),
-```
-
-MMOCR has also made conventions on the return value of `Gatherer`. `Gatherer` returns a tuple with two elements. The first element is a list of image paths (including all image paths) or the folder containing all images. The second element is a list of annotation file paths (including all annotation file paths) or the path of the annotation file (the annotation file contains all image annotation information). Specifically, the return value of `PairGatherer` is (list of image paths, list of annotation file paths), as shown below:
-
-```python
- (['{taskname}_imgs/{split}/img_1.jpg', '{taskname}_imgs/{split}/img_2.jpg', '{taskname}_imgs/{split}/img_3.JPG'],
- ['annotations/{split}/gt_img_1.txt', 'annotations/{split}/gt_img_2.txt', 'annotations/{split}/gt_img_3.txt'])
-```
-
-`MonoGatherer` returns a tuple containing the path to the image directory and the path to the annotation file, as follows:
-
-```python
- ('{taskname}/{split}', 'annotations/gt.txt')
-```
-
-#### Dataset parsing (Parser)
-
-`Parser` is mainly used to parse the original annotation files. Since the original annotation formats vary greatly, MMOCR provides `BaseParser` as a base class, which users can inherit to implement their own `Parser`. In `BaseParser`, MMOCR has designed two interfaces: `parse_files` and `parse_file`, where the annotation parsing is conventionally carried out. For the two different input situations of `Gatherer` (many-to-many, one-to-many), the implementations of these two interfaces should be different.
-
-- `BaseParser` by default handles the many-to-many situation. Among them, `parse_files` distributes the data in parallel to multiple `parse_file` processes, and each `parse_file` parses the annotation of a single image separately.
-- For the one-to-many situation, the user needs to override `parse_files` to implement loading the annotation and returning standardized results.
-
-The interface of `BaseParser` is defined as follows:
-
-```python
-class BaseParser:
- def __call__(self, img_paths, ann_paths):
- return self.parse_files(img_paths, ann_paths)
-
- def parse_files(self, img_paths: Union[List[str], str],
- ann_paths: Union[List[str], str]) -> List[Tuple]:
- samples = track_parallel_progress_multi_args(
- self.parse_file, (img_paths, ann_paths), nproc=self.nproc)
- return samples
-
- @abstractmethod
- def parse_file(self, img_path: str, ann_path: str) -> Tuple:
-
- raise NotImplementedError
-```
-
-In order to ensure the uniformity of subsequent modules, MMOCR has made conventions for the return values of `parse_files` and `parse_file`. The return value of `parse_file` is a tuple, the first element of which is the image path, and the second element is the annotation information. The annotation information is a list, each element of which is a dictionary with the fields `poly`, `text`, and `ignore`, as shown below:
-
-```python
-# An example of returned values:
-(
- 'imgs/train/xxx.jpg',
- [
- dict(
- poly=[0, 1, 1, 1, 1, 0, 0, 0],
- text='hello',
- ignore=False),
- ...
- ]
-)
-```
-
-The output of `parse_files` is a list, and each element in the list is the return value of `parse_file`. An example is:
-
-```python
-[
- (
- 'imgs/train/xxx.jpg',
- [
- dict(
- poly=[0, 1, 1, 1, 1, 0, 0, 0],
- text='hello',
- ignore=False),
- ...
- ]
- ),
- ...
-]
-```
-
-#### Dataset Conversion (Packer)
-
-`Packer` is mainly used to convert data into a unified annotation format, because the input data is the output of parsers and the format has been fixed. Therefore, the packer only needs to convert the input format into a unified annotation format for each task. Currently, MMOCR supports tasks such as text detection, text recognition, end-to-end OCR, and key information extraction, and MMOCR has a corresponding packer for each task, as shown below:
-
-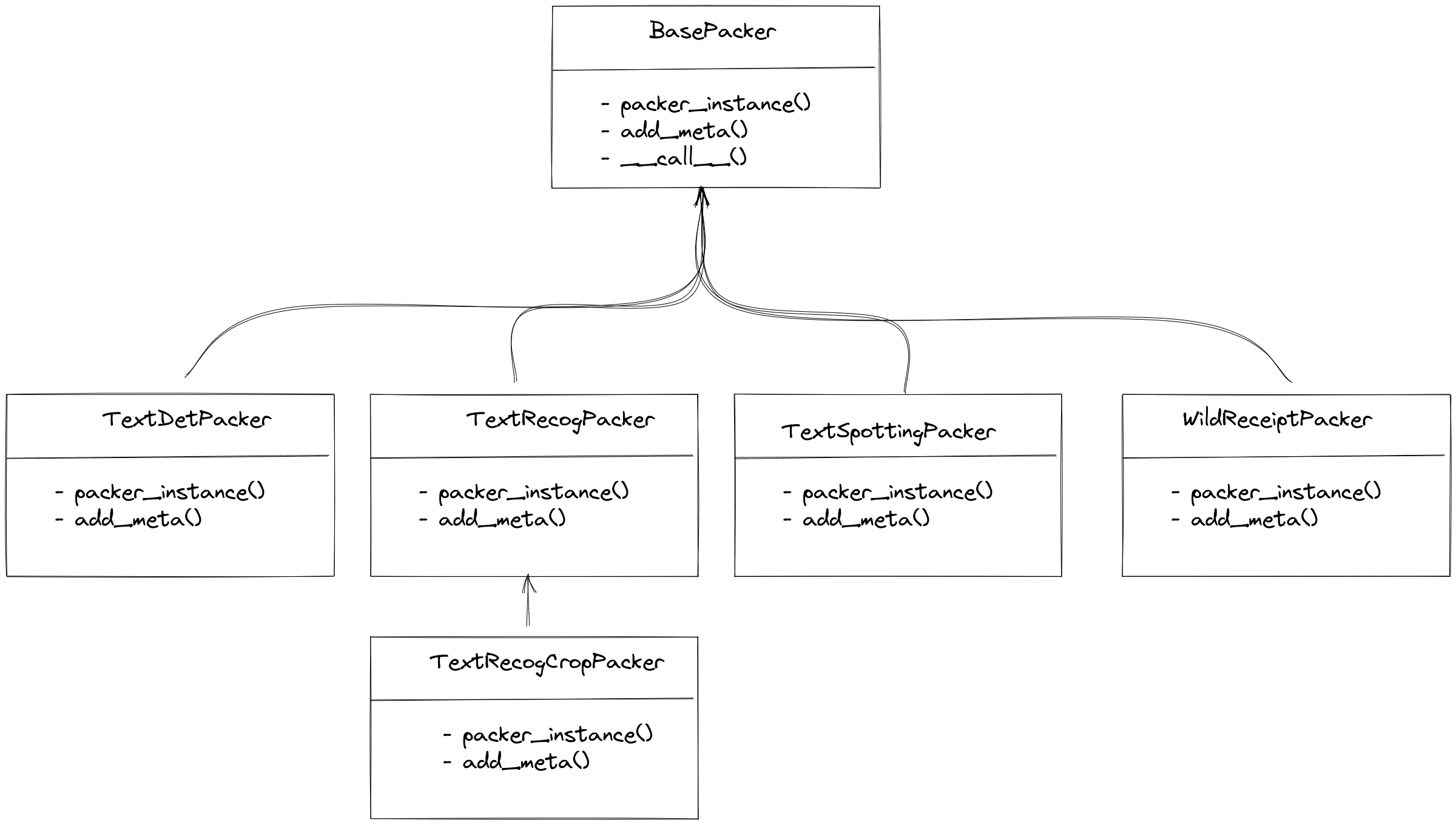
-
-For text detection, end-to-end OCR, and key information extraction, MMOCR has a unique corresponding `Packer`. However, for text recognition, MMOCR provides two `Packer` options: `TextRecogPacker` and `TextRecogCropPacker`, due to the existence of two types of datasets:
-
-- Each image is a recognition sample, and the annotation information returned by the `parser` is only a `dict(text='xxx')`. In this case, `TextRecogPacker` can be used.
-- The dataset does not crop text from the image, and it essentially contains end-to-end OCR annotations that include the position information of the text and the corresponding text information. `TextRecogCropPacker` will crop the text from the image and then convert it into the unified format for text recognition.
-
-#### Annotation Saving (Dumper)
-
-The `dumper` module is used to determine what format the data should be saved in. Currently, MMOCR supports `JsonDumper`, `WildreceiptOpensetDumper`, and `TextRecogLMDBDumper`. They are used to save data in the standard MMOCR JSON format, the Wildreceipt format, and the LMDB format commonly used in the academic community for text recognition, respectively.
-
-#### Delete files (Delete)
-
-When processing a dataset, temporary files that are not needed may be generated. Here, a list of such files or folders can be passed in, which will be deleted when the conversion is finished.
-
-#### Generate the configuration file for the dataset (ConfigGenerator)
-
-In order to automatically generate basic configuration files after preparing the dataset, MMOCR has implemented `TextDetConfigGenerator`, `TextRecogConfigGenerator`, and `TextSpottingConfigGenerator` for each task. The main parameters supported by these generators are as follows:
-
-| Field Name | Meaning |
-| ----------- | --------------------------------------------------------------------------------------------------------------------------------------------------------------------------------------------- |
-| data_root | Root directory where the dataset is stored. |
-| train_anns | Path to the training set annotations in the configuration file. If not specified, it defaults to `[dict(ann_file='{taskname}_train.json', dataset_postfix='']`. |
-| val_anns | Path to the validation set annotations in the configuration file. If not specified, it defaults to an empty string. |
-| test_anns | Path to the test set annotations in the configuration file. If not specified, it defaults to `[dict(ann_file='{taskname}_test.json', dataset_postfix='']`. |
-| config_path | Path to the directory where the configuration files for the algorithm are stored. The configuration generator will write the default configuration to `{config_path}/{taskname}/_base_/datasets/{dataset_name}.py`. If not specified, it defaults to `configs/`. |
-
-After preparing all the files for the dataset, the configuration generator will automatically generate the basic configuration files required to call the dataset. Below is a minimal example of a `TextDetConfigGenerator` configuration:
-
-```python
-config_generator = dict(type='TextDetConfigGenerator')
-```
-
-The generated file will be placed by default under `configs/{task}/_base_/datasets/`. In this example, the basic configuration file for the ICDAR 2015 dataset will be generated at `configs/textdet/_base_/datasets/icdar2015.py`.
-
-```python
-icdar2015_textdet_data_root = 'data/icdar2015'
-
-icdar2015_textdet_train = dict(
- type='OCRDataset',
- data_root=icdar2015_textdet_data_root,
- ann_file='textdet_train.json',
- filter_cfg=dict(filter_empty_gt=True, min_size=32),
- pipeline=None)
-
-icdar2015_textdet_test = dict(
- type='OCRDataset',
- data_root=icdar2015_textdet_data_root,
- ann_file='textdet_test.json',
- test_mode=True,
- pipeline=None)
-```
-
-If the dataset is special and there are several variants of the annotations, the configuration generator also supports generating variables pointing to each variant in the base configuration. However, this requires users to differentiate them by using different `dataset_postfix` when setting up. For example, the ICDAR 2015 text recognition dataset has two annotation versions for the test set, the original version and the 1811 version, which can be specified in `test_anns` as follows:
-
-```python
-config_generator = dict(
- type='TextRecogConfigGenerator',
- test_anns=[
- dict(ann_file='textrecog_test.json'),
- dict(dataset_postfix='857', ann_file='textrecog_test_857.json')
- ])
-```
-
-The configuration generator will generate the following configurations:
-
-```python
-icdar2015_textrecog_data_root = 'data/icdar2015'
-
-icdar2015_textrecog_train = dict(
- type='OCRDataset',
- data_root=icdar2015_textrecog_data_root,
- ann_file='textrecog_train.json',
- pipeline=None)
-
-icdar2015_textrecog_test = dict(
- type='OCRDataset',
- data_root=icdar2015_textrecog_data_root,
- ann_file='textrecog_test.json',
- test_mode=True,
- pipeline=None)
-
-icdar2015_1811_textrecog_test = dict(
- type='OCRDataset',
- data_root=icdar2015_textrecog_data_root,
- ann_file='textrecog_test_1811.json',
- test_mode=True,
- pipeline=None)
-```
-
-With this file, MMOCR can directly import this dataset into the `dataloader` from the model configuration file (the following sample is excerpted from [`configs/textdet/dbnet/dbnet_resnet18_fpnc_1200e_icdar2015.py`](/configs/textdet/dbnet/dbnet_resnet18_fpnc_1200e_icdar2015.py)):
-
-```python
-_base_ = [
- '../_base_/datasets/icdar2015.py',
- # ...
-]
-
-# dataset settings
-icdar2015_textdet_train = _base_.icdar2015_textdet_train
-icdar2015_textdet_test = _base_.icdar2015_textdet_test
-# ...
-
-train_dataloader = dict(
- dataset=icdar2015_textdet_train)
-
-val_dataloader = dict(
- dataset=icdar2015_textdet_test)
-
-test_dataloader = val_dataloader
-```
-
-```{note}
-By default, the configuration generator does not overwrite existing base configuration files unless the user manually specifies `overwrite-cfg` when running the script.
-```
-
-## Adding a new dataset to Dataset Preparer
-
-### Adding Public Datasets
-
-MMOCR has already supported many [commonly used public datasets](./datasetzoo.md). If the dataset you want to use has not been supported yet and you are willing to [contribute to the MMOCR](../../notes/contribution_guide.md) open-source community, you can follow the steps below to add a new dataset.
-
-In the following example, we will show you how to add the **ICDAR2013** dataset step by step.
-
-#### Adding `metafile.yml`
-
-First, make sure that the dataset you want to add does not already exist in `dataset_zoo/`. Then, create a new folder named after the dataset you want to add, such as `icdar2013/` (usually, use lowercase alphanumeric characters without symbols to name the dataset). In the `icdar2013/` folder, create a `metafile.yml` file and fill in the basic information of the dataset according to the following template:
-
-```yaml
-Name: 'Incidental Scene Text IC13'
-Paper:
- Title: ICDAR 2013 Robust Reading Competition
- URL: https://www.imlab.jp/publication_data/1352/icdar_competition_report.pdf
- Venue: ICDAR
- Year: '2013'
- BibTeX: '@inproceedings{karatzas2013icdar,
- title={ICDAR 2013 robust reading competition},
- author={Karatzas, Dimosthenis and Shafait, Faisal and Uchida, Seiichi and Iwamura, Masakazu and i Bigorda, Lluis Gomez and Mestre, Sergi Robles and Mas, Joan and Mota, David Fernandez and Almazan, Jon Almazan and De Las Heras, Lluis Pere},
- booktitle={2013 12th international conference on document analysis and recognition},
- pages={1484--1493},
- year={2013},
- organization={IEEE}}'
-Data:
- Website: https://rrc.cvc.uab.es/?ch=2
- Language:
- - English
- Scene:
- - Natural Scene
- Granularity:
- - Word
- Tasks:
- - textdet
- - textrecog
- - textspotting
- License:
- Type: N/A
- Link: N/A
- Format: .txt
- Keywords:
- - Horizontal
-```
-
-#### Add Annotation Examples
-
-Finally, you can add an annotation example file `sample_anno.md` under the `dataset_zoo/icdar2013/` directory to help the documentation script add annotation examples when generating documentation. The annotation example file is a Markdown file that typically contains the raw data format of a single sample. For example, the following code block shows a sample data file for the ICDAR2013 dataset:
-
-````markdown
- **Text Detection**
-
- ```text
- # train split
- # x1 y1 x2 y2 "transcript"
-
- 158 128 411 181 "Footpath"
- 443 128 501 169 "To"
- 64 200 363 243 "Colchester"
-
- # test split
- # x1, y1, x2, y2, "transcript"
-
- 38, 43, 920, 215, "Tiredness"
- 275, 264, 665, 450, "kills"
- 0, 699, 77, 830, "A"
-````
-
-#### Add configuration files for corresponding tasks
-
-In the `dataset_zoo/icdar2013` directory, add a `.py` configuration file named after the task. For example, `textdet.py`, `textrecog.py`, `textspotting.py`, `kie.py`, etc. The configuration template is shown below:
-
-```python
-data_root = ''
-data_cache = 'data/cache'
-train_prepare = dict(
- obtainer=dict(
- type='NaiveObtainer',
- data_cache=data_cache,
- files=[
- dict(
- url='xx',
- md5='',
- save_name='xxx',
- mapping=list())
- ]),
- gatherer=dict(type='xxxGatherer', **kwargs),
- parser=dict(type='xxxParser', **kwargs),
- packer=dict(type='TextxxxPacker'), # Packer for the task
- dumper=dict(type='JsonDumper'),
-)
-test_prepare = dict(
- obtainer=dict(
- type='NaiveObtainer',
- data_cache=data_cache,
- files=[
- dict(
- url='xx',
- md5='',
- save_name='xxx',
- mapping=list())
- ]),
- gatherer=dict(type='xxxGatherer', **kwargs),
- parser=dict(type='xxxParser', **kwargs),
- packer=dict(type='TextxxxPacker'), # Packer for the task
- dumper=dict(type='JsonDumper'),
-)
-```
-
-Taking the file detection task as an example, let's introduce the specific content of the configuration file. In general, users do not need to implement new `obtainer`, `gatherer`, `packer`, or `dumper`, but usually need to implement a new `parser` according to the annotation format of the dataset.
-
-Regarding the configuration of `obtainer`, we will not go into detail here, and you can refer to [Data set download, extraction, and movement (Obtainer)](#Dataset-download-extraction-and-movement-obtainer).
-
-For the `gatherer`, by observing the obtained ICDAR2013 dataset files, we found that each image has a corresponding `.txt` format annotation file:
-
-```text
-data_root
-โโโ textdet_imgs/train/
-โ โโโ img_1.jpg
-โ โโโ img_2.jpg
-โ โโโ ...
-โโโ annotations/train/
-โ โโโ gt_img_1.txt
-โ โโโ gt_img_2.txt
-โ โโโ ...
-```
-
-Moreover, the name of each annotation file corresponds to the image: `gt_img_1.txt` corresponds to `img_1.jpg`, and so on. Therefore, `PairGatherer` can be used to match them.
-
-```python
-gatherer=dict(
- type='PairGatherer',
- img_suffixes=['.jpg'],
- rule=[r'(\w+)\.jpg', r'gt_\1.txt'])
-```
-
-The first regular expression in the rule is used to match the image file name, and the second regular expression is used to match the annotation file name. Here, `(\w+)` is used to match the image file name, and `gt_\1.txt` is used to match the annotation file name, where `\1` represents the content matched by the first regular expression. That is, it replaces `img_xx.jpg` with `gt_img_xx.txt`.
-
-Next, you need to implement a `parser` to parse the original annotation files into a standard format. Usually, before adding a new dataset, users can browse the [details page](./datasetzoo.md) of the supported datasets and check if there is a dataset with the same format. If there is, you can use the parser of that dataset directly. Otherwise, you need to implement a new format parser.
-
-Data format parsers are stored in the `mmocr/datasets/preparers/parsers` directory. All parsers need to inherit from `BaseParser` and implement the `parse_file` or `parse_files` method. For more information, please refer to [Parsing original annotations (Parser)](#dataset-parsing-parser).
-
-By observing the annotation files of the ICDAR2013 dataset:
-
-```text
-158 128 411 181 "Footpath"
-443 128 501 169 "To"
-64 200 363 243 "Colchester"
-542, 710, 938, 841, "break"
-87, 884, 457, 1021, "could"
-517, 919, 831, 1024, "save"
-```
-
-We found that the built-in `ICDARTxtTextDetAnnParser` already meets the requirements, so we can directly use this parser and configure it in the `preparer`.
-
-```python
-parser=dict(
- type='ICDARTxtTextDetAnnParser',
- remove_strs=[',', '"'],
- encoding='utf-8',
- format='x1 y1 x2 y2 trans',
- separator=' ',
- mode='xyxy')
-```
-
-In the configuration for the `ICDARTxtTextDetAnnParser`, `remove_strs=[',', '"']` is specified to remove extra quotes and commas in the annotation files. In the `format` section, `x1 y1 x2 y2 trans` indicates that each line in the annotation file contains four coordinates and a text content separated by spaces (`separator`=' '). Also, `mode` is set to `xyxy`, which means that the coordinates in the annotation file are the coordinates of the top-left and bottom-right corners, so that `ICDARTxtTextDetAnnParser` can parse the annotations into a unified format.
-
-For the `packer`, taking the file detection task as an example, its `packer` is `TextDetPacker`, and its configuration is as follows:
-
-```python
-packer=dict(type='TextDetPacker')
-```
-
-Finally, specify the `dumper`, which is generally saved in json format. Its configuration is as follows:
-
-```python
-dumper=dict(type='JsonDumper')
-```
-
-After the above configuration, the configuration file for the ICDAR2013 training set is as follows:
-
-```python
-train_preparer = dict(
- obtainer=dict(
- type='NaiveDataObtainer',
- cache_path=cache_path,
- files=[
- dict(
- url='https://rrc.cvc.uab.es/downloads/'
- 'Challenge2_Training_Task12_Images.zip',
- save_name='ic13_textdet_train_img.zip',
- md5='a443b9649fda4229c9bc52751bad08fb',
- content=['image'],
- mapping=[['ic13_textdet_train_img', 'textdet_imgs/train']]),
- dict(
- url='https://rrc.cvc.uab.es/downloads/'
- 'Challenge2_Training_Task1_GT.zip',
- save_name='ic13_textdet_train_gt.zip',
- md5='f3a425284a66cd67f455d389c972cce4',
- content=['annotation'],
- mapping=[['ic13_textdet_train_gt', 'annotations/train']]),
- ]),
- gatherer=dict(
- type='PairGatherer',
- img_suffixes=['.jpg'],
- rule=[r'(\w+)\.jpg', r'gt_\1.txt']),
- parser=dict(
- type='ICDARTxtTextDetAnnParser',
- remove_strs=[',', '"'],
- format='x1 y1 x2 y2 trans',
- separator=' ',
- mode='xyxy'),
- packer=dict(type='TextDetPacker'),
- dumper=dict(type='JsonDumper'),
-)
-```
-
-To automatically generate the basic configuration after the dataset is prepared, you also need to configure the corresponding task's `config_generator`.
-
-In this example, since it is a text detection task, you only need to set the generator to `TextDetConfigGenerator`.
-
-```python
-config_generator = dict(type='TextDetConfigGenerator')
-```
-
-### Use DataPreparer to prepare customized dataset
-
-\[Coming Soon\]
diff --git a/pipeline/mmocr/docs/en/user_guides/data_prepare/det.md b/pipeline/mmocr/docs/en/user_guides/data_prepare/det.md
deleted file mode 100644
index 8221215000d13e747495f09aaa11398f1aa1d774..0000000000000000000000000000000000000000
--- a/pipeline/mmocr/docs/en/user_guides/data_prepare/det.md
+++ /dev/null
@@ -1,635 +0,0 @@
-# Text Detection
-
-```{note}
-This page is a manual preparation guide for datasets not yet supported by [Dataset Preparer](./dataset_preparer.md), which all these scripts will be eventually migrated into.
-```
-
-## Overview
-
-| Dataset | Images | | Annotation Files | | |
-| :---------------: | :------------------------------------------------------: | :------------------------------------------------: | :-----------------------------------------------------------------: | :-----: | :-: |
-| | | training | validation | testing | |
-| ICDAR2011 | [homepage](https://rrc.cvc.uab.es/?ch=1) | - | - | | |
-| ICDAR2017 | [homepage](https://rrc.cvc.uab.es/?ch=8&com=downloads) | [instances_training.json](https://download.openmmlab.com/mmocr/data/icdar2017/instances_training.json) | [instances_val.json](https://download.openmmlab.com/mmocr/data/icdar2017/instances_val.json) | - | |
-| CurvedSynText150k | [homepage](https://github.com/aim-uofa/AdelaiDet/blob/master/datasets/README.md) \| [Part1](https://drive.google.com/file/d/1OSJ-zId2h3t_-I7g_wUkrK-VqQy153Kj/view?usp=sharing) \| [Part2](https://drive.google.com/file/d/1EzkcOlIgEp5wmEubvHb7-J5EImHExYgY/view?usp=sharing) | [instances_training.json](https://download.openmmlab.com/mmocr/data/curvedsyntext/instances_training.json) | - | - | |
-| DeText | [homepage](https://rrc.cvc.uab.es/?ch=9) | - | - | - | |
-| Lecture Video DB | [homepage](https://cvit.iiit.ac.in/research/projects/cvit-projects/lecturevideodb) | - | - | - | |
-| LSVT | [homepage](https://rrc.cvc.uab.es/?ch=16) | - | - | - | |
-| IMGUR | [homepage](https://github.com/facebookresearch/IMGUR5K-Handwriting-Dataset) | - | - | - | |
-| KAIST | [homepage](http://www.iapr-tc11.org/mediawiki/index.php/KAIST_Scene_Text_Database) | - | - | - | |
-| MTWI | [homepage](https://tianchi.aliyun.com/competition/entrance/231685/information?lang=en-us) | - | - | - | |
-| ReCTS | [homepage](https://rrc.cvc.uab.es/?ch=12) | - | - | - | |
-| IIIT-ILST | [homepage](http://cvit.iiit.ac.in/research/projects/cvit-projects/iiit-ilst) | - | - | - | |
-| VinText | [homepage](https://github.com/VinAIResearch/dict-guided) | - | - | - | |
-| BID | [homepage](https://github.com/ricardobnjunior/Brazilian-Identity-Document-Dataset) | - | - | - | |
-| RCTW | [homepage](https://rctw.vlrlab.net/index.html) | - | - | - | |
-| HierText | [homepage](https://github.com/google-research-datasets/hiertext) | - | - | - | |
-| ArT | [homepage](https://rrc.cvc.uab.es/?ch=14) | - | - | - | |
-
-### Install AWS CLI (optional)
-
-- Since there are some datasets that require the [AWS CLI](https://docs.aws.amazon.com/cli/latest/userguide/getting-started-install.html) to be installed in advance, we provide a quick installation guide here:
-
- ```bash
- curl "https://awscli.amazonaws.com/awscli-exe-linux-x86_64.zip" -o "awscliv2.zip"
- unzip awscliv2.zip
- sudo ./aws/install
- ./aws/install -i /usr/local/aws-cli -b /usr/local/bin
- !aws configure
- # this command will require you to input keys, you can skip them except
- # for the Default region name
- # AWS Access Key ID [None]:
- # AWS Secret Access Key [None]:
- # Default region name [None]: us-east-1
- # Default output format [None]
- ```
-
-For users in China, these datasets can also be downloaded from [OpenDataLab](https://opendatalab.com/) with high speed:
-
-- [CTW1500](https://opendatalab.com/SCUT-CTW1500?source=OpenMMLab%20GitHub)
-- [ICDAR2013](https://opendatalab.com/ICDAR_2013?source=OpenMMLab%20GitHub)
-- [ICDAR2015](https://opendatalab.com/ICDAR2015?source=OpenMMLab%20GitHub)
-- [Totaltext](https://opendatalab.com/TotalText?source=OpenMMLab%20GitHub)
-- [MSRA-TD500](https://opendatalab.com/MSRA-TD500?source=OpenMMLab%20GitHub)
-
-## Important Note
-
-```{note}
-**For users who want to train models on CTW1500, ICDAR 2015/2017, and Totaltext dataset,** there might be some images containing orientation info in EXIF data. The default OpenCV
-backend used in MMCV would read them and apply the rotation on the images. However, their gold annotations are made on the raw pixels, and such
-inconsistency results in false examples in the training set. Therefore, users should use `dict(type='LoadImageFromFile', color_type='color_ignore_orientation')` in pipelines to change MMCV's default loading behaviour. (see [DBNet's pipeline config](https://github.com/open-mmlab/mmocr/blob/main/configs/_base_/det_pipelines/dbnet_pipeline.py) for example)
-```
-
-## ICDAR 2011 (Born-Digital Images)
-
-- Step1: Download `Challenge1_Training_Task12_Images.zip`, `Challenge1_Training_Task1_GT.zip`, `Challenge1_Test_Task12_Images.zip`, and `Challenge1_Test_Task1_GT.zip` from [homepage](https://rrc.cvc.uab.es/?ch=1&com=downloads) `Task 1.1: Text Localization (2013 edition)`.
-
- ```bash
- mkdir icdar2011 && cd icdar2011
- mkdir imgs && mkdir annotations
-
- # Download ICDAR 2011
- wget https://rrc.cvc.uab.es/downloads/Challenge1_Training_Task12_Images.zip --no-check-certificate
- wget https://rrc.cvc.uab.es/downloads/Challenge1_Training_Task1_GT.zip --no-check-certificate
- wget https://rrc.cvc.uab.es/downloads/Challenge1_Test_Task12_Images.zip --no-check-certificate
- wget https://rrc.cvc.uab.es/downloads/Challenge1_Test_Task1_GT.zip --no-check-certificate
-
- # For images
- unzip -q Challenge1_Training_Task12_Images.zip -d imgs/training
- unzip -q Challenge1_Test_Task12_Images.zip -d imgs/test
- # For annotations
- unzip -q Challenge1_Training_Task1_GT.zip -d annotations/training
- unzip -q Challenge1_Test_Task1_GT.zip -d annotations/test
-
- rm Challenge1_Training_Task12_Images.zip && rm Challenge1_Test_Task12_Images.zip && rm Challenge1_Training_Task1_GT.zip && rm Challenge1_Test_Task1_GT.zip
- ```
-
-- Step 2: Generate `instances_training.json` and `instances_test.json` with the following command:
-
- ```bash
- python tools/dataset_converters/textdet/ic11_converter.py PATH/TO/icdar2011 --nproc 4
- ```
-
-- After running the above codes, the directory structure should be as follows:
-
- ```text
- โโโ icdar2011
- โ โโโ imgs
- โ โโโ instances_test.json
- โ โโโ instances_training.json
- ```
-
-## ICDAR 2017
-
-- Follow similar steps as [ICDAR 2015](#icdar-2015).
-
-- The resulting directory structure looks like the following:
-
- ```text
- โโโ icdar2017
- โย ย โโโ imgs
- โย ย โโโ annotations
- โย ย โโโ instances_training.json
- โย ย โโโ instances_val.json
- ```
-
-## CurvedSynText150k
-
-- Step1: Download [syntext1.zip](https://drive.google.com/file/d/1OSJ-zId2h3t_-I7g_wUkrK-VqQy153Kj/view?usp=sharing) and [syntext2.zip](https://drive.google.com/file/d/1EzkcOlIgEp5wmEubvHb7-J5EImHExYgY/view?usp=sharing) to `CurvedSynText150k/`.
-
-- Step2:
-
- ```bash
- unzip -q syntext1.zip
- mv train.json train1.json
- unzip images.zip
- rm images.zip
-
- unzip -q syntext2.zip
- mv train.json train2.json
- unzip images.zip
- rm images.zip
- ```
-
-- Step3: Download [instances_training.json](https://download.openmmlab.com/mmocr/data/curvedsyntext/instances_training.json) to `CurvedSynText150k/`
-
-- Or, generate `instances_training.json` with following command:
-
- ```bash
- python tools/dataset_converters/common/curvedsyntext_converter.py PATH/TO/CurvedSynText150k --nproc 4
- ```
-
-- The resulting directory structure looks like the following:
-
- ```text
- โโโ CurvedSynText150k
- โย ย โโโ syntext_word_eng
- โย ย โโโ emcs_imgs
- โย ย โโโ instances_training.json
- ```
-
-## DeText
-
-- Step1: Download `ch9_training_images.zip`, `ch9_training_localization_transcription_gt.zip`, `ch9_validation_images.zip`, and `ch9_validation_localization_transcription_gt.zip` from **Task 3: End to End** on the [homepage](https://rrc.cvc.uab.es/?ch=9).
-
- ```bash
- mkdir detext && cd detext
- mkdir imgs && mkdir annotations && mkdir imgs/training && mkdir imgs/val && mkdir annotations/training && mkdir annotations/val
-
- # Download DeText
- wget https://rrc.cvc.uab.es/downloads/ch9_training_images.zip --no-check-certificate
- wget https://rrc.cvc.uab.es/downloads/ch9_training_localization_transcription_gt.zip --no-check-certificate
- wget https://rrc.cvc.uab.es/downloads/ch9_validation_images.zip --no-check-certificate
- wget https://rrc.cvc.uab.es/downloads/ch9_validation_localization_transcription_gt.zip --no-check-certificate
-
- # Extract images and annotations
- unzip -q ch9_training_images.zip -d imgs/training && unzip -q ch9_training_localization_transcription_gt.zip -d annotations/training && unzip -q ch9_validation_images.zip -d imgs/val && unzip -q ch9_validation_localization_transcription_gt.zip -d annotations/val
-
- # Remove zips
- rm ch9_training_images.zip && rm ch9_training_localization_transcription_gt.zip && rm ch9_validation_images.zip && rm ch9_validation_localization_transcription_gt.zip
- ```
-
-- Step2: Generate `instances_training.json` and `instances_val.json` with following command:
-
- ```bash
- python tools/dataset_converters/textdet/detext_converter.py PATH/TO/detext --nproc 4
- ```
-
-- After running the above codes, the directory structure should be as follows:
-
- ```text
- โโโ detext
- โย ย โโโ annotations
- โย ย โโโ imgs
- โย ย โโโ instances_test.json
- โย ย โโโ instances_training.json
- ```
-
-## Lecture Video DB
-
-- Step1: Download [IIIT-CVid.zip](http://cdn.iiit.ac.in/cdn/preon.iiit.ac.in/~kartik/IIIT-CVid.zip) to `lv/`.
-
- ```bash
- mkdir lv && cd lv
-
- # Download LV dataset
- wget http://cdn.iiit.ac.in/cdn/preon.iiit.ac.in/~kartik/IIIT-CVid.zip
- unzip -q IIIT-CVid.zip
-
- mv IIIT-CVid/Frames imgs
-
- rm IIIT-CVid.zip
- ```
-
-- Step2: Generate `instances_training.json`, `instances_val.json`, and `instances_test.json` with following command:
-
- ```bash
- python tools/dataset_converters/textdet/lv_converter.py PATH/TO/lv --nproc 4
- ```
-
-- The resulting directory structure looks like the following:
-
- ```text
- โโโ lv
- โย ย โโโ imgs
- โย ย โโโ instances_test.json
- โย ย โโโ instances_training.json
- โย ย โโโ instances_val.json
- ```
-
-## LSVT
-
-- Step1: Download [train_full_images_0.tar.gz](https://dataset-bj.cdn.bcebos.com/lsvt/train_full_images_0.tar.gz), [train_full_images_1.tar.gz](https://dataset-bj.cdn.bcebos.com/lsvt/train_full_images_1.tar.gz), and [train_full_labels.json](https://dataset-bj.cdn.bcebos.com/lsvt/train_full_labels.json) to `lsvt/`.
-
- ```bash
- mkdir lsvt && cd lsvt
-
- # Download LSVT dataset
- wget https://dataset-bj.cdn.bcebos.com/lsvt/train_full_images_0.tar.gz
- wget https://dataset-bj.cdn.bcebos.com/lsvt/train_full_images_1.tar.gz
- wget https://dataset-bj.cdn.bcebos.com/lsvt/train_full_labels.json
-
- mkdir annotations
- tar -xf train_full_images_0.tar.gz && tar -xf train_full_images_1.tar.gz
- mv train_full_labels.json annotations/ && mv train_full_images_1/*.jpg train_full_images_0/
- mv train_full_images_0 imgs
-
- rm train_full_images_0.tar.gz && rm train_full_images_1.tar.gz && rm -rf train_full_images_1
- ```
-
-- Step2: Generate `instances_training.json` and `instances_val.json` (optional) with the following command:
-
- ```bash
- # Annotations of LSVT test split is not publicly available, split a validation
- # set by adding --val-ratio 0.2
- python tools/dataset_converters/textdet/lsvt_converter.py PATH/TO/lsvt
- ```
-
-- After running the above codes, the directory structure should be as follows:
-
- ```text
- |โโ lsvt
- โย ย โโโ imgs
- โย ย โโโ instances_training.json
- โย ย โโโ instances_val.json (optional)
- ```
-
-## IMGUR
-
-- Step1: Run `download_imgur5k.py` to download images. You can merge [PR#5](https://github.com/facebookresearch/IMGUR5K-Handwriting-Dataset/pull/5) in your local repository to enable a **much faster** parallel execution of image download.
-
- ```bash
- mkdir imgur && cd imgur
-
- git clone https://github.com/facebookresearch/IMGUR5K-Handwriting-Dataset.git
-
- # Download images from imgur.com. This may take SEVERAL HOURS!
- python ./IMGUR5K-Handwriting-Dataset/download_imgur5k.py --dataset_info_dir ./IMGUR5K-Handwriting-Dataset/dataset_info/ --output_dir ./imgs
-
- # For annotations
- mkdir annotations
- mv ./IMGUR5K-Handwriting-Dataset/dataset_info/*.json annotations
-
- rm -rf IMGUR5K-Handwriting-Dataset
- ```
-
-- Step2: Generate `instances_train.json`, `instance_val.json` and `instances_test.json` with the following command:
-
- ```bash
- python tools/dataset_converters/textdet/imgur_converter.py PATH/TO/imgur
- ```
-
-- After running the above codes, the directory structure should be as follows:
-
- ```text
- โโโ imgur
- โ โโโ annotations
- โ โโโ imgs
- โ โโโ instances_test.json
- โ โโโ instances_training.json
- โ โโโ instances_val.json
- ```
-
-## KAIST
-
-- Step1: Complete download [KAIST_all.zip](http://www.iapr-tc11.org/mediawiki/index.php/KAIST_Scene_Text_Database) to `kaist/`.
-
- ```bash
- mkdir kaist && cd kaist
- mkdir imgs && mkdir annotations
-
- # Download KAIST dataset
- wget http://www.iapr-tc11.org/dataset/KAIST_SceneText/KAIST_all.zip
- unzip -q KAIST_all.zip
-
- rm KAIST_all.zip
- ```
-
-- Step2: Extract zips:
-
- ```bash
- python tools/dataset_converters/common/extract_kaist.py PATH/TO/kaist
- ```
-
-- Step3: Generate `instances_training.json` and `instances_val.json` (optional) with following command:
-
- ```bash
- # Since KAIST does not provide an official split, you can split the dataset by adding --val-ratio 0.2
- python tools/dataset_converters/textdet/kaist_converter.py PATH/TO/kaist --nproc 4
- ```
-
-- After running the above codes, the directory structure should be as follows:
-
- ```text
- โโโ kaist
- โ โโโ annotations
- โ โโโ imgs
- โ โโโ instances_training.json
- โ โโโ instances_val.json (optional)
- ```
-
-## MTWI
-
-- Step1: Download `mtwi_2018_train.zip` from [homepage](https://tianchi.aliyun.com/competition/entrance/231685/information?lang=en-us).
-
- ```bash
- mkdir mtwi && cd mtwi
-
- unzip -q mtwi_2018_train.zip
- mv image_train imgs && mv txt_train annotations
-
- rm mtwi_2018_train.zip
- ```
-
-- Step2: Generate `instances_training.json` and `instance_val.json` (optional) with the following command:
-
- ```bash
- # Annotations of MTWI test split is not publicly available, split a validation
- # set by adding --val-ratio 0.2
- python tools/dataset_converters/textdet/mtwi_converter.py PATH/TO/mtwi --nproc 4
- ```
-
-- After running the above codes, the directory structure should be as follows:
-
- ```text
- โโโ mtwi
- โ โโโ annotations
- โ โโโ imgs
- โ โโโ instances_training.json
- โ โโโ instances_val.json (optional)
- ```
-
-## ReCTS
-
-- Step1: Download [ReCTS.zip](https://datasets.cvc.uab.es/rrc/ReCTS.zip) to `rects/` from the [homepage](https://rrc.cvc.uab.es/?ch=12&com=downloads).
-
- ```bash
- mkdir rects && cd rects
-
- # Download ReCTS dataset
- # You can also find Google Drive link on the dataset homepage
- wget https://datasets.cvc.uab.es/rrc/ReCTS.zip --no-check-certificate
- unzip -q ReCTS.zip
-
- mv img imgs && mv gt_unicode annotations
-
- rm ReCTS.zip && rm -rf gt
- ```
-
-- Step2: Generate `instances_training.json` and `instances_val.json` (optional) with following command:
-
- ```bash
- # Annotations of ReCTS test split is not publicly available, split a validation
- # set by adding --val-ratio 0.2
- python tools/dataset_converters/textdet/rects_converter.py PATH/TO/rects --nproc 4 --val-ratio 0.2
- ```
-
-- After running the above codes, the directory structure should be as follows:
-
- ```text
- โโโ rects
- โ โโโ annotations
- โ โโโ imgs
- โ โโโ instances_val.json (optional)
- โ โโโ instances_training.json
- ```
-
-## ILST
-
-- Step1: Download `IIIT-ILST` from [onedrive](https://iiitaphyd-my.sharepoint.com/:f:/g/personal/minesh_mathew_research_iiit_ac_in/EtLvCozBgaBIoqglF4M-lHABMgNcCDW9rJYKKWpeSQEElQ?e=zToXZP)
-
-- Step2: Run the following commands
-
- ```bash
- unzip -q IIIT-ILST.zip && rm IIIT-ILST.zip
- cd IIIT-ILST
-
- # rename files
- cd Devanagari && for i in `ls`; do mv -f $i `echo "devanagari_"$i`; done && cd ..
- cd Malayalam && for i in `ls`; do mv -f $i `echo "malayalam_"$i`; done && cd ..
- cd Telugu && for i in `ls`; do mv -f $i `echo "telugu_"$i`; done && cd ..
-
- # transfer image path
- mkdir imgs && mkdir annotations
- mv Malayalam/{*jpg,*jpeg} imgs/ && mv Malayalam/*xml annotations/
- mv Devanagari/*jpg imgs/ && mv Devanagari/*xml annotations/
- mv Telugu/*jpeg imgs/ && mv Telugu/*xml annotations/
-
- # remove unnecessary files
- rm -rf Devanagari && rm -rf Malayalam && rm -rf Telugu && rm -rf README.txt
- ```
-
-- Step3: Generate `instances_training.json` and `instances_val.json` (optional). Since the original dataset doesn't have a validation set, you may specify `--val-ratio` to split the dataset. E.g., if val-ratio is 0.2, then 20% of the data are left out as the validation set in this example.
-
- ```bash
- python tools/dataset_converters/textdet/ilst_converter.py PATH/TO/IIIT-ILST --nproc 4
- ```
-
-- After running the above codes, the directory structure should be as follows:
-
- ```text
- โโโ IIIT-ILST
- โย ย โโโ annotations
- โย ย โโโ imgs
- โย ย โโโ instances_val.json (optional)
- โย ย โโโ instances_training.json
- ```
-
-## VinText
-
-- Step1: Download [vintext.zip](https://drive.google.com/drive/my-drive) to `vintext`
-
- ```bash
- mkdir vintext && cd vintext
-
- # Download dataset from google drive
- wget --load-cookies /tmp/cookies.txt "https://docs.google.com/uc?export=download&confirm=$(wget --quiet --save-cookies /tmp/cookies.txt --keep-session-cookies --no-check-certificate 'https://docs.google.com/uc?export=download&id=1UUQhNvzgpZy7zXBFQp0Qox-BBjunZ0ml' -O- โ sed -rn 's/.*confirm=([0-9A-Za-z_]+).*/\1\n/p')&id=1UUQhNvzgpZy7zXBFQp0Qox-BBjunZ0ml" -O vintext.zip && rm -rf /tmp/cookies.txt
-
- # Extract images and annotations
- unzip -q vintext.zip && rm vintext.zip
- mv vietnamese/labels ./ && mv vietnamese/test_image ./ && mv vietnamese/train_images ./ && mv vietnamese/unseen_test_images ./
- rm -rf vietnamese
-
- # Rename files
- mv labels annotations && mv test_image test && mv train_images training && mv unseen_test_images unseen_test
- mkdir imgs
- mv training imgs/ && mv test imgs/ && mv unseen_test imgs/
- ```
-
-- Step2: Generate `instances_training.json`, `instances_test.json` and `instances_unseen_test.json`
-
- ```bash
- python tools/dataset_converters/textdet/vintext_converter.py PATH/TO/vintext --nproc 4
- ```
-
-- After running the above codes, the directory structure should be as follows:
-
- ```text
- โโโ vintext
- โย ย โโโ annotations
- โย ย โโโ imgs
- โย ย โโโ instances_test.json
- โย ย โโโ instances_unseen_test.json
- โย ย โโโ instances_training.json
- ```
-
-## BID
-
-- Step1: Download [BID Dataset.zip](https://drive.google.com/file/d/1Oi88TRcpdjZmJ79WDLb9qFlBNG8q2De6/view)
-
-- Step2: Run the following commands to preprocess the dataset
-
- ```bash
- # Rename
- mv BID\ Dataset.zip BID_Dataset.zip
-
- # Unzip and Rename
- unzip -q BID_Dataset.zip && rm BID_Dataset.zip
- mv BID\ Dataset BID
-
- # The BID dataset has a problem of permission, and you may
- # add permission for this file
- chmod -R 777 BID
- cd BID
- mkdir imgs && mkdir annotations
-
- # For images and annotations
- mv CNH_Aberta/*in.jpg imgs && mv CNH_Aberta/*txt annotations && rm -rf CNH_Aberta
- mv CNH_Frente/*in.jpg imgs && mv CNH_Frente/*txt annotations && rm -rf CNH_Frente
- mv CNH_Verso/*in.jpg imgs && mv CNH_Verso/*txt annotations && rm -rf CNH_Verso
- mv CPF_Frente/*in.jpg imgs && mv CPF_Frente/*txt annotations && rm -rf CPF_Frente
- mv CPF_Verso/*in.jpg imgs && mv CPF_Verso/*txt annotations && rm -rf CPF_Verso
- mv RG_Aberto/*in.jpg imgs && mv RG_Aberto/*txt annotations && rm -rf RG_Aberto
- mv RG_Frente/*in.jpg imgs && mv RG_Frente/*txt annotations && rm -rf RG_Frente
- mv RG_Verso/*in.jpg imgs && mv RG_Verso/*txt annotations && rm -rf RG_Verso
-
- # Remove unnecessary files
- rm -rf desktop.ini
- ```
-
-- Step3: - Step3: Generate `instances_training.json` and `instances_val.json` (optional). Since the original dataset doesn't have a validation set, you may specify `--val-ratio` to split the dataset. E.g., if val-ratio is 0.2, then 20% of the data are left out as the validation set in this example.
-
- ```bash
- python tools/dataset_converters/textdet/bid_converter.py PATH/TO/BID --nproc 4
- ```
-
-- After running the above codes, the directory structure should be as follows:
-
- ```text
- โโโ BID
- โย ย โโโ annotations
- โย ย โโโ imgs
- โย ย โโโ instances_training.json
- โย ย โโโ instances_val.json (optional)
- ```
-
-## RCTW
-
-- Step1: Download `train_images.zip.001`, `train_images.zip.002`, and `train_gts.zip` from the [homepage](https://rctw.vlrlab.net/dataset.html), extract the zips to `rctw/imgs` and `rctw/annotations`, respectively.
-
-- Step2: Generate `instances_training.json` and `instances_val.json` (optional). Since the test annotations are not publicly available, you may specify `--val-ratio` to split the dataset. E.g., if val-ratio is 0.2, then 20% of the data are left out as the validation set in this example.
-
- ```bash
- # Annotations of RCTW test split is not publicly available, split a validation set by adding --val-ratio 0.2
- python tools/dataset_converters/textdet/rctw_converter.py PATH/TO/rctw --nproc 4
- ```
-
-- After running the above codes, the directory structure should be as follows:
-
- ```text
- โโโ rctw
- โย ย โโโ annotations
- โย ย โโโ imgs
- โย ย โโโ instances_training.json
- โย ย โโโ instances_val.json (optional)
- ```
-
-## HierText
-
-- Step1 (optional): Install [AWS CLI](https://mmocr.readthedocs.io/en/latest/datasets/det.html#install-aws-cli-optional).
-
-- Step2: Clone [HierText](https://github.com/google-research-datasets/hiertext) repo to get annotations
-
- ```bash
- mkdir HierText
- git clone https://github.com/google-research-datasets/hiertext.git
- ```
-
-- Step3: Download `train.tgz`, `validation.tgz` from aws
-
- ```bash
- aws s3 --no-sign-request cp s3://open-images-dataset/ocr/train.tgz .
- aws s3 --no-sign-request cp s3://open-images-dataset/ocr/validation.tgz .
- ```
-
-- Step4: Process raw data
-
- ```bash
- # process annotations
- mv hiertext/gt ./
- rm -rf hiertext
- mv gt annotations
- gzip -d annotations/train.jsonl.gz
- gzip -d annotations/validation.jsonl.gz
- # process images
- mkdir imgs
- mv train.tgz imgs/
- mv validation.tgz imgs/
- tar -xzvf imgs/train.tgz
- tar -xzvf imgs/validation.tgz
- ```
-
-- Step5: Generate `instances_training.json` and `instance_val.json`. HierText includes different levels of annotation, from paragraph, line, to word. Check the original [paper](https://arxiv.org/pdf/2203.15143.pdf) for details. E.g. set `--level paragraph` to get paragraph-level annotation. Set `--level line` to get line-level annotation. set `--level word` to get word-level annotation.
-
- ```bash
- # Collect word annotation from HierText --level word
- python tools/dataset_converters/textdet/hiertext_converter.py PATH/TO/HierText --level word --nproc 4
- ```
-
-- After running the above codes, the directory structure should be as follows:
-
- ```text
- โโโ HierText
- โย ย โโโ annotations
- โย ย โโโ imgs
- โย ย โโโ instances_training.json
- โย ย โโโ instances_val.json
- ```
-
-## ArT
-
-- Step1: Download `train_images.tar.gz`, and `train_labels.json` from the [homepage](https://rrc.cvc.uab.es/?ch=14&com=downloads) to `art/`
-
- ```bash
- mkdir art && cd art
- mkdir annotations
-
- # Download ArT dataset
- wget https://dataset-bj.cdn.bcebos.com/art/train_images.tar.gz --no-check-certificate
- wget https://dataset-bj.cdn.bcebos.com/art/train_labels.json --no-check-certificate
-
- # Extract
- tar -xf train_images.tar.gz
- mv train_images imgs
- mv train_labels.json annotations/
-
- # Remove unnecessary files
- rm train_images.tar.gz
- ```
-
-- Step2: Generate `instances_training.json` and `instances_val.json` (optional). Since the test annotations are not publicly available, you may specify `--val-ratio` to split the dataset. E.g., if val-ratio is 0.2, then 20% of the data are left out as the validation set in this example.
-
- ```bash
- # Annotations of ArT test split is not publicly available, split a validation set by adding --val-ratio 0.2
- python tools/data/textdet/art_converter.py PATH/TO/art --nproc 4
- ```
-
-- After running the above codes, the directory structure should be as follows:
-
- ```text
- โโโ art
- โย ย โโโ annotations
- โย ย โโโ imgs
- โย ย โโโ instances_training.json
- โย ย โโโ instances_val.json (optional)
- ```
diff --git a/pipeline/mmocr/docs/en/user_guides/data_prepare/kie.md b/pipeline/mmocr/docs/en/user_guides/data_prepare/kie.md
deleted file mode 100644
index 9d324383c45726c564ed86d040a93e0c6fcc2d80..0000000000000000000000000000000000000000
--- a/pipeline/mmocr/docs/en/user_guides/data_prepare/kie.md
+++ /dev/null
@@ -1,42 +0,0 @@
-# Key Information Extraction
-
-```{note}
-This page is a manual preparation guide for datasets not yet supported by [Dataset Preparer](./dataset_preparer.md), which all these scripts will be eventually migrated into.
-```
-
-## Overview
-
-The structure of the key information extraction dataset directory is organized as follows.
-
-```text
-โโโ wildreceipt
- โโโ class_list.txt
- โโโ dict.txt
- โโโ image_files
- โโโ openset_train.txt
- โโโ openset_test.txt
- โโโ test.txt
- โโโ train.txt
-```
-
-## Preparation Steps
-
-### WildReceipt
-
-- Just download and extract [wildreceipt.tar](https://download.openmmlab.com/mmocr/data/wildreceipt.tar).
-
-### WildReceiptOpenset
-
-- Step0: have [WildReceipt](#WildReceipt) prepared.
-- Step1: Convert annotation files to OpenSet format:
-
-```bash
-# You may find more available arguments by running
-# python tools/data/kie/closeset_to_openset.py -h
-python tools/data/kie/closeset_to_openset.py data/wildreceipt/train.txt data/wildreceipt/openset_train.txt
-python tools/data/kie/closeset_to_openset.py data/wildreceipt/test.txt data/wildreceipt/openset_test.txt
-```
-
-```{note}
-You can learn more about the key differences between CloseSet and OpenSet annotations in our [tutorial](../tutorials/kie_closeset_openset.md).
-```
diff --git a/pipeline/mmocr/docs/en/user_guides/data_prepare/recog.md b/pipeline/mmocr/docs/en/user_guides/data_prepare/recog.md
deleted file mode 100644
index e4a021581c770d8455eec1f69cb42320dc67c555..0000000000000000000000000000000000000000
--- a/pipeline/mmocr/docs/en/user_guides/data_prepare/recog.md
+++ /dev/null
@@ -1,784 +0,0 @@
-# Text Recognition
-
-```{note}
-This page is a manual preparation guide for datasets not yet supported by [Dataset Preparer](./dataset_preparer.md), which all these scripts will be eventually migrated into.
-```
-
-## Overview
-
-| Dataset | images | annotation file | annotation file |
-| :--------------: | :-----------------------------------------------------: | :--------------------------------------------------------------: | :---------------------------------------------------------------: |
-| | | training | test |
-| coco_text | [homepage](https://rrc.cvc.uab.es/?ch=5&com=downloads) | [train_labels.json](#TODO) | - |
-| ICDAR2011 | [homepage](https://rrc.cvc.uab.es/?ch=1) | - | - |
-| SynthAdd | [SynthText_Add.zip](https://pan.baidu.com/s/1uV0LtoNmcxbO-0YA7Ch4dg) (code:627x) | [train_labels.json](https://download.openmmlab.com/mmocr/data/1.x/recog/synthtext_add/train_labels.json) | - |
-| OpenVINO | [Open Images](https://github.com/cvdfoundation/open-images-dataset) | [annotations](https://storage.openvinotoolkit.org/repositories/openvino_training_extensions/datasets/open_images_v5_text) | [annotations](https://storage.openvinotoolkit.org/repositories/openvino_training_extensions/datasets/open_images_v5_text) |
-| DeText | [homepage](https://rrc.cvc.uab.es/?ch=9) | - | - |
-| Lecture Video DB | [homepage](https://cvit.iiit.ac.in/research/projects/cvit-projects/lecturevideodb) | - | - |
-| LSVT | [homepage](https://rrc.cvc.uab.es/?ch=16) | - | - |
-| IMGUR | [homepage](https://github.com/facebookresearch/IMGUR5K-Handwriting-Dataset) | - | - |
-| KAIST | [homepage](http://www.iapr-tc11.org/mediawiki/index.php/KAIST_Scene_Text_Database) | - | - |
-| MTWI | [homepage](https://tianchi.aliyun.com/competition/entrance/231685/information?lang=en-us) | - | - |
-| ReCTS | [homepage](https://rrc.cvc.uab.es/?ch=12) | - | - |
-| IIIT-ILST | [homepage](http://cvit.iiit.ac.in/research/projects/cvit-projects/iiit-ilst) | - | - |
-| VinText | [homepage](https://github.com/VinAIResearch/dict-guided) | - | - |
-| BID | [homepage](https://github.com/ricardobnjunior/Brazilian-Identity-Document-Dataset) | - | - |
-| RCTW | [homepage](https://rctw.vlrlab.net/index.html) | - | - |
-| HierText | [homepage](https://github.com/google-research-datasets/hiertext) | - | - |
-| ArT | [homepage](https://rrc.cvc.uab.es/?ch=14) | - | - |
-
-(\*) Since the official homepage is unavailable now, we provide an alternative for quick reference. However, we do not guarantee the correctness of the dataset.
-
-### Install AWS CLI (optional)
-
-- Since there are some datasets that require the [AWS CLI](https://docs.aws.amazon.com/cli/latest/userguide/getting-started-install.html) to be installed in advance, we provide a quick installation guide here:
-
- ```bash
- curl "https://awscli.amazonaws.com/awscli-exe-linux-x86_64.zip" -o "awscliv2.zip"
- unzip awscliv2.zip
- sudo ./aws/install
- ./aws/install -i /usr/local/aws-cli -b /usr/local/bin
- !aws configure
- # this command will require you to input keys, you can skip them except
- # for the Default region name
- # AWS Access Key ID [None]:
- # AWS Secret Access Key [None]:
- # Default region name [None]: us-east-1
- # Default output format [None]
- ```
-
-For users in China, these datasets can also be downloaded from [OpenDataLab](https://opendatalab.com/) with high speed:
-
-- [icdar_2013](https://opendatalab.com/ICDAR_2013?source=OpenMMLab%20GitHub)
-- [icdar_2015](https://opendatalab.com/ICDAR2015?source=OpenMMLab%20GitHub)
-- [IIIT5K](https://opendatalab.com/IIIT_5K?source=OpenMMLab%20GitHub)
-- [ct80](https://opendatalab.com/CUTE_80?source=OpenMMLab%20GitHub)
-- [svt](https://opendatalab.com/SVT?source=OpenMMLab%20GitHub)
-- [Totaltext](https://opendatalab.com/TotalText?source=OpenMMLab%20GitHub)
-- [IAM](https://opendatalab.com/IAM_Handwriting?source=OpenMMLab%20GitHub)
-
-## ICDAR 2011 (Born-Digital Images)
-
-- Step1: Download `Challenge1_Training_Task3_Images_GT.zip`, `Challenge1_Test_Task3_Images.zip`, and `Challenge1_Test_Task3_GT.txt` from [homepage](https://rrc.cvc.uab.es/?ch=1&com=downloads) `Task 1.3: Word Recognition (2013 edition)`.
-
- ```bash
- mkdir icdar2011 && cd icdar2011
- mkdir annotations
-
- # Download ICDAR 2011
- wget https://rrc.cvc.uab.es/downloads/Challenge1_Training_Task3_Images_GT.zip --no-check-certificate
- wget https://rrc.cvc.uab.es/downloads/Challenge1_Test_Task3_Images.zip --no-check-certificate
- wget https://rrc.cvc.uab.es/downloads/Challenge1_Test_Task3_GT.txt --no-check-certificate
-
- # For images
- mkdir crops
- unzip -q Challenge1_Training_Task3_Images_GT.zip -d crops/train
- unzip -q Challenge1_Test_Task3_Images.zip -d crops/test
-
- # For annotations
- mv Challenge1_Test_Task3_GT.txt annotations && mv crops/train/gt.txt annotations/Challenge1_Train_Task3_GT.txt
- ```
-
-- Step2: Convert original annotations to `train_labels.json` and `test_labels.json` with the following command:
-
- ```bash
- python tools/dataset_converters/textrecog/ic11_converter.py PATH/TO/icdar2011
- ```
-
-- After running the above codes, the directory structure should be as follows:
-
- ```text
- โโโ icdar2011
- โ โโโ crops
- โ โโโ train_labels.json
- โ โโโ test_labels.json
- ```
-
-## coco_text
-
-- Step1: Download from [homepage](https://rrc.cvc.uab.es/?ch=5&com=downloads)
-
-- Step2: Download [train_labels.json](https://download.openmmlab.com/mmocr/data/mixture/coco_text/train_labels.json)
-
-- After running the above codes, the directory structure
- should be as follows:
-
- ```text
- โโโ coco_text
- โ โโโ train_labels.json
- โ โโโ train_words
- ```
-
-## SynthAdd
-
-- Step1: Download `SynthText_Add.zip` from [SynthAdd](https://pan.baidu.com/s/1uV0LtoNmcxbO-0YA7Ch4dg) (code:627x))
-
-- Step2: Download [train_labels.json](https://download.openmmlab.com/mmocr/data/1.x/recog/synthtext_add/train_labels.json)
-
-- Step3:
-
- ```bash
- mkdir SynthAdd && cd SynthAdd
-
- mv /path/to/SynthText_Add.zip .
-
- unzip SynthText_Add.zip
-
- mv /path/to/train_labels.json .
-
- # create soft link
- cd /path/to/mmocr/data/recog
-
- ln -s /path/to/SynthAdd SynthAdd
-
- ```
-
-- After running the above codes, the directory structure
- should be as follows:
-
- ```text
- โโโ SynthAdd
- โ โโโ train_labels.json
- โ โโโ SynthText_Add
- ```
-
-## OpenVINO
-
-- Step1 (optional): Install [AWS CLI](https://mmocr.readthedocs.io/en/latest/datasets/recog.html#install-aws-cli-optional).
-
-- Step2: Download [Open Images](https://github.com/cvdfoundation/open-images-dataset#download-images-with-bounding-boxes-annotations) subsets `train_1`, `train_2`, `train_5`, `train_f`, and `validation` to `openvino/`.
-
- ```bash
- mkdir openvino && cd openvino
-
- # Download Open Images subsets
- for s in 1 2 5 f; do
- aws s3 --no-sign-request cp s3://open-images-dataset/tar/train_${s}.tar.gz .
- done
- aws s3 --no-sign-request cp s3://open-images-dataset/tar/validation.tar.gz .
-
- # Download annotations
- for s in 1 2 5 f; do
- wget https://storage.openvinotoolkit.org/repositories/openvino_training_extensions/datasets/open_images_v5_text/text_spotting_openimages_v5_train_${s}.json
- done
- wget https://storage.openvinotoolkit.org/repositories/openvino_training_extensions/datasets/open_images_v5_text/text_spotting_openimages_v5_validation.json
-
- # Extract images
- mkdir -p openimages_v5/val
- for s in 1 2 5 f; do
- tar zxf train_${s}.tar.gz -C openimages_v5
- done
- tar zxf validation.tar.gz -C openimages_v5/val
- ```
-
-- Step3: Generate `train_{1,2,5,f}_labels.json`, `val_labels.json` and crop images using 4 processes with the following command:
-
- ```bash
- python tools/dataset_converters/textrecog/openvino_converter.py /path/to/openvino 4
- ```
-
-- After running the above codes, the directory structure
- should be as follows:
-
- ```text
- โโโ OpenVINO
- โ โโโ image_1
- โ โโโ image_2
- โ โโโ image_5
- โ โโโ image_f
- โ โโโ image_val
- โ โโโ train_1_labels.json
- โ โโโ train_2_labels.json
- โ โโโ train_5_labels.json
- โ โโโ train_f_labels.json
- โ โโโ val_labels.json
- ```
-
-## DeText
-
-- Step1: Download `ch9_training_images.zip`, `ch9_training_localization_transcription_gt.zip`, `ch9_validation_images.zip`, and `ch9_validation_localization_transcription_gt.zip` from **Task 3: End to End** on the [homepage](https://rrc.cvc.uab.es/?ch=9).
-
- ```bash
- mkdir detext && cd detext
- mkdir imgs && mkdir annotations && mkdir imgs/training && mkdir imgs/val && mkdir annotations/training && mkdir annotations/val
-
- # Download DeText
- wget https://rrc.cvc.uab.es/downloads/ch9_training_images.zip --no-check-certificate
- wget https://rrc.cvc.uab.es/downloads/ch9_training_localization_transcription_gt.zip --no-check-certificate
- wget https://rrc.cvc.uab.es/downloads/ch9_validation_images.zip --no-check-certificate
- wget https://rrc.cvc.uab.es/downloads/ch9_validation_localization_transcription_gt.zip --no-check-certificate
-
- # Extract images and annotations
- unzip -q ch9_training_images.zip -d imgs/training && unzip -q ch9_training_localization_transcription_gt.zip -d annotations/training && unzip -q ch9_validation_images.zip -d imgs/val && unzip -q ch9_validation_localization_transcription_gt.zip -d annotations/val
-
- # Remove zips
- rm ch9_training_images.zip && rm ch9_training_localization_transcription_gt.zip && rm ch9_validation_images.zip && rm ch9_validation_localization_transcription_gt.zip
- ```
-
-- Step2: Generate `train_labels.json` and `test_labels.json` with following command:
-
- ```bash
- # Add --preserve-vertical to preserve vertical texts for training, otherwise
- # vertical images will be filtered and stored in PATH/TO/detext/ignores
- python tools/dataset_converters/textrecog/detext_converter.py PATH/TO/detext --nproc 4
- ```
-
-- After running the above codes, the directory structure should be as follows:
-
- ```text
- โโโ detext
- โ โโโ crops
- โ โโโ ignores
- โ โโโ train_labels.json
- โ โโโ test_labels.json
- ```
-
-## NAF
-
-- Step1: Download [labeled_images.tar.gz](https://github.com/herobd/NAF_dataset/releases/tag/v1.0) to `naf/`.
-
- ```bash
- mkdir naf && cd naf
-
- # Download NAF dataset
- wget https://github.com/herobd/NAF_dataset/releases/download/v1.0/labeled_images.tar.gz
- tar -zxf labeled_images.tar.gz
-
- # For images
- mkdir annotations && mv labeled_images imgs
-
- # For annotations
- git clone https://github.com/herobd/NAF_dataset.git
- mv NAF_dataset/train_valid_test_split.json annotations/ && mv NAF_dataset/groups annotations/
-
- rm -rf NAF_dataset && rm labeled_images.tar.gz
- ```
-
-- Step2: Generate `train_labels.json`, `val_labels.json`, and `test_labels.json` with following command:
-
- ```bash
- # Add --preserve-vertical to preserve vertical texts for training, otherwise
- # vertical images will be filtered and stored in PATH/TO/naf/ignores
- python tools/dataset_converters/textrecog/naf_converter.py PATH/TO/naf --nproc 4
-
- ```
-
-- After running the above codes, the directory structure should be as follows:
-
- ```text
- โโโ naf
- โ โโโ crops
- โ โโโ train_labels.json
- โ โโโ val_labels.json
- โ โโโ test_labels.json
- ```
-
-## Lecture Video DB
-
-```{warning}
-This section is not fully tested yet.
-```
-
-```{note}
-The LV dataset has already provided cropped images and the corresponding annotations
-```
-
-- Step1: Download [IIIT-CVid.zip](http://cdn.iiit.ac.in/cdn/preon.iiit.ac.in/~kartik/IIIT-CVid.zip) to `lv/`.
-
- ```bash
- mkdir lv && cd lv
-
- # Download LV dataset
- wget http://cdn.iiit.ac.in/cdn/preon.iiit.ac.in/~kartik/IIIT-CVid.zip
- unzip -q IIIT-CVid.zip
-
- # For image
- mv IIIT-CVid/Crops ./
-
- # For annotation
- mv IIIT-CVid/train.txt train_labels.json && mv IIIT-CVid/val.txt val_label.txt && mv IIIT-CVid/test.txt test_labels.json
-
- rm IIIT-CVid.zip
- ```
-
-- Step2: Generate `train_labels.json`, `val.json`, and `test.json` with following command:
-
- ```bash
- python tools/dataset_converters/textdreog/lv_converter.py PATH/TO/lv
- ```
-
-- After running the above codes, the directory structure should be as follows:
-
- ```text
- โโโ lv
- โ โโโ Crops
- โ โโโ train_labels.json
- โ โโโ test_labels.json
- ```
-
-## LSVT
-
-```{warning}
-This section is not fully tested yet.
-```
-
-- Step1: Download [train_full_images_0.tar.gz](https://dataset-bj.cdn.bcebos.com/lsvt/train_full_images_0.tar.gz), [train_full_images_1.tar.gz](https://dataset-bj.cdn.bcebos.com/lsvt/train_full_images_1.tar.gz), and [train_full_labels.json](https://dataset-bj.cdn.bcebos.com/lsvt/train_full_labels.json) to `lsvt/`.
-
- ```bash
- mkdir lsvt && cd lsvt
-
- # Download LSVT dataset
- wget https://dataset-bj.cdn.bcebos.com/lsvt/train_full_images_0.tar.gz
- wget https://dataset-bj.cdn.bcebos.com/lsvt/train_full_images_1.tar.gz
- wget https://dataset-bj.cdn.bcebos.com/lsvt/train_full_labels.json
-
- mkdir annotations
- tar -xf train_full_images_0.tar.gz && tar -xf train_full_images_1.tar.gz
- mv train_full_labels.json annotations/ && mv train_full_images_1/*.jpg train_full_images_0/
- mv train_full_images_0 imgs
-
- rm train_full_images_0.tar.gz && rm train_full_images_1.tar.gz && rm -rf train_full_images_1
- ```
-
-- Step2: Generate `train_labels.json` and `val_label.json` (optional) with the following command:
-
- ```bash
- # Annotations of LSVT test split is not publicly available, split a validation
- # set by adding --val-ratio 0.2
- # Add --preserve-vertical to preserve vertical texts for training, otherwise
- # vertical images will be filtered and stored in PATH/TO/lsvt/ignores
- python tools/dataset_converters/textdrecog/lsvt_converter.py PATH/TO/lsvt --nproc 4
- ```
-
-- After running the above codes, the directory structure should be as follows:
-
- ```text
- โโโ lsvt
- โ โโโ crops
- โ โโโ ignores
- โ โโโ train_labels.json
- โ โโโ val_label.json (optional)
- ```
-
-## IMGUR
-
-```{warning}
-This section is not fully tested yet.
-```
-
-- Step1: Run `download_imgur5k.py` to download images. You can merge [PR#5](https://github.com/facebookresearch/IMGUR5K-Handwriting-Dataset/pull/5) in your local repository to enable a **much faster** parallel execution of image download.
-
- ```bash
- mkdir imgur && cd imgur
-
- git clone https://github.com/facebookresearch/IMGUR5K-Handwriting-Dataset.git
-
- # Download images from imgur.com. This may take SEVERAL HOURS!
- python ./IMGUR5K-Handwriting-Dataset/download_imgur5k.py --dataset_info_dir ./IMGUR5K-Handwriting-Dataset/dataset_info/ --output_dir ./imgs
-
- # For annotations
- mkdir annotations
- mv ./IMGUR5K-Handwriting-Dataset/dataset_info/*.json annotations
-
- rm -rf IMGUR5K-Handwriting-Dataset
- ```
-
-- Step2: Generate `train_labels.json`, `val_label.txt` and `test_labels.json` and crop images with the following command:
-
- ```bash
- python tools/dataset_converters/textrecog/imgur_converter.py PATH/TO/imgur
- ```
-
-- After running the above codes, the directory structure should be as follows:
-
- ```text
- โโโ imgur
- โ โโโ crops
- โ โโโ train_labels.json
- โ โโโ test_labels.json
- โ โโโ val_label.json
- ```
-
-## KAIST
-
-```{warning}
-This section is not fully tested yet.
-```
-
-- Step1: Download [KAIST_all.zip](http://www.iapr-tc11.org/mediawiki/index.php/KAIST_Scene_Text_Database) to `kaist/`.
-
- ```bash
- mkdir kaist && cd kaist
- mkdir imgs && mkdir annotations
-
- # Download KAIST dataset
- wget http://www.iapr-tc11.org/dataset/KAIST_SceneText/KAIST_all.zip
- unzip -q KAIST_all.zip && rm KAIST_all.zip
- ```
-
-- Step2: Extract zips:
-
- ```bash
- python tools/dataset_converters/common/extract_kaist.py PATH/TO/kaist
- ```
-
-- Step3: Generate `train_labels.json` and `val_label.json` (optional) with following command:
-
- ```bash
- # Since KAIST does not provide an official split, you can split the dataset by adding --val-ratio 0.2
- # Add --preserve-vertical to preserve vertical texts for training, otherwise
- # vertical images will be filtered and stored in PATH/TO/kaist/ignores
- python tools/dataset_converters/textrecog/kaist_converter.py PATH/TO/kaist --nproc 4
- ```
-
-- After running the above codes, the directory structure should be as follows:
-
- ```text
- โโโ kaist
- โ โโโ crops
- โ โโโ ignores
- โ โโโ train_labels.json
- โ โโโ val_label.json (optional)
- ```
-
-## MTWI
-
-```{warning}
-This section is not fully tested yet.
-```
-
-- Step1: Download `mtwi_2018_train.zip` from [homepage](https://tianchi.aliyun.com/competition/entrance/231685/information?lang=en-us).
-
- ```bash
- mkdir mtwi && cd mtwi
-
- unzip -q mtwi_2018_train.zip
- mv image_train imgs && mv txt_train annotations
-
- rm mtwi_2018_train.zip
- ```
-
-- Step2: Generate `train_labels.json` and `val_label.json` (optional) with the following command:
-
- ```bash
- # Annotations of MTWI test split is not publicly available, split a validation
- # set by adding --val-ratio 0.2
- # Add --preserve-vertical to preserve vertical texts for training, otherwise
- # vertical images will be filtered and stored in PATH/TO/mtwi/ignores
- python tools/dataset_converters/textrecog/mtwi_converter.py PATH/TO/mtwi --nproc 4
- ```
-
-- After running the above codes, the directory structure should be as follows:
-
- ```text
- โโโ mtwi
- โ โโโ crops
- โ โโโ train_labels.json
- โ โโโ val_label.json (optional)
- ```
-
-## ReCTS
-
-```{warning}
-This section is not fully tested yet.
-```
-
-- Step1: Download [ReCTS.zip](https://datasets.cvc.uab.es/rrc/ReCTS.zip) to `rects/` from the [homepage](https://rrc.cvc.uab.es/?ch=12&com=downloads).
-
- ```bash
- mkdir rects && cd rects
-
- # Download ReCTS dataset
- # You can also find Google Drive link on the dataset homepage
- wget https://datasets.cvc.uab.es/rrc/ReCTS.zip --no-check-certificate
- unzip -q ReCTS.zip
-
- mv img imgs && mv gt_unicode annotations
-
- rm ReCTS.zip -f && rm -rf gt
- ```
-
-- Step2: Generate `train_labels.json` and `val_label.json` (optional) with the following command:
-
- ```bash
- # Annotations of ReCTS test split is not publicly available, split a validation
- # set by adding --val-ratio 0.2
- # Add --preserve-vertical to preserve vertical texts for training, otherwise
- # vertical images will be filtered and stored in PATH/TO/rects/ignores
- python tools/dataset_converters/textrecog/rects_converter.py PATH/TO/rects --nproc 4
- ```
-
-- After running the above codes, the directory structure should be as follows:
-
- ```text
- โโโ rects
- โ โโโ crops
- โ โโโ ignores
- โ โโโ train_labels.json
- โ โโโ val_label.json (optional)
- ```
-
-## ILST
-
-```{warning}
-This section is not fully tested yet.
-```
-
-- Step1: Download `IIIT-ILST.zip` from [onedrive link](https://iiitaphyd-my.sharepoint.com/:f:/g/personal/minesh_mathew_research_iiit_ac_in/EtLvCozBgaBIoqglF4M-lHABMgNcCDW9rJYKKWpeSQEElQ?e=zToXZP)
-
-- Step2: Run the following commands
-
- ```bash
- unzip -q IIIT-ILST.zip && rm IIIT-ILST.zip
- cd IIIT-ILST
-
- # rename files
- cd Devanagari && for i in `ls`; do mv -f $i `echo "devanagari_"$i`; done && cd ..
- cd Malayalam && for i in `ls`; do mv -f $i `echo "malayalam_"$i`; done && cd ..
- cd Telugu && for i in `ls`; do mv -f $i `echo "telugu_"$i`; done && cd ..
-
- # transfer image path
- mkdir imgs && mkdir annotations
- mv Malayalam/{*jpg,*jpeg} imgs/ && mv Malayalam/*xml annotations/
- mv Devanagari/*jpg imgs/ && mv Devanagari/*xml annotations/
- mv Telugu/*jpeg imgs/ && mv Telugu/*xml annotations/
-
- # remove unnecessary files
- rm -rf Devanagari && rm -rf Malayalam && rm -rf Telugu && rm -rf README.txt
- ```
-
-- Step3: Generate `train_labels.json` and `val_label.json` (optional) and crop images using 4 processes with the following command (add `--preserve-vertical` if you wish to preserve the images containing vertical texts). Since the original dataset doesn't have a validation set, you may specify `--val-ratio` to split the dataset. E.g., if val-ratio is 0.2, then 20% of the data are left out as the validation set in this example.
-
- ```bash
- python tools/dataset_converters/textrecog/ilst_converter.py PATH/TO/IIIT-ILST --nproc 4
- ```
-
-- After running the above codes, the directory structure should be as follows:
-
- ```text
- โโโ IIIT-ILST
- โ โโโ crops
- โ โโโ ignores
- โ โโโ train_labels.json
- โ โโโ val_label.json (optional)
- ```
-
-## VinText
-
-```{warning}
-This section is not fully tested yet.
-```
-
-- Step1: Download [vintext.zip](https://drive.google.com/drive/my-drive) to `vintext`
-
- ```bash
- mkdir vintext && cd vintext
-
- # Download dataset from google drive
- wget --load-cookies /tmp/cookies.txt "https://docs.google.com/uc?export=download&confirm=$(wget --quiet --save-cookies /tmp/cookies.txt --keep-session-cookies --no-check-certificate 'https://docs.google.com/uc?export=download&id=1UUQhNvzgpZy7zXBFQp0Qox-BBjunZ0ml' -O- | sed -rn 's/.*confirm=([0-9A-Za-z_]+).*/\1\n/p')&id=1UUQhNvzgpZy7zXBFQp0Qox-BBjunZ0ml" -O vintext.zip && rm -rf /tmp/cookies.txt
-
- # Extract images and annotations
- unzip -q vintext.zip && rm vintext.zip
- mv vietnamese/labels ./ && mv vietnamese/test_image ./ && mv vietnamese/train_images ./ && mv vietnamese/unseen_test_images ./
- rm -rf vietnamese
-
- # Rename files
- mv labels annotations && mv test_image test && mv train_images training && mv unseen_test_images unseen_test
- mkdir imgs
- mv training imgs/ && mv test imgs/ && mv unseen_test imgs/
- ```
-
-- Step2: Generate `train_labels.json`, `test_labels.json`, `unseen_test_labels.json`, and crop images using 4 processes with the following command (add `--preserve-vertical` if you wish to preserve the images containing vertical texts).
-
- ```bash
- python tools/dataset_converters/textrecog/vintext_converter.py PATH/TO/vietnamese --nproc 4
- ```
-
-- After running the above codes, the directory structure should be as follows:
-
- ```text
- โโโ vintext
- โ โโโ crops
- โ โโโ ignores
- โ โโโ train_labels.json
- โ โโโ test_labels.json
- โ โโโ unseen_test_labels.json
- ```
-
-## BID
-
-```{warning}
-This section is not fully tested yet.
-```
-
-- Step1: Download [BID Dataset.zip](https://drive.google.com/file/d/1Oi88TRcpdjZmJ79WDLb9qFlBNG8q2De6/view)
-
-- Step2: Run the following commands to preprocess the dataset
-
- ```bash
- # Rename
- mv BID\ Dataset.zip BID_Dataset.zip
-
- # Unzip and Rename
- unzip -q BID_Dataset.zip && rm BID_Dataset.zip
- mv BID\ Dataset BID
-
- # The BID dataset has a problem of permission, and you may
- # add permission for this file
- chmod -R 777 BID
- cd BID
- mkdir imgs && mkdir annotations
-
- # For images and annotations
- mv CNH_Aberta/*in.jpg imgs && mv CNH_Aberta/*txt annotations && rm -rf CNH_Aberta
- mv CNH_Frente/*in.jpg imgs && mv CNH_Frente/*txt annotations && rm -rf CNH_Frente
- mv CNH_Verso/*in.jpg imgs && mv CNH_Verso/*txt annotations && rm -rf CNH_Verso
- mv CPF_Frente/*in.jpg imgs && mv CPF_Frente/*txt annotations && rm -rf CPF_Frente
- mv CPF_Verso/*in.jpg imgs && mv CPF_Verso/*txt annotations && rm -rf CPF_Verso
- mv RG_Aberto/*in.jpg imgs && mv RG_Aberto/*txt annotations && rm -rf RG_Aberto
- mv RG_Frente/*in.jpg imgs && mv RG_Frente/*txt annotations && rm -rf RG_Frente
- mv RG_Verso/*in.jpg imgs && mv RG_Verso/*txt annotations && rm -rf RG_Verso
-
- # Remove unnecessary files
- rm -rf desktop.ini
- ```
-
-- Step3: Generate `train_labels.json` and `val_label.json` (optional) and crop images using 4 processes with the following command (add `--preserve-vertical` if you wish to preserve the images containing vertical texts). Since the original dataset doesn't have a validation set, you may specify `--val-ratio` to split the dataset. E.g., if test-ratio is 0.2, then 20% of the data are left out as the validation set in this example.
-
- ```bash
- python tools/dataset_converters/textrecog/bid_converter.py PATH/TO/BID --nproc 4
- ```
-
-- After running the above codes, the directory structure should be as follows:
-
- ```text
- โโโ BID
- โ โโโ crops
- โ โโโ ignores
- โ โโโ train_labels.json
- โ โโโ val_label.json (optional)
- ```
-
-## RCTW
-
-```{warning}
-This section is not fully tested yet.
-```
-
-- Step1: Download `train_images.zip.001`, `train_images.zip.002`, and `train_gts.zip` from the [homepage](https://rctw.vlrlab.net/dataset.html), extract the zips to `rctw/imgs` and `rctw/annotations`, respectively.
-
-- Step2: Generate `train_labels.json` and `val_label.json` (optional). Since the original dataset doesn't have a validation set, you may specify `--val-ratio` to split the dataset. E.g., if val-ratio is 0.2, then 20% of the data are left out as the validation set in this example.
-
- ```bash
- # Annotations of RCTW test split is not publicly available, split a validation set by adding --val-ratio 0.2
- # Add --preserve-vertical to preserve vertical texts for training, otherwise vertical images will be filtered and stored in PATH/TO/rctw/ignores
- python tools/dataset_converters/textrecog/rctw_converter.py PATH/TO/rctw --nproc 4
- ```
-
-- After running the above codes, the directory structure should be as follows:
-
- ```text
- โโโ rctw
- โย ย โโโ crops
- โย ย โโโ ignores
- โย ย โโโ train_labels.json
- โย ย โโโ val_label.json (optional)
- ```
-
-## HierText
-
-```{warning}
-This section is not fully tested yet.
-```
-
-- Step1 (optional): Install [AWS CLI](https://mmocr.readthedocs.io/en/latest/datasets/recog.html#install-aws-cli-optional).
-
-- Step2: Clone [HierText](https://github.com/google-research-datasets/hiertext) repo to get annotations
-
- ```bash
- mkdir HierText
- git clone https://github.com/google-research-datasets/hiertext.git
- ```
-
-- Step3: Download `train.tgz`, `validation.tgz` from aws
-
- ```bash
- aws s3 --no-sign-request cp s3://open-images-dataset/ocr/train.tgz .
- aws s3 --no-sign-request cp s3://open-images-dataset/ocr/validation.tgz .
- ```
-
-- Step4: Process raw data
-
- ```bash
- # process annotations
- mv hiertext/gt ./
- rm -rf hiertext
- mv gt annotations
- gzip -d annotations/train.json.gz
- gzip -d annotations/validation.json.gz
- # process images
- mkdir imgs
- mv train.tgz imgs/
- mv validation.tgz imgs/
- tar -xzvf imgs/train.tgz
- tar -xzvf imgs/validation.tgz
- ```
-
-- Step5: Generate `train_labels.json` and `val_label.json`. HierText includes different levels of annotation, including `paragraph`, `line`, and `word`. Check the original [paper](https://arxiv.org/pdf/2203.15143.pdf) for details. E.g. set `--level paragraph` to get paragraph-level annotation. Set `--level line` to get line-level annotation. set `--level word` to get word-level annotation.
-
- ```bash
- # Collect word annotation from HierText --level word
- # Add --preserve-vertical to preserve vertical texts for training, otherwise vertical images will be filtered and stored in PATH/TO/HierText/ignores
- python tools/dataset_converters/textrecog/hiertext_converter.py PATH/TO/HierText --level word --nproc 4
- ```
-
-- After running the above codes, the directory structure should be as follows:
-
- ```text
- โโโ HierText
- โย ย โโโ crops
- โย ย โโโ ignores
- โย ย โโโ train_labels.json
- โย ย โโโ val_label.json
- ```
-
-## ArT
-
-```{warning}
-This section is not fully tested yet.
-```
-
-- Step1: Download `train_images.tar.gz`, and `train_labels.json` from the [homepage](https://rrc.cvc.uab.es/?ch=14&com=downloads) to `art/`
-
- ```bash
- mkdir art && cd art
- mkdir annotations
-
- # Download ArT dataset
- wget https://dataset-bj.cdn.bcebos.com/art/train_task2_images.tar.gz
- wget https://dataset-bj.cdn.bcebos.com/art/train_task2_labels.json
-
- # Extract
- tar -xf train_task2_images.tar.gz
- mv train_task2_images crops
- mv train_task2_labels.json annotations/
-
- # Remove unnecessary files
- rm train_images.tar.gz
- ```
-
-- Step2: Generate `train_labels.json` and `val_label.json` (optional). Since the test annotations are not publicly available, you may specify `--val-ratio` to split the dataset. E.g., if val-ratio is 0.2, then 20% of the data are left out as the validation set in this example.
-
- ```bash
- # Annotations of ArT test split is not publicly available, split a validation set by adding --val-ratio 0.2
- python tools/dataset_converters/textrecog/art_converter.py PATH/TO/art
- ```
-
-- After running the above codes, the directory structure should be as follows:
-
- ```text
- โโโ art
- โย ย โโโ crops
- โย ย โโโ train_labels.json
- โย ย โโโ val_label.json (optional)
- ```
diff --git a/pipeline/mmocr/docs/en/user_guides/dataset_prepare.md b/pipeline/mmocr/docs/en/user_guides/dataset_prepare.md
deleted file mode 100644
index 02c3ac0f914264754539918554e3708d35e05ace..0000000000000000000000000000000000000000
--- a/pipeline/mmocr/docs/en/user_guides/dataset_prepare.md
+++ /dev/null
@@ -1,153 +0,0 @@
-# Dataset Preparation
-
-## Introduction
-
-After decades of development, the OCR community has produced a series of related datasets that often provide annotations of text in a variety of styles, making it necessary for users to convert these datasets to the required format when using them. MMOCR supports dozens of commonly used text-related datasets and provides a [data preparation script](./data_prepare/dataset_preparer.md) to help users prepare the datasets with only one command.
-
-In this section, we will introduce a typical process of preparing a dataset for MMOCR:
-
-1. [Download datasets and convert its format to the suggested one](#downloading-datasets-and-converting-format)
-2. [Modify the config file](#dataset-configuration)
-
-However, the first step is not necessary if you already have a dataset in the format that MMOCR supports. You can read [Dataset Classes](../basic_concepts/datasets.md#dataset-classes-and-annotation-formats) for more details.
-
-## Downloading Datasets and Converting Format
-
-As an example of the data preparation steps, you can use the following command to prepare the ICDAR 2015 dataset for text detection task.
-
-```shell
-python tools/dataset_converters/prepare_dataset.py icdar2015 --task textdet
-```
-
-Then, the dataset has been downloaded and converted to MMOCR format, and the file directory structure is as follows:
-
-```text
-data/icdar2015
-โโโ textdet_imgs
-โ โโโ test
-โ โโโ train
-โโโ textdet_test.json
-โโโ textdet_train.json
-```
-
-Once your dataset has been prepared, you can use the [browse_dataset.py](./useful_tools.md#dataset-visualization-tool) to visualize the dataset and check if the annotations are correct.
-
-```bash
-python tools/analysis_tools/browse_dataset.py configs/textdet/_base_/datasets/icdar2015.py
-```
-
-## Dataset Configuration
-
-### Single Dataset Training
-
-When training or evaluating a model on new datasets, we need to write the dataset config where the image path, annotation path, and image prefix are set. The path `configs/xxx/_base_/datasets/` is pre-configured with the commonly used datasets in MMOCR (if you use `prepare_dataset.py` to prepare dataset, this config will be generated automatically), here we take the ICDAR 2015 dataset as an example (see `configs/textdet/_base_/datasets/icdar2015.py`).
-
-```Python
-icdar2015_textdet_data_root = 'data/icdar2015' # dataset root path
-
-# Train set config
-icdar2015_textdet_train = dict(
- type='OCRDataset',
- data_root=icdar2015_textdet_data_root, # dataset root path
- ann_file='textdet_train.json', # name of annotation
- filter_cfg=dict(filter_empty_gt=True, min_size=32), # filtering empty images
- pipeline=None)
-# Test set config
-icdar2015_textdet_test = dict(
- type='OCRDataset',
- data_root=icdar2015_textdet_data_root,
- ann_file='textdet_test.json',
- test_mode=True,
- pipeline=None)
-```
-
-After configuring the dataset, we can import it in the corresponding model configs. For example, to train the "DBNet_R18" model on the ICDAR 2015 dataset.
-
-```Python
-_base_ = [
- '_base_dbnet_r18_fpnc.py',
- '../_base_/datasets/icdar2015.py', # import the dataset config
- '../_base_/default_runtime.py',
- '../_base_/schedules/schedule_sgd_1200e.py',
-]
-
-icdar2015_textdet_train = _base_.icdar2015_textdet_train # specify the training set
-icdar2015_textdet_train.pipeline = _base_.train_pipeline # specify the training pipeline
-icdar2015_textdet_test = _base_.icdar2015_textdet_test # specify the testing set
-icdar2015_textdet_test.pipeline = _base_.test_pipeline # specify the testing pipeline
-
-train_dataloader = dict(
- batch_size=16,
- num_workers=8,
- persistent_workers=True,
- sampler=dict(type='DefaultSampler', shuffle=True),
- dataset=icdar2015_textdet_train) # specify the dataset in train_dataloader
-
-val_dataloader = dict(
- batch_size=1,
- num_workers=4,
- persistent_workers=True,
- sampler=dict(type='DefaultSampler', shuffle=False),
- dataset=icdar2015_textdet_test) # specify the dataset in val_dataloader
-
-test_dataloader = val_dataloader
-```
-
-### Multi-dataset Training
-
-In addition, [`ConcatDataset`](mmocr.datasets.ConcatDataset) enables users to train or test the model on a combination of multiple datasets. You just need to set the dataset type in the dataloader to `ConcatDataset` in the configuration file and specify the corresponding list of datasets.
-
-```Python
-train_list = [ic11, ic13, ic15]
-train_dataloader = dict(
- dataset=dict(
- type='ConcatDataset', datasets=train_list, pipeline=train_pipeline))
-```
-
-For example, the following configuration uses the MJSynth dataset for training and 6 academic datasets (CUTE80, IIIT5K, SVT, SVTP, ICDAR2013, ICDAR2015) for testing.
-
-```Python
-_base_ = [ # Import all dataset configurations you want to use
- '../_base_/datasets/mjsynth.py',
- '../_base_/datasets/cute80.py',
- '../_base_/datasets/iiit5k.py',
- '../_base_/datasets/svt.py',
- '../_base_/datasets/svtp.py',
- '../_base_/datasets/icdar2013.py',
- '../_base_/datasets/icdar2015.py',
- '../_base_/default_runtime.py',
- '../_base_/schedules/schedule_adadelta_5e.py',
- '_base_crnn_mini-vgg.py',
-]
-
-# List of training datasets
-train_list = [_base_.mjsynth_textrecog_train]
-# List of testing datasets
-test_list = [
- _base_.cute80_textrecog_test, _base_.iiit5k_textrecog_test, _base_.svt_textrecog_test,
- _base_.svtp_textrecog_test, _base_.icdar2013_textrecog_test, _base_.icdar2015_textrecog_test
-]
-
-# Use ConcatDataset to combine the datasets in the list
-train_dataset = dict(
- type='ConcatDataset', datasets=train_list, pipeline=_base_.train_pipeline)
-test_dataset = dict(
- type='ConcatDataset', datasets=test_list, pipeline=_base_.test_pipeline)
-
-train_dataloader = dict(
- batch_size=192 * 4,
- num_workers=32,
- persistent_workers=True,
- sampler=dict(type='DefaultSampler', shuffle=True),
- dataset=train_dataset)
-
-test_dataloader = dict(
- batch_size=1,
- num_workers=4,
- persistent_workers=True,
- drop_last=False,
- sampler=dict(type='DefaultSampler', shuffle=False),
- dataset=test_dataset)
-
-val_dataloader = test_dataloader
-```
diff --git a/pipeline/mmocr/docs/en/user_guides/inference.md b/pipeline/mmocr/docs/en/user_guides/inference.md
deleted file mode 100644
index 0687a327320017b9a4e268c8947644aa95aa4ed5..0000000000000000000000000000000000000000
--- a/pipeline/mmocr/docs/en/user_guides/inference.md
+++ /dev/null
@@ -1,538 +0,0 @@
-# Inference
-
-In OpenMMLab, all the inference operations are unified into a new interface - `Inferencer`. `Inferencer` is designed to expose a neat and simple API to users, and shares very similar interface across different OpenMMLab libraries.
-
-In MMOCR, Inferencers are constructed in different levels of task abstraction.
-
-- Standard Inferencer: Following OpenMMLab's convention, each fundamental task in MMOCR has a standard Inferencer, namely `TextDetInferencer` (text detection), `TextRecInferencer` (text recognition), `TextSpottingInferencer` (end-to-end OCR), and `KIEInferencer` (key information extraction). They are designed to perform inference on a single task, and can be chained together to perform inference on a series of tasks. They also share very similar interface, have standard input/output protocol, and overall follow the OpenMMLab design.
-- **MMOCRInferencer**: We also provide `MMOCRInferencer`, a convenient inference interface only designed for MMOCR. It encapsulates and chains all the Inferencers in MMOCR, so users can use this Inferencer to perform a series of tasks on an image and directly get the final result in an end-to-end manner. *However, it has a relatively different interface from other standard Inferencers, and some of standard Inferencer functionalities might be sacrificed for the sake of simplicity.*
-
-For new users, we recommend using **MMOCRInferencer** to test out different combinations of models.
-
-If you are a developer and wish to integrate the models into your own project, we recommend using **standard Inferencers**, as they are more flexible and standardized, equipped with full functionalities.
-
-## Basic Usage
-
-`````{tabs}
-
-````{group-tab} MMOCRInferencer
-
-As of now, `MMOCRInferencer` can perform inference on the following tasks:
-
-- Text detection
-- Text recognition
-- OCR (text detection + text recognition)
-- Key information extraction (text detection + text recognition + key information extraction)
-- *OCR (text spotting)* (coming soon)
-
-For convenience, `MMOCRInferencer` provides both Python and command line interfaces. For example, if you want to perform OCR inference on `demo/demo_text_ocr.jpg` with `DBNet` as the text detection model and `CRNN` as the text recognition model, you can simply run the following command:
-
-::::{tabs}
-
-:::{code-tab} python
->>> from mmocr.apis import MMOCRInferencer
->>> # Load models into memory
->>> ocr = MMOCRInferencer(det='DBNet', rec='SAR')
->>> # Perform inference
->>> ocr('demo/demo_text_ocr.jpg', show=True)
-:::
-
-:::{code-tab} bash
-python tools/infer.py demo/demo_text_ocr.jpg --det DBNet --rec SAR --show
-:::
-::::
-
-The resulting OCR output will be displayed in a new window:
-
-
-
-
-
-```{note}
-If you are running MMOCR on a server without GUI or via SSH tunnel with X11 forwarding disabled, the `show` option will not work. However, you can still save visualizations to files by setting `out_dir` and `save_vis=True` arguments. Read [Dumping Results](#dumping-results) for details.
-```
-
-Depending on the initialization arguments, `MMOCRInferencer` can run in different modes. For example, it can run in KIE mode if it is initialized with `det`, `rec` and `kie` specified.
-
-::::{tabs}
-
-:::{code-tab} python
->>> kie = MMOCRInferencer(det='DBNet', rec='SAR', kie='SDMGR')
->>> kie('demo/demo_kie.jpeg', show=True)
-:::
-
-:::{code-tab} bash
-python tools/infer.py demo/demo_kie.jpeg --det DBNet --rec SAR --kie SDMGR --show
-:::
-
-::::
-
-The output image should look like this:
-
-
-
-
-
-
-You may have found that the Python interface and the command line interface of `MMOCRInferencer` are very similar. The following sections will use the Python interface as an example to introduce the usage of `MMOCRInferencer`. For more information about the command line interface, please refer to [Command Line Interface](#command-line-interface).
-
-````
-
-````{group-tab} Standard Inferencer
-
-In general, all the standard Inferencers across OpenMMLab share a very similar interface. The following example shows how to use `TextDetInferencer` to perform inference on a single image.
-
-```python
->>> from mmocr.apis import TextDetInferencer
->>> # Load models into memory
->>> inferencer = TextDetInferencer(model='DBNet')
->>> # Inference
->>> inferencer('demo/demo_text_ocr.jpg', show=True)
-```
-
-The visualization result should look like:
-
-
-
-
-
-````
-
-`````
-
-## Initialization
-
-Each Inferencer must be initialized with a model. You can also choose the inference device during initialization.
-
-### Model Initialization
-
-`````{tabs}
-
-````{group-tab} MMOCRInferencer
-
-For each task, `MMOCRInferencer` takes two arguments in the form of `xxx` and `xxx_weights` (e.g. `det` and `det_weights`) for initialization, and there are many ways to initialize a model for inference. We will take `det` and `det_weights` as an example to illustrate some typical ways to initialize a model.
-
-- To infer with MMOCR's pre-trained model, passing its name to the argument `det` can work. The weights will be automatically downloaded and loaded from OpenMMLab's model zoo. Check [Weights](../modelzoo.md#weights) for available model names.
-
- ```python
- >>> MMOCRInferencer(det='DBNet')
- ```
-
-- To load custom config and weight, you can pass the path to the config file to `det` and the path to the weight to `det_weights`.
-
- ```python
- >>> MMOCRInferencer(det='path/to/dbnet_config.py', det_weights='path/to/dbnet.pth')
- ```
-
-You may click on the "Standard Inferencer" tab to find more initialization methods.
-
-````
-
-````{group-tab} Standard Inferencer
-
-Every standard `Inferencer` accepts two parameters, `model` and `weights`. (In `MMOCRInferencer`, they are referred to as `xxx` and `xxx_weights`)
-
-- `model` takes either the name of a model, or the path to a config file as input. The name of a model is obtained from the model's metafile ([Example](https://github.com/open-mmlab/mmocr/blob/1.x/configs/textdet/dbnet/metafile.yml)) indexed from [model-index.yml](https://github.com/open-mmlab/mmocr/blob/1.x/model-index.yml). You can find the list of available weights [here](../modelzoo.md#weights).
-
-- `weights` accepts the path to a weight file.
-
-
-
-There are various ways to initialize a model.
-
-- To infer with MMOCR's pre-trained model, you can pass its name to `model`. The weights will be automatically downloaded and loaded from OpenMMLab's model zoo.
-
- ```python
- >>> from mmocr.apis import TextDetInferencer
- >>> inferencer = TextDetInferencer(model='DBNet')
- ```
-
- ```{note}
- The model type must match the Inferencer type.
- ```
-
- You can load another weight by passing its path/url to `weights`.
-
- ```python
- >>> inferencer = TextDetInferencer(model='DBNet', weights='path/to/dbnet.pth')
- ```
-
-- To load custom config and weight, you can pass the path to the config file to `model` and the path to the weight to `weights`.
-
- ```python
- >>> inferencer = TextDetInferencer(model='path/to/dbnet_config.py', weights='path/to/dbnet.pth')
- ```
-
-- By default, [MMEngine](https://github.com/open-mmlab/mmengine/) dumps config to the weight. If you have a weight trained on MMEngine, you can also pass the path to the weight file to `weights` without specifying `model`:
-
- ```python
- >>> # It will raise an error if the config file cannot be found in the weight
- >>> inferencer = TextDetInferencer(weights='path/to/dbnet.pth')
- ```
-
-- Passing config file to `model` without specifying `weight` will result in a randomly initialized model.
-
-````
-`````
-
-### Device
-
-Each Inferencer instance is bound to a device.
-By default, the best device is automatically decided by [MMEngine](https://github.com/open-mmlab/mmengine/). You can also alter the device by specifying the `device` argument. For example, you can use the following code to create an Inferencer on GPU 1.
-
-`````{tabs}
-
-````{group-tab} MMOCRInferencer
-
-```python
->>> inferencer = MMOCRInferencer(det='DBNet', device='cuda:1')
-```
-
-````
-
-````{group-tab} Standard Inferencer
-
-```python
->>> inferencer = TextDetInferencer(model='DBNet', device='cuda:1')
-```
-
-````
-
-`````
-
-To create an Inferencer on CPU:
-
-`````{tabs}
-
-````{group-tab} MMOCRInferencer
-
-```python
->>> inferencer = MMOCRInferencer(det='DBNet', device='cpu')
-```
-
-````
-
-````{group-tab} Standard Inferencer
-
-```python
->>> inferencer = TextDetInferencer(model='DBNet', device='cpu')
-```
-
-````
-
-`````
-
-Refer to [torch.device](torch.device) for all the supported forms.
-
-## Inference
-
-Once the Inferencer is initialized, you can directly pass in the raw data to be inferred and get the inference results from return values.
-
-### Input
-
-`````{tabs}
-
-````{tab} MMOCRInferencer / TextDetInferencer / TextRecInferencer / TextSpottingInferencer
-
-Input can be either of these types:
-
-- str: Path/URL to the image.
-
- ```python
- >>> inferencer('demo/demo_text_ocr.jpg')
- ```
-
-- array: Image in numpy array. It should be in BGR order.
-
- ```python
- >>> import mmcv
- >>> array = mmcv.imread('demo/demo_text_ocr.jpg')
- >>> inferencer(array)
- ```
-
-- list: A list of basic types above. Each element in the list will be processed separately.
-
- ```python
- >>> inferencer(['img_1.jpg', 'img_2.jpg])
- >>> # You can even mix the types
- >>> inferencer(['img_1.jpg', array])
- ```
-
-- str: Path to the directory. All images in the directory will be processed.
-
- ```python
- >>> inferencer('tests/data/det_toy_dataset/imgs/test/')
- ```
-
-````
-
-````{tab} KIEInferencer
-
-Input can be a dict or list[dict], where each dictionary contains
-following keys:
-
-- `img` (str or ndarray): Path to the image or the image itself. If KIE Inferencer is used in no-visual mode, this key is not required.
-If it's an numpy array, it should be in BGR order.
-- `img_shape` (tuple(int, int)): Image shape in (H, W). Only required when KIE Inferencer is used in no-visual mode and no `img` is provided.
-- `instances` (list[dict]): A list of instances.
-
-Each `instance` looks like the following:
-
-```python
-{
- # A nested list of 4 numbers representing the bounding box of
- # the instance, in (x1, y1, x2, y2) order.
- "bbox": np.array([[x1, y1, x2, y2], [x1, y1, x2, y2], ...],
- dtype=np.int32),
-
- # List of texts.
- "texts": ['text1', 'text2', ...],
-}
-```
-
-````
-`````
-
-### Output
-
-By default, each `Inferencer` returns the prediction results in a dictionary format.
-
-- `visualization` contains the visualized predictions. But it's an empty list by default unless `return_vis=True`.
-
-- `predictions` contains the predictions results in a json-serializable format. As presented below, the contents are slightly different depending on the task type.
-
- `````{tabs}
-
- :::{group-tab} MMOCRInferencer
-
- ```python
- {
- 'predictions' : [
- # Each instance corresponds to an input image
- {
- 'det_polygons': [...], # 2d list of length (N,), format: [x1, y1, x2, y2, ...]
- 'det_scores': [...], # float list of length (N,)
- 'det_bboxes': [...], # 2d list of shape (N, 4), format: [min_x, min_y, max_x, max_y]
- 'rec_texts': [...], # str list of length (N,)
- 'rec_scores': [...], # float list of length (N,)
- 'kie_labels': [...], # node labels, length (N, )
- 'kie_scores': [...], # node scores, length (N, )
- 'kie_edge_scores': [...], # edge scores, shape (N, N)
- 'kie_edge_labels': [...] # edge labels, shape (N, N)
- },
- ...
- ],
- 'visualization' : [
- array(..., dtype=uint8),
- ]
- }
- ```
-
- :::
-
- :::{group-tab} Standard Inferencer
-
- ````{tabs}
- ```{code-tab} python TextDetInferencer
-
- {
- 'predictions' : [
- # Each instance corresponds to an input image
- {
- 'polygons': [...], # 2d list of len (N,) in the format of [x1, y1, x2, y2, ...]
- 'bboxes': [...], # 2d list of shape (N, 4), in the format of [min_x, min_y, max_x, max_y]
- 'scores': [...] # list of float, len (N, )
- },
- ]
- 'visualization' : [
- array(..., dtype=uint8),
- ]
- }
- ```
-
- ```{code-tab} python TextRecInferencer
- {
- 'predictions' : [
- # Each instance corresponds to an input image
- {
- 'text': '...', # a string
- 'scores': 0.1, # a float
- },
- ...
- ]
- 'visualization' : [
- array(..., dtype=uint8),
- ]
- }
- ```
-
- ```{code-tab} python TextSpottingInferencer
- {
- 'predictions' : [
- # Each instance corresponds to an input image
- {
- 'polygons': [...], # 2d list of len (N,) in the format of [x1, y1, x2, y2, ...]
- 'bboxes': [...], # 2d list of shape (N, 4), in the format of [min_x, min_y, max_x, max_y]
- 'scores': [...] # list of float, len (N, )
- 'texts': ['...',] # list of texts, len (N, )
- },
- ]
- 'visualization' : [
- array(..., dtype=uint8),
- ]
- }
- ```
-
- ```{code-tab} python KIEInferencer
- {
- 'predictions' : [
- # Each instance corresponds to an input image
- {
- 'labels': [...], # node label, len (N,)
- 'scores': [...], # node scores, len (N, )
- 'edge_scores': [...], # edge scores, shape (N, N)
- 'edge_labels': [...], # edge labels, shape (N, N)
- },
- ]
- 'visualization' : [
- array(..., dtype=uint8),
- ]
- }
- ```
- ````
-
- :::
-
- `````
-
-If you wish to get the raw outputs from the model, you can set `return_datasamples` to `True` to get the original [DataSample](structures.md), which will be stored in `predictions`.
-
-### Dumping Results
-
-Apart from obtaining predictions from the return value, you can also export the predictions/visualizations to files by setting `out_dir` and `save_pred`/`save_vis` arguments.
-
-```python
->>> inferencer('img_1.jpg', out_dir='outputs/', save_pred=True, save_vis=True)
-```
-
-Results in the directory structure like:
-
-```text
-outputs
-โโโ preds
-โ โโโ img_1.json
-โโโ vis
- โโโ img_1.jpg
-```
-
-The filename of each file is the same as the corresponding input image filename. If the input image is an array, the filename will be a number starting from 0.
-
-### Batch Inference
-
-You can customize the batch size by setting `batch_size`. The default batch size is 1.
-
-## API
-
-Here are extensive lists of parameters that you can use.
-
-````{tabs}
-
-```{group-tab} MMOCRInferencer
-
-**MMOCRInferencer.\_\_init\_\_():**
-
-| Arguments | Type | Default | Description |
-| ------------- | ---------------------------------------------------- | ------- | -------------------------------------------------------------------------------------------------------------------------------------------------------------------------------- |
-| `det` | str or [Weights](../modelzoo.html#weights), optional | None | Pretrained text detection algorithm. It's the path to the config file or the model name defined in metafile. |
-| `det_weights` | str, optional | None | Path to the custom checkpoint file of the selected det model. If it is not specified and "det" is a model name of metafile, the weights will be loaded from metafile. |
-| `rec` | str or [Weights](../modelzoo.html#weights), optional | None | Pretrained text recognition algorithm. Itโs the path to the config file or the model name defined in metafile. |
-| `rec_weights` | str, optional | None | Path to the custom checkpoint file of the selected rec model. If it is not specified and โrecโ is a model name of metafile, the weights will be loaded from metafile. |
-| `kie` \[1\] | str or [Weights](../modelzoo.html#weights), optional | None | Pretrained key information extraction algorithm. Itโs the path to the config file or the model name defined in metafile. |
-| `kie_weights` | str, optional | None | Path to the custom checkpoint file of the selected kie model. If it is not specified and โkieโ is a model name of metafile, the weights will be loaded from metafile. |
-| `device` | str, optional | None | Device used for inference, accepting all allowed strings by `torch.device`. E.g., 'cuda:0' or 'cpu'. If None, the available device will be automatically used. Defaults to None. |
-
-\[1\]: `kie` is only effective when both text detection and recognition models are specified.
-
-**MMOCRInferencer.\_\_call\_\_()**
-
-| Arguments | Type | Default | Description |
-| -------------------- | ----------------------- | ------------ | ------------------------------------------------------------------------------------------------ |
-| `inputs` | str/list/tuple/np.array | **required** | It can be a path to an image/a folder, an np array or a list/tuple (with img paths or np arrays) |
-| `return_datasamples` | bool | False | Whether to return results as DataSamples. If False, the results will be packed into a dict. |
-| `batch_size` | int | 1 | Inference batch size. |
-| `det_batch_size` | int, optional | None | Inference batch size for text detection model. Overwrite batch_size if it is not None. |
-| `rec_batch_size` | int, optional | None | Inference batch size for text recognition model. Overwrite batch_size if it is not None. |
-| `kie_batch_size` | int, optional | None | Inference batch size for KIE model. Overwrite batch_size if it is not None. |
-| `return_vis` | bool | False | Whether to return the visualization result. |
-| `print_result` | bool | False | Whether to print the inference result to the console. |
-| `show` | bool | False | Whether to display the visualization results in a popup window. |
-| `wait_time` | float | 0 | The interval of show(s). |
-| `out_dir` | str | `results/` | Output directory of results. |
-| `save_vis` | bool | False | Whether to save the visualization results to `out_dir`. |
-| `save_pred` | bool | False | Whether to save the inference results to `out_dir`. |
-
-```
-
-```{group-tab} Standard Inferencer
-
-**Inferencer.\_\_init\_\_():**
-
-| Arguments | Type | Default | Description |
-| --------- | ---------------------------------------------------- | ------- | -------------------------------------------------------------------------------------------------------------------------------------------------------------------------------- |
-| `model` | str or [Weights](../modelzoo.html#weights), optional | None | Path to the config file or the model name defined in metafile. |
-| `weights` | str, optional | None | Path to the custom checkpoint file of the selected det model. If it is not specified and "det" is a model name of metafile, the weights will be loaded from metafile. |
-| `device` | str, optional | None | Device used for inference, accepting all allowed strings by `torch.device`. E.g., 'cuda:0' or 'cpu'. If None, the available device will be automatically used. Defaults to None. |
-
-**Inferencer.\_\_call\_\_()**
-
-| Arguments | Type | Default | Description |
-| -------------------- | ----------------------- | ------------ | ---------------------------------------------------------------------------------------------------------------- |
-| `inputs` | str/list/tuple/np.array | **required** | It can be a path to an image/a folder, an np array or a list/tuple (with img paths or np arrays) |
-| `return_datasamples` | bool | False | Whether to return results as DataSamples. If False, the results will be packed into a dict. |
-| `batch_size` | int | 1 | Inference batch size. |
-| `progress_bar` | bool | True | Whether to show a progress bar. |
-| `return_vis` | bool | False | Whether to return the visualization result. |
-| `print_result` | bool | False | Whether to print the inference result to the console. |
-| `show` | bool | False | Whether to display the visualization results in a popup window. |
-| `wait_time` | float | 0 | The interval of show(s). |
-| `draw_pred` | bool | True | Whether to draw predicted bounding boxes. *Only applicable on `TextDetInferencer` and `TextSpottingInferencer`.* |
-| `out_dir` | str | `results/` | Output directory of results. |
-| `save_vis` | bool | False | Whether to save the visualization results to `out_dir`. |
-| `save_pred` | bool | False | Whether to save the inference results to `out_dir`. |
-
-```
-````
-
-## Command Line Interface
-
-```{note}
-This section is only applicable to `MMOCRInferencer`.
-```
-
-You can use `tools/infer.py` to perform inference through `MMOCRInferencer`.
-Its general usage is as follows:
-
-```bash
-python tools/infer.py INPUT_PATH [--det DET] [--det-weights ...] ...
-```
-
-where `INPUT_PATH` is a required field, which should be a path to an image or a folder. Command-line parameters follow the mapping relationship with the Python interface parameters as follows:
-
-- To convert the Python interface parameters to the command line ones, you need to add two `--` in front of the Python interface parameters, and replace the underscore `_` with the hyphen `-`. For example, `out_dir` becomes `--out-dir`.
-- For boolean type parameters, putting the parameter in the command is equivalent to specifying it as True. For example, `--show` will specify the `show` parameter as True.
-
-In addition, the command line will not display the inference result by default. You can use the `--print-result` parameter to view the inference result.
-
-Here is an example:
-
-```bash
-python tools/infer.py demo/demo_text_ocr.jpg --det DBNet --rec SAR --show --print-result
-```
-
-Running this command will give the following result:
-
-```bash
-{'predictions': [{'rec_texts': ['CBank', 'Docbcba', 'GROUP', 'MAUN', 'CROBINSONS', 'AOCOC', '916M3', 'BOO9', 'Oven', 'BRANDS', 'ARETAIL', '14', '70S', 'ROUND', 'SALE', 'YEAR', 'ALLY', 'SALE', 'SALE'],
-'rec_scores': [0.9753464579582214, ...], 'det_polygons': [[551.9930285844646, 411.9138765335083, 553.6153911653112,
-383.53195309638977, 620.2410061195247, 387.33785033226013, 618.6186435386782, 415.71977376937866], ...], 'det_scores': [0.8230461478233337, ...]}]}
-```
diff --git a/pipeline/mmocr/docs/en/user_guides/train_test.md b/pipeline/mmocr/docs/en/user_guides/train_test.md
deleted file mode 100644
index 0e825217f89017ea04ece03f170eef4f9c53a4bc..0000000000000000000000000000000000000000
--- a/pipeline/mmocr/docs/en/user_guides/train_test.md
+++ /dev/null
@@ -1,323 +0,0 @@
-# Training and Testing
-
-To meet diverse requirements, MMOCR supports training and testing models on various devices, including PCs, work stations, computation clusters, etc.
-
-## Single GPU Training and Testing
-
-### Training
-
-`tools/train.py` provides the basic training service. MMOCR recommends using GPUs for model training and testing, but it still enables CPU-Only training and testing. For example, the following commands demonstrate how to train a DBNet model using a single GPU or CPU.
-
-```bash
-# Train the specified MMOCR model by calling tools/train.py
-CUDA_VISIBLE_DEVICES= python tools/train.py ${CONFIG_FILE} [PY_ARGS]
-
-# Training
-# Example 1: Training DBNet with CPU
-CUDA_VISIBLE_DEVICES=-1 python tools/train.py configs/textdet/dbnet/dbnet_resnet50-dcnv2_fpnc_1200e_icdar2015.py
-
-# Example 2: Specify to train DBNet with gpu:0, specify the working directory as dbnet/, and turn on mixed precision (amp) training
-CUDA_VISIBLE_DEVICES=0 python tools/train.py configs/textdet/dbnet/dbnet_resnet50-dcnv2_fpnc_1200e_icdar2015.py --work-dir dbnet/ --amp
-```
-
-```{note}
-If multiple GPUs are available, you can specify a certain GPU, e.g. the third one, by setting CUDA_VISIBLE_DEVICES=3.
-```
-
-The following table lists all the arguments supported by `train.py`. Args without the `--` prefix are mandatory, while others are optional.
-
-| ARGS | Type | Description |
-| --------------- | ---- | --------------------------------------------------------------------------- |
-| config | str | (required) Path to config. |
-| --work-dir | str | Specify the working directory for the training logs and models checkpoints. |
-| --resume | bool | Whether to resume training from the latest checkpoint. |
-| --amp | bool | Whether to use automatic mixture precision for training. |
-| --auto-scale-lr | bool | Whether to use automatic learning rate scaling. |
-| --cfg-options | str | Override some settings in the configs. [Example](<>) |
-| --launcher | str | Option for launcher๏ผ\['none', 'pytorch', 'slurm', 'mpi'\]. |
-| --local_rank | int | Rank of local machine๏ผused for distributed training๏ผdefaults to 0ใ |
-| --tta | bool | Whether to use test time augmentation. |
-
-### Test
-
-`tools/test.py` provides the basic testing service, which is used in a similar way to the training script. For example, the following command demonstrates test a DBNet model on a single GPU or CPU.
-
-```bash
-# Test a pretrained MMOCR model by calling tools/test.py
-CUDA_VISIBLE_DEVICES= python tools/test.py ${CONFIG_FILE} ${CHECKPOINT_FILE} [PY_ARGS]
-
-# Test
-# Example 1: Testing DBNet with CPU
-CUDA_VISIBLE_DEVICES=-1 python tools/test.py configs/textdet/dbnet/dbnet_resnet50-dcnv2_fpnc_1200e_icdar2015.py dbnet_r50.pth
-
-# Example 2: Testing DBNet on gpu:0
-CUDA_VISIBLE_DEVICES=0 python tools/test.py configs/textdet/dbnet/dbnet_resnet50-dcnv2_fpnc_1200e_icdar2015.py dbnet_r50.pth
-```
-
-The following table lists all the arguments supported by `test.py`. Args without the `--` prefix are mandatory, while others are optional.
-
-| ARGS | Type | Description |
-| ------------- | ----- | -------------------------------------------------------------------- |
-| config | str | (required) Path to config. |
-| checkpoint | str | (required) The model to be tested. |
-| --work-dir | str | Specify the working directory for the logs. |
-| --save-preds | bool | Whether to save the predictions to a pkl file. |
-| --show | bool | Whether to visualize the predictions. |
-| --show-dir | str | Path to save the visualization results. |
-| --wait-time | float | Interval of visualization (s), defaults to 2. |
-| --cfg-options | str | Override some settings in the configs. [Example](<>) |
-| --launcher | str | Option for launcher๏ผ\['none', 'pytorch', 'slurm', 'mpi'\]. |
-| --local_rank | int | Rank of local machine๏ผused for distributed training๏ผdefaults to 0. |
-
-## Training and Testing with Multiple GPUs
-
-For large models, distributed training or testing significantly improves the efficiency. For this purpose, MMOCR provides distributed scripts `tools/dist_train.sh` and `tools/dist_test.sh` implemented based on [MMDistributedDataParallel](mmengine.model.wrappers.MMDistributedDataParallel).
-
-```bash
-# Training
-NNODES=${NNODES} NODE_RANK=${NODE_RANK} PORT=${MASTER_PORT} MASTER_ADDR=${MASTER_ADDR} ./tools/dist_train.sh ${CONFIG_FILE} ${GPU_NUM} [PY_ARGS]
-
-# Testing
-NNODES=${NNODES} NODE_RANK=${NODE_RANK} PORT=${MASTER_PORT} MASTER_ADDR=${MASTER_ADDR} ./tools/dist_test.sh ${CONFIG_FILE} ${CHECKPOINT_FILE} ${GPU_NUM} [PY_ARGS]
-```
-
-The following table lists the arguments supported by `dist_*.sh`.
-
-| ARGS | Type | Description |
-| --------------- | ---- | --------------------------------------------------------------------------------------------- |
-| NNODES | int | The number of nodes. Defaults to 1. |
-| NODE_RANK | int | The rank of current node. Defaults to 0. |
-| PORT | int | The master port that will be used by rank 0 node, ranging from 0 to 65535. Defaults to 29500. |
-| MASTER_ADDR | str | The address of rank 0 node. Defaults to "127.0.0.1". |
-| CONFIG_FILE | str | (required) The path to config. |
-| CHECKPOINT_FILE | str | (required๏ผonly used in dist_test.sh)The path to checkpoint to be tested. |
-| GPU_NUM | int | (required) The number of GPUs to be used per node. |
-| \[PY_ARGS\] | str | Arguments to be parsed by tools/train.py and tools/test.py. |
-
-These two scripts enable training and testing on **single-machine multi-GPU** or **multi-machine multi-GPU**. See the following example for usage.
-
-### Single-machine Multi-GPU
-
-The following commands demonstrate how to train and test with a specified number of GPUs on a **single machine** with multiple GPUs.
-
-1. **Training**
-
- Training DBNet using 4 GPUs on a single machine.
-
- ```bash
- tools/dist_train.sh configs/textdet/dbnet/dbnet_r50dcnv2_fpnc_1200e_icdar2015.py 4
- ```
-
-2. **Testing**
-
- Testing DBNet using 4 GPUs on a single machine.
-
- ```bash
- tools/dist_test.sh configs/textdet/dbnet/dbnet_r50dcnv2_fpnc_1200e_icdar2015.py dbnet_r50.pth 4
- ```
-
-### Launching Multiple Tasks on Single Machine
-
-For a workstation equipped with multiple GPUs, the user can launch multiple tasks simultaneously by specifying the GPU IDs. For example, the following command demonstrates how to test DBNet with GPU `[0, 1, 2, 3]` and train CRNN on GPU `[4, 5, 6, 7]`.
-
-```bash
-# Specify gpu:0,1,2,3 for testing and assign port number 29500
-CUDA_VISIBLE_DEVICES=0,1,2,3 PORT=29500 ./tools/dist_test.sh configs/textdet/dbnet/dbnet_r50dcnv2_fpnc_1200e_icdar2015.py dbnet_r50.pth 4
-
-# Specify gpu:4,5,6,7 for training and assign port number 29501
-CUDA_VISIBLE_DEVICES=4,5,6,7 PORT=29501 ./tools/dist_train.sh configs/textrecog/crnn/crnn_academic_dataset.py 4
-```
-
-```{note}
-`dist_train.sh` sets `MASTER_PORT` to `29500` by default. When other processes already occupy this port, the program will get a runtime error `RuntimeError: Address already in use`. In this case, you need to set `MASTER_PORT` to another free port number in the range of `(0~65535)`.
-```
-
-### Multi-machine Multi-GPU Training and Testing
-
-You can launch a task on multiple machines connected to the same network. MMOCR relies on `torch.distributed` package for distributed training. Find more information at PyTorchโs [launch utility](https://pytorch.org/docs/stable/distributed.html#launch-utility).
-
-1. **Training**
-
- The following command demonstrates how to train DBNet on two machines with a total of 4 GPUs.
-
- ```bash
- # Say that you want to launch the training job on two machines
- # On the first machine:
- NNODES=2 NODE_RANK=0 PORT=29500 MASTER_ADDR=10.140.0.169 tools/dist_train.sh configs/textdet/dbnet/dbnet_r50dcnv2_fpnc_1200e_icdar2015.py 2
- # On the second machine:
- NNODES=2 NODE_RANK=1 PORT=29501 MASTER_ADDR=10.140.0.169 tools/dist_train.sh configs/textdet/dbnet/dbnet_r50dcnv2_fpnc_1200e_icdar2015.py 2
- ```
-
-2. **Testing**
-
- The following command demonstrates how to test DBNet on two machines with a total of 4 GPUs.
-
- ```bash
- # Say that you want to launch the testing job on two machines
- # On the first machine:
- NNODES=2 NODE_RANK=0 PORT=29500 MASTER_ADDR=10.140.0.169 tools/dist_test.sh configs/textdet/dbnet/dbnet_r50dcnv2_fpnc_1200e_icdar2015.py dbnet_r50.pth 2
- # On the second machine:
- NNODES=2 NODE_RANK=1 PORT=29501 MASTER_ADDR=10.140.0.169 tools/dist_test.sh configs/textdet/dbnet/dbnet_r50dcnv2_fpnc_1200e_icdar2015.py dbnet_r50.pth 2
- ```
-
- ```{note}
- The speed of the network could be the bottleneck of training.
- ```
-
-## Training and Testing with Slurm Cluster
-
-If you run MMOCR on a cluster managed with [Slurm](https://slurm.schedmd.com/), you can use the script `tools/slurm_train.sh` and `tools/slurm_test.sh`.
-
-```bash
-# tools/slurm_train.sh provides scripts for submitting training tasks on clusters managed by the slurm
-GPUS=${GPUS} GPUS_PER_NODE=${GPUS_PER_NODE} CPUS_PER_TASK=${CPUS_PER_TASK} SRUN_ARGS=${SRUN_ARGS} ./tools/slurm_train.sh ${PARTITION} ${JOB_NAME} ${CONFIG_FILE} ${WORK_DIR} [PY_ARGS]
-
-# tools/slurm_test.sh provides scripts for submitting testing tasks on clusters managed by the slurm
-GPUS=${GPUS} GPUS_PER_NODE=${GPUS_PER_NODE} CPUS_PER_TASK=${CPUS_PER_TASK} SRUN_ARGS=${SRUN_ARGS} ./tools/slurm_test.sh ${PARTITION} ${JOB_NAME} ${CONFIG_FILE} ${CHECKPOINT_FILE} ${WORK_DIR} [PY_ARGS]
-```
-
-| ARGS | Type | Description |
-| --------------- | ---- | ----------------------------------------------------------------------------------------------------------- |
-| GPUS | int | The number of GPUs to be used by this task. Defaults to 8. |
-| GPUS_PER_NODE | int | The number of GPUs to be allocated per node. Defaults to 8. |
-| CPUS_PER_TASK | int | The number of CPUs to be allocated per task. Defaults to 5. |
-| SRUN_ARGS | str | Arguments to be parsed by srun. Available options can be found [here](https://slurm.schedmd.com/srun.html). |
-| PARTITION | str | (required) Specify the partition on cluster. |
-| JOB_NAME | str | (required) Name of the submitted job. |
-| WORK_DIR | str | (required) Specify the working directory for saving the logs and checkpoints. |
-| CHECKPOINT_FILE | str | (required๏ผonly used in slurm_test.sh)Path to the checkpoint to be tested. |
-| PY_ARGS | str | Arguments to be parsed by `tools/train.py` and `tools/test.py`. |
-
-These scripts enable training and testing on slurm clusters, see the following examples.
-
-1. Training
-
- Here is an example of using 1 GPU to train a DBNet model on the `dev` partition.
-
- ```bash
- # Example: Request 1 GPU resource on dev partition for DBNet training task
- GPUS=1 GPUS_PER_NODE=1 CPUS_PER_TASK=5 tools/slurm_train.sh dev db_r50 configs/textdet/dbnet/dbnet_r50dcnv2_fpnc_1200e_icdar2015.py work_dir
- ```
-
-2. Testing
-
- Similarly, the following example requests 1 GPU for testing.
-
- ```bash
- # Example: Request 1 GPU resource on dev partition for DBNet testing task
- GPUS=1 GPUS_PER_NODE=1 CPUS_PER_TASK=5 tools/slurm_test.sh dev db_r50 configs/textdet/dbnet/dbnet_r50dcnv2_fpnc_1200e_icdar2015.py dbnet_r50.pth work_dir
- ```
-
-## Advanced Tips
-
-### Resume Training from a Checkpoint
-
-`tools/train.py` allows users to resume training from a checkpoint by specifying the `--resume` parameter, where it will automatically resume training from the latest saved checkpoint.
-
-```bash
-# Example: Resuming training from the latest checkpoint
-python tools/train.py configs/textdet/dbnet/dbnet_r50dcnv2_fpnc_1200e_icdar2015.py 4 --resume
-```
-
-By default, the program will automatically resume training from the last successfully saved checkpoint in the last training session, i.e. `latest.pth`. However,
-
-```python
-# Example: Set the path of the checkpoint you want to load in the configuration file
-load_from = 'work_dir/dbnet/models/epoch_10000.pth'
-```
-
-### Mixed Precision Training
-
-Mixed precision training offers significant computational speedup by performing operations in half-precision format, while storing minimal information in single-precision to retain as much information as possible in critical parts of the network. In MMOCR, the users can enable the automatic mixed precision training by simply add `--amp`.
-
-```bash
-# Example: Using automatic mixed precision training
-python tools/train.py configs/textdet/dbnet/dbnet_r50dcnv2_fpnc_1200e_icdar2015.py 4 --amp
-```
-
-The following table shows the support of each algorithm in MMOCR for automatic mixed precision training.
-
-| | Whether support AMP | Description |
-| ------------- | :-----------------: | :-------------------------------------: |
-| | Text Detection | |
-| DBNet | Y | |
-| DBNetpp | Y | |
-| DRRG | N | roi_align_rotated does not support fp16 |
-| FCENet | N | BCELoss does not support fp16 |
-| Mask R-CNN | Y | |
-| PANet | Y | |
-| PSENet | Y | |
-| TextSnake | N | |
-| | Text Recognition | |
-| ABINet | Y | |
-| CRNN | Y | |
-| MASTER | Y | |
-| NRTR | Y | |
-| RobustScanner | Y | |
-| SAR | Y | |
-| SATRN | Y | |
-
-### Automatic Learning Rate Scaling
-
-MMOCR sets default initial learning rates for each model in the configuration file. However, these initial learning rates may not be applicable when the user uses a different `batch_size` than our preset `base_batch_size`. Therefore, we provide a tool to automatically scale the learning rate, which can be called by adding the `--auto-scale-lr`.
-
-```bash
-# Example: Using automatic learning rate scaling
-python tools/train.py configs/textdet/dbnet/dbnet_r50dcnv2_fpnc_1200e_icdar2015.py 4 --auto-scale-lr
-```
-
-### Visualize the Predictions
-
-`tools/test.py` provides the visualization interface to facilitate the qualitative analysis of the OCR models.
-
-
-
-
-
-(Green boxes are GTs, while red boxes are predictions)
-
-
-
-
-
-
-
-(Green font is the GT, red font is the prediction)
-
-
-
-
-
-
-
-(From left to right: original image, text detection and recognition result, text classification result, relationship)
-
-
-
-```bash
-# Example 1: Show the visualization results per 2 seconds
-python tools/test.py configs/textdet/dbnet/dbnet_r50dcnv2_fpnc_1200e_icdar2015.py dbnet_r50.pth --show --wait-time 2
-
-# Example 2: For systems that do not support graphical interfaces (such as computing clusters, etc.), the visualization results can be dumped in the specified path
-python tools/test.py configs/textdet/dbnet/dbnet_r50dcnv2_fpnc_1200e_icdar2015.py dbnet_r50.pth --show-dir ./vis_results
-```
-
-The visualization-related parameters in `tools/test.py` are described as follows.
-
-| ARGS | Type | Description |
-| ----------- | ----- | --------------------------------------------- |
-| --show | bool | Whether to show the visualization results. |
-| --show-dir | str | Path to save the visualization results. |
-| --wait-time | float | Interval of visualization (s), defaults to 2. |
-
-### Test Time Augmentation
-
-Test time augmentation (TTA) is a technique that is used to improve the performance of a model by performing data augmentation on the input image at test time. It is a simple yet effective method to improve the performance of a model. In MMOCR, we support TTA in the following ways:
-
-```{note}
-TTA is only supported for text recognition models.
-```
-
-```bash
-python tools/test.py configs/textrecog/crnn/crnn_mini-vgg_5e_mj.py checkpoints/crnn_mini-vgg_5e_mj.pth --tta
-```
diff --git a/pipeline/mmocr/docs/en/user_guides/useful_tools.md b/pipeline/mmocr/docs/en/user_guides/useful_tools.md
deleted file mode 100644
index 9828198f62fcd818946cfa19459b0d841d7cd4e4..0000000000000000000000000000000000000000
--- a/pipeline/mmocr/docs/en/user_guides/useful_tools.md
+++ /dev/null
@@ -1,241 +0,0 @@
-# Useful Tools
-
-## Visualization Tools
-
-### Dataset Visualization Tool
-
-MMOCR provides a dataset visualization tool `tools/visualizations/browse_datasets.py` to help users troubleshoot possible dataset-related problems. You just need to specify the path to the training config (usually stored in `configs/textdet/dbnet/xxx.py`) or the dataset config (usually stored in `configs/textdet/_base_/datasets/xxx.py`), and the tool will automatically plots the transformed (or original) images and labels.
-
-#### Usage
-
-```bash
-python tools/visualizations/browse_dataset.py \
- ${CONFIG_FILE} \
- [-o, --output-dir ${OUTPUT_DIR}] \
- [-p, --phase ${DATASET_PHASE}] \
- [-m, --mode ${DISPLAY_MODE}] \
- [-t, --task ${DATASET_TASK}] \
- [-n, --show-number ${NUMBER_IMAGES_DISPLAY}] \
- [-i, --show-interval ${SHOW_INTERRVAL}] \
- [--cfg-options ${CFG_OPTIONS}]
-```
-
-| ARGS | Type | Description |
-| ------------------- | ------------------------------------- | ------------------------------------------------------------------------------------------------------------------------------------------------ |
-| config | str | (required) Path to the config. |
-| -o, --output-dir | str | If GUI is not available, specifying an output path to save the visualization results. |
-| -p, --phase | str | Phase of dataset to visualize. Use "train", "test" or "val" if you just want to visualize the default split. It's also possible to be a dataset variable name, which might be useful when a dataset split has multiple variants in the config. |
-| -m, --mode | `original`, `transformed`, `pipeline` | Display mode: display original pictures or transformed pictures or comparison pictures.`original` only visualizes the original dataset & annotations; `transformed` shows the resulting images processed through all the transforms; `pipeline` shows all the intermediate images. Defaults to "transformed". |
-| -t, --task | `auto`, `textdet`, `textrecog` | Specify the task type of the dataset. If `auto`, the task type will be inferred from the config. If the script is unable to infer the task type, you need to specify it manually. Defaults to `auto`. |
-| -n, --show-number | int | The number of samples to visualized. If not specified, display all images in the dataset. |
-| -i, --show-interval | float | Interval of visualization (s), defaults to 2. |
-| --cfg-options | float | Override configs.[Example](./config.md#command-line-modification) |
-
-#### Examples
-
-The following example demonstrates how to use the tool to visualize the training data used by the "DBNet_R50_icdar2015" model.
-
-```Bash
-# Example: Visualizing the training data used by dbnet_r50dcn_v2_fpnc_1200e_icadr2015 model
-python tools/visualizations/browse_dataset.py configs/textdet/dbnet/dbnet_resnet50-dcnv2_fpnc_1200e_icdar2015.py
-```
-
-By default, the visualization mode is "transformed", and you will see the images & annotations being transformed by the pipeline:
-
-
-
-
-
-If you just want to visualize the original dataset, simply set the mode to "original":
-
-```Bash
-python tools/visualizations/browse_dataset.py configs/textdet/dbnet/dbnet_resnet50-dcnv2_fpnc_1200e_icdar2015.py -m original
-```
-
-
-
-Or, to visualize the entire pipeline:
-
-```Bash
-python tools/visualizations/browse_dataset.py configs/textdet/dbnet/dbnet_resnet50-dcnv2_fpnc_1200e_icdar2015.py -m pipeline
-```
-
-
-
-In addition, users can also visualize the original images and their corresponding labels of the dataset by specifying the path to the dataset config file, for example:
-
-```Bash
-python tools/visualizations/browse_dataset.py configs/textrecog/_base_/datasets/icdar2015.py
-```
-
-Some datasets might have multiple variants. For example, the test split of `icdar2015` textrecog dataset has two variants, which the [base dataset config](/configs/textrecog/_base_/datasets/icdar2015.py) defines as follows:
-
-```python
-icdar2015_textrecog_test = dict(
- ann_file='textrecog_test.json',
- # ...
- )
-
-icdar2015_1811_textrecog_test = dict(
- ann_file='textrecog_test_1811.json',
- # ...
-)
-```
-
-In this case, you can specify the variant name to visualize the corresponding dataset:
-
-```Bash
-python tools/visualizations/browse_dataset.py configs/textrecog/_base_/datasets/icdar2015.py -p icdar2015_1811_textrecog_test
-```
-
-Based on this tool, users can easily verify if the annotation of a custom dataset is correct.
-
-### Hyper-parameter Scheduler Visualization
-
-This tool aims to help the user to check the hyper-parameter scheduler of the optimizer (without training), which support the "learning rate" or "momentum"
-
-#### Introduce the scheduler visualization tool
-
-```bash
-python tools/visualizations/vis_scheduler.py \
- ${CONFIG_FILE} \
- [-p, --parameter ${PARAMETER_NAME}] \
- [-d, --dataset-size ${DATASET_SIZE}] \
- [-n, --ngpus ${NUM_GPUs}] \
- [-s, --save-path ${SAVE_PATH}] \
- [--title ${TITLE}] \
- [--style ${STYLE}] \
- [--window-size ${WINDOW_SIZE}] \
- [--cfg-options]
-```
-
-**Description of all arguments**๏ผ
-
-- `config`: The path of a model config file.
-- **`-p, --parameter`**: The param to visualize its change curve, choose from "lr" and "momentum". Default to use "lr".
-- **`-d, --dataset-size`**: The size of the datasets. If set๏ผ`build_dataset` will be skipped and `${DATASET_SIZE}` will be used as the size. Default to use the function `build_dataset`.
-- **`-n, --ngpus`**: The number of GPUs used in training, default to be 1.
-- **`-s, --save-path`**: The learning rate curve plot save path, default not to save.
-- `--title`: Title of figure. If not set, default to be config file name.
-- `--style`: Style of plt. If not set, default to be `whitegrid`.
-- `--window-size`: The shape of the display window. If not specified, it will be set to `12*7`. If used, it must be in the format `'W*H'`.
-- `--cfg-options`: Modifications to the configuration file, refer to [Learn about Configs](../user_guides/config.md).
-
-```{note}
-Loading annotations maybe consume much time, you can directly specify the size of the dataset with `-d, dataset-size` to save time.
-```
-
-#### How to plot the learning rate curve without training
-
-You can use the following command to plot the step learning rate schedule used in the config `configs/textdet/dbnet/dbnet_resnet50-dcnv2_fpnc_1200e_icdar2015.py`:
-
-```bash
-python tools/visualizations/vis_scheduler.py configs/textdet/dbnet/dbnet_resnet50-dcnv2_fpnc_1200e_icdar2015.py -d 100
-```
-
-
-
-## Analysis Tools
-
-### Offline Evaluation Tool
-
-For saved prediction results, we provide an offline evaluation script `tools/analysis_tools/offline_eval.py`. The following example demonstrates how to use this tool to evaluate the output of the "PSENet" model offline.
-
-```Bash
-# When running the test script for the first time, you can save the output of the model by specifying the --save-preds parameter
-python tools/test.py ${CONFIG_FILE} ${CHECKPOINT_FILE} --save-preds
-# Example: Testing on PSENet
-python tools/test.py configs/textdet/psenet/psenet_r50_fpnf_600e_icdar2015.py epoch_600.pth --save-preds
-
-# Then, using the saved outputs for offline evaluation
-python tools/analysis_tool/offline_eval.py ${CONFIG_FILE} ${PRED_FILE}
-# Example: Offline evaluation of saved PSENet results
-python tools/analysis_tools/offline_eval.py configs/textdet/psenet/psenet_r50_fpnf_600e_icdar2015.py work_dirs/psenet_r50_fpnf_600e_icdar2015/epoch_600.pth_predictions.pkl
-```
-
-`-save-preds` saves the output to `work_dir/CONFIG_NAME/MODEL_NAME_predictions.pkl` by default
-
-In addition, based on this tool, users can also convert predictions obtained from other libraries into MMOCR-supported formats, then use MMOCR's built-in metrics to evaluate them.
-
-| ARGS | Type | Description |
-| ------------- | ----- | ----------------------------------------------------------------- |
-| config | str | (required) Path to the config. |
-| pkl_results | str | (required) The saved predictions. |
-| --cfg-options | float | Override configs.[Example](./config.md#command-line-modification) |
-
-### Calculate FLOPs and the Number of Parameters
-
-We provide a method to calculate the FLOPs and the number of parameters, first we install the dependencies using the following command.
-
-```shell
-pip install fvcore
-```
-
-The usage of the script to calculate FLOPs and the number of parameters is as follows.
-
-```shell
-python tools/analysis_tools/get_flops.py ${config} --shape ${IMAGE_SHAPE}
-```
-
-| ARGS | Type | Description |
-| ------- | ---- | ----------------------------------------------------------------------------------------- |
-| config | str | (required) Path to the config. |
-| --shape | int | Image size to use when calculating FLOPs, such as `--shape 320 320`. Default is `640 640` |
-
-For example, you can run the following command to get FLOPs and the number of parameters of `dbnet_resnet18_fpnc_100k_synthtext.py`:
-
-```shell
-python tools/analysis_tools/get_flops.py configs/textdet/dbnet/dbnet_resnet18_fpnc_100k_synthtext.py --shape 1024 1024
-```
-
-The output is as follows:
-
-```shell
-input shape is (1, 3, 1024, 1024)
-| module | #parameters or shape | #flops |
-| :------------------------ | :------------------- | :------ |
-| model | 12.341M | 63.955G |
-| backbone | 11.177M | 38.159G |
-| backbone.conv1 | 9.408K | 2.466G |
-| backbone.conv1.weight | (64, 3, 7, 7) | |
-| backbone.bn1 | 0.128K | 83.886M |
-| backbone.bn1.weight | (64,) | |
-| backbone.bn1.bias | (64,) | |
-| backbone.layer1 | 0.148M | 9.748G |
-| backbone.layer1.0 | 73.984K | 4.874G |
-| backbone.layer1.1 | 73.984K | 4.874G |
-| backbone.layer2 | 0.526M | 8.642G |
-| backbone.layer2.0 | 0.23M | 3.79G |
-| backbone.layer2.1 | 0.295M | 4.853G |
-| backbone.layer3 | 2.1M | 8.616G |
-| backbone.layer3.0 | 0.919M | 3.774G |
-| backbone.layer3.1 | 1.181M | 4.842G |
-| backbone.layer4 | 8.394M | 8.603G |
-| backbone.layer4.0 | 3.673M | 3.766G |
-| backbone.layer4.1 | 4.721M | 4.837G |
-| neck | 0.836M | 14.887G |
-| neck.lateral_convs | 0.246M | 2.013G |
-| neck.lateral_convs.0.conv | 16.384K | 1.074G |
-| neck.lateral_convs.1.conv | 32.768K | 0.537G |
-| neck.lateral_convs.2.conv | 65.536K | 0.268G |
-| neck.lateral_convs.3.conv | 0.131M | 0.134G |
-| neck.smooth_convs | 0.59M | 12.835G |
-| neck.smooth_convs.0.conv | 0.147M | 9.664G |
-| neck.smooth_convs.1.conv | 0.147M | 2.416G |
-| neck.smooth_convs.2.conv | 0.147M | 0.604G |
-| neck.smooth_convs.3.conv | 0.147M | 0.151G |
-| det_head | 0.329M | 10.909G |
-| det_head.binarize | 0.164M | 10.909G |
-| det_head.binarize.0 | 0.147M | 9.664G |
-| det_head.binarize.1 | 0.128K | 20.972M |
-| det_head.binarize.3 | 16.448K | 1.074G |
-| det_head.binarize.4 | 0.128K | 83.886M |
-| det_head.binarize.6 | 0.257K | 67.109M |
-| det_head.threshold | 0.164M | |
-| det_head.threshold.0 | 0.147M | |
-| det_head.threshold.1 | 0.128K | |
-| det_head.threshold.3 | 16.448K | |
-| det_head.threshold.4 | 0.128K | |
-| det_head.threshold.6 | 0.257K | |
-!!!Please be cautious if you use the results in papers. You may need to check if all ops are supported and verify that the flops computation is correct.
-```
diff --git a/pipeline/mmocr/docs/en/user_guides/visualization.md b/pipeline/mmocr/docs/en/user_guides/visualization.md
deleted file mode 100644
index 2ce21cf30fb6798f198206fb41aed17fc61afe3d..0000000000000000000000000000000000000000
--- a/pipeline/mmocr/docs/en/user_guides/visualization.md
+++ /dev/null
@@ -1,107 +0,0 @@
-# Visualization
-
-Before reading this tutorial, it is recommended to read MMEngine's {external+mmengine:doc}`MMEngine: Visualization ` documentation to get a first glimpse of the `Visualizer` definition and usage.
-
-In brief, the [`Visualizer`](mmengine.visualization.Visualizer) is implemented in MMEngine to meet the daily visualization needs, and contains three main functions:
-
-- Implement common drawing APIs, such as [`draw_bboxes`](mmengine.visualization.Visualizer.draw_bboxes) which implements bounding box drawing functions, [`draw_lines`](mmengine.visualization.Visualizer.draw_lines) implements the line drawing function.
-- Support writing visualization results, learning rate curves, loss function curves, and verification accuracy curves to various backends, including local disks and common deep learning training logging tools such as [TensorBoard](https://www.tensorflow.org/tensorboard) and [Wandb](https://wandb.ai/site).
-- Support calling anywhere in the code to visualize or record intermediate states of the model during training or testing, such as feature maps and validation results.
-
-Based on MMEngine's Visualizer, MMOCR comes with a variety of pre-built visualization tools that can be used by the user by simply modifying the following configuration files.
-
-- The `tools/analysis_tools/browse_dataset.py` script provides a dataset visualization function that draws images and corresponding annotations after Data Transforms, as described in [`browse_dataset.py`](useful_tools.md).
-- MMEngine implements `LoggerHook`, which uses `Visualizer` to write the learning rate, loss and evaluation results to the backend set by `Visualizer`. Therefore, by modifying the `Visualizer` backend in the configuration file, for example to ` TensorBoardVISBackend` or `WandbVISBackend`, you can implement logging to common training logging tools such as `TensorBoard` or `WandB`, thus making it easy for users to use these visualization tools to analyze and monitor the training process.
-- The `VisualizerHook` is implemented in MMOCR, which uses the `Visualizer` to visualize or store the prediction results of the validation or prediction phase into the backend set by the `Visualizer`, so by modifying the `Visualizer` backend in the configuration file, for example, to ` TensorBoardVISBackend` or `WandbVISBackend`, you can implement storing the predicted images to `TensorBoard` or `Wandb`.
-
-## Configuration
-
-Thanks to the use of the registration mechanism, in MMOCR we can set the behavior of the `Visualizer` by modifying the configuration file. Usually, we define the default configuration for the visualizer in `task/_base_/default_runtime.py`, see [configuration tutorial](config.md) for details.
-
-```Python
-vis_backends = [dict(type='LocalVisBackend')]
-visualizer = dict(
- type='TextxxxLocalVisualizer', # use different visualizers for different tasks
- vis_backends=vis_backends,
- name='visualizer')
-```
-
-Based on the above example, we can see that the configuration of `Visualizer` consists of two main parts, namely, the type of `Visualizer` and the visualization backend `vis_backends` it uses.
-
-- For different OCR tasks, various visualizers are pre-configured in MMOCR, including [`TextDetLocalVisualizer`](mmocr.visualization.TextDetLocalVisualizer), [`TextRecogLocalVisualizer`](mmocr.visualization.TextRecogLocalVisualizer), [`TextSpottingLocalVisualizer`](mmocr.visualization.TextSpottingLocalVisualizer) and [`KIELocalVisualizer`](mmocr.visualization.KIELocalVisualizer). These visualizers extend the basic Visulizer API according to the characteristics of their tasks and implement the corresponding tag information interface `add_datasamples`. For example, users can directly use `TextDetLocalVisualizer` to visualize labels or predictions for text detection tasks.
-- MMOCR sets the visualization backend `vis_backend` to the local visualization backend `LocalVisBackend` by default, saving all visualization results and other training information in a local folder.
-
-## Storage
-
-MMOCR uses the local visualization backend [`LocalVisBackend`](mmengine.visualization.LocalVisBackend) by default, and the model loss, learning rate, model evaluation accuracy and visualization The information stored in `VisualizerHook` and `LoggerHook`, including loss, learning rate, evaluation accuracy will be saved to the `{work_dir}/{config_name}/{time}/{vis_data}` folder by default. In addition, MMOCR also supports other common visualization backends, such as `TensorboardVisBackend` and `WandbVisBackend`, and you only need to change the `vis_backends` type in the configuration file to the corresponding visualization backend. For example, you can store data to `TensorBoard` and `Wandb` by simply inserting the following code block into the configuration file.
-
-```Python
-_base_.visualizer.vis_backends = [
- dict(type='LocalVisBackend'),
- dict(type='TensorboardVisBackend'),
- dict(type='WandbVisBackend'),]
-```
-
-## Plot
-
-### Plot the prediction results
-
-MMOCR mainly uses [`VisualizationHook`](mmocr.engine.hooks.VisualizationHook) to plot the prediction results of validation and test, by default `VisualizationHook` is off, and the default configuration is as follows.
-
-```Python
-visualization=dict( # user visualization of validation and test results
- type='VisualizationHook',
- enable=False,
- interval=1,
- show=False,
- draw_gt=False,
- draw_pred=False)
-```
-
-The following table shows the parameters supported by `VisualizationHook`.
-
-| Parameters | Description |
-| :--------: | :-----------------------------------------------------------------------------------------------------------: |
-| enable | The VisualizationHook is turned on and off by the enable parameter, which is the default state. |
-| interval | Controls how much iteration to store or display the results of a val or test if VisualizationHook is enabled. |
-| show | Controls whether to visualize the results of val or test. |
-| draw_gt | Whether the results of val or test are drawn with or without labeling information |
-| draw_pred | whether to draw predictions for val or test results |
-
-If you want to enable `VisualizationHook` related functions and configurations during training or testing, you only need to modify the configuration, take `dbnet_resnet18_fpnc_1200e_icdar2015.py` as an example, draw annotations and predictions at the same time, and display the images, the configuration can be modified as follows
-
-```Python
-visualization = _base_.default_hooks.visualization
-visualization.update(
- dict(enable=True, show=True, draw_gt=True, draw_pred=True))
-```
-
-
-
-
-
-If you only want to see the predicted result information you can just let `draw_pred=True`
-
-```Python
-visualization = _base_.default_hooks.visualization
-visualization.update(
- dict(enable=True, show=True, draw_gt=False, draw_pred=True))
-```
-
-
-
-
-
-The `test.py` procedure is further simplified by providing the `--show` and `--show-dir` parameters to visualize the annotation and prediction results during the test without modifying the configuration.
-
-```Shell
-# Show test results
-python tools/test.py configs/textdet/dbnet/dbnet_resnet18_fpnc_1200e_icdar2015.py dbnet_r18_fpnc_1200e_icdar2015/epoch_400.pth --show
-
-# Specify where to store the prediction results
-python tools/test.py configs/textdet/dbnet/dbnet_resnet18_fpnc_1200e_icdar2015.py dbnet_r18_fpnc_1200e_icdar2015/epoch_400.pth --show-dir imgs/
-```
-
-
-
-
diff --git a/pipeline/mmocr/docs/en/weight_list.py b/pipeline/mmocr/docs/en/weight_list.py
deleted file mode 100644
index 0d7fee5678c4162cadf7ac7e2670821ec29205e6..0000000000000000000000000000000000000000
--- a/pipeline/mmocr/docs/en/weight_list.py
+++ /dev/null
@@ -1,115 +0,0 @@
-import os.path as osp
-
-from mmengine.fileio import load
-from tabulate import tabulate
-
-
-class BaseWeightList:
- """Class for generating model list in markdown format.
-
- Args:
- dataset_list (list[str]): List of dataset names.
- table_header (list[str]): List of table header.
- msg (str): Message to be displayed.
- task_abbr (str): Abbreviation of task name.
- metric_name (str): Metric name.
- """
-
- base_url: str = 'https://github.com/open-mmlab/mmocr/blob/1.x/'
- table_cfg: dict = dict(
- tablefmt='pipe', floatfmt='.2f', numalign='right', stralign='center')
- dataset_list: list
- table_header: list
- msg: str
- task_abbr: str
- metric_name: str
-
- def __init__(self):
- data = (d + f' ({self.metric_name})' for d in self.dataset_list)
- self.table_header = ['Model', 'README', *data]
-
- def _get_model_info(self, task_name: str):
- meta_indexes = load('../../model-index.yml')
- for meta_path in meta_indexes['Import']:
- meta_path = osp.join('../../', meta_path)
- metainfo = load(meta_path)
- collection2md = {}
- for item in metainfo['Collections']:
- url = self.base_url + item['README']
- collection2md[item['Name']] = f'[link]({url})'
- for item in metainfo['Models']:
- if task_name not in item['Config']:
- continue
- name = f'`{item["Name"]}`'
- if item.get('Alias', None):
- if isinstance(item['Alias'], str):
- item['Alias'] = [item['Alias']]
- aliases = [f'`{alias}`' for alias in item['Alias']]
- aliases.append(name)
- name = ' / '.join(aliases)
- readme = collection2md[item['In Collection']]
- eval_res = self._get_eval_res(item)
- yield (name, readme, *eval_res)
-
- def _get_eval_res(self, item):
- eval_res = {k: '-' for k in self.dataset_list}
- for res in item['Results']:
- if res['Dataset'] in self.dataset_list:
- eval_res[res['Dataset']] = res['Metrics'][self.metric_name]
- return (eval_res[k] for k in self.dataset_list)
-
- def gen_model_list(self):
- content = f'\n{self.msg}\n'
- content += '```{table}\n:class: model-summary nowrap field-list '
- content += 'table table-hover\n'
- content += tabulate(
- self._get_model_info(self.task_abbr), self.table_header,
- **self.table_cfg)
- content += '\n```\n'
- return content
-
-
-class TextDetWeightList(BaseWeightList):
-
- dataset_list = ['ICDAR2015', 'CTW1500', 'Totaltext']
- msg = '### Text Detection'
- task_abbr = 'textdet'
- metric_name = 'hmean-iou'
-
-
-class TextRecWeightList(BaseWeightList):
-
- dataset_list = [
- 'Avg', 'IIIT5K', 'SVT', 'ICDAR2013', 'ICDAR2015', 'SVTP', 'CT80'
- ]
- msg = ('### Text Recognition\n'
- '```{note}\n'
- 'Avg is the average on IIIT5K, SVT, ICDAR2013, ICDAR2015, SVTP,'
- ' CT80.\n```\n')
- task_abbr = 'textrecog'
- metric_name = 'word_acc'
-
- def _get_eval_res(self, item):
- eval_res = {k: '-' for k in self.dataset_list}
- avg = []
- for res in item['Results']:
- if res['Dataset'] in self.dataset_list:
- eval_res[res['Dataset']] = res['Metrics'][self.metric_name]
- avg.append(res['Metrics'][self.metric_name])
- eval_res['Avg'] = sum(avg) / len(avg)
- return (eval_res[k] for k in self.dataset_list)
-
-
-class KIEWeightList(BaseWeightList):
-
- dataset_list = ['wildreceipt']
- msg = '### Key Information Extraction'
- task_abbr = 'kie'
- metric_name = 'macro_f1'
-
-
-def gen_weight_list():
- content = TextDetWeightList().gen_model_list()
- content += TextRecWeightList().gen_model_list()
- content += KIEWeightList().gen_model_list()
- return content
diff --git a/pipeline/mmocr/docs/zh_cn/Makefile b/pipeline/mmocr/docs/zh_cn/Makefile
deleted file mode 100644
index d4bb2cbb9eddb1bb1b4f366623044af8e4830919..0000000000000000000000000000000000000000
--- a/pipeline/mmocr/docs/zh_cn/Makefile
+++ /dev/null
@@ -1,20 +0,0 @@
-# Minimal makefile for Sphinx documentation
-#
-
-# You can set these variables from the command line, and also
-# from the environment for the first two.
-SPHINXOPTS ?=
-SPHINXBUILD ?= sphinx-build
-SOURCEDIR = .
-BUILDDIR = _build
-
-# Put it first so that "make" without argument is like "make help".
-help:
- @$(SPHINXBUILD) -M help "$(SOURCEDIR)" "$(BUILDDIR)" $(SPHINXOPTS) $(O)
-
-.PHONY: help Makefile
-
-# Catch-all target: route all unknown targets to Sphinx using the new
-# "make mode" option. $(O) is meant as a shortcut for $(SPHINXOPTS).
-%: Makefile
- @$(SPHINXBUILD) -M $@ "$(SOURCEDIR)" "$(BUILDDIR)" $(SPHINXOPTS) $(O)
diff --git a/pipeline/mmocr/docs/zh_cn/_static/css/readthedocs.css b/pipeline/mmocr/docs/zh_cn/_static/css/readthedocs.css
deleted file mode 100644
index c4736f9dc728b2b0a49fd8e10d759c5d58e506d1..0000000000000000000000000000000000000000
--- a/pipeline/mmocr/docs/zh_cn/_static/css/readthedocs.css
+++ /dev/null
@@ -1,6 +0,0 @@
-.header-logo {
- background-image: url("../images/mmocr.png");
- background-size: 110px 40px;
- height: 40px;
- width: 110px;
-}
diff --git a/pipeline/mmocr/docs/zh_cn/_static/images/mmocr.png b/pipeline/mmocr/docs/zh_cn/_static/images/mmocr.png
deleted file mode 100644
index 363e34989e376b23b78ca4c31933542f15ec78ee..0000000000000000000000000000000000000000
Binary files a/pipeline/mmocr/docs/zh_cn/_static/images/mmocr.png and /dev/null differ
diff --git a/pipeline/mmocr/docs/zh_cn/_static/js/collapsed.js b/pipeline/mmocr/docs/zh_cn/_static/js/collapsed.js
deleted file mode 100644
index bedebadc0183105fe5c5978fb6e07d4afca2a149..0000000000000000000000000000000000000000
--- a/pipeline/mmocr/docs/zh_cn/_static/js/collapsed.js
+++ /dev/null
@@ -1 +0,0 @@
-var collapsedSections = ['MMOCR 0.x ่ฟ็งปๆๅ', 'API ๆๆกฃ']
diff --git a/pipeline/mmocr/docs/zh_cn/_static/js/table.js b/pipeline/mmocr/docs/zh_cn/_static/js/table.js
deleted file mode 100644
index 8dacf477f33e81bba3a0c0edc11b135f648b1f0a..0000000000000000000000000000000000000000
--- a/pipeline/mmocr/docs/zh_cn/_static/js/table.js
+++ /dev/null
@@ -1,31 +0,0 @@
-$(document).ready(function () {
- table = $('.model-summary').DataTable({
- "stateSave": false,
- "lengthChange": false,
- "pageLength": 10,
- "order": [],
- "scrollX": true,
- "columnDefs": [
- { "type": "summary", targets: '_all' },
- ]
- });
- // Override the default sorting for the summary columns, which
- // never takes the "-" character into account.
- jQuery.extend(jQuery.fn.dataTableExt.oSort, {
- "summary-asc": function (str1, str2) {
- if (str1 == "-
")
- return 1;
- if (str2 == "-
")
- return -1;
- return ((str1 < str2) ? -1 : ((str1 > str2) ? 1 : 0));
- },
-
- "summary-desc": function (str1, str2) {
- if (str1 == "-
")
- return 1;
- if (str2 == "-
")
- return -1;
- return ((str1 < str2) ? 1 : ((str1 > str2) ? -1 : 0));
- }
- });
-})
diff --git a/pipeline/mmocr/docs/zh_cn/_templates/classtemplate.rst b/pipeline/mmocr/docs/zh_cn/_templates/classtemplate.rst
deleted file mode 100644
index 4f74842394ec9807fb1ae2d8f05a8a57e9a2e24c..0000000000000000000000000000000000000000
--- a/pipeline/mmocr/docs/zh_cn/_templates/classtemplate.rst
+++ /dev/null
@@ -1,14 +0,0 @@
-.. role:: hidden
- :class: hidden-section
-.. currentmodule:: {{ module }}
-
-
-{{ name | underline}}
-
-.. autoclass:: {{ name }}
- :members:
-
-
-..
- autogenerated from source/_templates/classtemplate.rst
- note it does not have :inherited-members:
diff --git a/pipeline/mmocr/docs/zh_cn/api/apis.rst b/pipeline/mmocr/docs/zh_cn/api/apis.rst
deleted file mode 100644
index fcca6a24ea6147a55010e4f3d6a5141a36d2d295..0000000000000000000000000000000000000000
--- a/pipeline/mmocr/docs/zh_cn/api/apis.rst
+++ /dev/null
@@ -1,26 +0,0 @@
-.. role:: hidden
- :class: hidden-section
-
-mmocr.apis
-===================================
-
-.. contents:: mmocr.apis
- :depth: 2
- :local:
- :backlinks: top
-
-.. currentmodule:: mmocr.apis.inferencers
-
-Inferencers
----------------------------------------------
-
-.. autosummary::
- :toctree: generated
- :nosignatures:
- :template: classtemplate.rst
-
- MMOCRInferencer
- TextDetInferencer
- TextRecInferencer
- TextSpotInferencer
- KIEInferencer
diff --git a/pipeline/mmocr/docs/zh_cn/api/datasets.rst b/pipeline/mmocr/docs/zh_cn/api/datasets.rst
deleted file mode 100644
index 8b63debf9a88a68fe97930066c2e1b8090859854..0000000000000000000000000000000000000000
--- a/pipeline/mmocr/docs/zh_cn/api/datasets.rst
+++ /dev/null
@@ -1,57 +0,0 @@
-.. role:: hidden
- :class: hidden-section
-
-mmocr.datasets
-===================================
-
-.. contents:: mmocr.datasets
- :depth: 2
- :local:
- :backlinks: top
-
-.. currentmodule:: mmocr.datasets.samplers
-
-Samplers
----------------------------------------------
-
-.. autosummary::
- :toctree: generated
- :nosignatures:
- :template: classtemplate.rst
-
- BatchAugSampler
-
-.. currentmodule:: mmocr.datasets
-
-Datasets
----------------------------------------------
-
-.. autosummary::
- :toctree: generated
- :nosignatures:
- :template: classtemplate.rst
-
- OCRDataset
- WildReceiptDataset
-
-Compatible Datasets
----------------------------------------------
-
-.. autosummary::
- :toctree: generated
- :nosignatures:
- :template: classtemplate.rst
-
- IcdarDataset
- RecogLMDBDataset
- RecogTextDataset
-
-Dataset Wrapper
----------------------------------------------
-
-.. autosummary::
- :toctree: generated
- :nosignatures:
- :template: classtemplate.rst
-
- ConcatDataset
diff --git a/pipeline/mmocr/docs/zh_cn/api/engine.rst b/pipeline/mmocr/docs/zh_cn/api/engine.rst
deleted file mode 100644
index ecc8fec238acd8a4be2f2453502aac2b8e3cb7ff..0000000000000000000000000000000000000000
--- a/pipeline/mmocr/docs/zh_cn/api/engine.rst
+++ /dev/null
@@ -1,22 +0,0 @@
-.. role:: hidden
- :class: hidden-section
-
-mmocr.engine
-===================================
-
-.. contents:: mmocr.engine
- :depth: 2
- :local:
- :backlinks: top
-
-.. currentmodule:: mmocr.engine.hooks
-
-Hooks
----------------------------------------------
-
-.. autosummary::
- :toctree: generated
- :nosignatures:
- :template: classtemplate.rst
-
- VisualizationHook
diff --git a/pipeline/mmocr/docs/zh_cn/api/evaluation.rst b/pipeline/mmocr/docs/zh_cn/api/evaluation.rst
deleted file mode 100644
index d92d51a4ffbdeb6c7b13559e6a927682c1f246ef..0000000000000000000000000000000000000000
--- a/pipeline/mmocr/docs/zh_cn/api/evaluation.rst
+++ /dev/null
@@ -1,57 +0,0 @@
-.. role:: hidden
- :class: hidden-section
-
-mmocr.evaluation
-===================================
-
-.. contents:: mmocr.evaluation
- :depth: 2
- :local:
- :backlinks: top
-
-.. currentmodule:: mmocr.evaluation.evaluator
-
-Evaluator
----------------------------------------------
-
-.. autosummary::
- :toctree: generated
- :nosignatures:
- :template: classtemplate.rst
-
- MultiDatasetsEvaluator
-
-.. currentmodule:: mmocr.evaluation.metrics
-
-TextDet Metric
----------------------------------------------
-
-.. autosummary::
- :toctree: generated
- :nosignatures:
- :template: classtemplate.rst
-
- HmeanIOUMetric
-
-TextRecog Metric
----------------------------------------------
-
-.. autosummary::
- :toctree: generated
- :nosignatures:
- :template: classtemplate.rst
-
- WordMetric
- CharMetric
- OneMinusNEDMetric
-
-
-KIE Metric
----------------------------------------------
-
-.. autosummary::
- :toctree: generated
- :nosignatures:
- :template: classtemplate.rst
-
- F1Metric
diff --git a/pipeline/mmocr/docs/zh_cn/api/models.rst b/pipeline/mmocr/docs/zh_cn/api/models.rst
deleted file mode 100644
index 9ac53908debea467cc0a23ff6e6d71b670e2534e..0000000000000000000000000000000000000000
--- a/pipeline/mmocr/docs/zh_cn/api/models.rst
+++ /dev/null
@@ -1,423 +0,0 @@
-.. role:: hidden
- :class: hidden-section
-
-mmocr.models
-===================================
-
-- :mod:`~mmocr.models.common`
-
- - :ref:`commombackbones`
- - :ref:`commomdictionary`
- - :ref:`commomlayers`
- - :ref:`commomlosses`
- - :ref:`commommodules`
-
-- :mod:`~mmocr.models.textdet`
-
- - :ref:`detdetectors`
- - :ref:`detdatapreprocessors`
- - :ref:`detnecks`
- - :ref:`detheads`
- - :ref:`detmodulelosses`
- - :ref:`detpostprocessors`
-
-- :mod:`~mmocr.models.textrecog`
-
- - :ref:`recrecognizers`
- - :ref:`recdatapreprocessors`
- - :ref:`recpreprocessors`
- - :ref:`recencoders`
- - :ref:`recdecoders`
- - :ref:`recmodulelosses`
- - :ref:`recpostprocessors`
- - :ref:`reclayers`
-
-- :mod:`~mmocr.models.kie`
-
- - :ref:`kieextractors`
- - :ref:`kieheads`
- - :ref:`kiemodulelosses`
- - :ref:`kiepostprocessors`
-
-
-.. module:: mmocr.models.common
-models.common
----------------------------------------------
-.. currentmodule:: mmocr.models.common
-
-.. _commombackbones:
-
-BackBones
-^^^^^^^^^^^^^^^^^^^^^^^^^^^^^^^^^^^^^^^^^^^^^
-.. autosummary::
- :toctree: generated
- :nosignatures:
- :template: classtemplate.rst
-
- UNet
-
-.. _commomdictionary:
-
-Dictionary
-^^^^^^^^^^^^^^^^^^^^^^^^^^^^^^^^^^^^^^^^^^^^^
-.. autosummary::
- :toctree: generated
- :nosignatures:
- :template: classtemplate.rst
-
- Dictionary
-
-.. _commomlosses:
-
-Losses
-^^^^^^^^^^^^^^^^^^^^^^^^^^^^^^^^^^^^^^^^^^^^^
-.. autosummary::
- :toctree: generated
- :nosignatures:
- :template: classtemplate.rst
-
- MaskedBalancedBCEWithLogitsLoss
- MaskedDiceLoss
- MaskedSmoothL1Loss
- MaskedSquareDiceLoss
- MaskedBCEWithLogitsLoss
- SmoothL1Loss
- CrossEntropyLoss
- MaskedBalancedBCELoss
- MaskedBCELoss
-
-.. _commomlayers:
-
-Layers
-^^^^^^^^^^^^^^^^^^^^^^^^^^^^^^^^^^^^^^^^^^^^^
-.. autosummary::
- :toctree: generated
- :nosignatures:
- :template: classtemplate.rst
-
- TFEncoderLayer
- TFDecoderLayer
-
-.. _commommodules:
-
-Modules
-^^^^^^^^^^^^^^^^^^^^^^^^^^^^^^^^^^^^^^^^^^^^^
-.. autosummary::
- :toctree: generated
- :nosignatures:
- :template: classtemplate.rst
-
- ScaledDotProductAttention
- MultiHeadAttention
- PositionwiseFeedForward
- PositionalEncoding
-
-
-.. module:: mmocr.models.textdet
-models.textdet
----------------------------------------------
-.. currentmodule:: mmocr.models.textdet
-
-.. _detdetectors:
-
-Detectors
-^^^^^^^^^^^^^^^^^^^^^^^^^^^^^^^^^^^^^^^^^^^^^
-.. autosummary::
- :toctree: generated
- :nosignatures:
- :template: classtemplate.rst
-
- SingleStageTextDetector
- DBNet
- PANet
- PSENet
- TextSnake
- FCENet
- DRRG
- MMDetWrapper
-
-
-.. _detdatapreprocessors:
-
-Data Preprocessors
-^^^^^^^^^^^^^^^^^^^^^^^^^^^^^^^^^^^^^^^^^^^^^
-.. autosummary::
- :toctree: generated
- :nosignatures:
- :template: classtemplate.rst
-
- TextDetDataPreprocessor
-
-
-.. _detnecks:
-
-Necks
-^^^^^^^^^^^^^^^^^^^^^^^^^^^^^^^^^^^^^^^^^^^^^
-.. autosummary::
- :toctree: generated
- :nosignatures:
- :template: classtemplate.rst
-
- FPEM_FFM
- FPNF
- FPNC
- FPN_UNet
-
-
-.. _detheads:
-
-Heads
-^^^^^^^^^^^^^^^^^^^^^^^^^^^^^^^^^^^^^^^^^^^^^
-.. autosummary::
- :toctree: generated
- :nosignatures:
- :template: classtemplate.rst
-
- BaseTextDetHead
- PSEHead
- PANHead
- DBHead
- FCEHead
- TextSnakeHead
- DRRGHead
-
-
-.. _detmodulelosses:
-
-Module Losses
-^^^^^^^^^^^^^^^^^^^^^^^^^^^^^^^^^^^^^^^^^^^^^
-.. autosummary::
- :toctree: generated
- :nosignatures:
- :template: classtemplate.rst
-
- SegBasedModuleLoss
- PANModuleLoss
- PSEModuleLoss
- DBModuleLoss
- TextSnakeModuleLoss
- FCEModuleLoss
- DRRGModuleLoss
-
-
-.. _detpostprocessors:
-
-Postprocessors
-^^^^^^^^^^^^^^^^^^^^^^^^^^^^^^^^^^^^^^^^^^^^^
-.. autosummary::
- :toctree: generated
- :nosignatures:
- :template: classtemplate.rst
-
- BaseTextDetPostProcessor
- PSEPostprocessor
- PANPostprocessor
- DBPostprocessor
- DRRGPostprocessor
- FCEPostprocessor
- TextSnakePostprocessor
-
-
-
-.. module:: mmocr.models.textrecog
-models.textrecog
----------------------------------------------
-.. currentmodule:: mmocr.models.textrecog
-
-.. _recrecognizers:
-
-
-Recognizers
-^^^^^^^^^^^^^^^^^^^^^^^^^^^^^^^^^^^^^^^^^^^^^
-
-.. autosummary::
- :toctree: generated
- :nosignatures:
- :template: classtemplate.rst
-
- BaseRecognizer
- EncoderDecoderRecognizer
- CRNN
- SARNet
- NRTR
- RobustScanner
- SATRN
- ABINet
- MASTER
- ASTER
-
-.. _recdatapreprocessors:
-
-Data Preprocessors
-^^^^^^^^^^^^^^^^^^^^^^^^^^^^^^^^^^^^^^^^^^^^^
-.. autosummary::
- :toctree: generated
- :nosignatures:
- :template: classtemplate.rst
-
- TextRecogDataPreprocessor
-
-.. _recpreprocessors:
-
-Preprocessors
-^^^^^^^^^^^^^^^^^^^^^^^^^^^^^^^^^^^^^^^^^^^^^
-.. autosummary::
- :toctree: generated
- :nosignatures:
- :template: classtemplate.rst
-
- STN
-
-.. _recbackbones:
-
-BackBones
-^^^^^^^^^^^^^^^^^^^^^^^^^^^^^^^^^^^^^^^^^^^^^
-.. autosummary::
- :toctree: generated
- :nosignatures:
- :template: classtemplate.rst
-
- ResNet31OCR
- MiniVGG
- NRTRModalityTransform
- ShallowCNN
- ResNetABI
- ResNet
- MobileNetV2
-
-
-.. _recencoders:
-
-Encoders
-^^^^^^^^^^^^^^^^^^^^^^^^^^^^^^^^^^^^^^^^^^^^^
-.. autosummary::
- :toctree: generated
- :nosignatures:
- :template: classtemplate.rst
-
- SAREncoder
- NRTREncoder
- BaseEncoder
- ChannelReductionEncoder
- SATRNEncoder
- ABIEncoder
- ASTEREncoder
-
-.. _recdecoders:
-
-Decoders
-^^^^^^^^^^^^^^^^^^^^^^^^^^^^^^^^^^^^^^^^^^^^^
-.. autosummary::
- :toctree: generated
- :nosignatures:
- :template: classtemplate.rst
-
- BaseDecoder
- ABILanguageDecoder
- ABIVisionDecoder
- ABIFuser
- CRNNDecoder
- ParallelSARDecoder
- SequentialSARDecoder
- ParallelSARDecoderWithBS
- NRTRDecoder
- SequenceAttentionDecoder
- PositionAttentionDecoder
- RobustScannerFuser
- MasterDecoder
- ASTERDecoder
-
-.. _recmodulelosses:
-
-Module Losses
-^^^^^^^^^^^^^^^^^^^^^^^^^^^^^^^^^^^^^^^^^^^^^
-.. autosummary::
- :toctree: generated
- :nosignatures:
- :template: classtemplate.rst
-
- BaseTextRecogModuleLoss
- CEModuleLoss
- CTCModuleLoss
- ABIModuleLoss
-
-.. _recpostprocessors:
-
-Postprocessors
-^^^^^^^^^^^^^^^^^^^^^^^^^^^^^^^^^^^^^^^^^^^^^
-.. autosummary::
- :toctree: generated
- :nosignatures:
- :template: classtemplate.rst
-
- BaseTextRecogPostprocessor
- AttentionPostprocessor
- CTCPostProcessor
-
-.. _reclayers:
-
-Layers
-^^^^^^^^^^^^^^^^^^^^^^^^^^^^^^^^^^^^^^^^^^^^^
-.. autosummary::
- :toctree: generated
- :nosignatures:
- :template: classtemplate.rst
-
- BidirectionalLSTM
- Adaptive2DPositionalEncoding
- BasicBlock
- Bottleneck
- RobustScannerFusionLayer
- DotProductAttentionLayer
- PositionAwareLayer
- SATRNEncoderLayer
-
-
-.. module:: mmocr.models.kie
-models.kie
----------------------------------------------
-.. currentmodule:: mmocr.models.kie
-
-.. _kieextractors:
-
-Extractors
-^^^^^^^^^^^^^^^^^^^^^^^^^^^^^^^^^^^^^^^^^^^^^
-.. autosummary::
- :toctree: generated
- :nosignatures:
- :template: classtemplate.rst
-
- SDMGR
-
-.. _kieheads:
-
-Heads
-^^^^^^^^^^^^^^^^^^^^^^^^^^^^^^^^^^^^^^^^^^^^^
-.. autosummary::
- :toctree: generated
- :nosignatures:
- :template: classtemplate.rst
-
- SDMGRHead
-
-.. _kiemodulelosses:
-
-Module Losses
-^^^^^^^^^^^^^^^^^^^^^^^^^^^^^^^^^^^^^^^^^^^^^
-.. autosummary::
- :toctree: generated
- :nosignatures:
- :template: classtemplate.rst
-
- SDMGRModuleLoss
-
-.. _kiepostprocessors:
-
-Postprocessors
-^^^^^^^^^^^^^^^^^^^^^^^^^^^^^^^^^^^^^^^^^^^^^
-.. autosummary::
- :toctree: generated
- :nosignatures:
- :template: classtemplate.rst
-
- SDMGRPostProcessor
diff --git a/pipeline/mmocr/docs/zh_cn/api/structures.rst b/pipeline/mmocr/docs/zh_cn/api/structures.rst
deleted file mode 100644
index 920c4d440d65861b380427c8d077936b49515d00..0000000000000000000000000000000000000000
--- a/pipeline/mmocr/docs/zh_cn/api/structures.rst
+++ /dev/null
@@ -1,15 +0,0 @@
-.. role:: hidden
- :class: hidden-section
-
-mmocr.structures
-===================================
-
-.. currentmodule:: mmocr.structures
-.. autosummary::
- :toctree: generated
- :nosignatures:
- :template: classtemplate.rst
-
- TextDetDataSample
- TextRecogDataSample
- KIEDataSample
diff --git a/pipeline/mmocr/docs/zh_cn/api/transforms.rst b/pipeline/mmocr/docs/zh_cn/api/transforms.rst
deleted file mode 100644
index 86f2848d6e9fd31b3b23393841200e576ee89954..0000000000000000000000000000000000000000
--- a/pipeline/mmocr/docs/zh_cn/api/transforms.rst
+++ /dev/null
@@ -1,110 +0,0 @@
-.. role:: hidden
- :class: hidden-section
-
-mmocr.datasets
-===================================
-
-.. contents:: mmocr.datasets.transforms
- :depth: 2
- :local:
- :backlinks: top
-
-.. currentmodule:: mmocr.datasets.transforms
-
-Loading
----------------------------------------------
-
-.. autosummary::
- :toctree: generated
- :nosignatures:
- :template: classtemplate.rst
-
- LoadImageFromFile
- LoadOCRAnnotations
- LoadKIEAnnotations
-
-
-TextDet Transforms
----------------------------------------------
-
-.. autosummary::
- :toctree: generated
- :nosignatures:
- :template: classtemplate.rst
-
- BoundedScaleAspectJitter
- RandomFlip
- SourceImagePad
- ShortScaleAspectJitter
- TextDetRandomCrop
- TextDetRandomCropFlip
-
-
-TextRecog Transforms
----------------------------------------------
-
-.. autosummary::
- :toctree: generated
- :nosignatures:
- :template: classtemplate.rst
-
- TextRecogGeneralAug
- CropHeight
- ImageContentJitter
- ReversePixels
- PyramidRescale
- PadToWidth
- RescaleToHeight
-
-
-OCR Transforms
----------------------------------------------
-
-.. autosummary::
- :toctree: generated
- :nosignatures:
- :template: classtemplate.rst
-
- RandomCrop
- RandomRotate
- Resize
- FixInvalidPolygon
- RemoveIgnored
-
-
-
-Formatting
----------------------------------------------
-
-.. autosummary::
- :toctree: generated
- :nosignatures:
- :template: classtemplate.rst
-
- PackTextDetInputs
- PackTextRecogInputs
- PackKIEInputs
-
-
-Transform Wrapper
----------------------------------------------
-
-.. autosummary::
- :toctree: generated
- :nosignatures:
- :template: classtemplate.rst
-
- ImgAugWrapper
- TorchVisionWrapper
-
-
-Adapter
----------------------------------------------
-
-.. autosummary::
- :toctree: generated
- :nosignatures:
- :template: classtemplate.rst
-
- MMDet2MMOCR
- MMOCR2MMDet
diff --git a/pipeline/mmocr/docs/zh_cn/api/utils.rst b/pipeline/mmocr/docs/zh_cn/api/utils.rst
deleted file mode 100644
index 06cc5c64383e094d5ea9d5edbc955a4f4acbfc4d..0000000000000000000000000000000000000000
--- a/pipeline/mmocr/docs/zh_cn/api/utils.rst
+++ /dev/null
@@ -1,101 +0,0 @@
-.. role:: hidden
- :class: hidden-section
-
-mmocr.utils
-===================================
-
-.. contents:: mmocr.utils
- :depth: 2
- :local:
- :backlinks: top
-
-.. currentmodule:: mmocr.utils
-
-Image Utils
----------------------------------------------
-.. autosummary::
- :toctree: generated
- :nosignatures:
- crop_img
- warp_img
-
-
-Box Utils
----------------------------------------------
-.. autosummary::
- :toctree: generated
- :nosignatures:
-
- bbox2poly
- bbox_center_distance
- bbox_diag_distance
- bezier2polygon
- is_on_same_line
- rescale_bboxes
-
- stitch_boxes_into_lines
-
-
-Point Utils
----------------------------------------------
-.. autosummary::
- :toctree: generated
- :nosignatures:
-
- point_distance
- points_center
-
-Polygon Utils
----------------------------------------------
-.. autosummary::
- :toctree: generated
- :nosignatures:
-
- boundary_iou
- crop_polygon
- is_poly_inside_rect
- offset_polygon
- poly2bbox
- poly2shapely
- poly_intersection
- poly_iou
- poly_make_valid
- poly_union
- polys2shapely
- rescale_polygon
- rescale_polygons
- shapely2poly
- sort_points
- sort_vertex
- sort_vertex8
-
-
-Mask Utils
----------------------------------------------
-.. autosummary::
- :toctree: generated
- :nosignatures:
-
- fill_hole
-
-
-Misc Utils
----------------------------------------------
-.. autosummary::
- :toctree: generated
- :nosignatures:
-
- equal_len
- is_2dlist
- is_3dlist
- is_none_or_type
- is_type_list
-
-
-Setup Env
----------------------------------------------
-.. autosummary::
- :toctree: generated
- :nosignatures:
-
- register_all_modules
diff --git a/pipeline/mmocr/docs/zh_cn/api/visualization.rst b/pipeline/mmocr/docs/zh_cn/api/visualization.rst
deleted file mode 100644
index e48469226cd9dac973ea7d5739af78bbe98e1992..0000000000000000000000000000000000000000
--- a/pipeline/mmocr/docs/zh_cn/api/visualization.rst
+++ /dev/null
@@ -1,18 +0,0 @@
-.. role:: hidden
- :class: hidden-section
-
-mmocr.visualization
-===================================
-
-.. currentmodule:: mmocr.visualization
-
-.. autosummary::
- :toctree: generated
- :nosignatures:
- :template: classtemplate.rst
-
- BaseLocalVisualizer
- TextDetLocalVisualizer
- TextRecogLocalVisualizer
- TextSpottingLocalVisualizer
- KIELocalVisualizer
diff --git a/pipeline/mmocr/docs/zh_cn/basic_concepts/convention.md b/pipeline/mmocr/docs/zh_cn/basic_concepts/convention.md
deleted file mode 100644
index a094beccac59874fb4299d27663548329190d1ee..0000000000000000000000000000000000000000
--- a/pipeline/mmocr/docs/zh_cn/basic_concepts/convention.md
+++ /dev/null
@@ -1,3 +0,0 @@
-# ๅผๅ้ป่ฎค็บฆๅฎ\[ๅพ
ๆดๆฐ\]
-
-ๅพ
ๆดๆฐ
diff --git a/pipeline/mmocr/docs/zh_cn/basic_concepts/data_flow.md b/pipeline/mmocr/docs/zh_cn/basic_concepts/data_flow.md
deleted file mode 100644
index a07a158b177e6ae5de9d57ed12a2382c379a8edc..0000000000000000000000000000000000000000
--- a/pipeline/mmocr/docs/zh_cn/basic_concepts/data_flow.md
+++ /dev/null
@@ -1,3 +0,0 @@
-# ๆฐๆฎๆต\[ๅพ
ๆดๆฐ\]
-
-ๅพ
ๆดๆฐ
diff --git a/pipeline/mmocr/docs/zh_cn/basic_concepts/datasets.md b/pipeline/mmocr/docs/zh_cn/basic_concepts/datasets.md
deleted file mode 100644
index 16e6162d867258ff30fa5c7e92cb123042251f0d..0000000000000000000000000000000000000000
--- a/pipeline/mmocr/docs/zh_cn/basic_concepts/datasets.md
+++ /dev/null
@@ -1,489 +0,0 @@
-# ๆฐๆฎ้็ฑป
-
-## ๆฆ่ง
-
-ๅจ MMOCR ไธญ๏ผๆๆ็ๆฐๆฎ้้ฝ้่ฟไธๅ็ๅบไบ [mmengine.BaseDataset](mmengine.dataset.BaseDataset) ็ Dataset ็ฑป่ฟ่กๅค็ใ Dataset ็ฑป่ด่ดฃๅ ่ฝฝๆฐๆฎๅนถ่ฟ่กๅๅง่งฃๆ๏ผ็ถๅๅฐๅ
ถ้ฆ้ๅฐ [ๆฐๆฎๆตๆฐด็บฟ](./transforms.md) ่ฟ่กๆฐๆฎ้ขๅค็ใๅขๅผบใๆ ผๅผๅ็ญๆไฝใ
-
-
-
-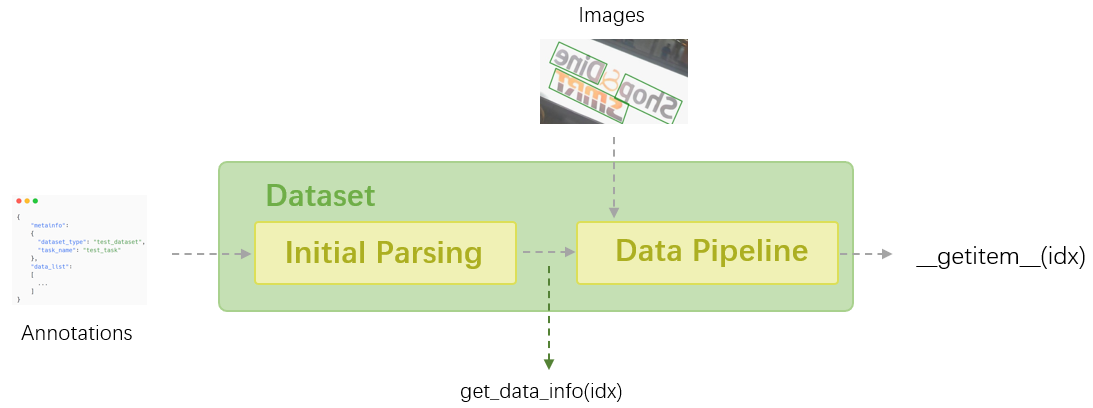
-
-
-
-ๅจๆฌๆ็จไธญ๏ผๆไปฌๅฐไป็ป Dataset ็ฑป็ไธไบๅธธ่งๆฅๅฃ๏ผไปฅๅ MMOCR ไธญ Dataset ๅฎ็ฐ็ไฝฟ็จไปฅๅๅฎไปฌๆฏๆ็ๆณจ้็ฑปๅใ
-
-```{tip}
-Dataset ็ฑปๆฏๆไธไบ้ซ็บงๅ่ฝ๏ผไพๅฆๆๅ ่ฝฝใๆฐๆฎๅบๅๅใๅฉ็จๅ็งๆฐๆฎ้ๅ
่ฃ
ๅจๆง่กๆฐๆฎ่ฟๆฅใ้ๅคๅ็ฑปๅซๅนณ่กกใ่ฟไบๅ
ๅฎนๅฐไธๅจๆฌๆ็จไธญไป็ป๏ผไฝๆจๅฏไปฅ้
่ฏป {external+mmengine:doc}`MMEngine: BaseDataset ` ไบ่งฃๆดๅค่ฏฆ็ปไฟกๆฏใ
-```
-
-## ๅธธ่งๆฅๅฃ
-
-็ฐๅจ๏ผ่ฎฉๆไปฌ็ไธไธชๅ
ทไฝ็็คบไพๅนถๅญฆไน Dataset ็ฑป็ไธไบๅ
ธๅๆฅๅฃใ`OCRDataset` ๆฏ MMOCR ไธญ้ป่ฎคไฝฟ็จ็ Dataset ๅฎ็ฐ๏ผๅ ไธบๅฎ็ๆ ๆณจๆ ผๅผ่ถณๅค็ตๆดป๏ผๆฏๆ *ๆๆ* OCR ไปปๅก๏ผ่ฏฆ่ง [OCRDataset](#ocrdataset)๏ผใ็ฐๅจๆไปฌๅฐๅฎไพๅไธไธช `OCRDataset` ๅฏน่ฑก๏ผๅ
ถไธญๅฐๅ ่ฝฝ `tests/data/det_toy_dataset` ไธญ็็ฉๅ
ทๆฐๆฎ้ใ
-
-```python
-from mmocr.datasets import OCRDataset
-from mmengine.registry import init_default_scope
-init_default_scope('mmocr')
-
-train_pipeline = [
- dict(
- type='LoadImageFromFile'),
- dict(
- type='LoadOCRAnnotations',
- with_polygon=True,
- with_bbox=True,
- with_label=True,
- ),
- dict(type='RandomCrop', min_side_ratio=0.1),
- dict(type='Resize', scale=(640, 640), keep_ratio=True),
- dict(type='Pad', size=(640, 640)),
- dict(
- type='PackTextDetInputs',
- meta_keys=('img_path', 'ori_shape', 'img_shape'))
-]
-dataset = OCRDataset(
- data_root='tests/data/det_toy_dataset',
- ann_file='textdet_test.json',
- test_mode=False,
- pipeline=train_pipeline)
-
-```
-
-่ฎฉๆไปฌๆฅ็ไธไธ่ฟไธชๆฐๆฎ้็ๅคงๅฐ๏ผ
-
-```python
->>> print(len(dataset))
-
-10
-```
-
-้ๅธธ๏ผDataset ็ฑปๅ ่ฝฝๅนถๅญๅจไธค็ง็ฑปๅ็ไฟกๆฏ๏ผ๏ผ1๏ผ**ๅ
ไฟกๆฏ**๏ผๅจๅญๆฐๆฎ้็ๅฑๆง๏ผไพๅฆๆญคๆฐๆฎ้ไธญๅฏ็จ็ๅฏน่ฑก็ฑปๅซใ ๏ผ2๏ผ**ๆ ๆณจ**๏ผๅพๅ็่ทฏๅพๅๅ
ถๆ ็ญพใๆไปฌๅฏไปฅ้่ฟ `dataset.metainfo` ่ฎฟ้ฎๅ
ไฟกๆฏ๏ผ
-
-```python
->>> from pprint import pprint
->>> pprint(dataset.metainfo)
-
-{'category': [{'id': 0, 'name': 'text'}],
- 'dataset_type': 'TextDetDataset',
- 'task_name': 'textdet'}
-```
-
-ๅฏนไบๆ ๆณจ๏ผๆไปฌๅฏไปฅ้่ฟ `dataset.get_data_info(idx)` ่ฎฟ้ฎๅฎใ่ฏฅๆนๆณ่ฟๅไธไธชๅญๅ
ธ๏ผๅ
ถไธญๅ
ๅซๆฐๆฎ้ไธญ็ฌฌ `idx` ไธชๆ ทๆฌ็ไฟกๆฏใ่ฏฅๆ ทๆฌๅทฒ็ป็ป่ฟๅๆญฅ่งฃๆ๏ผไฝๅฐๆช็ฑ [ๆฐๆฎๆตๆฐด็บฟ](./transforms.md) ๅค็ใ
-
-```python
->>> from pprint import pprint
->>> pprint(dataset.get_data_info(0))
-
-{'height': 720,
- 'img_path': 'tests/data/det_toy_dataset/test/img_10.jpg',
- 'instances': [{'bbox': [260.0, 138.0, 284.0, 158.0],
- 'bbox_label': 0,
- 'ignore': True,
- 'polygon': [261, 138, 284, 140, 279, 158, 260, 158]},
- ...,
- {'bbox': [1011.0, 157.0, 1079.0, 173.0],
- 'bbox_label': 0,
- 'ignore': True,
- 'polygon': [1011, 157, 1079, 160, 1076, 173, 1011, 170]}],
- 'sample_idx': 0,
- 'seg_map': 'test/gt_img_10.txt',
- 'width': 1280}
-```
-
-ๅฆไธๆน้ข๏ผๆไปฌๅฏไปฅ้่ฟ `dataset[idx]` ๆ `dataset.__getitem__(idx)` ่ทๅ็ฑๆฐๆฎๆตๆฐด็บฟๅฎๆดๅค็่ฟๅ็ๆ ทๆฌ๏ผ่ฏฅๆ ทๆฌๅฏไปฅ็ดๆฅ้ฆๅ
ฅๆจกๅๅนถๆง่กๅฎๆด็่ฎญ็ป/ๆต่ฏๅพช็ฏใๅฎๆไธคไธชๅญๆฎต๏ผ
-
-- `inputs`๏ผ็ป่ฟๆฐๆฎๅขๅผบๅ็ๅพๅ๏ผ
-- `data_samples`๏ผๅ
ๅซ็ป่ฟๆฐๆฎๅขๅผบๅ็ๆ ๆณจๅๅ
ไฟกๆฏ็ [DataSample](./structures.md)๏ผ่ฟไบๅ
ไฟกๆฏๅฏ่ฝ็ฑไธไบๆฐๆฎๅๆขไบง็๏ผๅนถ็จไปฅ่ฎฐๅฝ่ฏฅๆ ทๆฌ็ๆไบๅ
ณ้ฎๅฑๆงใ
-
-```python
->>> pprint(dataset[0])
-
-{'data_samples':
-) at 0x7f735a0508e0>,
- 'inputs': tensor([[[129, 111, 131, ..., 0, 0, 0], ...
- [ 19, 18, 15, ..., 0, 0, 0]]], dtype=torch.uint8)}
-```
-
-## ๆฐๆฎ้็ฑปๅๆ ๆณจๆ ผๅผ
-
-ๆฏไธชๆฐๆฎ้ๅฎ็ฐๅช่ฝๅ ่ฝฝ็นๅฎๆ ผๅผ็ๆฐๆฎ้ใ่ฟ้ๅๅบไบๆๆๆฏๆ็ๆฐๆฎ้็ฑปๅๅ
ถๅ
ผๅฎน็ๆ ผๅผ๏ผไปฅๅไธไธช็คบไพ้
็ฝฎ๏ผไปฅๆผ็คบๅฆไฝๅจๅฎ่ทตไธญไฝฟ็จๅฎไปฌใ
-
-```{note}
-ๅฆๆๆจไธ็ๆ้
็ฝฎ็ณป็ป๏ผๅฏไปฅ้
่ฏป [ๆฐๆฎ้้
็ฝฎๆไปถ](../user_guides/dataset_prepare.md#ๆฐๆฎ้้
็ฝฎๆไปถ)ใ
-```
-
-### OCRDataset
-
-้ๅธธ๏ผOCR ๆฐๆฎ้ไธญๆ่ฎธๅคไธๅ็ฑปๅ็ๆ ๆณจ๏ผๅจไธๅ็ๅญไปปๅก๏ผๅฆๆๆฌๆฃๆตๅๆๆฌ่ฏๅซ๏ผไธญ๏ผๆ ผๅผไน็ปๅธธไผๆๆไธๅใ่ฟไบๅทฎๅผๅฏ่ฝไผๅฏผ่ดๅจไฝฟ็จไธๅๆฐๆฎ้ๆถ้่ฆไธๅ็ๆฐๆฎๅ ่ฝฝไปฃ็ ๏ผๅขๅ ไบ็จๆท็ๅญฆไน ๅ็ปดๆคๆๆฌใ
-
-ๅจ MMOCR ไธญ๏ผๆไปฌๆๅบไบไธ็ง็ปไธ็ๆฐๆฎ้ๆ ผๅผ๏ผๅฏไปฅ้ๅบ OCR ็ๆๆไธไธชๅญไปปๅก๏ผๆๆฌๆฃๆตใๆๆฌ่ฏๅซๅ็ซฏๅฐ็ซฏ OCRใ่ฟ็ง่ฎพ่ฎกๆๅคง็จๅบฆๅฐๆ้ซไบๆฐๆฎ้็ไธ่ดๆง๏ผๅ
่ฎธๅจไธๅไปปๅกไน้ด้ๅคไฝฟ็จๆฐๆฎๆ ๆณจ๏ผไนไฝฟๅพๆฐๆฎ้็ฎก็ๆดๅ ๆนไพฟใ่่ๅฐๆต่ก็ๆฐๆฎ้ๆ ผๅผๅนถไธไธ่ด๏ผMMOCR ๆไพไบ [Dataset Preparer](../user_guides/data_prepare/dataset_preparer.md) ๆฅๅธฎๅฉ็จๆทๅฐๅ
ถๆฐๆฎ้่ฝฌๆขไธบ MMOCR ๆ ผๅผใๆไปฌไนๅๅ้ผๅฑ็ ็ฉถไบบๅๅบไบๆญคๆฐๆฎๆ ผๅผๅผๅ่ชๅทฑ็ๆฐๆฎ้ใ
-
-#### ๆ ๆณจๆ ผๅผ
-
-ๆญคๆ ๆณจๆไปถๆฏไธไธช `.json` ๆไปถ๏ผๅญๅจไธไธชๅ
ๅซ `metainfo` ๅ `data_list` ็ `dict`๏ผๅ่
ๅ
ๆฌๆๅ
ณๆฐๆฎ้็ๅบๆฌไฟกๆฏ๏ผๅ่
็ฑๆฏไธชๅพ็็ๆ ๆณจ็ปๆใ่ฟ้ๅ็ฐไบๆ ๆณจๆไปถไธญ็ๆๆๅญๆฎต็ๅ่กจ๏ผไฝๅ
ถไธญๆไบๅญๆฎตไป
ไผๅจ็นๅฎไปปๅกไธญ่ขซ็จๅฐใ
-
-```python
-{
- "metainfo":
- {
- "dataset_type": "TextDetDataset", # ๅฏ้้กน: TextDetDataset/TextRecogDataset/TextSpotterDataset
- "task_name": "textdet", # ๅฏ้้กน: textdet/textspotter/textrecog
- "category": [{"id": 0, "name": "text"}] # ๅจ textdet/textspotter ้็จๅฐ
- },
- "data_list":
- [
- {
- "img_path": "test_img.jpg",
- "height": 604,
- "width": 640,
- "instances": # ไธๅพๅ
็ๅคไธชๅฎไพ
- [
- {
- "bbox": [0, 0, 10, 20], # textdet/textspotter ๅ
็จๅฐ, [x1, y1, x2, y2]ใ
- "bbox_label": 0, # ๅฏน่ฑก็ฑปๅซ, ๅจ MMOCR ไธญๆไธบ 0 (ๆๆฌ)
- "polygon": [0, 0, 0, 10, 10, 20, 20, 0], # textdet/textspotter ๅ
็จๅฐใ [x1, y1, x2, y2, ....]
- "text": "mmocr", # textspotter/textrecog ๅ
็จๅฐ
- "ignore": False # textspotter/textdet ๅ
็จๅฐ๏ผๅณๅฎๆฏๅฆๅจ่ฎญ็ปๆถๅฟฝ็ฅ่ฏฅๅฎไพ
- },
- #...
- ],
- }
- #... ๅคๅพ็
- ]
-}
-```
-
-#### ็คบไพ้
็ฝฎ
-
-ไปฅไธๆฏ้
็ฝฎ็ไธ้จๅ๏ผๆไปฌๅจ `train_dataloader` ไธญไฝฟ็จ `OCRDataset` ๅ ่ฝฝ็จไบๆๆฌๆฃๆตๆจกๅ็ ICDAR2015 ๆฐๆฎ้ใ่ฏทๆณจๆ๏ผ`OCRDataset` ๅฏไปฅๅ ่ฝฝ็ฑ Dataset Preparer ๅๅค็ไปปไฝ OCR ๆฐๆฎ้ใไนๅฐฑๆฏ่ฏด๏ผๆจๅฏไปฅๅฐๅ
ถ็จไบๆๆฌ่ฏๅซๅๆๆฌๆฃๆต๏ผไฝๆจไป็ถ้่ฆๆ นๆฎไธๅไปปๅก็้ๆฑไฟฎๆน `pipeline` ไธญ็ๆฐๆฎๅๆขใ
-
-```python
-pipeline = [
- dict(
- type='LoadImageFromFile'),
- dict(
- type='LoadOCRAnnotations',
- with_polygon=True,
- with_bbox=True,
- with_label=True,
- ),
- dict(
- type='PackTextDetInputs',
- meta_keys=('img_path', 'ori_shape', 'img_shape'))
-]
-
-icdar2015_textdet_train = dict(
- type='OCRDataset',
- data_root='data/icdar2015',
- ann_file='textdet_train.json',
- filter_cfg=dict(filter_empty_gt=True, min_size=32),
- pipeline=pipeline)
-
-train_dataloader = dict(
- batch_size=16,
- num_workers=8,
- persistent_workers=True,
- sampler=dict(type='DefaultSampler', shuffle=True),
- dataset=icdar2015_textdet_train)
-```
-
-### RecogLMDBDataset
-
-ๅฝๆฐๆฎ้้ๅธธๅคงๆถ๏ผไปๆไปถไธญ่ฏปๅๅพๅๆๆ ็ญพๅฏ่ฝไผๅพๆ
ขใๆญคๅค๏ผๅจๅญฆๆฏ็๏ผๅคงๅคๆฐๅบๆฏๆๆฌ่ฏๅซๆฐๆฎ้็ๅพๅๅๆ ็ญพ้ฝไปฅ lmdb ๆ ผๅผๅญๅจใ๏ผ[็คบไพ](https://github.com/clovaai/deep-text-recognition-benchmark)๏ผ
-
-ไธบไบๆดๆฅ่ฟไธปๆตๅฎ่ทตๅนถๆ้ซๆฐๆฎๅญๅจๆ็๏ผMMOCRๆฏๆ้่ฟ `RecogLMDBDataset` ไป lmdb ๆฐๆฎ้ๅ ่ฝฝๅพๅๅๆ ็ญพใ
-
-#### ๆ ๆณจๆ ผๅผ
-
-MMOCR ไผ่ฏปๅ lmdb ๆฐๆฎ้ไธญ็ไปฅไธ้ฎ๏ผ
-
-- `num_samples`๏ผๆ่ฟฐๆฐๆฎ้็ๆฐๆฎ้็ๅๆฐใ
-- ๅพๅๅๆ ็ญพ็้ฎๅๅซไปฅ `image-000000001` ๅ `label-000000001` ็ๆ ผๅผๅฝๅ๏ผ็ดขๅผไป1ๅผๅงใ
-
-MMOCR ๅจ `tests/data/rec_toy_dataset/imgs.lmdb` ไธญๆไพไบไธไธช toy lmdb ๆฐๆฎ้ใๆจๅฏไปฅไฝฟ็จไปฅไธไปฃ็ ็ๆฎตไบ่งฃๅ
ถๆ ผๅผใ
-
-```python
->>> import lmdb
->>>
->>> env = lmdb.open('tests/data/rec_toy_dataset/imgs.lmdb')
->>> txn = env.begin()
->>> for k, v in txn.cursor():
->>> print(k, v)
-
-b'image-000000001' b'\xff...'
-b'image-000000002' b'\xff...'
-b'image-000000003' b'\xff...'
-b'image-000000004' b'\xff...'
-b'image-000000005' b'\xff...'
-b'image-000000006' b'\xff...'
-b'image-000000007' b'\xff...'
-b'image-000000008' b'\xff...'
-b'image-000000009' b'\xff...'
-b'image-000000010' b'\xff...'
-b'label-000000001' b'GRAND'
-b'label-000000002' b'HOTEL'
-b'label-000000003' b'HOTEL'
-b'label-000000004' b'PACIFIC'
-b'label-000000005' b'03/09/2009'
-b'label-000000006' b'ANING'
-b'label-000000007' b'Virgin'
-b'label-000000008' b'america'
-b'label-000000009' b'ATTACK'
-b'label-000000010' b'DAVIDSON'
-b'num-samples' b'10'
-
-```
-
-#### ็คบไพ้
็ฝฎ
-
-ไปฅไธๆฏ็คบไพ้
็ฝฎ็ไธ้จๅ๏ผๆไปฌๅจๅ
ถไธญไฝฟ็จ `RecogLMDBDataset` ๅ ่ฝฝ toy ๆฐๆฎ้ใ็ฑไบ `RecogLMDBDataset` ไผๅฐๅพๅๅ ่ฝฝไธบ numpy ๆฐ็ป๏ผๅ ๆญคๅฆๆ่ฆๅจๆฐๆฎ็ฎก้ไธญๆๅๅ ่ฝฝๅพๅ๏ผๅบ่ฏฅ่ฎฐๅพๆ`LoadImageFromFile` ๆฟๆขๆ `LoadImageFromNDArray` ใ
-
-```python
-pipeline = [
- dict(
- type='LoadImageFromNDArray'),
- dict(
- type='LoadOCRAnnotations',
- with_text=True,
- ),
- dict(
- type='PackTextRecogInputs',
- meta_keys=('img_path', 'ori_shape', 'img_shape'))
-]
-
-toy_textrecog_train = dict(
- type='RecogLMDBDataset',
- data_root='tests/data/rec_toy_dataset/',
- ann_file='imgs.lmdb',
- pipeline=pipeline)
-
-train_dataloader = dict(
- batch_size=16,
- num_workers=8,
- persistent_workers=True,
- sampler=dict(type='DefaultSampler', shuffle=True),
- dataset=toy_textrecog_train)
-```
-
-### RecogTextDataset
-
-ๅจ MMOCR 1.0 ไนๅ๏ผMMOCR 0.x ็ๆๆฌ่ฏๅซไปปๅก็่พๅ
ฅๆฏๆๆฌๆไปถใ่ฟไบๆ ผๅผๅทฒๅจ MMOCR 1.0 ไธญๅผ็จ๏ผ่ฟไธช็ฑป้ๆถๅฏ่ฝ่ขซๅ ้คใ[ๆดๅคไฟกๆฏ](../migration/dataset.md)
-
-#### ๆ ๆณจๆ ผๅผ
-
-ๆๆฌๆไปถๅฏไปฅๆฏ `txt` ๆ ผๅผๆ `jsonl` ๆ ผๅผใ็ฎๅ็ `.txt` ๆ ๆณจ้่ฟ็ฉบๆ ผๅฐๅพๅๅ็งฐๅ่ฏ่ฏญๆ ๆณจๅ้ๅผ๏ผๅ ๆญค่ฟ็งๆ ผๅผๅนถๆ ๆณๅค็ๆๆฌๅฎไพไธญๅ
ๅซ็ฉบๆ ผ็ๆ
ๅตใ
-
-```text
-img1.jpg OpenMMLab
-img2.jpg MMOCR
-```
-
-`jsonl` ๆ ผๅผไฝฟ็จ็ฑปไผผๅญๅ
ธ็็ปๆๆฅ่กจ็คบๆ ๆณจ๏ผๅ
ถไธญ้ฎ `filename` ๅ `text` ๅญๅจๅพๅๅ็งฐๅๅ่ฏๆ ็ญพใ
-
-```json
-{"filename": "img1.jpg", "text": "OpenMMLab"}
-{"filename": "img2.jpg", "text": "MMOCR"}
-```
-
-#### ็คบไพ้
็ฝฎ
-
-ไปฅไธๆฏไธไธช็คบไพ้
็ฝฎ๏ผๆไปฌๅจ่ฎญ็ปไธญไฝฟ็จ `RecogTextDataset` ๅ ่ฝฝ txt ๆ ็ญพ๏ผ่ๅจๆต่ฏไธญไฝฟ็จ jsonl ๆ ็ญพใ
-
-```python
-pipeline = [
- dict(
- type='LoadImageFromFile'),
- dict(
- type='LoadOCRAnnotations',
- with_polygon=True,
- with_bbox=True,
- with_label=True,
- ),
- dict(
- type='PackTextDetInputs',
- meta_keys=('img_path', 'ori_shape', 'img_shape'))
-]
-
- # loading 0.x txt format annos
- txt_dataset = dict(
- type='RecogTextDataset',
- data_root=data_root,
- ann_file='old_label.txt',
- data_prefix=dict(img_path='imgs'),
- parser_cfg=dict(
- type='LineStrParser',
- keys=['filename', 'text'],
- keys_idx=[0, 1]),
- pipeline=pipeline)
-
-
-train_dataloader = dict(
- batch_size=16,
- num_workers=8,
- persistent_workers=True,
- sampler=dict(type='DefaultSampler', shuffle=True),
- dataset=txt_dataset)
-
- # loading 0.x json line format annos
- jsonl_dataset = dict(
- type='RecogTextDataset',
- data_root=data_root,
- ann_file='old_label.jsonl',
- data_prefix=dict(img_path='imgs'),
- parser_cfg=dict(
- type='LineJsonParser',
- keys=['filename', 'text'],
- pipeline=pipeline))
-
-test_dataloader = dict(
- batch_size=16,
- num_workers=8,
- persistent_workers=True,
- sampler=dict(type='DefaultSampler', shuffle=False),
- dataset=jsonl_dataset)
-```
-
-### IcdarDataset
-
-ๅจ MMOCR 1.0 ไนๅ๏ผMMOCR 0.x ็ๆๆฌๆฃๆต่พๅ
ฅ้็จไบ็ฑปไผผ COCO ๆ ผๅผ็ๆณจ้ใ่ฟไบๆ ผๅผๅทฒๅจ MMOCR 1.0 ไธญๅผ็จ๏ผ่ฟไธช็ฑปๅจๅฐๆฅ็ไปปไฝๆถๅ้ฝๅฏ่ฝ่ขซๅ ้คใ[ๆดๅคไฟกๆฏ](../migration/dataset.md)
-
-#### ๆ ๆณจๆ ผๅผ
-
-```json
-{
- "images": [
- {
- "id": 1,
- "width": 800,
- "height": 600,
- "file_name": "test.jpg"
- }
- ],
- "annotations": [
- {
- "id": 1,
- "image_id": 1,
- "category_id": 1,
- "bbox": [0,0,10,10],
- "segmentation": [
- [0,0,10,0,10,10,0,10]
- ],
- "area": 100,
- "iscrowd": 0
- }
- ]
-}
-
-```
-
-#### ้
็ฝฎ็คบไพ
-
-่ฟๆฏ้
็ฝฎ็คบไพ็ไธ้จๅ๏ผๅ
ถไธญๆไปฌไปค `train_dataloader` ไฝฟ็จ `IcdarDataset` ๆฅๅ ่ฝฝๆงๆ ็ญพใ
-
-```python
-pipeline = [
- dict(
- type='LoadImageFromFile'),
- dict(
- type='LoadOCRAnnotations',
- with_polygon=True,
- with_bbox=True,
- with_label=True,
- ),
- dict(
- type='PackTextDetInputs',
- meta_keys=('img_path', 'ori_shape', 'img_shape'))
-]
-
-icdar2015_textdet_train = dict(
- type='IcdarDatasetDataset',
- data_root='data/det/icdar2015',
- ann_file='instances_training.json',
- filter_cfg=dict(filter_empty_gt=True, min_size=32),
- pipeline=pipeline)
-
-train_dataloader = dict(
- batch_size=16,
- num_workers=8,
- persistent_workers=True,
- sampler=dict(type='DefaultSampler', shuffle=True),
- dataset=icdar2015_textdet_train)
-```
-
-### WildReceiptDataset
-
-่ฏฅ็ฑปไธบ [WildReceipt](https://mmocr.readthedocs.io/en/dev-1.x/user_guides/data_prepare/datasetzoo.html#wildreceipt) ๆฐๆฎ้ๅฎๅถใ
-
-#### ๆ ๆณจๆ ผๅผ
-
-```json
-// Close Set
-{
- "file_name": "image_files/Image_16/11/d5de7f2a20751e50b84c747c17a24cd98bed3554.jpeg",
- "height": 1200,
- "width": 1600,
- "annotations":
- [
- {
- "box": [550.0, 190.0, 937.0, 190.0, 937.0, 104.0, 550.0, 104.0],
- "text": "SAFEWAY",
- "label": 1
- },
- {
- "box": [1048.0, 211.0, 1074.0, 211.0, 1074.0, 196.0, 1048.0, 196.0],
- "text": "TM",
- "label": 25
- }
- ], //...
-}
-
-// Open Set
-{
- "file_name": "image_files/Image_12/10/845be0dd6f5b04866a2042abd28d558032ef2576.jpeg",
- "height": 348,
- "width": 348,
- "annotations":
- [
- {
- "box": [114.0, 19.0, 230.0, 19.0, 230.0, 1.0, 114.0, 1.0],
- "text": "CHOEUN",
- "label": 2,
- "edge": 1
- },
- {
- "box": [97.0, 35.0, 236.0, 35.0, 236.0, 19.0, 97.0, 19.0],
- "text": "KOREANRESTAURANT",
- "label": 2,
- "edge": 1
- }
- ]
-}
-```
-
-#### ้
็ฝฎ็คบไพ
-
-่ฏทๅ่ [SDMGR ็้
็ฝฎ](https://github.com/open-mmlab/mmocr/blob/f30c16ce96bd2393570c04eeb9cf48a7916315cc/configs/kie/sdmgr/sdmgr_novisual_60e_wildreceipt.py)ใ
diff --git a/pipeline/mmocr/docs/zh_cn/basic_concepts/engine.md b/pipeline/mmocr/docs/zh_cn/basic_concepts/engine.md
deleted file mode 100644
index 57cb62ae9d5f35d6dccd4467e5fba09353972a49..0000000000000000000000000000000000000000
--- a/pipeline/mmocr/docs/zh_cn/basic_concepts/engine.md
+++ /dev/null
@@ -1,3 +0,0 @@
-# ๅผๆ\[ๅพ
ๆดๆฐ\]
-
-ๅพ
ๆดๆฐ
diff --git a/pipeline/mmocr/docs/zh_cn/basic_concepts/evaluation.md b/pipeline/mmocr/docs/zh_cn/basic_concepts/evaluation.md
deleted file mode 100644
index 15eab4daebcb4a835ca915a18ee827826ea03b1e..0000000000000000000000000000000000000000
--- a/pipeline/mmocr/docs/zh_cn/basic_concepts/evaluation.md
+++ /dev/null
@@ -1,198 +0,0 @@
-# ๆจกๅ่ฏๆต
-
-```{note}
-้
่ฏปๆญคๆๆกฃๅ๏ผๅปบ่ฎฎๆจๅ
ไบ่งฃ {external+mmengine:doc}`MMEngine: ๆจกๅ็ฒพๅบฆ่ฏๆตๅบๆฌๆฆๅฟต `ใ
-```
-
-## ่ฏๆตๆๆ
-
-MMOCR ๅบไบ {external+mmengine:doc}`MMEngine: BaseMetric ` ๅบ็ฑปๅฎ็ฐไบๅธธ็จ็ๆๆฌๆฃๆตใๆๆฌ่ฏๅซไปฅๅๅ
ณ้ฎไฟกๆฏๆฝๅไปปๅก็่ฏๆตๆๆ ๏ผ็จๆทๅฏไปฅ้่ฟไฟฎๆน้
็ฝฎๆไปถไธญ็ `val_evaluator` ไธ `test_evaluator` ๅญๆฎตๆฅไพฟๆทๅฐๆๅฎ้ช่ฏไธๆต่ฏ้ถๆฎต้็จ็่ฏๆตๆนๆณใไพๅฆ๏ผไปฅไธ้
็ฝฎๅฑ็คบไบๅฆไฝๅจๆๆฌๆฃๆต็ฎๆณไธญไฝฟ็จ `HmeanIOUMetric` ๆฅ่ฏๆตๆจกๅๆง่ฝใ
-
-```python
-# ๆๆฌๆฃๆตไปปๅกไธญ้ๅธธไฝฟ็จ HmeanIOUMetric ๆฅ่ฏๆตๆจกๅๆง่ฝ
-val_evaluator = [dict(type='HmeanIOUMetric')]
-
-# ๆญคๅค๏ผMMOCR ไนๆฏๆ็ธๅไปปๅกไธ็ๅค็งๆๆ ็ปๅ่ฏๆต๏ผๅฆๅๆถไฝฟ็จ WordMetric ๅ CharMetric
-val_evaluator = [
- dict(type='WordMetric', mode=['exact', 'ignore_case', 'ignore_case_symbol']),
- dict(type='CharMetric')
-]
-```
-
-```{tip}
-ๆดๅค่ฏๆต็ธๅ
ณ้
็ฝฎ่ฏทๅ่[่ฏๆต้
็ฝฎๆ็จ](../user_guides/config.md#่ฏๆต้
็ฝฎ)ใ
-```
-
-ๅฆไธ่กจๆ็คบ๏ผMMOCR ็ฎๅ้ๅฏนๆๆฌๆฃๆตใ่ฏๅซใๅๅ
ณ้ฎไฟกๆฏๆฝๅ็ญไปปๅกๅ
ฑๅ
็ฝฎไบ 5 ็ง่ฏๆตๆๆ ๏ผๅๅซไธบ `HmeanIOUMetric`๏ผ`WordMetric`๏ผ`CharMetric`๏ผ`OneMinusNEDMetric`๏ผๅ `F1Metric`ใ
-
-| | | | |
-| --------------------------------------- | ------------ | ------------------------------------------------- | --------------------------------------------------------------------- |
-| ่ฏๆตๆๆ | ไปปๅก็ฑปๅ | ่พๅ
ฅๅญๆฎต | ่พๅบๅญๆฎต |
-| [HmeanIOUMetric](#hmeanioumetric) | ๆๆฌๆฃๆต | `pred_polygons` `pred_scores` `gt_polygons` | `recall` `precision` `hmean` |
-| [WordMetric](#wordmetric) | ๆๆฌ่ฏๅซ | `pred_text` `gt_text` | `word_acc` `word_acc_ignore_case` `word_acc_ignore_case_symbol` |
-| [CharMetric](#charmetric) | ๆๆฌ่ฏๅซ | `pred_text` `gt_text` | `char_recall` `char_precision` |
-| [OneMinusNEDMetric](#oneminusnedmetric) | ๆๆฌ่ฏๅซ | `pred_text` `gt_text` | `1-N.E.D` |
-| [F1Metric](#f1metric) | ๅ
ณ้ฎไฟกๆฏๆฝๅ | `pred_labels` `gt_labels` | `macro_f1` `micro_f1` |
-
-้ๅธธๆฅ่ฏด๏ผๆฏไธ็ฑปไปปๅกๆ้็จ็่ฏๆตๆ ๅๆฏ็บฆๅฎไฟๆ็๏ผ็จๆทไธ่ฌๆ ้กปๆทฑๅ
ฅไบ่งฃๆๆๅจไฟฎๆน่ฏๆตๆนๆณ็ๅ
้จๅฎ็ฐใ็ถ่๏ผไธบไบๆนไพฟ็จๆทๅฎ็ฐๆดๅ ๅฎๅถๅ็้ๆฑ๏ผๆฌๆๆกฃๅฐ่ฟไธๆญฅไป็ปไบ MMOCR ๅ
็ฝฎ่ฏๆต็ฎๆณ็ๅ
ทไฝๅฎ็ฐ็ญ็ฅ๏ผไปฅๅๅฏ้
็ฝฎๅๆฐใ
-
-### HmeanIOUMetric
-
-[HmeanIOUMetric](mmocr.evaluation.metrics.hmean_iou_metric.HmeanIOUMetric) ๆฏๆๆฌๆฃๆตไปปๅกไธญๅบ็จๆๅนฟๆณ็่ฏๆตๆๆ ไนไธ๏ผๅ ๅ
ถ่ฎก็ฎไบๆฃๆต็ฒพๅบฆ๏ผPrecision๏ผไธๅฌๅ็๏ผRecall๏ผไน้ด็่ฐๅๅนณๅๆฐ๏ผHarmonic mean, H-mean๏ผ๏ผๆ
ๅพๅ `HmeanIOUMetric`ใ่ฎฐ็ฒพๅบฆไธบ *P*๏ผๅฌๅ็ไธบ *R*๏ผๅ `HmeanIOUMetric` ๅฏ็ฑไธๅผ่ฎก็ฎๅพๅฐ๏ผ
-
-```{math}
-H = \frac{2}{\frac{1}{P} + \frac{1}{R}} = \frac{2PR}{P+R}
-```
-
-ๅฆๅค๏ผ็ฑไบๅ
ถ็ญไปทไบ {math}`\beta = 1` ๆถ็ F-score (ๅ็งฐ F-measure ๆ F-metric)๏ผ`HmeanIOUMetric` ๆๆถไน่ขซๅไฝ `F1Metric` ๆ `f1-score` ็ญ๏ผ
-
-```{math}
-F_1=(1+\beta^2)\cdot\frac{PR}{\beta^2\cdot P+R} = \frac{2PR}{P+R}
-```
-
-ๅจ MMOCR ็่ฎพ่ฎกไธญ๏ผ`HmeanIOUMetric` ็่ฎก็ฎๅฏไปฅๆฆๆฌไธบไปฅไธๅ ไธชๆญฅ้ชค๏ผ
-
-1. ่ฟๆปคๆ ๆ็้ขๆต่พน็็
-
- - ไพๆฎ็ฝฎไฟกๅบฆ้ๅผ `pred_score_thrs` ่ฟๆปคๆๅพๅ่พไฝ็้ขๆต่พน็็
- - ไพๆฎ `ignore_precision_thr` ้ๅผ่ฟๆปคๆไธ `ignored` ๆ ทๆฌ้ๅๅบฆ่ฟ้ซ็้ขๆต่พน็็
-
- ๅผๅพๆณจๆ็ๆฏ๏ผ`pred_score_thrs` ้ป่ฎคๅฐ**่ชๅจๆ็ดข**ไธๅฎ่ๅดๅ
็**ๆไฝณ้ๅผ**๏ผ็จๆทไนๅฏไปฅ้่ฟๆๅจไฟฎๆน้
็ฝฎๆไปถๆฅ่ชๅฎไนๆ็ดข่ๅด๏ผ
-
- ```python
- # HmeanIOUMetric ้ป่ฎคไปฅ 0.1 ไธบๆญฅ้ฟๆ็ดข [0.3, 0.9] ่ๅดๅ
็ๆไฝณๅพๅ้ๅผ
- val_evaluator = dict(type='HmeanIOUMetric', pred_score_thrs=dict(start=0.3, stop=0.9, step=0.1))
- ```
-
-2. ่ฎก็ฎ IoU ็ฉ้ต
-
- - ๅจๆฐๆฎๅค็้ถๆฎต๏ผ`HmeanIOUMetric` ไผ่ฎก็ฎๅนถ็ปดๆคไธไธช {math}`M \times N` ็ IoU ็ฉ้ต `iou_metric`๏ผไปฅๆนไพฟๅ็ปญ็่พน็็้
ๅฏนๆญฅ้ชคใๅ
ถไธญ๏ผM ๅ N ๅๅซไธบๆ ็ญพ่พน็็ไธ่ฟๆปคๅ้ขๆต่พน็็็ๆฐ้ใ็ฑๆญค๏ผ่ฏฅ็ฉ้ต็ๆฏไธชๅ
็ด ้ฝๅญๆพไบ็ฌฌ m ไธชๆ ็ญพ่พน็็ไธ็ฌฌ n ไธช้ขๆต่พน็็ไน้ด็ไบคๅนถๆฏ๏ผIoU๏ผใ
-
-3. ๅบไบ็ธๅบ็้
ๅฏน็ญ็ฅ็ป่ฎก่ฝ่ขซๅ็กฎๅน้
็ GT ๆ ทๆฌๆฐ
-
- ๅฐฝ็ฎก `HmeanIOUMetric` ๅฏไปฅ็ฑๅบๅฎ็ๅ
ฌๅผ่ฎก็ฎๅๅพ๏ผไธๅ็ไปปๅกๆ็ฎๆณๅบๅ
้จ็ๅ
ทไฝๅฎ็ฐไปๅฏ่ฝๅญๅจไธไบ็ปๅพฎๅทฎๅซใ่ฟไบๅทฎๅผไธป่ฆไฝ็ฐๅจ้็จไธๅ็็ญ็ฅๆฅๅน้
็ๅฎไธ้ขๆต่พน็็๏ผไป่ๅฏผ่ดๆ็ปๅพๅ็ๅทฎ่ทใ็ฎๅ๏ผMMOCR ๅ
้จ็ `HmeanIOUMetric` ๅ
ฑๆฏๆไธค็งไธๅ็ๅน้
็ญ็ฅ๏ผๅณ `vanilla` ไธ `max_matching`ใๅฆไธๆ็คบ๏ผ็จๆทๅฏไปฅ้่ฟไฟฎๆน้
็ฝฎๆไปถๆฅๆๅฎไธๅ็ๅน้
็ญ็ฅใ
-
- - `vanilla` ๅน้
็ญ็ฅ
-
- `HmeanIOUMetric` ้ป่ฎค้็จ `vanilla` ๅน้
็ญ็ฅ๏ผ่ฏฅๅฎ็ฐไธ MMOCR 0.x ็ๆฌไธญ็ `hmean-iou` ๅ ICDAR ็ณปๅ**ๅฎๆนๆๆฌๆฃๆต็ซ่ต็่ฏๆตๆ ๅไฟๆไธ่ด**๏ผ้็จๅ
ๅฐๅ
ๅพ็ๅน้
ๆนๅผๅฏนๆ ็ญพ่พน็็๏ผGround-truth bbox๏ผไธ้ขๆต่พน็็๏ผPredicted bbox๏ผ่ฟ่ก้
ๅฏนใ
-
- ```python
- # ไธๆๅฎ strategy ๆถ๏ผHmeanIOUMetric ้ป่ฎค้็จ 'vanilla' ๅน้
็ญ็ฅ
- val_evaluator = dict(type='HmeanIOUMetric')
- ```
-
- - `max_matching` ๅน้
็ญ็ฅ
-
- ้ๅฏน็ฐๆๅน้
ๆบๅถไธญ็ไธๅฎๅไนๅค๏ผMMOCR ็ฎๆณๅบๅฎ็ฐไบไธๅฅๆด้ซๆ็ๅน้
็ญ็ฅ๏ผ็จไปฅๆๅคงๅๅน้
ๆฐ็ฎใ
-
- ```python
- # ๆๅฎ้็จ 'max_matching' ๅน้
็ญ็ฅ
- val_evaluator = dict(type='HmeanIOUMetric', strategy='max_matching')
- ```
-
- ```{note}
- ๆไปฌๅปบ่ฎฎ้ขๅๅญฆๆฏ็ ็ฉถ็ๅผๅ็จๆท้็จ้ป่ฎค็ `vanilla` ๅน้
็ญ็ฅ๏ผไปฅไฟ่ฏไธๅ
ถไป่ฎบๆ็ๅฏนๆฏ็ปๆไฟๆไธ่ดใ่้ขๅๅทฅไธๅบ็จ็ๅผๅ็จๆทๅๅฏไปฅ้็จ `max_matching` ๅน้
็ญ็ฅ๏ผไปฅ่ทๅพ็ฒพๅ็็ปๆใ
- ```
-
-4. ๆ นๆฎไธๆไป็ป็ `HmeanIOUMetric` ๅ
ฌๅผ่ฎก็ฎๆ็ป็่ฏๆตๅพๅ
-
-### WordMetric
-
-[WordMetric](mmocr.evaluation.metrics.recog_metric.WordMetric) ๅฎ็ฐไบ**ๅ่ฏ็บงๅซ**็ๆๆฌ่ฏๅซ่ฏๆตๆๆ ๏ผๅนถๅ
็ฝฎไบ `exact`๏ผ`ignore_case`๏ผๅ `ignore_case_symbol` ไธ็งๆๆฌๅน้
ๆจกๅผ๏ผ็จๆทๅฏไปฅๅจ้
็ฝฎๆไปถไธญไฟฎๆน `mode` ๅญๆฎตๆฅ่ช็ฑ็ปๅ่พๅบไธ็งๆๅค็งๆๆฌๅน้
ๆจกๅผไธ็ `WordMetric` ๅพๅใ
-
-```python
-# ๅจๆๆฌ่ฏๅซไปปๅกไธญไฝฟ็จ WordMetric ่ฏๆต
-val_evaluator = [
- dict(type='WordMetric', mode=['exact', 'ignore_case', 'ignore_case_symbol'])
-]
-```
-
-- `exact`๏ผๅ
จๅน้
ๆจกๅผ๏ผๅณ๏ผ้ขๆตไธๆ ็ญพๅฎๅ
จไธ่ดๆ่ฝ่ขซ่ฎฐๅฝไธบๆญฃ็กฎๆ ทๆฌใ
-- `ignore_case`๏ผๅฟฝ็ฅๅคงๅฐๅ็ๅน้
ๆจกๅผใ
-- `ignore_case_symbol`๏ผๅฟฝ็ฅๅคงๅฐๅๅ็ฌฆๅท็ๅน้
ๆจกๅผ๏ผ่ฟไนๆฏๅคง้จๅๅญฆๆฏ่ฎบๆไธญๆฅๅ็ๆๆฌ่ฏๅซๅ็กฎ็๏ผMMOCR ๆฅๅ็่ฏๅซๆจกๅๆง่ฝ้ป่ฎค้็จ่ฏฅๅน้
ๆจกๅผใ
-
-ๅ่ฎพ็ๅฎๆ ็ญพไธบ `MMOCR!`๏ผๆจกๅ็่พๅบ็ปๆไธบ `mmocr`๏ผๅไธ็งๅน้
ๆจกๅผไธ็ `WordMetric` ๅพๅๅๅซไธบ๏ผ`{'exact': 0, 'ignore_case': 0, 'ignore_case_symbol': 1}`ใ
-
-### CharMetric
-
-[CharMetric](mmocr.evaluation.metrics.recog_metric.CharMetric) ๅฎ็ฐไบ**ไธๅบๅๅคงๅฐๅ**็**ๅญ็ฌฆ็บงๅซ**็ๆๆฌ่ฏๅซ่ฏๆตๆๆ ใ
-
-```python
-# ๅจๆๆฌ่ฏๅซไปปๅกไธญไฝฟ็จ CharMetric ่ฏๆต
-val_evaluator = [dict(type='CharMetric')]
-```
-
-ๅ
ทไฝ่่จ๏ผ`CharMetric` ไผ่พๅบไธคไธช่ฏๆต่ฏๆตๆๆ ๏ผๅณๅญ็ฌฆ็ฒพๅบฆ `char_precision` ๅๅญ็ฌฆๅฌๅ็ `char_recall`ใ่ฎพๆญฃ็กฎ้ขๆต็ๅญ็ฌฆ๏ผTrue Positive๏ผๆฐ้ไธบ {math}`\sigma_{tp}`๏ผๅ็ฒพๅบฆ *P* ๅๅฌๅ็ *R* ๅฏ็ฑไธๅผ่ฎก็ฎๅๅพ๏ผ
-
-```{math}
-P=\frac{\sigma_{tp}}{\sigma_{pred}}, R = \frac{\sigma_{tp}}{\sigma_{gt}}
-```
-
-ๅ
ถไธญ๏ผ{math}`\sigma_{gt}` ไธ {math}`\sigma_{pred}` ๅๅซไธบๆ ็ญพๆๆฌไธ้ขๆตๆๆฌๆๅ
ๅซ็ๅญ็ฌฆๆปๆฐใ
-
-ไพๅฆ๏ผๅ่ฎพๆ ็ญพๆๆฌไธบ "MM**O**CR"๏ผ้ขๆตๆๆฌไธบ "mm**0**cR**1**"๏ผๅไฝฟ็จ `CharMetric` ่ฏๆตๆๆ ็ๅพๅไธบ๏ผ
-
-```{math}
-P=\frac{4}{6}, R=\frac{4}{5}
-```
-
-### OneMinusNEDMetric
-
-[`OneMinusNEDMetric(1-N.E.D)`](mmocr.evaluation.metrics.recog_metric.OneMinusNEDMetric) ๅธธ็จไบไธญๆๆ่ฑๆ**ๆๆฌ่ก็บงๅซ**ๆ ๆณจ็ๆๆฌ่ฏๅซ่ฏๆต๏ผไธๅไบๅ
จๅน้
็่ฏๆตๆ ๅ่ฆๆฑ้ขๆตไธ็ๅฎๆ ทๆฌๅฎๅ
จไธ่ด๏ผ่ฏฅ่ฏๆตๆๆ ไฝฟ็จๅฝไธๅ็[็ผ่พ่ท็ฆป](https://en.wikipedia.org/wiki/Edit_distance)๏ผEdit Distance๏ผๅๅ่ฑๆธฉๆฏๅฆ่ท็ฆป Levenshtein Distance๏ผๆฅๆต้้ขๆตๆๆฌไธ็ๅฎๆๆฌไน้ด็ๅทฎๅผๆง๏ผไป่ๅจ่ฏๆต้ฟๆๆฌๆ ทๆฌๆถ่ฝๅคๆดๅฅฝๅฐๅบๅๅบๆจกๅ็ๆง่ฝๅทฎๅผใๅ่ฎพ็ๅฎๅ้ขๆตๆๆฌๅๅซไธบ {math}`s_i` ๅ {math}`\hat{s_i}`๏ผๅ
ถ้ฟๅบฆๅๅซไธบ {math}`l_{i}` ๅ {math}`\hat{l_i}`๏ผๅ `OneMinusNEDMetric` ๅพๅๅฏ็ฑไธๅผ่ฎก็ฎๅพๅฐ๏ผ
-
-```{math}
-score = 1 - \frac{1}{N}\sum_{i=1}^{N}\frac{D(s_i, \hat{s_{i}})}{max(l_{i},\hat{l_{i}})}
-```
-
-ๅ
ถไธญ๏ผ*N* ๆฏๆ ทๆฌๆปๆฐ๏ผ{math}`D(s_1, s_2)` ไธบไธคไธชๅญ็ฌฆไธฒไน้ด็็ผ่พ่ท็ฆปใ
-
-ไพๅฆ๏ผๅ่ฎพ็ๅฎๆ ็ญพไธบ "OpenMMLabMMOCR"๏ผๆจกๅ A ็้ขๆต็ปๆไธบ "0penMMLabMMOCR", ๆจกๅ B ็้ขๆต็ปๆไธบ "uvwxyz"๏ผๅ้็จๅ
จๅน้
ๅ `OneMinusNEDMetric` ่ฏๆตๆๆ ็็ปๆๅๅซไธบ:
-
-| | | |
-| ------ | ------ | ---------- |
-| | ๅ
จๅน้
| 1 - N.E.D. |
-| ๆจกๅ A | 0 | 0.92857 |
-| ๆจกๅ B | 0 | 0 |
-
-็ฑไธ่กจๅฏไปฅๅ็ฐ๏ผๅฐฝ็ฎกๆจกๅ A ไป
้ขๆต้ไบไธไธชๅญๆฏ๏ผ่ๆจกๅ B ๅ
จ้จ้ขๆต้่ฏฏ๏ผๅจไฝฟ็จๅ
จๅน้
็่ฏๆตๆๆ ๆถ๏ผ่ฟไธคไธชๆจกๅ็ๅพๅ้ฝไธบ0๏ผ่ไฝฟ็จ `OneMinuesNEDMetric` ็่ฏๆตๆๆ ๅ่ฝๅคๆดๅฅฝๅฐๅบๅๆจกๅๅจ**้ฟๆๆฌ**ไธ็ๆง่ฝๅทฎๅผใ
-
-### F1Metric
-
-[F1Metric](mmocr.evaluation.metrics.f_metric.F1Metric) ๅฎ็ฐไบ้ๅฏน KIE ไปปๅก็ F1-Metric ่ฏๆตๆๆ ๏ผๅนถๆไพไบ `micro` ๅ `macro` ไธค็ง่ฏๆตๆจกๅผใ
-
-```python
-val_evaluator = [
- dict(type='F1Metric', mode=['micro', 'macro'],
-]
-```
-
-- `micro` ๆจกๅผ๏ผไพๆฎ True Positive๏ผFalse Negative๏ผๅ False Positive ๆปๆฐๆฅ่ฎก็ฎๅ
จๅฑ F1-Metric ๅพๅใ
-
-- `macro` ๆจกๅผ๏ผไพๆฎ็ฑปๅซๆ ็ญพ่ฎก็ฎๆฏไธ็ฑป็ F1-Metric๏ผๅนถๆฑๅนณๅๅผใ
-
-### ่ชๅฎไน่ฏๆตๆๆ
-
-ๅฏนไบ่ฟฝๆฑๆด้ซๅฎๅถๅๅ่ฝ็็จๆท๏ผMMOCR ไนๆฏๆ่ชๅฎไนๅฎ็ฐไธๅ็ฑปๅ็่ฏๆตๆๆ ใไธ่ฌๆฅ่ฏด๏ผ็จๆทๅช้่ฆๆฐๅปบ่ชๅฎไน่ฏๆตๆๆ ็ฑป `CustomizedMetric` ๅนถ็ปงๆฟ {external+mmengine:doc}`MMEngine: BaseMetric `๏ผ็ถๅๅๅซ้ๅๆฐๆฎๆ ผๅผๅค็ๆนๆณ `process` ไปฅๅๆๆ ่ฎก็ฎๆนๆณ `compute_metrics`ใๆๅ๏ผๅฐๅ
ถๅ ๅ
ฅ `METRICS` ๆณจๅๅจๅณๅฏๅฎ็ฐไปปๆๅฎๅถๅ็่ฏๆตๆๆ ใ
-
-```python
-from mmengine.evaluator import BaseMetric
-from mmocr.registry import METRICS
-
-@METRICS.register_module()
-class CustomizedMetric(BaseMetric):
-
- def process(self, data_batch: Sequence[Dict], predictions: Sequence[Dict]):
- """ process ๆฅๆถไธคไธชๅๆฐ๏ผๅๅซไธบ data_batch ๅญๆพ็ๅฎๆ ็ญพไฟกๆฏ๏ผไปฅๅ predictions
- ๅญๆพ้ขๆต็ปๆใprocess ๆนๆณ่ด่ดฃๅฐๆ ็ญพไฟกๆฏ่ฝฌๆขๅนถๅญๆพ่ณ self.results ๅ้ไธญ
- """
- pass
-
- def compute_metrics(self, results: List):
- """ compute_metric ไฝฟ็จ็ป่ฟ process ๆนๆณๅค็่ฟ็ๆ ็ญพๆฐๆฎ่ฎก็ฎๆ็ป่ฏๆตๅพๅ
- """
- pass
-```
-
-```{note}
-ๆดๅคๅ
ๅฎนๅฏๅ่ง {external+mmengine:doc}`MMEngine ๆๆกฃ: BaseMetric `ใ
-```
diff --git a/pipeline/mmocr/docs/zh_cn/basic_concepts/models.md b/pipeline/mmocr/docs/zh_cn/basic_concepts/models.md
deleted file mode 100644
index 7ec449d5c7015e73a4cef161988e0fe57c8706ef..0000000000000000000000000000000000000000
--- a/pipeline/mmocr/docs/zh_cn/basic_concepts/models.md
+++ /dev/null
@@ -1,3 +0,0 @@
-# ๆจกๅ\[ๅพ
ๆดๆฐ\]
-
-ๅพ
ๆดๆฐ
diff --git a/pipeline/mmocr/docs/zh_cn/basic_concepts/overview.md b/pipeline/mmocr/docs/zh_cn/basic_concepts/overview.md
deleted file mode 100644
index bbd721395c5075bc7564dbd206c21bef27353fe5..0000000000000000000000000000000000000000
--- a/pipeline/mmocr/docs/zh_cn/basic_concepts/overview.md
+++ /dev/null
@@ -1,3 +0,0 @@
-# ่ฎพ่ฎก็ๅฟตไธ็นๆง\[ๅพ
ๆดๆฐ\]
-
-ๅพ
ๆดๆฐ
diff --git a/pipeline/mmocr/docs/zh_cn/basic_concepts/structures.md b/pipeline/mmocr/docs/zh_cn/basic_concepts/structures.md
deleted file mode 100644
index 857a356f8b42950c1d941115b2f4d2f257d04078..0000000000000000000000000000000000000000
--- a/pipeline/mmocr/docs/zh_cn/basic_concepts/structures.md
+++ /dev/null
@@ -1,219 +0,0 @@
-# ๆฐๆฎๅ
็ด ไธๆฐๆฎ็ปๆ
-
-MMOCR ๅบไบ {external+mmengine:doc}`MMEngine: ๆฝ่ฑกๆฐๆฎๆฅๅฃ ` ๅฐๅไปปๅกๆ้็ๆฐๆฎ็ปไธๅฐ่ฃ
ๅ
ฅ `data_sample` ไธญใMMEngine ็ๆฝ่ฑกๆฐๆฎๆฅๅฃๅฎ็ฐไบๅบ็ก็ๅข/ๅ /ๆน/ๆฅๅ่ฝ๏ผไธๆฏๆไธๅ่ฎพๅค้ด็ๆฐๆฎ่ฟ็งป๏ผไนๆฏๆไบ็ฑปๅญๅ
ธๅๅผ ้็ๆไฝ๏ผๅ
ๅๆปก่ถณไบๆฐๆฎ็ๆฅๅธธไฝฟ็จ้ๆฑ๏ผ่ฟไนไฝฟๅพไธๅ็ฎๆณ็ๆฐๆฎๆฅๅฃๅฏไปฅๅพๅฐ็ปไธใ
-
-ๅพ็ไบ็ปไธ็ๆฐๆฎๅฐ่ฃ
๏ผ็ฎๆณๅบๅ
็ [`visualizer`](./visualizers.md)๏ผ[`evaluator`](./evaluation.md)๏ผ[`dataset`](./datasets.md) ็ญๅไธชๆจกๅ้ด็ๆฐๆฎๆต้้ฝๅพๅฐไบๆๅคง็็ฎๅใๅจ MMOCR ไธญ๏ผๆไปฌๅฏนๆฐๆฎๆฅๅฃ็ฑปๅไฝๅบไปฅไธ็บฆๅฎ๏ผ
-
-- **xxxData**: ๅไธ็ฒๅบฆ็ๆฐๆฎๆ ๆณจๆๆจกๅ่พๅบใ็ฎๅ MMEngine ๅ
็ฝฎไบไธ็ง็ฒๅบฆ็{external+mmengine:doc}`ๆฐๆฎๅ
็ด `๏ผๅ
ๆฌๅฎไพ็บงๆฐๆฎ๏ผ`InstanceData`๏ผ๏ผๅ็ด ็บงๆฐๆฎ๏ผ`PixelData`๏ผไปฅๅๅพๅ็บง็ๆ ็ญพๆฐๆฎ๏ผ`LabelData`๏ผใๅจ MMOCR ็ฎๅๆฏๆ็ไปปๅกไธญ๏ผๆๆฌๆฃๆตไปฅๅๅ
ณ้ฎไฟกๆฏๆฝๅไปปๅกไฝฟ็จ `InstanceData` ๆฅๅฐ่ฃ
ๆๆฌๅฎไพ็ๆฃๆตๆกๅๅฏนๅบๆ ็ญพ๏ผ่ๆๆฌ่ฏๅซไปปๅกๅไฝฟ็จไบ `LabelData` ๆฅๅฐ่ฃ
ๆๆฌๅ
ๅฎนใ
-- **xxxDataSample**: ็ปงๆฟ่ช {external+mmengine:doc}`MMEngine: ๆฐๆฎๅบ็ฑป ` `BaseDataElement`๏ผ็จไบไฟๅญๅไธชไปปๅก็่ฎญ็ปๆๆต่ฏๆ ทๆฌ็**ๆๆ**ๆ ๆณจๅ้ขๆตไฟกๆฏใๅฆๆๆฌๆฃๆตไปปๅก็ๆฐๆฎๆ ทๆฌ็ฑป [`TextDetDataSample`](mmocr.structures.textdet_data_sample.TextDetDataSample)๏ผๆๆฌ่ฏๅซไปปๅก็ๆฐๆฎๆ ทๆฌ็ฑป [`TextRecogDataSample`](mmocr.structures.textrecog_data_sample.TextRecogDataSample)๏ผไปฅๅๅ
ณ้ฎไฟกๆฏๆฝไปปๅก็ๆฐๆฎๆ ทๆฌ็ฑป [`KIEDataSample`](mmocr.structures.kie_data_sample.KIEDataSample)ใ
-
-ไธ้ข๏ผๆไปฌๅฐๅๅซไป็ปๆฐๆฎๅ
็ด **xxxData** ไธๆฐๆฎๆ ทๆฌ **xxxDataSample** ๅจ MMOCR ไธญ็ๅฎ้
ๅบ็จใ
-
-## ๆฐๆฎๅ
็ด xxxData
-
-`InstanceData` ๅ `LabelData` ๆฏ `MMEngine`ไธญๅฎไน็ๅบ็กๆฐๆฎๅ
็ด ๏ผ็จไบๅฐ่ฃ
ไธๅ็ฒๅบฆ็ๆ ๆณจๆฐๆฎๆๆจกๅ่พๅบใๅจ MMOCR ไธญ๏ผๆไปฌ้ๅฏนไธๅไปปๅกไธญๅฎ้
ไฝฟ็จ็ๆฐๆฎ็ฑปๅ๏ผๅๅซ้็จไบ `InstanceData` ไธ `LabelData` ่ฟ่กไบๅฐ่ฃ
ใ
-
-### InstanceData
-
-ๅจ**ๆๆฌๆฃๆต**ไปปๅกไธญ๏ผๆฃๆตๅจๅ
ณๆณจ็ๆฏๅฎไพ็บงๅซ็ๆๅญๆ ทๆฌ๏ผๅ ๆญคๆไปฌไฝฟ็จ `InstanceData` ๆฅๅฐ่ฃ
่ฏฅไปปๅกๆ้็ๆฐๆฎใๅ
ถๆ้็่ฎญ็ปๆ ๆณจๅ้ขๆต่พๅบ้ๅธธๅ
ๅซไบ็ฉๅฝขๆๅค่พนๅฝข่พน็็๏ผไปฅๅ่พน็็ๆ ็ญพใ็ฑไบๆๆฌๆฃๆตไปปๅกๅชๆไธ็งๆญฃๆ ทๆฌ็ฑป๏ผๅณ โtextโ๏ผๅจ MMOCR ไธญๆไปฌ้ป่ฎคไฝฟ็จ `0` ๆฅ็ผๅท่ฏฅ็ฑปๅซใไปฅไธไปฃ็ ็คบไพๅฑ็คบไบๅฆไฝไฝฟ็จ `InstanceData` ๆฐๆฎๆฝ่ฑกๆฅๅฃๆฅๅฐ่ฃ
ๆๆฌๆฃๆตไปปๅกไธญไฝฟ็จ็ๆฐๆฎ็ฑปๅใ
-
-```python
-import torch
-from mmengine.structures import InstanceData
-
-# ๅฎไน gt_instance ็จไบๅฐ่ฃ
่พน็็็ๆ ๆณจไฟกๆฏ
-gt_instance = InstanceData()
-gt_instance.bbox = torch.Tensor([[0, 0, 10, 10], [10, 10, 20, 20]])
-gt_instance.polygons = torch.Tensor([[[0, 0], [10, 0], [10, 10], [0, 10]],
- [[10, 10], [20, 10], [20, 20], [10, 20]]])
-gt_instance.label = torch.Tensor([0, 0])
-
-# ๅฎไน pred_instance ็จไบๅฐ่ฃ
ๆจกๅ็่พๅบไฟกๆฏ
-pred_instances = InstanceData()
-pred_polygons, scores = model(input)
-pred_instances.polygons = pred_polygons
-pred_instances.scores = scores
-```
-
-MMOCR ไธญๅฏน `InstanceData` ๅญๆฎต็็บฆๅฎๅฆไธ่กจๆ็คบใๅผๅพๆณจๆ็ๆฏ๏ผ`InstanceData` ไธญ็ๅๅญๆฎต็้ฟๅบฆๅฟ
้กปไธบไธๆ ทๆฌไธญ็ๅฎไพไธชๆฐ `N` ็ธ็ญใ
-
-| | | |
-| ----------- | ---------------------------------- | ------------------------------------------------------------------------------------------------------------------------------------ |
-| ๅญๆฎต | ็ฑปๅ | ่ฏดๆ |
-| bboxes | `torch.FloatTensor` | ๆๆฌ่พน็ๆก `[x1, y1, x2, y2]`๏ผๅฝข็ถไธบ `(N, 4)`ใ |
-| labels | `torch.LongTensor` | ๅฎไพ็็ฑปๅซ๏ผ้ฟๅบฆไธบ `(N, )`ใMMOCR ไธญ้ป่ฎคไฝฟ็จ `0` ๆฅ่กจ็คบๆญฃๆ ทๆฌ็ฑป๏ผๅณ โtextโ ็ฑปใ |
-| polygons | `list[np.array(dtype=np.float32)]` | ่กจ็คบๆๆฌๅฎไพ็ๅค่พนๅฝข๏ผๅ่กจ้ฟๅบฆไธบ `(N, )`ใ |
-| scores | `torch.Tensor` | ๆๆฌๅฎไพๆฃๆตๆก็็ฝฎไฟกๅบฆ๏ผ้ฟๅบฆไธบ `(N, )`ใ |
-| ignored | `torch.BoolTensor` | ๆฏๅฆๅจ่ฎญ็ปไธญๅฟฝ็ฅๅฝๅๆๆฌๅฎไพ๏ผ้ฟๅบฆไธบ `(N, )`ใ |
-| texts | `list[str]` | ๅฎไพๅฏนๅบ็ๆๆฌ๏ผ้ฟๅบฆไธบ `(N, )`๏ผ็จไบ็ซฏๅฐ็ซฏ OCR ไปปๅกๅ KIEใ |
-| text_scores | `torch.FloatTensor` | ๆๆฌ้ขๆต็็ฝฎไฟกๅบฆ๏ผ้ฟๅบฆไธบ`(N, )`๏ผ็จไบ็ซฏๅฐ็ซฏ OCR ไปปๅกใ |
-| edge_labels | `torch.IntTensor` | ่็น็้ปๆฅ็ฉ้ต๏ผๅฝข็ถไธบ `(N, N)`ใๅจ KIE ไปปๅกไธญ๏ผ่็นไน้ด็ถๆ็ๅฏ้ๅผไธบ `-1` ๏ผๅฟฝ็ฅ๏ผไธๅไธ loss ่ฎก็ฎ๏ผ๏ผ`0` ๏ผๆญๅผ๏ผๅ `1`๏ผ่ฟๆฅ๏ผใ |
-| edge_scores | `torch.FloatTensor` | ็จไบ KIE ไปปๅกไธญๆฏๆก่พน็้ขๆต็ฝฎไฟกๅบฆ๏ผๅฝข็ถไธบ `(N, N)`ใ |
-
-### LabelData
-
-ๅฏนไบ**ๆๅญ่ฏๅซ**ไปปๅก๏ผๆ ๆณจๅ
ๅฎนๅ้ขๆตๅ
ๅฎน้ฝไผไฝฟ็จ `LabelData` ่ฟ่กๅฐ่ฃ
ใ
-
-```python
-import torch
-from mmengine.data import LabelData
-
-# ๅฎไนไธไธช gt_text ็จไบๅฐ่ฃ
ๆ ็ญพๆๆฌๅ
ๅฎน
-gt_text = LabelData()
-gt_text.item = 'MMOCR'
-
-# ๅฎไนไธไธช pred_text ๅฏน่ฑก็จไบๅฐ่ฃ
้ขๆตๆๆฌไปฅๅ็ฝฎไฟกๅบฆ
-pred_text = LabelData()
-index, score = model(input)
-text = dictionary.idx2str(index)
-pred_text.score = score
-pred_text.item = text
-```
-
-MMOCR ไธญๅฏน `LabelData` ๅญๆฎต็็บฆๅฎๅฆไธ่กจๆ็คบ๏ผ
-
-| | | |
-| -------------- | ------------------ | -------------------------------------------------------------------------------------------------------------------------- |
-| ๅญๆฎต | ็ฑปๅ | ่ฏดๆ |
-| item | `str` | ๆๆฌๅ
ๅฎนใ |
-| score | `list[float]` | ้ขๆต็ๆๆฌๅ
ๅฎน็็ฝฎไฟกๅบฆใ |
-| indexes | `torch.LongTensor` | ๆๆฌๅญ็ฌฆ็ป่ฟ[ๅญๅ
ธ](../basic_concepts/models.md#dictionary)็ผ็ ๅ็ๅบๅ๏ผไธๅ
ๅซไบ้ค `` ไปฅๅค็ๆๆ็นๆฎๅญ็ฌฆใ |
-| padded_indexes | `torch.LongTensor` | ๅฆๆ indexes ็้ฟๅบฆๅฐไบๆๅคงๅบๅ้ฟๅบฆ๏ผไธ `pad_idx` ๅญๅจๆถ๏ผ่ฏฅๅญๆฎตไฟๅญไบๅกซๅ
่ณๆๅคงๅบๅ้ฟๅบฆ `max_seq_len`็็ผ็ ๅ็ๆๆฌๅบๅใ |
-
-## ๆฐๆฎๆ ทๆฌ xxxDataSample
-
-้่ฟๅฎไน็ปไธ็ๆฐๆฎ็ปๆ๏ผๆไปฌๅฏไปฅๆนไพฟๅฐๅฐๆ ๆณจๆฐๆฎๅ้ขๆต็ปๆ่ฟ่ก็ปไธๅฐ่ฃ
๏ผไฝฟไปฃ็ ๅบไธๅๆจกๅ้ด็ๆฐๆฎไผ ้ๆดๅ ไพฟๆทใๅจ MMOCR ไธญ๏ผๆไปฌๅบไบ็ฐๅจๆฏๆ็ไธไธชไปปๅกๅๅ
ถๆ้่ฆ็ๆฐๆฎๅๅซๅฐ่ฃ
ไบไธ็งๆฐๆฎๆฝ่ฑก๏ผๅ
ๆฌๆๆฌๆฃๆตไปปๅกๆฐๆฎๆฝ่ฑก [`TextDetDataSample`](mmocr.structures.textdet_data_sample.TextDetDataSample)๏ผๆๆฌ่ฏๅซไปปๅกๆฐๆฎๆฝ่ฑก [`TextRecogDataSample`](mmocr.structures.textrecog_data_sample.TextRecogDataSample)๏ผไปฅๅๅ
ณ้ฎไฟกๆฏๆฝๅไปปๅกๆฐๆฎๆฝ่ฑก [`KIEDataSample`](mmocr.structures.kie_data_sample.KIEDataSample)ใ่ฟไบๆฐๆฎๆฝ่ฑกๅ็ปงๆฟ่ช {external+mmengine:doc}`MMEngine: ๆฐๆฎๅบ็ฑป ` `BaseDataElement`๏ผ็จไบไฟๅญๅไธชไปปๅก็่ฎญ็ปๆๆต่ฏๆ ทๆฌ็ๆๆๆ ๆณจๅ้ขๆตไฟกๆฏใ
-
-### ๆๆฌๆฃๆตไปปๅกๆฐๆฎๆฝ่ฑก TextDetDataSample
-
-[TextDetDataSample](mmocr.structures.textdet_data_sample.TextDetDataSample) ็จไบๅฐ่ฃ
ๆๅญๆฃๆตไปปๅกๆ้็ๆฐๆฎ๏ผๅ
ถไธป่ฆๅ
ๅซไบไธคไธชๅญๆฎต `gt_instances` ไธ `pred_instances`๏ผๅๅซ็จไบๅญๆพๆ ๆณจไฟกๆฏไธ้ขๆต็ปๆใ
-
-| | | |
-| -------------- | ------------------------------- | ---------- |
-| ๅญๆฎต | ็ฑปๅ | ่ฏดๆ |
-| gt_instances | [`InstanceData`](#instancedata) | ๆ ๆณจไฟกๆฏใ |
-| pred_instances | [`InstanceData`](#instancedata) | ้ขๆต็ปๆใ |
-
-ๅ
ถไธญไผ็จๅฐ็ [`InstanceData`](#instancedata) ็บฆๅฎๅญๆฎตๆ๏ผ
-
-| | | |
-| -------- | ---------------------------------- | -------------------------------------------------------------------------------- |
-| ๅญๆฎต | ็ฑปๅ | ่ฏดๆ |
-| bboxes | `torch.FloatTensor` | ๆๆฌ่พน็ๆก `[x1, y1, x2, y2]`๏ผๅฝข็ถไธบ `(N, 4)`ใ |
-| labels | `torch.LongTensor` | ๅฎไพ็็ฑปๅซ๏ผ้ฟๅบฆไธบ `(N, )`ใๅจ MMOCR ไธญ้ๅธธไฝฟ็จ `0` ๆฅ่กจ็คบๆญฃๆ ทๆฌ็ฑป๏ผๅณ โtextโ ็ฑป |
-| polygons | `list[np.array(dtype=np.float32)]` | ่กจ็คบๆๆฌๅฎไพ็ๅค่พนๅฝข๏ผๅ่กจ้ฟๅบฆไธบ `(N, )`ใ |
-| scores | `torch.Tensor` | ๆๆฌๅฎไพไปปๅก้ขๆต็ๆฃๆตๆก็็ฝฎไฟกๅบฆ๏ผ้ฟๅบฆไธบ `(N, )`ใ |
-| ignored | `torch.BoolTensor` | ๆฏๅฆๅจ่ฎญ็ปไธญๅฟฝ็ฅๅฝๅๆๆฌๅฎไพ๏ผ้ฟๅบฆไธบ `(N, )`ใ |
-
-็ฑไบๆๆฌๆฃๆตๆจกๅ้ๅธธๅชไผ่พๅบ bboxes/polygons ไธญ็ไธ้กน๏ผๅ ๆญคๆไปฌๅช้็กฎไฟ่ฟไธค้กนไธญ็ไธไธช่ขซ่ตๅผๅณๅฏใ
-
-ไปฅไธ็คบไพไปฃ็ ๅฑ็คบไบ `TextDetDataSample` ็ไฝฟ็จๆนๆณ๏ผ
-
-```python
-import torch
-from mmengine.data import TextDetDataSample
-
-data_sample = TextDetDataSample()
-# ๆๅฎๅฝๅๅพ็็ๆ ๆณจไฟกๆฏ
-img_meta = dict(img_shape=(800, 1196, 3), pad_shape=(800, 1216, 3))
-gt_instances = InstanceData(metainfo=img_meta)
-gt_instances.bboxes = torch.rand((5, 4))
-gt_instances.labels = torch.zeros((5,), dtype=torch.long)
-data_sample.gt_instances = gt_instances
-
-# ๆๅฎๅฝๅๅพ็็้ขๆตไฟกๆฏ
-pred_instances = InstanceData()
-pred_instances.bboxes = torch.rand((5, 4))
-pred_instances.labels = torch.zeros((5,), dtype=torch.long)
-data_sample.pred_instances = pred_instances
-```
-
-### ๆๆฌ่ฏๅซไปปๅกๆฐๆฎๆฝ่ฑก TextRecogDataSample
-
-[`TextRecogDataSample`](mmocr.structures.textrecog_data_sample.TextRecogDataSample) ็จไบๅฐ่ฃ
ๆๅญ่ฏๅซไปปๅก็ๆฐๆฎใๅฎๆไธคไธชๅฑๆง๏ผ`gt_text` ๅ `pred_text` , ๅๅซ็จไบๅญๆพๆ ๆณจไฟกๆฏๅ้ขๆต็ปๆใ
-
-| | | |
-| --------- | ------------------------- | ---------- |
-| ๅญๆฎต | ็ฑปๅ | ่ฏดๆ |
-| gt_text | [`LabelData`](#labeldata) | ๆ ๆณจไฟกๆฏใ |
-| pred_text | [`LabelData`](#labeldata) | ้ขๆต็ปๆใ |
-
-ไปฅไธ็คบไพไปฃ็ ๅฑ็คบไบ [`TextRecogDataSample`](mmocr.structures.textrecog_data_sample.TextRecogDataSample) ็ไฝฟ็จๆนๆณ๏ผ
-
-```python
-import torch
-from mmengine.data import TextRecogDataSample
-
-data_sample = TextRecogDataSample()
-# ๆๅฎๅฝๅๅพ็็ๆ ๆณจไฟกๆฏ
-img_meta = dict(img_shape=(800, 1196, 3), pad_shape=(800, 1216, 3))
-gt_text = LabelData(metainfo=img_meta)
-gt_text.item = 'mmocr'
-data_sample.gt_text = gt_text
-
-# ๆๅฎๅฝๅๅพ็็้ขๆต็ปๆ
-pred_text = LabelData(metainfo=img_meta)
-pred_text.item = 'mmocr'
-data_sample.pred_text = pred_text
-```
-
-ๅ
ถไธญไผ็จๅฐ็ `LabelData` ๅญๆฎตๆ๏ผ
-
-| | | |
-| -------------- | ------------------- | -------------------------------------------------------------------------------------------------------------------------- |
-| ๅญๆฎต | ็ฑปๅ | ่ฏดๆ |
-| item | `list[str]` | ๅฎไพๅฏนๅบ็ๆๆฌ๏ผ้ฟๅบฆไธบ (N, ) ๏ผ็จไบ็ซฏๅฐ็ซฏ OCR ไปปๅกๅ KIE |
-| score | `torch.FloatTensor` | ๆๆฌ้ขๆต็็ฝฎไฟกๅบฆ๏ผ้ฟๅบฆไธบ (N, )๏ผ็จไบ็ซฏๅฐ็ซฏ OCR ไปปๅก |
-| indexes | `torch.LongTensor` | ๆๆฌๅญ็ฌฆ็ป่ฟ[ๅญๅ
ธ](../basic_concepts/models.md#dictionary)็ผ็ ๅ็ๅบๅ๏ผไธๅ
ๅซไบ้ค `` ไปฅๅค็ๆๆ็นๆฎๅญ็ฌฆใ |
-| padded_indexes | `torch.LongTensor` | ๅฆๆ indexes ็้ฟๅบฆๅฐไบๆๅคงๅบๅ้ฟๅบฆ๏ผไธ `pad_idx` ๅญๅจๆถ๏ผ่ฏฅๅญๆฎตไฟๅญไบๅกซๅ
่ณๆๅคงๅบๅ้ฟๅบฆ `max_seq_len`็็ผ็ ๅ็ๆๆฌๅบๅใ |
-
-### ๅ
ณ้ฎไฟกๆฏๆฝๅไปปๅกๆฐๆฎๆฝ่ฑก KIEDataSample
-
-[`KIEDataSample`](mmocr.structures.kie_data_sample.KIEDataSample) ็จไบๅฐ่ฃ
KIE ไปปๅกๆ้็ๆฐๆฎ๏ผๅ
ถๅๆ ท็บฆๅฎไบไธคไธชๅฑๆง๏ผๅณ `gt_instances` ไธ `pred_instances`๏ผๅๅซ็จไบๅญๆพๆ ๆณจไฟกๆฏไธ้ขๆต็ปๆใ
-
-| | | |
-| -------------- | ------------------------------- | ---------- |
-| ๅญๆฎต | ็ฑปๅ | ่ฏดๆ |
-| gt_instances | [`InstanceData`](#instancedata) | ๆ ๆณจไฟกๆฏใ |
-| pred_instances | [`InstanceData`](#instancedata) | ้ขๆต็ปๆใ |
-
-่ฏฅไปปๅกไผ็จๅฐ็ [`InstanceData`](#instancedata) ๅญๆฎตๅฆไธ่กจๆ็คบ๏ผ
-
-| | | |
-| ----------- | ------------------- | --------------------------------------------------------------------------------------------------------------------------------------------- |
-| ๅญๆฎต | ็ฑปๅ | ่ฏดๆ |
-| bboxes | `torch.Tensor` | ๆๆฌ่พน็ๆก `[x1, y1, x2, y2]`๏ผๅฝข็ถไธบ `(N, 4)`ใ |
-| labels | `torch.LongTensor` | ๅฎไพ็็ฑปๅซ๏ผ้ฟๅบฆไธบ `(N, )`ใๅจ MMOCR ไธญ้ๅธธไธบ 0๏ผๅณ โtextโ ็ฑปใ |
-| texts | `list[str]` | ๅฎไพๅฏนๅบ็ๆๆฌ๏ผ้ฟๅบฆไธบ `(N, )` ๏ผ็จไบ็ซฏๅฐ็ซฏ OCR ไปปๅกๅ KIE ไปปๅกใ |
-| edge_labels | `torch.IntTensor` | ่็นไน้ด็้ปๆฅ็ฉ้ต๏ผๅฝข็ถไธบ `(N, N)`ใๅจ KIE ไปปๅกไธญ๏ผ่็นไน้ด็ถๆ็ๅฏ้ๅผไธบ `-1` ๏ผไธๅ
ณๅฟ๏ผไธไธๅไธ loss ่ฎก็ฎ๏ผ๏ผ`0` ๏ผๆญๅผ๏ผๅ `1` ๏ผ่ฟๆฅ๏ผใ |
-| edge_scores | `torch.FloatTensor` | ๆฏๆก่พน็้ขๆต็ฝฎไฟกๅบฆ๏ผๅฝข็ถไธบ `(N, N)`ใ |
-| scores | `torch.FloatTensor` | ่็นๆ ็ญพ็้ขๆต็ฝฎไฟกๅบฆ, ๅฝข็ถไธบ `(N,)`ใ |
-
-```{warning}
-็ฑไบ KIE ไปปๅก็ๆจกๅๅฎ็ฐๅฐๆชๆ็ปไธๆ ๅ๏ผ่ฏฅ่ฎพ่ฎก็ฎๅไป
่่ไบ [SDMGR](../../../configs/kie/sdmgr/README.md) ๆจกๅ็ไฝฟ็จๅบๆฏใๅ ๆญค๏ผ่ฏฅ่ฎพ่ฎกๆๅฏ่ฝๅจๆไปฌๆฏๆๆดๅค KIE ๆจกๅๅไบง็ๅๅจใ
-```
-
-ไปฅไธ็คบไพไปฃ็ ๅฑ็คบไบ [`KIEDataSample`](mmocr.structures.kie_data_sample.KIEDataSample) ็ไฝฟ็จๆนๆณใ
-
-```python
-import torch
-from mmengine.data import KIEDataSample
-
-data_sample = KIEDataSample()
-# ๆๅฎๅฝๅๅพ็็ๆ ๆณจไฟกๆฏ
-img_meta = dict(img_shape=(800, 1196, 3),pad_shape=(800, 1216, 3))
-gt_instances = InstanceData(metainfo=img_meta)
-gt_instances.bboxes = torch.rand((5, 4))
-gt_instances.labels = torch.zeros((5,), dtype=torch.long)
-gt_instances.texts = ['text1', 'text2', 'text3', 'text4', 'text5']
-gt_instances.edge_lebels = torch.randint(-1, 2, (5, 5))
-data_sample.gt_instances = gt_instances
-
-# ๆๅฎๅฝๅๅพ็็้ขๆตไฟกๆฏ
-pred_instances = InstanceData()
-pred_instances.bboxes = torch.rand((5, 4))
-pred_instances.labels = torch.rand((5,))
-pred_instances.edge_labels = torch.randint(-1, 2, (10, 10))
-pred_instances.edge_scores = torch.rand((10, 10))
-data_sample.pred_instances = pred_instances
-```
diff --git a/pipeline/mmocr/docs/zh_cn/basic_concepts/transforms.md b/pipeline/mmocr/docs/zh_cn/basic_concepts/transforms.md
deleted file mode 100644
index cc07c986d713089585aa28d19e85e1b397025ff8..0000000000000000000000000000000000000000
--- a/pipeline/mmocr/docs/zh_cn/basic_concepts/transforms.md
+++ /dev/null
@@ -1,227 +0,0 @@
-# ๆฐๆฎๅๆขไธๆตๆฐด็บฟ
-
-ๅจ MMOCR ็่ฎพ่ฎกไธญ๏ผๆฐๆฎ้็ๆๅปบไธๆฐๆฎๅๅคๆฏ็ธไบ่งฃ่ฆ็ใไนๅฐฑๆฏ่ฏด๏ผ[`OCRDataset`](mmocr.datasets.ocr_dataset.OCRDataset) ็ญๆฐๆฎ้ๆๅปบ็ฑป่ด่ดฃๅฎๆๆ ๆณจๆไปถ็่ฏปๅไธ่งฃๆๅ่ฝ๏ผ่ๆฐๆฎๅๆขๆนๆณ๏ผData Transforms๏ผๅ่ฟไธๆญฅๅฎ็ฐไบๆฐๆฎ้ขๅค็ใๆฐๆฎๅขๅผบใๆฐๆฎๆ ผๅผๅ็ญ็ธๅ
ณๅ่ฝใ็ฎๅ๏ผๅฆไธ่กจๆ็คบ๏ผMMOCR ไธญๅ
ฑๅฎ็ฐไบ 5 ็ฑปๆฐๆฎๅๆขๆนๆณ๏ผ
-
-| | | |
-| -------------- | --------------------------------------------------------------------- | ------------------------------------------------------------------- |
-| ๆฐๆฎๅๆข็ฑปๅ | ๅฏนๅบๆไปถ | ๅ่ฝ่ฏดๆ |
-| ๆฐๆฎ่ฏปๅ | loading.py | ๅฎ็ฐไบไธๅๆ ผๅผๆฐๆฎ็่ฏปๅๅ่ฝใ |
-| ๆฐๆฎๆ ผๅผๅ | formatting.py | ๅฎๆไธๅไปปๅกๆ้ๆฐๆฎ็ๆ ผๅผๅๅ่ฝใ |
-| ่ทจๅบๆฐๆฎ้้
ๅจ | adapters.py | ่ด่ดฃ OpenMMLab ้กน็ฎๅ
่ทจๅบ่ฐ็จ็ๆฐๆฎๆ ผๅผ่ฝฌๆขๅ่ฝใ |
-| ๆฐๆฎๅขๅผบ | ocr_transforms.py textdet_transforms.py textrecog_transforms.py | ๅฎ็ฐไบไธๅไปปๅกไธ็ๅ็ฑปๆฐๆฎๅขๅผบๆนๆณใ |
-| ๅ
่ฃ
็ฑป | wrappers.py | ๅฎ็ฐไบๅฏน ImgAug ็ญๅธธ็จ็ฎๆณๅบ็ๅ
่ฃ
๏ผไฝฟๅ
ถ้้
MMOCR ็ๅ
้จๆฐๆฎๆ ผๅผใ |
-
-็ฑไบๆฏไธไธชๆฐๆฎๅๆข็ฑปไน้ด้ฝๆฏ็ธไบ็ฌ็ซ็๏ผๅ ๆญค๏ผๅจ็บฆๅฎๅฅฝๅบๅฎ็ๆฐๆฎๅญๅจๅญๆฎตๅ๏ผๆไปฌๅฏไปฅไพฟๆทๅฐ้็จไปปๆ็ๆฐๆฎๅๆข็ปๅๆฅๆๅปบๆฐๆฎๆตๆฐด็บฟ๏ผPipeline๏ผใๅฆไธๅพๆ็คบ๏ผๅจ MMOCR ไธญ๏ผไธไธชๅ
ธๅ็่ฎญ็ปๆฐๆฎๆตๆฐด็บฟไธป่ฆ็ฑ**ๆฐๆฎ่ฏปๅ**ใ**ๅพๅๅขๅผบ**ไปฅๅ**ๆฐๆฎๆ ผๅผๅ**ไธ้จๅๆๆ๏ผ็จๆทๅช้่ฆๅจ้
็ฝฎๆไปถไธญๅฎไน็ธๅ
ณ็ๆฐๆฎๆตๆฐด็บฟๅ่กจ๏ผๅนถๆๅฎๅ
ทไฝๆ้็ๆฐๆฎๅๆข็ฑปๅๅ
ถๅๆฐๅณๅฏ๏ผ
-
-
-
-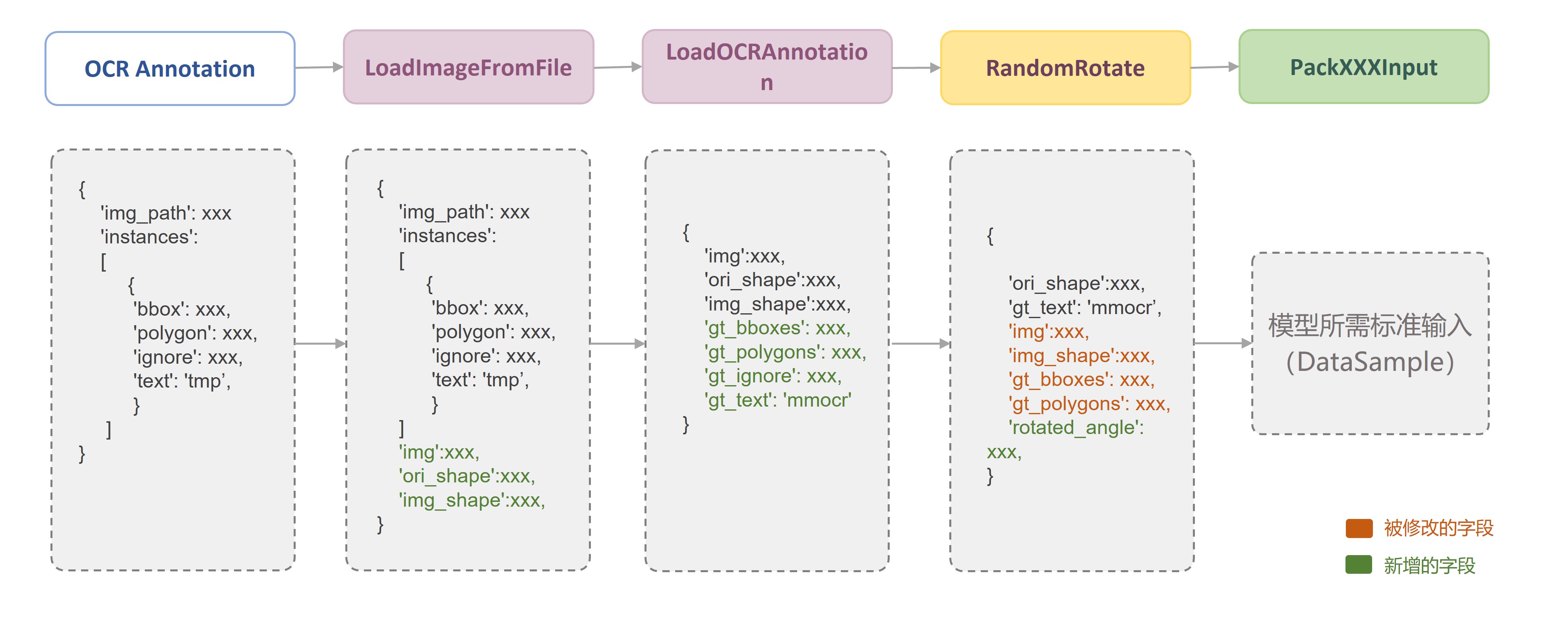
-
-
-
-```python
-train_pipeline_r18 = [
- # ๆฐๆฎ่ฏปๅ๏ผๅพๅ๏ผ
- dict(
- type='LoadImageFromFile',
- color_type='color_ignore_orientation'),
- # ๆฐๆฎ่ฏปๅ๏ผๆ ๆณจ๏ผ
- dict(
- type='LoadOCRAnnotations',
- with_polygon=True,
- with_bbox=True,
- with_label=True,
- ),
- # ไฝฟ็จ ImgAug ไฝๆฐๆฎๅขๅผบ
- dict(
- type='ImgAugWrapper',
- args=[['Fliplr', 0.5],
- dict(cls='Affine', rotate=[-10, 10]), ['Resize', [0.5, 3.0]]]),
- # ไฝฟ็จ MMOCR ๅ
็ฝฎ็ๅพๅๅขๅผบ
- dict(type='RandomCrop', min_side_ratio=0.1),
- dict(type='Resize', scale=(640, 640), keep_ratio=True),
- dict(type='Pad', size=(640, 640)),
- # ๆฐๆฎๆ ผๅผๅ
- dict(
- type='PackTextDetInputs',
- meta_keys=('img_path', 'ori_shape', 'img_shape'))
-]
-```
-
-```{tip}
-ๆดๅคๆๅ
ณๆฐๆฎๆตๆฐด็บฟ้
็ฝฎ็ๆ็จๅฏ่ง[้
็ฝฎๆๆกฃ](../user_guides/config.md#ๆฐๆฎๆตๆฐด็บฟ้
็ฝฎ)ใไธ้ข๏ผๆไปฌๅฐ็ฎๅไป็ป MMOCR ไธญๅทฒๆฏๆ็ๆฐๆฎๅๆข็ฑปๅใ
-```
-
-ๅฏนไบๆฏไธไธชๆฐๆฎๅๆขๆนๆณ๏ผMMOCR ้ฝไธฅๆ ผๆ็
งๆๆกฃๅญ็ฌฆไธฒ๏ผdocstring๏ผ่ง่ๅจๆบ็ ไธญๆไพไบ่ฏฆ็ป็ไปฃ็ ๆณจ้ใไพๅฆ๏ผๆฏไธไธชๆฐๆฎ่ฝฌๆข็ฑป็ๅคด้จๆไปฌ้ฝๆณจ้ไบ โ้ๆฑๅญๆฎตโ๏ผ`Required keys`๏ผ๏ผ โไฟฎๆนๅญๆฎตโ๏ผ`Modified Keys`๏ผไธ โๆทปๅ ๅญๆฎตโ๏ผ`Added Keys`๏ผใๅ
ถไธญ๏ผโ้ๆฑๅญๆฎตโไปฃ่กจ่ฏฅๆฐๆฎ่ฝฌๆขๆนๆณๅฏนไบ่พๅ
ฅๆฐๆฎๆ้ๅ
ๅซๅญๆฎต็ๅผบๅถ้ๆฑ๏ผ่โไฟฎๆนๅญๆฎตโไธโๆทปๅ ๅญๆฎตโๅ่กจๆ่ฏฅๆนๆณๅฏ่ฝไผๅจๅๆๆฐๆฎๅบ็กไนไธไฟฎๆนๆๆทปๅ ็ๅญๆฎตใไพๅฆ๏ผ`LoadImageFromFile` ๅฎ็ฐไบๅพ็็่ฏปๅๅ่ฝ๏ผๅ
ถ้ๆฑๅญๆฎตไธบๅพๅ็ๅญๅจ่ทฏๅพ `img_path`๏ผ่ไฟฎๆนๅญๆฎตๅๅ
ๆฌไบ่ฏปๅ
ฅ็ๅพๅไฟกๆฏ `img`๏ผไปฅๅๅพ็ๅฝๅๅฐบๅฏธ `img_shape`๏ผๅพ็ๅๅงๅฐบๅฏธ `ori_shape` ็ญๅพ็ๅฑๆงใ
-
-```python
-@TRANSFORMS.register_module()
-class LoadImageFromFile(MMCV_LoadImageFromFile):
- # ๅจๆฏไธไธชๆฐๆฎๅๆขๆนๆณ็ๅคด้จ๏ผๆไปฌ้ฝๆไพไบ่ฏฆ็ป็ไปฃ็ ๆณจ้ใ
- """Load an image from file.
-
- Required Keys:
-
- - img_path
-
- Modified Keys:
-
- - img
- - img_shape
- - ori_shape
- """
-```
-
-```{note}
-ๅจ MMOCR ็ๆฐๆฎๆตๆฐด็บฟไธญ๏ผๅพๅๅๆ ็ญพ็ญไฟกๆฏ่ขซ็ปไธไฟๅญๅจๅญๅ
ธไธญใ้่ฟ็ปไธ็ๅญๆฎตๅ๏ผๆไปฌๅฏไปฅๅจไธๅ็ๆฐๆฎๅๆขๆนๆณ้ด็ตๆดปๅฐไผ ้ๆฐๆฎใๅ ๆญค๏ผไบ่งฃ MMOCR ไธญๅธธ็จ็็บฆๅฎๅญๆฎตๅๆฏ้ๅธธ้่ฆ็ใ
-```
-
-ไธบๆนไพฟ็จๆทๆฅ่ฏข๏ผไธ่กจๅๅบไบ MMOCR ไธญๅๆฐๆฎ่ฝฌๆข๏ผData Transform๏ผ็ฑปๅธธ็จ็ๅญๆฎต็บฆๅฎๅ่ฏดๆใ
-
-| | | |
-| ---------------- | --------------------------------- | ------------------------------------------------------------------------------------------------------------------------------------ |
-| ๅญๆฎต | ็ฑปๅ | ่ฏดๆ |
-| img | `np.array(dtype=np.uint8)` | ๅพๅไฟกๆฏ๏ผๅฝข็ถไธบ `(h, w, c)`ใ |
-| img_shape | `tuple(int, int)` | ๅฝๅๅพๅๅฐบๅฏธ `(h, w)`ใ |
-| ori_shape | `tuple(int, int)` | ๅพๅๅจๅๅงๅๆถ็ๅฐบๅฏธ `(h, w)`ใ |
-| scale | `tuple(int, int)` | ๅญๆพ็จๆทๅจ Resize ็ณปๅๆฐๆฎๅๆข๏ผTransform๏ผไธญๆๅฎ็็ฎๆ ๅพๅๅฐบๅฏธ `(h, w)`ใๆณจๆ๏ผ่ฏฅๅผๆชๅฟ
ไธๅๆขๅ็ๅฎ้
ๅพๅๅฐบๅฏธ็ธ็ฌฆใ |
-| scale_factor | `tuple(float, float)` | ๅญๆพ็จๆทๅจ Resize ็ณปๅๆฐๆฎๅๆข๏ผTransform๏ผไธญๆๅฎ็็ฎๆ ๅพๅ็ผฉๆพๅ ๅญ `(w_scale, h_scale)`ใๆณจๆ๏ผ่ฏฅๅผๆชๅฟ
ไธๅๆขๅ็ๅฎ้
ๅพๅๅฐบๅฏธ็ธ็ฌฆใ |
-| keep_ratio | `bool` | ๆฏๅฆๆ็ญๆฏไพๅฏนๅพๅ่ฟ่ก็ผฉๆพใ |
-| flip | `bool` | ๅพๅๆฏๅฆ่ขซ็ฟป่ฝฌใ |
-| flip_direction | `str` | ็ฟป่ฝฌๆนๅใๅฏ้้กนไธบ `horizontal`, `vertical`, `diagonal`ใ |
-| gt_bboxes | `np.array(dtype=np.float32)` | ๆๆฌๅฎไพ่พน็ๆก็็ๅฎๆ ็ญพใ |
-| gt_polygons | `list[np.array(dtype=np.float32)` | ๆๆฌๅฎไพ่พน็ๅค่พนๅฝข็็ๅฎๆ ็ญพใ |
-| gt_bboxes_labels | `np.array(dtype=np.int64)` | ๆๆฌๅฎไพๅฏนๅบ็็ฑปๅซๆ ็ญพใๅจ MMOCR ไธญ้ๅธธไธบ 0๏ผไปฃๆ "text" ็ฑปๅซใ |
-| gt_texts | `list[str]` | ไธๆๆฌๅฎไพๅฏนๅบ็ๅญ็ฌฆไธฒๆ ๆณจใ |
-| gt_ignored | `np.array(dtype=np.bool_)` | ๆฏๅฆ่ฆๅจ่ฎก็ฎ็ฎๆ ๆถๅฟฝ็ฅ่ฏฅๅฎไพ๏ผ็จไบๆฃๆตไปปๅกไธญ๏ผใ |
-
-## ๆฐๆฎ่ฏปๅ - loading.py
-
-ๆฐๆฎ่ฏปๅ็ฑปไธป่ฆๅฎ็ฐไบไธๅๆไปถๆ ผๅผใๅ็ซฏ่ฏปๅๅพ็ๅๅ ่ฝฝๆ ๆณจไฟกๆฏ็ๅ่ฝใ็ฎๅ๏ผMMOCR ๅ
้จๅ
ฑๅฎ็ฐไบไปฅไธๆฐๆฎ่ฏปๅ็ฑป็ Data Transforms๏ผ
-
-| | | | |
-| ------------------ | --------------------------------------------------------- | -------------------------------------------------------------- | --------------------------------------------------------------- |
-| ๆฐๆฎ่ฝฌๆข็ฑปๅ็งฐ | ้ๆฑๅญๆฎต | ไฟฎๆน/ๆทปๅ ๅญๆฎต | ่ฏดๆ |
-| LoadImageFromFile | `img_path` | `img` `img_shape` `ori_shape` | ไปๅพ็่ทฏๅพ่ฏปๅๅพ็๏ผๆฏๆๅค็งๆไปถๅญๅจๅ็ซฏ๏ผๅฆ `disk`, `http`, `petrel` ็ญ๏ผๅๅพ็่งฃ็ ๅ็ซฏ๏ผๅฆ `cv2`, `turbojpeg`, `pillow`, `tifffile`็ญ๏ผใ |
-| LoadOCRAnnotations | `bbox` `bbox_label` `polygon` `ignore` `text` | `gt_bboxes` `gt_bboxes_labels` `gt_polygons` `gt_ignored` `gt_texts` | ่งฃๆ OCR ไปปๅกๆ้็ๆ ๆณจไฟกๆฏใ |
-| LoadKIEAnnotations | `bboxes` `bbox_labels` `edge_labels` `texts` | `gt_bboxes` `gt_bboxes_labels` `gt_edge_labels` `gt_texts` `ori_shape` | ่งฃๆ KIE ไปปๅกๆ้็ๆ ๆณจไฟกๆฏใ |
-
-## ๆฐๆฎๅขๅผบ - xxx_transforms.py
-
-ๆฐๆฎๅขๅผบๆฏๆๆฌๆฃๆตใ่ฏๅซ็ญไปปๅกไธญๅฟ
ไธๅฏๅฐ็ๆต็จไนไธใ็ฎๅ๏ผMMOCR ไธญๅ
ฑๅฎ็ฐไบๆฐๅ็งๆๆฌ้ขๅๅ
ๅธธ็จ็ๆฐๆฎๅขๅผบๆจกๅ๏ผไพๆฎๅ
ถไปปๅก็ฑปๅ๏ผๅๅซไธบ้็จ OCR ๆฐๆฎๅขๅผบๆจกๅ [ocr_transforms.py](/mmocr/datasets/transforms/ocr_transforms.py)๏ผๆๆฌๆฃๆตๆฐๆฎๅขๅผบๆจกๅ [textdet_transforms.py](/mmocr/datasets/transforms/textdet_transforms.py)๏ผไปฅๅๆๆฌ่ฏๅซๆฐๆฎๅขๅผบๆจกๅ [textrecog_transforms.py](/mmocr/datasets/transforms/textrecog_transforms.py)ใ
-
-ๅ
ทไฝ่่จ๏ผ`ocr_transforms.py` ไธญๅฎ็ฐไบ้ๆบๅช่ฃใ้ๆบๆ่ฝฌ็ญๅไปปๅก้็จ็ๆฐๆฎๅขๅผบๆจกๅ๏ผ
-
-| | | | |
-| -------------- | -------------------------------------------------------------- | -------------------------------------------------------------- | -------------------------------------------------------------- |
-| ๆฐๆฎ่ฝฌๆข็ฑปๅ็งฐ | ้ๆฑๅญๆฎต | ไฟฎๆน/ๆทปๅ ๅญๆฎต | ่ฏดๆ |
-| RandomCrop | `img` `gt_bboxes` `gt_bboxes_labels` `gt_polygons` `gt_ignored` `gt_texts` (optional) | `img` `img_shape` `gt_bboxes` `gt_bboxes_labels` `gt_polygons` `gt_ignored` `gt_texts` (optional) | ้ๆบ่ฃๅช๏ผๅนถ็กฎไฟ่ฃๅชๅ็ๅพ็่ณๅฐๅ
ๅซไธไธชๆๆฌๅฎไพใๅฏ้ๅๆฐไธบ `min_side_ratio`๏ผ็จไปฅๆงๅถ่ฃๅชๅพ็็็ญ่พนๅ ๅๅงๅพ็็ๆฏไพ๏ผ้ป่ฎคๅผไธบ `0.4`ใ |
-| RandomRotate | `img` `img_shape` `gt_bboxes` (optional) `gt_polygons` (optional) | `img` `img_shape` `gt_bboxes` (optional) `gt_polygons` (optional) `rotated_angle` | ้ๆบๆ่ฝฌ๏ผๅนถๅฏ้ๆฉๅฏนๆ่ฝฌๅๅพๅ็้ป่พน่ฟ่กๅกซๅ
ใ |
-| | | | |
-
-`textdet_transforms.py` ๅๅฎ็ฐไบๆๆฌๆฃๆตไปปๅกไธญๅธธ็จ็ๆฐๆฎๅขๅผบๆจกๅ๏ผ
-
-| | | | |
-| ----------------- | ------------------------------------- | ------------------------------------------------------------------- | -------------------------------------------------------------------- |
-| ๆฐๆฎ่ฝฌๆข็ฑปๅ็งฐ | ้ๆฑๅญๆฎต | ไฟฎๆน/ๆทปๅ ๅญๆฎต | ่ฏดๆ |
-| RandomFlip | `img` `gt_bboxes` `gt_polygons` | `img` `gt_bboxes` `gt_polygons` `flip` `flip_direction` | ้ๆบ็ฟป่ฝฌ๏ผๆฏๆๆฐดๅนณใๅ็ดๅๅฏน่งไธ็งๆนๅ็ๅพๅ็ฟป่ฝฌใ้ป่ฎคไฝฟ็จๆฐดๅนณ็ฟป่ฝฌใ |
-| FixInvalidPolygon | `gt_polygons` `gt_ignored` | `gt_polygons` `gt_ignored` | ่ชๅจไฟฎๅคๆๅฟฝ็ฅ้ๆณๅค่พนๅฝขๆ ๆณจใ |
-
-`textrecog_transforms.py` ไธญๅฎ็ฐไบๆๆฌ่ฏๅซไปปๅกไธญๅธธ็จ็ๆฐๆฎๅขๅผบๆจกๅ๏ผ
-
-| | | | |
-| --------------- | -------- | ----------------------------------------------------------------- | ---------------------------------------------------------------------------------------------------------- |
-| ๆฐๆฎ่ฝฌๆข็ฑปๅ็งฐ | ้ๆฑๅญๆฎต | ไฟฎๆน/ๆทปๅ ๅญๆฎต | ่ฏดๆ |
-| RescaleToHeight | `img` | `img` `img_shape` `scale` `scale_factor` `keep_ratio` | ็ผฉๆพๅพๅ่ณๆๅฎ้ซๅบฆ๏ผๅนถๅฐฝๅฏ่ฝไฟๆ้ฟๅฎฝๆฏไธๅใๅฝ `min_width` ๅ `max_width` ่ขซๆๅฎๆถ๏ผ้ฟๅฎฝๆฏๅๅฏ่ฝไผ่ขซๆนๅใ |
-| | | | |
-
-```{warning}
-ไปฅไธ่กจๆ ผไป
้ๆฉๆงๅฐๅฏน้จๅๆฐๆฎๅขๅผบๆนๆณไฝ็ฎ่ฆไป็ป๏ผๆดๅคๆฐๆฎๅขๅผบๆนๆณไป็ป่ฏทๅ่[API ๆๆกฃ](../api.rst)ๆ้
่ฏปไปฃ็ ๅ
็ๆๆกฃๆณจ้ใ
-```
-
-## ๆฐๆฎๆ ผๅผๅ - formatting.py
-
-ๆฐๆฎๆ ผๅผๅ่ด่ดฃๅฐๅพๅใ็ๅฎๆ ็ญพไปฅๅๅ
ถๅฎๅธธ็จไฟกๆฏ็ญๆๅ
ๆไธไธชๅญๅ
ธใไธๅ็ไปปๅก้ๅธธไพ่ตไบไธๅ็ๆฐๆฎๆ ผๅผๅๆฐๆฎๅๆข็ฑปใไพๅฆ๏ผ
-
-| | | | |
-| ------------------- | -------- | ------------- | ------------------------------------------ |
-| ๆฐๆฎ่ฝฌๆข็ฑปๅ็งฐ | ้ๆฑๅญๆฎต | ไฟฎๆน/ๆทปๅ ๅญๆฎต | ่ฏดๆ |
-| PackTextDetInputs | - | - | ็จไบๆๅ
ๆๆฌๆฃๆตไปปๅกๆ้่ฆ็่พๅ
ฅไฟกๆฏใ |
-| PackTextRecogInputs | - | - | ็จไบๆๅ
ๆๆฌ่ฏๅซไปปๅกๆ้่ฆ็่พๅ
ฅไฟกๆฏใ |
-| PackKIEInputs | - | - | ็จไบๆๅ
ๅ
ณ้ฎไฟกๆฏๆฝๅไปปๅกๆ้่ฆ็่พๅ
ฅไฟกๆฏใ |
-
-## ่ทจๅบๆฐๆฎ้้
ๅจ - adapters.py
-
-่ทจๅบๆฐๆฎ้้
ๅจๆ้ไบ MMOCR ไธๅ
ถไป OpenMMLab ็ณปๅ็ฎๆณๅบๅฆ [MMDetection](https://github.com/open-mmlab/mmdetection) ไน้ด็ๆฐๆฎๆ ผๅผ๏ผไฝฟๅพ่ทจ้กน็ฎ่ฐ็จๅ
ถๅฎๅผๆบ็ฎๆณๅบ็้
็ฝฎๆไปถๅ็ฎๆณๆไธบไบๅฏ่ฝใ็ฎๅ๏ผMMOCR ๅฎ็ฐไบ `MMDet2MMOCR` ไปฅๅ `MMOCR2MMDet`๏ผไฝฟๅพๆฐๆฎๅฏไปฅๅจ MMDetection ไธ MMOCR ็ๆ ผๅผไน้ด่ช็ฑ่ฝฌๆข๏ผๅๅฉ่ฟไบ้้
่ฝฌๆขๅจ๏ผ็จๆทๅฏไปฅๅจ MMOCR ็ฎๆณๅบๅ
้จ่ฝปๆพ่ฐ็จไปปไฝ MMDetection ๅทฒๆฏๆ็ๆฃๆต็ฎๆณ๏ผๅนถๅจ OCR ็ธๅ
ณๆฐๆฎ้ไธ่ฟ่ก่ฎญ็ปใไพๅฆ๏ผๆไปฌไปฅ Mask R-CNN ไธบไพๆไพไบ[ๆ็จ](#todo)๏ผๅฑ็คบไบๅฆไฝๅจ MMOCR ไธญไฝฟ็จ MMDetection ็ๆฃๆต็ฎๆณ่ฎญ็ปๆๆฌๆฃๆตๅจใ
-
-| | | | |
-| -------------- | -------------------------------------------- | ----------------------------- | ---------------------------------------------- |
-| ๆฐๆฎ่ฝฌๆข็ฑปๅ็งฐ | ้ๆฑๅญๆฎต | ไฟฎๆน/ๆทปๅ ๅญๆฎต | ่ฏดๆ |
-| MMDet2MMOCR | `gt_masks` `gt_ignore_flags` | `gt_polygons` `gt_ignored` | ๅฐ MMDet ไธญ้็จ็ๅญๆฎต่ฝฌๆขไธบๅฏนๅบ็ MMOCR ๅญๆฎตใ |
-| MMOCR2MMDet | `img_shape` `gt_polygons` `gt_ignored` | `gt_masks` `gt_ignore_flags` | ๅฐ MMOCR ไธญ้็จ็ๅญๆฎต่ฝฌๆขไธบๅฏนๅบ็ MMDet ๅญๆฎตใ |
-
-## ๅ
่ฃ
็ฑป - wrappers.py
-
-ไธบไบๆนไพฟ็จๆทๅจ MMOCR ๅ
้จๆ ็ผ่ฐ็จๅธธ็จ็ CV ็ฎๆณๅบ๏ผๆไปฌๅจ wrappers.py ไธญๆไพไบ็ธๅบ็ๅ
่ฃ
็ฑปใๅ
ถไธป่ฆๆ้ไบ MMOCR ไธๅ
ถๅฎ็ฌฌไธๆน็ฎๆณๅบไน้ด็ๆฐๆฎๆ ผๅผๅ่ฝฌๆขๆ ๅ๏ผไฝฟๅพ็จๆทๅฏไปฅๅจ MMOCR ็้
็ฝฎๆไปถๅ
็ดๆฅ้
็ฝฎไฝฟ็จ่ฟไบ็ฌฌไธๆนๅบๆไพ็ๆฐๆฎๅๆขๆนๆณใ็ฎๅๆฏๆ็ๅ
่ฃ
็ฑปๆ๏ผ
-
-| | | | |
-| ------------------ | ------------------------------------------------------------ | ------------------------------------------------------------- | ------------------------------------------------------------- |
-| ๆฐๆฎ่ฝฌๆข็ฑปๅ็งฐ | ้ๆฑๅญๆฎต | ไฟฎๆน/ๆทปๅ ๅญๆฎต | ่ฏดๆ |
-| ImgAugWrapper | `img` `gt_polygons` (optional for text recognition) `gt_bboxes` (optional for text recognition) `gt_bboxes_labels` (optional for text recognition) `gt_ignored` (optional for text recognition) `gt_texts` (optional) | `img` `gt_polygons` (optional for text recognition) `gt_bboxes` (optional for text recognition) `gt_bboxes_labels` (optional for text recognition) `gt_ignored` (optional for text recognition) `img_shape` (optional) `gt_texts` (optional) | [ImgAug](https://github.com/aleju/imgaug) ๅ
่ฃ
็ฑป๏ผ็จไบๆ้ ImgAug ไธ MMOCR ็ๆฐๆฎๆ ผๅผๅ้
็ฝฎ๏ผๆนไพฟ็จๆท่ฐ็จ ImgAug ๅฎ็ฐ็ไธ็ณปๅๆฐๆฎๅขๅผบๆนๆณใ |
-| TorchVisionWrapper | `img` | `img` `img_shape` | [TorchVision](https://github.com/pytorch/vision) ๅ
่ฃ
็ฑป๏ผ็จไบๆ้ TorchVision ไธ MMOCR ็ๆฐๆฎๆ ผๅผๅ้
็ฝฎ๏ผๆนไพฟ็จๆท่ฐ็จ `torchvision.transforms` ไธญๅฎ็ฐ็ไธ็ณปๅๆฐๆฎๅๆขๆนๆณใ |
-
-### `ImgAugWrapper` ็คบไพ
-
-ไพๅฆ๏ผๅจๅ็็ ImgAug ไธญ๏ผๆไปฌๅฏไปฅๆ็
งๅฆไธไปฃ็ ๅฎไนไธไธช `Sequential` ็ฑปๅ็ๆฐๆฎๅขๅผบๆต็จ๏ผๅฏนๅพๅๅๅซ่ฟ่ก้ๆบ็ฟป่ฝฌใ้ๆบๆ่ฝฌๅ้ๆบ็ผฉๆพ๏ผ
-
-```python
-import imgaug.augmenters as iaa
-
-aug = iaa.Sequential(
- iaa.Fliplr(0.5), # ไปฅๆฆ็ 0.5 ่ฟ่กๆฐดๅนณ็ฟป่ฝฌ
- iaa.Affine(rotate=(-10, 10)), # ้ๆบๆ่ฝฌ -10 ๅฐ 10 ๅบฆ
- iaa.Resize((0.5, 3.0)) # ้ๆบ็ผฉๆพๅฐ 50% ๅฐ 300% ็ๅฐบๅฏธ
-)
-```
-
-่ๅจ MMOCR ไธญ๏ผๆไปฌๅฏไปฅ้่ฟ `ImgAugWrapper` ๅ
่ฃ
็ฑป๏ผๅฐไธ่ฟฐๆฐๆฎๅขๅผบๆต็จ็ดๆฅ้
็ฝฎๅฐ `train_pipeline` ไธญ๏ผ
-
-```python
-dict(
- type='ImgAugWrapper',
- args=[
- ['Fliplr', 0.5],
- dict(cls='Affine', rotate=[-10, 10]),
- ['Resize', [0.5, 3.0]],
- ]
-)
-```
-
-ๅ
ถไธญ๏ผ`args` ๅๆฐๆฅๆถไธไธชๅ่กจ๏ผๅ่กจไธญ็ๆฏไธชๅ
็ด ๅฏไปฅๆฏไธไธชๅ่กจ๏ผไนๅฏไปฅๆฏไธไธชๅญๅ
ธใๅฆๆๆฏๅ่กจ๏ผๅๅ่กจ็็ฌฌไธไธชๅ
็ด ไธบ `imgaug.augmenters` ไธญ็็ฑปๅ๏ผๅ้ข็ๅ
็ด ไธบ่ฏฅ็ฑป็ๅๅงๅๅๆฐ๏ผๅฆๆๆฏๅญๅ
ธ๏ผๅๅญๅ
ธ็ `cls` ้ฎๅฏนๅบ `imgaug.augmenters` ไธญ็็ฑปๅ๏ผๅ
ถไป้ฎๅผๅฏนๅๅฏนๅบ่ฏฅ็ฑป็ๅๅงๅๅๆฐใ
-
-### `TorchVisionWrapper` ็คบไพ
-
-ไพๅฆ๏ผๅจๅ็็ TorchVision ไธญ๏ผๆไปฌๅฏไปฅๆ็
งๅฆไธไปฃ็ ๅฎไนไธไธช `Compose` ็ฑปๅ็ๆฐๆฎๅๆขๆต็จ๏ผๅฏนๅพๅ่ฟ่ก่ฒๅฝฉๆๅจ๏ผ
-
-```python
-import torchvision.transforms as transforms
-
-aug = transforms.Compose([
- transforms.ColorJitter(
- brightness=32.0 / 255, # ไบฎๅบฆๆๅจ่ๅด
- saturation=0.5) # ้ฅฑๅๅบฆๆๅจ่ๅด
-])
-```
-
-่ๅจ MMOCR ไธญ๏ผๆไปฌๅฏไปฅ้่ฟ `TorchVisionWrapper` ๅ
่ฃ
็ฑป๏ผๅฐไธ่ฟฐๆฐๆฎๅๆขๆต็จ็ดๆฅ้
็ฝฎๅฐ `train_pipeline` ไธญ๏ผ
-
-```python
-dict(
- type='TorchVisionWrapper',
- op='ColorJitter',
- brightness=32.0 / 255,
- saturation=0.5
-)
-```
-
-ๅ
ถไธญ๏ผ`op` ๅๆฐไธบ `torchvision.transforms` ไธญ็็ฑปๅ๏ผๅ้ข็ๅๆฐๅๅฏนๅบ่ฏฅ็ฑป็ๅๅงๅๅๆฐใ
diff --git a/pipeline/mmocr/docs/zh_cn/basic_concepts/visualizers.md b/pipeline/mmocr/docs/zh_cn/basic_concepts/visualizers.md
deleted file mode 100644
index 323dc0a28aa7cb128dc39003b257802022cc933c..0000000000000000000000000000000000000000
--- a/pipeline/mmocr/docs/zh_cn/basic_concepts/visualizers.md
+++ /dev/null
@@ -1,3 +0,0 @@
-# ๅฏ่งๅ็ปไปถ\[ๅพ
ๆดๆฐ\]
-
-ๅพ
ๆดๆฐ
diff --git a/pipeline/mmocr/docs/zh_cn/conf.py b/pipeline/mmocr/docs/zh_cn/conf.py
deleted file mode 100644
index dc1980716f7cc71270220b7d82ef3c455b4e5895..0000000000000000000000000000000000000000
--- a/pipeline/mmocr/docs/zh_cn/conf.py
+++ /dev/null
@@ -1,173 +0,0 @@
-# Copyright (c) OpenMMLab. All rights reserved.
-# Configuration file for the Sphinx documentation builder.
-#
-# This file only contains a selection of the most common options. For a full
-# list see the documentation:
-# https://www.sphinx-doc.org/en/master/usage/configuration.html
-
-# -- Path setup --------------------------------------------------------------
-
-# If extensions (or modules to document with autodoc) are in another directory,
-# add these directories to sys.path here. If the directory is relative to the
-# documentation root, use os.path.abspath to make it absolute, like shown here.
-
-import os
-import subprocess
-import sys
-
-import pytorch_sphinx_theme
-
-sys.path.insert(0, os.path.abspath('../../'))
-
-# -- Project information -----------------------------------------------------
-
-project = 'MMOCR'
-copyright = '2020-2030, OpenMMLab'
-author = 'OpenMMLab'
-
-# The full version, including alpha/beta/rc tags
-version_file = '../../mmocr/version.py'
-with open(version_file) as f:
- exec(compile(f.read(), version_file, 'exec'))
-__version__ = locals()['__version__']
-release = __version__
-
-# -- General configuration ---------------------------------------------------
-
-# Add any Sphinx extension module names here, as strings. They can be
-# extensions coming with Sphinx (named 'sphinx.ext.*') or your custom
-# ones.
-extensions = [
- 'sphinx.ext.autodoc',
- 'sphinx.ext.napoleon',
- 'sphinx.ext.viewcode',
- 'sphinx_markdown_tables',
- 'sphinx_copybutton',
- 'myst_parser',
- 'sphinx.ext.intersphinx',
- 'sphinx.ext.autodoc.typehints',
- 'sphinx.ext.autosummary',
- 'sphinx.ext.autosectionlabel',
- 'sphinx_tabs.tabs',
-]
-autodoc_typehints = 'description'
-
-autodoc_mock_imports = ['mmcv._ext']
-autosummary_generate = True # Turn on sphinx.ext.autosummary
-# Ignore >>> when copying code
-copybutton_prompt_text = r'>>> |\.\.\. '
-copybutton_prompt_is_regexp = True
-
-myst_enable_extensions = ['colon_fence']
-
-# Add any paths that contain templates here, relative to this directory.
-templates_path = ['_templates']
-
-# The suffix(es) of source filenames.
-# You can specify multiple suffix as a list of string:
-#
-source_suffix = {
- '.rst': 'restructuredtext',
- '.md': 'markdown',
-}
-
-# The master toctree document.
-master_doc = 'index'
-
-# List of patterns, relative to source directory, that match files and
-# directories to ignore when looking for source files.
-# This pattern also affects html_static_path and html_extra_path.
-exclude_patterns = ['_build', 'Thumbs.db', '.DS_Store']
-
-# -- Options for HTML output -------------------------------------------------
-
-# The theme to use for HTML and HTML Help pages. See the documentation for
-# a list of builtin themes.
-#
-# html_theme = 'sphinx_rtd_theme'
-html_theme = 'pytorch_sphinx_theme'
-html_theme_path = [pytorch_sphinx_theme.get_html_theme_path()]
-html_theme_options = {
- 'logo_url':
- 'https://mmocr.readthedocs.io/zh_CN/dev-1.x/',
- 'menu': [
- {
- 'name':
- 'ๆ็จ Notebook',
- 'url':
- 'https://colab.research.google.com/github/open-mmlab/mmocr/blob/'
- 'dev-1.x/demo/tutorial.ipynb'
- },
- {
- 'name': 'GitHub',
- 'url': 'https://github.com/open-mmlab/mmocr'
- },
- {
- 'name':
- 'ไธๆธธๅบ',
- 'children': [
- {
- 'name': 'MMEngine',
- 'url': 'https://github.com/open-mmlab/mmengine',
- 'description': 'ๆทฑๅบฆๅญฆไน ๆจกๅ่ฎญ็ปๅบ็กๅบ'
- },
- {
- 'name': 'MMCV',
- 'url': 'https://github.com/open-mmlab/mmcv',
- 'description': 'ๅบ็ก่ง่งๅบ'
- },
- {
- 'name': 'MMDetection',
- 'url': 'https://github.com/open-mmlab/mmdetection',
- 'description': '็ฎๆ ๆฃๆตๅทฅๅ
ท็ฎฑ'
- },
- ]
- },
- ],
- # Specify the language of shared menu
- 'menu_lang':
- 'cn',
-}
-
-language = 'zh_CN'
-
-master_doc = 'index'
-
-# Add any paths that contain custom static files (such as style sheets) here,
-# relative to this directory. They are copied after the builtin static files,
-# so a file named "default.css" will overwrite the builtin "default.css".
-html_static_path = ['_static']
-html_css_files = [
- 'https://cdn.datatables.net/1.13.2/css/dataTables.bootstrap5.min.css',
- 'css/readthedocs.css'
-]
-html_js_files = [
- 'https://cdn.datatables.net/1.13.2/js/jquery.dataTables.min.js',
- 'https://cdn.datatables.net/1.13.2/js/dataTables.bootstrap5.min.js',
- 'js/collapsed.js',
- 'js/table.js',
-]
-
-myst_heading_anchors = 4
-
-# Configuration for intersphinx
-intersphinx_mapping = {
- 'python': ('https://docs.python.org/3', None),
- 'numpy': ('https://numpy.org/doc/stable', None),
- 'torch': ('https://pytorch.org/docs/stable/', None),
- 'mmcv': ('https://mmcv.readthedocs.io/zh_CN/2.x/', None),
- 'mmengine': ('https://mmengine.readthedocs.io/zh_CN/latest/', None),
- 'mmdetection': ('https://mmdetection.readthedocs.io/zh_CN/dev-3.x/', None),
-}
-
-
-def builder_inited_handler(app):
- subprocess.run(['./cp_origin_docs.sh'])
- subprocess.run(['./merge_docs.sh'])
- subprocess.run(['./stats.py'])
- subprocess.run(['./dataset_zoo.py'])
- subprocess.run(['./project_zoo.py'])
-
-
-def setup(app):
- app.connect('builder-inited', builder_inited_handler)
diff --git a/pipeline/mmocr/docs/zh_cn/contact.md b/pipeline/mmocr/docs/zh_cn/contact.md
deleted file mode 100644
index aafea7776c1b499da08d3e1ea47f25043e1a254b..0000000000000000000000000000000000000000
--- a/pipeline/mmocr/docs/zh_cn/contact.md
+++ /dev/null
@@ -1,18 +0,0 @@
-## ๆฌข่ฟๅ ๅ
ฅ OpenMMLab ็คพๅบ
-
-ๆซๆไธๆน็ไบ็ปด็ ๅฏๅ
ณๆณจ OpenMMLab ๅข้็ [็ฅไนๅฎๆน่ดฆๅท](https://www.zhihu.com/people/openmmlab)๏ผๅ ๅ
ฅ OpenMMLab ๅข้็ [ๅฎๆนไบคๆต QQ ็พค](https://jq.qq.com/?_wv=1027&k=aCvMxdr3)๏ผๆ้่ฟๆทปๅ ๅพฎไฟกโOpenๅฐๅตLabโๅ ๅ
ฅๅฎๆนไบคๆตๅพฎไฟก็พค๏ผ ๆ่
ๅ ๅ
ฅๆไปฌ็ [Slack ็คพๅบ](https://join.slack.com/t/mmocrworkspace/shared_invite/zt-1ifqhfla8-yKnLO_aKhVA2h71OrK8GZw)
-
-
-
-ๆไปฌไผๅจ OpenMMLab ็คพๅบไธบๅคงๅฎถ
-
-- ๐ข ๅไบซ AI ๆกๆถ็ๅๆฒฟๆ ธๅฟๆๆฏ
-- ๐ป ่งฃ่ฏป PyTorch ๅธธ็จๆจกๅๆบ็
-- ๐ฐ ๅๅธ OpenMMLab ็็ธๅ
ณๆฐ้ป
-- ๐ ไป็ป OpenMMLab ๅผๅ็ๅๆฒฟ็ฎๆณ
-- ๐ ่ทๅๆด้ซๆ็้ฎ้ข็ญ็ๅๆ่งๅ้ฆ
-- ๐ฅ ๆไพไธๅ่กๅไธๅผๅ่
ๅ
ๅไบคๆต็ๅนณๅฐ
-
-ๅนฒ่ดงๆปกๆปก ๐๏ผ็ญไฝ ๆฅๆฉ ๐๏ผOpenMMLab ็คพๅบๆๅพ
ๆจ็ๅ ๅ
ฅ ๐ฌ
diff --git a/pipeline/mmocr/docs/zh_cn/cp_origin_docs.sh b/pipeline/mmocr/docs/zh_cn/cp_origin_docs.sh
deleted file mode 100644
index 1e728323684a0aad1571eb392871d6c5de6644fc..0000000000000000000000000000000000000000
--- a/pipeline/mmocr/docs/zh_cn/cp_origin_docs.sh
+++ /dev/null
@@ -1,9 +0,0 @@
-#!/usr/bin/env bash
-
-# Copy *.md files from docs/ if it doesn't have a Chinese translation
-
-for filename in $(find ../en/ -name '*.md' -printf "%P\n");
-do
- mkdir -p $(dirname $filename)
- cp -n ../en/$filename ./$filename
-done
diff --git a/pipeline/mmocr/docs/zh_cn/dataset_zoo.py b/pipeline/mmocr/docs/zh_cn/dataset_zoo.py
deleted file mode 100644
index 2d5f22604d2154068305f5bedd43e06b3a62f906..0000000000000000000000000000000000000000
--- a/pipeline/mmocr/docs/zh_cn/dataset_zoo.py
+++ /dev/null
@@ -1,67 +0,0 @@
-#!/usr/bin/env python
-import os
-import os.path as osp
-import re
-
-import yaml
-
-dataset_zoo_path = '../../dataset_zoo'
-datasets = os.listdir(dataset_zoo_path)
-datasets.sort()
-
-table = '# ๆฏๆๆฐๆฎ้ไธ่ง\n'
-table += '## ๆฏๆ็ๆฐๆฎ้\n'
-table += '| ๆฐๆฎ้ๅ็งฐ | ๆๆฌๆฃๆต | ๆๆฌ่ฏๅซ | ็ซฏๅฐ็ซฏๆๆฌๆฃๆต่ฏๅซ | ๅ
ณ้ฎไฟกๆฏๆฝๅ |\n' \
- '|----------|---------|--------|------------------|-----------|\n'
-details = '## ๆฐๆฎ้่ฏฆๆ
\n'
-
-for dataset in datasets:
- meta = yaml.safe_load(
- open(osp.join(dataset_zoo_path, dataset, 'metafile.yml')))
- dataset_name = meta['Name']
- detail_link = re.sub('[^A-Za-z0-9- ]', '',
- dataset_name).replace(' ', '-').lower()
- paper = meta['Paper']
- data = meta['Data']
-
- table += '| [{}](#{}) | {} | {} | {} | {} |\n'.format(
- dataset,
- detail_link,
- 'โ' if 'textdet' in data['Tasks'] else '',
- 'โ' if 'textrecog' in data['Tasks'] else '',
- 'โ' if 'textspotting' in data['Tasks'] else '',
- 'โ' if 'kie' in data['Tasks'] else '',
- )
-
- details += '### {}\n'.format(dataset_name)
- details += "> \"{}\", *{}*, {}. [PDF]({})\n\n".format(
- paper['Title'], paper['Venue'], paper['Year'], paper['URL'])
- # Basic Info
- details += 'A. ๆฐๆฎ้ๅบ็กไฟกๆฏ\n'
- details += ' - ๅฎๆน็ฝๅ: [{}]({})\n'.format(dataset, data['Website'])
- details += ' - ๅๅธๅนดไปฝ: {}\n'.format(paper['Year'])
- details += ' - ่ฏญ่จ: {}\n'.format(data['Language'])
- details += ' - ๅบๆฏ: {}\n'.format(data['Scene'])
- details += ' - ๆ ๆณจ็ฒๅบฆ: {}\n'.format(data['Granularity'])
- details += ' - ๆฏๆไปปๅก: {}\n'.format(data['Tasks'])
- details += ' - ๆฐๆฎ้่ฎธๅฏ่ฏ: [{}]({})\n\n'.format(data['License']['Type'],
- data['License']['Link'])
-
- # Format
- details += ' B. ๆ ๆณจๆ ผๅผ \n\n'
- sample_path = osp.join(dataset_zoo_path, dataset, 'sample_anno.md')
- if osp.exists(sample_path):
- with open(sample_path, 'r') as f:
- samples = f.readlines()
- samples = ''.join(samples)
- details += samples
- details += ' \n\n'
-
- # Reference
- details += 'C. ๅ่ๆ็ฎ\n'
- details += '```bibtex\n{}\n```\n'.format(paper['BibTeX'])
-
-datasetzoo = table + details
-
-with open('user_guides/data_prepare/datasetzoo.md', 'w') as f:
- f.write(datasetzoo)
diff --git a/pipeline/mmocr/docs/zh_cn/docutils.conf b/pipeline/mmocr/docs/zh_cn/docutils.conf
deleted file mode 100644
index 0c00c84688701117f231fd0c8ec295fb747b7d8f..0000000000000000000000000000000000000000
--- a/pipeline/mmocr/docs/zh_cn/docutils.conf
+++ /dev/null
@@ -1,2 +0,0 @@
-[html writers]
-table_style: colwidths-auto
diff --git a/pipeline/mmocr/docs/zh_cn/get_started/install.md b/pipeline/mmocr/docs/zh_cn/get_started/install.md
deleted file mode 100644
index a0f94424eba315fcd888b9fc3b85bb0496ca4887..0000000000000000000000000000000000000000
--- a/pipeline/mmocr/docs/zh_cn/get_started/install.md
+++ /dev/null
@@ -1,243 +0,0 @@
-# ๅฎ่ฃ
-
-## ็ฏๅขไพ่ต
-
-- Linux | Windows | macOS
-- Python 3.7
-- PyTorch 1.6 ๆๆด้ซ็ๆฌ
-- torchvision 0.7.0
-- CUDA 10.1
-- NCCL 2
-- GCC 5.4.0 ๆๆด้ซ็ๆฌ
-
-## ๅๅค็ฏๅข
-
-```{note}
-ๅฆๆไฝ ๅทฒ็ปๅจๆฌๅฐๅฎ่ฃ
ไบ PyTorch๏ผ่ฏท็ดๆฅ่ทณ่ฝฌๅฐ[ๅฎ่ฃ
ๆญฅ้ชค](#ๅฎ่ฃ
ๆญฅ้ชค)ใ
-```
-
-**็ฌฌไธๆญฅ** ไธ่ฝฝๅนถๅฎ่ฃ
[Miniconda](https://docs.conda.io/en/latest/miniconda.html).
-
-**็ฌฌไบๆญฅ** ๅๅปบๅนถๆฟๆดปไธไธช conda ็ฏๅข๏ผ
-
-```shell
-conda create --name openmmlab python=3.8 -y
-conda activate openmmlab
-```
-
-**็ฌฌไธๆญฅ** ไพ็
ง[ๅฎๆนๆๅ](https://pytorch.org/get-started/locally/)๏ผๅฎ่ฃ
PyTorchใ
-
-````{tabs}
-
-```{code-tab} shell GPU ๅนณๅฐ
-conda install pytorch torchvision -c pytorch
-```
-
-```{code-tab} shell CPU ๅนณๅฐ
-conda install pytorch torchvision cpuonly -c pytorch
-```
-
-````
-
-## ๅฎ่ฃ
ๆญฅ้ชค
-
-ๆไปฌๅปบ่ฎฎๅคงๅคๆฐ็จๆท้็จๆไปฌ็ๆจ่ๆนๅผๅฎ่ฃ
MMOCRใๅ่ฅไฝ ้่ฆๆด็ตๆดป็ๅฎ่ฃ
่ฟ็จ๏ผๅๅฏไปฅๅ่[่ชๅฎไนๅฎ่ฃ
](#่ชๅฎไนๅฎ่ฃ
)ไธ่ใ
-
-### ๆจ่ๆญฅ้ชค
-
-**็ฌฌไธๆญฅ** ไฝฟ็จ [MIM](https://github.com/open-mmlab/mim) ๅฎ่ฃ
[MMEngine](https://github.com/open-mmlab/mmengine)๏ผ [MMCV](https://github.com/open-mmlab/mmcv) ๅ [MMDetection](https://github.com/open-mmlab/mmdetection)ใ
-
-```shell
-pip install -U openmim
-mim install mmengine
-mim install mmcv
-mim install mmdet
-```
-
-**็ฌฌไบๆญฅ** ๅฎ่ฃ
MMOCR.
-
-่ฅไฝ ้่ฆ็ดๆฅ่ฟ่ก MMOCR ๆๅจๅ
ถๅบ็กไธ่ฟ่กๅผๅ๏ผๅ้่ฟๆบ็ ๅฎ่ฃ
๏ผๆจ่๏ผใ
-
-ๅฆๆไฝ ๅฐ MMOCR ไฝไธบไธไธชๅค็ฝฎไพ่ตๅบไฝฟ็จ๏ผๅๅฏไปฅ้่ฟ MIM ๅฎ่ฃ
ใ
-
-`````{tabs}
-
-````{group-tab} ๆบ็ ๅฎ่ฃ
-
-```shell
-git clone https://github.com/open-mmlab/mmocr.git
-cd mmocr
-pip install -v -e .
-# "-v" ไผ่ฎฉๅฎ่ฃ
่ฟ็จไบง็ๆด่ฏฆ็ป็่พๅบ
-# "-e" ไผไปฅๅฏ็ผ่พ็ๆนๅผๅฎ่ฃ
่ฏฅไปฃ็ ๅบ๏ผไฝ ๅฏน่ฏฅไปฃ็ ๅบๆไฝ็ไปปไฝๆดๆน้ฝไผ็ซๅณ็ๆ
-```
-
-````
-
-````{group-tab} MIM ๅฎ่ฃ
-
-```shell
-
-mim install mmocr
-
-```
-
-````
-
-`````
-
-**็ฌฌไธๆญฅ๏ผๅฏ้๏ผ** ๅฆๆไฝ ้่ฆไฝฟ็จไธ `albumentations` ๆๅ
ณ็ๅๆข๏ผๅฆ ABINet ๆฐๆฎๆตๆฐด็บฟไธญ็ `Albu`๏ผ๏ผๆ้่ฆๆๅปบๆๆกฃใ่ฟ่กๅๅ
ๆต่ฏ็ไพ่ต๏ผ่ฏทไฝฟ็จไปฅไธๅฝไปคๅฎ่ฃ
ไพ่ต๏ผ
-
-`````{tabs}
-
-````{group-tab} ๆบ็ ๅฎ่ฃ
-
-```shell
-# ๅฎ่ฃ
albu
-pip install -r requirements/albu.txt
-# ๅฎ่ฃ
ๆๆกฃใๆต่ฏ็ญไพ่ต
-pip install -r requirements.txt
-```
-
-````
-
-````{group-tab} MIM ๅฎ่ฃ
-
-```shell
-pip install albumentations>=1.1.0 --no-binary qudida,albumentations
-```
-
-````
-
-`````
-
-```{note}
-
-ๆไปฌๅปบ่ฎฎๅจๅฎ่ฃ
`albumentations` ไนๅๆฃๆฅๅฝๅ็ฏๅข๏ผ็กฎไฟ `opencv-python` ๅ `opencv-python-headless` ๆฒกๆๅๆถ่ขซๅฎ่ฃ
๏ผๅฆๅๆๅฏ่ฝไผไบง็ไธไบๆ ๆณ้ข็ฅ็้่ฏฏใๅฆๆๅฎไปฌไธๅทงๅๆถๅญๅจไบ็ฏๅขๅฝไธญ๏ผ่ฏทๅธ่ฝฝ `opencv-python-headless` ไปฅ็กฎไฟ MMOCR ็ๅฏ่งๅๅทฅๅ
ทๅฏไปฅๆญฃๅธธ่ฟ่กใ
-
-ๆฅ็ [`albumentations` ็ๅฎๆนๆๆกฃ](https://albumentations.ai/docs/getting_started/installation/#note-on-opencv-dependencies)ไปฅ่ท็ฅ่ฏฆๆ
ใ
-
-```
-
-### ๆฃ้ช
-
-ไฝ ๅฏไปฅ้่ฟ่ฟ่กไธไธช็ฎๅ็ๆจ็ไปปๅกๆฅๆฃ้ช MMOCR ็ๅฎ่ฃ
ๆฏๅฆๆๅใ
-
-`````{tabs}
-
-````{tab} Python
-
-ๅจ Python ไธญ่ฟ่กไปฅไธไปฃ็ ๏ผ
-
-```python
->>> from mmocr.apis import MMOCRInferencer
->>> ocr = MMOCRInferencer(det='DBNet', rec='CRNN')
->>> ocr('demo/demo_text_ocr.jpg', show=True, print_result=True)
-```
-````
-
-````{tab} Shell
-
-ๅฆๆไฝ ๆฏ้่ฟๆบ็ ๅฎ่ฃ
็ MMOCR๏ผไฝ ๅฏไปฅๅจ MMOCR ็ๆ น็ฎๅฝไธ่ฟ่กไปฅไธๅฝไปค๏ผ
-
-```shell
-python tools/infer.py demo/demo_text_ocr.jpg --det DBNet --rec CRNN --show --print-result
-```
-````
-
-`````
-
-่ฅ MMOCR ็ๅฎ่ฃ
ๆ ่ฏฏ๏ผไฝ ๅจ่ฟไธ่ๅฎๆๅๅบๅฝ่ฝ็ๅฐไปฅๅพ็ๅๆๅญๅฝขๅผ่กจ็คบ็่ฏๅซ็ปๆ๏ผ
-
-
-
-
-
-
-```bash
-# ่ฏๅซ็ปๆ
-{'predictions': [{'rec_texts': ['cbanks', 'docecea', 'grouf', 'pwate', 'chobnsonsg', 'soxee', 'oeioh', 'c', 'sones', 'lbrandec', 'sretalg', '11', 'to8', 'round', 'sale', 'year',
-'ally', 'sie', 'sall'], 'rec_scores': [...], 'det_polygons': [...], 'det_scores':
-[...]}]}
-```
-
-```{note}
-ๅฆๆไฝ ๅจๆฒกๆ GUI ็ๆๅกๅจไธ่ฟ่ก MMOCR๏ผๆ่
้่ฟๆฒกๆๅผๅฏ X11 ่ฝฌๅ็ SSH ้ง้่ฟ่ก MMOCR๏ผไฝ ๅฏ่ฝๆ ๆณ็ๅฐๅผนๅบ็็ชๅฃใ
-```
-
-## ่ชๅฎไนๅฎ่ฃ
-
-### CUDA ็ๆฌ
-
-ๅฎ่ฃ
PyTorch ๆถ๏ผ้่ฆๆๅฎ CUDA ็ๆฌใๅฆๆๆจไธๆธ
ๆฅ้ๆฉๅชไธช๏ผ่ฏท้ตๅพชๆไปฌ็ๅปบ่ฎฎ๏ผ
-
-- ๅฏนไบ Ampere ๆถๆ็ NVIDIA GPU๏ผไพๅฆ GeForce 30 series ไปฅๅ NVIDIA A100๏ผCUDA 11 ๆฏๅฟ
้็ใ
-- ๅฏนไบๆดๆฉ็ NVIDIA GPU๏ผCUDA 11 ๆฏๅๅๅ
ผๅฎน็๏ผไฝ CUDA 10.2 ่ฝๅคๆไพๆดๅฅฝ็ๅ
ผๅฎนๆง๏ผไนๆดๅ ่ฝป้ใ
-
-่ฏท็กฎไฟไฝ ็ GPU ้ฉฑๅจ็ๆฌๆปก่ถณๆไฝ็็ๆฌ้ๆฑ๏ผๅ้
[่ฟๅผ ่กจ](https://docs.nvidia.com/cuda/cuda-toolkit-release-notes/index.html#cuda-major-component-versions__table-cuda-toolkit-driver-versions)ใ
-
-```{note}
-ๅฆๆๆ็
งๆไปฌ็ๆไฝณๅฎ่ทต่ฟ่กๅฎ่ฃ
๏ผCUDA ่ฟ่กๆถๅบๅฐฑ่ถณๅคไบ๏ผๅ ไธบๆไปฌๆไพ็ธๅ
ณ CUDA ไปฃ็ ็้ข็ผ่ฏ๏ผไฝ ไธ้่ฆ่ฟ่กๆฌๅฐ็ผ่ฏใ
-ไฝๅฆๆไฝ ๅธๆไปๆบ็ ่ฟ่ก MMCV ็็ผ่ฏ๏ผๆๆฏ่ฟ่กๅ
ถไป CUDA ็ฎๅญ็ๅผๅ๏ผ้ฃไนๅฐฑๅฟ
้กปๅฎ่ฃ
ๅฎๆด็ CUDA ๅทฅๅ
ท้พ๏ผๅ่ง
-[NVIDIA ๅฎ็ฝ](https://developer.nvidia.com/cuda-downloads)๏ผๅฆๅค่ฟ้่ฆ็กฎไฟ่ฏฅ CUDA ๅทฅๅ
ท้พ็็ๆฌไธ PyTorch ๅฎ่ฃ
ๆถ
-็้
็ฝฎ็ธๅน้
๏ผๅฆ็จ `conda install` ๅฎ่ฃ
PyTorch ๆถๆๅฎ็ cudatoolkit ็ๆฌ๏ผใ
-```
-
-### ไธไฝฟ็จ MIM ๅฎ่ฃ
MMCV
-
-MMCV ๅ
ๅซ C++ ๅ CUDA ๆฉๅฑ๏ผๅ ๆญคๅ
ถๅฏน PyTorch ็ไพ่ตๆฏ่พๅคๆใMIM ไผ่ชๅจ่งฃๆ่ฟไบ
-ไพ่ต๏ผ้ๆฉๅ้็ MMCV ้ข็ผ่ฏๅ
๏ผไฝฟๅฎ่ฃ
ๆด็ฎๅ๏ผไฝๅฎๅนถไธๆฏๅฟ
้็ใ
-
-่ฆไฝฟ็จ pip ่ไธๆฏ MIM ๆฅๅฎ่ฃ
MMCV๏ผ่ฏท้ต็
ง [MMCV ๅฎ่ฃ
ๆๅ](https://mmcv.readthedocs.io/zh_CN/latest/get_started/installation.html)ใ
-ๅฎ้่ฆไฝ ็จๆๅฎ url ็ๅฝขๅผๆๅจๆๅฎๅฏนๅบ็ PyTorch ๅ CUDA ็ๆฌใ
-
-ไธพไธชไพๅญ๏ผๅฆไธๅฝไปคๅฐไผๅฎ่ฃ
ๅบไบ PyTorch 1.10.x ๅ CUDA 11.3 ็ผ่ฏ็ mmcv-fullใ
-
-```shell
-pip install 'mmcv>=2.0.0rc1' -f https://download.openmmlab.com/mmcv/dist/cu113/torch1.10/index.html
-```
-
-### ๅจ CPU ็ฏๅขไธญๅฎ่ฃ
-
-MMOCR ๅฏไปฅไป
ๅจ CPU ็ฏๅขไธญๅฎ่ฃ
๏ผๅจ CPU ๆจกๅผไธ๏ผไฝ ๅฏไปฅๅฎๆ่ฎญ็ป๏ผ้่ฆ MMCV ็ๆฌ >= 1.4.4๏ผใๆต่ฏๅๆจกๅๆจ็็ญๆๆๆไฝใ
-
-ๅจ CPU ๆจกๅผไธ๏ผMMCV ไธญ็ไปฅไธ็ฎๅญๅฐไธๅฏ็จ๏ผ
-
-- Deformable Convolution
-- Modulated Deformable Convolution
-- ROI pooling
-- SyncBatchNorm
-
-ๅฆๆไฝ ๅฐ่ฏไฝฟ็จ็จๅฐไบไปฅไธ็ฎๅญ็ๆจกๅ่ฟ่ก่ฎญ็ปใๆต่ฏๆๆจ็๏ผ็จๅบๅฐไผๆฅ้ใไปฅไธไธบๅฏ่ฝๅๅฐๅฝฑๅ็ๆจกๅๅ่กจ๏ผ
-
-| ็ฎๅญ | ๆจกๅ |
-| :-----------------------------------------------------: | :-----------------------------------------------------: |
-| Deformable Convolution/Modulated Deformable Convolution | DBNet (r50dcnv2), DBNet++ (r50dcnv2), FCENet (r50dcnv2) |
-| SyncBatchNorm | PANet, PSENet |
-
-### ้่ฟ Docker ไฝฟ็จ MMOCR
-
-ๆไปฌๆไพไบไธไธช [Dockerfile](https://github.com/open-mmlab/mmocr/blob/master/docker/Dockerfile) ๆไปถไปฅๅปบ็ซ docker ้ๅ ใ
-
-```shell
-# build an image with PyTorch 1.6, CUDA 10.1
-docker build -t mmocr docker/
-```
-
-ไฝฟ็จไปฅไธๅฝไปค่ฟ่กใ
-
-```shell
-docker run --gpus all --shm-size=8g -it -v {ๅฎ้
ๆฐๆฎ็ฎๅฝ}:/mmocr/data mmocr
-```
-
-## ๅฏน MMEngineใMMCV ๅ MMDetection ็็ๆฌไพ่ต
-
-ไธบไบ็กฎไฟไปฃ็ ๅฎ็ฐ็ๆญฃ็กฎๆง๏ผMMOCR ๆฏไธช็ๆฌ้ฝๆๅฏ่ฝๆนๅๅฏน MMEngineใMMCV ๅ MMDetection ็ๆฌ็ไพ่ตใ่ฏทๆ นๆฎไปฅไธ่กจๆ ผ็กฎไฟ็ๆฌไน้ด็็ธไบๅน้
ใ
-
-| MMOCR | MMEngine | MMCV | MMDetection |
-| -------------- | --------------------------- | -------------------------- | --------------------------- |
-| dev-1.x | 0.7.1 \<= mmengine \< 1.0.0 | 2.0.0rc4 \<= mmcv \< 2.1.0 | 3.0.0rc5 \<= mmdet \< 3.1.0 |
-| 1.0.0 | 0.7.1 \<= mmengine \< 1.0.0 | 2.0.0rc4 \<= mmcv \< 2.1.0 | 3.0.0rc5 \<= mmdet \< 3.1.0 |
-| 1.0.0rc6 | 0.6.0 \<= mmengine \< 1.0.0 | 2.0.0rc4 \<= mmcv \< 2.1.0 | 3.0.0rc5 \<= mmdet \< 3.1.0 |
-| 1.0.0rc\[4-5\] | 0.1.0 \<= mmengine \< 1.0.0 | 2.0.0rc1 \<= mmcv \< 2.1.0 | 3.0.0rc0 \<= mmdet \< 3.1.0 |
-| 1.0.0rc\[0-3\] | 0.0.0 \<= mmengine \< 0.2.0 | 2.0.0rc1 \<= mmcv \< 2.1.0 | 3.0.0rc0 \<= mmdet \< 3.1.0 |
diff --git a/pipeline/mmocr/docs/zh_cn/get_started/overview.md b/pipeline/mmocr/docs/zh_cn/get_started/overview.md
deleted file mode 100644
index 02dc798b910fc0342ceaf2e64a95e8e32b8d9621..0000000000000000000000000000000000000000
--- a/pipeline/mmocr/docs/zh_cn/get_started/overview.md
+++ /dev/null
@@ -1,18 +0,0 @@
-# ๆฆ่ง
-
-MMOCR ๆฏไธไธชๅบไบ [PyTorch](https://pytorch.org/) ๅ [MMDetection](https://github.com/open-mmlab/mmdetection) ็ๅผๆบๅทฅๅ
ท็ฎฑ๏ผๆฏๆไผๅค OCR ็ธๅ
ณ็ๆจกๅ๏ผๆถต็ไบๆๆฌๆฃๆตใๆๆฌ่ฏๅซไปฅๅๅ
ณ้ฎไฟกๆฏๆๅ็ญๅคไธชไธป่ฆๆนๅใๅฎ่ฟๆฏๆไบๅคงๅคๆฐๆต่ก็ๅญฆๆฏๆฐๆฎ้๏ผๅนถๆไพไบ่ฎธๅคๅฎ็จๅทฅๅ
ทๅธฎๅฉ็จๆทๅฏนๆฐๆฎ้ๅๆจกๅ่ฟ่กๅคๆน้ข็ๆข็ดขๅ่ฐ่ฏ๏ผๅฉๅไผ่ดจๆจกๅ็ไบงๅบๅ่ฝๅฐใๅฎๅ
ทๆไปฅไธ็น็น๏ผ
-
-- **ๅ
จๆต็จ๏ผๅคๆจกๅ**๏ผๆฏๆไบๅ
จๆต็จ็ OCR ไปปๅก๏ผๅ
ๆฌๆๆฌๆฃๆตใๆๆฌ่ฏๅซๅๅ
ณ้ฎไฟกๆฏๆๅ็ๅ็งๆๆฐๆจกๅใ
-- **ๆจกๅๅ่ฎพ่ฎก**๏ผMMOCR ็ๆจกๅๅ่ฎพ่ฎกไฝฟ็จๆทๅฏไปฅๆ้ๅฎไนๅๅค็จๆจกๅไธญ็ๅไธชๆจกๅใ
-- **ๅฎ็จๅทฅๅ
ทไผๅค**๏ผMMOCR ๆไพไบๅ
จ้ข็ๅฏ่งๅๅทฅๅ
ทใ้ช่ฏๅทฅๅ
ทๅๆง่ฝ่ฏๆตๅทฅๅ
ท๏ผๅธฎๅฉ็จๆทๅฏนๆจกๅ่ฟ่กๆ้ใ่ฐไผๆๅฎข่งๆฏ่พใ
-- **็ฑ [OpenMMLab](https://openmmlab.com/) ๅผบๅ้ฉฑๅจ**๏ผไธๅฎถๆๅ
็ๅ
ถๅฎ็ฎๆณๅบไธๆ ท๏ผMMOCR ้ตๅพช็ OpenMMLab ไธฅ่ฐจ็ๅผๅๅๅๅๆฅๅฃ็บฆๅฎ๏ผๆๅคงๅฐ้ไฝไบ็จๆทๅๆขๅ็ฎๆณๅบๆถ็ๅญฆไน ๆๆฌใๅๆถ๏ผMMOCR ไนๅฏไปฅ้ๅธธไพฟๆทๅฐไธๅฎถๆๅ
ๅ
ถไป็ฎๆณๅบ่ทจๅบ่ๅจ๏ผไป่ๆปก่ถณ็จๆท่ทจ้ขๅ็ ็ฉถๅ่ฝๅฐ็้ๆฑใ
-
-้็ OpenMMLab ๅฎถๆๆถๆ็ๆดไฝๅ็บง๏ผ MMOCR ไน็ธๅบๅฐ่ฟ่กไบๅคงๅน
ๅบฆ็ๅ็บงๅไฟฎๆนใๅจ่ฟไธชๅคง็ๆฌ็ๆดๆฐไธญ๏ผMMOCR ไธญๅคง้็ๅไฝไปฃ็ ๅ้ๅคๅฎ็ฐ่ขซ็งป้ค๏ผๅคไธชๅ
ณ้ฎๆนๆณ็่ฟ่กๆ็ๅพๅฐไบๆๅ๏ผไธๆดไฝๆกๆถ่ฎพ่ฎกไธๅๅพๆดไธบ็ปไธใ่่ๅฐ่ฏฅ็ๆฌ็ธ่พไบ 0.x ๅญๅจไธไบๅๅไธๅ
ผๅฎน็ไฟฎๆน๏ผๆไปฌๅๅคไบไธไปฝ่ฏฆ็ป็[่ฟ็งปๆๅ](../migration/overview.md)๏ผๅนถๅจ้้ขๅๅบไบๆฐ็ๆฌๆไฝๅบ็ๆๆๆนๅจๅ่ฟ็งปๆ้็ๆญฅ้ชค๏ผๅๆฑๅธฎๅฉ็ๆๆง็ๆกๆถ็็จๆทๅฐฝๅฟซๅฎๆๅ็บงใๅฐฝ็ฎก่ฟๅฏ่ฝ้่ฆไธๅฎๆถ้ด๏ผไฝๆไปฌ็ธไฟก็ฑ MMOCR ๅ OpenMMLab ็ๆ็ณป็ปๆดไฝๅธฆๆฅ็ๆฐ็นๆงไผ่ฎฉ่ฟไธๅๅๅพๅฐคไธบๅผๅพใ๐
-
-ๆฅไธๆฅ๏ผ่ฏทๆ นๆฎๅฎ้
้ๆฑ้ๆฉไฝ ้่ฆ้
่ฏป็็ซ ่ใ
-
-- ๆไปฌๆจ่ๅๅญฆ่
้่ฟใ[ๅฟซ้่ฟ่ก](quick_run.md)ใๆฅ็ๆ MMOCR ็ๅบๆฌ็จๆณ๏ผๅนถไปใ็จๆทๆๅใๆไพ็ๆกไพไธญ้ๆญฅๆๆก MMOCR ็็จๆณใ
-- ไธญ้ซ็บงๅผๅ่
ๅๅฏไปฅไปใๅบ็กๆฆๅฟตใไธญไบ่งฃๅไธช็ปไปถ็่ๆฏใ็บฆๅฎๅๆจ่ๅฎ็ฐใ
-- ่ฏท้
่ฏป [FAQ](faq.md) ๆฅๆฅๆพๅธธ่ง้ฎ้ข็็ญๆกใ
-- ๅๆถ๏ผๅฆๆไฝ ๅจๆๆกฃไธญๆช่ฝๆพๅฐ้่ฆ็็ญๆก๏ผๆฌข่ฟ้่ฟ [issue](https://github.com/open-mmlab/mmocr/issues) ่ฟ่กๅ้ฆใ
-- ๆไปฌไนๆฌข่ฟๆฏไธไฝ็จๆทๆไธบ่ดก็ฎ่
๏ผ่ฏท้
่ฏป [่ดก็ฎๆๅ](../notes/contribution_guide.md) ๆฅไบ่งฃๅฆไฝไธบ MMOCR ๅๅบ่ดก็ฎใ
diff --git a/pipeline/mmocr/docs/zh_cn/get_started/quick_run.md b/pipeline/mmocr/docs/zh_cn/get_started/quick_run.md
deleted file mode 100644
index bcca73704d250176b3e911ba0aac55933454e12e..0000000000000000000000000000000000000000
--- a/pipeline/mmocr/docs/zh_cn/get_started/quick_run.md
+++ /dev/null
@@ -1,201 +0,0 @@
-# ๅฟซ้่ฟ่ก
-
-่ฟไธช็ซ ่ไผไป็ป MMOCR ็ไธไบๅบๆฌๅ่ฝใๆไปฌๅ่ฎพไฝ ๅทฒ็ป[ไปๆบ็ ๅฎ่ฃ
ไบ MMOCR](install.md#best-practices)ใๆญคๅค๏ผไฝ ไนๅฏไปฅ้่ฟ[ๆ็จ Notebook](https://colab.research.google.com/github/open-mmlab/mmocr/blob/dev-1.x/demo/tutorial.ipynb)ๆฅไบ่งฃๅฆไฝๅจไบคไบๅผ็ฏๅขไธๅฎ็ฐๆจ็ใ่ฎญ็ปๅๆต่ฏใ
-
-## ๆจ็
-
-ๅจ MMOCR ็ๆ น็ฎๅฝไธ่ฟ่กไปฅไธๅฝไปค๏ผ
-
-```shell
-python tools/infer.py demo/demo_text_ocr.jpg --det DBNet --rec CRNN --show --print-result
-```
-
-ไฝ ๅฏไปฅ็ๅฐๅผนๅบ็้ขๆต็ปๆ๏ผไปฅๅๅจๆงๅถๅฐไธญๆๅฐๅบ็ๆจ็็ปๆใ
-
-
-
-
-
-
-```bash
-# ่ฏๅซ็ปๆ
-{'predictions': [{'rec_texts': ['cbanks', 'docecea', 'grouf', 'pwate', 'chobnsonsg', 'soxee', 'oeioh', 'c', 'sones', 'lbrandec', 'sretalg', '11', 'to8', 'round', 'sale', 'year',
-'ally', 'sie', 'sall'], 'rec_scores': [...], 'det_polygons': [...], 'det_scores':
-[...]}]}
-```
-
-```{note}
-ๅฆๆไฝ ๅจๆฒกๆ GUI ็ๆๅกๅจไธ่ฟ่ก MMOCR๏ผๆ่
้่ฟๆฒกๆๅผๅฏ X11 ่ฝฌๅ็ SSH ้ง้่ฟ่ก MMOCR๏ผไฝ ๅฏ่ฝๆ ๆณ็ๅฐๅผนๅบ็็ชๅฃใ
-```
-
-ๅฏน MMOCR ไธญๆจ็ๆฅๅฃๆดไธบ่ฏฆ็ป็่ฏดๆ๏ผๅฏไปฅๅจ[่ฟ้](../user_guides/inference.md)ๆพๅฐใ
-
-้คไบไฝฟ็จๆไปฌๆไพๅฅฝ็้ข่ฎญ็ปๆจกๅ๏ผ็จๆทไนๅฏไปฅๅจ่ชๅทฑ็ๆฐๆฎ้ไธ่ฎญ็ปๆต่กๆจกๅใๆฅไธๆฅๆไปฌไปฅๅจ่ฟทไฝ ็ [ICDAR 2015](https://rrc.cvc.uab.es/?ch=4&com=downloads) ๆฐๆฎ้ไธ่ฎญ็ป DBNet ไธบไพ๏ผๅธฆๅคงๅฎถ็ๆ MMOCR ็ๅบๆฌๅ่ฝใ
-
-## ๅๅคๆฐๆฎ้
-
-็ฑไบ OCR ไปปๅก็ๆฐๆฎ้็ง็ฑปๅคๆ ท๏ผๆ ผๅผไธไธ๏ผไธๅฉไบๅคๆฐๆฎ้็ๅๆขๅ่ๅ่ฎญ็ป๏ผๅ ๆญค MMOCR ็บฆๅฎไบไธ็ง[็ปไธ็ๆฐๆฎๆ ผๅผ](../user_guides/dataset_prepare.md)๏ผๅนถ้ๅฏนๅธธ็จ็ OCR ๆฐๆฎ้ๆไพไบ[ไธ้ฎๅผๆฐๆฎๅๅค่ๆฌ](../user_guides/data_prepare/dataset_preparer.md)ใ้ๅธธ๏ผ่ฆๅจ MMOCR ไธญไฝฟ็จๆฐๆฎ้๏ผไฝ ๅช้่ฆๆ็
งๅฏนๅบๆญฅ้ชค่ฟ่กๆไปคๅณๅฏใ
-
-```{note}
-ไฝๆไปฌไบฆๆทฑ็ฅ๏ผๆ็ๅฐฑๆฏ็ๅฝโโๅฐคๅ
ถๅฏนๆณ่ฆๅฟซ้ไธๆ MMOCR ็ไฝ ๆฅ่ฏดใ
-```
-
-ๅจ่ฟ้๏ผๆไปฌๅๅคไบไธไธช็จไบๆผ็คบ็็ฒพ็ฎ็ ICDAR 2015 ๆฐๆฎ้ใไธ่ฝฝๆไปฌ้ขๅ
ๅๅคๅฅฝ็[ๅ็ผฉๅ
](https://download.openmmlab.com/mmocr/data/icdar2015/mini_icdar2015.tar.gz)๏ผ่งฃๅๅฐ mmocr ็ `data/` ็ฎๅฝไธ๏ผๅฐฑ่ฝๅพๅฐๆไปฌๅๅคๅฅฝ็ๅพ็ๅๆ ๆณจๆไปถใ
-
-```Bash
-wget https://download.openmmlab.com/mmocr/data/icdar2015/mini_icdar2015.tar.gz
-mkdir -p data/
-tar xzvf mini_icdar2015.tar.gz -C data/
-```
-
-## ไฟฎๆน้
็ฝฎ
-
-ๅๅคๅฅฝๆฐๆฎ้ๅ๏ผๆไปฌๆฅไธๆฅๅฐฑ้่ฆ้่ฟไฟฎๆน้
็ฝฎ็ๆนๅผๆๅฎ่ฎญ็ป้็ไฝ็ฝฎๅ่ฎญ็ปๅๆฐใ
-
-ๅจ่ฟไธชไพๅญไธญ๏ผๆไปฌๅฐไผ่ฎญ็ปไธไธชไปฅ resnet18 ไฝไธบ้ชจๅนฒ็ฝ็ป๏ผbackbone๏ผ็ DBNetใ็ฑไบ MMOCR ๅทฒ็ปๆ้ๅฏนๅฎๆด ICDAR 2015 ๆฐๆฎ้็้
็ฝฎ ๏ผ`configs/textdet/dbnet/dbnet_resnet18_fpnc_1200e_icdar2015.py`๏ผ๏ผๆไปฌๅช้่ฆๅจๅฎ็ๅบ็กไธไฝๅบไธ็นไฟฎๆนใ
-
-ๆไปฌ้ฆๅ
้่ฆไฟฎๆนๆฐๆฎ้็่ทฏๅพใๅจ่ฟไธช้
็ฝฎไธญ๏ผๅคง้จๅๅ
ณ้ฎ็้
็ฝฎๆไปถ้ฝๅจ `_base_` ไธญ่ขซๅฏผๅ
ฅ๏ผๅฆๆฐๆฎๅบ็้
็ฝฎๅฐฑๆฅ่ช `configs/textdet/_base_/datasets/icdar2015.py`ใๆๅผ่ฏฅๆไปถ๏ผๆ็ฌฌไธ่ก `icdar2015_textdet_data_root` ๆๅ็่ทฏๅพๆฟๆข๏ผ
-
-```Python
-icdar2015_textdet_data_root = 'data/mini_icdar2015'
-```
-
-ๅฆๅค๏ผๅ ไธบๆฐๆฎ้ๅฐบๅฏธ็ผฉๅฐไบ๏ผๆไปฌไน่ฆ็ธๅบๅฐๅๅฐ่ฎญ็ป็่ฝฎๆฌกๅฐ 400๏ผ็ผฉ็ญ้ช่ฏๅๅจๅญๆ้็้ด้ๅฐ10่ฝฎ๏ผๅนถๆพๅผๅญฆไน ็่กฐๅ็ญ็ฅใ็ดๆฅๆไปฅไธๅ ่ก้
็ฝฎๆพๅ
ฅ `configs/textdet/dbnet/dbnet_resnet18_fpnc_1200e_icdar2015.py`ๅณๅฏ็ๆ๏ผ
-
-```Python
-# ๆฏ 10 ไธช epoch ๅจๅญไธๆฌกๆ้๏ผไธๅชไฟ็ๆๅไธไธชๆ้
-default_hooks = dict(
- checkpoint=dict(
- type='CheckpointHook',
- interval=10,
- max_keep_ckpts=1,
- ))
-# ่ฎพ็ฝฎๆๅคง epoch ๆฐไธบ 400๏ผๆฏ 10 ไธช epoch ่ฟ่กไธๆฌก้ช่ฏ
-train_cfg = dict(type='EpochBasedTrainLoop', max_epochs=400, val_interval=10)
-# ไปคๅญฆไน ็ไธบๅธธ้๏ผๅณไธ่ฟ่กๅญฆไน ็่กฐๅ
-param_scheduler = [dict(type='ConstantLR', factor=1.0),]
-```
-
-่ฟ้๏ผๆไปฌ้่ฟ้
็ฝฎ็็ปงๆฟ ({external+mmengine:doc}`MMEngine: Config `) ๆบๅถๅฐๅบ็ก้
็ฝฎไธญ็็ธๅบๅๆฐ็ดๆฅ่ฟ่กไบๆนๅใๅๆฌ็ๅญๆฎตๅๅธๅจ `configs/textdet/_base_/schedules/schedule_sgd_1200e.py` ๅ `configs/textdet/_base_/default_runtime.py` ไธญ๏ผๆๅ
ด่ถฃ็่ฏป่
ๅฏไปฅ่ช่กๆฅ็ใ
-
-```{note}
-ๅ
ณไบ้
็ฝฎๆไปถๆดๅ ่ฏฆๅฐฝ็่ฏดๆ๏ผ่ฏทๅ่[ๆญคๅค](../user_guides/config.md)ใ
-```
-
-## ๅฏ่งๅๆฐๆฎ้
-
-ๅจๆญฃๅผๅผๅง่ฎญ็ปๅ๏ผๆไปฌ่ฟๅฏไปฅๅฏ่งๅไธไธ็ป่ฟ่ฎญ็ป่ฟ็จไธญ[ๆฐๆฎๅๆข๏ผtransforms๏ผ](../basic_concepts/transforms.md)ๅ็ๅพๅใๆนๆณไนๅพ็ฎๅ๏ผๆๆไปฌ้่ฆๅฏ่งๅ็้
็ฝฎไผ ๅ
ฅ [browse_dataset.py](/tools/analysis_tools/browse_dataset.py) ่ๆฌๅณๅฏ๏ผ
-
-```Bash
-python tools/analysis_tools/browse_dataset.py configs/textdet/dbnet/dbnet_resnet18_fpnc_1200e_icdar2015.py
-```
-
-ๆฐๆฎๅๆขๅ็ๅพ็ๅๆ ็ญพไผๅจๅผน็ชไธญ้ๅผ ่ขซๅฑ็คบๅบๆฅใ
-
-
-
-
-
-```{note}
-ๆๅ
ณ่ฏฅ่ๆฌๆด่ฏฆ็ป็ๆๅ๏ผ่ฏทๅ่[ๆญคๅค](../user_guides/useful_tools.md).
-```
-
-```{tip}
-้คไบๆปก่ถณๅฅฝๅฅๅฟไนๅค๏ผๅฏ่งๅ่ฟๅฏไปฅๅธฎๅฉๆไปฌๅจ่ฎญ็ปๅๆฃๆฅๅฏ่ฝๅฝฑๅๅฐๆจกๅ่กจ็ฐ็้จๅ๏ผๅฆ้
็ฝฎๆไปถใๆฐๆฎ้ๅๆฐๆฎๅๆขไธญ็้ฎ้ขใ
-```
-
-## ่ฎญ็ป
-
-ไธไบไฟฑๅค๏ผๅชๆฌ ไธ้ฃใ่ฟ่กไปฅไธๅฝไปคๅฏๅจ่ฎญ็ป๏ผ
-
-```Bash
-python tools/train.py configs/textdet/dbnet/dbnet_resnet18_fpnc_1200e_icdar2015.py
-```
-
-ๆ นๆฎ็ณป็ปๆ
ๅต๏ผMMOCR ไผ่ชๅจไฝฟ็จๆไฝณ็่ฎพๅค่ฟ่ก่ฎญ็ปใๅฆๆๆ GPU๏ผๅไผ้ป่ฎคๅจ็ฌฌไธๅผ ๅกๅฏๅจๅๅก่ฎญ็ปใๅฝๅผๅง็ๅฐ loss ็่พๅบ๏ผๅฐฑ่ฏดๆไฝ ๅทฒ็ปๆๅๅฏๅจไบ่ฎญ็ปใ
-
-```Bash
-2022/08/22 18:42:22 - mmengine - INFO - Epoch(train) [1][5/7] lr: 7.0000e-03 memory: 7730 data_time: 0.4496 loss_prob: 14.6061 loss_thr: 2.2904 loss_db: 0.9879 loss: 17.8843 time: 1.8666
-2022/08/22 18:42:24 - mmengine - INFO - Exp name: dbnet_resnet18_fpnc_1200e_icdar2015
-2022/08/22 18:42:28 - mmengine - INFO - Epoch(train) [2][5/7] lr: 7.0000e-03 memory: 6695 data_time: 0.2052 loss_prob: 6.7840 loss_thr: 1.4114 loss_db: 0.9855 loss: 9.1809 time: 0.7506
-2022/08/22 18:42:29 - mmengine - INFO - Exp name: dbnet_resnet18_fpnc_1200e_icdar2015
-2022/08/22 18:42:33 - mmengine - INFO - Epoch(train) [3][5/7] lr: 7.0000e-03 memory: 6690 data_time: 0.2101 loss_prob: 3.0700 loss_thr: 1.1800 loss_db: 0.9967 loss: 5.2468 time: 0.6244
-2022/08/22 18:42:33 - mmengine - INFO - Exp name: dbnet_resnet18_fpnc_1200e_icdar2015
-```
-
-ๅจไธๆๅฎ้ขๅคๅๆฐๆถ๏ผ่ฎญ็ป็ๆ้้ป่ฎคไผ่ขซไฟๅญๅฐ `work_dirs/dbnet_resnet18_fpnc_1200e_icdar2015/` ไธ้ข๏ผ่ๆฅๅฟๅไผไฟๅญๅจ`work_dirs/dbnet_resnet18_fpnc_1200e_icdar2015/ๅผๅง่ฎญ็ป็ๆถ้ดๆณ/`้ใๆฅไธๆฅ๏ผๆไปฌๅช้่ฆ่ๅฟ็ญๅพ
ๆจกๅ่ฎญ็ปๅฎๆๅณๅฏใ
-
-```{note}
-่ฅ้่ฆไบ่งฃ่ฎญ็ป็้ซ็บง็จๆณ๏ผๅฆ CPU ่ฎญ็ปใๅคๅก่ฎญ็ปๅ้็พค่ฎญ็ป็ญ๏ผ่ฏทๆฅ้
[่ฎญ็ปไธๆต่ฏ](../user_guides/train_test.md)ใ
-```
-
-## ๆต่ฏ
-
-็ป่ฟๆฐๅๅ้็็ญๅพ
๏ผๆจกๅ้กบๅฉๅฎๆไบ400 epochs็่ฎญ็ปใๆไปฌ้่ฟๆงๅถๅฐ็่พๅบ๏ผ่งๅฏๅฐ DBNet ๅจๆๅไธไธช epoch ็่กจ็ฐๆๅฅฝ๏ผ`hmean` ่พพๅฐไบ 60.86๏ผไฝ ๅฏ่ฝไผๅพๅฐไธไธชไธๅคชไธๆ ท็็ปๆ๏ผ๏ผ
-
-```Bash
-08/22 19:24:52 - mmengine - INFO - Epoch(val) [400][100/100] icdar/precision: 0.7285 icdar/recall: 0.5226 icdar/hmean: 0.6086
-```
-
-```{note}
-ๅฎๆ่ฎธ่ฟๆฒก่ขซ่ฎญ็ปๅฐๆไผ็ถๆ๏ผไฝๅฏนไบไธไธชๆผ็คบ่่จๅทฒ็ป่ถณๅคไบใ
-```
-
-็ถ่๏ผ่ฟไธชๆฐๅผๅชๅๆ ไบ DBNet ๅจ่ฟทไฝ ICDAR 2015 ๆฐๆฎ้ไธ็ๆง่ฝใ่ฆๆณๆดๅ ๅฎข่งๅฐ่ฏๅคๅฎ็ๆฃๆต่ฝๅ๏ผๆไปฌ่ฟ่ฆ็็ๅฎๅจๅๅธๅคๆฐๆฎ้ไธ็่กจ็ฐใไพๅฆ๏ผ`tests/data/det_toy_dataset` ๅฐฑๆฏไธไธชๅพๅฐ็็ๅฎๆฐๆฎ้๏ผๆไปฌๅฏไปฅ็จๅฎๆฅ้ช่ฏไธไธ DBNet ็ๅฎ้
ๆง่ฝใ
-
-ๅจๆต่ฏๅ๏ผๆไปฌๅๆ ท้่ฆๅฏนๆฐๆฎ้็ไฝ็ฝฎๅไธไธไฟฎๆนใๆๅผ `configs/textdet/_base_/datasets/icdar2015.py`๏ผไฟฎๆน `icdar2015_textdet_test` ็ `data_root` ไธบ `tests/data/det_toy_dataset`:
-
-```Python
-# ...
-icdar2015_textdet_test = dict(
- type='OCRDataset',
- data_root='tests/data/det_toy_dataset',
- # ...
- )
-```
-
-ไฟฎๆนๅฎๆฏ๏ผ่ฟ่กๅฝไปคๅฏๅจๆต่ฏใ
-
-```Bash
-python tools/test.py configs/textdet/dbnet/dbnet_resnet18_fpnc_1200e_icdar2015.py work_dirs/dbnet_resnet18_fpnc_1200e_icdar2015/epoch_400.pth
-```
-
-ๅพๅฐ่พๅบ๏ผ
-
-```Bash
-08/21 21:45:59 - mmengine - INFO - Epoch(test) [5/10] memory: 8562
-08/21 21:45:59 - mmengine - INFO - Epoch(test) [10/10] eta: 0:00:00 time: 0.4893 data_time: 0.0191 memory: 283
-08/21 21:45:59 - mmengine - INFO - Evaluating hmean-iou...
-08/21 21:45:59 - mmengine - INFO - prediction score threshold: 0.30, recall: 0.6190, precision: 0.4815, hmean: 0.5417
-08/21 21:45:59 - mmengine - INFO - prediction score threshold: 0.40, recall: 0.6190, precision: 0.5909, hmean: 0.6047
-08/21 21:45:59 - mmengine - INFO - prediction score threshold: 0.50, recall: 0.6190, precision: 0.6842, hmean: 0.6500
-08/21 21:45:59 - mmengine - INFO - prediction score threshold: 0.60, recall: 0.6190, precision: 0.7222, hmean: 0.6667
-08/21 21:45:59 - mmengine - INFO - prediction score threshold: 0.70, recall: 0.3810, precision: 0.8889, hmean: 0.5333
-08/21 21:45:59 - mmengine - INFO - prediction score threshold: 0.80, recall: 0.0000, precision: 0.0000, hmean: 0.0000
-08/21 21:45:59 - mmengine - INFO - prediction score threshold: 0.90, recall: 0.0000, precision: 0.0000, hmean: 0.0000
-08/21 21:45:59 - mmengine - INFO - Epoch(test) [10/10] icdar/precision: 0.7222 icdar/recall: 0.6190 icdar/hmean: 0.6667
-```
-
-ๅฏไปฅๅ็ฐ๏ผๆจกๅๅจ่ฟไธชๆฐๆฎ้ไธ่ฝ่พพๅฐ็ hmean ไธบ 0.6667๏ผๆๆ่ฟๆฏไธ้็ใ
-
-```{note}
-่ฅ้่ฆไบ่งฃๆต่ฏ็้ซ็บง็จๆณ๏ผๅฆ CPU ๆต่ฏใๅคๅกๆต่ฏๅ้็พคๆต่ฏ็ญ๏ผ่ฏทๆฅ้
[่ฎญ็ปไธๆต่ฏ](../user_guides/train_test.md)ใ
-```
-
-## ๅฏ่งๅ่พๅบ
-
-ไธบไบๅฏนๆจกๅ็่พๅบๆไธไธชๆด็ด่ง็ๆๅ๏ผๆไปฌ่ฟๅฏไปฅ็ดๆฅๅฏ่งๅๅฎ็้ขๆต่พๅบใๅจ `test.py` ไธญ๏ผ็จๆทๅฏไปฅ้่ฟ `show` ๅๆฐๆๅผๅผน็ชๅฏ่งๅ๏ผไนๅฏไปฅ้่ฟ `show-dir` ๅๆฐๆๅฎ้ขๆต็ปๆๅพๅฏผๅบ็็ฎๅฝใ
-
-```Bash
-python tools/test.py configs/textdet/dbnet/dbnet_resnet18_fpnc_1200e_icdar2015.py work_dirs/dbnet_resnet18_fpnc_1200e_icdar2015/epoch_400.pth --show-dir imgs/
-```
-
-็ๅฎๆ ็ญพๅ้ขๆตๅผไผๅจๅฏ่งๅ็ปๆไธญไปฅๅนณ้บ็ๆนๅผๅฑ็คบใๅทฆๅพ็็ปฟๆก่กจ็คบ็ๅฎๆ ็ญพ๏ผๅณๅพ็็บขๆก่กจ็คบ้ขๆตๅผใ
-
-
-
-
-
-```{note}
-ๆๅ
ณๆดๅคๅฏ่งๅๅ่ฝ็ไป็ป๏ผ่ฏทๅ้
[่ฟ้](../user_guides/visualization.md)ใ
-```
diff --git a/pipeline/mmocr/docs/zh_cn/index.rst b/pipeline/mmocr/docs/zh_cn/index.rst
deleted file mode 100644
index 5ed0a2530583b53344ffc910c6c4f8356daa09f8..0000000000000000000000000000000000000000
--- a/pipeline/mmocr/docs/zh_cn/index.rst
+++ /dev/null
@@ -1,112 +0,0 @@
-ๆฌข่ฟๆฅๅฐ MMOCR ็ไธญๆๆๆกฃ!
-=======================================
-
-ๆจๅฏไปฅๅจ้กต้ขๅทฆไธ่งๅๆขไธญ่ฑๆๆๆกฃใ
-
-.. toctree::
- :maxdepth: 2
- :caption: ๆฐๆๅ
ฅ้จ
-
- get_started/overview.md
- get_started/install.md
- get_started/quick_run.md
- get_started/faq.md
-
-.. toctree::
- :maxdepth: 2
- :caption: ็จๆทๆๅ
-
- user_guides/inference.md
- user_guides/config.md
- user_guides/dataset_prepare.md
- user_guides/train_test.md
- user_guides/visualization.md
- user_guides/useful_tools.md
-
-.. toctree::
- :maxdepth: 2
- :caption: ๅบ็กๆฆๅฟต
-
- basic_concepts/structures.md
- basic_concepts/transforms.md
- basic_concepts/evaluation.md
- basic_concepts/datasets.md
- basic_concepts/overview.md
- basic_concepts/data_flow.md
- basic_concepts/models.md
- basic_concepts/visualizers.md
- basic_concepts/convention.md
- basic_concepts/engine.md
-
-
-.. toctree::
- :maxdepth: 2
- :caption: ๆฐๆฎ้ๆฏๆ
-
- user_guides/data_prepare/datasetzoo.md
- user_guides/data_prepare/dataset_preparer.md
- user_guides/data_prepare/det.md
- user_guides/data_prepare/recog.md
- user_guides/data_prepare/kie.md
-
-.. toctree::
- :maxdepth: 2
- :caption: ๆจกๅๆฏๆ
-
- modelzoo.md
- projectzoo.md
- backbones.md
- textdet_models.md
- textrecog_models.md
- kie_models.md
-
-.. toctree::
- :maxdepth: 2
- :caption: ๅ
ถๅฎ
-
- notes/branches.md
- notes/contribution_guide.md
- notes/changelog.md
-
-.. toctree::
- :maxdepth: 2
- :caption: MMOCR 0.x ่ฟ็งปๆๅ
-
- migration/overview.md
- migration/news.md
- migration/branches.md
- migration/code.md
- migration/dataset.md
- migration/model.md
- migration/transforms.md
-
-.. toctree::
- :maxdepth: 2
- :caption: API ๆๆกฃ
-
- mmocr.apis
- mmocr.structures
- mmocr.datasets
- mmocr.transforms
- mmocr.models
- mmocr.evaluation
- mmocr.visualization
- mmocr.engine
- mmocr.utils
-
-.. toctree::
- :maxdepth: 2
- :caption: ่็ณปๆไปฌ
-
- contact.md
-
-.. toctree::
- :caption: ๅๆข่ฏญ่จ
-
- switch_language.md
-
-ๅฏผๅผ
-==================
-
-* :ref:`genindex`
-* :ref:`search`
diff --git a/pipeline/mmocr/docs/zh_cn/make.bat b/pipeline/mmocr/docs/zh_cn/make.bat
deleted file mode 100644
index 8a3a0e25b49a52ade52c4f69ddeb0bc3d12527ff..0000000000000000000000000000000000000000
--- a/pipeline/mmocr/docs/zh_cn/make.bat
+++ /dev/null
@@ -1,36 +0,0 @@
-@ECHO OFF
-
-pushd %~dp0
-
-REM Command file for Sphinx documentation
-
-if "%SPHINXBUILD%" == "" (
- set SPHINXBUILD=sphinx-build
-)
-set SOURCEDIR=.
-set BUILDDIR=_build
-
-if "%1" == "" goto help
-
-%SPHINXBUILD% >NUL 2>NUL
-if errorlevel 9009 (
- echo.
- echo.The 'sphinx-build' command was not found. Make sure you have Sphinx
- echo.installed, then set the SPHINXBUILD environment variable to point
- echo.to the full path of the 'sphinx-build' executable. Alternatively you
- echo.may add the Sphinx directory to PATH.
- echo.
- echo.If you don't have Sphinx installed, grab it from
- echo.http://sphinx-doc.org/
- exit /b 1
-)
-
-
-%SPHINXBUILD% -M %1 %SOURCEDIR% %BUILDDIR% %SPHINXOPTS% %O%
-goto end
-
-:help
-%SPHINXBUILD% -M help %SOURCEDIR% %BUILDDIR% %SPHINXOPTS% %O%
-
-:end
-popd
diff --git a/pipeline/mmocr/docs/zh_cn/merge_docs.sh b/pipeline/mmocr/docs/zh_cn/merge_docs.sh
deleted file mode 100644
index a13706d5d61b065fd83d66d663303969f5e9c930..0000000000000000000000000000000000000000
--- a/pipeline/mmocr/docs/zh_cn/merge_docs.sh
+++ /dev/null
@@ -1,7 +0,0 @@
-#!/usr/bin/env bash
-
-# gather models
-sed -e '$a\\n' -s ../../configs/kie/*/*.md | sed "s/md###t/html#t/g" | sed "s/#/#&/" | sed '1i\# ๅ
ณ้ฎไฟกๆฏๆๅๆจกๅ' | sed 's/](\/docs\//](/g' | sed 's=](/=](https://github.com/open-mmlab/mmocr/tree/master/=g' >kie_models.md
-sed -e '$a\\n' -s ../../configs/textdet/*/*.md | sed "s/md###t/html#t/g" | sed "s/#/#&/" | sed '1i\# ๆๆฌๆฃๆตๆจกๅ' | sed 's/](\/docs\//](/g' | sed 's=](/=](https://github.com/open-mmlab/mmocr/tree/master/=g' >textdet_models.md
-sed -e '$a\\n' -s ../../configs/textrecog/*/*.md | sed "s/md###t/html#t/g" | sed "s/#/#&/" | sed '1i\# ๆๆฌ่ฏๅซๆจกๅ' | sed 's/](\/docs\//](/g' | sed 's=](/=](https://github.com/open-mmlab/mmocr/tree/master/=g' >textrecog_models.md
-sed -e '$a\\n' -s ../../configs/backbone/*/*.md | sed "s/md###t/html#t/g" | sed "s/#/#&/" | sed '1i\# ้ชจๅนฒ็ฝ็ป' | sed 's/](\/docs\//](/g' | sed 's=](/=](https://github.com/open-mmlab/mmocr/tree/master/=g' >backbones.md
diff --git a/pipeline/mmocr/docs/zh_cn/migration/branches.md b/pipeline/mmocr/docs/zh_cn/migration/branches.md
deleted file mode 100644
index 1d7f8d2fca14605f59484a703793fb69f98013b7..0000000000000000000000000000000000000000
--- a/pipeline/mmocr/docs/zh_cn/migration/branches.md
+++ /dev/null
@@ -1,38 +0,0 @@
-# ๅๆฏ่ฟ็งป
-
-ๅจๆฉๆ้ถๆฎต๏ผMMOCR ๆไธไธชๅๆฏ๏ผ`main`ใ`1.x` ๅ `dev-1.x`ใ้็ MMOCR 1.0.0 ๆญฃๅผ็็ๅๅธ๏ผๆไปฌไน้ๅฝๅไบๅ
ถไธญไธไบๅๆฏ๏ผไธ้ขๆไพไบๆฐๆงๅๆฏ็ๅฏน็
งใ
-
-- `main` ๅๆฏๅ
ๆฌไบ MMOCR 0.x๏ผไพๅฆ v0.6.3๏ผ็ไปฃ็ ใ็ฐๅจๅทฒ็ป่ขซ้ๅฝๅไธบ `0.x`ใ
-- `1.x` ๅ
ๅซไบ MMOCR 1.x๏ผไพๅฆ 1.0.0rc6๏ผ็ไปฃ็ ใ็ฐๅจๅฎๆฏ `main` ๅๆฏ็ๅซๅ๏ผไผๅจ 2023 ็ๅนดไธญๅ ้คใ
-- `dev-1.x` ๆฏ MMOCR 1.x ็ๅผๅๅๆฏใ็ฐๅจไฟๆไธๅใ
-
-ๆๅ
ณๅๆฏ็ๆดๅคไฟกๆฏ๏ผ่ฏทๆฅ็[ๅๆฏ](../notes/branches.md)ใ
-
-## ๅ็บง `main` ๅๆฏๆถ่งฃๅณๅฒ็ช
-
-ๅฏนไบๅธๆไปๆง `main` ๅๆฏ๏ผๅ
ๅซ MMOCR 0.x ไปฃ็ ๏ผๅ็บง็็จๆท๏ผไปฃ็ ๅฏ่ฝไผๅฏผ่ดๅฒ็ชใ่ฆ้ฟๅ
่ฟไบๅฒ็ช๏ผ่ฏทๆ็
งไปฅไธๆญฅ้ชคๆไฝ๏ผ
-
-1. ่ฏท commit ๅจ `main` ไธ็ๆๆๆดๆน๏ผ่ฅๆ๏ผ๏ผๅนถๅคไปฝๆจๅฝๅ็ `main` ๅๆฏใ
-
- ```bash
- git checkout main
- git add --all
- git commit -m 'backup'
- git checkout -b main_backup
- ```
-
-2. ไป่ฟ็จๅญๅจๅบ่ทๅๆๆฐๆดๆนใ
-
- ```bash
- git remote add openmmlab git@github.com:open-mmlab/mmocr.git
- git fetch openmmlab
- ```
-
-3. ้่ฟ่ฟ่ก `git reset --hard openmmlab/main` ๅฐ `main` ๅๆฏ้็ฝฎไธบ่ฟ็จๅญๅจๅบไธ็ๆๆฐ `main` ๅๆฏใ
-
- ```bash
- git checkout main
- git reset --hard openmmlab/main
- ```
-
-ๆ็
ง่ฟไบๆญฅ้ชค๏ผๆจๅฏไปฅๆๅๅ็บงๆจ็ `main` ๅๆฏใ
diff --git a/pipeline/mmocr/docs/zh_cn/migration/code.md b/pipeline/mmocr/docs/zh_cn/migration/code.md
deleted file mode 100644
index ee63dd9523b6b3a5b3a7f9e1f96f1ed870610455..0000000000000000000000000000000000000000
--- a/pipeline/mmocr/docs/zh_cn/migration/code.md
+++ /dev/null
@@ -1,151 +0,0 @@
-# ไปฃ็ ็ปๆๅๅจ
-
-MMOCR ไธบไบๅ
ผ้กพๆๆฌๆฃๆตใ่ฏๅซๅๅ
ณ้ฎไฟกๆฏๆๅ็ญไปปๅก๏ผๅจๅ็่ฎพ่ฎกๆถๅญๅจ่ฎธๅคๆฌ ็ผบ่่็ๅฐๆนใๅจๆฌๆฌก 1.0 ็ๆฌ็ๅ็บงไธญ๏ผMMOCR ๅๆญฅๆๅบไบๆฐ็ๆจกๅๆถๆ๏ผๆจๅจๅฐฝ้ไธ OpenMMLab ๆดไฝ็่ฎพ่ฎกๅฏน้ฝ๏ผไธๅจ็ฎๆณๅบๅ
้จ่พพๆ็ปๆไธ็็ปไธใ่ฝ็ถๆฌๆฌกๅ็บงๅนถ้ๅฎๅ
จๅๅๅ
ผๅฎน๏ผไฝๆๆ็ๅๅจ้ฝๆฏๆ่ฟนๅฏๅพช็ใๅ ๆญค๏ผๆไปฌๅจๆฌ็ซ ่ๆป็ปๅบไบๅผๅ่
ๅฏ่ฝไผๅ
ณๅฟ็ๆนๅจ๏ผไพๆ้่ฆ็็จๆทๅ่ใ
-
-## ๆดไฝๆนๅจ
-
-MMOCR 0.x ๅญๅจ็ๅฏนๆจกๅๅ่ฝ่พน็ๅฎไนไธๆธ
ๆฐ็้ฎ้ขใๅจ MMOCR 1.0 ไธญ๏ผๆไปฌ้ๆไบๆจกๅๆจกๅ็่ฎพ่ฎก๏ผๅนถๅฎไนไบๅฎไปฌ็ๆจกๅ่พน็ใ
-
-- ่่ๅฐๆนๅๅทฎๅผ่ฟๅคง๏ผMMOCR 1.0 ไธญๅๆถไบๅฏนๅฝๅๅฎไฝ่ฏๅซ็ๆฏๆใ
-
-- ๆจกๅไธญ่ฎก็ฎๆๅคฑ๏ผloss๏ผ็้จๅๆจกๅ่ขซๆฝ่ฑกๅไธบ Module Loss๏ผ่ฝฌๆขๅๅงๆ ๆณจไธบๆๅคฑ็ฎๆ ๏ผloss target๏ผ็ๅ่ฝไน่ขซๅ
ๆฌๅจๅ
ใๅฆไธไธชๆจกๅๆฝ่ฑก Postprocessor ๅ่ด่ดฃๅจ้ขๆตๆถ่งฃ็ ๆจกๅๅๅง่พๅบไธบๅฏนๅบไปปๅก็ `DataSample`ใ
-
-- ๆๆๆจกๅ็่พๅ
ฅ็ฎๅไธบๅ
ๅซๅพๅๅๅง็นๅพ็ `inputs` ๅๅพ็ๅ
ไฟกๆฏ็ `List[DataSample]`ใ่พๅบๆ ผๅผไนๅพๅฐ็ปไธ๏ผ่ฎญ็ปๆถๆฏๅ
ๅซ loss ็ๅญๅ
ธ๏ผๆต่ฏๆถ็่พๅบไธบๅ
ๅซ้ขๆต็ปๆ็ๅฏนๅบไปปๅก็ [`DataSample`](<>)ใ
-
-- Module Loss ๆฅๆบไบ 0.x ็ๆฌไธญๅฎ็ฐไธๅไธชๆจกๅๅผบ็ธๅ
ณ็ `XXLoss` ็ฑป๏ผๅฎไปฌๅจ 1.0 ไธญๅ่ขซ็ปไธ้ๅฝๅไธบ`XXModuleLoss`็ๅฝขๅผ๏ผๅฆ`DBLoss` ่ขซ้ๅฝๅไธบ `DBModuleLoss`๏ผ, `head` ไผ ๅ
ฅ็ loss ้
็ฝฎๅๆฐๅไนไป `loss` ๆนไธบ `module_loss`ใ
-
-- ไธๆจกๅๅฎ็ฐๆ ๅ
ณ็้็จๆๅคฑ็ฑปๅ็งฐไฟๆ `XXLoss` ็ๅฝขๅผ๏ผๅนถๆพ็ฝฎไบ `mmocr/models/common/losses` ไธ๏ผๅฆ [`MaskedBCELoss`](mmocr.models.common.losses.MaskedBCELoss)ใ
-
-- `mmocr/models/common/losses` ไธ็ๆนๅจ๏ผ0.x ไธญ `DiceLoss` ่ขซ้ๅไธบ [`MaskedDiceLoss`](mmocr.models.common.losses.MaskedDiceLoss)ใ`FocalLoss` ่ขซ็งป้คใ
-
-- ๅขๅ ไบ่ตทๆบไบ label converter ็ Dictionary ๆจกๅ๏ผๅฎไผๅจๆๆฌ่ฏๅซๅๅ
ณ้ฎไฟกๆฏๆๅไปปๅกไธญ่ขซ็จๅฐใ
-
-## ๆๆฌๆฃๆต
-
-### ๅ
ณ้ฎๆนๅจ๏ผๅคช้ฟไธ็็๏ผ
-
-- ๆง็็ๆจกๅๆ้ไป็ถ้็จไบๆฐ็๏ผไฝ้่ฆๅฐๆ้ๅญๅ
ธ `state_dict` ไธญไปฅ `bbox_head` ๅผๅคด็ๅญๆฎต้ๅฝๅไธบ `det_head`ใ
-
-- ่ฎก็ฎ target ๆๅ
ณ็ๅๆข `XXTargets` ่ขซ่ฝฌ็งปๅฐไบ `XXModuleLoss` ไธญใ
-
-### SingleStageTextDetector
-
-- ๅๆฌ็ปงๆฟ้พไธบ `mmdet.BaseDetector->SingleStageDetector->SingleStageTextDetector`๏ผ็ฐๅจๆนไธบ็ดๆฅ็ปงๆฟ่ช `BaseDetector`, ไธญ้ด็ `SingleStageDetector` ่ขซๅ ้คใ
-
-- `bbox_head` ๆนๅไธบ `det_head`ใ
-
-- `train_cfg`ใ`test_cfg`ๅ`pretrained`ๅญๆฎต่ขซ็งป้คใ
-
-- `forward_train()` ไธ `simple_test()` ๅๅซ่ขซ้ๆไธบ `loss()` ไธ `predict()` ๆนๆณใๅ
ถไธญ `simple_test()` ไธญ่ด่ดฃๅฐๆจกๅๅๅง่พๅบๆๅๅนถ่พๅ
ฅ `head.get_bounary()` ็้จๅ่ขซๆดๅ่ฟไบ `BaseTextDetPostProcessor` ไธญใ
-
-- `TextDetectorMixin` ไธญๅชๅฎ็ฐไบ `show_result()`ๆนๆณ๏ผๅฎ็ฐไธ `TextDetLocalVisualizer` ้ๅ๏ผๅ ๆญคๅทฒ็ป่ขซ็งป้คใ
-
-### Head
-
-- `HeadMixin` ไธบ`XXXHead` ๅจ 0.x ็ๆฌไธญๅฟ
้กป็ปงๆฟ็ๅบ็ฑป๏ผ็ฐๅจ่ขซ `BaseTextDetHead` ไปฃๆฟใ้้ข็ `get_boundary()` ๅ `resize_boundary()` ๆนๆณ่ขซ้ๅไธบ `BaseTextDetPostProcessor` ็ `__call__()` ๅ `rescale()` ๆนๆณใ
-
-### ModuleLoss
-
-- ๆๆฌๆฃๆตไธญ็นๆ็ๆฐๆฎๅๆข `XXXTargets` ๅ
จ้จ็งปๅจๅฐ `XXXModuleLoss._get_target_single` ไธญ๏ผไธ็ๆ target ็ธๅ
ณ็้
็ฝฎไธๅๅจๆฐๆฎๆตๆฐด็บฟ๏ผpipeline๏ผไธญ่ฎพ็ฝฎ๏ผ่ฝฌ่ๅจ `XXXLoss` ไธญ่ขซ้
็ฝฎใไพๅฆ๏ผ`DBNetTargets` ็ๅฎ็ฐ่ขซ็งปๅจๅฐ `DBModuleLoss._get_target_single()`ไธญ๏ผ่็จๆทๅฏไปฅ้่ฟ่ฎพ็ฝฎ `DBModuleLoss` ็ๅๅงๅๅๆฐๆฅๆงๅถๆๅคฑ็ฎๆ ็็ๆใ
-
-### Postprocessor
-
-- ๅๆฌ็ `XXXPostprocessor.__call__()` ไธญ็้ป่พ่ฝฌ็งปๅฐ้ๆๅ็ `XXXPostprocessor.get_text_instances()` ใ
-
-- `BasePostprocessor` ้ๆไธบ `BaseTextDetPostProcessor`๏ผๆญคๅบ็ฑปไผๅฐๆจกๅ่พๅบ็้ขๆต็ปๆๆๅๅนถ้ไธช่ฟ่กๅค็๏ผๅนถๆฏๆๆ นๆฎ `scale_factor` ่ชๅจ็ผฉๆพ่พๅบ็ๅค่พนๅฝข๏ผpolygon๏ผๆ็ๅฎๆก๏ผbounding box๏ผใ
-
-## ๆๆฌ่ฏๅซ
-
-### ๅ
ณ้ฎๆนๅจ๏ผๅคช้ฟไธ็็๏ผ
-
-- ็ฑไบๅญๅ
ธๅบๅ็ไบๅๅ๏ผไธๅญๅจ้จๅๆจกๅๆถๆไธ็ bug ่ขซไฟฎๅค๏ผๆง็็่ฏๅซๆจกๅๆ้ๅทฒ็ปไธๅ่ฝ็ดๆฅๅบ็จไบ 1.0 ไธญ๏ผๆไปฌๅฐไผๅจๅ็ปญไธบๆ้่ฆ็็จๆทๆจๅบ่ฟ็งป่ๆฌๆ็จใ
-
-- 0.x ็ๆฌไธญ็ SegOCR ๆฏๆๆๆถ็งป้ค๏ผTPS-CRNN ไผๅจๅ็ปญ็ๆฌไธญ่ขซๆฏๆใ
-
-- ๆต่ฏๆถๅขๅผบ๏ผtest time augmentation๏ผๅจๆญค็ๆฌไธญๆๆชๆฏๆ๏ผไฝๅฐไผๅจๅ็ปญ็ๆฌไธญๆดๆฐใ
-
-- Label converter ๆจกๅ่ขซ็งป้ค๏ผ้้ข็ๅ่ฝ่ขซๆๅ่ณ Dictionary, ModuleLoss ๅ Postprocessor ๆจกๅไธญใ
-
-- ็ปไธๆจกๅไธญๅฏน `max_seq_len` ็ๅฎไนไธบๆจกๅ็ๅๅง่พๅบ้ฟๅบฆใ
-
-### Label Converter
-
-- ๅๆ็ label converter ๅญๅจๆผๅ้่ฏฏ (label convertor)๏ผๆไปฌ้่ฟๅ ้คๆ่ฟไธช็ฑป่ง้ฟไบ่ฟไธช้ฎ้ขใ
-
-- ่ด่ดฃๅฏนๅญ็ฌฆ/ๅญ็ฌฆไธฒไธๆฐๅญ็ดขๅผไบ็ธ่ฝฌๆข็้จๅ่ขซๆๅ่ณ [`Dictionary`](mmocr.models.common.Dictionary) ็ฑปไธญใ
-
-- ๅจๆง็ๆฌไธญ๏ผไธๅ็ label converter ไผๆไธไธๆ ท็็นๆฎๅญ็ฌฆ้ๅๅญ็ฌฆๅบใๅจ 0.x ็ๆฌไธญ๏ผๅญ็ฌฆๅบๅฆไธ๏ผ
-
- | Converter | ๅญ็ฌฆๅบ |
- | ------------------------------- | ----------------------------------------- |
- | `AttnConvertor`, `ABIConvertor` | ``, ``, ``, characters |
- | `CTCConvertor` | ``, ``, characters |
-
-ๅจ 1.0 ไธญ๏ผๆไปฌไธๅไปฅไปปๅกไธบ่พน็่ฎพ่ฎกไธๅ็ๅญๅ
ธๅๅญ็ฌฆๅบ๏ผๅ่ไปฃไน็ๆฏ็ปไธไบๅญ็ฌฆๅบ็ Dictionary๏ผๅ
ถๅญ็ฌฆๅบไธบ characters, \, \, \ใ`CTCConvertor` ไธญ \ ่ขซ็ญไปทๆฟๆขไธบ \ใ
-
-- `label_convertor` ไธญๅๆฌๆฏๆไธ็งๆนๅผๅๅงๅๅญๅ
ธ๏ผ`dict_type`ใ`dict_file` ๅ `dict_list`๏ผ็ฐๅจๅจ `Dictionary` ไธญ่ขซ็ฎๅไธบ `dict_file` ไธ็งใๅๆถ๏ผๆไปฌไนๆๅๆฌๅจ `dict_type` ไธญๆฏๆ็ๅญๅ
ธๆ ผๅผ่ฝฌๅไธบ็ฐๅจ `dicts/` ็ฎๅฝไธ็้ข่ฎพๅญๅ
ธๆไปถใๅฏนๅบๆ ๅฐๅฆไธ๏ผ
-
- | MMOCR 0.x: `dict_type` | MMOCR 1.0: ๅญๅ
ธ่ทฏๅพ |
- | ---------------------- | -------------------------------------- |
- | DICT90 | dicts/english_digits_symbols.txt |
- | DICT91 | dicts/english_digits_symbols_space.txt |
- | DICT36 | dicts/lower_english_digits.txt |
- | DICT37 | dicts/lower_english_digits_space.txt |
-
-- `label_converter` ไธญ `str2tensor()` ็ๅฎ็ฐ่ขซ่ฝฌ็งปๅฐ `ModuleLoss.get_targets()` ไธญใไธ้ข็่กจๆ ผๅๅบไบๆง็ไธๆฐ็ๆนๆณๅฎ็ฐ็ๅฏนๅบๅ
ณ็ณปใๆณจๆ๏ผๆฐๆง็็ๅฎ็ฐๅนถ้ๅฎๅ
จไธ่ดใ
-
- | MMOCR 0.x | MMOCR 1.0 | ๅคๆณจ |
- | --------------------------------------------------------- | --------------------------------------- | -------------------------------------------- |
- | `ABIConvertor.str2tensor()`, `AttnConvertor.str2tensor()` | `BaseTextRecogModuleLoss.get_targets()` | ๅๆฌไธคไธช็ฑปไธญ็ๅฎ็ฐๅญๅจ็ๅทฎๅผๅจๆฐ็ๆฌไธญ่ขซ็ปไธ |
- | `CTCConvertor.str2tensor()` | `CTCModuleLoss.get_targets()` | |
-
-- `label_converter` ไธญ `tensor2idx()` ็ๅฎ็ฐ่ขซ่ฝฌ็งปๅฐ `Postprocessor.get_single_prediction()` ไธญใไธ้ข็่กจๆ ผๅๅบไบๆง็ไธๆฐ็ๆนๆณๅฎ็ฐ็ๅฏนๅบๅ
ณ็ณปใๆณจๆ๏ผๆฐๆง็็ๅฎ็ฐๅนถ้ๅฎๅ
จไธ่ดใ
-
- | MMOCR 0.x | MMOCR 1.0 |
- | --------------------------------------------------------- | ------------------------------------------------ |
- | `ABIConvertor.tensor2idx()`, `AttnConvertor.tensor2idx()` | `AttentionPostprocessor.get_single_prediction()` |
- | `CTCConvertor.tensor2idx()` | `CTCPostProcessor.get_single_prediction()` |
-
-## ๅ
ณ้ฎไฟกๆฏๆๅ
-
-### ๅ
ณ้ฎๆนๅจ๏ผๅคช้ฟไธ็็๏ผ
-
-- ็ฑไบๆจกๅ็่พๅ
ฅๅ็ไบๅๅ๏ผๆง็ๆจกๅ็ๆ้ๅทฒ็ปไธๅ่ฝ็ดๆฅๅบ็จไบ 1.0 ไธญใ
-
-### KIEDataset & OpensetKIEDataset
-
-- ่ฏปๅๆฐๆฎ็้จๅ่ขซ็ฎๅๅฐ `WildReceiptDataset` ไธญใ
-
-- ๅฏน่็นๅ่พนไฝ้ขๅคๅค็็้จๅ่ขซ่ฝฌ็งปๅฐไบ `LoadKIEAnnotation` ไธญใ
-
-- ไฝฟ็จๅญๅ
ธๅฏนๆๆฌ่ฟ่ก่ฝฌๅ็้จๅ่ขซ่ฝฌ็งปๅฐไบ `SDMGRHead.convert_text()` ไธญ๏ผไฝฟ็จ `Dictionary` ๅฎ็ฐใ
-
-- ่ฎก็ฎๆๆฌๆกไน้ดๅ
ณ็ณป็้จๅ`compute_relation()` ่ขซ่ฝฌ็งปๅฐ `SDMGRHead.compute_relations()` ไธญ๏ผๅจๆจกๅๅ
่ฟ่กใ
-
-- ่ฏไผฐๆจกๅ่กจ็ฐ็้จๅ่ขซ็ฎๅไธบ `F1Metric`ใ
-
-- `OpensetKIEDataset` ไธญๅค็ๆจกๅ่พน่พๅบ็้จๅ่ขซๆด็ๅฐ `SDMGRPostProcessor`ไธญใ
-
-### SDMGR
-
-- `show_result()` ่ขซๆดๅๅฐ `KIEVisualizer` ไธญใ
-
-- `forward_test()` ไธญๅฏน่พๅบ่ฟ่กๅๅค็็้จๅ่ขซๆด็ๅฐ `SDMGRPostProcessor`ไธญใ
-
-## Utils ๅๅจ
-
-ๅๆฌๆฃๅธๅจๅๅค็ๅ่ฝๅฝๆฐ็ฐๅทฒ่ขซ็ปไธๅฝ็ฑปๅจ `mmocr/utils/` ไธใไปฅไธไธบ่ฏฅ็ฎๅฝไธๅๆไปถ็ไฝ็จๅ๏ผ
-
-- bbox_utils.py๏ผๅ่พน็ๅฎๆก๏ผbounding box๏ผๆๅ
ณ็ๅ่ฝๅฝๆฐใ
-- check_argument.py๏ผๆฃๆฅๅๆฐ็ฑปๅ็ๅ่ฝๅฝๆฐใ
-- collect_env.py๏ผๆถ้่ฟ่ก็ฏๅข็ๅ่ฝๅฝๆฐใ
-- data_converter_utils.py๏ผ็จไบๆฐๆฎ้่ฝฌๆข็ๅ่ฝๅฝๆฐใ
-- fileio.py๏ผ่พๅ
ฅ/่พๅบๆๅ
ณ็ๅ่ฝๅฝๆฐใ
-- img_utils.py๏ผๅค็ๅพ็็ๅ่ฝๅฝๆฐใ
-- mask_utils.py๏ผไธๆฉ็ ๆๅ
ณ็ๅ่ฝๅฝๆฐใ
-- ocr.py๏ผ็จไบ MMOCR ๆจ็็ๅ่ฝๅฝๆฐใ
-- parsers.py๏ผ่งฃ็ ๆไปถ็ๅ่ฝๅฝๆฐใ
-- polygon_utils.py๏ผๅค่พนๅฝข็ๅ่ฝๅฝๆฐใ
-- setup_env.py๏ผๅญๆพๅๅงๅ MMOCR ็ๅ่ฝๅฝๆฐใ
-- string_utils.py๏ผๅญๆพๅญ็ฌฆไธฒ็ๅ่ฝๅฝๆฐใ
-- typing.py๏ผๅญๆพ MMOCR ไธญๅธธ็จๆฐๆฎ็ฑปๅ็็ผฉๅใ
diff --git a/pipeline/mmocr/docs/zh_cn/migration/dataset.md b/pipeline/mmocr/docs/zh_cn/migration/dataset.md
deleted file mode 100644
index ac69703be3b62bed0c964d657ea09f51a1266551..0000000000000000000000000000000000000000
--- a/pipeline/mmocr/docs/zh_cn/migration/dataset.md
+++ /dev/null
@@ -1,254 +0,0 @@
-# ๆฐๆฎ้่ฟ็งป
-
-ๅจ OpenMMLab 2.0 ็ณปๅ็ฎๆณๅบๅบไบ [MMEngine](https://github.com/open-mmlab/mmengine) ่ฎพ่ฎกไบ็ปไธ็ๆฐๆฎ้ๅบ็ฑป [BaseDataset](mmengine.dataset.BaseDataset)๏ผๅนถๅถๅฎไบๆฐๆฎ้ๆ ๆณจๆไปถ่ง่ใๅบไบๆญค๏ผๆไปฌๅจ MMOCR 1.0 ็ๆฌไธญ้ๆไบ OCR ไปปๅกๆฐๆฎ้ๅบ็ฑป [`OCRDataset`](mmocr.datasets.OCRDataset)ใไปฅไธๆๆกฃๅฐไป็ป MMOCR ไธญๆฐๆงๆฐๆฎ้ๆ ผๅผ็ๅบๅซ๏ผไปฅๅๅฆไฝๅฐๆงๆฐๆฎ้่ฟ็งป่ณๆฐ็ๆฌไธญใๅฏนไบๆไธๆนไพฟ่ฟ่กๆฐๆฎ่ฟ็งป็็จๆท๏ผๆไปฌไนๅจ[็ฌฌไธ่](#ๅ
ผๅฎนๆง)ๆไพไบไธดๆถ็ไปฃ็ ๅ
ผๅฎนๆนๆกใ
-
-```{note}
-ๅ
ณ้ฎไฟกๆฏๆฝๅไปปๅกไป้็จๅๆ็ WildReceipt ๆฐๆฎ้ๆ ๆณจๆ ผๅผใ
-```
-
-## ๆง็ๆฐๆฎๆ ผๅผๅ้กพ
-
-้ๅฏนไธๅไปปๅก๏ผMMOCR 0.x ็ๆฌๅฎ็ฐไบๅค็งไธๅ็ๆฐๆฎ้็ฑปๅ๏ผๅฆๆๆฌๆฃๆตไปปๅก็ `IcdarDataset`๏ผ`TextDetDataset`๏ผๆๆฌ่ฏๅซไปปๅก็ `OCRDataset`๏ผ`OCRSegDataset` ็ญใ่ไธๅ็ๆฐๆฎ้็ฑปๅๅๆถ่ฟๅฏ่ฝๅญๅจๅค็งไธๅ็ๆ ๆณจๅๆไปถๅญๅจๅ็ซฏ๏ผๅฆ `.txt`ใ`.json`ใ`.jsonl` ็ญ๏ผไฝฟๅพ็จๆทๅจ่ชๅฎไนๆฐๆฎ้ๆถ้่ฆ้
็ฝฎๅ็ฑปๆฐๆฎๅ ่ฝฝๅจ (`Loader`) ไปฅๅๆฐๆฎ่งฃๆๅจ (`Parser`)ใ่ฟไธไป
ๅขๅ ไบ็จๆท็ไฝฟ็จ้พๅบฆ๏ผไนๅธฆๆฅไบ่ฎธๅค้ฎ้ขๅ้ๆฃใไพๅฆ๏ผไปฅ `.txt` ๆ ผๅผๅญๅจ็็ฎๅ `OCDDataset` ๅจ้ๅฐๅ
ๅซ็ฉบๆ ผ็ๆๆฌๆ ๆณจๆถๅฐไผๆฅ้ใ
-
-### ๆๆฌๆฃๆต
-
-ๆๆฌๆฃๆตไปปๅกไธญ๏ผ`IcdarDataset` ้็จไบไธ้็จ็ฎๆ ๆฃๆต COCO ๆฐๆฎ้ไธ่ด็ๆ ๆณจๆ ผๅผใ
-
-```json
-{
- "images": [
- {
- "id": 1,
- "width": 800,
- "height": 600,
- "file_name": "test.jpg"
- }
- ],
- "annotations": [
- {
- "id": 1,
- "image_id": 1,
- "category_id": 1,
- "bbox": [0,0,10,10],
- "segmentation": [
- [0,0,10,0,10,10,0,10]
- ],
- "area": 100,
- "iscrowd": 0
- }
- ]
-}
-```
-
-่ `TextDetDataset` ๅ้็จไบ JSON Line ็ๅญๅจๆ ผๅผ๏ผๅฐ็ฑปไผผ COCO ๆ ผๅผ็ๆ ็ญพ่ฝฌๆขๆๆๆฌๅญๆพๅจ `.txt` ๆ `.jsonl` ๆ ผๅผๆไปถไธญใ
-
-```text
-{"file_name": "test/img_2.jpg", "height": 720, "width": 1280, "annotations": [{"iscrowd": 0, "category_id": 1, "bbox": [602.0, 173.0, 33.0, 24.0], "segmentation": [[602, 173, 635, 175, 634, 197, 602, 196]]}, {"iscrowd": 0, "category_id": 1, "bbox": [734.0, 310.0, 58.0, 54.0], "segmentation": [[734, 310, 792, 320, 792, 364, 738, 361]]}]}
-{"file_name": "test/img_5.jpg", "height": 720, "width": 1280, "annotations": [{"iscrowd": 1, "category_id": 1, "bbox": [405.0, 409.0, 32.0, 52.0], "segmentation": [[408, 409, 437, 436, 434, 461, 405, 433]]}, {"iscrowd": 1, "category_id": 1, "bbox": [435.0, 434.0, 8.0, 33.0], "segmentation": [[437, 434, 443, 440, 441, 467, 435, 462]]}]}
-```
-
-### ๆๆฌ่ฏๅซ
-
-ๅฏนไบๆๆฌ่ฏๅซไปปๅก๏ผMMOCR 0.x ็ๆฌไธญๅญๅจไธค็งๆฐๆฎๆ ๆณจๆ ผๅผใๅ
ถไธญ `.txt` ๆ ผๅผ็ๆ ๆณจๆไปถๆฏไธ่กๅ
ฑๆไธคไธชๅญๆฎต๏ผๅๅซๅญๆพไบๅพ็ๅไปฅๅๆ ๆณจ็ๆๆฌๅ
ๅฎน๏ผๅนถไปฅ็ฉบๆ ผๅ้ใ
-
-```text
-img1.jpg OpenMMLab
-img2.jpg MMOCR
-```
-
-่ JSON Line ๆ ผๅผๅไฝฟ็จ `json.dumps` ๅฐ JSON ๆ ผๅผ็ๆ ๆณจ่ฝฌๆขไธบๆๆฌๅ
ๅฎนๅๅญๆพๅจ .jsonl ๆไปถไธญ๏ผๅ
ถๅ
ๅฎนๅฝขไผผไธไธชๅญๅ
ธ๏ผๅฐๆไปถๅๅๆๆฌๆ ๆณจไฟกๆฏๅๅซๅญๆพๅจ `filename` ๅ `text` ๅญๆฎตไธญใ
-
-```json
-{"filename": "img1.jpg", "text": "OpenMMLab"}
-{"filename": "img2.jpg", "text": "MMOCR"}
-```
-
-## ๆฐ็ๆฐๆฎๆ ผๅผ
-
-ไธบ่งฃๅณ 0.x ็ๆฌไธญๆฐๆฎ้ๆ ผๅผ่ฟไบๆททๆ็ๆ
ๅต๏ผMMOCR 1.x ้็จไบๅบไบ MMEngine ่ฎพ่ฎก็็ปไธๆฐๆฎๆ ๅใๆฏไธไธชๆฐๆฎๆ ๆณจๆไปถๅญๆพๅจ `.json` ๆไปถไธญ๏ผๅนถไฝฟ็จ็ฑปไผผๅญๅ
ธ็ๆ ผๅผๅๅซๅญๆพไบๆฐๆฎ้็ๅ
ไฟกๆฏ๏ผ`metainfo`๏ผไธๅ
ทไฝ็ๆ ๆณจๅ
ๅฎน๏ผ`data_list`๏ผใ
-
-```json
-{
- "metainfo":
- {
- "classes": ("cat", "dog"),
- // ...
- },
- "data_list":
- [
- {
- "img_path": "xxx/xxx_0.jpg",
- "img_label": 0,
- // ...
- },
- // ...
- ]
-}
-```
-
-ๅบไบๆญค๏ผๆไปฌ้ๅฏน MMOCR ็นๆ็ไปปๅก่ฎพ่ฎกไบ `TextDetDataset`ใ`TextRecogDataset`ใ
-
-### ๆๆฌๆฃๆต
-
-#### ๆฐ็ๆ ผๅผไป็ป
-
-`TextDetDataset` ไธญๅญๆพไบๆๆฌๆฃๆตไปปๅกๆ้็่พน็็ๆ ๆณจใๆไปถๅ็ญไฟกๆฏใ็ฑไบๆๆฌๆฃๆตไปปๅกไธญๅชๆ 1 ไธช็ฑปๅซ๏ผๅ ๆญคๆไปฌๅฐๅ
ถ็ฑปๅซ id ้ป่ฎค่ฎพ็ฝฎไธบ 0๏ผ่่ๆฏ็ฑปๅไธบ 1ใ`tests/data/det_toy_dataset/instances_test.json` ไธญๅญๆพไบไธไธชๆๆฌๆฃๆตไปปๅก็ๆฐๆฎๆ ๆณจ็คบไพ๏ผ็จๆทๅฏไปฅๅ่่ฏฅๆไปถๆฅๅฐ่ชๅทฑ็ๆฐๆฎ้่ฝฌๆขไธบๆไปฌๆฏๆ็ๆ ผๅผใ
-
-```json
-{
- "metainfo":
- {
- "dataset_type": "TextDetDataset",
- "task_name": "textdet",
- "category": [{"id": 0, "name": "text"}]
- },
- "data_list":
- [
- {
- "img_path": "test_img.jpg",
- "height": 640,
- "width": 640,
- "instances":
- [
- {
- "polygon": [0, 0, 0, 10, 10, 20, 20, 0],
- "bbox": [0, 0, 10, 20],
- "bbox_label": 0,
- "ignore": False
- },
- // ...
- ]
- }
- ]
-}
-```
-
-ๅ
ถไธญ๏ผ`bbox` ๅญๆฎต็ๆ ผๅผไธบ `[min_x, min_y, max_x, max_y]`ใ
-
-#### ่ฟ็งป่ๆฌ
-
-ไธบๅธฎๅฉ็จๆทๅฐๆง็ๆฌๆ ๆณจๆไปถ่ฟ็งป่ณๆฐๆ ผๅผ๏ผๆไปฌๆไพไบ่ฟ็งป่ๆฌใไฝฟ็จๆนๆณๅฆไธ๏ผ
-
-```bash
-python tools/dataset_converters/textdet/data_migrator.py ${IN_PATH} ${OUT_PATH}
-```
-
-| ๅๆฐ | ็ฑปๅ | ่ฏดๆ |
-| -------- | -------------------------------- | -------------------------------------------------------------------------------------------------------------------------------------------------- |
-| in_path | str | ๏ผๅฟ
้กป๏ผๆง็ๆ ๆณจ็่ทฏๅพ |
-| out_path | str | ๏ผๅฟ
้กป๏ผๆฐ็ๆ ๆณจ็่ทฏๅพ |
-| --task | 'auto', 'textdet', 'textspotter' | ๆๅฎ่พๅบๆฐๆฎ้ๆ ๆณจ็ๆๅ
ผๅฎน็ไปปๅกใ่ฅๆๅฎไธบ textdet ๏ผๅไธไผ่ฝฌๅญ coco ๆ ผๅผไธญ็ text ๅญๆฎตใ้ป่ฎคไธบ auto๏ผๅณๆ นๆฎๆง็ๆ ๆณจ็ๆ ผๅผ่ชๅจๅณๅฎ่พๅบ็ๆ ๆณจๆ ผๅผใ |
-
-### ๆๆฌ่ฏๅซ
-
-#### ๆฐ็ๆ ผๅผไป็ป
-
-`TextRecogDataset` ไธญๅญๆพไบๆๆฌ่ฏๅซไปปๅกๆ้็ๆๆฌๅ
ๅฎน๏ผ้ๅธธ่่จ๏ผๆๆฌ่ฏๅซๆฐๆฎ้ไธญ็ๆฏไธๅผ ๅพ็้ฝไป
ๅ
ๅซไธไธชๆๆฌๅฎไพใๆไปฌๅจ `tests/data/rec_toy_dataset/labels.json` ๆไพไบไธไธช็ฎๅ็่ฏๅซๆฐๆฎๆ ผๅผ็คบไพ๏ผ็จๆทๅฏไปฅๅ่่ฏฅๆไปถไปฅ่ฟไธๆญฅไบ่งฃๅ
ถไธญ็็ป่ใ
-
-```json
-{
- "metainfo":
- {
- "dataset_type": "TextRecogDataset",
- "task_name": "textrecog",
- },
- "data_list":
- [
- {
- "img_path": "test_img.jpg",
- "instances":
- [
- {
- "text": "GRAND"
- }
- ]
- }
- ]
-}
-```
-
-#### ่ฟ็งป่ๆฌ
-
-ไธบๅธฎๅฉ็จๆทๅฐๆง็ๆฌๆ ๆณจๆไปถ่ฟ็งป่ณๆฐๆ ผๅผ๏ผๆไปฌๆไพไบ่ฟ็งป่ๆฌใไฝฟ็จๆนๆณๅฆไธ๏ผ
-
-```bash
-python tools/dataset_converters/textrecog/data_migrator.py ${IN_PATH} ${OUT_PATH} --format ${txt, jsonl, lmdb}
-```
-
-| ๅๆฐ | ็ฑปๅ | ่ฏดๆ |
-| -------- | ---------------------- | -------------------------- |
-| in_path | str | ๏ผๅฟ
้กป๏ผๆง็ๆ ๆณจ็่ทฏๅพ |
-| out_path | str | ๏ผๅฟ
้กป๏ผๆฐ็ๆ ๆณจ็่ทฏๅพ |
-| --format | 'txt', 'jsonl', 'lmdb' | ๆๅฎๆง็ๆฐๆฎ้ๆ ๆณจ็ๆ ผๅผใ |
-
-## ๅ
ผๅฎนๆง
-
-่่ๅฐ็จๆทๅฏนๆฐๆฎ่ฟ็งปๆ้็ๆๆฌ๏ผๆไปฌๅจ MMOCR 1.x ็ๆฌไธญๆๆถๅฏน MMOCR 0.x ๆง็ๆฌๆ ผๅผ่ฟ่กไบๅ
ผๅฎนใ
-
-```{note}
-็จไบๅ
ผๅฎนๆงๆฐๆฎๆ ผๅผ็ไปฃ็ ๅ็ปไปถๅฏ่ฝๅจๆชๆฅ็็ๆฌไธญ่ขซๅฎๅ
จ็งป้คใๅ ๆญค๏ผๆไปฌๅผบ็ๅปบ่ฎฎ็จๆทๅฐๆฐๆฎ้่ฟ็งป่ณๆฐ็ๆฐๆฎๆ ผๅผๆ ๅใ
-```
-
-ๅ
ทไฝ่่จ๏ผๆไปฌๆไพไบไธไธชไธดๆถ็ๆฐๆฎ้็ฑป [IcdarDataset](mmocr.datasets.IcdarDataset), [RecogTextDataset](mmocr.datasets.RecogTextDataset), [RecogLMDBDataset](mmocr.datasets.RecogLMDBDataset) ๆฅๅ
ผๅฎนๆงๆ ผๅผ็ๆ ๆณจๆไปถใๅๅซๅฏนๅบไบ MMOCR 0.x ็ๆฌไธญ็ๆๆฌๆฃๆตๆฐๆฎ้ `IcdarDataset`๏ผ`.txt`ใ`.jsonl` ๅ `LMDB` ๆ ผๅผ็ๆๆฌ่ฏๅซๆฐๆฎๆ ๆณจใๅ
ถไฝฟ็จๆนๅผไธ 0.x ็ๆฌไธ่ดใ
-
-1. [IcdarDataset](mmocr.datasets.IcdarDataset) ๆฏๆ 0.x ็ๆฌๆๆฌๆฃๆตไปปๅก็ COCO ๆ ๆณจๆ ผๅผใๅช้่ฆๅจ `configs/textdet/_base_/datasets` ไธญๆทปๅ ๆฐ็ๆฐๆฎ้้
็ฝฎๆไปถ๏ผๅนถๆๅฎๅ
ถๆฐๆฎ้็ฑปๅไธบ `IcdarDataset` ๅณๅฏใ
-
- ```python
- data_root = 'data/det/icdar2015'
-
- train_dataset = dict(
- type='IcdarDataset',
- data_root=data_root,
- ann_file='instances_training.json',
- data_prefix=dict(img_path='imgs/'),
- filter_cfg=dict(filter_empty_gt=True, min_size=32),
- pipeline=None)
- ```
-
-2. [RecogTextDataset](mmocr.datasets.RecogTextDataset) ๆฏๆ 0.x ็ๆฌๆๆฌ่ฏๅซไปปๅก็ `txt` ๅ `jsonl` ๆ ๆณจๆ ผๅผใๅช้่ฆๅจ `configs/textrecog/_base_/datasets` ไธญๆทปๅ ๆฐ็ๆฐๆฎ้้
็ฝฎๆไปถ๏ผๅนถๆๅฎๅ
ถๆฐๆฎ้็ฑปๅไธบ `RecogTextDataset` ๅณๅฏใไพๅฆ๏ผไปฅไธ็คบไพๅฑ็คบไบๅฆไฝ้
็ฝฎๅนถ่ฏปๅ toy dataset ไธญ็ๆงๆ ผๅผๆ ็ญพ `old_label.txt` ไปฅๅ `old_label.jsonl`ใ
-
- ```python
- data_root = 'tests/data/rec_toy_dataset/'
-
- # ่ฏปๅๆง็ txt ๆ ผๅผ่ฏๅซๆฐๆฎๆ ็ญพ
- txt_dataset = dict(
- type='RecogTextDataset',
- data_root=data_root,
- ann_file='old_label.txt',
- data_prefix=dict(img_path='imgs'),
- parser_cfg=dict(
- type='LineStrParser',
- keys=['filename', 'text'],
- keys_idx=[0, 1]),
- pipeline=[])
-
- # ่ฏปๅๆง็ json line ๆ ผๅผ่ฏๅซๆฐๆฎๆ ็ญพ
- jsonl_dataset = dict(
- type='RecogTextDataset',
- data_root=data_root,
- ann_file='old_label.jsonl',
- data_prefix=dict(img_path='imgs'),
- parser_cfg=dict(
- type='LineJsonParser',
- keys=['filename', 'text'],
- pipeline=[])
- ```
-
-3. [RecogLMDBDataset](mmocr.datasets.RecogLMDBDataset) ๆฏๆ 0.x ็ๆฌๆๆฌ่ฏๅซไปปๅก**ๅพๅ+ๆๅญ**็ `LMDB` ๆ ๆณจๆ ผๅผใๅช้่ฆๅจ `configs/textrecog/_base_/datasets` ไธญๆทปๅ ๆฐ็ๆฐๆฎ้้
็ฝฎๆไปถ๏ผๅนถๆๅฎๅ
ถๆฐๆฎ้็ฑปๅไธบ `RecogLMDBDataset` ๅณๅฏใไพๅฆ๏ผไปฅไธ็คบไพๅฑ็คบไบๅฆไฝ้
็ฝฎๅนถ่ฏปๅ toy dataset ไธญ็ `imgs.lmdb`๏ผ่ฏฅ `lmdb` ๆไปถ**ๅ
ๅซๆ ็ญพๅๅพๅ**ใ
-
- ```python
- # ๅฐๆฐๆฎ้็ฑปๅ่ฎพๅฎไธบ RecogLMDBDataset
- data_root = 'tests/data/rec_toy_dataset/'
-
- lmdb_dataset = dict(
- type='RecogLMDBDataset',
- data_root=data_root,
- ann_file='imgs.lmdb',
- pipeline=None)
- ```
-
- ่ฟ้ๆ `train_pipeline` ๅ `test_pipeline` ไธญ็ๆฐๆฎ่ฏปๅๆนๆณๅฆ [`LoadImageFromFile`](mmocr.datasets.transforms.LoadImageFromFile) ๆฟๆขไธบ [`LoadImageFromNDArray`](mmocr.datasets.transforms.LoadImageFromNDArray)๏ผ
-
- ```python
- train_pipeline = [dict(type='LoadImageFromNDArray')]
- ```
diff --git a/pipeline/mmocr/docs/zh_cn/migration/model.md b/pipeline/mmocr/docs/zh_cn/migration/model.md
deleted file mode 100644
index 0e276513df0e3704c36f1986451791c00d1f18e5..0000000000000000000000000000000000000000
--- a/pipeline/mmocr/docs/zh_cn/migration/model.md
+++ /dev/null
@@ -1,5 +0,0 @@
-# ้ข่ฎญ็ปๆจกๅ่ฟ็งปๆๅ
-
-็ฑไบๅจๆฐ็ๆฌไธญๆไปฌๅฏนๆจกๅ็็ปๆ่ฟ่กไบๅคง้็้ๆๅไฟฎๅค๏ผMMOCR 1.x ๅนถไธ่ฝ็ดๆฅ่ฏปๅ
ฅๆง็็้ข่ฎญ็ปๆ้ใๆไปฌๅจ็ฝ็ซไธๅๆญฅๆดๆฐไบๆๆๆจกๅ็้ข่ฎญ็ปๆ้ๅlog๏ผไพๆ้่ฆ็็จๆทไฝฟ็จใ
-
-ๆญคๅค๏ผๆไปฌๆญฃๅจ่ฟ่ก้ๅฏนๆๆฌๆฃๆตไปปๅก็ๆ้่ฟ็งปๅทฅๅ
ท็ๅผๅ๏ผๅนถ่ฎกๅไบ่ฟๆ็ๆฌๅ
ๅๅธใ็ฑไบๆๆฌ่ฏๅซๅๅ
ณ้ฎไฟกๆฏๆๅๆจกๅๆนๅจ่ฟๅคง๏ผไธ่ฟ็งปๆฏๆๆ็๏ผๆไปฌๆๆถไธ่ฎกๅไฝ็ธๅบๆฏๆใๅฆๆๆจๆๅ
ทไฝ็้ๆฑ๏ผๆฌข่ฟ้่ฟ [Issue](https://github.com/open-mmlab/mmocr/issues) ๅๆไปฌๆ้ฎใ
diff --git a/pipeline/mmocr/docs/zh_cn/migration/news.md b/pipeline/mmocr/docs/zh_cn/migration/news.md
deleted file mode 100644
index e1ca6f91ac0bc3b0db1ef83af63250923fedad66..0000000000000000000000000000000000000000
--- a/pipeline/mmocr/docs/zh_cn/migration/news.md
+++ /dev/null
@@ -1,19 +0,0 @@
-# MMOCR 1.x ๆดๆฐๆฑๆป
-
-ๆญคๅคๅๅบไบ MMOCR 1.x ็ธๅฏนไบ 0.x ็ๆฌ็้ๅคงๆดๆฐใ
-
-1. ๆถๆๅ็บง๏ผMMOCR 1.x ๆฏๅบไบ [MMEngine](https://github.com/open-mmlab/mmengine)๏ผๆไพไบไธไธช้็จ็ใๅผบๅคง็ๆง่กๅจ๏ผๅ
่ฎธๆด็ตๆดป็ๅฎๅถ๏ผๆไพไบ็ปไธ็่ฎญ็ปๅๆต่ฏๅ
ฅๅฃใ
-
-2. ็ปไธๆฅๅฃ๏ผMMOCR 1.x ็ปไธไบๆฐๆฎ้ใๆจกๅใ่ฏไผฐๅๅฏ่งๅ็ๆฅๅฃๅๅ
้จ้ป่พใๆฏๆๆดๅผบ็ๆฉๅฑๆงใ
-
-3. ่ทจ้กน็ฎ่ฐ็จ๏ผๅ็ไบ็ปไธ็่ฎพ่ฎก๏ผไฝ ๅฏไปฅไฝฟ็จๅ
ถไปOpenMMLab้กน็ฎไธญๅฎ็ฐ็ๆจกๅ๏ผๅฆMMDetใ ๆไปฌๆไพไบไธไธชไพๅญ๏ผ่ฏดๆๅฆไฝ้่ฟMMDetWrapperไฝฟ็จMMDetection็Mask R-CNNใๆฅ็ๆไปฌ็ๆๆกฃไปฅไบ่งฃๆดๅค็ป่ใๆดๅค็ๅ
่ฃ
ๅจๅฐๅจๆชๆฅๅๅธใ
-
-4. ๆดๅผบ็ๅฏ่งๅ๏ผๆไปฌๆไพไบไธ็ณปๅๅฏ่งๅๅทฅๅ
ท๏ผ ็จๆท็ฐๅจๅฏไปฅๆดๆนไพฟๅฏ่งๅๆฐๆฎใ
-
-5. ๆดๅค็ๆๆกฃๅๆ็จ๏ผๆไปฌๅขๅ ไบๆดๅค็ๆ็จ๏ผ้ไฝ็จๆท็ๅญฆไน ้จๆงใ
-
-6. ไธ็ซๅผๆฐๆฎๅๅค๏ผๅๅคๆฐๆฎ้ๅทฒ็ปไธๅๆฏ้พไบใไฝฟ็จๆไปฌ็ [Dataset Preparer](https://mmocr.readthedocs.io/zh_CN/dev-1.x/user_guides/data_prepare/dataset_preparer.html)๏ผไธ่กๅฝไปคๅณๅฏ่ฎฉๅคไธชๆฐๆฎ้ๅๅคๅฐฑ็ปชใ
-
-7. ๆฅๆฑๆดๅค `projects/`: ๆไปฌๆจๅบไบ `projects/` ๆไปถๅคน๏ผ็จไบๅญๆพไธไบๅฎ้ชๆง็ๆฐ็นๆงใๆกๆถๅๆจกๅใๆไปฌๅฏน่ฟไธชๆไปถๅคนไธ็ไปฃ็ ่ง่ไธไฝ่ฟๅค่ฆๆฑ๏ผๅๆฑ่ฎฉ็คพๅบ็ๆๆๆณๆณ็ฌฌไธๆถ้ดๅพๅฐๅฎ็ฐๅๅฑ็คบใ่ฏทๆฅ็ๆไปฌ็[ๆ ทไพ project](https://github.com/open-mmlab/mmocr/blob/dev-1.x/projects/example_project/) ไปฅไบ่งฃๆดๅคใ
-
-8. ๆดๅคๆฐๆจกๅ๏ผMMOCR 1.0 ๆฏๆไบๆดๅคๆจกๅๅๆจกๅ็ง็ฑปใ
diff --git a/pipeline/mmocr/docs/zh_cn/migration/overview.md b/pipeline/mmocr/docs/zh_cn/migration/overview.md
deleted file mode 100644
index 987774a446c6b28967ed22fa778b7fb9c6966792..0000000000000000000000000000000000000000
--- a/pipeline/mmocr/docs/zh_cn/migration/overview.md
+++ /dev/null
@@ -1,18 +0,0 @@
-# ๆฆ่ง
-
-ไผด้็ OpenMMLab 2.0 ็ๅๅธ๏ผMMOCR 1.0 ๆฌ่บซไนไฝๅบไบ่ฎธๅค็ช็ ดๆง็ๆนๅ๏ผไฝฟๅพไปฃ็ ็ๅไฝๅบฆ้ไฝ๏ผไปฃ็ ๆ็ๆ้ซ๏ผๆดไฝ่ฎพ่ฎกไธไนๅๅพๆดไธบไธ่ดใ็ถ่๏ผ่ฟไบๆนๅไฝฟๅพๅฎ็พ็ๅๅๅ
ผๅฎนไธๅๅฏ่ฝใๆไปฌไนๆทฑ็ฅๅจ่ฟๆ ทๅทจๅคง็ๅๅจไนไธ๏ผ่็จๆทๆณ็ฌฌไธๆถ้ด้ๅบๆฐ็ๆฌไน็ป้ๆไบใๅ ๆญค๏ผๆไปฌๆจๅบไบ่ฏฆ็ป็่ฟ็งปๆๅ๏ผๆจๅจ่ฎฉ่็จๆทไปฌๅฐฝๅฏ่ฝๅนณๆปๅฐ่ฟๆธกๅฐๅ
จๆฐ็ๆกๆถ๏ผๆ็ป่ฝไบซๅๅฐๅ
จๆฐ็ MMOCR ๅๆดไธชOpenMMLab 2.0 ็ๆ็ณป็ปไธบ็ไบงๅๅธฆๆฅ็ๅทจๅคงไผๅฟใ
-
-```{warning}
-MMOCR 1.0 ไพ่ตไบๆฐ็ๅบ็ก่ฎญ็ปๆกๆถ [MMEngine](https://github.com/open-mmlab/mmengine)๏ผๅ ่ๆ็ไธ MMOCR 0.x ๅฎๅ
จไธๅ็ไพ่ต้พใๅฐฝ็ฎกไฝ ๅฏ่ฝๅทฒ็ปๆฅๆไบไธไธชๅฏไปฅๆญฃๅธธ่ฟ่ก MMOCR 0.x ็็ฏๅข๏ผไฝไฝ ไป็ถ้่ฆๅๅปบไธไธชๆฐ็ python ็ฏๅขๆฅๅฎ่ฃ
MMOCR 1.0 ็ๆฌๆ้่ฆ็ไพ่ตๅบใๆไปฌๆไพไบ่ฏฆ็ป็[ๅฎ่ฃ
ๆๆกฃ](../get_started/install.md)ไปฅไพๅ่ใ
-```
-
-ๆฅไธๆฅ๏ผ่ฏทๆ นๆฎไฝ ็ๅฎ้
้ๆฑ๏ผ้
่ฏป้่ฆ็็ซ ่๏ผ
-
-- ่ฅ้่ฆไบ่งฃ MMOCR 1.0 ็ไธป่ฆๅๅ๏ผ่ฏท้
่ฏป [MMOCR 1.x ๆดๆฐๆฑๆป](./news.md)
-- ๅฆๆไฝ ้่ฆๆ 0.x ็ๆฌไธญ่ฎญ็ป็ๆจกๅ็ดๆฅ่ฟ็งปๅฐ 1.0 ็ๆฌไธญไฝฟ็จ๏ผ่ฏท้
่ฏป [้ข่ฎญ็ปๆจกๅ่ฟ็งป](./model.md)
-- ๅฆๆไฝ ้่ฆ่ฎญ็ปๆจกๅ๏ผ่ฏท้
่ฏป [ๆฐๆฎ้่ฟ็งป](./dataset.md) ๅ [ๆฐๆฎๅขๅผบ่ฟ็งป](./transforms.md)
-- ๅฆๆไฝ ้่ฆๅจ MMOCR ไธ่ฟ่กๅผๅ๏ผ่ฏท้
่ฏป [ไปฃ็ ่ฟ็งป](code.md) ๏ผ[ๅๆฏ่ฟ็งป](branches.md) ๅ [ไธๆธธไพ่ตๅบๅๆด](https://github.com/open-mmlab/mmengine/tree/main/docs/zh_cn/migration)
-
-ๅฆไธๅพๆ็คบ๏ผMMOCR 1.x ็ๆฌ็็ปดๆค่ฎกๅไธป่ฆๅไธบไธไธช้ถๆฎต๏ผๅณโๅ
ฌๆตๆโ๏ผโๅ
ผๅฎนๆโไปฅๅโ็ปดๆคๆโใๅฏนไบๆง็ๆฌ๏ผๆไปฌๅฐไธๅๅขๅ ไธป่ฆๆฐๅ่ฝใๅ ๆญค๏ผๆไปฌๅผบ็ๅปบ่ฎฎ็จๆทๅฐฝๆฉ่ฟ็งป่ณ MMOCR 1.x ็ๆฌใ
-
-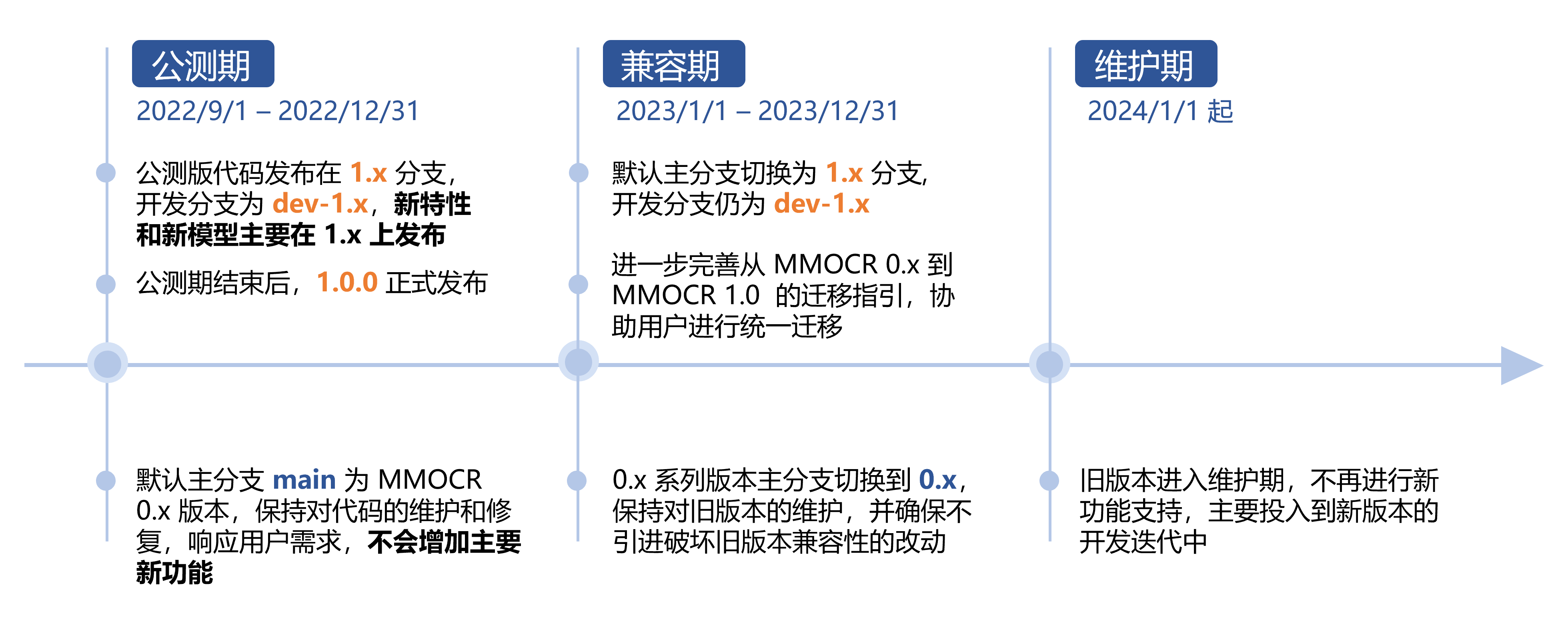
diff --git a/pipeline/mmocr/docs/zh_cn/migration/transforms.md b/pipeline/mmocr/docs/zh_cn/migration/transforms.md
deleted file mode 100644
index 57acd3d386d83c088d6434dc351b7462464671b2..0000000000000000000000000000000000000000
--- a/pipeline/mmocr/docs/zh_cn/migration/transforms.md
+++ /dev/null
@@ -1,583 +0,0 @@
-# ๆฐๆฎๅๆข่ฟ็งป
-
-## ็ฎไป
-
-MMOCR 0.x ็ๆฌไธญ๏ผๆไปฌๅจ `mmocr/datasets/pipelines/xxx_transforms.py` ไธญๅฎ็ฐไบไธ็ณปๅ็ๆฐๆฎๅๆข๏ผData Transforms๏ผๆนๆณใ็ถ่๏ผ่ฟไบๆจกๅๅๆฃๅจๅๅค๏ผไธ็ผบไน่ง่็ปไธ็่ฎพ่ฎกใๅ ๆญค๏ผๆไปฌๅจ MMOCR 1.x ็ๆฌไธญๅฏนๆๆ็ๆฐๆฎๅขๅผบๆจกๅ่ฟ่กไบ้ๆ๏ผๅนถไพ็
งไปปๅก็ฑปๅๅๅซๅญๆพๅจ `mmocr/datasets/transforms` ็ฎๅฝไธ็ `ocr_transforms.py`๏ผ`textdet_transforms.py` ๅ `textrecog_transforms.py` ไธญใๅ
ถไธญ๏ผ`ocr_transforms.py` ไธญๅฎ็ฐไบ OCR ็ธๅ
ณไปปๅก้็จ็ๆฐๆฎๅขๅผบๆจกๅ๏ผ่ `textdet_transforms.py` ๅ `textrecog_transforms.py` ๅๅๅซๅฎ็ฐไบๆๆฌๆฃๆตไปปๅกไธๆๆฌ่ฏๅซไปปๅก็ธๅ
ณ็ๆฐๆฎๅขๅผบๆจก็ปใ
-
-็ฑไบๅจ้ๆ่ฟ็จไธญๆไปฌๅฏน้จๅๆจกๅ่ฟ่กไบ้ๅฝๅใๅๅนถๆๆๅ๏ผไฝฟๅพๆฐ็่ฐ็จๆฅๅฃไธ้ป่ฎคๅๆฐๅฏ่ฝไธๆง็ๆฌๅญๅจไธไธ่ดใๅ ๆญค๏ผๆฌๆๆกฃๅฐ่ฏฆ็ปไป็ปๅฆไฝๅฏนๆฐๆฎๅขๅผบๆจกๅ่ฟ่ก่ฟ็งป๏ผๅณ๏ผๅฆไฝ้
็ฝฎ็ฐๆ็ๆฐๆฎๅๆขๆฅ่พพๅฐไธๆง็ไธ่ด็่กไธบใ
-
-## ้
็ฝฎ่ฟ็งปๆๅ
-
-### ๆฐๆฎๆ ผๅผๅ็ธๅ
ณๆฐๆฎๅๆข
-
-1. `Collect` + `CustomFormatBundle` -> [`PackTextDetInputs`](mmocr.datasets.transforms.PackTextDetInputs)/[`PackTextRecogInputs`](mmocr.datasets.transforms.PackTextRecogInputs)
-
-`PackxxxInputs` ๅๆถๅๆฌไบ `Collect` ๅ `CustomFormatBundle` ไธคไธชๅ่ฝ๏ผไธไธๅๆ `key` ๅๆฐ๏ผ่่ฎญ็ป็ฎๆ target ็็ๆ็ฐๅจ่ขซ่ฝฌ็งป่ณๅจ `loss` ไธญๅฎๆใ
-
-
-
-
- MMOCR 0.x ้
็ฝฎ
- MMOCR 1.x ้
็ฝฎ
-
-
-
-
-```python
-dict(
- type='CustomFormatBundle',
- keys=['gt_shrink', 'gt_shrink_mask', 'gt_thr', 'gt_thr_mask'],
- meta_keys=['img_path', 'ori_shape', 'img_shape'],
- visualize=dict(flag=False, boundary_key='gt_shrink')),
-dict(
- type='Collect',
- keys=['img', 'gt_shrink', 'gt_shrink_mask', 'gt_thr', 'gt_thr_mask'])
-```
-
-
-
-```python
-dict(
- type='PackTextDetInputs',
- meta_keys=('img_path', 'ori_shape', 'img_shape'))
-```
-
-
-
-
-
-### ๆฐๆฎๅขๅผบ็ธๅ
ณๆฐๆฎๅๆข
-
-1. `ResizeOCR` -> [`Resize`](mmocr.datasets.transforms.Resize), [`RescaleToHeight`](mmocr.datasets.transforms.RescaleToHeight), [`PadToWidth`](mmocr.datasets.transforms.PadToWidth)
-
- ๅๆ็ `ResizeOCR` ็ฐๅจ่ขซๆๅไธบไธไธช็ฌ็ซ็ๆฐๆฎๅขๅผบๆจกๅใ
-
- `keep_aspect_ratio=False` ๆถ๏ผ็ญไปทไธบ 1.x ็ๆฌไธญ็ `Resize`๏ผๅ
ถ้
็ฝฎๅฏๆๅฆไธๆนๅผไฟฎๆนใ
-
-
-
-
- MMOCR 0.x ้
็ฝฎ
- MMOCR 1.x ้
็ฝฎ
-
-
-
-
-```python
-dict(
- type='ResizeOCR',
- height=32,
- min_width=100,
- max_width=100,
- keep_aspect_ratio=False)
-```
-
-
-
-```python
-dict(
- type='Resize',
- scale=(100, 32),
- keep_ratio=False)
-```
-
-
-
-
-
-`keep_aspect_ratio=True`๏ผไธ `max_width=None` ๆถใๅฐๅพ็็้ซ็ผฉๆพ่ณๅบๅฎๅผ๏ผๅนถ็ญๆฏไพ็ผฉๆพๅพๅ็ๅฎฝใ
-
-
-
-
- MMOCR 0.x ้
็ฝฎ
- MMOCR 1.x ้
็ฝฎ
-
-
-
-
-```python
-dict(
- type='ResizeOCR',
- height=32,
- min_width=32,
- max_width=None,
- width_downsample_ratio = 1.0 / 16
- keep_aspect_ratio=True)
-```
-
-
-
-```python
-dict(
- type='RescaleToHeight',
- height=32,
- min_width=32,
- max_width=None,
- width_divisor=16),
-```
-
-
-
-
-
-`keep_aspect_ratio=True`๏ผไธ `max_width` ไธบๅบๅฎๅผๆถใๅฐๅพ็็้ซ็ผฉๆพ่ณๅบๅฎๅผ๏ผๅนถ็ญๆฏไพ็ผฉๆพๅพๅ็ๅฎฝใ่ฅ็ผฉๆพๅ็ๅพๅๅฎฝๅฐไบ `max_width`, ๅๅฐๅ
ถๅกซๅ
่ณ `max_width`, ๅไนๅๅฐๅ
ถ่ฃๅช่ณ `max_width`ใๅณ๏ผ่พๅบๅพๅ็ๅฐบๅฏธๅบๅฎไธบ `(height, max_width)`ใ
-
-
-
-
- MMOCR 0.x ้
็ฝฎ
- MMOCR 1.x ้
็ฝฎ
-
-
-
-
-```python
-dict(
- type='ResizeOCR',
- height=32,
- min_width=32,
- max_width=100,
- width_downsample_ratio = 1.0 / 16,
- keep_aspect_ratio=True)
-```
-
-
-
-```python
-dict(
- type='RescaleToHeight',
- height=32,
- min_width=32,
- max_width=100,
- width_divisor=16),
-dict(
- type='PadToWidth',
- width=100)
-```
-
-
-
-
-
-2. `RandomRotateTextDet` & `RandomRotatePolyInstances` -> [`RandomRotate`](mmocr.datasets.transforms.RandomRotate)
-
- ้ๆบๆ่ฝฌๆฐๆฎๅขๅผบ็ญ็ฅๅทฒ่ขซๆดๅ่ณ `RanomRotate`ใ่ฏฅๆนๆณ็้ป่ฎค่กไธบไธ 0.x ็ๆฌไธญ็ `RandomRotateTextDet` ไฟๆไธ่ดใๆญคๆถไป
้ๆๅฎๆๅคงๆ่ฝฌ่งๅบฆ `max_angle` ๅณๅฏใ
-
-```{note}
- ๆฐๆง็ๆฌ "max_angle" ็้ป่ฎคๅผไธๅ๏ผๅ ๆญค้่ฆ้ๆฐ่ฟ่กๆๅฎใ
-```
-
-
-
-
- MMOCR 0.x ้
็ฝฎ
- MMOCR 1.x ้
็ฝฎ
-
-
-
-
-```python
-dict(type='RandomRotateTextDet')
-```
-
-
-
-```python
-dict(type='RandomRotate', max_angle=10)
-```
-
-
-
-
-
-ๅฏนไบ `RandomRotatePolyInstances`๏ผๅ้่ฆๆๅฎๅๆฐ `use_canvas=True`ใ
-
-
-
-
- MMOCR 0.x ้
็ฝฎ
- MMOCR 1.x ้
็ฝฎ
-
-
-
-
-```python
-dict(
- type='RandomRotatePolyInstances',
- rotate_ratio=0.5, # ๆๅฎๆฆ็ไธบ0.5
- max_angle=60,
- pad_with_fixed_color=False)
-```
-
-
-
-```python
-# ็จ RandomApply ๅฏนๆฐๆฎๅๆข่ฟ่กๅ
่ฃ
๏ผๅนถๆๅฎๆง่กๆฆ็
-dict(
- type='RandomApply',
- transforms=[
- dict(type='RandomRotate',
- max_angle=60,
- pad_with_fixed_color=False,
- use_canvas=True)],
- prob=0.5) # ่ฎพ็ฝฎๆง่กๆฆ็ไธบ 0.5
-```
-
-
-
-
-
-```{note}
-ๅจ 0.x ็ๆฌไธญ๏ผ้จๅๆฐๆฎๅขๅผบๆนๆณ้่ฟๅฎไนไธไธชๅ
้จๅ้ "xxx_ratio" ๆฅๆๅฎๆง่กๆฆ็๏ผๅฆ "rotate_ratio", "crop_ratio" ็ญใๅจ 1.x ็ๆฌไธญ๏ผ่ฟไบๅๆฐๅทฒ่ขซ็ปไธๅ ้คใ็ฐๅจ๏ผๆไปฌๅฏไปฅ้่ฟ "RandomApply" ๆฅๅฏนไธๅ็ๆฐๆฎๅๆขๆนๆณ่ฟ่กๅ
่ฃ
๏ผๅนถๆๅฎๅ
ถๆง่กๆฆ็ใ
-```
-
-3. `RandomCropFlip` -> [`TextDetRandomCropFlip`](mmocr.datasets.transforms.TextDetRandomCropFlip)
-
- ็ฎๅไป
ๅฏนๆนๆณๅ่ฟ่กไบๆดๆน๏ผๅ
ถไปๅๆฐไฟๆไธ่ดใ
-
-4. `RandomCropPolyInstances` -> [`RandomCrop`](mmocr.datasets.transforms.RandomCrop)
-
- ๆฐ็ๆฌ็งป้คไบ `crop_ratio` ไปฅๅ `instance_key`๏ผๅนถ็ปไธไฝฟ็จ `gt_polygons` ไธบ็ฎๆ ่ฟ่ก่ฃๅชใ
-
-
-
-
- MMOCR 0.x ้
็ฝฎ
- MMOCR 1.x ้
็ฝฎ
-
-
-
-
-```python
-dict(
- type='RandomCropPolyInstances',
- instance_key='gt_masks',
- crop_ratio=0.8, # ๆๅฎๆฆ็ไธบ 0.8
- min_side_ratio=0.3)
-```
-
-
-
-```python
-# ็จ RandomApply ๅฏนๆฐๆฎๅๆข่ฟ่กๅ
่ฃ
๏ผๅนถๆๅฎๆง่กๆฆ็
-dict(
- type='RandomApply',
- transforms=[dict(type='RandomCrop', min_side_ratio=0.3)],
- prob=0.8) # ่ฎพ็ฝฎๆง่กๆฆ็ไธบ 0.8
-```
-
-
-
-
-
-5. `RandomCropInstances` -> [`TextDetRandomCrop`](mmocr.datasets.transforms.TextDetRandomCrop)
-
- ๆฐ็ๆฌ็งป้คไบ `instance_key` ๅ `mask_type`๏ผๅนถ็ปไธไฝฟ็จ `gt_polygons` ไธบ็ฎๆ ่ฟ่ก่ฃๅชใ
-
-
-
-
- MMOCR 0.x ้
็ฝฎ
- MMOCR 1.x ้
็ฝฎ
-
-
-
-
-```python
-dict(
- type='RandomCropInstances',
- target_size=(800๏ผ800),
- instance_key='gt_kernels')
-```
-
-
-
-```python
-dict(
- type='TextDetRandomCrop',
- target_size=(800๏ผ800))
-```
-
-
-
-
-
-6. `EastRandomCrop` -> [`RandomCrop`](mmocr.datasets.transforms.RandomCrop) + [`Resize`](mmocr.datasets.transforms.Resize) + [`mmengine.Pad`](mmcv.transforms.Pad)
-
- ๅๆ็ `EastRandomCrop` ๅ
ๅๆถๅฏนๅพๅ่ฟ่กไบๅช่ฃใ็ผฉๆพไปฅๅๅกซๅ
ใๅจๆฐ็ๆฌไธญ๏ผๆไปฌๅฏไปฅ้่ฟ็ปๅไธ็งๆฐๆฎๅขๅผบ็ญ็ฅๆฅ่พพๅฐ็ธๅ็ๆๆใ
-
-
-
-
- MMOCR 0.x ้
็ฝฎ
- MMOCR 1.x ้
็ฝฎ
-
-
-
-
-```python
-dict(
- type='EastRandomCrop',
- max_tries=10,
- min_crop_side_ratio=0.1,
- target_size=(640, 640))
-```
-
-
-
-```python
-dict(type='RandomCrop', min_side_ratio=0.1),
-dict(type='Resize', scale=(640,640), keep_ratio=True),
-dict(type='Pad', size=(640,640))
-```
-
-
-
-
-
-7. `RandomScaling` -> [`mmengine.RandomResize`](mmcv.transforms.RandomResize)
-
- ๅจๆฐ็ๆฌไธญ๏ผๆไปฌ็ดๆฅไฝฟ็จ MMEngine ไธญๅฎ็ฐ็ `RandomResize` ๆฅไปฃๆฟๅๆ็ๅฎ็ฐใ
-
-
-
-
- MMOCR 0.x ้
็ฝฎ
- MMOCR 1.x ้
็ฝฎ
-
-
-
-
-```python
- dict(
- type='RandomScaling',
- size=800,
- scale=(0.75, 2.5))
-```
-
-
-
-```python
-dict(
- type='RandomResize',
- scale=(800, 800),
- ratio_range=(0.75, 2.5),
- keep_ratio=True)
-```
-
-
-
-
-
-```{note}
-้ป่ฎคๅฐ๏ผๆฐๆฎๆตๆฐด็บฟไผไปๅฝๅ *scope* ็ๆณจๅๅจไธญๆ็ดขๅฏนๅบ็ๆฐๆฎๅๆข๏ผๅฆๆไธๅญๅจ่ฏฅๆฐๆฎๅๆข๏ผๅๅฐ็ปง็ปญๅจไธๆธธๅบ๏ผๅฆ MMCV ๅ MMEngine ไธญ่ฟ่กๆ็ดขใไพๅฆ๏ผMMOCR ไธญๅนถๆชๅฎ็ฐ `RandomResize` ๆนๆณ๏ผไฝๆไปฌไป็ถๅฏไปฅๅจ้
็ฝฎไธญ็ดๆฅๅผ็จ่ฏฅๆฐๆฎๅขๅผบๆนๆณ๏ผๅ ไธบ็จๅบๅฐ่ชๅจไปไธๆธธ็ MMCV ไธญๆ็ดข่ฏฅๆนๆณใๆญคๅค๏ผ็จๆทไนๅฏไปฅ้่ฟๆทปๅ ๅ็ผ็ๅฝขๅผๆฅๆๅฎ *scope*ใไพๅฆ๏ผ`mmengine.RandomResize` ๅฐๅผบๅถๆๅฎไฝฟ็จ MMCV ๅบไธญๅฎ็ฐ็ `RandomResize`๏ผๅฝไธไธๆธธๅบไธญๅญๅจๅๅๆนๆณๆถ๏ผๅๅฏไปฅ้่ฟ่ฟ็งๅฝขๅผๅผบๅถไฝฟ็จ็นๅฎ็็ๆฌใๅฆๅค้่ฆๆณจๆ็ๆฏ๏ผMMCV ไธญๆๆ็ๆฐๆฎๅๆขๆนๆณ้ฝ่ขซๆณจๅ่ณ MMEngine ไธญ๏ผๅ ๆญคๆไปฌไฝฟ็จ `mmengine.RandomResize` ่ไธๆฏ `mmcv.RandomResize`ใ
-```
-
-8. `SquareResizePad` -> [`Resize`](mmocr.datasets.transforms.Resize) + [`SourceImagePad`](mmocr.datasets.transforms.SourceImagePad)
-
- ๅๆ็ `SquareResizePad` ๅ
้จๅฎ็ฐไบไธคไธชๅๆฏ๏ผๅนถไพๆฎๆฆ็ `pad_ratio` ้ๆบไฝฟ็จๅ
ถไธญ็ไธไธชๅๆฏ่ฟ่กๆฐๆฎๅขๅผบใๅ
ทไฝ่่จ๏ผไธไธชๅๆฏๅ
ๅฏนๅพๅ็ผฉๆพๅๅกซๅ
๏ผๅฆไธไธชๅๆฏๅ็ดๆฅๅฏนๅพๅ่ฟ่ก็ผฉๆพใไธบๅขๅผบไธๅๆจกๅ็ๅค็จๆง๏ผๆไปฌๅจ 1.x ็ๆฌไธญๅฐ่ฏฅๆนๆณๆๅๆไบ `Resize` + `SourceImagePad` ็็ปๅๅฝขๅผ๏ผๅนถ้่ฟ MMCV ไธญ็ `RandomChoice` ๆฅๆงๅถๅๆฏใ
-
-
-
-
- MMOCR 0.x ้
็ฝฎ
- MMOCR 1.x ้
็ฝฎ
-
-
-
-
-```python
-dict(
- type='SquareResizePad',
- target_size=800,
- pad_ratio=0.6)
-```
-
-
-
-```python
-dict(
- type='RandomChoice',
- transforms=[
- [
- dict(
- type='Resize',
- scale=800,
- keep_ratio=True),
- dict(
- type='SourceImagePad',
- target_scale=800)
- ],
- [
- dict(
- type='Resize',
- scale=800,
- keep_ratio=False)
- ]
- ],
- prob=[0.4, 0.6]), # ไธค็ง็ปๅ็้็จๆฆ็
-```
-
-
-
-
-
-```{note}
-ๅจ 1.x ็ๆฌไธญ๏ผ้ๆบ้ๆฉๅ
่ฃ
ๅจ "RandomChoice" ไปฃๆฟไบ "OneOfWrapper"๏ผๅฏไปฅไปไธ็ณปๅๆฐๆฎๅๆข็ปๅไธญ้ๆบๆฝๅไธ็ปๅนถๅบ็จใ
-```
-
-9. `RandomWrapper` -> [`mmegnine.RandomApply`](mmcv.transforms.RandomApply)
-
- ๅจ 1.x ็ๆฌไธญ๏ผ`RandomWrapper` ๅ
่ฃ
ๅจ่ขซๆฟๆขไธบ็ฑ MMCV ๅฎ็ฐ็ `RandomApply`๏ผ็จไปฅๆๅฎๆฐๆฎๅๆข็ๆง่กๆฆ็ใๅ
ถไธญๆฆ็ `p` ็ฐๅจ่ขซๅฝๅไธบ `prob`ใ
-
-
-
-
- MMOCR 0.x ้
็ฝฎ
- MMOCR 1.x ้
็ฝฎ
-
-
-
-
-```python
- dict(
- type='RandomWrapper',
- p=0.25,
- transforms=[
- dict(type='PyramidRescale'),
- ])
-```
-
-
-
-```python
-dict(
- type='RandomApply',
- prob=0.25,
- transforms=[
- dict(type='PyramidRescale'),
- ])
-```
-
-
-
-
-
-10. `OneOfWrapper` -> [`mmegnine.RandomChoice`](mmcv.transforms.RandomChoice)
-
-้ๆบ้ๆฉๅ
่ฃ
ๅจ็ฐๅจ่ขซ้ๅฝๅไธบ `RandomChoice`๏ผๅนถไธไฝฟ็จๆนๆณๅๅๆฅๅฎๅ
จไธ่ดใ
-
-11. `ScaleAspectJitter` -> [`ShortScaleAspectJitter`](mmocr.datasets.transforms.ShortScaleAspectJitter), [`BoundedScaleAspectJitter`](mmocr.datasets.transforms.BoundedScaleAspectJitter)
-
-ๅๆ็ `ScaleAspectJitter` ๅฎ็ฐไบๅค็งไธๅ็ๅพๅๅฐบๅฏธๆๅจๆฐๆฎๅขๅผบ็ญ็ฅ๏ผๅจๆฐ็ๆฌไธญ๏ผๆไปฌๅฐๅ
ถๆๅไธบๆฐไธช้ป่พๆดๅ ๆธ
ๆฐ็็ฌ็ซๆฐๆฎๅๅๆนๆณใ
-
-`resize_type='indep_sample_in_range'` ๆถ๏ผๅ
ถ็ญไปทไบๅพๅๅจๆๅฎ่ๅดๅ
็้ๆบ็ผฉๆพใ
-
-
-
-
- MMOCR 0.x ้
็ฝฎ
- MMOCR 1.x ้
็ฝฎ
-
-
-
-
-```python
-dict(
- type='ScaleAspectJitter',
- img_scale=None,
- keep_ratio=False,
- resize_type='indep_sample_in_range',
- scale_range=(640, 2560))
-```
-
-
-
-```python
- dict(
- type='RandomResize',
- scale=(640, 640),
- ratio_range=(1.0, 4.125),
- resize_type='Resize',
- keep_ratio=True))
-```
-
-
-
-
-
-`resize_type='long_short_bound'` ๆถ๏ผๅฐๅพๅ็ผฉๆพ่ณๆๅฎๅคงๅฐ๏ผๅๅฏนๅ
ถ้ฟๅฎฝๆฏ่ฟ่กๆๅจใ่ฟไธ้ป่พ็ฐๅจ็ฑๆฐ็ๆฐๆฎๅๆข็ฑป `BoundedScaleAspectJitter` ๅฎ็ฐใ
-
-
-
-
- MMOCR 0.x ้
็ฝฎ
- MMOCR 1.x ้
็ฝฎ
-
-
-
-
-```python
-dict(
- type='ScaleAspectJitter',
- img_scale=[(3000, 736)], # Unused
- ratio_range=(0.7, 1.3),
- aspect_ratio_range=(0.9, 1.1),
- multiscale_mode='value',
- long_size_bound=800,
- short_size_bound=480,
- resize_type='long_short_bound',
- keep_ratio=False)
-```
-
-
-
-```python
-dict(
- type='BoundedScaleAspectJitter',
- long_size_bound=800,
- short_size_bound=480,
- ratio_range=(0.7, 1.3),
- aspect_ratio_range=(0.9, 1.1))
-```
-
-
-
-
-
-`resize_type='around_min_img_scale'` ๏ผ้ป่ฎคๅๆฐ๏ผๆถ๏ผๅฐๅพๅ็็ญ่พน็ผฉๆพ่ณๆๅฎๅคงๅฐ๏ผๅๅจๆๅฎ่ๅดๅ
ๅฏน้ฟๅฎฝๆฏ่ฟ่กๆๅจใๆๅ๏ผ็กฎไฟๅ
ถ่พน้ฟ่ฝ่ขซ `scale_divisor` ๆด้คใ่ฟไธ้ป่พ็ฑๆฐ็ๆฐๆฎๅๆข็ฑป `ShortScaleAspectJitter` ๅฎ็ฐใ
-
-
-
-
- MMOCR 0.x ้
็ฝฎ
- MMOCR 1.x ้
็ฝฎ
-
-
-
-
-```python
-dict(
- type='ScaleAspectJitter',
- img_scale=[(3000, 640)],
- ratio_range=(0.7, 1.3),
- aspect_ratio_range=(0.9, 1.1),
- multiscale_mode='value',
- keep_ratio=False)
-```
-
-
-
-```python
-dict(
- type='ShortScaleAspectJitter',
- short_size=640,
- ratio_range=(0.7, 1.3),
- aspect_ratio_range=(0.9, 1.1),
- scale_divisor=32),
-```
-
-
-
-
diff --git a/pipeline/mmocr/docs/zh_cn/notes/branches.md b/pipeline/mmocr/docs/zh_cn/notes/branches.md
deleted file mode 100644
index ddcc54cdfe870c8cc623583456e01e341cb047ba..0000000000000000000000000000000000000000
--- a/pipeline/mmocr/docs/zh_cn/notes/branches.md
+++ /dev/null
@@ -1,25 +0,0 @@
-# ๅๆฏ
-
-ๆฌๆๆกฃๆจๅจๅ
จ้ข่งฃ้ MMOCR ไธญๆฏไธชๅๆฏ็็ฎ็ๅๅ่ฝใ
-
-## ๅๆฏๆฆ่ฟฐ
-
-### 1. `main`
-
-`main` ๅๆฏๆฏ MMOCR ้กน็ฎ็้ป่ฎคๅๆฏใๅฎๅ
ๅซไบ MMOCR ็ๆๆฐ็จณๅฎ็ๆฌ๏ผ็ฎๅๅ
ๅซไบ MMOCR 1.x๏ผไพๅฆ v1.0.0๏ผ็ไปฃ็ ใ`main` ๅๆฏ็กฎไฟ็จๆท่ฝๅคไฝฟ็จๆๆฐๅๆๅฏ้ ็่ฝฏไปถ็ๆฌใ
-
-### 2. `dev-1.x`
-
-`dev-1.x` ๅๆฏ็จไบๅผๅ MMOCR ็ไธไธไธช็ๆฌใๆญคๅๆฏๅฐๅจๅ็ๅ่ฟ่กไพ่ตๆงๆต่ฏ๏ผ้่ฟ็ๆไบคๅฐไผๅๆๅฐๆฐ็ๆฌไธญ๏ผๅนถ่ขซๅๅธๅฐ `main` ๅๆฏใ้่ฟ่ฎพ็ฝฎๅ็ฌ็ๅผๅๅๆฏ๏ผ้กน็ฎๅฏไปฅๅจไธๅฝฑๅ `main` ๅๆฏ็จณๅฎๆง็ๆ
ๅตไธ็ปง็ปญๅๅฑใ**ๆๆ PR ๅบๅๅนถๅฐ `dev-1.x` ๅๆฏใ**
-
-### 3. `0.x`
-
-`0.x` ๅๆฏ็จไฝ MMOCR 0.x๏ผไพๅฆ v0.6.3๏ผ็ๅญๆกฃใๆญคๅๆฏๅฐไธๅ็งฏๆๆฅๅๆดๆฐๆๆน่ฟ๏ผไฝๅฎไปๅฏไฝไธบๅๅฒๅ่๏ผๆไพๅฐๆชๅ็บงๅฐ MMOCR 1.x ็็จๆทไฝฟ็จใ
-
-### 4. `1.x`
-
-ๅฎๆฏ `main` ๅๆฏ็ๅซๅ๏ผๆจๅจๅฎ็ฐไปๅ
ผๅฎนๆงๆถๆๅนณ็จณ่ฟๆธกใๅฎๅฐๅจ 2023 ๅนด็ๅนดไธญๅ ้คใ
-
-```{note}
-ๅๆฏๆ ๅฐๅจ 2023.04.06 ๅ็ไบๅๅใๆๅ
ณๆงๅๆฏๆ ๅฐๅ่ฟ็งปๆๅ๏ผ่ฏทๅ้
[ๅๆฏ่ฟ็งปๆๅ](../migration/branches.md)ใ
-```
diff --git a/pipeline/mmocr/docs/zh_cn/notes/contribution_guide.md b/pipeline/mmocr/docs/zh_cn/notes/contribution_guide.md
deleted file mode 100644
index 90611e8ac8d5778d58242c0fa97f9db88f83e36f..0000000000000000000000000000000000000000
--- a/pipeline/mmocr/docs/zh_cn/notes/contribution_guide.md
+++ /dev/null
@@ -1,135 +0,0 @@
-# ่ดก็ฎๆๅ
-
-OpenMMLab ๆฌข่ฟๆๆไบบๅไธๆไปฌ้กน็ฎ็ๅ
ฑๅปบใๆฌๆๆกฃๅฐๆๅฏผๆจๅฆไฝ้่ฟๆๅ่ฏทๆฑไธบ OpenMMLab ้กน็ฎไฝๅบ่ดก็ฎใ
-
-## ไปไนๆฏๆๅ่ฏทๆฑ๏ผ
-
-`ๆๅ่ฏทๆฑ` (Pull Request), [GitHub ๅฎๆนๆๆกฃ](https://docs.github.com/en/github/collaborating-with-pull-requests/proposing-changes-to-your-work-with-pull-requests/about-pull-requests)ๅฎไนๅฆไธใ
-
-```
-ๆๅ่ฏทๆฑๆฏไธ็ง้็ฅๆบๅถใไฝ ไฟฎๆนไบไปไบบ็ไปฃ็ ๏ผๅฐไฝ ็ไฟฎๆน้็ฅๅๆฅไฝ่
๏ผๅธๆไปๅๅนถไฝ ็ไฟฎๆนใ
-```
-
-## ๅบๆฌ็ๅทฅไฝๆต๏ผ
-
-1. ่ทๅๆๆฐ็ไปฃ็ ๅบ
-2. ไปๆๆฐ็ `dev-1.x` ๅๆฏๅๅปบๅๆฏ่ฟ่กๅผๅ
-3. ๆไบคไฟฎๆน ([ไธ่ฆๅฟ่ฎฐไฝฟ็จ pre-commit hooks!](#3-ๆไบคไฝ ็ไฟฎๆน))
-4. ๆจ้ไฝ ็ไฟฎๆนๅนถๅๅปบไธไธช `ๆๅ่ฏทๆฑ`
-5. ่ฎจ่ฎบใๅฎกๆ ธไปฃ็
-6. ๅฐๅผๅๅๆฏๅๅนถๅฐ `dev-1.x` ๅๆฏ
-
-## ๅ
ทไฝๆญฅ้ชค
-
-### 1. ่ทๅๆๆฐ็ไปฃ็ ๅบ
-
-- ๅฝไฝ ็ฌฌไธๆฌกๆ PR ๆถ
-
- ๅคๅป OpenMMLab ๅไปฃ็ ๅบ๏ผ็นๅป GitHub ้กต้ขๅณไธ่ง็ **Fork** ๆ้ฎๅณๅฏ
- 
-
- ๅ
้ๅคๅป็ไปฃ็ ๅบๅฐๆฌๅฐ
-
- ```bash
- git clone git@github.com:XXX/mmocr.git
- ```
-
- ๆทปๅ ๅไปฃ็ ๅบไธบไธๆธธไปฃ็ ๅบ
-
- ```bash
- git remote add upstream git@github.com:open-mmlab/mmocr
- ```
-
-- ไป็ฌฌไบไธช PR ่ตท
-
- ๆฃๅบๆฌๅฐไปฃ็ ๅบ็ไธปๅๆฏ๏ผ็ถๅไปๆๆฐ็ๅไปฃ็ ๅบ็ไธปๅๆฏๆๅๆดๆฐใ่ฟ้ๅ่ฎพไฝ ๆญฃๅบไบ `dev-1.x` ๅผๅใ
-
- ```bash
- git checkout dev-1.x
- git pull upstream dev-1.x
- ```
-
-### 2. ไป `dev-1.x` ๅๆฏๅๅปบไธไธชๆฐ็ๅผๅๅๆฏ
-
-```bash
-git checkout -b branchname
-```
-
-```{tip}
-ไธบไบไฟ่ฏๆไบคๅๅฒๆธ
ๆฐๅฏ่ฏป๏ผๆไปฌๅผบ็ๆจ่ๆจๅ
ๅๆขๅฐ `dev-1.x` ๅๆฏ๏ผๅๅๅปบๆฐ็ๅๆฏใ
-```
-
-### 3. ๆไบคไฝ ็ไฟฎๆน
-
-- ๅฆๆไฝ ๆฏ็ฌฌไธๆฌกๅฐ่ฏ่ดก็ฎ๏ผ่ฏทๅจ MMOCR ็็ฎๅฝไธๅฎ่ฃ
ๅนถๅๅงๅ pre-commit hooksใ
-
- ```bash
- pip install -U pre-commit
- pre-commit install
- ```
-
-- ๆไบคไฟฎๆนใๅจๆฏๆฌกๆไบคๅ๏ผpre-commit hooks ้ฝไผ่ขซ่งฆๅๅนถ่ง่ๅไฝ ็ไปฃ็ ๆ ผๅผใ
-
- ```bash
- # coding
- git add [files]
- git commit -m 'messages'
- ```
-
- ```{note}
- ๆๆถไฝ ็ๆไปถๅฏ่ฝไผๅจๆไบคๆถ่ขซ pre-commit hooks ่ชๅจไฟฎๆนใ่ฟๆถ่ฏท้ๆฐๆทปๅ ๅนถๆไบคไฟฎๆนๅ็ๆไปถใ
- ```
-
-### 4. ๆจ้ไฝ ็ไฟฎๆนๅฐๅคๅป็ไปฃ็ ๅบ๏ผๅนถๅๅปบไธไธชๆๅ่ฏทๆฑ
-
-- ๆจ้ๅฝๅๅๆฏๅฐ่ฟ็ซฏๅคๅป็ไปฃ็ ๅบ
-
- ```bash
- git push origin branchname
- ```
-
-- ๅๅปบไธไธชๆๅ่ฏทๆฑ
-
- 
-
-- ไฟฎๆนๆๅ่ฏทๆฑไฟกๆฏๆจกๆฟ๏ผๆ่ฟฐไฟฎๆนๅๅ ๅไฟฎๆนๅ
ๅฎนใ่ฟๅฏไปฅๅจ PR ๆ่ฟฐไธญ๏ผๆๅจๅ
ณ่ๅฐ็ธๅ
ณ็่ฎฎ้ข (issue),๏ผๆดๅค็ป่๏ผ่ฏทๅ่[ๅฎๆนๆๆกฃ](https://docs.github.com/en/issues/tracking-your-work-with-issues/linking-a-pull-request-to-an-issue)๏ผใ
-
-- ๅฆๅค๏ผๅฆๆไฝ ๆญฃๅจๅพ `dev-1.x` ๅๆฏๆไบคไปฃ็ ๏ผไฝ ่ฟ้่ฆๅจๅๅปบ PR ็็้ขไธญๅฐๅบ็กๅๆฏๆนไธบ `dev-1.x`๏ผๅ ไธบ็ฐๅจ้ป่ฎค็ๅบ็กๅๆฏๆฏ `main`ใ
-
- 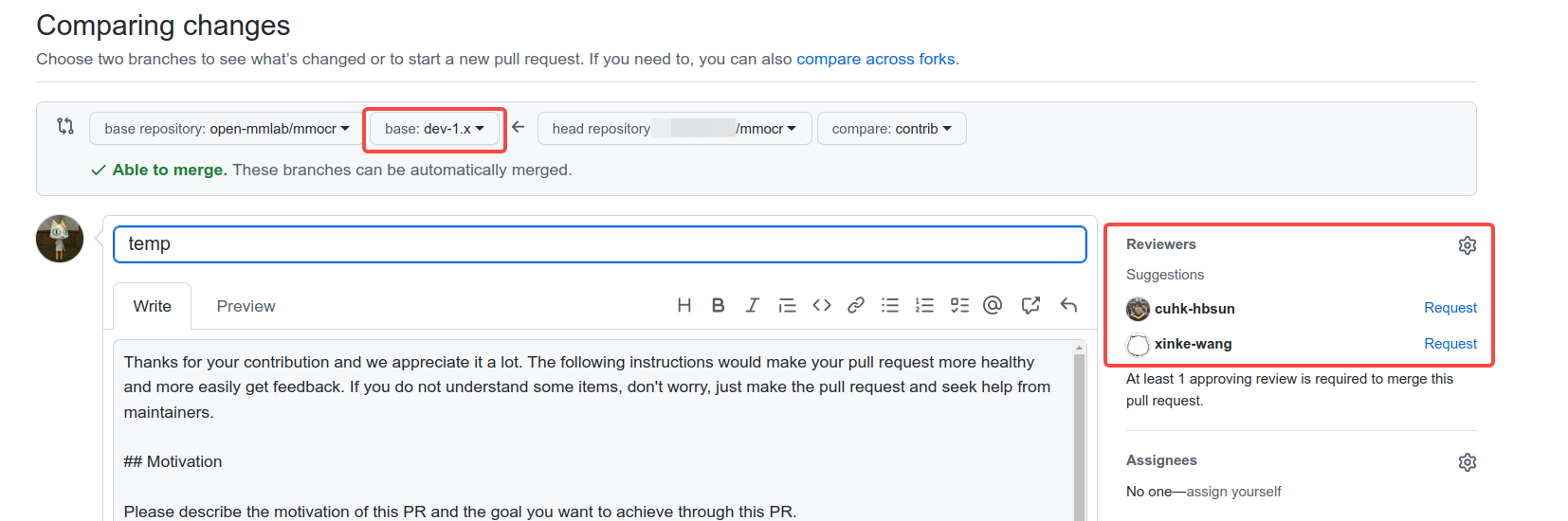
-
-- ไฝ ๅๆ ทๅฏไปฅๆ PR ๅ
ณ่็ป็ธๅ
ณไบบๅ่ฟ่ก่ฏๅฎกใ
-
-### 5. ่ฎจ่ฎบๅนถ่ฏๅฎกไฝ ็ไปฃ็
-
-- ๆ นๆฎ่ฏๅฎกไบบๅ็ๆ่งไฟฎๆนไปฃ็ ๏ผๅนถๆจ้ไฟฎๆน
-
-### 6. `ๆๅ่ฏทๆฑ`ๅๅนถไนๅๅ ้ค่ฏฅๅๆฏ
-
-- ๅจ PR ๅๅนถไนๅ๏ผไฝ ๅฐฑๅฏไปฅๅ ้ค่ฏฅๅๆฏไบใ
-
- ```bash
- git branch -d branchname # ๅ ้คๆฌๅฐๅๆฏ
- git push origin --delete branchname # ๅ ้ค่ฟ็จๅๆฏ
- ```
-
-## PR ่ง่
-
-1. ไฝฟ็จ [pre-commit hook](https://pre-commit.com)๏ผๅฐฝ้ๅๅฐไปฃ็ ้ฃๆ ผ็ธๅ
ณ้ฎ้ข
-
-2. ไธไธช PR ๅฏนๅบไธไธช็ญๆๅๆฏ
-
-3. ็ฒๅบฆ่ฆ็ป๏ผไธไธชPRๅชๅไธไปถไบๆ
๏ผ้ฟๅ
่ถ
ๅคง็PR
-
- - Bad๏ผๅฎ็ฐ Faster R-CNN
- - Acceptable๏ผ็ป Faster R-CNN ๆทปๅ ไธไธช box head
- - Good๏ผ็ป box head ๅขๅ ไธไธชๅๆฐๆฅๆฏๆ่ชๅฎไน็ conv ๅฑๆฐ
-
-4. ๆฏๆฌก Commit ๆถ้่ฆๆไพๆธ
ๆฐไธๆๆไน commit ไฟกๆฏ
-
-5. ๆไพๆธ
ๆฐไธๆๆไน็`ๆๅ่ฏทๆฑ`ๆ่ฟฐ
-
- - ๆ ้ขๅๆ็ฝไปปๅกๅ็งฐ๏ผไธ่ฌๆ ผๅผ:\[Prefix\] Short description of the pull request (Suffix)
- - prefix: ๆฐๅขๅ่ฝ \[Feature\], ไฟฎ bug \[Fix\], ๆๆกฃ็ธๅ
ณ \[Docs\], ๅผๅไธญ \[WIP\] (ๆๆถไธไผ่ขซreview)
- - ๆ่ฟฐ้ไป็ป`ๆๅ่ฏทๆฑ`็ไธป่ฆไฟฎๆนๅ
ๅฎน๏ผ็ปๆ๏ผไปฅๅๅฏนๅ
ถไป้จๅ็ๅฝฑๅ, ๅ่`ๆๅ่ฏทๆฑ`ๆจกๆฟ
- - ๅ
ณ่็ธๅ
ณ็`่ฎฎ้ข` (issue) ๅๅ
ถไป`ๆๅ่ฏทๆฑ`
diff --git a/pipeline/mmocr/docs/zh_cn/project_zoo.py b/pipeline/mmocr/docs/zh_cn/project_zoo.py
deleted file mode 100644
index cc403f8419e619a7d00bf5b9d5af50d086160717..0000000000000000000000000000000000000000
--- a/pipeline/mmocr/docs/zh_cn/project_zoo.py
+++ /dev/null
@@ -1,51 +0,0 @@
-#!/usr/bin/env python
-import os.path as osp
-import re
-
-# This script reads /projects/selected.txt and generate projectzoo.md
-
-files = []
-
-project_zoo = """
-# ๅๆฒฟๆจกๅ
-
-่ฟ้ๆฏไธไบๅทฒ็ปๅค็ฐ๏ผไฝๆฏๅฐๆชๅ
ๅซๅจ MMOCR ๅ
ไธญ็ๅๆฒฟๆจกๅใ
-
-"""
-
-files = open('../../projects/selected.txt').readlines()
-
-for file in files:
- file = file.strip()
- with open(osp.join('../../', file)) as f:
- content = f.read()
-
- # Extract title
- expr = '# (.*?)\n'
- title = re.search(expr, content).group(1)
- project_zoo += f'## {title}\n\n'
-
- # Locate the description
- expr = '## Description\n(.*?)##'
- description = re.search(expr, content, re.DOTALL).group(1)
- project_zoo += f'{description}\n'
-
- # check milestone 1
- expr = r'- \[(.?)\] Milestone 1'
- state = re.search(expr, content, re.DOTALL).group(1)
- infer_state = 'โ' if state == 'x' else 'โ'
-
- # check milestone 2
- expr = r'- \[(.?)\] Milestone 2'
- state = re.search(expr, content, re.DOTALL).group(1)
- training_state = 'โ' if state == 'x' else 'โ'
-
- # add table
- readme_link = f'https://github.com/open-mmlab/mmocr/blob/dev-1.x/{file}'
- project_zoo += '### ๆจกๅ็ถๆ \n'
- project_zoo += '| ๆจ็ | ่ฎญ็ป | README |\n'
- project_zoo += '| --------- | -------- | ------ |\n'
- project_zoo += f'|๏ธ{infer_state}|{training_state}|[link]({readme_link})|\n'
-
-with open('projectzoo.md', 'w') as f:
- f.write(project_zoo)
diff --git a/pipeline/mmocr/docs/zh_cn/stats.py b/pipeline/mmocr/docs/zh_cn/stats.py
deleted file mode 100644
index 96f814fcf8226f27c2b49b1136c25271d80371a5..0000000000000000000000000000000000000000
--- a/pipeline/mmocr/docs/zh_cn/stats.py
+++ /dev/null
@@ -1,128 +0,0 @@
-#!/usr/bin/env python
-# Copyright (c) OpenMMLab. All rights reserved.
-import functools as func
-import re
-from os.path import basename, splitext
-
-import numpy as np
-import titlecase
-from weight_list import gen_weight_list
-
-
-def title2anchor(name):
- return re.sub(r'-+', '-', re.sub(r'[^a-zA-Z0-9]', '-',
- name.strip().lower())).strip('-')
-
-
-# Count algorithms
-
-files = [
- 'backbones.md', 'textdet_models.md', 'textrecog_models.md', 'kie_models.md'
-]
-
-stats = []
-
-for f in files:
- with open(f) as content_file:
- content = content_file.read()
-
- # Remove the blackquote notation from the paper link under the title
- # for better layout in readthedocs
- expr = r'(^## \s*?.*?\s+?)>\s*?(\[.*?\]\(.*?\))'
- content = re.sub(expr, r'\1\2', content, flags=re.MULTILINE)
- with open(f, 'w') as content_file:
- content_file.write(content)
-
- # title
- title = content.split('\n')[0].replace('#', '')
-
- # count papers
- exclude_papertype = ['ABSTRACT', 'IMAGE']
- exclude_expr = ''.join(f'(?!{s})' for s in exclude_papertype)
- expr = rf''\
- r'\s*\n.*?\btitle\s*=\s*{(.*?)}'
- papers = {(papertype, titlecase.titlecase(paper.lower().strip()))
- for (papertype, paper) in re.findall(expr, content, re.DOTALL)}
- print(papers)
- # paper links
- revcontent = '\n'.join(list(reversed(content.splitlines())))
- paperlinks = {}
- for _, p in papers:
- q = p.replace('\\', '\\\\').replace('?', '\\?')
- paper_link = title2anchor(
- re.search(
- rf'\btitle\s*=\s*{{\s*{q}\s*}}.*?\n## (.*?)\s*[,;]?\s*\n',
- revcontent, re.DOTALL | re.IGNORECASE).group(1))
- paperlinks[p] = f'[{p}]({splitext(basename(f))[0]}.md#{paper_link})'
- paperlist = '\n'.join(
- sorted(f' - [{t}] {paperlinks[x]}' for t, x in papers))
- # count configs
- configs = {
- x.lower().strip()
- for x in re.findall(r'https.*configs/.*\.py', content)
- }
-
- # count ckpts
- ckpts = {
- x.lower().strip()
- for x in re.findall(r'https://download.*\.pth', content)
- if 'mmocr' in x
- }
-
- statsmsg = f"""
-## [{title}]({f})
-
-* ๆจกๅๆ้ๆไปถๆฐ้๏ผ {len(ckpts)}
-* ้
็ฝฎๆไปถๆฐ้๏ผ {len(configs)}
-* ่ฎบๆๆฐ้๏ผ {len(papers)}
-{paperlist}
-
- """
-
- stats.append((papers, configs, ckpts, statsmsg))
-
-allpapers = func.reduce(lambda a, b: a.union(b), [p for p, _, _, _ in stats])
-allconfigs = func.reduce(lambda a, b: a.union(b), [c for _, c, _, _ in stats])
-allckpts = func.reduce(lambda a, b: a.union(b), [c for _, _, c, _ in stats])
-msglist = '\n'.join(x for _, _, _, x in stats)
-
-papertypes, papercounts = np.unique([t for t, _ in allpapers],
- return_counts=True)
-countstr = '\n'.join(
- [f' - {t}: {c}' for t, c in zip(papertypes, papercounts)])
-
-# get model list
-weight_list = gen_weight_list()
-
-modelzoo = f"""
-# ๆป่ง
-
-## ๆ้
-ไปฅไธๆฏๅฏ็จไบ[ๆจ็](user_guides/inference.md)็ๆ้ๅ่กจใ
-
-ไธบไบไพฟไบไฝฟ็จ๏ผๆ็ๆ้ๅฏ่ฝไผๅญๅจๅคไธช่พ็ญ็ๅซๅ๏ผ่ฟๅจ่กจๆ ผไธญๅฐ็จโ/โๅ้ใ
-
-ไพๅฆ๏ผ่กจๆ ผไธญๅฑ็คบ็ `DB_r18 / dbnet_resnet18_fpnc_1200e_icdar2015` ่กจ็คบๆจๅฏไปฅไฝฟ็จ
-`DB_r18` ๆ `dbnet_resnet18_fpnc_1200e_icdar2015` ๆฅๅๅงๅๆจ็ๅจ๏ผ
-
-```python
->>> from mmocr.apis import TextDetInferencer
->>> inferencer = TextDetInferencer(model='DB_r18')
->>> # ็ญไปทไบ
->>> inferencer = TextDetInferencer(model='dbnet_resnet18_fpnc_1200e_icdar2015')
-```
-
-{weight_list}
-
-## ็ป่ฎกๆฐๆฎ
-
-* ๆจกๅๆ้ๆไปถๆฐ้๏ผ {len(allckpts)}
-* ้
็ฝฎๆไปถๆฐ้๏ผ {len(allconfigs)}
-* ่ฎบๆๆฐ้๏ผ {len(allpapers)}
-{countstr}
-
-{msglist}
-""" # noqa
-
-with open('modelzoo.md', 'w') as f:
- f.write(modelzoo)
diff --git a/pipeline/mmocr/docs/zh_cn/switch_language.md b/pipeline/mmocr/docs/zh_cn/switch_language.md
deleted file mode 100644
index 7baa29992eb3b36ab2804b577d3bb76db8cc4233..0000000000000000000000000000000000000000
--- a/pipeline/mmocr/docs/zh_cn/switch_language.md
+++ /dev/null
@@ -1,3 +0,0 @@
-## English
-
-## ็ฎไฝไธญๆ
diff --git a/pipeline/mmocr/docs/zh_cn/user_guides/config.md b/pipeline/mmocr/docs/zh_cn/user_guides/config.md
deleted file mode 100644
index fd16af58e17dd85e76e037687a86f0bebfe70083..0000000000000000000000000000000000000000
--- a/pipeline/mmocr/docs/zh_cn/user_guides/config.md
+++ /dev/null
@@ -1,719 +0,0 @@
-# ้
็ฝฎๆไปถ
-
-MMOCR ไธป่ฆไฝฟ็จ Python ๆไปถไฝไธบ้
็ฝฎๆไปถใๅ
ถ้
็ฝฎๆไปถ็ณป็ป็่ฎพ่ฎกๆดๅไบๆจกๅๅไธ็ปงๆฟ็ๆๆณ๏ผๆนไพฟ็จๆท่ฟ่กๅ็งๅฎ้ชใ
-
-## ๅธธ่ง็จๆณ
-
-```{note}
-ๆฌๅฐ่ๅปบ่ฎฎ็ปๅ {external+mmengine:doc}`MMEngine: ้
็ฝฎ(Config) ` ไธญ็ๅ็บง็จๆณๅ
ฑๅ้
่ฏปใ
-```
-
-MMOCR ๆๅธธ็จ็ๆไฝไธบไธ็ง๏ผ้
็ฝฎๆไปถ็็ปงๆฟ๏ผๅฏน `_base_` ๅ้็ๅผ็จไปฅๅๅฏน `_base_` ๅ้็ไฟฎๆนใๅฏนไบ `_base_` ็็ปงๆฟไธไฟฎๆน, MMEngine.Config ๆไพไบไธค็ง่ฏญๆณ๏ผไธ็งๆฏ้ๅฏน Python๏ผJson๏ผ Yaml ๅๅฏไฝฟ็จ็ๆไฝ๏ผๅฆไธ็งๅไป
้็จไบ Python ้
็ฝฎๆไปถใๅจ MMOCR ไธญ๏ผๆไปฌ**ๆดๆจ่ไฝฟ็จๅช้ๅฏนPython็่ฏญๆณ**๏ผๅ ๆญคไธๆๅฐไปฅๆญคไธบๅบ็กไฝ่ฟไธๆญฅไป็ปใ
-
-่ฟ้ไปฅ `configs/textdet/dbnet/dbnet_resnet18_fpnc_1200e_icdar2015.py` ไธบไพ๏ผ่ฏดๆๅธธ็จ็ไธ็ง็จๆณใ
-
-```Python
-_base_ = [
- '_base_dbnet_resnet18_fpnc.py',
- '../_base_/datasets/icdar2015.py',
- '../_base_/default_runtime.py',
- '../_base_/schedules/schedule_sgd_1200e.py',
-]
-
-# dataset settings
-icdar2015_textdet_train = _base_.icdar2015_textdet_train
-icdar2015_textdet_train.pipeline = _base_.train_pipeline
-icdar2015_textdet_test = _base_.icdar2015_textdet_test
-icdar2015_textdet_test.pipeline = _base_.test_pipeline
-
-train_dataloader = dict(
- batch_size=16,
- num_workers=8,
- persistent_workers=True,
- sampler=dict(type='DefaultSampler', shuffle=True),
- dataset=icdar2015_textdet_train)
-
-val_dataloader = dict(
- batch_size=1,
- num_workers=4,
- persistent_workers=True,
- sampler=dict(type='DefaultSampler', shuffle=False),
- dataset=icdar2015_textdet_test)
-```
-
-### ้
็ฝฎๆไปถ็็ปงๆฟ
-
-้
็ฝฎๆไปถๅญๅจ็ปงๆฟ็ๆบๅถ๏ผๅณไธไธช้
็ฝฎๆไปถ A ๅฏไปฅๅฐๅฆไธไธช้
็ฝฎๆไปถ B ไฝไธบ่ชๅทฑ็ๅบ็กๅนถ็ดๆฅ็ปงๆฟๅ
ถไธญ็ๆๆๅญๆฎต๏ผไป่้ฟๅ
ไบๅคง้็ๅคๅถ็ฒ่ดดใ
-
-ๅจ dbnet_resnet18_fpnc_1200e_icdar2015.py ไธญๅฏไปฅ็ๅฐ๏ผ
-
-```Python
-_base_ = [
- '_base_dbnet_resnet18_fpnc.py',
- '../_base_/datasets/icdar2015.py',
- '../_base_/default_runtime.py',
- '../_base_/schedules/schedule_sgd_1200e.py',
-]
-```
-
-ไธ่ฟฐ่ฏญๅฅไผ่ฏปๅๅ่กจไธญ็ๆๆๅบ็ก้
็ฝฎๆไปถ๏ผๅฎไปฌไธญ็ๆๆๅญๆฎต้ฝไผ่ขซ่ฝฝๅ
ฅๅฐ dbnet_resnet18_fpnc_1200e_icdar2015.py ไธญใๆไปฌๅฏไปฅ้่ฟๅจ Python ่งฃ้ไธญ่ฟ่กไปฅไธ่ฏญๅฅ๏ผไบ่งฃ้
็ฝฎๆไปถ่ขซ่งฃๆๅ็็ปๆ๏ผ
-
-```Python
-from mmengine import Config
-db_config = Config.fromfile('configs/textdet/dbnet/dbnet_resnet18_fpnc_1200e_icdar2015.py')
-print(db_config)
-```
-
-ๅฏไปฅๅ็ฐ๏ผ่ขซ่งฃๆ็้
็ฝฎๅ
ๅซไบๆๆbase้
็ฝฎไธญ็ๅญๆฎตๅไฟกๆฏใ
-
-```{note}
-่ฏทๆณจๆ๏ผๅ _base_ ้
็ฝฎๆไปถไธญไธ่ฝๅญๅจๅๅๅ้ใ
-```
-
-### `_base_` ๅ้็ๅผ็จ
-
-ๆๆถ๏ผๆไปฌๅฏ่ฝ้่ฆ็ดๆฅๅผ็จ `_base_` ้
็ฝฎไธญ็ๆไบๅญๆฎต๏ผไปฅ้ฟๅ
้ๅคๅฎไนใๅ่ฎพๆไปฌๆณ่ฆ่ทๅ `_base_` ้
็ฝฎไธญ็ๅ้ `pseudo`๏ผๅฐฑๅฏไปฅ็ดๆฅ้่ฟ `_base_.pseudo` ่ทๅพ `_base_` ้
็ฝฎไธญ็ๅ้ใ
-
-่ฏฅ่ฏญๆณๅทฒๅนฟๆณ็จไบ MMOCR ็้
็ฝฎไธญใMMOCR ไธญๅไธชๆจกๅ็ๆฐๆฎ้ๅ็ฎก้๏ผpipeline๏ผ้
็ฝฎ้ฝๅผ็จไบ*ๅบๆฌ*้
็ฝฎใๅฆๅจ
-
-```Python
-icdar2015_textdet_train = _base_.icdar2015_textdet_train
-# ...
-train_dataloader = dict(
- # ...
- dataset=icdar2015_textdet_train)
-```
-
-### `_base_` ๅ้็ไฟฎๆน
-
-ๅจ MMOCR ไธญไธๅ็ฎๆณๅจไธๅๆฐๆฎ้้ๅธธๆไธๅ็ๆฐๆฎๆตๆฐด็บฟ๏ผpipeline)๏ผๅ ๆญค็ปๅธธไผไผๅญๅจไฟฎๆนๆฐๆฎ้ไธญ `pipeline` ็ๅบๆฏใๅๆถ่ฟๅญๅจๅพๅคๅบๆฏ้่ฆไฟฎๆน `_base_` ้
็ฝฎไธญ็ๅ้๏ผไพๅฆๆณไฟฎๆนๆไธช็ฎๆณ็่ฎญ็ป็ญ็ฅ๏ผๆไธชๆจกๅ็ๆไบ็ฎๆณๆจกๅ๏ผๆดๆข backbone ็ญ๏ผใ็จๆทๅฏไปฅ็ดๆฅๅฉ็จ Python ็่ฏญๆณ็ดๆฅไฟฎๆนๅผ็จ็ `_base_` ๅ้ใ้ๅฏน dict๏ผๆไปฌไนๆไพไบไธ็ฑปๅฑๆงไฟฎๆน็ฑปไผผ็ๆนๆณ๏ผๅฏไปฅ็ดๆฅไฟฎๆน็ฑปๅฑๆงไฟฎๆนๅญๅ
ธๅ
็ๅ
ๅฎนใ
-
-1. ๅญๅ
ธ
-
- ่ฟ้ไปฅไฟฎๆนๆฐๆฎ้ไธญ็ `pipeline` ไธบไพ๏ผ
-
- ๅฏไปฅๅฉ็จ Python ่ฏญๆณไฟฎๆนๅญๅ
ธ๏ผ
-
- ```python
- # ่ทๅ _base_ ไธญ็ๆฐๆฎ้
- icdar2015_textdet_train = _base_.icdar2015_textdet_train
- # ๅฏไปฅ็ดๆฅๅฉ็จ Python ็ update ไฟฎๆนๅ้
- icdar2015_textdet_train.update(pipeline=_base_.train_pipeline)
- ```
-
- ไนๅฏไปฅไฝฟ็จ็ฑปๅฑๆง็ๆนๆณ่ฟ่กไฟฎๆน๏ผ
-
- ```Python
- # ่ทๅ _base_ ไธญ็ๆฐๆฎ้
- icdar2015_textdet_train = _base_.icdar2015_textdet_train
- # ็ฑปๅฑๆงๆนๆณไฟฎๆน
- icdar2015_textdet_train.pipeline = _base_.train_pipeline
- ```
-
-2. ๅ่กจ
-
- ๅ่ฎพ `_base_` ้
็ฝฎไธญ็ๅ้ `pseudo = [1, 2, 3]`๏ผ ้่ฆไฟฎๆนไธบ `[1, 2, 4]`:
-
- ```Python
- # pseudo.py
- pseudo = [1, 2, 3]
- ```
-
- ๅฏไปฅ็ดๆฅ้ๅ๏ผ
-
- ```Python
- _base_ = ['pseudo.py']
- pseudo = [1, 2, 4]
- ```
-
- ๆ่
ๅฉ็จ Python ่ฏญๆณไฟฎๆนๅ่กจ๏ผ
-
- ```Python
- _base_ = ['pseudo.py']
- pseudo = _base_.pseudo
- pseudo[2] = 4
- ```
-
-### ๅฝไปค่กไฟฎๆน้
็ฝฎ
-
-ๆๆถๅๆไปฌๅชๅธๆไฟฎ้จๅ้
็ฝฎ๏ผ่ไธๆณไฟฎๆน้
็ฝฎๆไปถๆฌ่บซใไพๅฆๅฎ้ช่ฟ็จไธญๆณๆดๆขๅญฆไน ็๏ผไฝๆฏๅไธๆณ้ๆฐๅไธไธช้
็ฝฎๆไปถ๏ผๅฏไปฅ้่ฟๅฝไปค่กไผ ๅ
ฅๅๆฐๆฅ่ฆ็็ธๅ
ณ้
็ฝฎใ
-
-ๆไปฌๅฏไปฅๅจๅฝไปค่ก้ไผ ๅ
ฅ `--cfg-options`๏ผๅนถๅจๅ
ถไนๅ็ๅๆฐ็ดๆฅไฟฎๆนๅฏนๅบๅญๆฎต๏ผไพๅฆๆไปฌๆณๅจ่ฟ่ก train ็ๆถๅไฟฎๆนๅญฆไน ็๏ผๅช้่ฆๅจๅฝไปค่กๆง่ก๏ผ
-
-```Shell
-python tools/train.py example.py --cfg-options optim_wrapper.optimizer.lr=1
-```
-
-ๆดๅค่ฏฆ็ป็จๆณๅ่ {external+mmengine:ref}`MMEngine: ๅฝไปค่กไฟฎๆน้
็ฝฎ <ๅฝไปค่กไฟฎๆน้
็ฝฎ>`.
-
-## ้
็ฝฎๅ
ๅฎน
-
-้่ฟ้
็ฝฎๆไปถไธๆณจๅๅจ็้
ๅ๏ผMMOCR ๅฏไปฅๅจไธไพตๅ
ฅไปฃ็ ็ๅๆไธไฟฎๆน่ฎญ็ปๅๆฐไปฅๅๆจกๅ้
็ฝฎใๅ
ทไฝ่่จ๏ผ็จๆทๅฏไปฅๅจ้
็ฝฎๆไปถไธญๅฏนๅฆไธๆจกๅ่ฟ่ก่ชๅฎไนไฟฎๆน๏ผ็ฏๅข้
็ฝฎใHook ้
็ฝฎใๆฅๅฟ้
็ฝฎใ่ฎญ็ป็ญ็ฅ้
็ฝฎใๆฐๆฎ็ธๅ
ณ้
็ฝฎใๆจกๅ็ธๅ
ณ้
็ฝฎใ่ฏๆต้
็ฝฎใๅฏ่งๅ้
็ฝฎใ
-
-ๆฌๆๆกฃๅฐไปฅๆๅญๆฃๆต็ฎๆณ `DBNet` ๅๆๅญ่ฏๅซ็ฎๆณ `CRNN` ไธบไพๆฅ่ฏฆ็ปไป็ป Config ไธญ็ๅ
ๅฎนใ
-
-
-
-### ็ฏๅข้
็ฝฎ
-
-```Python
-default_scope = 'mmocr'
-env_cfg = dict(
- cudnn_benchmark=True,
- mp_cfg=dict(mp_start_method='fork', opencv_num_threads=0),
- dist_cfg=dict(backend='nccl'))
-randomness = dict(seed=None)
-```
-
-ไธป่ฆๅ
ๅซไธไธช้จๅ๏ผ
-
-- ่ฎพ็ฝฎๆๆๆณจๅๅจ็้ป่ฎค `scope` ไธบ `mmocr`๏ผ ไฟ่ฏๆๆ็ๆจกๅ้ฆๅ
ไป `MMOCR` ไปฃ็ ๅบไธญ่ฟ่กๆ็ดขใ่ฅๆ่ฏฅๆจกๅไธๅญๅจ๏ผๅ็ปง็ปญไปไธๆธธ็ฎๆณๅบ `MMEngine` ๅ `MMCV` ไธญ่ฟ่กๆ็ดข๏ผ่ฏฆ่ง {external+mmengine:doc}`MMEngine: ๆณจๅๅจ `ใ
-
-- `env_cfg` ่ฎพ็ฝฎๅๅธๅผ็ฏๅข้
็ฝฎ๏ผ ๆดๅค้
็ฝฎๅฏไปฅ่ฏฆ่ง {external+mmengine:doc}`MMEngine: Runner `ใ
-
-- `randomness` ่ฎพ็ฝฎ numpy๏ผ torch๏ผcudnn ็ญ้ๆบ็งๅญ๏ผๆดๅค้
็ฝฎ่ฏฆ่ง {external+mmengine:doc}`MMEngine: Runner `ใ
-
-
-
-### Hook ้
็ฝฎ
-
-Hook ไธป่ฆๅไธบไธคไธช้จๅ๏ผ้ป่ฎค hook ไปฅๅ่ชๅฎไน hookใ้ป่ฎค hook ไธบๆๆไปปๅกๆณ่ฆ่ฟ่กๆๅฟ
้กป็้
็ฝฎ๏ผ่ชๅฎไน hook ไธ่ฌๆๅกไบ็นๅฎ็็ฎๆณๆๆไบ็นๅฎไปปๅก๏ผ็ฎๅไธบๆญข MMOCR ไธญๆฒกๆ่ชๅฎไน็ Hook๏ผใ
-
-```Python
-default_hooks = dict(
- timer=dict(type='IterTimerHook'), # ๆถ้ด่ฎฐๅฝ๏ผๅ
ๆฌๆฐๆฎๅขๅผบๆถ้ดไปฅๅๆจกๅๆจ็ๆถ้ด
- logger=dict(type='LoggerHook', interval=1), # ๆฅๅฟๆๅฐ้ด้
- param_scheduler=dict(type='ParamSchedulerHook'), # ๆดๆฐๅญฆไน ็็ญ่ถ
ๅ
- checkpoint=dict(type='CheckpointHook', interval=1),# ไฟๅญ checkpoint๏ผ intervalๆงๅถไฟๅญ้ด้
- sampler_seed=dict(type='DistSamplerSeedHook'), # ๅคๆบๆ
ๅตไธ่ฎพ็ฝฎ็งๅญ
- sync_buffer=dict(type='SyncBuffersHook'), # ๅคๅกๆ
ๅตไธ๏ผๅๆญฅbuffer
- visualization=dict( # ๅฏ่งๅval ๅ test ็็ปๆ
- type='VisualizationHook',
- interval=1,
- enable=False,
- show=False,
- draw_gt=False,
- draw_pred=False))
- custom_hooks = []
-```
-
-่ฟ้็ฎๅไป็ปๅ ไธช็ปๅธธๅฏ่ฝไผๅๅจ็ hook๏ผ้็จ็ไฟฎๆนๆนๆณๅ่[ไฟฎๆน้
็ฝฎ](#base-ๅ้็ไฟฎๆน)ใ
-
-- `LoggerHook`๏ผ็จไบ้
็ฝฎๆฅๅฟ่ฎฐๅฝๅจ็่กไธบใไพๅฆ๏ผ้่ฟไฟฎๆน `interval` ๅฏไปฅๆงๅถๆฅๅฟๆๅฐ็้ด้๏ผๆฏ `interval` ๆฌก่ฟญไปฃ (iteration) ๆๅฐไธๆฌกๆฅๅฟ๏ผๆดๅค่ฎพ็ฝฎๅฏๅ่ [LoggerHook API](mmengine.hooks.LoggerHook)ใ
-
-- `CheckpointHook`๏ผ็จไบ้
็ฝฎๆจกๅๆญ็นไฟๅญ็ธๅ
ณ็่กไธบ๏ผๅฆไฟๅญๆไผๆ้๏ผไฟๅญๆๆฐๆ้็ญใๅๆ ทๅฏไปฅไฟฎๆน `interval` ๆงๅถไฟๅญ checkpoint ็้ด้ใๆดๅค่ฎพ็ฝฎๅฏๅ่ [CheckpointHook API](mmengine.hooks.CheckpointHook)
-
-- `VisualizationHook`๏ผ็จไบ้
็ฝฎๅฏ่งๅ็ธๅ
ณ่กไธบ๏ผไพๅฆๅจ้ช่ฏๆๆต่ฏๆถๅฏ่งๅ้ขๆต็ปๆ๏ผ**้ป่ฎคไธบๅ
ณ**ใๅๆถ่ฏฅ Hook ไพ่ต[ๅฏ่งๅ้
็ฝฎ](#ๅฏ่งๅ้
็ฝฎ)ใๆณ่ฆไบ่งฃ่ฏฆ็ปๅ่ฝๅฏไปฅๅ่ [Visualizer](visualization.md)ใๆดๅค้
็ฝฎๅฏไปฅๅ่ [VisualizationHook API](mmocr.engine.hooks.VisualizationHook)ใ
-
-ๅฆๆๆณ่ฟไธๆญฅไบ่งฃ้ป่ฎค hook ็้
็ฝฎไปฅๅๅ่ฝ๏ผๅฏไปฅๅ่ {external+mmengine:doc}`MMEngine: ้ฉๅญ(Hook) `ใ
-
-
-
-### ๆฅๅฟ้
็ฝฎ
-
-ๆญค้จๅไธป่ฆ็จๆฅ้
็ฝฎๆฅๅฟ้
็ฝฎ็ญ็บงไปฅๅๆฅๅฟๅค็ๅจใ
-
-```Python
-log_level = 'INFO' # ๆฅๅฟ่ฎฐๅฝ็ญ็บง
-log_processor = dict(type='LogProcessor',
- window_size=10,
- by_epoch=True)
-```
-
-- ๆฅๅฟ้
็ฝฎ็ญ็บงไธ {external+python:doc}`Python: logging ` ็้
็ฝฎไธ่ด๏ผ
-
-- ๆฅๅฟๅค็ๅจไธป่ฆ็จๆฅๆงๅถ่พๅบ็ๆ ผๅผ๏ผ่ฏฆ็ปๅ่ฝๅฏๅ่ {external+mmengine:doc}`MMEngine: ่ฎฐๅฝๆฅๅฟ `๏ผ
-
- - `by_epoch=True` ่กจ็คบๆ็
งepoch่พๅบๆฅๅฟ๏ผๆฅๅฟๆ ผๅผ้่ฆๅ `train_cfg` ไธญ็ `type='EpochBasedTrainLoop'` ๅๆฐไฟๆไธ่ดใไพๅฆๆณๆ่ฟญไปฃๆฌกๆฐ่พๅบๆฅๅฟ๏ผๅฐฑ้่ฆไปค `log_processor` ไธญ็ ` by_epoch=False` ็ๅๆถ `train_cfg` ไธญ็ `type = 'IterBasedTrainLoop'`ใ
-
- - `window_size` ่กจ็คบๆๅคฑ็ๅนณๆป็ชๅฃ๏ผๅณๆ่ฟ `window_size` ๆฌก่ฟญไปฃ็ๅ็งๆๅคฑ็ๅๅผใlogger ไธญๆ็ปๆๅฐ็ loss ๅผไธบๅ็งๆๅคฑ็ๅนณๅๅผใ
-
-
-
-### ่ฎญ็ป็ญ็ฅ้
็ฝฎ
-
-ๆญค้จๅไธป่ฆๅ
ๅซไผๅๅจ่ฎพ็ฝฎใๅญฆไน ็็ญ็ฅๅ `Loop` ่ฎพ็ฝฎใ
-
-ๅฏนไธๅ็ฎๆณไปปๅก(ๆๅญๆฃๆต๏ผๆๅญ่ฏๅซ๏ผๅ
ณ้ฎไฟกๆฏๆๅ)๏ผ้ๅธธๆ่ชๅทฑไปปๅกๅธธ็จ็่ฐๅ็ญ็ฅใ่ฟ้ๅๅบไบๆๅญ่ฏๅซไธญ็ `CRNN` ๆ็จๆถๅ็็ธๅบ้
็ฝฎใ
-
-```Python
-# ไผๅๅจ
-optim_wrapper = dict(
- type='OptimWrapper', optimizer=dict(type='Adadelta', lr=1.0))
-param_scheduler = [dict(type='ConstantLR', factor=1.0)]
-train_cfg = dict(type='EpochBasedTrainLoop',
- max_epochs=5, # ่ฎญ็ป่ฝฎๆฐ
- val_interval=1) # ่ฏๆต้ด้
-val_cfg = dict(type='ValLoop')
-test_cfg = dict(type='TestLoop')
-```
-
-- `optim_wrapper` : ไธป่ฆๅ
ๅซไธคไธช้จๅ๏ผไผๅๅจๅฐ่ฃ
(OptimWrapper) ไปฅๅไผๅๅจ (Optimizer)ใ่ฏฆๆ
ไฝฟ็จไฟกๆฏๅฏ่ง {external+mmengine:doc}`MMEngine: ไผๅๅจๅฐ่ฃ
`
-
- - ไผๅๅจๅฐ่ฃ
ๆฏๆไธๅ็่ฎญ็ป็ญ็ฅ๏ผๅ
ๆฌๆททๅ็ฒพๅบฆ่ฎญ็ป(AMP)ใๆขฏๅบฆ็ดฏๅ ๅๆขฏๅบฆๆชๆญใ
-
- - ไผๅๅจ่ฎพ็ฝฎไธญๆฏๆไบ PyTorch ๆๆ็ไผๅๅจ๏ผๆๆๆฏๆ็ไผๅๅจ่ง {external+torch:ref}`PyTorch ไผๅๅจๅ่กจ `ใ
-
-- `param_scheduler` : ๅญฆไน ็่ฐๆด็ญ็ฅ๏ผๆฏๆๅคง้จๅ PyTorch ไธญ็ๅญฆไน ็่ฐๅบฆๅจ๏ผไพๅฆ `ExponentialLR`๏ผ`LinearLR`๏ผ`StepLR`๏ผ`MultiStepLR` ็ญ๏ผไฝฟ็จๆนๅผไนๅบๆฌไธ่ด๏ผๆๆๆฏๆ็่ฐๅบฆๅจ่ง[่ฐๅบฆๅจๆฅๅฃๆๆกฃ](mmengine.optim.scheduler), ๆดๅคๅ่ฝๅฏไปฅๅ่ {external+mmengine:doc}`MMEngine: ไผๅๅจๅๆฐ่ฐๆด็ญ็ฅ `ใ
-
-- `train/test/val_cfg` : ไปปๅก็ๆง่กๆต็จ๏ผMMEngine ๆไพไบๅ็งๆต็จ๏ผ`EpochBasedTrainLoop`, `IterBasedTrainLoop`, `ValLoop`, `TestLoop` ๆดๅคๅฏไปฅๅ่ {external+mmengine:doc}`MMEngine: ๅพช็ฏๆงๅถๅจ `ใ
-
-### ๆฐๆฎ็ธๅ
ณ้
็ฝฎ
-
-
-
-#### ๆฐๆฎ้้
็ฝฎ
-
-ไธป่ฆ็จไบ้
็ฝฎไธคไธชๆนๅ๏ผ
-
-- ๆฐๆฎ้็ๅพๅไธๆ ๆณจๆไปถ็ไฝ็ฝฎใ
-
-- ๆฐๆฎๅขๅผบ็ธๅ
ณ็้
็ฝฎใๅจ OCR ้ขๅไธญ๏ผๆฐๆฎๅขๅผบ้ๅธธไธๆจกๅๅผบ็ธๅ
ณใ
-
-ๆดๅคๅๆฐ้
็ฝฎๅฏไปฅๅ่[ๆฐๆฎๅบ็ฑป](#TODO)ใ
-
-ๆฐๆฎ้ๅญๆฎต็ๅฝๅ่งๅๅจ MMOCR ไธญไธบ๏ผ
-
-```Python
-{ๆฐๆฎ้ๅ็งฐ็ผฉๅ}_{็ฎๆณไปปๅก}_{่ฎญ็ป/ๆต่ฏ/้ช่ฏ} = dict(...)
-```
-
-- ๆฐๆฎ้็ผฉๅ๏ผ่ง [ๆฐๆฎ้ๅ็งฐๅฏนๅบ่กจ](#TODO)
-
-- ็ฎๆณไปปๅก๏ผๆๆฌๆฃๆต-det๏ผๆๅญ่ฏๅซ-rec๏ผๅ
ณ้ฎไฟกๆฏๆๅ-kie
-
-- ่ฎญ็ป/ๆต่ฏ/้ช่ฏ๏ผๆฐๆฎ้็จไบ่ฎญ็ป๏ผๆต่ฏ่ฟๆฏ้ช่ฏ
-
-ไปฅ่ฏๅซไธบไพ๏ผไฝฟ็จ Syn90k ไฝไธบ่ฎญ็ป้๏ผไปฅ icdar2013 ๅ icdar2015 ไฝไธบๆต่ฏ้้
็ฝฎๅฆไธ๏ผ
-
-```Python
-# ่ฏๅซๆฐๆฎ้้
็ฝฎ
-mjsynth_textrecog_train = dict(
- type='OCRDataset',
- data_root='data/rec/Syn90k/',
- data_prefix=dict(img_path='mnt/ramdisk/max/90kDICT32px'),
- ann_file='train_labels.json',
- test_mode=False,
- pipeline=None)
-
-icdar2013_textrecog_test = dict(
- type='OCRDataset',
- data_root='data/rec/icdar_2013/',
- data_prefix=dict(img_path='Challenge2_Test_Task3_Images/'),
- ann_file='test_labels.json',
- test_mode=True,
- pipeline=None)
-
-icdar2015_textrecog_test = dict(
- type='OCRDataset',
- data_root='data/rec/icdar_2015/',
- data_prefix=dict(img_path='ch4_test_word_images_gt/'),
- ann_file='test_labels.json',
- test_mode=True,
- pipeline=None)
-```
-
-
-
-#### ๆฐๆฎๆตๆฐด็บฟ้
็ฝฎ
-
-MMOCR ไธญ๏ผๆฐๆฎ้็ๆๅปบไธๆฐๆฎๅๅคๆฏ็ธไบ่งฃ่ฆ็ใไนๅฐฑๆฏ่ฏด๏ผ`OCRDataset` ็ญๆฐๆฎ้ๆๅปบ็ฑป่ด่ดฃๅฎๆๆ ๆณจๆไปถ็่ฏปๅไธ่งฃๆๅ่ฝ๏ผ่ๆฐๆฎๅๆขๆนๆณ๏ผData Transforms๏ผๅ่ฟไธๆญฅๅฎ็ฐไบๆฐๆฎ่ฏปๅใๆฐๆฎๅขๅผบใๆฐๆฎๆ ผๅผๅ็ญ็ธๅ
ณๅ่ฝใ
-
-ๅๆถไธ่ฌๆ
ๅตไธ่ฎญ็ปๅๆต่ฏไผๅญๅจไธๅ็ๅขๅผบ็ญ็ฅ๏ผๅ ๆญคไธ่ฌไผๅญๅจ่ฎญ็ปๆตๆฐด็บฟ๏ผtrain_pipeline๏ผๅๆต่ฏๆตๆฐด็บฟ๏ผtest_pipeline๏ผใๆดๅคไฟกๆฏๅฏไปฅๅ่[ๆฐๆฎๆตๆฐด็บฟ](../basic_concepts/transforms.md)
-
-- ่ฎญ็ปๆตๆฐด็บฟ็ๆฐๆฎๅขๅผบๆต็จ้ๅธธไธบ๏ผๆฐๆฎ่ฏปๅ(LoadImageFromFile)->ๆ ๆณจไฟกๆฏ่ฏปๅ(LoadXXXAnntation)->ๆฐๆฎๅขๅผบ->ๆฐๆฎๆ ผๅผๅ(PackXXXInputs)ใ
-
-- ๆต่ฏๆตๆฐด็บฟ็ๆฐๆฎๅขๅผบๆต็จ้ๅธธไธบ๏ผๆฐๆฎ่ฏปๅ(LoadImageFromFile)->ๆฐๆฎๅขๅผบ->ๆ ๆณจไฟกๆฏ่ฏปๅ(LoadXXXAnntation)->ๆฐๆฎๆ ผๅผๅ(PackXXXInputs)ใ
-
-็ฑไบ OCR ไปปๅก็็นๆฎๆง๏ผไธ่ฌๆ
ๅตไธไธๅๆจกๅๆไธๅๆฐๆฎๅขๅผบ็ๆนๅผ๏ผ็ธๅๆจกๅๅจไธๅๆฐๆฎ้ไธ่ฌไนไผๆไธๅ็ๆฐๆฎๅขๅผบๆนๅผใไปฅ CRNN ไธบไพ๏ผ
-
-```Python
-# ๆฐๆฎๅขๅผบ
-train_pipeline = [
- dict(
- type='LoadImageFromFile',
- color_type='grayscale',
- ignore_empty=True,
- min_size=5),
- dict(type='LoadOCRAnnotations', with_text=True),
- dict(type='Resize', scale=(100, 32), keep_ratio=False),
- dict(
- type='PackTextRecogInputs',
- meta_keys=('img_path', 'ori_shape', 'img_shape', 'valid_ratio'))
-]
-test_pipeline = [
- dict(
- type='LoadImageFromFile',
- color_type='grayscale'),
- dict(
- type='RescaleToHeight',
- height=32,
- min_width=32,
- max_width=None,
- width_divisor=16),
- dict(type='LoadOCRAnnotations', with_text=True),
- dict(
- type='PackTextRecogInputs',
- meta_keys=('img_path', 'ori_shape', 'img_shape', 'valid_ratio'))
-]
-```
-
-
-
-#### Dataloader ้
็ฝฎ
-
-ไธป่ฆไธบๆ้ ๆฐๆฎ้ๅ ่ฝฝๅจ(dataloader)ๆ้็้
็ฝฎไฟกๆฏ๏ผๆดๅคๆ็จ็ๅ่ {external+torch:doc}`PyTorch ๆฐๆฎๅ ่ฝฝๅจ
`ใ
-
-```Python
-# Dataloader ้จๅ
-train_dataloader = dict(
- batch_size=64,
- num_workers=8,
- persistent_workers=True,
- sampler=dict(type='DefaultSampler', shuffle=True),
- dataset=dict(
- type='ConcatDataset',
- datasets=[mjsynth_textrecog_train],
- pipeline=train_pipeline))
-val_dataloader = dict(
- batch_size=1,
- num_workers=4,
- persistent_workers=True,
- drop_last=False,
- sampler=dict(type='DefaultSampler', shuffle=False),
- dataset=dict(
- type='ConcatDataset',
- datasets=[icdar2013_textrecog_test, icdar2015_textrecog_test],
- pipeline=test_pipeline))
-test_dataloader = val_dataloader
-```
-
-### ๆจกๅ็ธๅ
ณ้
็ฝฎ
-
-
-
-#### ็ฝ็ป้
็ฝฎ
-
-็จไบ้
็ฝฎๆจกๅ็็ฝ็ป็ปๆ๏ผไธๅ็็ฎๆณไปปๅกๆไธๅ็็ฝ็ป็ปๆใๆดๅคไฟกๆฏๅฏไปฅๅ่[็ฝ็ป็ปๆ](../basic_concepts/structures.md)
-
-##### ๆๆฌๆฃๆต
-
-ๆๆฌๆฃๆตไธป่ฆๅ
ๅซๅ ไธช้จๅ:
-
-- `data_preprocessor`: [ๆฐๆฎๅค็ๅจ](mmocr.models.textdet.data_preprocessors.TextDetDataPreprocessor)
-- `backbone`: ็นๅพๆๅ็ฝ็ป
-- `neck`: ้ข็ฝ็ป้
็ฝฎ
-- `det_head`: ๆฃๆตๅคด็ฝ็ป้
็ฝฎ
- - `module_loss`: ๆจกๅๆๅคฑๅฝๆฐ้
็ฝฎ
- - `postprocessor`: ๆจกๅ้ขๆต็ปๆๅๅค็้
็ฝฎ
-
-ๆไปฌไปฅ DBNet ไธบไพ๏ผไป็ปๆๅญๆฃๆตไธญๆจกๅ้
็ฝฎ๏ผ
-
-```Python
-model = dict(
- type='DBNet',
- data_preprocessor=dict(
- type='TextDetDataPreprocessor',
- mean=[123.675, 116.28, 103.53],
- std=[58.395, 57.12, 57.375],
- bgr_to_rgb=True,
- pad_size_divisor=32)
- backbone=dict(
- type='mmdet.ResNet',
- depth=18,
- num_stages=4,
- out_indices=(0, 1, 2, 3),
- frozen_stages=-1,
- norm_cfg=dict(type='BN', requires_grad=True),
- init_cfg=dict(type='Pretrained', checkpoint='torchvision://resnet18'),
- norm_eval=False,
- style='caffe'),
- neck=dict(
- type='FPNC', in_channels=[64, 128, 256, 512], lateral_channels=256),
- det_head=dict(
- type='DBHead',
- in_channels=256,
- module_loss=dict(type='DBModuleLoss'),
- postprocessor=dict(type='DBPostprocessor', text_repr_type='quad')))
-```
-
-##### ๆๆฌ่ฏๅซ
-
-ๆๆฌ่ฏๅซไธป่ฆๅ
ๅซ๏ผ
-
-- `data_processor`: [ๆฐๆฎ้ขๅค็้
็ฝฎ](mmocr.models.textrec.data_processors.TextRecDataPreprocessor)
-- `preprocessor`: ็ฝ็ป้ขๅค็้
็ฝฎ๏ผๅฆTPS็ญ
-- `backbone`:็นๅพๆๅ้
็ฝฎ
-- `encoder`: ็ผ็ ๅจ้
็ฝฎ
-- `decoder`: ่งฃ็ ๅจ้
็ฝฎ
- - `module_loss`: ่งฃ็ ๅจๆๅคฑ
- - `postprocessor`: ่งฃ็ ๅจๅๅค็
- - `dictionary`: ๅญๅ
ธ้
็ฝฎ
-
-ไปฅ CRNN ไธบไพ๏ผ
-
-```Python
-# ๆจกๅ้จๅ
-model = dict(
- type='CRNN',
- data_preprocessor=dict(
- type='TextRecogDataPreprocessor', mean=[127], std=[127])
- preprocessor=None,
- backbone=dict(type='VeryDeepVgg', leaky_relu=False, input_channels=1),
- encoder=None,
- decoder=dict(
- type='CRNNDecoder',
- in_channels=512,
- rnn_flag=True,
- module_loss=dict(type='CTCModuleLoss', letter_case='lower'),
- postprocessor=dict(type='CTCPostProcessor'),
- dictionary=dict(
- type='Dictionary',
- dict_file='dicts/lower_english_digits.txt',
- with_padding=True)))
-```
-
-
-
-#### ๆ้ๅ ่ฝฝ้
็ฝฎ
-
-ๅฏไปฅ้่ฟ `load_from` ๅๆฐๅ ่ฝฝๆฃๆฅ็น๏ผcheckpoint๏ผๆไปถไธญ็ๆจกๅๆ้๏ผๅช้่ฆๅฐ `load_from` ๅๆฐ่ฎพ็ฝฎไธบๆฃๆฅ็นๆไปถ็่ทฏๅพๅณๅฏใ
-
-็จๆทไนๅฏ้่ฟ่ฎพ็ฝฎ `resume=True` ๏ผๅ ่ฝฝๆฃๆฅ็นไธญ็่ฎญ็ป็ถๆไฟกๆฏๆฅๆขๅค่ฎญ็ปใๅฝ `load_from` ๅ `resume=True` ๅๆถ่ขซ่ฎพ็ฝฎๆถ๏ผๆง่กๅจๅฐๅ ่ฝฝ `load_from` ่ทฏๅพๅฏนๅบ็ๆฃๆฅ็นๆไปถไธญ็่ฎญ็ป็ถๆใ
-
-ๅฆๆไป
่ฎพ็ฝฎ `resume=True`๏ผๆง่กๅจๅฐไผๅฐ่ฏไป `work_dir` ๆไปถๅคนไธญๅฏปๆพๅนถ่ฏปๅๆๆฐ็ๆฃๆฅ็นๆไปถ
-
-```Python
-load_from = None # ๅ ่ฝฝcheckpoint็่ทฏๅพ
-resume = False # ๆฏๅฆ resume
-```
-
-ๆดๅคๅฏไปฅๅ่ {external+mmengine:ref}`MMEngine: ๅ ่ฝฝๆ้ๆๆขๅค่ฎญ็ป <ๅ ่ฝฝๆ้ๆๆขๅค่ฎญ็ป>` ไธ [OCR ่ฟ้ถๆๅทง-ๆญ็นๆขๅค่ฎญ็ป](train_test.md#ไปๆญ็นๆขๅค่ฎญ็ป)ใ
-
-
-
-### ่ฏๆต้
็ฝฎ
-
-ๅจๆจกๅ้ช่ฏๅๆจกๅๆต่ฏไธญ๏ผ้ๅธธ้่ฆๅฏนๆจกๅ็ฒพๅบฆๅๅฎ้่ฏๆตใMMOCR ้่ฟ่ฏๆตๆๆ (Metric)ๅ่ฏๆตๅจ(Evaluator)ๆฅๅฎๆ่ฟไธๅ่ฝใๆดๅคๅฏไปฅๅ่{external+mmengine:doc}`MMEngine: ่ฏๆตๆๆ (Metric)ๅ่ฏๆตๅจ(Evaluator)
` ๅ [่ฏๆตๅจ](../basic_concepts/evaluation.md)
-
-่ฏๆต้จๅๅ
ๅซไธคไธช้จๅ๏ผ่ฏๆตๅจๅ่ฏๆตๆๆ ใๆฅไธๆฅๆไปฌๅ้จๅๅฑๅผ่ฎฒ่งฃใ
-
-#### ่ฏๆตๅจ
-
-่ฏๆตๅจไธป่ฆ็จๆฅ็ฎก็ๅคไธชๆฐๆฎ้ไปฅๅๅคไธช `Metric`ใ้ๅฏนๅๆฐๆฎ้ไธๅคๆฐๆฎ้ๆ
ๅต๏ผ่ฏๆตๅจๅไธบไบๅๆฐๆฎ้่ฏๆตๅจไธๅคๆฐๆฎ้่ฏๆตๅจ๏ผ่ฟไธค็ง่ฏๆตๅจๅๅฏ็ฎก็ๅคไธช `Metric`.
-
-ๅๆฐๆฎ้่ฏๆตๅจ้
็ฝฎๅฆไธ๏ผ
-
-```Python
-# ๅไธชๆฐๆฎ้ ๅไธช Metric ๆ
ๅต
-val_evaluator = dict(
- type='Evaluator',
- metrics=dict())
-
-# ๅไธชๆฐๆฎ้ ๅคไธช Metric ๆ
ๅต
-val_evaluator = dict(
- type='Evaluator',
- metrics=[...])
-```
-
-ๅจๅฎ็ฐไธญ้ป่ฎคไธบๅๆฐๆฎ้่ฏๆตๅจ๏ผๅ ๆญคๅฏนๅๆฐๆฎ้่ฏๆตๆ
ๅตไธ๏ผไธ่ฌๆ
ๅตไธๅช้้
็ฝฎ่ฏๆตๅจ๏ผๅณไธบ
-
-```Python
-# ๅไธชๆฐๆฎ้ ๅไธช Metric ๆ
ๅต
-val_evaluator = dict()
-
-# ๅไธชๆฐๆฎ้ ๅคไธช Metric ๆ
ๅต
-val_evaluator = [...]
-```
-
-ๅคๆฐๆฎ้่ฏๆตไธๅๆฐๆฎ้่ฏๆตๅญๅจไธคไธชไฝ็ฝฎไธ็ไธๅ๏ผ่ฏๆตๅจ็ฑปๅซไธๅ็ผใ่ฏๆตๅจ็ฑปๅซๅฟ
้กปไธบ`MultiDatasetsEvaluator`ไธไธ่ฝ็็ฅ๏ผๅ็ผไธป่ฆ็จๆฅๅบๅไธๅๆฐๆฎ้ๅจ็ธๅ่ฏๆตๆๆ ไธ็็ปๆ๏ผ่ฏทๅ่[ๅคๆฐๆฎ้่ฏๆต](../basic_concepts/evaluation.md)ใ
-
-ๅ่ฎพๆไปฌ้่ฆๅจ IC13 ๅ IC15 ๆ
ๅตไธๆต่ฏ็ฒพๅบฆ๏ผๅ้
็ฝฎๅฆไธ๏ผ
-
-```Python
-# ๅคไธชๆฐๆฎ้๏ผๅไธช Metric ๆ
ๅต
-val_evaluator = dict(
- type='MultiDatasetsEvaluator',
- metrics=dict(),
- dataset_prefixes=['IC13', 'IC15'])
-
-# ๅคไธชๆฐๆฎ้๏ผๅคไธช Metric ๆ
ๅต
-val_evaluator = dict(
- type='MultiDatasetsEvaluator',
- metrics=[...],
- dataset_prefixes=['IC13', 'IC15'])
-```
-
-#### ่ฏๆตๆๆ
-
-่ฏๆตๆๆ ๆไธๅๅบฆ้็ฒพๅบฆ็ๆนๆณ๏ผๅๆถๅฏไปฅๅคไธช่ฏๆตๆๆ ๅ
ฑๅไฝฟ็จ๏ผๆดๅค่ฏๆตๆๆ ๅ็ๅ่ {external+mmengine:doc}`MMEngine: ่ฏๆตๆๆ `๏ผๅจ MMOCR ไธญไธๅ็ฎๆณไปปๅกๆไธๅ็่ฏๆตๆๆ ใ ๆดๅค OCR ็ธๅ
ณ็่ฏๆตๆๆ ๅฏไปฅๅ่ [่ฏๆตๆๆ ](../basic_concepts/evaluation.md)ใ
-
-ๆๅญๆฃๆต: [`HmeanIOUMetric`](mmocr.evaluation.metrics.HmeanIOUMetric)
-
-ๆๅญ่ฏๅซ: [`WordMetric`](mmocr.evaluation.metrics.WordMetric)๏ผ[`CharMetric`](mmocr.evaluation.metrics.CharMetric)๏ผ [`OneMinusNEDMetric`](mmocr.evaluation.metrics.OneMinusNEDMetric)
-
-ๅ
ณ้ฎไฟกๆฏๆๅ: [`F1Metric`](mmocr.evaluation.metrics.F1Metric)
-
-ไปฅๆๆฌๆฃๆตไธบไพ่ฏดๆ๏ผๅจๅๆฐๆฎ้่ฏๆตๆ
ๅตไธ๏ผไฝฟ็จๅไธช `Metric`๏ผ
-
-```Python
-val_evaluator = dict(type='HmeanIOUMetric')
-```
-
-ไปฅๆๆฌ่ฏๅซไธบไพ๏ผๅฏนๅคไธชๆฐๆฎ้(IC13 ๅ IC15)็จๅคไธช `Metric` (`WordMetric` ๅ `CharMetric`)่ฟ่ก่ฏๆต๏ผ
-
-```Python
-# ่ฏๆต้จๅ
-val_evaluator = dict(
- type='MultiDatasetsEvaluator',
- metrics=[
- dict(
- type='WordMetric',
- mode=['exact', 'ignore_case', 'ignore_case_symbol']),
- dict(type='CharMetric')
- ],
- dataset_prefixes=['IC13', 'IC15'])
-test_evaluator = val_evaluator
-```
-
-
-
-### ๅฏ่งๅ้
็ฝฎ
-
-ๆฏไธชไปปๅก้
็ฝฎ่ฏฅไปปๅกๅฏนๅบ็ๅฏ่งๅๅจใๅฏ่งๅๅจไธป่ฆ็จไบ็จๆทๆจกๅไธญ้ด็ปๆ็ๅฏ่งๅๆๅญๅจ๏ผๅ val ๅ test ้ขๆต็ปๆ็ๅฏ่งๅใๅๆถๅฏ่งๅ็็ปๆๅฏไปฅ้่ฟๅฏ่งๅๅ็ซฏๅจๅญๅฐไธๅ็ๅ็ซฏ๏ผๆฏๅฆ WandB๏ผTensorBoard ็ญใๅธธ็จไฟฎๆนๆไฝๅฏ่ง[ๅฏ่งๅ](visualization.md)ใ
-
-ๆๆฌๆฃๆต็ๅฏ่งๅ้ป่ฎค้
็ฝฎๅฆไธ๏ผ
-
-```Python
-vis_backends = [dict(type='LocalVisBackend')]
-visualizer = dict(
- type='TextDetLocalVisualizer', # ไธๅไปปๅกๆไธๅ็ๅฏ่งๅๅจ
- vis_backends=vis_backends,
- name='visualizer')
-```
-
-## ็ฎๅฝ็ปๆ
-
-`MMOCR` ๆๆ้
็ฝฎๆไปถ้ฝๆพ็ฝฎๅจ `configs` ๆไปถๅคนไธใไธบไบ้ฟๅ
้
็ฝฎๆไปถ่ฟ้ฟ๏ผๅๆถๆ้ซ้
็ฝฎๆไปถ็ๅฏๅค็จๆงไปฅๅๆธ
ๆฐๆง๏ผMMOCR ๅฉ็จ Config ๆไปถ็็ปงๆฟ็นๆง๏ผๅฐ้
็ฝฎๅ
ๅฎน็ๅ
ซไธช้จๅๅไบๆๅใๅ ไธบๆฏ้จๅๅไธ็ฎๆณไปปๅก็ธๅ
ณ๏ผๅ ๆญค MMOCR ๅฏนๆฏไธชไปปๅกๅจ Config ไธญๆไพไบไธไธชไปปๅกๆไปถๅคน๏ผๅณ `textdet` (ๆๅญๆฃๆตไปปๅก)ใ`textrecog` (ๆๅญ่ฏๅซไปปๅก)ใ`kie` (ๅ
ณ้ฎไฟกๆฏๆๅ)ใๅๆถๅไธชไปปๅก็ฎๆณ้
็ฝฎๆไปถๅคนไธ่ฟไธๆญฅๅๅไธบไธคไธช้จๅ๏ผ`_base_` ๆไปถๅคนไธ่ฏธๅค็ฎๆณๆไปถๅคน๏ผ
-
-1. `_base_` ๆไปถๅคนไธไธป่ฆๅญๆพไธๅ
ทไฝ็ฎๆณๆ ๅ
ณ็ไธไบ้็จ้
็ฝฎๆไปถ๏ผๅ้จๅไพ็ฎๅฝๅไธบๅธธ็จ็ๆฐๆฎ้ใๅธธ็จ็่ฎญ็ป็ญ็ฅไปฅๅ้็จ็่ฟ่ก้
็ฝฎใ
-
-2. ็ฎๆณ้
็ฝฎๆไปถๅคนไธญๅญๆพไธ็ฎๆณๅผบ็ธๅ
ณ็้
็ฝฎ้กนใ็ฎๆณ้
็ฝฎๆไปถๅคนไธป่ฆๅไธบไธค้จๅ๏ผ
-
- 1. ็ฎๆณ็ๆจกๅไธๆฐๆฎๆตๆฐด็บฟ๏ผOCR ้ขๅไธญไธ่ฌๆ
ๅตไธๆฐๆฎๅขๅผบ็ญ็ฅไธ็ฎๆณๅผบ็ธๅ
ณ๏ผๅ ๆญคๆจกๅไธๆฐๆฎๆตๆฐด็บฟ้ๅธธ็ฝฎไบ็ปไธไฝ็ฝฎใ
-
- 2. ็ฎๆณๅจๅถๅฎๆฐๆฎ้ไธ็็นๅฎ้
็ฝฎ๏ผ็จไบ่ฎญ็ปๅๆต่ฏ็้
็ฝฎ๏ผๅฐๅๆฃๅจไธๅไฝ็ฝฎ็ *base* ้
็ฝฎๆฑๆปใๅๆถๅฏ่ฝไผไฟฎๆนไธไบ`_base_`ไธญ็ๅ้๏ผๅฆbatch size, ๆฐๆฎๆตๆฐด็บฟ๏ผ่ฎญ็ป็ญ็ฅ็ญ
-
-ๆๅ็ๅฐ้
็ฝฎๅ
ๅฎนไธญ็ๅไธชๆจกๅๅๅธๅจไธๅ้
็ฝฎๆไปถไธญ๏ผๆ็ปๅ้
็ฝฎๆไปถๅ
ๅฎนๅฆไธ:
-
-
-
-
-
-ๆ็ป็ฎๅฝ็ปๆๅฆไธ๏ผ
-
-```Python
-configs
-โโโ textdet
-โ โโโ _base_
-โ โ โโโ datasets
-โ โ โ โโโ icdar2015.py
-โ โ โ โโโ icdar2017.py
-โ โ โ โโโ totaltext.py
-โ โ โโโ schedules
-โ โ โ โโโ schedule_adam_600e.py
-โ โ โโโ default_runtime.py
-โ โโโ dbnet
-โ โโโ _base_dbnet_resnet18_fpnc.py
-โ โโโ dbnet_resnet18_fpnc_1200e_icdar2015.py
-โโโ textrecog
-โ โโโ _base_
-โ โ โโโ datasets
-โ โ โ โโโ icdar2015.py
-โ โ โ โโโ icdar2017.py
-โ โ โ โโโ totaltext.py
-โ โ โโโ schedules
-โ โ โ โโโ schedule_adam_base.py
-โ โ โโโ default_runtime.py
-โ โโโ crnn
-โ โโโ _base_crnn_mini-vgg.py
-โ โโโ crnn_mini-vgg_5e_mj.py
-โโโ kie
- โโโ _base_
- โ โโโdatasets
- โ โโโ default_runtime.py
- โโโ sgdmr
- โโโ sdmgr_novisual_60e_wildreceipt_openset.py
-```
-
-## ้
็ฝฎๆไปถไปฅๅๆ้ๅฝๅ่งๅ
-
-MMOCR ๆ็
งไปฅไธ้ฃๆ ผ่ฟ่ก้
็ฝฎๆไปถๅฝๅ๏ผไปฃ็ ๅบ็่ดก็ฎ่
้่ฆ้ตๅพช็ธๅ็ๅฝๅ่งๅใๆไปถๅๆปไฝๅไธบๅ้จๅ๏ผ็ฎๆณไฟกๆฏ๏ผๆจกๅไฟกๆฏ๏ผ่ฎญ็ปไฟกๆฏๅๆฐๆฎไฟกๆฏใ้ป่พไธๅฑไบไธๅ้จๅ็ๅ่ฏไน้ด็จไธๅ็บฟ `'_'` ่ฟๆฅ๏ผๅไธ้จๅๆๅคไธชๅ่ฏ็จ็ญๆจช็บฟ `'-'` ่ฟๆฅใ
-
-```Python
-{{็ฎๆณไฟกๆฏ}}_{{ๆจกๅไฟกๆฏ}}_{{่ฎญ็ปไฟกๆฏ}}_{{ๆฐๆฎไฟกๆฏ}}.py
-```
-
-- ็ฎๆณไฟกๆฏ(algorithm info)๏ผ็ฎๆณๅ็งฐ๏ผๅฆ dbnet, crnn ็ญ
-
-- ๆจกๅไฟกๆฏ(module info)๏ผๆ็
งๆฐๆฎๆต็้กบๅบๅไธพไธไบไธญ้ด็ๆจกๅ๏ผๅ
ถๅ
ๅฎนไพ่ตไบ็ฎๆณไปปๅก๏ผๅๆถไธบไบ้ฟๅ
Config่ฟ้ฟ๏ผไผ็็ฅไธไบไธๆจกๅๅผบ็ธๅ
ณ็ๆจกๅใไธ้ขไธพไพ่ฏดๆ๏ผ
-
- - ๅฏนไบๆๅญๆฃๆตไปปๅกๅๅ
ณ้ฎไฟกๆฏๆๅไปปๅก:
-
- ```Python
- {{็ฎๆณไฟกๆฏ}}_{{backbone}}_{{neck}}_{{head}}_{{่ฎญ็ปไฟกๆฏ}}_{{ๆฐๆฎไฟกๆฏ}}.py
- ```
-
- ไธ่ฌๆ
ๅตไธ head ไฝ็ฝฎไธ่ฌไธบ็ฎๆณไธๆ็ head๏ผๅ ๆญคไธ่ฌ็็ฅใ
-
- - ๅฏนไบๆๆฌ่ฏๅซไปปๅก๏ผ
-
- ```Python
- {{็ฎๆณไฟกๆฏ}}_{{backbone}}_{{encoder}}_{{decoder}}_{{่ฎญ็ปไฟกๆฏ}}_{{ๆฐๆฎไฟกๆฏ}}.py
- ```
-
- ไธ่ฌๆ
ๅตไธ encoder ๅ decoder ไฝ็ฝฎไธ่ฌไธบ็ฎๆณไธๆ๏ผๅ ๆญคไธ่ฌ็็ฅใ
-
-- ่ฎญ็ปไฟกๆฏ(training info)๏ผ่ฎญ็ป็ญ็ฅ็ไธไบ่ฎพ็ฝฎ๏ผๅ
ๆฌ batch size๏ผschedule ็ญ
-
-- ๆฐๆฎไฟกๆฏ(data info)๏ผๆฐๆฎ้ๅ็งฐใๆจกๆใ่พๅ
ฅๅฐบๅฏธ็ญ๏ผๅฆ icdar2015๏ผsynthtext ็ญ
diff --git a/pipeline/mmocr/docs/zh_cn/user_guides/data_prepare/dataset_preparer.md b/pipeline/mmocr/docs/zh_cn/user_guides/data_prepare/dataset_preparer.md
deleted file mode 100644
index f755833672b83cb380cf8edc1923f7a1b9d88472..0000000000000000000000000000000000000000
--- a/pipeline/mmocr/docs/zh_cn/user_guides/data_prepare/dataset_preparer.md
+++ /dev/null
@@ -1,780 +0,0 @@
-# ๆฐๆฎๅๅค (Beta)
-
-```{note}
-Dataset Preparer ็ฎๅไปๅคๅจๅ
ฌๆต้ถๆฎต๏ผๆฌข่ฟๅฐ้ฒ่ฏ็จ๏ผๅฆ้ๅฐไปปไฝ้ฎ้ข๏ผ่ฏทๅๆถๅๆไปฌๅ้ฆใ
-```
-
-## ไธ้ฎๅผๆฐๆฎๅๅค่ๆฌ
-
-MMOCR ๆไพไบ็ปไธ็ไธ็ซๅผๆฐๆฎ้ๅๅค่ๆฌ `prepare_dataset.py`ใ
-
-ไป
้ไธ่กๅฝไปคๅณๅฏๅฎๆๆฐๆฎ็ไธ่ฝฝใ่งฃๅใๆ ผๅผ่ฝฌๆข๏ผๅๅบ็ก้
็ฝฎ็็ๆใ
-
-```bash
-python tools/dataset_converters/prepare_dataset.py [-h] [--nproc NPROC] [--task {textdet,textrecog,textspotting,kie}] [--splits SPLITS [SPLITS ...]] [--lmdb] [--overwrite-cfg] [--dataset-zoo-path DATASET_ZOO_PATH] datasets [datasets ...]
-```
-
-| ๅๆฐ | ็ฑปๅ | ่ฏดๆ |
-| ------------------ | -------------------------- | ----------------------------------------------------------------------------------------------------- |
-| dataset_name | str | ๏ผๅฟ
้กป๏ผ้่ฆๅๅค็ๆฐๆฎ้ๅ็งฐใ |
-| --nproc | str | ไฝฟ็จ็่ฟ็จๆฐ๏ผ้ป่ฎคไธบ 4ใ |
-| --task | str | ๅฐๆฐๆฎ้ๆ ผๅผ่ฝฌๆขไธบๆๅฎไปปๅก็ MMOCR ๆ ผๅผใๅฏ้้กนไธบ๏ผ 'textdet', 'textrecog', 'textspotting' ๅ 'kie'ใ |
-| --splits | \['train', 'val', 'test'\] | ๅธๆๅๅค็ๆฐๆฎ้ๅๅฒ๏ผๅฏไปฅๆฅๅๅคไธชๅๆฐใ้ป่ฎคไธบ `train val test`ใ |
-| --lmdb | str | ๆๆฐๆฎๅจๅญไธบ LMDB ๆ ผๅผ๏ผไป
ๅฝไปปๅกไธบ `textrecog` ๆถ็ๆใ |
-| --overwrite-cfg | str | ่ฅๆฐๆฎ้็ๅบ็ก้
็ฝฎๅทฒ็ปๅจ `configs/{task}/_base_/datasets` ไธญๅญๅจ๏ผไพ็ถ้ๅ่ฏฅ้
็ฝฎ |
-| --dataset-zoo-path | str | ๅญๆพๆฐๆฎๅบ้
็ฝฎๆไปถ็่ทฏๅพใ่ฅไธๆๅฎ๏ผๅ้ป่ฎคไธบ `./dataset_zoo` |
-
-ไพๅฆ๏ผไปฅไธๅฝไปคๅฑ็คบไบๅฆไฝไฝฟ็จ่ฏฅ่ๆฌไธบ ICDAR2015 ๆฐๆฎ้ๅๅคๆๆฌๆฃๆตไปปๅกๆ้็ๆฐๆฎใ
-
-```bash
-python tools/dataset_converters/prepare_dataset.py icdar2015 --task textdet --overwrite-cfg
-```
-
-่ฏฅ่ๆฌไนๆฏๆๅๆถๅๅคๅคไธชๆฐๆฎ้๏ผไพๅฆ๏ผไปฅไธๅฝไปคๅฑ็คบไบๅฆไฝไฝฟ็จ่ฏฅ่ๆฌๅๆถไธบ ICDAR2015 ๅ TotalText ๆฐๆฎ้ๅๅคๆๆฌ่ฏๅซไปปๅกๆ้็ๆฐๆฎใ
-
-```bash
-python tools/dataset_converters/prepare_dataset.py icdar2015 totaltext --task textrecog --overwrite-cfg
-```
-
-่ฟไธๆญฅไบ่งฃ Dataset Preparer ๆฏๆ็ๆฐๆฎ้๏ผๆจๅฏไปฅๆต่ง[ๆฏๆ็ๆฐๆฎ้ๆๆกฃ](./datasetzoo.md)ใไธไบ้่ฆๆๅจๅๅค็ๆฐๆฎ้ไนๅๅจไบ [ๆๅญๆฃๆต](./det.md) ๅ [ๆๅญ่ฏๅซ](./recog.md) ๅ
ใ
-
-ๅฏนไบไธญๅฝๅขๅ
็็จๆท๏ผๆไปฌไนๆจ่้่ฟๅผๆบๆฐๆฎๅนณๅฐ[OpenDataLab](https://opendatalab.com/)ๆฅไธ่ฝฝๆฐๆฎ๏ผไปฅ่ทๅพๆดๅฅฝ็ไธ่ฝฝไฝ้ชใๆฐๆฎไธ่ฝฝๅ๏ผๅ่่ๆฌไธญ `data_obtainer` ็ `save_name` ๅญๆฎต๏ผๅฐๆไปถๆพๅจ `data/cache/` ไธๅนถ้ๆฐ่ฟ่ก่ๆฌๅณๅฏใ
-
-## ่ฟ้ถ็จๆณ
-
-### LMDB ๆ ผๅผ
-
-ๅจๆๆฌ่ฏๅซไปปๅกไธญ๏ผ้ๅธธไฝฟ็จ LMDB ๆ ผๅผๆฅๅญๅจๆฐๆฎ๏ผไปฅๅ ๅฟซๆฐๆฎ็่ฏปๅ้ๅบฆใๅจไฝฟ็จ `prepare_dataset.py` ่ๆฌๅๅคๆฐๆฎๆถ๏ผๅฏไปฅ้่ฟ `--lmdb` ๅๆฐๆฅๆๅฎๅฐๆฐๆฎ่ฝฌๆขไธบ LMDB ๆ ผๅผใไพๅฆ๏ผ
-
-```bash
-python tools/dataset_converters/prepare_dataset.py icdar2015 --task textrecog --lmdb
-```
-
-ๆฐๆฎ้ๅๅคๅฎๆๅ๏ผDataset Preparer ไผๅจ `configs/textrecog/_base_/datasets/` ไธญ็ๆ `icdar2015_lmdb.py` ้
็ฝฎใไฝ ๅฏไปฅ็ปงๆฟ่ฏฅ้
็ฝฎ๏ผๅนถๅฐ `dataloader` ๆๅ LMDB ๆฐๆฎ้ใ็ถ่๏ผLMDB ๆฐๆฎ้็่ฏปๅ้่ฆ้
ๅ [`LoadImageFromNDArray`](mmocr.datasets.transforms.LoadImageFromNDArray)๏ผๅ ๆญคไฝ ไนๅๆ ท้่ฆไฟฎๆน `pipeline`ใ
-
-ไพๅฆ๏ผๆณ่ฆๅฐ `configs/textrecog/crnn/crnn_mini-vgg_5e_mj.py` ็่ฎญ็ป้ๆนไธบๅๅ็ๆ็ icdar2015๏ผๅ้่ฆไฝๅฆไธไฟฎๆน๏ผ
-
-1. ไฟฎๆน `configs/textrecog/crnn/crnn_mini-vgg_5e_mj.py`:
-
- ```python
- _base_ = [
- '../_base_/datasets/icdar2015_lmdb.py', # ๆๅ icdar2015 lmdb ๆฐๆฎ้
- ... # ็็ฅ
- ]
-
- train_list = [_base_.icdar2015_lmdb_textrecog_train]
- ...
- ```
-
-2. ไฟฎๆน `configs/textrecog/crnn/_base_crnn_mini-vgg.py` ไธญ็ `train_pipeline`, ๅฐ `LoadImageFromFile` ๆนไธบ `LoadImageFromNDArray`๏ผ
-
- ```python
- train_pipeline = [
- dict(
- type='LoadImageFromNDArray',
- color_type='grayscale',
- file_client_args=file_client_args,
- ignore_empty=True,
- min_size=2),
- ...
- ]
- ```
-
-## ่ฎพ่ฎก
-
-OCR ๆฐๆฎ้ๆฐ้ไผๅค๏ผไธๅ็ๆฐๆฎ้ๆ็ไธๅ็่ฏญ่จ๏ผไธๅ็ๆ ๆณจๆ ผๅผ๏ผไธๅ็ๅบๆฏ็ญใ ๆฐๆฎ้็ไฝฟ็จๆ
ๅตไธ่ฌๆไธค็ง๏ผไธ็งๆฏๅฟซ้็ไบ่งฃๆฐๆฎ้็็ธๅ
ณไฟกๆฏ๏ผๅฆไธ็งๆฏๅจไฝฟ็จๆฐๆฎ้่ฎญ็ปๆจกๅใไธบไบๆปก่ถณ่ฟไธค็งไฝฟ็จๅบๆฏMMOCR ๆไพๆฐๆฎ้่ชๅจๅๅๅค่ๆฌ๏ผๆฐๆฎ้่ชๅจๅๅๅค่ๆฌไฝฟ็จไบๆจกๅๅ็่ฎพ่ฎก๏ผๆๅคงๅฐๅขๅผบไบๆฉๅฑๆง๏ผ็จๆท่ฝๅคๅพๆนไพฟๅฐ้
็ฝฎๅ
ถไปๅ
ฌๅผๆฐๆฎ้ๆ็งๆๆฐๆฎ้ใๆฐๆฎ้่ชๅจๅๅๅค่ๆฌ็้
็ฝฎๆไปถ่ขซ็ปไธๅญๅจๅจ `dataset_zoo/` ็ฎๅฝไธ๏ผ็จๆทๅฏไปฅๅจ่ฏฅ็ฎๅฝไธๆพๅฐๆๆๅทฒ็ฑ MMOCR ๅฎๆนๆฏๆ็ๆฐๆฎ้ๅๅค่ๆฌ้
็ฝฎๆไปถใ่ฏฅๆไปถๅคน็็ฎๅฝ็ปๆๅฆไธ๏ผ
-
-```text
-dataset_zoo/
-โโโ icdar2015
-โ โโโ metafile.yml
-โ โโโ sample_anno.md
-โ โโโ textdet.py
-โ โโโ textrecog.py
-โ โโโ textspotting.py
-โโโ wildreceipt
- โโโ metafile.yml
- โโโ sample_anno.md
- โโโ kie.py
- โโโ textdet.py
- โโโ textrecog.py
- โโโ textspotting.py
-```
-
-### ๆฐๆฎ้็ธๅ
ณไฟกๆฏ
-
-ๆฐๆฎ้็็ธๅ
ณไฟกๆฏๅ
ๆฌๆฐๆฎ้็ๆ ๆณจๆ ผๅผใๆฐๆฎ้็ๆ ๆณจ็คบไพใๆฐๆฎ้็ๅบๆฌ็ป่ฎกไฟกๆฏ็ญใ่ฝ็ถๅจๆฏไธชๆฐๆฎ้็ๅฎ็ฝไธญ้ฝๆ่ฟไบไฟกๆฏ๏ผไฝๆฏ่ฟไบไฟกๆฏๅๆฃๅจๅไธชๆฐๆฎ้็ๅฎ็ฝไธญ๏ผ็จๆท้่ฆ่ฑ่ดนๅคง้็ๆถ้ดๆฅๆๆๆฐๆฎ้็ๅบๆฌไฟกๆฏใๅ ๆญค๏ผMMOCR ่ฎพ่ฎกไบไธไบ่ๅผ๏ผๅฎๅฏไปฅๅธฎๅฉ็จๆทๅฟซ้ไบ่งฃๆฐๆฎ้็ๅบๆฌไฟกๆฏใ MMOCR ๅฐๆฐๆฎ้็็ธๅ
ณไฟกๆฏๅไธบไธคไธช้จๅ๏ผไธ้จๅๆฏๆฐๆฎ้็ๅบๆฌไฟกๆฏๅ
ๆฌๅ
ๆฌๅๅธๅนดไปฝ๏ผ่ฎบๆไฝ่
๏ผไปฅๅ็ๆ็ญๅ
ถไปไฟกๆฏ๏ผๅฆไธ้จๅๆฏๆฐๆฎ้็ๆ ๆณจไฟกๆฏ๏ผๅ
ๆฌๆฐๆฎ้็ๆ ๆณจๆ ผๅผใๆฐๆฎ้็ๆ ๆณจ็คบไพใๆฏไธ้จๅ MMOCR ้ฝไผๆไพไธไธช่ๅผ๏ผ่ดก็ฎ่
ๅฏไปฅๆ นๆฎ่ๅผๆฅๅกซๅๆฐๆฎ้็ๅบๆฌไฟกๆฏ๏ผไฝฟ็จ็จๆทๅฐฑๅฏไปฅๅฟซ้ไบ่งฃๆฐๆฎ้็ๅบๆฌไฟกๆฏใ ๆ นๆฎๆฐๆฎ้็ๅบๆฌไฟกๆฏ MMOCR ๆไพไบไธไธช `metafile.yml` ๆไปถ๏ผๅ
ถไธญๅญๆพไบๅฏนๅบๆฐๆฎ้็ๅบๆฌไฟกๆฏ๏ผๅ
ๆฌๅๅธๅนดไปฝ๏ผ่ฎบๆไฝ่
๏ผไปฅๅ็ๆ็ญๅ
ถไปไฟกๆฏ๏ผ่ฟๆ ท็จๆทๅฐฑๅฏไปฅๅฟซ้ไบ่งฃๆฐๆฎ้็ๅบๆฌไฟกๆฏใ่ฏฅๆไปถๅจๆฐๆฎ้ๅๅค่ฟ็จไธญๅนถไธๆฏๅผบๅถ่ฆๆฑ็๏ผๅ ๆญค็จๆทๅจไฝฟ็จๆทปๅ ่ชๅทฑ็็งๆๆฐๆฎ้ๆถๅฏไปฅๅฟฝ็ฅ่ฏฅๆไปถ๏ผ๏ผไฝไธบไบ็จๆทๆดๅฅฝๅฐไบ่งฃๅไธชๅ
ฌๅผๆฐๆฎ้็ไฟกๆฏ๏ผMMOCR ๅปบ่ฎฎ็จๆทๅจไฝฟ็จๆฐๆฎ้ๅๅค่ๆฌๅ้
่ฏปๅฏนๅบ็ๅ
ๆไปถไฟกๆฏ๏ผไปฅไบ่งฃ่ฏฅๆฐๆฎ้็็นๅพๆฏๅฆ็ฌฆๅ็จๆท้ๆฑใMMOCR ไปฅ ICDAR2015 ไฝไธบ็คบไพ๏ผ ๅ
ถ็คบไพๅ
ๅฎนๅฆไธๆ็คบ๏ผ
-
-```yaml
-Name: 'Incidental Scene Text IC15'
-Paper:
- Title: ICDAR 2015 Competition on Robust Reading
- URL: https://rrc.cvc.uab.es/files/short_rrc_2015.pdf
- Venue: ICDAR
- Year: '2015'
- BibTeX: '@inproceedings{karatzas2015icdar,
- title={ICDAR 2015 competition on robust reading},
- author={Karatzas, Dimosthenis and Gomez-Bigorda, Lluis and Nicolaou, Anguelos and Ghosh, Suman and Bagdanov, Andrew and Iwamura, Masakazu and Matas, Jiri and Neumann, Lukas and Chandrasekhar, Vijay Ramaseshan and Lu, Shijian and others},
- booktitle={2015 13th international conference on document analysis and recognition (ICDAR)},
- pages={1156--1160},
- year={2015},
- organization={IEEE}}'
-Data:
- Website: https://rrc.cvc.uab.es/?ch=4
- Language:
- - English
- Scene:
- - Natural Scene
- Granularity:
- - Word
- Tasks:
- - textdet
- - textrecog
- - textspotting
- License:
- Type: CC BY 4.0
- Link: https://creativecommons.org/licenses/by/4.0/
-```
-
-ๅ
ทไฝๅฐ๏ผMMOCR ๅจไธ่กจไธญๅๅบๆฏไธชๅญๆฎตๅฏนๅบ็ๅซไน๏ผ
-
-| ๅญๆฎตๅ | ๅซไน |
-| :--------------- | :-------------------------------------------------------------------- |
-| Name | ๆฐๆฎ้็ๅ็งฐ |
-| Paper.Title | ๆฐๆฎ้่ฎบๆ็ๆ ้ข |
-| Paper.URL | ๆฐๆฎ้่ฎบๆ็้พๆฅ |
-| Paper.Venue | ๆฐๆฎ้่ฎบๆๅ่กจ็ไผ่ฎฎ/ๆๅๅ็งฐ |
-| Paper.Year | ๆฐๆฎ้่ฎบๆๅ่กจ็ๅนดไปฝ |
-| Paper.BibTeX | ๆฐๆฎ้่ฎบๆ็ๅผ็จ็ BibTex |
-| Data.Website | ๆฐๆฎ้็ๅฎๆน็ฝ็ซ |
-| Data.Language | ๆฐๆฎ้ๆฏๆ็่ฏญ่จ |
-| Data.Scene | ๆฐๆฎ้ๆฏๆ็ๅบๆฏ๏ผๅฆ `Natural Scene`, `Document`, `Handwritten` ็ญ |
-| Data.Granularity | ๆฐๆฎ้ๆฏๆ็็ฒๅบฆ๏ผๅฆ `Character`, `Word`, `Line` ็ญ |
-| Data.Tasks | ๆฐๆฎ้ๆฏๆ็ไปปๅก๏ผๅฆ `textdet`, `textrecog`, `textspotting`, `kie` ็ญ |
-| Data.License | ๆฐๆฎ้็่ฎธๅฏ่ฏไฟกๆฏ๏ผๅฆๆไธๅญๅจ่ฎธๅฏ่ฏ๏ผๅไฝฟ็จ `N/A` ๅกซๅ
|
-| Data.Format | ๆฐๆฎ้ๆ ๆณจๆไปถ็ๆ ผๅผ๏ผๅฆ `.txt`, `.xml`, `.json` ็ญ |
-| Data.Keywords | ๆฐๆฎ้็็นๆงๅ
ณ้ฎ่ฏ๏ผๅฆ `Horizontal`, `Vertical`, `Curved` ็ญ |
-
-ๅฏนไบๆฐๆฎ้็ๆ ๆณจไฟกๆฏ๏ผMMOCR ๆไพไบไธไธช `sample_anno.md` ๆไปถ๏ผ็จๆทๅฏไปฅๆ นๆฎ่ๅผๆฅๅกซๅๆฐๆฎ้็ๆ ๆณจไฟกๆฏ๏ผ่ฟๆ ท็จๆทๅฐฑๅฏไปฅๅฟซ้ไบ่งฃๆฐๆฎ้็ๆ ๆณจไฟกๆฏใMMOCR ไปฅ ICDAR2015 ไฝไธบ็คบไพ๏ผ ๅ
ถ็คบไพๅ
ๅฎนๅฆไธๆ็คบ๏ผ
-
-````markdown
- **Text Detection**
-
- ```text
- # x1,y1,x2,y2,x3,y3,x4,y4,trans
-
- 377,117,463,117,465,130,378,130,Genaxis Theatre
- 493,115,519,115,519,131,493,131,[06]
- 374,155,409,155,409,170,374,170,###
- ```
-````
-
-`sample_anno.md` ไธญๅ
ๅซๆฐๆฎ้้ๅฏนไธๅไปปๅก็ๆ ๆณจไฟกๆฏ๏ผๅ
ๅซๆ ๆณจๆไปถ็ๆ ผๅผ(text ๅฏนๅบ็ๆฏ txt ๆไปถ๏ผๆ ๆณจๆไปถ็ๆ ผๅผไนๅฏไปฅๅจ meta.yml ไธญๆพๅฐ)๏ผๆ ๆณจ็็คบไพใ
-
-้่ฟไธ่ฟฐไธคไธชๆไปถ็ไฟกๆฏ๏ผ็จๆทๅฐฑๅฏไปฅๅฟซ้ไบ่งฃๆฐๆฎ้็ๅบๆฌไฟกๆฏ๏ผๅๆถ MMOCR ๆฑๆปไบๆๆๆฐๆฎ้็ๅบๆฌไฟกๆฏ๏ผ็จๆทๅฏไปฅๅจ [Overview](.overview.md) ไธญๆฅ็ๆๆๆฐๆฎ้็ๅบๆฌไฟกๆฏใ
-
-### ๆฐๆฎ้ไฝฟ็จ
-
-็ป่ฟๆฐๅๅนด็ๅๅฑ๏ผOCR ้ขๅๆถ็ฐๅบไบไธ็ณปๅ็็ธๅ
ณๆฐๆฎ้๏ผ่ฟไบๆฐๆฎ้ๅพๅพ้็จ้ฃๆ ผๅๅผ็ๆ ผๅผๆฅๆไพๆๆฌ็ๆ ๆณจๆไปถ๏ผไฝฟๅพ็จๆทๅจไฝฟ็จ่ฟไบๆฐๆฎ้ๆถไธๅพไธ่ฟ่กๆ ผๅผ่ฝฌๆขใๅ ๆญค๏ผไธบไบๆนไพฟ็จๆท่ฟ่กๆฐๆฎ้ๅๅค๏ผๆไปฌ่ฎพ่ฎกไบ Dataset Preaprer๏ผๅธฎๅฉ็จๆทๅฟซ้ๅฐๆฐๆฎ้ๅๅคไธบ MMOCR ๆฏๆ็ๆ ผๅผ, ่ฏฆ่ง[ๆฐๆฎๆ ผๅผๆๆกฃ](../../basic_concepts/datasets.md)ใไธๅพๅฑ็คบไบ Dataset Preparer ็ๅ
ธๅ่ฟ่กๆต็จใ
-
-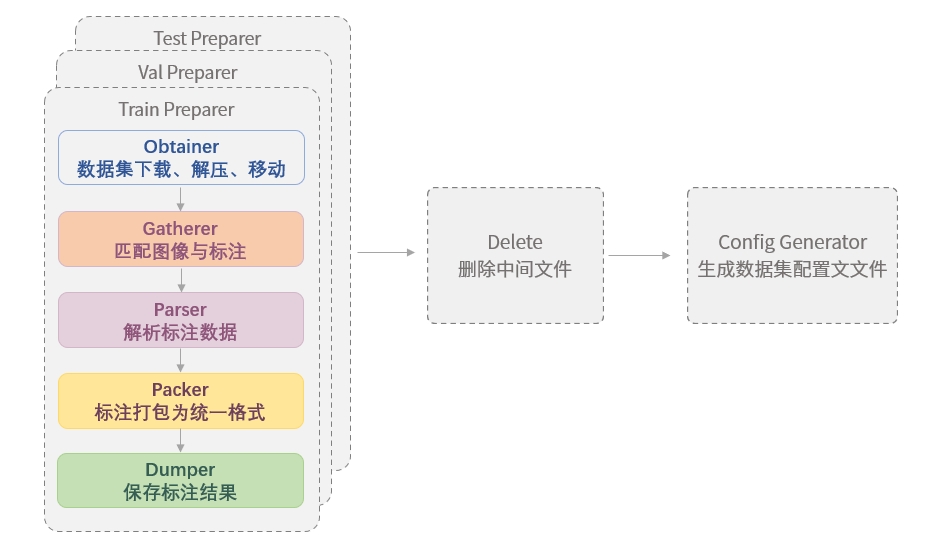
-
-็ฑๅพๅฏ่ง๏ผDataset Preparer ๅจ่ฟ่กๆถ๏ผไผไพๆฌกๆง่กไปฅไธๆไฝ๏ผ
-
-1. ๅฏน่ฎญ็ป้ใ้ช่ฏ้ๅๆต่ฏ้๏ผ็ฑๅ preparer ่ฟ่ก๏ผ
-
- 1. [ๆฐๆฎ้็ไธ่ฝฝใ่งฃๅใ็งปๅจ๏ผObtainer๏ผ](#ๆฐๆฎ้ไธ่ฝฝ่งฃๅ็งปๅจ-obtainer)
- 2. [ๅน้
ๆ ๆณจไธๅพๅ๏ผGatherer๏ผ](#ๆฐๆฎ้ๆถ้-gatherer)
- 3. [่งฃๆๅๆ ๆณจ๏ผParser๏ผ](#ๆฐๆฎ้่งฃๆ-parser)
- 4. [ๆๅ
ๆ ๆณจไธบ็ปไธๆ ผๅผ๏ผPacker๏ผ](#ๆฐๆฎ้่ฝฌๆข-packer)
- 5. [ไฟๅญๆ ๆณจ๏ผDumper๏ผ](#ๆ ๆณจไฟๅญ-dumper)
-
-2. ๅ ้คๆไปถ๏ผDelete๏ผ
-
-3. ็ๆๆฐๆฎ้็้
็ฝฎๆไปถ๏ผConfig Generator๏ผ
-
-ไธบไบไพฟไบๅบๅฏนๅ็งๆฐๆฎ้็ๆ
ๅต๏ผMMOCR ๅฐๆฏไธช้จๅๅ่ฎพ่ฎกไธบๅฏๆๆ็ๆจกๅ๏ผๅนถๅ
่ฎธ็จๆท้่ฟ dataset_zoo/ ไธ็้
็ฝฎๆไปถๅฏนๆฐๆฎ้ๅๅคๆต็จ่ฟ่ก้
็ฝฎใ่ฟไบ้
็ฝฎๆไปถ้็จไบ Python ๆ ผๅผ๏ผๅ
ถไฝฟ็จๆนๆณไธ MMOCR ็ฎๆณๅบ็ๅ
ถไป้
็ฝฎๆไปถๅฎๅ
จไธ่ด๏ผ่ฏฆ่ง[้
็ฝฎๆไปถๆๆกฃ](../config.md)ใ
-
-ๅจ `dataset_zoo/` ไธ๏ผๆฏไธชๆฐๆฎ้ๅๅ ๆไธไธชๆไปถๅคน๏ผๆไปถๅคนไธไผไปฅไปปๅกๅๅฝๅ้
็ฝฎๆไปถ๏ผไปฅๅบๅไธๅไปปๅกไธ็้
็ฝฎใไปฅ ICDAR2015 ๆๅญๆฃๆต้จๅไธบไพ๏ผ็คบไพ้
็ฝฎ `dataset_zoo/icdar2015/textdet.py` ๅฆไธๆ็คบ๏ผ
-
-```python
-data_root = 'data/icdar2015'
-cache_path = 'data/cache'
-train_preparer = dict(
- obtainer=dict(
- type='NaiveDataObtainer',
- cache_path=cache_path,
- files=[
- dict(
- url='https://rrc.cvc.uab.es/downloads/ch4_training_images.zip',
- save_name='ic15_textdet_train_img.zip',
- md5='c51cbace155dcc4d98c8dd19d378f30d',
- content=['image'],
- mapping=[['ic15_textdet_train_img', 'textdet_imgs/train']]),
- dict(
- url='https://rrc.cvc.uab.es/downloads/'
- 'ch4_training_localization_transcription_gt.zip',
- save_name='ic15_textdet_train_gt.zip',
- md5='3bfaf1988960909014f7987d2343060b',
- content=['annotation'],
- mapping=[['ic15_textdet_train_gt', 'annotations/train']]),
- ]),
- gatherer=dict(
- type='PairGatherer',
- img_suffixes=['.jpg', '.JPG'],
- rule=[r'img_(\d+)\.([jJ][pP][gG])', r'gt_img_\1.txt']),
- parser=dict(type='ICDARTxtTextDetAnnParser', encoding='utf-8-sig'),
- packer=dict(type='TextDetPacker'),
- dumper=dict(type='JsonDumper'),
-)
-
-test_preparer = dict(
- obtainer=dict(
- type='NaiveDataObtainer',
- cache_path=cache_path,
- files=[
- dict(
- url='https://rrc.cvc.uab.es/downloads/ch4_test_images.zip',
- save_name='ic15_textdet_test_img.zip',
- md5='97e4c1ddcf074ffcc75feff2b63c35dd',
- content=['image'],
- mapping=[['ic15_textdet_test_img', 'textdet_imgs/test']]),
- dict(
- url='https://rrc.cvc.uab.es/downloads/'
- 'Challenge4_Test_Task4_GT.zip',
- save_name='ic15_textdet_test_gt.zip',
- md5='8bce173b06d164b98c357b0eb96ef430',
- content=['annotation'],
- mapping=[['ic15_textdet_test_gt', 'annotations/test']]),
- ]),
- gatherer=dict(
- type='PairGatherer',
- img_suffixes=['.jpg', '.JPG'],
- rule=[r'img_(\d+)\.([jJ][pP][gG])', r'gt_img_\1.txt']),
- parser=dict(type='ICDARTxtTextDetAnnParser', encoding='utf-8-sig'),
- packer=dict(type='TextDetPacker'),
- dumper=dict(type='JsonDumper'),
-)
-
-delete = ['annotations', 'ic15_textdet_test_img', 'ic15_textdet_train_img']
-config_generator = dict(type='TextDetConfigGenerator')
-```
-
-#### ๆฐๆฎ้ไธ่ฝฝใ่งฃๅใ็งปๅจ (Obtainer)
-
-Dataset Preparer ไธญ๏ผ`obtainer` ๆจกๅ่ด่ดฃไบๆฐๆฎ้็ไธ่ฝฝใ่งฃๅๅ็งปๅจใๅฆไป๏ผMMOCR ๆๆถๅชๆไพไบ `NaiveDataObtainer`ใ้ๅธธๆฅ่ฏด๏ผๅ
็ฝฎ็ `NaiveDataObtainer` ๅณๅฏๅฎๆ็ปๅคง้จๅๅฏไปฅ้่ฟ็ด้พ่ฎฟ้ฎ็ๆฐๆฎ้็ไธ่ฝฝ๏ผๅนถๆฏๆ่งฃๅใ็งปๅจๆไปถๅ้ๅฝๅ็ญๆไฝใ็ถ่๏ผMMOCR ๆๆถไธๆฏๆ่ชๅจไธ่ฝฝๅญๅจๅจ็พๅบฆๆ่ฐทๆญ็ฝ็็ญ้่ฆ็ป้ๆ่ฝ่ฎฟ้ฎ่ตๆบ็ๆฐๆฎ้ใ ่ฟ้็ฎ่ฆไป็ปไธไธ `NaiveDataObtainer`.
-
-| ๅญๆฎตๅ | ๅซไน |
-| ---------- | ---------------------------------------------------------- |
-| cache_path | ๆฐๆฎ้็ผๅญ่ทฏๅพ๏ผ็จไบๅญๅจๆฐๆฎ้ๅๅค่ฟ็จไธญไธ่ฝฝ็ๅ็ผฉๅ
็ญๆไปถ |
-| data_root | ๆฐๆฎ้ๅญๅจ็ๆ น็ฎๅฝ |
-| files | ๆฐๆฎ้ๆไปถๅ่กจ๏ผ็จไบๆ่ฟฐๆฐๆฎ้็ไธ่ฝฝไฟกๆฏ |
-
-`files` ๅญๆฎตๆฏไธไธชๅ่กจ๏ผๅ่กจไธญ็ๆฏไธชๅ
็ด ้ฝๆฏไธไธชๅญๅ
ธ๏ผ็จไบๆ่ฟฐไธไธชๆฐๆฎ้ๆไปถ็ไธ่ฝฝไฟกๆฏใๅฆไธ่กจๆ็คบ๏ผ
-
-| ๅญๆฎตๅ | ๅซไน |
-| ----------------- | -------------------------------------------------------------------- |
-| url | ๆฐๆฎ้ๆไปถ็ไธ่ฝฝ้พๆฅ |
-| save_name | ๆฐๆฎ้ๆไปถ็ไฟๅญๅ็งฐ |
-| md5 (ๅฏ้) | ๆฐๆฎ้ๆไปถ็ md5 ๅผ๏ผ็จไบๆ ก้ชไธ่ฝฝ็ๆไปถๆฏๅฆๅฎๆด |
-| split ๏ผๅฏ้๏ผ | ๆฐๆฎ้ๆไปถๆๅฑ็ๆฐๆฎ้ๅๅ๏ผๅฆ `train`๏ผ`test` ็ญ๏ผ่ฏฅๅญๆฎตๅฏไปฅ็ฉบ็ผบ |
-| content ๏ผๅฏ้๏ผ | ๆฐๆฎ้ๆไปถ็ๅ
ๅฎน๏ผๅฆ `image`๏ผ`annotation` ็ญ๏ผ่ฏฅๅญๆฎตๅฏไปฅ็ฉบ็ผบ |
-| mapping ๏ผๅฏ้๏ผ | ๆฐๆฎ้ๆไปถ็่งฃๅๆ ๅฐ๏ผ็จไบๆๅฎ่งฃๅๅ็ๆไปถๅญๅจ็ไฝ็ฝฎ๏ผ่ฏฅๅญๆฎตๅฏไปฅ็ฉบ็ผบ |
-
-ๅๆถ๏ผDataset Preparer ๅญๅจไปฅไธ็บฆๅฎ๏ผ
-
-- ไธๅ็ฑปๅ็ๆฐๆฎ้็ๅพ็็ปไธ็งปๅจๅฐๅฏนๅบ็ฑปๅซ `{taskname}_imgs/{split}/`ๆไปถๅคนไธ๏ผๅฆ `textdet_imgs/train/`ใ
-- ๅฏนไบไธไธชๆ ๆณจๆไปถๅ
ๅซๆๆๅพๅ็ๆ ๆณจไฟกๆฏ็ๆ
ๅต๏ผๆ ๆณจ็งปๅฐๅฐ`annotations/{split}.*`ๆไปถไธญใ ๅฆ `annotations/train.json`ใ
-- ๅฏนไบไธไธชๆ ๆณจๆไปถๅ
ๅซไธไธชๅพๅ็ๆ ๆณจไฟกๆฏ็ๆ
ๅต๏ผๆๆ็ๆ ๆณจๆไปถ็งปๅจๅฐ`annotations/{split}/`ๆไปถไธญใ ๅฆ `annotations/train/`ใ
-- ๅฏนไบไธไบๅ
ถไป็็นๆฎๆ
ๅต๏ผๆฏๅฆๆๆ่ฎญ็ปใๆต่ฏใ้ช่ฏ็ๅพๅ้ฝๅจไธไธชๆไปถๅคนไธ๏ผๅฏไปฅๅฐๅพๅ็งปๅจๅฐ่ชๅทฑ่ฎพๅฎ็ๆไปถๅคนไธ๏ผๆฏๅฆ `{taskname}_imgs/imgs/`๏ผๅๆถ่ฆๅจๅ็ปญ็ `gatherer` ๆจกๅไธญๆๅฎๅพๅ็ๅญๅจไฝ็ฝฎใ
-
-็คบไพ้
็ฝฎๅฆไธ๏ผ
-
-```python
- obtainer=dict(
- type='NaiveDataObtainer',
- cache_path=cache_path,
- files=[
- dict(
- url='https://rrc.cvc.uab.es/downloads/ch4_training_images.zip',
- save_name='ic15_textdet_train_img.zip',
- md5='c51cbace155dcc4d98c8dd19d378f30d',
- content=['image'],
- mapping=[['ic15_textdet_train_img', 'textdet_imgs/train']]),
- dict(
- url='https://rrc.cvc.uab.es/downloads/'
- 'ch4_training_localization_transcription_gt.zip',
- save_name='ic15_textdet_train_gt.zip',
- md5='3bfaf1988960909014f7987d2343060b',
- content=['annotation'],
- mapping=[['ic15_textdet_train_gt', 'annotations/train']]),
- ]),
-```
-
-#### ๆฐๆฎ้ๆถ้ (Gatherer)
-
-`gatherer` ้ๅๆฐๆฎ้็ฎๅฝไธ็ๆไปถ๏ผๅฐๅพๅไธๆ ๆณจๆไปถไธไธๅฏนๅบ๏ผๅนถๆด็ๅบไธไปฝๆไปถๅ่กจไพ `parser` ่ฏปๅใๅ ๆญค๏ผ้ฆๅ
้่ฆ็ฅ้ๅฝๅๆฐๆฎ้ไธ๏ผๅพ็ๆไปถไธๆ ๆณจๆไปถๅน้
็่งๅใOCR ๆฐๆฎ้ๆไธค็งๅธธ็จๆ ๆณจไฟๅญๅฝขๅผ๏ผไธ็งไธบๅคไธชๆ ๆณจๆไปถๅฏนๅบๅคๅผ ๅพ็๏ผไธ็งๅไธบๅไธชๆ ๆณจๆไปถๅฏนๅบๅคๅผ ๅพ็๏ผๅฆ๏ผ
-
-```text
-ๅคๅฏนๅค
-โโโ {taskname}_imgs/{split}/img_img_1.jpg
-โโโ annotations/{split}/gt_img_1.txt
-โโโ {taskname}_imgs/{split}/img_2.jpg
-โโโ annotations/{split}/gt_img_2.txt
-โโโ {taskname}_imgs/{split}/img_3.JPG
-โโโ annotations/{split}/gt_img_3.txt
-
-ๅๅฏนๅค
-โโโ {taskname}/{split}/img_1.jpg
-โโโ {taskname}/{split}/img_2.jpg
-โโโ {taskname}/{split}/img_3.JPG
-โโโ annotations/gt.txt
-```
-
-ๅ
ทไฝ่ฎพ่ฎกๅฆไธๆ็คบ
-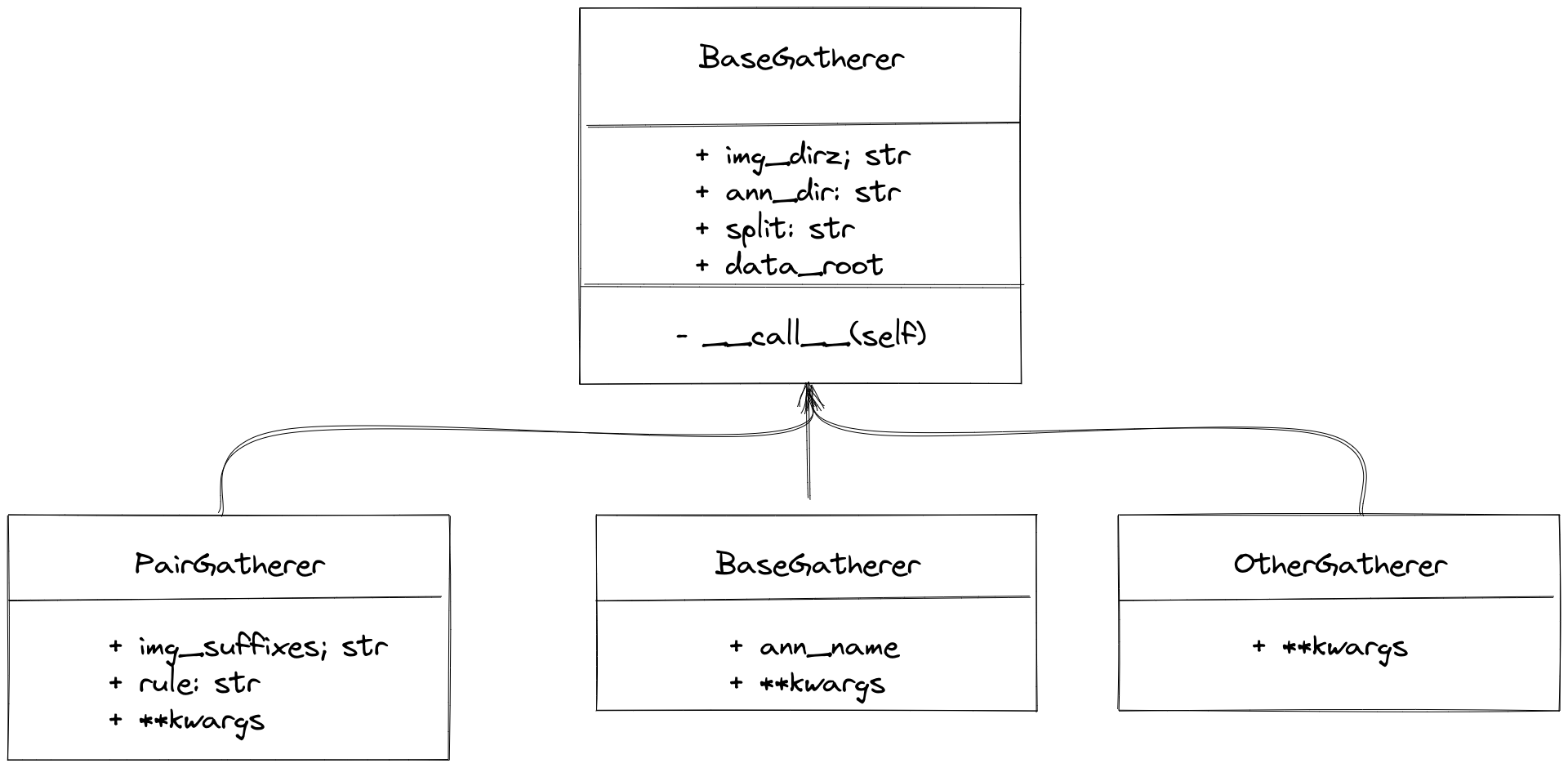
-
-MMOCR ๅ
็ฝฎไบ `PairGatherer` ไธ `MonoGatherer` ๆฅๅค็ไปฅไธ่ฟไธค็งๅธธ็จๆ
ๅตใๅ
ถไธญ `PairGatherer` ็จไบๅคๅฏนๅค็ๆ
ๅต๏ผ`MonoGatherer` ็จไบๅๅฏนๅค็ๆ
ๅตใ
-
-```{note}
-ไธบไบ็ฎๅๅค็๏ผgatherer ็บฆๅฎๆฐๆฎ้็ๅพ็ๅๆ ๆณจ้่ฆๅๅซๅจๅญๅจ `{taskname}_imgs/{split}/` ๅ `annotations/` ไธใ็นๅซๅฐ๏ผๅฏนไบๅคๅฏนๅค็ๆ
ๅต๏ผๆ ๆณจๆไปถ้่ฆๆพ็ฝฎไบ `annotations/{split}`ใ
-```
-
-- ๅจๅคๅฏนๅค็ๆ
ๅตไธ๏ผ`PairGatherer` ้่ฆๆ็
งไธๅฎ็ๅฝๅ่งๅๆพๅฐๅพ็ๆไปถๅๅฏนๅบ็ๆ ๆณจๆไปถใ้ฆๅ
๏ผ้่ฆ้่ฟ `img_suffixes` ๅๆฐๆๅฎๅพ็็ๅ็ผๅ๏ผๅฆไธ่ฟฐไพๅญไธญ็ `img_suffixes=[.jpg,.JPG]`ใๆญคๅค๏ผ่ฟ้่ฆ้่ฟ[ๆญฃๅ่กจ่พพๅผ](https://docs.python.org/3/library/re.html) `rule`, ๆฅๆๅฎๅพ็ไธๆ ๆณจๆไปถ็ๅฏนๅบๅ
ณ็ณป๏ผๅ
ถไธญ๏ผ่งๅ `rule` ๆฏไธไธช**ๆญฃๅ่กจ่พพๅผๅฏน**๏ผไพๅฆ `rule=[r'img_(\d+)\.([jJ][pP][gG])'๏ผr'gt_img_\1.txt']`ใ ็ฌฌไธไธชๆญฃๅ่กจ่พพๅผ็จไบๅน้
ๅพ็ๆไปถๅ๏ผ`\d+` ็จไบๅน้
ๅพ็็ๅบๅท๏ผ`([jJ][pP][gG])` ็จไบๅน้
ๅพ็็ๅ็ผๅใ ็ฌฌไบไธชๆญฃๅ่กจ่พพๅผ็จไบๅน้
ๆ ๆณจๆไปถๅ๏ผๅ
ถไธญ `\1` ๅๅฐๅน้
ๅฐ็ๅพ็ๅบๅทไธๆ ๆณจๆไปถๅบๅทๅฏนๅบ่ตทๆฅใ็คบไพ้
็ฝฎไธบ
-
-```python
- gatherer=dict(
- type='PairGatherer',
- img_suffixes=['.jpg', '.JPG'],
- rule=[r'img_(\d+)\.([jJ][pP][gG])', r'gt_img_\1.txt']),
-```
-
-- ๅๅฏนๅค็ๆ
ๅต้ๅธธๆฏ่พ็ฎๅ๏ผ็จๆทๅช้่ฆๆๅฎๆ ๆณจๆไปถๅๅณๅฏใๅฏนไบ่ฎญ็ป้็คบไพ้
็ฝฎไธบ
-
-```python
- gatherer=dict(type='MonoGatherer', ann_name='train.txt'),
-```
-
-MMOCR ๅๆ ทๅฏน `Gatherer` ็่ฟๅๅผๅไบ็บฆๅฎ๏ผ`Gatherer` ไผ่ฟๅไธคไธชๅ
็ด ็ๅ
็ป๏ผ็ฌฌไธไธชๅ
็ด ไธบๅพๅ่ทฏๅพๅ่กจ(ๅ
ๅซๆๆๅพๅ่ทฏๅพ) ๆ่
ๆๆๅพๅๆๅจ็ๆไปถๅคน๏ผ ็ฌฌไบไธชๅ
็ด ไธบๆ ๆณจๆไปถ่ทฏๅพๅ่กจ(ๅ
ๅซๆๆๆ ๆณจๆไปถ่ทฏๅพ)ๆ่
ๆ ๆณจๆไปถ็่ทฏๅพ(่ฏฅๆ ๆณจๆไปถๅ
ๅซๆๆๅพๅๆ ๆณจไฟกๆฏ)ใ
-ๅ
ทไฝ่่จ๏ผ`PairGatherer` ็่ฟๅๅผไธบ(ๅพๅ่ทฏๅพๅ่กจ๏ผ ๆ ๆณจๆไปถ่ทฏๅพๅ่กจ)๏ผ็คบไพๅฆไธ๏ผ
-
-```python
- (['{taskname}_imgs/{split}/img_1.jpg', '{taskname}_imgs/{split}/img_2.jpg', '{taskname}_imgs/{split}/img_3.JPG'],
- ['annotations/{split}/gt_img_1.txt', 'annotations/{split}/gt_img_2.txt', 'annotations/{split}/gt_img_3.txt'])
-```
-
-`MonoGatherer` ็่ฟๅๅผไธบ(ๅพๅๆไปถๅคน่ทฏๅพ๏ผ ๆ ๆณจๆไปถ่ทฏๅพ)๏ผ ็คบไพไธบ๏ผ
-
-```python
- ('{taskname}/{split}', 'annotations/gt.txt')
-```
-
-#### ๆฐๆฎ้่งฃๆ (Parser)
-
-`Parser` ไธป่ฆ็จไบ่งฃๆๅๅง็ๆ ๆณจๆไปถ๏ผๅ ไธบๅๅงๆ ๆณจๆ
ๅตๅค็งๅคๆ ท๏ผๅ ๆญค MMOCR ๆไพไบ `BaseParser` ไฝไธบๅบ็ฑป๏ผ็จๆทๅฏไปฅ็ปงๆฟ่ฏฅ็ฑปๆฅๅฎ็ฐ่ชๅทฑ็ `Parser`ใๅจ `BaseParser` ไธญ๏ผMMOCR ่ฎพ่ฎกไบไธคไธชๆฅๅฃ๏ผ`parse_files` ๅ `parse_file`๏ผ็บฆๅฎๅจๅ
ถไธญ่ฟ่กๆ ๆณจ็่งฃๆใ่ๅฏนไบ `Gatherer` ็ไธค็งไธๅ่พๅ
ฅๆ
ๅต๏ผๅคๅฏนๅคใๅๅฏนๅค๏ผ๏ผ่ฟไธคไธชๆฅๅฃ็ๅฎ็ฐๅๅบๆๆไธๅใ
-
-- `BaseParser` ้ป่ฎคๅค็**ๅคๅฏนๅค**็ๆ
ๅตใๅ
ถไธญ๏ผ็ฑ `parer_files` ๅฐๆฐๆฎๅนถ่กๅๅ่ณๅคไธช `parse_file` ่ฟ็จ๏ผๅนถ็ฑๆฏไธช `parse_file` ๅๅซ่ฟ่กๅไธชๅพๅๆ ๆณจ็่งฃๆใ
-- ๅฏนไบ**ๅๅฏนๅค**็ๆ
ๅต๏ผ็จๆทๅ้่ฆ้ๅ `parse_files`๏ผไปฅๅฎ็ฐๅ ่ฝฝๆ ๆณจ๏ผๅนถ่ฟๅ่ง่็็ปๆใ
-
-`BaseParser` ็ๆฅๅฃๅฎไนๅฆไธๆ็คบ๏ผ
-
-```python
-class BaseParser:
-
- def __call__(self, img_paths, ann_paths):
- return self.parse_files(img_paths, ann_paths)
-
- def parse_files(self, img_paths: Union[List[str], str],
- ann_paths: Union[List[str], str]) -> List[Tuple]:
- samples = track_parallel_progress_multi_args(
- self.parse_file, (img_paths, ann_paths), nproc=self.nproc)
- return samples
-
- @abstractmethod
- def parse_file(self, img_path: str, ann_path: str) -> Tuple:
-
- raise NotImplementedError
-```
-
-ไธบไบไฟ่ฏๅ็ปญๆจกๅ็็ปไธๆง๏ผMMOCR ๅฏน `parse_files` ไธ `parse_file` ็่ฟๅๅผๅไบ็บฆๅฎใ `parse_file` ็่ฟๅๅผไธบไธไธชๅ
็ป๏ผๅ
็ปไธญ็็ฌฌไธไธชๅ
็ด ไธบๅพๅ่ทฏๅพ๏ผ็ฌฌไบไธชๅ
็ด ไธบๆ ๆณจไฟกๆฏใๆ ๆณจไฟกๆฏไธบไธไธชๅ่กจ๏ผๅ่กจไธญ็ๆฏไธชๅ
็ด ไธบไธไธชๅญๅ
ธ๏ผๅญๅ
ธไธญ็ๅญๆฎตไธบ`poly`, `text`, `ignore`๏ผๅฆไธๆ็คบ๏ผ
-
-```python
-# An example of returned values:
-(
- 'imgs/train/xxx.jpg',
- [
- dict(
- poly=[0, 1, 1, 1, 1, 0, 0, 0],
- text='hello',
- ignore=False),
- ...
- ]
-)
-```
-
-`parse_files` ็่พๅบไธบไธไธชๅ่กจ๏ผๅ่กจไธญ็ๆฏไธชๅ
็ด ไธบ `parse_file` ็่ฟๅๅผใ ็คบไพไธบ๏ผ
-
-```python
-[
- (
- 'imgs/train/xxx.jpg',
- [
- dict(
- poly=[0, 1, 1, 1, 1, 0, 0, 0],
- text='hello',
- ignore=False),
- ...
- ]
- ),
- ...
-]
-```
-
-#### ๆฐๆฎ้่ฝฌๆข (Packer)
-
-`packer` ไธป่ฆๆฏๅฐๆฐๆฎ่ฝฌๅๅฐ็ปไธ็ๆ ๆณจๆ ผๅผ, ๅ ไธบ่พๅ
ฅ็ๆฐๆฎไธบ Parsers ็่พๅบ๏ผๆ ผๅผๅทฒ็ปๅบๅฎ๏ผ ๅ ๆญค Packer ๅช้่ฆๅฐ่พๅ
ฅ็ๆ ผๅผ่ฝฌๅไธบๆฏ็งไปปๅก็ปไธ็ๆ ๆณจๆ ผๅผๅณๅฏใๅฆไป MMOCR ๆฏๆ็ไปปๅกๆๆๆฌๆฃๆตใๆๆฌ่ฏๅซใ็ซฏๅฏน็ซฏOCR ไปฅๅๅ
ณ้ฎไฟกๆฏๆๅ๏ผMMOCR ้ๅฏนๆฏไธชไปปๅกๅๆๅฏนๅบ็ Packer๏ผๅฆไธๆ็คบ๏ผ
-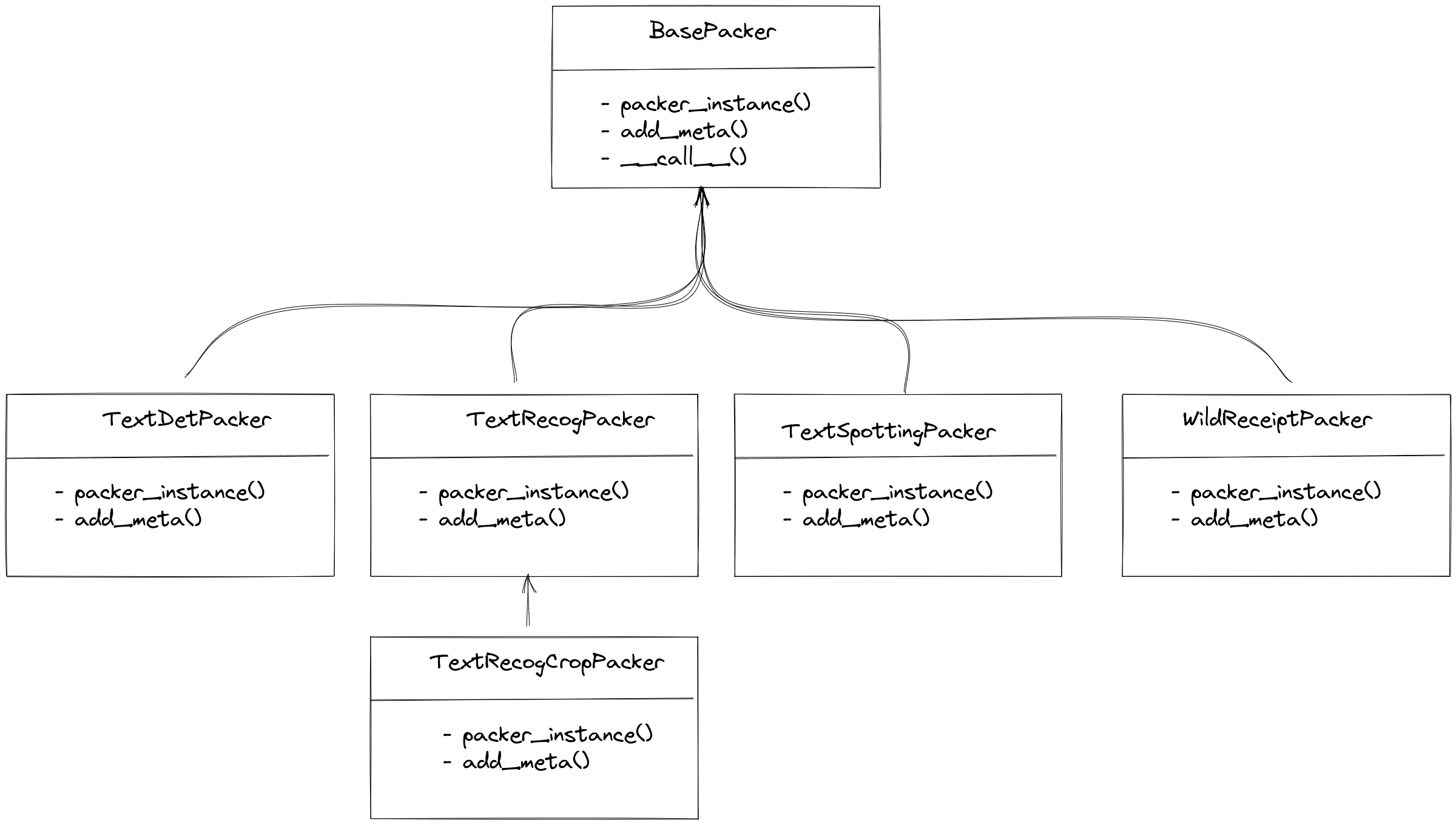
-
-ๅฏนไบๆๅญๆฃๆตใ็ซฏๅฏน็ซฏOCRๅๅ
ณ้ฎไฟกๆฏๆๅ๏ผMMOCR ๅๆๅฏไธๅฏนๅบ็ `Packer`ใ่ๅจๆๅญ่ฏๅซ้ขๅ๏ผ MMOCR ๅๆไพไบไธค็ง `Packer`๏ผๅๅซไธบ `TextRecogPacker` ๅ `TextRecogCropPacker`๏ผๅ
ถๅๅ ๅจไธๆๅญ่ฏๅซ็ๆฐๆฎ้ๅญๅจไธค็งๆ
ๅต๏ผ
-
-- ๆฏไธชๅพๅๅไธบไธไธช่ฏๅซๆ ทๆฌ๏ผ`parser` ่ฟๅ็ๆ ๆณจไฟกๆฏไป
ไธบไธไธช`dict(text='xxx')`๏ผๆญคๆถไฝฟ็จ `TextRecogPacker` ๅณๅฏใ
-- ๆฐๆฎ้ๆฒกๆๅฐๆๅญไปๅพๅไธญ่ฃๅชๅบๆฅ๏ผๆฌ่ดจๆฏไธไธช็ซฏๅฏน็ซฏOCR็ๆ ๆณจ๏ผๅ
ๅซไบๆๅญ็ไฝ็ฝฎไฟกๆฏไปฅๅๅฏนๅบ็ๆๆฌไฟกๆฏ๏ผ`TextRecogCropPacker` ไผๅฐๆๅญไปๅพๅไธญ่ฃๅชๅบๆฅ๏ผ็ถๅๅ่ฝฌๅๆๆๅญ่ฏๅซ็็ปไธๆ ผๅผใ
-
-#### ๆ ๆณจไฟๅญ (Dumper)
-
-`dumper` ๆฅๅณๅฎ่ฆๅฐๆฐๆฎไฟๅญไธบไฝ็งๆ ผๅผใ็ฎๅ๏ผMMOCR ๆฏๆ `JsonDumper`๏ผ `WildreceiptOpensetDumper`๏ผๅ `TextRecogLMDBDumper`ใไปไปฌๅๅซ็จไบๅฐๆฐๆฎไฟๅญไธบๆ ๅ็ MMOCR Json ๆ ผๅผใWildreceipt ๆ ผๅผ๏ผๅๆๆฌ่ฏๅซ้ขๅๅญฆๆฏ็ๅธธ็จ็ LMDB ๆ ผๅผใ
-
-#### ไธดๆถๆไปถๆธ
็ (Delete)
-
-ๅจๅค็ๆฐๆฎ้ๆถ๏ผๅพๅพไผไบง็ไธไบไธ้่ฆ็ไธดๆถๆไปถใ่ฟ้ๅฏไปฅไปฅๅ่กจ็ๅฝขๅผไผ ๅ
ฅ่ฟไบๆไปถๆๆไปถๅคน๏ผๅจ็ปๆ่ฝฌๆขๆถๅณไผๅ ้คใ
-
-#### ็ๆๅบ็ก้
็ฝฎ (ConfigGenerator)
-
-ไธบไบๅจๆฐๆฎ้ๅๅคๅฎๆฏๅๅฏไปฅ่ชๅจ็ๆๅบ็ก้
็ฝฎ๏ผ็ฎๅ๏ผMMOCR ๆไปปๅกๅฎ็ฐไบ `TextDetConfigGenerator`ใ`TextRecogConfigGenerator` ๅ `TextSpottingConfigGenerator`ใๅฎไปฌๆฏๆ็ไธป่ฆๅๆฐๅฆไธ๏ผ
-
-| ๅญๆฎตๅ | ๅซไน |
-| ----------- | --------------------------------------------------------------------------------------------------------------------------------------------------- |
-| data_root | ๆฐๆฎ้ๅญๅจ็ๆ น็ฎๅฝ |
-| train_anns | ้
็ฝฎๆไปถๅ
่ฎญ็ป้ๆ ๆณจ็่ทฏๅพใ่ฅไธๆๅฎ๏ผๅ้ป่ฎคไธบ `[dict(ann_file='{taskname}_train.json', dataset_postfix='']`ใ |
-| val_anns | ้
็ฝฎๆไปถๅ
้ช่ฏ้ๆ ๆณจ็่ทฏๅพใ่ฅไธๆๅฎ๏ผๅ้ป่ฎคไธบ็ฉบใ |
-| test_anns | ้
็ฝฎๆไปถๅ
ๆต่ฏ้ๆ ๆณจ็่ทฏๅพใ่ฅไธๆๅฎ๏ผๅ้ป่ฎคๆๅ `[dict(ann_file='{taskname}_test.json', dataset_postfix='']`ใ |
-| config_path | ็ฎๆณๅบๅญๆพ้
็ฝฎๆไปถ็่ทฏๅพ๏ผ้
็ฝฎ็ๆๅจไผๅฐ้ป่ฎค้
็ฝฎๅๅ
ฅ `{config_path}/{taskname}/_base_/datasets/{dataset_name}.py` ไธใ่ฅไธๆๅฎ๏ผๅ้ป่ฎคไธบ `configs/` |
-
-ๅจๅๅคๅฅฝๆฐๆฎ้็ๆๆๆไปถๅ๏ผ้
็ฝฎ็ๆๅจๅฐฑไผ่ชๅจ็ๆ่ฐ็จ่ฏฅๆฐๆฎ้ๆ้่ฆ็ๅบ็ก้
็ฝฎๆไปถใไธ้ข็ปๅบไบไธไธชๆๅฐๅ็ `TextDetConfigGenerator` ้
็ฝฎ็คบไพ๏ผ
-
-```python
-config_generator = dict(type='TextDetConfigGenerator')
-```
-
-็ๆๅ็ๆไปถ้ป่ฎคไผ่ขซ็ฝฎไบ `configs/{task}/_base_/datasets/` ไธใไพๅฆ๏ผๆฌไพไธญ๏ผicdar 2015 ็ๅบ็ก้
็ฝฎๆไปถๅฐฑไผ่ขซ็ๆๅจ `configs/textdet/_base_/datasets/icdar2015.py` ไธ๏ผ
-
-```python
-icdar2015_textdet_data_root = 'data/icdar2015'
-
-icdar2015_textdet_train = dict(
- type='OCRDataset',
- data_root=icdar2015_textdet_data_root,
- ann_file='textdet_train.json',
- filter_cfg=dict(filter_empty_gt=True, min_size=32),
- pipeline=None)
-
-icdar2015_textdet_test = dict(
- type='OCRDataset',
- data_root=icdar2015_textdet_data_root,
- ann_file='textdet_test.json',
- test_mode=True,
- pipeline=None)
-```
-
-ๅๅฆๆฐๆฎ้ๆฏ่พ็นๆฎ๏ผๆ ๆณจๅญๅจ็ๅ ไธชๅไฝ๏ผ้
็ฝฎ็ๆๅจไนๆฏๆๅจๅบ็ก้
็ฝฎไธญ็ๆๆๅๅ่ชๅไฝ็ๅ้๏ผไฝ่ฟ้่ฆ็จๆทๅจ่ฎพ็ฝฎๆถ็จไธๅ็ `dataset_postfix` ๅบๅใไพๅฆ๏ผICDAR 2015 ๆๅญ่ฏๅซๆฐๆฎ็ๆต่ฏ้ๅฐฑๅญๅจ็ๅ็ๅ 1811 ไธค็งๆ ๆณจ็ๆฌ๏ผๅฏไปฅๅจ `test_anns` ไธญๆๅฎๅฎไปฌ๏ผๅฆไธๆ็คบ๏ผ
-
-```python
-config_generator = dict(
- type='TextRecogConfigGenerator',
- test_anns=[
- dict(ann_file='textrecog_test.json'),
- dict(dataset_postfix='857', ann_file='textrecog_test_857.json')
- ])
-```
-
-้
็ฝฎ็ๆๅจไผ็ๆไปฅไธ้
็ฝฎ๏ผ
-
-```python
-icdar2015_textrecog_data_root = 'data/icdar2015'
-
-icdar2015_textrecog_train = dict(
- type='OCRDataset',
- data_root=icdar2015_textrecog_data_root,
- ann_file='textrecog_train.json',
- pipeline=None)
-
-icdar2015_textrecog_test = dict(
- type='OCRDataset',
- data_root=icdar2015_textrecog_data_root,
- ann_file='textrecog_test.json',
- test_mode=True,
- pipeline=None)
-
-icdar2015_1811_textrecog_test = dict(
- type='OCRDataset',
- data_root=icdar2015_textrecog_data_root,
- ann_file='textrecog_test_1811.json',
- test_mode=True,
- pipeline=None)
-```
-
-ๆไบ่ฏฅๆไปถๅ๏ผMMOCR ๅฐฑ่ฝไปๆจกๅ็้
็ฝฎๆไปถไธญ็ดๆฅๅฏผๅ
ฅ่ฏฅๆฐๆฎ้ๅฐ `dataloader` ไธญไฝฟ็จ๏ผไปฅไธๆ ทไพ่้่ช [`configs/textdet/dbnet/dbnet_resnet18_fpnc_1200e_icdar2015.py`](/configs/textdet/dbnet/dbnet_resnet18_fpnc_1200e_icdar2015.py)๏ผ๏ผ
-
-```python
-_base_ = [
- '../_base_/datasets/icdar2015.py',
- # ...
-]
-
-# dataset settings
-icdar2015_textdet_train = _base_.icdar2015_textdet_train
-icdar2015_textdet_test = _base_.icdar2015_textdet_test
-# ...
-
-train_dataloader = dict(
- dataset=icdar2015_textdet_train)
-
-val_dataloader = dict(
- dataset=icdar2015_textdet_test)
-
-test_dataloader = val_dataloader
-```
-
-```{note}
-้ค้็จๆทๅจ่ฟ่ก่ๆฌ็ๆถๅๆๅจๆๅฎไบ `overwrite-cfg`๏ผ้
็ฝฎ็ๆๅจ้ป่ฎคไธไผ่ชๅจ่ฆ็ๅทฒ็ปๅญๅจ็ๅบ็ก้
็ฝฎๆไปถใ
-```
-
-## ๅ Dataset Preparer ๆทปๅ ๆฐ็ๆฐๆฎ้
-
-### ๆทปๅ ๅ
ฌๅผๆฐๆฎ้
-
-MMOCR ๅทฒ็ปๆฏๆไบ่ฎธๅค[ๅธธ็จ็ๅ
ฌๅผๆฐๆฎ้](./datasetzoo.md)ใๅฆๆไฝ ๆณ็จ็ๆฐๆฎ้่ฟๆฒกๆ่ขซๆฏๆ๏ผๅนถไธไฝ ไนๆฟๆไธบ MMOCR ๅผๆบ็คพๅบ[่ดก็ฎไปฃ็ ](../../notes/contribution_guide.md)๏ผไฝ ๅฏไปฅๆ็
งไปฅไธๆญฅ้ชคๆฅๆทปๅ ไธไธชๆฐ็ๆฐๆฎ้ใ
-
-ๆฅไธๆฅไปฅๆทปๅ **ICDAR2013** ๆฐๆฎ้ไธบไพ๏ผๅฑ็คบๅฆไฝไธๆญฅไธๆญฅๅฐๆทปๅ ไธไธชๆฐ็ๅ
ฌๅผๆฐๆฎ้ใ
-
-#### ๆทปๅ `metafile.yml`
-
-้ฆๅ
๏ผ็กฎ่ฎค `dataset_zoo/` ไธญไธๅญๅจๅๅคๆทปๅ ็ๆฐๆฎ้ใ็ถๅๆไปฌๅ
ๆฐๅปบไปฅๅพ
ๆทปๅ ๆฐๆฎ้ๅฝๅ็ๆไปถๅคน๏ผๅฆ `icdar2013/`๏ผ้ๅธธ๏ผไฝฟ็จไธๅ
ๅซ็ฌฆๅท็ๅฐๅ่ฑๆๅญๆฏๅๆฐๅญๆฅๅฝๅๆฐๆฎ้๏ผใๅจ `icdar2013/` ๆไปถๅคนไธญ๏ผๆฐๅปบ `metafile.yml` ๆไปถ๏ผๅนถๆ็
งไปฅไธๆจกๆฟๆฅๅกซๅ
ๆฐๆฎ้็ๅบๆฌไฟกๆฏ๏ผ
-
-```yaml
-Name: 'Incidental Scene Text IC13'
-Paper:
- Title: ICDAR 2013 Robust Reading Competition
- URL: https://www.imlab.jp/publication_data/1352/icdar_competition_report.pdf
- Venue: ICDAR
- Year: '2013'
- BibTeX: '@inproceedings{karatzas2013icdar,
- title={ICDAR 2013 robust reading competition},
- author={Karatzas, Dimosthenis and Shafait, Faisal and Uchida, Seiichi and Iwamura, Masakazu and i Bigorda, Lluis Gomez and Mestre, Sergi Robles and Mas, Joan and Mota, David Fernandez and Almazan, Jon Almazan and De Las Heras, Lluis Pere},
- booktitle={2013 12th international conference on document analysis and recognition},
- pages={1484--1493},
- year={2013},
- organization={IEEE}}'
-Data:
- Website: https://rrc.cvc.uab.es/?ch=2
- Language:
- - English
- Scene:
- - Natural Scene
- Granularity:
- - Word
- Tasks:
- - textdet
- - textrecog
- - textspotting
- License:
- Type: N/A
- Link: N/A
- Format: .txt
- Keywords:
- - Horizontal
-```
-
-#### ๆทปๅ ๆ ๆณจ็คบไพ
-
-ๆๅ๏ผๅฏไปฅๅจ `dataset_zoo/icdar2013/` ็ฎๅฝไธๆทปๅ ๆ ๆณจ็คบไพๆไปถ `sample_anno.md` ไปฅๅธฎๅฉๆๆกฃ่ๆฌๅจ็ๆๆๆกฃๆถๆทปๅ ๆ ๆณจ็คบไพ๏ผๆ ๆณจ็คบไพๆไปถๆฏไธไธช Markdown ๆไปถ๏ผๅ
ถๅ
ๅฎน้ๅธธๅ
ๅซไบๅไธชๆ ทๆฌ็ๅๅงๆฐๆฎๆ ผๅผใไพๅฆ๏ผไปฅไธไปฃ็ ๅๅฑ็คบไบ ICDAR2013 ๆฐๆฎ้็ๆฐๆฎๆ ทไพๆไปถ๏ผ
-
-````markdown
- **Text Detection**
-
- ```text
- # train split
- # x1 y1 x2 y2 "transcript"
-
- 158 128 411 181 "Footpath"
- 443 128 501 169 "To"
- 64 200 363 243 "Colchester"
-
- # test split
- # x1, y1, x2, y2, "transcript"
-
- 38, 43, 920, 215, "Tiredness"
- 275, 264, 665, 450, "kills"
- 0, 699, 77, 830, "A"
- ```
-````
-
-#### ๆทปๅ ๅฏนๅบไปปๅก็้
็ฝฎๆไปถ
-
-ๅจ `dataset_zoo/icdar2013` ไธญ๏ผๆฅ็ๆทปๅ ไปฅไปปๅกๅ็งฐๅฝๅ็ `.py` ้
็ฝฎๆไปถใๅฆ `textdet.py`๏ผ`textrecog.py`๏ผ`textspotting.py`๏ผ`kie.py` ็ญใ้
็ฝฎๆจกๆฟๅฆไธๆ็คบ๏ผ
-
-```python
-data_root = ''
-data_cache = 'data/cache'
-train_prepare = dict(
- obtainer=dict(
- type='NaiveObtainer',
- data_cache=data_cache,
- files=[
- dict(
- url='xx',
- md5='',
- save_name='xxx',
- mapping=list())
- ]),
- gatherer=dict(type='xxxGatherer', **kwargs),
- parser=dict(type='xxxParser', **kwargs),
- packer=dict(type='TextxxxPacker'), # ๅฏนๅบไปปๅก็ Packer
- dumper=dict(type='JsonDumper'),
-)
-test_prepare = dict(
- obtainer=dict(
- type='NaiveObtainer',
- data_cache=data_cache,
- files=[
- dict(
- url='xx',
- md5='',
- save_name='xxx',
- mapping=list())
- ]),
- gatherer=dict(type='xxxGatherer', **kwargs),
- parser=dict(type='xxxParser', **kwargs),
- packer=dict(type='TextxxxPacker'), # ๅฏนๅบไปปๅก็ Packer
- dumper=dict(type='JsonDumper'),
-)
-```
-
-ไปฅๆไปถๆฃๆตไปปๅกไธบไพ๏ผๆฅไป็ป้
็ฝฎๆไปถ็ๅ
ทไฝๅ
ๅฎนใ
-ไธ่ฌๆ
ๅตไธ็จๆทๆ ้้ๆฐๅฎ็ฐๆฐ็ `obtainer`, `gatherer`, `packer` ๆ `dumper`๏ผไฝๆฏ้ๅธธ้่ฆๆ นๆฎๆฐๆฎ้็ๆ ๆณจๆ ผๅผๅฎ็ฐๆฐ็ `parser`ใ
-ๅฏนไบ `obtainer` ็้
็ฝฎ่ฟ้ไธๅจๅ่ฟ็ไป็ป๏ผๅฏไปฅๅ่ [ๆฐๆฎ้ไธ่ฝฝใ่งฃๅใ็งปๅจ](#ๆฐๆฎ้ไธ่ฝฝ่งฃๅ็งปๅจ-obtainer)ใ
-้ๅฏน `gatherer`๏ผ้่ฟ่งๅฏ่ทๅ็ ICDAR2013 ๆฐๆฎ้ๆไปถๅ็ฐ๏ผๅ
ถๆฏไธๅผ ๅพ็้ฝๆไธไธชๅฏนๅบ็ `.txt` ๆ ผๅผ็ๆ ๆณจๆไปถ๏ผ
-
-```text
-data_root
-โโโ textdet_imgs/train/
-โ โโโ img_1.jpg
-โ โโโ img_2.jpg
-โ โโโ ...
-โโโ annotations/train/
-โ โโโ gt_img_1.txt
-โ โโโ gt_img_2.txt
-โ โโโ ...
-```
-
-ไธๆฏไธชๆ ๆณจๆไปถๅไธๅพ็็ๅฏนๅบๅ
ณ็ณปไธบ๏ผ`gt_img_1.txt` ๅฏนๅบ `img_1.jpg`๏ผไปฅๆญค็ฑปๆจใๅ ๆญคๅฏไปฅไฝฟ็จ `PairGatherer` ๆฅ่ฟ่กๅน้
ใ
-
-```python
-gatherer=dict(
- type='PairGatherer',
- img_suffixes=['.jpg'],
- rule=[r'(\w+)\.jpg', r'gt_\1.txt'])
-```
-
-่งๅ `rule` ็ฌฌไธไธชๆญฃๅ่กจ่พพๅผ็จไบๅน้
ๅพ็ๆไปถๅ๏ผ็ฌฌไบไธชๆญฃๅ่กจ่พพๅผ็จไบๅน้
ๆ ๆณจๆไปถๅใๅจ่ฟ้๏ผไฝฟ็จ `(\w+)` ๆฅๅน้
ๅพ็ๆไปถๅ๏ผไฝฟ็จ `gt_\1.txt` ๆฅๅน้
ๆ ๆณจๆไปถๅ๏ผๅ
ถไธญ `\1` ่กจ็คบ็ฌฌไธไธชๆญฃๅ่กจ่พพๅผๅน้
ๅฐ็ๅ
ๅฎนใๅณ๏ผๅฎ็ฐไบๅฐ `img_xx.jpg` ๆฟๆขไธบ `gt_img_xx.txt` ็ๅ่ฝใ
-
-ๆฅไธๆฅ๏ผ้่ฆๅฎ็ฐ `parser`๏ผๅณๅฐๅๅงๆ ๆณจๆไปถ่งฃๆไธบๆ ๅๆ ผๅผใ้ๅธธๆฅ่ฏด๏ผ็จๆทๅจๆทปๅ ๆฐ็ๆฐๆฎ้ๅ๏ผๅฏไปฅๆต่งๅทฒๆฏๆๆฐๆฎ้็[่ฏฆๆ
้กต](./datasetzoo.md)๏ผๅนถๆฅ็ๆฏๅฆๅทฒๆ็ธๅๆ ผๅผ็ๆฐๆฎ้ใๅฆๆๅทฒๆ็ธๅๆ ผๅผ็ๆฐๆฎ้๏ผๅๅฏไปฅ็ดๆฅไฝฟ็จ่ฏฅๆฐๆฎ้็ `parser`ใๅฆๅ๏ผๅ้่ฆๅฎ็ฐๆฐ็ๆ ผๅผ่งฃๆๅจใ
-
-ๆฐๆฎๆ ผๅผ่งฃๆๅจ่ขซ็ปไธๅญๅจๅจ `mmocr/datasets/preparers/parsers` ็ฎๅฝไธใๆๆ็ `parser` ้ฝ้่ฆ็ปงๆฟ `BaseParser`๏ผๅนถๅฎ็ฐ `parse_file` ๆ `parse_files` ๆนๆณใๅ
ทไฝๅฏไปฅๅ่[ๆฐๆฎ้่งฃๆ](#ๆฐๆฎ้่งฃๆ)
-
-้่ฟ่งๅฏ ICDAR2013 ๆฐๆฎ้็ๆ ๆณจๆไปถ๏ผ
-
-```text
-158 128 411 181 "Footpath"
-443 128 501 169 "To"
-64 200 363 243 "Colchester"
-542, 710, 938, 841, "break"
-87, 884, 457, 1021, "could"
-517, 919, 831, 1024, "save"
-```
-
-ๆไปฌๅ็ฐๅ
็ฝฎ็ `ICDARTxtTextDetAnnParser` ๅทฒ็ปๅฏไปฅๆปก่ถณ้ๆฑ๏ผๅ ๆญคๅฏไปฅ็ดๆฅไฝฟ็จ่ฏฅ `parser`๏ผๅนถๅฐๅ
ถ้
็ฝฎๅฐ `preparer` ไธญใ
-
-```python
-parser=dict(
- type='ICDARTxtTextDetAnnParser',
- remove_strs=[',', '"'],
- encoding='utf-8',
- format='x1 y1 x2 y2 trans',
- separator=' ',
- mode='xyxy')
-```
-
-ๅ
ถไธญ๏ผ็ฑไบๆ ๆณจๆไปถไธญๆททๆไบๅคไฝ็ๅผๅท `โโ` ๅ้ๅท `,`๏ผๅฏไปฅ้่ฟๆๅฎ `remove_strs=[',', '"']` ๆฅ่ฟ่ก็งป้คใๅฆๅคๅจ `format` ไธญๆๅฎไบๆ ๆณจๆไปถ็ๆ ผๅผ๏ผๅ
ถไธญ `x1 y1 x2 y2 trans` ่กจ็คบๆ ๆณจๆไปถไธญ็ๆฏไธ่กๅ
ๅซไบๅไธชๅๆ ๅไธไธชๆๆฌๅ
ๅฎน๏ผไธๅๆ ๅๆๆฌๅ
ๅฎนไน้ดไฝฟ็จ็ฉบๆ ผๅ้๏ผ`separator`=' '๏ผใๅฆๅค๏ผ้่ฆๆๅฎ `mode` ไธบ `xyxy`๏ผ่กจ็คบๆ ๆณจๆไปถไธญ็ๅๆ ๆฏๅทฆไธ่งๅๅณไธ่ง็ๅๆ ๏ผ่ฟๆ ทไปฅๆฅ๏ผ`ICDARTxtTextDetAnnParser` ๅณๅฏๅฐ่ฏฅๆ ผๅผ็ๆ ๆณจ่งฃๆไธบ็ปไธๆ ผๅผใ
-
-ๅฏนไบ `packer`๏ผไปฅๆไปถๆฃๆตไปปๅกไธบไพ๏ผๅ
ถ `packer` ไธบ `TextDetPacker`๏ผๅ
ถ้
็ฝฎๅฆไธ๏ผ
-
-```python
-packer=dict(type='TextDetPacker')
-```
-
-ๆๅ๏ผๆๅฎ `dumper`๏ผ่ฟ้ไธ่ฌๆ
ๅตไธไฟๅญไธบjsonๆ ผๅผ๏ผๅ
ถ้
็ฝฎๅฆไธ๏ผ
-
-```python
-dumper=dict(type='JsonDumper')
-```
-
-็ป่ฟไธ่ฟฐ้
็ฝฎๅ๏ผ้ๅฏน ICDAR2013 ่ฎญ็ป้็้
็ฝฎๆไปถๅฆไธ๏ผ
-
-```python
-train_preparer = dict(
- obtainer=dict(
- type='NaiveDataObtainer',
- cache_path=cache_path,
- files=[
- dict(
- url='https://rrc.cvc.uab.es/downloads/'
- 'Challenge2_Training_Task12_Images.zip',
- save_name='ic13_textdet_train_img.zip',
- md5='a443b9649fda4229c9bc52751bad08fb',
- content=['image'],
- mapping=[['ic13_textdet_train_img', 'textdet_imgs/train']]),
- dict(
- url='https://rrc.cvc.uab.es/downloads/'
- 'Challenge2_Training_Task1_GT.zip',
- save_name='ic13_textdet_train_gt.zip',
- md5='f3a425284a66cd67f455d389c972cce4',
- content=['annotation'],
- mapping=[['ic13_textdet_train_gt', 'annotations/train']]),
- ]),
- gatherer=dict(
- type='PairGatherer',
- img_suffixes=['.jpg'],
- rule=[r'(\w+)\.jpg', r'gt_\1.txt']),
- parser=dict(
- type='ICDARTxtTextDetAnnParser',
- remove_strs=[',', '"'],
- format='x1 y1 x2 y2 trans',
- separator=' ',
- mode='xyxy'),
- packer=dict(type='TextDetPacker'),
- dumper=dict(type='JsonDumper'),
-)
-```
-
-ไธบไบๅจๆฐๆฎ้ๅๅคๅฎๆฏๅๅฏไปฅ่ชๅจ็ๆๅบ็ก้
็ฝฎ๏ผ ่ฟ้่ฆ้
็ฝฎไธไธๅฏนๅบไปปๅก็ `config_generator`ใ
-
-ๅจๆฌไพไธญ๏ผๅ ไธบไธบๆๅญๆฃๆตไปปๅก๏ผไป
้่ฆ่ฎพ็ฝฎ Generator ไธบ `TextDetConfigGenerator`ๅณๅฏ
-
-```python
-config_generator = dict(type='TextDetConfigGenerator', )
-```
-
-### ๆทปๅ ็งๆๆฐๆฎ้
-
-ๅพ
ๆดๆฐ...
diff --git a/pipeline/mmocr/docs/zh_cn/user_guides/data_prepare/kie.md b/pipeline/mmocr/docs/zh_cn/user_guides/data_prepare/kie.md
deleted file mode 100644
index ce8d146272dd287465885bf0ed78439d34c7e18a..0000000000000000000000000000000000000000
--- a/pipeline/mmocr/docs/zh_cn/user_guides/data_prepare/kie.md
+++ /dev/null
@@ -1,40 +0,0 @@
-# ๅ
ณ้ฎไฟกๆฏๆๅ
-
-```{note}
-ๆไปฌๆญฃๅชๅๅพ [Dataset Preparer](./dataset_preparer.md) ไธญๅขๅ ๆดๅคๆฐๆฎ้ใๅฏนไบ [Dataset Preparer](./dataset_preparer.md) ๆๆช่ฝๅฎๆดๆฏๆ็ๆฐๆฎ้๏ผๆฌ้กตๆไพไบไธ็ณปๅๆๅจไธ่ฝฝ็ๆญฅ้ชค๏ผไพๆ้่ฆ็็จๆทไฝฟ็จใ
-```
-
-## ๆฆ่ง
-
-ๅ
ณ้ฎไฟกๆฏๆๅไปปๅก็ๆฐๆฎ้๏ผๆไปถ็ฎๅฝๅบๆๅฆไธ้
็ฝฎ๏ผ
-
-```text
-โโโ wildreceipt
- โโโ class_list.txt
- โโโ dict.txt
- โโโ image_files
- โโโ test.txt
- โโโ train.txt
-```
-
-## ๅๅคๆญฅ้ชค
-
-### WildReceipt
-
-- ไธ่ฝฝๅนถ่งฃๅ [wildreceipt.tar](https://download.openmmlab.com/mmocr/data/wildreceipt.tar)
-
-### WildReceiptOpenset
-
-- ๅๅคๅฅฝ [WildReceipt](#WildReceipt)ใ
-- ่ฝฌๆข WildReceipt ๆ OpenSet ๆ ผๅผ:
-
-```bash
-# ไฝ ๅฏไปฅ่ฟ่กไปฅไธๅฝไปคไปฅ่ทๅๆดๅคๅฏ็จๅๆฐ๏ผ
-# python tools/data/kie/closeset_to_openset.py -h
-python tools/data/kie/closeset_to_openset.py data/wildreceipt/train.txt data/wildreceipt/openset_train.txt
-python tools/data/kie/closeset_to_openset.py data/wildreceipt/test.txt data/wildreceipt/openset_test.txt
-```
-
-```{note}
-[่ฟ็ฏๆ็จ](../tutorials/kie_closeset_openset.md)้่ฎฒ่ฟฐไบๆดๅค CloseSet ๅ OpenSet ๆฐๆฎๆ ผๅผไน้ด็ๅบๅซใ
-```
diff --git a/pipeline/mmocr/docs/zh_cn/user_guides/dataset_prepare.md b/pipeline/mmocr/docs/zh_cn/user_guides/dataset_prepare.md
deleted file mode 100644
index a0e3d0bad86a0f2eb130fb333e7a8047e5d6f533..0000000000000000000000000000000000000000
--- a/pipeline/mmocr/docs/zh_cn/user_guides/dataset_prepare.md
+++ /dev/null
@@ -1,153 +0,0 @@
-# ๆฐๆฎ้ๅๅค
-
-## ๅ่จ
-
-็ป่ฟๆฐๅๅนด็ๅๅฑ๏ผOCR ้ขๅๆถ็ฐๅบไบไธ็ณปๅ็็ธๅ
ณๆฐๆฎ้๏ผ่ฟไบๆฐๆฎ้ๅพๅพ้็จ้ฃๆ ผๅๅผ็ๆ ผๅผๆฅๆไพๆๆฌ็ๆ ๆณจๆไปถ๏ผไฝฟๅพ็จๆทๅจไฝฟ็จ่ฟไบๆฐๆฎ้ๆถไธๅพไธ่ฟ่กๆ ผๅผ่ฝฌๆขใๅ ๆญค๏ผไธบไบๆนไพฟ็จๆท่ฟ่กๆฐๆฎ้ๅๅค๏ผๆไปฌๆไพไบ[ไธ้ฎๅผ็ๆฐๆฎๅๅค่ๆฌ](./data_prepare/dataset_preparer.md)๏ผไฝฟๅพ็จๆทไป
้ไฝฟ็จไธ่กๅฝไปคๅณๅฏๅฎๆๆฐๆฎ้ๅๅค็ๅ
จ้จๆญฅ้ชคใ
-
-ๅจ่ฟไธ่๏ผๆไปฌๅฐไป็ปไธไธชๅ
ธๅ็ๆฐๆฎ้ๅๅคๆต็จ๏ผ
-
-1. [ไธ่ฝฝๆฐๆฎ้ๅนถๅฐๅ
ถๆ ผๅผ่ฝฌๆขไธบ MMOCR ๆฏๆ็ๆ ผๅผ](#ๆฐๆฎ้ไธ่ฝฝๅๆ ผๅผ่ฝฌๆข)
-2. [ไฟฎๆน้
็ฝฎๆไปถ](#ไฟฎๆน้
็ฝฎๆไปถ)
-
-็ถ่๏ผๅฆๆไฝ ๅทฒ็ปๆไบ MMOCR ๆฏๆ็ๆ ผๅผ็ๆฐๆฎ้๏ผ้ฃไน็ฌฌไธๆญฅๅฐฑไธๆฏๅฟ
้กป็ใไฝ ๅฏไปฅ้
่ฏป[ๆฐๆฎ้็ฑปๅๆ ๆณจๆ ผๅผ](../basic_concepts/datasets.md#ๆฐๆฎ้็ฑปๅๆ ๆณจๆ ผๅผ)ๆฅไบ่งฃๆดๅค็ป่ใ
-
-## ๆฐๆฎ้ไธ่ฝฝๅๆ ผๅผ่ฝฌๆข
-
-ไปฅ ICDAR 2015 ๆฐๆฎ้็ๆๆฌๆฃๆตไปปๅกๅๅคๆญฅ้ชคไธบไพ๏ผไฝ ๅฏไปฅๆง่กไปฅไธๅฝไปคๆฅๅฎๆๆฐๆฎ้ๅๅค๏ผ
-
-```shell
-python tools/dataset_converters/prepare_dataset.py icdar2015 --task textdet
-```
-
-ๅฝไปคๆง่กๅฎๆๅ๏ผๆฐๆฎ้ๅฐ่ขซไธ่ฝฝๅนถ่ฝฌๆข่ณ MMOCR ๆ ผๅผ๏ผๆไปถ็ฎๅฝ็ปๆๅฆไธ๏ผ
-
-```text
-data/icdar2015
-โโโ textdet_imgs
-โ โโโ test
-โ โโโ train
-โโโ textdet_test.json
-โโโ textdet_train.json
-```
-
-ๆฐๆฎๅๅคๅฎๆฏไปฅๅ๏ผไฝ ไนๅฏไปฅ้่ฟไฝฟ็จๆไปฌๆไพ็ๆฐๆฎ้ๆต่งๅทฅๅ
ท [browse_dataset.py](./useful_tools.md#ๆฐๆฎ้ๅฏ่งๅๅทฅๅ
ท) ๆฅๅฏ่งๅๆฐๆฎ้็ๆ ็ญพๆฏๅฆ่ขซๆญฃ็กฎ็ๆ๏ผไพๅฆ๏ผ
-
-```bash
-python tools/analysis_tools/browse_dataset.py configs/textdet/_base_/datasets/icdar2015.py
-```
-
-## ไฟฎๆน้
็ฝฎๆไปถ
-
-### ๅๆฐๆฎ้่ฎญ็ป
-
-ๅจไฝฟ็จๆฐ็ๆฐๆฎ้ๆถ๏ผๆไปฌ้่ฆๅฏนๅ
ถๅพๅใๆ ๆณจๆไปถ็่ทฏๅพ็ญๅบ็กไฟกๆฏ่ฟ่ก้
็ฝฎใ`configs/xxx/_base_/datasets/` ่ทฏๅพไธๅทฒ้ขๅ
้
็ฝฎไบ MMOCR ไธญๅธธ็จ็ๆฐๆฎ้๏ผๅฝไฝ ไฝฟ็จ `prepare_dataset.py` ๆฅๅๅคๆฐๆฎ้ๆถ๏ผ่ฟไธช้
็ฝฎๆไปถ้ๅธธไผๅจๆฐๆฎ้ๅๅคๅฐฑ็ปชๅ่ชๅจ็ๆ๏ผ๏ผ่ฟ้ๆไปฌไปฅ ICDAR 2015 ๆฐๆฎ้ไธบไพ๏ผ่ง `configs/textdet/_base_/datasets/icdar2015.py`๏ผ๏ผ
-
-```Python
-icdar2015_textdet_data_root = 'data/icdar2015' # ๆฐๆฎ้ๆ น็ฎๅฝ
-
-# ่ฎญ็ป้้
็ฝฎ
-icdar2015_textdet_train = dict(
- type='OCRDataset',
- data_root=icdar2015_textdet_data_root, # ๆฐๆฎๆ น็ฎๅฝ
- ann_file='textdet_train.json', # ๆ ๆณจๆไปถๅ็งฐ
- filter_cfg=dict(filter_empty_gt=True, min_size=32), # ๆฐๆฎ่ฟๆปค
- pipeline=None)
-# ๆต่ฏ้้
็ฝฎ
-icdar2015_textdet_test = dict(
- type='OCRDataset',
- data_root=icdar2015_textdet_data_root,
- ann_file='textdet_test.json',
- test_mode=True,
- pipeline=None)
-```
-
-ๅจ้
็ฝฎๅฅฝๆฐๆฎ้ๅ๏ผๆไปฌ่ฟ้่ฆๅจ็ธๅบ็็ฎๆณๆจกๅ้
็ฝฎๆไปถไธญๅฏผๅ
ฅๆณ่ฆไฝฟ็จ็ๆฐๆฎ้ใไพๅฆ๏ผๅจ ICDAR 2015 ๆฐๆฎ้ไธ่ฎญ็ป "DBNet_R18" ๆจกๅ๏ผ
-
-```Python
-_base_ = [
- '_base_dbnet_r18_fpnc.py',
- '../_base_/datasets/icdar2015.py', # ๅฏผๅ
ฅๆฐๆฎ้้
็ฝฎๆไปถ
- '../_base_/default_runtime.py',
- '../_base_/schedules/schedule_sgd_1200e.py',
-]
-
-icdar2015_textdet_train = _base_.icdar2015_textdet_train # ๆๅฎ่ฎญ็ป้
-icdar2015_textdet_train.pipeline = _base_.train_pipeline # ๆๅฎ่ฎญ็ป้ไฝฟ็จ็ๆฐๆฎๆตๆฐด็บฟ
-icdar2015_textdet_test = _base_.icdar2015_textdet_test # ๆๅฎๆต่ฏ้
-icdar2015_textdet_test.pipeline = _base_.test_pipeline # ๆๅฎๆต่ฏ้ไฝฟ็จ็ๆฐๆฎๆตๆฐด็บฟ
-
-train_dataloader = dict(
- batch_size=16,
- num_workers=8,
- persistent_workers=True,
- sampler=dict(type='DefaultSampler', shuffle=True),
- dataset=icdar2015_textdet_train) # ๅจ train_dataloader ไธญๆๅฎไฝฟ็จ็่ฎญ็ปๆฐๆฎ้
-
-val_dataloader = dict(
- batch_size=1,
- num_workers=4,
- persistent_workers=True,
- sampler=dict(type='DefaultSampler', shuffle=False),
- dataset=icdar2015_textdet_test) # ๅจ val_dataloader ไธญๆๅฎไฝฟ็จ็้ช่ฏๆฐๆฎ้
-
-test_dataloader = val_dataloader
-```
-
-### ๅคๆฐๆฎ้่ฎญ็ป
-
-ๆญคๅค๏ผๅบไบ [`ConcatDataset`](mmocr.datasets.ConcatDataset)๏ผ็จๆท่ฟๅฏไปฅไฝฟ็จๅคไธชๆฐๆฎ้็ปๅๆฅ่ฎญ็ปๆๆต่ฏๆจกๅใ็จๆทๅช้ๅจ้
็ฝฎๆไปถไธญๅฐ dataloader ไธญ็ dataset ็ฑปๅ่ฎพ็ฝฎไธบ `ConcatDataset`๏ผๅนถๆๅฎๅฏนๅบ็ๆฐๆฎ้ๅ่กจๅณๅฏใ
-
-```Python
-train_list = [ic11, ic13, ic15]
-train_dataloader = dict(
- dataset=dict(
- type='ConcatDataset', datasets=train_list, pipeline=train_pipeline))
-```
-
-ไพๅฆ๏ผไปฅไธ้
็ฝฎไฝฟ็จไบ MJSynth ๆฐๆฎ้่ฟ่ก่ฎญ็ป๏ผๅนถไฝฟ็จ 6 ไธชๅญฆๆฏๆฐๆฎ้๏ผCUTE80, IIIT5K, SVT, SVTP, ICDAR2013, ICDAR2015๏ผ่ฟ่กๆต่ฏใ
-
-```Python
-_base_ = [ # ๅฏผๅ
ฅๆๆ้่ฆไฝฟ็จ็ๆฐๆฎ้้
็ฝฎ
- '../_base_/datasets/mjsynth.py',
- '../_base_/datasets/cute80.py',
- '../_base_/datasets/iiit5k.py',
- '../_base_/datasets/svt.py',
- '../_base_/datasets/svtp.py',
- '../_base_/datasets/icdar2013.py',
- '../_base_/datasets/icdar2015.py',
- '../_base_/default_runtime.py',
- '../_base_/schedules/schedule_adadelta_5e.py',
- '_base_crnn_mini-vgg.py',
-]
-
-# ่ฎญ็ป้ๅ่กจ
-train_list = [_base_.mjsynth_textrecog_train]
-# ๆต่ฏ้ๅ่กจ
-test_list = [
- _base_.cute80_textrecog_test, _base_.iiit5k_textrecog_test, _base_.svt_textrecog_test,
- _base_.svtp_textrecog_test, _base_.icdar2013_textrecog_test, _base_.icdar2015_textrecog_test
-]
-
-# ไฝฟ็จ ConcatDataset ๆฅ็บง่ๅ่กจไธญ็ๅคไธชๆฐๆฎ้
-train_dataset = dict(
- type='ConcatDataset', datasets=train_list, pipeline=_base_.train_pipeline)
-test_dataset = dict(
- type='ConcatDataset', datasets=test_list, pipeline=_base_.test_pipeline)
-
-train_dataloader = dict(
- batch_size=192 * 4,
- num_workers=32,
- persistent_workers=True,
- sampler=dict(type='DefaultSampler', shuffle=True),
- dataset=train_dataset)
-
-test_dataloader = dict(
- batch_size=1,
- num_workers=4,
- persistent_workers=True,
- drop_last=False,
- sampler=dict(type='DefaultSampler', shuffle=False),
- dataset=test_dataset)
-
-val_dataloader = test_dataloader
-```
diff --git a/pipeline/mmocr/docs/zh_cn/user_guides/inference.md b/pipeline/mmocr/docs/zh_cn/user_guides/inference.md
deleted file mode 100644
index 7b69dcee8c964aa60f06e4912467e9d5e9a9f011..0000000000000000000000000000000000000000
--- a/pipeline/mmocr/docs/zh_cn/user_guides/inference.md
+++ /dev/null
@@ -1,534 +0,0 @@
-# ๆจ็
-
-ๅจ OpenMMLab ไธญ๏ผๆๆ็ๆจ็ๆไฝ้ฝ่ขซ็ปไธๅฐไบๆจ็ๅจ `Inferencer` ไธญใๆจ็ๅจ่ขซ่ฎพ่ฎกๆไธบไธไธช็ฎๆดๆ็จ็ API๏ผๅฎๅจไธๅ็ OpenMMLab ๅบไธญ้ฝๆ็้ๅธธ็ธไผผ็ๆฅๅฃใ
-
-MMOCR ไธญๅญๅจไธค็งไธๅ็ๆจ็ๅจ๏ผ
-
-- **ๆ ๅๆจ็ๅจ**๏ผMMOCR ไธญ็ๆฏไธชๅบๆฌไปปๅก้ฝๆไธไธชๆ ๅๆจ็ๅจ๏ผๅณ `TextDetInferencer`๏ผๆๆฌๆฃๆต๏ผ๏ผ`TextRecInferencer`๏ผๆๆฌ่ฏๅซ๏ผ๏ผ`TextSpottingInferencer`๏ผ็ซฏๅฐ็ซฏ OCR๏ผ ๅ `KIEInferencer`๏ผๅ
ณ้ฎไฟกๆฏๆๅ๏ผใๅฎไปฌๅ
ทๆ้ๅธธ็ธไผผ็ๆฅๅฃ๏ผๅ
ทๆๆ ๅ็่พๅ
ฅ/่พๅบๅ่ฎฎ๏ผๅนถไธๆปไฝ้ตๅพช OpenMMLab ็่ฎพ่ฎกใ่ฟไบๆจ็ๅจไนๅฏไปฅ่ขซไธฒ่ๅจไธ่ตท๏ผไปฅไพฟๅฏนไธ็ณปๅไปปๅก่ฟ่กๆจ็ใ
-- **MMOCRInferencer**๏ผๆไปฌ่ฟๆไพไบ `MMOCRInferencer`๏ผไธไธชไธ้จไธบ MMOCR ่ฎพ่ฎก็ไพฟๆทๆจ็ๆฅๅฃใๅฎๅฐ่ฃ
ๅ้พๆฅไบ MMOCR ไธญ็ๆๆๆจ็ๅจ๏ผๅ ๆญค็จๆทๅฏไปฅไฝฟ็จๆญคๆจ็ๅจๅฏนๅพๅๆง่กไธ็ณปๅไปปๅก๏ผๅนถ็ดๆฅ่ทๅพๆ็ป็ปๆใ*ไฝๆฏ๏ผๅฎ็ๆฅๅฃไธๆ ๅๆจ็ๅจๆไธไบไธๅ๏ผๅนถไธไธบไบ็ฎๅ่ตท่ง๏ผๅฏ่ฝไผ็บ็ฒไธไบๆ ๅ็ๆจ็ๅจๅ่ฝใ*
-
-ๅฏนไบๆฐ็จๆท๏ผๆไปฌๅปบ่ฎฎไฝฟ็จ **MMOCRInferencer** ๆฅๆต่ฏไธๅๆจกๅ็็ปๅใ
-
-ๅฆๆไฝ ๆฏๅผๅไบบๅๅนถๅธๆๅฐๆจกๅ้ๆๅฐ่ชๅทฑ็้กน็ฎไธญ๏ผๆไปฌๅปบ่ฎฎไฝฟ็จ**ๆ ๅๆจ็ๅจ**๏ผๅ ไธบๅฎไปฌๆด็ตๆดปไธๆ ๅๅ๏ผๅนถๅ
ทๆๅฎๆด็ๅ่ฝใ
-
-## ๅบ็ก็จๆณ
-
-`````{tabs}
-
-````{group-tab} MMOCRInferencer
-
-็ฎๅ๏ผ`MMOCRInferencer` ๅฏไปฅๅฏนไปฅไธไปปๅก่ฟ่กๆจ็๏ผ
-
-- ๆๆฌๆฃๆต
-- ๆๆฌ่ฏๅซ
-- OCR๏ผๆๆฌๆฃๆต + ๆๆฌ่ฏๅซ๏ผ
-- ๅ
ณ้ฎไฟกๆฏๆๅ๏ผๆๆฌๆฃๆต + ๆๆฌ่ฏๅซ + ๅ
ณ้ฎไฟกๆฏๆๅ๏ผ
-- *OCR๏ผtext spotting๏ผ*๏ผๅณๅฐๆจๅบ๏ผ
-
-ไธบไบไพฟไบไฝฟ็จ๏ผ`MMOCRInferencer` ๅ็จๆทๆไพไบ Python ๆฅๅฃๅๅฝไปค่กๆฅๅฃใไพๅฆ๏ผๅฆๆไฝ ๆณ่ฆๅฏน demo/demo_text_ocr.jpg ่ฟ่ก OCR ๆจ็๏ผไฝฟ็จ `DBNet` ไฝไธบๆๆฌๆฃๆตๆจกๅ๏ผ`CRNN` ไฝไธบๆๆฌ่ฏๅซๆจกๅ๏ผๅช้ๆง่กไปฅไธๅฝไปค:
-
-::::{tabs}
-
-:::{code-tab} python
->>> from mmocr.apis import MMOCRInferencer
->>> # ่ฏปๅๆจกๅ
->>> ocr = MMOCRInferencer(det='DBNet', rec='SAR')
->>> # ่ฟ่กๆจ็ๅนถๅฏ่งๅ็ปๆ
->>> ocr('demo/demo_text_ocr.jpg', show=True)
-:::
-
-:::{code-tab} bash ๅฝไปค่ก
-python tools/infer.py demo/demo_text_ocr.jpg --det DBNet --rec SAR --show
-:::
-::::
-
-ๅฏ่งๅ็ปๆๅฐ่ขซๆพ็คบๅจไธไธชๆฐ็ชๅฃไธญ๏ผ
-
-
-
-
-
-```{note}
-ๅฆๆไฝ ๅจๆฒกๆ GUI ็ๆๅกๅจไธ่ฟ่ก MMOCR๏ผๆ่
ๆฏ้่ฟ็ฆ็จ X11 ่ฝฌๅ็ SSH ้ง้่ฟ่ก่ฏฅๆไปค๏ผ`show` ้้กนๅฐไธ่ตทไฝ็จใ็ถ่๏ผไฝ ไป็ถๅฏไปฅ้่ฟ่ฎพ็ฝฎ `out_dir` ๅ `save_vis=True` ๅๆฐๅฐๅฏ่งๅๆฐๆฎไฟๅญๅฐๆไปถใ้
่ฏป [ๅจๅญ็ปๆ](#ๅจๅญ็ปๆ) ไบ่งฃ่ฏฆๆ
ใ
-```
-
-ๆ นๆฎๅๅงๅๅๆฐ๏ผ`MMOCRInferencer`ๅฏไปฅๅจไธๅๆจกๅผไธ่ฟ่กใไพๅฆ๏ผๅฆๆๅๅงๅๆถๆๅฎไบ `det`ใ`rec` ๅ `kie`๏ผๅฎๅฏไปฅๅจ KIE ๆจกๅผไธ่ฟ่กใ
-
-::::{tabs}
-
-:::{code-tab} python
->>> kie = MMOCRInferencer(det='DBNet', rec='SAR', kie='SDMGR')
->>> kie('demo/demo_kie.jpeg', show=True)
-:::
-
-:::{code-tab} bash ๅฝไปค่ก
-python tools/infer.py demo/demo_kie.jpeg --det DBNet --rec SAR --kie SDMGR --show
-:::
-
-::::
-
-ๅฏ่งๅ็ปๆๅฆไธ๏ผ
-
-
-
-
-
-
-ๅฏไปฅ่งๅฐ๏ผMMOCRInferencer ็ Python ๆฅๅฃไธๅฝไปค่กๆฅๅฃ็ไฝฟ็จๆนๆณ้ๅธธ็ธไผผใไธๆๅฐไปฅ Python ๆฅๅฃไธบไพ๏ผไป็ป MMOCRInferencer ็ๅ
ทไฝ็จๆณใๅ
ณไบๅฝไปค่กๆฅๅฃ็ๆดๅคไฟกๆฏ๏ผ่ฏทๅ่ [ๅฝไปค่กๆฅๅฃ](#ๅฝไปค่กๆฅๅฃ)ใ
-
-
-````
-
-````{group-tab} ๆ ๅๆจ็ๅจ
-
-้ๅธธ๏ผOpenMMLab ไธญ็ๆๆๆ ๅๆจ็ๅจ้ฝๅ
ทๆ้ๅธธ็ธไผผ็ๆฅๅฃใไธ้ข็ไพๅญๅฑ็คบไบๅฆไฝไฝฟ็จ `TextDetInferencer` ๅฏนๅไธชๅพๅ่ฟ่กๆจ็ใ
-
-```python
->>> from mmocr.apis import TextDetInferencer
->>> # ่ฏปๅๆจกๅ
->>> inferencer = TextDetInferencer(model='DBNet')
->>> # ๆจ็
->>> inferencer('demo/demo_text_ocr.jpg', show=True)
-```
-
-ๅฏ่งๅ็ปๆๅฆๅพ๏ผ
-
-
-
-
-
-````
-
-`````
-
-## ๅๅงๅ
-
-ๆฏไธชๆจ็ๅจๅฟ
้กปไฝฟ็จไธไธชๆจกๅ่ฟ่กๅๅงๅใๅๅงๅๆถ๏ผๅฏไปฅๆๅจ้ๆฉๆจ็่ฎพๅคใ
-
-### ๆจกๅๅๅงๅ
-
-`````{tabs}
-
-````{group-tab} MMOCRInferencer
-
-ๅฏนไบๆฏไธชไปปๅก๏ผ`MMOCRInferencer` ้่ฆไธคไธชๅๆฐ `xxx` ๅ `xxx_weights` ๏ผไพๅฆ `det` ๅ `det_weights`๏ผไปฅๅฏนๆจกๅ่ฟ่กๅๅงๅใๆญคๅคๅฐไปฅ`det`ๅ`det_weights`ไธบไพๆฅ่ฏดๆไธไบๅ
ธๅ็ๅๅงๅๆจกๅ็ๆนๆณใ
-
-- ่ฆ็จ MMOCR ็้ข่ฎญ็ปๆจกๅ่ฟ่กๆจ็๏ผๅช้่ฆๆๅฎ็ๅๅญไผ ็ปๅๆฐ `det`๏ผๆ้ๅฐ่ชๅจไป OpenMMLab ็ๆจกๅๅบไธญไธ่ฝฝๅๅ ่ฝฝใ[ๆญคๅค](../modelzoo.md#ๆ้)่ฎฐๅฝไบ MMOCR ไธญๅฏไปฅ้่ฟ่ฏฅๆนๆณๅๅงๅ็ๆๆๆจกๅใ
-
- ```python
- >>> MMOCRInferencer(det='DBNet')
- ```
-
-- ่ฆๅ ่ฝฝ่ชๅฎไน็้
็ฝฎๅๆ้๏ผไฝ ๅฏไปฅๆ้
็ฝฎๆไปถ็่ทฏๅพไผ ็ป `det`๏ผๆๆ้็่ทฏๅพไผ ็ป `det_weights`ใ
-
- ```python
- >>> MMOCRInferencer(det='path/to/dbnet_config.py', det_weights='path/to/dbnet.pth')
- ```
-
-ๅฆๆ้่ฆๆฅ็ๆดๅค็ๅๅงๅๆนๆณ๏ผ่ฏท็นๅปโๆ ๅๆจ็ๅจโ้้กนๅกใ
-
-````
-
-````{group-tab} ๆ ๅๆจ็ๅจ
-
-ๆฏไธชๆ ๅ็ `Inferencer` ้ฝๆฅๅไธคไธชๅๆฐ๏ผ`model` ๅ `weights` ใๅจ MMOCRInferencer ไธญ๏ผ่ฟไธคไธชๅๆฐๅๅซๅฏนๅบ `xxx` ๅ `xxx_weights` ๏ผไพๅฆ `det` ๅ `det_weights`๏ผใ
-
-- `model` ๆฅๅๆจกๅ็ๅ็งฐๆ้
็ฝฎๆไปถ็่ทฏๅพไฝไธบ่พๅ
ฅใๆจกๅ็ๅ็งฐไป [model-index.yml](https://github.com/open-mmlab/mmocr/blob/1.x/model-index.yml) ไธญ็ๆจกๅ็ๅ
ๆไปถ๏ผ[็คบไพ](https://github.com/open-mmlab/mmocr/blob/1.x/configs/textdet/dbnet/metafile.yml) ๏ผไธญ่ทๅใไฝ ๅฏไปฅๅจ[ๆญคๅค](../modelzoo.md#ๆ้)ๆพๅฐๅฏ็จๆ้็ๅ่กจใ
-
-- `weights` ๆฅๅๆ้ๆไปถ็่ทฏๅพใ
-
-
-
-ๆญคๅคๅไธพไบไธไบๅธธ่ง็ๅๅงๅๆจกๅ็ๆนๆณใ
-
-- ไฝ ๅฏไปฅ้่ฟไผ ้ๆจกๅ็ๅ็งฐ็ป `model` ๆฅๆจ็ MMOCR ็้ข่ฎญ็ปๆจกๅใๆ้ๅฐไผ่ชๅจไป OpenMMLab ็ๆจกๅๅบไธญไธ่ฝฝๅนถๅ ่ฝฝใ
-
- ```python
- >>> from mmocr.apis import TextDetInferencer
- >>> inferencer = TextDetInferencer(model='DBNet')
- ```
-
- ```{note}
- ๆจกๅไธๆจ็ๅจ็ไปปๅก็ง็ฑปๅฟ
้กปๅน้
ใ
- ```
-
- ไฝ ๅฏไปฅ้่ฟๅฐๆ้็่ทฏๅพๆ URL ไผ ้็ป `weights` ๆฅ่ฎฉๆจ็ๅจๅ ่ฝฝ่ชๅฎไน็ๆ้ใ
-
- ```python
- >>> inferencer = TextDetInferencer(model='DBNet', weights='path/to/dbnet.pth')
- ```
-
-- ๅฆๆๆ่ชๅฎไน็้
็ฝฎๅๆ้๏ผไฝ ๅฏไปฅๅฐ้
็ฝฎๆไปถ็่ทฏๅพไผ ้็ป `model`๏ผๅฐๆ้็่ทฏๅพไผ ้็ป `weights`ใ
-
- ```python
- >>> inferencer = TextDetInferencer(model='path/to/dbnet_config.py', weights='path/to/dbnet.pth')
- ```
-
-- ้ป่ฎคๆ
ๅตไธ๏ผ[MMEngine](https://github.com/open-mmlab/mmengine/) ไผๅจ่ฎญ็ปๆจกๅๆถ่ชๅจๅฐ้
็ฝฎๆไปถ่ฝฌๅจๅฐๆ้ๆไปถไธญใๅฆๆไฝ ๆไธไธชๅจ MMEngine ไธ่ฎญ็ป็ๆ้๏ผไฝ ไนๅฏไปฅๅฐๆ้ๆไปถ็่ทฏๅพไผ ้็ป `weights`๏ผ่ไธ้่ฆๆๅฎ `model`๏ผ
-
- ```python
- >>> # ๅฆๆๆ ๆณๅจๆ้ไธญๆพๅฐ้
็ฝฎๆไปถ๏ผๅไผๅผๅ้่ฏฏ
- >>> inferencer = TextDetInferencer(weights='path/to/dbnet.pth')
- ```
-
-- ไผ ้้
็ฝฎๆไปถๅฐ `model` ่ไธๆๅฎ `weight` ๅไผไบง็ไธไธช้ๆบๅๅงๅ็ๆจกๅใ
-
-````
-`````
-
-### ๆจ็่ฎพๅค
-
-ๆฏไธชๆจ็ๅจๅฎไพ้ฝไผ่ทไธไธช่ฎพๅค็ปๅฎใ้ป่ฎคๆ
ๅตไธ๏ผๆไฝณ่ฎพๅคๆฏ็ฑ [MMEngine](https://github.com/open-mmlab/mmengine/) ่ชๅจๅณๅฎ็ใไฝ ไนๅฏไปฅ้่ฟๆๅฎ `device` ๅๆฐๆฅๆนๅ่ฎพๅคใไพๅฆ๏ผไฝ ๅฏไปฅไฝฟ็จไปฅไธไปฃ็ ๅจ GPU 1ไธๅๅปบไธไธชๆจ็ๅจใ
-
-`````{tabs}
-
-````{group-tab} MMOCRInferencer
-
-```python
->>> inferencer = MMOCRInferencer(det='DBNet', device='cuda:1')
-```
-
-````
-
-````{group-tab} ๆ ๅๆจ็ๅจ
-
-```python
->>> inferencer = TextDetInferencer(model='DBNet', device='cuda:1')
-```
-
-````
-
-`````
-
-ๅฆ่ฆๅจ CPU ไธๅๅปบไธไธชๆจ็ๅจ๏ผ
-
-`````{tabs}
-
-````{group-tab} MMOCRInferencer
-
-```python
->>> inferencer = MMOCRInferencer(det='DBNet', device='cpu')
-```
-
-````
-
-````{group-tab} ๆ ๅๆจ็ๅจ
-
-```python
->>> inferencer = TextDetInferencer(model='DBNet', device='cpu')
-```
-
-````
-
-`````
-
-่ฏทๅ่ [torch.device](torch.device) ไบ่งฃ `device` ๅๆฐๆฏๆ็ๆๆๅฝขๅผใ
-
-## ๆจ็
-
-ๅฝๆจ็ๅจๅๅงๅๅ๏ผไฝ ๅฏไปฅ็ดๆฅไผ ๅ
ฅ่ฆๆจ็็ๅๅงๆฐๆฎ๏ผไป่ฟๅๅผไธญ่ทๅๆจ็็ปๆใ
-
-### ่พๅ
ฅ
-
-`````{tabs}
-
-````{tab} MMOCRInferencer / TextDetInferencer / TextRecInferencer / TextSpottingInferencer
-
-่พๅ
ฅๅฏไปฅๆฏไปฅไธไปปๆไธ็งๆ ผๅผ๏ผ
-
-- str: ๅพๅ็่ทฏๅพ/URLใ
-
- ```python
- >>> inferencer('demo/demo_text_ocr.jpg')
- ```
-
-- array: ๅพๅ็ numpy ๆฐ็ปใๅฎๅบ่ฏฅๆฏ BGR ๆ ผๅผใ
-
- ```python
- >>> import mmcv
- >>> array = mmcv.imread('demo/demo_text_ocr.jpg')
- >>> inferencer(array)
- ```
-
-- list: ๅบๆฌ็ฑปๅ็ๅ่กจใๅ่กจไธญ็ๆฏไธชๅ
็ด ้ฝๅฐๅ็ฌๅค็ใ
-
- ```python
- >>> inferencer(['img_1.jpg', 'img_2.jpg])
- >>> # ๅ่กจๅ
ๆททๅ็ฑปๅไนๆฏๅ
่ฎธ็
- >>> inferencer(['img_1.jpg', array])
- ```
-
-- str: ็ฎๅฝ็่ทฏๅพใ็ฎๅฝไธญ็ๆๆๅพๅ้ฝๅฐ่ขซๅค็ใ
-
- ```python
- >>> inferencer('tests/data/det_toy_dataset/imgs/test/')
- ```
-
-````
-
-````{tab} KIEInferencer
-
-่พๅ
ฅๅฏไปฅๆฏไธไธชๅญๅ
ธๆ่
ไธไธชๅญๅ
ธๅ่กจ๏ผๅ
ถไธญๆฏไธชๅญๅ
ธๅ
ๅซไปฅไธ้ฎ๏ผ
-
-- `img` (str ๆ่
ndarray): ๅพๅ็่ทฏๅพๆๅพๅๆฌ่บซใๅฆๆ KIE ๆจ็ๅจๅจๆ ๅฏ่งๆจกๅผไธไฝฟ็จ๏ผๅไธ้่ฆๆญค้ฎใๅฆๆๅฎๆฏไธไธช numpy ๆฐ็ป๏ผๅๅบ่ฏฅๆฏ BGR ้กบๅบ็ผ็ ็ๅพ็ใ
-- `img_shape` (tuple(int, int)): ๅพๅ็ๅฝข็ถ (H, W)ใไป
ๅจ KIE ๆจ็ๅจๅจๆ ๅฏ่งๆจกๅผไธไฝฟ็จไธๆฒกๆๆไพ `img` ๆถๆ้่ฆใ
-- `instances` (list[dict]): ๅฎไพๅ่กจใ
-
-ๆฏไธช `instance` ้ฝๅบ่ฏฅๅ
ๅซไปฅไธ้ฎ๏ผ
-
-```python
-{
- # ไธไธชๅตๅฅๅ่กจ๏ผๅ
ถไธญๅ
ๅซ 4 ไธชๆฐๅญ๏ผ่กจ็คบๅฎไพ็่พน็ๆก๏ผ้กบๅบไธบ (x1, y1, x2, y2)
- "bbox": np.array([[x1, y1, x2, y2], [x1, y1, x2, y2], ...],
- dtype=np.int32),
-
- # ๆๆฌๅ่กจ
- "texts": ['text1', 'text2', ...],
-}
-```
-
-````
-`````
-
-### ่พๅบ
-
-้ป่ฎคๆ
ๅตไธ๏ผๆฏไธชๆจ็ๅจ้ฝไปฅๅญๅ
ธๆ ผๅผ่ฟๅ้ขๆต็ปๆใ
-
-- `visualization` ๅ
ๅซๅฏ่งๅ็้ขๆต็ปๆใไฝ้ป่ฎคๆ
ๅตไธ๏ผๅฎๆฏไธไธช็ฉบๅ่กจ๏ผ้ค้ `return_vis=True`ใ
-
-- `predictions` ๅ
ๅซไปฅ json-ๅฏๅบๅๅๆ ผๅผ่ฟๅ็้ขๆต็ปๆใๅฆไธๆ็คบ๏ผๅ
ๅฎนๅ ไปปๅก็ฑปๅ่ๅผใ
-
- `````{tabs}
-
- :::{group-tab} MMOCRInferencer
-
- ```python
- {
- 'predictions' : [
- # ๆฏไธชๅฎไพ้ฝๅฏนๅบไบไธไธช่พๅ
ฅๅพๅ
- {
- 'det_polygons': [...], # 2d ๅ่กจ๏ผ้ฟๅบฆไธบ (N,)๏ผๆ ผๅผไธบ [x1, y1, x2, y2, ...]
- 'det_scores': [...], # ๆตฎ็นๅ่กจ๏ผ้ฟๅบฆไธบ๏ผN, ๏ผ
- 'det_bboxes': [...], # 2d ๅ่กจ๏ผๅฝข็ถไธบ (N, 4)๏ผๆ ผๅผไธบ [min_x, min_y, max_x, max_y]
- 'rec_texts': [...], # ๅญ็ฌฆไธฒๅ่กจ๏ผ้ฟๅบฆไธบ๏ผN, ๏ผ
- 'rec_scores': [...], # ๆตฎ็นๅ่กจ๏ผ้ฟๅบฆไธบ๏ผN, ๏ผ
- 'kie_labels': [...], # ่็นๆ ็ญพ๏ผ้ฟๅบฆไธบ (N, )
- 'kie_scores': [...], # ่็น็ฝฎไฟกๅบฆ๏ผ้ฟๅบฆไธบ (N, )
- 'kie_edge_scores': [...], # ่พน้ขๆต็ฝฎไฟกๅบฆ, ๅฝข็ถไธบ (N, N)
- 'kie_edge_labels': [...] # ่พนๆ ็ญพ, ๅฝข็ถไธบ (N, N)
- },
- ...
- ],
- 'visualization' : [
- array(..., dtype=uint8),
- ]
- }
- ```
-
- :::
-
- ````{group-tab} ๆ ๅๆจ็ๅจ
-
- ::::{tabs}
- :::{code-tab} python TextDetInferencer
-
- {
- 'predictions' : [
- # ๆฏไธชๅฎไพ้ฝๅฏนๅบไบไธไธช่พๅ
ฅๅพๅ
- {
- 'polygons': [...], # 2d ๅ่กจ๏ผ้ฟๅบฆไธบ (N,)๏ผๆ ผๅผไธบ [x1, y1, x2, y2, ...]
- 'bboxes': [...], # 2d ๅ่กจ๏ผๅฝข็ถไธบ (N, 4)๏ผๆ ผๅผไธบ [min_x, min_y, max_x, max_y]
- 'scores': [...] # ๆตฎ็นๅ่กจ๏ผ้ฟๅบฆไธบ๏ผN, ๏ผ
- },
- ...
- ]
- 'visualization' : [
- array(..., dtype=uint8),
- ]
- }
- :::
-
- :::{code-tab} python TextRecInferencer
- {
- 'predictions' : [
- # ๆฏไธชๅฎไพ้ฝๅฏนๅบไบไธไธช่พๅ
ฅๅพๅ
- {
- 'text': '...', # ๅญ็ฌฆไธฒ
- 'scores': 0.1, # ๆตฎ็น
- },
- ...
- ]
- 'visualization' : [
- array(..., dtype=uint8),
- ]
- }
- :::
-
- :::{code-tab} python TextSpottingInferencer
- {
- 'predictions' : [
- # ๆฏไธชๅฎไพ้ฝๅฏนๅบไบไธไธช่พๅ
ฅๅพๅ
- {
- 'polygons': [...], # 2d ๅ่กจ๏ผ้ฟๅบฆไธบ (N,)๏ผๆ ผๅผไธบ [x1, y1, x2, y2, ...]
- 'bboxes': [...], # 2d ๅ่กจ๏ผๅฝข็ถไธบ (N, 4)๏ผๆ ผๅผไธบ [min_x, min_y, max_x, max_y]
- 'scores': [...] # ๆตฎ็นๅ่กจ๏ผ้ฟๅบฆไธบ๏ผN, ๏ผ
- 'texts': ['...',] # ๅญ็ฌฆไธฒๅ่กจ๏ผ้ฟๅบฆไธบ๏ผN, ๏ผ
- },
- ]
- 'visualization' : [
- array(..., dtype=uint8),
- ]
- }
- :::
-
- :::{code-tab} python KIEInferencer
- {
- 'predictions' : [
- # ๆฏไธชๅฎไพ้ฝๅฏนๅบไบไธไธช่พๅ
ฅๅพๅ
- {
- 'labels': [...], # ่็นๆ ็ญพ๏ผ้ฟๅบฆไธบ (N, )
- 'scores': [...], # ่็น็ฝฎไฟกๅบฆ๏ผ้ฟๅบฆไธบ (N, )
- 'edge_scores': [...], # ่พน้ขๆต็ฝฎไฟกๅบฆ, ๅฝข็ถไธบ (N, N)
- 'edge_labels': [...], # ่พนๆ ็ญพ, ๅฝข็ถไธบ (N, N)
- },
- ]
- 'visualization' : [
- array(..., dtype=uint8),
- ]
- }
- :::
- ::::
- ````
-
- `````
-
-ๅฆๆไฝ ๆณ่ฆไปๆจกๅไธญ่ทๅๅๅง่พๅบ๏ผๅฏไปฅๅฐ `return_datasamples` ่ฎพ็ฝฎไธบ `True` ๆฅ่ทๅๅๅง็ [DataSample](structures.md)๏ผๅฎๅฐๅญๅจๅจ `predictions` ไธญใ
-
-### ๅจๅญ็ปๆ
-
-้คไบไป่ฟๅๅผไธญ่ทๅ้ขๆต็ปๆ๏ผไฝ ่ฟๅฏไปฅ้่ฟ่ฎพ็ฝฎ `out_dir` ๅ `save_pred`/`save_vis` ๅๆฐๅฐ้ขๆต็ปๆๅๅฏ่งๅ็ปๆๅฏผๅบๅฐๆไปถไธญใ
-
-```python
->>> inferencer('img_1.jpg', out_dir='outputs/', save_pred=True, save_vis=True)
-```
-
-็ปๆ็ฎๅฝ็ปๆๅฆไธ๏ผ
-
-```text
-outputs
-โโโ preds
-โ โโโ img_1.json
-โโโ vis
- โโโ img_1.jpg
-```
-
-ๆไปถๅไธๅฏนๅบ็่พๅ
ฅๅพๅๆไปถๅ็ธๅใ ๅฆๆ่พๅ
ฅๅพๅๆฏๆฐ็ป๏ผๅๆไปถๅๅฐๆฏไป0ๅผๅง็ๆฐๅญใ
-
-### ๆน้ๆจ็
-
-ไฝ ๅฏไปฅ้่ฟ่ฎพ็ฝฎ `batch_size` ๆฅ่ชๅฎไนๆน้ๆจ็็ๆนๅคงๅฐใ ้ป่ฎคๆนๅคงๅฐไธบ 1ใ
-
-## API
-
-่ฟ้ๅๅบไบๆจ็ๅจ่ฏฆๅฐฝ็ๅๆฐๅ่กจใ
-
-````{tabs}
-
-```{group-tab} MMOCRInferencer
-
-**MMOCRInferencer.\_\_init\_\_():**
-
-| ๅๆฐ | ็ฑปๅ | ้ป่ฎคๅผ | ๆ่ฟฐ |
-| ------------- | ----------------------------------------- | ------ | ------------------------------------------------------------------------------------------------------------------------------ |
-| `det` | str ๆ [ๆ้](../modelzoo.html#id2), ๅฏ้ | None | ้ข่ฎญ็ป็ๆๆฌๆฃๆต็ฎๆณใๅฎๆฏ้
็ฝฎๆไปถ็่ทฏๅพๆ่
ๆฏ metafile ไธญๅฎไน็ๆจกๅๅ็งฐใ |
-| `det_weights` | str, ๅฏ้ | None | det ๆจกๅ็ๆ้ๆไปถ็่ทฏๅพใ |
-| `rec` | str ๆ [ๆ้](../modelzoo.html#id2), ๅฏ้ | None | ้ข่ฎญ็ป็ๆๆฌ่ฏๅซ็ฎๆณใๅฎๆฏ้
็ฝฎๆไปถ็่ทฏๅพๆ่
ๆฏ metafile ไธญๅฎไน็ๆจกๅๅ็งฐใ |
-| `rec_weights` | str, ๅฏ้ | None | rec ๆจกๅ็ๆ้ๆไปถ็่ทฏๅพใ |
-| `kie` \[1\] | str ๆ [ๆ้](../modelzoo.html#id2), ๅฏ้ | None | ้ข่ฎญ็ป็ๅ
ณ้ฎไฟกๆฏๆๅ็ฎๆณใๅฎๆฏ้
็ฝฎๆไปถ็่ทฏๅพๆ่
ๆฏ metafile ไธญๅฎไน็ๆจกๅๅ็งฐใ |
-| `kie_weights` | str, ๅฏ้ | None | kie ๆจกๅ็ๆ้ๆไปถ็่ทฏๅพใ |
-| `device` | str, ๅฏ้ | None | ๆจ็ไฝฟ็จ็่ฎพๅค๏ผๆฅๅ `torch.device` ๅ
่ฎธ็ๆๆๅญ็ฌฆไธฒใไพๅฆ๏ผ'cuda:0' ๆ 'cpu'ใๅฆๆไธบ None๏ผๅฐ่ชๅจไฝฟ็จๅฏ็จ่ฎพๅคใ ้ป่ฎคไธบ Noneใ |
-
-\[1\]: ๅฝๅๆถๆๅฎไบๆๆฌๆฃๆตๅ่ฏๅซๆจกๅๆถ๏ผ`kie` ๆไผ็ๆใ
-
-**MMOCRInferencer.\_\_call\_\_()**
-
-| ๅๆฐ | ็ฑปๅ | ้ป่ฎคๅผ | ๆ่ฟฐ |
-| -------------------- | ----------------------- | ---------- | ---------------------------------------------------------------------------------------------- |
-| `inputs` | str/list/tuple/np.array | **ๅฟ
้** | ๅฎๅฏไปฅๆฏไธไธชๅพ็/ๆไปถๅคน็่ทฏๅพ๏ผไธไธช numpy ๆฐ็ป๏ผๆ่
ๆฏไธไธชๅ
ๅซๅพ็่ทฏๅพๆ numpy ๆฐ็ป็ๅ่กจ/ๅ
็ป |
-| `return_datasamples` | bool | False | ๆฏๅฆๅฐ็ปๆไฝไธบ DataSample ่ฟๅใๅฆๆไธบ False๏ผ็ปๆๅฐ่ขซๆๅ
ๆไธไธชๅญๅ
ธใ |
-| `batch_size` | int | 1 | ๆจ็็ๆนๅคงๅฐใ |
-| `det_batch_size` | int, ๅฏ้ | None | ๆจ็็ๆนๅคงๅฐ (ๆๆฌๆฃๆตๆจกๅ)ใๅฆๆไธไธบ None๏ผๅ่ฆ็ batch_sizeใ |
-| `rec_batch_size` | int, ๅฏ้ | None | ๆจ็็ๆนๅคงๅฐ (ๆๆฌ่ฏๅซๆจกๅ)ใๅฆๆไธไธบ None๏ผๅ่ฆ็ batch_sizeใ |
-| `kie_batch_size` | int, ๅฏ้ | None | ๆจ็็ๆนๅคงๅฐ (ๅ
ณ้ฎไฟกๆฏๆๅๆจกๅ)ใๅฆๆไธไธบ None๏ผๅ่ฆ็ batch_sizeใ |
-| `return_vis` | bool | False | ๆฏๅฆ่ฟๅๅฏ่งๅ็ปๆใ |
-| `print_result` | bool | False | ๆฏๅฆๅฐๆจ็็ปๆๆๅฐๅฐๆงๅถๅฐใ |
-| `show` | bool | False | ๆฏๅฆๅจๅผนๅบ็ชๅฃไธญๆพ็คบๅฏ่งๅ็ปๆใ |
-| `wait_time` | float | 0 | ๅผน็ชๅฑ็คบๅฏ่งๅ็ปๆ็ๆถ้ด้ด้ใ |
-| `out_dir` | str | `results/` | ็ปๆ็่พๅบ็ฎๅฝใ |
-| `save_vis` | bool | False | ๆฏๅฆๅฐๅฏ่งๅ็ปๆไฟๅญๅฐ `out_dir`ใ |
-| `save_pred` | bool | False | ๆฏๅฆๅฐๆจ็็ปๆไฟๅญๅฐ `out_dir`ใ |
-
-```
-
-```{group-tab} ๆ ๅๆจ็ๅจ
-
-**Inferencer.\_\_init\_\_():**
-
-| ๅๆฐ | ็ฑปๅ | ้ป่ฎคๅผ | ๆ่ฟฐ |
-| --------- | ----------------------------------------- | ------ | ---------------------------------------------------------------------------------------------------------------------------------- |
-| `model` | str ๆ [ๆ้](../modelzoo.html#id2), ๅฏ้ | None | ่ทฏๅพๅฐ้
็ฝฎๆไปถๆ่
ๅจ metafile ไธญๅฎไน็ๆจกๅๅ็งฐใ |
-| `weights` | str, ๅฏ้ | None | ๆ้ๆไปถ็่ทฏๅพใ |
-| `device` | str, ๅฏ้ | None | ๆจ็ไฝฟ็จ็่ฎพๅค๏ผๆฅๅ `torch.device` ๅ
่ฎธ็ๆๆๅญ็ฌฆไธฒใ ไพๅฆ๏ผ'cuda:0' ๆ 'cpu'ใ ๅฆๆไธบ None๏ผๅๅฐ่ชๅจไฝฟ็จๅฏ็จ่ฎพๅคใ ้ป่ฎคไธบ Noneใ |
-
-**Inferencer.\_\_call\_\_()**
-
-| ๅๆฐ | ็ฑปๅ | ้ป่ฎคๅผ | ๆ่ฟฐ |
-| -------------------- | ----------------------- | ---------- | ----------------------------------------------------------------------------------- |
-| `inputs` | str/list/tuple/np.array | **ๅฟ
้** | ๅฏไปฅๆฏๅพๅ็่ทฏๅพ/ๆไปถๅคน๏ผnp ๆฐ็ปๆๅ่กจ/ๅ
็ป๏ผๅธฆๆๅพๅ่ทฏๅพๆ np ๆฐ็ป๏ผ |
-| `return_datasamples` | bool | False | ๆฏๅฆๅฐ็ปๆไฝไธบ DataSamples ่ฟๅใ ๅฆๆไธบ False๏ผๅ็ปๆๅฐ่ขซๆๅ
ๅฐไธไธช dict ไธญใ |
-| `batch_size` | int | 1 | ๆจ็ๆนๅคงๅฐใ |
-| `progress_bar` | bool | True | ๆฏๅฆๆพ็คบ่ฟๅบฆๆกใ |
-| `return_vis` | bool | False | ๆฏๅฆ่ฟๅๅฏ่งๅ็ปๆใ |
-| `print_result` | bool | False | ๆฏๅฆๅฐๆจ็็ปๆๆๅฐๅฐๆงๅถๅฐใ |
-| `show` | bool | False | ๆฏๅฆๅจๅผนๅบ็ชๅฃไธญๆพ็คบๅฏ่งๅ็ปๆใ |
-| `wait_time` | float | 0 | ๅผน็ชๅฑ็คบๅฏ่งๅ็ปๆ็ๆถ้ด้ด้ใ |
-| `draw_pred` | bool | True | ๆฏๅฆ็ปๅถ้ขๆต็่พน็ๆกใ *ไป
้็จไบ `TextDetInferencer` ๅ `TextSpottingInferencer`ใ* |
-| `out_dir` | str | `results/` | ็ปๆ็่พๅบ็ฎๅฝใ |
-| `save_vis` | bool | False | ๆฏๅฆๅฐๅฏ่งๅ็ปๆไฟๅญๅฐ `out_dir`ใ |
-| `save_pred` | bool | False | ๆฏๅฆๅฐๆจ็็ปๆไฟๅญๅฐ `out_dir`ใ |
-
-```
-````
-
-## ๅฝไปค่กๆฅๅฃ
-
-```{note}
-่ฏฅ่ไป
้็จไบ `MMOCRInferencer`.
-```
-
-`MMOCRInferencer` ็ๅฝไปค่กๅฝขๅผๅฏไปฅ้่ฟ `tools/infer.py` ่ฐ็จ๏ผๅคง่ดๅฝขๅผๅฆไธ๏ผ
-
-```bash
-python tools/infer.py INPUT_PATH [--det DET] [--det-weights ...] ...
-```
-
-ๅ
ถไธญ๏ผ`INPUT_PATH` ไธบๅฟ
้กปๅญๆฎต๏ผๅ
ๅฎนๅบๅฝไธบๆๅๅพ็ๆๆไปถ็ฎๅฝ็่ทฏๅพใๅ
ถไปๅๆฐไธ Python ๆฅๅฃ้ตๅพช็ๆ ๅฐๅ
ณ็ณปๅฆไธ๏ผ
-
-- ๅจๅฝไปค่กไธญ่ฐ็จๅๆฐๆถ๏ผ้่ฆๅจ Python ๆฅๅฃ็ๅๆฐๅ้ขๅ ไธไธคไธช`-`๏ผ็ถๅๆไธๅ็บฟ`_`ๆฟๆขๆ่ฟๅญ็ฌฆ`-`ใไพๅฆ๏ผ `out_dir` ไผๅๆ `--out-dir`ใ
-- ๅฏนไบๅธๅฐ็ฑปๅ็ๅๆฐ๏ผๅฐๅๆฐๆพๅจๅฝไปคไธญๅฐฑ็ธๅฝไบๅฐๅ
ถๆๅฎไธบ Trueใไพๅฆ๏ผ `--show` ไผๅฐ `show` ๅๆฐๆๅฎไธบ Trueใ
-
-ๆญคๅค๏ผๅฝไปค่กไธญ้ป่ฎคไธไผๅๆพๆจ็็ปๆ๏ผไฝ ๅฏไปฅ้่ฟ `--print-result` ๅๆฐๆฅๆฅ็ๆจ็็ปๆใ
-
-ไธ้ขๆฏไธไธชไพๅญ๏ผ
-
-```bash
-python tools/infer.py demo/demo_text_ocr.jpg --det DBNet --rec SAR --show --print-result
-```
-
-่ฟ่ก่ฏฅๅฝไปค๏ผๅฏไปฅๅพๅฐๅฆไธ็ปๆ๏ผ
-
-```bash
-{'predictions': [{'rec_texts': ['CBank', 'Docbcba', 'GROUP', 'MAUN', 'CROBINSONS', 'AOCOC', '916M3', 'BOO9', 'Oven', 'BRANDS', 'ARETAIL', '14', '70S', 'ROUND', 'SALE', 'YEAR', 'ALLY', 'SALE', 'SALE'],
-'rec_scores': [0.9753464579582214, ...], 'det_polygons': [[551.9930285844646, 411.9138765335083, 553.6153911653112,
-383.53195309638977, 620.2410061195247, 387.33785033226013, 618.6186435386782, 415.71977376937866], ...], 'det_scores': [0.8230461478233337, ...]}]}
-```
diff --git a/pipeline/mmocr/docs/zh_cn/user_guides/train_test.md b/pipeline/mmocr/docs/zh_cn/user_guides/train_test.md
deleted file mode 100644
index 7ab634f8b362e1e9387ac1d4585c3253b577ea1d..0000000000000000000000000000000000000000
--- a/pipeline/mmocr/docs/zh_cn/user_guides/train_test.md
+++ /dev/null
@@ -1,324 +0,0 @@
-# ่ฎญ็ปไธๆต่ฏ
-
-ไธบไบ้้
ๅคๆ ทๅ็็จๆท้ๆฑ๏ผMMOCR ๅฎ็ฐไบๅค็งไธๅๆไฝ็ณป็ปๅ่ฎพๅคไธ็ๆจกๅ่ฎญ็ปๅๆต่ฏใๆ ่ฎบๆฏไฝฟ็จๆฌๅฐๆบๅจ่ฟ่กๅๆบๅๅก่ฎญ็ปๆต่ฏ๏ผ่ฟๆฏๅจ้จ็ฝฒไบ slurm ็ณป็ป็ๅคง่งๆจก้็พคไธ่ฟ่ก่ฎญ็ปๆต่ฏ๏ผMMOCR ้ฝๆไพไบไพฟๆท็่งฃๅณๆนๆกใ
-
-## ๅๅกๆบๅจ่ฎญ็ปๅๆต่ฏ
-
-### ่ฎญ็ป
-
-`tools/train.py` ๅฎ็ฐไบๅบ็ก็่ฎญ็ปๆๅกใMMOCR ๆจ่็จๆทไฝฟ็จ GPU ่ฟ่กๆจกๅ่ฎญ็ปๅๆต่ฏ๏ผไฝๆฏ๏ผ็จๆทไนๅฏไปฅ้่ฟๆๅฎ `CUDA_VISIBLE_DEVICES=-1` ๆฅไฝฟ็จ CPU ่ฎพๅค่ฟ่กๆจกๅ่ฎญ็ปๅๆต่ฏใไพๅฆ๏ผไปฅไธๅฝไปคๆผ็คบไบๅฆไฝไฝฟ็จ CPU ๆๅๅก GPU ๆฅ่ฎญ็ป DBNet ๆๆฌๆฃๆตๅจใ
-
-```bash
-# ้่ฟ่ฐ็จ tools/train.py ๆฅ่ฎญ็ปๆๅฎ็ MMOCR ๆจกๅ
-CUDA_VISIBLE_DEVICES= python tools/train.py ${CONFIG_FILE} [PY_ARGS]
-
-# ่ฎญ็ป
-# ็คบไพ 1๏ผไฝฟ็จ CPU ่ฎญ็ป DBNet
-CUDA_VISIBLE_DEVICES=-1 python tools/train.py configs/textdet/dbnet/dbnet_resnet50-dcnv2_fpnc_1200e_icdar2015.py
-
-# ็คบไพ 2๏ผๆๅฎไฝฟ็จ gpu:0 ่ฎญ็ป DBNet๏ผๆๅฎๅทฅไฝ็ฎๅฝไธบ dbnet/๏ผๅนถๆๅผๆททๅ็ฒพๅบฆ๏ผamp๏ผ่ฎญ็ป
-CUDA_VISIBLE_DEVICES=0 python tools/train.py configs/textdet/dbnet/dbnet_resnet50-dcnv2_fpnc_1200e_icdar2015.py --work-dir dbnet/ --amp
-```
-
-```{note}
-ๆญคๅค๏ผๅฆ้ไฝฟ็จๆๅฎ็ผๅท็ GPU ่ฟ่ก่ฎญ็ปๆๆต่ฏ๏ผไพๅฆไฝฟ็จ3ๅท GPU๏ผๅๅฏไปฅ้่ฟ่ฎพๅฎ CUDA_VISIBLE_DEVICES=3 ๆฅๅฎ็ฐใ
-```
-
-ไธ่กจๅๅบไบ `train.py` ๆฏๆ็ๆๆๅๆฐใๅ
ถไธญ๏ผไธๅธฆ `--` ๅ็ผ็ๅๆฐไธบๅฟ
้กป็ไฝ็ฝฎๅๆฐ๏ผๅธฆ `--` ๅ็ผ็ๅๆฐไธบๅฏ้ๅๆฐใ
-
-| ๅๆฐ | ็ฑปๅ | ่ฏดๆ |
-| --------------- | ---- | -------------------------------------------------------------- |
-| config | str | ๏ผๅฟ
้กป๏ผ้
็ฝฎๆไปถ่ทฏๅพใ |
-| --work-dir | str | ๆๅฎๅทฅไฝ็ฎๅฝ๏ผ็จไบๅญๆพ่ฎญ็ปๆฅๅฟไปฅๅๆจกๅ checkpointsใ |
-| --resume | bool | ๆฏๅฆไปๆญ็นๅคๆขๅค่ฎญ็ปใ |
-| --amp | bool | ๆฏๅฆไฝฟ็จๆททๅ็ฒพๅบฆใ |
-| --auto-scale-lr | bool | ๆฏๅฆไฝฟ็จๅญฆไน ็่ชๅจ็ผฉๆพใ |
-| --cfg-options | str | ็จไบ่ฆๅ้
็ฝฎๆไปถไธญ็ๆๅฎๅๆฐใ[็คบไพ](#ๆทปๅ ็คบไพ) |
-| --launcher | str | ๅฏๅจๅจ้้กน๏ผๅฏ้้กน็ฎไธบ \['none', 'pytorch', 'slurm', 'mpi'\]ใ |
-| --local_rank | int | ๆฌๅฐๆบๅจ็ผๅท๏ผ็จไบๅคๆบๅคๅกๅๅธๅผ่ฎญ็ป๏ผ้ป่ฎคไธบ 0ใ |
-
-### ๆต่ฏ
-
-`tools/test.py` ๆไพไบๅบ็ก็ๆต่ฏๆๅก๏ผๅ
ถไฝฟ็จๅ็ๅ่ฎญ็ป่ๆฌ็ฑปไผผใไพๅฆ๏ผไปฅไธๅฝไปคๆผ็คบไบ CPU ๆ GPU ๅๅกๆต่ฏ DBNet ๆจกๅใ
-
-```bash
-# ้่ฟ่ฐ็จ tools/test.py ๆฅๆต่ฏๆๅฎ็ MMOCR ๆจกๅ
-CUDA_VISIBLE_DEVICES= python tools/test.py ${CONFIG_FILE} ${CHECKPOINT_FILE} [PY_ARGS]
-
-# ๆต่ฏ
-# ็คบไพ 1๏ผไฝฟ็จ CPU ๆต่ฏ DBNet
-CUDA_VISIBLE_DEVICES=-1 python tools/test.py configs/textdet/dbnet/dbnet_resnet50-dcnv2_fpnc_1200e_icdar2015.py dbnet_r50.pth
-# ็คบไพ 2๏ผไฝฟ็จ gpu:0 ๆต่ฏ DBNet
-CUDA_VISIBLE_DEVICES=0 python tools/test.py configs/textdet/dbnet/dbnet_resnet50-dcnv2_fpnc_1200e_icdar2015.py dbnet_r50.pth
-```
-
-ไธ่กจๅๅบไบ `test.py` ๆฏๆ็ๆๆๅๆฐใๅ
ถไธญ๏ผไธๅธฆ `--` ๅ็ผ็ๅๆฐไธบๅฟ
้กป็ไฝ็ฝฎๅๆฐ๏ผๅธฆ `--` ๅ็ผ็ๅๆฐไธบๅฏ้ๅๆฐใ
-
-| ๅๆฐ | ็ฑปๅ | ่ฏดๆ |
-| ------------- | ----- | -------------------------------------------------------------- |
-| config | str | ๏ผๅฟ
้กป๏ผ้
็ฝฎๆไปถ่ทฏๅพใ |
-| checkpoint | str | ๏ผๅฟ
้กป๏ผๅพ
ๆต่ฏๆจกๅ่ทฏๅพใ |
-| --work-dir | str | ๅทฅไฝ็ฎๅฝ๏ผ็จไบๅญๆพ่ฎญ็ปๆฅๅฟไปฅๅๆจกๅ checkpointsใ |
-| --save-preds | bool | ๆฏๅฆๅฐ้ขๆต็ปๆๅๅ
ฅ pkl ๆไปถๅนถไฟๅญใ |
-| --show | bool | ๆฏๅฆๅฏ่งๅ้ขๆต็ปๆใ |
-| --show-dir | str | ๅฐๅฏ่งๅ็้ขๆต็ปๆไฟๅญ่ณๆๅฎ่ทฏๅพใ |
-| --wait-time | float | ๅฏ่งๅ้ด้ๆถ้ด๏ผ็ง๏ผ๏ผ้ป่ฎคไธบ 2 ็งใ |
-| --cfg-options | str | ็จไบ่ฆๅ้
็ฝฎๆไปถไธญ็ๆๅฎๅๆฐใ[็คบไพ](#ๆทปๅ ็คบไพ) |
-| --launcher | str | ๅฏๅจๅจ้้กน๏ผๅฏ้้กน็ฎไธบ \['none', 'pytorch', 'slurm', 'mpi'\]ใ |
-| --local_rank | int | ๆฌๅฐๆบๅจ็ผๅท๏ผ็จไบๅคๆบๅคๅกๅๅธๅผ่ฎญ็ป๏ผ้ป่ฎคไธบ 0ใ |
-| --tta | bool | ๆฏๅฆไฝฟ็จๆต่ฏๆถๆฐๆฎๅขๅผบ |
-
-## ๅคๅกๆบๅจ่ฎญ็ปๅๆต่ฏ
-
-ๅฏนไบๅคง่งๆจกๆจกๅ๏ผ้็จๅค GPU ่ฎญ็ปๅๆต่ฏๅฏไปฅๆๅคงๅฐๆๅๆไฝ็ๆ็ใไธบๆญค๏ผMMOCR ๆไพไบๅบไบ [MMDistributedDataParallel](mmengine.model.wrappers.MMDistributedDataParallel) ๅฎ็ฐ็ๅๅธๅผ่ๆฌ `tools/dist_train.sh` ๅ `tools/dist_test.sh`ใ
-
-```bash
-# ่ฎญ็ป
-NNODES=${NNODES} NODE_RANK=${NODE_RANK} PORT=${MASTER_PORT} MASTER_ADDR=${MASTER_ADDR} ./tools/dist_train.sh ${CONFIG_FILE} ${GPU_NUM} [PY_ARGS]
-# ๆต่ฏ
-NNODES=${NNODES} NODE_RANK=${NODE_RANK} PORT=${MASTER_PORT} MASTER_ADDR=${MASTER_ADDR} ./tools/dist_test.sh ${CONFIG_FILE} ${CHECKPOINT_FILE} ${GPU_NUM} [PY_ARGS]
-```
-
-ไธ่กจๅๅบไบ `dist_*.sh` ๆฏๆ็ๅๆฐ๏ผ
-
-| ๅๆฐ | ็ฑปๅ | ่ฏดๆ |
-| --------------- | ---- | ---------------------------------------------------------------------------------- |
-| NNODES | int | ๆปๅ
ฑไฝฟ็จ็ๆบๅจ่็นไธชๆฐ๏ผ้ป่ฎคไธบ 1ใ |
-| NODE_RANK | int | ่็น็ผๅท๏ผ้ป่ฎคไธบ 0ใ |
-| PORT | int | ๅจ RANK 0 ๆบๅจไธไฝฟ็จ็ MASTER_PORT ็ซฏๅฃๅท๏ผๅๅผ่ๅดๆฏ 0 ่ณ 65535๏ผ้ป่ฎคๅผไธบ 29500ใ |
-| MASTER_ADDR | str | RANK 0 ๆบๅจ็ IP ๅฐๅ๏ผ้ป่ฎคๅผไธบ 127.0.0.1ใ |
-| CONFIG_FILE | str | ๏ผๅฟ
้กป๏ผๆๅฎ้
็ฝฎๆไปถ็ๅฐๅใ |
-| CHECKPOINT_FILE | str | ๏ผๅฟ
้กป๏ผไป
ๅจ dist_test.sh ไธญ้็จ๏ผๆๅฎๆจกๅๆ้็ๅฐๅใ |
-| GPU_NUM | int | ๏ผๅฟ
้กป๏ผๆๅฎ GPU ็ๆฐ้ใ |
-| \[PY_ARGS\] | str | ่ฏฅ้จๅไธๅ็ๅๆฐ้ฝไผ่ขซ็ดๆฅไผ ๅ
ฅ tools/train.py ๆ tools/test.py ไธญใ |
-
-่ฟไธคไธช่ๆฌๅฏไปฅๅฎ็ฐ**ๅๆบๅคๅก**ๆ**ๅคๆบๅคๅก**็่ฎญ็ปๅๆต่ฏ๏ผไธ้ขๆผ็คบไบๅฎไปฌๅจไธๅๅบๆฏไธ็็จๆณใ
-
-### ๅๆบๅคๅก
-
-ไปฅไธๅฝไปคๆผ็คบไบๅฆไฝๅจๆญ่ฝฝๅคๅ GPU ็**ๅๅฐๆบๅจ**ไธไฝฟ็จๆๅฎๆฐ็ฎ็ GPU ่ฟ่ก่ฎญ็ปๅๆต่ฏ๏ผ
-
-1. **่ฎญ็ป**
-
- ไฝฟ็จๅๅฐๆบๅจไธ็ 4 ๅ GPU ่ฎญ็ป DBNetใ
-
- ```bash
- # ๅๆบ 4 ๅก่ฎญ็ป DBNet
- tools/dist_train.sh configs/textdet/dbnet/dbnet_r50dcnv2_fpnc_1200e_icdar2015.py 4
- ```
-
-2. **ๆต่ฏ**
-
- ไฝฟ็จๅๅฐๆบๅจไธ็ 4 ๅ GPU ๆต่ฏ DBNetใ
-
- ```bash
- # ๅๆบ 4 ๅกๆต่ฏ DBNet
- tools/dist_test.sh configs/textdet/dbnet/dbnet_r50dcnv2_fpnc_1200e_icdar2015.py dbnet_r50.pth 4
- ```
-
-### ๅๆบๅคไปปๅก่ฎญ็ปๅๆต่ฏ
-
-ๅฏนไบๆญ่ฝฝๅคๅ GPU ็ๅๅฐๆๅกๅจ่่จ๏ผ็จๆทๅฏไปฅ้่ฟๆๅฎ GPU ็ๅฝขๅผๆฅๅๆถๆง่กไธๅ็่ฎญ็ปไปปๅกใไพๅฆ๏ผไปฅไธๅฝไปคๆผ็คบไบๅฆไฝๅจไธๅฐ 8 ๅก GPU ๆๅกๅจไธๅๅซไฝฟ็จ `[0, 1, 2, 3]` ๅกๆต่ฏ DBNet ๅ `[4, 5, 6, 7]` ๅก่ฎญ็ป CRNN๏ผ
-
-```bash
-# ๆๅฎไฝฟ็จ gpu:0,1,2,3 ๆต่ฏ DBNet๏ผๅนถๅ้
็ซฏๅฃๅท 29500
-CUDA_VISIBLE_DEVICES=0,1,2,3 PORT=29500 ./tools/dist_test.sh configs/textdet/dbnet/dbnet_r50dcnv2_fpnc_1200e_icdar2015.py dbnet_r50.pth 4
-# ๆๅฎไฝฟ็จ gpu:4,5,6,7 ่ฎญ็ป CRNN๏ผๅนถๅ้
็ซฏๅฃๅท 29501
-CUDA_VISIBLE_DEVICES=4,5,6,7 PORT=29501 ./tools/dist_train.sh configs/textrecog/crnn/crnn_academic_dataset.py 4
-```
-
-```{note}
-`dist_train.sh` ้ป่ฎคๅฐ `MASTER_PORT` ่ฎพ็ฝฎไธบ `29500`๏ผๅฝๅๅฐๆบๅจไธๆๅ
ถๅฎ่ฟ็จๅทฒๅ ็จ่ฏฅ็ซฏๅฃๆถ๏ผ็จๅบๅไผๅบ็ฐ่ฟ่กๆถ้่ฏฏ `RuntimeError: Address already in use`ใๆญคๆถ๏ผ็จๆท้่ฆๅฐ `MASTER_PORT` ่ฎพ็ฝฎไธบ `(0~65535)` ่ๅดๅ
็ๅ
ถๅฎ็ฉบ้ฒ็ซฏๅฃๅทใ
-```
-
-### ๅคๆบๅคๅก่ฎญ็ปๅๆต่ฏ
-
-MMOCR ๅบไบ[torch.distributed](https://pytorch.org/docs/stable/distributed.html#launch-utility) ๆไพไบ็ธๅๅฑๅ็ฝไธ็ๅคๅฐๆบๅจ้ด็ๅคๅกๅๅธๅผ่ฎญ็ปใ
-
-1. **่ฎญ็ป**
-
- ไปฅไธๅฝไปคๆผ็คบไบๅฆไฝๅจไธคๅฐๆบๅจไธๅๅซไฝฟ็จ 2 ๅผ GPU ๅ่ฎก 4 ๅก่ฎญ็ป DBNet๏ผ
-
- ```bash
- # ็คบไพ๏ผๅจไธคๅฐๆบๅจไธๅๅซไฝฟ็จ 2 ๅผ GPU ๅ่ฎก 4 ๅก่ฎญ็ป DBNet
- # ๅจ โๆบๅจ1โ ไธ่ฟ่กไปฅไธๅฝไปค
- NNODES=2 NODE_RANK=0 PORT=29501 MASTER_ADDR=10.140.0.169 tools/dist_train.sh configs/textdet/dbnet/dbnet_r50dcnv2_fpnc_1200e_icdar2015.py 2
- # ๅจ โๆบๅจ2โ ไธ่ฟ่กไปฅไธๅฝไปค
- NNODES=2 NODE_RANK=1 PORT=29501 MASTER_ADDR=10.140.0.169 tools/dist_train.sh configs/textdet/dbnet/dbnet_r50dcnv2_fpnc_1200e_icdar2015.py 2
- ```
-
-2. **ๆต่ฏ**
-
- ไปฅไธๅฝไปคๆผ็คบไบๅฆไฝๅจไธคๅฐๆบๅจไธๅๅซไฝฟ็จ 2 ๅผ GPU ๅ่ฎก 4 ๅกๆต่ฏ๏ผ
-
- ```bash
- # ็คบไพ๏ผๅจไธคๅฐๆบๅจไธๅๅซไฝฟ็จ 2 ๅผ GPU ๅ่ฎก 4 ๅกๆต่ฏ
- # ๅจ โๆบๅจ1โ ไธ่ฟ่กไปฅไธๅฝไปค
- NNODES=2 NODE_RANK=0 PORT=29500 MASTER_ADDR=10.140.0.169 tools/dist_test.sh configs/textdet/dbnet/dbnet_r50dcnv2_fpnc_1200e_icdar2015.py dbnet_r50.pth 2
- # ๅจ โๆบๅจ2โ ไธ่ฟ่กไปฅไธๅฝไปค
- NNODES=2 NODE_RANK=1 PORT=29501 MASTER_ADDR=10.140.0.169 tools/dist_test.sh configs/textdet/dbnet/dbnet_r50dcnv2_fpnc_1200e_icdar2015.py dbnet_r50.pth 2
- ```
-
- ```{note}
- ้่ฆๆณจๆ็ๆฏ๏ผ้็จๅคๆบๅคๅก่ฎญ็ปๆถ๏ผๆบๅจ้ด็็ฝ็ปไผ ่พ้ๅบฆๅฏ่ฝๆไธบ่ฎญ็ป้ๅบฆ็็ถ้ขใ
- ```
-
-## ้็พค่ฎญ็ปๅๆต่ฏ
-
-้ๅฏน [Slurm](https://slurm.schedmd.com/) ่ฐๅบฆ็ณป็ป็ฎก็็่ฎก็ฎ้็พค๏ผMMOCR ๆไพไบๅฏนๅบ็่ฎญ็ปๅๆต่ฏไปปๅกๆไบค่ๆฌ `tools/slurm_train.sh` ๅ `tools/slurm_test.sh`ใ
-
-```bash
-# tools/slurm_train.sh ๆไพๅบไบ slurm ่ฐๅบฆ็ณป็ป็ฎก็็่ฎก็ฎ้็พคไธๆไบค่ฎญ็ปไปปๅก็่ๆฌ
-GPUS=${GPUS} GPUS_PER_NODE=${GPUS_PER_NODE} CPUS_PER_TASK=${CPUS_PER_TASK} SRUN_ARGS=${SRUN_ARGS} ./tools/slurm_train.sh ${PARTITION} ${JOB_NAME} ${CONFIG_FILE} ${WORK_DIR} [PY_ARGS]
-
-# tools/slurm_test.sh ๆไพๅบไบ slurm ่ฐๅบฆ็ณป็ป็ฎก็็่ฎก็ฎ้็พคไธๆไบคๆต่ฏไปปๅก็่ๆฌ
-GPUS=${GPUS} GPUS_PER_NODE=${GPUS_PER_NODE} CPUS_PER_TASK=${CPUS_PER_TASK} SRUN_ARGS=${SRUN_ARGS} ./tools/slurm_test.sh ${PARTITION} ${JOB_NAME} ${CONFIG_FILE} ${CHECKPOINT_FILE} ${WORK_DIR} [PY_ARGS]
-```
-
-| ๅๆฐ | ็ฑปๅ | ่ฏดๆ |
-| --------------- | ---- | ------------------------------------------------------------------------- |
-| GPUS | int | ไฝฟ็จ็ GPU ๆฐ็ฎ๏ผ้ป่ฎคไธบ8ใ |
-| GPUS_PER_NODE | int | ๆฏๅฐ่็นๆบๅจไธๆญ่ฝฝ็ GPU ๆฐ็ฎ๏ผ้ป่ฎคไธบ8ใ |
-| CPUS_PER_TASK | int | ไปปๅกไฝฟ็จ็ CPU ไธชๆฐ๏ผ้ป่ฎคไธบ5ใ |
-| SRUN_ARGS | str | ๅ
ถไป srun ๆฏๆ็ๅๆฐใ่ฏฆ่ง[่ฟ้](https://slurm.schedmd.com/srun.html) |
-| PARTITION | str | ๏ผๅฟ
้กป๏ผๆๅฎไฝฟ็จ็้็พคๅๅบใ |
-| JOB_NAME | str | ๏ผๅฟ
้กป๏ผๆไบคไปปๅก็ๅ็งฐใ |
-| WORK_DIR | str | ๏ผๅฟ
้กป๏ผไปปๅก็ๅทฅไฝ็ฎๅฝ๏ผ่ฎญ็ปๆฅๅฟไปฅๅๆจกๅ็ checkpoints ๅฐ่ขซไฟๅญ่ณ่ฏฅ็ฎๅฝใ |
-| CHECKPOINT_FILE | str | ๏ผๅฟ
้กป๏ผไป
ๅจ slurm_test.sh ไธญ้็จ๏ผๆๅๆจกๅๆ้็ๅฐๅใ |
-| \[PY_ARGS\] | str | tools/train.py ไปฅๅ tools/test.py ๆฏๆ็ๅๆฐใ |
-
-่ฟไธคไธช่ๆฌๅฏไปฅๅฎ็ฐ slurm ้็พคไธ็่ฎญ็ปๅๆต่ฏ๏ผไธ้ขๆผ็คบไบๅฎไปฌๅจไธๅๅบๆฏไธ็็จๆณใ
-
-1. ่ฎญ็ป
-
- ไปฅไธ็คบไพไธบๅจ slurm ้็พค dev ๅๅบ็ณ่ฏท 1 ๅ GPU ่ฟ่ก DBNet ่ฎญ็ปใ
-
-```bash
-# ็คบไพ๏ผๅจ slurm ้็พค dev ๅๅบ็ณ่ฏท 1ๅ GPU ่ตๆบ่ฟ่ก DBNet ่ฎญ็ปไปปๅก
-GPUS=1 GPUS_PER_NODE=1 CPUS_PER_TASK=5 tools/slurm_train.sh dev db_r50 configs/textdet/dbnet/dbnet_r50dcnv2_fpnc_1200e_icdar2015.py work_dir
-```
-
-2. ๆต่ฏ
-
- ๅ็๏ผ ๅๆไพไบๆต่ฏไปปๅกๆไบค่ๆฌใไปฅไธ็คบไพไธบๅจ slurm ้็พค dev ๅๅบ็ณ่ฏท 1 ๅ GPU ่ตๆบ่ฟ่ก DBNet ๆต่ฏใ
-
-```bash
-# ็คบไพ๏ผๅจ slurm ้็พค dev ๅๅบ็ณ่ฏท 1ๅ GPU ่ตๆบ่ฟ่ก DBNet ๆต่ฏไปปๅก
-GPUS=1 GPUS_PER_NODE=1 CPUS_PER_TASK=5 tools/slurm_test.sh dev db_r50 configs/textdet/dbnet/dbnet_r50dcnv2_fpnc_1200e_icdar2015.py dbnet_r50.pth work_dir
-```
-
-## ่ฟ้ถๆๅทง
-
-### ไปๆญ็นๆขๅค่ฎญ็ป
-
-`tools/train.py` ๆไพไบไปๆญ็นๆขๅค่ฎญ็ป็ๅ่ฝ๏ผ็จๆทไป
้ๅจๅฝไปคไธญๆๅฎ `--resume` ๅๆฐ๏ผๅณๅฏ่ชๅจไปๆญ็นๆขๅค่ฎญ็ปใ
-
-```bash
-# ็คบไพ๏ผไปๆญ็นๆขๅค่ฎญ็ป
-python tools/train.py configs/textdet/dbnet/dbnet_r50dcnv2_fpnc_1200e_icdar2015.py 4 --resume
-```
-
-้ป่ฎคๅฐ๏ผ็จๅบๅฐ่ชๅจไปไธๆฌก่ฎญ็ป่ฟ็จไธญๆๅๆๅไฟๅญ็ๆญ็น๏ผๅณ `latest.pth` ๅคๅผๅง็ปง็ปญ่ฎญ็ปใๅฆๆ็จๆทๅธๆๆๅฎไป็นๅฎ็ๆญ็นๅคๅผๅงๆขๅค่ฎญ็ป๏ผๅๅฏไปฅๆๅฆไธๆ ผๅผๅจๆจกๅ็้
็ฝฎๆไปถไธญ่ฎพๅฎ่ฏฅๆญ็น็่ทฏๅพใ
-
-```python
-# ็คบไพ๏ผๅจ้
็ฝฎๆไปถไธญ่ฎพ็ฝฎๆณ่ฆๅ ่ฝฝ็ๆญ็น่ทฏๅพ
-load_from = 'work_dir/dbnet/models/epoch_10000.pth'
-```
-
-### ๆททๅ็ฒพๅบฆ่ฎญ็ป
-
-ๆททๅ็ฒพๅบฆ่ฎญ็ปๅฏไปฅๅจ็ผฉๅๅ
ๅญๅ ็จ็ๅๆถๆๅ่ฎญ็ป้ๅบฆ๏ผไธบๆญค๏ผMMOCR ๆไพไบไธ้ฎๅผ็ๆททๅ็ฒพๅบฆ่ฎญ็ปๆนๆก๏ผไป
้ๅจ่ฎญ็ปๆถๆทปๅ `--amp` ๅๆฐๅณๅฏใ
-
-```bash
-# ็คบไพ๏ผไฝฟ็จ่ชๅจๆททๅ็ฒพๅบฆ่ฎญ็ป
-python tools/train.py configs/textdet/dbnet/dbnet_r50dcnv2_fpnc_1200e_icdar2015.py 4 --amp
-```
-
-ไธ่กจๅๅบไบ MMOCR ไธญๅ็ฎๆณๅฏน่ชๅจๆททๅ็ฒพๅบฆ่ฎญ็ป็ๆฏๆๆ
ๅต๏ผ
-
-| | ๆฏๅฆๆฏๆๆททๅ็ฒพๅบฆ่ฎญ็ป | ๅคๆณจ |
-| ------------- | :------------------: | :---------------------------: |
-| | ๆๆฌๆฃๆต | |
-| DBNet | ๆฏ | |
-| DBNetpp | ๆฏ | |
-| DRRG | ๅฆ | roi_align_rotated ไธๆฏๆ fp16 |
-| FCENet | ๅฆ | BCELoss ไธๆฏๆ fp16 |
-| Mask R-CNN | ๆฏ | |
-| PANet | ๆฏ | |
-| PSENet | ๆฏ | |
-| TextSnake | ๅฆ | |
-| | ๆๆฌ่ฏๅซ | |
-| ABINet | ๆฏ | |
-| ASTER | ๆฏ | |
-| CRNN | ๆฏ | |
-| MASTER | ๆฏ | |
-| NRTR | ๆฏ | |
-| RobustScanner | ๆฏ | |
-| SAR | ๆฏ | |
-| SATRN | ๆฏ | |
-
-### ่ชๅจๅญฆไน ็็ผฉๆพ
-
-MMOCR ๅจ้
็ฝฎๆไปถไธญไธบๆฏไธไธชๆจกๅ่ฎพ็ฝฎไบ้ป่ฎค็ๅๅงๅญฆไน ็๏ผ็ถ่๏ผๅฝ็จๆทไฝฟ็จ็ `batch_size` ไธๅไบๆไปฌ้ข่ฎพ็ `base_batch_size` ๆถ๏ผ่ฟไบๅๅงๅญฆไน ็ๅฏ่ฝไธๅๅฎๅ
จ้็จใๅ ๆญค๏ผๆไปฌๆไพไบ่ชๅจๅญฆไน ็็ผฉๆพๅทฅๅ
ทใๅฝไฝฟ็จไธๅไบ MMOCR ้ข่ฎพ็ `base_batch_size` ่ฟ่ก่ฎญ็ปๆถ๏ผ็จๆทไป
้ๆทปๅ `--auto-scale-lr` ๅๆฐๅณๅฏ่ชๅจไพๆฎๆฐ็ `batch_size` ๅฐๅญฆไน ็็ผฉๆพ่ณๅฏนๅบๅฐบๅบฆใ
-
-```bash
-# ็คบไพ๏ผไฝฟ็จ่ชๅจๅญฆไน ็็ผฉๆพ
-python tools/train.py configs/textdet/dbnet/dbnet_r50dcnv2_fpnc_1200e_icdar2015.py 4 --auto-scale-lr
-```
-
-### ๅฏ่งๅๆจกๅๆต่ฏ็ปๆ
-
-`tools/test.py` ๆไพไบๅฏ่งๅๆฅๅฃ๏ผไปฅๆนไพฟ็จๆทๅฏนๆจกๅ่ฟ่กๅฎๆงๅๆใ
-
-
-
-
-
-(็ปฟ่ฒๆกไธบ็ๅฎๆ ๆณจ๏ผ็บข่ฒๆกไธบ้ขๆต็ปๆ)
-
-
-
-
-
-
-
-๏ผ็ปฟ่ฒๅญไฝไธบ็ๅฎๆ ๆณจ๏ผ็บข่ฒๅญไฝไธบ้ขๆต็ปๆ๏ผ
-
-
-
-
-
-
-
-๏ผไปๅทฆ่ณๅณๅๅซไธบ๏ผๅๅพ๏ผๆๆฌๆฃๆตๅ่ฏๅซ็ปๆ๏ผๆๆฌๅ็ฑป็ปๆ๏ผๅ
ณ็ณปๅพ๏ผ
-
-
-
-```bash
-# ็คบไพ1๏ผๆฏ้ด้ 2 ็ง็ปๅถๅบ
-python tools/test.py configs/textdet/dbnet/dbnet_r50dcnv2_fpnc_1200e_icdar2015.py dbnet_r50.pth --show --wait-time 2
-
-# ็คบไพ2๏ผๅฏนไบไธๆฏๆๅพๅฝขๅ็้ข็็ณป็ป๏ผๅฆ่ฎก็ฎ้็พค็ญ๏ผ๏ผๅฏไปฅๅฐๅฏ่งๅ็ปๆๅญๅ
ฅๆๅฎ่ทฏๅพ
-python tools/test.py configs/textdet/dbnet/dbnet_r50dcnv2_fpnc_1200e_icdar2015.py dbnet_r50.pth --show-dir ./vis_results
-```
-
-`tools/test.py` ไธญๅฏ่งๅ็ธๅ
ณๅๆฐ่ฏดๆ๏ผ
-
-| ๅๆฐ | ็ฑปๅ | ่ฏดๆ |
-| ----------- | ----- | -------------------------------- |
-| --show | bool | ๆฏๅฆ็ปๅถๅฏ่งๅ็ปๆใ |
-| --show-dir | str | ๅฏ่งๅๅพ็ๅญๅจ่ทฏๅพใ |
-| --wait-time | float | ๅฏ่งๅ้ด้ๆถ้ด๏ผ็ง๏ผ๏ผ้ป่ฎคไธบ 2ใ |
-
-### ๆต่ฏๆถๆฐๆฎๅขๅผบ
-
-ๆต่ฏๆถๅขๅผบ๏ผๆ็ๆฏๅจๆจ็๏ผ้ขๆต๏ผ้ถๆฎต๏ผๅฐๅๅงๅพ็่ฟ่กๆฐดๅนณ็ฟป่ฝฌใๅ็ด็ฟป่ฝฌใๅฏน่ง็บฟ็ฟป่ฝฌใๆ่ฝฌ่งๅบฆ็ญๆฐๆฎๅขๅผบๆไฝ๏ผๅพๅฐๅคๅผ ๅพ๏ผๅๅซ่ฟ่กๆจ็๏ผๅๅฏนๅคไธช็ปๆ่ฟ่ก็ปผๅๅๆ๏ผๅพๅฐๆ็ป่พๅบ็ปๆใ
-ไธบๆญค๏ผMMOCR ๆไพไบไธ้ฎๅผๆต่ฏๆถๆฐๆฎๅขๅผบ๏ผไป
้ๅจๆต่ฏๆถๆทปๅ `--tta` ๅๆฐๅณๅฏใ
-
-```{note}
-TTA ไป
ๆฏๆๆๆฌ่ฏๅซๆจกๅใ
-```
-
-```bash
-python tools/test.py configs/textrecog/crnn/crnn_mini-vgg_5e_mj.py checkpoints/crnn_mini-vgg_5e_mj.pth --tta
-```
diff --git a/pipeline/mmocr/docs/zh_cn/user_guides/useful_tools.md b/pipeline/mmocr/docs/zh_cn/user_guides/useful_tools.md
deleted file mode 100644
index 2a607245a300e9c93ae7388da481dfa347c3a2e9..0000000000000000000000000000000000000000
--- a/pipeline/mmocr/docs/zh_cn/user_guides/useful_tools.md
+++ /dev/null
@@ -1,241 +0,0 @@
-# ๅธธ็จๅทฅๅ
ท
-
-## ๅฏ่งๅๅทฅๅ
ท
-
-### ๆฐๆฎ้ๅฏ่งๅๅทฅๅ
ท
-
-MMOCR ๆไพไบๆฐๆฎ้ๅฏ่งๅๅทฅๅ
ท `tools/visualizations/browse_datasets.py` ไปฅ่พ
ๅฉ็จๆทๆๆฅๅฏ่ฝ้ๅฐ็ๆฐๆฎ้็ธๅ
ณ็้ฎ้ขใ็จๆทๅช้่ฆๆๅฎๆไฝฟ็จ็่ฎญ็ป้
็ฝฎๆไปถ๏ผ้ๅธธๅญๆพๅจๅฆ `configs/textdet/dbnet/xxx.py` ๆไปถไธญ๏ผๆๆฐๆฎ้้
็ฝฎ๏ผ้ๅธธๅญๆพๅจ `configs/textdet/_base_/datasets/xxx.py` ๆไปถไธญ๏ผ่ทฏๅพใ่ฏฅๅทฅๅ
ทๅฐไพๆฎ่พๅ
ฅ็้
็ฝฎๆไปถ็ฑปๅ่ชๅจๅฐ็ป่ฟๆฐๆฎๆตๆฐด็บฟ๏ผdata pipeline๏ผๅค็่ฟ็ๅพๅๅๅ
ถๅฏนๅบ็ๆ ็ญพ๏ผๆๅๅงๅพ็ๅๅ
ถๅฏนๅบ็ๆ ็ญพ็ปๅถๅบๆฅใ
-
-#### ๆฏๆๅๆฐ
-
-```bash
-python tools/visualizations/browse_dataset.py \
- ${CONFIG_FILE} \
- [-o, --output-dir ${OUTPUT_DIR}] \
- [-p, --phase ${DATASET_PHASE}] \
- [-m, --mode ${DISPLAY_MODE}] \
- [-t, --task ${DATASET_TASK}] \
- [-n, --show-number ${NUMBER_IMAGES_DISPLAY}] \
- [-i, --show-interval ${SHOW_INTERRVAL}] \
- [--cfg-options ${CFG_OPTIONS}]
-```
-
-| ๅๆฐๅ | ็ฑปๅ | ๆ่ฟฐ |
-| ------------------- | ------------------------------------- | ------------------------------------------------------------------------------------------------------------------------------------------------ |
-| config | str | (ๅฟ
้กป) ้
็ฝฎๆไปถ่ทฏๅพใ |
-| -o, --output-dir | str | ๅฆๆๅพๅฝขๅ็้ขไธๅฏ็จ๏ผ่ฏทๆๅฎไธไธช่พๅบ่ทฏๅพๆฅไฟๅญๅฏ่งๅ็ปๆใ |
-| -p, --phase | str | ็จไบๆๅฎ้่ฆๅฏ่งๅ็ๆฐๆฎ้ๅ็๏ผๅฆ "train", "test", "val"ใๅฝๆฐๆฎ้ๅญๅจๅคไธชๅ็งๆถ๏ผไนๅฏไปฅ้่ฟ่ฏฅๅๆฐๆฅๆๅฎๅพ
ๅฏ่งๅ็ๅ็ใ |
-| -m, --mode | `original`, `transformed`, `pipeline` | ็จไบๆๅฎๆฐๆฎๅฏ่งๅ็ๆจกๅผใ`original`๏ผๅๅงๆจกๅผ๏ผไป
ๅฏ่งๅๆฐๆฎ้็ๅๅงๆ ๆณจ๏ผ`transformed`๏ผๅๆขๆจกๅผ๏ผๅฑ็คบ็ป่ฟๆๆๆฐๆฎๅๆขๆญฅ้ชค็ๆ็ปๅพๅ๏ผ`pipeline`๏ผๆตๆฐด็บฟๆจกๅผ๏ผๅฑ็คบๆฐๆฎๅๆข่ฟ็จไธญๆฏไธไธชไธญ้ดๆญฅ้ชค็ๅๆขๅพๅใ้ป่ฎคไฝฟ็จ `transformed` ๅๆขๆจกๅผใ |
-| -t, --task | `auto`, `textdet`, `textrecog` | ็จไบๆๅฎๅฏ่งๅๆฐๆฎ้็ไปปๅก็ฑปๅใ`auto`๏ผ่ชๅจๆจกๅผ๏ผๅฐไพๆฎ็ปๅฎ็้
็ฝฎๆไปถ่ชๅจ้ๆฉๅ้็ไปปๅก็ฑปๅ๏ผๅฆๆๆ ๆณ่ชๅจ่ทๅไปปๅก็ฑปๅ๏ผๅ้่ฆ็จๆทๆๅจๆๅฎไธบ `textdet` ๆๆฌๆฃๆตไปปๅก ๆ `textrecog` ๆๆฌ่ฏๅซไปปๅกใ้ป่ฎค้็จ `auto` ่ชๅจๆจกๅผใ |
-| -n, --show-number | int | ๆๅฎ้่ฆๅฏ่งๅ็ๆ ทๆฌๆฐ้ใ่ฅ่ฏฅๅๆฐ็ผบ็ๅ้ป่ฎคๅฐๅฏ่งๅๅ
จ้จๅพ็ใ |
-| -i, --show-interval | float | ๅฏ่งๅๅพๅ้ด้ๆถ้ด๏ผ้ป่ฎคไธบ 2 ็งใ |
-| --cfg-options | float | ็จไบ่ฆ็้
็ฝฎๆไปถไธญ็ๅๆฐ๏ผ่ฏฆ่ง[็คบไพ](./config.md#command-line-modification)ใ |
-
-#### ็จๆณ็คบไพ
-
-ไปฅไธ็คบไพๆผ็คบไบๅฆไฝไฝฟ็จ่ฏฅๅทฅๅ
ทๅฏ่งๅ "DBNet_R50_icdar2015" ๆจกๅไฝฟ็จ็่ฎญ็ปๆฐๆฎใ
-
-```Bash
-# ไฝฟ็จ้ป่ฎคๅๆฐๅฏ่งๅ "dbnet_r50dcn_v2_fpnc_1200e_icadr2015" ๆจกๅ็่ฎญ็ปๆฐๆฎ
-python tools/visualizations/browse_dataset.py configs/textdet/dbnet/dbnet_resnet50-dcnv2_fpnc_1200e_icdar2015.py
-```
-
-้ป่ฎคๆ
ๅตไธ๏ผๅฏ่งๅๆจกๅผไธบ "transformed"๏ผๆจๅฐ็ๅฐ็ป็ฑๆฐๆฎๆตๆฐด็บฟๅๆข่ฟๅ็ๅพๅๅๆ ๆณจ๏ผ
-
-
-
-
-
-ๅฆๆๆจๅชๆณๅฏ่งๅๅๅงๆฐๆฎ้๏ผๅช้ๅฐๆจกๅผ่ฎพ็ฝฎไธบ "original"๏ผ
-
-```Bash
-python tools/visualizations/browse_dataset.py configs/textdet/dbnet/dbnet_resnet50-dcnv2_fpnc_1200e_icdar2015.py -m original
-```
-
-
-
-ๆ่
๏ผๆจไนๅฏไปฅไฝฟ็จ "pipeline" ๆจกๅผๆฅๅฏ่งๅๆดไธชๆฐๆฎๆตๆฐด็บฟ็ไธญ้ด็ปๆ๏ผ
-
-```Bash
-python tools/visualizations/browse_dataset.py configs/textdet/dbnet/dbnet_resnet50-dcnv2_fpnc_1200e_icdar2015.py -m pipeline
-```
-
-
-
-ๅฆๅค๏ผ็จๆท่ฟๅฏไปฅ้่ฟๆๅฎๆฐๆฎ้้
็ฝฎๆไปถ็่ทฏๅพๆฅๅฏ่งๅๆฐๆฎ้็ๅๅงๅพๅๅๅ
ถๅฏนๅบ็ๆ ๆณจ๏ผไพๅฆ๏ผ
-
-```Bash
-python tools/visualizations/browse_dataset.py configs/textrecog/_base_/datasets/icdar2015.py
-```
-
-้จๅๆฐๆฎ้ๅฏ่ฝๆๅคไธชๅไฝใไพๅฆ๏ผ`icdar2015` ๆๆฌ่ฏๅซๆฐๆฎ้็[้
็ฝฎๆไปถ](/configs/textrecog/_base_/datasets/icdar2015.py)ไธญๅ
ๅซไธคไธชๆต่ฏ้ๅไฝ๏ผๅๅซไธบ `icdar2015_textrecog_test` ๅ `icdar2015_1811_textrecog_test`๏ผๅฆไธๆ็คบ๏ผ
-
-```python
-icdar2015_textrecog_test = dict(
- ann_file='textrecog_test.json',
- # ...
- )
-
-icdar2015_1811_textrecog_test = dict(
- ann_file='textrecog_test_1811.json',
- # ...
-)
-```
-
-ๅจ่ฟ็งๆ
ๅตไธ๏ผ็จๆทๅฏไปฅ้่ฟๆๅฎ `-p` ๅๆฐๆฅๅฏ่งๅไธๅ็ๅไฝ๏ผไพๅฆ๏ผไฝฟ็จไปฅไธๅฝไปคๅฏ่งๅ `icdar2015_1811_textrecog_test` ๅไฝ๏ผ
-
-```Bash
-python tools/visualizations/browse_dataset.py configs/textrecog/_base_/datasets/icdar2015.py -p icdar2015_1811_textrecog_test
-```
-
-ๅบไบ่ฏฅๅทฅๅ
ท๏ผ็จๆทๅฏไปฅ่ฝปๆพๅฐๆฅ็ๆฐๆฎ้็ๅๅงๅพๅๅๅ
ถๅฏนๅบ็ๆ ๆณจ๏ผไปฅไพฟไบๆฃๆฅๆฐๆฎ้็ๆ ๆณจๆฏๅฆๆญฃ็กฎใ
-
-### ไผๅๅจๅๆฐ็ญ็ฅๅฏ่งๅๅทฅๅ
ท
-
-MMOCRๆไพไบไผๅๅจๅๆฐๅฏ่งๅๅทฅๅ
ท `tools/visualizations/vis_scheduler.py` ไปฅ่พ
ๅฉ็จๆทๆๆฅไผๅๅจ็่ถ
ๅๆฐ่ฐๅบฆๅจ๏ผๆ ้่ฎญ็ป๏ผ๏ผๆฏๆๅญฆไน ็๏ผlearning rate๏ผๅๅจ้๏ผmomentum๏ผใ
-
-#### ๅทฅๅ
ท็ฎไป
-
-```bash
-python tools/visualizations/vis_scheduler.py \
- ${CONFIG_FILE} \
- [-p, --parameter ${PARAMETER_NAME}] \
- [-d, --dataset-size ${DATASET_SIZE}] \
- [-n, --ngpus ${NUM_GPUs}] \
- [-s, --save-path ${SAVE_PATH}] \
- [--title ${TITLE}] \
- [--style ${STYLE}] \
- [--window-size ${WINDOW_SIZE}] \
- [--cfg-options]
-```
-
-**ๆๆๅๆฐ็่ฏดๆ**๏ผ
-
-- `config` : ๆจกๅ้
็ฝฎๆไปถ็่ทฏๅพใ
-- **`-p, parameter`**: ๅฏ่งๅๅๆฐๅ๏ผๅช่ฝไธบ `["lr", "momentum"]` ไนไธ๏ผ ้ป่ฎคไธบ `"lr"`.
-- **`-d, --dataset-size`**: ๆฐๆฎ้็ๅคงๅฐใๅฆๆๆๅฎ๏ผ`build_dataset` ๅฐ่ขซ่ทณ่ฟๅนถไฝฟ็จ่ฟไธชๅคงๅฐไฝไธบๆฐๆฎ้ๅคงๅฐ๏ผ้ป่ฎคไฝฟ็จ `build_dataset` ๆๅพๆฐๆฎ้็ๅคงๅฐใ
-- **`-n, --ngpus`**: ไฝฟ็จ GPU ็ๆฐ้, ้ป่ฎคไธบ1ใ
-- **`-s, --save-path`**: ไฟๅญ็ๅฏ่งๅๅพ็็่ทฏๅพ๏ผ้ป่ฎคไธไฟๅญใ
-- `--title`: ๅฏ่งๅๅพ็็ๆ ้ข๏ผ้ป่ฎคไธบ้
็ฝฎๆไปถๅใ
-- `--style`: ๅฏ่งๅๅพ็็้ฃๆ ผ๏ผ้ป่ฎคไธบ `whitegrid`ใ
-- `--window-size`: ๅฏ่งๅ็ชๅฃๅคงๅฐ๏ผๅฆๆๆฒกๆๆๅฎ๏ผ้ป่ฎคไธบ `12*7`ใๅฆๆ้่ฆๆๅฎ๏ผๆ็
งๆ ผๅผ \`W\*H'ใ
-- `--cfg-options`: ๅฏน้
็ฝฎๆไปถ็ไฟฎๆน๏ผๅ่[ๅญฆไน ้
็ฝฎๆไปถ](../user_guides/config.md)ใ
-
-```{note}
-้จๅๆฐๆฎ้ๅจ่งฃๆๆ ๆณจ้ถๆฎตๆฏ่พ่ๆถ๏ผๅฏ็ดๆฅๅฐ `-d, dataset-size` ๆๅฎๆฐๆฎ้็ๅคงๅฐ๏ผไปฅ่็บฆๆถ้ดใ
-```
-
-#### ๅฆไฝๅจๅผๅง่ฎญ็ปๅๅฏ่งๅๅญฆไน ็ๆฒ็บฟ
-
-ไฝ ๅฏไปฅไฝฟ็จๅฆไธๅฝไปคๆฅ็ปๅถ้
็ฝฎๆไปถ `configs/textdet/dbnet/dbnet_resnet50-dcnv2_fpnc_1200e_icdar2015.py` ๅฐไผไฝฟ็จ็ๅๅ็ๆฒ็บฟ๏ผ
-
-```bash
-python tools/visualizations/vis_scheduler.py configs/textdet/dbnet/dbnet_resnet50-dcnv2_fpnc_1200e_icdar2015.py -d 100
-```
-
-
-
-## ๅๆๅทฅๅ
ท
-
-### ็ฆป็บฟ่ฏๆตๅทฅๅ
ท
-
-ๅฏนไบๅทฒไฟๅญ็้ขๆต็ปๆ๏ผๆไปฌๆไพไบ็ฆป็บฟ่ฏๆต่ๆฌ `tools/analysis_tools/offline_eval.py`ใไพๅฆ๏ผไปฅไธไปฃ็ ๆผ็คบไบๅฆไฝไฝฟ็จ่ฏฅๅทฅๅ
ทๅฏน "PSENet" ๆจกๅ็่พๅบ็ปๆ่ฟ่ก็ฆป็บฟ่ฏไผฐ๏ผ
-
-```Bash
-# ๅๆฌก่ฟ่กๆต่ฏ่ๆฌๆถ๏ผ็จๆทๅฏไปฅ้่ฟๆๅฎ --save-preds ๅๆฐๆฅไฟๅญๆจกๅ็่พๅบ็ปๆ
-python tools/test.py ${CONFIG_FILE} ${CHECKPOINT_FILE} --save-preds
-# ็คบไพ๏ผๅฏน PSENet ่ฟ่กๆต่ฏ
-python tools/test.py configs/textdet/psenet/psenet_r50_fpnf_600e_icdar2015.py epoch_600.pth --save-preds
-
-# ไนๅๅณๅฏไฝฟ็จๅทฒไฟๅญ็่พๅบๆไปถ่ฟ่ก็ฆป็บฟ่ฏไผฐ
-python tools/analysis_tool/offline_eval.py ${CONFIG_FILE} ${PRED_FILE}
-# ็คบไพ๏ผๅฏนๅทฒไฟๅญ็ PSENet ็ปๆ่ฟ่ก็ฆป็บฟ่ฏไผฐ
-python tools/analysis_tools/offline_eval.py configs/textdet/psenet/psenet_r50_fpnf_600e_icdar2015.py work_dirs/psenet_r50_fpnf_600e_icdar2015/epoch_600.pth_predictions.pkl
-```
-
-`--save-preds` ้ป่ฎคๅฐ่พๅบ็ปๆไฟๅญ่ณ `work_dir/CONFIG_NAME/MODEL_NAME_predictions.pkl`
-
-ๆญคๅค๏ผๅบไบๆญคๅทฅๅ
ท๏ผ็จๆทไนๅฏไปฅๅฐๅ
ถไป็ฎๆณๅบ่ทๅ็้ขๆต็ปๆ่ฝฌๆขๆ MMOCR ๆฏๆ็ๆ ผๅผ๏ผไป่ไฝฟ็จ MMOCR ๅ
็ฝฎ็่ฏไผฐๆๆ ๆฅๅฏนๅ
ถไป็ฎๆณๅบ็ๆจกๅ่ฟ่ก่ฏๆตใ
-
-| ๅๆฐ | ็ฑปๅ | ่ฏดๆ |
-| ------------- | ----- | ---------------------------------------------------------------- |
-| config | str | ๏ผๅฟ
้กป๏ผ้
็ฝฎๆไปถ่ทฏๅพใ |
-| pkl_results | str | ๏ผๅฟ
้กป๏ผ้ขๅ
ไฟๅญ็้ขๆต็ปๆๆไปถใ |
-| --cfg-options | float | ็จไบ่ฆๅ้
็ฝฎๆไปถไธญ็ๆๅฎๅๆฐใ[็คบไพ](./config.md#ๅฝไปค่กไฟฎๆน้
็ฝฎ) |
-
-### ่ฎก็ฎ FLOPs ๅๅๆฐ้
-
-ๆไปฌๆไพไธไธช่ฎก็ฎ FLOPs ๅๅๆฐ้็ๆนๆณ๏ผ้ฆๅ
ๆไปฌไฝฟ็จไปฅไธๅฝไปคๅฎ่ฃ
ไพ่ตใ
-
-```shell
-pip install fvcore
-```
-
-่ฎก็ฎ FLOPs ๅๅๆฐ้็่ๆฌไฝฟ็จๆนๆณๅฆไธ๏ผ
-
-```shell
-python tools/analysis_tools/get_flops.py ${config} --shape ${IMAGE_SHAPE}
-```
-
-| ๅๆฐ | ็ฑปๅ | ่ฏดๆ |
-| ------- | ------ | ------------------------------------------------------------------ |
-| config | str | ๏ผๅฟ
้กป) ้
็ฝฎๆไปถ่ทฏๅพใ |
-| --shape | int\*2 | ่ฎก็ฎ FLOPs ไฝฟ็จ็ๅพ็ๅฐบๅฏธ๏ผๅฆ `--shape 320 320`ใ ้ป่ฎคไธบ `640 640` |
-
-่ทๅ `dbnet_resnet18_fpnc_100k_synthtext.py` FLOPs ๅๅๆฐ้็็คบไพๅฝไปคๅฆไธใ
-
-```shell
-python tools/analysis_tools/get_flops.py configs/textdet/dbnet/dbnet_resnet18_fpnc_100k_synthtext.py --shape 1024 1024
-```
-
-่พๅบๅฆไธ๏ผ
-
-```shell
-input shape is (1, 3, 1024, 1024)
-| module | #parameters or shape | #flops |
-| :------------------------ | :------------------- | :------ |
-| model | 12.341M | 63.955G |
-| backbone | 11.177M | 38.159G |
-| backbone.conv1 | 9.408K | 2.466G |
-| backbone.conv1.weight | (64, 3, 7, 7) | |
-| backbone.bn1 | 0.128K | 83.886M |
-| backbone.bn1.weight | (64,) | |
-| backbone.bn1.bias | (64,) | |
-| backbone.layer1 | 0.148M | 9.748G |
-| backbone.layer1.0 | 73.984K | 4.874G |
-| backbone.layer1.1 | 73.984K | 4.874G |
-| backbone.layer2 | 0.526M | 8.642G |
-| backbone.layer2.0 | 0.23M | 3.79G |
-| backbone.layer2.1 | 0.295M | 4.853G |
-| backbone.layer3 | 2.1M | 8.616G |
-| backbone.layer3.0 | 0.919M | 3.774G |
-| backbone.layer3.1 | 1.181M | 4.842G |
-| backbone.layer4 | 8.394M | 8.603G |
-| backbone.layer4.0 | 3.673M | 3.766G |
-| backbone.layer4.1 | 4.721M | 4.837G |
-| neck | 0.836M | 14.887G |
-| neck.lateral_convs | 0.246M | 2.013G |
-| neck.lateral_convs.0.conv | 16.384K | 1.074G |
-| neck.lateral_convs.1.conv | 32.768K | 0.537G |
-| neck.lateral_convs.2.conv | 65.536K | 0.268G |
-| neck.lateral_convs.3.conv | 0.131M | 0.134G |
-| neck.smooth_convs | 0.59M | 12.835G |
-| neck.smooth_convs.0.conv | 0.147M | 9.664G |
-| neck.smooth_convs.1.conv | 0.147M | 2.416G |
-| neck.smooth_convs.2.conv | 0.147M | 0.604G |
-| neck.smooth_convs.3.conv | 0.147M | 0.151G |
-| det_head | 0.329M | 10.909G |
-| det_head.binarize | 0.164M | 10.909G |
-| det_head.binarize.0 | 0.147M | 9.664G |
-| det_head.binarize.1 | 0.128K | 20.972M |
-| det_head.binarize.3 | 16.448K | 1.074G |
-| det_head.binarize.4 | 0.128K | 83.886M |
-| det_head.binarize.6 | 0.257K | 67.109M |
-| det_head.threshold | 0.164M | |
-| det_head.threshold.0 | 0.147M | |
-| det_head.threshold.1 | 0.128K | |
-| det_head.threshold.3 | 16.448K | |
-| det_head.threshold.4 | 0.128K | |
-| det_head.threshold.6 | 0.257K | |
-!!!Please be cautious if you use the results in papers. You may need to check if all ops are supported and verify that the flops computation is correct.
-```
diff --git a/pipeline/mmocr/docs/zh_cn/user_guides/visualization.md b/pipeline/mmocr/docs/zh_cn/user_guides/visualization.md
deleted file mode 100644
index 79a9d7e39b3f1828b2407a4add268e50647b2b72..0000000000000000000000000000000000000000
--- a/pipeline/mmocr/docs/zh_cn/user_guides/visualization.md
+++ /dev/null
@@ -1,107 +0,0 @@
-# ๅฏ่งๅ
-
-้
่ฏปๆฌๆๅๅปบ่ฎฎๅ
้
่ฏป {external+mmengine:doc}`MMEngine: ๅฏ่งๅ ` ไปฅๅๆญฅไบ่งฃ Visualizer ็ๅฎไนๅ็ธๅ
ณ็จๆณใ
-
-็ฎๅๆฅ่ฏด๏ผMMEngine ไธญๅฎ็ฐไบ็จไบๆปก่ถณๆฅๅธธๅฏ่งๅ้ๆฑ็ๅฏ่งๅๅจไปถ [`Visualizer`](mmengine.visualization.Visualizer)๏ผๅ
ถไธป่ฆๅ
ๅซไธไธชๅ่ฝ๏ผ
-
-- ๅฎ็ฐไบๅธธ็จ็็ปๅพ API๏ผไพๅฆ [`draw_bboxes`](mmengine.visualization.Visualizer.draw_bboxes) ๅฎ็ฐไบ่พน็็็็ปๅถๅ่ฝ๏ผ[`draw_lines`](mmengine.visualization.Visualizer.draw_lines) ๅฎ็ฐไบ็บฟๆก็็ปๅถๅ่ฝใ
-- ๆฏๆๅฐๅฏ่งๅ็ปๆใๅญฆไน ็ๆฒ็บฟใๆๅคฑๅฝๆฐๆฒ็บฟไปฅๅ้ช่ฏ็ฒพๅบฆๆฒ็บฟ็ญๅๅ
ฅๅค็งๅ็ซฏไธญ๏ผๅ
ๆฌๆฌๅฐ็ฃ็ไปฅๅๅธธ็จ็ๆทฑๅบฆๅญฆไน ่ฎญ็ปๆฅๅฟ่ฎฐๅฝๅทฅๅ
ท๏ผๅฆ [TensorBoard](https://www.tensorflow.org/tensorboard) ๅ [WandB](https://wandb.ai/site)ใ
-- ๆฏๆๅจไปฃ็ ไธญ็ไปปๆไฝ็ฝฎ่ฟ่ก่ฐ็จ๏ผไพๅฆๅจ่ฎญ็ปๆๆต่ฏ่ฟ็จไธญๅฏ่งๅๆ่ฎฐๅฝๆจกๅ็ไธญ้ด็ถๆ๏ผๅฆ็นๅพๅพๅ้ช่ฏ็ปๆ็ญใ
-
-ๅบไบ MMEngine ็ Visualizer๏ผMMOCR ๅ
้ข็ฝฎไบๅค็งๅฏ่งๅๅทฅๅ
ท๏ผ็จๆทไป
้็ฎๅไฟฎๆน้
็ฝฎๆไปถๅณๅฏไฝฟ็จ๏ผ
-
-- `tools/analysis_tools/browse_dataset.py` ่ๆฌๆไพไบๆฐๆฎ้ๅฏ่งๅๅ่ฝ๏ผๅ
ถๅฏไปฅ็ปๅถ็ป่ฟๆฐๆฎๅๆข๏ผData Transforms๏ผไนๅ็ๅพๅๅๅฏนๅบ็ๆ ๆณจๅ
ๅฎน๏ผ่ฏฆ่ง [`browse_dataset.py`](useful_tools.md)ใ
-- MMEngine ไธญๅฎ็ฐไบ `LoggerHook`๏ผ่ฏฅ Hook ๅฉ็จ `Visualizer` ๅฐๅญฆไน ็ใๆๅคฑไปฅๅ่ฏไผฐ็ปๆ็ญๆฐๆฎๅๅ
ฅ `Visualizer` ่ฎพ็ฝฎ็ๅ็ซฏไธญ๏ผๅ ๆญค้่ฟไฟฎๆน้
็ฝฎๆไปถไธญ็ `Visualizer` ๅ็ซฏ๏ผๆฏๅฆไฟฎๆนไธบ`TensorBoardVISBackend` ๆ `WandbVISBackend`๏ผๅฏไปฅๅฎ็ฐๅฐๆฅๅฟๅฐ `TensorBoard` ๆ `WandB` ็ญๅธธ่ง็่ฎญ็ปๆฅๅฟ่ฎฐๅฝๅทฅๅ
ทไธญ๏ผไป่ๆนไพฟ็จๆทไฝฟ็จ่ฟไบๅฏ่งๅๅทฅๅ
ทๆฅๅๆๅ็ๆง่ฎญ็ปๆต็จใ
-- MMOCR ไธญๅฎ็ฐไบ`VisualizerHook`๏ผ่ฏฅ Hook ๅฉ็จ `Visualizer` ๅฐ้ช่ฏ้ถๆฎตๆ้ขๆต้ถๆฎต็้ขๆต็ปๆ่ฟ่กๅฏ่งๅๆๅจๅญ่ณ `Visualizer` ่ฎพ็ฝฎ็ๅ็ซฏไธญ๏ผๅ ๆญค้่ฟไฟฎๆน้
็ฝฎๆไปถไธญ็ `Visualizer` ๅ็ซฏ๏ผๆฏๅฆไฟฎๆนไธบ`TensorBoardVISBackend` ๆ `WandbVISBackend`๏ผๅฏไปฅๅฎ็ฐๅฐ้ขๆต็ๅพๅๅญๅจๅฐ `TensorBoard` ๆ `Wandb`ไธญใ
-
-## ้
็ฝฎ
-
-ๅพ็ไบๆณจๅๆบๅถ็ไฝฟ็จ๏ผๅจ MMOCR ไธญ๏ผๆไปฌๅฏไปฅ้่ฟไฟฎๆน้
็ฝฎๆไปถๆฅ่ฎพ็ฝฎๅฏ่งๅๅจไปถ `Visualizer` ็่กไธบใ้ๅธธ๏ผๆไปฌๅจ `task/_base_/default_runtime.py` ไธญๅฎไนๅฏ่งๅ็ธๅ
ณ็้ป่ฎค้
็ฝฎ๏ผ ่ฏฆ่ง[้
็ฝฎๆ็จ](config.md)ใ
-
-```Python
-vis_backends = [dict(type='LocalVisBackend')]
-visualizer = dict(
- type='TextxxxLocalVisualizer', # ไธๅไปปๅกไฝฟ็จไธๅ็ๅฏ่งๅๅจ
- vis_backends=vis_backends,
- name='visualizer')
-```
-
-ไพๆฎไปฅไธ็คบไพ๏ผๆไปฌๅฏไปฅ็ๅบ `Visualizer` ็้
็ฝฎไธป่ฆ็ฑไธคไธช้จๅ็ปๆ๏ผๅณ๏ผ`Visualizer`็็ฑปๅไปฅๅๅ
ถ้็จ็ๅฏ่งๅๅ็ซฏ `vis_backends`ใ
-
-- ้ๅฏนไธๅ็ OCR ไปปๅก๏ผMMOCR ไธญ้ข็ฝฎไบๅค็งๅฏ่งๅๅจไปถ๏ผๅ
ๆฌ [`TextDetLocalVisualizer`](mmocr.visualization.TextDetLocalVisualizer)๏ผ[`TextRecogLocalVisualizer`](mmocr.visualization.TextRecogLocalVisualizer)๏ผ[`TextSpottingLocalVisualizer`](mmocr.visualization.TextSpottingLocalVisualizer) ไปฅๅ[`KIELocalVisualizer`](mmocr.visualization.KIELocalVisualizer)ใ่ฟไบๅฏ่งๅๅจไปถไพ็
ง่ช่บซไปปๅก็็น็นๅฏนๅบ็ก็ Visulizer API ่ฟ่กไบๆๅฑ๏ผๅนถๅฎ็ฐไบ็ธๅบ็ๆ ็ญพไฟกๆฏๆฅๅฃ `add_datasamples`ใไพๅฆ๏ผ็จๆทๅฏไปฅ็ดๆฅไฝฟ็จ `TextDetLocalVisualizer` ๆฅๅฏ่งๅๆๆฌๆฃๆตไปปๅก็ๆ ็ญพๆ้ขๆต็ปๆใ
-- MMOCR ้ป่ฎคๅฐๅฏ่งๅๅ็ซฏ `vis_backend` ่ฎพ็ฝฎไธบๆฌๅฐๅฏ่งๅๅ็ซฏ `LocalVisBackend`๏ผๅฐๆๆๅฏ่งๅ็ปๆๅๅ
ถไป่ฎญ็ปไฟกๆฏไฟๅญๅจๆฌๅฐๆไปถๅคนไธญใ
-
-## ๅญๅจ
-
-MMOCR ้ป่ฎคไฝฟ็จๆฌๅฐๅฏ่งๅๅ็ซฏ [`LocalVisBackend`](mmengine.visualization.LocalVisBackend)๏ผ`VisualizerHook` ๅ`LoggerHook` ไธญๅญๅจ็ๆจกๅๆๅคฑใๅญฆไน ็ใๆจกๅ่ฏไผฐ็ฒพๅบฆไปฅๅๅฏ่งๅ็ปๆ็ญไฟกๆฏๅฐ่ขซ้ป่ฎคไฟๅญ่ณ`{work_dir}/{config_name}/{time}/{vis_data}` ๆไปถๅคนใๆญคๅค๏ผMMOCR ไนๆฏๆๅ
ถๅฎๅธธ็จ็ๅฏ่งๅๅ็ซฏ๏ผๅฆ `TensorboardVisBackend` ไปฅๅ `WandbVisBackend`็จๆทๅช้่ฆๅฐ้
็ฝฎๆไปถไธญ็ `vis_backends` ็ฑปๅไฟฎๆนไธบๅฏนๅบ็ๅฏ่งๅๅ็ซฏๅณๅฏใไพๅฆ๏ผ็จๆทๅช้่ฆๅจ้
็ฝฎๆไปถไธญๆๅ
ฅไปฅไธไปฃ็ ๅ๏ผๅณๅฏๅฐๆฐๆฎๅญๅจ่ณ `TensorBoard` ไปฅๅ `WandB`ไธญใ
-
-```Python
-_base_.visualizer.vis_backends = [
- dict(type='LocalVisBackend'),
- dict(type='TensorboardVisBackend'),
- dict(type='WandbVisBackend'),]
-```
-
-## ็ปๅถ
-
-### ็ปๅถ้ขๆต็ปๆไฟกๆฏ
-
-MMOCR ไธป่ฆๅฉ็จ [`VisualizationHook`](mmocr.engine.hooks.VisualizationHook)validation ๅ test ็้ขๆต็ปๆ, ้ป่ฎคๆ
ๅตไธ `VisualizationHook`ไธบๅ
ณ้ญ็ถๆ๏ผ้ป่ฎค้
็ฝฎๅฆไธ๏ผ
-
-```Python
-visualization=dict( # ็จๆทๅฏ่งๅ validation ๅ test ็็ปๆ
- type='VisualizationHook',
- enable=False,
- interval=1,
- show=False,
- draw_gt=False,
- draw_pred=False)
-```
-
-ไธ่กจไธบ `VisualizationHook` ๆฏๆ็ๅๆฐ๏ผ
-
-| ๅๆฐ | ่ฏดๆ |
-| :-------: | :---------------------------------------------------------------------------------: |
-| enable | VisualizationHook ็ๅผๅฏๅๅ
ณ้ญ็ฑๅๆฐenableๆงๅถ้ป่ฎคๆฏๅ
ณ้ญ็็ถๆ๏ผ |
-| interval | ๅจVisualizationHookๅผๅฏ็ๆ
ๅตไธ,็จไปฅๆงๅถๅคๅฐiteration ๅญๅจๆๅฑ็คบ val ๆ test ็็ปๆ |
-| show | ๆงๅถๆฏๅฆๅฏ่งๅ val ๆ test ็็ปๆ |
-| draw_gt | val ๆ test ็็ปๆๆฏๅฆ็ปๅถๆ ๆณจไฟกๆฏ |
-| draw_pred | val ๆ test ็็ปๆๆฏๅฆ็ปๅถ้ขๆต็ปๆ |
-
-ๅฆๆๅจ่ฎญ็ปๆ่
ๆต่ฏ่ฟ็จไธญๆณๅผๅฏ `VisualizationHook` ็ธๅ
ณๅ่ฝๅ้
็ฝฎ๏ผไป
้ไฟฎๆน้
็ฝฎๅณๅฏ๏ผไปฅ `dbnet_resnet18_fpnc_1200e_icdar2015.py`ไธบไพ๏ผ ๅๆถ็ปๅถๆ ๆณจๅ้ขๆต๏ผๅนถไธๅฐๅพๅๅฑ็คบ๏ผ้
็ฝฎๅฏ่ฟ่กๅฆไธไฟฎๆน
-
-```Python
-visualization = _base_.default_hooks.visualization
-visualization.update(
- dict(enable=True, show=True, draw_gt=True, draw_pred=True))
-```
-
-
-
-
-
-ๅฆๆๅชๆณๆฅ็้ขๆต็ปๆไฟกๆฏๅฏไปฅๅช่ฎฉ`draw_pred=True`
-
-```Python
-visualization = _base_.default_hooks.visualization
-visualization.update(
- dict(enable=True, show=True, draw_gt=False, draw_pred=True))
-```
-
-
-
-
-
-ๅจ `test.py` ่ฟ็จไธญ่ฟไธๆญฅ็ฎๅ๏ผๆไพไบ `--show` ๅ `--show-dir`ไธคไธชๅๆฐ๏ผๆ ้ไฟฎๆน้
็ฝฎๅณๅฏ่งๅๆต่ฏ่ฟ็จไธญ็ปๅถๆ ๆณจๅ้ขๆต็ปๆใ
-
-```Shell
-# ๅฑ็คบtest ็ปๆ
-python tools/test.py configs/textdet/dbnet/dbnet_resnet18_fpnc_1200e_icdar2015.py dbnet_r18_fpnc_1200e_icdar2015/epoch_400.pth --show
-
-# ๆๅฎ้ขๆต็ปๆ็ๅญๅจไฝ็ฝฎ
-python tools/test.py configs/textdet/dbnet/dbnet_resnet18_fpnc_1200e_icdar2015.py dbnet_r18_fpnc_1200e_icdar2015/epoch_400.pth --show-dir imgs/
-```
-
-
-
-
diff --git a/pipeline/mmocr/docs/zh_cn/weight_list.py b/pipeline/mmocr/docs/zh_cn/weight_list.py
deleted file mode 100644
index 11a540208e6ad187fbe30f306d585274d283220f..0000000000000000000000000000000000000000
--- a/pipeline/mmocr/docs/zh_cn/weight_list.py
+++ /dev/null
@@ -1,115 +0,0 @@
-import os.path as osp
-
-from mmengine.fileio import load
-from tabulate import tabulate
-
-
-class BaseWeightList:
- """Class for generating model list in markdown format.
-
- Args:
- dataset_list (list[str]): List of dataset names.
- table_header (list[str]): List of table header.
- msg (str): Message to be displayed.
- task_abbr (str): Abbreviation of task name.
- metric_name (str): Metric name.
- """
-
- base_url: str = 'https://github.com/open-mmlab/mmocr/blob/1.x/'
- table_cfg: dict = dict(
- tablefmt='pipe', floatfmt='.2f', numalign='right', stralign='center')
- dataset_list: list
- table_header: list
- msg: str
- task_abbr: str
- metric_name: str
-
- def __init__(self):
- data = (d + f' ({self.metric_name})' for d in self.dataset_list)
- self.table_header = ['ๆจกๅ', 'README', *data]
-
- def _get_model_info(self, task_name: str):
- meta_indexes = load('../../model-index.yml')
- for meta_path in meta_indexes['Import']:
- meta_path = osp.join('../../', meta_path)
- metainfo = load(meta_path)
- collection2md = {}
- for item in metainfo['Collections']:
- url = self.base_url + item['README']
- collection2md[item['Name']] = f'[้พๆฅ]({url})'
- for item in metainfo['Models']:
- if task_name not in item['Config']:
- continue
- name = f'`{item["Name"]}`'
- if item.get('Alias', None):
- if isinstance(item['Alias'], str):
- item['Alias'] = [item['Alias']]
- aliases = [f'`{alias}`' for alias in item['Alias']]
- aliases.append(name)
- name = ' / '.join(aliases)
- readme = collection2md[item['In Collection']]
- eval_res = self._get_eval_res(item)
- yield (name, readme, *eval_res)
-
- def _get_eval_res(self, item):
- eval_res = {k: '-' for k in self.dataset_list}
- for res in item['Results']:
- if res['Dataset'] in self.dataset_list:
- eval_res[res['Dataset']] = res['Metrics'][self.metric_name]
- return (eval_res[k] for k in self.dataset_list)
-
- def gen_model_list(self):
- content = f'\n{self.msg}\n'
- content += '```{table}\n:class: model-summary nowrap field-list '
- content += 'table table-hover\n'
- content += tabulate(
- self._get_model_info(self.task_abbr), self.table_header,
- **self.table_cfg)
- content += '\n```\n'
- return content
-
-
-class TextDetWeightList(BaseWeightList):
-
- dataset_list = ['ICDAR2015', 'CTW1500', 'Totaltext']
- msg = '### ๆๅญๆฃๆต'
- task_abbr = 'textdet'
- metric_name = 'hmean-iou'
-
-
-class TextRecWeightList(BaseWeightList):
-
- dataset_list = [
- 'Avg', 'IIIT5K', 'SVT', 'ICDAR2013', 'ICDAR2015', 'SVTP', 'CT80'
- ]
- msg = ('### ๆๅญ่ฏๅซ\n'
- '```{note}\n'
- 'Avg ๆ่ฏฅๆจกๅๅจ IIIT5KใSVTใICDAR2013ใICDAR2015ใSVTPใ'
- 'CT80 ไธ็ๅนณๅ็ปๆใ\n```\n')
- task_abbr = 'textrecog'
- metric_name = 'word_acc'
-
- def _get_eval_res(self, item):
- eval_res = {k: '-' for k in self.dataset_list}
- avg = []
- for res in item['Results']:
- if res['Dataset'] in self.dataset_list:
- eval_res[res['Dataset']] = res['Metrics'][self.metric_name]
- avg.append(res['Metrics'][self.metric_name])
- eval_res['Avg'] = sum(avg) / len(avg)
- return (eval_res[k] for k in self.dataset_list)
-
-
-class KIEWeightList(BaseWeightList):
-
- dataset_list = ['wildreceipt']
- task_abbr = 'kie'
- metric_name = 'macro_f1'
- msg = '### ๅ
ณ้ฎไฟกๆฏๆๅ'
-
-
-def gen_weight_list():
- content = TextDetWeightList().gen_model_list()
- content += TextRecWeightList().gen_model_list()
- content += KIEWeightList().gen_model_list()
- return content
diff --git a/pipeline/mmocr/infer.py b/pipeline/mmocr/infer.py
deleted file mode 100644
index acb21ddb0d198734fb39808575f844660748057e..0000000000000000000000000000000000000000
--- a/pipeline/mmocr/infer.py
+++ /dev/null
@@ -1,108 +0,0 @@
-# Copyright (c) OpenMMLab. All rights reserved.
-from argparse import ArgumentParser
-# import sys
-# sys.path.append("..")
-# import mmocr
-from mmocr.apis.inferencers import MMOCRInferencer
-
-
-def parse_args():
- parser = ArgumentParser()
- parser.add_argument(
- 'inputs', type=str, help='Input image file or folder path.')
- parser.add_argument(
- '--out-dir',
- type=str,
- default='results/',
- help='Output directory of results.')
- parser.add_argument(
- '--det',
- type=str,
- default=None,
- help='Pretrained text detection algorithm. It\'s the path to the '
- 'config file or the model name defined in metafile.')
- parser.add_argument(
- '--det-weights',
- type=str,
- default=None,
- help='Path to the custom checkpoint file of the selected det model. '
- 'If it is not specified and "det" is a model name of metafile, the '
- 'weights will be loaded from metafile.')
- parser.add_argument(
- '--rec',
- type=str,
- default=None,
- help='Pretrained text recognition algorithm. It\'s the path to the '
- 'config file or the model name defined in metafile.')
- parser.add_argument(
- '--rec-weights',
- type=str,
- default=None,
- help='Path to the custom checkpoint file of the selected recog model. '
- 'If it is not specified and "rec" is a model name of metafile, the '
- 'weights will be loaded from metafile.')
- parser.add_argument(
- '--kie',
- type=str,
- default=None,
- help='Pretrained key information extraction algorithm. It\'s the path'
- 'to the config file or the model name defined in metafile.')
- parser.add_argument(
- '--kie-weights',
- type=str,
- default=None,
- help='Path to the custom checkpoint file of the selected kie model. '
- 'If it is not specified and "kie" is a model name of metafile, the '
- 'weights will be loaded from metafile.')
- parser.add_argument(
- '--device',
- type=str,
- default=None,
- help='Device used for inference. '
- 'If not specified, the available device will be automatically used.')
- parser.add_argument(
- '--batch-size', type=int, default=1, help='Inference batch size.')
- parser.add_argument(
- '--show',
- action='store_true',
- help='Display the image in a popup window.')
- parser.add_argument(
- '--print-result',
- action='store_true',
- help='Whether to print the results.')
- parser.add_argument(
- '--save_pred',
- action='store_true',
- help='Save the inference results to out_dir.')
- parser.add_argument(
- '--save_vis',
- action='store_true',
- help='Save the visualization results to out_dir.')
-
- call_args = vars(parser.parse_args())
-
- init_kws = [
- 'det', 'det_weights', 'rec', 'rec_weights', 'kie', 'kie_weights',
- 'device'
- ]
- init_args = {}
- for init_kw in init_kws:
- init_args[init_kw] = call_args.pop(init_kw)
-
- return init_args, call_args
-
-
-def main():
- init_args, call_args = parse_args()
- ocr = MMOCRInferencer(**init_args)
- # (det="configs/textdet/dbnetpp/dbnetpp_resnet50-oclip_fpnc_1200e_icdar2015.py",
- # det_weights="/newdisk3/wcx/dbnetpp.pth",
- # rec="configs/textrecog/maerec/maerec_b_union14m.py",
- # rec_weights="/newdisk3/wcx/maerec_b.pth ",
- # device="cuda:0")
- res = ocr(**call_args)
- print(res)
-
-
-if __name__ == '__main__':
- main()
diff --git a/pipeline/mmocr/mmocr.egg-info/PKG-INFO b/pipeline/mmocr/mmocr.egg-info/PKG-INFO
deleted file mode 100644
index 4d80464818bde3f3917b118345de42161f33cc89..0000000000000000000000000000000000000000
--- a/pipeline/mmocr/mmocr.egg-info/PKG-INFO
+++ /dev/null
@@ -1,279 +0,0 @@
-Metadata-Version: 2.1
-Name: mmocr
-Version: 1.0.0
-Summary: OpenMMLab Text Detection, OCR, and NLP Toolbox
-Home-page: https://github.com/open-mmlab/mmocr
-Maintainer: MMOCR Authors
-Maintainer-email: openmmlab@gmail.com
-License: Apache License 2.0
-Keywords: Text Detection,OCR,KIE,NLP
-Platform: UNKNOWN
-Classifier: Development Status :: 4 - Beta
-Classifier: License :: OSI Approved :: Apache Software License
-Classifier: Operating System :: OS Independent
-Classifier: Programming Language :: Python :: 3
-Classifier: Programming Language :: Python :: 3.6
-Classifier: Programming Language :: Python :: 3.7
-Classifier: Programming Language :: Python :: 3.8
-Classifier: Programming Language :: Python :: 3.9
-Description-Content-Type: text/markdown
-Provides-Extra: all
-Provides-Extra: tests
-Provides-Extra: build
-Provides-Extra: optional
-Provides-Extra: mim
-License-File: LICENSE
-
-
-
-
-
-
-
-[](https://github.com/open-mmlab/mmocr/actions)
-[](https://mmocr.readthedocs.io/en/dev-1.x/?badge=dev-1.x)
-[](https://codecov.io/gh/open-mmlab/mmocr)
-[](https://github.com/open-mmlab/mmocr/blob/main/LICENSE)
-[](https://pypi.org/project/mmocr/)
-[](https://github.com/open-mmlab/mmocr/issues)
-[](https://github.com/open-mmlab/mmocr/issues)
-
-
-[๐Documentation](https://mmocr.readthedocs.io/en/dev-1.x/) |
-[๐ ๏ธInstallation](https://mmocr.readthedocs.io/en/dev-1.x/get_started/install.html) |
-[๐Model Zoo](https://mmocr.readthedocs.io/en/dev-1.x/modelzoo.html) |
-[๐Update News](https://mmocr.readthedocs.io/en/dev-1.x/notes/changelog.html) |
-[๐คReporting Issues](https://github.com/open-mmlab/mmocr/issues/new/choose)
-
-
-
-
-
-English | [็ฎไฝไธญๆ](README_zh-CN.md)
-
-
-
-
-
-
-
-
-
-
-
-
-
-
-
-
-
-
-
-
-
-
-## Latest Updates
-
-**The default branch is now `main` and the code on the branch has been upgraded to v1.0.0. The old `main` branch (v0.6.3) code now exists on the `0.x` branch.** If you have been using the `main` branch and encounter upgrade issues, please read the [Migration Guide](https://mmocr.readthedocs.io/en/dev-1.x/migration/overview.html) and notes on [Branches](https://mmocr.readthedocs.io/en/dev-1.x/migration/branches.html) .
-
-v1.0.0 was released in 2023-04-06. Major updates from 1.0.0rc6 include:
-
-1. Support for SCUT-CTW1500, SynthText, and MJSynth datasets in Dataset Preparer
-2. Updated FAQ and documentation
-3. Deprecation of file_client_args in favor of backend_args
-4. Added a new MMOCR tutorial notebook
-
-To know more about the updates in MMOCR 1.0, please refer to [What's New in MMOCR 1.x](https://mmocr.readthedocs.io/en/dev-1.x/migration/news.html), or
-Read [Changelog](https://mmocr.readthedocs.io/en/dev-1.x/notes/changelog.html) for more details!
-
-## Introduction
-
-MMOCR is an open-source toolbox based on PyTorch and mmdetection for text detection, text recognition, and the corresponding downstream tasks including key information extraction. It is part of the [OpenMMLab](https://openmmlab.com/) project.
-
-The main branch works with **PyTorch 1.6+**.
-
-
-
-
-
-### Major Features
-
-- **Comprehensive Pipeline**
-
- The toolbox supports not only text detection and text recognition, but also their downstream tasks such as key information extraction.
-
-- **Multiple Models**
-
- The toolbox supports a wide variety of state-of-the-art models for text detection, text recognition and key information extraction.
-
-- **Modular Design**
-
- The modular design of MMOCR enables users to define their own optimizers, data preprocessors, and model components such as backbones, necks and heads as well as losses. Please refer to [Overview](https://mmocr.readthedocs.io/en/dev-1.x/get_started/overview.html) for how to construct a customized model.
-
-- **Numerous Utilities**
-
- The toolbox provides a comprehensive set of utilities which can help users assess the performance of models. It includes visualizers which allow visualization of images, ground truths as well as predicted bounding boxes, and a validation tool for evaluating checkpoints during training. It also includes data converters to demonstrate how to convert your own data to the annotation files which the toolbox supports.
-
-## Installation
-
-MMOCR depends on [PyTorch](https://pytorch.org/), [MMEngine](https://github.com/open-mmlab/mmengine), [MMCV](https://github.com/open-mmlab/mmcv) and [MMDetection](https://github.com/open-mmlab/mmdetection).
-Below are quick steps for installation.
-Please refer to [Install Guide](https://mmocr.readthedocs.io/en/dev-1.x/get_started/install.html) for more detailed instruction.
-
-```shell
-conda create -n open-mmlab python=3.8 pytorch=1.10 cudatoolkit=11.3 torchvision -c pytorch -y
-conda activate open-mmlab
-pip3 install openmim
-git clone https://github.com/open-mmlab/mmocr.git
-cd mmocr
-mim install -e .
-```
-
-## Get Started
-
-Please see [Quick Run](https://mmocr.readthedocs.io/en/dev-1.x/get_started/quick_run.html) for the basic usage of MMOCR.
-
-## [Model Zoo](https://mmocr.readthedocs.io/en/dev-1.x/modelzoo.html)
-
-Supported algorithms:
-
-
-BackBone
-
-- [x] [oCLIP](configs/backbone/oclip/README.md) (ECCV'2022)
-
-
-
-
-Text Detection
-
-- [x] [DBNet](configs/textdet/dbnet/README.md) (AAAI'2020) / [DBNet++](configs/textdet/dbnetpp/README.md) (TPAMI'2022)
-- [x] [Mask R-CNN](configs/textdet/maskrcnn/README.md) (ICCV'2017)
-- [x] [PANet](configs/textdet/panet/README.md) (ICCV'2019)
-- [x] [PSENet](configs/textdet/psenet/README.md) (CVPR'2019)
-- [x] [TextSnake](configs/textdet/textsnake/README.md) (ECCV'2018)
-- [x] [DRRG](configs/textdet/drrg/README.md) (CVPR'2020)
-- [x] [FCENet](configs/textdet/fcenet/README.md) (CVPR'2021)
-
-
-
-
-Text Recognition
-
-- [x] [ABINet](configs/textrecog/abinet/README.md) (CVPR'2021)
-- [x] [ASTER](configs/textrecog/aster/README.md) (TPAMI'2018)
-- [x] [CRNN](configs/textrecog/crnn/README.md) (TPAMI'2016)
-- [x] [MASTER](configs/textrecog/master/README.md) (PR'2021)
-- [x] [NRTR](configs/textrecog/nrtr/README.md) (ICDAR'2019)
-- [x] [RobustScanner](configs/textrecog/robust_scanner/README.md) (ECCV'2020)
-- [x] [SAR](configs/textrecog/sar/README.md) (AAAI'2019)
-- [x] [SATRN](configs/textrecog/satrn/README.md) (CVPR'2020 Workshop on Text and Documents in the Deep Learning Era)
-- [x] [SVTR](configs/textrecog/svtr/README.md) (IJCAI'2022)
-
-
-
-
-Key Information Extraction
-
-- [x] [SDMG-R](configs/kie/sdmgr/README.md) (ArXiv'2021)
-
-
-
-
-Text Spotting
-
-- [x] [ABCNet](projects/ABCNet/README.md) (CVPR'2020)
-- [x] [ABCNetV2](projects/ABCNet/README_V2.md) (TPAMI'2021)
-- [x] [SPTS](projects/SPTS/README.md) (ACM MM'2022)
-
-
-
-Please refer to [model_zoo](https://mmocr.readthedocs.io/en/dev-1.x/modelzoo.html) for more details.
-
-## Projects
-
-[Here](projects/README.md) are some implementations of SOTA models and solutions built on MMOCR, which are supported and maintained by community users. These projects demonstrate the best practices based on MMOCR for research and product development. We welcome and appreciate all the contributions to OpenMMLab ecosystem.
-
-## Contributing
-
-We appreciate all contributions to improve MMOCR. Please refer to [CONTRIBUTING.md](.github/CONTRIBUTING.md) for the contributing guidelines.
-
-## Acknowledgement
-
-MMOCR is an open-source project that is contributed by researchers and engineers from various colleges and companies. We appreciate all the contributors who implement their methods or add new features, as well as users who give valuable feedbacks.
-We hope the toolbox and benchmark could serve the growing research community by providing a flexible toolkit to reimplement existing methods and develop their own new OCR methods.
-
-## Citation
-
-If you find this project useful in your research, please consider cite:
-
-```bibtex
-@article{mmocr2021,
- title={MMOCR: A Comprehensive Toolbox for Text Detection, Recognition and Understanding},
- author={Kuang, Zhanghui and Sun, Hongbin and Li, Zhizhong and Yue, Xiaoyu and Lin, Tsui Hin and Chen, Jianyong and Wei, Huaqiang and Zhu, Yiqin and Gao, Tong and Zhang, Wenwei and Chen, Kai and Zhang, Wayne and Lin, Dahua},
- journal= {arXiv preprint arXiv:2108.06543},
- year={2021}
-}
-```
-
-## License
-
-This project is released under the [Apache 2.0 license](LICENSE).
-
-## OpenMMLab Family
-
-- [MMEngine](https://github.com/open-mmlab/mmengine): OpenMMLab foundational library for training deep learning models
-- [MMCV](https://github.com/open-mmlab/mmcv): OpenMMLab foundational library for computer vision.
-- [MIM](https://github.com/open-mmlab/mim): MIM installs OpenMMLab packages.
-- [MMClassification](https://github.com/open-mmlab/mmclassification): OpenMMLab image classification toolbox and benchmark.
-- [MMDetection](https://github.com/open-mmlab/mmdetection): OpenMMLab detection toolbox and benchmark.
-- [MMDetection3D](https://github.com/open-mmlab/mmdetection3d): OpenMMLab's next-generation platform for general 3D object detection.
-- [MMRotate](https://github.com/open-mmlab/mmrotate): OpenMMLab rotated object detection toolbox and benchmark.
-- [MMSegmentation](https://github.com/open-mmlab/mmsegmentation): OpenMMLab semantic segmentation toolbox and benchmark.
-- [MMOCR](https://github.com/open-mmlab/mmocr): OpenMMLab text detection, recognition, and understanding toolbox.
-- [MMPose](https://github.com/open-mmlab/mmpose): OpenMMLab pose estimation toolbox and benchmark.
-- [MMHuman3D](https://github.com/open-mmlab/mmhuman3d): OpenMMLab 3D human parametric model toolbox and benchmark.
-- [MMSelfSup](https://github.com/open-mmlab/mmselfsup): OpenMMLab self-supervised learning toolbox and benchmark.
-- [MMRazor](https://github.com/open-mmlab/mmrazor): OpenMMLab model compression toolbox and benchmark.
-- [MMFewShot](https://github.com/open-mmlab/mmfewshot): OpenMMLab fewshot learning toolbox and benchmark.
-- [MMAction2](https://github.com/open-mmlab/mmaction2): OpenMMLab's next-generation action understanding toolbox and benchmark.
-- [MMTracking](https://github.com/open-mmlab/mmtracking): OpenMMLab video perception toolbox and benchmark.
-- [MMFlow](https://github.com/open-mmlab/mmflow): OpenMMLab optical flow toolbox and benchmark.
-- [MMEditing](https://github.com/open-mmlab/mmediting): OpenMMLab image and video editing toolbox.
-- [MMGeneration](https://github.com/open-mmlab/mmgeneration): OpenMMLab image and video generative models toolbox.
-- [MMDeploy](https://github.com/open-mmlab/mmdeploy): OpenMMLab model deployment framework.
-
-## Welcome to the OpenMMLab community
-
-Scan the QR code below to follow the OpenMMLab team's [**Zhihu Official Account**](https://www.zhihu.com/people/openmmlab) and join the OpenMMLab team's [**QQ Group**](https://jq.qq.com/?_wv=1027&k=aCvMxdr3), or join the official communication WeChat group by adding the WeChat, or join our [**Slack**](https://join.slack.com/t/mmocrworkspace/shared_invite/zt-1ifqhfla8-yKnLO_aKhVA2h71OrK8GZw)
-
-
-
-We will provide you with the OpenMMLab community
-
-- ๐ข share the latest core technologies of AI frameworks
-- ๐ป Explaining PyTorch common module source Code
-- ๐ฐ News related to the release of OpenMMLab
-- ๐ Introduction of cutting-edge algorithms developed by OpenMMLab
- ๐ Get the more efficient answer and feedback
-- ๐ฅ Provide a platform for communication with developers from all walks of life
-
-The OpenMMLab community looks forward to your participation! ๐ฌ
-
-
diff --git a/pipeline/mmocr/mmocr.egg-info/SOURCES.txt b/pipeline/mmocr/mmocr.egg-info/SOURCES.txt
deleted file mode 100644
index 661e6f75fedca014df78a64abbe75f0c3ed1f0b5..0000000000000000000000000000000000000000
--- a/pipeline/mmocr/mmocr.egg-info/SOURCES.txt
+++ /dev/null
@@ -1,483 +0,0 @@
-LICENSE
-MANIFEST.in
-README.md
-setup.cfg
-setup.py
-mmocr/__init__.py
-mmocr/registry.py
-mmocr/version.py
-mmocr.egg-info/PKG-INFO
-mmocr.egg-info/SOURCES.txt
-mmocr.egg-info/dependency_links.txt
-mmocr.egg-info/not-zip-safe
-mmocr.egg-info/requires.txt
-mmocr.egg-info/top_level.txt
-mmocr/.mim/model-index.yml
-mmocr/.mim/configs/backbone/oclip/metafile.yml
-mmocr/.mim/configs/kie/_base_/default_runtime.py
-mmocr/.mim/configs/kie/_base_/datasets/wildreceipt-openset.py
-mmocr/.mim/configs/kie/_base_/datasets/wildreceipt.py
-mmocr/.mim/configs/kie/_base_/schedules/schedule_adam_60e.py
-mmocr/.mim/configs/kie/sdmgr/_base_sdmgr_novisual.py
-mmocr/.mim/configs/kie/sdmgr/_base_sdmgr_unet16.py
-mmocr/.mim/configs/kie/sdmgr/metafile.yml
-mmocr/.mim/configs/kie/sdmgr/sdmgr_novisual_60e_wildreceipt-openset.py
-mmocr/.mim/configs/kie/sdmgr/sdmgr_novisual_60e_wildreceipt.py
-mmocr/.mim/configs/kie/sdmgr/sdmgr_unet16_60e_wildreceipt.py
-mmocr/.mim/configs/textdet/_base_/default_runtime.py
-mmocr/.mim/configs/textdet/_base_/pretrain_runtime.py
-mmocr/.mim/configs/textdet/_base_/datasets/ctw1500.py
-mmocr/.mim/configs/textdet/_base_/datasets/icdar2015.py
-mmocr/.mim/configs/textdet/_base_/datasets/icdar2017.py
-mmocr/.mim/configs/textdet/_base_/datasets/synthtext.py
-mmocr/.mim/configs/textdet/_base_/datasets/totaltext.py
-mmocr/.mim/configs/textdet/_base_/datasets/toy_data.py
-mmocr/.mim/configs/textdet/_base_/schedules/schedule_adam_600e.py
-mmocr/.mim/configs/textdet/_base_/schedules/schedule_sgd_100k.py
-mmocr/.mim/configs/textdet/_base_/schedules/schedule_sgd_1200e.py
-mmocr/.mim/configs/textdet/_base_/schedules/schedule_sgd_base.py
-mmocr/.mim/configs/textdet/dbnet/_base_dbnet_resnet18_fpnc.py
-mmocr/.mim/configs/textdet/dbnet/_base_dbnet_resnet50-dcnv2_fpnc.py
-mmocr/.mim/configs/textdet/dbnet/dbnet_resnet18_fpnc_100k_synthtext.py
-mmocr/.mim/configs/textdet/dbnet/dbnet_resnet18_fpnc_1200e_icdar2015.py
-mmocr/.mim/configs/textdet/dbnet/dbnet_resnet18_fpnc_1200e_totaltext.py
-mmocr/.mim/configs/textdet/dbnet/dbnet_resnet50-dcnv2_fpnc_100k_synthtext.py
-mmocr/.mim/configs/textdet/dbnet/dbnet_resnet50-dcnv2_fpnc_1200e_icdar2015.py
-mmocr/.mim/configs/textdet/dbnet/dbnet_resnet50-oclip_1200e_icdar2015.py
-mmocr/.mim/configs/textdet/dbnet/dbnet_resnet50_1200e_icdar2015.py
-mmocr/.mim/configs/textdet/dbnet/metafile.yml
-mmocr/.mim/configs/textdet/dbnetpp/_base_dbnetpp_resnet50-dcnv2_fpnc.py
-mmocr/.mim/configs/textdet/dbnetpp/dbnetpp_resnet50-dcnv2_fpnc_100k_synthtext.py
-mmocr/.mim/configs/textdet/dbnetpp/dbnetpp_resnet50-dcnv2_fpnc_1200e_icdar2015.py
-mmocr/.mim/configs/textdet/dbnetpp/dbnetpp_resnet50-oclip_fpnc_1200e_icdar2015.py
-mmocr/.mim/configs/textdet/dbnetpp/dbnetpp_resnet50_fpnc_1200e_icdar2015.py
-mmocr/.mim/configs/textdet/dbnetpp/metafile.yml
-mmocr/.mim/configs/textdet/drrg/_base_drrg_resnet50_fpn-unet.py
-mmocr/.mim/configs/textdet/drrg/drrg_resnet50-oclip_fpn-unet_1200e_ctw1500.py
-mmocr/.mim/configs/textdet/drrg/drrg_resnet50_fpn-unet_1200e_ctw1500.py
-mmocr/.mim/configs/textdet/drrg/metafile.yml
-mmocr/.mim/configs/textdet/fcenet/_base_fcenet_resnet50-dcnv2_fpn.py
-mmocr/.mim/configs/textdet/fcenet/_base_fcenet_resnet50_fpn.py
-mmocr/.mim/configs/textdet/fcenet/fcenet_resnet50-dcnv2_fpn_1500e_ctw1500.py
-mmocr/.mim/configs/textdet/fcenet/fcenet_resnet50-oclip_fpn_1500e_ctw1500.py
-mmocr/.mim/configs/textdet/fcenet/fcenet_resnet50-oclip_fpn_1500e_icdar2015.py
-mmocr/.mim/configs/textdet/fcenet/fcenet_resnet50_fpn_1500e_icdar2015.py
-mmocr/.mim/configs/textdet/fcenet/fcenet_resnet50_fpn_1500e_totaltext.py
-mmocr/.mim/configs/textdet/fcenet/metafile.yml
-mmocr/.mim/configs/textdet/maskrcnn/_base_mask-rcnn_resnet50_fpn.py
-mmocr/.mim/configs/textdet/maskrcnn/mask-rcnn_resnet50-oclip_fpn_160e_ctw1500.py
-mmocr/.mim/configs/textdet/maskrcnn/mask-rcnn_resnet50-oclip_fpn_160e_icdar2015.py
-mmocr/.mim/configs/textdet/maskrcnn/mask-rcnn_resnet50_fpn_160e_ctw1500.py
-mmocr/.mim/configs/textdet/maskrcnn/mask-rcnn_resnet50_fpn_160e_icdar2015.py
-mmocr/.mim/configs/textdet/maskrcnn/mask-rcnn_resnet50_fpn_160e_icdar2017.py
-mmocr/.mim/configs/textdet/maskrcnn/metafile.yml
-mmocr/.mim/configs/textdet/panet/_base_panet_resnet18_fpem-ffm.py
-mmocr/.mim/configs/textdet/panet/_base_panet_resnet50_fpem-ffm.py
-mmocr/.mim/configs/textdet/panet/metafile.yml
-mmocr/.mim/configs/textdet/panet/panet_resnet18_fpem-ffm_600e_ctw1500.py
-mmocr/.mim/configs/textdet/panet/panet_resnet18_fpem-ffm_600e_icdar2015.py
-mmocr/.mim/configs/textdet/panet/panet_resnet50_fpem-ffm_600e_icdar2017.py
-mmocr/.mim/configs/textdet/psenet/_base_psenet_resnet50_fpnf.py
-mmocr/.mim/configs/textdet/psenet/metafile.yml
-mmocr/.mim/configs/textdet/psenet/psenet_resnet50-oclip_fpnf_600e_ctw1500.py
-mmocr/.mim/configs/textdet/psenet/psenet_resnet50-oclip_fpnf_600e_icdar2015.py
-mmocr/.mim/configs/textdet/psenet/psenet_resnet50_fpnf_600e_ctw1500.py
-mmocr/.mim/configs/textdet/psenet/psenet_resnet50_fpnf_600e_icdar2015.py
-mmocr/.mim/configs/textdet/psenet/psenet_resnet50_fpnf_600e_icdar2017.py
-mmocr/.mim/configs/textdet/textsnake/_base_textsnake_resnet50_fpn-unet.py
-mmocr/.mim/configs/textdet/textsnake/metafile.yml
-mmocr/.mim/configs/textdet/textsnake/textsnake_resnet50-oclip_fpn-unet_1200e_ctw1500.py
-mmocr/.mim/configs/textdet/textsnake/textsnake_resnet50_fpn-unet_1200e_ctw1500.py
-mmocr/.mim/configs/textrecog/_base_/default_runtime.py
-mmocr/.mim/configs/textrecog/_base_/datasets/coco_text_v1.py
-mmocr/.mim/configs/textrecog/_base_/datasets/cute80.py
-mmocr/.mim/configs/textrecog/_base_/datasets/icdar2011.py
-mmocr/.mim/configs/textrecog/_base_/datasets/icdar2013.py
-mmocr/.mim/configs/textrecog/_base_/datasets/icdar2015.py
-mmocr/.mim/configs/textrecog/_base_/datasets/iiit5k.py
-mmocr/.mim/configs/textrecog/_base_/datasets/mjsynth.py
-mmocr/.mim/configs/textrecog/_base_/datasets/svt.py
-mmocr/.mim/configs/textrecog/_base_/datasets/svtp.py
-mmocr/.mim/configs/textrecog/_base_/datasets/synthtext.py
-mmocr/.mim/configs/textrecog/_base_/datasets/synthtext_add.py
-mmocr/.mim/configs/textrecog/_base_/datasets/totaltext.py
-mmocr/.mim/configs/textrecog/_base_/datasets/toy_data.py
-mmocr/.mim/configs/textrecog/_base_/datasets/union14m_benchmark.py
-mmocr/.mim/configs/textrecog/_base_/datasets/union14m_train.py
-mmocr/.mim/configs/textrecog/_base_/schedules/schedule_adadelta_5e.py
-mmocr/.mim/configs/textrecog/_base_/schedules/schedule_adam_base.py
-mmocr/.mim/configs/textrecog/_base_/schedules/schedule_adam_step_5e.py
-mmocr/.mim/configs/textrecog/_base_/schedules/schedule_adamw_cos_10e.py
-mmocr/.mim/configs/textrecog/_base_/schedules/schedule_adamw_cos_6e.py
-mmocr/.mim/configs/textrecog/abinet/_base_abinet-vision.py
-mmocr/.mim/configs/textrecog/abinet/_base_abinet.py
-mmocr/.mim/configs/textrecog/abinet/abinet-vision_20e_st-an_mj.py
-mmocr/.mim/configs/textrecog/abinet/abinet_10e_union14m.py
-mmocr/.mim/configs/textrecog/abinet/abinet_20e_st-an_mj.py
-mmocr/.mim/configs/textrecog/abinet/metafile.yml
-mmocr/.mim/configs/textrecog/aster/_base_aster.py
-mmocr/.mim/configs/textrecog/aster/aster_resnet45_6e_st_mj.py
-mmocr/.mim/configs/textrecog/aster/aster_resnet45_6e_union14m.py
-mmocr/.mim/configs/textrecog/aster/metafile.yml
-mmocr/.mim/configs/textrecog/crnn/_base_crnn_mini-vgg.py
-mmocr/.mim/configs/textrecog/crnn/crnn_mini-vgg_5e_mj.py
-mmocr/.mim/configs/textrecog/crnn/crnn_mini-vgg_5e_toy.py
-mmocr/.mim/configs/textrecog/crnn/metafile.yml
-mmocr/.mim/configs/textrecog/maerec/_base_marec_vit_s.py
-mmocr/.mim/configs/textrecog/maerec/maerec_b_lora_union14m.py
-mmocr/.mim/configs/textrecog/maerec/maerec_b_union14m.py
-mmocr/.mim/configs/textrecog/maerec/maerec_s_lora_union14m.py
-mmocr/.mim/configs/textrecog/maerec/maerec_s_union14m.py
-mmocr/.mim/configs/textrecog/master/_base_master_resnet31.py
-mmocr/.mim/configs/textrecog/master/master_resnet31_12e_st_mj_sa.py
-mmocr/.mim/configs/textrecog/master/master_resnet31_12e_toy.py
-mmocr/.mim/configs/textrecog/master/metafile.yml
-mmocr/.mim/configs/textrecog/nrtr/_base_nrtr_modality-transform.py
-mmocr/.mim/configs/textrecog/nrtr/_base_nrtr_resnet31.py
-mmocr/.mim/configs/textrecog/nrtr/metafile.yml
-mmocr/.mim/configs/textrecog/nrtr/nrtr_modality-transform_6e_st_mj.py
-mmocr/.mim/configs/textrecog/nrtr/nrtr_modality-transform_6e_toy.py
-mmocr/.mim/configs/textrecog/nrtr/nrtr_resnet31-1by16-1by8_6e_st_mj.py
-mmocr/.mim/configs/textrecog/nrtr/nrtr_resnet31-1by8-1by4_6e_st_mj.py
-mmocr/.mim/configs/textrecog/nrtr/nrtr_resnet31-1by8-1by4_union14m.py
-mmocr/.mim/configs/textrecog/robust_scanner/_base_robustscanner_resnet31.py
-mmocr/.mim/configs/textrecog/robust_scanner/metafile.yml
-mmocr/.mim/configs/textrecog/robust_scanner/robustscanner_resnet31_5e_st-sub_mj-sub_sa_real.py
-mmocr/.mim/configs/textrecog/robust_scanner/robustscanner_resnet31_5e_toy.py
-mmocr/.mim/configs/textrecog/sar/_base_sar_resnet31_parallel-decoder.py
-mmocr/.mim/configs/textrecog/sar/metafile.yml
-mmocr/.mim/configs/textrecog/sar/sar_resnet31_parallel-decoder_5e_st-sub_mj-sub_sa_real.py
-mmocr/.mim/configs/textrecog/sar/sar_resnet31_parallel-decoder_5e_toy.py
-mmocr/.mim/configs/textrecog/sar/sar_resnet31_sequential-decoder_5e_st-sub_mj-sub_sa_real.py
-mmocr/.mim/configs/textrecog/sar/sar_resnet31_sequential-decoder_5e_union14m.py
-mmocr/.mim/configs/textrecog/satrn/_base_satrn_shallow.py
-mmocr/.mim/configs/textrecog/satrn/metafile.yml
-mmocr/.mim/configs/textrecog/satrn/satrn_shallow-small_5e_st_mj.py
-mmocr/.mim/configs/textrecog/satrn/satrn_shallow_5e_st_mj.py
-mmocr/.mim/configs/textrecog/satrn/satrn_shallow_5e_union14m.py
-mmocr/.mim/configs/textrecog/svtr/_base_svtr-tiny.py
-mmocr/.mim/configs/textrecog/svtr/metafile.yml
-mmocr/.mim/configs/textrecog/svtr/svtr-base_20e_st_mj.py
-mmocr/.mim/configs/textrecog/svtr/svtr-large_20e_st_mj.py
-mmocr/.mim/configs/textrecog/svtr/svtr-small_20e_st_mj.py
-mmocr/.mim/configs/textrecog/svtr/svtr-tiny_20e_st_mj.py
-mmocr/.mim/dicts/chinese_english_digits.txt
-mmocr/.mim/dicts/english_digits_symbols.txt
-mmocr/.mim/dicts/english_digits_symbols_space.txt
-mmocr/.mim/dicts/korean_english_digits_symbols.txt
-mmocr/.mim/dicts/lower_english_digits.txt
-mmocr/.mim/dicts/lower_english_digits_space.txt
-mmocr/.mim/dicts/sdmgr_dict.txt
-mmocr/.mim/tools/dist_test.sh
-mmocr/.mim/tools/dist_train.sh
-mmocr/.mim/tools/infer.py
-mmocr/.mim/tools/slurm_test.sh
-mmocr/.mim/tools/slurm_train.sh
-mmocr/.mim/tools/test.py
-mmocr/.mim/tools/train.py
-mmocr/.mim/tools/analysis_tools/get_flops.py
-mmocr/.mim/tools/analysis_tools/offline_eval.py
-mmocr/.mim/tools/analysis_tools/print_config.py
-mmocr/.mim/tools/dataset_converters/prepare_dataset.py
-mmocr/.mim/tools/dataset_converters/common/curvedsyntext_converter.py
-mmocr/.mim/tools/dataset_converters/common/extract_kaist.py
-mmocr/.mim/tools/dataset_converters/kie/closeset_to_openset.py
-mmocr/.mim/tools/dataset_converters/textdet/art_converter.py
-mmocr/.mim/tools/dataset_converters/textdet/bid_converter.py
-mmocr/.mim/tools/dataset_converters/textdet/coco_to_line_dict.py
-mmocr/.mim/tools/dataset_converters/textdet/cocotext_converter.py
-mmocr/.mim/tools/dataset_converters/textdet/data_migrator.py
-mmocr/.mim/tools/dataset_converters/textdet/detext_converter.py
-mmocr/.mim/tools/dataset_converters/textdet/funsd_converter.py
-mmocr/.mim/tools/dataset_converters/textdet/hiertext_converter.py
-mmocr/.mim/tools/dataset_converters/textdet/ic11_converter.py
-mmocr/.mim/tools/dataset_converters/textdet/ilst_converter.py
-mmocr/.mim/tools/dataset_converters/textdet/imgur_converter.py
-mmocr/.mim/tools/dataset_converters/textdet/kaist_converter.py
-mmocr/.mim/tools/dataset_converters/textdet/lsvt_converter.py
-mmocr/.mim/tools/dataset_converters/textdet/lv_converter.py
-mmocr/.mim/tools/dataset_converters/textdet/mtwi_converter.py
-mmocr/.mim/tools/dataset_converters/textdet/naf_converter.py
-mmocr/.mim/tools/dataset_converters/textdet/rctw_converter.py
-mmocr/.mim/tools/dataset_converters/textdet/rects_converter.py
-mmocr/.mim/tools/dataset_converters/textdet/sroie_converter.py
-mmocr/.mim/tools/dataset_converters/textdet/vintext_converter.py
-mmocr/.mim/tools/dataset_converters/textrecog/art_converter.py
-mmocr/.mim/tools/dataset_converters/textrecog/bid_converter.py
-mmocr/.mim/tools/dataset_converters/textrecog/cocotext_converter.py
-mmocr/.mim/tools/dataset_converters/textrecog/data_migrator.py
-mmocr/.mim/tools/dataset_converters/textrecog/detext_converter.py
-mmocr/.mim/tools/dataset_converters/textrecog/funsd_converter.py
-mmocr/.mim/tools/dataset_converters/textrecog/hiertext_converter.py
-mmocr/.mim/tools/dataset_converters/textrecog/ic11_converter.py
-mmocr/.mim/tools/dataset_converters/textrecog/ilst_converter.py
-mmocr/.mim/tools/dataset_converters/textrecog/imgur_converter.py
-mmocr/.mim/tools/dataset_converters/textrecog/kaist_converter.py
-mmocr/.mim/tools/dataset_converters/textrecog/lmdb_converter.py
-mmocr/.mim/tools/dataset_converters/textrecog/lsvt_converter.py
-mmocr/.mim/tools/dataset_converters/textrecog/lv_converter.py
-mmocr/.mim/tools/dataset_converters/textrecog/mtwi_converter.py
-mmocr/.mim/tools/dataset_converters/textrecog/naf_converter.py
-mmocr/.mim/tools/dataset_converters/textrecog/openvino_converter.py
-mmocr/.mim/tools/dataset_converters/textrecog/rctw_converter.py
-mmocr/.mim/tools/dataset_converters/textrecog/rects_converter.py
-mmocr/.mim/tools/dataset_converters/textrecog/sroie_converter.py
-mmocr/.mim/tools/dataset_converters/textrecog/vintext_converter.py
-mmocr/.mim/tools/model_converters/publish_model.py
-mmocr/.mim/tools/visualizations/browse_dataset.py
-mmocr/.mim/tools/visualizations/vis_scheduler.py
-mmocr/apis/__init__.py
-mmocr/apis/inferencers/__init__.py
-mmocr/apis/inferencers/base_mmocr_inferencer.py
-mmocr/apis/inferencers/kie_inferencer.py
-mmocr/apis/inferencers/mmocr_inferencer.py
-mmocr/apis/inferencers/textdet_inferencer.py
-mmocr/apis/inferencers/textrec_inferencer.py
-mmocr/apis/inferencers/textspot_inferencer.py
-mmocr/datasets/__init__.py
-mmocr/datasets/dataset_wrapper.py
-mmocr/datasets/icdar_dataset.py
-mmocr/datasets/ocr_dataset.py
-mmocr/datasets/recog_lmdb_dataset.py
-mmocr/datasets/recog_text_dataset.py
-mmocr/datasets/wildreceipt_dataset.py
-mmocr/datasets/preparers/__init__.py
-mmocr/datasets/preparers/data_preparer.py
-mmocr/datasets/preparers/config_generators/__init__.py
-mmocr/datasets/preparers/config_generators/base.py
-mmocr/datasets/preparers/config_generators/textdet_config_generator.py
-mmocr/datasets/preparers/config_generators/textrecog_config_generator.py
-mmocr/datasets/preparers/config_generators/textspotting_config_generator.py
-mmocr/datasets/preparers/dumpers/__init__.py
-mmocr/datasets/preparers/dumpers/base.py
-mmocr/datasets/preparers/dumpers/json_dumper.py
-mmocr/datasets/preparers/dumpers/lmdb_dumper.py
-mmocr/datasets/preparers/dumpers/wild_receipt_openset_dumper.py
-mmocr/datasets/preparers/gatherers/__init__.py
-mmocr/datasets/preparers/gatherers/base.py
-mmocr/datasets/preparers/gatherers/mono_gatherer.py
-mmocr/datasets/preparers/gatherers/naf_gatherer.py
-mmocr/datasets/preparers/gatherers/pair_gatherer.py
-mmocr/datasets/preparers/obtainers/__init__.py
-mmocr/datasets/preparers/obtainers/naive_data_obtainer.py
-mmocr/datasets/preparers/packers/__init__.py
-mmocr/datasets/preparers/packers/base.py
-mmocr/datasets/preparers/packers/textdet_packer.py
-mmocr/datasets/preparers/packers/textrecog_packer.py
-mmocr/datasets/preparers/packers/textspotting_packer.py
-mmocr/datasets/preparers/packers/wildreceipt_packer.py
-mmocr/datasets/preparers/parsers/__init__.py
-mmocr/datasets/preparers/parsers/base.py
-mmocr/datasets/preparers/parsers/coco_parser.py
-mmocr/datasets/preparers/parsers/ctw1500_parser.py
-mmocr/datasets/preparers/parsers/funsd_parser.py
-mmocr/datasets/preparers/parsers/icdar_txt_parser.py
-mmocr/datasets/preparers/parsers/mjsynth_parser.py
-mmocr/datasets/preparers/parsers/naf_parser.py
-mmocr/datasets/preparers/parsers/sroie_parser.py
-mmocr/datasets/preparers/parsers/svt_parser.py
-mmocr/datasets/preparers/parsers/synthtext_parser.py
-mmocr/datasets/preparers/parsers/totaltext_parser.py
-mmocr/datasets/preparers/parsers/wildreceipt_parser.py
-mmocr/datasets/samplers/__init__.py
-mmocr/datasets/samplers/batch_aug.py
-mmocr/datasets/transforms/__init__.py
-mmocr/datasets/transforms/adapters.py
-mmocr/datasets/transforms/formatting.py
-mmocr/datasets/transforms/loading.py
-mmocr/datasets/transforms/ocr_transforms.py
-mmocr/datasets/transforms/textdet_transforms.py
-mmocr/datasets/transforms/textrecog_transforms.py
-mmocr/datasets/transforms/wrappers.py
-mmocr/engine/__init__.py
-mmocr/engine/hooks/__init__.py
-mmocr/engine/hooks/visualization_hook.py
-mmocr/evaluation/__init__.py
-mmocr/evaluation/evaluator/__init__.py
-mmocr/evaluation/evaluator/multi_datasets_evaluator.py
-mmocr/evaluation/functional/__init__.py
-mmocr/evaluation/functional/hmean.py
-mmocr/evaluation/metrics/__init__.py
-mmocr/evaluation/metrics/f_metric.py
-mmocr/evaluation/metrics/hmean_iou_metric.py
-mmocr/evaluation/metrics/recog_metric.py
-mmocr/models/__init__.py
-mmocr/models/common/__init__.py
-mmocr/models/common/backbones/__init__.py
-mmocr/models/common/backbones/clip_resnet.py
-mmocr/models/common/backbones/unet.py
-mmocr/models/common/backbones/vit.py
-mmocr/models/common/dictionary/__init__.py
-mmocr/models/common/dictionary/dictionary.py
-mmocr/models/common/layers/__init__.py
-mmocr/models/common/layers/transformer_layers.py
-mmocr/models/common/losses/__init__.py
-mmocr/models/common/losses/bce_loss.py
-mmocr/models/common/losses/ce_loss.py
-mmocr/models/common/losses/dice_loss.py
-mmocr/models/common/losses/l1_loss.py
-mmocr/models/common/modules/__init__.py
-mmocr/models/common/modules/transformer_module.py
-mmocr/models/common/plugins/__init__.py
-mmocr/models/common/plugins/common.py
-mmocr/models/kie/__init__.py
-mmocr/models/kie/extractors/__init__.py
-mmocr/models/kie/extractors/sdmgr.py
-mmocr/models/kie/heads/__init__.py
-mmocr/models/kie/heads/sdmgr_head.py
-mmocr/models/kie/module_losses/__init__.py
-mmocr/models/kie/module_losses/sdmgr_module_loss.py
-mmocr/models/kie/postprocessors/__init__.py
-mmocr/models/kie/postprocessors/sdmgr_postprocessor.py
-mmocr/models/textdet/__init__.py
-mmocr/models/textdet/data_preprocessors/__init__.py
-mmocr/models/textdet/data_preprocessors/data_preprocessor.py
-mmocr/models/textdet/detectors/__init__.py
-mmocr/models/textdet/detectors/base.py
-mmocr/models/textdet/detectors/dbnet.py
-mmocr/models/textdet/detectors/drrg.py
-mmocr/models/textdet/detectors/fcenet.py
-mmocr/models/textdet/detectors/mmdet_wrapper.py
-mmocr/models/textdet/detectors/panet.py
-mmocr/models/textdet/detectors/psenet.py
-mmocr/models/textdet/detectors/single_stage_text_detector.py
-mmocr/models/textdet/detectors/textsnake.py
-mmocr/models/textdet/heads/__init__.py
-mmocr/models/textdet/heads/base.py
-mmocr/models/textdet/heads/db_head.py
-mmocr/models/textdet/heads/drrg_head.py
-mmocr/models/textdet/heads/fce_head.py
-mmocr/models/textdet/heads/pan_head.py
-mmocr/models/textdet/heads/pse_head.py
-mmocr/models/textdet/heads/textsnake_head.py
-mmocr/models/textdet/module_losses/__init__.py
-mmocr/models/textdet/module_losses/base.py
-mmocr/models/textdet/module_losses/db_module_loss.py
-mmocr/models/textdet/module_losses/drrg_module_loss.py
-mmocr/models/textdet/module_losses/fce_module_loss.py
-mmocr/models/textdet/module_losses/pan_module_loss.py
-mmocr/models/textdet/module_losses/pse_module_loss.py
-mmocr/models/textdet/module_losses/seg_based_module_loss.py
-mmocr/models/textdet/module_losses/textsnake_module_loss.py
-mmocr/models/textdet/necks/__init__.py
-mmocr/models/textdet/necks/fpem_ffm.py
-mmocr/models/textdet/necks/fpn_cat.py
-mmocr/models/textdet/necks/fpn_unet.py
-mmocr/models/textdet/necks/fpnf.py
-mmocr/models/textdet/postprocessors/__init__.py
-mmocr/models/textdet/postprocessors/base.py
-mmocr/models/textdet/postprocessors/db_postprocessor.py
-mmocr/models/textdet/postprocessors/drrg_postprocessor.py
-mmocr/models/textdet/postprocessors/fce_postprocessor.py
-mmocr/models/textdet/postprocessors/pan_postprocessor.py
-mmocr/models/textdet/postprocessors/pse_postprocessor.py
-mmocr/models/textdet/postprocessors/textsnake_postprocessor.py
-mmocr/models/textrecog/__init__.py
-mmocr/models/textrecog/backbones/__init__.py
-mmocr/models/textrecog/backbones/mini_vgg.py
-mmocr/models/textrecog/backbones/mobilenet_v2.py
-mmocr/models/textrecog/backbones/nrtr_modality_transformer.py
-mmocr/models/textrecog/backbones/resnet.py
-mmocr/models/textrecog/backbones/resnet31_ocr.py
-mmocr/models/textrecog/backbones/resnet_abi.py
-mmocr/models/textrecog/backbones/shallow_cnn.py
-mmocr/models/textrecog/data_preprocessors/__init__.py
-mmocr/models/textrecog/data_preprocessors/data_preprocessor.py
-mmocr/models/textrecog/decoders/__init__.py
-mmocr/models/textrecog/decoders/abi_fuser.py
-mmocr/models/textrecog/decoders/abi_language_decoder.py
-mmocr/models/textrecog/decoders/abi_vision_decoder.py
-mmocr/models/textrecog/decoders/aster_decoder.py
-mmocr/models/textrecog/decoders/base.py
-mmocr/models/textrecog/decoders/crnn_decoder.py
-mmocr/models/textrecog/decoders/maerec_decoder.py
-mmocr/models/textrecog/decoders/master_decoder.py
-mmocr/models/textrecog/decoders/nrtr_decoder.py
-mmocr/models/textrecog/decoders/position_attention_decoder.py
-mmocr/models/textrecog/decoders/robust_scanner_fuser.py
-mmocr/models/textrecog/decoders/sar_decoder.py
-mmocr/models/textrecog/decoders/sar_decoder_with_bs.py
-mmocr/models/textrecog/decoders/sequence_attention_decoder.py
-mmocr/models/textrecog/decoders/svtr_decoder.py
-mmocr/models/textrecog/encoders/__init__.py
-mmocr/models/textrecog/encoders/abi_encoder.py
-mmocr/models/textrecog/encoders/aster_encoder.py
-mmocr/models/textrecog/encoders/base.py
-mmocr/models/textrecog/encoders/channel_reduction_encoder.py
-mmocr/models/textrecog/encoders/nrtr_encoder.py
-mmocr/models/textrecog/encoders/sar_encoder.py
-mmocr/models/textrecog/encoders/satrn_encoder.py
-mmocr/models/textrecog/encoders/svtr_encoder.py
-mmocr/models/textrecog/layers/__init__.py
-mmocr/models/textrecog/layers/conv_layer.py
-mmocr/models/textrecog/layers/dot_product_attention_layer.py
-mmocr/models/textrecog/layers/lstm_layer.py
-mmocr/models/textrecog/layers/position_aware_layer.py
-mmocr/models/textrecog/layers/robust_scanner_fusion_layer.py
-mmocr/models/textrecog/layers/satrn_layers.py
-mmocr/models/textrecog/module_losses/__init__.py
-mmocr/models/textrecog/module_losses/abi_module_loss.py
-mmocr/models/textrecog/module_losses/base.py
-mmocr/models/textrecog/module_losses/ce_module_loss.py
-mmocr/models/textrecog/module_losses/ctc_module_loss.py
-mmocr/models/textrecog/plugins/__init__.py
-mmocr/models/textrecog/plugins/common.py
-mmocr/models/textrecog/postprocessors/__init__.py
-mmocr/models/textrecog/postprocessors/attn_postprocessor.py
-mmocr/models/textrecog/postprocessors/base.py
-mmocr/models/textrecog/postprocessors/ctc_postprocessor.py
-mmocr/models/textrecog/preprocessors/__init__.py
-mmocr/models/textrecog/preprocessors/base.py
-mmocr/models/textrecog/preprocessors/tps_preprocessor.py
-mmocr/models/textrecog/recognizers/__init__.py
-mmocr/models/textrecog/recognizers/abinet.py
-mmocr/models/textrecog/recognizers/aster.py
-mmocr/models/textrecog/recognizers/base.py
-mmocr/models/textrecog/recognizers/crnn.py
-mmocr/models/textrecog/recognizers/encoder_decoder_recognizer.py
-mmocr/models/textrecog/recognizers/encoder_decoder_recognizer_tta.py
-mmocr/models/textrecog/recognizers/maerec.py
-mmocr/models/textrecog/recognizers/master.py
-mmocr/models/textrecog/recognizers/nrtr.py
-mmocr/models/textrecog/recognizers/robust_scanner.py
-mmocr/models/textrecog/recognizers/sar.py
-mmocr/models/textrecog/recognizers/satrn.py
-mmocr/models/textrecog/recognizers/svtr.py
-mmocr/structures/__init__.py
-mmocr/structures/kie_data_sample.py
-mmocr/structures/textdet_data_sample.py
-mmocr/structures/textrecog_data_sample.py
-mmocr/structures/textspotting_data_sample.py
-mmocr/testing/__init__.py
-mmocr/testing/data.py
-mmocr/utils/__init__.py
-mmocr/utils/bbox_utils.py
-mmocr/utils/bezier_utils.py
-mmocr/utils/check_argument.py
-mmocr/utils/collect_env.py
-mmocr/utils/data_converter_utils.py
-mmocr/utils/fileio.py
-mmocr/utils/img_utils.py
-mmocr/utils/mask_utils.py
-mmocr/utils/parsers.py
-mmocr/utils/point_utils.py
-mmocr/utils/polygon_utils.py
-mmocr/utils/processing.py
-mmocr/utils/setup_env.py
-mmocr/utils/string_utils.py
-mmocr/utils/transform_utils.py
-mmocr/utils/typing_utils.py
-mmocr/visualization/__init__.py
-mmocr/visualization/base_visualizer.py
-mmocr/visualization/kie_visualizer.py
-mmocr/visualization/textdet_visualizer.py
-mmocr/visualization/textrecog_visualizer.py
-mmocr/visualization/textspotting_visualizer.py
-requirements/albu.txt
-requirements/build.txt
-requirements/docs.txt
-requirements/mminstall.txt
-requirements/optional.txt
-requirements/readthedocs.txt
-requirements/runtime.txt
-requirements/tests.txt
\ No newline at end of file
diff --git a/pipeline/mmocr/mmocr.egg-info/dependency_links.txt b/pipeline/mmocr/mmocr.egg-info/dependency_links.txt
deleted file mode 100644
index 8b137891791fe96927ad78e64b0aad7bded08bdc..0000000000000000000000000000000000000000
--- a/pipeline/mmocr/mmocr.egg-info/dependency_links.txt
+++ /dev/null
@@ -1 +0,0 @@
-
diff --git a/pipeline/mmocr/mmocr.egg-info/not-zip-safe b/pipeline/mmocr/mmocr.egg-info/not-zip-safe
deleted file mode 100644
index 8b137891791fe96927ad78e64b0aad7bded08bdc..0000000000000000000000000000000000000000
--- a/pipeline/mmocr/mmocr.egg-info/not-zip-safe
+++ /dev/null
@@ -1 +0,0 @@
-
diff --git a/pipeline/mmocr/mmocr.egg-info/requires.txt b/pipeline/mmocr/mmocr.egg-info/requires.txt
deleted file mode 100644
index a82d08ef86b90c376e2db15ec132d0ddc7b0a5e6..0000000000000000000000000000000000000000
--- a/pipeline/mmocr/mmocr.egg-info/requires.txt
+++ /dev/null
@@ -1,65 +0,0 @@
-imgaug
-lmdb
-matplotlib
-numpy
-opencv-python!=4.5.5.*,>=4.2.0.32
-pyclipper
-pycocotools
-rapidfuzz>=2.0.0
-scikit-image
-
-[all]
-numpy
-pyclipper
-torch>=1.1
-imgaug
-lmdb
-matplotlib
-numpy
-opencv-python!=4.5.5.*,>=4.2.0.32
-pyclipper
-pycocotools
-rapidfuzz>=2.0.0
-scikit-image
-asynctest
-codecov
-flake8
-interrogate
-isort
-kwarray
-lanms-neo==1.0.2
-parameterized
-pytest
-pytest-cov
-pytest-runner
-ubelt
-xdoctest>=0.10.0
-yapf
-
-[build]
-numpy
-pyclipper
-torch>=1.1
-
-[mim]
-mmcv<2.1.0,>=2.0.0rc4
-mmdet<3.1.0,>=3.0.0rc5
-mmengine<1.0.0,>=0.7.0
-
-[optional]
-
-[tests]
-asynctest
-codecov
-flake8
-interrogate
-isort
-kwarray
-lanms-neo==1.0.2
-parameterized
-pytest
-pytest-cov
-pytest-runner
-ubelt
-xdoctest>=0.10.0
-yapf
diff --git a/pipeline/mmocr/mmocr.egg-info/top_level.txt b/pipeline/mmocr/mmocr.egg-info/top_level.txt
deleted file mode 100644
index cf9933f3400301cc296c6abab55a3080eca2aa24..0000000000000000000000000000000000000000
--- a/pipeline/mmocr/mmocr.egg-info/top_level.txt
+++ /dev/null
@@ -1 +0,0 @@
-mmocr
diff --git a/pipeline/mmocr/mmocr/.mim/configs/backbone/oclip/README.md b/pipeline/mmocr/mmocr/.mim/configs/backbone/oclip/README.md
deleted file mode 100644
index e29cf971f6f8e6ba6c4fc640e6d06c5583d2909d..0000000000000000000000000000000000000000
--- a/pipeline/mmocr/mmocr/.mim/configs/backbone/oclip/README.md
+++ /dev/null
@@ -1,41 +0,0 @@
-# oCLIP
-
-> [Language Matters: A Weakly Supervised Vision-Language Pre-training Approach for Scene Text Detection and Spotting](https://www.ecva.net/papers/eccv_2022/papers_ECCV/papers/136880282.pdf)
-
-
-
-## Abstract
-
-Recently, Vision-Language Pre-training (VLP) techniques have greatly benefited various vision-language tasks by jointly learning visual and textual representations, which intuitively helps in Optical Character Recognition (OCR) tasks due to the rich visual and textual information in scene text images. However, these methods cannot well cope with OCR tasks because of the difficulty in both instance-level text encoding and image-text pair acquisition (i.e. images and captured texts in them). This paper presents a weakly supervised pre-training method, oCLIP, which can acquire effective scene text representations by jointly learning and aligning visual and textual information. Our network consists of an image encoder and a character-aware text encoder that extract visual and textual features, respectively, as well as a visual-textual decoder that models the interaction among textual and visual features for learning effective scene text representations. With the learning of textual features, the pre-trained model can attend texts in images well with character awareness. Besides, these designs enable the learning from weakly annotated texts (i.e. partial texts in images without text bounding boxes) which mitigates the data annotation constraint greatly. Experiments over the weakly annotated images in ICDAR2019-LSVT show that our pre-trained model improves F-score by +2.5% and +4.8% while transferring its weights to other text detection and spotting networks, respectively. In addition, the proposed method outperforms existing pre-training techniques consistently across multiple public datasets (e.g., +3.2% and +1.3% for Total-Text and CTW1500).
-
-
-
-
-
-## Models
-
-| Backbone | Pre-train Data | Model |
-| :-------: | :------------: | :-------------------------------------------------------------------------------: |
-| ResNet-50 | SynthText | [Link](https://download.openmmlab.com/mmocr/backbone/resnet50-oclip-7ba0c533.pth) |
-
-```{note}
-The model is converted from the official [oCLIP](https://github.com/bytedance/oclip.git).
-```
-
-## Supported Text Detection Models
-
-| | [DBNet](https://mmocr.readthedocs.io/en/dev-1.x/textdet_models.html#dbnet) | [DBNet++](https://mmocr.readthedocs.io/en/dev-1.x/textdet_models.html#dbnetpp) | [FCENet](https://mmocr.readthedocs.io/en/dev-1.x/textdet_models.html#fcenet) | [TextSnake](https://mmocr.readthedocs.io/en/dev-1.x/textdet_models.html#fcenet) | [PSENet](https://mmocr.readthedocs.io/en/dev-1.x/textdet_models.html#psenet) | [DRRG](https://mmocr.readthedocs.io/en/dev-1.x/textdet_models.html#drrg) | [Mask R-CNN](https://mmocr.readthedocs.io/en/dev-1.x/textdet_models.html#mask-r-cnn) |
-| :-------: | :------------------------------------------------------------------------: | :----------------------------------------------------------------------------: | :--------------------------------------------------------------------------: | :-----------------------------------------------------------------------------: | :--------------------------------------------------------------------------: | :----------------------------------------------------------------------: | :----------------------------------------------------------------------------------: |
-| ICDAR2015 | โ | โ | โ | | โ | | โ |
-| CTW1500 | | | โ | โ | โ | โ | โ |
-
-## Citation
-
-```bibtex
-@article{xue2022language,
- title={Language Matters: A Weakly Supervised Vision-Language Pre-training Approach for Scene Text Detection and Spotting},
- author={Xue, Chuhui and Zhang, Wenqing and Hao, Yu and Lu, Shijian and Torr, Philip and Bai, Song},
- journal={Proceedings of the European Conference on Computer Vision (ECCV)},
- year={2022}
-}
-```
diff --git a/pipeline/mmocr/mmocr/.mim/configs/backbone/oclip/metafile.yml b/pipeline/mmocr/mmocr/.mim/configs/backbone/oclip/metafile.yml
deleted file mode 100644
index 8953af1b6b3c7b6190602be0af9e07753ed67518..0000000000000000000000000000000000000000
--- a/pipeline/mmocr/mmocr/.mim/configs/backbone/oclip/metafile.yml
+++ /dev/null
@@ -1,13 +0,0 @@
-Collections:
-- Name: oCLIP
- Metadata:
- Training Data: SynthText
- Architecture:
- - CLIPResNet
- Paper:
- URL: https://arxiv.org/abs/2203.03911
- Title: 'Language Matters: A Weakly Supervised Vision-Language Pre-training Approach for Scene Text Detection and Spotting'
- README: configs/backbone/oclip/README.md
-
-Models:
- Weights: https://download.openmmlab.com/mmocr/backbone/resnet50-oclip-7ba0c533.pth
diff --git a/pipeline/mmocr/mmocr/.mim/configs/kie/_base_/datasets/wildreceipt-openset.py b/pipeline/mmocr/mmocr/.mim/configs/kie/_base_/datasets/wildreceipt-openset.py
deleted file mode 100644
index f82512839cdea57e559bd375be2a3f4146558af3..0000000000000000000000000000000000000000
--- a/pipeline/mmocr/mmocr/.mim/configs/kie/_base_/datasets/wildreceipt-openset.py
+++ /dev/null
@@ -1,26 +0,0 @@
-wildreceipt_openset_data_root = 'data/wildreceipt/'
-
-wildreceipt_openset_train = dict(
- type='WildReceiptDataset',
- data_root=wildreceipt_openset_data_root,
- metainfo=dict(category=[
- dict(id=0, name='bg'),
- dict(id=1, name='key'),
- dict(id=2, name='value'),
- dict(id=3, name='other')
- ]),
- ann_file='openset_train.txt',
- pipeline=None)
-
-wildreceipt_openset_test = dict(
- type='WildReceiptDataset',
- data_root=wildreceipt_openset_data_root,
- metainfo=dict(category=[
- dict(id=0, name='bg'),
- dict(id=1, name='key'),
- dict(id=2, name='value'),
- dict(id=3, name='other')
- ]),
- ann_file='openset_test.txt',
- test_mode=True,
- pipeline=None)
diff --git a/pipeline/mmocr/mmocr/.mim/configs/kie/_base_/datasets/wildreceipt.py b/pipeline/mmocr/mmocr/.mim/configs/kie/_base_/datasets/wildreceipt.py
deleted file mode 100644
index 9c1122edd53c5c8df4bad55ad764c12e1714026a..0000000000000000000000000000000000000000
--- a/pipeline/mmocr/mmocr/.mim/configs/kie/_base_/datasets/wildreceipt.py
+++ /dev/null
@@ -1,16 +0,0 @@
-wildreceipt_data_root = 'data/wildreceipt/'
-
-wildreceipt_train = dict(
- type='WildReceiptDataset',
- data_root=wildreceipt_data_root,
- metainfo=wildreceipt_data_root + 'class_list.txt',
- ann_file='train.txt',
- pipeline=None)
-
-wildreceipt_test = dict(
- type='WildReceiptDataset',
- data_root=wildreceipt_data_root,
- metainfo=wildreceipt_data_root + 'class_list.txt',
- ann_file='test.txt',
- test_mode=True,
- pipeline=None)
diff --git a/pipeline/mmocr/mmocr/.mim/configs/kie/_base_/default_runtime.py b/pipeline/mmocr/mmocr/.mim/configs/kie/_base_/default_runtime.py
deleted file mode 100644
index bcc5b3fa02a0f3259f701cddecbc307988424a6b..0000000000000000000000000000000000000000
--- a/pipeline/mmocr/mmocr/.mim/configs/kie/_base_/default_runtime.py
+++ /dev/null
@@ -1,33 +0,0 @@
-default_scope = 'mmocr'
-env_cfg = dict(
- cudnn_benchmark=False,
- mp_cfg=dict(mp_start_method='fork', opencv_num_threads=0),
- dist_cfg=dict(backend='nccl'),
-)
-randomness = dict(seed=None)
-
-default_hooks = dict(
- timer=dict(type='IterTimerHook'),
- logger=dict(type='LoggerHook', interval=100),
- param_scheduler=dict(type='ParamSchedulerHook'),
- checkpoint=dict(type='CheckpointHook', interval=1),
- sampler_seed=dict(type='DistSamplerSeedHook'),
- sync_buffer=dict(type='SyncBuffersHook'),
- visualization=dict(
- type='VisualizationHook',
- interval=1,
- enable=False,
- show=False,
- draw_gt=False,
- draw_pred=False),
-)
-
-# Logging
-log_level = 'INFO'
-log_processor = dict(type='LogProcessor', window_size=10, by_epoch=True)
-
-load_from = None
-resume = False
-
-visualizer = dict(
- type='KIELocalVisualizer', name='visualizer', is_openset=False)
diff --git a/pipeline/mmocr/mmocr/.mim/configs/kie/_base_/schedules/schedule_adam_60e.py b/pipeline/mmocr/mmocr/.mim/configs/kie/_base_/schedules/schedule_adam_60e.py
deleted file mode 100644
index fd7147e2b86a8640966617bae1eb86d3347057f9..0000000000000000000000000000000000000000
--- a/pipeline/mmocr/mmocr/.mim/configs/kie/_base_/schedules/schedule_adam_60e.py
+++ /dev/null
@@ -1,10 +0,0 @@
-# optimizer
-optim_wrapper = dict(
- type='OptimWrapper', optimizer=dict(type='Adam', weight_decay=0.0001))
-train_cfg = dict(type='EpochBasedTrainLoop', max_epochs=60, val_interval=1)
-val_cfg = dict(type='ValLoop')
-test_cfg = dict(type='TestLoop')
-# learning rate
-param_scheduler = [
- dict(type='MultiStepLR', milestones=[40, 50], end=60),
-]
diff --git a/pipeline/mmocr/mmocr/.mim/configs/kie/sdmgr/README.md b/pipeline/mmocr/mmocr/.mim/configs/kie/sdmgr/README.md
deleted file mode 100644
index 921af5310e46803c937168c6e1c0bdf17a372798..0000000000000000000000000000000000000000
--- a/pipeline/mmocr/mmocr/.mim/configs/kie/sdmgr/README.md
+++ /dev/null
@@ -1,41 +0,0 @@
-# SDMGR
-
-> [Spatial Dual-Modality Graph Reasoning for Key Information Extraction](https://arxiv.org/abs/2103.14470)
-
-
-
-## Abstract
-
-Key information extraction from document images is of paramount importance in office automation. Conventional template matching based approaches fail to generalize well to document images of unseen templates, and are not robust against text recognition errors. In this paper, we propose an end-to-end Spatial Dual-Modality Graph Reasoning method (SDMG-R) to extract key information from unstructured document images. We model document images as dual-modality graphs, nodes of which encode both the visual and textual features of detected text regions, and edges of which represent the spatial relations between neighboring text regions. The key information extraction is solved by iteratively propagating messages along graph edges and reasoning the categories of graph nodes. In order to roundly evaluate our proposed method as well as boost the future research, we release a new dataset named WildReceipt, which is collected and annotated tailored for the evaluation of key information extraction from document images of unseen templates in the wild. It contains 25 key information categories, a total of about 69000 text boxes, and is about 2 times larger than the existing public datasets. Extensive experiments validate that all information including visual features, textual features and spatial relations can benefit key information extraction. It has been shown that SDMG-R can effectively extract key information from document images of unseen templates, and obtain new state-of-the-art results on the recent popular benchmark SROIE and our WildReceipt. Our code and dataset will be publicly released.
-
-
-
-
-
-## Results and models
-
-### WildReceipt
-
-| Method | Modality | Macro F1-Score | Download |
-| :--------------------------------------------------------------------: | :--------------: | :------------: | :--------------------------------------------------------------------------------------------------: |
-| [sdmgr_unet16](/configs/kie/sdmgr/sdmgr_unet16_60e_wildreceipt.py) | Visual + Textual | 0.890 | [model](https://download.openmmlab.com/mmocr/kie/sdmgr/sdmgr_unet16_60e_wildreceipt/sdmgr_unet16_60e_wildreceipt_20220825_151648-22419f37.pth) \| [log](https://download.openmmlab.com/mmocr/kie/sdmgr/sdmgr_unet16_60e_wildreceipt/20220825_151648.log) |
-| [sdmgr_novisual](/configs/kie/sdmgr/sdmgr_novisual_60e_wildreceipt.py) | Textual | 0.873 | [model](https://download.openmmlab.com/mmocr/kie/sdmgr/sdmgr_novisual_60e_wildreceipt/sdmgr_novisual_60e_wildreceipt_20220831_193317-827649d8.pth) \| [log](https://download.openmmlab.com/mmocr/kie/sdmgr/sdmgr_novisual_60e_wildreceipt/20220831_193317.log) |
-
-### WildReceiptOpenset
-
-| Method | Modality | Edge F1-Score | Node Macro F1-Score | Node Micro F1-Score | Download |
-| :-------------------------------------------------------------------: | :------: | :-----------: | :-----------------: | :-----------------: | :----------------------------------------------------------------------: |
-| [sdmgr_novisual_openset](/configs/kie/sdmgr/sdmgr_novisual_60e_wildreceipt-openset.py) | Textual | 0.792 | 0.931 | 0.940 | [model](https://download.openmmlab.com/mmocr/kie/sdmgr/sdmgr_novisual_60e_wildreceipt-openset/sdmgr_novisual_60e_wildreceipt-openset_20220831_200807-dedf15ec.pth) \| [log](https://download.openmmlab.com/mmocr/kie/sdmgr/sdmgr_novisual_60e_wildreceipt-openset/20220831_200807.log) |
-
-## Citation
-
-```bibtex
-@misc{sun2021spatial,
- title={Spatial Dual-Modality Graph Reasoning for Key Information Extraction},
- author={Hongbin Sun and Zhanghui Kuang and Xiaoyu Yue and Chenhao Lin and Wayne Zhang},
- year={2021},
- eprint={2103.14470},
- archivePrefix={arXiv},
- primaryClass={cs.CV}
-}
-```
diff --git a/pipeline/mmocr/mmocr/.mim/configs/kie/sdmgr/_base_sdmgr_novisual.py b/pipeline/mmocr/mmocr/.mim/configs/kie/sdmgr/_base_sdmgr_novisual.py
deleted file mode 100644
index 5e85de2f78f020bd5695858098ad143dbbd09ed0..0000000000000000000000000000000000000000
--- a/pipeline/mmocr/mmocr/.mim/configs/kie/sdmgr/_base_sdmgr_novisual.py
+++ /dev/null
@@ -1,35 +0,0 @@
-num_classes = 26
-
-model = dict(
- type='SDMGR',
- kie_head=dict(
- type='SDMGRHead',
- visual_dim=16,
- num_classes=num_classes,
- module_loss=dict(type='SDMGRModuleLoss'),
- postprocessor=dict(type='SDMGRPostProcessor')),
- dictionary=dict(
- type='Dictionary',
- dict_file='{{ fileDirname }}/../../../dicts/sdmgr_dict.txt',
- with_padding=True,
- with_unknown=True,
- unknown_token=None),
-)
-
-train_pipeline = [
- dict(type='LoadKIEAnnotations'),
- dict(type='Resize', scale=(1024, 512), keep_ratio=True),
- dict(type='PackKIEInputs')
-]
-test_pipeline = [
- dict(type='LoadKIEAnnotations'),
- dict(type='Resize', scale=(1024, 512), keep_ratio=True),
- dict(type='PackKIEInputs'),
-]
-
-val_evaluator = dict(
- type='F1Metric',
- mode='macro',
- num_classes=num_classes,
- ignored_classes=[0, 2, 4, 6, 8, 10, 12, 14, 16, 18, 20, 22, 24, 25])
-test_evaluator = val_evaluator
diff --git a/pipeline/mmocr/mmocr/.mim/configs/kie/sdmgr/_base_sdmgr_unet16.py b/pipeline/mmocr/mmocr/.mim/configs/kie/sdmgr/_base_sdmgr_unet16.py
deleted file mode 100644
index 76aa631bdfbbf29013d27ac76c0e160d232d1500..0000000000000000000000000000000000000000
--- a/pipeline/mmocr/mmocr/.mim/configs/kie/sdmgr/_base_sdmgr_unet16.py
+++ /dev/null
@@ -1,28 +0,0 @@
-_base_ = '_base_sdmgr_novisual.py'
-
-model = dict(
- backbone=dict(type='UNet', base_channels=16),
- roi_extractor=dict(
- type='mmdet.SingleRoIExtractor',
- roi_layer=dict(type='RoIAlign', output_size=7),
- featmap_strides=[1]),
- data_preprocessor=dict(
- type='ImgDataPreprocessor',
- mean=[123.675, 116.28, 103.53],
- std=[58.395, 57.12, 57.375],
- bgr_to_rgb=True,
- pad_size_divisor=32),
-)
-
-train_pipeline = [
- dict(type='LoadImageFromFile'),
- dict(type='LoadKIEAnnotations'),
- dict(type='Resize', scale=(1024, 512), keep_ratio=True),
- dict(type='PackKIEInputs')
-]
-test_pipeline = [
- dict(type='LoadImageFromFile'),
- dict(type='LoadKIEAnnotations'),
- dict(type='Resize', scale=(1024, 512), keep_ratio=True),
- dict(type='PackKIEInputs', meta_keys=('img_path', )),
-]
diff --git a/pipeline/mmocr/mmocr/.mim/configs/kie/sdmgr/metafile.yml b/pipeline/mmocr/mmocr/.mim/configs/kie/sdmgr/metafile.yml
deleted file mode 100644
index da430e3d87ab7fe02a9560f7d0e441cce2ccf929..0000000000000000000000000000000000000000
--- a/pipeline/mmocr/mmocr/.mim/configs/kie/sdmgr/metafile.yml
+++ /dev/null
@@ -1,52 +0,0 @@
-Collections:
-- Name: SDMGR
- Metadata:
- Training Data: KIEDataset
- Training Techniques:
- - Adam
- Training Resources: 1x NVIDIA A100-SXM4-80GB
- Architecture:
- - UNet
- - SDMGRHead
- Paper:
- URL: https://arxiv.org/abs/2103.14470.pdf
- Title: 'Spatial Dual-Modality Graph Reasoning for Key Information Extraction'
- README: configs/kie/sdmgr/README.md
-
-Models:
- - Name: sdmgr_unet16_60e_wildreceipt
- Alias: SDMGR
- In Collection: SDMGR
- Config: configs/kie/sdmgr/sdmgr_unet16_60e_wildreceipt.py
- Metadata:
- Training Data: wildreceipt
- Results:
- - Task: Key Information Extraction
- Dataset: wildreceipt
- Metrics:
- macro_f1: 0.890
- Weights: https://download.openmmlab.com/mmocr/kie/sdmgr/sdmgr_unet16_60e_wildreceipt/sdmgr_unet16_60e_wildreceipt_20220825_151648-22419f37.pth
- - Name: sdmgr_novisual_60e_wildreceipt
- In Collection: SDMGR
- Config: configs/kie/sdmgr/sdmgr_novisual_60e_wildreceipt.py
- Metadata:
- Training Data: wildreceipt
- Results:
- - Task: Key Information Extraction
- Dataset: wildreceipt
- Metrics:
- macro_f1: 0.873
- Weights: https://download.openmmlab.com/mmocr/kie/sdmgr/sdmgr_novisual_60e_wildreceipt/sdmgr_novisual_60e_wildreceipt_20220831_193317-827649d8.pth
- - Name: sdmgr_novisual_60e_wildreceipt_openset
- In Collection: SDMGR
- Config: configs/kie/sdmgr/sdmgr_novisual_60e_wildreceipt-openset.py
- Metadata:
- Training Data: wildreceipt-openset
- Results:
- - Task: Key Information Extraction
- Dataset: wildreceipt
- Metrics:
- macro_f1: 0.931
- micro_f1: 0.940
- edge_micro_f1: 0.792
- Weights: https://download.openmmlab.com/mmocr/kie/sdmgr/sdmgr_novisual_60e_wildreceipt-openset/sdmgr_novisual_60e_wildreceipt-openset_20220831_200807-dedf15ec.pth
diff --git a/pipeline/mmocr/mmocr/.mim/configs/kie/sdmgr/sdmgr_novisual_60e_wildreceipt-openset.py b/pipeline/mmocr/mmocr/.mim/configs/kie/sdmgr/sdmgr_novisual_60e_wildreceipt-openset.py
deleted file mode 100644
index bc3d52a1ce93d4baf267edc923c71f2b9482e767..0000000000000000000000000000000000000000
--- a/pipeline/mmocr/mmocr/.mim/configs/kie/sdmgr/sdmgr_novisual_60e_wildreceipt-openset.py
+++ /dev/null
@@ -1,71 +0,0 @@
-_base_ = [
- '../_base_/default_runtime.py',
- '../_base_/datasets/wildreceipt-openset.py',
- '../_base_/schedules/schedule_adam_60e.py',
- '_base_sdmgr_novisual.py',
-]
-
-node_num_classes = 4 # 4 classes: bg, key, value and other
-edge_num_classes = 2 # edge connectivity
-key_node_idx = 1
-value_node_idx = 2
-
-model = dict(
- type='SDMGR',
- kie_head=dict(
- num_classes=node_num_classes,
- postprocessor=dict(
- link_type='one-to-many',
- key_node_idx=key_node_idx,
- value_node_idx=value_node_idx)),
-)
-
-test_pipeline = [
- dict(
- type='LoadKIEAnnotations',
- key_node_idx=key_node_idx,
- value_node_idx=value_node_idx), # Keep key->value edges for evaluation
- dict(type='Resize', scale=(1024, 512), keep_ratio=True),
- dict(type='PackKIEInputs'),
-]
-
-wildreceipt_openset_train = _base_.wildreceipt_openset_train
-wildreceipt_openset_train.pipeline = _base_.train_pipeline
-wildreceipt_openset_test = _base_.wildreceipt_openset_test
-wildreceipt_openset_test.pipeline = test_pipeline
-
-train_dataloader = dict(
- batch_size=4,
- num_workers=1,
- persistent_workers=True,
- sampler=dict(type='DefaultSampler', shuffle=True),
- dataset=wildreceipt_openset_train)
-val_dataloader = dict(
- batch_size=1,
- num_workers=1,
- persistent_workers=True,
- sampler=dict(type='DefaultSampler', shuffle=False),
- dataset=wildreceipt_openset_test)
-test_dataloader = val_dataloader
-
-val_evaluator = [
- dict(
- type='F1Metric',
- prefix='node',
- key='labels',
- mode=['micro', 'macro'],
- num_classes=node_num_classes,
- cared_classes=[key_node_idx, value_node_idx]),
- dict(
- type='F1Metric',
- prefix='edge',
- mode='micro',
- key='edge_labels',
- cared_classes=[1], # Collapse to binary F1 score
- num_classes=edge_num_classes)
-]
-test_evaluator = val_evaluator
-
-visualizer = dict(
- type='KIELocalVisualizer', name='visualizer', is_openset=True)
-auto_scale_lr = dict(base_batch_size=4)
diff --git a/pipeline/mmocr/mmocr/.mim/configs/kie/sdmgr/sdmgr_novisual_60e_wildreceipt.py b/pipeline/mmocr/mmocr/.mim/configs/kie/sdmgr/sdmgr_novisual_60e_wildreceipt.py
deleted file mode 100644
index b56c2b9b665b1bd5c2734aa41fa1e563feda5a81..0000000000000000000000000000000000000000
--- a/pipeline/mmocr/mmocr/.mim/configs/kie/sdmgr/sdmgr_novisual_60e_wildreceipt.py
+++ /dev/null
@@ -1,28 +0,0 @@
-_base_ = [
- '../_base_/default_runtime.py',
- '../_base_/datasets/wildreceipt.py',
- '../_base_/schedules/schedule_adam_60e.py',
- '_base_sdmgr_novisual.py',
-]
-
-wildreceipt_train = _base_.wildreceipt_train
-wildreceipt_train.pipeline = _base_.train_pipeline
-wildreceipt_test = _base_.wildreceipt_test
-wildreceipt_test.pipeline = _base_.test_pipeline
-
-train_dataloader = dict(
- batch_size=4,
- num_workers=1,
- persistent_workers=True,
- sampler=dict(type='DefaultSampler', shuffle=True),
- dataset=wildreceipt_train)
-
-val_dataloader = dict(
- batch_size=1,
- num_workers=1,
- persistent_workers=True,
- sampler=dict(type='DefaultSampler', shuffle=False),
- dataset=wildreceipt_test)
-test_dataloader = val_dataloader
-
-auto_scale_lr = dict(base_batch_size=4)
diff --git a/pipeline/mmocr/mmocr/.mim/configs/kie/sdmgr/sdmgr_unet16_60e_wildreceipt.py b/pipeline/mmocr/mmocr/.mim/configs/kie/sdmgr/sdmgr_unet16_60e_wildreceipt.py
deleted file mode 100644
index d49cbbc33798e815a24cb29cf3bc008460948c88..0000000000000000000000000000000000000000
--- a/pipeline/mmocr/mmocr/.mim/configs/kie/sdmgr/sdmgr_unet16_60e_wildreceipt.py
+++ /dev/null
@@ -1,29 +0,0 @@
-_base_ = [
- '../_base_/default_runtime.py',
- '../_base_/datasets/wildreceipt.py',
- '../_base_/schedules/schedule_adam_60e.py',
- '_base_sdmgr_unet16.py',
-]
-
-wildreceipt_train = _base_.wildreceipt_train
-wildreceipt_train.pipeline = _base_.train_pipeline
-wildreceipt_test = _base_.wildreceipt_test
-wildreceipt_test.pipeline = _base_.test_pipeline
-
-train_dataloader = dict(
- batch_size=4,
- num_workers=4,
- persistent_workers=True,
- sampler=dict(type='DefaultSampler', shuffle=True),
- dataset=wildreceipt_train)
-
-val_dataloader = dict(
- batch_size=1,
- num_workers=1,
- persistent_workers=True,
- sampler=dict(type='DefaultSampler', shuffle=False),
- dataset=wildreceipt_test)
-
-test_dataloader = val_dataloader
-
-auto_scale_lr = dict(base_batch_size=4)
diff --git a/pipeline/mmocr/mmocr/.mim/configs/textdet/_base_/datasets/ctw1500.py b/pipeline/mmocr/mmocr/.mim/configs/textdet/_base_/datasets/ctw1500.py
deleted file mode 100644
index 3361f734d0d92752336d13b60f293b785a92e927..0000000000000000000000000000000000000000
--- a/pipeline/mmocr/mmocr/.mim/configs/textdet/_base_/datasets/ctw1500.py
+++ /dev/null
@@ -1,15 +0,0 @@
-ctw1500_textdet_data_root = 'data/ctw1500'
-
-ctw1500_textdet_train = dict(
- type='OCRDataset',
- data_root=ctw1500_textdet_data_root,
- ann_file='textdet_train.json',
- filter_cfg=dict(filter_empty_gt=True, min_size=32),
- pipeline=None)
-
-ctw1500_textdet_test = dict(
- type='OCRDataset',
- data_root=ctw1500_textdet_data_root,
- ann_file='textdet_test.json',
- test_mode=True,
- pipeline=None)
diff --git a/pipeline/mmocr/mmocr/.mim/configs/textdet/_base_/datasets/icdar2015.py b/pipeline/mmocr/mmocr/.mim/configs/textdet/_base_/datasets/icdar2015.py
deleted file mode 100644
index 958cb4fa17f50ed7dc967ccceb11cfb9426cd867..0000000000000000000000000000000000000000
--- a/pipeline/mmocr/mmocr/.mim/configs/textdet/_base_/datasets/icdar2015.py
+++ /dev/null
@@ -1,15 +0,0 @@
-icdar2015_textdet_data_root = 'data/icdar2015'
-
-icdar2015_textdet_train = dict(
- type='OCRDataset',
- data_root=icdar2015_textdet_data_root,
- ann_file='textdet_train.json',
- filter_cfg=dict(filter_empty_gt=True, min_size=32),
- pipeline=None)
-
-icdar2015_textdet_test = dict(
- type='OCRDataset',
- data_root=icdar2015_textdet_data_root,
- ann_file='textdet_test.json',
- test_mode=True,
- pipeline=None)
diff --git a/pipeline/mmocr/mmocr/.mim/configs/textdet/_base_/datasets/icdar2017.py b/pipeline/mmocr/mmocr/.mim/configs/textdet/_base_/datasets/icdar2017.py
deleted file mode 100644
index 804cb26f96f2bcfb3fdf9803cf36d79e997c57a8..0000000000000000000000000000000000000000
--- a/pipeline/mmocr/mmocr/.mim/configs/textdet/_base_/datasets/icdar2017.py
+++ /dev/null
@@ -1,17 +0,0 @@
-icdar2017_textdet_data_root = 'data/det/icdar_2017'
-
-icdar2017_textdet_train = dict(
- type='OCRDataset',
- data_root=icdar2017_textdet_data_root,
- ann_file='instances_training.json',
- data_prefix=dict(img_path='imgs/'),
- filter_cfg=dict(filter_empty_gt=True, min_size=32),
- pipeline=None)
-
-icdar2017_textdet_test = dict(
- type='OCRDataset',
- data_root=icdar2017_textdet_data_root,
- ann_file='instances_test.json',
- data_prefix=dict(img_path='imgs/'),
- test_mode=True,
- pipeline=None)
diff --git a/pipeline/mmocr/mmocr/.mim/configs/textdet/_base_/datasets/synthtext.py b/pipeline/mmocr/mmocr/.mim/configs/textdet/_base_/datasets/synthtext.py
deleted file mode 100644
index 9b2310c36fbd89be9a99d2ecba6f823d28532e35..0000000000000000000000000000000000000000
--- a/pipeline/mmocr/mmocr/.mim/configs/textdet/_base_/datasets/synthtext.py
+++ /dev/null
@@ -1,8 +0,0 @@
-synthtext_textdet_data_root = 'data/synthtext'
-
-synthtext_textdet_train = dict(
- type='OCRDataset',
- data_root=synthtext_textdet_data_root,
- ann_file='textdet_train.json',
- filter_cfg=dict(filter_empty_gt=True, min_size=32),
- pipeline=None)
diff --git a/pipeline/mmocr/mmocr/.mim/configs/textdet/_base_/datasets/totaltext.py b/pipeline/mmocr/mmocr/.mim/configs/textdet/_base_/datasets/totaltext.py
deleted file mode 100644
index 29efc842fb0c558b98c1b8e805973360013b804e..0000000000000000000000000000000000000000
--- a/pipeline/mmocr/mmocr/.mim/configs/textdet/_base_/datasets/totaltext.py
+++ /dev/null
@@ -1,15 +0,0 @@
-totaltext_textdet_data_root = 'data/totaltext'
-
-totaltext_textdet_train = dict(
- type='OCRDataset',
- data_root=totaltext_textdet_data_root,
- ann_file='textdet_train.json',
- filter_cfg=dict(filter_empty_gt=True, min_size=32),
- pipeline=None)
-
-totaltext_textdet_test = dict(
- type='OCRDataset',
- data_root=totaltext_textdet_data_root,
- ann_file='textdet_test.json',
- test_mode=True,
- pipeline=None)
diff --git a/pipeline/mmocr/mmocr/.mim/configs/textdet/_base_/datasets/toy_data.py b/pipeline/mmocr/mmocr/.mim/configs/textdet/_base_/datasets/toy_data.py
deleted file mode 100644
index 50138769b7bfd99babafcc2aa6e85593c2b0dbf1..0000000000000000000000000000000000000000
--- a/pipeline/mmocr/mmocr/.mim/configs/textdet/_base_/datasets/toy_data.py
+++ /dev/null
@@ -1,17 +0,0 @@
-toy_det_data_root = 'tests/data/det_toy_dataset'
-
-toy_det_train = dict(
- type='OCRDataset',
- data_root=toy_det_data_root,
- ann_file='instances_training.json',
- data_prefix=dict(img_path='imgs/'),
- filter_cfg=dict(filter_empty_gt=True, min_size=32),
- pipeline=None)
-
-toy_det_test = dict(
- type='OCRDataset',
- data_root=toy_det_data_root,
- ann_file='instances_test.json',
- data_prefix=dict(img_path='imgs/'),
- test_mode=True,
- pipeline=None)
diff --git a/pipeline/mmocr/mmocr/.mim/configs/textdet/_base_/default_runtime.py b/pipeline/mmocr/mmocr/.mim/configs/textdet/_base_/default_runtime.py
deleted file mode 100644
index 81480273b5a7b30d5d7113fb1cb9380b16de5e8f..0000000000000000000000000000000000000000
--- a/pipeline/mmocr/mmocr/.mim/configs/textdet/_base_/default_runtime.py
+++ /dev/null
@@ -1,41 +0,0 @@
-default_scope = 'mmocr'
-env_cfg = dict(
- cudnn_benchmark=False,
- mp_cfg=dict(mp_start_method='fork', opencv_num_threads=0),
- dist_cfg=dict(backend='nccl'),
-)
-randomness = dict(seed=None)
-
-default_hooks = dict(
- timer=dict(type='IterTimerHook'),
- logger=dict(type='LoggerHook', interval=5),
- param_scheduler=dict(type='ParamSchedulerHook'),
- checkpoint=dict(type='CheckpointHook', interval=20),
- sampler_seed=dict(type='DistSamplerSeedHook'),
- sync_buffer=dict(type='SyncBuffersHook'),
- visualization=dict(
- type='VisualizationHook',
- interval=1,
- enable=False,
- show=False,
- draw_gt=False,
- draw_pred=False),
-)
-
-# Logging
-log_level = 'INFO'
-log_processor = dict(type='LogProcessor', window_size=10, by_epoch=True)
-
-load_from = None
-resume = False
-
-# Evaluation
-val_evaluator = dict(type='HmeanIOUMetric')
-test_evaluator = val_evaluator
-
-# Visualization
-vis_backends = [dict(type='LocalVisBackend')]
-visualizer = dict(
- type='TextDetLocalVisualizer',
- name='visualizer',
- vis_backends=vis_backends)
diff --git a/pipeline/mmocr/mmocr/.mim/configs/textdet/_base_/pretrain_runtime.py b/pipeline/mmocr/mmocr/.mim/configs/textdet/_base_/pretrain_runtime.py
deleted file mode 100644
index cb2800d50a570881475035e3b0da9c81e88712d1..0000000000000000000000000000000000000000
--- a/pipeline/mmocr/mmocr/.mim/configs/textdet/_base_/pretrain_runtime.py
+++ /dev/null
@@ -1,14 +0,0 @@
-_base_ = 'default_runtime.py'
-
-default_hooks = dict(
- logger=dict(type='LoggerHook', interval=1000),
- checkpoint=dict(
- type='CheckpointHook',
- interval=10000,
- by_epoch=False,
- max_keep_ckpts=1),
-)
-
-# Evaluation
-val_evaluator = None
-test_evaluator = None
diff --git a/pipeline/mmocr/mmocr/.mim/configs/textdet/_base_/schedules/schedule_adam_600e.py b/pipeline/mmocr/mmocr/.mim/configs/textdet/_base_/schedules/schedule_adam_600e.py
deleted file mode 100644
index eb61f7b9ee1b2ab18c8f75f24e7a204a9f90ee54..0000000000000000000000000000000000000000
--- a/pipeline/mmocr/mmocr/.mim/configs/textdet/_base_/schedules/schedule_adam_600e.py
+++ /dev/null
@@ -1,9 +0,0 @@
-# optimizer
-optim_wrapper = dict(type='OptimWrapper', optimizer=dict(type='Adam', lr=1e-3))
-train_cfg = dict(type='EpochBasedTrainLoop', max_epochs=600, val_interval=20)
-val_cfg = dict(type='ValLoop')
-test_cfg = dict(type='TestLoop')
-# learning rate
-param_scheduler = [
- dict(type='PolyLR', power=0.9, end=600),
-]
diff --git a/pipeline/mmocr/mmocr/.mim/configs/textdet/_base_/schedules/schedule_sgd_100k.py b/pipeline/mmocr/mmocr/.mim/configs/textdet/_base_/schedules/schedule_sgd_100k.py
deleted file mode 100644
index f760774b7b2e21886fc3bbe0746fe3bf843d3471..0000000000000000000000000000000000000000
--- a/pipeline/mmocr/mmocr/.mim/configs/textdet/_base_/schedules/schedule_sgd_100k.py
+++ /dev/null
@@ -1,12 +0,0 @@
-# optimizer
-optim_wrapper = dict(
- type='OptimWrapper',
- optimizer=dict(type='SGD', lr=0.007, momentum=0.9, weight_decay=0.0001))
-
-train_cfg = dict(type='IterBasedTrainLoop', max_iters=100000)
-test_cfg = None
-val_cfg = None
-# learning policy
-param_scheduler = [
- dict(type='PolyLR', power=0.9, eta_min=1e-7, by_epoch=False, end=100000),
-]
diff --git a/pipeline/mmocr/mmocr/.mim/configs/textdet/_base_/schedules/schedule_sgd_1200e.py b/pipeline/mmocr/mmocr/.mim/configs/textdet/_base_/schedules/schedule_sgd_1200e.py
deleted file mode 100644
index f8555e468bccaa6e5dbca23c9d2821164e21e516..0000000000000000000000000000000000000000
--- a/pipeline/mmocr/mmocr/.mim/configs/textdet/_base_/schedules/schedule_sgd_1200e.py
+++ /dev/null
@@ -1,11 +0,0 @@
-# optimizer
-optim_wrapper = dict(
- type='OptimWrapper',
- optimizer=dict(type='SGD', lr=0.007, momentum=0.9, weight_decay=0.0001))
-train_cfg = dict(type='EpochBasedTrainLoop', max_epochs=1200, val_interval=20)
-val_cfg = dict(type='ValLoop')
-test_cfg = dict(type='TestLoop')
-# learning policy
-param_scheduler = [
- dict(type='PolyLR', power=0.9, eta_min=1e-7, end=1200),
-]
diff --git a/pipeline/mmocr/mmocr/.mim/configs/textdet/_base_/schedules/schedule_sgd_base.py b/pipeline/mmocr/mmocr/.mim/configs/textdet/_base_/schedules/schedule_sgd_base.py
deleted file mode 100644
index baf559de231db06382529079be7d5bba071b209e..0000000000000000000000000000000000000000
--- a/pipeline/mmocr/mmocr/.mim/configs/textdet/_base_/schedules/schedule_sgd_base.py
+++ /dev/null
@@ -1,15 +0,0 @@
-# Note: This schedule config serves as a base config for other schedules.
-# Users would have to at least fill in "max_epochs" and "val_interval"
-# in order to use this config in their experiments.
-
-# optimizer
-optim_wrapper = dict(
- type='OptimWrapper',
- optimizer=dict(type='SGD', lr=0.007, momentum=0.9, weight_decay=0.0001))
-train_cfg = dict(type='EpochBasedTrainLoop', max_epochs=None, val_interval=20)
-val_cfg = dict(type='ValLoop')
-test_cfg = dict(type='TestLoop')
-# learning policy
-param_scheduler = [
- dict(type='ConstantLR', factor=1.0),
-]
diff --git a/pipeline/mmocr/mmocr/.mim/configs/textdet/dbnet/README.md b/pipeline/mmocr/mmocr/.mim/configs/textdet/dbnet/README.md
deleted file mode 100644
index 07c91edbaf8c8bbe96ae59fc8d17725314da47c8..0000000000000000000000000000000000000000
--- a/pipeline/mmocr/mmocr/.mim/configs/textdet/dbnet/README.md
+++ /dev/null
@@ -1,47 +0,0 @@
-# DBNet
-
-> [Real-time Scene Text Detection with Differentiable Binarization](https://arxiv.org/abs/1911.08947)
-
-
-
-## Abstract
-
-Recently, segmentation-based methods are quite popular in scene text detection, as the segmentation results can more accurately describe scene text of various shapes such as curve text. However, the post-processing of binarization is essential for segmentation-based detection, which converts probability maps produced by a segmentation method into bounding boxes/regions of text. In this paper, we propose a module named Differentiable Binarization (DB), which can perform the binarization process in a segmentation network. Optimized along with a DB module, a segmentation network can adaptively set the thresholds for binarization, which not only simplifies the post-processing but also enhances the performance of text detection. Based on a simple segmentation network, we validate the performance improvements of DB on five benchmark datasets, which consistently achieves state-of-the-art results, in terms of both detection accuracy and speed. In particular, with a light-weight backbone, the performance improvements by DB are significant so that we can look for an ideal tradeoff between detection accuracy and efficiency. Specifically, with a backbone of ResNet-18, our detector achieves an F-measure of 82.8, running at 62 FPS, on the MSRA-TD500 dataset.
-
-
-
-
-
-## Results and models
-
-### SynthText
-
-| Method | Backbone | Training set | #iters | Download |
-| :-----------------------------------------------------------------------: | :------: | :----------: | :-----: | :--------------------------------------------------------------------------------------------------: |
-| [DBNet_r18](/configs/textdet/dbnet/dbnet_resnet18_fpnc_100k_synthtext.py) | ResNet18 | SynthText | 100,000 | [model](https://download.openmmlab.com/mmocr/textdet/dbnet/dbnet_resnet18_fpnc_100k_synthtext/dbnet_resnet18_fpnc_100k_synthtext-2e9bf392.pth) \| [log](https://download.openmmlab.com/mmocr/textdet/dbnet/dbnet_resnet18_fpnc_100k_synthtext/20221214_150351.log) |
-
-### ICDAR2015
-
-| Method | Backbone | Pretrained Model | Training set | Test set | #epochs | Test size | Precision | Recall | Hmean | Download |
-| :----------------------------: | :------------------------------: | :--------------------------------------: | :-------------: | :------------: | :-----: | :-------: | :-------: | :----: | :----: | :------------------------------: |
-| [DBNet_r18](/configs/textdet/dbnet/dbnet_resnet18_fpnc_1200e_icdar2015.py) | ResNet18 | - | ICDAR2015 Train | ICDAR2015 Test | 1200 | 736 | 0.8853 | 0.7583 | 0.8169 | [model](https://download.openmmlab.com/mmocr/textdet/dbnet/dbnet_resnet18_fpnc_1200e_icdar2015/dbnet_resnet18_fpnc_1200e_icdar2015_20220825_221614-7c0e94f2.pth) \| [log](https://download.openmmlab.com/mmocr/textdet/dbnet/dbnet_resnet18_fpnc_1200e_icdar2015/20220825_221614.log) |
-| [DBNet_r50](/configs/textdet/dbnet/dbnet_resnet50_1200e_icdar2015.py) | ResNet50 | - | ICDAR2015 Train | ICDAR2015 Test | 1200 | 1024 | 0.8744 | 0.8276 | 0.8504 | [model](https://download.openmmlab.com/mmocr/textdet/dbnet/dbnet_resnet50_1200e_icdar2015/dbnet_resnet50_1200e_icdar2015_20221102_115917-54f50589.pth) \| [log](https://download.openmmlab.com/mmocr/textdet/dbnet/dbnet_resnet50_1200e_icdar2015/20221102_115917.log) |
-| [DBNet_r50dcn](/configs/textdet/dbnet/dbnet_resnet50-dcnv2_fpnc_1200e_icdar2015.py) | ResNet50-DCN | [Synthtext](https://download.openmmlab.com/mmocr/textdet/dbnet/tmp_1.0_pretrain/dbnet_r50dcnv2_fpnc_sbn_2e_synthtext_20210325-ed322016.pth) | ICDAR2015 Train | ICDAR2015 Test | 1200 | 1024 | 0.8784 | 0.8315 | 0.8543 | [model](https://download.openmmlab.com/mmocr/textdet/dbnet/dbnet_resnet50-dcnv2_fpnc_1200e_icdar2015/dbnet_resnet50-dcnv2_fpnc_1200e_icdar2015_20220828_124917-452c443c.pth) \| [log](https://download.openmmlab.com/mmocr/textdet/dbnet/dbnet_resnet50-dcnv2_fpnc_1200e_icdar2015/20220828_124917.log) |
-| [DBNet_r50-oclip](/configs/textdet/dbnet/dbnet_resnet50-oclip_1200e_icdar2015.py) | [ResNet50-oCLIP](https://download.openmmlab.com/mmocr/backbone/resnet50-oclip-7ba0c533.pth) | - | ICDAR2015 Train | ICDAR2015 Test | 1200 | 1024 | 0.9052 | 0.8272 | 0.8644 | [model](https://download.openmmlab.com/mmocr/textdet/dbnet/dbnet_resnet50-oclip_1200e_icdar2015/dbnet_resnet50-oclip_1200e_icdar2015_20221102_115917-bde8c87a.pth) \| [log](https://download.openmmlab.com/mmocr/textdet/dbnet/dbnet_resnet50-oclip_1200e_icdar2015/20221102_115917.log) |
-
-### Total Text
-
-| Method | Backbone | Pretrained Model | Training set | Test set | #epochs | Test size | Precision | Recall | Hmean | Download |
-| :----------------------------------------------------: | :------: | :--------------: | :-------------: | :------------: | :-----: | :-------: | :-------: | :----: | :----: | :------------------------------------------------------: |
-| [DBNet_r18](/configs/textdet/dbnet/dbnet_resnet18_fpnc_1200e_totaltext.py) | ResNet18 | - | Totaltext Train | Totaltext Test | 1200 | 736 | 0.8640 | 0.7770 | 0.8182 | [model](https://download.openmmlab.com/mmocr/textdet/dbnet/dbnet_resnet18_fpnc_1200e_totaltext/dbnet_resnet18_fpnc_1200e_totaltext-3ed3233c.pth) \| [log](https://download.openmmlab.com/mmocr/textdet/dbnet/dbnet_resnet18_fpnc_1200e_totaltext/20221219_201038.log) |
-
-## Citation
-
-```bibtex
-@article{Liao_Wan_Yao_Chen_Bai_2020,
- title={Real-Time Scene Text Detection with Differentiable Binarization},
- journal={Proceedings of the AAAI Conference on Artificial Intelligence},
- author={Liao, Minghui and Wan, Zhaoyi and Yao, Cong and Chen, Kai and Bai, Xiang},
- year={2020},
- pages={11474-11481}}
-```
diff --git a/pipeline/mmocr/mmocr/.mim/configs/textdet/dbnet/_base_dbnet_resnet18_fpnc.py b/pipeline/mmocr/mmocr/.mim/configs/textdet/dbnet/_base_dbnet_resnet18_fpnc.py
deleted file mode 100644
index 44907100b05b2544e27ce476a6368feef1a178da..0000000000000000000000000000000000000000
--- a/pipeline/mmocr/mmocr/.mim/configs/textdet/dbnet/_base_dbnet_resnet18_fpnc.py
+++ /dev/null
@@ -1,64 +0,0 @@
-model = dict(
- type='DBNet',
- backbone=dict(
- type='mmdet.ResNet',
- depth=18,
- num_stages=4,
- out_indices=(0, 1, 2, 3),
- frozen_stages=-1,
- norm_cfg=dict(type='BN', requires_grad=True),
- init_cfg=dict(type='Pretrained', checkpoint='torchvision://resnet18'),
- norm_eval=False,
- style='caffe'),
- neck=dict(
- type='FPNC', in_channels=[64, 128, 256, 512], lateral_channels=256),
- det_head=dict(
- type='DBHead',
- in_channels=256,
- module_loss=dict(type='DBModuleLoss'),
- postprocessor=dict(type='DBPostprocessor', text_repr_type='quad')),
- data_preprocessor=dict(
- type='TextDetDataPreprocessor',
- mean=[123.675, 116.28, 103.53],
- std=[58.395, 57.12, 57.375],
- bgr_to_rgb=True,
- pad_size_divisor=32))
-
-train_pipeline = [
- dict(type='LoadImageFromFile', color_type='color_ignore_orientation'),
- dict(
- type='LoadOCRAnnotations',
- with_polygon=True,
- with_bbox=True,
- with_label=True,
- ),
- dict(
- type='TorchVisionWrapper',
- op='ColorJitter',
- brightness=32.0 / 255,
- saturation=0.5),
- dict(
- type='ImgAugWrapper',
- args=[['Fliplr', 0.5],
- dict(cls='Affine', rotate=[-10, 10]), ['Resize', [0.5, 3.0]]]),
- dict(type='RandomCrop', min_side_ratio=0.1),
- dict(type='Resize', scale=(640, 640), keep_ratio=True),
- dict(type='Pad', size=(640, 640)),
- dict(
- type='PackTextDetInputs',
- meta_keys=('img_path', 'ori_shape', 'img_shape'))
-]
-
-test_pipeline = [
- dict(type='LoadImageFromFile', color_type='color_ignore_orientation'),
- dict(type='Resize', scale=(1333, 736), keep_ratio=True),
- dict(
- type='LoadOCRAnnotations',
- with_polygon=True,
- with_bbox=True,
- with_label=True,
- ),
- dict(
- type='PackTextDetInputs',
- meta_keys=('img_path', 'ori_shape', 'img_shape', 'scale_factor'))
-]
diff --git a/pipeline/mmocr/mmocr/.mim/configs/textdet/dbnet/_base_dbnet_resnet50-dcnv2_fpnc.py b/pipeline/mmocr/mmocr/.mim/configs/textdet/dbnet/_base_dbnet_resnet50-dcnv2_fpnc.py
deleted file mode 100644
index 952f079d478586516c28ddafea63ebc45ab7aa80..0000000000000000000000000000000000000000
--- a/pipeline/mmocr/mmocr/.mim/configs/textdet/dbnet/_base_dbnet_resnet50-dcnv2_fpnc.py
+++ /dev/null
@@ -1,66 +0,0 @@
-model = dict(
- type='DBNet',
- backbone=dict(
- type='mmdet.ResNet',
- depth=50,
- num_stages=4,
- out_indices=(0, 1, 2, 3),
- frozen_stages=-1,
- norm_cfg=dict(type='BN', requires_grad=True),
- norm_eval=False,
- style='pytorch',
- dcn=dict(type='DCNv2', deform_groups=1, fallback_on_stride=False),
- init_cfg=dict(type='Pretrained', checkpoint='torchvision://resnet50'),
- stage_with_dcn=(False, True, True, True)),
- neck=dict(
- type='FPNC', in_channels=[256, 512, 1024, 2048], lateral_channels=256),
- det_head=dict(
- type='DBHead',
- in_channels=256,
- module_loss=dict(type='DBModuleLoss'),
- postprocessor=dict(type='DBPostprocessor', text_repr_type='quad')),
- data_preprocessor=dict(
- type='TextDetDataPreprocessor',
- mean=[123.675, 116.28, 103.53],
- std=[58.395, 57.12, 57.375],
- bgr_to_rgb=True,
- pad_size_divisor=32))
-
-train_pipeline = [
- dict(type='LoadImageFromFile', color_type='color_ignore_orientation'),
- dict(
- type='LoadOCRAnnotations',
- with_bbox=True,
- with_polygon=True,
- with_label=True,
- ),
- dict(
- type='TorchVisionWrapper',
- op='ColorJitter',
- brightness=32.0 / 255,
- saturation=0.5),
- dict(
- type='ImgAugWrapper',
- args=[['Fliplr', 0.5],
- dict(cls='Affine', rotate=[-10, 10]), ['Resize', [0.5, 3.0]]]),
- dict(type='RandomCrop', min_side_ratio=0.1),
- dict(type='Resize', scale=(640, 640), keep_ratio=True),
- dict(type='Pad', size=(640, 640)),
- dict(
- type='PackTextDetInputs',
- meta_keys=('img_path', 'ori_shape', 'img_shape'))
-]
-
-test_pipeline = [
- dict(type='LoadImageFromFile', color_type='color_ignore_orientation'),
- dict(type='Resize', scale=(4068, 1024), keep_ratio=True),
- dict(
- type='LoadOCRAnnotations',
- with_polygon=True,
- with_bbox=True,
- with_label=True,
- ),
- dict(
- type='PackTextDetInputs',
- meta_keys=('img_path', 'ori_shape', 'img_shape', 'scale_factor'))
-]
diff --git a/pipeline/mmocr/mmocr/.mim/configs/textdet/dbnet/dbnet_resnet18_fpnc_100k_synthtext.py b/pipeline/mmocr/mmocr/.mim/configs/textdet/dbnet/dbnet_resnet18_fpnc_100k_synthtext.py
deleted file mode 100644
index 839146dd380a5b6f2a24280bdab123662b0d8476..0000000000000000000000000000000000000000
--- a/pipeline/mmocr/mmocr/.mim/configs/textdet/dbnet/dbnet_resnet18_fpnc_100k_synthtext.py
+++ /dev/null
@@ -1,45 +0,0 @@
-_base_ = [
- '_base_dbnet_resnet18_fpnc.py',
- '../_base_/datasets/synthtext.py',
- '../_base_/pretrain_runtime.py',
- '../_base_/schedules/schedule_sgd_100k.py',
-]
-
-train_pipeline = [
- dict(type='LoadImageFromFile', color_type='color_ignore_orientation'),
- dict(
- type='LoadOCRAnnotations',
- with_polygon=True,
- with_bbox=True,
- with_label=True,
- ),
- dict(type='FixInvalidPolygon'),
- dict(
- type='TorchVisionWrapper',
- op='ColorJitter',
- brightness=32.0 / 255,
- saturation=0.5),
- dict(
- type='ImgAugWrapper',
- args=[['Fliplr', 0.5],
- dict(cls='Affine', rotate=[-10, 10]), ['Resize', [0.5, 3.0]]]),
- dict(type='RandomCrop', min_side_ratio=0.1),
- dict(type='Resize', scale=(640, 640), keep_ratio=True),
- dict(type='Pad', size=(640, 640)),
- dict(
- type='PackTextDetInputs',
- meta_keys=('img_path', 'ori_shape', 'img_shape'))
-]
-
-# dataset settings
-synthtext_textdet_train = _base_.synthtext_textdet_train
-synthtext_textdet_train.pipeline = train_pipeline
-
-train_dataloader = dict(
- batch_size=16,
- num_workers=8,
- persistent_workers=True,
- sampler=dict(type='DefaultSampler', shuffle=True),
- dataset=synthtext_textdet_train)
-
-auto_scale_lr = dict(base_batch_size=16)
diff --git a/pipeline/mmocr/mmocr/.mim/configs/textdet/dbnet/dbnet_resnet18_fpnc_1200e_icdar2015.py b/pipeline/mmocr/mmocr/.mim/configs/textdet/dbnet/dbnet_resnet18_fpnc_1200e_icdar2015.py
deleted file mode 100644
index feea2004b158fa3787b9a9f9d1c2b32e1bb8ae1d..0000000000000000000000000000000000000000
--- a/pipeline/mmocr/mmocr/.mim/configs/textdet/dbnet/dbnet_resnet18_fpnc_1200e_icdar2015.py
+++ /dev/null
@@ -1,30 +0,0 @@
-_base_ = [
- '_base_dbnet_resnet18_fpnc.py',
- '../_base_/datasets/icdar2015.py',
- '../_base_/default_runtime.py',
- '../_base_/schedules/schedule_sgd_1200e.py',
-]
-
-# dataset settings
-icdar2015_textdet_train = _base_.icdar2015_textdet_train
-icdar2015_textdet_train.pipeline = _base_.train_pipeline
-icdar2015_textdet_test = _base_.icdar2015_textdet_test
-icdar2015_textdet_test.pipeline = _base_.test_pipeline
-
-train_dataloader = dict(
- batch_size=16,
- num_workers=8,
- persistent_workers=True,
- sampler=dict(type='DefaultSampler', shuffle=True),
- dataset=icdar2015_textdet_train)
-
-val_dataloader = dict(
- batch_size=1,
- num_workers=4,
- persistent_workers=True,
- sampler=dict(type='DefaultSampler', shuffle=False),
- dataset=icdar2015_textdet_test)
-
-test_dataloader = val_dataloader
-
-auto_scale_lr = dict(base_batch_size=16)
diff --git a/pipeline/mmocr/mmocr/.mim/configs/textdet/dbnet/dbnet_resnet18_fpnc_1200e_totaltext.py b/pipeline/mmocr/mmocr/.mim/configs/textdet/dbnet/dbnet_resnet18_fpnc_1200e_totaltext.py
deleted file mode 100644
index 9728db946b0419ae1825a986c9918c7e0f70bb55..0000000000000000000000000000000000000000
--- a/pipeline/mmocr/mmocr/.mim/configs/textdet/dbnet/dbnet_resnet18_fpnc_1200e_totaltext.py
+++ /dev/null
@@ -1,73 +0,0 @@
-_base_ = [
- '_base_dbnet_resnet18_fpnc.py',
- '../_base_/datasets/totaltext.py',
- '../_base_/default_runtime.py',
- '../_base_/schedules/schedule_sgd_1200e.py',
-]
-
-train_pipeline = [
- dict(type='LoadImageFromFile', color_type='color_ignore_orientation'),
- dict(
- type='LoadOCRAnnotations',
- with_polygon=True,
- with_bbox=True,
- with_label=True,
- ),
- dict(type='FixInvalidPolygon', min_poly_points=4),
- dict(
- type='TorchVisionWrapper',
- op='ColorJitter',
- brightness=32.0 / 255,
- saturation=0.5),
- dict(
- type='ImgAugWrapper',
- args=[['Fliplr', 0.5],
- dict(cls='Affine', rotate=[-10, 10]), ['Resize', [0.5, 3.0]]]),
- dict(type='RandomCrop', min_side_ratio=0.1),
- dict(type='Resize', scale=(640, 640), keep_ratio=True),
- dict(type='Pad', size=(640, 640)),
- dict(
- type='PackTextDetInputs',
- meta_keys=('img_path', 'ori_shape', 'img_shape'))
-]
-
-test_pipeline = [
- dict(type='LoadImageFromFile', color_type='color_ignore_orientation'),
- dict(type='Resize', scale=(1333, 736), keep_ratio=True),
- dict(
- type='LoadOCRAnnotations',
- with_polygon=True,
- with_bbox=True,
- with_label=True,
- ),
- dict(type='FixInvalidPolygon', min_poly_points=4),
- dict(
- type='PackTextDetInputs',
- meta_keys=('img_path', 'ori_shape', 'img_shape', 'scale_factor'))
-]
-
-# dataset settings
-totaltext_textdet_train = _base_.totaltext_textdet_train
-totaltext_textdet_test = _base_.totaltext_textdet_test
-totaltext_textdet_train.pipeline = train_pipeline
-totaltext_textdet_test.pipeline = test_pipeline
-
-train_dataloader = dict(
- batch_size=16,
- num_workers=16,
- pin_memory=True,
- persistent_workers=True,
- sampler=dict(type='DefaultSampler', shuffle=True),
- dataset=totaltext_textdet_train)
-
-val_dataloader = dict(
- batch_size=1,
- num_workers=1,
- pin_memory=True,
- persistent_workers=True,
- sampler=dict(type='DefaultSampler', shuffle=False),
- dataset=totaltext_textdet_test)
-
-test_dataloader = val_dataloader
-
-auto_scale_lr = dict(base_batch_size=16)
diff --git a/pipeline/mmocr/mmocr/.mim/configs/textdet/dbnet/dbnet_resnet50-dcnv2_fpnc_100k_synthtext.py b/pipeline/mmocr/mmocr/.mim/configs/textdet/dbnet/dbnet_resnet50-dcnv2_fpnc_100k_synthtext.py
deleted file mode 100644
index 567e5984e54e9747f044715078d2a6f69bcfc792..0000000000000000000000000000000000000000
--- a/pipeline/mmocr/mmocr/.mim/configs/textdet/dbnet/dbnet_resnet50-dcnv2_fpnc_100k_synthtext.py
+++ /dev/null
@@ -1,30 +0,0 @@
-_base_ = [
- '_base_dbnet_resnet50-dcnv2_fpnc.py',
- '../_base_/default_runtime.py',
- '../_base_/datasets/synthtext.py',
- '../_base_/schedules/schedule_sgd_100k.py',
-]
-
-# dataset settings
-synthtext_textdet_train = _base_.synthtext_textdet_train
-synthtext_textdet_train.pipeline = _base_.train_pipeline
-synthtext_textdet_test = _base_.synthtext_textdet_test
-synthtext_textdet_test.pipeline = _base_.test_pipeline
-
-train_dataloader = dict(
- batch_size=16,
- num_workers=8,
- persistent_workers=True,
- sampler=dict(type='DefaultSampler', shuffle=True),
- dataset=synthtext_textdet_train)
-
-val_dataloader = dict(
- batch_size=1,
- num_workers=4,
- persistent_workers=True,
- sampler=dict(type='DefaultSampler', shuffle=False),
- dataset=synthtext_textdet_test)
-
-test_dataloader = val_dataloader
-
-auto_scale_lr = dict(base_batch_size=16)
diff --git a/pipeline/mmocr/mmocr/.mim/configs/textdet/dbnet/dbnet_resnet50-dcnv2_fpnc_1200e_icdar2015.py b/pipeline/mmocr/mmocr/.mim/configs/textdet/dbnet/dbnet_resnet50-dcnv2_fpnc_1200e_icdar2015.py
deleted file mode 100644
index f961a2e70c9a17d0bfbfbc5963bd8a0da79427b1..0000000000000000000000000000000000000000
--- a/pipeline/mmocr/mmocr/.mim/configs/textdet/dbnet/dbnet_resnet50-dcnv2_fpnc_1200e_icdar2015.py
+++ /dev/null
@@ -1,33 +0,0 @@
-_base_ = [
- '_base_dbnet_resnet50-dcnv2_fpnc.py',
- '../_base_/datasets/icdar2015.py',
- '../_base_/default_runtime.py',
- '../_base_/schedules/schedule_sgd_1200e.py',
-]
-
-# TODO: Replace the link
-load_from = 'https://download.openmmlab.com/mmocr/textdet/dbnet/tmp_1.0_pretrain/dbnet_r50dcnv2_fpnc_sbn_2e_synthtext_20210325-ed322016.pth' # noqa
-
-# dataset settings
-icdar2015_textdet_train = _base_.icdar2015_textdet_train
-icdar2015_textdet_train.pipeline = _base_.train_pipeline
-icdar2015_textdet_test = _base_.icdar2015_textdet_test
-icdar2015_textdet_test.pipeline = _base_.test_pipeline
-
-train_dataloader = dict(
- batch_size=16,
- num_workers=8,
- persistent_workers=True,
- sampler=dict(type='DefaultSampler', shuffle=True),
- dataset=icdar2015_textdet_train)
-
-val_dataloader = dict(
- batch_size=1,
- num_workers=4,
- persistent_workers=True,
- sampler=dict(type='DefaultSampler', shuffle=False),
- dataset=icdar2015_textdet_test)
-
-test_dataloader = val_dataloader
-
-auto_scale_lr = dict(base_batch_size=16)
diff --git a/pipeline/mmocr/mmocr/.mim/configs/textdet/dbnet/dbnet_resnet50-oclip_1200e_icdar2015.py b/pipeline/mmocr/mmocr/.mim/configs/textdet/dbnet/dbnet_resnet50-oclip_1200e_icdar2015.py
deleted file mode 100644
index 1c67883c63b601990bea7292d4fe22819b31e91e..0000000000000000000000000000000000000000
--- a/pipeline/mmocr/mmocr/.mim/configs/textdet/dbnet/dbnet_resnet50-oclip_1200e_icdar2015.py
+++ /dev/null
@@ -1,20 +0,0 @@
-_base_ = [
- 'dbnet_resnet50-dcnv2_fpnc_1200e_icdar2015.py',
-]
-
-load_from = None
-
-_base_.model.backbone = dict(
- type='CLIPResNet',
- init_cfg=dict(
- type='Pretrained',
- checkpoint='https://download.openmmlab.com/'
- 'mmocr/backbone/resnet50-oclip-7ba0c533.pth'))
-
-_base_.train_dataloader.num_workers = 24
-_base_.optim_wrapper.optimizer.lr = 0.002
-
-param_scheduler = [
- dict(type='LinearLR', end=100, start_factor=0.001),
- dict(type='PolyLR', power=0.9, eta_min=1e-7, begin=100, end=1200),
-]
diff --git a/pipeline/mmocr/mmocr/.mim/configs/textdet/dbnet/dbnet_resnet50_1200e_icdar2015.py b/pipeline/mmocr/mmocr/.mim/configs/textdet/dbnet/dbnet_resnet50_1200e_icdar2015.py
deleted file mode 100644
index 38a876b2583e50c5b99d271383492e7f05d429a7..0000000000000000000000000000000000000000
--- a/pipeline/mmocr/mmocr/.mim/configs/textdet/dbnet/dbnet_resnet50_1200e_icdar2015.py
+++ /dev/null
@@ -1,24 +0,0 @@
-_base_ = [
- 'dbnet_resnet50-dcnv2_fpnc_1200e_icdar2015.py',
-]
-
-load_from = None
-
-_base_.model.backbone = dict(
- type='mmdet.ResNet',
- depth=50,
- num_stages=4,
- out_indices=(0, 1, 2, 3),
- frozen_stages=-1,
- norm_cfg=dict(type='BN', requires_grad=True),
- norm_eval=True,
- style='pytorch',
- init_cfg=dict(type='Pretrained', checkpoint='torchvision://resnet50'))
-
-_base_.train_dataloader.num_workers = 24
-_base_.optim_wrapper.optimizer.lr = 0.002
-
-param_scheduler = [
- dict(type='LinearLR', end=100, start_factor=0.001),
- dict(type='PolyLR', power=0.9, eta_min=1e-7, begin=100, end=1200),
-]
diff --git a/pipeline/mmocr/mmocr/.mim/configs/textdet/dbnet/metafile.yml b/pipeline/mmocr/mmocr/.mim/configs/textdet/dbnet/metafile.yml
deleted file mode 100644
index bdcb89faeeadc944a1466f1a4cfa46f7d910330c..0000000000000000000000000000000000000000
--- a/pipeline/mmocr/mmocr/.mim/configs/textdet/dbnet/metafile.yml
+++ /dev/null
@@ -1,80 +0,0 @@
-Collections:
-- Name: DBNet
- Metadata:
- Training Data: ICDAR2015
- Training Techniques:
- - SGD with Momentum
- - Weight Decay
- Training Resources: 1x NVIDIA A100-SXM4-80GB
- Architecture:
- - ResNet
- - FPNC
- Paper:
- URL: https://arxiv.org/pdf/1911.08947.pdf
- Title: 'Real-time Scene Text Detection with Differentiable Binarization'
- README: configs/textdet/dbnet/README.md
-
-Models:
- - Name: dbnet_resnet18_fpnc_1200e_icdar2015
- Alias: DB_r18
- In Collection: DBNet
- Config: configs/textdet/dbnet/dbnet_resnet18_fpnc_1200e_icdar2015.py
- Metadata:
- Training Data: ICDAR2015
- Results:
- - Task: Text Detection
- Dataset: ICDAR2015
- Metrics:
- hmean-iou: 0.8169
- Weights: https://download.openmmlab.com/mmocr/textdet/dbnet/dbnet_resnet18_fpnc_1200e_icdar2015/dbnet_resnet18_fpnc_1200e_icdar2015_20220825_221614-7c0e94f2.pth
-
- - Name: dbnet_resnet50_fpnc_1200e_icdar2015
- In Collection: DBNet
- Config: configs/textdet/dbnet/dbnet_resnet50_fpnc_1200e_icdar2015.py
- Metadata:
- Training Data: ICDAR2015
- Results:
- - Task: Text Detection
- Dataset: ICDAR2015
- Metrics:
- hmean-iou: 0.8504
- Weights: https://download.openmmlab.com/mmocr/textdet/dbnet/dbnet_resnet50_1200e_icdar2015/dbnet_resnet50_1200e_icdar2015_20221102_115917-54f50589.pth
-
- - Name: dbnet_resnet50-dcnv2_fpnc_1200e_icdar2015
- In Collection: DBNet
- Config: configs/textdet/dbnet/dbnet_resnet50-dcnv2_fpnc_1200e_icdar2015.py
- Metadata:
- Training Data: ICDAR2015
- Results:
- - Task: Text Detection
- Dataset: ICDAR2015
- Metrics:
- hmean-iou: 0.8543
- Weights: https://download.openmmlab.com/mmocr/textdet/dbnet/dbnet_resnet50-dcnv2_fpnc_1200e_icdar2015/dbnet_resnet50-dcnv2_fpnc_1200e_icdar2015_20220828_124917-452c443c.pth
-
- - Name: dbnet_resnet50-oclip_fpnc_1200e_icdar2015
- In Collection: DBNet
- Alias:
- - DB_r50
- - DBNet
- Config: configs/textdet/dbnet/dbnet_resnet50-oclip_1200e_icdar2015.py
- Metadata:
- Training Data: ICDAR2015
- Results:
- - Task: Text Detection
- Dataset: ICDAR2015
- Metrics:
- hmean-iou: 0.8644
- Weights: https://download.openmmlab.com/mmocr/textdet/dbnet/dbnet_resnet50-oclip_1200e_icdar2015/dbnet_resnet50-oclip_1200e_icdar2015_20221102_115917-bde8c87a.pth
-
- - Name: dbnet_resnet18_fpnc_1200e_totaltext
- In Collection: DBNet
- Config: configs/textdet/dbnet/dbnet_resnet18_fpnc_1200e_totaltext.py
- Metadata:
- Training Data: Totaltext
- Results:
- - Task: Text Detection
- Dataset: Totaltext
- Metrics:
- hmean-iou: 0.8182
- Weights: https://download.openmmlab.com/mmocr/textdet/dbnet/dbnet_resnet18_fpnc_1200e_totaltext/dbnet_resnet18_fpnc_1200e_totaltext-3ed3233c.pth
diff --git a/pipeline/mmocr/mmocr/.mim/configs/textdet/dbnetpp/README.md b/pipeline/mmocr/mmocr/.mim/configs/textdet/dbnetpp/README.md
deleted file mode 100644
index 7f9b668a45de9314de24b790316ece859aea9e11..0000000000000000000000000000000000000000
--- a/pipeline/mmocr/mmocr/.mim/configs/textdet/dbnetpp/README.md
+++ /dev/null
@@ -1,41 +0,0 @@
-# DBNetpp
-
-> [Real-Time Scene Text Detection with Differentiable Binarization and Adaptive Scale Fusion](https://arxiv.org/abs/2202.10304)
-
-
-
-## Abstract
-
-Recently, segmentation-based scene text detection methods have drawn extensive attention in the scene text detection field, because of their superiority in detecting the text instances of arbitrary shapes and extreme aspect ratios, profiting from the pixel-level descriptions. However, the vast majority of the existing segmentation-based approaches are limited to their complex post-processing algorithms and the scale robustness of their segmentation models, where the post-processing algorithms are not only isolated to the model optimization but also time-consuming and the scale robustness is usually strengthened by fusing multi-scale feature maps directly. In this paper, we propose a Differentiable Binarization (DB) module that integrates the binarization process, one of the most important steps in the post-processing procedure, into a segmentation network. Optimized along with the proposed DB module, the segmentation network can produce more accurate results, which enhances the accuracy of text detection with a simple pipeline. Furthermore, an efficient Adaptive Scale Fusion (ASF) module is proposed to improve the scale robustness by fusing features of different scales adaptively. By incorporating the proposed DB and ASF with the segmentation network, our proposed scene text detector consistently achieves state-of-the-art results, in terms of both detection accuracy and speed, on five standard benchmarks.
-
-
-
-
-
-## Results and models
-
-### SynthText
-
-| Method | BackBone | Training set | #iters | Download |
-| :--------------------------------------------------------------------------------: | :------------: | :----------: | :-----: | :-----------------------------------------------------------------------------------: |
-| [DBNetpp_r50dcn](/configs/textdet/dbnetpp/dbnetpp_resnet50-dcnv2_fpnc_100k_synthtext.py) | ResNet50-dcnv2 | SynthText | 100,000 | [model](https://download.openmmlab.com/mmocr/textdet/dbnetpp/dbnetpp_resnet50-dcnv2_fpnc_100k_synthtext/dbnetpp_resnet50-dcnv2_fpnc_100k_synthtext-00f0a80b.pth) \| [log](https://download.openmmlab.com/mmocr/textdet/dbnetpp/dbnetpp_resnet50-dcnv2_fpnc_100k_synthtext/20221215_013531.log) |
-
-### ICDAR2015
-
-| Method | BackBone | Pretrained Model | Training set | Test set | #epochs | Test size | Precision | Recall | Hmean | Download |
-| :----------------------------: | :------------------------------: | :--------------------------------------: | :-------------: | :------------: | :-----: | :-------: | :-------: | :----: | :----: | :------------------------------: |
-| [DBNetpp_r50](/configs/textdet/dbnetpp/dbnetpp_resnet50_fpnc_1200e_icdar2015.py) | ResNet50 | - | ICDAR2015 Train | ICDAR2015 Test | 1200 | 1024 | 0.9079 | 0.8209 | 0.8622 | [model](https://download.openmmlab.com/mmocr/textdet/dbnetpp/dbnetpp_resnet50_fpnc_1200e_icdar2015/dbnetpp_resnet50_fpnc_1200e_icdar2015_20221025_185550-013730aa.pth) \| [log](https://download.openmmlab.com/mmocr/textdet/dbnetpp/dbnetpp_resnet50_fpnc_1200e_icdar2015/20221025_185550.log) |
-| [DBNetpp_r50dcn](/configs/textdet/dbnetpp/dbnetpp_resnet50-dcnv2_fpnc_1200e_icdar2015.py) | ResNet50-dcnv2 | [Synthtext](/configs/textdet/dbnetpp/dbnetpp_resnet50-dcnv2_fpnc_100k_synthtext.py) ([model](https://download.openmmlab.com/mmocr/textdet/dbnetpp/tmp_1.0_pretrain/dbnetpp_r50dcnv2_fpnc_100k_iter_synthtext-20220502-352fec8a.pth)) | ICDAR2015 Train | ICDAR2015 Test | 1200 | 1024 | 0.9116 | 0.8291 | 0.8684 | [model](https://download.openmmlab.com/mmocr/textdet/dbnetpp/dbnetpp_resnet50-dcnv2_fpnc_1200e_icdar2015/dbnetpp_resnet50-dcnv2_fpnc_1200e_icdar2015_20220829_230108-f289bd20.pth) \| [log](https://download.openmmlab.com/mmocr/textdet/dbnetpp/dbnetpp_resnet50-dcnv2_fpnc_1200e_icdar2015/20220829_230108.log) |
-| [DBNetpp_r50-oclip](/configs/textdet/dbnetpp/dbnetpp_resnet50-oclip_fpnc_1200e_icdar2015.py) | [ResNet50-oCLIP](https://download.openmmlab.com/mmocr/backbone/resnet50-oclip-7ba0c533.pth) | - | ICDAR2015 Train | ICDAR2015 Test | 1200 | 1024 | 0.9174 | 0.8609 | 0.8882 | [model](https://download.openmmlab.com/mmocr/textdet/dbnetpp/dbnetpp_resnet50-oclip_fpnc_1200e_icdar2015/dbnetpp_resnet50-oclip_fpnc_1200e_icdar2015_20221101_124139-4ecb39ac.pth) \| [log](https://download.openmmlab.com/mmocr/textdet/dbnetpp/dbnetpp_resnet50-oclip_fpnc_1200e_icdar2015/20221101_124139.log) |
-
-## Citation
-
-```bibtex
-@article{liao2022real,
- title={Real-Time Scene Text Detection with Differentiable Binarization and Adaptive Scale Fusion},
- author={Liao, Minghui and Zou, Zhisheng and Wan, Zhaoyi and Yao, Cong and Bai, Xiang},
- journal={IEEE Transactions on Pattern Analysis and Machine Intelligence},
- year={2022},
- publisher={IEEE}
-}
-```
diff --git a/pipeline/mmocr/mmocr/.mim/configs/textdet/dbnetpp/_base_dbnetpp_resnet50-dcnv2_fpnc.py b/pipeline/mmocr/mmocr/.mim/configs/textdet/dbnetpp/_base_dbnetpp_resnet50-dcnv2_fpnc.py
deleted file mode 100644
index ec4d1bcc5624d32db8bcf7ba96015d4780118925..0000000000000000000000000000000000000000
--- a/pipeline/mmocr/mmocr/.mim/configs/textdet/dbnetpp/_base_dbnetpp_resnet50-dcnv2_fpnc.py
+++ /dev/null
@@ -1,72 +0,0 @@
-model = dict(
- type='DBNet',
- backbone=dict(
- type='mmdet.ResNet',
- depth=50,
- num_stages=4,
- out_indices=(0, 1, 2, 3),
- frozen_stages=-1,
- norm_cfg=dict(type='BN', requires_grad=True),
- norm_eval=False,
- style='pytorch',
- dcn=dict(type='DCNv2', deform_groups=1, fallback_on_stride=False),
- init_cfg=dict(type='Pretrained', checkpoint='torchvision://resnet50'),
- stage_with_dcn=(False, True, True, True)),
- neck=dict(
- type='FPNC',
- in_channels=[256, 512, 1024, 2048],
- lateral_channels=256,
- asf_cfg=dict(attention_type='ScaleChannelSpatial')),
- det_head=dict(
- type='DBHead',
- in_channels=256,
- module_loss=dict(type='DBModuleLoss'),
- postprocessor=dict(
- type='DBPostprocessor', text_repr_type='quad',
- epsilon_ratio=0.002)),
- data_preprocessor=dict(
- type='TextDetDataPreprocessor',
- mean=[123.675, 116.28, 103.53],
- std=[58.395, 57.12, 57.375],
- bgr_to_rgb=True,
- pad_size_divisor=32))
-
-train_pipeline = [
- dict(type='LoadImageFromFile', color_type='color_ignore_orientation'),
- dict(
- type='LoadOCRAnnotations',
- with_bbox=True,
- with_polygon=True,
- with_label=True,
- ),
- dict(
- type='TorchVisionWrapper',
- op='ColorJitter',
- brightness=32.0 / 255,
- saturation=0.5),
- dict(
- type='ImgAugWrapper',
- args=[['Fliplr', 0.5],
- dict(cls='Affine', rotate=[-10, 10]), ['Resize', [0.5, 3.0]]]),
- dict(type='RandomCrop', min_side_ratio=0.1),
- dict(type='Resize', scale=(640, 640), keep_ratio=True),
- dict(type='Pad', size=(640, 640)),
- dict(
- type='PackTextDetInputs',
- meta_keys=('img_path', 'ori_shape', 'img_shape'))
-]
-
-test_pipeline = [
- dict(type='LoadImageFromFile', color_type='color_ignore_orientation'),
- dict(type='Resize', scale=(4068, 1024), keep_ratio=True),
- dict(
- type='LoadOCRAnnotations',
- with_polygon=True,
- with_bbox=True,
- with_label=True,
- ),
- dict(
- type='PackTextDetInputs',
- meta_keys=('img_path', 'ori_shape', 'img_shape', 'scale_factor',
- 'instances'))
-]
diff --git a/pipeline/mmocr/mmocr/.mim/configs/textdet/dbnetpp/dbnetpp_resnet50-dcnv2_fpnc_100k_synthtext.py b/pipeline/mmocr/mmocr/.mim/configs/textdet/dbnetpp/dbnetpp_resnet50-dcnv2_fpnc_100k_synthtext.py
deleted file mode 100644
index 7174055dae61e8e4406e891359aa38957acf6a24..0000000000000000000000000000000000000000
--- a/pipeline/mmocr/mmocr/.mim/configs/textdet/dbnetpp/dbnetpp_resnet50-dcnv2_fpnc_100k_synthtext.py
+++ /dev/null
@@ -1,44 +0,0 @@
-_base_ = [
- '_base_dbnetpp_resnet50-dcnv2_fpnc.py',
- '../_base_/pretrain_runtime.py',
- '../_base_/datasets/synthtext.py',
- '../_base_/schedules/schedule_sgd_100k.py',
-]
-
-train_pipeline = [
- dict(type='LoadImageFromFile', color_type='color_ignore_orientation'),
- dict(
- type='LoadOCRAnnotations',
- with_bbox=True,
- with_polygon=True,
- with_label=True,
- ),
- dict(type='FixInvalidPolygon'),
- dict(
- type='TorchVisionWrapper',
- op='ColorJitter',
- brightness=32.0 / 255,
- saturation=0.5),
- dict(
- type='ImgAugWrapper',
- args=[['Fliplr', 0.5],
- dict(cls='Affine', rotate=[-10, 10]), ['Resize', [0.5, 3.0]]]),
- dict(type='RandomCrop', min_side_ratio=0.1),
- dict(type='Resize', scale=(640, 640), keep_ratio=True),
- dict(type='Pad', size=(640, 640)),
- dict(
- type='PackTextDetInputs',
- meta_keys=('img_path', 'ori_shape', 'img_shape'))
-]
-
-synthtext_textdet_train = _base_.synthtext_textdet_train
-synthtext_textdet_train.pipeline = train_pipeline
-
-train_dataloader = dict(
- batch_size=16,
- num_workers=8,
- persistent_workers=True,
- sampler=dict(type='DefaultSampler', shuffle=True),
- dataset=synthtext_textdet_train)
-
-auto_scale_lr = dict(base_batch_size=16)
diff --git a/pipeline/mmocr/mmocr/.mim/configs/textdet/dbnetpp/dbnetpp_resnet50-dcnv2_fpnc_1200e_icdar2015.py b/pipeline/mmocr/mmocr/.mim/configs/textdet/dbnetpp/dbnetpp_resnet50-dcnv2_fpnc_1200e_icdar2015.py
deleted file mode 100644
index c4682b440320db97af808704fb8c3606937ee235..0000000000000000000000000000000000000000
--- a/pipeline/mmocr/mmocr/.mim/configs/textdet/dbnetpp/dbnetpp_resnet50-dcnv2_fpnc_1200e_icdar2015.py
+++ /dev/null
@@ -1,36 +0,0 @@
-_base_ = [
- '_base_dbnetpp_resnet50-dcnv2_fpnc.py',
- '../_base_/default_runtime.py',
- '../_base_/datasets/icdar2015.py',
- '../_base_/schedules/schedule_sgd_1200e.py',
-]
-
-load_from = 'https://download.openmmlab.com/mmocr/textdet/dbnetpp/tmp_1.0_pretrain/dbnetpp_r50dcnv2_fpnc_100k_iter_synthtext-20220502-352fec8a.pth' # noqa
-
-# dataset settings
-train_list = [_base_.icdar2015_textdet_train]
-test_list = [_base_.icdar2015_textdet_test]
-
-train_dataloader = dict(
- batch_size=16,
- num_workers=8,
- persistent_workers=True,
- sampler=dict(type='DefaultSampler', shuffle=True),
- dataset=dict(
- type='ConcatDataset',
- datasets=train_list,
- pipeline=_base_.train_pipeline))
-
-val_dataloader = dict(
- batch_size=16,
- num_workers=8,
- persistent_workers=True,
- sampler=dict(type='DefaultSampler', shuffle=False),
- dataset=dict(
- type='ConcatDataset',
- datasets=test_list,
- pipeline=_base_.test_pipeline))
-
-test_dataloader = val_dataloader
-
-auto_scale_lr = dict(base_batch_size=16)
diff --git a/pipeline/mmocr/mmocr/.mim/configs/textdet/dbnetpp/dbnetpp_resnet50-oclip_fpnc_1200e_icdar2015.py b/pipeline/mmocr/mmocr/.mim/configs/textdet/dbnetpp/dbnetpp_resnet50-oclip_fpnc_1200e_icdar2015.py
deleted file mode 100644
index 737985241484fa1d2649d4da698a3bcf0e83321b..0000000000000000000000000000000000000000
--- a/pipeline/mmocr/mmocr/.mim/configs/textdet/dbnetpp/dbnetpp_resnet50-oclip_fpnc_1200e_icdar2015.py
+++ /dev/null
@@ -1,20 +0,0 @@
-_base_ = [
- 'dbnetpp_resnet50-dcnv2_fpnc_1200e_icdar2015.py',
-]
-
-load_from = None
-
-_base_.model.backbone = dict(
- type='CLIPResNet',
- init_cfg=dict(
- type='Pretrained',
- checkpoint='https://download.openmmlab.com/'
- 'mmocr/backbone/resnet50-oclip-7ba0c533.pth'))
-
-_base_.train_dataloader.num_workers = 24
-_base_.optim_wrapper.optimizer.lr = 0.002
-
-param_scheduler = [
- dict(type='LinearLR', end=200, start_factor=0.001),
- dict(type='PolyLR', power=0.9, eta_min=1e-7, begin=200, end=1200),
-]
diff --git a/pipeline/mmocr/mmocr/.mim/configs/textdet/dbnetpp/dbnetpp_resnet50_fpnc_1200e_icdar2015.py b/pipeline/mmocr/mmocr/.mim/configs/textdet/dbnetpp/dbnetpp_resnet50_fpnc_1200e_icdar2015.py
deleted file mode 100644
index 0e2f2789c953238b04b3d42a6da1a8c5887b13d7..0000000000000000000000000000000000000000
--- a/pipeline/mmocr/mmocr/.mim/configs/textdet/dbnetpp/dbnetpp_resnet50_fpnc_1200e_icdar2015.py
+++ /dev/null
@@ -1,24 +0,0 @@
-_base_ = [
- 'dbnetpp_resnet50-dcnv2_fpnc_1200e_icdar2015.py',
-]
-
-load_from = None
-
-_base_.model.backbone = dict(
- type='mmdet.ResNet',
- depth=50,
- num_stages=4,
- out_indices=(0, 1, 2, 3),
- frozen_stages=-1,
- norm_cfg=dict(type='BN', requires_grad=True),
- norm_eval=True,
- style='pytorch',
- init_cfg=dict(type='Pretrained', checkpoint='torchvision://resnet50'))
-
-_base_.train_dataloader.num_workers = 24
-_base_.optim_wrapper.optimizer.lr = 0.003
-
-param_scheduler = [
- dict(type='LinearLR', end=200, start_factor=0.001),
- dict(type='PolyLR', power=0.9, eta_min=1e-7, begin=200, end=1200),
-]
diff --git a/pipeline/mmocr/mmocr/.mim/configs/textdet/dbnetpp/metafile.yml b/pipeline/mmocr/mmocr/.mim/configs/textdet/dbnetpp/metafile.yml
deleted file mode 100644
index bb78ffac9c06a8a47c183123fa6d94eea1534102..0000000000000000000000000000000000000000
--- a/pipeline/mmocr/mmocr/.mim/configs/textdet/dbnetpp/metafile.yml
+++ /dev/null
@@ -1,56 +0,0 @@
-Collections:
-- Name: DBNetpp
- Metadata:
- Training Data: ICDAR2015
- Training Techniques:
- - SGD with Momentum
- - Weight Decay
- Training Resources: 1x NVIDIA A100-SXM4-80GB
- Architecture:
- - ResNet
- - FPNC
- Paper:
- URL: https://arxiv.org/abs/2202.10304
- Title: 'Real-Time Scene Text Detection with Differentiable Binarization and Adaptive Scale Fusion'
- README: configs/textdet/dbnetpp/README.md
-
-Models:
- - Name: dbnetpp_resnet50_fpnc_1200e_icdar2015
- In Collection: DBNetpp
- Alias:
- - DBPP_r50
- Config: configs/textdet/dbnetpp/dbnetpp_resnet50_fpnc_1200e_icdar2015.py
- Metadata:
- Training Data: ICDAR2015
- Results:
- - Task: Text Detection
- Dataset: ICDAR2015
- Metrics:
- hmean-iou: 0.8622
- Weights: https://download.openmmlab.com/mmocr/textdet/dbnetpp/dbnetpp_resnet50_fpnc_1200e_icdar2015/dbnetpp_resnet50_fpnc_1200e_icdar2015_20221025_185550-013730aa.pth
-
- - Name: dbnetpp_resnet50-dcnv2_fpnc_1200e_icdar2015
- In Collection: DBNetpp
- Config: configs/textdet/dbnetpp/dbnetpp_resnet50-dcnv2_fpnc_1200e_icdar2015.py
- Metadata:
- Training Data: ICDAR2015
- Results:
- - Task: Text Detection
- Dataset: ICDAR2015
- Metrics:
- hmean-iou: 0.8684
- Weights: https://download.openmmlab.com/mmocr/textdet/dbnetpp/dbnetpp_resnet50-dcnv2_fpnc_1200e_icdar2015/dbnetpp_resnet50-dcnv2_fpnc_1200e_icdar2015_20220829_230108-f289bd20.pth
-
- - Name: dbnetpp_resnet50-oclip_fpnc_1200e_icdar2015
- Alias:
- - DBNetpp
- In Collection: DBNetpp
- Config: configs/textdet/dbnetpp/dbnetpp_resnet50-oclip_fpnc_1200e_icdar2015.py
- Metadata:
- Training Data: ICDAR2015
- Results:
- - Task: Text Detection
- Dataset: ICDAR2015
- Metrics:
- hmean-iou: 0.8882
- Weights: https://download.openmmlab.com/mmocr/textdet/dbnetpp/dbnetpp_resnet50-oclip_fpnc_1200e_icdar2015/dbnetpp_resnet50-oclip_fpnc_1200e_icdar2015_20221101_124139-4ecb39ac.pth
diff --git a/pipeline/mmocr/mmocr/.mim/configs/textdet/drrg/README.md b/pipeline/mmocr/mmocr/.mim/configs/textdet/drrg/README.md
deleted file mode 100644
index 0a056fc5f17a56de4f2c461f05883dfb2b97dadb..0000000000000000000000000000000000000000
--- a/pipeline/mmocr/mmocr/.mim/configs/textdet/drrg/README.md
+++ /dev/null
@@ -1,34 +0,0 @@
-# DRRG
-
-> [Deep relational reasoning graph network for arbitrary shape text detection](https://arxiv.org/abs/2003.07493)
-
-
-
-## Abstract
-
-Arbitrary shape text detection is a challenging task due to the high variety and complexity of scenes texts. In this paper, we propose a novel unified relational reasoning graph network for arbitrary shape text detection. In our method, an innovative local graph bridges a text proposal model via Convolutional Neural Network (CNN) and a deep relational reasoning network via Graph Convolutional Network (GCN), making our network end-to-end trainable. To be concrete, every text instance will be divided into a series of small rectangular components, and the geometry attributes (e.g., height, width, and orientation) of the small components will be estimated by our text proposal model. Given the geometry attributes, the local graph construction model can roughly establish linkages between different text components. For further reasoning and deducing the likelihood of linkages between the component and its neighbors, we adopt a graph-based network to perform deep relational reasoning on local graphs. Experiments on public available datasets demonstrate the state-of-the-art performance of our method.
-
-
-
-
-
-## Results and models
-
-### CTW1500
-
-| Method | BackBone | Pretrained Model | Training set | Test set | #epochs | Test size | Precision | Recall | Hmean | Download |
-| :-------------------------------------: | :---------------------------------------: | :--------------: | :-----------: | :----------: | :-----: | :-------: | :-------: | :----: | :----: | :----------------------------------------: |
-| [DRRG](/configs/textdet/drrg/drrg_resnet50_fpn-unet_1200e_ctw1500.py) | ResNet50 | - | CTW1500 Train | CTW1500 Test | 1200 | 640 | 0.8775 | 0.8179 | 0.8467 | [model](https://download.openmmlab.com/mmocr/textdet/drrg/drrg_resnet50_fpn-unet_1200e_ctw1500/drrg_resnet50_fpn-unet_1200e_ctw1500_20220827_105233-d5c702dd.pth) \\ [log](https://download.openmmlab.com/mmocr/textdet/drrg/drrg_resnet50_fpn-unet_1200e_ctw1500/20220827_105233.log) |
-| [DRRG_r50-oclip](/configs/textdet/drrg/drrg_resnet50-oclip_fpn-unet_1200e_ctw1500.py) | [ResNet50-oCLIP](https://download.openmmlab.com/mmocr/backbone/resnet50-oclip-7ba0c533.pth) | - | CTW1500 Train | CTW1500 Test | 1200 | | | | | [model](<>) \\ [log](<>) |
-
-## Citation
-
-```bibtex
-@article{zhang2020drrg,
- title={Deep relational reasoning graph network for arbitrary shape text detection},
- author={Zhang, Shi-Xue and Zhu, Xiaobin and Hou, Jie-Bo and Liu, Chang and Yang, Chun and Wang, Hongfa and Yin, Xu-Cheng},
- booktitle={CVPR},
- pages={9699-9708},
- year={2020}
-}
-```
diff --git a/pipeline/mmocr/mmocr/.mim/configs/textdet/drrg/_base_drrg_resnet50_fpn-unet.py b/pipeline/mmocr/mmocr/.mim/configs/textdet/drrg/_base_drrg_resnet50_fpn-unet.py
deleted file mode 100644
index 4d6c230d22406f02590241b864d949c6a67f54de..0000000000000000000000000000000000000000
--- a/pipeline/mmocr/mmocr/.mim/configs/textdet/drrg/_base_drrg_resnet50_fpn-unet.py
+++ /dev/null
@@ -1,92 +0,0 @@
-model = dict(
- type='DRRG',
- backbone=dict(
- type='mmdet.ResNet',
- depth=50,
- num_stages=4,
- out_indices=(0, 1, 2, 3),
- frozen_stages=-1,
- norm_cfg=dict(type='BN', requires_grad=True),
- init_cfg=dict(type='Pretrained', checkpoint='torchvision://resnet50'),
- norm_eval=True,
- style='caffe'),
- neck=dict(
- type='FPN_UNet', in_channels=[256, 512, 1024, 2048], out_channels=32),
- det_head=dict(
- type='DRRGHead',
- in_channels=32,
- text_region_thr=0.3,
- center_region_thr=0.4,
- module_loss=dict(type='DRRGModuleLoss'),
- postprocessor=dict(type='DRRGPostprocessor', link_thr=0.80)),
- data_preprocessor=dict(
- type='TextDetDataPreprocessor',
- mean=[123.675, 116.28, 103.53],
- std=[58.395, 57.12, 57.375],
- bgr_to_rgb=True,
- pad_size_divisor=32))
-
-train_pipeline = [
- dict(type='LoadImageFromFile', color_type='color_ignore_orientation'),
- dict(
- type='LoadOCRAnnotations',
- with_bbox=True,
- with_polygon=True,
- with_label=True),
- dict(
- type='TorchVisionWrapper',
- op='ColorJitter',
- brightness=32.0 / 255,
- saturation=0.5),
- dict(
- type='RandomResize',
- scale=(800, 800),
- ratio_range=(0.75, 2.5),
- keep_ratio=True),
- dict(
- type='TextDetRandomCropFlip',
- crop_ratio=0.5,
- iter_num=1,
- min_area_ratio=0.2),
- dict(
- type='RandomApply',
- transforms=[dict(type='RandomCrop', min_side_ratio=0.3)],
- prob=0.8),
- dict(
- type='RandomApply',
- transforms=[
- dict(
- type='RandomRotate',
- max_angle=60,
- use_canvas=True,
- pad_with_fixed_color=False)
- ],
- prob=0.5),
- dict(
- type='RandomChoice',
- transforms=[[
- dict(type='Resize', scale=800, keep_ratio=True),
- dict(type='SourceImagePad', target_scale=800)
- ],
- dict(type='Resize', scale=800, keep_ratio=False)],
- prob=[0.4, 0.6]),
- dict(type='RandomFlip', prob=0.5, direction='horizontal'),
- dict(
- type='PackTextDetInputs',
- meta_keys=('img_path', 'ori_shape', 'img_shape'))
-]
-
-test_pipeline = [
- dict(type='LoadImageFromFile', color_type='color_ignore_orientation'),
- dict(type='Resize', scale=(1024, 640), keep_ratio=True),
- # add loading annotation after ``Resize`` because ground truth
- # does not need to do resize data transform
- dict(
- type='LoadOCRAnnotations',
- with_polygon=True,
- with_bbox=True,
- with_label=True),
- dict(
- type='PackTextDetInputs',
- meta_keys=('img_path', 'ori_shape', 'img_shape', 'scale_factor'))
-]
diff --git a/pipeline/mmocr/mmocr/.mim/configs/textdet/drrg/drrg_resnet50-oclip_fpn-unet_1200e_ctw1500.py b/pipeline/mmocr/mmocr/.mim/configs/textdet/drrg/drrg_resnet50-oclip_fpn-unet_1200e_ctw1500.py
deleted file mode 100644
index f7a721ecf9863f1c0ea95ba9a24174c305b30104..0000000000000000000000000000000000000000
--- a/pipeline/mmocr/mmocr/.mim/configs/textdet/drrg/drrg_resnet50-oclip_fpn-unet_1200e_ctw1500.py
+++ /dev/null
@@ -1,17 +0,0 @@
-_base_ = [
- 'drrg_resnet50_fpn-unet_1200e_ctw1500.py',
-]
-
-load_from = None
-
-_base_.model.backbone = dict(
- type='CLIPResNet',
- init_cfg=dict(
- type='Pretrained',
- checkpoint='https://download.openmmlab.com/'
- 'mmocr/backbone/resnet50-oclip-7ba0c533.pth'))
-
-param_scheduler = [
- dict(type='LinearLR', end=100, start_factor=0.001),
- dict(type='PolyLR', power=0.9, eta_min=1e-7, begin=100, end=1200),
-]
diff --git a/pipeline/mmocr/mmocr/.mim/configs/textdet/drrg/drrg_resnet50_fpn-unet_1200e_ctw1500.py b/pipeline/mmocr/mmocr/.mim/configs/textdet/drrg/drrg_resnet50_fpn-unet_1200e_ctw1500.py
deleted file mode 100644
index c35030997193d2c54b125d540e646c3f1ef9e997..0000000000000000000000000000000000000000
--- a/pipeline/mmocr/mmocr/.mim/configs/textdet/drrg/drrg_resnet50_fpn-unet_1200e_ctw1500.py
+++ /dev/null
@@ -1,30 +0,0 @@
-_base_ = [
- '_base_drrg_resnet50_fpn-unet.py',
- '../_base_/datasets/ctw1500.py',
- '../_base_/default_runtime.py',
- '../_base_/schedules/schedule_sgd_1200e.py',
-]
-
-# dataset settings
-ctw1500_textdet_train = _base_.ctw1500_textdet_train
-ctw1500_textdet_train.pipeline = _base_.train_pipeline
-ctw1500_textdet_test = _base_.ctw1500_textdet_test
-ctw1500_textdet_test.pipeline = _base_.test_pipeline
-
-train_dataloader = dict(
- batch_size=4,
- num_workers=4,
- persistent_workers=True,
- sampler=dict(type='DefaultSampler', shuffle=True),
- dataset=ctw1500_textdet_train)
-
-val_dataloader = dict(
- batch_size=1,
- num_workers=1,
- persistent_workers=True,
- sampler=dict(type='DefaultSampler', shuffle=False),
- dataset=ctw1500_textdet_test)
-
-test_dataloader = val_dataloader
-
-auto_scale_lr = dict(base_batch_size=16)
diff --git a/pipeline/mmocr/mmocr/.mim/configs/textdet/drrg/metafile.yml b/pipeline/mmocr/mmocr/.mim/configs/textdet/drrg/metafile.yml
deleted file mode 100644
index 435a7c43bb6ecfb2d1c1cf162b24bb7edff7c4b5..0000000000000000000000000000000000000000
--- a/pipeline/mmocr/mmocr/.mim/configs/textdet/drrg/metafile.yml
+++ /dev/null
@@ -1,28 +0,0 @@
-Collections:
-- Name: DRRG
- Metadata:
- Training Data: SCUT-CTW1500
- Training Techniques:
- - SGD with Momentum
- Training Resources: 4x NVIDIA A100-SXM4-80GB
- Architecture:
- - ResNet
- - FPN_UNet
- Paper:
- URL: https://arxiv.org/abs/2003.07493.pdf
- Title: 'Deep Relational Reasoning Graph Network for Arbitrary Shape Text Detection'
- README: configs/textdet/drrg/README.md
-
-Models:
- - Name: drrg_resnet50_fpn-unet_1200e_ctw1500
- Alias: DRRG
- In Collection: DRRG
- Config: configs/textdet/drrg/drrg_resnet50_fpn-unet_1200e_ctw1500.py
- Metadata:
- Training Data: CTW1500
- Results:
- - Task: Text Detection
- Dataset: CTW1500
- Metrics:
- hmean-iou: 0.8467
- Weights: https://download.openmmlab.com/mmocr/textdet/drrg/drrg_resnet50_fpn-unet_1200e_ctw1500/drrg_resnet50_fpn-unet_1200e_ctw1500_20220827_105233-d5c702dd.pth
diff --git a/pipeline/mmocr/mmocr/.mim/configs/textdet/fcenet/README.md b/pipeline/mmocr/mmocr/.mim/configs/textdet/fcenet/README.md
deleted file mode 100644
index 34beec1e27c5b98f9d89e7c6bbe2c9e75ae2fdc5..0000000000000000000000000000000000000000
--- a/pipeline/mmocr/mmocr/.mim/configs/textdet/fcenet/README.md
+++ /dev/null
@@ -1,46 +0,0 @@
-# FCENet
-
-> [Fourier Contour Embedding for Arbitrary-Shaped Text Detection](https://arxiv.org/abs/2104.10442)
-
-
-
-## Abstract
-
-One of the main challenges for arbitrary-shaped text detection is to design a good text instance representation that allows networks to learn diverse text geometry variances. Most of existing methods model text instances in image spatial domain via masks or contour point sequences in the Cartesian or the polar coordinate system. However, the mask representation might lead to expensive post-processing, while the point sequence one may have limited capability to model texts with highly-curved shapes. To tackle these problems, we model text instances in the Fourier domain and propose one novel Fourier Contour Embedding (FCE) method to represent arbitrary shaped text contours as compact signatures. We further construct FCENet with a backbone, feature pyramid networks (FPN) and a simple post-processing with the Inverse Fourier Transformation (IFT) and Non-Maximum Suppression (NMS). Different from previous methods, FCENet first predicts compact Fourier signatures of text instances, and then reconstructs text contours via IFT and NMS during test. Extensive experiments demonstrate that FCE is accurate and robust to fit contours of scene texts even with highly-curved shapes, and also validate the effectiveness and the good generalization of FCENet for arbitrary-shaped text detection. Furthermore, experimental results show that our FCENet is superior to the state-of-the-art (SOTA) methods on CTW1500 and Total-Text, especially on challenging highly-curved text subset.
-
-
-
-
-
-## Results and models
-
-### CTW1500
-
-| Method | Backbone | Pretrained Model | Training set | Test set | #epochs | Test size | Precision | Recall | Hmean | Download |
-| :------------------------------------: | :---------------------------------------: | :--------------: | :-----------: | :----------: | :-----: | :---------: | :-------: | :----: | :----: | :---------------------------------------: |
-| [FCENet_r50dcn](/configs/textdet/fcenet/fcenet_resnet50-dcnv2_fpn_1500e_ctw1500.py) | ResNet50 + DCNv2 | - | CTW1500 Train | CTW1500 Test | 1500 | (736, 1080) | 0.8689 | 0.8296 | 0.8488 | [model](https://download.openmmlab.com/mmocr/textdet/fcenet/fcenet_resnet50-dcnv2_fpn_1500e_ctw1500/fcenet_resnet50-dcnv2_fpn_1500e_ctw1500_20220825_221510-4d705392.pth) \| [log](https://download.openmmlab.com/mmocr/textdet/fcenet/fcenet_resnet50-dcnv2_fpn_1500e_ctw1500/20220825_221510.log) |
-| [FCENet_r50-oclip](/configs/textdet/fcenet/fcenet_resnet50-oclip-dcnv2_fpn_1500e_ctw1500.py) | [ResNet50-oCLIP](https://download.openmmlab.com/mmocr/backbone/resnet50-oclip-7ba0c533.pth) | - | CTW1500 Train | CTW1500 Test | 1500 | (736, 1080) | 0.8383 | 0.801 | 0.8192 | [model](https://download.openmmlab.com/mmocr/textdet/fcenet/fcenet_resnet50-oclip_fpn_1500e_ctw1500/fcenet_resnet50-oclip_fpn_1500e_ctw1500_20221102_121909-101df7e6.pth) \| [log](https://download.openmmlab.com/mmocr/textdet/fcenet/fcenet_resnet50-oclip_fpn_1500e_ctw1500/20221102_121909.log) |
-
-### ICDAR2015
-
-| Method | Backbone | Pretrained Model | Training set | Test set | #epochs | Test size | Precision | Recall | Hmean | Download |
-| :---------------------------------------------------: | :------------: | :--------------: | :----------: | :-------: | :-----: | :----------: | :-------: | :----: | :----: | :------------------------------------------------------: |
-| [FCENet_r50](/configs/textdet/fcenet/fcenet_resnet50_fpn_1500e_icdar2015.py) | ResNet50 | - | IC15 Train | IC15 Test | 1500 | (2260, 2260) | 0.8243 | 0.8834 | 0.8528 | [model](https://download.openmmlab.com/mmocr/textdet/fcenet/fcenet_resnet50_fpn_1500e_icdar2015/fcenet_resnet50_fpn_1500e_icdar2015_20220826_140941-167d9042.pth) \| [log](https://download.openmmlab.com/mmocr/textdet/fcenet/fcenet_resnet50_fpn_1500e_icdar2015/20220826_140941.log) |
-| [FCENet_r50-oclip](/configs/textdet/fcenet/fcenet_resnet50-oclip_fpn_1500e_icdar2015.py) | ResNet50-oCLIP | - | IC15 Train | IC15 Test | 1500 | (2260, 2260) | 0.9176 | 0.8098 | 0.8604 | [model](https://download.openmmlab.com/mmocr/textdet/fcenet/fcenet_resnet50-oclip_fpn_1500e_icdar2015/fcenet_resnet50-oclip_fpn_1500e_icdar2015_20221101_150145-5a6fc412.pth) \| [log](https://download.openmmlab.com/mmocr/textdet/fcenet/fcenet_resnet50-oclip_fpn_1500e_icdar2015/20221101_150145.log) |
-
-### Total Text
-
-| Method | Backbone | Pretrained Model | Training set | Test set | #epochs | Test size | Precision | Recall | Hmean | Download |
-| :---------------------------------------------------: | :------: | :--------------: | :-------------: | :------------: | :-----: | :---------: | :-------: | :----: | :----: | :-----------------------------------------------------: |
-| [FCENet_r50](/configs/textdet/fcenet/fcenet_resnet50_fpn_1500e_totaltext.py) | ResNet50 | - | Totaltext Train | Totaltext Test | 1500 | (1280, 960) | 0.8485 | 0.7810 | 0.8134 | [model](https://download.openmmlab.com/mmocr/textdet/fcenet/fcenet_resnet50_fpn_1500e_totaltext/fcenet_resnet50_fpn_1500e_totaltext-91bd37af.pth) \| [log](https://download.openmmlab.com/mmocr/textdet/fcenet/fcenet_resnet50_fpn_1500e_totaltext/20221219_201107.log) |
-
-## Citation
-
-```bibtex
-@InProceedings{zhu2021fourier,
- title={Fourier Contour Embedding for Arbitrary-Shaped Text Detection},
- author={Yiqin Zhu and Jianyong Chen and Lingyu Liang and Zhanghui Kuang and Lianwen Jin and Wayne Zhang},
- year={2021},
- booktitle = {CVPR}
- }
-```
diff --git a/pipeline/mmocr/mmocr/.mim/configs/textdet/fcenet/_base_fcenet_resnet50-dcnv2_fpn.py b/pipeline/mmocr/mmocr/.mim/configs/textdet/fcenet/_base_fcenet_resnet50-dcnv2_fpn.py
deleted file mode 100644
index 39dd981c21cb07e00ae51527fcc0c31162e705cf..0000000000000000000000000000000000000000
--- a/pipeline/mmocr/mmocr/.mim/configs/textdet/fcenet/_base_fcenet_resnet50-dcnv2_fpn.py
+++ /dev/null
@@ -1,16 +0,0 @@
-_base_ = [
- '_base_fcenet_resnet50_fpn.py',
-]
-
-model = dict(
- backbone=dict(
- norm_eval=True,
- style='pytorch',
- dcn=dict(type='DCNv2', deform_groups=2, fallback_on_stride=False),
- stage_with_dcn=(False, True, True, True)),
- det_head=dict(
- module_loss=dict(
- type='FCEModuleLoss',
- num_sample=50,
- level_proportion_range=((0, 0.25), (0.2, 0.65), (0.55, 1.0))),
- postprocessor=dict(text_repr_type='poly', alpha=1.0, beta=2.0)))
diff --git a/pipeline/mmocr/mmocr/.mim/configs/textdet/fcenet/_base_fcenet_resnet50_fpn.py b/pipeline/mmocr/mmocr/.mim/configs/textdet/fcenet/_base_fcenet_resnet50_fpn.py
deleted file mode 100644
index 44267d256834a8aa4ae7e6b574f6c87d5a795394..0000000000000000000000000000000000000000
--- a/pipeline/mmocr/mmocr/.mim/configs/textdet/fcenet/_base_fcenet_resnet50_fpn.py
+++ /dev/null
@@ -1,106 +0,0 @@
-model = dict(
- type='FCENet',
- backbone=dict(
- type='mmdet.ResNet',
- depth=50,
- num_stages=4,
- out_indices=(1, 2, 3),
- frozen_stages=-1,
- norm_cfg=dict(type='BN', requires_grad=True),
- init_cfg=dict(type='Pretrained', checkpoint='torchvision://resnet50'),
- norm_eval=False,
- style='pytorch'),
- neck=dict(
- type='mmdet.FPN',
- in_channels=[512, 1024, 2048],
- out_channels=256,
- add_extra_convs='on_output',
- num_outs=3,
- relu_before_extra_convs=True,
- act_cfg=None),
- det_head=dict(
- type='FCEHead',
- in_channels=256,
- fourier_degree=5,
- module_loss=dict(type='FCEModuleLoss', num_sample=50),
- postprocessor=dict(
- type='FCEPostprocessor',
- scales=(8, 16, 32),
- text_repr_type='quad',
- num_reconstr_points=50,
- alpha=1.2,
- beta=1.0,
- score_thr=0.3)),
- data_preprocessor=dict(
- type='TextDetDataPreprocessor',
- mean=[123.675, 116.28, 103.53],
- std=[58.395, 57.12, 57.375],
- bgr_to_rgb=True,
- pad_size_divisor=32))
-
-train_pipeline = [
- dict(type='LoadImageFromFile', color_type='color_ignore_orientation'),
- dict(
- type='LoadOCRAnnotations',
- with_polygon=True,
- with_bbox=True,
- with_label=True,
- ),
- dict(
- type='RandomResize',
- scale=(800, 800),
- ratio_range=(0.75, 2.5),
- keep_ratio=True),
- dict(
- type='TextDetRandomCropFlip',
- crop_ratio=0.5,
- iter_num=1,
- min_area_ratio=0.2),
- dict(
- type='RandomApply',
- transforms=[dict(type='RandomCrop', min_side_ratio=0.3)],
- prob=0.8),
- dict(
- type='RandomApply',
- transforms=[
- dict(
- type='RandomRotate',
- max_angle=30,
- pad_with_fixed_color=False,
- use_canvas=True)
- ],
- prob=0.5),
- dict(
- type='RandomChoice',
- transforms=[[
- dict(type='Resize', scale=800, keep_ratio=True),
- dict(type='SourceImagePad', target_scale=800)
- ],
- dict(type='Resize', scale=800, keep_ratio=False)],
- prob=[0.6, 0.4]),
- dict(type='RandomFlip', prob=0.5, direction='horizontal'),
- dict(
- type='TorchVisionWrapper',
- op='ColorJitter',
- brightness=32.0 / 255,
- saturation=0.5,
- contrast=0.5),
- dict(
- type='PackTextDetInputs',
- meta_keys=('img_path', 'ori_shape', 'img_shape', 'scale_factor'))
-]
-
-test_pipeline = [
- dict(type='LoadImageFromFile', color_type='color_ignore_orientation'),
- dict(type='Resize', scale=(2260, 2260), keep_ratio=True),
- # add loading annotation after ``Resize`` because ground truth
- # does not need to do resize data transform
- dict(
- type='LoadOCRAnnotations',
- with_polygon=True,
- with_bbox=True,
- with_label=True),
- dict(
- type='PackTextDetInputs',
- meta_keys=('img_path', 'ori_shape', 'img_shape', 'scale_factor'))
-]
diff --git a/pipeline/mmocr/mmocr/.mim/configs/textdet/fcenet/fcenet_resnet50-dcnv2_fpn_1500e_ctw1500.py b/pipeline/mmocr/mmocr/.mim/configs/textdet/fcenet/fcenet_resnet50-dcnv2_fpn_1500e_ctw1500.py
deleted file mode 100644
index 9e54bea571e15a485187ae908578ccff625aacf7..0000000000000000000000000000000000000000
--- a/pipeline/mmocr/mmocr/.mim/configs/textdet/fcenet/fcenet_resnet50-dcnv2_fpn_1500e_ctw1500.py
+++ /dev/null
@@ -1,54 +0,0 @@
-_base_ = [
- '_base_fcenet_resnet50-dcnv2_fpn.py',
- '../_base_/datasets/ctw1500.py',
- '../_base_/default_runtime.py',
- '../_base_/schedules/schedule_sgd_base.py',
-]
-
-optim_wrapper = dict(optimizer=dict(lr=1e-3, weight_decay=5e-4))
-train_cfg = dict(max_epochs=1500)
-# learning policy
-param_scheduler = [
- dict(type='PolyLR', power=0.9, eta_min=1e-7, end=1500),
-]
-
-# dataset settings
-ctw1500_textdet_train = _base_.ctw1500_textdet_train
-ctw1500_textdet_test = _base_.ctw1500_textdet_test
-
-# test pipeline for CTW1500
-ctw_test_pipeline = [
- dict(type='LoadImageFromFile', color_type='color_ignore_orientation'),
- dict(type='Resize', scale=(1080, 736), keep_ratio=True),
- # add loading annotation after ``Resize`` because ground truth
- # does not need to do resize data transform
- dict(
- type='LoadOCRAnnotations',
- with_polygon=True,
- with_bbox=True,
- with_label=True),
- dict(
- type='PackTextDetInputs',
- meta_keys=('img_path', 'ori_shape', 'img_shape', 'scale_factor'))
-]
-
-ctw1500_textdet_train.pipeline = _base_.train_pipeline
-ctw1500_textdet_test.pipeline = ctw_test_pipeline
-
-train_dataloader = dict(
- batch_size=8,
- num_workers=4,
- persistent_workers=True,
- sampler=dict(type='DefaultSampler', shuffle=True),
- dataset=ctw1500_textdet_train)
-
-val_dataloader = dict(
- batch_size=1,
- num_workers=1,
- persistent_workers=True,
- sampler=dict(type='DefaultSampler', shuffle=False),
- dataset=ctw1500_textdet_test)
-
-test_dataloader = val_dataloader
-
-auto_scale_lr = dict(base_batch_size=8)
diff --git a/pipeline/mmocr/mmocr/.mim/configs/textdet/fcenet/fcenet_resnet50-oclip_fpn_1500e_ctw1500.py b/pipeline/mmocr/mmocr/.mim/configs/textdet/fcenet/fcenet_resnet50-oclip_fpn_1500e_ctw1500.py
deleted file mode 100644
index 3bc13090fbfffddd01ed5698fcf22d6ad82832ef..0000000000000000000000000000000000000000
--- a/pipeline/mmocr/mmocr/.mim/configs/textdet/fcenet/fcenet_resnet50-oclip_fpn_1500e_ctw1500.py
+++ /dev/null
@@ -1,16 +0,0 @@
-_base_ = [
- 'fcenet_resnet50-dcnv2_fpn_1500e_ctw1500.py',
-]
-
-load_from = None
-
-_base_.model.backbone = dict(
- type='CLIPResNet',
- out_indices=(1, 2, 3),
- init_cfg=dict(
- type='Pretrained',
- checkpoint='https://download.openmmlab.com/'
- 'mmocr/backbone/resnet50-oclip-7ba0c533.pth'))
-
-_base_.train_dataloader.num_workers = 24
-_base_.optim_wrapper.optimizer.lr = 0.0005
diff --git a/pipeline/mmocr/mmocr/.mim/configs/textdet/fcenet/fcenet_resnet50-oclip_fpn_1500e_icdar2015.py b/pipeline/mmocr/mmocr/.mim/configs/textdet/fcenet/fcenet_resnet50-oclip_fpn_1500e_icdar2015.py
deleted file mode 100644
index 87d87de5d1ae38deef32dcca42018eeab57cf359..0000000000000000000000000000000000000000
--- a/pipeline/mmocr/mmocr/.mim/configs/textdet/fcenet/fcenet_resnet50-oclip_fpn_1500e_icdar2015.py
+++ /dev/null
@@ -1,16 +0,0 @@
-_base_ = [
- 'fcenet_resnet50_fpn_1500e_icdar2015.py',
-]
-load_from = None
-
-_base_.model.backbone = dict(
- type='CLIPResNet',
- out_indices=(1, 2, 3),
- init_cfg=dict(
- type='Pretrained',
- checkpoint='https://download.openmmlab.com/'
- 'mmocr/backbone/resnet50-oclip-7ba0c533.pth'))
-
-_base_.train_dataloader.batch_size = 16
-_base_.train_dataloader.num_workers = 24
-_base_.optim_wrapper.optimizer.lr = 0.0005
diff --git a/pipeline/mmocr/mmocr/.mim/configs/textdet/fcenet/fcenet_resnet50_fpn_1500e_icdar2015.py b/pipeline/mmocr/mmocr/.mim/configs/textdet/fcenet/fcenet_resnet50_fpn_1500e_icdar2015.py
deleted file mode 100644
index 8257a046314dc7d671eb28714e42fb6d70f2b8e0..0000000000000000000000000000000000000000
--- a/pipeline/mmocr/mmocr/.mim/configs/textdet/fcenet/fcenet_resnet50_fpn_1500e_icdar2015.py
+++ /dev/null
@@ -1,37 +0,0 @@
-_base_ = [
- '_base_fcenet_resnet50_fpn.py',
- '../_base_/datasets/icdar2015.py',
- '../_base_/default_runtime.py',
- '../_base_/schedules/schedule_sgd_base.py',
-]
-
-optim_wrapper = dict(optimizer=dict(lr=1e-3, weight_decay=5e-4))
-train_cfg = dict(max_epochs=1500)
-# learning policy
-param_scheduler = [
- dict(type='PolyLR', power=0.9, eta_min=1e-7, end=1500),
-]
-
-# dataset settings
-icdar2015_textdet_train = _base_.icdar2015_textdet_train
-icdar2015_textdet_test = _base_.icdar2015_textdet_test
-icdar2015_textdet_train.pipeline = _base_.train_pipeline
-icdar2015_textdet_test.pipeline = _base_.test_pipeline
-
-train_dataloader = dict(
- batch_size=8,
- num_workers=4,
- persistent_workers=True,
- sampler=dict(type='DefaultSampler', shuffle=True),
- dataset=icdar2015_textdet_train)
-
-val_dataloader = dict(
- batch_size=1,
- num_workers=1,
- persistent_workers=True,
- sampler=dict(type='DefaultSampler', shuffle=False),
- dataset=icdar2015_textdet_test)
-
-test_dataloader = val_dataloader
-
-auto_scale_lr = dict(base_batch_size=8)
diff --git a/pipeline/mmocr/mmocr/.mim/configs/textdet/fcenet/fcenet_resnet50_fpn_1500e_totaltext.py b/pipeline/mmocr/mmocr/.mim/configs/textdet/fcenet/fcenet_resnet50_fpn_1500e_totaltext.py
deleted file mode 100644
index cc63975e2a86cd8a0fbc6b08adf3d1ccde6e6cf3..0000000000000000000000000000000000000000
--- a/pipeline/mmocr/mmocr/.mim/configs/textdet/fcenet/fcenet_resnet50_fpn_1500e_totaltext.py
+++ /dev/null
@@ -1,117 +0,0 @@
-_base_ = [
- '_base_fcenet_resnet50_fpn.py',
- '../_base_/datasets/totaltext.py',
- '../_base_/default_runtime.py',
- '../_base_/schedules/schedule_sgd_base.py',
-]
-
-default_hooks = dict(
- checkpoint=dict(
- type='CheckpointHook',
- save_best='icdar/hmean',
- rule='greater',
- _delete_=True))
-
-train_pipeline = [
- dict(type='LoadImageFromFile', color_type='color_ignore_orientation'),
- dict(
- type='LoadOCRAnnotations',
- with_polygon=True,
- with_bbox=True,
- with_label=True,
- ),
- dict(type='FixInvalidPolygon'),
- dict(
- type='RandomResize',
- scale=(800, 800),
- ratio_range=(0.75, 2.5),
- keep_ratio=True),
- dict(
- type='TextDetRandomCropFlip',
- crop_ratio=0.5,
- iter_num=1,
- min_area_ratio=0.2),
- dict(
- type='RandomApply',
- transforms=[dict(type='RandomCrop', min_side_ratio=0.3)],
- prob=0.8),
- dict(
- type='RandomApply',
- transforms=[
- dict(
- type='RandomRotate',
- max_angle=30,
- pad_with_fixed_color=False,
- use_canvas=True)
- ],
- prob=0.5),
- dict(
- type='RandomChoice',
- transforms=[[
- dict(type='Resize', scale=800, keep_ratio=True),
- dict(type='SourceImagePad', target_scale=800)
- ],
- dict(type='Resize', scale=800, keep_ratio=False)],
- prob=[0.6, 0.4]),
- dict(type='RandomFlip', prob=0.5, direction='horizontal'),
- dict(
- type='TorchVisionWrapper',
- op='ColorJitter',
- brightness=32.0 / 255,
- saturation=0.5,
- contrast=0.5),
- dict(
- type='PackTextDetInputs',
- meta_keys=('img_path', 'ori_shape', 'img_shape', 'scale_factor'))
-]
-
-test_pipeline = [
- dict(type='LoadImageFromFile', color_type='color_ignore_orientation'),
- dict(type='Resize', scale=(1280, 960), keep_ratio=True),
- # add loading annotation after ``Resize`` because ground truth
- # does not need to do resize data transform
- dict(
- type='LoadOCRAnnotations',
- with_polygon=True,
- with_bbox=True,
- with_label=True),
- dict(type='FixInvalidPolygon'),
- dict(
- type='PackTextDetInputs',
- meta_keys=('img_path', 'ori_shape', 'img_shape', 'scale_factor'))
-]
-
-optim_wrapper = dict(optimizer=dict(lr=1e-3, weight_decay=5e-4))
-train_cfg = dict(max_epochs=1500)
-# learning policy
-param_scheduler = [
- dict(type='StepLR', gamma=0.8, step_size=200, end=1200),
-]
-
-# dataset settings
-totaltext_textdet_train = _base_.totaltext_textdet_train
-totaltext_textdet_test = _base_.totaltext_textdet_test
-totaltext_textdet_train.pipeline = train_pipeline
-totaltext_textdet_test.pipeline = test_pipeline
-
-train_dataloader = dict(
- batch_size=16,
- num_workers=16,
- persistent_workers=True,
- pin_memory=True,
- sampler=dict(type='DefaultSampler', shuffle=True),
- dataset=totaltext_textdet_train)
-
-val_dataloader = dict(
- batch_size=1,
- num_workers=1,
- persistent_workers=True,
- pin_memory=True,
- sampler=dict(type='DefaultSampler', shuffle=False),
- dataset=totaltext_textdet_test)
-
-test_dataloader = val_dataloader
-
-auto_scale_lr = dict(base_batch_size=16)
-
-find_unused_parameters = True
diff --git a/pipeline/mmocr/mmocr/.mim/configs/textdet/fcenet/metafile.yml b/pipeline/mmocr/mmocr/.mim/configs/textdet/fcenet/metafile.yml
deleted file mode 100644
index 7cc6c6b806e75aa3d677e14f119758f3e5932c58..0000000000000000000000000000000000000000
--- a/pipeline/mmocr/mmocr/.mim/configs/textdet/fcenet/metafile.yml
+++ /dev/null
@@ -1,79 +0,0 @@
-Collections:
-- Name: FCENet
- Metadata:
- Training Data: SCUT-CTW1500
- Training Techniques:
- - SGD with Momentum
- Training Resources: 1x NVIDIA A100-SXM4-80GB
- Architecture:
- - ResNet50 with DCNv2
- - FPN
- - FCEHead
- Paper:
- URL: https://arxiv.org/abs/2002.02709.pdf
- Title: 'FourierNet: Compact mask representation for instance segmentation using differentiable shape decoders'
- README: configs/textdet/fcenet/README.md
-
-Models:
- - Name: fcenet_resnet50-dcnv2_fpn_1500e_ctw1500
- Alias: FCE_CTW_DCNv2
- In Collection: FCENet
- Config: configs/textdet/fcenet/fcenet_resnet50-dcnv2_fpn_1500e_ctw1500.py
- Metadata:
- Training Data: CTW1500
- Results:
- - Task: Text Detection
- Dataset: CTW1500
- Metrics:
- hmean-iou: 0.8488
- Weights: https://download.openmmlab.com/mmocr/textdet/fcenet/fcenet_resnet50-dcnv2_fpn_1500e_ctw1500/fcenet_resnet50-dcnv2_fpn_1500e_ctw1500_20220825_221510-4d705392.pth
-
- - Name: fcenet_resnet50-oclip_fpn_1500e_ctw1500
- In Collection: FCENet
- Config: configs/textdet/fcenet/fcenet_resnet50-oclip_fpn_1500e_ctw1500.py
- Metadata:
- Training Data: CTW1500
- Results:
- - Task: Text Detection
- Dataset: CTW1500
- Metrics:
- hmean-iou: 0.8192
- Weights: https://download.openmmlab.com/mmocr/textdet/fcenet/fcenet_resnet50-oclip_fpn_1500e_ctw1500/fcenet_resnet50-oclip_fpn_1500e_ctw1500_20221102_121909-101df7e6.pth
-
- - Name: fcenet_resnet50_fpn_1500e_icdar2015
- Alias: FCE_IC15
- In Collection: FCENet
- Config: configs/textdet/fcenet/fcenet_resnet50_fpn_1500e_icdar2015.py
- Metadata:
- Training Data: ICDAR2015
- Results:
- - Task: Text Detection
- Dataset: ICDAR2015
- Metrics:
- hmean-iou: 0.8528
- Weights: https://download.openmmlab.com/mmocr/textdet/fcenet/fcenet_resnet50_fpn_1500e_icdar2015/fcenet_resnet50_fpn_1500e_icdar2015_20220826_140941-167d9042.pth
-
- - Name: fcenet_resnet50-oclip_fpn_1500e_icdar2015
- Alias: FCENet
- In Collection: FCENet
- Config: configs/textdet/fcenet/fcenet_resnet50-oclip_fpn_1500e_icdar2015.py
- Metadata:
- Training Data: ICDAR2015
- Results:
- - Task: Text Detection
- Dataset: ICDAR2015
- Metrics:
- hmean-iou: 0.8604
- Weights: https://download.openmmlab.com/mmocr/textdet/fcenet/fcenet_resnet50-oclip_fpn_1500e_icdar2015/fcenet_resnet50-oclip_fpn_1500e_icdar2015_20221101_150145-5a6fc412.pth
-
- - Name: fcenet_resnet50_fpn_1500e_totaltext
- In Collection: FCENet
- Config: configs/textdet/fcenet/fcenet_resnet50_fpn_1500e_totaltext.py
- Metadata:
- Training Data: Totaltext
- Results:
- - Task: Text Detection
- Dataset: Totaltext
- Metrics:
- hmean-iou: 0.8134
- Weights: https://download.openmmlab.com/mmocr/textdet/fcenet/fcenet_resnet50_fpn_1500e_totaltext/fcenet_resnet50_fpn_1500e_totaltext-91bd37af.pth
diff --git a/pipeline/mmocr/mmocr/.mim/configs/textdet/maskrcnn/README.md b/pipeline/mmocr/mmocr/.mim/configs/textdet/maskrcnn/README.md
deleted file mode 100644
index d520d7370c48f200cdf24fea74d979b57593941e..0000000000000000000000000000000000000000
--- a/pipeline/mmocr/mmocr/.mim/configs/textdet/maskrcnn/README.md
+++ /dev/null
@@ -1,41 +0,0 @@
-# Mask R-CNN
-
-> [Mask R-CNN](https://arxiv.org/abs/1703.06870)
-
-
-
-## Abstract
-
-We present a conceptually simple, flexible, and general framework for object instance segmentation. Our approach efficiently detects objects in an image while simultaneously generating a high-quality segmentation mask for each instance. The method, called Mask R-CNN, extends Faster R-CNN by adding a branch for predicting an object mask in parallel with the existing branch for bounding box recognition. Mask R-CNN is simple to train and adds only a small overhead to Faster R-CNN, running at 5 fps. Moreover, Mask R-CNN is easy to generalize to other tasks, e.g., allowing us to estimate human poses in the same framework. We show top results in all three tracks of the COCO suite of challenges, including instance segmentation, bounding-box object detection, and person keypoint detection. Without bells and whistles, Mask R-CNN outperforms all existing, single-model entries on every task, including the COCO 2016 challenge winners. We hope our simple and effective approach will serve as a solid baseline and help ease future research in instance-level recognition.
-
-
-
-
-
-## Results and models
-
-### CTW1500
-
-| Method | BackBone | Pretrained Model | Training set | Test set | #epochs | Test size | Precision | Recall | Hmean | Download |
-| :-------------------------------------: | :---------------------------------------: | :--------------: | :-----------: | :----------: | :-----: | :-------: | :-------: | :----: | :----: | :----------------------------------------: |
-| [MaskRCNN](/configs/textdet/maskrcnn/mask-rcnn_resnet50_fpn_160e_ctw1500.py) | - | - | CTW1500 Train | CTW1500 Test | 160 | 1600 | 0.7165 | 0.7776 | 0.7458 | [model](https://download.openmmlab.com/mmocr/textdet/maskrcnn/mask-rcnn_resnet50_fpn_160e_ctw1500/mask-rcnn_resnet50_fpn_160e_ctw1500_20220826_154755-ce68ee8e.pth) \| [log](https://download.openmmlab.com/mmocr/textdet/maskrcnn/mask-rcnn_resnet50_fpn_160e_ctw1500/20220826_154755.log) |
-| [MaskRCNN_r50-oclip](/configs/textdet/maskrcnn/mask-rcnn_resnet50-oclip_fpn_160e_ctw1500.py) | [ResNet50-oCLIP](https://download.openmmlab.com/mmocr/backbone/resnet50-oclip-7ba0c533.pth) | - | CTW1500 Train | CTW1500 Test | 160 | 1600 | 0.753 | 0.7593 | 0.7562 | [model](https://download.openmmlab.com/mmocr/textdet/maskrcnn/mask-rcnn_resnet50-oclip_fpn_160e_ctw1500/mask-rcnn_resnet50-oclip_fpn_160e_ctw1500_20221101_154448-6e9e991c.pth) \| [log](https://download.openmmlab.com/mmocr/textdet/maskrcnn/mask-rcnn_resnet50-oclip_fpn_160e_ctw1500/20221101_154448.log) |
-
-### ICDAR2015
-
-| Method | BackBone | Pretrained Model | Training set | Test set | #epochs | Test size | Precision | Recall | Hmean | Download |
-| :------------------------------------: | :--------------------------------------: | :--------------: | :-------------: | :------------: | :-----: | :-------: | :-------: | :----: | :----: | :--------------------------------------: |
-| [MaskRCNN](/configs/textdet/maskrcnn/mask-rcnn_resnet50_fpn_160e_icdar2015.py) | ResNet50 | - | ICDAR2015 Train | ICDAR2015 Test | 160 | 1920 | 0.8644 | 0.7766 | 0.8182 | [model](https://download.openmmlab.com/mmocr/textdet/maskrcnn/mask-rcnn_resnet50_fpn_160e_icdar2015/mask-rcnn_resnet50_fpn_160e_icdar2015_20220826_154808-ff5c30bf.pth) \| [log](https://download.openmmlab.com/mmocr/textdet/maskrcnn/mask-rcnn_resnet50_fpn_160e_icdar2015/20220826_154808.log) |
-| [MaskRCNN_r50-oclip](/configs/textdet/maskrcnn/mask-rcnn_resnet50-oclip_fpn_160e_icdar2015.py) | [ResNet50-oCLIP](https://download.openmmlab.com/mmocr/backbone/resnet50-oclip-7ba0c533.pth) | - | ICDAR2015 Train | ICDAR2015 Test | 160 | 1920 | 0.8695 | 0.8339 | 0.8513 | [model](https://download.openmmlab.com/mmocr/textdet/maskrcnn/mask-rcnn_resnet50-oclip_fpn_160e_icdar2015/mask-rcnn_resnet50-oclip_fpn_160e_icdar2015_20221101_131357-a19f7802.pth) \| [log](https://download.openmmlab.com/mmocr/textdet/maskrcnn/mask-rcnn_resnet50-oclip_fpn_160e_icdar2015/20221101_131357.log) |
-
-## Citation
-
-```bibtex
-@INPROCEEDINGS{8237584,
- author={K. {He} and G. {Gkioxari} and P. {Dollรกr} and R. {Girshick}},
- booktitle={2017 IEEE International Conference on Computer Vision (ICCV)},
- title={Mask R-CNN},
- year={2017},
- pages={2980-2988},
- doi={10.1109/ICCV.2017.322}}
-```
diff --git a/pipeline/mmocr/mmocr/.mim/configs/textdet/maskrcnn/_base_mask-rcnn_resnet50_fpn.py b/pipeline/mmocr/mmocr/.mim/configs/textdet/maskrcnn/_base_mask-rcnn_resnet50_fpn.py
deleted file mode 100644
index be3af65379d45afa3b07c64944d33a7a7e852c0b..0000000000000000000000000000000000000000
--- a/pipeline/mmocr/mmocr/.mim/configs/textdet/maskrcnn/_base_mask-rcnn_resnet50_fpn.py
+++ /dev/null
@@ -1,57 +0,0 @@
-_base_ = ['mmdet::_base_/models/mask-rcnn_r50_fpn.py']
-
-mask_rcnn = _base_.pop('model')
-# Adapt Mask R-CNN model to OCR task
-mask_rcnn.update(
- dict(
- data_preprocessor=dict(pad_mask=False),
- rpn_head=dict(
- anchor_generator=dict(
- scales=[4], ratios=[0.17, 0.44, 1.13, 2.90, 7.46])),
- roi_head=dict(
- bbox_head=dict(num_classes=1),
- mask_head=dict(num_classes=1),
- )))
-
-model = dict(type='MMDetWrapper', text_repr_type='poly', cfg=mask_rcnn)
-
-train_pipeline = [
- dict(type='LoadImageFromFile', color_type='color_ignore_orientation'),
- dict(
- type='LoadOCRAnnotations',
- with_polygon=True,
- with_bbox=True,
- with_label=True,
- ),
- dict(
- type='TorchVisionWrapper',
- op='ColorJitter',
- brightness=32.0 / 255,
- saturation=0.5,
- contrast=0.5),
- dict(
- type='RandomResize',
- scale=(640, 640),
- ratio_range=(1.0, 4.125),
- keep_ratio=True),
- dict(type='RandomFlip', prob=0.5),
- dict(type='TextDetRandomCrop', target_size=(640, 640)),
- dict(type='MMOCR2MMDet', poly2mask=True),
- dict(
- type='mmdet.PackDetInputs',
- meta_keys=('img_path', 'ori_shape', 'img_shape', 'flip',
- 'scale_factor', 'flip_direction'))
-]
-
-test_pipeline = [
- dict(type='LoadImageFromFile', color_type='color_ignore_orientation'),
- dict(type='Resize', scale=(1920, 1920), keep_ratio=True),
- dict(
- type='LoadOCRAnnotations',
- with_polygon=True,
- with_bbox=True,
- with_label=True),
- dict(
- type='PackTextDetInputs',
- meta_keys=('img_path', 'ori_shape', 'img_shape', 'scale_factor'))
-]
diff --git a/pipeline/mmocr/mmocr/.mim/configs/textdet/maskrcnn/mask-rcnn_resnet50-oclip_fpn_160e_ctw1500.py b/pipeline/mmocr/mmocr/.mim/configs/textdet/maskrcnn/mask-rcnn_resnet50-oclip_fpn_160e_ctw1500.py
deleted file mode 100644
index 8abc008a9b46f79a6ec59b471a710ff3179c6f5c..0000000000000000000000000000000000000000
--- a/pipeline/mmocr/mmocr/.mim/configs/textdet/maskrcnn/mask-rcnn_resnet50-oclip_fpn_160e_ctw1500.py
+++ /dev/null
@@ -1,15 +0,0 @@
-_base_ = [
- 'mask-rcnn_resnet50_fpn_160e_ctw1500.py',
-]
-
-load_from = None
-
-_base_.model.cfg.backbone = dict(
- _scope_='mmocr',
- type='CLIPResNet',
- init_cfg=dict(
- type='Pretrained',
- checkpoint='https://download.openmmlab.com/'
- 'mmocr/backbone/resnet50-oclip-7ba0c533.pth'))
-
-_base_.optim_wrapper.optimizer.lr = 0.02
diff --git a/pipeline/mmocr/mmocr/.mim/configs/textdet/maskrcnn/mask-rcnn_resnet50-oclip_fpn_160e_icdar2015.py b/pipeline/mmocr/mmocr/.mim/configs/textdet/maskrcnn/mask-rcnn_resnet50-oclip_fpn_160e_icdar2015.py
deleted file mode 100644
index 57bf9b6a8d8383645233729596a5cf419621e281..0000000000000000000000000000000000000000
--- a/pipeline/mmocr/mmocr/.mim/configs/textdet/maskrcnn/mask-rcnn_resnet50-oclip_fpn_160e_icdar2015.py
+++ /dev/null
@@ -1,15 +0,0 @@
-_base_ = [
- 'mask-rcnn_resnet50_fpn_160e_icdar2015.py',
-]
-
-load_from = None
-
-_base_.model.cfg.backbone = dict(
- _scope_='mmocr',
- type='CLIPResNet',
- init_cfg=dict(
- type='Pretrained',
- checkpoint='https://download.openmmlab.com/'
- 'mmocr/backbone/resnet50-oclip-7ba0c533.pth'))
-
-_base_.optim_wrapper.optimizer.lr = 0.02
diff --git a/pipeline/mmocr/mmocr/.mim/configs/textdet/maskrcnn/mask-rcnn_resnet50_fpn_160e_ctw1500.py b/pipeline/mmocr/mmocr/.mim/configs/textdet/maskrcnn/mask-rcnn_resnet50_fpn_160e_ctw1500.py
deleted file mode 100644
index 547a4212e23e7f3ee188960a7c4858d3bba0d414..0000000000000000000000000000000000000000
--- a/pipeline/mmocr/mmocr/.mim/configs/textdet/maskrcnn/mask-rcnn_resnet50_fpn_160e_ctw1500.py
+++ /dev/null
@@ -1,56 +0,0 @@
-_base_ = [
- '_base_mask-rcnn_resnet50_fpn.py',
- '../_base_/datasets/ctw1500.py',
- '../_base_/default_runtime.py',
- '../_base_/schedules/schedule_sgd_base.py',
-]
-
-# optimizer
-optim_wrapper = dict(optimizer=dict(lr=0.08))
-train_cfg = dict(max_epochs=160)
-# learning policy
-param_scheduler = [
- dict(type='LinearLR', end=500, start_factor=0.001, by_epoch=False),
- dict(type='MultiStepLR', milestones=[80, 128], end=160),
-]
-
-# dataset settings
-ctw1500_textdet_train = _base_.ctw1500_textdet_train
-ctw1500_textdet_test = _base_.ctw1500_textdet_test
-
-# test pipeline for CTW1500
-ctw_test_pipeline = [
- dict(type='LoadImageFromFile', color_type='color_ignore_orientation'),
- dict(type='Resize', scale=(1600, 1600), keep_ratio=True),
- # add loading annotation after ``Resize`` because ground truth
- # does not need to do resize data transform
- dict(
- type='LoadOCRAnnotations',
- with_polygon=True,
- with_bbox=True,
- with_label=True),
- dict(
- type='PackTextDetInputs',
- meta_keys=('img_path', 'ori_shape', 'img_shape', 'scale_factor'))
-]
-
-ctw1500_textdet_train.pipeline = _base_.train_pipeline
-ctw1500_textdet_test.pipeline = ctw_test_pipeline
-
-train_dataloader = dict(
- batch_size=8,
- num_workers=4,
- persistent_workers=True,
- sampler=dict(type='DefaultSampler', shuffle=True),
- dataset=ctw1500_textdet_train)
-
-val_dataloader = dict(
- batch_size=1,
- num_workers=1,
- persistent_workers=True,
- sampler=dict(type='DefaultSampler', shuffle=False),
- dataset=ctw1500_textdet_test)
-
-test_dataloader = val_dataloader
-
-auto_scale_lr = dict(base_batch_size=8)
diff --git a/pipeline/mmocr/mmocr/.mim/configs/textdet/maskrcnn/mask-rcnn_resnet50_fpn_160e_icdar2015.py b/pipeline/mmocr/mmocr/.mim/configs/textdet/maskrcnn/mask-rcnn_resnet50_fpn_160e_icdar2015.py
deleted file mode 100644
index 41509ac17785bcfb93726c16139dd11bddb6020b..0000000000000000000000000000000000000000
--- a/pipeline/mmocr/mmocr/.mim/configs/textdet/maskrcnn/mask-rcnn_resnet50_fpn_160e_icdar2015.py
+++ /dev/null
@@ -1,39 +0,0 @@
-_base_ = [
- '_base_mask-rcnn_resnet50_fpn.py',
- '../_base_/datasets/icdar2015.py',
- '../_base_/default_runtime.py',
- '../_base_/schedules/schedule_sgd_base.py',
-]
-
-# optimizer
-optim_wrapper = dict(optimizer=dict(lr=0.08))
-train_cfg = dict(max_epochs=160)
-# learning policy
-param_scheduler = [
- dict(type='LinearLR', end=500, start_factor=0.001, by_epoch=False),
- dict(type='MultiStepLR', milestones=[80, 128], end=160),
-]
-
-# dataset settings
-icdar2015_textdet_train = _base_.icdar2015_textdet_train
-icdar2015_textdet_test = _base_.icdar2015_textdet_test
-icdar2015_textdet_train.pipeline = _base_.train_pipeline
-icdar2015_textdet_test.pipeline = _base_.test_pipeline
-
-train_dataloader = dict(
- batch_size=8,
- num_workers=4,
- persistent_workers=True,
- sampler=dict(type='DefaultSampler', shuffle=True),
- dataset=icdar2015_textdet_train)
-
-val_dataloader = dict(
- batch_size=1,
- num_workers=1,
- persistent_workers=True,
- sampler=dict(type='DefaultSampler', shuffle=False),
- dataset=icdar2015_textdet_test)
-
-test_dataloader = val_dataloader
-
-auto_scale_lr = dict(base_batch_size=8)
diff --git a/pipeline/mmocr/mmocr/.mim/configs/textdet/maskrcnn/mask-rcnn_resnet50_fpn_160e_icdar2017.py b/pipeline/mmocr/mmocr/.mim/configs/textdet/maskrcnn/mask-rcnn_resnet50_fpn_160e_icdar2017.py
deleted file mode 100644
index 17bda5a99906829bb5ac2bce560194a459a2d143..0000000000000000000000000000000000000000
--- a/pipeline/mmocr/mmocr/.mim/configs/textdet/maskrcnn/mask-rcnn_resnet50_fpn_160e_icdar2017.py
+++ /dev/null
@@ -1,14 +0,0 @@
-_base_ = [
- 'mask-rcnn_resnet50_fpn_160e_icdar2015.py',
- '../_base_/datasets/icdar2017.py',
-]
-
-icdar2017_textdet_train = _base_.icdar2017_textdet_train
-icdar2017_textdet_test = _base_.icdar2017_textdet_test
-# use the same pipeline as icdar2015
-icdar2017_textdet_train.pipeline = _base_.train_pipeline
-icdar2017_textdet_test.pipeline = _base_.test_pipeline
-
-train_dataloader = dict(dataset=icdar2017_textdet_train)
-val_dataloader = dict(dataset=icdar2017_textdet_test)
-test_dataloader = val_dataloader
diff --git a/pipeline/mmocr/mmocr/.mim/configs/textdet/maskrcnn/metafile.yml b/pipeline/mmocr/mmocr/.mim/configs/textdet/maskrcnn/metafile.yml
deleted file mode 100644
index 626f059c0986eecec538ad9f7037983e864c75bf..0000000000000000000000000000000000000000
--- a/pipeline/mmocr/mmocr/.mim/configs/textdet/maskrcnn/metafile.yml
+++ /dev/null
@@ -1,68 +0,0 @@
-Collections:
-- Name: Mask R-CNN
- Metadata:
- Training Data: ICDAR2015 SCUT-CTW1500
- Training Techniques:
- - SGD with Momentum
- - Weight Decay
- Training Resources: 1x NVIDIA A100-SXM4-80GB
- Architecture:
- - ResNet
- - FPN
- - RPN
- Paper:
- URL: https://arxiv.org/pdf/1703.06870.pdf
- Title: 'Mask R-CNN'
- README: configs/textdet/maskrcnn/README.md
-
-Models:
- - Name: mask-rcnn_resnet50_fpn_160e_ctw1500
- In Collection: Mask R-CNN
- Alias: MaskRCNN_CTW
- Config: configs/textdet/maskrcnn/mask-rcnn_resnet50_fpn_160e_ctw1500.py
- Metadata:
- Training Data: CTW1500
- Results:
- - Task: Text Detection
- Dataset: CTW1500
- Metrics:
- hmean-iou: 0.7458
- Weights: https://download.openmmlab.com/mmocr/textdet/maskrcnn/mask-rcnn_resnet50_fpn_160e_ctw1500/mask-rcnn_resnet50_fpn_160e_ctw1500_20220826_154755-ce68ee8e.pth
-
- - Name: mask-rcnn_resnet50-oclip_fpn_160e_ctw1500
- In Collection: Mask R-CNN
- Config: configs/textdet/maskrcnn/mask-rcnn_resnet50-oclip_fpn_160e_ctw1500.py
- Metadata:
- Training Data: CTW1500
- Results:
- - Task: Text Detection
- Dataset: CTW1500
- Metrics:
- hmean-iou: 0.7562
- Weights: https://download.openmmlab.com/mmocr/textdet/maskrcnn/mask-rcnn_resnet50-oclip_fpn_160e_ctw1500/mask-rcnn_resnet50-oclip_fpn_160e_ctw1500_20221101_154448-6e9e991c.pth
-
- - Name: mask-rcnn_resnet50_fpn_160e_icdar2015
- In Collection: Mask R-CNN
- Alias: MaskRCNN_IC15
- Config: configs/textdet/maskrcnn/mask-rcnn_resnet50_fpn_160e_icdar2015.py
- Metadata:
- Training Data: ICDAR2015
- Results:
- - Task: Text Detection
- Dataset: ICDAR2015
- Metrics:
- hmean-iou: 0.8182
- Weights: https://download.openmmlab.com/mmocr/textdet/maskrcnn/mask-rcnn_resnet50_fpn_160e_icdar2015/mask-rcnn_resnet50_fpn_160e_icdar2015_20220826_154808-ff5c30bf.pth
-
- - Name: mask-rcnn_resnet50-oclip_fpn_160e_icdar2015
- In Collection: Mask R-CNN
- Alias: MaskRCNN
- Config: configs/textdet/maskrcnn/mask-rcnn_resnet50-oclip_fpn_160e_icdar2015.py
- Metadata:
- Training Data: ICDAR2015
- Results:
- - Task: Text Detection
- Dataset: ICDAR2015
- Metrics:
- hmean-iou: 0.8513
- Weights: https://download.openmmlab.com/mmocr/textdet/maskrcnn/mask-rcnn_resnet50-oclip_fpn_160e_icdar2015/mask-rcnn_resnet50-oclip_fpn_160e_icdar2015_20221101_131357-a19f7802.pth
diff --git a/pipeline/mmocr/mmocr/.mim/configs/textdet/panet/README.md b/pipeline/mmocr/mmocr/.mim/configs/textdet/panet/README.md
deleted file mode 100644
index 571539057252e8225c91d5aa4a666e762bc127b0..0000000000000000000000000000000000000000
--- a/pipeline/mmocr/mmocr/.mim/configs/textdet/panet/README.md
+++ /dev/null
@@ -1,39 +0,0 @@
-# PANet
-
-> [Efficient and Accurate Arbitrary-Shaped Text Detection with Pixel Aggregation Network](https://arxiv.org/abs/1908.05900)
-
-
-
-## Abstract
-
-Scene text detection, an important step of scene text reading systems, has witnessed rapid development with convolutional neural networks. Nonetheless, two main challenges still exist and hamper its deployment to real-world applications. The first problem is the trade-off between speed and accuracy. The second one is to model the arbitrary-shaped text instance. Recently, some methods have been proposed to tackle arbitrary-shaped text detection, but they rarely take the speed of the entire pipeline into consideration, which may fall short in practical this http URL this paper, we propose an efficient and accurate arbitrary-shaped text detector, termed Pixel Aggregation Network (PAN), which is equipped with a low computational-cost segmentation head and a learnable post-processing. More specifically, the segmentation head is made up of Feature Pyramid Enhancement Module (FPEM) and Feature Fusion Module (FFM). FPEM is a cascadable U-shaped module, which can introduce multi-level information to guide the better segmentation. FFM can gather the features given by the FPEMs of different depths into a final feature for segmentation. The learnable post-processing is implemented by Pixel Aggregation (PA), which can precisely aggregate text pixels by predicted similarity vectors. Experiments on several standard benchmarks validate the superiority of the proposed PAN. It is worth noting that our method can achieve a competitive F-measure of 79.9% at 84.2 FPS on CTW1500.
-
-
-
-
-
-## Results and models
-
-### CTW1500
-
-| Method | Pretrained Model | Training set | Test set | #epochs | Test size | Precision | Recall | Hmean | Download |
-| :----------------------------------------------------------: | :--------------: | :-----------: | :----------: | :-----: | :-------: | :-------: | :----: | :----: | :------------------------------------------------------------: |
-| [PANet](/configs/textdet/panet/panet_resnet18_fpem-ffm_600e_ctw1500.py) | ImageNet | CTW1500 Train | CTW1500 Test | 600 | 640 | 0.8208 | 0.7376 | 0.7770 | [model](https://download.openmmlab.com/mmocr/textdet/panet/panet_resnet18_fpem-ffm_600e_ctw1500/panet_resnet18_fpem-ffm_600e_ctw1500_20220826_144818-980f32d0.pth) \| [log](https://download.openmmlab.com/mmocr/textdet/panet/panet_resnet18_fpem-ffm_600e_ctw1500/20220826_144818.log) |
-
-### ICDAR2015
-
-| Method | Pretrained Model | Training set | Test set | #epochs | Test size | Precision | Recall | Hmean | Download |
-| :--------------------------------------------------------: | :--------------: | :-------------: | :------------: | :-----: | :-------: | :-------: | :----: | :----: | :----------------------------------------------------------: |
-| [PANet](/configs/textdet/panet/panet_resnet18_fpem-ffm_600e_icdar2015.py) | ImageNet | ICDAR2015 Train | ICDAR2015 Test | 600 | 736 | 0.8455 | 0.7323 | 0.7848 | [model](https://download.openmmlab.com/mmocr/textdet/panet/panet_resnet18_fpem-ffm_600e_icdar2015/panet_resnet18_fpem-ffm_600e_icdar2015_20220826_144817-be2acdb4.pth) \| [log](https://download.openmmlab.com/mmocr/textdet/panet/panet_resnet18_fpem-ffm_600e_icdar2015/20220826_144817.log) |
-
-## Citation
-
-```bibtex
-@inproceedings{WangXSZWLYS19,
- author={Wenhai Wang and Enze Xie and Xiaoge Song and Yuhang Zang and Wenjia Wang and Tong Lu and Gang Yu and Chunhua Shen},
- title={Efficient and Accurate Arbitrary-Shaped Text Detection With Pixel Aggregation Network},
- booktitle={ICCV},
- pages={8439--8448},
- year={2019}
- }
-```
diff --git a/pipeline/mmocr/mmocr/.mim/configs/textdet/panet/_base_panet_resnet18_fpem-ffm.py b/pipeline/mmocr/mmocr/.mim/configs/textdet/panet/_base_panet_resnet18_fpem-ffm.py
deleted file mode 100644
index 49b66da4afec5245883c40116d35e018e8935e71..0000000000000000000000000000000000000000
--- a/pipeline/mmocr/mmocr/.mim/configs/textdet/panet/_base_panet_resnet18_fpem-ffm.py
+++ /dev/null
@@ -1,77 +0,0 @@
-# BasicBlock has a little difference from official PANet
-# BasicBlock in mmdet lacks RELU in the last convolution.
-model = dict(
- type='PANet',
- data_preprocessor=dict(
- type='TextDetDataPreprocessor',
- mean=[123.675, 116.28, 103.53],
- std=[58.395, 57.12, 57.375],
- bgr_to_rgb=True,
- pad_size_divisor=32),
- backbone=dict(
- type='mmdet.ResNet',
- depth=18,
- num_stages=4,
- stem_channels=128,
- deep_stem=True,
- out_indices=(0, 1, 2, 3),
- frozen_stages=-1,
- norm_eval=False,
- init_cfg=dict(type='Pretrained', checkpoint='torchvision://resnet18'),
- style='pytorch'),
- neck=dict(type='FPEM_FFM', in_channels=[64, 128, 256, 512]),
- det_head=dict(
- type='PANHead',
- in_channels=[128, 128, 128, 128],
- hidden_dim=128,
- out_channel=6,
- module_loss=dict(
- type='PANModuleLoss',
- loss_text=dict(type='MaskedSquareDiceLoss'),
- loss_kernel=dict(type='MaskedSquareDiceLoss'),
- ),
- postprocessor=dict(type='PANPostprocessor', text_repr_type='quad')))
-
-train_pipeline = [
- dict(type='LoadImageFromFile', color_type='color_ignore_orientation'),
- dict(
- type='LoadOCRAnnotations',
- with_polygon=True,
- with_bbox=True,
- with_label=True,
- ),
- dict(type='ShortScaleAspectJitter', short_size=736, scale_divisor=32),
- dict(type='RandomFlip', prob=0.5, direction='horizontal'),
- dict(type='RandomRotate', max_angle=10),
- dict(type='TextDetRandomCrop', target_size=(736, 736)),
- dict(type='Pad', size=(736, 736)),
- dict(
- type='TorchVisionWrapper',
- op='ColorJitter',
- brightness=32.0 / 255,
- saturation=0.5),
- dict(
- type='PackTextDetInputs',
- meta_keys=('img_path', 'ori_shape', 'img_shape', 'scale_factor'))
-]
-
-test_pipeline = [
- dict(type='LoadImageFromFile', color_type='color_ignore_orientation'),
- # TODO Replace with mmcv.RescaleToShort when it's ready
- dict(
- type='ShortScaleAspectJitter',
- short_size=736,
- scale_divisor=1,
- ratio_range=(1.0, 1.0),
- aspect_ratio_range=(1.0, 1.0)),
- # add loading annotation after ``Resize`` because ground truth
- # does not need to do resize data transform
- dict(
- type='LoadOCRAnnotations',
- with_polygon=True,
- with_bbox=True,
- with_label=True),
- dict(
- type='PackTextDetInputs',
- meta_keys=('img_path', 'ori_shape', 'img_shape', 'scale_factor'))
-]
diff --git a/pipeline/mmocr/mmocr/.mim/configs/textdet/panet/_base_panet_resnet50_fpem-ffm.py b/pipeline/mmocr/mmocr/.mim/configs/textdet/panet/_base_panet_resnet50_fpem-ffm.py
deleted file mode 100644
index 223d1c9adf25bbc4d59f22e0ef29cb99e61655cc..0000000000000000000000000000000000000000
--- a/pipeline/mmocr/mmocr/.mim/configs/textdet/panet/_base_panet_resnet50_fpem-ffm.py
+++ /dev/null
@@ -1,18 +0,0 @@
-_base_ = '_base_panet_resnet18_fpem-ffm.py'
-
-model = dict(
- type='PANet',
- backbone=dict(
- _delete_=True,
- type='mmdet.ResNet',
- depth=50,
- num_stages=4,
- out_indices=(0, 1, 2, 3),
- frozen_stages=1,
- norm_cfg=dict(type='BN', requires_grad=True),
- norm_eval=True,
- style='caffe',
- init_cfg=dict(type='Pretrained', checkpoint='torchvision://resnet50'),
- ),
- neck=dict(in_channels=[256, 512, 1024, 2048]),
- det_head=dict(postprocessor=dict(text_repr_type='poly')))
diff --git a/pipeline/mmocr/mmocr/.mim/configs/textdet/panet/metafile.yml b/pipeline/mmocr/mmocr/.mim/configs/textdet/panet/metafile.yml
deleted file mode 100644
index f2f0e9d72850a1930e0e744022560323acf99a06..0000000000000000000000000000000000000000
--- a/pipeline/mmocr/mmocr/.mim/configs/textdet/panet/metafile.yml
+++ /dev/null
@@ -1,41 +0,0 @@
-Collections:
-- Name: PANet
- Metadata:
- Training Data: ICDAR2015 SCUT-CTW1500
- Training Techniques:
- - Adam
- Training Resources: 1x NVIDIA A100-SXM4-80GB
- Architecture:
- - ResNet
- - FPEM_FFM
- Paper:
- URL: https://arxiv.org/pdf/1803.01534.pdf
- Title: 'Path Aggregation Network for Instance Segmentation'
- README: configs/textdet/panet/README.md
-
-Models:
- - Name: panet_resnet18_fpem-ffm_600e_ctw1500
- Alias: PANet_CTW
- In Collection: PANet
- Config: configs/textdet/panet/panet_resnet18_fpem-ffm_600e_ctw1500.py
- Metadata:
- Training Data: CTW1500
- Results:
- - Task: Text Detection
- Dataset: CTW1500
- Metrics:
- hmean-iou: 0.7770
- Weights: https://download.openmmlab.com/mmocr/textdet/panet/panet_resnet18_fpem-ffm_600e_ctw1500/panet_resnet18_fpem-ffm_600e_ctw1500_20220826_144818-980f32d0.pth
-
- - Name: panet_resnet18_fpem-ffm_600e_icdar2015
- Alias: PANet_IC15
- In Collection: PANet
- Config: configs/textdet/panet/panet_resnet18_fpem-ffm_600e_icdar2015.py
- Metadata:
- Training Data: ICDAR2015
- Results:
- - Task: Text Detection
- Dataset: ICDAR2015
- Metrics:
- hmean-iou: 0.7848
- Weights: https://download.openmmlab.com/mmocr/textdet/panet/panet_resnet18_fpem-ffm_600e_icdar2015/panet_resnet18_fpem-ffm_600e_icdar2015_20220826_144817-be2acdb4.pth
diff --git a/pipeline/mmocr/mmocr/.mim/configs/textdet/panet/panet_resnet18_fpem-ffm_600e_ctw1500.py b/pipeline/mmocr/mmocr/.mim/configs/textdet/panet/panet_resnet18_fpem-ffm_600e_ctw1500.py
deleted file mode 100644
index 98f28f1a16f9113e9d7c263fae6669e988b56668..0000000000000000000000000000000000000000
--- a/pipeline/mmocr/mmocr/.mim/configs/textdet/panet/panet_resnet18_fpem-ffm_600e_ctw1500.py
+++ /dev/null
@@ -1,79 +0,0 @@
-_base_ = [
- '../_base_/datasets/ctw1500.py',
- '../_base_/default_runtime.py',
- '../_base_/schedules/schedule_adam_600e.py',
- '_base_panet_resnet18_fpem-ffm.py',
-]
-
-model = dict(det_head=dict(module_loss=dict(shrink_ratio=(1, 0.7))))
-
-default_hooks = dict(checkpoint=dict(type='CheckpointHook', interval=20), )
-
-train_pipeline = [
- dict(type='LoadImageFromFile', color_type='color_ignore_orientation'),
- dict(
- type='LoadOCRAnnotations',
- with_polygon=True,
- with_bbox=True,
- with_label=True,
- ),
- dict(type='ShortScaleAspectJitter', short_size=640, scale_divisor=32),
- dict(type='RandomFlip', prob=0.5, direction='horizontal'),
- dict(type='RandomRotate', max_angle=10),
- dict(type='TextDetRandomCrop', target_size=(640, 640)),
- dict(type='Pad', size=(640, 640)),
- dict(
- type='TorchVisionWrapper',
- op='ColorJitter',
- brightness=32.0 / 255,
- saturation=0.5),
- dict(
- type='PackTextDetInputs',
- meta_keys=('img_path', 'ori_shape', 'img_shape', 'scale_factor'))
-]
-
-test_pipeline = [
- dict(type='LoadImageFromFile', color_type='color_ignore_orientation'),
- # TODO Replace with mmcv.RescaleToShort when it's ready
- dict(
- type='ShortScaleAspectJitter',
- short_size=640,
- scale_divisor=1,
- ratio_range=(1.0, 1.0),
- aspect_ratio_range=(1.0, 1.0)),
- dict(
- type='LoadOCRAnnotations',
- with_polygon=True,
- with_bbox=True,
- with_label=True),
- dict(
- type='PackTextDetInputs',
- meta_keys=('img_path', 'ori_shape', 'img_shape', 'scale_factor'))
-]
-
-# dataset settings
-ctw1500_textdet_train = _base_.ctw1500_textdet_train
-ctw1500_textdet_test = _base_.ctw1500_textdet_test
-# pipeline settings
-ctw1500_textdet_train.pipeline = train_pipeline
-ctw1500_textdet_test.pipeline = test_pipeline
-
-train_dataloader = dict(
- batch_size=16,
- num_workers=4,
- persistent_workers=True,
- sampler=dict(type='DefaultSampler', shuffle=True),
- dataset=ctw1500_textdet_train)
-val_dataloader = dict(
- batch_size=1,
- num_workers=4,
- persistent_workers=True,
- sampler=dict(type='DefaultSampler', shuffle=False),
- dataset=ctw1500_textdet_test)
-test_dataloader = val_dataloader
-
-val_evaluator = dict(
- type='HmeanIOUMetric', pred_score_thrs=dict(start=0.3, stop=1, step=0.05))
-test_evaluator = val_evaluator
-
-auto_scale_lr = dict(base_batch_size=16)
diff --git a/pipeline/mmocr/mmocr/.mim/configs/textdet/panet/panet_resnet18_fpem-ffm_600e_icdar2015.py b/pipeline/mmocr/mmocr/.mim/configs/textdet/panet/panet_resnet18_fpem-ffm_600e_icdar2015.py
deleted file mode 100644
index 1f5bf0e22d13c7bc79c83024a73182ae46cc3ffa..0000000000000000000000000000000000000000
--- a/pipeline/mmocr/mmocr/.mim/configs/textdet/panet/panet_resnet18_fpem-ffm_600e_icdar2015.py
+++ /dev/null
@@ -1,35 +0,0 @@
-_base_ = [
- '../_base_/datasets/icdar2015.py',
- '../_base_/default_runtime.py',
- '../_base_/schedules/schedule_adam_600e.py',
- '_base_panet_resnet18_fpem-ffm.py',
-]
-
-default_hooks = dict(checkpoint=dict(type='CheckpointHook', interval=20), )
-
-# dataset settings
-icdar2015_textdet_train = _base_.icdar2015_textdet_train
-icdar2015_textdet_test = _base_.icdar2015_textdet_test
-# pipeline settings
-icdar2015_textdet_train.pipeline = _base_.train_pipeline
-icdar2015_textdet_test.pipeline = _base_.test_pipeline
-
-train_dataloader = dict(
- batch_size=64,
- num_workers=8,
- persistent_workers=True,
- sampler=dict(type='DefaultSampler', shuffle=True),
- dataset=icdar2015_textdet_train)
-val_dataloader = dict(
- batch_size=1,
- num_workers=4,
- persistent_workers=True,
- sampler=dict(type='DefaultSampler', shuffle=False),
- dataset=icdar2015_textdet_test)
-test_dataloader = val_dataloader
-
-val_evaluator = dict(
- type='HmeanIOUMetric', pred_score_thrs=dict(start=0.3, stop=1, step=0.05))
-test_evaluator = val_evaluator
-
-auto_scale_lr = dict(base_batch_size=64)
diff --git a/pipeline/mmocr/mmocr/.mim/configs/textdet/panet/panet_resnet50_fpem-ffm_600e_icdar2017.py b/pipeline/mmocr/mmocr/.mim/configs/textdet/panet/panet_resnet50_fpem-ffm_600e_icdar2017.py
deleted file mode 100644
index d5947bbe5356a63452afa2e078c57293cc2911ef..0000000000000000000000000000000000000000
--- a/pipeline/mmocr/mmocr/.mim/configs/textdet/panet/panet_resnet50_fpem-ffm_600e_icdar2017.py
+++ /dev/null
@@ -1,74 +0,0 @@
-_base_ = [
- '../_base_/datasets/icdar2017.py',
- '../_base_/default_runtime.py',
- '../_base_/schedules/schedule_adam_600e.py',
- '_base_panet_resnet50_fpem-ffm.py',
-]
-
-default_hooks = dict(checkpoint=dict(type='CheckpointHook', interval=20), )
-
-train_pipeline = [
- dict(type='LoadImageFromFile', color_type='color_ignore_orientation'),
- dict(
- type='LoadOCRAnnotations',
- with_polygon=True,
- with_bbox=True,
- with_label=True,
- ),
- dict(type='ShortScaleAspectJitter', short_size=800, scale_divisor=32),
- dict(type='RandomFlip', prob=0.5, direction='horizontal'),
- dict(type='RandomRotate', max_angle=10),
- dict(type='TextDetRandomCrop', target_size=(800, 800)),
- dict(type='Pad', size=(800, 800)),
- dict(
- type='TorchVisionWrapper',
- op='ColorJitter',
- brightness=32.0 / 255,
- saturation=0.5),
- dict(
- type='PackTextDetInputs',
- meta_keys=('img_path', 'ori_shape', 'img_shape', 'scale_factor'))
-]
-
-test_pipeline = [
- dict(type='LoadImageFromFile', color_type='color_ignore_orientation'),
- # TODO Replace with mmcv.RescaleToShort when it's ready
- dict(
- type='ShortScaleAspectJitter',
- short_size=800,
- scale_divisor=1,
- ratio_range=(1.0, 1.0),
- aspect_ratio_range=(1.0, 1.0)),
- dict(
- type='LoadOCRAnnotations',
- with_polygon=True,
- with_bbox=True,
- with_label=True),
- dict(
- type='PackTextDetInputs',
- meta_keys=('img_path', 'ori_shape', 'img_shape', 'scale_factor'))
-]
-icdar2017_textdet_train = _base_.icdar2017_textdet_train
-icdar2017_textdet_test = _base_.icdar2017_textdet_test
-# pipeline settings
-icdar2017_textdet_train.pipeline = train_pipeline
-icdar2017_textdet_test.pipeline = test_pipeline
-train_dataloader = dict(
- batch_size=64,
- num_workers=8,
- persistent_workers=True,
- sampler=dict(type='DefaultSampler', shuffle=True),
- dataset=icdar2017_textdet_train)
-val_dataloader = dict(
- batch_size=1,
- num_workers=4,
- persistent_workers=True,
- sampler=dict(type='DefaultSampler', shuffle=False),
- dataset=icdar2017_textdet_test)
-test_dataloader = val_dataloader
-
-val_evaluator = dict(
- type='HmeanIOUMetric', pred_score_thrs=dict(start=0.3, stop=1, step=0.05))
-test_evaluator = val_evaluator
-
-auto_scale_lr = dict(base_batch_size=64)
diff --git a/pipeline/mmocr/mmocr/.mim/configs/textdet/psenet/README.md b/pipeline/mmocr/mmocr/.mim/configs/textdet/psenet/README.md
deleted file mode 100644
index b389f71f8b79a31fc6d3f023b8eb31998f775d05..0000000000000000000000000000000000000000
--- a/pipeline/mmocr/mmocr/.mim/configs/textdet/psenet/README.md
+++ /dev/null
@@ -1,41 +0,0 @@
-# PSENet
-
-> [Shape robust text detection with progressive scale expansion network](https://arxiv.org/abs/1903.12473)
-
-
-
-## Abstract
-
-Scene text detection has witnessed rapid progress especially with the recent development of convolutional neural networks. However, there still exists two challenges which prevent the algorithm into industry applications. On the one hand, most of the state-of-art algorithms require quadrangle bounding box which is in-accurate to locate the texts with arbitrary shape. On the other hand, two text instances which are close to each other may lead to a false detection which covers both instances. Traditionally, the segmentation-based approach can relieve the first problem but usually fail to solve the second challenge. To address these two challenges, in this paper, we propose a novel Progressive Scale Expansion Network (PSENet), which can precisely detect text instances with arbitrary shapes. More specifically, PSENet generates the different scale of kernels for each text instance, and gradually expands the minimal scale kernel to the text instance with the complete shape. Due to the fact that there are large geometrical margins among the minimal scale kernels, our method is effective to split the close text instances, making it easier to use segmentation-based methods to detect arbitrary-shaped text instances. Extensive experiments on CTW1500, Total-Text, ICDAR 2015 and ICDAR 2017 MLT validate the effectiveness of PSENet. Notably, on CTW1500, a dataset full of long curve texts, PSENet achieves a F-measure of 74.3% at 27 FPS, and our best F-measure (82.2%) outperforms state-of-art algorithms by 6.6%. The code will be released in the future.
-
-
-
-
-
-## Results and models
-
-### CTW1500
-
-| Method | Backbone | Pretrained Model | Training set | Test set | #epochs | Test size | Precision | Recall | Hmean | Download |
-| :-------------------------------------: | :---------------------------------------: | :--------------: | :-----------: | :----------: | :-----: | :-------: | :-------: | :----: | :----: | :----------------------------------------: |
-| [PSENet](/configs/textdet/psenet/psenet_resnet50_fpnf_600e_ctw1500.py) | ResNet50 | - | CTW1500 Train | CTW1500 Test | 600 | 1280 | 0.7705 | 0.7883 | 0.7793 | [model](https://download.openmmlab.com/mmocr/textdet/psenet/psenet_resnet50_fpnf_600e_ctw1500/psenet_resnet50_fpnf_600e_ctw1500_20220825_221459-7f974ac8.pth) \| [log](https://download.openmmlab.com/mmocr/textdet/psenet/psenet_resnet50_fpnf_600e_ctw1500/20220825_221459.log) |
-| [PSENet_r50-oclip](/configs/textdet/psenet/psenet_resnet50-oclip_fpnf_600e_ctw1500.py) | [ResNet50-oCLIP](https://download.openmmlab.com/mmocr/backbone/resnet50-oclip-7ba0c533.pth) | - | CTW1500 Train | CTW1500 Test | 600 | 1280 | 0.8483 | 0.7636 | 0.8037 | [model](https://download.openmmlab.com/mmocr/textdet/psenet/psenet_resnet50-oclip_fpnf_600e_ctw1500/psenet_resnet50-oclip_fpnf_600e_ctw1500_20221101_140406-d431710d.pth) \| [log](https://download.openmmlab.com/mmocr/textdet/psenet/psenet_resnet50-oclip_fpnf_600e_ctw1500/20221101_140406.log) |
-
-### ICDAR2015
-
-| Method | Backbone | Pretrained Model | Training set | Test set | #epochs | Test size | Precision | Recall | Hmean | Download |
-| :--------------------------------------: | :-----------------------------------------: | :--------------: | :----------: | :-------: | :-----: | :-------: | :-------: | :----: | :----: | :-----------------------------------------: |
-| [PSENet](/configs/textdet/psenet/psenet_resnet50_fpnf_600e_icdar2015.py) | ResNet50 | - | IC15 Train | IC15 Test | 600 | 2240 | 0.8396 | 0.7636 | 0.7998 | [model](https://download.openmmlab.com/mmocr/textdet/psenet/psenet_resnet50_fpnf_600e_icdar2015/psenet_resnet50_fpnf_600e_icdar2015_20220825_222709-b6741ec3.pth) \| [log](https://download.openmmlab.com/mmocr/textdet/psenet/psenet_resnet50_fpnf_600e_icdar2015/20220825_222709.log) |
-| [PSENet_r50-oclip](/configs/textdet/psenet/psenet_resnet50-oclip_fpnf_600e_icdar2015.py) | [ResNet50-oCLIP](https://download.openmmlab.com/mmocr/backbone/resnet50-oclip-7ba0c533.pth) | - | IC15 Train | IC15 Test | 600 | 2240 | 0.8895 | 0.8098 | 0.8478 | [model](https://download.openmmlab.com/mmocr/textdet/psenet/psenet_resnet50-oclip_fpnf_600e_icdar2015/psenet_resnet50-oclip_fpnf_600e_icdar2015_20221101_131357-2bdca389.pth) \| [log](https://download.openmmlab.com/mmocr/textdet/psenet/psenet_resnet50-oclip_fpnf_600e_icdar2015/20221101_131357.log) |
-
-## Citation
-
-```bibtex
-@inproceedings{wang2019shape,
- title={Shape robust text detection with progressive scale expansion network},
- author={Wang, Wenhai and Xie, Enze and Li, Xiang and Hou, Wenbo and Lu, Tong and Yu, Gang and Shao, Shuai},
- booktitle={Proceedings of the IEEE/CVF Conference on Computer Vision and Pattern Recognition},
- pages={9336--9345},
- year={2019}
-}
-```
diff --git a/pipeline/mmocr/mmocr/.mim/configs/textdet/psenet/_base_psenet_resnet50_fpnf.py b/pipeline/mmocr/mmocr/.mim/configs/textdet/psenet/_base_psenet_resnet50_fpnf.py
deleted file mode 100644
index 2a73423b6deedcfc863e0c2b8845e1c3e490dfa9..0000000000000000000000000000000000000000
--- a/pipeline/mmocr/mmocr/.mim/configs/textdet/psenet/_base_psenet_resnet50_fpnf.py
+++ /dev/null
@@ -1,66 +0,0 @@
-model = dict(
- type='PSENet',
- backbone=dict(
- type='mmdet.ResNet',
- depth=50,
- num_stages=4,
- out_indices=(0, 1, 2, 3),
- frozen_stages=-1,
- norm_cfg=dict(type='SyncBN', requires_grad=True),
- init_cfg=dict(type='Pretrained', checkpoint='torchvision://resnet50'),
- norm_eval=True,
- style='caffe'),
- neck=dict(
- type='FPNF',
- in_channels=[256, 512, 1024, 2048],
- out_channels=256,
- fusion_type='concat'),
- det_head=dict(
- type='PSEHead',
- in_channels=[256],
- hidden_dim=256,
- out_channel=7,
- module_loss=dict(type='PSEModuleLoss'),
- postprocessor=dict(type='PSEPostprocessor', text_repr_type='poly')),
- data_preprocessor=dict(
- type='TextDetDataPreprocessor',
- mean=[123.675, 116.28, 103.53],
- std=[58.395, 57.12, 57.375],
- bgr_to_rgb=True,
- pad_size_divisor=32))
-
-train_pipeline = [
- dict(type='LoadImageFromFile', color_type='color_ignore_orientation'),
- dict(
- type='LoadOCRAnnotations',
- with_polygon=True,
- with_bbox=True,
- with_label=True),
- dict(
- type='TorchVisionWrapper',
- op='ColorJitter',
- brightness=32.0 / 255,
- saturation=0.5),
- dict(type='FixInvalidPolygon'),
- dict(type='ShortScaleAspectJitter', short_size=736, scale_divisor=32),
- dict(type='RandomFlip', prob=0.5, direction='horizontal'),
- dict(type='RandomRotate', max_angle=10),
- dict(type='TextDetRandomCrop', target_size=(736, 736)),
- dict(type='Pad', size=(736, 736)),
- dict(
- type='PackTextDetInputs',
- meta_keys=('img_path', 'ori_shape', 'img_shape', 'scale_factor'))
-]
-
-test_pipeline = [
- dict(type='LoadImageFromFile', color_type='color_ignore_orientation'),
- dict(type='Resize', scale=(2240, 2240), keep_ratio=True),
- dict(
- type='LoadOCRAnnotations',
- with_polygon=True,
- with_bbox=True,
- with_label=True),
- dict(
- type='PackTextDetInputs',
- meta_keys=('img_path', 'ori_shape', 'img_shape', 'scale_factor'))
-]
diff --git a/pipeline/mmocr/mmocr/.mim/configs/textdet/psenet/metafile.yml b/pipeline/mmocr/mmocr/.mim/configs/textdet/psenet/metafile.yml
deleted file mode 100644
index bd7a11f0ee13927215c1eeef1084199208cb8f6c..0000000000000000000000000000000000000000
--- a/pipeline/mmocr/mmocr/.mim/configs/textdet/psenet/metafile.yml
+++ /dev/null
@@ -1,67 +0,0 @@
-Collections:
-- Name: PSENet
- Metadata:
- Training Data: ICDAR2015 SCUT-CTW1500
- Training Techniques:
- - Adam
- Training Resources: 1x NVIDIA A100-SXM4-80GB
- Architecture:
- - ResNet
- - FPNF
- - PSEHead
- Paper:
- URL: https://arxiv.org/abs/1806.02559.pdf
- Title: 'Shape Robust Text Detection with Progressive Scale Expansion Network'
- README: configs/textdet/psenet/README.md
-
-Models:
- - Name: psenet_resnet50_fpnf_600e_ctw1500
- Alias: PS_CTW
- In Collection: PSENet
- Config: configs/textdet/psenet/psenet_resnet50_fpnf_600e_ctw1500.py
- Metadata:
- Training Data: CTW1500
- Results:
- - Task: Text Detection
- Dataset: CTW1500
- Metrics:
- hmean-iou: 0.7793
- Weights: https://download.openmmlab.com/mmocr/textdet/psenet/psenet_resnet50_fpnf_600e_ctw1500/psenet_resnet50_fpnf_600e_ctw1500_20220825_221459-7f974ac8.pth
-
- - Name: psenet_resnet50-oclip_fpnf_600e_ctw1500
- In Collection: PSENet
- Config: configs/textdet/psenet/psenet_resnet50-oclip_fpnf_600e_ctw1500.py
- Metadata:
- Training Data: CTW1500
- Results:
- - Task: Text Detection
- Dataset: CTW1500
- Metrics:
- hmean-iou: 0.8037
- Weights: https://download.openmmlab.com/mmocr/textdet/psenet/psenet_resnet50-oclip_fpnf_600e_ctw1500/psenet_resnet50-oclip_fpnf_600e_ctw1500_20221101_140406-d431710d.pth
-
- - Name: psenet_resnet50_fpnf_600e_icdar2015
- Alias: PS_IC15
- In Collection: PSENet
- Config: configs/textdet/psenet/psenet_resnet50_fpnf_600e_icdar2015.py
- Metadata:
- Training Data: ICDAR2015
- Results:
- - Task: Text Detection
- Dataset: ICDAR2015
- Metrics:
- hmean-iou: 0.7998
- Weights: https://download.openmmlab.com/mmocr/textdet/psenet/psenet_resnet50_fpnf_600e_icdar2015/psenet_resnet50_fpnf_600e_icdar2015_20220825_222709-b6741ec3.pth
-
- - Name: psenet_resnet50-oclip_fpnf_600e_icdar2015
- Alias: PSENet
- In Collection: PSENet
- Config: configs/textdet/psenet/psenet_resnet50-oclip_fpnf_600e_icdar2015.py
- Metadata:
- Training Data: ICDAR2015
- Results:
- - Task: Text Detection
- Dataset: ICDAR2015
- Metrics:
- hmean-iou: 0.8478
- Weights: https://download.openmmlab.com/mmocr/textdet/psenet/psenet_resnet50-oclip_fpnf_600e_icdar2015/psenet_resnet50-oclip_fpnf_600e_icdar2015_20221101_131357-2bdca389.pth
diff --git a/pipeline/mmocr/mmocr/.mim/configs/textdet/psenet/psenet_resnet50-oclip_fpnf_600e_ctw1500.py b/pipeline/mmocr/mmocr/.mim/configs/textdet/psenet/psenet_resnet50-oclip_fpnf_600e_ctw1500.py
deleted file mode 100644
index 255e6885e7dc049c9f7e922e869ff9f7b0d63d00..0000000000000000000000000000000000000000
--- a/pipeline/mmocr/mmocr/.mim/configs/textdet/psenet/psenet_resnet50-oclip_fpnf_600e_ctw1500.py
+++ /dev/null
@@ -1,10 +0,0 @@
-_base_ = [
- 'psenet_resnet50_fpnf_600e_ctw1500.py',
-]
-
-_base_.model.backbone = dict(
- type='CLIPResNet',
- init_cfg=dict(
- type='Pretrained',
- checkpoint='https://download.openmmlab.com/'
- 'mmocr/backbone/resnet50-oclip-7ba0c533.pth'))
diff --git a/pipeline/mmocr/mmocr/.mim/configs/textdet/psenet/psenet_resnet50-oclip_fpnf_600e_icdar2015.py b/pipeline/mmocr/mmocr/.mim/configs/textdet/psenet/psenet_resnet50-oclip_fpnf_600e_icdar2015.py
deleted file mode 100644
index 9871f98013b11209a76d680d185bdc271b4fdf27..0000000000000000000000000000000000000000
--- a/pipeline/mmocr/mmocr/.mim/configs/textdet/psenet/psenet_resnet50-oclip_fpnf_600e_icdar2015.py
+++ /dev/null
@@ -1,10 +0,0 @@
-_base_ = [
- 'psenet_resnet50_fpnf_600e_icdar2015.py',
-]
-
-_base_.model.backbone = dict(
- type='CLIPResNet',
- init_cfg=dict(
- type='Pretrained',
- checkpoint='https://download.openmmlab.com/'
- 'mmocr/backbone/resnet50-oclip-7ba0c533.pth'))
diff --git a/pipeline/mmocr/mmocr/.mim/configs/textdet/psenet/psenet_resnet50_fpnf_600e_ctw1500.py b/pipeline/mmocr/mmocr/.mim/configs/textdet/psenet/psenet_resnet50_fpnf_600e_ctw1500.py
deleted file mode 100644
index a6d97b99bbcb12008433851356e67b6dcd779b15..0000000000000000000000000000000000000000
--- a/pipeline/mmocr/mmocr/.mim/configs/textdet/psenet/psenet_resnet50_fpnf_600e_ctw1500.py
+++ /dev/null
@@ -1,52 +0,0 @@
-_base_ = [
- '_base_psenet_resnet50_fpnf.py',
- '../_base_/datasets/ctw1500.py',
- '../_base_/default_runtime.py',
- '../_base_/schedules/schedule_adam_600e.py',
-]
-
-# optimizer
-optim_wrapper = dict(optimizer=dict(lr=1e-4))
-train_cfg = dict(val_interval=40)
-param_scheduler = [
- dict(type='MultiStepLR', milestones=[200, 400], end=600),
-]
-
-# dataset settings
-ctw1500_textdet_train = _base_.ctw1500_textdet_train
-ctw1500_textdet_test = _base_.ctw1500_textdet_test
-
-test_pipeline_ctw = [
- dict(type='LoadImageFromFile', color_type='color_ignore_orientation'),
- dict(type='Resize', scale=(1280, 1280), keep_ratio=True),
- dict(
- type='LoadOCRAnnotations',
- with_polygon=True,
- with_bbox=True,
- with_label=True),
- dict(
- type='PackTextDetInputs',
- meta_keys=('img_path', 'ori_shape', 'img_shape', 'scale_factor'))
-]
-
-# pipeline settings
-ctw1500_textdet_train.pipeline = _base_.train_pipeline
-ctw1500_textdet_test.pipeline = test_pipeline_ctw
-
-train_dataloader = dict(
- batch_size=16,
- num_workers=8,
- persistent_workers=False,
- sampler=dict(type='DefaultSampler', shuffle=True),
- dataset=ctw1500_textdet_train)
-
-val_dataloader = dict(
- batch_size=1,
- num_workers=1,
- persistent_workers=False,
- sampler=dict(type='DefaultSampler', shuffle=False),
- dataset=ctw1500_textdet_test)
-
-test_dataloader = val_dataloader
-
-auto_scale_lr = dict(base_batch_size=64 * 4)
diff --git a/pipeline/mmocr/mmocr/.mim/configs/textdet/psenet/psenet_resnet50_fpnf_600e_icdar2015.py b/pipeline/mmocr/mmocr/.mim/configs/textdet/psenet/psenet_resnet50_fpnf_600e_icdar2015.py
deleted file mode 100644
index d5610c0dd91a0651cd44b1c1839cb810b57a0c5a..0000000000000000000000000000000000000000
--- a/pipeline/mmocr/mmocr/.mim/configs/textdet/psenet/psenet_resnet50_fpnf_600e_icdar2015.py
+++ /dev/null
@@ -1,44 +0,0 @@
-_base_ = [
- '_base_psenet_resnet50_fpnf.py',
- '../_base_/datasets/icdar2015.py',
- '../_base_/default_runtime.py',
- '../_base_/schedules/schedule_adam_600e.py',
-]
-
-# optimizer
-optim_wrapper = dict(optimizer=dict(lr=1e-4))
-train_cfg = dict(val_interval=40)
-param_scheduler = [
- dict(type='MultiStepLR', milestones=[200, 400], end=600),
-]
-
-# dataset settings
-icdar2015_textdet_train = _base_.icdar2015_textdet_train
-icdar2015_textdet_test = _base_.icdar2015_textdet_test
-
-# use quadrilaterals for icdar2015
-model = dict(
- backbone=dict(style='pytorch'),
- det_head=dict(postprocessor=dict(text_repr_type='quad')))
-
-# pipeline settings
-icdar2015_textdet_train.pipeline = _base_.train_pipeline
-icdar2015_textdet_test.pipeline = _base_.test_pipeline
-
-train_dataloader = dict(
- batch_size=16,
- num_workers=8,
- persistent_workers=False,
- sampler=dict(type='DefaultSampler', shuffle=True),
- dataset=icdar2015_textdet_train)
-
-val_dataloader = dict(
- batch_size=1,
- num_workers=1,
- persistent_workers=False,
- sampler=dict(type='DefaultSampler', shuffle=False),
- dataset=icdar2015_textdet_test)
-
-test_dataloader = val_dataloader
-
-auto_scale_lr = dict(base_batch_size=64 * 4)
diff --git a/pipeline/mmocr/mmocr/.mim/configs/textdet/psenet/psenet_resnet50_fpnf_600e_icdar2017.py b/pipeline/mmocr/mmocr/.mim/configs/textdet/psenet/psenet_resnet50_fpnf_600e_icdar2017.py
deleted file mode 100644
index a1bec586e96a51ddf2efa9b74d6b7354d32e8053..0000000000000000000000000000000000000000
--- a/pipeline/mmocr/mmocr/.mim/configs/textdet/psenet/psenet_resnet50_fpnf_600e_icdar2017.py
+++ /dev/null
@@ -1,16 +0,0 @@
-_base_ = [
- 'psenet_resnet50_fpnf_600e_icdar2015.py',
- '../_base_/datasets/icdar2017.py',
-]
-
-icdar2017_textdet_train = _base_.icdar2017_textdet_train
-icdar2017_textdet_test = _base_.icdar2017_textdet_test
-# use the same pipeline as icdar2015
-icdar2017_textdet_train.pipeline = _base_.train_pipeline
-icdar2017_textdet_test.pipeline = _base_.test_pipeline
-
-train_dataloader = dict(dataset=icdar2017_textdet_train)
-val_dataloader = dict(dataset=icdar2017_textdet_test)
-test_dataloader = val_dataloader
-
-auto_scale_lr = dict(base_batch_size=64 * 4)
diff --git a/pipeline/mmocr/mmocr/.mim/configs/textdet/textsnake/README.md b/pipeline/mmocr/mmocr/.mim/configs/textdet/textsnake/README.md
deleted file mode 100644
index e1cd5d39d08e3f1f5b67e761452245cf0c4d9ef9..0000000000000000000000000000000000000000
--- a/pipeline/mmocr/mmocr/.mim/configs/textdet/textsnake/README.md
+++ /dev/null
@@ -1,34 +0,0 @@
-# Textsnake
-
-> [TextSnake: A Flexible Representation for Detecting Text of Arbitrary Shapes](https://arxiv.org/abs/1807.01544)
-
-
-
-## Abstract
-
-Driven by deep neural networks and large scale datasets, scene text detection methods have progressed substantially over the past years, continuously refreshing the performance records on various standard benchmarks. However, limited by the representations (axis-aligned rectangles, rotated rectangles or quadrangles) adopted to describe text, existing methods may fall short when dealing with much more free-form text instances, such as curved text, which are actually very common in real-world scenarios. To tackle this problem, we propose a more flexible representation for scene text, termed as TextSnake, which is able to effectively represent text instances in horizontal, oriented and curved forms. In TextSnake, a text instance is described as a sequence of ordered, overlapping disks centered at symmetric axes, each of which is associated with potentially variable radius and orientation. Such geometry attributes are estimated via a Fully Convolutional Network (FCN) model. In experiments, the text detector based on TextSnake achieves state-of-the-art or comparable performance on Total-Text and SCUT-CTW1500, the two newly published benchmarks with special emphasis on curved text in natural images, as well as the widely-used datasets ICDAR 2015 and MSRA-TD500. Specifically, TextSnake outperforms the baseline on Total-Text by more than 40% in F-measure.
-
-
-
-
-
-## Results and models
-
-### CTW1500
-
-| Method | BackBone | Pretrained Model | Training set | Test set | #epochs | Test size | Precision | Recall | Hmean | Download |
-| :-------------------------------------: | :---------------------------------------: | :--------------: | :-----------: | :----------: | :-----: | :-------: | :-------: | :----: | :----: | :----------------------------------------: |
-| [TextSnake](/configs/textdet/textsnake/textsnake_resnet50_fpn-unet_1200e_ctw1500.py) | ResNet50 | - | CTW1500 Train | CTW1500 Test | 1200 | 736 | 0.8535 | 0.8052 | 0.8286 | [model](https://download.openmmlab.com/mmocr/textdet/textsnake/textsnake_resnet50_fpn-unet_1200e_ctw1500/textsnake_resnet50_fpn-unet_1200e_ctw1500_20220825_221459-c0b6adc4.pth) \| [log](https://download.openmmlab.com/mmocr/textdet/textsnake/textsnake_resnet50_fpn-unet_1200e_ctw1500/20220825_221459.log) |
-| [TextSnake_r50-oclip](/configs/textdet/textsnake/textsnake_resnet50-oclip_fpn-unet_1200e_ctw1500.py) | [ResNet50-oCLIP](https://download.openmmlab.com/mmocr/backbone/resnet50-oclip-7ba0c533.pth) | - | CTW1500 Train | CTW1500 Test | 1200 | 736 | 0.8869 | 0.8215 | 0.8529 | [model](https://download.openmmlab.com/mmocr/textdet/textsnake/textsnake_resnet50-oclip_fpn-unet_1200e_ctw1500/textsnake_resnet50-oclip_fpn-unet_1200e_ctw1500_20221101_134814-a216e5b2.pth) \| [log](https://download.openmmlab.com/mmocr/textdet/textsnake/textsnake_resnet50-oclip_fpn-unet_1200e_ctw1500/20221101_134814.log) |
-
-## Citation
-
-```bibtex
-@article{long2018textsnake,
- title={TextSnake: A Flexible Representation for Detecting Text of Arbitrary Shapes},
- author={Long, Shangbang and Ruan, Jiaqiang and Zhang, Wenjie and He, Xin and Wu, Wenhao and Yao, Cong},
- booktitle={ECCV},
- pages={20-36},
- year={2018}
-}
-```
diff --git a/pipeline/mmocr/mmocr/.mim/configs/textdet/textsnake/_base_textsnake_resnet50_fpn-unet.py b/pipeline/mmocr/mmocr/.mim/configs/textdet/textsnake/_base_textsnake_resnet50_fpn-unet.py
deleted file mode 100644
index f1586d61f9886bcb08fe43c95764f944dfd3e099..0000000000000000000000000000000000000000
--- a/pipeline/mmocr/mmocr/.mim/configs/textdet/textsnake/_base_textsnake_resnet50_fpn-unet.py
+++ /dev/null
@@ -1,82 +0,0 @@
-model = dict(
- type='TextSnake',
- backbone=dict(
- type='mmdet.ResNet',
- depth=50,
- num_stages=4,
- out_indices=(0, 1, 2, 3),
- frozen_stages=-1,
- norm_cfg=dict(type='BN', requires_grad=True),
- init_cfg=dict(type='Pretrained', checkpoint='torchvision://resnet50'),
- norm_eval=True,
- style='caffe'),
- neck=dict(
- type='FPN_UNet', in_channels=[256, 512, 1024, 2048], out_channels=32),
- det_head=dict(
- type='TextSnakeHead',
- in_channels=32,
- module_loss=dict(type='TextSnakeModuleLoss'),
- postprocessor=dict(
- type='TextSnakePostprocessor', text_repr_type='poly')),
- data_preprocessor=dict(
- type='TextDetDataPreprocessor',
- mean=[123.675, 116.28, 103.53],
- std=[58.395, 57.12, 57.375],
- bgr_to_rgb=True,
- pad_size_divisor=32))
-
-train_pipeline = [
- dict(type='LoadImageFromFile', color_type='color_ignore_orientation'),
- dict(
- type='LoadOCRAnnotations',
- with_bbox=True,
- with_polygon=True,
- with_label=True),
- dict(
- type='TorchVisionWrapper',
- op='ColorJitter',
- brightness=32.0 / 255,
- saturation=0.5),
- dict(
- type='RandomApply',
- transforms=[dict(type='RandomCrop', min_side_ratio=0.3)],
- prob=0.65),
- dict(
- type='RandomRotate',
- max_angle=20,
- pad_with_fixed_color=False,
- use_canvas=True),
- dict(
- type='BoundedScaleAspectJitter',
- long_size_bound=800,
- short_size_bound=480,
- ratio_range=(0.7, 1.3),
- aspect_ratio_range=(0.9, 1.1)),
- dict(
- type='RandomChoice',
- transforms=[[
- dict(type='Resize', scale=800, keep_ratio=True),
- dict(type='SourceImagePad', target_scale=800)
- ],
- dict(type='Resize', scale=800, keep_ratio=False)],
- prob=[0.4, 0.6]),
- dict(type='RandomFlip', prob=0.5, direction='horizontal'),
- dict(
- type='PackTextDetInputs',
- meta_keys=('img_path', 'ori_shape', 'img_shape'))
-]
-
-test_pipeline = [
- dict(type='LoadImageFromFile', color_type='color_ignore_orientation'),
- dict(type='Resize', scale=(1333, 736), keep_ratio=True),
- # add loading annotation after ``Resize`` because ground truth
- # does not need to do resize data transform
- dict(
- type='LoadOCRAnnotations',
- with_polygon=True,
- with_bbox=True,
- with_label=True),
- dict(
- type='PackTextDetInputs',
- meta_keys=('img_path', 'ori_shape', 'img_shape', 'scale_factor'))
-]
diff --git a/pipeline/mmocr/mmocr/.mim/configs/textdet/textsnake/metafile.yml b/pipeline/mmocr/mmocr/.mim/configs/textdet/textsnake/metafile.yml
deleted file mode 100644
index cdb69fbe0c18c0147f0df5afef03bfe66f02cb00..0000000000000000000000000000000000000000
--- a/pipeline/mmocr/mmocr/.mim/configs/textdet/textsnake/metafile.yml
+++ /dev/null
@@ -1,40 +0,0 @@
-Collections:
-- Name: TextSnake
- Metadata:
- Training Data: SCUT-CTW1500
- Training Techniques:
- - SGD with Momentum
- Training Resources: 1x NVIDIA A100-SXM4-80GB
- Architecture:
- - ResNet
- - FPN_UNet
- Paper:
- URL: https://arxiv.org/abs/1807.01544.pdf
- Title: 'TextSnake: A Flexible Representation for Detecting Text of Arbitrary Shapes'
- README: configs/textdet/textsnake/README.md
-
-Models:
- - Name: textsnake_resnet50_fpn-unet_1200e_ctw1500
- In Collection: TextSnake
- Config: configs/textdet/textsnake/textsnake_resnet50_fpn-unet_1200e_ctw1500.py
- Metadata:
- Training Data: CTW1500
- Results:
- - Task: Text Detection
- Dataset: CTW1500
- Metrics:
- hmean-iou: 0.8286
- Weights: https://download.openmmlab.com/mmocr/textdet/textsnake/textsnake_resnet50_fpn-unet_1200e_ctw1500/textsnake_resnet50_fpn-unet_1200e_ctw1500_20220825_221459-c0b6adc4.pth
-
- - Name: textsnake_resnet50-oclip_fpn-unet_1200e_ctw1500
- Alias: TextSnake
- In Collection: TextSnake
- Config: configs/textdet/textsnake/textsnake_resnet50-oclip_fpn-unet_1200e_ctw1500.py
- Metadata:
- Training Data: CTW1500
- Results:
- - Task: Text Detection
- Dataset: CTW1500
- Metrics:
- hmean-iou: 0.8529
- Weights: https://download.openmmlab.com/mmocr/textdet/textsnake/textsnake_resnet50-oclip_fpn-unet_1200e_ctw1500/textsnake_resnet50-oclip_fpn-unet_1200e_ctw1500_20221101_134814-a216e5b2.pth
diff --git a/pipeline/mmocr/mmocr/.mim/configs/textdet/textsnake/textsnake_resnet50-oclip_fpn-unet_1200e_ctw1500.py b/pipeline/mmocr/mmocr/.mim/configs/textdet/textsnake/textsnake_resnet50-oclip_fpn-unet_1200e_ctw1500.py
deleted file mode 100644
index 3c7142e427351d5b9294bd8df0184613ef34cdd6..0000000000000000000000000000000000000000
--- a/pipeline/mmocr/mmocr/.mim/configs/textdet/textsnake/textsnake_resnet50-oclip_fpn-unet_1200e_ctw1500.py
+++ /dev/null
@@ -1,10 +0,0 @@
-_base_ = [
- 'textsnake_resnet50_fpn-unet_1200e_ctw1500.py',
-]
-
-_base_.model.backbone = dict(
- type='CLIPResNet',
- init_cfg=dict(
- type='Pretrained',
- checkpoint='https://download.openmmlab.com/'
- 'mmocr/backbone/resnet50-oclip-7ba0c533.pth'))
diff --git a/pipeline/mmocr/mmocr/.mim/configs/textdet/textsnake/textsnake_resnet50_fpn-unet_1200e_ctw1500.py b/pipeline/mmocr/mmocr/.mim/configs/textdet/textsnake/textsnake_resnet50_fpn-unet_1200e_ctw1500.py
deleted file mode 100644
index 5e3158630bc047172ce50dc27ba23faf2f1606f1..0000000000000000000000000000000000000000
--- a/pipeline/mmocr/mmocr/.mim/configs/textdet/textsnake/textsnake_resnet50_fpn-unet_1200e_ctw1500.py
+++ /dev/null
@@ -1,30 +0,0 @@
-_base_ = [
- '_base_textsnake_resnet50_fpn-unet.py',
- '../_base_/datasets/ctw1500.py',
- '../_base_/default_runtime.py',
- '../_base_/schedules/schedule_sgd_1200e.py',
-]
-
-# dataset settings
-ctw1500_textdet_train = _base_.ctw1500_textdet_train
-ctw1500_textdet_train.pipeline = _base_.train_pipeline
-ctw1500_textdet_test = _base_.ctw1500_textdet_test
-ctw1500_textdet_test.pipeline = _base_.test_pipeline
-
-train_dataloader = dict(
- batch_size=4,
- num_workers=4,
- persistent_workers=True,
- sampler=dict(type='DefaultSampler', shuffle=True),
- dataset=ctw1500_textdet_train)
-
-val_dataloader = dict(
- batch_size=1,
- num_workers=1,
- persistent_workers=True,
- sampler=dict(type='DefaultSampler', shuffle=False),
- dataset=ctw1500_textdet_test)
-
-test_dataloader = val_dataloader
-
-auto_scale_lr = dict(base_batch_size=4)
diff --git a/pipeline/mmocr/mmocr/.mim/configs/textrecog/_base_/datasets/coco_text_v1.py b/pipeline/mmocr/mmocr/.mim/configs/textrecog/_base_/datasets/coco_text_v1.py
deleted file mode 100644
index b88bcd3d391ad73b0db5ae49fa36dbb04af06761..0000000000000000000000000000000000000000
--- a/pipeline/mmocr/mmocr/.mim/configs/textrecog/_base_/datasets/coco_text_v1.py
+++ /dev/null
@@ -1,8 +0,0 @@
-cocotextv1_textrecog_data_root = 'data/rec/coco_text_v1'
-
-cocotextv1_textrecog_train = dict(
- type='OCRDataset',
- data_root=cocotextv1_textrecog_data_root,
- ann_file='train_labels.json',
- test_mode=False,
- pipeline=None)
diff --git a/pipeline/mmocr/mmocr/.mim/configs/textrecog/_base_/datasets/cute80.py b/pipeline/mmocr/mmocr/.mim/configs/textrecog/_base_/datasets/cute80.py
deleted file mode 100644
index 7e3a6fad84bc121209f9c6d3042f5cee3dc89f6b..0000000000000000000000000000000000000000
--- a/pipeline/mmocr/mmocr/.mim/configs/textrecog/_base_/datasets/cute80.py
+++ /dev/null
@@ -1,8 +0,0 @@
-cute80_textrecog_data_root = '../data/common_benchmarks/CUTE80'
-
-cute80_textrecog_test = dict(
- type='OCRDataset',
- data_root=cute80_textrecog_data_root,
- ann_file='annotation.json',
- test_mode=True,
- pipeline=None)
diff --git a/pipeline/mmocr/mmocr/.mim/configs/textrecog/_base_/datasets/icdar2011.py b/pipeline/mmocr/mmocr/.mim/configs/textrecog/_base_/datasets/icdar2011.py
deleted file mode 100644
index 6071c251cd7e3e0ea7fcbcf190262526b9bff910..0000000000000000000000000000000000000000
--- a/pipeline/mmocr/mmocr/.mim/configs/textrecog/_base_/datasets/icdar2011.py
+++ /dev/null
@@ -1,8 +0,0 @@
-icdar2011_textrecog_data_root = 'data/rec/icdar_2011/'
-
-icdar2011_textrecog_train = dict(
- type='OCRDataset',
- data_root=icdar2011_textrecog_data_root,
- ann_file='train_labels.json',
- test_mode=False,
- pipeline=None)
diff --git a/pipeline/mmocr/mmocr/.mim/configs/textrecog/_base_/datasets/icdar2013.py b/pipeline/mmocr/mmocr/.mim/configs/textrecog/_base_/datasets/icdar2013.py
deleted file mode 100644
index e3756f0cf3deb98900fcd2fde61c43b9e7c0ad45..0000000000000000000000000000000000000000
--- a/pipeline/mmocr/mmocr/.mim/configs/textrecog/_base_/datasets/icdar2013.py
+++ /dev/null
@@ -1,21 +0,0 @@
-icdar2013_textrecog_data_root = '../data/common_benchmarks/IC13'
-
-icdar2013_textrecog_train = dict(
- type='OCRDataset',
- data_root=icdar2013_textrecog_data_root,
- ann_file='textrecog_train.json',
- pipeline=None)
-
-icdar2013_textrecog_test = dict(
- type='OCRDataset',
- data_root=icdar2013_textrecog_data_root,
- ann_file='annotation.json',
- test_mode=True,
- pipeline=None)
-
-icdar2013_857_textrecog_test = dict(
- type='OCRDataset',
- data_root=icdar2013_textrecog_data_root,
- ann_file='textrecog_test_857.json',
- test_mode=True,
- pipeline=None)
diff --git a/pipeline/mmocr/mmocr/.mim/configs/textrecog/_base_/datasets/icdar2015.py b/pipeline/mmocr/mmocr/.mim/configs/textrecog/_base_/datasets/icdar2015.py
deleted file mode 100644
index b6ed92d7a54d0757c0afbbea891acf59a2daf137..0000000000000000000000000000000000000000
--- a/pipeline/mmocr/mmocr/.mim/configs/textrecog/_base_/datasets/icdar2015.py
+++ /dev/null
@@ -1,21 +0,0 @@
-icdar2015_textrecog_data_root = '../data/common_benchmarks/IC15'
-
-icdar2015_textrecog_train = dict(
- type='OCRDataset',
- data_root=icdar2015_textrecog_data_root,
- ann_file='textrecog_train.json',
- pipeline=None)
-
-icdar2015_textrecog_test = dict(
- type='OCRDataset',
- data_root=icdar2015_textrecog_data_root,
- ann_file='annotation.json',
- test_mode=True,
- pipeline=None)
-
-icdar2015_1811_textrecog_test = dict(
- type='OCRDataset',
- data_root=icdar2015_textrecog_data_root,
- ann_file='textrecog_test_1811.json',
- test_mode=True,
- pipeline=None)
diff --git a/pipeline/mmocr/mmocr/.mim/configs/textrecog/_base_/datasets/iiit5k.py b/pipeline/mmocr/mmocr/.mim/configs/textrecog/_base_/datasets/iiit5k.py
deleted file mode 100644
index 11d1183955e893585323321ca0a23bb655074715..0000000000000000000000000000000000000000
--- a/pipeline/mmocr/mmocr/.mim/configs/textrecog/_base_/datasets/iiit5k.py
+++ /dev/null
@@ -1,14 +0,0 @@
-iiit5k_textrecog_data_root = '../data/common_benchmarks/IIIT5K'
-
-iiit5k_textrecog_train = dict(
- type='OCRDataset',
- data_root=iiit5k_textrecog_data_root,
- ann_file='textrecog_train.json',
- pipeline=None)
-
-iiit5k_textrecog_test = dict(
- type='OCRDataset',
- data_root=iiit5k_textrecog_data_root,
- ann_file='annotation.json',
- test_mode=True,
- pipeline=None)
diff --git a/pipeline/mmocr/mmocr/.mim/configs/textrecog/_base_/datasets/mjsynth.py b/pipeline/mmocr/mmocr/.mim/configs/textrecog/_base_/datasets/mjsynth.py
deleted file mode 100644
index defe84a8db4e2e7341f6c386c2f72560be045a2e..0000000000000000000000000000000000000000
--- a/pipeline/mmocr/mmocr/.mim/configs/textrecog/_base_/datasets/mjsynth.py
+++ /dev/null
@@ -1,13 +0,0 @@
-mjsynth_textrecog_data_root = 'data/mjsynth'
-
-mjsynth_textrecog_train = dict(
- type='OCRDataset',
- data_root=mjsynth_textrecog_data_root,
- ann_file='textrecog_train.json',
- pipeline=None)
-
-mjsynth_sub_textrecog_train = dict(
- type='OCRDataset',
- data_root=mjsynth_textrecog_data_root,
- ann_file='subset_textrecog_train.json',
- pipeline=None)
diff --git a/pipeline/mmocr/mmocr/.mim/configs/textrecog/_base_/datasets/svt.py b/pipeline/mmocr/mmocr/.mim/configs/textrecog/_base_/datasets/svt.py
deleted file mode 100644
index 60dbd7a19808d074212d8973d8cb78b879e8b841..0000000000000000000000000000000000000000
--- a/pipeline/mmocr/mmocr/.mim/configs/textrecog/_base_/datasets/svt.py
+++ /dev/null
@@ -1,14 +0,0 @@
-svt_textrecog_data_root = '../data/common_benchmarks/SVT'
-
-svt_textrecog_train = dict(
- type='OCRDataset',
- data_root=svt_textrecog_data_root,
- ann_file='textrecog_train.json',
- pipeline=None)
-
-svt_textrecog_test = dict(
- type='OCRDataset',
- data_root=svt_textrecog_data_root,
- ann_file='annotation.json',
- test_mode=True,
- pipeline=None)
diff --git a/pipeline/mmocr/mmocr/.mim/configs/textrecog/_base_/datasets/svtp.py b/pipeline/mmocr/mmocr/.mim/configs/textrecog/_base_/datasets/svtp.py
deleted file mode 100644
index 38301d1bb8de9b056e4cd0bcaf16d86200cd4a7d..0000000000000000000000000000000000000000
--- a/pipeline/mmocr/mmocr/.mim/configs/textrecog/_base_/datasets/svtp.py
+++ /dev/null
@@ -1,14 +0,0 @@
-svtp_textrecog_data_root = '../data/common_benchmarks/SVTP'
-
-svtp_textrecog_train = dict(
- type='OCRDataset',
- data_root=svtp_textrecog_data_root,
- ann_file='textrecog_train.json',
- pipeline=None)
-
-svtp_textrecog_test = dict(
- type='OCRDataset',
- data_root=svtp_textrecog_data_root,
- ann_file='annotation.json',
- test_mode=True,
- pipeline=None)
diff --git a/pipeline/mmocr/mmocr/.mim/configs/textrecog/_base_/datasets/synthtext.py b/pipeline/mmocr/mmocr/.mim/configs/textrecog/_base_/datasets/synthtext.py
deleted file mode 100644
index 94fc3049b3a1832ccff20571a7b7fda88383b767..0000000000000000000000000000000000000000
--- a/pipeline/mmocr/mmocr/.mim/configs/textrecog/_base_/datasets/synthtext.py
+++ /dev/null
@@ -1,19 +0,0 @@
-synthtext_textrecog_data_root = 'data/synthtext'
-
-synthtext_textrecog_train = dict(
- type='OCRDataset',
- data_root=synthtext_textrecog_data_root,
- ann_file='textrecog_train.json',
- pipeline=None)
-
-synthtext_sub_textrecog_train = dict(
- type='OCRDataset',
- data_root=synthtext_textrecog_data_root,
- ann_file='subset_textrecog_train.json',
- pipeline=None)
-
-synthtext_an_textrecog_train = dict(
- type='OCRDataset',
- data_root=synthtext_textrecog_data_root,
- ann_file='alphanumeric_textrecog_train.json',
- pipeline=None)
diff --git a/pipeline/mmocr/mmocr/.mim/configs/textrecog/_base_/datasets/synthtext_add.py b/pipeline/mmocr/mmocr/.mim/configs/textrecog/_base_/datasets/synthtext_add.py
deleted file mode 100644
index f31e41f6e58712c0521abf03617a47a138c1f4fb..0000000000000000000000000000000000000000
--- a/pipeline/mmocr/mmocr/.mim/configs/textrecog/_base_/datasets/synthtext_add.py
+++ /dev/null
@@ -1,8 +0,0 @@
-synthtext_add_textrecog_data_root = 'data/rec/synthtext_add/'
-
-synthtext_add_textrecog_train = dict(
- type='OCRDataset',
- data_root=synthtext_add_textrecog_data_root,
- ann_file='train_labels.json',
- test_mode=False,
- pipeline=None)
diff --git a/pipeline/mmocr/mmocr/.mim/configs/textrecog/_base_/datasets/totaltext.py b/pipeline/mmocr/mmocr/.mim/configs/textrecog/_base_/datasets/totaltext.py
deleted file mode 100644
index 07743439b1dcb688b7bcf5c918609d4e018bc4b7..0000000000000000000000000000000000000000
--- a/pipeline/mmocr/mmocr/.mim/configs/textrecog/_base_/datasets/totaltext.py
+++ /dev/null
@@ -1,15 +0,0 @@
-totaltext_textrecog_data_root = 'data/totaltext/'
-
-totaltext_textrecog_train = dict(
- type='OCRDataset',
- data_root=totaltext_textrecog_data_root,
- ann_file='textrecog_train.json',
- test_mode=False,
- pipeline=None)
-
-totaltext_textrecog_test = dict(
- type='OCRDataset',
- data_root=totaltext_textrecog_data_root,
- ann_file='textrecog_test.json',
- test_mode=True,
- pipeline=None)
diff --git a/pipeline/mmocr/mmocr/.mim/configs/textrecog/_base_/datasets/toy_data.py b/pipeline/mmocr/mmocr/.mim/configs/textrecog/_base_/datasets/toy_data.py
deleted file mode 100644
index ca73d196184cf59d076327100cdafe1503a92b9e..0000000000000000000000000000000000000000
--- a/pipeline/mmocr/mmocr/.mim/configs/textrecog/_base_/datasets/toy_data.py
+++ /dev/null
@@ -1,17 +0,0 @@
-toy_data_root = 'tests/data/rec_toy_dataset/'
-
-toy_rec_train = dict(
- type='OCRDataset',
- data_root=toy_data_root,
- data_prefix=dict(img_path='imgs/'),
- ann_file='labels.json',
- pipeline=None,
- test_mode=False)
-
-toy_rec_test = dict(
- type='OCRDataset',
- data_root=toy_data_root,
- data_prefix=dict(img_path='imgs/'),
- ann_file='labels.json',
- pipeline=None,
- test_mode=True)
diff --git a/pipeline/mmocr/mmocr/.mim/configs/textrecog/_base_/datasets/union14m_benchmark.py b/pipeline/mmocr/mmocr/.mim/configs/textrecog/_base_/datasets/union14m_benchmark.py
deleted file mode 100644
index cbcc26b86e2291057eb97cd5a6b8c6a869a89e89..0000000000000000000000000000000000000000
--- a/pipeline/mmocr/mmocr/.mim/configs/textrecog/_base_/datasets/union14m_benchmark.py
+++ /dev/null
@@ -1,65 +0,0 @@
-union14m_root = '../data/Union14M-L/'
-union14m_benchmark_root = '../data/Union14M-L/Union14M-Benchmarks'
-
-union14m_benchmark_artistic = dict(
- type='OCRDataset',
- data_prefix=dict(img_path=f'{union14m_benchmark_root}/artistic'),
- ann_file=f'{union14m_benchmark_root}/artistic/annotation.json',
- test_mode=True,
- pipeline=None)
-
-union14m_benchmark_contextless = dict(
- type='OCRDataset',
- data_prefix=dict(img_path=f'{union14m_benchmark_root}/contextless'),
- ann_file=f'{union14m_benchmark_root}/contextless/annotation.json',
- test_mode=True,
- pipeline=None)
-
-union14m_benchmark_curve = dict(
- type='OCRDataset',
- data_prefix=dict(img_path=f'{union14m_benchmark_root}/curve'),
- ann_file=f'{union14m_benchmark_root}/curve/annotation.json',
- test_mode=True,
- pipeline=None)
-
-union14m_benchmark_incomplete = dict(
- type='OCRDataset',
- data_prefix=dict(img_path=f'{union14m_benchmark_root}/incomplete'),
- ann_file=f'{union14m_benchmark_root}/incomplete/annotation.json',
- test_mode=True,
- pipeline=None)
-
-union14m_benchmark_incomplete_ori = dict(
- type='OCRDataset',
- data_prefix=dict(img_path=f'{union14m_benchmark_root}/incomplete_ori'),
- ann_file=f'{union14m_benchmark_root}/incomplete_ori/annotation.json',
- test_mode=True,
- pipeline=None)
-
-union14m_benchmark_multi_oriented = dict(
- type='OCRDataset',
- data_prefix=dict(img_path=f'{union14m_benchmark_root}/multi_oriented'),
- ann_file=f'{union14m_benchmark_root}/multi_oriented/annotation.json',
- test_mode=True,
- pipeline=None)
-
-union14m_benchmark_multi_words = dict(
- type='OCRDataset',
- data_prefix=dict(img_path=f'{union14m_benchmark_root}/multi_words'),
- ann_file=f'{union14m_benchmark_root}/multi_words/annotation.json',
- test_mode=True,
- pipeline=None)
-
-union14m_benchmark_salient = dict(
- type='OCRDataset',
- data_prefix=dict(img_path=f'{union14m_benchmark_root}/salient'),
- ann_file=f'{union14m_benchmark_root}/salient/annotation.json',
- test_mode=True,
- pipeline=None)
-
-union14m_benchmark_general = dict(
- type='OCRDataset',
- data_prefix=dict(img_path=f'{union14m_root}/'),
- ann_file=f'{union14m_benchmark_root}/general/annotation.json',
- test_mode=True,
- pipeline=None)
diff --git a/pipeline/mmocr/mmocr/.mim/configs/textrecog/_base_/datasets/union14m_train.py b/pipeline/mmocr/mmocr/.mim/configs/textrecog/_base_/datasets/union14m_train.py
deleted file mode 100644
index e0ec77c07fc1aa2c7a9921e3693966a0818e3392..0000000000000000000000000000000000000000
--- a/pipeline/mmocr/mmocr/.mim/configs/textrecog/_base_/datasets/union14m_train.py
+++ /dev/null
@@ -1,38 +0,0 @@
-union14m_data_root = '../data/Union14M-L/'
-
-union14m_challenging = dict(
- type='OCRDataset',
- data_root=union14m_data_root,
- ann_file='train_annos/mmocr1.0/train_challenging.json',
- test_mode=True,
- pipeline=None)
-
-union14m_hard = dict(
- type='OCRDataset',
- data_root=union14m_data_root,
- ann_file='train_annos/mmocr1.0/train_hard.json',
- pipeline=None)
-
-union14m_medium = dict(
- type='OCRDataset',
- data_root=union14m_data_root,
- ann_file='train_annos/mmocr1.0/train_medium.json',
- pipeline=None)
-
-union14m_normal = dict(
- type='OCRDataset',
- data_root=union14m_data_root,
- ann_file='train_annos/mmocr1.0/train_normal.json',
- pipeline=None)
-
-union14m_easy = dict(
- type='OCRDataset',
- data_root=union14m_data_root,
- ann_file='train_annos/mmocr1.0/train_easy.json',
- pipeline=None)
-
-union14m_val = dict(
- type='OCRDataset',
- data_root=union14m_data_root,
- ann_file='train_annos/mmocr1.0/val_annos.json',
- pipeline=None)
diff --git a/pipeline/mmocr/mmocr/.mim/configs/textrecog/_base_/default_runtime.py b/pipeline/mmocr/mmocr/.mim/configs/textrecog/_base_/default_runtime.py
deleted file mode 100644
index f3ce4e1a43a0811db084ccfdc6787761fb62b13b..0000000000000000000000000000000000000000
--- a/pipeline/mmocr/mmocr/.mim/configs/textrecog/_base_/default_runtime.py
+++ /dev/null
@@ -1,50 +0,0 @@
-default_scope = 'mmocr'
-env_cfg = dict(
- cudnn_benchmark=False,
- mp_cfg=dict(mp_start_method='fork', opencv_num_threads=0),
- dist_cfg=dict(backend='nccl'),
-)
-randomness = dict(seed=None)
-
-default_hooks = dict(
- timer=dict(type='IterTimerHook'),
- logger=dict(type='LoggerHook', interval=100),
- param_scheduler=dict(type='ParamSchedulerHook'),
- checkpoint=dict(type='CheckpointHook', interval=1),
- sampler_seed=dict(type='DistSamplerSeedHook'),
- sync_buffer=dict(type='SyncBuffersHook'),
- visualization=dict(
- type='VisualizationHook',
- interval=1,
- enable=False,
- show=False,
- draw_gt=False,
- draw_pred=False),
-)
-# Logging
-log_level = 'INFO'
-log_processor = dict(type='LogProcessor', window_size=10, by_epoch=True)
-
-load_from = None
-resume = False
-
-# Evaluation
-val_evaluator = dict(
- type='MultiDatasetsEvaluator',
- metrics=[
- dict(
- type='WordMetric',
- mode=['exact', 'ignore_case', 'ignore_case_symbol']),
- dict(type='CharMetric')
- ],
- dataset_prefixes=None)
-test_evaluator = val_evaluator
-
-# Visualization
-vis_backends = [dict(type='LocalVisBackend')]
-visualizer = dict(
- type='TextRecogLocalVisualizer',
- name='visualizer',
- vis_backends=vis_backends)
-
-tta_model = dict(type='EncoderDecoderRecognizerTTAModel')
diff --git a/pipeline/mmocr/mmocr/.mim/configs/textrecog/_base_/schedules/schedule_adadelta_5e.py b/pipeline/mmocr/mmocr/.mim/configs/textrecog/_base_/schedules/schedule_adadelta_5e.py
deleted file mode 100644
index 465072eb3746670a6bfe5077733a5cd8bf635766..0000000000000000000000000000000000000000
--- a/pipeline/mmocr/mmocr/.mim/configs/textrecog/_base_/schedules/schedule_adadelta_5e.py
+++ /dev/null
@@ -1,9 +0,0 @@
-optim_wrapper = dict(
- type='OptimWrapper', optimizer=dict(type='Adadelta', lr=1.0))
-train_cfg = dict(type='EpochBasedTrainLoop', max_epochs=5, val_interval=1)
-val_cfg = dict(type='ValLoop')
-test_cfg = dict(type='TestLoop')
-# learning rate
-param_scheduler = [
- dict(type='ConstantLR', factor=1.0),
-]
diff --git a/pipeline/mmocr/mmocr/.mim/configs/textrecog/_base_/schedules/schedule_adam_base.py b/pipeline/mmocr/mmocr/.mim/configs/textrecog/_base_/schedules/schedule_adam_base.py
deleted file mode 100644
index 744f32858e0fdf2722472e3f467444f5ffdd9577..0000000000000000000000000000000000000000
--- a/pipeline/mmocr/mmocr/.mim/configs/textrecog/_base_/schedules/schedule_adam_base.py
+++ /dev/null
@@ -1,13 +0,0 @@
-# Note: This schedule config serves as a base config for other schedules.
-# Users would have to at least fill in "max_epochs" and "val_interval"
-# in order to use this config in their experiments.
-
-# optimizer
-optim_wrapper = dict(type='OptimWrapper', optimizer=dict(type='Adam', lr=3e-4))
-train_cfg = dict(type='EpochBasedTrainLoop', max_epochs=None, val_interval=1)
-val_cfg = dict(type='ValLoop')
-test_cfg = dict(type='TestLoop')
-# learning policy
-param_scheduler = [
- dict(type='ConstantLR', factor=1.0),
-]
diff --git a/pipeline/mmocr/mmocr/.mim/configs/textrecog/_base_/schedules/schedule_adam_step_5e.py b/pipeline/mmocr/mmocr/.mim/configs/textrecog/_base_/schedules/schedule_adam_step_5e.py
deleted file mode 100644
index 73aad763608c78fa5c818ddc557b12f9f34056c8..0000000000000000000000000000000000000000
--- a/pipeline/mmocr/mmocr/.mim/configs/textrecog/_base_/schedules/schedule_adam_step_5e.py
+++ /dev/null
@@ -1,9 +0,0 @@
-# optimizer
-optim_wrapper = dict(type='OptimWrapper', optimizer=dict(type='Adam', lr=1e-3))
-train_cfg = dict(type='EpochBasedTrainLoop', max_epochs=5, val_interval=1)
-val_cfg = dict(type='ValLoop')
-test_cfg = dict(type='TestLoop')
-# learning policy
-param_scheduler = [
- dict(type='MultiStepLR', milestones=[3, 4], end=5),
-]
diff --git a/pipeline/mmocr/mmocr/.mim/configs/textrecog/_base_/schedules/schedule_adamw_cos_10e.py b/pipeline/mmocr/mmocr/.mim/configs/textrecog/_base_/schedules/schedule_adamw_cos_10e.py
deleted file mode 100644
index a497104e95822424cbbae45f9d587d260351346b..0000000000000000000000000000000000000000
--- a/pipeline/mmocr/mmocr/.mim/configs/textrecog/_base_/schedules/schedule_adamw_cos_10e.py
+++ /dev/null
@@ -1,21 +0,0 @@
-# optimizer
-optim_wrapper = dict(
- type='OptimWrapper',
- optimizer=dict(
- type='AdamW',
- lr=1e-4,
- betas=(0.9, 0.999),
- eps=1e-08,
- weight_decay=0.01))
-train_cfg = dict(type='EpochBasedTrainLoop', max_epochs=10, val_interval=1)
-val_cfg = dict(type='ValLoop')
-test_cfg = dict(type='TestLoop')
-
-# learning policy
-param_scheduler = [
- dict(
- type='CosineAnnealingLR',
- T_max=10,
- eta_min=1e-8,
- convert_to_iter_based=True)
-]
diff --git a/pipeline/mmocr/mmocr/.mim/configs/textrecog/_base_/schedules/schedule_adamw_cos_6e.py b/pipeline/mmocr/mmocr/.mim/configs/textrecog/_base_/schedules/schedule_adamw_cos_6e.py
deleted file mode 100644
index cd9d29323583c5db51fa3fc8aba2e2aa3a0ed618..0000000000000000000000000000000000000000
--- a/pipeline/mmocr/mmocr/.mim/configs/textrecog/_base_/schedules/schedule_adamw_cos_6e.py
+++ /dev/null
@@ -1,21 +0,0 @@
-# optimizer
-optim_wrapper = dict(
- type='OptimWrapper',
- optimizer=dict(
- type='AdamW',
- lr=4e-4,
- betas=(0.9, 0.999),
- eps=1e-08,
- weight_decay=0.05))
-train_cfg = dict(type='EpochBasedTrainLoop', max_epochs=6, val_interval=1)
-val_cfg = dict(type='ValLoop')
-test_cfg = dict(type='TestLoop')
-
-# learning policy
-param_scheduler = [
- dict(
- type='CosineAnnealingLR',
- T_max=6,
- eta_min=4e-6,
- convert_to_iter_based=True)
-]
diff --git a/pipeline/mmocr/mmocr/.mim/configs/textrecog/abinet/README.md b/pipeline/mmocr/mmocr/.mim/configs/textrecog/abinet/README.md
deleted file mode 100644
index 6a7faadb37d17699123c15184d63f7afcb73dc55..0000000000000000000000000000000000000000
--- a/pipeline/mmocr/mmocr/.mim/configs/textrecog/abinet/README.md
+++ /dev/null
@@ -1,59 +0,0 @@
-# ABINet
-
-> [Read Like Humans: Autonomous, Bidirectional and Iterative Language Modeling for Scene Text Recognition](https://arxiv.org/abs/2103.06495)
-
-
-
-## Abstract
-
-Linguistic knowledge is of great benefit to scene text recognition. However, how to effectively model linguistic rules in end-to-end deep networks remains a research challenge. In this paper, we argue that the limited capacity of language models comes from: 1) implicitly language modeling; 2) unidirectional feature representation; and 3) language model with noise input. Correspondingly, we propose an autonomous, bidirectional and iterative ABINet for scene text recognition. Firstly, the autonomous suggests to block gradient flow between vision and language models to enforce explicitly language modeling. Secondly, a novel bidirectional cloze network (BCN) as the language model is proposed based on bidirectional feature representation. Thirdly, we propose an execution manner of iterative correction for language model which can effectively alleviate the impact of noise input. Additionally, based on the ensemble of iterative predictions, we propose a self-training method which can learn from unlabeled images effectively. Extensive experiments indicate that ABINet has superiority on low-quality images and achieves state-of-the-art results on several mainstream benchmarks. Besides, the ABINet trained with ensemble self-training shows promising improvement in realizing human-level recognition.
-
-
-
-
-
-## Dataset
-
-### Train Dataset
-
-| trainset | instance_num | repeat_num | note |
-| :-------: | :----------: | :--------: | :----------: |
-| Syn90k | 8919273 | 1 | synth |
-| SynthText | 7239272 | 1 | alphanumeric |
-
-### Test Dataset
-
-| testset | instance_num | note |
-| :-----: | :----------: | :-------: |
-| IIIT5K | 3000 | regular |
-| SVT | 647 | regular |
-| IC13 | 1015 | regular |
-| IC15 | 2077 | irregular |
-| SVTP | 645 | irregular |
-| CT80 | 288 | irregular |
-
-## Results and models
-
-| methods | pretrained | | Regular Text | | | Irregular Text | | download |
-| :--------------------------------------------: | :------------------------------------------------: | :----: | :----------: | :-------: | :-------: | :------------: | :----: | :----------------------------------------------- |
-| | | IIIT5K | SVT | IC13-1015 | IC15-2077 | SVTP | CT80 | |
-| [ABINet-Vision](/configs/textrecog/abinet/abinet-vision_20e_st-an_mj.py) | - | 0.9523 | 0.9196 | 0.9369 | 0.7896 | 0.8403 | 0.8437 | [model](https://download.openmmlab.com/mmocr/textrecog/abinet/abinet-vision_20e_st-an_mj/abinet-vision_20e_st-an_mj_20220915_152445-85cfb03d.pth) \| [log](https://download.openmmlab.com/mmocr/textrecog/abinet/abinet-vision_20e_st-an_mj/20220915_152445.log) |
-| [ABINet-Vision-TTA](/configs/textrecog/abinet/abinet-vision_20e_st-an_mj.py) | - | 0.9523 | 0.9196 | 0.9360 | 0.8175 | 0.8450 | 0.8542 | |
-| [ABINet](/configs/textrecog/abinet/abinet_20e_st-an_mj.py) | [Pretrained](https://download.openmmlab.com/mmocr/textrecog/abinet/abinet_pretrain-45deac15.pth) | 0.9603 | 0.9397 | 0.9557 | 0.8146 | 0.8868 | 0.8785 | [model](https://download.openmmlab.com/mmocr/textrecog/abinet/abinet_20e_st-an_mj/abinet_20e_st-an_mj_20221005_012617-ead8c139.pth) \| [log](https://download.openmmlab.com/mmocr/textrecog/abinet/abinet_20e_st-an_mj/20221005_012617.log) |
-| [ABINet-TTA](/configs/textrecog/abinet/abinet_20e_st-an_mj.py) | [Pretrained](https://download.openmmlab.com/mmocr/textrecog/abinet/abinet_pretrain-45deac15.pth) | 0.9597 | 0.9397 | 0.9527 | 0.8426 | 0.8930 | 0.8854 | |
-
-```{note}
-1. ABINet allows its encoder to run and be trained without decoder and fuser. Its encoder is designed to recognize texts as a stand-alone model and therefore can work as an independent text recognizer. We release it as ABINet-Vision.
-2. Facts about the pretrained model: MMOCR does not have a systematic pipeline to pretrain the language model (LM) yet, thus the weights of LM are converted from [the official pretrained model](https://github.com/FangShancheng/ABINet). The weights of ABINet-Vision are directly used as the vision model of ABINet.
-```
-
-## Citation
-
-```bibtex
-@article{fang2021read,
- title={Read Like Humans: Autonomous, Bidirectional and Iterative Language Modeling for Scene Text Recognition},
- author={Fang, Shancheng and Xie, Hongtao and Wang, Yuxin and Mao, Zhendong and Zhang, Yongdong},
- booktitle={Proceedings of the IEEE/CVF Conference on Computer Vision and Pattern Recognition},
- year={2021}
-}
-```
diff --git a/pipeline/mmocr/mmocr/.mim/configs/textrecog/abinet/_base_abinet-vision.py b/pipeline/mmocr/mmocr/.mim/configs/textrecog/abinet/_base_abinet-vision.py
deleted file mode 100644
index b43736b413990fda8ea421e55956d329063ef98c..0000000000000000000000000000000000000000
--- a/pipeline/mmocr/mmocr/.mim/configs/textrecog/abinet/_base_abinet-vision.py
+++ /dev/null
@@ -1,159 +0,0 @@
-dictionary = dict(
- type='Dictionary',
- dict_file='{{ fileDirname }}/../../../dicts/lower_english_digits.txt',
- with_start=True,
- with_end=True,
- same_start_end=True,
- with_padding=False,
- with_unknown=False)
-
-model = dict(
- type='ABINet',
- backbone=dict(type='ResNetABI'),
- encoder=dict(
- type='ABIEncoder',
- n_layers=3,
- n_head=8,
- d_model=512,
- d_inner=2048,
- dropout=0.1,
- max_len=8 * 32,
- ),
- decoder=dict(
- type='ABIFuser',
- vision_decoder=dict(
- type='ABIVisionDecoder',
- in_channels=512,
- num_channels=64,
- attn_height=8,
- attn_width=32,
- attn_mode='nearest',
- init_cfg=dict(type='Xavier', layer='Conv2d')),
- module_loss=dict(type='ABIModuleLoss', letter_case='lower'),
- postprocessor=dict(type='AttentionPostprocessor'),
- dictionary=dictionary,
- max_seq_len=26,
- ),
- data_preprocessor=dict(
- type='TextRecogDataPreprocessor',
- mean=[123.675, 116.28, 103.53],
- std=[58.395, 57.12, 57.375]))
-
-train_pipeline = [
- dict(type='LoadImageFromFile', ignore_empty=True, min_size=2),
- dict(type='LoadOCRAnnotations', with_text=True),
- dict(type='Resize', scale=(128, 32)),
- dict(
- type='RandomApply',
- prob=0.5,
- transforms=[
- dict(
- type='RandomChoice',
- transforms=[
- dict(
- type='RandomRotate',
- max_angle=15,
- ),
- dict(
- type='TorchVisionWrapper',
- op='RandomAffine',
- degrees=15,
- translate=(0.3, 0.3),
- scale=(0.5, 2.),
- shear=(-45, 45),
- ),
- dict(
- type='TorchVisionWrapper',
- op='RandomPerspective',
- distortion_scale=0.5,
- p=1,
- ),
- ])
- ],
- ),
- dict(
- type='RandomApply',
- prob=0.25,
- transforms=[
- dict(type='PyramidRescale'),
- dict(
- type='mmdet.Albu',
- transforms=[
- dict(type='GaussNoise', var_limit=(20, 20), p=0.5),
- dict(type='MotionBlur', blur_limit=7, p=0.5),
- ]),
- ]),
- dict(
- type='RandomApply',
- prob=0.25,
- transforms=[
- dict(
- type='TorchVisionWrapper',
- op='ColorJitter',
- brightness=0.5,
- saturation=0.5,
- contrast=0.5,
- hue=0.1),
- ]),
- dict(
- type='PackTextRecogInputs',
- meta_keys=('img_path', 'ori_shape', 'img_shape', 'valid_ratio'))
-]
-
-test_pipeline = [
- dict(type='LoadImageFromFile'),
- dict(type='Resize', scale=(128, 32)),
- # add loading annotation after ``Resize`` because ground truth
- # does not need to do resize data transform
- dict(type='LoadOCRAnnotations', with_text=True),
- dict(
- type='PackTextRecogInputs',
- meta_keys=('img_path', 'ori_shape', 'img_shape', 'valid_ratio'))
-]
-
-tta_pipeline = [
- dict(type='LoadImageFromFile'),
- dict(
- type='TestTimeAug',
- transforms=[
- [
- dict(
- type='ConditionApply',
- true_transforms=[
- dict(
- type='ImgAugWrapper',
- args=[dict(cls='Rot90', k=0, keep_size=False)])
- ],
- condition="results['img_shape'][1] [ASTER: An Attentional Scene Text Recognizer with Flexible Rectification](https://ieeexplore.ieee.org/abstract/document/8395027/)
-
-
-
-## Abstract
-
-A challenging aspect of scene text recognition is to handle text with distortions or irregular layout. In particular, perspective text and curved text are common in natural scenes and are difficult to recognize. In this work, we introduce ASTER, an end-to-end neural network model that comprises a rectification network and a recognition network. The rectification network adaptively transforms an input image into a new one, rectifying the text in it. It is powered by a flexible Thin-Plate Spline transformation which handles a variety of text irregularities and is trained without human annotations. The recognition network is an attentional sequence-to-sequence model that predicts a character sequence directly from the rectified image. The whole model is trained end to end, requiring only images and their groundtruth text. Through extensive experiments, we verify the effectiveness of the rectification and demonstrate the state-of-the-art recognition performance of ASTER. Furthermore, we demonstrate that ASTER is a powerful component in end-to-end recognition systems, for its ability to enhance the detector.
-
-
-
-
-
-## Dataset
-
-### Train Dataset
-
-| trainset | instance_num | repeat_num | note |
-| :-------: | :----------: | :--------: | :----------: |
-| Syn90k | 8919273 | 1 | synth |
-| SynthText | 7239272 | 1 | alphanumeric |
-
-### Test Dataset
-
-| testset | instance_num | note |
-| :-----: | :----------: | :-------: |
-| IIIT5K | 3000 | regular |
-| SVT | 647 | regular |
-| IC13 | 1015 | regular |
-| IC15 | 2077 | irregular |
-| SVTP | 645 | irregular |
-| CT80 | 288 | irregular |
-
-## Results and models
-
-| Methods | Backbone | | Regular Text | | | | Irregular Text | | download |
-| :--------------------------------------------------------------: | :------: | :----: | :----------: | :-------: | :-: | :-------: | :------------: | :----: | :-------------------------------------------------------------------: |
-| | | IIIT5K | SVT | IC13-1015 | | IC15-2077 | SVTP | CT80 | |
-| [ASTER](/configs/textrecog/aster/aster_resnet45_6e_st_mj.py) | ResNet45 | 0.9357 | 0.8949 | 0.9281 | | 0.7665 | 0.8062 | 0.8507 | [model](https://download.openmmlab.com/mmocr/textrecog/aster/aster_resnet45_6e_st_mj/aster_resnet45_6e_st_mj-cc56eca4.pth) \| [log](https://download.openmmlab.com/mmocr/textrecog/aster/aster_resnet45_6e_st_mj/20221214_232605.log) |
-| [ASTER-TTA](/configs/textrecog/aster/aster_resnet45_6e_st_mj.py) | ResNet45 | 0.9337 | 0.8949 | 0.9251 | | 0.7925 | 0.8109 | 0.8507 | |
-
-## Citation
-
-```bibtex
-@article{shi2018aster,
- title={Aster: An attentional scene text recognizer with flexible rectification},
- author={Shi, Baoguang and Yang, Mingkun and Wang, Xinggang and Lyu, Pengyuan and Yao, Cong and Bai, Xiang},
- journal={IEEE transactions on pattern analysis and machine intelligence},
- volume={41},
- number={9},
- pages={2035--2048},
- year={2018},
- publisher={IEEE}
-}
-```
diff --git a/pipeline/mmocr/mmocr/.mim/configs/textrecog/aster/_base_aster.py b/pipeline/mmocr/mmocr/.mim/configs/textrecog/aster/_base_aster.py
deleted file mode 100644
index 5f011522ca9858484d1633e67fc14c4f91fdaf9f..0000000000000000000000000000000000000000
--- a/pipeline/mmocr/mmocr/.mim/configs/textrecog/aster/_base_aster.py
+++ /dev/null
@@ -1,104 +0,0 @@
-dictionary = dict(
- type='Dictionary',
- dict_file='{{ fileDirname }}/../../../dicts/english_digits_symbols.txt',
- with_padding=True,
- with_unknown=True,
- same_start_end=True,
- with_start=True,
- with_end=True)
-
-model = dict(
- type='ASTER',
- preprocessor=dict(
- type='STN',
- in_channels=3,
- resized_image_size=(32, 64),
- output_image_size=(32, 100),
- num_control_points=20),
- backbone=dict(
- type='ResNet',
- in_channels=3,
- stem_channels=[32],
- block_cfgs=dict(type='BasicBlock', use_conv1x1='True'),
- arch_layers=[3, 4, 6, 6, 3],
- arch_channels=[32, 64, 128, 256, 512],
- strides=[(2, 2), (2, 2), (2, 1), (2, 1), (2, 1)],
- init_cfg=[
- dict(type='Kaiming', layer='Conv2d'),
- dict(type='Constant', val=1, layer='BatchNorm2d'),
- ]),
- encoder=dict(type='ASTEREncoder', in_channels=512),
- decoder=dict(
- type='ASTERDecoder',
- max_seq_len=25,
- in_channels=512,
- emb_dims=512,
- attn_dims=512,
- hidden_size=512,
- postprocessor=dict(type='AttentionPostprocessor'),
- module_loss=dict(
- type='CEModuleLoss', flatten=True, ignore_first_char=True),
- dictionary=dictionary,
- ),
- data_preprocessor=dict(
- type='TextRecogDataPreprocessor',
- mean=[127.5, 127.5, 127.5],
- std=[127.5, 127.5, 127.5]))
-
-train_pipeline = [
- dict(type='LoadImageFromFile', ignore_empty=True, min_size=0),
- dict(type='LoadOCRAnnotations', with_text=True),
- dict(type='Resize', scale=(256, 64)),
- dict(
- type='PackTextRecogInputs',
- meta_keys=('img_path', 'ori_shape', 'img_shape', 'valid_ratio'))
-]
-
-test_pipeline = [
- dict(type='LoadImageFromFile'),
- dict(type='Resize', scale=(256, 64)),
- dict(type='LoadOCRAnnotations', with_text=True),
- dict(
- type='PackTextRecogInputs',
- meta_keys=('img_path', 'ori_shape', 'img_shape', 'valid_ratio',
- 'instances'))
-]
-
-tta_pipeline = [
- dict(type='LoadImageFromFile'),
- dict(
- type='TestTimeAug',
- transforms=[[
- dict(
- type='ConditionApply',
- true_transforms=[
- dict(
- type='ImgAugWrapper',
- args=[dict(cls='Rot90', k=0, keep_size=False)])
- ],
- condition="results['img_shape'][1] [An end-to-end trainable neural network for image-based sequence recognition and its application to scene text recognition](https://arxiv.org/abs/1507.05717)
-
-
-
-## Abstract
-
-Image-based sequence recognition has been a long-standing research topic in computer vision. In this paper, we investigate the problem of scene text recognition, which is among the most important and challenging tasks in image-based sequence recognition. A novel neural network architecture, which integrates feature extraction, sequence modeling and transcription into a unified framework, is proposed. Compared with previous systems for scene text recognition, the proposed architecture possesses four distinctive properties: (1) It is end-to-end trainable, in contrast to most of the existing algorithms whose components are separately trained and tuned. (2) It naturally handles sequences in arbitrary lengths, involving no character segmentation or horizontal scale normalization. (3) It is not confined to any predefined lexicon and achieves remarkable performances in both lexicon-free and lexicon-based scene text recognition tasks. (4) It generates an effective yet much smaller model, which is more practical for real-world application scenarios. The experiments on standard benchmarks, including the IIIT-5K, Street View Text and ICDAR datasets, demonstrate the superiority of the proposed algorithm over the prior arts. Moreover, the proposed algorithm performs well in the task of image-based music score recognition, which evidently verifies the generality of it.
-
-
-
-
-
-## Dataset
-
-### Train Dataset
-
-| trainset | instance_num | repeat_num | note |
-| :------: | :----------: | :--------: | :---: |
-| Syn90k | 8919273 | 1 | synth |
-
-### Test Dataset
-
-| testset | instance_num | note |
-| :-----: | :----------: | :-------: |
-| IIIT5K | 3000 | regular |
-| SVT | 647 | regular |
-| IC13 | 1015 | regular |
-| IC15 | 2077 | irregular |
-| SVTP | 645 | irregular |
-| CT80 | 288 | irregular |
-
-## Results and models
-
-| methods | | Regular Text | | | | Irregular Text | | download |
-| :--------------------------------------------------------: | :----: | :----------: | :-------: | :-: | :-------: | :------------: | :----: | :---------------------------------------------------------------------------------: |
-| methods | IIIT5K | SVT | IC13-1015 | | IC15-2077 | SVTP | CT80 | |
-| [CRNN](/configs/textrecog/crnn/crnn_mini-vgg_5e_mj.py) | 0.8053 | 0.7991 | 0.8739 | | 0.5571 | 0.6093 | 0.5694 | [model](https://download.openmmlab.com/mmocr/textrecog/crnn/crnn_mini-vgg_5e_mj/crnn_mini-vgg_5e_mj_20220826_224120-8afbedbb.pth) \| [log](https://download.openmmlab.com/mmocr/textrecog/crnn/crnn_mini-vgg_5e_mj/20220826_224120.log) |
-| [CRNN-TTA](/configs/textrecog/crnn/crnn_mini-vgg_5e_mj.py) | 0.8013 | 0.7975 | 0.8631 | | 0.5763 | 0.6093 | 0.5764 | [model](https://download.openmmlab.com/mmocr/textrecog/crnn/crnn_mini-vgg_5e_mj/crnn_mini-vgg_5e_mj_20220826_224120-8afbedbb.pth) \| [log](https://download.openmmlab.com/mmocr/textrecog/crnn/crnn_mini-vgg_5e_mj/20220826_224120.log) |
-
-## Citation
-
-```bibtex
-@article{shi2016end,
- title={An end-to-end trainable neural network for image-based sequence recognition and its application to scene text recognition},
- author={Shi, Baoguang and Bai, Xiang and Yao, Cong},
- journal={IEEE transactions on pattern analysis and machine intelligence},
- year={2016}
-}
-```
diff --git a/pipeline/mmocr/mmocr/.mim/configs/textrecog/crnn/_base_crnn_mini-vgg.py b/pipeline/mmocr/mmocr/.mim/configs/textrecog/crnn/_base_crnn_mini-vgg.py
deleted file mode 100644
index 794bd63b120610004ac03239b1443114e871b805..0000000000000000000000000000000000000000
--- a/pipeline/mmocr/mmocr/.mim/configs/textrecog/crnn/_base_crnn_mini-vgg.py
+++ /dev/null
@@ -1,102 +0,0 @@
-dictionary = dict(
- type='Dictionary',
- dict_file='{{ fileDirname }}/../../../dicts/lower_english_digits.txt',
- with_padding=True)
-
-model = dict(
- type='CRNN',
- preprocessor=None,
- backbone=dict(type='MiniVGG', leaky_relu=False, input_channels=1),
- encoder=None,
- decoder=dict(
- type='CRNNDecoder',
- in_channels=512,
- rnn_flag=True,
- module_loss=dict(type='CTCModuleLoss', letter_case='lower'),
- postprocessor=dict(type='CTCPostProcessor'),
- dictionary=dictionary),
- data_preprocessor=dict(
- type='TextRecogDataPreprocessor', mean=[127], std=[127]))
-
-train_pipeline = [
- dict(
- type='LoadImageFromFile',
- color_type='grayscale',
- ignore_empty=True,
- min_size=2),
- dict(type='LoadOCRAnnotations', with_text=True),
- dict(type='Resize', scale=(100, 32), keep_ratio=False),
- dict(
- type='PackTextRecogInputs',
- meta_keys=('img_path', 'ori_shape', 'img_shape', 'valid_ratio'))
-]
-
-test_pipeline = [
- dict(type='LoadImageFromFile', color_type='grayscale'),
- dict(
- type='RescaleToHeight',
- height=32,
- min_width=32,
- max_width=None,
- width_divisor=16),
- # add loading annotation after ``Resize`` because ground truth
- # does not need to do resize data transform
- dict(type='LoadOCRAnnotations', with_text=True),
- dict(
- type='PackTextRecogInputs',
- meta_keys=('img_path', 'ori_shape', 'img_shape', 'valid_ratio'))
-]
-
-tta_pipeline = [
- dict(type='LoadImageFromFile', color_type='grayscale'),
- dict(
- type='TestTimeAug',
- transforms=[
- [
- dict(
- type='ConditionApply',
- true_transforms=[
- dict(
- type='ImgAugWrapper',
- args=[dict(cls='Rot90', k=0, keep_size=False)])
- ],
- condition="results['img_shape'][1] [MASTER: Multi-aspect non-local network for scene text recognition](https://arxiv.org/abs/1910.02562)
-
-
-
-## Abstract
-
-Attention-based scene text recognizers have gained huge success, which leverages a more compact intermediate representation to learn 1d- or 2d- attention by a RNN-based encoder-decoder architecture. However, such methods suffer from attention-drift problem because high similarity among encoded features leads to attention confusion under the RNN-based local attention mechanism. Moreover, RNN-based methods have low efficiency due to poor parallelization. To overcome these problems, we propose the MASTER, a self-attention based scene text recognizer that (1) not only encodes the input-output attention but also learns self-attention which encodes feature-feature and target-target relationships inside the encoder and decoder and (2) learns a more powerful and robust intermediate representation to spatial distortion, and (3) owns a great training efficiency because of high training parallelization and a high-speed inference because of an efficient memory-cache mechanism. Extensive experiments on various benchmarks demonstrate the superior performance of our MASTER on both regular and irregular scene text.
-
-
-
-
-
-## Dataset
-
-### Train Dataset
-
-| trainset | instance_num | repeat_num | source |
-| :-------: | :----------: | :--------: | :----: |
-| SynthText | 7266686 | 1 | synth |
-| SynthAdd | 1216889 | 1 | synth |
-| Syn90k | 8919273 | 1 | synth |
-
-### Test Dataset
-
-| testset | instance_num | type |
-| :-----: | :----------: | :-------: |
-| IIIT5K | 3000 | regular |
-| SVT | 647 | regular |
-| IC13 | 1015 | regular |
-| IC15 | 2077 | irregular |
-| SVTP | 645 | irregular |
-| CT80 | 288 | irregular |
-
-## Results and Models
-
-| Methods | Backbone | | Regular Text | | | | Irregular Text | | download |
-| :-------------------------------------------------------------: | :-----------: | :----: | :----------: | :-------: | :-: | :-------: | :------------: | :----: | :---------------------------------------------------------------: |
-| | | IIIT5K | SVT | IC13-1015 | | IC15-2077 | SVTP | CT80 | |
-| [MASTER](/configs/textrecog/master/master_resnet31_12e_st_mj_sa.py) | R31-GCAModule | 0.9490 | 0.8887 | 0.9517 | | 0.7650 | 0.8465 | 0.8889 | [model](https://download.openmmlab.com/mmocr/textrecog/master/master_resnet31_12e_st_mj_sa/master_resnet31_12e_st_mj_sa_20220915_152443-f4a5cabc.pth) \| [log](https://download.openmmlab.com/mmocr/textrecog/master/master_resnet31_12e_st_mj_sa/20220915_152443.log) |
-| [MASTER-TTA](/configs/textrecog/master/master_resnet31_12e_st_mj_sa.py) | R31-GCAModule | 0.9450 | 0.8887 | 0.9478 | | 0.7906 | 0.8481 | 0.8958 | |
-
-## Citation
-
-```bibtex
-@article{Lu2021MASTER,
- title={MASTER: Multi-Aspect Non-local Network for Scene Text Recognition},
- author={Ning Lu and Wenwen Yu and Xianbiao Qi and Yihao Chen and Ping Gong and Rong Xiao and Xiang Bai},
- journal={Pattern Recognition},
- year={2021}
-}
-```
diff --git a/pipeline/mmocr/mmocr/.mim/configs/textrecog/master/_base_master_resnet31.py b/pipeline/mmocr/mmocr/.mim/configs/textrecog/master/_base_master_resnet31.py
deleted file mode 100644
index 7214d2fbf03d92614dc129e4b74e02b23d64e8c5..0000000000000000000000000000000000000000
--- a/pipeline/mmocr/mmocr/.mim/configs/textrecog/master/_base_master_resnet31.py
+++ /dev/null
@@ -1,160 +0,0 @@
-dictionary = dict(
- type='Dictionary',
- dict_file='{{ fileDirname }}/../../../dicts/english_digits_symbols.txt',
- with_padding=True,
- with_unknown=True,
- same_start_end=True,
- with_start=True,
- with_end=True)
-
-model = dict(
- type='MASTER',
- backbone=dict(
- type='ResNet',
- in_channels=3,
- stem_channels=[64, 128],
- block_cfgs=dict(
- type='BasicBlock',
- plugins=dict(
- cfg=dict(
- type='GCAModule',
- ratio=0.0625,
- n_head=1,
- pooling_type='att',
- is_att_scale=False,
- fusion_type='channel_add'),
- position='after_conv2')),
- arch_layers=[1, 2, 5, 3],
- arch_channels=[256, 256, 512, 512],
- strides=[1, 1, 1, 1],
- plugins=[
- dict(
- cfg=dict(type='Maxpool2d', kernel_size=2, stride=(2, 2)),
- stages=(True, True, False, False),
- position='before_stage'),
- dict(
- cfg=dict(type='Maxpool2d', kernel_size=(2, 1), stride=(2, 1)),
- stages=(False, False, True, False),
- position='before_stage'),
- dict(
- cfg=dict(
- type='ConvModule',
- kernel_size=3,
- stride=1,
- padding=1,
- norm_cfg=dict(type='BN'),
- act_cfg=dict(type='ReLU')),
- stages=(True, True, True, True),
- position='after_stage')
- ],
- init_cfg=[
- dict(type='Kaiming', layer='Conv2d'),
- dict(type='Constant', val=1, layer='BatchNorm2d'),
- ]),
- encoder=None,
- decoder=dict(
- type='MasterDecoder',
- d_model=512,
- n_head=8,
- attn_drop=0.,
- ffn_drop=0.,
- d_inner=2048,
- n_layers=3,
- feat_pe_drop=0.2,
- feat_size=6 * 40,
- postprocessor=dict(type='AttentionPostprocessor'),
- module_loss=dict(
- type='CEModuleLoss', reduction='mean', ignore_first_char=True),
- max_seq_len=30,
- dictionary=dictionary),
- data_preprocessor=dict(
- type='TextRecogDataPreprocessor',
- mean=[127.5, 127.5, 127.5],
- std=[127.5, 127.5, 127.5]))
-
-train_pipeline = [
- dict(type='LoadImageFromFile', ignore_empty=True, min_size=2),
- dict(type='LoadOCRAnnotations', with_text=True),
- dict(
- type='RescaleToHeight',
- height=48,
- min_width=48,
- max_width=160,
- width_divisor=16),
- dict(type='PadToWidth', width=160),
- dict(
- type='PackTextRecogInputs',
- meta_keys=('img_path', 'ori_shape', 'img_shape', 'valid_ratio'))
-]
-
-test_pipeline = [
- dict(type='LoadImageFromFile'),
- dict(
- type='RescaleToHeight',
- height=48,
- min_width=48,
- max_width=160,
- width_divisor=16),
- dict(type='PadToWidth', width=160),
- # add loading annotation after ``Resize`` because ground truth
- # does not need to do resize data transform
- dict(type='LoadOCRAnnotations', with_text=True),
- dict(
- type='PackTextRecogInputs',
- meta_keys=('img_path', 'ori_shape', 'img_shape', 'valid_ratio'))
-]
-
-tta_pipeline = [
- dict(type='LoadImageFromFile'),
- dict(
- type='TestTimeAug',
- transforms=[
- [
- dict(
- type='ConditionApply',
- true_transforms=[
- dict(
- type='ImgAugWrapper',
- args=[dict(cls='Rot90', k=0, keep_size=False)])
- ],
- condition="results['img_shape'][1] [NRTR: A No-Recurrence Sequence-to-Sequence Model For Scene Text Recognition](https://arxiv.org/abs/1806.00926)
-
-
-
-## Abstract
-
-Scene text recognition has attracted a great many researches due to its importance to various applications. Existing methods mainly adopt recurrence or convolution based networks. Though have obtained good performance, these methods still suffer from two limitations: slow training speed due to the internal recurrence of RNNs, and high complexity due to stacked convolutional layers for long-term feature extraction. This paper, for the first time, proposes a no-recurrence sequence-to-sequence text recognizer, named NRTR, that dispenses with recurrences and convolutions entirely. NRTR follows the encoder-decoder paradigm, where the encoder uses stacked self-attention to extract image features, and the decoder applies stacked self-attention to recognize texts based on encoder output. NRTR relies solely on self-attention mechanism thus could be trained with more parallelization and less complexity. Considering scene image has large variation in text and background, we further design a modality-transform block to effectively transform 2D input images to 1D sequences, combined with the encoder to extract more discriminative features. NRTR achieves state-of-the-art or highly competitive performance on both regular and irregular benchmarks, while requires only a small fraction of training time compared to the best model from the literature (at least 8 times faster).
-
-
-
-
-
-## Dataset
-
-### Train Dataset
-
-| trainset | instance_num | repeat_num | source |
-| :-------: | :----------: | :--------: | :----: |
-| SynthText | 7266686 | 1 | synth |
-| Syn90k | 8919273 | 1 | synth |
-
-### Test Dataset
-
-| testset | instance_num | type |
-| :-----: | :----------: | :-------: |
-| IIIT5K | 3000 | regular |
-| SVT | 647 | regular |
-| IC13 | 1015 | regular |
-| IC15 | 2077 | irregular |
-| SVTP | 645 | irregular |
-| CT80 | 288 | irregular |
-
-## Results and Models
-
-| Methods | Backbone | | Regular Text | | | | Irregular Text | | download |
-| :---------------------------------------------------------: | :-------------------: | :----: | :----------: | :-------: | :-: | :-------: | :------------: | :----: | :-----------------------------------------------------------: |
-| | | IIIT5K | SVT | IC13-1015 | | IC15-2077 | SVTP | CT80 | |
-| [NRTR](/configs/textrecog/nrtr/nrtr_modality-transform_6e_st_mj.py) | NRTRModalityTransform | 0.9147 | 0.8841 | 0.9369 | | 0.7246 | 0.7783 | 0.7500 | [model](https://download.openmmlab.com/mmocr/textrecog/nrtr/nrtr_modality-transform_6e_st_mj/nrtr_modality-transform_6e_st_mj_20220916_103322-bd9425be.pth) \| [log](https://download.openmmlab.com/mmocr/textrecog/nrtr/nrtr_modality-transform_6e_st_mj/20220916_103322.log) |
-| [NRTR-TTA](/configs/textrecog/nrtr/nrtr_modality-transform_6e_st_mj.py) | NRTRModalityTransform | 0.9123 | 0.8825 | 0.9310 | | 0.7492 | 0.7798 | 0.7535 | |
-| [NRTR](/configs/textrecog/nrtr/nrtr_resnet31-1by8-1by4_6e_st_mj.py) | R31-1/8-1/4 | 0.9483 | 0.8918 | 0.9507 | | 0.7578 | 0.8016 | 0.8889 | [model](https://download.openmmlab.com/mmocr/textrecog/nrtr/nrtr_resnet31-1by8-1by4_6e_st_mj/nrtr_resnet31-1by8-1by4_6e_st_mj_20220916_103322-a6a2a123.pth) \| [log](https://download.openmmlab.com/mmocr/textrecog/nrtr/nrtr_resnet31-1by8-1by4_6e_st_mj/20220916_103322.log) |
-| [NRTR-TTA](/configs/textrecog/nrtr/nrtr_resnet31-1by8-1by4_6e_st_mj.py) | R31-1/8-1/4 | 0.9443 | 0.8903 | 0.9478 | | 0.7790 | 0.8078 | 0.8854 | |
-| [NRTR](/configs/textrecog/nrtr/nrtr_resnet31-1by16-1by8_6e_st_mj.py) | R31-1/16-1/8 | 0.9470 | 0.8918 | 0.9399 | | 0.7376 | 0.7969 | 0.8854 | [model](https://download.openmmlab.com/mmocr/textrecog/nrtr/nrtr_resnet31-1by16-1by8_6e_st_mj/nrtr_resnet31-1by16-1by8_6e_st_mj_20220920_143358-43767036.pth) \| [log](https://download.openmmlab.com/mmocr/textrecog/nrtr/nrtr_resnet31-1by16-1by8_6e_st_mj/20220920_143358.log) |
-| [NRTR-TTA](/configs/textrecog/nrtr/nrtr_resnet31-1by16-1by8_6e_st_mj.py) | R31-1/16-1/8 | 0.9423 | 0.8903 | 0.9360 | | 0.7641 | 0.8016 | 0.8854 | |
-
-## Citation
-
-```bibtex
-@inproceedings{sheng2019nrtr,
- title={NRTR: A no-recurrence sequence-to-sequence model for scene text recognition},
- author={Sheng, Fenfen and Chen, Zhineng and Xu, Bo},
- booktitle={2019 International Conference on Document Analysis and Recognition (ICDAR)},
- pages={781--786},
- year={2019},
- organization={IEEE}
-}
-```
diff --git a/pipeline/mmocr/mmocr/.mim/configs/textrecog/nrtr/_base_nrtr_modality-transform.py b/pipeline/mmocr/mmocr/.mim/configs/textrecog/nrtr/_base_nrtr_modality-transform.py
deleted file mode 100644
index 5b21549f8ab62ae72988ef5ebbe13dee14d13ece..0000000000000000000000000000000000000000
--- a/pipeline/mmocr/mmocr/.mim/configs/textrecog/nrtr/_base_nrtr_modality-transform.py
+++ /dev/null
@@ -1,111 +0,0 @@
-dictionary = dict(
- type='Dictionary',
- dict_file='{{ fileDirname }}/../../../dicts/english_digits_symbols.txt',
- with_padding=True,
- with_unknown=True,
- same_start_end=True,
- with_start=True,
- with_end=True)
-
-model = dict(
- type='NRTR',
- backbone=dict(type='NRTRModalityTransform'),
- encoder=dict(type='NRTREncoder', n_layers=12),
- decoder=dict(
- type='NRTRDecoder',
- module_loss=dict(
- type='CEModuleLoss', ignore_first_char=True, flatten=True),
- postprocessor=dict(type='AttentionPostprocessor'),
- dictionary=dictionary,
- max_seq_len=30),
- data_preprocessor=dict(
- type='TextRecogDataPreprocessor',
- mean=[123.675, 116.28, 103.53],
- std=[58.395, 57.12, 57.375]))
-
-train_pipeline = [
- dict(type='LoadImageFromFile', ignore_empty=True, min_size=2),
- dict(type='LoadOCRAnnotations', with_text=True),
- dict(
- type='RescaleToHeight',
- height=32,
- min_width=32,
- max_width=160,
- width_divisor=4),
- dict(type='PadToWidth', width=160),
- dict(
- type='PackTextRecogInputs',
- meta_keys=('img_path', 'ori_shape', 'img_shape', 'valid_ratio'))
-]
-
-test_pipeline = [
- dict(type='LoadImageFromFile'),
- dict(
- type='RescaleToHeight',
- height=32,
- min_width=32,
- max_width=160,
- width_divisor=16),
- dict(type='PadToWidth', width=160),
- # add loading annotation after ``Resize`` because ground truth
- # does not need to do resize data transform
- dict(type='LoadOCRAnnotations', with_text=True),
- dict(
- type='PackTextRecogInputs',
- meta_keys=('img_path', 'ori_shape', 'img_shape', 'valid_ratio'))
-]
-
-tta_pipeline = [
- dict(type='LoadImageFromFile'),
- dict(
- type='TestTimeAug',
- transforms=[
- [
- dict(
- type='ConditionApply',
- true_transforms=[
- dict(
- type='ImgAugWrapper',
- args=[dict(cls='Rot90', k=0, keep_size=False)])
- ],
- condition="results['img_shape'][1] [RobustScanner: Dynamically Enhancing Positional Clues for Robust Text Recognition](https://arxiv.org/abs/2007.07542)
-
-
-
-## Abstract
-
-The attention-based encoder-decoder framework has recently achieved impressive results for scene text recognition, and many variants have emerged with improvements in recognition quality. However, it performs poorly on contextless texts (e.g., random character sequences) which is unacceptable in most of real application scenarios. In this paper, we first deeply investigate the decoding process of the decoder. We empirically find that a representative character-level sequence decoder utilizes not only context information but also positional information. Contextual information, which the existing approaches heavily rely on, causes the problem of attention drift. To suppress such side-effect, we propose a novel position enhancement branch, and dynamically fuse its outputs with those of the decoder attention module for scene text recognition. Specifically, it contains a position aware module to enable the encoder to output feature vectors encoding their own spatial positions, and an attention module to estimate glimpses using the positional clue (i.e., the current decoding time step) only. The dynamic fusion is conducted for more robust feature via an element-wise gate mechanism. Theoretically, our proposed method, dubbed \\emph{RobustScanner}, decodes individual characters with dynamic ratio between context and positional clues, and utilizes more positional ones when the decoding sequences with scarce context, and thus is robust and practical. Empirically, it has achieved new state-of-the-art results on popular regular and irregular text recognition benchmarks while without much performance drop on contextless benchmarks, validating its robustness in both contextual and contextless application scenarios.
-
-
-
-
-
-## Dataset
-
-### Train Dataset
-
-| trainset | instance_num | repeat_num | source |
-| :--------: | :----------: | :--------: | :------------------------: |
-| icdar_2011 | 3567 | 20 | real |
-| icdar_2013 | 848 | 20 | real |
-| icdar2015 | 4468 | 20 | real |
-| coco_text | 42142 | 20 | real |
-| IIIT5K | 2000 | 20 | real |
-| SynthText | 2400000 | 1 | synth |
-| SynthAdd | 1216889 | 1 | synth, 1.6m in [\[1\]](#1) |
-| Syn90k | 2400000 | 1 | synth |
-
-### Test Dataset
-
-| testset | instance_num | type |
-| :-----: | :----------: | :---------------------------: |
-| IIIT5K | 3000 | regular |
-| SVT | 647 | regular |
-| IC13 | 1015 | regular |
-| IC15 | 2077 | irregular |
-| SVTP | 645 | irregular, 639 in [\[1\]](#1) |
-| CT80 | 288 | irregular |
-
-## Results and Models
-
-| Methods | GPUs | | Regular Text | | | | Irregular Text | | download |
-| :------------------------------------------------------------------: | :--: | :----: | :----------: | :-------: | :-: | :-------: | :------------: | :----: | :-------------------------------------------------------------------: |
-| | | IIIT5K | SVT | IC13-1015 | | IC15-2077 | SVTP | CT80 | |
-| [RobustScanner](/configs/textrecog/robust_scanner/robustscanner_resnet31_5e_st-sub_mj-sub_sa_real.py) | 4 | 0.9510 | 0.9011 | 0.9320 | | 0.7578 | 0.8078 | 0.8750 | [model](https://download.openmmlab.com/mmocr/textrecog/robust_scanner/robustscanner_resnet31_5e_st-sub_mj-sub_sa_real/robustscanner_resnet31_5e_st-sub_mj-sub_sa_real_20220915_152447-7fc35929.pth) \| [log](https://download.openmmlab.com/mmocr/textrecog/robust_scanner/robustscanner_resnet31_5e_st-sub_mj-sub_sa_real/20220915_152447.log) |
-| [RobustScanner-TTA](/configs/textrecog/robust_scanner/robustscanner_resnet31_5e_st-sub_mj-sub_sa_real.py) | 4 | 0.9487 | 0.9011 | 0.9261 | | 0.7805 | 0.8124 | 0.8819 | |
-
-## References
-
-\[1\] Li, Hui and Wang, Peng and Shen, Chunhua and Zhang, Guyu. Show, attend and read: A simple and strong baseline for irregular text recognition. In AAAI 2019.
-
-## Citation
-
-```bibtex
-@inproceedings{yue2020robustscanner,
- title={RobustScanner: Dynamically Enhancing Positional Clues for Robust Text Recognition},
- author={Yue, Xiaoyu and Kuang, Zhanghui and Lin, Chenhao and Sun, Hongbin and Zhang, Wayne},
- booktitle={European Conference on Computer Vision},
- year={2020}
-}
-```
diff --git a/pipeline/mmocr/mmocr/.mim/configs/textrecog/robust_scanner/_base_robustscanner_resnet31.py b/pipeline/mmocr/mmocr/.mim/configs/textrecog/robust_scanner/_base_robustscanner_resnet31.py
deleted file mode 100644
index 357794016f7891234d0e54bfd5fad96a09eed76c..0000000000000000000000000000000000000000
--- a/pipeline/mmocr/mmocr/.mim/configs/textrecog/robust_scanner/_base_robustscanner_resnet31.py
+++ /dev/null
@@ -1,117 +0,0 @@
-dictionary = dict(
- type='Dictionary',
- dict_file='{{ fileDirname }}/../../../dicts/english_digits_symbols.txt',
- with_start=True,
- with_end=True,
- same_start_end=True,
- with_padding=True,
- with_unknown=True)
-
-model = dict(
- type='RobustScanner',
- data_preprocessor=dict(
- type='TextRecogDataPreprocessor',
- mean=[127, 127, 127],
- std=[127, 127, 127]),
- backbone=dict(type='ResNet31OCR'),
- encoder=dict(
- type='ChannelReductionEncoder', in_channels=512, out_channels=128),
- decoder=dict(
- type='RobustScannerFuser',
- hybrid_decoder=dict(
- type='SequenceAttentionDecoder', dim_input=512, dim_model=128),
- position_decoder=dict(
- type='PositionAttentionDecoder', dim_input=512, dim_model=128),
- in_channels=[512, 512],
- postprocessor=dict(type='AttentionPostprocessor'),
- module_loss=dict(
- type='CEModuleLoss', ignore_first_char=True, reduction='mean'),
- dictionary=dictionary,
- max_seq_len=30))
-
-train_pipeline = [
- dict(type='LoadImageFromFile', ignore_empty=True, min_size=2),
- dict(type='LoadOCRAnnotations', with_text=True),
- dict(
- type='RescaleToHeight',
- height=48,
- min_width=48,
- max_width=160,
- width_divisor=4),
- dict(type='PadToWidth', width=160),
- dict(
- type='PackTextRecogInputs',
- meta_keys=('img_path', 'ori_shape', 'img_shape', 'valid_ratio'))
-]
-
-test_pipeline = [
- dict(type='LoadImageFromFile'),
- dict(
- type='RescaleToHeight',
- height=48,
- min_width=48,
- max_width=160,
- width_divisor=4),
- dict(type='PadToWidth', width=160),
- # add loading annotation after ``Resize`` because ground truth
- # does not need to do resize data transform
- dict(type='LoadOCRAnnotations', with_text=True),
- dict(
- type='PackTextRecogInputs',
- meta_keys=('img_path', 'ori_shape', 'img_shape', 'valid_ratio'))
-]
-
-tta_pipeline = [
- dict(type='LoadImageFromFile'),
- dict(
- type='TestTimeAug',
- transforms=[
- [
- dict(
- type='ConditionApply',
- true_transforms=[
- dict(
- type='ImgAugWrapper',
- args=[dict(cls='Rot90', k=0, keep_size=False)])
- ],
- condition="results['img_shape'][1] [Show, Attend and Read: A Simple and Strong Baseline for Irregular Text Recognition](https://arxiv.org/abs/1811.00751)
-
-
-
-## Abstract
-
-Recognizing irregular text in natural scene images is challenging due to the large variance in text appearance, such as curvature, orientation and distortion. Most existing approaches rely heavily on sophisticated model designs and/or extra fine-grained annotations, which, to some extent, increase the difficulty in algorithm implementation and data collection. In this work, we propose an easy-to-implement strong baseline for irregular scene text recognition, using off-the-shelf neural network components and only word-level annotations. It is composed of a 31-layer ResNet, an LSTM-based encoder-decoder framework and a 2-dimensional attention module. Despite its simplicity, the proposed method is robust and achieves state-of-the-art performance on both regular and irregular scene text recognition benchmarks.
-
-
-
-
-
-## Dataset
-
-### Train Dataset
-
-| trainset | instance_num | repeat_num | source |
-| :--------: | :----------: | :--------: | :------------------------: |
-| icdar_2011 | 3567 | 20 | real |
-| icdar_2013 | 848 | 20 | real |
-| icdar2015 | 4468 | 20 | real |
-| coco_text | 42142 | 20 | real |
-| IIIT5K | 2000 | 20 | real |
-| SynthText | 2400000 | 1 | synth |
-| SynthAdd | 1216889 | 1 | synth, 1.6m in [\[1\]](#1) |
-| Syn90k | 2400000 | 1 | synth |
-
-### Test Dataset
-
-| testset | instance_num | type |
-| :-----: | :----------: | :---------------------------: |
-| IIIT5K | 3000 | regular |
-| SVT | 647 | regular |
-| IC13 | 1015 | regular |
-| IC15 | 2077 | irregular |
-| SVTP | 645 | irregular, 639 in [\[1\]](#1) |
-| CT80 | 288 | irregular |
-
-## Results and Models
-
-| Methods | Backbone | Decoder | | Regular Text | | | | Irregular Text | | download |
-| :----------------------------------------------------: | :---------: | :------------------: | :----: | :----------: | :-------: | :-: | :-------: | :------------: | :----: | :------------------------------------------------------: |
-| | | | IIIT5K | SVT | IC13-1015 | | IC15-2077 | SVTP | CT80 | |
-| [SAR](/configs/textrecog/sar/sar_r31_parallel_decoder_academic.py) | R31-1/8-1/4 | ParallelSARDecoder | 0.9533 | 0.8964 | 0.9369 | | 0.7602 | 0.8326 | 0.9062 | [model](https://download.openmmlab.com/mmocr/textrecog/sar/sar_resnet31_parallel-decoder_5e_st-sub_mj-sub_sa_real/sar_resnet31_parallel-decoder_5e_st-sub_mj-sub_sa_real_20220915_171910-04eb4e75.pth) \| [log](https://download.openmmlab.com/mmocr/textrecog/sar/sar_resnet31_parallel-decoder_5e_st-sub_mj-sub_sa_real/20220915_171910.log) |
-| [SAR-TTA](/configs/textrecog/sar/sar_r31_parallel_decoder_academic.py) | R31-1/8-1/4 | ParallelSARDecoder | 0.9510 | 0.8964 | 0.9340 | | 0.7862 | 0.8372 | 0.9132 | |
-| [SAR](/configs/textrecog/sar/sar_r31_sequential_decoder_academic.py) | R31-1/8-1/4 | SequentialSARDecoder | 0.9553 | 0.9073 | 0.9409 | | 0.7761 | 0.8093 | 0.8958 | [model](https://download.openmmlab.com/mmocr/textrecog/sar/sar_resnet31_sequential-decoder_5e_st-sub_mj-sub_sa_real/sar_resnet31_sequential-decoder_5e_st-sub_mj-sub_sa_real_20220915_185451-1fd6b1fc.pth) \| [log](https://download.openmmlab.com/mmocr/textrecog/sar/sar_resnet31_sequential-decoder_5e_st-sub_mj-sub_sa_real/20220915_185451.log) |
-| [SAR-TTA](/configs/textrecog/sar/sar_r31_sequential_decoder_academic.py) | R31-1/8-1/4 | SequentialSARDecoder | 0.9530 | 0.9073 | 0.9389 | | 0.8002 | 0.8124 | 0.9028 | |
-
-## Citation
-
-```bibtex
-@inproceedings{li2019show,
- title={Show, attend and read: A simple and strong baseline for irregular text recognition},
- author={Li, Hui and Wang, Peng and Shen, Chunhua and Zhang, Guyu},
- booktitle={Proceedings of the AAAI Conference on Artificial Intelligence},
- volume={33},
- number={01},
- pages={8610--8617},
- year={2019}
-}
-```
diff --git a/pipeline/mmocr/mmocr/.mim/configs/textrecog/sar/_base_sar_resnet31_parallel-decoder.py b/pipeline/mmocr/mmocr/.mim/configs/textrecog/sar/_base_sar_resnet31_parallel-decoder.py
deleted file mode 100644
index 8e8df4850e323c3e88370612ae85d67c8a4d81fa..0000000000000000000000000000000000000000
--- a/pipeline/mmocr/mmocr/.mim/configs/textrecog/sar/_base_sar_resnet31_parallel-decoder.py
+++ /dev/null
@@ -1,123 +0,0 @@
-dictionary = dict(
- type='Dictionary',
- dict_file='{{ fileDirname }}/../../../dicts/english_digits_symbols.txt',
- with_start=True,
- with_end=True,
- same_start_end=True,
- with_padding=True,
- with_unknown=True)
-
-model = dict(
- type='SARNet',
- data_preprocessor=dict(
- type='TextRecogDataPreprocessor',
- mean=[127, 127, 127],
- std=[127, 127, 127]),
- backbone=dict(type='ResNet31OCR'),
- encoder=dict(
- type='SAREncoder',
- enc_bi_rnn=False,
- enc_do_rnn=0.1,
- enc_gru=False,
- ),
- decoder=dict(
- type='ParallelSARDecoder',
- enc_bi_rnn=False,
- dec_bi_rnn=False,
- dec_do_rnn=0,
- dec_gru=False,
- pred_dropout=0.1,
- d_k=512,
- pred_concat=True,
- postprocessor=dict(type='AttentionPostprocessor'),
- module_loss=dict(
- type='CEModuleLoss', ignore_first_char=True, reduction='mean'),
- dictionary=dictionary,
- max_seq_len=30))
-
-train_pipeline = [
- dict(type='LoadImageFromFile', ignore_empty=True, min_size=2),
- dict(type='LoadOCRAnnotations', with_text=True),
- dict(
- type='RescaleToHeight',
- height=48,
- min_width=48,
- max_width=160,
- width_divisor=4),
- dict(type='PadToWidth', width=160),
- dict(
- type='PackTextRecogInputs',
- meta_keys=('img_path', 'ori_shape', 'img_shape', 'valid_ratio'))
-]
-
-test_pipeline = [
- dict(type='LoadImageFromFile'),
- dict(
- type='RescaleToHeight',
- height=48,
- min_width=48,
- max_width=160,
- width_divisor=4),
- dict(type='PadToWidth', width=160),
- # add loading annotation after ``Resize`` because ground truth
- # does not need to do resize data transform
- dict(type='LoadOCRAnnotations', with_text=True),
- dict(
- type='PackTextRecogInputs',
- meta_keys=('img_path', 'ori_shape', 'img_shape', 'valid_ratio'))
-]
-
-tta_pipeline = [
- dict(type='LoadImageFromFile'),
- dict(
- type='TestTimeAug',
- transforms=[
- [
- dict(
- type='ConditionApply',
- true_transforms=[
- dict(
- type='ImgAugWrapper',
- args=[dict(cls='Rot90', k=0, keep_size=False)])
- ],
- condition="results['img_shape'][1] [On Recognizing Texts of Arbitrary Shapes with 2D Self-Attention](https://arxiv.org/abs/1910.04396)
-
-
-
-## Abstract
-
-Scene text recognition (STR) is the task of recognizing character sequences in natural scenes. While there have been great advances in STR methods, current methods still fail to recognize texts in arbitrary shapes, such as heavily curved or rotated texts, which are abundant in daily life (e.g. restaurant signs, product labels, company logos, etc). This paper introduces a novel architecture to recognizing texts of arbitrary shapes, named Self-Attention Text Recognition Network (SATRN), which is inspired by the Transformer. SATRN utilizes the self-attention mechanism to describe two-dimensional (2D) spatial dependencies of characters in a scene text image. Exploiting the full-graph propagation of self-attention, SATRN can recognize texts with arbitrary arrangements and large inter-character spacing. As a result, SATRN outperforms existing STR models by a large margin of 5.7 pp on average in "irregular text" benchmarks. We provide empirical analyses that illustrate the inner mechanisms and the extent to which the model is applicable (e.g. rotated and multi-line text). We will open-source the code.
-
-
-
-
-
-## Dataset
-
-### Train Dataset
-
-| trainset | instance_num | repeat_num | source |
-| :-------: | :----------: | :--------: | :----: |
-| SynthText | 7266686 | 1 | synth |
-| Syn90k | 8919273 | 1 | synth |
-
-### Test Dataset
-
-| testset | instance_num | type |
-| :-----: | :----------: | :-------: |
-| IIIT5K | 3000 | regular |
-| SVT | 647 | regular |
-| IC13 | 1015 | regular |
-| IC15 | 2077 | irregular |
-| SVTP | 645 | irregular |
-| CT80 | 288 | irregular |
-
-## Results and Models
-
-| Methods | | Regular Text | | | | Irregular Text | | download |
-| :--------------------------------------------------------------------: | :----: | :----------: | :-------: | :-: | :-------: | :------------: | :----: | :---------------------------------------------------------------------: |
-| | IIIT5K | SVT | IC13-1015 | | IC15-2077 | SVTP | CT80 | |
-| [Satrn](/configs/textrecog/satrn/satrn_shallow_5e_st_mj.py) | 0.9600 | 0.9181 | 0.9606 | | 0.8045 | 0.8837 | 0.8993 | [model](https://download.openmmlab.com/mmocr/textrecog/satrn/satrn_shallow_5e_st_mj/satrn_shallow_5e_st_mj_20220915_152443-5fd04a4c.pth) \| [log](https://download.openmmlab.com/mmocr/textrecog/satrn/satrn_shallow_5e_st_mj/20220915_152443.log) |
-| [Satrn-TTA](/configs/textrecog/satrn/satrn_shallow_5e_st_mj.py) | 0.9530 | 0.9181 | 0.9527 | | 0.8276 | 0.8884 | 0.9028 | |
-| [Satrn_small](/configs/textrecog/satrn/satrn_shallow-small_5e_st_mj.py) | 0.9423 | 0.9011 | 0.9567 | | 0.7886 | 0.8574 | 0.8472 | [model](https://download.openmmlab.com/mmocr/textrecog/satrn/satrn_shallow-small_5e_st_mj/satrn_shallow-small_5e_st_mj_20220915_152442-5591bf27.pth) \| [log](https://download.openmmlab.com/mmocr/textrecog/satrn/satrn_shallow-small_5e_st_mj/20220915_152442.log) |
-| [Satrn_small-TTA](/configs/textrecog/satrn/satrn_shallow-small_5e_st_mj.py) | 0.9380 | 0.8995 | 0.9488 | | 0.8122 | 0.8620 | 0.8507 | |
-
-## Citation
-
-```bibtex
-@article{junyeop2019recognizing,
- title={On Recognizing Texts of Arbitrary Shapes with 2D Self-Attention},
- author={Junyeop Lee, Sungrae Park, Jeonghun Baek, Seong Joon Oh, Seonghyeon Kim, Hwalsuk Lee},
- year={2019}
-}
-```
diff --git a/pipeline/mmocr/mmocr/.mim/configs/textrecog/satrn/_base_satrn_shallow.py b/pipeline/mmocr/mmocr/.mim/configs/textrecog/satrn/_base_satrn_shallow.py
deleted file mode 100644
index 2eb2a05a645c76f250f7453183cbef904985df18..0000000000000000000000000000000000000000
--- a/pipeline/mmocr/mmocr/.mim/configs/textrecog/satrn/_base_satrn_shallow.py
+++ /dev/null
@@ -1,107 +0,0 @@
-dictionary = dict(
- type='Dictionary',
- dict_file='{{ fileDirname }}/../../../dicts/english_digits_symbols.txt',
- with_padding=True,
- with_unknown=True,
- same_start_end=True,
- with_start=True,
- with_end=True)
-
-model = dict(
- type='SATRN',
- backbone=dict(type='ShallowCNN', input_channels=3, hidden_dim=512),
- encoder=dict(
- type='SATRNEncoder',
- n_layers=12,
- n_head=8,
- d_k=512 // 8,
- d_v=512 // 8,
- d_model=512,
- n_position=100,
- d_inner=512 * 4,
- dropout=0.1),
- decoder=dict(
- type='NRTRDecoder',
- n_layers=6,
- d_embedding=512,
- n_head=8,
- d_model=512,
- d_inner=512 * 4,
- d_k=512 // 8,
- d_v=512 // 8,
- module_loss=dict(
- type='CEModuleLoss', flatten=True, ignore_first_char=True),
- dictionary=dictionary,
- max_seq_len=25,
- postprocessor=dict(type='AttentionPostprocessor')),
- data_preprocessor=dict(
- type='TextRecogDataPreprocessor',
- mean=[123.675, 116.28, 103.53],
- std=[58.395, 57.12, 57.375]))
-
-train_pipeline = [
- dict(type='LoadImageFromFile', ignore_empty=True, min_size=0),
- dict(type='LoadOCRAnnotations', with_text=True),
- dict(type='Resize', scale=(100, 32), keep_ratio=False),
- dict(
- type='PackTextRecogInputs',
- meta_keys=('img_path', 'ori_shape', 'img_shape', 'valid_ratio'))
-]
-
-test_pipeline = [
- dict(type='LoadImageFromFile'),
- dict(type='Resize', scale=(100, 32), keep_ratio=False),
- # add loading annotation after ``Resize`` because ground truth
- # does not need to do resize data transform
- dict(type='LoadOCRAnnotations', with_text=True),
- dict(
- type='PackTextRecogInputs',
- meta_keys=('img_path', 'ori_shape', 'img_shape', 'valid_ratio'))
-]
-
-tta_pipeline = [
- dict(type='LoadImageFromFile'),
- dict(
- type='TestTimeAug',
- transforms=[
- [
- dict(
- type='ConditionApply',
- true_transforms=[
- dict(
- type='ImgAugWrapper',
- args=[dict(cls='Rot90', k=0, keep_size=False)])
- ],
- condition="results['img_shape'][1] [SVTR: Scene Text Recognition with a Single Visual Model](https://arxiv.org/abs/2205.00159)
-
-
-
-## Abstract
-
-Dominant scene text recognition models commonly contain two building blocks, a visual model for feature extraction and a sequence model for text transcription. This hybrid architecture, although accurate, is complex and less efficient. In this study, we propose a Single Visual model for Scene Text recognition within the patch-wise image tokenization framework, which dispenses with the sequential modeling entirely. The method, termed SVTR, firstly decomposes an image text into small patches named character components. Afterward, hierarchical stages are recurrently carried out by component-level mixing, merging and/or combining. Global and local mixing blocks are devised to perceive the inter-character and intra-character patterns, leading to a multi-grained character component perception. Thus, characters are recognized by a simple linear prediction. Experimental results on both English and Chinese scene text recognition tasks demonstrate the effectiveness of SVTR. SVTR-L (Large) achieves highly competitive accuracy in English and outperforms existing methods by a large margin in Chinese, while running faster. In addition, SVTR-T (Tiny) is an effective and much smaller model, which shows appealing speed at inference.
-
-
-
-
-
-## Dataset
-
-### Train Dataset
-
-| trainset | instance_num | repeat_num | source |
-| :-------: | :----------: | :--------: | :----: |
-| SynthText | 7266686 | 1 | synth |
-| Syn90k | 8919273 | 1 | synth |
-
-### Test Dataset
-
-| testset | instance_num | type |
-| :-----: | :----------: | :-------: |
-| IIIT5K | 3000 | regular |
-| SVT | 647 | regular |
-| IC13 | 1015 | regular |
-| IC15 | 2077 | irregular |
-| SVTP | 645 | irregular |
-| CT80 | 288 | irregular |
-
-## Results and Models
-
-| Methods | | Regular Text | | | | Irregular Text | | download |
-| :---------------------------------------------------------------: | :----: | :----------: | :-------: | :-: | :-------: | :------------: | :----: | :--------------------------------------------------------------------------: |
-| | IIIT5K | SVT | IC13-1015 | | IC15-2077 | SVTP | CT80 | |
-| [SVTR-tiny](/configs/textrecog/svtr/svtr-tiny_20e_st_mj.py) | - | - | - | | - | - | - | - |
-| [SVTR-small](/configs/textrecog/svtr/svtr-small_20e_st_mj.py) | 0.8553 | 0.9026 | 0.9448 | | 0.7496 | 0.8496 | 0.8854 | [model](https://download.openmmlab.com/mmocr/textrecog/svtr/svtr-small_20e_st_mj/svtr-small_20e_st_mj-35d800d6.pth) \| [log](https://download.openmmlab.com/mmocr/textrecog/svtr/svtr-small_20e_st_mj/20230105_184454.log) |
-| [SVTR-small-TTA](/configs/textrecog/svtr/svtr-small_20e_st_mj.py) | 0.8397 | 0.8964 | 0.9241 | | 0.7597 | 0.8124 | 0.8646 | |
-| [SVTR-base](/configs/textrecog/svtr/svtr-base_20e_st_mj.py) | 0.8570 | 0.9181 | 0.9438 | | 0.7448 | 0.8388 | 0.9028 | [model](https://download.openmmlab.com/mmocr/textrecog/svtr/svtr-base_20e_st_mj/svtr-base_20e_st_mj-ea500101.pth) \| [log](https://download.openmmlab.com/mmocr/textrecog/svtr/svtr-base_20e_st_mj/20221227_175415.log) |
-| [SVTR-base-TTA](/configs/textrecog/svtr/svtr-base_20e_st_mj.py) | 0.8517 | 0.9011 | 0.9379 | | 0.7569 | 0.8279 | 0.8819 | |
-| [SVTR-large](/configs/textrecog/svtr/svtr-large_20e_st_mj.py) | - | - | - | | - | - | - | - |
-
-```{note}
-The implementation and configuration follow the original code and paper, but there is still a gap between the reproduced results and the official ones. We appreciate any suggestions to improve its performance.
-```
-
-## Citation
-
-```bibtex
-@inproceedings{ijcai2022p124,
- title = {SVTR: Scene Text Recognition with a Single Visual Model},
- author = {Du, Yongkun and Chen, Zhineng and Jia, Caiyan and Yin, Xiaoting and Zheng, Tianlun and Li, Chenxia and Du, Yuning and Jiang, Yu-Gang},
- booktitle = {Proceedings of the Thirty-First International Joint Conference on
- Artificial Intelligence, {IJCAI-22}},
- publisher = {International Joint Conferences on Artificial Intelligence Organization},
- editor = {Lud De Raedt},
- pages = {884--890},
- year = {2022},
- month = {7},
- note = {Main Track},
- doi = {10.24963/ijcai.2022/124},
- url = {https://doi.org/10.24963/ijcai.2022/124},
-}
-
-```
diff --git a/pipeline/mmocr/mmocr/.mim/configs/textrecog/svtr/_base_svtr-tiny.py b/pipeline/mmocr/mmocr/.mim/configs/textrecog/svtr/_base_svtr-tiny.py
deleted file mode 100644
index 4f1fa69e29ef806705e7c48c461fc79069cab6d6..0000000000000000000000000000000000000000
--- a/pipeline/mmocr/mmocr/.mim/configs/textrecog/svtr/_base_svtr-tiny.py
+++ /dev/null
@@ -1,159 +0,0 @@
-dictionary = dict(
- type='Dictionary',
- dict_file='{{ fileDirname }}/../../../dicts/lower_english_digits.txt',
- with_padding=True,
- with_unknown=True,
-)
-
-model = dict(
- type='SVTR',
- preprocessor=dict(
- type='STN',
- in_channels=3,
- resized_image_size=(32, 64),
- output_image_size=(32, 100),
- num_control_points=20,
- margins=[0.05, 0.05]),
- encoder=dict(
- type='SVTREncoder',
- img_size=[32, 100],
- in_channels=3,
- out_channels=192,
- embed_dims=[64, 128, 256],
- depth=[3, 6, 3],
- num_heads=[2, 4, 8],
- mixer_types=['Local'] * 6 + ['Global'] * 6,
- window_size=[[7, 11], [7, 11], [7, 11]],
- merging_types='Conv',
- prenorm=False,
- max_seq_len=25),
- decoder=dict(
- type='SVTRDecoder',
- in_channels=192,
- module_loss=dict(
- type='CTCModuleLoss', letter_case='lower', zero_infinity=True),
- postprocessor=dict(type='CTCPostProcessor'),
- dictionary=dictionary),
- data_preprocessor=dict(
- type='TextRecogDataPreprocessor', mean=[127.5], std=[127.5]))
-
-train_pipeline = [
- dict(type='LoadImageFromFile', ignore_empty=True, min_size=5),
- dict(type='LoadOCRAnnotations', with_text=True),
- dict(
- type='RandomApply',
- prob=0.4,
- transforms=[
- dict(type='TextRecogGeneralAug', ),
- ],
- ),
- dict(
- type='RandomApply',
- prob=0.4,
- transforms=[
- dict(type='CropHeight', ),
- ],
- ),
- dict(
- type='ConditionApply',
- condition='min(results["img_shape"])>10',
- true_transforms=dict(
- type='RandomApply',
- prob=0.4,
- transforms=[
- dict(
- type='TorchVisionWrapper',
- op='GaussianBlur',
- kernel_size=5,
- sigma=1,
- ),
- ],
- )),
- dict(
- type='RandomApply',
- prob=0.4,
- transforms=[
- dict(
- type='TorchVisionWrapper',
- op='ColorJitter',
- brightness=0.5,
- saturation=0.5,
- contrast=0.5,
- hue=0.1),
- ]),
- dict(
- type='RandomApply',
- prob=0.4,
- transforms=[
- dict(type='ImageContentJitter', ),
- ],
- ),
- dict(
- type='RandomApply',
- prob=0.4,
- transforms=[
- dict(
- type='ImgAugWrapper',
- args=[dict(cls='AdditiveGaussianNoise', scale=0.1**0.5)]),
- ],
- ),
- dict(
- type='RandomApply',
- prob=0.4,
- transforms=[
- dict(type='ReversePixels', ),
- ],
- ),
- dict(type='Resize', scale=(256, 64)),
- dict(
- type='PackTextRecogInputs',
- meta_keys=('img_path', 'ori_shape', 'img_shape', 'valid_ratio'))
-]
-
-test_pipeline = [
- dict(type='LoadImageFromFile'),
- dict(type='Resize', scale=(256, 64)),
- dict(type='LoadOCRAnnotations', with_text=True),
- dict(
- type='PackTextRecogInputs',
- meta_keys=('img_path', 'ori_shape', 'img_shape', 'valid_ratio'))
-]
-
-tta_pipeline = [
- dict(type='LoadImageFromFile'),
- dict(
- type='TestTimeAug',
- transforms=[[
- dict(
- type='ConditionApply',
- true_transforms=[
- dict(
- type='ImgAugWrapper',
- args=[dict(cls='Rot90', k=0, keep_size=False)])
- ],
- condition="results['img_shape'][1]
-?
-@
-A
-B
-C
-D
-E
-F
-G
-H
-I
-J
-K
-L
-M
-N
-O
-P
-Q
-R
-S
-T
-U
-V
-W
-X
-Y
-Z
-[
-\
-]
-^
-_
-`
-a
-b
-c
-d
-e
-f
-g
-h
-i
-j
-k
-l
-m
-n
-o
-p
-q
-r
-s
-t
-u
-v
-w
-x
-y
-z
-{
-|
-}
-~
-ยฎ
-ยฐ
-ยฑ
-ยณ
-ยด
-ยท
-ร
-ร
-ร
-ร
-ร
-ร
-ร
-ร
-ร
-ร
-ร
-รค
-รจ
-รฉ
-รฌ
-รฒ
-รณ
-รด
-รถ
-รท
-รผ
-ล
-ษ
-ห
-ฮฉ
-ฮฒ
-ฮด
-ฮฟ
-ะ
-ะท
-ู
-โ
-โ
-โ
-โ
-โ
-โ
-โ
-โ
-โข
-โฅ
-โฆ
-โฐ
-โฒ
-โป
-โฌ
-โ
-โข
-โ
-โ
ก
-โ
ข
-โ
ฃ
-โ
ค
-โ
ฅ
-โ
-โ
-โ
-โ
-โ
-โ
-โถ
-โ
-โค
-โ
-โ
-โ
-โ
-โ
-โก
-โช
-โฒ
-โณ
-โถ
-โธ
-โผ
-โฝ
-โ
-โ
-โ
-โ
-โฅ
-โ
-โ
-โ
-โ
-โฅ
-โช
-โญ
-โ
-โค
-ใ
-ใ
-ใ
-ใ
-ใ
-ใ
-ใ
-ใ
-ใ
-ใ
-ใ
-ใ
-ใ
-ใ
-ใ
-ใ
-ใ
-ใ
-ใ
-ใ
-ใ
-ใ
-ใ
-ใ
-ใ
-ใ
-ใ
-ใ
-ใ
-ใ
-ใ
-ใ
-ใ
-ใ
-ใ
-ใ
-ใ
-ใ
-ใ
-ใ
-ใ
-ใ
-ใ
-ใ
-ใ
-ใ
-ใ
-ใ
-ใ
-ใ
-ใก
-ใข
-ใฃ
-ใค
-ใฅ
-ใฆ
-ใง
-ใจ
-ใฉ
-ใช
-ใซ
-ใฌ
-ใญ
-ใฎ
-ใฏ
-ใฐ
-ใฑ
-ใฒ
-ใณ
-ใด
-ใต
-ใถ
-ใท
-ใธ
-ใน
-ใบ
-ใป
-ใผ
-ใฝ
-ใพ
-ใฟ
-ใ
-ใ
-ใ
-ใ
-ใ
-ใ
-ใ
-ใ
-ใ
-ใ
-ใ
-ใ
-ใ
-ใ
-ใ
-ใ
-ใ
-ใ
-ใ
-ใก
-ใข
-ใฃ
-ใค
-ใฅ
-ใฆ
-ใง
-ใจ
-ใฉ
-ใช
-ใซ
-ใฌ
-ใญ
-ใฎ
-ใฏ
-ใฐ
-ใฑ
-ใฒ
-ใณ
-ใด
-ใต
-ใถ
-ใท
-ใธ
-ใน
-ใบ
-ใป
-ใผ
-ใฝ
-ใพ
-ใฟ
-ใ
-ใ
-ใ
-ใ
-ใ
-ใ
-ใ
-ใ
-ใ
-ใ
-ใ
-ใ
-ใ
-ใ
-ใ
-ใ
-ใ
-ใ
-ใ
-ใ
-ใ
-ใ
-ใ
-ใ
-ใ
-ใ
-ใ
-ใ
-ใ
-ใ
-ใ
-ใ
-ใก
-ใข
-ใฃ
-ใค
-ใฅ
-ใฆ
-ใง
-ใจ
-ใฉ
-ใช
-ใซ
-ใฌ
-ใญ
-ใฏ
-ใฑ
-ใฒ
-ใณ
-ใด
-ใต
-ใถ
-ใผ
-ใ
-ใก
-ใฅ
-ใ
-ใ
-ใง
-ใถ
-ใบ
-ไ
-ไฒ
-ไท
-ไธ
-ไน
-ไฝ
-ไ
-ไ
-ไ
-ไฃ
-ไ
-ไ
-ไฏ
-ได
-ได
-ได
-ได
-ไธ
-ไธ
-ไธ
-ไธ
-ไธ
-ไธ
-ไธ
-ไธ
-ไธ
-ไธ
-ไธ
-ไธ
-ไธ
-ไธ
-ไธ
-ไธ
-ไธ
-ไธ
-ไธ
-ไธ
-ไธ
-ไธ
-ไธ
-ไธก
-ไธข
-ไธฃ
-ไธค
-ไธฅ
-ไธง
-ไธจ
-ไธช
-ไธซ
-ไธฌ
-ไธญ
-ไธฐ
-ไธฑ
-ไธฒ
-ไธด
-ไธถ
-ไธธ
-ไธน
-ไธบ
-ไธป
-ไธผ
-ไธฝ
-ไธพ
-ไธฟ
-ไน
-ไน
-ไน
-ไน
-ไน
-ไน
-ไน
-ไน
-ไน
-ไน
-ไน
-ไน
-ไน
-ไน
-ไน
-ไน
-ไน
-ไน
-ไน
-ไน
-ไน
-ไน
-ไน
-ไน
-ไนก
-ไนฆ
-ไนฉ
-ไนฐ
-ไนฑ
-ไนณ
-ไนธ
-ไนพ
-ไบ
-ไบ
-ไบ
-ไบ
-ไบ
-ไบ
-ไบ
-ไบ
-ไบ
-ไบ
-ไบ
-ไบ
-ไบ
-ไบ
-ไบ
-ไบ
-ไบ
-ไบ
-ไบ
-ไบ
-ไบ
-ไบก
-ไบข
-ไบค
-ไบฅ
-ไบฆ
-ไบง
-ไบจ
-ไบฉ
-ไบซ
-ไบฌ
-ไบญ
-ไบฎ
-ไบฒ
-ไบณ
-ไบต
-ไบถ
-ไบธ
-ไบน
-ไบบ
-ไบป
-ไบฟ
-ไป
-ไป
-ไป
-ไป
-ไป
-ไป
-ไป
-ไป
-ไป
-ไป
-ไป
-ไป
-ไป
-ไป
-ไป
-ไป
-ไป
-ไป
-ไป
-ไป
-ไป
-ไป
-ไป
-ไป
-ไป
-ไป
-ไปก
-ไปฃ
-ไปค
-ไปฅ
-ไปจ
-ไปช
-ไปซ
-ไปฌ
-ไปญ
-ไปฎ
-ไปฐ
-ไปฑ
-ไปฒ
-ไปณ
-ไปต
-ไปถ
-ไปท
-ไปป
-ไปฝ
-ไปฟ
-ไผ
-ไผ
-ไผ
-ไผ
-ไผ
-ไผ
-ไผ
-ไผ
-ไผ
-ไผ
-ไผ
-ไผ
-ไผ
-ไผ
-ไผ
-ไผ
-ไผ
-ไผ
-ไผ
-ไผ
-ไผ
-ไผ
-ไผข
-ไผฃ
-ไผค
-ไผฅ
-ไผฆ
-ไผง
-ไผฉ
-ไผช
-ไผซ
-ไผฌ
-ไผฏ
-ไผฐ
-ไผฑ
-ไผฒ
-ไผด
-ไผถ
-ไผท
-ไผธ
-ไผบ
-ไผป
-ไผผ
-ไผฝ
-ไผพ
-ไฝ
-ไฝ
-ไฝ
-ไฝ
-ไฝ
-ไฝ
-ไฝ
-ไฝ
-ไฝ
-ไฝ
-ไฝ
-ไฝ
-ไฝ
-ไฝ
-ไฝ
-ไฝ
-ไฝ
-ไฝ
-ไฝ
-ไฝ
-ไฝ
-ไฝ
-ไฝ
-ไฝข
-ไฝฃ
-ไฝค
-ไฝฅ
-ไฝง
-ไฝฉ
-ไฝฌ
-ไฝฎ
-ไฝฏ
-ไฝฐ
-ไฝณ
-ไฝด
-ไฝต
-ไฝถ
-ไฝท
-ไฝธ
-ไฝน
-ไฝบ
-ไฝป
-ไฝผ
-ไฝฝ
-ไฝพ
-ไฝฟ
-ไพ
-ไพ
-ไพ
-ไพ
-ไพ
-ไพ
-ไพ
-ไพ
-ไพ
-ไพ
-ไพ
-ไพ
-ไพ
-ไพ
-ไพ
-ไพ
-ไพ
-ไพ
-ไพก
-ไพฃ
-ไพฅ
-ไพฆ
-ไพง
-ไพจ
-ไพฉ
-ไพช
-ไพฌ
-ไพญ
-ไพฎ
-ไพฏ
-ไพฒ
-ไพต
-ไพน
-ไพผ
-ไพฟ
-ไฟ
-ไฟ
-ไฟ
-ไฟ
-ไฟ
-ไฟ
-ไฟ
-ไฟ
-ไฟ
-ไฟ
-ไฟ
-ไฟ
-ไฟ
-ไฟ
-ไฟ
-ไฟ
-ไฟ
-ไฟ
-ไฟ
-ไฟ
-ไฟ
-ไฟก
-ไฟฃ
-ไฟค
-ไฟฆ
-ไฟง
-ไฟจ
-ไฟฉ
-ไฟช
-ไฟซ
-ไฟฌ
-ไฟญ
-ไฟฎ
-ไฟฏ
-ไฟฑ
-ไฟณ
-ไฟด
-ไฟต
-ไฟถ
-ไฟท
-ไฟธ
-ไฟบ
-ไฟพ
-ๅ
-ๅ
-ๅ
-ๅ
-ๅ
-ๅ
-ๅ
-ๅ
-ๅ
-ๅ
-ๅ
-ๅ
-ๅ
-ๅ
-ๅ
-ๅ
-ๅ
-ๅ
-ๅ
-ๅ
-ๅก
-ๅข
-ๅค
-ๅฅ
-ๅฆ
-ๅจ
-ๅฉ
-ๅช
-ๅซ
-ๅฌ
-ๅญ
-ๅฎ
-ๅณ
-ๅด
-ๅถ
-ๅท
-ๅธ
-ๅน
-ๅบ
-ๅผ
-ๅพ
-ๅ
-ๅ
-ๅ
-ๅ
-ๅ
-ๅ
-ๅ
-ๅ
-ๅ
-ๅ
-ๅ
-ๅ
-ๅ
-ๅ
-ๅข
-ๅฅ
-ๅช
-ๅฌ
-ๅญ
-ๅฐ
-ๅฑ
-ๅฒ
-ๅด
-ๅถ
-ๅท
-ๅป
-ๅพ
-ๅฟ
-ๅ
-ๅ
-ๅ
-ๅ
-ๅ
-ๅ
-ๅ
-ๅ
-ๅ
-ๅ
-ๅ
-ๅฃ
-ๅฅ
-ๅฆ
-ๅง
-ๅจ
-ๅฉ
-ๅช
-ๅซ
-ๅฌ
-ๅฏ
-ๅฒ
-ๅณ
-ๅต
-ๅท
-ๅบ
-ๅป
-ๅพ
-ๅ
-ๅ
-ๅ
-ๅ
-ๅ
-ๅ
-ๅ
-ๅ
-ๅ
-ๅ
-ๅ
-ๅ
-ๅ
-ๅ
-ๅก
-ๅค
-ๅฆ
-ๅง
-ๅช
-ๅฌ
-ๅญ
-ๅฎ
-ๅฐ
-ๅฑ
-ๅณ
-ๅด
-ๅต
-ๅถ
-ๅธ
-ๅน
-ๅป
-ๅฝ
-ๅพ
-ๅฟ
-ๅ
-ๅ
-ๅ
-ๅ
-ๅ
-ๅ
-ๅ
-ๅ
-ๅ
-ๅ
-ๅ
-ๅ
-ๅ
-ๅก
-ๅฃ
-ๅฆ
-ๅฒ
-ๅด
-ๅต
-ๅฝ
-ๅพ
-ๅฟ
-ๅ
-ๅ
-ๅ
-ๅ
-ๅ
-ๅ
-ๅ
-ๅ
-ๅ
-ๅ
-ๅ
-ๅ
-ๅ
-ๅ
-ๅ
-ๅ
-ๅ
-ๅ
-ๅ
-ๅ
-ๅ
ข
-ๅ
ฅ
-ๅ
ง
-ๅ
จ
-ๅ
ฉ
-ๅ
ซ
-ๅ
ฌ
-ๅ
ญ
-ๅ
ฎ
-ๅ
ฐ
-ๅ
ฑ
-ๅ
ณ
-ๅ
ด
-ๅ
ต
-ๅ
ถ
-ๅ
ท
-ๅ
ธ
-ๅ
น
-ๅ
ป
-ๅ
ผ
-ๅ
ฝ
-ๅ
ฟ
-ๅ
-ๅ
-ๅ
-ๅ
-ๅ
-ๅ
-ๅ
-ๅ
-ๅ
-ๅ
-ๅ
-ๅ
-ๅ
-ๅ
-ๅ
-ๅ
-ๅ
-ๅ
-ๅ
-ๅ
-ๅ
-ๅ
-ๅ
-ๅข
-ๅค
-ๅฅ
-ๅง
-ๅจ
-ๅฌ
-ๅฏ
-ๅฐ
-ๅฑ
-ๅฒ
-ๅณ
-ๅด
-ๅต
-ๅถ
-ๅท
-ๅป
-ๅผ
-ๅฝ
-ๅฟ
-ๅ
-ๅ
-ๅ
-ๅ
-ๅ
-ๅ
-ๅ
-ๅ
-ๅ
-ๅ
-ๅ
-ๅ
-ๅ
-ๅ
-ๅ
-ๅ
-ๅก
-ๅค
-ๅฆ
-ๅง
-ๅช
-ๅซ
-ๅญ
-ๅฏ
-ๅฐ
-ๅฑ
-ๅณ
-ๅถ
-ๅธ
-ๅน
-ๅบ
-ๅป
-ๅผ
-ๅฝ
-ๅฟ
-ๅ
-ๅ
-ๅ
-ๅ
-ๅ
-ๅ
-ๅ
-ๅ
-ๅ
-ๅ
-ๅ
-ๅ
-ๅ
-ๅ
-ๅ
-ๅ
-ๅ
-ๅ
-ๅ
-ๅ
-ๅ
-ๅ
-ๅค
-ๅฅ
-ๅจ
-ๅฉ
-ๅซ
-ๅฌ
-ๅญ
-ๅฎ
-ๅฐ
-ๅฒ
-ๅณ
-ๅต
-ๅถ
-ๅท
-ๅธ
-ๅน
-ๅบ
-ๅป
-ๅฝ
-ๅฟ
-ๅ
-ๅ
-ๅ
-ๅ
-ๅ
-ๅ
-ๅ
-ๅ
-ๅ
-ๅ
-ๅ
-ๅ
-ๅ
-ๅ
-ๅ
-ๅ
-ๅ
-ๅ
-ๅ
-ๅก
-ๅฃ
-ๅค
-ๅฅ
-ๅง
-ๅจ
-ๅฉ
-ๅช
-ๅฏ
-ๅฐ
-ๅฒ
-ๅธ
-ๅบ
-ๅฝ
-ๅฟ
-ๅ
-ๅ
-ๅ
-ๅ
-ๅ
-ๅ
-ๅ
-ๅ
-ๅ
-ๅ
-ๅ
-ๅ
-ๅ
-ๅก
-ๅข
-ๅฃ
-ๅจ
-ๅฉ
-ๅช
-ๅซ
-ๅฌ
-ๅญ
-ๅฑ
-ๅฒ
-ๅณ
-ๅด
-ๅต
-ๅท
-ๅน
-ๅป
-ๅผ
-ๅพ
-ๅฟ
-ๅ
-ๅ
-ๅ
-ๅ
-ๅ
-ๅ
-ๅ
-ๅ
-ๅ
-ๅ
-ๅ
-ๅ
-ๅ
-ๅ
-ๅ
-ๅ
-ๅข
-ๅค
-ๅง
-ๅฉ
-ๅฎ
-ๅฐ
-ๅฒ
-ๅท
-ๅธ
-ๅบ
-ๅผ
-ๅพ
-ๅฟ
-ๅ
-ๅ
-ๅ
-ๅ
-ๅ
-ๅ
-ๅ
-ๅ
-ๅ
-ๅ
-ๅ
-ๅ
-ๅ
-ๅ
-ๅ
-ๅ
-ๅ
-ๅ
-ๅ
-ๅก
-ๅฃ
-ๅค
-ๅฆ
-ๅช
-ๅฎ
-ๅฏ
-ๅณ
-ๅน
-ๅบ
-ๅป
-ๅผ
-ๅฝ
-ๅพ
-ๅฟ
-ๅ
-ๅ
-ๅ
-ๅ
-ๅ
-ๅ
-ๅ
-ๅ
-ๅ
-ๅ
-ๅ
-ๅ
-ๅ
-ๅ
-ๅ
-ๅ
-ๅ
-ๅ
-ๅ
-ๅ
-ๅ
-ๅ
-ๅ
-ๅ
-ๅก
-ๅข
-ๅฃ
-ๅค
-ๅฆ
-ๅง
-ๅจ
-ๅซ
-ๅฌ
-ๅญ
-ๅฎ
-ๅฏ
-ๅฐ
-ๅฑ
-ๅฒ
-ๅณ
-ๅด
-ๅต
-ๅท
-ๅธ
-ๅบ
-ๅป
-ๅผ
-ๅฟ
-ๅ
-ๅ
-ๅ
-ๅ
-ๅ
-ๅ
-ๅ
-ๅ
-ๅ
-ๅ
-ๅ
-ๅ
-ๅ
-ๅ
-ๅ
-ๅ
-ๅ
-ๅ
-ๅข
-ๅฃ
-ๅฅ
-ๅฆ
-ๅจ
-ๅฉ
-ๅญ
-ๅฎ
-ๅฒ
-ๅณ
-ๅน
-ๅป
-ๅพ
-ๅฟ
-ๅ
-ๅ
-ๅ
-ๅ
-ๅ
-ๅ
-ๅ
-ๅ
-ๅ
-ๅ
-ๅ
-ๅ
-ๅ
-ๅ
-ๅ
-ๅ
-ๅ
-ๅ
-ๅ
-ๅ
-ๅ
-ๅ
-ๅก
-ๅฃ
-ๅค
-ๅฅ
-ๅฆ
-ๅจ
-ๅฉ
-ๅช
-ๅซ
-ๅฌ
-ๅญ
-ๅฎ
-ๅฏ
-ๅฐ
-ๅฑ
-ๅฒ
-ๅณ
-ๅต
-ๅถ
-ๅท
-ๅธ
-ๅน
-ๅป
-ๅผ
-ๅฝ
-ๅ
-ๅ
-ๅ
-ๅ
-ๅ
-ๅ
-ๅ
-ๅ
-ๅ
-ๅ
-ๅ
-ๅ
-ๅ
-ๅ
-ๅ
-ๅ
-ๅ
-ๅ
-ๅ
-ๅ
-ๅ
-ๅ
-ๅ
-ๅ
-ๅ
-ๅก
-ๅฆ
-ๅง
-ๅจ
-ๅฉ
-ๅช
-ๅซ
-ๅฌ
-ๅญ
-ๅฎ
-ๅฏ
-ๅฐ
-ๅฑ
-ๅฒ
-ๅณ
-ๅด
-ๅต
-ๅท
-ๅธ
-ๅน
-ๅบ
-ๅป
-ๅผ
-ๅฝ
-ๅพ
-ๅฟ
-ๅ
-ๅ
-ๅ
-ๅ
-ๅ
-ๅ
-ๅ
-ๅ
-ๅ
-ๅ
-ๅ
-ๅ
-ๅ
-ๅ
-ๅ
-ๅ
-ๅ
-ๅ
-ๅ
-ๅ
-ๅข
-ๅฃ
-ๅค
-ๅฅ
-ๅฆ
-ๅจ
-ๅช
-ๅซ
-ๅฐ
-ๅฑ
-ๅฒ
-ๅณ
-ๅด
-ๅต
-ๅถ
-ๅท
-ๅธ
-ๅบ
-ๅป
-ๅผ
-ๅฝ
-ๅฟ
-ๅ
-ๅ
-ๅ
-ๅ
-ๅ
-ๅ
-ๅ
-ๅ
-ๅ
-ๅ
-ๅ
-ๅ
-ๅ
-ๅ
-ๅ
-ๅ
-ๅ
-ๅ
-ๅ
-ๅ
-ๅ
-ๅ
-ๅ
-ๅ
-ๅ
-ๅข
-ๅฃ
-ๅค
-ๅฅ
-ๅฆ
-ๅง
-ๅจ
-ๅฉ
-ๅช
-ๅซ
-ๅฌ
-ๅญ
-ๅฎ
-ๅฏ
-ๅฑ
-ๅฒ
-ๅณ
-ๅถ
-ๅท
-ๅธ
-ๅบ
-ๅป
-ๅฝ
-ๅพ
-ๅฟ
-ๅ
-ๅ
-ๅ
-ๅ
-ๅ
-ๅ
-ๅ
-ๅ
-ๅ
-ๅ
-ๅ
-ๅ
-ๅ
-ๅ
-ๅ
-ๅ
-ๅ
-ๅ
-ๅ
-ๅ
-ๅ
-ๅ
-ๅ
-ๅ
-ๅ
-ๅ
-ๅ
-ๅก
-ๅข
-ๅฃ
-ๅค
-ๅฅ
-ๅฆ
-ๅง
-ๅจ
-ๅฉ
-ๅช
-ๅญ
-ๅฎ
-ๅฒ
-ๅณ
-ๅบ
-ๅผ
-ๅฝ
-ๅฟ
-ๅ
-ๅ
-ๅ
-ๅ
-ๅ
-ๅ
-ๅ
-ๅ
-ๅ
-ๅ
-ๅ
-ๅ
-ๅ
-ๅ
-ๅ
-ๅ
-ๅก
-ๅข
-ๅฃ
-ๅค
-ๅฅ
-ๅฆ
-ๅง
-ๅช
-ๅซ
-ๅฌ
-ๅญ
-ๅฎ
-ๅฏ
-ๅฐ
-ๅฑ
-ๅฒ
-ๅณ
-ๅต
-ๅถ
-ๅท
-ๅธ
-ๅป
-ๅผ
-ๅฝ
-ๅพ
-ๅฟ
-ๅ
-ๅ
-ๅ
-ๅ
-ๅ
-ๅ
-ๅ
-ๅ
-ๅ
-ๅ
-ๅ
-ๅ
-ๅ
-ๅ
-ๅ
-ๅ
-ๅ
-ๅ
-ๅ
-ๅ
-ๅ
-ๅ
-ๅก
-ๅค
-ๅฅ
-ๅฆ
-ๅง
-ๅฉ
-ๅช
-ๅซ
-ๅฌ
-ๅญ
-ๅฎ
-ๅฐ
-ๅฑ
-ๅฒ
-ๅณ
-ๅด
-ๅต
-ๅถ
-ๅท
-ๅธ
-ๅป
-ๅผ
-ๅพ
-ๅ
-ๅ
-ๅ
-ๅ
-ๅ
-ๅ
-ๅ
-ๅ
-ๅ
-ๅ
-ๅ
-ๅ
-ๅ
-ๅ
-ๅ
-ๅ
-ๅ
-ๅ
-ๅ
-ๅ
-ๅ
-ๅ
-ๅข
-ๅฃ
-ๅค
-ๅฆ
-ๅง
-ๅจ
-ๅช
-ๅซ
-ๅฌ
-ๅญ
-ๅฎ
-ๅฏ
-ๅฐ
-ๅฑ
-ๅณ
-ๅต
-ๅถ
-ๅท
-ๅน
-ๅบ
-ๅป
-ๅผ
-ๅฝ
-ๅพ
-ๅ
-ๅ
-ๅ
-ๅ
-ๅ
-ๅ
-ๅ
-ๅ
-ๅ
-ๅ
-ๅ
-ๅ
-ๅ
-ๅ
-ๅ
-ๅ
-ๅ
-ๅ
-ๅ
-ๅ
-ๅก
-ๅข
-ๅฃ
-ๅค
-ๅฅ
-ๅฆ
-ๅจ
-ๅช
-ๅซ
-ๅฌ
-ๅฎ
-ๅฏ
-ๅฐ
-ๅฑ
-ๅฒ
-ๅณ
-ๅต
-ๅท
-ๅป
-ๅฝ
-ๅพ
-ๅฟ
-ๅ
-ๅ
-ๅ
-ๅ
-ๅ
-ๅ
-ๅ
-ๅ
-ๅ
-ๅ
-ๅ
-ๅ
-ๅ
-ๅ
-ๅ
-ๅ
-ๅ
-ๅ
-ๅ
-ๅก
-ๅข
-ๅฃ
-ๅค
-ๅฅ
-ๅง
-ๅฌ
-ๅญ
-ๅฑ
-ๅฒ
-ๅด
-ๅถ
-ๅท
-ๅน
-ๅป
-ๅฟ
-ๅ
-ๅ
-ๅ
-ๅ
-ๅ
-ๅ
-ๅ
-ๅ
-ๅ
-ๅ
-ๅ
-ๅ
-ๅ
-ๅ
-ๅ
-ๅ
-ๅ
-ๅ
-ๅข
-ๅฃ
-ๅค
-ๅจ
-ๅฉ
-ๅช
-ๅซ
-ๅฌ
-ๅญ
-ๅฑ
-ๅณ
-ๅถ
-ๅท
-ๅป
-ๅผ
-ๅ
-ๅ
-ๅ
-ๅ
-ๅ
-ๅ
-ๅ
-ๅ
-ๅ
-ๅ
-ๅ
-ๅ
-ๅก
-ๅข
-ๅฃ
-ๅค
-ๅฅ
-ๅฌ
-ๅญ
-ๅฏ
-ๅฐ
-ๅฑ
-ๅด
-ๅต
-ๅท
-ๅบ
-ๅผ
-ๅฟ
-ๅ
-ๅ
-ๅ
-ๅ
-ๅ
-ๅ
-ๅ
-ๅ
-ๅ
-ๅ
-ๅ
-ๅ
-ๅ
-ๅ
-ๅ
-ๅ
-ๅก
-ๅข
-ๅฃ
-ๅค
-ๅฆ
-ๅง
-ๅจ
-ๅซ
-ๅญ
-ๅฎ
-ๅฏ
-ๅฐ
-ๅฑ
-ๅฒ
-ๅณ
-ๅด
-ๅต
-ๅท
-ๅน
-ๅบ
-ๅฝ
-ๅพ
-ๅฟ
-ๅ
-ๅ
-ๅ
-ๅ
-ๅ
-ๅ
-ๅ
-ๅ
-ๅ
-ๅ
-ๅ
-ๅ
-ๅ
-ๅ
-ๅ
-ๅ
-ๅ
-ๅ
-ๅ
-ๅ
-ๅ
-ๅฃ
-ๅฅ
-ๅง
-ๅจ
-ๅฉ
-ๅช
-ๅฌ
-ๅญ
-ๅฎ
-ๅฏ
-ๅฐ
-ๅณ
-ๅด
-ๅน
-ๅบ
-ๅป
-ๅพ
-ๅฟ
-ๅ
-ๅ
-ๅ
-ๅ
-ๅ
-ๅ
-ๅ
-ๅ
-ๅ
-ๅ
-ๅ
-ๅ
-ๅ
-ๅ
-ๅ
-ๅ
-ๅ
-ๅ
-ๅก
-ๅฃ
-ๅค
-ๅฆ
-ๅจ
-ๅฉ
-ๅช
-ๅซ
-ๅฌ
-ๅญ
-ๅฏ
-ๅฐ
-ๅฑ
-ๅณ
-ๅต
-ๅท
-ๅบ
-ๅป
-ๅผ
-ๅพ
-ๅ
-ๅ
-ๅ
-ๅ
-ๅ
-ๅ
-ๅ
-ๅ
-ๅ
-ๅ
-ๅ
-ๅ
-ๅ
-ๅ
-ๅ
-ๅ
-ๅ
-ๅ
-ๅ
-ๅ
-ๅ
-ๅ
-ๅก
-ๅข
-ๅฃ
-ๅค
-ๅฆ
-ๅง
-ๅจ
-ๅฉ
-ๅซ
-ๅญ
-ๅฎ
-ๅฏ
-ๅฑ
-ๅฒ
-ๅด
-ๅต
-ๅธ
-ๅบ
-ๅพ
-ๅฟ
-ๅ
-ๅ
-ๅ
-ๅ
-ๅ
-ๅ
-ๅ
-ๅ
-ๅ
-ๅ
-ๅ
-ๅ
-ๅ
-ๅ
-ๅ
-ๅ
-ๅ
-ๅ
-ๅ
-ๅ
-ๅ
-ๅค
-ๅฅ
-ๅง
-ๅจ
-ๅฉ
-ๅช
-ๅซ
-ๅญ
-ๅฎ
-ๅฏ
-ๅณ
-ๅด
-ๅต
-ๅถ
-ๅท
-ๅธ
-ๅน
-ๅบ
-ๅผ
-ๅฝ
-ๅฟ
-ๅ
-ๅ
-ๅ
-ๅ
-ๅ
-ๅ
-ๅ
-ๅ
-ๅ
-ๅ
-ๅ
-ๅ
-ๅ
-ๅ
-ๅ
-ๅ
-ๅ ก
-ๅ ฃ
-ๅ ค
-ๅ ฆ
-ๅ ง
-ๅ จ
-ๅ ช
-ๅ ญ
-ๅ ฎ
-ๅ ฐ
-ๅ ฑ
-ๅ ณ
-ๅ ด
-ๅ ต
-ๅ ถ
-ๅ บ
-ๅ ผ
-ๅ ฝ
-ๅก
-ๅก
-ๅก
-ๅก
-ๅก
-ๅก
-ๅก
-ๅก
-ๅก
-ๅก
-ๅก
-ๅก
-ๅก
-ๅก
-ๅก
-ๅก
-ๅก
-ๅก
-ๅกจ
-ๅกฉ
-ๅกซ
-ๅกฌ
-ๅกญ
-ๅกฏ
-ๅกฑ
-ๅกธ
-ๅกผ
-ๅกฝ
-ๅกพ
-ๅกฟ
-ๅข
-ๅข
-ๅข
-ๅข
-ๅข
-ๅข
-ๅข
-ๅข
-ๅข
-ๅข
-ๅข
-ๅข
-ๅข
-ๅข
-ๅข
-ๅข
-ๅข
-ๅข
-ๅข
-ๅข
-ๅขก
-ๅขฆ
-ๅขจ
-ๅขฉ
-ๅขน
-ๅขบ
-ๅฃ
-ๅฃ
-ๅฃ
-ๅฃ
-ๅฃ
-ๅฃ
-ๅฃ
-ๅฃ
-ๅฃ
-ๅฃ
-ๅฃ
-ๅฃ
-ๅฃ
-ๅฃ
-ๅฃก
-ๅฃค
-ๅฃฉ
-ๅฃซ
-ๅฃฌ
-ๅฃฎ
-ๅฃฐ
-ๅฃฑ
-ๅฃฒ
-ๅฃณ
-ๅฃถ
-ๅฃธ
-ๅฃน
-ๅฃบ
-ๅฃผ
-ๅฃฝ
-ๅค
-ๅค
-ๅค
-ๅค
-ๅค
-ๅค
-ๅค
-ๅค
-ๅค
-ๅค
-ๅค
-ๅค
-ๅค
-ๅค
-ๅค
-ๅค
-ๅคก
-ๅคข
-ๅคค
-ๅคฅ
-ๅคง
-ๅคฉ
-ๅคช
-ๅคซ
-ๅคฌ
-ๅคญ
-ๅคฎ
-ๅคฏ
-ๅคฐ
-ๅคฑ
-ๅคด
-ๅคท
-ๅคธ
-ๅคน
-ๅคบ
-ๅคผ
-ๅคพ
-ๅคฟ
-ๅฅ
-ๅฅ
-ๅฅ
-ๅฅ
-ๅฅ
-ๅฅ
-ๅฅ
-ๅฅ
-ๅฅ
-ๅฅ
-ๅฅ
-ๅฅ
-ๅฅ
-ๅฅ
-ๅฅ
-ๅฅ
-ๅฅ
-ๅฅ
-ๅฅ
-ๅฅ
-ๅฅ
-ๅฅก
-ๅฅข
-ๅฅฃ
-ๅฅค
-ๅฅฅ
-ๅฅง
-ๅฅจ
-ๅฅซ
-ๅฅญ
-ๅฅฐ
-ๅฅณ
-ๅฅด
-ๅฅถ
-ๅฅธ
-ๅฅน
-ๅฅฝ
-ๅฆ
-ๅฆ
-ๅฆ
-ๅฆ
-ๅฆ
-ๅฆ
-ๅฆ
-ๅฆ
-ๅฆ
-ๅฆ
-ๅฆ
-ๅฆ
-ๅฆ
-ๅฆ
-ๅฆ
-ๅฆ
-ๅฆ
-ๅฆฃ
-ๅฆค
-ๅฆฅ
-ๅฆจ
-ๅฆฉ
-ๅฆช
-ๅฆซ
-ๅฆฌ
-ๅฆฎ
-ๅฆฏ
-ๅฆฒ
-ๅฆณ
-ๅฆน
-ๅฆป
-ๅฆผ
-ๅฆพ
-ๅง
-ๅง
-ๅง
-ๅง
-ๅง
-ๅง
-ๅง
-ๅง
-ๅง
-ๅง
-ๅง
-ๅง
-ๅง
-ๅง
-ๅง
-ๅง
-ๅง
-ๅง
-ๅงฃ
-ๅงค
-ๅงฅ
-ๅงจ
-ๅงช
-ๅงซ
-ๅงฌ
-ๅงฎ
-ๅงฑ
-ๅงต
-ๅงน
-ๅงบ
-ๅงป
-ๅงฟ
-ๅจ
-ๅจ
-ๅจ
-ๅจ
-ๅจ
-ๅจ
-ๅจ
-ๅจ
-ๅจ
-ๅจ
-ๅจ
-ๅจ
-ๅจ
-ๅจ
-ๅจ
-ๅจ
-ๅจ
-ๅจ
-ๅจ
-ๅจ
-ๅจก
-ๅจฃ
-ๅจฅ
-ๅจฉ
-ๅจญ
-ๅจฏ
-ๅจฑ
-ๅจฒ
-ๅจด
-ๅจต
-ๅจถ
-ๅจผ
-ๅฉ
-ๅฉ
-ๅฉ
-ๅฉ
-ๅฉ
-ๅฉ
-ๅฉ
-ๅฉ
-ๅฉ
-ๅฉ
-ๅฉ
-ๅฉ
-ๅฉข
-ๅฉฃ
-ๅฉฅ
-ๅฉฆ
-ๅฉง
-ๅฉจ
-ๅฉช
-ๅฉฒ
-ๅฉด
-ๅฉต
-ๅฉถ
-ๅฉท
-ๅฉบ
-ๅฉผ
-ๅฉฟ
-ๅช
-ๅช
-ๅช
-ๅช
-ๅช
-ๅช
-ๅช
-ๅช
-ๅช
-ๅชข
-ๅชฅ
-ๅชช
-ๅชญ
-ๅชฎ
-ๅชฒ
-ๅชณ
-ๅชต
-ๅชธ
-ๅชป
-ๅชฝ
-ๅชพ
-ๅชฟ
-ๅซ
-ๅซ
-ๅซ
-ๅซ
-ๅซ
-ๅซ
-ๅซ
-ๅซ
-ๅซ
-ๅซ
-ๅซ
-ๅซ
-ๅซ
-ๅซ
-ๅซ
-ๅซ
-ๅซก
-ๅซฃ
-ๅซฆ
-ๅซง
-ๅซฉ
-ๅซช
-ๅซซ
-ๅซญ
-ๅซฎ
-ๅซฐ
-ๅซฑ
-ๅซฒ
-ๅซณ
-ๅซถ
-ๅซฝ
-ๅฌ
-ๅฌ
-ๅฌ
-ๅฌ
-ๅฌ
-ๅฌ
-ๅฌ
-ๅฌ
-ๅฌ
-ๅฌ
-ๅฌข
-ๅฌฌ
-ๅฌฒ
-ๅฌด
-ๅฌท
-ๅฌพ
-ๅฌฟ
-ๅญ
-ๅญ
-ๅญ
-ๅญ
-ๅญ
-ๅญ
-ๅญ
-ๅญ
-ๅญ
-ๅญ
-ๅญ
-ๅญ
-ๅญ
-ๅญ
-ๅญ
-ๅญ
-ๅญ
-ๅญข
-ๅญฃ
-ๅญค
-ๅญฅ
-ๅญฆ
-ๅญฉ
-ๅญช
-ๅญฌ
-ๅญญ
-ๅญฐ
-ๅญฑ
-ๅญฒ
-ๅญณ
-ๅญต
-ๅญธ
-ๅญบ
-ๅญป
-ๅญฝ
-ๅฎ
-ๅฎ
-ๅฎ
-ๅฎ
-ๅฎ
-ๅฎ
-ๅฎ
-ๅฎ
-ๅฎ
-ๅฎ
-ๅฎ
-ๅฎ
-ๅฎ
-ๅฎ
-ๅฎ
-ๅฎ
-ๅฎ
-ๅฎ
-ๅฎ
-ๅฎ
-ๅฎ
-ๅฎ
-ๅฎ
-ๅฎ
-ๅฎ
-ๅฎก
-ๅฎข
-ๅฎฃ
-ๅฎค
-ๅฎฅ
-ๅฎฆ
-ๅฎช
-ๅฎซ
-ๅฎฌ
-ๅฎฎ
-ๅฎฐ
-ๅฎณ
-ๅฎด
-ๅฎต
-ๅฎถ
-ๅฎธ
-ๅฎน
-ๅฎผ
-ๅฎฝ
-ๅฎพ
-ๅฎฟ
-ๅฏ
-ๅฏ
-ๅฏ
-ๅฏ
-ๅฏ
-ๅฏ
-ๅฏ
-ๅฏ
-ๅฏ
-ๅฏ
-ๅฏ
-ๅฏ
-ๅฏ
-ๅฏ
-ๅฏ
-ๅฏ
-ๅฏ
-ๅฏ
-ๅฏ
-ๅฏ
-ๅฏ
-ๅฏก
-ๅฏข
-ๅฏค
-ๅฏฅ
-ๅฏฆ
-ๅฏง
-ๅฏจ
-ๅฏฉ
-ๅฏฎ
-ๅฏฏ
-ๅฏฐ
-ๅฏถ
-ๅฏธ
-ๅฏน
-ๅฏบ
-ๅฏป
-ๅฏผ
-ๅฏพ
-ๅฏฟ
-ๅฐ
-ๅฐ
-ๅฐ
-ๅฐ
-ๅฐ
-ๅฐ
-ๅฐ
-ๅฐ
-ๅฐ
-ๅฐ
-ๅฐ
-ๅฐ
-ๅฐ
-ๅฐ
-ๅฐ
-ๅฐ
-ๅฐ
-ๅฐ
-ๅฐ
-ๅฐ
-ๅฐ
-ๅฐ
-ๅฐ
-ๅฐค
-ๅฐฅ
-ๅฐง
-ๅฐจ
-ๅฐช
-ๅฐซ
-ๅฐฌ
-ๅฐญ
-ๅฐฐ
-ๅฐฑ
-ๅฐด
-ๅฐธ
-ๅฐน
-ๅฐบ
-ๅฐป
-ๅฐผ
-ๅฐฝ
-ๅฐพ
-ๅฐฟ
-ๅฑ
-ๅฑ
-ๅฑ
-ๅฑ
-ๅฑ
-ๅฑ
-ๅฑ
-ๅฑ
-ๅฑ
-ๅฑ
-ๅฑ
-ๅฑ
-ๅฑ
-ๅฑ
-ๅฑ
-ๅฑ
-ๅฑ
-ๅฑ
-ๅฑ
-ๅฑ
-ๅฑ
-ๅฑ
-ๅฑ
-ๅฑก
-ๅฑฃ
-ๅฑฅ
-ๅฑฆ
-ๅฑง
-ๅฑฉ
-ๅฑญ
-ๅฑฎ
-ๅฑฏ
-ๅฑฑ
-ๅฑณ
-ๅฑด
-ๅฑน
-ๅฑบ
-ๅฑผ
-ๅฑพ
-ๅฑฟ
-ๅฒ
-ๅฒ
-ๅฒ
-ๅฒ
-ๅฒ
-ๅฒ
-ๅฒ
-ๅฒ
-ๅฒ
-ๅฒ
-ๅฒ
-ๅฒ
-ๅฒ
-ๅฒ
-ๅฒ
-ๅฒ
-ๅฒ
-ๅฒ
-ๅฒ
-ๅฒ
-ๅฒก
-ๅฒข
-ๅฒฃ
-ๅฒง
-ๅฒจ
-ๅฒฉ
-ๅฒซ
-ๅฒฌ
-ๅฒญ
-ๅฒฑ
-ๅฒณ
-ๅฒต
-ๅฒท
-ๅฒธ
-ๅฒฝ
-ๅฒฟ
-ๅณ
-ๅณ
-ๅณ
-ๅณ
-ๅณ
-ๅณ
-ๅณ
-ๅณ
-ๅณ
-ๅณ
-ๅณ
-ๅณก
-ๅณฃ
-ๅณค
-ๅณฅ
-ๅณฆ
-ๅณง
-ๅณจ
-ๅณฉ
-ๅณช
-ๅณญ
-ๅณฏ
-ๅณฐ
-ๅณป
-ๅณฟ
-ๅด
-ๅด
-ๅด
-ๅด
-ๅด
-ๅด
-ๅด
-ๅด
-ๅด
-ๅด
-ๅด
-ๅด
-ๅด
-ๅด
-ๅด
-ๅด
-ๅด
-ๅด
-ๅด
-ๅด
-ๅด
-ๅด
-ๅด
-ๅดฃ
-ๅดค
-ๅดฆ
-ๅดง
-ๅดฉ
-ๅดญ
-ๅดฎ
-ๅดฑ
-ๅดด
-ๅดถ
-ๅดท
-ๅดฝ
-ๅดพ
-ๅดฟ
-ๅต
-ๅต
-ๅต
-ๅต
-ๅต
-ๅต
-ๅต
-ๅต
-ๅต
-ๅต
-ๅต
-ๅต
-ๅต
-ๅต
-ๅต
-ๅต
-ๅต
-ๅตก
-ๅตฅ
-ๅตฆ
-ๅตฉ
-ๅตซ
-ๅตฌ
-ๅตฏ
-ๅตฐ
-ๅตฒ
-ๅตด
-ๅตท
-ๅตธ
-ๅตบ
-ๅตฝ
-ๅตพ
-ๅถ
-ๅถ
-ๅถ
-ๅถ
-ๅถ
-ๅถ
-ๅถ
-ๅถ
-ๅถ
-ๅถ
-ๅถ
-ๅถ
-ๅถ
-ๅถจ
-ๅถช
-ๅถญ
-ๅถฎ
-ๅถฐ
-ๅถฑ
-ๅถฒ
-ๅถถ
-ๅถท
-ๅถฝ
-ๅถพ
-ๅท
-ๅท
-ๅท
-ๅท
-ๅท
-ๅท
-ๅท
-ๅท
-ๅท
-ๅท
-ๅท
-ๅท
-ๅท
-ๅท
-ๅท
-ๅทก
-ๅทข
-ๅทฃ
-ๅทฅ
-ๅทฆ
-ๅทง
-ๅทจ
-ๅทฉ
-ๅทซ
-ๅทฎ
-ๅทฏ
-ๅทฑ
-ๅทฒ
-ๅทณ
-ๅทด
-ๅทต
-ๅทท
-ๅทป
-ๅทฝ
-ๅทพ
-ๅทฟ
-ๅธ
-ๅธ
-ๅธ
-ๅธ
-ๅธ
-ๅธ
-ๅธ
-ๅธ
-ๅธ
-ๅธ
-ๅธ
-ๅธ
-ๅธ
-ๅธ
-ๅธ
-ๅธ
-ๅธ
-ๅธ
-ๅธ
-ๅธ
-ๅธ
-ๅธ
-ๅธก
-ๅธข
-ๅธฃ
-ๅธฆ
-ๅธง
-ๅธจ
-ๅธซ
-ๅธญ
-ๅธฎ
-ๅธฏ
-ๅธฐ
-ๅธฑ
-ๅธณ
-ๅธถ
-ๅธท
-ๅธธ
-ๅธป
-ๅธผ
-ๅธฝ
-ๅธฟ
-ๅน
-ๅน
-ๅน
-ๅน
-ๅน
-ๅน
-ๅน
-ๅน
-ๅน
-ๅน
-ๅน
-ๅน
-ๅนก
-ๅนข
-ๅนฃ
-ๅนค
-ๅนฅ
-ๅนจ
-ๅนฉ
-ๅนช
-ๅนญ
-ๅนฎ
-ๅนฐ
-ๅนฒ
-ๅนณ
-ๅนด
-ๅนต
-ๅนถ
-ๅนท
-ๅนธ
-ๅนน
-ๅนบ
-ๅนป
-ๅนผ
-ๅนฝ
-ๅนพ
-ๅนฟ
-ๅบ
-ๅบ
-ๅบ
-ๅบ
-ๅบ
-ๅบ
-ๅบ
-ๅบ
-ๅบ
-ๅบ
-ๅบ
-ๅบ
-ๅบ
-ๅบ
-ๅบ
-ๅบ
-ๅบ
-ๅบ
-ๅบ
-ๅบ
-ๅบ
-ๅบ
-ๅบค
-ๅบฅ
-ๅบฆ
-ๅบง
-ๅบช
-ๅบญ
-ๅบณ
-ๅบต
-ๅบถ
-ๅบท
-ๅบธ
-ๅบน
-ๅบพ
-ๅป
-ๅป
-ๅป
-ๅป
-ๅป
-ๅป
-ๅป
-ๅป
-ๅป
-ๅป
-ๅป
-ๅปข
-ๅปฃ
-ๅปฅ
-ๅปฆ
-ๅปง
-ๅปจ
-ๅปช
-ๅปญ
-ๅปถ
-ๅปท
-ๅปธ
-ๅปบ
-ๅปป
-ๅปผ
-ๅปฟ
-ๅผ
-ๅผ
-ๅผ
-ๅผ
-ๅผ
-ๅผ
-ๅผ
-ๅผ
-ๅผ
-ๅผ
-ๅผ
-ๅผ
-ๅผ
-ๅผ
-ๅผ
-ๅผ
-ๅผ
-ๅผ
-ๅผ
-ๅผ
-ๅผ
-ๅผ
-ๅผ
-ๅผข
-ๅผฅ
-ๅผฆ
-ๅผง
-ๅผจ
-ๅผฉ
-ๅผช
-ๅผญ
-ๅผฎ
-ๅผฏ
-ๅผฐ
-ๅผฑ
-ๅผต
-ๅผถ
-ๅผท
-ๅผธ
-ๅผน
-ๅผบ
-ๅผผ
-ๅผพ
-ๅฝ
-ๅฝ
-ๅฝ
-ๅฝ
-ๅฝ
-ๅฝ
-ๅฝ
-ๅฝ
-ๅฝ
-ๅฝ
-ๅฝ
-ๅฝ
-ๅฝ
-ๅฝ
-ๅฝก
-ๅฝข
-ๅฝค
-ๅฝฆ
-ๅฝง
-ๅฝฉ
-ๅฝช
-ๅฝซ
-ๅฝฌ
-ๅฝญ
-ๅฝฏ
-ๅฝฐ
-ๅฝฑ
-ๅฝณ
-ๅฝด
-ๅฝท
-ๅฝน
-ๅฝป
-ๅฝผ
-ๅฝฟ
-ๅพ
-ๅพ
-ๅพ
-ๅพ
-ๅพ
-ๅพ
-ๅพ
-ๅพ
-ๅพ
-ๅพ
-ๅพ
-ๅพ
-ๅพ
-ๅพ
-ๅพ
-ๅพ
-ๅพ
-ๅพ
-ๅพ
-ๅพ
-ๅพ
-ๅพก
-ๅพง
-ๅพจ
-ๅพช
-ๅพฌ
-ๅพญ
-ๅพฎ
-ๅพฏ
-ๅพณ
-ๅพด
-ๅพต
-ๅพถ
-ๅพท
-ๅพน
-ๅพผ
-ๅพฝ
-ๅฟ
-ๅฟ
-ๅฟ
-ๅฟ
-ๅฟ
-ๅฟ
-ๅฟ
-ๅฟ
-ๅฟ
-ๅฟ
-ๅฟ
-ๅฟ
-ๅฟ
-ๅฟ
-ๅฟ
-ๅฟ
-ๅฟ
-ๅฟ
-ๅฟ
-ๅฟ
-ๅฟก
-ๅฟค
-ๅฟง
-ๅฟช
-ๅฟซ
-ๅฟญ
-ๅฟฎ
-ๅฟฑ
-ๅฟณ
-ๅฟต
-ๅฟธ
-ๅฟบ
-ๅฟป
-ๅฟผ
-ๅฟฝ
-ๅฟพ
-ๅฟฟ
-ๆ
-ๆ
-ๆ
-ๆ
-ๆ
-ๆ
-ๆ
-ๆ
-ๆ
-ๆ
-ๆ
-ๆ
-ๆ
-ๆ
-ๆ
-ๆ
-ๆ
-ๆ
-ๆ
-ๆ
-ๆ
-ๆ
-ๆ
-ๆ
-ๆ
-ๆก
-ๆฅ
-ๆฆ
-ๆง
-ๆจ
-ๆฉ
-ๆช
-ๆซ
-ๆฏ
-ๆฑ
-ๆฒ
-ๆณ
-ๆต
-ๆป
-ๆผ
-ๆฟ
-ๆ
-ๆ
-ๆ
-ๆ
-ๆ
-ๆ
-ๆ
-ๆ
-ๆ
-ๆ
-ๆ
-ๆ
-ๆ
-ๆ
-ๆ
-ๆ
-ๆ
-ๆ
-ๆ
-ๆ
-ๆข
-ๆฃ
-ๆค
-ๆง
-ๆจ
-ๆฉ
-ๆช
-ๆซ
-ๆฌ
-ๆญ
-ๆฏ
-ๆฐ
-ๆณ
-ๆต
-ๆถ
-ๆธ
-ๆน
-ๆบ
-ๆป
-ๆผ
-ๆฝ
-ๆฟ
-ๆ
-ๆ
-ๆ
-ๆ
-ๆ
-ๆ
-ๆ
-ๆ
-ๆ
-ๆ
-ๆ
-ๆ
-ๆ
-ๆ
-ๆ
-ๆ
-ๆ
-ๆ
-ๆข
-ๆฃ
-ๆฆ
-ๆจ
-ๆฉ
-ๆช
-ๆซ
-ๆฌ
-ๆญ
-ๆฎ
-ๆฏ
-ๆฐ
-ๆฑ
-ๆฒ
-ๆด
-ๆธ
-ๆป
-ๆผ
-ๆ
-ๆ
-ๆ
-ๆ
-ๆ
-ๆ
-ๆ
-ๆ
-ๆ
-ๆ
-ๆ
-ๆ
-ๆ
-ๆ
-ๆ
-ๆ
-ๆ
-ๆ
-ๆ
-ๆ
-ๆ
-ๆก
-ๆฆ
-ๆง
-ๆจ
-ๆฉ
-ๆซ
-ๆฌ
-ๆญ
-ๆฎ
-ๆฏ
-ๆฐ
-ๆณ
-ๆด
-ๆต
-ๆถ
-ๆธ
-ๆน
-ๆบ
-ๆ
-ๆ
-ๆ
-ๆ
-ๆ
-ๆ
-ๆ
-ๆ
-ๆ
-ๆ
-ๆ
-ๆ
-ๆ
-ๆ
-ๆ
-ๆ
-ๆ
-ๆ
-ๆฃ
-ๆค
-ๆฅ
-ๆฆ
-ๆง
-ๆซ
-ๆฌ
-ๆต
-ๆฟ
-ๆ
-ๆ
-ๆ
-ๆ
-ๆ
-ๆ
-ๆ
-ๆ
-ๆ
-ๆ
-ๆ
-ๆ
-ๆ
-ๆ
-ๆ
-ๆ
-ๆ
-ๆ
ข
-ๆ
ฅ
-ๆ
ง
-ๆ
จ
-ๆ
ฌ
-ๆ
ญ
-ๆ
ฐ
-ๆ
ฑ
-ๆ
ด
-ๆ
ต
-ๆ
ถ
-ๆ
ท
-ๆ
ฝ
-ๆ
พ
-ๆ
-ๆ
-ๆ
-ๆ
-ๆ
-ๆ
-ๆ
-ๆ
-ๆ
-ๆ
-ๆ
-ๆ
-ๆง
-ๆจ
-ๆฉ
-ๆฌ
-ๆญ
-ๆฏ
-ๆฐ
-ๆฒ
-ๆท
-ๆธ
-ๆน
-ๆบ
-ๆพ
-ๆ
-ๆ
-ๆ
-ๆ
-ๆ
-ๆ
-ๆ
-ๆ
-ๆ
-ๆ
-ๆ
-ๆ
-ๆ
-ๆ
-ๆ
-ๆ
-ๆ
-ๆก
-ๆฆ
-ๆญ
-ๆฐ
-ๆฒ
-ๆต
-ๆน
-ๆป
-ๆฝ
-ๆฟ
-ๆ
-ๆ
-ๆ
-ๆ
-ๆ
-ๆ
-ๆ
-ๆ
-ๆ
-ๆ
-ๆ
-ๆ
-ๆ
-ๆ
-ๆ
-ๆ
-ๆ
-ๆ
-ๆ
-ๆ
-ๆ
-ๆ
-ๆ
-ๆก
-ๆข
-ๆฅ
-ๆฆ
-ๆช
-ๆซ
-ๆฌ
-ๆญ
-ๆฎ
-ๆฒ
-ๆณ
-ๆด
-ๆถ
-ๆท
-ๆธ
-ๆบ
-ๆป
-ๆฝ
-ๆพ
-ๆฟ
-ๆ
-ๆ
-ๆ
-ๆ
-ๆ
-ๆ
-ๆ
-ๆ
-ๆ
-ๆ
-ๆ
-ๆ
-ๆ
-ๆ
-ๆ
-ๆ
-ๆ
-ๆ
-ๆ
-ๆ
-ๆ
-ๆ
-ๆ
-ๆ
-ๆ
-ๆ
-ๆ
-ๆก
-ๆข
-ๆฃ
-ๆค
-ๆฅ
-ๆฆ
-ๆง
-ๆฉ
-ๆช
-ๆซ
-ๆฌ
-ๆญ
-ๆฎ
-ๆฏ
-ๆฐ
-ๆฑ
-ๆณ
-ๆถ
-ๆน
-ๆผ
-ๆฝ
-ๆพ
-ๆฟ
-ๆ
-ๆ
-ๆ
-ๆ
-ๆ
-ๆ
-ๆ
-ๆ
-ๆ
-ๆ
-ๆ
-ๆ
-ๆ
-ๆ
-ๆ
-ๆ
-ๆ
-ๆ
-ๆ
-ๆ
-ๆ
-ๆ
-ๆ
-ๆก
-ๆข
-ๆค
-ๆฅ
-ๆจ
-ๆซ
-ๆฌ
-ๆฑ
-ๆต
-ๆถ
-ๆน
-ๆป
-ๆผ
-ๆฝ
-ๆฟ
-ๆ
-ๆ
-ๆ
-ๆ
-ๆ
-ๆ
-ๆ
-ๆ
-ๆ
-ๆ
-ๆ
-ๆ
-ๆ
-ๆ
-ๆ
-ๆ
-ๆ
-ๆ
-ๆ
-ๆ
-ๆ
-ๆ
-ๆ
-ๆ
-ๆ
-ๆ
-ๆ
-ๆ
-ๆ
-ๆก
-ๆข
-ๆฃ
-ๆฅ
-ๆฆ
-ๆง
-ๆจ
-ๆฉ
-ๆซ
-ๆฌ
-ๆญ
-ๆฎ
-ๆฏ
-ๆฑ
-ๆณ
-ๆด
-ๆถ
-ๆท
-ๆผ
-ๆฝ
-ๆพ
-ๆฟ
-ๆ
-ๆ
-ๆ
-ๆ
-ๆ
-ๆ
-ๆ
-ๆ
-ๆ
-ๆ
-ๆ
-ๆ
-ๆ
-ๆ
-ๆ
-ๆ
-ๆ
-ๆ
-ๆ
-ๆ
-ๆก
-ๆข
-ๆฃ
-ๆค
-ๆฅ
-ๆฆ
-ๆจ
-ๆช
-ๆซ
-ๆญ
-ๆฏ
-ๆฑ
-ๆฒ
-ๆต
-ๆน
-ๆบ
-ๆผ
-ๆฝ
-ๆฟ
-ๆ
-ๆ
-ๆ
-ๆ
-ๆ
-ๆ
-ๆ
-ๆ
-ๆ
-ๆ
-ๆ
-ๆ
-ๆ
-ๆ
-ๆ
-ๆ
-ๆ
-ๆ
-ๆก
-ๆข
-ๆฃ
-ๆง
-ๆจ
-ๆฉ
-ๆญ
-ๆฎ
-ๆฐ
-ๆฑ
-ๆฒ
-ๆด
-ๆถ
-ๆท
-ๆบ
-ๆป
-ๆฝ
-ๆ
-ๆ
-ๆ
-ๆ
-ๆ
-ๆ
-ๆ
-ๆ
-ๆ
-ๆ
-ๆ
-ๆ
-ๆ
-ๆ
-ๆ
-ๆ
-ๆ
-ๆ
-ๆ
-ๆก
-ๆข
-ๆฃ
-ๆค
-ๆฅ
-ๆง
-ๆจ
-ๆฉ
-ๆช
-ๆฌ
-ๆฎ
-ๆฏ
-ๆฐ
-ๆฑ
-ๆฒ
-ๆณ
-ๆด
-ๆท
-ๆธ
-ๆน
-ๆบ
-ๆป
-ๆผ
-ๆพ
-ๆฟ
-ๆ
-ๆ
-ๆ
-ๆ
-ๆ
-ๆ
-ๆ
-ๆ
-ๆ
-ๆ
-ๆ
-ๆ
-ๆ
-ๆ
-ๆ
-ๆ
-ๆ
-ๆ
-ๆ
-ๆก
-ๆฃ
-ๆฅ
-ๆฆ
-ๆฉ
-ๆช
-ๆซ
-ๆญ
-ๆฐ
-ๆฒ
-ๆด
-ๆต
-ๆถ
-ๆธ
-ๆบ
-ๆผ
-ๆฝ
-ๆพ
-ๆฟ
-ๆ
-ๆ
-ๆ
-ๆ
-ๆ
-ๆ
-ๆ
-ๆ
-ๆ
-ๆ
-ๆ
-ๆ
-ๆ
-ๆ
-ๆ
-ๆ
-ๆ
-ๆ
-ๆ
-ๆ
-ๆ
-ๆก
-ๆข
-ๆฃ
-ๆค
-ๆฅ
-ๆฆ
-ๆง
-ๆจ
-ๆช
-ๆฌ
-ๆญ
-ๆฏ
-ๆฐ
-ๆด
-ๆต
-ๆบ
-ๆฝ
-ๆพ
-ๆ
-ๆ
-ๆ
-ๆ
-ๆ
-ๆ
-ๆ
-ๆ
-ๆ
-ๆ
-ๆ
-ๆ
-ๆ
-ๆ
-ๆ
-ๆ
-ๆ
-ๆ
-ๆ
-ๆง
-ๆฉ
-ๆญ
-ๆด
-ๆต
-ๆธ
-ๆน
-ๆบ
-ๆฝ
-ๆ
-ๆ
-ๆ
-ๆ
-ๆ
-ๆ
-ๆ
-ๆ
-ๆ
-ๆ
-ๆ
-ๆ
-ๆ
-ๆ
-ๆค
-ๆฅ
-ๆฆ
-ๆง
-ๆฉ
-ๆฌ
-ๆญ
-ๆฎ
-ๆฐ
-ๆฑ
-ๆด
-ๆต
-ๆถ
-ๆท
-ๆธ
-ๆบ
-ๆผ
-ๆ
-ๆ
-ๆ
-ๆ
-ๆ
-ๆ
-ๆ
-ๆ
-ๆ
-ๆ
-ๆ
-ๆ
-ๆ
-ๆ
-ๆ
-ๆข
-ๆค
-ๆฆ
-ๆฉ
-ๆซ
-ๆท
-ๆธ
-ๆฟ
-ๆ
-ๆ
-ๆ
-ๆ
-ๆ
-ๆ
-ๆ
-ๆ
-ๆ
-ๆ
-ๆฅ
-ๆง
-ๆฉ
-ๆซ
-ๆฎ
-ๆฏ
-ๆฐ
-ๆฒ
-ๆถ
-ๆธ
-ๆน
-ๆป
-ๆฝ
-ๆพ
-ๆฟ
-ๆ
-ๆ
-ๆ
-ๆ
-ๆ
-ๆ
-ๆ
-ๆ
-ๆ
-ๆ
-ๆ
-ๆ
-ๆข
-ๆฃ
-ๆฆ
-ๆง
-ๆฉ
-ๆซ
-ๆฌ
-ๆฐ
-ๆฑ
-ๆฒ
-ๆด
-ๆท
-ๆธ
-ๆบ
-ๆป
-ๆ
-ๆ
-ๆ
-ๆ
-ๆ
-ๆ
-ๆ
-ๆ
-ๆ
-ๆ
-ๆ
-ๆ
-ๆ
-ๆ
-ๆ
-ๆ
-ๆ
-ๆ
-ๆ
-ๆก
-ๆค
-ๆฅ
-ๆง
-ๆจ
-ๆฉ
-ๆซ
-ๆญ
-ๆฎ
-ๆฏ
-ๆฐ
-ๆฒ
-ๆด
-ๆถ
-ๆธ
-ๆน
-ๆผ
-ๆฝ
-ๆฟ
-ๆ
-ๆ
-ๆ
-ๆ
-ๆ
-ๆ
-ๆ
-ๆ
-ๆ
-ๆ
-ๆ
-ๆ
-ๆ
-ๆ
-ๆ
-ๆ
-ๆ
-ๆ
-ๆ
-ๆ
-ๆ
-ๆก
-ๆข
-ๆฅ
-ๆฆ
-ๆง
-ๆจ
-ๆฉ
-ๆฌ
-ๆญ
-ๆฎ
-ๆฏ
-ๆฐ
-ๆฑ
-ๆณ
-ๆด
-ๆต
-ๆถ
-ๆท
-ๆธ
-ๆบ
-ๆป
-ๆผ
-ๆฟ
-ๆ
-ๆ
-ๆ
-ๆ
-ๆ
-ๆ
-ๆ
-ๆ
-ๆ
-ๆ
-ๆ
-ๆ
-ๆ
-ๆ
-ๆ
-ๆ
-ๆ
-ๆ
-ๆ
-ๆ
-ๆ
-ๆ
-ๆฃ
-ๆค
-ๆฅ
-ๆง
-ๆจ
-ๆช
-ๆซ
-ๆฌ
-ๆญ
-ๆฏ
-ๆฐ
-ๆฑ
-ๆณ
-ๆด
-ๆต
-ๆถ
-ๆบ
-ๆผ
-ๆฝ
-ๆพ
-ๆ
-ๆ
-ๆ
-ๆ
-ๆ
-ๆ
-ๆ
-ๆ
-ๆ
-ๆ
-ๆ
-ๆ
-ๆ
-ๆ
-ๆ
-ๆ
-ๆ
-ๆ
-ๆ
-ๆก
-ๆข
-ๆฃ
-ๆค
-ๆฅ
-ๆฆ
-ๆง
-ๆจ
-ๆฉ
-ๆซ
-ๆฌ
-ๆฎ
-ๆฏ
-ๆฐ
-ๆณ
-ๆด
-ๆถ
-ๆท
-ๆน
-ๆบ
-ๆป
-ๆผ
-ๆพ
-ๆ
-ๆ
-ๆ
-ๆ
-ๆ
-ๆ
-ๆ
-ๆ
-ๆ
-ๆ
-ๆ
-ๆ
-ๆ
-ๆ
-ๆ
-ๆฃ
-ๆฆ
-ๆง
-ๆจ
-ๆฌ
-ๆฎ
-ๆฑ
-ๆฒ
-ๆด
-ๆต
-ๆถ
-ๆน
-ๆป
-ๆพ
-ๆ
-ๆ
-ๆ
-ๆ
-ๆ
-ๆ
-ๆ
-ๆ
-ๆ
-ๆข
-ๆฆ
-ๆง
-ๆจ
-ๆฉ
-ๆช
-ๆญ
-ๆฐ
-ๆฑ
-ๆฒ
-ๆณ
-ๆด
-ๆต
-ๆท
-ๆธ
-ๆน
-ๆผ
-ๆฝ
-ๆพ
-ๆฟ
-ๆ
-ๆ
-ๆ
-ๆ
-ๆ
-ๆ
-ๆ
-ๆ
-ๆ
-ๆ
-ๆ
-ๆ
-ๆ
-ๆ
-ๆ
-ๆ
-ๆ
-ๆ
-ๆ
-ๆ
-ๆ
-ๆฃ
-ๆฆ
-ๆจ
-ๆช
-ๆซ
-ๆฌ
-ๆญ
-ๆฎ
-ๆฏ
-ๆฑ
-ๆณ
-ๆด
-ๆต
-ๆถ
-ๆบ
-ๆฝ
-ๆฟ
-ๆ
-ๆ
-ๆ
-ๆ
-ๆ
-ๆ
-ๆ
-ๆ
-ๆ
-ๆ
-ๆ
-ๆ
-ๆ
-ๆ
-ๆ
-ๆ
-ๆ
-ๆ
-ๆ
-ๆ
-ๆก
-ๆข
-ๆฅ
-ๆง
-ๆจ
-ๆฉ
-ๆช
-ๆฌ
-ๆญ
-ๆฏ
-ๆฐ
-ๆฑ
-ๆฒ
-ๆณ
-ๆต
-ๆท
-ๆป
-ๆผ
-ๆพ
-ๆฟ
-ๆ
-ๆ
-ๆ
-ๆ
-ๆ
-ๆ
-ๆ
-ๆ
-ๆ
-ๆ
-ๆ
-ๆ
-ๆ
-ๆ
-ๆ
-ๆ
-ๆ
-ๆ
-ๆ
-ๆ
-ๆ
-ๆก
-ๆข
-ๆฃ
-ๆฅ
-ๆฆ
-ๆง
-ๆจ
-ๆช
-ๆซ
-ๆฌ
-ๆญ
-ๆฎ
-ๆฏ
-ๆฐ
-ๆฒ
-ๆณ
-ๆต
-ๆถ
-ๆท
-ๆธ
-ๆน
-ๆป
-ๆฟ
-ๆ
-ๆ
-ๆ
-ๆ
-ๆ
-ๆ
-ๆ
-ๆ
-ๆ
-ๆ
-ๆ
-ๆ
-ๆ
-ๆ
-ๆ
-ๆ
-ๆ
-ๆ
-ๆ
-ๆ
-ๆ
-ๆ
-ๆ
-ๆข
-ๆฃ
-ๆค
-ๆฅ
-ๆฉ
-ๆฌ
-ๆฎ
-ๆฏ
-ๆฐ
-ๆฑ
-ๆณ
-ๆด
-ๆท
-ๆน
-ๆบ
-ๆป
-ๆฝ
-ๆฟ
-ๆ
-ๆ
-ๆ
-ๆ
-ๆ
-ๆ
-ๆ
-ๆ
-ๆ
-ๆ
-ๆ
-ๆ
-ๆ
-ๆ
-ๆ
-ๆ
-ๆ
-ๆ
-ๆ
-ๆ
-ๆ
-ๆ
-ๆ
-ๆ
-ๆ ก
-ๆ ข
-ๆ ฉ
-ๆ ช
-ๆ ฏ
-ๆ ฑ
-ๆ ฒ
-ๆ ณ
-ๆ ด
-ๆ ต
-ๆ ท
-ๆ ธ
-ๆ น
-ๆ ป
-ๆ ผ
-ๆ ฝ
-ๆ พ
-ๆ ฟ
-ๆก
-ๆก
-ๆก
-ๆก
-ๆก
-ๆก
-ๆก
-ๆก
-ๆก
-ๆก
-ๆก
-ๆก
-ๆก
-ๆก
-ๆก
-ๆก
-ๆก
-ๆก
-ๆก
-ๆก
-ๆก
-ๆก
-ๆก
-ๆกก
-ๆกข
-ๆกฃ
-ๆกค
-ๆกฅ
-ๆกฆ
-ๆกง
-ๆกจ
-ๆกฉ
-ๆกซ
-ๆกฎ
-ๆกฏ
-ๆกฒ
-ๆกด
-ๆกถ
-ๆกท
-ๆกน
-ๆกป
-ๆข
-ๆข
-ๆข
-ๆข
-ๆข
-ๆข
-ๆข
-ๆข
-ๆข
-ๆข
-ๆข
-ๆขก
-ๆขข
-ๆขฃ
-ๆขฆ
-ๆขง
-ๆขจ
-ๆขฉ
-ๆขช
-ๆขซ
-ๆขฌ
-ๆขญ
-ๆขฏ
-ๆขฐ
-ๆขฑ
-ๆขฒ
-ๆขณ
-ๆขด
-ๆขต
-ๆขถ
-ๆขผ
-ๆขฝ
-ๆขพ
-ๆขฟ
-ๆฃ
-ๆฃ
-ๆฃ
-ๆฃ
-ๆฃ
-ๆฃ
-ๆฃ
-ๆฃ
-ๆฃ
-ๆฃ
-ๆฃ
-ๆฃ
-ๆฃ
-ๆฃ
-ๆฃ
-ๆฃ
-ๆฃ
-ๆฃ
-ๆฃ
-ๆฃ
-ๆฃ
-ๆฃก
-ๆฃฃ
-ๆฃจ
-ๆฃช
-ๆฃซ
-ๆฃฌ
-ๆฃฎ
-ๆฃฏ
-ๆฃฐ
-ๆฃฑ
-ๆฃฒ
-ๆฃต
-ๆฃธ
-ๆฃน
-ๆฃบ
-ๆฃป
-ๆฃผ
-ๆฃฝ
-ๆค
-ๆค
-ๆค
-ๆค
-ๆค
-ๆค
-ๆค
-ๆค
-ๆค
-ๆค
-ๆค
-ๆค
-ๆค
-ๆค
-ๆค
-ๆค
-ๆค
-ๆคค
-ๆคฉ
-ๆคซ
-ๆคญ
-ๆคฎ
-ๆคฐ
-ๆคฒ
-ๆคด
-ๆคธ
-ๆคน
-ๆคฝ
-ๆคฟ
-ๆฅ
-ๆฅ
-ๆฅ
-ๆฅ
-ๆฅ
-ๆฅ
-ๆฅ
-ๆฅ
-ๆฅ
-ๆฅ
-ๆฅ
-ๆฅ
-ๆฅ
-ๆฅ
-ๆฅ
-ๆฅ
-ๆฅข
-ๆฅฃ
-ๆฅค
-ๆฅฅ
-ๆฅฆ
-ๆฅจ
-ๆฅฉ
-ๆฅช
-ๆฅซ
-ๆฅญ
-ๆฅฎ
-ๆฅฏ
-ๆฅฐ
-ๆฅฑ
-ๆฅต
-ๆฅถ
-ๆฅท
-ๆฅธ
-ๆฅน
-ๆฅบ
-ๆฅผ
-ๆฅฝ
-ๆฆ
-ๆฆ
-ๆฆ
-ๆฆ
-ๆฆ
-ๆฆ
-ๆฆ
-ๆฆ
-ๆฆ
-ๆฆ
-ๆฆ
-ๆฆ
-ๆฆ
-ๆฆ
-ๆฆ
-ๆฆ
-ๆฆ
-ๆฆ
-ๆฆ
-ๆฆฅ
-ๆฆง
-ๆฆจ
-ๆฆซ
-ๆฆญ
-ๆฆฐ
-ๆฆฑ
-ๆฆด
-ๆฆท
-ๆฆบ
-ๆฆป
-ๆฆผ
-ๆฆพ
-ๆง
-ๆง
-ๆง
-ๆง
-ๆง
-ๆง
-ๆง
-ๆง
-ๆง
-ๆง
-ๆง
-ๆง
-ๆง
-ๆง
-ๆง
-ๆง
-ๆง
-ๆง
-ๆง
-ๆงข
-ๆงฅ
-ๆงฉ
-ๆงฌ
-ๆงญ
-ๆงฑ
-ๆงฒ
-ๆงต
-ๆงป
-ๆงฝ
-ๆงพ
-ๆงฟ
-ๆจ
-ๆจ
-ๆจ
-ๆจ
-ๆจ
-ๆจ
-ๆจ
-ๆจ
-ๆจ
-ๆจ
-ๆจ
-ๆจ
-ๆจ
-ๆจก
-ๆจฃ
-ๆจจ
-ๆจฉ
-ๆจช
-ๆจซ
-ๆจญ
-ๆจฏ
-ๆจฑ
-ๆจต
-ๆจน
-ๆจฝ
-ๆจพ
-ๆฉ
-ๆฉ
-ๆฉ
-ๆฉ
-ๆฉ
-ๆฉ
-ๆฉ
-ๆฉ
-ๆฉ
-ๆฉ
-ๆฉ
-ๆฉ
-ๆฉ
-ๆฉ
-ๆฉก
-ๆฉฆ
-ๆฉช
-ๆฉฑ
-ๆฉน
-ๆฉบ
-ๆฉผ
-ๆฉฟ
-ๆช
-ๆช
-ๆช
-ๆช
-ๆช
-ๆช
-ๆช
-ๆช
-ๆช
-ๆช
-ๆช
-ๆช
-ๆช
-ๆช
-ๆช
-ๆช
-ๆช
-ๆชฅ
-ๆชง
-ๆชจ
-ๆชฉ
-ๆชซ
-ๆชฌ
-ๆชฐ
-ๆชต
-ๆชป
-ๆชฟ
-ๆซ
-ๆซ
-ๆซ
-ๆซ
-ๆซ
-ๆซ
-ๆซ
-ๆซ
-ๆซ
-ๆซก
-ๆซฐ
-ๆซป
-ๆซผ
-ๆฌ
-ๆฌ
-ๆฌ
-ๆฌ
-ๆฌ
-ๆฌ
-ๆฌ
-ๆฌ
-ๆฌ
-ๆฌก
-ๆฌข
-ๆฌฃ
-ๆฌค
-ๆฌฆ
-ๆฌง
-ๆฌฌ
-ๆฌฑ
-ๆฌฒ
-ๆฌท
-ๆฌธ
-ๆฌน
-ๆฌบ
-ๆฌป
-ๆฌพ
-ๆฌฟ
-ๆญ
-ๆญ
-ๆญ
-ๆญ
-ๆญ
-ๆญ
-ๆญ
-ๆญ
-ๆญ
-ๆญ
-ๆญ
-ๆญ
-ๆญ
-ๆญ
-ๆญ
-ๆญ
-ๆญข
-ๆญฃ
-ๆญค
-ๆญฅ
-ๆญฆ
-ๆญง
-ๆญฉ
-ๆญช
-ๆญฏ
-ๆญณ
-ๆญด
-ๆญธ
-ๆญน
-ๆญป
-ๆญผ
-ๆฎ
-ๆฎ
-ๆฎ
-ๆฎ
-ๆฎ
-ๆฎ
-ๆฎ
-ๆฎ
-ๆฎ
-ๆฎ
-ๆฎ
-ๆฎ
-ๆฎ
-ๆฎ
-ๆฎ
-ๆฎ
-ๆฎ
-ๆฎก
-ๆฎข
-ๆฎฃ
-ๆฎช
-ๆฎญ
-ๆฎณ
-ๆฎด
-ๆฎต
-ๆฎท
-ๆฎฝ
-ๆฎฟ
-ๆฏ
-ๆฏ
-ๆฏ
-ๆฏ
-ๆฏ
-ๆฏ
-ๆฏ
-ๆฏ
-ๆฏ
-ๆฏ
-ๆฏ
-ๆฏ
-ๆฏ
-ๆฏ
-ๆฏ
-ๆฏ
-ๆฏ
-ๆฏ
-ๆฏ
-ๆฏ
-ๆฏก
-ๆฏจ
-ๆฏซ
-ๆฏฌ
-ๆฏฏ
-ๆฏฐ
-ๆฏณ
-ๆฏต
-ๆฏถ
-ๆฏธ
-ๆฏน
-ๆฏป
-ๆฏฝ
-ๆฐ
-ๆฐ
-ๆฐ
-ๆฐ
-ๆฐ
-ๆฐ
-ๆฐ
-ๆฐ
-ๆฐ
-ๆฐ
-ๆฐ
-ๆฐ
-ๆฐ
-ๆฐ
-ๆฐ
-ๆฐ
-ๆฐ
-ๆฐ
-ๆฐ
-ๆฐก
-ๆฐข
-ๆฐฃ
-ๆฐค
-ๆฐฆ
-ๆฐง
-ๆฐจ
-ๆฐฉ
-ๆฐช
-ๆฐฎ
-ๆฐฏ
-ๆฐฐ
-ๆฐฒ
-ๆฐด
-ๆฐต
-ๆฐท
-ๆฐธ
-ๆฐน
-ๆฐฝ
-ๆฐพ
-ๆฐฟ
-ๆฑ
-ๆฑ
-ๆฑ
-ๆฑ
-ๆฑ
-ๆฑ
-ๆฑ
-ๆฑ
-ๆฑ
-ๆฑ
-ๆฑ
-ๆฑ
-ๆฑ
-ๆฑ
-ๆฑ
-ๆฑ
-ๆฑ
-ๆฑ
-ๆฑ
-ๆฑ
-ๆฑ
-ๆฑ
-ๆฑก
-ๆฑฃ
-ๆฑค
-ๆฑง
-ๆฑจ
-ๆฑฉ
-ๆฑช
-ๆฑซ
-ๆฑญ
-ๆฑฏ
-ๆฑฐ
-ๆฑฒ
-ๆฑด
-ๆฑถ
-ๆฑธ
-ๆฑน
-ๆฑบ
-ๆฑฝ
-ๆฑพ
-ๆฒ
-ๆฒ
-ๆฒ
-ๆฒ
-ๆฒ
-ๆฒ
-ๆฒ
-ๆฒ
-ๆฒ
-ๆฒ
-ๆฒ
-ๆฒ
-ๆฒ
-ๆฒ
-ๆฒ
-ๆฒ
-ๆฒ
-ๆฒ
-ๆฒ
-ๆฒ
-ๆฒ
-ๆฒ
-ๆฒ
-ๆฒก
-ๆฒข
-ๆฒฃ
-ๆฒค
-ๆฒฅ
-ๆฒฆ
-ๆฒง
-ๆฒจ
-ๆฒฉ
-ๆฒช
-ๆฒซ
-ๆฒฌ
-ๆฒญ
-ๆฒฎ
-ๆฒฑ
-ๆฒฒ
-ๆฒณ
-ๆฒด
-ๆฒต
-ๆฒถ
-ๆฒธ
-ๆฒน
-ๆฒป
-ๆฒผ
-ๆฒฝ
-ๆฒพ
-ๆฒฟ
-ๆณ
-ๆณ
-ๆณ
-ๆณ
-ๆณ
-ๆณ
-ๆณ
-ๆณ
-ๆณ
-ๆณ
-ๆณ
-ๆณ
-ๆณ
-ๆณ
-ๆณ
-ๆณ
-ๆณ
-ๆณ
-ๆณ
-ๆณ
-ๆณ
-ๆณ
-ๆณ
-ๆณก
-ๆณข
-ๆณฃ
-ๆณฅ
-ๆณจ
-ๆณช
-ๆณซ
-ๆณฌ
-ๆณฎ
-ๆณฏ
-ๆณฐ
-ๆณฑ
-ๆณฒ
-ๆณณ
-ๆณต
-ๆณท
-ๆณธ
-ๆณบ
-ๆณป
-ๆณผ
-ๆณฝ
-ๆณพ
-ๆด
-ๆด
-ๆด
-ๆด
-ๆด
-ๆด
-ๆด
-ๆด
-ๆด
-ๆด
-ๆด
-ๆด
-ๆด
-ๆด
-ๆด
-ๆด
-ๆด
-ๆดฃ
-ๆดค
-ๆดฅ
-ๆดง
-ๆดจ
-ๆดฉ
-ๆดช
-ๆดซ
-ๆดญ
-ๆดฎ
-ๆดฑ
-ๆดฒ
-ๆดณ
-ๆดด
-ๆดต
-ๆดธ
-ๆดน
-ๆดบ
-ๆดป
-ๆดผ
-ๆดฝ
-ๆดพ
-ๆดฟ
-ๆต
-ๆต
-ๆต
-ๆต
-ๆต
-ๆต
-ๆต
-ๆต
-ๆต
-ๆต
-ๆต
-ๆต
-ๆต
-ๆต
-ๆต
-ๆต
-ๆต
-ๆต
-ๆต
-ๆต
-ๆต
-ๆต
-ๆต
-ๆต
-ๆต
-ๆต
-ๆตก
-ๆตฃ
-ๆตค
-ๆตฅ
-ๆตฆ
-ๆตฉ
-ๆตช
-ๆตฌ
-ๆตฎ
-ๆตฏ
-ๆตฐ
-ๆตฑ
-ๆตฒ
-ๆตด
-ๆตท
-ๆตธ
-ๆตผ
-ๆตฟ
-ๆถ
-ๆถ
-ๆถ
-ๆถ
-ๆถ
-ๆถ
-ๆถ
-ๆถ
-ๆถ
-ๆถ
-ๆถ
-ๆถ
-ๆถ
-ๆถ
-ๆถ
-ๆถ
-ๆถ
-ๆถ
-ๆถ
-ๆถ
-ๆถ
-ๆถก
-ๆถข
-ๆถฃ
-ๆถค
-ๆถฅ
-ๆถฆ
-ๆถง
-ๆถจ
-ๆถฉ
-ๆถช
-ๆถซ
-ๆถฌ
-ๆถฎ
-ๆถฏ
-ๆถฒ
-ๆถด
-ๆถต
-ๆถท
-ๆถธ
-ๆถผ
-ๆถฟ
-ๆท
-ๆท
-ๆท
-ๆท
-ๆท
-ๆท
-ๆท
-ๆท
-ๆท
-ๆท
-ๆท
-ๆท
-ๆท
-ๆท
-ๆท
-ๆท
-ๆท
-ๆท
-ๆท
-ๆท
-ๆท
-ๆทก
-ๆทข
-ๆทค
-ๆทฆ
-ๆทจ
-ๆทซ
-ๆทฌ
-ๆทฎ
-ๆทฏ
-ๆทฐ
-ๆทฑ
-ๆทณ
-ๆทท
-ๆทน
-ๆทป
-ๆทผ
-ๆธ
-ๆธ
-ๆธ
-ๆธ
-ๆธ
-ๆธ
-ๆธ
-ๆธ
-ๆธ
-ๆธ
-ๆธ
-ๆธ
-ๆธ
-ๆธ
-ๆธ
-ๆธ
-ๆธ
-ๆธ
-ๆธ
-ๆธ
-ๆธ
-ๆธ
-ๆธ
-ๆธ
-ๆธก
-ๆธข
-ๆธฃ
-ๆธค
-ๆธฅ
-ๆธง
-ๆธฉ
-ๆธซ
-ๆธฌ
-ๆธญ
-ๆธฏ
-ๆธฐ
-ๆธฑ
-ๆธฒ
-ๆธด
-ๆธถ
-ๆธธ
-ๆธบ
-ๆธป
-ๆธผ
-ๆน
-ๆน
-ๆน
-ๆน
-ๆน
-ๆน
-ๆน
-ๆน
-ๆน
-ๆน
-ๆน
-ๆน
-ๆน
-ๆน
-ๆน
-ๆน
-ๆน
-ๆนก
-ๆนข
-ๆนฃ
-ๆนฆ
-ๆนง
-ๆนฉ
-ๆนซ
-ๆนฎ
-ๆนฒ
-ๆนณ
-ๆนด
-ๆนผ
-ๆนพ
-ๆนฟ
-ๆบ
-ๆบ
-ๆบ
-ๆบ
-ๆบ
-ๆบ
-ๆบ
-ๆบ
-ๆบ
-ๆบ
-ๆบ
-ๆบ
-ๆบ
-ๆบ
-ๆบ
-ๆบ
-ๆบ
-ๆบ
-ๆบ
-ๆบ
-ๆบ
-ๆบ
-ๆบก
-ๆบข
-ๆบฅ
-ๆบฆ
-ๆบง
-ๆบช
-ๆบซ
-ๆบฏ
-ๆบฑ
-ๆบฒ
-ๆบณ
-ๆบด
-ๆบต
-ๆบถ
-ๆบท
-ๆบบ
-ๆบป
-ๆบฝ
-ๆป
-ๆป
-ๆป
-ๆป
-ๆป
-ๆป
-ๆป
-ๆป
-ๆป
-ๆป
-ๆป
-ๆป
-ๆป
-ๆป
-ๆป
-ๆป
-ๆป
-ๆป
-ๆป
-ๆป
-ๆป
-ๆป
-ๆป
-ๆปก
-ๆปข
-ๆปค
-ๆปฅ
-ๆปฆ
-ๆปง
-ๆปจ
-ๆปฉ
-ๆปช
-ๆปซ
-ๆปฎ
-ๆปด
-ๆปน
-ๆปบ
-ๆปฝ
-ๆผ
-ๆผ
-ๆผ
-ๆผ
-ๆผ
-ๆผ
-ๆผ
-ๆผ
-ๆผ
-ๆผ
-ๆผ
-ๆผ
-ๆผ
-ๆผ
-ๆผ
-ๆผข
-ๆผค
-ๆผฆ
-ๆผฉ
-ๆผช
-ๆผซ
-ๆผญ
-ๆผฏ
-ๆผฐ
-ๆผฑ
-ๆผณ
-ๆผด
-ๆผถ
-ๆผท
-ๆผน
-ๆผป
-ๆผผ
-ๆผพ
-ๆฝ
-ๆฝ
-ๆฝ
-ๆฝ
-ๆฝ
-ๆฝ
-ๆฝ
-ๆฝ
-ๆฝ
-ๆฝ
-ๆฝ
-ๆฝ
-ๆฝ
-ๆฝ
-ๆฝ
-ๆฝ
-ๆฝ
-ๆฝ
-ๆฝข
-ๆฝค
-ๆฝฆ
-ๆฝฉ
-ๆฝฌ
-ๆฝญ
-ๆฝฎ
-ๆฝฒ
-ๆฝด
-ๆฝต
-ๆฝธ
-ๆฝบ
-ๆฝผ
-ๆฝฝ
-ๆฝพ
-ๆพ
-ๆพ
-ๆพ
-ๆพ
-ๆพ
-ๆพ
-ๆพ
-ๆพ
-ๆพ
-ๆพ
-ๆพ
-ๆพ
-ๆพ
-ๆพ
-ๆพก
-ๆพฃ
-ๆพค
-ๆพฅ
-ๆพง
-ๆพจ
-ๆพช
-ๆพซ
-ๆพญ
-ๆพณ
-ๆพด
-ๆพถ
-ๆพน
-ๆพผ
-ๆพพ
-ๆฟ
-ๆฟ
-ๆฟ
-ๆฟ
-ๆฟ
-ๆฟ
-ๆฟ
-ๆฟ
-ๆฟ
-ๆฟ
-ๆฟ
-ๆฟ
-ๆฟ
-ๆฟ
-ๆฟ
-ๆฟ
-ๆฟก
-ๆฟฆ
-ๆฟฉ
-ๆฟซ
-ๆฟฌ
-ๆฟฎ
-ๆฟฏ
-ๆฟธ
-็
-็
-็
-็
-็
-็
-็
-็
-็
-็
-็
-็
-็ก
-็ฃ
-็ฉ
-็ฌ
-็ฐ
-็ฑ
-็ด
-็ต
-็น
-็บ
-็ผ
-็ฝ
-็
-็
-็
-็
-็
-็
-็
-็
-็
-็ฉ
-็ซ
-็ฌ
-็ญ
-็ฏ
-็ฐ
-็ต
-็ถ
-็ธ
-็บ
-็ผ
-็พ
-็ฟ
-็
-็
-็
-็
-็
-็
-็
-็
-็
-็
-็
-็
-็
-็
-็
-็
-็
-็ก
-็ค
-็ง
-็ซ
-็ฌ
-็ญ
-็ฎ
-็ฏ
-็ฐ
-็ฑ
-็ณ
-็ต
-็ท
-็ธ
-็น
-็บ
-็ผ
-็ฝ
-็ฟ
-็
-็
-็
-็
-็
-็
-็
-็
-็
-็
-็
-็
-็
-็
-็
-็
-็ค
-็ฆ
-็ง
-็จ
-็ฉ
-็ซ
-็ฌ
-็ญ
-็ฏ
-็ท
-็น
-็บ
-็ป
-็ฝ
-็
-็
-็
-็
-็
-็
-็
-็
-็
-็
-็
-็
-็
-็
-็
-็
-็
-็
-็ก
-็ฆ
-็ฉ
-็ซ
-็ฎ
-็ฏ
-็ฐ
-็ฑ
-็ฒ
-็ด
-็ถ
-็น
-็ป
-็ผ
-็
-็
-็
-็
-็
-็
-็
-็
-็
-็
-็
-็
-็
-็
-็
-็
-็
ค
-็
ฆ
-็
ง
-็
จ
-็
ฎ
-็
ฒ
-็
ณ
-็
ด
-็
ต
-็
ถ
-็
ธ
-็
น
-็
บ
-็
ฝ
-็
ฟ
-็
-็
-็
-็
-็
-็
-็
-็
-็
-็
-็
-็
-็
-็
-็
-็ค
-็จ
-็ฌ
-็ญ
-็ฏ
-็ฑ
-็ณ
-็ด
-็ต
-็ธ
-็น
-็ฟ
-็
-็
-็
-็
-็
-็
-็
-็
-็
-็
-็
-็
-็
-็
-็
-็
-็
-็
-็ข
-็ฅ
-็ง
-็ฌ
-็ฎ
-็น
-็ฟ
-็
-็
-็
-็
-็
-็
-็
-็
-็
-็
-็
-็ข
-็ง
-็จ
-็ช
-็ฌ
-็ญ
-็ฐ
-็ฑ
-็ต
-็ถ
-็ท
-็ธ
-็น
-็ป
-็ฝ
-็ฟ
-็
-็
-็
-็
-็
-็
-็
-็
-็
-็
-็
-็
-็
-็
-็ก
-็ข
-็ฃ
-็ค
-็ฅ
-็ฆ
-็ง
-็ฉ
-็ฎ
-็ฏ
-็ฒ
-็ด
-็ต
-็ท
-็ธ
-็น
-็บ
-็ป
-็พ
-็ฟ
-็
-็
-็
-็
-็
-็
-็
-็
-็
-็
-็
-็
-็
-็จ
-็ฌ
-็ญ
-็ฏ
-็ฐ
-็ด
-็ถ
-็ท
-็ธ
-็น
-็บ
-็ผ
-็ฝ
-็
-็
-็
-็
-็
-็
-็
-็
-็
-็
-็
-็
-็
-็
-็
-็
-็
-็
-็
-็ก
-็ข
-็ฅ
-็ง
-็จ
-็ฉ
-็ฌ
-็ญ
-็ฎ
-็ฏ
-็ฐ
-็ฑ
-็ฒ
-็ณ
-็ด
-็ถ
-็ท
-็ธ
-็บ
-็ป
-็ผ
-็
-็
-็
-็
-็
-็
-็
-็
-็
-็
-็
-็
-็
-็
-็
-็
-็ก
-็ข
-็ฅ
-็ง
-็ฉ
-็ช
-็ซ
-็ฌ
-็ฎ
-็ฐ
-็ฑ
-็ฒ
-็ณ
-็ด
-็ต
-็ถ
-็ท
-็ธ
-็พ
-็ฟ
-็
-็
-็
-็
-็
-็
-็
-็
-็
-็
-็
-็
-็ฃ
-็ฆ
-็จ
-็ฌ
-็ญ
-็ฎ
-็ฏ
-็ฒ
-็ด
-็ถ
-็ป
-็พ
-็
-็
-็
-็
-็
-็
-็
-็
-็
-็
-็
-็
-็
-็
-็
-็
-็
-็
-็
-็
-็
-็
-็ก
-็ข
-็ฅ
-็ฆ
-็ฉ
-็ซ
-็ญ
-็ฎ
-็ฏ
-็ฐ
-็ฑ
-็ฒ
-็ณ
-็ถ
-็ท
-็บ
-็ป
-็ผ
-็
-็
-็
-็
-็
-็
-็
-็
-็
-็
-็
-็
-็
-็
-็
-็
-็
-็
-็
-็ฃ
-็ฅ
-็ฆ
-็ง
-็ฉ
-็ช
-็ญ
-็ฎ
-็ฐ
-็ฒ
-็ต
-็ธ
-็น
-็บ
-็ฝ
-็พ
-็
-็
-็
-็
-็
-็
-็
-็
-็
-็
-็
-็
-็
-็
-็ข
-็ฃ
-็ค
-็ฅ
-็ฆ
-็จ
-็ช
-็ซ
-็ฌ
-็ญ
-็ฎ
-็ฏ
-็ฐ
-็ฑ
-็ฒ
-็ณ
-็ด
-็ต
-็ถ
-็ป
-็ผ
-็
-็
-็
-็
-็
-็
-็
-็
-็
-็
-็
-็
-็
-็
-็
-็
-็
-็
-็
-็
-็
-็ข
-็ง
-็จ
-็ช
-็ญ
-็ฎ
-็ฐ
-็ฑ
-็ณ
-็ด
-็ถ
-็ท
-็ธ
-็ฝ
-็พ
-็ฟ
-็
-็
-็
-็
-็
-็
-็
-็
-็
-็
-็
-็
-็
-็
-็
-็
-็
-็
-็
-็
-็
-็ค
-็ฅ
-็ง
-็จ
-็ฉ
-็ช
-็ฒ
-็ต
-็ท
-็ธ
-็น
-็บ
-็ฟ
-็
-็
-็
-็
-็
-็
-็
-็
-็
-็
-็
-็
-็
-็
-็ข
-็ฃ
-็ค
-็ฆ
-็จ
-็ฌ
-็ฎ
-็ฏ
-็ด
-็ถ
-็ท
-็น
-็ป
-็ฟ
-็
-็
-็
-็
-็
-็
-็
-็
-็
-็
-็
-็
-็
-็
-็
-็
-็
-็
-็
-็ก
-็ข
-็ฅ
-็ฆ
-็จ
-็ฉ
-็ช
-็ซ
-็ฌ
-็ญ
-็ฏ
-็ฐ
-็ฑ
-็ฒ
-็ณ
-็ด
-็ต
-็ท
-็ธ
-็บ
-็ป
-็ฝ
-็พ
-็ฟ
-็
-็
-็
-็
-็
-็
-็
-็
-็
-็
-็
-็
-็
-็
-็
-็
-็
-็
-็
-็ค
-็ฅ
-็ฆ
-็ง
-็ช
-็ซ
-็ฌ
-็ฏ
-็ฒ
-็ณ
-็ด
-็ถ
-็ท
-็ธ
-็น
-็ผ
-็ฝ
-็ฟ
-็
-็
-็
-็
-็
-็
-็
-็
-็
-็
-็
-็
-็
-็
-็
-็
-็
-็
-็ก
-็ข
-็ฃ
-็ค
-็ฅ
-็ง
-็ซ
-็ฌ
-็ญ
-็ฎ
-็ฏ
-็ฐ
-็ฑ
-็ฒ
-็ณ
-็ด
-็ต
-็ธ
-็น
-็ป
-็ผ
-็ฝ
-็พ
-็
-็
-็
-็
-็
-็
-็
-็
-็
-็
-็
-็
-็
-็
-็
-็
-็
-็
-็
-็
-็
-็
-็
-็ก
-็ข
-็ฃ
-็ค
-็ฆ
-็ง
-็จ
-็ฉ
-็ช
-็ซ
-็ฏ
-็ฐ
-็ฑ
-็ฒ
-็ด
-็น
-็บ
-็ป
-็ผ
-็พ
-็ฟ
-็
-็
-็
-็
-็
-็
-็
-็
-็
-็
-็
-็
-็
-็
-็
-็
-็
-็
-็
-็ข
-็ฃ
-็ค
-็ฅ
-็ฆ
-็จ
-็ฉ
-็ช
-็ซ
-็ญ
-็ฐ
-็ฑ
-็ณ
-็ด
-็ต
-็ธ
-็ผ
-็พ
-็ฟ
-็
-็
-็
-็
-็
-็
-็
-็
-็
-็
-็ข
-็ฃ
-็ฅ
-็ฆ
-็จ
-็ช
-็ซ
-็ฏ
-็ด
-็ถ
-็ธ
-็น
-็บ
-็ป
-็ผ
-็ฝ
-็พ
-็ฟ
-็
-็
-็
-็
-็
-็
-็
-็
-็
-็
-็
-็
-็
-็
-็
-็ค
-็ฆ
-็ช
-็ฎ
-็ฑ
-็ฒ
-็ด
-็ฟ
-็
-็
-็
-็
-็
-็
-็
-็
-็
-็
-็
-็
-็
-็
-็
-็
-็
-็
-็
-็ก
-็ข
-็ฃ
-็ฅ
-็ฉ
-็ฌ
-็ญ
-็ฎ
-็ฏ
-็ฑ
-็ฒ
-็ด
-็ต
-็ธ
-็น
-็ป
-็ผ
-็พ
-็
-็
-็
-็
-็
-็
-็
-็
-็
-็
-็
-็
-็
-็
-็
-็
-็
-็
-็ข
-็ฆ
-็จ
-็ฉ
-็ฌ
-็ญ
-็ฏ
-็ด
-็ต
-็ถ
-็ท
-็ธ
-็น
-็บ
-็ผ
-็ฝ
-็
-็
-็
-็
-็
-็
-็
-็
-็
-็
-็
-็
-็
-็
-็
-็
-็
-็ก
-็ข
-็ฃ
-็ฅ
-็ฆ
-็จ
-็ฉ
-็ช
-็ซ
-็ฌ
-็ญ
-็ฐ
-็ณ
-็ท
-็น
-็บ
-็ฝ
-็พ
-็ฟ
-็
-็
-็
-็
-็
-็
-็
-็
-็
-็
-็
-็
-็
-็
-็
-็ข
-็ค
-็ฅ
-็ง
-็ฉ
-็ช
-็ฌ
-็ญ
-็ฏ
-็ฐ
-็ณ
-็ต
-็ป
-็ฝ
-็พ
-็ฟ
-็
-็
-็
-็
-็
-็
-็
-็
-็
-็
-็
-็
-็ข
-็ฃ
-็ฅ
-็ง
-็จ
-็ฉ
-็ซ
-็ฌ
-็ญ
-็ฎ
-็ฐ
-็ฑ
-็ฒ
-็ณ
-็ถ
-็ธ
-็ป
-็ผ
-็ฝ
-็พ
-็ฟ
-็
-็
-็
-็
-็
-็
-็
-็
-็
-็
-็
-็
-็
-็
-็
-็
-็
-็
-็
-็
-็
-็ ข
-็ ฃ
-็ ฅ
-็ ฆ
-็ ง
-็ ฌ
-็ ญ
-็ ฎ
-็ ฏ
-็ ฐ
-็ ฒ
-็ ด
-็ ท
-็ ธ
-็ น
-็ บ
-็ ป
-็ ผ
-็ พ
-็ก
-็ก
-็ก
-็ก
-็ก
-็ก
-็ก
-็ก
-็ก
-็ก
-็ก
-็ก
-็ก
-็ก
-็ก
-็ก
-็ก
-็ก
-็กก
-็กช
-็กซ
-็กฌ
-็กฎ
-็กฐ
-็กต
-็กท
-็กผ
-็กพ
-็กฟ
-็ข
-็ข
-็ข
-็ข
-็ข
-็ข
-็ข
-็ข
-็ข
-็ข
-็ข
-็ข
-็ข
-็ข
-็ข
-็ข
-็ข
-็ข
-็ข
-็ข
-็ขก
-็ขฃ
-็ขฅ
-็ขง
-็ขจ
-็ขช
-็ขซ
-็ขฐ
-็ขฑ
-็ขฒ
-็ขณ
-็ขด
-็ขถ
-็ขบ
-็ขป
-็ขพ
-็ฃ
-็ฃ
-็ฃ
-็ฃ
-็ฃ
-็ฃ
-็ฃ
-็ฃ
-็ฃ
-็ฃ
-็ฃ
-็ฃ
-็ฃ
-็ฃ
-็ฃ
-็ฃ
-็ฃ
-็ฃ
-็ฃ
-็ฃก
-็ฃข
-็ฃจ
-็ฃฌ
-็ฃฒ
-็ฃณ
-็ฃด
-็ฃต
-็ฃท
-็ฃน
-็ฃบ
-็ฃป
-็ฃพ
-็ฃฟ
-็ค
-็ค
-็ค
-็ค
-็ค
-็ค
-็ค
-็ค
-็ค
-็คก
-็คค
-็คง
-็คจ
-็คฎ
-็คฒ
-็คด
-็คถ
-็คบ
-็คผ
-็คฝ
-็คพ
-็คฟ
-็ฅ
-็ฅ
-็ฅ
-็ฅ
-็ฅ
-็ฅ
-็ฅ
-็ฅ
-็ฅ
-็ฅ
-็ฅ
-็ฅ
-็ฅ
-็ฅ
-็ฅ
-็ฅ
-็ฅ
-็ฅ
-็ฅ
-็ฅ
-็ฅ
-็ฅ
-็ฅ
-็ฅ
-็ฅ
-็ฅ
-็ฅข
-็ฅค
-็ฅฅ
-็ฅง
-็ฅจ
-็ฅซ
-็ฅญ
-็ฅฏ
-็ฅฑ
-็ฅฒ
-็ฅด
-็ฅถ
-็ฅท
-็ฅธ
-็ฅบ
-็ฅผ
-็ฅพ
-็ฅฟ
-็ฆ
-็ฆ
-็ฆ
-็ฆ
-็ฆ
-็ฆ
-็ฆ
-็ฆ
-็ฆ
-็ฆ
-็ฆ
-็ฆ
-็ฆ
-็ฆ
-็ฆ
-็ฆ
-็ฆ
-็ฆ
-็ฆ
-็ฆค
-็ฆฅ
-็ฆง
-็ฆจ
-็ฆฉ
-็ฆซ
-็ฆฎ
-็ฆณ
-็ฆด
-็ฆต
-็ฆท
-็ฆน
-็ฆบ
-็ฆป
-็ฆฝ
-็ฆพ
-็ง
-็ง
-็ง
-็ง
-็ง
-็ง
-็ง
-็ง
-็ง
-็ง
-็ง
-็ง
-็ง
-็ง
-็ง
-็ง
-็ง
-็ง
-็ง
-็ง
-็ง
-็งฃ
-็งค
-็งฆ
-็งง
-็งฉ
-็งช
-็งซ
-็งฌ
-็งญ
-็งฏ
-็งฐ
-็งด
-็งธ
-็งป
-็งฝ
-็งพ
-็งฟ
-็จ
-็จ
-็จ
-็จ
-็จ
-็จ
-็จ
-็จ
-็จ
-็จ
-็จ
-็จ
-็จ
-็จ
-็จ
-็จ
-็จ
-็จ
-็จฃ
-็จญ
-็จฎ
-็จฑ
-็จฒ
-็จณ
-็จท
-็จน
-็จบ
-็จป
-็จผ
-็จฝ
-็จพ
-็จฟ
-็ฉ
-็ฉ
-็ฉ
-็ฉ
-็ฉ
-็ฉ
-็ฉ
-็ฉ
-็ฉ
-็ฉ
-็ฉ
-็ฉ
-็ฉ
-็ฉ
-็ฉ
-็ฉ
-็ฉก
-็ฉง
-็ฉจ
-็ฉฌ
-็ฉฐ
-็ฉด
-็ฉต
-็ฉถ
-็ฉท
-็ฉธ
-็ฉน
-็ฉบ
-็ฉผ
-็ฉฝ
-็ฉพ
-็ฉฟ
-็ช
-็ช
-็ช
-็ช
-็ช
-็ช
-็ช
-็ช
-็ช
-็ช
-็ช
-็ช
-็ช
-็ช
-็ช
-็ช
-็ช
-็ช
-็ช
-็ช
-็ช
-็ช
-็ช
-็ช
-็ช
-็ชก
-็ชฃ
-็ชฅ
-็ชฆ
-็ชจ
-็ชฉ
-็ชซ
-็ชฌ
-็ชญ
-็ชฐ
-็ชฑ
-็ชณ
-็ชด
-็ชธ
-็ชน
-็ชบ
-็ชป
-็ชฝ
-็ชพ
-็ชฟ
-็ซ
-็ซ
-็ซ
-็ซ
-็ซ
-็ซ
-็ซ
-็ซ
-็ซ
-็ซ
-็ซ
-็ซ
-็ซ
-็ซฃ
-็ซฅ
-็ซฆ
-็ซญ
-็ซฎ
-็ซฏ
-็ซน
-็ซบ
-็ซป
-็ซฝ
-็ซฟ
-็ฌ
-็ฌ
-็ฌ
-็ฌ
-็ฌ
-็ฌ
-็ฌ
-็ฌ
-็ฌ
-็ฌ
-็ฌ
-็ฌ
-็ฌ
-็ฌ
-็ฌ
-็ฌ
-็ฌก
-็ฌค
-็ฌฅ
-็ฌฆ
-็ฌจ
-็ฌช
-็ฌซ
-็ฌฌ
-็ฌญ
-็ฌฎ
-็ฌฏ
-็ฌฑ
-็ฌฒ
-็ฌณ
-็ฌด
-็ฌต
-็ฌธ
-็ฌน
-็ฌบ
-็ฌผ
-็ฌพ
-็ญ
-็ญ
-็ญ
-็ญ
-็ญ
-็ญ
-็ญ
-็ญ
-็ญ
-็ญ
-็ญ
-็ญ
-็ญ
-็ญ
-็ญ
-็ญ
-็ญ
-็ญข
-็ญค
-็ญฅ
-็ญฆ
-็ญฉ
-็ญญ
-็ญฎ
-็ญฏ
-็ญฐ
-็ญฑ
-็ญฒ
-็ญณ
-็ญด
-็ญต
-็ญท
-็ญน
-็ญป
-็ญผ
-็ญพ
-็ฎ
-็ฎ
-็ฎ
-็ฎ
-็ฎ
-็ฎ
-็ฎ
-็ฎ
-็ฎ
-็ฎ
-็ฎ
-็ฎ
-็ฎ
-็ฎ
-็ฎ
-็ฎ
-็ฎ
-็ฎ
-็ฎก
-็ฎข
-็ฎฃ
-็ฎฆ
-็ฎง
-็ฎจ
-็ฎฉ
-็ฎช
-็ฎซ
-็ฎฌ
-็ฎญ
-็ฎฏ
-็ฎฑ
-็ฎด
-็ฎต
-็ฎธ
-็ฎป
-็ฎผ
-็ฎพ
-็ฏ
-็ฏ
-็ฏ
-็ฏ
-็ฏ
-็ฏ
-็ฏ
-็ฏ
-็ฏ
-็ฏ
-็ฏ
-็ฏ
-็ฏก
-็ฏข
-็ฏฅ
-็ฏฆ
-็ฏจ
-็ฏช
-็ฏญ
-็ฏฎ
-็ฏฏ
-็ฏฑ
-็ฏฒ
-็ฏท
-็ฏธ
-็ฏน
-็ฏป
-็ฏผ
-็ฏพ
-็ฐ
-็ฐ
-็ฐ
-็ฐ
-็ฐ
-็ฐ
-็ฐ
-็ฐ
-็ฐ
-็ฐ
-็ฐ
-็ฐ
-็ฐ
-็ฐ
-็ฐก
-็ฐฆ
-็ฐง
-็ฐจ
-็ฐช
-็ฐฌ
-็ฐฐ
-็ฐณ
-็ฐด
-็ฐต
-็ฐธ
-็ฐฟ
-็ฑ
-็ฑ
-็ฑ
-็ฑ
-็ฑ
-็ฑ
-็ฑ
-็ฑ
-็ฑ
-็ฑ
-็ฑ
-็ฑ
-็ฑข
-็ฑฃ
-็ฑค
-็ฑฅ
-็ฑง
-็ฑฏ
-็ฑณ
-็ฑด
-็ฑน
-็ฑบ
-็ฑป
-็ฑผ
-็ฑฝ
-็ฑพ
-็ฒ
-็ฒ
-็ฒ
-็ฒ
-็ฒ
-็ฒ
-็ฒ
-็ฒ
-็ฒ
-็ฒ
-็ฒ
-็ฒ
-็ฒ
-็ฒ
-็ฒ
-็ฒ
-็ฒข
-็ฒค
-็ฒฅ
-็ฒง
-็ฒฉ
-็ฒช
-็ฒฎ
-็ฒฑ
-็ฒฒ
-็ฒณ
-็ฒถ
-็ฒน
-็ฒบ
-็ฒป
-็ฒผ
-็ฒฝ
-็ฒพ
-็ฒฟ
-็ณ
-็ณ
-็ณ
-็ณ
-็ณ
-็ณ
-็ณ
-็ณ
-็ณ
-็ณ
-็ณ
-็ณ
-็ณ
-็ณ
-็ณ
-็ณ
-็ณฆ
-็ณง
-็ณจ
-็ณฏ
-็ณต
-็ณธ
-็ณป
-็ด
-็ด
-็ด
-็ด
-็ด
-็ด
-็ด
-็ด
-็ด
-็ด
-็ด
-็ดข
-็ดง
-็ดซ
-็ดฏ
-็ดต
-็ดฝ
-็ดพ
-็ต
-็ต
-็ต
-็ต
-็ต
-็ต
-็ต
-็ต
-็ต
-็ต
-็ต
-็ต
-็ต
-็ตฃ
-็ตฆ
-็ตฉ
-็ตช
-็ตซ
-็ตฎ
-็ตต
-็ตท
-็ตบ
-็ตป
-็ตฟ
-็ถ
-็ถ
-็ถ
-็ถ
-็ถ
-็ถ
-็ถ
-็ถ
-็ถ
-็ถ
-็ถ
-็ถฆ
-็ถช
-็ถฎ
-็ถฏ
-็ถด
-็ถท
-็ท
-็ท
-็ท
-็ท
-็ท
-็ท
-็ท
-็ท
-็ทฃ
-็ทฉ
-็ทบ
-็ทผ
-็ธ
-็ธ
-็ธ
-็ธ
-็ธ
-็ธ
-็ธข
-็ธฆ
-็ธฉ
-็ธฎ
-็ธฏ
-็ธบ
-็ธป
-็ธฟ
-็น
-็น
-็น
-็น
-็น
-็น
-็น
-็น
-็น
-็น
-็น
-็น
-็น
-็นฃ
-็นณ
-็นธ
-็นป
-็บ
-็บ
-็บ
-็บ
-็บ
-็บ
-็บ
-็บ
-็บ
-็บ
-็บ
-็บก
-็บข
-็บฃ
-็บค
-็บฅ
-็บฆ
-็บง
-็บจ
-็บฉ
-็บช
-็บซ
-็บฌ
-็บญ
-็บฎ
-็บฏ
-็บฐ
-็บฑ
-็บฒ
-็บณ
-็บด
-็บต
-็บถ
-็บท
-็บธ
-็บน
-็บบ
-็บป
-็บผ
-็บฝ
-็บพ
-็บฟ
-็ป
-็ป
-็ป
-็ป
-็ป
-็ป
-็ป
-็ป
-็ป
-็ป
-็ป
-็ป
-็ป
-็ป
-็ป
-็ป
-็ป
-็ป
-็ป
-็ป
-็ป
-็ป
-็ป
-็ป
-็ป
-็ป
-็ป
-็ป
-็ป
-็ป
-็ป
-็ป
-็ป
-็ปก
-็ปข
-็ปฃ
-็ปค
-็ปฅ
-็ปฆ
-็ปง
-็ปจ
-็ปฉ
-็ปช
-็ปซ
-็ปญ
-็ปฎ
-็ปฏ
-็ปฐ
-็ปฑ
-็ปฒ
-็ปณ
-็ปด
-็ปต
-็ปถ
-็ปท
-็ปธ
-็ปน
-็ปบ
-็ปป
-็ปผ
-็ปฝ
-็ปพ
-็ปฟ
-็ผ
-็ผ
-็ผ
-็ผ
-็ผ
-็ผ
-็ผ
-็ผ
-็ผ
-็ผ
-็ผ
-็ผ
-็ผ
-็ผ
-็ผ
-็ผ
-็ผ
-็ผ
-็ผ
-็ผ
-็ผ
-็ผ
-็ผ
-็ผ
-็ผ
-็ผ
-็ผ
-็ผ
-็ผ
-็ผ
-็ผ
-็ผ
-็ผก
-็ผข
-็ผฃ
-็ผค
-็ผฅ
-็ผฆ
-็ผง
-็ผจ
-็ผฉ
-็ผช
-็ผซ
-็ผฌ
-็ผญ
-็ผฎ
-็ผฏ
-็ผฐ
-็ผฑ
-็ผฒ
-็ผณ
-็ผด
-็ผต
-็ผถ
-็ผธ
-็ผบ
-็ผผ
-็ผพ
-็ฝ
-็ฝ
-็ฝ
-็ฝ
-็ฝ
-็ฝ
-็ฝ
-็ฝ
-็ฝ
-็ฝ
-็ฝ
-็ฝ
-็ฝ
-็ฝ
-็ฝ
-็ฝ
-็ฝ
-็ฝ
-็ฝ
-็ฝก
-็ฝข
-็ฝฃ
-็ฝฅ
-็ฝฆ
-็ฝจ
-็ฝฉ
-็ฝช
-็ฝญ
-็ฝฎ
-็ฝฏ
-็ฝฒ
-็ฝณ
-็ฝด
-็ฝถ
-็ฝน
-็ฝป
-็ฝฝ
-็ฝพ
-็ฝฟ
-็พ
-็พ
-็พ
-็พ
-็พ
-็พ
-็พ
-็พ
-็พ
-็พ
-็พ
-็พ
-็พ
-็พ
-็พ
-็พ
-็พ
-็พ
-็พ
-็พ
-็พ
-็พ
-็พก
-็พฃ
-็พค
-็พง
-็พจ
-็พฉ
-็พซ
-็พญ
-็พฏ
-็พฐ
-็พฑ
-็พฒ
-็พด
-็พต
-็พถ
-็พธ
-็พน
-็พผ
-็พฝ
-็พพ
-็พฟ
-็ฟ
-็ฟ
-็ฟ
-็ฟ
-็ฟ
-็ฟ
-็ฟ
-็ฟ
-็ฟ
-็ฟ
-็ฟ
-็ฟ
-็ฟ
-็ฟ
-็ฟ
-็ฟ
-็ฟ
-็ฟ
-็ฟ
-็ฟ
-็ฟก
-็ฟฃ
-็ฟฅ
-็ฟฆ
-็ฟฉ
-็ฟซ
-็ฟฌ
-็ฟฎ
-็ฟฏ
-็ฟฐ
-็ฟฑ
-็ฟฒ
-็ฟณ
-็ฟถ
-็ฟป
-็ฟผ
-็ฟพ
-็ฟฟ
-่
-่
-่
-่
-่
-่
-่
-่
-่
-่
-่
-่
-่
-่
-่
-่
-่
-่
-่
-่
-่
-่ก
-่ข
-่ค
-่ฅ
-่ฆ
-่ง
-่จ
-่ฉ
-่ญ
-่ฐ
-่ฑ
-่ณ
-่ต
-่ถ
-่ท
-่ธ
-่ป
-่ฝ
-่ฟ
-่
-่
-่
-่
-่
-่
-่
-่
-่
-่
-่
-่
-่
-่ก
-่ฉ
-่ช
-่ฐ
-่ฑ
-่ฒ
-่ด
-่ป
-่ฝ
-่ฟ
-่
-่
-่
-่
-่
-่
-่
-่
-่
-่
-่
-่
-่
-่
-่
-่
-่
-่
-่
-่ก
-่ข
-่ฃ
-่ค
-่ฅ
-่ฆ
-่ฉ
-่ช
-่ซ
-่ฌ
-่ญ
-่ฎ
-่ฏ
-่ฑ
-่ฒ
-่ณ
-่ด
-่ถ
-่ธ
-่บ
-่ผ
-่ฝ
-่พ
-่ฟ
-่
-่
-่
-่
-่
-่
-่
-่
-่
-่
-่
-่
-่
-่
-่
-่
-่
-่
-่
-่
-่
-่
-่ก
-่ค
-่ฅ
-่ง
-่จ
-่ฉ
-่ช
-่ซ
-่ฌ
-่ญ
-่ฎ
-่ฏ
-่ฐ
-่ฑ
-่ฒ
-่ณ
-่ด
-่ถ
-่ธ
-่น
-่บ
-่ป
-่ผ
-่ฝ
-่พ
-่
-่
-่
-่
-่
-่
-่
-่
-่
-่
-่
-่
-่
-่
-่
-่
-่
-่
-่
-่
-่
-่
-่ก
-่ข
-่ฃ
-่ค
-่ฉ
-่ฌ
-่ฏ
-่ฐ
-่ฑ
-่ฒ
-่ณ
-่ถ
-่ท
-่ธ
-่น
-่ฝ
-่พ
-่ฟ
-่
-่
-่
-่
-่
-่
-่
-่
-่
-่
-่
-่
-่
-่
-่
-่
-่
-่
ฅ
-่
ง
-่
ฉ
-่
ฌ
-่
ญ
-่
ฎ
-่
ฏ
-่
ฐ
-่
ฑ
-่
ฒ
-่
ด
-่
ท
-่
น
-่
บ
-่
ป
-่
ผ
-่
ฝ
-่
พ
-่
ฟ
-่
-่
-่
-่
-่
-่
-่
-่
-่
-่
-่
-่
-่
-่
-่
-่ฃ
-่ฆ
-่จ
-่ช
-่ซ
-่ฐ
-่ณ
-่ด
-่ถ
-่บ
-่ป
-่
-่
-่
-่
-่
-่
-่
-่
-่
-่
-่
-่
-่
-่
-่
-่
-่
-่ก
-่ฃ
-่ง
-่จ
-่ฉ
-่ช
-่ฌ
-่ญ
-่ฏ
-่ฑ
-่ฒ
-่ณ
-่ด
-่บ
-่ป
-่ผ
-่พ
-่ฟ
-่
-่
-่
-่
-่
-่
-่
-่
-่
-่
-่
-่
-่
-่
-่
-่
-่
-่
-่
-่
-่
-่
-่
-่
-่
-่
-่ก
-่ข
-่ฃ
-่ฅ
-่ฆ
-่จ
-่ช
-่ซ
-่ฌ
-่ฐ
-่ฑ
-่ฒ
-่ณ
-่ด
-่ต
-่ถ
-่ท
-่ธ
-่น
-่บ
-่ป
-่ผ
-่
-่
-่
-่
-่
-่
-่
-่
-่
-่
-่
-่
-่
-่
-่จ
-่ฎ
-่ฏ
-่ฐ
-่ฒ
-่ณ
-่ด
-่น
-่บ
-่ป
-่ฝ
-่พ
-่ฟ
-่
-่
-่
-่
-่
-่
-่
-่
-่
-่
-่
-่
-่
-่
-่
-่
-่
-่
-่
-่
-่ก
-่ฃ
-่ค
-่ฅ
-่ฆ
-่ง
-่จ
-่ฉ
-่ช
-่ซ
-่ฌ
-่ญ
-่ฎ
-่ฏ
-่ฐ
-่ฑ
-่ณ
-่ด
-่ถ
-่ท
-่ธ
-่น
-่บ
-่ผ
-่ฝ
-่พ
-่
-่
-่
-่
-่
-่
-่
-่
-่
-่
-่
-่
-่
-่
-่
-่
-่
-่
-่
-่
-่
-่
-่
-่
-่
-่
-่ก
-่ฃ
-่ค
-่ฅ
-่ฆ
-่ง
-่จ
-่ซ
-่ฏ
-่ฑ
-่ณ
-่ด
-่ถ
-่ท
-่น
-่บ
-่ป
-่พ
-่
-่
-่
-่
-่
-่
-่
-่
-่
-่
-่
-่
-่
-่
-่
-่
-่
-่
-่
-่
-่
-่
-่
-่
-่
-่
-่ง
-่จ
-่ซ
-่ฌ
-่ญ
-่ฏ
-่ฑ
-่ฒ
-่ณ
-่ด
-่ต
-่ถ
-่ท
-่ธ
-่น
-่บ
-่ผ
-่
-่
-่
-่
-่
-่
-่
-่
-่
-่
-่
-่
-่
-่
-่
-่
-่
-่
-่
-่
-่
-่
-่
-่
-่
-่
-่ก
-่ฃ
-่ค
-่ฅ
-่ฆ
-่ง
-่จ
-่ฉ
-่ช
-่ซ
-่ฌ
-่ญ
-่ฎ
-่ฏ
-่ด
-่ต
-่ท
-่ธ
-่ป
-่ผ
-่ฝ
-่
-่
-่
-่
-่
-่
-่
-่
-่
-่
-่
-่
-่
-่
-่
-่
-่จ
-่ฉ
-่ช
-่ซ
-่ฐ
-่ฑ
-่ฒ
-่ณ
-่ด
-่ถ
-่ท
-่ธ
-่น
-่บ
-่ผ
-่ฝ
-่ฟ
-่
-่
-่
-่
-่
-่
-่
-่
-่
-่
-่
-่
-่
-่
-่
-่
-่
-่
-่
-่
-่
-่ก
-่ข
-่ฅ
-่ง
-่ฉ
-่ช
-่ซ
-่ญ
-่ฏ
-่ฐ
-่ฑ
-่ฒ
-่ด
-่ต
-่ถ
-่ท
-่ธ
-่น
-่บ
-่ผ
-่ฝ
-่พ
-่
-่
-่
-่
-่
-่
-่
-่
-่
-่
-่
-่
-่
-่
-่
-่ฃ
-่ค
-่ฅ
-่ฆ
-่ง
-่จ
-่ฉ
-่ฌ
-่ฎ
-่ฏ
-่ฑ
-่ถ
-่ท
-่ธ
-่น
-่ผ
-่ฝ
-่
-่
-่
-่
-่
-่
-่
-่
-่
-่
-่
-่
-่
-่
-่
-่
-่ก
-่ฃ
-่จ
-่ฉ
-่ซ
-่ฌ
-่ญ
-่ฐ
-่ฑ
-่ณ
-่ด
-่ต
-่ถ
-่ธ
-่น
-่บ
-่ฝ
-่
-่
-่
-่
-่
-่
-่
-่
-่
-่
-่
-่
-่
-่
-่
-่ก
-่ข
-่ง
-่จ
-่ฎ
-่ฏ
-่ฑ
-่ฒ
-่ด
-่ถ
-่ธ
-่น
-่บ
-่ป
-่ผ
-่ฝ
-่พ
-่ฟ
-่
-่
-่
-่
-่
-่
-่
-่
-่
-่
-่
-่
-่
-่
-่
-่
-่
-่ข
-่ฃ
-่ฅ
-่ฆ
-่ง
-่ช
-่ซ
-่ฌ
-่ฎ
-่ฐ
-่ฑ
-่ณ
-่ด
-่ต
-่ท
-่บ
-่ผ
-่ฟ
-่
-่
-่
-่
-่
-่
-่
-่
-่
-่
-่
-่
-่
-่
-่ก
-่ช
-่ซ
-่ฌ
-่ฏ
-่ต
-่ท
-่ธ
-่น
-่บ
-่ป
-่ผ
-่ฝ
-่
-่
-่
-่
-่
-่
-่
-่
-่
-่
-่
-่
-่ก
-่ฃ
-่ค
-่จ
-่ซ
-่ฐ
-่ฒ
-่ณ
-่ด
-่น
-่บ
-่ป
-่พ
-่
-่
-่
-่
-่
-่
-่
-่
-่
-่
-่
-่
-่
-่ก
-่ข
-่ค
-่ฆ
-่จ
-่ช
-่ซ
-่ฌ
-่ฎ
-่ฏ
-่ฐ
-่ถ
-่ท
-่ธ
-่น
-่ฟ
-่
-่
-่
-่
-่
-่
-่
-่
-่
-่
-่
-่
-่
-่
-่
-่
-่
-่
-่ข
-่ค
-่ฅ
-่ฆ
-่จ
-่ฉ
-่ซ
-่ญ
-่ณ
-่น
-่ป
-่พ
-่ฟ
-่
-่
-่
-่
-่
-่
-่
-่
-่
-่ก
-่ค
-่ง
-่ฉ
-่ญ
-่ต
-่ธ
-่ผ
-่ฝ
-่
-่
-่
-่
-่
-่
-่
-่
-่
-่
-่
-่
-่
-่
-่
-่
-่
-่ก
-่ข
-่ฅ
-่ง
-่ซ
-่ฌ
-่ฎ
-่ฑ
-่ต
-่น
-่บ
-่ป
-่ผ
-่ฝ
-่พ
-่ฟ
-่
-่
-่
-่
-่
-่
-่
-่
-่
-่
-่
-่
-่
-่
-่
-่
-่
-่ก
-่ข
-่ฃ
-่ค
-่ง
-่จ
-่ฉ
-่ช
-่ฌ
-่ฏ
-่ฐ
-่ฑ
-่ณ
-่ด
-่ต
-่ถ
-่ท
-่น
-่บ
-่ป
-่พ
-่ฟ
-่
-่
-่
-่
-่
-่
-่
-่
-่
-่
-่
-่
-่
-่
-่
-่
-่
-่
-่
-่
-่
-่ฃ
-่ค
-่ฆ
-่ฉ
-่ช
-่ซ
-่ญ
-่ฎ
-่ฐ
-่ฑ
-่ฒ
-่ณ
-่ด
-่ธ
-่น
-่พ
-่
-่
-่
-่
-่
-่
-่
-่
-่
-่
-่
-่
-่
-่
-่
-่
-่
-่
-่
-่
-่
-่
-่ก
-่ข
-่ฃ
-่ค
-่ฅ
-่จ
-่ฉ
-่ฎ
-่ฐ
-่ฑ
-่ด
-่ท
-่บ
-่ป
-่ผ
-่พ
-่ฟ
-่
-่
-่
-่
-่
-่
-่
-่
-่
-่
-่
-่
-่
-่
-่
-่
-่
-่
-่ก
-่ฃ
-่ค
-่ฅ
-่ฎ
-่ฏ
-่ฐ
-่ฑ
-่ฒ
-่ด
-่ถ
-่ป
-่ผ
-่ฝ
-่พ
-่
-่
-่
-่
-่
-่
-่
-่
-่
-่
-่
-่
-่
-่
-่
-่ฃ
-่จ
-่ฉ
-่ซ
-่ฌ
-่ญ
-่ฎ
-่ฏ
-่ณ
-่ต
-่น
-่บ
-่ฝ
-่พ
-่ฟ
-่
-่
-่
-่
-่
-่
-่
-่
-่
-่
-่
-่
-่
-่
-่
-่ข
-่ฅ
-่ฆ
-่ง
-่ฉ
-่ช
-่ซ
-่ญ
-่ฎ
-่ณ
-่น
-่บ
-่พ
-่
-่
-่
-่
-่
-่
-่
-่
-่
-่
-่
-่
-่
-่
-่ ก
-่ ข
-่ จ
-่ ช
-่ ญ
-่ ฒ
-่ น
-่ ป
-่ ผ
-่ก
-่ก
-่ก
-่ก
-่ก
-่ก
-่ก
-่ก
-่ก
-่ก
-่ก
-่ก
-่ก
-่ก
-่กก
-่กข
-่กฃ
-่กค
-่กฅ
-่กจ
-่กฉ
-่กซ
-่กฌ
-่กฎ
-่กฐ
-่กฑ
-่กฒ
-่กต
-่กท
-่กน
-่กฝ
-่กพ
-่กฟ
-่ข
-่ข
-่ข
-่ข
-่ข
-่ข
-่ข
-่ข
-่ข
-่ข
-่ข
-่ข
-่ข
-่ข
-่ข
-่ข
-่ข
-่ขข
-่ขค
-่ขจ
-่ขฉ
-่ขช
-่ขซ
-่ขญ
-่ขฏ
-่ขฑ
-่ขด
-่ขท
-่ขธ
-่ขบ
-่ขผ
-่ขฟ
-่ฃ
-่ฃ
-่ฃ
-่ฃ
-่ฃ
-่ฃ
-่ฃ
-่ฃ
-่ฃ
-่ฃ
-่ฃ
-่ฃ
-่ฃ
-่ฃ
-่ฃ
-่ฃ
-่ฃ
-่ฃ
-่ฃ
-่ฃ
-่ฃ
-่ฃข
-่ฃค
-่ฃฅ
-่ฃจ
-่ฃช
-่ฃฏ
-่ฃฐ
-่ฃฑ
-่ฃณ
-่ฃด
-่ฃธ
-่ฃน
-่ฃผ
-่ฃฝ
-่ฃพ
-่ค
-่ค
-่ค
-่ค
-่ค
-่ค
-่ค
-่ค
-่ค
-่ค
-่ค
-่ค
-่คก
-่คฃ
-่คฅ
-่คฆ
-่คง
-่คช
-่คซ
-่คญ
-่คฏ
-่คฐ
-่คฑ
-่คด
-่คต
-่คถ
-่คท
-่คพ
-่ฅ
-่ฅ
-่ฅ
-่ฅ
-่ฅ
-่ฅ
-่ฅ
-่ฅ
-่ฅ
-่ฅ
-่ฅ
-่ฅ
-่ฅฆ
-่ฅช
-่ฅซ
-่ฅญ
-่ฅฎ
-่ฅฐ
-่ฅต
-่ฅถ
-่ฅป
-่ฅผ
-่ฅฟ
-่ฆ
-่ฆ
-่ฆ
-่ฆ
-่ฆ
-่ฆ
-่ฆ
-่ฆ
-่ฆ
-่ฆ
-่ฆ
-่ฆ
-่ฆง
-่ฆช
-่ฆณ
-่ง
-่ง
-่ง
-่ง
-่ง
-่ง
-่ง
-่ง
-่ง
-่ง
-่ง
-่ง
-่ง
-่ง
-่ง
-่ง
-่ง
-่ง
-่ง
-่ง
-่ง
-่ง
-่งฃ
-่งฅ
-่งฆ
-่งฉ
-่งซ
-่งญ
-่งฏ
-่งฑ
-่งณ
-่งบ
-่งผ
-่งฝ
-่งฟ
-่จ
-่จ
-่จ
-่จ
-่จ
-่จ
-่จ
-่จ
-่จ
-่จ
-่จ
-่จฆ
-่จง
-่จช
-่จญ
-่จฑ
-่จณ
-่จด
-่จน
-่จป
-่จผ
-่จพ
-่จฟ
-่ฉ
-่ฉ
-่ฉ
-่ฉ
-่ฉ
-่ฉ
-่ฉข
-่ฉง
-่ฉฐ
-่ฉฑ
-่ฉน
-่ช
-่ช
-่ช
-่ช
-่ช
-่ช
-่ช
-่ชจ
-่ชญ
-่ชฐ
-่ชฒ
-่ชท
-่ชพ
-่ชฟ
-่ซ
-่ซ
-่ซ
-่ซ
-่ซ
-่ซ
-่ซก
-่ซฎ
-่ซฒ
-่ซด
-่ซต
-่ซพ
-่ฌ
-่ฌ
-่ฌ
-่ฌฅ
-่ฌฆ
-่ฌท
-่ญ
-่ญ
-่ญ
-่ญ
-่ญ
-่ญ
-่ญ
-่ญ
-่ญฆ
-่ญฉ
-่ญฌ
-่ญฐ
-่ญฒ
-่ญณ
-่ญถ
-่ญบ
-่ญพ
-่ฎ
-่ฎ
-่ฎ
-่ฎ
-่ฎ
-่ฎ
-่ฎก
-่ฎข
-่ฎฃ
-่ฎค
-่ฎฅ
-่ฎฆ
-่ฎง
-่ฎจ
-่ฎฉ
-่ฎช
-่ฎซ
-่ฎฌ
-่ฎญ
-่ฎฎ
-่ฎฏ
-่ฎฐ
-่ฎฑ
-่ฎฒ
-่ฎณ
-่ฎด
-่ฎต
-่ฎถ
-่ฎท
-่ฎธ
-่ฎน
-่ฎบ
-่ฎป
-่ฎผ
-่ฎฝ
-่ฎพ
-่ฎฟ
-่ฏ
-่ฏ
-่ฏ
-่ฏ
-่ฏ
-่ฏ
-่ฏ
-่ฏ
-่ฏ
-่ฏ
-่ฏ
-่ฏ
-่ฏ
-่ฏ
-่ฏ
-่ฏ
-่ฏ
-่ฏ
-่ฏ
-่ฏ
-่ฏ
-่ฏ
-่ฏ
-่ฏ
-่ฏ
-่ฏ
-่ฏ
-่ฏ
-่ฏ
-่ฏ
-่ฏ
-่ฏ
-่ฏ
-่ฏก
-่ฏข
-่ฏฃ
-่ฏค
-่ฏฅ
-่ฏฆ
-่ฏง
-่ฏจ
-่ฏฉ
-่ฏช
-่ฏซ
-่ฏฌ
-่ฏญ
-่ฏฎ
-่ฏฏ
-่ฏฐ
-่ฏฑ
-่ฏฒ
-่ฏณ
-่ฏด
-่ฏต
-่ฏถ
-่ฏท
-่ฏธ
-่ฏน
-่ฏบ
-่ฏป
-่ฏผ
-่ฏฝ
-่ฏพ
-่ฏฟ
-่ฐ
-่ฐ
-่ฐ
-่ฐ
-่ฐ
-่ฐ
-่ฐ
-่ฐ
-่ฐ
-่ฐ
-่ฐ
-่ฐ
-่ฐ
-่ฐ
-่ฐ
-่ฐ
-่ฐ
-่ฐ
-่ฐ
-่ฐ
-่ฐ
-่ฐ
-่ฐ
-่ฐ
-่ฐ
-่ฐ
-่ฐ
-่ฐ
-่ฐ
-่ฐ
-่ฐ
-่ฐ
-่ฐก
-่ฐข
-่ฐฃ
-่ฐค
-่ฐฅ
-่ฐฆ
-่ฐง
-่ฐจ
-่ฐฉ
-่ฐช
-่ฐซ
-่ฐฌ
-่ฐญ
-่ฐฎ
-่ฐฏ
-่ฐฐ
-่ฐฑ
-่ฐฒ
-่ฐณ
-่ฐด
-่ฐต
-่ฐถ
-่ฐท
-่ฐน
-่ฐบ
-่ฐผ
-่ฐฝ
-่ฐพ
-่ฐฟ
-่ฑ
-่ฑ
-่ฑ
-่ฑ
-่ฑ
-่ฑ
-่ฑ
-่ฑ
-่ฑ
-่ฑ
-่ฑ
-่ฑ
-่ฑ
-่ฑ
-่ฑ
-่ฑก
-่ฑข
-่ฑจ
-่ฑฉ
-่ฑช
-่ฑซ
-่ฑญ
-่ฑฏ
-่ฑฐ
-่ฑณ
-่ฑต
-่ฑธ
-่ฑน
-่ฑบ
-่ฑฟ
-่ฒ
-่ฒ
-่ฒ
-่ฒ
-่ฒ
-่ฒ
-่ฒ
-่ฒ
-่ฒ
-่ฒ
-่ฒ
-่ฒ
-่ฒ
-่ฒ
-่ฒ
-่ฒ
-่ฒ
-่ฒ
-่ฒก
-่ฒค
-่ฒจ
-่ฒฌ
-่ฒฎ
-่ฒณ
-่ฒด
-่ฒท
-่ฒธ
-่ฒป
-่ฒผ
-่ฒฟ
-่ณ
-่ณ
-่ณ
-่ณ
-่ณ
-่ณ
-่ณฃ
-่ณฆ
-่ณจ
-่ณธ
-่ณผ
-่ด
-่ด
-่ด
-่ด
-่ด
-่ด
-่ด
-่ด
-่ดก
-่ดข
-่ดฃ
-่ดค
-่ดฅ
-่ดฆ
-่ดง
-่ดจ
-่ดฉ
-่ดช
-่ดซ
-่ดฌ
-่ดญ
-่ดฎ
-่ดฏ
-่ดฐ
-่ดฑ
-่ดฒ
-่ดณ
-่ดด
-่ดต
-่ดถ
-่ดท
-่ดธ
-่ดน
-่ดบ
-่ดป
-่ดผ
-่ดฝ
-่ดพ
-่ดฟ
-่ต
-่ต
-่ต
-่ต
-่ต
-่ต
-่ต
-่ต
-่ต
-่ต
-่ต
-่ต
-่ต
-่ต
-่ต
-่ต
-่ต
-่ต
-่ต
-่ต
-่ต
-่ต
-่ต
-่ต
-่ต
-่ต
-่ต
-่ต
-่ต
-่ต
-่ต
-่ต
-่ตก
-่ตข
-่ตฃ
-่ตค
-่ตฅ
-่ตฆ
-่ตง
-่ตฉ
-่ตช
-่ตซ
-่ตญ
-่ตฐ
-่ตณ
-่ตด
-่ตต
-่ตถ
-่ตท
-่ตบ
-่ถ
-่ถ
-่ถ
-่ถ
-่ถ
-่ถ
-่ถ
-่ถ
-่ถ
-่ถ
-่ถ
-่ถ
-่ถ
-่ถข
-่ถฃ
-่ถจ
-่ถซ
-่ถฎ
-่ถฏ
-่ถฑ
-่ถณ
-่ถด
-่ถต
-่ถท
-่ถธ
-่ถน
-่ถบ
-่ถผ
-่ถพ
-่ถฟ
-่ท
-่ท
-่ท
-่ท
-่ท
-่ท
-่ท
-่ท
-่ท
-่ท
-่ท
-่ท
-่ท
-่ท
-่ท
-่ท
-่ท
-่ท
-่ท
-่ท
-่ท
-่ท
-่ทก
-่ทฃ
-่ทค
-่ทง
-่ทจ
-่ทฉ
-่ทช
-่ทซ
-่ทฌ
-่ทฏ
-่ทฑ
-่ทณ
-่ทต
-่ทถ
-่ทท
-่ทธ
-่ทน
-่ทบ
-่ทป
-่ทผ
-่ทฝ
-่ทฟ
-่ธ
-่ธ
-่ธ
-่ธ
-่ธ
-่ธ
-่ธ
-่ธ
-่ธ
-่ธ
-่ธ
-่ธ
-่ธ
-่ธ
-่ธ
-่ธ
-่ธ
-่ธก
-่ธข
-่ธฃ
-่ธฆ
-่ธง
-่ธฉ
-่ธช
-่ธฌ
-่ธญ
-่ธฎ
-่ธฏ
-่ธฐ
-่ธฑ
-่ธต
-่ธถ
-่ธธ
-่ธน
-่ธบ
-่ธผ
-่ธฝ
-่น
-่น
-่น
-่น
-่น
-่น
-่น
-่น
-่น
-่น
-่น
-่น
-่น
-่น
-่น
-่น
-่น
-่น
-่น
-่น
-่น
-่น
-่นก
-่นข
-่นฆ
-่นฉ
-่นฌ
-่นญ
-่นฎ
-่นฏ
-่นฐ
-่นฑ
-่นฒ
-่นณ
-่นด
-่นถ
-่นธ
-่นป
-่นผ
-่นฟ
-่บ
-่บ
-่บ
-่บ
-่บ
-่บ
-่บ
-่บ
-่บ
-่บ
-่บ
-่บ
-่บง
-่บจ
-่บฉ
-่บซ
-่บฌ
-่บญ
-่บฏ
-่บฒ
-่บด
-่บบ
-่บฟ
-่ป
-่ป
-่ป
-่ป
-่ป
-่ปข
-่ปฅ
-่ปง
-่ปจ
-่ปฑ
-่ปต
-่ปถ
-่ปท
-่ปฝ
-่ปฟ
-่ผ
-่ผ
-่ผ
-่ผ
-่ผ
-่ผฃ
-่ผค
-่ผง
-่ผฎ
-่ผด
-่ผต
-่ผถ
-่ผท
-่ผธ
-่ผผ
-่ฝ
-่ฝ
-่ฝ
-่ฝ
-่ฝ
-่ฝ
-่ฝ
-่ฝฃ
-่ฝฅ
-่ฝฆ
-่ฝง
-่ฝจ
-่ฝฉ
-่ฝช
-่ฝซ
-่ฝฌ
-่ฝญ
-่ฝฎ
-่ฝฏ
-่ฝฐ
-่ฝฑ
-่ฝฒ
-่ฝณ
-่ฝด
-่ฝต
-่ฝถ
-่ฝธ
-่ฝน
-่ฝบ
-่ฝป
-่ฝผ
-่ฝฝ
-่ฝพ
-่ฝฟ
-่พ
-่พ
-่พ
-่พ
-่พ
-่พ
-่พ
-่พ
-่พ
-่พ
-่พ
-่พ
-่พ
-่พ
-่พ
-่พ
-่พ
-่พ
-่พ
-่พ
-่พ
-่พ
-่พ
-่พ
-่พ
-่พ
-่พ
-่พ
-่พ
-่พฃ
-่พฆ
-่พจ
-่พฉ
-่พซ
-่พฐ
-่พฑ
-่พด
-่พถ
-่พท
-่พน
-่พบ
-่พป
-่พผ
-่พฝ
-่พพ
-่พฟ
-่ฟ
-่ฟ
-่ฟ
-่ฟ
-่ฟ
-่ฟ
-่ฟ
-่ฟ
-่ฟ
-่ฟ
-่ฟ
-่ฟ
-่ฟ
-่ฟ
-่ฟ
-่ฟ
-่ฟ
-่ฟ
-่ฟ
-่ฟ
-่ฟ
-่ฟ
-่ฟข
-่ฟค
-่ฟฅ
-่ฟฆ
-่ฟจ
-่ฟฉ
-่ฟช
-่ฟซ
-่ฟญ
-่ฟฎ
-่ฟฐ
-่ฟณ
-่ฟด
-่ฟต
-่ฟท
-่ฟธ
-่ฟน
-่ฟบ
-่ฟฝ
-่ฟพ
-้
-้
-้
-้
-้
-้
-้
-้
-้
-้
-้
-้
-้
-้
-้
-้
-้
-้
-้
-้
-้
-้
-้
-้
-้
-้
-้ก
-้ข
-้ฃ
-้ค
-้ฅ
-้ฆ
-้ญ
-้ฎ
-้ฏ
-้ฒ
-้ด
-้ต
-้ถ
-้ท
-้ธ
-้ป
-้ผ
-้พ
-้ฟ
-้
-้
-้
-้
-้
-้
-้
-้
-้
-้
-้
-้
-้
-้
-้
-้
-้
-้
-้
-้ก
-้ข
-้ฃ
-้ฅ
-้จ
-้ฉ
-้ซ
-้ญ
-้ฎ
-้ฏ
-้ฑ
-้ด
-้ต
-้ถ
-้น
-้บ
-้ฝ
-้ฟ
-้
-้
-้
-้
-้
-้
-้
-้
-้
-้
-้
-้
-้
-้
-้
-้
-้
-้
-้
-้ก
-้ข
-้ฃ
-้ค
-้ฅ
-้ฆ
-้จ
-้ช
-้ฌ
-้ฎ
-้ฏ
-้ฐ
-้ฑ
-้ฒ
-้ณ
-้ด
-้ต
-้ถ
-้ท
-้ธ
-้น
-้บ
-้ป
-้ฝ
-้พ
-้
-้
-้
-้
-้
-้
-้
-้
-้
-้
-้
-้
-้
-้
-้
-้
-้
-้
-้
-้
-้ก
-้ข
-้ค
-้ฆ
-้ง
-้จ
-้ฉ
-้ช
-้ซ
-้ญ
-้ฏ
-้ด
-้ท
-้ธ
-้ฝ
-้พ
-้ฟ
-้
-้
-้
-้
-้
-้
-้
-้
-้
-้
-้
-้
-้
-้
-้
-้ก
-้ข
-้ฃ
-้ค
-้ฆ
-้จ
-้ฉ
-้ช
-้ฌ
-้ฎ
-้ฏ
-้ฐ
-้ฑ
-้ณ
-้น
-้ผ
-้ฝ
-้
-้
-้
-้
-้
-้
-้
-้
-้
-้
-้
-้
-้
-้
-้
-้
-้
-้
-้
-้
-้
-้
-้
-้
-้
ก
-้
ข
-้
ฃ
-้
ค
-้
ฅ
-้
ฆ
-้
ง
-้
ฉ
-้
ช
-้
ฌ
-้
ญ
-้
ฎ
-้
ฏ
-้
ฐ
-้
ฑ
-้
ฒ
-้
ด
-้
ต
-้
ถ
-้
ท
-้
ธ
-้
น
-้
บ
-้
ฝ
-้
พ
-้
ฟ
-้
-้
-้
-้
-้
-้
-้
-้
-้
-้
-้
-้
-้
-้
-้
-้
-้
-้
-้
-้ก
-้ข
-้ค
-้ฅ
-้ฆ
-้จ
-้ช
-้ซ
-้ญ
-้ฎ
-้ฏ
-้ฒ
-้ณ
-้ด
-้ต
-้ธ
-้น
-้บ
-้ป
-้ฝ
-้พ
-้ฟ
-้
-้
-้
-้
-้
-้
-้
-้
-้
-้
-้
-้
-้
-้
-้ญ
-้ฑ
-้ด
-้
-้
-้
-้
-้
-้
-้
-้ก
-้ฃ
-้ด
-้ต
-้
-้
-้
-้
-้
-้
-้
-้
-้ข
-้ค
-้ฅ
-้ง
-้จ
-้ฑ
-้ฒ
-้ด
-้ผ
-้ฝ
-้
-้
-้
-้
-้
-้ญ
-้ฎ
-้ถ
-้บ
-้
-้
-้
-้
-้
-้
-้
-้
-้ฃ
-้ช
-้น
-้
-้
-้
-้ข
-้ค
-้ง
-้ซ
-้ฌ
-้ฏ
-้ฑ
-้บ
-้ป
-้ฝ
-้พ
-้
-้
-้
-้ง
-้ช
-้ญ
-้ฎ
-้ฑ
-้ผ
-้
-้
-้
-้
-้
-้
-้
-้
-้
-้
-้ฌ
-้ถ
-้ท
-้น
-้
-้
-้
-้ฃ
-้ฆ
-้ธ
-้
-้
-้
-้
-้
-้ป
-้
-้
-้
-้จ
-้ซ
-้ฏ
-้ด
-้
-้
-้
-้
-้
-้
-้
-้
-้
-้
-้
-้
-้
-้
-้
-้
-้
-้
-้
-้
-้
-้
-้
-้
-้
-้
-้
-้ก
-้ข
-้ฃ
-้ค
-้ฅ
-้ฆ
-้ง
-้จ
-้ฉ
-้ช
-้ซ
-้ฌ
-้ญ
-้ฎ
-้ฏ
-้ฐ
-้ฑ
-้ฒ
-้ณ
-้ด
-้ต
-้ธ
-้น
-้บ
-้ป
-้ผ
-้ฝ
-้พ
-้ฟ
-้
-้
-้
-้
-้
-้
-้
-้
-้
-้
-้
-้
-้
-้
-้
-้
-้
-้
-้
-้
-้
-้
-้
-้
-้
-้
-้
-้
-้
-้
-้
-้
-้ก
-้ข
-้ฃ
-้ค
-้ฅ
-้ฆ
-้ง
-้จ
-้ฉ
-้ช
-้ซ
-้ฌ
-้ญ
-้ฎ
-้ฏ
-้ฐ
-้ฑ
-้ฒ
-้ณ
-้ด
-้ต
-้ถ
-้ท
-้ธ
-้บ
-้ป
-้ผ
-้ฝ
-้พ
-้ฟ
-้
-้
-้
-้
-้
-้
-้
-้
-้
-้
-้
-้
-้
-้
-้
-้
-้
-้
-้
-้
-้
-้
-้
-้
-้
-้
-้
-้
-้
-้
-้
-้ก
-้ข
-้ฃ
-้ค
-้ฅ
-้ฆ
-้ง
-้จ
-้ช
-้ญ
-้ฎ
-้ฏ
-้ฐ
-้ฑ
-้ฒ
-้ด
-้ต
-้ถ
-้ท
-้ธ
-้น
-้บ
-้ป
-้ผ
-้ฝ
-้พ
-้
-้
-้
-้
-้
-้
-้
-้
-้
-้
-้
-้
-้
-้
-้
-้
-้
-้
-้
-้
-้
-้
-้
-้
-้
-้
-้
-้
-้
-้ก
-้ข
-้ฃ
-้ค
-้ฅ
-้ฆ
-้ง
-้จ
-้ฉ
-้ช
-้ซ
-้ฌ
-้ญ
-้ฎ
-้ฏ
-้ฐ
-้ฑ
-้ฒ
-้ณ
-้ด
-้ต
-้ถ
-้ท
-้ฟ
-้
-้
-้
-้
-้
-้
-้
-้
-้
-้
-้
-้ข
-้ค
-้ฆ
-้ง
-้ช
-้ด
-้
-้
-้
-้
-้
-้
-้
-้
-้
-้ข
-้จ
-้ฉ
-้ช
-้ซ
-้ฌ
-้ญ
-้ฎ
-้ฏ
-้ฐ
-้ฑ
-้ฒ
-้ณ
-้ด
-้ต
-้ท
-้ธ
-้น
-้บ
-้ป
-้ผ
-้ฝ
-้พ
-้ฟ
-้
-้
-้
-้
-้
-้
-้
-้
-้
-้
-้
-้
-้
-้
-้
-้
-้
-้
-้
-้
-้
-้
-้
-้
-้
-้
-้
-้
-้
-้
-้
-้
-้ก
-้จ
-้ช
-้ฌ
-้ฎ
-้ฏ
-้ฑ
-้ฒ
-้ณ
-้ด
-้ต
-้ถ
-้ป
-้ผ
-้ฝ
-้ฟ
-้
-้
-้
-้
-้
-้
-้
-้
-้
-้
-้
-้
-้
-้
-้
-้
-้
-้
-้
-้ก
-้ข
-้ค
-้ฅ
-้ง
-้จ
-้ฉ
-้ช
-้ฌ
-้ญ
-้ฒ
-้ด
-้ต
-้ถ
-้ท
-้บ
-้ป
-้ผ
-้ฝ
-้พ
-้
-้
-้
-้
-้
-้
-้
-้
-้
-้
-้
-้
-้
-้
-้
-้
-้
-้
-้
-้
-้ฃ
-้ค
-้ฅ
-้ฆ
-้ง
-้จ
-้ฉ
-้ช
-้ฎ
-้ฐ
-้ณ
-้ถ
-้ท
-้น
-้ป
-้ผ
-้ฝ
-้พ
-้
-้
-้
-้
-้
-้
-้
-้
-้
-้
-้
-้
-้
-้
-้
-้
-้
-้
-้
-้
-้ฃ
-้จ
-้ฉ
-้ช
-้ซ
-้ฏ
-้ฐ
-้ฑ
-้ฒ
-้ณ
-้ถ
-้ท
-้น
-้ป
-้พ
-้
-้
-้
-้
-้
-้
-้
-้
-้
-้
-้
-้
-้
-้
-้
-้
-้
-้
-้
-้
-้
-้
-้ก
-้ข
-้ฃ
-้ค
-้จ
-้ช
-้ญ
-้ฎ
-้ฐ
-้ฒ
-้ณ
-้ธ
-้น
-้พ
-้ฟ
-้
-้
-้
-้
-้
-้
-้
-้
-้
-้
-้ก
-้ข
-้ฅ
-้ฆ
-้ง
-้ฉ
-้ซ
-้ฎ
-้ฐ
-้ณ
-้ด
-้ถ
-้ท
-้ธ
-้บ
-้ผ
-้ฟ
-้
-้
-้
-้
-้
-้
-้
-้
-้
-้
-้
-้
-้
-้
-้
-้
-้
-้
-้
-้ฃ
-้จ
-้ซ
-้ฌ
-้ญ
-้ฎ
-้ฏ
-้ฒ
-้ณ
-้ด
-้ต
-้ถ
-้ธ
-้น
-้บ
-้พ
-้ฟ
-้
-้
-้
-้
-้
-้
-้
-้
-้
-้
-้ก
-้ฆ
-้ง
-้จ
-้ฉ
-้ช
-้ซ
-้ฌ
-้ญ
-้ฎ
-้ณ
-้ต
-้ถ
-้น
-้ป
-้
-้
-้
-้
-้
-้
-้
-้
-้
-้
-้
-้ ฃ
-้ ฉ
-้ ซ
-้ ฌ
-้ ญ
-้ ฏ
-้ ณ
-้ ต
-้ ผ
-้ก
-้ก
-้ก
-้ก
-้ก
-้ก
-้ก
-้ก
-้ก
-้กก
-้กฆ
-้กต
-้กถ
-้กท
-้กธ
-้กน
-้กบ
-้กป
-้กผ
-้กฝ
-้กพ
-้กฟ
-้ข
-้ข
-้ข
-้ข
-้ข
-้ข
-้ข
-้ข
-้ข
-้ข
-้ข
-้ข
-้ข
-้ข
-้ข
-้ข
-้ข
-้ข
-้ข
-้ข
-้ข
-้ข
-้ข
-้ข
-้ข
-้ข
-้ข
-้ข
-้ข
-้ข
-้ข
-้ข
-้ข
-้ขก
-้ขข
-้ขฃ
-้ขค
-้ขฅ
-้ขฆ
-้ขง
-้ขจ
-้ขพ
-้ขฟ
-้ฃ
-้ฃ
-้ฃ
-้ฃ
-้ฃ
-้ฃ
-้ฃ
-้ฃ
-้ฃ
-้ฃ
-้ฃ
-้ฃ
-้ฃ
-้ฃ
-้ฃ
-้ฃ
-้ฃ
-้ฃ
-้ฃ
-้ฃ
-้ฃก
-้ฃฃ
-้ฃง
-้ฃจ
-้ฃฏ
-้ฃฐ
-้ฃฑ
-้ฃถ
-้ฃฝ
-้ค
-้ค
-้ค
-้ค
-้ค
-้ค
-้ค
-้ค
-้คค
-้คง
-้คจ
-้คฌ
-้คฎ
-้คฒ
-้คด
-้คธ
-้คฝ
-้ฅ
-้ฅ
-้ฅ
-้ฅ
-้ฅ
-้ฅ
-้ฅ
-้ฅ
-้ฅ
-้ฅ
-้ฅ
-้ฅ
-้ฅ
-้ฅฃ
-้ฅค
-้ฅฅ
-้ฅฆ
-้ฅง
-้ฅจ
-้ฅฉ
-้ฅช
-้ฅซ
-้ฅฌ
-้ฅญ
-้ฅฎ
-้ฅฏ
-้ฅฐ
-้ฅฑ
-้ฅฒ
-้ฅด
-้ฅต
-้ฅถ
-้ฅท
-้ฅธ
-้ฅน
-้ฅบ
-้ฅผ
-้ฅฝ
-้ฅพ
-้ฅฟ
-้ฆ
-้ฆ
-้ฆ
-้ฆ
-้ฆ
-้ฆ
-้ฆ
-้ฆ
-้ฆ
-้ฆ
-้ฆ
-้ฆ
-้ฆ
-้ฆ
-้ฆ
-้ฆ
-้ฆ
-้ฆ
-้ฆ
-้ฆ
-้ฆ
-้ฆ
-้ฆ
-้ฆ
-้ฆ
-้ฆ
-้ฆ
-้ฆ
-้ฆก
-้ฆค
-้ฆฅ
-้ฆง
-้ฆจ
-้ฆฌ
-้ฆฏ
-้ฆน
-้ฆบ
-้ฆฝ
-้ง
-้ง
-้ง
-้ง
-้ง
-้ง
-้ง
-้ง
-้ง
-้ง
-้งช
-้งฌ
-้งฎ
-้งฐ
-้งฑ
-้งท
-้งธ
-้งน
-้งป
-้งผ
-้งฝ
-้งพ
-้งฟ
-้จ
-้จ
-้จ
-้จ
-้จ
-้จ
-้จ
-้จ
-้จ
-้จ
-้จ
-้จ
-้จ
-้จ
-้จก
-้จข
-้จฃ
-้จง
-้จช
-้จฐ
-้จฑ
-้จณ
-้จต
-้จน
-้จบ
-้ฉ
-้ฉ
-้ฉ
-้ฉ
-้ฉ
-้ฉ
-้ฉ
-้ฉฉ
-้ฉฌ
-้ฉญ
-้ฉฎ
-้ฉฏ
-้ฉฐ
-้ฉฑ
-้ฉฒ
-้ฉณ
-้ฉด
-้ฉต
-้ฉถ
-้ฉท
-้ฉธ
-้ฉน
-้ฉบ
-้ฉป
-้ฉผ
-้ฉฝ
-้ฉพ
-้ฉฟ
-้ช
-้ช
-้ช
-้ช
-้ช
-้ช
-้ช
-้ช
-้ช
-้ช
-้ช
-้ช
-้ช
-้ช
-้ช
-้ช
-้ช
-้ช
-้ช
-้ช
-้ช
-้ช
-้ช
-้ช
-้ช
-้ช
-้ช
-้ช
-้ช
-้ช
-้ช
-้ช
-้ชก
-้ชข
-้ชฃ
-้ชค
-้ชฅ
-้ชฆ
-้ชง
-้ชจ
-้ชซ
-้ชญ
-้ชฐ
-้ชฑ
-้ชถ
-้ชท
-้ชธ
-้ชน
-้ชบ
-้ชผ
-้ซ
-้ซ
-้ซ
-้ซ
-้ซ
-้ซ
-้ซ
-้ซ
-้ซ
-้ซ
-้ซ
-้ซ
-้ซ
-้ซ
-้ซ
-้ซ
-้ซ
-้ซ
-้ซก
-้ซข
-้ซค
-้ซฆ
-้ซง
-้ซช
-้ซซ
-้ซญ
-้ซฎ
-้ซฏ
-้ซฒ
-้ซณ
-้ซต
-้ซน
-้ซป
-้ซฝ
-้ซพ
-้ฌ
-้ฌ
-้ฌ
-้ฌ
-้ฌ
-้ฌ
-้ฌ
-้ฌ
-้ฌ
-้ฌ
-้ฌ
-้ฌ
-้ฌ
-้ฌ
-้ฌก
-้ฌฃ
-้ฌฌ
-้ฌฏ
-้ฌฑ
-้ฌฒ
-้ฌต
-้ฌท
-้ฌบ
-้ฌป
-้ฌผ
-้ฌฝ
-้ญ
-้ญ
-้ญ
-้ญ
-้ญ
-้ญ
-้ญ
-้ญ
-้ญ
-้ญ
-้ญ
-้ญ
-้ญ
-้ญ
-้ญ
-้ญ
-้ญ
-้ญ
-้ญ
-้ญฃ
-้ญญ
-้ญฎ
-้ญฏ
-้ญถ
-้ญผ
-้ญพ
-้ญฟ
-้ฎ
-้ฎ
-้ฎ
-้ฎ
-้ฎ
-้ฎ
-้ฎ
-้ฎ
-้ฎ
-้ฎ
-้ฎ
-้ฎก
-้ฎฃ
-้ฎง
-้ฎจ
-้ฎฎ
-้ฎฐ
-้ฎธ
-้ฎน
-้ฎป
-้ฎผ
-้ฏ
-้ฏ
-้ฏ
-้ฏ
-้ฏฅ
-้ฏฎ
-้ฏต
-้ฏถ
-้ฏบ
-้ฏป
-้ฏพ
-้ฐ
-้ฐ
-้ฐ
-้ฐ
-้ฐ
-้ฐ
-้ฐ
-้ฐค
-้ฐง
-้ฐฌ
-้ฐถ
-้ฐฝ
-้ฑ
-้ฑ
-้ฑ
-้ฑ
-้ฑ
-้ฑ
-้ฑ
-้ฑ
-้ฑ
-้ฑ
-้ฑฅ
-้ฑฎ
-้ฑฒ
-้ฑณ
-้ฑต
-้ฑป
-้ฑผ
-้ฑพ
-้ฑฟ
-้ฒ
-้ฒ
-้ฒ
-้ฒ
-้ฒ
-้ฒ
-้ฒ
-้ฒ
-้ฒ
-้ฒ
-้ฒ
-้ฒ
-้ฒ
-้ฒ
-้ฒ
-้ฒ
-้ฒ
-้ฒ
-้ฒ
-้ฒ
-้ฒ
-้ฒ
-้ฒ
-้ฒ
-้ฒ
-้ฒ
-้ฒ
-้ฒ
-้ฒ
-้ฒ
-้ฒก
-้ฒข
-้ฒฃ
-้ฒค
-้ฒฅ
-้ฒฆ
-้ฒง
-้ฒจ
-้ฒฉ
-้ฒช
-้ฒซ
-้ฒฌ
-้ฒญ
-้ฒฎ
-้ฒฏ
-้ฒฐ
-้ฒฑ
-้ฒฒ
-้ฒณ
-้ฒด
-้ฒต
-้ฒถ
-้ฒท
-้ฒธ
-้ฒน
-้ฒบ
-้ฒป
-้ฒผ
-้ฒฝ
-้ฒพ
-้ฒฟ
-้ณ
-้ณ
-้ณ
-้ณ
-้ณ
-้ณ
-้ณ
-้ณ
-้ณ
-้ณ
-้ณ
-้ณ
-้ณ
-้ณ
-้ณ
-้ณ
-้ณ
-้ณ
-้ณ
-้ณ
-้ณ
-้ณ
-้ณ
-้ณ
-้ณ
-้ณ
-้ณ
-้ณ
-้ณ
-้ณ
-้ณ
-้ณก
-้ณข
-้ณฃ
-้ณค
-้ณฅ
-้ณฆ
-้ณง
-้ณฉ
-้ณฒ
-้ณณ
-้ณด
-้ณถ
-้ณท
-้ณธ
-้ณบ
-้ณผ
-้ณฝ
-้ด
-้ด
-้ด
-้ด
-้ด
-้ด
-้ด
-้ด
-้ดฅ
-้ดณ
-้ดน
-้ดฝ
-้ต
-้ต
-้ต
-้ต
-้ต
-้ต
-้ต
-้ตฉ
-้ตฐ
-้ตฑ
-้ตป
-้ถ
-้ถ
-้ถ
-้ถ
-้ถ
-้ถ
-้ถ
-้ถ
-้ถก
-้ถข
-้ถค
-้ถฌ
-้ถฑ
-้ถต
-้ถน
-้ถบ
-้ท
-้ท
-้ท
-้ท
-้ท
-้ท
-้ท
-้ท
-้ท
-้ทข
-้ทฃ
-้ทค
-้ทฉ
-้ทซ
-้ทฎ
-้ทพ
-้ทฟ
-้ธ
-้ธ
-้ธ
-้ธ
-้ธ
-้ธ
-้ธ
-้ธ
-้ธ
-้ธ
-้ธ
-้ธ
-้ธ
-้ธก
-้ธข
-้ธฃ
-้ธค
-้ธฅ
-้ธฆ
-้ธง
-้ธจ
-้ธฉ
-้ธช
-้ธซ
-้ธฌ
-้ธญ
-้ธฎ
-้ธฏ
-้ธฐ
-้ธฑ
-้ธฒ
-้ธณ
-้ธด
-้ธต
-้ธถ
-้ธท
-้ธธ
-้ธน
-้ธบ
-้ธป
-้ธฝ
-้ธพ
-้ธฟ
-้น
-้น
-้น
-้น
-้น
-้น
-้น
-้น
-้น
-้น
-้น
-้น
-้น
-้น
-้น
-้น
-้น
-้น
-้น
-้น
-้น
-้น
-้น
-้น
-้น
-้น
-้น
-้น
-้น
-้น
-้น
-้น
-้น
-้นก
-้นข
-้นฃ
-้นค
-้นฅ
-้นฆ
-้นง
-้นจ
-้นฉ
-้นช
-้นซ
-้นฌ
-้นญ
-้นฎ
-้นฏ
-้นฐ
-้นฑ
-้นฒ
-้นณ
-้นด
-้นพ
-้นฟ
-้บ
-้บ
-้บ
-้บ
-้บ
-้บ
-้บ
-้บ
-้บ
-้บ
-้บ
-้บ
-้บ
-้บ
-้บ
-้บ
-้บ
-้บ
-้บ
-้บ
-้บค
-้บฆ
-้บง
-้บจ
-้บฏ
-้บฐ
-้บด
-้บธ
-้บน
-้บบ
-้บป
-้บผ
-้บฝ
-้บพ
-้บฟ
-้ป
-้ป
-้ป
-้ป
-้ป
-้ป
-้ป
-้ป
-้ป
-้ป
-้ป
-้ป
-้ป
-้ป
-้ป
-้ป
-้ป
-้ป
-้ป
-้ป
-้ป
-้ป
-้ป
-้ปก
-้ปข
-้ปค
-้ปฅ
-้ปฆ
-้ปง
-้ปจ
-้ปฉ
-้ปช
-้ปซ
-้ปฌ
-้ปฎ
-้ปฏ
-้ปณ
-้ปธ
-้ปป
-้ปผ
-้ปพ
-้ผ
-้ผ
-้ผ
-้ผ
-้ผ
-้ผ
-้ผ
-้ผ
-้ผ
-้ผ
-้ผ
-้ผ
-้ผข
-้ผฉ
-้ผช
-้ผซ
-้ผฌ
-้ผฎ
-้ผฏ
-้ผท
-้ผน
-้ผบ
-้ผป
-้ผฝ
-้ผพ
-้ผฟ
-้ฝ
-้ฝ
-้ฝ
-้ฝ
-้ฝ
-้ฝ
-้ฝ
-้ฝ
-้ฝ
-้ฝข
-้ฝง
-้ฝจ
-้ฝฎ
-้ฝฐ
-้ฝพ
-้ฝฟ
-้พ
-้พ
-้พ
-้พ
-้พ
-้พ
-้พ
-้พ
-้พ
-้พ
-้พ
-้พ
-้พ
-้พ
-้พ
-้พ
-้พ
-้พ
-้พ
-้พ
-้พ
-้พ
-๊ฑฐ
-๋
-๋ฌ
-๋ค
-๋
-๋
-๋ฆฌ
-๋งฅ
-๋ฒ
-์
-์
-ํ
-๎
-๎ข
-๏ฅน
-๏ธฐ
-๏น
-๏น
-๏น
-๏ผ
-๏ผ
-๏ผ
-๏ผ
-๏ผ
-๏ผ
-๏ผ
-๏ผ
-๏ผ
-๏ผ
-๏ผ
-๏ผ
-๏ผ
-๏ผ
-๏ผ
-๏ผฟ
-๏ฝ
-๏ฝ
-๏ฝ
-๏ฟฅ
-๐ก
-๐จฑ
\ No newline at end of file
diff --git a/pipeline/mmocr/mmocr/.mim/dicts/english_digits_symbols.txt b/pipeline/mmocr/mmocr/.mim/dicts/english_digits_symbols.txt
deleted file mode 100644
index a945ae9c526e4faa68852eb3fb47d078a2f3f6ce..0000000000000000000000000000000000000000
--- a/pipeline/mmocr/mmocr/.mim/dicts/english_digits_symbols.txt
+++ /dev/null
@@ -1,90 +0,0 @@
-0
-1
-2
-3
-4
-5
-6
-7
-8
-9
-a
-b
-c
-d
-e
-f
-g
-h
-i
-j
-k
-l
-m
-n
-o
-p
-q
-r
-s
-t
-u
-v
-w
-x
-y
-z
-A
-B
-C
-D
-E
-F
-G
-H
-I
-J
-K
-L
-M
-N
-O
-P
-Q
-R
-S
-T
-U
-V
-W
-X
-Y
-Z
-!
-"
-#
-$
-%
-&
-'
-(
-)
-*
-+
-,
--
-.
-/
-:
-;
-<
-=
->
-?
-@
-[
-\
-]
-_
-`
-~
\ No newline at end of file
diff --git a/pipeline/mmocr/mmocr/.mim/dicts/english_digits_symbols_space.txt b/pipeline/mmocr/mmocr/.mim/dicts/english_digits_symbols_space.txt
deleted file mode 100644
index 5bd66a185eeeb64e39b78f33ba96c8120ca28112..0000000000000000000000000000000000000000
--- a/pipeline/mmocr/mmocr/.mim/dicts/english_digits_symbols_space.txt
+++ /dev/null
@@ -1,91 +0,0 @@
-0
-1
-2
-3
-4
-5
-6
-7
-8
-9
-a
-b
-c
-d
-e
-f
-g
-h
-i
-j
-k
-l
-m
-n
-o
-p
-q
-r
-s
-t
-u
-v
-w
-x
-y
-z
-A
-B
-C
-D
-E
-F
-G
-H
-I
-J
-K
-L
-M
-N
-O
-P
-Q
-R
-S
-T
-U
-V
-W
-X
-Y
-Z
-!
-"
-#
-$
-%
-&
-'
-(
-)
-*
-+
-,
--
-.
-/
-:
-;
-<
-=
->
-?
-@
-[
-\
-]
-_
-`
-~
-
\ No newline at end of file
diff --git a/pipeline/mmocr/mmocr/.mim/dicts/korean_english_digits_symbols.txt b/pipeline/mmocr/mmocr/.mim/dicts/korean_english_digits_symbols.txt
deleted file mode 100644
index 764080d5486ebd77cb7b5db26ac82022eb84cc4e..0000000000000000000000000000000000000000
--- a/pipeline/mmocr/mmocr/.mim/dicts/korean_english_digits_symbols.txt
+++ /dev/null
@@ -1,1803 +0,0 @@
-0
-1
-2
-3
-4
-5
-6
-7
-8
-9
-!
-"
-#
-$
-%
-&
-\
-'
-(
-)
-*
-+
-,
--
-.
-/
-:
-;
-<
-=
->
-?
-@
-[
-]
-^
-_
-`
-{
-|
-}
-~
-
-a
-b
-c
-d
-e
-f
-g
-h
-i
-j
-k
-l
-m
-n
-o
-p
-q
-r
-s
-t
-u
-v
-w
-x
-y
-z
-A
-B
-C
-D
-E
-F
-G
-H
-I
-J
-K
-L
-M
-N
-O
-P
-Q
-R
-S
-T
-U
-V
-W
-X
-Y
-Z
-ใ
-๊ฐ
-๊ฐ
-๊ฐ
-๊ฐ
-๊ฐ
-๊ฐ
-๊ฐ
-๊ฐ
-๊ฐ
-๊ฐ
-๊ฐ
-๊ฐ
-๊ฐ
-๊ฐ
-๊ฐ
-๊ฐ
-๊ฐ
-๊ฐ
-๊ฐค
-๊ฐฌ
-๊ฐญ
-๊ฐฏ
-๊ฐฑ
-๊ฐธ
-๊ฑ
-๊ฑฐ
-๊ฑฑ
-๊ฑด
-๊ฑท
-๊ฑธ
-๊ฒ
-๊ฒ
-๊ฒ
-๊ฒ
-๊ฒ
-๊ฒ
-๊ฒ
-๊ฒ
-๊ฒ
-๊ฒ
-๊ฒจ
-๊ฒฉ
-๊ฒช
-๊ฒฌ
-๊ฒฐ
-๊ฒธ
-๊ฒน
-๊ฒผ
-๊ฒฝ
-๊ณ
-๊ณ
-๊ณ
-๊ณก
-๊ณค
-๊ณง
-๊ณจ
-๊ณฐ
-๊ณฑ
-๊ณณ
-๊ณต
-๊ณถ
-๊ณผ
-๊ณฝ
-๊ด
-๊ด
-๊ด
-๊ด
-๊ด
-๊ด
-๊ดญ
-๊ดด
-๊ดผ
-๊ต
-๊ต
-๊ต
-๊ตฌ
-๊ตญ
-๊ตฐ
-๊ตณ
-๊ตด
-๊ตต
-๊ตถ
-๊ตผ
-๊ตฝ
-๊ตฟ
-๊ถ
-๊ถ
-๊ถ
-๊ถ
-๊ถ
-๊ถค
-๊ท
-๊ท
-๊ท
-๊ท
-๊ท
-๊ทค
-๊ทธ
-๊ทน
-๊ทผ
-๊ธ
-๊ธ
-๊ธ
-๊ธ
-๊ธ
-๊ธ
-๊ธฐ
-๊ธด
-๊ธธ
-๊น
-๊น
-๊น
-๊น
-๊น
-๊น
-๊น
-๊น
-๊น
-๊น
-๊น
-๊น
-๊นก
-๊นฅ
-๊นจ
-๊นฌ
-๊นป
-๊นฝ
-๊บ
-๊บต
-๊บผ
-๊บฝ
-๊บพ
-๊ป
-๊ป
-๊ป
-๊ป
-๊ป
-๊ป
-๊ป
-๊ปด
-๊ผ
-๊ผฌ
-๊ผญ
-๊ผด
-๊ผผ
-๊ผฝ
-๊ฝ
-๊ฝ
-๊ฝ
-๊ฝ
-๊ฝ
-๊ฝ
-๊ฝ
-๊ฝค
-๊ฝฅ
-๊พ
-๊พธ
-๊พน
-๊พผ
-๊ฟ
-๊ฟ
-๊ฟ
-๊ฟ
-๊ฟ
-๊ฟ
-๊ฟจ
-๊ฟฉ
-๊ฟฐ
-๋
-๋
-๋
-๋
-๋
-๋
-๋
-๋
-๋
-๋
-๋
-๋
-๋ผ
-๋
-๋
-๋
-๋
-๋
-๋
-๋
-๋
-๋ก
-๋ข
-๋จ
-๋ฉ
-๋ซ
-๋ฌ
-๋ญ
-๋ฎ
-๋ฏ
-๋ฑ
-๋ณ
-๋ด
-๋ต
-๋ธ
-๋ผ
-๋
-๋
-๋
-๋
-๋
-๋
-๋
-๋
-๋ฅ
-๋
-๋
-๋
-๋
-๋
-๋
-๋
-๋
-๋
-๋ฃ
-๋ค
-๋ฅ
-๋จ
-๋ฌ
-๋ด
-๋ต
-๋ท
-๋
-๋
-๋
-๋
-๋
-๋
-๋
-๋
-๋
-๋
ธ
-๋
น
-๋
ผ
-๋
-๋
-๋
-๋
-๋
-๋
-๋
-๋
-๋จ
-๋
-๋จ
-๋ฐ
-๋ธ
-๋ฝ
-๋
-๋
-๋
-๋
-๋
-๋
-๋
-๋
-๋
-๋
-๋ด
-๋ผ
-๋
-๋
-๋
-๋
-๋
-๋
-๋
-๋ฅ
-๋ฆ
-๋ช
-๋ฌ
-๋
-๋
-๋
-๋
-๋
-๋
-๋
-๋
-๋ค
-๋ฅ
-๋ฆ
-๋จ
-๋ซ
-๋ฌ
-๋ญ
-๋ฎ
-๋ณ
-๋ด
-๋ต
-๋ท
-๋น
-๋ป
-๋ฟ
-๋
-๋
-๋
-๋
-๋
-๋
-๋
-๋
-๋
-๋
-๋
-๋
-๋
-๋
-๋ค
-๋ฅ
-๋ง
-๋ฉ
-๋ซ
-๋ฎ
-๋ฐ
-๋ฑ
-๋ด
-๋ธ
-๋
-๋
-๋
-๋ฌ
-๋
-๋
-๋
-๋
-๋
-๋
-๋
-๋
-๋
-๋
-๋ผ
-๋
-๋
-๋
-๋
-๋จ
-๋ฉ
-๋
-๋
-๋
-๋
-๋
-๋ก
-๋ฃ
-๋ฅ
-๋ฌ
-๋
-๋ค
-๋ท
-๋น
-๋
-๋
-๋
-๋
-๋
-๋
-๋ฃ
-๋ค
-๋ฆ
-๋ฌ
-๋ญ
-๋ฏ
-๋ฑ
-๋ธ
-๋
-๋
-๋
-๋
-๋
-๋
-๋
-๋ค
-๋ฅ
-๋ง
-๋ฉ
-๋ช
-๋ฐ
-๋ฑ
-๋ด
-๋ธ
-๋
-๋
-๋
-๋
-๋
-๋
-๋
-๋ก
-๋
-๋ก
-๋ค
-๋จ
-๋ด
-๋ป
-๋ผ
-๋ด
-๋
-๋
-๋
-๋
-๋ฃ
-๋ฅ
-๋
-๋
-๋ซ
-๋ฑ
-๋ฐ
-๋ด
-๋ธ
-๋
-๋จ
-๋ฉ
-๋ฌ
-๋ฏ
-๋ฐ
-๋ธ
-๋ป
-๋
-๋
-๋
-๋ค
-๋ต
-๋ผ
-๋ฝ
-๋
-๋
-๋
-๋
-๋
-๋
-๋
-๋
-๋
-๋
-๋
-๋
-๋จ
-๋ฉ
-๋ซ
-๋ฌ
-๋ญ
-๋ฒ
-๋ด
-๋ต
-๋ธ
-๋
-๋ฌ
-๋ญ
-๋ฐ
-๋ด
-๋ผ
-๋ฝ
-๋ฟ
-๋
-๋
-๋
-๋
-๋
-๋
-๋
-๋
-๋
-๋
-๋
-๋ ค
-๋ ฅ
-๋ จ
-๋ ฌ
-๋ ด
-๋ ต
-๋ ท
-๋ ธ
-๋ น
-๋ก
-๋ก
-๋ก
-๋ก
-๋ก
-๋กค
-๋กฌ
-๋กญ
-๋กฏ
-๋กฑ
-๋กธ
-๋ขฐ
-๋ฃ
-๋ฃ
-๋ฃก
-๋ฃจ
-๋ฃฉ
-๋ฃฌ
-๋ฃฐ
-๋ฃธ
-๋ฃน
-๋ฃป
-๋ฃฝ
-๋ค
-๋ค
-๋คผ
-๋คฝ
-๋ฅ
-๋ฅ
-๋ฅ
-๋ฅ
-๋ฅ
-๋ฅ
-๋ฅจ
-๋ฅซ
-๋ฅญ
-๋ฅด
-๋ฅต
-๋ฅธ
-๋ฅผ
-๋ฆ
-๋ฆ
-๋ฆ
-๋ฆ
-๋ฆ
-๋ฆฌ
-๋ฆญ
-๋ฆฐ
-๋ฆณ
-๋ฆด
-๋ฆผ
-๋ฆฝ
-๋ฆฟ
-๋ง
-๋ง
-๋ง
-๋ง
-๋ง
-๋ง
-๋ง
-๋ง
-๋ง
-๋ง
-๋ง
-๋ง
-๋ง
-๋งก
-๋งฃ
-๋งค
-๋งฅ
-๋งจ
-๋งฌ
-๋งด
-๋งต
-๋งท
-๋งน
-๋งบ
-๋จ
-๋จธ
-๋จน
-๋จผ
-๋ฉ
-๋ฉ
-๋ฉ
-๋ฉ
-๋ฉ
-๋ฉ
-๋ฉ
-๋ฉ
-๋ฉค
-๋ฉฅ
-๋ฉง
-๋ฉฉ
-๋ฉฐ
-๋ฉฑ
-๋ฉด
-๋ฉธ
-๋ช
-๋ช
-๋ช
-๋ชจ
-๋ชฉ
-๋ชซ
-๋ชฌ
-๋ชฐ
-๋ชธ
-๋ชน
-๋ชป
-๋ชฝ
-๋ซผ
-๋ฌ
-๋ฌ
-๋ฌด
-๋ฌต
-๋ฌถ
-๋ฌธ
-๋ฌป
-๋ฌผ
-๋ญ
-๋ญ
-๋ญ
-๋ญ
-๋ญ
-๋ญ
-๋ฎ
-๋ฎ
-๋ฎ
-๋ฎค
-๋ฎฌ
-๋ฏ
-๋ฏ
-๋ฏ
-๋ฏ
-๋ฏธ
-๋ฏน
-๋ฏผ
-๋ฏฟ
-๋ฐ
-๋ฐ
-๋ฐ
-๋ฐ
-๋ฐ
-๋ฐ
-๋ฐ
-๋ฐ
-๋ฐ
-๋ฐ
-๋ฐ
-๋ฐ
-๋ฐ
-๋ฐ
-๋ฐ
-๋ฐค
-๋ฐฅ
-๋ฐง
-๋ฐฉ
-๋ฐญ
-๋ฐฐ
-๋ฐฑ
-๋ฐด
-๋ฐธ
-๋ฑ
-๋ฑ
-๋ฑ
-๋ฑ
-๋ฑ
-๋ฒ
-๋ฒ
-๋ฒ
-๋ฒ
-๋ฒ
-๋ฒ
-๋ฒ
-๋ฒ
-๋ฒ
-๋ฒ
-๋ฒก
-๋ฒค
-๋ฒง
-๋ฒจ
-๋ฒฐ
-๋ฒณ
-๋ฒต
-๋ฒผ
-๋ฒฝ
-๋ณ
-๋ณ
-๋ณ
-๋ณ
-๋ณ
-๋ณด
-๋ณต
-๋ณถ
-๋ณธ
-๋ณผ
-๋ด
-๋ด
-๋ด
-๋ด
-๋ด
-๋ดค
-๋ต
-๋ต
-๋ตค
-๋ถ
-๋ถ
-๋ถ
-๋ถ
-๋ถ
-๋ถ
-๋ถ
-๋ถ
-๋ถ
-๋ถ
-๋ท
-๋ท
-๋ทฐ
-๋ธ
-๋ธ
-๋ธ
-๋ธ
-๋ธจ
-๋น
-๋น
-๋น
-๋น
-๋น
-๋น
-๋น
-๋น
-๋น
-๋น
-๋น
-๋นก
-๋นค
-๋นจ
-๋นต
-๋นป
-๋นผ
-๋นฝ
-๋บ
-๋บ
-๋บ
-๋บ
-๋บ
-๋บจ
-๋บด
-๋บต
-๋ป
-๋ป
-๋ป
-๋ป
-๋ป
-๋ปค
-๋ปฅ
-๋ปฌ
-๋ผ
-๋ผ
-๋ฝ
-๋ฝ
-๋ฝ
-๋ฝ
-๋ฝ
-๋ฝ
-๋พฐ
-๋ฟ
-๋ฟ
-๋ฟ
-๋ฟ
-๋ฟ
-๋ฟ
-์
-์
-์
-์จ
-์
-์
-์
-์ฌ
-์ญ
-์ฐ
-์ด
-์ถ
-์ผ
-์ฝ
-์ฟ
-์
-์
-์
-์
-์
-์
-์
-์
-์
-์ค
-์ฅ
-์จ
-์ฌ
-์ด
-์ต
-์ท
-์น
-์พ
-์
-์
-์
-์
-์
-์ฃ
-์ค
-์ฌ
-์ญ
-์ฏ
-์ฐ
-์ฑ
-์ธ
-์น
-์ผ
-์
-์
-์
-์
-์
-์
-์
-์
-์
ค
-์
ง
-์
จ
-์
ฐ
-์
ด
-์
ธ
-์
-์
-์
-์
-์
-์
-์
-์ก
-์ฅ
-์ฝ
-์
-์
-์ณ
-์ผ
-์
-์
-์
-์
-์
-์
-์
-์
-์
-์
-์
-์จ
-์ซ
-์ญ
-์ฎ
-์ฏ
-์ฑ
-์ฒ
-์ด
-์
-์
-์ฌ
-์ด
-์ผ
-์ฝ
-์ฟ
-์
-์
-์
-์
-์
-์ค
-์จ
-์ฌ
-์ญ
-์ด
-์ต
-์ท
-์น
-์
-์
-์
-์ฃ
-์ค
-์ซ
-์ฌ
-์ญ
-์ฏ
-์ฐ
-์ฑ
-์ถ
-์ธ
-์น
-์ผ
-์
-์
-์
-์
-์
-์
-์
-์ค
-์ฉ
-์
-์จ
-์ฉ
-์ฌ
-์ฐ
-์ธ
-์น
-์ผ
-์ฝ
-์
-์
-์
-์ด
-์
-์
-์
-์
-์
-์ฉ
-์ญ
-์ด
-์
-์
-์
-์
-์ค
-์ฅ
-์จ
-์ฌ
-์น
-์ฐ
-์
-์ฐ
-์ฑ
-์ด
-์ธ
-์
-์
-์
-์จ
-์ฉ
-์ฌ
-์ฐ
-์ท
-์น
-์ป
-์ฝ
-์
-์
-์
-์
-์
-์
-์
-์
-์
-์
-์
-์
-์
-์
-์ก
-์ค
-์จ
-์ฐ
-์ฑ
-์ณ
-์ด
-์ต
-์ผ
-์ฝ
-์
-์
-์
-์
-์
-์
-์
-์
-์
-์ด
-์ต
-์ธ
-์น
-์ป
-์ผ
-์ฝ
-์
-์
-์
-์
-์
-์
-์
-์
-์
-์
-์
-์
-์
-์ก
-์ฃ
-์ฅ
-์ฌ
-์ญ
-์ฎ
-์ฐ
-์ด
-์ท
-์ผ
-์ฝ
-์ฟ
-์
-์
-์
-์
-์
-์
-์
-์
-์
-์ค
-์ฅ
-์จ
-์ฌ
-์ญ
-์ฎ
-์ณ
-์ด
-์ต
-์ท
-์น
-์ป
-์
-์
-์
-์
-์
-์
-์
-์
-์
-์ธ
-์ผ
-์
-์
-์
-์
-์ค
-์ฉ
-์ฐ
-์ฑ
-์ด
-์ธ
-์
-์
-์
-์
-์
-์
-์
-์
-์
-์
-์จ
-์ฉ
-์ฌ
-์ฐ
-์ธ
-์น
-์
-์
-์
-์
-์
-์
-์
-์
-์ก
-์ค
-์จ
-์ฐ
-์ณ
-์ต
-์ผ
-์ฝ
-์
-์
-์
-์
-์
-์
-์
-์ด
-์ต
-์ธ
-์ผ
-์ฝ
-์
-์
-์
-์
-์
-์
-์
-์
-์
-์
-์
-์
-์
-์
-์
-์ก
-์ฃ
-์ฅ
-์ฆ
-์ฌ
-์ญ
-์ผ
-์ฟ
-์
-์
-์
-์
-์
-์
-์
-์
-์
-์
-์
-์
-์
-์
-์
-์
-์
-์
-์
-์ ค
-์ ฌ
-์ ญ
-์ ฏ
-์ ฑ
-์ ธ
-์ ผ
-์ก
-์กฐ
-์กฑ
-์กด
-์กธ
-์ข
-์ข
-์ข
-์ข
-์ข
-์ข
-์ขก
-์ฃ
-์ฃ
-์ฃค
-์ฃผ
-์ฃฝ
-์ค
-์ค
-์ค
-์ค
-์ค
-์ค
-์ค
-์คฌ
-์ฅ
-์ฅ
-์ฅ
-์ฅฌ
-์ฅฐ
-์ฅด
-์ฆ
-์ฆ
-์ฆ
-์ฆ
-์ฆ
-์ฆ
-์ฆ
-์ง
-์ง
-์ง
-์ง
-์ง
-์ง
-์ง
-์ง
-์ง
-์ง
-์ง
-์ง
-์ง
-์ง
-์ง
-์ง
-์งค
-์งง
-์งฌ
-์งญ
-์งฑ
-์งธ
-์จ
-์จ
-์จ
-์จฐ
-์ฉ
-์ฉ
-์ฉ
-์ฉ
-์ฉ
-์ฉก
-์ฉฐ
-์ช
-์ชผ
-์ชฝ
-์ซ
-์ซ
-์ซ
-์ซ
-์ฌ
-์ฌ
-์ญ
-์ญ
-์ญ
-์ญค
-์ฎธ
-์ฏ
-์ฏค
-์ฐ
-์ฐ
-์ฐ
-์ฐ
-์ฐ
-์ฐ
-์ฐก
-์ฐข
-์ฐง
-์ฐจ
-์ฐฉ
-์ฐฌ
-์ฐฎ
-์ฐฐ
-์ฐธ
-์ฐน
-์ฐป
-์ฐฝ
-์ฐพ
-์ฑ
-์ฑ
-์ฑ
-์ฑ
-์ฑ
-์ฑ
-์ฑ
-์ฑ
-์ฑ
-์ฑค
-์ฒ
-์ฒ
-์ฒ
-์ฒ
-์ฒจ
-์ฒฉ
-์ฒซ
-์ฒญ
-์ฒด
-์ฒต
-์ฒธ
-์ฒผ
-์ณ
-์ณ
-์ณค
-์ด
-์ด
-์ด
-์ด
-์ด
-์ด
-์ด
-์ดจ
-์ดฌ
-์ต
-์ตธ
-์ตน
-์ถ
-์ถ
-์ถ
-์ถ
-์ถค
-์ถฅ
-์ถง
-์ถฉ
-์ถฐ
-์ท
-์ท
-์ทจ
-์ธ
-์ธ
-์ธก
-์ธจ
-์ธฐ
-์ธณ
-์ธต
-์น
-์น
-์น
-์น
-์นก
-์นจ
-์นฉ
-์นซ
-์นญ
-์นฒ
-์นด
-์นต
-์นธ
-์นผ
-์บ
-์บ
-์บ
-์บ
-์บ
-์บ
-์บ
-์บ
-์บก
-์บฃ
-์บฅ
-์บฌ
-์ปค
-์ปฅ
-์ปจ
-์ปซ
-์ปฌ
-์ปด
-์ปต
-์ปท
-์ปธ
-์ปน
-์ผ
-์ผ
-์ผ
-์ผ
-์ผ
-์ผ
-์ผ
-์ผฐ
-์ฝ
-์ฝ
-์ฝ
-์ฝ
-์ฝค
-์ฝฅ
-์ฝง
-์ฝฉ
-์ฝฐ
-์ฝด
-์ฝธ
-์พ
-์พ
-์พจ
-์พฐ
-์ฟ
-์ฟ
-์ฟก
-์ฟค
-์ฟจ
-์ฟฐ
-์ฟต
-์ฟผ
-ํ
-ํ
-ํ
-ํด
-ํต
-ํธ
-ํผ
-ํ
-ํ
-ํ
-ํ
-ํฌ
-ํฐ
-ํด
-ํผ
-ํค
-ํฅ
-ํจ
-ํฌ
-ํด
-ํต
-ํท
-ํน
-ํ
-ํ
-ํ
-ํ
-ํ
-ํ
-ํ
-ํ
-ํ
-ํ
-ํ
-ํ
-ํ
-ํค
-ํฌ
-ํญ
-ํฑ
-ํธ
-ํฐ
-ํฑ
-ํด
-ํธ
-ํ
-ํ
-ํ
-ํ
-ํ
-ํ
-ํ
-ํ
-ํ
-ํ
-ํ
-ํ
ก
-ํ
จ
-ํ
-ํ
-ํก
-ํค
-ํจ
-ํฐ
-ํฑ
-ํณ
-ํต
-ํบ
-ํ
-ํด
-ํฌ
-ํญ
-ํฐ
-ํด
-ํผ
-ํ
-ํ
-ํ
-ํ
-ํ
-ํฌ
-ํธ
-ํน
-ํผ
-ํฟ
-ํ
-ํ
-ํ
-ํฐ
-ํฑ
-ํด
-ํธ
-ํ
-ํ
-ํ
-ํ
-ํ
-ํ
-ํ
-ํ
-ํ
-ํ
-ํ
-ํ
-ํก
-ํฅ
-ํจ
-ํฉ
-ํฌ
-ํฐ
-ํธ
-ํน
-ํป
-ํฝ
-ํผ
-ํฝ
-ํ
-ํ
-ํ
-ํ
-ํ
-ํ
-ํ
-ํ
-ํ
-ํ
-ํจ
-ํฉ
-ํซ
-ํญ
-ํด
-ํธ
-ํผ
-ํ
-ํ
-ํ
-ํ
-ํฌ
-ํญ
-ํฐ
-ํด
-ํผ
-ํ
-ํ
-ํ
-ํธ
-ํน
-ํผ
-ํ
-ํ
-ํ
-ํ
-ํ
-ํจ
-ํฐ
-ํธ
-ํ
-ํ
-ํ
-ํ
-ํผ
-ํฝ
-ํ
-ํ
-ํ
-ํ
-ํ
-ํ
-ํ
-ํ
-ํ
-ํ
-ํฅ
-ํจ
-ํฉ
-ํซ
-ํญ
-ํด
-ํต
-ํธ
-ํผ
-ํ
-ํ
-ํ
-ํ
-ํ
-ํ
-ํฅ
-ํ
-ํ
-ํ
-ํ
-ํ
-ํ
-ํ
-ํค
-ํฅ
-ํจ
-ํฌ
-ํด
-ํต
-ํธ
-ํน
-ํ
-ํ
-ํ
-ํ
-ํ
-ํ
-ํ
-ํ
-ํ
-ํ
-ํธ
-ํน
-ํผ
-ํ
-ํ
-ํ
-ํ
-ํ
-ํ
-ํ
-ํ
-ํ
-ํฉ
-ํฐ
-ํ
-ํ
-ํ
-ํ
-ํ
-ํก
-ํจ
-ํ
-ํ
-ํ
-ํ
-ํ
-ํ
-ํ
-ํ
-ํ
-ํค
-ํจ
-ํผ
-ํ
-ํ
-ํ
-ํ
-ํ
-ํฉ
-ํด
-ํ
-ํ
-ํ
-ํ
-ํ
-ํ
-ํ
-ํ
-ํก
-ํฅ
-ํฉ
-ํฌ
-ํฐ
-ํ
-ํ
-ํ
-ํ
-ํ
-ํ
-๊ณน
-๋
-์จ
-๋
-์ฑฑ
-์ฌฌ
-ํฌ
-์ดค
-ํค
-๊ฐ
-๋จ
-๋ฐ
-๊ฑ
-์ฅ
-๋ฝ
-๊ท
-๋ฝ
-์ด
-์ฟฑ
-์
-์ผ
-์ข
-๋ฝ
-์
-๋ฆ
-์ก
-์จ
-์ฝ
-๋ณฝ
-๋ข
-๊ผฐ
-๋ณ
-์ฐ
-๋ท
-์
-์ก
-์ธ
-์
-์ฅ
-์
-๋ค
-๋
-๋ผ
-์ผ
-๊ธฑ
-์ฃ
-์ฐ
-ํ
ฌ
-์ฐ
-๋ค
-๋คจ
-๋
-๋ทธ
-๋
-๋
-๋ฌ
-์
-์ฉจ
-๋นณ
-๊บณ
-์
-๋
-๊น
-๋ฅ
-์ฌ
-์ฉ
\ No newline at end of file
diff --git a/pipeline/mmocr/mmocr/.mim/dicts/lower_english_digits.txt b/pipeline/mmocr/mmocr/.mim/dicts/lower_english_digits.txt
deleted file mode 100644
index 474060366f8a2a00c108d5c743821c0a61867cd5..0000000000000000000000000000000000000000
--- a/pipeline/mmocr/mmocr/.mim/dicts/lower_english_digits.txt
+++ /dev/null
@@ -1,36 +0,0 @@
-0
-1
-2
-3
-4
-5
-6
-7
-8
-9
-a
-b
-c
-d
-e
-f
-g
-h
-i
-j
-k
-l
-m
-n
-o
-p
-q
-r
-s
-t
-u
-v
-w
-x
-y
-z
\ No newline at end of file
diff --git a/pipeline/mmocr/mmocr/.mim/dicts/lower_english_digits_space.txt b/pipeline/mmocr/mmocr/.mim/dicts/lower_english_digits_space.txt
deleted file mode 100644
index a80a4a20b6f47e0338d5cccb9b71504eb38f62bb..0000000000000000000000000000000000000000
--- a/pipeline/mmocr/mmocr/.mim/dicts/lower_english_digits_space.txt
+++ /dev/null
@@ -1,37 +0,0 @@
-0
-1
-2
-3
-4
-5
-6
-7
-8
-9
-a
-b
-c
-d
-e
-f
-g
-h
-i
-j
-k
-l
-m
-n
-o
-p
-q
-r
-s
-t
-u
-v
-w
-x
-y
-z
-
\ No newline at end of file
diff --git a/pipeline/mmocr/mmocr/.mim/dicts/sdmgr_dict.txt b/pipeline/mmocr/mmocr/.mim/dicts/sdmgr_dict.txt
deleted file mode 100644
index b68274119a13962dc989c7330edd371d5c43ced4..0000000000000000000000000000000000000000
--- a/pipeline/mmocr/mmocr/.mim/dicts/sdmgr_dict.txt
+++ /dev/null
@@ -1,91 +0,0 @@
-/
-\
-.
-$
-ยฃ
-โฌ
-ยฅ
-:
--
-,
-*
-#
-(
-)
-%
-@
-!
-'
-&
-=
->
-+
-"
-ร
-?
-<
-[
-]
-_
-0
-1
-2
-3
-4
-5
-6
-7
-8
-9
-a
-b
-c
-d
-e
-f
-g
-h
-i
-j
-k
-l
-m
-n
-o
-p
-q
-r
-s
-t
-u
-v
-w
-x
-y
-z
-A
-B
-C
-D
-E
-F
-G
-H
-I
-J
-K
-L
-M
-N
-O
-P
-Q
-R
-S
-T
-U
-V
-W
-X
-Y
-Z
\ No newline at end of file
diff --git a/pipeline/mmocr/mmocr/.mim/model-index.yml b/pipeline/mmocr/mmocr/.mim/model-index.yml
deleted file mode 100644
index 563372c2623fe797281a4a3d0b80ad8c559ea2ef..0000000000000000000000000000000000000000
--- a/pipeline/mmocr/mmocr/.mim/model-index.yml
+++ /dev/null
@@ -1,19 +0,0 @@
-Import:
- - configs/textdet/dbnet/metafile.yml
- - configs/textdet/dbnetpp/metafile.yml
- - configs/textdet/maskrcnn/metafile.yml
- - configs/textdet/drrg/metafile.yml
- - configs/textdet/fcenet/metafile.yml
- - configs/textdet/panet/metafile.yml
- - configs/textdet/psenet/metafile.yml
- - configs/textdet/textsnake/metafile.yml
- - configs/textrecog/abinet/metafile.yml
- - configs/textrecog/aster/metafile.yml
- - configs/textrecog/crnn/metafile.yml
- - configs/textrecog/master/metafile.yml
- - configs/textrecog/nrtr/metafile.yml
- - configs/textrecog/svtr/metafile.yml
- - configs/textrecog/robust_scanner/metafile.yml
- - configs/textrecog/sar/metafile.yml
- - configs/textrecog/satrn/metafile.yml
- - configs/kie/sdmgr/metafile.yml
diff --git a/pipeline/mmocr/mmocr/.mim/tools/analysis_tools/get_flops.py b/pipeline/mmocr/mmocr/.mim/tools/analysis_tools/get_flops.py
deleted file mode 100644
index caa97203aa1e077bd266ab64aa02c1d59f88ec7f..0000000000000000000000000000000000000000
--- a/pipeline/mmocr/mmocr/.mim/tools/analysis_tools/get_flops.py
+++ /dev/null
@@ -1,55 +0,0 @@
-# Copyright (c) OpenMMLab. All rights reserved.
-import argparse
-
-import torch
-from fvcore.nn import FlopCountAnalysis, flop_count_table
-from mmengine import Config
-from mmengine.registry import init_default_scope
-
-from mmocr.registry import MODELS
-
-
-def parse_args():
- parser = argparse.ArgumentParser(description='Train a detector')
- parser.add_argument('config', help='train config file path')
- parser.add_argument(
- '--shape',
- type=int,
- nargs='+',
- default=[640, 640],
- help='input image size')
- args = parser.parse_args()
- return args
-
-
-def main():
-
- args = parse_args()
-
- if len(args.shape) == 1:
- h = w = args.shape[0]
- elif len(args.shape) == 2:
- h, w = args.shape
- else:
- raise ValueError('invalid input shape, please use --shape h w')
-
- input_shape = (1, 3, h, w)
-
- cfg = Config.fromfile(args.config)
- init_default_scope(cfg.get('default_scope', 'mmocr'))
- model = MODELS.build(cfg.model)
-
- flops = FlopCountAnalysis(model, torch.ones(input_shape))
-
- # params = parameter_count_table(model)
- flops_data = flop_count_table(flops)
-
- print(flops_data)
-
- print('!!!Please be cautious if you use the results in papers. '
- 'You may need to check if all ops are supported and verify that the '
- 'flops computation is correct.')
-
-
-if __name__ == '__main__':
- main()
diff --git a/pipeline/mmocr/mmocr/.mim/tools/analysis_tools/offline_eval.py b/pipeline/mmocr/mmocr/.mim/tools/analysis_tools/offline_eval.py
deleted file mode 100644
index b454942238d59d4f07067896ca9f9742094d0d59..0000000000000000000000000000000000000000
--- a/pipeline/mmocr/mmocr/.mim/tools/analysis_tools/offline_eval.py
+++ /dev/null
@@ -1,49 +0,0 @@
-# Copyright (c) OpenMMLab. All rights reserved.
-import argparse
-import json
-
-import mmengine
-from mmengine.config import Config, DictAction
-from mmengine.evaluator import Evaluator
-from mmengine.registry import init_default_scope
-
-
-def parse_args():
- parser = argparse.ArgumentParser(description='Offline evaluation of the '
- 'prediction saved in pkl format')
- parser.add_argument('config', help='Config of the model')
- parser.add_argument(
- 'pkl_results', help='Path to the predictions in '
- 'pickle format')
- parser.add_argument(
- '--cfg-options',
- nargs='+',
- action=DictAction,
- help='Override some settings in the used config, the key-value pair '
- 'in xxx=yyy format will be merged into config file. If the value to '
- 'be overwritten is a list, it should be like key="[a,b]" or key=a,b '
- 'It also allows nested list/tuple values, e.g. key="[(a,b),(c,d)]" '
- 'Note that the quotation marks are necessary and that no white space '
- 'is allowed.')
- args = parser.parse_args()
- return args
-
-
-def main():
- args = parse_args()
-
- # load config
- cfg = Config.fromfile(args.config)
- init_default_scope(cfg.get('default_scope', 'mmocr'))
- if args.cfg_options is not None:
- cfg.merge_from_dict(args.cfg_options)
-
- predictions = mmengine.load(args.pkl_results)
-
- evaluator = Evaluator(cfg.test_evaluator)
- eval_results = evaluator.offline_evaluate(predictions)
- print(json.dumps(eval_results))
-
-
-if __name__ == '__main__':
- main()
diff --git a/pipeline/mmocr/mmocr/.mim/tools/analysis_tools/print_config.py b/pipeline/mmocr/mmocr/.mim/tools/analysis_tools/print_config.py
deleted file mode 100644
index 770bb6da216bd382751a8b20c323e87119afe4e6..0000000000000000000000000000000000000000
--- a/pipeline/mmocr/mmocr/.mim/tools/analysis_tools/print_config.py
+++ /dev/null
@@ -1,36 +0,0 @@
-# Copyright (c) OpenMMLab. All rights reserved.
-import argparse
-
-from mmengine import Config, DictAction
-
-
-def parse_args():
- parser = argparse.ArgumentParser(description='Print the whole config')
- parser.add_argument('config', help='config file path')
- parser.add_argument(
- '--cfg-options',
- nargs='+',
- action=DictAction,
- help='override some settings in the used config, the key-value pair '
- 'in xxx=yyy format will be merged into config file. If the value to '
- 'be overwritten is a list, it should be like key="[a,b]" or key=a,b '
- 'It also allows nested list/tuple values, e.g. key="[(a,b),(c,d)]" '
- 'Note that the quotation marks are necessary and that no white space '
- 'is allowed.')
- args = parser.parse_args()
-
- return args
-
-
-def main():
- args = parse_args()
-
- cfg = Config.fromfile(args.config)
-
- if args.cfg_options is not None:
- cfg.merge_from_dict(args.cfg_options)
- print(f'Config:\n{cfg.pretty_text}')
-
-
-if __name__ == '__main__':
- main()
diff --git a/pipeline/mmocr/mmocr/.mim/tools/dataset_converters/common/curvedsyntext_converter.py b/pipeline/mmocr/mmocr/.mim/tools/dataset_converters/common/curvedsyntext_converter.py
deleted file mode 100644
index 5fd8784a708c45591c00b97fc1e0c4fe96c88df7..0000000000000000000000000000000000000000
--- a/pipeline/mmocr/mmocr/.mim/tools/dataset_converters/common/curvedsyntext_converter.py
+++ /dev/null
@@ -1,129 +0,0 @@
-# Copyright (c) OpenMMLab. All rights reserved.
-import argparse
-import os.path as osp
-from functools import partial
-
-import mmengine
-import numpy as np
-
-from mmocr.utils import bezier2polygon, sort_points
-
-# The default dictionary used by CurvedSynthText
-dict95 = [
- ' ', '!', '"', '#', '$', '%', '&', '\'', '(', ')', '*', '+', ',', '-', '.',
- '/', '0', '1', '2', '3', '4', '5', '6', '7', '8', '9', ':', ';', '<', '=',
- '>', '?', '@', 'A', 'B', 'C', 'D', 'E', 'F', 'G', 'H', 'I', 'J', 'K', 'L',
- 'M', 'N', 'O', 'P', 'Q', 'R', 'S', 'T', 'U', 'V', 'W', 'X', 'Y', 'Z', '[',
- '\\', ']', '^', '_', '`', 'a', 'b', 'c', 'd', 'e', 'f', 'g', 'h', 'i', 'j',
- 'k', 'l', 'm', 'n', 'o', 'p', 'q', 'r', 's', 't', 'u', 'v', 'w', 'x', 'y',
- 'z', '{', '|', '}', '~'
-]
-UNK = len(dict95)
-EOS = UNK + 1
-
-
-def digit2text(rec):
- res = []
- for d in rec:
- assert d <= EOS
- if d == EOS:
- break
- if d == UNK:
- print('Warning: Has a UNK character')
- res.append('ๅฃ') # Or any special character not in the target dict
- res.append(dict95[d])
- return ''.join(res)
-
-
-def modify_annotation(ann, num_sample, start_img_id=0, start_ann_id=0):
- ann['text'] = digit2text(ann.pop('rec'))
- # Get hide egmentation points
- polygon_pts = bezier2polygon(ann['bezier_pts'], num_sample=num_sample)
- ann['segmentation'] = np.asarray(sort_points(polygon_pts)).reshape(
- 1, -1).tolist()
- ann['image_id'] += start_img_id
- ann['id'] += start_ann_id
- return ann
-
-
-def modify_image_info(image_info, path_prefix, start_img_id=0):
- image_info['file_name'] = osp.join(path_prefix, image_info['file_name'])
- image_info['id'] += start_img_id
- return image_info
-
-
-def parse_args():
- parser = argparse.ArgumentParser(
- description='Convert CurvedSynText150k to COCO format')
- parser.add_argument('root_path', help='CurvedSynText150k root path')
- parser.add_argument('-o', '--out-dir', help='Output path')
- parser.add_argument(
- '-n',
- '--num-sample',
- type=int,
- default=4,
- help='Number of sample points at each Bezier curve.')
- parser.add_argument(
- '--nproc', default=1, type=int, help='Number of processes')
- args = parser.parse_args()
- return args
-
-
-def convert_annotations(data,
- path_prefix,
- num_sample,
- nproc,
- start_img_id=0,
- start_ann_id=0):
- modify_image_info_with_params = partial(
- modify_image_info, path_prefix=path_prefix, start_img_id=start_img_id)
- modify_annotation_with_params = partial(
- modify_annotation,
- num_sample=num_sample,
- start_img_id=start_img_id,
- start_ann_id=start_ann_id)
- if nproc > 1:
- data['annotations'] = mmengine.track_parallel_progress(
- modify_annotation_with_params, data['annotations'], nproc=nproc)
- data['images'] = mmengine.track_parallel_progress(
- modify_image_info_with_params, data['images'], nproc=nproc)
- else:
- data['annotations'] = mmengine.track_progress(
- modify_annotation_with_params, data['annotations'])
- data['images'] = mmengine.track_progress(
- modify_image_info_with_params,
- data['images'],
- )
- data['categories'] = [{'id': 1, 'name': 'text'}]
- return data
-
-
-def main():
- args = parse_args()
- root_path = args.root_path
- out_dir = args.out_dir if args.out_dir else root_path
- mmengine.mkdir_or_exist(out_dir)
-
- anns = mmengine.load(osp.join(root_path, 'train1.json'))
- data1 = convert_annotations(anns, 'syntext_word_eng', args.num_sample,
- args.nproc)
-
- # Get the maximum image id from data1
- start_img_id = max(data1['images'], key=lambda x: x['id'])['id'] + 1
- start_ann_id = max(data1['annotations'], key=lambda x: x['id'])['id'] + 1
- anns = mmengine.load(osp.join(root_path, 'train2.json'))
- data2 = convert_annotations(
- anns,
- 'emcs_imgs',
- args.num_sample,
- args.nproc,
- start_img_id=start_img_id,
- start_ann_id=start_ann_id)
-
- data1['images'] += data2['images']
- data1['annotations'] += data2['annotations']
- mmengine.dump(data1, osp.join(out_dir, 'instances_training.json'))
-
-
-if __name__ == '__main__':
- main()
diff --git a/pipeline/mmocr/mmocr/.mim/tools/dataset_converters/common/extract_kaist.py b/pipeline/mmocr/mmocr/.mim/tools/dataset_converters/common/extract_kaist.py
deleted file mode 100644
index 76d2579ccbb59f9addc60bbbe9df9037fd543665..0000000000000000000000000000000000000000
--- a/pipeline/mmocr/mmocr/.mim/tools/dataset_converters/common/extract_kaist.py
+++ /dev/null
@@ -1,75 +0,0 @@
-# Copyright (c) OpenMMLab. All rights reserved.
-import argparse
-import os
-import os.path as osp
-import shutil
-import xml.etree.ElementTree as ET
-import zipfile
-from xml.etree.ElementTree import ParseError
-
-
-def extract(root_path):
- idx = 0
- for language in ['English', 'Korean', 'Mixed']:
- for camera in ['Digital_Camera', 'Mobile_Phone']:
- crt_path = osp.join(root_path, 'KAIST', language, camera)
- zips = os.listdir(crt_path)
- for zip in zips:
- extracted_path = osp.join(root_path, 'tmp', zip)
- extract_zipfile(osp.join(crt_path, zip), extracted_path)
- for file in os.listdir(extracted_path):
- if file.endswith('xml'):
- src_ann = os.path.join(extracted_path, file)
- # Filtering broken annotations
- try:
- ET.parse(src_ann)
- except ParseError:
- continue
- src_img = None
- img_names = [
- file.replace('xml', suffix)
- for suffix in ['jpg', 'JPG']
- ]
- for im in img_names:
- img_path = osp.join(extracted_path, im)
- if osp.exists(img_path):
- src_img = img_path
- if src_img:
- shutil.move(
- src_ann,
- osp.join(root_path, 'annotations',
- str(idx).zfill(5) + '.xml'))
- shutil.move(
- src_img,
- osp.join(root_path, 'imgs',
- str(idx).zfill(5) + '.jpg'))
- idx += 1
-
-
-def extract_zipfile(zip_path, dst_dir, delete=True):
-
- files = zipfile.ZipFile(zip_path)
- for file in files.namelist():
- files.extract(file, dst_dir)
- if delete:
- os.remove(zip_path)
-
-
-def parse_args():
- parser = argparse.ArgumentParser(description='Extract KAIST zips')
- parser.add_argument('root_path', help='Root path of KAIST')
- args = parser.parse_args()
- return args
-
-
-def main():
- args = parse_args()
- root_path = args.root_path
- assert osp.exists(root_path)
- extract(root_path)
- shutil.rmtree(osp.join(args.root_path, 'tmp'))
- shutil.rmtree(osp.join(args.root_path, 'KAIST'))
-
-
-if __name__ == '__main__':
- main()
diff --git a/pipeline/mmocr/mmocr/.mim/tools/dataset_converters/kie/closeset_to_openset.py b/pipeline/mmocr/mmocr/.mim/tools/dataset_converters/kie/closeset_to_openset.py
deleted file mode 100644
index 2057e9797bd0586fd8820ef3ae161486bea22d32..0000000000000000000000000000000000000000
--- a/pipeline/mmocr/mmocr/.mim/tools/dataset_converters/kie/closeset_to_openset.py
+++ /dev/null
@@ -1,122 +0,0 @@
-# Copyright (c) OpenMMLab. All rights reserved.
-import argparse
-import json
-from functools import partial
-
-import mmengine
-
-from mmocr.utils import list_from_file, list_to_file
-
-
-def convert(closeset_line, merge_bg_others=False, ignore_idx=0, others_idx=25):
- """Convert line-json str of closeset to line-json str of openset. Note that
- this function is designed for closeset-wildreceipt to openset-wildreceipt.
- It may not be suitable to your own dataset.
-
- Args:
- closeset_line (str): The string to be deserialized to
- the closeset dictionary object.
- merge_bg_others (bool): If True, give the same label to "background"
- class and "others" class.
- ignore_idx (int): Index for ``ignore`` class.
- others_idx (int): Index for ``others`` class.
- """
- # Two labels at the same index of the following two lists
- # make up a key-value pair. For example, in wildreceipt,
- # closeset_key_inds[0] maps to "Store_name_key"
- # and closeset_value_inds[0] maps to "Store_addr_value".
- closeset_key_inds = list(range(2, others_idx, 2))
- closeset_value_inds = list(range(1, others_idx, 2))
-
- openset_node_label_mapping = {'bg': 0, 'key': 1, 'value': 2, 'others': 3}
- if merge_bg_others:
- openset_node_label_mapping['others'] = openset_node_label_mapping['bg']
-
- closeset_obj = json.loads(closeset_line)
- openset_obj = {
- 'file_name': closeset_obj['file_name'],
- 'height': closeset_obj['height'],
- 'width': closeset_obj['width'],
- 'annotations': []
- }
-
- edge_idx = 1
- label_to_edge = {}
- for anno in closeset_obj['annotations']:
- label = anno['label']
- if label == ignore_idx:
- anno['label'] = openset_node_label_mapping['bg']
- anno['edge'] = edge_idx
- edge_idx += 1
- elif label == others_idx:
- anno['label'] = openset_node_label_mapping['others']
- anno['edge'] = edge_idx
- edge_idx += 1
- else:
- edge = label_to_edge.get(label, None)
- if edge is not None:
- anno['edge'] = edge
- if label in closeset_key_inds:
- anno['label'] = openset_node_label_mapping['key']
- elif label in closeset_value_inds:
- anno['label'] = openset_node_label_mapping['value']
- else:
- tmp_key = 'key'
- if label in closeset_key_inds:
- label_with_same_edge = closeset_value_inds[
- closeset_key_inds.index(label)]
- elif label in closeset_value_inds:
- label_with_same_edge = closeset_key_inds[
- closeset_value_inds.index(label)]
- tmp_key = 'value'
- edge_counterpart = label_to_edge.get(label_with_same_edge,
- None)
- if edge_counterpart is not None:
- anno['edge'] = edge_counterpart
- else:
- anno['edge'] = edge_idx
- edge_idx += 1
- anno['label'] = openset_node_label_mapping[tmp_key]
- label_to_edge[label] = anno['edge']
-
- openset_obj['annotations'] = closeset_obj['annotations']
-
- return json.dumps(openset_obj, ensure_ascii=False)
-
-
-def process(closeset_file, openset_file, merge_bg_others=False, n_proc=10):
- closeset_lines = list_from_file(closeset_file)
-
- convert_func = partial(convert, merge_bg_others=merge_bg_others)
-
- openset_lines = mmengine.track_parallel_progress(
- convert_func, closeset_lines, nproc=n_proc)
-
- list_to_file(openset_file, openset_lines)
-
-
-def parse_args():
- parser = argparse.ArgumentParser()
- parser.add_argument('in_file', help='Annotation file for closeset.')
- parser.add_argument('out_file', help='Annotation file for openset.')
- parser.add_argument(
- '--merge',
- action='store_true',
- help='Merge two classes: "background" and "others" in closeset '
- 'to one class in openset.')
- parser.add_argument(
- '--n_proc', type=int, default=10, help='Number of process.')
- args = parser.parse_args()
- return args
-
-
-def main():
- args = parse_args()
-
- process(args.in_file, args.out_file, args.merge, args.n_proc)
-
- print('finish')
-
-
-if __name__ == '__main__':
- main()
diff --git a/pipeline/mmocr/mmocr/.mim/tools/dataset_converters/prepare_dataset.py b/pipeline/mmocr/mmocr/.mim/tools/dataset_converters/prepare_dataset.py
deleted file mode 100644
index 84b8a0353c420adc696a628baa54829d28367020..0000000000000000000000000000000000000000
--- a/pipeline/mmocr/mmocr/.mim/tools/dataset_converters/prepare_dataset.py
+++ /dev/null
@@ -1,153 +0,0 @@
-# Copyright (c) OpenMMLab. All rights reserved.
-import argparse
-import os.path as osp
-import time
-import warnings
-
-from mmengine import Config
-
-from mmocr.datasets.preparers import DatasetPreparer
-
-
-def parse_args():
- parser = argparse.ArgumentParser(
- description='Preparing datasets used in MMOCR.')
- parser.add_argument(
- 'datasets',
- help='A list of the dataset names that would like to prepare.',
- nargs='+')
- parser.add_argument(
- '--nproc', help='Number of processes to run', default=4, type=int)
- parser.add_argument(
- '--task',
- default='textdet',
- choices=['textdet', 'textrecog', 'textspotting', 'kie'],
- help='Task type. Options are "textdet", "textrecog", "textspotting"'
- ' and "kie".')
- parser.add_argument(
- '--splits',
- default=['train', 'test', 'val'],
- help='A list of the split that would like to prepare.',
- nargs='+')
- parser.add_argument(
- '--lmdb',
- action='store_true',
- default=False,
- help='Whether to dump the textrecog dataset to LMDB format, It\'s a '
- 'shortcut to force the dataset to be dumped in lmdb format. '
- 'Applicable when --task=textrecog')
- parser.add_argument(
- '--overwrite-cfg',
- action='store_true',
- default=False,
- help='Whether to overwrite the dataset config file if it already'
- ' exists. If not specified, Dataset Preparer will not generate'
- ' new config for datasets whose configs are already in base.')
- parser.add_argument(
- '--dataset-zoo-path',
- default='./dataset_zoo',
- help='Path to dataset zoo config files.')
- args = parser.parse_args()
- return args
-
-
-def parse_meta(task: str, meta_path: str) -> None:
- """Parse meta file.
-
- Args:
- cfg_path (str): Path to meta file.
- """
- try:
- meta = Config.fromfile(meta_path)
- except FileNotFoundError:
- return
- assert task in meta['Data']['Tasks'], \
- f'Task {task} not supported!'
- # License related
- if meta['Data']['License']['Type']:
- print(f"\033[1;33;40mDataset Name: {meta['Name']}")
- print(f"License Type: {meta['Data']['License']['Type']}")
- print(f"License Link: {meta['Data']['License']['Link']}")
- print(f"BibTeX: {meta['Paper']['BibTeX']}\033[0m")
- print('\033[1;31;43mMMOCR does not own the dataset. Using this '
- 'dataset you must accept the license provided by the owners, '
- 'and cite the corresponding papers appropriately.')
- print('If you do not agree with the above license, please cancel '
- 'the progress immediately by pressing ctrl+c. Otherwise, '
- 'you are deemed to accept the terms and conditions.\033[0m')
- for i in range(5):
- print(f'{5-i}...')
- time.sleep(1)
-
-
-def force_lmdb(cfg):
- """Force the dataset to be dumped in lmdb format.
-
- Args:
- cfg (Config): Config object.
-
- Returns:
- Config: Config object.
- """
- for split in ['train', 'val', 'test']:
- preparer_cfg = cfg.get(f'{split}_preparer')
- if preparer_cfg:
- if preparer_cfg.get('dumper') is None:
- raise ValueError(
- f'{split} split does not come with a dumper, '
- 'so most likely the annotations are MMOCR-ready and do '
- 'not need any adaptation, and it '
- 'cannot be dumped in LMDB format.')
- preparer_cfg.dumper['type'] = 'TextRecogLMDBDumper'
-
- cfg.config_generator['dataset_name'] = f'{cfg.dataset_name}_lmdb'
-
- for split in ['train_anns', 'val_anns', 'test_anns']:
- if split in cfg.config_generator:
- # It can be None when users want to clear out the default
- # value
- if not cfg.config_generator[split]:
- continue
- ann_list = cfg.config_generator[split]
- for ann_dict in ann_list:
- ann_dict['ann_file'] = (
- osp.splitext(ann_dict['ann_file'])[0] + '.lmdb')
- else:
- if split == 'train_anns':
- ann_list = [dict(ann_file='textrecog_train.lmdb')]
- elif split == 'test_anns':
- ann_list = [dict(ann_file='textrecog_test.lmdb')]
- else:
- ann_list = []
- cfg.config_generator[split] = ann_list
-
- return cfg
-
-
-def main():
- args = parse_args()
- if args.lmdb and args.task != 'textrecog':
- raise ValueError('--lmdb only works with --task=textrecog')
- for dataset in args.datasets:
- if not osp.isdir(osp.join(args.dataset_zoo_path, dataset)):
- warnings.warn(f'{dataset} is not supported yet. Please check '
- 'dataset zoo for supported datasets.')
- continue
- meta_path = osp.join(args.dataset_zoo_path, dataset, 'metafile.yml')
- parse_meta(args.task, meta_path)
- cfg_path = osp.join(args.dataset_zoo_path, dataset, args.task + '.py')
- cfg = Config.fromfile(cfg_path)
- if args.overwrite_cfg and cfg.get('config_generator',
- None) is not None:
- cfg.config_generator.overwrite_cfg = args.overwrite_cfg
- cfg.nproc = args.nproc
- cfg.task = args.task
- cfg.dataset_name = dataset
- if args.lmdb:
- cfg = force_lmdb(cfg)
- preparer = DatasetPreparer.from_file(cfg)
- preparer.run(args.splits)
-
-
-if __name__ == '__main__':
- main()
diff --git a/pipeline/mmocr/mmocr/.mim/tools/dataset_converters/textdet/art_converter.py b/pipeline/mmocr/mmocr/.mim/tools/dataset_converters/textdet/art_converter.py
deleted file mode 100644
index 9d3b6a25132752887cd3beaf82d515c53d4cc083..0000000000000000000000000000000000000000
--- a/pipeline/mmocr/mmocr/.mim/tools/dataset_converters/textdet/art_converter.py
+++ /dev/null
@@ -1,145 +0,0 @@
-# Copyright (c) OpenMMLab. All rights reserved.
-import argparse
-import math
-import os.path as osp
-
-import mmcv
-import mmengine
-
-from mmocr.utils import convert_annotations
-
-
-def parse_args():
- parser = argparse.ArgumentParser(
- description='Generate training and validation set of ArT ')
- parser.add_argument('root_path', help='Root dir path of ArT')
- parser.add_argument(
- '--val-ratio', help='Split ratio for val set', default=0.0, type=float)
- args = parser.parse_args()
- return args
-
-
-def collect_art_info(root_path, split, ratio, print_every=1000):
- """Collect the annotation information.
-
- The annotation format is as the following:
- {
- 'gt_1726': # 'gt_1726' is file name
- [
- {
- 'transcription': '็็ณ้ๅข',
- 'points': [
- [141, 199],
- [237, 201],
- [313, 236],
- [357, 283],
- [359, 300],
- [309, 261],
- [233, 230],
- [140, 231]
- ],
- 'language': 'Chinese',
- 'illegibility': False
- },
- ...
- ],
- ...
- }
-
-
- Args:
- root_path (str): Root path to the dataset
- split (str): Dataset split, which should be 'train' or 'val'
- ratio (float): Split ratio for val set
- print_every (int): Print log info per iteration
-
- Returns:
- img_info (dict): The dict of the img and annotation information
- """
-
- annotation_path = osp.join(root_path, 'annotations/train_labels.json')
- if not osp.exists(annotation_path):
- raise Exception(
- f'{annotation_path} not exists, please check and try again.')
-
- annotation = mmengine.load(annotation_path)
- img_prefixes = annotation.keys()
-
- trn_files, val_files = [], []
- if ratio > 0:
- for i, file in enumerate(img_prefixes):
- if i % math.floor(1 / ratio):
- trn_files.append(file)
- else:
- val_files.append(file)
- else:
- trn_files, val_files = img_prefixes, []
- print(f'training #{len(trn_files)}, val #{len(val_files)}')
-
- if split == 'train':
- img_prefixes = trn_files
- elif split == 'val':
- img_prefixes = val_files
- else:
- raise NotImplementedError
-
- img_infos = []
- for i, prefix in enumerate(img_prefixes):
- if i > 0 and i % print_every == 0:
- print(f'{i}/{len(img_prefixes)}')
- img_file = osp.join(root_path, 'imgs', prefix + '.jpg')
- # Skip not exist images
- if not osp.exists(img_file):
- continue
- img = mmcv.imread(img_file)
-
- img_info = dict(
- file_name=osp.join(osp.basename(img_file)),
- height=img.shape[0],
- width=img.shape[1],
- segm_file=osp.join(osp.basename(annotation_path)))
-
- anno_info = []
- for ann in annotation[prefix]:
- segmentation = []
- for x, y in ann['points']:
- segmentation.append(max(0, x))
- segmentation.append(max(0, y))
- xs, ys = segmentation[::2], segmentation[1::2]
- x, y = min(xs), min(ys)
- w, h = max(xs) - x, max(ys) - y
- bbox = [x, y, w, h]
- if ann['transcription'] == '###' or ann['illegibility']:
- iscrowd = 1
- else:
- iscrowd = 0
- anno = dict(
- iscrowd=iscrowd,
- category_id=1,
- bbox=bbox,
- area=w * h,
- segmentation=[segmentation])
- anno_info.append(anno)
- img_info.update(anno_info=anno_info)
- img_infos.append(img_info)
-
- return img_infos
-
-
-def main():
- args = parse_args()
- root_path = args.root_path
- print('Processing training set...')
- training_infos = collect_art_info(root_path, 'train', args.val_ratio)
- convert_annotations(training_infos,
- osp.join(root_path, 'instances_training.json'))
- if args.val_ratio > 0:
- print('Processing validation set...')
- val_infos = collect_art_info(root_path, 'val', args.val_ratio)
- convert_annotations(val_infos, osp.join(root_path,
- 'instances_val.json'))
- print('Finish')
-
-
-if __name__ == '__main__':
- main()
diff --git a/pipeline/mmocr/mmocr/.mim/tools/dataset_converters/textdet/bid_converter.py b/pipeline/mmocr/mmocr/.mim/tools/dataset_converters/textdet/bid_converter.py
deleted file mode 100644
index a16a3439e5cf1802e24505d97b1e94a790010698..0000000000000000000000000000000000000000
--- a/pipeline/mmocr/mmocr/.mim/tools/dataset_converters/textdet/bid_converter.py
+++ /dev/null
@@ -1,185 +0,0 @@
-# Copyright (c) OpenMMLab. All rights reserved.
-import argparse
-import os
-import os.path as osp
-
-import mmcv
-import mmengine
-
-from mmocr.utils import dump_ocr_data
-
-
-def collect_files(img_dir, gt_dir):
- """Collect all images and their corresponding groundtruth files.
-
- Args:
- img_dir (str): The image directory
- gt_dir (str): The groundtruth directory
-
- Returns:
- files (list): The list of tuples (img_file, groundtruth_file)
- """
- assert isinstance(img_dir, str)
- assert img_dir
- assert isinstance(gt_dir, str)
- assert gt_dir
-
- ann_list, imgs_list = [], []
- for img_file in os.listdir(img_dir):
- ann_file = img_file.split('_')[0] + '_gt_ocr.txt'
- ann_list.append(osp.join(gt_dir, ann_file))
- imgs_list.append(osp.join(img_dir, img_file))
-
- files = list(zip(imgs_list, ann_list))
- assert len(files), f'No images found in {img_dir}'
- print(f'Loaded {len(files)} images from {img_dir}')
-
- return files
-
-
-def collect_annotations(files, nproc=1):
- """Collect the annotation information.
-
- Args:
- files (list): The list of tuples (image_file, groundtruth_file)
- nproc (int): The number of process to collect annotations
-
- Returns:
- images (list): The list of image information dicts
- """
- assert isinstance(files, list)
- assert isinstance(nproc, int)
-
- if nproc > 1:
- images = mmengine.track_parallel_progress(
- load_img_info, files, nproc=nproc)
- else:
- images = mmengine.track_progress(load_img_info, files)
-
- return images
-
-
-def load_img_info(files):
- """Load the information of one image.
-
- Args:
- files (tuple): The tuple of (img_file, groundtruth_file)
-
- Returns:
- img_info (dict): The dict of the img and annotation information
- """
- assert isinstance(files, tuple)
-
- img_file, gt_file = files
- assert osp.basename(gt_file).split('_')[0] == osp.basename(gt_file).split(
- '_')[0]
- # read imgs while ignoring orientations
- img = mmcv.imread(img_file, 'unchanged')
-
- img_info = dict(
- file_name=osp.basename(img_file),
- height=img.shape[0],
- width=img.shape[1],
- segm_file=osp.basename(gt_file))
-
- if osp.splitext(gt_file)[1] == '.txt':
- img_info = load_txt_info(gt_file, img_info)
- else:
- raise NotImplementedError
-
- return img_info
-
-
-def load_txt_info(gt_file, img_info):
- """Collect the annotation information.
-
- The annotation format is as the following:
- x, y, w, h, text
- 977, 152, 16, 49, NOME
- 962, 143, 12, 323, APPINHANESI BLAZEK PASSOTTO
- 906, 446, 12, 94, 206940361
- 905, 641, 12, 44, SPTC
-
- Args:
- gt_file (str): The path to ground-truth
- img_info (dict): The dict of the img and annotation information
-
- Returns:
- img_info (dict): The dict of the img and annotation information
- """
- with open(gt_file, encoding='latin1') as f:
- anno_info = []
- for line in f:
- line = line.strip('\n')
- if line[0] == '[' or line[0] == 'x':
- continue
- ann = line.split(',')
- bbox = ann[0:4]
- bbox = [int(coord) for coord in bbox]
- x, y, w, h = bbox
- segmentation = [x, y, x + w, y, x + w, y + h, x, y + h]
- anno = dict(
- iscrowd=0,
- category_id=1,
- bbox=bbox,
- area=w * h,
- segmentation=[segmentation])
- anno_info.append(anno)
-
- img_info.update(anno_info=anno_info)
-
- return img_info
-
-
-def split_train_val_list(full_list, val_ratio):
- """Split list by val_ratio.
-
- Args:
- full_list (list): list to be split
- val_ratio (float): split ratio for val set
-
- return:
- list(list, list): train_list and val_list
- """
- n_total = len(full_list)
- offset = int(n_total * val_ratio)
- if n_total == 0 or offset < 1:
- return [], full_list
- val_list = full_list[:offset]
- train_list = full_list[offset:]
- return [train_list, val_list]
-
-
-def parse_args():
- parser = argparse.ArgumentParser(
- description='Generate training and val set of BID ')
- parser.add_argument('root_path', help='Root dir path of BID')
- parser.add_argument(
- '--nproc', default=1, type=int, help='Number of processes')
- parser.add_argument(
- '--val-ratio', help='Split ratio for val set', default=0., type=float)
- args = parser.parse_args()
- return args
-
-
-def main():
- args = parse_args()
- root_path = args.root_path
- with mmengine.Timer(print_tmpl='It takes {}s to convert BID annotation'):
- files = collect_files(
- osp.join(root_path, 'imgs'), osp.join(root_path, 'annotations'))
- image_infos = collect_annotations(files, nproc=args.nproc)
- if args.val_ratio:
- image_infos = split_train_val_list(image_infos, args.val_ratio)
- splits = ['training', 'val']
- else:
- image_infos = [image_infos]
- splits = ['training']
- for i, split in enumerate(splits):
- dump_ocr_data(image_infos[i],
- osp.join(root_path, 'instances_' + split + '.json'),
- 'textdet')
-
-
-if __name__ == '__main__':
- main()
diff --git a/pipeline/mmocr/mmocr/.mim/tools/dataset_converters/textdet/coco_to_line_dict.py b/pipeline/mmocr/mmocr/.mim/tools/dataset_converters/textdet/coco_to_line_dict.py
deleted file mode 100644
index 7dcb5edb453edbc7904478de6d636b241a29336e..0000000000000000000000000000000000000000
--- a/pipeline/mmocr/mmocr/.mim/tools/dataset_converters/textdet/coco_to_line_dict.py
+++ /dev/null
@@ -1,67 +0,0 @@
-# Copyright (c) OpenMMLab. All rights reserved.
-import argparse
-import json
-
-import mmengine
-
-from mmocr.utils import list_to_file
-
-
-def parse_coco_json(in_path):
- json_obj = mmengine.load(in_path)
- image_infos = json_obj['images']
- annotations = json_obj['annotations']
- imgid2imgname = {}
- img_ids = []
- for image_info in image_infos:
- imgid2imgname[image_info['id']] = image_info
- img_ids.append(image_info['id'])
- imgid2anno = {}
- for img_id in img_ids:
- imgid2anno[img_id] = []
- for anno in annotations:
- img_id = anno['image_id']
- new_anno = {}
- new_anno['iscrowd'] = anno['iscrowd']
- new_anno['category_id'] = anno['category_id']
- new_anno['bbox'] = anno['bbox']
- new_anno['segmentation'] = anno['segmentation']
- if img_id in imgid2anno.keys():
- imgid2anno[img_id].append(new_anno)
-
- return imgid2imgname, imgid2anno
-
-
-def gen_line_dict_file(out_path, imgid2imgname, imgid2anno):
- lines = []
- for key, value in imgid2imgname.items():
- if key in imgid2anno:
- anno = imgid2anno[key]
- line_dict = {}
- line_dict['file_name'] = value['file_name']
- line_dict['height'] = value['height']
- line_dict['width'] = value['width']
- line_dict['annotations'] = anno
- lines.append(json.dumps(line_dict))
- list_to_file(out_path, lines)
-
-
-def parse_args():
- parser = argparse.ArgumentParser()
- parser.add_argument('--in-path', help='input json path with coco format')
- parser.add_argument(
- '--out-path', help='output txt path with line-json format')
-
- args = parser.parse_args()
- return args
-
-
-def main():
- args = parse_args()
- imgid2imgname, imgid2anno = parse_coco_json(args.in_path)
- gen_line_dict_file(args.out_path, imgid2imgname, imgid2anno)
- print('finish')
-
-
-if __name__ == '__main__':
- main()
diff --git a/pipeline/mmocr/mmocr/.mim/tools/dataset_converters/textdet/cocotext_converter.py b/pipeline/mmocr/mmocr/.mim/tools/dataset_converters/textdet/cocotext_converter.py
deleted file mode 100644
index e4ef78ee39ffe945e1a7e5cf3eba87b19c0fd002..0000000000000000000000000000000000000000
--- a/pipeline/mmocr/mmocr/.mim/tools/dataset_converters/textdet/cocotext_converter.py
+++ /dev/null
@@ -1,124 +0,0 @@
-# Copyright (c) OpenMMLab. All rights reserved.
-import argparse
-import math
-import os.path as osp
-
-import mmengine
-
-from mmocr.utils import dump_ocr_data
-
-
-def parse_args():
- parser = argparse.ArgumentParser(
- description='Generate training and validation set of COCO Text v2 ')
- parser.add_argument('root_path', help='Root dir path of COCO Text v2')
- args = parser.parse_args()
- return args
-
-
-def collect_cocotext_info(root_path, split, print_every=1000):
- """Collect the annotation information.
-
- The annotation format is as the following:
- {
- 'anns':{
- '45346':{
- 'mask': [468.9,286.7,468.9,295.2,493.0,295.8,493.0,287.2],
- 'class': 'machine printed',
- 'bbox': [468.9, 286.7, 24.1, 9.1], # x, y, w, h
- 'image_id': 217925,
- 'id': 45346,
- 'language': 'english', # 'english' or 'not english'
- 'area': 206.06,
- 'utf8_string': 'New',
- 'legibility': 'legible', # 'legible' or 'illegible'
- },
- ...
- }
- 'imgs':{
- '540965':{
- 'id': 540965,
- 'set': 'train', # 'train' or 'val'
- 'width': 640,
- 'height': 360,
- 'file_name': 'COCO_train2014_000000540965.jpg'
- },
- ...
- }
- 'imgToAnns':{
- '540965': [],
- '260932': [63993, 63994, 63995, 63996, 63997, 63998, 63999],
- ...
- }
- }
-
- Args:
- root_path (str): Root path to the dataset
- split (str): Dataset split, which should be 'train' or 'val'
- print_every (int): Print log information per iter
-
- Returns:
- img_info (dict): The dict of the img and annotation information
- """
-
- annotation_path = osp.join(root_path, 'annotations/cocotext.v2.json')
- if not osp.exists(annotation_path):
- raise Exception(
- f'{annotation_path} not exists, please check and try again.')
-
- annotation = mmengine.load(annotation_path)
-
- img_infos = []
- for i, img_info in enumerate(annotation['imgs'].values()):
- if i > 0 and i % print_every == 0:
- print(f'{i}/{len(annotation["imgs"].values())}')
-
- if img_info['set'] == split:
- img_info['segm_file'] = annotation_path
- ann_ids = annotation['imgToAnns'][str(img_info['id'])]
- # Filter out images without text
- if len(ann_ids) == 0:
- continue
- anno_info = []
- for ann_id in ann_ids:
- ann = annotation['anns'][str(ann_id)]
-
- # Ignore illegible or non-English words
- iscrowd = 1 if ann['language'] == 'not english' or ann[
- 'legibility'] == 'illegible' else 0
-
- x, y, w, h = ann['bbox']
- x, y = max(0, math.floor(x)), max(0, math.floor(y))
- w, h = math.ceil(w), math.ceil(h)
- bbox = [x, y, w, h]
- segmentation = [max(0, int(x)) for x in ann['mask']]
- if len(segmentation) < 8 or len(segmentation) % 2 != 0:
- segmentation = [x, y, x + w, y, x + w, y + h, x, y + h]
- anno = dict(
- iscrowd=iscrowd,
- category_id=1,
- bbox=bbox,
- area=ann['area'],
- segmentation=[segmentation])
- anno_info.append(anno)
- img_info.update(anno_info=anno_info)
- img_infos.append(img_info)
- return img_infos
-
-
-def main():
- args = parse_args()
- root_path = args.root_path
- print('Processing training set...')
- training_infos = collect_cocotext_info(root_path, 'train')
- dump_ocr_data(training_infos,
- osp.join(root_path, 'instances_training.json'), 'textdet')
- print('Processing validation set...')
- val_infos = collect_cocotext_info(root_path, 'val')
- dump_ocr_data(val_infos, osp.join(root_path, 'instances_val.json'),
- 'textdet')
- print('Finish')
-
-
-if __name__ == '__main__':
- main()
diff --git a/pipeline/mmocr/mmocr/.mim/tools/dataset_converters/textdet/data_migrator.py b/pipeline/mmocr/mmocr/.mim/tools/dataset_converters/textdet/data_migrator.py
deleted file mode 100644
index 38da8a04861aa5d4f80dbeb65a6be5fdcd55acaf..0000000000000000000000000000000000000000
--- a/pipeline/mmocr/mmocr/.mim/tools/dataset_converters/textdet/data_migrator.py
+++ /dev/null
@@ -1,70 +0,0 @@
-# Copyright (c) OpenMMLab. All rights reserved.
-import argparse
-from collections import defaultdict
-from copy import deepcopy
-from typing import Dict, List
-
-import mmengine
-
-from mmocr.utils import dump_ocr_data
-
-
-def parse_coco_json(in_path: str) -> List[Dict]:
- """Load coco annotations into image_infos parsable by dump_ocr_data().
-
- Args:
- in_path (str): COCO text annotation path.
-
- Returns:
- list[dict]: List of image information dicts. To be used by
- dump_ocr_data().
- """
- json_obj = mmengine.load(in_path)
- image_infos = json_obj['images']
- annotations = json_obj['annotations']
- imgid2annos = defaultdict(list)
- for anno in annotations:
- new_anno = deepcopy(anno)
- new_anno['category_id'] = 0 # Must be 0 for OCR tasks which stands
- # for "text" category
- imgid2annos[anno['image_id']].append(new_anno)
-
- results = []
- for image_info in image_infos:
- image_info['anno_info'] = imgid2annos[image_info['id']]
- results.append(image_info)
-
- return results
-
-
-def parse_args():
- parser = argparse.ArgumentParser()
- parser.add_argument('in_path', help='Input json path in coco format.')
- parser.add_argument(
- 'out_path', help='Output json path in openmmlab format.')
- parser.add_argument(
- '--task',
- type=str,
- default='auto',
- choices=['auto', 'textdet', 'textspotter'],
- help='Output annotation type, defaults to "auto", which decides the'
- 'best task type based on whether "text" is annotated. Other options'
- 'are "textdet" and "textspotter".')
- args = parser.parse_args()
- return args
-
-
-def main():
- args = parse_args()
- image_infos = parse_coco_json(args.in_path)
- task_name = args.task
- if task_name == 'auto':
- task_name = 'textdet'
- if 'text' in image_infos[0]['anno_info'][0]:
- task_name = 'textspotter'
- dump_ocr_data(image_infos, args.out_path, task_name)
- print('finish')
-
-
-if __name__ == '__main__':
- main()
diff --git a/pipeline/mmocr/mmocr/.mim/tools/dataset_converters/textdet/detext_converter.py b/pipeline/mmocr/mmocr/.mim/tools/dataset_converters/textdet/detext_converter.py
deleted file mode 100644
index d99378e44559222d0d32f1cccd4ccf673a33b6df..0000000000000000000000000000000000000000
--- a/pipeline/mmocr/mmocr/.mim/tools/dataset_converters/textdet/detext_converter.py
+++ /dev/null
@@ -1,162 +0,0 @@
-# Copyright (c) OpenMMLab. All rights reserved.
-import argparse
-import os
-import os.path as osp
-
-import mmcv
-import mmengine
-import numpy as np
-
-from mmocr.utils import dump_ocr_data
-
-
-def collect_files(img_dir, gt_dir):
- """Collect all images and their corresponding groundtruth files.
-
- Args:
- img_dir (str): The image directory
- gt_dir (str): The groundtruth directory
-
- Returns:
- files (list): The list of tuples (img_file, groundtruth_file)
- """
- assert isinstance(img_dir, str)
- assert img_dir
- assert isinstance(gt_dir, str)
- assert gt_dir
-
- ann_list, imgs_list = [], []
- for img in os.listdir(img_dir):
- imgs_list.append(osp.join(img_dir, img))
- ann_list.append(osp.join(gt_dir, 'gt_' + img.replace('jpg', 'txt')))
-
- files = list(zip(imgs_list, ann_list))
- assert len(files), f'No images found in {img_dir}'
- print(f'Loaded {len(files)} images from {img_dir}')
-
- return files
-
-
-def collect_annotations(files, nproc=1):
- """Collect the annotation information.
-
- Args:
- files (list): The list of tuples (image_file, groundtruth_file)
- nproc (int): The number of process to collect annotations
-
- Returns:
- images (list): The list of image information dicts
- """
- assert isinstance(files, list)
- assert isinstance(nproc, int)
-
- if nproc > 1:
- images = mmengine.track_parallel_progress(
- load_img_info, files, nproc=nproc)
- else:
- images = mmengine.track_progress(load_img_info, files)
-
- return images
-
-
-def load_img_info(files):
- """Load the information of one image.
-
- Args:
- files (tuple): The tuple of (img_file, groundtruth_file)
-
- Returns:
- img_info (dict): The dict of the img and annotation information
- """
- assert isinstance(files, tuple)
-
- img_file, gt_file = files
- # read imgs while ignoring orientations
- img = mmcv.imread(img_file, 'unchanged')
-
- img_info = dict(
- file_name=osp.join(osp.basename(img_file)),
- height=img.shape[0],
- width=img.shape[1],
- segm_file=osp.join(osp.basename(gt_file)))
-
- if osp.splitext(gt_file)[1] == '.txt':
- img_info = load_txt_info(gt_file, img_info)
- else:
- raise NotImplementedError
-
- return img_info
-
-
-def load_txt_info(gt_file, img_info):
- """Collect the annotation information.
-
- # Annotation Format
- # x1, y1, x2, y2, x3, y3, x4, y4, transcript
-
- Args:
- gt_file (str): The path to ground-truth
- img_info (dict): The dict of the img and annotation information
-
- Returns:
- img_info (dict): The dict of the img and annotation information
- """
-
- with open(gt_file) as f:
- anno_info = []
- annotations = f.readlines()
- for ann in annotations:
- try:
- ann_box = np.array(ann.split(',')[0:8]).astype(int).tolist()
- except ValueError:
- # skip invalid annotation line
- continue
- x = max(0, min(ann_box[0::2]))
- y = max(0, min(ann_box[1::2]))
- w, h = max(ann_box[0::2]) - x, max(ann_box[1::2]) - y
- bbox = [x, y, w, h]
- segmentation = ann_box
- word = ann.split(',')[-1].replace('\n', '').strip()
-
- anno = dict(
- iscrowd=0 if word != '###' else 1,
- category_id=1,
- bbox=bbox,
- area=w * h,
- segmentation=[segmentation])
- anno_info.append(anno)
-
- img_info.update(anno_info=anno_info)
-
- return img_info
-
-
-def parse_args():
- parser = argparse.ArgumentParser(
- description='Generate training and val set of DeText ')
- parser.add_argument('root_path', help='Root dir path of DeText')
- parser.add_argument(
- '--nproc', default=1, type=int, help='Number of process')
- args = parser.parse_args()
- return args
-
-
-def main():
- args = parse_args()
- root_path = args.root_path
-
- for split in ['training', 'val']:
- print(f'Processing {split} set...')
- with mmengine.Timer(
- print_tmpl='It takes {}s to convert DeText annotation'):
- files = collect_files(
- osp.join(root_path, 'imgs', split),
- osp.join(root_path, 'annotations', split))
- image_infos = collect_annotations(files, nproc=args.nproc)
- dump_ocr_data(image_infos,
- osp.join(root_path, 'instances_' + split + '.json'),
- 'textdet')
-
-
-if __name__ == '__main__':
- main()
diff --git a/pipeline/mmocr/mmocr/.mim/tools/dataset_converters/textdet/funsd_converter.py b/pipeline/mmocr/mmocr/.mim/tools/dataset_converters/textdet/funsd_converter.py
deleted file mode 100644
index 7be887d2637b99f0113f99f06a05f7591c061f39..0000000000000000000000000000000000000000
--- a/pipeline/mmocr/mmocr/.mim/tools/dataset_converters/textdet/funsd_converter.py
+++ /dev/null
@@ -1,159 +0,0 @@
-# Copyright (c) OpenMMLab. All rights reserved.
-import argparse
-import math
-import os
-import os.path as osp
-
-import mmcv
-import mmengine
-
-from mmocr.utils import dump_ocr_data
-
-
-def collect_files(img_dir, gt_dir):
- """Collect all images and their corresponding groundtruth files.
-
- Args:
- img_dir (str): The image directory
- gt_dir (str): The groundtruth directory
-
- Returns:
- files (list): The list of tuples (img_file, groundtruth_file)
- """
- assert isinstance(img_dir, str)
- assert img_dir
- assert isinstance(gt_dir, str)
- assert gt_dir
-
- ann_list, imgs_list = [], []
- for gt_file in os.listdir(gt_dir):
- ann_list.append(osp.join(gt_dir, gt_file))
- imgs_list.append(osp.join(img_dir, gt_file.replace('.json', '.png')))
-
- files = list(zip(sorted(imgs_list), sorted(ann_list)))
- assert len(files), f'No images found in {img_dir}'
- print(f'Loaded {len(files)} images from {img_dir}')
-
- return files
-
-
-def collect_annotations(files, nproc=1):
- """Collect the annotation information.
-
- Args:
- files (list): The list of tuples (image_file, groundtruth_file)
- nproc (int): The number of process to collect annotations
-
- Returns:
- images (list): The list of image information dicts
- """
- assert isinstance(files, list)
- assert isinstance(nproc, int)
-
- if nproc > 1:
- images = mmengine.track_parallel_progress(
- load_img_info, files, nproc=nproc)
- else:
- images = mmengine.track_progress(load_img_info, files)
-
- return images
-
-
-def load_img_info(files):
- """Load the information of one image.
-
- Args:
- files (tuple): The tuple of (img_file, groundtruth_file)
-
- Returns:
- img_info (dict): The dict of the img and annotation information
- """
- assert isinstance(files, tuple)
-
- img_file, gt_file = files
- assert osp.basename(gt_file).split('.')[0] == osp.basename(img_file).split(
- '.')[0]
- # read imgs while ignoring orientations
- img = mmcv.imread(img_file, 'unchanged')
-
- img_info = dict(
- file_name=osp.join(osp.basename(img_file)),
- height=img.shape[0],
- width=img.shape[1],
- segm_file=osp.join(osp.basename(gt_file)))
-
- if osp.splitext(gt_file)[1] == '.json':
- img_info = load_json_info(gt_file, img_info)
- else:
- raise NotImplementedError
-
- return img_info
-
-
-def load_json_info(gt_file, img_info):
- """Collect the annotation information.
-
- Args:
- gt_file (str): The path to ground-truth
- img_info (dict): The dict of the img and annotation information
-
- Returns:
- img_info (dict): The dict of the img and annotation information
- """
-
- annotation = mmengine.load(gt_file)
- anno_info = []
- for form in annotation['form']:
- for ann in form['words']:
-
- iscrowd = 1 if len(ann['text']) == 0 else 0
-
- x1, y1, x2, y2 = ann['box']
- x = max(0, min(math.floor(x1), math.floor(x2)))
- y = max(0, min(math.floor(y1), math.floor(y2)))
- w, h = math.ceil(abs(x2 - x1)), math.ceil(abs(y2 - y1))
- bbox = [x, y, w, h]
- segmentation = [x, y, x + w, y, x + w, y + h, x, y + h]
-
- anno = dict(
- iscrowd=iscrowd,
- category_id=1,
- bbox=bbox,
- area=w * h,
- segmentation=[segmentation])
- anno_info.append(anno)
-
- img_info.update(anno_info=anno_info)
-
- return img_info
-
-
-def parse_args():
- parser = argparse.ArgumentParser(
- description='Generate training and test set of FUNSD ')
- parser.add_argument('root_path', help='Root dir path of FUNSD')
- parser.add_argument(
- '--nproc', default=1, type=int, help='Number of process')
- args = parser.parse_args()
- return args
-
-
-def main():
- args = parse_args()
- root_path = args.root_path
-
- for split in ['training', 'test']:
- print(f'Processing {split} set...')
- with mmengine.Timer(
- print_tmpl='It takes {}s to convert FUNSD annotation'):
- files = collect_files(
- osp.join(root_path, 'imgs'),
- osp.join(root_path, 'annotations', split))
- image_infos = collect_annotations(files, nproc=args.nproc)
- dump_ocr_data(image_infos,
- osp.join(root_path, 'instances_' + split + '.json'),
- 'textdet')
-
-
-if __name__ == '__main__':
- main()
diff --git a/pipeline/mmocr/mmocr/.mim/tools/dataset_converters/textdet/hiertext_converter.py b/pipeline/mmocr/mmocr/.mim/tools/dataset_converters/textdet/hiertext_converter.py
deleted file mode 100644
index 9ca0163099c815382fe3362da1b0525d109bc23f..0000000000000000000000000000000000000000
--- a/pipeline/mmocr/mmocr/.mim/tools/dataset_converters/textdet/hiertext_converter.py
+++ /dev/null
@@ -1,152 +0,0 @@
-# Copyright (c) OpenMMLab. All rights reserved.
-import argparse
-import json
-import os.path as osp
-
-import numpy as np
-from shapely.geometry import Polygon
-
-from mmocr.utils import dump_ocr_data
-
-
-def collect_level_info(annotation):
- """Collect information from any level in HierText.
-
- Args:
- annotation (dict): dict at each level
-
- Return:
- anno (dict): dict containing annotations
- """
- iscrowd = 0 if annotation['legible'] else 1
- vertices = np.array(annotation['vertices'])
- polygon = Polygon(vertices)
- area = polygon.area
- min_x, min_y, max_x, max_y = polygon.bounds
- bbox = [min_x, min_y, max_x - min_x, max_y - min_y]
- segmentation = [i for j in vertices for i in j]
- anno = dict(
- iscrowd=iscrowd,
- category_id=1,
- bbox=bbox,
- area=area,
- segmentation=[segmentation])
- return anno
-
-
-def collect_hiertext_info(root_path, level, split, print_every=1000):
- """Collect the annotation information.
-
- The annotation format is as the following:
- {
- "info": {
- "date": "release date",
- "version": "current version"
- },
- "annotations": [ // List of dictionaries, one for each image.
- {
- "image_id": "the filename of corresponding image.",
- "image_width": image_width, // (int) The image width.
- "image_height": image_height, // (int) The image height.
- "paragraphs": [ // List of paragraphs.
- {
- "vertices": [[x1, y1], [x2, y2],...,[xn, yn]]
- "legible": true
- "lines": [
- {
- "vertices": [[x1, y1], [x2, y2],...,[x4, y4]]
- "text": L
- "legible": true,
- "handwritten": false
- "vertical": false,
- "words": [
- {
- "vertices": [[x1, y1], [x2, y2],...,[xm, ym]]
- "text": "the text content of this word",
- "legible": true
- "handwritten": false,
- "vertical": false,
- }, ...
- ]
- }, ...
- ]
- }, ...
- ]
- }, ...
- ]
- }
-
- Args:
- root_path (str): Root path to the dataset
- level (str): Level of annotations, which should be 'word', 'line',
- or 'paragraphs'
- split (str): Dataset split, which should be 'train' or 'validation'
- print_every (int): Print log information per iter
-
- Returns:
- img_info (dict): The dict of the img and annotation information
- """
-
- annotation_path = osp.join(root_path, 'annotations/' + split + '.jsonl')
- if not osp.exists(annotation_path):
- raise Exception(
- f'{annotation_path} not exists, please check and try again.')
-
- annotation = json.load(open(annotation_path))['annotations']
- img_infos = []
- for i, img_annos in enumerate(annotation):
- if i > 0 and i % print_every == 0:
- print(f'{i}/{len(annotation)}')
- img_info = {}
- img_info['file_name'] = img_annos['image_id'] + '.jpg'
- img_info['height'] = img_annos['image_height']
- img_info['width'] = img_annos['image_width']
- img_info['segm_file'] = annotation_path
- anno_info = []
- for paragraph in img_annos['paragraphs']:
- if level == 'paragraph':
- anno = collect_level_info(paragraph)
- anno_info.append(anno)
- elif level == 'line':
- for line in paragraph['lines']:
- anno = collect_level_info(line)
- anno_info.append(anno)
- elif level == 'word':
- for line in paragraph['lines']:
- for word in line['words']:
- anno = collect_level_info(line)
- anno_info.append(anno)
- img_info.update(anno_info=anno_info)
- img_infos.append(img_info)
- return img_infos
-
-
-def parse_args():
- parser = argparse.ArgumentParser(
- description='Generate training and validation set of HierText ')
- parser.add_argument('root_path', help='Root dir path of HierText')
- parser.add_argument(
- '--level',
- default='word',
- help='HierText provides three levels of annotation',
- choices=['word', 'line', 'paragraph'])
- args = parser.parse_args()
- return args
-
-
-def main():
- args = parse_args()
- root_path = args.root_path
- print('Processing training set...')
- training_infos = collect_hiertext_info(root_path, args.level, 'train')
- dump_ocr_data(training_infos,
- osp.join(root_path, 'instances_training.json'), 'textdet')
- print('Processing validation set...')
- val_infos = collect_hiertext_info(root_path, args.level, 'val')
- dump_ocr_data(val_infos, osp.join(root_path, 'instances_val.json'),
- 'textdet')
- print('Finish')
-
-
-if __name__ == '__main__':
- main()
diff --git a/pipeline/mmocr/mmocr/.mim/tools/dataset_converters/textdet/ic11_converter.py b/pipeline/mmocr/mmocr/.mim/tools/dataset_converters/textdet/ic11_converter.py
deleted file mode 100644
index 1a5683f4ae17e52fa8f13fc542a8424ae6cb488f..0000000000000000000000000000000000000000
--- a/pipeline/mmocr/mmocr/.mim/tools/dataset_converters/textdet/ic11_converter.py
+++ /dev/null
@@ -1,173 +0,0 @@
-# Copyright (c) OpenMMLab. All rights reserved.
-import argparse
-import os
-import os.path as osp
-
-import mmcv
-import mmengine
-from PIL import Image
-
-from mmocr.utils import dump_ocr_data
-
-
-def convert_gif(img_path):
- """Convert the gif image to png format.
-
- Args:
- img_path (str): The path to the gif image
- """
- img = Image.open(img_path)
- dst_path = img_path.replace('gif', 'png')
- img.save(dst_path)
- os.remove(img_path)
- print(f'Convert {img_path} to {dst_path}')
-
-
-def collect_files(img_dir, gt_dir):
- """Collect all images and their corresponding groundtruth files.
-
- Args:
- img_dir (str): The image directory
- gt_dir (str): The groundtruth directory
-
- Returns:
- files (list): The list of tuples (img_file, groundtruth_file)
- """
- assert isinstance(img_dir, str)
- assert img_dir
- assert isinstance(gt_dir, str)
- assert gt_dir
-
- ann_list, imgs_list = [], []
- for img in os.listdir(img_dir):
- img_path = osp.join(img_dir, img)
- # mmcv cannot read gif images, so convert them to png
- if img.endswith('gif'):
- convert_gif(img_path)
- img_path = img_path.replace('gif', 'png')
- imgs_list.append(img_path)
- ann_list.append(osp.join(gt_dir, 'gt_' + img.split('.')[0] + '.txt'))
-
- files = list(zip(sorted(imgs_list), sorted(ann_list)))
- assert len(files), f'No images found in {img_dir}'
- print(f'Loaded {len(files)} images from {img_dir}')
-
- return files
-
-
-def collect_annotations(files, nproc=1):
- """Collect the annotation information.
-
- Args:
- files (list): The list of tuples (image_file, groundtruth_file)
- nproc (int): The number of process to collect annotations
-
- Returns:
- images (list): The list of image information dicts
- """
- assert isinstance(files, list)
- assert isinstance(nproc, int)
-
- if nproc > 1:
- images = mmengine.track_parallel_progress(
- load_img_info, files, nproc=nproc)
- else:
- images = mmengine.track_progress(load_img_info, files)
-
- return images
-
-
-def load_img_info(files):
- """Load the information of one image.
-
- Args:
- files (tuple): The tuple of (img_file, groundtruth_file)
-
- Returns:
- img_info (dict): The dict of the img and annotation information
- """
- assert isinstance(files, tuple)
-
- img_file, gt_file = files
- # read imgs while ignoring orientations
- img = mmcv.imread(img_file, 'unchanged')
-
- img_info = dict(
- file_name=osp.join(osp.basename(img_file)),
- height=img.shape[0],
- width=img.shape[1],
- segm_file=osp.join(osp.basename(gt_file)))
-
- if osp.splitext(gt_file)[1] == '.txt':
- img_info = load_txt_info(gt_file, img_info)
- else:
- raise NotImplementedError
-
- return img_info
-
-
-def load_txt_info(gt_file, img_info):
- """Collect the annotation information.
-
- The annotation format is as the following:
- left, top, right, bottom, "transcription"
-
- Args:
- gt_file (str): The path to ground-truth
- img_info (dict): The dict of the img and annotation information
-
- Returns:
- img_info (dict): The dict of the img and annotation information
- """
- anno_info = []
- with open(gt_file) as f:
- lines = f.readlines()
- for line in lines:
- xmin, ymin, xmax, ymax = line.split(',')[0:4]
- x = max(0, int(xmin))
- y = max(0, int(ymin))
- w = int(xmax) - x
- h = int(ymax) - y
- bbox = [x, y, w, h]
- segmentation = [x, y, x + w, y, x + w, y + h, x, y + h]
-
- anno = dict(
- iscrowd=0,
- category_id=1,
- bbox=bbox,
- area=w * h,
- segmentation=[segmentation])
- anno_info.append(anno)
- img_info.update(anno_info=anno_info)
-
- return img_info
-
-
-def parse_args():
- parser = argparse.ArgumentParser(
- description='Generate training and test set of IC11')
- parser.add_argument('root_path', help='Root dir path of IC11')
- parser.add_argument(
- '--nproc', default=1, type=int, help='Number of process')
- args = parser.parse_args()
- return args
-
-
-def main():
- args = parse_args()
- root_path = args.root_path
-
- for split in ['training', 'test']:
- print(f'Processing {split} set...')
- with mmengine.Timer(print_tmpl='It takes {}s to convert annotation'):
- files = collect_files(
- osp.join(root_path, 'imgs', split),
- osp.join(root_path, 'annotations', split))
- image_infos = collect_annotations(files, nproc=args.nproc)
- dump_ocr_data(image_infos,
- osp.join(root_path, 'instances_' + split + '.json'),
- 'textdet')
-
-
-if __name__ == '__main__':
- main()
diff --git a/pipeline/mmocr/mmocr/.mim/tools/dataset_converters/textdet/ilst_converter.py b/pipeline/mmocr/mmocr/.mim/tools/dataset_converters/textdet/ilst_converter.py
deleted file mode 100644
index 56ac54e3e30ed95159b25bee69afe39c47896a2a..0000000000000000000000000000000000000000
--- a/pipeline/mmocr/mmocr/.mim/tools/dataset_converters/textdet/ilst_converter.py
+++ /dev/null
@@ -1,206 +0,0 @@
-# Copyright (c) OpenMMLab. All rights reserved.
-import argparse
-import os
-import os.path as osp
-import xml.etree.ElementTree as ET
-
-import mmcv
-import mmengine
-
-from mmocr.utils import dump_ocr_data
-
-
-def collect_files(img_dir, gt_dir):
- """Collect all images and their corresponding groundtruth files.
-
- Args:
- img_dir (str): The image directory
- gt_dir (str): The groundtruth directory
-
- Returns:
- files (list): The list of tuples (img_file, groundtruth_file)
- """
- assert isinstance(img_dir, str)
- assert img_dir
- assert isinstance(gt_dir, str)
- assert gt_dir
-
- ann_list, imgs_list = [], []
- for img_file in os.listdir(img_dir):
- ann_path = osp.join(gt_dir, img_file.split('.')[0] + '.xml')
- if os.path.exists(ann_path):
- ann_list.append(ann_path)
- imgs_list.append(osp.join(img_dir, img_file))
-
- files = list(zip(imgs_list, ann_list))
- assert len(files), f'No images found in {img_dir}'
- print(f'Loaded {len(files)} images from {img_dir}')
-
- return files
-
-
-def collect_annotations(files, nproc=1):
- """Collect the annotation information.
-
- Args:
- files (list): The list of tuples (image_file, groundtruth_file)
- nproc (int): The number of process to collect annotations
-
- Returns:
- images (list): The list of image information dicts
- """
- assert isinstance(files, list)
- assert isinstance(nproc, int)
-
- if nproc > 1:
- images = mmengine.track_parallel_progress(
- load_img_info, files, nproc=nproc)
- else:
- images = mmengine.track_progress(load_img_info, files)
-
- return images
-
-
-def load_img_info(files):
- """Load the information of one image.
-
- Args:
- files (tuple): The tuple of (img_file, groundtruth_file)
-
- Returns:
- img_info (dict): The dict of the img and annotation information
- """
- assert isinstance(files, tuple)
-
- img_file, gt_file = files
- assert osp.basename(gt_file).split('.')[0] == osp.basename(img_file).split(
- '.')[0]
- # read imgs while ignoring orientations
- img = mmcv.imread(img_file, 'unchanged')
-
- try:
- img_info = dict(
- file_name=osp.join(osp.basename(img_file)),
- height=img.shape[0],
- width=img.shape[1],
- segm_file=osp.join(osp.basename(gt_file)))
- except AttributeError:
- print(f'Skip broken img {img_file}')
- return None
-
- if osp.splitext(gt_file)[1] == '.xml':
- img_info = load_xml_info(gt_file, img_info)
- else:
- raise NotImplementedError
-
- return img_info
-
-
-def load_xml_info(gt_file, img_info):
- """Collect the annotation information.
-
- The annotation format is as the following:
-
- ...
-
- SMT
- Unspecified
- 0
- 0
-
- 157
- 294
- 237
- 357
-
-
-
- Args:
- gt_file (str): The path to ground-truth
- img_info (dict): The dict of the img and annotation information
-
- Returns:
- img_info (dict): The dict of the img and annotation information
- """
- obj = ET.parse(gt_file)
- root = obj.getroot()
- anno_info = []
- for object in root.iter('object'):
- word = object.find('name').text
- iscrowd = 1 if len(word) == 0 else 0
- x1 = int(object.find('bndbox').find('xmin').text)
- y1 = int(object.find('bndbox').find('ymin').text)
- x2 = int(object.find('bndbox').find('xmax').text)
- y2 = int(object.find('bndbox').find('ymax').text)
-
- x = max(0, min(x1, x2))
- y = max(0, min(y1, y2))
- w, h = abs(x2 - x1), abs(y2 - y1)
- bbox = [x1, y1, w, h]
- segmentation = [x, y, x + w, y, x + w, y + h, x, y + h]
- anno = dict(
- iscrowd=iscrowd,
- category_id=1,
- bbox=bbox,
- area=w * h,
- segmentation=[segmentation])
- anno_info.append(anno)
-
- img_info.update(anno_info=anno_info)
-
- return img_info
-
-
-def split_train_val_list(full_list, val_ratio):
- """Split list by val_ratio.
-
- Args:
- full_list (list): List to be split
- val_ratio (float): Split ratio for val set
-
- return:
- list(list, list): Train_list and val_list
- """
-
- n_total = len(full_list)
- offset = int(n_total * val_ratio)
- if n_total == 0 or offset < 1:
- return [], full_list
- val_list = full_list[:offset]
- train_list = full_list[offset:]
- return [train_list, val_list]
-
-
-def parse_args():
- parser = argparse.ArgumentParser(
- description='Generate training and val set of ILST ')
- parser.add_argument('root_path', help='Root dir path of ILST')
- parser.add_argument(
- '--val-ratio', help='Split ratio for val set', default=0., type=float)
- parser.add_argument(
- '--nproc', default=1, type=int, help='Number of processes')
- args = parser.parse_args()
- return args
-
-
-def main():
- args = parse_args()
- root_path = args.root_path
- with mmengine.Timer(print_tmpl='It takes {}s to convert ILST annotation'):
- files = collect_files(
- osp.join(root_path, 'imgs'), osp.join(root_path, 'annotations'))
- image_infos = collect_annotations(files, nproc=args.nproc)
- if args.val_ratio:
- image_infos = split_train_val_list(image_infos, args.val_ratio)
- splits = ['training', 'val']
- else:
- image_infos = [image_infos]
- splits = ['training']
- for i, split in enumerate(splits):
- dump_ocr_data(
- list(filter(None, image_infos[i])),
- osp.join(root_path, 'instances_' + split + '.json'), 'textdet')
-
-
-if __name__ == '__main__':
- main()
diff --git a/pipeline/mmocr/mmocr/.mim/tools/dataset_converters/textdet/imgur_converter.py b/pipeline/mmocr/mmocr/.mim/tools/dataset_converters/textdet/imgur_converter.py
deleted file mode 100644
index f6c19cd33cdf27bc085563992a126aa02028c43e..0000000000000000000000000000000000000000
--- a/pipeline/mmocr/mmocr/.mim/tools/dataset_converters/textdet/imgur_converter.py
+++ /dev/null
@@ -1,152 +0,0 @@
-# Copyright (c) OpenMMLab. All rights reserved.
-import argparse
-import math
-import os.path as osp
-
-import mmcv
-import mmengine
-import numpy as np
-
-from mmocr.utils import dump_ocr_data
-
-
-def parse_args():
- parser = argparse.ArgumentParser(
- description='Generate training, validation and test set of IMGUR ')
- parser.add_argument('root_path', help='Root dir path of IMGUR')
- args = parser.parse_args()
-
- return args
-
-
-def collect_imgur_info(root_path, annotation_filename, print_every=1000):
-
- annotation_path = osp.join(root_path, 'annotations', annotation_filename)
- if not osp.exists(annotation_path):
- raise Exception(
- f'{annotation_path} not exists, please check and try again.')
-
- annotation = mmengine.load(annotation_path)
- images = annotation['index_to_ann_map'].keys()
- img_infos = []
- for i, img_name in enumerate(images):
- if i >= 0 and i % print_every == 0:
- print(f'{i}/{len(images)}')
-
- img_path = osp.join(root_path, 'imgs', img_name + '.jpg')
-
- # Skip not exist images
- if not osp.exists(img_path):
- continue
-
- img = mmcv.imread(img_path, 'unchanged')
-
- # Skip broken images
- if img is None:
- continue
-
- img_info = dict(
- file_name=img_name + '.jpg',
- height=img.shape[0],
- width=img.shape[1])
-
- anno_info = []
- for ann_id in annotation['index_to_ann_map'][img_name]:
- ann = annotation['ann_id'][ann_id]
-
- # The original annotation is oriented rects [x, y, w, h, a]
- box = np.fromstring(
- ann['bounding_box'][1:-2], sep=',', dtype=float)
- quadrilateral = convert_oriented_box(box)
-
- xs, ys = quadrilateral[::2], quadrilateral[1::2]
- x = max(0, math.floor(min(xs)))
- y = max(0, math.floor(min(ys)))
- w = math.floor(max(xs)) - x
- h = math.floor(max(ys)) - y
- bbox = [x, y, w, h]
- segmentation = quadrilateral
-
- anno = dict(
- iscrowd=0,
- category_id=1,
- bbox=bbox,
- area=w * h,
- segmentation=[segmentation])
- anno_info.append(anno)
- img_info.update(anno_info=anno_info)
- img_infos.append(img_info)
-
- return img_infos
-
-
-def convert_oriented_box(box):
-
- x_ctr, y_ctr, width, height, angle = box[:5]
- angle = -angle * math.pi / 180
-
- tl_x, tl_y, br_x, br_y = -width / 2, -height / 2, width / 2, height / 2
- rect = np.array([[tl_x, br_x, br_x, tl_x], [tl_y, tl_y, br_y, br_y]])
- R = np.array([[np.cos(angle), -np.sin(angle)],
- [np.sin(angle), np.cos(angle)]])
- poly = R.dot(rect)
- x0, x1, x2, x3 = poly[0, :4] + x_ctr
- y0, y1, y2, y3 = poly[1, :4] + y_ctr
- poly = np.array([x0, y0, x1, y1, x2, y2, x3, y3], dtype=np.float32)
- poly = get_best_begin_point_single(poly)
-
- return poly.tolist()
-
-
-def get_best_begin_point_single(coordinate):
-
- x1, y1, x2, y2, x3, y3, x4, y4 = coordinate
- xmin = min(x1, x2, x3, x4)
- ymin = min(y1, y2, y3, y4)
- xmax = max(x1, x2, x3, x4)
- ymax = max(y1, y2, y3, y4)
- combine = [[[x1, y1], [x2, y2], [x3, y3], [x4, y4]],
- [[x2, y2], [x3, y3], [x4, y4], [x1, y1]],
- [[x3, y3], [x4, y4], [x1, y1], [x2, y2]],
- [[x4, y4], [x1, y1], [x2, y2], [x3, y3]]]
- dst_coordinate = [[xmin, ymin], [xmax, ymin], [xmax, ymax], [xmin, ymax]]
- force = 100000000.0
- force_flag = 0
- for i in range(4):
- temp_force = cal_line_length(combine[i][0], dst_coordinate[0]) \
- + cal_line_length(combine[i][1], dst_coordinate[1]) \
- + cal_line_length(combine[i][2], dst_coordinate[2]) \
- + cal_line_length(combine[i][3], dst_coordinate[3])
- if temp_force < force:
- force = temp_force
- force_flag = i
- if force_flag != 0:
- pass
-
- return np.array(combine[force_flag]).reshape(8)
-
-
-def cal_line_length(point1, point2):
-
- return math.sqrt(
- math.pow(point1[0] - point2[0], 2) +
- math.pow(point1[1] - point2[1], 2))
-
-
-def main():
- args = parse_args()
- root_path = args.root_path
-
- for split in ['train', 'val', 'test']:
- print(f'Processing {split} set...')
- with mmengine.Timer(
- print_tmpl='It takes {}s to convert IMGUR annotation'):
- anno_infos = collect_imgur_info(
- root_path, f'imgur5k_annotations_{split}.json')
- dump_ocr_data(anno_infos,
- osp.join(root_path, f'instances_{split}.json'),
- 'textdet')
-
-
-if __name__ == '__main__':
- main()
diff --git a/pipeline/mmocr/mmocr/.mim/tools/dataset_converters/textdet/kaist_converter.py b/pipeline/mmocr/mmocr/.mim/tools/dataset_converters/textdet/kaist_converter.py
deleted file mode 100644
index 3f95804d1dda27a88db247e177c3f7522361faf5..0000000000000000000000000000000000000000
--- a/pipeline/mmocr/mmocr/.mim/tools/dataset_converters/textdet/kaist_converter.py
+++ /dev/null
@@ -1,201 +0,0 @@
-# Copyright (c) OpenMMLab. All rights reserved.
-import argparse
-import math
-import os
-import os.path as osp
-import xml.etree.ElementTree as ET
-
-import mmcv
-import mmengine
-
-from mmocr.utils import dump_ocr_data
-
-
-def collect_files(img_dir, gt_dir, ratio):
- """Collect all images and their corresponding groundtruth files.
-
- Args:
- img_dir (str): The image directory
- gt_dir (str): The groundtruth directory
- ratio (float): Split ratio for val set
-
- Returns:
- files (list): The list of tuples (img_file, groundtruth_file)
- """
- assert isinstance(img_dir, str)
- assert img_dir
- assert isinstance(gt_dir, str)
- assert gt_dir
- assert isinstance(ratio, float)
- assert ratio < 1.0, 'val_ratio should be a float between 0.0 to 1.0'
-
- ann_list, imgs_list = [], []
- for img_file in os.listdir(img_dir):
- ann_list.append(osp.join(gt_dir, img_file.split('.')[0] + '.xml'))
- imgs_list.append(osp.join(img_dir, img_file))
-
- all_files = list(zip(sorted(imgs_list), sorted(ann_list)))
- assert len(all_files), f'No images found in {img_dir}'
- print(f'Loaded {len(all_files)} images from {img_dir}')
-
- trn_files, val_files = [], []
- if ratio > 0:
- for i, file in enumerate(all_files):
- if i % math.floor(1 / ratio):
- trn_files.append(file)
- else:
- val_files.append(file)
- else:
- trn_files, val_files = all_files, []
-
- print(f'training #{len(trn_files)}, val #{len(val_files)}')
-
- return trn_files, val_files
-
-
-def collect_annotations(files, nproc=1):
- """Collect the annotation information.
-
- Args:
- files (list): The list of tuples (image_file, groundtruth_file)
- nproc (int): The number of process to collect annotations
-
- Returns:
- images (list): The list of image information dicts
- """
- assert isinstance(files, list)
- assert isinstance(nproc, int)
-
- if nproc > 1:
- images = mmengine.track_parallel_progress(
- load_img_info, files, nproc=nproc)
- else:
- images = mmengine.track_progress(load_img_info, files)
-
- return images
-
-
-def load_img_info(files):
- """Load the information of one image.
-
- Args:
- files (tuple): The tuple of (img_file, groundtruth_file)
-
- Returns:
- img_info (dict): The dict of the img and annotation information
- """
- assert isinstance(files, tuple)
-
- img_file, gt_file = files
- assert osp.basename(gt_file).split('.')[0] == osp.basename(img_file).split(
- '.')[0]
- # read imgs while ignoring orientations
- img = mmcv.imread(img_file, 'unchanged')
-
- img_info = dict(
- file_name=osp.join(osp.basename(img_file)),
- height=img.shape[0],
- width=img.shape[1],
- segm_file=osp.join(osp.basename(gt_file)))
-
- if osp.splitext(gt_file)[1] == '.xml':
- img_info = load_xml_info(gt_file, img_info)
- else:
- raise NotImplementedError
-
- return img_info
-
-
-def load_xml_info(gt_file, img_info):
- """Collect the annotation information.
-
- Annotation Format
-
- DSC02306.JPG
-
-
-
-
-
-
-
-
-
-
-
-
- no
- 2
-
-
-
-
- Args:
- gt_file (str): The path to ground-truth
- img_info (dict): The dict of the img and annotation information
-
- Returns:
- img_info (dict): The dict of the img and annotation information
- """
-
- obj = ET.parse(gt_file)
- root = obj.getroot()
- anno_info = []
- for word in root.iter('word'):
- x, y = max(0, int(word.attrib['x'])), max(0, int(word.attrib['y']))
- w, h = int(word.attrib['width']), int(word.attrib['height'])
- bbox = [x, y, w, h]
- segmentation = [x, y, x + w, y, x + w, y + h, x, y + h]
-
- anno = dict(
- iscrowd=0,
- category_id=1,
- bbox=bbox,
- area=w * h,
- segmentation=[segmentation])
- anno_info.append(anno)
-
- img_info.update(anno_info=anno_info)
-
- return img_info
-
-
-def parse_args():
- parser = argparse.ArgumentParser(
- description='Generate training and val set of KAIST ')
- parser.add_argument('root_path', help='Root dir path of KAIST')
- parser.add_argument(
- '--val-ratio', help='Split ratio for val set', default=0.0, type=float)
- parser.add_argument(
- '--nproc', default=1, type=int, help='Number of process')
- args = parser.parse_args()
- return args
-
-
-def main():
- args = parse_args()
- root_path = args.root_path
- ratio = args.val_ratio
-
- trn_files, val_files = collect_files(
- osp.join(root_path, 'imgs'), osp.join(root_path, 'annotations'), ratio)
-
- # Train set
- trn_infos = collect_annotations(trn_files, nproc=args.nproc)
- with mmengine.Timer(
- print_tmpl='It takes {}s to convert KAIST Training annotation'):
- dump_ocr_data(trn_infos, osp.join(root_path,
- 'instances_training.json'),
- 'textdet')
-
- # Val set
- if len(val_files) > 0:
- val_infos = collect_annotations(val_files, nproc=args.nproc)
- with mmengine.Timer(
- print_tmpl='It takes {}s to convert KAIST Val annotation'):
- dump_ocr_data(val_infos, osp.join(root_path, 'instances_val.json'),
- 'textdet')
-
-
-if __name__ == '__main__':
- main()
diff --git a/pipeline/mmocr/mmocr/.mim/tools/dataset_converters/textdet/lsvt_converter.py b/pipeline/mmocr/mmocr/.mim/tools/dataset_converters/textdet/lsvt_converter.py
deleted file mode 100644
index aa44d10663e762ddbcccb354b65cfd349634a6ce..0000000000000000000000000000000000000000
--- a/pipeline/mmocr/mmocr/.mim/tools/dataset_converters/textdet/lsvt_converter.py
+++ /dev/null
@@ -1,130 +0,0 @@
-# Copyright (c) OpenMMLab. All rights reserved.
-import argparse
-import math
-import os.path as osp
-
-import mmcv
-import mmengine
-
-from mmocr.utils import dump_ocr_data
-
-
-def parse_args():
- parser = argparse.ArgumentParser(
- description='Generate training and validation set of LSVT ')
- parser.add_argument('root_path', help='Root dir path of LSVT')
- parser.add_argument(
- '--val-ratio', help='Split ratio for val set', default=0.0, type=float)
- args = parser.parse_args()
- return args
-
-
-def collect_lsvt_info(root_path, split, ratio, print_every=1000):
- """Collect the annotation information.
-
- The annotation format is as the following:
- [
- {'gt_1234': # 'gt_1234' is file name
- [
- {
- 'transcription': 'ไธ็ซๅผ่ดญ็ฉไธญๅฟ',
- 'points': [[45, 272], [215, 273], [212, 296], [45, 290]]
- 'illegibility': False
- }, ...
- ]
- }
- ]
-
-
- Args:
- root_path (str): Root path to the dataset
- split (str): Dataset split, which should be 'train' or 'val'
- ratio (float): Split ratio for val set
- print_every (int): Print log info per iteration
-
- Returns:
- img_info (dict): The dict of the img and annotation information
- """
-
- annotation_path = osp.join(root_path, 'annotations/train_full_labels.json')
- if not osp.exists(annotation_path):
- raise Exception(
- f'{annotation_path} not exists, please check and try again.')
-
- annotation = mmengine.load(annotation_path)
- img_prefixes = annotation.keys()
-
- trn_files, val_files = [], []
- if ratio > 0:
- for i, file in enumerate(img_prefixes):
- if i % math.floor(1 / ratio):
- trn_files.append(file)
- else:
- val_files.append(file)
- else:
- trn_files, val_files = img_prefixes, []
- print(f'training #{len(trn_files)}, val #{len(val_files)}')
-
- if split == 'train':
- img_prefixes = trn_files
- elif split == 'val':
- img_prefixes = val_files
- else:
- raise NotImplementedError
-
- img_infos = []
- for i, prefix in enumerate(img_prefixes):
- if i > 0 and i % print_every == 0:
- print(f'{i}/{len(img_prefixes)}')
- img_file = osp.join(root_path, 'imgs', prefix + '.jpg')
- # Skip not exist images
- if not osp.exists(img_file):
- continue
- img = mmcv.imread(img_file)
-
- img_info = dict(
- file_name=osp.join(osp.basename(img_file)),
- height=img.shape[0],
- width=img.shape[1],
- segm_file=osp.join(osp.basename(annotation_path)))
-
- anno_info = []
- for ann in annotation[prefix]:
- segmentation = []
- for x, y in ann['points']:
- segmentation.append(max(0, x))
- segmentation.append(max(0, y))
- xs, ys = segmentation[::2], segmentation[1::2]
- x, y = min(xs), min(ys)
- w, h = max(xs) - x, max(ys) - y
- bbox = [x, y, w, h]
- anno = dict(
- iscrowd=1 if ann['illegibility'] else 0,
- category_id=1,
- bbox=bbox,
- area=w * h,
- segmentation=[segmentation])
- anno_info.append(anno)
- img_info.update(anno_info=anno_info)
- img_infos.append(img_info)
-
- return img_infos
-
-
-def main():
- args = parse_args()
- root_path = args.root_path
- print('Processing training set...')
- training_infos = collect_lsvt_info(root_path, 'train', args.val_ratio)
- dump_ocr_data(training_infos,
- osp.join(root_path, 'instances_training.json'), 'textdet')
- if args.val_ratio > 0:
- print('Processing validation set...')
- val_infos = collect_lsvt_info(root_path, 'val', args.val_ratio)
- dump_ocr_data(val_infos, osp.join(root_path, 'instances_val.json'),
- 'textdet')
- print('Finish')
-
-
-if __name__ == '__main__':
- main()
diff --git a/pipeline/mmocr/mmocr/.mim/tools/dataset_converters/textdet/lv_converter.py b/pipeline/mmocr/mmocr/.mim/tools/dataset_converters/textdet/lv_converter.py
deleted file mode 100644
index 6efcc14317da87adc655f4096f5a4db3cbfb7558..0000000000000000000000000000000000000000
--- a/pipeline/mmocr/mmocr/.mim/tools/dataset_converters/textdet/lv_converter.py
+++ /dev/null
@@ -1,182 +0,0 @@
-# Copyright (c) OpenMMLab. All rights reserved.
-import argparse
-import os
-import os.path as osp
-import xml.etree.ElementTree as ET
-
-import mmcv
-import mmengine
-
-from mmocr.utils import dump_ocr_data
-
-
-def collect_files(data_dir):
- """Collect all images and their corresponding groundtruth files.
-
- Args:
- data_dir (str): The directory to dataset
-
- Returns:
- files (list): The list of tuples (img_file, groundtruth_file)
- """
- assert isinstance(data_dir, str)
- assert data_dir
-
- ann_list, imgs_list = [], []
- for video_dir in os.listdir(data_dir):
- for frame_dir in os.listdir(osp.join(data_dir, video_dir)):
- crt_dir = osp.join(data_dir, video_dir, frame_dir)
- if not osp.isdir(crt_dir):
- continue
- for crt_file in os.listdir(crt_dir):
- if crt_file.endswith('xml'):
- ann_path = osp.join(crt_dir, crt_file)
- img_path = osp.join(crt_dir,
- crt_file.replace('xml', 'png'))
- if os.path.exists(img_path):
- ann_list.append(ann_path)
- imgs_list.append(img_path)
- else:
- continue
-
- files = list(zip(imgs_list, ann_list))
- assert len(files), f'No images found in {data_dir}'
- print(f'Loaded {len(files)} images from {data_dir}')
-
- return files
-
-
-def collect_annotations(files, nproc=1):
- """Collect the annotation information.
-
- Args:
- files (list): The list of tuples (image_file, groundtruth_file)
- nproc (int): The number of process to collect annotations
-
- Returns:
- images (list): The list of image information dicts
- """
- assert isinstance(files, list)
- assert isinstance(nproc, int)
-
- if nproc > 1:
- images = mmengine.track_parallel_progress(
- load_img_info, files, nproc=nproc)
- else:
- images = mmengine.track_progress(load_img_info, files)
-
- return images
-
-
-def load_img_info(files):
- """Load the information of one image.
-
- Args:
- files (tuple): The tuple of (img_file, groundtruth_file)
-
- Returns:
- img_info (dict): The dict of the img and annotation information
- """
- assert isinstance(files, tuple)
-
- img_file, gt_file = files
- assert osp.basename(gt_file).split('.')[0] == osp.basename(img_file).split(
- '.')[0]
- # read imgs while ignoring orientations
- img = mmcv.imread(img_file, 'unchanged')
- img_file = os.path.split(img_file)[-1]
-
- img_info = dict(
- file_name=img_file,
- height=img.shape[0],
- width=img.shape[1],
- segm_file=osp.join(osp.basename(gt_file)))
-
- if osp.splitext(gt_file)[1] == '.xml':
- img_info = load_xml_info(gt_file, img_info)
- else:
- raise NotImplementedError
-
- return img_info
-
-
-def load_xml_info(gt_file, img_info):
- """Collect the annotation information.
-
- The annotation format is as the following:
-
-
- hierarchy
- Unspecified
- 0
- 0
-
- 657
- 467
- 839
- 557
-
-
-
-
- Args:
- gt_file (str): The path to ground-truth
- img_info (dict): The dict of the img and annotation information
-
- Returns:
- img_info (dict): The dict of the img and annotation information
- """
-
- obj = ET.parse(gt_file)
- root = obj.getroot()
- anno_info = []
- for obj in root.iter('object'):
- x = max(0, int(obj.find('bndbox').find('xmin').text))
- y = max(0, int(obj.find('bndbox').find('ymin').text))
- xmax = int(obj.find('bndbox').find('xmax').text)
- ymax = int(obj.find('bndbox').find('ymax').text)
-
- w, h = abs(xmax - x), abs(ymax - y)
- bbox = [x, y, w, h]
- segmentation = [x, y, x + w, y, x + w, y + h, x, y + h]
-
- anno = dict(
- iscrowd=0,
- category_id=1,
- bbox=bbox,
- area=w * h,
- segmentation=[segmentation])
- anno_info.append(anno)
-
- img_info.update(anno_info=anno_info)
-
- return img_info
-
-
-def parse_args():
- parser = argparse.ArgumentParser(
- description='Generate training, val and test set of Lecture Video DB ')
- parser.add_argument('root_path', help='Root dir path of Lecture Video DB')
- parser.add_argument(
- '--nproc', default=1, type=int, help='number of process')
- args = parser.parse_args()
- return args
-
-
-def main():
- args = parse_args()
- root_path = args.root_path
-
- for split in ['train', 'val', 'test']:
- print(f'Processing {split} set...')
- with mmengine.Timer(
- print_tmpl='It takes {}s to convert LV annotation'):
- files = collect_files(osp.join(root_path, 'imgs', split))
- image_infos = collect_annotations(files, nproc=args.nproc)
- dump_ocr_data(image_infos,
- osp.join(root_path, 'instances_' + split + '.json'),
- 'textdet')
-
-
-if __name__ == '__main__':
- main()
diff --git a/pipeline/mmocr/mmocr/.mim/tools/dataset_converters/textdet/mtwi_converter.py b/pipeline/mmocr/mmocr/.mim/tools/dataset_converters/textdet/mtwi_converter.py
deleted file mode 100644
index 1c9fde3bf870ffcd1ea482e3d73bfc138f51a381..0000000000000000000000000000000000000000
--- a/pipeline/mmocr/mmocr/.mim/tools/dataset_converters/textdet/mtwi_converter.py
+++ /dev/null
@@ -1,203 +0,0 @@
-# Copyright (c) OpenMMLab. All rights reserved.
-import argparse
-import math
-import os
-import os.path as osp
-
-import cv2
-import mmcv
-import mmengine
-from PIL import Image
-
-from mmocr.utils import dump_ocr_data
-
-
-def collect_files(img_dir, gt_dir, ratio):
- """Collect all images and their corresponding groundtruth files.
- Args:
- img_dir (str): The image directory
- gt_dir (str): The groundtruth directory
- ratio (float): Split ratio for val set
-
- Returns:
- files (list): The list of tuples (img_file, groundtruth_file)
- """
- assert isinstance(img_dir, str)
- assert img_dir
- assert isinstance(gt_dir, str)
- assert gt_dir
- assert isinstance(ratio, float)
- assert ratio < 1.0, 'val_ratio should be a float between 0.0 to 1.0'
-
- ann_list, imgs_list = [], []
- for ann_file in os.listdir(gt_dir):
- img_file = osp.join(img_dir, ann_file.replace('txt', 'jpg'))
- # This dataset contains some images obtained from .gif,
- # which cannot be loaded by mmcv.imread(), convert them
- # to RGB mode.
- try:
- if mmcv.imread(img_file) is None:
- print(f'Convert {img_file} to RGB mode.')
- img = Image.open(img_file)
- img = img.convert('RGB')
- img.save(img_file)
- except cv2.error:
- print(f'Skip broken img {img_file}')
- continue
-
- ann_list.append(osp.join(gt_dir, ann_file))
- imgs_list.append(img_file)
-
- all_files = list(zip(imgs_list, ann_list))
- assert len(all_files), f'No images found in {img_dir}'
- print(f'Loaded {len(all_files)} images from {img_dir}')
-
- trn_files, val_files = [], []
- if ratio > 0:
- for i, file in enumerate(all_files):
- if i % math.floor(1 / ratio):
- trn_files.append(file)
- else:
- val_files.append(file)
- else:
- trn_files, val_files = all_files, []
-
- print(f'training #{len(trn_files)}, val #{len(val_files)}')
-
- return trn_files, val_files
-
-
-def collect_annotations(files, nproc=1):
- """Collect the annotation information.
- Args:
- files (list): The list of tuples (image_file, groundtruth_file)
- nproc (int): The number of process to collect annotations
-
- Returns:
- images (list): The list of image information dicts
- """
- assert isinstance(files, list)
- assert isinstance(nproc, int)
-
- if nproc > 1:
- images = mmengine.track_parallel_progress(
- load_img_info, files, nproc=nproc)
- else:
- images = mmengine.track_progress(load_img_info, files)
-
- return images
-
-
-def load_img_info(files):
- """Load the information of one image.
- Args:
- files (tuple): The tuple of (img_file, groundtruth_file)
-
- Returns:
- img_info (dict): The dict of the img and annotation information
- """
- assert isinstance(files, tuple)
-
- img_file, gt_file = files
- assert osp.basename(gt_file).split('.')[0] == osp.basename(img_file).split(
- '.')[0]
- # read imgs while ignoring orientations
- img = mmcv.imread(img_file)
-
- img_info = dict(
- file_name=osp.join(osp.basename(img_file)),
- height=img.shape[0],
- width=img.shape[1],
- segm_file=osp.join(osp.basename(gt_file)))
-
- if osp.splitext(gt_file)[1] == '.txt':
- img_info = load_txt_info(gt_file, img_info)
- else:
- raise NotImplementedError
-
- return img_info
-
-
-def load_txt_info(gt_file, img_info):
- """Collect the annotation information.
-
- The annotation format is as the following:
- x1,y1,x2,y2,x3,y3,x4,y4,text
-
- 45.45,226.83,11.87,181.79,183.84,13.1,233.79,49.95,ๆถๅฐ่ข่ข
- 345.98,311.18,345.98,347.21,462.26,347.21,462.26,311.18,73774
- 462.26,292.34,461.44,299.71,502.39,299.71,502.39,292.34,73/74/737
-
- Args:
- gt_file (str): The path to ground-truth
- img_info (dict): The dict of the img and annotation information
-
- Returns:
- img_info (dict): The dict of the img and annotation information
- """
-
- anno_info = []
- with open(gt_file) as f:
- lines = f.readlines()
- for line in lines:
- points = line.split(',')[0:8]
- word = line.split(',')[8].rstrip('\n')
- segmentation = [math.floor(float(pt)) for pt in points]
- x = max(0, min(segmentation[0::2]))
- y = max(0, min(segmentation[1::2]))
- w = abs(max(segmentation[0::2]) - x)
- h = abs(max(segmentation[1::2]) - y)
- bbox = [x, y, w, h]
-
- anno = dict(
- iscrowd=1 if word == '###' else 0,
- category_id=1,
- bbox=bbox,
- area=w * h,
- segmentation=[segmentation])
- anno_info.append(anno)
-
- img_info.update(anno_info=anno_info)
-
- return img_info
-
-
-def parse_args():
- parser = argparse.ArgumentParser(
- description='Generate training and val set of MTWI.')
- parser.add_argument('root_path', help='Root dir path of MTWI')
- parser.add_argument(
- '--val-ratio', help='Split ratio for val set', default=0.0, type=float)
- parser.add_argument(
- '--nproc', default=1, type=int, help='Number of process')
- args = parser.parse_args()
- return args
-
-
-def main():
- args = parse_args()
- root_path = args.root_path
- ratio = args.val_ratio
-
- trn_files, val_files = collect_files(
- osp.join(root_path, 'imgs'), osp.join(root_path, 'annotations'), ratio)
-
- # Train set
- trn_infos = collect_annotations(trn_files, nproc=args.nproc)
- with mmengine.Timer(
- print_tmpl='It takes {}s to convert MTWI Training annotation'):
- dump_ocr_data(trn_infos, osp.join(root_path,
- 'instances_training.json'),
- 'textdet')
-
- # Val set
- if len(val_files) > 0:
- val_infos = collect_annotations(val_files, nproc=args.nproc)
- with mmengine.Timer(
- print_tmpl='It takes {}s to convert MTWI Val annotation'):
- dump_ocr_data(val_infos, osp.join(root_path, 'instances_val.json'),
- 'textdet')
-
-
-if __name__ == '__main__':
- main()
diff --git a/pipeline/mmocr/mmocr/.mim/tools/dataset_converters/textdet/naf_converter.py b/pipeline/mmocr/mmocr/.mim/tools/dataset_converters/textdet/naf_converter.py
deleted file mode 100644
index 2e43c8fba909723edd55f7b13b2a9cfa0b6c2e15..0000000000000000000000000000000000000000
--- a/pipeline/mmocr/mmocr/.mim/tools/dataset_converters/textdet/naf_converter.py
+++ /dev/null
@@ -1,197 +0,0 @@
-# Copyright (c) OpenMMLab. All rights reserved.
-import argparse
-import os.path as osp
-
-import mmcv
-import mmengine
-
-from mmocr.utils import dump_ocr_data
-
-
-def collect_files(img_dir, gt_dir, split_info):
- """Collect all images and their corresponding groundtruth files.
-
- Args:
- img_dir (str): The image directory
- gt_dir (str): The groundtruth directory
- split_info (dict): The split information for train/val/test
-
- Returns:
- files (list): The list of tuples (img_file, groundtruth_file)
- """
- assert isinstance(img_dir, str)
- assert img_dir
- assert isinstance(gt_dir, str)
- assert gt_dir
- assert isinstance(split_info, dict)
- assert split_info
-
- ann_list, imgs_list = [], []
- for group in split_info:
- for img in split_info[group]:
- image_path = osp.join(img_dir, img)
- anno_path = osp.join(gt_dir, 'groups', group,
- img.replace('jpg', 'json'))
-
- # Filtering out the missing images
- if not osp.exists(image_path) or not osp.exists(anno_path):
- continue
-
- imgs_list.append(image_path)
- ann_list.append(anno_path)
-
- files = list(zip(imgs_list, ann_list))
- assert len(files), f'No images found in {img_dir}'
- print(f'Loaded {len(files)} images from {img_dir}')
-
- return files
-
-
-def collect_annotations(files, nproc=1):
- """Collect the annotation information.
-
- Args:
- files (list): The list of tuples (image_file, groundtruth_file)
- nproc (int): The number of process to collect annotations
-
- Returns:
- images (list): The list of image information dicts
- """
- assert isinstance(files, list)
- assert isinstance(nproc, int)
-
- if nproc > 1:
- images = mmengine.track_parallel_progress(
- load_img_info, files, nproc=nproc)
- else:
- images = mmengine.track_progress(load_img_info, files)
-
- return images
-
-
-def load_img_info(files):
- """Load the information of one image.
-
- Args:
- files (tuple): The tuple of (img_file, groundtruth_file)
-
- Returns:
- img_info (dict): The dict of the img and annotation information
- """
- assert isinstance(files, tuple)
-
- img_file, gt_file = files
- assert osp.basename(gt_file).split('.')[0] == osp.basename(img_file).split(
- '.')[0]
- # Read imgs while ignoring orientations
- img = mmcv.imread(img_file, 'unchanged')
-
- img_info = dict(
- file_name=osp.join(osp.basename(img_file)),
- height=img.shape[0],
- width=img.shape[1],
- segm_file=osp.join(osp.basename(gt_file)))
-
- if osp.splitext(gt_file)[1] == '.json':
- img_info = load_json_info(gt_file, img_info)
- else:
- raise NotImplementedError
-
- return img_info
-
-
-def load_json_info(gt_file, img_info):
- """Collect the annotation information.
-
- Annotation Format
- {
- 'textBBs': [{
- 'poly_points': [[435,1406], [466,1406], [466,1439], [435,1439]],
- "type": "text",
- "id": "t1",
- }], ...
- }
-
- Some special characters are used in the transcription:
- "ยซtextยป" indicates that "text" had a strikethrough
- "ยฟ" indicates the transcriber could not read a character
- "ยง" indicates the whole line or word was illegible
- "" (empty string) is if the field was blank
-
- Args:
- gt_file (str): The path to ground-truth
- img_info (dict): The dict of the img and annotation information
-
- Returns:
- img_info (dict): The dict of the img and annotation information
- """
- assert isinstance(gt_file, str)
- assert isinstance(img_info, dict)
-
- annotation = mmengine.load(gt_file)
- anno_info = []
-
- # 'textBBs' contains the printed texts of the table while 'fieldBBs'
- # contains the text filled by human.
- for box_type in ['textBBs', 'fieldBBs']:
- for anno in annotation[box_type]:
- # Skip blanks
- if box_type == 'fieldBBs':
- if anno['type'] == 'blank':
- continue
-
- xs, ys, segmentation = [], [], []
- for p in anno['poly_points']:
- xs.append(p[0])
- ys.append(p[1])
- segmentation.append(p[0])
- segmentation.append(p[1])
- x, y = max(0, min(xs)), max(0, min(ys))
- w, h = max(xs) - x, max(ys) - y
- bbox = [x, y, w, h]
-
- anno = dict(
- iscrowd=0,
- category_id=1,
- bbox=bbox,
- area=w * h,
- segmentation=[segmentation])
- anno_info.append(anno)
-
- img_info.update(anno_info=anno_info)
-
- return img_info
-
-
-def parse_args():
- parser = argparse.ArgumentParser(
- description='Generate training, val, and test set of NAF ')
- parser.add_argument('root_path', help='Root dir path of NAF')
- parser.add_argument(
- '--nproc', default=1, type=int, help='Number of process')
- args = parser.parse_args()
- return args
-
-
-def main():
- args = parse_args()
- root_path = args.root_path
- split_info = mmengine.load(
- osp.join(root_path, 'annotations', 'train_valid_test_split.json'))
- split_info['training'] = split_info.pop('train')
- split_info['val'] = split_info.pop('valid')
- for split in ['training', 'val', 'test']:
- print(f'Processing {split} set...')
- with mmengine.Timer(
- print_tmpl='It takes {}s to convert NAF annotation'):
- files = collect_files(
- osp.join(root_path, 'imgs'),
- osp.join(root_path, 'annotations'), split_info[split])
- image_infos = collect_annotations(files, nproc=args.nproc)
- dump_ocr_data(image_infos,
- osp.join(root_path, 'instances_' + split + '.json'),
- 'textdet')
-
-
-if __name__ == '__main__':
- main()
diff --git a/pipeline/mmocr/mmocr/.mim/tools/dataset_converters/textdet/rctw_converter.py b/pipeline/mmocr/mmocr/.mim/tools/dataset_converters/textdet/rctw_converter.py
deleted file mode 100644
index cc46dd85999c616a89167a56de27ccf2f306ec4a..0000000000000000000000000000000000000000
--- a/pipeline/mmocr/mmocr/.mim/tools/dataset_converters/textdet/rctw_converter.py
+++ /dev/null
@@ -1,193 +0,0 @@
-# Copyright (c) OpenMMLab. All rights reserved.
-import argparse
-import math
-import os
-import os.path as osp
-
-import mmcv
-import mmengine
-
-from mmocr.utils import dump_ocr_data
-
-
-def collect_files(img_dir, gt_dir, ratio):
- """Collect all images and their corresponding groundtruth files.
- Args:
- img_dir (str): The image directory
- gt_dir (str): The groundtruth directory
- ratio (float): Split ratio for val set
-
- Returns:
- files (list): The list of tuples (img_file, groundtruth_file)
- """
- assert isinstance(img_dir, str)
- assert img_dir
- assert isinstance(gt_dir, str)
- assert gt_dir
- assert isinstance(ratio, float)
- assert ratio < 1.0, 'val_ratio should be a float between 0.0 to 1.0'
-
- ann_list, imgs_list = [], []
- for ann_file in os.listdir(gt_dir):
- ann_list.append(osp.join(gt_dir, ann_file))
- imgs_list.append(osp.join(img_dir, ann_file.replace('txt', 'jpg')))
-
- all_files = list(zip(imgs_list, ann_list))
- assert len(all_files), f'No images found in {img_dir}'
- print(f'Loaded {len(all_files)} images from {img_dir}')
-
- trn_files, val_files = [], []
- if ratio > 0:
- for i, file in enumerate(all_files):
- if i % math.floor(1 / ratio):
- trn_files.append(file)
- else:
- val_files.append(file)
- else:
- trn_files, val_files = all_files, []
-
- print(f'training #{len(trn_files)}, val #{len(val_files)}')
-
- return trn_files, val_files
-
-
-def collect_annotations(files, nproc=1):
- """Collect the annotation information.
- Args:
- files (list): The list of tuples (image_file, groundtruth_file)
- nproc (int): The number of process to collect annotations
-
- Returns:
- images (list): The list of image information dicts
- """
- assert isinstance(files, list)
- assert isinstance(nproc, int)
-
- if nproc > 1:
- images = mmengine.track_parallel_progress(
- load_img_info, files, nproc=nproc)
- else:
- images = mmengine.track_progress(load_img_info, files)
-
- return images
-
-
-def load_img_info(files):
- """Load the information of one image.
- Args:
- files (tuple): The tuple of (img_file, groundtruth_file)
-
- Returns:
- img_info (dict): The dict of the img and annotation information
- """
- assert isinstance(files, tuple)
-
- img_file, gt_file = files
- assert osp.basename(gt_file).split('.')[0] == osp.basename(img_file).split(
- '.')[0]
- # read imgs while ignoring orientations
- img = mmcv.imread(img_file)
-
- img_info = dict(
- file_name=osp.join(osp.basename(img_file)),
- height=img.shape[0],
- width=img.shape[1],
- segm_file=osp.join(osp.basename(gt_file)))
-
- if osp.splitext(gt_file)[1] == '.txt':
- img_info = load_txt_info(gt_file, img_info)
- else:
- raise NotImplementedError
-
- return img_info
-
-
-def load_txt_info(gt_file, img_info):
- """Collect the annotation information.
-
- The annotation format is as the following:
- x1, y1, x2, y2, x3, y3, x4, y4, difficult, text
-
- 390,902,1856,902,1856,1225,390,1225,0,"้ๆฐ็ผ้"
- 1875,1170,2149,1170,2149,1245,1875,1245,0,"ๅไบ1989"
- 2054,1277,2190,1277,2190,1323,2054,1323,0,"ๅๅปบๅบ"
-
- Args:
- gt_file (str): The path to ground-truth
- img_info (dict): The dict of the img and annotation information
-
- Returns:
- img_info (dict): The dict of the img and annotation information
- """
-
- anno_info = []
- with open(gt_file, encoding='utf-8-sig') as f:
- lines = f.readlines()
- for line in lines:
- points = line.split(',')[0:8]
- word = line.split(',')[9].rstrip('\n').strip('"')
- difficult = 1 if line.split(',')[8] != '0' else 0
- segmentation = [int(pt) for pt in points]
- x = max(0, min(segmentation[0::2]))
- y = max(0, min(segmentation[1::2]))
- w = abs(max(segmentation[0::2]) - x)
- h = abs(max(segmentation[1::2]) - y)
- bbox = [x, y, w, h]
-
- if word == '###' or difficult == 1:
- iscrowd = 1
- else:
- iscrowd = 0
-
- anno = dict(
- iscrowd=iscrowd,
- category_id=1,
- bbox=bbox,
- area=w * h,
- segmentation=[segmentation])
- anno_info.append(anno)
-
- img_info.update(anno_info=anno_info)
-
- return img_info
-
-
-def parse_args():
- parser = argparse.ArgumentParser(
- description='Generate training and val set of RCTW.')
- parser.add_argument('root_path', help='Root dir path of RCTW')
- parser.add_argument(
- '--val-ratio', help='Split ratio for val set', default=0.0, type=float)
- parser.add_argument(
- '--nproc', default=1, type=int, help='Number of process')
- args = parser.parse_args()
- return args
-
-
-def main():
- args = parse_args()
- root_path = args.root_path
- ratio = args.val_ratio
-
- trn_files, val_files = collect_files(
- osp.join(root_path, 'imgs'), osp.join(root_path, 'annotations'), ratio)
-
- # Train set
- with mmengine.Timer(
- print_tmpl='It takes {}s to convert RCTW Training annotation'):
- trn_infos = collect_annotations(trn_files, nproc=args.nproc)
- dump_ocr_data(trn_infos, osp.join(root_path,
- 'instances_training.json'),
- 'textdet')
-
- # Val set
- if len(val_files) > 0:
- with mmengine.Timer(
- print_tmpl='It takes {}s to convert RCTW Val annotation'):
- val_infos = collect_annotations(val_files, nproc=args.nproc)
- dump_ocr_data(val_infos, osp.join(root_path, 'instances_val.json'),
- 'textdet')
-
-
-if __name__ == '__main__':
- main()
diff --git a/pipeline/mmocr/mmocr/.mim/tools/dataset_converters/textdet/rects_converter.py b/pipeline/mmocr/mmocr/.mim/tools/dataset_converters/textdet/rects_converter.py
deleted file mode 100644
index 75f3b734607af2c44b78273df3401457166432c2..0000000000000000000000000000000000000000
--- a/pipeline/mmocr/mmocr/.mim/tools/dataset_converters/textdet/rects_converter.py
+++ /dev/null
@@ -1,207 +0,0 @@
-# Copyright (c) OpenMMLab. All rights reserved.
-import argparse
-import math
-import os
-import os.path as osp
-
-import mmcv
-import mmengine
-
-from mmocr.utils import dump_ocr_data
-
-
-def collect_files(img_dir, gt_dir, ratio):
- """Collect all images and their corresponding groundtruth files.
- Args:
- img_dir (str): The image directory
- gt_dir (str): The groundtruth directory
- ratio (float): Split ratio for val set
-
- Returns:
- files (list): The list of tuples (img_file, groundtruth_file)
- """
- assert isinstance(img_dir, str)
- assert img_dir
- assert isinstance(gt_dir, str)
- assert gt_dir
- assert isinstance(ratio, float)
- assert ratio < 1.0, 'val_ratio should be a float between 0.0 to 1.0'
-
- ann_list, imgs_list = [], []
- for ann_file in os.listdir(gt_dir):
- ann_list.append(osp.join(gt_dir, ann_file))
- imgs_list.append(osp.join(img_dir, ann_file.replace('json', 'jpg')))
-
- all_files = list(zip(imgs_list, ann_list))
- assert len(all_files), f'No images found in {img_dir}'
- print(f'Loaded {len(all_files)} images from {img_dir}')
-
- trn_files, val_files = [], []
- if ratio > 0:
- for i, file in enumerate(all_files):
- if i % math.floor(1 / ratio):
- trn_files.append(file)
- else:
- val_files.append(file)
- else:
- trn_files, val_files = all_files, []
-
- print(f'training #{len(trn_files)}, val #{len(val_files)}')
-
- return trn_files, val_files
-
-
-def collect_annotations(files, nproc=1):
- """Collect the annotation information.
- Args:
- files (list): The list of tuples (image_file, groundtruth_file)
- nproc (int): The number of process to collect annotations
-
- Returns:
- images (list): The list of image information dicts
- """
- assert isinstance(files, list)
- assert isinstance(nproc, int)
-
- if nproc > 1:
- images = mmengine.track_parallel_progress(
- load_img_info, files, nproc=nproc)
- else:
- images = mmengine.track_progress(load_img_info, files)
-
- return images
-
-
-def load_img_info(files):
- """Load the information of one image.
- Args:
- files (tuple): The tuple of (img_file, groundtruth_file)
-
- Returns:
- img_info (dict): The dict of the img and annotation information
- """
- assert isinstance(files, tuple)
-
- img_file, gt_file = files
- assert osp.basename(gt_file).split('.')[0] == osp.basename(img_file).split(
- '.')[0]
- # read imgs while ignoring orientations
- img = mmcv.imread(img_file)
-
- img_info = dict(
- file_name=osp.join(osp.basename(img_file)),
- height=img.shape[0],
- width=img.shape[1],
- segm_file=osp.join(osp.basename(gt_file)))
-
- if osp.splitext(gt_file)[1] == '.json':
- img_info = load_json_info(gt_file, img_info)
- else:
- raise NotImplementedError
-
- return img_info
-
-
-def load_json_info(gt_file, img_info):
- """Collect the annotation information.
-
- The annotation format is as the following:
-
- {
- "chars": [
- {
- "ignore": 0,
- "transcription": "H",
- "points": [25, 175, 112, 175, 112, 286, 25, 286]
- },
- {
- "ignore": 0,
- "transcription": "O",
- "points": [102, 182, 210, 182, 210, 273, 102, 273]
- }, ...
- ]
- "lines": [
- {
- "ignore": 0,
- "transcription": "HOKI",
- "points": [23, 173, 327, 180, 327, 290, 23, 283]
- },
- {
- "ignore": 0,
- "transcription": "TEA",
- "points": [368, 180, 621, 180, 621, 294, 368, 294]
- }, ...
- ]
- }
-
-
- Args:
- gt_file (str): The path to ground-truth
- img_info (dict): The dict of the img and annotation information
-
- Returns:
- img_info (dict): The dict of the img and annotation information
- """
-
- annotation = mmengine.load(gt_file)
- anno_info = []
- for line in annotation['lines']:
- segmentation = line['points']
- x = max(0, min(segmentation[0::2]))
- y = max(0, min(segmentation[1::2]))
- w = abs(max(segmentation[0::2]) - x)
- h = abs(max(segmentation[1::2]) - y)
- bbox = [x, y, w, h]
-
- anno = dict(
- iscrowd=line['ignore'],
- category_id=1,
- bbox=bbox,
- area=w * h,
- segmentation=[segmentation])
- anno_info.append(anno)
-
- img_info.update(anno_info=anno_info)
-
- return img_info
-
-
-def parse_args():
- parser = argparse.ArgumentParser(
- description='Generate training and val set of ReCTS.')
- parser.add_argument('root_path', help='Root dir path of ReCTS')
- parser.add_argument(
- '--val-ratio', help='Split ratio for val set', default=0.0, type=float)
- parser.add_argument(
- '--nproc', default=1, type=int, help='Number of process')
- args = parser.parse_args()
- return args
-
-
-def main():
- args = parse_args()
- root_path = args.root_path
- ratio = args.val_ratio
-
- trn_files, val_files = collect_files(
- osp.join(root_path, 'imgs'), osp.join(root_path, 'annotations'), ratio)
-
- # Train set
- trn_infos = collect_annotations(trn_files, nproc=args.nproc)
- with mmengine.Timer(
- print_tmpl='It takes {}s to convert ReCTS Training annotation'):
- dump_ocr_data(trn_infos, osp.join(root_path,
- 'instances_training.json'),
- 'textdet')
-
- # Val set
- if len(val_files) > 0:
- val_infos = collect_annotations(val_files, nproc=args.nproc)
- with mmengine.Timer(
- print_tmpl='It takes {}s to convert ReCTS Val annotation'):
- dump_ocr_data(val_infos, osp.join(root_path, 'instances_val.json'),
- 'textdet')
-
-
-if __name__ == '__main__':
- main()
diff --git a/pipeline/mmocr/mmocr/.mim/tools/dataset_converters/textdet/sroie_converter.py b/pipeline/mmocr/mmocr/.mim/tools/dataset_converters/textdet/sroie_converter.py
deleted file mode 100644
index 7ee0725e2fde0533248ed584c290377416bd5b46..0000000000000000000000000000000000000000
--- a/pipeline/mmocr/mmocr/.mim/tools/dataset_converters/textdet/sroie_converter.py
+++ /dev/null
@@ -1,168 +0,0 @@
-# Copyright (c) OpenMMLab. All rights reserved.
-import argparse
-import os
-import os.path as osp
-
-import mmcv
-import mmengine
-import numpy as np
-
-from mmocr.utils import dump_ocr_data
-
-
-def collect_files(img_dir, gt_dir):
- """Collect all images and their corresponding groundtruth files.
-
- Args:
- img_dir (str): The image directory
- gt_dir (str): The groundtruth directory
-
- Returns:
- files (list): The list of tuples (img_file, groundtruth_file)
- """
-
- assert isinstance(img_dir, str)
- assert img_dir
- assert isinstance(gt_dir, str)
- assert gt_dir
-
- ann_list, imgs_list = [], []
- for gt_file in os.listdir(gt_dir):
- # Filtering repeated and missing images
- if '(' in gt_file or gt_file == 'X51006619570.txt':
- continue
- ann_list.append(osp.join(gt_dir, gt_file))
- imgs_list.append(osp.join(img_dir, gt_file.replace('.txt', '.jpg')))
-
- files = list(zip(sorted(imgs_list), sorted(ann_list)))
- assert len(files), f'No images found in {img_dir}'
-
- print(f'Loaded {len(files)} images from {img_dir}')
-
- return files
-
-
-def collect_annotations(files, nproc=1):
- """Collect the annotation information.
-
- Args:
- files (list): The list of tuples (image_file, groundtruth_file)
- nproc (int): The number of process to collect annotations
-
- Returns:
- images (list): The list of image information dicts
- """
-
- assert isinstance(files, list)
- assert isinstance(nproc, int)
-
- if nproc > 1:
- images = mmengine.track_parallel_progress(
- load_img_info, files, nproc=nproc)
- else:
- images = mmengine.track_progress(load_img_info, files)
-
- return images
-
-
-def load_img_info(files):
- """Load the information of one image.
-
- Args:
- files (tuple): The tuple of (img_file, groundtruth_file)
-
- Returns:
- img_info (dict): The dict of the img and annotation information
- """
-
- assert isinstance(files, tuple)
-
- img_file, gt_file = files
- assert osp.basename(gt_file).split('.')[0] == osp.basename(img_file).split(
- '.')[0]
- # read imgs while ignoring orientations
- img = mmcv.imread(img_file, 'unchanged')
-
- img_info = dict(
- file_name=osp.join(osp.basename(img_file)),
- height=img.shape[0],
- width=img.shape[1],
- segm_file=osp.join(osp.basename(gt_file)))
-
- if osp.splitext(gt_file)[1] == '.txt':
- img_info = load_txt_info(gt_file, img_info)
- else:
- raise NotImplementedError
-
- return img_info
-
-
-def load_txt_info(gt_file, img_info):
- """Collect the annotation information.
-
- Args:
- gt_file (list): The list of tuples (image_file, groundtruth_file)
- img_info (int): The dict of the img and annotation information
-
- Returns:
- img_info (list): The dict of the img and annotation information
- """
-
- with open(gt_file, encoding='unicode_escape') as f:
- anno_info = []
- for ann in f.readlines():
-
- # annotation format [x1, y1, x2, y2, x3, y3, x4, y4, transcript]
- try:
- ann_box = np.array(ann.split(',')[0:8]).astype(int).tolist()
- except ValueError:
- # skip invalid annotation line
- continue
- x = max(0, min(ann_box[0::2]))
- y = max(0, min(ann_box[1::2]))
- w, h = max(ann_box[0::2]) - x, max(ann_box[1::2]) - y
- bbox = [x, y, w, h]
- segmentation = ann_box
-
- anno = dict(
- iscrowd=0,
- category_id=1,
- bbox=bbox,
- area=w * h,
- segmentation=[segmentation])
- anno_info.append(anno)
-
- img_info.update(anno_info=anno_info)
-
- return img_info
-
-
-def parse_args():
- parser = argparse.ArgumentParser(
- description='Generate training and test set of SROIE')
- parser.add_argument('root_path', help='Root dir path of SROIE')
- parser.add_argument(
- '--nproc', default=1, type=int, help='Number of process')
- args = parser.parse_args()
- return args
-
-
-def main():
- args = parse_args()
- root_path = args.root_path
-
- for split in ['training', 'test']:
- print(f'Processing {split} set...')
- with mmengine.Timer(
- print_tmpl='It takes {}s to convert SROIE annotation'):
- files = collect_files(
- osp.join(root_path, 'imgs', split),
- osp.join(root_path, 'annotations', split))
- image_infos = collect_annotations(files, nproc=args.nproc)
- dump_ocr_data(image_infos,
- osp.join(root_path, 'instances_' + split + '.json'),
- 'textdet')
-
-
-if __name__ == '__main__':
- main()
diff --git a/pipeline/mmocr/mmocr/.mim/tools/dataset_converters/textdet/vintext_converter.py b/pipeline/mmocr/mmocr/.mim/tools/dataset_converters/textdet/vintext_converter.py
deleted file mode 100644
index fb7a364d9591bec7785a73d571670121bb985978..0000000000000000000000000000000000000000
--- a/pipeline/mmocr/mmocr/.mim/tools/dataset_converters/textdet/vintext_converter.py
+++ /dev/null
@@ -1,171 +0,0 @@
-# Copyright (c) OpenMMLab. All rights reserved.
-import argparse
-import os
-import os.path as osp
-
-import mmcv
-import mmengine
-
-from mmocr.utils import dump_ocr_data
-
-
-def collect_files(img_dir, gt_dir):
- """Collect all images and their corresponding groundtruth files.
-
- Args:
- img_dir (str): The image directory
- gt_dir (str): The groundtruth directory
-
- Returns:
- files (list): The list of tuples (img_file, groundtruth_file)
- """
- assert isinstance(img_dir, str)
- assert img_dir
- assert isinstance(gt_dir, str)
- assert gt_dir
-
- ann_list, imgs_list = [], []
- for img_file in os.listdir(img_dir):
- ann_file = 'gt_' + str(int(img_file[2:6])) + '.txt'
- ann_list.append(osp.join(gt_dir, ann_file))
- imgs_list.append(osp.join(img_dir, img_file))
-
- files = list(zip(imgs_list, ann_list))
- assert len(files), f'No images found in {img_dir}'
- print(f'Loaded {len(files)} images from {img_dir}')
-
- return files
-
-
-def collect_annotations(files, nproc=1):
- """Collect the annotation information.
-
- Args:
- files (list): The list of tuples (image_file, groundtruth_file)
- nproc (int): The number of process to collect annotations
-
- Returns:
- images (list): The list of image information dicts
- """
- assert isinstance(files, list)
- assert isinstance(nproc, int)
-
- if nproc > 1:
- images = mmengine.track_parallel_progress(
- load_img_info, files, nproc=nproc)
- else:
- images = mmengine.track_progress(load_img_info, files)
-
- return images
-
-
-def load_img_info(files):
- """Load the information of one image.
-
- Args:
- files (tuple): The tuple of (img_file, groundtruth_file)
-
- Returns:
- img_info (dict): The dict of the img and annotation information
- """
- assert isinstance(files, tuple)
-
- img_file, gt_file = files
- assert int(osp.basename(gt_file)[3:-4]) == int(
- osp.basename(img_file)[2:-4])
- # read imgs while ignoring orientations
- img = mmcv.imread(img_file, 'unchanged')
-
- img_info = dict(
- file_name=osp.basename(img_file),
- height=img.shape[0],
- width=img.shape[1],
- segm_file=osp.basename(gt_file))
-
- if osp.splitext(gt_file)[1] == '.txt':
- img_info = load_txt_info(gt_file, img_info)
- else:
- raise NotImplementedError
-
- return img_info
-
-
-def load_txt_info(gt_file, img_info):
- """Collect the annotation information.
-
- The annotation format is as the following:
- x1,y1,x2,y2,x3,y3,x4,y4,text
- 118,15,147,15,148,46,118,46,LฦฏแปขNG
- 149,9,165,9,165,43,150,43,TแปT
- 167,9,180,9,179,43,167,42,ฤแป
- 181,12,193,12,193,43,181,43,Cร
- 195,13,215,14,215,46,196,46,VIแปC
- 217,13,237,14,239,47,217,46,LรM,
-
- Args:
- gt_file (str): The path to ground-truth
- img_info (dict): The dict of the img and annotation information
-
- Returns:
- img_info (dict): The dict of the img and annotation information
- """
-
- with open(gt_file, encoding='utf-8') as f:
- anno_info = []
- for line in f:
- line = line.strip('\n')
- ann = line.split(',')
- bbox = ann[0:8]
- word = line[len(','.join(bbox)) + 1:]
- bbox = [int(coord) for coord in bbox]
- segmentation = bbox
- x_min = min(bbox[0], bbox[2], bbox[4], bbox[6])
- x_max = max(bbox[0], bbox[2], bbox[4], bbox[6])
- y_min = min(bbox[1], bbox[3], bbox[5], bbox[7])
- y_max = max(bbox[1], bbox[3], bbox[5], bbox[7])
- w = x_max - x_min
- h = y_max - y_min
- bbox = [x_min, y_min, w, h]
- iscrowd = 1 if word == '###' else 0
-
- anno = dict(
- iscrowd=iscrowd,
- category_id=1,
- bbox=bbox,
- area=w * h,
- segmentation=[segmentation])
- anno_info.append(anno)
-
- img_info.update(anno_info=anno_info)
-
- return img_info
-
-
-def parse_args():
- parser = argparse.ArgumentParser(
- description='Generate training and test set of VinText ')
- parser.add_argument('root_path', help='Root dir path of VinText')
- parser.add_argument(
- '--nproc', default=1, type=int, help='Number of processes')
- args = parser.parse_args()
- return args
-
-
-def main():
- args = parse_args()
- root_path = args.root_path
- for split in ['training', 'test', 'unseen_test']:
- print(f'Processing {split} set...')
- with mmengine.Timer(
- print_tmpl='It takes {}s to convert VinText annotation'):
- files = collect_files(
- osp.join(root_path, 'imgs', split),
- osp.join(root_path, 'annotations'))
- image_infos = collect_annotations(files, nproc=args.nproc)
- dump_ocr_data(image_infos,
- osp.join(root_path, 'instances_' + split + '.json'),
- 'textdet')
-
-
-if __name__ == '__main__':
- main()
diff --git a/pipeline/mmocr/mmocr/.mim/tools/dataset_converters/textrecog/art_converter.py b/pipeline/mmocr/mmocr/.mim/tools/dataset_converters/textrecog/art_converter.py
deleted file mode 100644
index 24acaad289be221558701d19a95ea7ce24a7e0f9..0000000000000000000000000000000000000000
--- a/pipeline/mmocr/mmocr/.mim/tools/dataset_converters/textrecog/art_converter.py
+++ /dev/null
@@ -1,108 +0,0 @@
-# Copyright (c) OpenMMLab. All rights reserved.
-import argparse
-import math
-import os.path as osp
-
-import mmengine
-
-from mmocr.utils import dump_ocr_data
-
-
-def parse_args():
- parser = argparse.ArgumentParser(
- description='Generate training and validation set of ArT ')
- parser.add_argument('root_path', help='Root dir path of ArT')
- parser.add_argument(
- '--val-ratio', help='Split ratio for val set', default=0.0, type=float)
- parser.add_argument(
- '--nproc', default=1, type=int, help='Number of processes')
- args = parser.parse_args()
- return args
-
-
-def convert_art(root_path, split, ratio):
- """Collect the annotation information and crop the images.
-
- The annotation format is as the following:
- {
- "gt_2836_0": [
- {
- "transcription": "URDER",
- "points": [
- [25, 51],
- [0, 2],
- [21, 0],
- [42, 43]
- ],
- "language": "Latin",
- "illegibility": false
- }
- ], ...
- }
-
- Args:
- root_path (str): The root path of the dataset
- split (str): The split of dataset. Namely: training or val
- ratio (float): Split ratio for val set
-
- Returns:
- img_info (dict): The dict of the img and annotation information
- """
-
- annotation_path = osp.join(root_path,
- 'annotations/train_task2_labels.json')
- if not osp.exists(annotation_path):
- raise Exception(
- f'{annotation_path} not exists, please check and try again.')
-
- annotation = mmengine.load(annotation_path)
- img_prefixes = annotation.keys()
-
- trn_files, val_files = [], []
- if ratio > 0:
- for i, file in enumerate(img_prefixes):
- if i % math.floor(1 / ratio):
- trn_files.append(file)
- else:
- val_files.append(file)
- else:
- trn_files, val_files = img_prefixes, []
- print(f'training #{len(trn_files)}, val #{len(val_files)}')
-
- if split == 'train':
- img_prefixes = trn_files
- elif split == 'val':
- img_prefixes = val_files
- else:
- raise NotImplementedError
-
- img_info = []
- for prefix in img_prefixes:
- text_label = annotation[prefix][0]['transcription']
- dst_img_name = prefix + '.jpg'
-
- img_info.append({
- 'file_name': dst_img_name,
- 'anno_info': [{
- 'text': text_label
- }]
- })
-
- ensure_ascii = dict(ensure_ascii=False)
- dump_ocr_data(img_info, osp.join(root_path, f'{split.lower()}_label.json'),
- 'textrecog', **ensure_ascii)
-
-
-def main():
- args = parse_args()
- root_path = args.root_path
- print('Processing training set...')
- convert_art(root_path=root_path, split='train', ratio=args.val_ratio)
- if args.val_ratio > 0:
- print('Processing validation set...')
- convert_art(root_path=root_path, split='val', ratio=args.val_ratio)
- print('Finish')
-
-
-if __name__ == '__main__':
- main()
diff --git a/pipeline/mmocr/mmocr/.mim/tools/dataset_converters/textrecog/bid_converter.py b/pipeline/mmocr/mmocr/.mim/tools/dataset_converters/textrecog/bid_converter.py
deleted file mode 100644
index ec61b64bb42effc6194e1661a819224fa02b2c13..0000000000000000000000000000000000000000
--- a/pipeline/mmocr/mmocr/.mim/tools/dataset_converters/textrecog/bid_converter.py
+++ /dev/null
@@ -1,247 +0,0 @@
-# Copyright (c) OpenMMLab. All rights reserved.
-import argparse
-import os
-import os.path as osp
-
-import mmcv
-import mmengine
-
-from mmocr.utils import crop_img, dump_ocr_data
-
-
-def collect_files(img_dir, gt_dir):
- """Collect all images and their corresponding groundtruth files.
-
- Args:
- img_dir (str): The image directory
- gt_dir (str): The groundtruth directory
-
- Returns:
- files (list): The list of tuples (img_file, groundtruth_file)
- """
- assert isinstance(img_dir, str)
- assert img_dir
- assert isinstance(gt_dir, str)
- assert gt_dir
-
- ann_list, imgs_list = [], []
- for img_file in os.listdir(img_dir):
- ann_file = img_file.split('_')[0] + '_gt_ocr.txt'
- ann_list.append(osp.join(gt_dir, ann_file))
- imgs_list.append(osp.join(img_dir, img_file))
-
- files = list(zip(imgs_list, ann_list))
- assert len(files), f'No images found in {img_dir}'
- print(f'Loaded {len(files)} images from {img_dir}')
-
- return files
-
-
-def collect_annotations(files, nproc=1):
- """Collect the annotation information.
-
- Args:
- files (list): The list of tuples (image_file, groundtruth_file)
- nproc (int): The number of process to collect annotations
-
- Returns:
- images (list): The list of image information dicts
- """
- assert isinstance(files, list)
- assert isinstance(nproc, int)
-
- if nproc > 1:
- images = mmengine.track_parallel_progress(
- load_img_info, files, nproc=nproc)
- else:
- images = mmengine.track_progress(load_img_info, files)
-
- return images
-
-
-def load_img_info(files):
- """Load the information of one image.
-
- Args:
- files (tuple): The tuple of (img_file, groundtruth_file)
-
- Returns:
- img_info (dict): The dict of the img and annotation information
- """
- assert isinstance(files, tuple)
-
- img_file, gt_file = files
- assert osp.basename(gt_file).split('_')[0] == osp.basename(gt_file).split(
- '_')[0]
- # read imgs while ignoring orientations
- img = mmcv.imread(img_file, 'unchanged')
-
- img_info = dict(
- file_name=osp.basename(img_file),
- height=img.shape[0],
- width=img.shape[1],
- segm_file=osp.basename(gt_file))
-
- if osp.splitext(gt_file)[1] == '.txt':
- img_info = load_txt_info(gt_file, img_info)
- else:
- raise NotImplementedError
-
- return img_info
-
-
-def load_txt_info(gt_file, img_info):
- """Collect the annotation information.
-
- The annotation format is as the following:
- x, y, w, h, text
- 977, 152, 16, 49, NOME
- 962, 143, 12, 323, APPINHANESI BLAZEK PASSOTTO
- 906, 446, 12, 94, 206940361
- 905, 641, 12, 44, SPTC
-
- Args:
- gt_file (str): The path to ground-truth
- img_info (dict): The dict of the img and annotation information
-
- Returns:
- img_info (dict): The dict of the img and annotation information
- """
- with open(gt_file, encoding='latin1') as f:
- anno_info = []
- for line in f:
- line = line.strip('\n')
- # Ignore hard samples
- if line[0] == '[' or line[0] == 'x':
- continue
- ann = line.split(',')
- bbox = ann[0:4]
- bbox = [int(coord) for coord in bbox]
- x, y, w, h = bbox
- # in case ',' exists in label
- word = ','.join(ann[4:]) if len(ann[4:]) > 1 else ann[4]
- # remove the initial space
- word = word.strip()
- bbox = [x, y, x + w, y, x + w, y + h, x, y + h]
-
- anno = dict(bbox=bbox, word=word)
- anno_info.append(anno)
-
- img_info.update(anno_info=anno_info)
-
- return img_info
-
-
-def split_train_val_list(full_list, val_ratio):
- """Split list by val_ratio.
-
- Args:
- full_list (list): List to be splited
- val_ratio (float): Split ratio for val set
-
- return:
- list(list, list): Train_list and val_list
- """
- n_total = len(full_list)
- offset = int(n_total * val_ratio)
- if n_total == 0 or offset < 1:
- return [], full_list
- val_list = full_list[:offset]
- train_list = full_list[offset:]
- return [train_list, val_list]
-
-
-def generate_ann(root_path, image_infos, preserve_vertical, val_ratio, format):
- """Generate cropped annotations and label txt file.
-
- Args:
- root_path (str): The root path of the dataset
- image_infos (list[dict]): A list of dicts of the img and
- annotation information
- preserve_vertical (bool): Whether to preserve vertical texts
- val_ratio (float): Split ratio for val set
- format (str): Using jsonl(dict) or str to format annotations
- """
-
- assert val_ratio <= 1.
-
- if val_ratio:
- image_infos = split_train_val_list(image_infos, val_ratio)
- splits = ['training', 'val']
-
- else:
- image_infos = [image_infos]
- splits = ['training']
-
- for i, split in enumerate(splits):
- dst_image_root = osp.join(root_path, 'crops', split)
- ignore_image_root = osp.join(root_path, 'ignores', split)
- os.makedirs(dst_image_root, exist_ok=True)
-
- img_info = []
- for image_info in image_infos[i]:
- index = 1
- src_img_path = osp.join(root_path, 'imgs', image_info['file_name'])
- image = mmcv.imread(src_img_path)
- src_img_root = image_info['file_name'].split('.')[0]
-
- for anno in image_info['anno_info']:
- word = anno['word']
- dst_img = crop_img(image, anno['bbox'], 0, 0)
- h, w, _ = dst_img.shape
-
- dst_img_name = f'{src_img_root}_{index}.png'
- index += 1
- # Skip invalid annotations
- if min(dst_img.shape) == 0:
- continue
- # Skip vertical texts
- if not preserve_vertical and h / w > 2 and split == 'training':
- dst_img_path = osp.join(ignore_image_root, dst_img_name)
- mmcv.imwrite(dst_img, dst_img_path)
- continue
-
- dst_img_path = osp.join(dst_image_root, dst_img_name)
- mmcv.imwrite(dst_img, dst_img_path)
-
- img_info.append({
- 'file_name': dst_img_name,
- 'anno_info': [{
- 'text': word
- }]
- })
-
- dump_ocr_data(img_info,
- osp.join(root_path, f'{split.lower()}_label.json'),
- 'textrecog')
-
-
-def parse_args():
- parser = argparse.ArgumentParser(
- description='Generate training and val set of BID ')
- parser.add_argument('root_path', help='Root dir path of BID')
- parser.add_argument(
- '--preserve-vertical',
- help='Preserve samples containing vertical texts',
- action='store_true')
- parser.add_argument(
- '--val-ratio', help='Split ratio for val set', default=0., type=float)
- parser.add_argument(
- '--nproc', default=1, type=int, help='Number of processes')
- args = parser.parse_args()
- return args
-
-
-def main():
- args = parse_args()
- root_path = args.root_path
- with mmengine.Timer(print_tmpl='It takes {}s to convert BID annotation'):
- files = collect_files(
- osp.join(root_path, 'imgs'), osp.join(root_path, 'annotations'))
- image_infos = collect_annotations(files, nproc=args.nproc)
- generate_ann(root_path, image_infos, args.preserve_vertical,
- args.val_ratio, args.format)
-
-
-if __name__ == '__main__':
- main()
diff --git a/pipeline/mmocr/mmocr/.mim/tools/dataset_converters/textrecog/cocotext_converter.py b/pipeline/mmocr/mmocr/.mim/tools/dataset_converters/textrecog/cocotext_converter.py
deleted file mode 100644
index 413c09b6c32c7f31ec86fe46c42d69809986bbf9..0000000000000000000000000000000000000000
--- a/pipeline/mmocr/mmocr/.mim/tools/dataset_converters/textrecog/cocotext_converter.py
+++ /dev/null
@@ -1,174 +0,0 @@
-# Copyright (c) OpenMMLab. All rights reserved.
-import argparse
-import math
-import os.path as osp
-from functools import partial
-
-import mmcv
-import mmengine
-
-from mmocr.utils import dump_ocr_data
-
-
-def parse_args():
- parser = argparse.ArgumentParser(
- description='Generate training and validation set of COCO Text v2 ')
- parser.add_argument('root_path', help='Root dir path of COCO Text v2')
- parser.add_argument(
- '--nproc', default=1, type=int, help='Number of processes')
- parser.add_argument(
- '--preserve-vertical',
- help='Preserve samples containing vertical texts',
- action='store_true')
- args = parser.parse_args()
- return args
-
-
-def process_img(args, src_image_root, dst_image_root, ignore_image_root,
- preserve_vertical, split):
- # Dirty hack for multi-processing
- img_idx, img_info, anns = args
- src_img = mmcv.imread(osp.join(src_image_root, img_info['file_name']))
- label = []
- for ann_idx, ann in enumerate(anns):
- text_label = ann['utf8_string']
-
- # Ignore illegible or non-English words
- if ann['language'] == 'not english':
- continue
- if ann['legibility'] == 'illegible':
- continue
-
- x, y, w, h = ann['bbox']
- x, y = max(0, math.floor(x)), max(0, math.floor(y))
- w, h = math.ceil(w), math.ceil(h)
- dst_img = src_img[y:y + h, x:x + w]
- dst_img_name = f'img_{img_idx}_{ann_idx}.jpg'
-
- if not preserve_vertical and h / w > 2 and split == 'train':
- dst_img_path = osp.join(ignore_image_root, dst_img_name)
- mmcv.imwrite(dst_img, dst_img_path)
- continue
-
- dst_img_path = osp.join(dst_image_root, dst_img_name)
- mmcv.imwrite(dst_img, dst_img_path)
-
- label.append({
- 'file_name': dst_img_name,
- 'anno_info': [{
- 'text': text_label
- }]
- })
-
- return label
-
-
-def convert_cocotext(root_path,
- split,
- preserve_vertical,
- nproc,
- img_start_idx=0):
- """Collect the annotation information and crop the images.
-
- The annotation format is as the following:
- {
- 'anns':{
- '45346':{
- 'mask': [468.9,286.7,468.9,295.2,493.0,295.8,493.0,287.2],
- 'class': 'machine printed',
- 'bbox': [468.9, 286.7, 24.1, 9.1], # x, y, w, h
- 'image_id': 217925,
- 'id': 45346,
- 'language': 'english', # 'english' or 'not english'
- 'area': 206.06,
- 'utf8_string': 'New',
- 'legibility': 'legible', # 'legible' or 'illegible'
- },
- ...
- }
- 'imgs':{
- '540965':{
- 'id': 540965,
- 'set': 'train', # 'train' or 'val'
- 'width': 640,
- 'height': 360,
- 'file_name': 'COCO_train2014_000000540965.jpg'
- },
- ...
- }
- 'imgToAnns':{
- '540965': [],
- '260932': [63993, 63994, 63995, 63996, 63997, 63998, 63999],
- ...
- }
- }
-
- Args:
- root_path (str): Root path to the dataset
- split (str): Dataset split, which should be 'train' or 'val'
- preserve_vertical (bool): Whether to preserve vertical texts
- nproc (int): Number of processes
- img_start_idx (int): Index of start image
-
- Returns:
- img_info (dict): The dict of the img and annotation information
- """
-
- annotation_path = osp.join(root_path, 'annotations/cocotext.v2.json')
- if not osp.exists(annotation_path):
- raise Exception(
- f'{annotation_path} not exists, please check and try again.')
-
- annotation = mmengine.load(annotation_path)
- # outputs
- dst_label_file = osp.join(root_path, f'{split}_label.json')
- dst_image_root = osp.join(root_path, 'crops', split)
- ignore_image_root = osp.join(root_path, 'ignores', split)
- src_image_root = osp.join(root_path, 'imgs')
- mmengine.mkdir_or_exist(dst_image_root)
- mmengine.mkdir_or_exist(ignore_image_root)
-
- process_img_with_path = partial(
- process_img,
- src_image_root=src_image_root,
- dst_image_root=dst_image_root,
- ignore_image_root=ignore_image_root,
- preserve_vertical=preserve_vertical,
- split=split)
- tasks = []
- for img_idx, img_info in enumerate(annotation['imgs'].values()):
- if img_info['set'] == split:
- ann_ids = annotation['imgToAnns'][str(img_info['id'])]
- anns = [annotation['anns'][str(ann_id)] for ann_id in ann_ids]
- tasks.append((img_idx + img_start_idx, img_info, anns))
- labels_list = mmengine.track_parallel_progress(
- process_img_with_path, tasks, keep_order=True, nproc=nproc)
- final_labels = []
- for label_list in labels_list:
- final_labels += label_list
- dump_ocr_data(final_labels, dst_label_file, 'textrecog')
-
- return len(annotation['imgs'])
-
-
-def main():
- args = parse_args()
- root_path = args.root_path
- print('Processing training set...')
- num_train_imgs = convert_cocotext(
- root_path=root_path,
- split='train',
- preserve_vertical=args.preserve_vertical,
- nproc=args.nproc)
- print('Processing validation set...')
- convert_cocotext(
- root_path=root_path,
- split='val',
- preserve_vertical=args.preserve_vertical,
- nproc=args.nproc,
- img_start_idx=num_train_imgs)
- print('Finish')
-
-
-if __name__ == '__main__':
- main()
diff --git a/pipeline/mmocr/mmocr/.mim/tools/dataset_converters/textrecog/data_migrator.py b/pipeline/mmocr/mmocr/.mim/tools/dataset_converters/textrecog/data_migrator.py
deleted file mode 100644
index 9fb0f205b67a4d55bb1208feba4e4db65c0b78e8..0000000000000000000000000000000000000000
--- a/pipeline/mmocr/mmocr/.mim/tools/dataset_converters/textrecog/data_migrator.py
+++ /dev/null
@@ -1,98 +0,0 @@
-# Copyright (c) OpenMMLab. All rights reserved.
-import argparse
-import json
-from typing import List, Tuple
-
-from mmocr.datasets import RecogLMDBDataset
-from mmocr.utils import StringStripper, dump_ocr_data, recog_anno_to_imginfo
-
-
-def parse_legacy_data(in_path: str,
- format: str) -> Tuple[List[str], List[str]]:
- """Load legacy data and return a list of file paths and labels.
-
- Args:
- in_path (str): Path to annotation file.
- format (str): Annotation format. Choices are 'txt', 'json' and 'lmdb'.
- For 'lmdb' format, the lmdb file should only contains labels. For
- lmdb file with labels and images, the conversion is unnecessary.
- Returns:
- tuple(list[str], list[str]): File paths and labels.
- """
- file_paths = []
- labels = []
- strip_cls = StringStripper()
- if format == 'lmdb':
- dataset = RecogLMDBDataset(
- in_path,
- parser_cfg=dict(type='LineJsonParser', keys=['filename', 'text']))
- for data_info in dataset:
- file_path = data_info['img_path']
- label = data_info['instances'][0]['text']
- file_path = strip_cls(file_path)
- label = strip_cls(label)
- # MJ's file_path starts with './'
- if file_path.startswith('./'):
- file_path = file_path[2:]
-
- file_paths.append(file_path)
- labels.append(label)
- return file_paths, labels
- else:
- with open(in_path) as f:
- if format == 'txt':
- for line in f:
- line = strip_cls(line)
- file_path, label = line.split()[:2]
- # MJ's file_path starts with './'
- if file_path.startswith('./'):
- file_path = file_path[2:]
-
- file_paths.append(file_path)
- labels.append(label)
- elif format == 'jsonl':
- for line in f:
- datum = json.loads(line)
- file_path = datum['filename']
- # MJ's file_path starts with './'
- if file_path.startswith('./'):
- file_path = file_path[2:]
-
- file_paths.append(file_path)
- labels.append(datum['text'])
-
- return file_paths, labels
-
-
-def parse_args():
- """Parse input arguments."""
- parser = argparse.ArgumentParser(
- description='Convert annotations for'
- 'text recognition tasks in MMOCR 0.x into the latest openmmlab format.'
- )
- parser.add_argument(
- 'in_path', help='The path to legacy recognition data file')
- parser.add_argument(
- 'out_path', help='The output json path in openmmlab format')
- parser.add_argument(
- '--format',
- choices=['txt', 'jsonl', 'lmdb'],
- type=str,
- default='txt',
- help='Legacy data format')
- args = parser.parse_args()
- if args.out_path.split('.')[-1] != 'json':
- raise ValueError('The output path must be a json file.')
- return args
-
-
-def main():
- args = parse_args()
- file_paths, labels = parse_legacy_data(args.in_path, args.format)
- img_infos = recog_anno_to_imginfo(file_paths, labels)
- dump_ocr_data(img_infos, args.out_path, 'textrecog')
- print('finish')
-
-
-if __name__ == '__main__':
- main()
diff --git a/pipeline/mmocr/mmocr/.mim/tools/dataset_converters/textrecog/detext_converter.py b/pipeline/mmocr/mmocr/.mim/tools/dataset_converters/textrecog/detext_converter.py
deleted file mode 100644
index 360dc5a6f4555d45fb69533f033710eb58e12cad..0000000000000000000000000000000000000000
--- a/pipeline/mmocr/mmocr/.mim/tools/dataset_converters/textrecog/detext_converter.py
+++ /dev/null
@@ -1,212 +0,0 @@
-# Copyright (c) OpenMMLab. All rights reserved.
-import argparse
-import os
-import os.path as osp
-
-import mmcv
-import mmengine
-import numpy as np
-
-from mmocr.utils import crop_img, dump_ocr_data
-
-
-def collect_files(img_dir, gt_dir):
- """Collect all images and their corresponding groundtruth files.
-
- Args:
- img_dir (str): The image directory
- gt_dir (str): The groundtruth directory
-
- Returns:
- files (list): The list of tuples (img_file, groundtruth_file)
- """
- assert isinstance(img_dir, str)
- assert img_dir
- assert isinstance(gt_dir, str)
- assert gt_dir
-
- ann_list, imgs_list = [], []
- for img in os.listdir(img_dir):
- imgs_list.append(osp.join(img_dir, img))
- ann_list.append(osp.join(gt_dir, 'gt_' + img.replace('jpg', 'txt')))
-
- files = list(zip(imgs_list, ann_list))
- assert len(files), f'No images found in {img_dir}'
- print(f'Loaded {len(files)} images from {img_dir}')
-
- return files
-
-
-def collect_annotations(files, nproc=1):
- """Collect the annotation information.
-
- Args:
- files (list): The list of tuples (image_file, groundtruth_file)
- nproc (int): The number of process to collect annotations
-
- Returns:
- images (list): The list of image information dicts
- """
- assert isinstance(files, list)
- assert isinstance(nproc, int)
-
- if nproc > 1:
- images = mmengine.track_parallel_progress(
- load_img_info, files, nproc=nproc)
- else:
- images = mmengine.track_progress(load_img_info, files)
-
- return images
-
-
-def load_img_info(files):
- """Load the information of one image.
-
- Args:
- files (tuple): The tuple of (img_file, groundtruth_file)
-
- Returns:
- img_info (dict): The dict of the img and annotation information
- """
- assert isinstance(files, tuple)
-
- img_file, gt_file = files
- # read imgs while ignoring orientations
- img = mmcv.imread(img_file, 'unchanged')
-
- img_info = dict(
- file_name=osp.join(osp.basename(img_file)),
- height=img.shape[0],
- width=img.shape[1],
- segm_file=osp.join(osp.basename(gt_file)))
-
- if osp.splitext(gt_file)[1] == '.txt':
- img_info = load_txt_info(gt_file, img_info)
- else:
- raise NotImplementedError
-
- return img_info
-
-
-def load_txt_info(gt_file, img_info):
- """Collect the annotation information.
-
- Args:
- gt_file (str): The path to ground-truth
- img_info (dict): The dict of the img and annotation information
-
- Returns:
- img_info (dict): The dict of the img and annotation information
- """
-
- with open(gt_file) as f:
- anno_info = []
- annotations = f.readlines()
- for ann in annotations:
- # Annotation format [x1, y1, x2, y2, x3, y3, x4, y4, transcript]
- try:
- bbox = np.array(ann.split(',')[0:8]).astype(int).tolist()
- except ValueError:
- # Skip invalid annotation line
- continue
- word = ann.split(',')[-1].replace('\n', '').strip()
-
- # Skip samples without recog gt
- if word == '###':
- continue
- anno = dict(bbox=bbox, word=word)
- anno_info.append(anno)
-
- img_info.update(anno_info=anno_info)
-
- return img_info
-
-
-def generate_ann(root_path, split, image_infos, preserve_vertical):
- """Generate cropped annotations and label txt file.
-
- Args:
- root_path (str): The root path of the dataset
- split (str): The split of dataset. Namely: training or test
- image_infos (list[dict]): A list of dicts of the img and
- annotation information
- preserve_vertical (bool): Whether to preserve vertical texts
- """
-
- dst_image_root = osp.join(root_path, 'crops', split)
- ignore_image_root = osp.join(root_path, 'ignores', split)
- if split == 'training':
- dst_label_file = osp.join(root_path, 'train_label.json')
- elif split == 'val':
- dst_label_file = osp.join(root_path, 'val_label.json')
- mmengine.mkdir_or_exist(dst_image_root)
- mmengine.mkdir_or_exist(ignore_image_root)
-
- img_info = []
- for image_info in image_infos:
- index = 1
- src_img_path = osp.join(root_path, 'imgs', split,
- image_info['file_name'])
- image = mmcv.imread(src_img_path)
- src_img_root = image_info['file_name'].split('.')[0]
-
- for anno in image_info['anno_info']:
- word = anno['word']
- dst_img = crop_img(image, anno['bbox'], 0, 0)
- h, w, _ = dst_img.shape
-
- dst_img_name = f'{src_img_root}_{index}.png'
- index += 1
- # Skip invalid annotations
- if min(dst_img.shape) == 0 or len(word) == 0:
- continue
- # Filter out vertical texts
- if not preserve_vertical and h / w > 2 and split == 'training':
- dst_img_path = osp.join(ignore_image_root, dst_img_name)
- mmcv.imwrite(dst_img, dst_img_path)
- continue
-
- dst_img_path = osp.join(dst_image_root, dst_img_name)
- mmcv.imwrite(dst_img, dst_img_path)
-
- img_info.append({
- 'file_name': dst_img_name,
- 'anno_info': [{
- 'text': word
- }]
- })
-
- dump_ocr_data(img_info, dst_label_file, 'textrecog')
-
-
-def parse_args():
- parser = argparse.ArgumentParser(
- description='Generate training and val set of DeText ')
- parser.add_argument('root_path', help='Root dir path of DeText')
- parser.add_argument(
- '--preserve-vertical',
- help='Preserve samples containing vertical texts',
- action='store_true')
- parser.add_argument(
- '--nproc', default=1, type=int, help='Number of process')
- args = parser.parse_args()
- return args
-
-
-def main():
- args = parse_args()
- root_path = args.root_path
-
- for split in ['training', 'val']:
- print(f'Processing {split} set...')
- with mmengine.Timer(
- print_tmpl='It takes {}s to convert DeText annotation'):
- files = collect_files(
- osp.join(root_path, 'imgs', split),
- osp.join(root_path, 'annotations', split))
- image_infos = collect_annotations(files, nproc=args.nproc)
- generate_ann(root_path, split, image_infos, args.preserve_vertical)
-
-
-if __name__ == '__main__':
- main()
diff --git a/pipeline/mmocr/mmocr/.mim/tools/dataset_converters/textrecog/funsd_converter.py b/pipeline/mmocr/mmocr/.mim/tools/dataset_converters/textrecog/funsd_converter.py
deleted file mode 100644
index a8da8ab00183f20ec749585bde1bb6958a48f9d0..0000000000000000000000000000000000000000
--- a/pipeline/mmocr/mmocr/.mim/tools/dataset_converters/textrecog/funsd_converter.py
+++ /dev/null
@@ -1,210 +0,0 @@
-# Copyright (c) OpenMMLab. All rights reserved.
-import argparse
-import math
-import os
-import os.path as osp
-
-import mmcv
-import mmengine
-
-from mmocr.utils import crop_img, dump_ocr_data
-
-
-def collect_files(img_dir, gt_dir):
- """Collect all images and their corresponding groundtruth files.
-
- Args:
- img_dir (str): The image directory
- gt_dir (str): The groundtruth directory
-
- Returns:
- files (list): The list of tuples (img_file, groundtruth_file)
- """
- assert isinstance(img_dir, str)
- assert img_dir
- assert isinstance(gt_dir, str)
- assert gt_dir
-
- ann_list, imgs_list = [], []
- for gt_file in os.listdir(gt_dir):
- ann_list.append(osp.join(gt_dir, gt_file))
- imgs_list.append(osp.join(img_dir, gt_file.replace('.json', '.png')))
-
- files = list(zip(sorted(imgs_list), sorted(ann_list)))
- assert len(files), f'No images found in {img_dir}'
- print(f'Loaded {len(files)} images from {img_dir}')
-
- return files
-
-
-def collect_annotations(files, nproc=1):
- """Collect the annotation information.
-
- Args:
- files (list): The list of tuples (image_file, groundtruth_file)
- nproc (int): The number of process to collect annotations
-
- Returns:
- images (list): The list of image information dicts
- """
- assert isinstance(files, list)
- assert isinstance(nproc, int)
-
- if nproc > 1:
- images = mmengine.track_parallel_progress(
- load_img_info, files, nproc=nproc)
- else:
- images = mmengine.track_progress(load_img_info, files)
-
- return images
-
-
-def load_img_info(files):
- """Load the information of one image.
-
- Args:
- files (tuple): The tuple of (img_file, groundtruth_file)
-
- Returns:
- img_info (dict): The dict of the img and annotation information
- """
- assert isinstance(files, tuple)
-
- img_file, gt_file = files
- assert osp.basename(gt_file).split('.')[0] == osp.basename(img_file).split(
- '.')[0]
- # read imgs while ignoring orientations
- img = mmcv.imread(img_file, 'unchanged')
-
- img_info = dict(
- file_name=osp.join(osp.basename(img_file)),
- height=img.shape[0],
- width=img.shape[1],
- segm_file=osp.join(osp.basename(gt_file)))
-
- if osp.splitext(gt_file)[1] == '.json':
- img_info = load_json_info(gt_file, img_info)
- else:
- raise NotImplementedError
-
- return img_info
-
-
-def load_json_info(gt_file, img_info):
- """Collect the annotation information.
-
- Args:
- gt_file (str): The path to ground-truth
- img_info (dict): The dict of the img and annotation information
-
- Returns:
- img_info (dict): The dict of the img and annotation information
- """
-
- annotation = mmengine.load(gt_file)
- anno_info = []
- for form in annotation['form']:
- for ann in form['words']:
-
- # Ignore illegible samples
- if len(ann['text']) == 0:
- continue
-
- x1, y1, x2, y2 = ann['box']
- x = max(0, min(math.floor(x1), math.floor(x2)))
- y = max(0, min(math.floor(y1), math.floor(y2)))
- w, h = math.ceil(abs(x2 - x1)), math.ceil(abs(y2 - y1))
- bbox = [x, y, x + w, y, x + w, y + h, x, y + h]
- word = ann['text']
-
- anno = dict(bbox=bbox, word=word)
- anno_info.append(anno)
-
- img_info.update(anno_info=anno_info)
-
- return img_info
-
-
-def generate_ann(root_path, split, image_infos, preserve_vertical):
- """Generate cropped annotations and label txt file.
-
- Args:
- root_path (str): The root path of the dataset
- split (str): The split of dataset. Namely: training or test
- image_infos (list[dict]): A list of dicts of the img and
- annotation information
- preserve_vertical (bool): Whether to preserve vertical texts
- """
-
- dst_image_root = osp.join(root_path, 'crops', split)
- if split == 'training':
- dst_label_file = osp.join(root_path, 'train_label.json')
- elif split == 'test':
- dst_label_file = osp.join(root_path, 'test_label.json')
- os.makedirs(dst_image_root, exist_ok=True)
-
- img_info = []
- for image_info in image_infos:
- index = 1
- src_img_path = osp.join(root_path, 'imgs', image_info['file_name'])
- image = mmcv.imread(src_img_path)
- src_img_root = image_info['file_name'].split('.')[0]
-
- for anno in image_info['anno_info']:
- word = anno['word']
- dst_img = crop_img(image, anno['bbox'])
- h, w, _ = dst_img.shape
-
- # Skip invalid annotations
- if min(dst_img.shape) == 0:
- continue
- # Skip vertical texts
- if not preserve_vertical and h / w > 2:
- continue
-
- dst_img_name = f'{src_img_root}_{index}.png'
- index += 1
- dst_img_path = osp.join(dst_image_root, dst_img_name)
- mmcv.imwrite(dst_img, dst_img_path)
-
- img_info.append({
- 'file_name': dst_img_name,
- 'anno_info': [{
- 'text': word
- }]
- })
-
- dump_ocr_data(img_info, dst_label_file, 'textrecog')
-
-
-def parse_args():
- parser = argparse.ArgumentParser(
- description='Generate training and test set of FUNSD ')
- parser.add_argument('root_path', help='Root dir path of FUNSD')
- parser.add_argument(
- '--preserve_vertical',
- help='Preserve samples containing vertical texts',
- action='store_true')
- parser.add_argument(
- '--nproc', default=1, type=int, help='Number of processes')
- args = parser.parse_args()
- return args
-
-
-def main():
- args = parse_args()
- root_path = args.root_path
-
- for split in ['training', 'test']:
- print(f'Processing {split} set...')
- with mmengine.Timer(
- print_tmpl='It takes {}s to convert FUNSD annotation'):
- files = collect_files(
- osp.join(root_path, 'imgs'),
- osp.join(root_path, 'annotations', split))
- image_infos = collect_annotations(files, nproc=args.nproc)
- generate_ann(root_path, split, image_infos, args.preserve_vertical)
-
-
-if __name__ == '__main__':
- main()
diff --git a/pipeline/mmocr/mmocr/.mim/tools/dataset_converters/textrecog/hiertext_converter.py b/pipeline/mmocr/mmocr/.mim/tools/dataset_converters/textrecog/hiertext_converter.py
deleted file mode 100644
index 948142446e30ceea2c1b58cbcd7aec930a982482..0000000000000000000000000000000000000000
--- a/pipeline/mmocr/mmocr/.mim/tools/dataset_converters/textrecog/hiertext_converter.py
+++ /dev/null
@@ -1,236 +0,0 @@
-# Copyright (c) OpenMMLab. All rights reserved.
-import argparse
-import json
-import math
-import os.path as osp
-from functools import partial
-
-import mmcv
-import mmengine
-import numpy as np
-from shapely.geometry import Polygon
-
-from mmocr.utils import dump_ocr_data
-
-
-def seg2bbox(seg):
- """Convert segmentation to bbox.
-
- Args:
- seg (list(int | float)): A set of coordinates
- """
- if len(seg) == 4:
- min_x = min(seg[0], seg[2], seg[4], seg[6])
- max_x = max(seg[0], seg[2], seg[4], seg[6])
- min_y = min(seg[1], seg[3], seg[5], seg[7])
- max_y = max(seg[1], seg[3], seg[5], seg[7])
- else:
- seg = np.array(seg).reshape(-1, 2)
- polygon = Polygon(seg)
- min_x, min_y, max_x, max_y = polygon.bounds
- bbox = [min_x, min_y, max_x - min_x, max_y - min_y]
- return bbox
-
-
-def process_level(
- src_img,
- annotation,
- dst_image_root,
- ignore_image_root,
- preserve_vertical,
- split,
- para_idx,
- img_idx,
- line_idx,
- word_idx=None,
-):
- vertices = annotation['vertices']
- text_label = annotation['text']
- segmentation = [i for j in vertices for i in j]
- x, y, w, h = seg2bbox(segmentation)
- x, y = max(0, math.floor(x)), max(0, math.floor(y))
- w, h = math.ceil(w), math.ceil(h)
- dst_img = src_img[y:y + h, x:x + w]
- if word_idx:
- dst_img_name = f'img_{img_idx}_{para_idx}_{line_idx}_{word_idx}.jpg'
- else:
- dst_img_name = f'img_{img_idx}_{para_idx}_{line_idx}.jpg'
- if not preserve_vertical and h / w > 2 and split == 'train':
- dst_img_path = osp.join(ignore_image_root, dst_img_name)
- mmcv.imwrite(dst_img, dst_img_path)
- return None
-
- dst_img_path = osp.join(dst_image_root, dst_img_name)
- mmcv.imwrite(dst_img, dst_img_path)
-
- label = {'file_name': dst_img_name, 'anno_info': [{'text': text_label}]}
-
- return label
-
-
-def process_img(args, src_image_root, dst_image_root, ignore_image_root, level,
- preserve_vertical, split):
- # Dirty hack for multi-processing
- img_idx, img_annos = args
- src_img = mmcv.imread(
- osp.join(src_image_root, img_annos['image_id'] + '.jpg'))
- labels = []
- for para_idx, paragraph in enumerate(img_annos['paragraphs']):
- for line_idx, line in enumerate(paragraph['lines']):
- if level == 'line':
- # Ignore illegible words
- if line['legible']:
-
- label = process_level(src_img, line, dst_image_root,
- ignore_image_root, preserve_vertical,
- split, para_idx, img_idx, line_idx)
- if label is not None:
- labels.append(label)
- elif level == 'word':
- for word_idx, word in enumerate(line['words']):
- if not word['legible']:
- continue
- label = process_level(src_img, word, dst_image_root,
- ignore_image_root, preserve_vertical,
- split, para_idx, img_idx, line_idx,
- word_idx)
- if label is not None:
- labels.append(label)
- return labels
-
-
-def convert_hiertext(
- root_path,
- split,
- level,
- preserve_vertical,
- nproc,
-):
- """Collect the annotation information and crop the images.
-
- The annotation format is as the following:
- {
- "info": {
- "date": "release date",
- "version": "current version"
- },
- "annotations": [ // List of dictionaries, one for each image.
- {
- "image_id": "the filename of corresponding image.",
- "image_width": image_width, // (int) The image width.
- "image_height": image_height, // (int) The image height.
- "paragraphs": [ // List of paragraphs.
- {
- "vertices": [[x1, y1], [x2, y2],...,[xn, yn]]
- "legible": true
- "lines": [
- {
- "vertices": [[x1, y1], [x2, y2],...,[x4, y4]]
- "text": L
- "legible": true,
- "handwritten": false
- "vertical": false,
- "words": [
- {
- "vertices": [[x1, y1], [x2, y2],...,[xm, ym]]
- "text": "the text content of this word",
- "legible": true
- "handwritten": false,
- "vertical": false,
- }, ...
- ]
- }, ...
- ]
- }, ...
- ]
- }, ...
- ]
- }
-
- Args:
- root_path (str): Root path to the dataset
- split (str): Dataset split, which should be 'train' or 'val'
- level (str): Crop word or line level instances
- preserve_vertical (bool): Whether to preserve vertical texts
- nproc (int): Number of processes
-
- Returns:
- img_info (dict): The dict of the img and annotation information
- """
-
- annotation_path = osp.join(root_path, 'annotations/' + split + '.jsonl')
- if not osp.exists(annotation_path):
- raise Exception(
- f'{annotation_path} not exists, please check and try again.')
-
- annotation = json.load(open(annotation_path))['annotations']
- # outputs
- dst_label_file = osp.join(root_path, f'{split}_label.json')
- dst_image_root = osp.join(root_path, 'crops', split)
- ignore_image_root = osp.join(root_path, 'ignores', split)
- src_image_root = osp.join(root_path, 'imgs', split)
- mmengine.mkdir_or_exist(dst_image_root)
- mmengine.mkdir_or_exist(ignore_image_root)
-
- process_img_with_path = partial(
- process_img,
- src_image_root=src_image_root,
- dst_image_root=dst_image_root,
- ignore_image_root=ignore_image_root,
- level=level,
- preserve_vertical=preserve_vertical,
- split=split)
- tasks = []
- for img_idx, img_info in enumerate(annotation):
- tasks.append((img_idx, img_info))
- labels_list = mmengine.track_parallel_progress(
- process_img_with_path, tasks, keep_order=True, nproc=nproc)
-
- final_labels = []
- for label_list in labels_list:
- final_labels += label_list
-
- dump_ocr_data(final_labels, dst_label_file, 'textrecog')
-
-
-def parse_args():
- parser = argparse.ArgumentParser(
- description='Generate training and validation set of HierText')
- parser.add_argument('root_path', help='Root dir path of HierText')
- parser.add_argument(
- '--nproc', default=1, type=int, help='Number of processes')
- parser.add_argument(
- '--preserve-vertical',
- help='Preserve samples containing vertical texts',
- action='store_true')
- parser.add_argument(
- '--level',
- default='word',
- help='Crop word or line level instance',
- choices=['word', 'line'])
- args = parser.parse_args()
- return args
-
-
-def main():
- args = parse_args()
- root_path = args.root_path
- print('Processing training set...')
- convert_hiertext(
- root_path=root_path,
- split='train',
- level=args.level,
- preserve_vertical=args.preserve_vertical,
- nproc=args.nproc)
- print('Processing validation set...')
- convert_hiertext(
- root_path=root_path,
- split='val',
- level=args.level,
- preserve_vertical=args.preserve_vertical,
- nproc=args.nproc)
- print('Finish')
-
-
-if __name__ == '__main__':
- main()
diff --git a/pipeline/mmocr/mmocr/.mim/tools/dataset_converters/textrecog/ic11_converter.py b/pipeline/mmocr/mmocr/.mim/tools/dataset_converters/textrecog/ic11_converter.py
deleted file mode 100644
index 3de125d39bd87c137b2ed1d470fa6bcfd19836ba..0000000000000000000000000000000000000000
--- a/pipeline/mmocr/mmocr/.mim/tools/dataset_converters/textrecog/ic11_converter.py
+++ /dev/null
@@ -1,66 +0,0 @@
-# Copyright (c) OpenMMLab. All rights reserved.
-import argparse
-import os.path as osp
-
-from mmocr.utils import dump_ocr_data
-
-
-def convert_annotations(root_path, split):
- """Convert original annotations to mmocr format.
-
- The annotation format of this dataset is as the following:
- word_1.png, "flying"
- word_2.png, "today"
- word_3.png, "means"
- See the format of converted annotation in mmocr.utils.dump_ocr_data.
-
- Args:
- root_path (str): The root path of the dataset
- split (str): The split of dataset. Namely: Train or Test
- """
- assert isinstance(root_path, str)
- assert isinstance(split, str)
-
- img_info = []
- with open(
- osp.join(root_path, 'annotations',
- f'Challenge1_{split}_Task3_GT.txt'),
- encoding='"utf-8-sig') as f:
- annos = f.readlines()
- for anno in annos:
- # text may contain comma ','
- dst_img_name, word = anno.split(', "')
- word = word.replace('"\n', '')
-
- img_info.append({
- 'file_name': dst_img_name,
- 'anno_info': [{
- 'text': word
- }]
- })
-
- return img_info
-
-
-def parse_args():
- parser = argparse.ArgumentParser(
- description='Generate training and test set of IC11')
- parser.add_argument('root_path', help='Root dir path of IC11')
- args = parser.parse_args()
- return args
-
-
-def main():
- args = parse_args()
- root_path = args.root_path
-
- for split in ['Train', 'Test']:
- img_info = convert_annotations(root_path, split)
- dump_ocr_data(img_info,
- osp.join(root_path, f'{split.lower()}_label.json'),
- 'textrecog')
- print(f'{split} split converted.')
-
-
-if __name__ == '__main__':
- main()
diff --git a/pipeline/mmocr/mmocr/.mim/tools/dataset_converters/textrecog/ilst_converter.py b/pipeline/mmocr/mmocr/.mim/tools/dataset_converters/textrecog/ilst_converter.py
deleted file mode 100644
index 01ce4bd17f57bf1281fe3b254e39d37c0f92c7a7..0000000000000000000000000000000000000000
--- a/pipeline/mmocr/mmocr/.mim/tools/dataset_converters/textrecog/ilst_converter.py
+++ /dev/null
@@ -1,260 +0,0 @@
-# Copyright (c) OpenMMLab. All rights reserved.
-import argparse
-import os
-import os.path as osp
-import xml.etree.ElementTree as ET
-
-import mmcv
-import mmengine
-
-from mmocr.utils import crop_img, dump_ocr_data
-
-
-def collect_files(img_dir, gt_dir):
- """Collect all images and their corresponding groundtruth files.
-
- Args:
- img_dir (str): The image directory
- gt_dir (str): The groundtruth directory
-
- Returns:
- files (list): The list of tuples (img_file, groundtruth_file)
- """
- assert isinstance(img_dir, str)
- assert img_dir
- assert isinstance(gt_dir, str)
- assert gt_dir
-
- ann_list, imgs_list = [], []
- for img_file in os.listdir(img_dir):
- ann_path = osp.join(gt_dir, img_file.split('.')[0] + '.xml')
- if os.path.exists(ann_path):
- ann_list.append(ann_path)
- imgs_list.append(osp.join(img_dir, img_file))
-
- files = list(zip(imgs_list, ann_list))
- assert len(files), f'No images found in {img_dir}'
- print(f'Loaded {len(files)} images from {img_dir}')
-
- return files
-
-
-def collect_annotations(files, nproc=1):
- """Collect the annotation information.
-
- Args:
- files (list): The list of tuples (image_file, groundtruth_file)
- nproc (int): The number of process to collect annotations
-
- Returns:
- images (list): The list of image information dicts
- """
- assert isinstance(files, list)
- assert isinstance(nproc, int)
-
- if nproc > 1:
- images = mmengine.track_parallel_progress(
- load_img_info, files, nproc=nproc)
- else:
- images = mmengine.track_progress(load_img_info, files)
-
- return images
-
-
-def load_img_info(files):
- """Load the information of one image.
-
- Args:
- files (tuple): The tuple of (img_file, groundtruth_file)
-
- Returns:
- img_info (dict): The dict of the img and annotation information
- """
- assert isinstance(files, tuple)
-
- img_file, gt_file = files
- assert osp.basename(gt_file).split('.')[0] == osp.basename(img_file).split(
- '.')[0]
- # read imgs while ignoring orientations
- img = mmcv.imread(img_file, 'unchanged')
-
- try:
- img_info = dict(
- file_name=osp.join(osp.basename(img_file)),
- height=img.shape[0],
- width=img.shape[1],
- segm_file=osp.join(osp.basename(gt_file)))
- except AttributeError:
- print(f'Skip broken img {img_file}')
- return None
-
- if osp.splitext(gt_file)[1] == '.xml':
- img_info = load_xml_info(gt_file, img_info)
- else:
- raise NotImplementedError
-
- return img_info
-
-
-def load_xml_info(gt_file, img_info):
- """Collect the annotation information.
-
- The annotation format is as the following:
-
- ...
-
- SMT
- Unspecified
- 0
- 0
-
- 157
- 294
- 237
- 357
-
-
-
- Args:
- gt_file (str): The path to ground-truth
- img_info (dict): The dict of the img and annotation information
-
- Returns:
- img_info (dict): The dict of the img and annotation information
- """
- obj = ET.parse(gt_file)
- root = obj.getroot()
- anno_info = []
- for object in root.iter('object'):
- word = object.find('name').text
- x1 = int(object.find('bndbox').find('xmin').text)
- y1 = int(object.find('bndbox').find('ymin').text)
- x2 = int(object.find('bndbox').find('xmax').text)
- y2 = int(object.find('bndbox').find('ymax').text)
-
- x = max(0, min(x1, x2))
- y = max(0, min(y1, y2))
- w, h = abs(x2 - x1), abs(y2 - y1)
- bbox = [x, y, x + w, y, x + w, y + h, x, y + h]
- anno = dict(bbox=bbox, word=word)
- anno_info.append(anno)
-
- img_info.update(anno_info=anno_info)
-
- return img_info
-
-
-def split_train_val_list(full_list, val_ratio):
- """Split list by val_ratio.
-
- Args:
- full_list (list): List to be splited
- val_ratio (float): Split ratio for val set
-
- return:
- list(list, list): Train_list and val_list
- """
- n_total = len(full_list)
- offset = int(n_total * val_ratio)
- if n_total == 0 or offset < 1:
- return [], full_list
- val_list = full_list[:offset]
- train_list = full_list[offset:]
- return [train_list, val_list]
-
-
-def generate_ann(root_path, image_infos, preserve_vertical, val_ratio):
- """Generate cropped annotations and label txt file.
-
- Args:
- root_path (str): The root path of the dataset
- split (str): The split of dataset. Namely: training or test
- image_infos (list[dict]): A list of dicts of the img and
- annotation information
- preserve_vertical (bool): Whether to preserve vertical texts
- val_ratio (float): Split ratio for val set
- """
-
- assert val_ratio <= 1.
-
- if val_ratio:
- image_infos = split_train_val_list(image_infos, val_ratio)
- splits = ['training', 'val']
-
- else:
- image_infos = [image_infos]
- splits = ['training']
-
- for i, split in enumerate(splits):
- dst_image_root = osp.join(root_path, 'crops', split)
- ignore_image_root = osp.join(root_path, 'ignores', split)
- dst_label_file = osp.join(root_path, f'{split}_label.json')
- os.makedirs(dst_image_root, exist_ok=True)
-
- img_info = []
- for image_info in image_infos[i]:
- index = 1
- src_img_path = osp.join(root_path, 'imgs', image_info['file_name'])
- image = mmcv.imread(src_img_path)
- src_img_root = image_info['file_name'].split('.')[0]
-
- for anno in image_info['anno_info']:
- word = anno['word']
- dst_img = crop_img(image, anno['bbox'], 0, 0)
- h, w, _ = dst_img.shape
-
- dst_img_name = f'{src_img_root}_{index}.png'
- index += 1
- # Skip invalid annotations
- if min(dst_img.shape) == 0:
- continue
- # Skip vertical texts
- if not preserve_vertical and h / w > 2 and split == 'training':
- dst_img_path = osp.join(ignore_image_root, dst_img_name)
- mmcv.imwrite(dst_img, dst_img_path)
- continue
-
- dst_img_path = osp.join(dst_image_root, dst_img_name)
- mmcv.imwrite(dst_img, dst_img_path)
- img_info.append({
- 'file_name': dst_img_name,
- 'anno_info': [{
- 'text': word
- }]
- })
-
- ensure_ascii = dict(ensure_ascii=False)
- dump_ocr_data(img_info, dst_label_file, 'textrecog', **ensure_ascii)
-
-
-def parse_args():
- parser = argparse.ArgumentParser(
- description='Generate training and val set of ILST ')
- parser.add_argument('root_path', help='Root dir path of ILST')
- parser.add_argument(
- '--preserve-vertical',
- help='Preserve samples containing vertical texts',
- action='store_true')
- parser.add_argument(
- '--val-ratio', help='Split ratio for val set', default=0., type=float)
- parser.add_argument(
- '--nproc', default=1, type=int, help='Number of processes')
- args = parser.parse_args(['data/IIIT-ILST'])
- return args
-
-
-def main():
- args = parse_args()
- root_path = args.root_path
- with mmengine.Timer(print_tmpl='It takes {}s to convert ILST annotation'):
- files = collect_files(
- osp.join(root_path, 'imgs'), osp.join(root_path, 'annotations'))
- image_infos = collect_annotations(files, nproc=args.nproc)
- # filter broken images
- image_infos = list(filter(None, image_infos))
- generate_ann(root_path, image_infos, args.preserve_vertical,
- args.val_ratio)
-
-
-if __name__ == '__main__':
- main()
diff --git a/pipeline/mmocr/mmocr/.mim/tools/dataset_converters/textrecog/imgur_converter.py b/pipeline/mmocr/mmocr/.mim/tools/dataset_converters/textrecog/imgur_converter.py
deleted file mode 100644
index 34d161eb3d39674dc5a64d98248e00d8760e0c42..0000000000000000000000000000000000000000
--- a/pipeline/mmocr/mmocr/.mim/tools/dataset_converters/textrecog/imgur_converter.py
+++ /dev/null
@@ -1,175 +0,0 @@
-# Copyright (c) OpenMMLab. All rights reserved.
-import argparse
-import math
-import os
-import os.path as osp
-
-import mmcv
-import mmengine
-import numpy as np
-
-from mmocr.utils import crop_img, dump_ocr_data
-
-
-def parse_args():
- parser = argparse.ArgumentParser(
- description='Generate training, validation and test set of IMGUR ')
- parser.add_argument('root_path', help='Root dir path of IMGUR')
- args = parser.parse_args()
-
- return args
-
-
-def collect_imgur_info(root_path, annotation_filename, print_every=1000):
-
- annotation_path = osp.join(root_path, 'annotations', annotation_filename)
- if not osp.exists(annotation_path):
- raise Exception(
- f'{annotation_path} not exists, please check and try again.')
-
- annotation = mmengine.load(annotation_path)
- images = annotation['index_to_ann_map'].keys()
- img_infos = []
- for i, img_name in enumerate(images):
- if i >= 0 and i % print_every == 0:
- print(f'{i}/{len(images)}')
-
- img_path = osp.join(root_path, 'imgs', img_name + '.jpg')
-
- # Skip not exist images
- if not osp.exists(img_path):
- continue
-
- img = mmcv.imread(img_path, 'unchanged')
-
- # Skip broken images
- if img is None:
- continue
-
- img_info = dict(
- file_name=img_name + '.jpg',
- height=img.shape[0],
- width=img.shape[1])
-
- anno_info = []
- for ann_id in annotation['index_to_ann_map'][img_name]:
- ann = annotation['ann_id'][ann_id]
-
- # The original annotation is oriented rects [x, y, w, h, a]
- box = np.fromstring(
- ann['bounding_box'][1:-2], sep=',', dtype=float)
- bbox = convert_oriented_box(box)
- word = ann['word']
-
- anno = dict(bbox=bbox, word=word)
- anno_info.append(anno)
- img_info.update(anno_info=anno_info)
- img_infos.append(img_info)
-
- return img_infos
-
-
-def convert_oriented_box(box):
-
- x_ctr, y_ctr, width, height, angle = box[:5]
- angle = -angle * math.pi / 180
-
- tl_x, tl_y, br_x, br_y = -width / 2, -height / 2, width / 2, height / 2
- rect = np.array([[tl_x, br_x, br_x, tl_x], [tl_y, tl_y, br_y, br_y]])
- R = np.array([[np.cos(angle), -np.sin(angle)],
- [np.sin(angle), np.cos(angle)]])
- poly = R.dot(rect)
- x0, x1, x2, x3 = poly[0, :4] + x_ctr
- y0, y1, y2, y3 = poly[1, :4] + y_ctr
- poly = np.array([x0, y0, x1, y1, x2, y2, x3, y3], dtype=np.float32)
- poly = get_best_begin_point_single(poly)
-
- return poly.tolist()
-
-
-def get_best_begin_point_single(coordinate):
-
- x1, y1, x2, y2, x3, y3, x4, y4 = coordinate
- xmin = min(x1, x2, x3, x4)
- ymin = min(y1, y2, y3, y4)
- xmax = max(x1, x2, x3, x4)
- ymax = max(y1, y2, y3, y4)
- combine = [[[x1, y1], [x2, y2], [x3, y3], [x4, y4]],
- [[x2, y2], [x3, y3], [x4, y4], [x1, y1]],
- [[x3, y3], [x4, y4], [x1, y1], [x2, y2]],
- [[x4, y4], [x1, y1], [x2, y2], [x3, y3]]]
- dst_coordinate = [[xmin, ymin], [xmax, ymin], [xmax, ymax], [xmin, ymax]]
- force = 100000000.0
- force_flag = 0
- for i in range(4):
- temp_force = cal_line_length(combine[i][0], dst_coordinate[0]) \
- + cal_line_length(combine[i][1], dst_coordinate[1]) \
- + cal_line_length(combine[i][2], dst_coordinate[2]) \
- + cal_line_length(combine[i][3], dst_coordinate[3])
- if temp_force < force:
- force = temp_force
- force_flag = i
- if force_flag != 0:
- pass
-
- return np.array(combine[force_flag]).reshape(8)
-
-
-def cal_line_length(point1, point2):
-
- return math.sqrt(
- math.pow(point1[0] - point2[0], 2) +
- math.pow(point1[1] - point2[1], 2))
-
-
-def generate_ann(root_path, split, image_infos):
-
- dst_image_root = osp.join(root_path, 'crops', split)
- dst_label_file = osp.join(root_path, f'{split}_label.json')
- os.makedirs(dst_image_root, exist_ok=True)
-
- img_info = []
- for image_info in image_infos:
- index = 1
- src_img_path = osp.join(root_path, 'imgs', image_info['file_name'])
- image = mmcv.imread(src_img_path)
- src_img_root = image_info['file_name'].split('.')[0]
-
- for anno in image_info['anno_info']:
- word = anno['word']
- dst_img = crop_img(image, anno['bbox'], 0, 0)
-
- # Skip invalid annotations
- if min(dst_img.shape) == 0:
- continue
-
- dst_img_name = f'{src_img_root}_{index}.png'
- index += 1
- dst_img_path = osp.join(dst_image_root, dst_img_name)
- mmcv.imwrite(dst_img, dst_img_path)
-
- img_info.append({
- 'file_name': dst_img_name,
- 'anno_info': [{
- 'text': word
- }]
- })
-
- dump_ocr_data(img_info, dst_label_file, 'textrecog')
-
-
-def main():
- args = parse_args()
- root_path = args.root_path
-
- for split in ['train', 'val', 'test']:
- print(f'Processing {split} set...')
- with mmengine.Timer(
- print_tmpl='It takes {}s to convert IMGUR annotation'):
- anno_infos = collect_imgur_info(
- root_path, f'imgur5k_annotations_{split}.json')
- generate_ann(root_path, split, anno_infos)
-
-
-if __name__ == '__main__':
- main()
diff --git a/pipeline/mmocr/mmocr/.mim/tools/dataset_converters/textrecog/kaist_converter.py b/pipeline/mmocr/mmocr/.mim/tools/dataset_converters/textrecog/kaist_converter.py
deleted file mode 100644
index 525e9be96d3652746d074bb2e924c62e7bb5b421..0000000000000000000000000000000000000000
--- a/pipeline/mmocr/mmocr/.mim/tools/dataset_converters/textrecog/kaist_converter.py
+++ /dev/null
@@ -1,259 +0,0 @@
-# Copyright (c) OpenMMLab. All rights reserved.
-import argparse
-import math
-import os
-import os.path as osp
-import xml.etree.ElementTree as ET
-
-import mmcv
-import mmengine
-
-from mmocr.utils import crop_img, dump_ocr_data
-
-
-def collect_files(img_dir, gt_dir, ratio):
- """Collect all images and their corresponding groundtruth files.
-
- Args:
- img_dir (str): The image directory
- gt_dir (str): The groundtruth directory
- ratio (float): Split ratio for val set
-
- Returns:
- files (list): The list of tuples (img_file, groundtruth_file)
- """
- assert isinstance(img_dir, str)
- assert img_dir
- assert isinstance(gt_dir, str)
- assert gt_dir
- assert isinstance(ratio, float)
- assert ratio < 1.0, 'val_ratio should be a float between 0.0 to 1.0'
-
- ann_list, imgs_list = [], []
- for img_file in os.listdir(img_dir):
- ann_list.append(osp.join(gt_dir, img_file.split('.')[0] + '.xml'))
- imgs_list.append(osp.join(img_dir, img_file))
-
- all_files = list(zip(sorted(imgs_list), sorted(ann_list)))
- assert len(all_files), f'No images found in {img_dir}'
- print(f'Loaded {len(all_files)} images from {img_dir}')
-
- trn_files, val_files = [], []
- if ratio > 0:
- for i, file in enumerate(all_files):
- if i % math.floor(1 / ratio):
- trn_files.append(file)
- else:
- val_files.append(file)
- else:
- trn_files, val_files = all_files, []
-
- print(f'training #{len(trn_files)}, val #{len(val_files)}')
-
- return trn_files, val_files
-
-
-def collect_annotations(files, nproc=1):
- """Collect the annotation information.
-
- Args:
- files (list): The list of tuples (image_file, groundtruth_file)
- nproc (int): The number of process to collect annotations
-
- Returns:
- images (list): The list of image information dicts
- """
- assert isinstance(files, list)
- assert isinstance(nproc, int)
-
- if nproc > 1:
- images = mmengine.track_parallel_progress(
- load_img_info, files, nproc=nproc)
- else:
- images = mmengine.track_progress(load_img_info, files)
-
- return images
-
-
-def load_img_info(files):
- """Load the information of one image.
-
- Args:
- files (tuple): The tuple of (img_file, groundtruth_file)
-
- Returns:
- img_info (dict): The dict of the img and annotation information
- """
- assert isinstance(files, tuple)
-
- img_file, gt_file = files
- assert osp.basename(gt_file).split('.')[0] == osp.basename(img_file).split(
- '.')[0]
- # read imgs while ignoring orientations
- img = mmcv.imread(img_file, 'unchanged')
-
- img_info = dict(
- file_name=osp.join(osp.basename(img_file)),
- height=img.shape[0],
- width=img.shape[1],
- segm_file=osp.join(osp.basename(gt_file)))
-
- if osp.splitext(gt_file)[1] == '.xml':
- img_info = load_xml_info(gt_file, img_info)
- else:
- raise NotImplementedError
-
- return img_info
-
-
-def load_xml_info(gt_file, img_info):
- """Collect the annotation information.
-
- Annotation Format
-
- DSC02306.JPG
-
-
-
-
-
-
-
-
-
-
-
-
- no
- 2
-
-
-
-
- Args:
- gt_file (str): The path to ground-truth
- img_info (dict): The dict of the img and annotation information
-
- Returns:
- img_info (dict): The dict of the img and annotation information
- """
-
- obj = ET.parse(gt_file)
- root = obj.getroot()
- anno_info = []
- for word in root.iter('word'):
- x, y = max(0, int(word.attrib['x'])), max(0, int(word.attrib['y']))
- w, h = int(word.attrib['width']), int(word.attrib['height'])
- bbox = [x, y, x + w, y, x + w, y + h, x, y + h]
- chars = []
- for character in word.iter('character'):
- chars.append(character.attrib['char'])
- word = ''.join(chars)
- if len(word) == 0:
- continue
- anno = dict(bbox=bbox, word=word)
- anno_info.append(anno)
-
- img_info.update(anno_info=anno_info)
-
- return img_info
-
-
-def generate_ann(root_path, split, image_infos, preserve_vertical):
- """Generate cropped annotations and label txt file.
-
- Args:
- root_path (str): The root path of the dataset
- split (str): The split of dataset. Namely: training or test
- image_infos (list[dict]): A list of dicts of the img and
- annotation information
- preserve_vertical (bool): Whether to preserve vertical texts
- format (str): Annotation format, should be either 'txt' or 'jsonl'
- """
-
- dst_image_root = osp.join(root_path, 'crops', split)
- ignore_image_root = osp.join(root_path, 'ignores', split)
- if split == 'training':
- dst_label_file = osp.join(root_path, 'train_label.json')
- elif split == 'val':
- dst_label_file = osp.join(root_path, 'val_label.json')
- mmengine.mkdir_or_exist(dst_image_root)
- mmengine.mkdir_or_exist(ignore_image_root)
-
- img_info = []
- for image_info in image_infos:
- index = 1
- src_img_path = osp.join(root_path, 'imgs', image_info['file_name'])
- image = mmcv.imread(src_img_path)
- src_img_root = image_info['file_name'].split('.')[0]
-
- for anno in image_info['anno_info']:
- word = anno['word']
- dst_img = crop_img(image, anno['bbox'], 0, 0)
- h, w, _ = dst_img.shape
-
- dst_img_name = f'{src_img_root}_{index}.png'
- index += 1
- # Skip invalid annotations
- if min(dst_img.shape) == 0:
- continue
- # Filter out vertical texts
- if not preserve_vertical and h / w > 2:
- dst_img_path = osp.join(ignore_image_root, dst_img_name)
- mmcv.imwrite(dst_img, dst_img_path)
- continue
-
- dst_img_path = osp.join(dst_image_root, dst_img_name)
- mmcv.imwrite(dst_img, dst_img_path)
-
- img_info.append({
- 'file_name': dst_img_name,
- 'anno_info': [{
- 'text': word
- }]
- })
-
- ensure_ascii = dict(ensure_ascii=False)
- dump_ocr_data(img_info, dst_label_file, 'textrecog', **ensure_ascii)
-
-
-def parse_args():
- parser = argparse.ArgumentParser(
- description='Generate training and val set of KAIST ')
- parser.add_argument('root_path', help='Root dir path of KAIST')
- parser.add_argument(
- '--val-ratio', help='Split ratio for val set', default=0.0, type=float)
- parser.add_argument(
- '--preserve-vertical',
- help='Preserve samples containing vertical texts',
- action='store_true')
- parser.add_argument(
- '--nproc', default=1, type=int, help='Number of process')
- args = parser.parse_args()
- return args
-
-
-def main():
- args = parse_args()
- root_path = args.root_path
- ratio = args.val_ratio
-
- trn_files, val_files = collect_files(
- osp.join(root_path, 'imgs'), osp.join(root_path, 'annotations'), ratio)
-
- # Train set
- trn_infos = collect_annotations(trn_files, nproc=args.nproc)
- with mmengine.Timer(
- print_tmpl='It takes {}s to convert KAIST Training annotation'):
- generate_ann(root_path, 'training', trn_infos, args.preserve_vertical)
-
- # Val set
- if len(val_files) > 0:
- val_infos = collect_annotations(val_files, nproc=args.nproc)
- with mmengine.Timer(
- print_tmpl='It takes {}s to convert KAIST Val annotation'):
- generate_ann(root_path, 'val', val_infos, args.preserve_vertical)
-
-
-if __name__ == '__main__':
- main()
diff --git a/pipeline/mmocr/mmocr/.mim/tools/dataset_converters/textrecog/lmdb_converter.py b/pipeline/mmocr/mmocr/.mim/tools/dataset_converters/textrecog/lmdb_converter.py
deleted file mode 100644
index 68afd28f3cc41ad819faf478e5068e787c4fb32b..0000000000000000000000000000000000000000
--- a/pipeline/mmocr/mmocr/.mim/tools/dataset_converters/textrecog/lmdb_converter.py
+++ /dev/null
@@ -1,175 +0,0 @@
-# Copyright (c) OpenMMLab. All rights reserved.
-import argparse
-import json
-import os
-import os.path as osp
-
-import cv2
-import lmdb
-import numpy as np
-
-from mmocr.utils import list_from_file
-
-
-def parse_line(line, format):
- if format == 'txt':
- img_name, text = line.split(' ')
- else:
- line = json.loads(line)
- img_name = line['filename']
- text = line['text']
- return img_name, text
-
-
-def check_image_is_valid(imageBin):
- if imageBin is None:
- return False
- imageBuf = np.frombuffer(imageBin, dtype=np.uint8)
- img = cv2.imdecode(imageBuf, cv2.IMREAD_GRAYSCALE)
- imgH, imgW = img.shape[0], img.shape[1]
- if imgH * imgW == 0:
- return False
- return True
-
-
-def write_cache(env, cache):
- with env.begin(write=True) as txn:
- cursor = txn.cursor()
- cursor.putmulti(cache, dupdata=False, overwrite=True)
-
-
-def recog2lmdb(img_root,
- label_path,
- output,
- label_format='txt',
- label_only=False,
- batch_size=1000,
- encoding='utf-8',
- lmdb_map_size=1099511627776,
- verify=True):
- """Create text recognition dataset to LMDB format.
-
- Args:
- img_root (str): Path to images.
- label_path (str): Path to label file.
- output (str): LMDB output path.
- label_format (str): Format of the label file, either txt or jsonl.
- label_only (bool): Only convert label to lmdb format.
- batch_size (int): Number of files written to the cache each time.
- encoding (str): Label encoding method.
- lmdb_map_size (int): Maximum size database may grow to.
- verify (bool): If true, check the validity of
- every image.Defaults to True.
-
- E.g.
- This function supports MMOCR's recognition data format and the label file
- can be txt or jsonl, as follows:
-
- โโโimg_root
- | |โโ img1.jpg
- | |โโ img2.jpg
- | |โโ ...
- |โโlabel.txt (or label.jsonl)
-
- label.txt: img1.jpg HELLO
- img2.jpg WORLD
- ...
-
- label.jsonl: {'filename':'img1.jpg', 'text':'HELLO'}
- {'filename':'img2.jpg', 'text':'WORLD'}
- ...
- """
- # check label format
- assert osp.basename(label_path).split('.')[-1] == label_format
- # create lmdb env
- os.makedirs(output, exist_ok=True)
- env = lmdb.open(output, map_size=lmdb_map_size)
- # load label file
- anno_list = list_from_file(label_path, encoding=encoding)
- cache = []
- # index start from 1
- cnt = 1
- n_samples = len(anno_list)
- for anno in anno_list:
- label_key = 'label-%09d'.encode(encoding) % cnt
- img_name, text = parse_line(anno, label_format)
- if label_only:
- # convert only labels to lmdb
- line = json.dumps(
- dict(filename=img_name, text=text), ensure_ascii=False)
- cache.append((label_key, line.encode(encoding)))
- else:
- # convert both images and labels to lmdb
- img_path = osp.join(img_root, img_name)
- if not osp.exists(img_path):
- print('%s does not exist' % img_path)
- continue
- with open(img_path, 'rb') as f:
- image_bin = f.read()
- if verify:
- try:
- if not check_image_is_valid(image_bin):
- print('%s is not a valid image' % img_path)
- continue
- except Exception:
- print('error occurred at ', img_name)
- image_key = 'image-%09d'.encode(encoding) % cnt
- cache.append((image_key, image_bin))
- cache.append((label_key, text.encode(encoding)))
-
- if cnt % batch_size == 0:
- write_cache(env, cache)
- cache = []
- print('Written %d / %d' % (cnt, n_samples))
- cnt += 1
- n_samples = cnt - 1
- cache.append(
- ('num-samples'.encode(encoding), str(n_samples).encode(encoding)))
- write_cache(env, cache)
- print('Created lmdb dataset with %d samples' % n_samples)
-
-
-def main():
- parser = argparse.ArgumentParser()
- parser.add_argument('label_path', type=str, help='Path to label file')
- parser.add_argument('output', type=str, help='Output lmdb path')
- parser.add_argument(
- '--img-root', '-i', type=str, help='Input imglist path')
- parser.add_argument(
- '--label-only',
- action='store_true',
- help='Only converter label to lmdb')
- parser.add_argument(
- '--label-format',
- '-f',
- default='txt',
- choices=['txt', 'jsonl'],
- help='The format of the label file, either txt or jsonl')
- parser.add_argument(
- '--batch-size',
- '-b',
- type=int,
- default=1000,
- help='Processing batch size, defaults to 1000')
- parser.add_argument(
- '--encoding',
- '-e',
- type=str,
- default='utf8',
- help='Bytes coding scheme, defaults to utf8')
- parser.add_argument(
- '--lmdb-map-size',
- '-m',
- type=int,
- default=1099511627776,
- help='Maximum size database may grow to, '
- 'defaults to 1099511627776 bytes (1TB)')
- opt = parser.parse_args()
-
- assert opt.img_root or opt.label_only
- recog2lmdb(opt.img_root, opt.label_path, opt.output, opt.label_format,
- opt.label_only, opt.batch_size, opt.encoding, opt.lmdb_map_size)
-
-
-if __name__ == '__main__':
- main()
diff --git a/pipeline/mmocr/mmocr/.mim/tools/dataset_converters/textrecog/lsvt_converter.py b/pipeline/mmocr/mmocr/.mim/tools/dataset_converters/textrecog/lsvt_converter.py
deleted file mode 100644
index b1f581974967cc6eebb8491fd163bd026e925fbb..0000000000000000000000000000000000000000
--- a/pipeline/mmocr/mmocr/.mim/tools/dataset_converters/textrecog/lsvt_converter.py
+++ /dev/null
@@ -1,186 +0,0 @@
-# Copyright (c) OpenMMLab. All rights reserved.
-import argparse
-import math
-import os.path as osp
-from functools import partial
-
-import mmcv
-import mmengine
-
-from mmocr.utils import dump_ocr_data
-
-
-def parse_args():
- parser = argparse.ArgumentParser(
- description='Generate training and validation set of LSVT ')
- parser.add_argument('root_path', help='Root dir path of LSVT')
- parser.add_argument(
- '--val-ratio', help='Split ratio for val set', default=0.0, type=float)
- parser.add_argument(
- '--nproc', default=1, type=int, help='Number of processes')
- parser.add_argument(
- '--preserve-vertical',
- help='Preserve samples containing vertical texts',
- action='store_true')
- args = parser.parse_args()
- return args
-
-
-def process_img(args, dst_image_root, ignore_image_root, preserve_vertical,
- split):
- # Dirty hack for multi-processing
- img_idx, img_info, anns = args
- src_img = mmcv.imread(img_info['file_name'])
- img_info = []
- for ann_idx, ann in enumerate(anns):
- segmentation = []
- for x, y in ann['points']:
- segmentation.append(max(0, x))
- segmentation.append(max(0, y))
- xs, ys = segmentation[::2], segmentation[1::2]
- x, y = min(xs), min(ys)
- w, h = max(xs) - x, max(ys) - y
- text_label = ann['transcription']
-
- dst_img = src_img[y:y + h, x:x + w]
- dst_img_name = f'img_{img_idx}_{ann_idx}.jpg'
-
- if not preserve_vertical and h / w > 2 and split == 'train':
- dst_img_path = osp.join(ignore_image_root, dst_img_name)
- mmcv.imwrite(dst_img, dst_img_path)
- continue
-
- dst_img_path = osp.join(dst_image_root, dst_img_name)
- mmcv.imwrite(dst_img, dst_img_path)
-
- img_info.append({
- 'file_name': dst_img_name,
- 'anno_info': [{
- 'text': text_label
- }]
- })
-
- return img_info
-
-
-def convert_lsvt(root_path,
- split,
- ratio,
- preserve_vertical,
- nproc,
- img_start_idx=0):
- """Collect the annotation information and crop the images.
-
- The annotation format is as the following:
- [
- {'gt_1234': # 'gt_1234' is file name
- [
- {
- 'transcription': 'ไธ็ซๅผ่ดญ็ฉไธญๅฟ',
- 'points': [[45, 272], [215, 273], [212, 296], [45, 290]]
- 'illegibility': False
- }, ...
- ]
- }
- ]
-
-
- Args:
- root_path (str): The root path of the dataset
- split (str): The split of dataset. Namely: training or val
- ratio (float): Split ratio for val set
- preserve_vertical (bool): Whether to preserve vertical texts
- nproc (int): The number of process to collect annotations
- img_start_idx (int): Index of start image
-
- Returns:
- img_info (dict): The dict of the img and annotation information
- """
-
- annotation_path = osp.join(root_path, 'annotations/train_full_labels.json')
- if not osp.exists(annotation_path):
- raise Exception(
- f'{annotation_path} not exists, please check and try again.')
-
- annotation = mmengine.load(annotation_path)
- # outputs
- dst_label_file = osp.join(root_path, f'{split}_label.json')
- dst_image_root = osp.join(root_path, 'crops', split)
- ignore_image_root = osp.join(root_path, 'ignores', split)
- src_image_root = osp.join(root_path, 'imgs')
- mmengine.mkdir_or_exist(dst_image_root)
- mmengine.mkdir_or_exist(ignore_image_root)
-
- process_img_with_path = partial(
- process_img,
- dst_image_root=dst_image_root,
- ignore_image_root=ignore_image_root,
- preserve_vertical=preserve_vertical,
- split=split)
-
- img_prefixes = annotation.keys()
-
- trn_files, val_files = [], []
- if ratio > 0:
- for i, file in enumerate(img_prefixes):
- if i % math.floor(1 / ratio):
- trn_files.append(file)
- else:
- val_files.append(file)
- else:
- trn_files, val_files = img_prefixes, []
- print(f'training #{len(trn_files)}, val #{len(val_files)}')
-
- if split == 'train':
- img_prefixes = trn_files
- elif split == 'val':
- img_prefixes = val_files
- else:
- raise NotImplementedError
-
- tasks = []
- idx = 0
- for img_idx, prefix in enumerate(img_prefixes):
- img_file = osp.join(src_image_root, prefix + '.jpg')
- img_info = {'file_name': img_file}
- # Skip not exist images
- if not osp.exists(img_file):
- continue
- tasks.append((img_idx + img_start_idx, img_info, annotation[prefix]))
- idx = idx + 1
-
- labels_list = mmengine.track_parallel_progress(
- process_img_with_path, tasks, keep_order=True, nproc=nproc)
- final_labels = []
- for label_list in labels_list:
- final_labels += label_list
-
- dump_ocr_data(final_labels, dst_label_file, 'textrecog')
-
- return idx
-
-
-def main():
- args = parse_args()
- root_path = args.root_path
- print('Processing training set...')
- num_train_imgs = convert_lsvt(
- root_path=root_path,
- split='train',
- ratio=args.val_ratio,
- preserve_vertical=args.preserve_vertical,
- nproc=args.nproc)
- if args.val_ratio > 0:
- print('Processing validation set...')
- convert_lsvt(
- root_path=root_path,
- split='val',
- ratio=args.val_ratio,
- preserve_vertical=args.preserve_vertical,
- nproc=args.nproc,
- img_start_idx=num_train_imgs)
- print('Finish')
-
-
-if __name__ == '__main__':
- main()
diff --git a/pipeline/mmocr/mmocr/.mim/tools/dataset_converters/textrecog/lv_converter.py b/pipeline/mmocr/mmocr/.mim/tools/dataset_converters/textrecog/lv_converter.py
deleted file mode 100644
index d22c60b224d7fb122ebe26b2729650a961aac992..0000000000000000000000000000000000000000
--- a/pipeline/mmocr/mmocr/.mim/tools/dataset_converters/textrecog/lv_converter.py
+++ /dev/null
@@ -1,68 +0,0 @@
-# Copyright (c) OpenMMLab. All rights reserved.
-import argparse
-import os.path as osp
-
-from mmocr.utils import dump_ocr_data
-
-
-def convert_annotations(root_path, split):
- """Convert original annotations to mmocr format.
-
- The annotation format is as the following:
- Crops/val/11/1/1.png weighted
- Crops/val/11/1/2.png 26
- Crops/val/11/1/3.png casting
- Crops/val/11/1/4.png 28
- After this module, the annotation has been changed to the format below:
- jsonl:
- {'filename': 'Crops/val/11/1/1.png', 'text': 'weighted'}
- {'filename': 'Crops/val/11/1/1.png', 'text': '26'}
- {'filename': 'Crops/val/11/1/1.png', 'text': 'casting'}
- {'filename': 'Crops/val/11/1/1.png', 'text': '28'}
-
- Args:
- root_path (str): The root path of the dataset
- split (str): The split of dataset. Namely: training or test
- """
- assert isinstance(root_path, str)
- assert isinstance(split, str)
-
- img_info = []
- with open(
- osp.join(root_path, f'{split}_label.txt'),
- encoding='"utf-8-sig') as f:
- annos = f.readlines()
- for anno in annos:
- if anno:
- # Text may contain spaces
- dst_img_name, word = anno.split('png ')
- word = word.strip('\n')
- img_info.append({
- 'file_name': dst_img_name + 'png',
- 'anno_info': [{
- 'text': word
- }]
- })
- dump_ocr_data(img_info, osp.join(root_path, f'{split.lower()}_label.json'),
- 'textrecog')
-
-
-def parse_args():
- parser = argparse.ArgumentParser(
- description='Generate training and test set of Lecture Video DB')
- parser.add_argument('root_path', help='Root dir path of Lecture Video DB')
- args = parser.parse_args()
- return args
-
-
-def main():
- args = parse_args()
- root_path = args.root_path
-
- for split in ['train', 'val', 'test']:
- convert_annotations(root_path, split)
- print(f'{split} split converted.')
-
-
-if __name__ == '__main__':
- main()
diff --git a/pipeline/mmocr/mmocr/.mim/tools/dataset_converters/textrecog/mtwi_converter.py b/pipeline/mmocr/mmocr/.mim/tools/dataset_converters/textrecog/mtwi_converter.py
deleted file mode 100644
index 86d4e4e895b36a225fd2e916b675dcdf3abe8d17..0000000000000000000000000000000000000000
--- a/pipeline/mmocr/mmocr/.mim/tools/dataset_converters/textrecog/mtwi_converter.py
+++ /dev/null
@@ -1,251 +0,0 @@
-# Copyright (c) OpenMMLab. All rights reserved.
-import argparse
-import math
-import os
-import os.path as osp
-
-import cv2
-import mmcv
-import mmengine
-from PIL import Image
-
-from mmocr.utils import crop_img, dump_ocr_data
-
-
-def collect_files(img_dir, gt_dir, ratio):
- """Collect all images and their corresponding groundtruth files.
- Args:
- img_dir (str): The image directory
- gt_dir (str): The groundtruth directory
- ratio (float): Split ratio for val set
-
- Returns:
- files (list): The list of tuples (img_file, groundtruth_file)
- """
- assert isinstance(img_dir, str)
- assert img_dir
- assert isinstance(gt_dir, str)
- assert gt_dir
- assert isinstance(ratio, float)
- assert ratio < 1.0, 'val_ratio should be a float between 0.0 to 1.0'
-
- ann_list, imgs_list = [], []
- for ann_file in os.listdir(gt_dir):
- img_file = osp.join(img_dir, ann_file.replace('txt', 'jpg'))
- # This dataset contains some images obtained from .gif,
- # which cannot be loaded by mmcv.imread(), convert them
- # to RGB mode.
- try:
- if mmcv.imread(img_file) is None:
- print(f'Convert {img_file} to RGB mode.')
- img = Image.open(img_file)
- img = img.convert('RGB')
- img.save(img_file)
- except cv2.error:
- print(f'Skip broken img {img_file}')
- continue
-
- ann_list.append(osp.join(gt_dir, ann_file))
- imgs_list.append(img_file)
-
- all_files = list(zip(imgs_list, ann_list))
- assert len(all_files), f'No images found in {img_dir}'
- print(f'Loaded {len(all_files)} images from {img_dir}')
-
- trn_files, val_files = [], []
- if ratio > 0:
- for i, file in enumerate(all_files):
- if i % math.floor(1 / ratio):
- trn_files.append(file)
- else:
- val_files.append(file)
- else:
- trn_files, val_files = all_files, []
-
- print(f'training #{len(trn_files)}, val #{len(val_files)}')
-
- return trn_files, val_files
-
-
-def collect_annotations(files, nproc=1):
- """Collect the annotation information.
- Args:
- files (list): The list of tuples (image_file, groundtruth_file)
- nproc (int): The number of process to collect annotations
-
- Returns:
- images (list): The list of image information dicts
- """
- assert isinstance(files, list)
- assert isinstance(nproc, int)
-
- if nproc > 1:
- images = mmengine.track_parallel_progress(
- load_img_info, files, nproc=nproc)
- else:
- images = mmengine.track_progress(load_img_info, files)
-
- return images
-
-
-def load_img_info(files):
- """Load the information of one image.
- Args:
- files (tuple): The tuple of (img_file, groundtruth_file)
-
- Returns:
- img_info (dict): The dict of the img and annotation information
- """
- assert isinstance(files, tuple)
-
- img_file, gt_file = files
- assert osp.basename(gt_file).split('.')[0] == osp.basename(img_file).split(
- '.')[0]
- # read imgs while ignoring orientations
- img = mmcv.imread(img_file)
-
- img_info = dict(
- file_name=osp.join(osp.basename(img_file)),
- height=img.shape[0],
- width=img.shape[1],
- segm_file=osp.join(osp.basename(gt_file)))
-
- if osp.splitext(gt_file)[1] == '.txt':
- img_info = load_txt_info(gt_file, img_info)
- else:
- raise NotImplementedError
-
- return img_info
-
-
-def load_txt_info(gt_file, img_info):
- """Collect the annotation information.
-
- The annotation format is as the following:
- x1,y1,x2,y2,x3,y3,x4,y4,text
-
- 45.45,226.83,11.87,181.79,183.84,13.1,233.79,49.95,ๆถๅฐ่ข่ข
- 345.98,311.18,345.98,347.21,462.26,347.21,462.26,311.18,73774
- 462.26,292.34,461.44,299.71,502.39,299.71,502.39,292.34,73/74/737
-
- Args:
- gt_file (str): The path to ground-truth
- img_info (dict): The dict of the img and annotation information
-
- Returns:
- img_info (dict): The dict of the img and annotation information
- """
-
- anno_info = []
- with open(gt_file) as f:
- lines = f.readlines()
- for line in lines:
- points = line.split(',')[0:8]
- word = line.split(',')[8].rstrip('\n')
- if word == '###':
- continue
- bbox = [math.floor(float(pt)) for pt in points]
- anno = dict(bbox=bbox, word=word)
- anno_info.append(anno)
-
- img_info.update(anno_info=anno_info)
-
- return img_info
-
-
-def generate_ann(root_path, split, image_infos, preserve_vertical):
- """Generate cropped annotations and label txt file.
-
- Args:
- root_path (str): The root path of the dataset
- split (str): The split of dataset. Namely: training or test
- image_infos (list[dict]): A list of dicts of the img and
- annotation information
- preserve_vertical (bool): Whether to preserve vertical texts
- """
- print('Cropping images...')
- dst_image_root = osp.join(root_path, 'crops', split)
- ignore_image_root = osp.join(root_path, 'ignores', split)
- if split == 'training':
- dst_label_file = osp.join(root_path, 'train_label.json')
- elif split == 'val':
- dst_label_file = osp.join(root_path, 'val_label.json')
- mmengine.mkdir_or_exist(dst_image_root)
- mmengine.mkdir_or_exist(ignore_image_root)
-
- img_info = []
- for image_info in image_infos:
- index = 1
- src_img_path = osp.join(root_path, 'imgs', image_info['file_name'])
- image = mmcv.imread(src_img_path)
- src_img_root = image_info['file_name'].split('.')[0]
-
- for anno in image_info['anno_info']:
- word = anno['word']
- dst_img = crop_img(image, anno['bbox'], 0, 0)
- h, w, _ = dst_img.shape
-
- dst_img_name = f'{src_img_root}_{index}.png'
- index += 1
- # Skip invalid annotations
- if min(dst_img.shape) == 0:
- continue
- # Skip vertical texts
- if not preserve_vertical and h / w > 2 and split == 'training':
- dst_img_path = osp.join(ignore_image_root, dst_img_name)
- mmcv.imwrite(dst_img, dst_img_path)
- continue
-
- dst_img_path = osp.join(dst_image_root, dst_img_name)
- mmcv.imwrite(dst_img, dst_img_path)
-
- img_info.append({
- 'file_name': dst_img_name,
- 'anno_info': [{
- 'text': word
- }]
- })
-
- dump_ocr_data(img_info, dst_label_file, 'textrecog')
-
-
-def parse_args():
- parser = argparse.ArgumentParser(
- description='Generate training and val set of MTWI.')
- parser.add_argument('root_path', help='Root dir path of MTWI')
- parser.add_argument(
- '--val-ratio', help='Split ratio for val set', default=0.0, type=float)
- parser.add_argument(
- '--preserve-vertical',
- help='Preserve samples containing vertical texts',
- action='store_true')
- parser.add_argument(
- '--nproc', default=1, type=int, help='Number of process')
- args = parser.parse_args()
- return args
-
-
-def main():
- args = parse_args()
- root_path = args.root_path
- ratio = args.val_ratio
-
- trn_files, val_files = collect_files(
- osp.join(root_path, 'imgs'), osp.join(root_path, 'annotations'), ratio)
-
- # Train set
- trn_infos = collect_annotations(trn_files, nproc=args.nproc)
- with mmengine.Timer(
- print_tmpl='It takes {}s to convert MTWI Training annotation'):
- generate_ann(root_path, 'training', trn_infos, args.preserve_vertical)
-
- # Val set
- if len(val_files) > 0:
- val_infos = collect_annotations(val_files, nproc=args.nproc)
- with mmengine.Timer(
- print_tmpl='It takes {}s to convert MTWI Val annotation'):
- generate_ann(root_path, 'val', val_infos, args.preserve_vertical)
-
-
-if __name__ == '__main__':
- main()
diff --git a/pipeline/mmocr/mmocr/.mim/tools/dataset_converters/textrecog/naf_converter.py b/pipeline/mmocr/mmocr/.mim/tools/dataset_converters/textrecog/naf_converter.py
deleted file mode 100644
index 3c6d84ad2d9613606e767bdd67793f65ae0e5239..0000000000000000000000000000000000000000
--- a/pipeline/mmocr/mmocr/.mim/tools/dataset_converters/textrecog/naf_converter.py
+++ /dev/null
@@ -1,272 +0,0 @@
-# Copyright (c) OpenMMLab. All rights reserved.
-import argparse
-import os.path as osp
-
-import mmcv
-import mmengine
-import numpy as np
-
-from mmocr.utils import crop_img, dump_ocr_data
-
-
-def collect_files(img_dir, gt_dir, split_info):
- """Collect all images and their corresponding groundtruth files.
-
- Args:
- img_dir (str): The image directory
- gt_dir (str): The groundtruth directory
- split_info (dict): The split information for train/val/test
-
- Returns:
- files (list): The list of tuples (img_file, groundtruth_file)
- """
- assert isinstance(img_dir, str)
- assert img_dir
- assert isinstance(gt_dir, str)
- assert gt_dir
- assert isinstance(split_info, dict)
- assert split_info
-
- ann_list, imgs_list = [], []
- for group in split_info:
- for img in split_info[group]:
- image_path = osp.join(img_dir, img)
- anno_path = osp.join(gt_dir, 'groups', group,
- img.replace('jpg', 'json'))
-
- # Filtering out the missing images
- if not osp.exists(image_path) or not osp.exists(anno_path):
- continue
-
- imgs_list.append(image_path)
- ann_list.append(anno_path)
-
- files = list(zip(imgs_list, ann_list))
- assert len(files), f'No images found in {img_dir}'
- print(f'Loaded {len(files)} images from {img_dir}')
-
- return files
-
-
-def collect_annotations(files, nproc=1):
- """Collect the annotation information.
-
- Args:
- files (list): The list of tuples (image_file, groundtruth_file)
- nproc (int): The number of process to collect annotations
-
- Returns:
- images (list): The list of image information dicts
- """
- assert isinstance(files, list)
- assert isinstance(nproc, int)
-
- if nproc > 1:
- images = mmengine.track_parallel_progress(
- load_img_info, files, nproc=nproc)
- else:
- images = mmengine.track_progress(load_img_info, files)
-
- return images
-
-
-def load_img_info(files):
- """Load the information of one image.
-
- Args:
- files (tuple): The tuple of (img_file, groundtruth_file)
-
- Returns:
- img_info (dict): The dict of the img and annotation information
- """
- assert isinstance(files, tuple)
-
- img_file, gt_file = files
- assert osp.basename(gt_file).split('.')[0] == osp.basename(img_file).split(
- '.')[0]
- # Read imgs while ignoring orientations
- img = mmcv.imread(img_file, 'unchanged')
-
- img_info = dict(
- file_name=osp.join(osp.basename(img_file)),
- height=img.shape[0],
- width=img.shape[1],
- segm_file=osp.join(osp.basename(gt_file)))
-
- if osp.splitext(gt_file)[1] == '.json':
- img_info = load_json_info(gt_file, img_info)
- else:
- raise NotImplementedError
-
- return img_info
-
-
-def load_json_info(gt_file, img_info):
- """Collect the annotation information.
-
- Annotation Format
- {
- 'filedBBs': [{
- 'poly_points': [[435,1406], [466,1406], [466,1439], [435,1439]],
- "type": "fieldCheckBox",
- "id": "f0",
- "isBlank": 1, # 0:text,1:handwriting,2:print,3:blank,4:signature,
- }], ...
- "transcriptions":{
- "f38": "CASE NUMBER",
- "f29": "July 1, 1949",
- "t20": "RANK",
- "t19": "COMPANY",
- ...
- }
- }
-
- Some special characters are used in the transcription:
- "ยซtextยป" indicates that "text" had a strikethrough
- "ยฟ" indicates the transcriber could not read a character
- "ยง" indicates the whole line or word was illegible
- "" (empty string) is if the field was blank
-
- Args:
- gt_file (str): The path to ground-truth
- img_info (dict): The dict of the img and annotation information
-
- Returns:
- img_info (dict): The dict of the img and annotation information
- """
- assert isinstance(gt_file, str)
- assert isinstance(img_info, dict)
-
- annotation = mmengine.load(gt_file)
- anno_info = []
-
- # 'textBBs' contains the printed texts of the table while 'fieldBBs'
- # contains the text filled by human.
- for box_type in ['textBBs', 'fieldBBs']:
- # NAF dataset only provides transcription GT for 'filedBBs', the
- # 'textBBs' is only used for detection task.
- if box_type == 'textBBs':
- continue
- for anno in annotation[box_type]:
- # Skip images containing detection annotations only
- if 'transcriptions' not in annotation.keys():
- continue
- # Skip boxes without recognition GT
- if anno['id'] not in annotation['transcriptions'].keys():
- continue
-
- word = annotation['transcriptions'][anno['id']]
- # Skip blank boxes
- if len(word) == 0:
- continue
-
- bbox = np.array(anno['poly_points']).reshape(1, 8)[0].tolist()
-
- anno = dict(bbox=bbox, word=word)
- anno_info.append(anno)
-
- img_info.update(anno_info=anno_info)
-
- return img_info
-
-
-def generate_ann(root_path, split, image_infos, preserve_vertical):
- """Generate cropped annotations and label txt file.
-
- Args:
- root_path (str): The root path of the dataset
- split (str): The split of dataset. Namely: training or test
- image_infos (list[dict]): A list of dicts of the img and
- annotation information
- preserve_vertical (bool): Whether to preserve vertical texts
- """
-
- dst_image_root = osp.join(root_path, 'crops', split)
- ignore_image_root = osp.join(root_path, 'ignores', split)
- if split == 'training':
- dst_label_file = osp.join(root_path, 'train_label.json')
- elif split == 'val':
- dst_label_file = osp.join(root_path, 'val_label.json')
- elif split == 'test':
- dst_label_file = osp.join(root_path, 'test_label.json')
- else:
- raise NotImplementedError
- mmengine.mkdir_or_exist(dst_image_root)
- mmengine.mkdir_or_exist(ignore_image_root)
-
- img_info = []
- for image_info in image_infos:
- index = 1
- src_img_path = osp.join(root_path, 'imgs', image_info['file_name'])
- image = mmcv.imread(src_img_path)
- src_img_root = image_info['file_name'].split('.')[0]
-
- for anno in image_info['anno_info']:
- word = anno['word']
- word = word.strip('\u202a') # Remove unicode control character
- word = word.replace('ยป',
- '').replace('ยซ',
- '') # Remove strikethrough flag
- dst_img = crop_img(image, anno['bbox'], 0, 0)
- h, w, _ = dst_img.shape
-
- dst_img_name = f'{src_img_root}_{index}.png'
- index += 1
- # Skip invalid and illegible annotations
- if min(dst_img.shape) == 0 or 'ยง' in word or 'ยฟ' in word or len(
- word) == 0:
- continue
- # Skip vertical texts
- # (Do Not Filter For Val and Test Split)
- if (not preserve_vertical and h / w > 2) and split == 'training':
- dst_img_path = osp.join(ignore_image_root, dst_img_name)
- mmcv.imwrite(dst_img, dst_img_path)
- continue
-
- dst_img_path = osp.join(dst_image_root, dst_img_name)
- mmcv.imwrite(dst_img, dst_img_path)
-
- img_info.append({
- 'file_name': dst_img_name,
- 'anno_info': [{
- 'text': word
- }]
- })
-
- dump_ocr_data(img_info, dst_label_file, 'textrecog')
-
-
-def parse_args():
- parser = argparse.ArgumentParser(
- description='Generate training, val, and test set of NAF ')
- parser.add_argument('root_path', help='Root dir path of NAF')
- parser.add_argument(
- '--preserve-vertical',
- help='Preserve samples containing vertical texts',
- action='store_true')
- parser.add_argument(
- '--nproc', default=1, type=int, help='Number of process')
- args = parser.parse_args()
- return args
-
-
-def main():
- args = parse_args()
- root_path = args.root_path
- split_info = mmengine.load(
- osp.join(root_path, 'annotations', 'train_valid_test_split.json'))
- split_info['training'] = split_info.pop('train')
- split_info['val'] = split_info.pop('valid')
- for split in ['training', 'val', 'test']:
- print(f'Processing {split} set...')
- with mmengine.Timer(
- print_tmpl='It takes {}s to convert NAF annotation'):
- files = collect_files(
- osp.join(root_path, 'imgs'),
- osp.join(root_path, 'annotations'), split_info[split])
- image_infos = collect_annotations(files, nproc=args.nproc)
- generate_ann(root_path, split, image_infos, args.preserve_vertical)
-
-
-if __name__ == '__main__':
- main()
diff --git a/pipeline/mmocr/mmocr/.mim/tools/dataset_converters/textrecog/openvino_converter.py b/pipeline/mmocr/mmocr/.mim/tools/dataset_converters/textrecog/openvino_converter.py
deleted file mode 100644
index 79b962bbdaa9ff35e8f726234fdd2c007fb8f105..0000000000000000000000000000000000000000
--- a/pipeline/mmocr/mmocr/.mim/tools/dataset_converters/textrecog/openvino_converter.py
+++ /dev/null
@@ -1,120 +0,0 @@
-# Copyright (c) OpenMMLab. All rights reserved.
-import math
-import os
-import os.path as osp
-from argparse import ArgumentParser
-from functools import partial
-
-import mmengine
-from PIL import Image
-
-from mmocr.utils import dump_ocr_data
-
-
-def parse_args():
- parser = ArgumentParser(description='Generate training and validation set '
- 'of OpenVINO annotations for Open '
- 'Images by cropping box image.')
- parser.add_argument(
- 'root_path', help='Root dir containing images and annotations')
- parser.add_argument(
- 'n_proc', default=1, type=int, help='Number of processes to run')
- args = parser.parse_args()
- return args
-
-
-def process_img(args, src_image_root, dst_image_root):
- # Dirty hack for multi-processing
- img_idx, img_info, anns = args
- src_img = Image.open(osp.join(src_image_root, img_info['file_name']))
- labels = []
- for ann_idx, ann in enumerate(anns):
- attrs = ann['attributes']
- text_label = attrs['transcription']
-
- # Ignore illegible or non-English words
- if not attrs['legible'] or attrs['language'] != 'english':
- continue
-
- x, y, w, h = ann['bbox']
- x, y = max(0, math.floor(x)), max(0, math.floor(y))
- w, h = math.ceil(w), math.ceil(h)
- dst_img = src_img.crop((x, y, x + w, y + h))
- dst_img_name = f'img_{img_idx}_{ann_idx}.jpg'
- dst_img_path = osp.join(dst_image_root, dst_img_name)
- # Preserve JPEG quality
- dst_img.save(dst_img_path, qtables=src_img.quantization)
- labels.append({
- 'file_name': dst_img_name,
- 'anno_info': [{
- 'text': text_label
- }]
- })
- src_img.close()
- return labels
-
-
-def convert_openimages(root_path,
- dst_image_path,
- dst_label_filename,
- annotation_filename,
- img_start_idx=0,
- nproc=1):
- annotation_path = osp.join(root_path, annotation_filename)
- if not osp.exists(annotation_path):
- raise Exception(
- f'{annotation_path} not exists, please check and try again.')
- src_image_root = root_path
-
- # outputs
- dst_label_file = osp.join(root_path, dst_label_filename)
- dst_image_root = osp.join(root_path, dst_image_path)
- os.makedirs(dst_image_root, exist_ok=True)
-
- annotation = mmengine.load(annotation_path)
-
- process_img_with_path = partial(
- process_img,
- src_image_root=src_image_root,
- dst_image_root=dst_image_root)
- tasks = []
- anns = {}
- for ann in annotation['annotations']:
- anns.setdefault(ann['image_id'], []).append(ann)
- for img_idx, img_info in enumerate(annotation['images']):
- tasks.append((img_idx + img_start_idx, img_info, anns[img_info['id']]))
- labels_list = mmengine.track_parallel_progress(
- process_img_with_path, tasks, keep_order=True, nproc=nproc)
- final_labels = []
- for label_list in labels_list:
- final_labels += label_list
- dump_ocr_data(final_labels, dst_label_file, 'textrecog')
- return len(annotation['images'])
-
-
-def main():
- args = parse_args()
- root_path = args.root_path
- print('Processing training set...')
- num_train_imgs = 0
- for s in '125f':
- num_train_imgs = convert_openimages(
- root_path=root_path,
- dst_image_path=f'image_{s}',
- dst_label_filename=f'train_{s}_label.json',
- annotation_filename=f'text_spotting_openimages_v5_train_{s}.json',
- img_start_idx=num_train_imgs,
- nproc=args.n_proc)
- print('Processing validation set...')
- convert_openimages(
- root_path=root_path,
- dst_image_path='image_val',
- dst_label_filename='val_label.json',
- annotation_filename='text_spotting_openimages_v5_validation.json',
- img_start_idx=num_train_imgs,
- nproc=args.n_proc)
- print('Finish')
-
-
-if __name__ == '__main__':
- main()
diff --git a/pipeline/mmocr/mmocr/.mim/tools/dataset_converters/textrecog/rctw_converter.py b/pipeline/mmocr/mmocr/.mim/tools/dataset_converters/textrecog/rctw_converter.py
deleted file mode 100644
index e3bfd2baa2a31594850ae2cae82040fb6e8e0362..0000000000000000000000000000000000000000
--- a/pipeline/mmocr/mmocr/.mim/tools/dataset_converters/textrecog/rctw_converter.py
+++ /dev/null
@@ -1,238 +0,0 @@
-# Copyright (c) OpenMMLab. All rights reserved.
-import argparse
-import math
-import os
-import os.path as osp
-
-import mmcv
-import mmengine
-
-from mmocr.utils import crop_img, dump_ocr_data
-
-
-def collect_files(img_dir, gt_dir, ratio):
- """Collect all images and their corresponding groundtruth files.
- Args:
- img_dir (str): The image directory
- gt_dir (str): The groundtruth directory
- ratio (float): Split ratio for val set
-
- Returns:
- files (list): The list of tuples (img_file, groundtruth_file)
- """
- assert isinstance(img_dir, str)
- assert img_dir
- assert isinstance(gt_dir, str)
- assert gt_dir
- assert isinstance(ratio, float)
- assert ratio < 1.0, 'val_ratio should be a float between 0.0 to 1.0'
-
- ann_list, imgs_list = [], []
- for ann_file in os.listdir(gt_dir):
- ann_list.append(osp.join(gt_dir, ann_file))
- imgs_list.append(osp.join(img_dir, ann_file.replace('txt', 'jpg')))
-
- all_files = list(zip(imgs_list, ann_list))
- assert len(all_files), f'No images found in {img_dir}'
- print(f'Loaded {len(all_files)} images from {img_dir}')
-
- trn_files, val_files = [], []
- if ratio > 0:
- for i, file in enumerate(all_files):
- if i % math.floor(1 / ratio):
- trn_files.append(file)
- else:
- val_files.append(file)
- else:
- trn_files, val_files = all_files, []
-
- print(f'training #{len(trn_files)}, val #{len(val_files)}')
-
- return trn_files, val_files
-
-
-def collect_annotations(files, nproc=1):
- """Collect the annotation information.
- Args:
- files (list): The list of tuples (image_file, groundtruth_file)
- nproc (int): The number of process to collect annotations
-
- Returns:
- images (list): The list of image information dicts
- """
- assert isinstance(files, list)
- assert isinstance(nproc, int)
-
- if nproc > 1:
- images = mmengine.track_parallel_progress(
- load_img_info, files, nproc=nproc)
- else:
- images = mmengine.track_progress(load_img_info, files)
-
- return images
-
-
-def load_img_info(files):
- """Load the information of one image.
- Args:
- files (tuple): The tuple of (img_file, groundtruth_file)
-
- Returns:
- img_info (dict): The dict of the img and annotation information
- """
- assert isinstance(files, tuple)
-
- img_file, gt_file = files
- assert osp.basename(gt_file).split('.')[0] == osp.basename(img_file).split(
- '.')[0]
- # read imgs while ignoring orientations
- img = mmcv.imread(img_file)
-
- img_info = dict(
- file_name=osp.join(osp.basename(img_file)),
- height=img.shape[0],
- width=img.shape[1],
- segm_file=osp.join(osp.basename(gt_file)))
-
- if osp.splitext(gt_file)[1] == '.txt':
- img_info = load_txt_info(gt_file, img_info)
- else:
- raise NotImplementedError
-
- return img_info
-
-
-def load_txt_info(gt_file, img_info):
- """Collect the annotation information.
-
- The annotation format is as the following:
- x1, y1, x2, y2, x3, y3, x4, y4, difficult, text
-
- 390,902,1856,902,1856,1225,390,1225,0,"้ๆฐ็ผ้"
- 1875,1170,2149,1170,2149,1245,1875,1245,0,"ๅไบ1989"
- 2054,1277,2190,1277,2190,1323,2054,1323,0,"ๅๅปบๅบ"
-
- Args:
- gt_file (str): The path to ground-truth
- img_info (dict): The dict of the img and annotation information
-
- Returns:
- img_info (dict): The dict of the img and annotation information
- """
-
- anno_info = []
- with open(gt_file, encoding='utf-8-sig') as f:
- lines = f.readlines()
- for line in lines:
- points = line.split(',')[0:8]
- word = line.split(',')[9].rstrip('\n').strip('"')
- difficult = 1 if line.split(',')[8] != '0' else 0
- bbox = [int(pt) for pt in points]
-
- if word == '###' or difficult == 1:
- continue
-
- anno = dict(bbox=bbox, word=word)
- anno_info.append(anno)
-
- img_info.update(anno_info=anno_info)
-
- return img_info
-
-
-def generate_ann(root_path, split, image_infos, preserve_vertical):
- """Generate cropped annotations and label txt file.
-
- Args:
- root_path (str): The root path of the dataset
- split (str): The split of dataset. Namely: training or val
- image_infos (list[dict]): A list of dicts of the img and
- annotation information
- preserve_vertical (bool): Whether to preserve vertical texts
- """
-
- dst_image_root = osp.join(root_path, 'crops', split)
- ignore_image_root = osp.join(root_path, 'ignores', split)
- if split == 'training':
- dst_label_file = osp.join(root_path, 'train_label.json')
- elif split == 'val':
- dst_label_file = osp.join(root_path, 'val_label.json')
- mmengine.mkdir_or_exist(dst_image_root)
- mmengine.mkdir_or_exist(ignore_image_root)
-
- img_info = []
- for image_info in image_infos:
- index = 1
- src_img_path = osp.join(root_path, 'imgs', image_info['file_name'])
- image = mmcv.imread(src_img_path)
- src_img_root = image_info['file_name'].split('.')[0]
-
- for anno in image_info['anno_info']:
- word = anno['word']
- dst_img = crop_img(image, anno['bbox'], 0, 0)
- h, w, _ = dst_img.shape
-
- dst_img_name = f'{src_img_root}_{index}.png'
- index += 1
- # Skip invalid annotations
- if min(dst_img.shape) == 0:
- continue
- # Filter out vertical texts
- if not preserve_vertical and h / w > 2:
- dst_img_path = osp.join(ignore_image_root, dst_img_name)
- mmcv.imwrite(dst_img, dst_img_path)
- continue
-
- dst_img_path = osp.join(dst_image_root, dst_img_name)
- mmcv.imwrite(dst_img, dst_img_path)
-
- img_info.append({
- 'file_name': dst_img_name,
- 'anno_info': [{
- 'text': word
- }]
- })
-
- dump_ocr_data(img_info, dst_label_file, 'textrecog')
-
-
-def parse_args():
- parser = argparse.ArgumentParser(
- description='Generate training and val set of RCTW.')
- parser.add_argument('root_path', help='Root dir path of RCTW')
- parser.add_argument(
- '--val-ratio', help='Split ratio for val set', default=0.0, type=float)
- parser.add_argument(
- '--nproc', default=1, type=int, help='Number of process')
- parser.add_argument(
- '--preserve-vertical',
- help='Preserve samples containing vertical texts',
- action='store_true')
- args = parser.parse_args()
- return args
-
-
-def main():
- args = parse_args()
- root_path = args.root_path
- ratio = args.val_ratio
-
- trn_files, val_files = collect_files(
- osp.join(root_path, 'imgs'), osp.join(root_path, 'annotations'), ratio)
-
- # Train set
- with mmengine.Timer(
- print_tmpl='It takes {}s to convert RCTW Training annotation'):
- trn_infos = collect_annotations(trn_files, nproc=args.nproc)
- generate_ann(root_path, 'training', trn_infos, args.preserve_vertical)
-
- # Val set
- if len(val_files) > 0:
- with mmengine.Timer(
- print_tmpl='It takes {}s to convert RCTW Val annotation'):
- val_infos = collect_annotations(val_files, nproc=args.nproc)
- generate_ann(root_path, 'val', val_infos, args.preserve_vertical)
-
-
-if __name__ == '__main__':
- main()
diff --git a/pipeline/mmocr/mmocr/.mim/tools/dataset_converters/textrecog/rects_converter.py b/pipeline/mmocr/mmocr/.mim/tools/dataset_converters/textrecog/rects_converter.py
deleted file mode 100644
index 630e81509715ef67edcb7dbf77542b399962d551..0000000000000000000000000000000000000000
--- a/pipeline/mmocr/mmocr/.mim/tools/dataset_converters/textrecog/rects_converter.py
+++ /dev/null
@@ -1,256 +0,0 @@
-# Copyright (c) OpenMMLab. All rights reserved.
-import argparse
-import math
-import os
-import os.path as osp
-
-import mmcv
-import mmengine
-
-from mmocr.utils import crop_img, dump_ocr_data
-
-
-def collect_files(img_dir, gt_dir, ratio):
- """Collect all images and their corresponding groundtruth files.
- Args:
- img_dir (str): The image directory
- gt_dir (str): The groundtruth directory
- ratio (float): Split ratio for val set
-
- Returns:
- files (list): The list of tuples (img_file, groundtruth_file)
- """
- assert isinstance(img_dir, str)
- assert img_dir
- assert isinstance(gt_dir, str)
- assert gt_dir
- assert isinstance(ratio, float)
- assert ratio < 1.0, 'val_ratio should be a float between 0.0 to 1.0'
-
- ann_list, imgs_list = [], []
- for ann_file in os.listdir(gt_dir):
- ann_list.append(osp.join(gt_dir, ann_file))
- imgs_list.append(osp.join(img_dir, ann_file.replace('json', 'jpg')))
-
- all_files = list(zip(imgs_list, ann_list))
- assert len(all_files), f'No images found in {img_dir}'
- print(f'Loaded {len(all_files)} images from {img_dir}')
-
- trn_files, val_files = [], []
- if ratio > 0:
- for i, file in enumerate(all_files):
- if i % math.floor(1 / ratio):
- trn_files.append(file)
- else:
- val_files.append(file)
- else:
- trn_files, val_files = all_files, []
-
- print(f'training #{len(trn_files)}, val #{len(val_files)}')
-
- return trn_files, val_files
-
-
-def collect_annotations(files, nproc=1):
- """Collect the annotation information.
- Args:
- files (list): The list of tuples (image_file, groundtruth_file)
- nproc (int): The number of process to collect annotations
-
- Returns:
- images (list): The list of image information dicts
- """
- assert isinstance(files, list)
- assert isinstance(nproc, int)
-
- if nproc > 1:
- images = mmengine.track_parallel_progress(
- load_img_info, files, nproc=nproc)
- else:
- images = mmengine.track_progress(load_img_info, files)
-
- return images
-
-
-def load_img_info(files):
- """Load the information of one image.
- Args:
- files (tuple): The tuple of (img_file, groundtruth_file)
-
- Returns:
- img_info (dict): The dict of the img and annotation information
- """
- assert isinstance(files, tuple)
-
- img_file, gt_file = files
- assert osp.basename(gt_file).split('.')[0] == osp.basename(img_file).split(
- '.')[0]
- # read imgs while ignoring orientations
- img = mmcv.imread(img_file)
-
- img_info = dict(
- file_name=osp.join(osp.basename(img_file)),
- height=img.shape[0],
- width=img.shape[1],
- segm_file=osp.join(osp.basename(gt_file)))
-
- if osp.splitext(gt_file)[1] == '.json':
- img_info = load_json_info(gt_file, img_info)
- else:
- raise NotImplementedError
-
- return img_info
-
-
-def load_json_info(gt_file, img_info):
- """Collect the annotation information.
-
- The annotation format is as the following:
-
- {
- "chars": [
- {
- "ignore": 0,
- "transcription": "H",
- "points": [25, 175, 112, 175, 112, 286, 25, 286]
- },
- {
- "ignore": 0,
- "transcription": "O",
- "points": [102, 182, 210, 182, 210, 273, 102, 273]
- }, ...
- ]
- "lines": [
- {
- "ignore": 0,
- "transcription": "HOKI",
- "points": [23, 173, 327, 180, 327, 290, 23, 283]
- },
- {
- "ignore": 0,
- "transcription": "TEA",
- "points": [368, 180, 621, 180, 621, 294, 368, 294]
- }, ...
- ]
- }
-
-
- Args:
- gt_file (str): The path to ground-truth
- img_info (dict): The dict of the img and annotation information
-
- Returns:
- img_info (dict): The dict of the img and annotation information
- """
-
- annotation = mmengine.load(gt_file)
- anno_info = []
- for line in annotation['lines']:
- if line['ignore'] == 1:
- continue
- segmentation = line['points']
- word = line['transcription']
- anno = dict(bbox=segmentation, word=word)
- anno_info.append(anno)
-
- img_info.update(anno_info=anno_info)
-
- return img_info
-
-
-def generate_ann(root_path, split, image_infos, preserve_vertical):
- """Generate cropped annotations and label txt file.
-
- Args:
- root_path (str): The root path of the dataset
- split (str): The split of dataset. Namely: training or test
- image_infos (list[dict]): A list of dicts of the img and
- annotation information
- preserve_vertical (bool): Whether to preserve vertical texts
- """
- print('Cropping images...')
- dst_image_root = osp.join(root_path, 'crops', split)
- ignore_image_root = osp.join(root_path, 'ignores', split)
- if split == 'training':
- dst_label_file = osp.join(root_path, 'train_label.json')
- elif split == 'val':
- dst_label_file = osp.join(root_path, 'val_label.json')
- mmengine.mkdir_or_exist(dst_image_root)
- mmengine.mkdir_or_exist(ignore_image_root)
-
- img_info = []
- for image_info in image_infos:
- index = 1
- src_img_path = osp.join(root_path, 'imgs', image_info['file_name'])
- image = mmcv.imread(src_img_path)
- src_img_root = image_info['file_name'].split('.')[0]
-
- for anno in image_info['anno_info']:
- word = anno['word']
- dst_img = crop_img(image, anno['bbox'], 0, 0)
- h, w, _ = dst_img.shape
-
- dst_img_name = f'{src_img_root}_{index}.png'
- index += 1
- # Skip invalid annotations
- if min(dst_img.shape) == 0:
- continue
- # Skip vertical texts
- if not preserve_vertical and h / w > 2 and split == 'training':
- dst_img_path = osp.join(ignore_image_root, dst_img_name)
- mmcv.imwrite(dst_img, dst_img_path)
- continue
-
- dst_img_path = osp.join(dst_image_root, dst_img_name)
- mmcv.imwrite(dst_img, dst_img_path)
-
- img_info.append({
- 'file_name': dst_img_name,
- 'anno_info': [{
- 'text': word
- }]
- })
-
- dump_ocr_data(img_info, dst_label_file, 'textrecog')
-
-
-def parse_args():
- parser = argparse.ArgumentParser(
- description='Generate training and val set of ReCTS.')
- parser.add_argument('root_path', help='Root dir path of ReCTS')
- parser.add_argument(
- '--val-ratio', help='Split ratio for val set', default=0.0, type=float)
- parser.add_argument(
- '--nproc', default=1, type=int, help='Number of process')
- parser.add_argument(
- '--preserve-vertical',
- help='Preserve samples containing vertical texts',
- action='store_true')
- args = parser.parse_args()
- return args
-
-
-def main():
- args = parse_args()
- root_path = args.root_path
- ratio = args.val_ratio
-
- trn_files, val_files = collect_files(
- osp.join(root_path, 'imgs'), osp.join(root_path, 'annotations'), ratio)
-
- # Train set
- trn_infos = collect_annotations(trn_files, nproc=args.nproc)
- with mmengine.Timer(
- print_tmpl='It takes {}s to convert ReCTS Training annotation'):
- generate_ann(root_path, 'training', trn_infos, args.preserve_vertical)
-
- # Val set
- if len(val_files) > 0:
- val_infos = collect_annotations(val_files, nproc=args.nproc)
- with mmengine.Timer(
- print_tmpl='It takes {}s to convert ReCTS Val annotation'):
- generate_ann(root_path, 'val', val_infos, args.preserve_vertical)
-
-
-if __name__ == '__main__':
- main()
diff --git a/pipeline/mmocr/mmocr/.mim/tools/dataset_converters/textrecog/sroie_converter.py b/pipeline/mmocr/mmocr/.mim/tools/dataset_converters/textrecog/sroie_converter.py
deleted file mode 100644
index 1c380e49d42d7c09907f5c1642baa172e5dff1ed..0000000000000000000000000000000000000000
--- a/pipeline/mmocr/mmocr/.mim/tools/dataset_converters/textrecog/sroie_converter.py
+++ /dev/null
@@ -1,207 +0,0 @@
-# Copyright (c) OpenMMLab. All rights reserved.
-import argparse
-import os
-import os.path as osp
-
-import mmcv
-import mmengine
-import numpy as np
-
-from mmocr.utils import crop_img, dump_ocr_data
-
-
-def collect_files(img_dir, gt_dir):
- """Collect all images and their corresponding groundtruth files.
-
- Args:
- img_dir (str): The image directory
- gt_dir (str): The groundtruth directory
-
- Returns:
- files (list): The list of tuples (img_file, groundtruth_file)
- """
-
- assert isinstance(img_dir, str)
- assert img_dir
- assert isinstance(gt_dir, str)
- assert gt_dir
-
- ann_list, imgs_list = [], []
- for gt_file in os.listdir(gt_dir):
- # Filtering repeated and missing images
- if '(' in gt_file or gt_file == 'X51006619570.txt':
- continue
- ann_list.append(osp.join(gt_dir, gt_file))
- imgs_list.append(osp.join(img_dir, gt_file.replace('.txt', '.jpg')))
-
- files = list(zip(sorted(imgs_list), sorted(ann_list)))
- assert len(files), f'No images found in {img_dir}'
-
- print(f'Loaded {len(files)} images from {img_dir}')
-
- return files
-
-
-def collect_annotations(files, nproc=1):
- """Collect the annotation information.
-
- Args:
- files (list): The list of tuples (image_file, groundtruth_file)
- nproc (int): The number of process to collect annotations
-
- Returns:
- images (list): The list of image information dicts
- """
-
- assert isinstance(files, list)
- assert isinstance(nproc, int)
-
- if nproc > 1:
- images = mmengine.track_parallel_progress(
- load_img_info, files, nproc=nproc)
- else:
- images = mmengine.track_progress(load_img_info, files)
-
- return images
-
-
-def load_img_info(files):
- """Load the information of one image.
-
- Args:
- files (tuple): The tuple of (img_file, groundtruth_file)
-
- Returns:
- img_info (dict): The dict of the img and annotation information
- """
-
- assert isinstance(files, tuple)
-
- img_file, gt_file = files
- assert osp.basename(gt_file).split('.')[0] == osp.basename(img_file).split(
- '.')[0]
- # read imgs while ignoring orientations
- img = mmcv.imread(img_file, 'unchanged')
-
- img_info = dict(
- file_name=osp.join(osp.basename(img_file)),
- height=img.shape[0],
- width=img.shape[1],
- segm_file=osp.join(osp.basename(gt_file)))
-
- if osp.splitext(gt_file)[1] == '.txt':
- img_info = load_txt_info(gt_file, img_info)
- else:
- raise NotImplementedError
-
- return img_info
-
-
-def load_txt_info(gt_file, img_info):
- """Collect the annotation information.
-
- Annotation Format
- x1, y1, x2, y2, x3, y3, x4, y4, transcript
-
- Args:
- gt_file (list): The list of tuples (image_file, groundtruth_file)
- img_info (int): The dict of the img and annotation information
-
- Returns:
- img_info (list): The dict of the img and annotation information
- """
-
- with open(gt_file, encoding='unicode_escape') as f:
- anno_info = []
- for ann in f.readlines():
- # skip invalid annotation line
- try:
- bbox = np.array(ann.split(',')[0:8]).astype(int).tolist()
- except ValueError:
-
- continue
- word = ann.split(',')[-1].replace('\n', '').strip()
-
- anno = dict(bbox=bbox, word=word)
- anno_info.append(anno)
-
- img_info.update(anno_info=anno_info)
-
- return img_info
-
-
-def generate_ann(root_path, split, image_infos):
- """Generate cropped annotations and label txt file.
-
- Args:
- root_path (str): The root path of the dataset
- split (str): The split of dataset. Namely: training or test
- image_infos (list[dict]): A list of dicts of the img and
- annotation information
- """
-
- dst_image_root = osp.join(root_path, 'crops', split)
- if split == 'training':
- dst_label_file = osp.join(root_path, 'train_label.json')
- elif split == 'test':
- dst_label_file = osp.join(root_path, 'test_label.json')
- os.makedirs(dst_image_root, exist_ok=True)
-
- img_info = []
- for image_info in image_infos:
- index = 1
- src_img_path = osp.join(root_path, 'imgs', split,
- image_info['file_name'])
- image = mmcv.imread(src_img_path)
- src_img_root = image_info['file_name'].split('.')[0]
-
- for anno in image_info['anno_info']:
- word = anno['word']
- dst_img = crop_img(image, anno['bbox'], 0, 0)
-
- # Skip invalid annotations
- if min(dst_img.shape) == 0 or len(word) == 0:
- continue
-
- dst_img_name = f'{src_img_root}_{index}.png'
- index += 1
- dst_img_path = osp.join(dst_image_root, dst_img_name)
- mmcv.imwrite(dst_img, dst_img_path)
-
- img_info.append({
- 'file_name': dst_img_name,
- 'anno_info': [{
- 'text': word
- }]
- })
-
- dump_ocr_data(img_info, dst_label_file, 'textrecog')
-
-
-def parse_args():
- parser = argparse.ArgumentParser(
- description='Generate training and test set of SROIE')
- parser.add_argument('root_path', help='Root dir path of SROIE')
- parser.add_argument(
- '--nproc', default=1, type=int, help='Number of process')
- args = parser.parse_args()
- return args
-
-
-def main():
- args = parse_args()
- root_path = args.root_path
-
- for split in ['training', 'test']:
- print(f'Processing {split} set...')
- with mmengine.Timer(
- print_tmpl='It takes {}s to convert SROIE annotation'):
- files = collect_files(
- osp.join(root_path, 'imgs', split),
- osp.join(root_path, 'annotations', split))
- image_infos = collect_annotations(files, nproc=args.nproc)
- generate_ann(root_path, split, image_infos)
-
-
-if __name__ == '__main__':
- main()
diff --git a/pipeline/mmocr/mmocr/.mim/tools/dataset_converters/textrecog/vintext_converter.py b/pipeline/mmocr/mmocr/.mim/tools/dataset_converters/textrecog/vintext_converter.py
deleted file mode 100644
index ea9103da033c2d4bdbba3855f78a88d32aec9553..0000000000000000000000000000000000000000
--- a/pipeline/mmocr/mmocr/.mim/tools/dataset_converters/textrecog/vintext_converter.py
+++ /dev/null
@@ -1,217 +0,0 @@
-# Copyright (c) OpenMMLab. All rights reserved.
-import argparse
-import os
-import os.path as osp
-
-import mmcv
-import mmengine
-
-from mmocr.utils import crop_img, dump_ocr_data
-
-
-def collect_files(img_dir, gt_dir):
- """Collect all images and their corresponding groundtruth files.
-
- Args:
- img_dir (str): The image directory
- gt_dir (str): The groundtruth directory
-
- Returns:
- files (list): The list of tuples (img_file, groundtruth_file)
- """
- assert isinstance(img_dir, str)
- assert img_dir
- assert isinstance(gt_dir, str)
- assert gt_dir
-
- ann_list, imgs_list = [], []
- for img_file in os.listdir(img_dir):
- ann_file = 'gt_' + str(int(img_file[2:6])) + '.txt'
- ann_list.append(osp.join(gt_dir, ann_file))
- imgs_list.append(osp.join(img_dir, img_file))
-
- files = list(zip(imgs_list, ann_list))
- assert len(files), f'No images found in {img_dir}'
- print(f'Loaded {len(files)} images from {img_dir}')
-
- return files
-
-
-def collect_annotations(files, nproc=1):
- """Collect the annotation information.
-
- Args:
- files (list): The list of tuples (image_file, groundtruth_file)
- nproc (int): The number of process to collect annotations
-
- Returns:
- images (list): The list of image information dicts
- """
- assert isinstance(files, list)
- assert isinstance(nproc, int)
-
- if nproc > 1:
- images = mmengine.track_parallel_progress(
- load_img_info, files, nproc=nproc)
- else:
- images = mmengine.track_progress(load_img_info, files)
-
- return images
-
-
-def load_img_info(files):
- """Load the information of one image.
-
- Args:
- files (tuple): The tuple of (img_file, groundtruth_file)
-
- Returns:
- img_info (dict): The dict of the img and annotation information
- """
- assert isinstance(files, tuple)
-
- img_file, gt_file = files
- assert int(osp.basename(gt_file)[3:-4]) == int(
- osp.basename(img_file)[2:-4])
- # read imgs while ignoring orientations
- img = mmcv.imread(img_file, 'unchanged')
-
- img_info = dict(
- file_name=osp.join(osp.basename(img_file)),
- height=img.shape[0],
- width=img.shape[1],
- segm_file=osp.join(osp.basename(gt_file)))
-
- if osp.splitext(gt_file)[1] == '.txt':
- img_info = load_txt_info(gt_file, img_info)
- else:
- raise NotImplementedError
-
- return img_info
-
-
-def load_txt_info(gt_file, img_info):
- """Collect the annotation information.
-
- The annotation format is as the following:
- x1,y1,x2,y2,x3,y3,x4,y4,text
- 118,15,147,15,148,46,118,46,LฦฏแปขNG
- 149,9,165,9,165,43,150,43,TแปT
- 167,9,180,9,179,43,167,42,ฤแป
- 181,12,193,12,193,43,181,43,Cร
- 195,13,215,14,215,46,196,46,VIแปC
- 217,13,237,14,239,47,217,46,LรM,
-
- Args:
- gt_file (str): The path to ground-truth
- img_info (dict): The dict of the img and annotation information
-
- Returns:
- img_info (dict): The dict of the img and annotation information
- """
-
- with open(gt_file, encoding='utf-8') as f:
- anno_info = []
- for line in f:
- line = line.strip('\n')
- ann = line.split(',')
- bbox = ann[0:8]
- word = line[len(','.join(bbox)) + 1:]
- bbox = [int(coord) for coord in bbox]
- # Ignore hard samples
- if word == '###':
- continue
- assert len(bbox) == 8
- anno = dict(bbox=bbox, word=word)
- anno_info.append(anno)
-
- img_info.update(anno_info=anno_info)
-
- return img_info
-
-
-def generate_ann(root_path, split, image_infos, preserve_vertical):
- """Generate cropped annotations and label txt file.
-
- Args:
- root_path (str): The root path of the dataset
- split (str): The split of dataset. Namely: training or test
- image_infos (list[dict]): A list of dicts of the img and
- annotation information
- preserve_vertical (bool): Whether to preserve vertical texts
- """
- dst_image_root = osp.join(root_path, 'crops', split)
- ignore_image_root = osp.join(root_path, 'ignores', split)
- if split == 'training':
- dst_label_file = osp.join(root_path, 'train_label.json')
- elif split == 'test':
- dst_label_file = osp.join(root_path, 'test_label.json')
- elif split == 'unseen_test':
- dst_label_file = osp.join(root_path, 'unseen_test_label.json')
- os.makedirs(dst_image_root, exist_ok=True)
-
- img_info = []
- for image_info in image_infos:
- index = 1
- src_img_path = osp.join(root_path, 'imgs', split,
- image_info['file_name'])
- image = mmcv.imread(src_img_path)
- src_img_root = image_info['file_name'].split('.')[0]
-
- for anno in image_info['anno_info']:
- word = anno['word']
- dst_img = crop_img(image, anno['bbox'], 0, 0)
- h, w, _ = dst_img.shape
-
- dst_img_name = f'{src_img_root}_{index}.png'
- index += 1
- # Skip invalid annotations
- if min(dst_img.shape) == 0:
- continue
- # Skip vertical texts
- if not preserve_vertical and h / w > 2 and split == 'training':
- dst_img_path = osp.join(ignore_image_root, dst_img_name)
- mmcv.imwrite(dst_img, dst_img_path)
- continue
-
- dst_img_path = osp.join(dst_image_root, dst_img_name)
- mmcv.imwrite(dst_img, dst_img_path)
- img_info.append({
- 'file_name': dst_img_name,
- 'anno_info': [{
- 'text': word
- }]
- })
- dump_ocr_data(img_info, dst_label_file, 'textrecog')
-
-
-def parse_args():
- parser = argparse.ArgumentParser(
- description='Generate training and test set of VinText ')
- parser.add_argument('root_path', help='Root dir path of VinText')
- parser.add_argument(
- '--preserve-vertical',
- help='Preserve samples containing vertical texts',
- action='store_true')
- parser.add_argument(
- '--nproc', default=1, type=int, help='Number of processes')
- args = parser.parse_args()
- return args
-
-
-def main():
- args = parse_args()
- root_path = args.root_path
- for split in ['training', 'test', 'unseen_test']:
- print(f'Processing {split} set...')
- with mmengine.Timer(
- print_tmpl='It takes {}s to convert VinText annotation'):
- files = collect_files(
- osp.join(root_path, 'imgs', split),
- osp.join(root_path, 'annotations'))
- image_infos = collect_annotations(files, nproc=args.nproc)
- generate_ann(root_path, split, image_infos, args.preserve_vertical)
-
-
-if __name__ == '__main__':
- main()
diff --git a/pipeline/mmocr/mmocr/.mim/tools/dist_test.sh b/pipeline/mmocr/mmocr/.mim/tools/dist_test.sh
deleted file mode 100644
index dea131b43ea8f1222661d20603d40c18ea7f28a1..0000000000000000000000000000000000000000
--- a/pipeline/mmocr/mmocr/.mim/tools/dist_test.sh
+++ /dev/null
@@ -1,22 +0,0 @@
-#!/usr/bin/env bash
-
-CONFIG=$1
-CHECKPOINT=$2
-GPUS=$3
-NNODES=${NNODES:-1}
-NODE_RANK=${NODE_RANK:-0}
-PORT=${PORT:-29500}
-MASTER_ADDR=${MASTER_ADDR:-"127.0.0.1"}
-
-PYTHONPATH="$(dirname $0)/..":$PYTHONPATH \
-python -m torch.distributed.launch \
- --nnodes=$NNODES \
- --node_rank=$NODE_RANK \
- --master_addr=$MASTER_ADDR \
- --nproc_per_node=$GPUS \
- --master_port=$PORT \
- $(dirname "$0")/test.py \
- $CONFIG \
- $CHECKPOINT \
- --launcher pytorch \
- ${@:4}
diff --git a/pipeline/mmocr/mmocr/.mim/tools/dist_train.sh b/pipeline/mmocr/mmocr/.mim/tools/dist_train.sh
deleted file mode 100644
index 3f5b40b2318c6bd58504d9e570b90adf21825376..0000000000000000000000000000000000000000
--- a/pipeline/mmocr/mmocr/.mim/tools/dist_train.sh
+++ /dev/null
@@ -1,20 +0,0 @@
-
-#!/usr/bin/env bash
-
-CONFIG=$1
-GPUS=$2
-NNODES=${NNODES:-1}
-NODE_RANK=${NODE_RANK:-0}
-PORT=${PORT:-29500}
-MASTER_ADDR=${MASTER_ADDR:-"127.0.0.1"}
-
-PYTHONPATH="$(dirname $0)/..":$PYTHONPATH \
-python -m torch.distributed.launch \
- --nnodes=$NNODES \
- --node_rank=$NODE_RANK \
- --master_addr=$MASTER_ADDR \
- --nproc_per_node=$GPUS \
- --master_port=$PORT \
- $(dirname "$0")/train.py \
- $CONFIG \
- --launcher pytorch ${@:3}
diff --git a/pipeline/mmocr/mmocr/.mim/tools/infer.py b/pipeline/mmocr/mmocr/.mim/tools/infer.py
deleted file mode 100644
index 5881a7efdc514c03383ec9a2282bf0b355121518..0000000000000000000000000000000000000000
--- a/pipeline/mmocr/mmocr/.mim/tools/infer.py
+++ /dev/null
@@ -1,102 +0,0 @@
-# Copyright (c) OpenMMLab. All rights reserved.
-from argparse import ArgumentParser
-# import sys
-# sys.path.append("..")
-# import mmocr
-from mmocr.apis.inferencers import MMOCRInferencer
-
-
-def parse_args():
- parser = ArgumentParser()
- parser.add_argument(
- 'inputs', type=str, help='Input image file or folder path.')
- parser.add_argument(
- '--out-dir',
- type=str,
- default='results/',
- help='Output directory of results.')
- parser.add_argument(
- '--det',
- type=str,
- default=None,
- help='Pretrained text detection algorithm. It\'s the path to the '
- 'config file or the model name defined in metafile.')
- parser.add_argument(
- '--det-weights',
- type=str,
- default=None,
- help='Path to the custom checkpoint file of the selected det model. '
- 'If it is not specified and "det" is a model name of metafile, the '
- 'weights will be loaded from metafile.')
- parser.add_argument(
- '--rec',
- type=str,
- default=None,
- help='Pretrained text recognition algorithm. It\'s the path to the '
- 'config file or the model name defined in metafile.')
- parser.add_argument(
- '--rec-weights',
- type=str,
- default=None,
- help='Path to the custom checkpoint file of the selected recog model. '
- 'If it is not specified and "rec" is a model name of metafile, the '
- 'weights will be loaded from metafile.')
- parser.add_argument(
- '--kie',
- type=str,
- default=None,
- help='Pretrained key information extraction algorithm. It\'s the path'
- 'to the config file or the model name defined in metafile.')
- parser.add_argument(
- '--kie-weights',
- type=str,
- default=None,
- help='Path to the custom checkpoint file of the selected kie model. '
- 'If it is not specified and "kie" is a model name of metafile, the '
- 'weights will be loaded from metafile.')
- parser.add_argument(
- '--device',
- type=str,
- default=None,
- help='Device used for inference. '
- 'If not specified, the available device will be automatically used.')
- parser.add_argument(
- '--batch-size', type=int, default=1, help='Inference batch size.')
- parser.add_argument(
- '--show',
- action='store_true',
- help='Display the image in a popup window.')
- parser.add_argument(
- '--print-result',
- action='store_true',
- help='Whether to print the results.')
- parser.add_argument(
- '--save_pred',
- action='store_true',
- help='Save the inference results to out_dir.')
- parser.add_argument(
- '--save_vis',
- action='store_true',
- help='Save the visualization results to out_dir.')
-
- call_args = vars(parser.parse_args())
-
- init_kws = [
- 'det', 'det_weights', 'rec', 'rec_weights', 'kie', 'kie_weights',
- 'device'
- ]
- init_args = {}
- for init_kw in init_kws:
- init_args[init_kw] = call_args.pop(init_kw)
-
- return init_args, call_args
-
-
-def main():
- init_args, call_args = parse_args()
- ocr = MMOCRInferencer(**init_args)
- ocr(**call_args)
-
-
-if __name__ == '__main__':
- main()
diff --git a/pipeline/mmocr/mmocr/.mim/tools/model_converters/publish_model.py b/pipeline/mmocr/mmocr/.mim/tools/model_converters/publish_model.py
deleted file mode 100644
index 18fc3e15045dea63a74ed1a7727a388e9031ac8c..0000000000000000000000000000000000000000
--- a/pipeline/mmocr/mmocr/.mim/tools/model_converters/publish_model.py
+++ /dev/null
@@ -1,57 +0,0 @@
-# Copyright (c) OpenMMLab. All rights reserved.
-import argparse
-import subprocess
-
-import torch
-from mmengine.logging import print_log
-
-
-def parse_args():
- parser = argparse.ArgumentParser(
- description='Process a checkpoint to be published')
- parser.add_argument('in_file', help='input checkpoint filename')
- parser.add_argument('out_file', help='output checkpoint filename')
- parser.add_argument(
- '--save-keys',
- nargs='+',
- type=str,
- default=['meta', 'state_dict'],
- help='keys to save in the published checkpoint')
- args = parser.parse_args()
- return args
-
-
-def process_checkpoint(in_file, out_file, save_keys=['meta', 'state_dict']):
- checkpoint = torch.load(in_file, map_location='cpu')
-
- # only keep `meta` and `state_dict` for smaller file size
- ckpt_keys = list(checkpoint.keys())
- for k in ckpt_keys:
- if k not in save_keys:
- print_log(
- f'Key `{k}` will be removed because it is not in '
- f'save_keys. If you want to keep it, '
- f'please set --save-keys.',
- logger='current')
- checkpoint.pop(k, None)
-
- # if it is necessary to remove some sensitive data in checkpoint['meta'],
- # add the code here.
- if torch.__version__ >= '1.6':
- torch.save(checkpoint, out_file, _use_new_zipfile_serialization=False)
- else:
- torch.save(checkpoint, out_file)
- sha = subprocess.check_output(['sha256sum', out_file]).decode()
- final_file = out_file.rstrip('.pth') + f'-{sha[:8]}.pth'
- subprocess.Popen(['mv', out_file, final_file])
- print_log(
- f'The published model is saved at {final_file}.', logger='current')
-
-
-def main():
- args = parse_args()
- process_checkpoint(args.in_file, args.out_file, args.save_keys)
-
-
-if __name__ == '__main__':
- main()
diff --git a/pipeline/mmocr/mmocr/.mim/tools/slurm_test.sh b/pipeline/mmocr/mmocr/.mim/tools/slurm_test.sh
deleted file mode 100644
index 865f45599ad883d216f0df0248a3815700615c17..0000000000000000000000000000000000000000
--- a/pipeline/mmocr/mmocr/.mim/tools/slurm_test.sh
+++ /dev/null
@@ -1,22 +0,0 @@
-#!/usr/bin/env bash
-
-set -x
-export PYTHONPATH=`pwd`:$PYTHONPATH
-
-PARTITION=$1
-JOB_NAME=$2
-CONFIG=$3
-CHECKPOINT=$4
-GPUS=${GPUS:-8}
-GPUS_PER_NODE=${GPUS_PER_NODE:-8}
-PY_ARGS=${@:5}
-SRUN_ARGS=${SRUN_ARGS:-""}
-
-srun -p ${PARTITION} \
- --job-name=${JOB_NAME} \
- --gres=gpu:${GPUS_PER_NODE} \
- --ntasks=${GPUS} \
- --ntasks-per-node=${GPUS_PER_NODE} \
- --kill-on-bad-exit=1 \
- ${SRUN_ARGS} \
- python -u tools/test.py ${CONFIG} ${CHECKPOINT} --launcher="slurm" ${PY_ARGS}
diff --git a/pipeline/mmocr/mmocr/.mim/tools/slurm_train.sh b/pipeline/mmocr/mmocr/.mim/tools/slurm_train.sh
deleted file mode 100644
index 452b09454a08ac522a9df2304c3039487ea517bd..0000000000000000000000000000000000000000
--- a/pipeline/mmocr/mmocr/.mim/tools/slurm_train.sh
+++ /dev/null
@@ -1,25 +0,0 @@
-#!/usr/bin/env bash
-export MASTER_PORT=$((12000 + $RANDOM % 20000))
-
-set -x
-
-PARTITION=$1
-JOB_NAME=$2
-CONFIG=$3
-WORK_DIR=$4
-GPUS=${GPUS:-8}
-GPUS_PER_NODE=${GPUS_PER_NODE:-8}
-CPUS_PER_TASK=${CPUS_PER_TASK:-5}
-PY_ARGS=${@:5}
-SRUN_ARGS=${SRUN_ARGS:-""}
-
-PYTHONPATH="$(dirname $0)/..":$PYTHONPATH \
-srun -p ${PARTITION} \
- --job-name=${JOB_NAME} \
- --gres=gpu:${GPUS_PER_NODE} \
- --ntasks=${GPUS} \
- --ntasks-per-node=${GPUS_PER_NODE} \
- --cpus-per-task=${CPUS_PER_TASK} \
- --kill-on-bad-exit=1 \
- ${SRUN_ARGS} \
- python -u tools/train.py ${CONFIG} --work-dir=${WORK_DIR} --launcher="slurm" ${PY_ARGS}
diff --git a/pipeline/mmocr/mmocr/.mim/tools/test.py b/pipeline/mmocr/mmocr/.mim/tools/test.py
deleted file mode 100644
index 15645f2207ebdb61fd70293f2b2c9602e99b2c61..0000000000000000000000000000000000000000
--- a/pipeline/mmocr/mmocr/.mim/tools/test.py
+++ /dev/null
@@ -1,141 +0,0 @@
-# Copyright (c) OpenMMLab. All rights reserved.
-import argparse
-import os
-import os.path as osp
-
-from mmengine.config import Config, DictAction
-from mmengine.registry import RUNNERS
-from mmengine.runner import Runner
-
-
-def parse_args():
- parser = argparse.ArgumentParser(description='Test (and eval) a model')
- parser.add_argument('config', help='Test config file path')
- parser.add_argument('checkpoint', help='Checkpoint file')
- parser.add_argument(
- '--work-dir',
- help='The directory to save the file containing evaluation metrics')
- parser.add_argument(
- '--save-preds',
- action='store_true',
- help='Dump predictions to a pickle file for offline evaluation')
- parser.add_argument(
- '--show', action='store_true', help='Show prediction results')
- parser.add_argument(
- '--show-dir',
- help='Directory where painted images will be saved. '
- 'If specified, it will be automatically saved '
- 'to the work_dir/timestamp/show_dir')
- parser.add_argument(
- '--wait-time', type=float, default=2, help='The interval of show (s)')
- parser.add_argument(
- '--cfg-options',
- nargs='+',
- action=DictAction,
- help='Override some settings in the used config, the key-value pair '
- 'in xxx=yyy format will be merged into config file. If the value to '
- 'be overwritten is a list, it should be like key="[a,b]" or key=a,b '
- 'It also allows nested list/tuple values, e.g. key="[(a,b),(c,d)]" '
- 'Note that the quotation marks are necessary and that no white space '
- 'is allowed.')
- parser.add_argument(
- '--launcher',
- choices=['none', 'pytorch', 'slurm', 'mpi'],
- default='none',
- help='Job launcher')
- parser.add_argument(
- '--tta', action='store_true', help='Test time augmentation')
- # When using PyTorch version >= 2.0.0, the `torch.distributed.launch`
- # will pass the `--local-rank` parameter to `tools/test.py` instead
- # of `--local_rank`.
- parser.add_argument('--local_rank', '--local-rank', type=int, default=0)
- args = parser.parse_args()
- if 'LOCAL_RANK' not in os.environ:
- os.environ['LOCAL_RANK'] = str(args.local_rank)
- return args
-
-
-def trigger_visualization_hook(cfg, args):
- default_hooks = cfg.default_hooks
- if 'visualization' in default_hooks:
- visualization_hook = default_hooks['visualization']
- # Turn on visualization
- visualization_hook['enable'] = True
- visualization_hook['draw_gt'] = True
- visualization_hook['draw_pred'] = True
- if args.show:
- visualization_hook['show'] = True
- visualization_hook['wait_time'] = args.wait_time
- if args.show_dir:
- cfg.visualizer['save_dir'] = args.show_dir
- cfg.visualizer['vis_backends'] = [dict(type='LocalVisBackend')]
- else:
- raise RuntimeError(
- 'VisualizationHook must be included in default_hooks.'
- 'refer to usage '
- '"visualization=dict(type=\'VisualizationHook\')"')
-
- return cfg
-
-
-def main():
- args = parse_args()
-
- # load config
- cfg = Config.fromfile(args.config)
- cfg.launcher = args.launcher
- if args.cfg_options is not None:
- cfg.merge_from_dict(args.cfg_options)
-
- # work_dir is determined in this priority: CLI > segment in file > filename
- if args.work_dir is not None:
- # update configs according to CLI args if args.work_dir is not None
- cfg.work_dir = args.work_dir
- elif cfg.get('work_dir', None) is None:
- # use config filename as default work_dir if cfg.work_dir is None
- cfg.work_dir = osp.join('./work_dirs',
- osp.splitext(osp.basename(args.config))[0])
-
- cfg.load_from = args.checkpoint
-
- # TODO: It will be supported after refactoring the visualizer
- if args.show and args.show_dir:
- raise NotImplementedError('--show and --show-dir cannot be set '
- 'at the same time')
-
- if args.show or args.show_dir:
- cfg = trigger_visualization_hook(cfg, args)
-
- if args.tta:
- cfg.test_dataloader.dataset.pipeline = cfg.tta_pipeline
- cfg.tta_model.module = cfg.model
- cfg.model = cfg.tta_model
-
- # save predictions
- if args.save_preds:
- dump_metric = dict(
- type='DumpResults',
- out_file_path=osp.join(
- cfg.work_dir,
- f'{osp.basename(args.checkpoint)}_predictions.pkl'))
- if isinstance(cfg.test_evaluator, (list, tuple)):
- cfg.test_evaluator = list(cfg.test_evaluator)
- cfg.test_evaluator.append(dump_metric)
- else:
- cfg.test_evaluator = [cfg.test_evaluator, dump_metric]
-
- # build the runner from config
- if 'runner_type' not in cfg:
- # build the default runner
- runner = Runner.from_cfg(cfg)
- else:
- # build customized runner from the registry
- # if 'runner_type' is set in the cfg
- runner = RUNNERS.build(cfg)
-
- # start testing
- runner.test()
-
-
-if __name__ == '__main__':
- main()
diff --git a/pipeline/mmocr/mmocr/.mim/tools/train.py b/pipeline/mmocr/mmocr/.mim/tools/train.py
deleted file mode 100644
index 349cbb3ef4fc74c821c9bd4266ecacdd6acd8cc2..0000000000000000000000000000000000000000
--- a/pipeline/mmocr/mmocr/.mim/tools/train.py
+++ /dev/null
@@ -1,113 +0,0 @@
-# Copyright (c) OpenMMLab. All rights reserved.
-import argparse
-import logging
-import os
-import os.path as osp
-
-from mmengine.config import Config, DictAction
-from mmengine.logging import print_log
-from mmengine.registry import RUNNERS
-from mmengine.runner import Runner
-
-
-def parse_args():
- parser = argparse.ArgumentParser(description='Train a model')
- parser.add_argument('config', help='Train config file path')
- parser.add_argument('--work-dir', help='The dir to save logs and models')
- parser.add_argument(
- '--resume', action='store_true', help='Whether to resume checkpoint.')
- parser.add_argument(
- '--amp',
- action='store_true',
- default=False,
- help='Enable automatic-mixed-precision training')
- parser.add_argument(
- '--auto-scale-lr',
- action='store_true',
- help='Whether to scale the learning rate automatically. It requires '
- '`auto_scale_lr` in config, and `base_batch_size` in `auto_scale_lr`')
- parser.add_argument(
- '--cfg-options',
- nargs='+',
- action=DictAction,
- help='Override some settings in the used config, the key-value pair '
- 'in xxx=yyy format will be merged into config file. If the value to '
- 'be overwritten is a list, it should be like key="[a,b]" or key=a,b '
- 'It also allows nested list/tuple values, e.g. key="[(a,b),(c,d)]" '
- 'Note that the quotation marks are necessary and that no white space '
- 'is allowed.')
- parser.add_argument(
- '--launcher',
- choices=['none', 'pytorch', 'slurm', 'mpi'],
- default='none',
- help='Job launcher')
- # When using PyTorch version >= 2.0.0, the `torch.distributed.launch`
- # will pass the `--local-rank` parameter to `tools/train.py` instead
- # of `--local_rank`.
- parser.add_argument('--local_rank', '--local-rank', type=int, default=0)
- args = parser.parse_args()
- if 'LOCAL_RANK' not in os.environ:
- os.environ['LOCAL_RANK'] = str(args.local_rank)
-
- return args
-
-
-def main():
- args = parse_args()
- # load config
- cfg = Config.fromfile(args.config)
- cfg.launcher = args.launcher
- if args.cfg_options is not None:
- cfg.merge_from_dict(args.cfg_options)
-
- # work_dir is determined in this priority: CLI > segment in file > filename
- if args.work_dir is not None:
- # update configs according to CLI args if args.work_dir is not None
- cfg.work_dir = args.work_dir
- elif cfg.get('work_dir', None) is None:
- # use config filename as default work_dir if cfg.work_dir is None
- cfg.work_dir = osp.join('./work_dirs',
- osp.splitext(osp.basename(args.config))[0])
- # enable automatic-mixed-precision training
- if args.amp:
- optim_wrapper = cfg.optim_wrapper.type
- if optim_wrapper == 'AmpOptimWrapper':
- print_log(
- 'AMP training is already enabled in your config.',
- logger='current',
- level=logging.WARNING)
- else:
- assert optim_wrapper == 'OptimWrapper', (
- '`--amp` is only supported when the optimizer wrapper type is '
- f'`OptimWrapper` but got {optim_wrapper}.')
- cfg.optim_wrapper.type = 'AmpOptimWrapper'
- cfg.optim_wrapper.loss_scale = 'dynamic'
-
- if args.resume:
- cfg.resume = True
-
- # enable automatically scaling LR
- if args.auto_scale_lr:
- if 'auto_scale_lr' in cfg and \
- 'base_batch_size' in cfg.auto_scale_lr:
- cfg.auto_scale_lr.enable = True
- else:
- raise RuntimeError('Can not find "auto_scale_lr" or '
- '"auto_scale_lr.base_batch_size" in your'
- ' configuration file.')
-
- # build the runner from config
- if 'runner_type' not in cfg:
- # build the default runner
- runner = Runner.from_cfg(cfg)
- else:
- # build customized runner from the registry
- # if 'runner_type' is set in the cfg
- runner = RUNNERS.build(cfg)
-
- # start training
- runner.train()
-
-
-if __name__ == '__main__':
- main()
diff --git a/pipeline/mmocr/mmocr/.mim/tools/visualizations/browse_dataset.py b/pipeline/mmocr/mmocr/.mim/tools/visualizations/browse_dataset.py
deleted file mode 100644
index d92ee83f586005de5b14ed95066c778547baa0d4..0000000000000000000000000000000000000000
--- a/pipeline/mmocr/mmocr/.mim/tools/visualizations/browse_dataset.py
+++ /dev/null
@@ -1,415 +0,0 @@
-# Copyright (c) OpenMMLab. All rights reserved.
-import argparse
-import os.path as osp
-import sys
-from typing import Optional, Tuple
-
-import cv2
-import mmcv
-import numpy as np
-from mmengine.config import Config, DictAction
-from mmengine.dataset import Compose
-from mmengine.registry import init_default_scope
-from mmengine.utils import ProgressBar
-from mmengine.visualization import Visualizer
-
-from mmocr.registry import DATASETS, VISUALIZERS
-
-
-# TODO: Support for printing the change in key of results
-def parse_args():
- parser = argparse.ArgumentParser(description='Browse a dataset')
- parser.add_argument('config', help='Path to model or dataset config.')
- parser.add_argument(
- '--phase',
- '-p',
- default='train',
- type=str,
- help='Phase of dataset to visualize. Use "train", "test" or "val" if '
- "you just want to visualize the default split. It's also possible to "
- 'be a dataset variable name, which might be useful when a dataset '
- 'split has multiple variants in the config.')
- parser.add_argument(
- '--mode',
- '-m',
- default='transformed',
- type=str,
- choices=['original', 'transformed', 'pipeline'],
- help='Display mode: display original pictures or '
- 'transformed pictures or comparison pictures. "original" '
- 'only visualizes the original dataset & annotations; '
- '"transformed" shows the resulting images processed through all the '
- 'transforms; "pipeline" shows all the intermediate images. '
- 'Defaults to "transformed".')
- parser.add_argument(
- '--output-dir',
- '-o',
- default=None,
- type=str,
- help='If there is no display interface, you can save it.')
- parser.add_argument(
- '--task',
- '-t',
- default='auto',
- choices=['auto', 'textdet', 'textrecog'],
- type=str,
- help='Specify the task type of the dataset. If "auto", the task type '
- 'will be inferred from the config. If the script is unable to infer '
- 'the task type, you need to specify it manually. Defaults to "auto".')
- parser.add_argument('--not-show', default=False, action='store_true')
- parser.add_argument(
- '--show-number',
- '-n',
- type=int,
- default=sys.maxsize,
- help='number of images selected to visualize, '
- 'must bigger than 0. if the number is bigger than length '
- 'of dataset, show all the images in dataset; '
- 'default "sys.maxsize", show all images in dataset')
- parser.add_argument(
- '--show-interval',
- '-i',
- type=float,
- default=3,
- help='the interval of show (s)')
- parser.add_argument(
- '--cfg-options',
- nargs='+',
- action=DictAction,
- help='override some settings in the used config, the key-value pair '
- 'in xxx=yyy format will be merged into config file. If the value to '
- 'be overwritten is a list, it should be like key="[a,b]" or key=a,b '
- 'It also allows nested list/tuple values, e.g. key="[(a,b),(c,d)]" '
- 'Note that the quotation marks are necessary and that no white space '
- 'is allowed.')
- args = parser.parse_args()
- return args
-
-
-def _get_adaptive_scale(img_shape: Tuple[int, int],
- min_scale: float = 0.3,
- max_scale: float = 3.0) -> float:
- """Get adaptive scale according to image shape.
-
- The target scale depends on the the short edge length of the image. If the
- short edge length equals 224, the output is 1.0. And output linear
- scales according the short edge length. You can also specify the minimum
- scale and the maximum scale to limit the linear scale.
-
- Args:
- img_shape (Tuple[int, int]): The shape of the canvas image.
- min_scale (int): The minimum scale. Defaults to 0.3.
- max_scale (int): The maximum scale. Defaults to 3.0.
-
- Returns:
- int: The adaptive scale.
- """
- short_edge_length = min(img_shape)
- scale = short_edge_length / 224.
- return min(max(scale, min_scale), max_scale)
-
-
-def make_grid(imgs, infos):
- """Concat list of pictures into a single big picture, align height here."""
- visualizer = Visualizer.get_current_instance()
- names = [info['name'] for info in infos]
- ori_shapes = [
- info['dataset_sample'].metainfo['img_shape'] for info in infos
- ]
- max_height = int(max(img.shape[0] for img in imgs) * 1.1)
- min_width = min(img.shape[1] for img in imgs)
- horizontal_gap = min_width // 10
- img_scale = _get_adaptive_scale((max_height, min_width))
-
- texts = []
- text_positions = []
- start_x = 0
- for i, img in enumerate(imgs):
- pad_height = (max_height - img.shape[0]) // 2
- pad_width = horizontal_gap // 2
- # make border
- imgs[i] = cv2.copyMakeBorder(
- img,
- pad_height,
- max_height - img.shape[0] - pad_height + int(img_scale * 30 * 2),
- pad_width,
- pad_width,
- cv2.BORDER_CONSTANT,
- value=(255, 255, 255))
- texts.append(f'{"execution: "}{i}\n{names[i]}\n{ori_shapes[i]}')
- text_positions.append(
- [start_x + img.shape[1] // 2 + pad_width, max_height])
- start_x += img.shape[1] + horizontal_gap
-
- display_img = np.concatenate(imgs, axis=1)
- visualizer.set_image(display_img)
- img_scale = _get_adaptive_scale(display_img.shape[:2])
- visualizer.draw_texts(
- texts,
- positions=np.array(text_positions),
- font_sizes=img_scale * 7,
- colors='black',
- horizontal_alignments='center',
- font_families='monospace')
- return visualizer.get_image()
-
-
-class InspectCompose(Compose):
- """Compose multiple transforms sequentially.
-
- And record "img" field of all results in one list.
- """
-
- def __init__(self, transforms, intermediate_imgs):
- super().__init__(transforms=transforms)
- self.intermediate_imgs = intermediate_imgs
-
- def __call__(self, data):
- self.ptransforms = [
- self.transforms[i] for i in range(len(self.transforms) - 1)
- ]
- for t in self.ptransforms:
- data = t(data)
- # Keep the same meta_keys in the PackTextDetInputs
- # or PackTextRecogInputs
- self.transforms[-1].meta_keys = [key for key in data]
- data_sample = self.transforms[-1](data)
- if data is None:
- return None
- if 'img' in data:
- self.intermediate_imgs.append({
- 'name':
- t.__class__.__name__,
- 'dataset_sample':
- data_sample['data_samples']
- })
- return data
-
-
-def infer_dataset_task(task: str,
- dataset_cfg: Config,
- var_name: Optional[str] = None) -> str:
- """Try to infer the dataset's task type from the config and the variable
- name."""
- if task != 'auto':
- return task
-
- if dataset_cfg.pipeline is not None:
- if dataset_cfg.pipeline[-1].type == 'PackTextDetInputs':
- return 'textdet'
- elif dataset_cfg.pipeline[-1].type == 'PackTextRecogInputs':
- return 'textrecog'
-
- if var_name is not None:
- if 'det' in var_name:
- return 'textdet'
- elif 'rec' in var_name:
- return 'textrecog'
-
- raise ValueError(
- 'Unable to infer the task type from dataset pipeline '
- 'or variable name. Please specify the task type with --task argument '
- 'explicitly.')
-
-
-def obtain_dataset_cfg(cfg: Config, phase: str, mode: str, task: str) -> Tuple:
- """Obtain dataset and visualizer from config. Two modes are supported:
- 1. Model Config Mode:
- In this mode, the input config should be a complete model config, which
- includes a dataset within pipeline and a visualizer.
- 2. Dataset Config Mode:
- In this mode, the input config should be a complete dataset config,
- which only includes basic dataset information, and it may does not
- contain a visualizer and dataset pipeline.
-
- Examples:
- Typically, the model config files are stored in
- `configs/textdet/dbnet/xxx.py` and should look like:
- >>> train_dataloader = dict(
- >>> batch_size=16,
- >>> num_workers=8,
- >>> persistent_workers=True,
- >>> sampler=dict(type='DefaultSampler', shuffle=True),
- >>> dataset=icdar2015_textdet_train)
-
- while the dataset config files are stored in
- `configs/textdet/_base_/datasets/xxx.py` and should be like:
- >>> icdar2015_textdet_train = dict(
- >>> type='OCRDataset',
- >>> data_root=ic15_det_data_root,
- >>> ann_file='textdet_train.json',
- >>> filter_cfg=dict(filter_empty_gt=True, min_size=32),
- >>> pipeline=None)
-
- Args:
- cfg (Config): Config object.
- phase (str): The dataset phase to visualize.
- mode (str): Script mode.
- task (str): The current task type.
-
- Returns:
- Tuple: Tuple of (dataset, visualizer).
- """
- default_cfgs = dict(
- textdet=dict(
- visualizer=dict(
- type='TextDetLocalVisualizer',
- name='visualizer',
- vis_backends=[dict(type='LocalVisBackend')]),
- pipeline=[
- dict(
- type='LoadImageFromFile',
- color_type='color_ignore_orientation'),
- dict(
- type='LoadOCRAnnotations',
- with_polygon=True,
- with_bbox=True,
- with_label=True,
- ),
- dict(
- type='PackTextDetInputs',
- meta_keys=('img_path', 'ori_shape', 'img_shape'))
- ]),
- textrecog=dict(
- visualizer=dict(
- type='TextRecogLocalVisualizer',
- name='visualizer',
- vis_backends=[dict(type='LocalVisBackend')]),
- pipeline=[
- dict(type='LoadImageFromFile', ignore_empty=True, min_size=2),
- dict(type='LoadOCRAnnotations', with_text=True),
- dict(
- type='PackTextRecogInputs',
- meta_keys=('img_path', 'ori_shape', 'img_shape',
- 'valid_ratio'))
- ]),
- )
-
- # Model config mode
- dataloader_name = f'{phase}_dataloader'
- if dataloader_name in cfg:
- dataset = cfg.get(dataloader_name).dataset
- visualizer = cfg.visualizer
-
- if mode == 'original':
- default_cfg = default_cfgs[infer_dataset_task(task, dataset)]
- # Image can be stored in other methods, like LMDB,
- # which LoadImageFromFile can not handle
- if dataset.pipeline is not None:
- all_transform_types = [tfm['type'] for tfm in dataset.pipeline]
- if any([
- tfm_type.startswith('LoadImageFrom')
- for tfm_type in all_transform_types
- ]):
- for tfm in dataset.pipeline:
- if tfm['type'].startswith('LoadImageFrom'):
- # update LoadImageFrom** transform
- default_cfg['pipeline'][0] = tfm
- dataset.pipeline = default_cfg['pipeline']
- else:
- # In test_pipeline LoadOCRAnnotations is placed behind
- # other transforms. Transform will not be applied on
- # gt annotation.
- if phase == 'test':
- all_transform_types = [tfm['type'] for tfm in dataset.pipeline]
- load_ocr_ann_tfm_index = all_transform_types.index(
- 'LoadOCRAnnotations')
- load_ocr_ann_tfm = dataset.pipeline.pop(load_ocr_ann_tfm_index)
- dataset.pipeline.insert(1, load_ocr_ann_tfm)
-
- return dataset, visualizer
-
- # Dataset config mode
-
- for key in cfg.keys():
- if key.endswith(phase) and cfg[key]['type'].endswith('Dataset'):
- dataset = cfg[key]
- default_cfg = default_cfgs[infer_dataset_task(
- task, dataset, key.lower())]
- visualizer = default_cfg['visualizer']
- dataset['pipeline'] = default_cfg['pipeline'] if dataset[
- 'pipeline'] is None else dataset['pipeline']
-
- return dataset, visualizer
-
- raise ValueError(
- f'Unable to find "{phase}_dataloader" or any dataset variable ending '
- f'with "{phase}". Please check your config file or --phase argument '
- 'and try again. More details can be found in the docstring of '
- 'obtain_dataset_cfg function. Or, you may visit the documentation via '
- 'https://mmocr.readthedocs.io/en/dev-1.x/user_guides/useful_tools.html#dataset-visualization-tool' # noqa: E501
- )
-
-
-def main():
- args = parse_args()
- cfg = Config.fromfile(args.config)
- if args.cfg_options is not None:
- cfg.merge_from_dict(args.cfg_options)
-
- init_default_scope(cfg.get('default_scope', 'mmocr'))
-
- dataset_cfg, visualizer_cfg = obtain_dataset_cfg(cfg, args.phase,
- args.mode, args.task)
- dataset = DATASETS.build(dataset_cfg)
- visualizer = VISUALIZERS.build(visualizer_cfg)
- visualizer.dataset_meta = dataset.metainfo
-
- intermediate_imgs = []
-
- if dataset_cfg.type == 'ConcatDataset':
- for sub_dataset in dataset.datasets:
- sub_dataset.pipeline = InspectCompose(
- sub_dataset.pipeline.transforms, intermediate_imgs)
- else:
- dataset.pipeline = InspectCompose(dataset.pipeline.transforms,
- intermediate_imgs)
-
- # init visualization image number
- assert args.show_number > 0
- display_number = min(args.show_number, len(dataset))
-
- progress_bar = ProgressBar(display_number)
- # fetching items from dataset is a must for visualization
- for i, _ in zip(range(display_number), dataset):
- image_i = []
- result_i = [result['dataset_sample'] for result in intermediate_imgs]
- for k, datasample in enumerate(result_i):
- image = datasample.img
- if len(image.shape) == 3:
- image = image[..., [2, 1, 0]] # bgr to rgb
- image_show = visualizer.add_datasample(
- 'result',
- image,
- datasample,
- draw_pred=False,
- draw_gt=True,
- show=False)
- image_i.append(image_show)
-
- if args.mode == 'pipeline':
- image = make_grid(image_i, intermediate_imgs)
- else:
- image = image_i[-1]
-
- if hasattr(datasample, 'img_path'):
- filename = osp.basename(datasample.img_path)
- else:
- # some dataset have not image path
- filename = f'{i}.jpg'
- out_file = osp.join(args.output_dir,
- filename) if args.output_dir is not None else None
-
- if out_file is not None:
- mmcv.imwrite(image[..., ::-1], out_file)
-
- if not args.not_show:
- visualizer.show(
- image, win_name=filename, wait_time=args.show_interval)
-
- intermediate_imgs.clear()
- progress_bar.update()
-
-
-if __name__ == '__main__':
- main()
diff --git a/pipeline/mmocr/mmocr/.mim/tools/visualizations/vis_scheduler.py b/pipeline/mmocr/mmocr/.mim/tools/visualizations/vis_scheduler.py
deleted file mode 100644
index 4a2d4a3c7e75f9cba0b82456ec009acef214f5fc..0000000000000000000000000000000000000000
--- a/pipeline/mmocr/mmocr/.mim/tools/visualizations/vis_scheduler.py
+++ /dev/null
@@ -1,286 +0,0 @@
-# Copyright (c) OpenMMLab. All rights reserved.
-import argparse
-import json
-import os.path as osp
-import re
-from pathlib import Path
-from unittest.mock import MagicMock
-
-import matplotlib.pyplot as plt
-import rich
-import torch.nn as nn
-from mmengine.config import Config, DictAction
-from mmengine.hooks import Hook
-from mmengine.model import BaseModel
-from mmengine.registry import init_default_scope
-from mmengine.runner import Runner
-from mmengine.visualization import Visualizer
-from rich.progress import BarColumn, MofNCompleteColumn, Progress, TextColumn
-
-from mmocr.registry import DATASETS
-
-
-class SimpleModel(BaseModel):
- """simple model that do nothing in train_step."""
-
- def __init__(self):
- super(SimpleModel, self).__init__()
- self.data_preprocessor = nn.Identity()
- self.conv = nn.Conv2d(1, 1, 1)
-
- def forward(self, inputs, data_samples, mode='tensor'):
- pass
-
- def train_step(self, data, optim_wrapper):
- pass
-
-
-class ParamRecordHook(Hook):
-
- def __init__(self, by_epoch):
- super().__init__()
- self.by_epoch = by_epoch
- self.lr_list = []
- self.momentum_list = []
- self.wd_list = []
- self.task_id = 0
- self.progress = Progress(BarColumn(), MofNCompleteColumn(),
- TextColumn('{task.description}'))
-
- def before_train(self, runner):
- if self.by_epoch:
- total = runner.train_loop.max_epochs
- self.task_id = self.progress.add_task(
- 'epochs', start=True, total=total)
- else:
- total = runner.train_loop.max_iters
- self.task_id = self.progress.add_task(
- 'iters', start=True, total=total)
- self.progress.start()
-
- def after_train_epoch(self, runner):
- if self.by_epoch:
- self.progress.update(self.task_id, advance=1)
-
- def after_train_iter(self, runner, batch_idx, data_batch, outputs):
- if not self.by_epoch:
- self.progress.update(self.task_id, advance=1)
- self.lr_list.append(runner.optim_wrapper.get_lr()['lr'][0])
- self.momentum_list.append(
- runner.optim_wrapper.get_momentum()['momentum'][0])
- self.wd_list.append(
- runner.optim_wrapper.param_groups[0]['weight_decay'])
-
- def after_train(self, runner):
- self.progress.stop()
-
-
-def parse_args():
- parser = argparse.ArgumentParser(
- description='Visualize a Dataset Pipeline')
- parser.add_argument('config', help='config file path')
- parser.add_argument(
- '-p',
- '--parameter',
- type=str,
- default='lr',
- choices=['lr', 'momentum', 'wd'],
- help='The parameter to visualize its change curve, choose from'
- '"lr", "wd" and "momentum". Defaults to "lr".')
- parser.add_argument(
- '-d',
- '--dataset-size',
- type=int,
- help='The size of the dataset. If specify, `build_dataset` will '
- 'be skipped and use this size as the dataset size.')
- parser.add_argument(
- '-n',
- '--ngpus',
- type=int,
- default=1,
- help='The number of GPUs used in training.')
- parser.add_argument(
- '-s',
- '--save-path',
- type=Path,
- help='The learning rate curve plot save path')
- parser.add_argument(
- '--log-level',
- default='WARNING',
- help='The log level of the handler and logger. Defaults to '
- 'WARNING.')
- parser.add_argument('--title', type=str, help='title of figure')
- parser.add_argument(
- '--style', type=str, default='whitegrid', help='style of plt')
- parser.add_argument('--not-show', default=False, action='store_true')
- parser.add_argument(
- '--window-size',
- default='12*7',
- help='Size of the window to display images, in format of "$W*$H".')
- parser.add_argument(
- '--cfg-options',
- nargs='+',
- action=DictAction,
- help='override some settings in the used config, the key-value pair '
- 'in xxx=yyy format will be merged into config file. If the value to '
- 'be overwritten is a list, it should be like key="[a,b]" or key=a,b '
- 'It also allows nested list/tuple values, e.g. key="[(a,b),(c,d)]" '
- 'Note that the quotation marks are necessary and that no white space '
- 'is allowed.')
- args = parser.parse_args()
- if args.window_size != '':
- assert re.match(r'\d+\*\d+', args.window_size), \
- "'window-size' must be in format 'W*H'."
-
- return args
-
-
-def plot_curve(lr_list, args, param_name, iters_per_epoch, by_epoch=True):
- """Plot learning rate vs iter graph."""
- try:
- import seaborn as sns
- sns.set_style(args.style)
- except ImportError:
- pass
-
- wind_w, wind_h = args.window_size.split('*')
- wind_w, wind_h = int(wind_w), int(wind_h)
- plt.figure(figsize=(wind_w, wind_h))
-
- ax: plt.Axes = plt.subplot()
- ax.plot(lr_list, linewidth=1)
-
- if by_epoch:
- ax.xaxis.tick_top()
- ax.set_xlabel('Iters')
- ax.xaxis.set_label_position('top')
- sec_ax = ax.secondary_xaxis(
- 'bottom',
- functions=(lambda x: x / iters_per_epoch,
- lambda y: y * iters_per_epoch))
- sec_ax.set_xlabel('Epochs')
- else:
- plt.xlabel('Iters')
- plt.ylabel(param_name)
-
- if args.title is None:
- plt.title(f'{osp.basename(args.config)} {param_name} curve')
- else:
- plt.title(args.title)
-
-
-def simulate_train(data_loader, cfg, by_epoch):
- model = SimpleModel()
- param_record_hook = ParamRecordHook(by_epoch=by_epoch)
- default_hooks = dict(
- param_scheduler=cfg.default_hooks['param_scheduler'],
- runtime_info=None,
- timer=None,
- logger=None,
- checkpoint=None,
- sampler_seed=None,
- param_record=param_record_hook)
-
- runner = Runner(
- model=model,
- work_dir=cfg.work_dir,
- train_dataloader=data_loader,
- train_cfg=cfg.train_cfg,
- log_level=cfg.log_level,
- optim_wrapper=cfg.optim_wrapper,
- param_scheduler=cfg.param_scheduler,
- default_scope=cfg.default_scope,
- default_hooks=default_hooks,
- visualizer=MagicMock(spec=Visualizer),
- custom_hooks=cfg.get('custom_hooks', None))
-
- runner.train()
-
- param_dict = dict(
- lr=param_record_hook.lr_list,
- momentum=param_record_hook.momentum_list,
- wd=param_record_hook.wd_list)
-
- return param_dict
-
-
-def build_dataset(cfg):
- return DATASETS.build(cfg)
-
-
-def main():
- args = parse_args()
- cfg = Config.fromfile(args.config)
-
- init_default_scope(cfg.get('default_scope', 'mmocr'))
-
- if args.cfg_options is not None:
- cfg.merge_from_dict(args.cfg_options)
- if cfg.get('work_dir', None) is None:
- # use config filename as default work_dir if cfg.work_dir is None
- cfg.work_dir = osp.join('./work_dirs',
- osp.splitext(osp.basename(args.config))[0])
-
- cfg.log_level = args.log_level
-
- # make sure save_root exists
- if args.save_path and not args.save_path.parent.exists():
- raise FileNotFoundError(
- f'The save path is {args.save_path}, and directory '
- f"'{args.save_path.parent}' do not exist.")
-
- # init logger
- print('Param_scheduler :')
- rich.print_json(json.dumps(cfg.param_scheduler))
-
- # prepare data loader
- batch_size = cfg.train_dataloader.batch_size * args.ngpus
-
- if 'by_epoch' in cfg.train_cfg:
- by_epoch = cfg.train_cfg.get('by_epoch')
- elif 'type' in cfg.train_cfg:
- by_epoch = cfg.train_cfg.get('type') == 'EpochBasedTrainLoop'
- else:
- raise ValueError('please set `train_cfg`.')
-
- if args.dataset_size is None and by_epoch:
- dataset_size = len(build_dataset(cfg.train_dataloader.dataset))
- else:
- dataset_size = args.dataset_size or batch_size
-
- class FakeDataloader(list):
- dataset = MagicMock(metainfo=None)
-
- data_loader = FakeDataloader(range(dataset_size // batch_size))
- dataset_info = (
- f'\nDataset infos:'
- f'\n - Dataset size: {dataset_size}'
- f'\n - Batch size per GPU: {cfg.train_dataloader.batch_size}'
- f'\n - Number of GPUs: {args.ngpus}'
- f'\n - Total batch size: {batch_size}')
- if by_epoch:
- dataset_info += f'\n - Iterations per epoch: {len(data_loader)}'
- rich.print(dataset_info + '\n')
-
- # simulation training process
- param_dict = simulate_train(data_loader, cfg, by_epoch)
- param_list = param_dict[args.parameter]
-
- if args.parameter == 'lr':
- param_name = 'Learning Rate'
- elif args.parameter == 'momentum':
- param_name = 'Momentum'
- else:
- param_name = 'Weight Decay'
- plot_curve(param_list, args, param_name, len(data_loader), by_epoch)
-
- if args.save_path:
- plt.savefig(args.save_path)
- print(f'\nThe {param_name} graph is saved at {args.save_path}')
-
- if not args.not_show:
- plt.show()
-
-
-if __name__ == '__main__':
- main()
diff --git a/pipeline/mmocr/mmocr/__init__.py b/pipeline/mmocr/mmocr/__init__.py
deleted file mode 100644
index 335bfd04b961b96d2fcf6bdc0ea235d98066094f..0000000000000000000000000000000000000000
--- a/pipeline/mmocr/mmocr/__init__.py
+++ /dev/null
@@ -1,56 +0,0 @@
-# Copyright (c) OpenMMLab. All rights reserved.
-
-import mmcv
-import mmdet
-
-try:
- import mmengine
- from mmengine.utils import digit_version
-except ImportError:
- mmengine = None
- from mmcv import digit_version
-
-from .version import __version__, short_version
-
-mmcv_minimum_version = '2.0.0rc4'
-mmcv_maximum_version = '2.1.0'
-mmcv_version = digit_version(mmcv.__version__)
-if mmengine is not None:
- mmengine_minimum_version = '0.7.1'
- mmengine_maximum_version = '1.0.0'
- mmengine_version = digit_version(mmengine.__version__)
-
-if not mmengine or mmcv_version < digit_version('2.0.0rc0') or digit_version(
- mmdet.__version__) < digit_version('3.0.0rc0'):
- raise RuntimeError(
- 'MMOCR 1.0 only runs with MMEngine, MMCV 2.0.0rc0+ and '
- 'MMDetection 3.0.0rc0+, but got MMCV '
- f'{mmcv.__version__} and MMDetection '
- f'{mmdet.__version__}. For more information, please refer to '
- 'https://mmocr.readthedocs.io/en/dev-1.x/migration/overview.html'
- ) # noqa
-
-assert (mmcv_version >= digit_version(mmcv_minimum_version)
- and mmcv_version < digit_version(mmcv_maximum_version)), \
- f'MMCV {mmcv.__version__} is incompatible with MMOCR {__version__}. ' \
- f'Please use MMCV >= {mmcv_minimum_version}, ' \
- f'< {mmcv_maximum_version} instead.'
-
-assert (mmengine_version >= digit_version(mmengine_minimum_version)
- and mmengine_version < digit_version(mmengine_maximum_version)), \
- f'MMEngine=={mmengine.__version__} is used but incompatible. ' \
- f'Please install mmengine>={mmengine_minimum_version}, ' \
- f'<{mmengine_maximum_version}.'
-
-mmdet_minimum_version = '3.0.0rc5'
-mmdet_maximum_version = '3.1.0'
-mmdet_version = digit_version(mmdet.__version__)
-
-assert (mmdet_version >= digit_version(mmdet_minimum_version)
- and mmdet_version < digit_version(mmdet_maximum_version)), \
- f'MMDetection {mmdet.__version__} is incompatible ' \
- f'with MMOCR {__version__}. ' \
- f'Please use MMDetection >= {mmdet_minimum_version}, ' \
- f'< {mmdet_maximum_version} instead.'
-
-__all__ = ['__version__', 'short_version', 'digit_version']
diff --git a/pipeline/mmocr/mmocr/__pycache__/__init__.cpython-38.pyc b/pipeline/mmocr/mmocr/__pycache__/__init__.cpython-38.pyc
deleted file mode 100644
index 877cce0348ebfb10aa0514edbdb1c041fe73393f..0000000000000000000000000000000000000000
Binary files a/pipeline/mmocr/mmocr/__pycache__/__init__.cpython-38.pyc and /dev/null differ
diff --git a/pipeline/mmocr/mmocr/__pycache__/__init__.cpython-39.pyc b/pipeline/mmocr/mmocr/__pycache__/__init__.cpython-39.pyc
deleted file mode 100644
index 88d3990c90c3dbb6feb34abb0bc3f4d1427cc10f..0000000000000000000000000000000000000000
Binary files a/pipeline/mmocr/mmocr/__pycache__/__init__.cpython-39.pyc and /dev/null differ
diff --git a/pipeline/mmocr/mmocr/__pycache__/registry.cpython-38.pyc b/pipeline/mmocr/mmocr/__pycache__/registry.cpython-38.pyc
deleted file mode 100644
index 7268bc801886ffce96db4e4b27e0b04459ef07c2..0000000000000000000000000000000000000000
Binary files a/pipeline/mmocr/mmocr/__pycache__/registry.cpython-38.pyc and /dev/null differ
diff --git a/pipeline/mmocr/mmocr/__pycache__/registry.cpython-39.pyc b/pipeline/mmocr/mmocr/__pycache__/registry.cpython-39.pyc
deleted file mode 100644
index 8567fc65ad4e25c9df2cb15f1a9774ea201b6dfc..0000000000000000000000000000000000000000
Binary files a/pipeline/mmocr/mmocr/__pycache__/registry.cpython-39.pyc and /dev/null differ
diff --git a/pipeline/mmocr/mmocr/__pycache__/version.cpython-38.pyc b/pipeline/mmocr/mmocr/__pycache__/version.cpython-38.pyc
deleted file mode 100644
index 12484155c396b9d550e42738123a02f9eeea9a10..0000000000000000000000000000000000000000
Binary files a/pipeline/mmocr/mmocr/__pycache__/version.cpython-38.pyc and /dev/null differ
diff --git a/pipeline/mmocr/mmocr/__pycache__/version.cpython-39.pyc b/pipeline/mmocr/mmocr/__pycache__/version.cpython-39.pyc
deleted file mode 100644
index 40f052f0e609a0cea5a2502e8264e24ca588d711..0000000000000000000000000000000000000000
Binary files a/pipeline/mmocr/mmocr/__pycache__/version.cpython-39.pyc and /dev/null differ
diff --git a/pipeline/mmocr/mmocr/apis/__init__.py b/pipeline/mmocr/mmocr/apis/__init__.py
deleted file mode 100644
index 71141fb7a5962d851b250a5ad71877ef5f80fd4a..0000000000000000000000000000000000000000
--- a/pipeline/mmocr/mmocr/apis/__init__.py
+++ /dev/null
@@ -1,2 +0,0 @@
-# Copyright (c) OpenMMLab. All rights reserved.
-from .inferencers import * # NOQA
diff --git a/pipeline/mmocr/mmocr/apis/__pycache__/__init__.cpython-38.pyc b/pipeline/mmocr/mmocr/apis/__pycache__/__init__.cpython-38.pyc
deleted file mode 100644
index fdeeafbe50f680c57f20abd866bb85e97a5597f6..0000000000000000000000000000000000000000
Binary files a/pipeline/mmocr/mmocr/apis/__pycache__/__init__.cpython-38.pyc and /dev/null differ
diff --git a/pipeline/mmocr/mmocr/apis/__pycache__/__init__.cpython-39.pyc b/pipeline/mmocr/mmocr/apis/__pycache__/__init__.cpython-39.pyc
deleted file mode 100644
index 8dc5590f335ddbf2519a59137a23ec0420f47e62..0000000000000000000000000000000000000000
Binary files a/pipeline/mmocr/mmocr/apis/__pycache__/__init__.cpython-39.pyc and /dev/null differ
diff --git a/pipeline/mmocr/mmocr/apis/inferencers/__init__.py b/pipeline/mmocr/mmocr/apis/inferencers/__init__.py
deleted file mode 100644
index deb4950150fdf68a7dcbb5dcfd4cc5b33e324b41..0000000000000000000000000000000000000000
--- a/pipeline/mmocr/mmocr/apis/inferencers/__init__.py
+++ /dev/null
@@ -1,11 +0,0 @@
-# Copyright (c) OpenMMLab. All rights reserved.
-from .kie_inferencer import KIEInferencer
-from .mmocr_inferencer import MMOCRInferencer
-from .textdet_inferencer import TextDetInferencer
-from .textrec_inferencer import TextRecInferencer
-from .textspot_inferencer import TextSpotInferencer
-
-__all__ = [
- 'TextDetInferencer', 'TextRecInferencer', 'KIEInferencer',
- 'MMOCRInferencer', 'TextSpotInferencer'
-]
diff --git a/pipeline/mmocr/mmocr/apis/inferencers/__pycache__/__init__.cpython-38.pyc b/pipeline/mmocr/mmocr/apis/inferencers/__pycache__/__init__.cpython-38.pyc
deleted file mode 100644
index 109037ff1978d4ae65fc3e00ec3fc547a787d086..0000000000000000000000000000000000000000
Binary files a/pipeline/mmocr/mmocr/apis/inferencers/__pycache__/__init__.cpython-38.pyc and /dev/null differ
diff --git a/pipeline/mmocr/mmocr/apis/inferencers/__pycache__/__init__.cpython-39.pyc b/pipeline/mmocr/mmocr/apis/inferencers/__pycache__/__init__.cpython-39.pyc
deleted file mode 100644
index fee42cb119cb71112a36b6247ffaf876d728ecb3..0000000000000000000000000000000000000000
Binary files a/pipeline/mmocr/mmocr/apis/inferencers/__pycache__/__init__.cpython-39.pyc and /dev/null differ
diff --git a/pipeline/mmocr/mmocr/apis/inferencers/__pycache__/base_mmocr_inferencer.cpython-38.pyc b/pipeline/mmocr/mmocr/apis/inferencers/__pycache__/base_mmocr_inferencer.cpython-38.pyc
deleted file mode 100644
index 26cfd092cadc3c9b66e862962169a49fb99ff6c8..0000000000000000000000000000000000000000
Binary files a/pipeline/mmocr/mmocr/apis/inferencers/__pycache__/base_mmocr_inferencer.cpython-38.pyc and /dev/null differ
diff --git a/pipeline/mmocr/mmocr/apis/inferencers/__pycache__/base_mmocr_inferencer.cpython-39.pyc b/pipeline/mmocr/mmocr/apis/inferencers/__pycache__/base_mmocr_inferencer.cpython-39.pyc
deleted file mode 100644
index 479335fc5eec117230fcab074acce7d11b9bd904..0000000000000000000000000000000000000000
Binary files a/pipeline/mmocr/mmocr/apis/inferencers/__pycache__/base_mmocr_inferencer.cpython-39.pyc and /dev/null differ
diff --git a/pipeline/mmocr/mmocr/apis/inferencers/__pycache__/kie_inferencer.cpython-38.pyc b/pipeline/mmocr/mmocr/apis/inferencers/__pycache__/kie_inferencer.cpython-38.pyc
deleted file mode 100644
index ece0e470d161685b83810fcf04514831ffd1a5eb..0000000000000000000000000000000000000000
Binary files a/pipeline/mmocr/mmocr/apis/inferencers/__pycache__/kie_inferencer.cpython-38.pyc and /dev/null differ
diff --git a/pipeline/mmocr/mmocr/apis/inferencers/__pycache__/kie_inferencer.cpython-39.pyc b/pipeline/mmocr/mmocr/apis/inferencers/__pycache__/kie_inferencer.cpython-39.pyc
deleted file mode 100644
index ac64494a0fd9807b2102bcce5ccab72061af1c5c..0000000000000000000000000000000000000000
Binary files a/pipeline/mmocr/mmocr/apis/inferencers/__pycache__/kie_inferencer.cpython-39.pyc and /dev/null differ
diff --git a/pipeline/mmocr/mmocr/apis/inferencers/__pycache__/mmocr_inferencer.cpython-38.pyc b/pipeline/mmocr/mmocr/apis/inferencers/__pycache__/mmocr_inferencer.cpython-38.pyc
deleted file mode 100644
index 652a434128d91b6cceca3f72da6d77759b43c615..0000000000000000000000000000000000000000
Binary files a/pipeline/mmocr/mmocr/apis/inferencers/__pycache__/mmocr_inferencer.cpython-38.pyc and /dev/null differ
diff --git a/pipeline/mmocr/mmocr/apis/inferencers/__pycache__/mmocr_inferencer.cpython-39.pyc b/pipeline/mmocr/mmocr/apis/inferencers/__pycache__/mmocr_inferencer.cpython-39.pyc
deleted file mode 100644
index 88bca227d402d445a87ed3d578c91e8e92859327..0000000000000000000000000000000000000000
Binary files a/pipeline/mmocr/mmocr/apis/inferencers/__pycache__/mmocr_inferencer.cpython-39.pyc and /dev/null differ
diff --git a/pipeline/mmocr/mmocr/apis/inferencers/__pycache__/textdet_inferencer.cpython-38.pyc b/pipeline/mmocr/mmocr/apis/inferencers/__pycache__/textdet_inferencer.cpython-38.pyc
deleted file mode 100644
index 81bf72f78842c53f7fc241510f8b57659deb9ec4..0000000000000000000000000000000000000000
Binary files a/pipeline/mmocr/mmocr/apis/inferencers/__pycache__/textdet_inferencer.cpython-38.pyc and /dev/null differ
diff --git a/pipeline/mmocr/mmocr/apis/inferencers/__pycache__/textdet_inferencer.cpython-39.pyc b/pipeline/mmocr/mmocr/apis/inferencers/__pycache__/textdet_inferencer.cpython-39.pyc
deleted file mode 100644
index 585b9b4bec5841f87de271aa79e74719eb21c1ea..0000000000000000000000000000000000000000
Binary files a/pipeline/mmocr/mmocr/apis/inferencers/__pycache__/textdet_inferencer.cpython-39.pyc and /dev/null differ
diff --git a/pipeline/mmocr/mmocr/apis/inferencers/__pycache__/textrec_inferencer.cpython-38.pyc b/pipeline/mmocr/mmocr/apis/inferencers/__pycache__/textrec_inferencer.cpython-38.pyc
deleted file mode 100644
index fa21585cf39318b2981e56a3a2579926ded092ab..0000000000000000000000000000000000000000
Binary files a/pipeline/mmocr/mmocr/apis/inferencers/__pycache__/textrec_inferencer.cpython-38.pyc and /dev/null differ
diff --git a/pipeline/mmocr/mmocr/apis/inferencers/__pycache__/textrec_inferencer.cpython-39.pyc b/pipeline/mmocr/mmocr/apis/inferencers/__pycache__/textrec_inferencer.cpython-39.pyc
deleted file mode 100644
index 28c708a07a6e0fc80fbf1cf11fd9ae587daebc0c..0000000000000000000000000000000000000000
Binary files a/pipeline/mmocr/mmocr/apis/inferencers/__pycache__/textrec_inferencer.cpython-39.pyc and /dev/null differ
diff --git a/pipeline/mmocr/mmocr/apis/inferencers/__pycache__/textspot_inferencer.cpython-38.pyc b/pipeline/mmocr/mmocr/apis/inferencers/__pycache__/textspot_inferencer.cpython-38.pyc
deleted file mode 100644
index 0031bcb80eaceb4b7d7db961963400e08d0710d1..0000000000000000000000000000000000000000
Binary files a/pipeline/mmocr/mmocr/apis/inferencers/__pycache__/textspot_inferencer.cpython-38.pyc and /dev/null differ
diff --git a/pipeline/mmocr/mmocr/apis/inferencers/__pycache__/textspot_inferencer.cpython-39.pyc b/pipeline/mmocr/mmocr/apis/inferencers/__pycache__/textspot_inferencer.cpython-39.pyc
deleted file mode 100644
index 163b91d6d1c630a9def9b5a02286c802f73550d3..0000000000000000000000000000000000000000
Binary files a/pipeline/mmocr/mmocr/apis/inferencers/__pycache__/textspot_inferencer.cpython-39.pyc and /dev/null differ
diff --git a/pipeline/mmocr/mmocr/apis/inferencers/base_mmocr_inferencer.py b/pipeline/mmocr/mmocr/apis/inferencers/base_mmocr_inferencer.py
deleted file mode 100644
index 02ac643d9ffea8dddde098aa02038ebfdc1cce25..0000000000000000000000000000000000000000
--- a/pipeline/mmocr/mmocr/apis/inferencers/base_mmocr_inferencer.py
+++ /dev/null
@@ -1,405 +0,0 @@
-# Copyright (c) OpenMMLab. All rights reserved.
-import os.path as osp
-from typing import Dict, Iterable, List, Optional, Sequence, Tuple, Union
-
-import mmcv
-import mmengine
-import numpy as np
-from mmengine.dataset import Compose
-from mmengine.infer.infer import BaseInferencer, ModelType
-from mmengine.model.utils import revert_sync_batchnorm
-from mmengine.registry import init_default_scope
-from mmengine.structures import InstanceData
-from rich.progress import track
-from torch import Tensor
-
-from mmocr.utils import ConfigType
-
-InstanceList = List[InstanceData]
-InputType = Union[str, np.ndarray]
-InputsType = Union[InputType, Sequence[InputType]]
-PredType = Union[InstanceData, InstanceList]
-ImgType = Union[np.ndarray, Sequence[np.ndarray]]
-ResType = Union[Dict, List[Dict], InstanceData, List[InstanceData]]
-
-
-class BaseMMOCRInferencer(BaseInferencer):
- """Base inferencer.
-
- Args:
- model (str, optional): Path to the config file or the model name
- defined in metafile. For example, it could be
- "dbnet_resnet18_fpnc_1200e_icdar2015" or
- "configs/textdet/dbnet/dbnet_resnet18_fpnc_1200e_icdar2015.py".
- If model is not specified, user must provide the
- `weights` saved by MMEngine which contains the config string.
- Defaults to None.
- weights (str, optional): Path to the checkpoint. If it is not specified
- and model is a model name of metafile, the weights will be loaded
- from metafile. Defaults to None.
- device (str, optional): Device to run inference. If None, the available
- device will be automatically used. Defaults to None.
- scope (str, optional): The scope of the model. Defaults to "mmocr".
- """
-
- preprocess_kwargs: set = set()
- forward_kwargs: set = set()
- visualize_kwargs: set = {
- 'return_vis', 'show', 'wait_time', 'draw_pred', 'pred_score_thr',
- 'save_vis'
- }
- postprocess_kwargs: set = {
- 'print_result', 'return_datasample', 'save_pred'
- }
- loading_transforms: list = ['LoadImageFromFile', 'LoadImageFromNDArray']
-
- def __init__(self,
- model: Union[ModelType, str, None] = None,
- weights: Optional[str] = None,
- device: Optional[str] = None,
- scope: str = 'mmocr') -> None:
- # A global counter tracking the number of images given in the form
- # of ndarray, for naming the output images
- self.num_unnamed_imgs = 0
- init_default_scope(scope)
- super().__init__(
- model=model, weights=weights, device=device, scope=scope)
- self.model = revert_sync_batchnorm(self.model)
-
- def preprocess(self, inputs: InputsType, batch_size: int = 1, **kwargs):
- """Process the inputs into a model-feedable format.
-
- Args:
- inputs (InputsType): Inputs given by user.
- batch_size (int): batch size. Defaults to 1.
-
- Yields:
- Any: Data processed by the ``pipeline`` and ``collate_fn``.
- """
- chunked_data = self._get_chunk_data(inputs, batch_size)
- yield from map(self.collate_fn, chunked_data)
-
- def _get_chunk_data(self, inputs: Iterable, chunk_size: int):
- """Get batch data from inputs.
-
- Args:
- inputs (Iterable): An iterable dataset.
- chunk_size (int): Equivalent to batch size.
-
- Yields:
- list: batch data.
- """
- inputs_iter = iter(inputs)
- while True:
- try:
- chunk_data = []
- for _ in range(chunk_size):
- inputs_ = next(inputs_iter)
- pipe_out = self.pipeline(inputs_)
- if pipe_out['data_samples'].get('img_path') is None:
- pipe_out['data_samples'].set_metainfo(
- dict(img_path=f'{self.num_unnamed_imgs}.jpg'))
- self.num_unnamed_imgs += 1
- chunk_data.append((inputs_, pipe_out))
- yield chunk_data
- except StopIteration:
- if chunk_data:
- yield chunk_data
- break
-
- def __call__(self,
- inputs: InputsType,
- return_datasamples: bool = False,
- batch_size: int = 1,
- progress_bar: bool = True,
- return_vis: bool = False,
- show: bool = False,
- wait_time: int = 0,
- draw_pred: bool = True,
- pred_score_thr: float = 0.3,
- out_dir: str = 'results/',
- save_vis: bool = False,
- save_pred: bool = False,
- print_result: bool = False,
- **kwargs) -> dict:
- """Call the inferencer.
-
- Args:
- inputs (InputsType): Inputs for the inferencer. It can be a path
- to image / image directory, or an array, or a list of these.
- Note: If it's an numpy array, it should be in BGR order.
- return_datasamples (bool): Whether to return results as
- :obj:`BaseDataElement`. Defaults to False.
- batch_size (int): Inference batch size. Defaults to 1.
- progress_bar (bool): Whether to show a progress bar. Defaults to
- True.
- return_vis (bool): Whether to return the visualization result.
- Defaults to False.
- show (bool): Whether to display the visualization results in a
- popup window. Defaults to False.
- wait_time (float): The interval of show (s). Defaults to 0.
- draw_pred (bool): Whether to draw predicted bounding boxes.
- Defaults to True.
- pred_score_thr (float): Minimum score of bboxes to draw.
- Defaults to 0.3.
- out_dir (str): Output directory of results. Defaults to 'results/'.
- save_vis (bool): Whether to save the visualization results to
- "out_dir". Defaults to False.
- save_pred (bool): Whether to save the inference results to
- "out_dir". Defaults to False.
- print_result (bool): Whether to print the inference result w/o
- visualization to the console. Defaults to False.
-
- **kwargs: Other keyword arguments passed to :meth:`preprocess`,
- :meth:`forward`, :meth:`visualize` and :meth:`postprocess`.
- Each key in kwargs should be in the corresponding set of
- ``preprocess_kwargs``, ``forward_kwargs``, ``visualize_kwargs``
- and ``postprocess_kwargs``.
-
- Returns:
- dict: Inference and visualization results, mapped from
- "predictions" and "visualization".
- """
- if (save_vis or save_pred) and not out_dir:
- raise ValueError('out_dir must be specified when save_vis or '
- 'save_pred is True!')
- if out_dir:
- img_out_dir = osp.join(out_dir, 'vis')
- pred_out_dir = osp.join(out_dir, 'preds')
- else:
- img_out_dir, pred_out_dir = '', ''
- (
- preprocess_kwargs,
- forward_kwargs,
- visualize_kwargs,
- postprocess_kwargs,
- ) = self._dispatch_kwargs(
- return_vis=return_vis,
- show=show,
- wait_time=wait_time,
- draw_pred=draw_pred,
- pred_score_thr=pred_score_thr,
- save_vis=save_vis,
- save_pred=save_pred,
- print_result=print_result,
- **kwargs)
-
- ori_inputs = self._inputs_to_list(inputs)
- inputs = self.preprocess(
- ori_inputs, batch_size=batch_size, **preprocess_kwargs)
- results = {'predictions': [], 'visualization': []}
- for ori_inputs, data in track(
- inputs, description='Inference', disable=not progress_bar):
- preds = self.forward(data, **forward_kwargs)
- visualization = self.visualize(
- ori_inputs, preds, img_out_dir=img_out_dir, **visualize_kwargs)
- batch_res = self.postprocess(
- preds,
- visualization,
- return_datasamples,
- pred_out_dir=pred_out_dir,
- **postprocess_kwargs)
- results['predictions'].extend(batch_res['predictions'])
- if return_vis and batch_res['visualization'] is not None:
- results['visualization'].extend(batch_res['visualization'])
- return results
-
- def _init_pipeline(self, cfg: ConfigType) -> Compose:
- """Initialize the test pipeline."""
- pipeline_cfg = cfg.test_dataloader.dataset.pipeline
-
- # For inference, the key of ``instances`` is not used.
- if 'meta_keys' in pipeline_cfg[-1]:
- pipeline_cfg[-1]['meta_keys'] = tuple(
- meta_key for meta_key in pipeline_cfg[-1]['meta_keys']
- if meta_key != 'instances')
-
- # Loading annotations is also not applicable
- idx = self._get_transform_idx(pipeline_cfg, 'LoadOCRAnnotations')
- if idx != -1:
- del pipeline_cfg[idx]
-
- for transform in self.loading_transforms:
- load_img_idx = self._get_transform_idx(pipeline_cfg, transform)
- if load_img_idx != -1:
- pipeline_cfg[load_img_idx]['type'] = 'InferencerLoader'
- break
- if load_img_idx == -1:
- raise ValueError(
- f'None of {self.loading_transforms} is found in the test '
- 'pipeline')
-
- return Compose(pipeline_cfg)
-
- def _get_transform_idx(self, pipeline_cfg: ConfigType, name: str) -> int:
- """Returns the index of the transform in a pipeline.
-
- If the transform is not found, returns -1.
- """
- for i, transform in enumerate(pipeline_cfg):
- if transform['type'] == name:
- return i
- return -1
-
- def visualize(self,
- inputs: InputsType,
- preds: PredType,
- return_vis: bool = False,
- show: bool = False,
- wait_time: int = 0,
- draw_pred: bool = True,
- pred_score_thr: float = 0.3,
- save_vis: bool = False,
- img_out_dir: str = '') -> Union[List[np.ndarray], None]:
- """Visualize predictions.
-
- Args:
- inputs (List[Union[str, np.ndarray]]): Inputs for the inferencer.
- preds (List[Dict]): Predictions of the model.
- return_vis (bool): Whether to return the visualization result.
- Defaults to False.
- show (bool): Whether to display the image in a popup window.
- Defaults to False.
- wait_time (float): The interval of show (s). Defaults to 0.
- draw_pred (bool): Whether to draw predicted bounding boxes.
- Defaults to True.
- pred_score_thr (float): Minimum score of bboxes to draw.
- Defaults to 0.3.
- save_vis (bool): Whether to save the visualization result. Defaults
- to False.
- img_out_dir (str): Output directory of visualization results.
- If left as empty, no file will be saved. Defaults to ''.
-
- Returns:
- List[np.ndarray] or None: Returns visualization results only if
- applicable.
- """
- if self.visualizer is None or not (show or save_vis or return_vis):
- return None
-
- if getattr(self, 'visualizer') is None:
- raise ValueError('Visualization needs the "visualizer" term'
- 'defined in the config, but got None.')
-
- results = []
-
- for single_input, pred in zip(inputs, preds):
- if isinstance(single_input, str):
- img_bytes = mmengine.fileio.get(single_input)
- img = mmcv.imfrombytes(img_bytes, channel_order='rgb')
- elif isinstance(single_input, np.ndarray):
- img = single_input.copy()[:, :, ::-1] # to RGB
- else:
- raise ValueError('Unsupported input type: '
- f'{type(single_input)}')
- img_name = osp.splitext(osp.basename(pred.img_path))[0]
-
- if save_vis and img_out_dir:
- out_file = osp.splitext(img_name)[0]
- out_file = f'{out_file}.jpg'
- out_file = osp.join(img_out_dir, out_file)
- else:
- out_file = None
-
- visualization = self.visualizer.add_datasample(
- img_name,
- img,
- pred,
- show=show,
- wait_time=wait_time,
- draw_gt=False,
- draw_pred=draw_pred,
- pred_score_thr=pred_score_thr,
- out_file=out_file,
- )
- results.append(visualization)
-
- return results
-
- def postprocess(
- self,
- preds: PredType,
- visualization: Optional[List[np.ndarray]] = None,
- return_datasample: bool = False,
- print_result: bool = False,
- save_pred: bool = False,
- pred_out_dir: str = '',
- ) -> Union[ResType, Tuple[ResType, np.ndarray]]:
- """Process the predictions and visualization results from ``forward``
- and ``visualize``.
-
- This method should be responsible for the following tasks:
-
- 1. Convert datasamples into a json-serializable dict if needed.
- 2. Pack the predictions and visualization results and return them.
- 3. Dump or log the predictions.
-
- Args:
- preds (List[Dict]): Predictions of the model.
- visualization (Optional[np.ndarray]): Visualized predictions.
- return_datasample (bool): Whether to use Datasample to store
- inference results. If False, dict will be used.
- print_result (bool): Whether to print the inference result w/o
- visualization to the console. Defaults to False.
- save_pred (bool): Whether to save the inference result. Defaults to
- False.
- pred_out_dir: File to save the inference results w/o
- visualization. If left as empty, no file will be saved.
- Defaults to ''.
-
- Returns:
- dict: Inference and visualization results with key ``predictions``
- and ``visualization``.
-
- - ``visualization`` (Any): Returned by :meth:`visualize`.
- - ``predictions`` (dict or DataSample): Returned by
- :meth:`forward` and processed in :meth:`postprocess`.
- If ``return_datasample=False``, it usually should be a
- json-serializable dict containing only basic data elements such
- as strings and numbers.
- """
- result_dict = {}
- results = preds
- if not return_datasample:
- results = []
- for pred in preds:
- result = self.pred2dict(pred)
- if save_pred and pred_out_dir:
- pred_name = osp.splitext(osp.basename(pred.img_path))[0]
- pred_name = f'{pred_name}.json'
- pred_out_file = osp.join(pred_out_dir, pred_name)
- mmengine.dump(result, pred_out_file)
- results.append(result)
- # Add img to the results after printing and dumping
- result_dict['predictions'] = results
- if print_result:
- print(result_dict)
- result_dict['visualization'] = visualization
- return result_dict
-
- def pred2dict(self, data_sample: InstanceData) -> Dict:
- """Extract elements necessary to represent a prediction into a
- dictionary.
-
- It's better to contain only basic data elements such as strings and
- numbers in order to guarantee it's json-serializable.
- """
- raise NotImplementedError
-
- def _array2list(self, array: Union[Tensor, np.ndarray,
- List]) -> List[float]:
- """Convert a tensor or numpy array to a list.
-
- Args:
- array (Union[Tensor, np.ndarray]): The array to be converted.
-
- Returns:
- List[float]: The converted list.
- """
- if isinstance(array, Tensor):
- return array.detach().cpu().numpy().tolist()
- if isinstance(array, np.ndarray):
- return array.tolist()
- if isinstance(array, list):
- array = [self._array2list(arr) for arr in array]
- return array
diff --git a/pipeline/mmocr/mmocr/apis/inferencers/kie_inferencer.py b/pipeline/mmocr/mmocr/apis/inferencers/kie_inferencer.py
deleted file mode 100644
index c7865d5c9b756d3556538304023039a6648b07db..0000000000000000000000000000000000000000
--- a/pipeline/mmocr/mmocr/apis/inferencers/kie_inferencer.py
+++ /dev/null
@@ -1,285 +0,0 @@
-# Copyright (c) OpenMMLab. All rights reserved.
-import copy
-import os.path as osp
-from typing import Any, Dict, List, Optional, Sequence, Union
-
-import mmcv
-import mmengine
-import numpy as np
-from mmengine.dataset import Compose, pseudo_collate
-from mmengine.runner.checkpoint import _load_checkpoint
-
-from mmocr.registry import DATASETS
-from mmocr.structures import KIEDataSample
-from mmocr.utils import ConfigType
-from .base_mmocr_inferencer import BaseMMOCRInferencer, ModelType, PredType
-
-InputType = Dict
-InputsType = Sequence[Dict]
-
-
-class KIEInferencer(BaseMMOCRInferencer):
- """Key Information Extraction Inferencer.
-
- Args:
- model (str, optional): Path to the config file or the model name
- defined in metafile. For example, it could be
- "sdmgr_unet16_60e_wildreceipt" or
- "configs/kie/sdmgr/sdmgr_unet16_60e_wildreceipt.py".
- If model is not specified, user must provide the
- `weights` saved by MMEngine which contains the config string.
- Defaults to None.
- weights (str, optional): Path to the checkpoint. If it is not specified
- and model is a model name of metafile, the weights will be loaded
- from metafile. Defaults to None.
- device (str, optional): Device to run inference. If None, the available
- device will be automatically used. Defaults to None.
- scope (str, optional): The scope of the model. Defaults to "mmocr".
- """
-
- def __init__(self,
- model: Union[ModelType, str, None] = None,
- weights: Optional[str] = None,
- device: Optional[str] = None,
- scope: Optional[str] = 'mmocr') -> None:
- super().__init__(
- model=model, weights=weights, device=device, scope=scope)
- self._load_metainfo_to_visualizer(weights, self.cfg)
- self.collate_fn = self.kie_collate
-
- def _load_metainfo_to_visualizer(self, weights: Optional[str],
- cfg: ConfigType) -> None:
- """Load meta information to visualizer."""
- if hasattr(self, 'visualizer'):
- if weights is not None:
- w = _load_checkpoint(weights, map_location='cpu')
- if w and 'meta' in w and 'dataset_meta' in w['meta']:
- self.visualizer.dataset_meta = w['meta']['dataset_meta']
- return
- if 'test_dataloader' in cfg:
- dataset_cfg = copy.deepcopy(cfg.test_dataloader.dataset)
- dataset_cfg['lazy_init'] = True
- dataset_cfg['metainfo'] = None
- dataset = DATASETS.build(dataset_cfg)
- self.visualizer.dataset_meta = dataset.metainfo
- else:
- raise ValueError(
- 'KIEVisualizer requires meta information from weights or '
- 'test dataset, but none of them is provided.')
-
- def _init_pipeline(self, cfg: ConfigType) -> None:
- """Initialize the test pipeline."""
- pipeline_cfg = cfg.test_dataloader.dataset.pipeline
- idx = self._get_transform_idx(pipeline_cfg, 'LoadKIEAnnotations')
- if idx == -1:
- raise ValueError(
- 'LoadKIEAnnotations is not found in the test pipeline')
- pipeline_cfg[idx]['with_label'] = False
- self.novisual = all(
- self._get_transform_idx(pipeline_cfg, t) == -1
- for t in self.loading_transforms)
- # Remove Resize from test_pipeline, since SDMGR requires bbox
- # annotations to be resized together with pictures, but visualization
- # loads the original image from the disk.
- # TODO: find a more elegant way to fix this
- idx = self._get_transform_idx(pipeline_cfg, 'Resize')
- if idx != -1:
- pipeline_cfg.pop(idx)
- # If it's in non-visual mode, self.pipeline will be specified.
- # Otherwise, file_pipeline and ndarray_pipeline will be specified.
- if self.novisual:
- return Compose(pipeline_cfg)
- return super()._init_pipeline(cfg)
-
- @staticmethod
- def kie_collate(data_batch: Sequence) -> Any:
- """A collate function designed for KIE, where the first element (input)
- is a dict and we only want to keep it as-is instead of batching
- elements inside.
-
- Returns:
- Any: Transversed Data in the same format as the data_itement of
- ``data_batch``.
- """ # noqa: E501
- transposed = list(zip(*data_batch))
- for i in range(1, len(transposed)):
- transposed[i] = pseudo_collate(transposed[i])
- return transposed
-
- def _inputs_to_list(self, inputs: InputsType) -> list:
- """Preprocess the inputs to a list.
-
- Preprocess inputs to a list according to its type.
-
- The inputs can be a dict or list[dict], where each dictionary contains
- following keys:
-
- - img (str or ndarray): Path to the image or the image itself. If KIE
- Inferencer is used in no-visual mode, this key is not required.
- Note: If it's an numpy array, it should be in BGR order.
- - img_shape (tuple(int, int)): Image shape in (H, W). In
- - instances (list[dict]): A list of instances.
- - bbox (ndarray(dtype=np.float32)): Shape (4, ). Bounding box.
- - text (str): Annotation text.
-
- Each ``instance`` looks like the following:
-
- .. code-block:: python
-
- {
- # A nested list of 4 numbers representing the bounding box of
- # the instance, in (x1, y1, x2, y2) order.
- 'bbox': np.array([[x1, y1, x2, y2], [x1, y1, x2, y2], ...],
- dtype=np.int32),
-
- # List of texts.
- "texts": ['text1', 'text2', ...],
- }
-
- Args:
- inputs (InputsType): Inputs for the inferencer.
-
- Returns:
- list: List of input for the :meth:`preprocess`.
- """
-
- processed_inputs = []
-
- if not isinstance(inputs, (list, tuple)):
- inputs = [inputs]
-
- for single_input in inputs:
- if self.novisual:
- processed_input = copy.deepcopy(single_input)
- if 'img' not in single_input and \
- 'img_shape' not in single_input:
- raise ValueError(
- 'KIEInferencer in no-visual mode '
- 'requires input has "img" or "img_shape", but both are'
- ' not found.')
- if 'img' in single_input:
- img = single_input['img']
- if isinstance(img, str):
- img_bytes = mmengine.fileio.get(img)
- img = mmcv.imfrombytes(img_bytes)
- processed_input['img'] = img
- processed_input['img_shape'] = img.shape[:2]
- processed_inputs.append(processed_input)
- else:
- if 'img' not in single_input:
- raise ValueError(
- 'This inferencer is constructed to '
- 'accept image inputs, but the input does not contain '
- '"img" key.')
- if isinstance(single_input['img'], str):
- processed_input = {
- k: v
- for k, v in single_input.items() if k != 'img'
- }
- processed_input['img_path'] = single_input['img']
- processed_inputs.append(processed_input)
- elif isinstance(single_input['img'], np.ndarray):
- processed_inputs.append(copy.deepcopy(single_input))
- else:
- atype = type(single_input['img'])
- raise ValueError(f'Unsupported input type: {atype}')
-
- return processed_inputs
-
- def visualize(self,
- inputs: InputsType,
- preds: PredType,
- return_vis: bool = False,
- show: bool = False,
- wait_time: int = 0,
- draw_pred: bool = True,
- pred_score_thr: float = 0.3,
- save_vis: bool = False,
- img_out_dir: str = '') -> Union[List[np.ndarray], None]:
- """Visualize predictions.
-
- Args:
- inputs (List[Union[str, np.ndarray]]): Inputs for the inferencer.
- preds (List[Dict]): Predictions of the model.
- return_vis (bool): Whether to return the visualization result.
- Defaults to False.
- show (bool): Whether to display the image in a popup window.
- Defaults to False.
- wait_time (float): The interval of show (s). Defaults to 0.
- draw_pred (bool): Whether to draw predicted bounding boxes.
- Defaults to True.
- pred_score_thr (float): Minimum score of bboxes to draw.
- Defaults to 0.3.
- save_vis (bool): Whether to save the visualization result. Defaults
- to False.
- img_out_dir (str): Output directory of visualization results.
- If left as empty, no file will be saved. Defaults to ''.
-
- Returns:
- List[np.ndarray] or None: Returns visualization results only if
- applicable.
- """
- if self.visualizer is None or not (show or save_vis or return_vis):
- return None
-
- if getattr(self, 'visualizer') is None:
- raise ValueError('Visualization needs the "visualizer" term'
- 'defined in the config, but got None.')
-
- results = []
-
- for single_input, pred in zip(inputs, preds):
- assert 'img' in single_input or 'img_shape' in single_input
- if 'img' in single_input:
- if isinstance(single_input['img'], str):
- img_bytes = mmengine.fileio.get(single_input['img'])
- img = mmcv.imfrombytes(img_bytes, channel_order='rgb')
- elif isinstance(single_input['img'], np.ndarray):
- img = single_input['img'].copy()[:, :, ::-1] # To RGB
- elif 'img_shape' in single_input:
- img = np.zeros(single_input['img_shape'], dtype=np.uint8)
- else:
- raise ValueError('Input does not contain either "img" or '
- '"img_shape"')
- img_name = osp.splitext(osp.basename(pred.img_path))[0]
-
- if save_vis and img_out_dir:
- out_file = osp.splitext(img_name)[0]
- out_file = f'{out_file}.jpg'
- out_file = osp.join(img_out_dir, out_file)
- else:
- out_file = None
-
- visualization = self.visualizer.add_datasample(
- img_name,
- img,
- pred,
- show=show,
- wait_time=wait_time,
- draw_gt=False,
- draw_pred=draw_pred,
- pred_score_thr=pred_score_thr,
- out_file=out_file,
- )
- results.append(visualization)
-
- return results
-
- def pred2dict(self, data_sample: KIEDataSample) -> Dict:
- """Extract elements necessary to represent a prediction into a
- dictionary. It's better to contain only basic data elements such as
- strings and numbers in order to guarantee it's json-serializable.
-
- Args:
- data_sample (TextRecogDataSample): The data sample to be converted.
-
- Returns:
- dict: The output dictionary.
- """
- result = {}
- pred = data_sample.pred_instances
- result['scores'] = pred.scores.cpu().numpy().tolist()
- result['edge_scores'] = pred.edge_scores.cpu().numpy().tolist()
- result['edge_labels'] = pred.edge_labels.cpu().numpy().tolist()
- result['labels'] = pred.labels.cpu().numpy().tolist()
- return result
diff --git a/pipeline/mmocr/mmocr/apis/inferencers/mmocr_inferencer.py b/pipeline/mmocr/mmocr/apis/inferencers/mmocr_inferencer.py
deleted file mode 100644
index be7f74237875ed42ef5cb099957662c8a125d94c..0000000000000000000000000000000000000000
--- a/pipeline/mmocr/mmocr/apis/inferencers/mmocr_inferencer.py
+++ /dev/null
@@ -1,422 +0,0 @@
-# Copyright (c) OpenMMLab. All rights reserved.
-import os.path as osp
-from datetime import datetime
-from typing import Dict, List, Optional, Tuple, Union
-
-import mmcv
-import mmengine
-import numpy as np
-from rich.progress import track
-
-from mmocr.registry import VISUALIZERS
-from mmocr.structures import TextSpottingDataSample
-from mmocr.utils import ConfigType, bbox2poly, crop_img, poly2bbox
-from .base_mmocr_inferencer import (BaseMMOCRInferencer, InputsType, PredType,
- ResType)
-from .kie_inferencer import KIEInferencer
-from .textdet_inferencer import TextDetInferencer
-from .textrec_inferencer import TextRecInferencer
-
-
-class MMOCRInferencer(BaseMMOCRInferencer):
- """MMOCR Inferencer. It's a wrapper around three base task
- inferenecers: TextDetInferencer, TextRecInferencer and KIEInferencer,
- and it can be used to perform end-to-end OCR or KIE inference.
-
- Args:
- det (Optional[Union[ConfigType, str]]): Pretrained text detection
- algorithm. It's the path to the config file or the model name
- defined in metafile. Defaults to None.
- det_weights (Optional[str]): Path to the custom checkpoint file of
- the selected det model. If it is not specified and "det" is a model
- name of metafile, the weights will be loaded from metafile.
- Defaults to None.
- rec (Optional[Union[ConfigType, str]]): Pretrained text recognition
- algorithm. It's the path to the config file or the model name
- defined in metafile. Defaults to None.
- rec_weights (Optional[str]): Path to the custom checkpoint file of
- the selected rec model. If it is not specified and "rec" is a model
- name of metafile, the weights will be loaded from metafile.
- Defaults to None.
- kie (Optional[Union[ConfigType, str]]): Pretrained key information
- extraction algorithm. It's the path to the config file or the model
- name defined in metafile. Defaults to None.
- kie_weights (Optional[str]): Path to the custom checkpoint file of
- the selected kie model. If it is not specified and "kie" is a model
- name of metafile, the weights will be loaded from metafile.
- Defaults to None.
- device (Optional[str]): Device to run inference. If None, the available
- device will be automatically used. Defaults to None.
-
- """
-
- def __init__(self,
- det: Optional[Union[ConfigType, str]] = None,
- det_weights: Optional[str] = None,
- rec: Optional[Union[ConfigType, str]] = None,
- rec_weights: Optional[str] = None,
- kie: Optional[Union[ConfigType, str]] = None,
- kie_weights: Optional[str] = None,
- device: Optional[str] = None) -> None:
-
- if det is None and rec is None and kie is None:
- raise ValueError('At least one of det, rec and kie should be '
- 'provided.')
-
- self.visualizer = None
-
- if det is not None:
- self.textdet_inferencer = TextDetInferencer(
- det, det_weights, device)
- self.mode = 'det'
- if rec is not None:
- self.textrec_inferencer = TextRecInferencer(
- rec, rec_weights, device)
- if getattr(self, 'mode', None) == 'det':
- self.mode = 'det_rec'
- ts = str(datetime.timestamp(datetime.now()))
- self.visualizer = VISUALIZERS.build(
- dict(
- type='TextSpottingLocalVisualizer',
- name=f'inferencer{ts}',
- font_families=self.textrec_inferencer.visualizer.
- font_families))
- else:
- self.mode = 'rec'
- if kie is not None:
- if det is None or rec is None:
- raise ValueError(
- 'kie_config is only applicable when det_config and '
- 'rec_config are both provided')
- self.kie_inferencer = KIEInferencer(kie, kie_weights, device)
- self.mode = 'det_rec_kie'
-
- def _inputs2ndarrray(self, inputs: List[InputsType]) -> List[np.ndarray]:
- """Preprocess the inputs to a list of numpy arrays."""
- new_inputs = []
- for item in inputs:
- if isinstance(item, np.ndarray):
- new_inputs.append(item)
- elif isinstance(item, str):
- img_bytes = mmengine.fileio.get(item)
- new_inputs.append(mmcv.imfrombytes(img_bytes))
- else:
- raise NotImplementedError(f'The input type {type(item)} is not'
- 'supported yet.')
- return new_inputs
-
- def forward(self,
- inputs: InputsType,
- batch_size: int = 1,
- det_batch_size: Optional[int] = None,
- rec_batch_size: Optional[int] = None,
- kie_batch_size: Optional[int] = None,
- **forward_kwargs) -> PredType:
- """Forward the inputs to the model.
-
- Args:
- inputs (InputsType): The inputs to be forwarded.
- batch_size (int): Batch size. Defaults to 1.
- det_batch_size (Optional[int]): Batch size for text detection
- model. Overwrite batch_size if it is not None.
- Defaults to None.
- rec_batch_size (Optional[int]): Batch size for text recognition
- model. Overwrite batch_size if it is not None.
- Defaults to None.
- kie_batch_size (Optional[int]): Batch size for KIE model.
- Overwrite batch_size if it is not None.
- Defaults to None.
-
- Returns:
- Dict: The prediction results. Possibly with keys "det", "rec", and
- "kie"..
- """
- result = {}
- forward_kwargs['progress_bar'] = False
- if det_batch_size is None:
- det_batch_size = batch_size
- if rec_batch_size is None:
- rec_batch_size = batch_size
- if kie_batch_size is None:
- kie_batch_size = batch_size
- if self.mode == 'rec':
- # The extra list wrapper here is for the ease of postprocessing
- self.rec_inputs = inputs
- predictions = self.textrec_inferencer(
- self.rec_inputs,
- return_datasamples=True,
- batch_size=rec_batch_size,
- **forward_kwargs)['predictions']
- result['rec'] = [[p] for p in predictions]
- elif self.mode.startswith('det'): # 'det'/'det_rec'/'det_rec_kie'
- result['det'] = self.textdet_inferencer(
- inputs,
- return_datasamples=True,
- batch_size=det_batch_size,
- **forward_kwargs)['predictions']
- if self.mode.startswith('det_rec'): # 'det_rec'/'det_rec_kie'
- result['rec'] = []
- for img, det_data_sample in zip(
- self._inputs2ndarrray(inputs), result['det']):
- det_pred = det_data_sample.pred_instances
- self.rec_inputs = []
- for polygon in det_pred['polygons']:
- # Roughly convert the polygon to a quadangle with
- # 4 points
- quad = bbox2poly(poly2bbox(polygon)).tolist()
- self.rec_inputs.append(crop_img(img, quad))
- result['rec'].append(
- self.textrec_inferencer(
- self.rec_inputs,
- return_datasamples=True,
- batch_size=rec_batch_size,
- **forward_kwargs)['predictions'])
- if self.mode == 'det_rec_kie':
- self.kie_inputs = []
- # TODO: when the det output is empty, kie will fail
- # as no gt-instances can be provided. It's a known
- # issue but cannot be solved elegantly since we support
- # batch inference.
- for img, det_data_sample, rec_data_samples in zip(
- inputs, result['det'], result['rec']):
- det_pred = det_data_sample.pred_instances
- kie_input = dict(img=img)
- kie_input['instances'] = []
- for polygon, rec_data_sample in zip(
- det_pred['polygons'], rec_data_samples):
- kie_input['instances'].append(
- dict(
- bbox=poly2bbox(polygon),
- text=rec_data_sample.pred_text.item))
- self.kie_inputs.append(kie_input)
- result['kie'] = self.kie_inferencer(
- self.kie_inputs,
- return_datasamples=True,
- batch_size=kie_batch_size,
- **forward_kwargs)['predictions']
- return result
-
- def visualize(self, inputs: InputsType, preds: PredType,
- **kwargs) -> Union[List[np.ndarray], None]:
- """Visualize predictions.
-
- Args:
- inputs (List[Union[str, np.ndarray]]): Inputs for the inferencer.
- preds (List[Dict]): Predictions of the model.
- show (bool): Whether to display the image in a popup window.
- Defaults to False.
- wait_time (float): The interval of show (s). Defaults to 0.
- draw_pred (bool): Whether to draw predicted bounding boxes.
- Defaults to True.
- pred_score_thr (float): Minimum score of bboxes to draw.
- Defaults to 0.3.
- save_vis (bool): Whether to save the visualization result. Defaults
- to False.
- img_out_dir (str): Output directory of visualization results.
- If left as empty, no file will be saved. Defaults to ''.
-
- Returns:
- List[np.ndarray] or None: Returns visualization results only if
- applicable.
- """
-
- if 'kie' in self.mode:
- return self.kie_inferencer.visualize(self.kie_inputs, preds['kie'],
- **kwargs)
- elif 'rec' in self.mode:
- if 'det' in self.mode:
- return super().visualize(inputs,
- self._pack_e2e_datasamples(preds),
- **kwargs)
- else:
- return self.textrec_inferencer.visualize(
- self.rec_inputs, preds['rec'][0], **kwargs)
- else:
- return self.textdet_inferencer.visualize(inputs, preds['det'],
- **kwargs)
-
- def __call__(
- self,
- inputs: InputsType,
- batch_size: int = 1,
- det_batch_size: Optional[int] = None,
- rec_batch_size: Optional[int] = None,
- kie_batch_size: Optional[int] = None,
- out_dir: str = 'results/',
- return_vis: bool = False,
- save_vis: bool = False,
- save_pred: bool = False,
- **kwargs,
- ) -> dict:
- """Call the inferencer.
-
- Args:
- inputs (InputsType): Inputs for the inferencer. It can be a path
- to image / image directory, or an array, or a list of these.
- batch_size (int): Batch size. Defaults to 1.
- det_batch_size (Optional[int]): Batch size for text detection
- model. Overwrite batch_size if it is not None.
- Defaults to None.
- rec_batch_size (Optional[int]): Batch size for text recognition
- model. Overwrite batch_size if it is not None.
- Defaults to None.
- kie_batch_size (Optional[int]): Batch size for KIE model.
- Overwrite batch_size if it is not None.
- Defaults to None.
- out_dir (str): Output directory of results. Defaults to 'results/'.
- return_vis (bool): Whether to return the visualization result.
- Defaults to False.
- save_vis (bool): Whether to save the visualization results to
- "out_dir". Defaults to False.
- save_pred (bool): Whether to save the inference results to
- "out_dir". Defaults to False.
- **kwargs: Key words arguments passed to :meth:`preprocess`,
- :meth:`forward`, :meth:`visualize` and :meth:`postprocess`.
- Each key in kwargs should be in the corresponding set of
- ``preprocess_kwargs``, ``forward_kwargs``, ``visualize_kwargs``
- and ``postprocess_kwargs``.
-
- Returns:
- dict: Inference and visualization results, mapped from
- "predictions" and "visualization".
- """
- if (save_vis or save_pred) and not out_dir:
- raise ValueError('out_dir must be specified when save_vis or '
- 'save_pred is True!')
- if out_dir:
- img_out_dir = osp.join(out_dir, 'vis')
- pred_out_dir = osp.join(out_dir, 'preds')
- else:
- img_out_dir, pred_out_dir = '', ''
-
- (
- preprocess_kwargs,
- forward_kwargs,
- visualize_kwargs,
- postprocess_kwargs,
- ) = self._dispatch_kwargs(
- save_vis=save_vis,
- save_pred=save_pred,
- return_vis=return_vis,
- **kwargs)
-
- ori_inputs = self._inputs_to_list(inputs)
- if det_batch_size is None:
- det_batch_size = batch_size
- if rec_batch_size is None:
- rec_batch_size = batch_size
- if kie_batch_size is None:
- kie_batch_size = batch_size
-
- chunked_inputs = super(BaseMMOCRInferencer,
- self)._get_chunk_data(ori_inputs, batch_size)
- results = {'predictions': [], 'visualization': []}
- for ori_input in track(chunked_inputs, description='Inference'):
- preds = self.forward(
- ori_input,
- det_batch_size=det_batch_size,
- rec_batch_size=rec_batch_size,
- kie_batch_size=kie_batch_size,
- **forward_kwargs)
- visualization = self.visualize(
- ori_input, preds, img_out_dir=img_out_dir, **visualize_kwargs)
- batch_res = self.postprocess(
- preds,
- visualization,
- pred_out_dir=pred_out_dir,
- **postprocess_kwargs)
- results['predictions'].extend(batch_res['predictions'])
- if return_vis and batch_res['visualization'] is not None:
- results['visualization'].extend(batch_res['visualization'])
- return results
-
- def postprocess(self,
- preds: PredType,
- visualization: Optional[List[np.ndarray]] = None,
- print_result: bool = False,
- save_pred: bool = False,
- pred_out_dir: str = ''
- ) -> Union[ResType, Tuple[ResType, np.ndarray]]:
- """Process the predictions and visualization results from ``forward``
- and ``visualize``.
-
- This method should be responsible for the following tasks:
-
- 1. Convert datasamples into a json-serializable dict if needed.
- 2. Pack the predictions and visualization results and return them.
- 3. Dump or log the predictions.
-
- Args:
- preds (PredType): Predictions of the model.
- visualization (Optional[np.ndarray]): Visualized predictions.
- print_result (bool): Whether to print the result.
- Defaults to False.
- save_pred (bool): Whether to save the inference result. Defaults to
- False.
- pred_out_dir: File to save the inference results w/o
- visualization. If left as empty, no file will be saved.
- Defaults to ''.
-
- Returns:
- Dict: Inference and visualization results, mapped from
- "predictions" and "visualization".
- """
-
- result_dict = {}
- pred_results = [{} for _ in range(len(next(iter(preds.values()))))]
- if 'rec' in self.mode:
- for i, rec_pred in enumerate(preds['rec']):
- result = dict(rec_texts=[], rec_scores=[])
- for rec_pred_instance in rec_pred:
- rec_dict_res = self.textrec_inferencer.pred2dict(
- rec_pred_instance)
- result['rec_texts'].append(rec_dict_res['text'])
- result['rec_scores'].append(rec_dict_res['scores'])
- pred_results[i].update(result)
- if 'det' in self.mode:
- for i, det_pred in enumerate(preds['det']):
- det_dict_res = self.textdet_inferencer.pred2dict(det_pred)
- pred_results[i].update(
- dict(
- det_polygons=det_dict_res['polygons'],
- det_scores=det_dict_res['scores']))
- if 'kie' in self.mode:
- for i, kie_pred in enumerate(preds['kie']):
- kie_dict_res = self.kie_inferencer.pred2dict(kie_pred)
- pred_results[i].update(
- dict(
- kie_labels=kie_dict_res['labels'],
- kie_scores=kie_dict_res['scores']),
- kie_edge_scores=kie_dict_res['edge_scores'],
- kie_edge_labels=kie_dict_res['edge_labels'])
-
- if save_pred and pred_out_dir:
- pred_key = 'det' if 'det' in self.mode else 'rec'
- for pred, pred_result in zip(preds[pred_key], pred_results):
- img_path = (
- pred.img_path if pred_key == 'det' else pred[0].img_path)
- pred_name = osp.splitext(osp.basename(img_path))[0]
- pred_name = f'{pred_name}.json'
- pred_out_file = osp.join(pred_out_dir, pred_name)
- mmengine.dump(pred_result, pred_out_file)
-
- result_dict['predictions'] = pred_results
- if print_result:
- print(result_dict)
- result_dict['visualization'] = visualization
- return result_dict
-
- def _pack_e2e_datasamples(self,
- preds: Dict) -> List[TextSpottingDataSample]:
- """Pack text detection and recognition results into a list of
- TextSpottingDataSample."""
- results = []
-
- for det_data_sample, rec_data_samples in zip(preds['det'],
- preds['rec']):
- texts = []
- for rec_data_sample in rec_data_samples:
- texts.append(rec_data_sample.pred_text.item)
- det_data_sample.pred_instances.texts = texts
- results.append(det_data_sample)
- return results
diff --git a/pipeline/mmocr/mmocr/apis/inferencers/textdet_inferencer.py b/pipeline/mmocr/mmocr/apis/inferencers/textdet_inferencer.py
deleted file mode 100644
index 8c526d91a648f9117b5b59c51bd404a3534e5097..0000000000000000000000000000000000000000
--- a/pipeline/mmocr/mmocr/apis/inferencers/textdet_inferencer.py
+++ /dev/null
@@ -1,45 +0,0 @@
-# Copyright (c) OpenMMLab. All rights reserved.
-from typing import Dict
-
-from mmocr.structures import TextDetDataSample
-from .base_mmocr_inferencer import BaseMMOCRInferencer
-
-
-class TextDetInferencer(BaseMMOCRInferencer):
- """Text Detection inferencer.
-
- Args:
- model (str, optional): Path to the config file or the model name
- defined in metafile. For example, it could be
- "dbnet_resnet18_fpnc_1200e_icdar2015" or
- "configs/textdet/dbnet/dbnet_resnet18_fpnc_1200e_icdar2015.py".
- If model is not specified, user must provide the
- `weights` saved by MMEngine which contains the config string.
- Defaults to None.
- weights (str, optional): Path to the checkpoint. If it is not specified
- and model is a model name of metafile, the weights will be loaded
- from metafile. Defaults to None.
- device (str, optional): Device to run inference. If None, the available
- device will be automatically used. Defaults to None.
- scope (str, optional): The scope of the model. Defaults to "mmocr".
- """
-
- def pred2dict(self, data_sample: TextDetDataSample) -> Dict:
- """Extract elements necessary to represent a prediction into a
- dictionary. It's better to contain only basic data elements such as
- strings and numbers in order to guarantee it's json-serializable.
-
- Args:
- data_sample (TextDetDataSample): The data sample to be converted.
-
- Returns:
- dict: The output dictionary.
- """
- result = {}
- pred_instances = data_sample.pred_instances
- if 'polygons' in pred_instances:
- result['polygons'] = self._array2list(pred_instances.polygons)
- if 'bboxes' in pred_instances:
- result['bboxes'] = self._array2list(pred_instances.bboxes)
- result['scores'] = self._array2list(pred_instances.scores)
- return result
diff --git a/pipeline/mmocr/mmocr/apis/inferencers/textrec_inferencer.py b/pipeline/mmocr/mmocr/apis/inferencers/textrec_inferencer.py
deleted file mode 100644
index cc78a8fe6a165500fac31cf993c63868862a8954..0000000000000000000000000000000000000000
--- a/pipeline/mmocr/mmocr/apis/inferencers/textrec_inferencer.py
+++ /dev/null
@@ -1,44 +0,0 @@
-# Copyright (c) OpenMMLab. All rights reserved.
-from typing import Dict
-
-import numpy as np
-
-from mmocr.structures import TextRecogDataSample
-from .base_mmocr_inferencer import BaseMMOCRInferencer
-
-
-class TextRecInferencer(BaseMMOCRInferencer):
- """Text Recognition inferencer.
-
- Args:
- model (str, optional): Path to the config file or the model name
- defined in metafile. For example, it could be
- "crnn_mini-vgg_5e_mj" or
- "configs/textrecog/crnn/crnn_mini-vgg_5e_mj.py".
- If model is not specified, user must provide the
- `weights` saved by MMEngine which contains the config string.
- Defaults to None.
- weights (str, optional): Path to the checkpoint. If it is not specified
- and model is a model name of metafile, the weights will be loaded
- from metafile. Defaults to None.
- device (str, optional): Device to run inference. If None, the available
- device will be automatically used. Defaults to None.
- scope (str, optional): The scope of the model. Defaults to "mmocr".
- """
-
- def pred2dict(self, data_sample: TextRecogDataSample) -> Dict:
- """Extract elements necessary to represent a prediction into a
- dictionary. It's better to contain only basic data elements such as
- strings and numbers in order to guarantee it's json-serializable.
-
- Args:
- data_sample (TextRecogDataSample): The data sample to be converted.
-
- Returns:
- dict: The output dictionary.
- """
- result = {}
- result['text'] = data_sample.pred_text.item
- score = self._array2list(data_sample.pred_text.score)
- result['scores'] = float(np.mean(score))
- return result
diff --git a/pipeline/mmocr/mmocr/apis/inferencers/textspot_inferencer.py b/pipeline/mmocr/mmocr/apis/inferencers/textspot_inferencer.py
deleted file mode 100644
index 374894cbe37f0d6a90d04f772710a5d0a278a3a6..0000000000000000000000000000000000000000
--- a/pipeline/mmocr/mmocr/apis/inferencers/textspot_inferencer.py
+++ /dev/null
@@ -1,47 +0,0 @@
-# Copyright (c) OpenMMLab. All rights reserved.
-from typing import Dict
-
-from mmocr.structures import TextSpottingDataSample
-from .base_mmocr_inferencer import BaseMMOCRInferencer
-
-
-class TextSpotInferencer(BaseMMOCRInferencer):
- """Text Spotting inferencer.
-
- Args:
- model (str, optional): Path to the config file or the model name
- defined in metafile. For example, it could be
- "dbnet_resnet18_fpnc_1200e_icdar2015" or
- "configs/textdet/dbnet/dbnet_resnet18_fpnc_1200e_icdar2015.py".
- If model is not specified, user must provide the
- `weights` saved by MMEngine which contains the config string.
- Defaults to None.
- weights (str, optional): Path to the checkpoint. If it is not specified
- and model is a model name of metafile, the weights will be loaded
- from metafile. Defaults to None.
- device (str, optional): Device to run inference. If None, the available
- device will be automatically used. Defaults to None.
- scope (str, optional): The scope of the model. Defaults to "mmocr".
- """
-
- def pred2dict(self, data_sample: TextSpottingDataSample) -> Dict:
- """Extract elements necessary to represent a prediction into a
- dictionary. It's better to contain only basic data elements such as
- strings and numbers in order to guarantee it's json-serializable.
-
- Args:
- data_sample (TextSpottingDataSample): The data sample to be
- converted.
-
- Returns:
- dict: The output dictionary.
- """
- result = {}
- pred_instances = data_sample.pred_instances
- if 'polygons' in pred_instances:
- result['polygons'] = self._array2list(pred_instances.polygons)
- if 'bboxes' in pred_instances:
- result['bboxes'] = self._array2list(pred_instances.bboxes)
- result['scores'] = self._array2list(pred_instances.scores)
- result['texts'] = pred_instances.texts
- return result
diff --git a/pipeline/mmocr/mmocr/datasets/__init__.py b/pipeline/mmocr/mmocr/datasets/__init__.py
deleted file mode 100644
index 54a9ea7f02824c517d2529ce3ae0ff4a607ca70f..0000000000000000000000000000000000000000
--- a/pipeline/mmocr/mmocr/datasets/__init__.py
+++ /dev/null
@@ -1,14 +0,0 @@
-# Copyright (c) OpenMMLab. All rights reserved.
-from .dataset_wrapper import ConcatDataset
-from .icdar_dataset import IcdarDataset
-from .ocr_dataset import OCRDataset
-from .recog_lmdb_dataset import RecogLMDBDataset
-from .recog_text_dataset import RecogTextDataset
-from .samplers import * # NOQA
-from .transforms import * # NOQA
-from .wildreceipt_dataset import WildReceiptDataset
-
-__all__ = [
- 'IcdarDataset', 'OCRDataset', 'RecogLMDBDataset', 'RecogTextDataset',
- 'WildReceiptDataset', 'ConcatDataset'
-]
diff --git a/pipeline/mmocr/mmocr/datasets/__pycache__/__init__.cpython-38.pyc b/pipeline/mmocr/mmocr/datasets/__pycache__/__init__.cpython-38.pyc
deleted file mode 100644
index 89fb6de74a1b0caace5d80f0f540dabf8790d0a2..0000000000000000000000000000000000000000
Binary files a/pipeline/mmocr/mmocr/datasets/__pycache__/__init__.cpython-38.pyc and /dev/null differ
diff --git a/pipeline/mmocr/mmocr/datasets/__pycache__/__init__.cpython-39.pyc b/pipeline/mmocr/mmocr/datasets/__pycache__/__init__.cpython-39.pyc
deleted file mode 100644
index 8b25b3ea6b4b46e4869a926eeb2ae55bee757feb..0000000000000000000000000000000000000000
Binary files a/pipeline/mmocr/mmocr/datasets/__pycache__/__init__.cpython-39.pyc and /dev/null differ
diff --git a/pipeline/mmocr/mmocr/datasets/__pycache__/dataset_wrapper.cpython-38.pyc b/pipeline/mmocr/mmocr/datasets/__pycache__/dataset_wrapper.cpython-38.pyc
deleted file mode 100644
index a535e476ea0514b46bb8ee0093648131e659655e..0000000000000000000000000000000000000000
Binary files a/pipeline/mmocr/mmocr/datasets/__pycache__/dataset_wrapper.cpython-38.pyc and /dev/null differ
diff --git a/pipeline/mmocr/mmocr/datasets/__pycache__/dataset_wrapper.cpython-39.pyc b/pipeline/mmocr/mmocr/datasets/__pycache__/dataset_wrapper.cpython-39.pyc
deleted file mode 100644
index 31ffcf115b741ca88450406ffed67e93c5044515..0000000000000000000000000000000000000000
Binary files a/pipeline/mmocr/mmocr/datasets/__pycache__/dataset_wrapper.cpython-39.pyc and /dev/null differ
diff --git a/pipeline/mmocr/mmocr/datasets/__pycache__/icdar_dataset.cpython-38.pyc b/pipeline/mmocr/mmocr/datasets/__pycache__/icdar_dataset.cpython-38.pyc
deleted file mode 100644
index db5a47c277be3bb0693f3f6d0023ef302db4b1dc..0000000000000000000000000000000000000000
Binary files a/pipeline/mmocr/mmocr/datasets/__pycache__/icdar_dataset.cpython-38.pyc and /dev/null differ
diff --git a/pipeline/mmocr/mmocr/datasets/__pycache__/icdar_dataset.cpython-39.pyc b/pipeline/mmocr/mmocr/datasets/__pycache__/icdar_dataset.cpython-39.pyc
deleted file mode 100644
index 5ee33d538349fb156097f2262500c0c2fa7f912b..0000000000000000000000000000000000000000
Binary files a/pipeline/mmocr/mmocr/datasets/__pycache__/icdar_dataset.cpython-39.pyc and /dev/null differ
diff --git a/pipeline/mmocr/mmocr/datasets/__pycache__/ocr_dataset.cpython-38.pyc b/pipeline/mmocr/mmocr/datasets/__pycache__/ocr_dataset.cpython-38.pyc
deleted file mode 100644
index fbcd67a81b39c2fa7445e7fcb8cf39d1a1ba073a..0000000000000000000000000000000000000000
Binary files a/pipeline/mmocr/mmocr/datasets/__pycache__/ocr_dataset.cpython-38.pyc and /dev/null differ
diff --git a/pipeline/mmocr/mmocr/datasets/__pycache__/ocr_dataset.cpython-39.pyc b/pipeline/mmocr/mmocr/datasets/__pycache__/ocr_dataset.cpython-39.pyc
deleted file mode 100644
index 74f3bfd214f510e3da9e4bb8a097257ba688cf3e..0000000000000000000000000000000000000000
Binary files a/pipeline/mmocr/mmocr/datasets/__pycache__/ocr_dataset.cpython-39.pyc and /dev/null differ
diff --git a/pipeline/mmocr/mmocr/datasets/__pycache__/recog_lmdb_dataset.cpython-38.pyc b/pipeline/mmocr/mmocr/datasets/__pycache__/recog_lmdb_dataset.cpython-38.pyc
deleted file mode 100644
index 93112ff7ac26e56c77e40d3c279b027b38f55d0d..0000000000000000000000000000000000000000
Binary files a/pipeline/mmocr/mmocr/datasets/__pycache__/recog_lmdb_dataset.cpython-38.pyc and /dev/null differ
diff --git a/pipeline/mmocr/mmocr/datasets/__pycache__/recog_lmdb_dataset.cpython-39.pyc b/pipeline/mmocr/mmocr/datasets/__pycache__/recog_lmdb_dataset.cpython-39.pyc
deleted file mode 100644
index 4ec88d3f6a9c745978f5ceef38c544a6b10af5f9..0000000000000000000000000000000000000000
Binary files a/pipeline/mmocr/mmocr/datasets/__pycache__/recog_lmdb_dataset.cpython-39.pyc and /dev/null differ
diff --git a/pipeline/mmocr/mmocr/datasets/__pycache__/recog_text_dataset.cpython-38.pyc b/pipeline/mmocr/mmocr/datasets/__pycache__/recog_text_dataset.cpython-38.pyc
deleted file mode 100644
index f18239c516c2f31c5bb9477af445e72c78a3f112..0000000000000000000000000000000000000000
Binary files a/pipeline/mmocr/mmocr/datasets/__pycache__/recog_text_dataset.cpython-38.pyc and /dev/null differ
diff --git a/pipeline/mmocr/mmocr/datasets/__pycache__/recog_text_dataset.cpython-39.pyc b/pipeline/mmocr/mmocr/datasets/__pycache__/recog_text_dataset.cpython-39.pyc
deleted file mode 100644
index cf2a62096b0d6f5497dcf1336801dfd679f26fa2..0000000000000000000000000000000000000000
Binary files a/pipeline/mmocr/mmocr/datasets/__pycache__/recog_text_dataset.cpython-39.pyc and /dev/null differ
diff --git a/pipeline/mmocr/mmocr/datasets/__pycache__/wildreceipt_dataset.cpython-38.pyc b/pipeline/mmocr/mmocr/datasets/__pycache__/wildreceipt_dataset.cpython-38.pyc
deleted file mode 100644
index 3b04e806d59930c48312211fc787d7652b1c24d6..0000000000000000000000000000000000000000
Binary files a/pipeline/mmocr/mmocr/datasets/__pycache__/wildreceipt_dataset.cpython-38.pyc and /dev/null differ
diff --git a/pipeline/mmocr/mmocr/datasets/__pycache__/wildreceipt_dataset.cpython-39.pyc b/pipeline/mmocr/mmocr/datasets/__pycache__/wildreceipt_dataset.cpython-39.pyc
deleted file mode 100644
index 9bd32715461558e06c78262cd9e17fbb56e8353c..0000000000000000000000000000000000000000
Binary files a/pipeline/mmocr/mmocr/datasets/__pycache__/wildreceipt_dataset.cpython-39.pyc and /dev/null differ
diff --git a/pipeline/mmocr/mmocr/datasets/dataset_wrapper.py b/pipeline/mmocr/mmocr/datasets/dataset_wrapper.py
deleted file mode 100644
index c1b8bc5cfe9836e18d5166bfb53ee86799e02cf1..0000000000000000000000000000000000000000
--- a/pipeline/mmocr/mmocr/datasets/dataset_wrapper.py
+++ /dev/null
@@ -1,77 +0,0 @@
-# Copyright (c) OpenMMLab. All rights reserved.
-from typing import Callable, List, Sequence, Union
-
-from mmengine.dataset import BaseDataset, Compose
-from mmengine.dataset import ConcatDataset as MMENGINE_CONCATDATASET
-
-from mmocr.registry import DATASETS
-
-
-@DATASETS.register_module()
-class ConcatDataset(MMENGINE_CONCATDATASET):
- """A wrapper of concatenated dataset.
-
- Same as ``torch.utils.data.dataset.ConcatDataset`` and support lazy_init.
-
- Note:
- ``ConcatDataset`` should not inherit from ``BaseDataset`` since
- ``get_subset`` and ``get_subset_`` could produce ambiguous meaning
- sub-dataset which conflicts with original dataset. If you want to use
- a sub-dataset of ``ConcatDataset``, you should set ``indices``
- arguments for wrapped dataset which inherit from ``BaseDataset``.
-
- Args:
- datasets (Sequence[BaseDataset] or Sequence[dict]): A list of datasets
- which will be concatenated.
- pipeline (list, optional): Processing pipeline to be applied to all
- of the concatenated datasets. Defaults to [].
- verify_meta (bool): Whether to verify the consistency of meta
- information of the concatenated datasets. Defaults to True.
- force_apply (bool): Whether to force apply pipeline to all datasets if
- any of them already has the pipeline configured. Defaults to False.
- lazy_init (bool, optional): Whether to load annotation during
- instantiation. Defaults to False.
- """
-
- def __init__(self,
- datasets: Sequence[Union[BaseDataset, dict]],
- pipeline: List[Union[dict, Callable]] = [],
- verify_meta: bool = True,
- force_apply: bool = False,
- lazy_init: bool = False):
- self.datasets: List[BaseDataset] = []
-
- # Compose dataset
- pipeline = Compose(pipeline)
-
- for i, dataset in enumerate(datasets):
- if isinstance(dataset, dict):
- self.datasets.append(DATASETS.build(dataset))
- elif isinstance(dataset, BaseDataset):
- self.datasets.append(dataset)
- else:
- raise TypeError(
- 'elements in datasets sequence should be config or '
- f'`BaseDataset` instance, but got {type(dataset)}')
- if len(pipeline.transforms) > 0:
- if len(self.datasets[-1].pipeline.transforms
- ) > 0 and not force_apply:
- raise ValueError(
- f'The pipeline of dataset {i} is not empty, '
- 'please set `force_apply` to True.')
- self.datasets[-1].pipeline = pipeline
-
- self._metainfo = self.datasets[0].metainfo
-
- if verify_meta:
- # Only use metainfo of first dataset.
- for i, dataset in enumerate(self.datasets, 1):
- if self._metainfo != dataset.metainfo:
- raise ValueError(
- f'The meta information of the {i}-th dataset does not '
- 'match meta information of the first dataset')
-
- self._fully_initialized = False
- if not lazy_init:
- self.full_init()
- self._metainfo.update(dict(cumulative_sizes=self.cumulative_sizes))
diff --git a/pipeline/mmocr/mmocr/datasets/icdar_dataset.py b/pipeline/mmocr/mmocr/datasets/icdar_dataset.py
deleted file mode 100644
index 68fd911adf5dac4ca5c97421260cd12962fb3428..0000000000000000000000000000000000000000
--- a/pipeline/mmocr/mmocr/datasets/icdar_dataset.py
+++ /dev/null
@@ -1,93 +0,0 @@
-# Copyright (c) OpenMMLab. All rights reserved.
-import os.path as osp
-from typing import List, Union
-
-from mmdet.datasets.coco import CocoDataset
-
-from mmocr.registry import DATASETS
-
-
-@DATASETS.register_module()
-class IcdarDataset(CocoDataset):
- """Dataset for text detection while ann_file in coco format.
-
- Args:
- ann_file (str): Annotation file path. Defaults to ''.
- metainfo (dict, optional): Meta information for dataset, such as class
- information. Defaults to None.
- data_root (str): The root directory for ``data_prefix`` and
- ``ann_file``. Defaults to ''.
- data_prefix (dict): Prefix for training data. Defaults to
- dict(img_path='').
- filter_cfg (dict, optional): Config for filter data. Defaults to None.
- indices (int or Sequence[int], optional): Support using first few
- data in annotation file to facilitate training/testing on a smaller
- dataset. Defaults to None which means using all ``data_infos``.
- serialize_data (bool, optional): Whether to hold memory using
- serialized objects, when enabled, data loader workers can use
- shared RAM from master process instead of making a copy. Defaults
- to True.
- pipeline (list, optional): Processing pipeline. Defaults to [].
- test_mode (bool, optional): ``test_mode=True`` means in test phase.
- Defaults to False.
- lazy_init (bool, optional): Whether to load annotation during
- instantiation. In some cases, such as visualization, only the meta
- information of the dataset is needed, which is not necessary to
- load annotation file. ``Basedataset`` can skip load annotations to
- save time by set ``lazy_init=False``. Defaults to False.
- max_refetch (int, optional): If ``Basedataset.prepare_data`` get a
- None img. The maximum extra number of cycles to get a valid
- image. Defaults to 1000.
- """
- METAINFO = {'classes': ('text', )}
-
- def parse_data_info(self, raw_data_info: dict) -> Union[dict, List[dict]]:
- """Parse raw annotation to target format.
-
- Args:
- raw_data_info (dict): Raw data information loaded from ``ann_file``
-
- Returns:
- Union[dict, List[dict]]: Parsed annotation.
- """
- img_info = raw_data_info['raw_img_info']
- ann_info = raw_data_info['raw_ann_info']
-
- data_info = {}
-
- img_path = osp.join(self.data_prefix['img_path'],
- img_info['file_name'])
- data_info['img_path'] = img_path
- data_info['img_id'] = img_info['img_id']
- data_info['height'] = img_info['height']
- data_info['width'] = img_info['width']
-
- instances = []
- for ann in ann_info:
- instance = {}
-
- if ann.get('ignore', False):
- continue
- x1, y1, w, h = ann['bbox']
- inter_w = max(0, min(x1 + w, img_info['width']) - max(x1, 0))
- inter_h = max(0, min(y1 + h, img_info['height']) - max(y1, 0))
- if inter_w * inter_h == 0:
- continue
- if ann['area'] <= 0 or w < 1 or h < 1:
- continue
- if ann['category_id'] not in self.cat_ids:
- continue
- bbox = [x1, y1, x1 + w, y1 + h]
-
- if ann.get('iscrowd', False):
- instance['ignore'] = 1
- else:
- instance['ignore'] = 0
- instance['bbox'] = bbox
- instance['bbox_label'] = self.cat2label[ann['category_id']]
- if ann.get('segmentation', None):
- instance['polygon'] = ann['segmentation'][0]
-
- instances.append(instance)
- data_info['instances'] = instances
- return data_info
diff --git a/pipeline/mmocr/mmocr/datasets/ocr_dataset.py b/pipeline/mmocr/mmocr/datasets/ocr_dataset.py
deleted file mode 100644
index 826c3fe9892daa41bf24eaa565a4b11c8d3bc9d6..0000000000000000000000000000000000000000
--- a/pipeline/mmocr/mmocr/datasets/ocr_dataset.py
+++ /dev/null
@@ -1,94 +0,0 @@
-# Copyright (c) OpenMMLab. All rights reserved.
-from mmengine.dataset import BaseDataset
-
-from mmocr.registry import DATASETS
-
-
-@DATASETS.register_module()
-class OCRDataset(BaseDataset):
- r"""OCRDataset for text detection and text recognition.
-
- The annotation format is shown as follows.
-
- .. code-block:: none
-
- {
- "metainfo":
- {
- "dataset_type": "test_dataset",
- "task_name": "test_task"
- },
- "data_list":
- [
- {
- "img_path": "test_img.jpg",
- "height": 604,
- "width": 640,
- "instances":
- [
- {
- "bbox": [0, 0, 10, 20],
- "bbox_label": 1,
- "mask": [0,0,0,10,10,20,20,0],
- "text": '123'
- },
- {
- "bbox": [10, 10, 110, 120],
- "bbox_label": 2,
- "mask": [10,10],10,110,110,120,120,10]],
- "extra_anns": '456'
- }
- ]
- },
- ]
- }
-
- Args:
- ann_file (str): Annotation file path. Defaults to ''.
- metainfo (dict, optional): Meta information for dataset, such as class
- information. Defaults to None.
- data_root (str, optional): The root directory for ``data_prefix`` and
- ``ann_file``. Defaults to None.
- data_prefix (dict): Prefix for training data. Defaults to
- dict(img_path='').
- filter_cfg (dict, optional): Config for filter data. Defaults to None.
- indices (int or Sequence[int], optional): Support using first few
- data in annotation file to facilitate training/testing on a smaller
- dataset. Defaults to None which means using all ``data_infos``.
- serialize_data (bool, optional): Whether to hold memory using
- serialized objects, when enabled, data loader workers can use
- shared RAM from master process instead of making a copy. Defaults
- to True.
- pipeline (list, optional): Processing pipeline. Defaults to [].
- test_mode (bool, optional): ``test_mode=True`` means in test phase.
- Defaults to False.
- lazy_init (bool, optional): Whether to load annotation during
- instantiation. In some cases, such as visualization, only the meta
- information of the dataset is needed, which is not necessary to
- load annotation file. ``OCRdataset`` can skip load annotations to
- save time by set ``lazy_init=False``. Defaults to False.
- max_refetch (int, optional): If ``OCRdataset.prepare_data`` get a
- None img. The maximum extra number of cycles to get a valid
- image. Defaults to 1000.
-
- Note:
- OCRDataset collects meta information from `annotation file` (the
- lowest priority), ``OCRDataset.METAINFO``(medium) and `metainfo
- parameter` (highest) passed to constructors. The lower priority meta
- information will be overwritten by higher one.
-
- Examples:
- Assume the annotation file is given above.
- >>> class CustomDataset(OCRDataset):
- >>> METAINFO: dict = dict(task_name='custom_task',
- >>> dataset_type='custom_type')
- >>> metainfo=dict(task_name='custom_task_name')
- >>> custom_dataset = CustomDataset(
- >>> 'path/to/ann_file',
- >>> metainfo=metainfo)
- >>> # meta information of annotation file will be overwritten by
- >>> # `CustomDataset.METAINFO`. The merged meta information will
- >>> # further be overwritten by argument `metainfo`.
- >>> custom_dataset.metainfo
- {'task_name': custom_task_name, dataset_type: custom_type}
- """
diff --git a/pipeline/mmocr/mmocr/datasets/preparers/__init__.py b/pipeline/mmocr/mmocr/datasets/preparers/__init__.py
deleted file mode 100644
index e2323e3273d988c4e26443567a77dbd328e4f329..0000000000000000000000000000000000000000
--- a/pipeline/mmocr/mmocr/datasets/preparers/__init__.py
+++ /dev/null
@@ -1,10 +0,0 @@
-# Copyright (c) OpenMMLab. All rights reserved.
-from .config_generators import * # noqa
-from .data_preparer import DatasetPreparer
-from .dumpers import * # noqa
-from .gatherers import * # noqa
-from .obtainers import * # noqa
-from .packers import * # noqa
-from .parsers import * # noqa
-
-__all__ = ['DatasetPreparer']
diff --git a/pipeline/mmocr/mmocr/datasets/preparers/config_generators/__init__.py b/pipeline/mmocr/mmocr/datasets/preparers/config_generators/__init__.py
deleted file mode 100644
index 8e884c6d9d4cbd71e2e7c9625a87a7993839b75e..0000000000000000000000000000000000000000
--- a/pipeline/mmocr/mmocr/datasets/preparers/config_generators/__init__.py
+++ /dev/null
@@ -1,10 +0,0 @@
-# Copyright (c) OpenMMLab. All rights reserved.
-from .base import BaseDatasetConfigGenerator
-from .textdet_config_generator import TextDetConfigGenerator
-from .textrecog_config_generator import TextRecogConfigGenerator
-from .textspotting_config_generator import TextSpottingConfigGenerator
-
-__all__ = [
- 'BaseDatasetConfigGenerator', 'TextDetConfigGenerator',
- 'TextRecogConfigGenerator', 'TextSpottingConfigGenerator'
-]
diff --git a/pipeline/mmocr/mmocr/datasets/preparers/config_generators/base.py b/pipeline/mmocr/mmocr/datasets/preparers/config_generators/base.py
deleted file mode 100644
index ba3811a425f203d2e5a810dc6e57a0934fb13a93..0000000000000000000000000000000000000000
--- a/pipeline/mmocr/mmocr/datasets/preparers/config_generators/base.py
+++ /dev/null
@@ -1,131 +0,0 @@
-# Copyright (c) OpenMMLab. All rights reserved.
-import os.path as osp
-from abc import abstractmethod
-from typing import Dict, List, Optional
-
-from mmengine import mkdir_or_exist
-
-
-class BaseDatasetConfigGenerator:
- """Base class for dataset config generator.
-
- Args:
- data_root (str): The root path of the dataset.
- task (str): The task of the dataset.
- dataset_name (str): The name of the dataset.
- overwrite_cfg (bool): Whether to overwrite the dataset config file if
- it already exists. If False, config generator will not generate new
- config for datasets whose configs are already in base.
- train_anns (List[Dict], optional): A list of train annotation files
- to appear in the base configs. Defaults to None.
- Each element is typically a dict with the following fields:
- - ann_file (str): The path to the annotation file relative to
- data_root.
- - dataset_postfix (str, optional): Affects the postfix of the
- resulting variable in the generated config. If specified, the
- dataset variable will be named in the form of
- ``{dataset_name}_{dataset_postfix}_{task}_{split}``. Defaults to
- None.
- val_anns (List[Dict], optional): A list of val annotation files
- to appear in the base configs, similar to ``train_anns``. Defaults
- to None.
- test_anns (List[Dict], optional): A list of test annotation files
- to appear in the base configs, similar to ``train_anns``. Defaults
- to None.
- config_path (str): Path to the configs. Defaults to 'configs/'.
- """
-
- def __init__(
- self,
- data_root: str,
- task: str,
- dataset_name: str,
- overwrite_cfg: bool = False,
- train_anns: Optional[List[Dict]] = None,
- val_anns: Optional[List[Dict]] = None,
- test_anns: Optional[List[Dict]] = None,
- config_path: str = 'configs/',
- ) -> None:
- self.config_path = config_path
- self.data_root = data_root
- self.task = task
- self.dataset_name = dataset_name
- self.overwrite_cfg = overwrite_cfg
- self._prepare_anns(train_anns, val_anns, test_anns)
-
- def _prepare_anns(self, train_anns: Optional[List[Dict]],
- val_anns: Optional[List[Dict]],
- test_anns: Optional[List[Dict]]) -> None:
- """Preprocess input arguments and stores these information into
- ``self.anns``.
-
- ``self.anns`` is a dict that maps the name of a dataset config variable
- to a dict, which contains the following fields:
- - ann_file (str): The path to the annotation file relative to
- data_root.
- - split (str): The split the annotation belongs to. Usually
- it can be 'train', 'val' and 'test'.
- - dataset_postfix (str, optional): Affects the postfix of the
- resulting variable in the generated config. If specified, the
- dataset variable will be named in the form of
- ``{dataset_name}_{dataset_postfix}_{task}_{split}``. Defaults to
- None.
- """
- self.anns = {}
- for split, ann_list in zip(('train', 'val', 'test'),
- (train_anns, val_anns, test_anns)):
- if ann_list is None:
- continue
- if not isinstance(ann_list, list):
- raise ValueError(f'{split}_anns must be either a list or'
- ' None!')
- for ann_dict in ann_list:
- assert 'ann_file' in ann_dict
- suffix = ann_dict['ann_file'].split('.')[-1]
- if suffix == 'json':
- dataset_type = 'OCRDataset'
- elif suffix == 'lmdb':
- assert self.task == 'textrecog', \
- 'LMDB format only works for textrecog now.'
- dataset_type = 'RecogLMDBDataset'
- else:
- raise NotImplementedError(
- 'ann file only supports JSON file or LMDB file')
- ann_dict['dataset_type'] = dataset_type
- if ann_dict.get('dataset_postfix', ''):
- key = f'{self.dataset_name}_{ann_dict["dataset_postfix"]}_{self.task}_{split}' # noqa
- else:
- key = f'{self.dataset_name}_{self.task}_{split}'
- ann_dict['split'] = split
- if key in self.anns:
- raise ValueError(
- f'Duplicate dataset variable {key} found! '
- 'Please use different dataset_postfix to avoid '
- 'conflict.')
- self.anns[key] = ann_dict
-
- def __call__(self) -> None:
- """Generates the base dataset config."""
-
- dataset_config = self._gen_dataset_config()
-
- cfg_path = osp.join(self.config_path, self.task, '_base_', 'datasets',
- f'{self.dataset_name}.py')
- if osp.exists(cfg_path) and not self.overwrite_cfg:
- print(f'{cfg_path} found, skipping.')
- return
- mkdir_or_exist(osp.dirname(cfg_path))
- with open(cfg_path, 'w') as f:
- f.write(
- f'{self.dataset_name}_{self.task}_data_root = \'{self.data_root}\'\n' # noqa: E501
- )
- f.write(dataset_config)
-
- @abstractmethod
- def _gen_dataset_config(self) -> str:
- """Generate a full dataset config based on the annotation file
- dictionary.
-
- Returns:
- str: The generated dataset config.
- """
diff --git a/pipeline/mmocr/mmocr/datasets/preparers/config_generators/textdet_config_generator.py b/pipeline/mmocr/mmocr/datasets/preparers/config_generators/textdet_config_generator.py
deleted file mode 100644
index fcb8af4fb0e0fc031e81acf51ebe9526c0192439..0000000000000000000000000000000000000000
--- a/pipeline/mmocr/mmocr/datasets/preparers/config_generators/textdet_config_generator.py
+++ /dev/null
@@ -1,96 +0,0 @@
-# Copyright (c) OpenMMLab. All rights reserved.
-from typing import Dict, List, Optional
-
-from mmocr.registry import CFG_GENERATORS
-from .base import BaseDatasetConfigGenerator
-
-
-@CFG_GENERATORS.register_module()
-class TextDetConfigGenerator(BaseDatasetConfigGenerator):
- """Text detection config generator.
-
- Args:
- data_root (str): The root path of the dataset.
- dataset_name (str): The name of the dataset.
- overwrite_cfg (bool): Whether to overwrite the dataset config file if
- it already exists. If False, config generator will not generate new
- config for datasets whose configs are already in base.
- train_anns (List[Dict], optional): A list of train annotation files
- to appear in the base configs. Defaults to
- ``[dict(file='textdet_train.json', dataset_postfix='')]``.
- Each element is typically a dict with the following fields:
- - ann_file (str): The path to the annotation file relative to
- data_root.
- - dataset_postfix (str, optional): Affects the postfix of the
- resulting variable in the generated config. If specified, the
- dataset variable will be named in the form of
- ``{dataset_name}_{dataset_postfix}_{task}_{split}``. Defaults to
- None.
- val_anns (List[Dict], optional): A list of val annotation files
- to appear in the base configs, similar to ``train_anns``. Defaults
- to [].
- test_anns (List[Dict], optional): A list of test annotation files
- to appear in the base configs, similar to ``train_anns``. Defaults
- to ``[dict(file='textdet_test.json')]``.
- config_path (str): Path to the configs. Defaults to 'configs/'.
- """
-
- def __init__(
- self,
- data_root: str,
- dataset_name: str,
- overwrite_cfg: bool = False,
- train_anns: Optional[List[Dict]] = [
- dict(ann_file='textdet_train.json', dataset_postfix='')
- ],
- val_anns: Optional[List[Dict]] = [],
- test_anns: Optional[List[Dict]] = [
- dict(ann_file='textdet_test.json', dataset_postfix='')
- ],
- config_path: str = 'configs/',
- ) -> None:
- super().__init__(
- data_root=data_root,
- task='textdet',
- overwrite_cfg=overwrite_cfg,
- dataset_name=dataset_name,
- train_anns=train_anns,
- val_anns=val_anns,
- test_anns=test_anns,
- config_path=config_path,
- )
-
- def _gen_dataset_config(self) -> str:
- """Generate a full dataset config based on the annotation file
- dictionary.
-
- Args:
- ann_dict (dict[str, dict(str, str)]): A nested dictionary that maps
- a config variable name (such as icdar2015_textrecog_train) to
- its corresponding annotation information dict. Each dict
- contains following keys:
- - ann_file (str): The path to the annotation file relative to
- data_root.
- - dataset_postfix (str, optional): Affects the postfix of the
- resulting variable in the generated config. If specified, the
- dataset variable will be named in the form of
- ``{dataset_name}_{dataset_postfix}_{task}_{split}``. Defaults
- to None.
- - split (str): The split the annotation belongs to. Usually
- it can be 'train', 'val' and 'test'.
-
- Returns:
- str: The generated dataset config.
- """
- cfg = ''
- for key_name, ann_dict in self.anns.items():
- cfg += f'\n{key_name} = dict(\n'
- cfg += ' type=\'OCRDataset\',\n'
- cfg += ' data_root=' + f'{self.dataset_name}_{self.task}_data_root,\n' # noqa: E501
- cfg += f' ann_file=\'{ann_dict["ann_file"]}\',\n'
- if ann_dict['split'] == 'train':
- cfg += ' filter_cfg=dict(filter_empty_gt=True, min_size=32),\n' # noqa: E501
- elif ann_dict['split'] in ['test', 'val']:
- cfg += ' test_mode=True,\n'
- cfg += ' pipeline=None)\n'
- return cfg
diff --git a/pipeline/mmocr/mmocr/datasets/preparers/config_generators/textrecog_config_generator.py b/pipeline/mmocr/mmocr/datasets/preparers/config_generators/textrecog_config_generator.py
deleted file mode 100644
index bb8b62625884e0d135fbcf4c61abe8162b9f7df5..0000000000000000000000000000000000000000
--- a/pipeline/mmocr/mmocr/datasets/preparers/config_generators/textrecog_config_generator.py
+++ /dev/null
@@ -1,128 +0,0 @@
-# Copyright (c) OpenMMLab. All rights reserved.
-from typing import Dict, List, Optional
-
-from mmocr.registry import CFG_GENERATORS
-from .base import BaseDatasetConfigGenerator
-
-
-@CFG_GENERATORS.register_module()
-class TextRecogConfigGenerator(BaseDatasetConfigGenerator):
- """Text recognition config generator.
-
- Args:
- data_root (str): The root path of the dataset.
- dataset_name (str): The name of the dataset.
- overwrite_cfg (bool): Whether to overwrite the dataset config file if
- it already exists. If False, config generator will not generate new
- config for datasets whose configs are already in base.
- train_anns (List[Dict], optional): A list of train annotation files
- to appear in the base configs. Defaults to
- ``[dict(file='textrecog_train.json'), dataset_postfix='']``.
- Each element is typically a dict with the following fields:
- - ann_file (str): The path to the annotation file relative to
- data_root.
- - dataset_postfix (str, optional): Affects the postfix of the
- resulting variable in the generated config. If specified, the
- dataset variable will be named in the form of
- ``{dataset_name}_{dataset_postfix}_{task}_{split}``. Defaults to
- None.
- val_anns (List[Dict], optional): A list of val annotation files
- to appear in the base configs, similar to ``train_anns``. Defaults
- to [].
- test_anns (List[Dict], optional): A list of test annotation files
- to appear in the base configs, similar to ``train_anns``. Defaults
- to ``[dict(file='textrecog_test.json')]``.
- config_path (str): Path to the configs. Defaults to 'configs/'.
-
- Example:
- It generates a dataset config like:
- >>> icdar2015_textrecog_data_root = 'data/icdar2015/'
- >>> icdar2015_textrecog_train = dict(
- >>> type='OCRDataset',
- >>> data_root=icdar2015_textrecog_data_root,
- >>> ann_file='textrecog_train.json',
- >>> pipeline=None)
- >>> icdar2015_textrecog_test = dict(
- >>> type='OCRDataset',
- >>> data_root=icdar2015_textrecog_data_root,
- >>> ann_file='textrecog_test.json',
- >>> test_mode=True,
- >>> pipeline=None)
-
- It generates a lmdb format dataset config like:
- >>> icdar2015_lmdb_textrecog_data_root = 'data/icdar2015'
- >>> icdar2015_lmdb_textrecog_train = dict(
- >>> type='RecogLMDBDataset',
- >>> data_root=icdar2015_lmdb_textrecog_data_root,
- >>> ann_file='textrecog_train.lmdb',
- >>> pipeline=None)
- >>> icdar2015_lmdb_textrecog_test = dict(
- >>> type='RecogLMDBDataset',
- >>> data_root=icdar2015_lmdb_textrecog_data_root,
- >>> ann_file='textrecog_test.lmdb',
- >>> test_mode=True,
- >>> pipeline=None)
- >>> icdar2015_lmdb_1811_textrecog_test = dict(
- >>> type='RecogLMDBDataset',
- >>> data_root=icdar2015_lmdb_textrecog_data_root,
- >>> ann_file='textrecog_test_1811.lmdb',
- >>> test_mode=True,
- >>> pipeline=None)
- """
-
- def __init__(
- self,
- data_root: str,
- dataset_name: str,
- overwrite_cfg: bool = False,
- train_anns: Optional[List[Dict]] = [
- dict(ann_file='textrecog_train.json', dataset_postfix='')
- ],
- val_anns: Optional[List[Dict]] = [],
- test_anns: Optional[List[Dict]] = [
- dict(ann_file='textrecog_test.json', dataset_postfix='')
- ],
- config_path: str = 'configs/',
- ) -> None:
- super().__init__(
- data_root=data_root,
- task='textrecog',
- overwrite_cfg=overwrite_cfg,
- dataset_name=dataset_name,
- train_anns=train_anns,
- val_anns=val_anns,
- test_anns=test_anns,
- config_path=config_path)
-
- def _gen_dataset_config(self) -> str:
- """Generate a full dataset config based on the annotation file
- dictionary.
-
- Args:
- ann_dict (dict[str, dict(str, str)]): A nested dictionary that maps
- a config variable name (such as icdar2015_textrecog_train) to
- its corresponding annotation information dict. Each dict
- contains following keys:
- - ann_file (str): The path to the annotation file relative to
- data_root.
- - dataset_postfix (str, optional): Affects the postfix of the
- resulting variable in the generated config. If specified, the
- dataset variable will be named in the form of
- ``{dataset_name}_{dataset_postfix}_{task}_{split}``. Defaults
- to None.
- - split (str): The split the annotation belongs to. Usually
- it can be 'train', 'val' and 'test'.
-
- Returns:
- str: The generated dataset config.
- """
- cfg = ''
- for key_name, ann_dict in self.anns.items():
- cfg += f'\n{key_name} = dict(\n'
- cfg += f' type=\'{ann_dict["dataset_type"]}\',\n'
- cfg += f' data_root={self.dataset_name}_{self.task}_data_root,\n' # noqa: E501
- cfg += f' ann_file=\'{ann_dict["ann_file"]}\',\n'
- if ann_dict['split'] in ['test', 'val']:
- cfg += ' test_mode=True,\n'
- cfg += ' pipeline=None)\n'
- return cfg
diff --git a/pipeline/mmocr/mmocr/datasets/preparers/config_generators/textspotting_config_generator.py b/pipeline/mmocr/mmocr/datasets/preparers/config_generators/textspotting_config_generator.py
deleted file mode 100644
index b4c1db7b642d6b1fd56354a87508baf09dede64f..0000000000000000000000000000000000000000
--- a/pipeline/mmocr/mmocr/datasets/preparers/config_generators/textspotting_config_generator.py
+++ /dev/null
@@ -1,63 +0,0 @@
-# Copyright (c) OpenMMLab. All rights reserved.
-from typing import Dict, List, Optional
-
-from mmocr.registry import CFG_GENERATORS
-from .base import BaseDatasetConfigGenerator
-from .textdet_config_generator import TextDetConfigGenerator
-
-
-@CFG_GENERATORS.register_module()
-class TextSpottingConfigGenerator(TextDetConfigGenerator):
- """Text spotting config generator.
-
- Args:
- data_root (str): The root path of the dataset.
- dataset_name (str): The name of the dataset.
- overwrite_cfg (bool): Whether to overwrite the dataset config file if
- it already exists. If False, config generator will not generate new
- config for datasets whose configs are already in base.
- train_anns (List[Dict], optional): A list of train annotation files
- to appear in the base configs. Defaults to
- ``[dict(file='textspotting_train.json', dataset_postfix='')]``.
- Each element is typically a dict with the following fields:
- - ann_file (str): The path to the annotation file relative to
- data_root.
- - dataset_postfix (str, optional): Affects the postfix of the
- resulting variable in the generated config. If specified, the
- dataset variable will be named in the form of
- ``{dataset_name}_{dataset_postfix}_{task}_{split}``. Defaults to
- None.
- val_anns (List[Dict], optional): A list of val annotation files
- to appear in the base configs, similar to ``train_anns``. Defaults
- to [].
- test_anns (List[Dict], optional): A list of test annotation files
- to appear in the base configs, similar to ``train_anns``. Defaults
- to ``[dict(file='textspotting_test.json')]``.
- config_path (str): Path to the configs. Defaults to 'configs/'.
- """
-
- def __init__(
- self,
- data_root: str,
- dataset_name: str,
- overwrite_cfg: bool = False,
- train_anns: Optional[List[Dict]] = [
- dict(ann_file='textspotting_train.json', dataset_postfix='')
- ],
- val_anns: Optional[List[Dict]] = [],
- test_anns: Optional[List[Dict]] = [
- dict(ann_file='textspotting_test.json', dataset_postfix='')
- ],
- config_path: str = 'configs/',
- ) -> None:
- BaseDatasetConfigGenerator.__init__(
- self,
- data_root=data_root,
- task='textspotting',
- overwrite_cfg=overwrite_cfg,
- dataset_name=dataset_name,
- train_anns=train_anns,
- val_anns=val_anns,
- test_anns=test_anns,
- config_path=config_path,
- )
diff --git a/pipeline/mmocr/mmocr/datasets/preparers/data_preparer.py b/pipeline/mmocr/mmocr/datasets/preparers/data_preparer.py
deleted file mode 100644
index 7e64856254194d91ac03e2c43aaa5161151b0564..0000000000000000000000000000000000000000
--- a/pipeline/mmocr/mmocr/datasets/preparers/data_preparer.py
+++ /dev/null
@@ -1,200 +0,0 @@
-# Copyright (c) OpenMMLab. All rights reserved.
-import copy
-import os
-import os.path as osp
-import shutil
-from typing import List, Optional, Union
-
-from mmocr.registry import (CFG_GENERATORS, DATA_DUMPERS, DATA_GATHERERS,
- DATA_OBTAINERS, DATA_PACKERS, DATA_PARSERS)
-from mmocr.utils.typing_utils import ConfigType, OptConfigType
-
-
-class DatasetPreparer:
- """Base class of dataset preparer.
-
- Dataset preparer is used to prepare dataset for MMOCR. It mainly consists
- of three steps:
- 1. For each split:
- - Obtain the dataset
- - Download
- - Extract
- - Move/Rename
- - Gather the dataset
- - Parse the dataset
- - Pack the dataset to MMOCR format
- - Dump the dataset
- 2. Delete useless files
- 3. Generate the base config for this dataset
-
- After all these steps, the original datasets have been prepared for
- usage in MMOCR. Check out the dataset format used in MMOCR here:
- https://mmocr.readthedocs.io/en/dev-1.x/user_guides/dataset_prepare.html
-
- Args:
- data_root (str): Root directory of data.
- dataset_name (str): Dataset name.
- task (str): Task type. Options are 'textdet', 'textrecog',
- 'textspotter', and 'kie'. Defaults to 'textdet'.
- nproc (int): Number of parallel processes. Defaults to 4.
- train_preparer (OptConfigType): cfg for train data prepare. It contains
- the following keys:
- - obtainer: cfg for data obtainer.
- - gatherer: cfg for data gatherer.
- - parser: cfg for data parser.
- - packer: cfg for data packer.
- - dumper: cfg for data dumper.
- Defaults to None.
- test_preparer (OptConfigType): cfg for test data prepare. Defaults to
- None.
- val_preparer (OptConfigType): cfg for val data prepare. Defaults to
- None.
- config_generator (OptConfigType): cfg for config generator. Defaults to
- None.
- delete (list[str], optional): List of files to be deleted.
- Defaults to None.
- """
-
- def __init__(self,
- data_root: str,
- dataset_name: str = '',
- task: str = 'textdet',
- nproc: int = 4,
- train_preparer: OptConfigType = None,
- test_preparer: OptConfigType = None,
- val_preparer: OptConfigType = None,
- config_generator: OptConfigType = None,
- delete: Optional[List[str]] = None) -> None:
- self.data_root = data_root
- self.nproc = nproc
- self.task = task
- self.dataset_name = dataset_name
- self.train_preparer = train_preparer
- self.test_preparer = test_preparer
- self.val_preparer = val_preparer
- self.config_generator = config_generator
- self.delete = delete
-
- def run(self, splits: Union[str, List] = ['train', 'test', 'val']) -> None:
- """Prepare the dataset."""
- if isinstance(splits, str):
- splits = [splits]
- assert set(splits).issubset(set(['train', 'test',
- 'val'])), 'Invalid split name'
- for split in splits:
- self.loop(split, getattr(self, f'{split}_preparer'))
- self.clean()
- self.generate_config()
-
- @classmethod
- def from_file(cls, cfg: ConfigType) -> 'DatasetPreparer':
- """Create a DataPreparer from config file.
-
- Args:
- cfg (ConfigType): A config used for building runner. Keys of
- ``cfg`` can see :meth:`__init__`.
-
- Returns:
- Runner: A DatasetPreparer build from ``cfg``.
- """
-
- cfg = copy.deepcopy(cfg)
- data_preparer = cls(
- data_root=cfg['data_root'],
- dataset_name=cfg.get('dataset_name', ''),
- task=cfg.get('task', 'textdet'),
- nproc=cfg.get('nproc', 4),
- train_preparer=cfg.get('train_preparer', None),
- test_preparer=cfg.get('test_preparer', None),
- val_preparer=cfg.get('val_preparer', None),
- delete=cfg.get('delete', None),
- config_generator=cfg.get('config_generator', None))
- return data_preparer
-
- def loop(self, split: str, cfg: ConfigType) -> None:
- """Loop over the dataset.
-
- Args:
- split (str): The split of the dataset.
- cfg (ConfigType): A config used for building obtainer, gatherer,
- parser, packer and dumper.
- """
- if cfg is None:
- return
-
- # build obtainer and run
- obtainer = cfg.get('obtainer', None)
- if obtainer:
- print(f'Obtaining {split} Dataset...')
- obtainer.setdefault('task', default=self.task)
- obtainer.setdefault('data_root', default=self.data_root)
- obtainer = DATA_OBTAINERS.build(obtainer)
- obtainer()
-
- # build gatherer
- gatherer = cfg.get('gatherer', None)
- parser = cfg.get('parser', None)
- packer = cfg.get('packer', None)
- dumper = cfg.get('dumper', None)
- related = [gatherer, parser, packer, dumper]
- if all(item is None for item in related): # no data process
- return
- if not all(item is not None for item in related):
- raise ValueError('gatherer, parser, packer and dumper should be '
- 'either all None or not None')
-
- print(f'Gathering {split} Dataset...')
- gatherer.setdefault('split', default=split)
- gatherer.setdefault('data_root', default=self.data_root)
- gatherer.setdefault('ann_dir', default='annotations')
- gatherer.setdefault(
- 'img_dir', default=osp.join(f'{self.task}_imgs', split))
-
- gatherer = DATA_GATHERERS.build(gatherer)
- img_paths, ann_paths = gatherer()
-
- # build parser
- print(f'Parsing {split} Images and Annotations...')
- parser.setdefault('split', default=split)
- parser.setdefault('nproc', default=self.nproc)
- parser = DATA_PARSERS.build(parser)
- # Convert dataset annotations to MMOCR format
- samples = parser(img_paths, ann_paths)
-
- # build packer
- print(f'Packing {split} Annotations...')
- packer.setdefault('split', default=split)
- packer.setdefault('nproc', default=self.nproc)
- packer.setdefault('data_root', default=self.data_root)
- packer = DATA_PACKERS.build(packer)
- samples = packer(samples)
-
- # build dumper
- print(f'Dumping {split} Annotations...')
- # Dump annotation files
- dumper.setdefault('task', default=self.task)
- dumper.setdefault('split', default=split)
- dumper.setdefault('data_root', default=self.data_root)
- dumper = DATA_DUMPERS.build(dumper)
- dumper(samples)
-
- def generate_config(self):
- if self.config_generator is None:
- return
- self.config_generator.setdefault(
- 'dataset_name', default=self.dataset_name)
- self.config_generator.setdefault('data_root', default=self.data_root)
- config_generator = CFG_GENERATORS.build(self.config_generator)
- print('Generating base configs...')
- config_generator()
-
- def clean(self) -> None:
- if self.delete is None:
- return
- for d in self.delete:
- delete_file = osp.join(self.data_root, d)
- if osp.exists(delete_file):
- if osp.isdir(delete_file):
- shutil.rmtree(delete_file)
- else:
- os.remove(delete_file)
diff --git a/pipeline/mmocr/mmocr/datasets/preparers/dumpers/__init__.py b/pipeline/mmocr/mmocr/datasets/preparers/dumpers/__init__.py
deleted file mode 100644
index ed3dda486b568ea5b4c7f48100b2c32c0b8ec987..0000000000000000000000000000000000000000
--- a/pipeline/mmocr/mmocr/datasets/preparers/dumpers/__init__.py
+++ /dev/null
@@ -1,10 +0,0 @@
-# Copyright (c) OpenMMLab. All rights reserved.
-from .base import BaseDumper
-from .json_dumper import JsonDumper
-from .lmdb_dumper import TextRecogLMDBDumper
-from .wild_receipt_openset_dumper import WildreceiptOpensetDumper
-
-__all__ = [
- 'BaseDumper', 'JsonDumper', 'WildreceiptOpensetDumper',
- 'TextRecogLMDBDumper'
-]
diff --git a/pipeline/mmocr/mmocr/datasets/preparers/dumpers/base.py b/pipeline/mmocr/mmocr/datasets/preparers/dumpers/base.py
deleted file mode 100644
index 3b4416a8d9adb4352a4426e834ac87841fc12c9b..0000000000000000000000000000000000000000
--- a/pipeline/mmocr/mmocr/datasets/preparers/dumpers/base.py
+++ /dev/null
@@ -1,35 +0,0 @@
-# Copyright (c) OpenMMLab. All rights reserved.
-from typing import Any
-
-
-class BaseDumper:
- """Base class for data dumpers.
-
- Args:
- task (str): Task type. Options are 'textdet', 'textrecog',
- 'textspotter', and 'kie'. It is usually set automatically and users
- do not need to set it manually in config file in most cases.
- split (str): It' s the partition of the datasets. Options are 'train',
- 'val' or 'test'. It is usually set automatically and users do not
- need to set it manually in config file in most cases. Defaults to
- None.
- data_root (str): The root directory of the image and
- annotation. It is usually set automatically and users do not need
- to set it manually in config file in most cases. Defaults to None.
- """
-
- def __init__(self, task: str, split: str, data_root: str) -> None:
- self.task = task
- self.split = split
- self.data_root = data_root
-
- def __call__(self, data: Any) -> None:
- """Call function.
-
- Args:
- data (Any): Data to be dumped.
- """
- self.dump(data)
-
- def dump(self, data: Any) -> None:
- raise NotImplementedError
diff --git a/pipeline/mmocr/mmocr/datasets/preparers/dumpers/json_dumper.py b/pipeline/mmocr/mmocr/datasets/preparers/dumpers/json_dumper.py
deleted file mode 100644
index e1c8ab026df3b03231e2edd6e9bf39de7cf27e38..0000000000000000000000000000000000000000
--- a/pipeline/mmocr/mmocr/datasets/preparers/dumpers/json_dumper.py
+++ /dev/null
@@ -1,24 +0,0 @@
-# Copyright (c) OpenMMLab. All rights reserved.
-import os.path as osp
-from typing import Dict
-
-import mmengine
-
-from mmocr.registry import DATA_DUMPERS
-from .base import BaseDumper
-
-
-@DATA_DUMPERS.register_module()
-class JsonDumper(BaseDumper):
- """Dumper for json file."""
-
- def dump(self, data: Dict) -> None:
- """Dump data to json file.
-
- Args:
- data (Dict): Data to be dumped.
- """
-
- filename = f'{self.task}_{self.split}.json'
- dst_file = osp.join(self.data_root, filename)
- mmengine.dump(data, dst_file)
diff --git a/pipeline/mmocr/mmocr/datasets/preparers/dumpers/lmdb_dumper.py b/pipeline/mmocr/mmocr/datasets/preparers/dumpers/lmdb_dumper.py
deleted file mode 100644
index 9cd49d17ff17a8224e16284669e3d1206e0463ca..0000000000000000000000000000000000000000
--- a/pipeline/mmocr/mmocr/datasets/preparers/dumpers/lmdb_dumper.py
+++ /dev/null
@@ -1,140 +0,0 @@
-# Copyright (c) OpenMMLab. All rights reserved.
-import os.path as osp
-import warnings
-from typing import Dict, List
-
-import cv2
-import lmdb
-import mmengine
-import numpy as np
-
-from mmocr.registry import DATA_DUMPERS
-from .base import BaseDumper
-
-
-@DATA_DUMPERS.register_module()
-class TextRecogLMDBDumper(BaseDumper):
- """Text recognition LMDB format dataset dumper.
-
- Args:
- task (str): Task type. Options are 'textdet', 'textrecog',
- 'textspotter', and 'kie'. It is usually set automatically and users
- do not need to set it manually in config file in most cases.
- split (str): It' s the partition of the datasets. Options are 'train',
- 'val' or 'test'. It is usually set automatically and users do not
- need to set it manually in config file in most cases. Defaults to
- None.
- data_root (str): The root directory of the image and
- annotation. It is usually set automatically and users do not need
- to set it manually in config file in most cases. Defaults to None.
- batch_size (int): Number of files written to the cache each time.
- Defaults to 1000.
- encoding (str): Label encoding method. Defaults to 'utf-8'.
- lmdb_map_size (int): Maximum size database may grow to. Defaults to
- 1099511627776.
- verify (bool): Whether to check the validity of every image. Defaults
- to True.
- """
-
- def __init__(self,
- task: str,
- split: str,
- data_root: str,
- batch_size: int = 1000,
- encoding: str = 'utf-8',
- lmdb_map_size: int = 1099511627776,
- verify: bool = True) -> None:
- assert task == 'textrecog', \
- f'TextRecogLMDBDumper only works with textrecog, but got {task}'
- super().__init__(task=task, split=split, data_root=data_root)
- self.batch_size = batch_size
- self.encoding = encoding
- self.lmdb_map_size = lmdb_map_size
- self.verify = verify
-
- def check_image_is_valid(self, imageBin):
- if imageBin is None:
- return False
- imageBuf = np.frombuffer(imageBin, dtype=np.uint8)
- img = cv2.imdecode(imageBuf, cv2.IMREAD_GRAYSCALE)
- imgH, imgW = img.shape[0], img.shape[1]
- if imgH * imgW == 0:
- return False
- return True
-
- def write_cache(self, env, cache):
- with env.begin(write=True) as txn:
- cursor = txn.cursor()
- cursor.putmulti(cache, dupdata=False, overwrite=True)
-
- def parser_pack_instance(self, instance: Dict):
- """parser an packed MMOCR format textrecog instance.
- Args:
- instance (Dict): An packed MMOCR format textrecog instance.
- For example,
- {
- "instance": [
- {
- "text": "Hello"
- }
- ],
- "img_path": "img1.jpg"
- }
- """
- assert isinstance(instance,
- Dict), 'Element of data_list must be a dict'
- assert 'img_path' in instance and 'instances' in instance, \
- 'Element of data_list must have the following keys: ' \
- f'img_path and instances, but got {instance.keys()}'
- assert isinstance(instance['instances'], List) and len(
- instance['instances']) == 1
- assert 'text' in instance['instances'][0]
-
- img_path = instance['img_path']
- text = instance['instances'][0]['text']
- return img_path, text
-
- def dump(self, data: Dict) -> None:
- """Dump data to LMDB format."""
-
- # create lmdb env
- output_dirname = f'{self.task}_{self.split}.lmdb'
- output = osp.join(self.data_root, output_dirname)
- mmengine.mkdir_or_exist(output)
- env = lmdb.open(output, map_size=self.lmdb_map_size)
- # load data
- if 'data_list' not in data:
- raise ValueError('Dump data must have data_list key')
- data_list = data['data_list']
- cache = []
- # index start from 1
- cnt = 1
- n_samples = len(data_list)
- for d in data_list:
- # convert both images and labels to lmdb
- label_key = 'label-%09d'.encode(self.encoding) % cnt
- img_name, text = self.parser_pack_instance(d)
- img_path = osp.join(self.data_root, img_name)
- if not osp.exists(img_path):
- warnings.warn('%s does not exist' % img_path)
- continue
- with open(img_path, 'rb') as f:
- image_bin = f.read()
- if self.verify:
- if not self.check_image_is_valid(image_bin):
- warnings.warn('%s is not a valid image' % img_path)
- continue
- image_key = 'image-%09d'.encode(self.encoding) % cnt
- cache.append((image_key, image_bin))
- cache.append((label_key, text.encode(self.encoding)))
-
- if cnt % self.batch_size == 0:
- self.write_cache(env, cache)
- cache = []
- print('Written %d / %d' % (cnt, n_samples))
- cnt += 1
- n_samples = cnt - 1
- cache.append(('num-samples'.encode(self.encoding),
- str(n_samples).encode(self.encoding)))
- self.write_cache(env, cache)
- print('Created lmdb dataset with %d samples' % n_samples)
diff --git a/pipeline/mmocr/mmocr/datasets/preparers/dumpers/wild_receipt_openset_dumper.py b/pipeline/mmocr/mmocr/datasets/preparers/dumpers/wild_receipt_openset_dumper.py
deleted file mode 100644
index df6a462c8e29b04a877698ca96c9739579484874..0000000000000000000000000000000000000000
--- a/pipeline/mmocr/mmocr/datasets/preparers/dumpers/wild_receipt_openset_dumper.py
+++ /dev/null
@@ -1,22 +0,0 @@
-# Copyright (c) OpenMMLab. All rights reserved.
-import os.path as osp
-from typing import List
-
-from mmocr.registry import DATA_DUMPERS
-from mmocr.utils import list_to_file
-from .base import BaseDumper
-
-
-@DATA_DUMPERS.register_module()
-class WildreceiptOpensetDumper(BaseDumper):
-
- def dump(self, data: List):
- """Dump data to txt file.
-
- Args:
- data (List): Data to be dumped.
- """
-
- filename = f'openset_{self.split}.txt'
- dst_file = osp.join(self.data_root, filename)
- list_to_file(dst_file, data)
diff --git a/pipeline/mmocr/mmocr/datasets/preparers/gatherers/__init__.py b/pipeline/mmocr/mmocr/datasets/preparers/gatherers/__init__.py
deleted file mode 100644
index 9a05c79754e6a6392c97b1e7937b725d2d9df752..0000000000000000000000000000000000000000
--- a/pipeline/mmocr/mmocr/datasets/preparers/gatherers/__init__.py
+++ /dev/null
@@ -1,8 +0,0 @@
-# Copyright (c) OpenMMLab. All rights reserved.
-
-from .base import BaseGatherer
-from .mono_gatherer import MonoGatherer
-from .naf_gatherer import NAFGatherer
-from .pair_gatherer import PairGatherer
-
-__all__ = ['BaseGatherer', 'MonoGatherer', 'PairGatherer', 'NAFGatherer']
diff --git a/pipeline/mmocr/mmocr/datasets/preparers/gatherers/base.py b/pipeline/mmocr/mmocr/datasets/preparers/gatherers/base.py
deleted file mode 100644
index f982a1a5d62e5071646d621865e6e9fd1dad674f..0000000000000000000000000000000000000000
--- a/pipeline/mmocr/mmocr/datasets/preparers/gatherers/base.py
+++ /dev/null
@@ -1,49 +0,0 @@
-# Copyright (c) OpenMMLab. All rights reserved.
-import os.path as osp
-from typing import List, Optional, Tuple, Union
-
-
-class BaseGatherer:
- """Base class for gatherer.
-
- Note: Gatherer assumes that all the annotation file is in the same
- directory and all the image files are in the same directory.
-
- Args:
- img_dir(str): The directory of the images. It is usually set
- automatically to f'text{task}_imgs/split' and users do not need to
- set it manually in config file in most cases. When the image files
- is not in 'text{task}_imgs/split' directory, users should set it.
- Defaults to ''.
- ann_dir (str): The directory of the annotation files. It is usually set
- automatically to 'annotations' and users do not need to set it
- manually in config file in most cases. When the annotation files
- is not in 'annotations' directory, users should set it. Defaults to
- 'annotations'.
- split (str, optional): List of splits to gather. It' s the partition of
- the datasets. Options are 'train', 'val' or 'test'. It is usually
- set automatically and users do not need to set it manually in
- config file in most cases. Defaults to None.
- data_root (str, optional): The root directory of the image and
- annotation. It is usually set automatically and users do not need
- to set it manually in config file in most cases. Defaults to None.
- """
-
- def __init__(self,
- img_dir: str = '',
- ann_dir: str = 'annotations',
- split: Optional[str] = None,
- data_root: Optional[str] = None) -> None:
- self.split = split
- self.data_root = data_root
- self.ann_dir = osp.join(data_root, ann_dir)
- self.img_dir = osp.join(data_root, img_dir)
-
- def __call__(self) -> Union[Tuple[List[str], List[str]], Tuple[str, str]]:
- """The return value of the gatherer is a tuple of two lists or strings.
-
- The first element is the list of image paths or the directory of the
- images. The second element is the list of annotation paths or the path
- of the annotation file which contains all the annotations.
- """
- raise NotImplementedError
diff --git a/pipeline/mmocr/mmocr/datasets/preparers/gatherers/mono_gatherer.py b/pipeline/mmocr/mmocr/datasets/preparers/gatherers/mono_gatherer.py
deleted file mode 100644
index bad35fa2f1a46362ac3e515fbe5281621143118a..0000000000000000000000000000000000000000
--- a/pipeline/mmocr/mmocr/datasets/preparers/gatherers/mono_gatherer.py
+++ /dev/null
@@ -1,34 +0,0 @@
-# Copyright (c) OpenMMLab. All rights reserved.
-import os.path as osp
-from typing import Tuple
-
-from mmocr.registry import DATA_GATHERERS
-from .base import BaseGatherer
-
-
-@DATA_GATHERERS.register_module()
-class MonoGatherer(BaseGatherer):
- """Gather the dataset file. Specifically for the case that only one
- annotation file is needed. For example,
-
- img_001.jpg \
- img_002.jpg ---> train.json
- img_003.jpg /
-
- Args:
- ann_name (str): The name of the annotation file.
- """
-
- def __init__(self, ann_name: str, **kwargs) -> None:
- super().__init__(**kwargs)
-
- self.ann_name = ann_name
-
- def __call__(self) -> Tuple[str, str]:
- """
- Returns:
- tuple(str, str): The directory of the image and the path of
- annotation file.
- """
-
- return (self.img_dir, osp.join(self.ann_dir, self.ann_name))
diff --git a/pipeline/mmocr/mmocr/datasets/preparers/gatherers/naf_gatherer.py b/pipeline/mmocr/mmocr/datasets/preparers/gatherers/naf_gatherer.py
deleted file mode 100644
index 3251bde40ddd01885ee45c4ad21911156a3ecf07..0000000000000000000000000000000000000000
--- a/pipeline/mmocr/mmocr/datasets/preparers/gatherers/naf_gatherer.py
+++ /dev/null
@@ -1,66 +0,0 @@
-# Copyright (c) OpenMMLab. All rights reserved.
-import json
-import os
-import os.path as osp
-import shutil
-from typing import List, Tuple
-
-from mmocr.registry import DATA_GATHERERS
-from .base import BaseGatherer
-
-
-@DATA_GATHERERS.register_module()
-class NAFGatherer(BaseGatherer):
- """Gather the dataset file from NAF dataset. Specifically for the case that
- there is a split file that contains the names of different splits. For
- example,
-
- img_001.jpg train: img_001.jpg
- img_002.jpg ---> split_file ---> test: img_002.jpg
- img_003.jpg val: img_003.jpg
-
- Args:
- split_file (str, optional): The name of the split file. Defaults to
- "data_split.json".
- temp_dir (str, optional): The directory of the temporary images.
- Defaults to "temp_images".
- """
-
- def __init__(self,
- split_file='data_split.json',
- temp_dir: str = 'temp_images',
- **kwargs) -> None:
- super().__init__(**kwargs)
- self.temp_dir = temp_dir
- self.split_file = split_file
-
- def __call__(self) -> Tuple[List[str], List[str]]:
- """
- Returns:
- tuple(list[str], list[str]): The list of image paths and the list
- of annotation paths.
- """
-
- split_file = osp.join(self.data_root, self.split_file)
- with open(split_file, 'r') as f:
- split_data = json.load(f)
- img_list = list()
- ann_list = list()
- # Rename the key
- split_data['val'] = split_data.pop('valid')
- if not osp.exists(self.img_dir):
- os.makedirs(self.img_dir)
- current_split_data = split_data[self.split]
- for groups in current_split_data:
- for img_name in current_split_data[groups]:
- src_img = osp.join(self.data_root, self.temp_dir, img_name)
- dst_img = osp.join(self.img_dir, img_name)
- if not osp.exists(src_img):
- Warning(f'{src_img} does not exist!')
- continue
- # move the image to the new path
- shutil.move(src_img, dst_img)
- ann = osp.join(self.ann_dir, img_name.replace('.jpg', '.json'))
- img_list.append(dst_img)
- ann_list.append(ann)
- return img_list, ann_list
diff --git a/pipeline/mmocr/mmocr/datasets/preparers/gatherers/pair_gatherer.py b/pipeline/mmocr/mmocr/datasets/preparers/gatherers/pair_gatherer.py
deleted file mode 100644
index 63c11e0c121a6608a7a39769f8a9f09bdf3ba076..0000000000000000000000000000000000000000
--- a/pipeline/mmocr/mmocr/datasets/preparers/gatherers/pair_gatherer.py
+++ /dev/null
@@ -1,59 +0,0 @@
-# Copyright (c) OpenMMLab. All rights reserved.
-import os.path as osp
-import re
-from typing import List, Optional, Tuple
-
-from mmocr.registry import DATA_GATHERERS
-from mmocr.utils import list_files
-from .base import BaseGatherer
-
-
-@DATA_GATHERERS.register_module()
-class PairGatherer(BaseGatherer):
- """Gather the dataset files. Specifically for the paired annotations. That
- is to say, each image has a corresponding annotation file. For example,
-
- img_1.jpg <---> gt_img_1.txt
- img_2.jpg <---> gt_img_2.txt
- img_3.jpg <---> gt_img_3.txt
-
- Args:
- img_suffixes (List[str]): File suffixes that used for searching.
- rule (Sequence): The rule for pairing the files. The first element is
- the matching pattern for the file, and the second element is the
- replacement pattern, which should be a regular expression. For
- example, to map the image name img_1.jpg to the annotation name
- gt_img_1.txt, the rule is
- [r'img_(\d+)\.([jJ][pP][gG])', r'gt_img_\1.txt'] # noqa: W605 E501
-
- Note: PairGatherer assumes that each split annotation file is in the
- correspond split directory. For example, all the train annotation files are
- in {ann_dir}/train.
- """
-
- def __init__(self,
- img_suffixes: Optional[List[str]] = None,
- rule: Optional[List[str]] = None,
- **kwargs) -> None:
- super().__init__(**kwargs)
- self.rule = rule
- self.img_suffixes = img_suffixes
- # ann_dir = {ann_root}/{ann_dir}/{split}
- self.ann_dir = osp.join(self.ann_dir, self.split)
-
- def __call__(self) -> Tuple[List[str], List[str]]:
- """tuple(list, list): The list of image paths and the list of
- annotation paths."""
-
- img_list = list()
- ann_list = list()
- for img_path in list_files(self.img_dir, self.img_suffixes):
- if not re.match(self.rule[0], osp.basename(img_path)):
- continue
- ann_name = re.sub(self.rule[0], self.rule[1],
- osp.basename(img_path))
- ann_path = osp.join(self.ann_dir, ann_name)
- img_list.append(img_path)
- ann_list.append(ann_path)
-
- return img_list, ann_list
diff --git a/pipeline/mmocr/mmocr/datasets/preparers/obtainers/__init__.py b/pipeline/mmocr/mmocr/datasets/preparers/obtainers/__init__.py
deleted file mode 100644
index 55d484d981deb70e7a557ee310a36ab9f2c45d64..0000000000000000000000000000000000000000
--- a/pipeline/mmocr/mmocr/datasets/preparers/obtainers/__init__.py
+++ /dev/null
@@ -1,4 +0,0 @@
-# Copyright (c) OpenMMLab. All rights reserved.
-from .naive_data_obtainer import NaiveDataObtainer
-
-__all__ = ['NaiveDataObtainer']
diff --git a/pipeline/mmocr/mmocr/datasets/preparers/obtainers/naive_data_obtainer.py b/pipeline/mmocr/mmocr/datasets/preparers/obtainers/naive_data_obtainer.py
deleted file mode 100644
index 51b0d266c847771b403dea62de3b2d81d4d71b02..0000000000000000000000000000000000000000
--- a/pipeline/mmocr/mmocr/datasets/preparers/obtainers/naive_data_obtainer.py
+++ /dev/null
@@ -1,201 +0,0 @@
-# Copyright (c) OpenMMLab. All rights reserved.
-import glob
-import os
-import os.path as osp
-import shutil
-import ssl
-import urllib.request as request
-from typing import Dict, List, Optional, Tuple
-
-from mmengine import mkdir_or_exist
-
-from mmocr.registry import DATA_OBTAINERS
-from mmocr.utils import check_integrity, is_archive
-
-ssl._create_default_https_context = ssl._create_unverified_context
-
-
-@DATA_OBTAINERS.register_module()
-class NaiveDataObtainer:
- """A naive pipeline for obtaining dataset.
-
- download -> extract -> move
-
- Args:
- files (list[dict]): A list of file information.
- cache_path (str): The path to cache the downloaded files.
- data_root (str): The root path of the dataset. It is usually set auto-
- matically and users do not need to set it manually in config file
- in most cases.
- task (str): The task of the dataset. It is usually set automatically
- and users do not need to set it manually in config file
- in most cases.
- """
-
- def __init__(self, files: List[Dict], cache_path: str, data_root: str,
- task: str) -> None:
- self.files = files
- self.cache_path = cache_path
- self.data_root = data_root
- self.task = task
- mkdir_or_exist(self.data_root)
- mkdir_or_exist(osp.join(self.data_root, f'{task}_imgs'))
- mkdir_or_exist(osp.join(self.data_root, 'annotations'))
- mkdir_or_exist(self.cache_path)
-
- def __call__(self):
- for file in self.files:
- save_name = file.get('save_name', None)
- url = file.get('url', None)
- md5 = file.get('md5', None)
- download_path = osp.join(
- self.cache_path,
- osp.basename(url) if save_name is None else save_name)
- # Download required files
- if not check_integrity(download_path, md5):
- self.download(url=url, dst_path=download_path)
- # Extract downloaded zip files to data root
- self.extract(src_path=download_path, dst_path=self.data_root)
- # Move & Rename dataset files
- if 'mapping' in file:
- self.move(mapping=file['mapping'])
- self.clean()
-
- def download(self, url: Optional[str], dst_path: str) -> None:
- """Download file from given url with progress bar.
-
- Args:
- url (str): The url to download the file.
- dst_path (str): The destination path to save the file.
- """
-
- def progress(down: float, block: float, size: float) -> None:
- """Show download progress.
-
- Args:
- down (float): Downloaded size.
- block (float): Block size.
- size (float): Total size of the file.
- """
-
- percent = min(100. * down * block / size, 100)
- file_name = osp.basename(dst_path)
- print(f'\rDownloading {file_name}: {percent:.2f}%', end='')
-
- if url is None and not osp.exists(dst_path):
- raise FileNotFoundError(
- 'Direct url is not available for this dataset.'
- ' Please manually download the required files'
- ' following the guides.')
-
- if url.startswith('magnet'):
- raise NotImplementedError('Please use any BitTorrent client to '
- 'download the following magnet link to '
- f'{osp.abspath(dst_path)} and '
- f'try again.\nLink: {url}')
-
- print('Downloading...')
- print(f'URL: {url}')
- print(f'Destination: {osp.abspath(dst_path)}')
- print('If you stuck here for a long time, please check your network, '
- 'or manually download the file to the destination path and '
- 'run the script again.')
- request.urlretrieve(url, dst_path, progress)
- print('')
-
- def extract(self,
- src_path: str,
- dst_path: str,
- delete: bool = False) -> None:
- """Extract zip/tar.gz files.
-
- Args:
- src_path (str): Path to the zip file.
- dst_path (str): Path to the destination folder.
- delete (bool, optional): Whether to delete the zip file. Defaults
- to False.
- """
- if not is_archive(src_path):
- # Copy the file to the destination folder if it is not a zip
- if osp.isfile(src_path):
- shutil.copy(src_path, dst_path)
- else:
- shutil.copytree(src_path, dst_path)
- return
-
- zip_name = osp.basename(src_path).split('.')[0]
- if dst_path is None:
- dst_path = osp.join(osp.dirname(src_path), zip_name)
- else:
- dst_path = osp.join(dst_path, zip_name)
-
- extracted = False
- if osp.exists(dst_path):
- name = set(os.listdir(dst_path))
- if '.finish' in name:
- extracted = True
- elif '.finish' not in name and len(name) > 0:
- while True:
- c = input(f'{dst_path} already exists when extracting '
- '{zip_name}, unzip again? (y/N) ') or 'N'
- if c.lower() in ['y', 'n']:
- extracted = c == 'n'
- break
- if extracted:
- open(osp.join(dst_path, '.finish'), 'w').close()
- print(f'{zip_name} has been extracted. Skip')
- return
- mkdir_or_exist(dst_path)
- print(f'Extracting: {osp.basename(src_path)}')
- if src_path.endswith('.zip'):
- try:
- import zipfile
- except ImportError:
- raise ImportError(
- 'Please install zipfile by running "pip install zipfile".')
- with zipfile.ZipFile(src_path, 'r') as zip_ref:
- zip_ref.extractall(dst_path)
- elif src_path.endswith('.tar.gz') or src_path.endswith('.tar'):
- if src_path.endswith('.tar.gz'):
- mode = 'r:gz'
- elif src_path.endswith('.tar'):
- mode = 'r:'
- try:
- import tarfile
- except ImportError:
- raise ImportError(
- 'Please install tarfile by running "pip install tarfile".')
- with tarfile.open(src_path, mode) as tar_ref:
- tar_ref.extractall(dst_path)
-
- open(osp.join(dst_path, '.finish'), 'w').close()
- if delete:
- os.remove(src_path)
-
- def move(self, mapping: List[Tuple[str, str]]) -> None:
- """Rename and move dataset files one by one.
-
- Args:
- mapping (List[Tuple[str, str]]): A list of tuples, each
- tuple contains the source file name and the destination file name.
- """
- for src, dst in mapping:
- src = osp.join(self.data_root, src)
- dst = osp.join(self.data_root, dst)
-
- if '*' in src:
- mkdir_or_exist(dst)
- for f in glob.glob(src):
- if not osp.exists(
- osp.join(dst, osp.relpath(f, self.data_root))):
- shutil.move(f, dst)
-
- elif osp.exists(src) and not osp.exists(dst):
- mkdir_or_exist(osp.dirname(dst))
- shutil.move(src, dst)
-
- def clean(self) -> None:
- """Remove empty dirs."""
- for root, dirs, files in os.walk(self.data_root, topdown=False):
- if not files and not dirs:
- os.rmdir(root)
diff --git a/pipeline/mmocr/mmocr/datasets/preparers/packers/__init__.py b/pipeline/mmocr/mmocr/datasets/preparers/packers/__init__.py
deleted file mode 100644
index 78eb55dc4e16e34b69dc0fa784e9c1120d912d07..0000000000000000000000000000000000000000
--- a/pipeline/mmocr/mmocr/datasets/preparers/packers/__init__.py
+++ /dev/null
@@ -1,11 +0,0 @@
-# Copyright (c) OpenMMLab. All rights reserved.
-from .base import BasePacker
-from .textdet_packer import TextDetPacker
-from .textrecog_packer import TextRecogCropPacker, TextRecogPacker
-from .textspotting_packer import TextSpottingPacker
-from .wildreceipt_packer import WildReceiptPacker
-
-__all__ = [
- 'BasePacker', 'TextDetPacker', 'TextRecogPacker', 'TextRecogCropPacker',
- 'TextSpottingPacker', 'WildReceiptPacker'
-]
diff --git a/pipeline/mmocr/mmocr/datasets/preparers/packers/base.py b/pipeline/mmocr/mmocr/datasets/preparers/packers/base.py
deleted file mode 100644
index 4826fd32225b9445ff868a0c9774ee01ae3849e5..0000000000000000000000000000000000000000
--- a/pipeline/mmocr/mmocr/datasets/preparers/packers/base.py
+++ /dev/null
@@ -1,57 +0,0 @@
-# Copyright (c) OpenMMLab. All rights reserved.
-from abc import abstractmethod
-from typing import Dict, List, Tuple
-
-from mmengine import track_parallel_progress
-
-
-class BasePacker:
- """Base class for packing the parsed annotation info to MMOCR format.
-
- Args:
- data_root (str): The root path of the dataset. It is usually set auto-
- matically and users do not need to set it manually in config file
- in most cases.
- split (str): The split of the dataset. It is usually set automatically
- and users do not need to set it manually in config file in most
- cases.
- nproc (int): Number of processes to process the data. Defaults to 1.
- It is usually set automatically and users do not need to set it
- manually in config file in most cases.
- """
-
- def __init__(self, data_root: str, split: str, nproc: int = 1) -> None:
- self.data_root = data_root
- self.split = split
- self.nproc = nproc
-
- @abstractmethod
- def pack_instance(self, sample: Tuple, split: str) -> Dict:
- """Pack the parsed annotation info to an MMOCR format instance.
-
- Args:
- sample (Tuple): A tuple of (img_file, ann_file).
- - img_path (str): Path to image file.
- - instances (Sequence[Dict]): A list of converted annos.
- split (str): The split of the instance.
-
- Returns:
- Dict: An MMOCR format instance.
- """
-
- @abstractmethod
- def add_meta(self, sample: List) -> Dict:
- """Add meta information to the sample.
-
- Args:
- sample (List): A list of samples of the dataset.
-
- Returns:
- Dict: A dict contains the meta information and samples.
- """
-
- def __call__(self, samples) -> Dict:
- samples = track_parallel_progress(
- self.pack_instance, samples, nproc=self.nproc)
- samples = self.add_meta(samples)
- return samples
diff --git a/pipeline/mmocr/mmocr/datasets/preparers/packers/textdet_packer.py b/pipeline/mmocr/mmocr/datasets/preparers/packers/textdet_packer.py
deleted file mode 100644
index b9d4c230945fefaca9d6c90a1b99ed05b3956269..0000000000000000000000000000000000000000
--- a/pipeline/mmocr/mmocr/datasets/preparers/packers/textdet_packer.py
+++ /dev/null
@@ -1,110 +0,0 @@
-# Copyright (c) OpenMMLab. All rights reserved.
-import os.path as osp
-from typing import Dict, List, Tuple
-
-import mmcv
-
-from mmocr.registry import DATA_PACKERS
-from mmocr.utils import bbox2poly, poly2bbox
-from .base import BasePacker
-
-
-@DATA_PACKERS.register_module()
-class TextDetPacker(BasePacker):
- """Text detection packer. It is used to pack the parsed annotation info to.
-
- .. code-block:: python
-
- {
- "metainfo":
- {
- "dataset_type": "TextDetDataset",
- "task_name": "textdet",
- "category": [{"id": 0, "name": "text"}]
- },
- "data_list":
- [
- {
- "img_path": "test_img.jpg",
- "height": 640,
- "width": 640,
- "instances":
- [
- {
- "polygon": [0, 0, 0, 10, 10, 20, 20, 0],
- "bbox": [0, 0, 10, 20],
- "bbox_label": 0,
- "ignore": False
- },
- // ...
- ]
- }
- ]
- }
- """
-
- def pack_instance(self, sample: Tuple, bbox_label: int = 0) -> Dict:
- """Pack the parsed annotation info to an MMOCR format instance.
-
- Args:
- sample (Tuple): A tuple of (img_file, instances).
- - img_path (str): Path to the image file.
- - instances (Sequence[Dict]): A list of converted annos. Each
- element should be a dict with the following keys:
-
- - 'poly' or 'box'
- - 'ignore'
- - 'bbox_label' (optional)
- split (str): The split of the instance.
-
- Returns:
- Dict: An MMOCR format instance.
- """
-
- img_path, instances = sample
-
- img = mmcv.imread(img_path)
- h, w = img.shape[:2]
-
- packed_instances = list()
- for instance in instances:
- poly = instance.get('poly', None)
- box = instance.get('box', None)
- assert box or poly
- packed_sample = dict(
- polygon=poly if poly else list(
- bbox2poly(box).astype('float64')),
- bbox=box if box else list(poly2bbox(poly).astype('float64')),
- bbox_label=bbox_label,
- ignore=instance['ignore'])
- packed_instances.append(packed_sample)
-
- packed_instances = dict(
- instances=packed_instances,
- img_path=osp.relpath(img_path, self.data_root),
- height=h,
- width=w)
-
- return packed_instances
-
- def add_meta(self, sample: List) -> Dict:
- """Add meta information to the sample.
-
- Args:
- sample (List): A list of samples of the dataset.
-
- Returns:
- Dict: A dict contains the meta information and samples.
- """
- meta = {
- 'metainfo': {
- 'dataset_type': 'TextDetDataset',
- 'task_name': 'textdet',
- 'category': [{
- 'id': 0,
- 'name': 'text'
- }]
- },
- 'data_list': sample
- }
- return meta
diff --git a/pipeline/mmocr/mmocr/datasets/preparers/packers/textrecog_packer.py b/pipeline/mmocr/mmocr/datasets/preparers/packers/textrecog_packer.py
deleted file mode 100644
index 6af70064aa7303d494c6d51121ece8c6e4cd06da..0000000000000000000000000000000000000000
--- a/pipeline/mmocr/mmocr/datasets/preparers/packers/textrecog_packer.py
+++ /dev/null
@@ -1,175 +0,0 @@
-# Copyright (c) OpenMMLab. All rights reserved.
-import os.path as osp
-from typing import Dict, List, Tuple
-
-import mmcv
-from mmengine import mkdir_or_exist
-
-from mmocr.registry import DATA_PACKERS
-from mmocr.utils import bbox2poly, crop_img, poly2bbox, warp_img
-from .base import BasePacker
-
-
-@DATA_PACKERS.register_module()
-class TextRecogPacker(BasePacker):
- """Text recogntion packer. It is used to pack the parsed annotation info
- to:
-
- .. code-block:: python
-
- {
- "metainfo":
- {
- "dataset_type": "TextRecogDataset",
- "task_name": "textrecog",
- },
- "data_list":
- [
- {
- "img_path": "textrecog_imgs/train/test_img.jpg",
- "instances":
- [
- {
- "text": "GRAND"
- }
- ]
- }
- ]
- }
- """
-
- def pack_instance(self, sample: Tuple) -> Dict:
- """Pack the text info to a recognition instance.
-
- Args:
- samples (Tuple): A tuple of (img_name, text).
- split (str): The split of the instance.
-
- Returns:
- Dict: The packed instance.
- """
-
- img_name, text = sample
- img_name = osp.relpath(img_name, self.data_root)
- packed_instance = dict(instances=[dict(text=text)], img_path=img_name)
-
- return packed_instance
-
- def add_meta(self, sample: List) -> Dict:
- """Add meta information to the sample.
-
- Args:
- sample (List): A list of samples of the dataset.
-
- Returns:
- Dict: A dict contains the meta information and samples.
- """
- meta = {
- 'metainfo': {
- 'dataset_type': 'TextRecogDataset',
- 'task_name': 'textrecog'
- },
- 'data_list': sample
- }
- return meta
-
-
-@DATA_PACKERS.register_module()
-class TextRecogCropPacker(TextRecogPacker):
- """Text recognition packer with image cropper. It is used to pack the
- parsed annotation info and crop out the word images from the full-size
- ones.
-
- Args:
- crop_with_warp (bool): Whether to crop the text from the original
- image using opencv warpPerspective.
- jitter (bool): (Applicable when crop_with_warp=True)
- Whether to jitter the box.
- jitter_ratio_x (float): (Applicable when crop_with_warp=True)
- Horizontal jitter ratio relative to the height.
- jitter_ratio_y (float): (Applicable when crop_with_warp=True)
- Vertical jitter ratio relative to the height.
- long_edge_pad_ratio (float): (Applicable when crop_with_warp=False)
- The ratio of padding the long edge of the cropped image.
- Defaults to 0.1.
- short_edge_pad_ratio (float): (Applicable when crop_with_warp=False)
- The ratio of padding the short edge of the cropped image.
- Defaults to 0.05.
- """
-
- def __init__(self,
- crop_with_warp: bool = False,
- jitter: bool = False,
- jitter_ratio_x: float = 0.0,
- jitter_ratio_y: float = 0.0,
- long_edge_pad_ratio: float = 0.0,
- short_edge_pad_ratio: float = 0.0,
- **kwargs):
- super().__init__(**kwargs)
- self.crop_with_warp = crop_with_warp
- self.jitter = jitter
- self.jrx = jitter_ratio_x
- self.jry = jitter_ratio_y
- self.lepr = long_edge_pad_ratio
- self.sepr = short_edge_pad_ratio
- # Crop converter crops the images of textdet to patches
- self.cropped_img_dir = 'textrecog_imgs'
- self.crop_save_path = osp.join(self.data_root, self.cropped_img_dir)
- mkdir_or_exist(self.crop_save_path)
- mkdir_or_exist(osp.join(self.crop_save_path, self.split))
-
- def pack_instance(self, sample: Tuple) -> List:
- """Crop patches from image.
-
- Args:
- samples (Tuple): A tuple of (img_name, text).
-
- Return:
- List: The list of cropped patches.
- """
-
- def get_box(instance: Dict) -> List:
- if 'box' in instance:
- return bbox2poly(instance['box']).tolist()
- if 'poly' in instance:
- return bbox2poly(poly2bbox(instance['poly'])).tolist()
-
- def get_poly(instance: Dict) -> List:
- if 'poly' in instance:
- return instance['poly']
- if 'box' in instance:
- return bbox2poly(instance['box']).tolist()
-
- data_list = []
- img_path, instances = sample
- img = mmcv.imread(img_path)
- for i, instance in enumerate(instances):
- if instance['ignore']:
- continue
- if self.crop_with_warp:
- poly = get_poly(instance)
- patch = warp_img(img, poly, self.jitter, self.jrx, self.jry)
- else:
- box = get_box(instance)
- patch = crop_img(img, box, self.lepr, self.sepr)
- if patch.shape[0] == 0 or patch.shape[1] == 0:
- continue
- text = instance['text']
- patch_name = osp.splitext(
- osp.basename(img_path))[0] + f'_{i}' + osp.splitext(
- osp.basename(img_path))[1]
- dst_path = osp.join(self.crop_save_path, self.split, patch_name)
- mmcv.imwrite(patch, dst_path)
- rec_instance = dict(
- instances=[dict(text=text)],
- img_path=osp.join(self.cropped_img_dir, self.split,
- patch_name))
- data_list.append(rec_instance)
-
- return data_list
-
- def add_meta(self, sample: List) -> Dict:
- # Since the TextRecogCropConverter packs all of the patches in a single
- # image into a list, we need to flatten the list.
- sample = [item for sublist in sample for item in sublist]
- return super().add_meta(sample)
diff --git a/pipeline/mmocr/mmocr/datasets/preparers/packers/textspotting_packer.py b/pipeline/mmocr/mmocr/datasets/preparers/packers/textspotting_packer.py
deleted file mode 100644
index ee5467169a66f727d9052905f8a4c0d1731003fe..0000000000000000000000000000000000000000
--- a/pipeline/mmocr/mmocr/datasets/preparers/packers/textspotting_packer.py
+++ /dev/null
@@ -1,113 +0,0 @@
-# Copyright (c) OpenMMLab. All rights reserved.
-import os.path as osp
-from typing import Dict, List, Tuple
-
-import mmcv
-
-from mmocr.registry import DATA_PACKERS
-from mmocr.utils import bbox2poly, poly2bbox
-from .base import BasePacker
-
-
-@DATA_PACKERS.register_module()
-class TextSpottingPacker(BasePacker):
- """Text spotting packer. It is used to pack the parsed annotation info to:
-
- .. code-block:: python
-
- {
- "metainfo":
- {
- "dataset_type": "TextDetDataset",
- "task_name": "textdet",
- "category": [{"id": 0, "name": "text"}]
- },
- "data_list":
- [
- {
- "img_path": "test_img.jpg",
- "height": 640,
- "width": 640,
- "instances":
- [
- {
- "polygon": [0, 0, 0, 10, 10, 20, 20, 0],
- "bbox": [0, 0, 10, 20],
- "bbox_label": 0,
- "ignore": False,
- "text": "mmocr"
- },
- // ...
- ]
- }
- ]
- }
- """
-
- def pack_instance(self, sample: Tuple, bbox_label: int = 0) -> Dict:
- """Pack the parsed annotation info to an MMOCR format instance.
-
- Args:
- sample (Tuple): A tuple of (img_file, ann_file).
- - img_path (str): Path to image file.
- - instances (Sequence[Dict]): A list of converted annos. Each
- element should be a dict with the following keys:
- - 'poly' or 'box'
- - 'text'
- - 'ignore'
- - 'bbox_label' (optional)
- split (str): The split of the instance.
-
- Returns:
- Dict: An MMOCR format instance.
- """
-
- img_path, instances = sample
-
- img = mmcv.imread(img_path)
- h, w = img.shape[:2]
-
- packed_instances = list()
- for instance in instances:
- assert 'text' in instance, 'Text is not found in the instance.'
- poly = instance.get('poly', None)
- box = instance.get('box', None)
- assert box or poly
- packed_sample = dict(
- polygon=poly if poly else list(
- bbox2poly(box).astype('float64')),
- bbox=box if box else list(poly2bbox(poly).astype('float64')),
- bbox_label=bbox_label,
- ignore=instance['ignore'],
- text=instance['text'])
- packed_instances.append(packed_sample)
-
- packed_instances = dict(
- instances=packed_instances,
- img_path=osp.relpath(img_path, self.data_root),
- height=h,
- width=w)
-
- return packed_instances
-
- def add_meta(self, sample: List) -> Dict:
- """Add meta information to the sample.
-
- Args:
- sample (List): A list of samples of the dataset.
-
- Returns:
- Dict: A dict contains the meta information and samples.
- """
- meta = {
- 'metainfo': {
- 'dataset_type': 'TextSpottingDataset',
- 'task_name': 'textspotting',
- 'category': [{
- 'id': 0,
- 'name': 'text'
- }]
- },
- 'data_list': sample
- }
- return meta
diff --git a/pipeline/mmocr/mmocr/datasets/preparers/packers/wildreceipt_packer.py b/pipeline/mmocr/mmocr/datasets/preparers/packers/wildreceipt_packer.py
deleted file mode 100644
index df13bc66a3dd5c188d3fa093651521955b4e1630..0000000000000000000000000000000000000000
--- a/pipeline/mmocr/mmocr/datasets/preparers/packers/wildreceipt_packer.py
+++ /dev/null
@@ -1,112 +0,0 @@
-# Copyright (c) OpenMMLab. All rights reserved.
-import json
-from typing import List
-
-from mmocr.registry import DATA_PACKERS
-from .base import BasePacker
-
-
-@DATA_PACKERS.register_module()
-class WildReceiptPacker(BasePacker):
- """Pack the wildreceipt annotation to MMOCR format.
-
- Args:
- merge_bg_others (bool): If True, give the same label to "background"
- class and "others" class. Defaults to True.
- ignore_idx (int): Index for ``ignore`` class. Defaults to 0.
- others_idx (int): Index for ``others`` class. Defaults to 25.
- """
-
- def __init__(self,
- merge_bg_others: bool = False,
- ignore_idx: int = 0,
- others_idx: int = 25,
- **kwargs) -> None:
- super().__init__(**kwargs)
-
- self.ignore_idx = ignore_idx
- self.others_idx = others_idx
- self.merge_bg_others = merge_bg_others
-
- def add_meta(self, samples: List) -> List:
- """No meta info is required for the wildreceipt dataset."""
- return samples
-
- def pack_instance(self, sample: str):
- """Pack line-json str of close set to line-json str of open set.
-
- Args:
- sample (str): The string to be deserialized to
- the close set dictionary object.
- split (str): The split of the instance.
- """
- # Two labels at the same index of the following two lists
- # make up a key-value pair. For example, in wildreceipt,
- # closeset_key_inds[0] maps to "Store_name_key"
- # and closeset_value_inds[0] maps to "Store_addr_value".
- closeset_key_inds = list(range(2, self.others_idx, 2))
- closeset_value_inds = list(range(1, self.others_idx, 2))
-
- openset_node_label_mapping = {
- 'bg': 0,
- 'key': 1,
- 'value': 2,
- 'others': 3
- }
- if self.merge_bg_others:
- openset_node_label_mapping['others'] = openset_node_label_mapping[
- 'bg']
-
- closeset_obj = json.loads(sample)
- openset_obj = {
- 'file_name':
- closeset_obj['file_name'].replace(self.data_root + '/', ''),
- 'height':
- closeset_obj['height'],
- 'width':
- closeset_obj['width'],
- 'annotations': []
- }
-
- edge_idx = 1
- label_to_edge = {}
- for anno in closeset_obj['annotations']:
- label = anno['label']
- if label == self.ignore_idx:
- anno['label'] = openset_node_label_mapping['bg']
- anno['edge'] = edge_idx
- edge_idx += 1
- elif label == self.others_idx:
- anno['label'] = openset_node_label_mapping['others']
- anno['edge'] = edge_idx
- edge_idx += 1
- else:
- edge = label_to_edge.get(label, None)
- if edge is not None:
- anno['edge'] = edge
- if label in closeset_key_inds:
- anno['label'] = openset_node_label_mapping['key']
- elif label in closeset_value_inds:
- anno['label'] = openset_node_label_mapping['value']
- else:
- tmp_key = 'key'
- if label in closeset_key_inds:
- label_with_same_edge = closeset_value_inds[
- closeset_key_inds.index(label)]
- elif label in closeset_value_inds:
- label_with_same_edge = closeset_key_inds[
- closeset_value_inds.index(label)]
- tmp_key = 'value'
- edge_counterpart = label_to_edge.get(
- label_with_same_edge, None)
- if edge_counterpart is not None:
- anno['edge'] = edge_counterpart
- else:
- anno['edge'] = edge_idx
- edge_idx += 1
- anno['label'] = openset_node_label_mapping[tmp_key]
- label_to_edge[label] = anno['edge']
-
- openset_obj['annotations'] = closeset_obj['annotations']
-
- return json.dumps(openset_obj, ensure_ascii=False)
diff --git a/pipeline/mmocr/mmocr/datasets/preparers/parsers/__init__.py b/pipeline/mmocr/mmocr/datasets/preparers/parsers/__init__.py
deleted file mode 100644
index fd37947107eba2d2cd54630f5d44360a046d7d32..0000000000000000000000000000000000000000
--- a/pipeline/mmocr/mmocr/datasets/preparers/parsers/__init__.py
+++ /dev/null
@@ -1,22 +0,0 @@
-# Copyright (c) OpenMMLab. All rights reserved.
-from .base import BaseParser
-from .coco_parser import COCOTextDetAnnParser
-from .ctw1500_parser import CTW1500AnnParser
-from .funsd_parser import FUNSDTextDetAnnParser
-from .icdar_txt_parser import (ICDARTxtTextDetAnnParser,
- ICDARTxtTextRecogAnnParser)
-from .mjsynth_parser import MJSynthAnnParser
-from .naf_parser import NAFAnnParser
-from .sroie_parser import SROIETextDetAnnParser
-from .svt_parser import SVTTextDetAnnParser
-from .synthtext_parser import SynthTextAnnParser
-from .totaltext_parser import TotaltextTextDetAnnParser
-from .wildreceipt_parser import WildreceiptKIEAnnParser
-
-__all__ = [
- 'BaseParser', 'ICDARTxtTextDetAnnParser', 'ICDARTxtTextRecogAnnParser',
- 'TotaltextTextDetAnnParser', 'WildreceiptKIEAnnParser',
- 'COCOTextDetAnnParser', 'SVTTextDetAnnParser', 'FUNSDTextDetAnnParser',
- 'SROIETextDetAnnParser', 'NAFAnnParser', 'CTW1500AnnParser',
- 'SynthTextAnnParser', 'MJSynthAnnParser'
-]
diff --git a/pipeline/mmocr/mmocr/datasets/preparers/parsers/base.py b/pipeline/mmocr/mmocr/datasets/preparers/parsers/base.py
deleted file mode 100644
index dfe79e1549320e22ce9a631a6b2fe81d192917e3..0000000000000000000000000000000000000000
--- a/pipeline/mmocr/mmocr/datasets/preparers/parsers/base.py
+++ /dev/null
@@ -1,124 +0,0 @@
-# Copyright (c) OpenMMLab. All rights reserved.
-from abc import abstractmethod
-from typing import Dict, List, Tuple, Union
-
-from mmocr.utils import track_parallel_progress_multi_args
-
-
-class BaseParser:
- """Base class for parsing annotations.
-
- Args:
- split (str): The split of the dataset. It is usually set automatically
- and users do not need to set it manually in config file in most
- cases.
- nproc (int): Number of processes to process the data. Defaults to 1.
- It is usually set automatically and users do not need to set it
- manually in config file in most cases.
- """
-
- def __init__(self, split: str, nproc: int = 1) -> None:
- self.nproc = nproc
- self.split = split
-
- def __call__(self, img_paths: Union[List[str], str],
- ann_paths: Union[List[str], str]) -> List[Tuple]:
- """Parse annotations.
-
- Args:
- img_paths (str or list[str]): the list of image paths or the
- directory of the images.
- ann_paths (str or list[str]): the list of annotation paths or the
- path of the annotation file which contains all the annotations.
-
- Returns:
- List: A list of a tuple of (image_path, instances)
- """
- samples = self.parse_files(img_paths, ann_paths)
- return samples
-
- def parse_files(self, img_paths: Union[List[str], str],
- ann_paths: Union[List[str], str]) -> List[Tuple]:
- """Convert annotations to MMOCR format.
-
- Args:
- img_paths (str or list[str]): the list of image paths or the
- directory of the images.
- ann_paths (str or list[str]): the list of annotation paths or the
- path of the annotation file which contains all the annotations.
-
- Returns:
- List[Tuple]: A list of a tuple of (image_path, instances).
-
- - img_path (str): The path of image file, which can be read
- directly by opencv.
- - instance: instance is a list of dict containing parsed
- annotations, which should contain the following keys:
-
- - 'poly' or 'box' (textdet or textspotting)
- - 'text' (textspotting or textrecog)
- - 'ignore' (all task)
- """
- samples = track_parallel_progress_multi_args(
- self.parse_file, (img_paths, ann_paths), nproc=self.nproc)
- return samples
-
- @abstractmethod
- def parse_file(self, img_path: str, ann_path: str) -> Tuple:
- """Convert annotation for a single image.
-
- Args:
- img_path (str): The path of image.
- ann_path (str): The path of annotation.
-
- Returns:
- Tuple: A tuple of (img_path, instance).
-
- - img_path (str): The path of image file, which can be read
- directly by opencv.
- - instance: instance is a list of dict containing parsed
- annotations, which should contain the following keys:
-
- - 'poly' or 'box' (textdet or textspotting)
- - 'text' (textspotting or textrecog)
- - 'ignore' (all task)
-
- Examples:
- An example of returned values:
- >>> ('imgs/train/xxx.jpg',
- >>> dict(
- >>> poly=[[[0, 1], [1, 1], [1, 0], [0, 0]]],
- >>> text='hello',
- >>> ignore=False)
- >>> )
- """
- raise NotImplementedError
-
- def loader(self,
- file_path: str,
- separator: str = ',',
- format: str = 'x1,y1,x2,y2,x3,y3,x4,y4,trans',
- encoding='utf-8') -> Union[Dict, str]:
- """A basic loader designed for .txt format annotation. It greedily
- extracts information separated by separators.
-
- Args:
- file_path (str): Path to the txt file.
- separator (str, optional): Separator of data. Defaults to ','.
- format (str, optional): Annotation format.
- Defaults to 'x1,y1,x2,y2,x3,y3,x4,y4,trans'.
- encoding (str, optional): Encoding format. Defaults to 'utf-8'.
-
- Yields:
- Iterator[Union[Dict, str]]: Original text line or a dict containing
- the information of the text line.
- """
- keys = format.split(separator)
- with open(file_path, 'r', encoding=encoding) as f:
- for line in f.readlines():
- line = line.strip()
- values = line.split(separator)
- values = values[:len(keys) -
- 1] + [separator.join(values[len(keys) - 1:])]
- if line:
- yield dict(zip(keys, values))
diff --git a/pipeline/mmocr/mmocr/datasets/preparers/parsers/coco_parser.py b/pipeline/mmocr/mmocr/datasets/preparers/parsers/coco_parser.py
deleted file mode 100644
index 0d23bd00e523d3212ea1387bef7b30338adb2e45..0000000000000000000000000000000000000000
--- a/pipeline/mmocr/mmocr/datasets/preparers/parsers/coco_parser.py
+++ /dev/null
@@ -1,99 +0,0 @@
-# Copyright (c) OpenMMLab. All rights reserved.
-import os.path as osp
-from typing import List
-
-from mmdet.datasets.api_wrappers import COCO
-
-from mmocr.datasets.preparers.parsers.base import BaseParser
-from mmocr.registry import DATA_PARSERS
-
-
-@DATA_PARSERS.register_module()
-class COCOTextDetAnnParser(BaseParser):
- """COCO-like Format Text Detection Parser.
-
- Args:
- data_root (str): The root path of the dataset. Defaults to None.
- nproc (int): The number of processes to parse the annotation. Defaults
- to 1.
- variant (str): Variant of COCO dataset, options are ['standard',
- 'cocotext', 'textocr']. Defaults to 'standard'.
- """
-
- def __init__(self,
- split: str,
- nproc: int = 1,
- variant: str = 'standard') -> None:
-
- super().__init__(nproc=nproc, split=split)
- assert variant in ['standard', 'cocotext', 'textocr'], \
- f'variant {variant} is not supported'
- self.variant = variant
-
- def parse_files(self, img_dir: str, ann_path: str) -> List:
- """Parse single annotation."""
- samples = list()
- coco = COCO(ann_path)
- if self.variant == 'cocotext' or self.variant == 'textocr':
- # cocotext stores both 'train' and 'val' split in one annotation
- # file, and uses the 'set' field to distinguish them.
- if self.variant == 'cocotext':
- for img in coco.dataset['imgs']:
- if self.split == coco.dataset['imgs'][img]['set']:
- coco.imgs[img] = coco.dataset['imgs'][img]
- # textocr stores 'train' and 'val'split separately
- elif self.variant == 'textocr':
- coco.imgs = coco.dataset['imgs']
- # both cocotext and textocr stores the annotation ID in the
- # 'imgToAnns' field, so we need to convert it to the 'anns' field
- for img in coco.dataset['imgToAnns']:
- ann_ids = coco.dataset['imgToAnns'][img]
- anns = [
- coco.dataset['anns'][str(ann_id)] for ann_id in ann_ids
- ]
- coco.dataset['imgToAnns'][img] = anns
- coco.imgToAnns = coco.dataset['imgToAnns']
- coco.anns = coco.dataset['anns']
- img_ids = coco.get_img_ids()
- total_ann_ids = []
- for img_id in img_ids:
- img_info = coco.load_imgs([img_id])[0]
- img_info['img_id'] = img_id
- img_path = img_info['file_name']
- ann_ids = coco.get_ann_ids(img_ids=[img_id])
- if len(ann_ids) == 0:
- continue
- ann_ids = [str(ann_id) for ann_id in ann_ids]
- ann_info = coco.load_anns(ann_ids)
- total_ann_ids.extend(ann_ids)
- instances = list()
- for ann in ann_info:
- if self.variant == 'standard':
- # standard coco format use 'segmentation' field to store
- # the polygon and 'iscrowd' field to store the ignore flag,
- # and the 'text' field to store the text content.
- instances.append(
- dict(
- poly=ann['segmentation'][0],
- text=ann.get('text', None),
- ignore=ann.get('iscrowd', False)))
- elif self.variant == 'cocotext':
- # cocotext use 'utf8_string' field to store the text and
- # 'legibility' field to store the ignore flag, and the
- # 'mask' field to store the polygon.
- instances.append(
- dict(
- poly=ann['mask'],
- text=ann.get('utf8_string', None),
- ignore=ann['legibility'] == 'illegible'))
- elif self.variant == 'textocr':
- # textocr use 'utf8_string' field to store the text and
- # the 'points' field to store the polygon, '.' is used to
- # represent the ignored text.
- text = ann.get('utf8_string', None)
- instances.append(
- dict(
- poly=ann['points'], text=text, ignore=text == '.'))
- samples.append((osp.join(img_dir,
- osp.basename(img_path)), instances))
- return samples
diff --git a/pipeline/mmocr/mmocr/datasets/preparers/parsers/ctw1500_parser.py b/pipeline/mmocr/mmocr/datasets/preparers/parsers/ctw1500_parser.py
deleted file mode 100644
index 4c6bdbc59a82c485b6f62142b3cb31ae5874a795..0000000000000000000000000000000000000000
--- a/pipeline/mmocr/mmocr/datasets/preparers/parsers/ctw1500_parser.py
+++ /dev/null
@@ -1,102 +0,0 @@
-# Copyright (c) OpenMMLab. All rights reserved.
-import xml.etree.ElementTree as ET
-from typing import List, Tuple
-
-import numpy as np
-
-from mmocr.datasets.preparers.data_preparer import DATA_PARSERS
-from mmocr.datasets.preparers.parsers.base import BaseParser
-from mmocr.utils import list_from_file
-
-
-@DATA_PARSERS.register_module()
-class CTW1500AnnParser(BaseParser):
- """SCUT-CTW1500 dataset parser.
-
- Args:
- ignore (str): The text of the ignored instances. Defaults to
- '###'.
- """
-
- def __init__(self, ignore: str = '###', **kwargs) -> None:
- self.ignore = ignore
- super().__init__(**kwargs)
-
- def parse_file(self, img_path: str, ann_path: str) -> Tuple:
- """Convert annotation for a single image.
-
- Args:
- img_path (str): The path of image.
- ann_path (str): The path of annotation.
-
- Returns:
- Tuple: A tuple of (img_path, instance).
-
- - img_path (str): The path of image file, which can be read
- directly by opencv.
- - instance: instance is a list of dict containing parsed
- annotations, which should contain the following keys:
-
- - 'poly' or 'box' (textdet or textspotting)
- - 'text' (textspotting or textrecog)
- - 'ignore' (all task)
-
- Examples:
- An example of returned values:
- >>> ('imgs/train/xxx.jpg',
- >>> dict(
- >>> poly=[[[0, 1], [1, 1], [1, 0], [0, 0]]],
- >>> text='hello',
- >>> ignore=False)
- >>> )
- """
-
- if self.split == 'train':
- instances = self.load_xml_info(ann_path)
- elif self.split == 'test':
- instances = self.load_txt_info(ann_path)
- return img_path, instances
-
- def load_txt_info(self, anno_dir: str) -> List:
- """Load the annotation of the SCUT-CTW dataset (test split).
- Args:
- anno_dir (str): Path to the annotation file.
-
- Returns:
- list[Dict]: List of instances.
- """
- instances = list()
- for line in list_from_file(anno_dir):
- # each line has one ploygen (n vetices), and one text.
- # e.g., 695,885,866,888,867,1146,696,1143,####Latin 9
- line = line.strip()
- strs = line.split(',')
- assert strs[28][0] == '#'
- xy = [int(x) for x in strs[0:28]]
- assert len(xy) == 28
- poly = np.array(xy).reshape(-1).tolist()
- text = strs[28][4:]
- instances.append(
- dict(poly=poly, text=text, ignore=text == self.ignore))
- return instances
-
- def load_xml_info(self, anno_dir: str) -> List:
- """Load the annotation of the SCUT-CTW dataset (train split).
- Args:
- anno_dir (str): Path to the annotation file.
-
- Returns:
- list[Dict]: List of instances.
- """
- obj = ET.parse(anno_dir)
- instances = list()
- for image in obj.getroot(): # image
- for box in image: # image
- text = box[0].text
- segs = box[1].text
- pts = segs.strip().split(',')
- pts = [int(x) for x in pts]
- assert len(pts) == 28
- poly = np.array(pts).reshape(-1).tolist()
- instances.append(dict(poly=poly, text=text, ignore=0))
- return instances
diff --git a/pipeline/mmocr/mmocr/datasets/preparers/parsers/funsd_parser.py b/pipeline/mmocr/mmocr/datasets/preparers/parsers/funsd_parser.py
deleted file mode 100644
index bf6d2cd5f636b0c12ae0d4fc1744b128b302528f..0000000000000000000000000000000000000000
--- a/pipeline/mmocr/mmocr/datasets/preparers/parsers/funsd_parser.py
+++ /dev/null
@@ -1,36 +0,0 @@
-# Copyright (c) OpenMMLab. All rights reserved.
-import json
-from typing import Tuple
-
-from mmocr.registry import DATA_PARSERS
-from mmocr.utils import bbox2poly
-from .base import BaseParser
-
-
-@DATA_PARSERS.register_module()
-class FUNSDTextDetAnnParser(BaseParser):
- """FUNSD Text Detection Annotation Parser. See
- dataset_zoo/funsd/sample_anno.md for annotation example.
-
- Args:
- nproc (int): The number of processes to parse the annotation. Defaults
- to 1.
- """
-
- def parse_file(self, img_path: str, ann_path: str) -> Tuple:
- """Parse single annotation."""
- instances = list()
- for poly, text, ignore in self.loader(ann_path):
- instances.append(dict(poly=poly, text=text, ignore=ignore))
-
- return img_path, instances
-
- def loader(self, file_path: str):
- with open(file_path, 'r') as f:
- data = json.load(f)
- for form in data['form']:
- for word in form['words']:
- poly = bbox2poly(word['box']).tolist()
- text = word['text']
- ignore = len(text) == 0
- yield poly, text, ignore
diff --git a/pipeline/mmocr/mmocr/datasets/preparers/parsers/icdar_txt_parser.py b/pipeline/mmocr/mmocr/datasets/preparers/parsers/icdar_txt_parser.py
deleted file mode 100644
index e90d5d7b94a2345fbe803d254428326215de4fea..0000000000000000000000000000000000000000
--- a/pipeline/mmocr/mmocr/datasets/preparers/parsers/icdar_txt_parser.py
+++ /dev/null
@@ -1,127 +0,0 @@
-# Copyright (c) OpenMMLab. All rights reserved.
-import os.path as osp
-from typing import List, Optional, Tuple
-
-from mmocr.registry import DATA_PARSERS
-from mmocr.utils import bbox2poly
-from .base import BaseParser
-
-
-@DATA_PARSERS.register_module()
-class ICDARTxtTextDetAnnParser(BaseParser):
- """ICDAR Txt Format Text Detection Annotation Parser.
-
- The original annotation format of this dataset is stored in txt files,
- which is formed as the following format:
- x1, y1, x2, y2, x3, y3, x4, y4, transcription
-
- Args:
- separator (str): The separator between each element in a line. Defaults
- to ','.
- ignore (str): The text to be ignored. Defaults to '###'.
- format (str): The format of the annotation. Defaults to
- 'x1,y1,x2,y2,x3,y3,x4,trans'.
- encoding (str): The encoding of the annotation file. Defaults to
- 'utf-8-sig'.
- nproc (int): The number of processes to parse the annotation. Defaults
- to 1.
- remove_strs (List[str], Optional): Used to remove redundant strings in
- the transcription. Defaults to None.
- mode (str, optional): The mode of the box converter. Supported modes
- are 'xywh' and 'xyxy'. Defaults to None.
- """
-
- def __init__(self,
- separator: str = ',',
- ignore: str = '###',
- format: str = 'x1,y1,x2,y2,x3,y3,x4,y4,trans',
- encoding: str = 'utf-8',
- remove_strs: Optional[List[str]] = None,
- mode: str = None,
- **kwargs) -> None:
- self.sep = separator
- self.format = format
- self.encoding = encoding
- self.ignore = ignore
- self.mode = mode
- self.remove_strs = remove_strs
- super().__init__(**kwargs)
-
- def parse_file(self, img_path: str, ann_path: str) -> Tuple:
- """Parse single annotation."""
- instances = list()
- for anno in self.loader(ann_path, self.sep, self.format,
- self.encoding):
- anno = list(anno.values())
- if self.remove_strs is not None:
- for strs in self.remove_strs:
- for i in range(len(anno)):
- if strs in anno[i]:
- anno[i] = anno[i].replace(strs, '')
- poly = list(map(float, anno[0:-1]))
- if self.mode is not None:
- poly = bbox2poly(poly, self.mode)
- poly = poly.tolist()
- text = anno[-1]
- instances.append(
- dict(poly=poly, text=text, ignore=text == self.ignore))
-
- return img_path, instances
-
-
-@DATA_PARSERS.register_module()
-class ICDARTxtTextRecogAnnParser(BaseParser):
- """ICDAR Txt Format Text Recognition Annotation Parser.
-
- The original annotation format of this dataset is stored in txt files,
- which is formed as the following format:
- img_path, transcription
-
- Args:
- separator (str): The separator between each element in a line. Defaults
- to ','.
- ignore (str): The text to be ignored. Defaults to '#'.
- format (str): The format of the annotation. Defaults to 'img, text'.
- encoding (str): The encoding of the annotation file. Defaults to
- 'utf-8-sig'.
- nproc (int): The number of processes to parse the annotation. Defaults
- to 1.
- base_name (bool): Whether to use the basename of the image path as the
- image name. Defaults to False.
- remove_strs (List[str], Optional): Used to remove redundant strings in
- the transcription. Defaults to ['"'].
- """
-
- def __init__(self,
- separator: str = ',',
- ignore: str = '#',
- format: str = 'img,text',
- encoding: str = 'utf-8',
- remove_strs: Optional[List[str]] = ['"'],
- **kwargs) -> None:
- self.sep = separator
- self.format = format
- self.encoding = encoding
- self.ignore = ignore
- self.remove_strs = remove_strs
- super().__init__(**kwargs)
-
- def parse_files(self, img_dir: str, ann_path: str) -> List:
- """Parse annotations."""
- assert isinstance(ann_path, str)
- samples = list()
- for anno in self.loader(
- file_path=ann_path,
- format=self.format,
- encoding=self.encoding,
- separator=self.sep):
- text = anno['text'].strip()
- if self.remove_strs is not None:
- for strs in self.remove_strs:
- text = text.replace(strs, '')
- if text == self.ignore:
- continue
- img_name = anno['img']
- samples.append((osp.join(img_dir, img_name), text))
-
- return samples
diff --git a/pipeline/mmocr/mmocr/datasets/preparers/parsers/mjsynth_parser.py b/pipeline/mmocr/mmocr/datasets/preparers/parsers/mjsynth_parser.py
deleted file mode 100644
index 3eee6e29a373bfb9689de1845f7a22587750816c..0000000000000000000000000000000000000000
--- a/pipeline/mmocr/mmocr/datasets/preparers/parsers/mjsynth_parser.py
+++ /dev/null
@@ -1,50 +0,0 @@
-# Copyright (c) OpenMMLab. All rights reserved.
-import os.path as osp
-from typing import List
-
-from mmocr.registry import DATA_PARSERS
-from .icdar_txt_parser import ICDARTxtTextRecogAnnParser
-
-
-@DATA_PARSERS.register_module()
-class MJSynthAnnParser(ICDARTxtTextRecogAnnParser):
- """MJSynth Text Recognition Annotation Parser.
-
- The original annotation format of this dataset is stored in txt files,
- which is formed as the following format:
- img_path, transcription
-
- Args:
- separator (str): The separator between each element in a line. Defaults
- to ','.
- ignore (str): The text to be ignored. Defaults to '#'.
- format (str): The format of the annotation. Defaults to 'img, text'.
- encoding (str): The encoding of the annotation file. Defaults to
- 'utf-8-sig'.
- nproc (int): The number of processes to parse the annotation. Defaults
- to 1.
- base_name (bool): Whether to use the basename of the image path as the
- image name. Defaults to False.
- remove_strs (List[str], Optional): Used to remove redundant strings in
- the transcription. Defaults to ['"'].
- """
-
- def parse_files(self, img_dir: str, ann_path: str) -> List:
- """Parse annotations."""
- assert isinstance(ann_path, str)
- samples = list()
- for anno in self.loader(
- file_path=ann_path,
- format=self.format,
- encoding=self.encoding,
- separator=self.sep):
- text = osp.basename(anno['img']).split('_')[1]
- if self.remove_strs is not None:
- for strs in self.remove_strs:
- text = text.replace(strs, '')
- if text == self.ignore:
- continue
- img_name = anno['img']
- samples.append((osp.join(img_dir, img_name), text))
-
- return samples
diff --git a/pipeline/mmocr/mmocr/datasets/preparers/parsers/naf_parser.py b/pipeline/mmocr/mmocr/datasets/preparers/parsers/naf_parser.py
deleted file mode 100644
index 988b4b453b1aba44dca342a4be1f0258f583ca08..0000000000000000000000000000000000000000
--- a/pipeline/mmocr/mmocr/datasets/preparers/parsers/naf_parser.py
+++ /dev/null
@@ -1,106 +0,0 @@
-# Copyright (c) OpenMMLab. All rights reserved.
-import json
-from typing import List, Tuple
-
-import numpy as np
-
-from mmocr.registry import DATA_PARSERS
-from .base import BaseParser
-
-
-@DATA_PARSERS.register_module()
-class NAFAnnParser(BaseParser):
- """NAF dataset parser.
-
- The original annotation format of this dataset is stored in json files,
- which has the following keys that will be used here:
- - 'textBBs': List of text bounding box objects
- - 'poly_points': list of [x,y] pairs, the box corners going
- top-left,top-right,bottom-right,bottom-left
- - 'id': id of the textBB, used to match with the text
- - 'transcriptions': Dict of transcription objects, use the 'id' key
- to match with the textBB.
-
- Some special characters are used in the transcription:
- "ยซtextยป" indicates that "text" had a strikethrough
- "ยฟ" indicates the transcriber could not read a character
- "ยง" indicates the whole line or word was illegible
- "" (empty string) is if the field was blank
-
- Args:
- ignore (list(str)): The text of the ignored instances. Default: ['#'].
- det (bool): Whether to parse the detection annotation. Default: True.
- If False, the parser will consider special case in NAF dataset
- where the transcription is not available.
- """
-
- def __init__(self,
- ignore: List[str] = ['#'],
- det: bool = True,
- **kwargs) -> None:
- self.ignore = ignore
- self.det = det
- super().__init__(**kwargs)
-
- def parse_file(self, img_path: str, ann_path: str) -> Tuple:
- """Convert single annotation."""
- instances = list()
- for poly, text in self.loader(ann_path):
- instances.append(
- dict(poly=poly, text=text, ignore=text in self.ignore))
-
- return img_path, instances
-
- def loader(self, file_path: str) -> str:
- """Load the annotation of the NAF dataset.
-
- Args:
- file_path (str): Path to the json file
-
- Retyrb:
- str: Complete annotation of the json file
- """
- with open(file_path, 'r') as f:
- data = json.load(f)
-
- # 'textBBs' contains the printed texts of the table while 'fieldBBs'
- # contains the text filled by human.
- for box_type in ['textBBs', 'fieldBBs']:
- if not self.det:
- # 'textBBs' is only used for detection task.
- if box_type == 'textBBs':
- continue
- for anno in data[box_type]:
- # Skip blanks
- if self.det:
- if box_type == 'fieldBBs':
- if anno['type'] == 'blank':
- continue
- poly = np.array(anno['poly_points']).reshape(
- 1, 8)[0].tolist()
- # Since detection task only need poly, we can skip the
- # transcription part that can be empty.
- text = None
- else:
- # For tasks that need transcription, NAF dataset has
- # serval special cases:
- # 1. The transcription for the whole image is not
- # available.
- # 2. The transcription for the certain text is not
- # available.
- # 3. If the length of the transcription is 0, it should
- # be ignored.
- if 'transcriptions' not in data.keys():
- break
- if anno['id'] not in data['transcriptions'].keys():
- continue
- text = data['transcriptions'][anno['id']]
- text = text.strip(
- '\u202a') # Remove unicode control character
- text = text.replace('ยป', '').replace(
- 'ยซ', '') # Remove strikethrough flag
- if len(text) == 0:
- continue
- poly = np.array(anno['poly_points']).reshape(
- 1, 8)[0].tolist()
- yield poly, text
diff --git a/pipeline/mmocr/mmocr/datasets/preparers/parsers/sroie_parser.py b/pipeline/mmocr/mmocr/datasets/preparers/parsers/sroie_parser.py
deleted file mode 100644
index f89793e8c4aeed43d1cf462e8041cf38c8b08af3..0000000000000000000000000000000000000000
--- a/pipeline/mmocr/mmocr/datasets/preparers/parsers/sroie_parser.py
+++ /dev/null
@@ -1,74 +0,0 @@
-# Copyright (c) OpenMMLab. All rights reserved.
-from typing import List, Optional, Tuple
-
-from mmocr.registry import DATA_PARSERS
-from mmocr.utils import bbox2poly
-from .base import BaseParser
-
-
-@DATA_PARSERS.register_module()
-class SROIETextDetAnnParser(BaseParser):
- """SROIE Txt Format Text Detection Annotation Parser.
-
- The original annotation format of this dataset is stored in txt files,
- which is formed as the following format:
- x1, y1, x2, y2, x3, y3, x4, y4, transcription
-
- Args:
- separator (str): The separator between each element in a line. Defaults
- to ','.
- ignore (str): The text to be ignored. Defaults to '###'.
- format (str): The format of the annotation. Defaults to
- 'x1,y1,x2,y2,x3,y3,x4,trans'.
- encoding (str): The encoding of the annotation file. Defaults to
- 'utf-8-sig'.
- nproc (int): The number of processes to parse the annotation. Defaults
- to 1.
- remove_strs (List[str], Optional): Used to remove redundant strings in
- the transcription. Defaults to None.
- mode (str, optional): The mode of the box converter. Supported modes
- are 'xywh' and 'xyxy'. Defaults to None.
- """
-
- def __init__(self,
- split: str,
- separator: str = ',',
- ignore: str = '###',
- format: str = 'x1,y1,x2,y2,x3,y3,x4,y4,trans',
- encoding: str = 'utf-8-sig',
- nproc: int = 1,
- remove_strs: Optional[List[str]] = None,
- mode: str = None) -> None:
- self.sep = separator
- self.format = format
- self.encoding = encoding
- self.ignore = ignore
- self.mode = mode
- self.remove_strs = remove_strs
- super().__init__(nproc=nproc, split=split)
-
- def parse_file(self, img_path: str, ann_path: str) -> Tuple:
- """Parse single annotation."""
- instances = list()
- try:
- # there might be some illegal symbols in the annotation
- # which cannot be parsed by loader
- for anno in self.loader(ann_path, self.sep, self.format,
- self.encoding):
- anno = list(anno.values())
- if self.remove_strs is not None:
- for strs in self.remove_strs:
- for i in range(len(anno)):
- if strs in anno[i]:
- anno[i] = anno[i].replace(strs, '')
- poly = list(map(float, anno[0:-1]))
- if self.mode is not None:
- poly = bbox2poly(poly, self.mode)
- poly = poly.tolist()
- text = anno[-1]
- instances.append(
- dict(poly=poly, text=text, ignore=text == self.ignore))
- except Exception:
- pass
-
- return img_path, instances
diff --git a/pipeline/mmocr/mmocr/datasets/preparers/parsers/svt_parser.py b/pipeline/mmocr/mmocr/datasets/preparers/parsers/svt_parser.py
deleted file mode 100644
index 553f46fb0f83c6b0b8d65479de6c2f6d597c64a3..0000000000000000000000000000000000000000
--- a/pipeline/mmocr/mmocr/datasets/preparers/parsers/svt_parser.py
+++ /dev/null
@@ -1,62 +0,0 @@
-# Copyright (c) OpenMMLab. All rights reserved.
-import os.path as osp
-import xml.etree.ElementTree as ET
-from typing import List, Tuple
-
-from mmocr.registry import DATA_PARSERS
-from .base import BaseParser
-
-
-@DATA_PARSERS.register_module()
-class SVTTextDetAnnParser(BaseParser):
- """SVT Text Detection Parser.
-
- Args:
- data_root (str): The root of the dataset. Defaults to None.
- nproc (int): The number of processes to parse the annotation. Defaults
- to 1.
- """
-
- def parse_files(self, img_dir: str, ann_path: str) -> List:
- """Parse annotations."""
- assert isinstance(ann_path, str)
- samples = list()
- for img_name, instance in self.loader(ann_path):
- samples.append((osp.join(img_dir,
- osp.basename(img_name)), instance))
-
- return samples
-
- def loader(self, file_path: str) -> Tuple[str, List]:
- """Load annotation from SVT xml format file. See annotation example in
- dataset_zoo/svt/sample_anno.md.
-
- Args:
- file_path (str): The path of the annotation file.
-
- Returns:
- Tuple[str, List]: The image name and the annotation list.
-
- Yields:
- Iterator[Tuple[str, List]]: The image name and the annotation list.
- """
- tree = ET.parse(file_path)
- root = tree.getroot()
- for image in root.findall('image'):
- image_name = image.find('imageName').text
- instances = list()
- for rectangle in image.find('taggedRectangles'):
- x = int(rectangle.get('x'))
- y = int(rectangle.get('y'))
- w = int(rectangle.get('width'))
- h = int(rectangle.get('height'))
- # The text annotation of this dataset is not case sensitive.
- # All of the texts were labeled as upper case. We convert them
- # to lower case for convenience.
- text = rectangle.find('tag').text.lower()
- instances.append(
- dict(
- poly=[x, y, x + w, y, x + w, y + h, x, y + h],
- text=text,
- ignore=False))
- yield image_name, instances
diff --git a/pipeline/mmocr/mmocr/datasets/preparers/parsers/synthtext_parser.py b/pipeline/mmocr/mmocr/datasets/preparers/parsers/synthtext_parser.py
deleted file mode 100644
index 0764e0d8f1f5b00bdc7d2c8210b24d8bb2b87a53..0000000000000000000000000000000000000000
--- a/pipeline/mmocr/mmocr/datasets/preparers/parsers/synthtext_parser.py
+++ /dev/null
@@ -1,172 +0,0 @@
-# Copyright (c) OpenMMLab. All rights reserved.
-import os.path as osp
-from typing import List, Optional, Tuple, Union
-
-import numpy as np
-from mmengine import track_parallel_progress
-from scipy.io import loadmat
-
-from mmocr.utils import is_type_list
-from ..data_preparer import DATA_PARSERS
-from .base import BaseParser
-
-
-@DATA_PARSERS.register_module()
-class SynthTextAnnParser(BaseParser):
- """SynthText Text Detection Annotation Parser.
-
- Args:
- split (str): The split of the dataset. It is usually set automatically
- and users do not need to set it manually in config file in most
- cases.
- nproc (int): Number of processes to process the data. Defaults to 1.
- It is usually set automatically and users do not need to set it
- manually in config file in most cases.
- separator (str): The separator between each element in a line. Defaults
- to ','.
- ignore (str): The text to be ignored. Defaults to '###'.
- format (str): The format of the annotation. Defaults to
- 'x1,y1,x2,y2,x3,y3,x4,trans'.
- encoding (str): The encoding of the annotation file. Defaults to
- 'utf-8-sig'.
- remove_strs (List[str], Optional): Used to remove redundant strings in
- the transcription. Defaults to None.
- mode (str, optional): The mode of the box converter. Supported modes
- are 'xywh' and 'xyxy'. Defaults to None.
- """
-
- def __init__(self,
- split: str,
- nproc: int,
- separator: str = ',',
- ignore: str = '###',
- format: str = 'x1,y1,x2,y2,x3,y3,x4,y4,trans',
- encoding: str = 'utf-8',
- remove_strs: Optional[List[str]] = None,
- mode: str = None) -> None:
- self.sep = separator
- self.format = format
- self.encoding = encoding
- self.ignore = ignore
- self.mode = mode
- self.remove_strs = remove_strs
- super().__init__(split=split, nproc=nproc)
-
- def _trace_boundary(self, char_boxes: List[np.ndarray]) -> np.ndarray:
- """Trace the boundary point of text.
-
- Args:
- char_boxes (list[ndarray]): The char boxes for one text. Each
- element is 4x2 ndarray.
-
- Returns:
- ndarray: The boundary point sets with size nx2.
- """
- assert is_type_list(char_boxes, np.ndarray)
-
- # from top left to to right
- p_top = [box[0:2] for box in char_boxes]
- # from bottom right to bottom left
- p_bottom = [
- char_boxes[idx][[2, 3], :]
- for idx in range(len(char_boxes) - 1, -1, -1)
- ]
-
- p = p_top + p_bottom
-
- boundary = np.concatenate(p).astype(int)
-
- return boundary
-
- def _match_bbox_char_str(self, bboxes: np.ndarray, char_bboxes: np.ndarray,
- strs: np.ndarray
- ) -> Tuple[List[np.ndarray], List[str]]:
- """Match the bboxes, char bboxes, and strs.
-
- Args:
- bboxes (ndarray): The text boxes of size (2, 4, num_box).
- char_bboxes (ndarray): The char boxes of size (2, 4, num_char_box).
- strs (ndarray): The string of size (num_strs,)
-
- Returns:
- Tuple(List[ndarray], List[str]): Polygon & word list.
- """
- assert isinstance(bboxes, np.ndarray)
- assert isinstance(char_bboxes, np.ndarray)
- assert isinstance(strs, np.ndarray)
- # bboxes = bboxes.astype(np.int32)
- char_bboxes = char_bboxes.astype(np.int32)
-
- if len(char_bboxes.shape) == 2:
- char_bboxes = np.expand_dims(char_bboxes, axis=2)
- char_bboxes = np.transpose(char_bboxes, (2, 1, 0))
- num_boxes = 1 if len(bboxes.shape) == 2 else bboxes.shape[-1]
-
- poly_charbox_list = [[] for _ in range(num_boxes)]
-
- words = []
- for line in strs:
- words += line.split()
- words_len = [len(w) for w in words]
- words_end_inx = np.cumsum(words_len)
- start_inx = 0
- for word_inx, end_inx in enumerate(words_end_inx):
- for char_inx in range(start_inx, end_inx):
- poly_charbox_list[word_inx].append(char_bboxes[char_inx])
- start_inx = end_inx
-
- for box_inx in range(num_boxes):
- assert len(poly_charbox_list[box_inx]) > 0
-
- poly_boundary_list = []
- for item in poly_charbox_list:
- boundary = np.ndarray((0, 2))
- if len(item) > 0:
- boundary = self._trace_boundary(item)
- poly_boundary_list.append(boundary)
-
- return poly_boundary_list, words
-
- def parse_files(self, img_paths: Union[List[str], str],
- ann_paths: Union[List[str], str]) -> List[Tuple]:
- """Convert annotations to MMOCR format.
-
- Args:
- img_paths (str or list[str]): the list of image paths or the
- directory of the images.
- ann_paths (str or list[str]): the list of annotation paths or the
- path of the annotation file which contains all the annotations.
-
- Returns:
- List[Tuple]: A list of a tuple of (image_path, instances).
-
- - img_path (str): The path of image file, which can be read
- directly by opencv.
- - instance: instance is a list of dict containing parsed
- annotations, which should contain the following keys:
-
- - 'poly' or 'box' (textdet or textspotting)
- - 'text' (textspotting or textrecog)
- - 'ignore' (all task)
- """
- assert isinstance(ann_paths, str)
- gt = loadmat(ann_paths)
- self.img_dir = img_paths
- samples = track_parallel_progress(
- self.parse_file,
- list(
- zip(gt['imnames'][0], gt['wordBB'][0], gt['charBB'][0],
- gt['txt'][0])),
- nproc=self.nproc)
- return samples
-
- def parse_file(self, annotation: Tuple) -> Tuple:
- """Parse single annotation."""
- img_file, wordBB, charBB, txt = annotation
- polys_list, word_list = self._match_bbox_char_str(wordBB, charBB, txt)
-
- instances = list()
- for poly, word in zip(polys_list, word_list):
- instances.append(
- dict(poly=poly.flatten().tolist(), text=word, ignore=False))
- return osp.join(self.img_dir, img_file[0]), instances
diff --git a/pipeline/mmocr/mmocr/datasets/preparers/parsers/totaltext_parser.py b/pipeline/mmocr/mmocr/datasets/preparers/parsers/totaltext_parser.py
deleted file mode 100644
index 2255f2f1b1abb01601dde8c33af8cf4732340938..0000000000000000000000000000000000000000
--- a/pipeline/mmocr/mmocr/datasets/preparers/parsers/totaltext_parser.py
+++ /dev/null
@@ -1,99 +0,0 @@
-# Copyright (c) OpenMMLab. All rights reserved.
-import re
-from typing import Dict, Tuple
-
-import yaml
-
-from mmocr.registry import DATA_PARSERS
-from .base import BaseParser
-
-
-@DATA_PARSERS.register_module()
-class TotaltextTextDetAnnParser(BaseParser):
- """TotalText Text Detection Parser.
-
- The original annotation format of this dataset is stored in txt files,
- which is formed as the following format:
- x: [[x1 x2 x3 ... xn]], y: [[y1 y2 y3 ... yn]],
- ornt: [u'c'], transcriptions: [u'transcription']
-
- Args:
- data_root (str): Path to the dataset root.
- ignore (str): The text of the ignored instances. Default: '#'.
- nproc (int): Number of processes to load the data. Default: 1.
- """
-
- def __init__(self, ignore: str = '#', **kwargs) -> None:
- self.ignore = ignore
- super().__init__(**kwargs)
-
- def parse_file(self, img_path: str, ann_path: str) -> Dict:
- """Convert single annotation."""
- instances = list()
- for poly, text in self.loader(ann_path):
- instances.append(
- dict(poly=poly, text=text, ignore=text == self.ignore))
-
- return img_path, instances
-
- def loader(self, file_path: str) -> str:
- """The annotation of the totaltext dataset may be stored in multiple
- lines, this loader is designed for this special case.
-
- Args:
- file_path (str): Path to the txt file
-
- Yield:
- str: Complete annotation of the txt file
- """
-
- def parsing_line(line: str) -> Tuple:
- """Parsing a line of the annotation.
-
- Args:
- line (str): A line of the annotation.
-
- Returns:
- Tuple: A tuple of (polygon, transcription).
- """
- line = '{' + line.replace('[[', '[').replace(']]', ']') + '}'
- ann_dict = re.sub('([0-9]) +([0-9])', r'\1,\2', line)
- ann_dict = re.sub('([0-9]) +([ 0-9])', r'\1,\2', ann_dict)
- ann_dict = re.sub('([0-9]) -([0-9])', r'\1,-\2', ann_dict)
- ann_dict = ann_dict.replace("[u',']", "[u'#']")
- ann_dict = yaml.safe_load(ann_dict)
-
- # polygon
- xs, ys = ann_dict['x'], ann_dict['y']
- poly = []
- for x, y in zip(xs, ys):
- poly.append(x)
- poly.append(y)
- # text
- text = ann_dict['transcriptions']
- if len(text) == 0:
- text = '#'
- else:
- word = text[0]
- if len(text) > 1:
- for ann_word in text[1:]:
- word += ',' + ann_word
- text = str(eval(word))
-
- return poly, text
-
- with open(file_path, 'r') as f:
- for idx, line in enumerate(f):
- line = line.strip()
- if idx == 0:
- tmp_line = line
- continue
- if not line.startswith('x:'):
- tmp_line += ' ' + line
- continue
- complete_line = tmp_line
- tmp_line = line
- yield parsing_line(complete_line)
-
- if tmp_line != '':
- yield parsing_line(tmp_line)
diff --git a/pipeline/mmocr/mmocr/datasets/preparers/parsers/wildreceipt_parser.py b/pipeline/mmocr/mmocr/datasets/preparers/parsers/wildreceipt_parser.py
deleted file mode 100644
index 22a131888d06db41d095c27b0ab1fe434957188b..0000000000000000000000000000000000000000
--- a/pipeline/mmocr/mmocr/datasets/preparers/parsers/wildreceipt_parser.py
+++ /dev/null
@@ -1,91 +0,0 @@
-# Copyright (c) OpenMMLab. All rights reserved.
-import json
-import os.path as osp
-from typing import Dict
-
-from mmocr.registry import DATA_PARSERS
-from mmocr.utils import list_from_file
-from .base import BaseParser
-
-
-@DATA_PARSERS.register_module()
-class WildreceiptTextDetAnnParser(BaseParser):
- """Wildreceipt Text Detection Parser.
-
- The original annotation format of this dataset is stored in txt files,
- which is formed as the following json line format:
- {"file_name": "xxx/xxx/xx/xxxx.jpeg",
- "height": 1200,
- "width": 1600,
- "annotations": [
- "box": [x1, y1, x2, y2, x3, y3, x4, y4],
- "text": "xxx",
- "label": 25,
- ]}
-
- Args:
- data_root (str): The root path of the dataset.
- ignore (int): The label to be ignored. Defaults to 0.
- nproc (int): The number of processes to parse the annotation. Defaults
- to 1.
- """
-
- def __init__(self, ignore: int = 0, **kwargs) -> None:
- self.ignore = ignore
- super().__init__(**kwargs)
-
- def parse_files(self, img_dir: str, ann_path) -> Dict:
- """Convert single annotation."""
- closeset_lines = list_from_file(ann_path)
- samples = list()
- for line in closeset_lines:
- instances = list()
- line = json.loads(line)
- img_file = osp.join(img_dir, osp.basename(line['file_name']))
- for anno in line['annotations']:
- poly = anno['box']
- text = anno['text']
- label = anno['label']
- instances.append(
- dict(poly=poly, text=text, ignore=label == self.ignore))
- samples.append((img_file, instances))
-
- return samples
-
-
-@DATA_PARSERS.register_module()
-class WildreceiptKIEAnnParser(BaseParser):
- """Wildreceipt KIE Parser.
-
- The original annotation format of this dataset is stored in txt files,
- which is formed as the following json line format:
- {"file_name": "xxx/xxx/xx/xxxx.jpeg",
- "height": 1200,
- "width": 1600,
- "annotations": [
- "box": [x1, y1, x2, y2, x3, y3, x4, y4],
- "text": "xxx",
- "label": 25,
- ]}
-
- Args:
- ignore (int): The label to be ignored. Defaults to 0.
- nproc (int): The number of processes to parse the annotation. Defaults
- to 1.
- """
-
- def __init__(self, ignore: int = 0, **kwargs) -> None:
- self.ignore = ignore
- super().__init__(**kwargs)
-
- def parse_files(self, img_dir: str, ann_path: str) -> Dict:
- """Convert single annotation."""
- closeset_lines = list_from_file(ann_path)
- samples = list()
- for line in closeset_lines:
- json_line = json.loads(line)
- img_file = osp.join(img_dir, osp.basename(json_line['file_name']))
- json_line['file_name'] = img_file
- samples.append(json.dumps(json_line))
-
- return samples
diff --git a/pipeline/mmocr/mmocr/datasets/recog_lmdb_dataset.py b/pipeline/mmocr/mmocr/datasets/recog_lmdb_dataset.py
deleted file mode 100644
index 88512c62a7674cc61804e6b420d38a2173a5af51..0000000000000000000000000000000000000000
--- a/pipeline/mmocr/mmocr/datasets/recog_lmdb_dataset.py
+++ /dev/null
@@ -1,179 +0,0 @@
-# Copyright (c) OpenMMLab. All rights reserved.
-from typing import Any, Callable, List, Optional, Sequence, Tuple, Union
-
-import mmcv
-from mmengine.dataset import BaseDataset
-
-from mmocr.registry import DATASETS
-
-
-@DATASETS.register_module()
-class RecogLMDBDataset(BaseDataset):
- r"""RecogLMDBDataset for text recognition.
-
- The annotation format should be in lmdb format. The lmdb file should
- contain three keys: 'num-samples', 'label-xxxxxxxxx' and 'image-xxxxxxxxx',
- where 'xxxxxxxxx' is the index of the image. The value of 'num-samples' is
- the total number of images. The value of 'label-xxxxxxx' is the text label
- of the image, and the value of 'image-xxxxxxx' is the image data.
-
- following keys:
- Each item fetched from this dataset will be a dict containing the
- following keys:
-
- - img (ndarray): The loaded image.
- - img_path (str): The image key.
- - instances (list[dict]): The list of annotations for the image.
-
- Args:
- ann_file (str): Annotation file path. Defaults to ''.
- img_color_type (str): The flag argument for :func:``mmcv.imfrombytes``,
- which determines how the image bytes will be parsed. Defaults to
- 'color'.
- metainfo (dict, optional): Meta information for dataset, such as class
- information. Defaults to None.
- data_root (str): The root directory for ``data_prefix`` and
- ``ann_file``. Defaults to ''.
- data_prefix (dict): Prefix for training data. Defaults to
- ``dict(img_path='')``.
- filter_cfg (dict, optional): Config for filter data. Defaults to None.
- indices (int or Sequence[int], optional): Support using first few
- data in annotation file to facilitate training/testing on a smaller
- dataset. Defaults to None which means using all ``data_infos``.
- serialize_data (bool, optional): Whether to hold memory using
- serialized objects, when enabled, data loader workers can use
- shared RAM from master process instead of making a copy. Defaults
- to True.
- pipeline (list, optional): Processing pipeline. Defaults to [].
- test_mode (bool, optional): ``test_mode=True`` means in test phase.
- Defaults to False.
- lazy_init (bool, optional): Whether to load annotation during
- instantiation. In some cases, such as visualization, only the meta
- information of the dataset is needed, which is not necessary to
- load annotation file. ``RecogLMDBDataset`` can skip load
- annotations to save time by set ``lazy_init=False``.
- Defaults to False.
- max_refetch (int, optional): If ``RecogLMDBdataset.prepare_data`` get a
- None img. The maximum extra number of cycles to get a valid
- image. Defaults to 1000.
- """
-
- def __init__(
- self,
- ann_file: str = '',
- img_color_type: str = 'color',
- metainfo: Optional[dict] = None,
- data_root: Optional[str] = '',
- data_prefix: dict = dict(img_path=''),
- filter_cfg: Optional[dict] = None,
- indices: Optional[Union[int, Sequence[int]]] = None,
- serialize_data: bool = True,
- pipeline: List[Union[dict, Callable]] = [],
- test_mode: bool = False,
- lazy_init: bool = False,
- max_refetch: int = 1000,
- ) -> None:
-
- super().__init__(
- ann_file=ann_file,
- metainfo=metainfo,
- data_root=data_root,
- data_prefix=data_prefix,
- filter_cfg=filter_cfg,
- indices=indices,
- serialize_data=serialize_data,
- pipeline=pipeline,
- test_mode=test_mode,
- lazy_init=lazy_init,
- max_refetch=max_refetch)
-
- self.color_type = img_color_type
-
- def load_data_list(self) -> List[dict]:
- """Load annotations from an annotation file named as ``self.ann_file``
-
- Returns:
- List[dict]: A list of annotation.
- """
- if not hasattr(self, 'env'):
- self._make_env()
- with self.env.begin(write=False) as txn:
- self.total_number = int(
- txn.get(b'num-samples').decode('utf-8'))
-
- data_list = []
- with self.env.begin(write=False) as txn:
- for i in range(self.total_number):
- idx = i + 1
- label_key = f'label-{idx:09d}'
- img_key = f'image-{idx:09d}'
- text = txn.get(label_key.encode('utf-8')).decode('utf-8')
- line = [img_key, text]
- data_list.append(self.parse_data_info(line))
- return data_list
-
- def parse_data_info(self,
- raw_anno_info: Tuple[Optional[str],
- str]) -> Union[dict, List[dict]]:
- """Parse raw annotation to target format.
-
- Args:
- raw_anno_info (str): One raw data information loaded
- from ``ann_file``.
-
- Returns:
- (dict): Parsed annotation.
- """
- data_info = {}
- img_key, text = raw_anno_info
- data_info['img_key'] = img_key
- data_info['instances'] = [dict(text=text)]
- return data_info
-
- def prepare_data(self, idx) -> Any:
- """Get data processed by ``self.pipeline``.
-
- Args:
- idx (int): The index of ``data_info``.
-
- Returns:
- Any: Depends on ``self.pipeline``.
- """
- data_info = self.get_data_info(idx)
- with self.env.begin(write=False) as txn:
- img_bytes = txn.get(data_info['img_key'].encode('utf-8'))
- if img_bytes is None:
- return None
- data_info['img'] = mmcv.imfrombytes(
- img_bytes, flag=self.color_type)
- return self.pipeline(data_info)
-
- def _make_env(self):
- """Create lmdb environment from self.ann_file and save it to
- ``self.env``.
-
- Returns:
- Lmdb environment.
- """
- try:
- import lmdb
- except ImportError:
- raise ImportError(
- 'Please install lmdb to enable RecogLMDBDataset.')
- if hasattr(self, 'env'):
- return
-
- self.env = lmdb.open(
- self.ann_file,
- max_readers=1,
- readonly=True,
- lock=False,
- readahead=False,
- meminit=False,
- )
-
- def close(self):
- """Close lmdb environment."""
- if hasattr(self, 'env'):
- self.env.close()
- del self.env
diff --git a/pipeline/mmocr/mmocr/datasets/recog_text_dataset.py b/pipeline/mmocr/mmocr/datasets/recog_text_dataset.py
deleted file mode 100644
index 25cc54ff8e3639fc5a3ba3182749d0920bfc0a8b..0000000000000000000000000000000000000000
--- a/pipeline/mmocr/mmocr/datasets/recog_text_dataset.py
+++ /dev/null
@@ -1,134 +0,0 @@
-# Copyright (c) OpenMMLab. All rights reserved.
-import os.path as osp
-from typing import Callable, List, Optional, Sequence, Union
-
-from mmengine.dataset import BaseDataset
-from mmengine.fileio import list_from_file
-
-from mmocr.registry import DATASETS, TASK_UTILS
-
-
-@DATASETS.register_module()
-class RecogTextDataset(BaseDataset):
- r"""RecogTextDataset for text recognition.
-
- The annotation format can be both in jsonl and txt. If the annotation file
- is in jsonl format, it should be a list of dicts. If the annotation file
- is in txt format, it should be a list of lines.
-
- The annotation formats are shown as follows.
- - txt format
- .. code-block:: none
-
- ``test_img1.jpg OpenMMLab``
- ``test_img2.jpg MMOCR``
-
- - jsonl format
- .. code-block:: none
-
- ``{"filename": "test_img1.jpg", "text": "OpenMMLab"}``
- ``{"filename": "test_img2.jpg", "text": "MMOCR"}``
-
- Args:
- ann_file (str): Annotation file path. Defaults to ''.
- backend_args (dict, optional): Arguments to instantiate the
- prefix of uri corresponding backend. Defaults to None.
- parse_cfg (dict, optional): Config of parser for parsing annotations.
- Use ``LineJsonParser`` when the annotation file is in jsonl format
- with keys of ``filename`` and ``text``. The keys in parse_cfg
- should be consistent with the keys in jsonl annotations. The first
- key in parse_cfg should be the key of the path in jsonl
- annotations. The second key in parse_cfg should be the key of the
- text in jsonl Use ``LineStrParser`` when the annotation file is in
- txt format. Defaults to
- ``dict(type='LineJsonParser', keys=['filename', 'text'])``.
- metainfo (dict, optional): Meta information for dataset, such as class
- information. Defaults to None.
- data_root (str): The root directory for ``data_prefix`` and
- ``ann_file``. Defaults to ''.
- data_prefix (dict): Prefix for training data. Defaults to
- ``dict(img_path='')``.
- filter_cfg (dict, optional): Config for filter data. Defaults to None.
- indices (int or Sequence[int], optional): Support using first few
- data in annotation file to facilitate training/testing on a smaller
- dataset. Defaults to None which means using all ``data_infos``.
- serialize_data (bool, optional): Whether to hold memory using
- serialized objects, when enabled, data loader workers can use
- shared RAM from master process instead of making a copy. Defaults
- to True.
- pipeline (list, optional): Processing pipeline. Defaults to [].
- test_mode (bool, optional): ``test_mode=True`` means in test phase.
- Defaults to False.
- lazy_init (bool, optional): Whether to load annotation during
- instantiation. In some cases, such as visualization, only the meta
- information of the dataset is needed, which is not necessary to
- load annotation file. ``RecogTextDataset`` can skip load
- annotations to save time by set ``lazy_init=False``. Defaults to
- False.
- max_refetch (int, optional): If ``RecogTextDataset.prepare_data`` get a
- None img. The maximum extra number of cycles to get a valid
- image. Defaults to 1000.
- """
-
- def __init__(self,
- ann_file: str = '',
- backend_args=None,
- parser_cfg: Optional[dict] = dict(
- type='LineJsonParser', keys=['filename', 'text']),
- metainfo: Optional[dict] = None,
- data_root: Optional[str] = '',
- data_prefix: dict = dict(img_path=''),
- filter_cfg: Optional[dict] = None,
- indices: Optional[Union[int, Sequence[int]]] = None,
- serialize_data: bool = True,
- pipeline: List[Union[dict, Callable]] = [],
- test_mode: bool = False,
- lazy_init: bool = False,
- max_refetch: int = 1000) -> None:
-
- self.parser = TASK_UTILS.build(parser_cfg)
- self.backend_args = backend_args
- super().__init__(
- ann_file=ann_file,
- metainfo=metainfo,
- data_root=data_root,
- data_prefix=data_prefix,
- filter_cfg=filter_cfg,
- indices=indices,
- serialize_data=serialize_data,
- pipeline=pipeline,
- test_mode=test_mode,
- lazy_init=lazy_init,
- max_refetch=max_refetch)
-
- def load_data_list(self) -> List[dict]:
- """Load annotations from an annotation file named as ``self.ann_file``
-
- Returns:
- List[dict]: A list of annotation.
- """
- data_list = []
- raw_anno_infos = list_from_file(
- self.ann_file, backend_args=self.backend_args)
- for raw_anno_info in raw_anno_infos:
- data_list.append(self.parse_data_info(raw_anno_info))
- return data_list
-
- def parse_data_info(self, raw_anno_info: str) -> dict:
- """Parse raw annotation to target format.
-
- Args:
- raw_anno_info (str): One raw data information loaded
- from ``ann_file``.
-
- Returns:
- (dict): Parsed annotation.
- """
- data_info = {}
- parsed_anno = self.parser(raw_anno_info)
- img_path = osp.join(self.data_prefix['img_path'],
- parsed_anno[self.parser.keys[0]])
-
- data_info['img_path'] = img_path
- data_info['instances'] = [dict(text=parsed_anno[self.parser.keys[1]])]
- return data_info
diff --git a/pipeline/mmocr/mmocr/datasets/samplers/__init__.py b/pipeline/mmocr/mmocr/datasets/samplers/__init__.py
deleted file mode 100644
index 063a79cb1286282712d8530b87cdfa50ae06f71a..0000000000000000000000000000000000000000
--- a/pipeline/mmocr/mmocr/datasets/samplers/__init__.py
+++ /dev/null
@@ -1,4 +0,0 @@
-# Copyright (c) OpenMMLab. All rights reserved.
-from .batch_aug import BatchAugSampler
-
-__all__ = ['BatchAugSampler']
diff --git a/pipeline/mmocr/mmocr/datasets/samplers/__pycache__/__init__.cpython-38.pyc b/pipeline/mmocr/mmocr/datasets/samplers/__pycache__/__init__.cpython-38.pyc
deleted file mode 100644
index dccf512a091cea953e3f001e972b7bcc058da25a..0000000000000000000000000000000000000000
Binary files a/pipeline/mmocr/mmocr/datasets/samplers/__pycache__/__init__.cpython-38.pyc and /dev/null differ
diff --git a/pipeline/mmocr/mmocr/datasets/samplers/__pycache__/__init__.cpython-39.pyc b/pipeline/mmocr/mmocr/datasets/samplers/__pycache__/__init__.cpython-39.pyc
deleted file mode 100644
index 7e672bab8963441bf36b9eccebc153c9800f9685..0000000000000000000000000000000000000000
Binary files a/pipeline/mmocr/mmocr/datasets/samplers/__pycache__/__init__.cpython-39.pyc and /dev/null differ
diff --git a/pipeline/mmocr/mmocr/datasets/samplers/__pycache__/batch_aug.cpython-38.pyc b/pipeline/mmocr/mmocr/datasets/samplers/__pycache__/batch_aug.cpython-38.pyc
deleted file mode 100644
index 737aa07b6a8177955b8ba27c7e17130fe2c3ee72..0000000000000000000000000000000000000000
Binary files a/pipeline/mmocr/mmocr/datasets/samplers/__pycache__/batch_aug.cpython-38.pyc and /dev/null differ
diff --git a/pipeline/mmocr/mmocr/datasets/samplers/__pycache__/batch_aug.cpython-39.pyc b/pipeline/mmocr/mmocr/datasets/samplers/__pycache__/batch_aug.cpython-39.pyc
deleted file mode 100644
index a372bdea851995ae0bc1267ec300ed7ed308f338..0000000000000000000000000000000000000000
Binary files a/pipeline/mmocr/mmocr/datasets/samplers/__pycache__/batch_aug.cpython-39.pyc and /dev/null differ
diff --git a/pipeline/mmocr/mmocr/datasets/samplers/batch_aug.py b/pipeline/mmocr/mmocr/datasets/samplers/batch_aug.py
deleted file mode 100644
index 852fbc67fbbb5dc4a0c3c202a71a0b84f9c3832b..0000000000000000000000000000000000000000
--- a/pipeline/mmocr/mmocr/datasets/samplers/batch_aug.py
+++ /dev/null
@@ -1,98 +0,0 @@
-import math
-from typing import Iterator, Optional, Sized
-
-import torch
-from mmengine.dist import get_dist_info, sync_random_seed
-from torch.utils.data import Sampler
-
-from mmocr.registry import DATA_SAMPLERS
-
-
-@DATA_SAMPLERS.register_module()
-class BatchAugSampler(Sampler):
- """Sampler that repeats the same data elements for num_repeats times. The
- batch size should be divisible by num_repeats.
-
- It ensures that different each
- augmented version of a sample will be visible to a different process (GPU).
- Heavily based on torch.utils.data.DistributedSampler.
-
- This sampler was modified from
- https://github.com/facebookresearch/deit/blob/0c4b8f60/samplers.py
- Used in
- Copyright (c) 2015-present, Facebook, Inc.
-
- Args:
- dataset (Sized): The dataset.
- shuffle (bool): Whether shuffle the dataset or not. Defaults to True.
- num_repeats (int): The repeat times of every sample. Defaults to 3.
- seed (int, optional): Random seed used to shuffle the sampler if
- :attr:`shuffle=True`. This number should be identical across all
- processes in the distributed group. Defaults to None.
- """
-
- def __init__(self,
- dataset: Sized,
- shuffle: bool = True,
- num_repeats: int = 3,
- seed: Optional[int] = None):
- rank, world_size = get_dist_info()
- self.rank = rank
- self.world_size = world_size
-
- self.dataset = dataset
- self.shuffle = shuffle
-
- if seed is None:
- seed = sync_random_seed()
- self.seed = seed
- self.epoch = 0
- self.num_repeats = num_repeats
-
- # The number of repeated samples in the rank
- self.num_samples = math.ceil(
- len(self.dataset) * num_repeats / world_size)
- # The total number of repeated samples in all ranks.
- self.total_size = self.num_samples * world_size
- # The number of selected samples in the rank
- self.num_selected_samples = math.ceil(len(self.dataset) / world_size)
-
- def __iter__(self) -> Iterator[int]:
- """Iterate the indices."""
- # deterministically shuffle based on epoch and seed
- if self.shuffle:
- g = torch.Generator()
- g.manual_seed(self.seed + self.epoch)
- indices = torch.randperm(len(self.dataset), generator=g).tolist()
- else:
- indices = list(range(len(self.dataset)))
-
- # produce repeats e.g. [0, 0, 0, 1, 1, 1, 2, 2, 2....]
- indices = [x for x in indices for _ in range(self.num_repeats)]
- # add extra samples to make it evenly divisible
- indices = (indices *
- int(self.total_size / len(indices) + 1))[:self.total_size]
- assert len(indices) == self.total_size
-
- # subsample per rank
- indices = indices[self.rank:self.total_size:self.world_size]
- assert len(indices) == self.num_samples
-
- # return up to num selected samples
- return iter(indices)
-
- def __len__(self) -> int:
- """The number of samples in this rank."""
- return self.num_selected_samples
-
- def set_epoch(self, epoch: int) -> None:
- """Sets the epoch for this sampler.
-
- When :attr:`shuffle=True`, this ensures all replicas use a different
- random ordering for each epoch. Otherwise, the next iteration of this
- sampler will yield the same ordering.
-
- Args:
- epoch (int): Epoch number.
- """
- self.epoch = epoch
diff --git a/pipeline/mmocr/mmocr/datasets/transforms/__init__.py b/pipeline/mmocr/mmocr/datasets/transforms/__init__.py
deleted file mode 100644
index 61a15ec9609c65edee438679ff7c68ff33aabcf6..0000000000000000000000000000000000000000
--- a/pipeline/mmocr/mmocr/datasets/transforms/__init__.py
+++ /dev/null
@@ -1,27 +0,0 @@
-# Copyright (c) OpenMMLab. All rights reserved.
-from .adapters import MMDet2MMOCR, MMOCR2MMDet
-from .formatting import PackKIEInputs, PackTextDetInputs, PackTextRecogInputs
-from .loading import (InferencerLoader, LoadImageFromFile,
- LoadImageFromNDArray, LoadKIEAnnotations,
- LoadOCRAnnotations)
-from .ocr_transforms import (FixInvalidPolygon, RandomCrop, RandomRotate,
- RemoveIgnored, Resize)
-from .textdet_transforms import (BoundedScaleAspectJitter, RandomFlip,
- ShortScaleAspectJitter, SourceImagePad,
- TextDetRandomCrop, TextDetRandomCropFlip)
-from .textrecog_transforms import (CropHeight, ImageContentJitter, PadToWidth,
- PyramidRescale, RescaleToHeight,
- ReversePixels, TextRecogGeneralAug)
-from .wrappers import ConditionApply, ImgAugWrapper, TorchVisionWrapper
-
-__all__ = [
- 'LoadOCRAnnotations', 'RandomRotate', 'ImgAugWrapper', 'SourceImagePad',
- 'TextDetRandomCropFlip', 'PyramidRescale', 'TorchVisionWrapper', 'Resize',
- 'RandomCrop', 'TextDetRandomCrop', 'RandomCrop', 'PackTextDetInputs',
- 'PackTextRecogInputs', 'RescaleToHeight', 'PadToWidth',
- 'ShortScaleAspectJitter', 'RandomFlip', 'BoundedScaleAspectJitter',
- 'PackKIEInputs', 'LoadKIEAnnotations', 'FixInvalidPolygon', 'MMDet2MMOCR',
- 'MMOCR2MMDet', 'LoadImageFromFile', 'LoadImageFromNDArray', 'CropHeight',
- 'InferencerLoader', 'RemoveIgnored', 'ConditionApply', 'CropHeight',
- 'TextRecogGeneralAug', 'ImageContentJitter', 'ReversePixels'
-]
diff --git a/pipeline/mmocr/mmocr/datasets/transforms/__pycache__/__init__.cpython-38.pyc b/pipeline/mmocr/mmocr/datasets/transforms/__pycache__/__init__.cpython-38.pyc
deleted file mode 100644
index c3387ba6d02b577163103304dbc8497ada55463e..0000000000000000000000000000000000000000
Binary files a/pipeline/mmocr/mmocr/datasets/transforms/__pycache__/__init__.cpython-38.pyc and /dev/null differ
diff --git a/pipeline/mmocr/mmocr/datasets/transforms/__pycache__/__init__.cpython-39.pyc b/pipeline/mmocr/mmocr/datasets/transforms/__pycache__/__init__.cpython-39.pyc
deleted file mode 100644
index b8346adc312b44b91b20b2a75c85d76000ca53ef..0000000000000000000000000000000000000000
Binary files a/pipeline/mmocr/mmocr/datasets/transforms/__pycache__/__init__.cpython-39.pyc and /dev/null differ
diff --git a/pipeline/mmocr/mmocr/datasets/transforms/__pycache__/adapters.cpython-38.pyc b/pipeline/mmocr/mmocr/datasets/transforms/__pycache__/adapters.cpython-38.pyc
deleted file mode 100644
index ae8823356ee8b62f7eec63980bd73611964c3a93..0000000000000000000000000000000000000000
Binary files a/pipeline/mmocr/mmocr/datasets/transforms/__pycache__/adapters.cpython-38.pyc and /dev/null differ
diff --git a/pipeline/mmocr/mmocr/datasets/transforms/__pycache__/adapters.cpython-39.pyc b/pipeline/mmocr/mmocr/datasets/transforms/__pycache__/adapters.cpython-39.pyc
deleted file mode 100644
index e5079c4d366a31fbd6c975fb842e5804a7719ac1..0000000000000000000000000000000000000000
Binary files a/pipeline/mmocr/mmocr/datasets/transforms/__pycache__/adapters.cpython-39.pyc and /dev/null differ
diff --git a/pipeline/mmocr/mmocr/datasets/transforms/__pycache__/formatting.cpython-38.pyc b/pipeline/mmocr/mmocr/datasets/transforms/__pycache__/formatting.cpython-38.pyc
deleted file mode 100644
index b79dca7f051ed09a8df42db7d5652f3e18048309..0000000000000000000000000000000000000000
Binary files a/pipeline/mmocr/mmocr/datasets/transforms/__pycache__/formatting.cpython-38.pyc and /dev/null differ
diff --git a/pipeline/mmocr/mmocr/datasets/transforms/__pycache__/formatting.cpython-39.pyc b/pipeline/mmocr/mmocr/datasets/transforms/__pycache__/formatting.cpython-39.pyc
deleted file mode 100644
index 6f5eb70101be0538f95bced0ad1dab7e1f76d29e..0000000000000000000000000000000000000000
Binary files a/pipeline/mmocr/mmocr/datasets/transforms/__pycache__/formatting.cpython-39.pyc and /dev/null differ
diff --git a/pipeline/mmocr/mmocr/datasets/transforms/__pycache__/loading.cpython-38.pyc b/pipeline/mmocr/mmocr/datasets/transforms/__pycache__/loading.cpython-38.pyc
deleted file mode 100644
index 0844a139b69c96ed17c0608a28d875ee1dcea8e7..0000000000000000000000000000000000000000
Binary files a/pipeline/mmocr/mmocr/datasets/transforms/__pycache__/loading.cpython-38.pyc and /dev/null differ
diff --git a/pipeline/mmocr/mmocr/datasets/transforms/__pycache__/loading.cpython-39.pyc b/pipeline/mmocr/mmocr/datasets/transforms/__pycache__/loading.cpython-39.pyc
deleted file mode 100644
index 8348579d0eaeac53894a5815e70bfb86a20f96b1..0000000000000000000000000000000000000000
Binary files a/pipeline/mmocr/mmocr/datasets/transforms/__pycache__/loading.cpython-39.pyc and /dev/null differ
diff --git a/pipeline/mmocr/mmocr/datasets/transforms/__pycache__/ocr_transforms.cpython-38.pyc b/pipeline/mmocr/mmocr/datasets/transforms/__pycache__/ocr_transforms.cpython-38.pyc
deleted file mode 100644
index c89f9302e5ebe708f715e1ba81c2526ff02dda78..0000000000000000000000000000000000000000
Binary files a/pipeline/mmocr/mmocr/datasets/transforms/__pycache__/ocr_transforms.cpython-38.pyc and /dev/null differ
diff --git a/pipeline/mmocr/mmocr/datasets/transforms/__pycache__/ocr_transforms.cpython-39.pyc b/pipeline/mmocr/mmocr/datasets/transforms/__pycache__/ocr_transforms.cpython-39.pyc
deleted file mode 100644
index 8a4465392ad0fa30d7361b0b13e5f06d55b931e8..0000000000000000000000000000000000000000
Binary files a/pipeline/mmocr/mmocr/datasets/transforms/__pycache__/ocr_transforms.cpython-39.pyc and /dev/null differ
diff --git a/pipeline/mmocr/mmocr/datasets/transforms/__pycache__/textdet_transforms.cpython-38.pyc b/pipeline/mmocr/mmocr/datasets/transforms/__pycache__/textdet_transforms.cpython-38.pyc
deleted file mode 100644
index e1a76df8a3fd26210b09d03ec82317d708499c3f..0000000000000000000000000000000000000000
Binary files a/pipeline/mmocr/mmocr/datasets/transforms/__pycache__/textdet_transforms.cpython-38.pyc and /dev/null differ
diff --git a/pipeline/mmocr/mmocr/datasets/transforms/__pycache__/textdet_transforms.cpython-39.pyc b/pipeline/mmocr/mmocr/datasets/transforms/__pycache__/textdet_transforms.cpython-39.pyc
deleted file mode 100644
index 57ecf27232965ca56d7f0667231ef48538370546..0000000000000000000000000000000000000000
Binary files a/pipeline/mmocr/mmocr/datasets/transforms/__pycache__/textdet_transforms.cpython-39.pyc and /dev/null differ
diff --git a/pipeline/mmocr/mmocr/datasets/transforms/__pycache__/textrecog_transforms.cpython-38.pyc b/pipeline/mmocr/mmocr/datasets/transforms/__pycache__/textrecog_transforms.cpython-38.pyc
deleted file mode 100644
index 9c4cac67434dbf5fe291018cf6c94272259a6b94..0000000000000000000000000000000000000000
Binary files a/pipeline/mmocr/mmocr/datasets/transforms/__pycache__/textrecog_transforms.cpython-38.pyc and /dev/null differ
diff --git a/pipeline/mmocr/mmocr/datasets/transforms/__pycache__/textrecog_transforms.cpython-39.pyc b/pipeline/mmocr/mmocr/datasets/transforms/__pycache__/textrecog_transforms.cpython-39.pyc
deleted file mode 100644
index eef1f581dc5e239c52494f1f7ea5f1557809ca96..0000000000000000000000000000000000000000
Binary files a/pipeline/mmocr/mmocr/datasets/transforms/__pycache__/textrecog_transforms.cpython-39.pyc and /dev/null differ
diff --git a/pipeline/mmocr/mmocr/datasets/transforms/__pycache__/wrappers.cpython-38.pyc b/pipeline/mmocr/mmocr/datasets/transforms/__pycache__/wrappers.cpython-38.pyc
deleted file mode 100644
index 10fa636f664b0f3015ad64230308a941cbceb2dc..0000000000000000000000000000000000000000
Binary files a/pipeline/mmocr/mmocr/datasets/transforms/__pycache__/wrappers.cpython-38.pyc and /dev/null differ
diff --git a/pipeline/mmocr/mmocr/datasets/transforms/__pycache__/wrappers.cpython-39.pyc b/pipeline/mmocr/mmocr/datasets/transforms/__pycache__/wrappers.cpython-39.pyc
deleted file mode 100644
index 0f2c95d980a854b823e32b5a04bd3499e592bcdd..0000000000000000000000000000000000000000
Binary files a/pipeline/mmocr/mmocr/datasets/transforms/__pycache__/wrappers.cpython-39.pyc and /dev/null differ
diff --git a/pipeline/mmocr/mmocr/datasets/transforms/adapters.py b/pipeline/mmocr/mmocr/datasets/transforms/adapters.py
deleted file mode 100644
index 370174727ade4117a4857e8ec72a8c70c7a8950e..0000000000000000000000000000000000000000
--- a/pipeline/mmocr/mmocr/datasets/transforms/adapters.py
+++ /dev/null
@@ -1,121 +0,0 @@
-# Copyright (c) OpenMMLab. All rights reserved.
-from typing import Dict
-
-from mmcv.transforms.base import BaseTransform
-from mmdet.structures.mask import PolygonMasks, bitmap_to_polygon
-
-from mmocr.registry import TRANSFORMS
-
-
-@TRANSFORMS.register_module()
-class MMDet2MMOCR(BaseTransform):
- """Convert transforms's data format from MMDet to MMOCR.
-
- Required Keys:
-
- - gt_masks (PolygonMasks | BitmapMasks) (optional)
- - gt_ignore_flags (np.bool) (optional)
-
- Added Keys:
-
- - gt_polygons (list[np.ndarray])
- - gt_ignored (np.ndarray)
- """
-
- def transform(self, results: Dict) -> Dict:
- """Convert MMDet's data format to MMOCR's data format.
-
- Args:
- results (Dict): Result dict containing the data to transform.
-
- Returns:
- (Dict): The transformed data.
- """
- # gt_masks -> gt_polygons
- if 'gt_masks' in results.keys():
- gt_polygons = []
- gt_masks = results.pop('gt_masks')
- if len(gt_masks) > 0:
- # PolygonMasks
- if isinstance(gt_masks[0], PolygonMasks):
- gt_polygons = [mask[0] for mask in gt_masks.masks]
- # BitmapMasks
- else:
- polygons = []
- for mask in gt_masks.masks:
- contours, _ = bitmap_to_polygon(mask)
- polygons += [
- contour.reshape(-1) for contour in contours
- ]
- # filter invalid polygons
- gt_polygons = []
- for polygon in polygons:
- if len(polygon) < 6:
- continue
- gt_polygons.append(polygon)
-
- results['gt_polygons'] = gt_polygons
- # gt_ignore_flags -> gt_ignored
- if 'gt_ignore_flags' in results.keys():
- gt_ignored = results.pop('gt_ignore_flags')
- results['gt_ignored'] = gt_ignored
-
- return results
-
- def __repr__(self) -> str:
- repr_str = self.__class__.__name__
- return repr_str
-
-
-@TRANSFORMS.register_module()
-class MMOCR2MMDet(BaseTransform):
- """Convert transforms's data format from MMOCR to MMDet.
-
- Required Keys:
-
- - img_shape
- - gt_polygons (List[ndarray]) (optional)
- - gt_ignored (np.bool) (optional)
-
- Added Keys:
-
- - gt_masks (PolygonMasks | BitmapMasks) (optional)
- - gt_ignore_flags (np.bool) (optional)
-
- Args:
- poly2mask (bool): Whether to convert mask to bitmap. Default: True.
- """
-
- def __init__(self, poly2mask: bool = False) -> None:
- self.poly2mask = poly2mask
-
- def transform(self, results: Dict) -> Dict:
- """Convert MMOCR's data format to MMDet's data format.
-
- Args:
- results (Dict): Result dict containing the data to transform.
-
- Returns:
- (Dict): The transformed data.
- """
- # gt_polygons -> gt_masks
- if 'gt_polygons' in results.keys():
- gt_polygons = results.pop('gt_polygons')
- gt_polygons = [[gt_polygon] for gt_polygon in gt_polygons]
- gt_masks = PolygonMasks(gt_polygons, *results['img_shape'])
-
- if self.poly2mask:
- gt_masks = gt_masks.to_bitmap()
-
- results['gt_masks'] = gt_masks
- # gt_ignore_flags -> gt_ignored
- if 'gt_ignored' in results.keys():
- gt_ignored = results.pop('gt_ignored')
- results['gt_ignore_flags'] = gt_ignored
-
- return results
-
- def __repr__(self) -> str:
- repr_str = self.__class__.__name__
- repr_str += f'(poly2mask = {self.poly2mask})'
- return repr_str
diff --git a/pipeline/mmocr/mmocr/datasets/transforms/formatting.py b/pipeline/mmocr/mmocr/datasets/transforms/formatting.py
deleted file mode 100644
index b9b71437a6cc1de2396b17fe5c04909855f2ed86..0000000000000000000000000000000000000000
--- a/pipeline/mmocr/mmocr/datasets/transforms/formatting.py
+++ /dev/null
@@ -1,330 +0,0 @@
-# Copyright (c) OpenMMLab. All rights reserved.
-import numpy as np
-import torch
-from mmcv.transforms import to_tensor
-from mmcv.transforms.base import BaseTransform
-from mmengine.structures import InstanceData, LabelData
-
-from mmocr.registry import TRANSFORMS
-from mmocr.structures import (KIEDataSample, TextDetDataSample,
- TextRecogDataSample)
-
-
-@TRANSFORMS.register_module()
-class PackTextDetInputs(BaseTransform):
- """Pack the inputs data for text detection.
-
- The type of outputs is `dict`:
-
- - inputs: image converted to tensor, whose shape is (C, H, W).
- - data_samples: Two components of ``TextDetDataSample`` will be updated:
-
- - gt_instances (InstanceData): Depending on annotations, a subset of the
- following keys will be updated:
-
- - bboxes (torch.Tensor((N, 4), dtype=torch.float32)): The groundtruth
- of bounding boxes in the form of [x1, y1, x2, y2]. Renamed from
- 'gt_bboxes'.
- - labels (torch.LongTensor(N)): The labels of instances.
- Renamed from 'gt_bboxes_labels'.
- - polygons(list[np.array((2k,), dtype=np.float32)]): The
- groundtruth of polygons in the form of [x1, y1,..., xk, yk]. Each
- element in polygons may have different number of points. Renamed from
- 'gt_polygons'. Using numpy instead of tensor is that polygon usually
- is not the output of model and operated on cpu.
- - ignored (torch.BoolTensor((N,))): The flag indicating whether the
- corresponding instance should be ignored. Renamed from
- 'gt_ignored'.
- - texts (list[str]): The groundtruth texts. Renamed from 'gt_texts'.
-
- - metainfo (dict): 'metainfo' is always populated. The contents of the
- 'metainfo' depends on ``meta_keys``. By default it includes:
-
- - "img_path": Path to the image file.
- - "img_shape": Shape of the image input to the network as a tuple
- (h, w). Note that the image may be zero-padded afterward on the
- bottom/right if the batch tensor is larger than this shape.
- - "scale_factor": A tuple indicating the ratio of width and height
- of the preprocessed image to the original one.
- - "ori_shape": Shape of the preprocessed image as a tuple
- (h, w).
- - "pad_shape": Image shape after padding (if any Pad-related
- transform involved) as a tuple (h, w).
- - "flip": A boolean indicating if the image has been flipped.
- - ``flip_direction``: the flipping direction.
-
- Args:
- meta_keys (Sequence[str], optional): Meta keys to be converted to
- the metainfo of ``TextDetSample``. Defaults to ``('img_path',
- 'ori_shape', 'img_shape', 'scale_factor', 'flip',
- 'flip_direction')``.
- """
- mapping_table = {
- 'gt_bboxes': 'bboxes',
- 'gt_bboxes_labels': 'labels',
- 'gt_polygons': 'polygons',
- 'gt_texts': 'texts',
- 'gt_ignored': 'ignored'
- }
-
- def __init__(self,
- meta_keys=('img_path', 'ori_shape', 'img_shape',
- 'scale_factor', 'flip', 'flip_direction')):
- self.meta_keys = meta_keys
-
- def transform(self, results: dict) -> dict:
- """Method to pack the input data.
-
- Args:
- results (dict): Result dict from the data pipeline.
-
- Returns:
- dict:
-
- - 'inputs' (obj:`torch.Tensor`): Data for model forwarding.
- - 'data_samples' (obj:`DetDataSample`): The annotation info of the
- sample.
- """
- packed_results = dict()
- if 'img' in results:
- img = results['img']
- if len(img.shape) < 3:
- img = np.expand_dims(img, -1)
- # A simple trick to speedup formatting by 3-5 times when
- # OMP_NUM_THREADS != 1
- # Refer to https://github.com/open-mmlab/mmdetection/pull/9533
- # for more details
- if img.flags.c_contiguous:
- img = to_tensor(img)
- img = img.permute(2, 0, 1).contiguous()
- else:
- img = np.ascontiguousarray(img.transpose(2, 0, 1))
- img = to_tensor(img)
- packed_results['inputs'] = img
-
- data_sample = TextDetDataSample()
- instance_data = InstanceData()
- for key in self.mapping_table.keys():
- if key not in results:
- continue
- if key in ['gt_bboxes', 'gt_bboxes_labels', 'gt_ignored']:
- instance_data[self.mapping_table[key]] = to_tensor(
- results[key])
- else:
- instance_data[self.mapping_table[key]] = results[key]
- data_sample.gt_instances = instance_data
-
- img_meta = {}
- for key in self.meta_keys:
- img_meta[key] = results[key]
- data_sample.set_metainfo(img_meta)
- packed_results['data_samples'] = data_sample
-
- return packed_results
-
- def __repr__(self) -> str:
- repr_str = self.__class__.__name__
- repr_str += f'(meta_keys={self.meta_keys})'
- return repr_str
-
-
-@TRANSFORMS.register_module()
-class PackTextRecogInputs(BaseTransform):
- """Pack the inputs data for text recognition.
-
- The type of outputs is `dict`:
-
- - inputs: Image as a tensor, whose shape is (C, H, W).
- - data_samples: Two components of ``TextRecogDataSample`` will be updated:
-
- - gt_text (LabelData):
-
- - item(str): The groundtruth of text. Rename from 'gt_texts'.
-
- - metainfo (dict): 'metainfo' is always populated. The contents of the
- 'metainfo' depends on ``meta_keys``. By default it includes:
-
- - "img_path": Path to the image file.
- - "ori_shape": Shape of the preprocessed image as a tuple
- (h, w).
- - "img_shape": Shape of the image input to the network as a tuple
- (h, w). Note that the image may be zero-padded afterward on the
- bottom/right if the batch tensor is larger than this shape.
- - "valid_ratio": The proportion of valid (unpadded) content of image
- on the x-axis. It defaults to 1 if not set in pipeline.
-
- Args:
- meta_keys (Sequence[str], optional): Meta keys to be converted to
- the metainfo of ``TextRecogDataSampel``. Defaults to
- ``('img_path', 'ori_shape', 'img_shape', 'pad_shape',
- 'valid_ratio')``.
- """
-
- def __init__(self,
- meta_keys=('img_path', 'ori_shape', 'img_shape', 'pad_shape',
- 'valid_ratio')):
- self.meta_keys = meta_keys
-
- def transform(self, results: dict) -> dict:
- """Method to pack the input data.
-
- Args:
- results (dict): Result dict from the data pipeline.
-
- Returns:
- dict:
-
- - 'inputs' (obj:`torch.Tensor`): Data for model forwarding.
- - 'data_samples' (obj:`TextRecogDataSample`): The annotation info
- of the sample.
- """
- packed_results = dict()
- if 'img' in results:
- img = results['img']
- if len(img.shape) < 3:
- img = np.expand_dims(img, -1)
- # A simple trick to speedup formatting by 3-5 times when
- # OMP_NUM_THREADS != 1
- # Refer to https://github.com/open-mmlab/mmdetection/pull/9533
- # for more details
- if img.flags.c_contiguous:
- img = to_tensor(img)
- img = img.permute(2, 0, 1).contiguous()
- else:
- img = np.ascontiguousarray(img.transpose(2, 0, 1))
- img = to_tensor(img)
- packed_results['inputs'] = img
-
- data_sample = TextRecogDataSample()
- gt_text = LabelData()
-
- if results.get('gt_texts', None):
- assert len(
- results['gt_texts']
- ) == 1, 'Each image sample should have one text annotation only'
- gt_text.item = results['gt_texts'][0]
- data_sample.gt_text = gt_text
-
- img_meta = {}
- for key in self.meta_keys:
- if key == 'valid_ratio':
- img_meta[key] = results.get('valid_ratio', 1)
- else:
- img_meta[key] = results[key]
- data_sample.set_metainfo(img_meta)
-
- packed_results['data_samples'] = data_sample
-
- return packed_results
-
- def __repr__(self) -> str:
- repr_str = self.__class__.__name__
- repr_str += f'(meta_keys={self.meta_keys})'
- return repr_str
-
-
-@TRANSFORMS.register_module()
-class PackKIEInputs(BaseTransform):
- """Pack the inputs data for key information extraction.
-
- The type of outputs is `dict`:
-
- - inputs: image converted to tensor, whose shape is (C, H, W).
- - data_samples: Two components of ``TextDetDataSample`` will be updated:
-
- - gt_instances (InstanceData): Depending on annotations, a subset of the
- following keys will be updated:
-
- - bboxes (torch.Tensor((N, 4), dtype=torch.float32)): The groundtruth
- of bounding boxes in the form of [x1, y1, x2, y2]. Renamed from
- 'gt_bboxes'.
- - labels (torch.LongTensor(N)): The labels of instances.
- Renamed from 'gt_bboxes_labels'.
- - edge_labels (torch.LongTensor(N, N)): The edge labels.
- Renamed from 'gt_edges_labels'.
- - texts (list[str]): The groundtruth texts. Renamed from 'gt_texts'.
-
- - metainfo (dict): 'metainfo' is always populated. The contents of the
- 'metainfo' depends on ``meta_keys``. By default it includes:
-
- - "img_path": Path to the image file.
- - "img_shape": Shape of the image input to the network as a tuple
- (h, w). Note that the image may be zero-padded afterward on the
- bottom/right if the batch tensor is larger than this shape.
- - "scale_factor": A tuple indicating the ratio of width and height
- of the preprocessed image to the original one.
- - "ori_shape": Shape of the preprocessed image as a tuple
- (h, w).
-
- Args:
- meta_keys (Sequence[str], optional): Meta keys to be converted to
- the metainfo of ``TextDetSample``. Defaults to ``('img_path',
- 'ori_shape', 'img_shape', 'scale_factor', 'flip',
- 'flip_direction')``.
- """
- mapping_table = {
- 'gt_bboxes': 'bboxes',
- 'gt_bboxes_labels': 'labels',
- 'gt_edges_labels': 'edge_labels',
- 'gt_texts': 'texts',
- }
-
- def __init__(self, meta_keys=()):
- self.meta_keys = meta_keys
-
- def transform(self, results: dict) -> dict:
- """Method to pack the input data.
-
- Args:
- results (dict): Result dict from the data pipeline.
-
- Returns:
- dict:
-
- - 'inputs' (obj:`torch.Tensor`): Data for model forwarding.
- - 'data_samples' (obj:`DetDataSample`): The annotation info of the
- sample.
- """
- packed_results = dict()
- if 'img' in results:
- img = results['img']
- if len(img.shape) < 3:
- img = np.expand_dims(img, -1)
- # A simple trick to speedup formatting by 3-5 times when
- # OMP_NUM_THREADS != 1
- # Refer to https://github.com/open-mmlab/mmdetection/pull/9533
- # for more details
- if img.flags.c_contiguous:
- img = to_tensor(img)
- img = img.permute(2, 0, 1).contiguous()
- else:
- img = np.ascontiguousarray(img.transpose(2, 0, 1))
- img = to_tensor(img)
- packed_results['inputs'] = img
- else:
- packed_results['inputs'] = torch.FloatTensor().reshape(0, 0, 0)
-
- data_sample = KIEDataSample()
- instance_data = InstanceData()
- for key in self.mapping_table.keys():
- if key not in results:
- continue
- if key in ['gt_bboxes', 'gt_bboxes_labels', 'gt_edges_labels']:
- instance_data[self.mapping_table[key]] = to_tensor(
- results[key])
- else:
- instance_data[self.mapping_table[key]] = results[key]
- data_sample.gt_instances = instance_data
-
- img_meta = {}
- for key in self.meta_keys:
- img_meta[key] = results[key]
- data_sample.set_metainfo(img_meta)
- packed_results['data_samples'] = data_sample
-
- return packed_results
-
- def __repr__(self) -> str:
- repr_str = self.__class__.__name__
- repr_str += f'(meta_keys={self.meta_keys})'
- return repr_str
diff --git a/pipeline/mmocr/mmocr/datasets/transforms/loading.py b/pipeline/mmocr/mmocr/datasets/transforms/loading.py
deleted file mode 100644
index e9a3af8189edb4159a4676c6401a0364981bc4d7..0000000000000000000000000000000000000000
--- a/pipeline/mmocr/mmocr/datasets/transforms/loading.py
+++ /dev/null
@@ -1,572 +0,0 @@
-# Copyright (c) OpenMMLab. All rights reserved.
-import copy
-import warnings
-from typing import Optional, Union
-
-import mmcv
-import mmengine.fileio as fileio
-import numpy as np
-from mmcv.transforms import BaseTransform
-from mmcv.transforms import LoadAnnotations as MMCV_LoadAnnotations
-from mmcv.transforms import LoadImageFromFile as MMCV_LoadImageFromFile
-
-from mmocr.registry import TRANSFORMS
-
-
-@TRANSFORMS.register_module()
-class LoadImageFromFile(MMCV_LoadImageFromFile):
- """Load an image from file.
-
- Required Keys:
-
- - img_path
-
- Modified Keys:
-
- - img
- - img_shape
- - ori_shape
-
- Args:
- to_float32 (bool): Whether to convert the loaded image to a float32
- numpy array. If set to False, the loaded image is an uint8 array.
- Defaults to False.
- color_type (str): The flag argument for :func:``mmcv.imfrombytes``.
- Defaults to 'color'.
- imdecode_backend (str): The image decoding backend type. The backend
- argument for :func:``mmcv.imfrombytes``.
- See :func:``mmcv.imfrombytes`` for details.
- Defaults to 'cv2'.
- file_client_args (dict): Arguments to instantiate a FileClient.
- See :class:`mmengine.fileio.FileClient` for details.
- Defaults to None. It will be deprecated in future. Please use
- ``backend_args`` instead.
- Deprecated in version 1.0.0rc6.
- backend_args (dict, optional): Instantiates the corresponding file
- backend. It may contain `backend` key to specify the file
- backend. If it contains, the file backend corresponding to this
- value will be used and initialized with the remaining values,
- otherwise the corresponding file backend will be selected
- based on the prefix of the file path. Defaults to None.
- New in version 1.0.0rc6.
- ignore_empty (bool): Whether to allow loading empty image or file path
- not existent. Defaults to False.
- min_size (int): The minimum size of the image to be loaded. If the
- image is smaller than the minimum size, it will be regarded as a
- broken image. Defaults to 0.
- """
-
- def __init__(
- self,
- to_float32: bool = False,
- color_type: str = 'color',
- imdecode_backend: str = 'cv2',
- file_client_args: Optional[dict] = None,
- min_size: int = 0,
- ignore_empty: bool = False,
- *,
- backend_args: Optional[dict] = None,
- ) -> None:
- self.ignore_empty = ignore_empty
- self.to_float32 = to_float32
- self.color_type = color_type
- self.imdecode_backend = imdecode_backend
- self.min_size = min_size
- self.file_client_args = file_client_args
- self.backend_args = backend_args
- if file_client_args is not None:
- warnings.warn(
- '"file_client_args" will be deprecated in future. '
- 'Please use "backend_args" instead', DeprecationWarning)
- if backend_args is not None:
- raise ValueError(
- '"file_client_args" and "backend_args" cannot be set '
- 'at the same time.')
-
- self.file_client_args = file_client_args.copy()
- if backend_args is not None:
- self.backend_args = backend_args.copy()
-
- def transform(self, results: dict) -> Optional[dict]:
- """Functions to load image.
-
- Args:
- results (dict): Result dict from :obj:``mmcv.BaseDataset``.
-
- Returns:
- dict: The dict contains loaded image and meta information.
- """
-
- filename = results['img_path']
- try:
- if getattr(self, 'file_client_args', None) is not None:
- file_client = fileio.FileClient.infer_client(
- self.file_client_args, filename)
- img_bytes = file_client.get(filename)
- else:
- img_bytes = fileio.get(
- filename, backend_args=self.backend_args)
- img = mmcv.imfrombytes(
- img_bytes, flag=self.color_type, backend=self.imdecode_backend)
- except Exception as e:
- if self.ignore_empty:
- warnings.warn(f'Failed to load {filename} due to {e}')
- return None
- else:
- raise e
- if img is None or min(img.shape[:2]) < self.min_size:
- if self.ignore_empty:
- warnings.warn(f'Ignore broken image: {filename}')
- return None
- raise IOError(f'{filename} is broken')
-
- if self.to_float32:
- img = img.astype(np.float32)
-
- results['img'] = img
- results['img_shape'] = img.shape[:2]
- results['ori_shape'] = img.shape[:2]
- return results
-
- def __repr__(self):
- repr_str = (f'{self.__class__.__name__}('
- f'ignore_empty={self.ignore_empty}, '
- f'min_size={self.min_size}, '
- f'to_float32={self.to_float32}, '
- f"color_type='{self.color_type}', "
- f"imdecode_backend='{self.imdecode_backend}', ")
-
- if self.file_client_args is not None:
- repr_str += f'file_client_args={self.file_client_args})'
- else:
- repr_str += f'backend_args={self.backend_args})'
- return repr_str
-
-
-@TRANSFORMS.register_module()
-class LoadImageFromNDArray(LoadImageFromFile):
- """Load an image from ``results['img']``.
-
- Similar with :obj:`LoadImageFromFile`, but the image has been loaded as
- :obj:`np.ndarray` in ``results['img']``. Can be used when loading image
- from webcam.
-
- Required Keys:
-
- - img
-
- Modified Keys:
-
- - img
- - img_path
- - img_shape
- - ori_shape
-
- Args:
- to_float32 (bool): Whether to convert the loaded image to a float32
- numpy array. If set to False, the loaded image is an uint8 array.
- Defaults to False.
- """
-
- def transform(self, results: dict) -> dict:
- """Transform function to add image meta information.
-
- Args:
- results (dict): Result dict with Webcam read image in
- ``results['img']``.
-
- Returns:
- dict: The dict contains loaded image and meta information.
- """
-
- img = results['img']
- if self.to_float32:
- img = img.astype(np.float32)
- if self.color_type == 'grayscale':
- img = mmcv.image.rgb2gray(img)
- results['img'] = img
- if results.get('img_path', None) is None:
- results['img_path'] = None
- results['img_shape'] = img.shape[:2]
- results['ori_shape'] = img.shape[:2]
- return results
-
-
-@TRANSFORMS.register_module()
-class InferencerLoader(BaseTransform):
- """Load the image in Inferencer's pipeline.
-
- Modified Keys:
-
- - img
- - img_path
- - img_shape
- - ori_shape
-
- Args:
- to_float32 (bool): Whether to convert the loaded image to a float32
- numpy array. If set to False, the loaded image is an uint8 array.
- Defaults to False.
- """
-
- def __init__(self, **kwargs) -> None:
- super().__init__()
- self.from_file = TRANSFORMS.build(
- dict(type='LoadImageFromFile', **kwargs))
- self.from_ndarray = TRANSFORMS.build(
- dict(type='LoadImageFromNDArray', **kwargs))
-
- def transform(self, single_input: Union[str, np.ndarray, dict]) -> dict:
- """Transform function to add image meta information.
-
- Args:
- single_input (str or dict or np.ndarray): The raw input from
- inferencer.
-
- Returns:
- dict: The dict contains loaded image and meta information.
- """
- if isinstance(single_input, str):
- inputs = dict(img_path=single_input)
- elif isinstance(single_input, np.ndarray):
- inputs = dict(img=single_input)
- elif isinstance(single_input, dict):
- inputs = single_input
- else:
- raise NotImplementedError
-
- if 'img' in inputs:
- return self.from_ndarray(inputs)
-
- return self.from_file(inputs)
-
-
-@TRANSFORMS.register_module()
-class LoadOCRAnnotations(MMCV_LoadAnnotations):
- """Load and process the ``instances`` annotation provided by dataset.
-
- The annotation format is as the following:
-
- .. code-block:: python
-
- {
- 'instances':
- [
- {
- # List of 4 numbers representing the bounding box of the
- # instance, in (x1, y1, x2, y2) order.
- # used in text detection or text spotting tasks.
- 'bbox': [x1, y1, x2, y2],
-
- # Label of instance, usually it's 0.
- # used in text detection or text spotting tasks.
- 'bbox_label': 0,
-
- # List of n numbers representing the polygon of the
- # instance, in (xn, yn) order.
- # used in text detection/ textspotter.
- "polygon": [x1, y1, x2, y2, ... xn, yn],
-
- # The flag indicating whether the instance should be ignored.
- # used in text detection or text spotting tasks.
- "ignore": False,
-
- # The groundtruth of text.
- # used in text recognition or text spotting tasks.
- "text": 'tmp',
- }
- ]
- }
-
- After this module, the annotation has been changed to the format below:
-
- .. code-block:: python
-
- {
- # In (x1, y1, x2, y2) order, float type. N is the number of bboxes
- # in np.float32
- 'gt_bboxes': np.ndarray(N, 4)
- # In np.int64 type.
- 'gt_bboxes_labels': np.ndarray(N, )
- # In (x1, y1,..., xk, yk) order, float type.
- # in list[np.float32]
- 'gt_polygons': list[np.ndarray(2k, )]
- # In np.bool_ type.
- 'gt_ignored': np.ndarray(N, )
- # In list[str]
- 'gt_texts': list[str]
- }
-
- Required Keys:
-
- - instances
-
- - bbox (optional)
- - bbox_label (optional)
- - polygon (optional)
- - ignore (optional)
- - text (optional)
-
- Added Keys:
-
- - gt_bboxes (np.float32)
- - gt_bboxes_labels (np.int64)
- - gt_polygons (list[np.float32])
- - gt_ignored (np.bool_)
- - gt_texts (list[str])
-
- Args:
- with_bbox (bool): Whether to parse and load the bbox annotation.
- Defaults to False.
- with_label (bool): Whether to parse and load the label annotation.
- Defaults to False.
- with_polygon (bool): Whether to parse and load the polygon annotation.
- Defaults to False.
- with_text (bool): Whether to parse and load the text annotation.
- Defaults to False.
- """
-
- def __init__(self,
- with_bbox: bool = False,
- with_label: bool = False,
- with_polygon: bool = False,
- with_text: bool = False,
- **kwargs) -> None:
- super().__init__(with_bbox=with_bbox, with_label=with_label, **kwargs)
- self.with_polygon = with_polygon
- self.with_text = with_text
- self.with_ignore = with_bbox or with_polygon
-
- def _load_ignore_flags(self, results: dict) -> None:
- """Private function to load ignore annotations.
-
- Args:
- results (dict): Result dict from :obj:``OCRDataset``.
-
- Returns:
- dict: The dict contains loaded ignore annotations.
- """
- gt_ignored = []
- for instance in results['instances']:
- gt_ignored.append(instance['ignore'])
- results['gt_ignored'] = np.array(gt_ignored, dtype=np.bool_)
-
- def _load_polygons(self, results: dict) -> None:
- """Private function to load polygon annotations.
-
- Args:
- results (dict): Result dict from :obj:``OCRDataset``.
-
- Returns:
- dict: The dict contains loaded polygon annotations.
- """
-
- gt_polygons = []
- for instance in results['instances']:
- gt_polygons.append(np.array(instance['polygon'], dtype=np.float32))
- results['gt_polygons'] = gt_polygons
-
- def _load_texts(self, results: dict) -> None:
- """Private function to load text annotations.
-
- Args:
- results (dict): Result dict from :obj:``OCRDataset``.
-
- Returns:
- dict: The dict contains loaded text annotations.
- """
- gt_texts = []
- for instance in results['instances']:
- gt_texts.append(instance['text'])
- results['gt_texts'] = gt_texts
-
- def transform(self, results: dict) -> dict:
- """Function to load multiple types annotations.
-
- Args:
- results (dict): Result dict from :obj:``OCRDataset``.
-
- Returns:
- dict: The dict contains loaded bounding box, label polygon and
- text annotations.
- """
- results = super().transform(results)
- if self.with_polygon:
- self._load_polygons(results)
- if self.with_text:
- self._load_texts(results)
- if self.with_ignore:
- self._load_ignore_flags(results)
- return results
-
- def __repr__(self) -> str:
- repr_str = self.__class__.__name__
- repr_str += f'(with_bbox={self.with_bbox}, '
- repr_str += f'with_label={self.with_label}, '
- repr_str += f'with_polygon={self.with_polygon}, '
- repr_str += f'with_text={self.with_text}, '
- repr_str += f"imdecode_backend='{self.imdecode_backend}', "
-
- if self.file_client_args is not None:
- repr_str += f'file_client_args={self.file_client_args})'
- else:
- repr_str += f'backend_args={self.backend_args})'
- return repr_str
-
-
-@TRANSFORMS.register_module()
-class LoadKIEAnnotations(MMCV_LoadAnnotations):
- """Load and process the ``instances`` annotation provided by dataset.
-
- The annotation format is as the following:
-
- .. code-block:: python
-
- {
- # A nested list of 4 numbers representing the bounding box of the
- # instance, in (x1, y1, x2, y2) order.
- 'bbox': np.array([[x1, y1, x2, y2], [x1, y1, x2, y2], ...],
- dtype=np.int32),
-
- # Labels of boxes. Shape is (N,).
- 'bbox_labels': np.array([0, 2, ...], dtype=np.int32),
-
- # Labels of edges. Shape (N, N).
- 'edge_labels': np.array([0, 2, ...], dtype=np.int32),
-
- # List of texts.
- "texts": ['text1', 'text2', ...],
- }
-
- After this module, the annotation has been changed to the format below:
-
- .. code-block:: python
-
- {
- # In (x1, y1, x2, y2) order, float type. N is the number of bboxes
- # in np.float32
- 'gt_bboxes': np.ndarray(N, 4),
- # In np.int64 type.
- 'gt_bboxes_labels': np.ndarray(N, ),
- # In np.int32 type.
- 'gt_edges_labels': np.ndarray(N, N),
- # In list[str]
- 'gt_texts': list[str],
- # tuple(int)
- 'ori_shape': (H, W)
- }
-
- Required Keys:
-
- - bboxes
- - bbox_labels
- - edge_labels
- - texts
-
- Added Keys:
-
- - gt_bboxes (np.float32)
- - gt_bboxes_labels (np.int64)
- - gt_edges_labels (np.int64)
- - gt_texts (list[str])
- - ori_shape (tuple[int])
-
- Args:
- with_bbox (bool): Whether to parse and load the bbox annotation.
- Defaults to True.
- with_label (bool): Whether to parse and load the label annotation.
- Defaults to True.
- with_text (bool): Whether to parse and load the text annotation.
- Defaults to True.
- directed (bool): Whether build edges as a directed graph.
- Defaults to False.
- key_node_idx (int, optional): Key node label, used to mask out edges
- that are not connected from key nodes to value nodes. It has to be
- specified together with ``value_node_idx``. Defaults to None.
- value_node_idx (int, optional): Value node label, used to mask out
- edges that are not connected from key nodes to value nodes. It has
- to be specified together with ``key_node_idx``. Defaults to None.
- """
-
- def __init__(self,
- with_bbox: bool = True,
- with_label: bool = True,
- with_text: bool = True,
- directed: bool = False,
- key_node_idx: Optional[int] = None,
- value_node_idx: Optional[int] = None,
- **kwargs) -> None:
- super().__init__(with_bbox=with_bbox, with_label=with_label, **kwargs)
- self.with_text = with_text
- self.directed = directed
- if key_node_idx is not None or value_node_idx is not None:
- assert key_node_idx is not None and value_node_idx is not None
- self.key_node_idx = key_node_idx
- self.value_node_idx = value_node_idx
-
- def _load_texts(self, results: dict) -> None:
- """Private function to load text annotations.
-
- Args:
- results (dict): Result dict from :obj:``OCRDataset``.
- """
- gt_texts = []
- for instance in results['instances']:
- gt_texts.append(instance['text'])
- results['gt_texts'] = gt_texts
-
- def _load_labels(self, results: dict) -> None:
- """Private function to load label annotations.
-
- Args:
- results (dict): Result dict from :obj:``WildReceiptDataset``.
- """
- bbox_labels = []
- edge_labels = []
- for instance in results['instances']:
- bbox_labels.append(instance['bbox_label'])
- edge_labels.append(instance['edge_label'])
-
- bbox_labels = np.array(bbox_labels, np.int32)
- edge_labels = np.array(edge_labels)
- edge_labels = (edge_labels[:, None] == edge_labels[None, :]).astype(
- np.int32)
-
- if self.directed:
- edge_labels = (edge_labels & bbox_labels == 1).astype(np.int32)
-
- if hasattr(self, 'key_node_idx'):
- key_nodes_mask = bbox_labels == self.key_node_idx
- value_nodes_mask = bbox_labels == self.value_node_idx
- key2value_mask = key_nodes_mask[:,
- None] * value_nodes_mask[None, :]
- edge_labels[~key2value_mask] = -1
-
- np.fill_diagonal(edge_labels, -1)
-
- results['gt_edges_labels'] = edge_labels.astype(np.int64)
- results['gt_bboxes_labels'] = bbox_labels.astype(np.int64)
-
- def transform(self, results: dict) -> dict:
- """Function to load multiple types annotations.
-
- Args:
- results (dict): Result dict from :obj:``OCRDataset``.
-
- Returns:
- dict: The dict contains loaded bounding box, label polygon and
- text annotations.
- """
- if 'ori_shape' not in results:
- results['ori_shape'] = copy.deepcopy(results['img_shape'])
- results = super().transform(results)
- if self.with_text:
- self._load_texts(results)
- return results
-
- def __repr__(self) -> str:
- repr_str = self.__class__.__name__
- repr_str += f'(with_bbox={self.with_bbox}, '
- repr_str += f'with_label={self.with_label}, '
- repr_str += f'with_text={self.with_text})'
- return repr_str
diff --git a/pipeline/mmocr/mmocr/datasets/transforms/ocr_transforms.py b/pipeline/mmocr/mmocr/datasets/transforms/ocr_transforms.py
deleted file mode 100644
index a05984a78935a99de2c7eed92edf5f1f764c3997..0000000000000000000000000000000000000000
--- a/pipeline/mmocr/mmocr/datasets/transforms/ocr_transforms.py
+++ /dev/null
@@ -1,758 +0,0 @@
-# Copyright (c) OpenMMLab. All rights reserved.
-import math
-from typing import Dict, Tuple
-
-import cv2
-import mmcv
-import numpy as np
-from mmcv.transforms import Resize as MMCV_Resize
-from mmcv.transforms.base import BaseTransform
-from mmcv.transforms.utils import avoid_cache_randomness, cache_randomness
-
-from mmocr.registry import TRANSFORMS
-from mmocr.utils import (bbox2poly, crop_polygon, is_poly_inside_rect,
- poly2bbox, poly2shapely, poly_make_valid,
- remove_pipeline_elements, rescale_polygon,
- shapely2poly)
-from .wrappers import ImgAugWrapper
-
-
-@TRANSFORMS.register_module()
-@avoid_cache_randomness
-class RandomCrop(BaseTransform):
- """Randomly crop images and make sure to contain at least one intact
- instance.
-
- Required Keys:
-
- - img
- - gt_polygons
- - gt_bboxes
- - gt_bboxes_labels
- - gt_ignored
- - gt_texts (optional)
-
- Modified Keys:
-
- - img
- - img_shape
- - gt_polygons
- - gt_bboxes
- - gt_bboxes_labels
- - gt_ignored
- - gt_texts (optional)
-
- Args:
- min_side_ratio (float): The ratio of the shortest edge of the cropped
- image to the original image size.
- """
-
- def __init__(self, min_side_ratio: float = 0.4) -> None:
- if not 0. <= min_side_ratio <= 1.:
- raise ValueError('`min_side_ratio` should be in range [0, 1],')
- self.min_side_ratio = min_side_ratio
-
- def _sample_valid_start_end(self, valid_array: np.ndarray, min_len: int,
- max_start_idx: int,
- min_end_idx: int) -> Tuple[int, int]:
- """Sample a start and end idx on a given axis that contains at least
- one polygon. There should be at least one intact polygon bounded by
- max_start_idx and min_end_idx.
-
- Args:
- valid_array (ndarray): A 0-1 mask 1D array indicating valid regions
- on the axis. 0 indicates text regions which are not allowed to
- be sampled from.
- min_len (int): Minimum distance between two start and end points.
- max_start_idx (int): The maximum start index.
- min_end_idx (int): The minimum end index.
-
- Returns:
- tuple(int, int): Start and end index on a given axis, where
- 0 <= start < max_start_idx and
- min_end_idx <= end < len(valid_array).
- """
- assert isinstance(min_len, int)
- assert len(valid_array) > min_len
-
- start_array = valid_array.copy()
- max_start_idx = min(len(start_array) - min_len, max_start_idx)
- start_array[max_start_idx:] = 0
- start_array[0] = 1
- diff_array = np.hstack([0, start_array]) - np.hstack([start_array, 0])
- region_starts = np.where(diff_array < 0)[0]
- region_ends = np.where(diff_array > 0)[0]
- region_ind = np.random.randint(0, len(region_starts))
- start = np.random.randint(region_starts[region_ind],
- region_ends[region_ind])
-
- end_array = valid_array.copy()
- min_end_idx = max(start + min_len, min_end_idx)
- end_array[:min_end_idx] = 0
- end_array[-1] = 1
- diff_array = np.hstack([0, end_array]) - np.hstack([end_array, 0])
- region_starts = np.where(diff_array < 0)[0]
- region_ends = np.where(diff_array > 0)[0]
- region_ind = np.random.randint(0, len(region_starts))
- # Note that end index will never be region_ends[region_ind]
- # and therefore end index is always in range [0, w+1]
- end = np.random.randint(region_starts[region_ind],
- region_ends[region_ind])
- return start, end
-
- def _sample_crop_box(self, img_size: Tuple[int, int],
- results: Dict) -> np.ndarray:
- """Generate crop box which only contains intact polygon instances with
- the number >= 1.
-
- Args:
- img_size (tuple(int, int)): The image size (h, w).
- results (dict): The results dict.
-
- Returns:
- ndarray: Crop area in shape (4, ).
- """
- assert isinstance(img_size, tuple)
- h, w = img_size[:2]
-
- # Crop box can be represented by any integer numbers in
- # range [0, w] and [0, h]
- x_valid_array = np.ones(w + 1, dtype=np.int32)
- y_valid_array = np.ones(h + 1, dtype=np.int32)
-
- polygons = results['gt_polygons']
-
- # Randomly select a polygon that must be inside
- # the cropped region
- kept_poly_idx = np.random.randint(0, len(polygons))
- for i, polygon in enumerate(polygons):
- polygon = polygon.reshape((-1, 2))
-
- clip_x = np.clip(polygon[:, 0], 0, w)
- clip_y = np.clip(polygon[:, 1], 0, h)
- min_x = np.floor(np.min(clip_x)).astype(np.int32)
- min_y = np.floor(np.min(clip_y)).astype(np.int32)
- max_x = np.ceil(np.max(clip_x)).astype(np.int32)
- max_y = np.ceil(np.max(clip_y)).astype(np.int32)
-
- x_valid_array[min_x:max_x] = 0
- y_valid_array[min_y:max_y] = 0
-
- if i == kept_poly_idx:
- max_x_start = min_x
- min_x_end = max_x
- max_y_start = min_y
- min_y_end = max_y
-
- min_w = int(w * self.min_side_ratio)
- min_h = int(h * self.min_side_ratio)
-
- x1, x2 = self._sample_valid_start_end(x_valid_array, min_w,
- max_x_start, min_x_end)
- y1, y2 = self._sample_valid_start_end(y_valid_array, min_h,
- max_y_start, min_y_end)
-
- return np.array([x1, y1, x2, y2])
-
- def _crop_img(self, img: np.ndarray, bbox: np.ndarray) -> np.ndarray:
- """Crop image given a bbox region.
- Args:
- img (ndarray): Image.
- bbox (ndarray): Cropping region in shape (4, )
-
- Returns:
- ndarray: Cropped image.
- """
- assert img.ndim == 3
- h, w, _ = img.shape
- assert 0 <= bbox[1] < bbox[3] <= h
- assert 0 <= bbox[0] < bbox[2] <= w
- return img[bbox[1]:bbox[3], bbox[0]:bbox[2]]
-
- def transform(self, results: Dict) -> Dict:
- """Applying random crop on results.
- Args:
- results (dict): Result dict contains the data to transform.
-
- Returns:
- dict: The transformed data.
- """
- if len(results['gt_polygons']) < 1:
- return results
-
- crop_box = self._sample_crop_box(results['img'].shape, results)
- img = self._crop_img(results['img'], crop_box)
- results['img'] = img
- results['img_shape'] = img.shape[:2]
- crop_x = crop_box[0]
- crop_y = crop_box[1]
- crop_w = crop_box[2] - crop_box[0]
- crop_h = crop_box[3] - crop_box[1]
-
- labels = results['gt_bboxes_labels']
- valid_labels = []
- ignored = results['gt_ignored']
- valid_ignored = []
- if 'gt_texts' in results:
- valid_texts = []
- texts = results['gt_texts']
-
- polys = results['gt_polygons']
- valid_polys = []
- for idx, poly in enumerate(polys):
- poly = poly.reshape(-1, 2)
- poly = (poly - (crop_x, crop_y)).flatten()
- if is_poly_inside_rect(poly, [0, 0, crop_w, crop_h]):
- valid_polys.append(poly)
- valid_labels.append(labels[idx])
- valid_ignored.append(ignored[idx])
- if 'gt_texts' in results:
- valid_texts.append(texts[idx])
- results['gt_polygons'] = valid_polys
- results['gt_bboxes_labels'] = np.array(valid_labels, dtype=np.int64)
- results['gt_ignored'] = np.array(valid_ignored, dtype=bool)
- if 'gt_texts' in results:
- results['gt_texts'] = valid_texts
- valid_bboxes = [poly2bbox(poly) for poly in results['gt_polygons']]
- results['gt_bboxes'] = np.array(valid_bboxes).astype(
- np.float32).reshape(-1, 4)
-
- return results
-
- def __repr__(self) -> str:
- repr_str = self.__class__.__name__
- repr_str += f'(min_side_ratio = {self.min_side_ratio})'
- return repr_str
-
-
-@TRANSFORMS.register_module()
-class RandomRotate(BaseTransform):
- """Randomly rotate the image, boxes, and polygons. For recognition task,
- only the image will be rotated. If set ``use_canvas`` as True, the shape of
- rotated image might be modified based on the rotated angle size, otherwise,
- the image will keep the shape before rotation.
-
- Required Keys:
-
- - img
- - img_shape
- - gt_bboxes (optional)
- - gt_polygons (optional)
-
- Modified Keys:
-
- - img
- - img_shape (optional)
- - gt_bboxes (optional)
- - gt_polygons (optional)
-
- Added Keys:
-
- - rotated_angle
-
- Args:
- max_angle (int): The maximum rotation angle (can be bigger than 180 or
- a negative). Defaults to 10.
- pad_with_fixed_color (bool): The flag for whether to pad rotated
- image with fixed value. Defaults to False.
- pad_value (tuple[int, int, int]): The color value for padding rotated
- image. Defaults to (0, 0, 0).
- use_canvas (bool): Whether to create a canvas for rotated image.
- Defaults to False. If set true, the image shape may be modified.
- """
-
- def __init__(
- self,
- max_angle: int = 10,
- pad_with_fixed_color: bool = False,
- pad_value: Tuple[int, int, int] = (0, 0, 0),
- use_canvas: bool = False,
- ) -> None:
- if not isinstance(max_angle, int):
- raise TypeError('`max_angle` should be an integer'
- f', but got {type(max_angle)} instead')
- if not isinstance(pad_with_fixed_color, bool):
- raise TypeError('`pad_with_fixed_color` should be a bool, '
- f'but got {type(pad_with_fixed_color)} instead')
- if not isinstance(pad_value, (list, tuple)):
- raise TypeError('`pad_value` should be a list or tuple, '
- f'but got {type(pad_value)} instead')
- if len(pad_value) != 3:
- raise ValueError('`pad_value` should contain three integers')
- if not isinstance(pad_value[0], int) or not isinstance(
- pad_value[1], int) or not isinstance(pad_value[2], int):
- raise ValueError('`pad_value` should contain three integers')
-
- self.max_angle = max_angle
- self.pad_with_fixed_color = pad_with_fixed_color
- self.pad_value = pad_value
- self.use_canvas = use_canvas
-
- @cache_randomness
- def _sample_angle(self, max_angle: int) -> float:
- """Sampling a random angle for rotation.
-
- Args:
- max_angle (int): Maximum rotation angle
-
- Returns:
- float: The random angle used for rotation
- """
- angle = np.random.random_sample() * 2 * max_angle - max_angle
- return angle
-
- @staticmethod
- def _cal_canvas_size(ori_size: Tuple[int, int],
- degree: int) -> Tuple[int, int]:
- """Calculate the canvas size.
-
- Args:
- ori_size (Tuple[int, int]): The original image size (height, width)
- degree (int): The rotation angle
-
- Returns:
- Tuple[int, int]: The size of the canvas
- """
- assert isinstance(ori_size, tuple)
- angle = degree * math.pi / 180.0
- h, w = ori_size[:2]
-
- cos = math.cos(angle)
- sin = math.sin(angle)
- canvas_h = int(w * math.fabs(sin) + h * math.fabs(cos))
- canvas_w = int(w * math.fabs(cos) + h * math.fabs(sin))
-
- canvas_size = (canvas_h, canvas_w)
- return canvas_size
-
- @staticmethod
- def _rotate_points(center: Tuple[float, float],
- points: np.array,
- theta: float,
- center_shift: Tuple[int, int] = (0, 0)) -> np.array:
- """Rotating a set of points according to the given theta.
-
- Args:
- center (Tuple[float, float]): The coordinate of the canvas center
- points (np.array): A set of points needed to be rotated
- theta (float): Rotation angle
- center_shift (Tuple[int, int]): The shifting offset of the center
- coordinate
-
- Returns:
- np.array: The rotated coordinates of the input points
- """
- (center_x, center_y) = center
- center_y = -center_y
- x, y = points[::2], points[1::2]
- y = -y
-
- theta = theta / 180 * math.pi
- cos = math.cos(theta)
- sin = math.sin(theta)
-
- x = (x - center_x)
- y = (y - center_y)
-
- _x = center_x + x * cos - y * sin + center_shift[0]
- _y = -(center_y + x * sin + y * cos) + center_shift[1]
-
- points[::2], points[1::2] = _x, _y
- return points
-
- def _rotate_img(self, results: Dict) -> Tuple[int, int]:
- """Rotating the input image based on the given angle.
-
- Args:
- results (dict): Result dict containing the data to transform.
-
- Returns:
- Tuple[int, int]: The shifting offset of the center point.
- """
- if results.get('img', None) is not None:
- h = results['img'].shape[0]
- w = results['img'].shape[1]
- rotation_matrix = cv2.getRotationMatrix2D(
- (w / 2, h / 2), results['rotated_angle'], 1)
-
- canvas_size = self._cal_canvas_size((h, w),
- results['rotated_angle'])
- center_shift = (int(
- (canvas_size[1] - w) / 2), int((canvas_size[0] - h) / 2))
- rotation_matrix[0, 2] += int((canvas_size[1] - w) / 2)
- rotation_matrix[1, 2] += int((canvas_size[0] - h) / 2)
- if self.pad_with_fixed_color:
- rotated_img = cv2.warpAffine(
- results['img'],
- rotation_matrix, (canvas_size[1], canvas_size[0]),
- flags=cv2.INTER_NEAREST,
- borderValue=self.pad_value)
- else:
- mask = np.zeros_like(results['img'])
- (h_ind, w_ind) = (np.random.randint(0, h * 7 // 8),
- np.random.randint(0, w * 7 // 8))
- img_cut = results['img'][h_ind:(h_ind + h // 9),
- w_ind:(w_ind + w // 9)]
- img_cut = mmcv.imresize(img_cut,
- (canvas_size[1], canvas_size[0]))
- mask = cv2.warpAffine(
- mask,
- rotation_matrix, (canvas_size[1], canvas_size[0]),
- borderValue=[1, 1, 1])
- rotated_img = cv2.warpAffine(
- results['img'],
- rotation_matrix, (canvas_size[1], canvas_size[0]),
- borderValue=[0, 0, 0])
- rotated_img = rotated_img + img_cut * mask
-
- results['img'] = rotated_img
- else:
- raise ValueError('`img` is not found in results')
-
- return center_shift
-
- def _rotate_bboxes(self, results: Dict, center_shift: Tuple[int,
- int]) -> None:
- """Rotating the bounding boxes based on the given angle.
-
- Args:
- results (dict): Result dict containing the data to transform.
- center_shift (Tuple[int, int]): The shifting offset of the
- center point
- """
- if results.get('gt_bboxes', None) is not None:
- height, width = results['img_shape']
- box_list = []
- for box in results['gt_bboxes']:
- rotated_box = self._rotate_points((width / 2, height / 2),
- bbox2poly(box),
- results['rotated_angle'],
- center_shift)
- rotated_box = poly2bbox(rotated_box)
- box_list.append(rotated_box)
-
- results['gt_bboxes'] = np.array(
- box_list, dtype=np.float32).reshape(-1, 4)
-
- def _rotate_polygons(self, results: Dict,
- center_shift: Tuple[int, int]) -> None:
- """Rotating the polygons based on the given angle.
-
- Args:
- results (dict): Result dict containing the data to transform.
- center_shift (Tuple[int, int]): The shifting offset of the
- center point
- """
- if results.get('gt_polygons', None) is not None:
- height, width = results['img_shape']
- polygon_list = []
- for poly in results['gt_polygons']:
- rotated_poly = self._rotate_points(
- (width / 2, height / 2), poly, results['rotated_angle'],
- center_shift)
- polygon_list.append(rotated_poly)
- results['gt_polygons'] = polygon_list
-
- def transform(self, results: Dict) -> Dict:
- """Applying random rotate on results.
-
- Args:
- results (Dict): Result dict containing the data to transform.
- center_shift (Tuple[int, int]): The shifting offset of the
- center point
-
- Returns:
- dict: The transformed data
- """
- # TODO rotate char_quads & char_rects for SegOCR
- if self.use_canvas:
- results['rotated_angle'] = self._sample_angle(self.max_angle)
- # rotate image
- center_shift = self._rotate_img(results)
- # rotate gt_bboxes
- self._rotate_bboxes(results, center_shift)
- # rotate gt_polygons
- self._rotate_polygons(results, center_shift)
-
- results['img_shape'] = (results['img'].shape[0],
- results['img'].shape[1])
- else:
- args = [
- dict(
- cls='Affine',
- rotate=[-self.max_angle, self.max_angle],
- backend='cv2',
- order=0) # order=0 -> cv2.INTER_NEAREST
- ]
- imgaug_transform = ImgAugWrapper(args)
- results = imgaug_transform(results)
- return results
-
- def __repr__(self) -> str:
- repr_str = self.__class__.__name__
- repr_str += f'(max_angle = {self.max_angle}'
- repr_str += f', pad_with_fixed_color = {self.pad_with_fixed_color}'
- repr_str += f', pad_value = {self.pad_value}'
- repr_str += f', use_canvas = {self.use_canvas})'
- return repr_str
-
-
-@TRANSFORMS.register_module()
-class Resize(MMCV_Resize):
- """Resize image & bboxes & polygons.
-
- This transform resizes the input image according to ``scale`` or
- ``scale_factor``. Bboxes and polygons are then resized with the same
- scale factor. if ``scale`` and ``scale_factor`` are both set, it will use
- ``scale`` to resize.
-
- Required Keys:
-
- - img
- - img_shape
- - gt_bboxes
- - gt_polygons
-
-
- Modified Keys:
-
- - img
- - img_shape
- - gt_bboxes
- - gt_polygons
-
- Added Keys:
-
- - scale
- - scale_factor
- - keep_ratio
-
- Args:
- scale (int or tuple): Image scales for resizing. Defaults to None.
- scale_factor (float or tuple[float, float]): Scale factors for
- resizing. It's either a factor applicable to both dimensions or
- in the form of (scale_w, scale_h). Defaults to None.
- keep_ratio (bool): Whether to keep the aspect ratio when resizing the
- image. Defaults to False.
- clip_object_border (bool): Whether to clip the objects outside the
- border of the image. Defaults to True.
- backend (str): Image resize backend, choices are 'cv2' and 'pillow'.
- These two backends generates slightly different results. Defaults
- to 'cv2'.
- interpolation (str): Interpolation method, accepted values are
- "nearest", "bilinear", "bicubic", "area", "lanczos" for 'cv2'
- backend, "nearest", "bilinear" for 'pillow' backend. Defaults
- to 'bilinear'.
- """
-
- def _resize_img(self, results: dict) -> None:
- """Resize images with ``results['scale']``.
-
- If no image is provided, only resize ``results['img_shape']``.
- """
- if results.get('img', None) is not None:
- return super()._resize_img(results)
- h, w = results['img_shape']
- if self.keep_ratio:
- new_w, new_h = mmcv.rescale_size((w, h),
- results['scale'],
- return_scale=False)
- else:
- new_w, new_h = results['scale']
- w_scale = new_w / w
- h_scale = new_h / h
- results['img_shape'] = (new_h, new_w)
- results['scale'] = (new_w, new_h)
- results['scale_factor'] = (w_scale, h_scale)
- results['keep_ratio'] = self.keep_ratio
-
- def _resize_bboxes(self, results: dict) -> None:
- """Resize bounding boxes."""
- super()._resize_bboxes(results)
- if results.get('gt_bboxes', None) is not None:
- results['gt_bboxes'] = results['gt_bboxes'].astype(np.float32)
-
- def _resize_polygons(self, results: dict) -> None:
- """Resize polygons with ``results['scale_factor']``."""
- if results.get('gt_polygons', None) is not None:
- polygons = results['gt_polygons']
- polygons_resize = []
- for idx, polygon in enumerate(polygons):
- polygon = rescale_polygon(polygon, results['scale_factor'])
- if self.clip_object_border:
- crop_bbox = np.array([
- 0, 0, results['img_shape'][1], results['img_shape'][0]
- ])
- polygon = crop_polygon(polygon, crop_bbox)
- if polygon is not None:
- polygons_resize.append(polygon.astype(np.float32))
- else:
- polygons_resize.append(
- np.zeros_like(polygons[idx], dtype=np.float32))
- results['gt_polygons'] = polygons_resize
-
- def transform(self, results: dict) -> dict:
- """Transform function to resize images, bounding boxes and polygons.
-
- Args:
- results (dict): Result dict from loading pipeline.
-
- Returns:
- dict: Resized results, 'img', 'gt_bboxes', 'gt_polygons',
- 'scale', 'scale_factor', 'height', 'width', and 'keep_ratio' keys
- are updated in result dict.
- """
- results = super().transform(results)
- self._resize_polygons(results)
- return results
-
- def __repr__(self):
- repr_str = self.__class__.__name__
- repr_str += f'(scale={self.scale}, '
- repr_str += f'scale_factor={self.scale_factor}, '
- repr_str += f'keep_ratio={self.keep_ratio}, '
- repr_str += f'clip_object_border={self.clip_object_border}), '
- repr_str += f'backend={self.backend}), '
- repr_str += f'interpolation={self.interpolation})'
- return repr_str
-
-
-@TRANSFORMS.register_module()
-class RemoveIgnored(BaseTransform):
- """Removed ignored elements from the pipeline.
-
- Required Keys:
-
- - gt_ignored
- - gt_polygons (optional)
- - gt_bboxes (optional)
- - gt_bboxes_labels (optional)
- - gt_texts (optional)
-
- Modified Keys:
-
- - gt_ignored
- - gt_polygons (optional)
- - gt_bboxes (optional)
- - gt_bboxes_labels (optional)
- - gt_texts (optional)
- """
-
- def transform(self, results: Dict) -> Dict:
- remove_inds = np.where(results['gt_ignored'])[0]
- if len(remove_inds) == len(results['gt_ignored']):
- return None
- return remove_pipeline_elements(results, remove_inds)
-
-
-@TRANSFORMS.register_module()
-class FixInvalidPolygon(BaseTransform):
- """Fix invalid polygons in the dataset.
-
- Required Keys:
-
- - gt_polygons
- - gt_ignored (optional)
- - gt_bboxes (optional)
- - gt_bboxes_labels (optional)
- - gt_texts (optional)
-
- Modified Keys:
-
- - gt_polygons
- - gt_ignored (optional)
- - gt_bboxes (optional)
- - gt_bboxes_labels (optional)
- - gt_texts (optional)
-
- Args:
- mode (str): The mode of fixing invalid polygons. Options are 'fix' and
- 'ignore'.
- For the 'fix' mode, the transform will try to fix
- the invalid polygons to a valid one by eliminating the
- self-intersection or converting the bboxes to polygons. If
- it can't be fixed by any means (e.g. the polygon contains less
- than 3 points or it's actually a line/point), the annotation will
- be removed.
- For the 'ignore' mode, the invalid polygons
- will be set to "ignored" during training.
- Defaults to 'fix'.
- min_poly_points (int): Minimum number of the coordinate points in a
- polygon. Defaults to 4.
- fix_from_bbox (bool): Whether to convert the bboxes to polygons when
- the polygon is invalid and not directly fixable. Defaults to True.
- """
-
- def __init__(self,
- mode: str = 'fix',
- min_poly_points: int = 4,
- fix_from_bbox: bool = True) -> None:
- super().__init__()
- self.mode = mode
- assert min_poly_points >= 3, 'min_poly_points must be greater than 3.'
- self.min_poly_points = min_poly_points
- self.fix_from_bbox = fix_from_bbox
- assert self.mode in [
- 'fix', 'ignore'
- ], f"Supported modes are 'fix' and 'ignore', but got {self.mode}"
-
- def transform(self, results: Dict) -> Dict:
- """Fix invalid polygons.
-
- Args:
- results (dict): Result dict containing the data to transform.
-
- Returns:
- Optional[dict]: The transformed data. If all the polygons are
- unfixable, return None.
- """
- if results.get('gt_polygons', None) is not None:
- remove_inds = []
- for idx, polygon in enumerate(results['gt_polygons']):
- if self.mode == 'ignore':
- if results['gt_ignored'][idx]:
- continue
- if not (len(polygon) >= self.min_poly_points * 2
- and len(polygon) % 2
- == 0) or not poly2shapely(polygon).is_valid:
- results['gt_ignored'][idx] = True
- else:
- # If "polygon" contains less than 3 points
- if len(polygon) < 6:
- remove_inds.append(idx)
- continue
- try:
- shapely_polygon = poly2shapely(polygon)
- if shapely_polygon.is_valid and len(
- polygon) >= self.min_poly_points * 2:
- continue
- results['gt_polygons'][idx] = shapely2poly(
- poly_make_valid(shapely_polygon))
- # If an empty polygon is generated, it's still a bad
- # fix
- if len(results['gt_polygons'][idx]) == 0:
- raise ValueError
- # It's hard to fix, e.g. the "polygon" is a line or
- # a point
- except Exception:
- if self.fix_from_bbox and 'gt_bboxes' in results:
- bbox = results['gt_bboxes'][idx]
- bbox_polygon = bbox2poly(bbox)
- results['gt_polygons'][idx] = bbox_polygon
- shapely_polygon = poly2shapely(bbox_polygon)
- if (not shapely_polygon.is_valid
- or shapely_polygon.is_empty):
- remove_inds.append(idx)
- else:
- remove_inds.append(idx)
- if len(remove_inds) == len(results['gt_polygons']):
- return None
- results = remove_pipeline_elements(results, remove_inds)
- return results
-
- def __repr__(self) -> str:
- repr_str = self.__class__.__name__
- repr_str += f'(mode = "{self.mode}", '
- repr_str += f'min_poly_points = {self.min_poly_points}, '
- repr_str += f'fix_from_bbox = {self.fix_from_bbox})'
- return repr_str
diff --git a/pipeline/mmocr/mmocr/datasets/transforms/textdet_transforms.py b/pipeline/mmocr/mmocr/datasets/transforms/textdet_transforms.py
deleted file mode 100644
index 537c9bd323888e8906e287ce72a77d1af4d48582..0000000000000000000000000000000000000000
--- a/pipeline/mmocr/mmocr/datasets/transforms/textdet_transforms.py
+++ /dev/null
@@ -1,854 +0,0 @@
-# Copyright (c) OpenMMLab. All rights reserved.
-import math
-import random
-from typing import Dict, List, Sequence, Tuple, Union
-
-import cv2
-import mmcv
-import numpy as np
-from mmcv.transforms import RandomFlip as MMCV_RandomFlip
-from mmcv.transforms.base import BaseTransform
-from mmcv.transforms.utils import avoid_cache_randomness, cache_randomness
-from shapely.geometry import Polygon as plg
-
-from mmocr.registry import TRANSFORMS
-from mmocr.utils import crop_polygon, poly2bbox, poly_intersection
-
-
-@TRANSFORMS.register_module()
-@avoid_cache_randomness
-class BoundedScaleAspectJitter(BaseTransform):
- """First randomly rescale the image so that the longside and shortside of
- the image are around the bound; then jitter its aspect ratio.
-
- Required Keys:
-
- - img
- - img_shape
- - gt_bboxes (optional)
- - gt_polygons (optional)
-
- Modified Keys:
-
- - img
- - img_shape
- - gt_bboxes (optional)
- - gt_polygons (optional)
-
- Added Keys:
-
- - scale
- - scale_factor
- - keep_ratio
-
- Args:
- long_size_bound (int): The approximate bound for long size.
- short_size_bound (int): The approximate bound for short size.
- size_jitter_range (tuple(float, float)): Range of the ratio used
- to jitter the size. Defaults to (0.7, 1.3).
- aspect_ratio_jitter_range (tuple(float, float)): Range of the ratio
- used to jitter its aspect ratio. Defaults to (0.9, 1.1).
- resize_type (str): The type of resize class to use. Defaults to
- "Resize".
- **resize_kwargs: Other keyword arguments for the ``resize_type``.
- """
-
- def __init__(
- self,
- long_size_bound: int,
- short_size_bound: int,
- ratio_range: Tuple[float, float] = (0.7, 1.3),
- aspect_ratio_range: Tuple[float, float] = (0.9, 1.1),
- resize_type: str = 'Resize',
- **resize_kwargs,
- ) -> None:
- super().__init__()
- self.ratio_range = ratio_range
- self.aspect_ratio_range = aspect_ratio_range
- self.long_size_bound = long_size_bound
- self.short_size_bound = short_size_bound
- self.resize_cfg = dict(type=resize_type, **resize_kwargs)
- # create an empty Reisize object
- self.resize_cfg.update(dict(scale=0))
- self.resize = TRANSFORMS.build(self.resize_cfg)
-
- def _sample_from_range(self, range: Tuple[float, float]) -> float:
- """A ratio will be randomly sampled from the range specified by
- ``range``.
-
- Args:
- ratio_range (tuple[float]): The minimum and maximum ratio.
-
- Returns:
- float: A ratio randomly sampled from the range.
- """
- min_value, max_value = min(range), max(range)
- value = np.random.random_sample() * (max_value - min_value) + min_value
- return value
-
- def transform(self, results: Dict) -> Dict:
- h, w = results['img'].shape[:2]
- new_scale = 1
- if max(h, w) > self.long_size_bound:
- new_scale = self.long_size_bound / max(h, w)
- jitter_ratio = self._sample_from_range(self.ratio_range)
- jitter_ratio = new_scale * jitter_ratio
- if min(h, w) * jitter_ratio <= self.short_size_bound:
- jitter_ratio = (self.short_size_bound + 10) * 1.0 / min(h, w)
- aspect = self._sample_from_range(self.aspect_ratio_range)
- h_scale = jitter_ratio * math.sqrt(aspect)
- w_scale = jitter_ratio / math.sqrt(aspect)
- new_h = int(h * h_scale)
- new_w = int(w * w_scale)
-
- self.resize.scale = (new_w, new_h)
- return self.resize(results)
-
- def __repr__(self) -> str:
- repr_str = self.__class__.__name__
- repr_str += f'(long_size_bound = {self.long_size_bound}, '
- repr_str += f'short_size_bound = {self.short_size_bound}, '
- repr_str += f'ratio_range = {self.ratio_range}, '
- repr_str += f'aspect_ratio_range = {self.aspect_ratio_range}, '
- repr_str += f'resize_cfg = {self.resize_cfg})'
- return repr_str
-
-
-@TRANSFORMS.register_module()
-class RandomFlip(MMCV_RandomFlip):
- """Flip the image & bbox polygon.
-
- There are 3 flip modes:
-
- - ``prob`` is float, ``direction`` is string: the image will be
- ``direction``ly flipped with probability of ``prob`` .
- E.g., ``prob=0.5``, ``direction='horizontal'``,
- then image will be horizontally flipped with probability of 0.5.
- - ``prob`` is float, ``direction`` is list of string: the image will
- be ``direction[i]``ly flipped with probability of
- ``prob/len(direction)``.
- E.g., ``prob=0.5``, ``direction=['horizontal', 'vertical']``,
- then image will be horizontally flipped with probability of 0.25,
- vertically with probability of 0.25.
- - ``prob`` is list of float, ``direction`` is list of string:
- given ``len(prob) == len(direction)``, the image will
- be ``direction[i]``ly flipped with probability of ``prob[i]``.
- E.g., ``prob=[0.3, 0.5]``, ``direction=['horizontal',
- 'vertical']``, then image will be horizontally flipped with
- probability of 0.3, vertically with probability of 0.5.
-
- Required Keys:
- - img
- - gt_bboxes (optional)
- - gt_polygons (optional)
-
- Modified Keys:
- - img
- - gt_bboxes (optional)
- - gt_polygons (optional)
-
- Added Keys:
- - flip
- - flip_direction
- Args:
- prob (float | list[float], optional): The flipping probability.
- Defaults to None.
- direction(str | list[str]): The flipping direction. Options
- If input is a list, the length must equal ``prob``. Each
- element in ``prob`` indicates the flip probability of
- corresponding direction. Defaults to 'horizontal'.
- """
-
- def flip_polygons(self, polygons: Sequence[np.ndarray],
- img_shape: Tuple[int, int],
- direction: str) -> Sequence[np.ndarray]:
- """Flip polygons horizontally, vertically or diagonally.
-
- Args:
- polygons (list[numpy.ndarray): polygons.
- img_shape (tuple[int]): Image shape (height, width)
- direction (str): Flip direction. Options are 'horizontal',
- 'vertical' and 'diagonal'.
- Returns:
- list[numpy.ndarray]: Flipped polygons.
- """
-
- h, w = img_shape
- flipped_polygons = []
- if direction == 'horizontal':
- for polygon in polygons:
- flipped_polygon = polygon.copy()
- flipped_polygon[0::2] = w - polygon[0::2]
- flipped_polygons.append(flipped_polygon)
- elif direction == 'vertical':
- for polygon in polygons:
- flipped_polygon = polygon.copy()
- flipped_polygon[1::2] = h - polygon[1::2]
- flipped_polygons.append(flipped_polygon)
- elif direction == 'diagonal':
- for polygon in polygons:
- flipped_polygon = polygon.copy()
- flipped_polygon[0::2] = w - polygon[0::2]
- flipped_polygon[1::2] = h - polygon[1::2]
- flipped_polygons.append(flipped_polygon)
- else:
- raise ValueError(
- f"Flipping direction must be 'horizontal', 'vertical', \
- or 'diagnal', but got '{direction}'")
- return flipped_polygons
-
- def _flip(self, results: dict) -> None:
- """Flip images, bounding boxes and polygons.
-
- Args:
- results (dict): Result dict containing the data to transform.
- """
- super()._flip(results)
- # flip polygons
- if results.get('gt_polygons', None) is not None:
- results['gt_polygons'] = self.flip_polygons(
- results['gt_polygons'], results['img'].shape[:2],
- results['flip_direction'])
-
-
-@TRANSFORMS.register_module()
-class SourceImagePad(BaseTransform):
- """Pad Image to target size. It will randomly crop an area from the
- original image and resize it to the target size, then paste the original
- image to its top left corner.
-
- Required Keys:
-
- - img
-
- Modified Keys:
-
- - img
- - img_shape
-
- Added Keys:
- - pad_shape
- - pad_fixed_size
-
- Args:
- target_scale (int or tuple[int, int]]): The target size of padded
- image. If it's an integer, then the padding size would be
- (target_size, target_size). If it's tuple, then ``target_scale[0]``
- should be the width and ``target_scale[1]`` should be the height.
- The size of the padded image will be (target_scale[1],
- target_scale[0])
- crop_ratio (float or Tuple[float, float]): Relative size for the
- crop region. If ``crop_ratio`` is a float, then the initial crop
- size would be
- ``(crop_ratio * img.shape[0], crop_ratio * img.shape[1])`` . If
- ``crop_ratio`` is a tuple, then ``crop_ratio[0]`` is for the width
- and ``crop_ratio[1]`` is for the height. The initial crop size
- would be
- ``(crop_ratio[1] * img.shape[0], crop_ratio[0] * img.shape[1])``.
- Defaults to 1./9.
- """
-
- def __init__(self,
- target_scale: Union[int, Tuple[int, int]],
- crop_ratio: Union[float, Tuple[float,
- float]] = 1. / 9) -> None:
- self.target_scale = target_scale if isinstance(
- target_scale, tuple) else (target_scale, target_scale)
- self.crop_ratio = crop_ratio if isinstance(
- crop_ratio, tuple) else (crop_ratio, crop_ratio)
-
- def transform(self, results: Dict) -> Dict:
- """Pad Image to target size. It will randomly select a small area from
- the original image and resize it to the target size, then paste the
- original image to its top left corner.
-
- Args:
- results (Dict): Result dict containing the data to transform.
-
- Returns:
- (Dict): The transformed data.
- """
- img = results['img']
- h, w = img.shape[:2]
- assert h <= self.target_scale[1] and w <= self.target_scale[
- 0], 'image size should be smaller that the target size'
- h_ind = np.random.randint(0, int(h - h * self.crop_ratio[1]) + 1)
- w_ind = np.random.randint(0, int(w - w * self.crop_ratio[0]) + 1)
- img_cut = img[h_ind:int(h_ind + h * self.crop_ratio[1]),
- w_ind:int(w_ind + w * self.crop_ratio[1])]
- expand_img = mmcv.imresize(img_cut, self.target_scale)
- # paste img to the top left corner of the padding region
- expand_img[0:h, 0:w] = img
- results['img'] = expand_img
- results['img_shape'] = expand_img.shape[:2]
- results['pad_shape'] = expand_img.shape
- results['pad_fixed_size'] = self.target_scale
- return results
-
- def __repr__(self) -> str:
- repr_str = self.__class__.__name__
- repr_str += f'(target_scale = {self.target_scale}, '
- repr_str += f'crop_ratio = {self.crop_ratio})'
- return repr_str
-
-
-@TRANSFORMS.register_module()
-@avoid_cache_randomness
-class ShortScaleAspectJitter(BaseTransform):
- """First rescale the image for its shorter side to reach the short_size and
- then jitter its aspect ratio, final rescale the shape guaranteed to be
- divided by scale_divisor.
-
- Required Keys:
-
- - img
- - img_shape
- - gt_bboxes (optional)
- - gt_polygons (optional)
-
-
- Modified Keys:
-
- - img
- - img_shape
- - gt_bboxes (optional)
- - gt_polygons (optional)
-
- Added Keys:
-
- - scale
- - scale_factor
- - keep_ratio
-
- Args:
- short_size (int): Target shorter size before jittering the aspect
- ratio. Defaults to 736.
- short_size_jitter_range (tuple(float, float)): Range of the ratio used
- to jitter the target shorter size. Defaults to (0.7, 1.3).
- aspect_ratio_jitter_range (tuple(float, float)): Range of the ratio
- used to jitter its aspect ratio. Defaults to (0.9, 1.1).
- scale_divisor (int): The scale divisor. Defaults to 1.
- resize_type (str): The type of resize class to use. Defaults to
- "Resize".
- **resize_kwargs: Other keyword arguments for the ``resize_type``.
- """
-
- def __init__(self,
- short_size: int = 736,
- ratio_range: Tuple[float, float] = (0.7, 1.3),
- aspect_ratio_range: Tuple[float, float] = (0.9, 1.1),
- scale_divisor: int = 1,
- resize_type: str = 'Resize',
- **resize_kwargs) -> None:
-
- super().__init__()
- self.short_size = short_size
- self.ratio_range = ratio_range
- self.aspect_ratio_range = aspect_ratio_range
- self.resize_cfg = dict(type=resize_type, **resize_kwargs)
-
- # create a empty Reisize object
- self.resize_cfg.update(dict(scale=0))
- self.resize = TRANSFORMS.build(self.resize_cfg)
- self.scale_divisor = scale_divisor
-
- def _sample_from_range(self, range: Tuple[float, float]) -> float:
- """A ratio will be randomly sampled from the range specified by
- ``range``.
-
- Args:
- ratio_range (tuple[float]): The minimum and maximum ratio.
-
- Returns:
- float: A ratio randomly sampled from the range.
- """
- min_value, max_value = min(range), max(range)
- value = np.random.random_sample() * (max_value - min_value) + min_value
- return value
-
- def transform(self, results: Dict) -> Dict:
- """Short Scale Aspect Jitter.
- Args:
- results (dict): Result dict containing the data to transform.
-
- Returns:
- dict: The transformed data.
- """
- h, w = results['img'].shape[:2]
- ratio = self._sample_from_range(self.ratio_range)
- scale = (ratio * self.short_size) / min(h, w)
-
- aspect = self._sample_from_range(self.aspect_ratio_range)
- h_scale = scale * math.sqrt(aspect)
- w_scale = scale / math.sqrt(aspect)
- new_h = round(h * h_scale)
- new_w = round(w * w_scale)
-
- new_h = math.ceil(new_h / self.scale_divisor) * self.scale_divisor
- new_w = math.ceil(new_w / self.scale_divisor) * self.scale_divisor
- self.resize.scale = (new_w, new_h)
- return self.resize(results)
-
- def __repr__(self) -> str:
- repr_str = self.__class__.__name__
- repr_str += f'(short_size = {self.short_size}, '
- repr_str += f'ratio_range = {self.ratio_range}, '
- repr_str += f'aspect_ratio_range = {self.aspect_ratio_range}, '
- repr_str += f'scale_divisor = {self.scale_divisor}, '
- repr_str += f'resize_cfg = {self.resize_cfg})'
- return repr_str
-
-
-@TRANSFORMS.register_module()
-class TextDetRandomCropFlip(BaseTransform):
- # TODO Rename this transformer; Refactor the redundant code.
- """Random crop and flip a patch in the image. Only used in text detection
- task.
-
- Required Keys:
-
- - img
- - gt_bboxes
- - gt_polygons
-
- Modified Keys:
-
- - img
- - gt_bboxes
- - gt_polygons
-
- Args:
- pad_ratio (float): The ratio of padding. Defaults to 0.1.
- crop_ratio (float): The ratio of cropping. Defaults to 0.5.
- iter_num (int): Number of operations. Defaults to 1.
- min_area_ratio (float): Minimal area ratio between cropped patch
- and original image. Defaults to 0.2.
- epsilon (float): The threshold of polygon IoU between cropped area
- and polygon, which is used to avoid cropping text instances.
- Defaults to 0.01.
- """
-
- def __init__(self,
- pad_ratio: float = 0.1,
- crop_ratio: float = 0.5,
- iter_num: int = 1,
- min_area_ratio: float = 0.2,
- epsilon: float = 1e-2) -> None:
- if not isinstance(pad_ratio, float):
- raise TypeError('`pad_ratio` should be an float, '
- f'but got {type(pad_ratio)} instead')
- if not isinstance(crop_ratio, float):
- raise TypeError('`crop_ratio` should be a float, '
- f'but got {type(crop_ratio)} instead')
- if not isinstance(iter_num, int):
- raise TypeError('`iter_num` should be an integer, '
- f'but got {type(iter_num)} instead')
- if not isinstance(min_area_ratio, float):
- raise TypeError('`min_area_ratio` should be a float, '
- f'but got {type(min_area_ratio)} instead')
- if not isinstance(epsilon, float):
- raise TypeError('`epsilon` should be a float, '
- f'but got {type(epsilon)} instead')
-
- self.pad_ratio = pad_ratio
- self.epsilon = epsilon
- self.crop_ratio = crop_ratio
- self.iter_num = iter_num
- self.min_area_ratio = min_area_ratio
-
- @cache_randomness
- def _random_prob(self) -> float:
- """Get the random prob to decide whether apply the transform.
-
- Returns:
- float: The probability
- """
- return random.random()
-
- @cache_randomness
- def _random_flip_type(self) -> int:
- """Get the random flip type.
-
- Returns:
- int: The flip type index. (0: horizontal; 1: vertical; 2: both)
- """
- return np.random.randint(3)
-
- @cache_randomness
- def _random_choice(self, axis: np.ndarray) -> np.ndarray:
- """Randomly select two coordinates from the axis.
-
- Args:
- axis (np.ndarray): Result dict containing the data to transform
-
- Returns:
- np.ndarray: The selected coordinates
- """
- return np.random.choice(axis, size=2)
-
- def transform(self, results: Dict) -> Dict:
- """Applying random crop flip on results.
-
- Args:
- results (dict): Result dict containing the data to transform
-
- Returns:
- dict: The transformed data
- """
- assert 'img' in results, '`img` is not found in results'
- for _ in range(self.iter_num):
- results = self._random_crop_flip_polygons(results)
- bboxes = [poly2bbox(poly) for poly in results['gt_polygons']]
- results['gt_bboxes'] = np.array(
- bboxes, dtype=np.float32).reshape(-1, 4)
- return results
-
- def _random_crop_flip_polygons(self, results: Dict) -> Dict:
- """Applying random crop flip on polygons.
-
- Args:
- results (dict): Result dict containing the data to transform
-
- Returns:
- dict: The transformed data
- """
- if results.get('gt_polygons', None) is None:
- return results
-
- image = results['img']
- polygons = results['gt_polygons']
- if len(polygons) == 0 or self._random_prob() > self.crop_ratio:
- return results
-
- h, w = results['img_shape']
- area = h * w
- pad_h = int(h * self.pad_ratio)
- pad_w = int(w * self.pad_ratio)
- h_axis, w_axis = self._generate_crop_target(image, polygons, pad_h,
- pad_w)
- if len(h_axis) == 0 or len(w_axis) == 0:
- return results
-
- # At most 10 attempts
- for _ in range(10):
- polys_keep = []
- polys_new = []
- kept_idxs = []
- xx = self._random_choice(w_axis)
- yy = self._random_choice(h_axis)
- xmin = np.clip(np.min(xx) - pad_w, 0, w - 1)
- xmax = np.clip(np.max(xx) - pad_w, 0, w - 1)
- ymin = np.clip(np.min(yy) - pad_h, 0, h - 1)
- ymax = np.clip(np.max(yy) - pad_h, 0, h - 1)
- if (xmax - xmin) * (ymax - ymin) < area * self.min_area_ratio:
- # Skip when cropped area is too small
- continue
-
- pts = np.stack([[xmin, xmax, xmax, xmin],
- [ymin, ymin, ymax, ymax]]).T.astype(np.int32)
- pp = plg(pts)
- success_flag = True
- for poly_idx, polygon in enumerate(polygons):
- ppi = plg(polygon.reshape(-1, 2))
- ppiou = poly_intersection(ppi, pp)
- if np.abs(ppiou - float(ppi.area)) > self.epsilon and \
- np.abs(ppiou) > self.epsilon:
- success_flag = False
- break
- kept_idxs.append(poly_idx)
- if np.abs(ppiou - float(ppi.area)) < self.epsilon:
- polys_new.append(polygon)
- else:
- polys_keep.append(polygon)
-
- if success_flag:
- break
-
- cropped = image[ymin:ymax, xmin:xmax, :]
- select_type = self._random_flip_type()
- if select_type == 0:
- img = np.ascontiguousarray(cropped[:, ::-1])
- elif select_type == 1:
- img = np.ascontiguousarray(cropped[::-1, :])
- else:
- img = np.ascontiguousarray(cropped[::-1, ::-1])
- image[ymin:ymax, xmin:xmax, :] = img
- results['img'] = image
-
- if len(polys_new) != 0:
- height, width, _ = cropped.shape
- if select_type == 0:
- for idx, polygon in enumerate(polys_new):
- poly = polygon.reshape(-1, 2)
- poly[:, 0] = width - poly[:, 0] + 2 * xmin
- polys_new[idx] = poly.reshape(-1, )
- elif select_type == 1:
- for idx, polygon in enumerate(polys_new):
- poly = polygon.reshape(-1, 2)
- poly[:, 1] = height - poly[:, 1] + 2 * ymin
- polys_new[idx] = poly.reshape(-1, )
- else:
- for idx, polygon in enumerate(polys_new):
- poly = polygon.reshape(-1, 2)
- poly[:, 0] = width - poly[:, 0] + 2 * xmin
- poly[:, 1] = height - poly[:, 1] + 2 * ymin
- polys_new[idx] = poly.reshape(-1, )
- polygons = polys_keep + polys_new
- # ignored = polys_keep_ignore_idx + polys_new_ignore_idx
- results['gt_polygons'] = polygons
- results['gt_ignored'] = results['gt_ignored'][kept_idxs]
- results['gt_bboxes_labels'] = results['gt_bboxes_labels'][
- kept_idxs]
- return results
-
- def _generate_crop_target(self, image: np.ndarray,
- all_polys: List[np.ndarray], pad_h: int,
- pad_w: int) -> Tuple[np.ndarray, np.ndarray]:
- """Generate cropping target and make sure not to crop the polygon
- instances.
-
- Args:
- image (np.ndarray): The image waited to be crop.
- all_polys (list[np.ndarray]): Ground-truth polygons.
- pad_h (int): Padding length of height.
- pad_w (int): Padding length of width.
-
- Returns:
- (np.ndarray, np.ndarray): Returns a tuple ``(h_axis, w_axis)``,
- where ``h_axis`` is the vertical cropping range and ``w_axis``
- is the horizontal cropping range.
- """
- h, w, _ = image.shape
- h_array = np.zeros((h + pad_h * 2), dtype=np.int32)
- w_array = np.zeros((w + pad_w * 2), dtype=np.int32)
-
- text_polys = []
- for polygon in all_polys:
- rect = cv2.minAreaRect(polygon.astype(np.int32).reshape(-1, 2))
- box = cv2.boxPoints(rect)
- box = np.int0(box)
- text_polys.append([box[0], box[1], box[2], box[3]])
-
- polys = np.array(text_polys, dtype=np.int32)
- for poly in polys:
- poly = np.round(poly, decimals=0).astype(np.int32)
- minx, maxx = np.min(poly[:, 0]), np.max(poly[:, 0])
- miny, maxy = np.min(poly[:, 1]), np.max(poly[:, 1])
- w_array[minx + pad_w:maxx + pad_w] = 1
- h_array[miny + pad_h:maxy + pad_h] = 1
-
- h_axis = np.where(h_array == 0)[0]
- w_axis = np.where(w_array == 0)[0]
- return h_axis, w_axis
-
- def __repr__(self) -> str:
- repr_str = self.__class__.__name__
- repr_str += f'(pad_ratio = {self.pad_ratio}'
- repr_str += f', crop_ratio = {self.crop_ratio}'
- repr_str += f', iter_num = {self.iter_num}'
- repr_str += f', min_area_ratio = {self.min_area_ratio}'
- repr_str += f', epsilon = {self.epsilon})'
- return repr_str
-
-
-@TRANSFORMS.register_module()
-@avoid_cache_randomness
-class TextDetRandomCrop(BaseTransform):
- """Randomly select a region and crop images to a target size and make sure
- to contain text region. This transform may break up text instances, and for
- broken text instances, we will crop it's bbox and polygon coordinates. This
- transform is recommend to be used in segmentation-based network.
-
- Required Keys:
-
- - img
- - gt_polygons
- - gt_bboxes
- - gt_bboxes_labels
- - gt_ignored
-
- Modified Keys:
-
- - img
- - img_shape
- - gt_polygons
- - gt_bboxes
- - gt_bboxes_labels
- - gt_ignored
-
- Args:
- target_size (tuple(int, int) or int): Target size for the cropped
- image. If it's a tuple, then target width and target height will be
- ``target_size[0]`` and ``target_size[1]``, respectively. If it's an
- integer, them both target width and target height will be
- ``target_size``.
- positive_sample_ratio (float): The probability of sampling regions
- that go through text regions. Defaults to 5. / 8.
- """
-
- def __init__(self,
- target_size: Tuple[int, int] or int,
- positive_sample_ratio: float = 5.0 / 8.0) -> None:
- self.target_size = target_size if isinstance(
- target_size, tuple) else (target_size, target_size)
- self.positive_sample_ratio = positive_sample_ratio
-
- def _get_postive_prob(self) -> float:
- """Get the probability to do positive sample.
-
- Returns:
- float: The probability to do positive sample.
- """
- return np.random.random_sample()
-
- def _sample_num(self, start, end):
- """Sample a number in range [start, end].
-
- Args:
- start (int): Starting point.
- end (int): Ending point.
-
- Returns:
- (int): Sampled number.
- """
- return random.randint(start, end)
-
- def _sample_offset(self, gt_polygons: Sequence[np.ndarray],
- img_size: Tuple[int, int]) -> Tuple[int, int]:
- """Samples the top-left coordinate of a crop region, ensuring that the
- cropped region contains at least one polygon.
-
- Args:
- gt_polygons (list(ndarray)) : Polygons.
- img_size (tuple(int, int)) : Image size in the format of
- (height, width).
-
- Returns:
- tuple(int, int): Top-left coordinate of the cropped region.
- """
- h, w = img_size
- t_w, t_h = self.target_size
-
- # target size is bigger than origin size
- t_h = t_h if t_h < h else h
- t_w = t_w if t_w < w else w
- if (gt_polygons is not None and len(gt_polygons) > 0
- and self._get_postive_prob() < self.positive_sample_ratio):
-
- # make sure to crop the positive region
-
- # the minimum top left to crop positive region (h,w)
- tl = np.array([h + 1, w + 1], dtype=np.int32)
- for gt_polygon in gt_polygons:
- temp_point = np.min(gt_polygon.reshape(2, -1), axis=1)
- if temp_point[0] <= tl[0]:
- tl[0] = temp_point[0]
- if temp_point[1] <= tl[1]:
- tl[1] = temp_point[1]
- tl = tl - (t_h, t_w)
- tl[tl < 0] = 0
- # the maximum bottum right to crop positive region
- br = np.array([0, 0], dtype=np.int32)
- for gt_polygon in gt_polygons:
- temp_point = np.max(gt_polygon.reshape(2, -1), axis=1)
- if temp_point[0] > br[0]:
- br[0] = temp_point[0]
- if temp_point[1] > br[1]:
- br[1] = temp_point[1]
- br = br - (t_h, t_w)
- br[br < 0] = 0
-
- # if br is too big so that crop the outside region of img
- br[0] = min(br[0], h - t_h)
- br[1] = min(br[1], w - t_w)
- #
- h = self._sample_num(tl[0], br[0]) if tl[0] < br[0] else 0
- w = self._sample_num(tl[1], br[1]) if tl[1] < br[1] else 0
- else:
- # make sure not to crop outside of img
-
- h = self._sample_num(0, h - t_h) if h - t_h > 0 else 0
- w = self._sample_num(0, w - t_w) if w - t_w > 0 else 0
-
- return (h, w)
-
- def _crop_img(self, img: np.ndarray, offset: Tuple[int, int],
- target_size: Tuple[int, int]) -> np.ndarray:
- """Crop the image given an offset and a target size.
-
- Args:
- img (ndarray): Image.
- offset (Tuple[int. int]): Coordinates of the starting point.
- target_size: Target image size.
- """
- h, w = img.shape[:2]
- target_size = target_size[::-1]
- br = np.min(
- np.stack((np.array(offset) + np.array(target_size), np.array(
- (h, w)))),
- axis=0)
- return img[offset[0]:br[0], offset[1]:br[1]], np.array(
- [offset[1], offset[0], br[1], br[0]])
-
- def _crop_polygons(self, polygons: Sequence[np.ndarray],
- crop_bbox: np.ndarray) -> Sequence[np.ndarray]:
- """Crop polygons to be within a crop region. If polygon crosses the
- crop_bbox, we will keep the part left in crop_bbox by cropping its
- boardline.
-
- Args:
- polygons (list(ndarray)): List of polygons [(N1, ), (N2, ), ...].
- crop_bbox (ndarray): Cropping region. [x1, y1, x2, y1].
-
- Returns
- tuple(List(ArrayLike), list[int]):
- - (List(ArrayLike)): The rest of the polygons located in the
- crop region.
- - (list[int]): Index list of the reserved polygons.
- """
- polygons_cropped = []
- kept_idx = []
- for idx, polygon in enumerate(polygons):
- if polygon.size < 6:
- continue
- poly = crop_polygon(polygon, crop_bbox)
- if poly is not None:
- poly = poly.reshape(-1, 2) - (crop_bbox[0], crop_bbox[1])
- polygons_cropped.append(poly.reshape(-1))
- kept_idx.append(idx)
- return (polygons_cropped, kept_idx)
-
- def transform(self, results: Dict) -> Dict:
- """Applying random crop on results.
- Args:
- results (dict): Result dict contains the data to transform.
-
- Returns:
- dict: The transformed data
- """
- if self.target_size == results['img'].shape[:2][::-1]:
- return results
- gt_polygons = results['gt_polygons']
- crop_offset = self._sample_offset(gt_polygons,
- results['img'].shape[:2])
- img, crop_bbox = self._crop_img(results['img'], crop_offset,
- self.target_size)
- results['img'] = img
- results['img_shape'] = img.shape[:2]
- gt_polygons, polygon_kept_idx = self._crop_polygons(
- gt_polygons, crop_bbox)
- bboxes = [poly2bbox(poly) for poly in gt_polygons]
- results['gt_bboxes'] = np.array(
- bboxes, dtype=np.float32).reshape(-1, 4)
-
- results['gt_polygons'] = gt_polygons
- results['gt_bboxes_labels'] = results['gt_bboxes_labels'][
- polygon_kept_idx]
- results['gt_ignored'] = results['gt_ignored'][polygon_kept_idx]
- return results
-
- def __repr__(self) -> str:
- repr_str = self.__class__.__name__
- repr_str += f'(target_size = {self.target_size}, '
- repr_str += f'positive_sample_ratio = {self.positive_sample_ratio})'
- return repr_str
diff --git a/pipeline/mmocr/mmocr/datasets/transforms/textrecog_transforms.py b/pipeline/mmocr/mmocr/datasets/transforms/textrecog_transforms.py
deleted file mode 100644
index abb094c316cc88b4f288de84aba281f9cabc4dd8..0000000000000000000000000000000000000000
--- a/pipeline/mmocr/mmocr/datasets/transforms/textrecog_transforms.py
+++ /dev/null
@@ -1,724 +0,0 @@
-# Copyright (c) OpenMMLab. All rights reserved.
-import math
-import random
-from typing import Dict, List, Optional, Tuple
-
-import cv2
-import mmcv
-import numpy as np
-from mmcv.transforms.base import BaseTransform
-from mmcv.transforms.utils import cache_randomness
-
-from mmocr.registry import TRANSFORMS
-
-
-@TRANSFORMS.register_module()
-class PyramidRescale(BaseTransform):
- """Resize the image to the base shape, downsample it with gaussian pyramid,
- and rescale it back to original size.
-
- Adapted from https://github.com/FangShancheng/ABINet.
-
- Required Keys:
-
- - img (ndarray)
-
- Modified Keys:
-
- - img (ndarray)
-
- Args:
- factor (int): The decay factor from base size, or the number of
- downsampling operations from the base layer.
- base_shape (tuple[int, int]): The shape (width, height) of the base
- layer of the pyramid.
- randomize_factor (bool): If True, the final factor would be a random
- integer in [0, factor].
- """
-
- def __init__(self,
- factor: int = 4,
- base_shape: Tuple[int, int] = (128, 512),
- randomize_factor: bool = True) -> None:
- if not isinstance(factor, int):
- raise TypeError('`factor` should be an integer, '
- f'but got {type(factor)} instead')
- if not isinstance(base_shape, (list, tuple)):
- raise TypeError('`base_shape` should be a list or tuple, '
- f'but got {type(base_shape)} instead')
- if not len(base_shape) == 2:
- raise ValueError('`base_shape` should contain two integers')
- if not isinstance(base_shape[0], int) or not isinstance(
- base_shape[1], int):
- raise ValueError('`base_shape` should contain two integers')
- if not isinstance(randomize_factor, bool):
- raise TypeError('`randomize_factor` should be a bool, '
- f'but got {type(randomize_factor)} instead')
-
- self.factor = factor
- self.randomize_factor = randomize_factor
- self.base_w, self.base_h = base_shape
-
- @cache_randomness
- def get_random_factor(self) -> float:
- """Get the randomized factor.
-
- Returns:
- float: The randomized factor.
- """
- return np.random.randint(0, self.factor + 1)
-
- def transform(self, results: Dict) -> Dict:
- """Applying pyramid rescale on results.
-
- Args:
- results (dict): Result dict containing the data to transform.
-
- Returns:
- Dict: The transformed data.
- """
-
- assert 'img' in results, '`img` is not found in results'
- if self.randomize_factor:
- self.factor = self.get_random_factor()
- if self.factor == 0:
- return results
- img = results['img']
- src_h, src_w = img.shape[:2]
- scale_img = mmcv.imresize(img, (self.base_w, self.base_h))
- for _ in range(self.factor):
- scale_img = cv2.pyrDown(scale_img)
- scale_img = mmcv.imresize(scale_img, (src_w, src_h))
- results['img'] = scale_img
- return results
-
- def __repr__(self) -> str:
- repr_str = self.__class__.__name__
- repr_str += f'(factor = {self.factor}'
- repr_str += f', randomize_factor = {self.randomize_factor}'
- repr_str += f', base_w = {self.base_w}'
- repr_str += f', base_h = {self.base_h})'
- return repr_str
-
-
-@TRANSFORMS.register_module()
-class RescaleToHeight(BaseTransform):
- """Rescale the image to the height according to setting and keep the aspect
- ratio unchanged if possible. However, if any of ``min_width``,
- ``max_width`` or ``width_divisor`` are specified, aspect ratio may still be
- changed to ensure the width meets these constraints.
-
- Required Keys:
-
- - img
-
- Modified Keys:
-
- - img
- - img_shape
-
- Added Keys:
-
- - scale
- - scale_factor
- - keep_ratio
-
- Args:
- height (int): Height of rescaled image.
- min_width (int, optional): Minimum width of rescaled image. Defaults
- to None.
- max_width (int, optional): Maximum width of rescaled image. Defaults
- to None.
- width_divisor (int): The divisor of width size. Defaults to 1.
- resize_type (str): The type of resize class to use. Defaults to
- "Resize".
- **resize_kwargs: Other keyword arguments for the ``resize_type``.
- """
-
- def __init__(self,
- height: int,
- min_width: Optional[int] = None,
- max_width: Optional[int] = None,
- width_divisor: int = 1,
- resize_type: str = 'Resize',
- **resize_kwargs) -> None:
-
- super().__init__()
- assert isinstance(height, int)
- assert isinstance(width_divisor, int)
- if min_width is not None:
- assert isinstance(min_width, int)
- if max_width is not None:
- assert isinstance(max_width, int)
- self.width_divisor = width_divisor
- self.height = height
- self.min_width = min_width
- self.max_width = max_width
- self.resize_cfg = dict(type=resize_type, **resize_kwargs)
- self.resize_cfg.update(dict(scale=0))
- self.resize = TRANSFORMS.build(self.resize_cfg)
-
- def transform(self, results: Dict) -> Dict:
- """Transform function to resize images, bounding boxes and polygons.
-
- Args:
- results (dict): Result dict from loading pipeline.
-
- Returns:
- dict: Resized results.
- """
- ori_height, ori_width = results['img'].shape[:2]
- new_width = math.ceil(float(self.height) / ori_height * ori_width)
- if self.min_width is not None:
- new_width = max(self.min_width, new_width)
- if self.max_width is not None:
- new_width = min(self.max_width, new_width)
-
- if new_width % self.width_divisor != 0:
- new_width = round(
- new_width / self.width_divisor) * self.width_divisor
- # TODO replace up code after testing precision.
- # new_width = math.ceil(
- # new_width / self.width_divisor) * self.width_divisor
- scale = (new_width, self.height)
- self.resize.scale = scale
- results = self.resize(results)
- return results
-
- def __repr__(self) -> str:
- repr_str = self.__class__.__name__
- repr_str += f'(height={self.height}, '
- repr_str += f'min_width={self.min_width}, '
- repr_str += f'max_width={self.max_width}, '
- repr_str += f'width_divisor={self.width_divisor}, '
- repr_str += f'resize_cfg={self.resize_cfg})'
- return repr_str
-
-
-@TRANSFORMS.register_module()
-class PadToWidth(BaseTransform):
- """Only pad the image's width.
-
- Required Keys:
-
- - img
-
- Modified Keys:
-
- - img
- - img_shape
-
- Added Keys:
-
- - pad_shape
- - pad_fixed_size
- - pad_size_divisor
- - valid_ratio
-
- Args:
- width (int): Target width of padded image. Defaults to None.
- pad_cfg (dict): Config to construct the Resize transform. Refer to
- ``Pad`` for detail. Defaults to ``dict(type='Pad')``.
- """
-
- def __init__(self, width: int, pad_cfg: dict = dict(type='Pad')) -> None:
- super().__init__()
- assert isinstance(width, int)
- self.width = width
- self.pad_cfg = pad_cfg
- _pad_cfg = self.pad_cfg.copy()
- _pad_cfg.update(dict(size=0))
- self.pad = TRANSFORMS.build(_pad_cfg)
-
- def transform(self, results: Dict) -> Dict:
- """Call function to pad images.
-
- Args:
- results (dict): Result dict from loading pipeline.
-
- Returns:
- dict: Updated result dict.
- """
- ori_height, ori_width = results['img'].shape[:2]
- valid_ratio = min(1.0, 1.0 * ori_width / self.width)
- size = (self.width, ori_height)
- self.pad.size = size
- results = self.pad(results)
- results['valid_ratio'] = valid_ratio
- return results
-
- def __repr__(self) -> str:
- repr_str = self.__class__.__name__
- repr_str += f'(width={self.width}, '
- repr_str += f'pad_cfg={self.pad_cfg})'
- return repr_str
-
-
-@TRANSFORMS.register_module()
-class TextRecogGeneralAug(BaseTransform):
- """A general geometric augmentation tool for text images in the CVPR 2020
- paper "Learn to Augment: Joint Data Augmentation and Network Optimization
- for Text Recognition". It applies distortion, stretching, and perspective
- transforms to an image.
-
- This implementation is adapted from
- https://github.com/RubanSeven/Text-Image-Augmentation-python/blob/master/augment.py # noqa
-
- TODO: Split this transform into three transforms.
-
- Required Keys:
-
- - img
-
- Modified Keys:
-
- - img
- - img_shape
- """ # noqa
-
- def transform(self, results: Dict) -> Dict:
- """Call function to pad images.
-
- Args:
- results (dict): Result dict from loading pipeline.
-
- Returns:
- dict: Updated result dict.
- """
- h, w = results['img'].shape[:2]
- if h >= 20 and w >= 20:
- results['img'] = self.tia_distort(results['img'],
- random.randint(3, 6))
- results['img'] = self.tia_stretch(results['img'],
- random.randint(3, 6))
- h, w = results['img'].shape[:2]
- if h >= 5 and w >= 5:
- results['img'] = self.tia_perspective(results['img'])
- results['img_shape'] = results['img'].shape[:2]
- return results
-
- def __repr__(self) -> str:
- repr_str = self.__class__.__name__
- repr_str += '()'
- return repr_str
-
- def tia_distort(self, img: np.ndarray, segment: int = 4) -> np.ndarray:
- """Image distortion.
-
- Args:
- img (np.ndarray): The image.
- segment (int): The number of segments to divide the image along
- the width. Defaults to 4.
- """
- img_h, img_w = img.shape[:2]
-
- cut = img_w // segment
- thresh = cut // 3
-
- src_pts = list()
- dst_pts = list()
-
- src_pts.append([0, 0])
- src_pts.append([img_w, 0])
- src_pts.append([img_w, img_h])
- src_pts.append([0, img_h])
-
- dst_pts.append([np.random.randint(thresh), np.random.randint(thresh)])
- dst_pts.append(
- [img_w - np.random.randint(thresh),
- np.random.randint(thresh)])
- dst_pts.append([
- img_w - np.random.randint(thresh),
- img_h - np.random.randint(thresh)
- ])
- dst_pts.append(
- [np.random.randint(thresh), img_h - np.random.randint(thresh)])
-
- half_thresh = thresh * 0.5
-
- for cut_idx in np.arange(1, segment, 1):
- src_pts.append([cut * cut_idx, 0])
- src_pts.append([cut * cut_idx, img_h])
- dst_pts.append([
- cut * cut_idx + np.random.randint(thresh) - half_thresh,
- np.random.randint(thresh) - half_thresh
- ])
- dst_pts.append([
- cut * cut_idx + np.random.randint(thresh) - half_thresh,
- img_h + np.random.randint(thresh) - half_thresh
- ])
-
- dst = self.warp_mls(img, src_pts, dst_pts, img_w, img_h)
-
- return dst
-
- def tia_stretch(self, img: np.ndarray, segment: int = 4) -> np.ndarray:
- """Image stretching.
-
- Args:
- img (np.ndarray): The image.
- segment (int): The number of segments to divide the image along
- the width. Defaults to 4.
- """
- img_h, img_w = img.shape[:2]
-
- cut = img_w // segment
- thresh = cut * 4 // 5
-
- src_pts = list()
- dst_pts = list()
-
- src_pts.append([0, 0])
- src_pts.append([img_w, 0])
- src_pts.append([img_w, img_h])
- src_pts.append([0, img_h])
-
- dst_pts.append([0, 0])
- dst_pts.append([img_w, 0])
- dst_pts.append([img_w, img_h])
- dst_pts.append([0, img_h])
-
- half_thresh = thresh * 0.5
-
- for cut_idx in np.arange(1, segment, 1):
- move = np.random.randint(thresh) - half_thresh
- src_pts.append([cut * cut_idx, 0])
- src_pts.append([cut * cut_idx, img_h])
- dst_pts.append([cut * cut_idx + move, 0])
- dst_pts.append([cut * cut_idx + move, img_h])
-
- dst = self.warp_mls(img, src_pts, dst_pts, img_w, img_h)
-
- return dst
-
- def tia_perspective(self, img: np.ndarray) -> np.ndarray:
- """Image perspective transformation.
-
- Args:
- img (np.ndarray): The image.
- segment (int): The number of segments to divide the image along
- the width. Defaults to 4.
- """
- img_h, img_w = img.shape[:2]
-
- thresh = img_h // 2
-
- src_pts = list()
- dst_pts = list()
-
- src_pts.append([0, 0])
- src_pts.append([img_w, 0])
- src_pts.append([img_w, img_h])
- src_pts.append([0, img_h])
-
- dst_pts.append([0, np.random.randint(thresh)])
- dst_pts.append([img_w, np.random.randint(thresh)])
- dst_pts.append([img_w, img_h - np.random.randint(thresh)])
- dst_pts.append([0, img_h - np.random.randint(thresh)])
-
- dst = self.warp_mls(img, src_pts, dst_pts, img_w, img_h)
-
- return dst
-
- def warp_mls(self,
- src: np.ndarray,
- src_pts: List[int],
- dst_pts: List[int],
- dst_w: int,
- dst_h: int,
- trans_ratio: float = 1.) -> np.ndarray:
- """Warp the image."""
- rdx, rdy = self._calc_delta(dst_w, dst_h, src_pts, dst_pts, 100)
- return self._gen_img(src, rdx, rdy, dst_w, dst_h, 100, trans_ratio)
-
- def _calc_delta(self, dst_w: int, dst_h: int, src_pts: List[int],
- dst_pts: List[int],
- grid_size: int) -> Tuple[np.ndarray, np.ndarray]:
- """Compute delta."""
-
- pt_count = len(dst_pts)
- rdx = np.zeros((dst_h, dst_w))
- rdy = np.zeros((dst_h, dst_w))
- w = np.zeros(pt_count, dtype=np.float32)
-
- if pt_count < 2:
- return
-
- i = 0
- while True:
- if dst_w <= i < dst_w + grid_size - 1:
- i = dst_w - 1
- elif i >= dst_w:
- break
-
- j = 0
- while True:
- if dst_h <= j < dst_h + grid_size - 1:
- j = dst_h - 1
- elif j >= dst_h:
- break
-
- sw = 0
- swp = np.zeros(2, dtype=np.float32)
- swq = np.zeros(2, dtype=np.float32)
- new_pt = np.zeros(2, dtype=np.float32)
- cur_pt = np.array([i, j], dtype=np.float32)
-
- k = 0
- for k in range(pt_count):
- if i == dst_pts[k][0] and j == dst_pts[k][1]:
- break
-
- w[k] = 1. / ((i - dst_pts[k][0]) * (i - dst_pts[k][0]) +
- (j - dst_pts[k][1]) * (j - dst_pts[k][1]))
-
- sw += w[k]
- swp = swp + w[k] * np.array(dst_pts[k])
- swq = swq + w[k] * np.array(src_pts[k])
-
- if k == pt_count - 1:
- pstar = 1 / sw * swp
- qstar = 1 / sw * swq
-
- miu_s = 0
- for k in range(pt_count):
- if i == dst_pts[k][0] and j == dst_pts[k][1]:
- continue
- pt_i = dst_pts[k] - pstar
- miu_s += w[k] * np.sum(pt_i * pt_i)
-
- cur_pt -= pstar
- cur_pt_j = np.array([-cur_pt[1], cur_pt[0]])
-
- for k in range(pt_count):
- if i == dst_pts[k][0] and j == dst_pts[k][1]:
- continue
-
- pt_i = dst_pts[k] - pstar
- pt_j = np.array([-pt_i[1], pt_i[0]])
-
- tmp_pt = np.zeros(2, dtype=np.float32)
- tmp_pt[0] = (
- np.sum(pt_i * cur_pt) * src_pts[k][0] -
- np.sum(pt_j * cur_pt) * src_pts[k][1])
- tmp_pt[1] = (-np.sum(pt_i * cur_pt_j) * src_pts[k][0] +
- np.sum(pt_j * cur_pt_j) * src_pts[k][1])
- tmp_pt *= (w[k] / miu_s)
- new_pt += tmp_pt
-
- new_pt += qstar
- else:
- new_pt = src_pts[k]
-
- rdx[j, i] = new_pt[0] - i
- rdy[j, i] = new_pt[1] - j
-
- j += grid_size
- i += grid_size
- return rdx, rdy
-
- def _gen_img(self, src: np.ndarray, rdx: np.ndarray, rdy: np.ndarray,
- dst_w: int, dst_h: int, grid_size: int,
- trans_ratio: float) -> np.ndarray:
- """Generate the image based on delta."""
-
- src_h, src_w = src.shape[:2]
- dst = np.zeros_like(src, dtype=np.float32)
-
- for i in np.arange(0, dst_h, grid_size):
- for j in np.arange(0, dst_w, grid_size):
- ni = i + grid_size
- nj = j + grid_size
- w = h = grid_size
- if ni >= dst_h:
- ni = dst_h - 1
- h = ni - i + 1
- if nj >= dst_w:
- nj = dst_w - 1
- w = nj - j + 1
-
- di = np.reshape(np.arange(h), (-1, 1))
- dj = np.reshape(np.arange(w), (1, -1))
- delta_x = self._bilinear_interp(di / h, dj / w, rdx[i, j],
- rdx[i, nj], rdx[ni, j],
- rdx[ni, nj])
- delta_y = self._bilinear_interp(di / h, dj / w, rdy[i, j],
- rdy[i, nj], rdy[ni, j],
- rdy[ni, nj])
- nx = j + dj + delta_x * trans_ratio
- ny = i + di + delta_y * trans_ratio
- nx = np.clip(nx, 0, src_w - 1)
- ny = np.clip(ny, 0, src_h - 1)
- nxi = np.array(np.floor(nx), dtype=np.int32)
- nyi = np.array(np.floor(ny), dtype=np.int32)
- nxi1 = np.array(np.ceil(nx), dtype=np.int32)
- nyi1 = np.array(np.ceil(ny), dtype=np.int32)
-
- if len(src.shape) == 3:
- x = np.tile(np.expand_dims(ny - nyi, axis=-1), (1, 1, 3))
- y = np.tile(np.expand_dims(nx - nxi, axis=-1), (1, 1, 3))
- else:
- x = ny - nyi
- y = nx - nxi
- dst[i:i + h,
- j:j + w] = self._bilinear_interp(x, y, src[nyi, nxi],
- src[nyi, nxi1],
- src[nyi1, nxi], src[nyi1,
- nxi1])
-
- dst = np.clip(dst, 0, 255)
- dst = np.array(dst, dtype=np.uint8)
-
- return dst
-
- @staticmethod
- def _bilinear_interp(x, y, v11, v12, v21, v22):
- """Bilinear interpolation.
-
- TODO: Docs for args and put it into utils.
- """
- return (v11 * (1 - y) + v12 * y) * (1 - x) + (v21 *
- (1 - y) + v22 * y) * x
-
-
-@TRANSFORMS.register_module()
-class CropHeight(BaseTransform):
- """Randomly crop the image's height, either from top or bottom.
-
- Adapted from
- https://github.com/PaddlePaddle/PaddleOCR/blob/release%2F2.6/ppocr/data/imaug/rec_img_aug.py # noqa
-
- Required Keys:
-
- - img
-
- Modified Keys:
-
- - img
- - img_shape
-
- Args:
- crop_min (int): Minimum pixel(s) to crop. Defaults to 1.
- crop_max (int): Maximum pixel(s) to crop. Defaults to 8.
- """
-
- def __init__(
- self,
- min_pixels: int = 1,
- max_pixels: int = 8,
- ) -> None:
- super().__init__()
- assert max_pixels >= min_pixels
- self.min_pixels = min_pixels
- self.max_pixels = max_pixels
-
- @cache_randomness
- def get_random_vars(self):
- """Get all the random values used in this transform."""
- crop_pixels = int(random.randint(self.min_pixels, self.max_pixels))
- crop_top = random.randint(0, 1)
- return crop_pixels, crop_top
-
- def transform(self, results: Dict) -> Dict:
- """Transform function to crop images.
-
- Args:
- results (dict): Result dict from loading pipeline.
-
- Returns:
- dict: Cropped results.
- """
- h = results['img'].shape[0]
- crop_pixels, crop_top = self.get_random_vars()
- crop_pixels = min(crop_pixels, h - 1)
- img = results['img'].copy()
- if crop_top:
- img = img[crop_pixels:h, :, :]
- else:
- img = img[0:h - crop_pixels, :, :]
- results['img_shape'] = img.shape[:2]
- results['img'] = img
- return results
-
- def __repr__(self) -> str:
- repr_str = self.__class__.__name__
- repr_str += f'(min_pixels = {self.min_pixels}, '
- repr_str += f'max_pixels = {self.max_pixels})'
- return repr_str
-
-
-@TRANSFORMS.register_module()
-class ImageContentJitter(BaseTransform):
- """Jitter the image contents.
-
- Adapted from
- https://github.com/PaddlePaddle/PaddleOCR/blob/release%2F2.6/ppocr/data/imaug/rec_img_aug.py # noqa
-
- Required Keys:
-
- - img
-
- Modified Keys:
-
- - img
- """
-
- def transform(self, results: Dict, jitter_ratio: float = 0.01) -> Dict:
- """Transform function to jitter images.
-
- Args:
- results (dict): Result dict from loading pipeline.
- jitter_ratio (float): Controls the strength of jittering.
- Defaults to 0.01.
-
- Returns:
- dict: Jittered results.
- """
- h, w = results['img'].shape[:2]
- img = results['img'].copy()
- if h > 10 and w > 10:
- thres = min(h, w)
- jitter_range = int(random.random() * thres * 0.01)
- for i in range(jitter_range):
- img[i:, i:, :] = img[:h - i, :w - i, :]
- results['img'] = img
- return results
-
- def __repr__(self) -> str:
- repr_str = self.__class__.__name__
- repr_str += '()'
- return repr_str
-
-
-@TRANSFORMS.register_module()
-class ReversePixels(BaseTransform):
- """Reverse image pixels.
-
- Adapted from
- https://github.com/PaddlePaddle/PaddleOCR/blob/release%2F2.6/ppocr/data/imaug/rec_img_aug.py # noqa
-
- Required Keys:
-
- - img
-
- Modified Keys:
-
- - img
- """
-
- def transform(self, results: Dict) -> Dict:
- """Transform function to reverse image pixels.
-
- Args:
- results (dict): Result dict from loading pipeline.
-
- Returns:
- dict: Reversed results.
- """
- results['img'] = 255. - results['img'].copy()
- return results
-
- def __repr__(self) -> str:
- repr_str = self.__class__.__name__
- repr_str += '()'
- return repr_str
diff --git a/pipeline/mmocr/mmocr/datasets/transforms/wrappers.py b/pipeline/mmocr/mmocr/datasets/transforms/wrappers.py
deleted file mode 100644
index 086edb759b20c20a94fe8d7139350ba22a636c03..0000000000000000000000000000000000000000
--- a/pipeline/mmocr/mmocr/datasets/transforms/wrappers.py
+++ /dev/null
@@ -1,343 +0,0 @@
-# Copyright (c) OpenMMLab. All rights reserved.
-import warnings
-from typing import Any, Dict, List, Optional, Tuple, Union
-
-import imgaug
-import imgaug.augmenters as iaa
-import numpy as np
-import torchvision.transforms as torchvision_transforms
-from mmcv.transforms import Compose
-from mmcv.transforms.base import BaseTransform
-from PIL import Image
-
-from mmocr.registry import TRANSFORMS
-from mmocr.utils import poly2bbox
-
-
-@TRANSFORMS.register_module()
-class ImgAugWrapper(BaseTransform):
- """A wrapper around imgaug https://github.com/aleju/imgaug.
-
- Find available augmenters at
- https://imgaug.readthedocs.io/en/latest/source/overview_of_augmenters.html.
-
- Required Keys:
-
- - img
- - gt_polygons (optional for text recognition)
- - gt_bboxes (optional for text recognition)
- - gt_bboxes_labels (optional for text recognition)
- - gt_ignored (optional for text recognition)
- - gt_texts (optional)
-
- Modified Keys:
-
- - img
- - gt_polygons (optional for text recognition)
- - gt_bboxes (optional for text recognition)
- - gt_bboxes_labels (optional for text recognition)
- - gt_ignored (optional for text recognition)
- - img_shape (optional)
- - gt_texts (optional)
-
- Args:
- args (list[list or dict]], optional): The argumentation list. For
- details, please refer to imgaug document. Take
- args=[['Fliplr', 0.5], dict(cls='Affine', rotate=[-10, 10]),
- ['Resize', [0.5, 3.0]]] as an example. The args horizontally flip
- images with probability 0.5, followed by random rotation with
- angles in range [-10, 10], and resize with an independent scale in
- range [0.5, 3.0] for each side of images. Defaults to None.
- fix_poly_trans (dict): The transform configuration to fix invalid
- polygons. Set it to None if no fixing is needed.
- Defaults to dict(type='FixInvalidPolygon').
- """
-
- def __init__(
- self,
- args: Optional[List[Union[List, Dict]]] = None,
- fix_poly_trans: Optional[dict] = dict(type='FixInvalidPolygon')
- ) -> None:
- assert args is None or isinstance(args, list) and len(args) > 0
- if args is not None:
- for arg in args:
- assert isinstance(arg, (list, dict)), \
- 'args should be a list of list or dict'
- self.args = args
- self.augmenter = self._build_augmentation(args)
- self.fix_poly_trans = fix_poly_trans
- if fix_poly_trans is not None:
- self.fix = TRANSFORMS.build(fix_poly_trans)
-
- def transform(self, results: Dict) -> Dict:
- """Transform the image and annotation data.
-
- Args:
- results (dict): Result dict containing the data to transform.
-
- Returns:
- dict: The transformed data.
- """
- # img is bgr
- image = results['img']
- aug = None
- ori_shape = image.shape
-
- if self.augmenter:
- aug = self.augmenter.to_deterministic()
- if not self._augment_annotations(aug, ori_shape, results):
- return None
- results['img'] = aug.augment_image(image)
- results['img_shape'] = (results['img'].shape[0],
- results['img'].shape[1])
- if getattr(self, 'fix', None) is not None:
- results = self.fix(results)
- return results
-
- def _augment_annotations(self, aug: imgaug.augmenters.meta.Augmenter,
- ori_shape: Tuple[int,
- int], results: Dict) -> Dict:
- """Augment annotations following the pre-defined augmentation sequence.
-
- Args:
- aug (imgaug.augmenters.meta.Augmenter): The imgaug augmenter.
- ori_shape (tuple[int, int]): The ori_shape of the original image.
- results (dict): Result dict containing annotations to transform.
-
- Returns:
- bool: Whether the transformation has been successfully applied. If
- the transform results in empty polygon/bbox annotations, return
- False.
- """
- # Assume co-existence of `gt_polygons`, `gt_bboxes` and `gt_ignored`
- # for text detection
- if 'gt_polygons' in results:
-
- # augment polygons
- transformed_polygons, removed_poly_inds = self._augment_polygons(
- aug, ori_shape, results['gt_polygons'])
- if len(transformed_polygons) == 0:
- return False
- results['gt_polygons'] = transformed_polygons
-
- # remove instances that are no longer inside the augmented image
- results['gt_bboxes_labels'] = np.delete(
- results['gt_bboxes_labels'], removed_poly_inds, axis=0)
- results['gt_ignored'] = np.delete(
- results['gt_ignored'], removed_poly_inds, axis=0)
- # TODO: deal with gt_texts corresponding to clipped polygons
- if 'gt_texts' in results:
- results['gt_texts'] = [
- text for i, text in enumerate(results['gt_texts'])
- if i not in removed_poly_inds
- ]
-
- # Generate new bboxes
- bboxes = [poly2bbox(poly) for poly in transformed_polygons]
- results['gt_bboxes'] = np.zeros((0, 4), dtype=np.float32)
- if len(bboxes) > 0:
- results['gt_bboxes'] = np.stack(bboxes)
-
- return True
-
- def _augment_polygons(self, aug: imgaug.augmenters.meta.Augmenter,
- ori_shape: Tuple[int, int], polys: List[np.ndarray]
- ) -> Tuple[List[np.ndarray], List[int]]:
- """Augment polygons.
-
- Args:
- aug (imgaug.augmenters.meta.Augmenter): The imgaug augmenter.
- ori_shape (tuple[int, int]): The shape of the original image.
- polys (list[np.ndarray]): The polygons to be augmented.
-
- Returns:
- tuple(list[np.ndarray], list[int]): The augmented polygons, and the
- indices of polygons removed as they are out of the augmented image.
- """
- imgaug_polys = []
- for poly in polys:
- poly = poly.reshape(-1, 2)
- imgaug_polys.append(imgaug.Polygon(poly))
- imgaug_polys = aug.augment_polygons(
- [imgaug.PolygonsOnImage(imgaug_polys, shape=ori_shape)])[0]
-
- new_polys = []
- removed_poly_inds = []
- for i, poly in enumerate(imgaug_polys.polygons):
- # Sometimes imgaug may produce some invalid polygons with no points
- if not poly.is_valid or poly.is_out_of_image(imgaug_polys.shape):
- removed_poly_inds.append(i)
- continue
- new_poly = []
- try:
- poly = poly.clip_out_of_image(imgaug_polys.shape)[0]
- except Exception as e:
- warnings.warn(f'Failed to clip polygon out of image: {e}')
- for point in poly:
- new_poly.append(np.array(point, dtype=np.float32))
- new_poly = np.array(new_poly, dtype=np.float32).flatten()
- # Under some conditions, imgaug can generate "polygon" with only
- # two points, which is not a valid polygon.
- if len(new_poly) <= 4:
- removed_poly_inds.append(i)
- continue
- new_polys.append(new_poly)
-
- return new_polys, removed_poly_inds
-
- def _build_augmentation(self, args, root=True):
- """Build ImgAugWrapper augmentations.
-
- Args:
- args (dict): Arguments to be passed to imgaug.
- root (bool): Whether it's building the root augmenter.
-
- Returns:
- imgaug.augmenters.meta.Augmenter: The built augmenter.
- """
- if args is None:
- return None
- if isinstance(args, (int, float, str)):
- return args
- if isinstance(args, list):
- if root:
- sequence = [
- self._build_augmentation(value, root=False)
- for value in args
- ]
- return iaa.Sequential(sequence)
- arg_list = [self._to_tuple_if_list(a) for a in args[1:]]
- return getattr(iaa, args[0])(*arg_list)
- if isinstance(args, dict):
- if 'cls' in args:
- cls = getattr(iaa, args['cls'])
- return cls(
- **{
- k: self._to_tuple_if_list(v)
- for k, v in args.items() if not k == 'cls'
- })
- else:
- return {
- key: self._build_augmentation(value, root=False)
- for key, value in args.items()
- }
- raise RuntimeError('unknown augmenter arg: ' + str(args))
-
- def _to_tuple_if_list(self, obj: Any) -> Any:
- """Convert an object into a tuple if it is a list."""
- if isinstance(obj, list):
- return tuple(obj)
- return obj
-
- def __repr__(self):
- repr_str = self.__class__.__name__
- repr_str += f'(args = {self.args}, '
- repr_str += f'fix_poly_trans = {self.fix_poly_trans})'
- return repr_str
-
-
-@TRANSFORMS.register_module()
-class TorchVisionWrapper(BaseTransform):
- """A wrapper around torchvision transforms. It applies specific transform
- to ``img`` and updates ``height`` and ``width`` accordingly.
-
- Required Keys:
-
- - img (ndarray): The input image.
-
- Modified Keys:
-
- - img (ndarray): The modified image.
- - img_shape (tuple(int, int)): The shape of the image in (height, width).
-
-
- Warning:
- This transform only affects the image but not its associated
- annotations, such as word bounding boxes and polygons. Therefore,
- it may only be applicable to text recognition tasks.
-
- Args:
- op (str): The name of any transform class in
- :func:`torchvision.transforms`.
- **kwargs: Arguments that will be passed to initializer of torchvision
- transform.
- """
-
- def __init__(self, op: str, **kwargs) -> None:
- assert isinstance(op, str)
- obj_cls = getattr(torchvision_transforms, op)
- self.torchvision = obj_cls(**kwargs)
- self.op = op
- self.kwargs = kwargs
-
- def transform(self, results):
- """Transform the image.
-
- Args:
- results (dict): Result dict from the data loader.
-
- Returns:
- dict: Transformed results.
- """
- assert 'img' in results
- # BGR -> RGB
- img = results['img'][..., ::-1]
- img = Image.fromarray(img)
- img = self.torchvision(img)
- img = np.asarray(img)
- img = img[..., ::-1]
- results['img'] = img
- results['img_shape'] = img.shape[:2]
- return results
-
- def __repr__(self):
- repr_str = self.__class__.__name__
- repr_str += f'(op = {self.op}'
- for k, v in self.kwargs.items():
- repr_str += f', {k} = {v}'
- repr_str += ')'
- return repr_str
-
-
-@TRANSFORMS.register_module()
-class ConditionApply(BaseTransform):
- """Apply transforms according to the condition. If the condition is met,
- true_transforms will be applied, otherwise false_transforms will be
- applied.
-
- Args:
- condition (str): The string that can be evaluated to a boolean value.
- true_transforms (list[dict]): Transforms to be applied if the condition
- is met. Defaults to [].
- false_transforms (list[dict]): Transforms to be applied if the
- condition is not met. Defaults to [].
- """
-
- def __init__(self,
- condition: str,
- true_transforms: Union[Dict, List[Dict]] = [],
- false_transforms: Union[Dict, List[Dict]] = []):
- self.condition = condition
- self.true_transforms = Compose(true_transforms)
- self.false_transforms = Compose(false_transforms)
-
- def transform(self, results: Dict) -> Optional[Dict]:
- """Transform the image.
-
- Args:
- results (dict):Result dict containing the data to transform.
-
- Returns:
- dict: Transformed results.
- """
- if eval(self.condition):
- return self.true_transforms(results) # type: ignore
- else:
- return self.false_transforms(results)
-
- def __repr__(self):
- repr_str = self.__class__.__name__
- repr_str += f'(condition = {self.condition}, '
- repr_str += f'true_transforms = {self.true_transforms}, '
- repr_str += f'false_transforms = {self.false_transforms})'
- return repr_str
diff --git a/pipeline/mmocr/mmocr/datasets/wildreceipt_dataset.py b/pipeline/mmocr/mmocr/datasets/wildreceipt_dataset.py
deleted file mode 100644
index 5f8699a043b893be2f826a760b81e8d939719a99..0000000000000000000000000000000000000000
--- a/pipeline/mmocr/mmocr/datasets/wildreceipt_dataset.py
+++ /dev/null
@@ -1,286 +0,0 @@
-# Copyright (c) OpenMMLab. All rights reserved.
-import copy
-from typing import Callable, List, Optional, Sequence, Union
-
-import numpy as np
-from mmengine.dataset import BaseDataset
-from mmengine.fileio import list_from_file
-
-from mmocr.registry import DATASETS
-from mmocr.utils.parsers import LineJsonParser
-from mmocr.utils.polygon_utils import sort_vertex8
-
-
-@DATASETS.register_module()
-class WildReceiptDataset(BaseDataset):
- """WildReceipt Dataset for key information extraction. There are two files
- to be loaded: metainfo and annotation. The metainfo file contains the
- mapping between classes and labels. The annotation file contains the all
- necessary information about the image, such as bounding boxes, texts, and
- labels etc.
-
- The metainfo file is a text file with the following format:
-
- .. code-block:: none
-
- 0 Ignore
- 1 Store_name_value
- 2 Store_name_key
-
- The annotation format is shown as follows.
-
- .. code-block:: json
-
- {
- "file_name": "a.jpeg",
- "height": 348,
- "width": 348,
- "annotations": [
- {
- "box": [
- 114.0,
- 19.0,
- 230.0,
- 19.0,
- 230.0,
- 1.0,
- 114.0,
- 1.0
- ],
- "text": "CHOEUN",
- "label": 1
- },
- {
- "box": [
- 97.0,
- 35.0,
- 236.0,
- 35.0,
- 236.0,
- 19.0,
- 97.0,
- 19.0
- ],
- "text": "KOREANRESTAURANT",
- "label": 2
- }
- ]
- }
-
- Args:
- directed (bool): Whether to use directed graph. Defaults to False.
- ann_file (str): Annotation file path. Defaults to ''.
- metainfo (str or dict, optional): Meta information for dataset, such as
- class information. If it's a string, it will be treated as a path
- to the class file from which the class information will be loaded.
- Defaults to None.
- data_root (str, optional): The root directory for ``data_prefix`` and
- ``ann_file``. Defaults to ''.
- data_prefix (dict, optional): Prefix for training data. Defaults to
- dict(img_path='').
- filter_cfg (dict, optional): Config for filter data. Defaults to None.
- indices (int or Sequence[int], optional): Support using first few
- data in annotation file to facilitate training/testing on a smaller
- dataset. Defaults to None which means using all ``data_infos``.
- serialize_data (bool, optional): Whether to hold memory using
- serialized objects, when enabled, data loader workers can use
- shared RAM from master process instead of making a copy. Defaults
- to True.
- pipeline (list, optional): Processing pipeline. Defaults to [].
- test_mode (bool, optional): ``test_mode=True`` means in test phase.
- Defaults to False.
- lazy_init (bool, optional): Whether to load annotation during
- instantiation. In some cases, such as visualization, only the meta
- information of the dataset is needed, which is not necessary to
- load annotation file. ``Basedataset`` can skip load annotations to
- save time by set ``lazy_init=False``. Defaults to False.
- max_refetch (int, optional): If ``Basedataset.prepare_data`` get a
- None img. The maximum extra number of cycles to get a valid
- image. Defaults to 1000.
- """
- METAINFO = {
- 'category': [{
- 'id': '0',
- 'name': 'Ignore'
- }, {
- 'id': '1',
- 'name': 'Store_name_value'
- }, {
- 'id': '2',
- 'name': 'Store_name_key'
- }, {
- 'id': '3',
- 'name': 'Store_addr_value'
- }, {
- 'id': '4',
- 'name': 'Store_addr_key'
- }, {
- 'id': '5',
- 'name': 'Tel_value'
- }, {
- 'id': '6',
- 'name': 'Tel_key'
- }, {
- 'id': '7',
- 'name': 'Date_value'
- }, {
- 'id': '8',
- 'name': 'Date_key'
- }, {
- 'id': '9',
- 'name': 'Time_value'
- }, {
- 'id': '10',
- 'name': 'Time_key'
- }, {
- 'id': '11',
- 'name': 'Prod_item_value'
- }, {
- 'id': '12',
- 'name': 'Prod_item_key'
- }, {
- 'id': '13',
- 'name': 'Prod_quantity_value'
- }, {
- 'id': '14',
- 'name': 'Prod_quantity_key'
- }, {
- 'id': '15',
- 'name': 'Prod_price_value'
- }, {
- 'id': '16',
- 'name': 'Prod_price_key'
- }, {
- 'id': '17',
- 'name': 'Subtotal_value'
- }, {
- 'id': '18',
- 'name': 'Subtotal_key'
- }, {
- 'id': '19',
- 'name': 'Tax_value'
- }, {
- 'id': '20',
- 'name': 'Tax_key'
- }, {
- 'id': '21',
- 'name': 'Tips_value'
- }, {
- 'id': '22',
- 'name': 'Tips_key'
- }, {
- 'id': '23',
- 'name': 'Total_value'
- }, {
- 'id': '24',
- 'name': 'Total_key'
- }, {
- 'id': '25',
- 'name': 'Others'
- }]
- }
-
- def __init__(self,
- directed: bool = False,
- ann_file: str = '',
- metainfo: Optional[Union[dict, str]] = None,
- data_root: str = '',
- data_prefix: dict = dict(img_path=''),
- filter_cfg: Optional[dict] = None,
- indices: Optional[Union[int, Sequence[int]]] = None,
- serialize_data: bool = True,
- pipeline: List[Union[dict, Callable]] = ...,
- test_mode: bool = False,
- lazy_init: bool = False,
- max_refetch: int = 1000):
- self.directed = directed
- super().__init__(ann_file, metainfo, data_root, data_prefix,
- filter_cfg, indices, serialize_data, pipeline,
- test_mode, lazy_init, max_refetch)
- self._metainfo['dataset_type'] = 'WildReceiptDataset'
- self._metainfo['task_name'] = 'KIE'
-
- @classmethod
- def _load_metainfo(cls, metainfo: Union[str, dict] = None) -> dict:
- """Collect meta information from path to the class list or the
- dictionary of meta.
-
- Args:
- metainfo (str or dict): Path to the class list, or a meta
- information dict. If ``metainfo`` contains existed filename, it
- will be parsed by ``list_from_file``.
-
- Returns:
- dict: Parsed meta information.
- """
- cls_metainfo = copy.deepcopy(cls.METAINFO)
- if isinstance(metainfo, str):
- cls_metainfo['category'] = []
- for line in list_from_file(metainfo):
- k, v = line.split()
- cls_metainfo['category'].append({'id': k, 'name': v})
- return cls_metainfo
- else:
- return super()._load_metainfo(metainfo)
-
- def load_data_list(self) -> List[dict]:
- """Load data list from annotation file.
-
- Returns:
- List[dict]: A list of annotation dict.
- """
- parser = LineJsonParser(
- keys=['file_name', 'height', 'width', 'annotations'])
- data_list = []
- for line in list_from_file(self.ann_file):
- data_info = parser(line)
- data_info = self.parse_data_info(data_info)
- data_list.append(data_info)
- return data_list
-
- def parse_data_info(self, raw_data_info: dict) -> dict:
- """Parse data info from raw data info.
-
- Args:
- raw_data_info (dict): Raw data info.
-
- Returns:
- dict: Parsed data info.
-
- - img_path (str): Path to the image.
- - img_shape (tuple(int, int)): Image shape in (H, W).
- - instances (list[dict]): A list of instances.
- - bbox (ndarray(dtype=np.float32)): Shape (4, ). Bounding box.
- - text (str): Annotation text.
- - edge_label (int): Edge label.
- - bbox_label (int): Bounding box label.
- """
-
- raw_data_info['img_path'] = raw_data_info['file_name']
- data_info = super().parse_data_info(raw_data_info)
- annotations = data_info['annotations']
-
- assert 'box' in annotations[0]
- assert 'text' in annotations[0]
-
- instances = []
-
- for ann in annotations:
- instance = {}
- bbox = np.array(sort_vertex8(ann['box']), dtype=np.int32)
- bbox = np.array([
- bbox[0::2].min(), bbox[1::2].min(), bbox[0::2].max(),
- bbox[1::2].max()
- ],
- dtype=np.int32)
-
- instance['bbox'] = bbox
- instance['text'] = ann['text']
- instance['bbox_label'] = ann.get('label', 0)
- instance['edge_label'] = ann.get('edge', 0)
- instances.append(instance)
-
- return dict(
- instances=instances,
- img_path=data_info['img_path'],
- img_shape=(data_info['height'], data_info['width']))
diff --git a/pipeline/mmocr/mmocr/engine/__init__.py b/pipeline/mmocr/mmocr/engine/__init__.py
deleted file mode 100644
index 1944bc1e57726ec1922b1e97fb69a75df9c384fe..0000000000000000000000000000000000000000
--- a/pipeline/mmocr/mmocr/engine/__init__.py
+++ /dev/null
@@ -1,2 +0,0 @@
-# Copyright (c) OpenMMLab. All rights reserved.
-from .hooks import * # NOQA
diff --git a/pipeline/mmocr/mmocr/engine/hooks/__init__.py b/pipeline/mmocr/mmocr/engine/hooks/__init__.py
deleted file mode 100644
index 62d8c9e56449a003b0b8ad186c4c18e4743c0906..0000000000000000000000000000000000000000
--- a/pipeline/mmocr/mmocr/engine/hooks/__init__.py
+++ /dev/null
@@ -1,4 +0,0 @@
-# Copyright (c) OpenMMLab. All rights reserved.
-from .visualization_hook import VisualizationHook
-
-__all__ = ['VisualizationHook']
diff --git a/pipeline/mmocr/mmocr/engine/hooks/visualization_hook.py b/pipeline/mmocr/mmocr/engine/hooks/visualization_hook.py
deleted file mode 100644
index 2bbc6aaf490b1a1804afe54dd078a1f63224d391..0000000000000000000000000000000000000000
--- a/pipeline/mmocr/mmocr/engine/hooks/visualization_hook.py
+++ /dev/null
@@ -1,130 +0,0 @@
-# Copyright (c) OpenMMLab. All rights reserved.
-import os.path as osp
-from typing import Optional, Sequence, Union
-
-import mmcv
-import mmengine.fileio as fileio
-from mmengine.hooks import Hook
-from mmengine.runner import Runner
-from mmengine.visualization import Visualizer
-
-from mmocr.registry import HOOKS
-from mmocr.structures import TextDetDataSample, TextRecogDataSample
-
-
-# TODO Files with the same name will be overwritten for multi datasets
-@HOOKS.register_module()
-class VisualizationHook(Hook):
- """Detection Visualization Hook. Used to visualize validation and testing
- process prediction results.
-
- Args:
- enable (bool): Whether to enable this hook. Defaults to False.
- interval (int): The interval of visualization. Defaults to 50.
- score_thr (float): The threshold to visualize the bboxes
- and masks. It's only useful for text detection. Defaults to 0.3.
- show (bool): Whether to display the drawn image. Defaults to False.
- wait_time (float): The interval of show in seconds. Defaults
- to 0.
- backend_args (dict, optional): Instantiates the corresponding file
- backend. It may contain `backend` key to specify the file
- backend. If it contains, the file backend corresponding to this
- value will be used and initialized with the remaining values,
- otherwise the corresponding file backend will be selected
- based on the prefix of the file path. Defaults to None.
- """
-
- def __init__(
- self,
- enable: bool = False,
- interval: int = 50,
- score_thr: float = 0.3,
- show: bool = False,
- draw_pred: bool = False,
- draw_gt: bool = False,
- wait_time: float = 0.,
- backend_args: Optional[dict] = None,
- ) -> None:
- self._visualizer: Visualizer = Visualizer.get_current_instance()
- self.interval = interval
- self.score_thr = score_thr
- self.show = show
- self.draw_pred = draw_pred
- self.draw_gt = draw_gt
- self.wait_time = wait_time
- self.backend_args = backend_args
- self.enable = enable
-
- # TODO after MultiDatasetWrapper, rewrites this function and try to merge
- # with after_val_iter and after_test_iter
- def after_val_iter(self, runner: Runner, batch_idx: int,
- data_batch: Sequence[dict],
- outputs: Sequence[Union[TextDetDataSample,
- TextRecogDataSample]]) -> None:
- """Run after every ``self.interval`` validation iterations.
-
- Args:
- runner (:obj:`Runner`): The runner of the validation process.
- batch_idx (int): The index of the current batch in the val loop.
- data_batch (Sequence[dict]): Data from dataloader.
- outputs (Sequence[:obj:`TextDetDataSample` or
- :obj:`TextRecogDataSample`]): Outputs from model.
- """
- # TODO: data_batch does not include annotation information
- if self.enable is False:
- return
-
- # There is no guarantee that the same batch of images
- # is visualized for each evaluation.
- total_curr_iter = runner.iter + batch_idx
-
- # Visualize only the first data
- if total_curr_iter % self.interval == 0:
- for output in outputs:
- img_path = output.img_path
- img_bytes = fileio.get(
- img_path, backend_args=self.backend_args)
- img = mmcv.imfrombytes(img_bytes, channel_order='rgb')
- self._visualizer.add_datasample(
- osp.splitext(osp.basename(img_path))[0],
- img,
- data_sample=output,
- draw_gt=self.draw_gt,
- draw_pred=self.draw_pred,
- show=self.show,
- wait_time=self.wait_time,
- pred_score_thr=self.score_thr,
- step=total_curr_iter)
-
- def after_test_iter(self, runner: Runner, batch_idx: int,
- data_batch: Sequence[dict],
- outputs: Sequence[Union[TextDetDataSample,
- TextRecogDataSample]]) -> None:
- """Run after every testing iterations.
-
- Args:
- runner (:obj:`Runner`): The runner of the testing process.
- batch_idx (int): The index of the current batch in the val loop.
- data_batch (Sequence[dict]): Data from dataloader.
- outputs (Sequence[:obj:`TextDetDataSample` or
- :obj:`TextRecogDataSample`]): Outputs from model.
- """
-
- if self.enable is False:
- return
-
- for output in outputs:
- img_path = output.img_path
- img_bytes = fileio.get(img_path, backend_args=self.backend_args)
- img = mmcv.imfrombytes(img_bytes, channel_order='rgb')
-
- self._visualizer.add_datasample(
- osp.splitext(osp.basename(img_path))[0],
- img,
- data_sample=output,
- show=self.show,
- draw_gt=self.draw_gt,
- draw_pred=self.draw_pred,
- wait_time=self.wait_time,
- pred_score_thr=self.score_thr,
- step=batch_idx)
diff --git a/pipeline/mmocr/mmocr/evaluation/__init__.py b/pipeline/mmocr/mmocr/evaluation/__init__.py
deleted file mode 100644
index 40cd21686174fe2831ab8bc0693e283297955125..0000000000000000000000000000000000000000
--- a/pipeline/mmocr/mmocr/evaluation/__init__.py
+++ /dev/null
@@ -1,3 +0,0 @@
-# Copyright (c) OpenMMLab. All rights reserved.
-from .evaluator import * # NOQA
-from .metrics import * # NOQA
diff --git a/pipeline/mmocr/mmocr/evaluation/evaluator/__init__.py b/pipeline/mmocr/mmocr/evaluation/evaluator/__init__.py
deleted file mode 100644
index 6b13fe99548e7e2e4c6e196a2da22b9c8cbec8a3..0000000000000000000000000000000000000000
--- a/pipeline/mmocr/mmocr/evaluation/evaluator/__init__.py
+++ /dev/null
@@ -1,4 +0,0 @@
-# Copyright (c) OpenMMLab. All rights reserved.
-from .multi_datasets_evaluator import MultiDatasetsEvaluator
-
-__all__ = ['MultiDatasetsEvaluator']
diff --git a/pipeline/mmocr/mmocr/evaluation/evaluator/multi_datasets_evaluator.py b/pipeline/mmocr/mmocr/evaluation/evaluator/multi_datasets_evaluator.py
deleted file mode 100644
index f01aa70f645d5a9f61fe02386ff214dc72bcffb4..0000000000000000000000000000000000000000
--- a/pipeline/mmocr/mmocr/evaluation/evaluator/multi_datasets_evaluator.py
+++ /dev/null
@@ -1,100 +0,0 @@
-# Copyright (c) OpenMMLab. All rights reserved.
-import warnings
-from collections import OrderedDict
-from typing import Sequence, Union
-
-from mmengine.dist import (broadcast_object_list, collect_results,
- is_main_process)
-from mmengine.evaluator import BaseMetric, Evaluator
-from mmengine.evaluator.metric import _to_cpu
-
-from mmocr.registry import EVALUATOR
-from mmocr.utils.typing_utils import ConfigType
-
-
-@EVALUATOR.register_module()
-class MultiDatasetsEvaluator(Evaluator):
- """Wrapper class to compose class: `ConcatDataset` and multiple
- :class:`BaseMetric` instances.
- The metrics will be evaluated on each dataset slice separately. The name of
- the each metric is the concatenation of the dataset prefix, the metric
- prefix and the key of metric - e.g.
- `dataset_prefix/metric_prefix/accuracy`.
-
- Args:
- metrics (dict or BaseMetric or Sequence): The config of metrics.
- dataset_prefixes (Sequence[str]): The prefix of each dataset. The
- length of this sequence should be the same as the length of the
- datasets.
- """
-
- def __init__(self, metrics: Union[ConfigType, BaseMetric, Sequence],
- dataset_prefixes: Sequence[str]) -> None:
- super().__init__(metrics)
- self.dataset_prefixes = dataset_prefixes
-
- def evaluate(self, size: int) -> dict:
- """Invoke ``evaluate`` method of each metric and collect the metrics
- dictionary.
-
- Args:
- size (int): Length of the entire validation dataset. When batch
- size > 1, the dataloader may pad some data samples to make
- sure all ranks have the same length of dataset slice. The
- ``collect_results`` function will drop the padded data based on
- this size.
-
- Returns:
- dict: Evaluation results of all metrics. The keys are the names
- of the metrics, and the values are corresponding results.
- """
- metrics_results = OrderedDict()
- dataset_slices = self.dataset_meta.get('cumulative_sizes', [size])
- assert len(dataset_slices) == len(self.dataset_prefixes)
- for metric in self.metrics:
- if len(metric.results) == 0:
- warnings.warn(
- f'{metric.__class__.__name__} got empty `self.results`.'
- 'Please ensure that the processed results are properly '
- 'added into `self.results` in `process` method.')
-
- results = collect_results(metric.results, size,
- metric.collect_device)
-
- if is_main_process():
- # cast all tensors in results list to cpu
- results = _to_cpu(results)
- for start, end, dataset_prefix in zip([0] +
- dataset_slices[:-1],
- dataset_slices,
- self.dataset_prefixes):
- metric_results = metric.compute_metrics(
- results[start:end]) # type: ignore
- # Add prefix to metric names
-
- if metric.prefix:
- final_prefix = '/'.join(
- (dataset_prefix, metric.prefix))
- else:
- final_prefix = dataset_prefix
- metric_results = {
- '/'.join((final_prefix, k)): v
- for k, v in metric_results.items()
- }
-
- # Check metric name conflicts
- for name in metric_results.keys():
- if name in metrics_results:
- raise ValueError(
- 'There are multiple evaluation results with '
- f'the same metric name {name}. Please make '
- 'sure all metrics have different prefixes.')
- metrics_results.update(metric_results)
- metric.results.clear()
- if is_main_process():
- metrics_results = [metrics_results]
- else:
- metrics_results = [None] # type: ignore
- broadcast_object_list(metrics_results)
-
- return metrics_results[0]
diff --git a/pipeline/mmocr/mmocr/evaluation/functional/__init__.py b/pipeline/mmocr/mmocr/evaluation/functional/__init__.py
deleted file mode 100644
index 6aaf75768924bef3e7ad6dc1c9d6d0161aab9879..0000000000000000000000000000000000000000
--- a/pipeline/mmocr/mmocr/evaluation/functional/__init__.py
+++ /dev/null
@@ -1,4 +0,0 @@
-# Copyright (c) OpenMMLab. All rights reserved.
-from .hmean import compute_hmean
-
-__all__ = ['compute_hmean']
diff --git a/pipeline/mmocr/mmocr/evaluation/functional/hmean.py b/pipeline/mmocr/mmocr/evaluation/functional/hmean.py
deleted file mode 100644
index d3aabf4c2804ca4d6df43e2699890e682f4f713c..0000000000000000000000000000000000000000
--- a/pipeline/mmocr/mmocr/evaluation/functional/hmean.py
+++ /dev/null
@@ -1,42 +0,0 @@
-# Copyright (c) OpenMMLab. All rights reserved.
-
-
-def compute_hmean(accum_hit_recall, accum_hit_prec, gt_num, pred_num):
- # TODO Add typehints
- """Compute hmean given hit number, ground truth number and prediction
- number.
-
- Args:
- accum_hit_recall (int|float): Accumulated hits for computing recall.
- accum_hit_prec (int|float): Accumulated hits for computing precision.
- gt_num (int): Ground truth number.
- pred_num (int): Prediction number.
-
- Returns:
- recall (float): The recall value.
- precision (float): The precision value.
- hmean (float): The hmean value.
- """
-
- assert isinstance(accum_hit_recall, (float, int))
- assert isinstance(accum_hit_prec, (float, int))
-
- assert isinstance(gt_num, int)
- assert isinstance(pred_num, int)
- assert accum_hit_recall >= 0.0
- assert accum_hit_prec >= 0.0
- assert gt_num >= 0.0
- assert pred_num >= 0.0
-
- if gt_num == 0:
- recall = 1.0
- precision = 0.0 if pred_num > 0 else 1.0
- else:
- recall = float(accum_hit_recall) / gt_num
- precision = 0.0 if pred_num == 0 else float(accum_hit_prec) / pred_num
-
- denom = recall + precision
-
- hmean = 0.0 if denom == 0 else (2.0 * precision * recall / denom)
-
- return recall, precision, hmean
diff --git a/pipeline/mmocr/mmocr/evaluation/metrics/__init__.py b/pipeline/mmocr/mmocr/evaluation/metrics/__init__.py
deleted file mode 100644
index 3b10f4b2ac720e096db27b7e54dcc75611f92dfa..0000000000000000000000000000000000000000
--- a/pipeline/mmocr/mmocr/evaluation/metrics/__init__.py
+++ /dev/null
@@ -1,9 +0,0 @@
-# Copyright (c) OpenMMLab. All rights reserved.
-from .f_metric import F1Metric
-from .hmean_iou_metric import HmeanIOUMetric
-from .recog_metric import CharMetric, OneMinusNEDMetric, WordMetric
-
-__all__ = [
- 'WordMetric', 'CharMetric', 'OneMinusNEDMetric', 'HmeanIOUMetric',
- 'F1Metric'
-]
diff --git a/pipeline/mmocr/mmocr/evaluation/metrics/f_metric.py b/pipeline/mmocr/mmocr/evaluation/metrics/f_metric.py
deleted file mode 100644
index e021ed6b73d059cc15c5255e947c1ff0a5d895ea..0000000000000000000000000000000000000000
--- a/pipeline/mmocr/mmocr/evaluation/metrics/f_metric.py
+++ /dev/null
@@ -1,164 +0,0 @@
-# Copyright (c) OpenMMLab. All rights reserved.
-from typing import Dict, Optional, Sequence, Union
-
-import torch
-from mmengine.evaluator import BaseMetric
-
-from mmocr.registry import METRICS
-
-
-@METRICS.register_module()
-class F1Metric(BaseMetric):
- """Compute F1 scores.
-
- Args:
- num_classes (int): Number of labels.
- key (str): The key name of the predicted and ground truth labels.
- Defaults to 'labels'.
- mode (str or list[str]): Options are:
- - 'micro': Calculate metrics globally by counting the total true
- positives, false negatives and false positives.
- - 'macro': Calculate metrics for each label, and find their
- unweighted mean.
- If mode is a list, then metrics in mode will be calculated
- separately. Defaults to 'micro'.
- cared_classes (list[int]): The indices of the labels particpated in
- the metirc computing. If both ``cared_classes`` and
- ``ignored_classes`` are empty, all classes will be taken into
- account. Defaults to []. Note: ``cared_classes`` and
- ``ignored_classes`` cannot be specified together.
- ignored_classes (list[int]): The index set of labels that are ignored
- when computing metrics. If both ``cared_classes`` and
- ``ignored_classes`` are empty, all classes will be taken into
- account. Defaults to []. Note: ``cared_classes`` and
- ``ignored_classes`` cannot be specified together.
- collect_device (str): Device name used for collecting results from
- different ranks during distributed training. Must be 'cpu' or
- 'gpu'. Defaults to 'cpu'.
- prefix (str, optional): The prefix that will be added in the metric
- names to disambiguate homonymous metrics of different evaluators.
- If prefix is not provided in the argument, self.default_prefix
- will be used instead. Defaults to None.
-
- Warning:
- Only non-negative integer labels are involved in computing. All
- negative ground truth labels will be ignored.
- """
-
- default_prefix: Optional[str] = 'kie'
-
- def __init__(self,
- num_classes: int,
- key: str = 'labels',
- mode: Union[str, Sequence[str]] = 'micro',
- cared_classes: Sequence[int] = [],
- ignored_classes: Sequence[int] = [],
- collect_device: str = 'cpu',
- prefix: Optional[str] = None) -> None:
- super().__init__(collect_device, prefix)
- assert isinstance(num_classes, int)
- assert isinstance(cared_classes, (list, tuple))
- assert isinstance(ignored_classes, (list, tuple))
- assert isinstance(mode, (list, str))
- assert not (len(cared_classes) > 0 and len(ignored_classes) > 0), \
- 'cared_classes and ignored_classes cannot be both non-empty'
-
- if isinstance(mode, str):
- mode = [mode]
- assert set(mode).issubset({'micro', 'macro'})
- self.mode = mode
-
- if len(cared_classes) > 0:
- assert min(cared_classes) >= 0 and \
- max(cared_classes) < num_classes, \
- 'cared_classes must be a subset of [0, num_classes)'
- self.cared_labels = sorted(cared_classes)
- elif len(ignored_classes) > 0:
- assert min(ignored_classes) >= 0 and \
- max(ignored_classes) < num_classes, \
- 'ignored_classes must be a subset of [0, num_classes)'
- self.cared_labels = sorted(
- set(range(num_classes)) - set(ignored_classes))
- else:
- self.cared_labels = list(range(num_classes))
- self.num_classes = num_classes
- self.key = key
-
- def process(self, data_batch: Sequence[Dict],
- data_samples: Sequence[Dict]) -> None:
- """Process one batch of data_samples. The processed results should be
- stored in ``self.results``, which will be used to compute the metrics
- when all batches have been processed.
-
- Args:
- data_batch (Sequence[Dict]): A batch of gts.
- data_samples (Sequence[Dict]): A batch of outputs from the model.
- """
- for data_sample in data_samples:
- pred_labels = data_sample.get('pred_instances').get(self.key).cpu()
- gt_labels = data_sample.get('gt_instances').get(self.key).cpu()
-
- result = dict(
- pred_labels=pred_labels.flatten(),
- gt_labels=gt_labels.flatten())
- self.results.append(result)
-
- def compute_metrics(self, results: Sequence[Dict]) -> Dict:
- """Compute the metrics from processed results.
-
- Args:
- results (list[Dict]): The processed results of each batch.
-
- Returns:
- dict[str, float]: The f1 scores. The keys are the names of the
- metrics, and the values are corresponding results. Possible
- keys are 'micro_f1' and 'macro_f1'.
- """
-
- preds = []
- gts = []
- for result in results:
- preds.append(result['pred_labels'])
- gts.append(result['gt_labels'])
- preds = torch.cat(preds)
- gts = torch.cat(gts)
-
- assert preds.max() < self.num_classes
- assert gts.max() < self.num_classes
-
- cared_labels = preds.new_tensor(self.cared_labels, dtype=torch.long)
-
- hits = (preds == gts)[None, :]
- preds_per_label = cared_labels[:, None] == preds[None, :]
- gts_per_label = cared_labels[:, None] == gts[None, :]
-
- tp = (hits * preds_per_label).float()
- fp = (~hits * preds_per_label).float()
- fn = (~hits * gts_per_label).float()
-
- result = {}
- if 'macro' in self.mode:
- result['macro_f1'] = self._compute_f1(
- tp.sum(-1), fp.sum(-1), fn.sum(-1))
- if 'micro' in self.mode:
- result['micro_f1'] = self._compute_f1(tp.sum(), fp.sum(), fn.sum())
-
- return result
-
- def _compute_f1(self, tp: torch.Tensor, fp: torch.Tensor,
- fn: torch.Tensor) -> float:
- """Compute the F1-score based on the true positives, false positives
- and false negatives.
-
- Args:
- tp (Tensor): The true positives.
- fp (Tensor): The false positives.
- fn (Tensor): The false negatives.
-
- Returns:
- float: The F1-score.
- """
- precision = tp / (tp + fp).clamp(min=1e-8)
- recall = tp / (tp + fn).clamp(min=1e-8)
- f1 = 2 * precision * recall / (precision + recall).clamp(min=1e-8)
- return float(f1.mean())
diff --git a/pipeline/mmocr/mmocr/evaluation/metrics/hmean_iou_metric.py b/pipeline/mmocr/mmocr/evaluation/metrics/hmean_iou_metric.py
deleted file mode 100644
index c5d40971cd965d0f8fcac2247e4859c40bc1760e..0000000000000000000000000000000000000000
--- a/pipeline/mmocr/mmocr/evaluation/metrics/hmean_iou_metric.py
+++ /dev/null
@@ -1,225 +0,0 @@
-# Copyright (c) OpenMMLab. All rights reserved.
-from typing import Dict, List, Optional, Sequence
-
-import numpy as np
-import torch
-from mmengine.evaluator import BaseMetric
-from mmengine.logging import MMLogger
-from scipy.sparse import csr_matrix
-from scipy.sparse.csgraph import maximum_bipartite_matching
-from shapely.geometry import Polygon
-
-from mmocr.evaluation.functional import compute_hmean
-from mmocr.registry import METRICS
-from mmocr.utils import poly_intersection, poly_iou, polys2shapely
-
-
-@METRICS.register_module()
-class HmeanIOUMetric(BaseMetric):
- """HmeanIOU metric.
-
- This method computes the hmean iou metric, which is done in the
- following steps:
-
- - Filter the prediction polygon:
-
- - Scores is smaller than minimum prediction score threshold.
- - The proportion of the area that intersects with gt ignored polygon is
- greater than ignore_precision_thr.
-
- - Computing an M x N IoU matrix, where each element indexing
- E_mn represents the IoU between the m-th valid GT and n-th valid
- prediction.
- - Based on different prediction score threshold:
- - Obtain the ignored predictions according to prediction score.
- The filtered predictions will not be involved in the later metric
- computations.
- - Based on the IoU matrix, get the match metric according to
- ``match_iou_thr``.
- - Based on different `strategy`, accumulate the match number.
- - calculate H-mean under different prediction score threshold.
-
- Args:
- match_iou_thr (float): IoU threshold for a match. Defaults to 0.5.
- ignore_precision_thr (float): Precision threshold when prediction and\
- gt ignored polygons are matched. Defaults to 0.5.
- pred_score_thrs (dict): Best prediction score threshold searching
- space. Defaults to dict(start=0.3, stop=0.9, step=0.1).
- strategy (str): Polygon matching strategy. Options are 'max_matching'
- and 'vanilla'. 'max_matching' refers to the optimum strategy that
- maximizes the number of matches. Vanilla strategy matches gt and
- pred polygons if both of them are never matched before. It was used
- in MMOCR 0.x and and academia. Defaults to 'vanilla'.
- collect_device (str): Device name used for collecting results from
- different ranks during distributed training. Must be 'cpu' or
- 'gpu'. Defaults to 'cpu'.
- prefix (str, optional): The prefix that will be added in the metric
- names to disambiguate homonymous metrics of different evaluators.
- If prefix is not provided in the argument, self.default_prefix
- will be used instead. Defaults to None
- """
- default_prefix: Optional[str] = 'icdar'
-
- def __init__(self,
- match_iou_thr: float = 0.5,
- ignore_precision_thr: float = 0.5,
- pred_score_thrs: Dict = dict(start=0.3, stop=0.9, step=0.1),
- strategy: str = 'vanilla',
- collect_device: str = 'cpu',
- prefix: Optional[str] = None) -> None:
- super().__init__(collect_device=collect_device, prefix=prefix)
- self.match_iou_thr = match_iou_thr
- self.ignore_precision_thr = ignore_precision_thr
- self.pred_score_thrs = np.arange(**pred_score_thrs)
- assert strategy in ['max_matching', 'vanilla']
- self.strategy = strategy
-
- def process(self, data_batch: Sequence[Dict],
- data_samples: Sequence[Dict]) -> None:
- """Process one batch of data samples and predictions. The processed
- results should be stored in ``self.results``, which will be used to
- compute the metrics when all batches have been processed.
-
- Args:
- data_batch (Sequence[Dict]): A batch of data from dataloader.
- data_samples (Sequence[Dict]): A batch of outputs from
- the model.
- """
- for data_sample in data_samples:
-
- pred_instances = data_sample.get('pred_instances')
- pred_polygons = pred_instances.get('polygons')
- pred_scores = pred_instances.get('scores')
- if isinstance(pred_scores, torch.Tensor):
- pred_scores = pred_scores.cpu().numpy()
- pred_scores = np.array(pred_scores, dtype=np.float32)
-
- gt_instances = data_sample.get('gt_instances')
- gt_polys = gt_instances.get('polygons')
- gt_ignore_flags = gt_instances.get('ignored')
- if isinstance(gt_ignore_flags, torch.Tensor):
- gt_ignore_flags = gt_ignore_flags.cpu().numpy()
- gt_polys = polys2shapely(gt_polys)
- pred_polys = polys2shapely(pred_polygons)
-
- pred_ignore_flags = self._filter_preds(pred_polys, gt_polys,
- pred_scores,
- gt_ignore_flags)
-
- gt_num = np.sum(~gt_ignore_flags)
- pred_num = np.sum(~pred_ignore_flags)
- iou_metric = np.zeros([gt_num, pred_num])
-
- # Compute IoU scores amongst kept pred and gt polygons
- for pred_mat_id, pred_poly_id in enumerate(
- self._true_indexes(~pred_ignore_flags)):
- for gt_mat_id, gt_poly_id in enumerate(
- self._true_indexes(~gt_ignore_flags)):
- iou_metric[gt_mat_id, pred_mat_id] = poly_iou(
- gt_polys[gt_poly_id], pred_polys[pred_poly_id])
-
- result = dict(
- iou_metric=iou_metric,
- pred_scores=pred_scores[~pred_ignore_flags])
- self.results.append(result)
-
- def compute_metrics(self, results: List[Dict]) -> Dict:
- """Compute the metrics from processed results.
-
- Args:
- results (list[dict]): The processed results of each batch.
-
- Returns:
- dict: The computed metrics. The keys are the names of the metrics,
- and the values are corresponding results.
- """
- logger: MMLogger = MMLogger.get_current_instance()
-
- best_eval_results = dict(hmean=-1)
- logger.info('Evaluating hmean-iou...')
-
- dataset_pred_num = np.zeros_like(self.pred_score_thrs)
- dataset_hit_num = np.zeros_like(self.pred_score_thrs)
- dataset_gt_num = 0
-
- for result in results:
- iou_metric = result['iou_metric'] # (gt_num, pred_num)
- pred_scores = result['pred_scores'] # (pred_num)
- dataset_gt_num += iou_metric.shape[0]
-
- # Filter out predictions by IoU threshold
- for i, pred_score_thr in enumerate(self.pred_score_thrs):
- pred_ignore_flags = pred_scores < pred_score_thr
- # get the number of matched boxes
- matched_metric = iou_metric[:, ~pred_ignore_flags] \
- > self.match_iou_thr
- if self.strategy == 'max_matching':
- csr_matched_metric = csr_matrix(matched_metric)
- matched_preds = maximum_bipartite_matching(
- csr_matched_metric, perm_type='row')
- # -1 denotes unmatched pred polygons
- dataset_hit_num[i] += np.sum(matched_preds != -1)
- else:
- # first come first matched
- matched_gt_indexes = set()
- matched_pred_indexes = set()
- for gt_idx, pred_idx in zip(*np.nonzero(matched_metric)):
- if gt_idx in matched_gt_indexes or \
- pred_idx in matched_pred_indexes:
- continue
- matched_gt_indexes.add(gt_idx)
- matched_pred_indexes.add(pred_idx)
- dataset_hit_num[i] += len(matched_gt_indexes)
- dataset_pred_num[i] += np.sum(~pred_ignore_flags)
-
- for i, pred_score_thr in enumerate(self.pred_score_thrs):
- recall, precision, hmean = compute_hmean(
- int(dataset_hit_num[i]), int(dataset_hit_num[i]),
- int(dataset_gt_num), int(dataset_pred_num[i]))
- eval_results = dict(
- precision=precision, recall=recall, hmean=hmean)
- logger.info(f'prediction score threshold: {pred_score_thr:.2f}, '
- f'recall: {eval_results["recall"]:.4f}, '
- f'precision: {eval_results["precision"]:.4f}, '
- f'hmean: {eval_results["hmean"]:.4f}\n')
- if eval_results['hmean'] > best_eval_results['hmean']:
- best_eval_results = eval_results
- return best_eval_results
-
- def _filter_preds(self, pred_polys: List[Polygon], gt_polys: List[Polygon],
- pred_scores: List[float],
- gt_ignore_flags: np.ndarray) -> np.ndarray:
- """Filter out the predictions by score threshold and whether it
- overlaps ignored gt polygons.
-
- Args:
- pred_polys (list[Polygon]): Pred polygons.
- gt_polys (list[Polygon]): GT polygons.
- pred_scores (list[float]): Pred scores of polygons.
- gt_ignore_flags (np.ndarray): 1D boolean array indicating
- the positions of ignored gt polygons.
-
- Returns:
- np.ndarray: 1D boolean array indicating the positions of ignored
- pred polygons.
- """
-
- # Filter out predictions based on the minimum score threshold
- pred_ignore_flags = pred_scores < self.pred_score_thrs.min()
-
- # Filter out pred polygons which overlaps any ignored gt polygons
- for pred_id in self._true_indexes(~pred_ignore_flags):
- for gt_id in self._true_indexes(gt_ignore_flags):
- # Match pred with ignored gt
- precision = poly_intersection(
- gt_polys[gt_id], pred_polys[pred_id]) / (
- pred_polys[pred_id].area + 1e-5)
- if precision > self.ignore_precision_thr:
- pred_ignore_flags[pred_id] = True
- break
-
- return pred_ignore_flags
-
- def _true_indexes(self, array: np.ndarray) -> np.ndarray:
- """Get indexes of True elements from a 1D boolean array."""
- return np.where(array)[0]
diff --git a/pipeline/mmocr/mmocr/evaluation/metrics/recog_metric.py b/pipeline/mmocr/mmocr/evaluation/metrics/recog_metric.py
deleted file mode 100644
index a046951211c1c1b7027dce83f9b7b3b7428e2b02..0000000000000000000000000000000000000000
--- a/pipeline/mmocr/mmocr/evaluation/metrics/recog_metric.py
+++ /dev/null
@@ -1,292 +0,0 @@
-# Copyright (c) OpenMMLab. All rights reserved.
-import re
-from difflib import SequenceMatcher
-from typing import Dict, Optional, Sequence, Union
-
-import mmengine
-from mmengine.evaluator import BaseMetric
-from rapidfuzz.distance import Levenshtein
-
-from mmocr.registry import METRICS
-
-
-@METRICS.register_module()
-class WordMetric(BaseMetric):
- """Word metrics for text recognition task.
-
- Args:
- mode (str or list[str]): Options are:
- - 'exact': Accuracy at word level.
- - 'ignore_case': Accuracy at word level, ignoring letter
- case.
- - 'ignore_case_symbol': Accuracy at word level, ignoring
- letter case and symbol. (Default metric for academic evaluation)
- If mode is a list, then metrics in mode will be calculated
- separately. Defaults to 'ignore_case_symbol'
- valid_symbol (str): Valid characters. Defaults to
- '[^A-Z^a-z^0-9^\u4e00-\u9fa5]'
- collect_device (str): Device name used for collecting results from
- different ranks during distributed training. Must be 'cpu' or
- 'gpu'. Defaults to 'cpu'.
- prefix (str, optional): The prefix that will be added in the metric
- names to disambiguate homonymous metrics of different evaluators.
- If prefix is not provided in the argument, self.default_prefix
- will be used instead. Defaults to None.
- """
-
- default_prefix: Optional[str] = 'recog'
-
- def __init__(self,
- mode: Union[str, Sequence[str]] = 'ignore_case_symbol',
- valid_symbol: str = '[^A-Z^a-z^0-9^\u4e00-\u9fa5]',
- collect_device: str = 'cpu',
- prefix: Optional[str] = None) -> None:
- super().__init__(collect_device, prefix)
- self.valid_symbol = re.compile(valid_symbol)
- if isinstance(mode, str):
- mode = [mode]
- assert mmengine.is_seq_of(mode, str)
- assert set(mode).issubset(
- {'exact', 'ignore_case', 'ignore_case_symbol'})
- self.mode = set(mode)
-
- def process(self, data_batch: Sequence[Dict],
- data_samples: Sequence[Dict]) -> None:
- """Process one batch of data_samples. The processed results should be
- stored in ``self.results``, which will be used to compute the metrics
- when all batches have been processed.
-
- Args:
- data_batch (Sequence[Dict]): A batch of gts.
- data_samples (Sequence[Dict]): A batch of outputs from the model.
- """
- for data_sample in data_samples:
- match_num = 0
- match_ignore_case_num = 0
- match_ignore_case_symbol_num = 0
- pred_text = data_sample.get('pred_text').get('item')
- gt_text = data_sample.get('gt_text').get('item')
- if 'ignore_case' in self.mode or 'ignore_case_symbol' in self.mode:
- pred_text_lower = pred_text.lower()
- gt_text_lower = gt_text.lower()
- if 'ignore_case_symbol' in self.mode:
- gt_text_lower_ignore = self.valid_symbol.sub('', gt_text_lower)
- pred_text_lower_ignore = self.valid_symbol.sub(
- '', pred_text_lower)
- match_ignore_case_symbol_num =\
- gt_text_lower_ignore == pred_text_lower_ignore
- if 'ignore_case' in self.mode:
- match_ignore_case_num = pred_text_lower == gt_text_lower
- if 'exact' in self.mode:
- match_num = pred_text == gt_text
- result = dict(
- match_num=match_num,
- match_ignore_case_num=match_ignore_case_num,
- match_ignore_case_symbol_num=match_ignore_case_symbol_num)
- self.results.append(result)
-
- def compute_metrics(self, results: Sequence[Dict]) -> Dict:
- """Compute the metrics from processed results.
-
- Args:
- results (list[Dict]): The processed results of each batch.
-
- Returns:
- Dict: The computed metrics. The keys are the names of the metrics,
- and the values are corresponding results.
- """
-
- eps = 1e-8
- eval_res = {}
- gt_word_num = len(results)
- if 'exact' in self.mode:
- match_nums = [result['match_num'] for result in results]
- match_nums = sum(match_nums)
- eval_res['word_acc'] = 1.0 * match_nums / (eps + gt_word_num)
- if 'ignore_case' in self.mode:
- match_ignore_case_num = [
- result['match_ignore_case_num'] for result in results
- ]
- match_ignore_case_num = sum(match_ignore_case_num)
- eval_res['word_acc_ignore_case'] = 1.0 *\
- match_ignore_case_num / (eps + gt_word_num)
- if 'ignore_case_symbol' in self.mode:
- match_ignore_case_symbol_num = [
- result['match_ignore_case_symbol_num'] for result in results
- ]
- match_ignore_case_symbol_num = sum(match_ignore_case_symbol_num)
- eval_res['word_acc_ignore_case_symbol'] = 1.0 *\
- match_ignore_case_symbol_num / (eps + gt_word_num)
-
- for key, value in eval_res.items():
- eval_res[key] = float(f'{value:.4f}')
- return eval_res
-
-
-@METRICS.register_module()
-class CharMetric(BaseMetric):
- """Character metrics for text recognition task.
-
- Args:
- valid_symbol (str): Valid characters.
- Defaults to '[^A-Z^a-z^0-9^\u4e00-\u9fa5]'
- collect_device (str): Device name used for collecting results from
- different ranks during distributed training. Must be 'cpu' or
- 'gpu'. Defaults to 'cpu'.
- prefix (str, optional): The prefix that will be added in the metric
- names to disambiguate homonymous metrics of different evaluators.
- If prefix is not provided in the argument, self.default_prefix
- will be used instead. Defaults to None.
- """
-
- default_prefix: Optional[str] = 'recog'
-
- def __init__(self,
- valid_symbol: str = '[^A-Z^a-z^0-9^\u4e00-\u9fa5]',
- collect_device: str = 'cpu',
- prefix: Optional[str] = None) -> None:
- super().__init__(collect_device, prefix)
- self.valid_symbol = re.compile(valid_symbol)
-
- def process(self, data_batch: Sequence[Dict],
- data_samples: Sequence[Dict]) -> None:
- """Process one batch of data_samples. The processed results should be
- stored in ``self.results``, which will be used to compute the metrics
- when all batches have been processed.
-
- Args:
- data_batch (Sequence[Dict]): A batch of gts.
- data_samples (Sequence[Dict]): A batch of outputs from the model.
- """
- for data_sample in data_samples:
- pred_text = data_sample.get('pred_text').get('item')
- gt_text = data_sample.get('gt_text').get('item')
- gt_text_lower = gt_text.lower()
- pred_text_lower = pred_text.lower()
- gt_text_lower_ignore = self.valid_symbol.sub('', gt_text_lower)
- pred_text_lower_ignore = self.valid_symbol.sub('', pred_text_lower)
- # number to calculate char level recall & precision
- result = dict(
- gt_char_num=len(gt_text_lower_ignore),
- pred_char_num=len(pred_text_lower_ignore),
- true_positive_char_num=self._cal_true_positive_char(
- pred_text_lower_ignore, gt_text_lower_ignore))
- self.results.append(result)
-
- def compute_metrics(self, results: Sequence[Dict]) -> Dict:
- """Compute the metrics from processed results.
-
- Args:
- results (list[Dict]): The processed results of each batch.
-
- Returns:
- Dict: The computed metrics. The keys are the names of the
- metrics, and the values are corresponding results.
- """
- gt_char_num = [result['gt_char_num'] for result in results]
- pred_char_num = [result['pred_char_num'] for result in results]
- true_positive_char_num = [
- result['true_positive_char_num'] for result in results
- ]
- gt_char_num = sum(gt_char_num)
- pred_char_num = sum(pred_char_num)
- true_positive_char_num = sum(true_positive_char_num)
-
- eps = 1e-8
- char_recall = 1.0 * true_positive_char_num / (eps + gt_char_num)
- char_precision = 1.0 * true_positive_char_num / (eps + pred_char_num)
- eval_res = {}
- eval_res['char_recall'] = char_recall
- eval_res['char_precision'] = char_precision
-
- for key, value in eval_res.items():
- eval_res[key] = float(f'{value:.4f}')
- return eval_res
-
- def _cal_true_positive_char(self, pred: str, gt: str) -> int:
- """Calculate correct character number in prediction.
-
- Args:
- pred (str): Prediction text.
- gt (str): Ground truth text.
-
- Returns:
- true_positive_char_num (int): The true positive number.
- """
-
- all_opt = SequenceMatcher(None, pred, gt)
- true_positive_char_num = 0
- for opt, _, _, s2, e2 in all_opt.get_opcodes():
- if opt == 'equal':
- true_positive_char_num += (e2 - s2)
- else:
- pass
- return true_positive_char_num
-
-
-@METRICS.register_module()
-class OneMinusNEDMetric(BaseMetric):
- """One minus NED metric for text recognition task.
-
- Args:
- valid_symbol (str): Valid characters. Defaults to
- '[^A-Z^a-z^0-9^\u4e00-\u9fa5]'
- collect_device (str): Device name used for collecting results from
- different ranks during distributed training. Must be 'cpu' or
- 'gpu'. Defaults to 'cpu'.
- prefix (str, optional): The prefix that will be added in the metric
- names to disambiguate homonymous metrics of different evaluators.
- If prefix is not provided in the argument, self.default_prefix
- will be used instead. Defaults to None
- """
- default_prefix: Optional[str] = 'recog'
-
- def __init__(self,
- valid_symbol: str = '[^A-Z^a-z^0-9^\u4e00-\u9fa5]',
- collect_device: str = 'cpu',
- prefix: Optional[str] = None) -> None:
- super().__init__(collect_device, prefix)
- self.valid_symbol = re.compile(valid_symbol)
-
- def process(self, data_batch: Sequence[Dict],
- data_samples: Sequence[Dict]) -> None:
- """Process one batch of data_samples. The processed results should be
- stored in ``self.results``, which will be used to compute the metrics
- when all batches have been processed.
-
- Args:
- data_batch (Sequence[Dict]): A batch of gts.
- data_samples (Sequence[Dict]): A batch of outputs from the model.
- """
- for data_sample in data_samples:
- pred_text = data_sample.get('pred_text').get('item')
- gt_text = data_sample.get('gt_text').get('item')
- gt_text_lower = gt_text.lower()
- pred_text_lower = pred_text.lower()
- gt_text_lower_ignore = self.valid_symbol.sub('', gt_text_lower)
- pred_text_lower_ignore = self.valid_symbol.sub('', pred_text_lower)
- norm_ed = Levenshtein.normalized_distance(pred_text_lower_ignore,
- gt_text_lower_ignore)
- result = dict(norm_ed=norm_ed)
- self.results.append(result)
-
- def compute_metrics(self, results: Sequence[Dict]) -> Dict:
- """Compute the metrics from processed results.
-
- Args:
- results (list[Dict]): The processed results of each batch.
-
- Returns:
- Dict: The computed metrics. The keys are the names of the
- metrics, and the values are corresponding results.
- """
-
- gt_word_num = len(results)
- norm_ed = [result['norm_ed'] for result in results]
- norm_ed_sum = sum(norm_ed)
- normalized_edit_distance = norm_ed_sum / max(1, gt_word_num)
- eval_res = {}
- eval_res['1-N.E.D'] = 1.0 - normalized_edit_distance
- for key, value in eval_res.items():
- eval_res[key] = float(f'{value:.4f}')
- return eval_res
diff --git a/pipeline/mmocr/mmocr/models/__init__.py b/pipeline/mmocr/mmocr/models/__init__.py
deleted file mode 100644
index abea668b3d52be16b5fe41ab20e3494885bba297..0000000000000000000000000000000000000000
--- a/pipeline/mmocr/mmocr/models/__init__.py
+++ /dev/null
@@ -1,5 +0,0 @@
-# Copyright (c) OpenMMLab. All rights reserved.
-from .common import * # NOQA
-from .kie import * # NOQA
-from .textdet import * # NOQA
-from .textrecog import * # NOQA
diff --git a/pipeline/mmocr/mmocr/models/__pycache__/__init__.cpython-38.pyc b/pipeline/mmocr/mmocr/models/__pycache__/__init__.cpython-38.pyc
deleted file mode 100644
index 096f66d248be3c570c8ed66c8fe415c55b1a005d..0000000000000000000000000000000000000000
Binary files a/pipeline/mmocr/mmocr/models/__pycache__/__init__.cpython-38.pyc and /dev/null differ
diff --git a/pipeline/mmocr/mmocr/models/__pycache__/__init__.cpython-39.pyc b/pipeline/mmocr/mmocr/models/__pycache__/__init__.cpython-39.pyc
deleted file mode 100644
index fe1d8ab2c6d6c8c6e0e42a51ad8c585cb42467d6..0000000000000000000000000000000000000000
Binary files a/pipeline/mmocr/mmocr/models/__pycache__/__init__.cpython-39.pyc and /dev/null differ
diff --git a/pipeline/mmocr/mmocr/models/common/__init__.py b/pipeline/mmocr/mmocr/models/common/__init__.py
deleted file mode 100644
index 30fe928ceced2064bc4adabc5d36291872df4b29..0000000000000000000000000000000000000000
--- a/pipeline/mmocr/mmocr/models/common/__init__.py
+++ /dev/null
@@ -1,7 +0,0 @@
-# Copyright (c) OpenMMLab. All rights reserved.
-from .backbones import * # NOQA
-from .dictionary import * # NOQA
-from .layers import * # NOQA
-from .losses import * # NOQA
-from .modules import * # NOQA
-from .plugins import * # NOQA
diff --git a/pipeline/mmocr/mmocr/models/common/__pycache__/__init__.cpython-38.pyc b/pipeline/mmocr/mmocr/models/common/__pycache__/__init__.cpython-38.pyc
deleted file mode 100644
index 0a7853ab59496b157009abfc4163fb491d89a290..0000000000000000000000000000000000000000
Binary files a/pipeline/mmocr/mmocr/models/common/__pycache__/__init__.cpython-38.pyc and /dev/null differ
diff --git a/pipeline/mmocr/mmocr/models/common/__pycache__/__init__.cpython-39.pyc b/pipeline/mmocr/mmocr/models/common/__pycache__/__init__.cpython-39.pyc
deleted file mode 100644
index cfaa2341263563ef1b0a665b7ff6af5e8e8e9fba..0000000000000000000000000000000000000000
Binary files a/pipeline/mmocr/mmocr/models/common/__pycache__/__init__.cpython-39.pyc and /dev/null differ
diff --git a/pipeline/mmocr/mmocr/models/common/backbones/__init__.py b/pipeline/mmocr/mmocr/models/common/backbones/__init__.py
deleted file mode 100644
index 053ed524657ebf335ea622776687291931df2358..0000000000000000000000000000000000000000
--- a/pipeline/mmocr/mmocr/models/common/backbones/__init__.py
+++ /dev/null
@@ -1,5 +0,0 @@
-# Copyright (c) OpenMMLab. All rights reserved.
-from .clip_resnet import CLIPResNet
-from .unet import UNet
-from .vit import VisionTransformer, VisionTransformer_LoRA
-__all__ = ['UNet', 'CLIPResNet', 'VisionTransformer', 'VisionTransformer_LoRA']
diff --git a/pipeline/mmocr/mmocr/models/common/backbones/__pycache__/__init__.cpython-38.pyc b/pipeline/mmocr/mmocr/models/common/backbones/__pycache__/__init__.cpython-38.pyc
deleted file mode 100644
index abc1d7a6013b3dbfb9724eef133353cc095422ae..0000000000000000000000000000000000000000
Binary files a/pipeline/mmocr/mmocr/models/common/backbones/__pycache__/__init__.cpython-38.pyc and /dev/null differ
diff --git a/pipeline/mmocr/mmocr/models/common/backbones/__pycache__/__init__.cpython-39.pyc b/pipeline/mmocr/mmocr/models/common/backbones/__pycache__/__init__.cpython-39.pyc
deleted file mode 100644
index 40db8bef75f567a4859a2bc39fcf6c9afedebb3a..0000000000000000000000000000000000000000
Binary files a/pipeline/mmocr/mmocr/models/common/backbones/__pycache__/__init__.cpython-39.pyc and /dev/null differ
diff --git a/pipeline/mmocr/mmocr/models/common/backbones/__pycache__/clip_resnet.cpython-38.pyc b/pipeline/mmocr/mmocr/models/common/backbones/__pycache__/clip_resnet.cpython-38.pyc
deleted file mode 100644
index 5915142af3025ce145cf41f78fd30d77d435c0f3..0000000000000000000000000000000000000000
Binary files a/pipeline/mmocr/mmocr/models/common/backbones/__pycache__/clip_resnet.cpython-38.pyc and /dev/null differ
diff --git a/pipeline/mmocr/mmocr/models/common/backbones/__pycache__/clip_resnet.cpython-39.pyc b/pipeline/mmocr/mmocr/models/common/backbones/__pycache__/clip_resnet.cpython-39.pyc
deleted file mode 100644
index 7a586087407a51e1c11e6e79daabd9e3bd6b9628..0000000000000000000000000000000000000000
Binary files a/pipeline/mmocr/mmocr/models/common/backbones/__pycache__/clip_resnet.cpython-39.pyc and /dev/null differ
diff --git a/pipeline/mmocr/mmocr/models/common/backbones/__pycache__/unet.cpython-38.pyc b/pipeline/mmocr/mmocr/models/common/backbones/__pycache__/unet.cpython-38.pyc
deleted file mode 100644
index 3ff69bd65b8d0bb8de4251f2001332e1d524664d..0000000000000000000000000000000000000000
Binary files a/pipeline/mmocr/mmocr/models/common/backbones/__pycache__/unet.cpython-38.pyc and /dev/null differ
diff --git a/pipeline/mmocr/mmocr/models/common/backbones/__pycache__/unet.cpython-39.pyc b/pipeline/mmocr/mmocr/models/common/backbones/__pycache__/unet.cpython-39.pyc
deleted file mode 100644
index ec39236c72abaaecbb961e93d394bb55e59c52cf..0000000000000000000000000000000000000000
Binary files a/pipeline/mmocr/mmocr/models/common/backbones/__pycache__/unet.cpython-39.pyc and /dev/null differ
diff --git a/pipeline/mmocr/mmocr/models/common/backbones/__pycache__/vit.cpython-38.pyc b/pipeline/mmocr/mmocr/models/common/backbones/__pycache__/vit.cpython-38.pyc
deleted file mode 100644
index 6739832b21af4a5acc9efd07ce4ecf1655bba58d..0000000000000000000000000000000000000000
Binary files a/pipeline/mmocr/mmocr/models/common/backbones/__pycache__/vit.cpython-38.pyc and /dev/null differ
diff --git a/pipeline/mmocr/mmocr/models/common/backbones/__pycache__/vit.cpython-39.pyc b/pipeline/mmocr/mmocr/models/common/backbones/__pycache__/vit.cpython-39.pyc
deleted file mode 100644
index 986cf26253ba7d3b569b42df3a03cc2379e62d22..0000000000000000000000000000000000000000
Binary files a/pipeline/mmocr/mmocr/models/common/backbones/__pycache__/vit.cpython-39.pyc and /dev/null differ
diff --git a/pipeline/mmocr/mmocr/models/common/backbones/clip_resnet.py b/pipeline/mmocr/mmocr/models/common/backbones/clip_resnet.py
deleted file mode 100644
index 4de20986b7e4ab3031c20d7d1660c3fb5b6894df..0000000000000000000000000000000000000000
--- a/pipeline/mmocr/mmocr/models/common/backbones/clip_resnet.py
+++ /dev/null
@@ -1,100 +0,0 @@
-# Copyright (c) OpenMMLab. All rights reserved.
-
-import torch.nn as nn
-from mmdet.models.backbones import ResNet
-from mmdet.models.backbones.resnet import Bottleneck
-
-from mmocr.registry import MODELS
-
-
-class CLIPBottleneck(Bottleneck):
- """Bottleneck for CLIPResNet.
-
- It is a Bottleneck variant used in the ResNet variant of CLIP. After the
- second convolution layer, there is an additional average pooling layer with
- kernel_size 2 and stride 2, which is added as a plugin when the
- input stride > 1. The stride of each convolution layer is always set to 1.
-
- Args:
- **kwargs: Keyword arguments for
- :class:``mmdet.models.backbones.resnet.Bottleneck``.
- """
-
- def __init__(self, **kwargs):
- stride = kwargs.get('stride', 1)
- kwargs['stride'] = 1
- plugins = kwargs.get('plugins', None)
- if stride > 1:
- if plugins is None:
- plugins = []
-
- plugins.insert(
- 0,
- dict(
- cfg=dict(type='mmocr.AvgPool2d', kernel_size=2),
- position='after_conv2'))
- kwargs['plugins'] = plugins
- super().__init__(**kwargs)
-
-
-@MODELS.register_module()
-class CLIPResNet(ResNet):
- """Implement the ResNet variant used in `oCLIP.
-
- `_.
-
- It is also the official structure in
- `CLIP `_.
-
- Compared with ResNetV1d structure, CLIPResNet replaces the
- max pooling layer with an average pooling layer at the end
- of the input stem.
-
- In the Bottleneck of CLIPResNet, after the second convolution
- layer, there is an additional average pooling layer with
- kernel_size 2 and stride 2, which is added as a plugin
- when the input stride > 1.
- The stride of each convolution layer is always set to 1.
-
- Args:
- depth (int): Depth of resnet, options are [50]. Defaults to 50.
- strides (sequence(int)): Strides of the first block of each stage.
- Defaults to (1, 2, 2, 2).
- deep_stem (bool): Replace 7x7 conv in input stem with 3 3x3 conv.
- Defaults to True.
- avg_down (bool): Use AvgPool instead of stride conv at
- the downsampling stage in the bottleneck. Defaults to True.
- **kwargs: Keyword arguments for
- :class:``mmdet.models.backbones.resnet.ResNet``.
- """
- arch_settings = {
- 50: (CLIPBottleneck, (3, 4, 6, 3)),
- }
-
- def __init__(self,
- depth=50,
- strides=(1, 2, 2, 2),
- deep_stem=True,
- avg_down=True,
- **kwargs):
- super().__init__(
- depth=depth,
- strides=strides,
- deep_stem=deep_stem,
- avg_down=avg_down,
- **kwargs)
-
- def _make_stem_layer(self, in_channels: int, stem_channels: int):
- """Build stem layer for CLIPResNet used in `CLIP
- https://github.com/openai/CLIP>`_.
-
- It uses an average pooling layer rather than a max pooling
- layer at the end of the input stem.
-
- Args:
- in_channels (int): Number of input channels.
- stem_channels (int): Number of output channels.
- """
- super()._make_stem_layer(in_channels, stem_channels)
- if self.deep_stem:
- self.maxpool = nn.AvgPool2d(kernel_size=2)
diff --git a/pipeline/mmocr/mmocr/models/common/backbones/unet.py b/pipeline/mmocr/mmocr/models/common/backbones/unet.py
deleted file mode 100644
index d582551715fc20353d26745b9d1bb55892b7a10d..0000000000000000000000000000000000000000
--- a/pipeline/mmocr/mmocr/models/common/backbones/unet.py
+++ /dev/null
@@ -1,516 +0,0 @@
-# Copyright (c) OpenMMLab. All rights reserved.
-import torch
-import torch.nn as nn
-import torch.utils.checkpoint as cp
-from mmcv.cnn import ConvModule, build_norm_layer
-from mmengine.model import BaseModule
-from mmengine.utils.dl_utils.parrots_wrapper import _BatchNorm
-
-from mmocr.registry import MODELS
-
-
-class UpConvBlock(nn.Module):
- """Upsample convolution block in decoder for UNet.
-
- This upsample convolution block consists of one upsample module
- followed by one convolution block. The upsample module expands the
- high-level low-resolution feature map and the convolution block fuses
- the upsampled high-level low-resolution feature map and the low-level
- high-resolution feature map from encoder.
-
- Args:
- conv_block (nn.Sequential): Sequential of convolutional layers.
- in_channels (int): Number of input channels of the high-level
- skip_channels (int): Number of input channels of the low-level
- high-resolution feature map from encoder.
- out_channels (int): Number of output channels.
- num_convs (int): Number of convolutional layers in the conv_block.
- Default: 2.
- stride (int): Stride of convolutional layer in conv_block. Default: 1.
- dilation (int): Dilation rate of convolutional layer in conv_block.
- Default: 1.
- with_cp (bool): Use checkpoint or not. Using checkpoint will save some
- memory while slowing down the training speed. Default: False.
- conv_cfg (dict | None): Config dict for convolution layer.
- Default: None.
- norm_cfg (dict | None): Config dict for normalization layer.
- Default: dict(type='BN').
- act_cfg (dict | None): Config dict for activation layer in ConvModule.
- Default: dict(type='ReLU').
- upsample_cfg (dict): The upsample config of the upsample module in
- decoder. Default: dict(type='InterpConv'). If the size of
- high-level feature map is the same as that of skip feature map
- (low-level feature map from encoder), it does not need upsample the
- high-level feature map and the upsample_cfg is None.
- dcn (bool): Use deformable convolution in convolutional layer or not.
- Default: None.
- plugins (dict): plugins for convolutional layers. Default: None.
- """
-
- def __init__(self,
- conv_block,
- in_channels,
- skip_channels,
- out_channels,
- num_convs=2,
- stride=1,
- dilation=1,
- with_cp=False,
- conv_cfg=None,
- norm_cfg=dict(type='BN'),
- act_cfg=dict(type='ReLU'),
- upsample_cfg=dict(type='InterpConv'),
- dcn=None,
- plugins=None):
- super().__init__()
- assert dcn is None, 'Not implemented yet.'
- assert plugins is None, 'Not implemented yet.'
-
- self.conv_block = conv_block(
- in_channels=2 * skip_channels,
- out_channels=out_channels,
- num_convs=num_convs,
- stride=stride,
- dilation=dilation,
- with_cp=with_cp,
- conv_cfg=conv_cfg,
- norm_cfg=norm_cfg,
- act_cfg=act_cfg,
- dcn=None,
- plugins=None)
- if upsample_cfg is not None:
- upsample_cfg.update(
- dict(
- in_channels=in_channels,
- out_channels=skip_channels,
- with_cp=with_cp,
- norm_cfg=norm_cfg,
- act_cfg=act_cfg))
- self.upsample = MODELS.build(upsample_cfg)
- else:
- self.upsample = ConvModule(
- in_channels,
- skip_channels,
- kernel_size=1,
- stride=1,
- padding=0,
- conv_cfg=conv_cfg,
- norm_cfg=norm_cfg,
- act_cfg=act_cfg)
-
- def forward(self, skip, x):
- """Forward function."""
-
- x = self.upsample(x)
- out = torch.cat([skip, x], dim=1)
- out = self.conv_block(out)
-
- return out
-
-
-class BasicConvBlock(nn.Module):
- """Basic convolutional block for UNet.
-
- This module consists of several plain convolutional layers.
-
- Args:
- in_channels (int): Number of input channels.
- out_channels (int): Number of output channels.
- num_convs (int): Number of convolutional layers. Default: 2.
- stride (int): Whether use stride convolution to downsample
- the input feature map. If stride=2, it only uses stride convolution
- in the first convolutional layer to downsample the input feature
- map. Options are 1 or 2. Default: 1.
- dilation (int): Whether use dilated convolution to expand the
- receptive field. Set dilation rate of each convolutional layer and
- the dilation rate of the first convolutional layer is always 1.
- Default: 1.
- with_cp (bool): Use checkpoint or not. Using checkpoint will save some
- memory while slowing down the training speed. Default: False.
- conv_cfg (dict | None): Config dict for convolution layer.
- Default: None.
- norm_cfg (dict | None): Config dict for normalization layer.
- Default: dict(type='BN').
- act_cfg (dict | None): Config dict for activation layer in ConvModule.
- Default: dict(type='ReLU').
- dcn (bool): Use deformable convolution in convolutional layer or not.
- Default: None.
- plugins (dict): plugins for convolutional layers. Default: None.
- """
-
- def __init__(self,
- in_channels,
- out_channels,
- num_convs=2,
- stride=1,
- dilation=1,
- with_cp=False,
- conv_cfg=None,
- norm_cfg=dict(type='BN'),
- act_cfg=dict(type='ReLU'),
- dcn=None,
- plugins=None):
- super().__init__()
- assert dcn is None, 'Not implemented yet.'
- assert plugins is None, 'Not implemented yet.'
-
- self.with_cp = with_cp
- convs = []
- for i in range(num_convs):
- convs.append(
- ConvModule(
- in_channels=in_channels if i == 0 else out_channels,
- out_channels=out_channels,
- kernel_size=3,
- stride=stride if i == 0 else 1,
- dilation=1 if i == 0 else dilation,
- padding=1 if i == 0 else dilation,
- conv_cfg=conv_cfg,
- norm_cfg=norm_cfg,
- act_cfg=act_cfg))
-
- self.convs = nn.Sequential(*convs)
-
- def forward(self, x):
- """Forward function."""
-
- if self.with_cp and x.requires_grad:
- out = cp.checkpoint(self.convs, x)
- else:
- out = self.convs(x)
- return out
-
-
-@MODELS.register_module()
-class DeconvModule(nn.Module):
- """Deconvolution upsample module in decoder for UNet (2X upsample).
-
- This module uses deconvolution to upsample feature map in the decoder
- of UNet.
-
- Args:
- in_channels (int): Number of input channels.
- out_channels (int): Number of output channels.
- with_cp (bool): Use checkpoint or not. Using checkpoint will save some
- memory while slowing down the training speed. Default: False.
- norm_cfg (dict | None): Config dict for normalization layer.
- Default: dict(type='BN').
- act_cfg (dict | None): Config dict for activation layer in ConvModule.
- Default: dict(type='ReLU').
- kernel_size (int): Kernel size of the convolutional layer. Default: 4.
- """
-
- def __init__(self,
- in_channels,
- out_channels,
- with_cp=False,
- norm_cfg=dict(type='BN'),
- act_cfg=dict(type='ReLU'),
- *,
- kernel_size=4,
- scale_factor=2):
- super().__init__()
-
- assert (
- kernel_size - scale_factor >= 0
- and (kernel_size - scale_factor) % 2 == 0), (
- f'kernel_size should be greater than or equal to scale_factor '
- f'and (kernel_size - scale_factor) should be even numbers, '
- f'while the kernel size is {kernel_size} and scale_factor is '
- f'{scale_factor}.')
-
- stride = scale_factor
- padding = (kernel_size - scale_factor) // 2
- self.with_cp = with_cp
- deconv = nn.ConvTranspose2d(
- in_channels,
- out_channels,
- kernel_size=kernel_size,
- stride=stride,
- padding=padding)
-
- _, norm = build_norm_layer(norm_cfg, out_channels)
- activate = MODELS.build(act_cfg)
- self.deconv_upsamping = nn.Sequential(deconv, norm, activate)
-
- def forward(self, x):
- """Forward function."""
-
- if self.with_cp and x.requires_grad:
- out = cp.checkpoint(self.deconv_upsamping, x)
- else:
- out = self.deconv_upsamping(x)
- return out
-
-
-@MODELS.register_module()
-class InterpConv(nn.Module):
- """Interpolation upsample module in decoder for UNet.
-
- This module uses interpolation to upsample feature map in the decoder
- of UNet. It consists of one interpolation upsample layer and one
- convolutional layer. It can be one interpolation upsample layer followed
- by one convolutional layer (conv_first=False) or one convolutional layer
- followed by one interpolation upsample layer (conv_first=True).
-
- Args:
- in_channels (int): Number of input channels.
- out_channels (int): Number of output channels.
- with_cp (bool): Use checkpoint or not. Using checkpoint will save some
- memory while slowing down the training speed. Default: False.
- norm_cfg (dict | None): Config dict for normalization layer.
- Default: dict(type='BN').
- act_cfg (dict | None): Config dict for activation layer in ConvModule.
- Default: dict(type='ReLU').
- conv_cfg (dict | None): Config dict for convolution layer.
- Default: None.
- conv_first (bool): Whether convolutional layer or interpolation
- upsample layer first. Default: False. It means interpolation
- upsample layer followed by one convolutional layer.
- kernel_size (int): Kernel size of the convolutional layer. Default: 1.
- stride (int): Stride of the convolutional layer. Default: 1.
- padding (int): Padding of the convolutional layer. Default: 1.
- upsample_cfg (dict): Interpolation config of the upsample layer.
- Default: dict(
- scale_factor=2, mode='bilinear', align_corners=False).
- """
-
- def __init__(self,
- in_channels,
- out_channels,
- with_cp=False,
- norm_cfg=dict(type='BN'),
- act_cfg=dict(type='ReLU'),
- *,
- conv_cfg=None,
- conv_first=False,
- kernel_size=1,
- stride=1,
- padding=0,
- upsample_cfg=dict(
- scale_factor=2, mode='bilinear', align_corners=False)):
- super().__init__()
-
- self.with_cp = with_cp
- conv = ConvModule(
- in_channels,
- out_channels,
- kernel_size=kernel_size,
- stride=stride,
- padding=padding,
- conv_cfg=conv_cfg,
- norm_cfg=norm_cfg,
- act_cfg=act_cfg)
- upsample = nn.Upsample(**upsample_cfg)
- if conv_first:
- self.interp_upsample = nn.Sequential(conv, upsample)
- else:
- self.interp_upsample = nn.Sequential(upsample, conv)
-
- def forward(self, x):
- """Forward function."""
-
- if self.with_cp and x.requires_grad:
- out = cp.checkpoint(self.interp_upsample, x)
- else:
- out = self.interp_upsample(x)
- return out
-
-
-@MODELS.register_module()
-class UNet(BaseModule):
- """UNet backbone.
- U-Net: Convolutional Networks for Biomedical Image Segmentation.
- https://arxiv.org/pdf/1505.04597.pdf
-
- Args:
- in_channels (int): Number of input image channels. Default" 3.
- base_channels (int): Number of base channels of each stage.
- The output channels of the first stage. Default: 64.
- num_stages (int): Number of stages in encoder, normally 5. Default: 5.
- strides (Sequence[int 1 | 2]): Strides of each stage in encoder.
- len(strides) is equal to num_stages. Normally the stride of the
- first stage in encoder is 1. If strides[i]=2, it uses stride
- convolution to downsample in the correspondence encoder stage.
- Default: (1, 1, 1, 1, 1).
- enc_num_convs (Sequence[int]): Number of convolutional layers in the
- convolution block of the correspondence encoder stage.
- Default: (2, 2, 2, 2, 2).
- dec_num_convs (Sequence[int]): Number of convolutional layers in the
- convolution block of the correspondence decoder stage.
- Default: (2, 2, 2, 2).
- downsamples (Sequence[int]): Whether use MaxPool to downsample the
- feature map after the first stage of encoder
- (stages: [1, num_stages)). If the correspondence encoder stage use
- stride convolution (strides[i]=2), it will never use MaxPool to
- downsample, even downsamples[i-1]=True.
- Default: (True, True, True, True).
- enc_dilations (Sequence[int]): Dilation rate of each stage in encoder.
- Default: (1, 1, 1, 1, 1).
- dec_dilations (Sequence[int]): Dilation rate of each stage in decoder.
- Default: (1, 1, 1, 1).
- with_cp (bool): Use checkpoint or not. Using checkpoint will save some
- memory while slowing down the training speed. Default: False.
- conv_cfg (dict | None): Config dict for convolution layer.
- Default: None.
- norm_cfg (dict | None): Config dict for normalization layer.
- Default: dict(type='BN').
- act_cfg (dict | None): Config dict for activation layer in ConvModule.
- Default: dict(type='ReLU').
- upsample_cfg (dict): The upsample config of the upsample module in
- decoder. Default: dict(type='InterpConv').
- norm_eval (bool): Whether to set norm layers to eval mode, namely,
- freeze running stats (mean and var). Note: Effect on Batch Norm
- and its variants only. Default: False.
- dcn (bool): Use deformable convolution in convolutional layer or not.
- Default: None.
- plugins (dict): plugins for convolutional layers. Default: None.
-
- Notice:
- The input image size should be divisible by the whole downsample rate
- of the encoder. More detail of the whole downsample rate can be found
- in UNet._check_input_divisible.
-
- """
-
- def __init__(self,
- in_channels=3,
- base_channels=64,
- num_stages=5,
- strides=(1, 1, 1, 1, 1),
- enc_num_convs=(2, 2, 2, 2, 2),
- dec_num_convs=(2, 2, 2, 2),
- downsamples=(True, True, True, True),
- enc_dilations=(1, 1, 1, 1, 1),
- dec_dilations=(1, 1, 1, 1),
- with_cp=False,
- conv_cfg=None,
- norm_cfg=dict(type='BN'),
- act_cfg=dict(type='ReLU'),
- upsample_cfg=dict(type='InterpConv'),
- norm_eval=False,
- dcn=None,
- plugins=None,
- init_cfg=[
- dict(type='Kaiming', layer='Conv2d'),
- dict(
- type='Constant',
- layer=['_BatchNorm', 'GroupNorm'],
- val=1)
- ]):
- super().__init__(init_cfg=init_cfg)
- assert dcn is None, 'Not implemented yet.'
- assert plugins is None, 'Not implemented yet.'
- assert len(strides) == num_stages, (
- 'The length of strides should be equal to num_stages, '
- f'while the strides is {strides}, the length of '
- f'strides is {len(strides)}, and the num_stages is '
- f'{num_stages}.')
- assert len(enc_num_convs) == num_stages, (
- 'The length of enc_num_convs should be equal to num_stages, '
- f'while the enc_num_convs is {enc_num_convs}, the length of '
- f'enc_num_convs is {len(enc_num_convs)}, and the num_stages is '
- f'{num_stages}.')
- assert len(dec_num_convs) == (num_stages - 1), (
- 'The length of dec_num_convs should be equal to (num_stages-1), '
- f'while the dec_num_convs is {dec_num_convs}, the length of '
- f'dec_num_convs is {len(dec_num_convs)}, and the num_stages is '
- f'{num_stages}.')
- assert len(downsamples) == (num_stages - 1), (
- 'The length of downsamples should be equal to (num_stages-1), '
- f'while the downsamples is {downsamples}, the length of '
- f'downsamples is {len(downsamples)}, and the num_stages is '
- f'{num_stages}.')
- assert len(enc_dilations) == num_stages, (
- 'The length of enc_dilations should be equal to num_stages, '
- f'while the enc_dilations is {enc_dilations}, the length of '
- f'enc_dilations is {len(enc_dilations)}, and the num_stages is '
- f'{num_stages}.')
- assert len(dec_dilations) == (num_stages - 1), (
- 'The length of dec_dilations should be equal to (num_stages-1), '
- f'while the dec_dilations is {dec_dilations}, the length of '
- f'dec_dilations is {len(dec_dilations)}, and the num_stages is '
- f'{num_stages}.')
- self.num_stages = num_stages
- self.strides = strides
- self.downsamples = downsamples
- self.norm_eval = norm_eval
- self.base_channels = base_channels
-
- self.encoder = nn.ModuleList()
- self.decoder = nn.ModuleList()
-
- for i in range(num_stages):
- enc_conv_block = []
- if i != 0:
- if strides[i] == 1 and downsamples[i - 1]:
- enc_conv_block.append(nn.MaxPool2d(kernel_size=2))
- upsample = (strides[i] != 1 or downsamples[i - 1])
- self.decoder.append(
- UpConvBlock(
- conv_block=BasicConvBlock,
- in_channels=base_channels * 2**i,
- skip_channels=base_channels * 2**(i - 1),
- out_channels=base_channels * 2**(i - 1),
- num_convs=dec_num_convs[i - 1],
- stride=1,
- dilation=dec_dilations[i - 1],
- with_cp=with_cp,
- conv_cfg=conv_cfg,
- norm_cfg=norm_cfg,
- act_cfg=act_cfg,
- upsample_cfg=upsample_cfg if upsample else None,
- dcn=None,
- plugins=None))
-
- enc_conv_block.append(
- BasicConvBlock(
- in_channels=in_channels,
- out_channels=base_channels * 2**i,
- num_convs=enc_num_convs[i],
- stride=strides[i],
- dilation=enc_dilations[i],
- with_cp=with_cp,
- conv_cfg=conv_cfg,
- norm_cfg=norm_cfg,
- act_cfg=act_cfg,
- dcn=None,
- plugins=None))
- self.encoder.append(nn.Sequential(*enc_conv_block))
- in_channels = base_channels * 2**i
-
- def forward(self, x):
- self._check_input_divisible(x)
- enc_outs = []
- for enc in self.encoder:
- x = enc(x)
- enc_outs.append(x)
- dec_outs = [x]
- for i in reversed(range(len(self.decoder))):
- x = self.decoder[i](enc_outs[i], x)
- dec_outs.append(x)
-
- return dec_outs
-
- def train(self, mode=True):
- """Convert the model into training mode while keep normalization layer
- freezed."""
- super().train(mode)
- if mode and self.norm_eval:
- for m in self.modules():
- # trick: eval have effect on BatchNorm only
- if isinstance(m, _BatchNorm):
- m.eval()
-
- def _check_input_divisible(self, x):
- h, w = x.shape[-2:]
- whole_downsample_rate = 1
- for i in range(1, self.num_stages):
- if self.strides[i] == 2 or self.downsamples[i - 1]:
- whole_downsample_rate *= 2
- assert (
- h % whole_downsample_rate == 0 and w % whole_downsample_rate == 0
- ), (f'The input image size {(h, w)} should be divisible by the whole '
- f'downsample rate {whole_downsample_rate}, when num_stages is '
- f'{self.num_stages}, strides is {self.strides}, and downsamples '
- f'is {self.downsamples}.')
diff --git a/pipeline/mmocr/mmocr/models/common/backbones/vit.py b/pipeline/mmocr/mmocr/models/common/backbones/vit.py
deleted file mode 100644
index 745c185b8918a890e1cb76a06d37bdbdf267e33c..0000000000000000000000000000000000000000
--- a/pipeline/mmocr/mmocr/models/common/backbones/vit.py
+++ /dev/null
@@ -1,284 +0,0 @@
-# Copyright (c) Meta Platforms, Inc. and affiliates.
-# All rights reserved.
-
-# This source code is licensed under the license found in the
-# LICENSE file in the root directory of this source tree.
-# --------------------------------------------------------
-# References:
-# timm: https://github.com/rwightman/pytorch-image-models/tree/master/timm
-# DeiT: https://github.com/facebookresearch/deit
-# --------------------------------------------------------
-
-from functools import partial
-from typing import Tuple
-import timm.models.vision_transformer
-from safetensors import safe_open
-from safetensors.torch import save_file
-import torch
-import torch.nn as nn
-import math
-from mmocr.registry import MODELS
-
-
-@MODELS.register_module()
-class VisionTransformer(timm.models.vision_transformer.VisionTransformer):
- """ Vision Transformer.
-
- Args:
- global_pool (bool): If True, apply global pooling to the output
- of the last stage. Default: False.
- patch_size (int): Patch token size. Default: 8.
- img_size (tuple[int]): Input image size. Default: (32, 128).
- embed_dim (int): Number of linear projection output channels.
- Default: 192.
- depth (int): Number of blocks. Default: 12.
- num_heads (int): Number of attention heads. Default: 3.
- mlp_ratio (float): Ratio of mlp hidden dim to embedding dim.
- Default: 4.
- qkv_bias (bool): If True, add a learnable bias to query, key,
- value. Default: True.
- norm_layer (nn.Module): Normalization layer. Default:
- partial(nn.LayerNorm, eps=1e-6).
- pretrained (str): Path to pre-trained checkpoint. Default: None.
- """
-
- def __init__(self,
- global_pool: bool = False,
- patch_size: int = 8,
- img_size: Tuple[int, int] = (32, 128),
- embed_dim: int = 192,
- depth: int = 12,
- num_heads: int = 3,
- mlp_ratio: int = 4.,
- qkv_bias: bool = True,
- norm_layer=partial(nn.LayerNorm, eps=1e-6),
- pretrained: bool = None,
- **kwargs):
- super(VisionTransformer, self).__init__(
- patch_size=patch_size,
- img_size=img_size,
- embed_dim=embed_dim,
- depth=depth,
- num_heads=num_heads,
- mlp_ratio=mlp_ratio,
- qkv_bias=qkv_bias,
- norm_layer=norm_layer,
- **kwargs)
-
- self.global_pool = global_pool
- if self.global_pool:
- norm_layer = kwargs['norm_layer']
- embed_dim = kwargs['embed_dim']
- self.fc_norm = norm_layer(embed_dim)
-
- del self.norm # remove the original norm
- self.reset_classifier(0)
-
- if pretrained:
- checkpoint = torch.load(pretrained, map_location='cpu')
-
- print("Load pre-trained checkpoint from: %s" % pretrained)
- checkpoint_model = checkpoint['model']
- state_dict = self.state_dict()
- for k in ['head.weight', 'head.bias']:
- if k in checkpoint_model and checkpoint_model[
- k].shape != state_dict[k].shape:
- print(f"Removing key {k} from pretrained checkpoint")
- del checkpoint_model[k]
- # remove key with decoder
- for k in list(checkpoint_model.keys()):
- if 'decoder' in k:
- del checkpoint_model[k]
- msg = self.load_state_dict(checkpoint_model, strict=False)
- print(msg)
-
- def forward_features(self, x: torch.Tensor):
- B = x.shape[0]
- x = self.patch_embed(x)
-
- cls_tokens = self.cls_token.expand(
- B, -1, -1) # stole cls_tokens impl from Phil Wang, thanks
- x = torch.cat((cls_tokens, x), dim=1)
- x = x + self.pos_embed
- x = self.pos_drop(x)
-
- for blk in self.blocks:
- x = blk(x)
-
- x = self.norm(x)
- return x
-
- def forward(self, x):
- return self.forward_features(x)
-
-
-class _LoRA_qkv_timm(nn.Module):
- """LoRA layer for query and value projection in Vision Transformer of timm.
-
- Args:
- qkv (nn.Module): qkv projection layer in Vision Transformer of timm.
- linear_a_q (nn.Module): Linear layer for query projection.
- linear_b_q (nn.Module): Linear layer for query projection.
- linear_a_v (nn.Module): Linear layer for value projection.
- linear_b_v (nn.Module): Linear layer for value projection.
- """
-
- def __init__(
- self,
- qkv: nn.Module,
- linear_a_q: nn.Module,
- linear_b_q: nn.Module,
- linear_a_v: nn.Module,
- linear_b_v: nn.Module,
- ):
- super().__init__()
- self.qkv = qkv
- self.linear_a_q = linear_a_q
- self.linear_b_q = linear_b_q
- self.linear_a_v = linear_a_v
- self.linear_b_v = linear_b_v
- self.dim = qkv.in_features
-
- def forward(self, x):
- qkv = self.qkv(x) # B, N, 3*dim
- new_q = self.linear_b_q(self.linear_a_q(x))
- new_v = self.linear_b_v(self.linear_a_v(x))
- qkv[:, :, :self.dim] += new_q
- qkv[:, :, -self.dim:] += new_v
- return qkv
-
-
-@MODELS.register_module()
-class VisionTransformer_LoRA(nn.Module):
- """Vision Transformer with LoRA. For each block, we add a LoRA layer for
- the linear projection of query and value.
-
- Args:
- vit_config (dict): Config dict for VisionTransformer.
- rank (int): Rank of LoRA layer. Default: 4.
- lora_layers (int): Stages to add LoRA layer. Defaults None means
- add LoRA layer to all stages.
- pretrained_lora (str): Path to pre-trained checkpoint of LoRA layer.
- """
-
- def __init__(self,
- vit_config: dict,
- rank: int = 4,
- lora_layers: int = None,
- pretrained_lora: str = None):
- super(VisionTransformer_LoRA, self).__init__()
- self.vit = MODELS.build(vit_config)
- assert rank > 0
- if lora_layers:
- self.lora_layers = lora_layers
- else:
- self.lora_layers = list(range(len(self.vit.blocks)))
- # creat list of LoRA layers
- self.query_As = nn.Sequential() # matrix A for query linear projection
- self.query_Bs = nn.Sequential()
- self.value_As = nn.Sequential() # matrix B for value linear projection
- self.value_Bs = nn.Sequential()
-
- # freeze the original vit
- for param in self.vit.parameters():
- param.requires_grad = False
-
- # compose LoRA layers
- for block_idx, block in enumerate(self.vit.blocks):
- if block_idx not in self.lora_layers:
- continue
- # create LoRA layer
- w_qkv_linear = block.attn.qkv
- self.dim = w_qkv_linear.in_features
- w_a_linear_q = nn.Linear(self.dim, rank, bias=False)
- w_b_linear_q = nn.Linear(rank, self.dim, bias=False)
- w_a_linear_v = nn.Linear(self.dim, rank, bias=False)
- w_b_linear_v = nn.Linear(rank, self.dim, bias=False)
- self.query_As.append(w_a_linear_q)
- self.query_Bs.append(w_b_linear_q)
- self.value_As.append(w_a_linear_v)
- self.value_Bs.append(w_b_linear_v)
- # replace the original qkv layer with LoRA layer
- block.attn.qkv = _LoRA_qkv_timm(
- w_qkv_linear,
- w_a_linear_q,
- w_b_linear_q,
- w_a_linear_v,
- w_b_linear_v,
- )
- self._init_lora()
- if pretrained_lora is not None:
- self._load_lora(pretrained_lora)
-
- def _init_lora(self):
- """Initialize the LoRA layers to be identity mapping."""
- for query_A, query_B, value_A, value_B in zip(self.query_As,
- self.query_Bs,
- self.value_As,
- self.value_Bs):
- nn.init.kaiming_uniform_(query_A.weight, a=math.sqrt(5))
- nn.init.kaiming_uniform_(value_A.weight, a=math.sqrt(5))
- nn.init.zeros_(query_B.weight)
- nn.init.zeros_(value_B.weight)
-
- def _load_lora(self, checkpoint_lora: str):
- """Load pre-trained LoRA checkpoint.
-
- Args:
- checkpoint_lora (str): Path to pre-trained LoRA checkpoint.
- """
- assert checkpoint_lora.endswith(".safetensors")
- with safe_open(checkpoint_lora, framework="pt") as f:
- for i, q_A, q_B, v_A, v_B in zip(
- range(len(self.query_As)),
- self.query_As,
- self.query_Bs,
- self.value_As,
- self.value_Bs,
- ):
- q_A.weight = nn.Parameter(f.get_tensor(f"q_a_{i:03d}"))
- q_B.weight = nn.Parameter(f.get_tensor(f"q_b_{i:03d}"))
- v_A.weight = nn.Parameter(f.get_tensor(f"v_a_{i:03d}"))
- v_B.weight = nn.Parameter(f.get_tensor(f"v_b_{i:03d}"))
-
- def forward(self, x):
- x = self.vit(x)
- return x
-
-
-def extract_lora_from_vit(checkpoint_path: str,
- save_path: str,
- ckpt_key: str = None):
- """Given a checkpoint of VisionTransformer_LoRA, extract the LoRA weights
- and save them to a new checkpoint.
-
- Args:
- checkpoint_path (str): Path to checkpoint of VisionTransformer_LoRA.
- ckpt_key (str): Key of model in the checkpoint.
- save_path (str): Path to save the extracted LoRA checkpoint.
- """
- assert save_path.endswith(".safetensors")
- ckpt = torch.load(checkpoint_path, map_location="cpu")
- # travel throung the ckpt to find the LoRA layers
- query_As = []
- query_Bs = []
- value_As = []
- value_Bs = []
- ckpt = ckpt if ckpt_key is None else ckpt[ckpt_key]
- for k, v in ckpt.items():
- if k.startswith("query_As"):
- query_As.append(v)
- elif k.startswith("query_Bs"):
- query_Bs.append(v)
- elif k.startswith("value_As"):
- value_As.append(v)
- elif k.startswith("value_Bs"):
- value_Bs.append(v)
- # save the LoRA layers to a new checkpoint
- ckpt_dict = {}
- for i in range(len(query_As)):
- ckpt_dict[f"q_a_{i:03d}"] = query_As[i]
- ckpt_dict[f"q_b_{i:03d}"] = query_Bs[i]
- ckpt_dict[f"v_a_{i:03d}"] = value_As[i]
- ckpt_dict[f"v_b_{i:03d}"] = value_Bs[i]
- save_file(ckpt_dict, save_path)
diff --git a/pipeline/mmocr/mmocr/models/common/dictionary/__init__.py b/pipeline/mmocr/mmocr/models/common/dictionary/__init__.py
deleted file mode 100644
index 9ad0ab306f183192aa5c8464eee5947e13d294e6..0000000000000000000000000000000000000000
--- a/pipeline/mmocr/mmocr/models/common/dictionary/__init__.py
+++ /dev/null
@@ -1,5 +0,0 @@
-# Copyright (c) OpenMMLab. All rights reserved.
-
-from .dictionary import Dictionary
-
-__all__ = ['Dictionary']
diff --git a/pipeline/mmocr/mmocr/models/common/dictionary/__pycache__/__init__.cpython-38.pyc b/pipeline/mmocr/mmocr/models/common/dictionary/__pycache__/__init__.cpython-38.pyc
deleted file mode 100644
index e153cf243ab2e868d4f59f95de2fdf00e93f7977..0000000000000000000000000000000000000000
Binary files a/pipeline/mmocr/mmocr/models/common/dictionary/__pycache__/__init__.cpython-38.pyc and /dev/null differ
diff --git a/pipeline/mmocr/mmocr/models/common/dictionary/__pycache__/__init__.cpython-39.pyc b/pipeline/mmocr/mmocr/models/common/dictionary/__pycache__/__init__.cpython-39.pyc
deleted file mode 100644
index 8be0df2b18c0e2f9086df2b15caebb58a4823ff8..0000000000000000000000000000000000000000
Binary files a/pipeline/mmocr/mmocr/models/common/dictionary/__pycache__/__init__.cpython-39.pyc and /dev/null differ
diff --git a/pipeline/mmocr/mmocr/models/common/dictionary/__pycache__/dictionary.cpython-38.pyc b/pipeline/mmocr/mmocr/models/common/dictionary/__pycache__/dictionary.cpython-38.pyc
deleted file mode 100644
index bd4976589d64922d13036ab8622a236a2474e30d..0000000000000000000000000000000000000000
Binary files a/pipeline/mmocr/mmocr/models/common/dictionary/__pycache__/dictionary.cpython-38.pyc and /dev/null differ
diff --git a/pipeline/mmocr/mmocr/models/common/dictionary/__pycache__/dictionary.cpython-39.pyc b/pipeline/mmocr/mmocr/models/common/dictionary/__pycache__/dictionary.cpython-39.pyc
deleted file mode 100644
index 7c7951007687d3b3c03d72fdfad2b2c565715392..0000000000000000000000000000000000000000
Binary files a/pipeline/mmocr/mmocr/models/common/dictionary/__pycache__/dictionary.cpython-39.pyc and /dev/null differ
diff --git a/pipeline/mmocr/mmocr/models/common/dictionary/dictionary.py b/pipeline/mmocr/mmocr/models/common/dictionary/dictionary.py
deleted file mode 100644
index d16dc87582da52f0179fb2188646bf5e07a3df6d..0000000000000000000000000000000000000000
--- a/pipeline/mmocr/mmocr/models/common/dictionary/dictionary.py
+++ /dev/null
@@ -1,188 +0,0 @@
-# Copyright (c) OpenMMLab. All rights reserved.
-from typing import List, Sequence
-
-from mmocr.registry import TASK_UTILS
-from mmocr.utils import list_from_file
-
-
-@TASK_UTILS.register_module()
-class Dictionary:
- """The class generates a dictionary for recognition. It pre-defines four
- special tokens: ``start_token``, ``end_token``, ``pad_token``, and
- ``unknown_token``, which will be sequentially placed at the end of the
- dictionary when their corresponding flags are True.
-
- Args:
- dict_file (str): The path of Character dict file which a single
- character must occupies a line.
- with_start (bool): The flag to control whether to include the start
- token. Defaults to False.
- with_end (bool): The flag to control whether to include the end token.
- Defaults to False.
- same_start_end (bool): The flag to control whether the start token and
- end token are the same. It only works when both ``with_start`` and
- ``with_end`` are True. Defaults to False.
- with_padding (bool):The padding token may represent more than a
- padding. It can also represent tokens like the blank token in CTC
- or the background token in SegOCR. Defaults to False.
- with_unknown (bool): The flag to control whether to include the
- unknown token. Defaults to False.
- start_token (str): The start token as a string. Defaults to ''.
- end_token (str): The end token as a string. Defaults to ''.
- start_end_token (str): The start/end token as a string. if start and
- end is the same. Defaults to ''.
- padding_token (str): The padding token as a string.
- Defaults to ''.
- unknown_token (str, optional): The unknown token as a string. If it's
- set to None and ``with_unknown`` is True, the unknown token will be
- skipped when converting string to index. Defaults to ''.
- """
-
- def __init__(self,
- dict_file: str,
- with_start: bool = False,
- with_end: bool = False,
- same_start_end: bool = False,
- with_padding: bool = False,
- with_unknown: bool = False,
- start_token: str = '',
- end_token: str = '',
- start_end_token: str = '',
- padding_token: str = '',
- unknown_token: str = '') -> None:
- self.with_start = with_start
- self.with_end = with_end
- self.same_start_end = same_start_end
- self.with_padding = with_padding
- self.with_unknown = with_unknown
- self.start_end_token = start_end_token
- self.start_token = start_token
- self.end_token = end_token
- self.padding_token = padding_token
- self.unknown_token = unknown_token
-
- assert isinstance(dict_file, str)
- self._dict = []
- for line_num, line in enumerate(list_from_file(dict_file)):
- line = line.strip('\r\n')
- if len(line) > 1:
- raise ValueError('Expect each line has 0 or 1 character, '
- f'got {len(line)} characters '
- f'at line {line_num + 1}')
- if line != '':
- self._dict.append(line)
-
- self._char2idx = {char: idx for idx, char in enumerate(self._dict)}
-
- self._update_dict()
- assert len(set(self._dict)) == len(self._dict), \
- 'Invalid dictionary: Has duplicated characters.'
-
- @property
- def num_classes(self) -> int:
- """int: Number of output classes. Special tokens are counted.
- """
- return len(self._dict)
-
- @property
- def dict(self) -> list:
- """list: Returns a list of characters to recognize, where special
- tokens are counted."""
- return self._dict
-
- def char2idx(self, char: str, strict: bool = True) -> int:
- """Convert a character to an index via ``Dictionary.dict``.
-
- Args:
- char (str): The character to convert to index.
- strict (bool): The flag to control whether to raise an exception
- when the character is not in the dictionary. Defaults to True.
-
- Return:
- int: The index of the character.
- """
- char_idx = self._char2idx.get(char, None)
- if char_idx is None:
- if self.with_unknown:
- return self.unknown_idx
- elif not strict:
- return None
- else:
- raise Exception(f'Chararcter: {char} not in dict,'
- ' please check gt_label and use'
- ' custom dict file,'
- ' or set "with_unknown=True"')
- return char_idx
-
- def str2idx(self, string: str) -> List:
- """Convert a string to a list of indexes via ``Dictionary.dict``.
-
- Args:
- string (str): The string to convert to indexes.
-
- Return:
- list: The list of indexes of the string.
- """
- idx = list()
- for s in string:
- char_idx = self.char2idx(s)
- if char_idx is None:
- if self.with_unknown:
- continue
- raise Exception(f'Chararcter: {s} not in dict,'
- ' please check gt_label and use'
- ' custom dict file,'
- ' or set "with_unknown=True"')
- idx.append(char_idx)
- return idx
-
- def idx2str(self, index: Sequence[int]) -> str:
- """Convert a list of index to string.
-
- Args:
- index (list[int]): The list of indexes to convert to string.
-
- Return:
- str: The converted string.
- """
- assert isinstance(index, (list, tuple))
- string = ''
- for i in index:
- assert i < len(self._dict), f'Index: {i} out of range! Index ' \
- f'must be less than {len(self._dict)}'
- string += self._dict[i]
- return string
-
- def _update_dict(self):
- """Update the dict with tokens according to parameters."""
- # BOS/EOS
- self.start_idx = None
- self.end_idx = None
- if self.with_start and self.with_end and self.same_start_end:
- self._dict.append(self.start_end_token)
- self.start_idx = len(self._dict) - 1
- self.end_idx = self.start_idx
- else:
- if self.with_start:
- self._dict.append(self.start_token)
- self.start_idx = len(self._dict) - 1
- if self.with_end:
- self._dict.append(self.end_token)
- self.end_idx = len(self._dict) - 1
-
- # padding
- self.padding_idx = None
- if self.with_padding:
- self._dict.append(self.padding_token)
- self.padding_idx = len(self._dict) - 1
-
- # unknown
- self.unknown_idx = None
- if self.with_unknown and self.unknown_token is not None:
- self._dict.append(self.unknown_token)
- self.unknown_idx = len(self._dict) - 1
-
- # update char2idx
- self._char2idx = {}
- for idx, char in enumerate(self._dict):
- self._char2idx[char] = idx
diff --git a/pipeline/mmocr/mmocr/models/common/layers/__init__.py b/pipeline/mmocr/mmocr/models/common/layers/__init__.py
deleted file mode 100644
index 1d1a921fdc8b57e2de15cedd6a214df77d9bdb42..0000000000000000000000000000000000000000
--- a/pipeline/mmocr/mmocr/models/common/layers/__init__.py
+++ /dev/null
@@ -1,4 +0,0 @@
-# Copyright (c) OpenMMLab. All rights reserved.
-from .transformer_layers import TFDecoderLayer, TFEncoderLayer
-
-__all__ = ['TFEncoderLayer', 'TFDecoderLayer']
diff --git a/pipeline/mmocr/mmocr/models/common/layers/__pycache__/__init__.cpython-38.pyc b/pipeline/mmocr/mmocr/models/common/layers/__pycache__/__init__.cpython-38.pyc
deleted file mode 100644
index 3e139b528bf0e287bd1498c185f25e3e942484de..0000000000000000000000000000000000000000
Binary files a/pipeline/mmocr/mmocr/models/common/layers/__pycache__/__init__.cpython-38.pyc and /dev/null differ
diff --git a/pipeline/mmocr/mmocr/models/common/layers/__pycache__/__init__.cpython-39.pyc b/pipeline/mmocr/mmocr/models/common/layers/__pycache__/__init__.cpython-39.pyc
deleted file mode 100644
index f10ce3317870bb1edc030b8df64037e85c7fc756..0000000000000000000000000000000000000000
Binary files a/pipeline/mmocr/mmocr/models/common/layers/__pycache__/__init__.cpython-39.pyc and /dev/null differ
diff --git a/pipeline/mmocr/mmocr/models/common/layers/__pycache__/transformer_layers.cpython-38.pyc b/pipeline/mmocr/mmocr/models/common/layers/__pycache__/transformer_layers.cpython-38.pyc
deleted file mode 100644
index d48594906e5871f8627a4f86e9c9ccbf5f1c0bfb..0000000000000000000000000000000000000000
Binary files a/pipeline/mmocr/mmocr/models/common/layers/__pycache__/transformer_layers.cpython-38.pyc and /dev/null differ
diff --git a/pipeline/mmocr/mmocr/models/common/layers/__pycache__/transformer_layers.cpython-39.pyc b/pipeline/mmocr/mmocr/models/common/layers/__pycache__/transformer_layers.cpython-39.pyc
deleted file mode 100644
index 462bd4e4349574e278452daba49954b8b298970a..0000000000000000000000000000000000000000
Binary files a/pipeline/mmocr/mmocr/models/common/layers/__pycache__/transformer_layers.cpython-39.pyc and /dev/null differ
diff --git a/pipeline/mmocr/mmocr/models/common/layers/transformer_layers.py b/pipeline/mmocr/mmocr/models/common/layers/transformer_layers.py
deleted file mode 100644
index 8be138d5c5af89b96f27f3646b14a60302659105..0000000000000000000000000000000000000000
--- a/pipeline/mmocr/mmocr/models/common/layers/transformer_layers.py
+++ /dev/null
@@ -1,167 +0,0 @@
-# Copyright (c) OpenMMLab. All rights reserved.
-import torch.nn as nn
-from mmengine.model import BaseModule
-
-from mmocr.models.common.modules import (MultiHeadAttention,
- PositionwiseFeedForward)
-
-
-class TFEncoderLayer(BaseModule):
- """Transformer Encoder Layer.
-
- Args:
- d_model (int): The number of expected features
- in the decoder inputs (default=512).
- d_inner (int): The dimension of the feedforward
- network model (default=256).
- n_head (int): The number of heads in the
- multiheadattention models (default=8).
- d_k (int): Total number of features in key.
- d_v (int): Total number of features in value.
- dropout (float): Dropout layer on attn_output_weights.
- qkv_bias (bool): Add bias in projection layer. Default: False.
- act_cfg (dict): Activation cfg for feedforward module.
- operation_order (tuple[str]): The execution order of operation
- in transformer. Such as ('self_attn', 'norm', 'ffn', 'norm')
- or ('norm', 'self_attn', 'norm', 'ffn').
- Default๏ผNone.
- """
-
- def __init__(self,
- d_model=512,
- d_inner=256,
- n_head=8,
- d_k=64,
- d_v=64,
- dropout=0.1,
- qkv_bias=False,
- act_cfg=dict(type='mmengine.GELU'),
- operation_order=None):
- super().__init__()
- self.attn = MultiHeadAttention(
- n_head, d_model, d_k, d_v, qkv_bias=qkv_bias, dropout=dropout)
- self.norm1 = nn.LayerNorm(d_model)
- self.mlp = PositionwiseFeedForward(
- d_model, d_inner, dropout=dropout, act_cfg=act_cfg)
- self.norm2 = nn.LayerNorm(d_model)
-
- self.operation_order = operation_order
- if self.operation_order is None:
- self.operation_order = ('norm', 'self_attn', 'norm', 'ffn')
-
- assert self.operation_order in [('norm', 'self_attn', 'norm', 'ffn'),
- ('self_attn', 'norm', 'ffn', 'norm')]
-
- def forward(self, x, mask=None):
- if self.operation_order == ('self_attn', 'norm', 'ffn', 'norm'):
- residual = x
- x = residual + self.attn(x, x, x, mask)
- x = self.norm1(x)
-
- residual = x
- x = residual + self.mlp(x)
- x = self.norm2(x)
- elif self.operation_order == ('norm', 'self_attn', 'norm', 'ffn'):
- residual = x
- x = self.norm1(x)
- x = residual + self.attn(x, x, x, mask)
-
- residual = x
- x = self.norm2(x)
- x = residual + self.mlp(x)
-
- return x
-
-
-class TFDecoderLayer(nn.Module):
- """Transformer Decoder Layer.
-
- Args:
- d_model (int): The number of expected features
- in the decoder inputs (default=512).
- d_inner (int): The dimension of the feedforward
- network model (default=256).
- n_head (int): The number of heads in the
- multiheadattention models (default=8).
- d_k (int): Total number of features in key.
- d_v (int): Total number of features in value.
- dropout (float): Dropout layer on attn_output_weights.
- qkv_bias (bool): Add bias in projection layer. Default: False.
- act_cfg (dict): Activation cfg for feedforward module.
- operation_order (tuple[str]): The execution order of operation
- in transformer. Such as ('self_attn', 'norm', 'enc_dec_attn',
- 'norm', 'ffn', 'norm') or ('norm', 'self_attn', 'norm',
- 'enc_dec_attn', 'norm', 'ffn').
- Default๏ผNone.
- """
-
- def __init__(self,
- d_model=512,
- d_inner=256,
- n_head=8,
- d_k=64,
- d_v=64,
- dropout=0.1,
- qkv_bias=False,
- act_cfg=dict(type='mmengine.GELU'),
- operation_order=None):
- super().__init__()
-
- self.norm1 = nn.LayerNorm(d_model)
- self.norm2 = nn.LayerNorm(d_model)
- self.norm3 = nn.LayerNorm(d_model)
-
- self.self_attn = MultiHeadAttention(
- n_head, d_model, d_k, d_v, dropout=dropout, qkv_bias=qkv_bias)
-
- self.enc_attn = MultiHeadAttention(
- n_head, d_model, d_k, d_v, dropout=dropout, qkv_bias=qkv_bias)
-
- self.mlp = PositionwiseFeedForward(
- d_model, d_inner, dropout=dropout, act_cfg=act_cfg)
-
- self.operation_order = operation_order
- if self.operation_order is None:
- self.operation_order = ('norm', 'self_attn', 'norm',
- 'enc_dec_attn', 'norm', 'ffn')
- assert self.operation_order in [
- ('norm', 'self_attn', 'norm', 'enc_dec_attn', 'norm', 'ffn'),
- ('self_attn', 'norm', 'enc_dec_attn', 'norm', 'ffn', 'norm')
- ]
-
- def forward(self,
- dec_input,
- enc_output,
- self_attn_mask=None,
- dec_enc_attn_mask=None):
- if self.operation_order == ('self_attn', 'norm', 'enc_dec_attn',
- 'norm', 'ffn', 'norm'):
- dec_attn_out = self.self_attn(dec_input, dec_input, dec_input,
- self_attn_mask)
- dec_attn_out += dec_input
- dec_attn_out = self.norm1(dec_attn_out)
-
- enc_dec_attn_out = self.enc_attn(dec_attn_out, enc_output,
- enc_output, dec_enc_attn_mask)
- enc_dec_attn_out += dec_attn_out
- enc_dec_attn_out = self.norm2(enc_dec_attn_out)
-
- mlp_out = self.mlp(enc_dec_attn_out)
- mlp_out += enc_dec_attn_out
- mlp_out = self.norm3(mlp_out)
- elif self.operation_order == ('norm', 'self_attn', 'norm',
- 'enc_dec_attn', 'norm', 'ffn'):
- dec_input_norm = self.norm1(dec_input)
- dec_attn_out = self.self_attn(dec_input_norm, dec_input_norm,
- dec_input_norm, self_attn_mask)
- dec_attn_out += dec_input
-
- enc_dec_attn_in = self.norm2(dec_attn_out)
- enc_dec_attn_out = self.enc_attn(enc_dec_attn_in, enc_output,
- enc_output, dec_enc_attn_mask)
- enc_dec_attn_out += dec_attn_out
-
- mlp_out = self.mlp(self.norm3(enc_dec_attn_out))
- mlp_out += enc_dec_attn_out
-
- return mlp_out
diff --git a/pipeline/mmocr/mmocr/models/common/losses/__init__.py b/pipeline/mmocr/mmocr/models/common/losses/__init__.py
deleted file mode 100644
index 336d2ed81e35a886ace8c54046abe13e1685b1ec..0000000000000000000000000000000000000000
--- a/pipeline/mmocr/mmocr/models/common/losses/__init__.py
+++ /dev/null
@@ -1,12 +0,0 @@
-# Copyright (c) OpenMMLab. All rights reserved.
-from .bce_loss import (MaskedBalancedBCELoss, MaskedBalancedBCEWithLogitsLoss,
- MaskedBCELoss, MaskedBCEWithLogitsLoss)
-from .ce_loss import CrossEntropyLoss
-from .dice_loss import MaskedDiceLoss, MaskedSquareDiceLoss
-from .l1_loss import MaskedSmoothL1Loss, SmoothL1Loss
-
-__all__ = [
- 'MaskedBalancedBCEWithLogitsLoss', 'MaskedDiceLoss', 'MaskedSmoothL1Loss',
- 'MaskedSquareDiceLoss', 'MaskedBCEWithLogitsLoss', 'SmoothL1Loss',
- 'CrossEntropyLoss', 'MaskedBalancedBCELoss', 'MaskedBCELoss'
-]
diff --git a/pipeline/mmocr/mmocr/models/common/losses/__pycache__/__init__.cpython-38.pyc b/pipeline/mmocr/mmocr/models/common/losses/__pycache__/__init__.cpython-38.pyc
deleted file mode 100644
index 67a570e62ccd4b85d4dcf20adb697d26f1a3a0eb..0000000000000000000000000000000000000000
Binary files a/pipeline/mmocr/mmocr/models/common/losses/__pycache__/__init__.cpython-38.pyc and /dev/null differ
diff --git a/pipeline/mmocr/mmocr/models/common/losses/__pycache__/__init__.cpython-39.pyc b/pipeline/mmocr/mmocr/models/common/losses/__pycache__/__init__.cpython-39.pyc
deleted file mode 100644
index fa9c97b359e38d41b29b518bc18c65d7d73b1e4d..0000000000000000000000000000000000000000
Binary files a/pipeline/mmocr/mmocr/models/common/losses/__pycache__/__init__.cpython-39.pyc and /dev/null differ
diff --git a/pipeline/mmocr/mmocr/models/common/losses/__pycache__/bce_loss.cpython-38.pyc b/pipeline/mmocr/mmocr/models/common/losses/__pycache__/bce_loss.cpython-38.pyc
deleted file mode 100644
index c2f655c5d37c0bd1a589f916aa9e1b425834aadd..0000000000000000000000000000000000000000
Binary files a/pipeline/mmocr/mmocr/models/common/losses/__pycache__/bce_loss.cpython-38.pyc and /dev/null differ
diff --git a/pipeline/mmocr/mmocr/models/common/losses/__pycache__/bce_loss.cpython-39.pyc b/pipeline/mmocr/mmocr/models/common/losses/__pycache__/bce_loss.cpython-39.pyc
deleted file mode 100644
index 1075043e8782c3ac738e33f3f750c1d00e254e24..0000000000000000000000000000000000000000
Binary files a/pipeline/mmocr/mmocr/models/common/losses/__pycache__/bce_loss.cpython-39.pyc and /dev/null differ
diff --git a/pipeline/mmocr/mmocr/models/common/losses/__pycache__/ce_loss.cpython-38.pyc b/pipeline/mmocr/mmocr/models/common/losses/__pycache__/ce_loss.cpython-38.pyc
deleted file mode 100644
index e02ecaa936d7316291a83612cf990920d11ec59b..0000000000000000000000000000000000000000
Binary files a/pipeline/mmocr/mmocr/models/common/losses/__pycache__/ce_loss.cpython-38.pyc and /dev/null differ
diff --git a/pipeline/mmocr/mmocr/models/common/losses/__pycache__/ce_loss.cpython-39.pyc b/pipeline/mmocr/mmocr/models/common/losses/__pycache__/ce_loss.cpython-39.pyc
deleted file mode 100644
index db8dc3ad78ccbb200a14894c727807771a87a914..0000000000000000000000000000000000000000
Binary files a/pipeline/mmocr/mmocr/models/common/losses/__pycache__/ce_loss.cpython-39.pyc and /dev/null differ
diff --git a/pipeline/mmocr/mmocr/models/common/losses/__pycache__/dice_loss.cpython-38.pyc b/pipeline/mmocr/mmocr/models/common/losses/__pycache__/dice_loss.cpython-38.pyc
deleted file mode 100644
index c01b06c204a11ff5bc844fad570a1a9d7a34ec20..0000000000000000000000000000000000000000
Binary files a/pipeline/mmocr/mmocr/models/common/losses/__pycache__/dice_loss.cpython-38.pyc and /dev/null differ
diff --git a/pipeline/mmocr/mmocr/models/common/losses/__pycache__/dice_loss.cpython-39.pyc b/pipeline/mmocr/mmocr/models/common/losses/__pycache__/dice_loss.cpython-39.pyc
deleted file mode 100644
index 7d2f1e783e0a0c4b63ddc3288d00f038ba373490..0000000000000000000000000000000000000000
Binary files a/pipeline/mmocr/mmocr/models/common/losses/__pycache__/dice_loss.cpython-39.pyc and /dev/null differ
diff --git a/pipeline/mmocr/mmocr/models/common/losses/__pycache__/l1_loss.cpython-38.pyc b/pipeline/mmocr/mmocr/models/common/losses/__pycache__/l1_loss.cpython-38.pyc
deleted file mode 100644
index ed1d42f38bc1246f733ac2f4cc4acf9b1c8abc10..0000000000000000000000000000000000000000
Binary files a/pipeline/mmocr/mmocr/models/common/losses/__pycache__/l1_loss.cpython-38.pyc and /dev/null differ
diff --git a/pipeline/mmocr/mmocr/models/common/losses/__pycache__/l1_loss.cpython-39.pyc b/pipeline/mmocr/mmocr/models/common/losses/__pycache__/l1_loss.cpython-39.pyc
deleted file mode 100644
index e34a76ee54763dae04559618d273a2177662b4be..0000000000000000000000000000000000000000
Binary files a/pipeline/mmocr/mmocr/models/common/losses/__pycache__/l1_loss.cpython-39.pyc and /dev/null differ
diff --git a/pipeline/mmocr/mmocr/models/common/losses/bce_loss.py b/pipeline/mmocr/mmocr/models/common/losses/bce_loss.py
deleted file mode 100644
index df4ce140dc6adb84c42dc4533dc2240dd6ca34bb..0000000000000000000000000000000000000000
--- a/pipeline/mmocr/mmocr/models/common/losses/bce_loss.py
+++ /dev/null
@@ -1,227 +0,0 @@
-# Copyright (c) OpenMMLab. All rights reserved.
-from typing import Optional, Union
-
-import torch
-import torch.nn as nn
-
-from mmocr.registry import MODELS
-
-
-@MODELS.register_module()
-class MaskedBalancedBCEWithLogitsLoss(nn.Module):
- """This loss combines a Sigmoid layers and a masked balanced BCE loss in
- one single class. It's AMP-eligible.
-
- Args:
- reduction (str, optional): The method to reduce the loss.
- Options are 'none', 'mean' and 'sum'. Defaults to 'none'.
- negative_ratio (float or int, optional): Maximum ratio of negative
- samples to positive ones. Defaults to 3.
- fallback_negative_num (int, optional): When the mask contains no
- positive samples, the number of negative samples to be sampled.
- Defaults to 0.
- eps (float, optional): Eps to avoid zero-division error. Defaults to
- 1e-6.
- """
-
- def __init__(self,
- reduction: str = 'none',
- negative_ratio: Union[float, int] = 3,
- fallback_negative_num: int = 0,
- eps: float = 1e-6) -> None:
- super().__init__()
- assert reduction in ['none', 'mean', 'sum']
- assert isinstance(negative_ratio, (float, int))
- assert isinstance(fallback_negative_num, int)
- assert isinstance(eps, float)
- self.eps = eps
- self.negative_ratio = negative_ratio
- self.reduction = reduction
- self.fallback_negative_num = fallback_negative_num
- self.loss = nn.BCEWithLogitsLoss(reduction=reduction)
-
- def forward(self,
- pred: torch.Tensor,
- gt: torch.Tensor,
- mask: Optional[torch.Tensor] = None) -> torch.Tensor:
- """Forward function.
-
- Args:
- pred (torch.Tensor): The prediction in any shape.
- gt (torch.Tensor): The learning target of the prediction in the
- same shape as pred.
- mask (torch.Tensor, optional): Binary mask in the same shape of
- pred, indicating positive regions to calculate the loss. Whole
- region will be taken into account if not provided. Defaults to
- None.
-
- Returns:
- torch.Tensor: The loss value.
- """
-
- assert pred.size() == gt.size() and gt.numel() > 0
- if mask is None:
- mask = torch.ones_like(gt)
- assert mask.size() == gt.size()
-
- positive = (gt * mask).float()
- negative = ((1 - gt) * mask).float()
- positive_count = int(positive.sum())
- if positive_count == 0:
- negative_count = min(
- int(negative.sum()), self.fallback_negative_num)
- else:
- negative_count = min(
- int(negative.sum()), int(positive_count * self.negative_ratio))
-
- assert gt.max() <= 1 and gt.min() >= 0
- loss = self.loss(pred, gt)
- positive_loss = loss * positive
- negative_loss = loss * negative
-
- negative_loss, _ = torch.topk(negative_loss.view(-1), negative_count)
-
- balance_loss = (positive_loss.sum() + negative_loss.sum()) / (
- positive_count + negative_count + self.eps)
-
- return balance_loss
-
-
-@MODELS.register_module()
-class MaskedBalancedBCELoss(MaskedBalancedBCEWithLogitsLoss):
- """Masked Balanced BCE loss.
-
- Args:
- reduction (str, optional): The method to reduce the loss.
- Options are 'none', 'mean' and 'sum'. Defaults to 'none'.
- negative_ratio (float or int): Maximum ratio of negative
- samples to positive ones. Defaults to 3.
- fallback_negative_num (int): When the mask contains no
- positive samples, the number of negative samples to be sampled.
- Defaults to 0.
- eps (float): Eps to avoid zero-division error. Defaults to
- 1e-6.
- """
-
- def __init__(self,
- reduction: str = 'none',
- negative_ratio: Union[float, int] = 3,
- fallback_negative_num: int = 0,
- eps: float = 1e-6) -> None:
- super().__init__()
- assert reduction in ['none', 'mean', 'sum']
- assert isinstance(negative_ratio, (float, int))
- assert isinstance(fallback_negative_num, int)
- assert isinstance(eps, float)
- self.eps = eps
- self.negative_ratio = negative_ratio
- self.reduction = reduction
- self.fallback_negative_num = fallback_negative_num
- self.loss = nn.BCELoss(reduction=reduction)
-
- def forward(self,
- pred: torch.Tensor,
- gt: torch.Tensor,
- mask: Optional[torch.Tensor] = None) -> torch.Tensor:
- """Forward function.
-
- Args:
- pred (torch.Tensor): The prediction in any shape.
- gt (torch.Tensor): The learning target of the prediction in the
- same shape as pred.
- mask (torch.Tensor, optional): Binary mask in the same shape of
- pred, indicating positive regions to calculate the loss. Whole
- region will be taken into account if not provided. Defaults to
- None.
-
- Returns:
- torch.Tensor: The loss value.
- """
-
- assert pred.max() <= 1 and pred.min() >= 0
- return super().forward(pred, gt, mask)
-
-
-@MODELS.register_module()
-class MaskedBCEWithLogitsLoss(nn.Module):
- """This loss combines a Sigmoid layers and a masked BCE loss in one single
- class. It's AMP-eligible.
-
- Args:
- eps (float): Eps to avoid zero-division error. Defaults to
- 1e-6.
- """
-
- def __init__(self, eps: float = 1e-6) -> None:
- super().__init__()
- assert isinstance(eps, float)
- self.eps = eps
- self.loss = nn.BCEWithLogitsLoss(reduction='none')
-
- def forward(self,
- pred: torch.Tensor,
- gt: torch.Tensor,
- mask: Optional[torch.Tensor] = None) -> torch.Tensor:
- """Forward function.
-
- Args:
- pred (torch.Tensor): The prediction in any shape.
- gt (torch.Tensor): The learning target of the prediction in the
- same shape as pred.
- mask (torch.Tensor, optional): Binary mask in the same shape of
- pred, indicating positive regions to calculate the loss. Whole
- region will be taken into account if not provided. Defaults to
- None.
-
- Returns:
- torch.Tensor: The loss value.
- """
-
- assert pred.size() == gt.size() and gt.numel() > 0
- if mask is None:
- mask = torch.ones_like(gt)
- assert mask.size() == gt.size()
-
- assert gt.max() <= 1 and gt.min() >= 0
- loss = self.loss(pred, gt)
-
- return (loss * mask).sum() / (mask.sum() + self.eps)
-
-
-@MODELS.register_module()
-class MaskedBCELoss(MaskedBCEWithLogitsLoss):
- """Masked BCE loss.
-
- Args:
- eps (float): Eps to avoid zero-division error. Defaults to
- 1e-6.
- """
-
- def __init__(self, eps: float = 1e-6) -> None:
- super().__init__()
- assert isinstance(eps, float)
- self.eps = eps
- self.loss = nn.BCELoss(reduction='none')
-
- def forward(self,
- pred: torch.Tensor,
- gt: torch.Tensor,
- mask: Optional[torch.Tensor] = None) -> torch.Tensor:
- """Forward function.
-
- Args:
- pred (torch.Tensor): The prediction in any shape.
- gt (torch.Tensor): The learning target of the prediction in the
- same shape as pred.
- mask (torch.Tensor, optional): Binary mask in the same shape of
- pred, indicating positive regions to calculate the loss. Whole
- region will be taken into account if not provided. Defaults to
- None.
-
- Returns:
- torch.Tensor: The loss value.
- """
-
- assert pred.max() <= 1 and pred.min() >= 0
-
- return super().forward(pred, gt, mask)
diff --git a/pipeline/mmocr/mmocr/models/common/losses/ce_loss.py b/pipeline/mmocr/mmocr/models/common/losses/ce_loss.py
deleted file mode 100644
index 9ff498723d9cbae1d71808ab028cd870da86b3b1..0000000000000000000000000000000000000000
--- a/pipeline/mmocr/mmocr/models/common/losses/ce_loss.py
+++ /dev/null
@@ -1,9 +0,0 @@
-# Copyright (c) OpenMMLab. All rights reserved.
-import torch.nn as nn
-
-from mmocr.registry import MODELS
-
-
-@MODELS.register_module()
-class CrossEntropyLoss(nn.CrossEntropyLoss):
- """Cross entropy loss."""
diff --git a/pipeline/mmocr/mmocr/models/common/losses/dice_loss.py b/pipeline/mmocr/mmocr/models/common/losses/dice_loss.py
deleted file mode 100644
index 37d2d3d1926263e85c4fd4b98c8f98087405686e..0000000000000000000000000000000000000000
--- a/pipeline/mmocr/mmocr/models/common/losses/dice_loss.py
+++ /dev/null
@@ -1,112 +0,0 @@
-# Copyright (c) OpenMMLab. All rights reserved.
-from typing import Optional
-
-import torch
-import torch.nn as nn
-
-from mmocr.registry import MODELS
-
-
-@MODELS.register_module()
-class MaskedDiceLoss(nn.Module):
- """Masked dice loss.
-
- Args:
- eps (float, optional): Eps to avoid zero-divison error. Defaults to
- 1e-6.
- """
-
- def __init__(self, eps: float = 1e-6) -> None:
- super().__init__()
- assert isinstance(eps, float)
- self.eps = eps
-
- def forward(self,
- pred: torch.Tensor,
- gt: torch.Tensor,
- mask: Optional[torch.Tensor] = None) -> torch.Tensor:
- """Forward function.
-
- Args:
- pred (torch.Tensor): The prediction in any shape.
- gt (torch.Tensor): The learning target of the prediction in the
- same shape as pred.
- mask (torch.Tensor, optional): Binary mask in the same shape of
- pred, indicating positive regions to calculate the loss. Whole
- region will be taken into account if not provided. Defaults to
- None.
-
- Returns:
- torch.Tensor: The loss value.
- """
-
- assert pred.size() == gt.size() and gt.numel() > 0
- if mask is None:
- mask = torch.ones_like(gt)
- assert mask.size() == gt.size()
-
- pred = pred.contiguous().view(pred.size(0), -1)
- gt = gt.contiguous().view(gt.size(0), -1)
-
- mask = mask.contiguous().view(mask.size(0), -1)
- pred = pred * mask
- gt = gt * mask
-
- dice_coeff = (2 * (pred * gt).sum()) / (
- pred.sum() + gt.sum() + self.eps)
-
- return 1 - dice_coeff
-
-
-@MODELS.register_module()
-class MaskedSquareDiceLoss(nn.Module):
- """Masked square dice loss.
-
- Args:
- eps (float, optional): Eps to avoid zero-divison error. Defaults to
- 1e-3.
- """
-
- def __init__(self, eps: float = 1e-3) -> None:
- super().__init__()
- assert isinstance(eps, float)
- self.eps = eps
-
- def forward(self,
- pred: torch.Tensor,
- gt: torch.Tensor,
- mask: Optional[torch.Tensor] = None) -> torch.Tensor:
- """Forward function.
-
- Args:
- pred (torch.Tensor): The prediction in any shape.
- gt (torch.Tensor): The learning target of the prediction in the
- same shape as pred.
- mask (torch.Tensor, optional): Binary mask in the same shape of
- pred, indicating positive regions to calculate the loss. Whole
- region will be taken into account if not provided. Defaults to
- None.
-
- Returns:
- torch.Tensor: The loss value.
- """
- assert pred.size() == gt.size() and gt.numel() > 0
- if mask is None:
- mask = torch.ones_like(gt)
- assert mask.size() == gt.size()
- batch_size = pred.size(0)
- pred = pred.contiguous().view(batch_size, -1)
- gt = gt.contiguous().view(batch_size, -1).float()
- mask = mask.contiguous().view(batch_size, -1).float()
-
- pred = pred * mask
- gt = gt * mask
-
- a = torch.sum(pred * gt, dim=1)
- b = torch.sum(pred * pred, dim=1) + self.eps
- c = torch.sum(gt * gt, dim=1) + self.eps
- d = (2 * a) / (b + c)
- loss = 1 - d
-
- loss = torch.mean(loss)
- return loss
diff --git a/pipeline/mmocr/mmocr/models/common/losses/l1_loss.py b/pipeline/mmocr/mmocr/models/common/losses/l1_loss.py
deleted file mode 100644
index 68771a328ddedb4d0d4b925626a3abbb17ab9a7c..0000000000000000000000000000000000000000
--- a/pipeline/mmocr/mmocr/models/common/losses/l1_loss.py
+++ /dev/null
@@ -1,70 +0,0 @@
-# Copyright (c) OpenMMLab. All rights reserved.
-from typing import Optional, Union
-
-import torch
-import torch.nn as nn
-
-from mmocr import digit_version
-from mmocr.registry import MODELS
-
-
-@MODELS.register_module()
-class SmoothL1Loss(nn.SmoothL1Loss):
- """Smooth L1 loss."""
-
-
-@MODELS.register_module()
-class MaskedSmoothL1Loss(nn.Module):
- """Masked Smooth L1 loss.
-
- Args:
- beta (float, optional): The threshold in the piecewise function.
- Defaults to 1.
- eps (float, optional): Eps to avoid zero-division error. Defaults to
- 1e-6.
- """
-
- def __init__(self, beta: Union[float, int] = 1, eps: float = 1e-6) -> None:
- super().__init__()
- if digit_version(torch.__version__) > digit_version('1.6.0'):
- if digit_version(torch.__version__) >= digit_version(
- '1.13.0') and beta == 0:
- beta = beta + eps
- self.smooth_l1_loss = nn.SmoothL1Loss(beta=beta, reduction='none')
- self.eps = eps
- self.beta = beta
-
- def forward(self,
- pred: torch.Tensor,
- gt: torch.Tensor,
- mask: Optional[torch.Tensor] = None) -> torch.Tensor:
- """Forward function.
-
- Args:
- pred (torch.Tensor): The prediction in any shape.
- gt (torch.Tensor): The learning target of the prediction in the
- same shape as pred.
- mask (torch.Tensor, optional): Binary mask in the same shape of
- pred, indicating positive regions to calculate the loss. Whole
- region will be taken into account if not provided. Defaults to
- None.
-
- Returns:
- torch.Tensor: The loss value.
- """
-
- assert pred.size() == gt.size() and gt.numel() > 0
- if mask is None:
- mask = torch.ones_like(gt).bool()
- assert mask.size() == gt.size()
- x = pred * mask
- y = gt * mask
- if digit_version(torch.__version__) > digit_version('1.6.0'):
- loss = self.smooth_l1_loss(x, y)
- else:
- loss = torch.zeros_like(gt)
- diff = torch.abs(x - y)
- mask_beta = diff < self.beta
- loss[mask_beta] = 0.5 * torch.square(diff)[mask_beta] / self.beta
- loss[~mask_beta] = diff[~mask_beta] - 0.5 * self.beta
- return loss.sum() / (mask.sum() + self.eps)
diff --git a/pipeline/mmocr/mmocr/models/common/modules/__init__.py b/pipeline/mmocr/mmocr/models/common/modules/__init__.py
deleted file mode 100644
index 30960fd5dd45f069c4ae2f6c74ec66d5eecb13b8..0000000000000000000000000000000000000000
--- a/pipeline/mmocr/mmocr/models/common/modules/__init__.py
+++ /dev/null
@@ -1,9 +0,0 @@
-# Copyright (c) OpenMMLab. All rights reserved.
-from .transformer_module import (MultiHeadAttention, PositionalEncoding,
- PositionwiseFeedForward,
- ScaledDotProductAttention)
-
-__all__ = [
- 'ScaledDotProductAttention', 'MultiHeadAttention',
- 'PositionwiseFeedForward', 'PositionalEncoding'
-]
diff --git a/pipeline/mmocr/mmocr/models/common/modules/__pycache__/__init__.cpython-38.pyc b/pipeline/mmocr/mmocr/models/common/modules/__pycache__/__init__.cpython-38.pyc
deleted file mode 100644
index 20bbf896c21a908afa38f758007548b0d5f9718c..0000000000000000000000000000000000000000
Binary files a/pipeline/mmocr/mmocr/models/common/modules/__pycache__/__init__.cpython-38.pyc and /dev/null differ
diff --git a/pipeline/mmocr/mmocr/models/common/modules/__pycache__/__init__.cpython-39.pyc b/pipeline/mmocr/mmocr/models/common/modules/__pycache__/__init__.cpython-39.pyc
deleted file mode 100644
index 9e3a208b3c2a1d39083176eea6218370ddc1a992..0000000000000000000000000000000000000000
Binary files a/pipeline/mmocr/mmocr/models/common/modules/__pycache__/__init__.cpython-39.pyc and /dev/null differ
diff --git a/pipeline/mmocr/mmocr/models/common/modules/__pycache__/transformer_module.cpython-38.pyc b/pipeline/mmocr/mmocr/models/common/modules/__pycache__/transformer_module.cpython-38.pyc
deleted file mode 100644
index 710b0dbd22911ea4db7797d9339827fce66e7292..0000000000000000000000000000000000000000
Binary files a/pipeline/mmocr/mmocr/models/common/modules/__pycache__/transformer_module.cpython-38.pyc and /dev/null differ
diff --git a/pipeline/mmocr/mmocr/models/common/modules/__pycache__/transformer_module.cpython-39.pyc b/pipeline/mmocr/mmocr/models/common/modules/__pycache__/transformer_module.cpython-39.pyc
deleted file mode 100644
index 57595d221bb1cc987a56cff3493330509f498a16..0000000000000000000000000000000000000000
Binary files a/pipeline/mmocr/mmocr/models/common/modules/__pycache__/transformer_module.cpython-39.pyc and /dev/null differ
diff --git a/pipeline/mmocr/mmocr/models/common/modules/transformer_module.py b/pipeline/mmocr/mmocr/models/common/modules/transformer_module.py
deleted file mode 100644
index 89dde388ae98e6da736b874746ac722992e6d0b1..0000000000000000000000000000000000000000
--- a/pipeline/mmocr/mmocr/models/common/modules/transformer_module.py
+++ /dev/null
@@ -1,164 +0,0 @@
-# Copyright (c) OpenMMLab. All rights reserved.
-import numpy as np
-import torch
-import torch.nn as nn
-import torch.nn.functional as F
-
-from mmocr.registry import MODELS
-
-
-class ScaledDotProductAttention(nn.Module):
- """Scaled Dot-Product Attention Module. This code is adopted from
- https://github.com/jadore801120/attention-is-all-you-need-pytorch.
-
- Args:
- temperature (float): The scale factor for softmax input.
- attn_dropout (float): Dropout layer on attn_output_weights.
- """
-
- def __init__(self, temperature, attn_dropout=0.1):
- super().__init__()
- self.temperature = temperature
- self.dropout = nn.Dropout(attn_dropout)
-
- def forward(self, q, k, v, mask=None):
-
- attn = torch.matmul(q / self.temperature, k.transpose(2, 3))
-
- if mask is not None:
- attn = attn.masked_fill(mask == 0, float('-inf'))
-
- attn = self.dropout(F.softmax(attn, dim=-1))
- output = torch.matmul(attn, v)
-
- return output, attn
-
-
-class MultiHeadAttention(nn.Module):
- """Multi-Head Attention module.
-
- Args:
- n_head (int): The number of heads in the
- multiheadattention models (default=8).
- d_model (int): The number of expected features
- in the decoder inputs (default=512).
- d_k (int): Total number of features in key.
- d_v (int): Total number of features in value.
- dropout (float): Dropout layer on attn_output_weights.
- qkv_bias (bool): Add bias in projection layer. Default: False.
- """
-
- def __init__(self,
- n_head=8,
- d_model=512,
- d_k=64,
- d_v=64,
- dropout=0.1,
- qkv_bias=False):
- super().__init__()
- self.n_head = n_head
- self.d_k = d_k
- self.d_v = d_v
-
- self.dim_k = n_head * d_k
- self.dim_v = n_head * d_v
-
- self.linear_q = nn.Linear(self.dim_k, self.dim_k, bias=qkv_bias)
- self.linear_k = nn.Linear(self.dim_k, self.dim_k, bias=qkv_bias)
- self.linear_v = nn.Linear(self.dim_v, self.dim_v, bias=qkv_bias)
-
- self.attention = ScaledDotProductAttention(d_k**0.5, dropout)
-
- self.fc = nn.Linear(self.dim_v, d_model, bias=qkv_bias)
- self.proj_drop = nn.Dropout(dropout)
-
- def forward(self, q, k, v, mask=None):
- batch_size, len_q, _ = q.size()
- _, len_k, _ = k.size()
-
- q = self.linear_q(q).view(batch_size, len_q, self.n_head, self.d_k)
- k = self.linear_k(k).view(batch_size, len_k, self.n_head, self.d_k)
- v = self.linear_v(v).view(batch_size, len_k, self.n_head, self.d_v)
-
- q, k, v = q.transpose(1, 2), k.transpose(1, 2), v.transpose(1, 2)
-
- if mask is not None:
- if mask.dim() == 3:
- mask = mask.unsqueeze(1)
- elif mask.dim() == 2:
- mask = mask.unsqueeze(1).unsqueeze(1)
-
- attn_out, _ = self.attention(q, k, v, mask=mask)
-
- attn_out = attn_out.transpose(1, 2).contiguous().view(
- batch_size, len_q, self.dim_v)
-
- attn_out = self.fc(attn_out)
- attn_out = self.proj_drop(attn_out)
-
- return attn_out
-
-
-class PositionwiseFeedForward(nn.Module):
- """Two-layer feed-forward module.
-
- Args:
- d_in (int): The dimension of the input for feedforward
- network model.
- d_hid (int): The dimension of the feedforward
- network model.
- dropout (float): Dropout layer on feedforward output.
- act_cfg (dict): Activation cfg for feedforward module.
- """
-
- def __init__(self, d_in, d_hid, dropout=0.1, act_cfg=dict(type='Relu')):
- super().__init__()
- self.w_1 = nn.Linear(d_in, d_hid)
- self.w_2 = nn.Linear(d_hid, d_in)
- self.act = MODELS.build(act_cfg)
- self.dropout = nn.Dropout(dropout)
-
- def forward(self, x):
- x = self.w_1(x)
- x = self.act(x)
- x = self.w_2(x)
- x = self.dropout(x)
-
- return x
-
-
-class PositionalEncoding(nn.Module):
- """Fixed positional encoding with sine and cosine functions."""
-
- def __init__(self, d_hid=512, n_position=200, dropout=0):
- super().__init__()
- self.dropout = nn.Dropout(p=dropout)
-
- # Not a parameter
- # Position table of shape (1, n_position, d_hid)
- self.register_buffer(
- 'position_table',
- self._get_sinusoid_encoding_table(n_position, d_hid))
-
- def _get_sinusoid_encoding_table(self, n_position, d_hid):
- """Sinusoid position encoding table."""
- denominator = torch.Tensor([
- 1.0 / np.power(10000, 2 * (hid_j // 2) / d_hid)
- for hid_j in range(d_hid)
- ])
- denominator = denominator.view(1, -1)
- pos_tensor = torch.arange(n_position).unsqueeze(-1).float()
- sinusoid_table = pos_tensor * denominator
- sinusoid_table[:, 0::2] = torch.sin(sinusoid_table[:, 0::2])
- sinusoid_table[:, 1::2] = torch.cos(sinusoid_table[:, 1::2])
-
- return sinusoid_table.unsqueeze(0)
-
- def forward(self, x):
- """
- Args:
- x (Tensor): Tensor of shape (batch_size, pos_len, d_hid, ...)
- """
- self.device = x.device
- x = x + self.position_table[:, :x.size(1)].clone().detach()
- return self.dropout(x)
diff --git a/pipeline/mmocr/mmocr/models/common/plugins/__init__.py b/pipeline/mmocr/mmocr/models/common/plugins/__init__.py
deleted file mode 100644
index 1ad4c93c0dbdc9f95d23df30413c495261970bfd..0000000000000000000000000000000000000000
--- a/pipeline/mmocr/mmocr/models/common/plugins/__init__.py
+++ /dev/null
@@ -1,4 +0,0 @@
-# Copyright (c) OpenMMLab. All rights reserved.
-from .common import AvgPool2d
-
-__all__ = ['AvgPool2d']
diff --git a/pipeline/mmocr/mmocr/models/common/plugins/__pycache__/__init__.cpython-38.pyc b/pipeline/mmocr/mmocr/models/common/plugins/__pycache__/__init__.cpython-38.pyc
deleted file mode 100644
index fff0e906d5f89fb5658bd516f698e1e0c90c03b2..0000000000000000000000000000000000000000
Binary files a/pipeline/mmocr/mmocr/models/common/plugins/__pycache__/__init__.cpython-38.pyc and /dev/null differ
diff --git a/pipeline/mmocr/mmocr/models/common/plugins/__pycache__/__init__.cpython-39.pyc b/pipeline/mmocr/mmocr/models/common/plugins/__pycache__/__init__.cpython-39.pyc
deleted file mode 100644
index 8cb47b0a735a9efe7e8784dad8e3d399224b53fa..0000000000000000000000000000000000000000
Binary files a/pipeline/mmocr/mmocr/models/common/plugins/__pycache__/__init__.cpython-39.pyc and /dev/null differ
diff --git a/pipeline/mmocr/mmocr/models/common/plugins/__pycache__/common.cpython-38.pyc b/pipeline/mmocr/mmocr/models/common/plugins/__pycache__/common.cpython-38.pyc
deleted file mode 100644
index 86071543530b52f746477aa49d41f208d5fc43de..0000000000000000000000000000000000000000
Binary files a/pipeline/mmocr/mmocr/models/common/plugins/__pycache__/common.cpython-38.pyc and /dev/null differ
diff --git a/pipeline/mmocr/mmocr/models/common/plugins/__pycache__/common.cpython-39.pyc b/pipeline/mmocr/mmocr/models/common/plugins/__pycache__/common.cpython-39.pyc
deleted file mode 100644
index ce73a2985f3bda1fe96b02361c9d3e5e24dd1285..0000000000000000000000000000000000000000
Binary files a/pipeline/mmocr/mmocr/models/common/plugins/__pycache__/common.cpython-39.pyc and /dev/null differ
diff --git a/pipeline/mmocr/mmocr/models/common/plugins/common.py b/pipeline/mmocr/mmocr/models/common/plugins/common.py
deleted file mode 100644
index 722b53f568002720f28c1683a2304d335b94b883..0000000000000000000000000000000000000000
--- a/pipeline/mmocr/mmocr/models/common/plugins/common.py
+++ /dev/null
@@ -1,40 +0,0 @@
-# Copyright (c) OpenMMLab. All rights reserved.
-from typing import Optional, Tuple, Union
-
-import torch
-import torch.nn as nn
-
-from mmocr.registry import MODELS
-
-
-@MODELS.register_module()
-class AvgPool2d(nn.Module):
- """Applies a 2D average pooling over an input signal composed of several
- input planes.
-
- It can also be used as a network plugin.
-
- Args:
- kernel_size (int or tuple(int)): the size of the window.
- stride (int or tuple(int), optional): the stride of the window.
- Defaults to None.
- padding (int or tuple(int)): implicit zero padding. Defaults to 0.
- """
-
- def __init__(self,
- kernel_size: Union[int, Tuple[int]],
- stride: Optional[Union[int, Tuple[int]]] = None,
- padding: Union[int, Tuple[int]] = 0,
- **kwargs) -> None:
- super().__init__()
- self.model = nn.AvgPool2d(kernel_size, stride, padding)
-
- def forward(self, x: torch.Tensor) -> torch.Tensor:
- """Forward function.
- Args:
- x (Tensor): Input feature map.
-
- Returns:
- Tensor: Output tensor after Avgpooling layer.
- """
- return self.model(x)
diff --git a/pipeline/mmocr/mmocr/models/kie/__init__.py b/pipeline/mmocr/mmocr/models/kie/__init__.py
deleted file mode 100644
index 82660bae2c780c0150eee06df55f80a416ca3104..0000000000000000000000000000000000000000
--- a/pipeline/mmocr/mmocr/models/kie/__init__.py
+++ /dev/null
@@ -1,5 +0,0 @@
-# Copyright (c) OpenMMLab. All rights reserved.
-from .extractors import * # NOQA
-from .heads import * # NOQA
-from .module_losses import * # NOQA
-from .postprocessors import * # NOQA
diff --git a/pipeline/mmocr/mmocr/models/kie/__pycache__/__init__.cpython-38.pyc b/pipeline/mmocr/mmocr/models/kie/__pycache__/__init__.cpython-38.pyc
deleted file mode 100644
index 25385ed9ce58fcb692cd3f30df3dbbe7974d6132..0000000000000000000000000000000000000000
Binary files a/pipeline/mmocr/mmocr/models/kie/__pycache__/__init__.cpython-38.pyc and /dev/null differ
diff --git a/pipeline/mmocr/mmocr/models/kie/__pycache__/__init__.cpython-39.pyc b/pipeline/mmocr/mmocr/models/kie/__pycache__/__init__.cpython-39.pyc
deleted file mode 100644
index 4fe529f8ed8a1185b203a2eabc3ede1ec3bc3e50..0000000000000000000000000000000000000000
Binary files a/pipeline/mmocr/mmocr/models/kie/__pycache__/__init__.cpython-39.pyc and /dev/null differ
diff --git a/pipeline/mmocr/mmocr/models/kie/extractors/__init__.py b/pipeline/mmocr/mmocr/models/kie/extractors/__init__.py
deleted file mode 100644
index 914d0f6903cefec1236107346e59901ac9d64fd4..0000000000000000000000000000000000000000
--- a/pipeline/mmocr/mmocr/models/kie/extractors/__init__.py
+++ /dev/null
@@ -1,4 +0,0 @@
-# Copyright (c) OpenMMLab. All rights reserved.
-from .sdmgr import SDMGR
-
-__all__ = ['SDMGR']
diff --git a/pipeline/mmocr/mmocr/models/kie/extractors/__pycache__/__init__.cpython-38.pyc b/pipeline/mmocr/mmocr/models/kie/extractors/__pycache__/__init__.cpython-38.pyc
deleted file mode 100644
index a49fc803b94262d930f1720f9151e999544cfb91..0000000000000000000000000000000000000000
Binary files a/pipeline/mmocr/mmocr/models/kie/extractors/__pycache__/__init__.cpython-38.pyc and /dev/null differ
diff --git a/pipeline/mmocr/mmocr/models/kie/extractors/__pycache__/__init__.cpython-39.pyc b/pipeline/mmocr/mmocr/models/kie/extractors/__pycache__/__init__.cpython-39.pyc
deleted file mode 100644
index 0da4d4e022f73431b00bb2dbc47d666900cb8fc0..0000000000000000000000000000000000000000
Binary files a/pipeline/mmocr/mmocr/models/kie/extractors/__pycache__/__init__.cpython-39.pyc and /dev/null differ
diff --git a/pipeline/mmocr/mmocr/models/kie/extractors/__pycache__/sdmgr.cpython-38.pyc b/pipeline/mmocr/mmocr/models/kie/extractors/__pycache__/sdmgr.cpython-38.pyc
deleted file mode 100644
index 42c0b589a324d18f560586b40e23aaa7ebc03602..0000000000000000000000000000000000000000
Binary files a/pipeline/mmocr/mmocr/models/kie/extractors/__pycache__/sdmgr.cpython-38.pyc and /dev/null differ
diff --git a/pipeline/mmocr/mmocr/models/kie/extractors/__pycache__/sdmgr.cpython-39.pyc b/pipeline/mmocr/mmocr/models/kie/extractors/__pycache__/sdmgr.cpython-39.pyc
deleted file mode 100644
index af109786032f2b2b50f93ca443c618f5af26232e..0000000000000000000000000000000000000000
Binary files a/pipeline/mmocr/mmocr/models/kie/extractors/__pycache__/sdmgr.cpython-39.pyc and /dev/null differ
diff --git a/pipeline/mmocr/mmocr/models/kie/extractors/sdmgr.py b/pipeline/mmocr/mmocr/models/kie/extractors/sdmgr.py
deleted file mode 100644
index 670dcdf59827ffb2ea3926474cddbdef76bdb105..0000000000000000000000000000000000000000
--- a/pipeline/mmocr/mmocr/models/kie/extractors/sdmgr.py
+++ /dev/null
@@ -1,191 +0,0 @@
-# Copyright (c) OpenMMLab. All rights reserved.
-import warnings
-from typing import Dict, List, Optional, Sequence, Tuple
-
-import torch
-from mmdet.structures.bbox import bbox2roi
-from mmengine.model import BaseModel
-from torch import nn
-
-from mmocr.registry import MODELS, TASK_UTILS
-from mmocr.structures import KIEDataSample
-
-
-@MODELS.register_module()
-class SDMGR(BaseModel):
- """The implementation of the paper: Spatial Dual-Modality Graph Reasoning
- for Key Information Extraction. https://arxiv.org/abs/2103.14470.
-
- Args:
- backbone (dict, optional): Config of backbone. If None, None will be
- passed to kie_head during training and testing. Defaults to None.
- roi_extractor (dict, optional): Config of roi extractor. Only
- applicable when backbone is not None. Defaults to None.
- neck (dict, optional): Config of neck. Defaults to None.
- kie_head (dict): Config of KIE head. Defaults to None.
- dictionary (dict, optional): Config of dictionary. Defaults to None.
- data_preprocessor (dict or ConfigDict, optional): The pre-process
- config of :class:`BaseDataPreprocessor`. it usually includes,
- ``pad_size_divisor``, ``pad_value``, ``mean`` and ``std``. It has
- to be None when working in non-visual mode. Defaults to None.
- init_cfg (dict or list[dict], optional): Initialization configs.
- Defaults to None.
- """
-
- def __init__(self,
- backbone: Optional[Dict] = None,
- roi_extractor: Optional[Dict] = None,
- neck: Optional[Dict] = None,
- kie_head: Dict = None,
- dictionary: Optional[Dict] = None,
- data_preprocessor: Optional[Dict] = None,
- init_cfg: Optional[Dict] = None) -> None:
- super().__init__(
- data_preprocessor=data_preprocessor, init_cfg=init_cfg)
- if dictionary is not None:
- self.dictionary = TASK_UTILS.build(dictionary)
- if kie_head.get('dictionary', None) is None:
- kie_head.update(dictionary=self.dictionary)
- else:
- warnings.warn(f"Using dictionary {kie_head['dictionary']} "
- "in kie_head's config.")
- if backbone is not None:
- self.backbone = MODELS.build(backbone)
- self.extractor = MODELS.build({
- **roi_extractor, 'out_channels':
- self.backbone.base_channels
- })
- self.maxpool = nn.MaxPool2d(
- roi_extractor['roi_layer']['output_size'])
- if neck is not None:
- self.neck = MODELS.build(neck)
- self.kie_head = MODELS.build(kie_head)
-
- def extract_feat(self, img: torch.Tensor,
- gt_bboxes: List[torch.Tensor]) -> torch.Tensor:
- """Extract features from images if self.backbone is not None. It
- returns None otherwise.
-
- Args:
- img (torch.Tensor): The input image with shape (N, C, H, W).
- gt_bboxes (list[torch.Tensor)): A list of ground truth bounding
- boxes, each of shape :math:`(N_i, 4)`.
-
- Returns:
- torch.Tensor: The extracted features with shape (N, E).
- """
- if not hasattr(self, 'backbone'):
- return None
- x = self.backbone(img)
- if hasattr(self, 'neck'):
- x = self.neck(x)
- x = x[-1]
- feats = self.maxpool(self.extractor([x], bbox2roi(gt_bboxes)))
- return feats.view(feats.size(0), -1)
-
- def forward(self,
- inputs: torch.Tensor,
- data_samples: Sequence[KIEDataSample] = None,
- mode: str = 'tensor',
- **kwargs) -> torch.Tensor:
- """The unified entry for a forward process in both training and test.
-
- The method should accept three modes: "tensor", "predict" and "loss":
-
- - "tensor": Forward the whole network and return tensor or tuple of
- tensor without any post-processing, same as a common nn.Module.
- - "predict": Forward and return the predictions, which are fully
- processed to a list of :obj:`DetDataSample`.
- - "loss": Forward and return a dict of losses according to the given
- inputs and data samples.
-
- Note that this method doesn't handle neither back propagation nor
- optimizer updating, which are done in the :meth:`train_step`.
-
- Args:
- inputs (torch.Tensor): The input tensor with shape
- (N, C, ...) in general.
- data_samples (list[:obj:`DetDataSample`], optional): The
- annotation data of every samples. Defaults to None.
- mode (str): Return what kind of value. Defaults to 'tensor'.
-
- Returns:
- The return type depends on ``mode``.
-
- - If ``mode="tensor"``, return a tensor or a tuple of tensor.
- - If ``mode="predict"``, return a list of :obj:`DetDataSample`.
- - If ``mode="loss"``, return a dict of tensor.
- """
- if mode == 'loss':
- return self.loss(inputs, data_samples, **kwargs)
- elif mode == 'predict':
- return self.predict(inputs, data_samples, **kwargs)
- elif mode == 'tensor':
- return self._forward(inputs, data_samples, **kwargs)
- else:
- raise RuntimeError(f'Invalid mode "{mode}". '
- 'Only supports loss, predict and tensor mode')
-
- def loss(self, inputs: torch.Tensor, data_samples: Sequence[KIEDataSample],
- **kwargs) -> dict:
- """Calculate losses from a batch of inputs and data samples.
-
- Args:
- inputs (torch.Tensor): Input images of shape (N, C, H, W).
- Typically these should be mean centered and std scaled.
- data_samples (list[KIEDataSample]): A list of N datasamples,
- containing meta information and gold annotations for each of
- the images.
- Returns:
- dict[str, Tensor]: A dictionary of loss components.
- """
- x = self.extract_feat(
- inputs,
- [data_sample.gt_instances.bboxes for data_sample in data_samples])
- return self.kie_head.loss(x, data_samples)
-
- def predict(self, inputs: torch.Tensor,
- data_samples: Sequence[KIEDataSample],
- **kwargs) -> List[KIEDataSample]:
- """Predict results from a batch of inputs and data samples with post-
- processing.
- Args:
- inputs (torch.Tensor): Input images of shape (N, C, H, W).
- Typically these should be mean centered and std scaled.
- data_samples (list[KIEDataSample]): A list of N datasamples,
- containing meta information and gold annotations for each of
- the images.
-
- Returns:
- List[KIEDataSample]: A list of datasamples of prediction results.
- Results are stored in ``pred_instances.labels`` and
- ``pred_instances.edge_labels``.
- """
- x = self.extract_feat(
- inputs,
- [data_sample.gt_instances.bboxes for data_sample in data_samples])
- return self.kie_head.predict(x, data_samples)
-
- def _forward(self, inputs: torch.Tensor,
- data_samples: Sequence[KIEDataSample],
- **kwargs) -> Tuple[torch.Tensor, torch.Tensor]:
- """Get the raw tensor outputs from backbone and head without any post-
- processing.
-
- Args:
- inputs (torch.Tensor): Input images of shape (N, C, H, W).
- Typically these should be mean centered and std scaled.
- data_samples (list[KIEDataSample]): A list of N datasamples,
- containing meta information and gold annotations for each of
- the images.
-
- Returns:
- tuple(torch.Tensor, torch.Tensor): Tensor output from head.
-
- - node_cls (torch.Tensor): Node classification output.
- - edge_cls (torch.Tensor): Edge classification output.
- """
- x = self.extract_feat(
- inputs,
- [data_sample.gt_instances.bboxes for data_sample in data_samples])
- return self.kie_head(x, data_samples)
diff --git a/pipeline/mmocr/mmocr/models/kie/heads/__init__.py b/pipeline/mmocr/mmocr/models/kie/heads/__init__.py
deleted file mode 100644
index 1c08ed6ffa4f8b177c56a947da9b49980ab0a2c2..0000000000000000000000000000000000000000
--- a/pipeline/mmocr/mmocr/models/kie/heads/__init__.py
+++ /dev/null
@@ -1,4 +0,0 @@
-# Copyright (c) OpenMMLab. All rights reserved.
-from .sdmgr_head import SDMGRHead
-
-__all__ = ['SDMGRHead']
diff --git a/pipeline/mmocr/mmocr/models/kie/heads/__pycache__/__init__.cpython-38.pyc b/pipeline/mmocr/mmocr/models/kie/heads/__pycache__/__init__.cpython-38.pyc
deleted file mode 100644
index 1737d1cfe6c9c6aa4b2d244a1f85b856bc14a7df..0000000000000000000000000000000000000000
Binary files a/pipeline/mmocr/mmocr/models/kie/heads/__pycache__/__init__.cpython-38.pyc and /dev/null differ
diff --git a/pipeline/mmocr/mmocr/models/kie/heads/__pycache__/__init__.cpython-39.pyc b/pipeline/mmocr/mmocr/models/kie/heads/__pycache__/__init__.cpython-39.pyc
deleted file mode 100644
index fce9828f22e44edc7cd14e5053ff274fe07a006e..0000000000000000000000000000000000000000
Binary files a/pipeline/mmocr/mmocr/models/kie/heads/__pycache__/__init__.cpython-39.pyc and /dev/null differ
diff --git a/pipeline/mmocr/mmocr/models/kie/heads/__pycache__/sdmgr_head.cpython-38.pyc b/pipeline/mmocr/mmocr/models/kie/heads/__pycache__/sdmgr_head.cpython-38.pyc
deleted file mode 100644
index 5b636526eea2b998da99977e22f5555bd62e24b9..0000000000000000000000000000000000000000
Binary files a/pipeline/mmocr/mmocr/models/kie/heads/__pycache__/sdmgr_head.cpython-38.pyc and /dev/null differ
diff --git a/pipeline/mmocr/mmocr/models/kie/heads/__pycache__/sdmgr_head.cpython-39.pyc b/pipeline/mmocr/mmocr/models/kie/heads/__pycache__/sdmgr_head.cpython-39.pyc
deleted file mode 100644
index 844a40d7ceb044a065e4832f42ef6fc84a5117ea..0000000000000000000000000000000000000000
Binary files a/pipeline/mmocr/mmocr/models/kie/heads/__pycache__/sdmgr_head.cpython-39.pyc and /dev/null differ
diff --git a/pipeline/mmocr/mmocr/models/kie/heads/sdmgr_head.py b/pipeline/mmocr/mmocr/models/kie/heads/sdmgr_head.py
deleted file mode 100644
index 311e870941f212f26a504afd4b6c30ccc0d9cc7e..0000000000000000000000000000000000000000
--- a/pipeline/mmocr/mmocr/models/kie/heads/sdmgr_head.py
+++ /dev/null
@@ -1,377 +0,0 @@
-# Copyright (c) OpenMMLab. All rights reserved.
-from typing import Dict, List, Optional, Tuple, Union
-
-import torch
-from mmengine.model import BaseModule
-from torch import Tensor, nn
-from torch.nn import functional as F
-
-from mmocr.models.common.dictionary import Dictionary
-from mmocr.registry import MODELS, TASK_UTILS
-from mmocr.structures import KIEDataSample
-
-
-@MODELS.register_module()
-class SDMGRHead(BaseModule):
- """SDMGR Head.
-
- Args:
- dictionary (dict or :obj:`Dictionary`): The config for `Dictionary` or
- the instance of `Dictionary`.
- num_classes (int): Number of class labels. Defaults to 26.
- visual_dim (int): Dimension of visual features :math:`E`. Defaults to
- 64.
- fusion_dim (int): Dimension of fusion layer. Defaults to 1024.
- node_input (int): Dimension of raw node embedding. Defaults to 32.
- node_embed (int): Dimension of node embedding. Defaults to 256.
- edge_input (int): Dimension of raw edge embedding. Defaults to 5.
- edge_embed (int): Dimension of edge embedding. Defaults to 256.
- num_gnn (int): Number of GNN layers. Defaults to 2.
- bidirectional (bool): Whether to use bidirectional RNN to embed nodes.
- Defaults to False.
- relation_norm (float): Norm to map value from one range to another.=
- Defaults to 10.
- module_loss (dict): Module Loss config. Defaults to
- ``dict(type='SDMGRModuleLoss')``.
- postprocessor (dict): Postprocessor config. Defaults to
- ``dict(type='SDMGRPostProcessor')``.
- init_cfg (dict or list[dict], optional): Initialization configs.
- """
-
- def __init__(
- self,
- dictionary: Union[Dictionary, Dict],
- num_classes: int = 26,
- visual_dim: int = 64,
- fusion_dim: int = 1024,
- node_input: int = 32,
- node_embed: int = 256,
- edge_input: int = 5,
- edge_embed: int = 256,
- num_gnn: int = 2,
- bidirectional: bool = False,
- relation_norm: float = 10.,
- module_loss: Dict = dict(type='SDMGRModuleLoss'),
- postprocessor: Dict = dict(type='SDMGRPostProcessor'),
- init_cfg: Optional[Union[Dict, List[Dict]]] = dict(
- type='Normal', override=dict(name='edge_embed'), mean=0, std=0.01)
- ) -> None:
- super().__init__(init_cfg=init_cfg)
- assert isinstance(dictionary, (dict, Dictionary))
- if isinstance(dictionary, dict):
- self.dictionary = TASK_UTILS.build(dictionary)
- elif isinstance(dictionary, Dictionary):
- self.dictionary = dictionary
-
- self.fusion = FusionBlock([visual_dim, node_embed], node_embed,
- fusion_dim)
- self.node_embed = nn.Embedding(self.dictionary.num_classes, node_input,
- self.dictionary.padding_idx)
- hidden = node_embed // 2 if bidirectional else node_embed
- self.rnn = nn.LSTM(
- input_size=node_input,
- hidden_size=hidden,
- num_layers=1,
- batch_first=True,
- bidirectional=bidirectional)
- self.edge_embed = nn.Linear(edge_input, edge_embed)
- self.gnn_layers = nn.ModuleList(
- [GNNLayer(node_embed, edge_embed) for _ in range(num_gnn)])
- self.node_cls = nn.Linear(node_embed, num_classes)
- self.edge_cls = nn.Linear(edge_embed, 2)
- self.module_loss = MODELS.build(module_loss)
- self.postprocessor = MODELS.build(postprocessor)
- self.relation_norm = relation_norm
-
- def loss(self, inputs: Tensor, data_samples: List[KIEDataSample]) -> Dict:
- """Calculate losses from a batch of inputs and data samples.
- Args:
- inputs (torch.Tensor): Shape :math:`(N, E)`.
- data_samples (List[KIEDataSample]): List of data samples.
-
- Returns:
- dict[str, tensor]: A dictionary of loss components.
- """
- preds = self.forward(inputs, data_samples)
- return self.module_loss(preds, data_samples)
-
- def predict(self, inputs: Tensor,
- data_samples: List[KIEDataSample]) -> List[KIEDataSample]:
- """Predict results from a batch of inputs and data samples with post-
- processing.
-
- Args:
- inputs (torch.Tensor): Shape :math:`(N, E)`.
- data_samples (List[KIEDataSample]): List of data samples.
-
- Returns:
- List[KIEDataSample]: A list of datasamples of prediction results.
- Results are stored in ``pred_instances.labels``,
- ``pred_instances.scores``, ``pred_instances.edge_labels`` and
- ``pred_instances.edge_scores``.
-
- - labels (Tensor): An integer tensor of shape (N, ) indicating bbox
- labels for each image.
- - scores (Tensor): A float tensor of shape (N, ), indicating the
- confidence scores for node label predictions.
- - edge_labels (Tensor): An integer tensor of shape (N, N)
- indicating the connection between nodes. Options are 0, 1.
- - edge_scores (Tensor): A float tensor of shape (N, ), indicating
- the confidence scores for edge predictions.
- """
- preds = self.forward(inputs, data_samples)
- return self.postprocessor(preds, data_samples)
-
- def forward(self, inputs: Tensor,
- data_samples: List[KIEDataSample]) -> Tuple[Tensor, Tensor]:
- """
- Args:
- inputs (torch.Tensor): Shape :math:`(N, E)`.
- data_samples (List[KIEDataSample]): List of data samples.
-
- Returns:
- tuple(Tensor, Tensor):
-
- - node_cls (Tensor): Raw logits scores for nodes. Shape
- :math:`(N, C_{l})` where :math:`C_{l}` is number of classes.
- - edge_cls (Tensor): Raw logits scores for edges. Shape
- :math:`(N * N, 2)`.
- """
-
- device = self.node_embed.weight.device
-
- node_nums, char_nums, all_nodes = self.convert_texts(data_samples)
-
- embed_nodes = self.node_embed(all_nodes.to(device).long())
- rnn_nodes, _ = self.rnn(embed_nodes)
-
- nodes = rnn_nodes.new_zeros(*rnn_nodes.shape[::2])
- all_nums = torch.cat(char_nums).to(device)
- valid = all_nums > 0
- nodes[valid] = rnn_nodes[valid].gather(
- 1, (all_nums[valid] - 1).unsqueeze(-1).unsqueeze(-1).expand(
- -1, -1, rnn_nodes.size(-1))).squeeze(1)
-
- if inputs is not None:
- nodes = self.fusion([inputs, nodes])
-
- relations = self.compute_relations(data_samples)
- all_edges = torch.cat(
- [relation.view(-1, relation.size(-1)) for relation in relations],
- dim=0)
- embed_edges = self.edge_embed(all_edges.float())
- embed_edges = F.normalize(embed_edges)
-
- for gnn_layer in self.gnn_layers:
- nodes, embed_edges = gnn_layer(nodes, embed_edges, node_nums)
-
- node_cls, edge_cls = self.node_cls(nodes), self.edge_cls(embed_edges)
- return node_cls, edge_cls
-
- def convert_texts(
- self, data_samples: List[KIEDataSample]
- ) -> Tuple[List[Tensor], List[Tensor], Tensor]:
- """Extract texts in datasamples and pack them into a batch.
-
- Args:
- data_samples (List[KIEDataSample]): List of data samples.
-
- Returns:
- tuple(List[int], List[Tensor], Tensor):
-
- - node_nums (List[int]): A list of node numbers for each
- sample.
- - char_nums (List[Tensor]): A list of character numbers for each
- sample.
- - nodes (Tensor): A tensor of shape :math:`(N, C)` where
- :math:`C` is the maximum number of characters in a sample.
- """
- node_nums, char_nums = [], []
- max_len = -1
- text_idxs = []
- for data_sample in data_samples:
- node_nums.append(len(data_sample.gt_instances.texts))
- for text in data_sample.gt_instances.texts:
- text_idxs.append(self.dictionary.str2idx(text))
- max_len = max(max_len, len(text))
-
- nodes = torch.zeros((sum(node_nums), max_len),
- dtype=torch.long) + self.dictionary.padding_idx
- for i, text_idx in enumerate(text_idxs):
- nodes[i, :len(text_idx)] = torch.LongTensor(text_idx)
- char_nums = (nodes != self.dictionary.padding_idx).sum(-1).split(
- node_nums, dim=0)
- return node_nums, char_nums, nodes
-
- def compute_relations(self, data_samples: List[KIEDataSample]) -> Tensor:
- """Compute the relations between every two boxes for each datasample,
- then return the concatenated relations."""
-
- relations = []
- for data_sample in data_samples:
- bboxes = data_sample.gt_instances.bboxes
- x1, y1 = bboxes[:, 0:1], bboxes[:, 1:2]
- x2, y2 = bboxes[:, 2:3], bboxes[:, 3:4]
- w, h = torch.clamp(
- x2 - x1 + 1, min=1), torch.clamp(
- y2 - y1 + 1, min=1)
- dx = (x1.t() - x1) / self.relation_norm
- dy = (y1.t() - y1) / self.relation_norm
- xhh, xwh = h.T / h, w.T / h
- whs = w / h + torch.zeros_like(xhh)
- relation = torch.stack([dx, dy, whs, xhh, xwh], -1).float()
- relations.append(relation)
- return relations
-
-
-class GNNLayer(nn.Module):
- """GNN layer for SDMGR.
-
- Args:
- node_dim (int): Dimension of node embedding. Defaults to 256.
- edge_dim (int): Dimension of edge embedding. Defaults to 256.
- """
-
- def __init__(self, node_dim: int = 256, edge_dim: int = 256) -> None:
- super().__init__()
- self.in_fc = nn.Linear(node_dim * 2 + edge_dim, node_dim)
- self.coef_fc = nn.Linear(node_dim, 1)
- self.out_fc = nn.Linear(node_dim, node_dim)
- self.relu = nn.ReLU()
-
- def forward(self, nodes: Tensor, edges: Tensor,
- nums: List[int]) -> Tuple[Tensor, Tensor]:
- """Forward function.
-
- Args:
- nodes (Tensor): Concatenated node embeddings.
- edges (Tensor): Concatenated edge embeddings.
- nums (List[int]): List of number of nodes in each batch.
-
- Returns:
- tuple(Tensor, Tensor):
-
- - nodes (Tensor): New node embeddings.
- - edges (Tensor): New edge embeddings.
- """
- start, cat_nodes = 0, []
- for num in nums:
- sample_nodes = nodes[start:start + num]
- cat_nodes.append(
- torch.cat([
- sample_nodes.unsqueeze(1).expand(-1, num, -1),
- sample_nodes.unsqueeze(0).expand(num, -1, -1)
- ], -1).view(num**2, -1))
- start += num
- cat_nodes = torch.cat([torch.cat(cat_nodes), edges], -1)
- cat_nodes = self.relu(self.in_fc(cat_nodes))
- coefs = self.coef_fc(cat_nodes)
-
- start, residuals = 0, []
- for num in nums:
- residual = F.softmax(
- -torch.eye(num).to(coefs.device).unsqueeze(-1) * 1e9 +
- coefs[start:start + num**2].view(num, num, -1), 1)
- residuals.append(
- (residual *
- cat_nodes[start:start + num**2].view(num, num, -1)).sum(1))
- start += num**2
-
- nodes += self.relu(self.out_fc(torch.cat(residuals)))
- return nodes, cat_nodes
-
-
-class FusionBlock(nn.Module):
- """Fusion block of SDMGR.
-
- Args:
- input_dims (tuple(int, int)): Visual dimension and node embedding
- dimension.
- output_dim (int): Output dimension.
- mm_dim (int): Model dimension. Defaults to 1600.
- chunks (int): Number of chunks. Defaults to 20.
- rank (int): Rank number. Defaults to 15.
- shared (bool): Whether to share the project layer between visual and
- node embedding features. Defaults to False.
- dropout_input (float): Dropout rate after the first projection layer.
- Defaults to 0.
- dropout_pre_lin (float): Dropout rate before the final project layer.
- Defaults to 0.
- dropout_pre_lin (float): Dropout rate after the final project layer.
- Defaults to 0.
- pos_norm (str): The normalization position. Options are 'before_cat'
- and 'after_cat'. Defaults to 'before_cat'.
- """
-
- def __init__(self,
- input_dims: Tuple[int, int],
- output_dim: int,
- mm_dim: int = 1600,
- chunks: int = 20,
- rank: int = 15,
- shared: bool = False,
- dropout_input: float = 0.,
- dropout_pre_lin: float = 0.,
- dropout_output: float = 0.,
- pos_norm: str = 'before_cat') -> None:
- super().__init__()
- self.rank = rank
- self.dropout_input = dropout_input
- self.dropout_pre_lin = dropout_pre_lin
- self.dropout_output = dropout_output
- assert (pos_norm in ['before_cat', 'after_cat'])
- self.pos_norm = pos_norm
- # Modules
- self.linear0 = nn.Linear(input_dims[0], mm_dim)
- self.linear1 = (
- self.linear0 if shared else nn.Linear(input_dims[1], mm_dim))
- self.merge_linears0 = nn.ModuleList()
- self.merge_linears1 = nn.ModuleList()
- self.chunks = self.chunk_sizes(mm_dim, chunks)
- for size in self.chunks:
- ml0 = nn.Linear(size, size * rank)
- self.merge_linears0.append(ml0)
- ml1 = ml0 if shared else nn.Linear(size, size * rank)
- self.merge_linears1.append(ml1)
- self.linear_out = nn.Linear(mm_dim, output_dim)
-
- def forward(self, x: Tensor) -> Tensor:
- """Forward function."""
- x0 = self.linear0(x[0])
- x1 = self.linear1(x[1])
- bs = x1.size(0)
- if self.dropout_input > 0:
- x0 = F.dropout(x0, p=self.dropout_input, training=self.training)
- x1 = F.dropout(x1, p=self.dropout_input, training=self.training)
- x0_chunks = torch.split(x0, self.chunks, -1)
- x1_chunks = torch.split(x1, self.chunks, -1)
- zs = []
- for x0_c, x1_c, m0, m1 in zip(x0_chunks, x1_chunks,
- self.merge_linears0,
- self.merge_linears1):
- m = m0(x0_c) * m1(x1_c) # bs x split_size*rank
- m = m.view(bs, self.rank, -1)
- z = torch.sum(m, 1)
- if self.pos_norm == 'before_cat':
- z = torch.sqrt(F.relu(z)) - torch.sqrt(F.relu(-z))
- z = F.normalize(z)
- zs.append(z)
- z = torch.cat(zs, 1)
- if self.pos_norm == 'after_cat':
- z = torch.sqrt(F.relu(z)) - torch.sqrt(F.relu(-z))
- z = F.normalize(z)
-
- if self.dropout_pre_lin > 0:
- z = F.dropout(z, p=self.dropout_pre_lin, training=self.training)
- z = self.linear_out(z)
- if self.dropout_output > 0:
- z = F.dropout(z, p=self.dropout_output, training=self.training)
- return z
-
- @staticmethod
- def chunk_sizes(dim: int, chunks: int) -> List[int]:
- """Compute chunk sizes."""
- split_size = (dim + chunks - 1) // chunks
- sizes_list = [split_size] * chunks
- sizes_list[-1] = sizes_list[-1] - (sum(sizes_list) - dim)
- return sizes_list
diff --git a/pipeline/mmocr/mmocr/models/kie/module_losses/__init__.py b/pipeline/mmocr/mmocr/models/kie/module_losses/__init__.py
deleted file mode 100644
index 9af5550ae843622d0fa2ff81a23d7c825c3c43fd..0000000000000000000000000000000000000000
--- a/pipeline/mmocr/mmocr/models/kie/module_losses/__init__.py
+++ /dev/null
@@ -1,4 +0,0 @@
-# Copyright (c) OpenMMLab. All rights reserved.
-from .sdmgr_module_loss import SDMGRModuleLoss
-
-__all__ = ['SDMGRModuleLoss']
diff --git a/pipeline/mmocr/mmocr/models/kie/module_losses/__pycache__/__init__.cpython-38.pyc b/pipeline/mmocr/mmocr/models/kie/module_losses/__pycache__/__init__.cpython-38.pyc
deleted file mode 100644
index 62974aac43c50d99603fab87794cd1c81a1d35a7..0000000000000000000000000000000000000000
Binary files a/pipeline/mmocr/mmocr/models/kie/module_losses/__pycache__/__init__.cpython-38.pyc and /dev/null differ
diff --git a/pipeline/mmocr/mmocr/models/kie/module_losses/__pycache__/__init__.cpython-39.pyc b/pipeline/mmocr/mmocr/models/kie/module_losses/__pycache__/__init__.cpython-39.pyc
deleted file mode 100644
index 24cbd2d7cac767b7b890739e30d7608abb565ffa..0000000000000000000000000000000000000000
Binary files a/pipeline/mmocr/mmocr/models/kie/module_losses/__pycache__/__init__.cpython-39.pyc and /dev/null differ
diff --git a/pipeline/mmocr/mmocr/models/kie/module_losses/__pycache__/sdmgr_module_loss.cpython-38.pyc b/pipeline/mmocr/mmocr/models/kie/module_losses/__pycache__/sdmgr_module_loss.cpython-38.pyc
deleted file mode 100644
index a66f2e7e816c70d2bb92d22bc1f65e0c947cf06e..0000000000000000000000000000000000000000
Binary files a/pipeline/mmocr/mmocr/models/kie/module_losses/__pycache__/sdmgr_module_loss.cpython-38.pyc and /dev/null differ
diff --git a/pipeline/mmocr/mmocr/models/kie/module_losses/__pycache__/sdmgr_module_loss.cpython-39.pyc b/pipeline/mmocr/mmocr/models/kie/module_losses/__pycache__/sdmgr_module_loss.cpython-39.pyc
deleted file mode 100644
index c2bdf61cc3e46edf2596d0f4f03be3c036d2566b..0000000000000000000000000000000000000000
Binary files a/pipeline/mmocr/mmocr/models/kie/module_losses/__pycache__/sdmgr_module_loss.cpython-39.pyc and /dev/null differ
diff --git a/pipeline/mmocr/mmocr/models/kie/module_losses/sdmgr_module_loss.py b/pipeline/mmocr/mmocr/models/kie/module_losses/sdmgr_module_loss.py
deleted file mode 100644
index 5dc87ea32c28d3d4fdc411e35cda79e82eb3b676..0000000000000000000000000000000000000000
--- a/pipeline/mmocr/mmocr/models/kie/module_losses/sdmgr_module_loss.py
+++ /dev/null
@@ -1,65 +0,0 @@
-# Copyright (c) OpenMMLab. All rights reserved.
-from typing import Dict, List, Tuple
-
-import torch
-from mmdet.models.losses import accuracy
-from torch import Tensor, nn
-
-from mmocr.registry import MODELS
-from mmocr.structures import KIEDataSample
-
-
-@MODELS.register_module()
-class SDMGRModuleLoss(nn.Module):
- """The implementation the loss of key information extraction proposed in
- the paper: `Spatial Dual-Modality Graph Reasoning for Key Information
- Extraction `_.
-
- Args:
- weight_node (float): Weight of node loss. Defaults to 1.0.
- weight_edge (float): Weight of edge loss. Defaults to 1.0.
- ignore_idx (int): Node label to ignore. Defaults to -100.
- """
-
- def __init__(self,
- weight_node: float = 1.0,
- weight_edge: float = 1.0,
- ignore_idx: int = -100) -> None:
- super().__init__()
- # TODO: Use MODELS.build after DRRG loss has been merged
- self.loss_node = nn.CrossEntropyLoss(ignore_index=ignore_idx)
- self.loss_edge = nn.CrossEntropyLoss(ignore_index=-1)
- self.weight_node = weight_node
- self.weight_edge = weight_edge
- self.ignore_idx = ignore_idx
-
- def forward(self, preds: Tuple[Tensor, Tensor],
- data_samples: List[KIEDataSample]) -> Dict:
- """Forward function.
-
- Args:
- preds (tuple(Tensor, Tensor)):
- data_samples (list[KIEDataSample]): A list of datasamples
- containing ``gt_instances.labels`` and
- ``gt_instances.edge_labels``.
-
- Returns:
- dict(str, Tensor): Loss dict, containing ``loss_node``,
- ``loss_edge``, ``acc_node`` and ``acc_edge``.
- """
- node_preds, edge_preds = preds
- node_gts, edge_gts = [], []
- for data_sample in data_samples:
- node_gts.append(data_sample.gt_instances.labels)
- edge_gts.append(data_sample.gt_instances.edge_labels.reshape(-1))
- node_gts = torch.cat(node_gts).long()
- edge_gts = torch.cat(edge_gts).long()
-
- node_valids = torch.nonzero(
- node_gts != self.ignore_idx, as_tuple=False).reshape(-1)
- edge_valids = torch.nonzero(edge_gts != -1, as_tuple=False).reshape(-1)
- return dict(
- loss_node=self.weight_node * self.loss_node(node_preds, node_gts),
- loss_edge=self.weight_edge * self.loss_edge(edge_preds, edge_gts),
- acc_node=accuracy(node_preds[node_valids], node_gts[node_valids]),
- acc_edge=accuracy(edge_preds[edge_valids], edge_gts[edge_valids]))
diff --git a/pipeline/mmocr/mmocr/models/kie/postprocessors/__init__.py b/pipeline/mmocr/mmocr/models/kie/postprocessors/__init__.py
deleted file mode 100644
index 645904bc1beb0b8e1b4f169a8b5344de55e41f8f..0000000000000000000000000000000000000000
--- a/pipeline/mmocr/mmocr/models/kie/postprocessors/__init__.py
+++ /dev/null
@@ -1,4 +0,0 @@
-# Copyright (c) OpenMMLab. All rights reserved.
-from .sdmgr_postprocessor import SDMGRPostProcessor
-
-__all__ = ['SDMGRPostProcessor']
diff --git a/pipeline/mmocr/mmocr/models/kie/postprocessors/__pycache__/__init__.cpython-38.pyc b/pipeline/mmocr/mmocr/models/kie/postprocessors/__pycache__/__init__.cpython-38.pyc
deleted file mode 100644
index c4df4e2c44f23c5af4265f29a0a17bc1f4ab546a..0000000000000000000000000000000000000000
Binary files a/pipeline/mmocr/mmocr/models/kie/postprocessors/__pycache__/__init__.cpython-38.pyc and /dev/null differ
diff --git a/pipeline/mmocr/mmocr/models/kie/postprocessors/__pycache__/__init__.cpython-39.pyc b/pipeline/mmocr/mmocr/models/kie/postprocessors/__pycache__/__init__.cpython-39.pyc
deleted file mode 100644
index 6066caec0bfc6afad07a03110e7d87f2fb132a09..0000000000000000000000000000000000000000
Binary files a/pipeline/mmocr/mmocr/models/kie/postprocessors/__pycache__/__init__.cpython-39.pyc and /dev/null differ
diff --git a/pipeline/mmocr/mmocr/models/kie/postprocessors/__pycache__/sdmgr_postprocessor.cpython-38.pyc b/pipeline/mmocr/mmocr/models/kie/postprocessors/__pycache__/sdmgr_postprocessor.cpython-38.pyc
deleted file mode 100644
index 32f86d1f6e8dcd3a3d63cc02b0427ffacb880429..0000000000000000000000000000000000000000
Binary files a/pipeline/mmocr/mmocr/models/kie/postprocessors/__pycache__/sdmgr_postprocessor.cpython-38.pyc and /dev/null differ
diff --git a/pipeline/mmocr/mmocr/models/kie/postprocessors/__pycache__/sdmgr_postprocessor.cpython-39.pyc b/pipeline/mmocr/mmocr/models/kie/postprocessors/__pycache__/sdmgr_postprocessor.cpython-39.pyc
deleted file mode 100644
index dd75437e964240346ff63ea0c287e9faea87df87..0000000000000000000000000000000000000000
Binary files a/pipeline/mmocr/mmocr/models/kie/postprocessors/__pycache__/sdmgr_postprocessor.cpython-39.pyc and /dev/null differ
diff --git a/pipeline/mmocr/mmocr/models/kie/postprocessors/sdmgr_postprocessor.py b/pipeline/mmocr/mmocr/models/kie/postprocessors/sdmgr_postprocessor.py
deleted file mode 100644
index 977c4f94ad087f244c8648ccd1081494e8a38d6c..0000000000000000000000000000000000000000
--- a/pipeline/mmocr/mmocr/models/kie/postprocessors/sdmgr_postprocessor.py
+++ /dev/null
@@ -1,170 +0,0 @@
-# Copyright (c) OpenMMLab. All rights reserved.
-from typing import List, Optional, Tuple
-
-import numpy as np
-import torch
-from mmengine.structures import InstanceData
-from torch import Tensor, nn
-
-from mmocr.registry import MODELS
-from mmocr.structures import KIEDataSample
-
-
-@MODELS.register_module()
-class SDMGRPostProcessor:
- """Postprocessor for SDMGR. It converts the node and edge scores into
- labels and edge labels. If the link_type is not "none", it reconstructs the
- edge labels with different strategies specified by ``link_type``, which is
- generally known as the "openset" mode. In "openset" mode, only the edges
- connecting from "key" to "value" nodes will be constructed.
-
- Args:
- link_type (str): The type of link to be constructed.
- Defaults to 'none'. Options are:
-
- - 'none': The simplest link type involving no edge
- postprocessing. The edge prediction will be returned as-is.
- - 'one-to-one': One key node can be connected to one value node.
- - 'one-to-many': One key node can be connected to multiple value
- nodes.
- - 'many-to-one': Multiple key nodes can be connected to one value
- node.
- - 'many-to-many': No restrictions on the number of edges that a
- key/value node can have.
- key_node_idx (int, optional): The label index of the key node. It must
- be specified if ``link_type`` is not "none". Defaults to None.
- value_node_idx (int, optional): The index of the value node. It must be
- specified if ``link_type`` is not "none". Defaults to None.
- """
-
- def __init__(self,
- link_type: str = 'none',
- key_node_idx: Optional[int] = None,
- value_node_idx: Optional[int] = None):
- assert link_type in [
- 'one-to-one', 'one-to-many', 'many-to-one', 'many-to-many', 'none'
- ]
- self.link_type = link_type
- if link_type != 'none':
- assert key_node_idx is not None and value_node_idx is not None
- self.key_node_idx = key_node_idx
- self.value_node_idx = value_node_idx
- self.softmax = nn.Softmax(dim=-1)
-
- def __call__(self, preds: Tuple[Tensor, Tensor],
- data_samples: List[KIEDataSample]) -> List[KIEDataSample]:
- """Postprocess raw outputs from SDMGR heads and pack the results into a
- list of KIEDataSample.
-
- Args:
- preds (tuple[Tensor]): A tuple of raw outputs from SDMGR heads.
- data_samples (list[KIEDataSample]): A list of N datasamples,
- containing meta information and gold annotations for each of
- the images.
-
- Returns:
- List[KIEDataSample]: A list of datasamples of prediction results.
- Results are stored in ``pred_instances.labels``,
- ``pred_instances.scores``, ``pred_instances.edge_labels`` and
- ``pred_instances.edge_scores``.
-
- - labels (Tensor): An integer tensor of shape (N, ) indicating bbox
- labels for each image.
- - scores (Tensor): A float tensor of shape (N, ), indicating the
- confidence scores for node label predictions.
- - edge_labels (Tensor): An integer tensor of shape (N, N)
- indicating the connection between nodes. Options are 0, 1.
- - edge_scores (Tensor): A float tensor of shape (N, ), indicating
- the confidence scores for edge predictions.
- """
- node_preds, edge_preds = preds
- all_node_scores = self.softmax(node_preds)
- all_edge_scores = self.softmax(edge_preds)
- chunk_size = [
- data_sample.gt_instances.bboxes.shape[0]
- for data_sample in data_samples
- ]
- node_scores, node_preds = torch.max(all_node_scores, dim=-1)
- edge_scores, edge_preds = torch.max(all_edge_scores, dim=-1)
- node_preds = node_preds.split(chunk_size, dim=0)
- node_scores = node_scores.split(chunk_size, dim=0)
-
- sq_chunks = [chunk**2 for chunk in chunk_size]
- edge_preds = list(edge_preds.split(sq_chunks, dim=0))
- edge_scores = list(edge_scores.split(sq_chunks, dim=0))
- for i, chunk in enumerate(chunk_size):
- edge_preds[i] = edge_preds[i].reshape((chunk, chunk))
- edge_scores[i] = edge_scores[i].reshape((chunk, chunk))
-
- for i in range(len(data_samples)):
- data_samples[i].pred_instances = InstanceData()
- data_samples[i].pred_instances.labels = node_preds[i].cpu()
- data_samples[i].pred_instances.scores = node_scores[i].cpu()
- if self.link_type != 'none':
- edge_scores[i], edge_preds[i] = self.decode_edges(
- node_preds[i], edge_scores[i], edge_preds[i])
- data_samples[i].pred_instances.edge_labels = edge_preds[i].cpu()
- data_samples[i].pred_instances.edge_scores = edge_scores[i].cpu()
-
- return data_samples
-
- def decode_edges(self, node_labels: Tensor, edge_scores: Tensor,
- edge_labels: Tensor) -> Tuple[Tensor, Tensor]:
- """Reconstruct the edges and update edge scores according to
- ``link_type``.
-
- Args:
- data_sample (KIEDataSample): A datasample containing prediction
- results.
-
- Returns:
- tuple(Tensor, Tensor):
-
- - edge_scores (Tensor): A float tensor of shape (N, N)
- indicating the confidence scores for edge predictions.
- - edge_labels (Tensor): An integer tensor of shape (N, N)
- indicating the connection between nodes. Options are 0, 1.
- """
- # Obtain the scores of the existence of edges.
- pos_edges_scores = edge_scores.clone()
- edge_labels_mask = edge_labels.bool()
- pos_edges_scores[
- ~edge_labels_mask] = 1 - pos_edges_scores[~edge_labels_mask]
-
- # Temporarily convert the directed graph to undirected by adding
- # reversed edges to every pair of nodes if they were already connected
- # by an directed edge before.
- edge_labels = torch.max(edge_labels, edge_labels.T)
-
- # Maximize edge scores
- edge_labels_mask = edge_labels.bool()
- edge_scores[~edge_labels_mask] = pos_edges_scores[~edge_labels_mask]
- new_edge_scores = torch.max(edge_scores, edge_scores.T)
-
- # Only reconstruct the edges from key nodes to value nodes.
- key_nodes_mask = node_labels == self.key_node_idx
- value_nodes_mask = node_labels == self.value_node_idx
- key2value_mask = key_nodes_mask[:, None] * value_nodes_mask[None, :]
-
- if self.link_type == 'many-to-many':
- new_edge_labels = (key2value_mask * edge_labels).int()
- else:
- new_edge_labels = torch.zeros_like(edge_labels).int()
-
- tmp_edge_scores = new_edge_scores.clone().cpu()
- tmp_edge_scores[~edge_labels_mask] = -1
- tmp_edge_scores[~key2value_mask] = -1
- # Greedily extract valid edges
- while (tmp_edge_scores > -1).any():
- i, j = np.unravel_index(
- torch.argmax(tmp_edge_scores), tmp_edge_scores.shape)
- new_edge_labels[i, j] = 1
- if self.link_type == 'one-to-one':
- tmp_edge_scores[i, :] = -1
- tmp_edge_scores[:, j] = -1
- elif self.link_type == 'one-to-many':
- tmp_edge_scores[:, j] = -1
- elif self.link_type == 'many-to-one':
- tmp_edge_scores[i, :] = -1
-
- return new_edge_scores.cpu(), new_edge_labels.cpu()
diff --git a/pipeline/mmocr/mmocr/models/textdet/__init__.py b/pipeline/mmocr/mmocr/models/textdet/__init__.py
deleted file mode 100644
index b803a0d22e93cdfde7986b5fe111d2b061d9d9fb..0000000000000000000000000000000000000000
--- a/pipeline/mmocr/mmocr/models/textdet/__init__.py
+++ /dev/null
@@ -1,7 +0,0 @@
-# Copyright (c) OpenMMLab. All rights reserved.
-from .data_preprocessors import * # NOQA
-from .detectors import * # NOQA
-from .heads import * # NOQA
-from .module_losses import * # NOQA
-from .necks import * # NOQA
-from .postprocessors import * # NOQA
diff --git a/pipeline/mmocr/mmocr/models/textdet/__pycache__/__init__.cpython-38.pyc b/pipeline/mmocr/mmocr/models/textdet/__pycache__/__init__.cpython-38.pyc
deleted file mode 100644
index f709b312558a990b96eec0d1ab9777b90139595b..0000000000000000000000000000000000000000
Binary files a/pipeline/mmocr/mmocr/models/textdet/__pycache__/__init__.cpython-38.pyc and /dev/null differ
diff --git a/pipeline/mmocr/mmocr/models/textdet/__pycache__/__init__.cpython-39.pyc b/pipeline/mmocr/mmocr/models/textdet/__pycache__/__init__.cpython-39.pyc
deleted file mode 100644
index 9e3db51beaedc0a8a6e57b5ab47a5e0fdd6a6b1f..0000000000000000000000000000000000000000
Binary files a/pipeline/mmocr/mmocr/models/textdet/__pycache__/__init__.cpython-39.pyc and /dev/null differ
diff --git a/pipeline/mmocr/mmocr/models/textdet/data_preprocessors/__init__.py b/pipeline/mmocr/mmocr/models/textdet/data_preprocessors/__init__.py
deleted file mode 100644
index 056e8b6d5a06aff8502c0a36712f6d2a5f4ac4b5..0000000000000000000000000000000000000000
--- a/pipeline/mmocr/mmocr/models/textdet/data_preprocessors/__init__.py
+++ /dev/null
@@ -1,4 +0,0 @@
-# Copyright (c) OpenMMLab. All rights reserved.
-from .data_preprocessor import TextDetDataPreprocessor
-
-__all__ = ['TextDetDataPreprocessor']
diff --git a/pipeline/mmocr/mmocr/models/textdet/data_preprocessors/__pycache__/__init__.cpython-38.pyc b/pipeline/mmocr/mmocr/models/textdet/data_preprocessors/__pycache__/__init__.cpython-38.pyc
deleted file mode 100644
index 4ec7cbbd5dcdb8bb5fc89f58c2ddba5de78fb9de..0000000000000000000000000000000000000000
Binary files a/pipeline/mmocr/mmocr/models/textdet/data_preprocessors/__pycache__/__init__.cpython-38.pyc and /dev/null differ
diff --git a/pipeline/mmocr/mmocr/models/textdet/data_preprocessors/__pycache__/__init__.cpython-39.pyc b/pipeline/mmocr/mmocr/models/textdet/data_preprocessors/__pycache__/__init__.cpython-39.pyc
deleted file mode 100644
index a6bf962bc62670de3884768ed43c0c0c303a4783..0000000000000000000000000000000000000000
Binary files a/pipeline/mmocr/mmocr/models/textdet/data_preprocessors/__pycache__/__init__.cpython-39.pyc and /dev/null differ
diff --git a/pipeline/mmocr/mmocr/models/textdet/data_preprocessors/__pycache__/data_preprocessor.cpython-38.pyc b/pipeline/mmocr/mmocr/models/textdet/data_preprocessors/__pycache__/data_preprocessor.cpython-38.pyc
deleted file mode 100644
index f6a48bbd019eac2275cbfb27b0b8601df8701d0c..0000000000000000000000000000000000000000
Binary files a/pipeline/mmocr/mmocr/models/textdet/data_preprocessors/__pycache__/data_preprocessor.cpython-38.pyc and /dev/null differ
diff --git a/pipeline/mmocr/mmocr/models/textdet/data_preprocessors/__pycache__/data_preprocessor.cpython-39.pyc b/pipeline/mmocr/mmocr/models/textdet/data_preprocessors/__pycache__/data_preprocessor.cpython-39.pyc
deleted file mode 100644
index aade7805e0f077e97dc50b404bd6b6f2a394f68b..0000000000000000000000000000000000000000
Binary files a/pipeline/mmocr/mmocr/models/textdet/data_preprocessors/__pycache__/data_preprocessor.cpython-39.pyc and /dev/null differ
diff --git a/pipeline/mmocr/mmocr/models/textdet/data_preprocessors/data_preprocessor.py b/pipeline/mmocr/mmocr/models/textdet/data_preprocessors/data_preprocessor.py
deleted file mode 100644
index 990f0b146455cbf315d8f12f8f25915caa112f11..0000000000000000000000000000000000000000
--- a/pipeline/mmocr/mmocr/models/textdet/data_preprocessors/data_preprocessor.py
+++ /dev/null
@@ -1,99 +0,0 @@
-# Copyright (c) OpenMMLab. All rights reserved.
-from numbers import Number
-from typing import Dict, List, Optional, Sequence, Union
-
-import torch.nn as nn
-from mmengine.model import ImgDataPreprocessor
-
-from mmocr.registry import MODELS
-
-
-@MODELS.register_module()
-class TextDetDataPreprocessor(ImgDataPreprocessor):
- """Image pre-processor for detection tasks.
-
- Comparing with the :class:`mmengine.ImgDataPreprocessor`,
-
- 1. It supports batch augmentations.
- 2. It will additionally append batch_input_shape and pad_shape
- to data_samples considering the object detection task.
-
- It provides the data pre-processing as follows
-
- - Collate and move data to the target device.
- - Pad inputs to the maximum size of current batch with defined
- ``pad_value``. The padding size can be divisible by a defined
- ``pad_size_divisor``
- - Stack inputs to batch_inputs.
- - Convert inputs from bgr to rgb if the shape of input is (3, H, W).
- - Normalize image with defined std and mean.
- - Do batch augmentations during training.
-
- Args:
- mean (Sequence[Number], optional): The pixel mean of R, G, B channels.
- Defaults to None.
- std (Sequence[Number], optional): The pixel standard deviation of
- R, G, B channels. Defaults to None.
- pad_size_divisor (int): The size of padded image should be
- divisible by ``pad_size_divisor``. Defaults to 1.
- pad_value (Number): The padded pixel value. Defaults to 0.
- pad_mask (bool): Whether to pad instance masks. Defaults to False.
- mask_pad_value (int): The padded pixel value for instance masks.
- Defaults to 0.
- pad_seg (bool): Whether to pad semantic segmentation maps.
- Defaults to False.
- seg_pad_value (int): The padded pixel value for semantic
- segmentation maps. Defaults to 255.
- bgr_to_rgb (bool): whether to convert image from BGR to RGB.
- Defaults to False.
- rgb_to_bgr (bool): whether to convert image from RGB to RGB.
- Defaults to False.
- batch_augments (list[dict], optional): Batch-level augmentations
- """
-
- def __init__(self,
- mean: Sequence[Number] = None,
- std: Sequence[Number] = None,
- pad_size_divisor: int = 1,
- pad_value: Union[float, int] = 0,
- bgr_to_rgb: bool = False,
- rgb_to_bgr: bool = False,
- batch_augments: Optional[List[Dict]] = None) -> None:
- super().__init__(
- mean=mean,
- std=std,
- pad_size_divisor=pad_size_divisor,
- pad_value=pad_value,
- bgr_to_rgb=bgr_to_rgb,
- rgb_to_bgr=rgb_to_bgr)
- if batch_augments is not None:
- self.batch_augments = nn.ModuleList(
- [MODELS.build(aug) for aug in batch_augments])
- else:
- self.batch_augments = None
-
- def forward(self, data: Dict, training: bool = False) -> Dict:
- """Perform normalizationใpadding and bgr2rgb conversion based on
- ``BaseDataPreprocessor``.
-
- Args:
- data (dict): data sampled from dataloader.
- training (bool): Whether to enable training time augmentation.
-
- Returns:
- dict: Data in the same format as the model input.
- """
- data = super().forward(data=data, training=training)
- inputs, data_samples = data['inputs'], data['data_samples']
-
- if data_samples is not None:
- batch_input_shape = tuple(inputs[0].size()[-2:])
- for data_sample in data_samples:
- data_sample.set_metainfo(
- {'batch_input_shape': batch_input_shape})
-
- if training and self.batch_augments is not None:
- for batch_aug in self.batch_augments:
- inputs, data_samples = batch_aug(inputs, data_samples)
-
- return data
diff --git a/pipeline/mmocr/mmocr/models/textdet/detectors/__init__.py b/pipeline/mmocr/mmocr/models/textdet/detectors/__init__.py
deleted file mode 100644
index 00b95bdb9aaf708a96fb4afb6a44f8b89bf489a5..0000000000000000000000000000000000000000
--- a/pipeline/mmocr/mmocr/models/textdet/detectors/__init__.py
+++ /dev/null
@@ -1,14 +0,0 @@
-# Copyright (c) OpenMMLab. All rights reserved.
-from .dbnet import DBNet
-from .drrg import DRRG
-from .fcenet import FCENet
-from .mmdet_wrapper import MMDetWrapper
-from .panet import PANet
-from .psenet import PSENet
-from .single_stage_text_detector import SingleStageTextDetector
-from .textsnake import TextSnake
-
-__all__ = [
- 'SingleStageTextDetector', 'DBNet', 'PANet', 'PSENet', 'TextSnake',
- 'FCENet', 'DRRG', 'MMDetWrapper'
-]
diff --git a/pipeline/mmocr/mmocr/models/textdet/detectors/__pycache__/__init__.cpython-38.pyc b/pipeline/mmocr/mmocr/models/textdet/detectors/__pycache__/__init__.cpython-38.pyc
deleted file mode 100644
index 7cd7bee0c90e01cadde2ccc80bb115c0cb9da7ea..0000000000000000000000000000000000000000
Binary files a/pipeline/mmocr/mmocr/models/textdet/detectors/__pycache__/__init__.cpython-38.pyc and /dev/null differ
diff --git a/pipeline/mmocr/mmocr/models/textdet/detectors/__pycache__/__init__.cpython-39.pyc b/pipeline/mmocr/mmocr/models/textdet/detectors/__pycache__/__init__.cpython-39.pyc
deleted file mode 100644
index fbee1b50dc784031d891cd93229644854b180780..0000000000000000000000000000000000000000
Binary files a/pipeline/mmocr/mmocr/models/textdet/detectors/__pycache__/__init__.cpython-39.pyc and /dev/null differ
diff --git a/pipeline/mmocr/mmocr/models/textdet/detectors/__pycache__/base.cpython-38.pyc b/pipeline/mmocr/mmocr/models/textdet/detectors/__pycache__/base.cpython-38.pyc
deleted file mode 100644
index 65338136e3416ddd1ac120b726c7900db6a66b59..0000000000000000000000000000000000000000
Binary files a/pipeline/mmocr/mmocr/models/textdet/detectors/__pycache__/base.cpython-38.pyc and /dev/null differ
diff --git a/pipeline/mmocr/mmocr/models/textdet/detectors/__pycache__/base.cpython-39.pyc b/pipeline/mmocr/mmocr/models/textdet/detectors/__pycache__/base.cpython-39.pyc
deleted file mode 100644
index 5b056c8baa5bda1fedf9f055b8af7fda5fa9519e..0000000000000000000000000000000000000000
Binary files a/pipeline/mmocr/mmocr/models/textdet/detectors/__pycache__/base.cpython-39.pyc and /dev/null differ
diff --git a/pipeline/mmocr/mmocr/models/textdet/detectors/__pycache__/dbnet.cpython-38.pyc b/pipeline/mmocr/mmocr/models/textdet/detectors/__pycache__/dbnet.cpython-38.pyc
deleted file mode 100644
index 03f4537cbc54104a8a8446e07ae578181823f962..0000000000000000000000000000000000000000
Binary files a/pipeline/mmocr/mmocr/models/textdet/detectors/__pycache__/dbnet.cpython-38.pyc and /dev/null differ
diff --git a/pipeline/mmocr/mmocr/models/textdet/detectors/__pycache__/dbnet.cpython-39.pyc b/pipeline/mmocr/mmocr/models/textdet/detectors/__pycache__/dbnet.cpython-39.pyc
deleted file mode 100644
index 3bb07a7b9bc6e4048fdd2275052b810f2d32c47a..0000000000000000000000000000000000000000
Binary files a/pipeline/mmocr/mmocr/models/textdet/detectors/__pycache__/dbnet.cpython-39.pyc and /dev/null differ
diff --git a/pipeline/mmocr/mmocr/models/textdet/detectors/__pycache__/drrg.cpython-38.pyc b/pipeline/mmocr/mmocr/models/textdet/detectors/__pycache__/drrg.cpython-38.pyc
deleted file mode 100644
index b6edf3a994c7298662480ee1dcfc2f1b2373d89b..0000000000000000000000000000000000000000
Binary files a/pipeline/mmocr/mmocr/models/textdet/detectors/__pycache__/drrg.cpython-38.pyc and /dev/null differ
diff --git a/pipeline/mmocr/mmocr/models/textdet/detectors/__pycache__/drrg.cpython-39.pyc b/pipeline/mmocr/mmocr/models/textdet/detectors/__pycache__/drrg.cpython-39.pyc
deleted file mode 100644
index d78e9b35b667a25307875fc90cddbc8d667f3d5f..0000000000000000000000000000000000000000
Binary files a/pipeline/mmocr/mmocr/models/textdet/detectors/__pycache__/drrg.cpython-39.pyc and /dev/null differ
diff --git a/pipeline/mmocr/mmocr/models/textdet/detectors/__pycache__/fcenet.cpython-38.pyc b/pipeline/mmocr/mmocr/models/textdet/detectors/__pycache__/fcenet.cpython-38.pyc
deleted file mode 100644
index 3960cdf9f916d1451a47f94548079e4fd5e203e3..0000000000000000000000000000000000000000
Binary files a/pipeline/mmocr/mmocr/models/textdet/detectors/__pycache__/fcenet.cpython-38.pyc and /dev/null differ
diff --git a/pipeline/mmocr/mmocr/models/textdet/detectors/__pycache__/fcenet.cpython-39.pyc b/pipeline/mmocr/mmocr/models/textdet/detectors/__pycache__/fcenet.cpython-39.pyc
deleted file mode 100644
index 07850e1c431578b9cf0f32d7ebd9bbb3e4b44352..0000000000000000000000000000000000000000
Binary files a/pipeline/mmocr/mmocr/models/textdet/detectors/__pycache__/fcenet.cpython-39.pyc and /dev/null differ
diff --git a/pipeline/mmocr/mmocr/models/textdet/detectors/__pycache__/mmdet_wrapper.cpython-38.pyc b/pipeline/mmocr/mmocr/models/textdet/detectors/__pycache__/mmdet_wrapper.cpython-38.pyc
deleted file mode 100644
index 7ec2833cdd87000513d004db5ffb75acd3ec435a..0000000000000000000000000000000000000000
Binary files a/pipeline/mmocr/mmocr/models/textdet/detectors/__pycache__/mmdet_wrapper.cpython-38.pyc and /dev/null differ
diff --git a/pipeline/mmocr/mmocr/models/textdet/detectors/__pycache__/mmdet_wrapper.cpython-39.pyc b/pipeline/mmocr/mmocr/models/textdet/detectors/__pycache__/mmdet_wrapper.cpython-39.pyc
deleted file mode 100644
index 7cf7af361baa1685574f615a692caedbde4dceb0..0000000000000000000000000000000000000000
Binary files a/pipeline/mmocr/mmocr/models/textdet/detectors/__pycache__/mmdet_wrapper.cpython-39.pyc and /dev/null differ
diff --git a/pipeline/mmocr/mmocr/models/textdet/detectors/__pycache__/panet.cpython-38.pyc b/pipeline/mmocr/mmocr/models/textdet/detectors/__pycache__/panet.cpython-38.pyc
deleted file mode 100644
index 7ccba5dd9856b639a2f51e1c70fc8cbf074e7c72..0000000000000000000000000000000000000000
Binary files a/pipeline/mmocr/mmocr/models/textdet/detectors/__pycache__/panet.cpython-38.pyc and /dev/null differ
diff --git a/pipeline/mmocr/mmocr/models/textdet/detectors/__pycache__/panet.cpython-39.pyc b/pipeline/mmocr/mmocr/models/textdet/detectors/__pycache__/panet.cpython-39.pyc
deleted file mode 100644
index af3e70b42e7af43ec6dec09c5131f635344dbce2..0000000000000000000000000000000000000000
Binary files a/pipeline/mmocr/mmocr/models/textdet/detectors/__pycache__/panet.cpython-39.pyc and /dev/null differ
diff --git a/pipeline/mmocr/mmocr/models/textdet/detectors/__pycache__/psenet.cpython-38.pyc b/pipeline/mmocr/mmocr/models/textdet/detectors/__pycache__/psenet.cpython-38.pyc
deleted file mode 100644
index 2837fe4d4dc5ff57effe3d8c83e0302cc172ec82..0000000000000000000000000000000000000000
Binary files a/pipeline/mmocr/mmocr/models/textdet/detectors/__pycache__/psenet.cpython-38.pyc and /dev/null differ
diff --git a/pipeline/mmocr/mmocr/models/textdet/detectors/__pycache__/psenet.cpython-39.pyc b/pipeline/mmocr/mmocr/models/textdet/detectors/__pycache__/psenet.cpython-39.pyc
deleted file mode 100644
index 94212c32cd466db3c64f600aad48b09a650d1ea8..0000000000000000000000000000000000000000
Binary files a/pipeline/mmocr/mmocr/models/textdet/detectors/__pycache__/psenet.cpython-39.pyc and /dev/null differ
diff --git a/pipeline/mmocr/mmocr/models/textdet/detectors/__pycache__/single_stage_text_detector.cpython-38.pyc b/pipeline/mmocr/mmocr/models/textdet/detectors/__pycache__/single_stage_text_detector.cpython-38.pyc
deleted file mode 100644
index 65be2e630b07bf654a2aca349ae9092e70cd2a8d..0000000000000000000000000000000000000000
Binary files a/pipeline/mmocr/mmocr/models/textdet/detectors/__pycache__/single_stage_text_detector.cpython-38.pyc and /dev/null differ
diff --git a/pipeline/mmocr/mmocr/models/textdet/detectors/__pycache__/single_stage_text_detector.cpython-39.pyc b/pipeline/mmocr/mmocr/models/textdet/detectors/__pycache__/single_stage_text_detector.cpython-39.pyc
deleted file mode 100644
index 7861d75912c9716ce407318462d81d059ecd1a18..0000000000000000000000000000000000000000
Binary files a/pipeline/mmocr/mmocr/models/textdet/detectors/__pycache__/single_stage_text_detector.cpython-39.pyc and /dev/null differ
diff --git a/pipeline/mmocr/mmocr/models/textdet/detectors/__pycache__/textsnake.cpython-38.pyc b/pipeline/mmocr/mmocr/models/textdet/detectors/__pycache__/textsnake.cpython-38.pyc
deleted file mode 100644
index 9d582426446e5c127e92a6044fa919e94b6bb4ea..0000000000000000000000000000000000000000
Binary files a/pipeline/mmocr/mmocr/models/textdet/detectors/__pycache__/textsnake.cpython-38.pyc and /dev/null differ
diff --git a/pipeline/mmocr/mmocr/models/textdet/detectors/__pycache__/textsnake.cpython-39.pyc b/pipeline/mmocr/mmocr/models/textdet/detectors/__pycache__/textsnake.cpython-39.pyc
deleted file mode 100644
index fa6db09ea71344c94c9b165096ff1f8aa92fb2f1..0000000000000000000000000000000000000000
Binary files a/pipeline/mmocr/mmocr/models/textdet/detectors/__pycache__/textsnake.cpython-39.pyc and /dev/null differ
diff --git a/pipeline/mmocr/mmocr/models/textdet/detectors/base.py b/pipeline/mmocr/mmocr/models/textdet/detectors/base.py
deleted file mode 100644
index a81ba0214d6bec28c0807e8a60d6ff376a6727ec..0000000000000000000000000000000000000000
--- a/pipeline/mmocr/mmocr/models/textdet/detectors/base.py
+++ /dev/null
@@ -1,106 +0,0 @@
-# Copyright (c) OpenMMLab. All rights reserved.
-from abc import ABCMeta, abstractmethod
-from typing import Dict, Tuple, Union
-
-import torch
-from mmengine.model import BaseModel
-from torch import Tensor
-
-from mmocr.utils.typing_utils import (DetSampleList, OptConfigType,
- OptDetSampleList, OptMultiConfig)
-
-ForwardResults = Union[Dict[str, torch.Tensor], DetSampleList,
- Tuple[torch.Tensor], torch.Tensor]
-
-
-class BaseTextDetector(BaseModel, metaclass=ABCMeta):
- """Base class for detectors.
-
- Args:
- data_preprocessor (dict or ConfigDict, optional): The pre-process
- config of :class:`BaseDataPreprocessor`. it usually includes,
- ``pad_size_divisor``, ``pad_value``, ``mean`` and ``std``.
- init_cfg (dict or ConfigDict, optional): the config to control the
- initialization. Defaults to None.
- """
-
- def __init__(self,
- data_preprocessor: OptConfigType = None,
- init_cfg: OptMultiConfig = None):
- super().__init__(
- data_preprocessor=data_preprocessor, init_cfg=init_cfg)
-
- @property
- def with_neck(self) -> bool:
- """bool: whether the detector has a neck"""
- return hasattr(self, 'neck') and self.neck is not None
-
- def forward(self,
- inputs: torch.Tensor,
- data_samples: OptDetSampleList = None,
- mode: str = 'tensor') -> ForwardResults:
- """The unified entry for a forward process in both training and test.
-
- The method should accept three modes: "tensor", "predict" and "loss":
-
- - "tensor": Forward the whole network and return tensor or tuple of
- tensor without any post-processing, same as a common nn.Module.
- - "predict": Forward and return the predictions, which are fully
- processed to a list of :obj:`TextDetDataSample`.
- - "loss": Forward and return a dict of losses according to the given
- inputs and data samples.
-
- Note that this method doesn't handle either back propagation or
- parameter update, which are supposed to be done in :meth:`train_step`.
-
- Args:
- inputs (torch.Tensor): The input tensor with shape
- (N, C, ...) in general.
- data_samples (list[:obj:`TextDetDataSample`], optional): A batch of
- data samples that contain annotations and predictions.
- Defaults to None.
- mode (str): Return what kind of value. Defaults to 'tensor'.
-
- Returns:
- The return type depends on ``mode``.
-
- - If ``mode="tensor"``, return a tensor or a tuple of tensor.
- - If ``mode="predict"``, return a list of :obj:`TextDetDataSample`.
- - If ``mode="loss"``, return a dict of tensor.
- """
- if mode == 'loss':
- return self.loss(inputs, data_samples)
- elif mode == 'predict':
- return self.predict(inputs, data_samples)
- elif mode == 'tensor':
- return self._forward(inputs, data_samples)
- else:
- raise RuntimeError(f'Invalid mode "{mode}". '
- 'Only supports loss, predict and tensor mode')
-
- @abstractmethod
- def loss(self, inputs: Tensor,
- data_samples: DetSampleList) -> Union[dict, tuple]:
- """Calculate losses from a batch of inputs and data samples."""
- pass
-
- @abstractmethod
- def predict(self, inputs: Tensor,
- data_samples: DetSampleList) -> DetSampleList:
- """Predict results from a batch of inputs and data samples with post-
- processing."""
- pass
-
- @abstractmethod
- def _forward(self, inputs: Tensor, data_samples: OptDetSampleList = None):
- """Network forward process.
-
- Usually includes backbone, neck and head forward without any post-
- processing.
- """
- pass
-
- @abstractmethod
- def extract_feat(self, inputs: Tensor):
- """Extract features from images."""
- pass
diff --git a/pipeline/mmocr/mmocr/models/textdet/detectors/dbnet.py b/pipeline/mmocr/mmocr/models/textdet/detectors/dbnet.py
deleted file mode 100644
index eed95b9fabd24ff17ffcba05fb814c0f1cdc9b42..0000000000000000000000000000000000000000
--- a/pipeline/mmocr/mmocr/models/textdet/detectors/dbnet.py
+++ /dev/null
@@ -1,12 +0,0 @@
-# Copyright (c) OpenMMLab. All rights reserved.
-from mmocr.registry import MODELS
-from .single_stage_text_detector import SingleStageTextDetector
-
-
-@MODELS.register_module()
-class DBNet(SingleStageTextDetector):
- """The class for implementing DBNet text detector: Real-time Scene Text
- Detection with Differentiable Binarization.
-
- [https://arxiv.org/abs/1911.08947].
- """
diff --git a/pipeline/mmocr/mmocr/models/textdet/detectors/drrg.py b/pipeline/mmocr/mmocr/models/textdet/detectors/drrg.py
deleted file mode 100644
index 04ea2da5fef75c7b2bbb51a9a7361332534f816c..0000000000000000000000000000000000000000
--- a/pipeline/mmocr/mmocr/models/textdet/detectors/drrg.py
+++ /dev/null
@@ -1,12 +0,0 @@
-# Copyright (c) OpenMMLab. All rights reserved.
-from mmocr.registry import MODELS
-from .single_stage_text_detector import SingleStageTextDetector
-
-
-@MODELS.register_module()
-class DRRG(SingleStageTextDetector):
- """The class for implementing DRRG text detector. Deep Relational Reasoning
- Graph Network for Arbitrary Shape Text Detection.
-
- [https://arxiv.org/abs/2003.07493]
- """
diff --git a/pipeline/mmocr/mmocr/models/textdet/detectors/fcenet.py b/pipeline/mmocr/mmocr/models/textdet/detectors/fcenet.py
deleted file mode 100644
index 9b99f491ff8eedaeb37d64990f0c1dd8dc3c5e89..0000000000000000000000000000000000000000
--- a/pipeline/mmocr/mmocr/models/textdet/detectors/fcenet.py
+++ /dev/null
@@ -1,13 +0,0 @@
-# Copyright (c) OpenMMLab. All rights reserved.
-from mmocr.registry import MODELS
-from .single_stage_text_detector import SingleStageTextDetector
-
-
-@MODELS.register_module()
-class FCENet(SingleStageTextDetector):
- """The class for implementing FCENet text detector
- FCENet(CVPR2021): Fourier Contour Embedding for Arbitrary-shaped Text
- Detection
-
- [https://arxiv.org/abs/2104.10442]
- """
diff --git a/pipeline/mmocr/mmocr/models/textdet/detectors/mmdet_wrapper.py b/pipeline/mmocr/mmocr/models/textdet/detectors/mmdet_wrapper.py
deleted file mode 100644
index 1d6be8caa6469ab2da2e55eb1f645f9129037490..0000000000000000000000000000000000000000
--- a/pipeline/mmocr/mmocr/models/textdet/detectors/mmdet_wrapper.py
+++ /dev/null
@@ -1,156 +0,0 @@
-# Copyright (c) OpenMMLab. All rights reserved.
-from typing import Dict, List, Optional, Tuple, Union
-
-import cv2
-import torch
-from mmdet.structures import DetDataSample
-from mmdet.structures import SampleList as MMDET_SampleList
-from mmdet.structures.mask import bitmap_to_polygon
-from mmengine.model import BaseModel
-from mmengine.structures import InstanceData
-
-from mmocr.registry import MODELS
-from mmocr.utils.bbox_utils import bbox2poly
-from mmocr.utils.typing_utils import DetSampleList
-
-ForwardResults = Union[Dict[str, torch.Tensor], List[DetDataSample],
- Tuple[torch.Tensor], torch.Tensor]
-
-
-@MODELS.register_module()
-class MMDetWrapper(BaseModel):
- """A wrapper of MMDet's model.
-
- Args:
- cfg (dict): The config of the model.
- text_repr_type (str): The boundary encoding type 'poly' or 'quad'.
- Defaults to 'poly'.
- """
-
- def __init__(self, cfg: Dict, text_repr_type: str = 'poly') -> None:
- data_preprocessor = cfg.pop('data_preprocessor')
- data_preprocessor.update(_scope_='mmdet')
- super().__init__(data_preprocessor=data_preprocessor, init_cfg=None)
- cfg['_scope_'] = 'mmdet'
- self.wrapped_model = MODELS.build(cfg)
- self.text_repr_type = text_repr_type
-
- def forward(self,
- inputs: torch.Tensor,
- data_samples: Optional[Union[DetSampleList,
- MMDET_SampleList]] = None,
- mode: str = 'tensor',
- **kwargs) -> ForwardResults:
- """The unified entry for a forward process in both training and test.
-
- The method works in three modes: "tensor", "predict" and "loss":
-
- - "tensor": Forward the whole network and return tensor or tuple of
- tensor without any post-processing, same as a common nn.Module.
- - "predict": Forward and return the predictions, which are fully
- processed to a list of :obj:`DetDataSample`.
- - "loss": Forward and return a dict of losses according to the given
- inputs and data samples.
-
- Note that this method doesn't handle either back propagation or
- parameter update, which are supposed to be done in :meth:`train_step`.
-
- Args:
- inputs (torch.Tensor): The input tensor with shape
- (N, C, ...) in general.
- data_samples (list[:obj:`DetDataSample`] or
- list[:obj:`TextDetDataSample`]): The annotation data of every
- sample. When in "predict" mode, it should be a list of
- :obj:`TextDetDataSample`. Otherwise they are
- :obj:`DetDataSample`s. Defaults to None.
- mode (str): Running mode. Defaults to 'tensor'.
-
- Returns:
- The return type depends on ``mode``.
-
- - If ``mode="tensor"``, return a tensor or a tuple of tensor.
- - If ``mode="predict"``, return a list of :obj:`TextDetDataSample`.
- - If ``mode="loss"``, return a dict of tensor.
- """
- if mode == 'predict':
- ocr_data_samples = data_samples
- data_samples = []
- for i in range(len(ocr_data_samples)):
- data_samples.append(
- DetDataSample(metainfo=ocr_data_samples[i].metainfo))
-
- results = self.wrapped_model.forward(inputs, data_samples, mode,
- **kwargs)
-
- if mode == 'predict':
- results = self.adapt_predictions(results, ocr_data_samples)
-
- return results
-
- def adapt_predictions(self, data: MMDET_SampleList,
- data_samples: DetSampleList) -> DetSampleList:
- """Convert Instance datas from MMDet into MMOCR's format.
-
- Args:
- data: (list[DetDataSample]): Detection results of the
- input images. Each DetDataSample usually contain
- 'pred_instances'. And the ``pred_instances`` usually
- contains following keys.
- - scores (Tensor): Classification scores, has a shape
- (num_instance, )
- - labels (Tensor): Labels of bboxes, has a shape
- (num_instances, ).
- - bboxes (Tensor): Has a shape (num_instances, 4),
- the last dimension 4 arrange as (x1, y1, x2, y2).
- - masks (Tensor, Optional): Has a shape (num_instances, H, W).
- data_samples (list[:obj:`TextDetDataSample`]): The annotation data
- of every samples.
-
- Returns:
- list[TextDetDataSample]: A list of N datasamples containing ground
- truth and prediction results.
- The polygon results are saved in
- ``TextDetDataSample.pred_instances.polygons``
- The confidence scores are saved in
- ``TextDetDataSample.pred_instances.scores``.
- """
- for i, det_data_sample in enumerate(data):
- data_samples[i].pred_instances = InstanceData()
- # convert mask to polygons if mask exists
- if 'masks' in det_data_sample.pred_instances.keys():
- masks = det_data_sample.pred_instances.masks.cpu().numpy()
- polygons = []
- scores = []
- for mask_idx, mask in enumerate(masks):
- contours, _ = bitmap_to_polygon(mask)
- polygons += [contour.reshape(-1) for contour in contours]
- scores += [
- det_data_sample.pred_instances.scores[mask_idx].cpu()
- ] * len(contours)
- # filter invalid polygons
- filterd_polygons = []
- keep_idx = []
- for poly_idx, polygon in enumerate(polygons):
- if len(polygon) < 6:
- continue
- filterd_polygons.append(polygon)
- keep_idx.append(poly_idx)
- # convert by text_repr_type
- if self.text_repr_type == 'quad':
- for j, poly in enumerate(filterd_polygons):
- rect = cv2.minAreaRect(poly)
- vertices = cv2.boxPoints(rect)
- poly = vertices.flatten()
- filterd_polygons[j] = poly
-
- data_samples[i].pred_instances.polygons = filterd_polygons
- data_samples[i].pred_instances.scores = torch.FloatTensor(
- scores)[keep_idx]
- else:
- bboxes = det_data_sample.pred_instances.bboxes.cpu().numpy()
- polygons = [bbox2poly(bbox) for bbox in bboxes]
- data_samples[i].pred_instances.polygons = polygons
- data_samples[i].pred_instances.scores = torch.FloatTensor(
- det_data_sample.pred_instances.scores.cpu())
-
- return data_samples
diff --git a/pipeline/mmocr/mmocr/models/textdet/detectors/panet.py b/pipeline/mmocr/mmocr/models/textdet/detectors/panet.py
deleted file mode 100644
index 135ee1e9af33e8207286d4990bd513dfd441176e..0000000000000000000000000000000000000000
--- a/pipeline/mmocr/mmocr/models/textdet/detectors/panet.py
+++ /dev/null
@@ -1,12 +0,0 @@
-# Copyright (c) OpenMMLab. All rights reserved.
-from mmocr.registry import MODELS
-from .single_stage_text_detector import SingleStageTextDetector
-
-
-@MODELS.register_module()
-class PANet(SingleStageTextDetector):
- """The class for implementing PANet text detector:
-
- Efficient and Accurate Arbitrary-Shaped Text Detection with Pixel
- Aggregation Network [https://arxiv.org/abs/1908.05900].
- """
diff --git a/pipeline/mmocr/mmocr/models/textdet/detectors/psenet.py b/pipeline/mmocr/mmocr/models/textdet/detectors/psenet.py
deleted file mode 100644
index 0ccf10a13a50e04610b6022552139c8c1ebc0a17..0000000000000000000000000000000000000000
--- a/pipeline/mmocr/mmocr/models/textdet/detectors/psenet.py
+++ /dev/null
@@ -1,12 +0,0 @@
-# Copyright (c) OpenMMLab. All rights reserved.
-from mmocr.registry import MODELS
-from .single_stage_text_detector import SingleStageTextDetector
-
-
-@MODELS.register_module()
-class PSENet(SingleStageTextDetector):
- """The class for implementing PSENet text detector: Shape Robust Text
- Detection with Progressive Scale Expansion Network.
-
- [https://arxiv.org/abs/1806.02559].
- """
diff --git a/pipeline/mmocr/mmocr/models/textdet/detectors/single_stage_text_detector.py b/pipeline/mmocr/mmocr/models/textdet/detectors/single_stage_text_detector.py
deleted file mode 100644
index 5617e26ae0507da3ee4a23475325c4ea11f94ffd..0000000000000000000000000000000000000000
--- a/pipeline/mmocr/mmocr/models/textdet/detectors/single_stage_text_detector.py
+++ /dev/null
@@ -1,127 +0,0 @@
-# Copyright (c) OpenMMLab. All rights reserved.
-from typing import Dict, Optional, Sequence
-
-import torch
-
-from mmocr.registry import MODELS
-from mmocr.structures import TextDetDataSample
-from .base import BaseTextDetector
-
-
-@MODELS.register_module()
-class SingleStageTextDetector(BaseTextDetector):
- """The class for implementing single stage text detector.
-
- Single-stage text detectors directly and densely predict bounding boxes or
- polygons on the output features of the backbone + neck (optional).
-
- Args:
- backbone (dict): Backbone config.
- neck (dict, optional): Neck config. If None, the output from backbone
- will be directly fed into ``det_head``.
- det_head (dict): Head config.
- data_preprocessor (dict, optional): Model preprocessing config
- for processing the input image data. Keys allowed are
- ``to_rgb``(bool), ``pad_size_divisor``(int), ``pad_value``(int or
- float), ``mean``(int or float) and ``std``(int or float).
- Preprcessing order: 1. to rgb; 2. normalization 3. pad.
- Defaults to None.
- init_cfg (dict or list[dict], optional): Initialization configs.
- Defaults to None.
- """
-
- def __init__(self,
- backbone: Dict,
- det_head: Dict,
- neck: Optional[Dict] = None,
- data_preprocessor: Optional[Dict] = None,
- init_cfg: Optional[Dict] = None) -> None:
- super().__init__(
- data_preprocessor=data_preprocessor, init_cfg=init_cfg)
- assert det_head is not None, 'det_head cannot be None!'
- self.backbone = MODELS.build(backbone)
- if neck is not None:
- self.neck = MODELS.build(neck)
- self.det_head = MODELS.build(det_head)
-
- def extract_feat(self, inputs: torch.Tensor) -> torch.Tensor:
- """Extract features.
-
- Args:
- inputs (Tensor): Image tensor with shape (N, C, H ,W).
-
- Returns:
- Tensor or tuple[Tensor]: Multi-level features that may have
- different resolutions.
- """
- inputs = self.backbone(inputs)
- if self.with_neck:
- inputs = self.neck(inputs)
- return inputs
-
- def loss(self, inputs: torch.Tensor,
- data_samples: Sequence[TextDetDataSample]) -> Dict:
- """Calculate losses from a batch of inputs and data samples.
-
- Args:
- inputs (torch.Tensor): Input images of shape (N, C, H, W).
- Typically these should be mean centered and std scaled.
- data_samples (list[TextDetDataSample]): A list of N
- datasamples, containing meta information and gold annotations
- for each of the images.
- Returns:
- dict[str, Tensor]: A dictionary of loss components.
- """
- inputs = self.extract_feat(inputs)
- return self.det_head.loss(inputs, data_samples)
-
- def predict(self, inputs: torch.Tensor,
- data_samples: Sequence[TextDetDataSample]
- ) -> Sequence[TextDetDataSample]:
- """Predict results from a batch of inputs and data samples with post-
- processing.
-
- Args:
- inputs (torch.Tensor): Images of shape (N, C, H, W).
- data_samples (list[TextDetDataSample]): A list of N
- datasamples, containing meta information and gold annotations
- for each of the images.
-
- Returns:
- list[TextDetDataSample]: A list of N datasamples of prediction
- results. Each DetDataSample usually contain
- 'pred_instances'. And the ``pred_instances`` usually
- contains following keys.
-
- - scores (Tensor): Classification scores, has a shape
- (num_instance, )
- - labels (Tensor): Labels of bboxes, has a shape
- (num_instances, ).
- - bboxes (Tensor): Has a shape (num_instances, 4),
- the last dimension 4 arrange as (x1, y1, x2, y2).
- - polygons (list[np.ndarray]): The length is num_instances.
- Each element represents the polygon of the
- instance, in (xn, yn) order.
- """
- x = self.extract_feat(inputs)
- return self.det_head.predict(x, data_samples)
-
- def _forward(self,
- inputs: torch.Tensor,
- data_samples: Optional[Sequence[TextDetDataSample]] = None,
- **kwargs) -> torch.Tensor:
- """Network forward process. Usually includes backbone, neck and head
- forward without any post-processing.
-
- Args:
- inputs (Tensor): Inputs with shape (N, C, H, W).
- data_samples (list[TextDetDataSample]): A list of N
- datasamples, containing meta information and gold annotations
- for each of the images.
-
- Returns:
- Tensor or tuple[Tensor]: A tuple of features from ``det_head``
- forward.
- """
- x = self.extract_feat(inputs)
- return self.det_head(x, data_samples)
diff --git a/pipeline/mmocr/mmocr/models/textdet/detectors/textsnake.py b/pipeline/mmocr/mmocr/models/textdet/detectors/textsnake.py
deleted file mode 100644
index 6a001806cb9fe7d3003cfb8c728b5d72254d6726..0000000000000000000000000000000000000000
--- a/pipeline/mmocr/mmocr/models/textdet/detectors/textsnake.py
+++ /dev/null
@@ -1,12 +0,0 @@
-# Copyright (c) OpenMMLab. All rights reserved.
-from mmocr.registry import MODELS
-from .single_stage_text_detector import SingleStageTextDetector
-
-
-@MODELS.register_module()
-class TextSnake(SingleStageTextDetector):
- """The class for implementing TextSnake text detector: TextSnake: A
- Flexible Representation for Detecting Text of Arbitrary Shapes.
-
- [https://arxiv.org/abs/1807.01544]
- """
diff --git a/pipeline/mmocr/mmocr/models/textdet/heads/__init__.py b/pipeline/mmocr/mmocr/models/textdet/heads/__init__.py
deleted file mode 100644
index 5663ebebb88ab2ef0cf41e8beee86f0253288972..0000000000000000000000000000000000000000
--- a/pipeline/mmocr/mmocr/models/textdet/heads/__init__.py
+++ /dev/null
@@ -1,13 +0,0 @@
-# Copyright (c) OpenMMLab. All rights reserved.
-from .base import BaseTextDetHead
-from .db_head import DBHead
-from .drrg_head import DRRGHead
-from .fce_head import FCEHead
-from .pan_head import PANHead
-from .pse_head import PSEHead
-from .textsnake_head import TextSnakeHead
-
-__all__ = [
- 'PSEHead', 'PANHead', 'DBHead', 'FCEHead', 'TextSnakeHead', 'DRRGHead',
- 'BaseTextDetHead'
-]
diff --git a/pipeline/mmocr/mmocr/models/textdet/heads/__pycache__/__init__.cpython-38.pyc b/pipeline/mmocr/mmocr/models/textdet/heads/__pycache__/__init__.cpython-38.pyc
deleted file mode 100644
index 417ab215e738186086a178bd86cbf079af9ee12b..0000000000000000000000000000000000000000
Binary files a/pipeline/mmocr/mmocr/models/textdet/heads/__pycache__/__init__.cpython-38.pyc and /dev/null differ
diff --git a/pipeline/mmocr/mmocr/models/textdet/heads/__pycache__/__init__.cpython-39.pyc b/pipeline/mmocr/mmocr/models/textdet/heads/__pycache__/__init__.cpython-39.pyc
deleted file mode 100644
index cd3c056e43c60894d267e1d6d3723c3f47a39acb..0000000000000000000000000000000000000000
Binary files a/pipeline/mmocr/mmocr/models/textdet/heads/__pycache__/__init__.cpython-39.pyc and /dev/null differ
diff --git a/pipeline/mmocr/mmocr/models/textdet/heads/__pycache__/base.cpython-38.pyc b/pipeline/mmocr/mmocr/models/textdet/heads/__pycache__/base.cpython-38.pyc
deleted file mode 100644
index a088205494e74d20fa973c464c1db9e513b94715..0000000000000000000000000000000000000000
Binary files a/pipeline/mmocr/mmocr/models/textdet/heads/__pycache__/base.cpython-38.pyc and /dev/null differ
diff --git a/pipeline/mmocr/mmocr/models/textdet/heads/__pycache__/base.cpython-39.pyc b/pipeline/mmocr/mmocr/models/textdet/heads/__pycache__/base.cpython-39.pyc
deleted file mode 100644
index 5bbca46a25d920300db5e2ba150e91325df654f9..0000000000000000000000000000000000000000
Binary files a/pipeline/mmocr/mmocr/models/textdet/heads/__pycache__/base.cpython-39.pyc and /dev/null differ
diff --git a/pipeline/mmocr/mmocr/models/textdet/heads/__pycache__/db_head.cpython-38.pyc b/pipeline/mmocr/mmocr/models/textdet/heads/__pycache__/db_head.cpython-38.pyc
deleted file mode 100644
index 851ccdb43857be7f5d9389c7ba38df20e1f48304..0000000000000000000000000000000000000000
Binary files a/pipeline/mmocr/mmocr/models/textdet/heads/__pycache__/db_head.cpython-38.pyc and /dev/null differ
diff --git a/pipeline/mmocr/mmocr/models/textdet/heads/__pycache__/db_head.cpython-39.pyc b/pipeline/mmocr/mmocr/models/textdet/heads/__pycache__/db_head.cpython-39.pyc
deleted file mode 100644
index a1ccea3ad692fb2a5f6c08d5275a3839bef5d172..0000000000000000000000000000000000000000
Binary files a/pipeline/mmocr/mmocr/models/textdet/heads/__pycache__/db_head.cpython-39.pyc and /dev/null differ
diff --git a/pipeline/mmocr/mmocr/models/textdet/heads/__pycache__/drrg_head.cpython-38.pyc b/pipeline/mmocr/mmocr/models/textdet/heads/__pycache__/drrg_head.cpython-38.pyc
deleted file mode 100644
index 61212b4c1c5819c3552f72332ed41b099ec7d252..0000000000000000000000000000000000000000
Binary files a/pipeline/mmocr/mmocr/models/textdet/heads/__pycache__/drrg_head.cpython-38.pyc and /dev/null differ
diff --git a/pipeline/mmocr/mmocr/models/textdet/heads/__pycache__/drrg_head.cpython-39.pyc b/pipeline/mmocr/mmocr/models/textdet/heads/__pycache__/drrg_head.cpython-39.pyc
deleted file mode 100644
index 83768e36bf32e18116372b0f858e430ca334bbee..0000000000000000000000000000000000000000
Binary files a/pipeline/mmocr/mmocr/models/textdet/heads/__pycache__/drrg_head.cpython-39.pyc and /dev/null differ
diff --git a/pipeline/mmocr/mmocr/models/textdet/heads/__pycache__/fce_head.cpython-38.pyc b/pipeline/mmocr/mmocr/models/textdet/heads/__pycache__/fce_head.cpython-38.pyc
deleted file mode 100644
index 90f33b3d6aee5f870385c125a4d1457404a4032d..0000000000000000000000000000000000000000
Binary files a/pipeline/mmocr/mmocr/models/textdet/heads/__pycache__/fce_head.cpython-38.pyc and /dev/null differ
diff --git a/pipeline/mmocr/mmocr/models/textdet/heads/__pycache__/fce_head.cpython-39.pyc b/pipeline/mmocr/mmocr/models/textdet/heads/__pycache__/fce_head.cpython-39.pyc
deleted file mode 100644
index aafd9466062e42c564f99073a4f7572fc31b99ab..0000000000000000000000000000000000000000
Binary files a/pipeline/mmocr/mmocr/models/textdet/heads/__pycache__/fce_head.cpython-39.pyc and /dev/null differ
diff --git a/pipeline/mmocr/mmocr/models/textdet/heads/__pycache__/pan_head.cpython-38.pyc b/pipeline/mmocr/mmocr/models/textdet/heads/__pycache__/pan_head.cpython-38.pyc
deleted file mode 100644
index a2b9edb2564a6a6d065c89a1a127ff4ba40a0fc1..0000000000000000000000000000000000000000
Binary files a/pipeline/mmocr/mmocr/models/textdet/heads/__pycache__/pan_head.cpython-38.pyc and /dev/null differ
diff --git a/pipeline/mmocr/mmocr/models/textdet/heads/__pycache__/pan_head.cpython-39.pyc b/pipeline/mmocr/mmocr/models/textdet/heads/__pycache__/pan_head.cpython-39.pyc
deleted file mode 100644
index 5076028f801644f9747ed1a98dc774e84b11fd3e..0000000000000000000000000000000000000000
Binary files a/pipeline/mmocr/mmocr/models/textdet/heads/__pycache__/pan_head.cpython-39.pyc and /dev/null differ
diff --git a/pipeline/mmocr/mmocr/models/textdet/heads/__pycache__/pse_head.cpython-38.pyc b/pipeline/mmocr/mmocr/models/textdet/heads/__pycache__/pse_head.cpython-38.pyc
deleted file mode 100644
index b1fe7c2f9e6ae971a2272dd5cda3f45769e0cb8a..0000000000000000000000000000000000000000
Binary files a/pipeline/mmocr/mmocr/models/textdet/heads/__pycache__/pse_head.cpython-38.pyc and /dev/null differ
diff --git a/pipeline/mmocr/mmocr/models/textdet/heads/__pycache__/pse_head.cpython-39.pyc b/pipeline/mmocr/mmocr/models/textdet/heads/__pycache__/pse_head.cpython-39.pyc
deleted file mode 100644
index d07947567db52601f62c4060264149dbc09f0814..0000000000000000000000000000000000000000
Binary files a/pipeline/mmocr/mmocr/models/textdet/heads/__pycache__/pse_head.cpython-39.pyc and /dev/null differ
diff --git a/pipeline/mmocr/mmocr/models/textdet/heads/__pycache__/textsnake_head.cpython-38.pyc b/pipeline/mmocr/mmocr/models/textdet/heads/__pycache__/textsnake_head.cpython-38.pyc
deleted file mode 100644
index 23112a29652d2e74f545e581fa011716bc6d6ea4..0000000000000000000000000000000000000000
Binary files a/pipeline/mmocr/mmocr/models/textdet/heads/__pycache__/textsnake_head.cpython-38.pyc and /dev/null differ
diff --git a/pipeline/mmocr/mmocr/models/textdet/heads/__pycache__/textsnake_head.cpython-39.pyc b/pipeline/mmocr/mmocr/models/textdet/heads/__pycache__/textsnake_head.cpython-39.pyc
deleted file mode 100644
index e6d8a2d50a644e80ba709f156fcb32595c84f53c..0000000000000000000000000000000000000000
Binary files a/pipeline/mmocr/mmocr/models/textdet/heads/__pycache__/textsnake_head.cpython-39.pyc and /dev/null differ
diff --git a/pipeline/mmocr/mmocr/models/textdet/heads/base.py b/pipeline/mmocr/mmocr/models/textdet/heads/base.py
deleted file mode 100644
index 82dee4dfc23702e5948d2ebf2e8ee8ae12560397..0000000000000000000000000000000000000000
--- a/pipeline/mmocr/mmocr/models/textdet/heads/base.py
+++ /dev/null
@@ -1,133 +0,0 @@
-# Copyright (c) OpenMMLab. All rights reserved.
-from typing import Dict, List, Optional, Tuple, Union
-
-import torch
-from mmengine.model import BaseModule
-from torch import Tensor
-
-from mmocr.registry import MODELS
-from mmocr.utils.typing_utils import DetSampleList
-
-
-@MODELS.register_module()
-class BaseTextDetHead(BaseModule):
- """Base head for text detection, build the loss and postprocessor.
-
- 1. The ``init_weights`` method is used to initialize head's
- model parameters. After detector initialization, ``init_weights``
- is triggered when ``detector.init_weights()`` is called externally.
-
- 2. The ``loss`` method is used to calculate the loss of head,
- which includes two steps: (1) the head model performs forward
- propagation to obtain the feature maps (2) The ``module_loss`` method
- is called based on the feature maps to calculate the loss.
-
- .. code:: text
-
- loss(): forward() -> module_loss()
-
- 3. The ``predict`` method is used to predict detection results,
- which includes two steps: (1) the head model performs forward
- propagation to obtain the feature maps (2) The ``postprocessor`` method
- is called based on the feature maps to predict detection results including
- post-processing.
-
- .. code:: text
-
- predict(): forward() -> postprocessor()
-
- 4. The ``loss_and_predict`` method is used to return loss and detection
- results at the same time. It will call head's ``forward``,
- ``module_loss`` and ``postprocessor`` methods in order.
-
- .. code:: text
-
- loss_and_predict(): forward() -> module_loss() -> postprocessor()
-
-
- Args:
- loss (dict, optional): Config to build loss. Defaults to None.
- postprocessor (dict, optional): Config to build postprocessor. Defaults
- to None.
- init_cfg (dict or list[dict], optional): Initialization configs.
- Defaults to None.
- """
-
- def __init__(self,
- module_loss: Optional[Dict] = None,
- postprocessor: Optional[Dict] = None,
- init_cfg: Optional[Union[Dict, List[Dict]]] = None) -> None:
- super().__init__(init_cfg=init_cfg)
- if module_loss is not None:
- assert isinstance(module_loss, dict)
- self.module_loss = MODELS.build(module_loss)
- else:
- self.module_loss = module_loss
- if postprocessor is not None:
- assert isinstance(postprocessor, dict)
- self.postprocessor = MODELS.build(postprocessor)
- else:
- self.postprocessor = postprocessor
-
- def loss(self, x: Tuple[Tensor], data_samples: DetSampleList) -> dict:
- """Perform forward propagation and loss calculation of the detection
- head on the features of the upstream network.
-
- Args:
- x (tuple[Tensor]): Features from the upstream network, each is
- a 4D-tensor.
- data_samples (List[:obj:`DetDataSample`]): The Data
- Samples. It usually includes information such as
- `gt_instance`, `gt_panoptic_seg` and `gt_sem_seg`.
-
- Returns:
- dict: A dictionary of loss components.
- """
- outs = self(x, data_samples)
- losses = self.module_loss(outs, data_samples)
- return losses
-
- def loss_and_predict(self, x: Tuple[Tensor], data_samples: DetSampleList
- ) -> Tuple[dict, DetSampleList]:
- """Perform forward propagation of the head, then calculate loss and
- predictions from the features and data samples.
-
- Args:
- x (tuple[Tensor]): Features from FPN.
- data_samples (list[:obj:`DetDataSample`]): Each item contains
- the meta information of each image and corresponding
- annotations.
-
- Returns:
- tuple: the return value is a tuple contains:
-
- - losses: (dict[str, Tensor]): A dictionary of loss components.
- - predictions (list[:obj:`InstanceData`]): Detection
- results of each image after the post process.
- """
- outs = self(x, data_samples)
- losses = self.module_loss(outs, data_samples)
-
- predictions = self.postprocessor(outs, data_samples, self.training)
- return losses, predictions
-
- def predict(self, x: torch.Tensor,
- data_samples: DetSampleList) -> DetSampleList:
- """Perform forward propagation of the detection head and predict
- detection results on the features of the upstream network.
-
- Args:
- x (tuple[Tensor]): Multi-level features from the
- upstream network, each is a 4D-tensor.
- data_samples (List[:obj:`DetDataSample`]): The Data
- Samples. It usually includes information such as
- `gt_instance`, `gt_panoptic_seg` and `gt_sem_seg`.
-
- Returns:
- SampleList: Detection results of each image
- after the post process.
- """
- outs = self(x, data_samples)
-
- predictions = self.postprocessor(outs, data_samples)
- return predictions
diff --git a/pipeline/mmocr/mmocr/models/textdet/heads/db_head.py b/pipeline/mmocr/mmocr/models/textdet/heads/db_head.py
deleted file mode 100644
index 848843e87fb2d99d44a915f8929893d218fa7d1f..0000000000000000000000000000000000000000
--- a/pipeline/mmocr/mmocr/models/textdet/heads/db_head.py
+++ /dev/null
@@ -1,184 +0,0 @@
-# Copyright (c) OpenMMLab. All rights reserved.
-from typing import Dict, List, Optional, Tuple, Union
-
-import torch
-import torch.nn as nn
-from mmengine.model import Sequential
-from torch import Tensor
-
-from mmocr.models.textdet.heads import BaseTextDetHead
-from mmocr.registry import MODELS
-from mmocr.structures import TextDetDataSample
-from mmocr.utils.typing_utils import DetSampleList
-
-
-@MODELS.register_module()
-class DBHead(BaseTextDetHead):
- """The class for DBNet head.
-
- This was partially adapted from https://github.com/MhLiao/DB
-
- Args:
- in_channels (int): The number of input channels.
- with_bias (bool): Whether add bias in Conv2d layer. Defaults to False.
- module_loss (dict): Config of loss for dbnet. Defaults to
- ``dict(type='DBModuleLoss')``
- postprocessor (dict): Config of postprocessor for dbnet.
- init_cfg (dict or list[dict], optional): Initialization configs.
- """
-
- def __init__(
- self,
- in_channels: int,
- with_bias: bool = False,
- module_loss: Dict = dict(type='DBModuleLoss'),
- postprocessor: Dict = dict(
- type='DBPostprocessor', text_repr_type='quad'),
- init_cfg: Optional[Union[Dict, List[Dict]]] = [
- dict(type='Kaiming', layer='Conv'),
- dict(type='Constant', layer='BatchNorm', val=1., bias=1e-4)
- ]
- ) -> None:
- super().__init__(
- module_loss=module_loss,
- postprocessor=postprocessor,
- init_cfg=init_cfg)
-
- assert isinstance(in_channels, int)
- assert isinstance(with_bias, bool)
-
- self.in_channels = in_channels
- self.binarize = Sequential(
- nn.Conv2d(
- in_channels, in_channels // 4, 3, bias=with_bias, padding=1),
- nn.BatchNorm2d(in_channels // 4), nn.ReLU(inplace=True),
- nn.ConvTranspose2d(in_channels // 4, in_channels // 4, 2, 2),
- nn.BatchNorm2d(in_channels // 4), nn.ReLU(inplace=True),
- nn.ConvTranspose2d(in_channels // 4, 1, 2, 2))
- self.threshold = self._init_thr(in_channels)
- self.sigmoid = nn.Sigmoid()
-
- def _diff_binarize(self, prob_map: Tensor, thr_map: Tensor,
- k: int) -> Tensor:
- """Differential binarization.
-
- Args:
- prob_map (Tensor): Probability map.
- thr_map (Tensor): Threshold map.
- k (int): Amplification factor.
-
- Returns:
- Tensor: Binary map.
- """
- return torch.reciprocal(1.0 + torch.exp(-k * (prob_map - thr_map)))
-
- def _init_thr(self,
- inner_channels: int,
- bias: bool = False) -> nn.ModuleList:
- """Initialize threshold branch."""
- in_channels = inner_channels
- seq = Sequential(
- nn.Conv2d(
- in_channels, inner_channels // 4, 3, padding=1, bias=bias),
- nn.BatchNorm2d(inner_channels // 4), nn.ReLU(inplace=True),
- nn.ConvTranspose2d(inner_channels // 4, inner_channels // 4, 2, 2),
- nn.BatchNorm2d(inner_channels // 4), nn.ReLU(inplace=True),
- nn.ConvTranspose2d(inner_channels // 4, 1, 2, 2), nn.Sigmoid())
- return seq
-
- def forward(self,
- img: Tensor,
- data_samples: Optional[List[TextDetDataSample]] = None,
- mode: str = 'predict') -> Tuple[Tensor, Tensor, Tensor]:
- """
- Args:
- img (Tensor): Shape :math:`(N, C, H, W)`.
- data_samples (list[TextDetDataSample], optional): A list of data
- samples. Defaults to None.
- mode (str): Forward mode. It affects the return values. Options are
- "loss", "predict" and "both". Defaults to "predict".
-
- - ``loss``: Run the full network and return the prob
- logits, threshold map and binary map.
- - ``predict``: Run the binarzation part and return the prob
- map only.
- - ``both``: Run the full network and return prob logits,
- threshold map, binary map and prob map.
-
- Returns:
- Tensor or tuple(Tensor): Its type depends on ``mode``, read its
- docstring for details. Each has the shape of
- :math:`(N, 4H, 4W)`.
- """
- prob_logits = self.binarize(img).squeeze(1)
- prob_map = self.sigmoid(prob_logits)
- if mode == 'predict':
- return prob_map
- thr_map = self.threshold(img).squeeze(1)
- binary_map = self._diff_binarize(prob_map, thr_map, k=50).squeeze(1)
- if mode == 'loss':
- return prob_logits, thr_map, binary_map
- return prob_logits, thr_map, binary_map, prob_map
-
- def loss(self, x: Tuple[Tensor],
- batch_data_samples: DetSampleList) -> Dict:
- """Perform forward propagation and loss calculation of the detection
- head on the features of the upstream network.
-
- Args:
- x (tuple[Tensor]): Features from the upstream network, each is
- a 4D-tensor.
- batch_data_samples (List[:obj:`DetDataSample`]): The Data
- Samples. It usually includes information such as
- `gt_instance`, `gt_panoptic_seg` and `gt_sem_seg`.
-
- Returns:
- dict: A dictionary of loss components.
- """
- outs = self(x, batch_data_samples, mode='loss')
- losses = self.module_loss(outs, batch_data_samples)
- return losses
-
- def loss_and_predict(self, x: Tuple[Tensor],
- batch_data_samples: DetSampleList
- ) -> Tuple[dict, DetSampleList]:
- """Perform forward propagation of the head, then calculate loss and
- predictions from the features and data samples.
-
- Args:
- x (tuple[Tensor]): Features from FPN.
- batch_data_samples (list[:obj:`DetDataSample`]): Each item contains
- the meta information of each image and corresponding
- annotations.
-
- Returns:
- tuple: the return value is a tuple contains:
-
- - losses: (dict[str, Tensor]): A dictionary of loss components.
- - predictions (list[:obj:`InstanceData`]): Detection
- results of each image after the post process.
- """
- outs = self(x, batch_data_samples, mode='both')
- losses = self.module_loss(outs[:3], batch_data_samples)
- predictions = self.postprocessor(outs[3], batch_data_samples)
- return losses, predictions
-
- def predict(self, x: torch.Tensor,
- batch_data_samples: DetSampleList) -> DetSampleList:
- """Perform forward propagation of the detection head and predict
- detection results on the features of the upstream network.
-
- Args:
- x (tuple[Tensor]): Multi-level features from the
- upstream network, each is a 4D-tensor.
- batch_data_samples (List[:obj:`DetDataSample`]): The Data
- Samples. It usually includes information such as
- `gt_instance`, `gt_panoptic_seg` and `gt_sem_seg`.
-
- Returns:
- SampleList: Detection results of each image
- after the post process.
- """
- outs = self(x, batch_data_samples, mode='predict')
- predictions = self.postprocessor(outs, batch_data_samples)
- return predictions
diff --git a/pipeline/mmocr/mmocr/models/textdet/heads/drrg_head.py b/pipeline/mmocr/mmocr/models/textdet/heads/drrg_head.py
deleted file mode 100644
index 14f70858a7a80e6fa1f2ee2964b40ad3b6d2a935..0000000000000000000000000000000000000000
--- a/pipeline/mmocr/mmocr/models/textdet/heads/drrg_head.py
+++ /dev/null
@@ -1,1181 +0,0 @@
-# Copyright (c) OpenMMLab. All rights reserved.
-from typing import Dict, List, Optional, Sequence, Tuple, Union
-
-import cv2
-import numpy as np
-import torch
-import torch.nn as nn
-import torch.nn.functional as F
-
-try:
- from lanms import merge_quadrangle_n9 as la_nms
-except ImportError:
- la_nms = None
-from mmcv.ops import RoIAlignRotated
-from mmengine.model import BaseModule
-from numpy import ndarray
-from torch import Tensor
-from torch.nn import init
-
-from mmocr.models.textdet.heads import BaseTextDetHead
-from mmocr.registry import MODELS
-from mmocr.structures import TextDetDataSample
-from mmocr.utils import fill_hole
-
-
-def normalize_adjacent_matrix(mat: ndarray) -> ndarray:
- """Normalize adjacent matrix for GCN. This code was partially adapted from
- https://github.com/GXYM/DRRG licensed under the MIT license.
-
- Args:
- mat (ndarray): The adjacent matrix.
-
- returns:
- ndarray: The normalized adjacent matrix.
- """
- assert mat.ndim == 2
- assert mat.shape[0] == mat.shape[1]
-
- mat = mat + np.eye(mat.shape[0])
- d = np.sum(mat, axis=0)
- d = np.clip(d, 0, None)
- d_inv = np.power(d, -0.5).flatten()
- d_inv[np.isinf(d_inv)] = 0.0
- d_inv = np.diag(d_inv)
- norm_mat = mat.dot(d_inv).transpose().dot(d_inv)
- return norm_mat
-
-
-def euclidean_distance_matrix(mat_a: ndarray, mat_b: ndarray) -> ndarray:
- """Calculate the Euclidean distance matrix.
-
- Args:
- mat_a (ndarray): The point sequence.
- mat_b (ndarray): The point sequence with the same dimensions as mat_a.
-
- returns:
- ndarray: The Euclidean distance matrix.
- """
- assert mat_a.ndim == 2
- assert mat_b.ndim == 2
- assert mat_a.shape[1] == mat_b.shape[1]
-
- m = mat_a.shape[0]
- n = mat_b.shape[0]
-
- mat_a_dots = (mat_a * mat_a).sum(axis=1).reshape(
- (m, 1)) * np.ones(shape=(1, n))
- mat_b_dots = (mat_b * mat_b).sum(axis=1) * np.ones(shape=(m, 1))
- mat_d_squared = mat_a_dots + mat_b_dots - 2 * mat_a.dot(mat_b.T)
-
- zero_mask = np.less(mat_d_squared, 0.0)
- mat_d_squared[zero_mask] = 0.0
- mat_d = np.sqrt(mat_d_squared)
- return mat_d
-
-
-def feature_embedding(input_feats: ndarray, out_feat_len: int) -> ndarray:
- """Embed features. This code was partially adapted from
- https://github.com/GXYM/DRRG licensed under the MIT license.
-
- Args:
- input_feats (ndarray): The input features of shape (N, d), where N is
- the number of nodes in graph, d is the input feature vector length.
- out_feat_len (int): The length of output feature vector.
-
- Returns:
- ndarray: The embedded features.
- """
- assert input_feats.ndim == 2
- assert isinstance(out_feat_len, int)
- assert out_feat_len >= input_feats.shape[1]
-
- num_nodes = input_feats.shape[0]
- feat_dim = input_feats.shape[1]
- feat_repeat_times = out_feat_len // feat_dim
- residue_dim = out_feat_len % feat_dim
-
- if residue_dim > 0:
- embed_wave = np.array([
- np.power(1000, 2.0 * (j // 2) / feat_repeat_times + 1)
- for j in range(feat_repeat_times + 1)
- ]).reshape((feat_repeat_times + 1, 1, 1))
- repeat_feats = np.repeat(
- np.expand_dims(input_feats, axis=0), feat_repeat_times, axis=0)
- residue_feats = np.hstack([
- input_feats[:, 0:residue_dim],
- np.zeros((num_nodes, feat_dim - residue_dim))
- ])
- residue_feats = np.expand_dims(residue_feats, axis=0)
- repeat_feats = np.concatenate([repeat_feats, residue_feats], axis=0)
- embedded_feats = repeat_feats / embed_wave
- embedded_feats[:, 0::2] = np.sin(embedded_feats[:, 0::2])
- embedded_feats[:, 1::2] = np.cos(embedded_feats[:, 1::2])
- embedded_feats = np.transpose(embedded_feats, (1, 0, 2)).reshape(
- (num_nodes, -1))[:, 0:out_feat_len]
- else:
- embed_wave = np.array([
- np.power(1000, 2.0 * (j // 2) / feat_repeat_times)
- for j in range(feat_repeat_times)
- ]).reshape((feat_repeat_times, 1, 1))
- repeat_feats = np.repeat(
- np.expand_dims(input_feats, axis=0), feat_repeat_times, axis=0)
- embedded_feats = repeat_feats / embed_wave
- embedded_feats[:, 0::2] = np.sin(embedded_feats[:, 0::2])
- embedded_feats[:, 1::2] = np.cos(embedded_feats[:, 1::2])
- embedded_feats = np.transpose(embedded_feats, (1, 0, 2)).reshape(
- (num_nodes, -1)).astype(np.float32)
-
- return embedded_feats
-
-
-@MODELS.register_module()
-class DRRGHead(BaseTextDetHead):
- """The class for DRRG head: `Deep Relational Reasoning Graph Network for
- Arbitrary Shape Text Detection `_.
-
- Args:
- in_channels (int): The number of input channels.
- k_at_hops (tuple(int)): The number of i-hop neighbors, i = 1, 2.
- Defaults to (8, 4).
- num_adjacent_linkages (int): The number of linkages when constructing
- adjacent matrix. Defaults to 3.
- node_geo_feat_len (int): The length of embedded geometric feature
- vector of a component. Defaults to 120.
- pooling_scale (float): The spatial scale of rotated RoI-Align. Defaults
- to 1.0.
- pooling_output_size (tuple(int)): The output size of RRoI-Aligning.
- Defaults to (4, 3).
- nms_thr (float): The locality-aware NMS threshold of text components.
- Defaults to 0.3.
- min_width (float): The minimum width of text components. Defaults to
- 8.0.
- max_width (float): The maximum width of text components. Defaults to
- 24.0.
- comp_shrink_ratio (float): The shrink ratio of text components.
- Defaults to 1.03.
- comp_ratio (float): The reciprocal of aspect ratio of text components.
- Defaults to 0.4.
- comp_score_thr (float): The score threshold of text components.
- Defaults to 0.3.
- text_region_thr (float): The threshold for text region probability map.
- Defaults to 0.2.
- center_region_thr (float): The threshold for text center region
- probability map. Defaults to 0.2.
- center_region_area_thr (int): The threshold for filtering small-sized
- text center region. Defaults to 50.
- local_graph_thr (float): The threshold to filter identical local
- graphs. Defaults to 0.7.
- module_loss (dict): The config of loss that DRRGHead uses. Defaults to
- ``dict(type='DRRGModuleLoss')``.
- postprocessor (dict): Config of postprocessor for Drrg. Defaults to
- ``dict(type='DrrgPostProcessor', link_thr=0.85)``.
- init_cfg (dict or list[dict], optional): Initialization configs.
- Defaults to ``dict(type='Normal',
- override=dict(name='out_conv'), mean=0, std=0.01)``.
- """
-
- def __init__(
- self,
- in_channels: int,
- k_at_hops: Tuple[int, int] = (8, 4),
- num_adjacent_linkages: int = 3,
- node_geo_feat_len: int = 120,
- pooling_scale: float = 1.0,
- pooling_output_size: Tuple[int, int] = (4, 3),
- nms_thr: float = 0.3,
- min_width: float = 8.0,
- max_width: float = 24.0,
- comp_shrink_ratio: float = 1.03,
- comp_ratio: float = 0.4,
- comp_score_thr: float = 0.3,
- text_region_thr: float = 0.2,
- center_region_thr: float = 0.2,
- center_region_area_thr: int = 50,
- local_graph_thr: float = 0.7,
- module_loss: Dict = dict(type='DRRGModuleLoss'),
- postprocessor: Dict = dict(type='DRRGPostprocessor', link_thr=0.85),
- init_cfg: Optional[Union[Dict, List[Dict]]] = dict(
- type='Normal', override=dict(name='out_conv'), mean=0, std=0.01)
- ) -> None:
- super().__init__(
- module_loss=module_loss,
- postprocessor=postprocessor,
- init_cfg=init_cfg)
-
- assert isinstance(in_channels, int)
- assert isinstance(k_at_hops, tuple)
- assert isinstance(num_adjacent_linkages, int)
- assert isinstance(node_geo_feat_len, int)
- assert isinstance(pooling_scale, float)
- assert isinstance(pooling_output_size, tuple)
- assert isinstance(comp_shrink_ratio, float)
- assert isinstance(nms_thr, float)
- assert isinstance(min_width, float)
- assert isinstance(max_width, float)
- assert isinstance(comp_ratio, float)
- assert isinstance(comp_score_thr, float)
- assert isinstance(text_region_thr, float)
- assert isinstance(center_region_thr, float)
- assert isinstance(center_region_area_thr, int)
- assert isinstance(local_graph_thr, float)
-
- self.in_channels = in_channels
- self.out_channels = 6
- self.downsample_ratio = 1.0
- self.k_at_hops = k_at_hops
- self.num_adjacent_linkages = num_adjacent_linkages
- self.node_geo_feat_len = node_geo_feat_len
- self.pooling_scale = pooling_scale
- self.pooling_output_size = pooling_output_size
- self.comp_shrink_ratio = comp_shrink_ratio
- self.nms_thr = nms_thr
- self.min_width = min_width
- self.max_width = max_width
- self.comp_ratio = comp_ratio
- self.comp_score_thr = comp_score_thr
- self.text_region_thr = text_region_thr
- self.center_region_thr = center_region_thr
- self.center_region_area_thr = center_region_area_thr
- self.local_graph_thr = local_graph_thr
-
- self.out_conv = nn.Conv2d(
- in_channels=self.in_channels,
- out_channels=self.out_channels,
- kernel_size=1,
- stride=1,
- padding=0)
-
- self.graph_train = LocalGraphs(self.k_at_hops,
- self.num_adjacent_linkages,
- self.node_geo_feat_len,
- self.pooling_scale,
- self.pooling_output_size,
- self.local_graph_thr)
-
- self.graph_test = ProposalLocalGraphs(
- self.k_at_hops, self.num_adjacent_linkages, self.node_geo_feat_len,
- self.pooling_scale, self.pooling_output_size, self.nms_thr,
- self.min_width, self.max_width, self.comp_shrink_ratio,
- self.comp_ratio, self.comp_score_thr, self.text_region_thr,
- self.center_region_thr, self.center_region_area_thr)
-
- pool_w, pool_h = self.pooling_output_size
- node_feat_len = (pool_w * pool_h) * (
- self.in_channels + self.out_channels) + self.node_geo_feat_len
- self.gcn = GCN(node_feat_len)
-
- def loss(self, inputs: torch.Tensor, data_samples: List[TextDetDataSample]
- ) -> Tuple[Tensor, Tensor, Tensor]:
- """Loss function.
-
- Args:
- inputs (Tensor): Shape of :math:`(N, C, H, W)`.
- data_samples (List[TextDetDataSample]): List of data samples.
-
- Returns:
- tuple(pred_maps, gcn_pred, gt_labels):
-
- - pred_maps (Tensor): Prediction map with shape
- :math:`(N, 6, H, W)`.
- - gcn_pred (Tensor): Prediction from GCN module, with
- shape :math:`(N, 2)`.
- - gt_labels (Tensor): Ground-truth label of shape
- :math:`(m, n)` where :math:`m * n = N`.
- """
- targets = self.module_loss.get_targets(data_samples)
- gt_comp_attribs = targets[-1]
-
- pred_maps = self.out_conv(inputs)
- feat_maps = torch.cat([inputs, pred_maps], dim=1)
- node_feats, adjacent_matrices, knn_inds, gt_labels = self.graph_train(
- feat_maps, np.stack(gt_comp_attribs))
-
- gcn_pred = self.gcn(node_feats, adjacent_matrices, knn_inds)
-
- return self.module_loss((pred_maps, gcn_pred, gt_labels), data_samples)
-
- def forward(
- self,
- inputs: Tensor,
- data_samples: Optional[List[TextDetDataSample]] = None
- ) -> Tuple[Tensor, Tensor, Tensor]:
- r"""Run DRRG head in prediction mode, and return the raw tensors only.
- Args:
- inputs (Tensor): Shape of :math:`(1, C, H, W)`.
- data_samples (list[TextDetDataSample], optional): A list of data
- samples. Defaults to None.
-
- Returns:
- tuple: Returns (edge, score, text_comps).
-
- - edge (ndarray): The edge array of shape :math:`(N_{edges}, 2)`
- where each row is a pair of text component indices
- that makes up an edge in graph.
- - score (ndarray): The score array of shape :math:`(N_{edges},)`,
- corresponding to the edge above.
- - text_comps (ndarray): The text components of shape
- :math:`(M, 9)` where each row corresponds to one box and
- its score: (x1, y1, x2, y2, x3, y3, x4, y4, score).
- """
- pred_maps = self.out_conv(inputs)
- inputs = torch.cat([inputs, pred_maps], dim=1)
-
- none_flag, graph_data = self.graph_test(pred_maps, inputs)
-
- (local_graphs_node_feat, adjacent_matrices, pivots_knn_inds,
- pivot_local_graphs, text_comps) = graph_data
-
- if none_flag:
- return None, None, None
-
- gcn_pred = self.gcn(local_graphs_node_feat, adjacent_matrices,
- pivots_knn_inds)
- pred_labels = F.softmax(gcn_pred, dim=1)
-
- edges = []
- scores = []
- pivot_local_graphs = pivot_local_graphs.long().squeeze().cpu().numpy()
-
- for pivot_ind, pivot_local_graph in enumerate(pivot_local_graphs):
- pivot = pivot_local_graph[0]
- for k_ind, neighbor_ind in enumerate(pivots_knn_inds[pivot_ind]):
- neighbor = pivot_local_graph[neighbor_ind.item()]
- edges.append([pivot, neighbor])
- scores.append(
- pred_labels[pivot_ind * pivots_knn_inds.shape[1] + k_ind,
- 1].item())
-
- edges = np.asarray(edges)
- scores = np.asarray(scores)
-
- return edges, scores, text_comps
-
-
-class LocalGraphs:
- """Generate local graphs for GCN to classify the neighbors of a pivot for
- `DRRG: Deep Relational Reasoning Graph Network for Arbitrary Shape Text
- Detection <[https://arxiv.org/abs/2003.07493]>`_.
-
- This code was partially adapted from
- https://github.com/GXYM/DRRG licensed under the MIT license.
-
- Args:
- k_at_hops (tuple(int)): The number of i-hop neighbors, i = 1, 2.
- num_adjacent_linkages (int): The number of linkages when constructing
- adjacent matrix.
- node_geo_feat_len (int): The length of embedded geometric feature
- vector of a text component.
- pooling_scale (float): The spatial scale of rotated RoI-Align.
- pooling_output_size (tuple(int)): The output size of rotated RoI-Align.
- local_graph_thr(float): The threshold for filtering out identical local
- graphs.
- """
-
- def __init__(self, k_at_hops: Tuple[int, int], num_adjacent_linkages: int,
- node_geo_feat_len: int, pooling_scale: float,
- pooling_output_size: Sequence[int],
- local_graph_thr: float) -> None:
-
- assert len(k_at_hops) == 2
- assert all(isinstance(n, int) for n in k_at_hops)
- assert isinstance(num_adjacent_linkages, int)
- assert isinstance(node_geo_feat_len, int)
- assert isinstance(pooling_scale, float)
- assert all(isinstance(n, int) for n in pooling_output_size)
- assert isinstance(local_graph_thr, float)
-
- self.k_at_hops = k_at_hops
- self.num_adjacent_linkages = num_adjacent_linkages
- self.node_geo_feat_dim = node_geo_feat_len
- self.pooling = RoIAlignRotated(pooling_output_size, pooling_scale)
- self.local_graph_thr = local_graph_thr
-
- def generate_local_graphs(self, sorted_dist_inds: ndarray,
- gt_comp_labels: ndarray
- ) -> Tuple[List[List[int]], List[List[int]]]:
- """Generate local graphs for GCN to predict which instance a text
- component belongs to.
-
- Args:
- sorted_dist_inds (ndarray): The complete graph node indices, which
- is sorted according to the Euclidean distance.
- gt_comp_labels(ndarray): The ground truth labels define the
- instance to which the text components (nodes in graphs) belong.
-
- Returns:
- Tuple(pivot_local_graphs, pivot_knns):
-
- - pivot_local_graphs (list[list[int]]): The list of local graph
- neighbor indices of pivots.
- - pivot_knns (list[list[int]]): The list of k-nearest neighbor
- indices of pivots.
- """
-
- assert sorted_dist_inds.ndim == 2
- assert (sorted_dist_inds.shape[0] == sorted_dist_inds.shape[1] ==
- gt_comp_labels.shape[0])
-
- knn_graph = sorted_dist_inds[:, 1:self.k_at_hops[0] + 1]
- pivot_local_graphs = []
- pivot_knns = []
- for pivot_ind, knn in enumerate(knn_graph):
-
- local_graph_neighbors = set(knn)
-
- for neighbor_ind in knn:
- local_graph_neighbors.update(
- set(sorted_dist_inds[neighbor_ind,
- 1:self.k_at_hops[1] + 1]))
-
- local_graph_neighbors.discard(pivot_ind)
- pivot_local_graph = list(local_graph_neighbors)
- pivot_local_graph.insert(0, pivot_ind)
- pivot_knn = [pivot_ind] + list(knn)
-
- if pivot_ind < 1:
- pivot_local_graphs.append(pivot_local_graph)
- pivot_knns.append(pivot_knn)
- else:
- add_flag = True
- for graph_ind, added_knn in enumerate(pivot_knns):
- added_pivot_ind = added_knn[0]
- added_local_graph = pivot_local_graphs[graph_ind]
-
- union = len(
- set(pivot_local_graph[1:]).union(
- set(added_local_graph[1:])))
- intersect = len(
- set(pivot_local_graph[1:]).intersection(
- set(added_local_graph[1:])))
- local_graph_iou = intersect / (union + 1e-8)
-
- if (local_graph_iou > self.local_graph_thr
- and pivot_ind in added_knn
- and gt_comp_labels[added_pivot_ind]
- == gt_comp_labels[pivot_ind]
- and gt_comp_labels[pivot_ind] != 0):
- add_flag = False
- break
- if add_flag:
- pivot_local_graphs.append(pivot_local_graph)
- pivot_knns.append(pivot_knn)
-
- return pivot_local_graphs, pivot_knns
-
- def generate_gcn_input(
- self, node_feat_batch: List[Tensor], node_label_batch: List[ndarray],
- local_graph_batch: List[List[List[int]]],
- knn_batch: List[List[List[int]]], sorted_dist_ind_batch: List[ndarray]
- ) -> Tuple[Tensor, Tensor, Tensor, Tensor]:
- """Generate graph convolution network input data.
-
- Args:
- node_feat_batch (List[Tensor]): The batched graph node features.
- node_label_batch (List[ndarray]): The batched text component
- labels.
- local_graph_batch (List[List[List[int]]]): The local graph node
- indices of image batch.
- knn_batch (List[List[List[int]]]): The knn graph node indices of
- image batch.
- sorted_dist_ind_batch (List[ndarray]): The node indices sorted
- according to the Euclidean distance.
-
- Returns:
- Tuple(local_graphs_node_feat, adjacent_matrices, pivots_knn_inds,
- gt_linkage):
-
- - local_graphs_node_feat (Tensor): The node features of graph.
- - adjacent_matrices (Tensor): The adjacent matrices of local
- graphs.
- - pivots_knn_inds (Tensor): The k-nearest neighbor indices in
- local graph.
- - gt_linkage (Tensor): The surpervision signal of GCN for linkage
- prediction.
- """
- assert isinstance(node_feat_batch, list)
- assert isinstance(node_label_batch, list)
- assert isinstance(local_graph_batch, list)
- assert isinstance(knn_batch, list)
- assert isinstance(sorted_dist_ind_batch, list)
-
- num_max_nodes = max(
- len(pivot_local_graph) for pivot_local_graphs in local_graph_batch
- for pivot_local_graph in pivot_local_graphs)
-
- local_graphs_node_feat = []
- adjacent_matrices = []
- pivots_knn_inds = []
- pivots_gt_linkage = []
-
- for batch_ind, sorted_dist_inds in enumerate(sorted_dist_ind_batch):
- node_feats = node_feat_batch[batch_ind]
- pivot_local_graphs = local_graph_batch[batch_ind]
- pivot_knns = knn_batch[batch_ind]
- node_labels = node_label_batch[batch_ind]
- device = node_feats.device
-
- for graph_ind, pivot_knn in enumerate(pivot_knns):
- pivot_local_graph = pivot_local_graphs[graph_ind]
- num_nodes = len(pivot_local_graph)
- pivot_ind = pivot_local_graph[0]
- node2ind_map = {j: i for i, j in enumerate(pivot_local_graph)}
-
- knn_inds = torch.tensor(
- [node2ind_map[i] for i in pivot_knn[1:]])
- pivot_feats = node_feats[pivot_ind]
- normalized_feats = node_feats[pivot_local_graph] - pivot_feats
-
- adjacent_matrix = np.zeros((num_nodes, num_nodes),
- dtype=np.float32)
- for node in pivot_local_graph:
- neighbors = sorted_dist_inds[node,
- 1:self.num_adjacent_linkages +
- 1]
- for neighbor in neighbors:
- if neighbor in pivot_local_graph:
-
- adjacent_matrix[node2ind_map[node],
- node2ind_map[neighbor]] = 1
- adjacent_matrix[node2ind_map[neighbor],
- node2ind_map[node]] = 1
-
- adjacent_matrix = normalize_adjacent_matrix(adjacent_matrix)
- pad_adjacent_matrix = torch.zeros(
- (num_max_nodes, num_max_nodes),
- dtype=torch.float,
- device=device)
- pad_adjacent_matrix[:num_nodes, :num_nodes] = torch.from_numpy(
- adjacent_matrix)
-
- pad_normalized_feats = torch.cat([
- normalized_feats,
- torch.zeros(
- (num_max_nodes - num_nodes, normalized_feats.shape[1]),
- dtype=torch.float,
- device=device)
- ],
- dim=0)
-
- local_graph_labels = node_labels[pivot_local_graph]
- knn_labels = local_graph_labels[knn_inds]
- link_labels = ((node_labels[pivot_ind] == knn_labels) &
- (node_labels[pivot_ind] > 0)).astype(np.int64)
- link_labels = torch.from_numpy(link_labels)
-
- local_graphs_node_feat.append(pad_normalized_feats)
- adjacent_matrices.append(pad_adjacent_matrix)
- pivots_knn_inds.append(knn_inds)
- pivots_gt_linkage.append(link_labels)
-
- local_graphs_node_feat = torch.stack(local_graphs_node_feat, 0)
- adjacent_matrices = torch.stack(adjacent_matrices, 0)
- pivots_knn_inds = torch.stack(pivots_knn_inds, 0)
- pivots_gt_linkage = torch.stack(pivots_gt_linkage, 0)
-
- return (local_graphs_node_feat, adjacent_matrices, pivots_knn_inds,
- pivots_gt_linkage)
-
- def __call__(self, feat_maps: Tensor, comp_attribs: ndarray
- ) -> Tuple[Tensor, Tensor, Tensor, Tensor]:
- """Generate local graphs as GCN input.
-
- Args:
- feat_maps (Tensor): The feature maps to extract the content
- features of text components.
- comp_attribs (ndarray): The text component attributes.
-
- Returns:
- Tuple(local_graphs_node_feat, adjacent_matrices, pivots_knn_inds,
- gt_linkage):
-
- - local_graphs_node_feat (Tensor): The node features of graph.
- - adjacent_matrices (Tensor): The adjacent matrices of local
- graphs.
- - pivots_knn_inds (Tensor): The k-nearest neighbor indices in local
- graph.
- - gt_linkage (Tensor): The surpervision signal of GCN for linkage
- prediction.
- """
-
- assert isinstance(feat_maps, Tensor)
- assert comp_attribs.ndim == 3
- assert comp_attribs.shape[2] == 8
-
- sorted_dist_inds_batch = []
- local_graph_batch = []
- knn_batch = []
- node_feat_batch = []
- node_label_batch = []
- device = feat_maps.device
-
- for batch_ind in range(comp_attribs.shape[0]):
- num_comps = int(comp_attribs[batch_ind, 0, 0])
- comp_geo_attribs = comp_attribs[batch_ind, :num_comps, 1:7]
- node_labels = comp_attribs[batch_ind, :num_comps,
- 7].astype(np.int32)
-
- comp_centers = comp_geo_attribs[:, 0:2]
- distance_matrix = euclidean_distance_matrix(
- comp_centers, comp_centers)
-
- batch_id = np.zeros(
- (comp_geo_attribs.shape[0], 1), dtype=np.float32) * batch_ind
- comp_geo_attribs[:, -2] = np.clip(comp_geo_attribs[:, -2], -1, 1)
- angle = np.arccos(comp_geo_attribs[:, -2]) * np.sign(
- comp_geo_attribs[:, -1])
- angle = angle.reshape((-1, 1))
- rotated_rois = np.hstack(
- [batch_id, comp_geo_attribs[:, :-2], angle])
- rois = torch.from_numpy(rotated_rois).to(device)
- content_feats = self.pooling(feat_maps[batch_ind].unsqueeze(0),
- rois)
-
- content_feats = content_feats.view(content_feats.shape[0],
- -1).to(feat_maps.device)
- geo_feats = feature_embedding(comp_geo_attribs,
- self.node_geo_feat_dim)
- geo_feats = torch.from_numpy(geo_feats).to(device)
- node_feats = torch.cat([content_feats, geo_feats], dim=-1)
-
- sorted_dist_inds = np.argsort(distance_matrix, axis=1)
- pivot_local_graphs, pivot_knns = self.generate_local_graphs(
- sorted_dist_inds, node_labels)
-
- node_feat_batch.append(node_feats)
- node_label_batch.append(node_labels)
- local_graph_batch.append(pivot_local_graphs)
- knn_batch.append(pivot_knns)
- sorted_dist_inds_batch.append(sorted_dist_inds)
-
- (node_feats, adjacent_matrices, knn_inds, gt_linkage) = \
- self.generate_gcn_input(node_feat_batch,
- node_label_batch,
- local_graph_batch,
- knn_batch,
- sorted_dist_inds_batch)
-
- return node_feats, adjacent_matrices, knn_inds, gt_linkage
-
-
-class ProposalLocalGraphs:
- """Propose text components and generate local graphs for GCN to classify
- the k-nearest neighbors of a pivot in `DRRG: Deep Relational Reasoning
- Graph Network for Arbitrary Shape Text Detection.
-
- `_.
-
- This code was partially adapted from https://github.com/GXYM/DRRG licensed
- under the MIT license.
-
- Args:
- k_at_hops (tuple(int)): The number of i-hop neighbors, i = 1, 2.
- num_adjacent_linkages (int): The number of linkages when constructing
- adjacent matrix.
- node_geo_feat_len (int): The length of embedded geometric feature
- vector of a text component.
- pooling_scale (float): The spatial scale of rotated RoI-Align.
- pooling_output_size (tuple(int)): The output size of rotated RoI-Align.
- nms_thr (float): The locality-aware NMS threshold for text components.
- min_width (float): The minimum width of text components.
- max_width (float): The maximum width of text components.
- comp_shrink_ratio (float): The shrink ratio of text components.
- comp_w_h_ratio (float): The width to height ratio of text components.
- comp_score_thr (float): The score threshold of text component.
- text_region_thr (float): The threshold for text region probability map.
- center_region_thr (float): The threshold for text center region
- probability map.
- center_region_area_thr (int): The threshold for filtering small-sized
- text center region.
- """
-
- def __init__(self, k_at_hops: Tuple[int, int], num_adjacent_linkages: int,
- node_geo_feat_len: int, pooling_scale: float,
- pooling_output_size: Sequence[int], nms_thr: float,
- min_width: float, max_width: float, comp_shrink_ratio: float,
- comp_w_h_ratio: float, comp_score_thr: float,
- text_region_thr: float, center_region_thr: float,
- center_region_area_thr: int) -> None:
-
- assert len(k_at_hops) == 2
- assert isinstance(k_at_hops, tuple)
- assert isinstance(num_adjacent_linkages, int)
- assert isinstance(node_geo_feat_len, int)
- assert isinstance(pooling_scale, float)
- assert isinstance(pooling_output_size, tuple)
- assert isinstance(nms_thr, float)
- assert isinstance(min_width, float)
- assert isinstance(max_width, float)
- assert isinstance(comp_shrink_ratio, float)
- assert isinstance(comp_w_h_ratio, float)
- assert isinstance(comp_score_thr, float)
- assert isinstance(text_region_thr, float)
- assert isinstance(center_region_thr, float)
- assert isinstance(center_region_area_thr, int)
-
- self.k_at_hops = k_at_hops
- self.active_connection = num_adjacent_linkages
- self.local_graph_depth = len(self.k_at_hops)
- self.node_geo_feat_dim = node_geo_feat_len
- self.pooling = RoIAlignRotated(pooling_output_size, pooling_scale)
- self.nms_thr = nms_thr
- self.min_width = min_width
- self.max_width = max_width
- self.comp_shrink_ratio = comp_shrink_ratio
- self.comp_w_h_ratio = comp_w_h_ratio
- self.comp_score_thr = comp_score_thr
- self.text_region_thr = text_region_thr
- self.center_region_thr = center_region_thr
- self.center_region_area_thr = center_region_area_thr
-
- def propose_comps(self, score_map: ndarray, top_height_map: ndarray,
- bot_height_map: ndarray, sin_map: ndarray,
- cos_map: ndarray, comp_score_thr: float,
- min_width: float, max_width: float,
- comp_shrink_ratio: float,
- comp_w_h_ratio: float) -> ndarray:
- """Propose text components.
-
- Args:
- score_map (ndarray): The score map for NMS.
- top_height_map (ndarray): The predicted text height map from each
- pixel in text center region to top sideline.
- bot_height_map (ndarray): The predicted text height map from each
- pixel in text center region to bottom sideline.
- sin_map (ndarray): The predicted sin(theta) map.
- cos_map (ndarray): The predicted cos(theta) map.
- comp_score_thr (float): The score threshold of text component.
- min_width (float): The minimum width of text components.
- max_width (float): The maximum width of text components.
- comp_shrink_ratio (float): The shrink ratio of text components.
- comp_w_h_ratio (float): The width to height ratio of text
- components.
-
- Returns:
- ndarray: The text components.
- """
-
- comp_centers = np.argwhere(score_map > comp_score_thr)
- comp_centers = comp_centers[np.argsort(comp_centers[:, 0])]
- y = comp_centers[:, 0]
- x = comp_centers[:, 1]
-
- top_height = top_height_map[y, x].reshape((-1, 1)) * comp_shrink_ratio
- bot_height = bot_height_map[y, x].reshape((-1, 1)) * comp_shrink_ratio
- sin = sin_map[y, x].reshape((-1, 1))
- cos = cos_map[y, x].reshape((-1, 1))
-
- top_mid_pts = comp_centers + np.hstack(
- [top_height * sin, top_height * cos])
- bot_mid_pts = comp_centers - np.hstack(
- [bot_height * sin, bot_height * cos])
-
- width = (top_height + bot_height) * comp_w_h_ratio
- width = np.clip(width, min_width, max_width)
- r = width / 2
-
- tl = top_mid_pts[:, ::-1] - np.hstack([-r * sin, r * cos])
- tr = top_mid_pts[:, ::-1] + np.hstack([-r * sin, r * cos])
- br = bot_mid_pts[:, ::-1] + np.hstack([-r * sin, r * cos])
- bl = bot_mid_pts[:, ::-1] - np.hstack([-r * sin, r * cos])
- text_comps = np.hstack([tl, tr, br, bl]).astype(np.float32)
-
- score = score_map[y, x].reshape((-1, 1))
- text_comps = np.hstack([text_comps, score])
-
- return text_comps
-
- def propose_comps_and_attribs(self, text_region_map: ndarray,
- center_region_map: ndarray,
- top_height_map: ndarray,
- bot_height_map: ndarray, sin_map: ndarray,
- cos_map: ndarray) -> Tuple[ndarray, ndarray]:
- """Generate text components and attributes.
-
- Args:
- text_region_map (ndarray): The predicted text region probability
- map.
- center_region_map (ndarray): The predicted text center region
- probability map.
- top_height_map (ndarray): The predicted text height map from each
- pixel in text center region to top sideline.
- bot_height_map (ndarray): The predicted text height map from each
- pixel in text center region to bottom sideline.
- sin_map (ndarray): The predicted sin(theta) map.
- cos_map (ndarray): The predicted cos(theta) map.
-
- Returns:
- tuple(ndarray, ndarray):
-
- - comp_attribs (ndarray): The text component attributes.
- - text_comps (ndarray): The text components.
- """
-
- assert (text_region_map.shape == center_region_map.shape ==
- top_height_map.shape == bot_height_map.shape == sin_map.shape
- == cos_map.shape)
- text_mask = text_region_map > self.text_region_thr
- center_region_mask = (center_region_map >
- self.center_region_thr) * text_mask
-
- scale = np.sqrt(1.0 / (sin_map**2 + cos_map**2 + 1e-8))
- sin_map, cos_map = sin_map * scale, cos_map * scale
-
- center_region_mask = fill_hole(center_region_mask)
- center_region_contours, _ = cv2.findContours(
- center_region_mask.astype(np.uint8), cv2.RETR_TREE,
- cv2.CHAIN_APPROX_SIMPLE)
-
- mask_sz = center_region_map.shape
- comp_list = []
- for contour in center_region_contours:
- current_center_mask = np.zeros(mask_sz)
- cv2.drawContours(current_center_mask, [contour], -1, 1, -1)
- if current_center_mask.sum() <= self.center_region_area_thr:
- continue
- score_map = text_region_map * current_center_mask
-
- text_comps = self.propose_comps(score_map, top_height_map,
- bot_height_map, sin_map, cos_map,
- self.comp_score_thr,
- self.min_width, self.max_width,
- self.comp_shrink_ratio,
- self.comp_w_h_ratio)
-
- if la_nms is None:
- raise ImportError('lanms-neo is not installed, '
- 'please run "pip install lanms-neo==1.0.2".')
- text_comps = la_nms(text_comps, self.nms_thr)
- text_comp_mask = np.zeros(mask_sz)
- text_comp_boxes = text_comps[:, :8].reshape(
- (-1, 4, 2)).astype(np.int32)
-
- cv2.drawContours(text_comp_mask, text_comp_boxes, -1, 1, -1)
- if (text_comp_mask * text_mask).sum() < text_comp_mask.sum() * 0.5:
- continue
- if text_comps.shape[-1] > 0:
- comp_list.append(text_comps)
-
- if len(comp_list) <= 0:
- return None, None
-
- text_comps = np.vstack(comp_list)
- text_comp_boxes = text_comps[:, :8].reshape((-1, 4, 2))
- centers = np.mean(text_comp_boxes, axis=1).astype(np.int32)
- x = centers[:, 0]
- y = centers[:, 1]
-
- scores = []
- for text_comp_box in text_comp_boxes:
- text_comp_box[:, 0] = np.clip(text_comp_box[:, 0], 0,
- mask_sz[1] - 1)
- text_comp_box[:, 1] = np.clip(text_comp_box[:, 1], 0,
- mask_sz[0] - 1)
- min_coord = np.min(text_comp_box, axis=0).astype(np.int32)
- max_coord = np.max(text_comp_box, axis=0).astype(np.int32)
- text_comp_box = text_comp_box - min_coord
- box_sz = (max_coord - min_coord + 1)
- temp_comp_mask = np.zeros((box_sz[1], box_sz[0]), dtype=np.uint8)
- cv2.fillPoly(temp_comp_mask, [text_comp_box.astype(np.int32)], 1)
- temp_region_patch = text_region_map[min_coord[1]:(max_coord[1] +
- 1),
- min_coord[0]:(max_coord[0] +
- 1)]
- score = cv2.mean(temp_region_patch, temp_comp_mask)[0]
- scores.append(score)
- scores = np.array(scores).reshape((-1, 1))
- text_comps = np.hstack([text_comps[:, :-1], scores])
-
- h = top_height_map[y, x].reshape(
- (-1, 1)) + bot_height_map[y, x].reshape((-1, 1))
- w = np.clip(h * self.comp_w_h_ratio, self.min_width, self.max_width)
- sin = sin_map[y, x].reshape((-1, 1))
- cos = cos_map[y, x].reshape((-1, 1))
-
- x = x.reshape((-1, 1))
- y = y.reshape((-1, 1))
- comp_attribs = np.hstack([x, y, h, w, cos, sin])
-
- return comp_attribs, text_comps
-
- def generate_local_graphs(self, sorted_dist_inds: ndarray,
- node_feats: Tensor
- ) -> Tuple[Tensor, Tensor, Tensor, Tensor]:
- """Generate local graphs and graph convolution network input data.
-
- Args:
- sorted_dist_inds (ndarray): The node indices sorted according to
- the Euclidean distance.
- node_feats (tensor): The features of nodes in graph.
-
- Returns:
- Tuple(local_graphs_node_feat, adjacent_matrices, pivots_knn_inds,
- pivots_local_graphs):
-
- - local_graphs_node_feats (tensor): The features of nodes in local
- graphs.
- - adjacent_matrices (tensor): The adjacent matrices.
- - pivots_knn_inds (tensor): The k-nearest neighbor indices in
- local graphs.
- - pivots_local_graphs (tensor): The indices of nodes in local
- graphs.
- """
-
- assert sorted_dist_inds.ndim == 2
- assert (sorted_dist_inds.shape[0] == sorted_dist_inds.shape[1] ==
- node_feats.shape[0])
-
- knn_graph = sorted_dist_inds[:, 1:self.k_at_hops[0] + 1]
- pivot_local_graphs = []
- pivot_knns = []
- device = node_feats.device
-
- for pivot_ind, knn in enumerate(knn_graph):
-
- local_graph_neighbors = set(knn)
-
- for neighbor_ind in knn:
- local_graph_neighbors.update(
- set(sorted_dist_inds[neighbor_ind,
- 1:self.k_at_hops[1] + 1]))
-
- local_graph_neighbors.discard(pivot_ind)
- pivot_local_graph = list(local_graph_neighbors)
- pivot_local_graph.insert(0, pivot_ind)
- pivot_knn = [pivot_ind] + list(knn)
-
- pivot_local_graphs.append(pivot_local_graph)
- pivot_knns.append(pivot_knn)
-
- num_max_nodes = max(
- len(pivot_local_graph) for pivot_local_graph in pivot_local_graphs)
-
- local_graphs_node_feat = []
- adjacent_matrices = []
- pivots_knn_inds = []
- pivots_local_graphs = []
-
- for graph_ind, pivot_knn in enumerate(pivot_knns):
- pivot_local_graph = pivot_local_graphs[graph_ind]
- num_nodes = len(pivot_local_graph)
- pivot_ind = pivot_local_graph[0]
- node2ind_map = {j: i for i, j in enumerate(pivot_local_graph)}
-
- knn_inds = torch.tensor([node2ind_map[i]
- for i in pivot_knn[1:]]).long().to(device)
- pivot_feats = node_feats[pivot_ind]
- normalized_feats = node_feats[pivot_local_graph] - pivot_feats
-
- adjacent_matrix = np.zeros((num_nodes, num_nodes))
- for node in pivot_local_graph:
- neighbors = sorted_dist_inds[node,
- 1:self.active_connection + 1]
- for neighbor in neighbors:
- if neighbor in pivot_local_graph:
- adjacent_matrix[node2ind_map[node],
- node2ind_map[neighbor]] = 1
- adjacent_matrix[node2ind_map[neighbor],
- node2ind_map[node]] = 1
-
- adjacent_matrix = normalize_adjacent_matrix(adjacent_matrix)
- pad_adjacent_matrix = torch.zeros((num_max_nodes, num_max_nodes),
- dtype=torch.float,
- device=device)
- pad_adjacent_matrix[:num_nodes, :num_nodes] = torch.from_numpy(
- adjacent_matrix)
-
- pad_normalized_feats = torch.cat([
- normalized_feats,
- torch.zeros(
- (num_max_nodes - num_nodes, normalized_feats.shape[1]),
- dtype=torch.float,
- device=device)
- ],
- dim=0)
-
- local_graph_nodes = torch.tensor(pivot_local_graph)
- local_graph_nodes = torch.cat([
- local_graph_nodes,
- torch.zeros(num_max_nodes - num_nodes, dtype=torch.long)
- ],
- dim=-1)
-
- local_graphs_node_feat.append(pad_normalized_feats)
- adjacent_matrices.append(pad_adjacent_matrix)
- pivots_knn_inds.append(knn_inds)
- pivots_local_graphs.append(local_graph_nodes)
-
- local_graphs_node_feat = torch.stack(local_graphs_node_feat, 0)
- adjacent_matrices = torch.stack(adjacent_matrices, 0)
- pivots_knn_inds = torch.stack(pivots_knn_inds, 0)
- pivots_local_graphs = torch.stack(pivots_local_graphs, 0)
-
- return (local_graphs_node_feat, adjacent_matrices, pivots_knn_inds,
- pivots_local_graphs)
-
- def __call__(self, preds: Tensor, feat_maps: Tensor
- ) -> Tuple[bool, Tensor, Tensor, Tensor, Tensor, ndarray]:
- """Generate local graphs and graph convolutional network input data.
-
- Args:
- preds (tensor): The predicted maps.
- feat_maps (tensor): The feature maps to extract content feature of
- text components.
-
- Returns:
- Tuple(none_flag, local_graphs_node_feat, adjacent_matrices,
- pivots_knn_inds, pivots_local_graphs, text_comps):
-
- - none_flag (bool): The flag showing whether the number of proposed
- text components is 0.
- - local_graphs_node_feats (tensor): The features of nodes in local
- graphs.
- - adjacent_matrices (tensor): The adjacent matrices.
- - pivots_knn_inds (tensor): The k-nearest neighbor indices in
- local graphs.
- - pivots_local_graphs (tensor): The indices of nodes in local
- graphs.
- - text_comps (ndarray): The predicted text components.
- """
-
- if preds.ndim == 4:
- assert preds.shape[0] == 1
- preds = torch.squeeze(preds)
- pred_text_region = torch.sigmoid(preds[0]).data.cpu().numpy()
- pred_center_region = torch.sigmoid(preds[1]).data.cpu().numpy()
- pred_sin_map = preds[2].data.cpu().numpy()
- pred_cos_map = preds[3].data.cpu().numpy()
- pred_top_height_map = preds[4].data.cpu().numpy()
- pred_bot_height_map = preds[5].data.cpu().numpy()
- device = preds.device
-
- comp_attribs, text_comps = self.propose_comps_and_attribs(
- pred_text_region, pred_center_region, pred_top_height_map,
- pred_bot_height_map, pred_sin_map, pred_cos_map)
-
- if comp_attribs is None or len(comp_attribs) < 2:
- none_flag = True
- return none_flag, (0, 0, 0, 0, 0)
-
- comp_centers = comp_attribs[:, 0:2]
- distance_matrix = euclidean_distance_matrix(comp_centers, comp_centers)
-
- geo_feats = feature_embedding(comp_attribs, self.node_geo_feat_dim)
- geo_feats = torch.from_numpy(geo_feats).to(preds.device)
-
- batch_id = np.zeros((comp_attribs.shape[0], 1), dtype=np.float32)
- comp_attribs = comp_attribs.astype(np.float32)
- angle = np.arccos(comp_attribs[:, -2]) * np.sign(comp_attribs[:, -1])
- angle = angle.reshape((-1, 1))
- rotated_rois = np.hstack([batch_id, comp_attribs[:, :-2], angle])
- rois = torch.from_numpy(rotated_rois).to(device)
-
- content_feats = self.pooling(feat_maps, rois)
- content_feats = content_feats.view(content_feats.shape[0],
- -1).to(device)
- node_feats = torch.cat([content_feats, geo_feats], dim=-1)
-
- sorted_dist_inds = np.argsort(distance_matrix, axis=1)
- (local_graphs_node_feat, adjacent_matrices, pivots_knn_inds,
- pivots_local_graphs) = self.generate_local_graphs(
- sorted_dist_inds, node_feats)
-
- none_flag = False
- return none_flag, (local_graphs_node_feat, adjacent_matrices,
- pivots_knn_inds, pivots_local_graphs, text_comps)
-
-
-class GraphConv(BaseModule):
- """Graph convolutional neural network.
-
- Args:
- in_dim (int): The number of input channels.
- out_dim (int): The number of output channels.
- """
-
- class MeanAggregator(BaseModule):
- """Mean aggregator for graph convolutional network."""
-
- def forward(self, features: Tensor, A: Tensor) -> Tensor:
- """Forward function."""
- x = torch.bmm(A, features)
- return x
-
- def __init__(self, in_dim: int, out_dim: int) -> None:
- super().__init__()
- self.in_dim = in_dim
- self.out_dim = out_dim
- self.weight = nn.Parameter(torch.FloatTensor(in_dim * 2, out_dim))
- self.bias = nn.Parameter(torch.FloatTensor(out_dim))
- init.xavier_uniform_(self.weight)
- init.constant_(self.bias, 0)
- self.aggregator = self.MeanAggregator()
-
- def forward(self, features: Tensor, A: Tensor) -> Tensor:
- """Forward function."""
- _, _, d = features.shape
- assert d == self.in_dim
- agg_feats = self.aggregator(features, A)
- cat_feats = torch.cat([features, agg_feats], dim=2)
- out = torch.einsum('bnd,df->bnf', cat_feats, self.weight)
- out = F.relu(out + self.bias)
- return out
-
-
-class GCN(BaseModule):
- """Graph convolutional network for clustering. This was from repo
- https://github.com/Zhongdao/gcn_clustering licensed under the MIT license.
-
- Args:
- feat_len (int): The input node feature length.
- init_cfg (dict or list[dict], optional): Initialization configs.
- """
-
- def __init__(self,
- feat_len: int,
- init_cfg: Optional[Union[Dict, List[Dict]]] = None) -> None:
- super().__init__(init_cfg=init_cfg)
- self.bn0 = nn.BatchNorm1d(feat_len, affine=False).float()
- self.conv1 = GraphConv(feat_len, 512)
- self.conv2 = GraphConv(512, 256)
- self.conv3 = GraphConv(256, 128)
- self.conv4 = GraphConv(128, 64)
- self.classifier = nn.Sequential(
- nn.Linear(64, 32), nn.PReLU(32), nn.Linear(32, 2))
-
- def forward(self, node_feats: Tensor, adj_mats: Tensor,
- knn_inds: Tensor) -> Tensor:
- """Forward function.
-
- Args:
- local_graphs_node_feat (Tensor): The node features of graph.
- adjacent_matrices (Tensor): The adjacent matrices of local
- graphs.
- pivots_knn_inds (Tensor): The k-nearest neighbor indices in
- local graph.
-
- Returns:
- Tensor: The output feature.
- """
-
- num_local_graphs, num_max_nodes, feat_len = node_feats.shape
-
- node_feats = node_feats.view(-1, feat_len)
- node_feats = self.bn0(node_feats)
- node_feats = node_feats.view(num_local_graphs, num_max_nodes, feat_len)
-
- node_feats = self.conv1(node_feats, adj_mats)
- node_feats = self.conv2(node_feats, adj_mats)
- node_feats = self.conv3(node_feats, adj_mats)
- node_feats = self.conv4(node_feats, adj_mats)
- k = knn_inds.size(-1)
- mid_feat_len = node_feats.size(-1)
- edge_feat = torch.zeros((num_local_graphs, k, mid_feat_len),
- device=node_feats.device)
- for graph_ind in range(num_local_graphs):
- edge_feat[graph_ind, :, :] = node_feats[graph_ind,
- knn_inds[graph_ind]]
- edge_feat = edge_feat.view(-1, mid_feat_len)
- pred = self.classifier(edge_feat)
-
- return pred
diff --git a/pipeline/mmocr/mmocr/models/textdet/heads/fce_head.py b/pipeline/mmocr/mmocr/models/textdet/heads/fce_head.py
deleted file mode 100644
index 949a2835a8aa08ffb1d32f18d480efb1c62260e3..0000000000000000000000000000000000000000
--- a/pipeline/mmocr/mmocr/models/textdet/heads/fce_head.py
+++ /dev/null
@@ -1,117 +0,0 @@
-# Copyright (c) OpenMMLab. All rights reserved.
-from typing import Dict, List, Optional
-
-import torch
-import torch.nn as nn
-from mmdet.models.utils import multi_apply
-
-from mmocr.models.textdet.heads import BaseTextDetHead
-from mmocr.registry import MODELS
-from mmocr.structures import TextDetDataSample
-
-
-@MODELS.register_module()
-class FCEHead(BaseTextDetHead):
- """The class for implementing FCENet head.
-
- FCENet(CVPR2021): `Fourier Contour Embedding for Arbitrary-shaped Text
- Detection `_
-
- Args:
- in_channels (int): The number of input channels.
- fourier_degree (int) : The maximum Fourier transform degree k. Defaults
- to 5.
- module_loss (dict): Config of loss for FCENet. Defaults to
- ``dict(type='FCEModuleLoss', num_sample=50)``.
- postprocessor (dict): Config of postprocessor for FCENet.
- init_cfg (dict, optional): Initialization configs.
- """
-
- def __init__(
- self,
- in_channels: int,
- fourier_degree: int = 5,
- module_loss: Dict = dict(type='FCEModuleLoss', num_sample=50),
- postprocessor: Dict = dict(
- type='FCEPostprocessor',
- text_repr_type='poly',
- num_reconstr_points=50,
- alpha=1.0,
- beta=2.0,
- score_thr=0.3),
- init_cfg: Optional[Dict] = dict(
- type='Normal',
- mean=0,
- std=0.01,
- override=[dict(name='out_conv_cls'),
- dict(name='out_conv_reg')])
- ) -> None:
- module_loss['fourier_degree'] = fourier_degree
- postprocessor['fourier_degree'] = fourier_degree
- super().__init__(
- module_loss=module_loss,
- postprocessor=postprocessor,
- init_cfg=init_cfg)
-
- assert isinstance(in_channels, int)
- assert isinstance(fourier_degree, int)
-
- self.in_channels = in_channels
- self.fourier_degree = fourier_degree
- self.out_channels_cls = 4
- self.out_channels_reg = (2 * self.fourier_degree + 1) * 2
-
- self.out_conv_cls = nn.Conv2d(
- self.in_channels,
- self.out_channels_cls,
- kernel_size=3,
- stride=1,
- padding=1)
- self.out_conv_reg = nn.Conv2d(
- self.in_channels,
- self.out_channels_reg,
- kernel_size=3,
- stride=1,
- padding=1)
-
- def forward(self,
- inputs: List[torch.Tensor],
- data_samples: Optional[List[TextDetDataSample]] = None
- ) -> Dict:
- """
- Args:
- inputs (List[Tensor]): Each tensor has the shape of :math:`(N, C_i,
- H_i, W_i)`.
- data_samples (list[TextDetDataSample], optional): A list of data
- samples. Defaults to None.
-
- Returns:
- list[dict]: A list of dict with keys of ``cls_res``, ``reg_res``
- corresponds to the classification result and regression result
- computed from the input tensor with the same index. They have
- the shapes of :math:`(N, C_{cls,i}, H_i, W_i)` and :math:`(N,
- C_{out,i}, H_i, W_i)`.
- """
- cls_res, reg_res = multi_apply(self.forward_single, inputs)
- level_num = len(cls_res)
- preds = [
- dict(cls_res=cls_res[i], reg_res=reg_res[i])
- for i in range(level_num)
- ]
- return preds
-
- def forward_single(self, x: torch.Tensor) -> torch.Tensor:
- """Forward function for a single feature level.
-
- Args:
- x (Tensor): The input tensor with the shape of :math:`(N, C_i,
- H_i, W_i)`.
-
- Returns:
- Tensor: The classification and regression result with the shape of
- :math:`(N, C_{cls,i}, H_i, W_i)` and :math:`(N, C_{out,i}, H_i,
- W_i)`.
- """
- cls_predict = self.out_conv_cls(x)
- reg_predict = self.out_conv_reg(x)
- return cls_predict, reg_predict
diff --git a/pipeline/mmocr/mmocr/models/textdet/heads/pan_head.py b/pipeline/mmocr/mmocr/models/textdet/heads/pan_head.py
deleted file mode 100644
index a7d4f053d09049c21442d357f631c51ac2f3e41d..0000000000000000000000000000000000000000
--- a/pipeline/mmocr/mmocr/models/textdet/heads/pan_head.py
+++ /dev/null
@@ -1,83 +0,0 @@
-# Copyright (c) OpenMMLab. All rights reserved.
-from typing import List, Optional
-
-import torch
-import torch.nn as nn
-
-from mmocr.registry import MODELS
-from mmocr.structures import TextDetDataSample
-from mmocr.utils import check_argument
-from .base import BaseTextDetHead
-
-
-@MODELS.register_module()
-class PANHead(BaseTextDetHead):
- """The class for PANet head.
-
- Args:
- in_channels (list[int]): A list of 4 numbers of input channels.
- hidden_dim (int): The hidden dimension of the first convolutional
- layer.
- out_channel (int): Number of output channels.
- module_loss (dict): Configuration dictionary for loss type. Defaults
- to dict(type='PANModuleLoss')
- postprocessor (dict): Config of postprocessor for PANet. Defaults to
- dict(type='PANPostprocessor', text_repr_type='poly').
- init_cfg (list[dict]): Initialization configs. Defaults to
- [dict(type='Normal', mean=0, std=0.01, layer='Conv2d'),
- dict(type='Constant', val=1, bias=0, layer='BN')]
- """
-
- def __init__(
- self,
- in_channels: List[int],
- hidden_dim: int,
- out_channel: int,
- module_loss=dict(type='PANModuleLoss'),
- postprocessor=dict(type='PANPostprocessor', text_repr_type='poly'),
- init_cfg=[
- dict(type='Normal', mean=0, std=0.01, layer='Conv2d'),
- dict(type='Constant', val=1, bias=0, layer='BN')
- ]
- ) -> None:
- super().__init__(
- module_loss=module_loss,
- postprocessor=postprocessor,
- init_cfg=init_cfg)
-
- assert check_argument.is_type_list(in_channels, int)
- assert isinstance(out_channel, int)
- assert isinstance(hidden_dim, int)
-
- in_channels = sum(in_channels)
- self.conv1 = nn.Conv2d(
- in_channels, hidden_dim, kernel_size=3, stride=1, padding=1)
- self.bn1 = nn.BatchNorm2d(hidden_dim)
- self.relu1 = nn.ReLU(inplace=True)
- self.conv2 = nn.Conv2d(
- hidden_dim, out_channel, kernel_size=1, stride=1, padding=0)
-
- def forward(self,
- inputs: torch.Tensor,
- data_samples: Optional[List[TextDetDataSample]] = None
- ) -> torch.Tensor:
- r"""PAN head forward.
- Args:
- inputs (list[Tensor] | Tensor): Each tensor has the shape of
- :math:`(N, C_i, W, H)`, where :math:`\sum_iC_i=C_{in}` and
- :math:`C_{in}` is ``input_channels``.
- data_samples (list[TextDetDataSample], optional): A list of data
- samples. Defaults to None.
-
- Returns:
- Tensor: A tensor of shape :math:`(N, C_{out}, W, H)` where
- :math:`C_{out}` is ``output_channels``.
- """
- if isinstance(inputs, tuple):
- outputs = torch.cat(inputs, dim=1)
- else:
- outputs = inputs
- outputs = self.conv1(outputs)
- outputs = self.relu1(self.bn1(outputs))
- outputs = self.conv2(outputs)
- return outputs
diff --git a/pipeline/mmocr/mmocr/models/textdet/heads/pse_head.py b/pipeline/mmocr/mmocr/models/textdet/heads/pse_head.py
deleted file mode 100644
index 0aee6a07b4d6325d22a14dc76c2796391ce62eab..0000000000000000000000000000000000000000
--- a/pipeline/mmocr/mmocr/models/textdet/heads/pse_head.py
+++ /dev/null
@@ -1,39 +0,0 @@
-# Copyright (c) OpenMMLab. All rights reserved.
-from typing import Dict, List, Optional, Union
-
-from mmocr.registry import MODELS
-from . import PANHead
-
-
-@MODELS.register_module()
-class PSEHead(PANHead):
- """The class for PSENet head.
-
- Args:
- in_channels (list[int]): A list of numbers of input channels.
- hidden_dim (int): The hidden dimension of the first convolutional
- layer.
- out_channel (int): Number of output channels.
- module_loss (dict): Configuration dictionary for loss type. Supported
- loss types are "PANModuleLoss" and "PSEModuleLoss". Defaults to
- PSEModuleLoss.
- postprocessor (dict): Config of postprocessor for PSENet.
- init_cfg (dict or list[dict], optional): Initialization configs.
- """
-
- def __init__(self,
- in_channels: List[int],
- hidden_dim: int,
- out_channel: int,
- module_loss: Dict = dict(type='PSEModuleLoss'),
- postprocessor: Dict = dict(
- type='PSEPostprocessor', text_repr_type='poly'),
- init_cfg: Optional[Union[Dict, List[Dict]]] = None) -> None:
-
- super().__init__(
- in_channels=in_channels,
- hidden_dim=hidden_dim,
- out_channel=out_channel,
- module_loss=module_loss,
- postprocessor=postprocessor,
- init_cfg=init_cfg)
diff --git a/pipeline/mmocr/mmocr/models/textdet/heads/textsnake_head.py b/pipeline/mmocr/mmocr/models/textdet/heads/textsnake_head.py
deleted file mode 100644
index 6cda55e10f445ed77771eabcde6a8dc91986550d..0000000000000000000000000000000000000000
--- a/pipeline/mmocr/mmocr/models/textdet/heads/textsnake_head.py
+++ /dev/null
@@ -1,76 +0,0 @@
-# Copyright (c) OpenMMLab. All rights reserved.
-from typing import Dict, List, Optional, Union
-
-import torch
-import torch.nn as nn
-
-from mmocr.models.textdet.heads import BaseTextDetHead
-from mmocr.registry import MODELS
-from mmocr.structures import TextDetDataSample
-
-
-@MODELS.register_module()
-class TextSnakeHead(BaseTextDetHead):
- """The class for TextSnake head: TextSnake: A Flexible Representation for
- Detecting Text of Arbitrary Shapes.
-
- TextSnake: `A Flexible Representation for Detecting Text of Arbitrary
- Shapes `_.
-
- Args:
- in_channels (int): Number of input channels.
- out_channels (int): Number of output channels.
- downsample_ratio (float): Downsample ratio.
- module_loss (dict): Configuration dictionary for loss type.
- Defaults to ``dict(type='TextSnakeModuleLoss')``.
- postprocessor (dict): Config of postprocessor for TextSnake.
- init_cfg (dict or list[dict], optional): Initialization configs.
- """
-
- def __init__(
- self,
- in_channels: int,
- out_channels: int = 5,
- downsample_ratio: float = 1.0,
- module_loss: Dict = dict(type='TextSnakeModuleLoss'),
- postprocessor: Dict = dict(
- type='TextSnakePostprocessor', text_repr_type='poly'),
- init_cfg: Optional[Union[Dict, List[Dict]]] = dict(
- type='Normal', override=dict(name='out_conv'), mean=0, std=0.01)
- ) -> None:
- super().__init__(
- module_loss=module_loss,
- postprocessor=postprocessor,
- init_cfg=init_cfg)
- assert isinstance(in_channels, int)
- assert isinstance(out_channels, int)
- self.in_channels = in_channels
- self.out_channels = out_channels
- self.downsample_ratio = downsample_ratio
-
- self.out_conv = nn.Conv2d(
- in_channels=self.in_channels,
- out_channels=self.out_channels,
- kernel_size=1,
- stride=1,
- padding=0)
-
- def forward(self,
- inputs: torch.Tensor,
- data_samples: Optional[List[TextDetDataSample]] = None
- ) -> Dict:
- """
- Args:
- inputs (torch.Tensor): Shape :math:`(N, C_{in}, H, W)`, where
- :math:`C_{in}` is ``in_channels``. :math:`H` and :math:`W`
- should be the same as the input of backbone.
- data_samples (list[TextDetDataSample], optional): A list of data
- samples. Defaults to None.
-
- Returns:
- Tensor: A tensor of shape :math:`(N, 5, H, W)`, where the five
- channels represent [0]: text score, [1]: center score,
- [2]: sin, [3] cos, [4] radius, respectively.
- """
- outputs = self.out_conv(inputs)
- return outputs
diff --git a/pipeline/mmocr/mmocr/models/textdet/module_losses/__init__.py b/pipeline/mmocr/mmocr/models/textdet/module_losses/__init__.py
deleted file mode 100644
index 111c47990143147a8acaf6fdf75a36749042af0c..0000000000000000000000000000000000000000
--- a/pipeline/mmocr/mmocr/models/textdet/module_losses/__init__.py
+++ /dev/null
@@ -1,13 +0,0 @@
-# Copyright (c) OpenMMLab. All rights reserved.
-from .db_module_loss import DBModuleLoss
-from .drrg_module_loss import DRRGModuleLoss
-from .fce_module_loss import FCEModuleLoss
-from .pan_module_loss import PANModuleLoss
-from .pse_module_loss import PSEModuleLoss
-from .seg_based_module_loss import SegBasedModuleLoss
-from .textsnake_module_loss import TextSnakeModuleLoss
-
-__all__ = [
- 'PANModuleLoss', 'PSEModuleLoss', 'DBModuleLoss', 'TextSnakeModuleLoss',
- 'FCEModuleLoss', 'DRRGModuleLoss', 'SegBasedModuleLoss'
-]
diff --git a/pipeline/mmocr/mmocr/models/textdet/module_losses/__pycache__/__init__.cpython-38.pyc b/pipeline/mmocr/mmocr/models/textdet/module_losses/__pycache__/__init__.cpython-38.pyc
deleted file mode 100644
index 87e57b3cd905d29bd9533e02123f1f662f9831c6..0000000000000000000000000000000000000000
Binary files a/pipeline/mmocr/mmocr/models/textdet/module_losses/__pycache__/__init__.cpython-38.pyc and /dev/null differ
diff --git a/pipeline/mmocr/mmocr/models/textdet/module_losses/__pycache__/__init__.cpython-39.pyc b/pipeline/mmocr/mmocr/models/textdet/module_losses/__pycache__/__init__.cpython-39.pyc
deleted file mode 100644
index 285aefd78bdcaa13267194796613f6186dfb67e2..0000000000000000000000000000000000000000
Binary files a/pipeline/mmocr/mmocr/models/textdet/module_losses/__pycache__/__init__.cpython-39.pyc and /dev/null differ
diff --git a/pipeline/mmocr/mmocr/models/textdet/module_losses/__pycache__/base.cpython-38.pyc b/pipeline/mmocr/mmocr/models/textdet/module_losses/__pycache__/base.cpython-38.pyc
deleted file mode 100644
index 22356aa9158e41b9609373a1613ec5c849e425b3..0000000000000000000000000000000000000000
Binary files a/pipeline/mmocr/mmocr/models/textdet/module_losses/__pycache__/base.cpython-38.pyc and /dev/null differ
diff --git a/pipeline/mmocr/mmocr/models/textdet/module_losses/__pycache__/base.cpython-39.pyc b/pipeline/mmocr/mmocr/models/textdet/module_losses/__pycache__/base.cpython-39.pyc
deleted file mode 100644
index 315bacde95532920e1f30fbb853c639c2954c94b..0000000000000000000000000000000000000000
Binary files a/pipeline/mmocr/mmocr/models/textdet/module_losses/__pycache__/base.cpython-39.pyc and /dev/null differ
diff --git a/pipeline/mmocr/mmocr/models/textdet/module_losses/__pycache__/db_module_loss.cpython-38.pyc b/pipeline/mmocr/mmocr/models/textdet/module_losses/__pycache__/db_module_loss.cpython-38.pyc
deleted file mode 100644
index 7bc1d42901a208dc82b005c1d28f748285680366..0000000000000000000000000000000000000000
Binary files a/pipeline/mmocr/mmocr/models/textdet/module_losses/__pycache__/db_module_loss.cpython-38.pyc and /dev/null differ
diff --git a/pipeline/mmocr/mmocr/models/textdet/module_losses/__pycache__/db_module_loss.cpython-39.pyc b/pipeline/mmocr/mmocr/models/textdet/module_losses/__pycache__/db_module_loss.cpython-39.pyc
deleted file mode 100644
index 843cc5f4d36e608c703ecb7dc3389c1490594f43..0000000000000000000000000000000000000000
Binary files a/pipeline/mmocr/mmocr/models/textdet/module_losses/__pycache__/db_module_loss.cpython-39.pyc and /dev/null differ
diff --git a/pipeline/mmocr/mmocr/models/textdet/module_losses/__pycache__/drrg_module_loss.cpython-38.pyc b/pipeline/mmocr/mmocr/models/textdet/module_losses/__pycache__/drrg_module_loss.cpython-38.pyc
deleted file mode 100644
index 065e9ff56fcd3d568d89270cb4dd1991fefe146f..0000000000000000000000000000000000000000
Binary files a/pipeline/mmocr/mmocr/models/textdet/module_losses/__pycache__/drrg_module_loss.cpython-38.pyc and /dev/null differ
diff --git a/pipeline/mmocr/mmocr/models/textdet/module_losses/__pycache__/drrg_module_loss.cpython-39.pyc b/pipeline/mmocr/mmocr/models/textdet/module_losses/__pycache__/drrg_module_loss.cpython-39.pyc
deleted file mode 100644
index 3f519f2130254832e6a13b6c076ab4ec966b1516..0000000000000000000000000000000000000000
Binary files a/pipeline/mmocr/mmocr/models/textdet/module_losses/__pycache__/drrg_module_loss.cpython-39.pyc and /dev/null differ
diff --git a/pipeline/mmocr/mmocr/models/textdet/module_losses/__pycache__/fce_module_loss.cpython-38.pyc b/pipeline/mmocr/mmocr/models/textdet/module_losses/__pycache__/fce_module_loss.cpython-38.pyc
deleted file mode 100644
index 8911b4edc1169d3f343db9dad08300999bd9b665..0000000000000000000000000000000000000000
Binary files a/pipeline/mmocr/mmocr/models/textdet/module_losses/__pycache__/fce_module_loss.cpython-38.pyc and /dev/null differ
diff --git a/pipeline/mmocr/mmocr/models/textdet/module_losses/__pycache__/fce_module_loss.cpython-39.pyc b/pipeline/mmocr/mmocr/models/textdet/module_losses/__pycache__/fce_module_loss.cpython-39.pyc
deleted file mode 100644
index e0861fc1b8ad02afe84c131c1393f225e4518862..0000000000000000000000000000000000000000
Binary files a/pipeline/mmocr/mmocr/models/textdet/module_losses/__pycache__/fce_module_loss.cpython-39.pyc and /dev/null differ
diff --git a/pipeline/mmocr/mmocr/models/textdet/module_losses/__pycache__/pan_module_loss.cpython-38.pyc b/pipeline/mmocr/mmocr/models/textdet/module_losses/__pycache__/pan_module_loss.cpython-38.pyc
deleted file mode 100644
index 6687ed59e17882618665fb77a20786e925ff71fe..0000000000000000000000000000000000000000
Binary files a/pipeline/mmocr/mmocr/models/textdet/module_losses/__pycache__/pan_module_loss.cpython-38.pyc and /dev/null differ
diff --git a/pipeline/mmocr/mmocr/models/textdet/module_losses/__pycache__/pan_module_loss.cpython-39.pyc b/pipeline/mmocr/mmocr/models/textdet/module_losses/__pycache__/pan_module_loss.cpython-39.pyc
deleted file mode 100644
index aed7bb7426947a70f5750c56a03f1f9bc5aedf0b..0000000000000000000000000000000000000000
Binary files a/pipeline/mmocr/mmocr/models/textdet/module_losses/__pycache__/pan_module_loss.cpython-39.pyc and /dev/null differ
diff --git a/pipeline/mmocr/mmocr/models/textdet/module_losses/__pycache__/pse_module_loss.cpython-38.pyc b/pipeline/mmocr/mmocr/models/textdet/module_losses/__pycache__/pse_module_loss.cpython-38.pyc
deleted file mode 100644
index f90e011151721b695f795a687192ebfeb5dcccc8..0000000000000000000000000000000000000000
Binary files a/pipeline/mmocr/mmocr/models/textdet/module_losses/__pycache__/pse_module_loss.cpython-38.pyc and /dev/null differ
diff --git a/pipeline/mmocr/mmocr/models/textdet/module_losses/__pycache__/pse_module_loss.cpython-39.pyc b/pipeline/mmocr/mmocr/models/textdet/module_losses/__pycache__/pse_module_loss.cpython-39.pyc
deleted file mode 100644
index f45056a453ec0fa24588a5ed9c2edc767ae88c4c..0000000000000000000000000000000000000000
Binary files a/pipeline/mmocr/mmocr/models/textdet/module_losses/__pycache__/pse_module_loss.cpython-39.pyc and /dev/null differ
diff --git a/pipeline/mmocr/mmocr/models/textdet/module_losses/__pycache__/seg_based_module_loss.cpython-38.pyc b/pipeline/mmocr/mmocr/models/textdet/module_losses/__pycache__/seg_based_module_loss.cpython-38.pyc
deleted file mode 100644
index f34ce12aecb92772f306183244a25a74c9588267..0000000000000000000000000000000000000000
Binary files a/pipeline/mmocr/mmocr/models/textdet/module_losses/__pycache__/seg_based_module_loss.cpython-38.pyc and /dev/null differ
diff --git a/pipeline/mmocr/mmocr/models/textdet/module_losses/__pycache__/seg_based_module_loss.cpython-39.pyc b/pipeline/mmocr/mmocr/models/textdet/module_losses/__pycache__/seg_based_module_loss.cpython-39.pyc
deleted file mode 100644
index 04d5cba213255d9ca5996bde9a922aa435260b7e..0000000000000000000000000000000000000000
Binary files a/pipeline/mmocr/mmocr/models/textdet/module_losses/__pycache__/seg_based_module_loss.cpython-39.pyc and /dev/null differ
diff --git a/pipeline/mmocr/mmocr/models/textdet/module_losses/__pycache__/textsnake_module_loss.cpython-38.pyc b/pipeline/mmocr/mmocr/models/textdet/module_losses/__pycache__/textsnake_module_loss.cpython-38.pyc
deleted file mode 100644
index 2ad6623d4b13cb925b9fc91810da93608c967f1a..0000000000000000000000000000000000000000
Binary files a/pipeline/mmocr/mmocr/models/textdet/module_losses/__pycache__/textsnake_module_loss.cpython-38.pyc and /dev/null differ
diff --git a/pipeline/mmocr/mmocr/models/textdet/module_losses/__pycache__/textsnake_module_loss.cpython-39.pyc b/pipeline/mmocr/mmocr/models/textdet/module_losses/__pycache__/textsnake_module_loss.cpython-39.pyc
deleted file mode 100644
index 78df42d3d34237db70f0ce30c98828c822345d74..0000000000000000000000000000000000000000
Binary files a/pipeline/mmocr/mmocr/models/textdet/module_losses/__pycache__/textsnake_module_loss.cpython-39.pyc and /dev/null differ
diff --git a/pipeline/mmocr/mmocr/models/textdet/module_losses/base.py b/pipeline/mmocr/mmocr/models/textdet/module_losses/base.py
deleted file mode 100644
index b65c5c5ec77f683ca8feaad28f8a6931458c816a..0000000000000000000000000000000000000000
--- a/pipeline/mmocr/mmocr/models/textdet/module_losses/base.py
+++ /dev/null
@@ -1,51 +0,0 @@
-# Copyright (c) OpenMMLab. All rights reserved.
-from abc import ABCMeta, abstractmethod
-from typing import Dict, Sequence, Tuple, Union
-
-import torch
-from torch import nn
-
-from mmocr.registry import MODELS
-from mmocr.utils.typing_utils import DetSampleList
-
-INPUT_TYPES = Union[torch.Tensor, Sequence[torch.Tensor], Dict]
-
-
-@MODELS.register_module()
-class BaseTextDetModuleLoss(nn.Module, metaclass=ABCMeta):
- r"""Base class for text detection module loss.
- """
-
- def __init__(self) -> None:
- super().__init__()
-
- @abstractmethod
- def forward(self,
- inputs: INPUT_TYPES,
- data_samples: DetSampleList = None) -> Dict:
- """Calculates losses from a batch of inputs and data samples. Returns a
- dict of losses.
-
- Args:
- inputs (Tensor or list[Tensor] or dict): The raw tensor outputs
- from the model.
- data_samples (list(TextDetDataSample)): Datasamples containing
- ground truth data.
-
- Returns:
- dict: A dict of losses.
- """
- pass
-
- @abstractmethod
- def get_targets(self, data_samples: DetSampleList) -> Tuple:
- """Generates loss targets from data samples. Returns a tuple of target
- tensors.
-
- Args:
- data_samples (list(TextDetDataSample)): Ground truth data samples.
-
- Returns:
- tuple: A tuple of target tensors.
- """
- pass
diff --git a/pipeline/mmocr/mmocr/models/textdet/module_losses/db_module_loss.py b/pipeline/mmocr/mmocr/models/textdet/module_losses/db_module_loss.py
deleted file mode 100644
index ba8487310f2ce9592a2fa5b8b20621b870a9fe05..0000000000000000000000000000000000000000
--- a/pipeline/mmocr/mmocr/models/textdet/module_losses/db_module_loss.py
+++ /dev/null
@@ -1,300 +0,0 @@
-# Copyright (c) OpenMMLab. All rights reserved.
-from typing import Dict, List, Sequence, Tuple, Union
-
-import cv2
-import numpy as np
-import torch
-from mmdet.models.utils import multi_apply
-from shapely.geometry import Polygon
-from torch import Tensor
-
-from mmocr.registry import MODELS
-from mmocr.structures import TextDetDataSample
-from mmocr.utils import offset_polygon
-from mmocr.utils.typing_utils import ArrayLike
-from .seg_based_module_loss import SegBasedModuleLoss
-
-
-@MODELS.register_module()
-class DBModuleLoss(SegBasedModuleLoss):
- r"""The class for implementing DBNet loss.
-
- This is partially adapted from https://github.com/MhLiao/DB.
-
- Args:
- loss_prob (dict): The loss config for probability map. Defaults to
- dict(type='MaskedBalancedBCEWithLogitsLoss').
- loss_thr (dict): The loss config for threshold map. Defaults to
- dict(type='MaskedSmoothL1Loss', beta=0).
- loss_db (dict): The loss config for binary map. Defaults to
- dict(type='MaskedDiceLoss').
- weight_prob (float): The weight of probability map loss.
- Denoted as :math:`\alpha` in paper. Defaults to 5.
- weight_thr (float): The weight of threshold map loss.
- Denoted as :math:`\beta` in paper. Defaults to 10.
- shrink_ratio (float): The ratio of shrunk text region. Defaults to 0.4.
- thr_min (float): The minimum threshold map value. Defaults to 0.3.
- thr_max (float): The maximum threshold map value. Defaults to 0.7.
- min_sidelength (int or float): The minimum sidelength of the
- minimum rotated rectangle around any text region. Defaults to 8.
- """
-
- def __init__(self,
- loss_prob: Dict = dict(
- type='MaskedBalancedBCEWithLogitsLoss'),
- loss_thr: Dict = dict(type='MaskedSmoothL1Loss', beta=0),
- loss_db: Dict = dict(type='MaskedDiceLoss'),
- weight_prob: float = 5.,
- weight_thr: float = 10.,
- shrink_ratio: float = 0.4,
- thr_min: float = 0.3,
- thr_max: float = 0.7,
- min_sidelength: Union[int, float] = 8) -> None:
- super().__init__()
- self.loss_prob = MODELS.build(loss_prob)
- self.loss_thr = MODELS.build(loss_thr)
- self.loss_db = MODELS.build(loss_db)
- self.weight_prob = weight_prob
- self.weight_thr = weight_thr
- self.shrink_ratio = shrink_ratio
- self.thr_min = thr_min
- self.thr_max = thr_max
- self.min_sidelength = min_sidelength
-
- def forward(self, preds: Tuple[Tensor, Tensor, Tensor],
- data_samples: Sequence[TextDetDataSample]) -> Dict:
- """Compute DBNet loss.
-
- Args:
- preds (tuple(tensor)): Raw predictions from model, containing
- ``prob_logits``, ``thr_map`` and ``binary_map``.
- Each is a tensor of shape :math:`(N, H, W)`.
- data_samples (list[TextDetDataSample]): The data samples.
-
- Returns:
- results(dict): The dict for dbnet losses with loss_prob, \
- loss_db and loss_thr.
- """
- prob_logits, thr_map, binary_map = preds
- gt_shrinks, gt_shrink_masks, gt_thrs, gt_thr_masks = self.get_targets(
- data_samples)
- gt_shrinks = gt_shrinks.to(prob_logits.device)
- gt_shrink_masks = gt_shrink_masks.to(prob_logits.device)
- gt_thrs = gt_thrs.to(thr_map.device)
- gt_thr_masks = gt_thr_masks.to(thr_map.device)
- loss_prob = self.loss_prob(prob_logits, gt_shrinks, gt_shrink_masks)
-
- loss_thr = self.loss_thr(thr_map, gt_thrs, gt_thr_masks)
- loss_db = self.loss_db(binary_map, gt_shrinks, gt_shrink_masks)
-
- results = dict(
- loss_prob=self.weight_prob * loss_prob,
- loss_thr=self.weight_thr * loss_thr,
- loss_db=loss_db)
-
- return results
-
- def _is_poly_invalid(self, poly: np.ndarray) -> bool:
- """Check if the input polygon is invalid or not. It is invalid if its
- area is smaller than 1 or the shorter side of its minimum bounding box
- is smaller than min_sidelength.
-
- Args:
- poly (ndarray): The polygon.
-
- Returns:
- bool: Whether the polygon is invalid.
- """
- poly = poly.reshape(-1, 2)
- area = Polygon(poly).area
- if abs(area) < 1:
- return True
- rect_size = cv2.minAreaRect(poly)[1]
- len_shortest_side = min(rect_size)
- if len_shortest_side < self.min_sidelength:
- return True
-
- return False
-
- def _generate_thr_map(self, img_size: Tuple[int, int],
- polygons: ArrayLike) -> np.ndarray:
- """Generate threshold map.
-
- Args:
- img_size (tuple(int)): The image size (h, w)
- polygons (Sequence[ndarray]): 2-d array, representing all the
- polygons of the text region.
-
- Returns:
- tuple:
-
- - thr_map (ndarray): The generated threshold map.
- - thr_mask (ndarray): The effective mask of threshold map.
- """
- thr_map = np.zeros(img_size, dtype=np.float32)
- thr_mask = np.zeros(img_size, dtype=np.uint8)
-
- for polygon in polygons:
- self._draw_border_map(polygon, thr_map, mask=thr_mask)
- thr_map = thr_map * (self.thr_max - self.thr_min) + self.thr_min
-
- return thr_map, thr_mask
-
- def _draw_border_map(self, polygon: np.ndarray, canvas: np.ndarray,
- mask: np.ndarray) -> None:
- """Generate threshold map for one polygon.
-
- Args:
- polygon (np.ndarray): The polygon.
- canvas (np.ndarray): The generated threshold map.
- mask (np.ndarray): The generated threshold mask.
- """
-
- polygon = polygon.reshape(-1, 2)
- polygon_obj = Polygon(polygon)
- distance = (
- polygon_obj.area * (1 - np.power(self.shrink_ratio, 2)) /
- polygon_obj.length)
- expanded_polygon = offset_polygon(polygon, distance)
- if len(expanded_polygon) == 0:
- print(f'Padding {polygon} with {distance} gets {expanded_polygon}')
- expanded_polygon = polygon.copy().astype(np.int32)
- else:
- expanded_polygon = expanded_polygon.reshape(-1, 2).astype(np.int32)
-
- x_min = expanded_polygon[:, 0].min()
- x_max = expanded_polygon[:, 0].max()
- y_min = expanded_polygon[:, 1].min()
- y_max = expanded_polygon[:, 1].max()
-
- width = x_max - x_min + 1
- height = y_max - y_min + 1
-
- polygon[:, 0] = polygon[:, 0] - x_min
- polygon[:, 1] = polygon[:, 1] - y_min
-
- xs = np.broadcast_to(
- np.linspace(0, width - 1, num=width).reshape(1, width),
- (height, width))
- ys = np.broadcast_to(
- np.linspace(0, height - 1, num=height).reshape(height, 1),
- (height, width))
-
- distance_map = np.zeros((polygon.shape[0], height, width),
- dtype=np.float32)
- for i in range(polygon.shape[0]):
- j = (i + 1) % polygon.shape[0]
- absolute_distance = self._dist_points2line(xs, ys, polygon[i],
- polygon[j])
- distance_map[i] = np.clip(absolute_distance / distance, 0, 1)
- distance_map = distance_map.min(axis=0)
-
- x_min_valid = min(max(0, x_min), canvas.shape[1] - 1)
- x_max_valid = min(max(0, x_max), canvas.shape[1] - 1)
- y_min_valid = min(max(0, y_min), canvas.shape[0] - 1)
- y_max_valid = min(max(0, y_max), canvas.shape[0] - 1)
-
- if x_min_valid - x_min >= width or y_min_valid - y_min >= height:
- return
-
- cv2.fillPoly(mask, [expanded_polygon.astype(np.int32)], 1.0)
- canvas[y_min_valid:y_max_valid + 1,
- x_min_valid:x_max_valid + 1] = np.fmax(
- 1 - distance_map[y_min_valid - y_min:y_max_valid - y_max +
- height, x_min_valid - x_min:x_max_valid -
- x_max + width],
- canvas[y_min_valid:y_max_valid + 1,
- x_min_valid:x_max_valid + 1])
-
- def get_targets(self, data_samples: List[TextDetDataSample]) -> Tuple:
- """Generate loss targets from data samples.
-
- Args:
- data_samples (list(TextDetDataSample)): Ground truth data samples.
-
- Returns:
- tuple: A tuple of four tensors as DBNet targets.
- """
-
- gt_shrinks, gt_shrink_masks, gt_thrs, gt_thr_masks = multi_apply(
- self._get_target_single, data_samples)
- gt_shrinks = torch.cat(gt_shrinks)
- gt_shrink_masks = torch.cat(gt_shrink_masks)
- gt_thrs = torch.cat(gt_thrs)
- gt_thr_masks = torch.cat(gt_thr_masks)
- return gt_shrinks, gt_shrink_masks, gt_thrs, gt_thr_masks
-
- def _get_target_single(self, data_sample: TextDetDataSample) -> Tuple:
- """Generate loss target from a data sample.
-
- Args:
- data_sample (TextDetDataSample): The data sample.
-
- Returns:
- tuple: A tuple of four tensors as the targets of one prediction.
- """
-
- gt_instances = data_sample.gt_instances
- ignore_flags = gt_instances.ignored
- for idx, polygon in enumerate(gt_instances.polygons):
- if self._is_poly_invalid(polygon):
- ignore_flags[idx] = True
- gt_shrink, ignore_flags = self._generate_kernels(
- data_sample.img_shape,
- gt_instances.polygons,
- self.shrink_ratio,
- ignore_flags=ignore_flags)
-
- # Get boolean mask where Trues indicate text instance pixels
- gt_shrink = gt_shrink > 0
-
- gt_shrink_mask = self._generate_effective_mask(
- data_sample.img_shape, gt_instances[ignore_flags].polygons)
- gt_thr, gt_thr_mask = self._generate_thr_map(
- data_sample.img_shape, gt_instances[~ignore_flags].polygons)
-
- # to_tensor
- gt_shrink = torch.from_numpy(gt_shrink).unsqueeze(0).float()
- gt_shrink_mask = torch.from_numpy(gt_shrink_mask).unsqueeze(0).float()
- gt_thr = torch.from_numpy(gt_thr).unsqueeze(0).float()
- gt_thr_mask = torch.from_numpy(gt_thr_mask).unsqueeze(0).float()
- return gt_shrink, gt_shrink_mask, gt_thr, gt_thr_mask
-
- @staticmethod
- def _dist_points2line(xs: np.ndarray, ys: np.ndarray, pt1: np.ndarray,
- pt2: np.ndarray) -> np.ndarray:
- """Compute distances from points to a line. This is adapted from
- https://github.com/MhLiao/DB.
-
- Args:
- xs (ndarray): The x coordinates of points of size :math:`(N, )`.
- ys (ndarray): The y coordinates of size :math:`(N, )`.
- pt1 (ndarray): The first point on the line of size :math:`(2, )`.
- pt2 (ndarray): The second point on the line of size :math:`(2, )`.
-
- Returns:
- ndarray: The distance matrix of size :math:`(N, )`.
- """
- # suppose a triangle with three edge abc with c=point_1 point_2
- # a^2
- a_square = np.square(xs - pt1[0]) + np.square(ys - pt1[1])
- # b^2
- b_square = np.square(xs - pt2[0]) + np.square(ys - pt2[1])
- # c^2
- c_square = np.square(pt1[0] - pt2[0]) + np.square(pt1[1] - pt2[1])
- # -cosC=(c^2-a^2-b^2)/2(ab)
- neg_cos_c = (
- (c_square - a_square - b_square) /
- (np.finfo(np.float32).eps + 2 * np.sqrt(a_square * b_square)))
- # clip -cosC value to [-1, 1]
- neg_cos_c = np.clip(neg_cos_c, -1.0, 1.0)
- # sinC^2=1-cosC^2
- square_sin = 1 - np.square(neg_cos_c)
- square_sin = np.nan_to_num(square_sin)
- # distance=a*b*sinC/c=a*h/c=2*area/c
- result = np.sqrt(a_square * b_square * square_sin /
- (np.finfo(np.float32).eps + c_square))
- # set result to minimum edge if C`_.
-
- Args:
- ohem_ratio (float): The negative/positive ratio in ohem. Defaults to
- 3.0.
- downsample_ratio (float): Downsample ratio. Defaults to 1.0. TODO:
- remove it.
- orientation_thr (float): The threshold for distinguishing between
- head edge and tail edge among the horizontal and vertical edges
- of a quadrangle. Defaults to 2.0.
- resample_step (float): The step size for resampling the text center
- line. Defaults to 8.0.
- num_min_comps (int): The minimum number of text components, which
- should be larger than k_hop1 mentioned in paper. Defaults to 9.
- num_max_comps (int): The maximum number of text components. Defaults
- to 600.
- min_width (float): The minimum width of text components. Defaults to
- 8.0.
- max_width (float): The maximum width of text components. Defaults to
- 24.0.
- center_region_shrink_ratio (float): The shrink ratio of text center
- regions. Defaults to 0.3.
- comp_shrink_ratio (float): The shrink ratio of text components.
- Defaults to 1.0.
- comp_w_h_ratio (float): The width to height ratio of text components.
- Defaults to 0.3.
- min_rand_half_height(float): The minimum half-height of random text
- components. Defaults to 8.0.
- max_rand_half_height (float): The maximum half-height of random
- text components. Defaults to 24.0.
- jitter_level (float): The jitter level of text component geometric
- features. Defaults to 0.2.
- loss_text (dict): The loss config used to calculate the text loss.
- Defaults to ``dict(type='MaskedBalancedBCEWithLogitsLoss',
- fallback_negative_num=100, eps=1e-5)``.
- loss_center (dict): The loss config used to calculate the center loss.
- Defaults to ``dict(type='MaskedBCEWithLogitsLoss')``.
- loss_top (dict): The loss config used to calculate the top loss, which
- is a part of the height loss. Defaults to
- ``dict(type='SmoothL1Loss', reduction='none')``.
- loss_btm (dict): The loss config used to calculate the bottom loss,
- which is a part of the height loss. Defaults to
- ``dict(type='SmoothL1Loss', reduction='none')``.
- loss_sin (dict): The loss config used to calculate the sin loss.
- Defaults to ``dict(type='MaskedSmoothL1Loss')``.
- loss_cos (dict): The loss config used to calculate the cos loss.
- Defaults to ``dict(type='MaskedSmoothL1Loss')``.
- loss_gcn (dict): The loss config used to calculate the GCN loss.
- Defaults to ``dict(type='CrossEntropyLoss')``.
- """
-
- def __init__(
- self,
- ohem_ratio: float = 3.0,
- downsample_ratio: float = 1.0,
- orientation_thr: float = 2.0,
- resample_step: float = 8.0,
- num_min_comps: int = 9,
- num_max_comps: int = 600,
- min_width: float = 8.0,
- max_width: float = 24.0,
- center_region_shrink_ratio: float = 0.3,
- comp_shrink_ratio: float = 1.0,
- comp_w_h_ratio: float = 0.3,
- text_comp_nms_thr: float = 0.25,
- min_rand_half_height: float = 8.0,
- max_rand_half_height: float = 24.0,
- jitter_level: float = 0.2,
- loss_text: Dict = dict(
- type='MaskedBalancedBCEWithLogitsLoss',
- fallback_negative_num=100,
- eps=1e-5),
- loss_center: Dict = dict(type='MaskedBCEWithLogitsLoss'),
- loss_top: Dict = dict(type='SmoothL1Loss', reduction='none'),
- loss_btm: Dict = dict(type='SmoothL1Loss', reduction='none'),
- loss_sin: Dict = dict(type='MaskedSmoothL1Loss'),
- loss_cos: Dict = dict(type='MaskedSmoothL1Loss'),
- loss_gcn: Dict = dict(type='CrossEntropyLoss')
- ) -> None:
- super().__init__()
- self.ohem_ratio = ohem_ratio
- self.downsample_ratio = downsample_ratio
- self.orientation_thr = orientation_thr
- self.resample_step = resample_step
- self.num_max_comps = num_max_comps
- self.num_min_comps = num_min_comps
- self.min_width = min_width
- self.max_width = max_width
- self.center_region_shrink_ratio = center_region_shrink_ratio
- self.comp_shrink_ratio = comp_shrink_ratio
- self.comp_w_h_ratio = comp_w_h_ratio
- self.text_comp_nms_thr = text_comp_nms_thr
- self.min_rand_half_height = min_rand_half_height
- self.max_rand_half_height = max_rand_half_height
- self.jitter_level = jitter_level
- self.loss_text = MODELS.build(loss_text)
- self.loss_center = MODELS.build(loss_center)
- self.loss_top = MODELS.build(loss_top)
- self.loss_btm = MODELS.build(loss_btm)
- self.loss_sin = MODELS.build(loss_sin)
- self.loss_cos = MODELS.build(loss_cos)
- self.loss_gcn = MODELS.build(loss_gcn)
-
- def forward(self, preds: Tuple[Tensor, Tensor, Tensor],
- data_samples: Sequence[TextDetDataSample]) -> Dict:
- """Compute Drrg loss.
-
- Args:
- preds (tuple): The prediction
- tuple(pred_maps, gcn_pred, gt_labels), each of shape
- :math:`(N, 6, H, W)`, :math:`(N, 2)` and :math:`(m ,n)`, where
- :math:`m * n = N`.
- data_samples (list[TextDetDataSample]): The data samples.
-
- Returns:
- dict: A loss dict with ``loss_text``, ``loss_center``,
- ``loss_height``, ``loss_sin``, ``loss_cos``, and ``loss_gcn``.
- """
- assert isinstance(preds, tuple)
-
- (gt_text_masks, gt_center_region_masks, gt_masks, gt_top_height_maps,
- gt_bot_height_maps, gt_sin_maps, gt_cos_maps,
- _) = self.get_targets(data_samples)
- pred_maps, gcn_pred, gt_labels = preds
- pred_text_region = pred_maps[:, 0, :, :]
- pred_center_region = pred_maps[:, 1, :, :]
- pred_sin_map = pred_maps[:, 2, :, :]
- pred_cos_map = pred_maps[:, 3, :, :]
- pred_top_height_map = pred_maps[:, 4, :, :]
- pred_bot_height_map = pred_maps[:, 5, :, :]
- feature_sz = pred_maps.size()
- device = pred_maps.device
-
- # bitmask 2 tensor
- mapping = {
- 'gt_text_masks': gt_text_masks,
- 'gt_center_region_masks': gt_center_region_masks,
- 'gt_masks': gt_masks,
- 'gt_top_height_maps': gt_top_height_maps,
- 'gt_bot_height_maps': gt_bot_height_maps,
- 'gt_sin_maps': gt_sin_maps,
- 'gt_cos_maps': gt_cos_maps
- }
- gt = {}
- for key, value in mapping.items():
- gt[key] = value
- if abs(self.downsample_ratio - 1.0) < 1e-2:
- gt[key] = self._batch_pad(gt[key], feature_sz[2:])
- else:
- gt[key] = [
- imrescale(
- mask,
- scale=self.downsample_ratio,
- interpolation='nearest') for mask in gt[key]
- ]
- gt[key] = self._batch_pad(gt[key], feature_sz[2:])
- if key in ['gt_top_height_maps', 'gt_bot_height_maps']:
- gt[key] *= self.downsample_ratio
- gt[key] = torch.from_numpy(gt[key]).float().to(device)
-
- scale = torch.sqrt(1.0 / (pred_sin_map**2 + pred_cos_map**2 + 1e-8))
- pred_sin_map = pred_sin_map * scale
- pred_cos_map = pred_cos_map * scale
-
- loss_text = self.loss_text(pred_text_region, gt['gt_text_masks'],
- gt['gt_masks'])
-
- text_mask = (gt['gt_text_masks'] * gt['gt_masks']).float()
- negative_text_mask = ((1 - gt['gt_text_masks']) *
- gt['gt_masks']).float()
- loss_center_positive = self.loss_center(pred_center_region,
- gt['gt_center_region_masks'],
- text_mask)
- loss_center_negative = self.loss_center(pred_center_region,
- gt['gt_center_region_masks'],
- negative_text_mask)
- loss_center = loss_center_positive + 0.5 * loss_center_negative
-
- center_mask = (gt['gt_center_region_masks'] * gt['gt_masks']).float()
- map_sz = pred_top_height_map.size()
- ones = torch.ones(map_sz, dtype=torch.float, device=device)
- loss_top = self.loss_top(
- pred_top_height_map / (gt['gt_top_height_maps'] + 1e-2), ones)
- loss_btm = self.loss_btm(
- pred_bot_height_map / (gt['gt_bot_height_maps'] + 1e-2), ones)
- gt_height = gt['gt_top_height_maps'] + gt['gt_bot_height_maps']
- loss_height = torch.sum((torch.log(gt_height + 1) *
- (loss_top + loss_btm)) * center_mask) / (
- torch.sum(center_mask) + 1e-6)
-
- loss_sin = self.loss_sin(pred_sin_map, gt['gt_sin_maps'], center_mask)
- loss_cos = self.loss_cos(pred_cos_map, gt['gt_cos_maps'], center_mask)
-
- loss_gcn = self.loss_gcn(gcn_pred,
- gt_labels.view(-1).to(gcn_pred.device))
-
- results = dict(
- loss_text=loss_text,
- loss_center=loss_center,
- loss_height=loss_height,
- loss_sin=loss_sin,
- loss_cos=loss_cos,
- loss_gcn=loss_gcn)
-
- return results
-
- def get_targets(self, data_samples: List[TextDetDataSample]) -> Tuple:
- """Generate loss targets from data samples.
-
- Args:
- data_samples (list(TextDetDataSample)): Ground truth data samples.
-
- Returns:
- tuple: A tuple of 8 lists of tensors as DRRG targets. Read
- docstring of ``_get_target_single`` for more details.
- """
-
- # If data_samples points to same object as self.cached_data_samples, it
- # means that get_targets is called more than once in the same train
- # iteration, and pre-computed targets can be reused.
- if hasattr(self, 'targets') and \
- self.cache_data_samples is data_samples:
- return self.targets
-
- self.cache_data_samples = data_samples
- self.targets = multi_apply(self._get_target_single, data_samples)
- return self.targets
-
- def _get_target_single(self, data_sample: TextDetDataSample) -> Tuple:
- """Generate loss target from a data sample.
-
- Args:
- data_sample (TextDetDataSample): The data sample.
-
- Returns:
- tuple: A tuple of 8 tensors as DRRG targets.
-
- - gt_text_mask (ndarray): The text region mask.
- - gt_center_region_mask (ndarray): The text center region mask.
- - gt_mask (ndarray): The effective mask.
- - gt_top_height_map (ndarray): The map on which the distance from
- points to top side lines will be drawn for each pixel in text
- center regions.
- - gt_bot_height_map (ndarray): The map on which the distance from
- points to bottom side lines will be drawn for each pixel in text
- center regions.
- - gt_sin_map (ndarray): The sin(theta) map where theta is the angle
- between vector (top point - bottom point) and vector (1, 0).
- - gt_cos_map (ndarray): The cos(theta) map where theta is the angle
- between vector (top point - bottom point) and vector (1, 0).
- - gt_comp_attribs (ndarray): The padded text component attributes
- of a fixed size. Shape: (num_component, 8).
- """
-
- gt_instances = data_sample.gt_instances
- ignore_flags = gt_instances.ignored
-
- polygons = gt_instances[~ignore_flags].polygons
- ignored_polygons = gt_instances[ignore_flags].polygons
- h, w = data_sample.img_shape
-
- gt_text_mask = self._generate_text_region_mask((h, w), polygons)
- gt_mask = self._generate_effective_mask((h, w), ignored_polygons)
- (center_lines, gt_center_region_mask, gt_top_height_map,
- gt_bot_height_map, gt_sin_map,
- gt_cos_map) = self._generate_center_mask_attrib_maps((h, w), polygons)
-
- gt_comp_attribs = self._generate_comp_attribs(center_lines,
- gt_text_mask,
- gt_center_region_mask,
- gt_top_height_map,
- gt_bot_height_map,
- gt_sin_map, gt_cos_map)
-
- return (gt_text_mask, gt_center_region_mask, gt_mask,
- gt_top_height_map, gt_bot_height_map, gt_sin_map, gt_cos_map,
- gt_comp_attribs)
-
- def _generate_center_mask_attrib_maps(self, img_size: Tuple[int, int],
- text_polys: List[ndarray]) -> Tuple:
- """Generate text center region masks and geometric attribute maps.
-
- Args:
- img_size (tuple(int, int)): The image size (height, width).
- text_polys (list[ndarray]): The list of text polygons.
-
- Returns:
- tuple(center_lines, center_region_mask, top_height_map,
- bot_height_map,sin_map, cos_map):
-
- center_lines (list[ndarray]): The list of text center lines.
- center_region_mask (ndarray): The text center region mask.
- top_height_map (ndarray): The map on which the distance from points
- to top side lines will be drawn for each pixel in text center
- regions.
- bot_height_map (ndarray): The map on which the distance from points
- to bottom side lines will be drawn for each pixel in text
- center regions.
- sin_map (ndarray): The sin(theta) map where theta is the angle
- between vector (top point - bottom point) and vector (1, 0).
- cos_map (ndarray): The cos(theta) map where theta is the angle
- between vector (top point - bottom point) and vector (1, 0).
- """
-
- assert isinstance(img_size, tuple)
- assert check_argument.is_type_list(text_polys, ndarray)
-
- h, w = img_size
-
- center_lines = []
- center_region_mask = np.zeros((h, w), np.uint8)
- top_height_map = np.zeros((h, w), dtype=np.float32)
- bot_height_map = np.zeros((h, w), dtype=np.float32)
- sin_map = np.zeros((h, w), dtype=np.float32)
- cos_map = np.zeros((h, w), dtype=np.float32)
-
- for poly in text_polys:
- polygon_points = poly.reshape(-1, 2)
- _, _, top_line, bot_line = self._reorder_poly_edge(polygon_points)
- resampled_top_line, resampled_bot_line = self._resample_sidelines(
- top_line, bot_line, self.resample_step)
- resampled_bot_line = resampled_bot_line[::-1]
- center_line = (resampled_top_line + resampled_bot_line) / 2
-
- if self.vector_slope(center_line[-1] - center_line[0]) > 2:
- if (center_line[-1] - center_line[0])[1] < 0:
- center_line = center_line[::-1]
- resampled_top_line = resampled_top_line[::-1]
- resampled_bot_line = resampled_bot_line[::-1]
- else:
- if (center_line[-1] - center_line[0])[0] < 0:
- center_line = center_line[::-1]
- resampled_top_line = resampled_top_line[::-1]
- resampled_bot_line = resampled_bot_line[::-1]
-
- line_head_shrink_len = np.clip(
- (norm(top_line[0] - bot_line[0]) * self.comp_w_h_ratio),
- self.min_width, self.max_width) / 2
- line_tail_shrink_len = np.clip(
- (norm(top_line[-1] - bot_line[-1]) * self.comp_w_h_ratio),
- self.min_width, self.max_width) / 2
- num_head_shrink = int(line_head_shrink_len // self.resample_step)
- num_tail_shrink = int(line_tail_shrink_len // self.resample_step)
- if len(center_line) > num_head_shrink + num_tail_shrink + 2:
- center_line = center_line[num_head_shrink:len(center_line) -
- num_tail_shrink]
- resampled_top_line = resampled_top_line[
- num_head_shrink:len(resampled_top_line) - num_tail_shrink]
- resampled_bot_line = resampled_bot_line[
- num_head_shrink:len(resampled_bot_line) - num_tail_shrink]
- center_lines.append(center_line.astype(np.int32))
-
- self._draw_center_region_maps(resampled_top_line,
- resampled_bot_line, center_line,
- center_region_mask, top_height_map,
- bot_height_map, sin_map, cos_map,
- self.center_region_shrink_ratio)
-
- return (center_lines, center_region_mask, top_height_map,
- bot_height_map, sin_map, cos_map)
-
- def _generate_comp_attribs(self, center_lines: List[ndarray],
- text_mask: ndarray, center_region_mask: ndarray,
- top_height_map: ndarray,
- bot_height_map: ndarray, sin_map: ndarray,
- cos_map: ndarray) -> ndarray:
- """Generate text component attributes.
-
- Args:
- center_lines (list[ndarray]): The list of text center lines .
- text_mask (ndarray): The text region mask.
- center_region_mask (ndarray): The text center region mask.
- top_height_map (ndarray): The map on which the distance from points
- to top side lines will be drawn for each pixel in text center
- regions.
- bot_height_map (ndarray): The map on which the distance from points
- to bottom side lines will be drawn for each pixel in text
- center regions.
- sin_map (ndarray): The sin(theta) map where theta is the angle
- between vector (top point - bottom point) and vector (1, 0).
- cos_map (ndarray): The cos(theta) map where theta is the angle
- between vector (top point - bottom point) and vector (1, 0).
-
- Returns:
- ndarray: The padded text component attributes of a fixed size.
- """
-
- assert isinstance(center_lines, list)
- assert (text_mask.shape == center_region_mask.shape ==
- top_height_map.shape == bot_height_map.shape == sin_map.shape
- == cos_map.shape)
-
- center_lines_mask = np.zeros_like(center_region_mask)
- cv2.polylines(center_lines_mask, center_lines, 0, 1, 1)
- center_lines_mask = center_lines_mask * center_region_mask
- comp_centers = np.argwhere(center_lines_mask > 0)
-
- y = comp_centers[:, 0]
- x = comp_centers[:, 1]
-
- top_height = top_height_map[y, x].reshape(
- (-1, 1)) * self.comp_shrink_ratio
- bot_height = bot_height_map[y, x].reshape(
- (-1, 1)) * self.comp_shrink_ratio
- sin = sin_map[y, x].reshape((-1, 1))
- cos = cos_map[y, x].reshape((-1, 1))
-
- top_mid_points = comp_centers + np.hstack(
- [top_height * sin, top_height * cos])
- bot_mid_points = comp_centers - np.hstack(
- [bot_height * sin, bot_height * cos])
-
- width = (top_height + bot_height) * self.comp_w_h_ratio
- width = np.clip(width, self.min_width, self.max_width)
- r = width / 2
-
- tl = top_mid_points[:, ::-1] - np.hstack([-r * sin, r * cos])
- tr = top_mid_points[:, ::-1] + np.hstack([-r * sin, r * cos])
- br = bot_mid_points[:, ::-1] + np.hstack([-r * sin, r * cos])
- bl = bot_mid_points[:, ::-1] - np.hstack([-r * sin, r * cos])
- text_comps = np.hstack([tl, tr, br, bl]).astype(np.float32)
-
- score = np.ones((text_comps.shape[0], 1), dtype=np.float32)
- text_comps = np.hstack([text_comps, score])
- if la_nms is None:
- raise ImportError('lanms-neo is not installed, '
- 'please run "pip install lanms-neo==1.0.2".')
- text_comps = la_nms(text_comps, self.text_comp_nms_thr)
-
- if text_comps.shape[0] >= 1:
- img_h, img_w = center_region_mask.shape
- text_comps[:, 0:8:2] = np.clip(text_comps[:, 0:8:2], 0, img_w - 1)
- text_comps[:, 1:8:2] = np.clip(text_comps[:, 1:8:2], 0, img_h - 1)
-
- comp_centers = np.mean(
- text_comps[:, 0:8].reshape((-1, 4, 2)),
- axis=1).astype(np.int32)
- x = comp_centers[:, 0]
- y = comp_centers[:, 1]
-
- height = (top_height_map[y, x] + bot_height_map[y, x]).reshape(
- (-1, 1))
- width = np.clip(height * self.comp_w_h_ratio, self.min_width,
- self.max_width)
-
- cos = cos_map[y, x].reshape((-1, 1))
- sin = sin_map[y, x].reshape((-1, 1))
-
- _, comp_label_mask = cv2.connectedComponents(
- center_region_mask, connectivity=8)
- comp_labels = comp_label_mask[y, x].reshape(
- (-1, 1)).astype(np.float32)
-
- x = x.reshape((-1, 1)).astype(np.float32)
- y = y.reshape((-1, 1)).astype(np.float32)
- comp_attribs = np.hstack(
- [x, y, height, width, cos, sin, comp_labels])
- comp_attribs = self._jitter_comp_attribs(comp_attribs,
- self.jitter_level)
-
- if comp_attribs.shape[0] < self.num_min_comps:
- num_rand_comps = self.num_min_comps - comp_attribs.shape[0]
- rand_comp_attribs = self._generate_rand_comp_attribs(
- num_rand_comps, 1 - text_mask)
- comp_attribs = np.vstack([comp_attribs, rand_comp_attribs])
- else:
- comp_attribs = self._generate_rand_comp_attribs(
- self.num_min_comps, 1 - text_mask)
-
- num_comps = (
- np.ones((comp_attribs.shape[0], 1), dtype=np.float32) *
- comp_attribs.shape[0])
- comp_attribs = np.hstack([num_comps, comp_attribs])
-
- if comp_attribs.shape[0] > self.num_max_comps:
- comp_attribs = comp_attribs[:self.num_max_comps, :]
- comp_attribs[:, 0] = self.num_max_comps
-
- pad_comp_attribs = np.zeros(
- (self.num_max_comps, comp_attribs.shape[1]), dtype=np.float32)
- pad_comp_attribs[:comp_attribs.shape[0], :] = comp_attribs
-
- return pad_comp_attribs
-
- def _generate_rand_comp_attribs(self, num_rand_comps: int,
- center_sample_mask: ndarray) -> ndarray:
- """Generate random text components and their attributes to ensure the
- the number of text components in an image is larger than k_hop1, which
- is the number of one hop neighbors in KNN graph.
-
- Args:
- num_rand_comps (int): The number of random text components.
- center_sample_mask (ndarray): The region mask for sampling text
- component centers .
-
- Returns:
- ndarray: The random text component attributes
- (x, y, h, w, cos, sin, comp_label=0).
- """
-
- assert isinstance(num_rand_comps, int)
- assert num_rand_comps > 0
- assert center_sample_mask.ndim == 2
-
- h, w = center_sample_mask.shape
-
- max_rand_half_height = self.max_rand_half_height
- min_rand_half_height = self.min_rand_half_height
- max_rand_height = max_rand_half_height * 2
- max_rand_width = np.clip(max_rand_height * self.comp_w_h_ratio,
- self.min_width, self.max_width)
- margin = int(
- np.sqrt((max_rand_height / 2)**2 + (max_rand_width / 2)**2)) + 1
-
- if 2 * margin + 1 > min(h, w):
-
- assert min(h, w) > (np.sqrt(2) * (self.min_width + 1))
- max_rand_half_height = max(min(h, w) / 4, self.min_width / 2 + 1)
- min_rand_half_height = max(max_rand_half_height / 4,
- self.min_width / 2)
-
- max_rand_height = max_rand_half_height * 2
- max_rand_width = np.clip(max_rand_height * self.comp_w_h_ratio,
- self.min_width, self.max_width)
- margin = int(
- np.sqrt((max_rand_height / 2)**2 +
- (max_rand_width / 2)**2)) + 1
-
- inner_center_sample_mask = np.zeros_like(center_sample_mask)
- inner_center_sample_mask[margin:h - margin, margin:w - margin] = \
- center_sample_mask[margin:h - margin, margin:w - margin]
- kernel_size = int(np.clip(max_rand_half_height, 7, 21))
- inner_center_sample_mask = cv2.erode(
- inner_center_sample_mask,
- np.ones((kernel_size, kernel_size), np.uint8))
-
- center_candidates = np.argwhere(inner_center_sample_mask > 0)
- num_center_candidates = len(center_candidates)
- sample_inds = np.random.choice(num_center_candidates, num_rand_comps)
- rand_centers = center_candidates[sample_inds]
-
- rand_top_height = np.random.randint(
- min_rand_half_height,
- max_rand_half_height,
- size=(len(rand_centers), 1))
- rand_bot_height = np.random.randint(
- min_rand_half_height,
- max_rand_half_height,
- size=(len(rand_centers), 1))
-
- rand_cos = 2 * np.random.random(size=(len(rand_centers), 1)) - 1
- rand_sin = 2 * np.random.random(size=(len(rand_centers), 1)) - 1
- scale = np.sqrt(1.0 / (rand_cos**2 + rand_sin**2 + 1e-8))
- rand_cos = rand_cos * scale
- rand_sin = rand_sin * scale
-
- height = (rand_top_height + rand_bot_height)
- width = np.clip(height * self.comp_w_h_ratio, self.min_width,
- self.max_width)
-
- rand_comp_attribs = np.hstack([
- rand_centers[:, ::-1], height, width, rand_cos, rand_sin,
- np.zeros_like(rand_sin)
- ]).astype(np.float32)
-
- return rand_comp_attribs
-
- def _jitter_comp_attribs(self, comp_attribs: ndarray,
- jitter_level: float) -> ndarray:
- """Jitter text components attributes.
-
- Args:
- comp_attribs (ndarray): The text component attributes.
- jitter_level (float): The jitter level of text components
- attributes.
-
- Returns:
- ndarray: The jittered text component
- attributes (x, y, h, w, cos, sin, comp_label).
- """
-
- assert comp_attribs.shape[1] == 7
- assert comp_attribs.shape[0] > 0
- assert isinstance(jitter_level, float)
-
- x = comp_attribs[:, 0].reshape((-1, 1))
- y = comp_attribs[:, 1].reshape((-1, 1))
- h = comp_attribs[:, 2].reshape((-1, 1))
- w = comp_attribs[:, 3].reshape((-1, 1))
- cos = comp_attribs[:, 4].reshape((-1, 1))
- sin = comp_attribs[:, 5].reshape((-1, 1))
- comp_labels = comp_attribs[:, 6].reshape((-1, 1))
-
- x += (np.random.random(size=(len(comp_attribs), 1)) -
- 0.5) * (h * np.abs(cos) + w * np.abs(sin)) * jitter_level
- y += (np.random.random(size=(len(comp_attribs), 1)) -
- 0.5) * (h * np.abs(sin) + w * np.abs(cos)) * jitter_level
-
- h += (np.random.random(size=(len(comp_attribs), 1)) -
- 0.5) * h * jitter_level
- w += (np.random.random(size=(len(comp_attribs), 1)) -
- 0.5) * w * jitter_level
-
- cos += (np.random.random(size=(len(comp_attribs), 1)) -
- 0.5) * 2 * jitter_level
- sin += (np.random.random(size=(len(comp_attribs), 1)) -
- 0.5) * 2 * jitter_level
-
- scale = np.sqrt(1.0 / (cos**2 + sin**2 + 1e-8))
- cos = cos * scale
- sin = sin * scale
-
- jittered_comp_attribs = np.hstack([x, y, h, w, cos, sin, comp_labels])
-
- return jittered_comp_attribs
-
- def _draw_center_region_maps(self, top_line: ndarray, bot_line: ndarray,
- center_line: ndarray,
- center_region_mask: ndarray,
- top_height_map: ndarray,
- bot_height_map: ndarray, sin_map: ndarray,
- cos_map: ndarray,
- region_shrink_ratio: float) -> None:
- """Draw attributes of text components on text center regions.
-
- Args:
- top_line (ndarray): The points composing the top side lines of text
- polygons.
- bot_line (ndarray): The points composing bottom side lines of text
- polygons.
- center_line (ndarray): The points composing the center lines of
- text instances.
- center_region_mask (ndarray): The text center region mask.
- top_height_map (ndarray): The map on which the distance from points
- to top side lines will be drawn for each pixel in text center
- regions.
- bot_height_map (ndarray): The map on which the distance from points
- to bottom side lines will be drawn for each pixel in text
- center regions.
- sin_map (ndarray): The map of vector_sin(top_point - bot_point)
- that will be drawn on text center regions.
- cos_map (ndarray): The map of vector_cos(top_point - bot_point)
- will be drawn on text center regions.
- region_shrink_ratio (float): The shrink ratio of text center
- regions.
- """
-
- assert top_line.shape == bot_line.shape == center_line.shape
- assert (center_region_mask.shape == top_height_map.shape ==
- bot_height_map.shape == sin_map.shape == cos_map.shape)
- assert isinstance(region_shrink_ratio, float)
-
- h, w = center_region_mask.shape
- for i in range(0, len(center_line) - 1):
-
- top_mid_point = (top_line[i] + top_line[i + 1]) / 2
- bot_mid_point = (bot_line[i] + bot_line[i + 1]) / 2
-
- sin_theta = self.vector_sin(top_mid_point - bot_mid_point)
- cos_theta = self.vector_cos(top_mid_point - bot_mid_point)
-
- tl = center_line[i] + (top_line[i] -
- center_line[i]) * region_shrink_ratio
- tr = center_line[i + 1] + (
- top_line[i + 1] - center_line[i + 1]) * region_shrink_ratio
- br = center_line[i + 1] + (
- bot_line[i + 1] - center_line[i + 1]) * region_shrink_ratio
- bl = center_line[i] + (bot_line[i] -
- center_line[i]) * region_shrink_ratio
- current_center_box = np.vstack([tl, tr, br, bl]).astype(np.int32)
-
- cv2.fillPoly(center_region_mask, [current_center_box], color=1)
- cv2.fillPoly(sin_map, [current_center_box], color=sin_theta)
- cv2.fillPoly(cos_map, [current_center_box], color=cos_theta)
-
- current_center_box[:, 0] = np.clip(current_center_box[:, 0], 0,
- w - 1)
- current_center_box[:, 1] = np.clip(current_center_box[:, 1], 0,
- h - 1)
- min_coord = np.min(current_center_box, axis=0).astype(np.int32)
- max_coord = np.max(current_center_box, axis=0).astype(np.int32)
- current_center_box = current_center_box - min_coord
- box_sz = (max_coord - min_coord + 1)
-
- center_box_mask = np.zeros((box_sz[1], box_sz[0]), dtype=np.uint8)
- cv2.fillPoly(center_box_mask, [current_center_box], color=1)
-
- inds = np.argwhere(center_box_mask > 0)
- inds = inds + (min_coord[1], min_coord[0])
- inds_xy = np.fliplr(inds)
- top_height_map[(inds[:, 0], inds[:, 1])] = self._dist_point2line(
- inds_xy, (top_line[i], top_line[i + 1]))
- bot_height_map[(inds[:, 0], inds[:, 1])] = self._dist_point2line(
- inds_xy, (bot_line[i], bot_line[i + 1]))
-
- def _dist_point2line(self, point: ndarray,
- line: Tuple[ndarray, ndarray]) -> ndarray:
- """Calculate the distance from points to a line.
-
- TODO: Check its mergibility with the one in mmocr.utils.point_utils.
- """
-
- assert isinstance(line, tuple)
- point1, point2 = line
- d = abs(np.cross(point2 - point1, point - point1)) / (
- norm(point2 - point1) + 1e-8)
- return d
diff --git a/pipeline/mmocr/mmocr/models/textdet/module_losses/fce_module_loss.py b/pipeline/mmocr/mmocr/models/textdet/module_losses/fce_module_loss.py
deleted file mode 100644
index c833c17787c3584605f83b04b0394e10ac7f14d5..0000000000000000000000000000000000000000
--- a/pipeline/mmocr/mmocr/models/textdet/module_losses/fce_module_loss.py
+++ /dev/null
@@ -1,563 +0,0 @@
-# Copyright (c) OpenMMLab. All rights reserved.
-from typing import Dict, List, Optional, Sequence, Tuple, Union
-
-import cv2
-import numpy as np
-import torch
-from mmdet.models.utils import multi_apply
-from numpy.fft import fft
-from numpy.linalg import norm
-
-from mmocr.registry import MODELS
-from mmocr.structures import TextDetDataSample
-from mmocr.utils.typing_utils import ArrayLike
-from .textsnake_module_loss import TextSnakeModuleLoss
-
-
-@MODELS.register_module()
-class FCEModuleLoss(TextSnakeModuleLoss):
- """The class for implementing FCENet loss.
-
- FCENet(CVPR2021): `Fourier Contour Embedding for Arbitrary-shaped Text
- Detection `_
-
- Args:
- fourier_degree (int) : The maximum Fourier transform degree k.
- num_sample (int) : The sampling points number of regression
- loss. If it is too small, fcenet tends to be overfitting.
- negative_ratio (float or int): Maximum ratio of negative
- samples to positive ones in OHEM. Defaults to 3.
- resample_step (float): The step size for resampling the text center
- line (TCL). It's better not to exceed half of the minimum width.
- center_region_shrink_ratio (float): The shrink ratio of text center
- region.
- level_size_divisors (tuple(int)): The downsample ratio on each level.
- level_proportion_range (tuple(tuple(int))): The range of text sizes
- assigned to each level.
- loss_tr (dict) : The loss config used to calculate the text region
- loss. Defaults to dict(type='MaskedBalancedBCELoss').
- loss_tcl (dict) : The loss config used to calculate the text center
- line loss. Defaults to dict(type='MaskedBCELoss').
- loss_reg_x (dict) : The loss config used to calculate the regression
- loss on x axis. Defaults to dict(type='MaskedSmoothL1Loss').
- loss_reg_y (dict) : The loss config used to calculate the regression
- loss on y axis. Defaults to dict(type='MaskedSmoothL1Loss').
- """
-
- def __init__(
- self,
- fourier_degree: int,
- num_sample: int,
- negative_ratio: Union[float, int] = 3.,
- resample_step: float = 4.0,
- center_region_shrink_ratio: float = 0.3,
- level_size_divisors: Tuple[int] = (8, 16, 32),
- level_proportion_range: Tuple[Tuple[int]] = ((0, 0.4), (0.3, 0.7),
- (0.6, 1.0)),
- loss_tr: Dict = dict(type='MaskedBalancedBCELoss'),
- loss_tcl: Dict = dict(type='MaskedBCELoss'),
- loss_reg_x: Dict = dict(type='SmoothL1Loss', reduction='none'),
- loss_reg_y: Dict = dict(type='SmoothL1Loss', reduction='none'),
- ) -> None:
- super().__init__()
- self.fourier_degree = fourier_degree
- self.num_sample = num_sample
- self.resample_step = resample_step
- self.center_region_shrink_ratio = center_region_shrink_ratio
- self.level_size_divisors = level_size_divisors
- self.level_proportion_range = level_proportion_range
-
- loss_tr.update(negative_ratio=negative_ratio)
- self.loss_tr = MODELS.build(loss_tr)
- self.loss_tcl = MODELS.build(loss_tcl)
- self.loss_reg_x = MODELS.build(loss_reg_x)
- self.loss_reg_y = MODELS.build(loss_reg_y)
-
- def forward(self, preds: Sequence[Dict],
- data_samples: Sequence[TextDetDataSample]) -> Dict:
- """Compute FCENet loss.
-
- Args:
- preds (list[dict]): A list of dict with keys of ``cls_res``,
- ``reg_res`` corresponds to the classification result and
- regression result computed from the input tensor with the
- same index. They have the shapes of :math:`(N, C_{cls,i}, H_i,
- W_i)` and :math: `(N, C_{out,i}, H_i, W_i)`.
- data_samples (list[TextDetDataSample]): The data samples.
-
- Returns:
- dict: The dict for fcenet losses with loss_text, loss_center,
- loss_reg_x and loss_reg_y.
- """
- assert isinstance(preds, list) and len(preds) == 3
- p3_maps, p4_maps, p5_maps = self.get_targets(data_samples)
- device = preds[0]['cls_res'].device
- # to device
- gts = [p3_maps.to(device), p4_maps.to(device), p5_maps.to(device)]
-
- losses = multi_apply(self.forward_single, preds, gts)
-
- loss_tr = torch.tensor(0., device=device).float()
- loss_tcl = torch.tensor(0., device=device).float()
- loss_reg_x = torch.tensor(0., device=device).float()
- loss_reg_y = torch.tensor(0., device=device).float()
-
- for idx, loss in enumerate(losses):
- if idx == 0:
- loss_tr += sum(loss)
- elif idx == 1:
- loss_tcl += sum(loss)
- elif idx == 2:
- loss_reg_x += sum(loss)
- else:
- loss_reg_y += sum(loss)
-
- results = dict(
- loss_text=loss_tr,
- loss_center=loss_tcl,
- loss_reg_x=loss_reg_x,
- loss_reg_y=loss_reg_y,
- )
-
- return results
-
- def forward_single(self, pred: torch.Tensor,
- gt: torch.Tensor) -> Sequence[torch.Tensor]:
- """Compute loss for one feature level.
-
- Args:
- pred (dict): A dict with keys ``cls_res`` and ``reg_res``
- corresponds to the classification result and regression result
- from one feature level.
- gt (Tensor): Ground truth for one feature level. Cls and reg
- targets are concatenated along the channel dimension.
-
- Returns:
- list[Tensor]: A list of losses for each feature level.
- """
- assert isinstance(pred, dict) and isinstance(gt, torch.Tensor)
- cls_pred = pred['cls_res'].permute(0, 2, 3, 1).contiguous()
- reg_pred = pred['reg_res'].permute(0, 2, 3, 1).contiguous()
-
- gt = gt.permute(0, 2, 3, 1).contiguous()
-
- k = 2 * self.fourier_degree + 1
- tr_pred = cls_pred[:, :, :, :2].view(-1, 2)
- tcl_pred = cls_pred[:, :, :, 2:].view(-1, 2)
- x_pred = reg_pred[:, :, :, 0:k].view(-1, k)
- y_pred = reg_pred[:, :, :, k:2 * k].view(-1, k)
-
- tr_mask = gt[:, :, :, :1].view(-1)
- tcl_mask = gt[:, :, :, 1:2].view(-1)
- train_mask = gt[:, :, :, 2:3].view(-1)
- x_map = gt[:, :, :, 3:3 + k].view(-1, k)
- y_map = gt[:, :, :, 3 + k:].view(-1, k)
-
- tr_train_mask = (train_mask * tr_mask).float()
- # text region loss
- loss_tr = self.loss_tr(tr_pred.softmax(-1)[:, 1], tr_mask, train_mask)
-
- # text center line loss
- tr_neg_mask = 1 - tr_train_mask
- loss_tcl_positive = self.loss_center(
- tcl_pred.softmax(-1)[:, 1], tcl_mask, tr_train_mask)
- loss_tcl_negative = self.loss_center(
- tcl_pred.softmax(-1)[:, 1], tcl_mask, tr_neg_mask)
- loss_tcl = loss_tcl_positive + 0.5 * loss_tcl_negative
-
- # regression loss
- loss_reg_x = torch.tensor(0.).float().to(x_pred.device)
- loss_reg_y = torch.tensor(0.).float().to(x_pred.device)
- if tr_train_mask.sum().item() > 0:
- weight = (tr_mask[tr_train_mask.bool()].float() +
- tcl_mask[tr_train_mask.bool()].float()) / 2
- weight = weight.contiguous().view(-1, 1)
-
- ft_x, ft_y = self._fourier2poly(x_map, y_map)
- ft_x_pre, ft_y_pre = self._fourier2poly(x_pred, y_pred)
-
- loss_reg_x = torch.mean(weight * self.loss_reg_x(
- ft_x_pre[tr_train_mask.bool()], ft_x[tr_train_mask.bool()]))
- loss_reg_y = torch.mean(weight * self.loss_reg_x(
- ft_y_pre[tr_train_mask.bool()], ft_y[tr_train_mask.bool()]))
-
- return loss_tr, loss_tcl, loss_reg_x, loss_reg_y
-
- def get_targets(self, data_samples: List[TextDetDataSample]) -> Tuple:
- """Generate loss targets for fcenet from data samples.
-
- Args:
- data_samples (list(TextDetDataSample)): Ground truth data samples.
-
- Returns:
- tuple[Tensor]: A tuple of three tensors from three different
- feature level as FCENet targets.
- """
- p3_maps, p4_maps, p5_maps = multi_apply(self._get_target_single,
- data_samples)
- p3_maps = torch.cat(p3_maps, 0)
- p4_maps = torch.cat(p4_maps, 0)
- p5_maps = torch.cat(p5_maps, 0)
-
- return p3_maps, p4_maps, p5_maps
-
- def _get_target_single(self, data_sample: TextDetDataSample) -> Tuple:
- """Generate loss target for fcenet from a data sample.
-
- Args:
- data_sample (TextDetDataSample): The data sample.
-
- Returns:
- tuple[Tensor]: A tuple of three tensors from three different
- feature level as the targets of one prediction.
- """
- img_size = data_sample.img_shape[:2]
- text_polys = data_sample.gt_instances.polygons
- ignore_flags = data_sample.gt_instances.ignored
-
- p3_map, p4_map, p5_map = self._generate_level_targets(
- img_size, text_polys, ignore_flags)
- # to tesnor
- p3_map = torch.from_numpy(p3_map).unsqueeze(0).float()
- p4_map = torch.from_numpy(p4_map).unsqueeze(0).float()
- p5_map = torch.from_numpy(p5_map).unsqueeze(0).float()
- return p3_map, p4_map, p5_map
-
- def _generate_level_targets(self,
- img_size: Tuple[int, int],
- text_polys: List[ArrayLike],
- ignore_flags: Optional[torch.BoolTensor] = None
- ) -> Tuple[torch.Tensor]:
- """Generate targets for one feature level.
-
- Args:
- img_size (tuple(int, int)): The image size of (height, width).
- text_polys (List[ndarray]): 2D array of text polygons.
- ignore_flags (torch.BoolTensor, optional): Indicate whether the
- corresponding text polygon is ignored. Defaults to None.
-
- Returns:
- tuple[Tensor]: A tuple of three tensors from one feature level
- as the targets.
- """
- h, w = img_size
- lv_size_divs = self.level_size_divisors
- lv_proportion_range = self.level_proportion_range
-
- lv_size_divs = self.level_size_divisors
- lv_proportion_range = self.level_proportion_range
- lv_text_polys = [[] for i in range(len(lv_size_divs))]
- lv_ignore_polys = [[] for i in range(len(lv_size_divs))]
- level_maps = []
-
- for poly_ind, poly in enumerate(text_polys):
- poly = np.array(poly, dtype=np.int_).reshape((1, -1, 2))
- _, _, box_w, box_h = cv2.boundingRect(poly)
- proportion = max(box_h, box_w) / (h + 1e-8)
-
- for ind, proportion_range in enumerate(lv_proportion_range):
- if proportion_range[0] < proportion < proportion_range[1]:
- if ignore_flags is not None and ignore_flags[poly_ind]:
- lv_ignore_polys[ind].append(poly[0] /
- lv_size_divs[ind])
- else:
- lv_text_polys[ind].append(poly[0] / lv_size_divs[ind])
-
- for ind, size_divisor in enumerate(lv_size_divs):
- current_level_maps = []
- level_img_size = (h // size_divisor, w // size_divisor)
-
- text_region = self._generate_text_region_mask(
- level_img_size, lv_text_polys[ind])[None]
- current_level_maps.append(text_region)
-
- center_region = self._generate_center_region_mask(
- level_img_size, lv_text_polys[ind])[None]
- current_level_maps.append(center_region)
-
- effective_mask = self._generate_effective_mask(
- level_img_size, lv_ignore_polys[ind])[None]
- current_level_maps.append(effective_mask)
-
- fourier_real_map, fourier_image_maps = self._generate_fourier_maps(
- level_img_size, lv_text_polys[ind])
- current_level_maps.append(fourier_real_map)
- current_level_maps.append(fourier_image_maps)
-
- level_maps.append(np.concatenate(current_level_maps))
-
- return level_maps
-
- def _generate_center_region_mask(self, img_size: Tuple[int, int],
- text_polys: ArrayLike) -> np.ndarray:
- """Generate text center region mask.
-
- Args:
- img_size (tuple): The image size of (height, width).
- text_polys (list[ndarray]): The list of text polygons.
-
- Returns:
- ndarray: The text center region mask.
- """
-
- assert isinstance(img_size, tuple)
-
- h, w = img_size
-
- center_region_mask = np.zeros((h, w), np.uint8)
-
- center_region_boxes = []
- for poly in text_polys:
- polygon_points = poly.reshape(-1, 2)
- _, _, top_line, bot_line = self._reorder_poly_edge(polygon_points)
- resampled_top_line, resampled_bot_line = self._resample_sidelines(
- top_line, bot_line, self.resample_step)
- resampled_bot_line = resampled_bot_line[::-1]
- center_line = (resampled_top_line + resampled_bot_line) / 2
-
- line_head_shrink_len = norm(resampled_top_line[0] -
- resampled_bot_line[0]) / 4.0
- line_tail_shrink_len = norm(resampled_top_line[-1] -
- resampled_bot_line[-1]) / 4.0
- head_shrink_num = int(line_head_shrink_len // self.resample_step)
- tail_shrink_num = int(line_tail_shrink_len // self.resample_step)
- if len(center_line) > head_shrink_num + tail_shrink_num + 2:
- center_line = center_line[head_shrink_num:len(center_line) -
- tail_shrink_num]
- resampled_top_line = resampled_top_line[
- head_shrink_num:len(resampled_top_line) - tail_shrink_num]
- resampled_bot_line = resampled_bot_line[
- head_shrink_num:len(resampled_bot_line) - tail_shrink_num]
-
- for i in range(0, len(center_line) - 1):
- tl = center_line[i] + (resampled_top_line[i] - center_line[i]
- ) * self.center_region_shrink_ratio
- tr = center_line[i + 1] + (
- resampled_top_line[i + 1] -
- center_line[i + 1]) * self.center_region_shrink_ratio
- br = center_line[i + 1] + (
- resampled_bot_line[i + 1] -
- center_line[i + 1]) * self.center_region_shrink_ratio
- bl = center_line[i] + (resampled_bot_line[i] - center_line[i]
- ) * self.center_region_shrink_ratio
- current_center_box = np.vstack([tl, tr, br,
- bl]).astype(np.int32)
- center_region_boxes.append(current_center_box)
-
- cv2.fillPoly(center_region_mask, center_region_boxes, 1)
- return center_region_mask
-
- def _generate_fourier_maps(self, img_size: Tuple[int, int],
- text_polys: ArrayLike
- ) -> Tuple[np.ndarray, np.ndarray]:
- """Generate Fourier coefficient maps.
-
- Args:
- img_size (tuple): The image size of (height, width).
- text_polys (list[ndarray]): The list of text polygons.
-
- Returns:
- tuple(ndarray, ndarray):
-
- - fourier_real_map (ndarray): The Fourier coefficient real part
- maps.
- - fourier_image_map (ndarray): The Fourier coefficient image part
- maps.
- """
-
- assert isinstance(img_size, tuple)
-
- h, w = img_size
- k = self.fourier_degree
- real_map = np.zeros((k * 2 + 1, h, w), dtype=np.float32)
- imag_map = np.zeros((k * 2 + 1, h, w), dtype=np.float32)
-
- for poly in text_polys:
- mask = np.zeros((h, w), dtype=np.uint8)
- polygon = np.array(poly).reshape((1, -1, 2))
- cv2.fillPoly(mask, polygon.astype(np.int32), 1)
- fourier_coeff = self._cal_fourier_signature(polygon[0], k)
- for i in range(-k, k + 1):
- if i != 0:
- real_map[i + k, :, :] = mask * fourier_coeff[i + k, 0] + (
- 1 - mask) * real_map[i + k, :, :]
- imag_map[i + k, :, :] = mask * fourier_coeff[i + k, 1] + (
- 1 - mask) * imag_map[i + k, :, :]
- else:
- yx = np.argwhere(mask > 0.5)
- k_ind = np.ones((len(yx)), dtype=np.int64) * k
- y, x = yx[:, 0], yx[:, 1]
- real_map[k_ind, y, x] = fourier_coeff[k, 0] - x
- imag_map[k_ind, y, x] = fourier_coeff[k, 1] - y
-
- return real_map, imag_map
-
- def _cal_fourier_signature(self, polygon: ArrayLike,
- fourier_degree: int) -> np.ndarray:
- """Calculate Fourier signature from input polygon.
-
- Args:
- polygon (list[ndarray]): The input polygon.
- fourier_degree (int): The maximum Fourier degree K.
- Returns:
- ndarray: An array shaped (2k+1, 2) containing
- real part and image part of 2k+1 Fourier coefficients.
- """
- resampled_polygon = self._resample_polygon(polygon)
- resampled_polygon = self._normalize_polygon(resampled_polygon)
-
- fourier_coeff = self._poly2fourier(resampled_polygon, fourier_degree)
- fourier_coeff = self._clockwise(fourier_coeff, fourier_degree)
-
- real_part = np.real(fourier_coeff).reshape((-1, 1))
- image_part = np.imag(fourier_coeff).reshape((-1, 1))
- fourier_signature = np.hstack([real_part, image_part])
-
- return fourier_signature
-
- def _resample_polygon(self,
- polygon: ArrayLike,
- n: int = 400) -> np.ndarray:
- """Resample one polygon with n points on its boundary.
-
- Args:
- polygon (list[ndarray]): The input polygon.
- n (int): The number of resampled points. Defaults to 400.
- Returns:
- ndarray: The resampled polygon.
- """
- length = []
-
- for i in range(len(polygon)):
- p1 = polygon[i]
- if i == len(polygon) - 1:
- p2 = polygon[0]
- else:
- p2 = polygon[i + 1]
- length.append(((p1[0] - p2[0])**2 + (p1[1] - p2[1])**2)**0.5)
-
- total_length = sum(length)
- n_on_each_line = (np.array(length) / (total_length + 1e-8)) * n
- n_on_each_line = n_on_each_line.astype(np.int32)
- new_polygon = []
-
- for i in range(len(polygon)):
- num = n_on_each_line[i]
- p1 = polygon[i]
- if i == len(polygon) - 1:
- p2 = polygon[0]
- else:
- p2 = polygon[i + 1]
-
- if num == 0:
- continue
-
- dxdy = (p2 - p1) / num
- for j in range(num):
- point = p1 + dxdy * j
- new_polygon.append(point)
-
- return np.array(new_polygon)
-
- def _normalize_polygon(self, polygon: ArrayLike) -> np.ndarray:
- """Normalize one polygon so that its start point is at right most.
-
- Args:
- polygon (list[ndarray]): The origin polygon.
- Returns:
- ndarray: The polygon with start point at right.
- """
- temp_polygon = polygon - polygon.mean(axis=0)
- x = np.abs(temp_polygon[:, 0])
- y = temp_polygon[:, 1]
- index_x = np.argsort(x)
- index_y = np.argmin(y[index_x[:8]])
- index = index_x[index_y]
- new_polygon = np.concatenate([polygon[index:], polygon[:index]])
- return new_polygon
-
- def _clockwise(self, fourier_coeff: np.ndarray,
- fourier_degree: int) -> np.ndarray:
- """Make sure the polygon reconstructed from Fourier coefficients c in
- the clockwise direction.
-
- Args:
- fourier_coeff (ndarray[complex]): The Fourier coefficients.
- fourier_degree: The maximum Fourier degree K.
- Returns:
- lost[float]: The polygon in clockwise point order.
- """
- if np.abs(fourier_coeff[fourier_degree + 1]) > np.abs(
- fourier_coeff[fourier_degree - 1]):
- return fourier_coeff
- elif np.abs(fourier_coeff[fourier_degree + 1]) < np.abs(
- fourier_coeff[fourier_degree - 1]):
- return fourier_coeff[::-1]
- else:
- if np.abs(fourier_coeff[fourier_degree + 2]) > np.abs(
- fourier_coeff[fourier_degree - 2]):
- return fourier_coeff
- else:
- return fourier_coeff[::-1]
-
- def _poly2fourier(self, polygon: ArrayLike,
- fourier_degree: int) -> np.ndarray:
- """Perform Fourier transformation to generate Fourier coefficients ck
- from polygon.
-
- Args:
- polygon (list[ndarray]): An input polygon.
- fourier_degree (int): The maximum Fourier degree K.
- Returns:
- ndarray: Fourier coefficients.
- """
- points = polygon[:, 0] + polygon[:, 1] * 1j
- c_fft = fft(points) / len(points)
- c = np.hstack((c_fft[-fourier_degree:], c_fft[:fourier_degree + 1]))
- return c
-
- def _fourier2poly(self, real_maps: torch.Tensor,
- imag_maps: torch.Tensor) -> Sequence[torch.Tensor]:
- """Transform Fourier coefficient maps to polygon maps.
-
- Args:
- real_maps (tensor): A map composed of the real parts of the
- Fourier coefficients, whose shape is (-1, 2k+1)
- imag_maps (tensor):A map composed of the imag parts of the
- Fourier coefficients, whose shape is (-1, 2k+1)
-
- Returns
- tuple(tensor, tensor):
-
- - x_maps (tensor): A map composed of the x value of the polygon
- represented by n sample points (xn, yn), whose shape is (-1, n)
- - y_maps (tensor): A map composed of the y value of the polygon
- represented by n sample points (xn, yn), whose shape is (-1, n)
- """
-
- device = real_maps.device
-
- k_vect = torch.arange(
- -self.fourier_degree,
- self.fourier_degree + 1,
- dtype=torch.float,
- device=device).view(-1, 1)
- i_vect = torch.arange(
- 0, self.num_sample, dtype=torch.float, device=device).view(1, -1)
-
- transform_matrix = 2 * np.pi / self.num_sample * torch.mm(
- k_vect, i_vect)
-
- x1 = torch.einsum('ak, kn-> an', real_maps,
- torch.cos(transform_matrix))
- x2 = torch.einsum('ak, kn-> an', imag_maps,
- torch.sin(transform_matrix))
- y1 = torch.einsum('ak, kn-> an', real_maps,
- torch.sin(transform_matrix))
- y2 = torch.einsum('ak, kn-> an', imag_maps,
- torch.cos(transform_matrix))
-
- x_maps = x1 - x2
- y_maps = y1 + y2
-
- return x_maps, y_maps
diff --git a/pipeline/mmocr/mmocr/models/textdet/module_losses/pan_module_loss.py b/pipeline/mmocr/mmocr/models/textdet/module_losses/pan_module_loss.py
deleted file mode 100644
index 6a5a6685aa9514f5d9afbfbe9b5a7fe4029ab96d..0000000000000000000000000000000000000000
--- a/pipeline/mmocr/mmocr/models/textdet/module_losses/pan_module_loss.py
+++ /dev/null
@@ -1,347 +0,0 @@
-# Copyright (c) OpenMMLab. All rights reserved.
-import warnings
-from typing import Dict, Sequence, Tuple, Union
-
-import numpy as np
-import torch
-import torch.nn.functional as F
-from mmdet.models.utils import multi_apply
-from torch import nn
-
-from mmocr.registry import MODELS
-from mmocr.structures import TextDetDataSample
-from .seg_based_module_loss import SegBasedModuleLoss
-
-
-@MODELS.register_module()
-class PANModuleLoss(SegBasedModuleLoss):
- """The class for implementing PANet loss. This was partially adapted from
- https://github.com/whai362/pan_pp.pytorch and
- https://github.com/WenmuZhou/PAN.pytorch.
-
- PANet: `Efficient and Accurate Arbitrary-
- Shaped Text Detection with Pixel Aggregation Network
- `_.
-
- Args:
- loss_text (dict) The loss config for text map. Defaults to
- dict(type='MaskedSquareDiceLoss').
- loss_kernel (dict) The loss config for kernel map. Defaults to
- dict(type='MaskedSquareDiceLoss').
- loss_embedding (dict) The loss config for embedding map. Defaults to
- dict(type='PANEmbLossV1').
- weight_text (float): The weight of text loss. Defaults to 1.
- weight_kernel (float): The weight of kernel loss. Defaults to 0.5.
- weight_embedding (float): The weight of embedding loss.
- Defaults to 0.25.
- ohem_ratio (float): The negative/positive ratio in ohem. Defaults to 3.
- shrink_ratio (tuple[float]) : The ratio of shrinking kernel. Defaults
- to (1.0, 0.5).
- max_shrink_dist (int or float): The maximum shrinking distance.
- Defaults to 20.
- reduction (str): The way to reduce the loss. Available options are
- "mean" and "sum". Defaults to 'mean'.
- """
-
- def __init__(
- self,
- loss_text: Dict = dict(type='MaskedSquareDiceLoss'),
- loss_kernel: Dict = dict(type='MaskedSquareDiceLoss'),
- loss_embedding: Dict = dict(type='PANEmbLossV1'),
- weight_text: float = 1.0,
- weight_kernel: float = 0.5,
- weight_embedding: float = 0.25,
- ohem_ratio: Union[int, float] = 3, # TODO Find a better name
- shrink_ratio: Sequence[Union[int, float]] = (1.0, 0.5),
- max_shrink_dist: Union[int, float] = 20,
- reduction: str = 'mean') -> None:
- super().__init__()
- assert reduction in ['mean', 'sum'], "reduction must in ['mean','sum']"
- self.weight_text = weight_text
- self.weight_kernel = weight_kernel
- self.weight_embedding = weight_embedding
- self.shrink_ratio = shrink_ratio
- self.ohem_ratio = ohem_ratio
- self.reduction = reduction
- self.max_shrink_dist = max_shrink_dist
- self.loss_text = MODELS.build(loss_text)
- self.loss_kernel = MODELS.build(loss_kernel)
- self.loss_embedding = MODELS.build(loss_embedding)
-
- def forward(self, preds: torch.Tensor,
- data_samples: Sequence[TextDetDataSample]) -> Dict:
- """Compute PAN loss.
-
- Args:
- preds (dict): Raw predictions from model with
- shape :math:`(N, C, H, W)`.
- data_samples (list[TextDetDataSample]): The data samples.
-
- Returns:
- dict: The dict for pan losses with loss_text, loss_kernel,
- loss_aggregation and loss_discrimination.
- """
-
- gt_kernels, gt_masks = self.get_targets(data_samples)
- target_size = gt_kernels.size()[2:]
- preds = F.interpolate(preds, size=target_size, mode='bilinear')
- pred_texts = preds[:, 0, :, :]
- pred_kernels = preds[:, 1, :, :]
- inst_embed = preds[:, 2:, :, :]
- gt_kernels = gt_kernels.to(preds.device)
- gt_masks = gt_masks.to(preds.device)
-
- # compute embedding loss
- loss_emb = self.loss_embedding(inst_embed, gt_kernels[0],
- gt_kernels[1], gt_masks)
- gt_kernels[gt_kernels <= 0.5] = 0
- gt_kernels[gt_kernels > 0.5] = 1
- # compute text loss
- sampled_mask = self._ohem_batch(pred_texts.detach(), gt_kernels[0],
- gt_masks)
- pred_texts = torch.sigmoid(pred_texts)
- loss_texts = self.loss_text(pred_texts, gt_kernels[0], sampled_mask)
-
- # compute kernel loss
- pred_kernels = torch.sigmoid(pred_kernels)
- sampled_masks_kernel = (gt_kernels[0] > 0.5).float() * gt_masks
- loss_kernels = self.loss_kernel(pred_kernels, gt_kernels[1],
- sampled_masks_kernel)
-
- losses = [loss_texts, loss_kernels, loss_emb]
- if self.reduction == 'mean':
- losses = [item.mean() for item in losses]
- else:
- losses = [item.sum() for item in losses]
-
- results = dict()
- results.update(
- loss_text=self.weight_text * losses[0],
- loss_kernel=self.weight_kernel * losses[1],
- loss_embedding=self.weight_embedding * losses[2])
- return results
-
- def get_targets(
- self,
- data_samples: Sequence[TextDetDataSample],
- ) -> Tuple[torch.Tensor, torch.Tensor]:
- """Generate the gt targets for PANet.
-
- Args:
- results (dict): The input result dictionary.
-
- Returns:
- results (dict): The output result dictionary.
- """
- gt_kernels, gt_masks = multi_apply(self._get_target_single,
- data_samples)
- # gt_kernels: (N, kernel_number, H, W)->(kernel_number, N, H, W)
- gt_kernels = torch.stack(gt_kernels, dim=0).permute(1, 0, 2, 3)
- gt_masks = torch.stack(gt_masks, dim=0)
- return gt_kernels, gt_masks
-
- def _get_target_single(self, data_sample: TextDetDataSample
- ) -> Tuple[torch.Tensor, torch.Tensor]:
- """Generate loss target from a data sample.
-
- Args:
- data_sample (TextDetDataSample): The data sample.
-
- Returns:
- tuple: A tuple of four tensors as the targets of one prediction.
- """
- gt_polygons = data_sample.gt_instances.polygons
- gt_ignored = data_sample.gt_instances.ignored
-
- gt_kernels = []
- for ratio in self.shrink_ratio:
- # TODO pass `gt_ignored` to `_generate_kernels`
- gt_kernel, _ = self._generate_kernels(
- data_sample.img_shape,
- gt_polygons,
- ratio,
- ignore_flags=None,
- max_shrink_dist=self.max_shrink_dist)
- gt_kernels.append(gt_kernel)
- gt_polygons_ignored = data_sample.gt_instances[gt_ignored].polygons
- gt_mask = self._generate_effective_mask(data_sample.img_shape,
- gt_polygons_ignored)
-
- gt_kernels = np.stack(gt_kernels, axis=0)
- gt_kernels = torch.from_numpy(gt_kernels).float()
- gt_mask = torch.from_numpy(gt_mask).float()
- return gt_kernels, gt_mask
-
- def _ohem_batch(self, text_scores: torch.Tensor, gt_texts: torch.Tensor,
- gt_mask: torch.Tensor) -> torch.Tensor:
- """OHEM sampling for a batch of imgs.
-
- Args:
- text_scores (Tensor): The text scores of size :math:`(H, W)`.
- gt_texts (Tensor): The gt text masks of size :math:`(H, W)`.
- gt_mask (Tensor): The gt effective mask of size :math:`(H, W)`.
-
- Returns:
- Tensor: The sampled mask of size :math:`(H, W)`.
- """
- assert isinstance(text_scores, torch.Tensor)
- assert isinstance(gt_texts, torch.Tensor)
- assert isinstance(gt_mask, torch.Tensor)
- assert len(text_scores.shape) == 3
- assert text_scores.shape == gt_texts.shape
- assert gt_texts.shape == gt_mask.shape
-
- sampled_masks = []
- for i in range(text_scores.shape[0]):
- sampled_masks.append(
- self._ohem_single(text_scores[i], gt_texts[i], gt_mask[i]))
-
- sampled_masks = torch.stack(sampled_masks)
-
- return sampled_masks
-
- def _ohem_single(self, text_score: torch.Tensor, gt_text: torch.Tensor,
- gt_mask: torch.Tensor) -> torch.Tensor:
- """Sample the top-k maximal negative samples and all positive samples.
-
- Args:
- text_score (Tensor): The text score of size :math:`(H, W)`.
- gt_text (Tensor): The ground truth text mask of size
- :math:`(H, W)`.
- gt_mask (Tensor): The effective region mask of size :math:`(H, W)`.
-
- Returns:
- Tensor: The sampled pixel mask of size :math:`(H, W)`.
- """
- assert isinstance(text_score, torch.Tensor)
- assert isinstance(gt_text, torch.Tensor)
- assert isinstance(gt_mask, torch.Tensor)
- assert len(text_score.shape) == 2
- assert text_score.shape == gt_text.shape
- assert gt_text.shape == gt_mask.shape
-
- pos_num = (int)(torch.sum(gt_text > 0.5).item()) - (int)(
- torch.sum((gt_text > 0.5) * (gt_mask <= 0.5)).item())
- neg_num = (int)(torch.sum(gt_text <= 0.5).item())
- neg_num = (int)(min(pos_num * self.ohem_ratio, neg_num))
-
- if pos_num == 0 or neg_num == 0:
- warnings.warn('pos_num = 0 or neg_num = 0')
- return gt_mask.bool()
-
- neg_score = text_score[gt_text <= 0.5]
- neg_score_sorted, _ = torch.sort(neg_score, descending=True)
- threshold = neg_score_sorted[neg_num - 1]
- sampled_mask = (((text_score >= threshold) + (gt_text > 0.5)) > 0) * (
- gt_mask > 0.5)
- return sampled_mask
-
-
-@MODELS.register_module()
-class PANEmbLossV1(nn.Module):
- """The class for implementing EmbLossV1. This was partially adapted from
- https://github.com/whai362/pan_pp.pytorch.
-
- Args:
- feature_dim (int): The dimension of the feature. Defaults to 4.
- delta_aggregation (float): The delta for aggregation. Defaults to 0.5.
- delta_discrimination (float): The delta for discrimination.
- Defaults to 1.5.
- """
-
- def __init__(self,
- feature_dim: int = 4,
- delta_aggregation: float = 0.5,
- delta_discrimination: float = 1.5) -> None:
- super().__init__()
- self.feature_dim = feature_dim
- self.delta_aggregation = delta_aggregation
- self.delta_discrimination = delta_discrimination
- self.weights = (1.0, 1.0)
-
- def _forward_single(self, emb: torch.Tensor, instance: torch.Tensor,
- kernel: torch.Tensor,
- training_mask: torch.Tensor) -> torch.Tensor:
- """Compute the loss for a single image.
-
- Args:
- emb (torch.Tensor): The embedding feature.
- instance (torch.Tensor): The instance feature.
- kernel (torch.Tensor): The kernel feature.
- training_mask (torch.Tensor): The effective mask.
- """
- training_mask = (training_mask > 0.5).float()
- kernel = (kernel > 0.5).float()
- instance = instance * training_mask
- instance_kernel = (instance * kernel).view(-1)
- instance = instance.view(-1)
- emb = emb.view(self.feature_dim, -1)
-
- unique_labels, unique_ids = torch.unique(
- instance_kernel, sorted=True, return_inverse=True)
- num_instance = unique_labels.size(0)
- if num_instance <= 1:
- return 0
-
- emb_mean = emb.new_zeros((self.feature_dim, num_instance),
- dtype=torch.float32)
- for i, lb in enumerate(unique_labels):
- if lb == 0:
- continue
- ind_k = instance_kernel == lb
- emb_mean[:, i] = torch.mean(emb[:, ind_k], dim=1)
-
- l_agg = emb.new_zeros(num_instance, dtype=torch.float32)
- for i, lb in enumerate(unique_labels):
- if lb == 0:
- continue
- ind = instance == lb
- emb_ = emb[:, ind]
- dist = (emb_ - emb_mean[:, i:i + 1]).norm(p=2, dim=0)
- dist = F.relu(dist - self.delta_aggregation)**2
- l_agg[i] = torch.mean(torch.log(dist + 1.0))
- l_agg = torch.mean(l_agg[1:])
-
- if num_instance > 2:
- emb_interleave = emb_mean.permute(1, 0).repeat(num_instance, 1)
- emb_band = emb_mean.permute(1, 0).repeat(1, num_instance).view(
- -1, self.feature_dim)
-
- mask = (1 - torch.eye(num_instance, dtype=torch.int8)).view(
- -1, 1).repeat(1, self.feature_dim)
- mask = mask.view(num_instance, num_instance, -1)
- mask[0, :, :] = 0
- mask[:, 0, :] = 0
- mask = mask.view(num_instance * num_instance, -1)
-
- dist = emb_interleave - emb_band
- dist = dist[mask > 0].view(-1, self.feature_dim).norm(p=2, dim=1)
- dist = F.relu(2 * self.delta_discrimination - dist)**2
- l_dis = torch.mean(torch.log(dist + 1.0))
- else:
- l_dis = 0
-
- l_agg = self.weights[0] * l_agg
- l_dis = self.weights[1] * l_dis
- l_reg = torch.mean(torch.log(torch.norm(emb_mean, 2, 0) + 1.0)) * 0.001
- loss = l_agg + l_dis + l_reg
- return loss
-
- def forward(self, emb: torch.Tensor, instance: torch.Tensor,
- kernel: torch.Tensor,
- training_mask: torch.Tensor) -> torch.Tensor:
- """Compute the loss for a batch image.
-
- Args:
- emb (torch.Tensor): The embedding feature.
- instance (torch.Tensor): The instance feature.
- kernel (torch.Tensor): The kernel feature.
- training_mask (torch.Tensor): The effective mask.
- """
- loss_batch = emb.new_zeros((emb.size(0)), dtype=torch.float32)
-
- for i in range(loss_batch.size(0)):
- loss_batch[i] = self._forward_single(emb[i], instance[i],
- kernel[i], training_mask[i])
-
- return loss_batch
diff --git a/pipeline/mmocr/mmocr/models/textdet/module_losses/pse_module_loss.py b/pipeline/mmocr/mmocr/models/textdet/module_losses/pse_module_loss.py
deleted file mode 100644
index 902588c49cc642c059e86dc1a76c08658349295d..0000000000000000000000000000000000000000
--- a/pipeline/mmocr/mmocr/models/textdet/module_losses/pse_module_loss.py
+++ /dev/null
@@ -1,129 +0,0 @@
-# Copyright (c) OpenMMLab. All rights reserved.
-from typing import Dict, Sequence, Tuple, Union
-
-import torch
-import torch.nn.functional as F
-
-from mmocr.registry import MODELS
-from mmocr.structures import TextDetDataSample
-from . import PANModuleLoss
-
-
-@MODELS.register_module()
-class PSEModuleLoss(PANModuleLoss):
- """The class for implementing PSENet loss. This is partially adapted from
- https://github.com/whai362/PSENet.
-
- PSENet: `Shape Robust Text Detection with
- Progressive Scale Expansion Network `_.
-
- Args:
- weight_text (float): The weight of text loss. Defaults to 0.7.
- weight_kernel (float): The weight of text kernel. Defaults to 0.3.
- loss_text (dict): Loss type for text. Defaults to
- dict('MaskedSquareDiceLoss').
- loss_kernel (dict): Loss type for kernel. Defaults to
- dict('MaskedSquareDiceLoss').
- ohem_ratio (int or float): The negative/positive ratio in ohem.
- Defaults to 3.
- reduction (str): The way to reduce the loss. Defaults to 'mean'.
- Options are 'mean' and 'sum'.
- kernel_sample_type (str): The way to sample kernel. Defaults to
- adaptive. Options are 'adaptive' and 'hard'.
- shrink_ratio (tuple): The ratio for shirinking text instances.
- Defaults to (1.0, 0.9, 0.8, 0.7, 0.6, 0.5, 0.4).
- max_shrink_dist (int or float): The maximum shrinking distance.
- Defaults to 20.
- """
-
- def __init__(
- self,
- weight_text: float = 0.7,
- weight_kernel: float = 0.3,
- loss_text: Dict = dict(type='MaskedSquareDiceLoss'),
- loss_kernel: Dict = dict(type='MaskedSquareDiceLoss'),
- ohem_ratio: Union[int, float] = 3,
- reduction: str = 'mean',
- kernel_sample_type: str = 'adaptive',
- shrink_ratio: Tuple[float] = (1.0, 0.9, 0.8, 0.7, 0.6, 0.5, 0.4),
- max_shrink_dist: Union[int, float] = 20,
- ) -> None:
- super().__init__()
- assert reduction in ['mean', 'sum'
- ], "reduction must be either of ['mean','sum']"
- assert kernel_sample_type in [
- 'adaptive', 'hard'
- ], "kernel_sample_type must be either of ['hard', 'adaptive']"
- self.weight_text = weight_text
- self.weight_kernel = weight_kernel
- self.ohem_ratio = ohem_ratio
- self.reduction = reduction
- self.shrink_ratio = shrink_ratio
- self.kernel_sample_type = kernel_sample_type
- self.max_shrink_dist = max_shrink_dist
- self.loss_text = MODELS.build(loss_text)
- self.loss_kernel = MODELS.build(loss_kernel)
-
- def forward(self, preds: torch.Tensor,
- data_samples: Sequence[TextDetDataSample]) -> Dict:
- """Compute PSENet loss.
-
- Args:
- preds (torch.Tensor): Raw predictions from model with
- shape :math:`(N, C, H, W)`.
- data_samples (list[TextDetDataSample]): The data samples.
-
- Returns:
- dict: The dict for pse losses with loss_text, loss_kernel,
- loss_aggregation and loss_discrimination.
- """
- losses = []
-
- gt_kernels, gt_masks = self.get_targets(data_samples)
- target_size = gt_kernels.size()[2:]
- preds = F.interpolate(preds, size=target_size, mode='bilinear')
- pred_texts = preds[:, 0, :, :]
- pred_kernels = preds[:, 1:, :, :]
-
- gt_kernels = gt_kernels.to(preds.device)
- gt_kernels[gt_kernels <= 0.5] = 0
- gt_kernels[gt_kernels > 0.5] = 1
- gt_masks = gt_masks.to(preds.device)
-
- # compute text loss
- sampled_mask = self._ohem_batch(pred_texts.detach(), gt_kernels[0],
- gt_masks)
- loss_texts = self.loss_text(pred_texts.sigmoid(), gt_kernels[0],
- sampled_mask)
- losses.append(self.weight_text * loss_texts)
-
- # compute kernel loss
- if self.kernel_sample_type == 'hard':
- sampled_masks_kernel = (gt_kernels[0] >
- 0.5).float() * gt_masks.float()
- elif self.kernel_sample_type == 'adaptive':
- sampled_masks_kernel = (pred_texts > 0).float() * (
- gt_masks.float())
- else:
- raise NotImplementedError
-
- num_kernel = pred_kernels.shape[1]
- assert num_kernel == len(gt_kernels) - 1
- loss_list = []
- for idx in range(num_kernel):
- loss_kernels = self.loss_kernel(
- pred_kernels[:, idx, :, :].sigmoid(), gt_kernels[1 + idx],
- sampled_masks_kernel)
- loss_list.append(loss_kernels)
-
- losses.append(self.weight_kernel * sum(loss_list) / len(loss_list))
-
- if self.reduction == 'mean':
- losses = [item.mean() for item in losses]
- elif self.reduction == 'sum':
- losses = [item.sum() for item in losses]
- else:
- raise NotImplementedError
-
- results = dict(loss_text=losses[0], loss_kernel=losses[1])
- return results
diff --git a/pipeline/mmocr/mmocr/models/textdet/module_losses/seg_based_module_loss.py b/pipeline/mmocr/mmocr/models/textdet/module_losses/seg_based_module_loss.py
deleted file mode 100644
index 2f2166921a1a31e9cbe1bfb0be7b8a9d2252b3d4..0000000000000000000000000000000000000000
--- a/pipeline/mmocr/mmocr/models/textdet/module_losses/seg_based_module_loss.py
+++ /dev/null
@@ -1,100 +0,0 @@
-# Copyright (c) OpenMMLab. All rights reserved.
-import sys
-from typing import Optional, Sequence, Tuple, Union
-
-import cv2
-import numpy as np
-import torch
-from mmengine.logging import MMLogger
-from shapely.geometry import Polygon
-
-from mmocr.utils.polygon_utils import offset_polygon
-from .base import BaseTextDetModuleLoss
-
-
-class SegBasedModuleLoss(BaseTextDetModuleLoss):
- """Base class for the module loss of segmentation-based text detection
- algorithms with some handy utilities."""
-
- def _generate_kernels(
- self,
- img_size: Tuple[int, int],
- text_polys: Sequence[np.ndarray],
- shrink_ratio: float,
- max_shrink_dist: Union[float, int] = sys.maxsize,
- ignore_flags: Optional[torch.Tensor] = None
- ) -> Tuple[np.ndarray, np.ndarray]:
- """Generate text instance kernels according to a shrink ratio.
-
- Args:
- img_size (tuple(int, int)): The image size of (height, width).
- text_polys (Sequence[np.ndarray]): 2D array of text polygons.
- shrink_ratio (float or int): The shrink ratio of kernel.
- max_shrink_dist (float or int): The maximum shrinking distance.
- ignore_flags (torch.BoolTensor, optional): Indicate whether the
- corresponding text polygon is ignored. Defaults to None.
-
- Returns:
- tuple(ndarray, ndarray): The text instance kernels of shape
- (height, width) and updated ignorance flags.
- """
- assert isinstance(img_size, tuple)
- assert isinstance(shrink_ratio, (float, int))
-
- logger: MMLogger = MMLogger.get_current_instance()
-
- h, w = img_size
- text_kernel = np.zeros((h, w), dtype=np.float32)
-
- for text_ind, poly in enumerate(text_polys):
- if ignore_flags is not None and ignore_flags[text_ind]:
- continue
- poly = poly.reshape(-1, 2).astype(np.int32)
- poly_obj = Polygon(poly)
- area = poly_obj.area
- peri = poly_obj.length
- distance = min(
- int(area * (1 - shrink_ratio * shrink_ratio) / (peri + 0.001) +
- 0.5), max_shrink_dist)
- shrunk_poly = offset_polygon(poly, -distance)
-
- if len(shrunk_poly) == 0:
- if ignore_flags is not None:
- ignore_flags[text_ind] = True
- continue
-
- try:
- shrunk_poly = shrunk_poly.reshape(-1, 2)
- except Exception as e:
- logger.info(f'{shrunk_poly} with error {e}')
- if ignore_flags is not None:
- ignore_flags[text_ind] = True
- continue
-
- cv2.fillPoly(text_kernel, [shrunk_poly.astype(np.int32)],
- text_ind + 1)
-
- return text_kernel, ignore_flags
-
- def _generate_effective_mask(self, mask_size: Tuple[int, int],
- ignored_polygons: Sequence[np.ndarray]
- ) -> np.ndarray:
- """Generate effective mask by setting the invalid regions to 0 and 1
- otherwise.
-
- Args:
- mask_size (tuple(int, int)): The mask size.
- ignored_polygons (Sequence[ndarray]): 2-d array, representing all
- the ignored polygons of the text region.
-
- Returns:
- mask (ndarray): The effective mask of shape (height, width).
- """
-
- mask = np.ones(mask_size, dtype=np.uint8)
-
- for poly in ignored_polygons:
- instance = poly.astype(np.int32).reshape(1, -1, 2)
- cv2.fillPoly(mask, instance, 0)
-
- return mask
diff --git a/pipeline/mmocr/mmocr/models/textdet/module_losses/textsnake_module_loss.py b/pipeline/mmocr/mmocr/models/textdet/module_losses/textsnake_module_loss.py
deleted file mode 100644
index 651a74755cf44e4103721b7416c6455bf0438f05..0000000000000000000000000000000000000000
--- a/pipeline/mmocr/mmocr/models/textdet/module_losses/textsnake_module_loss.py
+++ /dev/null
@@ -1,648 +0,0 @@
-# Copyright (c) OpenMMLab. All rights reserved.
-from typing import Dict, List, Sequence, Tuple
-
-import cv2
-import numpy as np
-import torch
-from mmcv.image import impad, imrescale
-from mmdet.models.utils import multi_apply
-from numpy import ndarray
-from numpy.linalg import norm
-from torch import Tensor
-
-from mmocr.registry import MODELS
-from mmocr.structures import TextDetDataSample
-from .seg_based_module_loss import SegBasedModuleLoss
-
-
-@MODELS.register_module()
-class TextSnakeModuleLoss(SegBasedModuleLoss):
- """The class for implementing TextSnake loss. This is partially adapted
- from https://github.com/princewang1994/TextSnake.pytorch.
-
- TextSnake: `A Flexible Representation for Detecting Text of Arbitrary
- Shapes `_.
-
- Args:
- ohem_ratio (float): The negative/positive ratio in ohem.
- downsample_ratio (float): Downsample ratio. Defaults to 1.0. TODO:
- remove it.
- orientation_thr (float): The threshold for distinguishing between
- head edge and tail edge among the horizontal and vertical edges
- of a quadrangle.
- resample_step (float): The step of resampling.
- center_region_shrink_ratio (float): The shrink ratio of text center.
- loss_text (dict): The loss config used to calculate the text loss.
- loss_center (dict): The loss config used to calculate the center loss.
- loss_radius (dict): The loss config used to calculate the radius loss.
- loss_sin (dict): The loss config used to calculate the sin loss.
- loss_cos (dict): The loss config used to calculate the cos loss.
- """
-
- def __init__(
- self,
- ohem_ratio: float = 3.0,
- downsample_ratio: float = 1.0,
- orientation_thr: float = 2.0,
- resample_step: float = 4.0,
- center_region_shrink_ratio: float = 0.3,
- loss_text: Dict = dict(
- type='MaskedBalancedBCEWithLogitsLoss',
- fallback_negative_num=100,
- eps=1e-5),
- loss_center: Dict = dict(type='MaskedBCEWithLogitsLoss'),
- loss_radius: Dict = dict(type='MaskedSmoothL1Loss'),
- loss_sin: Dict = dict(type='MaskedSmoothL1Loss'),
- loss_cos: Dict = dict(type='MaskedSmoothL1Loss')
- ) -> None:
- super().__init__()
- self.ohem_ratio = ohem_ratio
- self.downsample_ratio = downsample_ratio
- self.orientation_thr = orientation_thr
- self.resample_step = resample_step
- self.center_region_shrink_ratio = center_region_shrink_ratio
- self.eps = 1e-8
- self.loss_text = MODELS.build(loss_text)
- self.loss_center = MODELS.build(loss_center)
- self.loss_radius = MODELS.build(loss_radius)
- self.loss_sin = MODELS.build(loss_sin)
- self.loss_cos = MODELS.build(loss_cos)
-
- def _batch_pad(self, masks: List[ndarray],
- target_sz: Tuple[int, int]) -> ndarray:
- """Pad the masks to the right and bottom side to the target size and
- pack them into a batch.
-
- Args:
- mask (list[ndarray]): The masks to be padded.
- target_sz (tuple(int, int)): The target tensor of size
- :math:`(H, W)`.
-
- Returns:
- ndarray: A batch of padded mask.
- """
- batch = []
- for mask in masks:
- # H x W
- mask_sz = mask.shape
- # left, top, right, bottom
- padding = (0, 0, target_sz[1] - mask_sz[1],
- target_sz[0] - mask_sz[0])
- padded_mask = impad(
- mask, padding=padding, padding_mode='constant', pad_val=0)
- batch.append(np.expand_dims(padded_mask, axis=0))
- return np.concatenate(batch)
-
- def forward(self, preds: Tensor,
- data_samples: Sequence[TextDetDataSample]) -> Dict:
- """
- Args:
- preds (Tensor): The prediction map of shape
- :math:`(N, 5, H, W)`, where each dimension is the map of
- "text_region", "center_region", "sin_map", "cos_map", and
- "radius_map" respectively.
- data_samples (list[TextDetDataSample]): The data samples.
-
- Returns:
- dict: A loss dict with ``loss_text``, ``loss_center``,
- ``loss_radius``, ``loss_sin`` and ``loss_cos``.
- """
-
- (gt_text_masks, gt_masks, gt_center_region_masks, gt_radius_maps,
- gt_sin_maps, gt_cos_maps) = self.get_targets(data_samples)
-
- pred_text_region = preds[:, 0, :, :]
- pred_center_region = preds[:, 1, :, :]
- pred_sin_map = preds[:, 2, :, :]
- pred_cos_map = preds[:, 3, :, :]
- pred_radius_map = preds[:, 4, :, :]
- feature_sz = preds.size()
- device = preds.device
-
- mapping = {
- 'gt_text_masks': gt_text_masks,
- 'gt_center_region_masks': gt_center_region_masks,
- 'gt_masks': gt_masks,
- 'gt_radius_maps': gt_radius_maps,
- 'gt_sin_maps': gt_sin_maps,
- 'gt_cos_maps': gt_cos_maps
- }
- gt = {}
- for key, value in mapping.items():
- gt[key] = value
- if abs(self.downsample_ratio - 1.0) < 1e-2:
- gt[key] = self._batch_pad(gt[key], feature_sz[2:])
- else:
- gt[key] = [
- imrescale(
- mask,
- scale=self.downsample_ratio,
- interpolation='nearest') for mask in gt[key]
- ]
- gt[key] = self._batch_pad(gt[key], feature_sz[2:])
- if key == 'gt_radius_maps':
- gt[key] *= self.downsample_ratio
- gt[key] = torch.from_numpy(gt[key]).float().to(device)
-
- scale = torch.sqrt(1.0 / (pred_sin_map**2 + pred_cos_map**2 + 1e-8))
- pred_sin_map = pred_sin_map * scale
- pred_cos_map = pred_cos_map * scale
-
- loss_text = self.loss_text(pred_text_region, gt['gt_text_masks'],
- gt['gt_masks'])
-
- text_mask = (gt['gt_text_masks'] * gt['gt_masks']).float()
- loss_center = self.loss_center(pred_center_region,
- gt['gt_center_region_masks'], text_mask)
-
- center_mask = (gt['gt_center_region_masks'] * gt['gt_masks']).float()
- map_sz = pred_radius_map.size()
- ones = torch.ones(map_sz, dtype=torch.float, device=device)
- loss_radius = self.loss_radius(
- pred_radius_map / (gt['gt_radius_maps'] + 1e-2), ones, center_mask)
- loss_sin = self.loss_sin(pred_sin_map, gt['gt_sin_maps'], center_mask)
- loss_cos = self.loss_cos(pred_cos_map, gt['gt_cos_maps'], center_mask)
-
- results = dict(
- loss_text=loss_text,
- loss_center=loss_center,
- loss_radius=loss_radius,
- loss_sin=loss_sin,
- loss_cos=loss_cos)
-
- return results
-
- def get_targets(self, data_samples: List[TextDetDataSample]) -> Tuple:
- """Generate loss targets from data samples.
-
- Args:
- data_samples (list(TextDetDataSample)): Ground truth data samples.
-
- Returns:
- tuple(gt_text_masks, gt_masks, gt_center_region_masks,
- gt_radius_maps, gt_sin_maps, gt_cos_maps):
- A tuple of six lists of ndarrays as the targets.
- """
- return multi_apply(self._get_target_single, data_samples)
-
- def _get_target_single(self, data_sample: TextDetDataSample) -> Tuple:
- """Generate loss target from a data sample.
-
- Args:
- data_sample (TextDetDataSample): The data sample.
-
- Returns:
- tuple(gt_text_mask, gt_mask, gt_center_region_mask, gt_radius_map,
- gt_sin_map, gt_cos_map):
- A tuple of six ndarrays as the targets of one prediction.
- """
-
- gt_instances = data_sample.gt_instances
- ignore_flags = gt_instances.ignored
-
- polygons = gt_instances[~ignore_flags].polygons
- ignored_polygons = gt_instances[ignore_flags].polygons
-
- gt_text_mask = self._generate_text_region_mask(data_sample.img_shape,
- polygons)
- gt_mask = self._generate_effective_mask(data_sample.img_shape,
- ignored_polygons)
-
- (gt_center_region_mask, gt_radius_map, gt_sin_map,
- gt_cos_map) = self._generate_center_mask_attrib_maps(
- data_sample.img_shape, polygons)
-
- return (gt_text_mask, gt_mask, gt_center_region_mask, gt_radius_map,
- gt_sin_map, gt_cos_map)
-
- def _generate_text_region_mask(self, img_size: Tuple[int, int],
- text_polys: List[ndarray]) -> ndarray:
- """Generate text center region mask and geometry attribute maps.
-
- Args:
- img_size (tuple): The image size (height, width).
- text_polys (list[ndarray]): The list of text polygons.
-
- Returns:
- text_region_mask (ndarray): The text region mask.
- """
-
- assert isinstance(img_size, tuple)
-
- text_region_mask = np.zeros(img_size, dtype=np.uint8)
-
- for poly in text_polys:
- polygon = np.array(poly, dtype=np.int32).reshape((1, -1, 2))
- cv2.fillPoly(text_region_mask, polygon, 1)
-
- return text_region_mask
-
- def _generate_center_mask_attrib_maps(
- self, img_size: Tuple[int, int], text_polys: List[ndarray]
- ) -> Tuple[ndarray, ndarray, ndarray, ndarray]:
- """Generate text center region mask and geometric attribute maps.
-
- Args:
- img_size (tuple(int, int)): The image size of (height, width).
- text_polys (list[ndarray]): The list of text polygons.
-
- Returns:
- Tuple(center_region_mask, radius_map, sin_map, cos_map):
-
- - center_region_mask (ndarray): The text center region mask.
- - radius_map (ndarray): The distance map from each pixel in text
- center region to top sideline.
- - sin_map (ndarray): The sin(theta) map where theta is the angle
- between vector (top point - bottom point) and vector (1, 0).
- - cos_map (ndarray): The cos(theta) map where theta is the angle
- between vector (top point - bottom point) and vector (1, 0).
- """
-
- assert isinstance(img_size, tuple)
-
- center_region_mask = np.zeros(img_size, np.uint8)
- radius_map = np.zeros(img_size, dtype=np.float32)
- sin_map = np.zeros(img_size, dtype=np.float32)
- cos_map = np.zeros(img_size, dtype=np.float32)
-
- for poly in text_polys:
- polygon_points = np.array(poly).reshape(-1, 2)
-
- n = len(polygon_points)
- keep_inds = []
- for i in range(n):
- if norm(polygon_points[i] -
- polygon_points[(i + 1) % n]) > 1e-5:
- keep_inds.append(i)
- polygon_points = polygon_points[keep_inds]
-
- _, _, top_line, bot_line = self._reorder_poly_edge(polygon_points)
- resampled_top_line, resampled_bot_line = self._resample_sidelines(
- top_line, bot_line, self.resample_step)
- resampled_bot_line = resampled_bot_line[::-1]
- center_line = (resampled_top_line + resampled_bot_line) / 2
-
- if self.vector_slope(center_line[-1] - center_line[0]) > 0.9:
- if (center_line[-1] - center_line[0])[1] < 0:
- center_line = center_line[::-1]
- resampled_top_line = resampled_top_line[::-1]
- resampled_bot_line = resampled_bot_line[::-1]
- else:
- if (center_line[-1] - center_line[0])[0] < 0:
- center_line = center_line[::-1]
- resampled_top_line = resampled_top_line[::-1]
- resampled_bot_line = resampled_bot_line[::-1]
-
- line_head_shrink_len = norm(resampled_top_line[0] -
- resampled_bot_line[0]) / 4.0
- line_tail_shrink_len = norm(resampled_top_line[-1] -
- resampled_bot_line[-1]) / 4.0
- head_shrink_num = int(line_head_shrink_len // self.resample_step)
- tail_shrink_num = int(line_tail_shrink_len // self.resample_step)
-
- if len(center_line) > head_shrink_num + tail_shrink_num + 2:
- center_line = center_line[head_shrink_num:len(center_line) -
- tail_shrink_num]
- resampled_top_line = resampled_top_line[
- head_shrink_num:len(resampled_top_line) - tail_shrink_num]
- resampled_bot_line = resampled_bot_line[
- head_shrink_num:len(resampled_bot_line) - tail_shrink_num]
-
- self._draw_center_region_maps(resampled_top_line,
- resampled_bot_line, center_line,
- center_region_mask, radius_map,
- sin_map, cos_map,
- self.center_region_shrink_ratio)
-
- return center_region_mask, radius_map, sin_map, cos_map
-
- def _reorder_poly_edge(self, points: ndarray
- ) -> Tuple[ndarray, ndarray, ndarray, ndarray]:
- """Get the respective points composing head edge, tail edge, top
- sideline and bottom sideline.
-
- Args:
- points (ndarray): The points composing a text polygon.
-
- Returns:
- Tuple(center_region_mask, radius_map, sin_map, cos_map):
-
- - head_edge (ndarray): The two points composing the head edge of
- text polygon.
- - tail_edge (ndarray): The two points composing the tail edge of
- text polygon.
- - top_sideline (ndarray): The points composing top curved sideline
- of text polygon.
- - bot_sideline (ndarray): The points composing bottom curved
- sideline of text polygon.
- """
-
- assert points.ndim == 2
- assert points.shape[0] >= 4
- assert points.shape[1] == 2
-
- head_inds, tail_inds = self._find_head_tail(points,
- self.orientation_thr)
- head_edge, tail_edge = points[head_inds], points[tail_inds]
-
- pad_points = np.vstack([points, points])
- if tail_inds[1] < 1:
- tail_inds[1] = len(points)
- sideline1 = pad_points[head_inds[1]:tail_inds[1]]
- sideline2 = pad_points[tail_inds[1]:(head_inds[1] + len(points))]
- sideline_mean_shift = np.mean(
- sideline1, axis=0) - np.mean(
- sideline2, axis=0)
-
- if sideline_mean_shift[1] > 0:
- top_sideline, bot_sideline = sideline2, sideline1
- else:
- top_sideline, bot_sideline = sideline1, sideline2
-
- return head_edge, tail_edge, top_sideline, bot_sideline
-
- def _find_head_tail(self, points: ndarray,
- orientation_thr: float) -> Tuple[List[int], List[int]]:
- """Find the head edge and tail edge of a text polygon.
-
- Args:
- points (ndarray): The points composing a text polygon.
- orientation_thr (float): The threshold for distinguishing between
- head edge and tail edge among the horizontal and vertical edges
- of a quadrangle.
-
- Returns:
- Tuple(head_inds, tail_inds):
-
- - head_inds (list[int]): The indexes of two points composing head
- edge.
- - tail_inds (list[int]): The indexes of two points composing tail
- edge.
- """
-
- assert points.ndim == 2
- assert points.shape[0] >= 4
- assert points.shape[1] == 2
- assert isinstance(orientation_thr, float)
-
- if len(points) > 4:
- pad_points = np.vstack([points, points[0]])
- edge_vec = pad_points[1:] - pad_points[:-1]
-
- theta_sum = []
- adjacent_vec_theta = []
- for i, edge_vec1 in enumerate(edge_vec):
- adjacent_ind = [x % len(edge_vec) for x in [i - 1, i + 1]]
- adjacent_edge_vec = edge_vec[adjacent_ind]
- temp_theta_sum = np.sum(
- self.vector_angle(edge_vec1, adjacent_edge_vec))
- temp_adjacent_theta = self.vector_angle(
- adjacent_edge_vec[0], adjacent_edge_vec[1])
- theta_sum.append(temp_theta_sum)
- adjacent_vec_theta.append(temp_adjacent_theta)
- theta_sum_score = np.array(theta_sum) / np.pi
- adjacent_theta_score = np.array(adjacent_vec_theta) / np.pi
- poly_center = np.mean(points, axis=0)
- edge_dist = np.maximum(
- norm(pad_points[1:] - poly_center, axis=-1),
- norm(pad_points[:-1] - poly_center, axis=-1))
- dist_score = edge_dist / (np.max(edge_dist) + self.eps)
- position_score = np.zeros(len(edge_vec))
- score = 0.5 * theta_sum_score + 0.15 * adjacent_theta_score
- score += 0.35 * dist_score
- if len(points) % 2 == 0:
- position_score[(len(score) // 2 - 1)] += 1
- position_score[-1] += 1
- score += 0.1 * position_score
- pad_score = np.concatenate([score, score])
- score_matrix = np.zeros((len(score), len(score) - 3))
- x = np.arange(len(score) - 3) / float(len(score) - 4)
- gaussian = 1. / (np.sqrt(2. * np.pi) * 0.5) * np.exp(-np.power(
- (x - 0.5) / 0.5, 2.) / 2)
- gaussian = gaussian / np.max(gaussian)
- for i in range(len(score)):
- score_matrix[i, :] = score[i] + pad_score[
- (i + 2):(i + len(score) - 1)] * gaussian * 0.3
-
- head_start, tail_increment = np.unravel_index(
- score_matrix.argmax(), score_matrix.shape)
- tail_start = (head_start + tail_increment + 2) % len(points)
- head_end = (head_start + 1) % len(points)
- tail_end = (tail_start + 1) % len(points)
-
- if head_end > tail_end:
- head_start, tail_start = tail_start, head_start
- head_end, tail_end = tail_end, head_end
- head_inds = [head_start, head_end]
- tail_inds = [tail_start, tail_end]
- else:
- if self.vector_slope(points[1] - points[0]) + self.vector_slope(
- points[3] - points[2]) < self.vector_slope(
- points[2] - points[1]) + self.vector_slope(points[0] -
- points[3]):
- horizontal_edge_inds = [[0, 1], [2, 3]]
- vertical_edge_inds = [[3, 0], [1, 2]]
- else:
- horizontal_edge_inds = [[3, 0], [1, 2]]
- vertical_edge_inds = [[0, 1], [2, 3]]
-
- vertical_len_sum = norm(points[vertical_edge_inds[0][0]] -
- points[vertical_edge_inds[0][1]]) + norm(
- points[vertical_edge_inds[1][0]] -
- points[vertical_edge_inds[1][1]])
- horizontal_len_sum = norm(
- points[horizontal_edge_inds[0][0]] -
- points[horizontal_edge_inds[0][1]]) + norm(
- points[horizontal_edge_inds[1][0]] -
- points[horizontal_edge_inds[1][1]])
-
- if vertical_len_sum > horizontal_len_sum * orientation_thr:
- head_inds = horizontal_edge_inds[0]
- tail_inds = horizontal_edge_inds[1]
- else:
- head_inds = vertical_edge_inds[0]
- tail_inds = vertical_edge_inds[1]
-
- return head_inds, tail_inds
-
- def _resample_line(self, line: ndarray, n: int) -> ndarray:
- """Resample n points on a line.
-
- Args:
- line (ndarray): The points composing a line.
- n (int): The resampled points number.
-
- Returns:
- resampled_line (ndarray): The points composing the resampled line.
- """
-
- assert line.ndim == 2
- assert line.shape[0] >= 2
- assert line.shape[1] == 2
- assert isinstance(n, int)
- assert n > 2
-
- edges_length, total_length = self._cal_curve_length(line)
- t_org = np.insert(np.cumsum(edges_length), 0, 0)
- unit_t = total_length / (n - 1)
- t_equidistant = np.arange(1, n - 1, dtype=np.float32) * unit_t
- edge_ind = 0
- points = [line[0]]
- for t in t_equidistant:
- while edge_ind < len(edges_length) - 1 and t > t_org[edge_ind + 1]:
- edge_ind += 1
- t_l, t_r = t_org[edge_ind], t_org[edge_ind + 1]
- weight = np.array([t_r - t, t - t_l], dtype=np.float32) / (
- t_r - t_l + self.eps)
- p_coords = np.dot(weight, line[[edge_ind, edge_ind + 1]])
- points.append(p_coords)
- points.append(line[-1])
- resampled_line = np.vstack(points)
-
- return resampled_line
-
- def _resample_sidelines(self, sideline1: ndarray, sideline2: ndarray,
- resample_step: float) -> Tuple[ndarray, ndarray]:
- """Resample two sidelines to be of the same points number according to
- step size.
-
- Args:
- sideline1 (ndarray): The points composing a sideline of a text
- polygon.
- sideline2 (ndarray): The points composing another sideline of a
- text polygon.
- resample_step (float): The resampled step size.
-
- Returns:
- Tuple(resampled_line1, resampled_line2):
-
- - resampled_line1 (ndarray): The resampled line 1.
- - resampled_line2 (ndarray): The resampled line 2.
- """
-
- assert sideline1.ndim == sideline2.ndim == 2
- assert sideline1.shape[1] == sideline2.shape[1] == 2
- assert sideline1.shape[0] >= 2
- assert sideline2.shape[0] >= 2
- assert isinstance(resample_step, float)
-
- _, length1 = self._cal_curve_length(sideline1)
- _, length2 = self._cal_curve_length(sideline2)
-
- avg_length = (length1 + length2) / 2
- resample_point_num = max(int(float(avg_length) / resample_step) + 1, 3)
-
- resampled_line1 = self._resample_line(sideline1, resample_point_num)
- resampled_line2 = self._resample_line(sideline2, resample_point_num)
-
- return resampled_line1, resampled_line2
-
- def _cal_curve_length(self, line: ndarray) -> Tuple[ndarray, float]:
- """Calculate the length of each edge on the discrete curve and the sum.
-
- Args:
- line (ndarray): The points composing a discrete curve.
-
- Returns:
- Tuple(edges_length, total_length):
-
- - edge_length (ndarray): The length of each edge on the
- discrete curve.
- - total_length (float): The total length of the discrete
- curve.
- """
-
- assert line.ndim == 2
- assert len(line) >= 2
-
- edges_length = np.sqrt((line[1:, 0] - line[:-1, 0])**2 +
- (line[1:, 1] - line[:-1, 1])**2)
- total_length = np.sum(edges_length)
- return edges_length, total_length
-
- def _draw_center_region_maps(self, top_line: ndarray, bot_line: ndarray,
- center_line: ndarray,
- center_region_mask: ndarray,
- radius_map: ndarray, sin_map: ndarray,
- cos_map: ndarray,
- region_shrink_ratio: float) -> None:
- """Draw attributes on text center region.
-
- Args:
- top_line (ndarray): The points composing top curved sideline of
- text polygon.
- bot_line (ndarray): The points composing bottom curved sideline
- of text polygon.
- center_line (ndarray): The points composing the center line of text
- instance.
- center_region_mask (ndarray): The text center region mask.
- radius_map (ndarray): The map where the distance from point to
- sidelines will be drawn on for each pixel in text center
- region.
- sin_map (ndarray): The map where vector_sin(theta) will be drawn
- on text center regions. Theta is the angle between tangent
- line and vector (1, 0).
- cos_map (ndarray): The map where vector_cos(theta) will be drawn on
- text center regions. Theta is the angle between tangent line
- and vector (1, 0).
- region_shrink_ratio (float): The shrink ratio of text center.
- """
-
- assert top_line.shape == bot_line.shape == center_line.shape
- assert (center_region_mask.shape == radius_map.shape == sin_map.shape
- == cos_map.shape)
- assert isinstance(region_shrink_ratio, float)
- for i in range(0, len(center_line) - 1):
-
- top_mid_point = (top_line[i] + top_line[i + 1]) / 2
- bot_mid_point = (bot_line[i] + bot_line[i + 1]) / 2
- radius = norm(top_mid_point - bot_mid_point) / 2
-
- text_direction = center_line[i + 1] - center_line[i]
- sin_theta = self.vector_sin(text_direction)
- cos_theta = self.vector_cos(text_direction)
-
- tl = center_line[i] + (top_line[i] -
- center_line[i]) * region_shrink_ratio
- tr = center_line[i + 1] + (
- top_line[i + 1] - center_line[i + 1]) * region_shrink_ratio
- br = center_line[i + 1] + (
- bot_line[i + 1] - center_line[i + 1]) * region_shrink_ratio
- bl = center_line[i] + (bot_line[i] -
- center_line[i]) * region_shrink_ratio
- current_center_box = np.vstack([tl, tr, br, bl]).astype(np.int32)
-
- cv2.fillPoly(center_region_mask, [current_center_box], color=1)
- cv2.fillPoly(sin_map, [current_center_box], color=sin_theta)
- cv2.fillPoly(cos_map, [current_center_box], color=cos_theta)
- cv2.fillPoly(radius_map, [current_center_box], color=radius)
-
- def vector_angle(self, vec1: ndarray, vec2: ndarray) -> ndarray:
- """Compute the angle between two vectors."""
- if vec1.ndim > 1:
- unit_vec1 = vec1 / (norm(vec1, axis=-1) + self.eps).reshape(
- (-1, 1))
- else:
- unit_vec1 = vec1 / (norm(vec1, axis=-1) + self.eps)
- if vec2.ndim > 1:
- unit_vec2 = vec2 / (norm(vec2, axis=-1) + self.eps).reshape(
- (-1, 1))
- else:
- unit_vec2 = vec2 / (norm(vec2, axis=-1) + self.eps)
- return np.arccos(
- np.clip(np.sum(unit_vec1 * unit_vec2, axis=-1), -1.0, 1.0))
-
- def vector_slope(self, vec: ndarray) -> float:
- """Compute the slope of a vector."""
- assert len(vec) == 2
- return abs(vec[1] / (vec[0] + self.eps))
-
- def vector_sin(self, vec: ndarray) -> float:
- """Compute the sin of the angle between vector and x-axis."""
- assert len(vec) == 2
- return vec[1] / (norm(vec) + self.eps)
-
- def vector_cos(self, vec: ndarray) -> float:
- """Compute the cos of the angle between vector and x-axis."""
- assert len(vec) == 2
- return vec[0] / (norm(vec) + self.eps)
diff --git a/pipeline/mmocr/mmocr/models/textdet/necks/__init__.py b/pipeline/mmocr/mmocr/models/textdet/necks/__init__.py
deleted file mode 100644
index 0b21bf192b93f8a09278989837f8b9b762052f7e..0000000000000000000000000000000000000000
--- a/pipeline/mmocr/mmocr/models/textdet/necks/__init__.py
+++ /dev/null
@@ -1,7 +0,0 @@
-# Copyright (c) OpenMMLab. All rights reserved.
-from .fpem_ffm import FPEM_FFM
-from .fpn_cat import FPNC
-from .fpn_unet import FPN_UNet
-from .fpnf import FPNF
-
-__all__ = ['FPEM_FFM', 'FPNF', 'FPNC', 'FPN_UNet']
diff --git a/pipeline/mmocr/mmocr/models/textdet/necks/__pycache__/__init__.cpython-38.pyc b/pipeline/mmocr/mmocr/models/textdet/necks/__pycache__/__init__.cpython-38.pyc
deleted file mode 100644
index f5b28121692c3473c8f5c5c6d93a7267abf64a29..0000000000000000000000000000000000000000
Binary files a/pipeline/mmocr/mmocr/models/textdet/necks/__pycache__/__init__.cpython-38.pyc and /dev/null differ
diff --git a/pipeline/mmocr/mmocr/models/textdet/necks/__pycache__/__init__.cpython-39.pyc b/pipeline/mmocr/mmocr/models/textdet/necks/__pycache__/__init__.cpython-39.pyc
deleted file mode 100644
index af38e74dbe83594719013b00fecfd67ae60a5952..0000000000000000000000000000000000000000
Binary files a/pipeline/mmocr/mmocr/models/textdet/necks/__pycache__/__init__.cpython-39.pyc and /dev/null differ
diff --git a/pipeline/mmocr/mmocr/models/textdet/necks/__pycache__/fpem_ffm.cpython-38.pyc b/pipeline/mmocr/mmocr/models/textdet/necks/__pycache__/fpem_ffm.cpython-38.pyc
deleted file mode 100644
index d9643b93e6537ad1e17fba5dc60fb4b3448d2f20..0000000000000000000000000000000000000000
Binary files a/pipeline/mmocr/mmocr/models/textdet/necks/__pycache__/fpem_ffm.cpython-38.pyc and /dev/null differ
diff --git a/pipeline/mmocr/mmocr/models/textdet/necks/__pycache__/fpem_ffm.cpython-39.pyc b/pipeline/mmocr/mmocr/models/textdet/necks/__pycache__/fpem_ffm.cpython-39.pyc
deleted file mode 100644
index 30883ef5efdc6aed258d9ded97b41c1439f38024..0000000000000000000000000000000000000000
Binary files a/pipeline/mmocr/mmocr/models/textdet/necks/__pycache__/fpem_ffm.cpython-39.pyc and /dev/null differ
diff --git a/pipeline/mmocr/mmocr/models/textdet/necks/__pycache__/fpn_cat.cpython-38.pyc b/pipeline/mmocr/mmocr/models/textdet/necks/__pycache__/fpn_cat.cpython-38.pyc
deleted file mode 100644
index 3ee0112a206cbe911c5f2ea79206c5b411317958..0000000000000000000000000000000000000000
Binary files a/pipeline/mmocr/mmocr/models/textdet/necks/__pycache__/fpn_cat.cpython-38.pyc and /dev/null differ
diff --git a/pipeline/mmocr/mmocr/models/textdet/necks/__pycache__/fpn_cat.cpython-39.pyc b/pipeline/mmocr/mmocr/models/textdet/necks/__pycache__/fpn_cat.cpython-39.pyc
deleted file mode 100644
index 4954a61ba9ac37542a0e62200710cf4f6fe37b5a..0000000000000000000000000000000000000000
Binary files a/pipeline/mmocr/mmocr/models/textdet/necks/__pycache__/fpn_cat.cpython-39.pyc and /dev/null differ
diff --git a/pipeline/mmocr/mmocr/models/textdet/necks/__pycache__/fpn_unet.cpython-38.pyc b/pipeline/mmocr/mmocr/models/textdet/necks/__pycache__/fpn_unet.cpython-38.pyc
deleted file mode 100644
index 95042ead7967f02bd32a5f200e4b01d52bb87f23..0000000000000000000000000000000000000000
Binary files a/pipeline/mmocr/mmocr/models/textdet/necks/__pycache__/fpn_unet.cpython-38.pyc and /dev/null differ
diff --git a/pipeline/mmocr/mmocr/models/textdet/necks/__pycache__/fpn_unet.cpython-39.pyc b/pipeline/mmocr/mmocr/models/textdet/necks/__pycache__/fpn_unet.cpython-39.pyc
deleted file mode 100644
index 5e61f95f837c0b8de9117c917f4ce5be2c9222e4..0000000000000000000000000000000000000000
Binary files a/pipeline/mmocr/mmocr/models/textdet/necks/__pycache__/fpn_unet.cpython-39.pyc and /dev/null differ
diff --git a/pipeline/mmocr/mmocr/models/textdet/necks/__pycache__/fpnf.cpython-38.pyc b/pipeline/mmocr/mmocr/models/textdet/necks/__pycache__/fpnf.cpython-38.pyc
deleted file mode 100644
index 04754211b192abe2563a34b55ef34a6258d7fb42..0000000000000000000000000000000000000000
Binary files a/pipeline/mmocr/mmocr/models/textdet/necks/__pycache__/fpnf.cpython-38.pyc and /dev/null differ
diff --git a/pipeline/mmocr/mmocr/models/textdet/necks/__pycache__/fpnf.cpython-39.pyc b/pipeline/mmocr/mmocr/models/textdet/necks/__pycache__/fpnf.cpython-39.pyc
deleted file mode 100644
index 41e64a650a488bf63c13307ce432df5de38aad54..0000000000000000000000000000000000000000
Binary files a/pipeline/mmocr/mmocr/models/textdet/necks/__pycache__/fpnf.cpython-39.pyc and /dev/null differ
diff --git a/pipeline/mmocr/mmocr/models/textdet/necks/fpem_ffm.py b/pipeline/mmocr/mmocr/models/textdet/necks/fpem_ffm.py
deleted file mode 100644
index 265fdaab674b29bba294a368e2a8683d1aa42da0..0000000000000000000000000000000000000000
--- a/pipeline/mmocr/mmocr/models/textdet/necks/fpem_ffm.py
+++ /dev/null
@@ -1,202 +0,0 @@
-# Copyright (c) OpenMMLab. All rights reserved.
-from typing import Dict, List, Optional, Tuple, Union
-
-import torch
-import torch.nn.functional as F
-from mmengine.model import BaseModule, ModuleList
-from torch import nn
-
-from mmocr.registry import MODELS
-
-
-class FPEM(BaseModule):
- """FPN-like feature fusion module in PANet.
-
- Args:
- in_channels (int): Number of input channels.
- init_cfg (dict or list[dict], optional): Initialization configs.
- """
-
- def __init__(self,
- in_channels: int = 128,
- init_cfg: Optional[Union[Dict, List[Dict]]] = None) -> None:
- super().__init__(init_cfg=init_cfg)
- self.up_add1 = SeparableConv2d(in_channels, in_channels, 1)
- self.up_add2 = SeparableConv2d(in_channels, in_channels, 1)
- self.up_add3 = SeparableConv2d(in_channels, in_channels, 1)
- self.down_add1 = SeparableConv2d(in_channels, in_channels, 2)
- self.down_add2 = SeparableConv2d(in_channels, in_channels, 2)
- self.down_add3 = SeparableConv2d(in_channels, in_channels, 2)
-
- def forward(self, c2: torch.Tensor, c3: torch.Tensor, c4: torch.Tensor,
- c5: torch.Tensor) -> List[torch.Tensor]:
- """
- Args:
- c2, c3, c4, c5 (Tensor): Each has the shape of
- :math:`(N, C_i, H_i, W_i)`.
-
- Returns:
- list[Tensor]: A list of 4 tensors of the same shape as input.
- """
- # upsample
- c4 = self.up_add1(self._upsample_add(c5, c4)) # c4 shape
- c3 = self.up_add2(self._upsample_add(c4, c3))
- c2 = self.up_add3(self._upsample_add(c3, c2))
-
- # downsample
- c3 = self.down_add1(self._upsample_add(c3, c2))
- c4 = self.down_add2(self._upsample_add(c4, c3))
- c5 = self.down_add3(self._upsample_add(c5, c4)) # c4 / 2
- return c2, c3, c4, c5
-
- def _upsample_add(self, x, y):
- return F.interpolate(x, size=y.size()[2:]) + y
-
-
-class SeparableConv2d(BaseModule):
- """Implementation of separable convolution, which is consisted of depthwise
- convolution and pointwise convolution.
-
- Args:
- in_channels (int): Number of input channels.
- out_channels (int): Number of output channels.
- stride (int): Stride of the depthwise convolution.
- init_cfg (dict or list[dict], optional): Initialization configs.
- """
-
- def __init__(self,
- in_channels: int,
- out_channels: int,
- stride: int = 1,
- init_cfg: Optional[Union[Dict, List[Dict]]] = None) -> None:
- super().__init__(init_cfg=init_cfg)
-
- self.depthwise_conv = nn.Conv2d(
- in_channels=in_channels,
- out_channels=in_channels,
- kernel_size=3,
- padding=1,
- stride=stride,
- groups=in_channels)
- self.pointwise_conv = nn.Conv2d(
- in_channels=in_channels, out_channels=out_channels, kernel_size=1)
- self.bn = nn.BatchNorm2d(out_channels)
- self.relu = nn.ReLU()
-
- def forward(self, x: torch.Tensor) -> torch.Tensor:
- """Forward function.
-
- Args:
- x (Tensor): Input tensor.
-
- Returns:
- Tensor: Output tensor.
- """
- x = self.depthwise_conv(x)
- x = self.pointwise_conv(x)
- x = self.bn(x)
- x = self.relu(x)
- return x
-
-
-@MODELS.register_module()
-class FPEM_FFM(BaseModule):
- """This code is from https://github.com/WenmuZhou/PAN.pytorch.
-
- Args:
- in_channels (list[int]): A list of 4 numbers of input channels.
- conv_out (int): Number of output channels.
- fpem_repeat (int): Number of FPEM layers before FFM operations.
- align_corners (bool): The interpolation behaviour in FFM operation,
- used in :func:`torch.nn.functional.interpolate`.
- init_cfg (dict or list[dict], optional): Initialization configs.
- """
-
- def __init__(
- self,
- in_channels: List[int],
- conv_out: int = 128,
- fpem_repeat: int = 2,
- align_corners: bool = False,
- init_cfg: Optional[Union[Dict, List[Dict]]] = dict(
- type='Xavier', layer='Conv2d', distribution='uniform')
- ) -> None:
- super().__init__(init_cfg=init_cfg)
- # reduce layers
- self.reduce_conv_c2 = nn.Sequential(
- nn.Conv2d(
- in_channels=in_channels[0],
- out_channels=conv_out,
- kernel_size=1), nn.BatchNorm2d(conv_out), nn.ReLU())
- self.reduce_conv_c3 = nn.Sequential(
- nn.Conv2d(
- in_channels=in_channels[1],
- out_channels=conv_out,
- kernel_size=1), nn.BatchNorm2d(conv_out), nn.ReLU())
- self.reduce_conv_c4 = nn.Sequential(
- nn.Conv2d(
- in_channels=in_channels[2],
- out_channels=conv_out,
- kernel_size=1), nn.BatchNorm2d(conv_out), nn.ReLU())
- self.reduce_conv_c5 = nn.Sequential(
- nn.Conv2d(
- in_channels=in_channels[3],
- out_channels=conv_out,
- kernel_size=1), nn.BatchNorm2d(conv_out), nn.ReLU())
- self.align_corners = align_corners
- self.fpems = ModuleList()
- for _ in range(fpem_repeat):
- self.fpems.append(FPEM(conv_out))
-
- def forward(self, x: List[torch.Tensor]) -> Tuple[torch.Tensor]:
- """
- Args:
- x (list[Tensor]): A list of four tensors of shape
- :math:`(N, C_i, H_i, W_i)`, representing C2, C3, C4, C5
- features respectively. :math:`C_i` should matches the number in
- ``in_channels``.
-
- Returns:
- tuple[Tensor]: Four tensors of shape
- :math:`(N, C_{out}, H_0, W_0)` where :math:`C_{out}` is
- ``conv_out``.
- """
- c2, c3, c4, c5 = x
- # reduce channel
- c2 = self.reduce_conv_c2(c2)
- c3 = self.reduce_conv_c3(c3)
- c4 = self.reduce_conv_c4(c4)
- c5 = self.reduce_conv_c5(c5)
-
- # FPEM
- for i, fpem in enumerate(self.fpems):
- c2, c3, c4, c5 = fpem(c2, c3, c4, c5)
- if i == 0:
- c2_ffm = c2
- c3_ffm = c3
- c4_ffm = c4
- c5_ffm = c5
- else:
- c2_ffm = c2_ffm + c2
- c3_ffm = c3_ffm + c3
- c4_ffm = c4_ffm + c4
- c5_ffm = c5_ffm + c5
-
- # FFM
- c5 = F.interpolate(
- c5_ffm,
- c2_ffm.size()[-2:],
- mode='bilinear',
- align_corners=self.align_corners)
- c4 = F.interpolate(
- c4_ffm,
- c2_ffm.size()[-2:],
- mode='bilinear',
- align_corners=self.align_corners)
- c3 = F.interpolate(
- c3_ffm,
- c2_ffm.size()[-2:],
- mode='bilinear',
- align_corners=self.align_corners)
- outs = [c2_ffm, c3, c4, c5]
- return tuple(outs)
diff --git a/pipeline/mmocr/mmocr/models/textdet/necks/fpn_cat.py b/pipeline/mmocr/mmocr/models/textdet/necks/fpn_cat.py
deleted file mode 100644
index c1c8efb354b3ca5598db76e785fdbe620ef147e6..0000000000000000000000000000000000000000
--- a/pipeline/mmocr/mmocr/models/textdet/necks/fpn_cat.py
+++ /dev/null
@@ -1,276 +0,0 @@
-# Copyright (c) OpenMMLab. All rights reserved.
-from typing import Dict, List, Optional, Union
-
-import torch
-import torch.nn as nn
-import torch.nn.functional as F
-from mmcv.cnn import ConvModule
-from mmengine.model import BaseModule, ModuleList, Sequential
-
-from mmocr.registry import MODELS
-
-
-@MODELS.register_module()
-class FPNC(BaseModule):
- """FPN-like fusion module in Real-time Scene Text Detection with
- Differentiable Binarization.
-
- This was partially adapted from https://github.com/MhLiao/DB and
- https://github.com/WenmuZhou/DBNet.pytorch.
-
- Args:
- in_channels (list[int]): A list of numbers of input channels.
- lateral_channels (int): Number of channels for lateral layers.
- out_channels (int): Number of output channels.
- bias_on_lateral (bool): Whether to use bias on lateral convolutional
- layers.
- bn_re_on_lateral (bool): Whether to use BatchNorm and ReLU
- on lateral convolutional layers.
- bias_on_smooth (bool): Whether to use bias on smoothing layer.
- bn_re_on_smooth (bool): Whether to use BatchNorm and ReLU on smoothing
- layer.
- asf_cfg (dict, optional): Adaptive Scale Fusion module configs. The
- attention_type can be 'ScaleChannelSpatial'.
- conv_after_concat (bool): Whether to add a convolution layer after
- the concatenation of predictions.
- init_cfg (dict or list[dict], optional): Initialization configs.
- """
-
- def __init__(
- self,
- in_channels: List[int],
- lateral_channels: int = 256,
- out_channels: int = 64,
- bias_on_lateral: bool = False,
- bn_re_on_lateral: bool = False,
- bias_on_smooth: bool = False,
- bn_re_on_smooth: bool = False,
- asf_cfg: Optional[Dict] = None,
- conv_after_concat: bool = False,
- init_cfg: Optional[Union[Dict, List[Dict]]] = [
- dict(type='Kaiming', layer='Conv'),
- dict(type='Constant', layer='BatchNorm', val=1., bias=1e-4)
- ]
- ) -> None:
- super().__init__(init_cfg=init_cfg)
- assert isinstance(in_channels, list)
- self.in_channels = in_channels
- self.lateral_channels = lateral_channels
- self.out_channels = out_channels
- self.num_ins = len(in_channels)
- self.bn_re_on_lateral = bn_re_on_lateral
- self.bn_re_on_smooth = bn_re_on_smooth
- self.asf_cfg = asf_cfg
- self.conv_after_concat = conv_after_concat
- self.lateral_convs = ModuleList()
- self.smooth_convs = ModuleList()
- self.num_outs = self.num_ins
-
- for i in range(self.num_ins):
- norm_cfg = None
- act_cfg = None
- if self.bn_re_on_lateral:
- norm_cfg = dict(type='BN')
- act_cfg = dict(type='ReLU')
- l_conv = ConvModule(
- in_channels[i],
- lateral_channels,
- 1,
- bias=bias_on_lateral,
- conv_cfg=None,
- norm_cfg=norm_cfg,
- act_cfg=act_cfg,
- inplace=False)
- norm_cfg = None
- act_cfg = None
- if self.bn_re_on_smooth:
- norm_cfg = dict(type='BN')
- act_cfg = dict(type='ReLU')
-
- smooth_conv = ConvModule(
- lateral_channels,
- out_channels,
- 3,
- bias=bias_on_smooth,
- padding=1,
- conv_cfg=None,
- norm_cfg=norm_cfg,
- act_cfg=act_cfg,
- inplace=False)
-
- self.lateral_convs.append(l_conv)
- self.smooth_convs.append(smooth_conv)
-
- if self.asf_cfg is not None:
- self.asf_conv = ConvModule(
- out_channels * self.num_outs,
- out_channels * self.num_outs,
- 3,
- padding=1,
- conv_cfg=None,
- norm_cfg=None,
- act_cfg=None,
- inplace=False)
- if self.asf_cfg['attention_type'] == 'ScaleChannelSpatial':
- self.asf_attn = ScaleChannelSpatialAttention(
- self.out_channels * self.num_outs,
- (self.out_channels * self.num_outs) // 4, self.num_outs)
- else:
- raise NotImplementedError
-
- if self.conv_after_concat:
- norm_cfg = dict(type='BN')
- act_cfg = dict(type='ReLU')
- self.out_conv = ConvModule(
- out_channels * self.num_outs,
- out_channels * self.num_outs,
- 3,
- padding=1,
- conv_cfg=None,
- norm_cfg=norm_cfg,
- act_cfg=act_cfg,
- inplace=False)
-
- def forward(self, inputs: List[torch.Tensor]) -> torch.Tensor:
- """
- Args:
- inputs (list[Tensor]): Each tensor has the shape of
- :math:`(N, C_i, H_i, W_i)`. It usually expects 4 tensors
- (C2-C5 features) from ResNet.
-
- Returns:
- Tensor: A tensor of shape :math:`(N, C_{out}, H_0, W_0)` where
- :math:`C_{out}` is ``out_channels``.
- """
- assert len(inputs) == len(self.in_channels)
- # build laterals
- laterals = [
- lateral_conv(inputs[i])
- for i, lateral_conv in enumerate(self.lateral_convs)
- ]
- used_backbone_levels = len(laterals)
- # build top-down path
- for i in range(used_backbone_levels - 1, 0, -1):
- prev_shape = laterals[i - 1].shape[2:]
- laterals[i - 1] = laterals[i - 1] + F.interpolate(
- laterals[i], size=prev_shape, mode='nearest')
- # build outputs
- # part 1: from original levels
- outs = [
- self.smooth_convs[i](laterals[i])
- for i in range(used_backbone_levels)
- ]
-
- for i, out in enumerate(outs):
- outs[i] = F.interpolate(
- outs[i], size=outs[0].shape[2:], mode='nearest')
-
- out = torch.cat(outs, dim=1)
- if self.asf_cfg is not None:
- asf_feature = self.asf_conv(out)
- attention = self.asf_attn(asf_feature)
- enhanced_feature = []
- for i, out in enumerate(outs):
- enhanced_feature.append(attention[:, i:i + 1] * outs[i])
- out = torch.cat(enhanced_feature, dim=1)
-
- if self.conv_after_concat:
- out = self.out_conv(out)
-
- return out
-
-
-class ScaleChannelSpatialAttention(BaseModule):
- """Spatial Attention module in Real-Time Scene Text Detection with
- Differentiable Binarization and Adaptive Scale Fusion.
-
- This was partially adapted from https://github.com/MhLiao/DB
-
- Args:
- in_channels (int): A numbers of input channels.
- c_wise_channels (int): Number of channel-wise attention channels.
- out_channels (int): Number of output channels.
- init_cfg (dict or list[dict], optional): Initialization configs.
- """
-
- def __init__(
- self,
- in_channels: int,
- c_wise_channels: int,
- out_channels: int,
- init_cfg: Optional[Union[Dict, List[Dict]]] = [
- dict(type='Kaiming', layer='Conv', bias=0)
- ]
- ) -> None:
- super().__init__(init_cfg=init_cfg)
- self.avg_pool = nn.AdaptiveAvgPool2d(1)
- # Channel Wise
- self.channel_wise = Sequential(
- ConvModule(
- in_channels,
- c_wise_channels,
- 1,
- bias=False,
- conv_cfg=None,
- norm_cfg=None,
- act_cfg=dict(type='ReLU'),
- inplace=False),
- ConvModule(
- c_wise_channels,
- in_channels,
- 1,
- bias=False,
- conv_cfg=None,
- norm_cfg=None,
- act_cfg=dict(type='Sigmoid'),
- inplace=False))
- # Spatial Wise
- self.spatial_wise = Sequential(
- ConvModule(
- 1,
- 1,
- 3,
- padding=1,
- bias=False,
- conv_cfg=None,
- norm_cfg=None,
- act_cfg=dict(type='ReLU'),
- inplace=False),
- ConvModule(
- 1,
- 1,
- 1,
- bias=False,
- conv_cfg=None,
- norm_cfg=None,
- act_cfg=dict(type='Sigmoid'),
- inplace=False))
- # Attention Wise
- self.attention_wise = ConvModule(
- in_channels,
- out_channels,
- 1,
- bias=False,
- conv_cfg=None,
- norm_cfg=None,
- act_cfg=dict(type='Sigmoid'),
- inplace=False)
-
- def forward(self, inputs: torch.Tensor) -> torch.Tensor:
- """
- Args:
- inputs (Tensor): A concat FPN feature tensor that has the shape of
- :math:`(N, C, H, W)`.
-
- Returns:
- Tensor: An attention map of shape :math:`(N, C_{out}, H, W)`
- where :math:`C_{out}` is ``out_channels``.
- """
- out = self.avg_pool(inputs)
- out = self.channel_wise(out)
- out = out + inputs
- inputs = torch.mean(out, dim=1, keepdim=True)
- out = self.spatial_wise(inputs) + out
- out = self.attention_wise(out)
-
- return out
diff --git a/pipeline/mmocr/mmocr/models/textdet/necks/fpn_unet.py b/pipeline/mmocr/mmocr/models/textdet/necks/fpn_unet.py
deleted file mode 100644
index 63e0d7fc794773263f97024d0392883022079858..0000000000000000000000000000000000000000
--- a/pipeline/mmocr/mmocr/models/textdet/necks/fpn_unet.py
+++ /dev/null
@@ -1,136 +0,0 @@
-# Copyright (c) OpenMMLab. All rights reserved.
-from typing import Dict, List, Optional, Tuple, Union
-
-import torch
-import torch.nn.functional as F
-from mmengine.model import BaseModule
-from torch import nn
-
-from mmocr.registry import MODELS
-
-
-class UpBlock(BaseModule):
- """Upsample block for DRRG and TextSnake.
-
- DRRG: `Deep Relational Reasoning Graph Network for Arbitrary Shape
- Text Detection `_.
-
- TextSnake: `A Flexible Representation for Detecting Text of Arbitrary
- Shapes `_.
-
- Args:
- in_channels (list[int]): Number of input channels at each scale. The
- length of the list should be 4.
- out_channels (int): The number of output channels.
- init_cfg (dict or list[dict], optional): Initialization configs.
- Defaults to None.
- """
-
- def __init__(self,
- in_channels: int,
- out_channels: int,
- init_cfg: Optional[Union[Dict, List[Dict]]] = None) -> None:
- super().__init__(init_cfg=init_cfg)
-
- assert isinstance(in_channels, int)
- assert isinstance(out_channels, int)
-
- self.conv1x1 = nn.Conv2d(
- in_channels, in_channels, kernel_size=1, stride=1, padding=0)
- self.conv3x3 = nn.Conv2d(
- in_channels, out_channels, kernel_size=3, stride=1, padding=1)
- self.deconv = nn.ConvTranspose2d(
- out_channels, out_channels, kernel_size=4, stride=2, padding=1)
-
- def forward(self, x: torch.Tensor) -> torch.Tensor:
- """Forward propagation."""
- x = F.relu(self.conv1x1(x))
- x = F.relu(self.conv3x3(x))
- x = self.deconv(x)
- return x
-
-
-@MODELS.register_module()
-class FPN_UNet(BaseModule):
- """The class for implementing DRRG and TextSnake U-Net-like FPN.
-
- DRRG: `Deep Relational Reasoning Graph Network for Arbitrary Shape
- Text Detection `_.
-
- TextSnake: `A Flexible Representation for Detecting Text of Arbitrary
- Shapes `_.
-
- Args:
- in_channels (list[int]): Number of input channels at each scale. The
- length of the list should be 4.
- out_channels (int): The number of output channels.
- init_cfg (dict or list[dict], optional): Initialization configs.
- """
-
- def __init__(
- self,
- in_channels: int,
- out_channels: int,
- init_cfg: Optional[Union[Dict, List[Dict]]] = dict(
- type='Xavier',
- layer=['Conv2d', 'ConvTranspose2d'],
- distribution='uniform')
- ) -> None:
- super().__init__(init_cfg=init_cfg)
-
- assert len(in_channels) == 4
- assert isinstance(out_channels, int)
-
- blocks_out_channels = [out_channels] + [
- min(out_channels * 2**i, 256) for i in range(4)
- ]
- blocks_in_channels = [blocks_out_channels[1]] + [
- in_channels[i] + blocks_out_channels[i + 2] for i in range(3)
- ] + [in_channels[3]]
-
- self.up4 = nn.ConvTranspose2d(
- blocks_in_channels[4],
- blocks_out_channels[4],
- kernel_size=4,
- stride=2,
- padding=1)
- self.up_block3 = UpBlock(blocks_in_channels[3], blocks_out_channels[3])
- self.up_block2 = UpBlock(blocks_in_channels[2], blocks_out_channels[2])
- self.up_block1 = UpBlock(blocks_in_channels[1], blocks_out_channels[1])
- self.up_block0 = UpBlock(blocks_in_channels[0], blocks_out_channels[0])
-
- def forward(self, x: List[Union[torch.Tensor,
- Tuple[torch.Tensor]]]) -> torch.Tensor:
- """
- Args:
- x (list[Tensor] | tuple[Tensor]): A list of four tensors of shape
- :math:`(N, C_i, H_i, W_i)`, representing C2, C3, C4, C5
- features respectively. :math:`C_i` should matches the number in
- ``in_channels``.
-
- Returns:
- Tensor: Shape :math:`(N, C, H, W)` where :math:`H=4H_0` and
- :math:`W=4W_0`.
- """
- c2, c3, c4, c5 = x
-
- x = F.relu(self.up4(c5))
-
- c4 = F.interpolate(
- c4, size=x.shape[2:], mode='bilinear', align_corners=True)
- x = torch.cat([x, c4], dim=1)
- x = F.relu(self.up_block3(x))
-
- c3 = F.interpolate(
- c3, size=x.shape[2:], mode='bilinear', align_corners=True)
- x = torch.cat([x, c3], dim=1)
- x = F.relu(self.up_block2(x))
-
- c2 = F.interpolate(
- c2, size=x.shape[2:], mode='bilinear', align_corners=True)
- x = torch.cat([x, c2], dim=1)
- x = F.relu(self.up_block1(x))
-
- x = self.up_block0(x)
- # the output should be of the same height and width as backbone input
- return x
diff --git a/pipeline/mmocr/mmocr/models/textdet/necks/fpnf.py b/pipeline/mmocr/mmocr/models/textdet/necks/fpnf.py
deleted file mode 100644
index 17887e66b8c74b1f60383479e5df8f01b528a40b..0000000000000000000000000000000000000000
--- a/pipeline/mmocr/mmocr/models/textdet/necks/fpnf.py
+++ /dev/null
@@ -1,136 +0,0 @@
-# Copyright (c) OpenMMLab. All rights reserved.
-from typing import Dict, List, Optional, Union
-
-import torch
-import torch.nn.functional as F
-from mmcv.cnn import ConvModule
-from mmengine.model import BaseModule, ModuleList
-from torch import Tensor
-
-from mmocr.registry import MODELS
-
-
-@MODELS.register_module()
-class FPNF(BaseModule):
- """FPN-like fusion module in Shape Robust Text Detection with Progressive
- Scale Expansion Network.
-
- Args:
- in_channels (list[int]): A list of number of input channels.
- Defaults to [256, 512, 1024, 2048].
- out_channels (int): The number of output channels.
- Defaults to 256.
- fusion_type (str): Type of the final feature fusion layer. Available
- options are "concat" and "add". Defaults to "concat".
- init_cfg (dict or list[dict], optional): Initialization configs.
- Defaults to
- dict(type='Xavier', layer='Conv2d', distribution='uniform')
- """
-
- def __init__(
- self,
- in_channels: List[int] = [256, 512, 1024, 2048],
- out_channels: int = 256,
- fusion_type: str = 'concat',
- init_cfg: Optional[Union[Dict, List[Dict]]] = dict(
- type='Xavier', layer='Conv2d', distribution='uniform')
- ) -> None:
- super().__init__(init_cfg=init_cfg)
- conv_cfg = None
- norm_cfg = dict(type='BN')
- act_cfg = dict(type='ReLU')
-
- self.in_channels = in_channels
- self.out_channels = out_channels
-
- self.lateral_convs = ModuleList()
- self.fpn_convs = ModuleList()
- self.backbone_end_level = len(in_channels)
- for i in range(self.backbone_end_level):
- l_conv = ConvModule(
- in_channels[i],
- out_channels,
- 1,
- conv_cfg=conv_cfg,
- norm_cfg=norm_cfg,
- act_cfg=act_cfg,
- inplace=False)
- self.lateral_convs.append(l_conv)
-
- if i < self.backbone_end_level - 1:
- fpn_conv = ConvModule(
- out_channels,
- out_channels,
- 3,
- padding=1,
- conv_cfg=conv_cfg,
- norm_cfg=norm_cfg,
- act_cfg=act_cfg,
- inplace=False)
- self.fpn_convs.append(fpn_conv)
-
- self.fusion_type = fusion_type
-
- if self.fusion_type == 'concat':
- feature_channels = 1024
- elif self.fusion_type == 'add':
- feature_channels = 256
- else:
- raise NotImplementedError
-
- self.output_convs = ConvModule(
- feature_channels,
- out_channels,
- 3,
- padding=1,
- conv_cfg=None,
- norm_cfg=norm_cfg,
- act_cfg=act_cfg,
- inplace=False)
-
- def forward(self, inputs: List[Tensor]) -> Tensor:
- """
- Args:
- inputs (list[Tensor]): Each tensor has the shape of
- :math:`(N, C_i, H_i, W_i)`. It usually expects 4 tensors
- (C2-C5 features) from ResNet.
-
- Returns:
- Tensor: A tensor of shape :math:`(N, C_{out}, H_0, W_0)` where
- :math:`C_{out}` is ``out_channels``.
- """
- assert len(inputs) == len(self.in_channels)
-
- # build laterals
- laterals = [
- lateral_conv(inputs[i])
- for i, lateral_conv in enumerate(self.lateral_convs)
- ]
-
- # build top-down path
- used_backbone_levels = len(laterals)
- for i in range(used_backbone_levels - 1, 0, -1):
- # step 1: upsample to level i-1 size and add level i-1
- prev_shape = laterals[i - 1].shape[2:]
- laterals[i - 1] = laterals[i - 1] + F.interpolate(
- laterals[i], size=prev_shape, mode='nearest')
- # step 2: smooth level i-1
- laterals[i - 1] = self.fpn_convs[i - 1](laterals[i - 1])
-
- # upsample and cat
- bottom_shape = laterals[0].shape[2:]
- for i in range(1, used_backbone_levels):
- laterals[i] = F.interpolate(
- laterals[i], size=bottom_shape, mode='nearest')
-
- if self.fusion_type == 'concat':
- out = torch.cat(laterals, 1)
- elif self.fusion_type == 'add':
- out = laterals[0]
- for i in range(1, used_backbone_levels):
- out += laterals[i]
- else:
- raise NotImplementedError
- out = self.output_convs(out)
-
- return out
diff --git a/pipeline/mmocr/mmocr/models/textdet/postprocessors/__init__.py b/pipeline/mmocr/mmocr/models/textdet/postprocessors/__init__.py
deleted file mode 100644
index 783958e518b3707736aef40be7c7720ad447424c..0000000000000000000000000000000000000000
--- a/pipeline/mmocr/mmocr/models/textdet/postprocessors/__init__.py
+++ /dev/null
@@ -1,14 +0,0 @@
-# Copyright (c) OpenMMLab. All rights reserved.
-from .base import BaseTextDetPostProcessor
-from .db_postprocessor import DBPostprocessor
-from .drrg_postprocessor import DRRGPostprocessor
-from .fce_postprocessor import FCEPostprocessor
-from .pan_postprocessor import PANPostprocessor
-from .pse_postprocessor import PSEPostprocessor
-from .textsnake_postprocessor import TextSnakePostprocessor
-
-__all__ = [
- 'PSEPostprocessor', 'PANPostprocessor', 'DBPostprocessor',
- 'DRRGPostprocessor', 'FCEPostprocessor', 'TextSnakePostprocessor',
- 'BaseTextDetPostProcessor'
-]
diff --git a/pipeline/mmocr/mmocr/models/textdet/postprocessors/__pycache__/__init__.cpython-38.pyc b/pipeline/mmocr/mmocr/models/textdet/postprocessors/__pycache__/__init__.cpython-38.pyc
deleted file mode 100644
index f96c71b5a5b1c5ecd2ba95b794eeacb17a2adf85..0000000000000000000000000000000000000000
Binary files a/pipeline/mmocr/mmocr/models/textdet/postprocessors/__pycache__/__init__.cpython-38.pyc and /dev/null differ
diff --git a/pipeline/mmocr/mmocr/models/textdet/postprocessors/__pycache__/__init__.cpython-39.pyc b/pipeline/mmocr/mmocr/models/textdet/postprocessors/__pycache__/__init__.cpython-39.pyc
deleted file mode 100644
index 0c5e9cd3619e4794078d593ddd9ccaad74586201..0000000000000000000000000000000000000000
Binary files a/pipeline/mmocr/mmocr/models/textdet/postprocessors/__pycache__/__init__.cpython-39.pyc and /dev/null differ
diff --git a/pipeline/mmocr/mmocr/models/textdet/postprocessors/__pycache__/base.cpython-38.pyc b/pipeline/mmocr/mmocr/models/textdet/postprocessors/__pycache__/base.cpython-38.pyc
deleted file mode 100644
index 86caddf43f888954e1f7aa7f8691070886e29852..0000000000000000000000000000000000000000
Binary files a/pipeline/mmocr/mmocr/models/textdet/postprocessors/__pycache__/base.cpython-38.pyc and /dev/null differ
diff --git a/pipeline/mmocr/mmocr/models/textdet/postprocessors/__pycache__/base.cpython-39.pyc b/pipeline/mmocr/mmocr/models/textdet/postprocessors/__pycache__/base.cpython-39.pyc
deleted file mode 100644
index f5217d03fe3c2deba45911bfe53bfb893903e8be..0000000000000000000000000000000000000000
Binary files a/pipeline/mmocr/mmocr/models/textdet/postprocessors/__pycache__/base.cpython-39.pyc and /dev/null differ
diff --git a/pipeline/mmocr/mmocr/models/textdet/postprocessors/__pycache__/db_postprocessor.cpython-38.pyc b/pipeline/mmocr/mmocr/models/textdet/postprocessors/__pycache__/db_postprocessor.cpython-38.pyc
deleted file mode 100644
index 7ab51809eb4d18cc2265d9b530c1115da00a4a77..0000000000000000000000000000000000000000
Binary files a/pipeline/mmocr/mmocr/models/textdet/postprocessors/__pycache__/db_postprocessor.cpython-38.pyc and /dev/null differ
diff --git a/pipeline/mmocr/mmocr/models/textdet/postprocessors/__pycache__/db_postprocessor.cpython-39.pyc b/pipeline/mmocr/mmocr/models/textdet/postprocessors/__pycache__/db_postprocessor.cpython-39.pyc
deleted file mode 100644
index c35d5566f159a39a5f73a8d0b3bb797b8ee80b49..0000000000000000000000000000000000000000
Binary files a/pipeline/mmocr/mmocr/models/textdet/postprocessors/__pycache__/db_postprocessor.cpython-39.pyc and /dev/null differ
diff --git a/pipeline/mmocr/mmocr/models/textdet/postprocessors/__pycache__/drrg_postprocessor.cpython-38.pyc b/pipeline/mmocr/mmocr/models/textdet/postprocessors/__pycache__/drrg_postprocessor.cpython-38.pyc
deleted file mode 100644
index e7dd22c16c66d78ed18a910a3df302d9b191923c..0000000000000000000000000000000000000000
Binary files a/pipeline/mmocr/mmocr/models/textdet/postprocessors/__pycache__/drrg_postprocessor.cpython-38.pyc and /dev/null differ
diff --git a/pipeline/mmocr/mmocr/models/textdet/postprocessors/__pycache__/drrg_postprocessor.cpython-39.pyc b/pipeline/mmocr/mmocr/models/textdet/postprocessors/__pycache__/drrg_postprocessor.cpython-39.pyc
deleted file mode 100644
index 492a460e87741a0d8548234c8d8029b2eebe7980..0000000000000000000000000000000000000000
Binary files a/pipeline/mmocr/mmocr/models/textdet/postprocessors/__pycache__/drrg_postprocessor.cpython-39.pyc and /dev/null differ
diff --git a/pipeline/mmocr/mmocr/models/textdet/postprocessors/__pycache__/fce_postprocessor.cpython-38.pyc b/pipeline/mmocr/mmocr/models/textdet/postprocessors/__pycache__/fce_postprocessor.cpython-38.pyc
deleted file mode 100644
index 37d2c56bcaab9052491269bbca3707acd37e74a3..0000000000000000000000000000000000000000
Binary files a/pipeline/mmocr/mmocr/models/textdet/postprocessors/__pycache__/fce_postprocessor.cpython-38.pyc and /dev/null differ
diff --git a/pipeline/mmocr/mmocr/models/textdet/postprocessors/__pycache__/fce_postprocessor.cpython-39.pyc b/pipeline/mmocr/mmocr/models/textdet/postprocessors/__pycache__/fce_postprocessor.cpython-39.pyc
deleted file mode 100644
index b4931da325478deb4636b715759d4917a5a90cb9..0000000000000000000000000000000000000000
Binary files a/pipeline/mmocr/mmocr/models/textdet/postprocessors/__pycache__/fce_postprocessor.cpython-39.pyc and /dev/null differ
diff --git a/pipeline/mmocr/mmocr/models/textdet/postprocessors/__pycache__/pan_postprocessor.cpython-38.pyc b/pipeline/mmocr/mmocr/models/textdet/postprocessors/__pycache__/pan_postprocessor.cpython-38.pyc
deleted file mode 100644
index e8ae0650c12e241b26d2594f6ad6c222adfe358c..0000000000000000000000000000000000000000
Binary files a/pipeline/mmocr/mmocr/models/textdet/postprocessors/__pycache__/pan_postprocessor.cpython-38.pyc and /dev/null differ
diff --git a/pipeline/mmocr/mmocr/models/textdet/postprocessors/__pycache__/pan_postprocessor.cpython-39.pyc b/pipeline/mmocr/mmocr/models/textdet/postprocessors/__pycache__/pan_postprocessor.cpython-39.pyc
deleted file mode 100644
index 94ea81f2c0b1ea62b9be78a08ce3254a8ca37a75..0000000000000000000000000000000000000000
Binary files a/pipeline/mmocr/mmocr/models/textdet/postprocessors/__pycache__/pan_postprocessor.cpython-39.pyc and /dev/null differ
diff --git a/pipeline/mmocr/mmocr/models/textdet/postprocessors/__pycache__/pse_postprocessor.cpython-38.pyc b/pipeline/mmocr/mmocr/models/textdet/postprocessors/__pycache__/pse_postprocessor.cpython-38.pyc
deleted file mode 100644
index df1758476463d95ee5f5fd531fe12a4ea54df8cc..0000000000000000000000000000000000000000
Binary files a/pipeline/mmocr/mmocr/models/textdet/postprocessors/__pycache__/pse_postprocessor.cpython-38.pyc and /dev/null differ
diff --git a/pipeline/mmocr/mmocr/models/textdet/postprocessors/__pycache__/pse_postprocessor.cpython-39.pyc b/pipeline/mmocr/mmocr/models/textdet/postprocessors/__pycache__/pse_postprocessor.cpython-39.pyc
deleted file mode 100644
index 1b1450c20e00e5d9409af0d6bca56260b9099c26..0000000000000000000000000000000000000000
Binary files a/pipeline/mmocr/mmocr/models/textdet/postprocessors/__pycache__/pse_postprocessor.cpython-39.pyc and /dev/null differ
diff --git a/pipeline/mmocr/mmocr/models/textdet/postprocessors/__pycache__/textsnake_postprocessor.cpython-38.pyc b/pipeline/mmocr/mmocr/models/textdet/postprocessors/__pycache__/textsnake_postprocessor.cpython-38.pyc
deleted file mode 100644
index f178c145e8a3ff3e285ffa73396031c948356647..0000000000000000000000000000000000000000
Binary files a/pipeline/mmocr/mmocr/models/textdet/postprocessors/__pycache__/textsnake_postprocessor.cpython-38.pyc and /dev/null differ
diff --git a/pipeline/mmocr/mmocr/models/textdet/postprocessors/__pycache__/textsnake_postprocessor.cpython-39.pyc b/pipeline/mmocr/mmocr/models/textdet/postprocessors/__pycache__/textsnake_postprocessor.cpython-39.pyc
deleted file mode 100644
index 680158e13665f51bf8ac6e651ecaa3d9d6d35b17..0000000000000000000000000000000000000000
Binary files a/pipeline/mmocr/mmocr/models/textdet/postprocessors/__pycache__/textsnake_postprocessor.cpython-39.pyc and /dev/null differ
diff --git a/pipeline/mmocr/mmocr/models/textdet/postprocessors/base.py b/pipeline/mmocr/mmocr/models/textdet/postprocessors/base.py
deleted file mode 100644
index 706b152672665c9500aeda5bab4cc5bd156fe678..0000000000000000000000000000000000000000
--- a/pipeline/mmocr/mmocr/models/textdet/postprocessors/base.py
+++ /dev/null
@@ -1,204 +0,0 @@
-# Copyright (c) OpenMMLab. All rights reserved.
-from functools import partial
-from typing import Dict, List, Optional, Sequence, Tuple, Union
-
-import mmengine
-import numpy as np
-from torch import Tensor
-
-from mmocr.structures import TextDetDataSample
-from mmocr.utils import boundary_iou, rescale_polygons
-
-
-class BaseTextDetPostProcessor:
- """Base postprocessor for text detection models.
-
- Args:
- text_repr_type (str): The boundary encoding type, 'poly' or 'quad'.
- Defaults to 'poly'.
- rescale_fields (list[str], optional): The bbox/polygon field names to
- be rescaled. If None, no rescaling will be performed.
- train_cfg (dict, optional): The parameters to be passed to
- ``self.get_text_instances`` in training. Defaults to None.
- test_cfg (dict, optional): The parameters to be passed to
- ``self.get_text_instances`` in testing. Defaults to None.
- """
-
- def __init__(self,
- text_repr_type: str = 'poly',
- rescale_fields: Optional[Sequence[str]] = None,
- train_cfg: Optional[Dict] = None,
- test_cfg: Optional[Dict] = None) -> None:
- assert text_repr_type in ['poly', 'quad']
- assert rescale_fields is None or isinstance(rescale_fields, list)
- assert train_cfg is None or isinstance(train_cfg, dict)
- assert test_cfg is None or isinstance(test_cfg, dict)
- self.text_repr_type = text_repr_type
- self.rescale_fields = rescale_fields
- self.train_cfg = train_cfg
- self.test_cfg = test_cfg
-
- def __call__(self,
- pred_results: Union[Tensor, List[Tensor]],
- data_samples: Sequence[TextDetDataSample],
- training: bool = False) -> Sequence[TextDetDataSample]:
- """Postprocess pred_results according to metainfos in data_samples.
-
- Args:
- pred_results (Union[Tensor, List[Tensor]]): The prediction results
- stored in a tensor or a list of tensor. Usually each item to
- be post-processed is expected to be a batched tensor.
- data_samples (list[TextDetDataSample]): Batch of data_samples,
- each corresponding to a prediction result.
- training (bool): Whether the model is in training mode. Defaults to
- False.
-
- Returns:
- list[TextDetDataSample]: Batch of post-processed datasamples.
- """
- cfg = self.train_cfg if training else self.test_cfg
- if cfg is None:
- cfg = {}
- pred_results = self.split_results(pred_results)
- process_single = partial(self._process_single, **cfg)
- results = list(map(process_single, pred_results, data_samples))
-
- return results
-
- def _process_single(self, pred_result: Union[Tensor, List[Tensor]],
- data_sample: TextDetDataSample,
- **kwargs) -> TextDetDataSample:
- """Process prediction results from one image.
-
- Args:
- pred_result (Union[Tensor, List[Tensor]]): Prediction results of an
- image.
- data_sample (TextDetDataSample): Datasample of an image.
- """
-
- results = self.get_text_instances(pred_result, data_sample, **kwargs)
-
- if self.rescale_fields and len(self.rescale_fields) > 0:
- assert isinstance(self.rescale_fields, list)
- assert set(self.rescale_fields).issubset(
- set(results.pred_instances.keys()))
- results = self.rescale(results, data_sample.scale_factor)
- return results
-
- def rescale(self, results: TextDetDataSample,
- scale_factor: Sequence[int]) -> TextDetDataSample:
- """Rescale results in ``results.pred_instances`` according to
- ``scale_factor``, whose keys are defined in ``self.rescale_fields``.
- Usually used to rescale bboxes and/or polygons.
-
- Args:
- results (TextDetDataSample): The post-processed prediction results.
- scale_factor (tuple(int)): (w_scale, h_scale)
-
- Returns:
- TextDetDataSample: Prediction results with rescaled results.
- """
- scale_factor = np.asarray(scale_factor)
- for key in self.rescale_fields:
- results.pred_instances[key] = rescale_polygons(
- results.pred_instances[key], scale_factor, mode='div')
- return results
-
- def get_text_instances(self, pred_results: Union[Tensor, List[Tensor]],
- data_sample: TextDetDataSample,
- **kwargs) -> TextDetDataSample:
- """Get text instance predictions of one image.
-
- Args:
- pred_result (tuple(Tensor)): Prediction results of an image.
- data_sample (TextDetDataSample): Datasample of an image.
- **kwargs: Other parameters. Configurable via ``__init__.train_cfg``
- and ``__init__.test_cfg``.
-
- Returns:
- TextDetDataSample: A new DataSample with predictions filled in.
- The polygon/bbox results are usually saved in
- ``TextDetDataSample.pred_instances.polygons`` or
- ``TextDetDataSample.pred_instances.bboxes``. The confidence scores
- are saved in ``TextDetDataSample.pred_instances.scores``.
- """
- raise NotImplementedError
-
- def split_results(
- self, pred_results: Union[Tensor, List[Tensor]]
- ) -> Union[List[Tensor], List[List[Tensor]]]:
- """Split batched tensor(s) along the first dimension pack split tensors
- into a list.
-
- Args:
- pred_results (tensor or list[tensor]): Raw result tensor(s) from
- detection head. Each tensor usually has the shape of (N, ...)
-
- Returns:
- list[tensor] or list[list[tensor]]: N tensors if ``pred_results``
- is a tensor, or a list of N lists of tensors if
- ``pred_results`` is a list of tensors.
- """
- assert isinstance(pred_results, Tensor) or mmengine.is_seq_of(
- pred_results, Tensor)
-
- if mmengine.is_seq_of(pred_results, Tensor):
- for i in range(1, len(pred_results)):
- assert pred_results[0].shape[0] == pred_results[i].shape[0], \
- 'The first dimension of all tensors should be the same'
-
- batch_num = len(pred_results) if isinstance(pred_results, Tensor) else\
- len(pred_results[0])
- results = []
- for i in range(batch_num):
- if isinstance(pred_results, Tensor):
- results.append(pred_results[i])
- else:
- results.append([])
- for tensor in pred_results:
- results[i].append(tensor[i])
- return results
-
- def poly_nms(self, polygons: List[np.ndarray], scores: List[float],
- threshold: float) -> Tuple[List[np.ndarray], List[float]]:
- """Non-maximum suppression for text detection.
-
- Args:
- polygons (list[ndarray]): List of polygons.
- scores (list[float]): List of scores.
- threshold (float): Threshold for NMS.
-
- Returns:
- tuple(keep_polys, keep_scores):
-
- - keep_polys (list[ndarray]): List of preserved polygons after NMS.
- - keep_scores (list[float]): List of preserved scores after NMS.
- """
- assert isinstance(polygons, list)
- assert isinstance(scores, list)
- assert len(polygons) == len(scores)
-
- polygons = [
- np.hstack((polygon, score))
- for polygon, score in zip(polygons, scores)
- ]
- polygons = np.array(sorted(polygons, key=lambda x: x[-1]))
- keep_polys = []
- keep_scores = []
- index = [i for i in range(len(polygons))]
-
- while len(index) > 0:
- keep_polys.append(polygons[index[-1]][:-1].tolist())
- keep_scores.append(polygons[index[-1]][-1])
- A = polygons[index[-1]][:-1]
- index = np.delete(index, -1)
-
- iou_list = np.zeros((len(index), ))
- for i in range(len(index)):
- B = polygons[index[i]][:-1]
-
- iou_list[i] = boundary_iou(A, B, 1)
- remove_index = np.where(iou_list > threshold)
- index = np.delete(index, remove_index)
-
- return keep_polys, keep_scores
diff --git a/pipeline/mmocr/mmocr/models/textdet/postprocessors/db_postprocessor.py b/pipeline/mmocr/mmocr/models/textdet/postprocessors/db_postprocessor.py
deleted file mode 100644
index 7ae3290e8645942a601d16ede54c6ba7146b8430..0000000000000000000000000000000000000000
--- a/pipeline/mmocr/mmocr/models/textdet/postprocessors/db_postprocessor.py
+++ /dev/null
@@ -1,169 +0,0 @@
-# Copyright (c) OpenMMLab. All rights reserved.
-from typing import Sequence
-
-import cv2
-import numpy as np
-import torch
-from mmengine.structures import InstanceData
-from shapely.geometry import Polygon
-from torch import Tensor
-
-from mmocr.registry import MODELS
-from mmocr.structures import TextDetDataSample
-from mmocr.utils import offset_polygon
-from .base import BaseTextDetPostProcessor
-
-
-@MODELS.register_module()
-class DBPostprocessor(BaseTextDetPostProcessor):
- """Decoding predictions of DbNet to instances. This is partially adapted
- from https://github.com/MhLiao/DB.
-
- Args:
- text_repr_type (str): The boundary encoding type 'poly' or 'quad'.
- Defaults to 'poly'.
- rescale_fields (list[str]): The bbox/polygon field names to
- be rescaled. If None, no rescaling will be performed. Defaults to
- ['polygons'].
- mask_thr (float): The mask threshold value for binarization. Defaults
- to 0.3.
- min_text_score (float): The threshold value for converting binary map
- to shrink text regions. Defaults to 0.3.
- min_text_width (int): The minimum width of boundary polygon/box
- predicted. Defaults to 5.
- unclip_ratio (float): The unclip ratio for text regions dilation.
- Defaults to 1.5.
- epsilon_ratio (float): The epsilon ratio for approximation accuracy.
- Defaults to 0.01.
- max_candidates (int): The maximum candidate number. Defaults to 3000.
- """
-
- def __init__(self,
- text_repr_type: str = 'poly',
- rescale_fields: Sequence[str] = ['polygons'],
- mask_thr: float = 0.3,
- min_text_score: float = 0.3,
- min_text_width: int = 5,
- unclip_ratio: float = 1.5,
- epsilon_ratio: float = 0.01,
- max_candidates: int = 3000,
- **kwargs) -> None:
- super().__init__(
- text_repr_type=text_repr_type,
- rescale_fields=rescale_fields,
- **kwargs)
- self.mask_thr = mask_thr
- self.min_text_score = min_text_score
- self.min_text_width = min_text_width
- self.unclip_ratio = unclip_ratio
- self.epsilon_ratio = epsilon_ratio
- self.max_candidates = max_candidates
-
- def get_text_instances(self, prob_map: Tensor,
- data_sample: TextDetDataSample
- ) -> TextDetDataSample:
- """Get text instance predictions of one image.
-
- Args:
- pred_result (Tensor): DBNet's output ``prob_map`` of shape
- :math:`(H, W)`.
- data_sample (TextDetDataSample): Datasample of an image.
-
- Returns:
- TextDetDataSample: A new DataSample with predictions filled in.
- Polygons and results are saved in
- ``TextDetDataSample.pred_instances.polygons``. The confidence
- scores are saved in ``TextDetDataSample.pred_instances.scores``.
- """
-
- data_sample.pred_instances = InstanceData()
- data_sample.pred_instances.polygons = []
- data_sample.pred_instances.scores = []
-
- text_mask = prob_map > self.mask_thr
-
- score_map = prob_map.data.cpu().numpy().astype(np.float32)
- text_mask = text_mask.data.cpu().numpy().astype(np.uint8) # to numpy
-
- contours, _ = cv2.findContours((text_mask * 255).astype(np.uint8),
- cv2.RETR_LIST, cv2.CHAIN_APPROX_SIMPLE)
-
- for i, poly in enumerate(contours):
- if i > self.max_candidates:
- break
- epsilon = self.epsilon_ratio * cv2.arcLength(poly, True)
- approx = cv2.approxPolyDP(poly, epsilon, True)
- poly_pts = approx.reshape((-1, 2))
- if poly_pts.shape[0] < 4:
- continue
- score = self._get_bbox_score(score_map, poly_pts)
- if score < self.min_text_score:
- continue
- poly = self._unclip(poly_pts)
- # If the result polygon does not exist, or it is split into
- # multiple polygons, skip it.
- if len(poly) == 0:
- continue
- poly = poly.reshape(-1, 2)
-
- if self.text_repr_type == 'quad':
- rect = cv2.minAreaRect(poly)
- vertices = cv2.boxPoints(rect)
- poly = vertices.flatten() if min(
- rect[1]) >= self.min_text_width else []
- elif self.text_repr_type == 'poly':
- poly = poly.flatten()
-
- if len(poly) < 8:
- poly = np.array([], dtype=np.float32)
-
- if len(poly) > 0:
- data_sample.pred_instances.polygons.append(poly)
- data_sample.pred_instances.scores.append(score)
-
- data_sample.pred_instances.scores = torch.FloatTensor(
- data_sample.pred_instances.scores)
-
- return data_sample
-
- def _get_bbox_score(self, score_map: np.ndarray,
- poly_pts: np.ndarray) -> float:
- """Compute the average score over the area of the bounding box of the
- polygon.
-
- Args:
- score_map (np.ndarray): The score map.
- poly_pts (np.ndarray): The polygon points.
-
- Returns:
- float: The average score.
- """
- h, w = score_map.shape[:2]
- poly_pts = poly_pts.copy()
- xmin = np.clip(
- np.floor(poly_pts[:, 0].min()).astype(np.int32), 0, w - 1)
- xmax = np.clip(
- np.ceil(poly_pts[:, 0].max()).astype(np.int32), 0, w - 1)
- ymin = np.clip(
- np.floor(poly_pts[:, 1].min()).astype(np.int32), 0, h - 1)
- ymax = np.clip(
- np.ceil(poly_pts[:, 1].max()).astype(np.int32), 0, h - 1)
-
- mask = np.zeros((ymax - ymin + 1, xmax - xmin + 1), dtype=np.uint8)
- poly_pts[:, 0] = poly_pts[:, 0] - xmin
- poly_pts[:, 1] = poly_pts[:, 1] - ymin
- cv2.fillPoly(mask, poly_pts.reshape(1, -1, 2).astype(np.int32), 1)
- return cv2.mean(score_map[ymin:ymax + 1, xmin:xmax + 1], mask)[0]
-
- def _unclip(self, poly_pts: np.ndarray) -> np.ndarray:
- """Unclip a polygon.
-
- Args:
- poly_pts (np.ndarray): The polygon points.
-
- Returns:
- np.ndarray: The expanded polygon points.
- """
- poly = Polygon(poly_pts)
- distance = poly.area * self.unclip_ratio / poly.length
- return offset_polygon(poly_pts, distance)
diff --git a/pipeline/mmocr/mmocr/models/textdet/postprocessors/drrg_postprocessor.py b/pipeline/mmocr/mmocr/models/textdet/postprocessors/drrg_postprocessor.py
deleted file mode 100644
index 1bf3dacdfa0ceaefddd7c946af2f2cbf862ac3d6..0000000000000000000000000000000000000000
--- a/pipeline/mmocr/mmocr/models/textdet/postprocessors/drrg_postprocessor.py
+++ /dev/null
@@ -1,447 +0,0 @@
-# Copyright (c) OpenMMLab. All rights reserved.
-import functools
-import operator
-from typing import Dict, List, Tuple, Union
-
-import cv2
-import numpy as np
-import torch
-from mmengine.structures import InstanceData
-from numpy import ndarray
-
-from mmocr.registry import MODELS
-from mmocr.structures import TextDetDataSample
-from .base import BaseTextDetPostProcessor
-
-
-class Node:
- """A simple graph node.
-
- Args:
- ind (int): The index of the node.
- """
-
- def __init__(self, ind: int) -> None:
- self.__ind = ind
- self.__links = set()
-
- @property
- def ind(self) -> int:
- """Current node index."""
- return self.__ind
-
- @property
- def links(self) -> set:
- """A set of links."""
- return set(self.__links)
-
- def add_link(self, link_node: 'Node') -> None:
- """Add a link to the node.
-
- Args:
- link_node (Node): The link node.
- """
- self.__links.add(link_node)
- link_node.__links.add(self)
-
-
-@MODELS.register_module()
-class DRRGPostprocessor(BaseTextDetPostProcessor):
- """Merge text components and construct boundaries of text instances.
-
- Args:
- link_thr (float): The edge score threshold. Defaults to 0.8.
- edge_len_thr (int or float): The edge length threshold. Defaults to 50.
- rescale_fields (list[str]): The bbox/polygon field names to
- be rescaled. If None, no rescaling will be performed. Defaults to
- [polygons'].
- """
-
- def __init__(self,
- link_thr: float = 0.8,
- edge_len_thr: Union[int, float] = 50.,
- rescale_fields=['polygons'],
- **kwargs) -> None:
- super().__init__(rescale_fields=rescale_fields)
- assert isinstance(link_thr, float)
- assert isinstance(edge_len_thr, (int, float))
- self.link_thr = link_thr
- self.edge_len_thr = edge_len_thr
-
- def get_text_instances(self, pred_results: Tuple[ndarray, ndarray,
- ndarray],
- data_sample: TextDetDataSample
- ) -> TextDetDataSample:
- """Get text instance predictions of one image.
-
- Args:
- pred_result (tuple(ndarray, ndarray, ndarray)): Prediction results
- edge, score and text_comps. Each of shape
- :math:`(N_{edges}, 2)`, :math:`(N_{edges},)` and
- :math:`(M, 9)`, respectively.
- data_sample (TextDetDataSample): Datasample of an image.
-
- Returns:
- TextDetDataSample: The original dataSample with predictions filled
- in. Polygons and results are saved in
- ``TextDetDataSample.pred_instances.polygons``. The confidence
- scores are saved in ``TextDetDataSample.pred_instances.scores``.
- """
-
- data_sample.pred_instances = InstanceData()
- polys = []
- scores = []
-
- pred_edges, pred_scores, text_comps = pred_results
-
- if pred_edges is not None:
- assert len(pred_edges) == len(pred_scores)
- assert text_comps.ndim == 2
- assert text_comps.shape[1] == 9
-
- vertices, score_dict = self._graph_propagation(
- pred_edges, pred_scores, text_comps)
- clusters = self._connected_components(vertices, score_dict)
- pred_labels = self._clusters2labels(clusters, text_comps.shape[0])
- text_comps, pred_labels = self._remove_single(
- text_comps, pred_labels)
- polys, scores = self._comps2polys(text_comps, pred_labels)
-
- data_sample.pred_instances.polygons = polys
- data_sample.pred_instances.scores = torch.FloatTensor(scores)
-
- return data_sample
-
- def split_results(self, pred_results: Tuple[ndarray, ndarray,
- ndarray]) -> List[Tuple]:
- """Split batched elements in pred_results along the first dimension
- into ``batch_num`` sub-elements and regather them into a list of dicts.
-
- However, DRRG only outputs one batch at inference time, so this
- function is a no-op.
- """
- return [pred_results]
-
- def _graph_propagation(self, edges: ndarray, scores: ndarray,
- text_comps: ndarray) -> Tuple[List[Node], Dict]:
- """Propagate edge score information and construct graph. This code was
- partially adapted from https://github.com/GXYM/DRRG licensed under the
- MIT license.
-
- Args:
- edges (ndarray): The edge array of shape N * 2, each row is a node
- index pair that makes up an edge in graph.
- scores (ndarray): The edge score array.
- text_comps (ndarray): The text components.
-
- Returns:
- tuple(vertices, score_dict):
-
- - vertices (list[Node]): The Nodes in graph.
- - score_dict (dict): The edge score dict.
- """
- assert edges.ndim == 2
- assert edges.shape[1] == 2
- assert edges.shape[0] == scores.shape[0]
- assert text_comps.ndim == 2
-
- edges = np.sort(edges, axis=1)
- score_dict = {}
- for i, edge in enumerate(edges):
- if text_comps is not None:
- box1 = text_comps[edge[0], :8].reshape(4, 2)
- box2 = text_comps[edge[1], :8].reshape(4, 2)
- center1 = np.mean(box1, axis=0)
- center2 = np.mean(box2, axis=0)
- distance = np.linalg.norm(center1 - center2)
- if distance > self.edge_len_thr:
- scores[i] = 0
- if (edge[0], edge[1]) in score_dict:
- score_dict[edge[0], edge[1]] = 0.5 * (
- score_dict[edge[0], edge[1]] + scores[i])
- else:
- score_dict[edge[0], edge[1]] = scores[i]
-
- nodes = np.sort(np.unique(edges.flatten()))
- mapping = -1 * np.ones((np.max(nodes) + 1), dtype=int)
- mapping[nodes] = np.arange(nodes.shape[0])
- order_inds = mapping[edges]
- vertices = [Node(node) for node in nodes]
- for ind in order_inds:
- vertices[ind[0]].add_link(vertices[ind[1]])
-
- return vertices, score_dict
-
- def _connected_components(self, nodes: List[Node],
- score_dict: Dict) -> List[List[Node]]:
- """Conventional connected components searching. This code was partially
- adapted from https://github.com/GXYM/DRRG licensed under the MIT
- license.
-
- Args:
- nodes (list[Node]): The list of Node objects.
- score_dict (dict): The edge score dict.
-
- Returns:
- List[list[Node]]: The clustered Node objects.
- """
- assert isinstance(nodes, list)
- assert all([isinstance(node, Node) for node in nodes])
- assert isinstance(score_dict, dict)
-
- clusters = []
- nodes = set(nodes)
- while nodes:
- node = nodes.pop()
- cluster = {node}
- node_queue = [node]
- while node_queue:
- node = node_queue.pop(0)
- neighbors = {
- neighbor
- for neighbor in node.links if score_dict[tuple(
- sorted([node.ind, neighbor.ind]))] >= self.link_thr
- }
- neighbors.difference_update(cluster)
- nodes.difference_update(neighbors)
- cluster.update(neighbors)
- node_queue.extend(neighbors)
- clusters.append(list(cluster))
- return clusters
-
- def _clusters2labels(self, clusters: List[List[Node]],
- num_nodes: int) -> ndarray:
- """Convert clusters of Node to text component labels. This code was
- partially adapted from https://github.com/GXYM/DRRG licensed under the
- MIT license.
-
- Args:
- clusters (List[list[Node]]): The clusters of Node objects.
- num_nodes (int): The total node number of graphs in an image.
-
- Returns:
- ndarray: The node label array.
- """
- assert isinstance(clusters, list)
- assert all([isinstance(cluster, list) for cluster in clusters])
- assert all([
- isinstance(node, Node) for cluster in clusters for node in cluster
- ])
- assert isinstance(num_nodes, int)
-
- node_labels = np.zeros(num_nodes)
- for cluster_ind, cluster in enumerate(clusters):
- for node in cluster:
- node_labels[node.ind] = cluster_ind
- return node_labels
-
- def _remove_single(self, text_comps: ndarray,
- comp_pred_labels: ndarray) -> Tuple[ndarray, ndarray]:
- """Remove isolated text components. This code was partially adapted
- from https://github.com/GXYM/DRRG licensed under the MIT license.
-
- Args:
- text_comps (ndarray): The text components.
- comp_pred_labels (ndarray): The clustering labels of text
- components.
-
- Returns:
- tuple(filtered_text_comps, comp_pred_labels):
-
- - filtered_text_comps (ndarray): The text components with isolated
- ones removed.
- - comp_pred_labels (ndarray): The clustering labels with labels of
- isolated text components removed.
- """
- assert text_comps.ndim == 2
- assert text_comps.shape[0] == comp_pred_labels.shape[0]
-
- single_flags = np.zeros_like(comp_pred_labels)
- pred_labels = np.unique(comp_pred_labels)
- for label in pred_labels:
- current_label_flag = (comp_pred_labels == label)
- if np.sum(current_label_flag) == 1:
- single_flags[np.where(current_label_flag)[0][0]] = 1
- keep_ind = [
- i for i in range(len(comp_pred_labels)) if not single_flags[i]
- ]
- filtered_text_comps = text_comps[keep_ind, :]
- filtered_labels = comp_pred_labels[keep_ind]
-
- return filtered_text_comps, filtered_labels
-
- def _comps2polys(self, text_comps: ndarray, comp_pred_labels: ndarray
- ) -> Tuple[List[ndarray], List[float]]:
- """Construct text instance boundaries from clustered text components.
- This code was partially adapted from https://github.com/GXYM/DRRG
- licensed under the MIT license.
-
- Args:
- text_comps (ndarray): The text components.
- comp_pred_labels (ndarray): The clustering labels of text
- components.
-
- Returns:
- tuple(boundaries, scores):
-
- - boundaries (list[ndarray]): The predicted boundaries of text
- instances.
- - scores (list[float]): The boundary scores.
- """
- assert text_comps.ndim == 2
- assert len(text_comps) == len(comp_pred_labels)
- boundaries = []
- scores = []
- if len(text_comps) < 1:
- return boundaries, scores
- for cluster_ind in range(0, int(np.max(comp_pred_labels)) + 1):
- cluster_comp_inds = np.where(comp_pred_labels == cluster_ind)
- text_comp_boxes = text_comps[cluster_comp_inds, :8].reshape(
- (-1, 4, 2)).astype(np.int32)
- score = np.mean(text_comps[cluster_comp_inds, -1])
-
- if text_comp_boxes.shape[0] < 1:
- continue
-
- elif text_comp_boxes.shape[0] > 1:
- centers = np.mean(
- text_comp_boxes, axis=1).astype(np.int32).tolist()
- shortest_path = self._min_connect_path(centers)
- text_comp_boxes = text_comp_boxes[shortest_path]
- top_line = np.mean(
- text_comp_boxes[:, 0:2, :],
- axis=1).astype(np.int32).tolist()
- bot_line = np.mean(
- text_comp_boxes[:, 2:4, :],
- axis=1).astype(np.int32).tolist()
- top_line, bot_line = self._fix_corner(top_line, bot_line,
- text_comp_boxes[0],
- text_comp_boxes[-1])
- boundary_points = top_line + bot_line[::-1]
-
- else:
- top_line = text_comp_boxes[0, 0:2, :].astype(np.int32).tolist()
- bot_line = text_comp_boxes[0, 2:4:-1, :].astype(
- np.int32).tolist()
- boundary_points = top_line + bot_line
-
- boundary = [p for coord in boundary_points for p in coord]
- boundaries.append(np.array(boundary, dtype=np.float32))
- scores.append(score)
-
- return boundaries, scores
-
- def _norm2(self, point1: List[int], point2: List[int]) -> float:
- """Calculate the norm of two points."""
- return ((point1[0] - point2[0])**2 + (point1[1] - point2[1])**2)**0.5
-
- def _min_connect_path(self, points: List[List[int]]) -> List[List[int]]:
- """Find the shortest path to traverse all points. This code was
- partially adapted from https://github.com/GXYM/DRRG licensed under the
- MIT license.
-
- Args:
- points(List[list[int]]): The point sequence
- [[x0, y0], [x1, y1], ...].
-
- Returns:
- List[list[int]]: The shortest index path.
- """
- assert isinstance(points, list)
- assert all([isinstance(point, list) for point in points])
- assert all(
- [isinstance(coord, int) for point in points for coord in point])
-
- points_queue = points.copy()
- shortest_path = []
- current_edge = [[], []]
-
- edge_dict0 = {}
- edge_dict1 = {}
- current_edge[0] = points_queue[0]
- current_edge[1] = points_queue[0]
- points_queue.remove(points_queue[0])
- while points_queue:
- for point in points_queue:
- length0 = self._norm2(point, current_edge[0])
- edge_dict0[length0] = [point, current_edge[0]]
- length1 = self._norm2(current_edge[1], point)
- edge_dict1[length1] = [current_edge[1], point]
- key0 = min(edge_dict0.keys())
- key1 = min(edge_dict1.keys())
-
- if key0 <= key1:
- start = edge_dict0[key0][0]
- end = edge_dict0[key0][1]
- shortest_path.insert(0,
- [points.index(start),
- points.index(end)])
- points_queue.remove(start)
- current_edge[0] = start
- else:
- start = edge_dict1[key1][0]
- end = edge_dict1[key1][1]
- shortest_path.append([points.index(start), points.index(end)])
- points_queue.remove(end)
- current_edge[1] = end
-
- edge_dict0 = {}
- edge_dict1 = {}
-
- shortest_path = functools.reduce(operator.concat, shortest_path)
- shortest_path = sorted(set(shortest_path), key=shortest_path.index)
-
- return shortest_path
-
- def _in_contour(self, contour: ndarray, point: ndarray) -> bool:
- """Whether a point is in a contour."""
- x, y = point
- return cv2.pointPolygonTest(contour, (int(x), int(y)), False) > 0.5
-
- def _fix_corner(self, top_line: List[List[int]], btm_line: List[List[int]],
- start_box: ndarray, end_box: ndarray
- ) -> Tuple[List[List[int]], List[List[int]]]:
- """Add corner points to predicted side lines. This code was partially
- adapted from https://github.com/GXYM/DRRG licensed under the MIT
- license.
-
- Args:
- top_line (List[list[int]]): The predicted top sidelines of text
- instance.
- btm_line (List[list[int]]): The predicted bottom sidelines of text
- instance.
- start_box (ndarray): The first text component box.
- end_box (ndarray): The last text component box.
-
- Returns:
- tuple(top_line, bot_line):
-
- - top_line (List[list[int]]): The top sidelines with corner point
- added.
- - bot_line (List[list[int]]): The bottom sidelines with corner
- point added.
- """
- assert isinstance(top_line, list)
- assert all(isinstance(point, list) for point in top_line)
- assert isinstance(btm_line, list)
- assert all(isinstance(point, list) for point in btm_line)
- assert start_box.shape == end_box.shape == (4, 2)
-
- contour = np.array(top_line + btm_line[::-1])
- start_left_mid = (start_box[0] + start_box[3]) / 2
- start_right_mid = (start_box[1] + start_box[2]) / 2
- end_left_mid = (end_box[0] + end_box[3]) / 2
- end_right_mid = (end_box[1] + end_box[2]) / 2
- if not self._in_contour(contour, start_left_mid):
- top_line.insert(0, start_box[0].tolist())
- btm_line.insert(0, start_box[3].tolist())
- elif not self._in_contour(contour, start_right_mid):
- top_line.insert(0, start_box[1].tolist())
- btm_line.insert(0, start_box[2].tolist())
- if not self._in_contour(contour, end_left_mid):
- top_line.append(end_box[0].tolist())
- btm_line.append(end_box[3].tolist())
- elif not self._in_contour(contour, end_right_mid):
- top_line.append(end_box[1].tolist())
- btm_line.append(end_box[2].tolist())
- return top_line, btm_line
diff --git a/pipeline/mmocr/mmocr/models/textdet/postprocessors/fce_postprocessor.py b/pipeline/mmocr/mmocr/models/textdet/postprocessors/fce_postprocessor.py
deleted file mode 100644
index b6c49bf433224284da715c1589a3041fe445bb97..0000000000000000000000000000000000000000
--- a/pipeline/mmocr/mmocr/models/textdet/postprocessors/fce_postprocessor.py
+++ /dev/null
@@ -1,239 +0,0 @@
-# Copyright (c) OpenMMLab. All rights reserved.
-from typing import Dict, List, Sequence
-
-import cv2
-import numpy as np
-import torch
-from mmengine.structures import InstanceData
-from numpy.fft import ifft
-
-from mmocr.registry import MODELS
-from mmocr.structures import TextDetDataSample
-from mmocr.utils import fill_hole
-from .base import BaseTextDetPostProcessor
-
-
-@MODELS.register_module()
-class FCEPostprocessor(BaseTextDetPostProcessor):
- """Decoding predictions of FCENet to instances.
-
- Args:
- fourier_degree (int): The maximum Fourier transform degree k.
- num_reconstr_points (int): The points number of the polygon
- reconstructed from predicted Fourier coefficients.
- rescale_fields (list[str]): The bbox/polygon field names to
- be rescaled. If None, no rescaling will be performed. Defaults to
- ['polygons'].
- scales (list[int]) : The down-sample scale of each layer. Defaults
- to [8, 16, 32].
- text_repr_type (str): Boundary encoding type 'poly' or 'quad'. Defaults
- to 'poly'.
- alpha (float): The parameter to calculate final scores
- :math:`Score_{final} = (Score_{text region} ^ alpha)
- * (Score_{text center_region}^ beta)`. Defaults to 1.0.
- beta (float): The parameter to calculate final score. Defaults to 2.0.
- score_thr (float): The threshold used to filter out the final
- candidates.Defaults to 0.3.
- nms_thr (float): The threshold of nms. Defaults to 0.1.
- """
-
- def __init__(self,
- fourier_degree: int,
- num_reconstr_points: int,
- rescale_fields: Sequence[str] = ['polygons'],
- scales: Sequence[int] = [8, 16, 32],
- text_repr_type: str = 'poly',
- alpha: float = 1.0,
- beta: float = 2.0,
- score_thr: float = 0.3,
- nms_thr: float = 0.1,
- **kwargs) -> None:
- super().__init__(
- text_repr_type=text_repr_type,
- rescale_fields=rescale_fields,
- **kwargs)
- self.fourier_degree = fourier_degree
- self.num_reconstr_points = num_reconstr_points
- self.scales = scales
- self.alpha = alpha
- self.beta = beta
- self.score_thr = score_thr
- self.nms_thr = nms_thr
-
- def split_results(self, pred_results: List[Dict]) -> List[List[Dict]]:
- """Split batched elements in pred_results along the first dimension
- into ``batch_num`` sub-elements and regather them into a list of dicts.
-
- Args:
- pred_results (list[dict]): A list of dict with keys of ``cls_res``,
- ``reg_res`` corresponding to the classification result and
- regression result computed from the input tensor with the
- same index. They have the shapes of :math:`(N, C_{cls,i},
- H_i, W_i)` and :math:`(N, C_{out,i}, H_i, W_i)`.
-
- Returns:
- list[list[dict]]: N lists. Each list contains three dicts from
- different feature level.
- """
- assert isinstance(pred_results, list) and len(pred_results) == len(
- self.scales)
-
- fields = list(pred_results[0].keys())
- batch_num = len(pred_results[0][fields[0]])
- level_num = len(pred_results)
- results = []
- for i in range(batch_num):
- batch_list = []
- for level in range(level_num):
- feat_dict = {}
- for field in fields:
- feat_dict[field] = pred_results[level][field][i]
- batch_list.append(feat_dict)
- results.append(batch_list)
- return results
-
- def get_text_instances(self, pred_results: Sequence[Dict],
- data_sample: TextDetDataSample
- ) -> TextDetDataSample:
- """Get text instance predictions of one image.
-
- Args:
- pred_results (List[dict]): A list of dict with keys of ``cls_res``,
- ``reg_res`` corresponding to the classification result and
- regression result computed from the input tensor with the
- same index. They have the shapes of :math:`(N, C_{cls,i}, H_i,
- W_i)` and :math:`(N, C_{out,i}, H_i, W_i)`.
- data_sample (TextDetDataSample): Datasample of an image.
-
- Returns:
- TextDetDataSample: A new DataSample with predictions filled in.
- Polygons and results are saved in
- ``TextDetDataSample.pred_instances.polygons``. The confidence
- scores are saved in ``TextDetDataSample.pred_instances.scores``.
- """
- assert len(pred_results) == len(self.scales)
- data_sample.pred_instances = InstanceData()
- data_sample.pred_instances.polygons = []
- data_sample.pred_instances.scores = []
-
- result_polys = []
- result_scores = []
- for idx, pred_result in enumerate(pred_results):
- # TODO: Scale can be calculated given image shape and feature
- # shape. This param can be removed in the future.
- polygons, scores = self._get_text_instances_single(
- pred_result, self.scales[idx])
- result_polys += polygons
- result_scores += scores
- result_polys, result_scores = self.poly_nms(result_polys,
- result_scores,
- self.nms_thr)
- for result_poly, result_score in zip(result_polys, result_scores):
- result_poly = np.array(result_poly, dtype=np.float32)
- data_sample.pred_instances.polygons.append(result_poly)
- data_sample.pred_instances.scores.append(result_score)
- data_sample.pred_instances.scores = torch.FloatTensor(
- data_sample.pred_instances.scores)
-
- return data_sample
-
- def _get_text_instances_single(self, pred_result: Dict, scale: int):
- """Get text instance predictions from one feature level.
-
- Args:
- pred_result (dict): A dict with keys of ``cls_res``, ``reg_res``
- corresponding to the classification result and regression
- result computed from the input tensor with the same index.
- They have the shapes of :math:`(1, C_{cls,i}, H_i, W_i)` and
- :math:`(1, C_{out,i}, H_i, W_i)`.
- scale (int): Scale of current feature map which equals to
- img_size / feat_size.
-
- Returns:
- result_polys (list[ndarray]): A list of polygons after postprocess.
- result_scores (list[ndarray]): A list of scores after postprocess.
- """
-
- cls_pred = pred_result['cls_res']
- tr_pred = cls_pred[0:2].softmax(dim=0).data.cpu().numpy()
- tcl_pred = cls_pred[2:].softmax(dim=0).data.cpu().numpy()
-
- reg_pred = pred_result['reg_res'].permute(1, 2, 0).data.cpu().numpy()
- x_pred = reg_pred[:, :, :2 * self.fourier_degree + 1]
- y_pred = reg_pred[:, :, 2 * self.fourier_degree + 1:]
-
- score_pred = (tr_pred[1]**self.alpha) * (tcl_pred[1]**self.beta)
- tr_pred_mask = (score_pred) > self.score_thr
- tr_mask = fill_hole(tr_pred_mask)
-
- tr_contours, _ = cv2.findContours(
- tr_mask.astype(np.uint8), cv2.RETR_TREE,
- cv2.CHAIN_APPROX_SIMPLE) # opencv4
-
- mask = np.zeros_like(tr_mask)
-
- result_polys = []
- result_scores = []
- for cont in tr_contours:
- deal_map = mask.copy().astype(np.int8)
- cv2.drawContours(deal_map, [cont], -1, 1, -1)
-
- score_map = score_pred * deal_map
- score_mask = score_map > 0
- xy_text = np.argwhere(score_mask)
- dxy = xy_text[:, 1] + xy_text[:, 0] * 1j
-
- x, y = x_pred[score_mask], y_pred[score_mask]
- c = x + y * 1j
- c[:, self.fourier_degree] = c[:, self.fourier_degree] + dxy
- c *= scale
-
- polygons = self._fourier2poly(c, self.num_reconstr_points)
- scores = score_map[score_mask].reshape(-1, 1).tolist()
- polygons, scores = self.poly_nms(polygons, scores, self.nms_thr)
- result_polys += polygons
- result_scores += scores
-
- result_polys, result_scores = self.poly_nms(result_polys,
- result_scores,
- self.nms_thr)
-
- if self.text_repr_type == 'quad':
- new_polys = []
- for poly in result_polys:
- poly = np.array(poly).reshape(-1, 2).astype(np.float32)
- points = cv2.boxPoints(cv2.minAreaRect(poly))
- points = np.int0(points)
- new_polys.append(points.reshape(-1))
-
- return new_polys, result_scores
- return result_polys, result_scores
-
- def _fourier2poly(self,
- fourier_coeff: np.ndarray,
- num_reconstr_points: int = 50):
- """ Inverse Fourier transform
- Args:
- fourier_coeff (ndarray): Fourier coefficients shaped (n, 2k+1),
- with n and k being candidates number and Fourier degree
- respectively.
- num_reconstr_points (int): Number of reconstructed polygon
- points. Defaults to 50.
-
- Returns:
- List[ndarray]: The reconstructed polygons.
- """
-
- a = np.zeros((len(fourier_coeff), num_reconstr_points),
- dtype='complex')
- k = (len(fourier_coeff[0]) - 1) // 2
-
- a[:, 0:k + 1] = fourier_coeff[:, k:]
- a[:, -k:] = fourier_coeff[:, :k]
-
- poly_complex = ifft(a) * num_reconstr_points
- polygon = np.zeros((len(fourier_coeff), num_reconstr_points, 2))
- polygon[:, :, 0] = poly_complex.real
- polygon[:, :, 1] = poly_complex.imag
- return polygon.astype('int32').reshape(
- (len(fourier_coeff), -1)).tolist()
diff --git a/pipeline/mmocr/mmocr/models/textdet/postprocessors/pan_postprocessor.py b/pipeline/mmocr/mmocr/models/textdet/postprocessors/pan_postprocessor.py
deleted file mode 100644
index 63676856bebd78dfc97156739a2745e51cb272da..0000000000000000000000000000000000000000
--- a/pipeline/mmocr/mmocr/models/textdet/postprocessors/pan_postprocessor.py
+++ /dev/null
@@ -1,159 +0,0 @@
-# Copyright (c) OpenMMLab. All rights reserved.
-from typing import List, Sequence
-
-import cv2
-import numpy as np
-import torch
-from mmcv.ops import pixel_group
-from mmengine.structures import InstanceData
-
-from mmocr.registry import MODELS
-from mmocr.structures import TextDetDataSample
-from .base import BaseTextDetPostProcessor
-
-
-@MODELS.register_module()
-class PANPostprocessor(BaseTextDetPostProcessor):
- """Convert scores to quadrangles via post processing in PANet. This is
- partially adapted from https://github.com/WenmuZhou/PAN.pytorch.
-
- Args:
- text_repr_type (str): The boundary encoding type 'poly' or 'quad'.
- Defaults to 'poly'.
- score_threshold (float): The minimal text score.
- Defaults to 0.3.
- rescale_fields (list[str]): The bbox/polygon field names to
- be rescaled. If None, no rescaling will be performed. Defaults to
- ['polygons'].
- min_text_confidence (float): The minimal text confidence.
- Defaults to 0.5.
- min_kernel_confidence (float): The minimal kernel confidence.
- Defaults to 0.5.
- distance_threshold (float): The minimal distance between the point to
- mean of text kernel. Defaults to 3.0.
- min_text_area (int): The minimal text instance region area.
- Defaults to 16.
- downsample_ratio (float): Downsample ratio. Defaults to 0.25.
- """
-
- def __init__(self,
- text_repr_type: str = 'poly',
- score_threshold: float = 0.3,
- rescale_fields: Sequence[str] = ['polygons'],
- min_text_confidence: float = 0.5,
- min_kernel_confidence: float = 0.5,
- distance_threshold: float = 3.0,
- min_text_area: int = 16,
- downsample_ratio: float = 0.25) -> None:
- super().__init__(text_repr_type, rescale_fields)
-
- self.min_text_confidence = min_text_confidence
- self.min_kernel_confidence = min_kernel_confidence
- self.score_threshold = score_threshold
- self.min_text_area = min_text_area
- self.distance_threshold = distance_threshold
- self.downsample_ratio = downsample_ratio
-
- def get_text_instances(self, pred_results: torch.Tensor,
- data_sample: TextDetDataSample,
- **kwargs) -> TextDetDataSample:
- """Get text instance predictions of one image.
-
- Args:
- pred_result (torch.Tensor): Prediction results of an image which
- is a tensor of shape :math:`(N, H, W)`.
- data_sample (TextDetDataSample): Datasample of an image.
-
- Returns:
- TextDetDataSample: A new DataSample with predictions filled in.
- Polygons and results are saved in
- ``TextDetDataSample.pred_instances.polygons``. The confidence
- scores are saved in ``TextDetDataSample.pred_instances.scores``.
- """
- assert pred_results.dim() == 3
-
- pred_results[:2, :, :] = torch.sigmoid(pred_results[:2, :, :])
- pred_results = pred_results.detach().cpu().numpy()
-
- text_score = pred_results[0].astype(np.float32)
- text = pred_results[0] > self.min_text_confidence
- kernel = (pred_results[1] > self.min_kernel_confidence) * text
- embeddings = pred_results[2:] * text.astype(np.float32)
- embeddings = embeddings.transpose((1, 2, 0)) # (h, w, 4)
-
- region_num, labels = cv2.connectedComponents(
- kernel.astype(np.uint8), connectivity=4)
- contours, _ = cv2.findContours((kernel * 255).astype(np.uint8),
- cv2.RETR_LIST, cv2.CHAIN_APPROX_NONE)
- kernel_contours = np.zeros(text.shape, dtype='uint8')
- cv2.drawContours(kernel_contours, contours, -1, 255)
- text_points = pixel_group(text_score, text, embeddings, labels,
- kernel_contours, region_num,
- self.distance_threshold)
-
- polygons = []
- scores = []
- for text_point in text_points:
- text_confidence = text_point[0]
- text_point = text_point[2:]
- text_point = np.array(text_point, dtype=int).reshape(-1, 2)
- area = text_point.shape[0]
- if (area < self.min_text_area
- or text_confidence <= self.score_threshold):
- continue
-
- polygon = self._points2boundary(text_point)
- if len(polygon) > 0:
- polygons.append(polygon)
- scores.append(text_confidence)
- pred_instances = InstanceData()
- pred_instances.polygons = polygons
- pred_instances.scores = torch.FloatTensor(scores)
- data_sample.pred_instances = pred_instances
- scale_factor = data_sample.scale_factor
- scale_factor = tuple(factor * self.downsample_ratio
- for factor in scale_factor)
- data_sample.set_metainfo(dict(scale_factor=scale_factor))
- return data_sample
-
- def _points2boundary(self,
- points: np.ndarray,
- min_width: int = 0) -> List[float]:
- """Convert a text mask represented by point coordinates sequence into a
- text boundary.
-
- Args:
- points (ndarray): Mask index of size (n, 2).
- min_width (int): Minimum bounding box width to be converted. Only
- applicable to 'quad' type. Defaults to 0.
-
- Returns:
- list[float]: The text boundary point coordinates (x, y) list.
- Return [] if no text boundary found.
- """
- assert isinstance(points, np.ndarray)
- assert points.shape[1] == 2
- assert self.text_repr_type in ['quad', 'poly']
-
- if self.text_repr_type == 'quad':
- rect = cv2.minAreaRect(points)
- vertices = cv2.boxPoints(rect)
- boundary = []
- if min(rect[1]) >= min_width:
- boundary = [p for p in vertices.flatten().tolist()]
- elif self.text_repr_type == 'poly':
-
- height = np.max(points[:, 1]) + 10
- width = np.max(points[:, 0]) + 10
-
- mask = np.zeros((height, width), np.uint8)
- mask[points[:, 1], points[:, 0]] = 255
-
- contours, _ = cv2.findContours(mask, cv2.RETR_EXTERNAL,
- cv2.CHAIN_APPROX_SIMPLE)
- boundary = list(contours[0].flatten().tolist())
-
- if len(boundary) < 8:
- return []
-
- return boundary
diff --git a/pipeline/mmocr/mmocr/models/textdet/postprocessors/pse_postprocessor.py b/pipeline/mmocr/mmocr/models/textdet/postprocessors/pse_postprocessor.py
deleted file mode 100644
index b0a1fb9f8a2dde54dfa71e0d531f0e85fb74d1c6..0000000000000000000000000000000000000000
--- a/pipeline/mmocr/mmocr/models/textdet/postprocessors/pse_postprocessor.py
+++ /dev/null
@@ -1,113 +0,0 @@
-# Copyright (c) OpenMMLab. All rights reserved.
-
-from typing import List
-
-import cv2
-import numpy as np
-import torch
-from mmcv.ops import contour_expand
-from mmengine.structures import InstanceData
-
-from mmocr.registry import MODELS
-from mmocr.structures import TextDetDataSample
-from .pan_postprocessor import PANPostprocessor
-
-
-@MODELS.register_module()
-class PSEPostprocessor(PANPostprocessor):
- """Decoding predictions of PSENet to instances. This is partially adapted
- from https://github.com/whai362/PSENet.
-
- Args:
- text_repr_type (str): The boundary encoding type 'poly' or 'quad'.
- Defaults to 'poly'.
- rescale_fields (list[str]): The bbox/polygon field names to
- be rescaled. If None, no rescaling will be performed. Defaults to
- ['polygons'].
- min_kernel_confidence (float): The minimal kernel confidence.
- Defaults to 0.5.
- score_threshold (float): The minimal text average confidence.
- Defaults to 0.3.
- min_kernel_area (int): The minimal text kernel area. Defaults to 0.
- min_text_area (int): The minimal text instance region area.
- Defaults to 16.
- downsample_ratio (float): Downsample ratio. Defaults to 0.25.
- """
-
- def __init__(self,
- text_repr_type: str = 'poly',
- rescale_fields: List[str] = ['polygons'],
- min_kernel_confidence: float = 0.5,
- score_threshold: float = 0.3,
- min_kernel_area: int = 0,
- min_text_area: int = 16,
- downsample_ratio: float = 0.25) -> None:
- super().__init__(
- text_repr_type=text_repr_type,
- rescale_fields=rescale_fields,
- min_kernel_confidence=min_kernel_confidence,
- score_threshold=score_threshold,
- min_text_area=min_text_area,
- downsample_ratio=downsample_ratio)
- self.min_kernel_area = min_kernel_area
-
- def get_text_instances(self, pred_results: torch.Tensor,
- data_sample: TextDetDataSample,
- **kwargs) -> TextDetDataSample:
- """
- Args:
- pred_result (torch.Tensor): Prediction results of an image which
- is a tensor of shape :math:`(N, H, W)`.
- data_sample (TextDetDataSample): Datasample of an image.
-
- Returns:
- TextDetDataSample: A new DataSample with predictions filled in.
- Polygons and results are saved in
- ``TextDetDataSample.pred_instances.polygons``. The confidence
- scores are saved in ``TextDetDataSample.pred_instances.scores``.
- """
- assert pred_results.dim() == 3
-
- pred_results = torch.sigmoid(pred_results) # text confidence
-
- masks = pred_results > self.min_kernel_confidence
- text_mask = masks[0, :, :]
- kernel_masks = masks[0:, :, :] * text_mask
- kernel_masks = kernel_masks.data.cpu().numpy().astype(np.uint8)
-
- score = pred_results[0, :, :]
- score = score.data.cpu().numpy().astype(np.float32)
-
- region_num, labels = cv2.connectedComponents(
- kernel_masks[-1], connectivity=4)
-
- labels = contour_expand(kernel_masks, labels, self.min_kernel_area,
- region_num)
- labels = np.array(labels)
- label_num = np.max(labels)
-
- polygons = []
- scores = []
- for i in range(1, label_num + 1):
- points = np.array(np.where(labels == i)).transpose((1, 0))[:, ::-1]
- area = points.shape[0]
- score_instance = np.mean(score[labels == i])
- if not (area >= self.min_text_area
- or score_instance > self.score_threshold):
- continue
-
- polygon = self._points2boundary(points)
- if polygon:
- polygons.append(polygon)
- scores.append(score_instance)
-
- pred_instances = InstanceData()
- pred_instances.polygons = polygons
- pred_instances.scores = torch.FloatTensor(scores)
- data_sample.pred_instances = pred_instances
- scale_factor = data_sample.scale_factor
- scale_factor = tuple(factor * self.downsample_ratio
- for factor in scale_factor)
- data_sample.set_metainfo(dict(scale_factor=scale_factor))
-
- return data_sample
diff --git a/pipeline/mmocr/mmocr/models/textdet/postprocessors/textsnake_postprocessor.py b/pipeline/mmocr/mmocr/models/textdet/postprocessors/textsnake_postprocessor.py
deleted file mode 100644
index a4f7ae02ee33688925d799df6ed303b61be59bd1..0000000000000000000000000000000000000000
--- a/pipeline/mmocr/mmocr/models/textdet/postprocessors/textsnake_postprocessor.py
+++ /dev/null
@@ -1,234 +0,0 @@
-# Copyright (c) OpenMMLab. All rights reserved.
-
-from typing import List, Sequence
-
-import cv2
-import numpy as np
-import torch
-from mmengine.structures import InstanceData
-from numpy.linalg import norm
-from skimage.morphology import skeletonize
-
-from mmocr.registry import MODELS
-from mmocr.structures import TextDetDataSample
-from mmocr.utils import fill_hole
-from .base import BaseTextDetPostProcessor
-
-
-@MODELS.register_module()
-class TextSnakePostprocessor(BaseTextDetPostProcessor):
- """Decoding predictions of TextSnake to instances. This was partially
- adapted from https://github.com/princewang1994/TextSnake.pytorch.
-
- Args:
- text_repr_type (str): The boundary encoding type 'poly' or 'quad'.
- min_text_region_confidence (float): The confidence threshold of text
- region in TextSnake.
- min_center_region_confidence (float): The confidence threshold of text
- center region in TextSnake.
- min_center_area (int): The minimal text center region area.
- disk_overlap_thr (float): The radius overlap threshold for merging
- disks.
- radius_shrink_ratio (float): The shrink ratio of ordered disks radii.
- rescale_fields (list[str], optional): The bbox/polygon field names to
- be rescaled. If None, no rescaling will be performed.
- """
-
- def __init__(self,
- text_repr_type: str = 'poly',
- min_text_region_confidence: float = 0.6,
- min_center_region_confidence: float = 0.2,
- min_center_area: int = 30,
- disk_overlap_thr: float = 0.03,
- radius_shrink_ratio: float = 1.03,
- rescale_fields: Sequence[str] = ['polygons'],
- **kwargs) -> None:
- super().__init__(
- text_repr_type=text_repr_type,
- rescale_fields=rescale_fields,
- **kwargs)
- assert text_repr_type == 'poly'
- self.min_text_region_confidence = min_text_region_confidence
- self.min_center_region_confidence = min_center_region_confidence
- self.min_center_area = min_center_area
- self.disk_overlap_thr = disk_overlap_thr
- self.radius_shrink_ratio = radius_shrink_ratio
-
- def get_text_instances(self, pred_results: torch.Tensor,
- data_sample: TextDetDataSample
- ) -> TextDetDataSample:
- """
- Args:
- pred_results (torch.Tensor): Prediction map with
- shape :math:`(C, H, W)`.
- data_sample (TextDetDataSample): Datasample of an image.
-
- Returns:
- list[list[float]]: The instance boundary and its confidence.
- """
- assert pred_results.dim() == 3
- data_sample.pred_instances = InstanceData()
- data_sample.pred_instances.polygons = []
- data_sample.pred_instances.scores = []
-
- pred_results[:2, :, :] = torch.sigmoid(pred_results[:2, :, :])
- pred_results = pred_results.detach().cpu().numpy()
-
- pred_text_score = pred_results[0]
- pred_text_mask = pred_text_score > self.min_text_region_confidence
- pred_center_score = pred_results[1] * pred_text_score
- pred_center_mask = \
- pred_center_score > self.min_center_region_confidence
- pred_sin = pred_results[2]
- pred_cos = pred_results[3]
- pred_radius = pred_results[4]
- mask_sz = pred_text_mask.shape
-
- scale = np.sqrt(1.0 / (pred_sin**2 + pred_cos**2 + 1e-8))
- pred_sin = pred_sin * scale
- pred_cos = pred_cos * scale
-
- pred_center_mask = fill_hole(pred_center_mask).astype(np.uint8)
- center_contours, _ = cv2.findContours(pred_center_mask, cv2.RETR_TREE,
- cv2.CHAIN_APPROX_SIMPLE)
-
- for contour in center_contours:
- if cv2.contourArea(contour) < self.min_center_area:
- continue
- instance_center_mask = np.zeros(mask_sz, dtype=np.uint8)
- cv2.drawContours(instance_center_mask, [contour], -1, 1, -1)
- skeleton = skeletonize(instance_center_mask)
- skeleton_yx = np.argwhere(skeleton > 0)
- y, x = skeleton_yx[:, 0], skeleton_yx[:, 1]
- cos = pred_cos[y, x].reshape((-1, 1))
- sin = pred_sin[y, x].reshape((-1, 1))
- radius = pred_radius[y, x].reshape((-1, 1))
-
- center_line_yx = self._centralize(skeleton_yx, cos, -sin, radius,
- instance_center_mask)
- y, x = center_line_yx[:, 0], center_line_yx[:, 1]
- radius = (pred_radius[y, x] * self.radius_shrink_ratio).reshape(
- (-1, 1))
- score = pred_center_score[y, x].reshape((-1, 1))
- instance_disks = np.hstack(
- [np.fliplr(center_line_yx), radius, score])
- instance_disks = self._merge_disks(instance_disks,
- self.disk_overlap_thr)
-
- instance_mask = np.zeros(mask_sz, dtype=np.uint8)
- for x, y, radius, score in instance_disks:
- if radius > 1:
- cv2.circle(instance_mask, (int(x), int(y)), int(radius), 1,
- -1)
- contours, _ = cv2.findContours(instance_mask, cv2.RETR_TREE,
- cv2.CHAIN_APPROX_SIMPLE)
-
- score = np.sum(instance_mask * pred_text_score) / (
- np.sum(instance_mask) + 1e-8)
- if (len(contours) > 0 and cv2.contourArea(contours[0]) > 0
- and contours[0].size > 8):
- polygon = contours[0].flatten().tolist()
- data_sample.pred_instances.polygons.append(polygon)
- data_sample.pred_instances.scores.append(score)
-
- data_sample.pred_instances.scores = torch.FloatTensor(
- data_sample.pred_instances.scores)
-
- return data_sample
-
- def split_results(self, pred_results: torch.Tensor) -> List[torch.Tensor]:
- """Split the prediction results into text score and kernel score.
-
- Args:
- pred_results (torch.Tensor): The prediction results.
-
- Returns:
- List[torch.Tensor]: The text score and kernel score.
- """
- pred_results = [pred_result for pred_result in pred_results]
- return pred_results
-
- @staticmethod
- def _centralize(points_yx: np.ndarray,
- normal_cos: torch.Tensor,
- normal_sin: torch.Tensor,
- radius: torch.Tensor,
- contour_mask: np.ndarray,
- step_ratio: float = 0.03) -> np.ndarray:
- """Centralize the points.
-
- Args:
- points_yx (np.array): The points in yx order.
- normal_cos (torch.Tensor): The normal cosine of the points.
- normal_sin (torch.Tensor): The normal sine of the points.
- radius (torch.Tensor): The radius of the points.
- contour_mask (np.array): The contour mask of the points.
- step_ratio (float): The step ratio of the centralization.
- Defaults to 0.03.
-
- Returns:
- np.ndarray: The centralized points.
- """
-
- h, w = contour_mask.shape
- top_yx = bot_yx = points_yx
- step_flags = np.ones((len(points_yx), 1), dtype=np.bool_)
- step = step_ratio * radius * np.hstack([normal_cos, normal_sin])
- while np.any(step_flags):
- next_yx = np.array(top_yx + step, dtype=np.int32)
- next_y, next_x = next_yx[:, 0], next_yx[:, 1]
- step_flags = (next_y >= 0) & (next_y < h) & (next_x > 0) & (
- next_x < w) & contour_mask[np.clip(next_y, 0, h - 1),
- np.clip(next_x, 0, w - 1)]
- top_yx = top_yx + step_flags.reshape((-1, 1)) * step
- step_flags = np.ones((len(points_yx), 1), dtype=np.bool_)
- while np.any(step_flags):
- next_yx = np.array(bot_yx - step, dtype=np.int32)
- next_y, next_x = next_yx[:, 0], next_yx[:, 1]
- step_flags = (next_y >= 0) & (next_y < h) & (next_x > 0) & (
- next_x < w) & contour_mask[np.clip(next_y, 0, h - 1),
- np.clip(next_x, 0, w - 1)]
- bot_yx = bot_yx - step_flags.reshape((-1, 1)) * step
- centers = np.array((top_yx + bot_yx) * 0.5, dtype=np.int32)
- return centers
-
- @staticmethod
- def _merge_disks(disks: np.ndarray, disk_overlap_thr: float) -> np.ndarray:
- """Merging overlapped disks.
-
- Args:
- disks (np.ndarray): The predicted disks.
- disk_overlap_thr (float): The radius overlap threshold for merging
- disks.
-
- Returns:
- np.ndarray: The merged disks.
- """
- xy = disks[:, 0:2]
- radius = disks[:, 2]
- scores = disks[:, 3]
- order = scores.argsort()[::-1]
-
- merged_disks = []
- while order.size > 0:
- if order.size == 1:
- merged_disks.append(disks[order])
- break
- i = order[0]
- d = norm(xy[i] - xy[order[1:]], axis=1)
- ri = radius[i]
- r = radius[order[1:]]
- d_thr = (ri + r) * disk_overlap_thr
-
- merge_inds = np.where(d <= d_thr)[0] + 1
- if merge_inds.size > 0:
- merge_order = np.hstack([i, order[merge_inds]])
- merged_disks.append(np.mean(disks[merge_order], axis=0))
- else:
- merged_disks.append(disks[i])
-
- inds = np.where(d > d_thr)[0] + 1
- order = order[inds]
- merged_disks = np.vstack(merged_disks)
-
- return merged_disks
diff --git a/pipeline/mmocr/mmocr/models/textrecog/__init__.py b/pipeline/mmocr/mmocr/models/textrecog/__init__.py
deleted file mode 100644
index e573c71efd65c3c94fe7e10c2031bae88cb9fc90..0000000000000000000000000000000000000000
--- a/pipeline/mmocr/mmocr/models/textrecog/__init__.py
+++ /dev/null
@@ -1,11 +0,0 @@
-# Copyright (c) OpenMMLab. All rights reserved.
-from .backbones import * # NOQA
-from .data_preprocessors import * # NOQA
-from .decoders import * # NOQA
-from .encoders import * # NOQA
-from .layers import * # NOQA
-from .module_losses import * # NOQA
-from .plugins import * # NOQA
-from .postprocessors import * # NOQA
-from .preprocessors import * # NOQA
-from .recognizers import * # NOQA
diff --git a/pipeline/mmocr/mmocr/models/textrecog/__pycache__/__init__.cpython-38.pyc b/pipeline/mmocr/mmocr/models/textrecog/__pycache__/__init__.cpython-38.pyc
deleted file mode 100644
index 537756010762a5fb8c2041db9b76dd29f5e34a20..0000000000000000000000000000000000000000
Binary files a/pipeline/mmocr/mmocr/models/textrecog/__pycache__/__init__.cpython-38.pyc and /dev/null differ
diff --git a/pipeline/mmocr/mmocr/models/textrecog/__pycache__/__init__.cpython-39.pyc b/pipeline/mmocr/mmocr/models/textrecog/__pycache__/__init__.cpython-39.pyc
deleted file mode 100644
index 6a7e0c0e925897fc539bf614141c91f86995348c..0000000000000000000000000000000000000000
Binary files a/pipeline/mmocr/mmocr/models/textrecog/__pycache__/__init__.cpython-39.pyc and /dev/null differ
diff --git a/pipeline/mmocr/mmocr/models/textrecog/backbones/__init__.py b/pipeline/mmocr/mmocr/models/textrecog/backbones/__init__.py
deleted file mode 100644
index 3201de3884b6582bd466daee1d0e8721075f5bac..0000000000000000000000000000000000000000
--- a/pipeline/mmocr/mmocr/models/textrecog/backbones/__init__.py
+++ /dev/null
@@ -1,13 +0,0 @@
-# Copyright (c) OpenMMLab. All rights reserved.
-from .mini_vgg import MiniVGG
-from .mobilenet_v2 import MobileNetV2
-from .nrtr_modality_transformer import NRTRModalityTransform
-from .resnet import ResNet
-from .resnet31_ocr import ResNet31OCR
-from .resnet_abi import ResNetABI
-from .shallow_cnn import ShallowCNN
-
-__all__ = [
- 'ResNet31OCR', 'MiniVGG', 'NRTRModalityTransform', 'ShallowCNN',
- 'ResNetABI', 'ResNet', 'MobileNetV2'
-]
diff --git a/pipeline/mmocr/mmocr/models/textrecog/backbones/__pycache__/__init__.cpython-38.pyc b/pipeline/mmocr/mmocr/models/textrecog/backbones/__pycache__/__init__.cpython-38.pyc
deleted file mode 100644
index e48901b5e9944824c0948f88428742245ea8f428..0000000000000000000000000000000000000000
Binary files a/pipeline/mmocr/mmocr/models/textrecog/backbones/__pycache__/__init__.cpython-38.pyc and /dev/null differ
diff --git a/pipeline/mmocr/mmocr/models/textrecog/backbones/__pycache__/__init__.cpython-39.pyc b/pipeline/mmocr/mmocr/models/textrecog/backbones/__pycache__/__init__.cpython-39.pyc
deleted file mode 100644
index 25eee33319e3641044bf5d0b17156df85e6a6896..0000000000000000000000000000000000000000
Binary files a/pipeline/mmocr/mmocr/models/textrecog/backbones/__pycache__/__init__.cpython-39.pyc and /dev/null differ
diff --git a/pipeline/mmocr/mmocr/models/textrecog/backbones/__pycache__/mini_vgg.cpython-38.pyc b/pipeline/mmocr/mmocr/models/textrecog/backbones/__pycache__/mini_vgg.cpython-38.pyc
deleted file mode 100644
index ad455e1cb17db02c7015c54288cbeff47fda00e4..0000000000000000000000000000000000000000
Binary files a/pipeline/mmocr/mmocr/models/textrecog/backbones/__pycache__/mini_vgg.cpython-38.pyc and /dev/null differ
diff --git a/pipeline/mmocr/mmocr/models/textrecog/backbones/__pycache__/mini_vgg.cpython-39.pyc b/pipeline/mmocr/mmocr/models/textrecog/backbones/__pycache__/mini_vgg.cpython-39.pyc
deleted file mode 100644
index c489ea8ed23c9b49ceb58c9ee0d213012fafa54b..0000000000000000000000000000000000000000
Binary files a/pipeline/mmocr/mmocr/models/textrecog/backbones/__pycache__/mini_vgg.cpython-39.pyc and /dev/null differ
diff --git a/pipeline/mmocr/mmocr/models/textrecog/backbones/__pycache__/mobilenet_v2.cpython-38.pyc b/pipeline/mmocr/mmocr/models/textrecog/backbones/__pycache__/mobilenet_v2.cpython-38.pyc
deleted file mode 100644
index bec716d8d0465b8e518fb7a116692cbfae28521a..0000000000000000000000000000000000000000
Binary files a/pipeline/mmocr/mmocr/models/textrecog/backbones/__pycache__/mobilenet_v2.cpython-38.pyc and /dev/null differ
diff --git a/pipeline/mmocr/mmocr/models/textrecog/backbones/__pycache__/mobilenet_v2.cpython-39.pyc b/pipeline/mmocr/mmocr/models/textrecog/backbones/__pycache__/mobilenet_v2.cpython-39.pyc
deleted file mode 100644
index 8ad10f4c4060c1a2a64e0c54b8a53e3a7993fb67..0000000000000000000000000000000000000000
Binary files a/pipeline/mmocr/mmocr/models/textrecog/backbones/__pycache__/mobilenet_v2.cpython-39.pyc and /dev/null differ
diff --git a/pipeline/mmocr/mmocr/models/textrecog/backbones/__pycache__/nrtr_modality_transformer.cpython-38.pyc b/pipeline/mmocr/mmocr/models/textrecog/backbones/__pycache__/nrtr_modality_transformer.cpython-38.pyc
deleted file mode 100644
index 746ceb6c235a29941b8b23ddb5a6b91b1280bac6..0000000000000000000000000000000000000000
Binary files a/pipeline/mmocr/mmocr/models/textrecog/backbones/__pycache__/nrtr_modality_transformer.cpython-38.pyc and /dev/null differ
diff --git a/pipeline/mmocr/mmocr/models/textrecog/backbones/__pycache__/nrtr_modality_transformer.cpython-39.pyc b/pipeline/mmocr/mmocr/models/textrecog/backbones/__pycache__/nrtr_modality_transformer.cpython-39.pyc
deleted file mode 100644
index 206936779dc483c13a8cf9c0cae8b70152f8f969..0000000000000000000000000000000000000000
Binary files a/pipeline/mmocr/mmocr/models/textrecog/backbones/__pycache__/nrtr_modality_transformer.cpython-39.pyc and /dev/null differ
diff --git a/pipeline/mmocr/mmocr/models/textrecog/backbones/__pycache__/resnet.cpython-38.pyc b/pipeline/mmocr/mmocr/models/textrecog/backbones/__pycache__/resnet.cpython-38.pyc
deleted file mode 100644
index 7f9199f804ec1fb60a5416e55778f225eba225c0..0000000000000000000000000000000000000000
Binary files a/pipeline/mmocr/mmocr/models/textrecog/backbones/__pycache__/resnet.cpython-38.pyc and /dev/null differ
diff --git a/pipeline/mmocr/mmocr/models/textrecog/backbones/__pycache__/resnet.cpython-39.pyc b/pipeline/mmocr/mmocr/models/textrecog/backbones/__pycache__/resnet.cpython-39.pyc
deleted file mode 100644
index 19fde531d289ae60b35f13eab0484b1f73fe537d..0000000000000000000000000000000000000000
Binary files a/pipeline/mmocr/mmocr/models/textrecog/backbones/__pycache__/resnet.cpython-39.pyc and /dev/null differ
diff --git a/pipeline/mmocr/mmocr/models/textrecog/backbones/__pycache__/resnet31_ocr.cpython-38.pyc b/pipeline/mmocr/mmocr/models/textrecog/backbones/__pycache__/resnet31_ocr.cpython-38.pyc
deleted file mode 100644
index dc701de12203fbf23d01bbb16a37b58a59e9053f..0000000000000000000000000000000000000000
Binary files a/pipeline/mmocr/mmocr/models/textrecog/backbones/__pycache__/resnet31_ocr.cpython-38.pyc and /dev/null differ
diff --git a/pipeline/mmocr/mmocr/models/textrecog/backbones/__pycache__/resnet31_ocr.cpython-39.pyc b/pipeline/mmocr/mmocr/models/textrecog/backbones/__pycache__/resnet31_ocr.cpython-39.pyc
deleted file mode 100644
index 48461d9fe0bce1b54939e259e9e5637b7be96a64..0000000000000000000000000000000000000000
Binary files a/pipeline/mmocr/mmocr/models/textrecog/backbones/__pycache__/resnet31_ocr.cpython-39.pyc and /dev/null differ
diff --git a/pipeline/mmocr/mmocr/models/textrecog/backbones/__pycache__/resnet_abi.cpython-38.pyc b/pipeline/mmocr/mmocr/models/textrecog/backbones/__pycache__/resnet_abi.cpython-38.pyc
deleted file mode 100644
index 36e20db9f5216690446884f8bec2e4cce1bcb7b7..0000000000000000000000000000000000000000
Binary files a/pipeline/mmocr/mmocr/models/textrecog/backbones/__pycache__/resnet_abi.cpython-38.pyc and /dev/null differ
diff --git a/pipeline/mmocr/mmocr/models/textrecog/backbones/__pycache__/resnet_abi.cpython-39.pyc b/pipeline/mmocr/mmocr/models/textrecog/backbones/__pycache__/resnet_abi.cpython-39.pyc
deleted file mode 100644
index 4e1dcd0b4df98433d1965e0a9893696144603d82..0000000000000000000000000000000000000000
Binary files a/pipeline/mmocr/mmocr/models/textrecog/backbones/__pycache__/resnet_abi.cpython-39.pyc and /dev/null differ
diff --git a/pipeline/mmocr/mmocr/models/textrecog/backbones/__pycache__/shallow_cnn.cpython-38.pyc b/pipeline/mmocr/mmocr/models/textrecog/backbones/__pycache__/shallow_cnn.cpython-38.pyc
deleted file mode 100644
index 5dfde0c68e6989d2f0c9c70a302010a37374147d..0000000000000000000000000000000000000000
Binary files a/pipeline/mmocr/mmocr/models/textrecog/backbones/__pycache__/shallow_cnn.cpython-38.pyc and /dev/null differ
diff --git a/pipeline/mmocr/mmocr/models/textrecog/backbones/__pycache__/shallow_cnn.cpython-39.pyc b/pipeline/mmocr/mmocr/models/textrecog/backbones/__pycache__/shallow_cnn.cpython-39.pyc
deleted file mode 100644
index 928dfc5e7352736084fbc090332d447445a427b6..0000000000000000000000000000000000000000
Binary files a/pipeline/mmocr/mmocr/models/textrecog/backbones/__pycache__/shallow_cnn.cpython-39.pyc and /dev/null differ
diff --git a/pipeline/mmocr/mmocr/models/textrecog/backbones/mini_vgg.py b/pipeline/mmocr/mmocr/models/textrecog/backbones/mini_vgg.py
deleted file mode 100644
index ed3601c1b4936cea459c6f0ac67042907fbc846c..0000000000000000000000000000000000000000
--- a/pipeline/mmocr/mmocr/models/textrecog/backbones/mini_vgg.py
+++ /dev/null
@@ -1,79 +0,0 @@
-# Copyright (c) OpenMMLab. All rights reserved.
-import torch.nn as nn
-from mmengine.model import BaseModule, Sequential
-
-from mmocr.registry import MODELS
-
-
-@MODELS.register_module()
-class MiniVGG(BaseModule):
- """A mini VGG backbone for text recognition, modified from `VGG-VeryDeep.
-
- `_
-
- Args:
- leaky_relu (bool): Use leakyRelu or not.
- input_channels (int): Number of channels of input image tensor.
- """
-
- def __init__(self,
- leaky_relu=True,
- input_channels=3,
- init_cfg=[
- dict(type='Xavier', layer='Conv2d'),
- dict(type='Uniform', layer='BatchNorm2d')
- ]):
- super().__init__(init_cfg=init_cfg)
-
- ks = [3, 3, 3, 3, 3, 3, 2]
- ps = [1, 1, 1, 1, 1, 1, 0]
- ss = [1, 1, 1, 1, 1, 1, 1]
- nm = [64, 128, 256, 256, 512, 512, 512]
-
- self.channels = nm
-
- # cnn = nn.Sequential()
- cnn = Sequential()
-
- def conv_relu(i, batch_normalization=False):
- n_in = input_channels if i == 0 else nm[i - 1]
- n_out = nm[i]
- cnn.add_module(f'conv{i}',
- nn.Conv2d(n_in, n_out, ks[i], ss[i], ps[i]))
- if batch_normalization:
- cnn.add_module(f'batchnorm{i}', nn.BatchNorm2d(n_out))
- if leaky_relu:
- cnn.add_module(f'relu{i}', nn.LeakyReLU(0.2, inplace=True))
- else:
- cnn.add_module(f'relu{i}', nn.ReLU(True))
-
- conv_relu(0)
- cnn.add_module(f'pooling{0}', nn.MaxPool2d(2, 2)) # 64x16x64
- conv_relu(1)
- cnn.add_module(f'pooling{1}', nn.MaxPool2d(2, 2)) # 128x8x32
- conv_relu(2, True)
- conv_relu(3)
- cnn.add_module(f'pooling{2}', nn.MaxPool2d((2, 2), (2, 1),
- (0, 1))) # 256x4x16
- conv_relu(4, True)
- conv_relu(5)
- cnn.add_module(f'pooling{3}', nn.MaxPool2d((2, 2), (2, 1),
- (0, 1))) # 512x2x16
- conv_relu(6, True) # 512x1x16
-
- self.cnn = cnn
-
- def out_channels(self):
- return self.channels[-1]
-
- def forward(self, x):
- """
- Args:
- x (Tensor): Images of shape :math:`(N, C, H, W)`.
-
- Returns:
- Tensor: The feature Tensor of shape :math:`(N, 512, H/32, (W/4+1)`.
- """
- output = self.cnn(x)
-
- return output
diff --git a/pipeline/mmocr/mmocr/models/textrecog/backbones/mobilenet_v2.py b/pipeline/mmocr/mmocr/models/textrecog/backbones/mobilenet_v2.py
deleted file mode 100644
index b0c671645f48773baa3df75a3ed868ca31c56a83..0000000000000000000000000000000000000000
--- a/pipeline/mmocr/mmocr/models/textrecog/backbones/mobilenet_v2.py
+++ /dev/null
@@ -1,43 +0,0 @@
-# Copyright (c) OpenMMLab. All rights reserved.
-from typing import List
-
-import torch.nn as nn
-from mmdet.models.backbones import MobileNetV2 as MMDet_MobileNetV2
-from torch import Tensor
-
-from mmocr.registry import MODELS
-from mmocr.utils.typing_utils import InitConfigType
-
-
-@MODELS.register_module()
-class MobileNetV2(MMDet_MobileNetV2):
- """See mmdet.models.backbones.MobileNetV2 for details.
-
- Args:
- pooling_layers (list): List of indices of pooling layers.
- init_cfg (InitConfigType, optional): Initialization config dict.
- """
- # Parameters to build layers. 4 parameters are needed to construct a
- # layer, from left to right: expand_ratio, channel, num_blocks, stride.
- arch_settings = [[1, 16, 1, 1], [6, 24, 2, 2], [6, 32, 3, 1],
- [6, 64, 4, 1], [6, 96, 3, 1], [6, 160, 3, 1],
- [6, 320, 1, 1]]
-
- def __init__(self,
- pooling_layers: List = [3, 4, 5],
- init_cfg: InitConfigType = None) -> None:
- super().__init__(init_cfg=init_cfg)
- self.pooling = nn.MaxPool2d((2, 2), (2, 1), (0, 1))
- self.pooling_layers = pooling_layers
-
- def forward(self, x: Tensor) -> Tensor:
- """Forward function."""
-
- x = self.conv1(x)
- for i, layer_name in enumerate(self.layers):
- layer = getattr(self, layer_name)
- x = layer(x)
- if i in self.pooling_layers:
- x = self.pooling(x)
-
- return x
diff --git a/pipeline/mmocr/mmocr/models/textrecog/backbones/nrtr_modality_transformer.py b/pipeline/mmocr/mmocr/models/textrecog/backbones/nrtr_modality_transformer.py
deleted file mode 100644
index 35f4f9c3f2e0e7f874620cfad643bfcbcb5cd0c5..0000000000000000000000000000000000000000
--- a/pipeline/mmocr/mmocr/models/textrecog/backbones/nrtr_modality_transformer.py
+++ /dev/null
@@ -1,75 +0,0 @@
-# Copyright (c) OpenMMLab. All rights reserved.
-from typing import Dict, Optional, Sequence, Union
-
-import torch
-import torch.nn as nn
-from mmengine.model import BaseModule
-
-from mmocr.registry import MODELS
-
-
-@MODELS.register_module()
-class NRTRModalityTransform(BaseModule):
- """Modality transform in NRTR.
-
- Args:
- in_channels (int): Input channel of image. Defaults to 3.
- init_cfg (dict or list[dict], optional): Initialization configs.
- """
-
- def __init__(
- self,
- in_channels: int = 3,
- init_cfg: Optional[Union[Dict, Sequence[Dict]]] = [
- dict(type='Kaiming', layer='Conv2d'),
- dict(type='Uniform', layer='BatchNorm2d')
- ]
- ) -> None:
- super().__init__(init_cfg=init_cfg)
-
- self.conv_1 = nn.Conv2d(
- in_channels=in_channels,
- out_channels=32,
- kernel_size=3,
- stride=2,
- padding=1)
- self.relu_1 = nn.ReLU(True)
- self.bn_1 = nn.BatchNorm2d(32)
-
- self.conv_2 = nn.Conv2d(
- in_channels=32,
- out_channels=64,
- kernel_size=3,
- stride=2,
- padding=1)
- self.relu_2 = nn.ReLU(True)
- self.bn_2 = nn.BatchNorm2d(64)
-
- self.linear = nn.Linear(512, 512)
-
- def forward(self, x: torch.Tensor) -> torch.Tensor:
- """Backbone forward.
-
- Args:
- x (torch.Tensor): Image tensor of shape :math:`(N, C, W, H)`. W, H
- is the width and height of image.
- Return:
- Tensor: Output tensor.
- """
- x = self.conv_1(x)
- x = self.relu_1(x)
- x = self.bn_1(x)
-
- x = self.conv_2(x)
- x = self.relu_2(x)
- x = self.bn_2(x)
-
- n, c, h, w = x.size()
-
- x = x.permute(0, 3, 2, 1).contiguous().view(n, w, h * c)
-
- x = self.linear(x)
-
- x = x.permute(0, 2, 1).contiguous().view(n, -1, 1, w)
-
- return x
diff --git a/pipeline/mmocr/mmocr/models/textrecog/backbones/resnet.py b/pipeline/mmocr/mmocr/models/textrecog/backbones/resnet.py
deleted file mode 100644
index bb17a8cdcbb732cc04674106fc043560555bec2e..0000000000000000000000000000000000000000
--- a/pipeline/mmocr/mmocr/models/textrecog/backbones/resnet.py
+++ /dev/null
@@ -1,264 +0,0 @@
-# Copyright (c) OpenMMLab. All rights reserved.
-from typing import Dict, List, Optional, Tuple, Union
-
-import torch
-from mmcv.cnn import ConvModule, build_plugin_layer
-from mmengine.model import BaseModule, Sequential
-
-import mmocr.utils as utils
-from mmocr.models.textrecog.layers import BasicBlock
-from mmocr.registry import MODELS
-
-
-@MODELS.register_module()
-class ResNet(BaseModule):
- """
- Args:
- in_channels (int): Number of channels of input image tensor.
- stem_channels (list[int]): List of channels in each stem layer. E.g.,
- [64, 128] stands for 64 and 128 channels in the first and second
- stem layers.
- block_cfgs (dict): Configs of block
- arch_layers (list[int]): List of Block number for each stage.
- arch_channels (list[int]): List of channels for each stage.
- strides (Sequence[int] or Sequence[tuple]): Strides of the first block
- of each stage.
- out_indices (Sequence[int], optional): Indices of output stages. If not
- specified, only the last stage will be returned.
- plugins (dict, optional): Configs of stage plugins
- init_cfg (dict or list[dict], optional): Initialization config dict.
- """
-
- def __init__(self,
- in_channels: int,
- stem_channels: List[int],
- block_cfgs: dict,
- arch_layers: List[int],
- arch_channels: List[int],
- strides: Union[List[int], List[Tuple]],
- out_indices: Optional[List[int]] = None,
- plugins: Optional[Dict] = None,
- init_cfg: Optional[Union[Dict, List[Dict]]] = [
- dict(type='Xavier', layer='Conv2d'),
- dict(type='Constant', val=1, layer='BatchNorm2d'),
- ]):
- super().__init__(init_cfg=init_cfg)
- assert isinstance(in_channels, int)
- assert isinstance(stem_channels, int) or utils.is_type_list(
- stem_channels, int)
- assert utils.is_type_list(arch_layers, int)
- assert utils.is_type_list(arch_channels, int)
- assert utils.is_type_list(strides, tuple) or utils.is_type_list(
- strides, int)
- assert len(arch_layers) == len(arch_channels) == len(strides)
- assert out_indices is None or isinstance(out_indices, (list, tuple))
-
- self.out_indices = out_indices
- self._make_stem_layer(in_channels, stem_channels)
- self.num_stages = len(arch_layers)
- self.use_plugins = False
- self.arch_channels = arch_channels
- self.res_layers = []
- if plugins is not None:
- self.plugin_ahead_names = []
- self.plugin_after_names = []
- self.use_plugins = True
- for i, num_blocks in enumerate(arch_layers):
- stride = strides[i]
- channel = arch_channels[i]
-
- if self.use_plugins:
- self._make_stage_plugins(plugins, stage_idx=i)
-
- res_layer = self._make_layer(
- block_cfgs=block_cfgs,
- inplanes=self.inplanes,
- planes=channel,
- blocks=num_blocks,
- stride=stride,
- )
- self.inplanes = channel
- layer_name = f'layer{i + 1}'
- self.add_module(layer_name, res_layer)
- self.res_layers.append(layer_name)
-
- def _make_layer(self, block_cfgs: Dict, inplanes: int, planes: int,
- blocks: int, stride: int) -> Sequential:
- """Build resnet layer.
-
- Args:
- block_cfgs (dict): Configs of blocks.
- inplanes (int): Number of input channels.
- planes (int): Number of output channels.
- blocks (int): Number of blocks.
- stride (int): Stride of the first block.
-
- Returns:
- Sequential: A sequence of blocks.
- """
- layers = []
- downsample = None
- block_cfgs_ = block_cfgs.copy()
- if isinstance(stride, int):
- stride = (stride, stride)
-
- if stride[0] != 1 or stride[1] != 1 or inplanes != planes:
- downsample = ConvModule(
- inplanes,
- planes,
- 1,
- stride,
- norm_cfg=dict(type='BN'),
- act_cfg=None)
-
- if block_cfgs_['type'] == 'BasicBlock':
- block = BasicBlock
- block_cfgs_.pop('type')
- else:
- raise ValueError('{} not implement yet'.format(block['type']))
-
- layers.append(
- block(
- inplanes,
- planes,
- stride=stride,
- downsample=downsample,
- **block_cfgs_))
- inplanes = planes
- for _ in range(1, blocks):
- layers.append(block(inplanes, planes, **block_cfgs_))
-
- return Sequential(*layers)
-
- def _make_stem_layer(self, in_channels: int,
- stem_channels: Union[int, List[int]]) -> None:
- """Make stem layers.
-
- Args:
- in_channels (int): Number of input channels.
- stem_channels (list[int] or int): List of channels in each stem
- layer. If int, only one stem layer will be created.
- """
- if isinstance(stem_channels, int):
- stem_channels = [stem_channels]
- stem_layers = []
- for _, channels in enumerate(stem_channels):
- stem_layer = ConvModule(
- in_channels,
- channels,
- kernel_size=3,
- stride=1,
- padding=1,
- bias=False,
- norm_cfg=dict(type='BN'),
- act_cfg=dict(type='ReLU'))
- in_channels = channels
- stem_layers.append(stem_layer)
- self.stem_layers = Sequential(*stem_layers)
- self.inplanes = stem_channels[-1]
-
- def _make_stage_plugins(self, plugins: List[Dict], stage_idx: int) -> None:
- """Make plugins for ResNet ``stage_idx``th stage.
-
- Currently we support inserting ``nn.Maxpooling``,
- ``mmcv.cnn.Convmodule``into the backbone. Originally designed
- for ResNet31-like architectures.
-
- Examples:
- >>> plugins=[
- ... dict(cfg=dict(type="Maxpooling", arg=(2,2)),
- ... stages=(True, True, False, False),
- ... position='before_stage'),
- ... dict(cfg=dict(type="Maxpooling", arg=(2,1)),
- ... stages=(False, False, True, Flase),
- ... position='before_stage'),
- ... dict(cfg=dict(
- ... type='ConvModule',
- ... kernel_size=3,
- ... stride=1,
- ... padding=1,
- ... norm_cfg=dict(type='BN'),
- ... act_cfg=dict(type='ReLU')),
- ... stages=(True, True, True, True),
- ... position='after_stage')]
-
- Suppose ``stage_idx=1``, the structure of stage would be:
-
- .. code-block:: none
-
- Maxpooling -> A set of Basicblocks -> ConvModule
-
- Args:
- plugins (list[dict]): List of plugin configs to build.
- stage_idx (int): Index of stage to build
- """
- in_channels = self.arch_channels[stage_idx]
- self.plugin_ahead_names.append([])
- self.plugin_after_names.append([])
- for plugin in plugins:
- plugin = plugin.copy()
- stages = plugin.pop('stages', None)
- position = plugin.pop('position', None)
- assert stages is None or len(stages) == self.num_stages
- if stages[stage_idx]:
- if position == 'before_stage':
- name, layer = build_plugin_layer(
- plugin['cfg'],
- f'_before_stage_{stage_idx+1}',
- in_channels=in_channels,
- out_channels=in_channels)
- self.plugin_ahead_names[stage_idx].append(name)
- self.add_module(name, layer)
- elif position == 'after_stage':
- name, layer = build_plugin_layer(
- plugin['cfg'],
- f'_after_stage_{stage_idx+1}',
- in_channels=in_channels,
- out_channels=in_channels)
- self.plugin_after_names[stage_idx].append(name)
- self.add_module(name, layer)
- else:
- raise ValueError('uncorrect plugin position')
-
- def forward_plugin(self, x: torch.Tensor,
- plugin_name: List[str]) -> torch.Tensor:
- """Forward tensor through plugin.
-
- Args:
- x (torch.Tensor): Input tensor.
- plugin_name (list[str]): Name of plugins.
-
- Returns:
- torch.Tensor: Output tensor.
- """
- out = x
- for name in plugin_name:
- out = getattr(self, name)(out)
- return out
-
- def forward(self,
- x: torch.Tensor) -> Union[torch.Tensor, List[torch.Tensor]]:
- """
- Args: x (Tensor): Image tensor of shape :math:`(N, 3, H, W)`.
-
- Returns:
- Tensor or list[Tensor]: Feature tensor. It can be a list of
- feature outputs at specific layers if ``out_indices`` is specified.
- """
- x = self.stem_layers(x)
-
- outs = []
- for i, layer_name in enumerate(self.res_layers):
- res_layer = getattr(self, layer_name)
- if not self.use_plugins:
- x = res_layer(x)
- if self.out_indices and i in self.out_indices:
- outs.append(x)
- else:
- x = self.forward_plugin(x, self.plugin_ahead_names[i])
- x = res_layer(x)
- x = self.forward_plugin(x, self.plugin_after_names[i])
- if self.out_indices and i in self.out_indices:
- outs.append(x)
-
- return tuple(outs) if self.out_indices else x
diff --git a/pipeline/mmocr/mmocr/models/textrecog/backbones/resnet31_ocr.py b/pipeline/mmocr/mmocr/models/textrecog/backbones/resnet31_ocr.py
deleted file mode 100644
index 96ca7b7af7ae9b1ed724d9ae783cf39df5aa6f57..0000000000000000000000000000000000000000
--- a/pipeline/mmocr/mmocr/models/textrecog/backbones/resnet31_ocr.py
+++ /dev/null
@@ -1,145 +0,0 @@
-# Copyright (c) OpenMMLab. All rights reserved.
-import torch.nn as nn
-from mmengine.model import BaseModule, Sequential
-
-import mmocr.utils as utils
-from mmocr.models.textrecog.layers import BasicBlock
-from mmocr.registry import MODELS
-
-
-@MODELS.register_module()
-class ResNet31OCR(BaseModule):
- """Implement ResNet backbone for text recognition, modified from
- `ResNet `_
- Args:
- base_channels (int): Number of channels of input image tensor.
- layers (list[int]): List of BasicBlock number for each stage.
- channels (list[int]): List of out_channels of Conv2d layer.
- out_indices (None | Sequence[int]): Indices of output stages.
- stage4_pool_cfg (dict): Dictionary to construct and configure
- pooling layer in stage 4.
- last_stage_pool (bool): If True, add `MaxPool2d` layer to last stage.
- """
-
- def __init__(self,
- base_channels=3,
- layers=[1, 2, 5, 3],
- channels=[64, 128, 256, 256, 512, 512, 512],
- out_indices=None,
- stage4_pool_cfg=dict(kernel_size=(2, 1), stride=(2, 1)),
- last_stage_pool=False,
- init_cfg=[
- dict(type='Kaiming', layer='Conv2d'),
- dict(type='Uniform', layer='BatchNorm2d')
- ]):
- super().__init__(init_cfg=init_cfg)
- assert isinstance(base_channels, int)
- assert utils.is_type_list(layers, int)
- assert utils.is_type_list(channels, int)
- assert out_indices is None or isinstance(out_indices, (list, tuple))
- assert isinstance(last_stage_pool, bool)
-
- self.out_indices = out_indices
- self.last_stage_pool = last_stage_pool
-
- # conv 1 (Conv, Conv)
- self.conv1_1 = nn.Conv2d(
- base_channels, channels[0], kernel_size=3, stride=1, padding=1)
- self.bn1_1 = nn.BatchNorm2d(channels[0])
- self.relu1_1 = nn.ReLU(inplace=True)
-
- self.conv1_2 = nn.Conv2d(
- channels[0], channels[1], kernel_size=3, stride=1, padding=1)
- self.bn1_2 = nn.BatchNorm2d(channels[1])
- self.relu1_2 = nn.ReLU(inplace=True)
-
- # conv 2 (Max-pooling, Residual block, Conv)
- self.pool2 = nn.MaxPool2d(
- kernel_size=2, stride=2, padding=0, ceil_mode=True)
- self.block2 = self._make_layer(channels[1], channels[2], layers[0])
- self.conv2 = nn.Conv2d(
- channels[2], channels[2], kernel_size=3, stride=1, padding=1)
- self.bn2 = nn.BatchNorm2d(channels[2])
- self.relu2 = nn.ReLU(inplace=True)
-
- # conv 3 (Max-pooling, Residual block, Conv)
- self.pool3 = nn.MaxPool2d(
- kernel_size=2, stride=2, padding=0, ceil_mode=True)
- self.block3 = self._make_layer(channels[2], channels[3], layers[1])
- self.conv3 = nn.Conv2d(
- channels[3], channels[3], kernel_size=3, stride=1, padding=1)
- self.bn3 = nn.BatchNorm2d(channels[3])
- self.relu3 = nn.ReLU(inplace=True)
-
- # conv 4 (Max-pooling, Residual block, Conv)
- self.pool4 = nn.MaxPool2d(padding=0, ceil_mode=True, **stage4_pool_cfg)
- self.block4 = self._make_layer(channels[3], channels[4], layers[2])
- self.conv4 = nn.Conv2d(
- channels[4], channels[4], kernel_size=3, stride=1, padding=1)
- self.bn4 = nn.BatchNorm2d(channels[4])
- self.relu4 = nn.ReLU(inplace=True)
-
- # conv 5 ((Max-pooling), Residual block, Conv)
- self.pool5 = None
- if self.last_stage_pool:
- self.pool5 = nn.MaxPool2d(
- kernel_size=2, stride=2, padding=0, ceil_mode=True) # 1/16
- self.block5 = self._make_layer(channels[4], channels[5], layers[3])
- self.conv5 = nn.Conv2d(
- channels[5], channels[5], kernel_size=3, stride=1, padding=1)
- self.bn5 = nn.BatchNorm2d(channels[5])
- self.relu5 = nn.ReLU(inplace=True)
-
- def _make_layer(self, input_channels, output_channels, blocks):
- layers = []
- for _ in range(blocks):
- downsample = None
- if input_channels != output_channels:
- downsample = Sequential(
- nn.Conv2d(
- input_channels,
- output_channels,
- kernel_size=1,
- stride=1,
- bias=False),
- nn.BatchNorm2d(output_channels),
- )
- layers.append(
- BasicBlock(
- input_channels, output_channels, downsample=downsample))
- input_channels = output_channels
-
- return Sequential(*layers)
-
- def forward(self, x):
-
- x = self.conv1_1(x)
- x = self.bn1_1(x)
- x = self.relu1_1(x)
-
- x = self.conv1_2(x)
- x = self.bn1_2(x)
- x = self.relu1_2(x)
-
- outs = []
- for i in range(4):
- layer_index = i + 2
- pool_layer = getattr(self, f'pool{layer_index}')
- block_layer = getattr(self, f'block{layer_index}')
- conv_layer = getattr(self, f'conv{layer_index}')
- bn_layer = getattr(self, f'bn{layer_index}')
- relu_layer = getattr(self, f'relu{layer_index}')
-
- if pool_layer is not None:
- x = pool_layer(x)
- x = block_layer(x)
- x = conv_layer(x)
- x = bn_layer(x)
- x = relu_layer(x)
-
- outs.append(x)
-
- if self.out_indices is not None:
- return tuple(outs[i] for i in self.out_indices)
-
- return x
diff --git a/pipeline/mmocr/mmocr/models/textrecog/backbones/resnet_abi.py b/pipeline/mmocr/mmocr/models/textrecog/backbones/resnet_abi.py
deleted file mode 100644
index ce79758501a34696e14005f0cf8b2cad68c6d7bb..0000000000000000000000000000000000000000
--- a/pipeline/mmocr/mmocr/models/textrecog/backbones/resnet_abi.py
+++ /dev/null
@@ -1,121 +0,0 @@
-# Copyright (c) OpenMMLab. All rights reserved.
-import torch.nn as nn
-from mmengine.model import BaseModule, Sequential
-
-import mmocr.utils as utils
-from mmocr.models.textrecog.layers import BasicBlock
-from mmocr.registry import MODELS
-
-
-@MODELS.register_module()
-class ResNetABI(BaseModule):
- """Implement ResNet backbone for text recognition, modified from `ResNet.
-
- `_ and
- ``_
-
- Args:
- in_channels (int): Number of channels of input image tensor.
- stem_channels (int): Number of stem channels.
- base_channels (int): Number of base channels.
- arch_settings (list[int]): List of BasicBlock number for each stage.
- strides (Sequence[int]): Strides of the first block of each stage.
- out_indices (None | Sequence[int]): Indices of output stages. If not
- specified, only the last stage will be returned.
- last_stage_pool (bool): If True, add `MaxPool2d` layer to last stage.
- """
-
- def __init__(self,
- in_channels=3,
- stem_channels=32,
- base_channels=32,
- arch_settings=[3, 4, 6, 6, 3],
- strides=[2, 1, 2, 1, 1],
- out_indices=None,
- last_stage_pool=False,
- init_cfg=[
- dict(type='Xavier', layer='Conv2d'),
- dict(type='Constant', val=1, layer='BatchNorm2d')
- ]):
- super().__init__(init_cfg=init_cfg)
- assert isinstance(in_channels, int)
- assert isinstance(stem_channels, int)
- assert utils.is_type_list(arch_settings, int)
- assert utils.is_type_list(strides, int)
- assert len(arch_settings) == len(strides)
- assert out_indices is None or isinstance(out_indices, (list, tuple))
- assert isinstance(last_stage_pool, bool)
-
- self.out_indices = out_indices
- self.last_stage_pool = last_stage_pool
- self.block = BasicBlock
- self.inplanes = stem_channels
-
- self._make_stem_layer(in_channels, stem_channels)
-
- self.res_layers = []
- planes = base_channels
- for i, num_blocks in enumerate(arch_settings):
- stride = strides[i]
- res_layer = self._make_layer(
- block=self.block,
- inplanes=self.inplanes,
- planes=planes,
- blocks=num_blocks,
- stride=stride)
- self.inplanes = planes * self.block.expansion
- planes *= 2
- layer_name = f'layer{i + 1}'
- self.add_module(layer_name, res_layer)
- self.res_layers.append(layer_name)
-
- def _make_layer(self, block, inplanes, planes, blocks, stride=1):
- layers = []
- downsample = None
- if stride != 1 or inplanes != planes:
- downsample = nn.Sequential(
- nn.Conv2d(inplanes, planes, 1, stride, bias=False),
- nn.BatchNorm2d(planes),
- )
- layers.append(
- block(
- inplanes,
- planes,
- use_conv1x1=True,
- stride=stride,
- downsample=downsample))
- inplanes = planes
- for _ in range(1, blocks):
- layers.append(block(inplanes, planes, use_conv1x1=True))
-
- return Sequential(*layers)
-
- def _make_stem_layer(self, in_channels, stem_channels):
- self.conv1 = nn.Conv2d(
- in_channels, stem_channels, kernel_size=3, stride=1, padding=1)
- self.bn1 = nn.BatchNorm2d(stem_channels)
- self.relu1 = nn.ReLU(inplace=True)
-
- def forward(self, x):
- """
- Args:
- x (Tensor): Image tensor of shape :math:`(N, 3, H, W)`.
-
- Returns:
- Tensor or list[Tensor]: Feature tensor. Its shape depends on
- ResNetABI's config. It can be a list of feature outputs at specific
- layers if ``out_indices`` is specified.
- """
-
- x = self.conv1(x)
- x = self.bn1(x)
- x = self.relu1(x)
-
- outs = []
- for i, layer_name in enumerate(self.res_layers):
- res_layer = getattr(self, layer_name)
- x = res_layer(x)
- if self.out_indices and i in self.out_indices:
- outs.append(x)
-
- return tuple(outs) if self.out_indices else x
diff --git a/pipeline/mmocr/mmocr/models/textrecog/backbones/shallow_cnn.py b/pipeline/mmocr/mmocr/models/textrecog/backbones/shallow_cnn.py
deleted file mode 100644
index 542b37bbc893c3bb0a01840a01ea81e6e259136a..0000000000000000000000000000000000000000
--- a/pipeline/mmocr/mmocr/models/textrecog/backbones/shallow_cnn.py
+++ /dev/null
@@ -1,75 +0,0 @@
-# Copyright (c) OpenMMLab. All rights reserved.
-from typing import Dict, List, Optional, Union
-
-import torch.nn as nn
-from mmcv.cnn import ConvModule
-from mmengine.model import BaseModule
-from torch import Tensor
-
-from mmocr.registry import MODELS
-
-
-@MODELS.register_module()
-class ShallowCNN(BaseModule):
- """Implement Shallow CNN block for SATRN.
-
- SATRN: `On Recognizing Texts of Arbitrary Shapes with 2D Self-Attention
- `_.
-
- Args:
- input_channels (int): Number of channels of input image tensor
- :math:`D_i`. Defaults to 1.
- hidden_dim (int): Size of hidden layers of the model :math:`D_m`.
- Defaults to 512.
- init_cfg (dict or list[dict], optional): Initialization configs.
- """
-
- def __init__(
- self,
- input_channels: int = 1,
- hidden_dim: int = 512,
- init_cfg: Optional[Union[Dict, List[Dict]]] = [
- dict(type='Kaiming', layer='Conv2d'),
- dict(type='Uniform', layer='BatchNorm2d')
- ]
- ) -> None:
- super().__init__(init_cfg=init_cfg)
- assert isinstance(input_channels, int)
- assert isinstance(hidden_dim, int)
-
- self.conv1 = ConvModule(
- input_channels,
- hidden_dim // 2,
- kernel_size=3,
- stride=1,
- padding=1,
- bias=False,
- norm_cfg=dict(type='BN'),
- act_cfg=dict(type='ReLU'))
- self.conv2 = ConvModule(
- hidden_dim // 2,
- hidden_dim,
- kernel_size=3,
- stride=1,
- padding=1,
- bias=False,
- norm_cfg=dict(type='BN'),
- act_cfg=dict(type='ReLU'))
- self.pool = nn.MaxPool2d(kernel_size=2, stride=2, padding=0)
-
- def forward(self, x: Tensor) -> Tensor:
- """
- Args:
- x (Tensor): Input image feature :math:`(N, D_i, H, W)`.
-
- Returns:
- Tensor: A tensor of shape :math:`(N, D_m, H/4, W/4)`.
- """
-
- x = self.conv1(x)
- x = self.pool(x)
-
- x = self.conv2(x)
- x = self.pool(x)
-
- return x
diff --git a/pipeline/mmocr/mmocr/models/textrecog/data_preprocessors/__init__.py b/pipeline/mmocr/mmocr/models/textrecog/data_preprocessors/__init__.py
deleted file mode 100644
index 43b65323c6baf512d358772df06b15dc1bf802da..0000000000000000000000000000000000000000
--- a/pipeline/mmocr/mmocr/models/textrecog/data_preprocessors/__init__.py
+++ /dev/null
@@ -1,4 +0,0 @@
-# Copyright (c) OpenMMLab. All rights reserved.
-from .data_preprocessor import TextRecogDataPreprocessor
-
-__all__ = ['TextRecogDataPreprocessor']
diff --git a/pipeline/mmocr/mmocr/models/textrecog/data_preprocessors/__pycache__/__init__.cpython-38.pyc b/pipeline/mmocr/mmocr/models/textrecog/data_preprocessors/__pycache__/__init__.cpython-38.pyc
deleted file mode 100644
index f7a2026783fade451c2de92a06804a1fb56eaf3d..0000000000000000000000000000000000000000
Binary files a/pipeline/mmocr/mmocr/models/textrecog/data_preprocessors/__pycache__/__init__.cpython-38.pyc and /dev/null differ
diff --git a/pipeline/mmocr/mmocr/models/textrecog/data_preprocessors/__pycache__/__init__.cpython-39.pyc b/pipeline/mmocr/mmocr/models/textrecog/data_preprocessors/__pycache__/__init__.cpython-39.pyc
deleted file mode 100644
index 82102f6adaf350cbbeaf2bd0395faa06d3470438..0000000000000000000000000000000000000000
Binary files a/pipeline/mmocr/mmocr/models/textrecog/data_preprocessors/__pycache__/__init__.cpython-39.pyc and /dev/null differ
diff --git a/pipeline/mmocr/mmocr/models/textrecog/data_preprocessors/__pycache__/data_preprocessor.cpython-38.pyc b/pipeline/mmocr/mmocr/models/textrecog/data_preprocessors/__pycache__/data_preprocessor.cpython-38.pyc
deleted file mode 100644
index ccf85d2785485e64f81463fe3cb9f0f337f73849..0000000000000000000000000000000000000000
Binary files a/pipeline/mmocr/mmocr/models/textrecog/data_preprocessors/__pycache__/data_preprocessor.cpython-38.pyc and /dev/null differ
diff --git a/pipeline/mmocr/mmocr/models/textrecog/data_preprocessors/__pycache__/data_preprocessor.cpython-39.pyc b/pipeline/mmocr/mmocr/models/textrecog/data_preprocessors/__pycache__/data_preprocessor.cpython-39.pyc
deleted file mode 100644
index 9518f76ab36431e28cfc40e5d9306372d74a855d..0000000000000000000000000000000000000000
Binary files a/pipeline/mmocr/mmocr/models/textrecog/data_preprocessors/__pycache__/data_preprocessor.cpython-39.pyc and /dev/null differ
diff --git a/pipeline/mmocr/mmocr/models/textrecog/data_preprocessors/data_preprocessor.py b/pipeline/mmocr/mmocr/models/textrecog/data_preprocessors/data_preprocessor.py
deleted file mode 100644
index 99ae1719ca9fcc722c0c4f2f8d01e14bdbfed13d..0000000000000000000000000000000000000000
--- a/pipeline/mmocr/mmocr/models/textrecog/data_preprocessors/data_preprocessor.py
+++ /dev/null
@@ -1,97 +0,0 @@
-# Copyright (c) OpenMMLab. All rights reserved.
-from numbers import Number
-from typing import Dict, List, Optional, Sequence, Union
-
-import torch.nn as nn
-from mmengine.model import ImgDataPreprocessor
-
-from mmocr.registry import MODELS
-
-
-@MODELS.register_module()
-class TextRecogDataPreprocessor(ImgDataPreprocessor):
- """Image pre-processor for recognition tasks.
-
- Comparing with the :class:`mmengine.ImgDataPreprocessor`,
-
- 1. It supports batch augmentations.
- 2. It will additionally append batch_input_shape and valid_ratio
- to data_samples considering the object recognition task.
-
- It provides the data pre-processing as follows
-
- - Collate and move data to the target device.
- - Pad inputs to the maximum size of current batch with defined
- ``pad_value``. The padding size can be divisible by a defined
- ``pad_size_divisor``
- - Stack inputs to inputs.
- - Convert inputs from bgr to rgb if the shape of input is (3, H, W).
- - Normalize image with defined std and mean.
- - Do batch augmentations during training.
-
- Args:
- mean (Sequence[Number], optional): The pixel mean of R, G, B channels.
- Defaults to None.
- std (Sequence[Number], optional): The pixel standard deviation of
- R, G, B channels. Defaults to None.
- pad_size_divisor (int): The size of padded image should be
- divisible by ``pad_size_divisor``. Defaults to 1.
- pad_value (Number): The padded pixel value. Defaults to 0.
- bgr_to_rgb (bool): whether to convert image from BGR to RGB.
- Defaults to False.
- rgb_to_bgr (bool): whether to convert image from RGB to RGB.
- Defaults to False.
- batch_augments (list[dict], optional): Batch-level augmentations
- """
-
- def __init__(self,
- mean: Sequence[Number] = None,
- std: Sequence[Number] = None,
- pad_size_divisor: int = 1,
- pad_value: Union[float, int] = 0,
- bgr_to_rgb: bool = False,
- rgb_to_bgr: bool = False,
- batch_augments: Optional[List[Dict]] = None) -> None:
- super().__init__(
- mean=mean,
- std=std,
- pad_size_divisor=pad_size_divisor,
- pad_value=pad_value,
- bgr_to_rgb=bgr_to_rgb,
- rgb_to_bgr=rgb_to_bgr)
- if batch_augments is not None:
- self.batch_augments = nn.ModuleList(
- [MODELS.build(aug) for aug in batch_augments])
- else:
- self.batch_augments = None
-
- def forward(self, data: Dict, training: bool = False) -> Dict:
- """Perform normalizationใpadding and bgr2rgb conversion based on
- ``BaseDataPreprocessor``.
-
- Args:
- data (dict): Data sampled from dataloader.
- training (bool): Whether to enable training time augmentation.
-
- Returns:
- dict: Data in the same format as the model input.
- """
- data = super().forward(data=data, training=training)
- inputs, data_samples = data['inputs'], data['data_samples']
-
- if data_samples is not None:
- batch_input_shape = tuple(inputs[0].size()[-2:])
- for data_sample in data_samples:
-
- valid_ratio = data_sample.valid_ratio * \
- data_sample.img_shape[1] / batch_input_shape[1]
- data_sample.set_metainfo(
- dict(
- valid_ratio=valid_ratio,
- batch_input_shape=batch_input_shape))
-
- if training and self.batch_augments is not None:
- for batch_aug in self.batch_augments:
- inputs, data_samples = batch_aug(inputs, data_samples)
-
- return data
diff --git a/pipeline/mmocr/mmocr/models/textrecog/decoders/__init__.py b/pipeline/mmocr/mmocr/models/textrecog/decoders/__init__.py
deleted file mode 100644
index ec4981fe891baebb6c623de124a7308192272abb..0000000000000000000000000000000000000000
--- a/pipeline/mmocr/mmocr/models/textrecog/decoders/__init__.py
+++ /dev/null
@@ -1,24 +0,0 @@
-# Copyright (c) OpenMMLab. All rights reserved.
-from .abi_fuser import ABIFuser
-from .abi_language_decoder import ABILanguageDecoder
-from .abi_vision_decoder import ABIVisionDecoder
-from .aster_decoder import ASTERDecoder
-from .base import BaseDecoder
-from .crnn_decoder import CRNNDecoder
-from .master_decoder import MasterDecoder
-from .nrtr_decoder import NRTRDecoder
-from .position_attention_decoder import PositionAttentionDecoder
-from .robust_scanner_fuser import RobustScannerFuser
-from .sar_decoder import ParallelSARDecoder, SequentialSARDecoder
-from .sar_decoder_with_bs import ParallelSARDecoderWithBS
-from .sequence_attention_decoder import SequenceAttentionDecoder
-from .svtr_decoder import SVTRDecoder
-from .maerec_decoder import MAERecDecoder
-__all__ = [
- 'CRNNDecoder', 'ParallelSARDecoder', 'SequentialSARDecoder',
- 'ParallelSARDecoderWithBS', 'NRTRDecoder', 'BaseDecoder',
- 'SequenceAttentionDecoder', 'PositionAttentionDecoder',
- 'ABILanguageDecoder', 'ABIVisionDecoder', 'MasterDecoder',
- 'RobustScannerFuser', 'ABIFuser', 'SVTRDecoder', 'ASTERDecoder',
- 'MAERecDecoder'
-]
diff --git a/pipeline/mmocr/mmocr/models/textrecog/decoders/__pycache__/__init__.cpython-38.pyc b/pipeline/mmocr/mmocr/models/textrecog/decoders/__pycache__/__init__.cpython-38.pyc
deleted file mode 100644
index fbab828e8121e4b4d8571642848f1fb7b42cfbfe..0000000000000000000000000000000000000000
Binary files a/pipeline/mmocr/mmocr/models/textrecog/decoders/__pycache__/__init__.cpython-38.pyc and /dev/null differ
diff --git a/pipeline/mmocr/mmocr/models/textrecog/decoders/__pycache__/__init__.cpython-39.pyc b/pipeline/mmocr/mmocr/models/textrecog/decoders/__pycache__/__init__.cpython-39.pyc
deleted file mode 100644
index 6cb9d32e6f9f0f3e0b3099189ba35f2205b5e067..0000000000000000000000000000000000000000
Binary files a/pipeline/mmocr/mmocr/models/textrecog/decoders/__pycache__/__init__.cpython-39.pyc and /dev/null differ
diff --git a/pipeline/mmocr/mmocr/models/textrecog/decoders/__pycache__/abi_fuser.cpython-38.pyc b/pipeline/mmocr/mmocr/models/textrecog/decoders/__pycache__/abi_fuser.cpython-38.pyc
deleted file mode 100644
index c2bb9d9a9578098ae206402d4771a71dec58941d..0000000000000000000000000000000000000000
Binary files a/pipeline/mmocr/mmocr/models/textrecog/decoders/__pycache__/abi_fuser.cpython-38.pyc and /dev/null differ
diff --git a/pipeline/mmocr/mmocr/models/textrecog/decoders/__pycache__/abi_fuser.cpython-39.pyc b/pipeline/mmocr/mmocr/models/textrecog/decoders/__pycache__/abi_fuser.cpython-39.pyc
deleted file mode 100644
index 6c46a1f3080a724eef63dfc88be48a3e46e5d9ba..0000000000000000000000000000000000000000
Binary files a/pipeline/mmocr/mmocr/models/textrecog/decoders/__pycache__/abi_fuser.cpython-39.pyc and /dev/null differ
diff --git a/pipeline/mmocr/mmocr/models/textrecog/decoders/__pycache__/abi_language_decoder.cpython-38.pyc b/pipeline/mmocr/mmocr/models/textrecog/decoders/__pycache__/abi_language_decoder.cpython-38.pyc
deleted file mode 100644
index 03c737b05656ba17b9e3c78a9539046631a5aac2..0000000000000000000000000000000000000000
Binary files a/pipeline/mmocr/mmocr/models/textrecog/decoders/__pycache__/abi_language_decoder.cpython-38.pyc and /dev/null differ
diff --git a/pipeline/mmocr/mmocr/models/textrecog/decoders/__pycache__/abi_language_decoder.cpython-39.pyc b/pipeline/mmocr/mmocr/models/textrecog/decoders/__pycache__/abi_language_decoder.cpython-39.pyc
deleted file mode 100644
index 833c3eba1ccb6dce9664a8442d61727a56bf3608..0000000000000000000000000000000000000000
Binary files a/pipeline/mmocr/mmocr/models/textrecog/decoders/__pycache__/abi_language_decoder.cpython-39.pyc and /dev/null differ
diff --git a/pipeline/mmocr/mmocr/models/textrecog/decoders/__pycache__/abi_vision_decoder.cpython-38.pyc b/pipeline/mmocr/mmocr/models/textrecog/decoders/__pycache__/abi_vision_decoder.cpython-38.pyc
deleted file mode 100644
index 7c4417dedfe9eea900a6b9e499d00be8493b2ee0..0000000000000000000000000000000000000000
Binary files a/pipeline/mmocr/mmocr/models/textrecog/decoders/__pycache__/abi_vision_decoder.cpython-38.pyc and /dev/null differ
diff --git a/pipeline/mmocr/mmocr/models/textrecog/decoders/__pycache__/abi_vision_decoder.cpython-39.pyc b/pipeline/mmocr/mmocr/models/textrecog/decoders/__pycache__/abi_vision_decoder.cpython-39.pyc
deleted file mode 100644
index 051a6ae120c07c32011b490889bcf5b7e7e04983..0000000000000000000000000000000000000000
Binary files a/pipeline/mmocr/mmocr/models/textrecog/decoders/__pycache__/abi_vision_decoder.cpython-39.pyc and /dev/null differ
diff --git a/pipeline/mmocr/mmocr/models/textrecog/decoders/__pycache__/aster_decoder.cpython-38.pyc b/pipeline/mmocr/mmocr/models/textrecog/decoders/__pycache__/aster_decoder.cpython-38.pyc
deleted file mode 100644
index 23d5645fd85e99dda348777c750d1c8205b4413c..0000000000000000000000000000000000000000
Binary files a/pipeline/mmocr/mmocr/models/textrecog/decoders/__pycache__/aster_decoder.cpython-38.pyc and /dev/null differ
diff --git a/pipeline/mmocr/mmocr/models/textrecog/decoders/__pycache__/aster_decoder.cpython-39.pyc b/pipeline/mmocr/mmocr/models/textrecog/decoders/__pycache__/aster_decoder.cpython-39.pyc
deleted file mode 100644
index 90fe0b5e56ba00c981b5cd1714e4447e24acccb0..0000000000000000000000000000000000000000
Binary files a/pipeline/mmocr/mmocr/models/textrecog/decoders/__pycache__/aster_decoder.cpython-39.pyc and /dev/null differ
diff --git a/pipeline/mmocr/mmocr/models/textrecog/decoders/__pycache__/base.cpython-38.pyc b/pipeline/mmocr/mmocr/models/textrecog/decoders/__pycache__/base.cpython-38.pyc
deleted file mode 100644
index be3261c59028019e6e08244db7f9638f899d6b73..0000000000000000000000000000000000000000
Binary files a/pipeline/mmocr/mmocr/models/textrecog/decoders/__pycache__/base.cpython-38.pyc and /dev/null differ
diff --git a/pipeline/mmocr/mmocr/models/textrecog/decoders/__pycache__/base.cpython-39.pyc b/pipeline/mmocr/mmocr/models/textrecog/decoders/__pycache__/base.cpython-39.pyc
deleted file mode 100644
index 91a1b23fe7e70b6f4357318c6d999603c7e848d3..0000000000000000000000000000000000000000
Binary files a/pipeline/mmocr/mmocr/models/textrecog/decoders/__pycache__/base.cpython-39.pyc and /dev/null differ
diff --git a/pipeline/mmocr/mmocr/models/textrecog/decoders/__pycache__/crnn_decoder.cpython-38.pyc b/pipeline/mmocr/mmocr/models/textrecog/decoders/__pycache__/crnn_decoder.cpython-38.pyc
deleted file mode 100644
index 329b23d7b7aefa5029baf60c0fb9dd5cee8e3082..0000000000000000000000000000000000000000
Binary files a/pipeline/mmocr/mmocr/models/textrecog/decoders/__pycache__/crnn_decoder.cpython-38.pyc and /dev/null differ
diff --git a/pipeline/mmocr/mmocr/models/textrecog/decoders/__pycache__/crnn_decoder.cpython-39.pyc b/pipeline/mmocr/mmocr/models/textrecog/decoders/__pycache__/crnn_decoder.cpython-39.pyc
deleted file mode 100644
index c9df4028b8a1ed86e101e3ff255f7769a5c8dacf..0000000000000000000000000000000000000000
Binary files a/pipeline/mmocr/mmocr/models/textrecog/decoders/__pycache__/crnn_decoder.cpython-39.pyc and /dev/null differ
diff --git a/pipeline/mmocr/mmocr/models/textrecog/decoders/__pycache__/maerec_decoder.cpython-38.pyc b/pipeline/mmocr/mmocr/models/textrecog/decoders/__pycache__/maerec_decoder.cpython-38.pyc
deleted file mode 100644
index 52d430651fb0819f6c5ceec8ee6989dab2bb39aa..0000000000000000000000000000000000000000
Binary files a/pipeline/mmocr/mmocr/models/textrecog/decoders/__pycache__/maerec_decoder.cpython-38.pyc and /dev/null differ
diff --git a/pipeline/mmocr/mmocr/models/textrecog/decoders/__pycache__/maerec_decoder.cpython-39.pyc b/pipeline/mmocr/mmocr/models/textrecog/decoders/__pycache__/maerec_decoder.cpython-39.pyc
deleted file mode 100644
index 27cd2b0a2a743eee424474cf97ee0537e5a6a289..0000000000000000000000000000000000000000
Binary files a/pipeline/mmocr/mmocr/models/textrecog/decoders/__pycache__/maerec_decoder.cpython-39.pyc and /dev/null differ
diff --git a/pipeline/mmocr/mmocr/models/textrecog/decoders/__pycache__/master_decoder.cpython-38.pyc b/pipeline/mmocr/mmocr/models/textrecog/decoders/__pycache__/master_decoder.cpython-38.pyc
deleted file mode 100644
index 35411cc2dc96c601e84189dd8ad663984d3f6216..0000000000000000000000000000000000000000
Binary files a/pipeline/mmocr/mmocr/models/textrecog/decoders/__pycache__/master_decoder.cpython-38.pyc and /dev/null differ
diff --git a/pipeline/mmocr/mmocr/models/textrecog/decoders/__pycache__/master_decoder.cpython-39.pyc b/pipeline/mmocr/mmocr/models/textrecog/decoders/__pycache__/master_decoder.cpython-39.pyc
deleted file mode 100644
index a29e6a684eca7e2952a715f23c185f506290c344..0000000000000000000000000000000000000000
Binary files a/pipeline/mmocr/mmocr/models/textrecog/decoders/__pycache__/master_decoder.cpython-39.pyc and /dev/null differ
diff --git a/pipeline/mmocr/mmocr/models/textrecog/decoders/__pycache__/nrtr_decoder.cpython-38.pyc b/pipeline/mmocr/mmocr/models/textrecog/decoders/__pycache__/nrtr_decoder.cpython-38.pyc
deleted file mode 100644
index c4e03b854e53532f45efed43283917b88a233534..0000000000000000000000000000000000000000
Binary files a/pipeline/mmocr/mmocr/models/textrecog/decoders/__pycache__/nrtr_decoder.cpython-38.pyc and /dev/null differ
diff --git a/pipeline/mmocr/mmocr/models/textrecog/decoders/__pycache__/nrtr_decoder.cpython-39.pyc b/pipeline/mmocr/mmocr/models/textrecog/decoders/__pycache__/nrtr_decoder.cpython-39.pyc
deleted file mode 100644
index e40c829fcc8c543527937fef688cba0ee3c0631d..0000000000000000000000000000000000000000
Binary files a/pipeline/mmocr/mmocr/models/textrecog/decoders/__pycache__/nrtr_decoder.cpython-39.pyc and /dev/null differ
diff --git a/pipeline/mmocr/mmocr/models/textrecog/decoders/__pycache__/position_attention_decoder.cpython-38.pyc b/pipeline/mmocr/mmocr/models/textrecog/decoders/__pycache__/position_attention_decoder.cpython-38.pyc
deleted file mode 100644
index cc7b56fe848b8153abdef89d4794a5ad8025fdc9..0000000000000000000000000000000000000000
Binary files a/pipeline/mmocr/mmocr/models/textrecog/decoders/__pycache__/position_attention_decoder.cpython-38.pyc and /dev/null differ
diff --git a/pipeline/mmocr/mmocr/models/textrecog/decoders/__pycache__/position_attention_decoder.cpython-39.pyc b/pipeline/mmocr/mmocr/models/textrecog/decoders/__pycache__/position_attention_decoder.cpython-39.pyc
deleted file mode 100644
index 4ff0295890689ef66ee0d3a3477a29b58937f0e1..0000000000000000000000000000000000000000
Binary files a/pipeline/mmocr/mmocr/models/textrecog/decoders/__pycache__/position_attention_decoder.cpython-39.pyc and /dev/null differ
diff --git a/pipeline/mmocr/mmocr/models/textrecog/decoders/__pycache__/robust_scanner_fuser.cpython-38.pyc b/pipeline/mmocr/mmocr/models/textrecog/decoders/__pycache__/robust_scanner_fuser.cpython-38.pyc
deleted file mode 100644
index 440a9099f81f08d9bf69822ad72b7ba007f0f6d5..0000000000000000000000000000000000000000
Binary files a/pipeline/mmocr/mmocr/models/textrecog/decoders/__pycache__/robust_scanner_fuser.cpython-38.pyc and /dev/null differ
diff --git a/pipeline/mmocr/mmocr/models/textrecog/decoders/__pycache__/robust_scanner_fuser.cpython-39.pyc b/pipeline/mmocr/mmocr/models/textrecog/decoders/__pycache__/robust_scanner_fuser.cpython-39.pyc
deleted file mode 100644
index 5c444f43b79b4d91a205f5cf19ba2aee639520bf..0000000000000000000000000000000000000000
Binary files a/pipeline/mmocr/mmocr/models/textrecog/decoders/__pycache__/robust_scanner_fuser.cpython-39.pyc and /dev/null differ
diff --git a/pipeline/mmocr/mmocr/models/textrecog/decoders/__pycache__/sar_decoder.cpython-38.pyc b/pipeline/mmocr/mmocr/models/textrecog/decoders/__pycache__/sar_decoder.cpython-38.pyc
deleted file mode 100644
index f1d4d64d15fc6ba72c85ffffacfe22cc6b13c949..0000000000000000000000000000000000000000
Binary files a/pipeline/mmocr/mmocr/models/textrecog/decoders/__pycache__/sar_decoder.cpython-38.pyc and /dev/null differ
diff --git a/pipeline/mmocr/mmocr/models/textrecog/decoders/__pycache__/sar_decoder.cpython-39.pyc b/pipeline/mmocr/mmocr/models/textrecog/decoders/__pycache__/sar_decoder.cpython-39.pyc
deleted file mode 100644
index 1607b910cf191a009a2f4794266451955380839b..0000000000000000000000000000000000000000
Binary files a/pipeline/mmocr/mmocr/models/textrecog/decoders/__pycache__/sar_decoder.cpython-39.pyc and /dev/null differ
diff --git a/pipeline/mmocr/mmocr/models/textrecog/decoders/__pycache__/sar_decoder_with_bs.cpython-38.pyc b/pipeline/mmocr/mmocr/models/textrecog/decoders/__pycache__/sar_decoder_with_bs.cpython-38.pyc
deleted file mode 100644
index 75b5d6888cda8d754274a3b18362b4fc2af9a16d..0000000000000000000000000000000000000000
Binary files a/pipeline/mmocr/mmocr/models/textrecog/decoders/__pycache__/sar_decoder_with_bs.cpython-38.pyc and /dev/null differ
diff --git a/pipeline/mmocr/mmocr/models/textrecog/decoders/__pycache__/sar_decoder_with_bs.cpython-39.pyc b/pipeline/mmocr/mmocr/models/textrecog/decoders/__pycache__/sar_decoder_with_bs.cpython-39.pyc
deleted file mode 100644
index 00c81fd97516bc55471c6331384fd990113bef97..0000000000000000000000000000000000000000
Binary files a/pipeline/mmocr/mmocr/models/textrecog/decoders/__pycache__/sar_decoder_with_bs.cpython-39.pyc and /dev/null differ
diff --git a/pipeline/mmocr/mmocr/models/textrecog/decoders/__pycache__/sequence_attention_decoder.cpython-38.pyc b/pipeline/mmocr/mmocr/models/textrecog/decoders/__pycache__/sequence_attention_decoder.cpython-38.pyc
deleted file mode 100644
index 7d7604fa0bab99fe2a1dcf40b10458bfe0739d53..0000000000000000000000000000000000000000
Binary files a/pipeline/mmocr/mmocr/models/textrecog/decoders/__pycache__/sequence_attention_decoder.cpython-38.pyc and /dev/null differ
diff --git a/pipeline/mmocr/mmocr/models/textrecog/decoders/__pycache__/sequence_attention_decoder.cpython-39.pyc b/pipeline/mmocr/mmocr/models/textrecog/decoders/__pycache__/sequence_attention_decoder.cpython-39.pyc
deleted file mode 100644
index e5eb6180c9db0e965ec74a8e6a2bc7faa02ea676..0000000000000000000000000000000000000000
Binary files a/pipeline/mmocr/mmocr/models/textrecog/decoders/__pycache__/sequence_attention_decoder.cpython-39.pyc and /dev/null differ
diff --git a/pipeline/mmocr/mmocr/models/textrecog/decoders/__pycache__/svtr_decoder.cpython-38.pyc b/pipeline/mmocr/mmocr/models/textrecog/decoders/__pycache__/svtr_decoder.cpython-38.pyc
deleted file mode 100644
index 436f57514f98d6c78978cbcfd1fd9deddfcecc89..0000000000000000000000000000000000000000
Binary files a/pipeline/mmocr/mmocr/models/textrecog/decoders/__pycache__/svtr_decoder.cpython-38.pyc and /dev/null differ
diff --git a/pipeline/mmocr/mmocr/models/textrecog/decoders/__pycache__/svtr_decoder.cpython-39.pyc b/pipeline/mmocr/mmocr/models/textrecog/decoders/__pycache__/svtr_decoder.cpython-39.pyc
deleted file mode 100644
index 02d551405b4abcc62920ed6ad90a096b29823891..0000000000000000000000000000000000000000
Binary files a/pipeline/mmocr/mmocr/models/textrecog/decoders/__pycache__/svtr_decoder.cpython-39.pyc and /dev/null differ
diff --git a/pipeline/mmocr/mmocr/models/textrecog/decoders/abi_fuser.py b/pipeline/mmocr/mmocr/models/textrecog/decoders/abi_fuser.py
deleted file mode 100644
index 43ecba41e87e72803525b38cf2c019bc1d2d7bba..0000000000000000000000000000000000000000
--- a/pipeline/mmocr/mmocr/models/textrecog/decoders/abi_fuser.py
+++ /dev/null
@@ -1,174 +0,0 @@
-# Copyright (c) OpenMMLab. All rights reserved.
-import warnings
-from typing import Dict, List, Optional, Sequence, Union
-
-import torch
-import torch.nn as nn
-
-from mmocr.models.common.dictionary import Dictionary
-from mmocr.registry import MODELS
-from mmocr.structures import TextRecogDataSample
-from .base import BaseDecoder
-
-
-@MODELS.register_module()
-class ABIFuser(BaseDecoder):
- r"""A special decoder responsible for mixing and aligning visual feature
- and linguistic feature. `ABINet `_
-
- Args:
- dictionary (dict or :obj:`Dictionary`): The config for `Dictionary` or
- the instance of `Dictionary`. The dictionary must have an end
- token.
- vision_decoder (dict): The config for vision decoder.
- language_decoder (dict, optional): The config for language decoder.
- num_iters (int): Rounds of iterative correction. Defaults to 1.
- d_model (int): Hidden size :math:`E` of model. Defaults to 512.
- max_seq_len (int): Maximum sequence length :math:`T`. The
- sequence is usually generated from decoder. Defaults to 40.
- module_loss (dict, optional): Config to build loss. Defaults to None.
- postprocessor (dict, optional): Config to build postprocessor.
- Defaults to None.
- init_cfg (dict or list[dict], optional): Initialization configs.
- Defaults to None.
- """
-
- def __init__(self,
- dictionary: Union[Dict, Dictionary],
- vision_decoder: Dict,
- language_decoder: Optional[Dict] = None,
- d_model: int = 512,
- num_iters: int = 1,
- max_seq_len: int = 40,
- module_loss: Optional[Dict] = None,
- postprocessor: Optional[Dict] = None,
- init_cfg: Optional[Union[Dict, List[Dict]]] = None,
- **kwargs) -> None:
- super().__init__(
- dictionary=dictionary,
- module_loss=module_loss,
- postprocessor=postprocessor,
- max_seq_len=max_seq_len,
- init_cfg=init_cfg)
-
- assert self.dictionary.end_idx is not None,\
- 'Dictionary must contain an end token! (with_end=True)'
-
- self.d_model = d_model
- self.num_iters = num_iters
- if language_decoder is not None:
- self.w_att = nn.Linear(2 * d_model, d_model)
- self.cls = nn.Linear(d_model, self.dictionary.num_classes)
-
- self.vision_decoder = vision_decoder
- self.language_decoder = language_decoder
- for cfg_name in ['vision_decoder', 'language_decoder']:
- if getattr(self, cfg_name, None) is not None:
- cfg = getattr(self, cfg_name)
- if cfg.get('dictionary', None) is None:
- cfg.update(dictionary=self.dictionary)
- else:
- warnings.warn(f"Using dictionary {cfg['dictionary']} "
- "in decoder's config.")
- if cfg.get('max_seq_len', None) is None:
- cfg.update(max_seq_len=max_seq_len)
- else:
- warnings.warn(f"Using max_seq_len {cfg['max_seq_len']} "
- "in decoder's config.")
- setattr(self, cfg_name, MODELS.build(cfg))
- self.softmax = nn.Softmax(dim=-1)
-
- def forward_train(
- self,
- feat: Optional[torch.Tensor] = None,
- out_enc: torch.Tensor = None,
- data_samples: Optional[Sequence[TextRecogDataSample]] = None
- ) -> Dict:
- """
- Args:
- feat (torch.Tensor, optional): Not required. Feature map
- placeholder. Defaults to None.
- out_enc (Tensor): Raw language logitis. Shape :math:`(N, T, C)`.
- Defaults to None.
- data_samples (list[TextRecogDataSample], optional): Not required.
- DataSample placeholder. Defaults to None.
-
- Returns:
- A dict with keys ``out_enc``, ``out_decs`` and ``out_fusers``.
-
- - out_vis (dict): Dict from ``self.vision_decoder`` with keys
- ``feature``, ``logits`` and ``attn_scores``.
- - out_langs (dict or list): Dict from ``self.vision_decoder`` with
- keys ``feature``, ``logits`` if applicable, or an empty list
- otherwise.
- - out_fusers (dict or list): Dict of fused visual and language
- features with keys ``feature``, ``logits`` if applicable, or
- an empty list otherwise.
- """
- out_vis = self.vision_decoder(feat, out_enc, data_samples)
- out_langs = []
- out_fusers = []
- if self.language_decoder is not None:
- text_logits = out_vis['logits']
- for _ in range(self.num_iters):
- out_dec = self.language_decoder(feat, text_logits,
- data_samples)
- out_langs.append(out_dec)
- out_fuser = self.fuse(out_vis['feature'], out_dec['feature'])
- text_logits = out_fuser['logits']
- out_fusers.append(out_fuser)
-
- outputs = dict(
- out_vis=out_vis, out_langs=out_langs, out_fusers=out_fusers)
-
- return outputs
-
- def forward_test(
- self,
- feat: Optional[torch.Tensor],
- logits: torch.Tensor,
- data_samples: Optional[Sequence[TextRecogDataSample]] = None
- ) -> torch.Tensor:
- """
- Args:
- feat (torch.Tensor, optional): Not required. Feature map
- placeholder. Defaults to None.
- logits (Tensor): Raw language logitis. Shape :math:`(N, T, C)`.
- data_samples (list[TextRecogDataSample], optional): Not required.
- DataSample placeholder. Defaults to None.
-
- Returns:
- Tensor: Character probabilities. of shape
- :math:`(N, self.max_seq_len, C)` where :math:`C` is
- ``num_classes``.
- """
- raw_result = self.forward_train(feat, logits, data_samples)
-
- if 'out_fusers' in raw_result and len(raw_result['out_fusers']) > 0:
- ret = raw_result['out_fusers'][-1]['logits']
- elif 'out_langs' in raw_result and len(raw_result['out_langs']) > 0:
- ret = raw_result['out_langs'][-1]['logits']
- else:
- ret = raw_result['out_vis']['logits']
-
- return self.softmax(ret)
-
- def fuse(self, l_feature: torch.Tensor, v_feature: torch.Tensor) -> Dict:
- """Mix and align visual feature and linguistic feature.
-
- Args:
- l_feature (torch.Tensor): (N, T, E) where T is length, N is batch
- size and E is dim of model.
- v_feature (torch.Tensor): (N, T, E) shape the same as l_feature.
-
- Returns:
- dict: A dict with key ``logits``. of shape :math:`(N, T, C)` where
- N is batch size, T is length and C is the number of characters.
- """
- f = torch.cat((l_feature, v_feature), dim=2)
- f_att = torch.sigmoid(self.w_att(f))
- output = f_att * v_feature + (1 - f_att) * l_feature
-
- logits = self.cls(output) # (N, T, C)
-
- return {'logits': logits}
diff --git a/pipeline/mmocr/mmocr/models/textrecog/decoders/abi_language_decoder.py b/pipeline/mmocr/mmocr/models/textrecog/decoders/abi_language_decoder.py
deleted file mode 100644
index 03492200fd1abb9ad9386f9578bae435a04bc6d0..0000000000000000000000000000000000000000
--- a/pipeline/mmocr/mmocr/models/textrecog/decoders/abi_language_decoder.py
+++ /dev/null
@@ -1,230 +0,0 @@
-# Copyright (c) OpenMMLab. All rights reserved.
-import copy
-from typing import Dict, List, Optional, Sequence, Union
-
-import torch
-import torch.nn as nn
-from mmcv.cnn.bricks.transformer import BaseTransformerLayer
-from mmengine.model import ModuleList
-
-from mmocr.models.common.dictionary import Dictionary
-from mmocr.models.common.modules import PositionalEncoding
-from mmocr.registry import MODELS
-from mmocr.structures import TextRecogDataSample
-from .base import BaseDecoder
-
-
-@MODELS.register_module()
-class ABILanguageDecoder(BaseDecoder):
- r"""Transformer-based language model responsible for spell correction.
- Implementation of language model of \
- `ABINet `_.
-
- Args:
- dictionary (dict or :obj:`Dictionary`): The config for `Dictionary` or
- the instance of `Dictionary`. The dictionary must have an end
- token.
- d_model (int): Hidden size :math:`E` of model. Defaults to 512.
- n_head (int): Number of multi-attention heads.
- d_inner (int): Hidden size of feedforward network model.
- n_layers (int): The number of similar decoding layers.
- dropout (float): Dropout rate.
- detach_tokens (bool): Whether to block the gradient flow at input
- tokens.
- use_self_attn (bool): If True, use self attention in decoder layers,
- otherwise cross attention will be used.
- max_seq_len (int): Maximum sequence length :math:`T`. The
- sequence is usually generated from decoder. Defaults to 40.
- module_loss (dict, optional): Config to build loss. Defaults to None.
- postprocessor (dict, optional): Config to build postprocessor.
- Defaults to None.
- init_cfg (dict or list[dict], optional): Initialization configs.
- Defaults to None.
- """
-
- def __init__(self,
- dictionary: Union[Dict, Dictionary],
- d_model: int = 512,
- n_head: int = 8,
- d_inner: int = 2048,
- n_layers: int = 4,
- dropout: float = 0.1,
- detach_tokens: bool = True,
- use_self_attn: bool = False,
- max_seq_len: int = 40,
- module_loss: Optional[Dict] = None,
- postprocessor: Optional[Dict] = None,
- init_cfg: Optional[Union[Dict, List[Dict]]] = None,
- **kwargs) -> None:
- super().__init__(
- dictionary=dictionary,
- module_loss=module_loss,
- postprocessor=postprocessor,
- max_seq_len=max_seq_len,
- init_cfg=init_cfg)
-
- assert self.dictionary.end_idx is not None,\
- 'Dictionary must contain an end token! (with_end=True)'
-
- self.detach_tokens = detach_tokens
- self.d_model = d_model
-
- self.proj = nn.Linear(self.dictionary.num_classes, d_model, False)
- self.token_encoder = PositionalEncoding(
- d_model, n_position=self.max_seq_len, dropout=0.1)
- self.pos_encoder = PositionalEncoding(
- d_model, n_position=self.max_seq_len)
-
- if use_self_attn:
- operation_order = ('self_attn', 'norm', 'cross_attn', 'norm',
- 'ffn', 'norm')
- else:
- operation_order = ('cross_attn', 'norm', 'ffn', 'norm')
-
- decoder_layer = BaseTransformerLayer(
- operation_order=operation_order,
- attn_cfgs=dict(
- type='MultiheadAttention',
- embed_dims=d_model,
- num_heads=n_head,
- attn_drop=dropout,
- dropout_layer=dict(type='Dropout', drop_prob=dropout),
- ),
- ffn_cfgs=dict(
- type='FFN',
- embed_dims=d_model,
- feedforward_channels=d_inner,
- ffn_drop=dropout,
- ),
- norm_cfg=dict(type='LN'),
- )
- self.decoder_layers = ModuleList(
- [copy.deepcopy(decoder_layer) for _ in range(n_layers)])
-
- self.cls = nn.Linear(d_model, self.dictionary.num_classes)
-
- def forward_train(
- self,
- feat: Optional[torch.Tensor] = None,
- out_enc: torch.Tensor = None,
- data_samples: Optional[Sequence[TextRecogDataSample]] = None
- ) -> Dict:
- """
- Args:
- feat (torch.Tensor, optional): Not required. Feature map
- placeholder. Defaults to None.
- out_enc (torch.Tensor): Logits with shape :math:`(N, T, C)`.
- Defaults to None.
- data_samples (list[TextRecogDataSample], optional): Not required.
- DataSample placeholder. Defaults to None.
-
- Returns:
- A dict with keys ``feature`` and ``logits``.
-
- - feature (Tensor): Shape :math:`(N, T, E)`. Raw textual features
- for vision language aligner.
- - logits (Tensor): Shape :math:`(N, T, C)`. The raw logits for
- characters after spell correction.
- """
- lengths = self._get_length(out_enc)
- lengths.clamp_(2, self.max_seq_len)
- tokens = torch.softmax(out_enc, dim=-1)
- if self.detach_tokens:
- tokens = tokens.detach()
- embed = self.proj(tokens) # (N, T, E)
- embed = self.token_encoder(embed) # (N, T, E)
- padding_mask = self._get_padding_mask(lengths, self.max_seq_len)
-
- zeros = embed.new_zeros(*embed.shape)
- query = self.pos_encoder(zeros)
- query = query.permute(1, 0, 2) # (T, N, E)
- embed = embed.permute(1, 0, 2)
- location_mask = self._get_location_mask(self.max_seq_len,
- tokens.device)
- output = query
- for m in self.decoder_layers:
- output = m(
- query=output,
- key=embed,
- value=embed,
- attn_masks=location_mask,
- key_padding_mask=padding_mask)
- output = output.permute(1, 0, 2) # (N, T, E)
-
- out_enc = self.cls(output) # (N, T, C)
- return {'feature': output, 'logits': out_enc}
-
- def forward_test(
- self,
- feat: Optional[torch.Tensor] = None,
- logits: torch.Tensor = None,
- data_samples: Optional[Sequence[TextRecogDataSample]] = None
- ) -> Dict:
- """
- Args:
- feat (torch.Tensor, optional): Not required. Feature map
- placeholder. Defaults to None.
- logits (Tensor): Raw language logitis. Shape :math:`(N, T, C)`.
- Defaults to None.
- data_samples (list[TextRecogDataSample], optional): Not required.
- DataSample placeholder. Defaults to None.
-
- Returns:
- A dict with keys ``feature`` and ``logits``.
-
- - feature (Tensor): Shape :math:`(N, T, E)`. Raw textual features
- for vision language aligner.
- - logits (Tensor): Shape :math:`(N, T, C)`. The raw logits for
- characters after spell correction.
- """
- return self.forward_train(feat, logits, data_samples)
-
- def _get_length(self, logit: torch.Tensor, dim: int = -1) -> torch.Tensor:
- """Greedy decoder to obtain length from logit.
-
- Returns the first location of padding index or the length of the entire
- tensor otherwise.
- """
- # out as a boolean vector indicating the existence of end token(s)
- out = (logit.argmax(dim=-1) == self.dictionary.end_idx)
- abn = out.any(dim)
- # Get the first index of end token
- out = ((out.cumsum(dim) == 1) & out).max(dim)[1]
- out = out + 1
- out = torch.where(abn, out, out.new_tensor(logit.shape[1]))
- return out
-
- @staticmethod
- def _get_location_mask(seq_len: int,
- device: Union[Optional[torch.device],
- str] = None) -> torch.Tensor:
- """Generate location masks given input sequence length.
-
- Args:
- seq_len (int): The length of input sequence to transformer.
- device (torch.device or str, optional): The device on which the
- masks will be placed.
-
- Returns:
- Tensor: A mask tensor of shape (seq_len, seq_len) with -infs on
- diagonal and zeros elsewhere.
- """
- mask = torch.eye(seq_len, device=device)
- mask = mask.float().masked_fill(mask == 1, float('-inf'))
- return mask
-
- @staticmethod
- def _get_padding_mask(length: int, max_length: int) -> torch.Tensor:
- """Generate padding masks.
-
- Args:
- length (Tensor): Shape :math:`(N,)`.
- max_length (int): The maximum sequence length :math:`T`.
-
- Returns:
- Tensor: A bool tensor of shape :math:`(N, T)` with Trues on
- elements located over the length, or Falses elsewhere.
- """
- length = length.unsqueeze(-1)
- grid = torch.arange(0, max_length, device=length.device).unsqueeze(0)
- return grid >= length
diff --git a/pipeline/mmocr/mmocr/models/textrecog/decoders/abi_vision_decoder.py b/pipeline/mmocr/mmocr/models/textrecog/decoders/abi_vision_decoder.py
deleted file mode 100644
index 7095e82209f24f9d30f68c979b504401ea514c05..0000000000000000000000000000000000000000
--- a/pipeline/mmocr/mmocr/models/textrecog/decoders/abi_vision_decoder.py
+++ /dev/null
@@ -1,238 +0,0 @@
-# Copyright (c) OpenMMLab. All rights reserved.
-from typing import Dict, List, Optional, Sequence, Tuple, Union
-
-import torch
-import torch.nn as nn
-from mmcv.cnn import ConvModule
-
-from mmocr.models.common.dictionary import Dictionary
-from mmocr.models.common.modules import PositionalEncoding
-from mmocr.registry import MODELS
-from mmocr.structures import TextRecogDataSample
-from .base import BaseDecoder
-
-
-@MODELS.register_module()
-class ABIVisionDecoder(BaseDecoder):
- """Converts visual features into text characters.
-
- Implementation of VisionEncoder in
- `ABINet `_.
-
- Args:
- dictionary (dict or :obj:`Dictionary`): The config for `Dictionary` or
- the instance of `Dictionary`.
- in_channels (int): Number of channels :math:`E` of input vector.
- Defaults to 512.
- num_channels (int): Number of channels of hidden vectors in mini U-Net.
- Defaults to 64.
- attn_height (int): Height :math:`H` of input image features. Defaults
- to 8.
- attn_width (int): Width :math:`W` of input image features. Defaults to
- 32.
- attn_mode (str): Upsampling mode for :obj:`torch.nn.Upsample` in mini
- U-Net. Defaults to 'nearest'.
- module_loss (dict, optional): Config to build loss. Defaults to None.
- postprocessor (dict, optional): Config to build postprocessor.
- Defaults to None.
- max_seq_len (int): Maximum sequence length. The
- sequence is usually generated from decoder. Defaults to 40.
- init_cfg (dict or list[dict], optional): Initialization configs.
- Defaults to dict(type='Xavier', layer='Conv2d').
- """
-
- def __init__(self,
- dictionary: Union[Dict, Dictionary],
- in_channels: int = 512,
- num_channels: int = 64,
- attn_height: int = 8,
- attn_width: int = 32,
- attn_mode: str = 'nearest',
- module_loss: Optional[Dict] = None,
- postprocessor: Optional[Dict] = None,
- max_seq_len: int = 40,
- init_cfg: Optional[Union[Dict, List[Dict]]] = dict(
- type='Xavier', layer='Conv2d'),
- **kwargs) -> None:
-
- super().__init__(
- dictionary=dictionary,
- module_loss=module_loss,
- postprocessor=postprocessor,
- max_seq_len=max_seq_len,
- init_cfg=init_cfg)
-
- # For mini-Unet
- self.k_encoder = nn.Sequential(
- self._encoder_layer(in_channels, num_channels, stride=(1, 2)),
- self._encoder_layer(num_channels, num_channels, stride=(2, 2)),
- self._encoder_layer(num_channels, num_channels, stride=(2, 2)),
- self._encoder_layer(num_channels, num_channels, stride=(2, 2)))
-
- self.k_decoder = nn.Sequential(
- self._decoder_layer(
- num_channels, num_channels, scale_factor=2, mode=attn_mode),
- self._decoder_layer(
- num_channels, num_channels, scale_factor=2, mode=attn_mode),
- self._decoder_layer(
- num_channels, num_channels, scale_factor=2, mode=attn_mode),
- self._decoder_layer(
- num_channels,
- in_channels,
- size=(attn_height, attn_width),
- mode=attn_mode))
-
- self.pos_encoder = PositionalEncoding(in_channels, max_seq_len)
- self.project = nn.Linear(in_channels, in_channels)
- self.cls = nn.Linear(in_channels, self.dictionary.num_classes)
-
- def forward_train(
- self,
- feat: Optional[torch.Tensor] = None,
- out_enc: torch.Tensor = None,
- data_samples: Optional[Sequence[TextRecogDataSample]] = None
- ) -> Dict:
- """
- Args:
- feat (Tensor, optional): Image features of shape (N, E, H, W).
- Defaults to None.
- out_enc (torch.Tensor): Encoder output. Defaults to None.
- data_samples (list[TextRecogDataSample], optional): Batch of
- TextRecogDataSample, containing gt_text information. Defaults
- to None.
-
- Returns:
- dict: A dict with keys ``feature``, ``logits`` and ``attn_scores``.
-
- - feature (Tensor): Shape (N, T, E). Raw visual features for
- language decoder.
- - logits (Tensor): Shape (N, T, C). The raw logits for
- characters.
- - attn_scores (Tensor): Shape (N, T, H, W). Intermediate result
- for vision-language aligner.
- """
- # Position Attention
- N, E, H, W = out_enc.size()
- k, v = out_enc, out_enc # (N, E, H, W)
-
- # Apply mini U-Net on k
- features = []
- for i in range(len(self.k_encoder)):
- k = self.k_encoder[i](k)
- features.append(k)
- for i in range(len(self.k_decoder) - 1):
- k = self.k_decoder[i](k)
- k = k + features[len(self.k_decoder) - 2 - i]
- k = self.k_decoder[-1](k)
-
- # q = positional encoding
- zeros = out_enc.new_zeros((N, self.max_seq_len, E)) # (N, T, E)
- q = self.pos_encoder(zeros) # (N, T, E)
- q = self.project(q) # (N, T, E)
-
- # Attention encoding
- attn_scores = torch.bmm(q, k.flatten(2, 3)) # (N, T, (H*W))
- attn_scores = attn_scores / (E**0.5)
- attn_scores = torch.softmax(attn_scores, dim=-1)
- v = v.permute(0, 2, 3, 1).view(N, -1, E) # (N, (H*W), E)
- attn_vecs = torch.bmm(attn_scores, v) # (N, T, E)
-
- out_enc = self.cls(attn_vecs)
- result = {
- 'feature': attn_vecs,
- 'logits': out_enc,
- 'attn_scores': attn_scores.view(N, -1, H, W)
- }
- return result
-
- def forward_test(
- self,
- feat: Optional[torch.Tensor] = None,
- out_enc: torch.Tensor = None,
- data_samples: Optional[Sequence[TextRecogDataSample]] = None
- ) -> Dict:
- """
- Args:
- feat (torch.Tensor, optional): Image features of shape
- (N, E, H, W). Defaults to None.
- out_enc (torch.Tensor): Encoder output. Defaults to None.
- data_samples (list[TextRecogDataSample], optional): Batch of
- TextRecogDataSample, containing gt_text information. Defaults
- to None.
-
- Returns:
- dict: A dict with keys ``feature``, ``logits`` and ``attn_scores``.
-
- - feature (Tensor): Shape (N, T, E). Raw visual features for
- language decoder.
- - logits (Tensor): Shape (N, T, C). The raw logits for
- characters.
- - attn_scores (Tensor): Shape (N, T, H, W). Intermediate result
- for vision-language aligner.
- """
- return self.forward_train(
- feat, out_enc=out_enc, data_samples=data_samples)
-
- def _encoder_layer(self,
- in_channels: int,
- out_channels: int,
- kernel_size: int = 3,
- stride: int = 2,
- padding: int = 1) -> nn.Sequential:
- """Generate encoder layer.
-
- Args:
- in_channels (int): Input channels.
- out_channels (int): Output channels.
- kernel_size (int, optional): Kernel size. Defaults to 3.
- stride (int, optional): Stride. Defaults to 2.
- padding (int, optional): Padding. Defaults to 1.
-
- Returns:
- nn.Sequential: Encoder layer.
- """
- return ConvModule(
- in_channels,
- out_channels,
- kernel_size=kernel_size,
- stride=stride,
- padding=padding,
- norm_cfg=dict(type='BN'),
- act_cfg=dict(type='ReLU'))
-
- def _decoder_layer(self,
- in_channels: int,
- out_channels: int,
- kernel_size: int = 3,
- stride: int = 1,
- padding: int = 1,
- mode: str = 'nearest',
- scale_factor: Optional[int] = None,
- size: Optional[Tuple[int, int]] = None):
- """Generate decoder layer.
-
- Args:
- in_channels (int): Input channels.
- out_channels (int): Output channels.
- kernel_size (int): Kernel size. Defaults to 3.
- stride (int): Stride. Defaults to 1.
- padding (int): Padding. Defaults to 1.
- mode (str): Interpolation mode. Defaults to 'nearest'.
- scale_factor (int, optional): Scale factor for upsampling.
- size (Tuple[int, int], optional): Output size. Defaults to None.
- """
- align_corners = None if mode == 'nearest' else True
- return nn.Sequential(
- nn.Upsample(
- size=size,
- scale_factor=scale_factor,
- mode=mode,
- align_corners=align_corners),
- ConvModule(
- in_channels,
- out_channels,
- kernel_size=kernel_size,
- stride=stride,
- padding=padding,
- norm_cfg=dict(type='BN'),
- act_cfg=dict(type='ReLU')))
diff --git a/pipeline/mmocr/mmocr/models/textrecog/decoders/aster_decoder.py b/pipeline/mmocr/mmocr/models/textrecog/decoders/aster_decoder.py
deleted file mode 100644
index 83e249b08c00acc06a7a31a5b5e44ba70ff3b712..0000000000000000000000000000000000000000
--- a/pipeline/mmocr/mmocr/models/textrecog/decoders/aster_decoder.py
+++ /dev/null
@@ -1,181 +0,0 @@
-# Copyright (c) OpenMMLab. All rights reserved.
-from typing import Dict, Optional, Sequence, Tuple, Union
-
-import torch
-import torch.nn as nn
-
-from mmocr.models.common.dictionary import Dictionary
-from mmocr.registry import MODELS
-from mmocr.structures import TextRecogDataSample
-from .base import BaseDecoder
-
-
-@MODELS.register_module()
-class ASTERDecoder(BaseDecoder):
- """Implement attention decoder.
-
- Args:
- in_channels (int): Number of input channels.
- emb_dims (int): Dims of char embedding. Defaults to 512.
- attn_dims (int): Dims of attention. Both hidden states and features
- will be projected to this dims. Defaults to 512.
- hidden_size (int): Dims of hidden state for GRU. Defaults to 512.
- dictionary (dict or :obj:`Dictionary`): The config for `Dictionary` or
- the instance of `Dictionary`. Defaults to None.
- max_seq_len (int): Maximum output sequence length :math:`T`. Defaults
- to 25.
- module_loss (dict, optional): Config to build loss. Defaults to None.
- postprocessor (dict, optional): Config to build postprocessor.
- Defaults to None.
- init_cfg (dict or list[dict], optional): Initialization configs.
- Defaults to None.
- """
-
- def __init__(self,
- in_channels: int,
- emb_dims: int = 512,
- attn_dims: int = 512,
- hidden_size: int = 512,
- dictionary: Union[Dictionary, Dict] = None,
- max_seq_len: int = 25,
- module_loss: Dict = None,
- postprocessor: Dict = None,
- init_cfg=dict(type='Xavier', layer='Conv2d')):
- super().__init__(
- init_cfg=init_cfg,
- dictionary=dictionary,
- module_loss=module_loss,
- postprocessor=postprocessor,
- max_seq_len=max_seq_len)
-
- self.start_idx = self.dictionary.start_idx
- self.num_classes = self.dictionary.num_classes
- self.in_channels = in_channels
- self.embedding_dim = emb_dims
- self.att_dims = attn_dims
- self.hidden_size = hidden_size
-
- # Projection layers
- self.proj_feat = nn.Linear(in_channels, attn_dims)
- self.proj_hidden = nn.Linear(hidden_size, attn_dims)
- self.proj_sum = nn.Linear(attn_dims, 1)
-
- # Decoder input embedding
- self.embedding = nn.Embedding(self.num_classes, self.att_dims)
-
- # GRU
- self.gru = nn.GRU(
- input_size=self.in_channels + self.embedding_dim,
- hidden_size=self.hidden_size,
- batch_first=True)
-
- # Prediction layer
- self.fc = nn.Linear(hidden_size, self.dictionary.num_classes)
- self.softmax = nn.Softmax(dim=-1)
-
- def _attention(self, feat: torch.Tensor, prev_hidden: torch.Tensor,
- prev_char: torch.Tensor
- ) -> Tuple[torch.Tensor, torch.Tensor]:
- """Implement the attention mechanism.
-
- Args:
- feat (Tensor): Feature map from encoder of shape :math:`(N, T, C)`.
- prev_hidden (Tensor): Previous hidden state from GRU of shape
- :math:`(1, N, self.hidden_size)`.
- prev_char (Tensor): Previous predicted character of shape
- :math:`(N, )`.
-
- Returns:
- tuple(Tensor, Tensor):
- - output (Tensor): Predicted character of current time step of
- shape :math:`(N, 1)`.
- - state (Tensor): Hidden state from GRU of current time step of
- shape :math:`(N, self.hidden_size)`.
- """
- # Calculate the attention weights
- B, T, _ = feat.size()
- feat_proj = self.proj_feat(feat) # [N, T, attn_dims]
- hidden_proj = self.proj_hidden(prev_hidden) # [1, N, attn_dims]
- hidden_proj = hidden_proj.squeeze(0).unsqueeze(1) # [N, 1, attn_dims]
- hidden_proj = hidden_proj.expand(B, T,
- self.att_dims) # [N, T, attn_dims]
-
- sum_tanh = torch.tanh(feat_proj + hidden_proj) # [N, T, attn_dims]
- sum_proj = self.proj_sum(sum_tanh).squeeze(-1) # [N, T]
- attn_weights = torch.softmax(sum_proj, dim=1) # [N, T]
-
- # GRU forward
- context = torch.bmm(attn_weights.unsqueeze(1), feat).squeeze(1)
- char_embed = self.embedding(prev_char.long()) # [N, emb_dims]
- output, state = self.gru(
- torch.cat([char_embed, context], 1).unsqueeze(1), prev_hidden)
- output = output.squeeze(1)
- output = self.fc(output)
- return output, state
-
- def forward_train(
- self,
- feat: torch.Tensor = None,
- out_enc: Optional[torch.Tensor] = None,
- data_samples: Optional[Sequence[TextRecogDataSample]] = None
- ) -> torch.Tensor:
- """
- Args:
- feat (Tensor): Feature from backbone. Unused in this decoder.
- out_enc (torch.Tensor, optional): Encoder output. Defaults to None.
- data_samples (list[TextRecogDataSample], optional): Batch of
- TextRecogDataSample, containing gt_text information. Defaults
- to None.
-
- Returns:
- Tensor: The raw logit tensor. Shape :math:`(N, T, C)` where
- :math:`C` is ``num_classes``.
- """
- B = out_enc.shape[0]
- state = torch.zeros(1, B, self.hidden_size).to(out_enc.device)
- padded_targets = [
- data_sample.gt_text.padded_indexes for data_sample in data_samples
- ]
- padded_targets = torch.stack(padded_targets, dim=0).to(out_enc.device)
- outputs = []
- for i in range(self.max_seq_len):
- prev_char = padded_targets[:, i].to(out_enc.device)
- output, state = self._attention(out_enc, state, prev_char)
- outputs.append(output)
- outputs = torch.cat([_.unsqueeze(1) for _ in outputs], 1)
- return outputs
-
- def forward_test(
- self,
- feat: Optional[torch.Tensor] = None,
- out_enc: Optional[torch.Tensor] = None,
- data_samples: Optional[Sequence[TextRecogDataSample]] = None
- ) -> torch.Tensor:
- """
- Args:
- feat (Tensor): Feature from backbone. Unused in this decoder.
- out_enc (torch.Tensor, optional): Encoder output. Defaults to None.
- data_samples (list[TextRecogDataSample], optional): Batch of
- TextRecogDataSample, containing gt_text information. Defaults
- to None. Unused in this decoder.
-
- Returns:
- Tensor: The raw logit tensor. Shape :math:`(N, T, C)` where
- :math:`C` is ``num_classes``.
- """
- B = out_enc.shape[0]
- predicted = []
- state = torch.zeros(1, B, self.hidden_size).to(out_enc.device)
- outputs = []
- for i in range(self.max_seq_len):
- if i == 0:
- prev_char = torch.zeros(B).fill_(self.start_idx).to(
- out_enc.device)
- else:
- prev_char = predicted
-
- output, state = self._attention(out_enc, state, prev_char)
- outputs.append(output)
- _, predicted = output.max(-1)
- outputs = torch.cat([_.unsqueeze(1) for _ in outputs], 1)
- return self.softmax(outputs)
diff --git a/pipeline/mmocr/mmocr/models/textrecog/decoders/base.py b/pipeline/mmocr/mmocr/models/textrecog/decoders/base.py
deleted file mode 100644
index 2c990ca0c1c9c1b6a2878ca05cb764b20e3d8fb1..0000000000000000000000000000000000000000
--- a/pipeline/mmocr/mmocr/models/textrecog/decoders/base.py
+++ /dev/null
@@ -1,166 +0,0 @@
-# Copyright (c) OpenMMLab. All rights reserved.
-from typing import Dict, List, Optional, Sequence, Union
-
-import torch
-from mmengine.model import BaseModule
-
-from mmocr.models.common.dictionary import Dictionary
-from mmocr.registry import MODELS, TASK_UTILS
-from mmocr.structures import TextRecogDataSample
-
-
-@MODELS.register_module()
-class BaseDecoder(BaseModule):
- """Base decoder for text recognition, build the loss and postprocessor.
-
- Args:
- dictionary (dict or :obj:`Dictionary`): The config for `Dictionary` or
- the instance of `Dictionary`.
- loss (dict, optional): Config to build loss. Defaults to None.
- postprocessor (dict, optional): Config to build postprocessor.
- Defaults to None.
- max_seq_len (int): Maximum sequence length. The
- sequence is usually generated from decoder. Defaults to 40.
- init_cfg (dict or list[dict], optional): Initialization configs.
- Defaults to None.
- """
-
- def __init__(self,
- dictionary: Union[Dict, Dictionary],
- module_loss: Optional[Dict] = None,
- postprocessor: Optional[Dict] = None,
- max_seq_len: int = 40,
- init_cfg: Optional[Union[Dict, List[Dict]]] = None) -> None:
- super().__init__(init_cfg=init_cfg)
- if isinstance(dictionary, dict):
- self.dictionary = TASK_UTILS.build(dictionary)
- elif isinstance(dictionary, Dictionary):
- self.dictionary = dictionary
- else:
- raise TypeError(
- 'The type of dictionary should be `Dictionary` or dict, '
- f'but got {type(dictionary)}')
- self.module_loss = None
- self.postprocessor = None
- self.max_seq_len = max_seq_len
-
- if module_loss is not None:
- assert isinstance(module_loss, dict)
- module_loss.update(dictionary=dictionary)
- module_loss.update(max_seq_len=max_seq_len)
- self.module_loss = MODELS.build(module_loss)
-
- if postprocessor is not None:
- assert isinstance(postprocessor, dict)
- postprocessor.update(dictionary=dictionary)
- postprocessor.update(max_seq_len=max_seq_len)
- self.postprocessor = MODELS.build(postprocessor)
-
- def forward_train(
- self,
- feat: Optional[torch.Tensor] = None,
- out_enc: Optional[torch.Tensor] = None,
- data_samples: Optional[Sequence[TextRecogDataSample]] = None
- ) -> torch.Tensor:
- """Forward for training.
-
- Args:
- feat (torch.Tensor, optional): The feature map from backbone of
- shape :math:`(N, E, H, W)`. Defaults to None.
- out_enc (torch.Tensor, optional): Encoder output. Defaults to None.
- data_samples (Sequence[TextRecogDataSample]): Batch of
- TextRecogDataSample, containing gt_text information. Defaults
- to None.
- """
- raise NotImplementedError
-
- def forward_test(
- self,
- feat: Optional[torch.Tensor] = None,
- out_enc: Optional[torch.Tensor] = None,
- data_samples: Optional[Sequence[TextRecogDataSample]] = None
- ) -> torch.Tensor:
- """Forward for testing.
-
- Args:
- feat (torch.Tensor, optional): The feature map from backbone of
- shape :math:`(N, E, H, W)`. Defaults to None.
- out_enc (torch.Tensor, optional): Encoder output. Defaults to None.
- data_samples (Sequence[TextRecogDataSample]): Batch of
- TextRecogDataSample, containing gt_text information. Defaults
- to None.
- """
- raise NotImplementedError
-
- def loss(self,
- feat: Optional[torch.Tensor] = None,
- out_enc: Optional[torch.Tensor] = None,
- data_samples: Optional[Sequence[TextRecogDataSample]] = None
- ) -> Dict:
- """Calculate losses from a batch of inputs and data samples.
-
- Args:
- feat (Tensor, optional): Features from the backbone. Defaults
- to None.
- out_enc (Tensor, optional): Features from the encoder.
- Defaults to None.
- data_samples (list[TextRecogDataSample], optional): A list of
- N datasamples, containing meta information and gold
- annotations for each of the images. Defaults to None.
-
- Returns:
- dict[str, tensor]: A dictionary of loss components.
- """
- out_dec = self(feat, out_enc, data_samples)
- return self.module_loss(out_dec, data_samples)
-
- def predict(
- self,
- feat: Optional[torch.Tensor] = None,
- out_enc: Optional[torch.Tensor] = None,
- data_samples: Optional[Sequence[TextRecogDataSample]] = None
- ) -> Sequence[TextRecogDataSample]:
- """Perform forward propagation of the decoder and postprocessor.
-
- Args:
- feat (Tensor, optional): Features from the backbone. Defaults
- to None.
- out_enc (Tensor, optional): Features from the encoder. Defaults
- to None.
- data_samples (list[TextRecogDataSample]): A list of N datasamples,
- containing meta information and gold annotations for each of
- the images. Defaults to None.
-
- Returns:
- list[TextRecogDataSample]: A list of N datasamples of prediction
- results. Results are stored in ``pred_text``.
- """
- out_dec = self(feat, out_enc, data_samples)
- return self.postprocessor(out_dec, data_samples)
-
- def forward(
- self,
- feat: Optional[torch.Tensor] = None,
- out_enc: Optional[torch.Tensor] = None,
- data_samples: Optional[Sequence[TextRecogDataSample]] = None
- ) -> torch.Tensor:
- """Decoder forward.
-
- Args:
- feat (Tensor, optional): Features from the backbone. Defaults
- to None.
- out_enc (Tensor, optional): Features from the encoder.
- Defaults to None.
- data_samples (list[TextRecogDataSample]): A list of N datasamples,
- containing meta information and gold annotations for each of
- the images. Defaults to None.
-
- Returns:
- Tensor: Features from ``decoder`` forward.
- """
- if self.training:
- if getattr(self, 'module_loss') is not None:
- data_samples = self.module_loss.get_targets(data_samples)
- return self.forward_train(feat, out_enc, data_samples)
- else:
- return self.forward_test(feat, out_enc, data_samples)
diff --git a/pipeline/mmocr/mmocr/models/textrecog/decoders/crnn_decoder.py b/pipeline/mmocr/mmocr/models/textrecog/decoders/crnn_decoder.py
deleted file mode 100644
index 7d29abbd86a9c5bd2cafe633efd1514eb1c97b96..0000000000000000000000000000000000000000
--- a/pipeline/mmocr/mmocr/models/textrecog/decoders/crnn_decoder.py
+++ /dev/null
@@ -1,109 +0,0 @@
-# Copyright (c) OpenMMLab. All rights reserved.
-from typing import Dict, Optional, Sequence, Union
-
-import torch
-import torch.nn as nn
-from mmengine.model import Sequential
-
-from mmocr.models.common.dictionary import Dictionary
-from mmocr.models.textrecog.layers import BidirectionalLSTM
-from mmocr.registry import MODELS
-from mmocr.structures import TextRecogDataSample
-from .base import BaseDecoder
-
-
-@MODELS.register_module()
-class CRNNDecoder(BaseDecoder):
- """Decoder for CRNN.
-
- Args:
- in_channels (int): Number of input channels.
- dictionary (dict or :obj:`Dictionary`): The config for `Dictionary` or
- the instance of `Dictionary`.
- rnn_flag (bool): Use RNN or CNN as the decoder. Defaults to False.
- module_loss (dict, optional): Config to build module_loss. Defaults
- to None.
- postprocessor (dict, optional): Config to build postprocessor.
- Defaults to None.
- init_cfg (dict or list[dict], optional): Initialization configs.
- Defaults to None.
- """
-
- def __init__(self,
- in_channels: int,
- dictionary: Union[Dictionary, Dict],
- rnn_flag: bool = False,
- module_loss: Dict = None,
- postprocessor: Dict = None,
- init_cfg=dict(type='Xavier', layer='Conv2d'),
- **kwargs):
- super().__init__(
- init_cfg=init_cfg,
- dictionary=dictionary,
- module_loss=module_loss,
- postprocessor=postprocessor)
- self.rnn_flag = rnn_flag
-
- if rnn_flag:
- self.decoder = Sequential(
- BidirectionalLSTM(in_channels, 256, 256),
- BidirectionalLSTM(256, 256, self.dictionary.num_classes))
- else:
- self.decoder = nn.Conv2d(
- in_channels,
- self.dictionary.num_classes,
- kernel_size=1,
- stride=1)
- self.softmax = nn.Softmax(dim=-1)
-
- def forward_train(
- self,
- feat: torch.Tensor,
- out_enc: Optional[torch.Tensor] = None,
- data_samples: Optional[Sequence[TextRecogDataSample]] = None
- ) -> torch.Tensor:
- """
- Args:
- feat (Tensor): A Tensor of shape :math:`(N, C, 1, W)`.
- out_enc (torch.Tensor, optional): Encoder output. Defaults to None.
- data_samples (list[TextRecogDataSample], optional): Batch of
- TextRecogDataSample, containing gt_text information. Defaults
- to None.
-
- Returns:
- Tensor: The raw logit tensor. Shape :math:`(N, W, C)` where
- :math:`C` is ``num_classes``.
- """
- assert feat.size(2) == 1, 'feature height must be 1'
- if self.rnn_flag:
- x = feat.squeeze(2) # [N, C, W]
- x = x.permute(2, 0, 1) # [W, N, C]
- x = self.decoder(x) # [W, N, C]
- outputs = x.permute(1, 0, 2).contiguous()
- else:
- x = self.decoder(feat)
- x = x.permute(0, 3, 1, 2).contiguous()
- n, w, c, h = x.size()
- outputs = x.view(n, w, c * h)
- return outputs
-
- def forward_test(
- self,
- feat: Optional[torch.Tensor] = None,
- out_enc: Optional[torch.Tensor] = None,
- data_samples: Optional[Sequence[TextRecogDataSample]] = None
- ) -> torch.Tensor:
- """
- Args:
- feat (Tensor): A Tensor of shape :math:`(N, C, 1, W)`.
- out_enc (torch.Tensor, optional): Encoder output. Defaults to None.
- data_samples (list[TextRecogDataSample]): Batch of
- TextRecogDataSample, containing ``gt_text`` information.
- Defaults to None.
-
- Returns:
- Tensor: Character probabilities. of shape
- :math:`(N, self.max_seq_len, C)` where :math:`C` is
- ``num_classes``.
- """
- return self.softmax(self.forward_train(feat, out_enc, data_samples))
diff --git a/pipeline/mmocr/mmocr/models/textrecog/decoders/maerec_decoder.py b/pipeline/mmocr/mmocr/models/textrecog/decoders/maerec_decoder.py
deleted file mode 100644
index e569b06f371769e32cb729e72ea65be64e4ecc5f..0000000000000000000000000000000000000000
--- a/pipeline/mmocr/mmocr/models/textrecog/decoders/maerec_decoder.py
+++ /dev/null
@@ -1,258 +0,0 @@
-# Copyright (c) OpenMMLab. All rights reserved.
-import math
-from typing import Dict, List, Optional, Sequence, Union
-
-import torch
-import torch.nn as nn
-from mmengine.model import ModuleList
-
-from mmocr.models.common import PositionalEncoding, TFDecoderLayer
-from mmocr.models.common.dictionary import Dictionary
-from mmocr.registry import MODELS
-from mmocr.structures import TextRecogDataSample
-from .base import BaseDecoder
-
-
-@MODELS.register_module()
-class MAERecDecoder(BaseDecoder):
- """Transformer Decoder block with self attention mechanism.
-
- Args:
- n_layers (int): Number of attention layers. Defaults to 6.
- d_embedding (int): Language embedding dimension. Defaults to 512.
- n_head (int): Number of parallel attention heads. Defaults to 8.
- d_k (int): Dimension of the key vector. Defaults to 64.
- d_v (int): Dimension of the value vector. Defaults to 64
- d_model (int): Dimension :math:`D_m` of the input from previous model.
- Defaults to 512.
- d_inner (int): Hidden dimension of feedforward layers. Defaults to 256.
- n_position (int): Length of the positional encoding vector. Must be
- greater than ``max_seq_len``. Defaults to 200.
- dropout (float): Dropout rate for text embedding, MHSA, FFN. Defaults
- to 0.1.
- module_loss (dict, optional): Config to build module_loss. Defaults
- to None.
- postprocessor (dict, optional): Config to build postprocessor.
- Defaults to None.
- dictionary (dict or :obj:`Dictionary`): The config for `Dictionary` or
- the instance of `Dictionary`.
- max_seq_len (int): Maximum output sequence length :math:`T`. Defaults
- to 30.
- init_cfg (dict or list[dict], optional): Initialization configs.
- """
-
- def __init__(self,
- n_layers: int = 6,
- d_embedding: int = 512,
- n_head: int = 8,
- d_k: int = 64,
- d_v: int = 64,
- d_model: int = 512,
- d_inner: int = 256,
- n_position: int = 200,
- dropout: float = 0.1,
- module_loss: Optional[Dict] = None,
- postprocessor: Optional[Dict] = None,
- dictionary: Optional[Union[Dict, Dictionary]] = None,
- max_seq_len: int = 30,
- init_cfg: Optional[Union[Dict, List[Dict]]] = None) -> None:
- super().__init__(
- module_loss=module_loss,
- postprocessor=postprocessor,
- dictionary=dictionary,
- init_cfg=init_cfg,
- max_seq_len=max_seq_len)
-
- self.padding_idx = self.dictionary.padding_idx
- self.start_idx = self.dictionary.start_idx
- self.max_seq_len = max_seq_len
-
- self.trg_word_emb = nn.Embedding(
- self.dictionary.num_classes,
- d_embedding,
- padding_idx=self.padding_idx)
-
- self.position_enc = PositionalEncoding(
- d_embedding, n_position=n_position)
- self.dropout = nn.Dropout(p=dropout)
-
- self.layer_stack = ModuleList([
- TFDecoderLayer(
- d_model, d_inner, n_head, d_k, d_v, dropout=dropout)
- for _ in range(n_layers)
- ])
- self.layer_norm = nn.LayerNorm(d_model, eps=1e-6)
-
- pred_num_class = self.dictionary.num_classes
- self.classifier = nn.Linear(d_model, pred_num_class)
- self.softmax = nn.Softmax(dim=-1)
-
- def _get_target_mask(self, trg_seq: torch.Tensor) -> torch.Tensor:
- """Generate mask for target sequence.
-
- Args:
- trg_seq (torch.Tensor): Input text sequence. Shape :math:`(N, T)`.
-
- Returns:
- Tensor: Target mask. Shape :math:`(N, T, T)`.
- E.g.:
- seq = torch.Tensor([[1, 2, 0, 0]]), pad_idx = 0, then
- target_mask =
- torch.Tensor([[[True, False, False, False],
- [True, True, False, False],
- [True, True, False, False],
- [True, True, False, False]]])
- """
-
- pad_mask = (trg_seq != self.padding_idx).unsqueeze(-2)
-
- len_s = trg_seq.size(1)
- subsequent_mask = 1 - torch.triu(
- torch.ones((len_s, len_s), device=trg_seq.device), diagonal=1)
- subsequent_mask = subsequent_mask.unsqueeze(0).bool()
-
- return pad_mask & subsequent_mask
-
- def _get_source_mask(self, src_seq: torch.Tensor,
- valid_ratios: Sequence[float]) -> torch.Tensor:
- """Generate mask for source sequence.
-
- Args:
- src_seq (torch.Tensor): Image sequence. Shape :math:`(N, T, C)`.
- valid_ratios (list[float]): The valid ratio of input image. For
- example, if the width of the original image is w1 and the width
- after padding is w2, then valid_ratio = w1/w2. Source mask is
- used to cover the area of the padding region.
-
- Returns:
- Tensor or None: Source mask. Shape :math:`(N, T)`. The region of
- padding area are False, and the rest are True.
- """
-
- N, T, _ = src_seq.size()
- mask = None
- if len(valid_ratios) > 0:
- mask = src_seq.new_zeros((N, T), device=src_seq.device)
- for i, valid_ratio in enumerate(valid_ratios):
- valid_width = min(T, math.ceil(T * valid_ratio))
- mask[i, :valid_width] = 1
-
- return mask
-
- def _attention(self,
- trg_seq: torch.Tensor,
- src: torch.Tensor,
- src_mask: Optional[torch.Tensor] = None) -> torch.Tensor:
- """A wrapped process for transformer based decoder including text
- embedding, position embedding, N x transformer decoder and a LayerNorm
- operation.
-
- Args:
- trg_seq (Tensor): Target sequence in. Shape :math:`(N, T)`.
- src (Tensor): Source sequence from encoder in shape
- Shape :math:`(N, T, D_m)` where :math:`D_m` is ``d_model``.
- src_mask (Tensor, Optional): Mask for source sequence.
- Shape :math:`(N, T)`. Defaults to None.
-
- Returns:
- Tensor: Output sequence from transformer decoder.
- Shape :math:`(N, T, D_m)` where :math:`D_m` is ``d_model``.
- """
-
- trg_embedding = self.trg_word_emb(trg_seq)
- trg_pos_encoded = self.position_enc(trg_embedding)
- trg_mask = self._get_target_mask(trg_seq)
- tgt_seq = self.dropout(trg_pos_encoded)
-
- output = tgt_seq
- for dec_layer in self.layer_stack:
- output = dec_layer(
- output,
- src,
- self_attn_mask=trg_mask,
- dec_enc_attn_mask=src_mask)
- output = self.layer_norm(output)
-
- return output
-
- def forward_train(
- self,
- feat: Optional[torch.Tensor] = None,
- out_enc: torch.Tensor = None,
- data_samples: Sequence[TextRecogDataSample] = None
- ) -> torch.Tensor:
- """Forward for training. Source mask will be used here.
-
- Args:
- feat (Tensor, optional): Unused.
- out_enc (Tensor): Encoder output of shape : math:`(N, T, D_m)`
- where :math:`D_m` is ``d_model``. Defaults to None.
- data_samples (list[TextRecogDataSample]): Batch of
- TextRecogDataSample, containing gt_text and valid_ratio
- information. Defaults to None.
-
- Returns:
- Tensor: The raw logit tensor. Shape :math:`(N, T, C)` where
- :math:`C` is ``num_classes``.
- """
- valid_ratios = []
- for data_sample in data_samples:
- valid_ratios.append(data_sample.get('valid_ratio'))
- src_mask = self._get_source_mask(feat, valid_ratios)
- trg_seq = []
- for data_sample in data_samples:
- trg_seq.append(data_sample.gt_text.padded_indexes.to(feat.device))
- trg_seq = torch.stack(trg_seq, dim=0)
- attn_output = self._attention(trg_seq, feat, src_mask=src_mask)
- outputs = self.classifier(attn_output)
-
- return outputs
-
- def forward_test(
- self,
- feat: Optional[torch.Tensor] = None,
- out_enc: torch.Tensor = None,
- data_samples: Sequence[TextRecogDataSample] = None
- ) -> torch.Tensor:
- """Forward for testing.
-
- Args:
- feat (Tensor, optional): Unused.
- out_enc (Tensor): Encoder output of shape:
- math:`(N, T, D_m)` where :math:`D_m` is ``d_model``.
- Defaults to None.
- data_samples (list[TextRecogDataSample]): Batch of
- TextRecogDataSample, containing gt_text and valid_ratio
- information. Defaults to None.
-
- Returns:
- Tensor: Character probabilities. of shape
- :math:`(N, self.max_seq_len, C)` where :math:`C` is
- ``num_classes``.
- """
- valid_ratios = []
- for data_sample in data_samples:
- valid_ratios.append(data_sample.get('valid_ratio'))
- src_mask = self._get_source_mask(feat, valid_ratios)
- N = feat.size(0)
- init_target_seq = torch.full((N, self.max_seq_len + 1),
- self.padding_idx,
- device=feat.device,
- dtype=torch.long)
- # bsz * seq_len
- init_target_seq[:, 0] = self.start_idx
-
- outputs = []
- for step in range(0, self.max_seq_len):
- decoder_output = self._attention(
- init_target_seq, feat, src_mask=src_mask)
- # bsz * seq_len * C
- step_result = self.classifier(decoder_output[:, step, :])
- # bsz * num_classes
- outputs.append(step_result)
- _, step_max_index = torch.max(step_result, dim=-1)
- init_target_seq[:, step + 1] = step_max_index
-
- outputs = torch.stack(outputs, dim=1)
-
- return self.softmax(outputs)
diff --git a/pipeline/mmocr/mmocr/models/textrecog/decoders/master_decoder.py b/pipeline/mmocr/mmocr/models/textrecog/decoders/master_decoder.py
deleted file mode 100644
index b92b4fc8f4538e1dd9f2485509e27f7036532ce7..0000000000000000000000000000000000000000
--- a/pipeline/mmocr/mmocr/models/textrecog/decoders/master_decoder.py
+++ /dev/null
@@ -1,275 +0,0 @@
-# Copyright (c) OpenMMLab. All rights reserved.
-import copy
-import math
-from typing import Dict, Optional, Sequence, Union
-
-import torch
-import torch.nn as nn
-from mmcv.cnn.bricks.transformer import BaseTransformerLayer
-from mmengine.model import ModuleList
-
-from mmocr.models.common.dictionary import Dictionary
-from mmocr.models.common.modules import PositionalEncoding
-from mmocr.registry import MODELS
-from mmocr.structures import TextRecogDataSample
-from .base import BaseDecoder
-
-
-def clones(module: nn.Module, N: int) -> nn.ModuleList:
- """Produce N identical layers.
-
- Args:
- module (nn.Module): A pytorch nn.module.
- N (int): Number of copies.
-
- Returns:
- nn.ModuleList: A pytorch nn.ModuleList with the copies.
- """
- return nn.ModuleList([copy.deepcopy(module) for _ in range(N)])
-
-
-class Embeddings(nn.Module):
- """Construct the word embeddings given vocab size and embed dim.
-
- Args:
- d_model (int): The embedding dimension.
- vocab (int): Vocablury size.
- """
-
- def __init__(self, d_model: int, vocab: int):
- super().__init__()
- self.lut = nn.Embedding(vocab, d_model)
- self.d_model = d_model
-
- def forward(self, *input: torch.Tensor) -> torch.Tensor:
- """Forward the embeddings.
-
- Args:
- input (torch.Tensor): The input tensors.
-
- Returns:
- torch.Tensor: The embeddings.
- """
- x = input[0]
- return self.lut(x) * math.sqrt(self.d_model)
-
-
-@MODELS.register_module()
-class MasterDecoder(BaseDecoder):
- """Decoder module in `MASTER `_.
-
- Code is partially modified from https://github.com/wenwenyu/MASTER-pytorch.
-
- Args:
- n_layers (int): Number of attention layers. Defaults to 3.
- n_head (int): Number of parallel attention heads. Defaults to 8.
- d_model (int): Dimension :math:`E` of the input from previous model.
- Defaults to 512.
- feat_size (int): The size of the input feature from previous model,
- usually :math:`H * W`. Defaults to 6 * 40.
- d_inner (int): Hidden dimension of feedforward layers.
- Defaults to 2048.
- attn_drop (float): Dropout rate of the attention layer. Defaults to 0.
- ffn_drop (float): Dropout rate of the feedforward layer. Defaults to 0.
- feat_pe_drop (float): Dropout rate of the feature positional encoding
- layer. Defaults to 0.2.
- dictionary (dict or :obj:`Dictionary`): The config for `Dictionary` or
- the instance of `Dictionary`. Defaults to None.
- module_loss (dict, optional): Config to build module_loss. Defaults
- to None.
- postprocessor (dict, optional): Config to build postprocessor.
- Defaults to None.
- max_seq_len (int): Maximum output sequence length :math:`T`. Defaults
- to 30.
- init_cfg (dict or list[dict], optional): Initialization configs.
- """
-
- def __init__(
- self,
- n_layers: int = 3,
- n_head: int = 8,
- d_model: int = 512,
- feat_size: int = 6 * 40,
- d_inner: int = 2048,
- attn_drop: float = 0.,
- ffn_drop: float = 0.,
- feat_pe_drop: float = 0.2,
- module_loss: Optional[Dict] = None,
- postprocessor: Optional[Dict] = None,
- dictionary: Optional[Union[Dict, Dictionary]] = None,
- max_seq_len: int = 30,
- init_cfg: Optional[Union[Dict, Sequence[Dict]]] = None,
- ):
- super().__init__(
- module_loss=module_loss,
- postprocessor=postprocessor,
- dictionary=dictionary,
- init_cfg=init_cfg,
- max_seq_len=max_seq_len)
- operation_order = ('norm', 'self_attn', 'norm', 'cross_attn', 'norm',
- 'ffn')
- decoder_layer = BaseTransformerLayer(
- operation_order=operation_order,
- attn_cfgs=dict(
- type='MultiheadAttention',
- embed_dims=d_model,
- num_heads=n_head,
- attn_drop=attn_drop,
- dropout_layer=dict(type='Dropout', drop_prob=attn_drop),
- ),
- ffn_cfgs=dict(
- type='FFN',
- embed_dims=d_model,
- feedforward_channels=d_inner,
- ffn_drop=ffn_drop,
- dropout_layer=dict(type='Dropout', drop_prob=ffn_drop),
- ),
- norm_cfg=dict(type='LN'),
- batch_first=True,
- )
- self.decoder_layers = ModuleList(
- [copy.deepcopy(decoder_layer) for _ in range(n_layers)])
-
- self.cls = nn.Linear(d_model, self.dictionary.num_classes)
-
- self.SOS = self.dictionary.start_idx
- self.PAD = self.dictionary.padding_idx
- self.max_seq_len = max_seq_len
- self.feat_size = feat_size
- self.n_head = n_head
-
- self.embedding = Embeddings(
- d_model=d_model, vocab=self.dictionary.num_classes)
-
- # TODO:
- self.positional_encoding = PositionalEncoding(
- d_hid=d_model, n_position=self.max_seq_len + 1)
- self.feat_positional_encoding = PositionalEncoding(
- d_hid=d_model, n_position=self.feat_size, dropout=feat_pe_drop)
- self.norm = nn.LayerNorm(d_model)
- self.softmax = nn.Softmax(dim=-1)
-
- def make_target_mask(self, tgt: torch.Tensor,
- device: torch.device) -> torch.Tensor:
- """Make target mask for self attention.
-
- Args:
- tgt (Tensor): Shape [N, l_tgt]
- device (torch.device): Mask device.
-
- Returns:
- Tensor: Mask of shape [N * self.n_head, l_tgt, l_tgt]
- """
-
- trg_pad_mask = (tgt != self.PAD).unsqueeze(1).unsqueeze(3).bool()
- tgt_len = tgt.size(1)
- trg_sub_mask = torch.tril(
- torch.ones((tgt_len, tgt_len), dtype=torch.bool, device=device))
- tgt_mask = trg_pad_mask & trg_sub_mask
-
- # inverse for mmcv's BaseTransformerLayer
- tril_mask = tgt_mask.clone()
- tgt_mask = tgt_mask.float().masked_fill_(tril_mask == 0, -1e9)
- tgt_mask = tgt_mask.masked_fill_(tril_mask, 0)
- tgt_mask = tgt_mask.repeat(1, self.n_head, 1, 1)
- tgt_mask = tgt_mask.view(-1, tgt_len, tgt_len)
- return tgt_mask
-
- def decode(self, tgt_seq: torch.Tensor, feature: torch.Tensor,
- src_mask: torch.BoolTensor,
- tgt_mask: torch.BoolTensor) -> torch.Tensor:
- """Decode the input sequence.
-
- Args:
- tgt_seq (Tensor): Target sequence of shape: math: `(N, T, C)`.
- feature (Tensor): Input feature map from encoder of
- shape: math: `(N, C, H, W)`
- src_mask (BoolTensor): The source mask of shape: math: `(N, H*W)`.
- tgt_mask (BoolTensor): The target mask of shape: math: `(N, T, T)`.
-
- Return:
- Tensor: The decoded sequence.
- """
- tgt_seq = self.embedding(tgt_seq)
- x = self.positional_encoding(tgt_seq)
- attn_masks = [tgt_mask, src_mask]
- for layer in self.decoder_layers:
- x = layer(
- query=x, key=feature, value=feature, attn_masks=attn_masks)
- x = self.norm(x)
- return self.cls(x)
-
- def forward_train(self,
- feat: Optional[torch.Tensor] = None,
- out_enc: torch.Tensor = None,
- data_samples: Sequence[TextRecogDataSample] = None
- ) -> torch.Tensor:
- """Forward for training. Source mask will not be used here.
-
- Args:
- feat (Tensor, optional): Input feature map from backbone.
- out_enc (Tensor): Unused.
- data_samples (list[TextRecogDataSample]): Batch of
- TextRecogDataSample, containing gt_text and valid_ratio
- information.
-
- Returns:
- Tensor: The raw logit tensor. Shape :math:`(N, T, C)` where
- :math:`C` is ``num_classes``.
- """
-
- # flatten 2D feature map
- if len(feat.shape) > 3:
- b, c, h, w = feat.shape
- feat = feat.view(b, c, h * w)
- feat = feat.permute((0, 2, 1))
- feat = self.feat_positional_encoding(feat)
-
- trg_seq = []
- for target in data_samples:
- trg_seq.append(target.gt_text.padded_indexes.to(feat.device))
-
- trg_seq = torch.stack(trg_seq, dim=0)
-
- src_mask = None
- tgt_mask = self.make_target_mask(trg_seq, device=feat.device)
- return self.decode(trg_seq, feat, src_mask, tgt_mask)
-
- def forward_test(self,
- feat: Optional[torch.Tensor] = None,
- out_enc: torch.Tensor = None,
- data_samples: Sequence[TextRecogDataSample] = None
- ) -> torch.Tensor:
- """Forward for testing.
-
- Args:
- feat (Tensor, optional): Input feature map from backbone.
- out_enc (Tensor): Unused.
- data_samples (list[TextRecogDataSample]): Unused.
-
- Returns:
- Tensor: Character probabilities. of shape
- :math:`(N, self.max_seq_len, C)` where :math:`C` is
- ``num_classes``.
- """
-
- # flatten 2D feature map
- if len(feat.shape) > 3:
- b, c, h, w = feat.shape
- feat = feat.view(b, c, h * w)
- feat = feat.permute((0, 2, 1))
- feat = self.feat_positional_encoding(feat)
-
- N = feat.shape[0]
- input = torch.full((N, 1),
- self.SOS,
- device=feat.device,
- dtype=torch.long)
- output = None
- for _ in range(self.max_seq_len):
- target_mask = self.make_target_mask(input, device=feat.device)
- out = self.decode(input, feat, None, target_mask)
- output = out
- _, next_word = torch.max(out, dim=-1)
- input = torch.cat([input, next_word[:, -1].unsqueeze(-1)], dim=1)
- return self.softmax(output)
diff --git a/pipeline/mmocr/mmocr/models/textrecog/decoders/nrtr_decoder.py b/pipeline/mmocr/mmocr/models/textrecog/decoders/nrtr_decoder.py
deleted file mode 100644
index dc986c48807e696b2001d3d91ae33a0312ae9044..0000000000000000000000000000000000000000
--- a/pipeline/mmocr/mmocr/models/textrecog/decoders/nrtr_decoder.py
+++ /dev/null
@@ -1,257 +0,0 @@
-# Copyright (c) OpenMMLab. All rights reserved.
-import math
-from typing import Dict, List, Optional, Sequence, Union
-
-import torch
-import torch.nn as nn
-from mmengine.model import ModuleList
-
-from mmocr.models.common import PositionalEncoding, TFDecoderLayer
-from mmocr.models.common.dictionary import Dictionary
-from mmocr.registry import MODELS
-from mmocr.structures import TextRecogDataSample
-from .base import BaseDecoder
-
-
-@MODELS.register_module()
-class NRTRDecoder(BaseDecoder):
- """Transformer Decoder block with self attention mechanism.
-
- Args:
- n_layers (int): Number of attention layers. Defaults to 6.
- d_embedding (int): Language embedding dimension. Defaults to 512.
- n_head (int): Number of parallel attention heads. Defaults to 8.
- d_k (int): Dimension of the key vector. Defaults to 64.
- d_v (int): Dimension of the value vector. Defaults to 64
- d_model (int): Dimension :math:`D_m` of the input from previous model.
- Defaults to 512.
- d_inner (int): Hidden dimension of feedforward layers. Defaults to 256.
- n_position (int): Length of the positional encoding vector. Must be
- greater than ``max_seq_len``. Defaults to 200.
- dropout (float): Dropout rate for text embedding, MHSA, FFN. Defaults
- to 0.1.
- module_loss (dict, optional): Config to build module_loss. Defaults
- to None.
- postprocessor (dict, optional): Config to build postprocessor.
- Defaults to None.
- dictionary (dict or :obj:`Dictionary`): The config for `Dictionary` or
- the instance of `Dictionary`.
- max_seq_len (int): Maximum output sequence length :math:`T`. Defaults
- to 30.
- init_cfg (dict or list[dict], optional): Initialization configs.
- """
-
- def __init__(self,
- n_layers: int = 6,
- d_embedding: int = 512,
- n_head: int = 8,
- d_k: int = 64,
- d_v: int = 64,
- d_model: int = 512,
- d_inner: int = 256,
- n_position: int = 200,
- dropout: float = 0.1,
- module_loss: Optional[Dict] = None,
- postprocessor: Optional[Dict] = None,
- dictionary: Optional[Union[Dict, Dictionary]] = None,
- max_seq_len: int = 30,
- init_cfg: Optional[Union[Dict, List[Dict]]] = None) -> None:
- super().__init__(
- module_loss=module_loss,
- postprocessor=postprocessor,
- dictionary=dictionary,
- init_cfg=init_cfg,
- max_seq_len=max_seq_len)
-
- self.padding_idx = self.dictionary.padding_idx
- self.start_idx = self.dictionary.start_idx
- self.max_seq_len = max_seq_len
-
- self.trg_word_emb = nn.Embedding(
- self.dictionary.num_classes,
- d_embedding,
- padding_idx=self.padding_idx)
-
- self.position_enc = PositionalEncoding(
- d_embedding, n_position=n_position)
- self.dropout = nn.Dropout(p=dropout)
-
- self.layer_stack = ModuleList([
- TFDecoderLayer(
- d_model, d_inner, n_head, d_k, d_v, dropout=dropout)
- for _ in range(n_layers)
- ])
- self.layer_norm = nn.LayerNorm(d_model, eps=1e-6)
-
- pred_num_class = self.dictionary.num_classes
- self.classifier = nn.Linear(d_model, pred_num_class)
- self.softmax = nn.Softmax(dim=-1)
-
- def _get_target_mask(self, trg_seq: torch.Tensor) -> torch.Tensor:
- """Generate mask for target sequence.
-
- Args:
- trg_seq (torch.Tensor): Input text sequence. Shape :math:`(N, T)`.
-
- Returns:
- Tensor: Target mask. Shape :math:`(N, T, T)`.
- E.g.:
- seq = torch.Tensor([[1, 2, 0, 0]]), pad_idx = 0, then
- target_mask =
- torch.Tensor([[[True, False, False, False],
- [True, True, False, False],
- [True, True, False, False],
- [True, True, False, False]]])
- """
-
- pad_mask = (trg_seq != self.padding_idx).unsqueeze(-2)
-
- len_s = trg_seq.size(1)
- subsequent_mask = 1 - torch.triu(
- torch.ones((len_s, len_s), device=trg_seq.device), diagonal=1)
- subsequent_mask = subsequent_mask.unsqueeze(0).bool()
-
- return pad_mask & subsequent_mask
-
- def _get_source_mask(self, src_seq: torch.Tensor,
- valid_ratios: Sequence[float]) -> torch.Tensor:
- """Generate mask for source sequence.
-
- Args:
- src_seq (torch.Tensor): Image sequence. Shape :math:`(N, T, C)`.
- valid_ratios (list[float]): The valid ratio of input image. For
- example, if the width of the original image is w1 and the width
- after padding is w2, then valid_ratio = w1/w2. Source mask is
- used to cover the area of the padding region.
-
- Returns:
- Tensor or None: Source mask. Shape :math:`(N, T)`. The region of
- padding area are False, and the rest are True.
- """
-
- N, T, _ = src_seq.size()
- mask = None
- if len(valid_ratios) > 0:
- mask = src_seq.new_zeros((N, T), device=src_seq.device)
- for i, valid_ratio in enumerate(valid_ratios):
- valid_width = min(T, math.ceil(T * valid_ratio))
- mask[i, :valid_width] = 1
-
- return mask
-
- def _attention(self,
- trg_seq: torch.Tensor,
- src: torch.Tensor,
- src_mask: Optional[torch.Tensor] = None) -> torch.Tensor:
- """A wrapped process for transformer based decoder including text
- embedding, position embedding, N x transformer decoder and a LayerNorm
- operation.
-
- Args:
- trg_seq (Tensor): Target sequence in. Shape :math:`(N, T)`.
- src (Tensor): Source sequence from encoder in shape
- Shape :math:`(N, T, D_m)` where :math:`D_m` is ``d_model``.
- src_mask (Tensor, Optional): Mask for source sequence.
- Shape :math:`(N, T)`. Defaults to None.
-
- Returns:
- Tensor: Output sequence from transformer decoder.
- Shape :math:`(N, T, D_m)` where :math:`D_m` is ``d_model``.
- """
-
- trg_embedding = self.trg_word_emb(trg_seq)
- trg_pos_encoded = self.position_enc(trg_embedding)
- trg_mask = self._get_target_mask(trg_seq)
- tgt_seq = self.dropout(trg_pos_encoded)
-
- output = tgt_seq
- for dec_layer in self.layer_stack:
- output = dec_layer(
- output,
- src,
- self_attn_mask=trg_mask,
- dec_enc_attn_mask=src_mask)
- output = self.layer_norm(output)
-
- return output
-
- def forward_train(self,
- feat: Optional[torch.Tensor] = None,
- out_enc: torch.Tensor = None,
- data_samples: Sequence[TextRecogDataSample] = None
- ) -> torch.Tensor:
- """Forward for training. Source mask will be used here.
-
- Args:
- feat (Tensor, optional): Unused.
- out_enc (Tensor): Encoder output of shape : math:`(N, T, D_m)`
- where :math:`D_m` is ``d_model``. Defaults to None.
- data_samples (list[TextRecogDataSample]): Batch of
- TextRecogDataSample, containing gt_text and valid_ratio
- information. Defaults to None.
-
- Returns:
- Tensor: The raw logit tensor. Shape :math:`(N, T, C)` where
- :math:`C` is ``num_classes``.
- """
- valid_ratios = []
- for data_sample in data_samples:
- valid_ratios.append(data_sample.get('valid_ratio'))
- src_mask = self._get_source_mask(out_enc, valid_ratios)
- trg_seq = []
- for data_sample in data_samples:
- trg_seq.append(
- data_sample.gt_text.padded_indexes.to(out_enc.device))
- trg_seq = torch.stack(trg_seq, dim=0)
- attn_output = self._attention(trg_seq, out_enc, src_mask=src_mask)
- outputs = self.classifier(attn_output)
-
- return outputs
-
- def forward_test(self,
- feat: Optional[torch.Tensor] = None,
- out_enc: torch.Tensor = None,
- data_samples: Sequence[TextRecogDataSample] = None
- ) -> torch.Tensor:
- """Forward for testing.
-
- Args:
- feat (Tensor, optional): Unused.
- out_enc (Tensor): Encoder output of shape:
- math:`(N, T, D_m)` where :math:`D_m` is ``d_model``.
- Defaults to None.
- data_samples (list[TextRecogDataSample]): Batch of
- TextRecogDataSample, containing gt_text and valid_ratio
- information. Defaults to None.
-
- Returns:
- Tensor: Character probabilities. of shape
- :math:`(N, self.max_seq_len, C)` where :math:`C` is
- ``num_classes``.
- """
- valid_ratios = []
- for data_sample in data_samples:
- valid_ratios.append(data_sample.get('valid_ratio'))
- src_mask = self._get_source_mask(out_enc, valid_ratios)
- N = out_enc.size(0)
- init_target_seq = torch.full((N, self.max_seq_len + 1),
- self.padding_idx,
- device=out_enc.device,
- dtype=torch.long)
- # bsz * seq_len
- init_target_seq[:, 0] = self.start_idx
-
- outputs = []
- for step in range(0, self.max_seq_len):
- decoder_output = self._attention(
- init_target_seq, out_enc, src_mask=src_mask)
- # bsz * seq_len * C
- step_result = self.classifier(decoder_output[:, step, :])
- # bsz * num_classes
- outputs.append(step_result)
- _, step_max_index = torch.max(step_result, dim=-1)
- init_target_seq[:, step + 1] = step_max_index
-
- outputs = torch.stack(outputs, dim=1)
-
- return self.softmax(outputs)
diff --git a/pipeline/mmocr/mmocr/models/textrecog/decoders/position_attention_decoder.py b/pipeline/mmocr/mmocr/models/textrecog/decoders/position_attention_decoder.py
deleted file mode 100644
index 7543c2b199814143fab916d811cc419c1163274a..0000000000000000000000000000000000000000
--- a/pipeline/mmocr/mmocr/models/textrecog/decoders/position_attention_decoder.py
+++ /dev/null
@@ -1,220 +0,0 @@
-# Copyright (c) OpenMMLab. All rights reserved.
-import math
-from typing import Dict, Optional, Sequence, Union
-
-import torch
-import torch.nn as nn
-
-from mmocr.models.common.dictionary import Dictionary
-from mmocr.models.textrecog.layers import (DotProductAttentionLayer,
- PositionAwareLayer)
-from mmocr.registry import MODELS
-from mmocr.structures import TextRecogDataSample
-from .base import BaseDecoder
-
-
-@MODELS.register_module()
-class PositionAttentionDecoder(BaseDecoder):
- """Position attention decoder for RobustScanner.
-
- RobustScanner: `RobustScanner: Dynamically Enhancing Positional Clues for
- Robust Text Recognition `_
-
- Args:
- dictionary (dict or :obj:`Dictionary`): The config for `Dictionary` or
- the instance of `Dictionary`.
- module_loss (dict, optional): Config to build module_loss. Defaults
- to None.
- postprocessor (dict, optional): Config to build postprocessor.
- Defaults to None.
- rnn_layers (int): Number of RNN layers. Defaults to 2.
- dim_input (int): Dimension :math:`D_i` of input vector ``feat``.
- Defaults to 512.
- dim_model (int): Dimension :math:`D_m` of the model. Should also be the
- same as encoder output vector ``out_enc``. Defaults to 128.
- max_seq_len (int): Maximum output sequence length :math:`T`. Defaults
- to 40.
- mask (bool): Whether to mask input features according to
- ``img_meta['valid_ratio']``. Defaults to True.
- return_feature (bool): Return feature or logits as the result. Defaults
- to True.
- encode_value (bool): Whether to use the output of encoder ``out_enc``
- as `value` of attention layer. If False, the original feature
- ``feat`` will be used. Defaults to False.
- init_cfg (dict or list[dict], optional): Initialization configs.
- Defaults to None.
- """
-
- def __init__(self,
- dictionary: Union[Dictionary, Dict],
- module_loss: Optional[Dict] = None,
- postprocessor: Optional[Dict] = None,
- rnn_layers: int = 2,
- dim_input: int = 512,
- dim_model: int = 128,
- max_seq_len: int = 40,
- mask: bool = True,
- return_feature: bool = True,
- encode_value: bool = False,
- init_cfg: Optional[Union[Dict,
- Sequence[Dict]]] = None) -> None:
- super().__init__(
- dictionary=dictionary,
- module_loss=module_loss,
- postprocessor=postprocessor,
- max_seq_len=max_seq_len,
- init_cfg=init_cfg)
-
- self.dim_input = dim_input
- self.dim_model = dim_model
- self.return_feature = return_feature
- self.encode_value = encode_value
- self.mask = mask
-
- self.embedding = nn.Embedding(self.max_seq_len + 1, self.dim_model)
-
- self.position_aware_module = PositionAwareLayer(
- self.dim_model, rnn_layers)
-
- self.attention_layer = DotProductAttentionLayer()
-
- self.prediction = None
- if not self.return_feature:
- self.prediction = nn.Linear(
- dim_model if encode_value else dim_input,
- self.dictionary.num_classes)
- self.softmax = nn.Softmax(dim=-1)
-
- def _get_position_index(self,
- length: int,
- batch_size: int,
- device: Optional[torch.device] = None
- ) -> torch.Tensor:
- """Get position index for position attention.
-
- Args:
- length (int): Length of the sequence.
- batch_size (int): Batch size.
- device (torch.device, optional): Device. Defaults to None.
-
- Returns:
- torch.Tensor: Position index.
- """
- position_index = torch.arange(0, length, device=device)
- position_index = position_index.repeat([batch_size, 1])
- position_index = position_index.long()
- return position_index
-
- def forward_train(self, feat: torch.Tensor, out_enc: torch.Tensor,
- data_samples: Sequence[TextRecogDataSample]
- ) -> torch.Tensor:
- """
- Args:
- feat (Tensor): Tensor of shape :math:`(N, D_i, H, W)`.
- out_enc (Tensor): Encoder output of shape
- :math:`(N, D_m, H, W)`.
- data_samples (list[TextRecogDataSample], optional): Batch of
- TextRecogDataSample, containing gt_text information. Defaults
- to None.
-
- Returns:
- Tensor: A raw logit tensor of shape :math:`(N, T, C)` if
- ``return_feature=False``. Otherwise it will be the hidden feature
- before the prediction projection layer, whose shape is
- :math:`(N, T, D_m)`.
- """
- valid_ratios = [
- data_sample.get('valid_ratio', 1.0) for data_sample in data_samples
- ] if self.mask else None
-
- #
- n, c_enc, h, w = out_enc.size()
- assert c_enc == self.dim_model
- _, c_feat, _, _ = feat.size()
- assert c_feat == self.dim_input
- position_index = self._get_position_index(self.max_seq_len, n,
- feat.device)
-
- position_out_enc = self.position_aware_module(out_enc)
-
- query = self.embedding(position_index)
- query = query.permute(0, 2, 1).contiguous()
- key = position_out_enc.view(n, c_enc, h * w)
- if self.encode_value:
- value = out_enc.view(n, c_enc, h * w)
- else:
- value = feat.view(n, c_feat, h * w)
-
- mask = None
- if valid_ratios is not None:
- mask = query.new_zeros((n, h, w))
- for i, valid_ratio in enumerate(valid_ratios):
- valid_width = min(w, math.ceil(w * valid_ratio))
- mask[i, :, valid_width:] = 1
- mask = mask.bool()
- mask = mask.view(n, h * w)
-
- attn_out = self.attention_layer(query, key, value, mask)
- attn_out = attn_out.permute(0, 2,
- 1).contiguous() # [n, max_seq_len, dim_v]
-
- if self.return_feature:
- return attn_out
-
- return self.prediction(attn_out)
-
- def forward_test(self, feat: torch.Tensor, out_enc: torch.Tensor,
- img_metas: Sequence[TextRecogDataSample]) -> torch.Tensor:
- """
- Args:
- feat (Tensor): Tensor of shape :math:`(N, D_i, H, W)`.
- out_enc (Tensor): Encoder output of shape
- :math:`(N, D_m, H, W)`.
- data_samples (list[TextRecogDataSample], optional): Batch of
- TextRecogDataSample, containing gt_text information. Defaults
- to None.
-
- Returns:
- Tensor: Character probabilities of shape :math:`(N, T, C)` if
- ``return_feature=False``. Otherwise it would be the hidden feature
- before the prediction projection layer, whose shape is
- :math:`(N, T, D_m)`.
- """
- valid_ratios = [
- img_meta.get('valid_ratio', 1.0) for img_meta in img_metas
- ] if self.mask else None
-
- seq_len = self.max_seq_len
- n, c_enc, h, w = out_enc.size()
- assert c_enc == self.dim_model
- _, c_feat, _, _ = feat.size()
- assert c_feat == self.dim_input
-
- position_index = self._get_position_index(seq_len, n, feat.device)
-
- position_out_enc = self.position_aware_module(out_enc)
-
- query = self.embedding(position_index)
- query = query.permute(0, 2, 1).contiguous()
- key = position_out_enc.view(n, c_enc, h * w)
- if self.encode_value:
- value = out_enc.view(n, c_enc, h * w)
- else:
- value = feat.view(n, c_feat, h * w)
-
- mask = None
- if valid_ratios is not None:
- mask = query.new_zeros((n, h, w))
- for i, valid_ratio in enumerate(valid_ratios):
- valid_width = min(w, math.ceil(w * valid_ratio))
- mask[i, :, valid_width:] = 1
- mask = mask.bool()
- mask = mask.view(n, h * w)
-
- attn_out = self.attention_layer(query, key, value, mask)
- attn_out = attn_out.permute(0, 2, 1).contiguous()
-
- if self.return_feature:
- return attn_out
-
- return self.softmax(self.prediction(attn_out))
diff --git a/pipeline/mmocr/mmocr/models/textrecog/decoders/robust_scanner_fuser.py b/pipeline/mmocr/mmocr/models/textrecog/decoders/robust_scanner_fuser.py
deleted file mode 100644
index be954e53fcfd13af59395ea911cb91f67c378c3f..0000000000000000000000000000000000000000
--- a/pipeline/mmocr/mmocr/models/textrecog/decoders/robust_scanner_fuser.py
+++ /dev/null
@@ -1,146 +0,0 @@
-# Copyright (c) OpenMMLab. All rights reserved.
-import warnings
-from typing import Dict, List, Optional, Sequence, Union
-
-import torch
-import torch.nn as nn
-
-from mmocr.models.common.dictionary import Dictionary
-from mmocr.registry import MODELS
-from mmocr.structures import TextRecogDataSample
-from .base import BaseDecoder
-
-
-@MODELS.register_module()
-class RobustScannerFuser(BaseDecoder):
- """Decoder for RobustScanner.
-
- Args:
- dictionary (dict or :obj:`Dictionary`): The config for `Dictionary` or
- the instance of `Dictionary`.
- module_loss (dict, optional): Config to build module_loss. Defaults
- to None.
- postprocessor (dict, optional): Config to build postprocessor.
- Defaults to None.
- hybrid_decoder (dict): Config to build hybrid_decoder. Defaults to
- dict(type='SequenceAttentionDecoder').
- position_decoder (dict): Config to build position_decoder. Defaults to
- dict(type='PositionAttentionDecoder').
- fuser (dict): Config to build fuser. Defaults to
- dict(type='RobustScannerFuser').
- max_seq_len (int): Maximum sequence length. The
- sequence is usually generated from decoder. Defaults to 30.
- in_channels (list[int]): List of input channels.
- Defaults to [512, 512].
- dim (int): The dimension on which to split the input. Defaults to -1.
- init_cfg (dict or list[dict], optional): Initialization configs.
- Defaults to None.
- """
-
- def __init__(self,
- dictionary: Union[Dict, Dictionary],
- module_loss: Optional[Dict] = None,
- postprocessor: Optional[Dict] = None,
- hybrid_decoder: Dict = dict(type='SequenceAttentionDecoder'),
- position_decoder: Dict = dict(
- type='PositionAttentionDecoder'),
- max_seq_len: int = 30,
- in_channels: List[int] = [512, 512],
- dim: int = -1,
- init_cfg: Optional[Union[Dict, List[Dict]]] = None) -> None:
- super().__init__(
- dictionary=dictionary,
- module_loss=module_loss,
- postprocessor=postprocessor,
- max_seq_len=max_seq_len,
- init_cfg=init_cfg)
-
- for cfg_name in ['hybrid_decoder', 'position_decoder']:
- cfg = eval(cfg_name)
- if cfg is not None:
- if cfg.get('dictionary', None) is None:
- cfg.update(dictionary=self.dictionary)
- else:
- warnings.warn(f"Using dictionary {cfg['dictionary']} "
- "in decoder's config.")
- if cfg.get('max_seq_len', None) is None:
- cfg.update(max_seq_len=max_seq_len)
- else:
- warnings.warn(f"Using max_seq_len {cfg['max_seq_len']} "
- "in decoder's config.")
- setattr(self, cfg_name, MODELS.build(cfg))
-
- in_channels = sum(in_channels)
- self.dim = dim
-
- self.linear_layer = nn.Linear(in_channels, in_channels)
- self.glu_layer = nn.GLU(dim=dim)
- self.prediction = nn.Linear(
- int(in_channels / 2), self.dictionary.num_classes)
- self.softmax = nn.Softmax(dim=-1)
-
- def forward_train(
- self,
- feat: Optional[torch.Tensor] = None,
- out_enc: Optional[torch.Tensor] = None,
- data_samples: Optional[Sequence[TextRecogDataSample]] = None
- ) -> torch.Tensor:
- """Forward for training.
-
- Args:
- feat (torch.Tensor, optional): The feature map from backbone of
- shape :math:`(N, E, H, W)`. Defaults to None.
- out_enc (torch.Tensor, optional): Encoder output. Defaults to None.
- data_samples (Sequence[TextRecogDataSample]): Batch of
- TextRecogDataSample, containing gt_text information. Defaults
- to None.
- """
- hybrid_glimpse = self.hybrid_decoder(feat, out_enc, data_samples)
- position_glimpse = self.position_decoder(feat, out_enc, data_samples)
- fusion_input = torch.cat([hybrid_glimpse, position_glimpse], self.dim)
- outputs = self.linear_layer(fusion_input)
- outputs = self.glu_layer(outputs)
- return self.prediction(outputs)
-
- def forward_test(
- self,
- feat: Optional[torch.Tensor] = None,
- out_enc: Optional[torch.Tensor] = None,
- data_samples: Optional[Sequence[TextRecogDataSample]] = None
- ) -> torch.Tensor:
- """Forward for testing.
-
- Args:
- feat (torch.Tensor, optional): The feature map from backbone of
- shape :math:`(N, E, H, W)`. Defaults to None.
- out_enc (torch.Tensor, optional): Encoder output. Defaults to None.
- data_samples (Sequence[TextRecogDataSample]): Batch of
- TextRecogDataSample, containing vaild_ratio information.
- Defaults to None.
-
- Returns:
- Tensor: Character probabilities. of shape
- :math:`(N, self.max_seq_len, C)` where :math:`C` is
- ``num_classes``.
- """
- position_glimpse = self.position_decoder(feat, out_enc, data_samples)
-
- batch_size = feat.size(0)
- decode_sequence = (feat.new_ones((batch_size, self.max_seq_len)) *
- self.dictionary.start_idx).long()
- outputs = []
- for step in range(self.max_seq_len):
- hybrid_glimpse_step = self.hybrid_decoder.forward_test_step(
- feat, out_enc, decode_sequence, step, data_samples)
-
- fusion_input = torch.cat(
- [hybrid_glimpse_step, position_glimpse[:, step, :]], self.dim)
- output = self.linear_layer(fusion_input)
- output = self.glu_layer(output)
- output = self.prediction(output)
- _, max_idx = torch.max(output, dim=1, keepdim=False)
- if step < self.max_seq_len - 1:
- decode_sequence[:, step + 1] = max_idx
- outputs.append(output)
- outputs = torch.stack(outputs, 1)
- return self.softmax(outputs)
diff --git a/pipeline/mmocr/mmocr/models/textrecog/decoders/sar_decoder.py b/pipeline/mmocr/mmocr/models/textrecog/decoders/sar_decoder.py
deleted file mode 100644
index d156c30fd144a5256965c7bc376ab5645c925792..0000000000000000000000000000000000000000
--- a/pipeline/mmocr/mmocr/models/textrecog/decoders/sar_decoder.py
+++ /dev/null
@@ -1,574 +0,0 @@
-# Copyright (c) OpenMMLab. All rights reserved.
-import math
-from typing import Dict, List, Optional, Sequence, Union
-
-import torch
-import torch.nn as nn
-import torch.nn.functional as F
-
-from mmocr.models.common.dictionary import Dictionary
-from mmocr.registry import MODELS
-from mmocr.structures import TextRecogDataSample
-from .base import BaseDecoder
-
-
-@MODELS.register_module()
-class ParallelSARDecoder(BaseDecoder):
- """Implementation Parallel Decoder module in `SAR.
-
- `_.
-
- Args:
- dictionary (dict or :obj:`Dictionary`): The config for `Dictionary` or
- the instance of `Dictionary`.
- module_loss (dict, optional): Config to build module_loss. Defaults
- to None.
- postprocessor (dict, optional): Config to build postprocessor.
- Defaults to None.
- enc_bi_rnn (bool): If True, use bidirectional RNN in encoder.
- Defaults to False.
- dec_bi_rnn (bool): If True, use bidirectional RNN in decoder.
- Defaults to False.
- dec_rnn_dropout (float): Dropout of RNN layer in decoder.
- Defaults to 0.0.
- dec_gru (bool): If True, use GRU, else LSTM in decoder. Defaults to
- False.
- d_model (int): Dim of channels from backbone :math:`D_i`. Defaults
- to 512.
- d_enc (int): Dim of encoder RNN layer :math:`D_m`. Defaults to 512.
- d_k (int): Dim of channels of attention module. Defaults to 64.
- pred_dropout (float): Dropout probability of prediction layer. Defaults
- to 0.0.
- max_seq_len (int): Maximum sequence length for decoding. Defaults to
- 30.
- mask (bool): If True, mask padding in feature map. Defaults to True.
- pred_concat (bool): If True, concat glimpse feature from
- attention with holistic feature and hidden state. Defaults to
- False.
- init_cfg (dict or list[dict], optional): Initialization configs.
- Defaults to None.
- """
-
- def __init__(self,
- dictionary: Union[Dict, Dictionary],
- module_loss: Optional[Dict] = None,
- postprocessor: Optional[Dict] = None,
- enc_bi_rnn: bool = False,
- dec_bi_rnn: bool = False,
- dec_rnn_dropout: Union[int, float] = 0.0,
- dec_gru: bool = False,
- d_model: int = 512,
- d_enc: int = 512,
- d_k: int = 64,
- pred_dropout: float = 0.0,
- max_seq_len: int = 30,
- mask: bool = True,
- pred_concat: bool = False,
- init_cfg: Optional[Union[Dict, List[Dict]]] = None,
- **kwargs) -> None:
- super().__init__(
- dictionary=dictionary,
- module_loss=module_loss,
- max_seq_len=max_seq_len,
- postprocessor=postprocessor,
- init_cfg=init_cfg)
-
- self.num_classes = self.dictionary.num_classes
- self.enc_bi_rnn = enc_bi_rnn
- self.d_k = d_k
- self.start_idx = self.dictionary.start_idx
- self.mask = mask
- self.pred_concat = pred_concat
-
- encoder_rnn_out_size = d_enc * (int(enc_bi_rnn) + 1)
- decoder_rnn_out_size = encoder_rnn_out_size * (int(dec_bi_rnn) + 1)
- # 2D attention layer
- self.conv1x1_1 = nn.Linear(decoder_rnn_out_size, d_k)
- self.conv3x3_1 = nn.Conv2d(
- d_model, d_k, kernel_size=3, stride=1, padding=1)
- self.conv1x1_2 = nn.Linear(d_k, 1)
-
- # Decoder RNN layer
- kwargs = dict(
- input_size=encoder_rnn_out_size,
- hidden_size=encoder_rnn_out_size,
- num_layers=2,
- batch_first=True,
- dropout=dec_rnn_dropout,
- bidirectional=dec_bi_rnn)
- if dec_gru:
- self.rnn_decoder = nn.GRU(**kwargs)
- else:
- self.rnn_decoder = nn.LSTM(**kwargs)
-
- # Decoder input embedding
- self.embedding = nn.Embedding(
- self.num_classes,
- encoder_rnn_out_size,
- padding_idx=self.dictionary.padding_idx)
-
- # Prediction layer
- self.pred_dropout = nn.Dropout(pred_dropout)
- if pred_concat:
- fc_in_channel = decoder_rnn_out_size + d_model + \
- encoder_rnn_out_size
- else:
- fc_in_channel = d_model
- self.prediction = nn.Linear(fc_in_channel, self.num_classes)
- self.softmax = nn.Softmax(dim=-1)
-
- def _2d_attention(self,
- decoder_input: torch.Tensor,
- feat: torch.Tensor,
- holistic_feat: torch.Tensor,
- valid_ratios: Optional[Sequence[float]] = None
- ) -> torch.Tensor:
- """2D attention layer.
-
- Args:
- decoder_input (torch.Tensor): Input of decoder RNN.
- feat (torch.Tensor): Feature map of encoder.
- holistic_feat (torch.Tensor): Feature map of holistic encoder.
- valid_ratios (Sequence[float]): Valid ratios of attention.
- Defaults to None.
-
- Returns:
- torch.Tensor: Output of 2D attention layer.
- """
- y = self.rnn_decoder(decoder_input)[0]
- # y: bsz * (seq_len + 1) * hidden_size
-
- attn_query = self.conv1x1_1(y) # bsz * (seq_len + 1) * attn_size
- bsz, seq_len, attn_size = attn_query.size()
- attn_query = attn_query.view(bsz, seq_len, attn_size, 1, 1)
-
- attn_key = self.conv3x3_1(feat)
- # bsz * attn_size * h * w
- attn_key = attn_key.unsqueeze(1)
- # bsz * 1 * attn_size * h * w
-
- attn_weight = torch.tanh(torch.add(attn_key, attn_query, alpha=1))
- # bsz * (seq_len + 1) * attn_size * h * w
- attn_weight = attn_weight.permute(0, 1, 3, 4, 2).contiguous()
- # bsz * (seq_len + 1) * h * w * attn_size
- attn_weight = self.conv1x1_2(attn_weight)
- # bsz * (seq_len + 1) * h * w * 1
- bsz, T, h, w, c = attn_weight.size()
- assert c == 1
-
- if valid_ratios is not None:
- # cal mask of attention weight
- attn_mask = torch.zeros_like(attn_weight)
- for i, valid_ratio in enumerate(valid_ratios):
- valid_width = min(w, math.ceil(w * valid_ratio))
- attn_mask[i, :, :, valid_width:, :] = 1
- attn_weight = attn_weight.masked_fill(attn_mask.bool(),
- float('-inf'))
-
- attn_weight = attn_weight.view(bsz, T, -1)
- attn_weight = F.softmax(attn_weight, dim=-1)
- attn_weight = attn_weight.view(bsz, T, h, w,
- c).permute(0, 1, 4, 2, 3).contiguous()
-
- attn_feat = torch.sum(
- torch.mul(feat.unsqueeze(1), attn_weight), (3, 4), keepdim=False)
- # bsz * (seq_len + 1) * C
-
- # linear transformation
- if self.pred_concat:
- hf_c = holistic_feat.size(-1)
- holistic_feat = holistic_feat.expand(bsz, seq_len, hf_c)
- y = self.prediction(torch.cat((y, attn_feat, holistic_feat), 2))
- else:
- y = self.prediction(attn_feat)
- # bsz * (seq_len + 1) * num_classes
- y = self.pred_dropout(y)
-
- return y
-
- def forward_train(self, feat: torch.Tensor, out_enc: torch.Tensor,
- data_samples: Sequence[TextRecogDataSample]
- ) -> torch.Tensor:
- """
- Args:
- feat (Tensor): Tensor of shape :math:`(N, D_i, H, W)`.
- out_enc (Tensor): Encoder output of shape
- :math:`(N, D_m, H, W)`.
- data_samples (list[TextRecogDataSample]): Batch of
- TextRecogDataSample, containing gt_text and valid_ratio
- information.
-
- Returns:
- Tensor: A raw logit tensor of shape :math:`(N, T, C)`.
- """
- if data_samples is not None:
- assert len(data_samples) == feat.size(0)
-
- valid_ratios = [
- img_meta.get('valid_ratio', 1.0) for img_meta in data_samples
- ] if self.mask else None
-
- padded_targets = [
- data_sample.gt_text.padded_indexes for data_sample in data_samples
- ]
- padded_targets = torch.stack(padded_targets, dim=0).to(feat.device)
- tgt_embedding = self.embedding(padded_targets)
- # bsz * seq_len * emb_dim
- out_enc = out_enc.unsqueeze(1)
- # bsz * 1 * emb_dim
- in_dec = torch.cat((out_enc, tgt_embedding), dim=1)
- # bsz * (seq_len + 1) * C
- out_dec = self._2d_attention(
- in_dec, feat, out_enc, valid_ratios=valid_ratios)
- # bsz * (seq_len + 1) * num_classes
-
- return out_dec[:, 1:, :] # bsz * seq_len * num_classes
-
- def forward_test(
- self,
- feat: torch.Tensor,
- out_enc: torch.Tensor,
- data_samples: Optional[Sequence[TextRecogDataSample]] = None
- ) -> torch.Tensor:
- """
- Args:
- feat (Tensor): Tensor of shape :math:`(N, D_i, H, W)`.
- out_enc (Tensor): Encoder output of shape
- :math:`(N, D_m, H, W)`.
- data_samples (list[TextRecogDataSample], optional): Batch of
- TextRecogDataSample, containing valid_ratio
- information. Defaults to None.
-
- Returns:
- Tensor: Character probabilities. of shape
- :math:`(N, self.max_seq_len, C)` where :math:`C` is
- ``num_classes``.
- """
- if data_samples is not None:
- assert len(data_samples) == feat.size(0)
-
- valid_ratios = None
- if data_samples is not None:
- valid_ratios = [
- data_sample.get('valid_ratio', 1.0)
- for data_sample in data_samples
- ] if self.mask else None
-
- seq_len = self.max_seq_len
-
- bsz = feat.size(0)
- start_token = torch.full((bsz, ),
- self.start_idx,
- device=feat.device,
- dtype=torch.long)
- # bsz
- start_token = self.embedding(start_token)
- # bsz * emb_dim
- start_token = start_token.unsqueeze(1).expand(-1, seq_len, -1)
- # bsz * seq_len * emb_dim
- out_enc = out_enc.unsqueeze(1)
- # bsz * 1 * emb_dim
- decoder_input = torch.cat((out_enc, start_token), dim=1)
- # bsz * (seq_len + 1) * emb_dim
-
- outputs = []
- for i in range(1, seq_len + 1):
- decoder_output = self._2d_attention(
- decoder_input, feat, out_enc, valid_ratios=valid_ratios)
- char_output = decoder_output[:, i, :] # bsz * num_classes
- outputs.append(char_output)
- _, max_idx = torch.max(char_output, dim=1, keepdim=False)
- char_embedding = self.embedding(max_idx) # bsz * emb_dim
- if i < seq_len:
- decoder_input[:, i + 1, :] = char_embedding
-
- outputs = torch.stack(outputs, 1) # bsz * seq_len * num_classes
-
- return self.softmax(outputs)
-
-
-@MODELS.register_module()
-class SequentialSARDecoder(BaseDecoder):
- """Implementation Sequential Decoder module in `SAR.
-
- `_.
-
- Args:
- dictionary (dict or :obj:`Dictionary`): The config for `Dictionary` or
- the instance of `Dictionary`.
- module_loss (dict, optional): Config to build module_loss. Defaults
- to None.
- postprocessor (dict, optional): Config to build postprocessor.
- Defaults to None.
- enc_bi_rnn (bool): If True, use bidirectional RNN in encoder. Defaults
- to False.
- dec_bi_rnn (bool): If True, use bidirectional RNN in decoder. Defaults
- to False.
- dec_do_rnn (float): Dropout of RNN layer in decoder. Defaults to 0.
- dec_gru (bool): If True, use GRU, else LSTM in decoder. Defaults to
- False.
- d_k (int): Dim of conv layers in attention module. Defaults to 64.
- d_model (int): Dim of channels from backbone :math:`D_i`. Defaults to
- 512.
- d_enc (int): Dim of encoder RNN layer :math:`D_m`. Defaults to 512.
- pred_dropout (float): Dropout probability of prediction layer. Defaults
- to 0.
- max_seq_len (int): Maximum sequence length during decoding. Defaults to
- 40.
- mask (bool): If True, mask padding in feature map. Defaults to False.
- pred_concat (bool): If True, concat glimpse feature from
- attention with holistic feature and hidden state. Defaults to
- False.
- init_cfg (dict or list[dict], optional): Initialization configs.
- Defaults to None.
- """
-
- def __init__(self,
- dictionary: Optional[Union[Dict, Dictionary]] = None,
- module_loss: Optional[Dict] = None,
- postprocessor: Optional[Dict] = None,
- enc_bi_rnn: bool = False,
- dec_bi_rnn: bool = False,
- dec_gru: bool = False,
- d_k: int = 64,
- d_model: int = 512,
- d_enc: int = 512,
- pred_dropout: float = 0.0,
- mask: bool = True,
- max_seq_len: int = 40,
- pred_concat: bool = False,
- init_cfg: Optional[Union[Dict, List[Dict]]] = None,
- **kwargs):
- super().__init__(
- dictionary=dictionary,
- module_loss=module_loss,
- postprocessor=postprocessor,
- max_seq_len=max_seq_len,
- init_cfg=init_cfg)
-
- self.num_classes = self.dictionary.num_classes
- self.enc_bi_rnn = enc_bi_rnn
- self.d_k = d_k
- self.start_idx = self.dictionary.start_idx
- self.dec_gru = dec_gru
- self.mask = mask
- self.pred_concat = pred_concat
-
- encoder_rnn_out_size = d_enc * (int(enc_bi_rnn) + 1)
- decoder_rnn_out_size = encoder_rnn_out_size * (int(dec_bi_rnn) + 1)
- # 2D attention layer
- self.conv1x1_1 = nn.Conv2d(
- decoder_rnn_out_size, d_k, kernel_size=1, stride=1)
- self.conv3x3_1 = nn.Conv2d(
- d_model, d_k, kernel_size=3, stride=1, padding=1)
- self.conv1x1_2 = nn.Conv2d(d_k, 1, kernel_size=1, stride=1)
-
- # Decoder rnn layer
- if dec_gru:
- self.rnn_decoder_layer1 = nn.GRUCell(encoder_rnn_out_size,
- encoder_rnn_out_size)
- self.rnn_decoder_layer2 = nn.GRUCell(encoder_rnn_out_size,
- encoder_rnn_out_size)
- else:
- self.rnn_decoder_layer1 = nn.LSTMCell(encoder_rnn_out_size,
- encoder_rnn_out_size)
- self.rnn_decoder_layer2 = nn.LSTMCell(encoder_rnn_out_size,
- encoder_rnn_out_size)
-
- # Decoder input embedding
- self.embedding = nn.Embedding(
- self.num_classes,
- encoder_rnn_out_size,
- padding_idx=self.dictionary.padding_idx)
-
- # Prediction layer
- self.pred_dropout = nn.Dropout(pred_dropout)
- if pred_concat:
- fc_in_channel = decoder_rnn_out_size + d_model + d_enc
- else:
- fc_in_channel = d_model
- self.prediction = nn.Linear(fc_in_channel, self.num_classes)
- self.softmax = nn.Softmax(dim=-1)
-
- def _2d_attention(self,
- y_prev: torch.Tensor,
- feat: torch.Tensor,
- holistic_feat: torch.Tensor,
- hx1: torch.Tensor,
- cx1: torch.Tensor,
- hx2: torch.Tensor,
- cx2: torch.Tensor,
- valid_ratios: Optional[Sequence[float]] = None
- ) -> torch.Tensor:
- """2D attention layer.
-
- Args:
- y_prev (torch.Tensor): Previous decoder hidden state.
- feat (torch.Tensor): Feature map.
- holistic_feat (torch.Tensor): Holistic feature map.
- hx1 (torch.Tensor): rnn decoder layer 1 hidden state.
- cx1 (torch.Tensor): rnn decoder layer 1 cell state.
- hx2 (torch.Tensor): rnn decoder layer 2 hidden state.
- cx2 (torch.Tensor): rnn decoder layer 2 cell state.
- valid_ratios (Optional[Sequence[float]]): Valid ratios of
- attention. Defaults to None.
- """
- _, _, h_feat, w_feat = feat.size()
- if self.dec_gru:
- hx1 = cx1 = self.rnn_decoder_layer1(y_prev, hx1)
- hx2 = cx2 = self.rnn_decoder_layer2(hx1, hx2)
- else:
- hx1, cx1 = self.rnn_decoder_layer1(y_prev, (hx1, cx1))
- hx2, cx2 = self.rnn_decoder_layer2(hx1, (hx2, cx2))
-
- tile_hx2 = hx2.view(hx2.size(0), hx2.size(1), 1, 1)
- attn_query = self.conv1x1_1(tile_hx2) # bsz * attn_size * 1 * 1
- attn_query = attn_query.expand(-1, -1, h_feat, w_feat)
- attn_key = self.conv3x3_1(feat)
- attn_weight = torch.tanh(torch.add(attn_key, attn_query, alpha=1))
- attn_weight = self.conv1x1_2(attn_weight)
- bsz, c, h, w = attn_weight.size()
- assert c == 1
-
- if valid_ratios is not None:
- # cal mask of attention weight
- attn_mask = torch.zeros_like(attn_weight)
- for i, valid_ratio in enumerate(valid_ratios):
- valid_width = min(w, math.ceil(w * valid_ratio))
- attn_mask[i, :, :, valid_width:] = 1
- attn_weight = attn_weight.masked_fill(attn_mask.bool(),
- float('-inf'))
-
- attn_weight = F.softmax(attn_weight.view(bsz, -1), dim=-1)
- attn_weight = attn_weight.view(bsz, c, h, w)
-
- attn_feat = torch.sum(
- torch.mul(feat, attn_weight), (2, 3), keepdim=False) # n * c
-
- # linear transformation
- if self.pred_concat:
- y = self.prediction(torch.cat((hx2, attn_feat, holistic_feat), 1))
- else:
- y = self.prediction(attn_feat)
-
- return y, hx1, hx1, hx2, hx2
-
- def forward_train(
- self,
- feat: torch.Tensor,
- out_enc: torch.Tensor,
- data_samples: Optional[Sequence[TextRecogDataSample]] = None
- ) -> torch.Tensor:
- """
- Args:
- feat (Tensor): Tensor of shape :math:`(N, D_i, H, W)`.
- out_enc (Tensor): Encoder output of shape
- :math:`(N, D_m, H, W)`.
- data_samples (list[TextRecogDataSample]): Batch of
- TextRecogDataSample, containing gt_text and valid_ratio
- information.
-
- Returns:
- Tensor: A raw logit tensor of shape :math:`(N, T, C)`.
- """
- valid_ratios = None
- if data_samples is not None:
- valid_ratios = [
- data_sample.get('valid_ratio', 1.0)
- for data_sample in data_samples
- ] if self.mask else None
-
- padded_targets = [
- data_sample.gt_text.padded_indexes for data_sample in data_samples
- ]
- padded_targets = torch.stack(padded_targets, dim=0).to(feat.device)
- tgt_embedding = self.embedding(padded_targets)
-
- outputs = []
- for i in range(-1, self.max_seq_len):
- if i == -1:
- if self.dec_gru:
- hx1 = cx1 = self.rnn_decoder_layer1(out_enc)
- hx2 = cx2 = self.rnn_decoder_layer2(hx1)
- else:
- hx1, cx1 = self.rnn_decoder_layer1(out_enc)
- hx2, cx2 = self.rnn_decoder_layer2(hx1)
- else:
- y_prev = tgt_embedding[:, i, :]
- y, hx1, cx1, hx2, cx2 = self._2d_attention(
- y_prev,
- feat,
- out_enc,
- hx1,
- cx1,
- hx2,
- cx2,
- valid_ratios=valid_ratios)
- y = self.pred_dropout(y)
-
- outputs.append(y)
-
- outputs = torch.stack(outputs, 1)
-
- return outputs
-
- def forward_test(
- self,
- feat: torch.Tensor,
- out_enc: torch.Tensor,
- data_samples: Optional[Sequence[TextRecogDataSample]] = None
- ) -> torch.Tensor:
- """
- Args:
- feat (Tensor): Tensor of shape :math:`(N, D_i, H, W)`.
- out_enc (Tensor): Encoder output of shape
- :math:`(N, D_m, H, W)`.
- data_samples (list[TextRecogDataSample]): Batch of
- TextRecogDataSample, containing valid_ratio
- information.
-
- Returns:
- Tensor: Character probabilities. of shape
- :math:`(N, self.max_seq_len, C)` where :math:`C` is
- ``num_classes``.
- """
- valid_ratios = None
- if data_samples is not None:
- valid_ratios = [
- data_sample.get('valid_ratio', 1.0)
- for data_sample in data_samples
- ] if self.mask else None
-
- outputs = []
- start_token = torch.full((feat.size(0), ),
- self.start_idx,
- device=feat.device,
- dtype=torch.long)
- start_token = self.embedding(start_token)
- for i in range(-1, self.max_seq_len):
- if i == -1:
- if self.dec_gru:
- hx1 = cx1 = self.rnn_decoder_layer1(out_enc)
- hx2 = cx2 = self.rnn_decoder_layer2(hx1)
- else:
- hx1, cx1 = self.rnn_decoder_layer1(out_enc)
- hx2, cx2 = self.rnn_decoder_layer2(hx1)
- y_prev = start_token
- else:
- y, hx1, cx1, hx2, cx2 = self._2d_attention(
- y_prev,
- feat,
- out_enc,
- hx1,
- cx1,
- hx2,
- cx2,
- valid_ratios=valid_ratios)
- _, max_idx = torch.max(y, dim=1, keepdim=False)
- char_embedding = self.embedding(max_idx)
- y_prev = char_embedding
- outputs.append(y)
-
- outputs = torch.stack(outputs, 1)
-
- return self.softmax(outputs)
diff --git a/pipeline/mmocr/mmocr/models/textrecog/decoders/sar_decoder_with_bs.py b/pipeline/mmocr/mmocr/models/textrecog/decoders/sar_decoder_with_bs.py
deleted file mode 100644
index 495b72fb1881f340b7cca7c70571bd669fd6a81b..0000000000000000000000000000000000000000
--- a/pipeline/mmocr/mmocr/models/textrecog/decoders/sar_decoder_with_bs.py
+++ /dev/null
@@ -1,162 +0,0 @@
-# Copyright (c) OpenMMLab. All rights reserved.
-from queue import PriorityQueue
-
-import torch
-import torch.nn.functional as F
-
-import mmocr.utils as utils
-from mmocr.registry import MODELS
-from . import ParallelSARDecoder
-
-
-class DecodeNode:
- """Node class to save decoded char indices and scores.
-
- Args:
- indexes (list[int]): Char indices that decoded yes.
- scores (list[float]): Char scores that decoded yes.
- """
-
- def __init__(self, indexes=[1], scores=[0.9]):
- assert utils.is_type_list(indexes, int)
- assert utils.is_type_list(scores, float)
- assert utils.equal_len(indexes, scores)
-
- self.indexes = indexes
- self.scores = scores
-
- def eval(self):
- """Calculate accumulated score."""
- accu_score = sum(self.scores)
- return accu_score
-
-
-@MODELS.register_module()
-class ParallelSARDecoderWithBS(ParallelSARDecoder):
- """Parallel Decoder module with beam-search in SAR.
-
- Args:
- beam_width (int): Width for beam search.
- """
-
- def __init__(self,
- beam_width=5,
- num_classes=37,
- enc_bi_rnn=False,
- dec_bi_rnn=False,
- dec_do_rnn=0,
- dec_gru=False,
- d_model=512,
- d_enc=512,
- d_k=64,
- pred_dropout=0.0,
- max_seq_len=40,
- mask=True,
- start_idx=0,
- padding_idx=0,
- pred_concat=False,
- init_cfg=None,
- **kwargs):
- super().__init__(
- num_classes,
- enc_bi_rnn,
- dec_bi_rnn,
- dec_do_rnn,
- dec_gru,
- d_model,
- d_enc,
- d_k,
- pred_dropout,
- max_seq_len,
- mask,
- start_idx,
- padding_idx,
- pred_concat,
- init_cfg=init_cfg)
- assert isinstance(beam_width, int)
- assert beam_width > 0
-
- self.beam_width = beam_width
-
- def forward_test(self, feat, out_enc, img_metas):
- assert utils.is_type_list(img_metas, dict)
- assert len(img_metas) == feat.size(0)
-
- valid_ratios = [
- img_meta.get('valid_ratio', 1.0) for img_meta in img_metas
- ] if self.mask else None
-
- seq_len = self.max_seq_len
- bsz = feat.size(0)
- assert bsz == 1, 'batch size must be 1 for beam search.'
-
- start_token = torch.full((bsz, ),
- self.start_idx,
- device=feat.device,
- dtype=torch.long)
- # bsz
- start_token = self.embedding(start_token)
- # bsz * emb_dim
- start_token = start_token.unsqueeze(1).expand(-1, seq_len, -1)
- # bsz * seq_len * emb_dim
- out_enc = out_enc.unsqueeze(1)
- # bsz * 1 * emb_dim
- decoder_input = torch.cat((out_enc, start_token), dim=1)
- # bsz * (seq_len + 1) * emb_dim
-
- # Initialize beam-search queue
- q = PriorityQueue()
- init_node = DecodeNode([self.start_idx], [0.0])
- q.put((-init_node.eval(), init_node))
-
- for i in range(1, seq_len + 1):
- next_nodes = []
- beam_width = self.beam_width if i > 1 else 1
- for _ in range(beam_width):
- _, node = q.get()
-
- input_seq = torch.clone(decoder_input) # bsz * T * emb_dim
- # fill previous input tokens (step 1...i) in input_seq
- for t, index in enumerate(node.indexes):
- input_token = torch.full((bsz, ),
- index,
- device=input_seq.device,
- dtype=torch.long)
- input_token = self.embedding(input_token) # bsz * emb_dim
- input_seq[:, t + 1, :] = input_token
-
- output_seq = self._2d_attention(
- input_seq, feat, out_enc, valid_ratios=valid_ratios)
-
- output_char = output_seq[:, i, :] # bsz * num_classes
- output_char = F.softmax(output_char, -1)
- topk_value, topk_idx = output_char.topk(self.beam_width, dim=1)
- topk_value, topk_idx = topk_value.squeeze(0), topk_idx.squeeze(
- 0)
-
- for k in range(self.beam_width):
- kth_score = topk_value[k].item()
- kth_idx = topk_idx[k].item()
- next_node = DecodeNode(node.indexes + [kth_idx],
- node.scores + [kth_score])
- delta = k * 1e-6
- next_nodes.append(
- (-node.eval() - kth_score - delta, next_node))
- # Use minus since priority queue sort
- # with ascending order
-
- while not q.empty():
- q.get()
-
- # Put all candidates to queue
- for next_node in next_nodes:
- q.put(next_node)
-
- best_node = q.get()
- num_classes = self.num_classes - 1 # ignore padding index
- outputs = torch.zeros(bsz, seq_len, num_classes)
- for i in range(seq_len):
- idx = best_node[1].indexes[i + 1]
- outputs[0, i, idx] = best_node[1].scores[i + 1]
-
- return outputs
diff --git a/pipeline/mmocr/mmocr/models/textrecog/decoders/sequence_attention_decoder.py b/pipeline/mmocr/mmocr/models/textrecog/decoders/sequence_attention_decoder.py
deleted file mode 100644
index dfbf293f730e7c729511afa6ea24d494b86fe1b2..0000000000000000000000000000000000000000
--- a/pipeline/mmocr/mmocr/models/textrecog/decoders/sequence_attention_decoder.py
+++ /dev/null
@@ -1,260 +0,0 @@
-# Copyright (c) OpenMMLab. All rights reserved.
-import math
-from typing import Dict, Optional, Sequence, Union
-
-import torch
-import torch.nn as nn
-
-from mmocr.models.common.dictionary import Dictionary
-from mmocr.models.textrecog.layers import DotProductAttentionLayer
-from mmocr.registry import MODELS
-from mmocr.structures import TextRecogDataSample
-from .base import BaseDecoder
-
-
-@MODELS.register_module()
-class SequenceAttentionDecoder(BaseDecoder):
- """Sequence attention decoder for RobustScanner.
-
- RobustScanner: `RobustScanner: Dynamically Enhancing Positional Clues for
- Robust Text Recognition `_
-
- Args:
- dictionary (dict or :obj:`Dictionary`): The config for `Dictionary` or
- the instance of `Dictionary`.
- module_loss (dict, optional): Config to build module_loss. Defaults
- to None.
- postprocessor (dict, optional): Config to build postprocessor.
- Defaults to None.
- rnn_layers (int): Number of RNN layers. Defaults to 2.
- dim_input (int): Dimension :math:`D_i` of input vector ``feat``.
- Defaults to 512.
- dim_model (int): Dimension :math:`D_m` of the model. Should also be the
- same as encoder output vector ``out_enc``. Defaults to 128.
- max_seq_len (int): Maximum output sequence length :math:`T`.
- Defaults to 40.
- mask (bool): Whether to mask input features according to
- ``data_sample.valid_ratio``. Defaults to True.
- dropout (float): Dropout rate for LSTM layer. Defaults to 0.
- return_feature (bool): Return feature or logic as the result.
- Defaults to True.
- encode_value (bool): Whether to use the output of encoder ``out_enc``
- as `value` of attention layer. If False, the original feature
- ``feat`` will be used. Defaults to False.
- init_cfg (dict or list[dict], optional): Initialization configs.
- Defaults to None.
- """
-
- def __init__(self,
- dictionary: Union[Dictionary, Dict],
- module_loss: Optional[Dict] = None,
- postprocessor: Optional[Dict] = None,
- rnn_layers: int = 2,
- dim_input: int = 512,
- dim_model: int = 128,
- max_seq_len: int = 40,
- mask: bool = True,
- dropout: int = 0,
- return_feature: bool = True,
- encode_value: bool = False,
- init_cfg: Optional[Union[Dict,
- Sequence[Dict]]] = None) -> None:
- super().__init__(
- dictionary=dictionary,
- module_loss=module_loss,
- postprocessor=postprocessor,
- max_seq_len=max_seq_len,
- init_cfg=init_cfg)
-
- self.dim_input = dim_input
- self.dim_model = dim_model
- self.return_feature = return_feature
- self.encode_value = encode_value
- self.mask = mask
-
- self.embedding = nn.Embedding(
- self.dictionary.num_classes,
- self.dim_model,
- padding_idx=self.dictionary.padding_idx)
-
- self.sequence_layer = nn.LSTM(
- input_size=dim_model,
- hidden_size=dim_model,
- num_layers=rnn_layers,
- batch_first=True,
- dropout=dropout)
-
- self.attention_layer = DotProductAttentionLayer()
-
- self.prediction = None
- if not self.return_feature:
- self.prediction = nn.Linear(
- dim_model if encode_value else dim_input,
- self.dictionary.num_classes)
- self.softmax = nn.Softmax(dim=-1)
-
- def forward_train(
- self,
- feat: torch.Tensor,
- out_enc: torch.Tensor,
- data_samples: Optional[Sequence[TextRecogDataSample]] = None
- ) -> torch.Tensor:
- """
- Args:
- feat (Tensor): Tensor of shape :math:`(N, D_i, H, W)`.
- out_enc (Tensor): Encoder output of shape
- :math:`(N, D_m, H, W)`.
- targets_dict (dict): A dict with the key ``padded_targets``, a
- tensor of shape :math:`(N, T)`. Each element is the index of a
- character.
- data_samples (list[TextRecogDataSample], optional): Batch of
- TextRecogDataSample, containing gt_text information. Defaults
- to None.
-
- Returns:
- Tensor: A raw logit tensor of shape :math:`(N, T, C)` if
- ``return_feature=False``. Otherwise it would be the hidden feature
- before the prediction projection layer, whose shape is
- :math:`(N, T, D_m)`.
- """
-
- valid_ratios = [
- data_sample.get('valid_ratio', 1.0) for data_sample in data_samples
- ] if self.mask else None
-
- padded_targets = [
- data_sample.gt_text.padded_indexes for data_sample in data_samples
- ]
- padded_targets = torch.stack(padded_targets, dim=0).to(feat.device)
- tgt_embedding = self.embedding(padded_targets)
-
- n, c_enc, h, w = out_enc.size()
- assert c_enc == self.dim_model
- _, c_feat, _, _ = feat.size()
- assert c_feat == self.dim_input
- _, len_q, c_q = tgt_embedding.size()
- assert c_q == self.dim_model
- assert len_q <= self.max_seq_len
-
- query, _ = self.sequence_layer(tgt_embedding)
- query = query.permute(0, 2, 1).contiguous()
- key = out_enc.view(n, c_enc, h * w)
- if self.encode_value:
- value = key
- else:
- value = feat.view(n, c_feat, h * w)
-
- mask = None
- if valid_ratios is not None:
- mask = query.new_zeros((n, h, w))
- for i, valid_ratio in enumerate(valid_ratios):
- valid_width = min(w, math.ceil(w * valid_ratio))
- mask[i, :, valid_width:] = 1
- mask = mask.bool()
- mask = mask.view(n, h * w)
-
- attn_out = self.attention_layer(query, key, value, mask)
- attn_out = attn_out.permute(0, 2, 1).contiguous()
-
- if self.return_feature:
- return attn_out
-
- out = self.prediction(attn_out)
-
- return out
-
- def forward_test(self, feat: torch.Tensor, out_enc: torch.Tensor,
- data_samples: Optional[Sequence[TextRecogDataSample]]
- ) -> torch.Tensor:
- """
- Args:
- feat (Tensor): Tensor of shape :math:`(N, D_i, H, W)`.
- out_enc (Tensor): Encoder output of shape
- :math:`(N, D_m, H, W)`.
- data_samples (list[TextRecogDataSample], optional): Batch of
- TextRecogDataSample, containing gt_text information. Defaults
- to None.
-
- Returns:
- Tensor: Character probabilities. of shape
- :math:`(N, self.max_seq_len, C)` where :math:`C` is
- ``num_classes``.
- """
- seq_len = self.max_seq_len
- batch_size = feat.size(0)
-
- decode_sequence = (feat.new_ones(
- (batch_size, seq_len)) * self.dictionary.start_idx).long()
- assert not self.return_feature
- outputs = []
- for i in range(seq_len):
- step_out = self.forward_test_step(feat, out_enc, decode_sequence,
- i, data_samples)
- outputs.append(step_out)
- _, max_idx = torch.max(step_out, dim=1, keepdim=False)
- if i < seq_len - 1:
- decode_sequence[:, i + 1] = max_idx
-
- outputs = torch.stack(outputs, 1)
-
- return self.softmax(outputs)
-
- def forward_test_step(self, feat: torch.Tensor, out_enc: torch.Tensor,
- decode_sequence: torch.Tensor, current_step: int,
- data_samples: Sequence[TextRecogDataSample]
- ) -> torch.Tensor:
- """
- Args:
- feat (Tensor): Tensor of shape :math:`(N, D_i, H, W)`.
- out_enc (Tensor): Encoder output of shape
- :math:`(N, D_m, H, W)`.
- decode_sequence (Tensor): Shape :math:`(N, T)`. The tensor that
- stores history decoding result.
- current_step (int): Current decoding step.
- data_samples (list[TextRecogDataSample], optional): Batch of
- TextRecogDataSample, containing gt_text information. Defaults
- to None.
-
- Returns:
- Tensor: Shape :math:`(N, C)`. The logit tensor of predicted
- tokens at current time step.
- """
- valid_ratios = [
- img_meta.get('valid_ratio', 1.0) for img_meta in data_samples
- ] if self.mask else None
-
- embed = self.embedding(decode_sequence)
-
- n, c_enc, h, w = out_enc.size()
- assert c_enc == self.dim_model
- _, c_feat, _, _ = feat.size()
- assert c_feat == self.dim_input
- _, _, c_q = embed.size()
- assert c_q == self.dim_model
-
- query, _ = self.sequence_layer(embed)
- query = query.permute(0, 2, 1).contiguous()
- key = out_enc.view(n, c_enc, h * w)
- if self.encode_value:
- value = key
- else:
- value = feat.view(n, c_feat, h * w)
-
- mask = None
- if valid_ratios is not None:
- mask = query.new_zeros((n, h, w))
- for i, valid_ratio in enumerate(valid_ratios):
- valid_width = min(w, math.ceil(w * valid_ratio))
- mask[i, :, valid_width:] = 1
- mask = mask.bool()
- mask = mask.view(n, h * w)
-
- # [n, c, l]
- attn_out = self.attention_layer(query, key, value, mask)
-
- out = attn_out[:, :, current_step]
-
- if not self.return_feature:
- out = self.prediction(out)
-
- return out
diff --git a/pipeline/mmocr/mmocr/models/textrecog/decoders/svtr_decoder.py b/pipeline/mmocr/mmocr/models/textrecog/decoders/svtr_decoder.py
deleted file mode 100644
index 122a51dc09b6c55d25ad80f3c763135317c6aca3..0000000000000000000000000000000000000000
--- a/pipeline/mmocr/mmocr/models/textrecog/decoders/svtr_decoder.py
+++ /dev/null
@@ -1,96 +0,0 @@
-# Copyright (c) OpenMMLab. All rights reserved.
-from typing import Dict, List, Optional, Sequence, Union
-
-import torch
-import torch.nn as nn
-
-from mmocr.models.common.dictionary import Dictionary
-from mmocr.registry import MODELS
-from mmocr.structures import TextRecogDataSample
-from .base import BaseDecoder
-
-
-@MODELS.register_module()
-class SVTRDecoder(BaseDecoder):
- """Decoder module in `SVTR `_.
-
- Args:
- in_channels (int): The num of input channels.
- dictionary (Union[Dict, Dictionary]): The config for `Dictionary` or
- the instance of `Dictionary`. Defaults to None.
- module_loss (Optional[Dict], optional): Cfg to build module_loss.
- Defaults to None.
- postprocessor (Optional[Dict], optional): Cfg to build postprocessor.
- Defaults to None.
- max_seq_len (int, optional): Maximum output sequence length :math:`T`.
- Defaults to 25.
- init_cfg (dict or list[dict], optional): Initialization configs.
- Defaults to None.
- """
-
- def __init__(self,
- in_channels: int,
- dictionary: Union[Dict, Dictionary] = None,
- module_loss: Optional[Dict] = None,
- postprocessor: Optional[Dict] = None,
- max_seq_len: int = 25,
- init_cfg: Optional[Union[Dict, List[Dict]]] = None) -> None:
-
- super().__init__(
- dictionary=dictionary,
- module_loss=module_loss,
- postprocessor=postprocessor,
- max_seq_len=max_seq_len,
- init_cfg=init_cfg)
-
- self.decoder = nn.Linear(
- in_features=in_channels, out_features=self.dictionary.num_classes)
- self.softmax = nn.Softmax(dim=-1)
-
- def forward_train(
- self,
- feat: Optional[torch.Tensor] = None,
- out_enc: Optional[torch.Tensor] = None,
- data_samples: Optional[Sequence[TextRecogDataSample]] = None
- ) -> torch.Tensor:
- """Forward for training.
-
- Args:
- feat (torch.Tensor, optional): The feature map. Defaults to None.
- out_enc (torch.Tensor, optional): Encoder output from encoder of
- shape :math:`(N, 1, H, W)`. Defaults to None.
- data_samples (Sequence[TextRecogDataSample]): Batch of
- TextRecogDataSample, containing gt_text information. Defaults
- to None.
-
- Returns:
- Tensor: The raw logit tensor. Shape :math:`(N, T, C)` where
- :math:`C` is ``num_classes``.
- """
- assert out_enc.size(2) == 1, 'feature height must be 1'
- x = out_enc.squeeze(2)
- x = x.permute(0, 2, 1)
- predicts = self.decoder(x)
- return predicts
-
- def forward_test(
- self,
- feat: Optional[torch.Tensor] = None,
- out_enc: Optional[torch.Tensor] = None,
- data_samples: Optional[Sequence[TextRecogDataSample]] = None
- ) -> torch.Tensor:
- """Forward for testing.
-
- Args:
- feat (torch.Tensor, optional): The feature map. Defaults to None.
- out_enc (torch.Tensor, optional): Encoder output from encoder of
- shape :math:`(N, 1, H, W)`. Defaults to None.
- data_samples (Sequence[TextRecogDataSample]): Batch of
- TextRecogDataSample, containing gt_text information. Defaults
- to None.
- Returns:
- Tensor: Character probabilities. of shape
- :math:`(N, self.max_seq_len, C)` where :math:`C` is
- ``num_classes``.
- """
- return self.softmax(self.forward_train(feat, out_enc, data_samples))
diff --git a/pipeline/mmocr/mmocr/models/textrecog/encoders/__init__.py b/pipeline/mmocr/mmocr/models/textrecog/encoders/__init__.py
deleted file mode 100644
index ceef10116baf4bf1bec14613af0bfbd1f28e86d0..0000000000000000000000000000000000000000
--- a/pipeline/mmocr/mmocr/models/textrecog/encoders/__init__.py
+++ /dev/null
@@ -1,14 +0,0 @@
-# Copyright (c) OpenMMLab. All rights reserved.
-from .abi_encoder import ABIEncoder
-from .aster_encoder import ASTEREncoder
-from .base import BaseEncoder
-from .channel_reduction_encoder import ChannelReductionEncoder
-from .nrtr_encoder import NRTREncoder
-from .sar_encoder import SAREncoder
-from .satrn_encoder import SATRNEncoder
-from .svtr_encoder import SVTREncoder
-
-__all__ = [
- 'SAREncoder', 'NRTREncoder', 'BaseEncoder', 'ChannelReductionEncoder',
- 'SATRNEncoder', 'ABIEncoder', 'SVTREncoder', 'ASTEREncoder'
-]
diff --git a/pipeline/mmocr/mmocr/models/textrecog/encoders/__pycache__/__init__.cpython-38.pyc b/pipeline/mmocr/mmocr/models/textrecog/encoders/__pycache__/__init__.cpython-38.pyc
deleted file mode 100644
index f63d979be3dce8564b25e708d6f67abd5749d7ec..0000000000000000000000000000000000000000
Binary files a/pipeline/mmocr/mmocr/models/textrecog/encoders/__pycache__/__init__.cpython-38.pyc and /dev/null differ
diff --git a/pipeline/mmocr/mmocr/models/textrecog/encoders/__pycache__/__init__.cpython-39.pyc b/pipeline/mmocr/mmocr/models/textrecog/encoders/__pycache__/__init__.cpython-39.pyc
deleted file mode 100644
index 3985590a376234e14819debe237b37c07f5ee6fd..0000000000000000000000000000000000000000
Binary files a/pipeline/mmocr/mmocr/models/textrecog/encoders/__pycache__/__init__.cpython-39.pyc and /dev/null differ
diff --git a/pipeline/mmocr/mmocr/models/textrecog/encoders/__pycache__/abi_encoder.cpython-38.pyc b/pipeline/mmocr/mmocr/models/textrecog/encoders/__pycache__/abi_encoder.cpython-38.pyc
deleted file mode 100644
index a5aaa95fa06f90f90e094ab1b8563523d2f0c781..0000000000000000000000000000000000000000
Binary files a/pipeline/mmocr/mmocr/models/textrecog/encoders/__pycache__/abi_encoder.cpython-38.pyc and /dev/null differ
diff --git a/pipeline/mmocr/mmocr/models/textrecog/encoders/__pycache__/abi_encoder.cpython-39.pyc b/pipeline/mmocr/mmocr/models/textrecog/encoders/__pycache__/abi_encoder.cpython-39.pyc
deleted file mode 100644
index 3c55fdf092440ea731bba4cbe39f96842c6e0ffe..0000000000000000000000000000000000000000
Binary files a/pipeline/mmocr/mmocr/models/textrecog/encoders/__pycache__/abi_encoder.cpython-39.pyc and /dev/null differ
diff --git a/pipeline/mmocr/mmocr/models/textrecog/encoders/__pycache__/aster_encoder.cpython-38.pyc b/pipeline/mmocr/mmocr/models/textrecog/encoders/__pycache__/aster_encoder.cpython-38.pyc
deleted file mode 100644
index 8ea4a1818b63776e4463a42c0fab9065fc60422e..0000000000000000000000000000000000000000
Binary files a/pipeline/mmocr/mmocr/models/textrecog/encoders/__pycache__/aster_encoder.cpython-38.pyc and /dev/null differ
diff --git a/pipeline/mmocr/mmocr/models/textrecog/encoders/__pycache__/aster_encoder.cpython-39.pyc b/pipeline/mmocr/mmocr/models/textrecog/encoders/__pycache__/aster_encoder.cpython-39.pyc
deleted file mode 100644
index 26d1d37d3e80f61ed751af3cd2461b83ece1a0dc..0000000000000000000000000000000000000000
Binary files a/pipeline/mmocr/mmocr/models/textrecog/encoders/__pycache__/aster_encoder.cpython-39.pyc and /dev/null differ
diff --git a/pipeline/mmocr/mmocr/models/textrecog/encoders/__pycache__/base.cpython-38.pyc b/pipeline/mmocr/mmocr/models/textrecog/encoders/__pycache__/base.cpython-38.pyc
deleted file mode 100644
index 39f88a8a17491775a74396c8f1be535bff924a49..0000000000000000000000000000000000000000
Binary files a/pipeline/mmocr/mmocr/models/textrecog/encoders/__pycache__/base.cpython-38.pyc and /dev/null differ
diff --git a/pipeline/mmocr/mmocr/models/textrecog/encoders/__pycache__/base.cpython-39.pyc b/pipeline/mmocr/mmocr/models/textrecog/encoders/__pycache__/base.cpython-39.pyc
deleted file mode 100644
index 80ec7bf594731f71a3c26d08c0399ba5d28884c7..0000000000000000000000000000000000000000
Binary files a/pipeline/mmocr/mmocr/models/textrecog/encoders/__pycache__/base.cpython-39.pyc and /dev/null differ
diff --git a/pipeline/mmocr/mmocr/models/textrecog/encoders/__pycache__/channel_reduction_encoder.cpython-38.pyc b/pipeline/mmocr/mmocr/models/textrecog/encoders/__pycache__/channel_reduction_encoder.cpython-38.pyc
deleted file mode 100644
index c60ce3718abf0c2e4a0124765c065e57b68b5970..0000000000000000000000000000000000000000
Binary files a/pipeline/mmocr/mmocr/models/textrecog/encoders/__pycache__/channel_reduction_encoder.cpython-38.pyc and /dev/null differ
diff --git a/pipeline/mmocr/mmocr/models/textrecog/encoders/__pycache__/channel_reduction_encoder.cpython-39.pyc b/pipeline/mmocr/mmocr/models/textrecog/encoders/__pycache__/channel_reduction_encoder.cpython-39.pyc
deleted file mode 100644
index 57fc3114f2d9f50ef64685eb4f088c981a3f83d0..0000000000000000000000000000000000000000
Binary files a/pipeline/mmocr/mmocr/models/textrecog/encoders/__pycache__/channel_reduction_encoder.cpython-39.pyc and /dev/null differ
diff --git a/pipeline/mmocr/mmocr/models/textrecog/encoders/__pycache__/nrtr_encoder.cpython-38.pyc b/pipeline/mmocr/mmocr/models/textrecog/encoders/__pycache__/nrtr_encoder.cpython-38.pyc
deleted file mode 100644
index eff25718735abab5516185e0698353a9b35a068c..0000000000000000000000000000000000000000
Binary files a/pipeline/mmocr/mmocr/models/textrecog/encoders/__pycache__/nrtr_encoder.cpython-38.pyc and /dev/null differ
diff --git a/pipeline/mmocr/mmocr/models/textrecog/encoders/__pycache__/nrtr_encoder.cpython-39.pyc b/pipeline/mmocr/mmocr/models/textrecog/encoders/__pycache__/nrtr_encoder.cpython-39.pyc
deleted file mode 100644
index 63d359cfdda95f046e1a56f3e9a3670a85d2d755..0000000000000000000000000000000000000000
Binary files a/pipeline/mmocr/mmocr/models/textrecog/encoders/__pycache__/nrtr_encoder.cpython-39.pyc and /dev/null differ
diff --git a/pipeline/mmocr/mmocr/models/textrecog/encoders/__pycache__/sar_encoder.cpython-38.pyc b/pipeline/mmocr/mmocr/models/textrecog/encoders/__pycache__/sar_encoder.cpython-38.pyc
deleted file mode 100644
index f88165800fb66336de2fcf2a734e5312a4c34303..0000000000000000000000000000000000000000
Binary files a/pipeline/mmocr/mmocr/models/textrecog/encoders/__pycache__/sar_encoder.cpython-38.pyc and /dev/null differ
diff --git a/pipeline/mmocr/mmocr/models/textrecog/encoders/__pycache__/sar_encoder.cpython-39.pyc b/pipeline/mmocr/mmocr/models/textrecog/encoders/__pycache__/sar_encoder.cpython-39.pyc
deleted file mode 100644
index 72e786f5e28ce38dd6c290e362eaa1863a7267a1..0000000000000000000000000000000000000000
Binary files a/pipeline/mmocr/mmocr/models/textrecog/encoders/__pycache__/sar_encoder.cpython-39.pyc and /dev/null differ
diff --git a/pipeline/mmocr/mmocr/models/textrecog/encoders/__pycache__/satrn_encoder.cpython-38.pyc b/pipeline/mmocr/mmocr/models/textrecog/encoders/__pycache__/satrn_encoder.cpython-38.pyc
deleted file mode 100644
index 0c8c396f2c9e58b3b3b34e50070537b636038f48..0000000000000000000000000000000000000000
Binary files a/pipeline/mmocr/mmocr/models/textrecog/encoders/__pycache__/satrn_encoder.cpython-38.pyc and /dev/null differ
diff --git a/pipeline/mmocr/mmocr/models/textrecog/encoders/__pycache__/satrn_encoder.cpython-39.pyc b/pipeline/mmocr/mmocr/models/textrecog/encoders/__pycache__/satrn_encoder.cpython-39.pyc
deleted file mode 100644
index 77d817ef2d04cc9f274d28bf3fc73d2b495f8fbb..0000000000000000000000000000000000000000
Binary files a/pipeline/mmocr/mmocr/models/textrecog/encoders/__pycache__/satrn_encoder.cpython-39.pyc and /dev/null differ
diff --git a/pipeline/mmocr/mmocr/models/textrecog/encoders/__pycache__/svtr_encoder.cpython-38.pyc b/pipeline/mmocr/mmocr/models/textrecog/encoders/__pycache__/svtr_encoder.cpython-38.pyc
deleted file mode 100644
index 654a28a4f73df806533add071787fd1bafb1afd4..0000000000000000000000000000000000000000
Binary files a/pipeline/mmocr/mmocr/models/textrecog/encoders/__pycache__/svtr_encoder.cpython-38.pyc and /dev/null differ
diff --git a/pipeline/mmocr/mmocr/models/textrecog/encoders/__pycache__/svtr_encoder.cpython-39.pyc b/pipeline/mmocr/mmocr/models/textrecog/encoders/__pycache__/svtr_encoder.cpython-39.pyc
deleted file mode 100644
index a35f8263f25fae04b74bc28ac0c5bff3bea35fb0..0000000000000000000000000000000000000000
Binary files a/pipeline/mmocr/mmocr/models/textrecog/encoders/__pycache__/svtr_encoder.cpython-39.pyc and /dev/null differ
diff --git a/pipeline/mmocr/mmocr/models/textrecog/encoders/abi_encoder.py b/pipeline/mmocr/mmocr/models/textrecog/encoders/abi_encoder.py
deleted file mode 100644
index a5f6a85c71bfe84c09fdc3d6d2eb560804f7564e..0000000000000000000000000000000000000000
--- a/pipeline/mmocr/mmocr/models/textrecog/encoders/abi_encoder.py
+++ /dev/null
@@ -1,83 +0,0 @@
-# Copyright (c) OpenMMLab. All rights reserved.
-import copy
-from typing import Dict, List, Optional, Union
-
-import torch
-from mmcv.cnn.bricks.transformer import BaseTransformerLayer
-from mmengine.model import BaseModule, ModuleList
-
-from mmocr.models.common.modules import PositionalEncoding
-from mmocr.registry import MODELS
-from mmocr.structures import TextRecogDataSample
-
-
-@MODELS.register_module()
-class ABIEncoder(BaseModule):
- """Implement transformer encoder for text recognition, modified from
- ``.
-
- Args:
- n_layers (int): Number of attention layers. Defaults to 2.
- n_head (int): Number of parallel attention heads. Defaults to 8.
- d_model (int): Dimension :math:`D_m` of the input from previous model.
- Defaults to 512.
- d_inner (int): Hidden dimension of feedforward layers. Defaults to
- 2048.
- dropout (float): Dropout rate. Defaults to 0.1.
- max_len (int): Maximum output sequence length :math:`T`. Defaults to
- 8 * 32.
- init_cfg (dict or list[dict], optional): Initialization configs.
- Defaults to None.
- """
-
- def __init__(self,
- n_layers: int = 2,
- n_head: int = 8,
- d_model: int = 512,
- d_inner: int = 2048,
- dropout: float = 0.1,
- max_len: int = 8 * 32,
- init_cfg: Optional[Union[Dict, List[Dict]]] = None):
- super().__init__(init_cfg=init_cfg)
-
- assert d_model % n_head == 0, 'd_model must be divisible by n_head'
-
- self.pos_encoder = PositionalEncoding(d_model, n_position=max_len)
- encoder_layer = BaseTransformerLayer(
- operation_order=('self_attn', 'norm', 'ffn', 'norm'),
- attn_cfgs=dict(
- type='MultiheadAttention',
- embed_dims=d_model,
- num_heads=n_head,
- attn_drop=dropout,
- dropout_layer=dict(type='Dropout', drop_prob=dropout),
- ),
- ffn_cfgs=dict(
- type='FFN',
- embed_dims=d_model,
- feedforward_channels=d_inner,
- ffn_drop=dropout,
- ),
- norm_cfg=dict(type='LN'),
- )
- self.transformer = ModuleList(
- [copy.deepcopy(encoder_layer) for _ in range(n_layers)])
-
- def forward(self, feature: torch.Tensor,
- data_samples: List[TextRecogDataSample]) -> torch.Tensor:
- """
- Args:
- feature (Tensor): Feature tensor of shape :math:`(N, D_m, H, W)`.
- data_samples (List[TextRecogDataSample]): List of data samples.
-
- Returns:
- Tensor: Features of shape :math:`(N, D_m, H, W)`.
- """
- n, c, h, w = feature.shape
- feature = feature.view(n, c, -1).transpose(1, 2) # (n, h*w, c)
- feature = self.pos_encoder(feature) # (n, h*w, c)
- feature = feature.transpose(0, 1) # (h*w, n, c)
- for m in self.transformer:
- feature = m(feature)
- feature = feature.permute(1, 2, 0).view(n, c, h, w)
- return feature
diff --git a/pipeline/mmocr/mmocr/models/textrecog/encoders/aster_encoder.py b/pipeline/mmocr/mmocr/models/textrecog/encoders/aster_encoder.py
deleted file mode 100644
index 521218153701c510478b1e4ac3912c89f8eecfd4..0000000000000000000000000000000000000000
--- a/pipeline/mmocr/mmocr/models/textrecog/encoders/aster_encoder.py
+++ /dev/null
@@ -1,43 +0,0 @@
-# Copyright (c) OpenMMLab. All rights reserved.
-import torch
-import torch.nn as nn
-
-from mmocr.registry import MODELS
-from .base import BaseEncoder
-
-
-@MODELS.register_module()
-class ASTEREncoder(BaseEncoder):
- """Implement BiLSTM encoder module in `ASTER: An Attentional Scene Text
- Recognizer with Flexible Rectification.
-
- None:
- super().__init__(init_cfg=init_cfg)
- self.bilstm = nn.LSTM(
- in_channels,
- in_channels // 2,
- num_layers=num_layers,
- bidirectional=True,
- batch_first=True)
-
- def forward(self, feat: torch.Tensor, img_metas=None) -> torch.Tensor:
- """
- Args:
- feat (Tensor): Feature of shape (N, C, 1, W).
- Returns:
- Tensor: Output of BiLSTM.
- """
- assert feat.dim() == 4
- assert feat.size(2) == 1, 'height must be 1'
- feat = feat.squeeze(2).permute(0, 2, 1)
- feat, _ = self.bilstm(feat)
- return feat.contiguous()
diff --git a/pipeline/mmocr/mmocr/models/textrecog/encoders/base.py b/pipeline/mmocr/mmocr/models/textrecog/encoders/base.py
deleted file mode 100644
index 26edafb79869c840ec9362faef7a871759d15d3b..0000000000000000000000000000000000000000
--- a/pipeline/mmocr/mmocr/models/textrecog/encoders/base.py
+++ /dev/null
@@ -1,12 +0,0 @@
-# Copyright (c) OpenMMLab. All rights reserved.
-from mmengine.model import BaseModule
-
-from mmocr.registry import MODELS
-
-
-@MODELS.register_module()
-class BaseEncoder(BaseModule):
- """Base Encoder class for text recognition."""
-
- def forward(self, feat, **kwargs):
- return feat
diff --git a/pipeline/mmocr/mmocr/models/textrecog/encoders/channel_reduction_encoder.py b/pipeline/mmocr/mmocr/models/textrecog/encoders/channel_reduction_encoder.py
deleted file mode 100644
index 49b40bf27406c0c1d1b46d4f7232bdeca50776f7..0000000000000000000000000000000000000000
--- a/pipeline/mmocr/mmocr/models/textrecog/encoders/channel_reduction_encoder.py
+++ /dev/null
@@ -1,50 +0,0 @@
-# Copyright (c) OpenMMLab. All rights reserved.
-from typing import Dict, Optional, Sequence
-
-import torch
-import torch.nn as nn
-
-from mmocr.registry import MODELS
-from mmocr.structures import TextRecogDataSample
-from .base import BaseEncoder
-
-
-@MODELS.register_module()
-class ChannelReductionEncoder(BaseEncoder):
- """Change the channel number with a one by one convoluational layer.
-
- Args:
- in_channels (int): Number of input channels.
- out_channels (int): Number of output channels.
- init_cfg (dict or list[dict], optional): Initialization configs.
- Defaults to dict(type='Xavier', layer='Conv2d').
- """
-
- def __init__(
- self,
- in_channels: int,
- out_channels: int,
- init_cfg: Dict = dict(type='Xavier', layer='Conv2d')
- ) -> None:
- super().__init__(init_cfg=init_cfg)
-
- self.layer = nn.Conv2d(
- in_channels, out_channels, kernel_size=1, stride=1, padding=0)
-
- def forward(
- self,
- feat: torch.Tensor,
- data_samples: Optional[Sequence[TextRecogDataSample]] = None
- ) -> torch.Tensor:
- """
- Args:
- feat (Tensor): Image features with the shape of
- :math:`(N, C_{in}, H, W)`.
- data_samples (list[TextRecogDataSample], optional): Batch of
- TextRecogDataSample, containing valid_ratio information.
- Defaults to None.
-
- Returns:
- Tensor: A tensor of shape :math:`(N, C_{out}, H, W)`.
- """
- return self.layer(feat)
diff --git a/pipeline/mmocr/mmocr/models/textrecog/encoders/nrtr_encoder.py b/pipeline/mmocr/mmocr/models/textrecog/encoders/nrtr_encoder.py
deleted file mode 100644
index e7d80778990dce9bd8f22eff9a32b6fc5b64fb5d..0000000000000000000000000000000000000000
--- a/pipeline/mmocr/mmocr/models/textrecog/encoders/nrtr_encoder.py
+++ /dev/null
@@ -1,108 +0,0 @@
-# Copyright (c) OpenMMLab. All rights reserved.
-import math
-from typing import Dict, Optional, Sequence, Union
-
-import torch
-import torch.nn as nn
-from mmengine.model import ModuleList
-
-from mmocr.models.common import TFEncoderLayer
-from mmocr.registry import MODELS
-from mmocr.structures import TextRecogDataSample
-from .base import BaseEncoder
-
-
-@MODELS.register_module()
-class NRTREncoder(BaseEncoder):
- """Transformer Encoder block with self attention mechanism.
-
- Args:
- n_layers (int): The number of sub-encoder-layers in the encoder.
- Defaults to 6.
- n_head (int): The number of heads in the multiheadattention models
- Defaults to 8.
- d_k (int): Total number of features in key. Defaults to 64.
- d_v (int): Total number of features in value. Defaults to 64.
- d_model (int): The number of expected features in the decoder inputs.
- Defaults to 512.
- d_inner (int): The dimension of the feedforward network model.
- Defaults to 256.
- dropout (float): Dropout rate for MHSA and FFN. Defaults to 0.1.
- init_cfg (dict or list[dict], optional): Initialization configs.
- """
-
- def __init__(self,
- n_layers: int = 6,
- n_head: int = 8,
- d_k: int = 64,
- d_v: int = 64,
- d_model: int = 512,
- d_inner: int = 256,
- dropout: float = 0.1,
- init_cfg: Optional[Union[Dict,
- Sequence[Dict]]] = None) -> None:
- super().__init__(init_cfg=init_cfg)
- self.d_model = d_model
- self.layer_stack = ModuleList([
- TFEncoderLayer(
- d_model, d_inner, n_head, d_k, d_v, dropout=dropout)
- for _ in range(n_layers)
- ])
- self.layer_norm = nn.LayerNorm(d_model)
-
- def _get_source_mask(self, src_seq: torch.Tensor,
- valid_ratios: Sequence[float]) -> torch.Tensor:
- """Generate mask for source sequence.
-
- Args:
- src_seq (torch.Tensor): Image sequence. Shape :math:`(N, T, C)`.
- valid_ratios (list[float]): The valid ratio of input image. For
- example, if the width of the original image is w1 and the width
- after pad is w2, then valid_ratio = w1/w2. source mask is used
- to cover the area of the pad region.
-
- Returns:
- Tensor or None: Source mask. Shape :math:`(N, T)`. The region of
- pad area are False, and the rest are True.
- """
-
- N, T, _ = src_seq.size()
- mask = None
- if len(valid_ratios) > 0:
- mask = src_seq.new_zeros((N, T), device=src_seq.device)
- for i, valid_ratio in enumerate(valid_ratios):
- valid_width = min(T, math.ceil(T * valid_ratio))
- mask[i, :valid_width] = 1
-
- return mask
-
- def forward(self,
- feat: torch.Tensor,
- data_samples: Sequence[TextRecogDataSample] = None
- ) -> torch.Tensor:
- """
- Args:
- feat (Tensor): Backbone output of shape :math:`(N, C, H, W)`.
- data_samples (list[TextRecogDataSample]): Batch of
- TextRecogDataSample, containing valid_ratio information.
- Defaults to None.
-
-
- Returns:
- Tensor: The encoder output tensor. Shape :math:`(N, T, C)`.
- """
- n, c, h, w = feat.size()
-
- feat = feat.view(n, c, h * w).permute(0, 2, 1).contiguous()
-
- valid_ratios = []
- for data_sample in data_samples:
- valid_ratios.append(data_sample.get('valid_ratio'))
- mask = self._get_source_mask(feat, valid_ratios)
-
- output = feat
- for enc_layer in self.layer_stack:
- output = enc_layer(output, mask)
- output = self.layer_norm(output)
-
- return output
diff --git a/pipeline/mmocr/mmocr/models/textrecog/encoders/sar_encoder.py b/pipeline/mmocr/mmocr/models/textrecog/encoders/sar_encoder.py
deleted file mode 100644
index 33d8c1ef8f5b8f57c5762d4449bc8baf06f8a380..0000000000000000000000000000000000000000
--- a/pipeline/mmocr/mmocr/models/textrecog/encoders/sar_encoder.py
+++ /dev/null
@@ -1,124 +0,0 @@
-# Copyright (c) OpenMMLab. All rights reserved.
-import math
-from typing import Dict, Optional, Sequence, Union
-
-import torch
-import torch.nn as nn
-import torch.nn.functional as F
-
-from mmocr.registry import MODELS
-from mmocr.structures import TextRecogDataSample
-from .base import BaseEncoder
-
-
-@MODELS.register_module()
-class SAREncoder(BaseEncoder):
- """Implementation of encoder module in `SAR.
-
- `_.
-
- Args:
- enc_bi_rnn (bool): If True, use bidirectional RNN in encoder.
- Defaults to False.
- rnn_dropout (float): Dropout probability of RNN layer in encoder.
- Defaults to 0.0.
- enc_gru (bool): If True, use GRU, else LSTM in encoder. Defaults
- to False.
- d_model (int): Dim :math:`D_i` of channels from backbone. Defaults
- to 512.
- d_enc (int): Dim :math:`D_m` of encoder RNN layer. Defaults to 512.
- mask (bool): If True, mask padding in RNN sequence. Defaults to
- True.
- init_cfg (dict or list[dict], optional): Initialization configs.
- Defaults to [dict(type='Xavier', layer='Conv2d'),
- dict(type='Uniform', layer='BatchNorm2d')].
- """
-
- def __init__(self,
- enc_bi_rnn: bool = False,
- rnn_dropout: Union[int, float] = 0.0,
- enc_gru: bool = False,
- d_model: int = 512,
- d_enc: int = 512,
- mask: bool = True,
- init_cfg: Sequence[Dict] = [
- dict(type='Xavier', layer='Conv2d'),
- dict(type='Uniform', layer='BatchNorm2d')
- ],
- **kwargs) -> None:
- super().__init__(init_cfg=init_cfg)
- assert isinstance(enc_bi_rnn, bool)
- assert isinstance(rnn_dropout, (int, float))
- assert 0 <= rnn_dropout < 1.0
- assert isinstance(enc_gru, bool)
- assert isinstance(d_model, int)
- assert isinstance(d_enc, int)
- assert isinstance(mask, bool)
-
- self.enc_bi_rnn = enc_bi_rnn
- self.rnn_dropout = rnn_dropout
- self.mask = mask
-
- # LSTM Encoder
- kwargs = dict(
- input_size=d_model,
- hidden_size=d_enc,
- num_layers=2,
- batch_first=True,
- dropout=rnn_dropout,
- bidirectional=enc_bi_rnn)
- if enc_gru:
- self.rnn_encoder = nn.GRU(**kwargs)
- else:
- self.rnn_encoder = nn.LSTM(**kwargs)
-
- # global feature transformation
- encoder_rnn_out_size = d_enc * (int(enc_bi_rnn) + 1)
- self.linear = nn.Linear(encoder_rnn_out_size, encoder_rnn_out_size)
-
- def forward(
- self,
- feat: torch.Tensor,
- data_samples: Optional[Sequence[TextRecogDataSample]] = None
- ) -> torch.Tensor:
- """
- Args:
- feat (Tensor): Tensor of shape :math:`(N, D_i, H, W)`.
- data_samples (list[TextRecogDataSample], optional): Batch of
- TextRecogDataSample, containing valid_ratio information.
- Defaults to None.
-
- Returns:
- Tensor: A tensor of shape :math:`(N, D_m)`.
- """
- if data_samples is not None:
- assert len(data_samples) == feat.size(0)
-
- valid_ratios = None
- if data_samples is not None:
- valid_ratios = [
- data_sample.get('valid_ratio', 1.0)
- for data_sample in data_samples
- ] if self.mask else None
-
- h_feat = feat.size(2)
- feat_v = F.max_pool2d(
- feat, kernel_size=(h_feat, 1), stride=1, padding=0)
- feat_v = feat_v.squeeze(2) # bsz * C * W
- feat_v = feat_v.permute(0, 2, 1).contiguous() # bsz * W * C
-
- holistic_feat = self.rnn_encoder(feat_v)[0] # bsz * T * C
-
- if valid_ratios is not None:
- valid_hf = []
- T = holistic_feat.size(1)
- for i, valid_ratio in enumerate(valid_ratios):
- valid_step = min(T, math.ceil(T * valid_ratio)) - 1
- valid_hf.append(holistic_feat[i, valid_step, :])
- valid_hf = torch.stack(valid_hf, dim=0)
- else:
- valid_hf = holistic_feat[:, -1, :] # bsz * C
-
- holistic_feat = self.linear(valid_hf) # bsz * C
-
- return holistic_feat
diff --git a/pipeline/mmocr/mmocr/models/textrecog/encoders/satrn_encoder.py b/pipeline/mmocr/mmocr/models/textrecog/encoders/satrn_encoder.py
deleted file mode 100644
index ec6613535f99ca233196adbeb9fec5cdfe2531c6..0000000000000000000000000000000000000000
--- a/pipeline/mmocr/mmocr/models/textrecog/encoders/satrn_encoder.py
+++ /dev/null
@@ -1,95 +0,0 @@
-# Copyright (c) OpenMMLab. All rights reserved.
-import math
-from typing import Dict, List, Optional, Union
-
-import torch.nn as nn
-from mmengine.model import ModuleList
-from torch import Tensor
-
-from mmocr.models.textrecog.layers import (Adaptive2DPositionalEncoding,
- SATRNEncoderLayer)
-from mmocr.registry import MODELS
-from mmocr.structures import TextRecogDataSample
-from .base import BaseEncoder
-
-
-@MODELS.register_module()
-class SATRNEncoder(BaseEncoder):
- """Implement encoder for SATRN, see `SATRN.
-
- `_.
-
- Args:
- n_layers (int): Number of attention layers. Defaults to 12.
- n_head (int): Number of parallel attention heads. Defaults to 8.
- d_k (int): Dimension of the key vector. Defaults to 64.
- d_v (int): Dimension of the value vector. Defaults to 64.
- d_model (int): Dimension :math:`D_m` of the input from previous model.
- Defaults to 512.
- n_position (int): Length of the positional encoding vector. Must be
- greater than ``max_seq_len``. Defaults to 100.
- d_inner (int): Hidden dimension of feedforward layers. Defaults to 256.
- dropout (float): Dropout rate. Defaults to 0.1.
- init_cfg (dict or list[dict], optional): Initialization configs.
- Defaults to None.
- """
-
- def __init__(self,
- n_layers: int = 12,
- n_head: int = 8,
- d_k: int = 64,
- d_v: int = 64,
- d_model: int = 512,
- n_position: int = 100,
- d_inner: int = 256,
- dropout: float = 0.1,
- init_cfg: Optional[Union[Dict, List[Dict]]] = None) -> None:
- super().__init__(init_cfg=init_cfg)
- self.d_model = d_model
- self.position_enc = Adaptive2DPositionalEncoding(
- d_hid=d_model,
- n_height=n_position,
- n_width=n_position,
- dropout=dropout)
- self.layer_stack = ModuleList([
- SATRNEncoderLayer(
- d_model, d_inner, n_head, d_k, d_v, dropout=dropout)
- for _ in range(n_layers)
- ])
- self.layer_norm = nn.LayerNorm(d_model)
-
- def forward(self,
- feat: Tensor,
- data_samples: List[TextRecogDataSample] = None) -> Tensor:
- """Forward propagation of encoder.
-
- Args:
- feat (Tensor): Feature tensor of shape :math:`(N, D_m, H, W)`.
- data_samples (list[TextRecogDataSample]): Batch of
- TextRecogDataSample, containing `valid_ratio` information.
- Defaults to None.
-
- Returns:
- Tensor: A tensor of shape :math:`(N, T, D_m)`.
- """
- valid_ratios = [1.0 for _ in range(feat.size(0))]
- if data_samples is not None:
- valid_ratios = [
- data_sample.get('valid_ratio', 1.0)
- for data_sample in data_samples
- ]
- feat = self.position_enc(feat)
- n, c, h, w = feat.size()
- mask = feat.new_zeros((n, h, w))
- for i, valid_ratio in enumerate(valid_ratios):
- valid_width = min(w, math.ceil(w * valid_ratio))
- mask[i, :, :valid_width] = 1
- mask = mask.view(n, h * w)
- feat = feat.view(n, c, h * w)
-
- output = feat.permute(0, 2, 1).contiguous()
- for enc_layer in self.layer_stack:
- output = enc_layer(output, h, w, mask)
- output = self.layer_norm(output)
-
- return output
diff --git a/pipeline/mmocr/mmocr/models/textrecog/encoders/svtr_encoder.py b/pipeline/mmocr/mmocr/models/textrecog/encoders/svtr_encoder.py
deleted file mode 100644
index aa27f42209c80fca9fdd58e1fae4566cbea9cc76..0000000000000000000000000000000000000000
--- a/pipeline/mmocr/mmocr/models/textrecog/encoders/svtr_encoder.py
+++ /dev/null
@@ -1,639 +0,0 @@
-# Copyright (c) OpenMMLab. All rights reserved.
-from typing import Dict, List, Optional, Tuple, Union
-
-import numpy as np
-import torch
-import torch.nn as nn
-import torch.nn.functional as F
-from mmcv.cnn import ConvModule
-from mmcv.cnn.bricks import DropPath
-from mmengine.model import BaseModule
-from mmengine.model.weight_init import trunc_normal_init
-
-from mmocr.registry import MODELS
-from mmocr.structures import TextRecogDataSample
-
-
-class OverlapPatchEmbed(BaseModule):
- """Image to the progressive overlapping Patch Embedding.
-
- Args:
- in_channels (int): Number of input channels. Defaults to 3.
- embed_dims (int): The dimensions of embedding. Defaults to 768.
- num_layers (int, optional): Number of Conv_BN_Layer. Defaults to 2 and
- limit to [2, 3].
- init_cfg (dict or list[dict], optional): Initialization configs.
- Defaults to None.
- """
-
- def __init__(self,
- in_channels: int = 3,
- embed_dims: int = 768,
- num_layers: int = 2,
- init_cfg: Optional[Union[Dict, List[Dict]]] = None):
-
- super().__init__(init_cfg=init_cfg)
-
- assert num_layers in [2, 3], \
- 'The number of layers must belong to [2, 3]'
- self.net = nn.Sequential()
- for num in range(num_layers, 0, -1):
- if (num == num_layers):
- _input = in_channels
- _output = embed_dims // (2**(num - 1))
- self.net.add_module(
- f'ConvModule{str(num_layers - num)}',
- ConvModule(
- in_channels=_input,
- out_channels=_output,
- kernel_size=3,
- stride=2,
- padding=1,
- norm_cfg=dict(type='BN'),
- act_cfg=dict(type='GELU')))
- _input = _output
-
- def forward(self, x: torch.Tensor) -> torch.Tensor:
- """Forward function.
-
- Args:
- x (Tensor): A Tensor of shape :math:`(N, C, H, W)`.
-
- Returns:
- Tensor: A tensor of shape math:`(N, HW//16, C)`.
- """
- x = self.net(x).flatten(2).permute(0, 2, 1)
- return x
-
-
-class ConvMixer(BaseModule):
- """The conv Mixer.
-
- Args:
- embed_dims (int): Number of character components.
- num_heads (int, optional): Number of heads. Defaults to 8.
- input_shape (Tuple[int, int], optional): The shape of input [H, W].
- Defaults to [8, 25].
- local_k (Tuple[int, int], optional): Window size. Defaults to [3, 3].
- init_cfg (dict or list[dict], optional): Initialization configs.
- Defaults to None.
- """
-
- def __init__(self,
- embed_dims: int,
- num_heads: int = 8,
- input_shape: Tuple[int, int] = [8, 25],
- local_k: Tuple[int, int] = [3, 3],
- init_cfg: Optional[Union[Dict, List[Dict]]] = None):
- super().__init__(init_cfg)
- self.input_shape = input_shape
- self.embed_dims = embed_dims
- self.local_mixer = nn.Conv2d(
- in_channels=embed_dims,
- out_channels=embed_dims,
- kernel_size=local_k,
- stride=1,
- padding=(local_k[0] // 2, local_k[1] // 2),
- groups=num_heads)
-
- def forward(self, x: torch.Tensor) -> torch.Tensor:
- """Forward function.
-
- Args:
- x (torch.Tensor): A Tensor of shape :math:`(N, HW, C)`.
-
- Returns:
- torch.Tensor: Tensor: A tensor of shape math:`(N, HW, C)`.
- """
- h, w = self.input_shape
- x = x.permute(0, 2, 1).reshape([-1, self.embed_dims, h, w])
- x = self.local_mixer(x)
- x = x.flatten(2).permute(0, 2, 1)
- return x
-
-
-class AttnMixer(BaseModule):
- """One of mixer of {'Global', 'Local'}. Defaults to Global Mixer.
-
- Args:
- embed_dims (int): Number of character components.
- num_heads (int, optional): Number of heads. Defaults to 8.
- mixer (str, optional): The mixer type, choices are 'Global' and
- 'Local'. Defaults to 'Global'.
- input_shape (Tuple[int, int], optional): The shape of input [H, W].
- Defaults to [8, 25].
- local_k (Tuple[int, int], optional): Window size. Defaults to [7, 11].
- qkv_bias (bool, optional): Whether a additive bias is required.
- Defaults to False.
- qk_scale (float, optional): A scaling factor. Defaults to None.
- attn_drop (float, optional): Attn dropout probability. Defaults to 0.0.
- proj_drop (float, optional): Proj dropout layer. Defaults to 0.0.
- init_cfg (dict or list[dict], optional): Initialization configs.
- Defaults to None.
- """
-
- def __init__(self,
- embed_dims: int,
- num_heads: int = 8,
- mixer: str = 'Global',
- input_shape: Tuple[int, int] = [8, 25],
- local_k: Tuple[int, int] = [7, 11],
- qkv_bias: bool = False,
- qk_scale: float = None,
- attn_drop: float = 0.,
- proj_drop: float = 0.,
- init_cfg: Optional[Union[Dict, List[Dict]]] = None):
- super().__init__(init_cfg)
- assert mixer in {'Global', 'Local'}, \
- "The type of mixer must belong to {'Global', 'Local'}"
- self.num_heads = num_heads
- head_dim = embed_dims // num_heads
- self.scale = qk_scale or head_dim**-0.5
- self.qkv = nn.Linear(embed_dims, embed_dims * 3, bias=qkv_bias)
- self.attn_drop = nn.Dropout(attn_drop)
- self.proj = nn.Linear(embed_dims, embed_dims)
- self.proj_drop = nn.Dropout(proj_drop)
- self.input_shape = input_shape
- if input_shape is not None:
- height, width = input_shape
- self.input_size = height * width
- self.embed_dims = embed_dims
- if mixer == 'Local' and input_shape is not None:
- hk = local_k[0]
- wk = local_k[1]
- mask = torch.ones(
- [height * width, height + hk - 1, width + wk - 1],
- dtype=torch.float32)
- for h in range(0, height):
- for w in range(0, width):
- mask[h * width + w, h:h + hk, w:w + wk] = 0.
- mask = mask[:, hk // 2:height + hk // 2,
- wk // 2:width + wk // 2].flatten(1)
- mask[mask >= 1] = -np.inf
- self.register_buffer('mask', mask[None, None, :, :])
- self.mixer = mixer
-
- def forward(self, x: torch.Tensor) -> torch.Tensor:
- """Forward function.
-
- Args:
- x (torch.Tensor): A Tensor of shape :math:`(N, H, W, C)`.
-
- Returns:
- torch.Tensor: A Tensor of shape :math:`(N, H, W, C)`.
- """
- if self.input_shape is not None:
- input_size, embed_dims = self.input_size, self.embed_dims
- else:
- _, input_size, embed_dims = x.shape
- qkv = self.qkv(x).reshape((-1, input_size, 3, self.num_heads,
- embed_dims // self.num_heads)).permute(
- (2, 0, 3, 1, 4))
- q, k, v = qkv[0] * self.scale, qkv[1], qkv[2]
- attn = q.matmul(k.permute(0, 1, 3, 2))
- if self.mixer == 'Local':
- attn += self.mask
- attn = F.softmax(attn, dim=-1)
- attn = self.attn_drop(attn)
-
- x = attn.matmul(v).permute(0, 2, 1, 3).reshape(-1, input_size,
- embed_dims)
- x = self.proj(x)
- x = self.proj_drop(x)
- return x
-
-
-class MLP(BaseModule):
- """The MLP block.
-
- Args:
- in_features (int): The input features.
- hidden_features (int, optional): The hidden features.
- Defaults to None.
- out_features (int, optional): The output features.
- Defaults to None.
- drop (float, optional): cfg of dropout function. Defaults to 0.0.
- init_cfg (dict or list[dict], optional): Initialization configs.
- Defaults to None.
- """
-
- def __init__(self,
- in_features: int,
- hidden_features: int = None,
- out_features: int = None,
- drop: float = 0.,
- init_cfg: Optional[Union[Dict, List[Dict]]] = None):
- super().__init__(init_cfg)
- hidden_features = hidden_features or in_features
- out_features = out_features or in_features
- self.fc1 = nn.Linear(in_features, hidden_features)
- self.act = nn.GELU()
- self.fc2 = nn.Linear(hidden_features, out_features)
- self.drop = nn.Dropout(drop)
-
- def forward(self, x: torch.Tensor) -> torch.Tensor:
- """Forward function.
-
- Args:
- x (torch.Tensor): A Tensor of shape :math:`(N, H, W, C)`.
-
- Returns:
- torch.Tensor: A Tensor of shape :math:`(N, H, W, C)`.
- """
- x = self.fc1(x)
- x = self.act(x)
- x = self.drop(x)
- x = self.fc2(x)
- x = self.drop(x)
- return x
-
-
-class MixingBlock(BaseModule):
- """The Mixing block.
-
- Args:
- embed_dims (int): Number of character components.
- num_heads (int): Number of heads
- mixer (str, optional): The mixer type. Defaults to 'Global'.
- window_size (Tuple[int ,int], optional): Local window size.
- Defaults to [7, 11].
- input_shape (Tuple[int, int], optional): The shape of input [H, W].
- Defaults to [8, 25].
- mlp_ratio (float, optional): The ratio of hidden features to input.
- Defaults to 4.0.
- qkv_bias (bool, optional): Whether a additive bias is required.
- Defaults to False.
- qk_scale (float, optional): A scaling factor. Defaults to None.
- drop (float, optional): cfg of Dropout. Defaults to 0..
- attn_drop (float, optional): cfg of Dropout. Defaults to 0.0.
- drop_path (float, optional): The probability of drop path.
- Defaults to 0.0.
- pernorm (bool, optional): Whether to place the MxingBlock before norm.
- Defaults to True.
- init_cfg (dict or list[dict], optional): Initialization configs.
- Defaults to None.
- """
-
- def __init__(self,
- embed_dims: int,
- num_heads: int,
- mixer: str = 'Global',
- window_size: Tuple[int, int] = [7, 11],
- input_shape: Tuple[int, int] = [8, 25],
- mlp_ratio: float = 4.,
- qkv_bias: bool = False,
- qk_scale: float = None,
- drop: float = 0.,
- attn_drop: float = 0.,
- drop_path=0.,
- prenorm: bool = True,
- init_cfg: Optional[Union[Dict, List[Dict]]] = None):
- super().__init__(init_cfg)
- self.norm1 = nn.LayerNorm(embed_dims, eps=1e-6)
- if mixer in {'Global', 'Local'}:
- self.mixer = AttnMixer(
- embed_dims,
- num_heads=num_heads,
- mixer=mixer,
- input_shape=input_shape,
- local_k=window_size,
- qkv_bias=qkv_bias,
- qk_scale=qk_scale,
- attn_drop=attn_drop,
- proj_drop=drop)
- elif mixer == 'Conv':
- self.mixer = ConvMixer(
- embed_dims,
- num_heads=num_heads,
- input_shape=input_shape,
- local_k=window_size)
- else:
- raise TypeError('The mixer must be one of [Global, Local, Conv]')
- self.drop_path = DropPath(
- drop_path) if drop_path > 0. else nn.Identity()
- self.norm2 = nn.LayerNorm(embed_dims, eps=1e-6)
- mlp_hidden_dim = int(embed_dims * mlp_ratio)
- self.mlp_ratio = mlp_ratio
- self.mlp = MLP(
- in_features=embed_dims, hidden_features=mlp_hidden_dim, drop=drop)
- self.prenorm = prenorm
-
- def forward(self, x: torch.Tensor) -> torch.Tensor:
- """Forward function.
-
- Args:
- x (torch.Tensor): A Tensor of shape :math:`(N, H*W, C)`.
-
- Returns:
- torch.Tensor: A Tensor of shape :math:`(N, H*W, C)`.
- """
- if self.prenorm:
- x = self.norm1(x + self.drop_path(self.mixer(x)))
- x = self.norm2(x + self.drop_path(self.mlp(x)))
- else:
- x = x + self.drop_path(self.mixer(self.norm1(x)))
- x = x + self.drop_path(self.mlp(self.norm2(x)))
- return x
-
-
-class MerigingBlock(BaseModule):
- """The last block of any stage, except for the last stage.
-
- Args:
- in_channels (int): The channels of input.
- out_channels (int): The channels of output.
- types (str, optional): Which downsample operation of ['Pool', 'Conv'].
- Defaults to 'Pool'.
- stride (Union[int, Tuple[int, int]], optional): Stride of the Conv.
- Defaults to [2, 1].
- act (bool, optional): activation function. Defaults to None.
- init_cfg (dict or list[dict], optional): Initialization configs.
- Defaults to None.
- """
-
- def __init__(self,
- in_channels: int,
- out_channels: int,
- types: str = 'Pool',
- stride: Union[int, Tuple[int, int]] = [2, 1],
- act: bool = None,
- init_cfg: Optional[Union[Dict, List[Dict]]] = None):
- super().__init__(init_cfg)
- self.types = types
- if types == 'Pool':
- self.avgpool = nn.AvgPool2d(
- kernel_size=[3, 5], stride=stride, padding=[1, 2])
- self.maxpool = nn.MaxPool2d(
- kernel_size=[3, 5], stride=stride, padding=[1, 2])
- self.proj = nn.Linear(in_channels, out_channels)
- else:
- self.conv = nn.Conv2d(
- in_channels,
- out_channels,
- kernel_size=3,
- stride=stride,
- padding=1)
- self.norm = nn.LayerNorm(out_channels)
- if act is not None:
- self.act = act()
- else:
- self.act = None
-
- def forward(self, x: torch.Tensor) -> torch.Tensor:
- """Forward function.
-
- Args:
- x (torch.Tensor): A Tensor of shape :math:`(N, H, W, C)`.
-
- Returns:
- torch.Tensor: A Tensor of shape :math:`(N, H/2, W, 2C)`.
- """
- if self.types == 'Pool':
- x = (self.avgpool(x) + self.maxpool(x)) * 0.5
- out = self.proj(x.flatten(2).permute(0, 2, 1))
-
- else:
- x = self.conv(x)
- out = x.flatten(2).permute(0, 2, 1)
- out = self.norm(out)
- if self.act is not None:
- out = self.act(out)
-
- return out
-
-
-@MODELS.register_module()
-class SVTREncoder(BaseModule):
- """A PyTorch implementation of `SVTR: Scene Text Recognition with a Single
- Visual Model `_
-
- Code is partially modified from https://github.com/PaddlePaddle/PaddleOCR.
-
- Args:
- img_size (Tuple[int, int], optional): The expected input image shape.
- Defaults to [32, 100].
- in_channels (int, optional): The num of input channels. Defaults to 3.
- embed_dims (Tuple[int, int, int], optional): Number of input channels.
- Defaults to [64, 128, 256].
- depth (Tuple[int, int, int], optional):
- The number of MixingBlock at each stage. Defaults to [3, 6, 3].
- num_heads (Tuple[int, int, int], optional): Number of attention heads.
- Defaults to [2, 4, 8].
- mixer_types (Tuple[str], optional): Mixing type in a MixingBlock.
- Defaults to ['Local']*6+['Global']*6.
- window_size (Tuple[Tuple[int, int]], optional):
- The height and width of the window at eeach stage.
- Defaults to [[7, 11], [7, 11], [7, 11]].
- merging_types (str, optional): The way of downsample in MergingBlock.
- Defaults to 'Conv'.
- mlp_ratio (int, optional): Ratio of hidden features to input in MLP.
- Defaults to 4.
- qkv_bias (bool, optional):
- Whether to add bias for qkv in attention modules. Defaults to True.
- qk_scale (float, optional): A scaling factor. Defaults to None.
- drop_rate (float, optional): Probability of an element to be zeroed.
- Defaults to 0.0.
- last_drop (float, optional): cfg of dropout at last stage.
- Defaults to 0.1.
- attn_drop_rate (float, optional): _description_. Defaults to 0..
- drop_path_rate (float, optional): stochastic depth rate.
- Defaults to 0.1.
- out_channels (int, optional): The num of output channels in backone.
- Defaults to 192.
- max_seq_len (int, optional): Maximum output sequence length :math:`T`.
- Defaults to 25.
- num_layers (int, optional): The num of conv in PatchEmbedding.
- Defaults to 2.
- prenorm (bool, optional): Whether to place the MixingBlock before norm.
- Defaults to True.
- init_cfg (dict or list[dict], optional): Initialization configs.
- Defaults to None.
- """
-
- def __init__(self,
- img_size: Tuple[int, int] = [32, 100],
- in_channels: int = 3,
- embed_dims: Tuple[int, int, int] = [64, 128, 256],
- depth: Tuple[int, int, int] = [3, 6, 3],
- num_heads: Tuple[int, int, int] = [2, 4, 8],
- mixer_types: Tuple[str] = ['Local'] * 6 + ['Global'] * 6,
- window_size: Tuple[Tuple[int, int]] = [[7, 11], [7, 11],
- [7, 11]],
- merging_types: str = 'Conv',
- mlp_ratio: int = 4,
- qkv_bias: bool = True,
- qk_scale: float = None,
- drop_rate: float = 0.,
- last_drop: float = 0.1,
- attn_drop_rate: float = 0.,
- drop_path_rate: float = 0.1,
- out_channels: int = 192,
- max_seq_len: int = 25,
- num_layers: int = 2,
- prenorm: bool = True,
- init_cfg: Optional[Union[Dict, List[Dict]]] = None):
- super().__init__(init_cfg)
- self.img_size = img_size
- self.embed_dims = embed_dims
- self.out_channels = out_channels
- self.prenorm = prenorm
- self.patch_embed = OverlapPatchEmbed(
- in_channels=in_channels,
- embed_dims=embed_dims[0],
- num_layers=num_layers)
- num_patches = (img_size[1] // (2**num_layers)) * (
- img_size[0] // (2**num_layers))
- self.input_shape = [
- img_size[0] // (2**num_layers), img_size[1] // (2**num_layers)
- ]
- self.absolute_pos_embed = nn.Parameter(
- torch.zeros([1, num_patches, embed_dims[0]], dtype=torch.float32),
- requires_grad=True)
- self.pos_drop = nn.Dropout(drop_rate)
- dpr = np.linspace(0, drop_path_rate, sum(depth))
-
- self.blocks1 = nn.ModuleList([
- MixingBlock(
- embed_dims=embed_dims[0],
- num_heads=num_heads[0],
- mixer=mixer_types[0:depth[0]][i],
- window_size=window_size[0],
- input_shape=self.input_shape,
- mlp_ratio=mlp_ratio,
- qkv_bias=qkv_bias,
- qk_scale=qk_scale,
- drop=drop_rate,
- attn_drop=attn_drop_rate,
- drop_path=dpr[0:depth[0]][i],
- prenorm=prenorm) for i in range(depth[0])
- ])
- self.downsample1 = MerigingBlock(
- in_channels=embed_dims[0],
- out_channels=embed_dims[1],
- types=merging_types,
- stride=[2, 1])
- input_shape = [self.input_shape[0] // 2, self.input_shape[1]]
- self.merging_types = merging_types
-
- self.blocks2 = nn.ModuleList([
- MixingBlock(
- embed_dims=embed_dims[1],
- num_heads=num_heads[1],
- mixer=mixer_types[depth[0]:depth[0] + depth[1]][i],
- window_size=window_size[1],
- input_shape=input_shape,
- mlp_ratio=mlp_ratio,
- qkv_bias=qkv_bias,
- qk_scale=qk_scale,
- drop=drop_rate,
- attn_drop=attn_drop_rate,
- drop_path=dpr[depth[0]:depth[0] + depth[1]][i],
- prenorm=prenorm) for i in range(depth[1])
- ])
- self.downsample2 = MerigingBlock(
- in_channels=embed_dims[1],
- out_channels=embed_dims[2],
- types=merging_types,
- stride=[2, 1])
- input_shape = [self.input_shape[0] // 4, self.input_shape[1]]
-
- self.blocks3 = nn.ModuleList([
- MixingBlock(
- embed_dims=embed_dims[2],
- num_heads=num_heads[2],
- mixer=mixer_types[depth[0] + depth[1]:][i],
- window_size=window_size[2],
- input_shape=input_shape,
- mlp_ratio=mlp_ratio,
- qkv_bias=qkv_bias,
- qk_scale=qk_scale,
- drop=drop_rate,
- attn_drop=attn_drop_rate,
- drop_path=dpr[depth[0] + depth[1]:][i],
- prenorm=prenorm) for i in range(depth[2])
- ])
- self.layer_norm = nn.LayerNorm(self.embed_dims[-1], eps=1e-6)
- self.avgpool = nn.AdaptiveAvgPool2d([1, max_seq_len])
- self.last_conv = nn.Conv2d(
- in_channels=embed_dims[2],
- out_channels=self.out_channels,
- kernel_size=1,
- bias=False,
- stride=1,
- padding=0)
- self.hardwish = nn.Hardswish()
- self.dropout = nn.Dropout(p=last_drop)
-
- trunc_normal_init(self.absolute_pos_embed, mean=0, std=0.02)
- self.apply(self._init_weights)
-
- def _init_weights(self, m):
- if isinstance(m, nn.Linear):
- trunc_normal_init(m.weight, mean=0, std=0.02)
- if isinstance(m, nn.Linear) and m.bias is not None:
- nn.init.zeros_(m.bias)
- if isinstance(m, nn.LayerNorm):
- nn.init.zeros_(m.bias)
- nn.init.ones_(m.weight)
- if isinstance(m, nn.Conv2d):
- nn.init.kaiming_normal_(
- m.weight, mode='fan_out', nonlinearity='relu')
-
- def forward_features(self, x: torch.Tensor) -> torch.Tensor:
- """Forward function except the last combing operation.
-
- Args:
- x (torch.Tensor): A Tensor of shape :math:`(N, H, W, C)`.
-
- Returns:
- torch.Tensor: A Tensor of shape :math:`(N, H/16, W/4, 256)`.
- """
- x = self.patch_embed(x)
- x = x + self.absolute_pos_embed
- x = self.pos_drop(x)
- for blk in self.blocks1:
- x = blk(x)
- x = self.downsample1(
- x.permute(0, 2, 1).reshape([
- -1, self.embed_dims[0], self.input_shape[0],
- self.input_shape[1]
- ]))
-
- for blk in self.blocks2:
- x = blk(x)
- x = self.downsample2(
- x.permute(0, 2, 1).reshape([
- -1, self.embed_dims[1], self.input_shape[0] // 2,
- self.input_shape[1]
- ]))
-
- for blk in self.blocks3:
- x = blk(x)
- if not self.prenorm:
- x = self.layer_norm(x)
- return x
-
- def forward(self,
- x: torch.Tensor,
- data_samples: List[TextRecogDataSample] = None
- ) -> torch.Tensor:
- """Forward function.
-
- Args:
- x (torch.Tensor): A Tensor of shape :math:`(N, H/16, W/4, 256)`.
- data_samples (list[TextRecogDataSample]): Batch of
- TextRecogDataSample. Defaults to None.
-
- Returns:
- torch.Tensor: A Tensor of shape :math:`(N, 1, W/4, 192)`.
- """
- x = self.forward_features(x)
- x = self.avgpool(
- x.permute(0, 2, 1).reshape([
- -1, self.embed_dims[2], self.input_shape[0] // 4,
- self.input_shape[1]
- ]))
- x = self.last_conv(x)
- x = self.hardwish(x)
- x = self.dropout(x)
- return x
diff --git a/pipeline/mmocr/mmocr/models/textrecog/layers/__init__.py b/pipeline/mmocr/mmocr/models/textrecog/layers/__init__.py
deleted file mode 100644
index a1fa8af5586145c8e31c463e6d0620c9f1af2e3b..0000000000000000000000000000000000000000
--- a/pipeline/mmocr/mmocr/models/textrecog/layers/__init__.py
+++ /dev/null
@@ -1,13 +0,0 @@
-# Copyright (c) OpenMMLab. All rights reserved.
-from .conv_layer import BasicBlock, Bottleneck
-from .dot_product_attention_layer import DotProductAttentionLayer
-from .lstm_layer import BidirectionalLSTM
-from .position_aware_layer import PositionAwareLayer
-from .robust_scanner_fusion_layer import RobustScannerFusionLayer
-from .satrn_layers import Adaptive2DPositionalEncoding, SATRNEncoderLayer
-
-__all__ = [
- 'BidirectionalLSTM', 'Adaptive2DPositionalEncoding', 'BasicBlock',
- 'Bottleneck', 'RobustScannerFusionLayer', 'DotProductAttentionLayer',
- 'PositionAwareLayer', 'SATRNEncoderLayer'
-]
diff --git a/pipeline/mmocr/mmocr/models/textrecog/layers/__pycache__/__init__.cpython-38.pyc b/pipeline/mmocr/mmocr/models/textrecog/layers/__pycache__/__init__.cpython-38.pyc
deleted file mode 100644
index ad7b3f9f1f33306f73f9e2169dcf0d0542b0bcaa..0000000000000000000000000000000000000000
Binary files a/pipeline/mmocr/mmocr/models/textrecog/layers/__pycache__/__init__.cpython-38.pyc and /dev/null differ
diff --git a/pipeline/mmocr/mmocr/models/textrecog/layers/__pycache__/__init__.cpython-39.pyc b/pipeline/mmocr/mmocr/models/textrecog/layers/__pycache__/__init__.cpython-39.pyc
deleted file mode 100644
index 7015b82bdfc0486b53d9681faf5413f99b21d2c4..0000000000000000000000000000000000000000
Binary files a/pipeline/mmocr/mmocr/models/textrecog/layers/__pycache__/__init__.cpython-39.pyc and /dev/null differ
diff --git a/pipeline/mmocr/mmocr/models/textrecog/layers/__pycache__/conv_layer.cpython-38.pyc b/pipeline/mmocr/mmocr/models/textrecog/layers/__pycache__/conv_layer.cpython-38.pyc
deleted file mode 100644
index 26b2e3de72f723d483fc3e8140feece692dbe825..0000000000000000000000000000000000000000
Binary files a/pipeline/mmocr/mmocr/models/textrecog/layers/__pycache__/conv_layer.cpython-38.pyc and /dev/null differ
diff --git a/pipeline/mmocr/mmocr/models/textrecog/layers/__pycache__/conv_layer.cpython-39.pyc b/pipeline/mmocr/mmocr/models/textrecog/layers/__pycache__/conv_layer.cpython-39.pyc
deleted file mode 100644
index d171641d3ec2b27588e231d02d5ce173bb993e1c..0000000000000000000000000000000000000000
Binary files a/pipeline/mmocr/mmocr/models/textrecog/layers/__pycache__/conv_layer.cpython-39.pyc and /dev/null differ
diff --git a/pipeline/mmocr/mmocr/models/textrecog/layers/__pycache__/dot_product_attention_layer.cpython-38.pyc b/pipeline/mmocr/mmocr/models/textrecog/layers/__pycache__/dot_product_attention_layer.cpython-38.pyc
deleted file mode 100644
index 0492495d672c77e29bd9d1389f625a97e5920175..0000000000000000000000000000000000000000
Binary files a/pipeline/mmocr/mmocr/models/textrecog/layers/__pycache__/dot_product_attention_layer.cpython-38.pyc and /dev/null differ
diff --git a/pipeline/mmocr/mmocr/models/textrecog/layers/__pycache__/dot_product_attention_layer.cpython-39.pyc b/pipeline/mmocr/mmocr/models/textrecog/layers/__pycache__/dot_product_attention_layer.cpython-39.pyc
deleted file mode 100644
index f25d6521e841fdec7facacdb0f7fb135587e69b3..0000000000000000000000000000000000000000
Binary files a/pipeline/mmocr/mmocr/models/textrecog/layers/__pycache__/dot_product_attention_layer.cpython-39.pyc and /dev/null differ
diff --git a/pipeline/mmocr/mmocr/models/textrecog/layers/__pycache__/lstm_layer.cpython-38.pyc b/pipeline/mmocr/mmocr/models/textrecog/layers/__pycache__/lstm_layer.cpython-38.pyc
deleted file mode 100644
index 0ef37165ab1c1e339c7546d46e5e90c37fdd223e..0000000000000000000000000000000000000000
Binary files a/pipeline/mmocr/mmocr/models/textrecog/layers/__pycache__/lstm_layer.cpython-38.pyc and /dev/null differ
diff --git a/pipeline/mmocr/mmocr/models/textrecog/layers/__pycache__/lstm_layer.cpython-39.pyc b/pipeline/mmocr/mmocr/models/textrecog/layers/__pycache__/lstm_layer.cpython-39.pyc
deleted file mode 100644
index d7f585eec448232b08fb8d6f05307cbbc337b714..0000000000000000000000000000000000000000
Binary files a/pipeline/mmocr/mmocr/models/textrecog/layers/__pycache__/lstm_layer.cpython-39.pyc and /dev/null differ
diff --git a/pipeline/mmocr/mmocr/models/textrecog/layers/__pycache__/position_aware_layer.cpython-38.pyc b/pipeline/mmocr/mmocr/models/textrecog/layers/__pycache__/position_aware_layer.cpython-38.pyc
deleted file mode 100644
index 81037a6969b0d2e9fda936439f790a27e791e571..0000000000000000000000000000000000000000
Binary files a/pipeline/mmocr/mmocr/models/textrecog/layers/__pycache__/position_aware_layer.cpython-38.pyc and /dev/null differ
diff --git a/pipeline/mmocr/mmocr/models/textrecog/layers/__pycache__/position_aware_layer.cpython-39.pyc b/pipeline/mmocr/mmocr/models/textrecog/layers/__pycache__/position_aware_layer.cpython-39.pyc
deleted file mode 100644
index 7a0a42781e7e1e78632512dc71802aab34fc6c11..0000000000000000000000000000000000000000
Binary files a/pipeline/mmocr/mmocr/models/textrecog/layers/__pycache__/position_aware_layer.cpython-39.pyc and /dev/null differ
diff --git a/pipeline/mmocr/mmocr/models/textrecog/layers/__pycache__/robust_scanner_fusion_layer.cpython-38.pyc b/pipeline/mmocr/mmocr/models/textrecog/layers/__pycache__/robust_scanner_fusion_layer.cpython-38.pyc
deleted file mode 100644
index 3f5c278ab37ed808f7621b27a21c8f9e5b160864..0000000000000000000000000000000000000000
Binary files a/pipeline/mmocr/mmocr/models/textrecog/layers/__pycache__/robust_scanner_fusion_layer.cpython-38.pyc and /dev/null differ
diff --git a/pipeline/mmocr/mmocr/models/textrecog/layers/__pycache__/robust_scanner_fusion_layer.cpython-39.pyc b/pipeline/mmocr/mmocr/models/textrecog/layers/__pycache__/robust_scanner_fusion_layer.cpython-39.pyc
deleted file mode 100644
index 2f02587e79c9bedd61745ed29b4379d4c9ac3f0d..0000000000000000000000000000000000000000
Binary files a/pipeline/mmocr/mmocr/models/textrecog/layers/__pycache__/robust_scanner_fusion_layer.cpython-39.pyc and /dev/null differ
diff --git a/pipeline/mmocr/mmocr/models/textrecog/layers/__pycache__/satrn_layers.cpython-38.pyc b/pipeline/mmocr/mmocr/models/textrecog/layers/__pycache__/satrn_layers.cpython-38.pyc
deleted file mode 100644
index dd0148bb2b8c594ce119ed1acf170a80e614ea6a..0000000000000000000000000000000000000000
Binary files a/pipeline/mmocr/mmocr/models/textrecog/layers/__pycache__/satrn_layers.cpython-38.pyc and /dev/null differ
diff --git a/pipeline/mmocr/mmocr/models/textrecog/layers/__pycache__/satrn_layers.cpython-39.pyc b/pipeline/mmocr/mmocr/models/textrecog/layers/__pycache__/satrn_layers.cpython-39.pyc
deleted file mode 100644
index ab5d85789e2d133b8eb72c3ad1c3cab5bf438652..0000000000000000000000000000000000000000
Binary files a/pipeline/mmocr/mmocr/models/textrecog/layers/__pycache__/satrn_layers.cpython-39.pyc and /dev/null differ
diff --git a/pipeline/mmocr/mmocr/models/textrecog/layers/conv_layer.py b/pipeline/mmocr/mmocr/models/textrecog/layers/conv_layer.py
deleted file mode 100644
index a60f2f5599318e29fd3e97b6079fa6db388a507e..0000000000000000000000000000000000000000
--- a/pipeline/mmocr/mmocr/models/textrecog/layers/conv_layer.py
+++ /dev/null
@@ -1,182 +0,0 @@
-# Copyright (c) OpenMMLab. All rights reserved.
-import torch.nn as nn
-from mmcv.cnn import build_plugin_layer
-
-
-def conv3x3(in_planes, out_planes, stride=1):
- """3x3 convolution with padding."""
- return nn.Conv2d(
- in_planes,
- out_planes,
- kernel_size=3,
- stride=stride,
- padding=1,
- bias=False)
-
-
-def conv1x1(in_planes, out_planes):
- """1x1 convolution with padding."""
- return nn.Conv2d(
- in_planes, out_planes, kernel_size=1, stride=1, padding=0, bias=False)
-
-
-class BasicBlock(nn.Module):
-
- expansion = 1
-
- def __init__(self,
- inplanes,
- planes,
- stride=1,
- downsample=None,
- use_conv1x1=False,
- plugins=None):
- super().__init__()
-
- if use_conv1x1:
- self.conv1 = conv1x1(inplanes, planes)
- self.conv2 = conv3x3(planes, planes * self.expansion, stride)
- else:
- self.conv1 = conv3x3(inplanes, planes, stride)
- self.conv2 = conv3x3(planes, planes * self.expansion)
-
- self.with_plugins = False
- if plugins:
- if isinstance(plugins, dict):
- plugins = [plugins]
- self.with_plugins = True
- # collect plugins for conv1/conv2/
- self.before_conv1_plugin = [
- plugin['cfg'] for plugin in plugins
- if plugin['position'] == 'before_conv1'
- ]
- self.after_conv1_plugin = [
- plugin['cfg'] for plugin in plugins
- if plugin['position'] == 'after_conv1'
- ]
- self.after_conv2_plugin = [
- plugin['cfg'] for plugin in plugins
- if plugin['position'] == 'after_conv2'
- ]
- self.after_shortcut_plugin = [
- plugin['cfg'] for plugin in plugins
- if plugin['position'] == 'after_shortcut'
- ]
-
- self.planes = planes
- self.bn1 = nn.BatchNorm2d(planes)
- self.relu = nn.ReLU(inplace=True)
- self.bn2 = nn.BatchNorm2d(planes * self.expansion)
- self.downsample = downsample
- self.stride = stride
-
- if self.with_plugins:
- self.before_conv1_plugin_names = self.make_block_plugins(
- inplanes, self.before_conv1_plugin)
- self.after_conv1_plugin_names = self.make_block_plugins(
- planes, self.after_conv1_plugin)
- self.after_conv2_plugin_names = self.make_block_plugins(
- planes, self.after_conv2_plugin)
- self.after_shortcut_plugin_names = self.make_block_plugins(
- planes, self.after_shortcut_plugin)
-
- def make_block_plugins(self, in_channels, plugins):
- """make plugins for block.
-
- Args:
- in_channels (int): Input channels of plugin.
- plugins (list[dict]): List of plugins cfg to build.
-
- Returns:
- list[str]: List of the names of plugin.
- """
- assert isinstance(plugins, list)
- plugin_names = []
- for plugin in plugins:
- plugin = plugin.copy()
- name, layer = build_plugin_layer(
- plugin,
- in_channels=in_channels,
- out_channels=in_channels,
- postfix=plugin.pop('postfix', ''))
- assert not hasattr(self, name), f'duplicate plugin {name}'
- self.add_module(name, layer)
- plugin_names.append(name)
- return plugin_names
-
- def forward_plugin(self, x, plugin_names):
- out = x
- for name in plugin_names:
- out = getattr(self, name)(x)
- return out
-
- def forward(self, x):
- if self.with_plugins:
- x = self.forward_plugin(x, self.before_conv1_plugin_names)
- residual = x
-
- out = self.conv1(x)
- out = self.bn1(out)
- out = self.relu(out)
-
- if self.with_plugins:
- out = self.forward_plugin(out, self.after_conv1_plugin_names)
-
- out = self.conv2(out)
- out = self.bn2(out)
-
- if self.with_plugins:
- out = self.forward_plugin(out, self.after_conv2_plugin_names)
-
- if self.downsample is not None:
- residual = self.downsample(x)
-
- out += residual
- out = self.relu(out)
-
- if self.with_plugins:
- out = self.forward_plugin(out, self.after_shortcut_plugin_names)
-
- return out
-
-
-class Bottleneck(nn.Module):
- expansion = 4
-
- def __init__(self, inplanes, planes, stride=1, downsample=False):
- super().__init__()
- self.conv1 = nn.Conv2d(inplanes, planes, kernel_size=1, bias=False)
- self.bn1 = nn.BatchNorm2d(planes)
- self.conv2 = nn.Conv2d(planes, planes, 3, stride, 1, bias=False)
- self.bn2 = nn.BatchNorm2d(planes)
- self.conv3 = nn.Conv2d(
- planes, planes * self.expansion, kernel_size=1, bias=False)
- self.bn3 = nn.BatchNorm2d(planes * self.expansion)
- self.relu = nn.ReLU(inplace=True)
- if downsample:
- self.downsample = nn.Sequential(
- nn.Conv2d(
- inplanes, planes * self.expansion, 1, stride, bias=False),
- nn.BatchNorm2d(planes * self.expansion),
- )
- else:
- self.downsample = nn.Sequential()
-
- def forward(self, x):
- residual = self.downsample(x)
-
- out = self.conv1(x)
- out = self.bn1(out)
- out = self.relu(out)
-
- out = self.conv2(out)
- out = self.bn2(out)
- out = self.relu(out)
-
- out = self.conv3(out)
- out = self.bn3(out)
-
- out += residual
- out = self.relu(out)
-
- return out
diff --git a/pipeline/mmocr/mmocr/models/textrecog/layers/dot_product_attention_layer.py b/pipeline/mmocr/mmocr/models/textrecog/layers/dot_product_attention_layer.py
deleted file mode 100644
index 6d9cdb6528d90d9ec6e0bf0ac2a2343bd7227cc2..0000000000000000000000000000000000000000
--- a/pipeline/mmocr/mmocr/models/textrecog/layers/dot_product_attention_layer.py
+++ /dev/null
@@ -1,28 +0,0 @@
-# Copyright (c) OpenMMLab. All rights reserved.
-import torch
-import torch.nn as nn
-import torch.nn.functional as F
-
-
-class DotProductAttentionLayer(nn.Module):
-
- def __init__(self, dim_model=None):
- super().__init__()
-
- self.scale = dim_model**-0.5 if dim_model is not None else 1.
-
- def forward(self, query, key, value, mask=None):
- n, seq_len = mask.size()
- logits = torch.matmul(query.permute(0, 2, 1), key) * self.scale
-
- if mask is not None:
- mask = mask.view(n, 1, seq_len)
- logits = logits.masked_fill(mask, float('-inf'))
-
- weights = F.softmax(logits, dim=2)
-
- glimpse = torch.matmul(weights, value.transpose(1, 2))
-
- glimpse = glimpse.permute(0, 2, 1).contiguous()
-
- return glimpse
diff --git a/pipeline/mmocr/mmocr/models/textrecog/layers/lstm_layer.py b/pipeline/mmocr/mmocr/models/textrecog/layers/lstm_layer.py
deleted file mode 100644
index 16d3c1a4e5285c238176d2e0be76463657f282e5..0000000000000000000000000000000000000000
--- a/pipeline/mmocr/mmocr/models/textrecog/layers/lstm_layer.py
+++ /dev/null
@@ -1,21 +0,0 @@
-# Copyright (c) OpenMMLab. All rights reserved.
-import torch.nn as nn
-
-
-class BidirectionalLSTM(nn.Module):
-
- def __init__(self, nIn, nHidden, nOut):
- super().__init__()
-
- self.rnn = nn.LSTM(nIn, nHidden, bidirectional=True)
- self.embedding = nn.Linear(nHidden * 2, nOut)
-
- def forward(self, input):
- recurrent, _ = self.rnn(input)
- T, b, h = recurrent.size()
- t_rec = recurrent.view(T * b, h)
-
- output = self.embedding(t_rec) # [T * b, nOut]
- output = output.view(T, b, -1)
-
- return output
diff --git a/pipeline/mmocr/mmocr/models/textrecog/layers/position_aware_layer.py b/pipeline/mmocr/mmocr/models/textrecog/layers/position_aware_layer.py
deleted file mode 100644
index 2c994e372782aa882e9c3a32cec4e9bf733008ae..0000000000000000000000000000000000000000
--- a/pipeline/mmocr/mmocr/models/textrecog/layers/position_aware_layer.py
+++ /dev/null
@@ -1,36 +0,0 @@
-# Copyright (c) OpenMMLab. All rights reserved.
-import torch.nn as nn
-
-
-class PositionAwareLayer(nn.Module):
-
- def __init__(self, dim_model, rnn_layers=2):
- super().__init__()
-
- self.dim_model = dim_model
-
- self.rnn = nn.LSTM(
- input_size=dim_model,
- hidden_size=dim_model,
- num_layers=rnn_layers,
- batch_first=True)
-
- self.mixer = nn.Sequential(
- nn.Conv2d(
- dim_model, dim_model, kernel_size=3, stride=1, padding=1),
- nn.ReLU(True),
- nn.Conv2d(
- dim_model, dim_model, kernel_size=3, stride=1, padding=1))
-
- def forward(self, img_feature):
- n, c, h, w = img_feature.size()
-
- rnn_input = img_feature.permute(0, 2, 3, 1).contiguous()
- rnn_input = rnn_input.view(n * h, w, c)
- rnn_output, _ = self.rnn(rnn_input)
- rnn_output = rnn_output.view(n, h, w, c)
- rnn_output = rnn_output.permute(0, 3, 1, 2).contiguous()
-
- out = self.mixer(rnn_output)
-
- return out
diff --git a/pipeline/mmocr/mmocr/models/textrecog/layers/robust_scanner_fusion_layer.py b/pipeline/mmocr/mmocr/models/textrecog/layers/robust_scanner_fusion_layer.py
deleted file mode 100644
index 126d119f3e3853c53d1a0a584c6cfbc0197ca90c..0000000000000000000000000000000000000000
--- a/pipeline/mmocr/mmocr/models/textrecog/layers/robust_scanner_fusion_layer.py
+++ /dev/null
@@ -1,24 +0,0 @@
-# Copyright (c) OpenMMLab. All rights reserved.
-import torch
-import torch.nn as nn
-from mmengine.model import BaseModule
-
-
-class RobustScannerFusionLayer(BaseModule):
-
- def __init__(self, dim_model, dim=-1, init_cfg=None):
- super().__init__(init_cfg=init_cfg)
-
- self.dim_model = dim_model
- self.dim = dim
-
- self.linear_layer = nn.Linear(dim_model * 2, dim_model * 2)
- self.glu_layer = nn.GLU(dim=dim)
-
- def forward(self, x0, x1):
- assert x0.size() == x1.size()
- fusion_input = torch.cat([x0, x1], self.dim)
- output = self.linear_layer(fusion_input)
- output = self.glu_layer(output)
-
- return output
diff --git a/pipeline/mmocr/mmocr/models/textrecog/layers/satrn_layers.py b/pipeline/mmocr/mmocr/models/textrecog/layers/satrn_layers.py
deleted file mode 100644
index a8441c1bcf0f98c10ff35ce270578016e003d1e6..0000000000000000000000000000000000000000
--- a/pipeline/mmocr/mmocr/models/textrecog/layers/satrn_layers.py
+++ /dev/null
@@ -1,230 +0,0 @@
-# Copyright (c) OpenMMLab. All rights reserved.
-from typing import Dict, List, Optional, Union
-
-import numpy as np
-import torch
-import torch.nn as nn
-from mmcv.cnn import ConvModule
-from mmengine.model import BaseModule
-from torch import Tensor
-
-from mmocr.models.common import MultiHeadAttention
-
-
-class SATRNEncoderLayer(BaseModule):
- """Implement encoder layer for SATRN, see `SATRN.
-
- `_.
-
- Args:
- d_model (int): Dimension :math:`D_m` of the input from previous model.
- Defaults to 512.
- d_inner (int): Hidden dimension of feedforward layers. Defaults to 256.
- n_head (int): Number of parallel attention heads. Defaults to 8.
- d_k (int): Dimension of the key vector. Defaults to 64.
- d_v (int): Dimension of the value vector. Defaults to 64.
- dropout (float): Dropout rate. Defaults to 0.1.
- qkv_bias (bool): Whether to use bias. Defaults to False.
- init_cfg (dict or list[dict], optional): Initialization configs.
- Defaults to None.
- """
-
- def __init__(self,
- d_model: int = 512,
- d_inner: int = 512,
- n_head: int = 8,
- d_k: int = 64,
- d_v: int = 64,
- dropout: float = 0.1,
- qkv_bias: bool = False,
- init_cfg: Optional[Union[Dict, List[Dict]]] = None) -> None:
- super().__init__(init_cfg=init_cfg)
- self.norm1 = nn.LayerNorm(d_model)
- self.attn = MultiHeadAttention(
- n_head, d_model, d_k, d_v, qkv_bias=qkv_bias, dropout=dropout)
- self.norm2 = nn.LayerNorm(d_model)
- self.feed_forward = LocalityAwareFeedforward(d_model, d_inner)
-
- def forward(self,
- x: Tensor,
- h: int,
- w: int,
- mask: Optional[Tensor] = None) -> Tensor:
- """Forward propagation of encoder.
-
- Args:
- x (Tensor): Feature tensor of shape :math:`(N, h*w, D_m)`.
- h (int): Height of the original feature.
- w (int): Width of the original feature.
- mask (Tensor, optional): Mask used for masked multi-head attention.
- Defaults to None.
-
- Returns:
- Tensor: A tensor of shape :math:`(N, h*w, D_m)`.
- """
- n, hw, c = x.size()
- residual = x
- x = self.norm1(x)
- x = residual + self.attn(x, x, x, mask)
- residual = x
- x = self.norm2(x)
- x = x.transpose(1, 2).contiguous().view(n, c, h, w)
- x = self.feed_forward(x)
- x = x.view(n, c, hw).transpose(1, 2)
- x = residual + x
- return x
-
-
-class LocalityAwareFeedforward(BaseModule):
- """Locality-aware feedforward layer in SATRN, see `SATRN.
-
- `_
-
- Args:
- d_in (int): Dimension of the input features.
- d_hid (int): Hidden dimension of feedforward layers.
- init_cfg (dict or list[dict], optional): Initialization configs.
- Defaults to [dict(type='Xavier', layer='Conv2d'),
- dict(type='Constant', layer='BatchNorm2d', val=1, bias=0)].
- """
-
- def __init__(
- self,
- d_in: int,
- d_hid: int,
- init_cfg: Optional[Union[Dict, List[Dict]]] = [
- dict(type='Xavier', layer='Conv2d'),
- dict(type='Constant', layer='BatchNorm2d', val=1, bias=0)
- ]
- ) -> None:
- super().__init__(init_cfg=init_cfg)
- self.conv1 = ConvModule(
- d_in,
- d_hid,
- kernel_size=1,
- padding=0,
- bias=False,
- norm_cfg=dict(type='BN'),
- act_cfg=dict(type='ReLU'))
-
- self.depthwise_conv = ConvModule(
- d_hid,
- d_hid,
- kernel_size=3,
- padding=1,
- bias=False,
- groups=d_hid,
- norm_cfg=dict(type='BN'),
- act_cfg=dict(type='ReLU'))
-
- self.conv2 = ConvModule(
- d_hid,
- d_in,
- kernel_size=1,
- padding=0,
- bias=False,
- norm_cfg=dict(type='BN'),
- act_cfg=dict(type='ReLU'))
-
- def forward(self, x: Tensor) -> Tensor:
- """Forward propagation of Locality Aware Feedforward module.
-
- Args:
- x (Tensor): Feature tensor.
-
- Returns:
- Tensor: Feature tensor after Locality Aware Feedforward.
- """
- x = self.conv1(x)
- x = self.depthwise_conv(x)
- x = self.conv2(x)
- return x
-
-
-class Adaptive2DPositionalEncoding(BaseModule):
- """Implement Adaptive 2D positional encoder for SATRN, see `SATRN.
-
- `_ Modified from
- https://github.com/Media-Smart/vedastr Licensed under the Apache License,
- Version 2.0 (the "License");
-
- Args:
- d_hid (int): Dimensions of hidden layer. Defaults to 512.
- n_height (int): Max height of the 2D feature output. Defaults to 100.
- n_width (int): Max width of the 2D feature output. Defaults to 100.
- dropout (float): Dropout rate. Defaults to 0.1.
- init_cfg (dict or list[dict], optional): Initialization configs.
- Defaults to [dict(type='Xavier', layer='Conv2d')]
- """
-
- def __init__(
- self,
- d_hid: int = 512,
- n_height: int = 100,
- n_width: int = 100,
- dropout: float = 0.1,
- init_cfg: Optional[Union[Dict, List[Dict]]] = [
- dict(type='Xavier', layer='Conv2d')
- ]
- ) -> None:
- super().__init__(init_cfg=init_cfg)
-
- h_position_encoder = self._get_sinusoid_encoding_table(n_height, d_hid)
- h_position_encoder = h_position_encoder.transpose(0, 1)
- h_position_encoder = h_position_encoder.view(1, d_hid, n_height, 1)
-
- w_position_encoder = self._get_sinusoid_encoding_table(n_width, d_hid)
- w_position_encoder = w_position_encoder.transpose(0, 1)
- w_position_encoder = w_position_encoder.view(1, d_hid, 1, n_width)
-
- self.register_buffer('h_position_encoder', h_position_encoder)
- self.register_buffer('w_position_encoder', w_position_encoder)
-
- self.h_scale = self._scale_factor_generate(d_hid)
- self.w_scale = self._scale_factor_generate(d_hid)
- self.pool = nn.AdaptiveAvgPool2d(1)
- self.dropout = nn.Dropout(p=dropout)
-
- @staticmethod
- def _get_sinusoid_encoding_table(n_position: int, d_hid: int) -> Tensor:
- """Generate sinusoid position encoding table."""
- denominator = torch.Tensor([
- 1.0 / np.power(10000, 2 * (hid_j // 2) / d_hid)
- for hid_j in range(d_hid)
- ])
- denominator = denominator.view(1, -1)
- pos_tensor = torch.arange(n_position).unsqueeze(-1).float()
- sinusoid_table = pos_tensor * denominator
- sinusoid_table[:, 0::2] = torch.sin(sinusoid_table[:, 0::2])
- sinusoid_table[:, 1::2] = torch.cos(sinusoid_table[:, 1::2])
-
- return sinusoid_table
-
- @staticmethod
- def _scale_factor_generate(d_hid: int) -> nn.Sequential:
- """Generate scale factor layers."""
- scale_factor = nn.Sequential(
- nn.Conv2d(d_hid, d_hid, kernel_size=1), nn.ReLU(inplace=True),
- nn.Conv2d(d_hid, d_hid, kernel_size=1), nn.Sigmoid())
-
- return scale_factor
-
- def forward(self, x: Tensor) -> Tensor:
- """Forward propagation of Locality Aware Feedforward module.
-
- Args:
- x (Tensor): Feature tensor.
-
- Returns:
- Tensor: Feature tensor after Locality Aware Feedforward.
- """
- _, _, h, w = x.size()
- avg_pool = self.pool(x)
- h_pos_encoding = \
- self.h_scale(avg_pool) * self.h_position_encoder[:, :, :h, :]
- w_pos_encoding = \
- self.w_scale(avg_pool) * self.w_position_encoder[:, :, :, :w]
- out = x + h_pos_encoding + w_pos_encoding
- out = self.dropout(out)
-
- return out
diff --git a/pipeline/mmocr/mmocr/models/textrecog/module_losses/__init__.py b/pipeline/mmocr/mmocr/models/textrecog/module_losses/__init__.py
deleted file mode 100644
index f5a81305d4fc345d6ce4c73a806aad551fac85b4..0000000000000000000000000000000000000000
--- a/pipeline/mmocr/mmocr/models/textrecog/module_losses/__init__.py
+++ /dev/null
@@ -1,9 +0,0 @@
-# Copyright (c) OpenMMLab. All rights reserved.
-from .abi_module_loss import ABIModuleLoss
-from .base import BaseTextRecogModuleLoss
-from .ce_module_loss import CEModuleLoss
-from .ctc_module_loss import CTCModuleLoss
-
-__all__ = [
- 'BaseTextRecogModuleLoss', 'CEModuleLoss', 'CTCModuleLoss', 'ABIModuleLoss'
-]
diff --git a/pipeline/mmocr/mmocr/models/textrecog/module_losses/__pycache__/__init__.cpython-38.pyc b/pipeline/mmocr/mmocr/models/textrecog/module_losses/__pycache__/__init__.cpython-38.pyc
deleted file mode 100644
index a8a328a1c99626ce5a9a1419ca4277d2a0241a9c..0000000000000000000000000000000000000000
Binary files a/pipeline/mmocr/mmocr/models/textrecog/module_losses/__pycache__/__init__.cpython-38.pyc and /dev/null differ
diff --git a/pipeline/mmocr/mmocr/models/textrecog/module_losses/__pycache__/__init__.cpython-39.pyc b/pipeline/mmocr/mmocr/models/textrecog/module_losses/__pycache__/__init__.cpython-39.pyc
deleted file mode 100644
index d325f340a8aaf852c9e5ee21ee8d283e90f31310..0000000000000000000000000000000000000000
Binary files a/pipeline/mmocr/mmocr/models/textrecog/module_losses/__pycache__/__init__.cpython-39.pyc and /dev/null differ
diff --git a/pipeline/mmocr/mmocr/models/textrecog/module_losses/__pycache__/abi_module_loss.cpython-38.pyc b/pipeline/mmocr/mmocr/models/textrecog/module_losses/__pycache__/abi_module_loss.cpython-38.pyc
deleted file mode 100644
index b907c5f703e109cede1da757ebbac1a5626b6561..0000000000000000000000000000000000000000
Binary files a/pipeline/mmocr/mmocr/models/textrecog/module_losses/__pycache__/abi_module_loss.cpython-38.pyc and /dev/null differ
diff --git a/pipeline/mmocr/mmocr/models/textrecog/module_losses/__pycache__/abi_module_loss.cpython-39.pyc b/pipeline/mmocr/mmocr/models/textrecog/module_losses/__pycache__/abi_module_loss.cpython-39.pyc
deleted file mode 100644
index 2afb8a330455628d76784c7ceb674d30c587b08c..0000000000000000000000000000000000000000
Binary files a/pipeline/mmocr/mmocr/models/textrecog/module_losses/__pycache__/abi_module_loss.cpython-39.pyc and /dev/null differ
diff --git a/pipeline/mmocr/mmocr/models/textrecog/module_losses/__pycache__/base.cpython-38.pyc b/pipeline/mmocr/mmocr/models/textrecog/module_losses/__pycache__/base.cpython-38.pyc
deleted file mode 100644
index 05470551b9629e462afb2c0a741a614b89f914f2..0000000000000000000000000000000000000000
Binary files a/pipeline/mmocr/mmocr/models/textrecog/module_losses/__pycache__/base.cpython-38.pyc and /dev/null differ
diff --git a/pipeline/mmocr/mmocr/models/textrecog/module_losses/__pycache__/base.cpython-39.pyc b/pipeline/mmocr/mmocr/models/textrecog/module_losses/__pycache__/base.cpython-39.pyc
deleted file mode 100644
index 42d6f1192078dfccf4f4007d45791683055a5a26..0000000000000000000000000000000000000000
Binary files a/pipeline/mmocr/mmocr/models/textrecog/module_losses/__pycache__/base.cpython-39.pyc and /dev/null differ
diff --git a/pipeline/mmocr/mmocr/models/textrecog/module_losses/__pycache__/ce_module_loss.cpython-38.pyc b/pipeline/mmocr/mmocr/models/textrecog/module_losses/__pycache__/ce_module_loss.cpython-38.pyc
deleted file mode 100644
index 00e58c6f656c9d4f35fb514ec9a0c218fcfac921..0000000000000000000000000000000000000000
Binary files a/pipeline/mmocr/mmocr/models/textrecog/module_losses/__pycache__/ce_module_loss.cpython-38.pyc and /dev/null differ
diff --git a/pipeline/mmocr/mmocr/models/textrecog/module_losses/__pycache__/ce_module_loss.cpython-39.pyc b/pipeline/mmocr/mmocr/models/textrecog/module_losses/__pycache__/ce_module_loss.cpython-39.pyc
deleted file mode 100644
index 79587b1e7b239a5367bdafd5d62c8b97b8709020..0000000000000000000000000000000000000000
Binary files a/pipeline/mmocr/mmocr/models/textrecog/module_losses/__pycache__/ce_module_loss.cpython-39.pyc and /dev/null differ
diff --git a/pipeline/mmocr/mmocr/models/textrecog/module_losses/__pycache__/ctc_module_loss.cpython-38.pyc b/pipeline/mmocr/mmocr/models/textrecog/module_losses/__pycache__/ctc_module_loss.cpython-38.pyc
deleted file mode 100644
index f09206eee2eadb03a85fe8ed73a6f241a9d0ab89..0000000000000000000000000000000000000000
Binary files a/pipeline/mmocr/mmocr/models/textrecog/module_losses/__pycache__/ctc_module_loss.cpython-38.pyc and /dev/null differ
diff --git a/pipeline/mmocr/mmocr/models/textrecog/module_losses/__pycache__/ctc_module_loss.cpython-39.pyc b/pipeline/mmocr/mmocr/models/textrecog/module_losses/__pycache__/ctc_module_loss.cpython-39.pyc
deleted file mode 100644
index 9f587400a5b6599868568a599bb4c148f774a86d..0000000000000000000000000000000000000000
Binary files a/pipeline/mmocr/mmocr/models/textrecog/module_losses/__pycache__/ctc_module_loss.cpython-39.pyc and /dev/null differ
diff --git a/pipeline/mmocr/mmocr/models/textrecog/module_losses/abi_module_loss.py b/pipeline/mmocr/mmocr/models/textrecog/module_losses/abi_module_loss.py
deleted file mode 100644
index 918847b9d02bcb7f5e9de9abbb2b0d0837dfe47c..0000000000000000000000000000000000000000
--- a/pipeline/mmocr/mmocr/models/textrecog/module_losses/abi_module_loss.py
+++ /dev/null
@@ -1,99 +0,0 @@
-# Copyright (c) OpenMMLab. All rights reserved.
-from typing import Dict, Sequence, Union
-
-import torch
-
-from mmocr.models.common.dictionary import Dictionary
-from mmocr.registry import MODELS
-from mmocr.structures import TextRecogDataSample
-from .base import BaseTextRecogModuleLoss
-from .ce_module_loss import CEModuleLoss
-
-
-@MODELS.register_module()
-class ABIModuleLoss(BaseTextRecogModuleLoss):
- """Implementation of ABINet multiloss that allows mixing different types of
- losses with weights.
-
- Args:
- dictionary (dict or :obj:`Dictionary`): The config for `Dictionary` or
- the instance of `Dictionary`.
- max_seq_len (int): Maximum sequence length. The sequence is usually
- generated from decoder. Defaults to 40.
- letter_case (str): There are three options to alter the letter cases
- of gt texts:
- - unchanged: Do not change gt texts.
- - upper: Convert gt texts into uppercase characters.
- - lower: Convert gt texts into lowercase characters.
- Usually, it only works for English characters. Defaults to
- 'unchanged'.
- weight_vis (float or int): The weight of vision decoder loss. Defaults
- to 1.0.
- weight_dec (float or int): The weight of language decoder loss.
- Defaults to 1.0.
- weight_fusion (float or int): The weight of fuser (aligner) loss.
- Defaults to 1.0.
- """
-
- def __init__(self,
- dictionary: Union[Dict, Dictionary],
- max_seq_len: int = 40,
- letter_case: str = 'unchanged',
- weight_vis: Union[float, int] = 1.0,
- weight_lang: Union[float, int] = 1.0,
- weight_fusion: Union[float, int] = 1.0,
- **kwargs) -> None:
- assert isinstance(weight_vis, (float, int))
- assert isinstance(weight_lang, (float, int))
- assert isinstance(weight_fusion, (float, int))
- super().__init__(
- dictionary=dictionary,
- max_seq_len=max_seq_len,
- letter_case=letter_case)
- self.weight_vis = weight_vis
- self.weight_lang = weight_lang
- self.weight_fusion = weight_fusion
- self._ce_loss = CEModuleLoss(
- self.dictionary,
- max_seq_len,
- letter_case,
- reduction='mean',
- ignore_first_char=True)
-
- def forward(self, outputs: Dict,
- data_samples: Sequence[TextRecogDataSample]) -> Dict:
- """
- Args:
- outputs (dict): The output dictionary with at least one of
- ``out_vis``, ``out_langs`` and ``out_fusers`` specified.
- data_samples (list[TextRecogDataSample]): List of
- ``TextRecogDataSample`` which are processed by ``get_target``.
-
- Returns:
- dict: A loss dictionary with ``loss_visual``, ``loss_lang`` and
- ``loss_fusion``. Each should either be the loss tensor or None if
- the output of its corresponding module is not given.
- """
- assert 'out_vis' in outputs or \
- 'out_langs' in outputs or 'out_fusers' in outputs
- losses = {}
-
- if outputs.get('out_vis', None):
- losses['loss_visual'] = self.weight_vis * self._ce_loss(
- outputs['out_vis']['logits'], data_samples)['loss_ce']
- if outputs.get('out_langs', None):
- lang_losses = []
- for out_lang in outputs['out_langs']:
- lang_losses.append(
- self._ce_loss(out_lang['logits'], data_samples)['loss_ce'])
- losses['loss_lang'] = self.weight_lang * torch.mean(
- torch.stack(lang_losses))
- if outputs.get('out_fusers', None):
- fuser_losses = []
- for out_fuser in outputs['out_fusers']:
- fuser_losses.append(
- self._ce_loss(out_fuser['logits'],
- data_samples)['loss_ce'])
- losses['loss_fusion'] = self.weight_fusion * torch.mean(
- torch.stack(fuser_losses))
- return losses
diff --git a/pipeline/mmocr/mmocr/models/textrecog/module_losses/base.py b/pipeline/mmocr/mmocr/models/textrecog/module_losses/base.py
deleted file mode 100644
index 5fbf83df9dbfe9d962d2e37af7edde7c833b603a..0000000000000000000000000000000000000000
--- a/pipeline/mmocr/mmocr/models/textrecog/module_losses/base.py
+++ /dev/null
@@ -1,132 +0,0 @@
-# Copyright (c) OpenMMLab. All rights reserved.
-
-from typing import Dict, Sequence, Union
-
-import torch
-import torch.nn as nn
-
-from mmocr.models.common.dictionary import Dictionary
-from mmocr.registry import TASK_UTILS
-from mmocr.structures import TextRecogDataSample
-
-
-class BaseTextRecogModuleLoss(nn.Module):
- """Base recognition loss.
-
- Args:
- dictionary (dict or :obj:`Dictionary`): The config for `Dictionary` or
- the instance of `Dictionary`.
- max_seq_len (int): Maximum sequence length. The sequence is usually
- generated from decoder. Defaults to 40.
- letter_case (str): There are three options to alter the letter cases
- of gt texts:
- - unchanged: Do not change gt texts.
- - upper: Convert gt texts into uppercase characters.
- - lower: Convert gt texts into lowercase characters.
- Usually, it only works for English characters. Defaults to
- 'unchanged'.
- pad_with (str): The padding strategy for ``gt_text.padded_indexes``.
- Defaults to 'auto'. Options are:
- - 'auto': Use dictionary.padding_idx to pad gt texts, or
- dictionary.end_idx if dictionary.padding_idx
- is None.
- - 'padding': Always use dictionary.padding_idx to pad gt texts.
- - 'end': Always use dictionary.end_idx to pad gt texts.
- - 'none': Do not pad gt texts.
- """
-
- def __init__(self,
- dictionary: Union[Dict, Dictionary],
- max_seq_len: int = 40,
- letter_case: str = 'unchanged',
- pad_with: str = 'auto',
- **kwargs) -> None:
- super().__init__()
- if isinstance(dictionary, dict):
- self.dictionary = TASK_UTILS.build(dictionary)
- elif isinstance(dictionary, Dictionary):
- self.dictionary = dictionary
- else:
- raise TypeError(
- 'The type of dictionary should be `Dictionary` or dict, '
- f'but got {type(dictionary)}')
- self.max_seq_len = max_seq_len
- assert letter_case in ['unchanged', 'upper', 'lower']
- self.letter_case = letter_case
-
- assert pad_with in ['auto', 'padding', 'end', 'none']
- if pad_with == 'auto':
- self.pad_idx = self.dictionary.padding_idx or \
- self.dictionary.end_idx
- elif pad_with == 'padding':
- self.pad_idx = self.dictionary.padding_idx
- elif pad_with == 'end':
- self.pad_idx = self.dictionary.end_idx
- else:
- self.pad_idx = None
- if self.pad_idx is None and pad_with != 'none':
- if pad_with == 'auto':
- raise ValueError('pad_with="auto", but dictionary.end_idx'
- ' and dictionary.padding_idx are both None')
- else:
- raise ValueError(
- f'pad_with="{pad_with}", but dictionary.{pad_with}_idx is'
- ' None')
-
- def get_targets(
- self, data_samples: Sequence[TextRecogDataSample]
- ) -> Sequence[TextRecogDataSample]:
- """Target generator.
-
- Args:
- data_samples (list[TextRecogDataSample]): It usually includes
- ``gt_text`` information.
-
- Returns:
- list[TextRecogDataSample]: Updated data_samples. Two keys will be
- added to data_sample:
-
- - indexes (torch.LongTensor): Character indexes representing gt
- texts. All special tokens are excluded, except for UKN.
- - padded_indexes (torch.LongTensor): Character indexes
- representing gt texts with BOS and EOS if applicable, following
- several padding indexes until the length reaches ``max_seq_len``.
- In particular, if ``pad_with='none'``, no padding will be
- applied.
- """
-
- for data_sample in data_samples:
- if data_sample.get('have_target', False):
- continue
- text = data_sample.gt_text.item
- if self.letter_case in ['upper', 'lower']:
- text = getattr(text, self.letter_case)()
- indexes = self.dictionary.str2idx(text)
- indexes = torch.LongTensor(indexes)
-
- # target indexes for loss
- src_target = torch.LongTensor(indexes.size(0) + 2).fill_(0)
- src_target[1:-1] = indexes
- if self.dictionary.start_idx is not None:
- src_target[0] = self.dictionary.start_idx
- slice_start = 0
- else:
- slice_start = 1
- if self.dictionary.end_idx is not None:
- src_target[-1] = self.dictionary.end_idx
- slice_end = src_target.size(0)
- else:
- slice_end = src_target.size(0) - 1
- src_target = src_target[slice_start:slice_end]
- if self.pad_idx is not None:
- padded_indexes = (torch.ones(self.max_seq_len) *
- self.pad_idx).long()
- char_num = min(src_target.size(0), self.max_seq_len)
- padded_indexes[:char_num] = src_target[:char_num]
- else:
- padded_indexes = src_target
- # put in DataSample
- data_sample.gt_text.indexes = indexes
- data_sample.gt_text.padded_indexes = padded_indexes
- data_sample.set_metainfo(dict(have_target=True))
- return data_samples
diff --git a/pipeline/mmocr/mmocr/models/textrecog/module_losses/ce_module_loss.py b/pipeline/mmocr/mmocr/models/textrecog/module_losses/ce_module_loss.py
deleted file mode 100644
index a351ea0c553bf1e1c7c9534630178904ba0f1a30..0000000000000000000000000000000000000000
--- a/pipeline/mmocr/mmocr/models/textrecog/module_losses/ce_module_loss.py
+++ /dev/null
@@ -1,138 +0,0 @@
-# Copyright (c) OpenMMLab. All rights reserved.
-import warnings
-from typing import Dict, Sequence, Union
-
-import torch
-import torch.nn as nn
-
-from mmocr.models.common.dictionary import Dictionary
-from mmocr.registry import MODELS
-from mmocr.structures import TextRecogDataSample
-from .base import BaseTextRecogModuleLoss
-
-
-@MODELS.register_module()
-class CEModuleLoss(BaseTextRecogModuleLoss):
- """Implementation of loss module for encoder-decoder based text recognition
- method with CrossEntropy loss.
-
- Args:
- dictionary (dict or :obj:`Dictionary`): The config for `Dictionary` or
- the instance of `Dictionary`.
- max_seq_len (int): Maximum sequence length. The sequence is usually
- generated from decoder. Defaults to 40.
- letter_case (str): There are three options to alter the letter cases
- of gt texts:
- - unchanged: Do not change gt texts.
- - upper: Convert gt texts into uppercase characters.
- - lower: Convert gt texts into lowercase characters.
- Usually, it only works for English characters. Defaults to
- 'unchanged'.
- pad_with (str): The padding strategy for ``gt_text.padded_indexes``.
- Defaults to 'auto'. Options are:
- - 'auto': Use dictionary.padding_idx to pad gt texts, or
- dictionary.end_idx if dictionary.padding_idx
- is None.
- - 'padding': Always use dictionary.padding_idx to pad gt texts.
- - 'end': Always use dictionary.end_idx to pad gt texts.
- - 'none': Do not pad gt texts.
- ignore_char (int or str): Specifies a target value that is
- ignored and does not contribute to the input gradient.
- ignore_char can be int or str. If int, it is the index of
- the ignored char. If str, it is the character to ignore.
- Apart from single characters, each item can be one of the
- following reversed keywords: 'padding', 'start', 'end',
- and 'unknown', which refer to their corresponding special
- tokens in the dictionary. It will not ignore any special
- tokens when ignore_char == -1 or 'none'. Defaults to 'padding'.
- flatten (bool): Whether to flatten the output and target before
- computing CE loss. Defaults to False.
- reduction (str): Specifies the reduction to apply to the output,
- should be one of the following: ('none', 'mean', 'sum'). Defaults
- to 'none'.
- ignore_first_char (bool): Whether to ignore the first token in target (
- usually the start token). If ``True``, the last token of the output
- sequence will also be removed to be aligned with the target length.
- Defaults to ``False``.
- flatten (bool): Whether to flatten the vectors for loss computation.
- Defaults to False.
- """
-
- def __init__(self,
- dictionary: Union[Dict, Dictionary],
- max_seq_len: int = 40,
- letter_case: str = 'unchanged',
- pad_with: str = 'auto',
- ignore_char: Union[int, str] = 'padding',
- flatten: bool = False,
- reduction: str = 'none',
- ignore_first_char: bool = False):
- super().__init__(
- dictionary=dictionary,
- max_seq_len=max_seq_len,
- letter_case=letter_case,
- pad_with=pad_with)
- assert isinstance(ignore_char, (int, str))
- assert isinstance(reduction, str)
- assert reduction in ['none', 'mean', 'sum']
- assert isinstance(ignore_first_char, bool)
- assert isinstance(flatten, bool)
- self.flatten = flatten
-
- self.ignore_first_char = ignore_first_char
-
- if isinstance(ignore_char, int):
- ignore_index = ignore_char
- else:
- mapping_table = {
- 'none': -1,
- 'start': self.dictionary.start_idx,
- 'padding': self.dictionary.padding_idx,
- 'end': self.dictionary.end_idx,
- 'unknown': self.dictionary.unknown_idx,
- }
-
- ignore_index = mapping_table.get(
- ignore_char,
- self.dictionary.char2idx(ignore_char, strict=False))
- if ignore_index is None or (ignore_index
- == self.dictionary.unknown_idx
- and ignore_char != 'unknown'):
- warnings.warn(
- f'{ignore_char} does not exist in the dictionary',
- UserWarning)
- ignore_index = -1
-
- self.ignore_char = ignore_char
- self.ignore_index = ignore_index
- self.loss_ce = nn.CrossEntropyLoss(
- ignore_index=ignore_index, reduction=reduction)
-
- def forward(self, outputs: torch.Tensor,
- data_samples: Sequence[TextRecogDataSample]) -> Dict:
- """
- Args:
- outputs (Tensor): A raw logit tensor of shape :math:`(N, T, C)`.
- data_samples (list[TextRecogDataSample]): List of
- ``TextRecogDataSample`` which are processed by ``get_target``.
-
- Returns:
- dict: A loss dict with the key ``loss_ce``.
- """
- targets = list()
- for data_sample in data_samples:
- targets.append(data_sample.gt_text.padded_indexes)
- targets = torch.stack(targets, dim=0).long()
- if self.ignore_first_char:
- targets = targets[:, 1:].contiguous()
- outputs = outputs[:, :-1, :].contiguous()
- if self.flatten:
- outputs = outputs.view(-1, outputs.size(-1))
- targets = targets.view(-1)
- else:
- outputs = outputs.permute(0, 2, 1).contiguous()
-
- loss_ce = self.loss_ce(outputs, targets.to(outputs.device))
- losses = dict(loss_ce=loss_ce)
-
- return losses
diff --git a/pipeline/mmocr/mmocr/models/textrecog/module_losses/ctc_module_loss.py b/pipeline/mmocr/mmocr/models/textrecog/module_losses/ctc_module_loss.py
deleted file mode 100644
index e98d7b4c905487d1158402dd00d82570207513b5..0000000000000000000000000000000000000000
--- a/pipeline/mmocr/mmocr/models/textrecog/module_losses/ctc_module_loss.py
+++ /dev/null
@@ -1,130 +0,0 @@
-# Copyright (c) OpenMMLab. All rights reserved.
-import math
-from typing import Dict, Sequence, Union
-
-import torch
-import torch.nn as nn
-
-from mmocr.models.common.dictionary import Dictionary
-from mmocr.registry import MODELS
-from mmocr.structures import TextRecogDataSample
-from .base import BaseTextRecogModuleLoss
-
-
-@MODELS.register_module()
-class CTCModuleLoss(BaseTextRecogModuleLoss):
- """Implementation of loss module for CTC-loss based text recognition.
-
- Args:
- dictionary (dict or :obj:`Dictionary`): The config for `Dictionary` or
- the instance of `Dictionary`.
- letter_case (str): There are three options to alter the letter cases
- of gt texts:
- - unchanged: Do not change gt texts.
- - upper: Convert gt texts into uppercase characters.
- - lower: Convert gt texts into lowercase characters.
- Usually, it only works for English characters. Defaults to
- 'unchanged'.
- flatten (bool): If True, use flattened targets, else padded targets.
- reduction (str): Specifies the reduction to apply to the output,
- should be one of the following: ('none', 'mean', 'sum').
- zero_infinity (bool): Whether to zero infinite losses and
- the associated gradients. Default: False.
- Infinite losses mainly occur when the inputs
- are too short to be aligned to the targets.
- """
-
- def __init__(self,
- dictionary: Union[Dict, Dictionary],
- letter_case: str = 'unchanged',
- flatten: bool = True,
- reduction: str = 'mean',
- zero_infinity: bool = False,
- **kwargs) -> None:
- super().__init__(dictionary=dictionary, letter_case=letter_case)
- assert isinstance(flatten, bool)
- assert isinstance(reduction, str)
- assert isinstance(zero_infinity, bool)
-
- self.flatten = flatten
- self.ctc_loss = nn.CTCLoss(
- blank=self.dictionary.padding_idx,
- reduction=reduction,
- zero_infinity=zero_infinity)
-
- def forward(self, outputs: torch.Tensor,
- data_samples: Sequence[TextRecogDataSample]) -> Dict:
- """
- Args:
- outputs (Tensor): A raw logit tensor of shape :math:`(N, T, C)`.
- data_samples (list[TextRecogDataSample]): List of
- ``TextRecogDataSample`` which are processed by ``get_target``.
-
- Returns:
- dict: The loss dict with key ``loss_ctc``.
- """
- valid_ratios = None
- if data_samples is not None:
- valid_ratios = [
- img_meta.get('valid_ratio', 1.0) for img_meta in data_samples
- ]
-
- outputs = torch.log_softmax(outputs, dim=2)
- bsz, seq_len = outputs.size(0), outputs.size(1)
- outputs_for_loss = outputs.permute(1, 0, 2).contiguous() # T * N * C
- targets = [
- data_sample.gt_text.indexes[:seq_len]
- for data_sample in data_samples
- ]
- target_lengths = torch.IntTensor([len(t) for t in targets])
- target_lengths = torch.clamp(target_lengths, max=seq_len).long()
- input_lengths = torch.full(
- size=(bsz, ), fill_value=seq_len, dtype=torch.long)
- if self.flatten:
- targets = torch.cat(targets)
- else:
- padded_targets = torch.full(
- size=(bsz, seq_len),
- fill_value=self.dictionary.padding_idx,
- dtype=torch.long)
- for idx, valid_len in enumerate(target_lengths):
- padded_targets[idx, :valid_len] = targets[idx][:valid_len]
- targets = padded_targets
-
- if valid_ratios is not None:
- input_lengths = [
- math.ceil(valid_ratio * seq_len)
- for valid_ratio in valid_ratios
- ]
- input_lengths = torch.Tensor(input_lengths).long()
- loss_ctc = self.ctc_loss(outputs_for_loss, targets, input_lengths,
- target_lengths)
- losses = dict(loss_ctc=loss_ctc)
-
- return losses
-
- def get_targets(
- self, data_samples: Sequence[TextRecogDataSample]
- ) -> Sequence[TextRecogDataSample]:
- """Target generator.
-
- Args:
- data_samples (list[TextRecogDataSample]): It usually includes
- ``gt_text`` information.
-
- Returns:
-
- list[TextRecogDataSample]: updated data_samples. It will add two
- key in data_sample:
-
- - indexes (torch.LongTensor): The index corresponding to the item.
- """
-
- for data_sample in data_samples:
- text = data_sample.gt_text.item
- if self.letter_case in ['upper', 'lower']:
- text = getattr(text, self.letter_case)()
- indexes = self.dictionary.str2idx(text)
- indexes = torch.IntTensor(indexes)
- data_sample.gt_text.indexes = indexes
- return data_samples
diff --git a/pipeline/mmocr/mmocr/models/textrecog/plugins/__init__.py b/pipeline/mmocr/mmocr/models/textrecog/plugins/__init__.py
deleted file mode 100644
index 053a33e2d647128fc7dcc60e85aea0b560103984..0000000000000000000000000000000000000000
--- a/pipeline/mmocr/mmocr/models/textrecog/plugins/__init__.py
+++ /dev/null
@@ -1,4 +0,0 @@
-# Copyright (c) OpenMMLab. All rights reserved.
-from .common import GCAModule, Maxpool2d
-
-__all__ = ['Maxpool2d', 'GCAModule']
diff --git a/pipeline/mmocr/mmocr/models/textrecog/plugins/__pycache__/__init__.cpython-38.pyc b/pipeline/mmocr/mmocr/models/textrecog/plugins/__pycache__/__init__.cpython-38.pyc
deleted file mode 100644
index 2515c67354613ff812d0dcf450671a67942aad29..0000000000000000000000000000000000000000
Binary files a/pipeline/mmocr/mmocr/models/textrecog/plugins/__pycache__/__init__.cpython-38.pyc and /dev/null differ
diff --git a/pipeline/mmocr/mmocr/models/textrecog/plugins/__pycache__/__init__.cpython-39.pyc b/pipeline/mmocr/mmocr/models/textrecog/plugins/__pycache__/__init__.cpython-39.pyc
deleted file mode 100644
index ba5c550a212bf52bf26dbcb30f36f46537e2bf7a..0000000000000000000000000000000000000000
Binary files a/pipeline/mmocr/mmocr/models/textrecog/plugins/__pycache__/__init__.cpython-39.pyc and /dev/null differ
diff --git a/pipeline/mmocr/mmocr/models/textrecog/plugins/__pycache__/common.cpython-38.pyc b/pipeline/mmocr/mmocr/models/textrecog/plugins/__pycache__/common.cpython-38.pyc
deleted file mode 100644
index 73742c6ad1bd17355c909d398ad267868015c92a..0000000000000000000000000000000000000000
Binary files a/pipeline/mmocr/mmocr/models/textrecog/plugins/__pycache__/common.cpython-38.pyc and /dev/null differ
diff --git a/pipeline/mmocr/mmocr/models/textrecog/plugins/__pycache__/common.cpython-39.pyc b/pipeline/mmocr/mmocr/models/textrecog/plugins/__pycache__/common.cpython-39.pyc
deleted file mode 100644
index 58c1bd30db34aef5500fb0a53fd526209b312c12..0000000000000000000000000000000000000000
Binary files a/pipeline/mmocr/mmocr/models/textrecog/plugins/__pycache__/common.cpython-39.pyc and /dev/null differ
diff --git a/pipeline/mmocr/mmocr/models/textrecog/plugins/common.py b/pipeline/mmocr/mmocr/models/textrecog/plugins/common.py
deleted file mode 100644
index 7a6e8c6de712978c571224b9e20ea881d1116211..0000000000000000000000000000000000000000
--- a/pipeline/mmocr/mmocr/models/textrecog/plugins/common.py
+++ /dev/null
@@ -1,194 +0,0 @@
-# Copyright (c) OpenMMLab. All rights reserved.
-from typing import Tuple, Union
-
-import torch
-import torch.nn as nn
-
-from mmocr.registry import MODELS
-
-
-@MODELS.register_module()
-class Maxpool2d(nn.Module):
- """A wrapper around nn.Maxpool2d().
-
- Args:
- kernel_size (int or tuple(int)): Kernel size for max pooling layer
- stride (int or tuple(int)): Stride for max pooling layer
- padding (int or tuple(int)): Padding for pooling layer
- """
-
- def __init__(self,
- kernel_size: Union[int, Tuple[int]],
- stride: Union[int, Tuple[int]],
- padding: Union[int, Tuple[int]] = 0,
- **kwargs) -> None:
- super().__init__()
- self.model = nn.MaxPool2d(kernel_size, stride, padding)
-
- def forward(self, x) -> torch.Tensor:
- """Forward function.
- Args:
- x (Tensor): Input feature map.
-
- Returns:
- Tensor: Output tensor after Maxpooling layer.
- """
- return self.model(x)
-
-
-@MODELS.register_module()
-class GCAModule(nn.Module):
- """GCAModule in MASTER.
-
- Args:
- in_channels (int): Channels of input tensor.
- ratio (float): Scale ratio of in_channels.
- n_head (int): Numbers of attention head.
- pooling_type (str): Spatial pooling type. Options are [``avg``,
- ``att``].
- scale_attn (bool): Whether to scale the attention map. Defaults to
- False.
- fusion_type (str): Fusion type of input and context. Options are
- [``channel_add``, ``channel_mul``, ``channel_concat``].
- """
-
- def __init__(self,
- in_channels: int,
- ratio: float,
- n_head: int,
- pooling_type: str = 'att',
- scale_attn: bool = False,
- fusion_type: str = 'channel_add',
- **kwargs) -> None:
- super().__init__()
-
- assert pooling_type in ['avg', 'att']
- assert fusion_type in ['channel_add', 'channel_mul', 'channel_concat']
-
- # in_channels must be divided by headers evenly
- assert in_channels % n_head == 0 and in_channels >= 8
-
- self.n_head = n_head
- self.in_channels = in_channels
- self.ratio = ratio
- self.planes = int(in_channels * ratio)
- self.pooling_type = pooling_type
- self.fusion_type = fusion_type
- self.scale_attn = scale_attn
- self.single_header_inplanes = int(in_channels / n_head)
-
- if pooling_type == 'att':
- self.conv_mask = nn.Conv2d(
- self.single_header_inplanes, 1, kernel_size=1)
- self.softmax = nn.Softmax(dim=2)
- else:
- self.avg_pool = nn.AdaptiveAvgPool2d(1)
-
- if fusion_type == 'channel_add':
- self.channel_add_conv = nn.Sequential(
- nn.Conv2d(self.in_channels, self.planes, kernel_size=1),
- nn.LayerNorm([self.planes, 1, 1]), nn.ReLU(inplace=True),
- nn.Conv2d(self.planes, self.in_channels, kernel_size=1))
- elif fusion_type == 'channel_concat':
- self.channel_concat_conv = nn.Sequential(
- nn.Conv2d(self.in_channels, self.planes, kernel_size=1),
- nn.LayerNorm([self.planes, 1, 1]), nn.ReLU(inplace=True),
- nn.Conv2d(self.planes, self.in_channels, kernel_size=1))
- # for concat
- self.cat_conv = nn.Conv2d(
- 2 * self.in_channels, self.in_channels, kernel_size=1)
- elif fusion_type == 'channel_mul':
- self.channel_mul_conv = nn.Sequential(
- nn.Conv2d(self.in_channels, self.planes, kernel_size=1),
- nn.LayerNorm([self.planes, 1, 1]), nn.ReLU(inplace=True),
- nn.Conv2d(self.planes, self.in_channels, kernel_size=1))
-
- def spatial_pool(self, x: torch.Tensor) -> torch.Tensor:
- """Spatial pooling function.
-
- Args:
- x (Tensor): Input feature map.
-
- Returns:
- Tensor: Output tensor after spatial pooling.
- """
- batch, channel, height, width = x.size()
- if self.pooling_type == 'att':
- # [N*headers, C', H , W] C = headers * C'
- x = x.view(batch * self.n_head, self.single_header_inplanes,
- height, width)
- input_x = x
-
- # [N*headers, C', H * W] C = headers * C'
- input_x = input_x.view(batch * self.n_head,
- self.single_header_inplanes, height * width)
-
- # [N*headers, 1, C', H * W]
- input_x = input_x.unsqueeze(1)
- # [N*headers, 1, H, W]
- context_mask = self.conv_mask(x)
- # [N*headers, 1, H * W]
- context_mask = context_mask.view(batch * self.n_head, 1,
- height * width)
-
- # scale variance
- if self.scale_attn and self.n_head > 1:
- context_mask = context_mask / \
- torch.sqrt(self.single_header_inplanes)
-
- # [N*headers, 1, H * W]
- context_mask = self.softmax(context_mask)
-
- # [N*headers, 1, H * W, 1]
- context_mask = context_mask.unsqueeze(-1)
- # [N*headers, 1, C', 1] =
- # [N*headers, 1, C', H * W] * [N*headers, 1, H * W, 1]
- context = torch.matmul(input_x, context_mask)
-
- # [N, headers * C', 1, 1]
- context = context.view(batch,
- self.n_head * self.single_header_inplanes,
- 1, 1)
- else:
- # [N, C, 1, 1]
- context = self.avg_pool(x)
-
- return context
-
- def forward(self, x: torch.Tensor) -> torch.Tensor:
- """Forward function.
-
- Args:
- x (Tensor): Input feature map.
-
- Returns:
- Tensor: Output tensor after GCAModule.
- """
- # [N, C, 1, 1]
- context = self.spatial_pool(x)
- out = x
-
- if self.fusion_type == 'channel_mul':
- # [N, C, 1, 1]
- channel_mul_term = torch.sigmoid(self.channel_mul_conv(context))
- out = out * channel_mul_term
- elif self.fusion_type == 'channel_add':
- # [N, C, 1, 1]
- channel_add_term = self.channel_add_conv(context)
- out = out + channel_add_term
- else:
- # [N, C, 1, 1]
- channel_concat_term = self.channel_concat_conv(context)
-
- # use concat
- _, C1, _, _ = channel_concat_term.shape
- N, C2, H, W = out.shape
-
- out = torch.cat([out,
- channel_concat_term.expand(-1, -1, H, W)],
- dim=1)
- out = self.cat_conv(out)
- out = nn.functional.layer_norm(out, [self.in_channels, H, W])
- out = nn.functional.relu(out)
-
- return out
diff --git a/pipeline/mmocr/mmocr/models/textrecog/postprocessors/__init__.py b/pipeline/mmocr/mmocr/models/textrecog/postprocessors/__init__.py
deleted file mode 100644
index 14b51daebd7dc398915ea733c7e257fd66313d80..0000000000000000000000000000000000000000
--- a/pipeline/mmocr/mmocr/models/textrecog/postprocessors/__init__.py
+++ /dev/null
@@ -1,8 +0,0 @@
-# Copyright (c) OpenMMLab. All rights reserved.
-from .attn_postprocessor import AttentionPostprocessor
-from .base import BaseTextRecogPostprocessor
-from .ctc_postprocessor import CTCPostProcessor
-
-__all__ = [
- 'BaseTextRecogPostprocessor', 'AttentionPostprocessor', 'CTCPostProcessor'
-]
diff --git a/pipeline/mmocr/mmocr/models/textrecog/postprocessors/__pycache__/__init__.cpython-38.pyc b/pipeline/mmocr/mmocr/models/textrecog/postprocessors/__pycache__/__init__.cpython-38.pyc
deleted file mode 100644
index e7b63dd4800b7b5a6c52abf38afe60d1d293e362..0000000000000000000000000000000000000000
Binary files a/pipeline/mmocr/mmocr/models/textrecog/postprocessors/__pycache__/__init__.cpython-38.pyc and /dev/null differ
diff --git a/pipeline/mmocr/mmocr/models/textrecog/postprocessors/__pycache__/__init__.cpython-39.pyc b/pipeline/mmocr/mmocr/models/textrecog/postprocessors/__pycache__/__init__.cpython-39.pyc
deleted file mode 100644
index 1ebc0587ffebedc36d1e3aae3c4e0b663218cd39..0000000000000000000000000000000000000000
Binary files a/pipeline/mmocr/mmocr/models/textrecog/postprocessors/__pycache__/__init__.cpython-39.pyc and /dev/null differ
diff --git a/pipeline/mmocr/mmocr/models/textrecog/postprocessors/__pycache__/attn_postprocessor.cpython-38.pyc b/pipeline/mmocr/mmocr/models/textrecog/postprocessors/__pycache__/attn_postprocessor.cpython-38.pyc
deleted file mode 100644
index b425367d011825b2280b20bebd0f8ae07d6587ee..0000000000000000000000000000000000000000
Binary files a/pipeline/mmocr/mmocr/models/textrecog/postprocessors/__pycache__/attn_postprocessor.cpython-38.pyc and /dev/null differ
diff --git a/pipeline/mmocr/mmocr/models/textrecog/postprocessors/__pycache__/attn_postprocessor.cpython-39.pyc b/pipeline/mmocr/mmocr/models/textrecog/postprocessors/__pycache__/attn_postprocessor.cpython-39.pyc
deleted file mode 100644
index 7891e2a19fb996dcf6489cc4355ba918267d403f..0000000000000000000000000000000000000000
Binary files a/pipeline/mmocr/mmocr/models/textrecog/postprocessors/__pycache__/attn_postprocessor.cpython-39.pyc and /dev/null differ
diff --git a/pipeline/mmocr/mmocr/models/textrecog/postprocessors/__pycache__/base.cpython-38.pyc b/pipeline/mmocr/mmocr/models/textrecog/postprocessors/__pycache__/base.cpython-38.pyc
deleted file mode 100644
index ecac5b00061702af75c52ff8b99a5ca90b14d89f..0000000000000000000000000000000000000000
Binary files a/pipeline/mmocr/mmocr/models/textrecog/postprocessors/__pycache__/base.cpython-38.pyc and /dev/null differ
diff --git a/pipeline/mmocr/mmocr/models/textrecog/postprocessors/__pycache__/base.cpython-39.pyc b/pipeline/mmocr/mmocr/models/textrecog/postprocessors/__pycache__/base.cpython-39.pyc
deleted file mode 100644
index 5d2b88bda29f1c8e5e415bfdc638097c8aa97270..0000000000000000000000000000000000000000
Binary files a/pipeline/mmocr/mmocr/models/textrecog/postprocessors/__pycache__/base.cpython-39.pyc and /dev/null differ
diff --git a/pipeline/mmocr/mmocr/models/textrecog/postprocessors/__pycache__/ctc_postprocessor.cpython-38.pyc b/pipeline/mmocr/mmocr/models/textrecog/postprocessors/__pycache__/ctc_postprocessor.cpython-38.pyc
deleted file mode 100644
index e31a7fb0bb6894518b7907d27fc1c245bbc23a48..0000000000000000000000000000000000000000
Binary files a/pipeline/mmocr/mmocr/models/textrecog/postprocessors/__pycache__/ctc_postprocessor.cpython-38.pyc and /dev/null differ
diff --git a/pipeline/mmocr/mmocr/models/textrecog/postprocessors/__pycache__/ctc_postprocessor.cpython-39.pyc b/pipeline/mmocr/mmocr/models/textrecog/postprocessors/__pycache__/ctc_postprocessor.cpython-39.pyc
deleted file mode 100644
index 546c23ec4953b8db3877de81a726e3337afce283..0000000000000000000000000000000000000000
Binary files a/pipeline/mmocr/mmocr/models/textrecog/postprocessors/__pycache__/ctc_postprocessor.cpython-39.pyc and /dev/null differ
diff --git a/pipeline/mmocr/mmocr/models/textrecog/postprocessors/attn_postprocessor.py b/pipeline/mmocr/mmocr/models/textrecog/postprocessors/attn_postprocessor.py
deleted file mode 100644
index e047a6a341ca90b874d993c0def6aed9a3af114e..0000000000000000000000000000000000000000
--- a/pipeline/mmocr/mmocr/models/textrecog/postprocessors/attn_postprocessor.py
+++ /dev/null
@@ -1,43 +0,0 @@
-# Copyright (c) OpenMMLab. All rights reserved.
-from typing import Optional, Sequence, Tuple
-
-import torch
-
-from mmocr.registry import MODELS
-from mmocr.structures import TextRecogDataSample
-from .base import BaseTextRecogPostprocessor
-
-
-@MODELS.register_module()
-class AttentionPostprocessor(BaseTextRecogPostprocessor):
- """PostProcessor for seq2seq."""
-
- def get_single_prediction(
- self,
- probs: torch.Tensor,
- data_sample: Optional[TextRecogDataSample] = None,
- ) -> Tuple[Sequence[int], Sequence[float]]:
- """Convert the output probabilities of a single image to index and
- score.
-
- Args:
- probs (torch.Tensor): Character probabilities with shape
- :math:`(T, C)`.
- data_sample (TextRecogDataSample, optional): Datasample of an
- image. Defaults to None.
-
- Returns:
- tuple(list[int], list[float]): index and score.
- """
- max_value, max_idx = torch.max(probs, -1)
- index, score = [], []
- output_index = max_idx.cpu().detach().numpy().tolist()
- output_score = max_value.cpu().detach().numpy().tolist()
- for char_index, char_score in zip(output_index, output_score):
- if char_index in self.ignore_indexes:
- continue
- if char_index == self.dictionary.end_idx:
- break
- index.append(char_index)
- score.append(char_score)
- return index, score
diff --git a/pipeline/mmocr/mmocr/models/textrecog/postprocessors/base.py b/pipeline/mmocr/mmocr/models/textrecog/postprocessors/base.py
deleted file mode 100644
index 818640a8ca572f55e8c819a14c496dd47a6b4e93..0000000000000000000000000000000000000000
--- a/pipeline/mmocr/mmocr/models/textrecog/postprocessors/base.py
+++ /dev/null
@@ -1,110 +0,0 @@
-# Copyright (c) OpenMMLab. All rights reserved.
-import warnings
-from typing import Dict, Optional, Sequence, Tuple, Union
-
-import mmengine
-import torch
-from mmengine.structures import LabelData
-
-from mmocr.models.common.dictionary import Dictionary
-from mmocr.registry import TASK_UTILS
-from mmocr.structures import TextRecogDataSample
-
-
-class BaseTextRecogPostprocessor:
- """Base text recognition postprocessor.
-
- Args:
- dictionary (dict or :obj:`Dictionary`): The config for `Dictionary` or
- the instance of `Dictionary`.
- max_seq_len (int): max_seq_len (int): Maximum sequence length. The
- sequence is usually generated from decoder. Defaults to 40.
- ignore_chars (list[str]): A list of characters to be ignored from the
- final results. Postprocessor will skip over these characters when
- converting raw indexes to characters. Apart from single characters,
- each item can be one of the following reversed keywords: 'padding',
- 'end' and 'unknown', which refer to their corresponding special
- tokens in the dictionary.
- """
-
- def __init__(self,
- dictionary: Union[Dictionary, Dict],
- max_seq_len: int = 40,
- ignore_chars: Sequence[str] = ['padding'],
- **kwargs) -> None:
-
- if isinstance(dictionary, dict):
- self.dictionary = TASK_UTILS.build(dictionary)
- elif isinstance(dictionary, Dictionary):
- self.dictionary = dictionary
- else:
- raise TypeError(
- 'The type of dictionary should be `Dictionary` or dict, '
- f'but got {type(dictionary)}')
- self.max_seq_len = max_seq_len
-
- mapping_table = {
- 'padding': self.dictionary.padding_idx,
- 'end': self.dictionary.end_idx,
- 'unknown': self.dictionary.unknown_idx,
- }
- if not mmengine.is_list_of(ignore_chars, str):
- raise TypeError('ignore_chars must be list of str')
- ignore_indexes = list()
- for ignore_char in ignore_chars:
- index = mapping_table.get(
- ignore_char,
- self.dictionary.char2idx(ignore_char, strict=False))
- if index is None or (index == self.dictionary.unknown_idx
- and ignore_char != 'unknown'):
- warnings.warn(
- f'{ignore_char} does not exist in the dictionary',
- UserWarning)
- continue
- ignore_indexes.append(index)
- self.ignore_indexes = ignore_indexes
-
- def get_single_prediction(
- self,
- probs: torch.Tensor,
- data_sample: Optional[TextRecogDataSample] = None,
- ) -> Tuple[Sequence[int], Sequence[float]]:
- """Convert the output probabilities of a single image to index and
- score.
-
- Args:
- probs (torch.Tensor): Character probabilities with shape
- :math:`(T, C)`.
- data_sample (TextRecogDataSample): Datasample of an image.
-
- Returns:
- tuple(list[int], list[float]): Index and scores per-character.
- """
- raise NotImplementedError
-
- def __call__(
- self, probs: torch.Tensor, data_samples: Sequence[TextRecogDataSample]
- ) -> Sequence[TextRecogDataSample]:
- """Convert outputs to strings and scores.
-
- Args:
- probs (torch.Tensor): Batched character probabilities, the model's
- softmaxed output in size: :math:`(N, T, C)`.
- data_samples (list[TextRecogDataSample]): The list of
- TextRecogDataSample.
-
- Returns:
- list(TextRecogDataSample): The list of TextRecogDataSample. It
- usually contain ``pred_text`` information.
- """
- batch_size = probs.size(0)
-
- for idx in range(batch_size):
- index, score = self.get_single_prediction(probs[idx, :, :],
- data_samples[idx])
- text = self.dictionary.idx2str(index)
- pred_text = LabelData()
- pred_text.score = score
- pred_text.item = text
- data_samples[idx].pred_text = pred_text
- return data_samples
diff --git a/pipeline/mmocr/mmocr/models/textrecog/postprocessors/ctc_postprocessor.py b/pipeline/mmocr/mmocr/models/textrecog/postprocessors/ctc_postprocessor.py
deleted file mode 100644
index 0fa28779abaf64e1d964ae05b4296e81308aab13..0000000000000000000000000000000000000000
--- a/pipeline/mmocr/mmocr/models/textrecog/postprocessors/ctc_postprocessor.py
+++ /dev/null
@@ -1,52 +0,0 @@
-# Copyright (c) OpenMMLab. All rights reserved.
-import math
-from typing import Sequence, Tuple
-
-import torch
-
-from mmocr.registry import MODELS
-from mmocr.structures import TextRecogDataSample
-from .base import BaseTextRecogPostprocessor
-
-
-# TODO support beam search
-@MODELS.register_module()
-class CTCPostProcessor(BaseTextRecogPostprocessor):
- """PostProcessor for CTC."""
-
- def get_single_prediction(self, probs: torch.Tensor,
- data_sample: TextRecogDataSample
- ) -> Tuple[Sequence[int], Sequence[float]]:
- """Convert the output probabilities of a single image to index and
- score.
-
- Args:
- probs (torch.Tensor): Character probabilities with shape
- :math:`(T, C)`.
- data_sample (TextRecogDataSample): Datasample of an image.
-
- Returns:
- tuple(list[int], list[float]): index and score.
- """
- feat_len = probs.size(0)
- max_value, max_idx = torch.max(probs, -1)
- valid_ratio = data_sample.get('valid_ratio', 1)
- decode_len = min(feat_len, math.ceil(feat_len * valid_ratio))
- index = []
- score = []
-
- prev_idx = self.dictionary.padding_idx
- for t in range(decode_len):
- tmp_value = max_idx[t].item()
- if tmp_value not in (prev_idx, *self.ignore_indexes):
- index.append(tmp_value)
- score.append(max_value[t].item())
- prev_idx = tmp_value
- return index, score
-
- def __call__(
- self, outputs: torch.Tensor,
- data_samples: Sequence[TextRecogDataSample]
- ) -> Sequence[TextRecogDataSample]:
- outputs = outputs.cpu().detach()
- return super().__call__(outputs, data_samples)
diff --git a/pipeline/mmocr/mmocr/models/textrecog/preprocessors/__init__.py b/pipeline/mmocr/mmocr/models/textrecog/preprocessors/__init__.py
deleted file mode 100644
index 15825f25fe22be1eb6d32a1555277d50ad5c5383..0000000000000000000000000000000000000000
--- a/pipeline/mmocr/mmocr/models/textrecog/preprocessors/__init__.py
+++ /dev/null
@@ -1,4 +0,0 @@
-# Copyright (c) OpenMMLab. All rights reserved.
-from .tps_preprocessor import STN, TPStransform
-
-__all__ = ['TPStransform', 'STN']
diff --git a/pipeline/mmocr/mmocr/models/textrecog/preprocessors/__pycache__/__init__.cpython-38.pyc b/pipeline/mmocr/mmocr/models/textrecog/preprocessors/__pycache__/__init__.cpython-38.pyc
deleted file mode 100644
index f3f9397f188f75a6771fa29a0df108c6d3f99d9e..0000000000000000000000000000000000000000
Binary files a/pipeline/mmocr/mmocr/models/textrecog/preprocessors/__pycache__/__init__.cpython-38.pyc and /dev/null differ
diff --git a/pipeline/mmocr/mmocr/models/textrecog/preprocessors/__pycache__/__init__.cpython-39.pyc b/pipeline/mmocr/mmocr/models/textrecog/preprocessors/__pycache__/__init__.cpython-39.pyc
deleted file mode 100644
index 5cc7891a32c746e2334279e0cb78648a61d5e5fe..0000000000000000000000000000000000000000
Binary files a/pipeline/mmocr/mmocr/models/textrecog/preprocessors/__pycache__/__init__.cpython-39.pyc and /dev/null differ
diff --git a/pipeline/mmocr/mmocr/models/textrecog/preprocessors/__pycache__/base.cpython-38.pyc b/pipeline/mmocr/mmocr/models/textrecog/preprocessors/__pycache__/base.cpython-38.pyc
deleted file mode 100644
index c0446a62ac62aaf8b78fbdccf2eaef4726e1ab5b..0000000000000000000000000000000000000000
Binary files a/pipeline/mmocr/mmocr/models/textrecog/preprocessors/__pycache__/base.cpython-38.pyc and /dev/null differ
diff --git a/pipeline/mmocr/mmocr/models/textrecog/preprocessors/__pycache__/base.cpython-39.pyc b/pipeline/mmocr/mmocr/models/textrecog/preprocessors/__pycache__/base.cpython-39.pyc
deleted file mode 100644
index 8e6b2b298609efb0e8e33ec027b3dbf0e21899a9..0000000000000000000000000000000000000000
Binary files a/pipeline/mmocr/mmocr/models/textrecog/preprocessors/__pycache__/base.cpython-39.pyc and /dev/null differ
diff --git a/pipeline/mmocr/mmocr/models/textrecog/preprocessors/__pycache__/tps_preprocessor.cpython-38.pyc b/pipeline/mmocr/mmocr/models/textrecog/preprocessors/__pycache__/tps_preprocessor.cpython-38.pyc
deleted file mode 100644
index 80526010b4bbe64ed68cf8111854b86246e31599..0000000000000000000000000000000000000000
Binary files a/pipeline/mmocr/mmocr/models/textrecog/preprocessors/__pycache__/tps_preprocessor.cpython-38.pyc and /dev/null differ
diff --git a/pipeline/mmocr/mmocr/models/textrecog/preprocessors/__pycache__/tps_preprocessor.cpython-39.pyc b/pipeline/mmocr/mmocr/models/textrecog/preprocessors/__pycache__/tps_preprocessor.cpython-39.pyc
deleted file mode 100644
index fdeda4b6d0a99f4ff5eaf555a3bedeedeed0c293..0000000000000000000000000000000000000000
Binary files a/pipeline/mmocr/mmocr/models/textrecog/preprocessors/__pycache__/tps_preprocessor.cpython-39.pyc and /dev/null differ
diff --git a/pipeline/mmocr/mmocr/models/textrecog/preprocessors/base.py b/pipeline/mmocr/mmocr/models/textrecog/preprocessors/base.py
deleted file mode 100644
index a1f138bed0eef9517f3e4b1e9f5e33c382a77292..0000000000000000000000000000000000000000
--- a/pipeline/mmocr/mmocr/models/textrecog/preprocessors/base.py
+++ /dev/null
@@ -1,12 +0,0 @@
-# Copyright (c) OpenMMLab. All rights reserved.
-from mmengine.model import BaseModule
-
-from mmocr.registry import MODELS
-
-
-@MODELS.register_module()
-class BasePreprocessor(BaseModule):
- """Base Preprocessor class for text recognition."""
-
- def forward(self, x, **kwargs):
- return x
diff --git a/pipeline/mmocr/mmocr/models/textrecog/preprocessors/tps_preprocessor.py b/pipeline/mmocr/mmocr/models/textrecog/preprocessors/tps_preprocessor.py
deleted file mode 100644
index 6a9e0ccc6b0d077b2e66a3e9d9df944b5f862d86..0000000000000000000000000000000000000000
--- a/pipeline/mmocr/mmocr/models/textrecog/preprocessors/tps_preprocessor.py
+++ /dev/null
@@ -1,272 +0,0 @@
-# Copyright (c) OpenMMLab. All rights reserved.
-import itertools
-from typing import Dict, List, Optional, Tuple, Union
-
-import numpy as np
-import torch
-import torch.nn as nn
-import torch.nn.functional as F
-from mmcv.cnn import ConvModule
-
-from mmocr.registry import MODELS
-from .base import BasePreprocessor
-
-
-class TPStransform(nn.Module):
- """Implement TPS transform.
-
- This was partially adapted from https://github.com/ayumiymk/aster.pytorch
-
- Args:
- output_image_size (tuple[int, int]): The size of the output image.
- Defaults to (32, 128).
- num_control_points (int): The number of control points. Defaults to 20.
- margins (tuple[float, float]): The margins for control points to the
- top and down side of the image. Defaults to [0.05, 0.05].
- """
-
- def __init__(self,
- output_image_size: Tuple[int, int] = (32, 100),
- num_control_points: int = 20,
- margins: Tuple[float, float] = [0.05, 0.05]) -> None:
- super().__init__()
- self.output_image_size = output_image_size
- self.num_control_points = num_control_points
- self.margins = margins
- self.target_height, self.target_width = output_image_size
-
- # build output control points
- target_control_points = self._build_output_control_points(
- num_control_points, margins)
- N = num_control_points
-
- # create padded kernel matrix
- forward_kernel = torch.zeros(N + 3, N + 3)
- target_control_partial_repr = self._compute_partial_repr(
- target_control_points, target_control_points)
- forward_kernel[:N, :N].copy_(target_control_partial_repr)
- forward_kernel[:N, -3].fill_(1)
- forward_kernel[-3, :N].fill_(1)
- forward_kernel[:N, -2:].copy_(target_control_points)
- forward_kernel[-2:, :N].copy_(target_control_points.transpose(0, 1))
-
- # compute inverse matrix
- inverse_kernel = torch.inverse(forward_kernel).contiguous()
-
- # create target coordinate matrix
- HW = self.target_height * self.target_width
- tgt_coord = list(
- itertools.product(
- range(self.target_height), range(self.target_width)))
- tgt_coord = torch.Tensor(tgt_coord)
- Y, X = tgt_coord.split(1, dim=1)
- Y = Y / (self.target_height - 1)
- X = X / (self.target_width - 1)
- tgt_coord = torch.cat([X, Y], dim=1)
- tgt_coord_partial_repr = self._compute_partial_repr(
- tgt_coord, target_control_points)
- tgt_coord_repr = torch.cat(
- [tgt_coord_partial_repr,
- torch.ones(HW, 1), tgt_coord], dim=1)
-
- # register precomputed matrices
- self.register_buffer('inverse_kernel', inverse_kernel)
- self.register_buffer('padding_matrix', torch.zeros(3, 2))
- self.register_buffer('target_coordinate_repr', tgt_coord_repr)
- self.register_buffer('target_control_points', target_control_points)
-
- def forward(self, input: torch.Tensor,
- source_control_points: torch.Tensor) -> torch.Tensor:
- """Forward function of the TPS block.
-
- Args:
- input (Tensor): The input image.
- source_control_points (Tensor): The control points of the source
- image of shape (N, self.num_control_points, 2).
- Returns:
- Tensor: The output image after TPS transform.
- """
- assert source_control_points.ndimension() == 3
- assert source_control_points.size(1) == self.num_control_points
- assert source_control_points.size(2) == 2
- batch_size = source_control_points.size(0)
-
- Y = torch.cat([
- source_control_points,
- self.padding_matrix.expand(batch_size, 3, 2)
- ], 1)
- mapping_matrix = torch.matmul(self.inverse_kernel, Y)
- source_coordinate = torch.matmul(self.target_coordinate_repr,
- mapping_matrix)
-
- grid = source_coordinate.view(-1, self.target_height,
- self.target_width, 2)
- grid = torch.clamp(grid, 0, 1)
- grid = 2.0 * grid - 1.0
- output_maps = self._grid_sample(input, grid, canvas=None)
- return output_maps
-
- def _grid_sample(self,
- input: torch.Tensor,
- grid: torch.Tensor,
- canvas: Optional[torch.Tensor] = None) -> torch.Tensor:
- """Sample the input image at the given grid.
-
- Args:
- input (Tensor): The input image.
- grid (Tensor): The grid to sample the input image.
- canvas (Optional[Tensor]): The canvas to store the output image.
- Returns:
- Tensor: The sampled image.
- """
- output = F.grid_sample(input, grid, align_corners=True)
- if canvas is None:
- return output
- else:
- input_mask = input.data.new(input.size()).fill_(1)
- output_mask = F.grid_sample(input_mask, grid, align_corners=True)
- padded_output = output * output_mask + canvas * (1 - output_mask)
- return padded_output
-
- def _compute_partial_repr(self, input_points: torch.Tensor,
- control_points: torch.Tensor) -> torch.Tensor:
- """Compute the partial representation matrix.
-
- Args:
- input_points (Tensor): The input points.
- control_points (Tensor): The control points.
- Returns:
- Tensor: The partial representation matrix.
- """
- N = input_points.size(0)
- M = control_points.size(0)
- pairwise_diff = input_points.view(N, 1, 2) - control_points.view(
- 1, M, 2)
- pairwise_diff_square = pairwise_diff * pairwise_diff
- pairwise_dist = pairwise_diff_square[:, :,
- 0] + pairwise_diff_square[:, :, 1]
- repr_matrix = 0.5 * pairwise_dist * torch.log(pairwise_dist)
- mask = repr_matrix != repr_matrix
- repr_matrix.masked_fill_(mask, 0)
- return repr_matrix
-
- # output_ctrl_pts are specified, according to our task.
- def _build_output_control_points(self, num_control_points: torch.Tensor,
- margins: Tuple[float,
- float]) -> torch.Tensor:
- """Build the output control points.
-
- The output points will be fix at
- top and down side of the image.
- Args:
- num_control_points (Tensor): The number of control points.
- margins (Tuple[float, float]): The margins for control points to
- the top and down side of the image.
- Returns:
- Tensor: The output control points.
- """
- margin_x, margin_y = margins
- num_ctrl_pts_per_side = num_control_points // 2
- ctrl_pts_x = np.linspace(margin_x, 1.0 - margin_x,
- num_ctrl_pts_per_side)
- ctrl_pts_y_top = np.ones(num_ctrl_pts_per_side) * margin_y
- ctrl_pts_y_bottom = np.ones(num_ctrl_pts_per_side) * (1.0 - margin_y)
- ctrl_pts_top = np.stack([ctrl_pts_x, ctrl_pts_y_top], axis=1)
- ctrl_pts_bottom = np.stack([ctrl_pts_x, ctrl_pts_y_bottom], axis=1)
- output_ctrl_pts_arr = np.concatenate([ctrl_pts_top, ctrl_pts_bottom],
- axis=0)
- output_ctrl_pts = torch.Tensor(output_ctrl_pts_arr)
- return output_ctrl_pts
-
-
-@MODELS.register_module()
-class STN(BasePreprocessor):
- """Implement STN module in ASTER: An Attentional Scene Text Recognizer with
- Flexible Rectification
- (https://ieeexplore.ieee.org/abstract/document/8395027/)
-
- Args:
- in_channels (int): The number of input channels.
- resized_image_size (Tuple[int, int]): The resized image size. The input
- image will be downsampled to have a better recitified result.
- output_image_size: The size of the output image for TPS. Defaults to
- (32, 100).
- num_control_points: The number of control points. Defaults to 20.
- margins: The margins for control points to the top and down side of the
- image for TPS. Defaults to [0.05, 0.05].
- """
-
- def __init__(self,
- in_channels: int,
- resized_image_size: Tuple[int, int] = (32, 64),
- output_image_size: Tuple[int, int] = (32, 100),
- num_control_points: int = 20,
- margins: Tuple[float, float] = [0.05, 0.05],
- init_cfg: Optional[Union[Dict, List[Dict]]] = [
- dict(type='Xavier', layer='Conv2d'),
- dict(type='Constant', val=1, layer='BatchNorm2d'),
- ]):
- super().__init__(init_cfg=init_cfg)
- self.resized_image_size = resized_image_size
- self.num_control_points = num_control_points
- self.tps = TPStransform(output_image_size, num_control_points, margins)
- self.stn_convnet = nn.Sequential(
- ConvModule(in_channels, 32, 3, 1, 1, norm_cfg=dict(type='BN')),
- nn.MaxPool2d(kernel_size=2, stride=2),
- ConvModule(32, 64, 3, 1, 1, norm_cfg=dict(type='BN')),
- nn.MaxPool2d(kernel_size=2, stride=2),
- ConvModule(64, 128, 3, 1, 1, norm_cfg=dict(type='BN')),
- nn.MaxPool2d(kernel_size=2, stride=2),
- ConvModule(128, 256, 3, 1, 1, norm_cfg=dict(type='BN')),
- nn.MaxPool2d(kernel_size=2, stride=2),
- ConvModule(256, 256, 3, 1, 1, norm_cfg=dict(type='BN')),
- nn.MaxPool2d(kernel_size=2, stride=2),
- ConvModule(256, 256, 3, 1, 1, norm_cfg=dict(type='BN')),
- )
-
- self.stn_fc1 = nn.Sequential(
- nn.Linear(2 * 256, 512), nn.BatchNorm1d(512),
- nn.ReLU(inplace=True))
- self.stn_fc2 = nn.Linear(512, num_control_points * 2)
- self.init_stn(self.stn_fc2)
-
- def init_stn(self, stn_fc2: nn.Linear) -> None:
- """Initialize the output linear layer of stn, so that the initial
- source point will be at the top and down side of the image, which will
- help to optimize.
-
- Args:
- stn_fc2 (nn.Linear): The output linear layer of stn.
- """
- margin = 0.01
- sampling_num_per_side = int(self.num_control_points / 2)
- ctrl_pts_x = np.linspace(margin, 1. - margin, sampling_num_per_side)
- ctrl_pts_y_top = np.ones(sampling_num_per_side) * margin
- ctrl_pts_y_bottom = np.ones(sampling_num_per_side) * (1 - margin)
- ctrl_pts_top = np.stack([ctrl_pts_x, ctrl_pts_y_top], axis=1)
- ctrl_pts_bottom = np.stack([ctrl_pts_x, ctrl_pts_y_bottom], axis=1)
- ctrl_points = np.concatenate([ctrl_pts_top, ctrl_pts_bottom],
- axis=0).astype(np.float32)
- stn_fc2.weight.data.zero_()
- stn_fc2.bias.data = torch.Tensor(ctrl_points).view(-1)
-
- def forward(self, img: torch.Tensor) -> torch.Tensor:
- """Forward function of STN.
-
- Args:
- img (Tensor): The input image tensor.
-
- Returns:
- Tensor: The rectified image tensor.
- """
- resize_img = F.interpolate(
- img, self.resized_image_size, mode='bilinear', align_corners=True)
- points = self.stn_convnet(resize_img)
- batch_size, _, _, _ = points.size()
- points = points.view(batch_size, -1)
- img_feat = self.stn_fc1(points)
- points = self.stn_fc2(0.1 * img_feat)
- points = points.view(-1, self.num_control_points, 2)
-
- transformd_image = self.tps(img, points)
- return transformd_image
diff --git a/pipeline/mmocr/mmocr/models/textrecog/recognizers/__init__.py b/pipeline/mmocr/mmocr/models/textrecog/recognizers/__init__.py
deleted file mode 100644
index f590e60dc695b21da4ed859e25a5dbecc0551601..0000000000000000000000000000000000000000
--- a/pipeline/mmocr/mmocr/models/textrecog/recognizers/__init__.py
+++ /dev/null
@@ -1,20 +0,0 @@
-# Copyright (c) OpenMMLab. All rights reserved.
-from .abinet import ABINet
-from .aster import ASTER
-from .base import BaseRecognizer
-from .crnn import CRNN
-from .encoder_decoder_recognizer import EncoderDecoderRecognizer
-from .encoder_decoder_recognizer_tta import EncoderDecoderRecognizerTTAModel
-from .master import MASTER
-from .nrtr import NRTR
-from .robust_scanner import RobustScanner
-from .sar import SARNet
-from .satrn import SATRN
-from .svtr import SVTR
-from .maerec import MAERec
-
-__all__ = [
- 'BaseRecognizer', 'EncoderDecoderRecognizer', 'CRNN', 'SARNet', 'NRTR',
- 'RobustScanner', 'SATRN', 'ABINet', 'MASTER', 'SVTR', 'ASTER',
- 'EncoderDecoderRecognizerTTAModel', 'MAERec'
-]
diff --git a/pipeline/mmocr/mmocr/models/textrecog/recognizers/__pycache__/__init__.cpython-38.pyc b/pipeline/mmocr/mmocr/models/textrecog/recognizers/__pycache__/__init__.cpython-38.pyc
deleted file mode 100644
index 335662b3ecab1f6df0e00590e4754c936e1c91cd..0000000000000000000000000000000000000000
Binary files a/pipeline/mmocr/mmocr/models/textrecog/recognizers/__pycache__/__init__.cpython-38.pyc and /dev/null differ
diff --git a/pipeline/mmocr/mmocr/models/textrecog/recognizers/__pycache__/__init__.cpython-39.pyc b/pipeline/mmocr/mmocr/models/textrecog/recognizers/__pycache__/__init__.cpython-39.pyc
deleted file mode 100644
index 5e56c4e8e67778d4708e934e1b25ad9e099d435a..0000000000000000000000000000000000000000
Binary files a/pipeline/mmocr/mmocr/models/textrecog/recognizers/__pycache__/__init__.cpython-39.pyc and /dev/null differ
diff --git a/pipeline/mmocr/mmocr/models/textrecog/recognizers/__pycache__/abinet.cpython-38.pyc b/pipeline/mmocr/mmocr/models/textrecog/recognizers/__pycache__/abinet.cpython-38.pyc
deleted file mode 100644
index 18b8adf0b9548c696c709f2e342cf7bb31b99646..0000000000000000000000000000000000000000
Binary files a/pipeline/mmocr/mmocr/models/textrecog/recognizers/__pycache__/abinet.cpython-38.pyc and /dev/null differ
diff --git a/pipeline/mmocr/mmocr/models/textrecog/recognizers/__pycache__/abinet.cpython-39.pyc b/pipeline/mmocr/mmocr/models/textrecog/recognizers/__pycache__/abinet.cpython-39.pyc
deleted file mode 100644
index c848442a2483200860b616eff254f7ee8523b48e..0000000000000000000000000000000000000000
Binary files a/pipeline/mmocr/mmocr/models/textrecog/recognizers/__pycache__/abinet.cpython-39.pyc and /dev/null differ
diff --git a/pipeline/mmocr/mmocr/models/textrecog/recognizers/__pycache__/aster.cpython-38.pyc b/pipeline/mmocr/mmocr/models/textrecog/recognizers/__pycache__/aster.cpython-38.pyc
deleted file mode 100644
index 222baaaf626f4713032b54e01331487fddfe3d29..0000000000000000000000000000000000000000
Binary files a/pipeline/mmocr/mmocr/models/textrecog/recognizers/__pycache__/aster.cpython-38.pyc and /dev/null differ
diff --git a/pipeline/mmocr/mmocr/models/textrecog/recognizers/__pycache__/aster.cpython-39.pyc b/pipeline/mmocr/mmocr/models/textrecog/recognizers/__pycache__/aster.cpython-39.pyc
deleted file mode 100644
index a4a71cd7b5cd9aa9682c71ee16e89e69bb398db1..0000000000000000000000000000000000000000
Binary files a/pipeline/mmocr/mmocr/models/textrecog/recognizers/__pycache__/aster.cpython-39.pyc and /dev/null differ
diff --git a/pipeline/mmocr/mmocr/models/textrecog/recognizers/__pycache__/base.cpython-38.pyc b/pipeline/mmocr/mmocr/models/textrecog/recognizers/__pycache__/base.cpython-38.pyc
deleted file mode 100644
index 11e55dd5db03818d4b7a5213cc952f6624a106a6..0000000000000000000000000000000000000000
Binary files a/pipeline/mmocr/mmocr/models/textrecog/recognizers/__pycache__/base.cpython-38.pyc and /dev/null differ
diff --git a/pipeline/mmocr/mmocr/models/textrecog/recognizers/__pycache__/base.cpython-39.pyc b/pipeline/mmocr/mmocr/models/textrecog/recognizers/__pycache__/base.cpython-39.pyc
deleted file mode 100644
index 3402a4bae8d4be4a290058a93ee8ba77c3a23681..0000000000000000000000000000000000000000
Binary files a/pipeline/mmocr/mmocr/models/textrecog/recognizers/__pycache__/base.cpython-39.pyc and /dev/null differ
diff --git a/pipeline/mmocr/mmocr/models/textrecog/recognizers/__pycache__/crnn.cpython-38.pyc b/pipeline/mmocr/mmocr/models/textrecog/recognizers/__pycache__/crnn.cpython-38.pyc
deleted file mode 100644
index 627ed56a8f38cd5f537cbdda8609b337878a5fe2..0000000000000000000000000000000000000000
Binary files a/pipeline/mmocr/mmocr/models/textrecog/recognizers/__pycache__/crnn.cpython-38.pyc and /dev/null differ
diff --git a/pipeline/mmocr/mmocr/models/textrecog/recognizers/__pycache__/crnn.cpython-39.pyc b/pipeline/mmocr/mmocr/models/textrecog/recognizers/__pycache__/crnn.cpython-39.pyc
deleted file mode 100644
index 761c660d504052b879fe778b83b3ed3746fea754..0000000000000000000000000000000000000000
Binary files a/pipeline/mmocr/mmocr/models/textrecog/recognizers/__pycache__/crnn.cpython-39.pyc and /dev/null differ
diff --git a/pipeline/mmocr/mmocr/models/textrecog/recognizers/__pycache__/encoder_decoder_recognizer.cpython-38.pyc b/pipeline/mmocr/mmocr/models/textrecog/recognizers/__pycache__/encoder_decoder_recognizer.cpython-38.pyc
deleted file mode 100644
index 7cae9431c2cf763a16895691b4bd984d1556bf17..0000000000000000000000000000000000000000
Binary files a/pipeline/mmocr/mmocr/models/textrecog/recognizers/__pycache__/encoder_decoder_recognizer.cpython-38.pyc and /dev/null differ
diff --git a/pipeline/mmocr/mmocr/models/textrecog/recognizers/__pycache__/encoder_decoder_recognizer.cpython-39.pyc b/pipeline/mmocr/mmocr/models/textrecog/recognizers/__pycache__/encoder_decoder_recognizer.cpython-39.pyc
deleted file mode 100644
index 37e56841b856f6e53c26afa61f5c2239c01dc790..0000000000000000000000000000000000000000
Binary files a/pipeline/mmocr/mmocr/models/textrecog/recognizers/__pycache__/encoder_decoder_recognizer.cpython-39.pyc and /dev/null differ
diff --git a/pipeline/mmocr/mmocr/models/textrecog/recognizers/__pycache__/encoder_decoder_recognizer_tta.cpython-38.pyc b/pipeline/mmocr/mmocr/models/textrecog/recognizers/__pycache__/encoder_decoder_recognizer_tta.cpython-38.pyc
deleted file mode 100644
index 683f75eee114024e6fc4e75bf8d0fc55ceeb2161..0000000000000000000000000000000000000000
Binary files a/pipeline/mmocr/mmocr/models/textrecog/recognizers/__pycache__/encoder_decoder_recognizer_tta.cpython-38.pyc and /dev/null differ
diff --git a/pipeline/mmocr/mmocr/models/textrecog/recognizers/__pycache__/encoder_decoder_recognizer_tta.cpython-39.pyc b/pipeline/mmocr/mmocr/models/textrecog/recognizers/__pycache__/encoder_decoder_recognizer_tta.cpython-39.pyc
deleted file mode 100644
index 574094a25f02b2612e0b297689761e32c0879137..0000000000000000000000000000000000000000
Binary files a/pipeline/mmocr/mmocr/models/textrecog/recognizers/__pycache__/encoder_decoder_recognizer_tta.cpython-39.pyc and /dev/null differ
diff --git a/pipeline/mmocr/mmocr/models/textrecog/recognizers/__pycache__/maerec.cpython-38.pyc b/pipeline/mmocr/mmocr/models/textrecog/recognizers/__pycache__/maerec.cpython-38.pyc
deleted file mode 100644
index d18d7a57a7b2d3337f19f4d88dd0a77b11f69582..0000000000000000000000000000000000000000
Binary files a/pipeline/mmocr/mmocr/models/textrecog/recognizers/__pycache__/maerec.cpython-38.pyc and /dev/null differ
diff --git a/pipeline/mmocr/mmocr/models/textrecog/recognizers/__pycache__/maerec.cpython-39.pyc b/pipeline/mmocr/mmocr/models/textrecog/recognizers/__pycache__/maerec.cpython-39.pyc
deleted file mode 100644
index bc3fde2547bf63365f57baae4cbaa3766aa05ffd..0000000000000000000000000000000000000000
Binary files a/pipeline/mmocr/mmocr/models/textrecog/recognizers/__pycache__/maerec.cpython-39.pyc and /dev/null differ
diff --git a/pipeline/mmocr/mmocr/models/textrecog/recognizers/__pycache__/master.cpython-38.pyc b/pipeline/mmocr/mmocr/models/textrecog/recognizers/__pycache__/master.cpython-38.pyc
deleted file mode 100644
index c391304c0a5e3425577f48124936d34d867b14ec..0000000000000000000000000000000000000000
Binary files a/pipeline/mmocr/mmocr/models/textrecog/recognizers/__pycache__/master.cpython-38.pyc and /dev/null differ
diff --git a/pipeline/mmocr/mmocr/models/textrecog/recognizers/__pycache__/master.cpython-39.pyc b/pipeline/mmocr/mmocr/models/textrecog/recognizers/__pycache__/master.cpython-39.pyc
deleted file mode 100644
index 6a5400b63f56462237d80c2afd3ec52d8c322e15..0000000000000000000000000000000000000000
Binary files a/pipeline/mmocr/mmocr/models/textrecog/recognizers/__pycache__/master.cpython-39.pyc and /dev/null differ
diff --git a/pipeline/mmocr/mmocr/models/textrecog/recognizers/__pycache__/nrtr.cpython-38.pyc b/pipeline/mmocr/mmocr/models/textrecog/recognizers/__pycache__/nrtr.cpython-38.pyc
deleted file mode 100644
index 75908bd824557a9484887f02ba703b019914a613..0000000000000000000000000000000000000000
Binary files a/pipeline/mmocr/mmocr/models/textrecog/recognizers/__pycache__/nrtr.cpython-38.pyc and /dev/null differ
diff --git a/pipeline/mmocr/mmocr/models/textrecog/recognizers/__pycache__/nrtr.cpython-39.pyc b/pipeline/mmocr/mmocr/models/textrecog/recognizers/__pycache__/nrtr.cpython-39.pyc
deleted file mode 100644
index 36bfee595ecf663c9c677b23a0fda609df88601d..0000000000000000000000000000000000000000
Binary files a/pipeline/mmocr/mmocr/models/textrecog/recognizers/__pycache__/nrtr.cpython-39.pyc and /dev/null differ
diff --git a/pipeline/mmocr/mmocr/models/textrecog/recognizers/__pycache__/robust_scanner.cpython-38.pyc b/pipeline/mmocr/mmocr/models/textrecog/recognizers/__pycache__/robust_scanner.cpython-38.pyc
deleted file mode 100644
index 5e0a4064d625db63c3a36ee9c03509113327322b..0000000000000000000000000000000000000000
Binary files a/pipeline/mmocr/mmocr/models/textrecog/recognizers/__pycache__/robust_scanner.cpython-38.pyc and /dev/null differ
diff --git a/pipeline/mmocr/mmocr/models/textrecog/recognizers/__pycache__/robust_scanner.cpython-39.pyc b/pipeline/mmocr/mmocr/models/textrecog/recognizers/__pycache__/robust_scanner.cpython-39.pyc
deleted file mode 100644
index 77284194dd853e7268743c1c8860b9a879f86e0c..0000000000000000000000000000000000000000
Binary files a/pipeline/mmocr/mmocr/models/textrecog/recognizers/__pycache__/robust_scanner.cpython-39.pyc and /dev/null differ
diff --git a/pipeline/mmocr/mmocr/models/textrecog/recognizers/__pycache__/sar.cpython-38.pyc b/pipeline/mmocr/mmocr/models/textrecog/recognizers/__pycache__/sar.cpython-38.pyc
deleted file mode 100644
index 95f7dbe7355260f35673b8221947fa3d226cfbe5..0000000000000000000000000000000000000000
Binary files a/pipeline/mmocr/mmocr/models/textrecog/recognizers/__pycache__/sar.cpython-38.pyc and /dev/null differ
diff --git a/pipeline/mmocr/mmocr/models/textrecog/recognizers/__pycache__/sar.cpython-39.pyc b/pipeline/mmocr/mmocr/models/textrecog/recognizers/__pycache__/sar.cpython-39.pyc
deleted file mode 100644
index 280688b3cfb2ecfab8b1b3c4f477a74c1c669089..0000000000000000000000000000000000000000
Binary files a/pipeline/mmocr/mmocr/models/textrecog/recognizers/__pycache__/sar.cpython-39.pyc and /dev/null differ
diff --git a/pipeline/mmocr/mmocr/models/textrecog/recognizers/__pycache__/satrn.cpython-38.pyc b/pipeline/mmocr/mmocr/models/textrecog/recognizers/__pycache__/satrn.cpython-38.pyc
deleted file mode 100644
index 2a84608dfbf8b445a42b47a310f5823195370528..0000000000000000000000000000000000000000
Binary files a/pipeline/mmocr/mmocr/models/textrecog/recognizers/__pycache__/satrn.cpython-38.pyc and /dev/null differ
diff --git a/pipeline/mmocr/mmocr/models/textrecog/recognizers/__pycache__/satrn.cpython-39.pyc b/pipeline/mmocr/mmocr/models/textrecog/recognizers/__pycache__/satrn.cpython-39.pyc
deleted file mode 100644
index 66ea8a92222f122aefde0b3dddd3edff5baf3e1e..0000000000000000000000000000000000000000
Binary files a/pipeline/mmocr/mmocr/models/textrecog/recognizers/__pycache__/satrn.cpython-39.pyc and /dev/null differ
diff --git a/pipeline/mmocr/mmocr/models/textrecog/recognizers/__pycache__/svtr.cpython-38.pyc b/pipeline/mmocr/mmocr/models/textrecog/recognizers/__pycache__/svtr.cpython-38.pyc
deleted file mode 100644
index 1b9b34c59b1af25720f9f7e2a4564460f18c3437..0000000000000000000000000000000000000000
Binary files a/pipeline/mmocr/mmocr/models/textrecog/recognizers/__pycache__/svtr.cpython-38.pyc and /dev/null differ
diff --git a/pipeline/mmocr/mmocr/models/textrecog/recognizers/__pycache__/svtr.cpython-39.pyc b/pipeline/mmocr/mmocr/models/textrecog/recognizers/__pycache__/svtr.cpython-39.pyc
deleted file mode 100644
index ab2bfa0c505616d54c3f10e5390ffc242b0bd53d..0000000000000000000000000000000000000000
Binary files a/pipeline/mmocr/mmocr/models/textrecog/recognizers/__pycache__/svtr.cpython-39.pyc and /dev/null differ
diff --git a/pipeline/mmocr/mmocr/models/textrecog/recognizers/abinet.py b/pipeline/mmocr/mmocr/models/textrecog/recognizers/abinet.py
deleted file mode 100644
index f8ee3a5cafd021d6072d33b1648a9722a91bcf10..0000000000000000000000000000000000000000
--- a/pipeline/mmocr/mmocr/models/textrecog/recognizers/abinet.py
+++ /dev/null
@@ -1,12 +0,0 @@
-# Copyright (c) OpenMMLab. All rights reserved.
-from mmocr.registry import MODELS
-from .encoder_decoder_recognizer import EncoderDecoderRecognizer
-
-
-@MODELS.register_module()
-class ABINet(EncoderDecoderRecognizer):
- """Implementation of `Read Like Humans: Autonomous, Bidirectional and
- Iterative LanguageModeling for Scene Text Recognition.
-
- `_
- """
diff --git a/pipeline/mmocr/mmocr/models/textrecog/recognizers/aster.py b/pipeline/mmocr/mmocr/models/textrecog/recognizers/aster.py
deleted file mode 100644
index ce6535448af0473fefee4d4289c88df36bf16707..0000000000000000000000000000000000000000
--- a/pipeline/mmocr/mmocr/models/textrecog/recognizers/aster.py
+++ /dev/null
@@ -1,12 +0,0 @@
-# Copyright (c) OpenMMLab. All rights reserved.
-from mmocr.registry import MODELS
-from .encoder_decoder_recognizer import EncoderDecoderRecognizer
-
-
-@MODELS.register_module()
-class ASTER(EncoderDecoderRecognizer):
- """Implement `ASTER: An Attentional Scene Text Recognizer with Flexible
- Rectification.
-
- torch.Tensor:
- """Extract features from images."""
- pass
-
- def forward(self,
- inputs: torch.Tensor,
- data_samples: OptRecSampleList = None,
- mode: str = 'tensor',
- **kwargs) -> RecForwardResults:
- """The unified entry for a forward process in both training and test.
-
- The method should accept three modes: "tensor", "predict" and "loss":
-
- - "tensor": Forward the whole network and return tensor or tuple of
- tensor without any post-processing, same as a common nn.Module.
- - "predict": Forward and return the predictions, which are fully
- processed to a list of :obj:`DetDataSample`.
- - "loss": Forward and return a dict of losses according to the given
- inputs and data samples.
-
- Note that this method doesn't handle neither back propagation nor
- optimizer updating, which are done in the :meth:`train_step`.
-
- Args:
- inputs (torch.Tensor): The input tensor with shape
- (N, C, ...) in general.
- data_samples (list[:obj:`DetDataSample`], optional): The
- annotation data of every samples. Defaults to None.
- mode (str): Return what kind of value. Defaults to 'tensor'.
-
- Returns:
- The return type depends on ``mode``.
-
- - If ``mode="tensor"``, return a tensor or a tuple of tensor.
- - If ``mode="predict"``, return a list of :obj:`DetDataSample`.
- - If ``mode="loss"``, return a dict of tensor.
- """
- if mode == 'loss':
- return self.loss(inputs, data_samples, **kwargs)
- elif mode == 'predict':
- return self.predict(inputs, data_samples, **kwargs)
- elif mode == 'tensor':
- return self._forward(inputs, data_samples, **kwargs)
- else:
- raise RuntimeError(f'Invalid mode "{mode}". '
- 'Only supports loss, predict and tensor mode')
-
- @abstractmethod
- def loss(self, inputs: torch.Tensor, data_samples: RecSampleList,
- **kwargs) -> Union[dict, tuple]:
- """Calculate losses from a batch of inputs and data samples."""
- pass
-
- @abstractmethod
- def predict(self, inputs: torch.Tensor, data_samples: RecSampleList,
- **kwargs) -> RecSampleList:
- """Predict results from a batch of inputs and data samples with post-
- processing."""
- pass
-
- @abstractmethod
- def _forward(self,
- inputs: torch.Tensor,
- data_samples: OptRecSampleList = None,
- **kwargs):
- """Network forward process.
-
- Usually includes backbone, neck and head forward without any post-
- processing.
- """
- pass
diff --git a/pipeline/mmocr/mmocr/models/textrecog/recognizers/crnn.py b/pipeline/mmocr/mmocr/models/textrecog/recognizers/crnn.py
deleted file mode 100644
index 61d6853d10c6fb1909b8b8cde2421b302cd8f52a..0000000000000000000000000000000000000000
--- a/pipeline/mmocr/mmocr/models/textrecog/recognizers/crnn.py
+++ /dev/null
@@ -1,8 +0,0 @@
-# Copyright (c) OpenMMLab. All rights reserved.
-from mmocr.registry import MODELS
-from .encoder_decoder_recognizer import EncoderDecoderRecognizer
-
-
-@MODELS.register_module()
-class CRNN(EncoderDecoderRecognizer):
- """CTC-loss based recognizer."""
diff --git a/pipeline/mmocr/mmocr/models/textrecog/recognizers/encoder_decoder_recognizer.py b/pipeline/mmocr/mmocr/models/textrecog/recognizers/encoder_decoder_recognizer.py
deleted file mode 100644
index 2696ac70ef3553e867d3be5a2a62b02923d3e3d3..0000000000000000000000000000000000000000
--- a/pipeline/mmocr/mmocr/models/textrecog/recognizers/encoder_decoder_recognizer.py
+++ /dev/null
@@ -1,130 +0,0 @@
-# Copyright (c) OpenMMLab. All rights reserved.
-
-from typing import Dict
-
-import torch
-
-from mmocr.registry import MODELS
-from mmocr.utils.typing_utils import (ConfigType, InitConfigType,
- OptConfigType, OptRecSampleList,
- RecForwardResults, RecSampleList)
-from .base import BaseRecognizer
-
-
-@MODELS.register_module()
-class EncoderDecoderRecognizer(BaseRecognizer):
- """Base class for encode-decode recognizer.
-
- Args:
- preprocessor (dict, optional): Config dict for preprocessor. Defaults
- to None.
- backbone (dict, optional): Backbone config. Defaults to None.
- encoder (dict, optional): Encoder config. If None, the output from
- backbone will be directly fed into ``decoder``. Defaults to None.
- decoder (dict, optional): Decoder config. Defaults to None.
- data_preprocessor (dict, optional): Model preprocessing config
- for processing the input image data. Keys allowed are
- ``to_rgb``(bool), ``pad_size_divisor``(int), ``pad_value``(int or
- float), ``mean``(int or float) and ``std``(int or float).
- Preprcessing order: 1. to rgb; 2. normalization 3. pad.
- Defaults to None.
- init_cfg (dict or list[dict], optional): Initialization configs.
- Defaults to None.
- """
-
- def __init__(self,
- preprocessor: OptConfigType = None,
- backbone: OptConfigType = None,
- encoder: OptConfigType = None,
- decoder: OptConfigType = None,
- data_preprocessor: ConfigType = None,
- init_cfg: InitConfigType = None) -> None:
-
- super().__init__(
- init_cfg=init_cfg, data_preprocessor=data_preprocessor)
-
- # Preprocessor module, e.g., TPS
- if preprocessor is not None:
- self.preprocessor = MODELS.build(preprocessor)
-
- # Backbone
- if backbone is not None:
- self.backbone = MODELS.build(backbone)
-
- # Encoder module
- if encoder is not None:
- self.encoder = MODELS.build(encoder)
-
- # Decoder module
- assert decoder is not None
- self.decoder = MODELS.build(decoder)
-
- def extract_feat(self, inputs: torch.Tensor) -> torch.Tensor:
- """Directly extract features from the backbone."""
- if self.with_preprocessor:
- inputs = self.preprocessor(inputs)
- if self.with_backbone:
- inputs = self.backbone(inputs)
- return inputs
-
- def loss(self, inputs: torch.Tensor, data_samples: RecSampleList,
- **kwargs) -> Dict:
- """Calculate losses from a batch of inputs and data samples.
- Args:
- inputs (tensor): Input images of shape (N, C, H, W).
- Typically these should be mean centered and std scaled.
- data_samples (list[TextRecogDataSample]): A list of N
- datasamples, containing meta information and gold
- annotations for each of the images.
-
- Returns:
- dict[str, tensor]: A dictionary of loss components.
- """
- feat = self.extract_feat(inputs)
- out_enc = None
- if self.with_encoder:
- out_enc = self.encoder(feat, data_samples)
- return self.decoder.loss(feat, out_enc, data_samples)
-
- def predict(self, inputs: torch.Tensor, data_samples: RecSampleList,
- **kwargs) -> RecSampleList:
- """Predict results from a batch of inputs and data samples with post-
- processing.
-
- Args:
- inputs (torch.Tensor): Image input tensor.
- data_samples (list[TextRecogDataSample]): A list of N datasamples,
- containing meta information and gold annotations for each of
- the images.
-
- Returns:
- list[TextRecogDataSample]: A list of N datasamples of prediction
- results. Results are stored in ``pred_text``.
- """
- feat = self.extract_feat(inputs)
- out_enc = None
- if self.with_encoder:
- out_enc = self.encoder(feat, data_samples)
- return self.decoder.predict(feat, out_enc, data_samples)
-
- def _forward(self,
- inputs: torch.Tensor,
- data_samples: OptRecSampleList = None,
- **kwargs) -> RecForwardResults:
- """Network forward process. Usually includes backbone, encoder and
- decoder forward without any post-processing.
-
- Args:
- inputs (Tensor): Inputs with shape (N, C, H, W).
- data_samples (list[TextRecogDataSample]): A list of N
- datasamples, containing meta information and gold
- annotations for each of the images.
-
- Returns:
- Tensor: A tuple of features from ``decoder`` forward.
- """
- feat = self.extract_feat(inputs)
- out_enc = None
- if self.with_encoder:
- out_enc = self.encoder(feat, data_samples)
- return self.decoder(feat, out_enc, data_samples)
diff --git a/pipeline/mmocr/mmocr/models/textrecog/recognizers/encoder_decoder_recognizer_tta.py b/pipeline/mmocr/mmocr/models/textrecog/recognizers/encoder_decoder_recognizer_tta.py
deleted file mode 100644
index 6ee7aa1c464e2d9efefd8d8cd50a3d4cf4c2ed50..0000000000000000000000000000000000000000
--- a/pipeline/mmocr/mmocr/models/textrecog/recognizers/encoder_decoder_recognizer_tta.py
+++ /dev/null
@@ -1,99 +0,0 @@
-# Copyright (c) OpenMMLab. All rights reserved.
-from typing import List
-
-import numpy as np
-from mmengine.model import BaseTTAModel
-
-from mmocr.registry import MODELS
-from mmocr.utils.typing_utils import RecSampleList
-
-
-@MODELS.register_module()
-class EncoderDecoderRecognizerTTAModel(BaseTTAModel):
- """Merge augmented recognition results. It will select the best result
- according average scores from all augmented results.
-
- Examples:
- >>> tta_model = dict(
- >>> type='EncoderDecoderRecognizerTTAModel')
- >>>
- >>> tta_pipeline = [
- >>> dict(
- >>> type='LoadImageFromFile',
- >>> color_type='grayscale'),
- >>> dict(
- >>> type='TestTimeAug',
- >>> transforms=[
- >>> [
- >>> dict(
- >>> type='ConditionApply',
- >>> true_transforms=[
- >>> dict(
- >>> type='ImgAugWrapper',
- >>> args=[dict(cls='Rot90', k=0, keep_size=False)]) # noqa: E501
- >>> ],
- >>> condition="results['img_shape'][1]>> ),
- >>> dict(
- >>> type='ConditionApply',
- >>> true_transforms=[
- >>> dict(
- >>> type='ImgAugWrapper',
- >>> args=[dict(cls='Rot90', k=1, keep_size=False)]) # noqa: E501
- >>> ],
- >>> condition="results['img_shape'][1]>> ),
- >>> dict(
- >>> type='ConditionApply',
- >>> true_transforms=[
- >>> dict(
- >>> type='ImgAugWrapper',
- >>> args=[dict(cls='Rot90', k=3, keep_size=False)])
- >>> ],
- >>> condition="results['img_shape'][1]>> ),
- >>> ],
- >>> [
- >>> dict(
- >>> type='RescaleToHeight',
- >>> height=32,
- >>> min_width=32,
- >>> max_width=None,
- >>> width_divisor=16)
- >>> ],
- >>> # add loading annotation after ``Resize`` because ground truth
- >>> # does not need to do resize data transform
- >>> [dict(type='LoadOCRAnnotations', with_text=True)],
- >>> [
- >>> dict(
- >>> type='PackTextRecogInputs',
- >>> meta_keys=('img_path', 'ori_shape', 'img_shape',
- >>> 'valid_ratio'))
- >>> ]
- >>> ])
- >>> ]
- """
-
- def merge_preds(self,
- data_samples_list: List[RecSampleList]) -> RecSampleList:
- """Merge predictions of enhanced data to one prediction.
-
- Args:
- data_samples_list (List[RecSampleList]): List of predictions of
- all enhanced data. The shape of data_samples_list is (B, M),
- where B is the batch size and M is the number of augmented
- data.
-
- Returns:
- RecSampleList: Merged prediction.
- """
- predictions = list()
- for data_samples in data_samples_list:
- scores = [
- data_sample.pred_text.score for data_sample in data_samples
- ]
- average_scores = np.array(
- [sum(score) / max(1, len(score)) for score in scores])
- max_idx = np.argmax(average_scores)
- predictions.append(data_samples[max_idx])
- return predictions
diff --git a/pipeline/mmocr/mmocr/models/textrecog/recognizers/maerec.py b/pipeline/mmocr/mmocr/models/textrecog/recognizers/maerec.py
deleted file mode 100644
index 6fdbfa246ce0d850a48af435359bbc54699c8ba3..0000000000000000000000000000000000000000
--- a/pipeline/mmocr/mmocr/models/textrecog/recognizers/maerec.py
+++ /dev/null
@@ -1,8 +0,0 @@
-# Copyright (c) OpenMMLab. All rights reserved.
-from mmocr.registry import MODELS
-from .encoder_decoder_recognizer import EncoderDecoderRecognizer
-
-
-@MODELS.register_module()
-class MAERec(EncoderDecoderRecognizer):
- """Implementation of MAERec"""
diff --git a/pipeline/mmocr/mmocr/models/textrecog/recognizers/master.py b/pipeline/mmocr/mmocr/models/textrecog/recognizers/master.py
deleted file mode 100644
index dbc059caadeb379e9d9514880187b5ee06367721..0000000000000000000000000000000000000000
--- a/pipeline/mmocr/mmocr/models/textrecog/recognizers/master.py
+++ /dev/null
@@ -1,8 +0,0 @@
-# Copyright (c) OpenMMLab. All rights reserved.
-from mmocr.registry import MODELS
-from .encoder_decoder_recognizer import EncoderDecoderRecognizer
-
-
-@MODELS.register_module()
-class MASTER(EncoderDecoderRecognizer):
- """Implementation of `MASTER `_"""
diff --git a/pipeline/mmocr/mmocr/models/textrecog/recognizers/nrtr.py b/pipeline/mmocr/mmocr/models/textrecog/recognizers/nrtr.py
deleted file mode 100644
index 9c57e02c0f828674cb47abc1b32bd870e6268c62..0000000000000000000000000000000000000000
--- a/pipeline/mmocr/mmocr/models/textrecog/recognizers/nrtr.py
+++ /dev/null
@@ -1,8 +0,0 @@
-# Copyright (c) OpenMMLab. All rights reserved.
-from mmocr.registry import MODELS
-from .encoder_decoder_recognizer import EncoderDecoderRecognizer
-
-
-@MODELS.register_module()
-class NRTR(EncoderDecoderRecognizer):
- """Implementation of `NRTR `_"""
diff --git a/pipeline/mmocr/mmocr/models/textrecog/recognizers/robust_scanner.py b/pipeline/mmocr/mmocr/models/textrecog/recognizers/robust_scanner.py
deleted file mode 100644
index 987ac965046ff14a5c6d1299dda3e394c1374a5f..0000000000000000000000000000000000000000
--- a/pipeline/mmocr/mmocr/models/textrecog/recognizers/robust_scanner.py
+++ /dev/null
@@ -1,11 +0,0 @@
-# Copyright (c) OpenMMLab. All rights reserved.
-from mmocr.registry import MODELS
-from .encoder_decoder_recognizer import EncoderDecoderRecognizer
-
-
-@MODELS.register_module()
-class RobustScanner(EncoderDecoderRecognizer):
- """Implementation of `RobustScanner.
-
-
- """
diff --git a/pipeline/mmocr/mmocr/models/textrecog/recognizers/sar.py b/pipeline/mmocr/mmocr/models/textrecog/recognizers/sar.py
deleted file mode 100644
index 8ba8306232b2598416c0149c8baf786338b07ab4..0000000000000000000000000000000000000000
--- a/pipeline/mmocr/mmocr/models/textrecog/recognizers/sar.py
+++ /dev/null
@@ -1,8 +0,0 @@
-# Copyright (c) OpenMMLab. All rights reserved.
-from mmocr.registry import MODELS
-from .encoder_decoder_recognizer import EncoderDecoderRecognizer
-
-
-@MODELS.register_module()
-class SARNet(EncoderDecoderRecognizer):
- """Implementation of `SAR `_"""
diff --git a/pipeline/mmocr/mmocr/models/textrecog/recognizers/satrn.py b/pipeline/mmocr/mmocr/models/textrecog/recognizers/satrn.py
deleted file mode 100644
index 9182d8bea829b5453dc8228d842b91c6d9915a9e..0000000000000000000000000000000000000000
--- a/pipeline/mmocr/mmocr/models/textrecog/recognizers/satrn.py
+++ /dev/null
@@ -1,8 +0,0 @@
-# Copyright (c) OpenMMLab. All rights reserved.
-from mmocr.registry import MODELS
-from .encoder_decoder_recognizer import EncoderDecoderRecognizer
-
-
-@MODELS.register_module()
-class SATRN(EncoderDecoderRecognizer):
- """Implementation of `SATRN `_"""
diff --git a/pipeline/mmocr/mmocr/models/textrecog/recognizers/svtr.py b/pipeline/mmocr/mmocr/models/textrecog/recognizers/svtr.py
deleted file mode 100644
index 6fc42b85d0beea3062e06f16ee3265c0763d32c6..0000000000000000000000000000000000000000
--- a/pipeline/mmocr/mmocr/models/textrecog/recognizers/svtr.py
+++ /dev/null
@@ -1,9 +0,0 @@
-# Copyright (c) OpenMMLab. All rights reserved.
-from mmocr.registry import MODELS
-from .encoder_decoder_recognizer import EncoderDecoderRecognizer
-
-
-@MODELS.register_module()
-class SVTR(EncoderDecoderRecognizer):
- """A PyTorch implementation of : `SVTR: Scene Text Recognition with a
- Single Visual Model `_"""
diff --git a/pipeline/mmocr/mmocr/registry.py b/pipeline/mmocr/mmocr/registry.py
deleted file mode 100644
index d1ed33552881316ab4dc151650243456e06e1c4f..0000000000000000000000000000000000000000
--- a/pipeline/mmocr/mmocr/registry.py
+++ /dev/null
@@ -1,157 +0,0 @@
-# Copyright (c) OpenMMLab. All rights reserved.
-"""MMOCR provides 20 registry nodes to support using modules across projects.
-Each node is a child of the root registry in MMEngine.
-
-More details can be found at
-https://mmengine.readthedocs.io/en/latest/tutorials/registry.html.
-"""
-
-from mmengine.registry import DATA_SAMPLERS as MMENGINE_DATA_SAMPLERS
-from mmengine.registry import DATASETS as MMENGINE_DATASETS
-from mmengine.registry import EVALUATOR as MMENGINE_EVALUATOR
-from mmengine.registry import HOOKS as MMENGINE_HOOKS
-from mmengine.registry import LOG_PROCESSORS as MMENGINE_LOG_PROCESSORS
-from mmengine.registry import LOOPS as MMENGINE_LOOPS
-from mmengine.registry import METRICS as MMENGINE_METRICS
-from mmengine.registry import MODEL_WRAPPERS as MMENGINE_MODEL_WRAPPERS
-from mmengine.registry import MODELS as MMENGINE_MODELS
-from mmengine.registry import \
- OPTIM_WRAPPER_CONSTRUCTORS as MMENGINE_OPTIM_WRAPPER_CONSTRUCTORS
-from mmengine.registry import OPTIM_WRAPPERS as MMENGINE_OPTIM_WRAPPERS
-from mmengine.registry import OPTIMIZERS as MMENGINE_OPTIMIZERS
-from mmengine.registry import PARAM_SCHEDULERS as MMENGINE_PARAM_SCHEDULERS
-from mmengine.registry import \
- RUNNER_CONSTRUCTORS as MMENGINE_RUNNER_CONSTRUCTORS
-from mmengine.registry import RUNNERS as MMENGINE_RUNNERS
-from mmengine.registry import TASK_UTILS as MMENGINE_TASK_UTILS
-from mmengine.registry import TRANSFORMS as MMENGINE_TRANSFORMS
-from mmengine.registry import VISBACKENDS as MMENGINE_VISBACKENDS
-from mmengine.registry import VISUALIZERS as MMENGINE_VISUALIZERS
-from mmengine.registry import \
- WEIGHT_INITIALIZERS as MMENGINE_WEIGHT_INITIALIZERS
-from mmengine.registry import Registry
-
-# manage all kinds of runners like `EpochBasedRunner` and `IterBasedRunner`
-RUNNERS = Registry(
- 'runner',
- parent=MMENGINE_RUNNERS,
- # TODO: update the location when mmocr has its own runner
- locations=['mmocr.engine'])
-# manage runner constructors that define how to initialize runners
-RUNNER_CONSTRUCTORS = Registry(
- 'runner constructor',
- parent=MMENGINE_RUNNER_CONSTRUCTORS,
- # TODO: update the location when mmocr has its own runner constructor
- locations=['mmocr.engine'])
-# manage all kinds of loops like `EpochBasedTrainLoop`
-LOOPS = Registry(
- 'loop',
- parent=MMENGINE_LOOPS,
- # TODO: update the location when mmocr has its own loop
- locations=['mmocr.engine'])
-# manage all kinds of hooks like `CheckpointHook`
-HOOKS = Registry(
- 'hook', parent=MMENGINE_HOOKS, locations=['mmocr.engine.hooks'])
-
-# manage data-related modules
-DATASETS = Registry(
- 'dataset', parent=MMENGINE_DATASETS, locations=['mmocr.datasets'])
-DATA_SAMPLERS = Registry(
- 'data sampler',
- parent=MMENGINE_DATA_SAMPLERS,
- locations=['mmocr.datasets.samplers'])
-TRANSFORMS = Registry(
- 'transform',
- parent=MMENGINE_TRANSFORMS,
- locations=['mmocr.datasets.transforms'])
-
-# manage all kinds of modules inheriting `nn.Module`
-MODELS = Registry('model', parent=MMENGINE_MODELS, locations=['mmocr.models'])
-# manage all kinds of model wrappers like 'MMDistributedDataParallel'
-MODEL_WRAPPERS = Registry(
- 'model wrapper',
- parent=MMENGINE_MODEL_WRAPPERS,
- locations=['mmocr.models'])
-# manage all kinds of weight initialization modules like `Uniform`
-WEIGHT_INITIALIZERS = Registry(
- 'weight initializer',
- parent=MMENGINE_WEIGHT_INITIALIZERS,
- locations=['mmocr.models'])
-
-# manage all kinds of optimizers like `SGD` and `Adam`
-OPTIMIZERS = Registry(
- 'optimizer',
- parent=MMENGINE_OPTIMIZERS,
- # TODO: update the location when mmocr has its own optimizer
- locations=['mmocr.engine'])
-# manage optimizer wrapper
-OPTIM_WRAPPERS = Registry(
- 'optimizer wrapper',
- parent=MMENGINE_OPTIM_WRAPPERS,
- # TODO: update the location when mmocr has its own optimizer wrapper
- locations=['mmocr.engine'])
-# manage constructors that customize the optimization hyperparameters.
-OPTIM_WRAPPER_CONSTRUCTORS = Registry(
- 'optimizer constructor',
- parent=MMENGINE_OPTIM_WRAPPER_CONSTRUCTORS,
- # TODO: update the location when mmocr has its own optimizer constructor
- locations=['mmocr.engine'])
-# manage all kinds of parameter schedulers like `MultiStepLR`
-PARAM_SCHEDULERS = Registry(
- 'parameter scheduler',
- parent=MMENGINE_PARAM_SCHEDULERS,
- # TODO: update the location when mmocr has its own parameter scheduler
- locations=['mmocr.engine'])
-# manage all kinds of metrics
-METRICS = Registry(
- 'metric', parent=MMENGINE_METRICS, locations=['mmocr.evaluation.metrics'])
-# manage evaluator
-EVALUATOR = Registry(
- 'evaluator',
- parent=MMENGINE_EVALUATOR,
- locations=['mmocr.evaluation.evaluator'])
-
-# manage task-specific modules like anchor generators and box coders
-TASK_UTILS = Registry(
- 'task util', parent=MMENGINE_TASK_UTILS, locations=['mmocr.models'])
-
-# manage visualizer
-VISUALIZERS = Registry(
- 'visualizer',
- parent=MMENGINE_VISUALIZERS,
- locations=['mmocr.visualization'])
-# manage visualizer backend
-VISBACKENDS = Registry(
- 'visualizer backend',
- parent=MMENGINE_VISBACKENDS,
- locations=['mmocr.visualization'])
-
-# manage logprocessor
-LOG_PROCESSORS = Registry(
- 'logger processor',
- parent=MMENGINE_LOG_PROCESSORS,
- # TODO: update the location when mmocr has its own log processor
- locations=['mmocr.engine'])
-# manage data obtainer
-DATA_OBTAINERS = Registry(
- 'data obtainer', locations=['mmocr.datasets.preparers.obtainers'])
-
-# manage data gatherer
-DATA_GATHERERS = Registry(
- 'data gatherer', locations=['mmocr.datasets.preparers.gatherers'])
-
-# manage data parser
-DATA_PARSERS = Registry(
- 'data parser', locations=['mmocr.datasets.preparers.parsers'])
-
-# manage data packer
-DATA_PACKERS = Registry(
- 'data packer', locations=['mmocr.datasets.preparers.packers'])
-
-# manage data dumper
-DATA_DUMPERS = Registry(
- 'data dumper', locations=['mmocr.datasets.preparers.dumpers'])
-
-# manage dataset config generator
-CFG_GENERATORS = Registry(
- 'cfg generator', locations=['mmocr.datasets.preparers.config_generators'])
diff --git a/pipeline/mmocr/mmocr/structures/__init__.py b/pipeline/mmocr/mmocr/structures/__init__.py
deleted file mode 100644
index 2b71ac262a07022d63faee8766a555933793da5e..0000000000000000000000000000000000000000
--- a/pipeline/mmocr/mmocr/structures/__init__.py
+++ /dev/null
@@ -1,10 +0,0 @@
-# Copyright (c) OpenMMLab. All rights reserved.
-from .kie_data_sample import KIEDataSample
-from .textdet_data_sample import TextDetDataSample
-from .textrecog_data_sample import TextRecogDataSample
-from .textspotting_data_sample import TextSpottingDataSample
-
-__all__ = [
- 'TextDetDataSample', 'TextRecogDataSample', 'KIEDataSample',
- 'TextSpottingDataSample'
-]
diff --git a/pipeline/mmocr/mmocr/structures/__pycache__/__init__.cpython-38.pyc b/pipeline/mmocr/mmocr/structures/__pycache__/__init__.cpython-38.pyc
deleted file mode 100644
index 984f296899e5fe8a5b19b5051805b7081586aefc..0000000000000000000000000000000000000000
Binary files a/pipeline/mmocr/mmocr/structures/__pycache__/__init__.cpython-38.pyc and /dev/null differ
diff --git a/pipeline/mmocr/mmocr/structures/__pycache__/__init__.cpython-39.pyc b/pipeline/mmocr/mmocr/structures/__pycache__/__init__.cpython-39.pyc
deleted file mode 100644
index 20ec12f00af4cec5ffc3083d9a6ef14ac5ba0b56..0000000000000000000000000000000000000000
Binary files a/pipeline/mmocr/mmocr/structures/__pycache__/__init__.cpython-39.pyc and /dev/null differ
diff --git a/pipeline/mmocr/mmocr/structures/__pycache__/kie_data_sample.cpython-38.pyc b/pipeline/mmocr/mmocr/structures/__pycache__/kie_data_sample.cpython-38.pyc
deleted file mode 100644
index fec393b494b92dcb72c6f18b1f8c829c7ba1d4a7..0000000000000000000000000000000000000000
Binary files a/pipeline/mmocr/mmocr/structures/__pycache__/kie_data_sample.cpython-38.pyc and /dev/null differ
diff --git a/pipeline/mmocr/mmocr/structures/__pycache__/kie_data_sample.cpython-39.pyc b/pipeline/mmocr/mmocr/structures/__pycache__/kie_data_sample.cpython-39.pyc
deleted file mode 100644
index 9196994959c175fe23dddac3cbc8055fb491746f..0000000000000000000000000000000000000000
Binary files a/pipeline/mmocr/mmocr/structures/__pycache__/kie_data_sample.cpython-39.pyc and /dev/null differ
diff --git a/pipeline/mmocr/mmocr/structures/__pycache__/textdet_data_sample.cpython-38.pyc b/pipeline/mmocr/mmocr/structures/__pycache__/textdet_data_sample.cpython-38.pyc
deleted file mode 100644
index 44fba3d72a422fe7d6f3204f318203286cbf841f..0000000000000000000000000000000000000000
Binary files a/pipeline/mmocr/mmocr/structures/__pycache__/textdet_data_sample.cpython-38.pyc and /dev/null differ
diff --git a/pipeline/mmocr/mmocr/structures/__pycache__/textdet_data_sample.cpython-39.pyc b/pipeline/mmocr/mmocr/structures/__pycache__/textdet_data_sample.cpython-39.pyc
deleted file mode 100644
index 8776b53b9e99e54718e6a8ea3037e0a011585d92..0000000000000000000000000000000000000000
Binary files a/pipeline/mmocr/mmocr/structures/__pycache__/textdet_data_sample.cpython-39.pyc and /dev/null differ
diff --git a/pipeline/mmocr/mmocr/structures/__pycache__/textrecog_data_sample.cpython-38.pyc b/pipeline/mmocr/mmocr/structures/__pycache__/textrecog_data_sample.cpython-38.pyc
deleted file mode 100644
index 18d38f3eb134d7134bb132855ec1093cc18fd795..0000000000000000000000000000000000000000
Binary files a/pipeline/mmocr/mmocr/structures/__pycache__/textrecog_data_sample.cpython-38.pyc and /dev/null differ
diff --git a/pipeline/mmocr/mmocr/structures/__pycache__/textrecog_data_sample.cpython-39.pyc b/pipeline/mmocr/mmocr/structures/__pycache__/textrecog_data_sample.cpython-39.pyc
deleted file mode 100644
index 3776dfbd27725c0a6cb5b595705eefa5a54dc4c8..0000000000000000000000000000000000000000
Binary files a/pipeline/mmocr/mmocr/structures/__pycache__/textrecog_data_sample.cpython-39.pyc and /dev/null differ
diff --git a/pipeline/mmocr/mmocr/structures/__pycache__/textspotting_data_sample.cpython-38.pyc b/pipeline/mmocr/mmocr/structures/__pycache__/textspotting_data_sample.cpython-38.pyc
deleted file mode 100644
index f060863c4e55c1ec1134cf7f094df0adfdd5712f..0000000000000000000000000000000000000000
Binary files a/pipeline/mmocr/mmocr/structures/__pycache__/textspotting_data_sample.cpython-38.pyc and /dev/null differ
diff --git a/pipeline/mmocr/mmocr/structures/__pycache__/textspotting_data_sample.cpython-39.pyc b/pipeline/mmocr/mmocr/structures/__pycache__/textspotting_data_sample.cpython-39.pyc
deleted file mode 100644
index 3750136a683ddd5fa1d63056128382b6b75c0bd0..0000000000000000000000000000000000000000
Binary files a/pipeline/mmocr/mmocr/structures/__pycache__/textspotting_data_sample.cpython-39.pyc and /dev/null differ
diff --git a/pipeline/mmocr/mmocr/structures/kie_data_sample.py b/pipeline/mmocr/mmocr/structures/kie_data_sample.py
deleted file mode 100644
index c681e5b2fd30a6f8cc52db90a4d3fe70df28fe1a..0000000000000000000000000000000000000000
--- a/pipeline/mmocr/mmocr/structures/kie_data_sample.py
+++ /dev/null
@@ -1,91 +0,0 @@
-# Copyright (c) OpenMMLab. All rights reserved.
-from mmengine.structures import BaseDataElement, InstanceData
-
-
-class KIEDataSample(BaseDataElement):
- """A data structure interface of MMOCR. They are used as interfaces between
- different components.
-
- The attributes in ``KIEDataSample`` are divided into two parts:
-
- - ``gt_instances``(InstanceData): Ground truth of instance annotations.
- - ``pred_instances``(InstanceData): Instances of model predictions.
-
- Examples:
- >>> import torch
- >>> import numpy as np
- >>> from mmengine.structures import InstanceData
- >>> from mmocr.data import KIEDataSample
- >>> # gt_instances
- >>> data_sample = KIEDataSample()
- >>> img_meta = dict(img_shape=(800, 1196, 3),
- ... pad_shape=(800, 1216, 3))
- >>> gt_instances = InstanceData(metainfo=img_meta)
- >>> gt_instances.bboxes = torch.rand((5, 4))
- >>> gt_instances.labels = torch.rand((5,))
- >>> data_sample.gt_instances = gt_instances
- >>> assert 'img_shape' in data_sample.gt_instances.metainfo_keys()
- >>> len(data_sample.gt_instances)
- 5
- >>> print(data_sample)
-
- ) at 0x7f21fb1b9880>
- >>> # pred_instances
- >>> pred_instances = InstanceData(metainfo=img_meta)
- >>> pred_instances.bboxes = torch.rand((5, 4))
- >>> pred_instances.scores = torch.rand((5,))
- >>> data_sample = KIEDataSample(pred_instances=pred_instances)
- >>> assert 'pred_instances' in data_sample
- >>> data_sample = KIEDataSample()
- >>> gt_instances_data = dict(
- ... bboxes=torch.rand(2, 4),
- ... labels=torch.rand(2))
- >>> gt_instances = InstanceData(**gt_instances_data)
- >>> data_sample.gt_instances = gt_instances
- >>> assert 'gt_instances' in data_sample
- """
-
- @property
- def gt_instances(self) -> InstanceData:
- """InstanceData: groundtruth instances."""
- return self._gt_instances
-
- @gt_instances.setter
- def gt_instances(self, value: InstanceData):
- """gt_instances setter."""
- self.set_field(value, '_gt_instances', dtype=InstanceData)
-
- @gt_instances.deleter
- def gt_instances(self):
- """gt_instances deleter."""
- del self._gt_instances
-
- @property
- def pred_instances(self) -> InstanceData:
- """InstanceData: prediction instances."""
- return self._pred_instances
-
- @pred_instances.setter
- def pred_instances(self, value: InstanceData):
- """pred_instances setter."""
- self.set_field(value, '_pred_instances', dtype=InstanceData)
-
- @pred_instances.deleter
- def pred_instances(self):
- """pred_instances deleter."""
- del self._pred_instances
diff --git a/pipeline/mmocr/mmocr/structures/textdet_data_sample.py b/pipeline/mmocr/mmocr/structures/textdet_data_sample.py
deleted file mode 100644
index 465967064b7b4038423b56cf7be49497663e7feb..0000000000000000000000000000000000000000
--- a/pipeline/mmocr/mmocr/structures/textdet_data_sample.py
+++ /dev/null
@@ -1,93 +0,0 @@
-# Copyright (c) OpenMMLab. All rights reserved.
-from mmengine.structures import BaseDataElement, InstanceData
-
-
-class TextDetDataSample(BaseDataElement):
- """A data structure interface of MMOCR. They are used as interfaces between
- different components.
-
- The attributes in ``TextDetDataSample`` are divided into two parts:
-
- - ``gt_instances``(InstanceData): Ground truth of instance annotations.
- - ``pred_instances``(InstanceData): Instances of model predictions.
-
- Examples:
- >>> import torch
- >>> import numpy as np
- >>> from mmengine.structures import InstanceData
- >>> from mmocr.data import TextDetDataSample
- >>> # gt_instances
- >>> data_sample = TextDetDataSample()
- >>> img_meta = dict(img_shape=(800, 1196, 3),
- ... pad_shape=(800, 1216, 3))
- >>> gt_instances = InstanceData(metainfo=img_meta)
- >>> gt_instances.bboxes = torch.rand((5, 4))
- >>> gt_instances.labels = torch.rand((5,))
- >>> data_sample.gt_instances = gt_instances
- >>> assert 'img_shape' in data_sample.gt_instances.metainfo_keys()
- >>> len(data_sample.gt_instances)
- 5
- >>> print(data_sample)
-
- ) at 0x7f21fb1b9880>
- >>> # pred_instances
- >>> pred_instances = InstanceData(metainfo=img_meta)
- >>> pred_instances.bboxes = torch.rand((5, 4))
- >>> pred_instances.scores = torch.rand((5,))
- >>> data_sample = TextDetDataSample(pred_instances=pred_instances)
- >>> assert 'pred_instances' in data_sample
- >>> data_sample = TextDetDataSample()
- >>> gt_instances_data = dict(
- ... bboxes=torch.rand(2, 4),
- ... labels=torch.rand(2),
- ... masks=np.random.rand(2, 2, 2))
- >>> gt_instances = InstanceData(**gt_instances_data)
- >>> data_sample.gt_instances = gt_instances
- >>> assert 'gt_instances' in data_sample
- >>> assert 'masks' in data_sample.gt_instances
- """
-
- @property
- def gt_instances(self) -> InstanceData:
- """InstanceData: groundtruth instances."""
- return self._gt_instances
-
- @gt_instances.setter
- def gt_instances(self, value: InstanceData):
- """gt_instances setter."""
- self.set_field(value, '_gt_instances', dtype=InstanceData)
-
- @gt_instances.deleter
- def gt_instances(self):
- """gt_instances deleter."""
- del self._gt_instances
-
- @property
- def pred_instances(self) -> InstanceData:
- """InstanceData: prediction instances."""
- return self._pred_instances
-
- @pred_instances.setter
- def pred_instances(self, value: InstanceData):
- """pred_instances setter."""
- self.set_field(value, '_pred_instances', dtype=InstanceData)
-
- @pred_instances.deleter
- def pred_instances(self):
- """pred_instances deleter."""
- del self._pred_instances
diff --git a/pipeline/mmocr/mmocr/structures/textrecog_data_sample.py b/pipeline/mmocr/mmocr/structures/textrecog_data_sample.py
deleted file mode 100644
index f40572b0282dd82d1bc67734dcfe52c0073fe5d4..0000000000000000000000000000000000000000
--- a/pipeline/mmocr/mmocr/structures/textrecog_data_sample.py
+++ /dev/null
@@ -1,82 +0,0 @@
-# Copyright (c) OpenMMLab. All rights reserved.
-from mmengine.structures import BaseDataElement, LabelData
-
-
-class TextRecogDataSample(BaseDataElement):
- """A data structure interface of MMOCR for text recognition. They are used
- as interfaces between different components.
-
- The attributes in ``TextRecogDataSample`` are divided into two parts:
-
- - ``gt_text``(LabelData): Ground truth text.
- - ``pred_text``(LabelData): predictions text.
-
- Examples:
- >>> import torch
- >>> import numpy as np
- >>> from mmengine.structures import LabelData
- >>> from mmocr.data import TextRecogDataSample
- >>> # gt_text
- >>> data_sample = TextRecogDataSample()
- >>> img_meta = dict(img_shape=(800, 1196, 3),
- ... pad_shape=(800, 1216, 3))
- >>> gt_text = LabelData(metainfo=img_meta)
- >>> gt_text.item = 'mmocr'
- >>> data_sample.gt_text = gt_text
- >>> assert 'img_shape' in data_sample.gt_text.metainfo_keys()
- >>> print(data_sample)
-
- ) at 0x7f21fb1b9880>
- >>> # pred_text
- >>> pred_text = LabelData(metainfo=img_meta)
- >>> pred_text.item = 'mmocr'
- >>> data_sample = TextRecogDataSample(pred_text=pred_text)
- >>> assert 'pred_text' in data_sample
- >>> data_sample = TextRecogDataSample()
- >>> gt_text_data = dict(item='mmocr')
- >>> gt_text = LabelData(**gt_text_data)
- >>> data_sample.gt_text = gt_text
- >>> assert 'gt_text' in data_sample
- >>> assert 'item' in data_sample.gt_text
- """
-
- @property
- def gt_text(self) -> LabelData:
- """LabelData: ground truth text.
- """
- return self._gt_text
-
- @gt_text.setter
- def gt_text(self, value: LabelData) -> None:
- """gt_text setter."""
- self.set_field(value, '_gt_text', dtype=LabelData)
-
- @gt_text.deleter
- def gt_text(self) -> None:
- """gt_text deleter."""
- del self._gt_text
-
- @property
- def pred_text(self) -> LabelData:
- """LabelData: prediction text.
- """
- return self._pred_text
-
- @pred_text.setter
- def pred_text(self, value: LabelData) -> None:
- """pred_text setter."""
- self.set_field(value, '_pred_text', dtype=LabelData)
-
- @pred_text.deleter
- def pred_text(self) -> None:
- """pred_text deleter."""
- del self._pred_text
diff --git a/pipeline/mmocr/mmocr/structures/textspotting_data_sample.py b/pipeline/mmocr/mmocr/structures/textspotting_data_sample.py
deleted file mode 100644
index 28478f516f96651d2e49c180cea4a97336fc5c97..0000000000000000000000000000000000000000
--- a/pipeline/mmocr/mmocr/structures/textspotting_data_sample.py
+++ /dev/null
@@ -1,64 +0,0 @@
-# Copyright (c) OpenMMLab. All rights reserved.
-from mmocr.structures import TextDetDataSample
-
-
-class TextSpottingDataSample(TextDetDataSample):
- """A data structure interface of MMOCR. They are used as interfaces between
- different components.
-
- The attributes in ``TextSpottingDataSample`` are divided into two parts:
-
- - ``gt_instances``(InstanceData): Ground truth of instance annotations.
- - ``pred_instances``(InstanceData): Instances of model predictions.
-
- Examples:
- >>> import torch
- >>> import numpy as np
- >>> from mmengine.structures import InstanceData
- >>> from mmocr.data import TextSpottingDataSample
- >>> # gt_instances
- >>> data_sample = TextSpottingDataSample()
- >>> img_meta = dict(img_shape=(800, 1196, 3),
- ... pad_shape=(800, 1216, 3))
- >>> gt_instances = InstanceData(metainfo=img_meta)
- >>> gt_instances.bboxes = torch.rand((5, 4))
- >>> gt_instances.labels = torch.rand((5,))
- >>> data_sample.gt_instances = gt_instances
- >>> assert 'img_shape' in data_sample.gt_instances.metainfo_keys()
- >>> len(data_sample.gt_instances)
- 5
- >>> print(data_sample)
-
- ) at 0x7f21fb1b9880>
- >>> # pred_instances
- >>> pred_instances = InstanceData(metainfo=img_meta)
- >>> pred_instances.bboxes = torch.rand((5, 4))
- >>> pred_instances.scores = torch.rand((5,))
- >>> data_sample = TextSpottingDataSample(
- ... pred_instances=pred_instances)
- >>> assert 'pred_instances' in data_sample
- >>> data_sample = TextSpottingDataSample()
- >>> gt_instances_data = dict(
- ... bboxes=torch.rand(2, 4),
- ... labels=torch.rand(2),
- ... masks=np.random.rand(2, 2, 2))
- >>> gt_instances = InstanceData(**gt_instances_data)
- >>> data_sample.gt_instances = gt_instances
- >>> assert 'gt_instances' in data_sample
- >>> assert 'masks' in data_sample.gt_instances
- """
diff --git a/pipeline/mmocr/mmocr/testing/__init__.py b/pipeline/mmocr/mmocr/testing/__init__.py
deleted file mode 100644
index 3000419b8fd971c4b05d87893e4d23df7459caf8..0000000000000000000000000000000000000000
--- a/pipeline/mmocr/mmocr/testing/__init__.py
+++ /dev/null
@@ -1,4 +0,0 @@
-# Copyright (c) OpenMMLab. All rights reserved.
-from .data import create_dummy_dict_file, create_dummy_textdet_inputs
-
-__all__ = ['create_dummy_dict_file', 'create_dummy_textdet_inputs']
diff --git a/pipeline/mmocr/mmocr/testing/data.py b/pipeline/mmocr/mmocr/testing/data.py
deleted file mode 100644
index fc0b4d2cddcda3e9200855853e58a8d2213c4194..0000000000000000000000000000000000000000
--- a/pipeline/mmocr/mmocr/testing/data.py
+++ /dev/null
@@ -1,110 +0,0 @@
-# Copyright (c) OpenMMLab. All rights reserved.
-from typing import Any, Dict, List, Optional, Sequence
-
-import numpy as np
-import torch
-from mmengine.structures import InstanceData
-
-from mmocr.structures import TextDetDataSample
-
-
-def create_dummy_textdet_inputs(input_shape: Sequence[int] = (1, 3, 300, 300),
- num_items: Optional[Sequence[int]] = None
- ) -> Dict[str, Any]:
- """Create dummy inputs to test text detectors.
-
- Args:
- input_shape (tuple(int)): 4-d shape of the input image. Defaults to
- (1, 3, 300, 300).
- num_items (list[int], optional): Number of bboxes to create for each
- image. If None, they will be randomly generated. Defaults to None.
-
- Returns:
- Dict[str, Any]: A dictionary of demo inputs.
- """
- (N, C, H, W) = input_shape
-
- rng = np.random.RandomState(0)
-
- imgs = rng.rand(*input_shape)
-
- metainfo = dict(
- img_shape=(H, W, C),
- ori_shape=(H, W, C),
- pad_shape=(H, W, C),
- filename='test.jpg',
- scale_factor=(1, 1),
- flip=False)
-
- gt_masks = []
- gt_kernels = []
- gt_effective_mask = []
-
- data_samples = []
-
- for batch_idx in range(N):
- if num_items is None:
- num_boxes = rng.randint(1, 10)
- else:
- num_boxes = num_items[batch_idx]
-
- data_sample = TextDetDataSample(
- metainfo=metainfo, gt_instances=InstanceData())
-
- cx, cy, bw, bh = rng.rand(num_boxes, 4).T
-
- tl_x = ((cx * W) - (W * bw / 2)).clip(0, W)
- tl_y = ((cy * H) - (H * bh / 2)).clip(0, H)
- br_x = ((cx * W) + (W * bw / 2)).clip(0, W)
- br_y = ((cy * H) + (H * bh / 2)).clip(0, H)
-
- boxes = np.vstack([tl_x, tl_y, br_x, br_y]).T
- class_idxs = [0] * num_boxes
-
- data_sample.gt_instances.bboxes = torch.FloatTensor(boxes)
- data_sample.gt_instances.labels = torch.LongTensor(class_idxs)
- data_sample.gt_instances.ignored = torch.BoolTensor([False] *
- num_boxes)
- data_samples.append(data_sample)
-
- # kernels = []
- # TODO: add support for multiple kernels (if necessary)
- # for _ in range(num_kernels):
- # kernel = np.random.rand(H, W)
- # kernels.append(kernel)
- gt_kernels.append(np.random.rand(H, W))
- gt_effective_mask.append(np.ones((H, W)))
-
- mask = np.random.randint(0, 2, (len(boxes), H, W), dtype=np.uint8)
- gt_masks.append(mask)
-
- mm_inputs = {
- 'imgs': torch.FloatTensor(imgs).requires_grad_(True),
- 'data_samples': data_samples,
- 'gt_masks': gt_masks,
- 'gt_kernels': gt_kernels,
- 'gt_mask': gt_effective_mask,
- 'gt_thr_mask': gt_effective_mask,
- 'gt_text_mask': gt_effective_mask,
- 'gt_center_region_mask': gt_effective_mask,
- 'gt_radius_map': gt_kernels,
- 'gt_sin_map': gt_kernels,
- 'gt_cos_map': gt_kernels,
- }
- return mm_inputs
-
-
-def create_dummy_dict_file(
- dict_file: str,
- chars: List[str] = list('0123456789abcdefghijklmnopqrstuvwxyz')
-) -> None: # NOQA
- """Create a dummy dictionary file.
-
- Args:
- dict_file (str): Path to the dummy dictionary file.
- chars (list[str]): List of characters in dictionary. Defaults to
- ``list('0123456789abcdefghijklmnopqrstuvwxyz')``.
- """
- with open(dict_file, 'w') as f:
- for char in chars:
- f.write(char + '\n')
diff --git a/pipeline/mmocr/mmocr/utils/__init__.py b/pipeline/mmocr/mmocr/utils/__init__.py
deleted file mode 100644
index 3e4fb6fb22fec6e35eb563547ff03b50354f4f2f..0000000000000000000000000000000000000000
--- a/pipeline/mmocr/mmocr/utils/__init__.py
+++ /dev/null
@@ -1,54 +0,0 @@
-# Copyright (c) OpenMMLab. All rights reserved.
-from .bbox_utils import (bbox2poly, bbox_center_distance, bbox_diag_distance,
- bezier2polygon, is_on_same_line, rescale_bbox,
- rescale_bboxes, stitch_boxes_into_lines)
-from .bezier_utils import bezier2poly, poly2bezier
-from .check_argument import (equal_len, is_2dlist, is_3dlist, is_none_or_type,
- is_type_list, valid_boundary)
-from .collect_env import collect_env
-from .data_converter_utils import dump_ocr_data, recog_anno_to_imginfo
-from .fileio import (check_integrity, get_md5, is_archive, list_files,
- list_from_file, list_to_file)
-from .img_utils import crop_img, warp_img
-from .mask_utils import fill_hole
-from .parsers import LineJsonParser, LineStrParser
-from .point_utils import point_distance, points_center
-from .polygon_utils import (boundary_iou, crop_polygon, is_poly_inside_rect,
- offset_polygon, poly2bbox, poly2shapely,
- poly_intersection, poly_iou, poly_make_valid,
- poly_union, polys2shapely, rescale_polygon,
- rescale_polygons, shapely2poly, sort_points,
- sort_vertex, sort_vertex8)
-from .processing import track_parallel_progress_multi_args
-from .setup_env import register_all_modules
-from .string_utils import StringStripper
-from .transform_utils import remove_pipeline_elements
-from .typing_utils import (ColorType, ConfigType, DetSampleList,
- InitConfigType, InstanceList, KIESampleList,
- LabelList, MultiConfig, OptConfigType,
- OptDetSampleList, OptInitConfigType,
- OptInstanceList, OptKIESampleList, OptLabelList,
- OptMultiConfig, OptRecSampleList, OptTensor,
- RangeType, RecForwardResults, RecSampleList)
-
-__all__ = [
- 'collect_env', 'is_3dlist', 'is_type_list', 'is_none_or_type', 'equal_len',
- 'is_2dlist', 'valid_boundary', 'list_to_file', 'list_from_file',
- 'is_on_same_line', 'stitch_boxes_into_lines', 'StringStripper',
- 'bezier2polygon', 'sort_points', 'dump_ocr_data', 'recog_anno_to_imginfo',
- 'rescale_polygons', 'rescale_polygon', 'rescale_bbox', 'rescale_bboxes',
- 'bbox2poly', 'crop_polygon', 'is_poly_inside_rect', 'poly2bbox',
- 'poly_intersection', 'poly_iou', 'poly_make_valid', 'poly_union',
- 'poly2shapely', 'polys2shapely', 'register_all_modules', 'offset_polygon',
- 'sort_vertex8', 'sort_vertex', 'bbox_center_distance',
- 'bbox_diag_distance', 'boundary_iou', 'point_distance', 'points_center',
- 'fill_hole', 'LineJsonParser', 'LineStrParser', 'shapely2poly', 'crop_img',
- 'warp_img', 'ConfigType', 'DetSampleList', 'RecForwardResults',
- 'InitConfigType', 'OptConfigType', 'OptDetSampleList', 'OptInitConfigType',
- 'OptMultiConfig', 'OptRecSampleList', 'RecSampleList', 'MultiConfig',
- 'OptTensor', 'ColorType', 'OptKIESampleList', 'KIESampleList',
- 'is_archive', 'check_integrity', 'list_files', 'get_md5', 'InstanceList',
- 'LabelList', 'OptInstanceList', 'OptLabelList', 'RangeType',
- 'remove_pipeline_elements', 'bezier2poly', 'poly2bezier',
- 'track_parallel_progress_multi_args'
-]
diff --git a/pipeline/mmocr/mmocr/utils/__pycache__/__init__.cpython-38.pyc b/pipeline/mmocr/mmocr/utils/__pycache__/__init__.cpython-38.pyc
deleted file mode 100644
index a70364bdc544b2f7c8e8571fb0e6d29d85445428..0000000000000000000000000000000000000000
Binary files a/pipeline/mmocr/mmocr/utils/__pycache__/__init__.cpython-38.pyc and /dev/null differ
diff --git a/pipeline/mmocr/mmocr/utils/__pycache__/__init__.cpython-39.pyc b/pipeline/mmocr/mmocr/utils/__pycache__/__init__.cpython-39.pyc
deleted file mode 100644
index 3c548d71c8c5afcf4693702920205d772a66a232..0000000000000000000000000000000000000000
Binary files a/pipeline/mmocr/mmocr/utils/__pycache__/__init__.cpython-39.pyc and /dev/null differ
diff --git a/pipeline/mmocr/mmocr/utils/__pycache__/bbox_utils.cpython-38.pyc b/pipeline/mmocr/mmocr/utils/__pycache__/bbox_utils.cpython-38.pyc
deleted file mode 100644
index 4b65c4b4dec32711515df13eb7f1e79745494a33..0000000000000000000000000000000000000000
Binary files a/pipeline/mmocr/mmocr/utils/__pycache__/bbox_utils.cpython-38.pyc and /dev/null differ
diff --git a/pipeline/mmocr/mmocr/utils/__pycache__/bbox_utils.cpython-39.pyc b/pipeline/mmocr/mmocr/utils/__pycache__/bbox_utils.cpython-39.pyc
deleted file mode 100644
index c56de68e92ea24e1ebf64f7ea3ad13ce54fd7d46..0000000000000000000000000000000000000000
Binary files a/pipeline/mmocr/mmocr/utils/__pycache__/bbox_utils.cpython-39.pyc and /dev/null differ
diff --git a/pipeline/mmocr/mmocr/utils/__pycache__/bezier_utils.cpython-38.pyc b/pipeline/mmocr/mmocr/utils/__pycache__/bezier_utils.cpython-38.pyc
deleted file mode 100644
index e2360cf6effc092bee114c3c814f315253c01819..0000000000000000000000000000000000000000
Binary files a/pipeline/mmocr/mmocr/utils/__pycache__/bezier_utils.cpython-38.pyc and /dev/null differ
diff --git a/pipeline/mmocr/mmocr/utils/__pycache__/bezier_utils.cpython-39.pyc b/pipeline/mmocr/mmocr/utils/__pycache__/bezier_utils.cpython-39.pyc
deleted file mode 100644
index 4c1e13d8b3fdf9a0af40133b09f2fc4831aaea17..0000000000000000000000000000000000000000
Binary files a/pipeline/mmocr/mmocr/utils/__pycache__/bezier_utils.cpython-39.pyc and /dev/null differ
diff --git a/pipeline/mmocr/mmocr/utils/__pycache__/check_argument.cpython-38.pyc b/pipeline/mmocr/mmocr/utils/__pycache__/check_argument.cpython-38.pyc
deleted file mode 100644
index db669ecb941689a42c5a529c9b9fff2e9728485d..0000000000000000000000000000000000000000
Binary files a/pipeline/mmocr/mmocr/utils/__pycache__/check_argument.cpython-38.pyc and /dev/null differ
diff --git a/pipeline/mmocr/mmocr/utils/__pycache__/check_argument.cpython-39.pyc b/pipeline/mmocr/mmocr/utils/__pycache__/check_argument.cpython-39.pyc
deleted file mode 100644
index 9f06fede6efc9d814dc6b01e6591cc03ce662b45..0000000000000000000000000000000000000000
Binary files a/pipeline/mmocr/mmocr/utils/__pycache__/check_argument.cpython-39.pyc and /dev/null differ
diff --git a/pipeline/mmocr/mmocr/utils/__pycache__/collect_env.cpython-38.pyc b/pipeline/mmocr/mmocr/utils/__pycache__/collect_env.cpython-38.pyc
deleted file mode 100644
index ab0f9f80d5b31d8b79dab9af95c789674c1d5109..0000000000000000000000000000000000000000
Binary files a/pipeline/mmocr/mmocr/utils/__pycache__/collect_env.cpython-38.pyc and /dev/null differ
diff --git a/pipeline/mmocr/mmocr/utils/__pycache__/collect_env.cpython-39.pyc b/pipeline/mmocr/mmocr/utils/__pycache__/collect_env.cpython-39.pyc
deleted file mode 100644
index 1b3f44d871d24e912065cc7e6c4ea8016d2354db..0000000000000000000000000000000000000000
Binary files a/pipeline/mmocr/mmocr/utils/__pycache__/collect_env.cpython-39.pyc and /dev/null differ
diff --git a/pipeline/mmocr/mmocr/utils/__pycache__/data_converter_utils.cpython-38.pyc b/pipeline/mmocr/mmocr/utils/__pycache__/data_converter_utils.cpython-38.pyc
deleted file mode 100644
index be922ef101c11162ab0e4b9c31a5d3b7f1a5739a..0000000000000000000000000000000000000000
Binary files a/pipeline/mmocr/mmocr/utils/__pycache__/data_converter_utils.cpython-38.pyc and /dev/null differ
diff --git a/pipeline/mmocr/mmocr/utils/__pycache__/data_converter_utils.cpython-39.pyc b/pipeline/mmocr/mmocr/utils/__pycache__/data_converter_utils.cpython-39.pyc
deleted file mode 100644
index 0438773610579d684008af342b2cd2978730d811..0000000000000000000000000000000000000000
Binary files a/pipeline/mmocr/mmocr/utils/__pycache__/data_converter_utils.cpython-39.pyc and /dev/null differ
diff --git a/pipeline/mmocr/mmocr/utils/__pycache__/fileio.cpython-38.pyc b/pipeline/mmocr/mmocr/utils/__pycache__/fileio.cpython-38.pyc
deleted file mode 100644
index 1b7ec06c6afc00e184864b1e7ca76257e016cfa3..0000000000000000000000000000000000000000
Binary files a/pipeline/mmocr/mmocr/utils/__pycache__/fileio.cpython-38.pyc and /dev/null differ
diff --git a/pipeline/mmocr/mmocr/utils/__pycache__/fileio.cpython-39.pyc b/pipeline/mmocr/mmocr/utils/__pycache__/fileio.cpython-39.pyc
deleted file mode 100644
index b81f6ba5d58a091282b6c1bfd1f89cb457fe0d5c..0000000000000000000000000000000000000000
Binary files a/pipeline/mmocr/mmocr/utils/__pycache__/fileio.cpython-39.pyc and /dev/null differ
diff --git a/pipeline/mmocr/mmocr/utils/__pycache__/img_utils.cpython-38.pyc b/pipeline/mmocr/mmocr/utils/__pycache__/img_utils.cpython-38.pyc
deleted file mode 100644
index 5c67d8b545f85e016264d9a6d2c462c47becdd99..0000000000000000000000000000000000000000
Binary files a/pipeline/mmocr/mmocr/utils/__pycache__/img_utils.cpython-38.pyc and /dev/null differ
diff --git a/pipeline/mmocr/mmocr/utils/__pycache__/img_utils.cpython-39.pyc b/pipeline/mmocr/mmocr/utils/__pycache__/img_utils.cpython-39.pyc
deleted file mode 100644
index a76f17cdb3ad0b144e04dd7f1a6ea3901f9adaa8..0000000000000000000000000000000000000000
Binary files a/pipeline/mmocr/mmocr/utils/__pycache__/img_utils.cpython-39.pyc and /dev/null differ
diff --git a/pipeline/mmocr/mmocr/utils/__pycache__/mask_utils.cpython-38.pyc b/pipeline/mmocr/mmocr/utils/__pycache__/mask_utils.cpython-38.pyc
deleted file mode 100644
index 78632b894a79b3cd18bd149aaeed693ff0fcc39e..0000000000000000000000000000000000000000
Binary files a/pipeline/mmocr/mmocr/utils/__pycache__/mask_utils.cpython-38.pyc and /dev/null differ
diff --git a/pipeline/mmocr/mmocr/utils/__pycache__/mask_utils.cpython-39.pyc b/pipeline/mmocr/mmocr/utils/__pycache__/mask_utils.cpython-39.pyc
deleted file mode 100644
index ce63a3b652502663bdea5355c88ba7fa6577fe7f..0000000000000000000000000000000000000000
Binary files a/pipeline/mmocr/mmocr/utils/__pycache__/mask_utils.cpython-39.pyc and /dev/null differ
diff --git a/pipeline/mmocr/mmocr/utils/__pycache__/parsers.cpython-38.pyc b/pipeline/mmocr/mmocr/utils/__pycache__/parsers.cpython-38.pyc
deleted file mode 100644
index 700cc87a4080f6e9d4c09593630a25b5a819c649..0000000000000000000000000000000000000000
Binary files a/pipeline/mmocr/mmocr/utils/__pycache__/parsers.cpython-38.pyc and /dev/null differ
diff --git a/pipeline/mmocr/mmocr/utils/__pycache__/parsers.cpython-39.pyc b/pipeline/mmocr/mmocr/utils/__pycache__/parsers.cpython-39.pyc
deleted file mode 100644
index 5eea2f303b761a1df6d32cec3a7b7e6644a4cd94..0000000000000000000000000000000000000000
Binary files a/pipeline/mmocr/mmocr/utils/__pycache__/parsers.cpython-39.pyc and /dev/null differ
diff --git a/pipeline/mmocr/mmocr/utils/__pycache__/point_utils.cpython-38.pyc b/pipeline/mmocr/mmocr/utils/__pycache__/point_utils.cpython-38.pyc
deleted file mode 100644
index 8f1d74508b8aa2efbc378d6b18da85439962ed58..0000000000000000000000000000000000000000
Binary files a/pipeline/mmocr/mmocr/utils/__pycache__/point_utils.cpython-38.pyc and /dev/null differ
diff --git a/pipeline/mmocr/mmocr/utils/__pycache__/point_utils.cpython-39.pyc b/pipeline/mmocr/mmocr/utils/__pycache__/point_utils.cpython-39.pyc
deleted file mode 100644
index 1faea5a234122071282f23fda81f8acc36cfa3d1..0000000000000000000000000000000000000000
Binary files a/pipeline/mmocr/mmocr/utils/__pycache__/point_utils.cpython-39.pyc and /dev/null differ
diff --git a/pipeline/mmocr/mmocr/utils/__pycache__/polygon_utils.cpython-38.pyc b/pipeline/mmocr/mmocr/utils/__pycache__/polygon_utils.cpython-38.pyc
deleted file mode 100644
index cb5d46cb9a8c5f2a16176086111736ade175cb8f..0000000000000000000000000000000000000000
Binary files a/pipeline/mmocr/mmocr/utils/__pycache__/polygon_utils.cpython-38.pyc and /dev/null differ
diff --git a/pipeline/mmocr/mmocr/utils/__pycache__/polygon_utils.cpython-39.pyc b/pipeline/mmocr/mmocr/utils/__pycache__/polygon_utils.cpython-39.pyc
deleted file mode 100644
index 5a7c006d76e1c7c6fac47286763477493510e34d..0000000000000000000000000000000000000000
Binary files a/pipeline/mmocr/mmocr/utils/__pycache__/polygon_utils.cpython-39.pyc and /dev/null differ
diff --git a/pipeline/mmocr/mmocr/utils/__pycache__/processing.cpython-38.pyc b/pipeline/mmocr/mmocr/utils/__pycache__/processing.cpython-38.pyc
deleted file mode 100644
index 0d7f6bd42791d0537cca89e404ceb29e9acaa423..0000000000000000000000000000000000000000
Binary files a/pipeline/mmocr/mmocr/utils/__pycache__/processing.cpython-38.pyc and /dev/null differ
diff --git a/pipeline/mmocr/mmocr/utils/__pycache__/processing.cpython-39.pyc b/pipeline/mmocr/mmocr/utils/__pycache__/processing.cpython-39.pyc
deleted file mode 100644
index bdd40396c2131cfea583d6a839a86a98182340cf..0000000000000000000000000000000000000000
Binary files a/pipeline/mmocr/mmocr/utils/__pycache__/processing.cpython-39.pyc and /dev/null differ
diff --git a/pipeline/mmocr/mmocr/utils/__pycache__/setup_env.cpython-38.pyc b/pipeline/mmocr/mmocr/utils/__pycache__/setup_env.cpython-38.pyc
deleted file mode 100644
index bf3e8a7738af0a60de76930bdc7c0e2cbc52f003..0000000000000000000000000000000000000000
Binary files a/pipeline/mmocr/mmocr/utils/__pycache__/setup_env.cpython-38.pyc and /dev/null differ
diff --git a/pipeline/mmocr/mmocr/utils/__pycache__/setup_env.cpython-39.pyc b/pipeline/mmocr/mmocr/utils/__pycache__/setup_env.cpython-39.pyc
deleted file mode 100644
index 50df868e57be2b7e539f3d8c26aaef0f914bfd8e..0000000000000000000000000000000000000000
Binary files a/pipeline/mmocr/mmocr/utils/__pycache__/setup_env.cpython-39.pyc and /dev/null differ
diff --git a/pipeline/mmocr/mmocr/utils/__pycache__/string_utils.cpython-38.pyc b/pipeline/mmocr/mmocr/utils/__pycache__/string_utils.cpython-38.pyc
deleted file mode 100644
index 4eb3ee0b9e436ea0caff6a28cc6df45731f54c51..0000000000000000000000000000000000000000
Binary files a/pipeline/mmocr/mmocr/utils/__pycache__/string_utils.cpython-38.pyc and /dev/null differ
diff --git a/pipeline/mmocr/mmocr/utils/__pycache__/string_utils.cpython-39.pyc b/pipeline/mmocr/mmocr/utils/__pycache__/string_utils.cpython-39.pyc
deleted file mode 100644
index b74c263e9fa321133b0598b008089019dfaafb49..0000000000000000000000000000000000000000
Binary files a/pipeline/mmocr/mmocr/utils/__pycache__/string_utils.cpython-39.pyc and /dev/null differ
diff --git a/pipeline/mmocr/mmocr/utils/__pycache__/transform_utils.cpython-38.pyc b/pipeline/mmocr/mmocr/utils/__pycache__/transform_utils.cpython-38.pyc
deleted file mode 100644
index dad7905cd0d13fa1342685bc4c16bf1dd66ab659..0000000000000000000000000000000000000000
Binary files a/pipeline/mmocr/mmocr/utils/__pycache__/transform_utils.cpython-38.pyc and /dev/null differ
diff --git a/pipeline/mmocr/mmocr/utils/__pycache__/transform_utils.cpython-39.pyc b/pipeline/mmocr/mmocr/utils/__pycache__/transform_utils.cpython-39.pyc
deleted file mode 100644
index ed52de0873c9673acc65558623eb19b04134e019..0000000000000000000000000000000000000000
Binary files a/pipeline/mmocr/mmocr/utils/__pycache__/transform_utils.cpython-39.pyc and /dev/null differ
diff --git a/pipeline/mmocr/mmocr/utils/__pycache__/typing_utils.cpython-38.pyc b/pipeline/mmocr/mmocr/utils/__pycache__/typing_utils.cpython-38.pyc
deleted file mode 100644
index fb8d58044d4ce6a59cd818deb9519e5d6970c2bb..0000000000000000000000000000000000000000
Binary files a/pipeline/mmocr/mmocr/utils/__pycache__/typing_utils.cpython-38.pyc and /dev/null differ
diff --git a/pipeline/mmocr/mmocr/utils/__pycache__/typing_utils.cpython-39.pyc b/pipeline/mmocr/mmocr/utils/__pycache__/typing_utils.cpython-39.pyc
deleted file mode 100644
index 4c609541935b6f25bb4d2fe2aeebae1cabf48651..0000000000000000000000000000000000000000
Binary files a/pipeline/mmocr/mmocr/utils/__pycache__/typing_utils.cpython-39.pyc and /dev/null differ
diff --git a/pipeline/mmocr/mmocr/utils/bbox_utils.py b/pipeline/mmocr/mmocr/utils/bbox_utils.py
deleted file mode 100644
index a91df91d79916c151c399b0489ae4662f6149ee7..0000000000000000000000000000000000000000
--- a/pipeline/mmocr/mmocr/utils/bbox_utils.py
+++ /dev/null
@@ -1,368 +0,0 @@
-# Copyright (c) OpenMMLab. All rights reserved.
-from typing import List, Tuple
-
-import numpy as np
-from shapely.geometry import LineString, Point
-
-from mmocr.utils.check_argument import is_type_list
-from mmocr.utils.point_utils import point_distance, points_center
-from mmocr.utils.typing_utils import ArrayLike
-
-
-def rescale_bbox(bbox: np.ndarray,
- scale_factor: Tuple[int, int],
- mode: str = 'mul') -> np.ndarray:
- """Rescale a bounding box according to scale_factor.
-
- The behavior is different depending on the mode. When mode is 'mul', the
- coordinates will be multiplied by scale_factor, which is usually used in
- preprocessing transforms such as :func:`Resize`.
- The coordinates will be divided by scale_factor if mode is 'div'. It can be
- used in postprocessors to recover the bbox in the original image size.
-
- Args:
- bbox (ndarray): A bounding box [x1, y1, x2, y2].
- scale_factor (tuple(int, int)): (w_scale, h_scale).
- model (str): Rescale mode. Can be 'mul' or 'div'. Defaults to 'mul'.
-
- Returns:
- np.ndarray: Rescaled bbox.
- """
- assert mode in ['mul', 'div']
- bbox = np.array(bbox, dtype=np.float32)
- bbox_shape = bbox.shape
- reshape_bbox = bbox.reshape(-1, 2)
- scale_factor = np.array(scale_factor, dtype=float)
- if mode == 'div':
- scale_factor = 1 / scale_factor
- bbox = (reshape_bbox * scale_factor[None]).reshape(bbox_shape)
- return bbox
-
-
-def rescale_bboxes(bboxes: np.ndarray,
- scale_factor: Tuple[int, int],
- mode: str = 'mul') -> np.ndarray:
- """Rescale bboxes according to scale_factor.
-
- The behavior is different depending on the mode. When mode is 'mul', the
- coordinates will be multiplied by scale_factor, which is usually used in
- preprocessing transforms such as :func:`Resize`.
- The coordinates will be divided by scale_factor if mode is 'div'. It can be
- used in postprocessors to recover the bboxes in the original
- image size.
-
- Args:
- bboxes (np.ndarray]): Bounding bboxes in shape (N, 4)
- scale_factor (tuple(int, int)): (w_scale, h_scale).
- model (str): Rescale mode. Can be 'mul' or 'div'. Defaults to 'mul'.
-
- Returns:
- list[np.ndarray]: Rescaled bboxes.
- """
- bboxes = rescale_bbox(bboxes, scale_factor, mode)
- return bboxes
-
-
-def bbox2poly(bbox: ArrayLike, mode: str = 'xyxy') -> np.array:
- """Converting a bounding box to a polygon.
-
- Args:
- bbox (ArrayLike): A bbox. In any form can be accessed by 1-D indices.
- E.g. list[float], np.ndarray, or torch.Tensor. bbox is written in
- [x1, y1, x2, y2].
- mode (str): Specify the format of bbox. Can be 'xyxy' or 'xywh'.
- Defaults to 'xyxy'.
-
- Returns:
- np.array: The converted polygon [x1, y1, x2, y1, x2, y2, x1, y2].
- """
- assert len(bbox) == 4
- if mode == 'xyxy':
- x1, y1, x2, y2 = bbox
- poly = np.array([x1, y1, x2, y1, x2, y2, x1, y2])
- elif mode == 'xywh':
- x, y, w, h = bbox
- poly = np.array([x, y, x + w, y, x + w, y + h, x, y + h])
- else:
- raise NotImplementedError('Not supported mode.')
-
- return poly
-
-
-def is_on_same_line(box_a, box_b, min_y_overlap_ratio=0.8):
- # TODO Check if it should be deleted after ocr.py refactored
- """Check if two boxes are on the same line by their y-axis coordinates.
-
- Two boxes are on the same line if they overlap vertically, and the length
- of the overlapping line segment is greater than min_y_overlap_ratio * the
- height of either of the boxes.
-
- Args:
- box_a (list), box_b (list): Two bounding boxes to be checked
- min_y_overlap_ratio (float): The minimum vertical overlapping ratio
- allowed for boxes in the same line
-
- Returns:
- The bool flag indicating if they are on the same line
- """
- a_y_min = np.min(box_a[1::2])
- b_y_min = np.min(box_b[1::2])
- a_y_max = np.max(box_a[1::2])
- b_y_max = np.max(box_b[1::2])
-
- # Make sure that box a is always the box above another
- if a_y_min > b_y_min:
- a_y_min, b_y_min = b_y_min, a_y_min
- a_y_max, b_y_max = b_y_max, a_y_max
-
- if b_y_min <= a_y_max:
- if min_y_overlap_ratio is not None:
- sorted_y = sorted([b_y_min, b_y_max, a_y_max])
- overlap = sorted_y[1] - sorted_y[0]
- min_a_overlap = (a_y_max - a_y_min) * min_y_overlap_ratio
- min_b_overlap = (b_y_max - b_y_min) * min_y_overlap_ratio
- return overlap >= min_a_overlap or \
- overlap >= min_b_overlap
- else:
- return True
- return False
-
-
-def stitch_boxes_into_lines(boxes, max_x_dist=10, min_y_overlap_ratio=0.8):
- # TODO Check if it should be deleted after ocr.py refactored
- """Stitch fragmented boxes of words into lines.
-
- Note: part of its logic is inspired by @Johndirr
- (https://github.com/faustomorales/keras-ocr/issues/22)
-
- Args:
- boxes (list): List of ocr results to be stitched
- max_x_dist (int): The maximum horizontal distance between the closest
- edges of neighboring boxes in the same line
- min_y_overlap_ratio (float): The minimum vertical overlapping ratio
- allowed for any pairs of neighboring boxes in the same line
-
- Returns:
- merged_boxes(list[dict]): List of merged boxes and texts
- """
-
- if len(boxes) <= 1:
- return boxes
-
- merged_boxes = []
-
- # sort groups based on the x_min coordinate of boxes
- x_sorted_boxes = sorted(boxes, key=lambda x: np.min(x['box'][::2]))
- # store indexes of boxes which are already parts of other lines
- skip_idxs = set()
-
- i = 0
- # locate lines of boxes starting from the leftmost one
- for i in range(len(x_sorted_boxes)):
- if i in skip_idxs:
- continue
- # the rightmost box in the current line
- rightmost_box_idx = i
- line = [rightmost_box_idx]
- for j in range(i + 1, len(x_sorted_boxes)):
- if j in skip_idxs:
- continue
- if is_on_same_line(x_sorted_boxes[rightmost_box_idx]['box'],
- x_sorted_boxes[j]['box'], min_y_overlap_ratio):
- line.append(j)
- skip_idxs.add(j)
- rightmost_box_idx = j
-
- # split line into lines if the distance between two neighboring
- # sub-lines' is greater than max_x_dist
- lines = []
- line_idx = 0
- lines.append([line[0]])
- rightmost = np.max(x_sorted_boxes[line[0]]['box'][::2])
- for k in range(1, len(line)):
- curr_box = x_sorted_boxes[line[k]]
- dist = np.min(curr_box['box'][::2]) - rightmost
- if dist > max_x_dist:
- line_idx += 1
- lines.append([])
- lines[line_idx].append(line[k])
- rightmost = max(rightmost, np.max(curr_box['box'][::2]))
-
- # Get merged boxes
- for box_group in lines:
- merged_box = {}
- merged_box['text'] = ' '.join(
- [x_sorted_boxes[idx]['text'] for idx in box_group])
- x_min, y_min = float('inf'), float('inf')
- x_max, y_max = float('-inf'), float('-inf')
- for idx in box_group:
- x_max = max(np.max(x_sorted_boxes[idx]['box'][::2]), x_max)
- x_min = min(np.min(x_sorted_boxes[idx]['box'][::2]), x_min)
- y_max = max(np.max(x_sorted_boxes[idx]['box'][1::2]), y_max)
- y_min = min(np.min(x_sorted_boxes[idx]['box'][1::2]), y_min)
- merged_box['box'] = [
- x_min, y_min, x_max, y_min, x_max, y_max, x_min, y_max
- ]
- merged_boxes.append(merged_box)
-
- return merged_boxes
-
-
-def bezier2polygon(bezier_points: np.ndarray,
- num_sample: int = 20) -> List[np.ndarray]:
- # TODO check test later
- """Sample points from the boundary of a polygon enclosed by two Bezier
- curves, which are controlled by ``bezier_points``.
-
- Args:
- bezier_points (ndarray): A :math:`(2, 4, 2)` array of 8 Bezeir points
- or its equalivance. The first 4 points control the curve at one
- side and the last four control the other side.
- num_sample (int): The number of sample points at each Bezeir curve.
- Defaults to 20.
-
- Returns:
- list[ndarray]: A list of 2*num_sample points representing the polygon
- extracted from Bezier curves.
-
- Warning:
- The points are not guaranteed to be ordered. Please use
- :func:`mmocr.utils.sort_points` to sort points if necessary.
- """
- assert num_sample > 0, 'The sampling number should greater than 0'
-
- bezier_points = np.asarray(bezier_points)
- assert np.prod(
- bezier_points.shape) == 16, 'Need 8 Bezier control points to continue!'
-
- bezier = bezier_points.reshape(2, 4, 2).transpose(0, 2, 1).reshape(4, 4)
- u = np.linspace(0, 1, num_sample)
-
- points = np.outer((1 - u) ** 3, bezier[:, 0]) \
- + np.outer(3 * u * ((1 - u) ** 2), bezier[:, 1]) \
- + np.outer(3 * (u ** 2) * (1 - u), bezier[:, 2]) \
- + np.outer(u ** 3, bezier[:, 3])
-
- # Convert points to polygon
- points = np.concatenate((points[:, :2], points[:, 2:]), axis=0)
- return points.tolist()
-
-
-def sort_vertex(points_x, points_y):
- # TODO Add typehints & docstring & test
- """Sort box vertices in clockwise order from left-top first.
-
- Args:
- points_x (list[float]): x of four vertices.
- points_y (list[float]): y of four vertices.
- Returns:
- sorted_points_x (list[float]): x of sorted four vertices.
- sorted_points_y (list[float]): y of sorted four vertices.
- """
- assert is_type_list(points_x, (float, int))
- assert is_type_list(points_y, (float, int))
- assert len(points_x) == 4
- assert len(points_y) == 4
- vertices = np.stack((points_x, points_y), axis=-1).astype(np.float32)
- vertices = _sort_vertex(vertices)
- sorted_points_x = list(vertices[:, 0])
- sorted_points_y = list(vertices[:, 1])
- return sorted_points_x, sorted_points_y
-
-
-def _sort_vertex(vertices):
- # TODO Add typehints & docstring & test
- assert vertices.ndim == 2
- assert vertices.shape[-1] == 2
- N = vertices.shape[0]
- if N == 0:
- return vertices
-
- center = np.mean(vertices, axis=0)
- directions = vertices - center
- angles = np.arctan2(directions[:, 1], directions[:, 0])
- sort_idx = np.argsort(angles)
- vertices = vertices[sort_idx]
-
- left_top = np.min(vertices, axis=0)
- dists = np.linalg.norm(left_top - vertices, axis=-1, ord=2)
- lefttop_idx = np.argmin(dists)
- indexes = (np.arange(N, dtype=np.int_) + lefttop_idx) % N
- return vertices[indexes]
-
-
-def sort_vertex8(points):
- # TODO Add typehints & docstring & test
- """Sort vertex with 8 points [x1 y1 x2 y2 x3 y3 x4 y4]"""
- assert len(points) == 8
- vertices = _sort_vertex(np.array(points, dtype=np.float32).reshape(-1, 2))
- sorted_box = list(vertices.flatten())
- return sorted_box
-
-
-def bbox_center_distance(box1: ArrayLike, box2: ArrayLike) -> float:
- """Calculate the distance between the center points of two bounding boxes.
-
- Args:
- box1 (ArrayLike): The first bounding box
- represented in [x1, y1, x2, y2].
- box2 (ArrayLike): The second bounding box
- represented in [x1, y1, x2, y2].
-
- Returns:
- float: The distance between the center points of two bounding boxes.
- """
- return point_distance(points_center(box1), points_center(box2))
-
-
-def bbox_diag_distance(box: ArrayLike) -> float:
- """Calculate the diagonal length of a bounding box (distance between the
- top-left and bottom-right).
-
- Args:
- box (ArrayLike): The bounding box represented in
- [x1, y1, x2, y2, x3, y3, x4, y4] or [x1, y1, x2, y2].
-
- Returns:
- float: The diagonal length of the bounding box.
- """
- box = np.array(box, dtype=np.float32)
- assert (box.size == 8 or box.size == 4)
-
- if box.size == 8:
- diag = point_distance(box[0:2], box[4:6])
- elif box.size == 4:
- diag = point_distance(box[0:2], box[2:4])
-
- return diag
-
-
-def bbox_jitter(points_x, points_y, jitter_ratio_x=0.5, jitter_ratio_y=0.1):
- """Jitter on the coordinates of bounding box.
-
- Args:
- points_x (list[float | int]): List of y for four vertices.
- points_y (list[float | int]): List of x for four vertices.
- jitter_ratio_x (float): Horizontal jitter ratio relative to the height.
- jitter_ratio_y (float): Vertical jitter ratio relative to the height.
- """
- assert len(points_x) == 4
- assert len(points_y) == 4
- assert isinstance(jitter_ratio_x, float)
- assert isinstance(jitter_ratio_y, float)
- assert 0 <= jitter_ratio_x < 1
- assert 0 <= jitter_ratio_y < 1
-
- points = [Point(points_x[i], points_y[i]) for i in range(4)]
- line_list = [
- LineString([points[i], points[i + 1 if i < 3 else 0]])
- for i in range(4)
- ]
-
- tmp_h = max(line_list[1].length, line_list[3].length)
-
- for i in range(4):
- jitter_pixel_x = (np.random.rand() - 0.5) * 2 * jitter_ratio_x * tmp_h
- jitter_pixel_y = (np.random.rand() - 0.5) * 2 * jitter_ratio_y * tmp_h
- points_x[i] += jitter_pixel_x
- points_y[i] += jitter_pixel_y
diff --git a/pipeline/mmocr/mmocr/utils/bezier_utils.py b/pipeline/mmocr/mmocr/utils/bezier_utils.py
deleted file mode 100644
index d93a6293926e2d807eb089bf92835e39a4ef5d84..0000000000000000000000000000000000000000
--- a/pipeline/mmocr/mmocr/utils/bezier_utils.py
+++ /dev/null
@@ -1,62 +0,0 @@
-# Copyright (c) OpenMMLab. All rights reserved.
-import numpy as np
-from scipy.special import comb as n_over_k
-
-from mmocr.utils.typing_utils import ArrayLike
-
-
-def bezier_coefficient(n, t, k):
- return t**k * (1 - t)**(n - k) * n_over_k(n, k)
-
-
-def bezier_coefficients(time, point_num, ratios):
- return [[bezier_coefficient(time, ratio, num) for num in range(point_num)]
- for ratio in ratios]
-
-
-def linear_interpolation(point1: np.ndarray,
- point2: np.ndarray,
- number: int = 2) -> np.ndarray:
- t = np.linspace(0, 1, number + 2).reshape(-1, 1)
- return point1 + (point2 - point1) * t
-
-
-def curve2bezier(curve: ArrayLike):
- curve = np.array(curve).reshape(-1, 2)
- if len(curve) == 2:
- return linear_interpolation(curve[0], curve[1])
- diff = curve[1:] - curve[:-1]
- distance = np.linalg.norm(diff, axis=-1)
- norm_distance = distance / distance.sum()
- norm_distance = np.hstack(([0], norm_distance))
- cum_norm_dis = norm_distance.cumsum()
- pseudo_inv = np.linalg.pinv(bezier_coefficients(3, 4, cum_norm_dis))
- control_points = pseudo_inv.dot(curve)
- return control_points
-
-
-def bezier2curve(bezier: np.ndarray, num_sample: int = 10):
- bezier = np.asarray(bezier)
- t = np.linspace(0, 1, num_sample)
- return np.array(bezier_coefficients(3, 4, t)).dot(bezier)
-
-
-def poly2bezier(poly):
- poly = np.array(poly).reshape(-1, 2)
- points_num = len(poly)
- up_curve = poly[:points_num // 2]
- down_curve = poly[points_num // 2:]
- up_bezier = curve2bezier(up_curve)
- down_bezier = curve2bezier(down_curve)
- up_bezier[0] = up_curve[0]
- up_bezier[-1] = up_curve[-1]
- down_bezier[0] = down_curve[0]
- down_bezier[-1] = down_curve[-1]
- return np.vstack((up_bezier, down_bezier)).flatten().tolist()
-
-
-def bezier2poly(bezier, num_sample=20):
- bezier = bezier.reshape(2, 4, 2)
- curve_top = bezier2curve(bezier[0], num_sample)
- curve_bottom = bezier2curve(bezier[1], num_sample)
- return np.vstack((curve_top, curve_bottom)).flatten().tolist()
diff --git a/pipeline/mmocr/mmocr/utils/check_argument.py b/pipeline/mmocr/mmocr/utils/check_argument.py
deleted file mode 100644
index 34cbe8dc2658d725c328eb5cd98652633a22aa24..0000000000000000000000000000000000000000
--- a/pipeline/mmocr/mmocr/utils/check_argument.py
+++ /dev/null
@@ -1,72 +0,0 @@
-# Copyright (c) OpenMMLab. All rights reserved.
-
-
-def is_3dlist(x):
- """check x is 3d-list([[[1], []]]) or 2d empty list([[], []]) or 1d empty
- list([]).
-
- Notice:
- The reason that it contains 1d or 2d empty list is because
- some arguments from gt annotation file or model prediction
- may be empty, but usually, it should be 3d-list.
- """
- if not isinstance(x, list):
- return False
- if len(x) == 0:
- return True
- for sub_x in x:
- if not is_2dlist(sub_x):
- return False
-
- return True
-
-
-def is_2dlist(x):
- """check x is 2d-list([[1], []]) or 1d empty list([]).
-
- Notice:
- The reason that it contains 1d empty list is because
- some arguments from gt annotation file or model prediction
- may be empty, but usually, it should be 2d-list.
- """
- if not isinstance(x, list):
- return False
- if len(x) == 0:
- return True
-
- return all(isinstance(item, list) for item in x)
-
-
-def is_type_list(x, type):
-
- if not isinstance(x, list):
- return False
-
- return all(isinstance(item, type) for item in x)
-
-
-def is_none_or_type(x, type):
-
- return isinstance(x, type) or x is None
-
-
-def equal_len(*argv):
- assert len(argv) > 0
-
- num_arg = len(argv[0])
- for arg in argv:
- if len(arg) != num_arg:
- return False
- return True
-
-
-def valid_boundary(x, with_score=True):
- num = len(x)
- if num < 8:
- return False
- if num % 2 == 0 and (not with_score):
- return True
- if num % 2 == 1 and with_score:
- return True
-
- return False
diff --git a/pipeline/mmocr/mmocr/utils/collect_env.py b/pipeline/mmocr/mmocr/utils/collect_env.py
deleted file mode 100644
index cf56ecc77902841220cb3e9040033de82fe81e2c..0000000000000000000000000000000000000000
--- a/pipeline/mmocr/mmocr/utils/collect_env.py
+++ /dev/null
@@ -1,17 +0,0 @@
-# Copyright (c) OpenMMLab. All rights reserved.
-from mmengine.utils import get_git_hash
-from mmengine.utils.dl_utils import collect_env as collect_base_env
-
-import mmocr
-
-
-def collect_env():
- """Collect the information of the running environments."""
- env_info = collect_base_env()
- env_info['MMOCR'] = mmocr.__version__ + '+' + get_git_hash()[:7]
- return env_info
-
-
-if __name__ == '__main__':
- for name, val in collect_env().items():
- print(f'{name}: {val}')
diff --git a/pipeline/mmocr/mmocr/utils/data_converter_utils.py b/pipeline/mmocr/mmocr/utils/data_converter_utils.py
deleted file mode 100644
index bbc4ad090a143c4acd705fdce8d45d2e3e73bf0d..0000000000000000000000000000000000000000
--- a/pipeline/mmocr/mmocr/utils/data_converter_utils.py
+++ /dev/null
@@ -1,189 +0,0 @@
-# Copyright (c) OpenMMLab. All rights reserved.
-import warnings
-from typing import Dict, Sequence
-
-import mmengine
-
-from mmocr.utils import is_type_list
-
-
-def dump_ocr_data(image_infos: Sequence[Dict], out_json_name: str,
- task_name: str, **kwargs) -> Dict:
- """Dump the annotation in openmmlab style.
-
- Args:
- image_infos (list): List of image information dicts. Read the example
- section for the format illustration.
- out_json_name (str): Output json filename.
- task_name (str): Task name. Options are 'textdet', 'textrecog' and
- 'textspotter'.
-
- Examples:
- Here is the general structure of image_infos for textdet/textspotter
- tasks:
-
- .. code-block:: python
-
- [ # A list of dicts. Each dict stands for a single image.
- {
- "file_name": "1.jpg",
- "height": 100,
- "width": 200,
- "segm_file": "seg.txt" # (optional) path to segmap
- "anno_info": [ # a list of dicts. Each dict
- # stands for a single text instance.
- {
- "iscrowd": 0, # 0: don't ignore this instance
- # 1: ignore
- "category_id": 0, # Instance class id. Must be 0
- # for OCR tasks to permanently
- # be mapped to 'text' category
- "bbox": [x, y, w, h],
- "segmentation": [x1, y1, x2, y2, ...],
- "text": "demo_text" # for textspotter only.
- }
- ]
- },
- ]
-
- The input for textrecog task is much simpler:
-
- .. code-block:: python
-
- [ # A list of dicts. Each dict stands for a single image.
- {
- "file_name": "1.jpg",
- "anno_info": [ # a list of dicts. Each dict
- # stands for a single text instance.
- # However, in textrecog, usually each
- # image only has one text instance.
- {
- "text": "demo_text"
- }
- ]
- },
- ]
-
-
- Returns:
- out_json(dict): The openmmlab-style annotation.
- """
-
- task2dataset = {
- 'textspotter': 'TextSpotterDataset',
- 'textdet': 'TextDetDataset',
- 'textrecog': 'TextRecogDataset'
- }
-
- assert isinstance(image_infos, list)
- assert isinstance(out_json_name, str)
- assert task_name in task2dataset.keys()
-
- dataset_type = task2dataset[task_name]
-
- out_json = dict(
- metainfo=dict(dataset_type=dataset_type, task_name=task_name),
- data_list=list())
- if task_name in ['textdet', 'textspotter']:
- out_json['metainfo']['category'] = [dict(id=0, name='text')]
-
- for image_info in image_infos:
-
- single_info = dict(instances=list())
- single_info['img_path'] = image_info['file_name']
- if task_name in ['textdet', 'textspotter']:
- single_info['height'] = image_info['height']
- single_info['width'] = image_info['width']
- if 'segm_file' in image_info:
- single_info['seg_map'] = image_info['segm_file']
-
- anno_infos = image_info['anno_info']
-
- for anno_info in anno_infos:
- instance = {}
- if task_name in ['textrecog', 'textspotter']:
- instance['text'] = anno_info['text']
- if task_name in ['textdet', 'textspotter']:
- mask = anno_info['segmentation']
- # TODO: remove this if-branch when all converters have been
- # verified
- if len(mask) == 1 and len(mask[0]) > 1:
- mask = mask[0]
- warnings.warn(
- 'Detected nested segmentation for a single'
- 'text instance, which should be a 1-d array now.'
- 'Please fix input accordingly.')
- instance['polygon'] = mask
- x, y, w, h = anno_info['bbox']
- instance['bbox'] = [x, y, x + w, y + h]
- instance['bbox_label'] = anno_info['category_id']
- instance['ignore'] = anno_info['iscrowd'] == 1
- single_info['instances'].append(instance)
-
- out_json['data_list'].append(single_info)
-
- mmengine.dump(out_json, out_json_name, **kwargs)
-
- return out_json
-
-
-def recog_anno_to_imginfo(
- file_paths: Sequence[str],
- labels: Sequence[str],
-) -> Sequence[Dict]:
- """Convert a list of file_paths and labels for recognition tasks into the
- format of image_infos acceptable by :func:`dump_ocr_data()`. It's meant to
- maintain compatibility with the legacy annotation format in MMOCR 0.x.
-
- In MMOCR 0.x, data converters for recognition usually converts the
- annotations into a list of file paths and a list of labels, which look
- like the following:
-
- .. code-block:: python
-
- file_paths = ['1.jpg', '2.jpg', ...]
- labels = ['aaa', 'bbb', ...]
-
- This utility merges them into a list of dictionaries parsable by
- :func:`dump_ocr_data()`:
-
- .. code-block:: python
-
- [ # A list of dicts. Each dict stands for a single image.
- {
- "file_name": "1.jpg",
- "anno_info": [
- {
- "text": "aaa"
- }
- ]
- },
- {
- "file_name": "2.jpg",
- "anno_info": [
- {
- "text": "bbb"
- }
- ]
- },
- ...
- ]
-
- Args:
- file_paths (list[str]): A list of file paths to images.
- labels (list[str]): A list of text labels.
-
- Returns:
- list[dict]: Annotations parsable by :func:`dump_ocr_data()`.
- """
- assert is_type_list(file_paths, str)
- assert is_type_list(labels, str)
- assert len(file_paths) == len(labels)
-
- results = []
- for i in range(len(file_paths)):
- result = dict(
- file_name=file_paths[i], anno_info=[dict(text=labels[i])])
- results.append(result)
-
- return results
diff --git a/pipeline/mmocr/mmocr/utils/fileio.py b/pipeline/mmocr/mmocr/utils/fileio.py
deleted file mode 100644
index cae4e58571c29a1f3573dc8053b7daf5b04c07cd..0000000000000000000000000000000000000000
--- a/pipeline/mmocr/mmocr/utils/fileio.py
+++ /dev/null
@@ -1,125 +0,0 @@
-# Copyright (c) OpenMMLab. All rights reserved.
-import hashlib
-import os.path as osp
-import sys
-import warnings
-from glob import glob
-from typing import List
-
-from mmengine import mkdir_or_exist
-
-
-def list_to_file(filename, lines):
- """Write a list of strings to a text file.
-
- Args:
- filename (str): The output filename. It will be created/overwritten.
- lines (list(str)): Data to be written.
- """
- mkdir_or_exist(osp.dirname(filename))
- with open(filename, 'w', encoding='utf-8') as fw:
- for line in lines:
- fw.write(f'{line}\n')
-
-
-def list_from_file(filename, encoding='utf-8'):
- """Load a text file and parse the content as a list of strings. The
- trailing "\\r" and "\\n" of each line will be removed.
-
- Note:
- This will be replaced by mmcv's version after it supports encoding.
-
- Args:
- filename (str): Filename.
- encoding (str): Encoding used to open the file. Default utf-8.
-
- Returns:
- list[str]: A list of strings.
- """
- item_list = []
- with open(filename, encoding=encoding) as f:
- for line in f:
- item_list.append(line.rstrip('\n\r'))
- return item_list
-
-
-def is_archive(file_path: str) -> bool:
- """Check whether the file is a supported archive format.
-
- Args:
- file_path (str): Path to the file.
-
- Returns:
- bool: Whether the file is an archive.
- """
-
- suffixes = ['zip', 'tar', 'tar.gz']
-
- for suffix in suffixes:
- if file_path.endswith(suffix):
- return True
- return False
-
-
-def check_integrity(file_path: str,
- md5: str,
- chunk_size: int = 1024 * 1024) -> bool:
- """Check if the file exist and match to the given md5 code.
-
- Args:
- file_path (str): Path to the file.
- md5 (str): MD5 to be matched.
- chunk_size (int, optional): Chunk size. Defaults to 1024*1024.
-
- Returns:
- bool: Whether the md5 is matched.
- """
- if md5 is None:
- warnings.warn('MD5 is None, skip the integrity check.')
- return True
- if not osp.exists(file_path):
- return False
-
- return get_md5(file_path=file_path, chunk_size=chunk_size) == md5
-
-
-def get_md5(file_path: str, chunk_size: int = 1024 * 1024) -> str:
- """Get the md5 of the file.
-
- Args:
- file_path (str): Path to the file.
- chunk_size (int, optional): Chunk size. Defaults to 1024*1024.
-
- Returns:
- str: MD5 of the file.
- """
- if not osp.exists(file_path):
- raise FileNotFoundError(f'{file_path} does not exist.')
-
- if sys.version_info >= (3, 9):
- hash = hashlib.md5(usedforsecurity=False)
- else:
- hash = hashlib.md5()
- with open(file_path, 'rb') as f:
- for chunk in iter(lambda: f.read(chunk_size), b''):
- hash.update(chunk)
-
- return hash.hexdigest()
-
-
-def list_files(path: str, suffixes: List) -> List:
- """Retrieve file list from the path.
-
- Args:
- path (str): Path to the directory.
- suffixes (list[str], optional): Suffixes to be retrieved.
-
- Returns:
- List: List of the files.
- """
-
- file_list = []
- for suffix in suffixes:
- file_list.extend(glob(osp.join(path, '*' + suffix)))
-
- return file_list
diff --git a/pipeline/mmocr/mmocr/utils/img_utils.py b/pipeline/mmocr/mmocr/utils/img_utils.py
deleted file mode 100644
index c96a05d2578ffc165d6323b37e3a7955b8ce68cf..0000000000000000000000000000000000000000
--- a/pipeline/mmocr/mmocr/utils/img_utils.py
+++ /dev/null
@@ -1,108 +0,0 @@
-# Copyright (c) OpenMMLab. All rights reserved.
-import cv2
-import numpy as np
-from mmengine.utils import is_seq_of
-from shapely.geometry import LineString, Point
-
-from .bbox_utils import bbox_jitter
-from .polygon_utils import sort_vertex
-
-
-def warp_img(src_img,
- box,
- jitter=False,
- jitter_ratio_x=0.5,
- jitter_ratio_y=0.1):
- """Crop box area from image using opencv warpPerspective.
-
- Args:
- src_img (np.array): Image before cropping.
- box (list[float | int]): Coordinates of quadrangle.
- jitter (bool): Whether to jitter the box.
- jitter_ratio_x (float): Horizontal jitter ratio relative to the height.
- jitter_ratio_y (float): Vertical jitter ratio relative to the height.
-
- Returns:
- np.array: The warped image.
- """
- assert is_seq_of(box, (float, int))
- assert len(box) == 8
-
- h, w = src_img.shape[:2]
- points_x = [min(max(x, 0), w) for x in box[0:8:2]]
- points_y = [min(max(y, 0), h) for y in box[1:9:2]]
-
- points_x, points_y = sort_vertex(points_x, points_y)
-
- if jitter:
- bbox_jitter(
- points_x,
- points_y,
- jitter_ratio_x=jitter_ratio_x,
- jitter_ratio_y=jitter_ratio_y)
-
- points = [Point(points_x[i], points_y[i]) for i in range(4)]
- edges = [
- LineString([points[i], points[i + 1 if i < 3 else 0]])
- for i in range(4)
- ]
-
- pts1 = np.float32([[points[i].x, points[i].y] for i in range(4)])
- box_width = max(edges[0].length, edges[2].length)
- box_height = max(edges[1].length, edges[3].length)
-
- pts2 = np.float32([[0, 0], [box_width, 0], [box_width, box_height],
- [0, box_height]])
- M = cv2.getPerspectiveTransform(pts1, pts2)
- dst_img = cv2.warpPerspective(src_img, M,
- (int(box_width), int(box_height)))
-
- return dst_img
-
-
-def crop_img(src_img, box, long_edge_pad_ratio=0.4, short_edge_pad_ratio=0.2):
- """Crop text region given the bounding box which might be slightly padded.
- The bounding box is assumed to be a quadrangle and tightly bound the text
- region.
-
- Args:
- src_img (np.array): The original image.
- box (list[float | int]): Points of quadrangle.
- long_edge_pad_ratio (float): The ratio of padding to the long edge. The
- padding will be the length of the short edge * long_edge_pad_ratio.
- Defaults to 0.4.
- short_edge_pad_ratio (float): The ratio of padding to the short edge.
- The padding will be the length of the long edge *
- short_edge_pad_ratio. Defaults to 0.2.
-
- Returns:
- np.array: The cropped image.
- """
- assert is_seq_of(box, (float, int))
- assert len(box) == 8
- assert 0. <= long_edge_pad_ratio < 1.0
- assert 0. <= short_edge_pad_ratio < 1.0
-
- h, w = src_img.shape[:2]
- points_x = np.clip(np.array(box[0::2]), 0, w)
- points_y = np.clip(np.array(box[1::2]), 0, h)
-
- box_width = np.max(points_x) - np.min(points_x)
- box_height = np.max(points_y) - np.min(points_y)
- shorter_size = min(box_height, box_width)
-
- if box_height < box_width:
- horizontal_pad = long_edge_pad_ratio * shorter_size
- vertical_pad = short_edge_pad_ratio * shorter_size
- else:
- horizontal_pad = short_edge_pad_ratio * shorter_size
- vertical_pad = long_edge_pad_ratio * shorter_size
-
- left = np.clip(int(np.min(points_x) - horizontal_pad), 0, w)
- top = np.clip(int(np.min(points_y) - vertical_pad), 0, h)
- right = np.clip(int(np.max(points_x) + horizontal_pad), 0, w)
- bottom = np.clip(int(np.max(points_y) + vertical_pad), 0, h)
-
- dst_img = src_img[top:bottom, left:right]
-
- return dst_img
diff --git a/pipeline/mmocr/mmocr/utils/mask_utils.py b/pipeline/mmocr/mmocr/utils/mask_utils.py
deleted file mode 100644
index a6903072f250766b876f1518be7c613e8c60cebc..0000000000000000000000000000000000000000
--- a/pipeline/mmocr/mmocr/utils/mask_utils.py
+++ /dev/null
@@ -1,40 +0,0 @@
-# Copyright (c) OpenMMLab. All rights reserved.
-import cv2
-import numpy as np
-
-from mmocr.utils.typing_utils import ArrayLike
-
-
-def fill_hole(input_mask: ArrayLike) -> np.array:
- """Fill holes in matrix.
-
- Input:
- [[0, 0, 0, 0, 0, 0, 0],
- [0, 1, 1, 1, 1, 1, 0],
- [0, 1, 0, 0, 0, 1, 0],
- [0, 1, 1, 1, 1, 1, 0],
- [0, 0, 0, 0, 0, 0, 0]]
- Output:
- [[0, 0, 0, 0, 0, 0, 0],
- [0, 1, 1, 1, 1, 1, 0],
- [0, 1, 1, 1, 1, 1, 0],
- [0, 1, 1, 1, 1, 1, 0],
- [0, 0, 0, 0, 0, 0, 0]]
-
- Args:
- input_mask (ArrayLike): The input mask.
-
- Returns:
- np.array: The output mask that has been filled.
- """
- input_mask = np.array(input_mask)
- h, w = input_mask.shape
- canvas = np.zeros((h + 2, w + 2), np.uint8)
- canvas[1:h + 1, 1:w + 1] = input_mask.copy()
-
- mask = np.zeros((h + 4, w + 4), np.uint8)
-
- cv2.floodFill(canvas, mask, (0, 0), 1)
- canvas = canvas[1:h + 1, 1:w + 1].astype(np.bool_)
-
- return ~canvas | input_mask
diff --git a/pipeline/mmocr/mmocr/utils/parsers.py b/pipeline/mmocr/mmocr/utils/parsers.py
deleted file mode 100644
index 87cc063de1252611cf662b5b62c312bbdcfca0c0..0000000000000000000000000000000000000000
--- a/pipeline/mmocr/mmocr/utils/parsers.py
+++ /dev/null
@@ -1,82 +0,0 @@
-# Copyright (c) OpenMMLab. All rights reserved.
-import json
-import warnings
-from typing import Dict, Tuple
-
-from mmocr.registry import TASK_UTILS
-from mmocr.utils.string_utils import StringStripper
-
-
-@TASK_UTILS.register_module()
-class LineStrParser:
- """Parse string of one line in annotation file to dict format.
-
- Args:
- keys (list[str]): Keys in result dict. Defaults to
- ['filename', 'text'].
- keys_idx (list[int]): Value index in sub-string list for each key
- above. Defaults to [0, 1].
- separator (str): Separator to separate string to list of sub-string.
- Defaults to ' '.
- """
-
- def __init__(self,
- keys: Tuple[str, str] = ['filename', 'text'],
- keys_idx: Tuple[int, int] = [0, 1],
- separator: str = ' ',
- **kwargs):
- assert isinstance(keys, list)
- assert isinstance(keys_idx, list)
- assert isinstance(separator, str)
- assert len(keys) > 0
- assert len(keys) == len(keys_idx)
- self.keys = keys
- self.keys_idx = keys_idx
- self.separator = separator
- self.strip_cls = StringStripper(**kwargs)
-
- def __call__(self, in_str: str) -> Dict:
- line_str = self.strip_cls(in_str)
- if len(line_str.split(' ')) > 2:
- msg = 'More than two blank spaces were detected. '
- msg += 'Please use LineJsonParser to handle '
- msg += 'annotations with blanks. '
- msg += 'Check Doc '
- msg += 'https://mmocr.readthedocs.io/en/latest/'
- msg += 'tutorials/blank_recog.html '
- msg += 'for details.'
- warnings.warn(msg, UserWarning)
- line_str = line_str.split(self.separator)
- if len(line_str) <= max(self.keys_idx):
- raise ValueError(
- f'key index: {max(self.keys_idx)} out of range: {line_str}')
-
- line_info = {}
- for i, key in enumerate(self.keys):
- line_info[key] = line_str[self.keys_idx[i]]
- return line_info
-
-
-@TASK_UTILS.register_module()
-class LineJsonParser:
- """Parse json-string of one line in annotation file to dict format.
-
- Args:
- keys (list[str]): Keys in both json-string and result dict. Defaults
- to ['filename', 'text'].
- """
-
- def __init__(self, keys: Tuple[str, str] = ['filename', 'text']) -> None:
- assert isinstance(keys, list)
- assert len(keys) > 0
- self.keys = keys
-
- def __call__(self, in_str: str) -> Dict:
- line_json_obj = json.loads(in_str)
- line_info = {}
- for key in self.keys:
- if key not in line_json_obj:
- raise Exception(f'key {key} not in line json {line_json_obj}')
- line_info[key] = line_json_obj[key]
-
- return line_info
diff --git a/pipeline/mmocr/mmocr/utils/point_utils.py b/pipeline/mmocr/mmocr/utils/point_utils.py
deleted file mode 100644
index 809805f2eaf44337c184216375428f07e99899b9..0000000000000000000000000000000000000000
--- a/pipeline/mmocr/mmocr/utils/point_utils.py
+++ /dev/null
@@ -1,40 +0,0 @@
-# Copyright (c) OpenMMLab. All rights reserved.
-import numpy as np
-
-from mmocr.utils.typing_utils import ArrayLike
-
-
-def points_center(points: ArrayLike) -> np.ndarray:
- """Calculate the center of a set of points.
-
- Args:
- points (ArrayLike): A set of points.
-
- Returns:
- np.ndarray: The coordinate of center point.
- """
- points = np.array(points, dtype=np.float32)
- assert points.size % 2 == 0
-
- points = points.reshape([-1, 2])
- return np.mean(points, axis=0)
-
-
-def point_distance(pt1: ArrayLike, pt2: ArrayLike) -> float:
- """Calculate the distance between two points.
-
- Args:
- pt1 (ArrayLike): The first point.
- pt2 (ArrayLike): The second point.
-
- Returns:
- float: The distance between two points.
- """
- pt1 = np.array(pt1)
- pt2 = np.array(pt2)
-
- assert (pt1.size == 2 and pt2.size == 2)
-
- dist = np.square(pt2 - pt1).sum()
- dist = np.sqrt(dist)
- return dist
diff --git a/pipeline/mmocr/mmocr/utils/polygon_utils.py b/pipeline/mmocr/mmocr/utils/polygon_utils.py
deleted file mode 100644
index 805404a6f49cdc26129cdad4197bab28a4da5556..0000000000000000000000000000000000000000
--- a/pipeline/mmocr/mmocr/utils/polygon_utils.py
+++ /dev/null
@@ -1,457 +0,0 @@
-# Copyright (c) OpenMMLab. All rights reserved.
-import math
-import operator
-from functools import reduce
-from typing import List, Optional, Sequence, Tuple, Union
-
-import numpy as np
-import pyclipper
-import shapely
-from mmengine.utils import is_list_of
-from shapely.geometry import MultiPolygon, Polygon
-
-from mmocr.utils import bbox2poly, valid_boundary
-from mmocr.utils.check_argument import is_2dlist
-from mmocr.utils.typing_utils import ArrayLike
-
-
-def rescale_polygon(polygon: ArrayLike,
- scale_factor: Tuple[int, int],
- mode: str = 'mul') -> np.ndarray:
- """Rescale a polygon according to scale_factor.
-
- The behavior is different depending on the mode. When mode is 'mul', the
- coordinates will be multiplied by scale_factor, which is usually used in
- preprocessing transforms such as :func:`Resize`.
- The coordinates will be divided by scale_factor if mode is 'div'. It can be
- used in postprocessors to recover the polygon in the original
- image size.
-
- Args:
- polygon (ArrayLike): A polygon. In any form can be converted
- to an 1-D numpy array. E.g. list[float], np.ndarray,
- or torch.Tensor. Polygon is written in
- [x1, y1, x2, y2, ...].
- scale_factor (tuple(int, int)): (w_scale, h_scale).
- model (str): Rescale mode. Can be 'mul' or 'div'. Defaults to 'mul'.
-
- Returns:
- np.ndarray: Rescaled polygon.
- """
- assert len(polygon) % 2 == 0
- assert mode in ['mul', 'div']
- polygon = np.array(polygon, dtype=np.float32)
- poly_shape = polygon.shape
- reshape_polygon = polygon.reshape(-1, 2)
- scale_factor = np.array(scale_factor, dtype=float)
- if mode == 'div':
- scale_factor = 1 / scale_factor
- polygon = (reshape_polygon * scale_factor[None]).reshape(poly_shape)
- return polygon
-
-
-def rescale_polygons(polygons: Union[ArrayLike, Sequence[ArrayLike]],
- scale_factor: Tuple[int, int],
- mode: str = 'mul'
- ) -> Union[ArrayLike, Sequence[np.ndarray]]:
- """Rescale polygons according to scale_factor.
-
- The behavior is different depending on the mode. When mode is 'mul', the
- coordinates will be multiplied by scale_factor, which is usually used in
- preprocessing transforms such as :func:`Resize`.
- The coordinates will be divided by scale_factor if mode is 'div'. It can be
- used in postprocessors to recover the polygon in the original
- image size.
-
- Args:
- polygons (list[ArrayLike] or ArrayLike): A list of polygons, each
- written in [x1, y1, x2, y2, ...] and in any form can be converted
- to an 1-D numpy array. E.g. list[list[float]],
- list[np.ndarray], or list[torch.Tensor].
- scale_factor (tuple(int, int)): (w_scale, h_scale).
- model (str): Rescale mode. Can be 'mul' or 'div'. Defaults to 'mul'.
-
- Returns:
- list[np.ndarray] or np.ndarray: Rescaled polygons. The type of the
- return value depends on the type of the input polygons.
- """
- results = []
- for polygon in polygons:
- results.append(rescale_polygon(polygon, scale_factor, mode))
- if isinstance(polygons, np.ndarray):
- results = np.array(results)
- return results
-
-
-def poly2bbox(polygon: ArrayLike) -> np.array:
- """Converting a polygon to a bounding box.
-
- Args:
- polygon (ArrayLike): A polygon. In any form can be converted
- to an 1-D numpy array. E.g. list[float], np.ndarray,
- or torch.Tensor. Polygon is written in
- [x1, y1, x2, y2, ...].
-
- Returns:
- np.array: The converted bounding box [x1, y1, x2, y2]
- """
- assert len(polygon) % 2 == 0
- polygon = np.array(polygon, dtype=np.float32)
- x = polygon[::2]
- y = polygon[1::2]
- return np.array([min(x), min(y), max(x), max(y)])
-
-
-def poly2shapely(polygon: ArrayLike) -> Polygon:
- """Convert a polygon to shapely.geometry.Polygon.
-
- Args:
- polygon (ArrayLike): A set of points of 2k shape.
-
- Returns:
- polygon (Polygon): A polygon object.
- """
- polygon = np.array(polygon, dtype=np.float32)
- assert polygon.size % 2 == 0 and polygon.size >= 6
-
- polygon = polygon.reshape([-1, 2])
- return Polygon(polygon)
-
-
-def polys2shapely(polygons: Sequence[ArrayLike]) -> Sequence[Polygon]:
- """Convert a nested list of boundaries to a list of Polygons.
-
- Args:
- polygons (list): The point coordinates of the instance boundary.
-
- Returns:
- list: Converted shapely.Polygon.
- """
- return [poly2shapely(polygon) for polygon in polygons]
-
-
-def shapely2poly(polygon: Polygon) -> np.array:
- """Convert a nested list of boundaries to a list of Polygons.
-
- Args:
- polygon (Polygon): A polygon represented by shapely.Polygon.
-
- Returns:
- np.array: Converted numpy array
- """
- return np.array(polygon.exterior.coords).reshape(-1, )
-
-
-def crop_polygon(polygon: ArrayLike,
- crop_box: np.ndarray) -> Union[np.ndarray, None]:
- """Crop polygon to be within a box region.
-
- Args:
- polygon (ndarray): polygon in shape (N, ).
- crop_box (ndarray): target box region in shape (4, ).
-
- Returns:
- np.array or None: Cropped polygon. If the polygon is not within the
- crop box, return None.
- """
- poly = poly_make_valid(poly2shapely(polygon))
- crop_poly = poly_make_valid(poly2shapely(bbox2poly(crop_box)))
- area, poly_cropped = poly_intersection(poly, crop_poly, return_poly=True)
- if area == 0 or area is None or not isinstance(
- poly_cropped, shapely.geometry.polygon.Polygon):
- return None
- else:
- poly_cropped = poly_make_valid(poly_cropped)
- poly_cropped = np.array(poly_cropped.boundary.xy, dtype=np.float32)
- poly_cropped = poly_cropped.T
- # reverse poly_cropped to have clockwise order
- poly_cropped = poly_cropped[::-1, :].reshape(-1)
- return poly_cropped
-
-
-def poly_make_valid(poly: Polygon) -> Polygon:
- """Convert a potentially invalid polygon to a valid one by eliminating
- self-crossing or self-touching parts. Note that if the input is a line, the
- returned polygon could be an empty one.
-
- Args:
- poly (Polygon): A polygon needed to be converted.
-
- Returns:
- Polygon: A valid polygon, which might be empty.
- """
- assert isinstance(poly, Polygon)
- fixed_poly = poly if poly.is_valid else poly.buffer(0)
- # Sometimes the fixed_poly is still a MultiPolygon,
- # so we need to find the convex hull of the MultiPolygon, which should
- # always be a Polygon (but could be empty).
- if not isinstance(fixed_poly, Polygon):
- fixed_poly = fixed_poly.convex_hull
- return fixed_poly
-
-
-def poly_intersection(poly_a: Polygon,
- poly_b: Polygon,
- invalid_ret: Optional[Union[float, int]] = None,
- return_poly: bool = False
- ) -> Tuple[float, Optional[Polygon]]:
- """Calculate the intersection area between two polygons.
-
- Args:
- poly_a (Polygon): Polygon a.
- poly_b (Polygon): Polygon b.
- invalid_ret (float or int, optional): The return value when the
- invalid polygon exists. If it is not specified, the function
- allows the computation to proceed with invalid polygons by
- cleaning the their self-touching or self-crossing parts.
- Defaults to None.
- return_poly (bool): Whether to return the polygon of the intersection
- Defaults to False.
-
- Returns:
- float or tuple(float, Polygon): Returns the intersection area or
- a tuple ``(area, Optional[poly_obj])``, where the `area` is the
- intersection area between two polygons and `poly_obj` is The Polygon
- object of the intersection area, which will be `None` if the input is
- invalid. `poly_obj` will be returned only if `return_poly` is `True`.
- """
- assert isinstance(poly_a, Polygon)
- assert isinstance(poly_b, Polygon)
- assert invalid_ret is None or isinstance(invalid_ret, (float, int))
-
- if invalid_ret is None:
- poly_a = poly_make_valid(poly_a)
- poly_b = poly_make_valid(poly_b)
-
- poly_obj = None
- area = invalid_ret
- if poly_a.is_valid and poly_b.is_valid:
- if poly_a.intersects(poly_b):
- poly_obj = poly_a.intersection(poly_b)
- area = poly_obj.area
- else:
- poly_obj = Polygon()
- area = 0.0
- return (area, poly_obj) if return_poly else area
-
-
-def poly_union(
- poly_a: Polygon,
- poly_b: Polygon,
- invalid_ret: Optional[Union[float, int]] = None,
- return_poly: bool = False
-) -> Tuple[float, Optional[Union[Polygon, MultiPolygon]]]:
- """Calculate the union area between two polygons.
-
- Args:
- poly_a (Polygon): Polygon a.
- poly_b (Polygon): Polygon b.
- invalid_ret (float or int, optional): The return value when the
- invalid polygon exists. If it is not specified, the function
- allows the computation to proceed with invalid polygons by
- cleaning the their self-touching or self-crossing parts.
- Defaults to False.
- return_poly (bool): Whether to return the polygon of the union.
- Defaults to False.
-
- Returns:
- tuple: Returns a tuple ``(area, Optional[poly_obj])``, where
- the `area` is the union between two polygons and `poly_obj` is the
- Polygon or MultiPolygon object of the union of the inputs. The type
- of object depends on whether they intersect or not. Set as `None`
- if the input is invalid. `poly_obj` will be returned only if
- `return_poly` is `True`.
- """
- assert isinstance(poly_a, Polygon)
- assert isinstance(poly_b, Polygon)
- assert invalid_ret is None or isinstance(invalid_ret, (float, int))
-
- if invalid_ret is None:
- poly_a = poly_make_valid(poly_a)
- poly_b = poly_make_valid(poly_b)
-
- poly_obj = None
- area = invalid_ret
- if poly_a.is_valid and poly_b.is_valid:
- poly_obj = poly_a.union(poly_b)
- area = poly_obj.area
- return (area, poly_obj) if return_poly else area
-
-
-def poly_iou(poly_a: Polygon,
- poly_b: Polygon,
- zero_division: float = 0.) -> float:
- """Calculate the IOU between two polygons.
-
- Args:
- poly_a (Polygon): Polygon a.
- poly_b (Polygon): Polygon b.
- zero_division (float): The return value when invalid polygon exists.
-
- Returns:
- float: The IoU between two polygons.
- """
- assert isinstance(poly_a, Polygon)
- assert isinstance(poly_b, Polygon)
- area_inters = poly_intersection(poly_a, poly_b)
- area_union = poly_union(poly_a, poly_b)
- return area_inters / area_union if area_union != 0 else zero_division
-
-
-def is_poly_inside_rect(poly: ArrayLike, rect: np.ndarray) -> bool:
- """Check if the polygon is inside the target region.
- Args:
- poly (ArrayLike): Polygon in shape (N, ).
- rect (ndarray): Target region [x1, y1, x2, y2].
-
- Returns:
- bool: Whether the polygon is inside the cropping region.
- """
-
- poly = poly2shapely(poly)
- rect = poly2shapely(bbox2poly(rect))
- return rect.contains(poly)
-
-
-def offset_polygon(poly: ArrayLike, distance: float) -> ArrayLike:
- """Offset (expand/shrink) the polygon by the target distance. It's a
- wrapper around pyclipper based on Vatti clipping algorithm.
-
- Warning:
- Polygon coordinates will be casted to int type in PyClipper. Mind the
- potential precision loss caused by the casting.
-
- Args:
- poly (ArrayLike): A polygon. In any form can be converted
- to an 1-D numpy array. E.g. list[float], np.ndarray,
- or torch.Tensor. Polygon is written in
- [x1, y1, x2, y2, ...].
- distance (float): The offset distance. Positive value means expanding,
- negative value means shrinking.
-
- Returns:
- np.array: 1-D Offsetted polygon ndarray in float32 type. If the
- result polygon is invalid or has been split into several parts,
- return an empty array.
- """
- poly = np.array(poly).reshape(-1, 2)
- pco = pyclipper.PyclipperOffset()
- pco.AddPath(poly, pyclipper.JT_ROUND, pyclipper.ET_CLOSEDPOLYGON)
- # Returned result will be in type of int32, convert it back to float32
- # following MMOCR's convention
- result = np.array(pco.Execute(distance), dtype=object)
- if len(result) > 0 and isinstance(result[0], list):
- # The processed polygon has been split into several parts
- result = np.array([])
- result = result.astype(np.float32)
- # Always use the first polygon since only one polygon is expected
- # But when the resulting polygon is invalid, return the empty array
- # as it is
- return result if len(result) == 0 else result[0].flatten()
-
-
-def boundary_iou(src: List,
- target: List,
- zero_division: Union[int, float] = 0) -> float:
- """Calculate the IOU between two boundaries.
-
- Args:
- src (list): Source boundary.
- target (list): Target boundary.
- zero_division (int or float): The return value when invalid
- boundary exists.
-
- Returns:
- float: The iou between two boundaries.
- """
- assert valid_boundary(src, False)
- assert valid_boundary(target, False)
- src_poly = poly2shapely(src)
- target_poly = poly2shapely(target)
-
- return poly_iou(src_poly, target_poly, zero_division=zero_division)
-
-
-def sort_points(points):
- # TODO Add typehints & test & docstring
- """Sort arbitrary points in clockwise order in Cartesian coordinate, you
- may need to reverse the output sequence if you are using OpenCV's image
- coordinate.
-
- Reference:
- https://github.com/novioleo/Savior/blob/master/Utils/GeometryUtils.py.
-
- Warning: This function can only sort convex polygons.
-
- Args:
- points (list[ndarray] or ndarray or list[list]): A list of unsorted
- boundary points.
-
- Returns:
- list[ndarray]: A list of points sorted in clockwise order.
- """
- assert is_list_of(points, np.ndarray) or isinstance(points, np.ndarray) \
- or is_2dlist(points)
- center_point = tuple(
- map(operator.truediv,
- reduce(lambda x, y: map(operator.add, x, y), points),
- [len(points)] * 2))
- return sorted(
- points,
- key=lambda coord: (180 + math.degrees(
- math.atan2(*tuple(map(operator.sub, coord, center_point))))) % 360)
-
-
-def sort_vertex(points_x, points_y):
- # TODO Add typehints & test
- """Sort box vertices in clockwise order from left-top first.
-
- Args:
- points_x (list[float]): x of four vertices.
- points_y (list[float]): y of four vertices.
-
- Returns:
- tuple[list[float], list[float]]: Sorted x and y of four vertices.
-
- - sorted_points_x (list[float]): x of sorted four vertices.
- - sorted_points_y (list[float]): y of sorted four vertices.
- """
- assert is_list_of(points_x, (float, int))
- assert is_list_of(points_y, (float, int))
- assert len(points_x) == 4
- assert len(points_y) == 4
- vertices = np.stack((points_x, points_y), axis=-1).astype(np.float32)
- vertices = _sort_vertex(vertices)
- sorted_points_x = list(vertices[:, 0])
- sorted_points_y = list(vertices[:, 1])
- return sorted_points_x, sorted_points_y
-
-
-def _sort_vertex(vertices):
- # TODO Add typehints & docstring & test
- assert vertices.ndim == 2
- assert vertices.shape[-1] == 2
- N = vertices.shape[0]
- if N == 0:
- return vertices
-
- center = np.mean(vertices, axis=0)
- directions = vertices - center
- angles = np.arctan2(directions[:, 1], directions[:, 0])
- sort_idx = np.argsort(angles)
- vertices = vertices[sort_idx]
-
- left_top = np.min(vertices, axis=0)
- dists = np.linalg.norm(left_top - vertices, axis=-1, ord=2)
- lefttop_idx = np.argmin(dists)
- indexes = (np.arange(N, dtype=np.int_) + lefttop_idx) % N
- return vertices[indexes]
-
-
-def sort_vertex8(points):
- # TODO Add typehints & docstring & test
- """Sort vertex with 8 points [x1 y1 x2 y2 x3 y3 x4 y4]"""
- assert len(points) == 8
- vertices = _sort_vertex(np.array(points, dtype=np.float32).reshape(-1, 2))
- sorted_box = list(vertices.flatten())
- return sorted_box
diff --git a/pipeline/mmocr/mmocr/utils/processing.py b/pipeline/mmocr/mmocr/utils/processing.py
deleted file mode 100644
index 2da6ff2c90d746c67c18fd1f22e6bd8d1f2bf887..0000000000000000000000000000000000000000
--- a/pipeline/mmocr/mmocr/utils/processing.py
+++ /dev/null
@@ -1,67 +0,0 @@
-# Copyright (c) OpenMMLab. All rights reserved.
-import sys
-from collections.abc import Iterable
-
-from mmengine.utils.progressbar import ProgressBar, init_pool
-
-
-def track_parallel_progress_multi_args(func,
- args,
- nproc,
- initializer=None,
- initargs=None,
- bar_width=50,
- chunksize=1,
- skip_first=False,
- file=sys.stdout):
- """Track the progress of parallel task execution with a progress bar.
-
- The built-in :mod:`multiprocessing` module is used for process pools and
- tasks are done with :func:`Pool.map` or :func:`Pool.imap_unordered`.
-
- Args:
- func (callable): The function to be applied to each task.
- tasks (tuple[Iterable]): A tuple of tasks.
- nproc (int): Process (worker) number.
- initializer (None or callable): Refer to :class:`multiprocessing.Pool`
- for details.
- initargs (None or tuple): Refer to :class:`multiprocessing.Pool` for
- details.
- chunksize (int): Refer to :class:`multiprocessing.Pool` for details.
- bar_width (int): Width of progress bar.
- skip_first (bool): Whether to skip the first sample for each worker
- when estimating fps, since the initialization step may takes
- longer.
- keep_order (bool): If True, :func:`Pool.imap` is used, otherwise
- :func:`Pool.imap_unordered` is used.
-
- Returns:
- list: The task results.
- """
- assert isinstance(args, tuple)
- for arg in args:
- assert isinstance(arg, Iterable)
- assert len(set([len(arg)
- for arg in args])) == 1, 'args must have same length'
- task_num = len(args[0])
- tasks = zip(*args)
-
- pool = init_pool(nproc, initializer, initargs)
- start = not skip_first
- task_num -= nproc * chunksize * int(skip_first)
- prog_bar = ProgressBar(task_num, bar_width, start, file=file)
- results = []
- gen = pool.starmap(func, tasks, chunksize)
- for result in gen:
- results.append(result)
- if skip_first:
- if len(results) < nproc * chunksize:
- continue
- elif len(results) == nproc * chunksize:
- prog_bar.start()
- continue
- prog_bar.update()
- prog_bar.file.write('\n')
- pool.close()
- pool.join()
- return results
diff --git a/pipeline/mmocr/mmocr/utils/setup_env.py b/pipeline/mmocr/mmocr/utils/setup_env.py
deleted file mode 100644
index 32206ecfa3fd847d37750411e3329af8a3a4703d..0000000000000000000000000000000000000000
--- a/pipeline/mmocr/mmocr/utils/setup_env.py
+++ /dev/null
@@ -1,41 +0,0 @@
-# Copyright (c) OpenMMLab. All rights reserved.
-import datetime
-import warnings
-
-from mmengine.registry import DefaultScope
-
-
-def register_all_modules(init_default_scope: bool = True) -> None:
- """Register all modules in mmocr into the registries.
-
- Args:
- init_default_scope (bool): Whether initialize the mmocr default scope.
- When `init_default_scope=True`, the global default scope will be
- set to `mmocr`, and all registries will build modules from mmocr's
- registry node. To understand more about the registry, please refer
- to https://github.com/open-mmlab/mmengine/blob/main/docs/en/tutorials/registry.md
- Defaults to True.
- """ # noqa
- import mmocr.apis # noqa: F401,F403
- import mmocr.datasets # noqa: F401,F403
- import mmocr.engine # noqa: F401,F403
- import mmocr.evaluation # noqa: F401,F403
- import mmocr.models # noqa: F401,F403
- import mmocr.structures # noqa: F401,F403
- import mmocr.visualization # noqa: F401,F403
- if init_default_scope:
- never_created = DefaultScope.get_current_instance() is None \
- or not DefaultScope.check_instance_created('mmocr')
- if never_created:
- DefaultScope.get_instance('mmocr', scope_name='mmocr')
- return
- current_scope = DefaultScope.get_current_instance()
- if current_scope.scope_name != 'mmocr':
- warnings.warn('The current default scope '
- f'"{current_scope.scope_name}" is not "mmocr", '
- '`register_all_modules` will force the current'
- 'default scope to be "mmocr". If this is not '
- 'expected, please set `init_default_scope=False`.')
- # avoid name conflict
- new_instance_name = f'mmocr-{datetime.datetime.now()}'
- DefaultScope.get_instance(new_instance_name, scope_name='mmocr')
diff --git a/pipeline/mmocr/mmocr/utils/string_utils.py b/pipeline/mmocr/mmocr/utils/string_utils.py
deleted file mode 100644
index 4c59740872dc9e086f7f672f9b0f58250d6512c6..0000000000000000000000000000000000000000
--- a/pipeline/mmocr/mmocr/utils/string_utils.py
+++ /dev/null
@@ -1,36 +0,0 @@
-# Copyright (c) OpenMMLab. All rights reserved.
-class StringStripper:
- """Removing the leading and/or the trailing characters based on the string
- argument passed.
-
- Args:
- strip (bool): Whether remove characters from both left and right of
- the string. Default: True.
- strip_pos (str): Which position for removing, can be one of
- ('both', 'left', 'right'), Default: 'both'.
- strip_str (str|None): A string specifying the set of characters
- to be removed from the left and right part of the string.
- If None, all leading and trailing whitespaces
- are removed from the string. Default: None.
- """
-
- def __init__(self, strip=True, strip_pos='both', strip_str=None):
- assert isinstance(strip, bool)
- assert strip_pos in ('both', 'left', 'right')
- assert strip_str is None or isinstance(strip_str, str)
-
- self.strip = strip
- self.strip_pos = strip_pos
- self.strip_str = strip_str
-
- def __call__(self, in_str):
-
- if not self.strip:
- return in_str
-
- if self.strip_pos == 'left':
- return in_str.lstrip(self.strip_str)
- elif self.strip_pos == 'right':
- return in_str.rstrip(self.strip_str)
- else:
- return in_str.strip(self.strip_str)
diff --git a/pipeline/mmocr/mmocr/utils/transform_utils.py b/pipeline/mmocr/mmocr/utils/transform_utils.py
deleted file mode 100644
index 4b45a82517212a67228eaad905d04bdf77d49afe..0000000000000000000000000000000000000000
--- a/pipeline/mmocr/mmocr/utils/transform_utils.py
+++ /dev/null
@@ -1,58 +0,0 @@
-# Copyright (c) OpenMMLab. All rights reserved.
-from typing import Dict, List, Union
-
-import numpy as np
-
-
-def remove_pipeline_elements(results: Dict,
- remove_inds: Union[List[int],
- np.ndarray]) -> Dict:
- """Remove elements in the pipeline given target indexes.
-
- Args:
- results (dict): Result dict from loading pipeline.
- remove_inds (list(int) or np.ndarray): The element indexes to be
- removed.
-
- Required Keys:
-
- - gt_polygons (optional)
- - gt_bboxes (optional)
- - gt_bboxes_labels (optional)
- - gt_ignored (optional)
- - gt_texts (optional)
-
- Modified Keys:
-
- - gt_polygons (optional)
- - gt_bboxes (optional)
- - gt_bboxes_labels (optional)
- - gt_ignored (optional)
- - gt_texts (optional)
-
- Returns:
- dict: The results with element removed.
- """
- keys = [
- 'gt_polygons', 'gt_bboxes', 'gt_bboxes_labels', 'gt_ignored',
- 'gt_texts'
- ]
- num_elements = -1
- for key in keys:
- if key in results:
- num_elements = len(results[key])
- break
- if num_elements == -1:
- return results
- kept_inds = np.array(
- [i for i in range(num_elements) if i not in remove_inds])
- for key in keys:
- if key in results:
- if isinstance(results[key], np.ndarray):
- results[key] = results[key][kept_inds]
- elif isinstance(results[key], list):
- results[key] = [results[key][i] for i in kept_inds]
- else:
- raise TypeError(
- f'Unsupported type {type(results[key])} for key {key}')
- return results
diff --git a/pipeline/mmocr/mmocr/utils/typing_utils.py b/pipeline/mmocr/mmocr/utils/typing_utils.py
deleted file mode 100644
index 592fb36e75ad17d282fe4fce70000227d7bcfa58..0000000000000000000000000000000000000000
--- a/pipeline/mmocr/mmocr/utils/typing_utils.py
+++ /dev/null
@@ -1,50 +0,0 @@
-# Copyright (c) OpenMMLab. All rights reserved.
-"""Collecting some commonly used type hint in MMOCR."""
-
-from typing import Dict, List, Optional, Sequence, Tuple, Union
-
-import numpy as np
-import torch
-from mmengine.config import ConfigDict
-from mmengine.structures import InstanceData, LabelData
-
-from mmocr import digit_version
-from mmocr.structures import (KIEDataSample, TextDetDataSample,
- TextRecogDataSample, TextSpottingDataSample)
-
-# Config
-ConfigType = Union[ConfigDict, Dict]
-OptConfigType = Optional[ConfigType]
-MultiConfig = Union[ConfigType, List[ConfigType]]
-OptMultiConfig = Optional[MultiConfig]
-InitConfigType = Union[Dict, List[Dict]]
-OptInitConfigType = Optional[InitConfigType]
-
-# Data
-InstanceList = List[InstanceData]
-OptInstanceList = Optional[InstanceList]
-LabelList = List[LabelData]
-OptLabelList = Optional[LabelList]
-E2ESampleList = List[TextSpottingDataSample]
-RecSampleList = List[TextRecogDataSample]
-DetSampleList = List[TextDetDataSample]
-KIESampleList = List[KIEDataSample]
-OptRecSampleList = Optional[RecSampleList]
-OptDetSampleList = Optional[DetSampleList]
-OptKIESampleList = Optional[KIESampleList]
-OptE2ESampleList = Optional[E2ESampleList]
-
-OptTensor = Optional[torch.Tensor]
-
-RecForwardResults = Union[Dict[str, torch.Tensor], List[TextRecogDataSample],
- Tuple[torch.Tensor], torch.Tensor]
-
-# Visualization
-ColorType = Union[str, Tuple, List[str], List[Tuple]]
-
-ArrayLike = 'ArrayLike'
-if digit_version(np.__version__) >= digit_version('1.20.0'):
- from numpy.typing import ArrayLike as NP_ARRAY_LIKE
- ArrayLike = NP_ARRAY_LIKE
-
-RangeType = Sequence[Tuple[int, int]]
diff --git a/pipeline/mmocr/mmocr/version.py b/pipeline/mmocr/mmocr/version.py
deleted file mode 100644
index e83928324b12ac13d2e2318fbcdb6b0935b354ec..0000000000000000000000000000000000000000
--- a/pipeline/mmocr/mmocr/version.py
+++ /dev/null
@@ -1,4 +0,0 @@
-# Copyright (c) Open-MMLab. All rights reserved.
-
-__version__ = '1.0.0'
-short_version = __version__
diff --git a/pipeline/mmocr/mmocr/visualization/__init__.py b/pipeline/mmocr/mmocr/visualization/__init__.py
deleted file mode 100644
index b070794bbd486e295520ba7bd141488e0574f92b..0000000000000000000000000000000000000000
--- a/pipeline/mmocr/mmocr/visualization/__init__.py
+++ /dev/null
@@ -1,11 +0,0 @@
-# Copyright (c) OpenMMLab. All rights reserved.
-from .base_visualizer import BaseLocalVisualizer
-from .kie_visualizer import KIELocalVisualizer
-from .textdet_visualizer import TextDetLocalVisualizer
-from .textrecog_visualizer import TextRecogLocalVisualizer
-from .textspotting_visualizer import TextSpottingLocalVisualizer
-
-__all__ = [
- 'BaseLocalVisualizer', 'KIELocalVisualizer', 'TextDetLocalVisualizer',
- 'TextRecogLocalVisualizer', 'TextSpottingLocalVisualizer'
-]
diff --git a/pipeline/mmocr/mmocr/visualization/__pycache__/__init__.cpython-38.pyc b/pipeline/mmocr/mmocr/visualization/__pycache__/__init__.cpython-38.pyc
deleted file mode 100644
index a036d7347082af4418fef02c63af6690fe6e3790..0000000000000000000000000000000000000000
Binary files a/pipeline/mmocr/mmocr/visualization/__pycache__/__init__.cpython-38.pyc and /dev/null differ
diff --git a/pipeline/mmocr/mmocr/visualization/__pycache__/__init__.cpython-39.pyc b/pipeline/mmocr/mmocr/visualization/__pycache__/__init__.cpython-39.pyc
deleted file mode 100644
index 8557521bd36ea4f79e8b368d539781daaea06164..0000000000000000000000000000000000000000
Binary files a/pipeline/mmocr/mmocr/visualization/__pycache__/__init__.cpython-39.pyc and /dev/null differ
diff --git a/pipeline/mmocr/mmocr/visualization/__pycache__/base_visualizer.cpython-38.pyc b/pipeline/mmocr/mmocr/visualization/__pycache__/base_visualizer.cpython-38.pyc
deleted file mode 100644
index 8ba78ad0feee4f7241a076e2fb1ae0b289c6ac53..0000000000000000000000000000000000000000
Binary files a/pipeline/mmocr/mmocr/visualization/__pycache__/base_visualizer.cpython-38.pyc and /dev/null differ
diff --git a/pipeline/mmocr/mmocr/visualization/__pycache__/base_visualizer.cpython-39.pyc b/pipeline/mmocr/mmocr/visualization/__pycache__/base_visualizer.cpython-39.pyc
deleted file mode 100644
index c78ea0a71fb810b06f2da2fb64f4174e6d24acd9..0000000000000000000000000000000000000000
Binary files a/pipeline/mmocr/mmocr/visualization/__pycache__/base_visualizer.cpython-39.pyc and /dev/null differ
diff --git a/pipeline/mmocr/mmocr/visualization/__pycache__/kie_visualizer.cpython-38.pyc b/pipeline/mmocr/mmocr/visualization/__pycache__/kie_visualizer.cpython-38.pyc
deleted file mode 100644
index 2306004964b77d226d0d7f71db56a0eda5eea009..0000000000000000000000000000000000000000
Binary files a/pipeline/mmocr/mmocr/visualization/__pycache__/kie_visualizer.cpython-38.pyc and /dev/null differ
diff --git a/pipeline/mmocr/mmocr/visualization/__pycache__/kie_visualizer.cpython-39.pyc b/pipeline/mmocr/mmocr/visualization/__pycache__/kie_visualizer.cpython-39.pyc
deleted file mode 100644
index debb804804638db5fe94b985fdc17de0a0fca3e4..0000000000000000000000000000000000000000
Binary files a/pipeline/mmocr/mmocr/visualization/__pycache__/kie_visualizer.cpython-39.pyc and /dev/null differ
diff --git a/pipeline/mmocr/mmocr/visualization/__pycache__/textdet_visualizer.cpython-38.pyc b/pipeline/mmocr/mmocr/visualization/__pycache__/textdet_visualizer.cpython-38.pyc
deleted file mode 100644
index 6246bacb7655ca570b77f165fa8c7c9cb7ec2597..0000000000000000000000000000000000000000
Binary files a/pipeline/mmocr/mmocr/visualization/__pycache__/textdet_visualizer.cpython-38.pyc and /dev/null differ
diff --git a/pipeline/mmocr/mmocr/visualization/__pycache__/textdet_visualizer.cpython-39.pyc b/pipeline/mmocr/mmocr/visualization/__pycache__/textdet_visualizer.cpython-39.pyc
deleted file mode 100644
index 33d0716e881c3c0c2da598f4a99981b5793fdc46..0000000000000000000000000000000000000000
Binary files a/pipeline/mmocr/mmocr/visualization/__pycache__/textdet_visualizer.cpython-39.pyc and /dev/null differ
diff --git a/pipeline/mmocr/mmocr/visualization/__pycache__/textrecog_visualizer.cpython-38.pyc b/pipeline/mmocr/mmocr/visualization/__pycache__/textrecog_visualizer.cpython-38.pyc
deleted file mode 100644
index 72ee2d0975f2552a35988c2cdf7706dc27ee2be6..0000000000000000000000000000000000000000
Binary files a/pipeline/mmocr/mmocr/visualization/__pycache__/textrecog_visualizer.cpython-38.pyc and /dev/null differ
diff --git a/pipeline/mmocr/mmocr/visualization/__pycache__/textrecog_visualizer.cpython-39.pyc b/pipeline/mmocr/mmocr/visualization/__pycache__/textrecog_visualizer.cpython-39.pyc
deleted file mode 100644
index b524432da9e3b4dd8d397ba2eef59daedad6e3e1..0000000000000000000000000000000000000000
Binary files a/pipeline/mmocr/mmocr/visualization/__pycache__/textrecog_visualizer.cpython-39.pyc and /dev/null differ
diff --git a/pipeline/mmocr/mmocr/visualization/__pycache__/textspotting_visualizer.cpython-38.pyc b/pipeline/mmocr/mmocr/visualization/__pycache__/textspotting_visualizer.cpython-38.pyc
deleted file mode 100644
index 1c9c68290536c23eb62301f647eae7ecd4d33183..0000000000000000000000000000000000000000
Binary files a/pipeline/mmocr/mmocr/visualization/__pycache__/textspotting_visualizer.cpython-38.pyc and /dev/null differ
diff --git a/pipeline/mmocr/mmocr/visualization/__pycache__/textspotting_visualizer.cpython-39.pyc b/pipeline/mmocr/mmocr/visualization/__pycache__/textspotting_visualizer.cpython-39.pyc
deleted file mode 100644
index 0ee56318a1c25e9cd8bef4f281e29137f7f1b64f..0000000000000000000000000000000000000000
Binary files a/pipeline/mmocr/mmocr/visualization/__pycache__/textspotting_visualizer.cpython-39.pyc and /dev/null differ
diff --git a/pipeline/mmocr/mmocr/visualization/base_visualizer.py b/pipeline/mmocr/mmocr/visualization/base_visualizer.py
deleted file mode 100644
index 38b4479d330bc7700ba8d66615719e76e7a1d8d0..0000000000000000000000000000000000000000
--- a/pipeline/mmocr/mmocr/visualization/base_visualizer.py
+++ /dev/null
@@ -1,261 +0,0 @@
-# Copyright (c) OpenMMLab. All rights reserved.
-import math
-from typing import List, Optional, Sequence, Union
-
-import numpy as np
-import torch
-from matplotlib.font_manager import FontProperties
-from mmengine.visualization import Visualizer
-
-from mmocr.registry import VISUALIZERS
-
-
-@VISUALIZERS.register_module()
-class BaseLocalVisualizer(Visualizer):
- """The MMOCR Text Detection Local Visualizer.
-
- Args:
- name (str): Name of the instance. Defaults to 'visualizer'.
- image (np.ndarray, optional): the origin image to draw. The format
- should be RGB. Defaults to None.
- vis_backends (list, optional): Visual backend config list.
- Default to None.
- save_dir (str, optional): Save file dir for all storage backends.
- If it is None, the backend storage will not save any data.
- fig_save_cfg (dict): Keyword parameters of figure for saving.
- Defaults to empty dict.
- fig_show_cfg (dict): Keyword parameters of figure for showing.
- Defaults to empty dict.
- is_openset (bool, optional): Whether the visualizer is used in
- OpenSet. Defaults to False.
- font_families (Union[str, List[str]]): The font families of labels.
- Defaults to 'sans-serif'.
- font_properties (Union[str, FontProperties], optional):
- The font properties of texts. The format should be a path str
- to font file or a `font_manager.FontProperties()` object.
- If you want to draw Chinese texts, you need to prepare
- a font file that can show Chinese characters properly.
- For example: `simhei.ttf`,`simsun.ttc`,`simkai.ttf` and so on.
- Then set font_properties=matplotlib.font_manager.FontProperties
- (fname='path/to/font_file') or font_properties='path/to/font_file'
- This function need mmengine version >=0.6.0.
- Defaults to None.
- """
- PALETTE = [(220, 20, 60), (119, 11, 32), (0, 0, 142), (0, 0, 230),
- (106, 0, 228), (0, 60, 100), (0, 80, 100), (0, 0, 70),
- (0, 0, 192), (250, 170, 30), (100, 170, 30), (220, 220, 0),
- (175, 116, 175), (250, 0, 30), (165, 42, 42), (255, 77, 255),
- (0, 226, 252), (182, 182, 255), (0, 82, 0), (120, 166, 157),
- (110, 76, 0), (174, 57, 255), (199, 100, 0), (72, 0, 118),
- (255, 179, 240), (0, 125, 92), (209, 0, 151), (188, 208, 182),
- (0, 220, 176), (255, 99, 164), (92, 0, 73), (133, 129, 255),
- (78, 180, 255), (0, 228, 0), (174, 255, 243), (45, 89, 255),
- (134, 134, 103), (145, 148, 174), (255, 208, 186),
- (197, 226, 255), (171, 134, 1), (109, 63, 54), (207, 138, 255),
- (151, 0, 95), (9, 80, 61), (84, 105, 51), (74, 65, 105),
- (166, 196, 102), (208, 195, 210), (255, 109, 65), (0, 143, 149),
- (179, 0, 194), (209, 99, 106), (5, 121, 0), (227, 255, 205),
- (147, 186, 208), (153, 69, 1), (3, 95, 161), (163, 255, 0),
- (119, 0, 170), (0, 182, 199), (0, 165, 120), (183, 130, 88),
- (95, 32, 0), (130, 114, 135), (110, 129, 133), (166, 74, 118),
- (219, 142, 185), (79, 210, 114), (178, 90, 62), (65, 70, 15),
- (127, 167, 115), (59, 105, 106), (142, 108, 45), (196, 172, 0),
- (95, 54, 80), (128, 76, 255), (201, 57, 1), (246, 0, 122),
- (191, 162, 208)]
-
- def __init__(self,
- name: str = 'visualizer',
- font_families: Union[str, List[str]] = 'sans-serif',
- font_properties: Optional[Union[str, FontProperties]] = None,
- **kwargs) -> None:
- super().__init__(name=name, **kwargs)
- self.font_families = font_families
- self.font_properties = self._set_font_properties(font_properties)
-
- def _set_font_properties(self,
- fp: Optional[Union[str, FontProperties]] = None):
- if fp is None:
- return None
- elif isinstance(fp, str):
- return FontProperties(fname=fp)
- elif isinstance(fp, FontProperties):
- return fp
- else:
- raise ValueError(
- 'font_properties argument type should be'
- ' `str` or `matplotlib.font_manager.FontProperties`')
-
- def get_labels_image(
- self,
- image: np.ndarray,
- labels: Union[np.ndarray, torch.Tensor],
- bboxes: Union[np.ndarray, torch.Tensor],
- colors: Union[str, Sequence[str]] = 'k',
- font_size: Union[int, float] = 10,
- auto_font_size: bool = False,
- font_families: Union[str, List[str]] = 'sans-serif',
- font_properties: Optional[Union[str, FontProperties]] = None
- ) -> np.ndarray:
- """Draw labels on image.
-
- Args:
- image (np.ndarray): The origin image to draw. The format
- should be RGB.
- labels (Union[np.ndarray, torch.Tensor]): The labels to draw.
- bboxes (Union[np.ndarray, torch.Tensor]): The bboxes to draw.
- colors (Union[str, Sequence[str]]): The colors of labels.
- ``colors`` can have the same length with labels or just single
- value. If ``colors`` is single value, all the labels will have
- the same colors. Refer to `matplotlib.colors` for full list of
- formats that are accepted. Defaults to 'k'.
- font_size (Union[int, float]): The font size of labels. Defaults
- to 10.
- auto_font_size (bool): Whether to automatically adjust font size.
- Defaults to False.
- font_families (Union[str, List[str]]): The font families of labels.
- Defaults to 'sans-serif'.
- font_properties (Union[str, FontProperties], optional):
- The font properties of texts. The format should be a path str
- to font file or a `font_manager.FontProperties()` object.
- If you want to draw Chinese texts, you need to prepare
- a font file that can show Chinese characters properly.
- For example: `simhei.ttf`,`simsun.ttc`,`simkai.ttf` and so on.
- Then set font_properties=matplotlib.font_manager.FontProperties
- (fname='path/to/font_file') or
- font_properties='path/to/font_file'.
- This function need mmengine version >=0.6.0.
- Defaults to None.
- """
- if not labels and not bboxes:
- return image
- if colors is not None and isinstance(colors, (list, tuple)):
- size = math.ceil(len(labels) / len(colors))
- colors = (colors * size)[:len(labels)]
- if auto_font_size:
- assert font_size is not None and isinstance(
- font_size, (int, float))
- font_size = (bboxes[:, 2:] - bboxes[:, :2]).min(-1) * font_size
- font_size = font_size.tolist()
- self.set_image(image)
- self.draw_texts(
- labels, (bboxes[:, :2] + bboxes[:, 2:]) / 2,
- vertical_alignments='center',
- horizontal_alignments='center',
- colors='k',
- font_sizes=font_size,
- font_families=font_families,
- font_properties=font_properties)
- return self.get_image()
-
- def get_polygons_image(self,
- image: np.ndarray,
- polygons: Sequence[np.ndarray],
- colors: Union[str, Sequence[str]] = 'g',
- filling: bool = False,
- line_width: Union[int, float] = 0.5,
- alpha: float = 0.5) -> np.ndarray:
- """Draw polygons on image.
-
- Args:
- image (np.ndarray): The origin image to draw. The format
- should be RGB.
- polygons (Sequence[np.ndarray]): The polygons to draw. The shape
- should be (N, 2).
- colors (Union[str, Sequence[str]]): The colors of polygons.
- ``colors`` can have the same length with polygons or just
- single value. If ``colors`` is single value, all the polygons
- will have the same colors. Refer to `matplotlib.colors` for
- full list of formats that are accepted. Defaults to 'g'.
- filling (bool): Whether to fill the polygons. Defaults to False.
- line_width (Union[int, float]): The line width of polygons.
- Defaults to 0.5.
- alpha (float): The alpha of polygons. Defaults to 0.5.
-
- Returns:
- np.ndarray: The image with polygons drawn.
- """
- if colors is not None and isinstance(colors, (list, tuple)):
- size = math.ceil(len(polygons) / len(colors))
- colors = (colors * size)[:len(polygons)]
- self.set_image(image)
- if filling:
- self.draw_polygons(
- polygons,
- face_colors=colors,
- edge_colors=colors,
- line_widths=line_width,
- alpha=alpha)
- else:
- self.draw_polygons(
- polygons,
- edge_colors=colors,
- line_widths=line_width,
- alpha=alpha)
- return self.get_image()
-
- def get_bboxes_image(self: Visualizer,
- image: np.ndarray,
- bboxes: Union[np.ndarray, torch.Tensor],
- colors: Union[str, Sequence[str]] = 'g',
- filling: bool = False,
- line_width: Union[int, float] = 0.5,
- alpha: float = 0.5) -> np.ndarray:
- """Draw bboxes on image.
-
- Args:
- image (np.ndarray): The origin image to draw. The format
- should be RGB.
- bboxes (Union[np.ndarray, torch.Tensor]): The bboxes to draw.
- colors (Union[str, Sequence[str]]): The colors of bboxes.
- ``colors`` can have the same length with bboxes or just single
- value. If ``colors`` is single value, all the bboxes will have
- the same colors. Refer to `matplotlib.colors` for full list of
- formats that are accepted. Defaults to 'g'.
- filling (bool): Whether to fill the bboxes. Defaults to False.
- line_width (Union[int, float]): The line width of bboxes.
- Defaults to 0.5.
- alpha (float): The alpha of bboxes. Defaults to 0.5.
-
- Returns:
- np.ndarray: The image with bboxes drawn.
- """
- if colors is not None and isinstance(colors, (list, tuple)):
- size = math.ceil(len(bboxes) / len(colors))
- colors = (colors * size)[:len(bboxes)]
- self.set_image(image)
- if filling:
- self.draw_bboxes(
- bboxes,
- face_colors=colors,
- edge_colors=colors,
- line_widths=line_width,
- alpha=alpha)
- else:
- self.draw_bboxes(
- bboxes,
- edge_colors=colors,
- line_widths=line_width,
- alpha=alpha)
- return self.get_image()
-
- def _draw_instances(self) -> np.ndarray:
- raise NotImplementedError
-
- def _cat_image(self, imgs: Sequence[np.ndarray], axis: int) -> np.ndarray:
- """Concatenate images.
-
- Args:
- imgs (Sequence[np.ndarray]): The images to concatenate.
- axis (int): The axis to concatenate.
-
- Returns:
- np.ndarray: The concatenated image.
- """
- cat_image = list()
- for img in imgs:
- if img is not None:
- cat_image.append(img)
- if len(cat_image):
- return np.concatenate(cat_image, axis=axis)
- else:
- return None
diff --git a/pipeline/mmocr/mmocr/visualization/kie_visualizer.py b/pipeline/mmocr/mmocr/visualization/kie_visualizer.py
deleted file mode 100644
index 753bac2e9b6387cf5c9908f19d4d15389269eb22..0000000000000000000000000000000000000000
--- a/pipeline/mmocr/mmocr/visualization/kie_visualizer.py
+++ /dev/null
@@ -1,402 +0,0 @@
-# Copyright (c) OpenMMLab. All rights reserved.
-import warnings
-from typing import Dict, List, Optional, Sequence, Union
-
-import mmcv
-import numpy as np
-import torch
-from matplotlib.collections import PatchCollection
-from matplotlib.patches import FancyArrow
-from mmengine.visualization import Visualizer
-from mmengine.visualization.utils import (check_type, check_type_and_length,
- color_val_matplotlib, tensor2ndarray,
- value2list)
-
-from mmocr.registry import VISUALIZERS
-from mmocr.structures import KIEDataSample
-from .base_visualizer import BaseLocalVisualizer
-
-
-@VISUALIZERS.register_module()
-class KIELocalVisualizer(BaseLocalVisualizer):
- """The MMOCR Text Detection Local Visualizer.
-
- Args:
- name (str): Name of the instance. Defaults to 'visualizer'.
- image (np.ndarray, optional): the origin image to draw. The format
- should be RGB. Defaults to None.
- vis_backends (list, optional): Visual backend config list.
- Default to None.
- save_dir (str, optional): Save file dir for all storage backends.
- If it is None, the backend storage will not save any data.
- fig_save_cfg (dict): Keyword parameters of figure for saving.
- Defaults to empty dict.
- fig_show_cfg (dict): Keyword parameters of figure for showing.
- Defaults to empty dict.
- is_openset (bool, optional): Whether the visualizer is used in
- OpenSet. Defaults to False.
- """
-
- def __init__(self,
- name: str = 'kie_visualizer',
- is_openset: bool = False,
- **kwargs) -> None:
- super().__init__(name=name, **kwargs)
- self.is_openset = is_openset
-
- def _draw_edge_label(self,
- image: np.ndarray,
- edge_labels: Union[np.ndarray, torch.Tensor],
- bboxes: Union[np.ndarray, torch.Tensor],
- texts: Sequence[str],
- arrow_colors: str = 'g') -> np.ndarray:
- """Draw edge labels on image.
-
- Args:
- image (np.ndarray): The origin image to draw. The format
- should be RGB.
- edge_labels (np.ndarray or torch.Tensor): The edge labels to draw.
- The shape of edge_labels should be (N, N), where N is the
- number of texts.
- bboxes (np.ndarray or torch.Tensor): The bboxes to draw. The shape
- of bboxes should be (N, 4), where N is the number of texts.
- texts (Sequence[str]): The texts to draw. The length of texts
- should be the same as the number of bboxes.
- arrow_colors (str, optional): The colors of arrows. Refer to
- `matplotlib.colors` for full list of formats that are accepted.
- Defaults to 'g'.
-
- Returns:
- np.ndarray: The image with edge labels drawn.
- """
- pairs = np.where(edge_labels > 0)
- if torch.is_tensor(pairs):
- pairs = pairs.cpu()
- key_bboxes = bboxes[pairs[0]]
- value_bboxes = bboxes[pairs[1]]
- x_data = np.stack([(key_bboxes[:, 2] + key_bboxes[:, 0]) / 2,
- (value_bboxes[:, 0] + value_bboxes[:, 2]) / 2],
- axis=-1)
- y_data = np.stack([(key_bboxes[:, 1] + key_bboxes[:, 3]) / 2,
- (value_bboxes[:, 1] + value_bboxes[:, 3]) / 2],
- axis=-1)
- key_index = np.array(list(set(pairs[0])))
- val_index = np.array(list(set(pairs[1])))
- key_texts = [texts[i] for i in key_index]
- val_texts = [texts[i] for i in val_index]
-
- self.set_image(image)
- if key_texts:
- self.draw_texts(
- key_texts, (bboxes[key_index, :2] + bboxes[key_index, 2:]) / 2,
- colors='k',
- horizontal_alignments='center',
- vertical_alignments='center',
- font_families=self.font_families,
- font_properties=self.font_properties)
- if val_texts:
- self.draw_texts(
- val_texts, (bboxes[val_index, :2] + bboxes[val_index, 2:]) / 2,
- colors='k',
- horizontal_alignments='center',
- vertical_alignments='center',
- font_families=self.font_families,
- font_properties=self.font_properties)
- self.draw_arrows(
- x_data,
- y_data,
- colors=arrow_colors,
- line_widths=0.3,
- arrow_tail_widths=0.05,
- arrow_head_widths=5,
- overhangs=1,
- arrow_shapes='full')
- return self.get_image()
-
- def _draw_instances(
- self,
- image: np.ndarray,
- bbox_labels: Union[np.ndarray, torch.Tensor],
- bboxes: Union[np.ndarray, torch.Tensor],
- polygons: Sequence[np.ndarray],
- edge_labels: Union[np.ndarray, torch.Tensor],
- texts: Sequence[str],
- class_names: Dict,
- is_openset: bool = False,
- arrow_colors: str = 'g',
- ) -> np.ndarray:
- """Draw instances on image.
-
- Args:
- image (np.ndarray): The origin image to draw. The format
- should be RGB.
- bbox_labels (np.ndarray or torch.Tensor): The bbox labels to draw.
- The shape of bbox_labels should be (N,), where N is the
- number of texts.
- bboxes (np.ndarray or torch.Tensor): The bboxes to draw. The shape
- of bboxes should be (N, 4), where N is the number of texts.
- polygons (Sequence[np.ndarray]): The polygons to draw. The length
- of polygons should be the same as the number of bboxes.
- edge_labels (np.ndarray or torch.Tensor): The edge labels to draw.
- The shape of edge_labels should be (N, N), where N is the
- number of texts.
- texts (Sequence[str]): The texts to draw. The length of texts
- should be the same as the number of bboxes.
- class_names (dict): The class names for bbox labels.
- is_openset (bool): Whether the dataset is openset. Defaults to
- False.
- arrow_colors (str, optional): The colors of arrows. Refer to
- `matplotlib.colors` for full list of formats that are accepted.
- Defaults to 'g'.
-
- Returns:
- np.ndarray: The image with instances drawn.
- """
- img_shape = image.shape[:2]
- empty_shape = (img_shape[0], img_shape[1], 3)
-
- text_image = np.full(empty_shape, 255, dtype=np.uint8)
- text_image = self.get_labels_image(
- text_image,
- texts,
- bboxes,
- font_families=self.font_families,
- font_properties=self.font_properties)
-
- classes_image = np.full(empty_shape, 255, dtype=np.uint8)
- bbox_classes = [class_names[int(i)]['name'] for i in bbox_labels]
- classes_image = self.get_labels_image(
- classes_image,
- bbox_classes,
- bboxes,
- font_families=self.font_families,
- font_properties=self.font_properties)
- if polygons:
- polygons = [polygon.reshape(-1, 2) for polygon in polygons]
- image = self.get_polygons_image(
- image, polygons, filling=True, colors=self.PALETTE)
- text_image = self.get_polygons_image(
- text_image, polygons, colors=self.PALETTE)
- classes_image = self.get_polygons_image(
- classes_image, polygons, colors=self.PALETTE)
- else:
- image = self.get_bboxes_image(
- image, bboxes, filling=True, colors=self.PALETTE)
- text_image = self.get_bboxes_image(
- text_image, bboxes, colors=self.PALETTE)
- classes_image = self.get_bboxes_image(
- classes_image, bboxes, colors=self.PALETTE)
- cat_image = [image, text_image, classes_image]
- if is_openset:
- edge_image = np.full(empty_shape, 255, dtype=np.uint8)
- edge_image = self._draw_edge_label(edge_image, edge_labels, bboxes,
- texts, arrow_colors)
- cat_image.append(edge_image)
- return self._cat_image(cat_image, axis=1)
-
- def add_datasample(self,
- name: str,
- image: np.ndarray,
- data_sample: Optional['KIEDataSample'] = None,
- draw_gt: bool = True,
- draw_pred: bool = True,
- show: bool = False,
- wait_time: int = 0,
- pred_score_thr: float = None,
- out_file: Optional[str] = None,
- step: int = 0) -> None:
- """Draw datasample and save to all backends.
-
- - If GT and prediction are plotted at the same time, they are
- displayed in a stitched image where the left image is the
- ground truth and the right image is the prediction.
- - If ``show`` is True, all storage backends are ignored, and
- the images will be displayed in a local window.
- - If ``out_file`` is specified, the drawn image will be
- saved to ``out_file``. This is usually used when the display
- is not available.
-
- Args:
- name (str): The image identifier.
- image (np.ndarray): The image to draw.
- data_sample (:obj:`KIEDataSample`, optional):
- KIEDataSample which contains gt and prediction. Defaults
- to None.
- draw_gt (bool): Whether to draw GT KIEDataSample.
- Defaults to True.
- draw_pred (bool): Whether to draw Predicted KIEDataSample.
- Defaults to True.
- show (bool): Whether to display the drawn image. Default to False.
- wait_time (float): The interval of show (s). Defaults to 0.
- pred_score_thr (float): The threshold to visualize the bboxes
- and masks. Defaults to 0.3.
- out_file (str): Path to output file. Defaults to None.
- step (int): Global step value to record. Defaults to 0.
- """
- cat_images = list()
-
- if draw_gt:
- gt_bboxes = data_sample.gt_instances.bboxes
- gt_labels = data_sample.gt_instances.labels
- gt_texts = data_sample.gt_instances.texts
- gt_polygons = data_sample.gt_instances.get('polygons', None)
- gt_edge_labels = data_sample.gt_instances.get('edge_labels', None)
- gt_img_data = self._draw_instances(image, gt_labels, gt_bboxes,
- gt_polygons, gt_edge_labels,
- gt_texts,
- self.dataset_meta['category'],
- self.is_openset, 'g')
- cat_images.append(gt_img_data)
- if draw_pred:
- gt_bboxes = data_sample.gt_instances.bboxes
- pred_labels = data_sample.pred_instances.labels
- gt_texts = data_sample.gt_instances.texts
- gt_polygons = data_sample.gt_instances.get('polygons', None)
- pred_edge_labels = data_sample.pred_instances.get(
- 'edge_labels', None)
- pred_img_data = self._draw_instances(image, pred_labels, gt_bboxes,
- gt_polygons, pred_edge_labels,
- gt_texts,
- self.dataset_meta['category'],
- self.is_openset, 'r')
- cat_images.append(pred_img_data)
-
- cat_images = self._cat_image(cat_images, axis=0)
- if cat_images is None:
- cat_images = image
-
- if show:
- self.show(cat_images, win_name=name, wait_time=wait_time)
- else:
- self.add_image(name, cat_images, step)
-
- if out_file is not None:
- mmcv.imwrite(cat_images[..., ::-1], out_file)
-
- self.set_image(cat_images)
- return self.get_image()
-
- def draw_arrows(self,
- x_data: Union[np.ndarray, torch.Tensor],
- y_data: Union[np.ndarray, torch.Tensor],
- colors: Union[str, tuple, List[str], List[tuple]] = 'C1',
- line_widths: Union[Union[int, float],
- List[Union[int, float]]] = 1,
- line_styles: Union[str, List[str]] = '-',
- arrow_tail_widths: Union[Union[int, float],
- List[Union[int, float]]] = 0.001,
- arrow_head_widths: Union[Union[int, float],
- List[Union[int, float]]] = None,
- arrow_head_lengths: Union[Union[int, float],
- List[Union[int, float]]] = None,
- arrow_shapes: Union[str, List[str]] = 'full',
- overhangs: Union[int, List[int]] = 0) -> 'Visualizer':
- """Draw single or multiple arrows.
-
- Args:
- x_data (np.ndarray or torch.Tensor): The x coordinate of
- each line' start and end points.
- y_data (np.ndarray, torch.Tensor): The y coordinate of
- each line' start and end points.
- colors (str or tuple or list[str or tuple]): The colors of
- lines. ``colors`` can have the same length with lines or just
- single value. If ``colors`` is single value, all the lines
- will have the same colors. Reference to
- https://matplotlib.org/stable/gallery/color/named_colors.html
- for more details. Defaults to 'g'.
- line_widths (int or float or list[int or float]):
- The linewidth of lines. ``line_widths`` can have
- the same length with lines or just single value.
- If ``line_widths`` is single value, all the lines will
- have the same linewidth. Defaults to 2.
- line_styles (str or list[str]]): The linestyle of lines.
- ``line_styles`` can have the same length with lines or just
- single value. If ``line_styles`` is single value, all the
- lines will have the same linestyle. Defaults to '-'.
- arrow_tail_widths (int or float or list[int, float]):
- The width of arrow tails. ``arrow_tail_widths`` can have
- the same length with lines or just single value. If
- ``arrow_tail_widths`` is single value, all the lines will
- have the same width. Defaults to 0.001.
- arrow_head_widths (int or float or list[int, float]):
- The width of arrow heads. ``arrow_head_widths`` can have
- the same length with lines or just single value. If
- ``arrow_head_widths`` is single value, all the lines will
- have the same width. Defaults to None.
- arrow_head_lengths (int or float or list[int, float]):
- The length of arrow heads. ``arrow_head_lengths`` can have
- the same length with lines or just single value. If
- ``arrow_head_lengths`` is single value, all the lines will
- have the same length. Defaults to None.
- arrow_shapes (str or list[str]]): The shapes of arrow heads.
- ``arrow_shapes`` can have the same length with lines or just
- single value. If ``arrow_shapes`` is single value, all the
- lines will have the same shape. Defaults to 'full'.
- overhangs (int or list[int]]): The overhangs of arrow heads.
- ``overhangs`` can have the same length with lines or just
- single value. If ``overhangs`` is single value, all the lines
- will have the same overhangs. Defaults to 0.
- """
- check_type('x_data', x_data, (np.ndarray, torch.Tensor))
- x_data = tensor2ndarray(x_data)
- check_type('y_data', y_data, (np.ndarray, torch.Tensor))
- y_data = tensor2ndarray(y_data)
- assert x_data.shape == y_data.shape, (
- '`x_data` and `y_data` should have the same shape')
- assert x_data.shape[-1] == 2, (
- f'The shape of `x_data` should be (N, 2), but got {x_data.shape}')
- if len(x_data.shape) == 1:
- x_data = x_data[None]
- y_data = y_data[None]
- number_arrow = x_data.shape[0]
- check_type_and_length('colors', colors, (str, tuple, list),
- number_arrow)
- colors = value2list(colors, (str, tuple), number_arrow)
- colors = color_val_matplotlib(colors) # type: ignore
- check_type_and_length('line_widths', line_widths, (int, float),
- number_arrow)
- line_widths = value2list(line_widths, (int, float), number_arrow)
- check_type_and_length('arrow_tail_widths', arrow_tail_widths,
- (int, float), number_arrow)
- check_type_and_length('line_styles', line_styles, str, number_arrow)
- line_styles = value2list(line_styles, str, number_arrow)
- arrow_tail_widths = value2list(arrow_tail_widths, (int, float),
- number_arrow)
- check_type_and_length('arrow_head_widths', arrow_head_widths,
- (int, float, type(None)), number_arrow)
- arrow_head_widths = value2list(arrow_head_widths,
- (int, float, type(None)), number_arrow)
- check_type_and_length('arrow_head_lengths', arrow_head_lengths,
- (int, float, type(None)), number_arrow)
- arrow_head_lengths = value2list(arrow_head_lengths,
- (int, float, type(None)), number_arrow)
- check_type_and_length('arrow_shapes', arrow_shapes, (str, list),
- number_arrow)
- arrow_shapes = value2list(arrow_shapes, (str, list), number_arrow)
- check_type('overhang', overhangs, int)
- overhangs = value2list(overhangs, int, number_arrow)
-
- lines = np.concatenate(
- (x_data.reshape(-1, 2, 1), y_data.reshape(-1, 2, 1)), axis=-1)
- if not self._is_posion_valid(lines):
- warnings.warn(
- 'Warning: The line is out of bounds,'
- ' the drawn line may not be in the image', UserWarning)
- arrows = []
- for i in range(number_arrow):
- arrows.append(
- FancyArrow(
- *tuple(lines[i, 0]),
- *tuple(lines[i, 1] - lines[i, 0]),
- linestyle=line_styles[i],
- color=colors[i],
- length_includes_head=True,
- width=arrow_tail_widths[i],
- head_width=arrow_head_widths[i],
- head_length=arrow_head_lengths[i],
- overhang=overhangs[i],
- shape=arrow_shapes[i],
- linewidth=line_widths[i]))
- p = PatchCollection(arrows, match_original=True)
- self.ax_save.add_collection(p)
- return self
diff --git a/pipeline/mmocr/mmocr/visualization/textdet_visualizer.py b/pipeline/mmocr/mmocr/visualization/textdet_visualizer.py
deleted file mode 100644
index 8b3f54da13984a77ec7ed7a13f3773bed00fc8e3..0000000000000000000000000000000000000000
--- a/pipeline/mmocr/mmocr/visualization/textdet_visualizer.py
+++ /dev/null
@@ -1,194 +0,0 @@
-# Copyright (c) OpenMMLab. All rights reserved.
-from typing import Dict, List, Optional, Sequence, Tuple, Union
-
-import mmcv
-import numpy as np
-import torch
-
-from mmocr.registry import VISUALIZERS
-from mmocr.structures import TextDetDataSample
-from .base_visualizer import BaseLocalVisualizer
-
-
-@VISUALIZERS.register_module()
-class TextDetLocalVisualizer(BaseLocalVisualizer):
- """The MMOCR Text Detection Local Visualizer.
-
- Args:
- name (str): Name of the instance. Defaults to 'visualizer'.
- image (np.ndarray, optional): The origin image to draw. The format
- should be RGB. Defaults to None.
- with_poly (bool): Whether to draw polygons. Defaults to True.
- with_bbox (bool): Whether to draw bboxes. Defaults to False.
- vis_backends (list, optional): Visual backend config list.
- Defaults to None.
- save_dir (str, optional): Save file dir for all storage backends.
- If it is None, the backend storage will not save any data.
- gt_color (Union[str, tuple, list[str], list[tuple]]): The
- colors of GT polygons and bboxes. ``colors`` can have the same
- length with lines or just single value. If ``colors`` is single
- value, all the lines will have the same colors. Refer to
- `matplotlib.colors` for full list of formats that are accepted.
- Defaults to 'g'.
- gt_ignored_color (Union[str, tuple, list[str], list[tuple]]): The
- colors of ignored GT polygons and bboxes. ``colors`` can have
- the same length with lines or just single value. If ``colors``
- is single value, all the lines will have the same colors. Refer
- to `matplotlib.colors` for full list of formats that are accepted.
- Defaults to 'b'.
- pred_color (Union[str, tuple, list[str], list[tuple]]): The
- colors of pred polygons and bboxes. ``colors`` can have the same
- length with lines or just single value. If ``colors`` is single
- value, all the lines will have the same colors. Refer to
- `matplotlib.colors` for full list of formats that are accepted.
- Defaults to 'r'.
- line_width (int, float): The linewidth of lines. Defaults to 2.
- alpha (float): The transparency of bboxes or polygons. Defaults to 0.8.
- """
-
- def __init__(self,
- name: str = 'visualizer',
- image: Optional[np.ndarray] = None,
- with_poly: bool = True,
- with_bbox: bool = False,
- vis_backends: Optional[Dict] = None,
- save_dir: Optional[str] = None,
- gt_color: Union[str, Tuple, List[str], List[Tuple]] = 'g',
- gt_ignored_color: Union[str, Tuple, List[str],
- List[Tuple]] = 'b',
- pred_color: Union[str, Tuple, List[str], List[Tuple]] = 'r',
- line_width: Union[int, float] = 2,
- alpha: float = 0.8) -> None:
- super().__init__(
- name=name,
- image=image,
- vis_backends=vis_backends,
- save_dir=save_dir)
- self.with_poly = with_poly
- self.with_bbox = with_bbox
- self.gt_color = gt_color
- self.gt_ignored_color = gt_ignored_color
- self.pred_color = pred_color
- self.line_width = line_width
- self.alpha = alpha
-
- def _draw_instances(
- self,
- image: np.ndarray,
- bboxes: Union[np.ndarray, torch.Tensor],
- polygons: Sequence[np.ndarray],
- color: Union[str, Tuple, List[str], List[Tuple]] = 'g',
- ) -> np.ndarray:
- """Draw bboxes and polygons on image.
-
- Args:
- image (np.ndarray): The origin image to draw.
- bboxes (Union[np.ndarray, torch.Tensor]): The bboxes to draw.
- polygons (Sequence[np.ndarray]): The polygons to draw.
- color (Union[str, tuple, list[str], list[tuple]]): The
- colors of polygons and bboxes. ``colors`` can have the same
- length with lines or just single value. If ``colors`` is
- single value, all the lines will have the same colors. Refer
- to `matplotlib.colors` for full list of formats that are
- accepted. Defaults to 'g'.
-
- Returns:
- np.ndarray: The image with bboxes and polygons drawn.
- """
- if polygons is not None and self.with_poly:
- polygons = [polygon.reshape(-1, 2) for polygon in polygons]
- image = self.get_polygons_image(
- image, polygons, filling=True, colors=color, alpha=self.alpha)
- if bboxes is not None and self.with_bbox:
- image = self.get_bboxes_image(
- image,
- bboxes,
- colors=color,
- line_width=self.line_width,
- alpha=self.alpha)
- return image
-
- def add_datasample(self,
- name: str,
- image: np.ndarray,
- data_sample: Optional['TextDetDataSample'] = None,
- draw_gt: bool = True,
- draw_pred: bool = True,
- show: bool = False,
- wait_time: int = 0,
- out_file: Optional[str] = None,
- pred_score_thr: float = 0.3,
- step: int = 0) -> None:
- """Draw datasample and save to all backends.
-
- - If GT and prediction are plotted at the same time, they are
- displayed in a stitched image where the left image is the
- ground truth and the right image is the prediction.
- - If ``show`` is True, all storage backends are ignored, and
- the images will be displayed in a local window.
- - If ``out_file`` is specified, the drawn image will be
- saved to ``out_file``. This is usually used when the display
- is not available.
-
- Args:
- name (str): The image identifier.
- image (np.ndarray): The image to draw.
- data_sample (:obj:`TextDetDataSample`, optional):
- TextDetDataSample which contains gt and prediction. Defaults
- to None.
- draw_gt (bool): Whether to draw GT TextDetDataSample.
- Defaults to True.
- draw_pred (bool): Whether to draw Predicted TextDetDataSample.
- Defaults to True.
- show (bool): Whether to display the drawn image. Default to False.
- wait_time (float): The interval of show (s). Defaults to 0.
- out_file (str): Path to output file. Defaults to None.
- pred_score_thr (float): The threshold to visualize the bboxes
- and masks. Defaults to 0.3.
- step (int): Global step value to record. Defaults to 0.
- """
- cat_images = []
- if data_sample is not None:
- if draw_gt and 'gt_instances' in data_sample:
- gt_instances = data_sample.gt_instances
- gt_img_data = image.copy()
- if gt_instances.get('ignored', None) is not None:
- ignore_flags = gt_instances.ignored
- gt_ignored_instances = gt_instances[ignore_flags]
- gt_ignored_polygons = gt_ignored_instances.get(
- 'polygons', None)
- gt_ignored_bboxes = gt_ignored_instances.get(
- 'bboxes', None)
- gt_img_data = self._draw_instances(gt_img_data,
- gt_ignored_bboxes,
- gt_ignored_polygons,
- self.gt_ignored_color)
- gt_instances = gt_instances[~ignore_flags]
- gt_polygons = gt_instances.get('polygons', None)
- gt_bboxes = gt_instances.get('bboxes', None)
- gt_img_data = self._draw_instances(gt_img_data, gt_bboxes,
- gt_polygons, self.gt_color)
- cat_images.append(gt_img_data)
- if draw_pred and 'pred_instances' in data_sample:
- pred_instances = data_sample.pred_instances
- pred_instances = pred_instances[
- pred_instances.scores > pred_score_thr].cpu()
- pred_polygons = pred_instances.get('polygons', None)
- pred_bboxes = pred_instances.get('bboxes', None)
- pred_img_data = self._draw_instances(image.copy(), pred_bboxes,
- pred_polygons,
- self.pred_color)
- cat_images.append(pred_img_data)
- cat_images = self._cat_image(cat_images, axis=1)
- if cat_images is None:
- cat_images = image
- if show:
- self.show(cat_images, win_name=name, wait_time=wait_time)
- else:
- self.add_image(name, cat_images, step)
-
- if out_file is not None:
- mmcv.imwrite(cat_images[..., ::-1], out_file)
-
- self.set_image(cat_images)
- return self.get_image()
diff --git a/pipeline/mmocr/mmocr/visualization/textrecog_visualizer.py b/pipeline/mmocr/mmocr/visualization/textrecog_visualizer.py
deleted file mode 100644
index d2f529b47f40b97d46ffdd73ee467da46e2e92c4..0000000000000000000000000000000000000000
--- a/pipeline/mmocr/mmocr/visualization/textrecog_visualizer.py
+++ /dev/null
@@ -1,144 +0,0 @@
-# Copyright (c) OpenMMLab. All rights reserved.
-from typing import Dict, Optional, Tuple, Union
-
-import cv2
-import mmcv
-import numpy as np
-
-from mmocr.registry import VISUALIZERS
-from mmocr.structures import TextRecogDataSample
-from .base_visualizer import BaseLocalVisualizer
-
-
-@VISUALIZERS.register_module()
-class TextRecogLocalVisualizer(BaseLocalVisualizer):
- """MMOCR Text Detection Local Visualizer.
-
- Args:
- name (str): Name of the instance. Defaults to 'visualizer'.
- image (np.ndarray, optional): The origin image to draw. The format
- should be RGB. Defaults to None.
- vis_backends (list, optional): Visual backend config list.
- Defaults to None.
- save_dir (str, optional): Save file dir for all storage backends.
- If it is None, the backend storage will not save any data.
- gt_color (str or tuple[int, int, int]): Colors of GT text. The tuple of
- color should be in RGB order. Or using an abbreviation of color,
- such as `'g'` for `'green'`. Defaults to 'g'.
- pred_color (str or tuple[int, int, int]): Colors of Predicted text.
- The tuple of color should be in RGB order. Or using an abbreviation
- of color, such as `'r'` for `'red'`. Defaults to 'r'.
- """
-
- def __init__(self,
- name: str = 'visualizer',
- image: Optional[np.ndarray] = None,
- vis_backends: Optional[Dict] = None,
- save_dir: Optional[str] = None,
- gt_color: Optional[Union[str, Tuple[int, int, int]]] = 'g',
- pred_color: Optional[Union[str, Tuple[int, int, int]]] = 'r',
- **kwargs) -> None:
- super().__init__(
- name=name,
- image=image,
- vis_backends=vis_backends,
- save_dir=save_dir,
- **kwargs)
- self.gt_color = gt_color
- self.pred_color = pred_color
-
- def _draw_instances(self, image: np.ndarray, text: str) -> np.ndarray:
- """Draw text on image.
-
- Args:
- image (np.ndarray): The image to draw.
- text (str): The text to draw.
-
- Returns:
- np.ndarray: The image with text drawn.
- """
- height, width = image.shape[:2]
- empty_img = np.full_like(image, 255)
- self.set_image(empty_img)
- font_size = min(0.5 * width / (len(text) + 1), 0.5 * height)
- self.draw_texts(
- text,
- np.array([width / 2, height / 2]),
- colors=self.gt_color,
- font_sizes=font_size,
- vertical_alignments='center',
- horizontal_alignments='center',
- font_families=self.font_families,
- font_properties=self.font_properties)
- text_image = self.get_image()
- return text_image
-
- def add_datasample(self,
- name: str,
- image: np.ndarray,
- data_sample: Optional['TextRecogDataSample'] = None,
- draw_gt: bool = True,
- draw_pred: bool = True,
- show: bool = False,
- wait_time: int = 0,
- pred_score_thr: float = None,
- out_file: Optional[str] = None,
- step=0) -> None:
- """Visualize datasample and save to all backends.
-
- - If GT and prediction are plotted at the same time, they are
- displayed in a stitched image where the left image is the
- ground truth and the right image is the prediction.
- - If ``show`` is True, all storage backends are ignored, and
- the images will be displayed in a local window.
- - If ``out_file`` is specified, the drawn image will be
- saved to ``out_file``. This is usually used when the display
- is not available.
-
- Args:
- name (str): The image title. Defaults to 'image'.
- image (np.ndarray): The image to draw.
- data_sample (:obj:`TextRecogDataSample`, optional):
- TextRecogDataSample which contains gt and prediction.
- Defaults to None.
- draw_gt (bool): Whether to draw GT TextRecogDataSample.
- Defaults to True.
- draw_pred (bool): Whether to draw Predicted TextRecogDataSample.
- Defaults to True.
- show (bool): Whether to display the drawn image. Defaults to False.
- wait_time (float): The interval of show (s). Defaults to 0.
- out_file (str): Path to output file. Defaults to None.
- step (int): Global step value to record. Defaults to 0.
- pred_score_thr (float): Threshold of prediction score. It's not
- used in this function. Defaults to None.
- """
- height, width = image.shape[:2]
- resize_height = 64
- resize_width = int(1.0 * width / height * resize_height)
- image = cv2.resize(image, (resize_width, resize_height))
-
- if image.ndim == 2:
- image = cv2.cvtColor(image, cv2.COLOR_GRAY2RGB)
-
- cat_images = [image]
- if (draw_gt and data_sample is not None and 'gt_text' in data_sample
- and 'item' in data_sample.gt_text):
- gt_text = data_sample.gt_text.item
- cat_images.append(self._draw_instances(image, gt_text))
- if (draw_pred and data_sample is not None
- and 'pred_text' in data_sample
- and 'item' in data_sample.pred_text):
- pred_text = data_sample.pred_text.item
- cat_images.append(self._draw_instances(image, pred_text))
- cat_images = self._cat_image(cat_images, axis=0)
-
- if show:
- self.show(cat_images, win_name=name, wait_time=wait_time)
- else:
- self.add_image(name, cat_images, step)
-
- if out_file is not None:
- mmcv.imwrite(cat_images[..., ::-1], out_file)
-
- self.set_image(cat_images)
- return self.get_image()
diff --git a/pipeline/mmocr/mmocr/visualization/textspotting_visualizer.py b/pipeline/mmocr/mmocr/visualization/textspotting_visualizer.py
deleted file mode 100644
index bd4038c35aadfc346e2b370d5a361462acdaf326..0000000000000000000000000000000000000000
--- a/pipeline/mmocr/mmocr/visualization/textspotting_visualizer.py
+++ /dev/null
@@ -1,144 +0,0 @@
-# Copyright (c) OpenMMLab. All rights reserved.
-from typing import Optional, Sequence, Union
-
-import mmcv
-import numpy as np
-import torch
-
-from mmocr.registry import VISUALIZERS
-from mmocr.structures import TextDetDataSample
-from mmocr.utils.polygon_utils import poly2bbox
-from .base_visualizer import BaseLocalVisualizer
-
-
-@VISUALIZERS.register_module()
-class TextSpottingLocalVisualizer(BaseLocalVisualizer):
-
- def _draw_instances(
- self,
- image: np.ndarray,
- bboxes: Union[np.ndarray, torch.Tensor],
- polygons: Sequence[np.ndarray],
- texts: Sequence[str],
- ) -> np.ndarray:
- """Draw instances on image.
-
- Args:
- image (np.ndarray): The origin image to draw. The format
- should be RGB.
- bboxes (np.ndarray, torch.Tensor): The bboxes to draw. The shape of
- bboxes should be (N, 4), where N is the number of texts.
- polygons (Sequence[np.ndarray]): The polygons to draw. The length
- of polygons should be the same as the number of bboxes.
- edge_labels (np.ndarray, torch.Tensor): The edge labels to draw.
- The shape of edge_labels should be (N, N), where N is the
- number of texts.
- texts (Sequence[str]): The texts to draw. The length of texts
- should be the same as the number of bboxes.
- class_names (dict): The class names for bbox labels.
- is_openset (bool): Whether the dataset is openset. Default: False.
-
- Returns:
- np.ndarray: The image with instances drawn.
- """
- img_shape = image.shape[:2]
- empty_shape = (img_shape[0], img_shape[1], 3)
- text_image = np.full(empty_shape, 255, dtype=np.uint8)
- if texts:
- text_image = self.get_labels_image(
- text_image,
- labels=texts,
- bboxes=bboxes,
- font_families=self.font_families,
- font_properties=self.font_properties)
- if polygons:
- polygons = [polygon.reshape(-1, 2) for polygon in polygons]
- image = self.get_polygons_image(
- image, polygons, filling=True, colors=self.PALETTE)
- text_image = self.get_polygons_image(
- text_image, polygons, colors=self.PALETTE)
- elif len(bboxes) > 0:
- image = self.get_bboxes_image(
- image, bboxes, filling=True, colors=self.PALETTE)
- text_image = self.get_bboxes_image(
- text_image, bboxes, colors=self.PALETTE)
- return np.concatenate([image, text_image], axis=1)
-
- def add_datasample(self,
- name: str,
- image: np.ndarray,
- data_sample: Optional['TextDetDataSample'] = None,
- draw_gt: bool = True,
- draw_pred: bool = True,
- show: bool = False,
- wait_time: int = 0,
- pred_score_thr: float = 0.5,
- out_file: Optional[str] = None,
- step: int = 0) -> None:
- """Draw datasample and save to all backends.
-
- - If GT and prediction are plotted at the same time, they are
- displayed in a stitched image where the left image is the
- ground truth and the right image is the prediction.
- - If ``show`` is True, all storage backends are ignored, and
- the images will be displayed in a local window.
- - If ``out_file`` is specified, the drawn image will be
- saved to ``out_file``. This is usually used when the display
- is not available.
-
- Args:
- name (str): The image identifier.
- image (np.ndarray): The image to draw.
- data_sample (:obj:`TextSpottingDataSample`, optional):
- TextDetDataSample which contains gt and prediction. Defaults
- to None.
- draw_gt (bool): Whether to draw GT TextDetDataSample.
- Defaults to True.
- draw_pred (bool): Whether to draw Predicted TextDetDataSample.
- Defaults to True.
- show (bool): Whether to display the drawn image. Default to False.
- wait_time (float): The interval of show (s). Defaults to 0.
- out_file (str): Path to output file. Defaults to None.
- pred_score_thr (float): The threshold to visualize the bboxes
- and masks. Defaults to 0.3.
- step (int): Global step value to record. Defaults to 0.
- """
- cat_images = []
-
- if data_sample is not None:
- if draw_gt and 'gt_instances' in data_sample:
- gt_bboxes = data_sample.gt_instances.get('bboxes', None)
- gt_texts = data_sample.gt_instances.texts
- gt_polygons = data_sample.gt_instances.get('polygons', None)
- gt_img_data = self._draw_instances(image, gt_bboxes,
- gt_polygons, gt_texts)
- cat_images.append(gt_img_data)
-
- if draw_pred and 'pred_instances' in data_sample:
- pred_instances = data_sample.pred_instances
- pred_instances = pred_instances[
- pred_instances.scores > pred_score_thr].cpu().numpy()
- pred_bboxes = pred_instances.get('bboxes', None)
- pred_texts = pred_instances.texts
- pred_polygons = pred_instances.get('polygons', None)
- if pred_bboxes is None:
- pred_bboxes = [poly2bbox(poly) for poly in pred_polygons]
- pred_bboxes = np.array(pred_bboxes)
- pred_img_data = self._draw_instances(image, pred_bboxes,
- pred_polygons, pred_texts)
- cat_images.append(pred_img_data)
-
- cat_images = self._cat_image(cat_images, axis=0)
- if cat_images is None:
- cat_images = image
-
- if show:
- self.show(cat_images, win_name=name, wait_time=wait_time)
- else:
- self.add_image(name, cat_images, step)
-
- if out_file is not None:
- mmcv.imwrite(cat_images[..., ::-1], out_file)
-
- self.set_image(cat_images)
- return self.get_image()
diff --git a/pipeline/mmocr/model-index.yml b/pipeline/mmocr/model-index.yml
deleted file mode 100644
index 563372c2623fe797281a4a3d0b80ad8c559ea2ef..0000000000000000000000000000000000000000
--- a/pipeline/mmocr/model-index.yml
+++ /dev/null
@@ -1,19 +0,0 @@
-Import:
- - configs/textdet/dbnet/metafile.yml
- - configs/textdet/dbnetpp/metafile.yml
- - configs/textdet/maskrcnn/metafile.yml
- - configs/textdet/drrg/metafile.yml
- - configs/textdet/fcenet/metafile.yml
- - configs/textdet/panet/metafile.yml
- - configs/textdet/psenet/metafile.yml
- - configs/textdet/textsnake/metafile.yml
- - configs/textrecog/abinet/metafile.yml
- - configs/textrecog/aster/metafile.yml
- - configs/textrecog/crnn/metafile.yml
- - configs/textrecog/master/metafile.yml
- - configs/textrecog/nrtr/metafile.yml
- - configs/textrecog/svtr/metafile.yml
- - configs/textrecog/robust_scanner/metafile.yml
- - configs/textrecog/sar/metafile.yml
- - configs/textrecog/satrn/metafile.yml
- - configs/kie/sdmgr/metafile.yml
diff --git a/pipeline/mmocr/projects/ABCNet/README.md b/pipeline/mmocr/projects/ABCNet/README.md
deleted file mode 100644
index 3606ffdafd0d9b7d17411e400356c748e8ed408c..0000000000000000000000000000000000000000
--- a/pipeline/mmocr/projects/ABCNet/README.md
+++ /dev/null
@@ -1,148 +0,0 @@
-# ABCNet: Real-time Scene Text Spotting with Adaptive Bezier-Curve Network
-
-
-
-## Description
-
-This is an implementation of [ABCNet](https://github.com/aim-uofa/AdelaiDet) based on [MMOCR](https://github.com/open-mmlab/mmocr/tree/dev-1.x), [MMCV](https://github.com/open-mmlab/mmcv), and [MMEngine](https://github.com/open-mmlab/mmengine).
-
-**ABCNet** is a conceptually novel, efficient, and fully convolutional framework for text spotting, which address the problem by proposing the Adaptive Bezier-Curve Network (ABCNet). Our contributions are three-fold: 1) For the first time, we adaptively fit arbitrarily-shaped text by a parameterized Bezier curve. 2) We design a novel BezierAlign layer for extracting accurate convolution features of a text instance with arbitrary shapes, significantly improving the precision compared with previous methods. 3) Compared with standard bounding box detection, our Bezier curve detection introduces negligible computation overhead, resulting in superiority of our method in both efficiency and accuracy. Experiments on arbitrarily-shaped benchmark datasets, namely Total-Text and CTW1500, demonstrate that ABCNet achieves state-of-the-art accuracy, meanwhile significantly improving the speed. In particular, on Total-Text, our realtime version is over 10 times faster than recent state-of-the-art methods with a competitive recognition accuracy.
-
-
-
-
-
-## Usage
-
-
-
-### Prerequisites
-
-- Python 3.7
-- PyTorch 1.6 or higher
-- [MIM](https://github.com/open-mmlab/mim)
-- [MMOCR](https://github.com/open-mmlab/mmocr)
-
-All the commands below rely on the correct configuration of `PYTHONPATH`, which should point to the project's directory so that Python can locate the module files. In `ABCNet/` root directory, run the following line to add the current directory to `PYTHONPATH`:
-
-```shell
-# Linux
-export PYTHONPATH=`pwd`:$PYTHONPATH
-# Windows PowerShell
-$env:PYTHONPATH=Get-Location
-```
-
-if the data is not in `ABCNet/`, you can link the data into `ABCNet/`:
-
-```shell
-# Linux
-ln -s ${DataPath} $PYTHONPATH
-# Windows PowerShell
-New-Item -ItemType SymbolicLink -Path $env:PYTHONPATH -Name data -Target ${DataPath}
-```
-
-### Training commands
-
-In the current directory, run the following command to train the model:
-
-```bash
-mim train mmocr config/abcnet/abcnet_resnet50_fpn_500e_icdar2015.py --work-dir work_dirs/
-```
-
-To train on multiple GPUs, e.g. 8 GPUs, run the following command:
-
-```bash
-mim train mmocr config/abcnet/abcnet_resnet50_fpn_500e_icdar2015.py --work-dir work_dirs/ --launcher pytorch --gpus 8
-```
-
-### Testing commands
-
-In the current directory, run the following command to test the model:
-
-```bash
-mim test mmocr config/abcnet/abcnet_resnet50_fpn_500e_icdar2015.py --work-dir work_dirs/ --checkpoint ${CHECKPOINT_PATH}
-```
-
-## Results
-
-Here we provide the baseline version of ABCNet with ResNet50 backbone.
-
-To find more variants, please visit the [official model zoo](https://github.com/aim-uofa/AdelaiDet/blob/master/configs/BAText/README.md).
-
-| Name | Pretrained Model | E2E-None-Hmean | det-Hmean | Download |
-| :-------------------: | :--------------------------------------------------------------------------------: | :------------: | :-------: | :------------------------------------------------------------------------: |
-| v1-icdar2015-finetune | [SynthText](https://download.openmmlab.com/mmocr/textspotting/abcnet/abcnet_resnet50_fpn_500e_icdar2015/abcnet_resnet50_fpn_pretrain-d060636c.pth) | 0.6127 | 0.8753 | [model](https://download.openmmlab.com/mmocr/textspotting/abcnet/abcnet_resnet50_fpn_500e_icdar2015/abcnet_resnet50_fpn_500e_icdar2015-326ac6f4.pth) \| [log](https://download.openmmlab.com/mmocr/textspotting/abcnet/abcnet_resnet50_fpn_500e_icdar2015/20221210_170401.log) |
-
-## Citation
-
-If you find ABCNet useful in your research or applications, please cite ABCNet with the following BibTeX entry.
-
-```BibTeX
-@inproceedings{liu2020abcnet,
- title = {{ABCNet}: Real-time Scene Text Spotting with Adaptive Bezier-Curve Network},
- author = {Liu, Yuliang and Chen, Hao and Shen, Chunhua and He, Tong and Jin, Lianwen and Wang, Liangwei},
- booktitle = {Proc. IEEE Conf. Computer Vision and Pattern Recognition (CVPR)},
- year = {2020}
-}
-```
-
-## Checklist
-
-
-
-- [x] Milestone 1: PR-ready, and acceptable to be one of the `projects/`.
-
- - [x] Finish the code
-
-
-
- - [x] Basic docstrings & proper citation
-
-
-
- - [x] Test-time correctness
-
-
-
- - [x] A full README
-
-
-
-- [x] Milestone 2: Indicates a successful model implementation.
-
- - [x] Training-time correctness
-
-
-
-- [ ] Milestone 3: Good to be a part of our core package!
-
- - [ ] Type hints and docstrings
-
-
-
- - [ ] Unit tests
-
-
-
- - [ ] Code polishing
-
-
-
- - [ ] Metafile.yml
-
-
-
-- [ ] Move your modules into the core package following the codebase's file hierarchy structure.
-
-
-
-- [ ] Refactor your modules into the core package following the codebase's file hierarchy structure.
diff --git a/pipeline/mmocr/projects/ABCNet/README_V2.md b/pipeline/mmocr/projects/ABCNet/README_V2.md
deleted file mode 100644
index 0e4580446dd61307a785de49742157e56acfc981..0000000000000000000000000000000000000000
--- a/pipeline/mmocr/projects/ABCNet/README_V2.md
+++ /dev/null
@@ -1,137 +0,0 @@
-# ABCNet v2: Adaptive Bezier-Curve Network for Real-time End-to-end Text Spotting
-
-
-
-## Description
-
-This is an implementation of [ABCNetV2](https://github.com/aim-uofa/AdelaiDet) based on [MMOCR](https://github.com/open-mmlab/mmocr/tree/dev-1.x), [MMCV](https://github.com/open-mmlab/mmcv), and [MMEngine](https://github.com/open-mmlab/mmengine).
-
-**ABCNetV2** contributions are four-fold: 1) For the first time, we adaptively fit arbitrarily-shaped text by a parameterized Bezier curve, which, compared with segmentation-based methods, can not only provide structured output but also controllable representation. 2) We design a novel BezierAlign layer for extracting accurate convolution features of a text instance of arbitrary shapes, significantly improving the precision of recognition over previous methods. 3) Different from previous methods, which often suffer from complex post-processing and sensitive hyper-parameters, our ABCNet v2 maintains a simple pipeline with the only post-processing non-maximum suppression (NMS). 4) As the performance of text recognition closely depends on feature alignment, ABCNet v2 further adopts a simple yet effective coordinate convolution to encode the position of the convolutional filters, which leads to a considerable improvement with negligible computation overhead. Comprehensive experiments conducted on various bilingual (English and Chinese) benchmark datasets demonstrate that ABCNet v2 can achieve state-of-the-art performance while maintaining very high efficiency.
-
-
-
-
-
-## Usage
-
-
-
-### Prerequisites
-
-- Python 3.7
-- PyTorch 1.6 or higher
-- [MIM](https://github.com/open-mmlab/mim)
-- [MMOCR](https://github.com/open-mmlab/mmocr)
-
-All the commands below rely on the correct configuration of `PYTHONPATH`, which should point to the project's directory so that Python can locate the module files. In `ABCNet/` root directory, run the following line to add the current directory to `PYTHONPATH`:
-
-```shell
-# Linux
-export PYTHONPATH=`pwd`:$PYTHONPATH
-# Windows PowerShell
-$env:PYTHONPATH=Get-Location
-```
-
-if the data is not in `ABCNet/`, you can link the data into `ABCNet/`:
-
-```shell
-# Linux
-ln -s ${DataPath} $PYTHONPATH
-# Windows PowerShell
-New-Item -ItemType SymbolicLink -Path $env:PYTHONPATH -Name data -Target ${DataPath}
-```
-
-### Testing commands
-
-In the current directory, run the following command to test the model:
-
-```bash
-mim test mmocr config/abcnet_v2/abcnet-v2_resnet50_bifpn_500e_icdar2015.py --work-dir work_dirs/ --checkpoint ${CHECKPOINT_PATH}
-```
-
-## Results
-
-Here we provide the baseline version of ABCNet with ResNet50 backbone.
-
-To find more variants, please visit the [official model zoo](https://github.com/aim-uofa/AdelaiDet/blob/master/configs/BAText/README.md).
-
-| Name | Pretrained Model | E2E-None-Hmean | det-Hmean | Download |
-| :-------------------: | :--------------: | :------------: | :-------: | :------------------------------------------------------------------------------------------------------------------------------------------: |
-| v2-icdar2015-finetune | SynthText | 0.6628 | 0.8886 | [model](https://download.openmmlab.com/mmocr/textspotting/abcnet-v2/abcnet-v2_resnet50_bifpn/abcnet-v2_resnet50_bifpn_500e_icdar2015-5e4cc7ed.pth) |
-
-## Citation
-
-If you find ABCNetV2 useful in your research or applications, please cite ABCNetV2 with the following BibTeX entry.
-
-```BibTeX
-@ARTICLE{9525302,
- author={Liu, Yuliang and Shen, Chunhua and Jin, Lianwen and He, Tong and Chen, Peng and Liu, Chongyu and Chen, Hao},
- journal={IEEE Transactions on Pattern Analysis and Machine Intelligence},
- title={ABCNet v2: Adaptive Bezier-Curve Network for Real-time End-to-end Text Spotting},
- year={2021},
- volume={},
- number={},
- pages={1-1},
- doi={10.1109/TPAMI.2021.3107437}}
-```
-
-## Checklist
-
-
-
-- [x] Milestone 1: PR-ready, and acceptable to be one of the `projects/`.
-
- - [x] Finish the code
-
-
-
- - [x] Basic docstrings & proper citation
-
-
-
- - [x] Test-time correctness
-
-
-
- - [x] A full README
-
-
-
-- [ ] Milestone 2: Indicates a successful model implementation.
-
- - [ ] Training-time correctness
-
-
-
-- [ ] Milestone 3: Good to be a part of our core package!
-
- - [ ] Type hints and docstrings
-
-
-
- - [ ] Unit tests
-
-
-
- - [ ] Code polishing
-
-
-
- - [ ] Metafile.yml
-
-
-
-- [ ] Move your modules into the core package following the codebase's file hierarchy structure.
-
-
-
-- [ ] Refactor your modules into the core package following the codebase's file hierarchy structure.
diff --git a/pipeline/mmocr/projects/ABCNet/abcnet/__init__.py b/pipeline/mmocr/projects/ABCNet/abcnet/__init__.py
deleted file mode 100644
index 79dc69bc8e1ce59b7396a733009f8df5d964722f..0000000000000000000000000000000000000000
--- a/pipeline/mmocr/projects/ABCNet/abcnet/__init__.py
+++ /dev/null
@@ -1,5 +0,0 @@
-# Copyright (c) OpenMMLab. All rights reserved.
-# Copyright (c) OpenMMLab. All rights reserved.
-from .metric import * # NOQA
-from .model import * # NOQA
-from .utils import * # NOQA
diff --git a/pipeline/mmocr/projects/ABCNet/abcnet/metric/__init__.py b/pipeline/mmocr/projects/ABCNet/abcnet/metric/__init__.py
deleted file mode 100644
index cbf8e944556c9c3c699958e54a9cb0e20fbe3134..0000000000000000000000000000000000000000
--- a/pipeline/mmocr/projects/ABCNet/abcnet/metric/__init__.py
+++ /dev/null
@@ -1,4 +0,0 @@
-# Copyright (c) OpenMMLab. All rights reserved.
-from .e2e_hmean_iou_metric import E2EHmeanIOUMetric
-
-__all__ = ['E2EHmeanIOUMetric']
diff --git a/pipeline/mmocr/projects/ABCNet/abcnet/metric/e2e_hmean_iou_metric.py b/pipeline/mmocr/projects/ABCNet/abcnet/metric/e2e_hmean_iou_metric.py
deleted file mode 100644
index bdab4375e41e3ff8051ebc1b873842ebc04f1e44..0000000000000000000000000000000000000000
--- a/pipeline/mmocr/projects/ABCNet/abcnet/metric/e2e_hmean_iou_metric.py
+++ /dev/null
@@ -1,370 +0,0 @@
-# Copyright (c) OpenMMLab. All rights reserved.
-from typing import Dict, List, Optional, Sequence
-
-import numpy as np
-import torch
-from mmengine.evaluator import BaseMetric
-from mmengine.logging import MMLogger
-from scipy.sparse import csr_matrix
-from scipy.sparse.csgraph import maximum_bipartite_matching
-from shapely.geometry import Polygon
-
-from mmocr.evaluation.functional import compute_hmean
-from mmocr.registry import METRICS
-from mmocr.utils import poly_intersection, poly_iou, polys2shapely
-
-
-@METRICS.register_module()
-class E2EHmeanIOUMetric(BaseMetric):
- # TODO docstring
- """HmeanIOU metric.
-
- This method computes the hmean iou metric, which is done in the
- following steps:
-
- - Filter the prediction polygon:
-
- - Scores is smaller than minimum prediction score threshold.
- - The proportion of the area that intersects with gt ignored polygon is
- greater than ignore_precision_thr.
-
- - Computing an M x N IoU matrix, where each element indexing
- E_mn represents the IoU between the m-th valid GT and n-th valid
- prediction.
- - Based on different prediction score threshold:
- - Obtain the ignored predictions according to prediction score.
- The filtered predictions will not be involved in the later metric
- computations.
- - Based on the IoU matrix, get the match metric according to
- ``match_iou_thr``.
- - Based on different `strategy`, accumulate the match number.
- - calculate H-mean under different prediction score threshold.
-
- Args:
- match_iou_thr (float): IoU threshold for a match. Defaults to 0.5.
- ignore_precision_thr (float): Precision threshold when prediction and\
- gt ignored polygons are matched. Defaults to 0.5.
- pred_score_thrs (dict): Best prediction score threshold searching
- space. Defaults to dict(start=0.3, stop=0.9, step=0.1).
- strategy (str): Polygon matching strategy. Options are 'max_matching'
- and 'vanilla'. 'max_matching' refers to the optimum strategy that
- maximizes the number of matches. Vanilla strategy matches gt and
- pred polygons if both of them are never matched before. It was used
- in MMOCR 0.x and and academia. Defaults to 'vanilla'.
- collect_device (str): Device name used for collecting results from
- different ranks during distributed training. Must be 'cpu' or
- 'gpu'. Defaults to 'cpu'.
- prefix (str, optional): The prefix that will be added in the metric
- names to disambiguate homonymous metrics of different evaluators.
- If prefix is not provided in the argument, self.default_prefix
- will be used instead. Defaults to None
- """
- default_prefix: Optional[str] = 'e2e_icdar'
-
- def __init__(self,
- match_iou_thr: float = 0.5,
- ignore_precision_thr: float = 0.5,
- pred_score_thrs: Dict = dict(start=0.3, stop=0.9, step=0.1),
- lexicon_path: Optional[str] = None,
- word_spotting: bool = False,
- min_length_case_word: int = 3,
- special_characters: str = "'!?.:,*\"()ยท[]/",
- strategy: str = 'vanilla',
- collect_device: str = 'cpu',
- prefix: Optional[str] = None) -> None:
- super().__init__(collect_device=collect_device, prefix=prefix)
- self.match_iou_thr = match_iou_thr
- self.ignore_precision_thr = ignore_precision_thr
- self.pred_score_thrs = np.arange(**pred_score_thrs)
- self.word_spotting = word_spotting
- self.min_length_case_word = min_length_case_word
- self.special_characters = special_characters
- assert strategy in ['max_matching', 'vanilla']
- self.strategy = strategy
-
- def process(self, data_batch: Sequence[Dict],
- data_samples: Sequence[Dict]) -> None:
- """Process one batch of data samples and predictions. The processed
- results should be stored in ``self.results``, which will be used to
- compute the metrics when all batches have been processed.
-
- Args:
- data_batch (Sequence[Dict]): A batch of data from dataloader.
- data_samples (Sequence[Dict]): A batch of outputs from
- the model.
- """
- for data_sample in data_samples:
-
- pred_instances = data_sample.get('pred_instances')
- pred_polygons = pred_instances.get('polygons')
- pred_scores = pred_instances.get('scores')
- if isinstance(pred_scores, torch.Tensor):
- pred_scores = pred_scores.cpu().numpy()
- pred_scores = np.array(pred_scores, dtype=np.float32)
- pred_texts = pred_instances.get('texts')
-
- gt_instances = data_sample.get('gt_instances')
- gt_polys = gt_instances.get('polygons')
- gt_ignore_flags = gt_instances.get('ignored')
- gt_texts = gt_instances.get('texts')
- if isinstance(gt_ignore_flags, torch.Tensor):
- gt_ignore_flags = gt_ignore_flags.cpu().numpy()
- gt_polys = polys2shapely(gt_polys)
- pred_polys = polys2shapely(pred_polygons)
- if self.word_spotting:
- gt_ignore_flags, gt_texts = self._word_spotting_filter(
- gt_ignore_flags, gt_texts)
- pred_ignore_flags = self._filter_preds(pred_polys, gt_polys,
- pred_scores,
- gt_ignore_flags)
- pred_indexes = self._true_indexes(~pred_ignore_flags)
- gt_indexes = self._true_indexes(~gt_ignore_flags)
- pred_texts = [pred_texts[i] for i in pred_indexes]
- gt_texts = [gt_texts[i] for i in gt_indexes]
-
- gt_num = np.sum(~gt_ignore_flags)
- pred_num = np.sum(~pred_ignore_flags)
- iou_metric = np.zeros([gt_num, pred_num])
-
- # Compute IoU scores amongst kept pred and gt polygons
- for pred_mat_id, pred_poly_id in enumerate(pred_indexes):
- for gt_mat_id, gt_poly_id in enumerate(gt_indexes):
- iou_metric[gt_mat_id, pred_mat_id] = poly_iou(
- gt_polys[gt_poly_id], pred_polys[pred_poly_id])
-
- result = dict(
- gt_texts=gt_texts,
- pred_texts=pred_texts,
- iou_metric=iou_metric,
- pred_scores=pred_scores[~pred_ignore_flags])
- self.results.append(result)
-
- def compute_metrics(self, results: List[Dict]) -> Dict:
- """Compute the metrics from processed results.
-
- Args:
- results (list[dict]): The processed results of each batch.
-
- Returns:
- dict: The computed metrics. The keys are the names of the metrics,
- and the values are corresponding results.
- """
- logger: MMLogger = MMLogger.get_current_instance()
-
- best_eval_results = dict(hmean=-1)
- logger.info('Evaluating hmean-iou...')
-
- dataset_pred_num = np.zeros_like(self.pred_score_thrs)
- dataset_hit_num = np.zeros_like(self.pred_score_thrs)
- dataset_gt_num = 0
- for result in results:
- iou_metric = result['iou_metric'] # (gt_num, pred_num)
- pred_scores = result['pred_scores'] # (pred_num)
- gt_texts = result['gt_texts']
- pred_texts = result['pred_texts']
- dataset_gt_num += iou_metric.shape[0]
-
- # Filter out predictions by IoU threshold
- for i, pred_score_thr in enumerate(self.pred_score_thrs):
- pred_ignore_flags = pred_scores < pred_score_thr
- # get the number of matched boxes
- pred_texts = [
- pred_texts[j]
- for j in self._true_indexes(~pred_ignore_flags)
- ]
- matched_metric = iou_metric[:, ~pred_ignore_flags] \
- > self.match_iou_thr
- if self.strategy == 'max_matching':
- csr_matched_metric = csr_matrix(matched_metric)
- matched_preds = maximum_bipartite_matching(
- csr_matched_metric, perm_type='row')
- # -1 denotes unmatched pred polygons
- dataset_hit_num[i] += np.sum(matched_preds != -1)
- else:
- # first come first matched
- matched_gt_indexes = set()
- matched_pred_indexes = set()
- matched_e2e_gt_indexes = set()
- for gt_idx, pred_idx in zip(*np.nonzero(matched_metric)):
- if gt_idx in matched_gt_indexes or \
- pred_idx in matched_pred_indexes:
- continue
- matched_gt_indexes.add(gt_idx)
- matched_pred_indexes.add(pred_idx)
- if self.word_spotting:
- if gt_texts[gt_idx] == pred_texts[pred_idx]:
- matched_e2e_gt_indexes.add(gt_idx)
- else:
- if self.text_match(gt_texts[gt_idx].upper(),
- pred_texts[pred_idx].upper()):
- matched_e2e_gt_indexes.add(gt_idx)
- dataset_hit_num[i] += len(matched_e2e_gt_indexes)
- dataset_pred_num[i] += np.sum(~pred_ignore_flags)
-
- for i, pred_score_thr in enumerate(self.pred_score_thrs):
- recall, precision, hmean = compute_hmean(
- int(dataset_hit_num[i]), int(dataset_hit_num[i]),
- int(dataset_gt_num), int(dataset_pred_num[i]))
- eval_results = dict(
- precision=precision, recall=recall, hmean=hmean)
- logger.info(f'prediction score threshold: {pred_score_thr:.2f}, '
- f'recall: {eval_results["recall"]:.4f}, '
- f'precision: {eval_results["precision"]:.4f}, '
- f'hmean: {eval_results["hmean"]:.4f}\n')
- if eval_results['hmean'] > best_eval_results['hmean']:
- best_eval_results = eval_results
- return best_eval_results
-
- def _filter_preds(self, pred_polys: List[Polygon], gt_polys: List[Polygon],
- pred_scores: List[float],
- gt_ignore_flags: np.ndarray) -> np.ndarray:
- """Filter out the predictions by score threshold and whether it
- overlaps ignored gt polygons.
-
- Args:
- pred_polys (list[Polygon]): Pred polygons.
- gt_polys (list[Polygon]): GT polygons.
- pred_scores (list[float]): Pred scores of polygons.
- gt_ignore_flags (np.ndarray): 1D boolean array indicating
- the positions of ignored gt polygons.
-
- Returns:
- np.ndarray: 1D boolean array indicating the positions of ignored
- pred polygons.
- """
-
- # Filter out predictions based on the minimum score threshold
- pred_ignore_flags = pred_scores < self.pred_score_thrs.min()
- pred_indexes = self._true_indexes(~pred_ignore_flags)
- gt_indexes = self._true_indexes(gt_ignore_flags)
- # Filter out pred polygons which overlaps any ignored gt polygons
- for pred_id in pred_indexes:
- for gt_id in gt_indexes:
- # Match pred with ignored gt
- precision = poly_intersection(
- gt_polys[gt_id], pred_polys[pred_id]) / (
- pred_polys[pred_id].area + 1e-5)
- if precision > self.ignore_precision_thr:
- pred_ignore_flags[pred_id] = True
- break
-
- return pred_ignore_flags
-
- def _true_indexes(self, array: np.ndarray) -> np.ndarray:
- """Get indexes of True elements from a 1D boolean array."""
- return np.where(array)[0]
-
- def _include_in_dictionary(self, text):
- """Function used in Word Spotting that finds if the Ground Truth text
- meets the rules to enter into the dictionary.
-
- If not, the text will be cared as don't care
- """
- # special case 's at final
- if text[len(text) - 2:] == "'s" or text[len(text) - 2:] == "'S":
- text = text[0:len(text) - 2]
-
- # hyphens at init or final of the word
- text = text.strip('-')
-
- for character in self.special_characters:
- text = text.replace(character, ' ')
-
- text = text.strip()
-
- if len(text) != len(text.replace(' ', '')):
- return False
-
- if len(text) < self.min_length_case_word:
- return False
-
- notAllowed = 'รรทฮ'
-
- range1 = [ord(u'a'), ord(u'z')]
- range2 = [ord(u'A'), ord(u'Z')]
- range3 = [ord(u'ร'), ord(u'ฦฟ')]
- range4 = [ord(u'ว'), ord(u'ษฟ')]
- range5 = [ord(u'ฮ'), ord(u'ฯฟ')]
- range6 = [ord(u'-'), ord(u'-')]
-
- for char in text:
- charCode = ord(char)
- if (notAllowed.find(char) != -1):
- return False
- # TODO: optimize it with for loop
- valid = (charCode >= range1[0] and charCode <= range1[1]) or (
- charCode >= range2[0] and charCode <= range2[1]
- ) or (charCode >= range3[0] and charCode <= range3[1]) or (
- charCode >= range4[0] and charCode <= range4[1]) or (
- charCode >= range5[0]
- and charCode <= range5[1]) or (charCode >= range6[0]
- and charCode <= range6[1])
- if not valid:
- return False
-
- return True
-
- def _include_in_dictionary_text(self, text):
- """Function applied to the Ground Truth texts used in Word Spotting.
-
- It removes special characters or terminations
- """
- # special case 's at final
- if text[len(text) - 2:] == "'s" or text[len(text) - 2:] == "'S":
- text = text[0:len(text) - 2]
-
- # hyphens at init or final of the word
- text = text.strip('-')
-
- for character in self.special_characters:
- text = text.replace(character, ' ')
-
- text = text.strip()
-
- return text
-
- def text_match(self,
- gt_text,
- pred_text,
- only_remove_first_end_character=True):
-
- if only_remove_first_end_character:
- # special characters in GT are allowed only at initial or final
- # position
- if (gt_text == pred_text):
- return True
-
- if self.special_characters.find(gt_text[0]) > -1:
- if gt_text[1:] == pred_text:
- return True
-
- if self.special_characters.find(gt_text[-1]) > -1:
- if gt_text[0:len(gt_text) - 1] == pred_text:
- return True
-
- if self.special_characters.find(
- gt_text[0]) > -1 and self.special_characters.find(
- gt_text[-1]) > -1:
- if gt_text[1:len(gt_text) - 1] == pred_text:
- return True
- return False
- else:
- # Special characters are removed from the beginning and the end of
- # both Detection and GroundTruth
- while len(gt_text) > 0 and self.special_characters.find(
- gt_text[0]) > -1:
- gt_text = gt_text[1:]
-
- while len(pred_text) > 0 and self.special_characters.find(
- pred_text[0]) > -1:
- pred_text = pred_text[1:]
-
- while len(gt_text) > 0 and self.special_characters.find(
- gt_text[-1]) > -1:
- gt_text = gt_text[0:len(gt_text) - 1]
-
- while len(pred_text) > 0 and self.special_characters.find(
- pred_text[-1]) > -1:
- pred_text = pred_text[0:len(pred_text) - 1]
-
- return gt_text == pred_text
diff --git a/pipeline/mmocr/projects/ABCNet/abcnet/model/__init__.py b/pipeline/mmocr/projects/ABCNet/abcnet/model/__init__.py
deleted file mode 100644
index f22d9b4f1f7999226030438b99569c14c6f2c4a8..0000000000000000000000000000000000000000
--- a/pipeline/mmocr/projects/ABCNet/abcnet/model/__init__.py
+++ /dev/null
@@ -1,21 +0,0 @@
-# Copyright (c) OpenMMLab. All rights reserved.
-from .abcnet import ABCNet
-from .abcnet_det_head import ABCNetDetHead
-from .abcnet_det_module_loss import ABCNetDetModuleLoss
-from .abcnet_det_postprocessor import ABCNetDetPostprocessor
-from .abcnet_postprocessor import ABCNetPostprocessor
-from .abcnet_rec import ABCNetRec
-from .abcnet_rec_backbone import ABCNetRecBackbone
-from .abcnet_rec_decoder import ABCNetRecDecoder
-from .abcnet_rec_encoder import ABCNetRecEncoder
-from .bezier_roi_extractor import BezierRoIExtractor
-from .bifpn import BiFPN
-from .coordinate_head import CoordinateHead
-from .rec_roi_head import RecRoIHead
-
-__all__ = [
- 'ABCNetDetHead', 'ABCNetDetPostprocessor', 'ABCNetRecBackbone',
- 'ABCNetRecDecoder', 'ABCNetRecEncoder', 'ABCNet', 'ABCNetRec',
- 'BezierRoIExtractor', 'RecRoIHead', 'ABCNetPostprocessor',
- 'ABCNetDetModuleLoss', 'BiFPN', 'CoordinateHead'
-]
diff --git a/pipeline/mmocr/projects/ABCNet/abcnet/model/abcnet.py b/pipeline/mmocr/projects/ABCNet/abcnet/model/abcnet.py
deleted file mode 100644
index 7a341226ac91cf4d9154bea8080a0ba6f808235f..0000000000000000000000000000000000000000
--- a/pipeline/mmocr/projects/ABCNet/abcnet/model/abcnet.py
+++ /dev/null
@@ -1,8 +0,0 @@
-# Copyright (c) OpenMMLab. All rights reserved.
-from mmocr.registry import MODELS
-from .two_stage_text_spotting import TwoStageTextSpotter
-
-
-@MODELS.register_module()
-class ABCNet(TwoStageTextSpotter):
- """CTC-loss based recognizer."""
diff --git a/pipeline/mmocr/projects/ABCNet/abcnet/model/abcnet_det_head.py b/pipeline/mmocr/projects/ABCNet/abcnet/model/abcnet_det_head.py
deleted file mode 100644
index 4eb45d905e56f937bfcfd2a6e74831468bbc2373..0000000000000000000000000000000000000000
--- a/pipeline/mmocr/projects/ABCNet/abcnet/model/abcnet_det_head.py
+++ /dev/null
@@ -1,197 +0,0 @@
-# Copyright (c) OpenMMLab. All rights reserved.
-import torch.nn as nn
-from mmcv.cnn import ConvModule, Scale
-from mmdet.models.utils import multi_apply
-
-from mmocr.models.textdet.heads.base import BaseTextDetHead
-from mmocr.registry import MODELS
-
-INF = 1e8
-
-
-@MODELS.register_module()
-class ABCNetDetHead(BaseTextDetHead):
-
- def __init__(self,
- in_channels,
- module_loss=dict(type='ABCNetLoss'),
- postprocessor=dict(type='ABCNetDetPostprocessor'),
- num_classes=1,
- strides=(4, 8, 16, 32, 64),
- feat_channels=256,
- stacked_convs=4,
- dcn_on_last_conv=False,
- conv_bias='auto',
- norm_on_bbox=False,
- centerness_on_reg=False,
- use_sigmoid_cls=True,
- with_bezier=False,
- use_scale=False,
- conv_cfg=None,
- norm_cfg=dict(type='GN', num_groups=32, requires_grad=True),
- init_cfg=dict(
- type='Normal',
- layer='Conv2d',
- std=0.01,
- override=dict(
- type='Normal',
- name='conv_cls',
- std=0.01,
- bias_prob=0.01))):
- super().__init__(
- module_loss=module_loss,
- postprocessor=postprocessor,
- init_cfg=init_cfg)
- self.num_classes = num_classes
- self.in_channels = in_channels
- self.strides = strides
- self.feat_channels = feat_channels
- self.stacked_convs = stacked_convs
- self.dcn_on_last_conv = dcn_on_last_conv
- assert conv_bias == 'auto' or isinstance(conv_bias, bool)
- self.conv_bias = conv_bias
- self.norm_on_bbox = norm_on_bbox
- self.centerness_on_reg = centerness_on_reg
- self.conv_cfg = conv_cfg
- self.norm_cfg = norm_cfg
- self.with_bezier = with_bezier
- self.use_scale = use_scale
- self.use_sigmoid_cls = use_sigmoid_cls
- if self.use_sigmoid_cls:
- self.cls_out_channels = num_classes
- else:
- self.cls_out_channels = num_classes + 1
-
- self._init_layers()
-
- def _init_layers(self):
- """Initialize layers of the head."""
- self._init_cls_convs()
- self._init_reg_convs()
- self._init_predictor()
- self.conv_centerness = nn.Conv2d(self.feat_channels, 1, 3, padding=1)
- # if self.use_scale:
- self.scales = nn.ModuleList([Scale(1.0) for _ in self.strides])
-
- def _init_cls_convs(self):
- """Initialize classification conv layers of the head."""
- self.cls_convs = nn.ModuleList()
- for i in range(self.stacked_convs):
- chn = self.in_channels if i == 0 else self.feat_channels
- if self.dcn_on_last_conv and i == self.stacked_convs - 1:
- conv_cfg = dict(type='DCNv2')
- else:
- conv_cfg = self.conv_cfg
- self.cls_convs.append(
- ConvModule(
- chn,
- self.feat_channels,
- 3,
- stride=1,
- padding=1,
- conv_cfg=conv_cfg,
- norm_cfg=self.norm_cfg,
- bias=self.conv_bias))
-
- def _init_reg_convs(self):
- """Initialize bbox regression conv layers of the head."""
- self.reg_convs = nn.ModuleList()
- for i in range(self.stacked_convs):
- chn = self.in_channels if i == 0 else self.feat_channels
- if self.dcn_on_last_conv and i == self.stacked_convs - 1:
- conv_cfg = dict(type='DCNv2')
- else:
- conv_cfg = self.conv_cfg
- self.reg_convs.append(
- ConvModule(
- chn,
- self.feat_channels,
- 3,
- stride=1,
- padding=1,
- conv_cfg=conv_cfg,
- norm_cfg=self.norm_cfg,
- bias=self.conv_bias))
-
- def _init_predictor(self):
- """Initialize predictor layers of the head."""
- self.conv_cls = nn.Conv2d(
- self.feat_channels, self.cls_out_channels, 3, padding=1)
- self.conv_reg = nn.Conv2d(self.feat_channels, 4, 3, padding=1)
- if self.with_bezier:
- self.conv_bezier = nn.Conv2d(
- self.feat_channels, 16, kernel_size=3, stride=1, padding=1)
-
- def forward(self, feats, data_samples=None):
- """Forward features from the upstream network.
-
- Args:
- feats (tuple[Tensor]): Features from the upstream network, each is
- a 4D-tensor.
-
- Returns:
- tuple:
- cls_scores (list[Tensor]): Box scores for each scale level, \
- each is a 4D-tensor, the channel number is \
- num_points * num_classes.
- bbox_preds (list[Tensor]): Box energies / deltas for each \
- scale level, each is a 4D-tensor, the channel number is \
- num_points * 4.
- centernesses (list[Tensor]): centerness for each scale level, \
- each is a 4D-tensor, the channel number is num_points * 1.
- """
-
- return multi_apply(self.forward_single, feats[1:], self.scales,
- self.strides)
-
- def forward_single(self, x, scale, stride):
- """Forward features of a single scale level.
-
- Args:
- x (Tensor): FPN feature maps of the specified stride.
- scale (:obj: `mmcv.cnn.Scale`): Learnable scale module to resize
- the bbox prediction.
- stride (int): The corresponding stride for feature maps, only
- used to normalize the bbox prediction when self.norm_on_bbox
- is True.
-
- Returns:
- tuple: scores for each class, bbox predictions and centerness \
- predictions of input feature maps. If ``with_bezier`` is True,
- Bezier prediction will also be returned.
- """
- cls_feat = x
- reg_feat = x
-
- for cls_layer in self.cls_convs:
- cls_feat = cls_layer(cls_feat)
- cls_score = self.conv_cls(cls_feat)
-
- for reg_layer in self.reg_convs:
- reg_feat = reg_layer(reg_feat)
- bbox_pred = self.conv_reg(reg_feat)
- if self.with_bezier:
- bezier_pred = self.conv_bezier(reg_feat)
-
- if self.centerness_on_reg:
- centerness = self.conv_centerness(reg_feat)
- else:
- centerness = self.conv_centerness(cls_feat)
- # scale the bbox_pred of different level
- # float to avoid overflow when enabling FP16
- if self.use_scale:
- bbox_pred = scale(bbox_pred).float()
- else:
- bbox_pred = bbox_pred.float()
- if self.norm_on_bbox:
- # bbox_pred needed for gradient computation has been modified
- # by F.relu(bbox_pred) when run with PyTorch 1.10. So replace
- # F.relu(bbox_pred) with bbox_pred.clamp(min=0)
- bbox_pred = bbox_pred.clamp(min=0)
- else:
- bbox_pred = bbox_pred.exp()
-
- if self.with_bezier:
- return cls_score, bbox_pred, centerness, bezier_pred
- else:
- return cls_score, bbox_pred, centerness
diff --git a/pipeline/mmocr/projects/ABCNet/abcnet/model/abcnet_det_module_loss.py b/pipeline/mmocr/projects/ABCNet/abcnet/model/abcnet_det_module_loss.py
deleted file mode 100644
index a8becc48dd381ec37c5a178508c6af5fec47337b..0000000000000000000000000000000000000000
--- a/pipeline/mmocr/projects/ABCNet/abcnet/model/abcnet_det_module_loss.py
+++ /dev/null
@@ -1,359 +0,0 @@
-# Copyright (c) OpenMMLab. All rights reserved.
-from typing import Dict, List, Tuple
-
-import torch
-from mmdet.models.task_modules.prior_generators import MlvlPointGenerator
-from mmdet.models.utils import multi_apply
-from mmdet.utils import reduce_mean
-from torch import Tensor
-
-from mmocr.models.textdet.module_losses.base import BaseTextDetModuleLoss
-from mmocr.registry import MODELS, TASK_UTILS
-from mmocr.structures import TextDetDataSample
-from mmocr.utils import ConfigType, DetSampleList, RangeType
-from ..utils import poly2bezier
-
-INF = 1e8
-
-
-@MODELS.register_module()
-class ABCNetDetModuleLoss(BaseTextDetModuleLoss):
- # TODO add docs
-
- def __init__(
- self,
- num_classes: int = 1,
- bbox_coder: ConfigType = dict(type='mmdet.DistancePointBBoxCoder'),
- regress_ranges: RangeType = ((-1, 64), (64, 128), (128, 256),
- (256, 512), (512, INF)),
- strides: List[int] = (8, 16, 32, 64, 128),
- center_sampling: bool = True,
- center_sample_radius: float = 1.5,
- norm_on_bbox: bool = True,
- loss_cls: ConfigType = dict(
- type='mmdet.FocalLoss',
- use_sigmoid=True,
- gamma=2.0,
- alpha=0.25,
- loss_weight=1.0),
- loss_bbox: ConfigType = dict(type='mmdet.GIoULoss', loss_weight=1.0),
- loss_centerness: ConfigType = dict(
- type='mmdet.CrossEntropyLoss', use_sigmoid=True, loss_weight=1.0),
- loss_bezier: ConfigType = dict(
- type='mmdet.SmoothL1Loss', reduction='mean', loss_weight=1.0)
- ) -> None:
- super().__init__()
- self.num_classes = num_classes
- self.strides = strides
- self.prior_generator = MlvlPointGenerator(strides)
- self.regress_ranges = regress_ranges
- self.center_sampling = center_sampling
- self.center_sample_radius = center_sample_radius
- self.norm_on_bbox = norm_on_bbox
- self.loss_centerness = MODELS.build(loss_centerness)
- self.loss_cls = MODELS.build(loss_cls)
- self.loss_bbox = MODELS.build(loss_bbox)
- self.loss_bezier = MODELS.build(loss_bezier)
- self.bbox_coder = TASK_UTILS.build(bbox_coder)
- use_sigmoid_cls = loss_cls.get('use_sigmoid', False)
- if use_sigmoid_cls:
- self.cls_out_channels = num_classes
- else:
- self.cls_out_channels = num_classes + 1
-
- def forward(self, inputs: Tuple[Tensor],
- data_samples: DetSampleList) -> Dict:
- """Compute ABCNet loss.
-
- Args:
- inputs (tuple(tensor)): Raw predictions from model, containing
- ``cls_scores``, ``bbox_preds``, ``beizer_preds`` and
- ``centernesses``.
- Each is a tensor of shape :math:`(N, H, W)`.
- data_samples (list[TextDetDataSample]): The data samples.
-
- Returns:
- dict: The dict for abcnet-det losses with loss_cls, loss_bbox,
- loss_centerness and loss_bezier.
- """
- cls_scores, bbox_preds, centernesses, beizer_preds = inputs
- assert len(cls_scores) == len(bbox_preds) == len(centernesses) == len(
- beizer_preds)
- featmap_sizes = [featmap.size()[-2:] for featmap in cls_scores]
- all_level_points = self.prior_generator.grid_priors(
- featmap_sizes,
- dtype=bbox_preds[0].dtype,
- device=bbox_preds[0].device)
- labels, bbox_targets, bezier_targets = self.get_targets(
- all_level_points, data_samples)
-
- num_imgs = cls_scores[0].size(0)
- # flatten cls_scores, bbox_preds and centerness
- flatten_cls_scores = [
- cls_score.permute(0, 2, 3, 1).reshape(-1, self.cls_out_channels)
- for cls_score in cls_scores
- ]
- flatten_bbox_preds = [
- bbox_pred.permute(0, 2, 3, 1).reshape(-1, 4)
- for bbox_pred in bbox_preds
- ]
- flatten_centerness = [
- centerness.permute(0, 2, 3, 1).reshape(-1)
- for centerness in centernesses
- ]
- flatten_bezier_preds = [
- bezier_pred.permute(0, 2, 3, 1).reshape(-1, 16)
- for bezier_pred in beizer_preds
- ]
- flatten_cls_scores = torch.cat(flatten_cls_scores)
- flatten_bbox_preds = torch.cat(flatten_bbox_preds)
- flatten_centerness = torch.cat(flatten_centerness)
- flatten_bezier_preds = torch.cat(flatten_bezier_preds)
- flatten_labels = torch.cat(labels)
- flatten_bbox_targets = torch.cat(bbox_targets)
- flatten_bezier_targets = torch.cat(bezier_targets)
- # repeat points to align with bbox_preds
- flatten_points = torch.cat(
- [points.repeat(num_imgs, 1) for points in all_level_points])
-
- # FG cat_id: [0, num_classes -1], BG cat_id: num_classes
- bg_class_ind = self.num_classes
- pos_inds = ((flatten_labels >= 0)
- & (flatten_labels < bg_class_ind)).nonzero().reshape(-1)
- num_pos = torch.tensor(
- len(pos_inds), dtype=torch.float, device=bbox_preds[0].device)
- num_pos = max(reduce_mean(num_pos), 1.0)
- loss_cls = self.loss_cls(
- flatten_cls_scores, flatten_labels, avg_factor=num_pos)
-
- pos_bbox_preds = flatten_bbox_preds[pos_inds]
- pos_centerness = flatten_centerness[pos_inds]
- pos_bezier_preds = flatten_bezier_preds[pos_inds]
- pos_bbox_targets = flatten_bbox_targets[pos_inds]
- pos_centerness_targets = self.centerness_target(pos_bbox_targets)
- pos_bezier_targets = flatten_bezier_targets[pos_inds]
- # centerness weighted iou loss
- centerness_denorm = max(
- reduce_mean(pos_centerness_targets.sum().detach()), 1e-6)
-
- if len(pos_inds) > 0:
- pos_points = flatten_points[pos_inds]
- pos_decoded_bbox_preds = self.bbox_coder.decode(
- pos_points, pos_bbox_preds)
- pos_decoded_target_preds = self.bbox_coder.decode(
- pos_points, pos_bbox_targets)
- loss_bbox = self.loss_bbox(
- pos_decoded_bbox_preds,
- pos_decoded_target_preds,
- weight=pos_centerness_targets,
- avg_factor=centerness_denorm)
- loss_centerness = self.loss_centerness(
- pos_centerness, pos_centerness_targets, avg_factor=num_pos)
- loss_bezier = self.loss_bezier(
- pos_bezier_preds,
- pos_bezier_targets,
- weight=pos_centerness_targets[:, None],
- avg_factor=centerness_denorm)
- else:
- loss_bbox = pos_bbox_preds.sum()
- loss_centerness = pos_centerness.sum()
- loss_bezier = pos_bezier_preds.sum()
-
- return dict(
- loss_cls=loss_cls,
- loss_bbox=loss_bbox,
- loss_centerness=loss_centerness,
- loss_bezier=loss_bezier)
-
- def get_targets(self, points: List[Tensor], data_samples: DetSampleList
- ) -> Tuple[List[Tensor], List[Tensor]]:
- """Compute regression, classification and centerness targets for points
- in multiple images.
-
- Args:
- points (list[Tensor]): Points of each fpn level, each has shape
- (num_points, 2).
- data_samples: Batch of data samples. Each data sample contains
- a gt_instance, which usually includes bboxes and labels
- attributes.
-
- Returns:
- tuple: Targets of each level.
-
- - concat_lvl_labels (list[Tensor]): Labels of each level.
- - concat_lvl_bbox_targets (list[Tensor]): BBox targets of each \
- level.
- """
- assert len(points) == len(self.regress_ranges)
- num_levels = len(points)
- # expand regress ranges to align with points
- expanded_regress_ranges = [
- points[i].new_tensor(self.regress_ranges[i])[None].expand_as(
- points[i]) for i in range(num_levels)
- ]
- # concat all levels points and regress ranges
- concat_regress_ranges = torch.cat(expanded_regress_ranges, dim=0)
- concat_points = torch.cat(points, dim=0)
-
- # the number of points per img, per lvl
- num_points = [center.size(0) for center in points]
-
- # get labels and bbox_targets of each image
- labels_list, bbox_targets_list, bezier_targets_list = multi_apply(
- self._get_targets_single,
- data_samples,
- points=concat_points,
- regress_ranges=concat_regress_ranges,
- num_points_per_lvl=num_points)
-
- # split to per img, per level
- labels_list = [labels.split(num_points, 0) for labels in labels_list]
- bbox_targets_list = [
- bbox_targets.split(num_points, 0)
- for bbox_targets in bbox_targets_list
- ]
- bezier_targets_list = [
- bezier_targets.split(num_points, 0)
- for bezier_targets in bezier_targets_list
- ]
- # concat per level image
- concat_lvl_labels = []
- concat_lvl_bbox_targets = []
- concat_lvl_bezier_targets = []
- for i in range(num_levels):
- concat_lvl_labels.append(
- torch.cat([labels[i] for labels in labels_list]))
- bbox_targets = torch.cat(
- [bbox_targets[i] for bbox_targets in bbox_targets_list])
- bezier_targets = torch.cat(
- [bezier_targets[i] for bezier_targets in bezier_targets_list])
- if self.norm_on_bbox:
- bbox_targets = bbox_targets / self.strides[i]
- bezier_targets = bezier_targets / self.strides[i]
- concat_lvl_bbox_targets.append(bbox_targets)
- concat_lvl_bezier_targets.append(bezier_targets)
- return (concat_lvl_labels, concat_lvl_bbox_targets,
- concat_lvl_bezier_targets)
-
- def _get_targets_single(self, data_sample: TextDetDataSample,
- points: Tensor, regress_ranges: Tensor,
- num_points_per_lvl: List[int]
- ) -> Tuple[Tensor, Tensor, Tensor]:
- """Compute regression and classification targets for a single image."""
- num_points = points.size(0)
- gt_instances = data_sample.gt_instances
- gt_instances = gt_instances[~gt_instances.ignored]
- num_gts = len(gt_instances)
- gt_bboxes = gt_instances.bboxes
- gt_labels = gt_instances.labels
- data_sample.gt_instances = gt_instances
- polygons = gt_instances.polygons
- beziers = gt_bboxes.new([poly2bezier(poly) for poly in polygons])
- gt_instances.beziers = beziers
- if num_gts == 0:
- return gt_labels.new_full((num_points,), self.num_classes), \
- gt_bboxes.new_zeros((num_points, 4)), \
- gt_bboxes.new_zeros((num_points, 16))
-
- areas = (gt_bboxes[:, 2] - gt_bboxes[:, 0]) * (
- gt_bboxes[:, 3] - gt_bboxes[:, 1])
- # TODO: figure out why these two are different
- # areas = areas[None].expand(num_points, num_gts)
- areas = areas[None].repeat(num_points, 1)
- regress_ranges = regress_ranges[:, None, :].expand(
- num_points, num_gts, 2)
- gt_bboxes = gt_bboxes[None].expand(num_points, num_gts, 4)
- xs, ys = points[:, 0], points[:, 1]
- xs = xs[:, None].expand(num_points, num_gts)
- ys = ys[:, None].expand(num_points, num_gts)
-
- left = xs - gt_bboxes[..., 0]
- right = gt_bboxes[..., 2] - xs
- top = ys - gt_bboxes[..., 1]
- bottom = gt_bboxes[..., 3] - ys
- bbox_targets = torch.stack((left, top, right, bottom), -1)
-
- beziers = beziers.reshape(-1, 8,
- 2)[None].expand(num_points, num_gts, 8, 2)
- beziers_left = beziers[..., 0] - xs[..., None]
- beziers_right = beziers[..., 1] - ys[..., None]
- bezier_targets = torch.stack((beziers_left, beziers_right), dim=-1)
- bezier_targets = bezier_targets.view(num_points, num_gts, 16)
- if self.center_sampling:
- # condition1: inside a `center bbox`
- radius = self.center_sample_radius
- center_xs = (gt_bboxes[..., 0] + gt_bboxes[..., 2]) / 2
- center_ys = (gt_bboxes[..., 1] + gt_bboxes[..., 3]) / 2
- center_gts = torch.zeros_like(gt_bboxes)
- stride = center_xs.new_zeros(center_xs.shape)
-
- # project the points on current lvl back to the `original` sizes
- lvl_begin = 0
- for lvl_idx, num_points_lvl in enumerate(num_points_per_lvl):
- lvl_end = lvl_begin + num_points_lvl
- stride[lvl_begin:lvl_end] = self.strides[lvl_idx] * radius
- lvl_begin = lvl_end
-
- x_mins = center_xs - stride
- y_mins = center_ys - stride
- x_maxs = center_xs + stride
- y_maxs = center_ys + stride
- center_gts[..., 0] = torch.where(x_mins > gt_bboxes[..., 0],
- x_mins, gt_bboxes[..., 0])
- center_gts[..., 1] = torch.where(y_mins > gt_bboxes[..., 1],
- y_mins, gt_bboxes[..., 1])
- center_gts[..., 2] = torch.where(x_maxs > gt_bboxes[..., 2],
- gt_bboxes[..., 2], x_maxs)
- center_gts[..., 3] = torch.where(y_maxs > gt_bboxes[..., 3],
- gt_bboxes[..., 3], y_maxs)
-
- cb_dist_left = xs - center_gts[..., 0]
- cb_dist_right = center_gts[..., 2] - xs
- cb_dist_top = ys - center_gts[..., 1]
- cb_dist_bottom = center_gts[..., 3] - ys
- center_bbox = torch.stack(
- (cb_dist_left, cb_dist_top, cb_dist_right, cb_dist_bottom), -1)
- inside_gt_bbox_mask = center_bbox.min(-1)[0] > 0
- else:
- # condition1: inside a gt bbox
- inside_gt_bbox_mask = bbox_targets.min(-1)[0] > 0
-
- # condition2: limit the regression range for each location
- max_regress_distance = bbox_targets.max(-1)[0]
- inside_regress_range = (
- (max_regress_distance >= regress_ranges[..., 0])
- & (max_regress_distance <= regress_ranges[..., 1]))
-
- # if there are still more than one objects for a location,
- # we choose the one with minimal area
- areas[inside_gt_bbox_mask == 0] = INF
- areas[inside_regress_range == 0] = INF
- min_area, min_area_inds = areas.min(dim=1)
-
- labels = gt_labels[min_area_inds]
- labels[min_area == INF] = self.num_classes # set as BG
- bbox_targets = bbox_targets[range(num_points), min_area_inds]
- bezier_targets = bezier_targets[range(num_points), min_area_inds]
-
- return labels, bbox_targets, bezier_targets
-
- def centerness_target(self, pos_bbox_targets: Tensor) -> Tensor:
- """Compute centerness targets.
-
- Args:
- pos_bbox_targets (Tensor): BBox targets of positive bboxes in shape
- (num_pos, 4)
-
- Returns:
- Tensor: Centerness target.
- """
- # only calculate pos centerness targets, otherwise there may be nan
- left_right = pos_bbox_targets[:, [0, 2]]
- top_bottom = pos_bbox_targets[:, [1, 3]]
- if len(left_right) == 0:
- centerness_targets = left_right[..., 0]
- else:
- centerness_targets = (
- left_right.min(dim=-1)[0] / left_right.max(dim=-1)[0]) * (
- top_bottom.min(dim=-1)[0] / top_bottom.max(dim=-1)[0])
- return torch.sqrt(centerness_targets)
diff --git a/pipeline/mmocr/projects/ABCNet/abcnet/model/abcnet_det_postprocessor.py b/pipeline/mmocr/projects/ABCNet/abcnet/model/abcnet_det_postprocessor.py
deleted file mode 100644
index db9a4d141c32ab840d8fe25640ad9c3fed00db5b..0000000000000000000000000000000000000000
--- a/pipeline/mmocr/projects/ABCNet/abcnet/model/abcnet_det_postprocessor.py
+++ /dev/null
@@ -1,228 +0,0 @@
-# Copyright (c) OpenMMLab. All rights reserved.
-from functools import partial
-from typing import List
-
-import numpy as np
-import torch
-from mmcv.ops import batched_nms
-from mmdet.models.task_modules.prior_generators import MlvlPointGenerator
-from mmdet.models.utils import (filter_scores_and_topk, multi_apply,
- select_single_mlvl)
-from mmengine.structures import InstanceData
-
-from mmocr.models.textdet.postprocessors.base import BaseTextDetPostProcessor
-from mmocr.registry import MODELS, TASK_UTILS
-
-
-@MODELS.register_module()
-class ABCNetDetPostprocessor(BaseTextDetPostProcessor):
- """Post-processing methods for ABCNet.
-
- Args:
- num_classes (int): Number of classes.
- use_sigmoid_cls (bool): Whether to use sigmoid for classification.
- strides (tuple): Strides of each feature map.
- norm_by_strides (bool): Whether to normalize the regression targets by
- the strides.
- bbox_coder (dict): Config dict for bbox coder.
- text_repr_type (str): Text representation type, 'poly' or 'quad'.
- with_bezier (bool): Whether to use bezier curve for text detection.
- train_cfg (dict): Config dict for training.
- test_cfg (dict): Config dict for testing.
- """
-
- def __init__(
- self,
- num_classes=1,
- use_sigmoid_cls=True,
- strides=(4, 8, 16, 32, 64),
- norm_by_strides=True,
- bbox_coder=dict(type='mmdet.DistancePointBBoxCoder'),
- text_repr_type='poly',
- rescale_fields=None,
- with_bezier=False,
- train_cfg=None,
- test_cfg=None,
- ):
- super().__init__(
- text_repr_type=text_repr_type,
- rescale_fields=rescale_fields,
- train_cfg=train_cfg,
- test_cfg=test_cfg,
- )
- self.strides = strides
- self.norm_by_strides = norm_by_strides
- self.prior_generator = MlvlPointGenerator(strides)
- self.bbox_coder = TASK_UTILS.build(bbox_coder)
- self.use_sigmoid_cls = use_sigmoid_cls
- self.with_bezier = with_bezier
- if self.use_sigmoid_cls:
- self.cls_out_channels = num_classes
- else:
- self.cls_out_channels = num_classes + 1
-
- def split_results(self, pred_results: List[torch.Tensor]):
- """Split the prediction results into multi-level features. The
- prediction results are concatenated in the first dimension.
- Args:
- pred_results (list[list[torch.Tensor]): Prediction results of all
- head with multi-level features.
- The first dimension of pred_results is the number of outputs of
- head. The second dimension is the number of level. The third
- dimension is the feature with (N, C, H, W).
-
- Returns:
- list[list[torch.Tensor]]:
- [Batch_size, Number of heads]
- """
-
- results = []
- num_levels = len(pred_results[0])
- bs = pred_results[0][0].size(0)
- featmap_sizes = [
- pred_results[0][i].shape[-2:] for i in range(num_levels)
- ]
- mlvl_priors = self.prior_generator.grid_priors(
- featmap_sizes,
- dtype=pred_results[0][0].dtype,
- device=pred_results[0][0].device)
- for img_id in range(bs):
- single_results = [mlvl_priors]
- for pred_result in pred_results:
- single_results.append(select_single_mlvl(pred_result, img_id))
- results.append(single_results)
- return results
-
- def get_text_instances(
- self,
- pred_results,
- data_sample,
- nms_pre=-1,
- score_thr=0,
- max_per_img=100,
- nms=dict(type='nms', iou_threshold=0.5),
- ):
- """Get text instance predictions of one image."""
- pred_instances = InstanceData()
-
- (mlvl_bboxes, mlvl_scores, mlvl_labels, mlvl_score_factors,
- mlvl_beziers) = multi_apply(
- self._get_preds_single_level,
- *pred_results,
- self.strides,
- img_shape=data_sample.get('img_shape'),
- nms_pre=nms_pre,
- score_thr=score_thr)
-
- mlvl_bboxes = torch.cat(mlvl_bboxes)
- mlvl_scores = torch.cat(mlvl_scores)
- mlvl_labels = torch.cat(mlvl_labels)
- if self.with_bezier:
- mlvl_beziers = torch.cat(mlvl_beziers)
-
- if mlvl_score_factors is not None:
- mlvl_score_factors = torch.cat(mlvl_score_factors)
- mlvl_scores = mlvl_scores * mlvl_score_factors
- mlvl_scores = torch.sqrt(mlvl_scores)
-
- if mlvl_bboxes.numel() == 0:
- pred_instances.bboxes = mlvl_bboxes.detach().cpu().numpy()
- pred_instances.scores = mlvl_scores.detach().cpu().numpy()
- pred_instances.labels = mlvl_labels.detach().cpu().numpy()
- if self.with_bezier:
- pred_instances.beziers = mlvl_beziers.detach().reshape(-1, 16)
- pred_instances.polygons = []
- data_sample.pred_instances = pred_instances
- return data_sample
- det_bboxes, keep_idxs = batched_nms(mlvl_bboxes, mlvl_scores,
- mlvl_labels, nms)
- det_bboxes, scores = np.split(det_bboxes, [-1], axis=1)
- pred_instances.bboxes = det_bboxes[:max_per_img].detach().cpu().numpy()
- pred_instances.scores = scores[:max_per_img].detach().cpu().numpy(
- ).squeeze(-1)
- pred_instances.labels = mlvl_labels[keep_idxs][:max_per_img].detach(
- ).cpu().numpy()
- if self.with_bezier:
- pred_instances.beziers = mlvl_beziers[
- keep_idxs][:max_per_img].detach().reshape(-1, 16)
- data_sample.pred_instances = pred_instances
- return data_sample
-
- def _get_preds_single_level(self,
- priors,
- cls_scores,
- bbox_preds,
- centernesses,
- bezier_preds=None,
- stride=1,
- score_thr=0,
- nms_pre=-1,
- img_shape=None):
- assert cls_scores.size()[-2:] == bbox_preds.size()[-2:]
- if self.norm_by_strides:
- bbox_preds = bbox_preds * stride
- bbox_preds = bbox_preds.permute(1, 2, 0).reshape(-1, 4)
- if self.with_bezier:
- if self.norm_by_strides:
- bezier_preds = bezier_preds * stride
- bezier_preds = bezier_preds.permute(1, 2, 0).reshape(-1, 8, 2)
- centernesses = centernesses.permute(1, 2, 0).reshape(-1).sigmoid()
- cls_scores = cls_scores.permute(1, 2,
- 0).reshape(-1, self.cls_out_channels)
- if self.use_sigmoid_cls:
- scores = cls_scores.sigmoid()
- else:
- # remind that we set FG labels to [0, num_class-1]
- # since mmdet v2.0
- # BG cat_id: num_class
- scores = cls_scores.softmax(-1)[:, :-1]
-
- # After https://github.com/open-mmlab/mmdetection/pull/6268/,
- # this operation keeps fewer bboxes under the same `nms_pre`.
- # There is no difference in performance for most models. If you
- # find a slight drop in performance, you can set a larger
- # `nms_pre` than before.
- results = filter_scores_and_topk(
- scores, score_thr, nms_pre,
- dict(bbox_preds=bbox_preds, priors=priors))
- scores, labels, keep_idxs, filtered_results = results
-
- bbox_preds = filtered_results['bbox_preds']
- priors = filtered_results['priors']
- centernesses = centernesses[keep_idxs]
- bboxes = self.bbox_coder.decode(
- priors, bbox_preds, max_shape=img_shape)
- if self.with_bezier:
- bezier_preds = bezier_preds[keep_idxs]
- bezier_preds = priors[:, None, :] + bezier_preds
- bezier_preds[:, :, 0].clamp_(min=0, max=img_shape[1])
- bezier_preds[:, :, 1].clamp_(min=0, max=img_shape[0])
- return bboxes, scores, labels, centernesses, bezier_preds
- else:
- return bboxes, scores, labels, centernesses
-
- def __call__(self, pred_results, data_samples, training: bool = False):
- """Postprocess pred_results according to metainfos in data_samples.
-
- Args:
- pred_results (Union[Tensor, List[Tensor]]): The prediction results
- stored in a tensor or a list of tensor. Usually each item to
- be post-processed is expected to be a batched tensor.
- data_samples (list[TextDetDataSample]): Batch of data_samples,
- each corresponding to a prediction result.
- training (bool): Whether the model is in training mode. Defaults to
- False.
-
- Returns:
- list[TextDetDataSample]: Batch of post-processed datasamples.
- """
- if training:
- return data_samples
- cfg = self.train_cfg if training else self.test_cfg
- if cfg is None:
- cfg = {}
- pred_results = self.split_results(pred_results)
- process_single = partial(self._process_single, **cfg)
- results = list(map(process_single, pred_results, data_samples))
-
- return results
diff --git a/pipeline/mmocr/projects/ABCNet/abcnet/model/abcnet_postprocessor.py b/pipeline/mmocr/projects/ABCNet/abcnet/model/abcnet_postprocessor.py
deleted file mode 100644
index 1f75635652a80b688884244a23a07e1b59ba53f4..0000000000000000000000000000000000000000
--- a/pipeline/mmocr/projects/ABCNet/abcnet/model/abcnet_postprocessor.py
+++ /dev/null
@@ -1,100 +0,0 @@
-# Copyright (c) OpenMMLab. All rights reserved.
-from mmocr.models.textdet.postprocessors.base import BaseTextDetPostProcessor
-from mmocr.registry import MODELS
-from ..utils import bezier2poly
-
-
-@MODELS.register_module()
-class ABCNetPostprocessor(BaseTextDetPostProcessor):
- """Post-processing methods for ABCNet.
-
- Args:
- num_classes (int): Number of classes.
- use_sigmoid_cls (bool): Whether to use sigmoid for classification.
- strides (tuple): Strides of each feature map.
- norm_by_strides (bool): Whether to normalize the regression targets by
- the strides.
- bbox_coder (dict): Config dict for bbox coder.
- text_repr_type (str): Text representation type, 'poly' or 'quad'.
- with_bezier (bool): Whether to use bezier curve for text detection.
- train_cfg (dict): Config dict for training.
- test_cfg (dict): Config dict for testing.
- """
-
- def __init__(
- self,
- text_repr_type='poly',
- rescale_fields=['beziers', 'polygons'],
- ):
- super().__init__(
- text_repr_type=text_repr_type, rescale_fields=rescale_fields)
-
- def merge_predict(self, spotting_data_samples, recog_data_samples):
- texts = [ds.pred_text.item for ds in recog_data_samples]
- start = 0
- for spotting_data_sample in spotting_data_samples:
- end = start + len(spotting_data_sample.pred_instances)
- spotting_data_sample.pred_instances.texts = texts[start:end]
- start = end
- return spotting_data_samples
-
- # TODO: fix docstr
- def __call__(self,
- spotting_data_samples,
- recog_data_samples,
- training: bool = False):
- """Postprocess pred_results according to metainfos in data_samples.
-
- Args:
- pred_results (Union[Tensor, List[Tensor]]): The prediction results
- stored in a tensor or a list of tensor. Usually each item to
- be post-processed is expected to be a batched tensor.
- data_samples (list[TextDetDataSample]): Batch of data_samples,
- each corresponding to a prediction result.
- training (bool): Whether the model is in training mode. Defaults to
- False.
-
- Returns:
- list[TextDetDataSample]: Batch of post-processed datasamples.
- """
- spotting_data_samples = list(
- map(self._process_single, spotting_data_samples))
- return self.merge_predict(spotting_data_samples, recog_data_samples)
-
- def _process_single(self, data_sample):
- """Process prediction results from one image.
-
- Args:
- pred_result (Union[Tensor, List[Tensor]]): Prediction results of an
- image.
- data_sample (TextDetDataSample): Datasample of an image.
- """
- data_sample = self.get_text_instances(data_sample)
- if self.rescale_fields and len(self.rescale_fields) > 0:
- assert isinstance(self.rescale_fields, list)
- assert set(self.rescale_fields).issubset(
- set(data_sample.pred_instances.keys()))
- data_sample = self.rescale(data_sample, data_sample.scale_factor)
- return data_sample
-
- def get_text_instances(self, data_sample, **kwargs):
- """Get text instance predictions of one image.
-
- Args:
- pred_result (tuple(Tensor)): Prediction results of an image.
- data_sample (TextDetDataSample): Datasample of an image.
- **kwargs: Other parameters. Configurable via ``__init__.train_cfg``
- and ``__init__.test_cfg``.
-
- Returns:
- TextDetDataSample: A new DataSample with predictions filled in.
- The polygon/bbox results are usually saved in
- ``TextDetDataSample.pred_instances.polygons`` or
- ``TextDetDataSample.pred_instances.bboxes``. The confidence scores
- are saved in ``TextDetDataSample.pred_instances.scores``.
- """
- data_sample = data_sample.cpu().numpy()
- pred_instances = data_sample.pred_instances
- data_sample.pred_instances.polygons = list(
- map(bezier2poly, pred_instances.beziers))
- return data_sample
diff --git a/pipeline/mmocr/projects/ABCNet/abcnet/model/abcnet_rec.py b/pipeline/mmocr/projects/ABCNet/abcnet/model/abcnet_rec.py
deleted file mode 100644
index 599a36d41f855a21ecf623389198162e03ee7d50..0000000000000000000000000000000000000000
--- a/pipeline/mmocr/projects/ABCNet/abcnet/model/abcnet_rec.py
+++ /dev/null
@@ -1,8 +0,0 @@
-# Copyright (c) OpenMMLab. All rights reserved.
-from mmocr.models.textrecog import EncoderDecoderRecognizer
-from mmocr.registry import MODELS
-
-
-@MODELS.register_module()
-class ABCNetRec(EncoderDecoderRecognizer):
- """CTC-loss based recognizer."""
diff --git a/pipeline/mmocr/projects/ABCNet/abcnet/model/abcnet_rec_backbone.py b/pipeline/mmocr/projects/ABCNet/abcnet/model/abcnet_rec_backbone.py
deleted file mode 100644
index 7d77cf2e6f07cd609df16a7feaf83da609b3da3a..0000000000000000000000000000000000000000
--- a/pipeline/mmocr/projects/ABCNet/abcnet/model/abcnet_rec_backbone.py
+++ /dev/null
@@ -1,52 +0,0 @@
-# Copyright (c) OpenMMLab. All rights reserved.
-import torch.nn as nn
-from mmcv.cnn import ConvModule
-from mmengine.model import BaseModule, Sequential
-
-from mmocr.registry import MODELS
-
-
-@MODELS.register_module()
-class ABCNetRecBackbone(BaseModule):
-
- def __init__(self, init_cfg=None):
- super().__init__(init_cfg)
-
- self.convs = Sequential(
- ConvModule(
- in_channels=256,
- out_channels=256,
- kernel_size=3,
- padding=1,
- bias='auto',
- norm_cfg=dict(type='BN'),
- act_cfg=dict(type='ReLU')),
- ConvModule(
- in_channels=256,
- out_channels=256,
- kernel_size=3,
- padding=1,
- bias='auto',
- norm_cfg=dict(type='BN'),
- act_cfg=dict(type='ReLU')),
- ConvModule(
- in_channels=256,
- out_channels=256,
- kernel_size=3,
- padding=1,
- stride=(2, 1),
- bias='auto',
- norm_cfg=dict(type='GN', num_groups=32),
- act_cfg=dict(type='ReLU')),
- ConvModule(
- in_channels=256,
- out_channels=256,
- kernel_size=3,
- padding=1,
- stride=(2, 1),
- bias='auto',
- norm_cfg=dict(type='GN', num_groups=32),
- act_cfg=dict(type='ReLU')), nn.AdaptiveAvgPool2d((1, None)))
-
- def forward(self, x):
- return self.convs(x)
diff --git a/pipeline/mmocr/projects/ABCNet/abcnet/model/abcnet_rec_decoder.py b/pipeline/mmocr/projects/ABCNet/abcnet/model/abcnet_rec_decoder.py
deleted file mode 100644
index e96f3a3b4fa6d33d79f8433320ee166da9ce0784..0000000000000000000000000000000000000000
--- a/pipeline/mmocr/projects/ABCNet/abcnet/model/abcnet_rec_decoder.py
+++ /dev/null
@@ -1,161 +0,0 @@
-# Copyright (c) OpenMMLab. All rights reserved.
-import random
-from typing import Dict, Optional, Sequence, Union
-
-import torch
-import torch.nn as nn
-from torch.nn import functional as F
-
-from mmocr.models.common.dictionary import Dictionary
-from mmocr.models.textrecog.decoders.base import BaseDecoder
-from mmocr.registry import MODELS
-from mmocr.structures import TextRecogDataSample
-
-
-@MODELS.register_module()
-class ABCNetRecDecoder(BaseDecoder):
- """Decoder for ABCNet.
-
- Args:
- in_channels (int): Number of input channels.
- dropout_prob (float): Probability of dropout. Default to 0.5.
- teach_prob (float): Probability of teacher forcing. Defaults to 0.5.
- dictionary (dict or :obj:`Dictionary`): The config for `Dictionary` or
- the instance of `Dictionary`.
- module_loss (dict, optional): Config to build module_loss. Defaults
- to None.
- postprocessor (dict, optional): Config to build postprocessor.
- Defaults to None.
- max_seq_len (int, optional): Max sequence length. Defaults to 30.
- init_cfg (dict or list[dict], optional): Initialization configs.
- Defaults to None.
- """
-
- def __init__(self,
- in_channels: int = 256,
- dropout_prob: float = 0.5,
- teach_prob: float = 0.5,
- dictionary: Union[Dictionary, Dict] = None,
- module_loss: Dict = None,
- postprocessor: Dict = None,
- max_seq_len: int = 30,
- init_cfg=dict(type='Xavier', layer='Conv2d'),
- **kwargs):
- super().__init__(
- init_cfg=init_cfg,
- dictionary=dictionary,
- module_loss=module_loss,
- postprocessor=postprocessor,
- max_seq_len=max_seq_len)
- self.in_channels = in_channels
- self.teach_prob = teach_prob
- self.embedding = nn.Embedding(self.dictionary.num_classes, in_channels)
- self.attn_combine = nn.Linear(in_channels * 2, in_channels)
- self.dropout = nn.Dropout(dropout_prob)
- self.gru = nn.GRU(in_channels, in_channels)
- self.out = nn.Linear(in_channels, self.dictionary.num_classes)
- self.vat = nn.Linear(in_channels, 1)
- self.softmax = nn.Softmax(dim=-1)
-
- def forward_train(
- self,
- feat: torch.Tensor,
- out_enc: Optional[torch.Tensor] = None,
- data_samples: Optional[Sequence[TextRecogDataSample]] = None
- ) -> torch.Tensor:
- """
- Args:
- feat (Tensor): A Tensor of shape :math:`(N, C, 1, W)`.
- out_enc (torch.Tensor, optional): Encoder output. Defaults to None.
- data_samples (list[TextRecogDataSample], optional): Batch of
- TextRecogDataSample, containing gt_text information. Defaults
- to None.
-
- Returns:
- Tensor: The raw logit tensor. Shape :math:`(N, W, C)` where
- :math:`C` is ``num_classes``.
- """
- bs = out_enc.size()[1]
- trg_seq = []
- for target in data_samples:
- trg_seq.append(target.gt_text.padded_indexes.to(feat.device))
- decoder_input = torch.zeros(bs).long().to(out_enc.device)
- trg_seq = torch.stack(trg_seq, dim=0)
- decoder_hidden = torch.zeros(1, bs,
- self.in_channels).to(out_enc.device)
- decoder_outputs = []
- for index in range(trg_seq.shape[1]):
- # decoder_output (nbatch, ncls)
- decoder_output, decoder_hidden = self._attention(
- decoder_input, decoder_hidden, out_enc)
- teach_forcing = True if random.random(
- ) > self.teach_prob else False
- if teach_forcing:
- decoder_input = trg_seq[:, index] # Teacher forcing
- else:
- _, topi = decoder_output.data.topk(1)
- decoder_input = topi.squeeze()
- decoder_outputs.append(decoder_output)
-
- return torch.stack(decoder_outputs, dim=1)
-
- def forward_test(
- self,
- feat: Optional[torch.Tensor] = None,
- out_enc: Optional[torch.Tensor] = None,
- data_samples: Optional[Sequence[TextRecogDataSample]] = None
- ) -> torch.Tensor:
- """
- Args:
- feat (Tensor): A Tensor of shape :math:`(N, C, 1, W)`.
- out_enc (torch.Tensor, optional): Encoder output. Defaults to None.
- data_samples (list[TextRecogDataSample]): Batch of
- TextRecogDataSample, containing ``gt_text`` information.
- Defaults to None.
-
- Returns:
- Tensor: Character probabilities. of shape
- :math:`(N, self.max_seq_len, C)` where :math:`C` is
- ``num_classes``.
- """
- bs = out_enc.size()[1]
- outputs = []
- decoder_input = torch.zeros(bs).long().to(out_enc.device)
- decoder_hidden = torch.zeros(1, bs,
- self.in_channels).to(out_enc.device)
- for _ in range(self.max_seq_len):
- # decoder_output (nbatch, ncls)
- decoder_output, decoder_hidden = self._attention(
- decoder_input, decoder_hidden, out_enc)
- _, topi = decoder_output.data.topk(1)
- decoder_input = topi.squeeze()
- outputs.append(decoder_output)
- outputs = torch.stack(outputs, dim=1)
- return self.softmax(outputs)
-
- def _attention(self, input, hidden, encoder_outputs):
- embedded = self.embedding(input)
- embedded = self.dropout(embedded)
-
- # test
- batch_size = encoder_outputs.shape[1]
-
- alpha = hidden + encoder_outputs
- alpha = alpha.view(-1, alpha.shape[-1]) # (T * n, hidden_size)
- attn_weights = self.vat(torch.tanh(alpha)) # (T * n, 1)
- attn_weights = attn_weights.view(-1, 1, batch_size).permute(
- (2, 1, 0)) # (T, 1, n) -> (n, 1, T)
- attn_weights = F.softmax(attn_weights, dim=2)
-
- attn_applied = torch.matmul(attn_weights,
- encoder_outputs.permute((1, 0, 2)))
-
- if embedded.dim() == 1:
- embedded = embedded.unsqueeze(0)
- output = torch.cat((embedded, attn_applied.squeeze(1)), 1)
- output = self.attn_combine(output).unsqueeze(0) # (1, n, hidden_size)
-
- output = F.relu(output)
- output, hidden = self.gru(output, hidden) # (1, n, hidden_size)
- output = self.out(output[0])
- return output, hidden
diff --git a/pipeline/mmocr/projects/ABCNet/abcnet/model/abcnet_rec_encoder.py b/pipeline/mmocr/projects/ABCNet/abcnet/model/abcnet_rec_encoder.py
deleted file mode 100644
index 5657ef096583efca964519dae36cc17c6ddf4034..0000000000000000000000000000000000000000
--- a/pipeline/mmocr/projects/ABCNet/abcnet/model/abcnet_rec_encoder.py
+++ /dev/null
@@ -1,54 +0,0 @@
-# Copyright (c) OpenMMLab. All rights reserved.
-from typing import Dict, Optional, Sequence
-
-import torch
-
-from mmocr.models.textrecog.encoders.base import BaseEncoder
-from mmocr.models.textrecog.layers import BidirectionalLSTM
-from mmocr.registry import MODELS
-from mmocr.structures import TextRecogDataSample
-
-
-@MODELS.register_module()
-class ABCNetRecEncoder(BaseEncoder):
- """Encoder for ABCNet.
-
- Args:
- in_channels (int): Number of input channels.
- out_channels (int): Number of output channels.
- init_cfg (dict or list[dict], optional): Initialization configs.
- Defaults to dict(type='Xavier', layer='Conv2d').
- """
-
- def __init__(self,
- in_channels: int = 256,
- hidden_channels: int = 256,
- out_channels: int = 256,
- init_cfg: Dict = None) -> None:
- super().__init__(init_cfg=init_cfg)
-
- self.layer = BidirectionalLSTM(in_channels, hidden_channels,
- out_channels)
-
- def forward(
- self,
- feat: torch.Tensor,
- data_samples: Optional[Sequence[TextRecogDataSample]] = None
- ) -> torch.Tensor:
- """
- Args:
- feat (Tensor): Image features with the shape of
- :math:`(N, C_{in}, H, W)`.
- data_samples (list[TextRecogDataSample], optional): Batch of
- TextRecogDataSample, containing valid_ratio information.
- Defaults to None.
-
- Returns:
- Tensor: A tensor of shape :math:`(N, C_{out}, H, W)`.
- """
- assert feat.size(2) == 1, 'feature height must be 1'
- feat = feat.squeeze(2)
- feat = feat.permute(2, 0, 1) # NxCxW -> WxNxC
- feat = self.layer(feat)
- # feat = feat.permute(1, 0, 2).contiguous()
- return feat
diff --git a/pipeline/mmocr/projects/ABCNet/abcnet/model/base_roi_extractor.py b/pipeline/mmocr/projects/ABCNet/abcnet/model/base_roi_extractor.py
deleted file mode 100644
index 372a23c2e428e2ca4364d134b726f6618d51af0e..0000000000000000000000000000000000000000
--- a/pipeline/mmocr/projects/ABCNet/abcnet/model/base_roi_extractor.py
+++ /dev/null
@@ -1,79 +0,0 @@
-# Copyright (c) OpenMMLab. All rights reserved.
-from abc import ABCMeta, abstractmethod
-from typing import List, Tuple
-
-import torch.nn as nn
-from mmcv import ops
-from mmengine.model import BaseModule
-from torch import Tensor
-
-from mmocr.utils import ConfigType, OptMultiConfig
-
-
-class BaseRoIExtractor(BaseModule, metaclass=ABCMeta):
- """Base class for RoI extractor.
-
- Args:
- roi_layer (:obj:`ConfigDict` or dict): Specify RoI layer type and
- arguments.
- out_channels (int): Output channels of RoI layers.
- featmap_strides (list[int]): Strides of input feature maps.
- init_cfg (:obj:`ConfigDict` or dict or list[:obj:`ConfigDict` or \
- dict], optional): Initialization config dict. Defaults to None.
- """
-
- def __init__(self,
- roi_layer: ConfigType,
- out_channels: int,
- featmap_strides: List[int],
- init_cfg: OptMultiConfig = None) -> None:
- super().__init__(init_cfg=init_cfg)
- self.roi_layers = self.build_roi_layers(roi_layer, featmap_strides)
- self.out_channels = out_channels
- self.featmap_strides = featmap_strides
-
- @property
- def num_inputs(self) -> int:
- """int: Number of input feature maps."""
- return len(self.featmap_strides)
-
- def build_roi_layers(self, layer_cfg: ConfigType,
- featmap_strides: List[int]) -> nn.ModuleList:
- """Build RoI operator to extract feature from each level feature map.
-
- Args:
- layer_cfg (:obj:`ConfigDict` or dict): Dictionary to construct and
- config RoI layer operation. Options are modules under
- ``mmcv/ops`` such as ``RoIAlign``.
- featmap_strides (list[int]): The stride of input feature map w.r.t
- to the original image size, which would be used to scale RoI
- coordinate (original image coordinate system) to feature
- coordinate system.
-
- Returns:
- :obj:`nn.ModuleList`: The RoI extractor modules for each level
- feature map.
- """
-
- cfg = layer_cfg.copy()
- layer_type = cfg.pop('type')
- assert hasattr(ops, layer_type)
- layer_cls = getattr(ops, layer_type)
- roi_layers = nn.ModuleList(
- [layer_cls(spatial_scale=1 / s, **cfg) for s in featmap_strides])
- return roi_layers
-
- @abstractmethod
- def forward(self, feats: Tuple[Tensor], data_samples) -> Tensor:
- """Extractor ROI feats.
-
- Args:
- feats (Tuple[Tensor]): Multi-scale features.
- data_samples (List[TextSpottingDataSample]):
-
- - proposals(InstanceData): The proposals of text detection.
-
- Returns:
- Tensor: RoI feature.
- """
- pass
diff --git a/pipeline/mmocr/projects/ABCNet/abcnet/model/base_roi_head.py b/pipeline/mmocr/projects/ABCNet/abcnet/model/base_roi_head.py
deleted file mode 100644
index 15652841fe6248ab9a81cf4e052ad67d7c93da5a..0000000000000000000000000000000000000000
--- a/pipeline/mmocr/projects/ABCNet/abcnet/model/base_roi_head.py
+++ /dev/null
@@ -1,58 +0,0 @@
-# Copyright (c) OpenMMLab. All rights reserved.
-from abc import ABCMeta, abstractmethod
-from typing import Tuple
-
-from mmengine.model import BaseModule
-from torch import Tensor
-
-from mmocr.utils import DetSampleList
-
-
-class BaseRoIHead(BaseModule, metaclass=ABCMeta):
- """Base class for RoIHeads."""
-
- @property
- def with_rec_head(self):
- """bool: whether the RoI head contains a `mask_head`"""
- return hasattr(self, 'rec_head') and self.rec_head is not None
-
- @property
- def with_extractor(self):
- """bool: whether the RoI head contains a `mask_head`"""
- return hasattr(self,
- 'roi_extractor') and self.roi_extractor is not None
-
- # @abstractmethod
- # def init_assigner_sampler(self, *args, **kwargs):
- # """Initialize assigner and sampler."""
- # pass
-
- @abstractmethod
- def loss(self, x: Tuple[Tensor], data_samples: DetSampleList):
- """Perform forward propagation and loss calculation of the roi head on
- the features of the upstream network."""
-
- @abstractmethod
- def predict(self, x: Tuple[Tensor],
- data_samples: DetSampleList) -> DetSampleList:
- """Perform forward propagation of the roi head and predict detection
- results on the features of the upstream network.
-
- Args:
- x (tuple[Tensor]): Features from upstream network. Each
- has shape (N, C, H, W).
- data_samples (List[:obj:`DetDataSample`]): The Data
- Samples. It usually includes `gt_instance`
-
- Returns:
- list[obj:`DetDataSample`]: Detection results of each image.
- Each item usually contains following keys in 'pred_instance'
-
- - scores (Tensor): Classification scores, has a shape
- (num_instance, )
- - labels (Tensor): Labels of bboxes, has a shape
- (num_instances, ).
- - bboxes (Tensor): Has a shape (num_instances, 4),
- the last dimension 4 arrange as (x1, y1, x2, y2).
- - polygon (List[Tensor]): Has a shape (num_instances, H, W).
- """
diff --git a/pipeline/mmocr/projects/ABCNet/abcnet/model/bezier_roi_extractor.py b/pipeline/mmocr/projects/ABCNet/abcnet/model/bezier_roi_extractor.py
deleted file mode 100644
index a4848d18e7c33eb6edad873eb376ed8f47480265..0000000000000000000000000000000000000000
--- a/pipeline/mmocr/projects/ABCNet/abcnet/model/bezier_roi_extractor.py
+++ /dev/null
@@ -1,120 +0,0 @@
-# Copyright (c) OpenMMLab. All rights reserved.
-from typing import List, Tuple
-
-import torch
-from mmengine.structures import InstanceData
-from torch import Tensor
-
-from mmocr.registry import MODELS
-from mmocr.utils import ConfigType, OptMultiConfig
-from .base_roi_extractor import BaseRoIExtractor
-
-
-@MODELS.register_module()
-class BezierRoIExtractor(BaseRoIExtractor):
- """Extract RoI features from a single level feature map.
-
- If there are multiple input feature levels, each RoI is mapped to a level
- according to its scale. The mapping rule is proposed in
- `FPN `_.
-
- Args:
- roi_layer (:obj:`ConfigDict` or dict): Specify RoI layer type and
- arguments.
- out_channels (int): Output channels of RoI layers.
- featmap_strides (List[int]): Strides of input feature maps.
- finest_scale (int): Scale threshold of mapping to level 0.
- Defaults to 56.
- init_cfg (:obj:`ConfigDict` or dict or list[:obj:`ConfigDict` or \
- dict], optional): Initialization config dict. Defaults to None.
- """
-
- def __init__(self,
- roi_layer: ConfigType,
- out_channels: int,
- featmap_strides: List[int],
- finest_scale: int = 96,
- init_cfg: OptMultiConfig = None) -> None:
- super().__init__(
- roi_layer=roi_layer,
- out_channels=out_channels,
- featmap_strides=featmap_strides,
- init_cfg=init_cfg)
- self.finest_scale = finest_scale
-
- def to_roi(self, beziers: Tensor) -> Tensor:
- rois_list = []
- for img_id, bezier in enumerate(beziers):
- img_inds = bezier.new_full((bezier.size(0), 1), img_id)
- rois = torch.cat([img_inds, bezier], dim=-1)
- rois_list.append(rois)
- rois = torch.cat(rois_list, 0)
- return rois
-
- def map_roi_levels(self, beziers: Tensor, num_levels: int) -> Tensor:
- """Map rois to corresponding feature levels by scales.
-
- - scale < finest_scale * 2: level 0
- - finest_scale * 2 <= scale < finest_scale * 4: level 1
- - finest_scale * 4 <= scale < finest_scale * 8: level 2
- - scale >= finest_scale * 8: level 3
- Args:
- beziers (Tensor): Input bezier control points, shape (k, 17).
- num_levels (int): Total level number.
- Returns:
- Tensor: Level index (0-based) of each RoI, shape (k, )
- """
-
- p1 = beziers[:, 1:3]
- p2 = beziers[:, 15:]
- scale = ((p1 - p2)**2).sum(dim=1).sqrt() * 2
- target_lvls = torch.floor(torch.log2(scale / self.finest_scale + 1e-6))
- target_lvls = target_lvls.clamp(min=0, max=num_levels - 1).long()
- return target_lvls
-
- def forward(self, feats: Tuple[Tensor],
- proposal_instances: List[InstanceData]) -> Tensor:
- """Extractor ROI feats.
-
- Args:
- feats (Tuple[Tensor]): Multi-scale features.
- proposal_instances(List[InstanceData]): Proposal instances.
-
- Returns:
- Tensor: RoI feature.
- """
- beziers = [p_i.beziers for p_i in proposal_instances]
- rois = self.to_roi(beziers)
- # convert fp32 to fp16 when amp is on
- rois = rois.type_as(feats[0])
- out_size = self.roi_layers[0].output_size
- feats = feats[:3]
- num_levels = len(feats)
- roi_feats = feats[0].new_zeros(
- rois.size(0), self.out_channels, *out_size)
-
- if num_levels == 1:
- if len(rois) == 0:
- return roi_feats
- return self.roi_layers[0](feats[0], rois)
-
- target_lvls = self.map_roi_levels(rois, num_levels)
-
- for i in range(num_levels):
- mask = target_lvls == i
- inds = mask.nonzero(as_tuple=False).squeeze(1)
- if inds.numel() > 0:
- rois_ = rois[inds]
- roi_feats_t = self.roi_layers[i](feats[i], rois_)
- roi_feats[inds] = roi_feats_t
- else:
- # Sometimes some pyramid levels will not be used for RoI
- # feature extraction and this will cause an incomplete
- # computation graph in one GPU, which is different from those
- # in other GPUs and will cause a hanging error.
- # Therefore, we add it to ensure each feature pyramid is
- # included in the computation graph to avoid runtime bugs.
- roi_feats += sum(
- x.view(-1)[0]
- for x in self.parameters()) * 0. + feats[i].sum() * 0.
- return roi_feats
diff --git a/pipeline/mmocr/projects/ABCNet/abcnet/model/bifpn.py b/pipeline/mmocr/projects/ABCNet/abcnet/model/bifpn.py
deleted file mode 100644
index 7f117dffe62bcb12f267df612abd56b22ad6e547..0000000000000000000000000000000000000000
--- a/pipeline/mmocr/projects/ABCNet/abcnet/model/bifpn.py
+++ /dev/null
@@ -1,242 +0,0 @@
-# Copyright (c) OpenMMLab. All rights reserved.
-from typing import List
-
-import torch
-import torch.nn as nn
-import torch.nn.functional as F
-from mmcv.cnn import ConvModule
-from mmengine.model import BaseModule
-
-from mmocr.registry import MODELS
-from mmocr.utils import ConfigType, MultiConfig, OptConfigType
-
-
-@MODELS.register_module()
-class BiFPN(BaseModule):
- """illustration of a minimal bifpn unit P7_0 ------------------------->
- P7_2 -------->
-
- |-------------| โ โ |
- P6_0 ---------> P6_1 ---------> P6_2 -------->
- |-------------|--------------โ โ โ | P5_0
- ---------> P5_1 ---------> P5_2 --------> |-------------|--------------โ
- โ โ | P4_0 ---------> P4_1 ---------> P4_2
- --------> |-------------|--------------โ โ
- |--------------โ | P3_0 -------------------------> P3_2 -------->
- """
-
- def __init__(self,
- in_channels: List[int],
- out_channels: int,
- num_outs: int,
- repeat_times: int = 2,
- start_level: int = 0,
- end_level: int = -1,
- add_extra_convs: bool = False,
- relu_before_extra_convs: bool = False,
- no_norm_on_lateral: bool = False,
- conv_cfg: OptConfigType = None,
- norm_cfg: OptConfigType = None,
- act_cfg: OptConfigType = None,
- laterial_conv1x1: bool = False,
- upsample_cfg: ConfigType = dict(mode='nearest'),
- pool_cfg: ConfigType = dict(),
- init_cfg: MultiConfig = dict(
- type='Xavier', layer='Conv2d', distribution='uniform')):
- super().__init__(init_cfg=init_cfg)
- assert isinstance(in_channels, list)
- self.in_channels = in_channels
- self.out_channels = out_channels
- self.num_ins = len(in_channels)
- self.num_outs = num_outs
- self.relu_before_extra_convs = relu_before_extra_convs
- self.no_norm_on_lateral = no_norm_on_lateral
- self.upsample_cfg = upsample_cfg.copy()
- self.repeat_times = repeat_times
- if end_level == -1 or end_level == self.num_ins - 1:
- self.backbone_end_level = self.num_ins
- assert num_outs >= self.num_ins - start_level
- else:
- # if end_level is not the last level, no extra level is allowed
- self.backbone_end_level = end_level + 1
- assert end_level < self.num_ins
- assert num_outs == end_level - start_level + 1
- self.start_level = start_level
- self.end_level = end_level
- self.add_extra_convs = add_extra_convs
-
- self.lateral_convs = nn.ModuleList()
- self.extra_convs = nn.ModuleList()
- self.bifpn_convs = nn.ModuleList()
- for i in range(self.start_level, self.backbone_end_level):
- if in_channels[i] == out_channels:
- l_conv = nn.Identity()
- else:
- l_conv = ConvModule(
- in_channels[i],
- out_channels,
- 1,
- conv_cfg=conv_cfg,
- norm_cfg=norm_cfg,
- bias=True,
- act_cfg=act_cfg,
- inplace=False)
- self.lateral_convs.append(l_conv)
-
- for _ in range(repeat_times):
- self.bifpn_convs.append(
- BiFPNLayer(
- channels=out_channels,
- levels=num_outs,
- conv_cfg=conv_cfg,
- norm_cfg=norm_cfg,
- act_cfg=act_cfg,
- pool_cfg=pool_cfg))
-
- # add extra conv layers (e.g., RetinaNet)
- extra_levels = num_outs - self.backbone_end_level + self.start_level
- if add_extra_convs and extra_levels >= 1:
- for i in range(extra_levels):
- if i == 0:
- in_channels = self.in_channels[self.backbone_end_level - 1]
- else:
- in_channels = out_channels
- if in_channels == out_channels:
- extra_fpn_conv = nn.MaxPool2d(
- kernel_size=3, stride=2, padding=1)
- else:
- extra_fpn_conv = nn.Sequential(
- ConvModule(
- in_channels=in_channels,
- out_channels=out_channels,
- kernel_size=1,
- norm_cfg=norm_cfg,
- act_cfg=act_cfg),
- nn.MaxPool2d(kernel_size=3, stride=2, padding=1))
- self.extra_convs.append(extra_fpn_conv)
-
- def forward(self, inputs):
-
- def extra_convs(inputs, extra_convs):
- outputs = list()
- for extra_conv in extra_convs:
- inputs = extra_conv(inputs)
- outputs.append(inputs)
- return outputs
-
- assert len(inputs) == len(self.in_channels)
-
- # build laterals
- laterals = [
- lateral_conv(inputs[i + self.start_level])
- for i, lateral_conv in enumerate(self.lateral_convs)
- ]
- if self.num_outs > len(laterals) and self.add_extra_convs:
- extra_source = inputs[self.backbone_end_level - 1]
- for extra_conv in self.extra_convs:
- extra_source = extra_conv(extra_source)
- laterals.append(extra_source)
-
- for bifpn_module in self.bifpn_convs:
- laterals = bifpn_module(laterals)
- outs = laterals
-
- return tuple(outs)
-
-
-def swish(x):
- return x * x.sigmoid()
-
-
-class BiFPNLayer(BaseModule):
-
- def __init__(self,
- channels,
- levels,
- init=0.5,
- conv_cfg=None,
- norm_cfg=None,
- act_cfg=None,
- upsample_cfg=None,
- pool_cfg=None,
- eps=0.0001,
- init_cfg=None):
- super().__init__(init_cfg=init_cfg)
- self.act_cfg = act_cfg
- self.upsample_cfg = upsample_cfg
- self.pool_cfg = pool_cfg
- self.eps = eps
- self.levels = levels
- self.bifpn_convs = nn.ModuleList()
- # weighted
- self.weight_two_nodes = nn.Parameter(
- torch.Tensor(2, levels).fill_(init))
- self.weight_three_nodes = nn.Parameter(
- torch.Tensor(3, levels - 2).fill_(init))
- self.relu = nn.ReLU()
- for _ in range(2):
- for _ in range(self.levels - 1): # 1,2,3
- fpn_conv = nn.Sequential(
- ConvModule(
- channels,
- channels,
- 3,
- padding=1,
- conv_cfg=conv_cfg,
- norm_cfg=norm_cfg,
- act_cfg=act_cfg,
- inplace=False))
- self.bifpn_convs.append(fpn_conv)
-
- def forward(self, inputs):
- assert len(inputs) == self.levels
- # build top-down and down-top path with stack
- levels = self.levels
- # w relu
- w1 = self.relu(self.weight_two_nodes)
- w1 /= torch.sum(w1, dim=0) + self.eps # normalize
- w2 = self.relu(self.weight_three_nodes)
- # w2 /= torch.sum(w2, dim=0) + self.eps # normalize
- # build top-down
- idx_bifpn = 0
- pathtd = inputs
- inputs_clone = []
- for in_tensor in inputs:
- inputs_clone.append(in_tensor.clone())
-
- for i in range(levels - 1, 0, -1):
- _, _, h, w = pathtd[i - 1].shape
- # pathtd[i - 1] = (
- # w1[0, i - 1] * pathtd[i - 1] + w1[1, i - 1] *
- # F.interpolate(pathtd[i], size=(h, w), mode='nearest')) / (
- # w1[0, i - 1] + w1[1, i - 1] + self.eps)
- pathtd[i -
- 1] = w1[0, i -
- 1] * pathtd[i - 1] + w1[1, i - 1] * F.interpolate(
- pathtd[i], size=(h, w), mode='nearest')
- pathtd[i - 1] = swish(pathtd[i - 1])
- pathtd[i - 1] = self.bifpn_convs[idx_bifpn](pathtd[i - 1])
- idx_bifpn = idx_bifpn + 1
- # build down-top
- for i in range(0, levels - 2, 1):
- tmp_path = torch.stack([
- inputs_clone[i + 1], pathtd[i + 1],
- F.max_pool2d(pathtd[i], kernel_size=3, stride=2, padding=1)
- ],
- dim=-1)
- norm_weight = w2[:, i] / (w2[:, i].sum() + self.eps)
- pathtd[i + 1] = (norm_weight * tmp_path).sum(dim=-1)
- # pathtd[i + 1] = w2[0, i] * inputs_clone[i + 1]
- # + w2[1, i] * pathtd[
- # i + 1] + w2[2, i] * F.max_pool2d(
- # pathtd[i], kernel_size=3, stride=2, padding=1)
- pathtd[i + 1] = swish(pathtd[i + 1])
- pathtd[i + 1] = self.bifpn_convs[idx_bifpn](pathtd[i + 1])
- idx_bifpn = idx_bifpn + 1
-
- pathtd[levels - 1] = w1[0, levels - 1] * pathtd[levels - 1] + w1[
- 1, levels - 1] * F.max_pool2d(
- pathtd[levels - 2], kernel_size=3, stride=2, padding=1)
- pathtd[levels - 1] = swish(pathtd[levels - 1])
- pathtd[levels - 1] = self.bifpn_convs[idx_bifpn](pathtd[levels - 1])
- return pathtd
diff --git a/pipeline/mmocr/projects/ABCNet/abcnet/model/coordinate_head.py b/pipeline/mmocr/projects/ABCNet/abcnet/model/coordinate_head.py
deleted file mode 100644
index dc31e88a628d0d8cd2f82cbdd4cc010eaea39938..0000000000000000000000000000000000000000
--- a/pipeline/mmocr/projects/ABCNet/abcnet/model/coordinate_head.py
+++ /dev/null
@@ -1,56 +0,0 @@
-import torch
-import torch.nn as nn
-from mmcv.cnn import ConvModule
-from mmengine.model import BaseModule
-
-from mmocr.registry import MODELS
-
-
-@MODELS.register_module()
-class CoordinateHead(BaseModule):
-
- def __init__(self,
- in_channel=256,
- conv_num=4,
- norm_cfg=dict(type='BN'),
- act_cfg=dict(type='ReLU'),
- init_cfg=None):
- super().__init__(init_cfg=init_cfg)
-
- mask_convs = list()
- for i in range(conv_num):
- if i == 0:
- mask_conv = ConvModule(
- in_channels=in_channel + 2, # 2 for coord
- out_channels=in_channel,
- kernel_size=3,
- padding=1,
- norm_cfg=norm_cfg,
- act_cfg=act_cfg)
- else:
- mask_conv = ConvModule(
- in_channels=in_channel,
- out_channels=in_channel,
- kernel_size=3,
- padding=1,
- norm_cfg=norm_cfg,
- act_cfg=act_cfg)
- mask_convs.append(mask_conv)
- self.mask_convs = nn.Sequential(*mask_convs)
-
- def forward(self, features):
- coord_features = list()
- for feature in features:
- x_range = torch.linspace(
- -1, 1, feature.shape[-1], device=feature.device)
- y_range = torch.linspace(
- -1, 1, feature.shape[-2], device=feature.device)
- y, x = torch.meshgrid(y_range, x_range)
- y = y.expand([feature.shape[0], 1, -1, -1])
- x = x.expand([feature.shape[0], 1, -1, -1])
- coord = torch.cat([x, y], 1)
- feature_with_coord = torch.cat([feature, coord], dim=1)
- feature_with_coord = self.mask_convs(feature_with_coord)
- feature_with_coord = feature_with_coord + feature
- coord_features.append(feature_with_coord)
- return coord_features
diff --git a/pipeline/mmocr/projects/ABCNet/abcnet/model/rec_roi_head.py b/pipeline/mmocr/projects/ABCNet/abcnet/model/rec_roi_head.py
deleted file mode 100644
index a102902c530dca85f2f87be1b5dec8882ac26b2b..0000000000000000000000000000000000000000
--- a/pipeline/mmocr/projects/ABCNet/abcnet/model/rec_roi_head.py
+++ /dev/null
@@ -1,70 +0,0 @@
-# Copyright (c) OpenMMLab. All rights reserved.
-from typing import Tuple
-
-from mmengine.structures import LabelData
-from torch import Tensor
-
-from mmocr.registry import MODELS, TASK_UTILS
-from mmocr.structures import TextRecogDataSample # noqa F401
-from mmocr.utils import DetSampleList, OptMultiConfig, RecSampleList
-from .base_roi_head import BaseRoIHead
-
-
-@MODELS.register_module()
-class RecRoIHead(BaseRoIHead):
- """Simplest base roi head including one bbox head and one mask head."""
-
- def __init__(self,
- neck=None,
- sampler: OptMultiConfig = None,
- roi_extractor: OptMultiConfig = None,
- rec_head: OptMultiConfig = None,
- init_cfg=None):
- super().__init__(init_cfg)
- if sampler is not None:
- self.sampler = TASK_UTILS.build(sampler)
- if neck is not None:
- self.neck = MODELS.build(neck)
- self.roi_extractor = MODELS.build(roi_extractor)
- self.rec_head = MODELS.build(rec_head)
-
- def loss(self, inputs: Tuple[Tensor], data_samples: DetSampleList) -> dict:
- """Perform forward propagation and loss calculation of the detection
- roi on the features of the upstream network.
-
- Args:
- x (tuple[Tensor]): List of multi-level img features.
- rpn_results_list (list[:obj:`InstanceData`]): List of region
- proposals.
- DetSampleList (list[:obj:`DetDataSample`]): The batch
- data samples. It usually includes information such
- as `gt_instance` or `gt_panoptic_seg` or `gt_sem_seg`.
-
- Returns:
- dict[str, Tensor]: A dictionary of loss components
- """
- proposals = [
- ds.gt_instances[~ds.gt_instances.ignored] for ds in data_samples
- ]
-
- proposals = [p for p in proposals if len(p) > 0]
- bbox_feats = self.roi_extractor(inputs, proposals)
- rec_data_samples = [
- TextRecogDataSample(gt_text=LabelData(item=text))
- for proposal in proposals for text in proposal.texts
- ]
- return self.rec_head.loss(bbox_feats, rec_data_samples)
-
- def predict(self, inputs: Tuple[Tensor],
- data_samples: DetSampleList) -> RecSampleList:
- if hasattr(self, 'neck') and self.neck is not None:
- inputs = self.neck(inputs)
- pred_instances = [ds.pred_instances for ds in data_samples]
- bbox_feats = self.roi_extractor(inputs, pred_instances)
- if bbox_feats.size(0) == 0:
- return []
- len_instance = sum(
- [len(instance_data) for instance_data in pred_instances])
- rec_data_samples = [TextRecogDataSample() for _ in range(len_instance)]
- rec_data_samples = self.rec_head.predict(bbox_feats, rec_data_samples)
- return rec_data_samples
diff --git a/pipeline/mmocr/projects/ABCNet/abcnet/model/two_stage_text_spotting.py b/pipeline/mmocr/projects/ABCNet/abcnet/model/two_stage_text_spotting.py
deleted file mode 100644
index 4a9bd8efc7f832b6fa3273af4eff9a6b670b3356..0000000000000000000000000000000000000000
--- a/pipeline/mmocr/projects/ABCNet/abcnet/model/two_stage_text_spotting.py
+++ /dev/null
@@ -1,93 +0,0 @@
-# Copyright (c) OpenMMLab. All rights reserved.
-from typing import Dict
-
-import torch
-
-from mmocr.models.textdet.detectors.base import BaseTextDetector
-from mmocr.registry import MODELS
-from mmocr.utils import OptConfigType, OptDetSampleList, OptMultiConfig
-
-
-@MODELS.register_module()
-class TwoStageTextSpotter(BaseTextDetector):
- """Two-stage text spotter.
-
- Args:
- backbone (dict, optional): Config dict for text spotter backbone.
- Defaults to None.
- neck (dict, optional): Config dict for text spotter neck. Defaults to
- None.
- det_head (dict, optional): Config dict for text spotter head. Defaults
- to None.
- roi_head (dict, optional): Config dict for text spotter roi head.
- Defaults to None.
- data_preprocessor (dict, optional): Config dict for text spotter data
- preprocessor. Defaults to None.
- init_cfg (dict, optional): Initialization config dict. Defaults to
- None.
- """
-
- def __init__(self,
- backbone: OptConfigType = None,
- neck: OptConfigType = None,
- det_head: OptConfigType = None,
- roi_head: OptConfigType = None,
- postprocessor: OptConfigType = None,
- data_preprocessor: OptConfigType = None,
- init_cfg: OptMultiConfig = None) -> None:
-
- super().__init__(
- data_preprocessor=data_preprocessor, init_cfg=init_cfg)
-
- self.backbone = MODELS.build(backbone)
- if neck is not None:
- self.neck = MODELS.build(neck)
- if det_head is not None:
- self.det_head = MODELS.build(det_head)
-
- if roi_head is not None:
- self.roi_head = MODELS.build(roi_head)
-
- if postprocessor is not None:
- self.postprocessor = MODELS.build(postprocessor)
-
- @property
- def with_det_head(self):
- """bool: whether the detector has RPN"""
- return hasattr(self, 'det_head') and self.det_head is not None
-
- @property
- def with_roi_head(self):
- """bool: whether the detector has a RoI head"""
- return hasattr(self, 'roi_head') and self.roi_head is not None
-
- def extract_feat(self, img):
- """Directly extract features from the backbone+neck."""
- x = self.backbone(img)
- if self.with_neck:
- x = self.neck(x)
- return x
-
- def loss(self, inputs: torch.Tensor,
- data_samples: OptDetSampleList) -> Dict:
- losses = dict()
- inputs = self.extract_feat(inputs)
- det_loss, data_samples = self.det_head.loss_and_predict(
- inputs, data_samples)
- roi_losses = self.roi_head.loss(inputs, data_samples)
- losses.update(det_loss)
- losses.update(roi_losses)
- return losses
-
- def predict(self, inputs: torch.Tensor,
- data_samples: OptDetSampleList) -> OptDetSampleList:
- """Predict results from a batch of inputs and data samples with post-
- processing."""
- inputs = self.extract_feat(inputs)
- data_samples = self.det_head.predict(inputs, data_samples)
- rec_data_samples = self.roi_head.predict(inputs, data_samples)
- return self.postprocessor(data_samples, rec_data_samples)
-
- def _forward(self, inputs: torch.Tensor,
- data_samples: OptDetSampleList) -> torch.Tensor:
- pass
diff --git a/pipeline/mmocr/projects/ABCNet/abcnet/utils/__init__.py b/pipeline/mmocr/projects/ABCNet/abcnet/utils/__init__.py
deleted file mode 100644
index d0007ffae850901ee62e43beebfe56fc2865cf73..0000000000000000000000000000000000000000
--- a/pipeline/mmocr/projects/ABCNet/abcnet/utils/__init__.py
+++ /dev/null
@@ -1,4 +0,0 @@
-# Copyright (c) OpenMMLab. All rights reserved.
-from .bezier_utils import bezier2poly, poly2bezier
-
-__all__ = ['poly2bezier', 'bezier2poly']
diff --git a/pipeline/mmocr/projects/ABCNet/abcnet/utils/bezier_utils.py b/pipeline/mmocr/projects/ABCNet/abcnet/utils/bezier_utils.py
deleted file mode 100644
index d93a6293926e2d807eb089bf92835e39a4ef5d84..0000000000000000000000000000000000000000
--- a/pipeline/mmocr/projects/ABCNet/abcnet/utils/bezier_utils.py
+++ /dev/null
@@ -1,62 +0,0 @@
-# Copyright (c) OpenMMLab. All rights reserved.
-import numpy as np
-from scipy.special import comb as n_over_k
-
-from mmocr.utils.typing_utils import ArrayLike
-
-
-def bezier_coefficient(n, t, k):
- return t**k * (1 - t)**(n - k) * n_over_k(n, k)
-
-
-def bezier_coefficients(time, point_num, ratios):
- return [[bezier_coefficient(time, ratio, num) for num in range(point_num)]
- for ratio in ratios]
-
-
-def linear_interpolation(point1: np.ndarray,
- point2: np.ndarray,
- number: int = 2) -> np.ndarray:
- t = np.linspace(0, 1, number + 2).reshape(-1, 1)
- return point1 + (point2 - point1) * t
-
-
-def curve2bezier(curve: ArrayLike):
- curve = np.array(curve).reshape(-1, 2)
- if len(curve) == 2:
- return linear_interpolation(curve[0], curve[1])
- diff = curve[1:] - curve[:-1]
- distance = np.linalg.norm(diff, axis=-1)
- norm_distance = distance / distance.sum()
- norm_distance = np.hstack(([0], norm_distance))
- cum_norm_dis = norm_distance.cumsum()
- pseudo_inv = np.linalg.pinv(bezier_coefficients(3, 4, cum_norm_dis))
- control_points = pseudo_inv.dot(curve)
- return control_points
-
-
-def bezier2curve(bezier: np.ndarray, num_sample: int = 10):
- bezier = np.asarray(bezier)
- t = np.linspace(0, 1, num_sample)
- return np.array(bezier_coefficients(3, 4, t)).dot(bezier)
-
-
-def poly2bezier(poly):
- poly = np.array(poly).reshape(-1, 2)
- points_num = len(poly)
- up_curve = poly[:points_num // 2]
- down_curve = poly[points_num // 2:]
- up_bezier = curve2bezier(up_curve)
- down_bezier = curve2bezier(down_curve)
- up_bezier[0] = up_curve[0]
- up_bezier[-1] = up_curve[-1]
- down_bezier[0] = down_curve[0]
- down_bezier[-1] = down_curve[-1]
- return np.vstack((up_bezier, down_bezier)).flatten().tolist()
-
-
-def bezier2poly(bezier, num_sample=20):
- bezier = bezier.reshape(2, 4, 2)
- curve_top = bezier2curve(bezier[0], num_sample)
- curve_bottom = bezier2curve(bezier[1], num_sample)
- return np.vstack((curve_top, curve_bottom)).flatten().tolist()
diff --git a/pipeline/mmocr/projects/ABCNet/config/_base_/datasets/icdar2015.py b/pipeline/mmocr/projects/ABCNet/config/_base_/datasets/icdar2015.py
deleted file mode 100644
index 240f1347fda7057aa20f009e493aca368d097954..0000000000000000000000000000000000000000
--- a/pipeline/mmocr/projects/ABCNet/config/_base_/datasets/icdar2015.py
+++ /dev/null
@@ -1,15 +0,0 @@
-icdar2015_textspotting_data_root = 'data/icdar2015'
-
-icdar2015_textspotting_train = dict(
- type='OCRDataset',
- data_root=icdar2015_textspotting_data_root,
- ann_file='textspotting_train.json',
- pipeline=None)
-
-icdar2015_textspotting_test = dict(
- type='OCRDataset',
- data_root=icdar2015_textspotting_data_root,
- ann_file='textspotting_test.json',
- test_mode=True,
- # indices=50,
- pipeline=None)
diff --git a/pipeline/mmocr/projects/ABCNet/config/_base_/default_runtime.py b/pipeline/mmocr/projects/ABCNet/config/_base_/default_runtime.py
deleted file mode 100644
index 4b9b72c53f6285ebb2a205982226066b4e21178e..0000000000000000000000000000000000000000
--- a/pipeline/mmocr/projects/ABCNet/config/_base_/default_runtime.py
+++ /dev/null
@@ -1,41 +0,0 @@
-default_scope = 'mmocr'
-env_cfg = dict(
- cudnn_benchmark=False,
- mp_cfg=dict(mp_start_method='fork', opencv_num_threads=0),
- dist_cfg=dict(backend='nccl'),
-)
-randomness = dict(seed=None)
-
-default_hooks = dict(
- timer=dict(type='IterTimerHook'),
- logger=dict(type='LoggerHook', interval=5),
- param_scheduler=dict(type='ParamSchedulerHook'),
- checkpoint=dict(type='CheckpointHook', interval=20),
- sampler_seed=dict(type='DistSamplerSeedHook'),
- sync_buffer=dict(type='SyncBuffersHook'),
- visualization=dict(
- type='VisualizationHook',
- interval=1,
- enable=False,
- show=False,
- draw_gt=False,
- draw_pred=False),
-)
-
-# Logging
-log_level = 'INFO'
-log_processor = dict(type='LogProcessor', window_size=10, by_epoch=True)
-
-load_from = None
-resume = False
-
-# Evaluation
-val_evaluator = [dict(type='E2EHmeanIOUMetric'), dict(type='HmeanIOUMetric')]
-test_evaluator = val_evaluator
-
-# Visualization
-vis_backends = [dict(type='LocalVisBackend')]
-visualizer = dict(
- type='TextSpottingLocalVisualizer',
- name='visualizer',
- vis_backends=vis_backends)
diff --git a/pipeline/mmocr/projects/ABCNet/config/_base_/schedules/schedule_sgd_500e.py b/pipeline/mmocr/projects/ABCNet/config/_base_/schedules/schedule_sgd_500e.py
deleted file mode 100644
index 431c48ff9ddfbcd25425007c633014d68f5a64e0..0000000000000000000000000000000000000000
--- a/pipeline/mmocr/projects/ABCNet/config/_base_/schedules/schedule_sgd_500e.py
+++ /dev/null
@@ -1,12 +0,0 @@
-# optimizer
-optim_wrapper = dict(
- type='OptimWrapper',
- optimizer=dict(type='SGD', lr=0.001, momentum=0.9, weight_decay=0.0001),
- clip_grad=dict(type='value', clip_value=1))
-train_cfg = dict(type='EpochBasedTrainLoop', max_epochs=500, val_interval=20)
-val_cfg = dict(type='ValLoop')
-test_cfg = dict(type='TestLoop')
-# learning policy
-param_scheduler = [
- dict(type='LinearLR', end=1000, start_factor=0.001, by_epoch=False),
-]
diff --git a/pipeline/mmocr/projects/ABCNet/config/abcnet/_base_abcnet_resnet50_fpn.py b/pipeline/mmocr/projects/ABCNet/config/abcnet/_base_abcnet_resnet50_fpn.py
deleted file mode 100644
index 05d570132485a43aa1afb8646f9aaa609a42f286..0000000000000000000000000000000000000000
--- a/pipeline/mmocr/projects/ABCNet/config/abcnet/_base_abcnet_resnet50_fpn.py
+++ /dev/null
@@ -1,165 +0,0 @@
-num_classes = 1
-strides = [8, 16, 32, 64, 128]
-bbox_coder = dict(type='mmdet.DistancePointBBoxCoder')
-with_bezier = True
-norm_on_bbox = True
-use_sigmoid_cls = True
-
-dictionary = dict(
- type='Dictionary',
- dict_file='{{ fileDirname }}/../../dicts/abcnet.txt',
- with_start=False,
- with_end=False,
- same_start_end=False,
- with_padding=True,
- with_unknown=True)
-
-model = dict(
- type='ABCNet',
- data_preprocessor=dict(
- type='TextDetDataPreprocessor',
- mean=[123.675, 116.28, 103.53][::-1],
- std=[1, 1, 1],
- bgr_to_rgb=False,
- pad_size_divisor=32),
- backbone=dict(
- type='mmdet.ResNet',
- depth=50,
- num_stages=4,
- out_indices=(0, 1, 2, 3),
- frozen_stages=1,
- norm_cfg=dict(type='BN', requires_grad=False),
- norm_eval=True,
- style='caffe',
- init_cfg=dict(
- type='Pretrained',
- checkpoint='open-mmlab://detectron2/resnet50_caffe')),
- neck=dict(
- type='mmdet.FPN',
- in_channels=[256, 512, 1024, 2048],
- out_channels=256,
- start_level=0,
- add_extra_convs='on_output', # use P5
- num_outs=6,
- relu_before_extra_convs=True),
- det_head=dict(
- type='ABCNetDetHead',
- num_classes=num_classes,
- in_channels=256,
- stacked_convs=4,
- feat_channels=256,
- strides=strides,
- norm_on_bbox=norm_on_bbox,
- use_sigmoid_cls=use_sigmoid_cls,
- centerness_on_reg=True,
- dcn_on_last_conv=False,
- conv_bias=True,
- use_scale=False,
- with_bezier=with_bezier,
- init_cfg=dict(
- type='Normal',
- layer='Conv2d',
- std=0.01,
- override=dict(
- type='Normal',
- name='conv_cls',
- std=0.01,
- bias=-4.59511985013459), # -log((1-p)/p) where p=0.01
- ),
- module_loss=dict(
- type='ABCNetDetModuleLoss',
- num_classes=num_classes,
- strides=strides,
- center_sampling=True,
- center_sample_radius=1.5,
- bbox_coder=bbox_coder,
- norm_on_bbox=norm_on_bbox,
- loss_cls=dict(
- type='mmdet.FocalLoss',
- use_sigmoid=use_sigmoid_cls,
- gamma=2.0,
- alpha=0.25,
- loss_weight=1.0),
- loss_bbox=dict(type='mmdet.GIoULoss', loss_weight=1.0),
- loss_centerness=dict(
- type='mmdet.CrossEntropyLoss',
- use_sigmoid=True,
- loss_weight=1.0)),
- postprocessor=dict(
- type='ABCNetDetPostprocessor',
- use_sigmoid_cls=use_sigmoid_cls,
- strides=[8, 16, 32, 64, 128],
- bbox_coder=dict(type='mmdet.DistancePointBBoxCoder'),
- with_bezier=True,
- test_cfg=dict(
- nms_pre=1000,
- nms=dict(type='nms', iou_threshold=0.5),
- score_thr=0.3))),
- roi_head=dict(
- type='RecRoIHead',
- roi_extractor=dict(
- type='BezierRoIExtractor',
- roi_layer=dict(
- type='BezierAlign', output_size=(8, 32), sampling_ratio=1.0),
- out_channels=256,
- featmap_strides=[4, 8, 16]),
- rec_head=dict(
- type='ABCNetRec',
- backbone=dict(type='ABCNetRecBackbone'),
- encoder=dict(type='ABCNetRecEncoder'),
- decoder=dict(
- type='ABCNetRecDecoder',
- dictionary=dictionary,
- postprocessor=dict(
- type='AttentionPostprocessor',
- ignore_chars=['padding', 'unknown']),
- module_loss=dict(
- type='CEModuleLoss',
- ignore_first_char=False,
- ignore_char=-1,
- reduction='mean'),
- max_seq_len=25))),
- postprocessor=dict(
- type='ABCNetPostprocessor',
- rescale_fields=['polygons', 'bboxes', 'beziers'],
- ))
-
-test_pipeline = [
- dict(type='LoadImageFromFile', color_type='color_ignore_orientation'),
- dict(type='Resize', scale=(2000, 4000), keep_ratio=True, backend='pillow'),
- dict(
- type='LoadOCRAnnotations',
- with_polygon=True,
- with_bbox=True,
- with_label=True,
- with_text=True),
- dict(
- type='PackTextDetInputs',
- meta_keys=('img_path', 'ori_shape', 'img_shape', 'scale_factor'))
-]
-
-train_pipeline = [
- dict(type='LoadImageFromFile', color_type='color_ignore_orientation'),
- dict(
- type='LoadOCRAnnotations',
- with_polygon=True,
- with_bbox=True,
- with_label=True,
- with_text=True),
- dict(type='RemoveIgnored'),
- dict(type='RandomCrop', min_side_ratio=0.1),
- dict(
- type='RandomRotate',
- max_angle=30,
- pad_with_fixed_color=True,
- use_canvas=True),
- dict(
- type='RandomChoiceResize',
- scales=[(980, 2900), (1044, 2900), (1108, 2900), (1172, 2900),
- (1236, 2900), (1300, 2900), (1364, 2900), (1428, 2900),
- (1492, 2900)],
- keep_ratio=True),
- dict(
- type='PackTextDetInputs',
- meta_keys=('img_path', 'ori_shape', 'img_shape', 'scale_factor'))
-]
diff --git a/pipeline/mmocr/projects/ABCNet/config/abcnet/abcnet_resnet50_fpn_500e_icdar2015.py b/pipeline/mmocr/projects/ABCNet/config/abcnet/abcnet_resnet50_fpn_500e_icdar2015.py
deleted file mode 100644
index 424a35254ebdd3050e8e13b506b7ee5d97a565fb..0000000000000000000000000000000000000000
--- a/pipeline/mmocr/projects/ABCNet/config/abcnet/abcnet_resnet50_fpn_500e_icdar2015.py
+++ /dev/null
@@ -1,37 +0,0 @@
-_base_ = [
- '_base_abcnet_resnet50_fpn.py',
- '../_base_/datasets/icdar2015.py',
- '../_base_/default_runtime.py',
- '../_base_/schedules/schedule_sgd_500e.py',
-]
-
-# dataset settings
-icdar2015_textspotting_train = _base_.icdar2015_textspotting_train
-icdar2015_textspotting_train.pipeline = _base_.train_pipeline
-icdar2015_textspotting_test = _base_.icdar2015_textspotting_test
-icdar2015_textspotting_test.pipeline = _base_.test_pipeline
-
-train_dataloader = dict(
- batch_size=2,
- num_workers=8,
- persistent_workers=True,
- sampler=dict(type='DefaultSampler', shuffle=True),
- dataset=icdar2015_textspotting_train)
-
-val_dataloader = dict(
- batch_size=1,
- num_workers=4,
- persistent_workers=True,
- sampler=dict(type='DefaultSampler', shuffle=False),
- dataset=icdar2015_textspotting_test)
-
-test_dataloader = val_dataloader
-
-val_cfg = dict(type='ValLoop')
-test_cfg = dict(type='TestLoop')
-
-custom_imports = dict(imports=['abcnet'], allow_failed_imports=False)
-
-load_from = 'https://download.openmmlab.com/mmocr/textspotting/abcnet/abcnet_resnet50_fpn_500e_icdar2015/abcnet_resnet50_fpn_pretrain-d060636c.pth' # noqa
-
-find_unused_parameters = True
diff --git a/pipeline/mmocr/projects/ABCNet/config/abcnet_v2/_base_abcnet-v2_resnet50_bifpn.py b/pipeline/mmocr/projects/ABCNet/config/abcnet_v2/_base_abcnet-v2_resnet50_bifpn.py
deleted file mode 100644
index b6bca5a6c292b663ba440df087265828f76a646a..0000000000000000000000000000000000000000
--- a/pipeline/mmocr/projects/ABCNet/config/abcnet_v2/_base_abcnet-v2_resnet50_bifpn.py
+++ /dev/null
@@ -1,118 +0,0 @@
-num_classes = 1
-strides = [8, 16, 32, 64, 128]
-bbox_coder = dict(type='mmdet.DistancePointBBoxCoder')
-with_bezier = True
-norm_on_bbox = True
-use_sigmoid_cls = True
-
-dictionary = dict(
- type='Dictionary',
- dict_file='{{ fileDirname }}/../../dicts/abcnet.txt',
- with_start=False,
- with_end=False,
- same_start_end=False,
- with_padding=True,
- with_unknown=True)
-
-model = dict(
- type='ABCNet',
- data_preprocessor=dict(
- type='TextDetDataPreprocessor',
- mean=[123.675, 116.28, 103.53][::-1],
- std=[1, 1, 1],
- bgr_to_rgb=False,
- pad_size_divisor=32),
- backbone=dict(
- type='mmdet.ResNet',
- depth=50,
- num_stages=4,
- out_indices=(0, 1, 2, 3),
- frozen_stages=1,
- norm_cfg=dict(type='BN', requires_grad=False),
- norm_eval=True,
- style='caffe',
- init_cfg=dict(
- type='Pretrained',
- checkpoint='open-mmlab://detectron2/resnet50_caffe')),
- neck=dict(
- type='BiFPN',
- in_channels=[256, 512, 1024, 2048],
- out_channels=256,
- start_level=0,
- add_extra_convs=True, # use P5
- norm_cfg=dict(type='BN'),
- num_outs=6,
- relu_before_extra_convs=True),
- det_head=dict(
- type='ABCNetDetHead',
- num_classes=num_classes,
- in_channels=256,
- stacked_convs=4,
- feat_channels=256,
- strides=strides,
- norm_on_bbox=norm_on_bbox,
- use_sigmoid_cls=use_sigmoid_cls,
- centerness_on_reg=True,
- dcn_on_last_conv=False,
- conv_bias=True,
- use_scale=False,
- with_bezier=with_bezier,
- init_cfg=dict(
- type='Normal',
- layer='Conv2d',
- std=0.01,
- override=dict(
- type='Normal',
- name='conv_cls',
- std=0.01,
- bias=-4.59511985013459), # -log((1-p)/p) where p=0.01
- ),
- module_loss=None,
- postprocessor=dict(
- type='ABCNetDetPostprocessor',
- # rescale_fields=['polygons', 'bboxes'],
- use_sigmoid_cls=use_sigmoid_cls,
- strides=[8, 16, 32, 64, 128],
- bbox_coder=dict(type='mmdet.DistancePointBBoxCoder'),
- with_bezier=True,
- test_cfg=dict(
- # rescale_fields=['polygon', 'bboxes', 'bezier'],
- nms_pre=1000,
- nms=dict(type='nms', iou_threshold=0.4),
- score_thr=0.3))),
- roi_head=dict(
- type='RecRoIHead',
- neck=dict(type='CoordinateHead'),
- roi_extractor=dict(
- type='BezierRoIExtractor',
- roi_layer=dict(
- type='BezierAlign', output_size=(16, 64), sampling_ratio=1.0),
- out_channels=256,
- featmap_strides=[4, 8, 16]),
- rec_head=dict(
- type='ABCNetRec',
- backbone=dict(type='ABCNetRecBackbone'),
- encoder=dict(type='ABCNetRecEncoder'),
- decoder=dict(
- type='ABCNetRecDecoder',
- dictionary=dictionary,
- postprocessor=dict(type='AttentionPostprocessor'),
- max_seq_len=25))),
- postprocessor=dict(
- type='ABCNetPostprocessor',
- rescale_fields=['polygons', 'bboxes', 'beziers'],
- ))
-
-test_pipeline = [
- dict(type='LoadImageFromFile', color_type='color_ignore_orientation'),
- dict(type='Resize', scale=(2000, 4000), keep_ratio=True, backend='pillow'),
- dict(
- type='LoadOCRAnnotations',
- with_polygon=True,
- with_bbox=True,
- with_label=True,
- with_text=True),
- dict(
- type='PackTextDetInputs',
- meta_keys=('img_path', 'ori_shape', 'img_shape', 'scale_factor'))
-]
diff --git a/pipeline/mmocr/projects/ABCNet/config/abcnet_v2/abcnet-v2_resnet50_bifpn_500e_icdar2015.py b/pipeline/mmocr/projects/ABCNet/config/abcnet_v2/abcnet-v2_resnet50_bifpn_500e_icdar2015.py
deleted file mode 100644
index 5b51f562438981299cd009349f795a4379eb9f96..0000000000000000000000000000000000000000
--- a/pipeline/mmocr/projects/ABCNet/config/abcnet_v2/abcnet-v2_resnet50_bifpn_500e_icdar2015.py
+++ /dev/null
@@ -1,23 +0,0 @@
-_base_ = [
- '_base_abcnet-v2_resnet50_bifpn.py',
- '../_base_/datasets/icdar2015.py',
- '../_base_/default_runtime.py',
-]
-
-# dataset settings
-icdar2015_textspotting_test = _base_.icdar2015_textspotting_test
-icdar2015_textspotting_test.pipeline = _base_.test_pipeline
-
-val_dataloader = dict(
- batch_size=1,
- num_workers=4,
- persistent_workers=True,
- sampler=dict(type='DefaultSampler', shuffle=False),
- dataset=icdar2015_textspotting_test)
-
-test_dataloader = val_dataloader
-
-val_cfg = dict(type='ValLoop')
-test_cfg = dict(type='TestLoop')
-
-custom_imports = dict(imports=['abcnet'], allow_failed_imports=False)
diff --git a/pipeline/mmocr/projects/ABCNet/dicts/abcnet.txt b/pipeline/mmocr/projects/ABCNet/dicts/abcnet.txt
deleted file mode 100644
index 173d6c4a7ad83dcb6cdb3d177456d0b4d553c01c..0000000000000000000000000000000000000000
--- a/pipeline/mmocr/projects/ABCNet/dicts/abcnet.txt
+++ /dev/null
@@ -1,95 +0,0 @@
-
-!
-"
-#
-$
-%
-&
-'
-(
-)
-*
-+
-,
--
-.
-/
-0
-1
-2
-3
-4
-5
-6
-7
-8
-9
-:
-;
-<
-=
->
-?
-@
-A
-B
-C
-D
-E
-F
-G
-H
-I
-J
-K
-L
-M
-N
-O
-P
-Q
-R
-S
-T
-U
-V
-W
-X
-Y
-Z
-[
-\
-]
-^
-_
-`
-a
-b
-c
-d
-e
-f
-g
-h
-i
-j
-k
-l
-m
-n
-o
-p
-q
-r
-s
-t
-u
-v
-w
-x
-y
-z
-{
-|
-}
-~
\ No newline at end of file
diff --git a/pipeline/mmocr/projects/README.md b/pipeline/mmocr/projects/README.md
deleted file mode 100644
index b9dc68752a1dd491eb2d3c43debe665fd00fa77a..0000000000000000000000000000000000000000
--- a/pipeline/mmocr/projects/README.md
+++ /dev/null
@@ -1,13 +0,0 @@
-# Projects
-
-The OpenMMLab ecosystem can only grow through the contributions of the community.
-Everyone is welcome to post their implementation of any great ideas in this folder! If you wish to start your own project, please go through the [example project](example_project/) for the best practice. For common questions about projects, please read our [faq](faq.md).
-
-## External Projects
-
-Here we lists some selected external projects released in the community built upon MMOCR:
-
-- [TableMASTER-mmocr](https://github.com/JiaquanYe/TableMASTER-mmocr)
-- [WordArt](https://github.com/xdxie/WordArt)
-
-Note: The core maintainers of MMOCR only ensure the results are reproducible and the code quality meets its claim at the time each project was submitted, but they may not be responsible for future maintenance. The original authors take responsibility for maintaining their own projects.
diff --git a/pipeline/mmocr/projects/SPTS/README.md b/pipeline/mmocr/projects/SPTS/README.md
deleted file mode 100644
index af4a4f9b3ba78979ff725c5ba2e58b8472984e3f..0000000000000000000000000000000000000000
--- a/pipeline/mmocr/projects/SPTS/README.md
+++ /dev/null
@@ -1,186 +0,0 @@
-# SPTS: Single-Point Text Spotting
-
-
-
-## Description
-
-This is an implementation of [SPTS](https://github.com/shannanyinxiang/SPTS) based on [MMOCR](https://github.com/open-mmlab/mmocr/tree/dev-1.x), [MMCV](https://github.com/open-mmlab/mmcv), and [MMEngine](https://github.com/open-mmlab/mmengine).
-
-Existing scene text spotting (i.e., end-to-end text detection and recognition) methods rely on costly bounding box annotations (e.g., text-line, word-level, or character-level bounding boxes). For the first time, we demonstrate that training scene text spotting models can be achieved with an extremely low-cost annotation of a single-point for each instance. We propose an end-to-end scene text spotting method that tackles scene text spotting as a sequence prediction task. Given an image as input, we formulate the desired detection and recognition results as a sequence of discrete tokens and use an auto-regressive Transformer to predict the sequence. The proposed method is simple yet effective, which can achieve state-of-the-art results on widely used benchmarks. Most significantly, we show that the performance is not very sensitive to the positions of the point annotation, meaning that it can be much easier to be annotated or even be automatically generated than the bounding box that requires precise positions. We believe that such a pioneer attempt indicates a significant opportunity for scene text spotting applications of a much larger scale than previously possible.
-
-
-
-
-
-## Usage
-
-
-
-### Prerequisites
-
-- Python 3.7
-- PyTorch 1.6 or higher
-- [MIM](https://github.com/open-mmlab/mim)
-- [MMOCR](https://github.com/open-mmlab/mmocr)
-
-All the commands below rely on the correct configuration of `PYTHONPATH`, which should point to the project's directory so that Python can locate the module files. In `SPTS/` root directory, run the following line to add the current directory to `PYTHONPATH`:
-
-```shell
-# Linux
-export PYTHONPATH=`pwd`:$PYTHONPATH
-# Windows PowerShell
-$env:PYTHONPATH=Get-Location
-```
-
-### Dataset
-
-As of now, the implementation uses datasets provided by SPTS for **pre-training**, and uses MMOCR's datasets for **fine-tuning and testing**. It's because the test split of SPTS's datasets does not contain enough information for e2e evaluation; and MMOCR's dataset preparer has not yet supported all the datasets used in SPTS. *We are working on this issue, and they will be available in MMOCR's dataset preparer very soon.*
-
-Please follow these steps to prepare the datasets:
-
-- Download and extract all the SPTS datasets into `spts-data/` following [SPTS official guide](https://github.com/shannanyinxiang/SPTS#dataset).
-
-- Use [Dataset Preparer](https://mmocr.readthedocs.io/en/dev-1.x/user_guides/data_prepare/dataset_preparer.html) to prepare `icdar2013`, `icdar2015` and `totaltext` for `textspotting` task.
-
- ```shell
- # Run in MMOCR's root directory
- python tools/dataset_converters/prepare_dataset.py icdar2013 icdar2015 totaltext --task textspotting
- ```
-
- Then create a soft link to `data/` directory in the project root directory:
-
- ```shell
- ln -s ../../data/ .
- ```
-
-### Training commands
-
-In the current directory, run the following command to train the model:
-
-#### Pretrain
-
-```bash
-mim train mmocr config/spts/spts_resnet50_8xb8-150e_pretrain-spts.py --work-dir work_dirs/ --amp
-```
-
-To train on multiple GPUs, e.g. 8 GPUs, run the following command:
-
-```bash
-mim train mmocr config/spts/spts_resnet50_8xb8-150e_pretrain-spts.py --work-dir work_dirs/ --launcher pytorch --gpus 8 --amp
-```
-
-#### Finetune
-
-Similarly, run the following command to finetune the model on a dataset (e.g. icdar2013):
-
-```bash
-mim train mmocr config/spts/spts_resnet50_8xb8-200e_icdar2013.py --work-dir work_dirs/ --cfg-options "load_from={CHECKPOINT_PATH}" --amp
-```
-
-To finetune on multiple GPUs, e.g. 8 GPUs, run the following command:
-
-```bash
-mim train mmocr config/spts/spts_resnet50_8xb8-200e_icdar2013.py --work-dir work_dirs/ --launcher pytorch --gpus 8 --cfg-options "load_from={CHECKPOINT_PATH}" --amp
-```
-
-### Testing commands
-
-In the current directory, run the following command to test the model on a dataset (e.g. icdar2013):
-
-```bash
-mim test mmocr config/spts/spts_resnet50_8xb8-200e_icdar2013.py --work-dir work_dirs/ --checkpoint ${CHECKPOINT_PATH}
-```
-
-## Convert Weights from Official Repo
-
-Users may download the weights from [SPTS](https://github.com/shannanyinxiang/SPTS#inference) and use the conversion script to convert them into MMOCR format.
-
-```bash
-python tools/ckpt_adapter.py [SPTS_WEIGHTS_PATH] [MMOCR_WEIGHTS_PATH]
-```
-
-## Results
-
-All the models are trained on 8x A100 GPUs with AMP on (`--amp`). The overall batch size is 64.
-
-| Name | Pretrained | Generic | Weak | Strong | Download |
-| ---------- | --------------------------------------------------------------------------------------- | ------- | ----- | ------ | ------------------------------------------------------------------------------------- |
-| ICDAR 2013 | [model](https://download.openmmlab.com/mmocr/textspotting/spts/spts_resnet50_150e_pretrain-spts/spts_resnet50_150e_pretrain-spts-c9fe4c78.pth)ย / [log](https://download.openmmlab.com/mmocr/textspotting/spts/spts_resnet50_150e_pretrain-spts/20230223_194550.log) | 87.10 | 91.46 | 93.41 | [model](https://download.openmmlab.com/mmocr/textspotting/spts/spts_resnet50_200e_icdar2013/spts_resnet50_200e_icdar2013-64cb4d31.pth)ย / [log](https://download.openmmlab.com/mmocr/textspotting/spts/spts_resnet50_200e_icdar2013/20230303_140316.log) |
-| ICDAR 2015 | [model](https://download.openmmlab.com/mmocr/textspotting/spts/spts_resnet50_150e_pretrain-spts/spts_resnet50_150e_pretrain-spts-c9fe4c78.pth)ย / [log](https://download.openmmlab.com/mmocr/textspotting/spts/spts_resnet50_150e_pretrain-spts/20230223_194550.log) | 69.09 | 73.45 | 79.19 | [model](https://download.openmmlab.com/mmocr/textspotting/spts/spts_resnet50_200e_icdar2015/spts_resnet50_200e_icdar2015-d6e8621c.pth)ย / [log](https://download.openmmlab.com/mmocr/textspotting/spts/spts_resnet50_200e_icdar2015/20230302_230026.log) |
-
-| Name | Pretrained | None-Hmean | Full-Hmean | Download |
-| :-------: | -------------------------------------------------------------------------------------- | :--------: | :--------: | ------------------------------------------------------------------------------------- |
-| Totaltext | [model](https://download.openmmlab.com/mmocr/textspotting/spts/spts_resnet50_150e_pretrain-spts/spts_resnet50_150e_pretrain-spts-c9fe4c78.pth)ย / [log](https://download.openmmlab.com/mmocr/textspotting/spts/spts_resnet50_150e_pretrain-spts/20230223_194550.log) | 73.99 | 82.34 | [model](https://download.openmmlab.com/mmocr/textspotting/spts/spts_resnet50_200e_totaltext/spts_resnet50_200e_totaltext-e3521af6.pth)ย / [log](https://download.openmmlab.com/mmocr/textspotting/spts/spts_resnet50_200e_totaltext/20230303_103040.log) |
-
-## Citation
-
-If you find SPTS useful in your research or applications, please cite SPTS with the following BibTeX entry.
-
-```BibTeX
-@inproceedings{peng2022spts,
- title={SPTS: Single-Point Text Spotting},
- author={Peng, Dezhi and Wang, Xinyu and Liu, Yuliang and Zhang, Jiaxin and Huang, Mingxin and Lai, Songxuan and Zhu, Shenggao and Li, Jing and Lin, Dahua and Shen, Chunhua and Bai, Xiang and Jin, Lianwen},
- booktitle={Proceedings of the 30th ACM International Conference on Multimedia},
- year={2022}
-}
-```
-
-## Checklist
-
-
-
-- [x] Milestone 1: PR-ready, and acceptable to be one of the `projects/`.
-
- - [x] Finish the code
-
-
-
- - [x] Basic docstrings & proper citation
-
-
-
- - [x] Test-time correctness
-
-
-
- - [x] A full README
-
-
-
-- [x] Milestone 2: Indicates a successful model implementation.
-
- - [x] Training-time correctness
-
-
-
-- [x] Milestone 3: Good to be a part of our core package!
-
- - [x] Type hints and docstrings
-
-
-
- - [ ] Unit tests
-
-
-
- - [ ] Code polishing
-
-
-
- - [ ] Metafile.yml
-
-
-
-- [ ] Move your modules into the core package following the codebase's file hierarchy structure.
-
-
-
-- [ ] Refactor your modules into the core package following the codebase's file hierarchy structure.
diff --git a/pipeline/mmocr/projects/SPTS/config/_base_/datasets/ctw1500-spts.py b/pipeline/mmocr/projects/SPTS/config/_base_/datasets/ctw1500-spts.py
deleted file mode 100644
index 13931a1bb40e47bada964e401cc3d82e0879e8c0..0000000000000000000000000000000000000000
--- a/pipeline/mmocr/projects/SPTS/config/_base_/datasets/ctw1500-spts.py
+++ /dev/null
@@ -1,17 +0,0 @@
-ctw1500_textspotting_data_root = 'data/CTW1500'
-
-ctw1500_textspotting_train = dict(
- type='AdelDataset',
- data_root=ctw1500_textspotting_data_root,
- ann_file='annotations/train_ctw1500_maxlen25_v2.json',
- data_prefix=dict(img_path='ctwtrain_text_image/'),
- filter_cfg=dict(filter_empty_gt=True, min_size=32),
- pipeline=None)
-
-ctw1500_textspotting_test = dict(
- type='AdelDataset',
- data_root=ctw1500_textspotting_data_root,
- ann_file='annotations/test_ctw1500_maxlen25.json',
- data_prefix=dict(img_path='ctwtest_text_image/'),
- test_mode=True,
- pipeline=None)
diff --git a/pipeline/mmocr/projects/SPTS/config/_base_/datasets/icdar2013-spts.py b/pipeline/mmocr/projects/SPTS/config/_base_/datasets/icdar2013-spts.py
deleted file mode 100644
index 61f57d44780d9238b60fc5c54b0c2bef20744cd0..0000000000000000000000000000000000000000
--- a/pipeline/mmocr/projects/SPTS/config/_base_/datasets/icdar2013-spts.py
+++ /dev/null
@@ -1,17 +0,0 @@
-icdar2013_textspotting_data_root = 'spts-data/icdar2013'
-
-icdar2013_textspotting_train = dict(
- type='AdelDataset',
- data_root=icdar2013_textspotting_data_root,
- ann_file='ic13_train.json',
- data_prefix=dict(img_path='train_images/'),
- filter_cfg=dict(filter_empty_gt=True, min_size=32),
- pipeline=None)
-
-icdar2013_textspotting_test = dict(
- type='AdelDataset',
- data_root=icdar2013_textspotting_data_root,
- data_prefix=dict(img_path='test_images/'),
- ann_file='ic13_test.json',
- test_mode=True,
- pipeline=None)
diff --git a/pipeline/mmocr/projects/SPTS/config/_base_/datasets/icdar2013.py b/pipeline/mmocr/projects/SPTS/config/_base_/datasets/icdar2013.py
deleted file mode 100644
index e4fea49c84b0e13d37a2e31f9ffa10aff9256038..0000000000000000000000000000000000000000
--- a/pipeline/mmocr/projects/SPTS/config/_base_/datasets/icdar2013.py
+++ /dev/null
@@ -1,15 +0,0 @@
-icdar2013_textspotting_data_root = 'data/icdar2013'
-
-icdar2013_textspotting_train = dict(
- type='OCRDataset',
- data_root=icdar2013_textspotting_data_root,
- ann_file='textspotting_train.json',
- filter_cfg=dict(filter_empty_gt=True, min_size=32),
- pipeline=None)
-
-icdar2013_textspotting_test = dict(
- type='OCRDataset',
- data_root=icdar2013_textspotting_data_root,
- ann_file='textspotting_test.json',
- test_mode=True,
- pipeline=None)
diff --git a/pipeline/mmocr/projects/SPTS/config/_base_/datasets/icdar2015-spts.py b/pipeline/mmocr/projects/SPTS/config/_base_/datasets/icdar2015-spts.py
deleted file mode 100644
index df0139ad198fce0dc7166b580709bd45dabedd36..0000000000000000000000000000000000000000
--- a/pipeline/mmocr/projects/SPTS/config/_base_/datasets/icdar2015-spts.py
+++ /dev/null
@@ -1,17 +0,0 @@
-icdar2015_textspotting_data_root = 'spts-data/icdar2015'
-
-icdar2015_textspotting_train = dict(
- type='AdelDataset',
- data_root=icdar2015_textspotting_data_root,
- ann_file='ic15_train.json',
- data_prefix=dict(img_path='train_images/'),
- filter_cfg=dict(filter_empty_gt=True, min_size=32),
- pipeline=None)
-
-icdar2015_textspotting_test = dict(
- type='AdelDataset',
- data_root=icdar2015_textspotting_data_root,
- data_prefix=dict(img_path='test_images/'),
- ann_file='ic15_test.json',
- test_mode=True,
- pipeline=None)
diff --git a/pipeline/mmocr/projects/SPTS/config/_base_/datasets/icdar2015.py b/pipeline/mmocr/projects/SPTS/config/_base_/datasets/icdar2015.py
deleted file mode 100644
index f71a721480814e60b4ab387f206b75e128ce136c..0000000000000000000000000000000000000000
--- a/pipeline/mmocr/projects/SPTS/config/_base_/datasets/icdar2015.py
+++ /dev/null
@@ -1,14 +0,0 @@
-icdar2015_textspotting_data_root = 'data/icdar2015'
-
-icdar2015_textspotting_train = dict(
- type='OCRDataset',
- data_root=icdar2015_textspotting_data_root,
- ann_file='textspotting_train.json',
- pipeline=None)
-
-icdar2015_textspotting_test = dict(
- type='OCRDataset',
- data_root=icdar2015_textspotting_data_root,
- ann_file='textspotting_test.json',
- test_mode=True,
- pipeline=None)
diff --git a/pipeline/mmocr/projects/SPTS/config/_base_/datasets/mlt-spts.py b/pipeline/mmocr/projects/SPTS/config/_base_/datasets/mlt-spts.py
deleted file mode 100644
index 22d45039bce71e2b9c14e7ff41b082a2bc48ecdb..0000000000000000000000000000000000000000
--- a/pipeline/mmocr/projects/SPTS/config/_base_/datasets/mlt-spts.py
+++ /dev/null
@@ -1,9 +0,0 @@
-mlt_textspotting_data_root = 'spts-data/mlt2017'
-
-mlt_textspotting_train = dict(
- type='AdelDataset',
- data_root=mlt_textspotting_data_root,
- ann_file='train.json',
- data_prefix=dict(img_path='MLT_train_images/'),
- filter_cfg=dict(filter_empty_gt=True, min_size=32),
- pipeline=None)
diff --git a/pipeline/mmocr/projects/SPTS/config/_base_/datasets/syntext1-spts.py b/pipeline/mmocr/projects/SPTS/config/_base_/datasets/syntext1-spts.py
deleted file mode 100644
index d30df532cba19661a1c6614c6ee20df716bede23..0000000000000000000000000000000000000000
--- a/pipeline/mmocr/projects/SPTS/config/_base_/datasets/syntext1-spts.py
+++ /dev/null
@@ -1,9 +0,0 @@
-syntext1_textspotting_data_root = 'spts-data/syntext1'
-
-syntext1_textspotting_train = dict(
- type='AdelDataset',
- data_root=syntext1_textspotting_data_root,
- ann_file='train.json',
- data_prefix=dict(img_path='syntext_word_eng/'),
- filter_cfg=dict(filter_empty_gt=True, min_size=32),
- pipeline=None)
diff --git a/pipeline/mmocr/projects/SPTS/config/_base_/datasets/syntext2-spts.py b/pipeline/mmocr/projects/SPTS/config/_base_/datasets/syntext2-spts.py
deleted file mode 100644
index 6fb06e30016a7bfbfc59aa1e72f33ee917471a42..0000000000000000000000000000000000000000
--- a/pipeline/mmocr/projects/SPTS/config/_base_/datasets/syntext2-spts.py
+++ /dev/null
@@ -1,9 +0,0 @@
-syntext2_textspotting_data_root = 'spts-data/syntext2'
-
-syntext2_textspotting_train = dict(
- type='AdelDataset',
- data_root=syntext2_textspotting_data_root,
- ann_file='train.json',
- data_prefix=dict(img_path='emcs_imgs/'),
- filter_cfg=dict(filter_empty_gt=True, min_size=32),
- pipeline=None)
diff --git a/pipeline/mmocr/projects/SPTS/config/_base_/datasets/totaltext-spts.py b/pipeline/mmocr/projects/SPTS/config/_base_/datasets/totaltext-spts.py
deleted file mode 100644
index 37bea881c6a1aad6cec9c81a265ab6979327cfe4..0000000000000000000000000000000000000000
--- a/pipeline/mmocr/projects/SPTS/config/_base_/datasets/totaltext-spts.py
+++ /dev/null
@@ -1,17 +0,0 @@
-totaltext_textspotting_data_root = 'spts-data/totaltext'
-
-totaltext_textspotting_train = dict(
- type='AdelDataset',
- data_root=totaltext_textspotting_data_root,
- ann_file='train.json',
- data_prefix=dict(img_path='train_images/'),
- filter_cfg=dict(filter_empty_gt=True, min_size=32),
- pipeline=None)
-
-totaltext_textspotting_test = dict(
- type='AdelDataset',
- data_root=totaltext_textspotting_data_root,
- ann_file='test.json',
- data_prefix=dict(img_path='test_images/'),
- test_mode=True,
- pipeline=None)
diff --git a/pipeline/mmocr/projects/SPTS/config/_base_/datasets/totaltext.py b/pipeline/mmocr/projects/SPTS/config/_base_/datasets/totaltext.py
deleted file mode 100644
index ddc8f32f69d084facfed395b9bac8b55eb3ba450..0000000000000000000000000000000000000000
--- a/pipeline/mmocr/projects/SPTS/config/_base_/datasets/totaltext.py
+++ /dev/null
@@ -1,15 +0,0 @@
-totaltext_textspotting_data_root = 'data/totaltext'
-
-totaltext_textspotting_train = dict(
- type='OCRDataset',
- data_root=totaltext_textspotting_data_root,
- ann_file='textspotting_train.json',
- filter_cfg=dict(filter_empty_gt=True, min_size=32),
- pipeline=None)
-
-totaltext_textspotting_test = dict(
- type='OCRDataset',
- data_root=totaltext_textspotting_data_root,
- ann_file='textspotting_test.json',
- test_mode=True,
- pipeline=None)
diff --git a/pipeline/mmocr/projects/SPTS/config/_base_/default_runtime.py b/pipeline/mmocr/projects/SPTS/config/_base_/default_runtime.py
deleted file mode 100644
index 22657075aec853cafa50a853f9a386f5e7c95bca..0000000000000000000000000000000000000000
--- a/pipeline/mmocr/projects/SPTS/config/_base_/default_runtime.py
+++ /dev/null
@@ -1,42 +0,0 @@
-default_scope = 'mmocr'
-env_cfg = dict(
- cudnn_benchmark=False,
- mp_cfg=dict(mp_start_method='fork', opencv_num_threads=0),
- dist_cfg=dict(backend='nccl'),
-)
-
-randomness = dict(seed=42)
-
-default_hooks = dict(
- timer=dict(type='IterTimerHook'),
- logger=dict(type='LoggerHook', interval=100),
- param_scheduler=dict(type='ParamSchedulerHook'),
- checkpoint=dict(type='CheckpointHook', interval=1, max_keep_ckpts=2),
- sampler_seed=dict(type='DistSamplerSeedHook'),
- sync_buffer=dict(type='SyncBuffersHook'),
- visualization=dict(
- type='VisualizationHook',
- interval=1,
- enable=False,
- show=False,
- draw_gt=False,
- draw_pred=False),
-)
-
-# Logging
-log_level = 'INFO'
-log_processor = dict(type='LogProcessor', window_size=10, by_epoch=True)
-
-load_from = None
-resume = False
-
-# Evaluation
-val_evaluator = dict(type='E2EPointMetric')
-test_evaluator = val_evaluator
-
-# Visualization
-vis_backends = [dict(type='LocalVisBackend')]
-visualizer = dict(
- type='TextSpottingLocalVisualizer',
- name='visualizer',
- vis_backends=vis_backends)
diff --git a/pipeline/mmocr/projects/SPTS/config/spts/_base_spts_resnet50.py b/pipeline/mmocr/projects/SPTS/config/spts/_base_spts_resnet50.py
deleted file mode 100644
index b07bcee1ba30ab0f91245ceb8783a6486ef7ae5d..0000000000000000000000000000000000000000
--- a/pipeline/mmocr/projects/SPTS/config/spts/_base_spts_resnet50.py
+++ /dev/null
@@ -1,115 +0,0 @@
-custom_imports = dict(
- imports=['projects.SPTS.spts'], allow_failed_imports=False)
-
-dictionary = dict(
- type='SPTSDictionary',
- dict_file='{{ fileDirname }}/../../dicts/spts.txt',
- with_start=True,
- with_end=True,
- with_seq_end=True,
- same_start_end=False,
- with_padding=True,
- with_unknown=True,
- unknown_token=None,
-)
-
-num_bins = 1000
-
-model = dict(
- type='SPTS',
- data_preprocessor=dict(
- type='TextDetDataPreprocessor',
- # mean=[123.675, 116.28, 103.53][::-1],
- # std=[1, 1, 1],
- mean=[0, 0, 0],
- std=[255, 255, 255],
- bgr_to_rgb=True),
- backbone=dict(
- type='mmdet.ResNet',
- depth=50,
- num_stages=4,
- out_indices=(3, ),
- frozen_stages=-1,
- norm_cfg=dict(type='BN', requires_grad=False), # freeze w & b
- norm_eval=True, # freeze running mean and var
- init_cfg=dict(type='Pretrained', checkpoint='torchvision://resnet50'),
- style='pytorch'),
- encoder=dict(
- type='SPTSEncoder',
- d_backbone=2048,
- d_model=256,
- ),
- decoder=dict(
- type='SPTSDecoder',
- dictionary=dictionary,
- num_bins=num_bins,
- d_model=256,
- dropout=0.1,
- max_num_text=60,
- module_loss=dict(
- type='SPTSModuleLoss', num_bins=num_bins, ignore_first_char=True),
- postprocessor=dict(type='SPTSPostprocessor', num_bins=num_bins)))
-
-test_pipeline = [
- dict(type='LoadImageFromFile', color_type='color_ignore_orientation'),
- # dict(type='Resize', scale=(1000, 1824), keep_ratio=True),
- dict(
- type='RescaleToShortSide',
- short_side_lens=[1000],
- long_side_bound=1824),
- dict(
- type='LoadOCRAnnotationsWithBezier',
- with_bbox=True,
- with_label=True,
- with_text=True),
- dict(
- type='PackTextDetInputs',
- meta_keys=('img_path', 'ori_shape', 'img_shape', 'scale_factor'))
-]
-
-train_pipeline = [
- dict(type='LoadImageFromFile', color_type='color_ignore_orientation'),
- dict(
- type='LoadOCRAnnotationsWithBezier',
- with_bbox=True,
- with_label=True,
- with_bezier=True,
- with_text=True),
- dict(type='Bezier2Polygon'),
- dict(type='FixInvalidPolygon'),
- dict(type='ConvertText', dictionary=dict(**dictionary, num_bins=0)),
- dict(type='RemoveIgnored'),
- dict(type='RandomCrop', min_side_ratio=0.5),
- dict(
- type='RandomApply',
- transforms=[
- dict(
- type='RandomRotate',
- max_angle=30,
- pad_with_fixed_color=True,
- use_canvas=True)
- ],
- prob=0.3),
- dict(type='FixInvalidPolygon'),
- dict(
- type='RandomChoiceResize',
- scales=[(640, 1600), (672, 1600), (704, 1600), (736, 1600),
- (768, 1600), (800, 1600), (832, 1600), (864, 1600),
- (896, 1600)],
- keep_ratio=True),
- dict(
- type='RandomApply',
- transforms=[
- dict(
- type='TorchVisionWrapper',
- op='ColorJitter',
- brightness=0.5,
- contrast=0.5,
- saturation=0.5,
- hue=0.5)
- ],
- prob=0.5),
- dict(
- type='PackTextDetInputs',
- meta_keys=('img_path', 'ori_shape', 'img_shape', 'scale_factor'))
-]
diff --git a/pipeline/mmocr/projects/SPTS/config/spts/_base_spts_resnet50_mmocr.py b/pipeline/mmocr/projects/SPTS/config/spts/_base_spts_resnet50_mmocr.py
deleted file mode 100644
index f4242e36e697b6109a2449b1270d326a7a0d6e15..0000000000000000000000000000000000000000
--- a/pipeline/mmocr/projects/SPTS/config/spts/_base_spts_resnet50_mmocr.py
+++ /dev/null
@@ -1,63 +0,0 @@
-_base_ = '_base_spts_resnet50.py'
-
-test_pipeline = [
- dict(type='LoadImageFromFile', color_type='color_ignore_orientation'),
- dict(
- type='RescaleToShortSide',
- short_side_lens=[1000],
- long_side_bound=1824),
- dict(
- type='LoadOCRAnnotations',
- with_bbox=True,
- with_label=True,
- with_polygon=True,
- with_text=True),
- dict(
- type='PackTextDetInputs',
- meta_keys=('img_path', 'ori_shape', 'img_shape', 'scale_factor'))
-]
-
-train_pipeline = [
- dict(type='LoadImageFromFile', color_type='color_ignore_orientation'),
- dict(
- type='LoadOCRAnnotations',
- with_bbox=True,
- with_label=True,
- with_polygon=True,
- with_text=True),
- dict(type='FixInvalidPolygon'),
- dict(type='RemoveIgnored'),
- dict(type='RandomCrop', min_side_ratio=0.5),
- dict(
- type='RandomApply',
- transforms=[
- dict(
- type='RandomRotate',
- max_angle=30,
- pad_with_fixed_color=True,
- use_canvas=True)
- ],
- prob=0.3),
- dict(type='FixInvalidPolygon'),
- dict(
- type='RandomChoiceResize',
- scales=[(640, 1600), (672, 1600), (704, 1600), (736, 1600),
- (768, 1600), (800, 1600), (832, 1600), (864, 1600),
- (896, 1600)],
- keep_ratio=True),
- dict(
- type='RandomApply',
- transforms=[
- dict(
- type='TorchVisionWrapper',
- op='ColorJitter',
- brightness=0.5,
- contrast=0.5,
- saturation=0.5,
- hue=0.5)
- ],
- prob=0.5),
- dict(
- type='PackTextDetInputs',
- meta_keys=('img_path', 'ori_shape', 'img_shape', 'scale_factor'))
-]
diff --git a/pipeline/mmocr/projects/SPTS/config/spts/spts_resnet50_8xb8-150e_pretrain-spts.py b/pipeline/mmocr/projects/SPTS/config/spts/spts_resnet50_8xb8-150e_pretrain-spts.py
deleted file mode 100644
index 42938f4354488f8d740a7f3b711a9e2eb1903b96..0000000000000000000000000000000000000000
--- a/pipeline/mmocr/projects/SPTS/config/spts/spts_resnet50_8xb8-150e_pretrain-spts.py
+++ /dev/null
@@ -1,58 +0,0 @@
-_base_ = [
- '_base_spts_resnet50.py',
- '../_base_/datasets/icdar2013-spts.py',
- '../_base_/datasets/icdar2015-spts.py',
- '../_base_/datasets/totaltext-spts.py',
- '../_base_/datasets/syntext1-spts.py',
- '../_base_/datasets/syntext2-spts.py',
- '../_base_/datasets/mlt-spts.py',
- '../_base_/default_runtime.py',
-]
-
-num_epochs = 150
-lr = 0.0005
-min_lr = 0.00001
-
-optim_wrapper = dict(
- type='OptimWrapper',
- optimizer=dict(type='AdamW', lr=lr, weight_decay=0.0001),
- paramwise_cfg=dict(custom_keys={
- 'backbone': dict(lr_mult=0.1),
- }))
-train_cfg = dict(
- type='EpochBasedTrainLoop', max_epochs=num_epochs, val_interval=5)
-# learning policy
-param_scheduler = [
- dict(type='LinearLR', end=5, start_factor=1 / 5, by_epoch=True),
- dict(
- type='LinearLR',
- begin=5,
- end=min(num_epochs,
- int((lr - min_lr) / (lr / num_epochs)) + 5),
- end_factor=min_lr / lr,
- by_epoch=True),
-]
-
-# dataset settings
-train_list = [
- _base_.icdar2013_textspotting_train,
- _base_.icdar2015_textspotting_train,
- _base_.mlt_textspotting_train,
- _base_.totaltext_textspotting_train,
- _base_.syntext1_textspotting_train,
- _base_.syntext2_textspotting_train,
-]
-
-train_dataset = dict(
- type='ConcatDataset', datasets=train_list, pipeline=_base_.train_pipeline)
-
-val_evaluator = None
-test_evaluator = None
-
-train_dataloader = dict(
- batch_size=8,
- num_workers=8,
- pin_memory=True,
- persistent_workers=True,
- sampler=dict(type='BatchAugSampler', shuffle=True, num_repeats=2),
- dataset=train_dataset)
diff --git a/pipeline/mmocr/projects/SPTS/config/spts/spts_resnet50_8xb8-200e_icdar2013.py b/pipeline/mmocr/projects/SPTS/config/spts/spts_resnet50_8xb8-200e_icdar2013.py
deleted file mode 100644
index 223c1c2458ce036387d94ce4d3d2fd7662c39a4c..0000000000000000000000000000000000000000
--- a/pipeline/mmocr/projects/SPTS/config/spts/spts_resnet50_8xb8-200e_icdar2013.py
+++ /dev/null
@@ -1,87 +0,0 @@
-_base_ = [
- '_base_spts_resnet50_mmocr.py',
- '../_base_/datasets/icdar2013.py',
- '../_base_/default_runtime.py',
-]
-
-load_from = 'work_dirs/spts_resnet50_150e_pretrain-spts/epoch_150.pth'
-
-num_epochs = 200
-lr = 0.00001
-
-default_hooks = dict(
- checkpoint=dict(
- type='CheckpointHook',
- save_best='generic/hmean',
- rule='greater',
- _delete_=True),
- logger=dict(type='LoggerHook', interval=1))
-
-optim_wrapper = dict(
- type='OptimWrapper',
- optimizer=dict(type='AdamW', lr=lr, weight_decay=0.0001),
- paramwise_cfg=dict(custom_keys={
- 'backbone': dict(lr_mult=0.1),
- }))
-
-train_cfg = dict(
- type='EpochBasedTrainLoop', max_epochs=num_epochs, val_interval=10)
-val_cfg = dict(type='ValLoop')
-test_cfg = dict(type='TestLoop')
-
-# dataset settings
-icdar2013_textspotting_train = _base_.icdar2013_textspotting_train
-icdar2013_textspotting_train.pipeline = _base_.train_pipeline
-icdar2013_textspotting_test = _base_.icdar2013_textspotting_test
-icdar2013_textspotting_test.pipeline = _base_.test_pipeline
-
-train_dataloader = dict(
- batch_size=8,
- num_workers=8,
- pin_memory=True,
- persistent_workers=True,
- sampler=dict(type='BatchAugSampler', shuffle=True, num_repeats=2),
- dataset=icdar2013_textspotting_train)
-
-val_dataloader = dict(
- batch_size=1,
- num_workers=4,
- pin_memory=True,
- persistent_workers=True,
- sampler=dict(type='DefaultSampler', shuffle=False),
- dataset=icdar2013_textspotting_test)
-
-test_dataloader = val_dataloader
-
-val_cfg = dict(type='ValLoop')
-test_cfg = dict(type='TestLoop')
-
-val_evaluator = [
- dict(
- type='E2EPointMetric',
- prefix='generic',
- lexicon_path='data/icdar2013/lexicons/GenericVocabulary_new.txt',
- pair_path='data/icdar2013/lexicons/'
- 'GenericVocabulary_pair_list.txt',
- match_dist_thr=None),
- dict(
- type='E2EPointMetric',
- prefix='weak',
- lexicon_path='data/icdar2013/lexicons/'
- 'ch2_test_vocabulary_new.txt',
- pair_path='data/icdar2013/lexicons/'
- 'ch2_test_vocabulary_pair_list.txt',
- match_dist_thr=0.4),
- dict(
- type='E2EPointMetric',
- prefix='strong',
- lexicon_path='data/icdar2013/lexicons/'
- 'new_strong_lexicon/lexicons/',
- lexicon_mapping=('(.*).jpg', r'new_voc_\1.txt'),
- pair_path='data/icdar2013/lexicons/'
- 'new_strong_lexicon/pairs/',
- pair_mapping=('(.*).jpg', r'pair_voc_\1.txt'),
- match_dist_thr=0.4),
-]
-
-test_evaluator = val_evaluator
diff --git a/pipeline/mmocr/projects/SPTS/config/spts/spts_resnet50_8xb8-200e_icdar2015.py b/pipeline/mmocr/projects/SPTS/config/spts/spts_resnet50_8xb8-200e_icdar2015.py
deleted file mode 100644
index 9f811a805539dbab09172cbf44fc57474eb3eb57..0000000000000000000000000000000000000000
--- a/pipeline/mmocr/projects/SPTS/config/spts/spts_resnet50_8xb8-200e_icdar2015.py
+++ /dev/null
@@ -1,87 +0,0 @@
-_base_ = [
- '_base_spts_resnet50_mmocr.py',
- '../_base_/datasets/icdar2015.py',
- '../_base_/default_runtime.py',
-]
-
-load_from = 'work_dirs/spts_resnet50_150e_pretrain-spts/epoch_150.pth'
-
-num_epochs = 200
-lr = 0.00001
-
-default_hooks = dict(
- checkpoint=dict(
- type='CheckpointHook',
- save_best='generic/hmean',
- rule='greater',
- _delete_=True),
- logger=dict(type='LoggerHook', interval=10))
-
-optim_wrapper = dict(
- type='OptimWrapper',
- optimizer=dict(type='AdamW', lr=lr, weight_decay=0.0001),
- paramwise_cfg=dict(custom_keys={
- 'backbone': dict(lr_mult=0.1),
- }))
-
-train_cfg = dict(
- type='EpochBasedTrainLoop', max_epochs=num_epochs, val_interval=10)
-val_cfg = dict(type='ValLoop')
-test_cfg = dict(type='TestLoop')
-
-# dataset settings
-icdar2015_textspotting_train = _base_.icdar2015_textspotting_train
-icdar2015_textspotting_train.pipeline = _base_.train_pipeline
-icdar2015_textspotting_test = _base_.icdar2015_textspotting_test
-icdar2015_textspotting_test.pipeline = _base_.test_pipeline
-
-train_dataloader = dict(
- batch_size=8,
- num_workers=8,
- pin_memory=True,
- persistent_workers=True,
- sampler=dict(type='BatchAugSampler', shuffle=True, num_repeats=2),
- dataset=icdar2015_textspotting_train)
-
-val_dataloader = dict(
- batch_size=1,
- num_workers=4,
- pin_memory=True,
- persistent_workers=True,
- sampler=dict(type='DefaultSampler', shuffle=False),
- dataset=icdar2015_textspotting_test)
-
-test_dataloader = val_dataloader
-
-val_cfg = dict(type='ValLoop')
-test_cfg = dict(type='TestLoop')
-
-val_evaluator = [
- dict(
- type='E2EPointMetric',
- prefix='generic',
- lexicon_path='data/icdar2015/lexicons/GenericVocabulary_new.txt',
- pair_path='data/icdar2015/lexicons/'
- 'GenericVocabulary_pair_list.txt',
- match_dist_thr=None),
- dict(
- type='E2EPointMetric',
- prefix='weak',
- lexicon_path='data/icdar2015/lexicons/'
- 'ch4_test_vocabulary_new.txt',
- pair_path='data/icdar2015/lexicons/'
- 'ch4_test_vocabulary_pair_list.txt',
- match_dist_thr=0.4),
- dict(
- type='E2EPointMetric',
- prefix='strong',
- lexicon_path='data/icdar2015/lexicons/'
- 'new_strong_lexicon/lexicons/',
- lexicon_mapping=('(.*).jpg', r'new_voc_\1.txt'),
- pair_path='data/icdar2015/lexicons/'
- 'new_strong_lexicon/pairs/',
- pair_mapping=('(.*).jpg', r'pair_voc_\1.txt'),
- match_dist_thr=0.4),
-]
-
-test_evaluator = val_evaluator
diff --git a/pipeline/mmocr/projects/SPTS/config/spts/spts_resnet50_8xb8-200e_totaltext.py b/pipeline/mmocr/projects/SPTS/config/spts/spts_resnet50_8xb8-200e_totaltext.py
deleted file mode 100644
index ed71f000bd3c1bc43041d5d7fa126ed066155ab1..0000000000000000000000000000000000000000
--- a/pipeline/mmocr/projects/SPTS/config/spts/spts_resnet50_8xb8-200e_totaltext.py
+++ /dev/null
@@ -1,75 +0,0 @@
-_base_ = [
- '_base_spts_resnet50_mmocr.py',
- '../_base_/datasets/totaltext.py',
- '../_base_/default_runtime.py',
-]
-
-load_from = 'work_dirs/spts_resnet50_150e_pretrain-spts/epoch_150.pth'
-
-num_epochs = 200
-lr = 0.00001
-
-default_hooks = dict(
- checkpoint=dict(
- type='CheckpointHook',
- save_best='none/hmean',
- rule='greater',
- _delete_=True),
- logger=dict(type='LoggerHook', interval=10))
-
-optim_wrapper = dict(
- type='OptimWrapper',
- optimizer=dict(type='AdamW', lr=lr, weight_decay=0.0001),
- paramwise_cfg=dict(custom_keys={
- 'backbone': dict(lr_mult=0.1),
- }))
-
-train_cfg = dict(
- type='EpochBasedTrainLoop', max_epochs=num_epochs, val_interval=10)
-val_cfg = dict(type='ValLoop')
-test_cfg = dict(type='TestLoop')
-
-# dataset settings
-totaltext_textspotting_train = _base_.totaltext_textspotting_train
-totaltext_textspotting_train.pipeline = _base_.train_pipeline
-totaltext_textspotting_test = _base_.totaltext_textspotting_test
-totaltext_textspotting_test.pipeline = _base_.test_pipeline
-
-train_dataloader = dict(
- batch_size=8,
- num_workers=8,
- persistent_workers=True,
- pin_memory=True,
- sampler=dict(type='BatchAugSampler', shuffle=True, num_repeats=2),
- dataset=totaltext_textspotting_train)
-
-val_dataloader = dict(
- batch_size=1,
- num_workers=4,
- persistent_workers=True,
- pin_memory=True,
- sampler=dict(type='DefaultSampler', shuffle=False),
- dataset=totaltext_textspotting_test)
-
-test_dataloader = val_dataloader
-
-val_cfg = dict(type='ValLoop')
-test_cfg = dict(type='TestLoop')
-
-val_evaluator = [
- dict(
- type='E2EPointMetric',
- prefix='none',
- word_spotting=True,
- match_dist_thr=0.4),
- dict(
- type='E2EPointMetric',
- prefix='full',
- lexicon_path='data/totaltext/lexicons/weak_voc_new.txt',
- pair_path='data/totaltext/lexicons/'
- 'weak_voc_pair_list.txt',
- word_spotting=True,
- match_dist_thr=0.4),
-]
-
-test_evaluator = val_evaluator
diff --git a/pipeline/mmocr/projects/SPTS/dicts/spts.txt b/pipeline/mmocr/projects/SPTS/dicts/spts.txt
deleted file mode 100644
index 173d6c4a7ad83dcb6cdb3d177456d0b4d553c01c..0000000000000000000000000000000000000000
--- a/pipeline/mmocr/projects/SPTS/dicts/spts.txt
+++ /dev/null
@@ -1,95 +0,0 @@
-
-!
-"
-#
-$
-%
-&
-'
-(
-)
-*
-+
-,
--
-.
-/
-0
-1
-2
-3
-4
-5
-6
-7
-8
-9
-:
-;
-<
-=
->
-?
-@
-A
-B
-C
-D
-E
-F
-G
-H
-I
-J
-K
-L
-M
-N
-O
-P
-Q
-R
-S
-T
-U
-V
-W
-X
-Y
-Z
-[
-\
-]
-^
-_
-`
-a
-b
-c
-d
-e
-f
-g
-h
-i
-j
-k
-l
-m
-n
-o
-p
-q
-r
-s
-t
-u
-v
-w
-x
-y
-z
-{
-|
-}
-~
\ No newline at end of file
diff --git a/pipeline/mmocr/projects/SPTS/spts/__init__.py b/pipeline/mmocr/projects/SPTS/spts/__init__.py
deleted file mode 100644
index 1952530c5624157ebaf1f83b999dee3257a71399..0000000000000000000000000000000000000000
--- a/pipeline/mmocr/projects/SPTS/spts/__init__.py
+++ /dev/null
@@ -1,5 +0,0 @@
-# Copyright (c) OpenMMLab. All rights reserved.
-# Copyright (c) OpenMMLab. All rights reserved.
-from .datasets import * # NOQA
-from .metric import * # NOQA
-from .model import * # NOQA
diff --git a/pipeline/mmocr/projects/SPTS/spts/datasets/__init__.py b/pipeline/mmocr/projects/SPTS/spts/datasets/__init__.py
deleted file mode 100644
index 9beaa869b7767bfdc5a6f03b8ba62975c7569442..0000000000000000000000000000000000000000
--- a/pipeline/mmocr/projects/SPTS/spts/datasets/__init__.py
+++ /dev/null
@@ -1,10 +0,0 @@
-# Copyright (c) OpenMMLab. All rights reserved.
-from .adel_dataset import AdelDataset
-from .transforms.spts_transforms import (Bezier2Polygon, ConvertText,
- LoadOCRAnnotationsWithBezier,
- Polygon2Bezier, RescaleToShortSide)
-
-__all__ = [
- 'AdelDataset', 'LoadOCRAnnotationsWithBezier', 'Bezier2Polygon',
- 'Polygon2Bezier', 'ConvertText', 'RescaleToShortSide'
-]
diff --git a/pipeline/mmocr/projects/SPTS/spts/datasets/adel_dataset.py b/pipeline/mmocr/projects/SPTS/spts/datasets/adel_dataset.py
deleted file mode 100644
index d1e3edda74f4e48d04b37b2d0139c98476c64a24..0000000000000000000000000000000000000000
--- a/pipeline/mmocr/projects/SPTS/spts/datasets/adel_dataset.py
+++ /dev/null
@@ -1,96 +0,0 @@
-# Copyright (c) OpenMMLab. All rights reserved.
-import os.path as osp
-from typing import List, Union
-
-import numpy as np
-from mmdet.datasets.coco import CocoDataset
-
-from mmocr.registry import DATASETS
-
-
-@DATASETS.register_module()
-class AdelDataset(CocoDataset):
- """Dataset for text detection while ann_file in Adelai's coco format.
-
- Args:
- ann_file (str): Annotation file path. Defaults to ''.
- metainfo (dict, optional): Meta information for dataset, such as class
- information. Defaults to None.
- data_root (str): The root directory for ``data_prefix`` and
- ``ann_file``. Defaults to ''.
- data_prefix (dict): Prefix for training data. Defaults to
- dict(img_path='').
- filter_cfg (dict, optional): Config for filter data. Defaults to None.
- indices (int or Sequence[int], optional): Support using first few
- data in annotation file to facilitate training/testing on a smaller
- dataset. Defaults to None which means using all ``data_infos``.
- serialize_data (bool, optional): Whether to hold memory using
- serialized objects, when enabled, data loader workers can use
- shared RAM from master process instead of making a copy. Defaults
- to True.
- pipeline (list, optional): Processing pipeline. Defaults to [].
- test_mode (bool, optional): ``test_mode=True`` means in test phase.
- Defaults to False.
- lazy_init (bool, optional): Whether to load annotation during
- instantiation. In some cases, such as visualization, only the meta
- information of the dataset is needed, which is not necessary to
- load annotation file. ``Basedataset`` can skip load annotations to
- save time by set ``lazy_init=False``. Defaults to False.
- max_refetch (int, optional): If ``Basedataset.prepare_data`` get a
- None img. The maximum extra number of cycles to get a valid
- image. Defaults to 1000.
- """
- METAINFO = {'classes': ('text', )}
-
- def parse_data_info(self, raw_data_info: dict) -> Union[dict, List[dict]]:
- """Parse raw annotation to target format.
-
- Args:
- raw_data_info (dict): Raw data information loaded from ``ann_file``
-
- Returns:
- Union[dict, List[dict]]: Parsed annotation.
- """
- img_info = raw_data_info['raw_img_info']
- ann_info = raw_data_info['raw_ann_info']
-
- data_info = {}
-
- img_path = osp.join(self.data_prefix['img_path'],
- img_info['file_name'])
- data_info['img_path'] = img_path
- data_info['img_id'] = img_info['img_id']
- data_info['height'] = img_info['height']
- data_info['width'] = img_info['width']
-
- instances = []
- for ann in ann_info:
- instance = {}
-
- if ann.get('ignore', False):
- continue
- x1, y1, w, h = ann['bbox']
- inter_w = max(0, min(x1 + w, img_info['width']) - max(x1, 0))
- inter_h = max(0, min(y1 + h, img_info['height']) - max(y1, 0))
- if inter_w * inter_h == 0:
- continue
- if ann['area'] <= 0 or w < 1 or h < 1:
- continue
- if ann['category_id'] not in self.cat_ids:
- continue
- bbox = [x1, y1, x1 + w, y1 + h]
-
- if ann.get('iscrowd', False):
- instance['ignore'] = 1
- else:
- instance['ignore'] = 0
- instance['bbox'] = bbox
- instance['bbox_label'] = self.cat2label[ann['category_id']]
- # instance['polygon'] = bezier2poly(
- # np.array(ann['bezier_pts'], dtype=np.float32))
- instance['beziers'] = np.array(ann['bezier_pts'], dtype=np.float32)
- instance['text'] = ann['rec']
-
- instances.append(instance)
- data_info['instances'] = instances
- return data_info
diff --git a/pipeline/mmocr/projects/SPTS/spts/datasets/transforms/spts_transforms.py b/pipeline/mmocr/projects/SPTS/spts/datasets/transforms/spts_transforms.py
deleted file mode 100644
index 91dd2289ddf5df1f5f52e58fb736386881aee6be..0000000000000000000000000000000000000000
--- a/pipeline/mmocr/projects/SPTS/spts/datasets/transforms/spts_transforms.py
+++ /dev/null
@@ -1,313 +0,0 @@
-import random
-from typing import Dict
-
-import numpy as np
-from mmcv.transforms.base import BaseTransform
-
-from mmocr.datasets.transforms import LoadOCRAnnotations
-from mmocr.registry import TASK_UTILS, TRANSFORMS
-from mmocr.utils import bezier2poly, poly2bezier
-
-
-@TRANSFORMS.register_module()
-class LoadOCRAnnotationsWithBezier(LoadOCRAnnotations):
- """Load and process the ``instances`` annotation provided by dataset.
-
- The annotation format is as the following:
-
- .. code-block:: python
-
- {
- 'instances':
- [
- {
- # List of 4 numbers representing the bounding box of the
- # instance, in (x1, y1, x2, y2) order.
- # used in text detection or text spotting tasks.
- 'bbox': [x1, y1, x2, y2],
-
- # Label of instance, usually it's 0.
- # used in text detection or text spotting tasks.
- 'bbox_label': 0,
-
- # List of n numbers representing the polygon of the
- # instance, in (xn, yn) order.
- # used in text detection/ textspotter.
- "polygon": [x1, y1, x2, y2, ... xn, yn],
-
- # The flag indicating whether the instance should be ignored.
- # used in text detection or text spotting tasks.
- "ignore": False,
-
- # The groundtruth of text.
- # used in text recognition or text spotting tasks.
- "text": 'tmp',
- }
- ]
- }
-
- After this module, the annotation has been changed to the format below:
-
- .. code-block:: python
-
- {
- # In (x1, y1, x2, y2) order, float type. N is the number of bboxes
- # in np.float32
- 'gt_bboxes': np.ndarray(N, 4)
- # In np.int64 type.
- 'gt_bboxes_labels': np.ndarray(N, )
- # In (x1, y1,..., xk, yk) order, float type.
- # in list[np.float32]
- 'gt_polygons': list[np.ndarray(2k, )]
- # In np.bool_ type.
- 'gt_ignored': np.ndarray(N, )
- # In list[str]
- 'gt_texts': list[str]
- }
-
- Required Keys:
-
- - instances
-
- - bbox (optional)
- - bbox_label (optional)
- - polygon (optional)
- - ignore (optional)
- - text (optional)
-
- Added Keys:
-
- - gt_bboxes (np.float32)
- - gt_bboxes_labels (np.int64)
- - gt_polygons (list[np.float32])
- - gt_ignored (np.bool_)
- - gt_texts (list[str])
-
- Args:
- with_bbox (bool): Whether to parse and load the bbox annotation.
- Defaults to False.
- with_label (bool): Whether to parse and load the label annotation.
- Defaults to False.
- with_polygon (bool): Whether to parse and load the polygon annotation.
- Defaults to False.
- with_bezier (bool): Whether to parse and load the bezier annotation.
- Defaults to False.
- with_text (bool): Whether to parse and load the text annotation.
- Defaults to False.
- """
-
- def __init__(self, with_bezier: bool = False, **kwargs) -> None:
- super().__init__(**kwargs)
- self.with_bezier = with_bezier
-
- def _load_beziers(self, results: dict) -> None:
- """Private function to load text annotations.
-
- Args:
- results (dict): Result dict from :obj:``OCRDataset``.
-
- Returns:
- dict: The dict contains loaded text annotations.
- """
- gt_beziers = []
- for instance in results['instances']:
- gt_beziers.append(instance['beziers'])
- results['gt_beziers'] = gt_beziers
-
- def transform(self, results: dict) -> dict:
- """Function to load multiple types annotations.
-
- Args:
- results (dict): Result dict from :obj:``OCRDataset``.
-
- Returns:
- dict: The dict contains loaded bounding box, label polygon and
- text annotations.
- """
- results = super().transform(results)
- if self.with_bezier:
- self._load_beziers(results)
- return results
-
- def __repr__(self) -> str:
- repr_str = self.__class__.__name__
- repr_str += f'(with_bbox={self.with_bbox}, '
- repr_str += f'with_label={self.with_label}, '
- repr_str += f'with_polygon={self.with_polygon}, '
- repr_str += f'with_bezier={self.with_bezier}, '
- repr_str += f'with_text={self.with_text}, '
- repr_str += f"imdecode_backend='{self.imdecode_backend}', "
-
- if self.file_client_args is not None:
- repr_str += f'file_client_args={self.file_client_args})'
- else:
- repr_str += f'backend_args={self.backend_args})'
-
- return repr_str
-
-
-@TRANSFORMS.register_module()
-class Bezier2Polygon(BaseTransform):
- """Convert bezier curves to polygon.
-
- Required Keys:
-
- - gt_beziers
-
- Modified Keys:
-
- - gt_polygons
- """
-
- def transform(self, results: Dict) -> Dict:
- """
-
- Args:
- results (dict): Result dict containing the data to transform.
-
- Returns:
- Optional[dict]: The transformed data. If all the polygons are
- unfixable, return None.
- """
- if results.get('gt_beziers', None) is not None:
- results['gt_polygons'] = [
- np.array(bezier2poly(poly), dtype=np.float32)
- for poly in results['gt_beziers']
- ]
- return results
-
- def __repr__(self) -> str:
- repr_str = self.__class__.__name__
- repr_str += '()'
- return repr_str
-
-
-@TRANSFORMS.register_module()
-class Polygon2Bezier(BaseTransform):
- """Convert polygons to bezier curves.
-
- Required Keys:
-
- - gt_polygons
-
- Added Keys:
-
- - gt_beziers
- """
-
- def transform(self, results: Dict) -> Dict:
- """
-
- Args:
- results (dict): Result dict containing the data to transform.
-
- Returns:
- Optional[dict]: The transformed data. If all the polygons are
- unfixable, return None.
- """
- if results.get('gt_polygons', None) is not None:
- beziers = [poly2bezier(poly) for poly in results['gt_polygons']]
- results['gt_beziers'] = np.array(beziers, dtype=np.float32)
- return results
-
- def __repr__(self) -> str:
- repr_str = self.__class__.__name__
- repr_str += '()'
- return repr_str
-
-
-@TRANSFORMS.register_module()
-class ConvertText(BaseTransform):
-
- def __init__(self, dictionary):
- if isinstance(dictionary, dict):
- self.dictionary = TASK_UTILS.build(dictionary)
- else:
- raise TypeError(
- 'The type of dictionary should be `Dictionary` or dict, '
- f'but got {type(dictionary)}')
-
- def transform(self, results: Dict) -> Dict:
- """
-
- Args:
- results (dict): Result dict containing the data to transform.
-
- Returns:
- Optional[dict]: The transformed data. If all the polygons are
- unfixable, return None.
- """
- new_gt_texts = []
- for gt_text in results['gt_texts']:
- if self.dictionary.end_idx in gt_text:
- gt_text = gt_text[:gt_text.index(self.dictionary.end_idx)]
- new_gt_texts.append(self.dictionary.idx2str(gt_text))
- results['gt_texts'] = new_gt_texts
- return results
-
- def __repr__(self) -> str:
- repr_str = self.__class__.__name__
- repr_str += '()'
- return repr_str
-
-
-@TRANSFORMS.register_module()
-class RescaleToShortSide(BaseTransform):
-
- def __init__(self,
- short_side_lens,
- long_side_bound,
- resize_type: str = 'Resize',
- **resize_kwargs):
- self.short_side_lens = short_side_lens
- self.long_side_bound = long_side_bound
- self.resize_cfg = dict(type=resize_type, **resize_kwargs)
-
- # create a empty Reisize object
- self.resize_cfg.update(dict(scale=0))
- self.resize = TRANSFORMS.build(self.resize_cfg)
-
- def transform(self, results: Dict) -> Dict:
- """Resize image.
-
- Args:
- results (dict): Result dict containing the data to transform.
-
- Returns:
- Optional[dict]: The transformed data. If all the polygons are
- unfixable, return None.
- """
- short_len = random.choice(self.short_side_lens)
- new_h, new_w = self.get_size_with_aspect_ratio(results['img_shape'],
- short_len,
- self.long_side_bound)
- self.resize.scale = (new_w, new_h)
- return self.resize(results)
-
- def get_size_with_aspect_ratio(self, image_size, size, max_size=None):
- h, w = image_size
- if max_size is not None:
- min_original_size = float(min((w, h)))
- max_original_size = float(max((w, h)))
- if max_original_size / min_original_size * size > max_size:
- size = int(
- round(max_size * min_original_size / max_original_size))
-
- if (w <= h and w == size) or (h <= w and h == size):
- return (h, w)
-
- if w < h:
- ow = size
- oh = int(size * h / w)
- else:
- oh = size
- ow = int(size * w / h)
-
- return (oh, ow)
-
- def __repr__(self) -> str:
- repr_str = self.__class__.__name__
- repr_str += f'(short_side_lens={self.short_side_lens}, '
- repr_str += f'long_side_bound={self.long_side_bound}, '
- repr_str += f'resize_cfg={self.resize_cfg})'
- return repr_str
diff --git a/pipeline/mmocr/projects/SPTS/spts/metric/__init__.py b/pipeline/mmocr/projects/SPTS/spts/metric/__init__.py
deleted file mode 100644
index 987669297cf00fe12f3a4f79f88bef3e31ad0b11..0000000000000000000000000000000000000000
--- a/pipeline/mmocr/projects/SPTS/spts/metric/__init__.py
+++ /dev/null
@@ -1,4 +0,0 @@
-# Copyright (c) OpenMMLab. All rights reserved.
-from .e2e_point_metric import E2EPointMetric
-
-__all__ = ['E2EPointMetric']
diff --git a/pipeline/mmocr/projects/SPTS/spts/metric/e2e_point_metric.py b/pipeline/mmocr/projects/SPTS/spts/metric/e2e_point_metric.py
deleted file mode 100644
index d219b4aa69b517fa737184a58c33eb78ed89a513..0000000000000000000000000000000000000000
--- a/pipeline/mmocr/projects/SPTS/spts/metric/e2e_point_metric.py
+++ /dev/null
@@ -1,315 +0,0 @@
-# Copyright (c) OpenMMLab. All rights reserved.
-import glob
-import os.path as osp
-import re
-from typing import Dict, List, Optional, Sequence, Tuple
-
-import numpy as np
-import torch
-from mmengine.evaluator import BaseMetric
-from mmengine.logging import MMLogger
-from rapidfuzz.distance import Levenshtein
-from shapely.geometry import Point
-
-from mmocr.registry import METRICS
-
-# TODO: CTW1500 read pair
-
-
-@METRICS.register_module()
-class E2EPointMetric(BaseMetric):
- """Point metric for textspotting. Proposed in SPTS.
-
- Args:
- text_score_thrs (dict): Best text score threshold searching
- space. Defaults to dict(start=0.8, stop=1, step=0.01).
- word_spotting (bool): Whether to work in word spotting mode. Defaults
- to False.
- lexicon_path (str, optional): Lexicon path for word spotting, which
- points to a lexicon file or a directory. Defaults to None.
- lexicon_mapping (tuple, optional): The rule to map test image name to
- its corresponding lexicon file. Only effective when lexicon path
- is a directory. Defaults to ('(.*).jpg', r'\1.txt').
- pair_path (str, optional): Pair path for word spotting, which points
- to a pair file or a directory. Defaults to None.
- pair_mapping (tuple, optional): The rule to map test image name to
- its corresponding pair file. Only effective when pair path is a
- directory. Defaults to ('(.*).jpg', r'\1.txt').
- match_dist_thr (float, optional): Matching distance threshold for
- word spotting. Defaults to None.
- collect_device (str): Device name used for collecting results from
- different ranks during distributed training. Must be 'cpu' or
- 'gpu'. Defaults to 'cpu'.
- prefix (str, optional): The prefix that will be added in the metric
- names to disambiguate homonymous metrics of different evaluators.
- If prefix is not provided in the argument, self.default_prefix
- will be used instead. Defaults to None
- """
- default_prefix: Optional[str] = 'e2e_icdar'
-
- def __init__(self,
- text_score_thrs: Dict = dict(start=0.8, stop=1, step=0.01),
- word_spotting: bool = False,
- lexicon_path: Optional[str] = None,
- lexicon_mapping: Tuple[str, str] = ('(.*).jpg', r'\1.txt'),
- pair_path: Optional[str] = None,
- pair_mapping: Tuple[str, str] = ('(.*).jpg', r'\1.txt'),
- match_dist_thr: Optional[float] = None,
- collect_device: str = 'cpu',
- prefix: Optional[str] = None) -> None:
- super().__init__(collect_device=collect_device, prefix=prefix)
- self.text_score_thrs = np.arange(**text_score_thrs)
- self.word_spotting = word_spotting
- self.match_dist_thr = match_dist_thr
- if lexicon_path:
- self.lexicon_mapping = lexicon_mapping
- self.pair_mapping = pair_mapping
- self.lexicons = self._read_lexicon(lexicon_path)
- self.pairs = self._read_pair(pair_path)
-
- def _read_lexicon(self, lexicon_path: str) -> List[str]:
- if lexicon_path.endswith('.txt'):
- lexicon = open(lexicon_path, 'r').read().splitlines()
- lexicon = [ele.strip() for ele in lexicon]
- else:
- lexicon = {}
- for file in glob.glob(osp.join(lexicon_path, '*.txt')):
- basename = osp.basename(file)
- lexicon[basename] = self._read_lexicon(file)
- return lexicon
-
- def _read_pair(self, pair_path: str) -> Dict[str, str]:
- pairs = {}
- if pair_path.endswith('.txt'):
- pair_lines = open(pair_path, 'r').read().splitlines()
- for line in pair_lines:
- line = line.strip()
- word = line.split(' ')[0].upper()
- word_gt = line[len(word) + 1:]
- pairs[word] = word_gt
- else:
- for file in glob.glob(osp.join(pair_path, '*.txt')):
- basename = osp.basename(file)
- pairs[basename] = self._read_pair(file)
- return pairs
-
- def poly_center(self, poly_pts):
- poly_pts = np.array(poly_pts).reshape(-1, 2)
- return poly_pts.mean(0)
-
- def process(self, data_batch: Sequence[Dict],
- data_samples: Sequence[Dict]) -> None:
- """Process one batch of data samples and predictions. The processed
- results should be stored in ``self.results``, which will be used to
- compute the metrics when all batches have been processed.
-
- Args:
- data_batch (Sequence[Dict]): A batch of data from dataloader.
- data_samples (Sequence[Dict]): A batch of outputs from
- the model.
- """
- for data_sample in data_samples:
-
- pred_instances = data_sample.get('pred_instances')
- pred_points = pred_instances.get('points')
- text_scores = pred_instances.get('text_scores')
- if isinstance(text_scores, torch.Tensor):
- text_scores = text_scores.cpu().numpy()
- text_scores = np.array(text_scores, dtype=np.float32)
- pred_texts = pred_instances.get('texts')
-
- gt_instances = data_sample.get('gt_instances')
- gt_polys = gt_instances.get('polygons')
- gt_ignore_flags = gt_instances.get('ignored')
- gt_texts = gt_instances.get('texts')
- if isinstance(gt_ignore_flags, torch.Tensor):
- gt_ignore_flags = gt_ignore_flags.cpu().numpy()
-
- gt_points = [self.poly_center(poly) for poly in gt_polys]
- if self.word_spotting:
- gt_ignore_flags, gt_texts = self._word_spotting_filter(
- gt_ignore_flags, gt_texts)
-
- pred_ignore_flags = text_scores < self.text_score_thrs.min()
- text_scores = text_scores[~pred_ignore_flags]
- pred_texts = self._get_true_elements(pred_texts,
- ~pred_ignore_flags)
- pred_points = self._get_true_elements(pred_points,
- ~pred_ignore_flags)
-
- result = dict(
- # reserved for image-level lexcions
- gt_img_name=osp.basename(data_sample.get('img_path', '')),
- text_scores=text_scores,
- pred_points=pred_points,
- gt_points=gt_points,
- pred_texts=pred_texts,
- gt_texts=gt_texts,
- gt_ignore_flags=gt_ignore_flags)
- self.results.append(result)
-
- def _get_true_elements(self, array: List, flags: np.ndarray) -> List:
- return [array[i] for i in self._true_indexes(flags)]
-
- def compute_metrics(self, results: List[Dict]) -> Dict:
- """Compute the metrics from processed results.
-
- Args:
- results (list[dict]): The processed results of each batch.
-
- Returns:
- dict: The computed metrics. The keys are the names of the metrics,
- and the values are corresponding results.
- """
- logger: MMLogger = MMLogger.get_current_instance()
-
- best_eval_results = dict(hmean=-1)
-
- num_thres = len(self.text_score_thrs)
- num_preds = np.zeros(
- num_thres, dtype=int) # the number of points actually predicted
- num_tp = np.zeros(num_thres, dtype=int) # number of true positives
- num_gts = np.zeros(num_thres, dtype=int) # number of valid gts
-
- for result in results:
- text_scores = result['text_scores']
- pred_points = result['pred_points']
- gt_points = result['gt_points']
- gt_texts = result['gt_texts']
- pred_texts = result['pred_texts']
- gt_ignore_flags = result['gt_ignore_flags']
- gt_img_name = result['gt_img_name']
-
- # Correct the words with lexicon
- pred_dist_flags = np.zeros(len(pred_texts), dtype=bool)
- if hasattr(self, 'lexicons'):
- for i, pred_text in enumerate(pred_texts):
- # If it's an image-level lexicon
- if isinstance(self.lexicons, dict):
- lexicon_name = self._map_img_name(
- gt_img_name, self.lexicon_mapping)
- pair_name = self._map_img_name(gt_img_name,
- self.pair_mapping)
- pred_texts[i], match_dist = self._match_word(
- pred_text, self.lexicons[lexicon_name],
- self.pairs[pair_name])
- else:
- pred_texts[i], match_dist = self._match_word(
- pred_text, self.lexicons, self.pairs)
- if (self.match_dist_thr
- and match_dist >= self.match_dist_thr):
- # won't even count this as a prediction
- pred_dist_flags[i] = True
-
- # Filter out predictions by IoU threshold
- for i, text_score_thr in enumerate(self.text_score_thrs):
- pred_ignore_flags = pred_dist_flags | (
- text_scores < text_score_thr)
- filtered_pred_texts = self._get_true_elements(
- pred_texts, ~pred_ignore_flags)
- filtered_pred_points = self._get_true_elements(
- pred_points, ~pred_ignore_flags)
- gt_matched = np.zeros(len(gt_texts), dtype=bool)
- num_gt = len(gt_texts) - np.sum(gt_ignore_flags)
- if num_gt == 0:
- continue
- num_gts[i] += num_gt
-
- for pred_text, pred_point in zip(filtered_pred_texts,
- filtered_pred_points):
- dists = [
- Point(pred_point).distance(Point(gt_point))
- for gt_point in gt_points
- ]
- min_idx = np.argmin(dists)
- if gt_texts[min_idx] == '###' or gt_ignore_flags[min_idx]:
- continue
- if not gt_matched[min_idx] and (
- pred_text.upper() == gt_texts[min_idx].upper()):
- gt_matched[min_idx] = True
- num_tp[i] += 1
- num_preds[i] += 1
-
- for i, text_score_thr in enumerate(self.text_score_thrs):
- if num_preds[i] == 0 or num_tp[i] == 0:
- recall, precision, hmean = 0, 0, 0
- else:
- recall = num_tp[i] / num_gts[i]
- precision = num_tp[i] / num_preds[i]
- hmean = 2 * recall * precision / (recall + precision)
- eval_results = dict(
- precision=precision, recall=recall, hmean=hmean)
- logger.info(f'text score threshold: {text_score_thr:.2f}, '
- f'recall: {eval_results["recall"]:.4f}, '
- f'precision: {eval_results["precision"]:.4f}, '
- f'hmean: {eval_results["hmean"]:.4f}\n')
- if eval_results['hmean'] > best_eval_results['hmean']:
- best_eval_results = eval_results
- return best_eval_results
-
- def _map_img_name(self, img_name: str, mapping: Tuple[str, str]) -> str:
- """Map the image name to the another one based on mapping."""
- return re.sub(mapping[0], mapping[1], img_name)
-
- def _true_indexes(self, array: np.ndarray) -> np.ndarray:
- """Get indexes of True elements from a 1D boolean array."""
- return np.where(array)[0]
-
- def _word_spotting_filter(self, gt_ignore_flags: np.ndarray,
- gt_texts: List[str]
- ) -> Tuple[np.ndarray, List[str]]:
- """Filter out gt instances that cannot be in a valid dictionary, and do
- some simple preprocessing to texts."""
-
- for i in range(len(gt_texts)):
- if gt_ignore_flags[i]:
- continue
- text = gt_texts[i]
- if text[-2:] in ["'s", "'S"]:
- text = text[:-2]
- text = text.strip('-')
- for char in "'!?.:,*\"()ยท[]/":
- text = text.replace(char, ' ')
- text = text.strip()
- gt_ignore_flags[i] = not self._include_in_dict(text)
- if not gt_ignore_flags[i]:
- gt_texts[i] = text
-
- return gt_ignore_flags, gt_texts
-
- def _include_in_dict(self, text: str) -> bool:
- """Check if the text could be in a valid dictionary."""
- if len(text) != len(text.replace(' ', '')) or len(text) < 3:
- return False
- not_allowed = 'รรทฮ'
- valid_ranges = [(ord(u'a'), ord(u'z')), (ord(u'A'), ord(u'Z')),
- (ord(u'ร'), ord(u'ฦฟ')), (ord(u'ว'), ord(u'ษฟ')),
- (ord(u'ฮ'), ord(u'ฯฟ')), (ord(u'-'), ord(u'-'))]
- for char in text:
- code = ord(char)
- if (not_allowed.find(char) != -1):
- return False
- valid = any(code >= r[0] and code <= r[1] for r in valid_ranges)
- if not valid:
- return False
- return True
-
- def _match_word(self,
- text: str,
- lexicons: List[str],
- pairs: Optional[Dict[str, str]] = None) -> Tuple[str, int]:
- """Match the text with the lexicons and pairs."""
- text = text.upper()
- matched_word = ''
- matched_dist = 100
- for lexicon in lexicons:
- lexicon = lexicon.upper()
- norm_dist = Levenshtein.distance(text, lexicon)
- norm_dist = Levenshtein.normalized_distance(text, lexicon)
- if norm_dist < matched_dist:
- matched_dist = norm_dist
- if pairs:
- matched_word = pairs[lexicon]
- else:
- matched_word = lexicon
- return matched_word, matched_dist
diff --git a/pipeline/mmocr/projects/SPTS/spts/model/__init__.py b/pipeline/mmocr/projects/SPTS/spts/model/__init__.py
deleted file mode 100644
index 133c38e45d9483847714e3e5153f8a5f5dc149d5..0000000000000000000000000000000000000000
--- a/pipeline/mmocr/projects/SPTS/spts/model/__init__.py
+++ /dev/null
@@ -1,12 +0,0 @@
-# Copyright (c) OpenMMLab. All rights reserved.
-from .spts import SPTS
-from .spts_decoder import SPTSDecoder
-from .spts_dictionary import SPTSDictionary
-from .spts_encoder import SPTSEncoder
-from .spts_module_loss import SPTSModuleLoss
-from .spts_postprocessor import SPTSPostprocessor
-
-__all__ = [
- 'SPTSEncoder', 'SPTSDecoder', 'SPTSPostprocessor', 'SPTS',
- 'SPTSDictionary', 'SPTSModuleLoss'
-]
diff --git a/pipeline/mmocr/projects/SPTS/spts/model/base_text_spotter.py b/pipeline/mmocr/projects/SPTS/spts/model/base_text_spotter.py
deleted file mode 100644
index 635f31ee9aa4f5c3648ac3ff4e2dd9fe602a7152..0000000000000000000000000000000000000000
--- a/pipeline/mmocr/projects/SPTS/spts/model/base_text_spotter.py
+++ /dev/null
@@ -1,122 +0,0 @@
-# Copyright (c) OpenMMLab. All rights reserved.
-from abc import ABCMeta, abstractmethod
-from typing import Union
-
-import torch
-from mmengine.model.base_model import BaseModel
-
-from mmocr.utils import (OptConfigType, OptMultiConfig, OptRecSampleList,
- RecForwardResults, RecSampleList)
-
-
-class BaseTextSpotter(BaseModel, metaclass=ABCMeta):
- """Base class for text spotter.
-
- TODO: Refine docstr & typehint
-
- Args:
- data_preprocessor (dict or ConfigDict, optional): The pre-process
- config of :class:`BaseDataPreprocessor`. it usually includes,
- ``pad_size_divisor``, ``pad_value``, ``mean`` and ``std``.
- init_cfg (dict or ConfigDict or List[dict], optional): the config
- to control the initialization. Defaults to None.
- """
-
- def __init__(self,
- data_preprocessor: OptConfigType = None,
- init_cfg: OptMultiConfig = None):
- super().__init__(
- data_preprocessor=data_preprocessor, init_cfg=init_cfg)
-
- @property
- def with_backbone(self):
- """bool: whether the recognizer has a backbone"""
- return hasattr(self, 'backbone')
-
- @property
- def with_encoder(self):
- """bool: whether the recognizer has an encoder"""
- return hasattr(self, 'encoder')
-
- @property
- def with_preprocessor(self):
- """bool: whether the recognizer has a preprocessor"""
- return hasattr(self, 'preprocessor')
-
- @property
- def with_decoder(self):
- """bool: whether the recognizer has a decoder"""
- return hasattr(self, 'decoder')
-
- @abstractmethod
- def extract_feat(self, inputs: torch.Tensor) -> torch.Tensor:
- """Extract features from images."""
- pass
-
- def forward(self,
- inputs: torch.Tensor,
- data_samples: OptRecSampleList = None,
- mode: str = 'tensor',
- **kwargs) -> RecForwardResults:
- """The unified entry for a forward process in both training and test.
-
- The method should accept three modes: "tensor", "predict" and "loss":
-
- - "tensor": Forward the whole network and return tensor or tuple of
- tensor without any post-processing, same as a common nn.Module.
- - "predict": Forward and return the predictions, which are fully
- processed to a list of :obj:`DetDataSample`.
- - "loss": Forward and return a dict of losses according to the given
- inputs and data samples.
-
- Note that this method doesn't handle neither back propagation nor
- optimizer updating, which are done in the :meth:`train_step`.
-
- Args:
- inputs (torch.Tensor): The input tensor with shape
- (N, C, ...) in general.
- data_samples (list[:obj:`DetDataSample`], optional): The
- annotation data of every samples. Defaults to None.
- mode (str): Return what kind of value. Defaults to 'tensor'.
-
- Returns:
- The return type depends on ``mode``.
-
- - If ``mode="tensor"``, return a tensor or a tuple of tensor.
- - If ``mode="predict"``, return a list of :obj:`DetDataSample`.
- - If ``mode="loss"``, return a dict of tensor.
- """
- if mode == 'loss':
- return self.loss(inputs, data_samples, **kwargs)
- elif mode == 'predict':
- return self.predict(inputs, data_samples, **kwargs)
- elif mode == 'tensor':
- return self._forward(inputs, data_samples, **kwargs)
- else:
- raise RuntimeError(f'Invalid mode "{mode}". '
- 'Only supports loss, predict and tensor mode')
-
- @abstractmethod
- def loss(self, inputs: torch.Tensor, data_samples: RecSampleList,
- **kwargs) -> Union[dict, tuple]:
- """Calculate losses from a batch of inputs and data samples."""
- pass
-
- @abstractmethod
- def predict(self, inputs: torch.Tensor, data_samples: RecSampleList,
- **kwargs) -> RecSampleList:
- """Predict results from a batch of inputs and data samples with post-
- processing."""
- pass
-
- @abstractmethod
- def _forward(self,
- inputs: torch.Tensor,
- data_samples: OptRecSampleList = None,
- **kwargs):
- """Network forward process.
-
- Usually includes backbone, neck and head forward without any post-
- processing.
- """
- pass
diff --git a/pipeline/mmocr/projects/SPTS/spts/model/encoder_decoder_text_spotter.py b/pipeline/mmocr/projects/SPTS/spts/model/encoder_decoder_text_spotter.py
deleted file mode 100644
index 81ebd2ff294a2ccc86dfe5612f72c2c4b7724953..0000000000000000000000000000000000000000
--- a/pipeline/mmocr/projects/SPTS/spts/model/encoder_decoder_text_spotter.py
+++ /dev/null
@@ -1,130 +0,0 @@
-# Copyright (c) OpenMMLab. All rights reserved.
-
-from typing import Dict
-
-import torch
-
-from mmocr.registry import MODELS
-from mmocr.utils.typing_utils import (ConfigType, InitConfigType,
- OptConfigType, OptRecSampleList,
- RecForwardResults, RecSampleList)
-from .base_text_spotter import BaseTextSpotter
-
-
-@MODELS.register_module()
-class EncoderDecoderTextSpotter(BaseTextSpotter):
- """Base class for encode-decode text spotter.
-
- Args:
- preprocessor (dict, optional): Config dict for preprocessor. Defaults
- to None.
- backbone (dict, optional): Backbone config. Defaults to None.
- encoder (dict, optional): Encoder config. If None, the output from
- backbone will be directly fed into ``decoder``. Defaults to None.
- decoder (dict, optional): Decoder config. Defaults to None.
- data_preprocessor (dict, optional): Model preprocessing config
- for processing the input image data. Keys allowed are
- ``to_rgb``(bool), ``pad_size_divisor``(int), ``pad_value``(int or
- float), ``mean``(int or float) and ``std``(int or float).
- Preprcessing order: 1. to rgb; 2. normalization 3. pad.
- Defaults to None.
- init_cfg (dict or list[dict], optional): Initialization configs.
- Defaults to None.
- """
-
- def __init__(self,
- preprocessor: OptConfigType = None,
- backbone: OptConfigType = None,
- encoder: OptConfigType = None,
- decoder: OptConfigType = None,
- data_preprocessor: ConfigType = None,
- init_cfg: InitConfigType = None) -> None:
-
- super().__init__(
- init_cfg=init_cfg, data_preprocessor=data_preprocessor)
-
- # Preprocessor module, e.g., TPS
- if preprocessor is not None:
- self.preprocessor = MODELS.build(preprocessor)
-
- # Backbone
- if backbone is not None:
- self.backbone = MODELS.build(backbone)
-
- # Encoder module
- if encoder is not None:
- self.encoder = MODELS.build(encoder)
-
- # Decoder module
- assert decoder is not None
- self.decoder = MODELS.build(decoder)
-
- def extract_feat(self, inputs: torch.Tensor) -> torch.Tensor:
- """Directly extract features from the backbone."""
- if self.with_preprocessor:
- inputs = self.preprocessor(inputs)
- if self.with_backbone:
- inputs = self.backbone(inputs)
- return inputs
-
- def loss(self, inputs: torch.Tensor, data_samples: RecSampleList,
- **kwargs) -> Dict:
- """Calculate losses from a batch of inputs and data samples.
- Args:
- inputs (tensor): Input images of shape (N, C, H, W).
- Typically these should be mean centered and std scaled.
- data_samples (list[TextRecogDataSample]): A list of N
- datasamples, containing meta information and gold
- annotations for each of the images.
-
- Returns:
- dict[str, tensor]: A dictionary of loss components.
- """
- feat = self.extract_feat(inputs)
- out_enc = None
- if self.with_encoder:
- out_enc = self.encoder(feat, data_samples)
- return self.decoder.loss(feat, out_enc, data_samples)
-
- def predict(self, inputs: torch.Tensor, data_samples: RecSampleList,
- **kwargs) -> RecSampleList:
- """Predict results from a batch of inputs and data samples with post-
- processing.
-
- Args:
- inputs (torch.Tensor): Image input tensor.
- data_samples (list[TextRecogDataSample]): A list of N datasamples,
- containing meta information and gold annotations for each of
- the images.
-
- Returns:
- list[TextRecogDataSample]: A list of N datasamples of prediction
- results. Results are stored in ``pred_text``.
- """
- feat = self.extract_feat(inputs)
- out_enc = None
- if self.with_encoder:
- out_enc = self.encoder(feat, data_samples)
- return self.decoder.predict(feat, out_enc, data_samples)
-
- def _forward(self,
- inputs: torch.Tensor,
- data_samples: OptRecSampleList = None,
- **kwargs) -> RecForwardResults:
- """Network forward process. Usually includes backbone, encoder and
- decoder forward without any post-processing.
-
- Args:
- inputs (Tensor): Inputs with shape (N, C, H, W).
- data_samples (list[TextRecogDataSample]): A list of N
- datasamples, containing meta information and gold
- annotations for each of the images.
-
- Returns:
- Tensor: A tuple of features from ``decoder`` forward.
- """
- feat = self.extract_feat(inputs)
- out_enc = None
- if self.with_encoder:
- out_enc = self.encoder(feat, data_samples)
- return self.decoder(feat, out_enc, data_samples)
diff --git a/pipeline/mmocr/projects/SPTS/spts/model/position_embedding.py b/pipeline/mmocr/projects/SPTS/spts/model/position_embedding.py
deleted file mode 100644
index b2836993825e779f0e136024d193d1d1836fa0db..0000000000000000000000000000000000000000
--- a/pipeline/mmocr/projects/SPTS/spts/model/position_embedding.py
+++ /dev/null
@@ -1,55 +0,0 @@
-import math
-
-import torch
-from torch import Tensor, nn
-
-
-class PositionEmbeddingSine(nn.Module):
- """This is a more standard version of the position embedding, very similar
- to the one used by the Attention is all you need paper, generalized to work
- on images.
-
- Adapted from https://github.com/shannanyinxiang/SPTS.
- """
-
- def __init__(self,
- num_pos_feats=64,
- temperature=10000,
- normalize=True,
- scale=None):
- super().__init__()
- self.num_pos_feats = num_pos_feats
- self.temperature = temperature
- self.normalize = normalize
- if scale is not None and normalize is False:
- raise ValueError('normalize should be True if scale is passed')
- if scale is None:
- scale = 2 * math.pi
- self.scale = scale
-
- def forward(self, mask: Tensor):
- assert mask is not None
- not_mask = ~mask
- y_embed = not_mask.cumsum(1, dtype=torch.float32)
- x_embed = not_mask.cumsum(2, dtype=torch.float32)
- if self.normalize:
- eps = 1e-6
- y_embed = y_embed / (y_embed[:, -1:, :] + eps) * self.scale
- x_embed = x_embed / (x_embed[:, :, -1:] + eps) * self.scale
-
- dim_t = torch.arange(
- self.num_pos_feats, dtype=torch.float32, device=mask.device)
- dim_t = self.temperature**(2 *
- torch.div(dim_t, 2, rounding_mode='floor') /
- self.num_pos_feats)
-
- pos_x = x_embed[:, :, :, None] / dim_t
- pos_y = y_embed[:, :, :, None] / dim_t
- pos_x = torch.stack(
- (pos_x[:, :, :, 0::2].sin(), pos_x[:, :, :, 1::2].cos()),
- dim=4).flatten(3)
- pos_y = torch.stack(
- (pos_y[:, :, :, 0::2].sin(), pos_y[:, :, :, 1::2].cos()),
- dim=4).flatten(3)
- pos = torch.cat((pos_y, pos_x), dim=3).permute(0, 3, 1, 2)
- return pos
diff --git a/pipeline/mmocr/projects/SPTS/spts/model/spts.py b/pipeline/mmocr/projects/SPTS/spts/model/spts.py
deleted file mode 100644
index 68890dab7a18018671ff692687d6593c07d06217..0000000000000000000000000000000000000000
--- a/pipeline/mmocr/projects/SPTS/spts/model/spts.py
+++ /dev/null
@@ -1,8 +0,0 @@
-# Copyright (c) OpenMMLab. All rights reserved.
-from mmocr.registry import MODELS
-from .encoder_decoder_text_spotter import EncoderDecoderTextSpotter
-
-
-@MODELS.register_module()
-class SPTS(EncoderDecoderTextSpotter):
- """SPTS."""
diff --git a/pipeline/mmocr/projects/SPTS/spts/model/spts_decoder.py b/pipeline/mmocr/projects/SPTS/spts/model/spts_decoder.py
deleted file mode 100644
index 374c65c649f07f573ca6fd080d0f2854fd216c37..0000000000000000000000000000000000000000
--- a/pipeline/mmocr/projects/SPTS/spts/model/spts_decoder.py
+++ /dev/null
@@ -1,539 +0,0 @@
-import copy
-from typing import Dict, List, Optional, Sequence, Union
-
-import torch
-import torch.nn.functional as F
-from torch import Tensor, nn
-
-from mmocr.models.common import Dictionary
-from mmocr.models.textrecog.decoders import BaseDecoder
-from mmocr.registry import MODELS
-from mmocr.utils.typing_utils import TextSpottingDataSample
-from .position_embedding import PositionEmbeddingSine
-
-
-@MODELS.register_module()
-class SPTSDecoder(BaseDecoder):
- """SPTS Decoder.
-
- Args:
- dictionary (dict or :obj:`Dictionary`): The config for `Dictionary` or
- the instance of `Dictionary`.
- num_bins (int): Number of bins dividing the image. Defaults to 1000.
- n_head (int): Number of parallel attention heads. Defaults to 8.
- d_model (int): Dimension :math:`D_m` of the input from previous model.
- Defaults to 256.
- d_feedforward (int): Dimension of the feedforward layer.
- Defaults to 1024.
- normalize_before (bool): Whether to normalize the input before
- encoding/decoding. Defaults to True.
- max_num_text (int): Maximum number of text instances in a sample.
- Defaults to 60.
- module_loss (dict, optional): Config to build loss. Defaults to None.
- postprocessor (dict, optional): Config to build postprocessor.
- Defaults to None.
- init_cfg (dict or list[dict], optional): Initialization configs.
- Defaults to None.
- """
-
- def __init__(self,
- dictionary: Union[Dict, Dictionary],
- num_bins: int = 1000,
- n_head: int = 8,
- d_model: int = 256,
- d_feedforward: int = 1024,
- normalize_before: bool = True,
- dropout: float = 0.1,
- max_num_text: int = 60,
- module_loss: Optional[Dict] = None,
- postprocessor: Optional[Dict] = None,
- init_cfg: Optional[Union[Dict, List[Dict]]] = None) -> None:
-
- # TODO: fix hardcode
- self.max_seq_len = (2 + 25) * max_num_text + 1
- super().__init__(
- dictionary=dictionary,
- module_loss=module_loss,
- postprocessor=postprocessor,
- max_seq_len=self.max_seq_len,
- init_cfg=init_cfg)
- self.num_bins = num_bins
-
- self.embedding = DecoderEmbeddings(self.dictionary.num_classes,
- self.dictionary.padding_idx,
- d_model, self.max_seq_len, dropout)
- self.pos_embedding = PositionEmbeddingSine(d_model // 2)
-
- self.vocab_embed = self._gen_vocab_embed(d_model, d_model,
- self.dictionary.num_classes,
- 3)
- encoder_layer = TransformerEncoderLayer(d_model, n_head, d_feedforward,
- dropout, 'relu',
- normalize_before)
- encoder_norm = nn.LayerNorm(d_model) if normalize_before else None
- num_encoder_layers = 6
- self.encoder = TransformerEncoder(encoder_layer, num_encoder_layers,
- encoder_norm)
-
- decoder_layer = TransformerDecoderLayer(d_model, n_head, d_feedforward,
- dropout, 'relu',
- normalize_before)
- decoder_norm = nn.LayerNorm(d_model)
- num_decoder_layers = 6
- self.decoder = TransformerDecoder(
- decoder_layer,
- num_decoder_layers,
- decoder_norm,
- return_intermediate=False)
- self._reset_parameters()
-
- def _reset_parameters(self):
- for p in self.parameters():
- if p.dim() > 1:
- nn.init.xavier_uniform_(p)
-
- def _gen_vocab_embed(self, input_dim: int, hidden_dim: int,
- output_dim: int, num_layers: int) -> nn.Module:
- """Generate vocab embedding layer."""
- net = nn.Sequential()
- h = [hidden_dim] * (num_layers - 1)
- for i, (n, k) in enumerate(zip([input_dim] + h, h + [output_dim])):
- net.add_module(f'layer-{i}', nn.Linear(n, k))
- if i < num_layers - 1:
- net.add_module(f'relu-{i}', nn.ReLU())
- return net
-
- def forward_train(
- self,
- feat: Optional[torch.Tensor] = None,
- out_enc: Optional[torch.Tensor] = None,
- data_samples: Optional[Sequence[TextSpottingDataSample]] = None
- ) -> torch.Tensor:
- """Forward for training.
-
- Args:
- feat (torch.Tensor, optional): The feature map from backbone of
- shape :math:`(N, E, H, W)`. Defaults to None.
- out_enc (torch.Tensor, optional): Encoder output. Defaults to None.
- data_samples (Sequence[TextRecogDataSample]): Batch of
- TextRecogDataSample, containing gt_text information. Defaults
- to None.
- """
- mask, pos_embed, memory, query_embed = self._embed(
- out_enc, data_samples)
-
- padded_targets = [
- data_sample.gt_instances.padded_indexes
- for data_sample in data_samples
- ]
- padded_targets = torch.stack(padded_targets, dim=0).to(out_enc.device)
- # we don't need eos here
- tgt = self.embedding(padded_targets[:, :-1]).permute(1, 0, 2)
- hs = self.decoder(
- tgt,
- memory,
- memory_key_padding_mask=mask,
- pos=pos_embed,
- query_pos=query_embed[:len(tgt)],
- tgt_mask=self._generate_square_subsequent_mask(len(tgt)).to(
- tgt.device))
- return self.vocab_embed(hs[-1].transpose(0, 1))
-
- def forward_test(
- self,
- feat: Optional[torch.Tensor] = None,
- out_enc: Optional[torch.Tensor] = None,
- data_samples: Optional[Sequence[TextSpottingDataSample]] = None
- ) -> torch.Tensor:
- """Forward for testing.
-
- Args:
- feat (torch.Tensor, optional): The feature map from backbone of
- shape :math:`(N, E, H, W)`. Defaults to None.
- out_enc (torch.Tensor, optional): Encoder output. Defaults to None.
- data_samples (Sequence[TextRecogDataSample]): Batch of
- TextRecogDataSample, containing gt_text information. Defaults
- to None.
- """
-
- batch_size = out_enc.shape[0]
- mask, pos_embed, memory, query_embed = self._embed(
- out_enc, data_samples)
-
- max_probs = []
- seq = torch.zeros(
- batch_size, 1, dtype=torch.long).to(
- out_enc.device) + self.dictionary.start_idx
- for i in range(self.max_seq_len):
- tgt = self.embedding(seq).permute(1, 0, 2)
- hs = self.decoder(
- tgt,
- memory,
- memory_key_padding_mask=mask,
- pos=pos_embed,
- query_pos=query_embed[:len(tgt)],
- tgt_mask=self._generate_square_subsequent_mask(len(tgt)).to(
- tgt.device)) # bs, 1, E ?
- out = self.vocab_embed(hs.transpose(1, 2)[-1, :, -1, :])
- out = out.softmax(-1)
-
- # bins chars unk eos seq_eos sos padding
- if i % 27 == 0: # coordinate or eos
- out[:, self.num_bins:self.dictionary.seq_end_idx] = 0
- out[:, self.dictionary.seq_end_idx + 1:] = 0
- elif i % 27 == 1: # coordinate
- out[:, self.num_bins:] = 0
- else: # chars
- out[:, :self.num_bins] = 0
- out[:, self.dictionary.seq_end_idx:] = 0
-
- max_prob, extra_seq = torch.max(out, dim=-1, keepdim=True)
- # prob, extra_seq = out.topk(dim=-1, k=1)
- # work for single batch only (original implementation)
- # TODO: optimize for multi-batch
- seq = torch.cat([seq, extra_seq], dim=-1)
- max_probs.append(max_prob)
- if extra_seq[0] == self.dictionary.seq_end_idx:
- break
-
- max_probs = torch.cat(max_probs, dim=-1)
- max_probs = max_probs[:, :-1] # remove seq_eos
- seq = seq[:, 1:-1] # remove start index and seq_eos
- return max_probs, seq
-
- def _embed(self, out_enc, data_samples):
- bs, c, h, w = out_enc.shape
- mask, pos_embed = self._gen_mask(out_enc, data_samples)
- out_enc = out_enc.flatten(2).permute(2, 0, 1)
- pos_embed = pos_embed.flatten(2).permute(2, 0, 1)
- mask = mask.flatten(1)
- # TODO move encoder to mmcv
- memory = self.encoder(
- out_enc, src_key_padding_mask=mask, pos=pos_embed.half())
-
- query_embed = self.embedding.position_embeddings.weight.unsqueeze(1)
- query_embed = query_embed.repeat(1, bs, 1)
- return mask, pos_embed, memory, query_embed
-
- def _generate_square_subsequent_mask(self, size):
- r"""Generate a square mask for the sequence. The masked positions are
- filled with float('-inf'). Unmasked positions are filled with
- float(0.0).
- """
- mask = (torch.triu(torch.ones(size, size)) == 1).transpose(0, 1)
- mask = mask.float().masked_fill(mask == 0, float('-inf')).masked_fill(
- mask == 1, float(0.0))
- return mask
-
- def _gen_mask(self, out_enc, data_samples):
- bs, _, h, w = out_enc.shape
- masks = torch.ones((bs, h, w), dtype=bool, device=out_enc.device)
- for i, data_sample in enumerate(data_samples):
- img_h, img_w = data_sample.img_shape
- masks[i, :img_h, :img_w] = False
- masks = F.interpolate(
- masks[None].float(), size=(h, w)).to(torch.bool)[0]
- return masks, self.pos_embedding(masks)
-
-
-class DecoderEmbeddings(nn.Module):
-
- def __init__(self, num_classes: int, padding_idx: int, hidden_dim,
- max_position_embeddings, dropout):
- super(DecoderEmbeddings, self).__init__()
- self.word_embeddings = nn.Embedding(
- num_classes, hidden_dim, padding_idx=padding_idx)
- self.position_embeddings = nn.Embedding(max_position_embeddings,
- hidden_dim)
-
- self.LayerNorm = torch.nn.LayerNorm(hidden_dim)
- self.dropout = nn.Dropout(dropout)
-
- def forward(self, x):
- input_shape = x.size()
- seq_length = input_shape[1]
- device = x.device
-
- position_ids = torch.arange(
- seq_length, dtype=torch.long, device=device)
- position_ids = position_ids.unsqueeze(0).expand(input_shape)
-
- input_embeds = self.word_embeddings(x)
- position_embeds = self.position_embeddings(position_ids)
-
- embeddings = input_embeds + position_embeds
- embeddings = self.LayerNorm(embeddings)
- embeddings = self.dropout(embeddings)
-
- return embeddings
-
-
-class TransformerEncoder(nn.Module):
-
- def __init__(self, encoder_layer, num_layers, norm=None):
- super(TransformerEncoder, self).__init__()
- self.layers = _get_clones(encoder_layer, num_layers)
- self.num_layers = num_layers
- self.norm = norm
-
- def forward(self,
- src,
- mask: Optional[Tensor] = None,
- src_key_padding_mask: Optional[Tensor] = None,
- pos: Optional[Tensor] = None):
- output = src
-
- for layer in self.layers:
- output = layer(
- output,
- src_mask=mask,
- src_key_padding_mask=src_key_padding_mask,
- pos=pos)
-
- if self.norm is not None:
- output = self.norm(output)
-
- return output
-
-
-class TransformerDecoder(nn.Module):
-
- def __init__(self,
- decoder_layer,
- num_layers,
- norm=None,
- return_intermediate=False):
- super(TransformerDecoder, self).__init__()
- self.layers = _get_clones(decoder_layer, num_layers)
- self.num_layers = num_layers
- self.norm = norm
- self.return_intermediate = return_intermediate
-
- def forward(self,
- tgt,
- memory,
- tgt_mask: Optional[Tensor] = None,
- memory_mask: Optional[Tensor] = None,
- tgt_key_padding_mask: Optional[Tensor] = None,
- memory_key_padding_mask: Optional[Tensor] = None,
- pos: Optional[Tensor] = None,
- query_pos: Optional[Tensor] = None):
- output = tgt
-
- for layer in self.layers:
- output = layer(
- output,
- memory,
- tgt_mask=tgt_mask,
- memory_mask=memory_mask,
- tgt_key_padding_mask=tgt_key_padding_mask,
- memory_key_padding_mask=memory_key_padding_mask,
- pos=pos,
- query_pos=query_pos)
-
- if self.norm is not None:
- # nn.LayerNorm(d_model)
- output = self.norm(output)
-
- return output.unsqueeze(0)
-
-
-class TransformerEncoderLayer(nn.Module):
-
- def __init__(self,
- d_model,
- nhead,
- dim_feedforward=2048,
- dropout=0.1,
- activation='relu',
- normalize_before=False):
- super(TransformerEncoderLayer, self).__init__()
- self.self_attn = nn.MultiheadAttention(d_model, nhead, dropout=dropout)
- # Implementation of Feedforward model
- self.linear1 = nn.Linear(d_model, dim_feedforward)
- self.dropout = nn.Dropout(dropout)
- self.linear2 = nn.Linear(dim_feedforward, d_model)
-
- self.norm1 = nn.LayerNorm(d_model)
- self.norm2 = nn.LayerNorm(d_model)
- self.dropout1 = nn.Dropout(dropout)
- self.dropout2 = nn.Dropout(dropout)
-
- self.activation = _get_activation_fn(activation)
- self.normalize_before = normalize_before
-
- def with_pos_embed(self, tensor, pos: Optional[Tensor]):
- return tensor if pos is None else tensor + pos
-
- def forward_post(self,
- src,
- src_mask: Optional[Tensor] = None,
- src_key_padding_mask: Optional[Tensor] = None,
- pos: Optional[Tensor] = None):
- q = k = self.with_pos_embed(src, pos)
- src2 = self.self_attn(
- q,
- k,
- value=src,
- attn_mask=src_mask,
- key_padding_mask=src_key_padding_mask)[0]
- src = src + self.dropout1(src2)
- src = self.norm1(src)
- src2 = self.linear2(self.dropout(self.activation(self.linear1(src))))
- src = src + self.dropout2(src2)
- src = self.norm2(src)
- return src
-
- def forward_pre(self,
- src,
- src_mask: Optional[Tensor] = None,
- src_key_padding_mask: Optional[Tensor] = None,
- pos: Optional[Tensor] = None):
- src2 = self.norm1(src)
- q = k = self.with_pos_embed(src2, pos)
- src2 = self.self_attn(
- q,
- k,
- value=src2,
- attn_mask=src_mask,
- key_padding_mask=src_key_padding_mask)[0]
- src = src + self.dropout1(src2)
- src2 = self.norm2(src)
- src2 = self.linear2(self.dropout(self.activation(self.linear1(src2))))
- src = src + self.dropout2(src2)
- return src
-
- def forward(self,
- src,
- src_mask: Optional[Tensor] = None,
- src_key_padding_mask: Optional[Tensor] = None,
- pos: Optional[Tensor] = None):
- if self.normalize_before:
- return self.forward_pre(src, src_mask, src_key_padding_mask, pos)
- return self.forward_post(src, src_mask, src_key_padding_mask, pos)
-
-
-class TransformerDecoderLayer(nn.Module):
-
- def __init__(self,
- d_model,
- nhead,
- dim_feedforward=2048,
- dropout=0.1,
- activation='relu',
- normalize_before=False):
- super(TransformerDecoderLayer, self).__init__()
- self.self_attn = nn.MultiheadAttention(d_model, nhead, dropout=dropout)
- self.multihead_attn = nn.MultiheadAttention(
- d_model, nhead, dropout=dropout)
- # Implementation of Feedforward model
- self.linear1 = nn.Linear(d_model, dim_feedforward)
- self.dropout = nn.Dropout(dropout)
- self.linear2 = nn.Linear(dim_feedforward, d_model)
-
- self.norm1 = nn.LayerNorm(d_model)
- self.norm2 = nn.LayerNorm(d_model)
- self.norm3 = nn.LayerNorm(d_model)
- self.dropout1 = nn.Dropout(dropout)
- self.dropout2 = nn.Dropout(dropout)
- self.dropout3 = nn.Dropout(dropout)
-
- self.activation = _get_activation_fn(activation)
- self.normalize_before = normalize_before
-
- def with_pos_embed(self, tensor, pos: Optional[Tensor]):
- return tensor if pos is None else tensor + pos
-
- def forward_post(self,
- tgt,
- memory,
- tgt_mask: Optional[Tensor] = None,
- memory_mask: Optional[Tensor] = None,
- tgt_key_padding_mask: Optional[Tensor] = None,
- memory_key_padding_mask: Optional[Tensor] = None,
- pos: Optional[Tensor] = None,
- query_pos: Optional[Tensor] = None):
- q = k = self.with_pos_embed(tgt, query_pos)
- tgt2 = self.self_attn(
- q,
- k,
- value=tgt,
- attn_mask=tgt_mask,
- key_padding_mask=tgt_key_padding_mask)[0]
- tgt = tgt + self.dropout1(tgt2)
- tgt = self.norm1(tgt)
- tgt2 = self.multihead_attn(
- query=self.with_pos_embed(tgt, query_pos),
- key=self.with_pos_embed(memory, pos),
- value=memory,
- attn_mask=memory_mask,
- key_padding_mask=memory_key_padding_mask)[0]
- tgt = tgt + self.dropout2(tgt2)
- tgt = self.norm2(tgt)
- tgt2 = self.linear2(self.dropout(self.activation(self.linear1(tgt))))
- tgt = tgt + self.dropout3(tgt2)
- tgt = self.norm3(tgt)
- return tgt
-
- def forward_pre(self,
- tgt,
- memory,
- tgt_mask: Optional[Tensor] = None,
- memory_mask: Optional[Tensor] = None,
- tgt_key_padding_mask: Optional[Tensor] = None,
- memory_key_padding_mask: Optional[Tensor] = None,
- pos: Optional[Tensor] = None,
- query_pos: Optional[Tensor] = None):
- tgt2 = self.norm1(tgt)
- q = k = self.with_pos_embed(tgt2, query_pos)
- tgt2 = self.self_attn(
- q,
- k,
- value=tgt2,
- attn_mask=tgt_mask,
- key_padding_mask=tgt_key_padding_mask)[0]
- tgt = tgt + self.dropout1(tgt2)
- tgt2 = self.norm2(tgt)
- tgt2 = self.multihead_attn(
- query=self.with_pos_embed(tgt2, query_pos),
- key=self.with_pos_embed(memory, pos),
- value=memory,
- attn_mask=memory_mask,
- key_padding_mask=memory_key_padding_mask)[0]
- tgt = tgt + self.dropout2(tgt2)
- tgt2 = self.norm3(tgt)
- tgt2 = self.linear2(self.dropout(self.activation(self.linear1(tgt2))))
- tgt = tgt + self.dropout3(tgt2)
- return tgt
-
- def forward(self,
- tgt,
- memory,
- tgt_mask: Optional[Tensor] = None,
- memory_mask: Optional[Tensor] = None,
- tgt_key_padding_mask: Optional[Tensor] = None,
- memory_key_padding_mask: Optional[Tensor] = None,
- pos: Optional[Tensor] = None,
- query_pos: Optional[Tensor] = None):
- if self.normalize_before:
- return self.forward_pre(tgt, memory, tgt_mask, memory_mask,
- tgt_key_padding_mask,
- memory_key_padding_mask, pos, query_pos)
- return self.forward_post(tgt, memory, tgt_mask, memory_mask,
- tgt_key_padding_mask, memory_key_padding_mask,
- pos, query_pos)
-
-
-def _get_clones(module, N):
- return nn.ModuleList([copy.deepcopy(module) for i in range(N)])
-
-
-def _get_activation_fn(activation):
- """Return an activation function given a string."""
- if activation == 'relu':
- return F.relu
- if activation == 'gelu':
- return F.gelu
- if activation == 'glu':
- return F.glu
- raise RuntimeError(F'activation should be relu/gelu, not {activation}.')
diff --git a/pipeline/mmocr/projects/SPTS/spts/model/spts_dictionary.py b/pipeline/mmocr/projects/SPTS/spts/model/spts_dictionary.py
deleted file mode 100644
index f204a71a5e06bd23b9c49b9ad33eda502f5b4dc5..0000000000000000000000000000000000000000
--- a/pipeline/mmocr/projects/SPTS/spts/model/spts_dictionary.py
+++ /dev/null
@@ -1,162 +0,0 @@
-# Copyright (c) OpenMMLab. All rights reserved.
-from typing import Sequence
-
-from mmocr.models.common.dictionary import Dictionary
-from mmocr.registry import TASK_UTILS
-
-
-@TASK_UTILS.register_module()
-class SPTSDictionary(Dictionary):
- """The class generates a dictionary for recognition. It pre-defines four
- special tokens: ``start_token``, ``end_token``, ``pad_token``, and
- ``unknown_token``, which will be sequentially placed at the end of the
- dictionary when their corresponding flags are True.
-
- Args:
- dict_file (str): The path of Character dict file which a single
- character must occupies a line.
- num_bins (int): Number of bins dividing the image, which is used to
- shift the character indexes. Defaults to 1000.
- with_start (bool): The flag to control whether to include the start
- token. Defaults to False.
- with_end (bool): The flag to control whether to include the end token.
- Defaults to False.
- with_seq end (bool): The flag to control whether to include the
- sequence end token. Defaults to False.
- same_start_end (bool): The flag to control whether the start token and
- end token are the same. It only works when both ``with_start`` and
- ``with_end`` are True. Defaults to False.
- with_padding (bool):The padding token may represent more than a
- padding. It can also represent tokens like the blank token in CTC
- or the background token in SegOCR. Defaults to False.
- with_unknown (bool): The flag to control whether to include the
- unknown token. Defaults to False.
- start_token (str): The start token as a string. Defaults to ''.
- end_token (str): The end token as a string. Defaults to ''.
- seq_end_token (str): The sequence end token as a string. Defaults to
- ''.
- start_end_token (str): The start/end token as a string. if start and
- end is the same. Defaults to ''.
- padding_token (str): The padding token as a string.
- Defaults to ''.
- unknown_token (str, optional): The unknown token as a string. If it's
- set to None and ``with_unknown`` is True, the unknown token will be
- skipped when converting string to index. Defaults to ''.
- """
-
- def __init__(
- self,
- dict_file: str,
- num_bins: int = 1000,
- with_start: bool = False,
- with_end: bool = False,
- with_seq_end: bool = False,
- same_start_end: bool = False,
- with_padding: bool = False,
- with_unknown: bool = False,
- start_token: str = '',
- end_token: str = '',
- seq_end_token: str = '',
- start_end_token: str = '',
- padding_token: str = '',
- unknown_token: str = '',
- ) -> None:
- self.with_seq_end = with_seq_end
- self.seq_end_token = seq_end_token
-
- super().__init__(
- dict_file=dict_file,
- with_start=with_start,
- with_end=with_end,
- same_start_end=same_start_end,
- with_padding=with_padding,
- with_unknown=with_unknown,
- start_token=start_token,
- end_token=end_token,
- start_end_token=start_end_token,
- padding_token=padding_token,
- unknown_token=unknown_token)
-
- self.num_bins = num_bins
- self._shift_idx()
-
- @property
- def num_classes(self) -> int:
- """int: Number of output classes. Special tokens are counted.
- """
- return len(self._dict) + self.num_bins
-
- def _shift_idx(self):
- idx_terms = [
- 'start_idx', 'end_idx', 'unknown_idx', 'seq_end_idx', 'padding_idx'
- ]
- for term in idx_terms:
- value = getattr(self, term)
- if value:
- setattr(self, term, value + self.num_bins)
- for char in self._dict:
- self._char2idx[char] += self.num_bins
-
- def _update_dict(self):
- """Update the dict with tokens according to parameters."""
- # BOS/EOS
- self.start_idx = None
- self.end_idx = None
- # unknown
- self.unknown_idx = None
- # TODO: Check if this line in Dictionary is correct and
- # work as expected
- # if self.with_unknown and self.unknown_token is not None:
- if self.with_unknown:
- self._dict.append(self.unknown_token)
- self.unknown_idx = len(self._dict) - 1
-
- if self.with_start and self.with_end and self.same_start_end:
- self._dict.append(self.start_end_token)
- self.start_idx = len(self._dict) - 1
- self.end_idx = self.start_idx
- if self.with_seq_end:
- self._dict.append(self.seq_end_token)
- self.seq_end_idx = len(self.dict) - 1
- else:
- if self.with_end:
- self._dict.append(self.end_token)
- self.end_idx = len(self._dict) - 1
- if self.with_seq_end:
- self._dict.append(self.seq_end_token)
- self.seq_end_idx = len(self.dict) - 1
- if self.with_start:
- self._dict.append(self.start_token)
- self.start_idx = len(self._dict) - 1
-
- # padding
- self.padding_idx = None
- if self.with_padding:
- self._dict.append(self.padding_token)
- self.padding_idx = len(self._dict) - 1
-
- # update char2idx
- self._char2idx = {}
- for idx, char in enumerate(self._dict):
- self._char2idx[char] = idx
-
- def idx2str(self, index: Sequence[int]) -> str:
- """Convert a list of index to string.
-
- Args:
- index (list[int]): The list of indexes to convert to string.
-
- Return:
- str: The converted string.
- """
- assert isinstance(index, (list, tuple))
- string = ''
- for i in index:
- assert i < self.num_classes, f'Index: {i} out of range! Index ' \
- f'must be less than {self.num_classes}'
- # TODO: find its difference from ignore_chars
- # in TextRecogPostprocessor
- shifted_i = i - self.num_bins
- if self._dict[shifted_i] is not None:
- string += self._dict[shifted_i]
- return string
diff --git a/pipeline/mmocr/projects/SPTS/spts/model/spts_encoder.py b/pipeline/mmocr/projects/SPTS/spts/model/spts_encoder.py
deleted file mode 100644
index ced078d0eb1f6d227eb0e7a2926937ac0cf963b4..0000000000000000000000000000000000000000
--- a/pipeline/mmocr/projects/SPTS/spts/model/spts_encoder.py
+++ /dev/null
@@ -1,44 +0,0 @@
-# Copyright (c) OpenMMLab. All rights reserved.
-from typing import Dict, List, Optional, Union
-
-import torch.nn as nn
-from torch import Tensor
-
-from mmocr.models.textrecog.encoders import BaseEncoder
-from mmocr.registry import MODELS
-from mmocr.structures import TextSpottingDataSample
-
-
-@MODELS.register_module()
-class SPTSEncoder(BaseEncoder):
- """SPTS Encoder.
-
- Args:
- d_backbone (int): Backbone output dimension.
- d_model (int): Model output dimension.
- init_cfg (dict or list[dict], optional): Initialization configs.
- Defaults to None.
- """
-
- def __init__(self,
- d_backbone: int = 2048,
- d_model: int = 256,
- init_cfg: Optional[Union[Dict, List[Dict]]] = None) -> None:
- super().__init__(init_cfg=init_cfg)
- self.input_proj = nn.Conv2d(d_backbone, d_model, kernel_size=1)
-
- def forward(self,
- feat: Tensor,
- data_samples: List[TextSpottingDataSample] = None) -> Tensor:
- """Forward propagation of encoder.
-
- Args:
- feat (Tensor): Feature tensor of shape :math:`(N, D_m, H, W)`.
- data_samples (list[TextSpottingDataSample]): Batch of
- TextSpottingDataSample.
- Defaults to None.
-
- Returns:
- Tensor: A tensor of shape :math:`(N, T, D_m)`.
- """
- return self.input_proj(feat[0])
diff --git a/pipeline/mmocr/projects/SPTS/spts/model/spts_module_loss.py b/pipeline/mmocr/projects/SPTS/spts/model/spts_module_loss.py
deleted file mode 100644
index 2847e14cc6739f85b4dd065098993da99cb564ae..0000000000000000000000000000000000000000
--- a/pipeline/mmocr/projects/SPTS/spts/model/spts_module_loss.py
+++ /dev/null
@@ -1,232 +0,0 @@
-# Copyright (c) OpenMMLab. All rights reserved.
-import random
-from typing import Dict, Sequence, Union
-
-import numpy as np
-import torch
-from torch import nn
-
-from mmocr.models.common.dictionary import Dictionary
-from mmocr.models.textrecog.module_losses import CEModuleLoss
-from mmocr.registry import MODELS
-from mmocr.structures import TextSpottingDataSample
-
-
-@MODELS.register_module()
-class SPTSModuleLoss(CEModuleLoss):
- """Implementation of loss module for SPTS with CrossEntropy loss.
-
- Args:
- dictionary (dict or :obj:`Dictionary`): The config for `Dictionary` or
- the instance of `Dictionary`.
- num_bins (int): Number of bins dividing the image. Defaults to 1000.
- seq_eos_coef (float): The loss weight coefficient of seq_eos token.
- Defaults to 0.01.
- max_seq_len (int): Maximum sequence length. In SPTS, a sequence
- encodes all the text instances in a sample. Defaults to 40, which
- will be overridden by SPTSDecoder.
- max_text_len (int): Maximum length for each text instance in a
- sequence. Defaults to 25.
- letter_case (str): There are three options to alter the letter cases
- of gt texts:
- - unchanged: Do not change gt texts.
- - upper: Convert gt texts into uppercase characters.
- - lower: Convert gt texts into lowercase characters.
- Usually, it only works for English characters. Defaults to
- 'unchanged'.
- pad_with (str): The padding strategy for ``gt_text.padded_indexes``.
- Defaults to 'auto'. Options are:
- - 'auto': Use dictionary.padding_idx to pad gt texts, or
- dictionary.end_idx if dictionary.padding_idx
- is None.
- - 'padding': Always use dictionary.padding_idx to pad gt texts.
- - 'end': Always use dictionary.end_idx to pad gt texts.
- - 'none': Do not pad gt texts.
- ignore_char (int or str): Specifies a target value that is
- ignored and does not contribute to the input gradient.
- ignore_char can be int or str. If int, it is the index of
- the ignored char. If str, it is the character to ignore.
- Apart from single characters, each item can be one of the
- following reversed keywords: 'padding', 'start', 'end',
- and 'unknown', which refer to their corresponding special
- tokens in the dictionary. It will not ignore any special
- tokens when ignore_char == -1 or 'none'. Defaults to 'padding'.
- flatten (bool): Whether to flatten the output and target before
- computing CE loss. Defaults to False.
- reduction (str): Specifies the reduction to apply to the output,
- should be one of the following: ('none', 'mean', 'sum'). Defaults
- to 'none'.
- ignore_first_char (bool): Whether to ignore the first token in target (
- usually the start token). Defaults to ``True``.
- flatten (bool): Whether to flatten the vectors for loss computation.
- Defaults to False.
- """
-
- def __init__(self,
- dictionary: Union[Dict, Dictionary],
- num_bins: int,
- seq_eos_coef: float = 0.01,
- max_seq_len: int = 40,
- max_text_len: int = 25,
- letter_case: str = 'unchanged',
- pad_with: str = 'auto',
- ignore_char: Union[int, str] = 'padding',
- flatten: bool = False,
- reduction: str = 'none',
- ignore_first_char: bool = True):
- super().__init__(dictionary, max_seq_len, letter_case, pad_with,
- ignore_char, flatten, reduction, ignore_first_char)
- # TODO: fix hardcode
- self.max_text_len = max_text_len
- self.max_num_text = (self.max_seq_len - 1) // (2 + max_text_len)
- self.num_bins = num_bins
-
- weights = torch.ones(self.dictionary.num_classes, dtype=torch.float32)
- weights[self.dictionary.seq_end_idx] = seq_eos_coef
- weights.requires_grad_ = False
- self.loss_ce = nn.CrossEntropyLoss(
- ignore_index=self.ignore_index,
- reduction=reduction,
- weight=weights)
-
- def get_targets(
- self, data_samples: Sequence[TextSpottingDataSample]
- ) -> Sequence[TextSpottingDataSample]:
- """Target generator.
-
- Args:
- data_samples (list[TextSpottingDataSample]): It usually includes
- ``gt_instances`` information.
-
- Returns:
- list[TextSpottingDataSample]: Updated data_samples. Two keys will
- be added to data_sample:
-
- - indexes (torch.LongTensor): Character indexes representing gt
- texts. All special tokens are excluded, except for UKN.
- - padded_indexes (torch.LongTensor): Character indexes
- representing gt texts with BOS and EOS if applicable, following
- several padding indexes until the length reaches ``max_seq_len``.
- In particular, if ``pad_with='none'``, no padding will be
- applied.
- """
-
- batch_max_len = 0
-
- for data_sample in data_samples:
- if data_sample.get('have_target', False):
- continue
-
- if len(data_sample.gt_instances) > self.max_num_text:
- keep = random.sample(
- range(len(data_sample.gt_instances)), self.max_num_text)
- data_sample.gt_instances = data_sample.gt_instances[keep]
-
- gt_instances = data_sample.gt_instances
-
- if len(gt_instances) > 0:
- center_pts = []
- # Slightly different from the original implementation
- # which gets the center points from bezier curves
- # for bezier_pt in gt_instances.beziers:
- # bezier_pt = bezier_pt.reshape(8, 2)
- # mid_pt1 = sample_bezier_curve(
- # bezier_pt[:4], mid_point=True)
- # mid_pt2 = sample_bezier_curve(
- # bezier_pt[4:], mid_point=True)
- # center_pt = (mid_pt1 + mid_pt2) / 2
- for polygon in gt_instances.polygons:
- center_pt = polygon.reshape(-1, 2).mean(0)
- center_pts.append(center_pt)
- center_pts = np.vstack(center_pts)
- center_pts /= data_sample.img_shape[::-1]
- center_pts = torch.from_numpy(center_pts).type(torch.float32)
- else:
- center_pts = torch.ones(0).reshape(-1, 2).type(torch.float32)
-
- center_pts = (center_pts * self.num_bins).floor().type(torch.long)
- center_pts = torch.clamp(center_pts, min=0, max=self.num_bins - 1)
-
- gt_indexes = []
- for text in gt_instances.texts:
- if self.letter_case in ['upper', 'lower']:
- text = getattr(text, self.letter_case)()
-
- indexes = self.dictionary.str2idx(text)
- indexes_tensor = torch.zeros(
- self.max_text_len,
- dtype=torch.long) + self.dictionary.end_idx
- max_len = min(self.max_text_len - 1, len(indexes))
- indexes_tensor[:max_len] = torch.LongTensor(indexes)[:max_len]
- indexes_tensor = indexes_tensor
- gt_indexes.append(indexes_tensor)
-
- if len(gt_indexes) == 0:
- gt_indexes = torch.ones(0).reshape(-1, self.max_text_len)
- else:
- gt_indexes = torch.vstack(gt_indexes)
- gt_indexes = torch.cat([center_pts, gt_indexes], dim=-1)
- gt_indexes = gt_indexes.flatten()
-
- if self.dictionary.start_idx is not None:
- gt_indexes = torch.cat([
- torch.LongTensor([self.dictionary.start_idx]), gt_indexes
- ])
- if self.dictionary.seq_end_idx is not None:
- gt_indexes = torch.cat([
- gt_indexes,
- torch.LongTensor([self.dictionary.seq_end_idx])
- ])
-
- batch_max_len = max(batch_max_len, len(gt_indexes))
-
- gt_instances.set_metainfo(dict(indexes=gt_indexes))
-
- # Here we have to have the second pass as we need to know the max
- # length of the batch to pad the indexes in order to save memory
- for data_sample in data_samples:
-
- if data_sample.get('have_target', False):
- continue
-
- indexes = data_sample.gt_instances.indexes
-
- padded_indexes = (
- torch.zeros(batch_max_len, dtype=torch.long) +
- self.dictionary.padding_idx)
- padded_indexes[:len(indexes)] = indexes
- data_sample.gt_instances.set_metainfo(
- dict(padded_indexes=padded_indexes))
- data_sample.set_metainfo(dict(have_target=True))
-
- return data_samples
-
- def forward(self, outputs: torch.Tensor,
- data_samples: Sequence[TextSpottingDataSample]) -> Dict:
- """
- Args:
- outputs (Tensor): A raw logit tensor of shape :math:`(N, T, C)`.
- data_samples (list[TextSpottingDataSample]): List of
- ``TextSpottingDataSample`` which are processed by
- ``get_targets``.
-
- Returns:
- dict: A loss dict with the key ``loss_ce``.
- """
- targets = list()
- for data_sample in data_samples:
- targets.append(data_sample.gt_instances.padded_indexes)
- targets = torch.stack(targets, dim=0).long()
- if self.ignore_first_char:
- targets = targets[:, 1:].contiguous()
- # outputs = outputs[:, :-1, :].contiguous()
- if self.flatten:
- outputs = outputs.view(-1, outputs.size(-1))
- targets = targets.view(-1)
- else:
- outputs = outputs.permute(0, 2, 1).contiguous()
-
- loss_ce = self.loss_ce(outputs, targets.to(outputs.device))
- losses = dict(loss_ce=loss_ce)
-
- return losses
diff --git a/pipeline/mmocr/projects/SPTS/spts/model/spts_postprocessor.py b/pipeline/mmocr/projects/SPTS/spts/model/spts_postprocessor.py
deleted file mode 100644
index 249c9694f95d90eb816208e8042febc8d176a781..0000000000000000000000000000000000000000
--- a/pipeline/mmocr/projects/SPTS/spts/model/spts_postprocessor.py
+++ /dev/null
@@ -1,173 +0,0 @@
-# Copyright (c) OpenMMLab. All rights reserved.
-from typing import Dict, List, Optional, Sequence, Tuple, Union
-
-import numpy as np
-import torch
-from mmengine.structures import InstanceData
-
-from mmocr.models import Dictionary
-from mmocr.models.textrecog.postprocessors import BaseTextRecogPostprocessor
-from mmocr.registry import MODELS
-from mmocr.structures import TextSpottingDataSample
-from mmocr.utils import rescale_polygons
-
-
-@MODELS.register_module()
-class SPTSPostprocessor(BaseTextRecogPostprocessor):
- """PostProcessor for SPTS.
-
- Args:
- dictionary (dict or :obj:`Dictionary`): The config for `Dictionary` or
- the instance of `Dictionary`.
- num_bins (int): Number of bins dividing the image. Defaults to 1000.
- rescale_fields (list[str], optional): The bbox/polygon field names to
- be rescaled. If None, no rescaling will be performed.
- max_seq_len (int): Maximum sequence length. In SPTS, a sequence
- encodes all the text instances in a sample. Defaults to 40, which
- will be overridden by SPTSDecoder.
- ignore_chars (list[str]): A list of characters to be ignored from the
- final results. Postprocessor will skip over these characters when
- converting raw indexes to characters. Apart from single characters,
- each item can be one of the following reversed keywords: 'padding',
- 'end' and 'unknown', which refer to their corresponding special
- tokens in the dictionary.
- """
-
- def __init__(self,
- dictionary: Union[Dictionary, Dict],
- num_bins: int,
- rescale_fields: Optional[Sequence[str]] = ['points'],
- max_seq_len: int = 40,
- ignore_chars: Sequence[str] = ['padding'],
- **kwargs) -> None:
- assert rescale_fields is None or isinstance(rescale_fields, list)
- self.num_bins = num_bins
- self.rescale_fields = rescale_fields
- super().__init__(
- dictionary=dictionary,
- num_bins=num_bins,
- max_seq_len=max_seq_len,
- ignore_chars=ignore_chars)
-
- def get_single_prediction(
- self,
- max_probs: torch.Tensor,
- seq: torch.Tensor,
- data_sample: Optional[TextSpottingDataSample] = None,
- ) -> Tuple[List[List[int]], List[List[float]], List[Tuple[float]],
- List[Tuple[float]]]:
- """Convert the output probabilities of a single image to character
- indexes, character scores, points and point scores.
-
- Args:
- max_probs (torch.Tensor): Character probabilities with shape
- :math:`(T)`.
- seq (torch.Tensor): Sequence indexes with shape
- :math:`(T)`.
-
- data_sample (TextSpottingDataSample, optional): Datasample of an
- image. Defaults to None.
-
- Returns:
- tuple(list[list[int]], list[list[float]], list[(float, float)],
- list(float, float)): character indexes, character scores, points
- and point scores. Each has len of max_seq_len.
- """
- h, w = data_sample.img_shape
- # the if is not a must since the softmaxed are masked out in decoder
- # if len(max_probs) % 27 != 0:
- # max_probs = max_probs[:-len(max_probs) % 27]
- # seq = seq[:-len(seq) % 27]
- # max_value, max_idx = torch.max(max_probs, -1)
- max_probs = max_probs.reshape(-1, 27)
- seq = seq.reshape(-1, 27)
-
- indexes, text_scores, points, pt_scores = [], [], [], []
- output_indexes = seq.cpu().detach().numpy().tolist()
- output_scores = max_probs.cpu().detach().numpy().tolist()
- for output_index, output_score in zip(output_indexes, output_scores):
- # work for multi-batch
- # if output_index[0] == self.dictionary.seq_end_idx +self.num_bins:
- # break
- point_x = output_index[0] / self.num_bins * w
- point_y = output_index[1] / self.num_bins * h
- points.append((point_x, point_y))
- pt_scores.append(
- np.mean([
- output_score[0],
- output_score[1],
- ]).item())
- indexes.append([])
- char_scores = []
- for char_index, char_score in zip(output_index[2:],
- output_score[2:]):
- # the first num_bins indexes are for points
- if char_index in self.ignore_indexes:
- continue
- if char_index == self.dictionary.end_idx:
- break
- indexes[-1].append(char_index)
- char_scores.append(char_score)
- text_scores.append(np.mean(char_scores).item())
- return indexes, text_scores, points, pt_scores
-
- def __call__(
- self, output: Tuple[torch.Tensor, torch.Tensor],
- data_samples: Sequence[TextSpottingDataSample]
- ) -> Sequence[TextSpottingDataSample]:
- """Convert outputs to strings and scores.
-
- Args:
- output (tuple(Tensor, Tensor)): A tuple of (probs, seq), each has
- the shape of :math:`(T,)`.
- data_samples (list[TextSpottingDataSample]): The list of
- TextSpottingDataSample.
-
- Returns:
- list(TextSpottingDataSample): The list of TextSpottingDataSample.
- """
- max_probs, seq = output
- batch_size = max_probs.size(0)
-
- for idx in range(batch_size):
- (char_idxs, text_scores, points,
- pt_scores) = self.get_single_prediction(max_probs[idx, :],
- seq[idx, :],
- data_samples[idx])
- texts = []
- scores = []
- for index, pt_score in zip(char_idxs, pt_scores):
- text = self.dictionary.idx2str(index)
- texts.append(text)
- # the "scores" field only accepts a float number
- scores.append(np.mean(pt_score).item())
- pred_instances = InstanceData()
- pred_instances.texts = texts
- pred_instances.scores = scores
- pred_instances.text_scores = text_scores
- pred_instances.points = points
- data_samples[idx].pred_instances = pred_instances
- pred_instances = self.rescale(data_samples[idx],
- data_samples[idx].scale_factor)
- return data_samples
-
- def rescale(self, results: TextSpottingDataSample,
- scale_factor: Sequence[int]) -> TextSpottingDataSample:
- """Rescale results in ``results.pred_instances`` according to
- ``scale_factor``, whose keys are defined in ``self.rescale_fields``.
- Usually used to rescale bboxes and/or polygons.
-
- Args:
- results (TextSpottingDataSample): The post-processed prediction
- results.
- scale_factor (tuple(int)): (w_scale, h_scale)
-
- Returns:
- TextDetDataSample: Prediction results with rescaled results.
- """
- scale_factor = np.asarray(scale_factor)
- for key in self.rescale_fields:
- # TODO: this util may need an alias or to be renamed
- results.pred_instances[key] = rescale_polygons(
- results.pred_instances[key], scale_factor, mode='div')
- return results
diff --git a/pipeline/mmocr/projects/SPTS/tools/ckpt_adapter.py b/pipeline/mmocr/projects/SPTS/tools/ckpt_adapter.py
deleted file mode 100644
index 154d29631bdaf383f5d77a4bf10c0f200fbd2c79..0000000000000000000000000000000000000000
--- a/pipeline/mmocr/projects/SPTS/tools/ckpt_adapter.py
+++ /dev/null
@@ -1,45 +0,0 @@
-# Copyright (c) OpenMMLab. All rights reserved.
-import argparse
-
-import torch
-
-prefix_mapping = {
- 'backbone.0.body': 'backbone',
- 'input_proj': 'encoder.input_proj',
- 'transformer': 'decoder',
- 'vocab_embed.layers.': 'decoder.vocab_embed.layer-'
-}
-
-
-def adapt(model_path, save_path):
- model = torch.load(model_path)
- model_dict = model['model']
- new_model_dict = model_dict.copy()
-
- for k, v in model_dict.items():
- for old_prefix, new_prefix in prefix_mapping.items():
- if k.startswith(old_prefix):
- new_k = k.replace(old_prefix, new_prefix)
- new_model_dict[new_k] = v
- del new_model_dict[k]
- break
- model['state_dict'] = new_model_dict
- del model['model']
- torch.save(model, save_path)
-
-
-def parse_args():
- parser = argparse.ArgumentParser(
- description='Adapt the pretrained checkpoints from SPTS official '
- 'implementation.')
- parser.add_argument(
- 'model_path', type=str, help='Path to the source model')
- parser.add_argument(
- 'out_path', type=str, help='Path to the converted model')
- args = parser.parse_args()
- return args
-
-
-if __name__ == '__main__':
- args = parse_args()
- adapt(args.model_path, args.out_path)
diff --git a/pipeline/mmocr/projects/example_project/README.md b/pipeline/mmocr/projects/example_project/README.md
deleted file mode 100644
index c2a22bfb27ceb25f39968591db875cf6ad868eb1..0000000000000000000000000000000000000000
--- a/pipeline/mmocr/projects/example_project/README.md
+++ /dev/null
@@ -1,143 +0,0 @@
-# Dummy ResNet Wrapper
-
-> This is a README template for community `projects/`.
-
-> All the fields in this README are **mandatory** for others to understand what you have achieved in this implementation. If you still feel unclear about the requirements, please read our [contribution guide](https://mmocr.readthedocs.io/en/dev-1.x/notes/contribution_guide.html), [projects FAQ](../faq.md), or approach us in [Discussions](https://github.com/open-mmlab/mmocr/discussions).
-
-## Description
-
-> Share any information you would like others to know. For example:
->
-> Author: @xxx.
->
-> This is an implementation of \[XXX\].
-
-This project implements a dummy ResNet wrapper, which literally does nothing new but prints "hello world" during initialization.
-
-## Usage
-
-> For a typical model, this section should contain the commands for training and testing. You are also suggested to dump your environment specification to env.yml by `conda env export > env.yml`.
-
-### Prerequisites
-
-- Python 3.7
-- PyTorch 1.6 or higher
-- [MIM](https://github.com/open-mmlab/mim)
-- [MMOCR](https://github.com/open-mmlab/mmocr)
-
-All the commands below rely on the correct configuration of `PYTHONPATH`, which should point to the project's directory so that Python can locate the module files. In `example_project/` root directory, run the following line to add the current directory to `PYTHONPATH`:
-
-```shell
-# Linux
-export PYTHONPATH=`pwd`:$PYTHONPATH
-# Windows PowerShell
-$env:PYTHONPATH=Get-Location
-```
-
-### Training commands
-
-In MMOCR's root directory, run the following command to train the model:
-
-```bash
-mim train mmocr configs/dbnet_dummy-resnet_fpnc_1200e_icdar2015.py --work-dir work_dirs/dummy_mae/
-```
-
-To train on multiple GPUs, e.g. 8 GPUs, run the following command:
-
-```bash
-mim train mmocr configs/dbnet_dummy-resnet_fpnc_1200e_icdar2015.py --work-dir work_dirs/dummy_mae/ --launcher pytorch --gpus 8
-```
-
-### Testing commands
-
-In MMOCR's root directory, run the following command to test the model:
-
-```bash
-mim test mmocr configs/dbnet_dummy-resnet_fpnc_1200e_icdar2015.py --work-dir work_dirs/dummy_mae/ --checkpoint ${CHECKPOINT_PATH}
-```
-
-## Results
-
-> List the results as usually done in other model's README. [Example](https://github.com/open-mmlab/mmocr/blob/1.x/configs/textdet/dbnet/README.md#results-and-models)
->
-> You should claim whether this is based on the pre-trained weights, which are converted from the official release; or it's a reproduced result obtained from retraining the model in this project.
-
-| Method | Backbone | Pretrained Model | Training set | Test set | #epoch | Test size | Precision | Recall | Hmean | Download |
-| :---------------------------------------------------------------: | :---------: | :--------------: | :-------------: | :------------: | :----: | :-------: | :-------: | :----: | :----: | :----------------------: |
-| [DBNet_dummy](configs/dbnet_dummy-resnet_fpnc_1200e_icdar2015.py) | DummyResNet | - | ICDAR2015 Train | ICDAR2015 Test | 1200 | 736 | 0.8853 | 0.7583 | 0.8169 | [model](<>) \| [log](<>) |
-
-## Citation
-
-> You may remove this section if not applicable.
-
-```bibtex
-@software{MMOCR_Contributors_OpenMMLab_Text_Detection_2020,
-author = {{MMOCR Contributors}},
-license = {Apache-2.0},
-month = {8},
-title = {{OpenMMLab Text Detection, Recognition and Understanding Toolbox}},
-url = {https://github.com/open-mmlab/mmocr},
-version = {0.3.0},
-year = {2020}
-}
-```
-
-## Checklist
-
-Here is a checklist illustrating a usual development workflow of a successful project, and also serves as an overview of this project's progress.
-
-> The PIC (person in charge) or contributors of this project should check all the items that they believe have been finished, which will further be verified by codebase maintainers via a PR.
->
-> OpenMMLab's maintainer will review the code to ensure the project's quality. Reaching the first milestone means that this project suffices the minimum requirement of being merged into 'projects/'. But this project is only eligible to become a part of the core package upon attaining the last milestone.
->
-> Note that keeping this section up-to-date is crucial not only for this project's developers but the entire community, since there might be some other contributors joining this project and deciding their starting point from this list. It also helps maintainers accurately estimate time and effort on further code polishing, if needed.
->
-> A project does not necessarily have to be finished in a single PR, but it's essential for the project to at least reach the first milestone in its very first PR.
-
-- [ ] Milestone 1: PR-ready, and acceptable to be one of the `projects/`.
-
- - [ ] Finish the code
-
- > The code's design shall follow existing interfaces and convention. For example, each model component should be registered into `mmocr.registry.MODELS` and configurable via a config file.
-
- - [ ] Basic docstrings & proper citation
-
- > Each major object should contain a docstring, describing its functionality and arguments. If you have adapted the code from other open-source projects, don't forget to cite the source project in docstring and make sure your behavior is not against its license. Typically, we do not accept any code snippet under GPL license. [A Short Guide to Open Source Licenses](https://medium.com/nationwide-technology/a-short-guide-to-open-source-licenses-cf5b1c329edd)
-
- - [ ] Test-time correctness
-
- > If you are reproducing the result from a paper, make sure your model's inference-time performance matches that in the original paper. The weights usually could be obtained by simply renaming the keys in the official pre-trained weights. This test could be skipped though, if you are able to prove the training-time correctness and check the second milestone.
-
- - [ ] A full README
-
- > As this template does.
-
-- [ ] Milestone 2: Indicates a successful model implementation.
-
- - [ ] Training-time correctness
-
- > If you are reproducing the result from a paper, checking this item means that you should have trained your model from scratch based on the original paper's specification and verified that the final result matches the report within a minor error range.
-
-- [ ] Milestone 3: Good to be a part of our core package!
-
- - [ ] Type hints and docstrings
-
- > Ideally *all* the methods should have [type hints](https://www.pythontutorial.net/python-basics/python-type-hints/) and [docstrings](https://google.github.io/styleguide/pyguide.html#381-docstrings). [Example](https://github.com/open-mmlab/mmocr/blob/76637a290507f151215d299707c57cea5120976e/mmocr/utils/polygon_utils.py#L80-L96)
-
- - [ ] Unit tests
-
- > Unit tests for each module are required. [Example](https://github.com/open-mmlab/mmocr/blob/76637a290507f151215d299707c57cea5120976e/tests/test_utils/test_polygon_utils.py#L97-L106)
-
- - [ ] Code polishing
-
- > Refactor your code according to reviewer's comment.
-
- - [ ] Metafile.yml
-
- > It will be parsed by MIM and Inferencer. [Example](https://github.com/open-mmlab/mmocr/blob/1.x/configs/textdet/dbnet/metafile.yml)
-
-- [ ] Move your modules into the core package following the codebase's file hierarchy structure.
-
- > In particular, you may have to refactor this README into a standard one. [Example](/configs/textdet/dbnet/README.md)
-
-- [ ] Refactor your modules into the core package following the codebase's file hierarchy structure.
diff --git a/pipeline/mmocr/projects/example_project/configs/dbnet_dummy-resnet_fpnc_1200e_icdar2015.py b/pipeline/mmocr/projects/example_project/configs/dbnet_dummy-resnet_fpnc_1200e_icdar2015.py
deleted file mode 100644
index 4e55154768f51d8d267217a8a5d190a55e63af29..0000000000000000000000000000000000000000
--- a/pipeline/mmocr/projects/example_project/configs/dbnet_dummy-resnet_fpnc_1200e_icdar2015.py
+++ /dev/null
@@ -1,5 +0,0 @@
-_base_ = ['mmocr::textdet/dbnet/dbnet_resnet18_fpnc_1200e_icdar2015.py']
-
-custom_imports = dict(imports=['dummy'])
-
-_base_.model.backbone.type = 'DummyResNet'
diff --git a/pipeline/mmocr/projects/example_project/dummy/__init__.py b/pipeline/mmocr/projects/example_project/dummy/__init__.py
deleted file mode 100644
index 70df7896d6ddb28688204a6402a2270e09ec255a..0000000000000000000000000000000000000000
--- a/pipeline/mmocr/projects/example_project/dummy/__init__.py
+++ /dev/null
@@ -1,3 +0,0 @@
-from .dummy_resnet import DummyResNet
-
-__all__ = ['DummyResNet']
diff --git a/pipeline/mmocr/projects/example_project/dummy/dummy_resnet.py b/pipeline/mmocr/projects/example_project/dummy/dummy_resnet.py
deleted file mode 100644
index 8ab3378218cd6707625c10c751df0f07b086fab7..0000000000000000000000000000000000000000
--- a/pipeline/mmocr/projects/example_project/dummy/dummy_resnet.py
+++ /dev/null
@@ -1,16 +0,0 @@
-from mmdet.models.backbones import ResNet
-
-from mmocr.registry import MODELS
-
-
-@MODELS.register_module()
-class DummyResNet(ResNet):
- """Implements a dummy ResNet wrapper for demonstration purpose.
-
- Args:
- **kwargs: All the arguments are passed to the parent class.
- """
-
- def __init__(self, **kwargs) -> None:
- print('Hello world!')
- super().__init__(**kwargs)
diff --git a/pipeline/mmocr/projects/faq.md b/pipeline/mmocr/projects/faq.md
deleted file mode 100644
index 80054b2cac008124df94c208b9a7782015a02183..0000000000000000000000000000000000000000
--- a/pipeline/mmocr/projects/faq.md
+++ /dev/null
@@ -1,21 +0,0 @@
-# Projects FAQ
-
-Q1: Why set up `projects/` folder?
-
-Implementing new models and features into OpenMMLab's algorithm libraries could be troublesome due to the rigorous requirements on code quality, which could hinder the fast iteration of SOTA models and might discourage our members from sharing their latest outcomes here. And that's why we have this `projects/` folder now, where some experimental features, frameworks and models are placed, only needed to satisfy the minimum requirement on the code quality, and can be used as standalone libraries. Users are welcome to use them if they [use MMOCR from source](https://mmocr.readthedocs.io/en/dev-1.x/get_started/install.html#best-practices).
-
-Q2: Why should there be a checklist for a project?
-
-This checklist is crucial not only for this project's developers but the entire community, since there might be some other contributors joining this project and deciding their starting point from this list. It also helps maintainers accurately estimate time and effort on further code polishing, if needed.
-
-Q3: What kind of PR will be merged?
-
-Reaching the first milestone means that this project suffices the minimum requirement of being merged into `projects/`. That is, the very first PR of a project must have all the terms in the first milestone checked. We do not have any extra requirements on the project's following PRs, so they can be a minor bug fix or update, and do not have to achieve one milestone at once. But keep in mind that this project is only eligible to become a part of the core package upon attaining the last milestone.
-
-Q4: Compared to other models in the core packages, why do the model implementations in projects have different training/testing commands?
-
-Projects are organized independently from the core package, and therefore their modules cannot be directly imported by train.py and test.py. Each model implementation in projects should either use `mim` for training/testing as suggested in the example project or provide a custom train.py/test.py.
-
-Q5: How to debug a project with a debugger?
-
-Debugger makes our lives easier, but using it becomes a bit tricky if we have to train/test a model via `mim`. The way to circumvent that is that we can take advantage of relative path to import these modules. Assuming that we are developing a project X and the core modules are placed under `projects/X/modules`, then simply adding `custom_imports = dict(imports='projects.X.modules')` to the config allows us to debug from usual entrypoints (e.g. `tools/train.py`) from the root directory of the algorithm library. Just don't forget to remove 'projects.X' before project publishment.
diff --git a/pipeline/mmocr/projects/selected.txt b/pipeline/mmocr/projects/selected.txt
deleted file mode 100644
index 544ad82c640fea0d7f496ce9db360450de453354..0000000000000000000000000000000000000000
--- a/pipeline/mmocr/projects/selected.txt
+++ /dev/null
@@ -1,3 +0,0 @@
-projects/ABCNet/README.md
-projects/ABCNet/README_V2.md
-projects/SPTS/README.md
diff --git a/pipeline/mmocr/requirements/albu.txt b/pipeline/mmocr/requirements/albu.txt
deleted file mode 100644
index ddcc3fb3b271dcad3526ef130e1267be3fc20b5b..0000000000000000000000000000000000000000
--- a/pipeline/mmocr/requirements/albu.txt
+++ /dev/null
@@ -1 +0,0 @@
-albumentations>=1.1.0 --no-binary qudida,albumentations
diff --git a/pipeline/mmocr/requirements/build.txt b/pipeline/mmocr/requirements/build.txt
deleted file mode 100644
index e06b090722e0079badeb07d094d39571754995e4..0000000000000000000000000000000000000000
--- a/pipeline/mmocr/requirements/build.txt
+++ /dev/null
@@ -1,4 +0,0 @@
-# These must be installed before building mmocr
-numpy
-pyclipper
-torch>=1.1
diff --git a/pipeline/mmocr/requirements/docs.txt b/pipeline/mmocr/requirements/docs.txt
deleted file mode 100644
index 16ddccda5db65197434a6c1b543f6c87395465a8..0000000000000000000000000000000000000000
--- a/pipeline/mmocr/requirements/docs.txt
+++ /dev/null
@@ -1,9 +0,0 @@
-docutils==0.16.0
-markdown>=3.4.0
-myst-parser
--e git+https://github.com/open-mmlab/pytorch_sphinx_theme.git#egg=pytorch_sphinx_theme
-sphinx==4.0.2
-sphinx-tabs
-sphinx_copybutton
-sphinx_markdown_tables>=0.0.16
-tabulate
diff --git a/pipeline/mmocr/requirements/mminstall.txt b/pipeline/mmocr/requirements/mminstall.txt
deleted file mode 100644
index fe6b6d945dd1e5593a2d3569a33f848aed864ec7..0000000000000000000000000000000000000000
--- a/pipeline/mmocr/requirements/mminstall.txt
+++ /dev/null
@@ -1,3 +0,0 @@
-mmcv>=2.0.0rc4,<2.1.0
-mmdet>=3.0.0rc5,<3.1.0
-mmengine>=0.7.0, <1.0.0
diff --git a/pipeline/mmocr/requirements/optional.txt b/pipeline/mmocr/requirements/optional.txt
deleted file mode 100644
index e69de29bb2d1d6434b8b29ae775ad8c2e48c5391..0000000000000000000000000000000000000000
diff --git a/pipeline/mmocr/requirements/readthedocs.txt b/pipeline/mmocr/requirements/readthedocs.txt
deleted file mode 100644
index 45edbc15ff0f9496f452c4d94764806640d0dc8c..0000000000000000000000000000000000000000
--- a/pipeline/mmocr/requirements/readthedocs.txt
+++ /dev/null
@@ -1,16 +0,0 @@
-imgaug
-kwarray
-lmdb
-matplotlib
-mmcv>=2.0.0rc1
-mmdet>=3.0.0rc0
-mmengine>=0.1.0
-pyclipper
-rapidfuzz>=2.0.0
-regex
-scikit-image
-scipy
-shapely
-titlecase
-torch
-torchvision
diff --git a/pipeline/mmocr/requirements/runtime.txt b/pipeline/mmocr/requirements/runtime.txt
deleted file mode 100644
index 52a9eec3c3bb54d6ae96d1293923c56c2399d690..0000000000000000000000000000000000000000
--- a/pipeline/mmocr/requirements/runtime.txt
+++ /dev/null
@@ -1,9 +0,0 @@
-imgaug
-lmdb
-matplotlib
-numpy
-opencv-python >=4.2.0.32, != 4.5.5.* # avoid Github security alert
-pyclipper
-pycocotools
-rapidfuzz>=2.0.0
-scikit-image
diff --git a/pipeline/mmocr/requirements/tests.txt b/pipeline/mmocr/requirements/tests.txt
deleted file mode 100644
index 19711e108cae25ce6e65f5493d3a4fe2646bc51c..0000000000000000000000000000000000000000
--- a/pipeline/mmocr/requirements/tests.txt
+++ /dev/null
@@ -1,15 +0,0 @@
-asynctest
-codecov
-flake8
-interrogate
-isort
-# Note: used for kwarray.group_items, this may be ported to mmcv in the future.
-kwarray
-lanms-neo==1.0.2
-parameterized
-pytest
-pytest-cov
-pytest-runner
-ubelt
-xdoctest >= 0.10.0
-yapf
diff --git a/pipeline/mmocr/resources/illustration.jpg b/pipeline/mmocr/resources/illustration.jpg
deleted file mode 100644
index b13d062221e44076248420803de1c34e4f28be52..0000000000000000000000000000000000000000
Binary files a/pipeline/mmocr/resources/illustration.jpg and /dev/null differ
diff --git a/pipeline/mmocr/resources/kie.jpg b/pipeline/mmocr/resources/kie.jpg
deleted file mode 100644
index f89865b38f2bd0d1d282291de208720d362fbbcd..0000000000000000000000000000000000000000
Binary files a/pipeline/mmocr/resources/kie.jpg and /dev/null differ
diff --git a/pipeline/mmocr/resources/mmocr-logo.png b/pipeline/mmocr/resources/mmocr-logo.png
deleted file mode 100644
index c81a3c8351748fa4d25e2d215b5997b930244aa6..0000000000000000000000000000000000000000
Binary files a/pipeline/mmocr/resources/mmocr-logo.png and /dev/null differ
diff --git a/pipeline/mmocr/resources/textdet.jpg b/pipeline/mmocr/resources/textdet.jpg
deleted file mode 100644
index 64b9b5b7fe032ffaf23ab4f679f04ede48b6c756..0000000000000000000000000000000000000000
Binary files a/pipeline/mmocr/resources/textdet.jpg and /dev/null differ
diff --git a/pipeline/mmocr/resources/textrecog.jpg b/pipeline/mmocr/resources/textrecog.jpg
deleted file mode 100644
index 24332f32bd0249c94c1c15f8b739c437d53044c9..0000000000000000000000000000000000000000
Binary files a/pipeline/mmocr/resources/textrecog.jpg and /dev/null differ
diff --git a/pipeline/mmocr/resources/verification.png b/pipeline/mmocr/resources/verification.png
deleted file mode 100644
index 6f0e5eb0a4ee99b88c130f9d15d2993891c34245..0000000000000000000000000000000000000000
Binary files a/pipeline/mmocr/resources/verification.png and /dev/null differ
diff --git a/pipeline/mmocr/setup.cfg b/pipeline/mmocr/setup.cfg
deleted file mode 100644
index e54ab9ea76642e8e4bbf5c8a7895d14d9ef9b637..0000000000000000000000000000000000000000
--- a/pipeline/mmocr/setup.cfg
+++ /dev/null
@@ -1,23 +0,0 @@
-[bdist_wheel]
-universal=1
-
-[yapf]
-based_on_style = pep8
-blank_line_before_nested_class_or_def = true
-split_before_expression_after_opening_paren = true
-split_penalty_import_names=0
-SPLIT_PENALTY_AFTER_OPENING_BRACKET=800
-
-[isort]
-line_length = 79
-multi_line_output = 0
-extra_standard_library = setuptools
-known_first_party = mmocr
-known_third_party = PIL,cv2,imgaug,lanms,lmdb,matplotlib,mmcv,mmdet,numpy,packaging,pyclipper,pytest,pytorch_sphinx_theme,rapidfuzz,requests,scipy,shapely,skimage,titlecase,torch,torchvision,ts,yaml,mmengine
-no_lines_before = STDLIB,LOCALFOLDER
-default_section = THIRDPARTY
-
-[style]
-BASED_ON_STYLE = pep8
-BLANK_LINE_BEFORE_NESTED_CLASS_OR_DEF = true
-SPLIT_BEFORE_EXPRESSION_AFTER_OPENING_PAREN = true
diff --git a/pipeline/mmocr/tests/models/textrecog/test_preprocessors/test_tps_preprocessor.py b/pipeline/mmocr/tests/models/textrecog/test_preprocessors/test_tps_preprocessor.py
deleted file mode 100644
index b069db8c2f1c07eb0344928a57a36344c0e4044b..0000000000000000000000000000000000000000
--- a/pipeline/mmocr/tests/models/textrecog/test_preprocessors/test_tps_preprocessor.py
+++ /dev/null
@@ -1,26 +0,0 @@
-# Copyright (c) OpenMMLab. All rights reserved.
-from unittest import TestCase
-
-import torch
-
-from mmocr.models.textrecog.preprocessors import STN, TPStransform
-
-
-class TestTPS(TestCase):
-
- def test_tps_transform(self):
- tps = TPStransform(output_image_size=(32, 100), num_control_points=20)
- image = torch.rand(2, 3, 32, 64)
- control_points = torch.rand(2, 20, 2)
- transformed_image = tps(image, control_points)
- self.assertEqual(transformed_image.shape, (2, 3, 32, 100))
-
- def test_stn(self):
- stn = STN(
- in_channels=3,
- resized_image_size=(32, 64),
- output_image_size=(32, 100),
- num_control_points=20)
- image = torch.rand(2, 3, 64, 256)
- transformed_image = stn(image)
- self.assertEqual(transformed_image.shape, (2, 3, 32, 100))
diff --git a/pipeline/mmocr/tests/test_apis/test_inferencers/test_kie_inferencer.py b/pipeline/mmocr/tests/test_apis/test_inferencers/test_kie_inferencer.py
deleted file mode 100644
index 5f4fa5450ef5502b6c09b35fa77cafaffeacc734..0000000000000000000000000000000000000000
--- a/pipeline/mmocr/tests/test_apis/test_inferencers/test_kie_inferencer.py
+++ /dev/null
@@ -1,175 +0,0 @@
-# Copyright (c) OpenMMLab. All rights reserved.
-import json
-import os.path as osp
-import random
-import tempfile
-from copy import deepcopy
-from unittest import TestCase, mock
-
-import mmcv
-import mmengine
-import numpy as np
-import torch
-
-from mmocr.apis.inferencers import KIEInferencer
-from mmocr.utils.check_argument import is_type_list
-from mmocr.utils.polygon_utils import poly2bbox
-from mmocr.utils.typing_utils import KIEDataSample
-
-
-class TestKIEInferencer(TestCase):
-
- @mock.patch('mmengine.infer.infer._load_checkpoint')
- def setUp(self, mock_load):
- mock_load.side_effect = lambda *x, **y: None
- seed = 1
- random.seed(seed)
- np.random.seed(seed)
- torch.manual_seed(seed)
- # init from alias
- self.inferencer = KIEInferencer('SDMGR')
- self.inferencer_novisual = KIEInferencer(
- 'sdmgr_novisual_60e_wildreceipt')
- with open('tests/data/kie_toy_dataset/wildreceipt/data.txt', 'r') as f:
- annos = [json.loads(anno) for anno in f.readlines()]
-
- self.data_novisual = []
- self.data_img_str = []
- self.data_img_ndarray = []
- self.data_img_woshape = []
-
- for anno in annos:
- datum_novisual = dict(img_shape=(anno['height'], anno['width']))
- datum_novisual['instances'] = []
- for ann in anno['annotations']:
- instance = {}
- instance['bbox'] = poly2bbox(
- np.array(ann['box'], dtype=np.float32))
- instance['text'] = ann['text']
- datum_novisual['instances'].append(instance)
- self.data_novisual.append(datum_novisual)
-
- datum_img_str = deepcopy(datum_novisual)
- datum_img_str['img'] = anno['file_name']
- self.data_img_str.append(datum_img_str)
-
- datum_img_ndarray = deepcopy(datum_novisual)
- datum_img_ndarray['img'] = mmcv.imread(anno['file_name'])
- self.data_img_ndarray.append(datum_img_ndarray)
-
- datum_img_woshape = deepcopy(datum_img_str)
- del datum_img_woshape['img_shape']
- self.data_img_woshape.append(datum_img_woshape)
-
- @mock.patch('mmengine.infer.infer._load_checkpoint')
- def test_init(self, mock_load):
- mock_load.side_effect = lambda *x, **y: None
- # init from metafile
- KIEInferencer('sdmgr_unet16_60e_wildreceipt')
- # init from cfg
- KIEInferencer(
- 'configs/kie/sdmgr/sdmgr_unet16_60e_wildreceipt.py',
- 'https://download.openmmlab.com/mmocr/kie/sdmgr/'
- 'sdmgr_unet16_60e_wildreceipt/'
- 'sdmgr_unet16_60e_wildreceipt_20220825_151648-22419f37.pth')
-
- def assert_predictions_equal(self, preds1, preds2):
- for pred1, pred2 in zip(preds1, preds2):
- self.assert_prediction_equal(pred1, pred2)
-
- def assert_prediction_equal(self, pred1, pred2):
- self.assertTrue(np.allclose(pred1['labels'], pred2['labels'], 0.1))
- self.assertTrue(
- np.allclose(pred1['edge_scores'], pred2['edge_scores'], 0.1))
- self.assertTrue(
- np.allclose(pred1['edge_labels'], pred2['edge_labels'], 0.1))
- self.assertTrue(np.allclose(pred1['scores'], pred2['scores'], 0.1))
-
- def test_call(self):
- # no visual, single input
- res_novis_1 = self.inferencer_novisual(
- self.data_novisual[0], return_vis=True)
- res_novis_2 = self.inferencer_novisual(
- self.data_img_woshape[0], return_vis=True)
- self.assert_predictions_equal(res_novis_1['predictions'],
- res_novis_2['predictions'])
- self.assertIn('visualization', res_novis_1)
- self.assertIn('visualization', res_novis_2)
-
- # no visual, multiple inputs
- res_novis_1 = self.inferencer_novisual(
- self.data_novisual, return_vis=True)
- res_novis_2 = self.inferencer_novisual(
- self.data_img_woshape, return_vis=True)
- self.assert_predictions_equal(res_novis_1['predictions'],
- res_novis_2['predictions'])
- self.assertIn('visualization', res_novis_1)
- self.assertIn('visualization', res_novis_2)
-
- # visual, single input
- res_ndarray = self.inferencer(
- self.data_img_ndarray[0], return_vis=True)
- # path
- res_path = self.inferencer(self.data_img_str[0], return_vis=True)
- self.assert_predictions_equal(res_path['predictions'],
- res_ndarray['predictions'])
- self.assertIn('visualization', res_path)
- self.assertIn('visualization', res_ndarray)
- self.assertTrue(
- np.allclose(res_ndarray['visualization'],
- res_path['visualization']))
-
- # visual, multiple inputs & different bs
- res_ndarray = self.inferencer(self.data_img_ndarray, return_vis=True)
- # path
- res_path = self.inferencer(self.data_img_str, return_vis=True)
- self.assert_predictions_equal(res_path['predictions'],
- res_ndarray['predictions'])
- for vis1, vis2 in zip(res_ndarray['visualization'],
- res_path['visualization']):
- self.assertTrue(np.allclose(vis1, vis2))
-
- def test_visualize(self):
-
- # img_out_dir
- with tempfile.TemporaryDirectory() as tmp_dir:
- self.inferencer(self.data_img_str, out_dir=tmp_dir, save_vis=True)
- for img_dir in ['1.jpg', '2.jpg']:
- self.assertTrue(osp.exists(osp.join(tmp_dir, 'vis', img_dir)))
-
- def test_postprocess(self):
- # return_datasample
- res = self.inferencer(self.data_img_ndarray, return_datasamples=True)
- self.assertTrue(is_type_list(res['predictions'], KIEDataSample))
-
- # pred_out_file
- with tempfile.TemporaryDirectory() as tmp_dir:
- res = self.inferencer(
- self.data_img_ndarray,
- print_result=True,
- out_dir=tmp_dir,
- save_pred=True)
- file_names = [
- f'{self.inferencer.num_unnamed_imgs - i}.json'
- for i in range(len(self.data_img_ndarray), 0, -1)
- ]
- for pred, file_name in zip(res['predictions'], file_names):
- dumped_res = mmengine.load(
- osp.join(tmp_dir, 'preds', file_name))
- self.assert_prediction_equal(dumped_res, pred)
-
- @mock.patch('mmocr.apis.inferencers.kie_inferencer._load_checkpoint')
- def test_load_metainfo_to_visualizer(self, mock_load):
- mock_load.side_effect = lambda *x, **y: {'meta': 'test'}
- with self.assertRaises(ValueError):
- self.inferencer._load_metainfo_to_visualizer('test', {})
-
- mock_load.side_effect = lambda *x, **y: {
- 'meta': {
- 'dataset_meta': 'test'
- }
- }
- self.inferencer._load_metainfo_to_visualizer('test', {})
-
- with self.assertRaises(ValueError):
- self.inferencer._load_metainfo_to_visualizer(None, {})
diff --git a/pipeline/mmocr/tests/test_apis/test_inferencers/test_mmocr_inferencer.py b/pipeline/mmocr/tests/test_apis/test_inferencers/test_mmocr_inferencer.py
deleted file mode 100644
index 8628cd44fcd25682197f4ef77214a36557668292..0000000000000000000000000000000000000000
--- a/pipeline/mmocr/tests/test_apis/test_inferencers/test_mmocr_inferencer.py
+++ /dev/null
@@ -1,255 +0,0 @@
-# Copyright (c) OpenMMLab. All rights reserved.
-import os.path as osp
-import random
-import tempfile
-from unittest import TestCase, mock
-
-import mmcv
-import mmengine
-import numpy as np
-import torch
-
-from mmocr.apis.inferencers import MMOCRInferencer
-
-
-class TestMMOCRInferencer(TestCase):
-
- def setUp(self):
- seed = 1
- random.seed(seed)
- np.random.seed(seed)
- torch.manual_seed(seed)
-
- def assert_predictions_equal(self, pred1, pred2):
- if 'det_polygons' in pred1:
- self.assertTrue(
- np.allclose(pred1['det_polygons'], pred2['det_polygons'], 0.1))
- if 'det_scores' in pred1:
- self.assertTrue(
- np.allclose(pred1['det_scores'], pred2['det_scores'], 0.1))
- if 'rec_texts' in pred1:
- self.assertEqual(pred1['rec_texts'], pred2['rec_texts'])
- if 'rec_scores' in pred1:
- self.assertTrue(
- np.allclose(pred1['rec_scores'], pred2['rec_scores'], 0.1))
- if 'kie_labels' in pred1:
- self.assertEqual(pred1['kie_labels'], pred2['kie_labels'])
- if 'kie_scores' in pred1:
- self.assertTrue(
- np.allclose(pred1['kie_scores'], pred2['kie_scores'], 0.1))
- if 'kie_edge_scores' in pred1:
- self.assertTrue(
- np.allclose(pred1['kie_edge_scores'], pred2['kie_edge_scores'],
- 0.1))
- if 'kie_edge_labels' in pred1:
- self.assertEqual(pred1['kie_edge_labels'],
- pred2['kie_edge_labels'])
-
- @mock.patch('mmengine.infer.infer._load_checkpoint')
- def test_init(self, mock_load):
- mock_load.side_effect = lambda *x, **y: None
- MMOCRInferencer(det='dbnet_resnet18_fpnc_1200e_icdar2015')
- MMOCRInferencer(
- det='configs/textdet/dbnet/dbnet_resnet18_fpnc_1200e_icdar2015.py',
- det_weights='https://download.openmmlab.com/mmocr/textdet/dbnet/'
- 'dbnet_resnet18_fpnc_1200e_icdar2015/'
- 'dbnet_resnet18_fpnc_1200e_icdar2015_20220825_221614-7c0e94f2.pth')
- MMOCRInferencer(rec='crnn_mini-vgg_5e_mj')
- with self.assertRaises(ValueError):
- MMOCRInferencer(det='dummy')
-
- @mock.patch('mmengine.infer.infer._load_checkpoint')
- def test_det(self, mock_load):
- mock_load.side_effect = lambda *x, **y: None
- inferencer = MMOCRInferencer(det='dbnet_resnet18_fpnc_1200e_icdar2015')
- img_path = 'tests/data/det_toy_dataset/imgs/test/img_1.jpg'
- res_img_path = inferencer(img_path, return_vis=True)
-
- img_paths = [
- 'tests/data/det_toy_dataset/imgs/test/img_1.jpg',
- 'tests/data/det_toy_dataset/imgs/test/img_2.jpg'
- ]
- res_img_paths = inferencer(img_paths, return_vis=True)
- self.assert_predictions_equal(res_img_path['predictions'][0],
- res_img_paths['predictions'][0])
- self.assertTrue(
- np.allclose(res_img_path['visualization'][0],
- res_img_paths['visualization'][0]))
-
- img_ndarray = mmcv.imread(img_path)
- res_img_ndarray = inferencer(img_ndarray, return_vis=True)
-
- img_ndarrays = [mmcv.imread(p) for p in img_paths]
- res_img_ndarrays = inferencer(img_ndarrays, return_vis=True)
- self.assert_predictions_equal(res_img_ndarray['predictions'][0],
- res_img_ndarrays['predictions'][0])
- self.assertTrue(
- np.allclose(res_img_ndarray['visualization'][0],
- res_img_ndarrays['visualization'][0]))
- # cross checking: ndarray <-> path
- self.assert_predictions_equal(res_img_ndarray['predictions'][0],
- res_img_path['predictions'][0])
- self.assertTrue(
- np.allclose(res_img_ndarray['visualization'][0],
- res_img_path['visualization'][0]))
-
- # test save_vis and save_pred
- with tempfile.TemporaryDirectory() as tmp_dir:
- res = inferencer(
- img_paths, out_dir=tmp_dir, save_vis=True, save_pred=True)
- for img_dir in ['img_1.jpg', 'img_2.jpg']:
- self.assertTrue(osp.exists(osp.join(tmp_dir, 'vis', img_dir)))
- for i, pred_dir in enumerate(['img_1.json', 'img_2.json']):
- dumped_res = mmengine.load(
- osp.join(tmp_dir, 'preds', pred_dir))
- self.assert_predictions_equal(res['predictions'][i],
- dumped_res)
-
- @mock.patch('mmengine.infer.infer._load_checkpoint')
- def test_rec(self, mock_load):
- mock_load.side_effect = lambda *x, **y: None
- inferencer = MMOCRInferencer(rec='crnn_mini-vgg_5e_mj')
- img_path = 'tests/data/rec_toy_dataset/imgs/1036169.jpg'
- res_img_path = inferencer(img_path, return_vis=True)
-
- img_paths = [
- 'tests/data/rec_toy_dataset/imgs/1036169.jpg',
- 'tests/data/rec_toy_dataset/imgs/1058891.jpg'
- ]
- res_img_paths = inferencer(img_paths, return_vis=True)
- self.assert_predictions_equal(res_img_path['predictions'][0],
- res_img_paths['predictions'][0])
- self.assertTrue(
- np.allclose(res_img_path['visualization'][0],
- res_img_paths['visualization'][0]))
- # cross checking: ndarray <-> path
- img_ndarray = mmcv.imread(img_path)
- res_img_ndarray = inferencer(img_ndarray, return_vis=True)
-
- img_ndarrays = [mmcv.imread(p) for p in img_paths]
- res_img_ndarrays = inferencer(img_ndarrays, return_vis=True)
- self.assert_predictions_equal(res_img_ndarray['predictions'][0],
- res_img_ndarrays['predictions'][0])
- self.assertTrue(
- np.allclose(res_img_ndarray['visualization'][0],
- res_img_ndarrays['visualization'][0]))
- self.assert_predictions_equal(res_img_ndarray['predictions'][0],
- res_img_path['predictions'][0])
- self.assertTrue(
- np.allclose(res_img_ndarray['visualization'][0],
- res_img_path['visualization'][0]))
-
- # test save_vis and save_pred
- with tempfile.TemporaryDirectory() as tmp_dir:
- res = inferencer(
- img_paths, out_dir=tmp_dir, save_vis=True, save_pred=True)
- for img_dir in ['1036169.jpg', '1058891.jpg']:
- self.assertTrue(osp.exists(osp.join(tmp_dir, 'vis', img_dir)))
- for i, pred_dir in enumerate(['1036169.json', '1058891.json']):
- dumped_res = mmengine.load(
- osp.join(tmp_dir, 'preds', pred_dir))
- self.assert_predictions_equal(res['predictions'][i],
- dumped_res)
-
- @mock.patch('mmengine.infer.infer._load_checkpoint')
- def test_det_rec(self, mock_load):
- mock_load.side_effect = lambda *x, **y: None
- inferencer = MMOCRInferencer(
- det='dbnet_resnet18_fpnc_1200e_icdar2015',
- rec='crnn_mini-vgg_5e_mj')
- img_path = 'tests/data/det_toy_dataset/imgs/test/img_1.jpg'
- res_img_path = inferencer(img_path, return_vis=True)
-
- img_paths = [
- 'tests/data/det_toy_dataset/imgs/test/img_1.jpg',
- 'tests/data/det_toy_dataset/imgs/test/img_2.jpg'
- ]
- res_img_paths = inferencer(img_paths, return_vis=True)
- self.assert_predictions_equal(res_img_path['predictions'][0],
- res_img_paths['predictions'][0])
- self.assertTrue(
- np.allclose(res_img_path['visualization'][0],
- res_img_paths['visualization'][0]))
-
- img_ndarray = mmcv.imread(img_path)
- res_img_ndarray = inferencer(img_ndarray, return_vis=True)
-
- img_ndarrays = [mmcv.imread(p) for p in img_paths]
- res_img_ndarrays = inferencer(img_ndarrays, return_vis=True)
- self.assert_predictions_equal(res_img_ndarray['predictions'][0],
- res_img_ndarrays['predictions'][0])
- self.assertTrue(
- np.allclose(res_img_ndarray['visualization'][0],
- res_img_ndarrays['visualization'][0]))
- # cross checking: ndarray <-> path
- self.assert_predictions_equal(res_img_ndarray['predictions'][0],
- res_img_path['predictions'][0])
- self.assertTrue(
- np.allclose(res_img_ndarray['visualization'][0],
- res_img_path['visualization'][0]))
-
- # test save_vis and save_pred
- with tempfile.TemporaryDirectory() as tmp_dir:
- res = inferencer(
- img_paths, out_dir=tmp_dir, save_vis=True, save_pred=True)
- for img_dir in ['img_1.jpg', 'img_2.jpg']:
- self.assertTrue(osp.exists(osp.join(tmp_dir, 'vis', img_dir)))
- for i, pred_dir in enumerate(['img_1.json', 'img_2.json']):
- dumped_res = mmengine.load(
- osp.join(tmp_dir, 'preds', pred_dir))
- self.assert_predictions_equal(res['predictions'][i],
- dumped_res)
-
- # corner case: when the det model cannot detect any texts
- inferencer(np.zeros((100, 100, 3)), return_vis=True)
-
- @mock.patch('mmengine.infer.infer._load_checkpoint')
- def test_dec_rec_kie(self, mock_load):
- mock_load.side_effect = lambda *x, **y: None
- inferencer = MMOCRInferencer(
- det='dbnet_resnet18_fpnc_1200e_icdar2015',
- rec='crnn_mini-vgg_5e_mj',
- kie='sdmgr_unet16_60e_wildreceipt')
- img_path = 'tests/data/kie_toy_dataset/wildreceipt/1.jpeg'
- res_img_path = inferencer(img_path, return_vis=True)
-
- img_paths = [
- 'tests/data/kie_toy_dataset/wildreceipt/1.jpeg',
- 'tests/data/kie_toy_dataset/wildreceipt/2.jpeg'
- ]
- res_img_paths = inferencer(img_paths, return_vis=True)
- self.assert_predictions_equal(res_img_path['predictions'][0],
- res_img_paths['predictions'][0])
- self.assertTrue(
- np.allclose(res_img_path['visualization'][0],
- res_img_paths['visualization'][0]))
-
- img_ndarray = mmcv.imread(img_path)
- res_img_ndarray = inferencer(img_ndarray, return_vis=True)
-
- img_ndarrays = [mmcv.imread(p) for p in img_paths]
- res_img_ndarrays = inferencer(img_ndarrays, return_vis=True)
-
- self.assert_predictions_equal(res_img_ndarray['predictions'][0],
- res_img_ndarrays['predictions'][0])
- self.assertTrue(
- np.allclose(res_img_ndarray['visualization'][0],
- res_img_ndarrays['visualization'][0]))
- # cross checking: ndarray <-> path
- self.assert_predictions_equal(res_img_ndarray['predictions'][0],
- res_img_path['predictions'][0])
- self.assertTrue(
- np.allclose(res_img_ndarray['visualization'][0],
- res_img_path['visualization'][0]))
-
- # test save_vis and save_pred
- with tempfile.TemporaryDirectory() as tmp_dir:
- res = inferencer(
- img_paths, out_dir=tmp_dir, save_vis=True, save_pred=True)
- for img_dir in ['1.jpg', '2.jpg']:
- self.assertTrue(osp.exists(osp.join(tmp_dir, 'vis', img_dir)))
- for i, pred_dir in enumerate(['1.json', '2.json']):
- dumped_res = mmengine.load(
- osp.join(tmp_dir, 'preds', pred_dir))
- self.assert_predictions_equal(res['predictions'][i],
- dumped_res)
diff --git a/pipeline/mmocr/tests/test_apis/test_inferencers/test_textdet_inferencer.py b/pipeline/mmocr/tests/test_apis/test_inferencers/test_textdet_inferencer.py
deleted file mode 100644
index badb4eaae45248a96cecdacd091e9d7365196ac6..0000000000000000000000000000000000000000
--- a/pipeline/mmocr/tests/test_apis/test_inferencers/test_textdet_inferencer.py
+++ /dev/null
@@ -1,138 +0,0 @@
-# Copyright (c) OpenMMLab. All rights reserved.
-import os.path as osp
-import random
-import tempfile
-from unittest import TestCase, mock
-
-import mmcv
-import mmengine
-import numpy as np
-import torch
-from mmengine.structures import InstanceData
-
-from mmocr.apis.inferencers import TextDetInferencer
-from mmocr.utils.check_argument import is_type_list
-from mmocr.utils.typing_utils import TextDetDataSample
-
-
-class TestTextDetinferencer(TestCase):
-
- @mock.patch('mmengine.infer.infer._load_checkpoint')
- def setUp(self, mock_load):
- mock_load.side_effect = lambda *x, **y: None
- self.inferencer = TextDetInferencer('DB_r18')
- seed = 1
- random.seed(seed)
- np.random.seed(seed)
- torch.manual_seed(seed)
-
- @mock.patch('mmengine.infer.infer._load_checkpoint')
- def test_init(self, mock_load):
- mock_load.side_effect = lambda *x, **y: None
- # init from metafile
- TextDetInferencer('dbnet_resnet18_fpnc_1200e_icdar2015')
- # init from cfg
- TextDetInferencer(
- 'configs/textdet/dbnet/'
- 'dbnet_resnet18_fpnc_1200e_icdar2015.py',
- 'https://download.openmmlab.com/mmocr/textdet/dbnet/'
- 'dbnet_resnet18_fpnc_1200e_icdar2015/'
- 'dbnet_resnet18_fpnc_1200e_icdar2015_20220825_221614-7c0e94f2.pth')
-
- def assert_predictions_equal(self, preds1, preds2):
- for pred1, pred2 in zip(preds1, preds2):
- self.assert_prediction_equal(pred1, pred2)
-
- def assert_prediction_equal(self, pred1, pred2):
- self.assertTrue(np.allclose(pred1['polygons'], pred2['polygons'], 0.1))
- self.assertTrue(np.allclose(pred1['scores'], pred2['scores'], 0.1))
-
- def test_call(self):
- # single img
- img_path = 'tests/data/det_toy_dataset/imgs/test/img_1.jpg'
- res_path = self.inferencer(img_path, return_vis=True)
- # ndarray
- img = mmcv.imread(img_path)
- res_ndarray = self.inferencer(img, return_vis=True)
- self.assert_predictions_equal(res_path['predictions'],
- res_ndarray['predictions'])
- self.assertTrue(
- np.allclose(res_path['visualization'],
- res_ndarray['visualization']))
-
- # multiple images
- img_paths = [
- 'tests/data/det_toy_dataset/imgs/test/img_1.jpg',
- 'tests/data/det_toy_dataset/imgs/test/img_2.jpg'
- ]
- res_path = self.inferencer(img_paths, return_vis=True)
- # list of ndarray
- imgs = [mmcv.imread(p) for p in img_paths]
- res_ndarray = self.inferencer(imgs, return_vis=True)
- self.assert_predictions_equal(res_path['predictions'],
- res_ndarray['predictions'])
- for i in range(len(img_paths)):
- self.assertTrue(
- np.allclose(res_path['visualization'][i],
- res_ndarray['visualization'][i]))
-
- # img dir, test different batch sizes
- img_dir = 'tests/data/det_toy_dataset/imgs/test/'
- res_bs1 = self.inferencer(img_dir, batch_size=1, return_vis=True)
- res_bs3 = self.inferencer(img_dir, batch_size=3, return_vis=True)
- self.assert_predictions_equal(res_bs1['predictions'],
- res_bs3['predictions'])
- self.assertTrue(
- np.array_equal(res_bs1['visualization'], res_bs3['visualization']))
-
- def test_visualize(self):
- img_paths = [
- 'tests/data/det_toy_dataset/imgs/test/img_1.jpg',
- 'tests/data/det_toy_dataset/imgs/test/img_2.jpg'
- ]
-
- # img_out_dir
- with tempfile.TemporaryDirectory() as tmp_dir:
- self.inferencer(img_paths, out_dir=tmp_dir, save_vis=True)
- for img_dir in ['img_1.jpg', 'img_2.jpg']:
- self.assertTrue(osp.exists(osp.join(tmp_dir, 'vis', img_dir)))
-
- def test_postprocess(self):
- # return_datasample
- img_path = 'tests/data/det_toy_dataset/imgs/test/img_1.jpg'
- res = self.inferencer(img_path, return_datasamples=True)
- self.assertTrue(is_type_list(res['predictions'], TextDetDataSample))
-
- # dump predictions
- with tempfile.TemporaryDirectory() as tmp_dir:
- res = self.inferencer(
- img_path, print_result=True, out_dir=tmp_dir, save_pred=True)
- dumped_res = mmengine.load(
- osp.join(tmp_dir, 'preds', 'img_1.json'))
- self.assert_prediction_equal(res['predictions'][0], dumped_res)
-
- def test_pred2dict(self):
- data_sample = TextDetDataSample()
- data_sample.pred_instances = InstanceData()
-
- data_sample.pred_instances.scores = np.array([0.9])
- data_sample.pred_instances.polygons = [
- np.array([0, 0, 0, 1, 1, 1, 1, 0])
- ]
- res = self.inferencer.pred2dict(data_sample)
- self.assertListAlmostEqual(res['polygons'], [[0, 0, 0, 1, 1, 1, 1, 0]])
- self.assertListAlmostEqual(res['scores'], [0.9])
-
- data_sample.pred_instances.bboxes = np.array([[0, 0, 1, 1]])
- data_sample.pred_instances.scores = torch.FloatTensor([0.9])
- res = self.inferencer.pred2dict(data_sample)
- self.assertListAlmostEqual(res['polygons'], [[0, 0, 0, 1, 1, 1, 1, 0]])
- self.assertListAlmostEqual(res['bboxes'], [[0, 0, 1, 1]])
- self.assertListAlmostEqual(res['scores'], [0.9])
-
- def assertListAlmostEqual(self, list1, list2, places=7):
- for i in range(len(list1)):
- if isinstance(list1[i], list):
- self.assertListAlmostEqual(list1[i], list2[i], places=places)
- else:
- self.assertAlmostEqual(list1[i], list2[i], places=places)
diff --git a/pipeline/mmocr/tests/test_apis/test_inferencers/test_textrec_inferencer.py b/pipeline/mmocr/tests/test_apis/test_inferencers/test_textrec_inferencer.py
deleted file mode 100644
index 1e89e4a9a31d6d3a1e4a929c32a4ae3836b2054a..0000000000000000000000000000000000000000
--- a/pipeline/mmocr/tests/test_apis/test_inferencers/test_textrec_inferencer.py
+++ /dev/null
@@ -1,108 +0,0 @@
-# Copyright (c) OpenMMLab. All rights reserved.
-import os.path as osp
-import random
-import tempfile
-from unittest import TestCase, mock
-
-import mmcv
-import mmengine
-import numpy as np
-import torch
-
-from mmocr.apis.inferencers import TextRecInferencer
-from mmocr.utils.check_argument import is_type_list
-from mmocr.utils.typing_utils import TextRecogDataSample
-
-
-class TestTextRecinferencer(TestCase):
-
- @mock.patch('mmengine.infer.infer._load_checkpoint')
- def setUp(self, mock_load):
- mock_load.side_effect = lambda *x, **y: None
- # init from alias
- self.inferencer = TextRecInferencer('CRNN')
- seed = 1
- random.seed(seed)
- np.random.seed(seed)
- torch.manual_seed(seed)
-
- @mock.patch('mmengine.infer.infer._load_checkpoint')
- def test_init(self, mock_load):
- mock_load.side_effect = lambda *x, **y: None
- # init from metafile
- TextRecInferencer('crnn_mini-vgg_5e_mj')
- # init from cfg
- TextRecInferencer(
- 'configs/textrecog/crnn/crnn_mini-vgg_5e_mj.py',
- 'https://download.openmmlab.com/mmocr/textrecog/crnn/'
- 'crnn_mini-vgg_5e_mj/'
- 'crnn_mini-vgg_5e_mj_20220826_224120-8afbedbb.pth')
-
- def assert_predictions_equal(self, preds1, preds2):
- for pred1, pred2 in zip(preds1, preds2):
- self.assert_prediction_equal(pred1, pred2)
-
- def assert_prediction_equal(self, pred1, pred2):
- self.assertEqual(pred1['text'], pred2['text'])
- self.assertTrue(np.allclose(pred1['scores'], pred2['scores'], 0.1))
-
- def test_call(self):
- # single img
- img_path = 'tests/data/rec_toy_dataset/imgs/1036169.jpg'
- res_path = self.inferencer(img_path, return_vis=True)
- # ndarray
- img = mmcv.imread(img_path)
- res_ndarray = self.inferencer(img, return_vis=True)
- self.assert_predictions_equal(res_path['predictions'],
- res_ndarray['predictions'])
- self.assertTrue(
- np.allclose(res_path['visualization'],
- res_ndarray['visualization']))
-
- # multiple images
- img_paths = [
- 'tests/data/rec_toy_dataset/imgs/1036169.jpg',
- 'tests/data/rec_toy_dataset/imgs/1058891.jpg'
- ]
- res_path = self.inferencer(img_paths, return_vis=True)
- # list of ndarray
- imgs = [mmcv.imread(p) for p in img_paths]
- res_ndarray = self.inferencer(imgs, return_vis=True)
- self.assert_predictions_equal(res_path['predictions'],
- res_ndarray['predictions'])
- for i in range(len(img_paths)):
- self.assertTrue(
- np.allclose(res_path['visualization'][i],
- res_ndarray['visualization'][i]))
-
- # img dir, test different batch sizes
- img_dir = 'tests/data/rec_toy_dataset/imgs'
- res_bs3 = self.inferencer(img_dir, batch_size=3, return_vis=True)
- self.assertIn('visualization', res_bs3)
- self.assertIn('predictions', res_bs3)
-
- def test_visualize(self):
- img_paths = [
- 'tests/data/rec_toy_dataset/imgs/1036169.jpg',
- 'tests/data/rec_toy_dataset/imgs/1058891.jpg'
- ]
-
- # img_out_dir
- with tempfile.TemporaryDirectory() as tmp_dir:
- self.inferencer(img_paths, out_dir=tmp_dir, save_vis=True)
- for img_dir in ['1036169.jpg', '1058891.jpg']:
- self.assertTrue(osp.exists(osp.join(tmp_dir, 'vis', img_dir)))
-
- def test_postprocess(self):
- # return_datasample
- img_path = 'tests/data/rec_toy_dataset/imgs/1036169.jpg'
- res = self.inferencer(img_path, return_datasamples=True)
- self.assertTrue(is_type_list(res['predictions'], TextRecogDataSample))
-
- # pred_out_file
- with tempfile.TemporaryDirectory() as tmp_dir:
- res = self.inferencer(
- img_path, print_result=True, out_dir=tmp_dir, save_pred=True)
- dumped_res = mmengine.load(
- osp.join(tmp_dir, 'preds', '1036169.json'))
- self.assert_prediction_equal(res['predictions'][0], dumped_res)
diff --git a/pipeline/mmocr/tests/test_datasets/test_dataset_wrapper.py b/pipeline/mmocr/tests/test_datasets/test_dataset_wrapper.py
deleted file mode 100644
index b9c4c2682ebd988258badcef364b6317309a827c..0000000000000000000000000000000000000000
--- a/pipeline/mmocr/tests/test_datasets/test_dataset_wrapper.py
+++ /dev/null
@@ -1,118 +0,0 @@
-# Copyright (c) OpenMMLab. All rights reserved.
-import os.path as osp
-from copy import deepcopy
-from unittest import TestCase
-from unittest.mock import MagicMock
-
-from mmengine.registry import init_default_scope
-
-from mmocr.datasets import ConcatDataset, OCRDataset
-from mmocr.registry import TRANSFORMS
-
-
-class TestConcatDataset(TestCase):
-
- @TRANSFORMS.register_module()
- class MockTransform:
-
- def __init__(self, return_value):
- self.return_value = return_value
-
- def __call__(self, *args, **kwargs):
- return self.return_value
-
- def setUp(self):
-
- init_default_scope('mmocr')
- dataset = OCRDataset
-
- # create dataset_a
- data_info = dict(filename='img_1.jpg', height=720, width=1280)
- dataset.parse_data_info = MagicMock(return_value=data_info)
-
- self.dataset_a = dataset(
- data_root=osp.join(
- osp.dirname(__file__), '../data/det_toy_dataset'),
- data_prefix=dict(img_path='imgs'),
- ann_file='textdet_test.json')
-
- self.dataset_a_with_pipeline = dataset(
- data_root=osp.join(
- osp.dirname(__file__), '../data/det_toy_dataset'),
- data_prefix=dict(img_path='imgs'),
- ann_file='textdet_test.json',
- pipeline=[dict(type='MockTransform', return_value=1)])
-
- # create dataset_b
- data_info = dict(filename='img_2.jpg', height=720, width=1280)
- dataset.parse_data_info = MagicMock(return_value=data_info)
- self.dataset_b = dataset(
- data_root=osp.join(
- osp.dirname(__file__), '../data/det_toy_dataset'),
- data_prefix=dict(img_path='imgs'),
- ann_file='textdet_test.json')
- self.dataset_b_with_pipeline = dataset(
- data_root=osp.join(
- osp.dirname(__file__), '../data/det_toy_dataset'),
- data_prefix=dict(img_path='imgs'),
- ann_file='textdet_test.json',
- pipeline=[dict(type='MockTransform', return_value=2)])
-
- def test_init(self):
- with self.assertRaises(TypeError):
- ConcatDataset(datasets=[0])
- with self.assertRaises(ValueError):
- ConcatDataset(
- datasets=[
- deepcopy(self.dataset_a_with_pipeline),
- deepcopy(self.dataset_b)
- ],
- pipeline=[dict(type='MockTransform', return_value=3)])
-
- with self.assertRaises(ValueError):
- ConcatDataset(
- datasets=[
- deepcopy(self.dataset_a),
- deepcopy(self.dataset_b_with_pipeline)
- ],
- pipeline=[dict(type='MockTransform', return_value=3)])
- with self.assertRaises(ValueError):
- dataset_a = deepcopy(self.dataset_a)
- dataset_b = OCRDataset(
- metainfo=dict(dummy='dummy'),
- data_root=osp.join(
- osp.dirname(__file__), '../data/det_toy_dataset'),
- data_prefix=dict(img_path='imgs'),
- ann_file='textdet_test.json')
- ConcatDataset(datasets=[dataset_a, dataset_b])
- # test lazy init
- ConcatDataset(
- datasets=[deepcopy(self.dataset_a),
- deepcopy(self.dataset_b)],
- pipeline=[dict(type='MockTransform', return_value=3)],
- lazy_init=True)
-
- def test_getitem(self):
- cat_datasets = ConcatDataset(
- datasets=[deepcopy(self.dataset_a),
- deepcopy(self.dataset_b)],
- pipeline=[dict(type='MockTransform', return_value=3)])
- for datum in cat_datasets:
- self.assertEqual(datum, 3)
-
- cat_datasets = ConcatDataset(
- datasets=[
- deepcopy(self.dataset_a_with_pipeline),
- deepcopy(self.dataset_b)
- ],
- pipeline=[dict(type='MockTransform', return_value=3)],
- force_apply=True)
- for datum in cat_datasets:
- self.assertEqual(datum, 3)
-
- cat_datasets = ConcatDataset(datasets=[
- deepcopy(self.dataset_a_with_pipeline),
- deepcopy(self.dataset_b_with_pipeline)
- ])
- self.assertEqual(cat_datasets[0], 1)
- self.assertEqual(cat_datasets[-1], 2)
diff --git a/pipeline/mmocr/tests/test_datasets/test_icdar_dataset.py b/pipeline/mmocr/tests/test_datasets/test_icdar_dataset.py
deleted file mode 100644
index 575c50619f9a1e48f4f58aba23de3eeba1e5165a..0000000000000000000000000000000000000000
--- a/pipeline/mmocr/tests/test_datasets/test_icdar_dataset.py
+++ /dev/null
@@ -1,145 +0,0 @@
-# Copyright (c) OpenMMLab. All rights reserved.
-import os.path as osp
-import tempfile
-from unittest import TestCase
-
-import mmengine
-
-from mmocr.datasets.icdar_dataset import IcdarDataset
-
-
-class TestIcdarDataset(TestCase):
-
- def _create_dummy_icdar_json(self, json_name):
- image_1 = {
- 'id': 0,
- 'width': 640,
- 'height': 640,
- 'file_name': 'fake_name.jpg',
- }
- image_2 = {
- 'id': 1,
- 'width': 640,
- 'height': 640,
- 'file_name': 'fake_name1.jpg',
- }
-
- annotation_1 = {
- 'id': 1,
- 'image_id': 0,
- 'category_id': 0,
- 'area': 400,
- 'bbox': [50, 60, 20, 20],
- 'iscrowd': 0,
- 'segmentation': [[50, 60, 70, 60, 70, 80, 50, 80]]
- }
-
- annotation_2 = {
- 'id': 2,
- 'image_id': 0,
- 'category_id': 0,
- 'area': 900,
- 'bbox': [100, 120, 30, 30],
- 'iscrowd': 0,
- 'segmentation': [[100, 120, 130, 120, 120, 150, 100, 150]]
- }
-
- annotation_3 = {
- 'id': 3,
- 'image_id': 0,
- 'category_id': 0,
- 'area': 1600,
- 'bbox': [150, 160, 40, 40],
- 'iscrowd': 1,
- 'segmentation': [[150, 160, 190, 160, 190, 200, 150, 200]]
- }
-
- annotation_4 = {
- 'id': 4,
- 'image_id': 0,
- 'category_id': 0,
- 'area': 10000,
- 'bbox': [250, 260, 100, 100],
- 'iscrowd': 1,
- 'segmentation': [[250, 260, 350, 260, 350, 360, 250, 360]]
- }
- annotation_5 = {
- 'id': 5,
- 'image_id': 1,
- 'category_id': 0,
- 'area': 10000,
- 'bbox': [250, 260, 100, 100],
- 'iscrowd': 1,
- 'segmentation': [[250, 260, 350, 260, 350, 360, 250, 360]]
- }
- annotation_6 = {
- 'id': 6,
- 'image_id': 1,
- 'category_id': 0,
- 'area': 0,
- 'bbox': [0, 0, 0, 0],
- 'iscrowd': 1,
- 'segmentation': [[250, 260, 350, 260, 350, 360, 250, 360]]
- }
- annotation_7 = {
- 'id': 7,
- 'image_id': 1,
- 'category_id': 2,
- 'area': 10000,
- 'bbox': [250, 260, 100, 100],
- 'iscrowd': 1,
- 'segmentation': [[250, 260, 350, 260, 350, 360, 250, 360]]
- }
- annotation_8 = {
- 'id': 8,
- 'image_id': 1,
- 'category_id': 0,
- 'area': 10000,
- 'bbox': [250, 260, 100, 100],
- 'iscrowd': 1,
- 'segmentation': [[250, 260, 350, 260, 350, 360, 250, 360]]
- }
-
- categories = [{
- 'id': 0,
- 'name': 'text',
- 'supercategory': 'text',
- }]
-
- fake_json = {
- 'images': [image_1, image_2],
- 'annotations': [
- annotation_1, annotation_2, annotation_3, annotation_4,
- annotation_5, annotation_6, annotation_7, annotation_8
- ],
- 'categories':
- categories
- }
- self.metainfo = dict(classes=('text'))
- mmengine.dump(fake_json, json_name)
-
- def test_icdar_dataset(self):
- tmp_dir = tempfile.TemporaryDirectory()
- # create dummy data
- fake_json_file = osp.join(tmp_dir.name, 'fake_data.json')
- self._create_dummy_icdar_json(fake_json_file)
-
- # test initialization
- dataset = IcdarDataset(
- ann_file=fake_json_file,
- data_prefix=dict(img_path='imgs'),
- metainfo=self.metainfo,
- pipeline=[])
- self.assertEqual(dataset.metainfo['classes'], self.metainfo['classes'])
- dataset.full_init()
- self.assertEqual(len(dataset), 2)
- self.assertEqual(len(dataset.load_data_list()), 2)
-
- # test load_data_list
- anno = dataset.load_data_list()[0]
- self.assertEqual(len(anno['instances']), 4)
- self.assertTrue('ignore' in anno['instances'][0])
- self.assertTrue('bbox' in anno['instances'][0])
- self.assertEqual(anno['instances'][0]['bbox_label'], 0)
- self.assertTrue('polygon' in anno['instances'][0])
- tmp_dir.cleanup()
diff --git a/pipeline/mmocr/tests/test_datasets/test_preparers/test_config_generators/test_textdet_config_generator.py b/pipeline/mmocr/tests/test_datasets/test_preparers/test_config_generators/test_textdet_config_generator.py
deleted file mode 100644
index 988e9a58a3fb52d375e5db5fc5f32e99dcd76d51..0000000000000000000000000000000000000000
--- a/pipeline/mmocr/tests/test_datasets/test_preparers/test_config_generators/test_textdet_config_generator.py
+++ /dev/null
@@ -1,59 +0,0 @@
-# Copyright (c) OpenMMLab. All rights reserved.
-import os.path as osp
-import tempfile
-import unittest
-
-from mmocr.datasets.preparers import TextDetConfigGenerator
-
-
-class TestTextDetConfigGenerator(unittest.TestCase):
-
- def setUp(self) -> None:
- self.root = tempfile.TemporaryDirectory()
-
- def test_textdet_config_generator(self):
- config_generator = TextDetConfigGenerator(
- data_root=self.root.name,
- dataset_name='dummy',
- train_anns=[
- dict(ann_file='textdet_train.json', dataset_postfix='')
- ],
- val_anns=[],
- test_anns=[
- dict(ann_file='textdet_test.json', dataset_postfix='fake')
- ],
- config_path=self.root.name,
- )
- cfg_path = osp.join(self.root.name, 'textdet', '_base_', 'datasets',
- 'dummy.py')
- config_generator()
- self.assertTrue(osp.exists(cfg_path))
- f = open(cfg_path, 'r')
- lines = ''.join(f.readlines())
-
- self.assertEquals(
- lines, (f"dummy_textdet_data_root = '{self.root.name}'\n"
- '\n'
- 'dummy_textdet_train = dict(\n'
- " type='OCRDataset',\n"
- ' data_root=dummy_textdet_data_root,\n'
- " ann_file='textdet_train.json',\n"
- ' filter_cfg=dict(filter_empty_gt=True, min_size=32),\n'
- ' pipeline=None)\n'
- '\n'
- 'dummy_fake_textdet_test = dict(\n'
- " type='OCRDataset',\n"
- ' data_root=dummy_textdet_data_root,\n'
- " ann_file='textdet_test.json',\n"
- ' test_mode=True,\n'
- ' pipeline=None)\n'))
- with self.assertRaises(ValueError):
- TextDetConfigGenerator(
- data_root=self.root.name,
- dataset_name='dummy',
- train_anns=[
- dict(ann_file='textdet_train.json', dataset_postfix='1'),
- dict(ann_file='textdet_train_1.json', dataset_postfix='1')
- ],
- config_path=self.root.name,
- )
diff --git a/pipeline/mmocr/tests/test_datasets/test_preparers/test_config_generators/test_textrecog_config_generator.py b/pipeline/mmocr/tests/test_datasets/test_preparers/test_config_generators/test_textrecog_config_generator.py
deleted file mode 100644
index 7a5cc83d1e07164ce3e8acf67be51e149f998d02..0000000000000000000000000000000000000000
--- a/pipeline/mmocr/tests/test_datasets/test_preparers/test_config_generators/test_textrecog_config_generator.py
+++ /dev/null
@@ -1,59 +0,0 @@
-# Copyright (c) OpenMMLab. All rights reserved.
-import os.path as osp
-import tempfile
-import unittest
-
-from mmocr.datasets.preparers import TextRecogConfigGenerator
-
-
-class TestTextRecogConfigGenerator(unittest.TestCase):
-
- def setUp(self) -> None:
- self.root = tempfile.TemporaryDirectory()
-
- def test_textrecog_config_generator(self):
- config_generator = TextRecogConfigGenerator(
- data_root=self.root.name,
- dataset_name='dummy',
- train_anns=[
- dict(ann_file='textrecog_train.json', dataset_postfix='')
- ],
- val_anns=[],
- test_anns=[
- dict(ann_file='textrecog_test.json', dataset_postfix='fake')
- ],
- config_path=self.root.name,
- )
- cfg_path = osp.join(self.root.name, 'textrecog', '_base_', 'datasets',
- 'dummy.py')
- config_generator()
- self.assertTrue(osp.exists(cfg_path))
- f = open(cfg_path, 'r')
- lines = ''.join(f.readlines())
-
- self.assertEquals(lines,
- (f"dummy_textrecog_data_root = '{self.root.name}'\n"
- '\n'
- 'dummy_textrecog_train = dict(\n'
- " type='OCRDataset',\n"
- ' data_root=dummy_textrecog_data_root,\n'
- " ann_file='textrecog_train.json',\n"
- ' pipeline=None)\n'
- '\n'
- 'dummy_fake_textrecog_test = dict(\n'
- " type='OCRDataset',\n"
- ' data_root=dummy_textrecog_data_root,\n'
- " ann_file='textrecog_test.json',\n"
- ' test_mode=True,\n'
- ' pipeline=None)\n'))
- with self.assertRaises(ValueError):
- TextRecogConfigGenerator(
- data_root=self.root.name,
- dataset_name='dummy',
- train_anns=[
- dict(ann_file='textrecog_train.json', dataset_postfix='1'),
- dict(
- ann_file='textrecog_train_1.json', dataset_postfix='1')
- ],
- config_path=self.root.name,
- )
diff --git a/pipeline/mmocr/tests/test_datasets/test_preparers/test_config_generators/test_textspotting_config_generator.py b/pipeline/mmocr/tests/test_datasets/test_preparers/test_config_generators/test_textspotting_config_generator.py
deleted file mode 100644
index cab434cfb78ca51a31a9e08bf72c4433358423cf..0000000000000000000000000000000000000000
--- a/pipeline/mmocr/tests/test_datasets/test_preparers/test_config_generators/test_textspotting_config_generator.py
+++ /dev/null
@@ -1,64 +0,0 @@
-# Copyright (c) OpenMMLab. All rights reserved.
-import os.path as osp
-import tempfile
-import unittest
-
-from mmocr.datasets.preparers import TextSpottingConfigGenerator
-
-
-class TestTextSpottingConfigGenerator(unittest.TestCase):
-
- def setUp(self) -> None:
- self.root = tempfile.TemporaryDirectory()
-
- def test_textspotting_config_generator(self):
- config_generator = TextSpottingConfigGenerator(
- data_root=self.root.name,
- dataset_name='dummy',
- train_anns=[
- dict(ann_file='textspotting_train.json', dataset_postfix='')
- ],
- val_anns=[],
- test_anns=[
- dict(
- ann_file='textspotting_test.json', dataset_postfix='fake')
- ],
- config_path=self.root.name,
- )
- cfg_path = osp.join(self.root.name, 'textspotting', '_base_',
- 'datasets', 'dummy.py')
- config_generator()
- self.assertTrue(osp.exists(cfg_path))
- f = open(cfg_path, 'r')
- lines = ''.join(f.readlines())
-
- self.assertEquals(
- lines, (f"dummy_textspotting_data_root = '{self.root.name}'\n"
- '\n'
- 'dummy_textspotting_train = dict(\n'
- " type='OCRDataset',\n"
- ' data_root=dummy_textspotting_data_root,\n'
- " ann_file='textspotting_train.json',\n"
- ' filter_cfg=dict(filter_empty_gt=True, min_size=32),\n'
- ' pipeline=None)\n'
- '\n'
- 'dummy_fake_textspotting_test = dict(\n'
- " type='OCRDataset',\n"
- ' data_root=dummy_textspotting_data_root,\n'
- " ann_file='textspotting_test.json',\n"
- ' test_mode=True,\n'
- ' pipeline=None)\n'))
- with self.assertRaises(ValueError):
- TextSpottingConfigGenerator(
- data_root=self.root.name,
- dataset_name='dummy',
- train_anns=[
- dict(
- ann_file='textspotting_train.json',
- dataset_postfix='1'),
- dict(
- ann_file='textspotting_train_1.json',
- dataset_postfix='1')
- ],
- config_path=self.root.name,
- )
diff --git a/pipeline/mmocr/tests/test_datasets/test_preparers/test_data_preparer.py b/pipeline/mmocr/tests/test_datasets/test_preparers/test_data_preparer.py
deleted file mode 100644
index 59ee0af14ec43f45b87abcb259338da075d31fac..0000000000000000000000000000000000000000
--- a/pipeline/mmocr/tests/test_datasets/test_preparers/test_data_preparer.py
+++ /dev/null
@@ -1,60 +0,0 @@
-# Copyright (c) OpenMMLab. All rights reserved.
-import os.path as osp
-import unittest
-
-from mmengine import Config
-
-from mmocr.datasets.preparers import DatasetPreparer
-from mmocr.datasets.preparers.data_preparer import (CFG_GENERATORS,
- DATA_DUMPERS,
- DATA_GATHERERS,
- DATA_OBTAINERS,
- DATA_PACKERS, DATA_PARSERS)
-
-
-class Fake:
-
- def __init__(self, *args, **kwargs):
- pass
-
- def __call__(self, *args, **kwargs):
- return None, None
-
-
-DATA_OBTAINERS.register_module(module=Fake)
-DATA_GATHERERS.register_module(module=Fake)
-DATA_PARSERS.register_module(module=Fake)
-DATA_DUMPERS.register_module(module=Fake)
-DATA_PACKERS.register_module(module=Fake)
-CFG_GENERATORS.register_module(module=Fake)
-
-
-class TestDataPreparer(unittest.TestCase):
-
- def _create_config(self):
- cfg_path = 'config.py'
- cfg = ''
- cfg += "data_root = ''\n"
- cfg += 'train_preparer=dict(\n'
- cfg += ' obtainer=dict(type="Fake"),\n'
- cfg += ' gatherer=dict(type="Fake"),\n'
- cfg += ' parser=dict(type="Fake"),\n'
- cfg += ' packer=dict(type="Fake"),\n'
- cfg += ' dumper=dict(type="Fake"),\n'
- cfg += ')\n'
- cfg += 'test_preparer=dict(\n'
- cfg += ' obtainer=dict(type="Fake"),\n'
- cfg += ')\n'
- cfg += 'cfg_generator=dict(type="Fake")\n'
- cfg += f"delete = ['{cfg_path}']\n"
-
- with open(cfg_path, 'w') as f:
- f.write(cfg)
- return cfg_path
-
- def test_dataset_preparer(self):
- cfg_path = self._create_config()
- cfg = Config.fromfile(cfg_path)
- preparer = DatasetPreparer.from_file(cfg)
- preparer.run()
- self.assertFalse(osp.exists(cfg_path))
diff --git a/pipeline/mmocr/tests/test_datasets/test_preparers/test_dumpers/test_dumpers.py b/pipeline/mmocr/tests/test_datasets/test_preparers/test_dumpers/test_dumpers.py
deleted file mode 100644
index fe6a6118ebc66cb952230c23801d9b93c649c7b6..0000000000000000000000000000000000000000
--- a/pipeline/mmocr/tests/test_datasets/test_preparers/test_dumpers/test_dumpers.py
+++ /dev/null
@@ -1,38 +0,0 @@
-# Copyright (c) OpenMMLab. All rights reserved.
-import json
-import os.path as osp
-import tempfile
-import unittest
-
-from mmocr.datasets.preparers.dumpers import (JsonDumper,
- WildreceiptOpensetDumper)
-
-
-class TestDumpers(unittest.TestCase):
-
- def setUp(self) -> None:
- self.root = tempfile.TemporaryDirectory()
-
- def test_json_dumpers(self):
- task, split = 'textdet', 'train'
- fake_data = dict(
- metainfo=dict(
- dataset_type='TextDetDataset',
- task_name='textdet',
- category=[dict(id=0, name='text')]))
-
- dumper = JsonDumper(task, split, self.root.name)
- dumper.dump(fake_data)
- with open(osp.join(self.root.name, f'{task}_{split}.json'), 'r') as f:
- data = json.load(f)
- self.assertEqual(data, fake_data)
-
- def test_wildreceipt_dumper(self):
- task, split = 'kie', 'train'
- fake_data = ['test1', 'test2']
-
- dumper = WildreceiptOpensetDumper(task, split, self.root.name)
- dumper.dump(fake_data)
- with open(osp.join(self.root.name, f'openset_{split}.txt'), 'r') as f:
- data = f.read().splitlines()
- self.assertEqual(data, fake_data)
diff --git a/pipeline/mmocr/tests/test_datasets/test_preparers/test_gatherers/test_mono_gatherer.py b/pipeline/mmocr/tests/test_datasets/test_preparers/test_gatherers/test_mono_gatherer.py
deleted file mode 100644
index 848184314cbdd72b99d43f758d231e4301c1590b..0000000000000000000000000000000000000000
--- a/pipeline/mmocr/tests/test_datasets/test_preparers/test_gatherers/test_mono_gatherer.py
+++ /dev/null
@@ -1,24 +0,0 @@
-# Copyright (c) OpenMMLab. All rights reserved.
-import os.path as osp
-import unittest
-
-from mmocr.datasets.preparers.gatherers import MonoGatherer
-
-
-class TestMonoGatherer(unittest.TestCase):
-
- def test_mono_text_gatherer(self):
- data_root = 'dummpy'
- img_dir = 'dummy_img'
- ann_dir = 'dummy_ann'
- ann_name = 'dummy_ann.json'
- split = 'train'
- gatherer = MonoGatherer(
- data_root=data_root,
- img_dir=img_dir,
- ann_dir=ann_dir,
- ann_name=ann_name,
- split=split)
- gather_img_dir, ann_path = gatherer()
- self.assertEqual(gather_img_dir, osp.join(data_root, img_dir))
- self.assertEqual(ann_path, osp.join(data_root, ann_dir, ann_name))
diff --git a/pipeline/mmocr/tests/test_datasets/test_preparers/test_gatherers/test_pair_gatherer.py b/pipeline/mmocr/tests/test_datasets/test_preparers/test_gatherers/test_pair_gatherer.py
deleted file mode 100644
index 7a9d448bcdb4ed0e520b9ae9a9664b4ff25f0056..0000000000000000000000000000000000000000
--- a/pipeline/mmocr/tests/test_datasets/test_preparers/test_gatherers/test_pair_gatherer.py
+++ /dev/null
@@ -1,42 +0,0 @@
-# Copyright (c) OpenMMLab. All rights reserved.
-import os
-import os.path as osp
-import tempfile
-import unittest
-
-import cv2
-import numpy as np
-
-from mmocr.datasets.preparers.gatherers import PairGatherer
-
-
-class TestPairGatherer(unittest.TestCase):
-
- def test_pair_text_gatherer(self):
- root = tempfile.TemporaryDirectory()
- data_root = root.name
- img_dir = 'dummy_img'
- ann_dir = 'dummy_ann'
- split = 'train'
- img = np.random.randint(0, 100, size=(100, 100, 3))
- os.makedirs(osp.join(data_root, img_dir))
- os.makedirs(osp.join(data_root, ann_dir))
- for i in range(10):
- cv2.imwrite(osp.join(data_root, img_dir, f'img_{i}.jpg'), img)
- f = open(osp.join(data_root, ann_dir, f'img_{i}.txt'), 'w')
- f.close()
- f = open(osp.join(data_root, ann_dir, 'img_10.mmocr'), 'w')
- f.close()
- gatherer = PairGatherer(
- data_root=data_root,
- img_dir=img_dir,
- ann_dir=ann_dir,
- split=split,
- img_suffixes=['.jpg'],
- rule=[r'img_(\d+)\.([jJ][pP][gG])', r'img_\1.txt'])
- img_list, ann_list = gatherer()
- self.assertEqual(len(img_list), 10)
- self.assertEqual(len(ann_list), 10)
- self.assertNotIn(
- osp.join(data_root, ann_dir, 'img_10.mmocr'), ann_list)
- root.cleanup()
diff --git a/pipeline/mmocr/tests/test_datasets/test_preparers/test_packers/test_textdet_packer.py b/pipeline/mmocr/tests/test_datasets/test_preparers/test_packers/test_textdet_packer.py
deleted file mode 100644
index 5e70d87de95f6057513b6f583af83395a192d6a9..0000000000000000000000000000000000000000
--- a/pipeline/mmocr/tests/test_datasets/test_preparers/test_packers/test_textdet_packer.py
+++ /dev/null
@@ -1,62 +0,0 @@
-# Copyright (c) OpenMMLab. All rights reserved.
-import os.path as osp
-import tempfile
-import unittest
-
-import cv2
-import numpy as np
-
-from mmocr.datasets.preparers import TextDetPacker
-
-
-class TestTextDetPacker(unittest.TestCase):
-
- def setUp(self) -> None:
- self.root = tempfile.TemporaryDirectory()
- img = np.random.randint(0, 255, (30, 20, 3), dtype=np.uint8)
- cv2.imwrite(osp.join(self.root.name, 'test_img.jpg'), img)
- self.instance = [{
- 'poly': [0, 0, 0, 10, 10, 20, 20, 0],
- 'ignore': False
- }, {
- 'box': [0, 0, 10, 20],
- 'ignore': False
- }]
- self.img_path = osp.join(self.root.name, 'test_img.jpg')
- self.sample = (self.img_path, self.instance)
-
- def test_pack_instance(self):
- packer = TextDetPacker(data_root=self.root.name, split='test')
- instance = packer.pack_instance(self.sample)
- self.assertEquals(instance['img_path'], 'test_img.jpg')
- self.assertEquals(instance['height'], 30)
- self.assertEquals(instance['width'], 20)
- self.assertEquals(instance['instances'][0]['polygon'],
- [0, 0, 0, 10, 10, 20, 20, 0])
- self.assertEquals(instance['instances'][0]['bbox'],
- [float(x) for x in [0, 0, 20, 20]])
- self.assertEquals(instance['instances'][0]['bbox_label'], 0)
- self.assertEquals(instance['instances'][0]['ignore'], False)
- self.assertEquals(instance['instances'][1]['polygon'],
- [0.0, 0.0, 10.0, 0.0, 10.0, 20.0, 0.0, 20.0])
- self.assertEquals(instance['instances'][1]['bbox'],
- [float(x) for x in [0, 0, 10, 20]])
- self.assertEquals(instance['instances'][1]['bbox_label'], 0)
- self.assertEquals(instance['instances'][1]['ignore'], False)
-
- def test_add_meta(self):
- packer = TextDetPacker(data_root=self.root.name, split='test')
- instance = packer.pack_instance(self.sample)
- meta = packer.add_meta(instance)
- self.assertDictEqual(
- meta['metainfo'], {
- 'dataset_type': 'TextDetDataset',
- 'task_name': 'textdet',
- 'category': [{
- 'id': 0,
- 'name': 'text'
- }]
- })
-
- def tearDown(self) -> None:
- self.root.cleanup()
diff --git a/pipeline/mmocr/tests/test_datasets/test_preparers/test_packers/test_textrecog_packer.py b/pipeline/mmocr/tests/test_datasets/test_preparers/test_packers/test_textrecog_packer.py
deleted file mode 100644
index 5e875af323f37913df6d0db5d523bdc348b8313d..0000000000000000000000000000000000000000
--- a/pipeline/mmocr/tests/test_datasets/test_preparers/test_packers/test_textrecog_packer.py
+++ /dev/null
@@ -1,76 +0,0 @@
-# Copyright (c) OpenMMLab. All rights reserved.
-import os.path as osp
-import tempfile
-import unittest
-
-import cv2
-import numpy as np
-
-from mmocr.datasets.preparers import TextRecogCropPacker, TextRecogPacker
-
-
-class TestTextRecogPacker(unittest.TestCase):
-
- def test_pack_instance(self):
-
- packer = TextRecogPacker(data_root='data/test/', split='test')
- sample = ('data/test/test.jpg', 'text')
- results = packer.pack_instance(sample)
- self.assertDictEqual(
- results, dict(img_path='test.jpg', instances=[dict(text='text')]))
-
- def test_add_meta(self):
- packer = TextRecogPacker(data_root='', split='test')
- sample = [dict(img_path='test.jpg', instances=[dict(text='text')])]
- results = packer.add_meta(sample)
- self.assertDictEqual(
- results,
- dict(
- metainfo=dict(
- dataset_type='TextRecogDataset', task_name='textrecog'),
- data_list=sample))
-
-
-class TestTextRecogCropPacker(unittest.TestCase):
-
- def setUp(self) -> None:
- self.root = tempfile.TemporaryDirectory()
- img = np.random.randint(0, 255, (30, 40, 3), dtype=np.uint8)
- cv2.imwrite(osp.join(self.root.name, 'test_img.jpg'), img)
- self.instance = [{
- 'poly': [0, 0, 0, 10, 10, 20, 20, 0],
- 'ignore': False,
- 'text': 'text1'
- }, {
- 'box': [0, 0, 10, 20],
- 'ignore': False,
- 'text': 'text2'
- }]
- self.img_path = osp.join(self.root.name, 'test_img.jpg')
- self.sample = (self.img_path, self.instance)
-
- def test_pack_instance(self):
- packer = TextRecogCropPacker(data_root=self.root.name, split='test')
- instance = packer.pack_instance(self.sample)
- self.assertListEqual(instance, [
- dict(
- img_path=osp.join('textrecog_imgs', 'test', 'test_img_0.jpg'),
- instances=[dict(text='text1')]),
- dict(
- img_path=osp.join('textrecog_imgs', 'test', 'test_img_1.jpg'),
- instances=[dict(text='text2')])
- ])
-
- def test_add_meta(self):
- packer = TextRecogCropPacker(data_root=self.root.name, split='test')
- instance = packer.pack_instance(self.sample)
- results = packer.add_meta([instance])
- self.assertDictEqual(
- results,
- dict(
- metainfo=dict(
- dataset_type='TextRecogDataset', task_name='textrecog'),
- data_list=instance))
-
- def tearDown(self) -> None:
- self.root.cleanup()
diff --git a/pipeline/mmocr/tests/test_datasets/test_preparers/test_packers/test_textspotting_packer.py b/pipeline/mmocr/tests/test_datasets/test_preparers/test_packers/test_textspotting_packer.py
deleted file mode 100644
index e3d4a85d02655916a50eef2354dedf4935726073..0000000000000000000000000000000000000000
--- a/pipeline/mmocr/tests/test_datasets/test_preparers/test_packers/test_textspotting_packer.py
+++ /dev/null
@@ -1,69 +0,0 @@
-# Copyright (c) OpenMMLab. All rights reserved.
-import os.path as osp
-import tempfile
-import unittest
-
-import cv2
-import numpy as np
-
-from mmocr.datasets.preparers import TextSpottingPacker
-
-
-class TestTextSpottingPacker(unittest.TestCase):
-
- def setUp(self) -> None:
- self.root = tempfile.TemporaryDirectory()
- img = np.random.randint(0, 255, (30, 20, 3), dtype=np.uint8)
- cv2.imwrite(osp.join(self.root.name, 'test_img.jpg'), img)
- self.instance = [{
- 'poly': [0, 0, 0, 10, 10, 20, 20, 0],
- 'ignore': False,
- 'text': 'text1'
- }, {
- 'box': [0, 0, 10, 20],
- 'ignore': False,
- 'text': 'text2'
- }]
- self.img_path = osp.join(self.root.name, 'test_img.jpg')
- self.sample = (self.img_path, self.instance)
-
- def test_pack_instance(self):
- packer = TextSpottingPacker(data_root=self.root.name, split='test')
- instance = packer.pack_instance(self.sample)
- self.assertEquals(instance['img_path'], 'test_img.jpg')
- self.assertEquals(instance['height'], 30)
- self.assertEquals(instance['width'], 20)
- self.assertEquals(instance['instances'][0]['polygon'],
- [0, 0, 0, 10, 10, 20, 20, 0])
- self.assertEquals(instance['instances'][0]['bbox'],
- [float(x) for x in [0, 0, 20, 20]])
- self.assertEquals(instance['instances'][0]['bbox_label'], 0)
- self.assertEquals(instance['instances'][0]['ignore'], False)
- self.assertEquals(instance['instances'][0]['text'], 'text1')
- self.assertEquals(instance['instances'][1]['polygon'],
- [0.0, 0.0, 10.0, 0.0, 10.0, 20.0, 0.0, 20.0])
- self.assertEquals(instance['instances'][1]['bbox'],
- [float(x) for x in [0, 0, 10, 20]])
- self.assertEquals(instance['instances'][1]['bbox_label'], 0)
- self.assertEquals(instance['instances'][1]['ignore'], False)
- self.assertEquals(instance['instances'][1]['text'], 'text2')
-
- def test_add_meta(self):
- packer = TextSpottingPacker(data_root=self.root.name, split='test')
- instance = packer.pack_instance(self.sample)
- meta = packer.add_meta(instance)
- self.assertDictEqual(
- meta, {
- 'metainfo': {
- 'dataset_type': 'TextSpottingDataset',
- 'task_name': 'textspotting',
- 'category': [{
- 'id': 0,
- 'name': 'text'
- }]
- },
- 'data_list': instance
- })
-
- def tearDown(self) -> None:
- self.root.cleanup()
diff --git a/pipeline/mmocr/tests/test_datasets/test_preparers/test_parsers/test_ctw1500_parser.py b/pipeline/mmocr/tests/test_datasets/test_preparers/test_parsers/test_ctw1500_parser.py
deleted file mode 100644
index c6d5d52e87b68e7b01cbec1a3eaa62443f6fd078..0000000000000000000000000000000000000000
--- a/pipeline/mmocr/tests/test_datasets/test_preparers/test_parsers/test_ctw1500_parser.py
+++ /dev/null
@@ -1,72 +0,0 @@
-# Copyright (c) OpenMMLab. All rights reserved.
-import os.path as osp
-import tempfile
-import unittest
-
-from mmocr.datasets.preparers.parsers import CTW1500AnnParser
-from mmocr.utils import list_to_file
-
-
-class TestCTW1500AnnParser(unittest.TestCase):
-
- def setUp(self) -> None:
- self.root = tempfile.TemporaryDirectory()
-
- def _create_dummy_ctw1500_det(self):
- fake_train_anno = [
- '',
- ' ',
- ' ',
- ' OLATHE ',
- ' 131,58,208,49,279,56,346,76,412,101,473,141,530,192,510,246,458,210,405,175,350,151,291,137,228,133,165,134 ', # noqa: E501
- ' ',
- ' ',
- ' ',
- ' ',
- ' ',
- ' ',
- ' ',
- ' ',
- ' ',
- ]
- train_ann_file = osp.join(self.root.name, 'ctw1500_train.xml')
- list_to_file(train_ann_file, fake_train_anno)
-
- fake_test_anno = [
- '48,84,61,79,75,73,88,68,102,74,116,79,130,84,135,73,119,67,104,60,89,56,74,61,59,67,45,73,#######', # noqa: E501
- '51,137,58,137,66,137,74,137,82,137,90,137,98,137,98,119,90,119,82,119,74,119,66,119,58,119,50,119,####E-313', # noqa: E501
- '41,155,49,155,57,155,65,155,73,155,81,155,89,155,87,136,79,136,71,136,64,136,56,136,48,136,41,137,#######', # noqa: E501
- '41,193,57,193,74,194,90,194,107,195,123,195,140,196,146,168,128,167,110,167,92,167,74,166,56,166,39,166,####F.D.N.Y.', # noqa: E501
- ]
- test_ann_file = osp.join(self.root.name, 'ctw1500_test.txt')
- list_to_file(test_ann_file, fake_test_anno)
- return (osp.join(self.root.name,
- 'ctw1500.jpg'), train_ann_file, test_ann_file)
-
- def test_textdet_parsers(self):
- parser = CTW1500AnnParser(split='train')
- img_path, train_file, test_file = self._create_dummy_ctw1500_det()
- img_path, instances = parser.parse_file(img_path, train_file)
- self.assertEqual(img_path, osp.join(self.root.name, 'ctw1500.jpg'))
- self.assertEqual(len(instances), 1)
- self.assertEqual(instances[0]['text'], 'OLATHE')
- self.assertEqual(instances[0]['poly'], [
- 131, 58, 208, 49, 279, 56, 346, 76, 412, 101, 473, 141, 530, 192,
- 510, 246, 458, 210, 405, 175, 350, 151, 291, 137, 228, 133, 165,
- 134
- ])
- self.assertEqual(instances[0]['ignore'], False)
-
- parser = CTW1500AnnParser(split='test')
- img_path, instances = parser.parse_file(img_path, test_file)
- self.assertEqual(img_path, osp.join(self.root.name, 'ctw1500.jpg'))
- self.assertEqual(len(instances), 4)
- self.assertEqual(instances[0]['ignore'], True)
- self.assertEqual(instances[1]['text'], 'E-313')
- self.assertEqual(instances[3]['poly'], [
- 41, 193, 57, 193, 74, 194, 90, 194, 107, 195, 123, 195, 140, 196,
- 146, 168, 128, 167, 110, 167, 92, 167, 74, 166, 56, 166, 39, 166
- ])
-
- def tearDown(self) -> None:
- self.root.cleanup()
diff --git a/pipeline/mmocr/tests/test_datasets/test_preparers/test_parsers/test_funsd_parser.py b/pipeline/mmocr/tests/test_datasets/test_preparers/test_parsers/test_funsd_parser.py
deleted file mode 100644
index 078a0e84747c8cbab0f08aecb1a5598456202d58..0000000000000000000000000000000000000000
--- a/pipeline/mmocr/tests/test_datasets/test_preparers/test_parsers/test_funsd_parser.py
+++ /dev/null
@@ -1,56 +0,0 @@
-# Copyright (c) OpenMMLab. All rights reserved.
-import json
-import os.path as osp
-import tempfile
-import unittest
-
-from mmocr.datasets.preparers import FUNSDTextDetAnnParser
-
-
-class TestFUNSDTextDetAnnParser(unittest.TestCase):
-
- def setUp(self) -> None:
- self.root = tempfile.TemporaryDirectory()
-
- def _create_fake_sample(self):
- fake_sample = {
- 'form': [{
- 'box': [91, 279, 123, 294],
- 'text': 'Date:',
- 'label': 'question',
- 'words': [{
- 'box': [91, 279, 123, 294],
- 'text': 'Date:'
- }],
- 'linking': [[0, 16]],
- 'id': 0
- }, {
- 'box': [92, 310, 130, 324],
- 'text': 'From:',
- 'label': 'question',
- 'words': [{
- 'box': [92, 310, 130, 324],
- 'text': ''
- }],
- 'linking': [[1, 22]],
- 'id': 1
- }]
- }
- ann_path = osp.join(self.root.name, 'funsd.json')
- with open(ann_path, 'w') as f:
- json.dump(fake_sample, f)
- return ann_path
-
- def test_textdet_parsers(self):
- ann_path = self._create_fake_sample()
- parser = FUNSDTextDetAnnParser(split='train')
- _, instances = parser.parse_file('fake.jpg', ann_path)
- self.assertEqual(len(instances), 2)
- self.assertEqual(instances[0]['text'], 'Date:')
- self.assertEqual(instances[0]['ignore'], False)
- self.assertEqual(instances[1]['ignore'], True)
- self.assertListEqual(instances[0]['poly'],
- [91, 279, 123, 279, 123, 294, 91, 294])
-
- def tearDown(self) -> None:
- self.root.cleanup()
diff --git a/pipeline/mmocr/tests/test_datasets/test_preparers/test_parsers/test_icdar_txt_parsers.py b/pipeline/mmocr/tests/test_datasets/test_preparers/test_parsers/test_icdar_txt_parsers.py
deleted file mode 100644
index edcdfba26783c2dd1689d777cc5b025382c71495..0000000000000000000000000000000000000000
--- a/pipeline/mmocr/tests/test_datasets/test_preparers/test_parsers/test_icdar_txt_parsers.py
+++ /dev/null
@@ -1,62 +0,0 @@
-# Copyright (c) OpenMMLab. All rights reserved.
-import os.path as osp
-import tempfile
-import unittest
-
-from mmocr.datasets.preparers.parsers.icdar_txt_parser import (
- ICDARTxtTextDetAnnParser, ICDARTxtTextRecogAnnParser)
-from mmocr.utils import list_to_file
-
-
-class TestIC15Parsers(unittest.TestCase):
-
- def setUp(self) -> None:
- self.root = tempfile.TemporaryDirectory()
-
- def _create_dummy_ic15_det(self):
- fake_anno = [
- '377,117,463,117,465,130,378,130,Genaxis Theatre',
- '493,115,519,115,519,131,493,131,[06]',
- '374,155,409,155,409,170,374,170,###',
- '374,155,409,155,409,170,374,170,100,000', ' '
- ]
- ann_file = osp.join(self.root.name, 'ic15_det.txt')
- list_to_file(ann_file, fake_anno)
- return (osp.join(self.root.name, 'ic15_det.jpg'), ann_file)
-
- def _create_dummy_ic15_recog(self):
- fake_anno = [
- 'word_1.png, "Genaxis Theatre"', 'word_2.png, "[06]"',
- 'word_3.png, "62-03"', 'word_4.png, "62-,03"', ''
- ]
- ann_file = osp.join(self.root.name, 'ic15_recog.txt')
- list_to_file(ann_file, fake_anno)
- return ann_file
-
- def test_textdet_parsers(self):
- file = self._create_dummy_ic15_det()
- parser = ICDARTxtTextDetAnnParser(split='train')
-
- img, instances = parser.parse_file(*file)
- self.assertEqual(img, file[0])
- self.assertEqual(len(instances), 4)
- self.assertIn('poly', instances[0])
- self.assertIn('text', instances[0])
- self.assertIn('ignore', instances[0])
- self.assertEqual(instances[0]['text'], 'Genaxis Theatre')
- self.assertEqual(instances[2]['ignore'], True)
- self.assertEqual(instances[3]['text'], '100,000')
-
- def test_textrecog_parsers(self):
- parser = ICDARTxtTextRecogAnnParser(split='train')
- file = self._create_dummy_ic15_recog()
- samples = parser.parse_files(self.root.name, file)
- self.assertEqual(len(samples), 4)
- img, text = samples[0]
- self.assertEqual(img, osp.join(self.root.name, 'word_1.png'))
- self.assertEqual(text, 'Genaxis Theatre')
- img, text = samples[3]
- self.assertEqual(text, '62-,03')
-
- def tearDown(self) -> None:
- self.root.cleanup()
diff --git a/pipeline/mmocr/tests/test_datasets/test_preparers/test_parsers/test_naf_parser.py b/pipeline/mmocr/tests/test_datasets/test_preparers/test_parsers/test_naf_parser.py
deleted file mode 100644
index 2d70ff20771fc914847ed6d9e19794cb6b36bb8b..0000000000000000000000000000000000000000
--- a/pipeline/mmocr/tests/test_datasets/test_preparers/test_parsers/test_naf_parser.py
+++ /dev/null
@@ -1,81 +0,0 @@
-# Copyright (c) OpenMMLab. All rights reserved.
-import json
-import os.path as osp
-import tempfile
-import unittest
-
-from mmocr.datasets.preparers import NAFAnnParser
-
-
-class TestNAFAnnParser(unittest.TestCase):
-
- def setUp(self) -> None:
- self.root = tempfile.TemporaryDirectory()
-
- def _create_fake_sample(self):
- fake_sample = {
- 'fieldBBs': [{
- 'poly_points': [[1357, 322], [1636, 324], [1636, 402],
- [1357, 400]],
- 'type':
- 'field',
- 'id':
- 'f0',
- 'isBlank':
- 1
- }, {
- 'poly_points': [[1831, 352], [1908, 353], [1908, 427],
- [1830, 427]],
- 'type':
- 'blank',
- 'id':
- 'f1',
- 'isBlank':
- 1
- }],
- 'textBBs': [{
- 'poly_points': [[1388, 80], [2003, 82], [2003, 133],
- [1388, 132]],
- 'type':
- 'text',
- 'id':
- 't0'
- }, {
- 'poly_points': [[1065, 366], [1320, 366], [1320, 413],
- [1065, 412]],
- 'type':
- 'text',
- 'id':
- 't1'
- }],
- 'imageFilename':
- '004173988_00005.jpg',
- 'transcriptions': {
- 'f0': '7/24',
- 'f1': '9',
- 't0': 'REGISTRY RETURN RECEIPT.',
- 't1': 'Date of delivery',
- }
- }
- ann_path = osp.join(self.root.name, 'naf.json')
- with open(ann_path, 'w') as f:
- json.dump(fake_sample, f)
- return ann_path
-
- def test_parsers(self):
- ann_path = self._create_fake_sample()
- parser = NAFAnnParser(split='train')
- _, instances = parser.parse_file('fake.jpg', ann_path)
- self.assertEqual(len(instances), 3)
- self.assertEqual(instances[0]['ignore'], False)
- self.assertEqual(instances[1]['ignore'], False)
- self.assertListEqual(instances[2]['poly'],
- [1357, 322, 1636, 324, 1636, 402, 1357, 400])
-
- parser = NAFAnnParser(split='train', det=False)
- _, instances = parser.parse_file('fake.jpg', ann_path)
- self.assertEqual(len(instances), 2)
- self.assertEqual(instances[0]['text'], '7/24')
-
- def tearDown(self) -> None:
- self.root.cleanup()
diff --git a/pipeline/mmocr/tests/test_datasets/test_preparers/test_parsers/test_sroie_parser.py b/pipeline/mmocr/tests/test_datasets/test_preparers/test_parsers/test_sroie_parser.py
deleted file mode 100644
index 93c0bc362aff883e023ebc2c9f0c71aa1f344472..0000000000000000000000000000000000000000
--- a/pipeline/mmocr/tests/test_datasets/test_preparers/test_parsers/test_sroie_parser.py
+++ /dev/null
@@ -1,43 +0,0 @@
-# Copyright (c) OpenMMLab. All rights reserved.
-import os.path as osp
-import tempfile
-import unittest
-
-from mmocr.datasets.preparers import SROIETextDetAnnParser
-from mmocr.utils import list_to_file
-
-
-class TestSROIETextDetAnnParser(unittest.TestCase):
-
- def setUp(self) -> None:
- self.root = tempfile.TemporaryDirectory()
-
- def _create_dummy_sroie_det(self):
- fake_anno = [
- '114,54,326,54,326,92,114,92,TAN CHAY YEE',
- '60,119,300,119,300,136,60,136,###',
- '100,139,267,139,267,162,100,162,ROC NO: 538358-H',
- '83,163,277,163,277,183,83,183,NO 2 & 4, JALAN BAYU 4,',
- ]
- ann_file = osp.join(self.root.name, 'sroie_det.txt')
- list_to_file(ann_file, fake_anno)
- return (osp.join(self.root.name, 'sroie_det.jpg'), ann_file)
-
- def test_textdet_parsers(self):
- file = self._create_dummy_sroie_det()
- parser = SROIETextDetAnnParser(split='train')
-
- img, instances = parser.parse_file(*file)
- self.assertEqual(img, file[0])
- self.assertEqual(len(instances), 4)
- self.assertIn('poly', instances[0])
- self.assertIn('text', instances[0])
- self.assertIn('ignore', instances[0])
- self.assertEqual(instances[0]['text'], 'TAN CHAY YEE')
- self.assertEqual(instances[1]['ignore'], True)
- self.assertEqual(instances[3]['text'], 'NO 2 & 4, JALAN BAYU 4,')
- self.assertListEqual(instances[2]['poly'],
- [100, 139, 267, 139, 267, 162, 100, 162])
-
- def tearDown(self) -> None:
- self.root.cleanup()
diff --git a/pipeline/mmocr/tests/test_datasets/test_preparers/test_parsers/test_svt_parsers.py b/pipeline/mmocr/tests/test_datasets/test_preparers/test_parsers/test_svt_parsers.py
deleted file mode 100644
index 1f7ddb659660b635e06733015ac4f50c9648832d..0000000000000000000000000000000000000000
--- a/pipeline/mmocr/tests/test_datasets/test_preparers/test_parsers/test_svt_parsers.py
+++ /dev/null
@@ -1,55 +0,0 @@
-# Copyright (c) OpenMMLab. All rights reserved.
-import os.path as osp
-import tempfile
-import unittest
-
-from mmocr.datasets.preparers.parsers.svt_parser import SVTTextDetAnnParser
-from mmocr.utils import list_to_file
-
-
-class TestSVTParsers(unittest.TestCase):
-
- def setUp(self) -> None:
- self.root = tempfile.TemporaryDirectory()
-
- def _create_dummy_svt_det(self):
- fake_anno = [
- '',
- '',
- ' ',
- ' img/test.jpg ',
- ' ',
- ' ',
- ' ', # noqa: E501
- ' LIVING ',
- ' ',
- ' ', # noqa: E501
- ' ROOM ',
- ' ',
- ' ', # noqa: E501
- ' THEATERS ',
- ' ',
- ' ',
- ' ',
- ' ',
- ]
- ann_file = osp.join(self.root.name, 'svt_det.xml')
- list_to_file(ann_file, fake_anno)
- return ann_file
-
- def test_textdet_parsers(self):
- parser = SVTTextDetAnnParser(split='train')
- file = self._create_dummy_svt_det()
- samples = parser.parse_files(self.root.name, file)
- self.assertEqual(len(samples), 1)
- self.assertEqual(samples[0][0], osp.join(self.root.name, 'test.jpg'))
- self.assertEqual(len(samples[0][1]), 3)
- self.assertEqual(samples[0][1][0]['text'], 'living')
- self.assertEqual(samples[0][1][1]['text'], 'room')
- self.assertEqual(samples[0][1][2]['text'], 'theaters')
- self.assertEqual(samples[0][1][0]['poly'],
- [375, 253, 611, 253, 611, 328, 375, 328])
- self.assertEqual(samples[0][1][0]['ignore'], False)
-
- def tearDown(self) -> None:
- self.root.cleanup()
diff --git a/pipeline/mmocr/tests/test_datasets/test_preparers/test_parsers/test_tt_parsers.py b/pipeline/mmocr/tests/test_datasets/test_preparers/test_parsers/test_tt_parsers.py
deleted file mode 100644
index 5f933e04337ca3404a27c2b25a650004fc1d1af0..0000000000000000000000000000000000000000
--- a/pipeline/mmocr/tests/test_datasets/test_preparers/test_parsers/test_tt_parsers.py
+++ /dev/null
@@ -1,39 +0,0 @@
-# Copyright (c) OpenMMLab. All rights reserved.
-import os.path as osp
-import tempfile
-import unittest
-
-from mmocr.datasets.preparers.parsers.totaltext_parser import \
- TotaltextTextDetAnnParser
-from mmocr.utils import list_to_file
-
-
-class TestTTParsers(unittest.TestCase):
-
- def setUp(self) -> None:
- self.root = tempfile.TemporaryDirectory()
-
- def _create_dummy_tt_det(self):
- fake_anno = [
- "x: [[ 53 120 121 56]], y: [[446 443 456 458]], ornt: [u'h'], transcriptions: [u'PERUNDING']", # noqa: E501
- "x: [[123 165 166 125]], y: [[443 440 453 455]], ornt: [u'h'], transcriptions: [u'PENILAI']", # noqa: E501
- "x: [[168 179 179 167]], y: [[439 439 452 453]], ornt: [u'#'], transcriptions: [u'#']", # noqa: E501
- ]
- ann_file = osp.join(self.root.name, 'tt_det.txt')
- list_to_file(ann_file, fake_anno)
- return (osp.join(self.root.name, 'tt_det.jpg'), ann_file)
-
- def test_textdet_parsers(self):
- parser = TotaltextTextDetAnnParser(split='train')
- file = self._create_dummy_tt_det()
- img, instances = parser.parse_file(*file)
- self.assertEqual(img, file[0])
- self.assertEqual(len(instances), 3)
- self.assertIn('poly', instances[0])
- self.assertIn('text', instances[0])
- self.assertIn('ignore', instances[0])
- self.assertEqual(instances[0]['text'], 'PERUNDING')
- self.assertEqual(instances[2]['ignore'], True)
-
- def tearDown(self) -> None:
- self.root.cleanup()
diff --git a/pipeline/mmocr/tests/test_datasets/test_preparers/test_parsers/test_wildreceipt_parsers.py b/pipeline/mmocr/tests/test_datasets/test_preparers/test_parsers/test_wildreceipt_parsers.py
deleted file mode 100644
index 045333d453f248ffdb0b06ef48dce3f1bea47eff..0000000000000000000000000000000000000000
--- a/pipeline/mmocr/tests/test_datasets/test_preparers/test_parsers/test_wildreceipt_parsers.py
+++ /dev/null
@@ -1,60 +0,0 @@
-# Copyright (c) OpenMMLab. All rights reserved.
-import json
-import os.path as osp
-import tempfile
-import unittest
-
-from mmocr.datasets.preparers.parsers.wildreceipt_parser import (
- WildreceiptKIEAnnParser, WildreceiptTextDetAnnParser)
-from mmocr.utils import list_to_file
-
-
-class TestWildReceiptParsers(unittest.TestCase):
-
- def setUp(self) -> None:
- self.root = tempfile.TemporaryDirectory()
- fake_sample = dict(
- file_name='test.jpg',
- height=100,
- width=100,
- annotations=[
- dict(
- box=[
- 550.0, 190.0, 937.0, 190.0, 937.0, 104.0, 550.0, 104.0
- ],
- text='test',
- label=1,
- ),
- dict(
- box=[
- 1048.0, 211.0, 1074.0, 211.0, 1074.0, 196.0, 1048.0,
- 196.0
- ],
- text='ATOREMGRTOMMILAZZO',
- label=0,
- )
- ])
- fake_sample = [json.dumps(fake_sample)]
- self.anno = osp.join(self.root.name, 'wildreceipt.txt')
- list_to_file(self.anno, fake_sample)
-
- def test_textdet_parsers(self):
- parser = WildreceiptTextDetAnnParser(split='train')
- samples = parser.parse_files(self.root.name, self.anno)
- self.assertEqual(len(samples), 1)
- self.assertEqual(osp.basename(samples[0][0]), 'test.jpg')
- instances = samples[0][1]
- self.assertEqual(len(instances), 2)
- self.assertIn('poly', instances[0])
- self.assertIn('text', instances[0])
- self.assertIn('ignore', instances[0])
- self.assertEqual(instances[0]['text'], 'test')
- self.assertEqual(instances[1]['ignore'], True)
-
- def test_kie_parsers(self):
- parser = WildreceiptKIEAnnParser(split='train')
- samples = parser.parse_files(self.root.name, self.anno)
- self.assertEqual(len(samples), 1)
-
- def tearDown(self) -> None:
- self.root.cleanup()
diff --git a/pipeline/mmocr/tests/test_datasets/test_recog_lmdb_dataset.py b/pipeline/mmocr/tests/test_datasets/test_recog_lmdb_dataset.py
deleted file mode 100644
index aa06db5b4ebac9fc57d8edf6dea0dea89065175d..0000000000000000000000000000000000000000
--- a/pipeline/mmocr/tests/test_datasets/test_recog_lmdb_dataset.py
+++ /dev/null
@@ -1,20 +0,0 @@
-# Copyright (c) OpenMMLab. All rights reserved.
-from unittest import TestCase
-
-from mmocr.datasets import RecogLMDBDataset
-
-
-class TestRecogLMDBDataset(TestCase):
-
- def test_label_and_image_dataset(self):
-
- # test initialization
- dataset = RecogLMDBDataset(
- ann_file='tests/data/rec_toy_dataset/imgs.lmdb', pipeline=[])
- dataset.full_init()
- self.assertEqual(len(dataset), 10)
- self.assertEqual(len(dataset.load_data_list()), 10)
- self.assertEqual(dataset[0]['img'].shape, (26, 67, 3))
- self.assertEqual(dataset[0]['instances'][0]['text'], 'GRAND')
- self.assertEqual(dataset[1]['img'].shape, (17, 37, 3))
- self.assertEqual(dataset[1]['instances'][0]['text'], 'HOTEL')
diff --git a/pipeline/mmocr/tests/test_datasets/test_recog_text_dataset.py b/pipeline/mmocr/tests/test_datasets/test_recog_text_dataset.py
deleted file mode 100644
index f9550d17a7be038b9654910a2fbf05c85d1aa978..0000000000000000000000000000000000000000
--- a/pipeline/mmocr/tests/test_datasets/test_recog_text_dataset.py
+++ /dev/null
@@ -1,51 +0,0 @@
-# Copyright (c) OpenMMLab. All rights reserved.
-from unittest import TestCase
-
-from mmocr.datasets import RecogTextDataset
-
-
-class TestRecogTextDataset(TestCase):
-
- def test_txt_dataset(self):
-
- # test initialization
- dataset = RecogTextDataset(
- ann_file='tests/data/rec_toy_dataset/old_label.txt',
- data_prefix=dict(img_path='imgs'),
- parser_cfg=dict(
- type='LineStrParser',
- keys=['filename', 'text'],
- keys_idx=[0, 1]),
- pipeline=[])
- dataset.full_init()
- self.assertEqual(len(dataset), 10)
- self.assertEqual(len(dataset.load_data_list()), 10)
-
- # test load_data_list
- anno = dataset.load_data_list()
- self.assertIn(anno[0]['img_path'],
- ['imgs/1223731.jpg', 'imgs\\1223731.jpg'])
- self.assertEqual(anno[0]['instances'][0]['text'], 'GRAND')
- self.assertIn(anno[1]['img_path'],
- ['imgs/1223733.jpg', 'imgs\\1223733.jpg'])
-
- self.assertEqual(anno[1]['instances'][0]['text'], 'HOTEL')
-
- def test_jsonl_dataset(self):
- dataset = RecogTextDataset(
- ann_file='tests/data/rec_toy_dataset/old_label.jsonl',
- data_prefix=dict(img_path='imgs'),
- parser_cfg=dict(type='LineJsonParser', keys=['filename', 'text']),
- pipeline=[])
- dataset.full_init()
- self.assertEqual(len(dataset), 10)
- self.assertEqual(len(dataset.load_data_list()), 10)
-
- # test load_data_list
- anno = dataset.load_data_list()
- self.assertIn(anno[0]['img_path'],
- ['imgs/1223731.jpg', 'imgs\\1223731.jpg'])
- self.assertEqual(anno[0]['instances'][0]['text'], 'GRAND')
- self.assertIn(anno[1]['img_path'],
- ['imgs/1223733.jpg', 'imgs\\1223733.jpg'])
- self.assertEqual(anno[1]['instances'][0]['text'], 'HOTEL')
diff --git a/pipeline/mmocr/tests/test_datasets/test_samplers/test_batch_aug.py b/pipeline/mmocr/tests/test_datasets/test_samplers/test_batch_aug.py
deleted file mode 100644
index 1c9da5812182269318b5c4f0ee7702a778754de4..0000000000000000000000000000000000000000
--- a/pipeline/mmocr/tests/test_datasets/test_samplers/test_batch_aug.py
+++ /dev/null
@@ -1,85 +0,0 @@
-# Copyright (c) OpenMMLab. All rights reserved.
-
-from unittest import TestCase
-from unittest.mock import patch
-
-import torch
-from mmengine.logging import MMLogger
-
-from mmocr.datasets import BatchAugSampler
-
-file = 'mmocr.datasets.samplers.batch_aug.'
-
-
-class MockDist:
-
- def __init__(self, dist_info=(0, 1), seed=7):
- self.dist_info = dist_info
- self.seed = seed
-
- def get_dist_info(self):
- return self.dist_info
-
- def sync_random_seed(self):
- return self.seed
-
- def is_main_process(self):
- return self.dist_info[0] == 0
-
-
-class TestBatchAugSampler(TestCase):
-
- def setUp(self):
- self.data_length = 100
- self.dataset = list(range(self.data_length))
-
- @patch(file + 'get_dist_info', return_value=(0, 1))
- def test_non_dist(self, mock):
- sampler = BatchAugSampler(self.dataset, num_repeats=3, shuffle=False)
- self.assertEqual(sampler.world_size, 1)
- self.assertEqual(sampler.rank, 0)
- self.assertEqual(sampler.total_size, self.data_length * 3)
- self.assertEqual(sampler.num_samples, self.data_length * 3)
- indices = [x for x in range(self.data_length) for _ in range(3)]
- self.assertEqual(list(sampler), indices)
-
- @patch(file + 'get_dist_info', return_value=(2, 3))
- def test_dist(self, mock):
- sampler = BatchAugSampler(self.dataset, num_repeats=3, shuffle=False)
- self.assertEqual(sampler.world_size, 3)
- self.assertEqual(sampler.rank, 2)
- self.assertEqual(sampler.num_samples, self.data_length)
- self.assertEqual(sampler.total_size, self.data_length * 3)
-
- logger = MMLogger.get_current_instance()
- with patch.object(logger, 'warning') as mock_log:
- sampler = BatchAugSampler(self.dataset, shuffle=False)
- mock_log.assert_not_called()
-
- @patch(file + 'get_dist_info', return_value=(0, 1))
- @patch(file + 'sync_random_seed', return_value=7)
- def test_shuffle(self, mock1, mock2):
- # test seed=None
- sampler = BatchAugSampler(self.dataset, seed=None)
- self.assertEqual(sampler.seed, 7)
-
- # test random seed
- sampler = BatchAugSampler(self.dataset, shuffle=True, seed=0)
- sampler.set_epoch(10)
- g = torch.Generator()
- g.manual_seed(10)
- indices = [
- x for x in torch.randperm(len(self.dataset), generator=g)
- for _ in range(3)
- ]
- self.assertEqual(list(sampler), indices)
-
- sampler = BatchAugSampler(self.dataset, shuffle=True, seed=42)
- sampler.set_epoch(10)
- g = torch.Generator()
- g.manual_seed(42 + 10)
- indices = [
- x for x in torch.randperm(len(self.dataset), generator=g)
- for _ in range(3)
- ]
- self.assertEqual(list(sampler), indices)
diff --git a/pipeline/mmocr/tests/test_datasets/test_transforms/test_adapters.py b/pipeline/mmocr/tests/test_datasets/test_transforms/test_adapters.py
deleted file mode 100644
index 6a1ba3ef4d640a03bc3c5335b224919ca2776388..0000000000000000000000000000000000000000
--- a/pipeline/mmocr/tests/test_datasets/test_transforms/test_adapters.py
+++ /dev/null
@@ -1,137 +0,0 @@
-# Copyright (c) OpenMMLab. All rights reserved.
-import unittest
-
-import numpy as np
-from mmdet.structures.mask import PolygonMasks, bitmap_to_polygon
-
-from mmocr.datasets import MMDet2MMOCR, MMOCR2MMDet, Resize
-from mmocr.utils import poly2shapely
-
-
-class TestMMDet2MMOCR(unittest.TestCase):
-
- def setUp(self):
- img = np.zeros((15, 30, 3))
- img_shape = (15, 30)
- polygons = [
- np.array([10., 5., 20., 5., 20., 10., 10., 10.]),
- np.array([10., 5., 20., 5., 20., 10., 10., 10., 8., 7.])
- ]
- ignores = np.array([True, False])
- bboxes = np.array([[10., 5., 20., 10.], [0., 0., 10., 10.]])
- self.data_info_ocr = dict(
- img=img,
- gt_polygons=polygons,
- gt_bboxes=bboxes,
- img_shape=img_shape,
- gt_ignored=ignores)
-
- _polygons = [[polygon] for polygon in polygons]
- masks = PolygonMasks(_polygons, *img_shape)
- self.data_info_det_polygon = dict(
- img=img,
- gt_masks=masks,
- gt_bboxes=bboxes,
- gt_ignore_flags=ignores,
- img_shape=img_shape)
-
- masks = masks.to_bitmap()
- self.data_info_det_mask = dict(
- img=img,
- gt_masks=masks,
- gt_bboxes=bboxes,
- gt_ignore_flags=ignores,
- img_shape=img_shape)
-
- def test_ocr2det_polygonmasks(self):
- transform = MMOCR2MMDet()
- results = transform(self.data_info_ocr.copy())
- self.assertEqual(results['img'].shape, (15, 30, 3))
- self.assertEqual(results['img_shape'], (15, 30))
- self.assertTrue(
- np.allclose(results['gt_masks'].masks[0][0],
- self.data_info_det_polygon['gt_masks'].masks[0][0]))
- self.assertTrue(
- np.allclose(results['gt_masks'].masks[0][0],
- self.data_info_det_polygon['gt_masks'].masks[0][0]))
- self.assertTrue(
- np.allclose(results['gt_bboxes'],
- self.data_info_det_polygon['gt_bboxes']))
- self.assertTrue(
- np.allclose(results['gt_ignore_flags'],
- self.data_info_det_polygon['gt_ignore_flags']))
-
- def test_ocr2det_bitmapmasks(self):
- transform = MMOCR2MMDet(poly2mask=True)
- results = transform(self.data_info_ocr.copy())
- self.assertEqual(results['img'].shape, (15, 30, 3))
- self.assertEqual(results['img_shape'], (15, 30))
- self.assertTrue(
- poly2shapely(
- bitmap_to_polygon(
- results['gt_masks'].masks[0])[0][0].flatten()).equals(
- poly2shapely(
- bitmap_to_polygon(
- self.data_info_det_mask['gt_masks'].masks[0])
- [0][0].flatten())))
-
- self.assertTrue(
- np.allclose(results['gt_bboxes'],
- self.data_info_det_mask['gt_bboxes']))
- self.assertTrue(
- np.allclose(results['gt_ignore_flags'],
- self.data_info_det_mask['gt_ignore_flags']))
-
- def test_det2ocr_polygonmasks(self):
- transform = MMDet2MMOCR()
- results = transform(self.data_info_det_polygon.copy())
- self.assertEqual(results['img'].shape, (15, 30, 3))
- self.assertEqual(results['img_shape'], (15, 30))
- self.assertTrue(
- np.allclose(results['gt_polygons'][0],
- self.data_info_ocr['gt_polygons'][0]))
- self.assertTrue(
- np.allclose(results['gt_polygons'][1],
- self.data_info_ocr['gt_polygons'][1]))
- self.assertTrue(
- np.allclose(results['gt_bboxes'], self.data_info_ocr['gt_bboxes']))
- self.assertTrue(
- np.allclose(results['gt_ignored'],
- self.data_info_ocr['gt_ignored']))
-
- def test_det2ocr_bitmapmasks(self):
- transform = MMDet2MMOCR()
- results = transform(self.data_info_det_mask.copy())
- self.assertEqual(results['img'].shape, (15, 30, 3))
- self.assertEqual(results['img_shape'], (15, 30))
- self.assertTrue(
- np.allclose(results['gt_bboxes'], self.data_info_ocr['gt_bboxes']))
- self.assertTrue(
- np.allclose(results['gt_ignored'],
- self.data_info_ocr['gt_ignored']))
-
- def test_ocr2det2ocr(self):
- from mmdet.datasets.transforms import Resize as MMDet_Resize
- t1 = MMOCR2MMDet()
- t2 = MMDet_Resize(scale=(60, 60))
- t3 = MMDet2MMOCR()
- t4 = Resize(scale=(30, 15))
- results = t4(t3(t2(t1(self.data_info_ocr.copy()))))
- self.assertEqual(results['img'].shape, (15, 30, 3))
- for i in range(2):
- self.assertTrue(
- poly2shapely(results['gt_polygons'][i]).equals(
- poly2shapely(self.data_info_ocr['gt_polygons'][i])))
- self.assertTrue(
- np.allclose(results['gt_bboxes'], self.data_info_ocr['gt_bboxes']))
- self.assertTrue(
- np.array_equal(results['gt_ignored'],
- self.data_info_ocr['gt_ignored']))
-
- def test_repr_det2ocr(self):
- transform = MMDet2MMOCR()
- self.assertEqual(repr(transform), ('MMDet2MMOCR'))
-
- def test_repr_ocr2det(self):
- transform = MMOCR2MMDet(poly2mask=True)
- self.assertEqual(repr(transform), ('MMOCR2MMDet(poly2mask = True)'))
diff --git a/pipeline/mmocr/tests/test_datasets/test_transforms/test_formatting.py b/pipeline/mmocr/tests/test_datasets/test_transforms/test_formatting.py
deleted file mode 100644
index 21e9d10f2ef05ef0b84895d1064bf0119a364004..0000000000000000000000000000000000000000
--- a/pipeline/mmocr/tests/test_datasets/test_transforms/test_formatting.py
+++ /dev/null
@@ -1,214 +0,0 @@
-# Copyright (c) OpenMMLab. All rights reserved.
-import copy
-from unittest import TestCase
-
-import numpy as np
-import torch
-
-from mmocr.datasets.transforms import (PackKIEInputs, PackTextDetInputs,
- PackTextRecogInputs)
-
-
-class TestPackTextDetInputs(TestCase):
-
- def test_packdetinput(self):
- datainfo = dict(
- img=np.random.random((10, 10)),
- img_shape=(10, 10),
- ori_shape=(10, 10),
- pad_shape=(10, 10),
- scale_factor=(1, 1),
- img_path='tmp/tmp.jpg',
- flip=True,
- flip_direction='left',
- gt_bboxes=np.array([[0, 0, 10, 10], [5, 5, 15, 15]],
- dtype=np.float32),
- gt_bboxes_labels=np.array([0, 0], np.int64),
- gt_polygons=[
- np.array([0, 0, 0, 10, 10, 10, 10, 0], dtype=np.float32),
- np.array([5, 5, 5, 15, 15, 15, 15, 5], dtype=np.float32)
- ],
- gt_texts=['mmocr', 'mmocr_ignore'],
- gt_ignored=np.bool_([False, True]))
- with self.assertRaises(KeyError):
- transform = PackTextDetInputs(meta_keys=('tmp', ))
- transform(copy.deepcopy(datainfo))
- transform = PackTextDetInputs()
- results = transform(copy.deepcopy(datainfo))
- self.assertIn('inputs', results)
- self.assertEqual(results['inputs'].shape, torch.Size([1, 10, 10]))
- self.assertTupleEqual(tuple(results['inputs'].shape), (1, 10, 10))
- self.assertIn('data_samples', results)
-
- # test non-contiugous img
- nc_datainfo = copy.deepcopy(datainfo)
- nc_datainfo['img'] = nc_datainfo['img'].transpose(1, 0)
- results = transform(nc_datainfo)
- self.assertIn('inputs', results)
- self.assertEqual(results['inputs'].shape, torch.Size([1, 10, 10]))
-
- data_sample = results['data_samples']
- self.assertIn('bboxes', data_sample.gt_instances)
- self.assertIsInstance(data_sample.gt_instances.bboxes, torch.Tensor)
- self.assertEqual(data_sample.gt_instances.bboxes.dtype, torch.float32)
- self.assertIsInstance(data_sample.gt_instances.polygons[0], np.ndarray)
- self.assertEqual(data_sample.gt_instances.polygons[0].dtype,
- np.float32)
- self.assertEqual(data_sample.gt_instances.ignored.dtype, torch.bool)
- self.assertEqual(data_sample.gt_instances.labels.dtype, torch.int64)
- self.assertIsInstance(data_sample.gt_instances.texts, list)
-
- self.assertIn('img_path', data_sample)
- self.assertIn('flip', data_sample)
-
- transform = PackTextDetInputs(meta_keys=('img_path', ))
- results = transform(copy.deepcopy(datainfo))
- self.assertIn('inputs', results)
- self.assertIn('data_samples', results)
-
- data_sample = results['data_samples']
- self.assertIn('bboxes', data_sample.gt_instances)
- self.assertIn('img_path', data_sample)
- self.assertNotIn('flip', data_sample)
-
- datainfo.pop('gt_texts')
- transform = PackTextDetInputs()
- results = transform(copy.deepcopy(datainfo))
- data_sample = results['data_samples']
- self.assertNotIn('texts', data_sample.gt_instances)
-
- datainfo = dict(img_shape=(10, 10))
- transform = PackTextDetInputs(meta_keys=('img_shape', ))
- results = transform(copy.deepcopy(datainfo))
- self.assertNotIn('inputs', results)
- data_sample = results['data_samples']
- self.assertNotIn('texts', data_sample.gt_instances)
-
- def test_repr(self):
- transform = PackTextDetInputs()
- self.assertEqual(
- repr(transform),
- ("PackTextDetInputs(meta_keys=('img_path', 'ori_shape', "
- "'img_shape', 'scale_factor', 'flip', 'flip_direction'))"))
-
-
-class TestPackTextRecogInputs(TestCase):
-
- def test_packrecogtinput(self):
- datainfo = dict(
- img=np.random.random((10, 10)),
- img_shape=(10, 10),
- ori_shape=(10, 10),
- pad_shape=(10, 10),
- scale_factor=(1, 1),
- img_path='tmp/tmp.jpg',
- flip=True,
- flip_direction='left',
- gt_bboxes=np.array([[0, 0, 10, 10]]),
- gt_labels=np.array([0]),
- gt_polygons=[[0, 0, 0, 10, 10, 10, 10, 0]],
- gt_texts=['mmocr'],
- )
- with self.assertRaises(KeyError):
- transform = PackTextRecogInputs(meta_keys=('tmp', ))
- transform(copy.deepcopy(datainfo))
- transform = PackTextRecogInputs()
- results = transform(copy.deepcopy(datainfo))
- self.assertIn('inputs', results)
- self.assertTupleEqual(tuple(results['inputs'].shape), (1, 10, 10))
- self.assertIn('data_samples', results)
- data_sample = results['data_samples']
- self.assertEqual(data_sample.gt_text.item, 'mmocr')
- self.assertIn('img_path', data_sample)
- self.assertIn('valid_ratio', data_sample)
- self.assertIn('pad_shape', data_sample)
-
- # test non-contiugous img
- nc_datainfo = copy.deepcopy(datainfo)
- nc_datainfo['img'] = nc_datainfo['img'].transpose(1, 0)
- results = transform(nc_datainfo)
- self.assertIn('inputs', results)
- self.assertEqual(results['inputs'].shape, torch.Size([1, 10, 10]))
-
- transform = PackTextRecogInputs(meta_keys=('img_path', ))
- results = transform(copy.deepcopy(datainfo))
- self.assertIn('inputs', results)
- self.assertIn('data_samples', results)
- data_sample = results['data_samples']
- self.assertEqual(data_sample.gt_text.item, 'mmocr')
- self.assertIn('img_path', data_sample)
- self.assertNotIn('valid_ratio', data_sample)
- self.assertNotIn('pad_shape', data_sample)
-
- datainfo = dict(img_shape=(10, 10))
- transform = PackTextRecogInputs(meta_keys=('img_shape', ))
- results = transform(copy.deepcopy(datainfo))
- self.assertNotIn('inputs', results)
- data_sample = results['data_samples']
- self.assertNotIn('item', data_sample.gt_text)
-
- def test_repr(self):
- transform = PackTextRecogInputs()
- self.assertEqual(
- repr(transform),
- ("PackTextRecogInputs(meta_keys=('img_path', 'ori_shape', "
- "'img_shape', 'pad_shape', 'valid_ratio'))"))
-
-
-class TestPackKIEInputs(TestCase):
-
- def setUp(self) -> None:
- self.transform = PackKIEInputs()
-
- def test_transform(self):
- datainfo = dict(
- img=np.random.random((10, 10)),
- img_shape=(10, 10),
- ori_shape=(10, 10),
- scale_factor=(1, 1),
- img_path='tmp/tmp.jpg',
- gt_bboxes=np.array([[0, 0, 10, 10], [5, 5, 15, 15]],
- dtype=np.float32),
- gt_bboxes_labels=np.array([0, 0], np.int64),
- gt_edges_labels=np.array([[0, 0], [0, 0]], np.int64),
- gt_texts=['text1', 'text2'])
-
- with self.assertRaises(KeyError):
- transform = PackKIEInputs(meta_keys=('tmp', ))
- transform(copy.deepcopy(datainfo))
-
- results = self.transform(copy.deepcopy(datainfo))
- self.assertIn('inputs', results)
- self.assertTupleEqual(tuple(results['inputs'].shape), (1, 10, 10))
- self.assertIn('data_samples', results)
- data_sample = results['data_samples']
- self.assertIsInstance(data_sample.gt_instances.bboxes, torch.Tensor)
- self.assertEqual(data_sample.gt_instances.bboxes.dtype, torch.float32)
- self.assertEqual(data_sample.gt_instances.labels.dtype, torch.int64)
- self.assertEqual(data_sample.gt_instances.edge_labels.dtype,
- torch.int64)
- self.assertIsInstance(data_sample.gt_instances.texts, list)
-
- # test non-contiugous img
- nc_datainfo = copy.deepcopy(datainfo)
- nc_datainfo['img'] = nc_datainfo['img'].transpose(1, 0)
- results = self.transform(nc_datainfo)
- self.assertIn('inputs', results)
- self.assertEqual(results['inputs'].shape, torch.Size([1, 10, 10]))
-
- transform = PackKIEInputs(meta_keys=('img_path', ))
- results = transform(copy.deepcopy(datainfo))
- self.assertIn('inputs', results)
- self.assertIn('data_samples', results)
-
- data_sample = results['data_samples']
- self.assertIn('bboxes', data_sample.gt_instances)
- self.assertIn('img_path', data_sample)
-
- datainfo.pop('img')
- results = self.transform(copy.deepcopy(datainfo))
- self.assertIn('inputs', results)
- self.assertEqual(results['inputs'].shape, torch.Size((0, 0, 0)))
-
- def test_repr(self):
- self.assertEqual(repr(self.transform), ('PackKIEInputs(meta_keys=())'))
diff --git a/pipeline/mmocr/tests/test_datasets/test_transforms/test_loading.py b/pipeline/mmocr/tests/test_datasets/test_transforms/test_loading.py
deleted file mode 100644
index 197d8a301980cc264da2bbb4dc0251468c96ad62..0000000000000000000000000000000000000000
--- a/pipeline/mmocr/tests/test_datasets/test_transforms/test_loading.py
+++ /dev/null
@@ -1,217 +0,0 @@
-# Copyright (c) OpenMMLab. All rights reserved.
-import copy
-import os.path as osp
-from unittest import TestCase
-
-import mmcv
-import numpy as np
-
-from mmocr.datasets.transforms import (InferencerLoader, LoadImageFromFile,
- LoadKIEAnnotations, LoadOCRAnnotations)
-
-
-class TestLoadImageFromFile(TestCase):
-
- def test_load_img(self):
- data_prefix = osp.join(
- osp.dirname(__file__), '../../data/rec_toy_dataset/imgs/')
-
- results = dict(img_path=osp.join(data_prefix, '1036169.jpg'))
- transform = LoadImageFromFile(min_size=0)
- results = transform(copy.deepcopy(results))
- self.assertEquals(results['img_path'],
- osp.join(data_prefix, '1036169.jpg'))
- self.assertEquals(results['img'].shape, (25, 119, 3))
- self.assertEquals(results['img'].dtype, np.uint8)
- self.assertEquals(results['img_shape'], (25, 119))
- self.assertEquals(results['ori_shape'], (25, 119))
- self.assertEquals(
- repr(transform),
- ('LoadImageFromFile(ignore_empty=False, min_size=0, '
- "to_float32=False, color_type='color', imdecode_backend='cv2', "
- 'backend_args=None)'))
-
- # to_float32
- transform = LoadImageFromFile(to_float32=True)
- results = transform(copy.deepcopy(results))
- self.assertEquals(results['img'].dtype, np.float32)
-
- # min_size
- transform = LoadImageFromFile(min_size=26, ignore_empty=True)
- self.assertIsNone(transform(copy.deepcopy(results)))
- transform = LoadImageFromFile(min_size=26)
- with self.assertRaises(IOError):
- transform(copy.deepcopy(results))
-
- # test load empty
- fake_img_path = osp.join(data_prefix, 'fake.jpg')
- results = dict(img_path=fake_img_path)
- transform = LoadImageFromFile(ignore_empty=False)
- with self.assertRaises(FileNotFoundError):
- transform(copy.deepcopy(results))
- transform = LoadImageFromFile(ignore_empty=True)
- results = transform(copy.deepcopy(results))
- self.assertIsNone(results)
-
- data_prefix = osp.join(osp.dirname(__file__), '../../data')
- broken_img_path = osp.join(data_prefix, 'broken.jpg')
- results = dict(img_path=broken_img_path)
- transform = LoadImageFromFile(ignore_empty=False)
- with self.assertRaises(IOError):
- transform(copy.deepcopy(results))
- transform = LoadImageFromFile(ignore_empty=True)
- results = transform(copy.deepcopy(results))
- self.assertIsNone(results)
-
-
-class TestLoadOCRAnnotations(TestCase):
-
- def setUp(self):
- self.results = {
- 'height':
- 288,
- 'width':
- 512,
- 'instances': [{
- 'bbox': [0, 0, 10, 20],
- 'bbox_label': 1,
- 'polygon': [0, 0, 0, 20, 10, 20, 10, 0],
- 'text': 'tmp1',
- 'ignore': False
- }, {
- 'bbox': [10, 10, 110, 120],
- 'bbox_label': 2,
- 'polygon': [10, 10, 10, 120, 110, 120, 110, 10],
- 'text': 'tmp2',
- 'ignore': False
- }, {
- 'bbox': [0, 0, 10, 20],
- 'bbox_label': 1,
- 'polygon': [0, 0, 0, 20, 10, 20, 10, 0],
- 'text': 'tmp3',
- 'ignore': True
- }, {
- 'bbox': [10, 10, 110, 120],
- 'bbox_label': 2,
- 'polygon': [10, 10, 10, 120, 110, 120, 110, 10],
- 'text': 'tmp4',
- 'ignore': True
- }]
- }
-
- def test_load_polygon(self):
- transform = LoadOCRAnnotations(
- with_bbox=False, with_label=False, with_polygon=True)
- results = transform(copy.deepcopy(self.results))
- self.assertIn('gt_polygons', results)
- self.assertIsInstance(results['gt_polygons'], list)
- self.assertEqual(len(results['gt_polygons']), 4)
- for gt_polygon in results['gt_polygons']:
- self.assertIsInstance(gt_polygon, np.ndarray)
- self.assertEqual(gt_polygon.dtype, np.float32)
-
- self.assertIn('gt_ignored', results)
- self.assertEqual(results['gt_ignored'].dtype, np.bool_)
- self.assertTrue((results['gt_ignored'],
- np.array([False, False, True, True], dtype=np.bool_)))
-
- def test_load_text(self):
- transform = LoadOCRAnnotations(
- with_bbox=False, with_label=False, with_text=True)
- results = transform(copy.deepcopy(self.results))
- self.assertIn('gt_texts', results)
- self.assertListEqual(results['gt_texts'],
- ['tmp1', 'tmp2', 'tmp3', 'tmp4'])
-
- def test_repr(self):
- transform = LoadOCRAnnotations(
- with_bbox=True, with_label=True, with_polygon=True, with_text=True)
- self.assertEqual(
- repr(transform),
- ('LoadOCRAnnotations(with_bbox=True, with_label=True, '
- 'with_polygon=True, with_text=True, '
- "imdecode_backend='cv2', backend_args=None)"))
-
-
-class TestLoadKIEAnnotations(TestCase):
-
- def setUp(self):
- self.results = {
- 'bboxes': np.random.rand(2, 4).astype(np.float32),
- 'bbox_labels': np.random.randint(0, 10, (2, )),
- 'edge_labels': np.random.randint(0, 10, (2, 2)),
- 'texts': ['text1', 'text2'],
- 'ori_shape': (288, 512)
- }
- self.results = {
- 'img_shape': (288, 512),
- 'ori_shape': (288, 512),
- 'instances': [{
- 'bbox': [0, 0, 10, 20],
- 'bbox_label': 1,
- 'edge_label': 1,
- 'text': 'tmp1',
- }, {
- 'bbox': [10, 10, 110, 120],
- 'bbox_label': 2,
- 'edge_label': 1,
- 'text': 'tmp2',
- }]
- }
- self.load = LoadKIEAnnotations()
-
- def test_transform(self):
- results = self.load(copy.deepcopy(self.results))
-
- self.assertIn('gt_bboxes', results)
- self.assertIsInstance(results['gt_bboxes'], np.ndarray)
- self.assertEqual(results['gt_bboxes'].shape, (2, 4))
- self.assertEqual(results['gt_bboxes'].dtype, np.float32)
-
- self.assertIn('gt_bboxes_labels', results)
- self.assertIsInstance(results['gt_bboxes_labels'], np.ndarray)
- self.assertEqual(results['gt_bboxes_labels'].shape, (2, ))
- self.assertEqual(results['gt_bboxes_labels'].dtype, np.int64)
-
- self.assertIn('gt_edges_labels', results)
- self.assertIsInstance(results['gt_edges_labels'], np.ndarray)
- self.assertEqual(results['gt_edges_labels'].shape, (2, 2))
- self.assertEqual(results['gt_edges_labels'].dtype, np.int64)
-
- self.assertIn('ori_shape', results)
- self.assertEqual(results['ori_shape'], (288, 512))
-
- load = LoadKIEAnnotations(key_node_idx=1, value_node_idx=2)
- results = load(copy.deepcopy(self.results))
- self.assertEqual(results['gt_edges_labels'][0, 1], 1)
- self.assertEqual(results['gt_edges_labels'][1, 0], -1)
-
- def test_repr(self):
- self.assertEqual(
- repr(self.load),
- 'LoadKIEAnnotations(with_bbox=True, with_label=True, '
- 'with_text=True)')
-
-
-class TestInferencerLoader(TestCase):
-
- def test_transform(self):
- loader = InferencerLoader()
-
- # load from path
- img_path = 'tests/data/det_toy_dataset/imgs/test/img_1.jpg'
- res = loader(img_path)
- self.assertIsInstance(res['img'], np.ndarray)
-
- # load from ndarray
- img = mmcv.imread(img_path)
- res = loader(img)
- self.assertIsInstance(res['img'], np.ndarray)
-
- # load from dict
- res = loader(dict(img=img))
- self.assertIsInstance(res['img'], np.ndarray)
-
- # invalid input
- with self.assertRaises(NotImplementedError):
- loader(['hello'])
diff --git a/pipeline/mmocr/tests/test_datasets/test_transforms/test_ocr_transforms.py b/pipeline/mmocr/tests/test_datasets/test_transforms/test_ocr_transforms.py
deleted file mode 100644
index 35a39779368c2f3e7be127b6a37a67a705c18635..0000000000000000000000000000000000000000
--- a/pipeline/mmocr/tests/test_datasets/test_transforms/test_ocr_transforms.py
+++ /dev/null
@@ -1,348 +0,0 @@
-# Copyright (c) OpenMMLab. All rights reserved.
-import copy
-import unittest
-import unittest.mock as mock
-
-import numpy as np
-
-from mmocr.datasets.transforms import (FixInvalidPolygon, RandomCrop,
- RandomRotate, RemoveIgnored, Resize)
-from mmocr.utils import poly2shapely
-
-
-class TestRandomCrop(unittest.TestCase):
-
- def setUp(self):
- img = np.zeros((30, 30, 3))
- gt_polygons = [
- np.array([5., 5., 25., 5., 25., 10., 5., 10.]),
- np.array([5., 20., 25., 20., 25., 25., 5., 25.])
- ]
- gt_bboxes = np.array([[5, 5, 25, 10], [5, 20, 25, 25]])
- labels = np.array([0, 1])
- gt_ignored = np.array([True, False], dtype=bool)
- texts = ['text1', 'text2']
- self.data_info = dict(
- img=img,
- gt_polygons=gt_polygons,
- gt_bboxes=gt_bboxes,
- gt_bboxes_labels=labels,
- gt_ignored=gt_ignored,
- gt_texts=texts)
-
- @mock.patch('mmocr.datasets.transforms.ocr_transforms.np.random.randint')
- def test_sample_crop_box(self, mock_randint):
-
- def rand_min(low, high):
- return low
-
- trans = RandomCrop(min_side_ratio=0.3)
- mock_randint.side_effect = rand_min
- crop_box = trans._sample_crop_box((30, 30), self.data_info.copy())
- assert np.allclose(np.array(crop_box), np.array([0, 0, 25, 10]))
-
- def rand_max(low, high):
- return high - 1
-
- mock_randint.side_effect = rand_max
- crop_box = trans._sample_crop_box((30, 30), self.data_info.copy())
- assert np.allclose(np.array(crop_box), np.array([4, 19, 30, 30]))
-
- @mock.patch('mmocr.datasets.transforms.ocr_transforms.np.random.randint')
- def test_transform(self, mock_randint):
-
- def rand_min(low, high):
- return low
-
- # mock_randint.side_effect = [0, 0, 0, 0, 30, 0, 0, 0, 15]
- mock_randint.side_effect = rand_min
- trans = RandomCrop(min_side_ratio=0.3)
- polygon_target = np.array([5., 5., 25., 5., 25., 10., 5., 10.])
- bbox_target = np.array([[5., 5., 25., 10.]])
- results = trans(self.data_info)
-
- self.assertEqual(results['img'].shape, (10, 25, 3))
- self.assertEqual(results['img_shape'], (10, 25))
- self.assertTrue(np.allclose(results['gt_bboxes'], bbox_target))
- self.assertEqual(results['gt_bboxes'].shape, (1, 4))
- self.assertEqual(len(results['gt_polygons']), 1)
- self.assertTrue(np.allclose(results['gt_polygons'][0], polygon_target))
- self.assertEqual(results['gt_bboxes_labels'][0], 0)
- self.assertEqual(results['gt_ignored'][0], True)
- self.assertEqual(results['gt_texts'][0], 'text1')
-
- def rand_max(low, high):
- return high - 1
-
- mock_randint.side_effect = rand_max
- trans = RandomCrop(min_side_ratio=0.3)
- polygon_target = np.array([1, 1, 21, 1, 21, 6, 1, 6])
- bbox_target = np.array([[1, 1, 21, 6]])
- results = trans(self.data_info)
-
- self.assertEqual(results['img'].shape, (6, 21, 3))
- self.assertEqual(results['img_shape'], (6, 21))
- self.assertTrue(np.allclose(results['gt_bboxes'], bbox_target))
- self.assertEqual(results['gt_bboxes'].shape, (1, 4))
- self.assertEqual(len(results['gt_polygons']), 1)
- self.assertTrue(np.allclose(results['gt_polygons'][0], polygon_target))
- self.assertEqual(results['gt_bboxes_labels'][0], 0)
- self.assertTrue(results['gt_ignored'][0])
- self.assertEqual(results['gt_texts'][0], 'text1')
-
- def test_repr(self):
- transform = RandomCrop(min_side_ratio=0.4)
- self.assertEqual(repr(transform), ('RandomCrop(min_side_ratio = 0.4)'))
-
-
-class TestRandomRotate(unittest.TestCase):
-
- def setUp(self):
- img = np.random.random((5, 5))
- self.data_info1 = dict(img=img.copy(), img_shape=img.shape[:2])
- self.data_info2 = dict(
- img=np.random.random((30, 30, 3)),
- gt_bboxes=np.array([[10, 10, 20, 20], [5, 5, 10, 10]]),
- img_shape=(30, 30))
- self.data_info3 = dict(
- img=np.random.random((30, 30, 3)),
- gt_polygons=[np.array([10., 10., 20., 10., 20., 20., 10., 20.])],
- img_shape=(30, 30))
-
- def test_init(self):
- # max angle is float
- with self.assertRaisesRegex(TypeError,
- '`max_angle` should be an integer'):
- RandomRotate(max_angle=16.8)
- # invalid pad value
- with self.assertRaisesRegex(
- ValueError, '`pad_value` should contain three integers'):
- RandomRotate(pad_value=[16.8, 0.1])
-
- def test_transform(self):
- self._test_recog()
- self._test_bboxes()
- self._test_polygons()
-
- def _test_recog(self):
- # test random rotate for recognition (image only) input
- transform = RandomRotate(max_angle=10)
- results = transform(copy.deepcopy(self.data_info1))
- self.assertTrue(np.allclose(results['img'], self.data_info1['img']))
-
- @mock.patch(
- 'mmocr.datasets.transforms.ocr_transforms.np.random.random_sample')
- def _test_bboxes(self, mock_sample):
- # test random rotate for bboxes
- # returns 1. for random_sample() in _sample_angle(), i.e., angle = 90
- mock_sample.side_effect = [1.]
- transform = RandomRotate(max_angle=90, use_canvas=True)
- results = transform(copy.deepcopy(self.data_info2))
- self.assertTrue(
- np.allclose(results['gt_bboxes'][0], np.array([10, 10, 20, 20])))
- self.assertTrue(
- np.allclose(results['gt_bboxes'][1], np.array([5, 20, 10, 25])))
- self.assertEqual(results['img'].shape, self.data_info2['img'].shape)
-
- @mock.patch(
- 'mmocr.datasets.transforms.ocr_transforms.np.random.random_sample')
- def _test_polygons(self, mock_sample):
- # test random rotate for polygons
- # returns 1. for random_sample() in _sample_angle(), i.e., angle = 90
- mock_sample.side_effect = [1.]
- transform = RandomRotate(max_angle=90, use_canvas=True)
- results = transform(copy.deepcopy(self.data_info3))
- self.assertTrue(
- np.allclose(results['gt_polygons'][0],
- np.array([10., 20., 10., 10., 20., 10., 20., 20.])))
- self.assertEqual(results['img'].shape, self.data_info3['img'].shape)
-
- def test_repr(self):
- transform = RandomRotate(
- max_angle=10,
- pad_with_fixed_color=False,
- pad_value=(0, 0, 0),
- use_canvas=False)
- self.assertEqual(
- repr(transform),
- ('RandomRotate(max_angle = 10, '
- 'pad_with_fixed_color = False, pad_value = (0, 0, 0), '
- 'use_canvas = False)'))
-
-
-class TestResize(unittest.TestCase):
-
- def test_resize_wo_img(self):
- # keep_ratio = True
- dummy_result = dict(img_shape=(10, 20))
- resize = Resize(scale=(40, 30), keep_ratio=True)
- result = resize(dummy_result)
- self.assertEqual(result['img_shape'], (20, 40))
- self.assertEqual(result['scale'], (40, 20))
- self.assertEqual(result['scale_factor'], (2., 2.))
- self.assertEqual(result['keep_ratio'], True)
-
- # keep_ratio = False
- dummy_result = dict(img_shape=(10, 20))
- resize = Resize(scale=(40, 30), keep_ratio=False)
- result = resize(dummy_result)
- self.assertEqual(result['img_shape'], (30, 40))
- self.assertEqual(result['scale'], (40, 30))
- self.assertEqual(result['scale_factor'], (
- 2.,
- 3.,
- ))
- self.assertEqual(result['keep_ratio'], False)
-
- def test_resize_bbox(self):
- # keep_ratio = True
- dummy_result = dict(
- img_shape=(10, 20),
- gt_bboxes=np.array([[0, 0, 1, 1]], dtype=np.float32))
- resize = Resize(scale=(40, 30))
- result = resize(dummy_result)
- self.assertEqual(result['gt_bboxes'].dtype, np.float32)
-
-
-class TestFixInvalidPolygon(unittest.TestCase):
-
- def setUp(self):
- # All polygons are invalid w/o gt_bboxes
- self.data_info = dict(
- img=np.random.random((30, 40, 3)),
- gt_polygons=[
- np.array([0., 0., 10., 10., 10., 0., 0., 10.]),
- np.array([0., 0., 10., 0., 0., 10., 5., 10.]),
- np.array([0, 10]),
- np.array([0, 10, 0, 10, 10, 0, 0, 10]),
- ],
- gt_ignored=np.array([False, False, False, False], dtype=bool))
- # All polygons are invalid with gt_bboxes
- # the third one can be recovered from gt_bboxes
- # the fourth one has no valid polygon and bbox
- self.data_info2 = dict(
- img=np.random.random((30, 40, 3)),
- gt_polygons=[
- np.array([0., 0., 10., 10., 10., 0.]),
- np.array([0., 0., 10., 0., 0., 10.]),
- np.array([0, 10, 0, 10, 10, 0, 0, 10]),
- np.array([0, 10, 0, 10, 10, 0, 0, 10]),
- ],
- gt_bboxes=np.array([[0., 0., 10., 10.], [0., 0., 10., 10.],
- [0, 0, 10, 10], [0, 0, 0, 0]]),
- gt_ignored=np.array([False, False, False, False], dtype=bool))
- # Contains all unfixable polygons
- self.data_info3 = dict(
- img=np.random.random((30, 40, 3)),
- gt_polygons=[
- np.array([0, 10]),
- np.array([0, 10, 0, 10, 10, 0, 0, 10]),
- ],
- gt_ignored=np.array([False, False], dtype=bool))
- # The first one is valid, and the second one is invalid
- self.data_info4 = dict(
- img=np.random.random((30, 40, 3)),
- gt_polygons=[
- np.array([0., 0., 10., 0., 10., 10., 0., 10.]),
- np.array([0, 10, 0, 10, 10, 0, 0, 10]),
- ],
- gt_ignored=np.array([False, False], dtype=bool))
- # no gt_polygons
- self.data_info5 = dict(
- img=np.random.random((30, 40, 3)),
- gt_bboxes=np.array([[0., 0., 10., 10.], [0., 0., 10., 10.],
- [0, 0, 10, 10], [0, 0, 0, 0]]),
- gt_ignored=np.array([False, False, False, False], dtype=bool))
-
- def test_transform_fix(self):
- transform = FixInvalidPolygon(mode='fix', min_poly_points=4)
- results = transform(copy.deepcopy(self.data_info))
- # The third one is removed because it doesn't have enough points
- # The fourth one is removed because it is a line
- assert len(
- results['gt_polygons']) == len(self.data_info['gt_polygons']) - 2
- for poly in results['gt_polygons']:
- self.assertTrue(poly2shapely(poly).is_valid)
- results = transform(copy.deepcopy(self.data_info2))
- # The fourth one is removed because it is a line, and its bbox is also
- # invalid
- assert len(
- results['gt_polygons']) == len(self.data_info['gt_polygons']) - 1
- for poly in results['gt_polygons']:
- self.assertTrue(len(poly) >= 8 and len(poly) % 2 == 0)
- # test not fixing the invalid polygons from bboxes
- transform_wo_bbox = FixInvalidPolygon(
- mode='fix', min_poly_points=4, fix_from_bbox=False)
- results = transform_wo_bbox(copy.deepcopy(self.data_info2))
- # The fourth one is removed because it is a line, and its bbox is also
- # invalid
- assert len(
- results['gt_polygons']) == len(self.data_info['gt_polygons']) - 2
- for poly in results['gt_polygons']:
- self.assertTrue(len(poly) >= 8 and len(poly) % 2 == 0)
- # Fixing all invalid polygons would result in an empty result dict,
- # and therefore the transform would return None
- results = transform(copy.deepcopy(self.data_info3))
- self.assertIsNone(results)
- # If no gt_polygon inside
- results = transform(copy.deepcopy(self.data_info5))
- for k, v in results.items():
- self.assertTrue(np.array_equal(v, self.data_info5[k]))
-
- def test_transform_ignore(self):
- transform = FixInvalidPolygon(mode='ignore')
- results = transform(copy.deepcopy(self.data_info))
- self.assertTrue(
- np.array_equal(results['gt_ignored'],
- np.array([True, True, True, True], dtype=bool)))
- results = transform(copy.deepcopy(self.data_info4))
- self.assertTrue(
- np.array_equal(results['gt_ignored'],
- np.array([False, True], dtype=bool)))
- for poly, ignored in zip(results['gt_polygons'],
- results['gt_ignored']):
- if not ignored:
- self.assertTrue(poly2shapely(poly).is_valid)
- # If no gt_polygon inside
- results = transform(copy.deepcopy(self.data_info5))
- for k, v in results.items():
- self.assertTrue(np.array_equal(v, self.data_info5[k]))
-
- def test_repr(self):
- transform = FixInvalidPolygon()
- print(repr(transform))
- self.assertEqual(
- repr(transform),
- 'FixInvalidPolygon(mode = "fix", min_poly_points = 4, '
- 'fix_from_bbox = True)')
-
-
-class TestRemoveIgnored(unittest.TestCase):
-
- def setUp(self):
- self.data_info = dict(
- img=np.random.random((30, 40, 3)),
- gt_polygons=[
- np.array([0., 0., 10., 10., 10., 0.]),
- np.array([0., 0., 10., 0., 0., 10.]),
- np.array([0, 10, 0, 10, 1, 2, 3, 4]),
- np.array([0, 10, 0, 10, 10, 0, 0, 10]),
- ],
- gt_bboxes=np.array([[1, 2, 3, 4], [5, 6, 7, 8], [0, 0, 10, 10],
- [0, 0, 0, 0]]),
- gt_ignored=np.array([False, True, True, False], dtype=bool),
- gt_texts=['t1', 't2', 't3', 't4'],
- gt_bboxes_labels=np.array([0, 1, 2, 3]))
- self.keys = [
- 'gt_polygons', 'gt_bboxes', 'gt_ignored', 'gt_texts',
- 'gt_bboxes_labels'
- ]
-
- def test_transform(self):
- transform = RemoveIgnored()
- results = transform(copy.deepcopy(self.data_info))
- for original_idx, new_idx in enumerate([0, 3]):
- for key in self.keys:
- self.assertTrue(
- np.array_equal(results[key][original_idx],
- self.data_info[key][new_idx]))
diff --git a/pipeline/mmocr/tests/test_datasets/test_transforms/test_textdet_transforms.py b/pipeline/mmocr/tests/test_datasets/test_transforms/test_textdet_transforms.py
deleted file mode 100644
index 92f01856b797a6eab38cc631c1d609dff0a61304..0000000000000000000000000000000000000000
--- a/pipeline/mmocr/tests/test_datasets/test_transforms/test_textdet_transforms.py
+++ /dev/null
@@ -1,493 +0,0 @@
-# Copyright (c) OpenMMLab. All rights reserved.
-import copy
-import unittest
-import unittest.mock as mock
-
-import numpy as np
-from mmcv.transforms import Pad, RandomResize
-
-from mmocr.datasets.transforms import (BoundedScaleAspectJitter, RandomCrop,
- RandomFlip, Resize,
- ShortScaleAspectJitter, SourceImagePad,
- TextDetRandomCrop,
- TextDetRandomCropFlip)
-from mmocr.utils import bbox2poly, poly2shapely
-
-
-class TestBoundedScaleAspectJitter(unittest.TestCase):
-
- @mock.patch(
- 'mmocr.datasets.transforms.textdet_transforms.np.random.random_sample')
- def test_transform(self, mock_random):
- mock_random.side_effect = [1.0, 1.0]
- data_info = dict(img=np.random.random((16, 25, 3)), img_shape=(16, 25))
- # test size and size_divisor are both set
- transform = BoundedScaleAspectJitter(10, 5)
- result = transform(data_info)
- print(result['img'].shape)
- self.assertEqual(result['img'].shape, (8, 12, 3))
- self.assertEqual(result['img_shape'], (8, 12))
-
- def test_repr(self):
- transform = BoundedScaleAspectJitter(10, 5)
- print(repr(transform))
- self.assertEqual(
- repr(transform),
- ('BoundedScaleAspectJitter(long_size_bound = 10, '
- 'short_size_bound = 5, ratio_range = (0.7, 1.3), '
- 'aspect_ratio_range = (0.9, 1.1), '
- "resize_cfg = {'type': 'Resize', 'scale': 0})"))
-
-
-class TestEastRandomCrop(unittest.TestCase):
-
- def setUp(self):
- img = np.ones((30, 30, 3))
- gt_polygons = [
- np.array([5., 5., 25., 5., 25., 10., 5., 10.]),
- np.array([5., 20., 25., 20., 25., 25., 5., 25.])
- ]
- gt_bboxes = np.array([[5, 5, 25, 10], [5, 20, 25, 25]])
- labels = np.array([0, 1])
- gt_ignored = np.array([True, False], dtype=bool)
- texts = ['text1', 'text2']
- self.data_info = dict(
- img=img,
- gt_polygons=gt_polygons,
- gt_bboxes=gt_bboxes,
- gt_bboxes_labels=labels,
- gt_ignored=gt_ignored,
- gt_texts=texts)
-
- @mock.patch('mmocr.datasets.transforms.ocr_transforms.np.random.randint')
- def test_east_random_crop(self, mock_randint):
-
- # test randomcrop
- randcrop = RandomCrop(min_side_ratio=0.5)
- mock_randint.side_effect = [0, 0, 0, 0, 30, 0, 0, 0, 15]
- crop_results = randcrop(self.data_info)
- polygon_target = np.array([5., 5., 25., 5., 25., 10., 5., 10.])
- bbox_target = np.array([[5., 5., 25., 10.]])
- self.assertEqual(crop_results['img'].shape, (15, 30, 3))
- self.assertEqual(crop_results['img_shape'], (15, 30))
- self.assertTrue(np.allclose(crop_results['gt_bboxes'], bbox_target))
- self.assertEqual(crop_results['gt_bboxes'].shape, (1, 4))
- self.assertEqual(len(crop_results['gt_polygons']), 1)
- self.assertTrue(
- np.allclose(crop_results['gt_polygons'][0], polygon_target))
- self.assertEqual(crop_results['gt_bboxes_labels'][0], 0)
- self.assertTrue(crop_results['gt_ignored'][0])
- self.assertEqual(crop_results['gt_texts'][0], 'text1')
-
- # test resize
- resize = Resize(scale=(30, 30), keep_ratio=True)
- resize_results = resize(crop_results)
- self.assertEqual(resize_results['img'].shape, (15, 30, 3))
- self.assertEqual(crop_results['img_shape'], (15, 30))
- self.assertEqual(crop_results['scale'], (30, 30))
- self.assertEqual(crop_results['scale_factor'], (1., 1.))
- self.assertTrue(crop_results['keep_ratio'])
-
- # test pad
- pad = Pad(size=(30, 30))
- pad_results = pad(resize_results)
- self.assertEqual(pad_results['img'].shape, (30, 30, 3))
- self.assertEqual(pad_results['pad_shape'], (30, 30, 3))
- self.assertEqual(pad_results['img'].sum(), 15 * 30 * 3)
-
-
-class TestRandomFlip(unittest.TestCase):
-
- def setUp(self):
- img = np.random.random((30, 40, 3))
- gt_polygons = [np.array([10., 5., 20., 5., 20., 10., 10., 10.])]
- self.data_info = dict(
- img_shape=(30, 40), img=img, gt_polygons=gt_polygons)
-
- def test_flip_polygons(self):
- t = RandomFlip(prob=1.0, direction='horizontal')
- results = t.flip_polygons(self.data_info['gt_polygons'], (30, 40),
- 'horizontal')
- self.assertIsInstance(results, list)
- self.assertIsInstance(results[0], np.ndarray)
- self.assertTrue(
- (results[0] == np.array([30., 5., 20., 5., 20., 10., 30.,
- 10.])).all())
-
- results = t.flip_polygons(self.data_info['gt_polygons'], (30, 40),
- 'vertical')
- self.assertIsInstance(results, list)
- self.assertIsInstance(results[0], np.ndarray)
- self.assertTrue(
- (results[0] == np.array([10., 25., 20., 25., 20., 20., 10.,
- 20.])).all())
- results = t.flip_polygons(self.data_info['gt_polygons'], (30, 40),
- 'diagonal')
- self.assertIsInstance(results, list)
- self.assertIsInstance(results[0], np.ndarray)
- self.assertTrue(
- (results[0] == np.array([30., 25., 20., 25., 20., 20., 30.,
- 20.])).all())
- with self.assertRaises(ValueError):
- t.flip_polygons(self.data_info['gt_polygons'], (30, 40), 'mmocr')
-
- def test_flip(self):
- t = RandomFlip(prob=1.0, direction='horizontal')
- results = t(self.data_info.copy())
- self.assertEqual(results['img'].shape, (30, 40, 3))
- self.assertEqual(results['img_shape'], (30, 40))
- self.assertTrue((results['gt_polygons'][0] == np.array(
- [30., 5., 20., 5., 20., 10., 30., 10.])).all())
-
-
-class TestRandomResize(unittest.TestCase):
-
- def setUp(self):
- self.data_info1 = dict(
- img=np.random.random((300, 400, 3)),
- gt_bboxes=np.array([[0, 0, 60, 100]]),
- gt_polygons=[np.array([0, 0, 200, 0, 200, 100, 0, 100])])
-
- @mock.patch('mmcv.transforms.processing.np.random.random_sample')
- def test_random_resize(self, mock_sample):
- randresize = RandomResize(
- scale=(500, 500),
- ratio_range=(0.8, 1.2),
- resize_type='mmocr.Resize',
- keep_ratio=True)
- target_bboxes = np.array([0, 0, 90, 150])
- target_polygons = [np.array([0, 0, 300, 0, 300, 150, 0, 150])]
-
- mock_sample.side_effect = [1.0]
- results = randresize(self.data_info1)
-
- self.assertEqual(results['img'].shape, (450, 600, 3))
- self.assertEqual(results['img_shape'], (450, 600))
- self.assertEqual(results['keep_ratio'], True)
- self.assertEqual(results['scale'], (600, 600))
- self.assertEqual(results['scale_factor'], (600. / 400., 450. / 300.))
-
- self.assertTrue(
- poly2shapely(bbox2poly(results['gt_bboxes'][0])).equals(
- poly2shapely(bbox2poly(target_bboxes))))
- self.assertTrue(
- poly2shapely(results['gt_polygons'][0]).equals(
- poly2shapely(target_polygons[0])))
-
-
-class TestShortScaleAspectJitter(unittest.TestCase):
-
- @mock.patch(
- 'mmocr.datasets.transforms.textdet_transforms.np.random.random_sample')
- def test_transform(self, mock_random):
- ratio_range = (0.5, 1.5)
- aspect_ratio_range = (0.9, 1.1)
- mock_random.side_effect = [0.5, 0.5]
- img = np.zeros((15, 20, 3))
- polygon = [np.array([10., 5., 20., 5., 20., 10., 10., 10.])]
- bbox = np.array([[10., 5., 20., 10.]])
- data_info = dict(img=img, gt_polygons=polygon, gt_bboxes=bbox)
- t = ShortScaleAspectJitter(
- short_size=40,
- ratio_range=ratio_range,
- aspect_ratio_range=aspect_ratio_range,
- scale_divisor=4)
- results = t(data_info)
- self.assertEqual(results['img'].shape, (40, 56, 3))
- self.assertEqual(results['img_shape'], (40, 56))
-
- def test_repr(self):
- transform = ShortScaleAspectJitter(
- short_size=40,
- ratio_range=(0.5, 1.5),
- aspect_ratio_range=(0.9, 1.1),
- scale_divisor=4,
- resize_type='Resize')
- self.assertEqual(
- repr(transform), ('ShortScaleAspectJitter('
- 'short_size = 40, '
- 'ratio_range = (0.5, 1.5), '
- 'aspect_ratio_range = (0.9, 1.1), '
- 'scale_divisor = 4, '
- "resize_cfg = {'type': 'Resize', 'scale': 0})"))
-
-
-class TestSourceImagePad(unittest.TestCase):
-
- def setUp(self):
- img = np.zeros((15, 30, 3))
- polygon = [np.array([10., 5., 20., 5., 20., 10., 10., 10.])]
- bbox = np.array([[10., 5., 20., 10.]])
- self.data_info = dict(img=img, gt_polygons=polygon, gt_bboxes=bbox)
-
- def test_source_image_pad(self):
- # test image size equals to target size
- trans = SourceImagePad(target_scale=(30, 15))
- target_polygon = self.data_info['gt_polygons'][0]
- target_bbox = self.data_info['gt_bboxes']
- results = trans(self.data_info.copy())
- self.assertEqual(results['img'].shape, (15, 30, 3))
- self.assertEqual(results['img_shape'], (15, 30))
- self.assertEqual(results['pad_shape'], (15, 30, 3))
- self.assertEqual(results['pad_fixed_size'], (30, 15))
- self.assertTrue(np.allclose(results['gt_polygons'][0], target_polygon))
- self.assertTrue(np.allclose(results['gt_bboxes'][0], target_bbox))
-
- # test pad to square
- trans = SourceImagePad(target_scale=30)
- target_polygon = np.array([10., 5., 20., 5., 20., 10., 10., 10.])
- target_bbox = np.array([[10., 5., 20., 10.]])
- results = trans(self.data_info.copy())
- self.assertEqual(results['img'].shape, (30, 30, 3))
- self.assertEqual(results['img_shape'], (30, 30))
- self.assertEqual(results['pad_shape'], (30, 30, 3))
- self.assertEqual(results['pad_fixed_size'], (30, 30))
- self.assertTrue(np.allclose(results['gt_polygons'][0], target_polygon))
- self.assertTrue(np.allclose(results['gt_bboxes'][0], target_bbox))
-
- # test pad to different shape
- trans = SourceImagePad(target_scale=(40, 60))
- target_polygon = np.array([10., 5., 20., 5., 20., 10., 10., 10.])
- target_bbox = np.array([[10., 5., 20., 10.]])
- results = trans(self.data_info.copy())
- self.assertEqual(results['img'].shape, (60, 40, 3))
- self.assertEqual(results['img_shape'], (60, 40))
- self.assertEqual(results['pad_shape'], (60, 40, 3))
- self.assertEqual(results['pad_fixed_size'], (40, 60))
- self.assertTrue(np.allclose(results['gt_polygons'][0], target_polygon))
- self.assertTrue(np.allclose(results['gt_bboxes'][0], target_bbox))
-
- # test pad with different crop_ratio
- trans = SourceImagePad(target_scale=30, crop_ratio=1.0)
- target_polygon = np.array([10., 5., 20., 5., 20., 10., 10., 10.])
- target_bbox = np.array([[10., 5., 20., 10.]])
- results = trans(self.data_info.copy())
- self.assertEqual(results['img'].shape, (30, 30, 3))
- self.assertEqual(results['img_shape'], (30, 30))
- self.assertEqual(results['pad_shape'], (30, 30, 3))
- self.assertEqual(results['pad_fixed_size'], (30, 30))
- self.assertTrue(np.allclose(results['gt_polygons'][0], target_polygon))
- self.assertTrue(np.allclose(results['gt_bboxes'][0], target_bbox))
-
- def test_repr(self):
- transform = SourceImagePad(target_scale=30, crop_ratio=0.1)
- self.assertEqual(
- repr(transform),
- ('SourceImagePad(target_scale = (30, 30), crop_ratio = (0.1, 0.1))'
- ))
-
-
-class TestTextDetRandomCrop(unittest.TestCase):
-
- def setUp(self):
- img = np.array([[[1, 2, 3, 4, 5], [1, 2, 3, 4, 5], [1, 2, 3, 4, 5],
- [1, 2, 3, 4, 5], [1, 2, 3, 4,
- 5]]]).transpose(1, 2, 0)
- gt_polygons = [np.array([2, 2, 5, 2, 5, 5, 2, 5])]
- gt_bboxes = np.array([[2, 2, 5, 5]])
- gt_bboxes_labels = np.array([0])
- gt_ignored = np.array([True], dtype=bool)
- self.data_info = dict(
- img=img,
- gt_polygons=gt_polygons,
- gt_bboxes=gt_bboxes,
- gt_bboxes_labels=gt_bboxes_labels,
- gt_ignored=gt_ignored)
-
- @mock.patch(
- 'mmocr.datasets.transforms.textdet_transforms.np.random.random_sample')
- @mock.patch('mmocr.datasets.transforms.textdet_transforms.random.randint')
- def test_sample_offset(self, mock_randint, mock_sample):
- # test target size is bigger than image size
- mock_sample.side_effect = [1]
- trans = TextDetRandomCrop(target_size=(6, 6))
- offset = trans._sample_offset(self.data_info['gt_polygons'],
- self.data_info['img'].shape[:2])
- self.assertEqual(offset, (0, 0))
-
- # test the first bracnh in sample_offset
- mock_sample.side_effect = [0.1]
- mock_randint.side_effect = [0, 2]
- trans = TextDetRandomCrop(target_size=(3, 3))
- offset = trans._sample_offset(self.data_info['gt_polygons'],
- self.data_info['img'].shape[:2])
- self.assertEqual(offset, (0, 2))
-
- # test the second branch in sample_offset
- mock_sample.side_effect = [1]
- mock_randint.side_effect = [1, 2]
- trans = TextDetRandomCrop(target_size=(3, 3))
- offset = trans._sample_offset(self.data_info['gt_polygons'],
- self.data_info['img'].shape[:2])
- self.assertEqual(offset, (1, 2))
-
- mock_sample.side_effect = [1]
- mock_randint.side_effect = [1, 2]
- trans = TextDetRandomCrop(target_size=(5, 5))
- offset = trans._sample_offset(self.data_info['gt_polygons'],
- self.data_info['img'].shape[:2])
- self.assertEqual(offset, (0, 0))
-
- def test_crop_image(self):
- img = self.data_info['img']
- offset = [0, 0]
- target = [6, 6]
- trans = TextDetRandomCrop(target_size=(3, 3))
- crop, _ = trans._crop_img(img, offset, target)
- self.assertEqual(img.shape, crop.shape)
-
- target = [3, 2]
- crop = trans._crop_img(img, offset, target)
- self.assertTrue(
- np.allclose(
- np.array([[[1, 2, 3], [1, 2, 3]]]).transpose(1, 2, 0),
- crop[0]))
- self.assertTrue(np.allclose(crop[1], np.array([0, 0, 3, 2])))
-
- def test_crop_polygons(self):
- trans = TextDetRandomCrop(target_size=(3, 3))
- crop_box = np.array([2, 3, 5, 5])
- polygons = [
- bbox2poly([2, 3, 4, 4]),
- bbox2poly([0, 0, 1, 1]),
- bbox2poly([1, 2, 4, 4]),
- bbox2poly([0, 0, 10, 10])
- ]
- kept_polygons, kept_idx = trans._crop_polygons(polygons, crop_box)
- target_polygons = [
- bbox2poly([0, 0, 2, 1]),
- bbox2poly([0, 0, 2, 1]),
- bbox2poly([0, 0, 3, 2]),
- ]
- self.assertEqual(len(kept_polygons), 3)
- self.assertEqual(kept_idx, [0, 2, 3])
- self.assertTrue(
- poly2shapely(target_polygons[0]).equals(
- poly2shapely(kept_polygons[0])))
- self.assertTrue(
- poly2shapely(target_polygons[1]).equals(
- poly2shapely(kept_polygons[1])))
- self.assertTrue(
- poly2shapely(target_polygons[2]).equals(
- poly2shapely(kept_polygons[2])))
-
- @mock.patch(
- 'mmocr.datasets.transforms.textdet_transforms.np.random.random_sample')
- @mock.patch('mmocr.datasets.transforms.textdet_transforms.random.randint')
- def test_transform(self, mock_randint, mock_sample):
- # test target size is equal to image size
- trans = TextDetRandomCrop(target_size=(5, 5))
- data_info = self.data_info.copy()
- results = trans(data_info)
- self.assertDictEqual(results, data_info)
-
- mock_sample.side_effect = [0.1]
- mock_randint.side_effect = [1, 1]
- trans = TextDetRandomCrop(target_size=(3, 3))
- data_info = self.data_info.copy()
- results = trans(data_info)
- box_target = np.array([1, 1, 3, 3])
- polygon_target = np.array([1, 1, 3, 1, 3, 3, 1, 3])
- self.assertEqual(results['img'].shape, (3, 3, 1))
- self.assertEqual(results['img_shape'], (3, 3))
- self.assertTrue(
- poly2shapely(bbox2poly(box_target)).equals(
- poly2shapely(bbox2poly(results['gt_bboxes'][0]))))
- self.assertTrue(
- poly2shapely(polygon_target).equals(
- poly2shapely(results['gt_polygons'][0])))
-
- self.assertTrue(results['gt_bboxes_labels'] == np.array([0]))
- self.assertTrue(results['gt_ignored'][0])
-
- def test_repr(self):
- transform = TextDetRandomCrop(
- target_size=(512, 512), positive_sample_ratio=0.4)
- self.assertEqual(
- repr(transform), ('TextDetRandomCrop(target_size = (512, 512), '
- 'positive_sample_ratio = 0.4)'))
-
-
-class TestTextDetRandomCropFlip(unittest.TestCase):
-
- def setUp(self):
- img = np.ones((10, 10, 3))
- img[0, 0, :] = 0
- self.data_info1 = dict(
- img=copy.deepcopy(img),
- gt_polygons=[np.array([0., 0., 0., 10., 10., 10., 10., 0.])],
- img_shape=[10, 10])
- self.data_info2 = dict(
- img=copy.deepcopy(img),
- gt_polygons=[np.array([1., 1., 1., 9., 9., 9., 9., 1.])],
- gt_bboxes_labels=np.array([0], dtype=np.int64),
- gt_ignored=np.array([True], dtype=np.bool_),
- img_shape=[10, 10])
- self.data_info3 = dict(
- img=copy.deepcopy(img),
- gt_polygons=[
- np.array([0., 0., 4., 0., 4., 4., 0., 4.]),
- np.array([4., 0., 8., 0., 8., 4., 4., 4.])
- ],
- gt_bboxes_labels=np.array([0, 0], dtype=np.int64),
- gt_ignored=np.array([True, True], dtype=np.bool_),
- img_shape=[10, 10])
-
- def test_init(self):
- # iter_num is int
- transform = TextDetRandomCropFlip(iter_num=1)
- self.assertEqual(transform.iter_num, 1)
- # iter_num is float
- with self.assertRaisesRegex(TypeError,
- '`iter_num` should be an integer'):
- transform = TextDetRandomCropFlip(iter_num=1.5)
-
- @mock.patch(
- 'mmocr.datasets.transforms.textdet_transforms.np.random.randint')
- def test_transforms(self, mock_sample):
- mock_sample.side_effect = [0, 1, 2]
- transform = TextDetRandomCropFlip(crop_ratio=1.0, iter_num=3)
- results = transform(self.data_info2)
- self.assertTrue(np.allclose(results['img'], self.data_info2['img']))
- self.assertTrue(
- np.allclose(results['gt_polygons'],
- self.data_info2['gt_polygons']))
- self.assertEqual(
- len(results['gt_bboxes']), len(results['gt_polygons']))
- self.assertTrue(
- poly2shapely(results['gt_polygons'][0]).equals(
- poly2shapely(bbox2poly(results['gt_bboxes'][0]))))
-
- def test_size(self):
- transform = TextDetRandomCropFlip(crop_ratio=1.0, iter_num=3)
- results = transform(self.data_info3)
- self.assertEqual(
- len(results['gt_bboxes']), len(results['gt_polygons']))
- self.assertEqual(
- len(results['gt_polygons']), len(results['gt_ignored']))
- self.assertEqual(
- len(results['gt_ignored']), len(results['gt_bboxes_labels']))
-
- def test_generate_crop_target(self):
- transform = TextDetRandomCropFlip(
- crop_ratio=1.0, iter_num=3, pad_ratio=0.1)
- h, w = self.data_info1['img_shape']
- pad_h = int(h * transform.pad_ratio)
- pad_w = int(w * transform.pad_ratio)
- h_axis, w_axis = transform._generate_crop_target(
- self.data_info1['img'], self.data_info1['gt_polygons'], pad_h,
- pad_w)
- self.assertTrue(np.allclose(h_axis, (0, 11)))
- self.assertTrue(np.allclose(w_axis, (0, 11)))
-
- def test_repr(self):
- transform = TextDetRandomCropFlip(
- pad_ratio=0.1,
- crop_ratio=0.5,
- iter_num=1,
- min_area_ratio=0.2,
- epsilon=1e-2)
- self.assertEqual(
- repr(transform),
- ('TextDetRandomCropFlip(pad_ratio = 0.1, crop_ratio = 0.5, '
- 'iter_num = 1, min_area_ratio = 0.2, epsilon = 0.01)'))
diff --git a/pipeline/mmocr/tests/test_datasets/test_transforms/test_textrecog_transforms.py b/pipeline/mmocr/tests/test_datasets/test_transforms/test_textrecog_transforms.py
deleted file mode 100644
index b602bc6c57c54e9708e15e706404f35b561bc887..0000000000000000000000000000000000000000
--- a/pipeline/mmocr/tests/test_datasets/test_transforms/test_textrecog_transforms.py
+++ /dev/null
@@ -1,212 +0,0 @@
-# Copyright (c) OpenMMLab. All rights reserved.
-import copy
-import unittest
-
-import numpy as np
-from parameterized import parameterized
-
-from mmocr.datasets.transforms import (CropHeight, ImageContentJitter,
- PadToWidth, PyramidRescale,
- RescaleToHeight, ReversePixels,
- TextRecogGeneralAug)
-
-
-class TestPadToWidth(unittest.TestCase):
-
- def test_pad_to_width(self):
- data_info = dict(img=np.random.random((16, 25, 3)))
- # test size and size_divisor are both set
- with self.assertRaises(AssertionError):
- PadToWidth(width=10.5)
-
- transform = PadToWidth(width=100)
- results = transform(copy.deepcopy(data_info))
- self.assertTupleEqual(results['img'].shape[:2], (16, 100))
- self.assertEqual(results['valid_ratio'], 25 / 100)
-
- def test_repr(self):
- transform = PadToWidth(width=100)
- self.assertEqual(
- repr(transform),
- ("PadToWidth(width=100, pad_cfg={'type': 'Pad'})"))
-
-
-class TestPyramidRescale(unittest.TestCase):
-
- def setUp(self):
- self.data_info = dict(img=np.random.random((128, 100, 3)))
-
- def test_init(self):
- # factor is int
- transform = PyramidRescale(factor=4, randomize_factor=False)
- self.assertEqual(transform.factor, 4)
- # factor is float
- with self.assertRaisesRegex(TypeError,
- '`factor` should be an integer'):
- PyramidRescale(factor=4.0)
- # invalid base_shape
- with self.assertRaisesRegex(TypeError,
- '`base_shape` should be a list or tuple'):
- PyramidRescale(base_shape=128)
- with self.assertRaisesRegex(
- ValueError, '`base_shape` should contain two integers'):
- PyramidRescale(base_shape=(128, ))
- with self.assertRaisesRegex(
- ValueError, '`base_shape` should contain two integers'):
- PyramidRescale(base_shape=(128.0, 2.0))
- # invalid randomize_factor
- with self.assertRaisesRegex(TypeError,
- '`randomize_factor` should be a bool'):
- PyramidRescale(randomize_factor=None)
-
- def test_transform(self):
- # test if the rescale keeps the original size
- transform = PyramidRescale()
- results = transform(copy.deepcopy(self.data_info))
- self.assertEqual(results['img'].shape, (128, 100, 3))
- # test factor = 0
- transform = PyramidRescale(factor=0, randomize_factor=False)
- results = transform(copy.deepcopy(self.data_info))
- self.assertTrue(np.all(results['img'] == self.data_info['img']))
-
- def test_repr(self):
- transform = PyramidRescale(
- factor=4, base_shape=(128, 512), randomize_factor=False)
- self.assertEqual(
- repr(transform),
- ('PyramidRescale(factor = 4, randomize_factor = False, '
- 'base_w = 128, base_h = 512)'))
-
-
-class TestRescaleToHeight(unittest.TestCase):
-
- def test_rescale_height(self):
- data_info = dict(
- img=np.random.random((16, 25, 3)),
- gt_seg_map=np.random.random((16, 25, 3)),
- gt_bboxes=np.array([[0, 0, 10, 10]]),
- gt_keypoints=np.array([[[10, 10, 1]]]))
- with self.assertRaises(AssertionError):
- RescaleToHeight(height=20.9)
- with self.assertRaises(AssertionError):
- RescaleToHeight(height=20, min_width=20.9)
- with self.assertRaises(AssertionError):
- RescaleToHeight(height=20, max_width=20.9)
- with self.assertRaises(AssertionError):
- RescaleToHeight(height=20, width_divisor=0.5)
- transform = RescaleToHeight(height=32)
- results = transform(copy.deepcopy(data_info))
- self.assertTupleEqual(results['img'].shape[:2], (32, 50))
- self.assertTupleEqual(results['scale'], (50, 32))
- self.assertTupleEqual(results['scale_factor'], (50 / 25, 32 / 16))
-
- # test min_width
- transform = RescaleToHeight(height=32, min_width=60)
- results = transform(copy.deepcopy(data_info))
- self.assertTupleEqual(results['img'].shape[:2], (32, 60))
- self.assertTupleEqual(results['scale'], (60, 32))
- self.assertTupleEqual(results['scale_factor'], (60 / 25, 32 / 16))
-
- # test max_width
- transform = RescaleToHeight(height=32, max_width=45)
- results = transform(copy.deepcopy(data_info))
- self.assertTupleEqual(results['img'].shape[:2], (32, 45))
- self.assertTupleEqual(results['scale'], (45, 32))
- self.assertTupleEqual(results['scale_factor'], (45 / 25, 32 / 16))
-
- # test width_divisor
- transform = RescaleToHeight(height=32, width_divisor=4)
- results = transform(copy.deepcopy(data_info))
- self.assertTupleEqual(results['img'].shape[:2], (32, 48))
- self.assertTupleEqual(results['scale'], (48, 32))
- self.assertTupleEqual(results['scale_factor'], (48 / 25, 32 / 16))
-
- def test_repr(self):
- transform = RescaleToHeight(height=32)
- self.assertEqual(
- repr(transform), ('RescaleToHeight(height=32, '
- 'min_width=None, max_width=None, '
- 'width_divisor=1, '
- "resize_cfg={'type': 'Resize', 'scale': 0})"))
-
-
-class TestTextRecogGeneralAug(unittest.TestCase):
-
- def setUp(self) -> None:
- self.transform = TextRecogGeneralAug()
-
- @parameterized.expand([(np.random.random((3, 3, 3)), ),
- (np.random.random((10, 10, 3)), ),
- (np.random.random((30, 30, 3)), )])
- def test_transform(self, img):
- data_info = dict(img=img)
- results = self.transform(copy.deepcopy(data_info))
- self.assertEqual(results['img'].shape[:2], results['img_shape'])
-
- def test_repr(self):
- repr_str = self.transform.__repr__()
- self.assertEqual(repr_str, 'TextRecogGeneralAug()')
-
-
-class TestCropHeight(unittest.TestCase):
-
- def setUp(self) -> None:
- self.data_info = dict(img=np.random.random((20, 20, 3)))
-
- @parameterized.expand([
- (3, 3),
- (5, 10),
- ])
- def test_transform(self, min_pixels, max_pixels):
- self.transform = CropHeight(
- min_pixels=min_pixels, max_pixels=max_pixels)
- results = self.transform(copy.deepcopy(self.data_info))
- self.assertEqual(results['img'].shape[:2], results['img_shape'])
- h_diff = self.data_info['img'].shape[0] - results['img_shape'][0]
- self.assertGreaterEqual(h_diff, min_pixels)
- self.assertLessEqual(h_diff, max_pixels)
-
- def test_invalid(self):
- with self.assertRaises(AssertionError):
- self.transform = CropHeight(min_pixels=10, max_pixels=9)
-
- def test_repr(self):
- transform = CropHeight(min_pixels=2, max_pixels=10)
- repr_str = transform.__repr__()
- self.assertEqual(repr_str, 'CropHeight(min_pixels = 2, '
- 'max_pixels = 10)')
-
-
-class TestImageContentJitter(unittest.TestCase):
-
- def setUp(self) -> None:
- self.transform = ImageContentJitter()
-
- @parameterized.expand([(np.random.random((3, 3, 3)), ),
- (np.random.random((10, 10, 3)), ),
- (np.random.random((30, 30, 3)), )])
- def test_transform(self, img):
- data_info = dict(img=img)
- self.transform(copy.deepcopy(data_info))
-
- def test_repr(self):
- repr_str = self.transform.__repr__()
- self.assertEqual(repr_str, 'ImageContentJitter()')
-
-
-class TestReversePixels(unittest.TestCase):
-
- def setUp(self) -> None:
- self.transform = ReversePixels()
-
- @parameterized.expand([(np.random.random((3, 3, 3)), ),
- (np.random.random((10, 10, 3)), ),
- (np.random.random((30, 30, 3)), )])
- def test_transform(self, img):
- data_info = dict(img=img)
- results = self.transform(copy.deepcopy(data_info))
- self.assertTrue(np.array_equal(results['img'], 255. - img))
-
- def test_repr(self):
- repr_str = self.transform.__repr__()
- self.assertEqual(repr_str, 'ReversePixels()')
diff --git a/pipeline/mmocr/tests/test_datasets/test_transforms/test_wrappers.py b/pipeline/mmocr/tests/test_datasets/test_transforms/test_wrappers.py
deleted file mode 100644
index 5195364069078c76568684031c66fe7425019b4b..0000000000000000000000000000000000000000
--- a/pipeline/mmocr/tests/test_datasets/test_transforms/test_wrappers.py
+++ /dev/null
@@ -1,196 +0,0 @@
-# Copyright (c) OpenMMLab. All rights reserved.
-import copy
-import unittest
-from typing import Dict, List, Optional
-
-import numpy as np
-from shapely.geometry import Polygon
-
-from mmocr.datasets.transforms import (ConditionApply, ImgAugWrapper,
- TorchVisionWrapper)
-
-
-class TestImgAug(unittest.TestCase):
-
- def test_init(self):
- with self.assertRaises(AssertionError):
- ImgAugWrapper(args=[])
- with self.assertRaises(AssertionError):
- ImgAugWrapper(args=['test'])
-
- def _create_dummy_data(self):
- img = np.random.rand(50, 50, 3)
- poly = np.array([[[0, 0, 50, 0, 50, 50, 0, 50]],
- [[20, 20, 50, 20, 50, 50, 20, 50]]])
- box = np.array([[0, 0, 50, 50], [20, 20, 50, 50]])
- # It shall always be 0 in MMOCR, but we assign different labels to
- # dummy instances for testing
- labels = np.array([0, 1], dtype=np.int64)
- ignored = np.array([False, True], dtype=bool)
- texts = ['text1', 'text2']
- return dict(
- img=img,
- img_shape=(50, 50),
- gt_polygons=poly,
- gt_bboxes=box,
- gt_bboxes_labels=labels,
- gt_ignored=ignored,
- gt_texts=texts)
-
- def assertPolyEqual(self, poly1: List[np.ndarray],
- poly2: List[np.ndarray]) -> None:
- for p1, p2 in zip(poly1, poly2):
- self.assertTrue(
- Polygon(p1.reshape(-1, 2)).equals(Polygon(p2.reshape(-1, 2))))
-
- def assert_result_equal(self,
- results: Dict,
- poly_targets: List[np.ndarray],
- bbox_targets: np.ndarray,
- bbox_label_targets: np.ndarray,
- ignore_targets: np.ndarray,
- text_targets: Optional[List[str]] = None) -> None:
- self.assertPolyEqual(poly_targets, results['gt_polygons'])
- self.assertTrue(np.array_equal(bbox_targets, results['gt_bboxes']))
- self.assertTrue(
- np.array_equal(bbox_label_targets, results['gt_bboxes_labels']))
- self.assertTrue(np.array_equal(ignore_targets, results['gt_ignored']))
- self.assertEqual(text_targets, results['gt_texts'])
- self.assertEqual(results['img_shape'],
- (results['img'].shape[0], results['img'].shape[1]))
-
- def test_transform(self):
-
- # Test empty transform
- imgaug_transform = ImgAugWrapper(fix_poly_trans=None)
- results = self._create_dummy_data()
- origin_results = copy.deepcopy(results)
- results = imgaug_transform(results)
- self.assert_result_equal(results, origin_results['gt_polygons'],
- origin_results['gt_bboxes'],
- origin_results['gt_bboxes_labels'],
- origin_results['gt_ignored'],
- origin_results['gt_texts'])
-
- args = [dict(cls='Affine', translate_px=dict(x=-10, y=-10))]
- imgaug_transform = ImgAugWrapper(args, fix_poly_trans=None)
- results = self._create_dummy_data()
- results = imgaug_transform(results)
-
- # Polygons and bboxes are partially outside the image after
- # transformation
- poly_target = [
- np.array([0, 0, 40, 0, 40, 40, 0, 40]),
- np.array([10, 10, 40, 10, 40, 40, 10, 40])
- ]
- box_target = np.array([[0, 0, 40, 40], [10, 10, 40, 40]])
- label_target = np.array([0, 1], dtype=np.int64)
- ignored = np.array([False, True], dtype=bool)
- texts = ['text1', 'text2']
- self.assert_result_equal(results, poly_target, box_target,
- label_target, ignored, texts)
-
- # Some polygons and bboxes are no longer inside the image after
- # transformation
- args = [
- dict(cls='Affine', translate_px=dict(x=30, y=30)), ['Fliplr', 1]
- ]
- poly_target = [np.array([0, 30, 20, 30, 20, 50, 0, 50])]
- box_target = np.array([[0, 30, 20, 50]])
- label_target = np.array([0], dtype=np.int64)
- ignored = np.array([False], dtype=bool)
- texts = ['text1']
- imgaug_transform = ImgAugWrapper(args, fix_poly_trans=None)
- results = self._create_dummy_data()
- results = imgaug_transform(results)
- self.assert_result_equal(results, poly_target, box_target,
- label_target, ignored, texts)
-
- # All polygons and bboxes are no longer inside the image after
- # transformation
-
- # When some transforms result in empty polygons
- args = [dict(cls='Affine', translate_px=dict(x=100, y=100))]
- results = self._create_dummy_data()
- invalid_transform = ImgAugWrapper(args)
- results = invalid_transform(results)
- self.assertIsNone(results)
-
- # Everything should work well without gt_texts
- results = self._create_dummy_data()
- del results['gt_texts']
- results = imgaug_transform(results)
- self.assertNotIn('gt_texts', results)
-
- # Everything should work well without keys required from text detection
- results = imgaug_transform(
- dict(
- img=np.random.rand(10, 20, 3),
- img_shape=(10, 20),
- gt_texts=['text1', 'text2']))
- self.assertEqual(results['gt_texts'], ['text1', 'text2'])
-
- def test_repr(self):
- args = [['Resize', [0.5, 3.0]], ['Fliplr', 0.5]]
- transform = ImgAugWrapper(args)
- print(repr(transform))
- self.assertEqual(
- repr(transform),
- ("ImgAugWrapper(args = [['Resize', [0.5, 3.0]], ['Fliplr', 0.5]], "
- "fix_poly_trans = {'type': 'FixInvalidPolygon'})"))
-
-
-class TestTorchVisionWrapper(unittest.TestCase):
-
- def test_transform(self):
- x = {'img': np.ones((128, 100, 3), dtype=np.uint8)}
- # object not found error
- with self.assertRaises(Exception):
- TorchVisionWrapper(op='NonExist')
- with self.assertRaises(TypeError):
- TorchVisionWrapper()
- f = TorchVisionWrapper('Grayscale')
- with self.assertRaises(AssertionError):
- f({})
- results = f(x)
- assert results['img'].shape == (128, 100)
- assert results['img_shape'] == (128, 100)
-
- def test_repr(self):
- f = TorchVisionWrapper('Grayscale', num_output_channels=3)
- self.assertEqual(
- repr(f),
- 'TorchVisionWrapper(op = Grayscale, num_output_channels = 3)')
-
-
-class TestConditionApply(unittest.TestCase):
-
- def test_transform(self):
- dummy_result = dict(img_shape=(100, 200), img=np.zeros((100, 200, 3)))
- resize = dict(type='Resize', scale=(40, 50), keep_ratio=False)
-
- trans = ConditionApply(
- "results['img_shape'][0] > 80", true_transforms=resize)
- results = trans(dummy_result)
- self.assertEqual(results['img_shape'], (50, 40))
- dummy_result = dict(img_shape=(100, 200), img=np.zeros((100, 200, 3)))
- trans = ConditionApply(
- "results['img_shape'][0] < 80", false_transforms=resize)
- results = trans(dummy_result)
- self.assertEqual(results['img_shape'], (50, 40))
- dummy_result = dict(img_shape=(100, 200), img=np.zeros((100, 200, 3)))
- trans = ConditionApply("results['img_shape'][0] < 80")
- results = trans(dummy_result)
- self.assertEqual(results['img_shape'], (100, 200))
-
- def test_repr(self):
- resize = dict(type='Resize', scale=(40, 50), keep_ratio=False)
- trans = ConditionApply(
- "results['img_shape'][0] < 80", true_transforms=resize)
- self.assertEqual(
- repr(trans),
- "ConditionApply(condition = results['img_shape'][0] < 80, "
- 'true_transforms = Compose(\n Resize(scale=(40, 50), '
- 'scale_factor=None, keep_ratio=False, clip_object_border=True), '
- 'backend=cv2), interpolation=bilinear)\n), '
- 'false_transforms = Compose(\n))')
diff --git a/pipeline/mmocr/tests/test_datasets/test_wildreceipt_dataset.py b/pipeline/mmocr/tests/test_datasets/test_wildreceipt_dataset.py
deleted file mode 100644
index 6e9eda753698d2b7bbce249192568ebfe6899059..0000000000000000000000000000000000000000
--- a/pipeline/mmocr/tests/test_datasets/test_wildreceipt_dataset.py
+++ /dev/null
@@ -1,40 +0,0 @@
-# Copyright (c) OpenMMLab. All rights reserved.
-import unittest
-
-from mmocr.datasets import WildReceiptDataset
-
-
-class TestWildReceiptDataset(unittest.TestCase):
-
- def setUp(self):
- metainfo = 'tests/data/kie_toy_dataset/wildreceipt/class_list.txt'
- self.dataset = WildReceiptDataset(
- data_prefix=dict(img_path='data/'),
- ann_file='tests/data/kie_toy_dataset/wildreceipt/data.txt',
- metainfo=metainfo,
- pipeline=[],
- serialize_data=False,
- lazy_init=False)
-
- def test_init(self):
- self.assertEqual(self.dataset.metainfo['category'][0], {
- 'id': '0',
- 'name': 'Ignore'
- })
- self.assertEqual(self.dataset.metainfo['task_name'], 'KIE')
- self.assertEqual(self.dataset.metainfo['dataset_type'],
- 'WildReceiptDataset')
-
- def test_getitem(self):
- data = self.dataset.__getitem__(0)
-
- instance = data['instances'][0]
- self.assertIsInstance(instance['bbox_label'], int)
- self.assertIsInstance(instance['edge_label'], int)
- self.assertIsInstance(instance['text'], str)
- self.assertEqual(instance['bbox'].shape, (4, ))
- self.assertEqual(data['img_shape'], (1200, 1600))
- self.assertEqual(
- data['img_path'],
- 'data/tests/data/kie_toy_dataset/wildreceipt/1.jpeg' # noqa
- )
diff --git a/pipeline/mmocr/tests/test_engine/test_hooks/test_visualization_hook.py b/pipeline/mmocr/tests/test_engine/test_hooks/test_visualization_hook.py
deleted file mode 100644
index 5b4591794123ffa83a45c1ab9b71bc151a31f9ae..0000000000000000000000000000000000000000
--- a/pipeline/mmocr/tests/test_engine/test_hooks/test_visualization_hook.py
+++ /dev/null
@@ -1,79 +0,0 @@
-# Copyright (c) OpenMMLab. All rights reserved.
-import os.path as osp
-import shutil
-import time
-from unittest import TestCase
-from unittest.mock import Mock
-
-import torch
-from mmengine.structures import InstanceData
-
-from mmocr.engine.hooks import VisualizationHook
-from mmocr.structures import TextDetDataSample
-from mmocr.visualization import TextDetLocalVisualizer
-
-
-def _rand_bboxes(num_boxes, h, w):
- cx, cy, bw, bh = torch.rand(num_boxes, 4).T
-
- tl_x = ((cx * w) - (w * bw / 2)).clamp(0, w).unsqueeze(0)
- tl_y = ((cy * h) - (h * bh / 2)).clamp(0, h).unsqueeze(0)
- br_x = ((cx * w) + (w * bw / 2)).clamp(0, w).unsqueeze(0)
- br_y = ((cy * h) + (h * bh / 2)).clamp(0, h).unsqueeze(0)
-
- bboxes = torch.cat([tl_x, tl_y, br_x, br_y], dim=0).T
- return bboxes
-
-
-class TestVisualizationHook(TestCase):
-
- def setUp(self) -> None:
-
- data_sample = TextDetDataSample()
- data_sample.set_metainfo({
- 'img_path':
- osp.join(
- osp.dirname(__file__),
- '../../data/det_toy_dataset/imgs/test/img_1.jpg')
- })
-
- pred_instances = InstanceData()
- pred_instances.bboxes = _rand_bboxes(5, 10, 12)
- pred_instances.labels = torch.randint(0, 2, (5, ))
- pred_instances.scores = torch.rand((5, ))
-
- data_sample.pred_instances = pred_instances
- self.outputs = [data_sample] * 2
- self.data_batch = None
-
- def test_after_val_iter(self):
- timestamp = time.strftime('%Y%m%d_%H%M%S', time.localtime())
- TextDetLocalVisualizer.get_instance(
- 'visualizer_val',
- vis_backends=[dict(type='LocalVisBackend', img_save_dir='')],
- save_dir=timestamp)
- runner = Mock()
- runner.iter = 1
- hook = VisualizationHook(enable=True, interval=1)
- self.assertFalse(osp.exists(timestamp))
- hook.after_val_iter(runner, 1, self.data_batch, self.outputs)
- self.assertTrue(osp.exists(timestamp))
- shutil.rmtree(timestamp)
-
- def test_after_test_iter(self):
- timestamp = time.strftime('%Y%m%d_%H%M%S', time.localtime())
- TextDetLocalVisualizer.get_instance(
- 'visualizer_test',
- vis_backends=[dict(type='LocalVisBackend', img_save_dir='')],
- save_dir=timestamp)
- runner = Mock()
- runner.iter = 1
-
- hook = VisualizationHook(enable=False)
- hook.after_test_iter(runner, 1, self.data_batch, self.outputs)
- self.assertFalse(osp.exists(timestamp))
-
- hook = VisualizationHook(enable=True)
- hook.after_test_iter(runner, 1, self.data_batch, self.outputs)
- self.assertTrue(osp.exists(timestamp))
- shutil.rmtree(timestamp)
diff --git a/pipeline/mmocr/tests/test_evaluation/test_evaluator/test_multi_datasets_evaluator.py b/pipeline/mmocr/tests/test_evaluation/test_evaluator/test_multi_datasets_evaluator.py
deleted file mode 100644
index 0b2ae3d7323cef792c5531f5c15001d9ca8b9e67..0000000000000000000000000000000000000000
--- a/pipeline/mmocr/tests/test_evaluation/test_evaluator/test_multi_datasets_evaluator.py
+++ /dev/null
@@ -1,128 +0,0 @@
-# Copyright (c) OpenMMLab. All rights reserved.
-
-import math
-from typing import Dict, List, Optional
-from unittest import TestCase
-
-import numpy as np
-from mmengine.evaluator import BaseMetric
-from mmengine.registry import METRICS, DefaultScope
-from mmengine.structures import BaseDataElement
-
-from mmocr.evaluation import MultiDatasetsEvaluator
-
-
-@METRICS.register_module()
-class ToyMetric(BaseMetric):
- """Evaluator that calculates the metric `accuracy` from predictions and
- labels. Alternatively, this evaluator can return arbitrary dummy metrics
- set in the config.
-
- Default prefix: Toy
-
- Metrics:
- - accuracy (float): The classification accuracy. Only when
- `dummy_metrics` is None.
- - size (int): The number of test samples. Only when `dummy_metrics`
- is None.
-
- If `dummy_metrics` is set as a dict in the config, it will be
- returned as the metrics and override `accuracy` and `size`.
- """
-
- default_prefix = None
-
- def __init__(self,
- collect_device: str = 'cpu',
- prefix: Optional[str] = 'Toy',
- dummy_metrics: Optional[Dict] = None):
- super().__init__(collect_device=collect_device, prefix=prefix)
- self.dummy_metrics = dummy_metrics
-
- def process(self, data_batch, predictions):
- results = [{
- 'pred': prediction['pred'],
- 'label': prediction['label']
- } for prediction in predictions]
- self.results.extend(results)
-
- def compute_metrics(self, results: List):
- if self.dummy_metrics is not None:
- assert isinstance(self.dummy_metrics, dict)
- return self.dummy_metrics.copy()
-
- pred = np.array([result['pred'] for result in results])
- label = np.array([result['label'] for result in results])
- acc = (pred == label).sum() / pred.size
-
- metrics = {
- 'accuracy': acc,
- 'size': pred.size, # To check the number of testing samples
- }
-
- return metrics
-
-
-def generate_test_results(size, batch_size, pred, label):
- num_batch = math.ceil(size / batch_size)
- bs_residual = size % batch_size
- for i in range(num_batch):
- bs = bs_residual if i == num_batch - 1 else batch_size
- data_batch = {
- 'inputs': [np.zeros((3, 10, 10)) for _ in range(bs)],
- 'data_samples': [BaseDataElement(label=label) for _ in range(bs)]
- }
- predictions = [
- BaseDataElement(pred=pred, label=label) for _ in range(bs)
- ]
- yield (data_batch, predictions)
-
-
-class TestMultiDatasetsEvaluator(TestCase):
-
- def test_composed_metrics(self):
- DefaultScope.get_instance('mmocr_metric', scope_name='mmocr')
- cfg = [
- dict(type='ToyMetric'),
- dict(type='ToyMetric', dummy_metrics=dict(mAP=0.0))
- ]
-
- evaluator = MultiDatasetsEvaluator(cfg, dataset_prefixes=['Fake'])
- evaluator.dataset_meta = {}
- size = 10
- batch_size = 4
-
- for data_samples, predictions in generate_test_results(
- size, batch_size, pred=1, label=1):
- evaluator.process(predictions, data_samples)
-
- metrics = evaluator.evaluate(size=size)
-
- self.assertAlmostEqual(metrics['Fake/Toy/accuracy'], 1.0)
- self.assertAlmostEqual(metrics['Fake/Toy/mAP'], 0.0)
- self.assertEqual(metrics['Fake/Toy/size'], size)
- with self.assertWarns(Warning):
- evaluator.evaluate(size=0)
-
- cfg = [dict(type='ToyMetric'), dict(type='ToyMetric')]
-
- evaluator = MultiDatasetsEvaluator(cfg, dataset_prefixes=['Fake'])
- evaluator.dataset_meta = {}
-
- for data_samples, predictions in generate_test_results(
- size, batch_size, pred=1, label=1):
- evaluator.process(predictions, data_samples)
- with self.assertRaises(ValueError):
- evaluator.evaluate(size=size)
-
- cfg = [dict(type='ToyMetric'), dict(type='ToyMetric', prefix=None)]
-
- evaluator = MultiDatasetsEvaluator(cfg, dataset_prefixes=['Fake'])
- evaluator.dataset_meta = {}
-
- for data_samples, predictions in generate_test_results(
- size, batch_size, pred=1, label=1):
- evaluator.process(predictions, data_samples)
- metrics = evaluator.evaluate(size=size)
- self.assertIn('Fake/Toy/accuracy', metrics)
- self.assertIn('Fake/accuracy', metrics)
diff --git a/pipeline/mmocr/tests/test_evaluation/test_functional/test_hmean.py b/pipeline/mmocr/tests/test_evaluation/test_functional/test_hmean.py
deleted file mode 100644
index 9fdde22809394e7a12ab5621080a54f1455a1236..0000000000000000000000000000000000000000
--- a/pipeline/mmocr/tests/test_evaluation/test_functional/test_hmean.py
+++ /dev/null
@@ -1,23 +0,0 @@
-# Copyright (c) OpenMMLab. All rights reserved.
-from unittest import TestCase
-
-from mmocr.evaluation.functional import compute_hmean
-
-
-class TestHmean(TestCase):
-
- def test_compute_hmean(self):
- with self.assertRaises(AssertionError):
- compute_hmean(0, 0, 0.0, 0)
- with self.assertRaises(AssertionError):
- compute_hmean(0, 0, 0, 0.0)
- with self.assertRaises(AssertionError):
- compute_hmean([1], 0, 0, 0)
- with self.assertRaises(AssertionError):
- compute_hmean(0, [1], 0, 0)
-
- _, _, hmean = compute_hmean(2, 2, 2, 2)
- self.assertEqual(hmean, 1)
-
- _, _, hmean = compute_hmean(0, 0, 2, 2)
- self.assertEqual(hmean, 0)
diff --git a/pipeline/mmocr/tests/test_evaluation/test_metrics/test_f_metric.py b/pipeline/mmocr/tests/test_evaluation/test_metrics/test_f_metric.py
deleted file mode 100644
index 5529580b166e3040bb2b02930d72499eb967bc2e..0000000000000000000000000000000000000000
--- a/pipeline/mmocr/tests/test_evaluation/test_metrics/test_f_metric.py
+++ /dev/null
@@ -1,157 +0,0 @@
-# Copyright (c) OpenMMLab. All rights reserved.
-import unittest
-
-import torch
-from mmengine.structures import InstanceData
-
-from mmocr.evaluation import F1Metric
-from mmocr.structures import KIEDataSample
-
-
-class TestF1Metric(unittest.TestCase):
-
- def test_init(self):
- with self.assertRaises(AssertionError):
- F1Metric(num_classes='3')
-
- with self.assertRaises(AssertionError):
- F1Metric(num_classes=3, ignored_classes=[1], cared_classes=[0])
-
- with self.assertRaises(AssertionError):
- F1Metric(num_classes=3, ignored_classes=1)
-
- with self.assertRaises(AssertionError):
- F1Metric(num_classes=2, mode=1)
-
- with self.assertRaises(AssertionError):
- F1Metric(num_classes=1, mode='1')
-
- def test_macro_f1(self):
- mode = 'macro'
- preds_cases = [
- [
- KIEDataSample(
- pred_instances=InstanceData(
- labels=torch.LongTensor([0, 1, 2])),
- gt_instances=InstanceData(
- labels=torch.LongTensor([0, 1, 4])))
- ],
- [
- KIEDataSample(
- gt_instances=InstanceData(labels=torch.LongTensor([0, 1])),
- pred_instances=InstanceData(
- labels=torch.LongTensor([0, 1]))),
- KIEDataSample(
- gt_instances=InstanceData(labels=torch.LongTensor([4])),
- pred_instances=InstanceData(labels=torch.LongTensor([2])))
- ]
- ]
-
- # num_classes < the maximum label index
- metric = F1Metric(num_classes=3, ignored_classes=[1])
- metric.process(None, preds_cases[0])
- with self.assertRaises(AssertionError):
- metric.evaluate(size=1)
-
- for preds in preds_cases:
- metric = F1Metric(num_classes=5, mode=mode)
- metric.process(None, preds)
- result = metric.evaluate(size=len(preds))
- self.assertAlmostEqual(result['kie/macro_f1'], 0.4)
-
- # Test ignored_classes
- metric = F1Metric(num_classes=5, ignored_classes=[1], mode=mode)
- metric.process(None, preds)
- result = metric.evaluate(size=len(preds))
- self.assertAlmostEqual(result['kie/macro_f1'], 0.25)
-
- # Test cared_classes
- metric = F1Metric(
- num_classes=5, cared_classes=[0, 2, 3, 4], mode=mode)
- metric.process(None, preds)
- result = metric.evaluate(size=len(preds))
- self.assertAlmostEqual(result['kie/macro_f1'], 0.25)
-
- def test_micro_f1(self):
- mode = 'micro'
- preds_cases = [[
- KIEDataSample(
- gt_instances=InstanceData(
- labels=torch.LongTensor([0, 1, 0, 1, 2])),
- pred_instances=InstanceData(
- labels=torch.LongTensor([0, 1, 2, 2, 0])))
- ],
- [
- KIEDataSample(
- gt_instances=InstanceData(
- labels=torch.LongTensor([0, 1, 2])),
- pred_instances=InstanceData(
- labels=torch.LongTensor([0, 1, 0]))),
- KIEDataSample(
- gt_instances=InstanceData(
- labels=torch.LongTensor([0, 1])),
- pred_instances=InstanceData(
- labels=torch.LongTensor([2, 2])))
- ]]
-
- # num_classes < the maximum label index
- metric = F1Metric(num_classes=1, ignored_classes=[0], mode=mode)
- metric.process(None, preds_cases[0])
- with self.assertRaises(AssertionError):
- metric.evaluate(size=1)
-
- for preds in preds_cases:
- # class 0: tp: 1, fp: 1, fn: 1
- # class 1: tp: 1, fp: 1, fn: 0
- # class 2: tp: 0, fp: 1, fn: 2
- # overall: tp: 2, fp: 3, fn: 3
- # f1: 0.4
-
- metric = F1Metric(num_classes=3, mode=mode)
- metric.process(None, preds)
- result = metric.evaluate(size=len(preds))
- self.assertAlmostEqual(result['kie/micro_f1'], 0.4, delta=0.01)
-
- metric = F1Metric(num_classes=5, mode=mode)
- metric.process(None, preds)
- result = metric.evaluate(size=len(preds))
- self.assertAlmostEqual(result['kie/micro_f1'], 0.4, delta=0.01)
-
- # class 0: tp: 1, fp: 1, fn: 1
- # class 2: tp: 0, fp: 1, fn: 2
- # overall: tp: 1, fp: 2, fn: 3
- # f1: 0.285
-
- metric = F1Metric(num_classes=5, ignored_classes=[1], mode=mode)
- metric.process(None, preds)
- result = metric.evaluate(size=len(preds))
- self.assertAlmostEqual(result['kie/micro_f1'], 0.285, delta=0.001)
-
- metric = F1Metric(
- num_classes=5, cared_classes=[0, 2, 3, 4], mode=mode)
- metric.process(None, preds)
- result = metric.evaluate(size=len(preds))
- self.assertAlmostEqual(result['kie/micro_f1'], 0.285, delta=0.001)
-
- def test_arguments(self):
- mode = ['micro', 'macro']
- preds = [
- KIEDataSample(
- gt_instances=InstanceData(
- test_labels=torch.LongTensor([0, 1, 0, 1, 2])),
- pred_instances=InstanceData(
- test_labels=torch.LongTensor([0, 1, 2, 2, 0])))
- ]
-
- # class 0: tp: 1, fp: 1, fn: 1
- # class 1: tp: 1, fp: 1, fn: 0
- # class 2: tp: 0, fp: 1, fn: 2
- # overall: tp: 2, fp: 3, fn: 3
- # micro_f1: 0.4
- # macro_f1:
-
- metric = F1Metric(num_classes=3, mode=mode, key='test_labels')
- metric.process(None, preds)
- result = metric.evaluate(size=1)
- self.assertAlmostEqual(result['kie/micro_f1'], 0.4, delta=0.01)
- self.assertAlmostEqual(result['kie/macro_f1'], 0.39, delta=0.01)
diff --git a/pipeline/mmocr/tests/test_evaluation/test_metrics/test_hmean_iou_metric.py b/pipeline/mmocr/tests/test_evaluation/test_metrics/test_hmean_iou_metric.py
deleted file mode 100644
index 6aa31666d599eec46c3dadcded88af1938e1120f..0000000000000000000000000000000000000000
--- a/pipeline/mmocr/tests/test_evaluation/test_metrics/test_hmean_iou_metric.py
+++ /dev/null
@@ -1,100 +0,0 @@
-# Copyright (c) OpenMMLab. All rights reserved.
-import unittest
-
-import numpy as np
-import torch
-from mmengine.structures import InstanceData
-
-from mmocr.evaluation import HmeanIOUMetric
-from mmocr.structures import TextDetDataSample
-
-
-class TestHmeanIOU(unittest.TestCase):
-
- def setUp(self):
- """Create dummy test data.
-
- We denote the polygons as the following.
- gt_polys: gt_a, gt_b, gt_c, gt_d_ignored
- pred_polys: pred_a, pred_b, pred_c, pred_d
-
- There are two pairs of matches: (gt_a, pred_a) and (gt_b, pred_b),
- because the IoU > threshold.
-
- gt_c and pred_c do not match any of the polygons.
-
- pred_d is ignored in the recall computation since it overlaps
- gt_d_ignored and the precision > ignore_precision_thr.
- """
- data_sample = TextDetDataSample()
- gt_instances = InstanceData()
- gt_instances.polygons = [
- torch.FloatTensor([0, 0, 1, 0, 1, 1, 0, 1]),
- torch.FloatTensor([2, 0, 3, 0, 3, 1, 2, 1]),
- torch.FloatTensor([10, 0, 11, 0, 11, 1, 10, 1]),
- torch.FloatTensor([1, 0, 2, 0, 2, 1, 1, 1]),
- ]
- gt_instances.ignored = np.bool_([False, False, False, True])
- pred_instances = InstanceData()
- pred_instances.polygons = [
- torch.FloatTensor([0, 0, 1, 0, 1, 1, 0, 1]),
- torch.FloatTensor([2, 0.1, 3, 0.1, 3, 1.1, 2, 1.1]),
- torch.FloatTensor([1, 1, 2, 1, 2, 2, 1, 2]),
- torch.FloatTensor([1, -0.5, 2, -0.5, 2, 0.5, 1, 0.5]),
- ]
- pred_instances.scores = torch.FloatTensor([1, 1, 1, 0.001])
- data_sample.gt_instances = gt_instances
- data_sample.pred_instances = pred_instances
- predictions = [data_sample.to_dict()]
-
- data_sample = TextDetDataSample()
- gt_instances = InstanceData()
- gt_instances.polygons = [torch.FloatTensor([0, 0, 1, 0, 1, 1, 0, 1])]
- gt_instances.ignored = np.bool_([False])
- pred_instances = InstanceData()
- pred_instances.polygons = [
- torch.FloatTensor([0, 0, 1, 0, 1, 1, 0, 1]),
- torch.FloatTensor([0, 0, 1, 0, 1, 1, 0, 1])
- ]
- pred_instances.scores = torch.FloatTensor([1, 0.95])
- data_sample.gt_instances = gt_instances
- data_sample.pred_instances = pred_instances
- predictions.append(data_sample.to_dict())
-
- self.predictions = predictions
-
- def test_hmean_iou(self):
-
- metric = HmeanIOUMetric(prefix='mmocr')
- metric.process(None, self.predictions)
- eval_results = metric.evaluate(size=2)
-
- precision = 3 / 5
- recall = 3 / 4
- hmean = 2 * precision * recall / (precision + recall)
- target_result = {
- 'mmocr/precision': precision,
- 'mmocr/recall': recall,
- 'mmocr/hmean': hmean
- }
- self.assertDictEqual(target_result, eval_results)
-
- def test_compute_metrics(self):
- # Test different strategies
- fake_results = [
- dict(
- iou_metric=np.array([[1, 1], [1, 0]]),
- pred_scores=np.array([1., 1.]))
- ]
-
- # Vanilla
- metric = HmeanIOUMetric(strategy='vanilla')
- eval_results = metric.compute_metrics(fake_results)
- target_result = {'precision': 0.5, 'recall': 0.5, 'hmean': 0.5}
- self.assertDictEqual(target_result, eval_results)
-
- # Max matching
- metric = HmeanIOUMetric(strategy='max_matching')
- eval_results = metric.compute_metrics(fake_results)
- target_result = {'precision': 1, 'recall': 1, 'hmean': 1}
- self.assertDictEqual(target_result, eval_results)
diff --git a/pipeline/mmocr/tests/test_evaluation/test_metrics/test_recog_metric.py b/pipeline/mmocr/tests/test_evaluation/test_metrics/test_recog_metric.py
deleted file mode 100644
index cd982b160aa2c9277e5ef0f234d1a73bf2fd075f..0000000000000000000000000000000000000000
--- a/pipeline/mmocr/tests/test_evaluation/test_metrics/test_recog_metric.py
+++ /dev/null
@@ -1,124 +0,0 @@
-# Copyright (c) OpenMMLab. All rights reserved.
-import unittest
-
-from mmengine.structures import LabelData
-
-from mmocr.evaluation import CharMetric, OneMinusNEDMetric, WordMetric
-from mmocr.structures import TextRecogDataSample
-
-
-class TestWordMetric(unittest.TestCase):
-
- def setUp(self):
-
- self.pred = []
- data_sample = TextRecogDataSample()
- pred_text = LabelData()
- pred_text.item = 'hello'
- data_sample.pred_text = pred_text
- gt_text = LabelData()
- gt_text.item = 'hello'
- data_sample.gt_text = gt_text
- self.pred.append(data_sample)
- data_sample = TextRecogDataSample()
- pred_text = LabelData()
- pred_text.item = 'hello'
- data_sample.pred_text = pred_text
- gt_text = LabelData()
- gt_text.item = 'HELLO'
- data_sample.gt_text = gt_text
- self.pred.append(data_sample)
- data_sample = TextRecogDataSample()
- pred_text = LabelData()
- pred_text.item = 'hello'
- data_sample.pred_text = pred_text
- gt_text = LabelData()
- gt_text.item = '$HELLO$'
- data_sample.gt_text = gt_text
- self.pred.append(data_sample)
-
- def test_word_acc_metric(self):
- metric = WordMetric(mode='exact')
- metric.process(None, self.pred)
- eval_res = metric.evaluate(size=3)
- self.assertAlmostEqual(eval_res['recog/word_acc'], 1. / 3, 4)
-
- def test_word_acc_ignore_case_metric(self):
- metric = WordMetric(mode='ignore_case')
- metric.process(None, self.pred)
- eval_res = metric.evaluate(size=3)
- self.assertAlmostEqual(eval_res['recog/word_acc_ignore_case'], 2. / 3,
- 4)
-
- def test_word_acc_ignore_case_symbol_metric(self):
- metric = WordMetric(mode='ignore_case_symbol')
- metric.process(None, self.pred)
- eval_res = metric.evaluate(size=3)
- self.assertEqual(eval_res['recog/word_acc_ignore_case_symbol'], 1.0)
-
- def test_all_metric(self):
- metric = WordMetric(
- mode=['exact', 'ignore_case', 'ignore_case_symbol'])
- metric.process(None, self.pred)
- eval_res = metric.evaluate(size=3)
- self.assertAlmostEqual(eval_res['recog/word_acc'], 1. / 3, 4)
- self.assertAlmostEqual(eval_res['recog/word_acc_ignore_case'], 2. / 3,
- 4)
- self.assertEqual(eval_res['recog/word_acc_ignore_case_symbol'], 1.0)
-
-
-class TestCharMetric(unittest.TestCase):
-
- def setUp(self):
- self.pred = []
- data_sample = TextRecogDataSample()
- pred_text = LabelData()
- pred_text.item = 'helL'
- data_sample.pred_text = pred_text
- gt_text = LabelData()
- gt_text.item = 'hello'
- data_sample.gt_text = gt_text
- self.pred.append(data_sample)
- data_sample = TextRecogDataSample()
- pred_text = LabelData()
- pred_text.item = 'HEL'
- data_sample.pred_text = pred_text
- gt_text = LabelData()
- gt_text.item = 'HELLO'
- data_sample.gt_text = gt_text
- self.pred.append(data_sample)
-
- def test_char_recall_precision_metric(self):
- metric = CharMetric()
- metric.process(None, self.pred)
- eval_res = metric.evaluate(size=2)
- self.assertEqual(eval_res['recog/char_recall'], 0.7)
- self.assertEqual(eval_res['recog/char_precision'], 1)
-
-
-class TestOneMinusNED(unittest.TestCase):
-
- def setUp(self):
- self.pred = []
- data_sample = TextRecogDataSample()
- pred_text = LabelData()
- pred_text.item = 'pred_helL'
- data_sample.pred_text = pred_text
- gt_text = LabelData()
- gt_text.item = 'hello'
- data_sample.gt_text = gt_text
- self.pred.append(data_sample)
- data_sample = TextRecogDataSample()
- pred_text = LabelData()
- pred_text.item = 'HEL'
- data_sample.pred_text = pred_text
- gt_text = LabelData()
- gt_text.item = 'HELLO'
- data_sample.gt_text = gt_text
- self.pred.append(data_sample)
-
- def test_one_minus_ned_metric(self):
- metric = OneMinusNEDMetric()
- metric.process(None, self.pred)
- eval_res = metric.evaluate(size=2)
- self.assertEqual(eval_res['recog/1-N.E.D'], 0.4875)
diff --git a/pipeline/mmocr/tests/test_models/test_textdet/test_heads/test_base_head.py b/pipeline/mmocr/tests/test_models/test_textdet/test_heads/test_base_head.py
deleted file mode 100644
index 4693ccab47c9fcac66289aed81d18001e51fb5cb..0000000000000000000000000000000000000000
--- a/pipeline/mmocr/tests/test_models/test_textdet/test_heads/test_base_head.py
+++ /dev/null
@@ -1,62 +0,0 @@
-# Copyright (c) OpenMMLab. All rights reserved.
-from unittest import TestCase, mock
-
-from mmocr.models.textdet import BaseTextDetHead
-from mmocr.registry import MODELS
-
-
-@MODELS.register_module()
-class FakeModule:
-
- def __init__(self) -> None:
- pass
-
- def get_targets(self, datasamples):
- return None
-
- def __call__(self, *args):
- return None
-
-
-class TestBaseTextDetHead(TestCase):
-
- def test_init(self):
- cfg = dict(type='FakeModule')
-
- with self.assertRaises(AssertionError):
- BaseTextDetHead([], cfg)
- with self.assertRaises(AssertionError):
- BaseTextDetHead(cfg, [])
-
- decoder = BaseTextDetHead(None, None)
- self.assertIsNone(decoder.module_loss)
- self.assertIsNone(decoder.postprocessor)
- decoder = BaseTextDetHead(cfg, cfg)
- self.assertIsInstance(decoder.module_loss, FakeModule)
- self.assertIsInstance(decoder.postprocessor, FakeModule)
-
- @mock.patch(f'{__name__}.BaseTextDetHead.forward')
- def test_forward(self, mock_forward):
-
- def mock_forward(feat, out_enc, datasamples):
-
- return True
-
- mock_forward.side_effect = mock_forward
- cfg = dict(type='FakeModule')
- decoder = BaseTextDetHead(cfg, cfg)
- # test loss
- loss = decoder.loss(None, None)
- self.assertIsNone(loss)
-
- # test predict
- predict = decoder.predict(None, None)
- self.assertIsNone(predict)
-
- # test forward
- tensor = decoder(None, None)
- self.assertTrue(tensor)
-
- loss, predict = decoder.loss_and_predict(None, None)
- self.assertIsNone(loss)
- self.assertIsNone(predict)
diff --git a/pipeline/mmocr/tests/test_models/test_textdet/test_heads/test_db_head.py b/pipeline/mmocr/tests/test_models/test_textdet/test_heads/test_db_head.py
deleted file mode 100644
index 6f39927f906528cfc8b75a66a845c5989102056f..0000000000000000000000000000000000000000
--- a/pipeline/mmocr/tests/test_models/test_textdet/test_heads/test_db_head.py
+++ /dev/null
@@ -1,45 +0,0 @@
-# Copyright (c) OpenMMLab. All rights reserved.
-from unittest import TestCase
-
-import torch
-
-from mmocr.models.textdet.heads import DBHead
-from mmocr.registry import MODELS
-
-
-class TestDBHead(TestCase):
-
- # Use to replace module loss and postprocessors
- @MODELS.register_module(name='DBDummy')
- class DummyModule:
-
- def __call__(self, x, data_samples):
- return x
-
- def setUp(self) -> None:
- self.db_head = DBHead(
- in_channels=10,
- module_loss=dict(type='DBDummy'),
- postprocessor=dict(type='DBDummy'))
-
- def test_init(self):
- with self.assertRaises(AssertionError):
- DBHead(in_channels='test', with_bias=False)
-
- with self.assertRaises(AssertionError):
- DBHead(in_channels=1, with_bias='Text')
-
- def test_forward(self):
- data = torch.randn((2, 10, 40, 50))
-
- results = self.db_head(data, None, 'loss')
- for i in range(3):
- self.assertEqual(results[i].shape, (2, 160, 200))
-
- results = self.db_head(data, None, 'predict')
- self.assertEqual(results.shape, (2, 160, 200))
-
- results = self.db_head(data, None, 'both')
- for i in range(4):
- self.assertEqual(results[i].shape, (2, 160, 200))
- self.assertTrue(torch.allclose(results[3], results[0].sigmoid()))
diff --git a/pipeline/mmocr/tests/test_models/test_textdet/test_heads/test_drrg_head.py b/pipeline/mmocr/tests/test_models/test_textdet/test_heads/test_drrg_head.py
deleted file mode 100644
index 9ec3681b96e86cfb00d8efa5866ebf9df15edb27..0000000000000000000000000000000000000000
--- a/pipeline/mmocr/tests/test_models/test_textdet/test_heads/test_drrg_head.py
+++ /dev/null
@@ -1,192 +0,0 @@
-# Copyright (c) OpenMMLab. All rights reserved.
-from unittest import TestCase, mock
-
-import numpy as np
-import torch
-
-from mmocr.models.textdet.heads.drrg_head import (GCN, DRRGHead, LocalGraphs,
- ProposalLocalGraphs,
- feature_embedding,
- normalize_adjacent_matrix)
-
-
-class TestDRRGHead(TestCase):
-
- def setUp(self) -> None:
- self.drrg_head = DRRGHead(in_channels=10)
-
- @mock.patch(
- 'mmocr.models.textdet.module_losses.drrg_module_loss.DRRGModuleLoss.'
- 'get_targets')
- @mock.patch(
- 'mmocr.models.textdet.module_losses.drrg_module_loss.DRRGModuleLoss.'
- 'forward')
- def test_loss(self, mock_forward, mock_get_targets):
- num_rois = 16
- feature_maps = torch.randn((2, 10, 128, 128), dtype=torch.float)
- x = np.random.randint(4, 124, (num_rois, 1))
- y = np.random.randint(4, 124, (num_rois, 1))
- h = 4 * np.ones((num_rois, 1))
- w = 4 * np.ones((num_rois, 1))
- angle = (np.random.random_sample((num_rois, 1)) * 2 - 1) * np.pi / 2
- cos, sin = np.cos(angle), np.sin(angle)
- comp_labels = np.random.randint(1, 3, (num_rois, 1))
- num_rois = num_rois * np.ones((num_rois, 1))
- comp_attribs = np.hstack([num_rois, x, y, h, w, cos, sin, comp_labels])
- comp_attribs = comp_attribs.astype(np.float32)
- comp_attribs_ = comp_attribs.copy()
- comp_attribs = np.stack([comp_attribs, comp_attribs_])
- mock_get_targets.return_value = (None, None, None, None, None, None,
- None, comp_attribs)
- mock_forward.side_effect = lambda *args: args[0]
- # It returns the tensor input to module loss
- pred_maps, pred_labels, gt_labels = self.drrg_head.loss(
- feature_maps, None)
- self.assertEqual(pred_maps.size(), (2, 6, 128, 128))
- self.assertTrue(pred_labels.ndim == gt_labels.ndim == 2)
- self.assertEqual(gt_labels.size()[0] * gt_labels.size()[1],
- pred_labels.size()[0])
- self.assertEqual(pred_labels.size()[1], 2)
-
- def test_predict(self):
- with torch.no_grad():
- feat_maps = torch.zeros((1, 10, 128, 128))
- self.drrg_head.out_conv.bias.data.fill_(-10)
- preds = self.drrg_head(feat_maps)
- self.assertTrue(all([pred is None for pred in preds]))
-
-
-class TestLocalGraphs(TestCase):
-
- def test_call(self):
- geo_feat_len = 24
- pooling_h, pooling_w = pooling_out_size = (2, 2)
- num_rois = 32
-
- local_graph_generator = LocalGraphs((4, 4), 3, geo_feat_len, 1.0,
- pooling_out_size, 0.5)
-
- feature_maps = torch.randn((2, 3, 128, 128), dtype=torch.float)
- x = np.random.randint(4, 124, (num_rois, 1))
- y = np.random.randint(4, 124, (num_rois, 1))
- h = 4 * np.ones((num_rois, 1))
- w = 4 * np.ones((num_rois, 1))
- angle = (np.random.random_sample((num_rois, 1)) * 2 - 1) * np.pi / 2
- cos, sin = np.cos(angle), np.sin(angle)
- comp_labels = np.random.randint(1, 3, (num_rois, 1))
- num_rois = num_rois * np.ones((num_rois, 1))
- comp_attribs = np.hstack([num_rois, x, y, h, w, cos, sin, comp_labels])
- comp_attribs = comp_attribs.astype(np.float32)
- comp_attribs_ = comp_attribs.copy()
- comp_attribs = np.stack([comp_attribs, comp_attribs_])
-
- (node_feats, adjacent_matrix, knn_inds,
- linkage_labels) = local_graph_generator(feature_maps, comp_attribs)
- feat_len = geo_feat_len + \
- feature_maps.size()[1] * pooling_h * pooling_w
-
- self.assertTrue(node_feats.dim() == adjacent_matrix.dim() == 3)
- self.assertEqual(node_feats.size()[-1], feat_len)
- self.assertEqual(knn_inds.size()[-1], 4)
- self.assertEqual(linkage_labels.size()[-1], 4)
- self.assertTrue(node_feats.size()[0] == adjacent_matrix.size()[0] ==
- knn_inds.size()[0] == linkage_labels.size()[0])
- self.assertTrue(node_feats.size()[1] == adjacent_matrix.size()[1] ==
- adjacent_matrix.size()[2])
-
-
-class TestProposalLocalGraphs(TestCase):
-
- def test_call(self):
- geo_feat_len = 24
- pooling_h, pooling_w = pooling_out_size = (2, 2)
-
- local_graph_generator = ProposalLocalGraphs(
- (4, 4), 2, geo_feat_len, 1., pooling_out_size, 0.1, 3., 6., 1.,
- 0.5, 0.3, 0.5, 0.5, 2)
-
- maps = torch.zeros((1, 6, 224, 224), dtype=torch.float)
- maps[:, 0:2, :, :] = -10.
- maps[:, 0, 60:100, 50:170] = 10.
- maps[:, 1, 75:85, 60:160] = 10.
- maps[:, 2, 75:85, 60:160] = 0.
- maps[:, 3, 75:85, 60:160] = 1.
- maps[:, 4, 75:85, 60:160] = 10.
- maps[:, 5, 75:85, 60:160] = 10.
- feature_maps = torch.randn((2, 6, 224, 224), dtype=torch.float)
- feat_len = geo_feat_len + \
- feature_maps.size()[1] * pooling_h * pooling_w
-
- none_flag, graph_data = local_graph_generator(maps, feature_maps)
- (node_feats, adjacent_matrices, knn_inds, local_graphs,
- text_comps) = graph_data
-
- self.assertFalse(none_flag, False)
- self.assertEqual(text_comps.ndim, 2)
- self.assertGreater(text_comps.shape[0], 0)
- self.assertEqual(text_comps.shape[1], 9)
- self.assertTrue(
- node_feats.size()[0] == adjacent_matrices.size()[0] == knn_inds.
- size()[0] == local_graphs.size()[0] == text_comps.shape[0])
- self.assertTrue(node_feats.size()[1] == adjacent_matrices.size()[1] ==
- adjacent_matrices.size()[2] == local_graphs.size()[1])
- self.assertEqual(node_feats.size()[-1], feat_len)
-
- # test proposal local graphs with area of center region less than
- # threshold
- maps[:, 1, 75:85, 60:160] = -10.
- maps[:, 1, 80, 80] = 10.
- none_flag, _ = local_graph_generator(maps, feature_maps)
- self.assertTrue(none_flag)
-
- # test proposal local graphs with one text component
- local_graph_generator = ProposalLocalGraphs(
- (4, 4), 2, geo_feat_len, 1., pooling_out_size, 0.1, 8., 20., 1.,
- 0.5, 0.3, 0.5, 0.5, 2)
- maps[:, 1, 78:82, 78:82] = 10.
- none_flag, _ = local_graph_generator(maps, feature_maps)
- self.assertTrue(none_flag)
-
- # test proposal local graphs with text components out of text region
- maps[:, 0, 60:100, 50:170] = -10.
- maps[:, 0, 78:82, 78:82] = 10.
- none_flag, _ = local_graph_generator(maps, feature_maps)
- self.assertTrue(none_flag)
-
-
-class TestUtils(TestCase):
-
- def test_normalize_adjacent_matrix(self):
- adjacent_matrix = np.random.randint(0, 2, (16, 16))
- normalized_matrix = normalize_adjacent_matrix(adjacent_matrix)
- self.assertEqual(normalized_matrix.shape, adjacent_matrix.shape)
-
- def test_feature_embedding(self):
- out_feat_len = 48
-
- # test without residue dimensions
- feats = np.random.randn(10, 8)
- embed_feats = feature_embedding(feats, out_feat_len)
- self.assertEqual(embed_feats.shape, (10, out_feat_len))
-
- # test with residue dimensions
- feats = np.random.randn(10, 9)
- embed_feats = feature_embedding(feats, out_feat_len)
- self.assertEqual(embed_feats.shape, (10, out_feat_len))
-
-
-class TestGCN(TestCase):
-
- def test_forward(self):
- num_local_graphs = 32
- num_max_graph_nodes = 16
- input_feat_len = 512
- k = 8
- gcn = GCN(input_feat_len)
- node_feat = torch.randn(
- (num_local_graphs, num_max_graph_nodes, input_feat_len))
- adjacent_matrix = torch.rand(
- (num_local_graphs, num_max_graph_nodes, num_max_graph_nodes))
- knn_inds = torch.randint(1, num_max_graph_nodes, (num_local_graphs, k))
- output = gcn(node_feat, adjacent_matrix, knn_inds)
- self.assertEqual(output.size(), (num_local_graphs * k, 2))
diff --git a/pipeline/mmocr/tests/test_models/test_textdet/test_heads/test_fce_head.py b/pipeline/mmocr/tests/test_models/test_textdet/test_heads/test_fce_head.py
deleted file mode 100644
index 8cf11e79ccb33ecb7d571081dd7ead591c77cb89..0000000000000000000000000000000000000000
--- a/pipeline/mmocr/tests/test_models/test_textdet/test_heads/test_fce_head.py
+++ /dev/null
@@ -1,33 +0,0 @@
-# Copyright (c) OpenMMLab. All rights reserved.
-from unittest import TestCase
-
-import torch
-
-from mmocr.models.textdet.heads import FCEHead
-
-
-class TestFCEHead(TestCase):
-
- def test_init(self):
- with self.assertRaises(AssertionError):
- FCEHead(in_channels='test', fourier_degree=5)
-
- with self.assertRaises(AssertionError):
- FCEHead(in_channels=1, fourier_degree='Text')
-
- def test_forward(self):
- fce_head = FCEHead(in_channels=10, fourier_degree=5)
- data = [
- torch.randn(2, 10, 20, 20),
- torch.randn(2, 10, 30, 30),
- torch.randn(2, 10, 40, 40)
- ]
- results = fce_head(data)
- self.assertIn('cls_res', results[0])
- self.assertIn('reg_res', results[0])
- self.assertEqual(results[0]['cls_res'].shape, (2, 4, 20, 20))
- self.assertEqual(results[0]['reg_res'].shape, (2, 22, 20, 20))
- self.assertEqual(results[1]['cls_res'].shape, (2, 4, 30, 30))
- self.assertEqual(results[1]['reg_res'].shape, (2, 22, 30, 30))
- self.assertEqual(results[2]['cls_res'].shape, (2, 4, 40, 40))
- self.assertEqual(results[2]['reg_res'].shape, (2, 22, 40, 40))
diff --git a/pipeline/mmocr/tests/test_models/test_textdet/test_heads/test_pan_head.py b/pipeline/mmocr/tests/test_models/test_textdet/test_heads/test_pan_head.py
deleted file mode 100644
index 242bfa29fdc5164d7f50a50a7f8743e4ef91b437..0000000000000000000000000000000000000000
--- a/pipeline/mmocr/tests/test_models/test_textdet/test_heads/test_pan_head.py
+++ /dev/null
@@ -1,25 +0,0 @@
-# Copyright (c) OpenMMLab. All rights reserved.
-from unittest import TestCase
-
-import torch
-
-from mmocr.models.textdet.heads import PANHead
-
-
-class TestPANHead(TestCase):
-
- def test_init(self):
- with self.assertRaises(AssertionError):
- PANHead(in_channels='test', hidden_dim=128, out_channel=6)
- with self.assertRaises(AssertionError):
- PANHead(in_channels=['test'], hidden_dim=128, out_channel=6)
- with self.assertRaises(AssertionError):
- PANHead(in_channels=[128, 128], hidden_dim='test', out_channel=6)
- with self.assertRaises(AssertionError):
- PANHead(in_channels=[128, 128], hidden_dim=128, out_channel='test')
-
- def test_forward(self):
- pan_head = PANHead(in_channels=[10], hidden_dim=128, out_channel=6)
- data = torch.randn((2, 10, 40, 50))
- results = pan_head(data)
- self.assertEqual(results.shape, (2, 6, 40, 50))
diff --git a/pipeline/mmocr/tests/test_models/test_textdet/test_heads/test_pse_head.py b/pipeline/mmocr/tests/test_models/test_textdet/test_heads/test_pse_head.py
deleted file mode 100644
index 4a433000618ca17024609709fa5f81b26430ca99..0000000000000000000000000000000000000000
--- a/pipeline/mmocr/tests/test_models/test_textdet/test_heads/test_pse_head.py
+++ /dev/null
@@ -1,24 +0,0 @@
-# Copyright (c) OpenMMLab. All rights reserved.
-from unittest import TestCase
-
-import torch
-
-from mmocr.models.textdet.heads import PSEHead
-
-
-class TestPSEHead(TestCase):
-
- def setUp(self):
- self.feature = torch.randn((2, 10, 40, 50))
-
- def test_init(self):
- with self.assertRaises(TypeError):
- PSEHead(in_channels=1)
-
- with self.assertRaises(TypeError):
- PSEHead(out_channels='out')
-
- def test_forward(self):
- pse_head = PSEHead(in_channels=[10], hidden_dim=128, out_channel=7)
- results = pse_head(self.feature)
- self.assertEqual(results.shape, (2, 7, 40, 50))
diff --git a/pipeline/mmocr/tests/test_models/test_textdet/test_heads/test_textsnake_head.py b/pipeline/mmocr/tests/test_models/test_textdet/test_heads/test_textsnake_head.py
deleted file mode 100644
index 57d65170403e6ce365658ca5a0973fa852c87363..0000000000000000000000000000000000000000
--- a/pipeline/mmocr/tests/test_models/test_textdet/test_heads/test_textsnake_head.py
+++ /dev/null
@@ -1,19 +0,0 @@
-# Copyright (c) OpenMMLab. All rights reserved.
-from unittest import TestCase
-
-import torch
-
-from mmocr.models.textdet.heads import TextSnakeHead
-
-
-class TestTextSnakeHead(TestCase):
-
- def test_init(self):
- with self.assertRaises(AssertionError):
- TextSnakeHead(in_channels='test')
-
- def test_forward(self):
- ts_head = TextSnakeHead(in_channels=10)
- data = torch.randn((2, 10, 40, 50))
- results = ts_head(data, None)
- self.assertEqual(results.shape, (2, 5, 40, 50))
diff --git a/pipeline/mmocr/tests/test_models/test_textdet/test_necks/test_fpem_ffm.py b/pipeline/mmocr/tests/test_models/test_textdet/test_necks/test_fpem_ffm.py
deleted file mode 100644
index 86f3115c783734673fd895275d55a5b514f25fe3..0000000000000000000000000000000000000000
--- a/pipeline/mmocr/tests/test_models/test_textdet/test_necks/test_fpem_ffm.py
+++ /dev/null
@@ -1,46 +0,0 @@
-# Copyright (c) OpenMMLab. All rights reserved.
-import unittest
-
-import torch
-
-from mmocr.models.textdet.necks.fpem_ffm import FPEM, FPEM_FFM
-
-
-class TestFPEM(unittest.TestCase):
-
- def setUp(self):
- self.c2 = torch.Tensor(1, 8, 64, 64)
- self.c3 = torch.Tensor(1, 8, 32, 32)
- self.c4 = torch.Tensor(1, 8, 16, 16)
- self.c5 = torch.Tensor(1, 8, 8, 8)
- self.fpem = FPEM(in_channels=8)
-
- def test_forward(self):
- neck = FPEM(in_channels=8)
- neck.init_weights()
- out = neck(self.c2, self.c3, self.c4, self.c5)
- self.assertTrue(out[0].shape == self.c2.shape)
- self.assertTrue(out[1].shape == self.c3.shape)
- self.assertTrue(out[2].shape == self.c4.shape)
- self.assertTrue(out[3].shape == self.c5.shape)
-
-
-class TestFPEM_FFM(unittest.TestCase):
-
- def setUp(self):
- self.c2 = torch.Tensor(1, 8, 64, 64)
- self.c3 = torch.Tensor(1, 16, 32, 32)
- self.c4 = torch.Tensor(1, 32, 16, 16)
- self.c5 = torch.Tensor(1, 64, 8, 8)
- self.in_channels = [8, 16, 32, 64]
- self.conv_out = 8
- self.features = [self.c2, self.c3, self.c4, self.c5]
-
- def test_forward(self):
- neck = FPEM_FFM(in_channels=self.in_channels, conv_out=self.conv_out)
- neck.init_weights()
- out = neck(self.features)
- self.assertTrue(out[0].shape == torch.Size([1, 8, 64, 64]))
- self.assertTrue(out[1].shape == out[0].shape)
- self.assertTrue(out[2].shape == out[0].shape)
- self.assertTrue(out[3].shape == out[0].shape)
diff --git a/pipeline/mmocr/tests/test_models/test_textdet/test_necks/test_fpn_cat.py b/pipeline/mmocr/tests/test_models/test_textdet/test_necks/test_fpn_cat.py
deleted file mode 100644
index e69de29bb2d1d6434b8b29ae775ad8c2e48c5391..0000000000000000000000000000000000000000
diff --git a/pipeline/mmocr/tests/test_models/test_textdet/test_necks/test_fpnf.py b/pipeline/mmocr/tests/test_models/test_textdet/test_necks/test_fpnf.py
deleted file mode 100644
index 0eeb1f44a3d669958f6f67361c6c9dbc0521c8e4..0000000000000000000000000000000000000000
--- a/pipeline/mmocr/tests/test_models/test_textdet/test_necks/test_fpnf.py
+++ /dev/null
@@ -1,24 +0,0 @@
-# Copyright (c) OpenMMLab. All rights reserved.
-import unittest
-
-import torch
-from parameterized import parameterized
-
-from mmocr.models.textdet.necks import FPNF
-
-
-class TestFPNF(unittest.TestCase):
-
- def setUp(self):
- in_channels = [256, 512, 1024, 2048]
- size = [112, 56, 28, 14]
- inputs = []
- for i in range(4):
- inputs.append(torch.rand(1, in_channels[i], size[i], size[i]))
- self.inputs = inputs
-
- @parameterized.expand([('concat'), ('add')])
- def test_forward(self, fusion_type):
- fpnf = FPNF(fusion_type=fusion_type)
- outputs = fpnf.forward(self.inputs)
- self.assertListEqual(list(outputs.size()), [1, 256, 112, 112])
diff --git a/pipeline/mmocr/tests/test_models/test_textrecog/test_backbones/test_mini_vgg.py b/pipeline/mmocr/tests/test_models/test_textrecog/test_backbones/test_mini_vgg.py
deleted file mode 100644
index bc8d188f94492140aa9bc85f4ce94001c7ca75f2..0000000000000000000000000000000000000000
--- a/pipeline/mmocr/tests/test_models/test_textrecog/test_backbones/test_mini_vgg.py
+++ /dev/null
@@ -1,19 +0,0 @@
-# Copyright (c) OpenMMLab. All rights reserved.
-from unittest import TestCase
-
-import torch
-
-from mmocr.models.textrecog.backbones import MiniVGG
-
-
-class TestMiniVGG(TestCase):
-
- def test_forward(self):
-
- model = MiniVGG()
- model.init_weights()
- model.train()
-
- imgs = torch.randn(1, 3, 32, 160)
- feats = model(imgs)
- self.assertEqual(feats.shape, torch.Size([1, 512, 1, 41]))
diff --git a/pipeline/mmocr/tests/test_models/test_textrecog/test_backbones/test_mobilenet_v2.py b/pipeline/mmocr/tests/test_models/test_textrecog/test_backbones/test_mobilenet_v2.py
deleted file mode 100644
index 7f55ef44027dadc7c79f4957f5d97106ffde2491..0000000000000000000000000000000000000000
--- a/pipeline/mmocr/tests/test_models/test_textrecog/test_backbones/test_mobilenet_v2.py
+++ /dev/null
@@ -1,17 +0,0 @@
-# Copyright (c) OpenMMLab. All rights reserved.
-from unittest import TestCase
-
-import torch
-
-from mmocr.models.textrecog.backbones import MobileNetV2
-
-
-class TestMobileNetV2(TestCase):
-
- def setUp(self) -> None:
- self.img = torch.rand(1, 3, 32, 160)
-
- def test_mobilenetv2(self):
- mobilenet_v2 = MobileNetV2()
- self.assertEqual(
- mobilenet_v2(self.img).shape, torch.Size([1, 1280, 1, 43]))
diff --git a/pipeline/mmocr/tests/test_models/test_textrecog/test_backbones/test_nrtr_modality_transformer.py b/pipeline/mmocr/tests/test_models/test_textrecog/test_backbones/test_nrtr_modality_transformer.py
deleted file mode 100644
index 3243cd40b000ddce1d43dffbee46146e20f15b36..0000000000000000000000000000000000000000
--- a/pipeline/mmocr/tests/test_models/test_textrecog/test_backbones/test_nrtr_modality_transformer.py
+++ /dev/null
@@ -1,19 +0,0 @@
-# Copyright (c) OpenMMLab. All rights reserved.
-import unittest
-
-import torch
-
-from mmocr.models.textrecog.backbones import NRTRModalityTransform
-
-
-class TestNRTRBackbone(unittest.TestCase):
-
- def setUp(self):
- self.img = torch.randn(2, 3, 32, 100)
-
- def test_encoder(self):
- nrtr_backbone = NRTRModalityTransform()
- nrtr_backbone.init_weights()
- nrtr_backbone.train()
- out_enc = nrtr_backbone(self.img)
- self.assertEqual(out_enc.shape, torch.Size([2, 512, 1, 25]))
diff --git a/pipeline/mmocr/tests/test_models/test_textrecog/test_backbones/test_resnet.py b/pipeline/mmocr/tests/test_models/test_textrecog/test_backbones/test_resnet.py
deleted file mode 100644
index 1a7904fbbdd0fea44d895546cf56ca429ee35ff1..0000000000000000000000000000000000000000
--- a/pipeline/mmocr/tests/test_models/test_textrecog/test_backbones/test_resnet.py
+++ /dev/null
@@ -1,104 +0,0 @@
-# Copyright (c) OpenMMLab. All rights reserved.
-from unittest import TestCase
-
-import torch
-from mmengine.registry import init_default_scope
-
-from mmocr.models.textrecog.backbones import ResNet
-
-
-class TestResNet(TestCase):
-
- def setUp(self) -> None:
- self.img = torch.rand(1, 3, 32, 100)
- init_default_scope('mmocr')
-
- def test_resnet45_aster(self):
- resnet45_aster = ResNet(
- in_channels=3,
- stem_channels=[64, 128],
- block_cfgs=dict(type='BasicBlock', use_conv1x1='True'),
- arch_layers=[3, 4, 6, 6, 3],
- arch_channels=[32, 64, 128, 256, 512],
- strides=[(2, 2), (2, 2), (2, 1), (2, 1), (2, 1)])
- self.assertEqual(
- resnet45_aster(self.img).shape, torch.Size([1, 512, 1, 25]))
-
- def test_resnet45_abi(self):
- resnet45_abi = ResNet(
- in_channels=3,
- stem_channels=32,
- block_cfgs=dict(type='BasicBlock', use_conv1x1='True'),
- arch_layers=[3, 4, 6, 6, 3],
- arch_channels=[32, 64, 128, 256, 512],
- strides=[2, 1, 2, 1, 1])
- self.assertEqual(
- resnet45_abi(self.img).shape, torch.Size([1, 512, 8, 25]))
-
- def test_resnet31_master(self):
- resnet31_master = ResNet(
- in_channels=3,
- stem_channels=[64, 128],
- block_cfgs=dict(type='BasicBlock'),
- arch_layers=[1, 2, 5, 3],
- arch_channels=[256, 256, 512, 512],
- strides=[1, 1, 1, 1],
- plugins=[
- dict(
- cfg=dict(type='Maxpool2d', kernel_size=2, stride=(2, 2)),
- stages=(True, True, False, False),
- position='before_stage'),
- dict(
- cfg=dict(
- type='Maxpool2d', kernel_size=(2, 1), stride=(2, 1)),
- stages=(False, False, True, False),
- position='before_stage'),
- dict(
- cfg=dict(type='GCAModule', ratio=0.0625, n_head=1),
- stages=[True, True, True, True],
- position='after_stage'),
- dict(
- cfg=dict(
- type='ConvModule',
- kernel_size=3,
- stride=1,
- padding=1,
- norm_cfg=dict(type='BN'),
- act_cfg=dict(type='ReLU')),
- stages=(True, True, True, True),
- position='after_stage')
- ])
- self.assertEqual(
- resnet31_master(self.img).shape, torch.Size([1, 512, 4, 25]))
-
- def test_resnet31(self):
- resnet_31 = ResNet(
- in_channels=3,
- stem_channels=[64, 128],
- block_cfgs=dict(type='BasicBlock'),
- arch_layers=[1, 2, 5, 3],
- arch_channels=[256, 256, 512, 512],
- strides=[1, 1, 1, 1],
- plugins=[
- dict(
- cfg=dict(type='Maxpool2d', kernel_size=2, stride=(2, 2)),
- stages=(True, True, False, False),
- position='before_stage'),
- dict(
- cfg=dict(
- type='Maxpool2d', kernel_size=(2, 1), stride=(2, 1)),
- stages=(False, False, True, False),
- position='before_stage'),
- dict(
- cfg=dict(
- type='ConvModule',
- kernel_size=3,
- stride=1,
- padding=1,
- norm_cfg=dict(type='BN'),
- act_cfg=dict(type='ReLU')),
- stages=(True, True, True, True),
- position='after_stage')
- ])
- self.assertEqual(
- resnet_31(self.img).shape, torch.Size([1, 512, 4, 25]))
diff --git a/pipeline/mmocr/tests/test_models/test_textrecog/test_backbones/test_resnet31_ocr.py b/pipeline/mmocr/tests/test_models/test_textrecog/test_backbones/test_resnet31_ocr.py
deleted file mode 100644
index a6bc566cc1be959194d776eb83411a5f9a4e115b..0000000000000000000000000000000000000000
--- a/pipeline/mmocr/tests/test_models/test_textrecog/test_backbones/test_resnet31_ocr.py
+++ /dev/null
@@ -1,29 +0,0 @@
-# Copyright (c) OpenMMLab. All rights reserved.
-from unittest import TestCase
-
-import torch
-
-from mmocr.models.textrecog.backbones import ResNet31OCR
-
-
-class TestResNet31OCR(TestCase):
-
- def test_forward(self):
- """Test resnet backbone."""
- with self.assertRaises(AssertionError):
- ResNet31OCR(2.5)
-
- with self.assertRaises(AssertionError):
- ResNet31OCR(3, layers=5)
-
- with self.assertRaises(AssertionError):
- ResNet31OCR(3, channels=5)
-
- # Test ResNet18 forward
- model = ResNet31OCR()
- model.init_weights()
- model.train()
-
- imgs = torch.randn(1, 3, 32, 160)
- feat = model(imgs)
- self.assertEqual(feat.shape, torch.Size([1, 512, 4, 40]))
diff --git a/pipeline/mmocr/tests/test_models/test_textrecog/test_backbones/test_resnet_abi.py b/pipeline/mmocr/tests/test_models/test_textrecog/test_backbones/test_resnet_abi.py
deleted file mode 100644
index 950e9a8a726bb8cc6c545d75802e6c79396a9614..0000000000000000000000000000000000000000
--- a/pipeline/mmocr/tests/test_models/test_textrecog/test_backbones/test_resnet_abi.py
+++ /dev/null
@@ -1,31 +0,0 @@
-# Copyright (c) OpenMMLab. All rights reserved.
-from unittest import TestCase
-
-import torch
-
-from mmocr.models.textrecog.backbones import ResNetABI
-
-
-class TestResNetABI(TestCase):
-
- def test_forward(self):
- """Test resnet backbone."""
- with self.assertRaises(AssertionError):
- ResNetABI(2.5)
-
- with self.assertRaises(AssertionError):
- ResNetABI(3, arch_settings=5)
-
- with self.assertRaises(AssertionError):
- ResNetABI(3, stem_channels=None)
-
- with self.assertRaises(AssertionError):
- ResNetABI(arch_settings=[3, 4, 6, 6], strides=[1, 2, 1, 2, 1])
-
- # Test forwarding
- model = ResNetABI()
- model.train()
-
- imgs = torch.randn(1, 3, 32, 160)
- feat = model(imgs)
- self.assertEqual(feat.shape, torch.Size([1, 512, 8, 40]))
diff --git a/pipeline/mmocr/tests/test_models/test_textrecog/test_backbones/test_shallow_cnn.py b/pipeline/mmocr/tests/test_models/test_textrecog/test_backbones/test_shallow_cnn.py
deleted file mode 100644
index 2d90bbc0dfb3bf26614032dce0ac047315b61cf8..0000000000000000000000000000000000000000
--- a/pipeline/mmocr/tests/test_models/test_textrecog/test_backbones/test_shallow_cnn.py
+++ /dev/null
@@ -1,21 +0,0 @@
-# Copyright (c) OpenMMLab. All rights reserved.
-import unittest
-
-import torch
-
-from mmocr.models.textrecog.backbones import ShallowCNN
-
-
-class TestShallowCNN(unittest.TestCase):
-
- def setUp(self):
- self.imgs = torch.randn(1, 1, 32, 100)
-
- def test_shallow_cnn(self):
-
- model = ShallowCNN()
- model.init_weights()
- model.train()
-
- feat = model(self.imgs)
- self.assertEqual(feat.shape, torch.Size([1, 512, 8, 25]))
diff --git a/pipeline/mmocr/tests/test_models/test_textrecog/test_data_preprocessors/test_data_preprocessor.py b/pipeline/mmocr/tests/test_models/test_textrecog/test_data_preprocessors/test_data_preprocessor.py
deleted file mode 100644
index 2216aba005d96d09304e349e8bb51e6d71d437a9..0000000000000000000000000000000000000000
--- a/pipeline/mmocr/tests/test_models/test_textrecog/test_data_preprocessors/test_data_preprocessor.py
+++ /dev/null
@@ -1,113 +0,0 @@
-# Copyright (c) OpenMMLab. All rights reserved.
-from unittest import TestCase
-
-import torch
-
-from mmocr.models.textrecog.data_preprocessors import TextRecogDataPreprocessor
-from mmocr.registry import MODELS
-from mmocr.structures import TextRecogDataSample
-
-
-@MODELS.register_module()
-class Augment(torch.nn.Module):
-
- def forward(self, inputs, data_samples):
- return inputs, data_samples
-
-
-class TestTextRecogDataPreprocessor(TestCase):
-
- def test_init(self):
- # test mean is None
- processor = TextRecogDataPreprocessor()
- self.assertTrue(not hasattr(processor, 'mean'))
- self.assertTrue(processor._enable_normalize is False)
-
- # test mean is not None
- processor = TextRecogDataPreprocessor(mean=[0, 0, 0], std=[1, 1, 1])
- self.assertTrue(hasattr(processor, 'mean'))
- self.assertTrue(hasattr(processor, 'std'))
- self.assertTrue(processor._enable_normalize)
-
- # please specify both mean and std
- with self.assertRaises(AssertionError):
- TextRecogDataPreprocessor(mean=[0, 0, 0])
-
- # bgr2rgb and rgb2bgr cannot be set to True at the same time
- with self.assertRaises(AssertionError):
- TextRecogDataPreprocessor(bgr_to_rgb=True, rgb_to_bgr=True)
-
- aug_cfg = [dict(type='Augment')]
- processor = TextRecogDataPreprocessor()
- self.assertIsNone(processor.batch_augments)
- processor = TextRecogDataPreprocessor(batch_augments=aug_cfg)
- self.assertIsInstance(processor.batch_augments, torch.nn.ModuleList)
- self.assertIsInstance(processor.batch_augments[0], Augment)
-
- def test_forward(self):
- processor = TextRecogDataPreprocessor(mean=[0, 0, 0], std=[1, 1, 1])
-
- data = {
- 'inputs': [
- torch.randint(0, 256, (3, 11, 10)),
- ],
- 'data_samples': [
- TextRecogDataSample(
- metainfo=dict(img_shape=(11, 10), valid_ratio=1.0)),
- ]
- }
- out = processor(data)
- inputs, data_samples = out['inputs'], out['data_samples']
- print(inputs.dtype)
- self.assertEqual(inputs.shape, (1, 3, 11, 10))
- self.assertEqual(len(data_samples), 1)
-
- # test channel_conversion
- processor = TextRecogDataPreprocessor(
- mean=[0., 0., 0.], std=[1., 1., 1.], bgr_to_rgb=True)
- out = processor(data)
- inputs, data_samples = out['inputs'], out['data_samples']
- self.assertEqual(inputs.shape, (1, 3, 11, 10))
- self.assertEqual(len(data_samples), 1)
-
- # test padding
- data = {
- 'inputs': [
- torch.randint(0, 256, (3, 10, 11)),
- torch.randint(0, 256, (3, 9, 14))
- ]
- }
- processor = TextRecogDataPreprocessor(
- mean=[0., 0., 0.], std=[1., 1., 1.], bgr_to_rgb=True)
- out = processor(data)
- inputs, data_samples = out['inputs'], out['data_samples']
- self.assertEqual(inputs.shape, (2, 3, 10, 14))
- self.assertIsNone(data_samples)
-
- # test pad_size_divisor
- data = {
- 'inputs': [
- torch.randint(0, 256, (3, 10, 11)),
- torch.randint(0, 256, (3, 9, 24)),
- ],
- 'data_samples': [
- TextRecogDataSample(
- metainfo=dict(img_shape=(10, 11), valid_ratio=1.0)),
- TextRecogDataSample(
- metainfo=dict(img_shape=(9, 24), valid_ratio=1.0))
- ]
- }
- aug_cfg = [dict(type='Augment')]
- processor = TextRecogDataPreprocessor(
- mean=[0., 0., 0.],
- std=[1., 1., 1.],
- pad_size_divisor=5,
- batch_augments=aug_cfg)
- out = processor(data)
- inputs, data_samples = out['inputs'], out['data_samples']
- self.assertEqual(inputs.shape, (2, 3, 10, 25))
- self.assertEqual(len(data_samples), 2)
- for data_sample, expected_shape, expected_ratio in zip(
- data_samples, [(10, 25), (10, 25)], [11 / 25., 24 / 25.]):
- self.assertEqual(data_sample.batch_input_shape, expected_shape)
- self.assertEqual(data_sample.valid_ratio, expected_ratio)
diff --git a/pipeline/mmocr/tests/test_models/test_textrecog/test_decoders/test_abi_fuser.py b/pipeline/mmocr/tests/test_models/test_textrecog/test_decoders/test_abi_fuser.py
deleted file mode 100644
index 208d863780f5a49364b619651f692bfadbe1139e..0000000000000000000000000000000000000000
--- a/pipeline/mmocr/tests/test_models/test_textrecog/test_decoders/test_abi_fuser.py
+++ /dev/null
@@ -1,121 +0,0 @@
-# Copyright (c) OpenMMLab. All rights reserved.
-import os.path as osp
-import tempfile
-from unittest import TestCase
-
-import torch
-
-from mmocr.models.textrecog.decoders import ABIFuser
-from mmocr.testing import create_dummy_dict_file
-
-
-class TestABINetFuser(TestCase):
-
- def setUp(self):
-
- self.tmp_dir = tempfile.TemporaryDirectory()
- self.dict_file = osp.join(self.tmp_dir.name, 'fake_chars.txt')
- create_dummy_dict_file(self.dict_file)
- self.dict_cfg = dict(
- type='Dictionary',
- dict_file=self.dict_file,
- with_start=True,
- with_end=True,
- same_start_end=False,
- with_padding=False,
- with_unknown=False)
-
- # both max_seq_len has been set
- with self.assertWarns(Warning):
- ABIFuser(
- self.dict_cfg,
- max_seq_len=10,
- vision_decoder=dict(
- type='ABIVisionDecoder',
- in_channels=2,
- num_channels=2,
- max_seq_len=5),
- language_decoder=dict(
- type='ABILanguageDecoder',
- d_model=2,
- n_head=2,
- d_inner=16,
- n_layers=1,
- max_seq_len=5))
-
- # both dictionaries have been set
- with self.assertWarns(Warning):
- ABIFuser(
- self.dict_cfg,
- max_seq_len=10,
- vision_decoder=dict(
- type='ABIVisionDecoder',
- in_channels=2,
- num_channels=2,
- dictionary=self.dict_cfg),
- language_decoder=dict(
- type='ABILanguageDecoder',
- d_model=2,
- n_head=2,
- d_inner=16,
- n_layers=1,
- dictionary=self.dict_cfg))
-
- def tearDown(self):
- self.tmp_dir.cleanup()
-
- def test_init(self):
- # No ending idx
- with self.assertRaises(AssertionError):
- dict_cfg = dict(
- type='Dictionary', dict_file=self.dict_file, with_end=False)
- ABIFuser(dict_cfg, None)
-
- def test_forward_full_model(self):
- # Full model
- model = ABIFuser(
- self.dict_cfg,
- max_seq_len=10,
- vision_decoder=dict(
- type='ABIVisionDecoder', in_channels=2, num_channels=2),
- language_decoder=dict(
- type='ABILanguageDecoder',
- d_model=2,
- n_head=2,
- d_inner=16,
- n_layers=1,
- ),
- d_model=2)
- model.train()
- result = model(None, torch.randn(1, 2, 8, 32))
- self.assertIsInstance(result, dict)
- self.assertIn('out_vis', result)
- self.assertIn('out_langs', result)
- self.assertIsInstance(result['out_langs'], list)
- self.assertIn('out_fusers', result)
- self.assertIsInstance(result['out_fusers'], list)
-
- model.eval()
- result = model(None, torch.randn(1, 2, 8, 32))
- self.assertIsInstance(result, torch.Tensor)
-
- def test_forward_vision_model(self):
- # Full model
- model = ABIFuser(
- self.dict_cfg,
- vision_decoder=dict(
- type='ABIVisionDecoder', in_channels=2, num_channels=2))
- model.train()
- result = model(None, torch.randn(1, 2, 8, 32))
- self.assertIsInstance(result, dict)
- self.assertIn('out_vis', result)
- self.assertIn('out_langs', result)
- self.assertIsInstance(result['out_langs'], list)
- self.assertEqual(len(result['out_langs']), 0)
- self.assertIn('out_fusers', result)
- self.assertIsInstance(result['out_fusers'], list)
- self.assertEqual(len(result['out_fusers']), 0)
-
- model.eval()
- result = model(None, torch.randn(1, 2, 8, 32))
- self.assertIsInstance(result, torch.Tensor)
diff --git a/pipeline/mmocr/tests/test_models/test_textrecog/test_decoders/test_abi_language_decoder.py b/pipeline/mmocr/tests/test_models/test_textrecog/test_decoders/test_abi_language_decoder.py
deleted file mode 100644
index 73efb164ff74a6f3b9b0a89bc17a1fed0d9cfed2..0000000000000000000000000000000000000000
--- a/pipeline/mmocr/tests/test_models/test_textrecog/test_decoders/test_abi_language_decoder.py
+++ /dev/null
@@ -1,58 +0,0 @@
-# Copyright (c) OpenMMLab. All rights reserved.
-import os.path as osp
-import tempfile
-from unittest import TestCase
-
-import torch
-
-from mmocr.models.textrecog.decoders import ABILanguageDecoder
-from mmocr.testing import create_dummy_dict_file
-
-
-class TestABILanguageDecoder(TestCase):
-
- def test_init(self):
- with tempfile.TemporaryDirectory() as tmp_dir:
- dict_file = osp.join(tmp_dir, 'fake_chars.txt')
- create_dummy_dict_file(dict_file)
- dict_cfg = dict(
- type='Dictionary', dict_file=dict_file, with_end=False)
- # No padding token
- with self.assertRaises(AssertionError):
- ABILanguageDecoder(dict_cfg)
-
- def test_forward(self):
- with tempfile.TemporaryDirectory() as tmp_dir:
- dict_file = osp.join(tmp_dir, 'fake_chars.txt')
- create_dummy_dict_file(dict_file)
- # test diction cfg
- dict_cfg = dict(
- type='Dictionary',
- dict_file=dict_file,
- with_start=True,
- with_end=True,
- same_start_end=False,
- with_padding=True,
- with_unknown=False)
- decoder = ABILanguageDecoder(
- dict_cfg, d_model=16, d_inner=16, max_seq_len=10)
- logits = torch.randn(2, 10, 39)
- result = decoder.forward_train(None, logits, None)
- self.assertIn('feature', result)
- self.assertIn('logits', result)
- self.assertEqual(result['feature'].shape, torch.Size([2, 10, 16]))
- self.assertEqual(result['logits'].shape, torch.Size([2, 10, 39]))
-
- decoder = ABILanguageDecoder(
- dict_cfg,
- d_model=16,
- d_inner=16,
- max_seq_len=10,
- with_self_attn=True,
- detach_tokens=False)
- logits = torch.randn(2, 10, 39)
- result = decoder.forward_test(None, logits, None)
- self.assertIn('feature', result)
- self.assertIn('logits', result)
- self.assertEqual(result['feature'].shape, torch.Size([2, 10, 16]))
- self.assertEqual(result['logits'].shape, torch.Size([2, 10, 39]))
diff --git a/pipeline/mmocr/tests/test_models/test_textrecog/test_decoders/test_abi_vision_decoder.py b/pipeline/mmocr/tests/test_models/test_textrecog/test_decoders/test_abi_vision_decoder.py
deleted file mode 100644
index db94ce86f63471e031590ca383c490ccbc9e42f4..0000000000000000000000000000000000000000
--- a/pipeline/mmocr/tests/test_models/test_textrecog/test_decoders/test_abi_vision_decoder.py
+++ /dev/null
@@ -1,50 +0,0 @@
-# Copyright (c) OpenMMLab. All rights reserved.
-import os.path as osp
-import tempfile
-from unittest import TestCase
-
-import torch
-
-from mmocr.models.textrecog.decoders import ABIVisionDecoder
-from mmocr.testing import create_dummy_dict_file
-
-
-class TestABIVisionDecoder(TestCase):
-
- def test_forward(self):
- with tempfile.TemporaryDirectory() as tmp_dir:
- dict_file = osp.join(tmp_dir, 'fake_chars.txt')
- create_dummy_dict_file(dict_file)
- # test diction cfg
- dict_cfg = dict(
- type='Dictionary',
- dict_file=dict_file,
- with_start=True,
- with_end=True,
- same_start_end=False,
- with_padding=False,
- with_unknown=False)
-
- decoder = ABIVisionDecoder(
- dict_cfg, in_channels=32, num_channels=16, max_seq_len=10)
-
- # training
- out_enc = torch.randn(2, 32, 8, 32)
- result = decoder.forward_train(None, out_enc)
- self.assertIn('feature', result)
- self.assertIn('logits', result)
- self.assertIn('attn_scores', result)
- self.assertEqual(result['feature'].shape, torch.Size([2, 10, 32]))
- self.assertEqual(result['logits'].shape, torch.Size([2, 10, 38]))
- self.assertEqual(result['attn_scores'].shape,
- torch.Size([2, 10, 8, 32]))
-
- # testing
- result = decoder.forward_test(None, out_enc)
- self.assertIn('feature', result)
- self.assertIn('logits', result)
- self.assertIn('attn_scores', result)
- self.assertEqual(result['feature'].shape, torch.Size([2, 10, 32]))
- self.assertEqual(result['logits'].shape, torch.Size([2, 10, 38]))
- self.assertEqual(result['attn_scores'].shape,
- torch.Size([2, 10, 8, 32]))
diff --git a/pipeline/mmocr/tests/test_models/test_textrecog/test_decoders/test_aster_decoder.py b/pipeline/mmocr/tests/test_models/test_textrecog/test_decoders/test_aster_decoder.py
deleted file mode 100644
index 0517b1492e6b93565cd4217919e5948d9d781b01..0000000000000000000000000000000000000000
--- a/pipeline/mmocr/tests/test_models/test_textrecog/test_decoders/test_aster_decoder.py
+++ /dev/null
@@ -1,100 +0,0 @@
-# Copyright (c) OpenMMLab. All rights reserved.
-import os.path as osp
-import tempfile
-from unittest import TestCase
-
-import torch
-from mmengine.structures import LabelData
-
-from mmocr.models.textrecog.decoders import ASTERDecoder
-from mmocr.structures import TextRecogDataSample
-
-
-class TestASTERDecoder(TestCase):
-
- def setUp(self):
- gt_text_sample1 = TextRecogDataSample()
- gt_text = LabelData()
- gt_text.item = 'Hello'
- gt_text_sample1.gt_text = gt_text
-
- gt_text_sample2 = TextRecogDataSample()
- gt_text = LabelData()
- gt_text = LabelData()
- gt_text.item = 'World1'
- gt_text_sample2.gt_text = gt_text
-
- self.data_info = [gt_text_sample1, gt_text_sample2]
-
- def _create_dummy_dict_file(
- self, dict_file,
- chars=list('0123456789abcdefghijklmnopqrstuvwxyz')): # NOQA
- with open(dict_file, 'w') as f:
- for char in chars:
- f.write(char + '\n')
-
- def test_init(self):
- tmp_dir = tempfile.TemporaryDirectory()
- dict_file = osp.join(tmp_dir.name, 'fake_chars.txt')
- self._create_dummy_dict_file(dict_file)
- dict_cfg = dict(
- type='Dictionary',
- dict_file=dict_file,
- with_start=True,
- with_end=True,
- same_start_end=True,
- with_padding=True,
- with_unknown=True)
- loss_cfg = dict(type='CEModuleLoss')
- ASTERDecoder(
- in_channels=512, dictionary=dict_cfg, module_loss=loss_cfg)
- tmp_dir.cleanup()
-
- def test_forward_train(self):
- encoder_out = torch.randn(2, 25, 512)
- tmp_dir = tempfile.TemporaryDirectory()
- dict_file = osp.join(tmp_dir.name, 'fake_chars.txt')
- self._create_dummy_dict_file(dict_file)
- # test diction cfg
- dict_cfg = dict(
- type='Dictionary',
- dict_file=dict_file,
- with_start=True,
- with_end=True,
- same_start_end=True,
- with_padding=True,
- with_unknown=True)
- loss_cfg = dict(type='CEModuleLoss')
- decoder = ASTERDecoder(
- in_channels=512,
- dictionary=dict_cfg,
- module_loss=loss_cfg,
- max_seq_len=25)
- data_samples = decoder.module_loss.get_targets(self.data_info)
- output = decoder.forward_train(
- out_enc=encoder_out, data_samples=data_samples)
- self.assertTupleEqual(tuple(output.shape), (2, 25, 39))
-
- def test_forward_test(self):
- encoder_out = torch.randn(2, 25, 512)
- tmp_dir = tempfile.TemporaryDirectory()
- dict_file = osp.join(tmp_dir.name, 'fake_chars.txt')
- self._create_dummy_dict_file(dict_file)
- # test diction cfg
- dict_cfg = dict(
- type='Dictionary',
- dict_file=dict_file,
- with_start=True,
- with_end=True,
- same_start_end=True,
- with_padding=True,
- with_unknown=True)
- loss_cfg = dict(type='CEModuleLoss')
- decoder = ASTERDecoder(
- in_channels=512,
- dictionary=dict_cfg,
- module_loss=loss_cfg,
- max_seq_len=25)
- output = decoder.forward_test(
- out_enc=encoder_out, data_samples=self.data_info)
- self.assertTupleEqual(tuple(output.shape), (2, 25, 39))
diff --git a/pipeline/mmocr/tests/test_models/test_textrecog/test_decoders/test_base_decoder.py b/pipeline/mmocr/tests/test_models/test_textrecog/test_decoders/test_base_decoder.py
deleted file mode 100644
index 30854666a3b9dd5fe904f924f16ec97b54c5a464..0000000000000000000000000000000000000000
--- a/pipeline/mmocr/tests/test_models/test_textrecog/test_decoders/test_base_decoder.py
+++ /dev/null
@@ -1,135 +0,0 @@
-# Copyright (c) OpenMMLab. All rights reserved.
-import os.path as osp
-import tempfile
-from unittest import TestCase, mock
-
-from mmocr.models.common.dictionary import Dictionary
-from mmocr.models.textrecog.decoders import BaseDecoder
-from mmocr.registry import MODELS, TASK_UTILS
-from mmocr.testing import create_dummy_dict_file
-
-
-@MODELS.register_module()
-class Tmp:
-
- def __init__(self, max_seq_len, dictionary) -> None:
- pass
-
- def get_targets(self, datasamples):
- return None
-
- def __call__(self, *args):
- return None
-
-
-class TestBaseDecoder(TestCase):
-
- def test_init(self):
- cfg = dict(type='Tmp')
- tmp_dir = tempfile.TemporaryDirectory()
- dict_file = osp.join(tmp_dir.name, 'fake_chars.txt')
- create_dummy_dict_file(dict_file)
- # test diction cfg
- dict_cfg = dict(
- type='Dictionary',
- dict_file=dict_file,
- with_start=True,
- with_end=True,
- same_start_end=False,
- with_padding=True,
- with_unknown=True)
- with self.assertRaises(AssertionError):
- BaseDecoder(dict_cfg, [], cfg)
- with self.assertRaises(AssertionError):
- BaseDecoder(dict_cfg, cfg, [])
- with self.assertRaises(TypeError):
- BaseDecoder([], cfg, cfg)
- decoder = BaseDecoder(dictionary=dict_cfg)
- self.assertIsNone(decoder.module_loss)
- self.assertIsNone(decoder.postprocessor)
- self.assertIsInstance(decoder.dictionary, Dictionary)
- decoder = BaseDecoder(dict_cfg, cfg, cfg)
- self.assertIsInstance(decoder.module_loss, Tmp)
- self.assertIsInstance(decoder.postprocessor, Tmp)
- dictionary = TASK_UTILS.build(dict_cfg)
- decoder = BaseDecoder(dictionary, cfg, cfg)
- self.assertIsInstance(decoder.dictionary, Dictionary)
- tmp_dir.cleanup()
-
- def test_forward_train(self):
- tmp_dir = tempfile.TemporaryDirectory()
- dict_file = osp.join(tmp_dir.name, 'fake_chars.txt')
- create_dummy_dict_file(dict_file)
- # test diction cfg
- dict_cfg = dict(
- type='Dictionary',
- dict_file=dict_file,
- with_start=True,
- with_end=True,
- same_start_end=False,
- with_padding=True,
- with_unknown=True)
- decoder = BaseDecoder(dictionary=dict_cfg)
- with self.assertRaises(NotImplementedError):
- decoder.forward_train(None, None, None)
- tmp_dir.cleanup()
-
- def test_forward_test(self):
- tmp_dir = tempfile.TemporaryDirectory()
- dict_file = osp.join(tmp_dir.name, 'fake_chars.txt')
- create_dummy_dict_file(dict_file)
- dict_cfg = dict(
- type='Dictionary',
- dict_file=dict_file,
- with_start=True,
- with_end=True,
- same_start_end=False,
- with_padding=True,
- with_unknown=True)
- decoder = BaseDecoder(dictionary=dict_cfg)
- with self.assertRaises(NotImplementedError):
- decoder.forward_test(None, None, None)
- tmp_dir.cleanup()
-
- @mock.patch(f'{__name__}.BaseDecoder.forward_test')
- @mock.patch(f'{__name__}.BaseDecoder.forward_train')
- def test_forward(self, mock_forward_train, mock_forward_test):
-
- def mock_func_train(feat, out_enc, datasamples):
-
- return True
-
- def mock_func_test(feat, out_enc, datasamples):
-
- return False
-
- tmp_dir = tempfile.TemporaryDirectory()
- dict_file = osp.join(tmp_dir.name, 'fake_chars.txt')
- create_dummy_dict_file(dict_file)
- dict_cfg = dict(
- type='Dictionary',
- dict_file=dict_file,
- with_start=True,
- with_end=True,
- same_start_end=False,
- with_padding=True,
- with_unknown=True)
- mock_forward_train.side_effect = mock_func_train
- mock_forward_test.side_effect = mock_func_test
- cfg = dict(type='Tmp')
- decoder = BaseDecoder(dict_cfg, cfg, cfg)
- # test loss
- loss = decoder.loss(None, None, None)
- self.assertIsNone(loss)
-
- # test predict
- predict = decoder.predict(None, None, None)
- self.assertIsNone(predict)
-
- # test forward
- tensor = decoder(None, None, None)
- self.assertTrue(tensor)
- decoder.eval()
- tensor = decoder(None, None, None)
- self.assertFalse(tensor)
- tmp_dir.cleanup()
diff --git a/pipeline/mmocr/tests/test_models/test_textrecog/test_decoders/test_crnn_decoder.py b/pipeline/mmocr/tests/test_models/test_textrecog/test_decoders/test_crnn_decoder.py
deleted file mode 100644
index 37149594b1169e4863c63d9d6ac585844f5ea8db..0000000000000000000000000000000000000000
--- a/pipeline/mmocr/tests/test_models/test_textrecog/test_decoders/test_crnn_decoder.py
+++ /dev/null
@@ -1,56 +0,0 @@
-# Copyright (c) OpenMMLab. All rights reserved.
-import os.path as osp
-import tempfile
-from unittest import TestCase
-
-import torch
-import torch.nn as nn
-
-from mmocr.models.textrecog.decoders import CRNNDecoder
-from mmocr.testing import create_dummy_dict_file
-
-
-class TestCRNNDecoder(TestCase):
-
- def test_init(self):
- with tempfile.TemporaryDirectory() as tmp_dir:
- dict_file = osp.join(tmp_dir, 'fake_chars.txt')
- create_dummy_dict_file(dict_file)
- # test diction cfg
- dict_cfg = dict(
- type='Dictionary',
- dict_file=dict_file,
- with_start=True,
- with_end=True,
- same_start_end=False,
- with_padding=True,
- with_unknown=True)
- # test rnn flag false
- decoder = CRNNDecoder(in_channels=3, dictionary=dict_cfg)
- self.assertIsInstance(decoder.decoder, nn.Conv2d)
-
- decoder = CRNNDecoder(
- in_channels=3, dictionary=dict_cfg, rnn_flag=True)
- self.assertIsInstance(decoder.decoder, nn.Sequential)
-
- def test_forward(self):
- inputs = torch.randn(3, 10, 1, 100)
- with tempfile.TemporaryDirectory() as tmp_dir:
- dict_file = osp.join(tmp_dir, 'fake_chars.txt')
- create_dummy_dict_file(dict_file)
- # test diction cfg
- dict_cfg = dict(
- type='Dictionary',
- dict_file=dict_file,
- with_start=False,
- with_end=False,
- same_start_end=False,
- with_padding=True,
- with_unknown=False)
- decoder = CRNNDecoder(in_channels=10, dictionary=dict_cfg)
- output = decoder.forward_train(inputs)
- self.assertTupleEqual(tuple(output.shape), (3, 100, 37))
- decoder = CRNNDecoder(
- in_channels=10, dictionary=dict_cfg, rnn_flag=True)
- output = decoder.forward_test(inputs)
- self.assertTupleEqual(tuple(output.shape), (3, 100, 37))
diff --git a/pipeline/mmocr/tests/test_models/test_textrecog/test_decoders/test_master_decoder.py b/pipeline/mmocr/tests/test_models/test_textrecog/test_decoders/test_master_decoder.py
deleted file mode 100644
index 77400955bac674011c89c48d96d98879341af3cf..0000000000000000000000000000000000000000
--- a/pipeline/mmocr/tests/test_models/test_textrecog/test_decoders/test_master_decoder.py
+++ /dev/null
@@ -1,87 +0,0 @@
-# Copyright (c) OpenMMLab. All rights reserved.
-import os.path as osp
-import tempfile
-from unittest import TestCase
-
-import torch
-from mmengine.structures import LabelData
-
-from mmocr.models.textrecog.decoders import MasterDecoder
-from mmocr.structures import TextRecogDataSample
-from mmocr.testing import create_dummy_dict_file
-
-
-class TestMasterDecoder(TestCase):
-
- def setUp(self):
- gt_text_sample1 = TextRecogDataSample()
- gt_text = LabelData()
- gt_text.item = 'Hello'
- gt_text_sample1.gt_text = gt_text
-
- gt_text_sample2 = TextRecogDataSample()
- gt_text = LabelData()
- gt_text = LabelData()
- gt_text.item = 'World1'
- gt_text_sample2.gt_text = gt_text
-
- self.data_info = [gt_text_sample1, gt_text_sample2]
-
- def test_init(self):
- tmp_dir = tempfile.TemporaryDirectory()
- dict_file = osp.join(tmp_dir.name, 'fake_chars.txt')
- create_dummy_dict_file(dict_file)
- dict_cfg = dict(
- type='Dictionary',
- dict_file=dict_file,
- with_start=True,
- with_end=True,
- same_start_end=True,
- with_padding=True,
- with_unknown=True)
- loss_cfg = dict(type='CEModuleLoss')
- MasterDecoder(dictionary=dict_cfg, module_loss=loss_cfg)
- tmp_dir.cleanup()
-
- def test_forward_train(self):
- encoder_out = torch.randn(2, 512, 6, 40)
- tmp_dir = tempfile.TemporaryDirectory()
- dict_file = osp.join(tmp_dir.name, 'fake_chars.txt')
- create_dummy_dict_file(dict_file)
- # test diction cfg
- dict_cfg = dict(
- type='Dictionary',
- dict_file=dict_file,
- with_start=True,
- with_end=True,
- same_start_end=True,
- with_padding=True,
- with_unknown=True)
- loss_cfg = dict(type='CEModuleLoss')
- decoder = MasterDecoder(
- dictionary=dict_cfg, module_loss=loss_cfg, max_seq_len=30)
- data_samples = decoder.module_loss.get_targets(self.data_info)
- output = decoder.forward_train(
- feat=encoder_out, data_samples=data_samples)
- self.assertTupleEqual(tuple(output.shape), (2, 30, 39))
-
- def test_forward_test(self):
- encoder_out = torch.randn(2, 512, 6, 40)
- tmp_dir = tempfile.TemporaryDirectory()
- dict_file = osp.join(tmp_dir.name, 'fake_chars.txt')
- create_dummy_dict_file(dict_file)
- # test diction cfg
- dict_cfg = dict(
- type='Dictionary',
- dict_file=dict_file,
- with_start=True,
- with_end=True,
- same_start_end=True,
- with_padding=True,
- with_unknown=True)
- loss_cfg = dict(type='CEModuleLoss')
- decoder = MasterDecoder(
- dictionary=dict_cfg, module_loss=loss_cfg, max_seq_len=30)
- output = decoder.forward_test(
- feat=encoder_out, data_samples=self.data_info)
- self.assertTupleEqual(tuple(output.shape), (2, 30, 39))
diff --git a/pipeline/mmocr/tests/test_models/test_textrecog/test_decoders/test_nrtr_decoder.py b/pipeline/mmocr/tests/test_models/test_textrecog/test_decoders/test_nrtr_decoder.py
deleted file mode 100644
index d4675a5dce48666a26da28ebd491f45bafd1b658..0000000000000000000000000000000000000000
--- a/pipeline/mmocr/tests/test_models/test_textrecog/test_decoders/test_nrtr_decoder.py
+++ /dev/null
@@ -1,90 +0,0 @@
-# Copyright (c) OpenMMLab. All rights reserved.
-import os.path as osp
-import tempfile
-from unittest import TestCase
-
-import torch
-from mmengine.structures import LabelData
-
-from mmocr.models.textrecog.decoders import NRTRDecoder
-from mmocr.structures import TextRecogDataSample
-from mmocr.testing import create_dummy_dict_file
-
-
-class TestNRTRDecoder(TestCase):
-
- def setUp(self):
- gt_text_sample1 = TextRecogDataSample()
- gt_text = LabelData()
- gt_text.item = 'Hello'
- gt_text_sample1.gt_text = gt_text
- gt_text_sample1.set_metainfo(dict(valid_ratio=0.9))
-
- gt_text_sample2 = TextRecogDataSample()
- gt_text = LabelData()
- gt_text = LabelData()
- gt_text.item = 'World'
- gt_text_sample2.gt_text = gt_text
- gt_text_sample2.set_metainfo(dict(valid_ratio=1.0))
-
- self.data_info = [gt_text_sample1, gt_text_sample2]
-
- def test_init(self):
- tmp_dir = tempfile.TemporaryDirectory()
- dict_file = osp.join(tmp_dir.name, 'fake_chars.txt')
- create_dummy_dict_file(dict_file)
- dict_cfg = dict(
- type='Dictionary',
- dict_file=dict_file,
- with_start=True,
- with_end=True,
- same_start_end=True,
- with_padding=True,
- with_unknown=True)
- loss_cfg = dict(type='CEModuleLoss')
- NRTRDecoder(dictionary=dict_cfg, module_loss=loss_cfg)
- tmp_dir.cleanup()
-
- def test_forward_train(self):
- encoder_out = torch.randn(2, 25, 512)
- tmp_dir = tempfile.TemporaryDirectory()
- max_seq_len = 40
- dict_file = osp.join(tmp_dir.name, 'fake_chars.txt')
- create_dummy_dict_file(dict_file)
- # test diction cfg
- dict_cfg = dict(
- type='Dictionary',
- dict_file=dict_file,
- with_start=True,
- with_end=True,
- same_start_end=True,
- with_padding=True,
- with_unknown=True)
- loss_cfg = dict(type='CEModuleLoss')
- decoder = NRTRDecoder(
- dictionary=dict_cfg, module_loss=loss_cfg, max_seq_len=max_seq_len)
- data_samples = decoder.module_loss.get_targets(self.data_info)
- output = decoder.forward_train(
- out_enc=encoder_out, data_samples=data_samples)
- self.assertTupleEqual(tuple(output.shape), (2, max_seq_len, 39))
-
- def test_forward_test(self):
- encoder_out = torch.randn(2, 25, 512)
- tmp_dir = tempfile.TemporaryDirectory()
- dict_file = osp.join(tmp_dir.name, 'fake_chars.txt')
- create_dummy_dict_file(dict_file)
- # test diction cfg
- dict_cfg = dict(
- type='Dictionary',
- dict_file=dict_file,
- with_start=True,
- with_end=True,
- same_start_end=True,
- with_padding=True,
- with_unknown=True)
- loss_cfg = dict(type='CEModuleLoss')
- decoder = NRTRDecoder(
- dictionary=dict_cfg, module_loss=loss_cfg, max_seq_len=40)
- output = decoder.forward_test(
- out_enc=encoder_out, data_samples=self.data_info)
- self.assertTupleEqual(tuple(output.shape), (2, 40, 39))
diff --git a/pipeline/mmocr/tests/test_models/test_textrecog/test_decoders/test_position_attention_decoder.py b/pipeline/mmocr/tests/test_models/test_textrecog/test_decoders/test_position_attention_decoder.py
deleted file mode 100644
index 490126363d2ce5a647e6e6f0a0e2fcb01057adbe..0000000000000000000000000000000000000000
--- a/pipeline/mmocr/tests/test_models/test_textrecog/test_decoders/test_position_attention_decoder.py
+++ /dev/null
@@ -1,78 +0,0 @@
-# Copyright (c) OpenMMLab. All rights reserved.
-from unittest import TestCase
-
-import torch
-from mmengine.structures import LabelData
-
-from mmocr.models.textrecog.decoders import PositionAttentionDecoder
-from mmocr.structures import TextRecogDataSample
-
-
-class TestPositionAttentionDecoder(TestCase):
-
- def setUp(self):
- gt_text_sample1 = TextRecogDataSample()
- gt_text = LabelData()
- gt_text.item = 'Hello'
- gt_text_sample1.gt_text = gt_text
- gt_text_sample1.set_metainfo(dict(valid_ratio=0.9))
-
- gt_text_sample2 = TextRecogDataSample()
- gt_text = LabelData()
- gt_text = LabelData()
- gt_text.item = 'World'
- gt_text_sample2.gt_text = gt_text
- gt_text_sample2.set_metainfo(dict(valid_ratio=1.0))
-
- self.data_info = [gt_text_sample1, gt_text_sample2]
- self.dict_cfg = dict(
- type='Dictionary',
- dict_file='dicts/lower_english_digits.txt',
- with_start=True,
- with_end=True,
- same_start_end=True,
- with_padding=True,
- with_unknown=True)
-
- def test_init(self):
-
- module_loss_cfg = dict(type='CEModuleLoss')
- decoder = PositionAttentionDecoder(
- dictionary=self.dict_cfg,
- module_loss=module_loss_cfg,
- return_feature=False)
- self.assertIsInstance(decoder.prediction, torch.nn.Linear)
-
- def test_forward_train(self):
- feat = torch.randn(2, 512, 8, 8)
- encoder_out = torch.randn(2, 128, 8, 8)
- module_loss_cfg = dict(type='CEModuleLoss')
- decoder = PositionAttentionDecoder(
- dictionary=self.dict_cfg,
- module_loss=module_loss_cfg,
- return_feature=False)
- output = decoder.forward_train(
- feat=feat, out_enc=encoder_out, data_samples=self.data_info)
- self.assertTupleEqual(tuple(output.shape), (2, 40, 39))
-
- decoder = PositionAttentionDecoder(
- dictionary=self.dict_cfg, module_loss=module_loss_cfg)
- output = decoder.forward_train(
- feat=feat, out_enc=encoder_out, data_samples=self.data_info)
- self.assertTupleEqual(tuple(output.shape), (2, 40, 512))
-
- feat_new = torch.randn(2, 256, 8, 8)
- with self.assertRaises(AssertionError):
- decoder.forward_train(feat_new, encoder_out, self.data_info)
- encoder_out_new = torch.randn(2, 256, 8, 8)
- with self.assertRaises(AssertionError):
- decoder.forward_train(feat, encoder_out_new, self.data_info)
-
- def test_forward_test(self):
- feat = torch.randn(2, 512, 8, 8)
- encoder_out = torch.randn(2, 128, 8, 8)
- module_loss_cfg = dict(type='CEModuleLoss')
- decoder = PositionAttentionDecoder(
- dictionary=self.dict_cfg, module_loss=module_loss_cfg)
- output = decoder.forward_test(feat, encoder_out, self.data_info)
- self.assertTupleEqual(tuple(output.shape), (2, 40, 512))
diff --git a/pipeline/mmocr/tests/test_models/test_textrecog/test_decoders/test_robust_scanner_fuser.py b/pipeline/mmocr/tests/test_models/test_textrecog/test_decoders/test_robust_scanner_fuser.py
deleted file mode 100644
index b3b140bcf1f0404d0038b4f34338c009c35a3033..0000000000000000000000000000000000000000
--- a/pipeline/mmocr/tests/test_models/test_textrecog/test_decoders/test_robust_scanner_fuser.py
+++ /dev/null
@@ -1,88 +0,0 @@
-# Copyright (c) OpenMMLab. All rights reserved.
-from unittest import TestCase
-
-import torch
-from mmengine.structures import LabelData
-
-from mmocr.models.textrecog.decoders import (PositionAttentionDecoder,
- RobustScannerFuser,
- SequenceAttentionDecoder)
-from mmocr.structures import TextRecogDataSample
-
-
-class TestRobustScannerFuser(TestCase):
-
- def setUp(self) -> None:
- gt_text_sample1 = TextRecogDataSample()
- gt_text = LabelData()
- gt_text.item = 'Hello'
- gt_text_sample1.gt_text = gt_text
- gt_text_sample1.set_metainfo(dict(valid_ratio=0.9))
-
- gt_text_sample2 = TextRecogDataSample()
- gt_text = LabelData()
- gt_text = LabelData()
- gt_text.item = 'World'
- gt_text_sample2.gt_text = gt_text
- gt_text_sample2.set_metainfo(dict(valid_ratio=1.0))
-
- self.data_info = [gt_text_sample1, gt_text_sample2]
- self.dict_cfg = dict(
- type='Dictionary',
- dict_file='dicts/lower_english_digits.txt',
- with_start=True,
- with_end=True,
- same_start_end=True,
- with_padding=True,
- with_unknown=True)
-
- self.loss_cfg = dict(type='CEModuleLoss')
- hybrid_decoder = dict(type='SequenceAttentionDecoder')
- position_decoder = dict(type='PositionAttentionDecoder')
- self.decoder = RobustScannerFuser(
- dictionary=self.dict_cfg,
- module_loss=self.loss_cfg,
- hybrid_decoder=hybrid_decoder,
- position_decoder=position_decoder,
- max_seq_len=40)
-
- def test_init(self):
-
- self.assertIsInstance(self.decoder.hybrid_decoder,
- SequenceAttentionDecoder)
- self.assertIsInstance(self.decoder.position_decoder,
- PositionAttentionDecoder)
- hybrid_decoder = dict(type='SequenceAttentionDecoder', max_seq_len=40)
- position_decoder = dict(type='PositionAttentionDecoder')
- with self.assertWarns(Warning):
- RobustScannerFuser(
- dictionary=self.dict_cfg,
- module_loss=self.loss_cfg,
- hybrid_decoder=hybrid_decoder,
- position_decoder=position_decoder,
- max_seq_len=40)
- hybrid_decoder = dict(
- type='SequenceAttentionDecoder', dictionary=self.dict_cfg)
- with self.assertWarns(Warning):
- RobustScannerFuser(
- dictionary=self.dict_cfg,
- module_loss=self.loss_cfg,
- hybrid_decoder=hybrid_decoder,
- position_decoder=position_decoder,
- max_seq_len=40)
-
- def test_forward_train(self):
- feat = torch.randn(2, 512, 8, 8)
- encoder_out = torch.randn(2, 128, 8, 8)
- self.decoder.train()
- output = self.decoder(
- feat=feat, out_enc=encoder_out, data_samples=self.data_info)
- self.assertTupleEqual(tuple(output.shape), (2, 40, 39))
-
- def test_forward_test(self):
- feat = torch.randn(2, 512, 8, 8)
- encoder_out = torch.randn(2, 128, 8, 8)
- self.decoder.eval()
- output = self.decoder(
- feat=feat, out_enc=encoder_out, data_samples=self.data_info)
- self.assertTupleEqual(tuple(output.shape), (2, 40, 39))
diff --git a/pipeline/mmocr/tests/test_models/test_textrecog/test_decoders/test_sar_decoder.py b/pipeline/mmocr/tests/test_models/test_textrecog/test_decoders/test_sar_decoder.py
deleted file mode 100644
index f6c4d3618a720c833ade5bee7e1c4f5bc44e4393..0000000000000000000000000000000000000000
--- a/pipeline/mmocr/tests/test_models/test_textrecog/test_decoders/test_sar_decoder.py
+++ /dev/null
@@ -1,130 +0,0 @@
-# Copyright (c) OpenMMLab. All rights reserved.
-from unittest import TestCase
-
-import torch
-from mmengine.structures import LabelData
-
-from mmocr.models.textrecog.decoders import (ParallelSARDecoder,
- SequentialSARDecoder)
-from mmocr.structures import TextRecogDataSample
-
-
-class TestParallelSARDecoder(TestCase):
-
- def setUp(self):
- gt_text_sample1 = TextRecogDataSample()
- gt_text = LabelData()
- gt_text.item = 'Hello'
- gt_text_sample1.gt_text = gt_text
- gt_text_sample1.set_metainfo(dict(valid_ratio=0.9))
-
- gt_text_sample2 = TextRecogDataSample()
- gt_text = LabelData()
- gt_text = LabelData()
- gt_text.item = 'World'
- gt_text_sample2.gt_text = gt_text
- gt_text_sample2.set_metainfo(dict(valid_ratio=1.0))
-
- self.data_info = [gt_text_sample1, gt_text_sample2]
- self.dict_cfg = dict(
- type='Dictionary',
- dict_file='dicts/lower_english_digits.txt',
- with_start=True,
- with_end=True,
- same_start_end=True,
- with_padding=True,
- with_unknown=True)
- self.max_seq_len = 40
-
- def test_init(self):
- decoder = ParallelSARDecoder(self.dict_cfg)
- self.assertIsInstance(decoder.rnn_decoder, torch.nn.LSTM)
- decoder = ParallelSARDecoder(
- self.dict_cfg, dec_gru=True, pred_concat=True)
- self.assertIsInstance(decoder.rnn_decoder, torch.nn.GRU)
-
- def test_forward_train(self):
- # test parallel sar decoder
- loss_cfg = dict(type='CEModuleLoss')
- decoder = ParallelSARDecoder(
- self.dict_cfg, module_loss=loss_cfg, max_seq_len=self.max_seq_len)
- decoder.init_weights()
- decoder.train()
- feat = torch.rand(2, 512, 4, self.max_seq_len)
- out_enc = torch.rand(2, 512)
- data_samples = decoder.module_loss.get_targets(self.data_info)
- decoder.train_mode = True
- out_train = decoder.forward_train(
- feat, out_enc, data_samples=data_samples)
- self.assertEqual(out_train.shape, torch.Size([2, self.max_seq_len,
- 39]))
-
- def test_forward_test(self):
- decoder = ParallelSARDecoder(
- self.dict_cfg, max_seq_len=self.max_seq_len)
- feat = torch.rand(2, 512, 4, self.max_seq_len)
- out_enc = torch.rand(2, 512)
- decoder.train_mode = False
- out_test = decoder.forward_test(feat, out_enc, self.data_info)
- assert out_test.shape == torch.Size([2, self.max_seq_len, 39])
- out_test = decoder.forward_test(feat, out_enc, None)
- assert out_test.shape == torch.Size([2, self.max_seq_len, 39])
-
-
-class TestSequentialSARDecoder(TestCase):
-
- def setUp(self):
- gt_text_sample1 = TextRecogDataSample()
- gt_text = LabelData()
- gt_text.item = 'Hello'
- gt_text_sample1.gt_text = gt_text
- gt_text_sample1.set_metainfo(dict(valid_ratio=0.9))
-
- gt_text_sample2 = TextRecogDataSample()
- gt_text = LabelData()
- gt_text = LabelData()
- gt_text.item = 'World'
- gt_text_sample2.gt_text = gt_text
- gt_text_sample2.set_metainfo(dict(valid_ratio=1.0))
-
- self.data_info = [gt_text_sample1, gt_text_sample2]
- self.dict_cfg = dict(
- type='Dictionary',
- dict_file='dicts/lower_english_digits.txt',
- with_start=True,
- with_end=True,
- same_start_end=True,
- with_padding=True,
- with_unknown=True)
-
- def test_init(self):
- decoder = SequentialSARDecoder(self.dict_cfg)
- self.assertIsInstance(decoder.rnn_decoder_layer1, torch.nn.LSTMCell)
- decoder = SequentialSARDecoder(
- self.dict_cfg, dec_gru=True, pred_concat=True)
- self.assertIsInstance(decoder.rnn_decoder_layer1, torch.nn.GRUCell)
-
- def test_forward_train(self):
- # test parallel sar decoder
- loss_cfg = dict(type='CEModuleLoss')
- decoder = SequentialSARDecoder(self.dict_cfg, module_loss=loss_cfg)
- decoder.init_weights()
- decoder.train()
- feat = torch.rand(2, 512, 4, 40)
- out_enc = torch.rand(2, 512)
- data_samples = decoder.module_loss.get_targets(self.data_info)
- out_train = decoder.forward_train(feat, out_enc, data_samples)
- self.assertEqual(out_train.shape, torch.Size([2, 40, 39]))
-
- def test_forward_test(self):
- # test parallel sar decoder
- loss_cfg = dict(type='CEModuleLoss')
- decoder = SequentialSARDecoder(self.dict_cfg, module_loss=loss_cfg)
- decoder.init_weights()
- decoder.train()
- feat = torch.rand(2, 512, 4, 40)
- out_enc = torch.rand(2, 512)
- out_test = decoder.forward_test(feat, out_enc, self.data_info)
- self.assertEqual(out_test.shape, torch.Size([2, 40, 39]))
- out_test = decoder.forward_test(feat, out_enc, None)
- self.assertEqual(out_test.shape, torch.Size([2, 40, 39]))
diff --git a/pipeline/mmocr/tests/test_models/test_textrecog/test_decoders/test_sequence_attention_decoder.py b/pipeline/mmocr/tests/test_models/test_textrecog/test_decoders/test_sequence_attention_decoder.py
deleted file mode 100644
index 909840e674121d0627104c8a8c86c279363a2641..0000000000000000000000000000000000000000
--- a/pipeline/mmocr/tests/test_models/test_textrecog/test_decoders/test_sequence_attention_decoder.py
+++ /dev/null
@@ -1,81 +0,0 @@
-# Copyright (c) OpenMMLab. All rights reserved.
-from unittest import TestCase
-
-import torch
-from mmengine.structures import LabelData
-
-from mmocr.models.textrecog.decoders import SequenceAttentionDecoder
-from mmocr.structures import TextRecogDataSample
-
-
-class TestSequenceAttentionDecoder(TestCase):
-
- def setUp(self):
- gt_text_sample1 = TextRecogDataSample()
- gt_text = LabelData()
- gt_text.item = 'Hello'
- gt_text_sample1.gt_text = gt_text
- gt_text_sample1.set_metainfo(dict(valid_ratio=0.9))
-
- gt_text_sample2 = TextRecogDataSample()
- gt_text = LabelData()
- gt_text = LabelData()
- gt_text.item = 'World'
- gt_text_sample2.gt_text = gt_text
- gt_text_sample2.set_metainfo(dict(valid_ratio=1.0))
-
- self.data_info = [gt_text_sample1, gt_text_sample2]
- self.dict_cfg = dict(
- type='Dictionary',
- dict_file='dicts/lower_english_digits.txt',
- with_start=True,
- with_end=True,
- same_start_end=True,
- with_padding=True,
- with_unknown=True)
-
- def test_init(self):
-
- module_loss_cfg = dict(type='CEModuleLoss')
- decoder = SequenceAttentionDecoder(
- dictionary=self.dict_cfg,
- module_loss=module_loss_cfg,
- return_feature=False)
- self.assertIsInstance(decoder.prediction, torch.nn.Linear)
-
- def test_forward_train(self):
- feat = torch.randn(2, 512, 8, 8)
- encoder_out = torch.randn(2, 128, 8, 8)
- module_loss_cfg = dict(type='CEModuleLoss')
- decoder = SequenceAttentionDecoder(
- dictionary=self.dict_cfg,
- module_loss=module_loss_cfg,
- return_feature=False)
- data_samples = decoder.module_loss.get_targets(self.data_info)
- output = decoder.forward_train(
- feat=feat, out_enc=encoder_out, data_samples=data_samples)
- self.assertTupleEqual(tuple(output.shape), (2, 40, 39))
-
- decoder = SequenceAttentionDecoder(
- dictionary=self.dict_cfg, module_loss=module_loss_cfg)
- output = decoder.forward_train(
- feat=feat, out_enc=encoder_out, data_samples=data_samples)
- self.assertTupleEqual(tuple(output.shape), (2, 40, 512))
-
- feat_new = torch.randn(2, 256, 8, 8)
- with self.assertRaises(AssertionError):
- decoder.forward_train(feat_new, encoder_out, self.data_info)
- encoder_out_new = torch.randn(2, 256, 8, 8)
- with self.assertRaises(AssertionError):
- decoder.forward_train(feat, encoder_out_new, self.data_info)
-
- def test_forward_test(self):
- feat = torch.randn(2, 512, 8, 8)
- encoder_out = torch.randn(2, 128, 8, 8)
- module_loss_cfg = dict(type='CEModuleLoss')
- decoder = SequenceAttentionDecoder(
- dictionary=self.dict_cfg,
- module_loss=module_loss_cfg,
- return_feature=False)
- output = decoder.forward_test(feat, encoder_out, self.data_info)
- self.assertTupleEqual(tuple(output.shape), (2, 40, 39))
diff --git a/pipeline/mmocr/tests/test_models/test_textrecog/test_decoders/test_svtr_decoder.py b/pipeline/mmocr/tests/test_models/test_textrecog/test_decoders/test_svtr_decoder.py
deleted file mode 100644
index 63396511393f7e85e5916cd9a20c8edd9dd3cad5..0000000000000000000000000000000000000000
--- a/pipeline/mmocr/tests/test_models/test_textrecog/test_decoders/test_svtr_decoder.py
+++ /dev/null
@@ -1,96 +0,0 @@
-# Copyright (c) OpenMMLab. All rights reserved.
-import os.path as osp
-import tempfile
-from unittest import TestCase
-
-import torch
-from mmengine.structures import LabelData
-
-from mmocr.models.textrecog.decoders.svtr_decoder import SVTRDecoder
-from mmocr.structures import TextRecogDataSample
-from mmocr.testing import create_dummy_dict_file
-
-
-class TestSVTRDecoder(TestCase):
-
- def setUp(self):
- gt_text_sample1 = TextRecogDataSample()
- gt_text = LabelData()
- gt_text.item = 'Hello'
- gt_text_sample1.gt_text = gt_text
- gt_text_sample1.set_metainfo(dict(valid_ratio=0.9))
-
- gt_text_sample2 = TextRecogDataSample()
- gt_text = LabelData()
- gt_text = LabelData()
- gt_text.item = 'World'
- gt_text_sample2.gt_text = gt_text
- gt_text_sample2.set_metainfo(dict(valid_ratio=1.0))
-
- self.data_info = [gt_text_sample1, gt_text_sample2]
-
- def test_init(self):
- with tempfile.TemporaryDirectory() as tmp_dir:
- dict_file = osp.join(tmp_dir, 'fake_chars.txt')
- create_dummy_dict_file(dict_file)
- dict_cfg = dict(
- type='Dictionary',
- dict_file=dict_file,
- with_start=True,
- with_end=True,
- same_start_end=True,
- with_padding=True,
- with_unknown=True)
- loss_cfg = dict(type='CTCModuleLoss', letter_case='lower')
- SVTRDecoder(
- in_channels=192, dictionary=dict_cfg, module_loss=loss_cfg)
-
- def test_forward_train(self):
- out_enc = torch.randn(1, 192, 1, 25)
- tmp_dir = tempfile.TemporaryDirectory()
- max_seq_len = 25
- dict_file = osp.join(tmp_dir.name, 'fake_chars.txt')
- create_dummy_dict_file(dict_file)
- dict_cfg = dict(
- type='Dictionary',
- dict_file=dict_file,
- with_start=True,
- with_end=True,
- same_start_end=True,
- with_padding=True,
- with_unknown=True)
- loss_cfg = dict(type='CTCModuleLoss', letter_case='lower')
- decoder = SVTRDecoder(
- in_channels=192,
- dictionary=dict_cfg,
- module_loss=loss_cfg,
- max_seq_len=max_seq_len,
- )
- data_samples = decoder.module_loss.get_targets(self.data_info)
- output = decoder.forward_train(
- out_enc=out_enc, data_samples=data_samples)
- self.assertTupleEqual(tuple(output.shape), (1, max_seq_len, 39))
-
- def test_forward_test(self):
- out_enc = torch.randn(1, 192, 1, 25)
- tmp_dir = tempfile.TemporaryDirectory()
- dict_file = osp.join(tmp_dir.name, 'fake_chars.txt')
- create_dummy_dict_file(dict_file)
- # test diction cfg
- dict_cfg = dict(
- type='Dictionary',
- dict_file=dict_file,
- with_start=True,
- with_end=True,
- same_start_end=True,
- with_padding=True,
- with_unknown=True)
- loss_cfg = dict(type='CTCModuleLoss', letter_case='lower')
- decoder = SVTRDecoder(
- in_channels=192,
- dictionary=dict_cfg,
- module_loss=loss_cfg,
- max_seq_len=25)
- output = decoder.forward_test(
- out_enc=out_enc, data_samples=self.data_info)
- self.assertTupleEqual(tuple(output.shape), (1, 25, 39))
diff --git a/pipeline/mmocr/tests/test_models/test_textrecog/test_dictionary/test_dictionary.py b/pipeline/mmocr/tests/test_models/test_textrecog/test_dictionary/test_dictionary.py
deleted file mode 100644
index d6e24d2abde94de2ccb901a7f6539d233f42b8a4..0000000000000000000000000000000000000000
--- a/pipeline/mmocr/tests/test_models/test_textrecog/test_dictionary/test_dictionary.py
+++ /dev/null
@@ -1,143 +0,0 @@
-# Copyright (c) OpenMMLab. All rights reserved.
-import os.path as osp
-import tempfile
-from unittest import TestCase
-
-from mmocr.models.common import Dictionary
-from mmocr.testing import create_dummy_dict_file
-
-
-class TestDictionary(TestCase):
-
- def test_init(self):
- tmp_dir = tempfile.TemporaryDirectory()
-
- # create dummy data
- dict_file = osp.join(tmp_dir.name, 'fake_chars.txt')
- create_dummy_dict_file(dict_file)
- # with start
- dict_gen = Dictionary(
- dict_file=dict_file,
- with_start=True,
- with_end=True,
- same_start_end=False,
- with_padding=True,
- with_unknown=True)
- self.assertEqual(dict_gen.num_classes, 40)
- self.assertListEqual(
- dict_gen.dict,
- list('0123456789abcdefghijklmnopqrstuvwxyz') +
- ['', '', '', ''])
- dict_gen = Dictionary(
- dict_file=dict_file,
- with_start=True,
- with_end=True,
- same_start_end=True,
- with_padding=True,
- with_unknown=True)
- assert dict_gen.num_classes == 39
- assert dict_gen.dict == list('0123456789abcdefghijklmnopqrstuvwxyz'
- ) + ['', '', '']
- self.assertEqual(dict_gen.num_classes, 39)
- self.assertListEqual(
- dict_gen.dict,
- list('0123456789abcdefghijklmnopqrstuvwxyz') +
- ['', '', ''])
- dict_gen = Dictionary(
- dict_file=dict_file,
- with_start=True,
- with_end=True,
- same_start_end=False,
- with_padding=True,
- with_unknown=True,
- start_token='',
- end_token='',
- padding_token='',
- unknown_token='')
- assert dict_gen.num_classes == 40
- assert dict_gen.dict[-4:] == ['', '', '', '']
- self.assertEqual(dict_gen.num_classes, 40)
- self.assertListEqual(dict_gen.dict[-4:],
- ['', '', '', ''])
- dict_gen = Dictionary(
- dict_file=dict_file,
- with_start=True,
- with_end=True,
- same_start_end=True,
- with_padding=True,
- with_unknown=True,
- start_end_token='')
- self.assertEqual(dict_gen.num_classes, 39)
- self.assertListEqual(dict_gen.dict[-3:], ['', '', ''])
- # test len(line) > 1
- create_dummy_dict_file(dict_file, chars=['12', '3', '4'])
- with self.assertRaises(ValueError):
- dict_gen = Dictionary(dict_file=dict_file)
-
- # test duplicated dict
- create_dummy_dict_file(dict_file, chars=['1', '1', '2'])
- with self.assertRaises(AssertionError):
- dict_gen = Dictionary(dict_file=dict_file)
-
- tmp_dir.cleanup()
-
- def test_num_classes(self):
- with tempfile.TemporaryDirectory() as tmp_dir:
- # create dummy data
- dict_file = osp.join(tmp_dir, 'fake_chars.txt')
- create_dummy_dict_file(dict_file)
- dict_gen = Dictionary(dict_file=dict_file)
- assert dict_gen.num_classes == 36
-
- def test_char2idx(self):
- with tempfile.TemporaryDirectory() as tmp_dir:
-
- # create dummy data
- dict_file = osp.join(tmp_dir, 'fake_chars.txt')
- create_dummy_dict_file(dict_file)
- dict_gen = Dictionary(dict_file=dict_file, with_unknown=False)
- self.assertEqual(dict_gen.char2idx('0'), 0)
-
- dict_gen = Dictionary(dict_file=dict_file, with_unknown=True)
- self.assertEqual(dict_gen.char2idx('H'), dict_gen.unknown_idx)
-
- dict_gen = Dictionary(
- dict_file=dict_file, with_unknown=True, unknown_token=None)
- self.assertEqual(dict_gen.char2idx('H'), None)
-
- # Test strict
- dict_gen = Dictionary(dict_file=dict_file, with_unknown=False)
- with self.assertRaises(Exception):
- dict_gen.char2idx('H', strict=True)
- self.assertEqual(dict_gen.char2idx('H', strict=False), None)
-
- def test_str2idx(self):
- with tempfile.TemporaryDirectory() as tmp_dir:
-
- # create dummy data
- dict_file = osp.join(tmp_dir, 'fake_chars.txt')
- create_dummy_dict_file(dict_file)
- dict_gen = Dictionary(dict_file=dict_file)
- self.assertEqual(dict_gen.str2idx('01234'), [0, 1, 2, 3, 4])
- with self.assertRaises(Exception):
- dict_gen.str2idx('H')
-
- dict_gen = Dictionary(dict_file=dict_file, with_unknown=True)
- self.assertListEqual(dict_gen.str2idx('H'), [dict_gen.unknown_idx])
-
- dict_gen = Dictionary(
- dict_file=dict_file, with_unknown=True, unknown_token=None)
- self.assertListEqual(dict_gen.str2idx('H'), [])
-
- def test_idx2str(self):
- with tempfile.TemporaryDirectory() as tmp_dir:
-
- # create dummy data
- dict_file = osp.join(tmp_dir, 'fake_chars.txt')
- create_dummy_dict_file(dict_file)
- dict_gen = Dictionary(dict_file=dict_file)
- self.assertEqual(dict_gen.idx2str([0, 1, 2, 3, 4]), '01234')
- with self.assertRaises(AssertionError):
- dict_gen.idx2str('01234')
- with self.assertRaises(AssertionError):
- dict_gen.idx2str([40])
diff --git a/pipeline/mmocr/tests/test_models/test_textrecog/test_encoders/test_abi_encoder.py b/pipeline/mmocr/tests/test_models/test_textrecog/test_encoders/test_abi_encoder.py
deleted file mode 100644
index 7b108856bfe5f86e366d696c89efa7b772812ba5..0000000000000000000000000000000000000000
--- a/pipeline/mmocr/tests/test_models/test_textrecog/test_encoders/test_abi_encoder.py
+++ /dev/null
@@ -1,18 +0,0 @@
-# Copyright (c) OpenMMLab. All rights reserved.
-from unittest import TestCase
-
-import torch
-
-from mmocr.models.textrecog.encoders.abi_encoder import ABIEncoder
-
-
-class TestABIEncoder(TestCase):
-
- def test_init(self):
- with self.assertRaises(AssertionError):
- ABIEncoder(d_model=512, n_head=10)
-
- def test_forward(self):
- model = ABIEncoder()
- x = torch.randn(10, 512, 8, 32)
- self.assertEqual(model(x, None).shape, torch.Size([10, 512, 8, 32]))
diff --git a/pipeline/mmocr/tests/test_models/test_textrecog/test_encoders/test_aster_encoder.py b/pipeline/mmocr/tests/test_models/test_textrecog/test_encoders/test_aster_encoder.py
deleted file mode 100644
index b279c97f4d73726280ff897611e249055eca529e..0000000000000000000000000000000000000000
--- a/pipeline/mmocr/tests/test_models/test_textrecog/test_encoders/test_aster_encoder.py
+++ /dev/null
@@ -1,15 +0,0 @@
-# Copyright (c) OpenMMLab. All rights reserved.
-import unittest
-
-import torch
-
-from mmocr.models.textrecog.encoders import ASTEREncoder
-
-
-class TestASTEREncoder(unittest.TestCase):
-
- def test_encoder(self):
- encoder = ASTEREncoder(10)
- feat = torch.randn(2, 10, 1, 25)
- out = encoder(feat)
- self.assertEqual(out.shape, torch.Size([2, 25, 10]))
diff --git a/pipeline/mmocr/tests/test_models/test_textrecog/test_encoders/test_channel_reduction_encoder.py b/pipeline/mmocr/tests/test_models/test_textrecog/test_encoders/test_channel_reduction_encoder.py
deleted file mode 100644
index ec82f3e74c5b6ecffa05008bb1797381b6988fef..0000000000000000000000000000000000000000
--- a/pipeline/mmocr/tests/test_models/test_textrecog/test_encoders/test_channel_reduction_encoder.py
+++ /dev/null
@@ -1,26 +0,0 @@
-# Copyright (c) OpenMMLab. All rights reserved.
-import unittest
-
-import torch
-
-from mmocr.models.textrecog.encoders import ChannelReductionEncoder
-from mmocr.structures import TextRecogDataSample
-
-
-class TestChannelReductionEncoder(unittest.TestCase):
-
- def setUp(self):
- self.feat = torch.randn(2, 512, 8, 25)
- gt_text_sample1 = TextRecogDataSample()
- gt_text_sample1.set_metainfo(dict(valid_ratio=0.9))
-
- gt_text_sample2 = TextRecogDataSample()
- gt_text_sample2.set_metainfo(dict(valid_ratio=1.0))
-
- self.data_info = [gt_text_sample1, gt_text_sample2]
-
- def test_encoder(self):
- encoder = ChannelReductionEncoder(512, 256)
- encoder.train()
- out_enc = encoder(self.feat, self.data_info)
- self.assertEqual(out_enc.shape, torch.Size([2, 256, 8, 25]))
diff --git a/pipeline/mmocr/tests/test_models/test_textrecog/test_encoders/test_nrtr_encoder.py b/pipeline/mmocr/tests/test_models/test_textrecog/test_encoders/test_nrtr_encoder.py
deleted file mode 100644
index fbe20efb0581b14f3d713abeae398eeeffc70496..0000000000000000000000000000000000000000
--- a/pipeline/mmocr/tests/test_models/test_textrecog/test_encoders/test_nrtr_encoder.py
+++ /dev/null
@@ -1,27 +0,0 @@
-# Copyright (c) OpenMMLab. All rights reserved.
-import unittest
-
-import torch
-
-from mmocr.models.textrecog.encoders import NRTREncoder
-from mmocr.structures import TextRecogDataSample
-
-
-class TestNRTREncoder(unittest.TestCase):
-
- def setUp(self):
- self.feat = torch.randn(2, 512, 8, 25)
- gt_text_sample1 = TextRecogDataSample()
- gt_text_sample1.set_metainfo(dict(valid_ratio=0.9))
-
- gt_text_sample2 = TextRecogDataSample()
- gt_text_sample2.set_metainfo(dict(valid_ratio=1.0))
-
- self.data_info = [gt_text_sample1, gt_text_sample2]
-
- def test_encoder(self):
- nrtr_encoder = NRTREncoder()
- nrtr_encoder.init_weights()
- nrtr_encoder.train()
- out_enc = nrtr_encoder(self.feat, self.data_info)
- self.assertEqual(out_enc.shape, torch.Size([2, 200, 512]))
diff --git a/pipeline/mmocr/tests/test_models/test_textrecog/test_encoders/test_sar_encoder.py b/pipeline/mmocr/tests/test_models/test_textrecog/test_encoders/test_sar_encoder.py
deleted file mode 100644
index f36d9736eaa4f7b40d68165bd60b4eb42b13dd35..0000000000000000000000000000000000000000
--- a/pipeline/mmocr/tests/test_models/test_textrecog/test_encoders/test_sar_encoder.py
+++ /dev/null
@@ -1,44 +0,0 @@
-# Copyright (c) OpenMMLab. All rights reserved.
-from unittest import TestCase
-
-import torch
-
-from mmocr.models.textrecog.encoders import SAREncoder
-from mmocr.structures import TextRecogDataSample
-
-
-class TestSAREncoder(TestCase):
-
- def setUp(self):
- gt_text_sample1 = TextRecogDataSample()
- gt_text_sample1.set_metainfo(dict(valid_ratio=0.9))
-
- gt_text_sample2 = TextRecogDataSample()
- gt_text_sample2.set_metainfo(dict(valid_ratio=1.0))
-
- self.data_info = [gt_text_sample1, gt_text_sample2]
-
- def test_init(self):
- with self.assertRaises(AssertionError):
- SAREncoder(enc_bi_rnn='bi')
- with self.assertRaises(AssertionError):
- SAREncoder(rnn_dropout=2)
- with self.assertRaises(AssertionError):
- SAREncoder(enc_gru='gru')
- with self.assertRaises(AssertionError):
- SAREncoder(d_model=512.5)
- with self.assertRaises(AssertionError):
- SAREncoder(d_enc=200.5)
- with self.assertRaises(AssertionError):
- SAREncoder(mask='mask')
-
- def test_forward(self):
- encoder = SAREncoder()
- encoder.init_weights()
- encoder.train()
-
- feat = torch.randn(2, 512, 4, 40)
- with self.assertRaises(AssertionError):
- encoder(feat, self.data_info * 2)
- out_enc = encoder(feat, self.data_info)
- self.assertEqual(out_enc.shape, torch.Size([2, 512]))
diff --git a/pipeline/mmocr/tests/test_models/test_textrecog/test_encoders/test_satrn_decoder.py b/pipeline/mmocr/tests/test_models/test_textrecog/test_encoders/test_satrn_decoder.py
deleted file mode 100644
index 6d7e7ddc4ba22c02899a6effa8e3f86e60ee10d7..0000000000000000000000000000000000000000
--- a/pipeline/mmocr/tests/test_models/test_textrecog/test_encoders/test_satrn_decoder.py
+++ /dev/null
@@ -1,25 +0,0 @@
-# Copyright (c) OpenMMLab. All rights reserved.
-import unittest
-
-import torch
-
-from mmocr.models.textrecog.encoders import SATRNEncoder
-from mmocr.structures import TextRecogDataSample
-
-
-class TestSATRNEncoder(unittest.TestCase):
-
- def setUp(self):
- self.feat = torch.randn(1, 512, 8, 25)
- data_info = TextRecogDataSample()
- data_info.set_metainfo(dict(valid_ratio=1.0))
- self.data_info = [data_info]
-
- def test_encoder(self):
- satrn_encoder = SATRNEncoder()
- satrn_encoder.init_weights()
- satrn_encoder.train()
- out_enc = satrn_encoder(self.feat)
- self.assertEqual(out_enc.shape, torch.Size([1, 200, 512]))
- out_enc = satrn_encoder(self.feat, self.data_info)
- self.assertEqual(out_enc.shape, torch.Size([1, 200, 512]))
diff --git a/pipeline/mmocr/tests/test_models/test_textrecog/test_encoders/test_svtr_encoder.py b/pipeline/mmocr/tests/test_models/test_textrecog/test_encoders/test_svtr_encoder.py
deleted file mode 100644
index 8dab69459ac2588d6a246b3feb4289a2eefde060..0000000000000000000000000000000000000000
--- a/pipeline/mmocr/tests/test_models/test_textrecog/test_encoders/test_svtr_encoder.py
+++ /dev/null
@@ -1,85 +0,0 @@
-# Copyright (c) OpenMMLab. All rights reserved.
-from unittest import TestCase
-
-import torch
-
-from mmocr.models.textrecog.encoders.svtr_encoder import (AttnMixer, ConvMixer,
- MerigingBlock,
- MixingBlock,
- OverlapPatchEmbed,
- SVTREncoder)
-
-
-class TestOverlapPatchEmbed(TestCase):
-
- def setUp(self) -> None:
- self.img = torch.rand(1, 3, 32, 100)
-
- def test_overlap_patch_embed(self):
- Overlap_Patch_Embed = OverlapPatchEmbed(in_channels=self.img.shape[1])
- self.assertEqual(
- Overlap_Patch_Embed(self.img).shape, torch.Size([1, 8 * 25, 768]))
-
-
-class TestConvMixer(TestCase):
-
- def setUp(self) -> None:
- self.img = torch.rand(1, 8 * 25, 768)
-
- def test_conv_mixer(self):
- conv_mixer = ConvMixer(embed_dims=self.img.shape[-1])
- self.assertEqual(
- conv_mixer(self.img).shape, torch.Size([1, 8 * 25, 768]))
-
-
-class TestAttnMixer(TestCase):
-
- def setUp(self) -> None:
- self.img = torch.rand(1, 8 * 25, 768)
-
- def test_attn_mixer(self):
- attn_mixer = AttnMixer(embed_dims=self.img.shape[-1])
- self.assertEqual(
- attn_mixer(self.img).shape, torch.Size([1, 8 * 25, 768]))
-
-
-class TestMixingBlock(TestCase):
-
- def setUp(self) -> None:
- self.img = torch.rand(1, 8 * 25, 768)
-
- def test_mixing_block(self):
- mixing_block = MixingBlock(self.img.shape[-1], num_heads=8)
- self.assertEqual(
- mixing_block(self.img).shape, torch.Size([1, 8 * 25, 768]))
-
-
-class TestMergingBlock(TestCase):
-
- def setUp(self) -> None:
- self.img = torch.rand(1, 64, 8, 25)
-
- def test_mergingblock(self):
- mergingblock1 = MerigingBlock(
- self.img.shape[1], self.img.shape[1] * 2, types='Pool')
- mergingblock2 = MerigingBlock(
- self.img.shape[1], self.img.shape[1] * 2, types='Conv')
- self.assertEqual(
- [mergingblock1(self.img).shape,
- mergingblock2(self.img).shape],
- [torch.Size([1, 4 * 25, 64 * 2]),
- torch.Size([1, 4 * 25, 64 * 2])])
-
-
-class TestSVTREncoder(TestCase):
-
- def setUp(self) -> None:
- self.img = torch.rand(1, 3, 32, 100)
-
- def test_svtr_encoder(self):
- model = SVTREncoder(
- img_size=self.img.shape[-2:],
- in_channels=self.img.shape[1],
- )
- model.train()
- self.assertEqual(model(self.img).shape, torch.Size([1, 192, 1, 25]))
diff --git a/pipeline/mmocr/tests/test_models/test_textrecog/test_layers/test_conv_layer.py b/pipeline/mmocr/tests/test_models/test_textrecog/test_layers/test_conv_layer.py
deleted file mode 100644
index bf65d86cc97a023d486209039f744d7f3f2b2227..0000000000000000000000000000000000000000
--- a/pipeline/mmocr/tests/test_models/test_textrecog/test_layers/test_conv_layer.py
+++ /dev/null
@@ -1,42 +0,0 @@
-# Copyright (c) OpenMMLab. All rights reserved.
-from unittest import TestCase
-
-import torch
-
-from mmocr.models.textrecog.layers.conv_layer import (BasicBlock, Bottleneck,
- conv1x1, conv3x3)
-
-
-class TestUtils(TestCase):
-
- def test_conv3x3(self):
- conv = conv3x3(3, 6)
- self.assertEqual(conv.in_channels, 3)
- self.assertEqual(conv.out_channels, 6)
- self.assertEqual(conv.kernel_size, (3, 3))
-
- def test_conv1x1(self):
- conv = conv1x1(3, 6)
- self.assertEqual(conv.in_channels, 3)
- self.assertEqual(conv.out_channels, 6)
- self.assertEqual(conv.kernel_size, (1, 1))
-
-
-class TestBasicBlock(TestCase):
-
- def test_forward(self):
- x = torch.rand(1, 64, 224, 224)
- basic_block = BasicBlock(64, 64)
- self.assertEqual(basic_block.expansion, 1)
- out = basic_block(x)
- self.assertEqual(out.shape, torch.Size([1, 64, 224, 224]))
-
-
-class TestBottleneck(TestCase):
-
- def test_forward(self):
- x = torch.rand(1, 64, 224, 224)
- bottle_neck = Bottleneck(64, 64, downsample=True)
- self.assertEqual(bottle_neck.expansion, 4)
- out = bottle_neck(x)
- self.assertEqual(out.shape, torch.Size([1, 256, 224, 224]))
diff --git a/pipeline/mmocr/tests/test_models/test_textrecog/test_module_losses/test_abi_module_loss.py b/pipeline/mmocr/tests/test_models/test_textrecog/test_module_losses/test_abi_module_loss.py
deleted file mode 100644
index f0f7c43e2bb61ad4f086fde1152e32ab3617f1a3..0000000000000000000000000000000000000000
--- a/pipeline/mmocr/tests/test_models/test_textrecog/test_module_losses/test_abi_module_loss.py
+++ /dev/null
@@ -1,65 +0,0 @@
-# Copyright (c) OpenMMLab. All rights reserved.
-from unittest import TestCase
-
-import numpy as np
-import torch
-from mmengine.structures import LabelData
-
-from mmocr.models.textrecog.module_losses import ABIModuleLoss
-from mmocr.structures import TextRecogDataSample
-
-
-class TestABIModuleLoss(TestCase):
-
- def setUp(self) -> None:
-
- data_sample1 = TextRecogDataSample()
- data_sample1.gt_text = LabelData(item='hello')
- data_sample2 = TextRecogDataSample()
- data_sample2.gt_text = LabelData(item='123')
- self.gt = [data_sample1, data_sample2]
-
- def _equal(self, a, b):
- if isinstance(a, (torch.Tensor, np.ndarray)):
- return (a == b).all()
- else:
- return a == b
-
- def test_forward(self):
- dict_cfg = dict(
- type='Dictionary',
- dict_file='dicts/lower_english_digits.txt',
- with_start=True,
- with_end=True,
- same_start_end=True,
- with_padding=True,
- with_unknown=False)
- abi_loss = ABIModuleLoss(dict_cfg, max_seq_len=10)
- abi_loss.get_targets(self.gt)
- outputs = dict(
- out_vis=dict(logits=torch.randn(2, 10, 38)),
- out_langs=[
- dict(logits=torch.randn(2, 10, 38)),
- dict(logits=torch.randn(2, 10, 38))
- ],
- out_fusers=[
- dict(logits=torch.randn(2, 10, 38)),
- dict(logits=torch.randn(2, 10, 38))
- ])
- losses = abi_loss(outputs, self.gt)
- self.assertIsInstance(losses, dict)
- self.assertIn('loss_visual', losses)
- self.assertIn('loss_lang', losses)
- self.assertIn('loss_fusion', losses)
- print(losses['loss_lang'])
- print(losses['loss_fusion'])
-
- outputs.pop('out_vis')
- abi_loss(outputs, self.gt)
- out_langs = outputs.pop('out_langs')
- abi_loss(outputs, self.gt)
- outputs.pop('out_fusers')
- with self.assertRaises(AssertionError):
- abi_loss(outputs, self.gt)
- outputs['out_langs'] = out_langs
- abi_loss(outputs, self.gt)
diff --git a/pipeline/mmocr/tests/test_models/test_textrecog/test_module_losses/test_base_recog_module_loss.py b/pipeline/mmocr/tests/test_models/test_textrecog/test_module_losses/test_base_recog_module_loss.py
deleted file mode 100644
index e7cc5f12807da924c8c64d30cd59be59001d94c8..0000000000000000000000000000000000000000
--- a/pipeline/mmocr/tests/test_models/test_textrecog/test_module_losses/test_base_recog_module_loss.py
+++ /dev/null
@@ -1,140 +0,0 @@
-# Copyright (c) OpenMMLab. All rights reserved.
-import os.path as osp
-import tempfile
-from unittest import TestCase
-
-import numpy as np
-import torch
-from mmengine.structures import LabelData
-
-from mmocr.models.common.dictionary import Dictionary
-from mmocr.models.textrecog.module_losses import BaseTextRecogModuleLoss
-from mmocr.structures import TextRecogDataSample
-from mmocr.testing import create_dummy_dict_file
-
-
-class TestBaseRecogModuleLoss(TestCase):
-
- def _equal(self, a, b):
- if isinstance(a, (torch.Tensor, np.ndarray)):
- return (a == b).all()
- else:
- return a == b
-
- def test_init(self):
- tmp_dir = tempfile.TemporaryDirectory()
- dict_file = osp.join(tmp_dir.name, 'fake_chars.txt')
- create_dummy_dict_file(dict_file)
- # test diction cfg
- dict_cfg = dict(
- type='Dictionary',
- dict_file=dict_file,
- with_start=True,
- with_end=True,
- same_start_end=False,
- with_padding=True,
- with_unknown=True)
- base_recog_loss = BaseTextRecogModuleLoss(dict_cfg)
- self.assertIsInstance(base_recog_loss.dictionary, Dictionary)
- # test case mode
- with self.assertRaises(AssertionError):
- base_recog_loss = BaseTextRecogModuleLoss(
- dict_cfg, letter_case='no')
- # test invalid pad_with
- with self.assertRaises(AssertionError):
- base_recog_loss = BaseTextRecogModuleLoss(
- dict_cfg, pad_with='test')
- # test invalid combination of dictionary and pad_with
- dict_cfg = dict(type='Dictionary', dict_file=dict_file, with_end=False)
- for pad_with in ['end', 'padding']:
- with self.assertRaisesRegex(
- ValueError, f'pad_with="{pad_with}", but'
- f' dictionary.{pad_with}_idx is None'):
- base_recog_loss = BaseTextRecogModuleLoss(
- dict_cfg, pad_with=pad_with)
- with self.assertRaisesRegex(
- ValueError, 'pad_with="auto", but'
- ' dictionary.end_idx and dictionary.padding_idx are both'
- ' None'):
- base_recog_loss = BaseTextRecogModuleLoss(
- dict_cfg, pad_with='auto')
-
- # test dictionary is invalid type
- dict_cfg = ['tmp']
- with self.assertRaisesRegex(
- TypeError, ('The type of dictionary should be `Dictionary`'
- ' or dict, '
- f'but got {type(dict_cfg)}')):
- base_recog_loss = BaseTextRecogModuleLoss(dict_cfg)
-
- tmp_dir.cleanup()
-
- def test_get_targets(self):
- label_data = LabelData(item='0123')
- data_sample = TextRecogDataSample()
- data_sample.gt_text = label_data
- tmp_dir = tempfile.TemporaryDirectory()
- dict_file = osp.join(tmp_dir.name, 'fake_chars.txt')
- create_dummy_dict_file(dict_file)
- # test diction cfg
- dictionary = Dictionary(
- dict_file=dict_file,
- with_start=True,
- with_end=True,
- same_start_end=False,
- with_padding=True,
- with_unknown=True)
- base_recog_loss = BaseTextRecogModuleLoss(dictionary, max_seq_len=10)
- target_data_samples = base_recog_loss.get_targets([data_sample])
- assert self._equal(target_data_samples[0].gt_text.indexes,
- torch.LongTensor([0, 1, 2, 3]))
- padding_idx = dictionary.padding_idx
- assert self._equal(
- target_data_samples[0].gt_text.padded_indexes,
- torch.LongTensor([
- dictionary.start_idx, 0, 1, 2, 3, dictionary.end_idx,
- padding_idx, padding_idx, padding_idx, padding_idx
- ]))
- self.assertTrue(target_data_samples[0].have_target)
-
- target_data_samples = base_recog_loss.get_targets(target_data_samples)
- data_sample.set_metainfo(dict(have_target=False))
-
- dictionary = Dictionary(
- dict_file=dict_file,
- with_start=False,
- with_end=False,
- same_start_end=False,
- with_padding=True,
- with_unknown=True)
- base_recog_loss = BaseTextRecogModuleLoss(dictionary, max_seq_len=3)
- data_sample.gt_text.item = '0123'
- target_data_samples = base_recog_loss.get_targets([data_sample])
- assert self._equal(target_data_samples[0].gt_text.indexes,
- torch.LongTensor([0, 1, 2, 3]))
- padding_idx = dictionary.padding_idx
- assert self._equal(target_data_samples[0].gt_text.padded_indexes,
- torch.LongTensor([0, 1, 2]))
- data_sample.set_metainfo(dict(have_target=False))
-
- dict_cfg = dict(
- type='Dictionary',
- dict_file='dicts/lower_english_digits.txt',
- with_start=False,
- with_end=True,
- same_start_end=False,
- with_padding=True,
- with_unknown=True)
- base_recog_loss = BaseTextRecogModuleLoss(
- dict_cfg, max_seq_len=10, letter_case='lower', pad_with='none')
- data_sample.gt_text.item = '0123'
- target_data_samples = base_recog_loss.get_targets([data_sample])
- assert self._equal(target_data_samples[0].gt_text.indexes,
- torch.LongTensor([0, 1, 2, 3]))
- assert self._equal(target_data_samples[0].gt_text.padded_indexes,
- torch.LongTensor([0, 1, 2, 3, 36]))
-
- target_data_samples = base_recog_loss.get_targets([])
- self.assertListEqual(target_data_samples, [])
-
- tmp_dir.cleanup()
diff --git a/pipeline/mmocr/tests/test_models/test_textrecog/test_module_losses/test_ce_module_loss.py b/pipeline/mmocr/tests/test_models/test_textrecog/test_module_losses/test_ce_module_loss.py
deleted file mode 100644
index f979ed0ddd88f62f52816b0e617eb9b269014386..0000000000000000000000000000000000000000
--- a/pipeline/mmocr/tests/test_models/test_textrecog/test_module_losses/test_ce_module_loss.py
+++ /dev/null
@@ -1,109 +0,0 @@
-# Copyright (c) OpenMMLab. All rights reserved.
-from unittest import TestCase
-
-import torch
-from mmengine.structures import LabelData
-
-from mmocr.models.textrecog.module_losses import CEModuleLoss
-from mmocr.structures import TextRecogDataSample
-
-
-class TestCEModuleLoss(TestCase):
-
- def setUp(self) -> None:
-
- data_sample1 = TextRecogDataSample()
- data_sample1.gt_text = LabelData(item='hello')
- data_sample2 = TextRecogDataSample()
- data_sample2.gt_text = LabelData(item='01abyz')
- data_sample3 = TextRecogDataSample()
- data_sample3.gt_text = LabelData(item='123456789')
- self.gt = [data_sample1, data_sample2, data_sample3]
-
- def test_init(self):
- dict_file = 'dicts/lower_english_digits.txt'
- dict_cfg = dict(
- type='Dictionary',
- dict_file=dict_file,
- with_start=True,
- with_end=True,
- same_start_end=True,
- with_padding=True,
- with_unknown=False)
-
- with self.assertRaises(AssertionError):
- CEModuleLoss(dict_cfg, reduction=1)
- with self.assertRaises(AssertionError):
- CEModuleLoss(dict_cfg, reduction='avg')
- with self.assertRaises(AssertionError):
- CEModuleLoss(dict_cfg, flatten=1)
- with self.assertRaises(AssertionError):
- CEModuleLoss(dict_cfg, ignore_first_char=1)
- with self.assertRaises(AssertionError):
- CEModuleLoss(dict_cfg, ignore_char=['ignore'])
- ce_loss = CEModuleLoss(dict_cfg)
- self.assertEqual(ce_loss.ignore_index, 37)
- ce_loss = CEModuleLoss(dict_cfg, ignore_char=-1)
- self.assertEqual(ce_loss.ignore_index, -1)
- # with self.assertRaises(ValueError):
- with self.assertWarns(UserWarning):
- ce_loss = CEModuleLoss(dict_cfg, ignore_char='ignore')
- with self.assertWarns(UserWarning):
- ce_loss = CEModuleLoss(
- dict(
- type='Dictionary', dict_file=dict_file, with_unknown=True),
- ignore_char='M',
- pad_with='none')
- with self.assertWarns(UserWarning):
- ce_loss = CEModuleLoss(
- dict(
- type='Dictionary', dict_file=dict_file,
- with_unknown=False),
- ignore_char='M',
- pad_with='none')
- with self.assertWarns(UserWarning):
- ce_loss = CEModuleLoss(
- dict(
- type='Dictionary', dict_file=dict_file,
- with_unknown=False),
- ignore_char='unknown',
- pad_with='none')
- ce_loss = CEModuleLoss(dict_cfg, ignore_char='1')
- self.assertEqual(ce_loss.ignore_index, 1)
-
- def test_forward(self):
- dict_cfg = dict(
- type='Dictionary',
- dict_file='dicts/lower_english_digits.txt',
- with_start=True,
- with_end=True,
- same_start_end=True,
- with_padding=True,
- with_unknown=False)
- max_seq_len = 40
- ce_loss = CEModuleLoss(dict_cfg)
- ce_loss.get_targets(self.gt)
- outputs = torch.rand(3, max_seq_len, ce_loss.dictionary.num_classes)
- losses = ce_loss(outputs, self.gt)
- self.assertIsInstance(losses, dict)
- self.assertIn('loss_ce', losses)
- self.assertEqual(losses['loss_ce'].size(1), max_seq_len)
-
- # test ignore_first_char
- ce_loss = CEModuleLoss(dict_cfg, ignore_first_char=True)
- ignore_first_char_losses = ce_loss(outputs, self.gt)
- self.assertEqual(ignore_first_char_losses['loss_ce'].shape,
- torch.Size([3, max_seq_len - 1]))
-
- # test flatten
- ce_loss = CEModuleLoss(dict_cfg, flatten=True)
- flatten_losses = ce_loss(outputs, self.gt)
- self.assertEqual(flatten_losses['loss_ce'].shape,
- torch.Size([3 * max_seq_len]))
-
- self.assertTrue(
- torch.isclose(
- losses['loss_ce'].view(-1),
- flatten_losses['loss_ce'],
- atol=1e-6,
- rtol=0).all())
diff --git a/pipeline/mmocr/tests/test_models/test_textrecog/test_module_losses/test_ctc_module_loss.py b/pipeline/mmocr/tests/test_models/test_textrecog/test_module_losses/test_ctc_module_loss.py
deleted file mode 100644
index e52a3ccbff794b82dfd528a7a20ca85c02d32e7e..0000000000000000000000000000000000000000
--- a/pipeline/mmocr/tests/test_models/test_textrecog/test_module_losses/test_ctc_module_loss.py
+++ /dev/null
@@ -1,80 +0,0 @@
-# Copyright (c) OpenMMLab. All rights reserved.
-import os.path as osp
-import tempfile
-from unittest import TestCase
-
-import torch
-from mmengine.structures import LabelData
-
-from mmocr.models.common.dictionary import Dictionary
-from mmocr.models.textrecog.module_losses import CTCModuleLoss
-from mmocr.structures import TextRecogDataSample
-from mmocr.testing import create_dummy_dict_file
-
-
-class TestCTCModuleLoss(TestCase):
-
- def test_ctc_loss(self):
- tmp_dir = tempfile.TemporaryDirectory()
- # create dummy data
- dict_file = osp.join(tmp_dir.name, 'fake_chars.txt')
- create_dummy_dict_file(dict_file)
-
- dictionary = Dictionary(dict_file=dict_file, with_padding=True)
- with self.assertRaises(AssertionError):
- CTCModuleLoss(dictionary=dictionary, flatten='flatten')
- with self.assertRaises(AssertionError):
- CTCModuleLoss(dictionary=dictionary, reduction=1)
- with self.assertRaises(AssertionError):
- CTCModuleLoss(dictionary=dictionary, zero_infinity='zero')
-
- outputs = torch.zeros(2, 40, 37)
- datasample1 = TextRecogDataSample()
- gt_text1 = LabelData(item='hell')
- datasample1.gt_text = gt_text1
- datasample2 = datasample1.clone()
- gt_text2 = LabelData(item='owrd')
- datasample2.gt_text = gt_text2
- data_samples = [datasample1, datasample2]
- ctc_loss = CTCModuleLoss(dictionary=dictionary)
- data_samples = ctc_loss.get_targets(data_samples)
- losses = ctc_loss(outputs, data_samples)
- assert isinstance(losses, dict)
- assert 'loss_ctc' in losses
- assert torch.allclose(losses['loss_ctc'],
- torch.tensor(losses['loss_ctc'].item()).float())
- # test flatten = False
- ctc_loss = CTCModuleLoss(dictionary=dictionary, flatten=False)
- losses = ctc_loss(outputs, data_samples)
- assert isinstance(losses, dict)
- assert 'loss_ctc' in losses
- assert torch.allclose(losses['loss_ctc'],
- torch.tensor(losses['loss_ctc'].item()).float())
- tmp_dir.cleanup()
-
- def test_get_targets(self):
- tmp_dir = tempfile.TemporaryDirectory()
- # create dummy data
- dict_file = osp.join(tmp_dir.name, 'fake_chars.txt')
- create_dummy_dict_file(dict_file, list('helowrd'))
-
- dictionary = Dictionary(dict_file=dict_file, with_padding=True)
- loss = CTCModuleLoss(dictionary=dictionary, letter_case='lower')
- # test encode str to tensor
- datasample1 = TextRecogDataSample()
- gt_text1 = LabelData(item='hell')
- datasample1.gt_text = gt_text1
- datasample2 = datasample1.clone()
- gt_text2 = LabelData(item='owrd')
- datasample2.gt_text = gt_text2
-
- data_samples = [datasample1, datasample2]
- expect_tensor1 = torch.IntTensor([0, 1, 2, 2])
- expect_tensor2 = torch.IntTensor([3, 4, 5, 6])
-
- data_samples = loss.get_targets(data_samples)
- self.assertTrue(
- torch.allclose(data_samples[0].gt_text.indexes, expect_tensor1))
- self.assertTrue(
- torch.allclose(data_samples[1].gt_text.indexes, expect_tensor2))
- tmp_dir.cleanup()
diff --git a/pipeline/mmocr/tests/test_models/test_textrecog/test_plugins/test_gcamodule.py b/pipeline/mmocr/tests/test_models/test_textrecog/test_plugins/test_gcamodule.py
deleted file mode 100644
index 17e8ea660c13dab1b2c26f3f367a171c6082387f..0000000000000000000000000000000000000000
--- a/pipeline/mmocr/tests/test_models/test_textrecog/test_plugins/test_gcamodule.py
+++ /dev/null
@@ -1,38 +0,0 @@
-# Copyright (c) OpenMMLab. All rights reserved.
-from unittest import TestCase
-
-import torch
-from parameterized import parameterized
-
-from mmocr.models.textrecog.plugins import GCAModule
-
-
-class TestGCAModule(TestCase):
-
- def setUp(self) -> None:
- self.img = torch.rand(1, 32, 32, 100)
-
- @parameterized.expand([('att'), ('avg')])
- def test_gca_module_pooling(self, pooling_type):
- gca_module = GCAModule(
- in_channels=32,
- ratio=0.0625,
- n_head=1,
- pooling_type=pooling_type,
- is_att_scale=False,
- fusion_type='channel_add')
- self.assertEqual(
- gca_module(self.img).shape, torch.Size([1, 32, 32, 100]))
-
- @parameterized.expand([('channel_add'), ('channel_mul'),
- ('channel_concat')])
- def test_gca_module_fusion(self, fusion_type):
- gca_module = GCAModule(
- in_channels=32,
- ratio=0.0625,
- n_head=1,
- pooling_type='att',
- is_att_scale=False,
- fusion_type=fusion_type)
- self.assertEqual(
- gca_module(self.img).shape, torch.Size([1, 32, 32, 100]))
diff --git a/pipeline/mmocr/tests/test_models/test_textrecog/test_plugins/test_maxpool.py b/pipeline/mmocr/tests/test_models/test_textrecog/test_plugins/test_maxpool.py
deleted file mode 100644
index 1800632f53bbce50416f54b8fe55f2da651b24c0..0000000000000000000000000000000000000000
--- a/pipeline/mmocr/tests/test_models/test_textrecog/test_plugins/test_maxpool.py
+++ /dev/null
@@ -1,16 +0,0 @@
-# Copyright (c) OpenMMLab. All rights reserved.
-from unittest import TestCase
-
-import torch
-
-from mmocr.models.textrecog.plugins import Maxpool2d
-
-
-class TestMaxpool2d(TestCase):
-
- def setUp(self) -> None:
- self.img = torch.rand(1, 3, 32, 100)
-
- def test_maxpool2d(self):
- maxpool2d = Maxpool2d(kernel_size=2, stride=2)
- self.assertEqual(maxpool2d(self.img).shape, torch.Size([1, 3, 16, 50]))
diff --git a/pipeline/mmocr/tests/test_models/test_textrecog/test_postprocessors/test_attn_postprocessor.py b/pipeline/mmocr/tests/test_models/test_textrecog/test_postprocessors/test_attn_postprocessor.py
deleted file mode 100644
index c0b0c18c42499b87b187928af5b5a109e84449d1..0000000000000000000000000000000000000000
--- a/pipeline/mmocr/tests/test_models/test_textrecog/test_postprocessors/test_attn_postprocessor.py
+++ /dev/null
@@ -1,43 +0,0 @@
-# Copyright (c) OpenMMLab. All rights reserved.
-
-import os.path as osp
-import tempfile
-from unittest import TestCase
-
-import torch
-
-from mmocr.models.common.dictionary import Dictionary
-from mmocr.models.textrecog.postprocessors.attn_postprocessor import \
- AttentionPostprocessor
-from mmocr.structures import TextRecogDataSample
-from mmocr.testing import create_dummy_dict_file
-
-
-class TestAttentionPostprocessor(TestCase):
-
- def test_call(self):
- tmp_dir = tempfile.TemporaryDirectory()
- dict_file = osp.join(tmp_dir.name, 'fake_chars.txt')
- create_dummy_dict_file(dict_file)
- dict_gen = Dictionary(
- dict_file=dict_file,
- with_start=True,
- with_end=True,
- same_start_end=True,
- with_padding=True,
- with_unknown=False)
- data_samples = [TextRecogDataSample()]
- postprocessor = AttentionPostprocessor(
- max_seq_len=None, dictionary=dict_gen, ignore_chars=['0'])
- dict_gen.end_idx = 3
- # test decode output to index
- dummy_output = torch.Tensor([[[1, 100, 3, 4, 5, 6, 7, 8],
- [100, 2, 3, 4, 5, 6, 7, 8],
- [1, 2, 100, 4, 5, 6, 7, 8],
- [1, 2, 100, 4, 5, 6, 7, 8],
- [100, 2, 3, 4, 5, 6, 7, 8],
- [1, 2, 3, 100, 5, 6, 7, 8],
- [100, 2, 3, 4, 5, 6, 7, 8],
- [1, 2, 3, 100, 5, 6, 7, 8]]])
- data_samples = postprocessor(dummy_output, data_samples)
- self.assertEqual(data_samples[0].pred_text.item, '122')
diff --git a/pipeline/mmocr/tests/test_models/test_textrecog/test_postprocessors/test_base_textrecog_postprocessor.py b/pipeline/mmocr/tests/test_models/test_textrecog/test_postprocessors/test_base_textrecog_postprocessor.py
deleted file mode 100644
index 1fb163c64d55caf4025c61e7b271b71ee90740b2..0000000000000000000000000000000000000000
--- a/pipeline/mmocr/tests/test_models/test_textrecog/test_postprocessors/test_base_textrecog_postprocessor.py
+++ /dev/null
@@ -1,146 +0,0 @@
-# Copyright (c) OpenMMLab. All rights reserved.
-import os.path as osp
-import tempfile
-from unittest import TestCase, mock
-
-import torch
-
-from mmocr.models.common.dictionary import Dictionary
-from mmocr.models.textrecog.postprocessors import BaseTextRecogPostprocessor
-from mmocr.structures import TextRecogDataSample
-from mmocr.testing import create_dummy_dict_file
-
-
-class TestBaseTextRecogPostprocessor(TestCase):
-
- def test_init(self):
- tmp_dir = tempfile.TemporaryDirectory()
- dict_file = osp.join(tmp_dir.name, 'fake_chars.txt')
- create_dummy_dict_file(dict_file)
- # test diction cfg
- dict_cfg = dict(
- type='Dictionary',
- dict_file=dict_file,
- with_start=True,
- with_end=True,
- same_start_end=False,
- with_padding=True,
- with_unknown=True)
- base_postprocessor = BaseTextRecogPostprocessor(dict_cfg)
- self.assertIsInstance(base_postprocessor.dictionary, Dictionary)
- self.assertListEqual(base_postprocessor.ignore_indexes,
- [base_postprocessor.dictionary.padding_idx])
-
- base_postprocessor = BaseTextRecogPostprocessor(
- dict_cfg, ignore_chars=['1', '2', '3'])
-
- self.assertListEqual(base_postprocessor.ignore_indexes, [1, 2, 3])
-
- # test ignore_chars
- with self.assertRaisesRegex(TypeError,
- 'ignore_chars must be list of str'):
- base_postprocessor = BaseTextRecogPostprocessor(
- dict_cfg, ignore_chars=[1, 2, 3])
- with self.assertWarnsRegex(Warning,
- 'M does not exist in the dictionary'):
- base_postprocessor = BaseTextRecogPostprocessor(
- dict_cfg, ignore_chars=['M'])
-
- base_postprocessor = BaseTextRecogPostprocessor(
- dict_cfg, ignore_chars=['1', '2', '3'])
- # test dictionary is invalid type
- dict_cfg = ['tmp']
- with self.assertRaisesRegex(
- TypeError, ('The type of dictionary should be `Dictionary`'
- ' or dict, '
- f'but got {type(dict_cfg)}')):
- base_postprocessor = BaseTextRecogPostprocessor(dict_cfg)
- # test diction cfg with with_unknown=False
- dict_cfg = dict(
- type='Dictionary',
- dict_file=dict_file,
- with_start=True,
- with_end=True,
- same_start_end=False,
- with_padding=True,
- with_unknown=False)
- base_postprocessor = BaseTextRecogPostprocessor(
- dict_cfg, ignore_chars=['1', '2', '3'])
-
- self.assertListEqual(base_postprocessor.ignore_indexes, [1, 2, 3])
-
- # test ignore_chars
- with self.assertRaisesRegex(TypeError,
- 'ignore_chars must be list of str'):
- base_postprocessor = BaseTextRecogPostprocessor(
- dict_cfg, ignore_chars=[1, 2, 3])
-
- with self.assertWarnsRegex(Warning,
- 'M does not exist in the dictionary'):
- base_postprocessor = BaseTextRecogPostprocessor(
- dict_cfg, ignore_chars=['M'])
-
- with self.assertWarnsRegex(Warning,
- 'M does not exist in the dictionary'):
- base_postprocessor = BaseTextRecogPostprocessor(
- dict(
- type='Dictionary',
- dict_file=dict_file,
- with_unknown=True,
- unknown_token=None),
- ignore_chars=['M'])
-
- with self.assertWarnsRegex(Warning,
- 'M does not exist in the dictionary'):
- base_postprocessor = BaseTextRecogPostprocessor(
- dict(
- type='Dictionary', dict_file=dict_file, with_unknown=True),
- ignore_chars=['M'])
-
- with self.assertWarnsRegex(Warning,
- 'unknown does not exist in the dictionary'):
- base_postprocessor = BaseTextRecogPostprocessor(
- dict(
- type='Dictionary', dict_file=dict_file,
- with_unknown=False),
- ignore_chars=['unknown'])
-
- base_postprocessor = BaseTextRecogPostprocessor(
- dict_cfg, ignore_chars=['1', '2', '3'])
- # test dictionary is invalid type
- dict_cfg = ['tmp']
- with self.assertRaisesRegex(
- TypeError, ('The type of dictionary should be `Dictionary`'
- ' or dict, '
- f'but got {type(dict_cfg)}')):
- base_postprocessor = BaseTextRecogPostprocessor(dict_cfg)
-
- tmp_dir.cleanup()
-
- @mock.patch(f'{__name__}.BaseTextRecogPostprocessor.get_single_prediction')
- def test_call(self, mock_get_single_prediction):
-
- def mock_func(output, data_sample):
- return [0, 1, 2], [0.8, 0.7, 0.9]
-
- tmp_dir = tempfile.TemporaryDirectory()
- dict_file = osp.join(tmp_dir.name, 'fake_chars.txt')
- create_dummy_dict_file(dict_file)
- dict_cfg = dict(
- type='Dictionary',
- dict_file=dict_file,
- with_start=True,
- with_end=True,
- same_start_end=False,
- with_padding=True,
- with_unknown=True)
- mock_get_single_prediction.side_effect = mock_func
- data_samples = [TextRecogDataSample()]
- postprocessor = BaseTextRecogPostprocessor(
- max_seq_len=None, dictionary=dict_cfg)
-
- # test decode output to index
- dummy_output = torch.Tensor([[[1, 100, 3, 4, 5, 6, 7, 8]]])
- data_samples = postprocessor(dummy_output, data_samples)
- self.assertEqual(data_samples[0].pred_text.item, '012')
- tmp_dir.cleanup()
diff --git a/pipeline/mmocr/tests/test_models/test_textrecog/test_postprocessors/test_ctc_postprocessor.py b/pipeline/mmocr/tests/test_models/test_textrecog/test_postprocessors/test_ctc_postprocessor.py
deleted file mode 100644
index 359744b3cc04c59f91fb64eeb5de799e665a308e..0000000000000000000000000000000000000000
--- a/pipeline/mmocr/tests/test_models/test_textrecog/test_postprocessors/test_ctc_postprocessor.py
+++ /dev/null
@@ -1,77 +0,0 @@
-# Copyright (c) OpenMMLab. All rights reserved.
-
-import os.path as osp
-import tempfile
-from unittest import TestCase
-
-import torch
-
-from mmocr.models.common.dictionary import Dictionary
-from mmocr.models.textrecog.postprocessors.ctc_postprocessor import \
- CTCPostProcessor
-from mmocr.structures import TextRecogDataSample
-from mmocr.testing import create_dummy_dict_file
-
-
-class TestCTCPostProcessor(TestCase):
-
- def test_get_single_prediction(self):
-
- tmp_dir = tempfile.TemporaryDirectory()
- dict_file = osp.join(tmp_dir.name, 'fake_chars.txt')
- create_dummy_dict_file(dict_file)
- dict_gen = Dictionary(
- dict_file=dict_file,
- with_start=False,
- with_end=False,
- with_padding=True,
- with_unknown=False)
- data_samples = [TextRecogDataSample()]
- postprocessor = CTCPostProcessor(max_seq_len=None, dictionary=dict_gen)
-
- # test decode output to index
- dummy_output = torch.Tensor([[[1, 100, 3, 4, 5, 6, 7, 8],
- [100, 2, 3, 4, 5, 6, 7, 8],
- [1, 2, 100, 4, 5, 6, 7, 8],
- [1, 2, 100, 4, 5, 6, 7, 8],
- [100, 2, 3, 4, 5, 6, 7, 8],
- [1, 2, 3, 100, 5, 6, 7, 8],
- [100, 2, 3, 4, 5, 6, 7, 8],
- [1, 2, 3, 100, 5, 6, 7, 8]]])
- index, score = postprocessor.get_single_prediction(
- dummy_output[0], data_samples[0])
- self.assertListEqual(index, [1, 0, 2, 0, 3, 0, 3])
- self.assertListEqual(score,
- [100.0, 100.0, 100.0, 100.0, 100.0, 100.0, 100.0])
- postprocessor = CTCPostProcessor(
- max_seq_len=None, dictionary=dict_gen, ignore_chars=['0'])
- index, score = postprocessor.get_single_prediction(
- dummy_output[0], data_samples[0])
- self.assertListEqual(index, [1, 2, 3, 3])
- self.assertListEqual(score, [100.0, 100.0, 100.0, 100.0])
- tmp_dir.cleanup()
-
- def test_call(self):
- tmp_dir = tempfile.TemporaryDirectory()
- dict_file = osp.join(tmp_dir.name, 'fake_chars.txt')
- create_dummy_dict_file(dict_file)
- dict_gen = Dictionary(
- dict_file=dict_file,
- with_start=False,
- with_end=False,
- with_padding=True,
- with_unknown=False)
- data_samples = [TextRecogDataSample()]
- postprocessor = CTCPostProcessor(max_seq_len=None, dictionary=dict_gen)
-
- # test decode output to index
- dummy_output = torch.Tensor([[[1, 100, 3, 4, 5, 6, 7, 8],
- [100, 2, 3, 4, 5, 6, 7, 8],
- [1, 2, 100, 4, 5, 6, 7, 8],
- [1, 2, 100, 4, 5, 6, 7, 8],
- [100, 2, 3, 4, 5, 6, 7, 8],
- [1, 2, 3, 100, 5, 6, 7, 8],
- [100, 2, 3, 4, 5, 6, 7, 8],
- [1, 2, 3, 100, 5, 6, 7, 8]]])
- data_samples = postprocessor(dummy_output, data_samples)
- self.assertEqual(data_samples[0].pred_text.item, '1020303')
diff --git a/pipeline/mmocr/tests/test_models/test_textrecog/test_recognizers/test_encoder_decoder_recognizer.py b/pipeline/mmocr/tests/test_models/test_textrecog/test_recognizers/test_encoder_decoder_recognizer.py
deleted file mode 100644
index 5c2165d983c2424f697f4609f980c0b5d89f371f..0000000000000000000000000000000000000000
--- a/pipeline/mmocr/tests/test_models/test_textrecog/test_recognizers/test_encoder_decoder_recognizer.py
+++ /dev/null
@@ -1,135 +0,0 @@
-# Copyright (c) OpenMMLab. All rights reserved.
-from unittest import TestCase
-
-import torch
-
-from mmocr.models.textrecog.recognizers import EncoderDecoderRecognizer
-from mmocr.registry import MODELS
-
-
-class TestEncoderDecoderRecognizer(TestCase):
-
- @MODELS.register_module()
- class DummyModule:
-
- def __init__(self, value):
- self.value = value
-
- def __call__(self, x, *args, **kwargs):
- return x + self.value
-
- def predict(self, x, y, *args, **kwargs):
- if y is None:
- return x
- return x + y
-
- def loss(self, x, y, *args, **kwargs):
- if y is None:
- return x
- return x * y
-
- def test_init(self):
- # Decoder is not allowed to be None
- with self.assertRaises(AssertionError):
- EncoderDecoderRecognizer()
-
- for attr in ['backbone', 'preprocessor', 'encoder']:
- recognizer = EncoderDecoderRecognizer(
- **{
- attr: dict(type='DummyModule', value=1),
- 'decoder': dict(type='DummyModule', value=2)
- })
- self.assertTrue(hasattr(recognizer, attr))
- self.assertFalse(
- any(
- hasattr(recognizer, t)
- for t in ['backbone', 'preprocessor', 'encoder']
- if t != attr))
-
- def test_extract_feat(self):
- model = EncoderDecoderRecognizer(
- decoder=dict(type='DummyModule', value=1))
- self.assertEqual(
- model.extract_feat(torch.tensor([1])), torch.Tensor([1]))
- model = EncoderDecoderRecognizer(
- backbone=dict(type='DummyModule', value=1),
- decoder=dict(type='DummyModule', value=1))
- self.assertEqual(
- model.extract_feat(torch.tensor([1])), torch.Tensor([2]))
- model = EncoderDecoderRecognizer(
- preprocessor=dict(type='DummyModule', value=2),
- decoder=dict(type='DummyModule', value=1))
- self.assertEqual(
- model.extract_feat(torch.tensor([1])), torch.Tensor([3]))
- model = EncoderDecoderRecognizer(
- preprocessor=dict(type='DummyModule', value=2),
- backbone=dict(type='DummyModule', value=1),
- decoder=dict(type='DummyModule', value=1))
- self.assertEqual(
- model.extract_feat(torch.tensor([1])), torch.Tensor([4]))
-
- def test_loss(self):
- model = EncoderDecoderRecognizer(
- decoder=dict(type='DummyModule', value=1))
- self.assertEqual(
- model.loss(torch.tensor([1]), None), torch.Tensor([1]))
- model = EncoderDecoderRecognizer(
- encoder=dict(type='DummyModule', value=2),
- decoder=dict(type='DummyModule', value=1))
- self.assertEqual(
- model.loss(torch.tensor([1]), None), torch.Tensor([3]))
- model = EncoderDecoderRecognizer(
- backbone=dict(type='DummyModule', value=1),
- encoder=dict(type='DummyModule', value=2),
- decoder=dict(type='DummyModule', value=1))
- self.assertEqual(
- model.loss(torch.tensor([1]), None), torch.Tensor([8]))
- model = EncoderDecoderRecognizer(
- backbone=dict(type='DummyModule', value=1),
- decoder=dict(type='DummyModule', value=1))
- self.assertEqual(
- model.loss(torch.tensor([1]), None), torch.Tensor([2]))
-
- def test_predict(self):
- model = EncoderDecoderRecognizer(
- decoder=dict(type='DummyModule', value=1))
- self.assertEqual(
- model.predict(torch.tensor([1]), None), torch.Tensor([1]))
- model = EncoderDecoderRecognizer(
- encoder=dict(type='DummyModule', value=2),
- decoder=dict(type='DummyModule', value=1))
- self.assertEqual(
- model.predict(torch.tensor([1]), None), torch.Tensor([4]))
- model = EncoderDecoderRecognizer(
- backbone=dict(type='DummyModule', value=1),
- encoder=dict(type='DummyModule', value=2),
- decoder=dict(type='DummyModule', value=1))
- self.assertEqual(
- model.predict(torch.tensor([1]), None), torch.Tensor([6]))
- model = EncoderDecoderRecognizer(
- backbone=dict(type='DummyModule', value=1),
- decoder=dict(type='DummyModule', value=1))
- self.assertEqual(
- model.loss(torch.tensor([1]), None), torch.Tensor([2]))
-
- def test_forward(self):
- model = EncoderDecoderRecognizer(
- decoder=dict(type='DummyModule', value=1))
- self.assertEqual(
- model._forward(torch.tensor([1]), None), torch.Tensor([2]))
- model = EncoderDecoderRecognizer(
- encoder=dict(type='DummyModule', value=2),
- decoder=dict(type='DummyModule', value=1))
- self.assertEqual(
- model._forward(torch.tensor([1]), None), torch.Tensor([2]))
- model = EncoderDecoderRecognizer(
- backbone=dict(type='DummyModule', value=1),
- encoder=dict(type='DummyModule', value=2),
- decoder=dict(type='DummyModule', value=1))
- self.assertEqual(
- model._forward(torch.tensor([1]), None), torch.Tensor([3]))
- model = EncoderDecoderRecognizer(
- backbone=dict(type='DummyModule', value=1),
- decoder=dict(type='DummyModule', value=1))
- self.assertEqual(
- model._forward(torch.tensor([1]), None), torch.Tensor([3]))
diff --git a/pipeline/mmocr/tests/test_models/test_textrecog/test_recognizers/test_encoder_decoder_recognizer_tta.py b/pipeline/mmocr/tests/test_models/test_textrecog/test_recognizers/test_encoder_decoder_recognizer_tta.py
deleted file mode 100644
index 2c2da3f8617cdd4acf2e927a732b14f2999edc83..0000000000000000000000000000000000000000
--- a/pipeline/mmocr/tests/test_models/test_textrecog/test_recognizers/test_encoder_decoder_recognizer_tta.py
+++ /dev/null
@@ -1,42 +0,0 @@
-# Copyright (c) OpenMMLab. All rights reserved.
-from unittest import TestCase
-
-import torch
-import torch.nn as nn
-from mmengine.structures import LabelData
-
-from mmocr.models.textrecog.recognizers import EncoderDecoderRecognizerTTAModel
-from mmocr.structures import TextRecogDataSample
-
-
-class DummyModel(nn.Module):
-
- def __init__(self):
- super().__init__()
-
- def forward(self, x):
- return x
-
- def test_step(self, x):
- return self.forward(x)
-
-
-class TestEncoderDecoderRecognizerTTAModel(TestCase):
-
- def test_merge_preds(self):
-
- data_sample1 = TextRecogDataSample(
- pred_text=LabelData(
- score=torch.tensor([0.1, 0.2, 0.3, 0.4, 0.5]), text='abcde'))
- data_sample2 = TextRecogDataSample(
- pred_text=LabelData(
- score=torch.tensor([0.2, 0.3, 0.4, 0.5, 0.6]), text='bcdef'))
- data_sample3 = TextRecogDataSample(
- pred_text=LabelData(
- score=torch.tensor([0.3, 0.4, 0.5, 0.6, 0.7]), text='cdefg'))
- aug_data_samples = [data_sample1, data_sample2, data_sample3]
- batch_aug_data_samples = [aug_data_samples] * 3
- model = EncoderDecoderRecognizerTTAModel(module=DummyModel())
- preds = model.merge_preds(batch_aug_data_samples)
- for pred in preds:
- self.assertEqual(pred.pred_text.text, 'cdefg')
diff --git a/pipeline/mmocr/tests/test_structures/test_kie_data_sample.py b/pipeline/mmocr/tests/test_structures/test_kie_data_sample.py
deleted file mode 100644
index 48c37cb9e61fdf917ed6cea43c1eb6c912283edb..0000000000000000000000000000000000000000
--- a/pipeline/mmocr/tests/test_structures/test_kie_data_sample.py
+++ /dev/null
@@ -1,105 +0,0 @@
-# Copyright (c) OpenMMLab. All rights reserved.
-from unittest import TestCase
-
-import numpy as np
-import torch
-from mmengine.structures import InstanceData
-
-from mmocr.structures import KIEDataSample
-
-
-class TestTextDetDataSample(TestCase):
-
- def _equal(self, a, b):
- if isinstance(a, (torch.Tensor, np.ndarray)):
- return (a == b).all()
- else:
- return a == b
-
- def test_init(self):
- meta_info = dict(
- img_size=[256, 256],
- scale_factor=np.array([1.5, 1.5]),
- img_shape=torch.rand(4))
-
- kie_data_sample = KIEDataSample(metainfo=meta_info)
- assert 'img_size' in kie_data_sample
-
- self.assertListEqual(kie_data_sample.img_size, [256, 256])
- self.assertListEqual(kie_data_sample.get('img_size'), [256, 256])
-
- def test_setter(self):
- kie_data_sample = KIEDataSample()
- # test gt_instances
- gt_instances_data = dict(
- bboxes=torch.rand(4, 4),
- labels=torch.rand(4),
- texts=['t1', 't2', 't3', 't4'],
- relations=torch.rand(4, 4),
- edge_labels=torch.randint(0, 4, (4, )))
- gt_instances = InstanceData(**gt_instances_data)
- kie_data_sample.gt_instances = gt_instances
- self.assertIn('gt_instances', kie_data_sample)
- self.assertTrue(
- self._equal(kie_data_sample.gt_instances.bboxes,
- gt_instances_data['bboxes']))
- self.assertTrue(
- self._equal(kie_data_sample.gt_instances.labels,
- gt_instances_data['labels']))
- self.assertTrue(
- self._equal(kie_data_sample.gt_instances.texts,
- gt_instances_data['texts']))
- self.assertTrue(
- self._equal(kie_data_sample.gt_instances.relations,
- gt_instances_data['relations']))
- self.assertTrue(
- self._equal(kie_data_sample.gt_instances.edge_labels,
- gt_instances_data['edge_labels']))
-
- # test pred_instances
- pred_instances_data = dict(
- bboxes=torch.rand(4, 4),
- labels=torch.rand(4),
- texts=['t1', 't2', 't3', 't4'],
- relations=torch.rand(4, 4),
- edge_labels=torch.randint(0, 4, (4, )))
- pred_instances = InstanceData(**pred_instances_data)
- kie_data_sample.pred_instances = pred_instances
- assert 'pred_instances' in kie_data_sample
- assert self._equal(kie_data_sample.pred_instances.bboxes,
- pred_instances_data['bboxes'])
- assert self._equal(kie_data_sample.pred_instances.labels,
- pred_instances_data['labels'])
- self.assertTrue(
- self._equal(kie_data_sample.pred_instances.texts,
- pred_instances_data['texts']))
- self.assertTrue(
- self._equal(kie_data_sample.pred_instances.relations,
- pred_instances_data['relations']))
- self.assertTrue(
- self._equal(kie_data_sample.pred_instances.edge_labels,
- pred_instances_data['edge_labels']))
-
- # test type error
- with self.assertRaises(AssertionError):
- kie_data_sample.gt_instances = torch.rand(2, 4)
- with self.assertRaises(AssertionError):
- kie_data_sample.pred_instances = torch.rand(2, 4)
-
- def test_deleter(self):
- gt_instances_data = dict(
- bboxes=torch.rand(4, 4),
- labels=torch.rand(4),
- )
-
- kie_data_sample = KIEDataSample()
- gt_instances = InstanceData(data=gt_instances_data)
- kie_data_sample.gt_instances = gt_instances
- assert 'gt_instances' in kie_data_sample
- del kie_data_sample.gt_instances
- assert 'gt_instances' not in kie_data_sample
-
- kie_data_sample.pred_instances = gt_instances
- assert 'pred_instances' in kie_data_sample
- del kie_data_sample.pred_instances
- assert 'pred_instances' not in kie_data_sample
diff --git a/pipeline/mmocr/tests/test_structures/test_textdet_data_sample.py b/pipeline/mmocr/tests/test_structures/test_textdet_data_sample.py
deleted file mode 100644
index f5b79439bc9d7d0ff819155eeb40f84792b64a0c..0000000000000000000000000000000000000000
--- a/pipeline/mmocr/tests/test_structures/test_textdet_data_sample.py
+++ /dev/null
@@ -1,85 +0,0 @@
-# Copyright (c) OpenMMLab. All rights reserved.
-from unittest import TestCase
-
-import numpy as np
-import torch
-from mmengine.structures import InstanceData
-
-from mmocr.structures import TextDetDataSample
-
-
-class TestTextDetDataSample(TestCase):
-
- def _equal(self, a, b):
- if isinstance(a, (torch.Tensor, np.ndarray)):
- return (a == b).all()
- else:
- return a == b
-
- def test_init(self):
- meta_info = dict(
- img_size=[256, 256],
- scale_factor=np.array([1.5, 1.5]),
- img_shape=torch.rand(4))
-
- det_data_sample = TextDetDataSample(metainfo=meta_info)
- assert 'img_size' in det_data_sample
-
- self.assertListEqual(det_data_sample.img_size, [256, 256])
- self.assertListEqual(det_data_sample.get('img_size'), [256, 256])
-
- def test_setter(self):
- det_data_sample = TextDetDataSample()
- # test gt_instances
- gt_instances_data = dict(
- bboxes=torch.rand(4, 4),
- labels=torch.rand(4),
- masks=np.random.rand(4, 2, 2))
- gt_instances = InstanceData(**gt_instances_data)
- det_data_sample.gt_instances = gt_instances
- assert 'gt_instances' in det_data_sample
- assert self._equal(det_data_sample.gt_instances.bboxes,
- gt_instances_data['bboxes'])
- assert self._equal(det_data_sample.gt_instances.labels,
- gt_instances_data['labels'])
- assert self._equal(det_data_sample.gt_instances.masks,
- gt_instances_data['masks'])
-
- # test pred_instances
- pred_instances_data = dict(
- bboxes=torch.rand(2, 4),
- labels=torch.rand(2),
- masks=np.random.rand(2, 2, 2))
- pred_instances = InstanceData(**pred_instances_data)
- det_data_sample.pred_instances = pred_instances
- assert 'pred_instances' in det_data_sample
- assert self._equal(det_data_sample.pred_instances.bboxes,
- pred_instances_data['bboxes'])
- assert self._equal(det_data_sample.pred_instances.labels,
- pred_instances_data['labels'])
- assert self._equal(det_data_sample.pred_instances.masks,
- pred_instances_data['masks'])
-
- # test type error
- with self.assertRaises(AssertionError):
- det_data_sample.gt_instances = torch.rand(2, 4)
- with self.assertRaises(AssertionError):
- det_data_sample.pred_instances = torch.rand(2, 4)
-
- def test_deleter(self):
- gt_instances_data = dict(
- bboxes=torch.rand(4, 4),
- labels=torch.rand(4),
- masks=np.random.rand(4, 2, 2))
-
- det_data_sample = TextDetDataSample()
- gt_instances = InstanceData(data=gt_instances_data)
- det_data_sample.gt_instances = gt_instances
- assert 'gt_instances' in det_data_sample
- del det_data_sample.gt_instances
- assert 'gt_instances' not in det_data_sample
-
- det_data_sample.pred_instances = gt_instances
- assert 'pred_instances' in det_data_sample
- del det_data_sample.pred_instances
- assert 'pred_instances' not in det_data_sample
diff --git a/pipeline/mmocr/tests/test_structures/test_textrecog_data_sample.py b/pipeline/mmocr/tests/test_structures/test_textrecog_data_sample.py
deleted file mode 100644
index a489200c40051e891236b7477c0e3b15e9cab1f3..0000000000000000000000000000000000000000
--- a/pipeline/mmocr/tests/test_structures/test_textrecog_data_sample.py
+++ /dev/null
@@ -1,59 +0,0 @@
-# Copyright (c) OpenMMLab. All rights reserved.
-from unittest import TestCase
-
-import numpy as np
-import torch
-from mmengine.structures import LabelData
-
-from mmocr.structures import TextRecogDataSample
-
-
-class TestTextRecogDataSample(TestCase):
-
- def test_init(self):
- meta_info = dict(
- img_size=[256, 256],
- scale_factor=np.array([1.5, 1.5]),
- img_shape=torch.rand(4))
-
- recog_data_sample = TextRecogDataSample(metainfo=meta_info)
- assert 'img_size' in recog_data_sample
-
- self.assertListEqual(recog_data_sample.img_size, [256, 256])
- self.assertListEqual(recog_data_sample.get('img_size'), [256, 256])
-
- def test_setter(self):
- recog_data_sample = TextRecogDataSample()
- # test gt_text
- gt_label_data = dict(item='mmocr')
- gt_text = LabelData(**gt_label_data)
- recog_data_sample.gt_text = gt_text
- assert 'gt_text' in recog_data_sample
- self.assertEqual(recog_data_sample.gt_text.item, gt_text.item)
-
- # test pred_text
- pred_label_data = dict(item='mmocr')
- pred_text = LabelData(**pred_label_data)
- recog_data_sample.pred_text = pred_text
- assert 'pred_text' in recog_data_sample
- self.assertEqual(recog_data_sample.pred_text.item, pred_text.item)
- # test type error
- with self.assertRaises(AssertionError):
- recog_data_sample.gt_text = torch.rand(2, 4)
- with self.assertRaises(AssertionError):
- recog_data_sample.pred_text = torch.rand(2, 4)
-
- def test_deleter(self):
- recog_data_sample = TextRecogDataSample()
- # test gt_text
- gt_label_data = dict(item='mmocr')
- gt_text = LabelData(**gt_label_data)
- recog_data_sample.gt_text = gt_text
- assert 'gt_text' in recog_data_sample
- del recog_data_sample.gt_text
- assert 'gt_text' not in recog_data_sample
-
- recog_data_sample.pred_text = gt_text
- assert 'pred_text' in recog_data_sample
- del recog_data_sample.pred_text
- assert 'pred_text' not in recog_data_sample
diff --git a/pipeline/mmocr/tests/test_structures/test_textspotting_data_sample.py b/pipeline/mmocr/tests/test_structures/test_textspotting_data_sample.py
deleted file mode 100644
index 2acf1fbb82731910cfe34a61e2c0831e99b888d0..0000000000000000000000000000000000000000
--- a/pipeline/mmocr/tests/test_structures/test_textspotting_data_sample.py
+++ /dev/null
@@ -1,85 +0,0 @@
-# Copyright (c) OpenMMLab. All rights reserved.
-from unittest import TestCase
-
-import numpy as np
-import torch
-from mmengine.structures import InstanceData
-
-from mmocr.structures import TextSpottingDataSample
-
-
-class TestTextSpottingDataSample(TestCase):
-
- def _equal(self, a, b):
- if isinstance(a, (torch.Tensor, np.ndarray)):
- return (a == b).all()
- else:
- return a == b
-
- def test_init(self):
- meta_info = dict(
- img_size=[256, 256],
- scale_factor=np.array([1.5, 1.5]),
- img_shape=torch.rand(4))
-
- e2e_data_sample = TextSpottingDataSample(metainfo=meta_info)
- assert 'img_size' in e2e_data_sample
-
- self.assertListEqual(e2e_data_sample.img_size, [256, 256])
- self.assertListEqual(e2e_data_sample.get('img_size'), [256, 256])
-
- def test_setter(self):
- e2e_data_sample = TextSpottingDataSample()
- # test gt_instances
- gt_instances_data = dict(
- bboxes=torch.rand(4, 4),
- labels=torch.rand(4),
- masks=np.random.rand(4, 2, 2))
- gt_instances = InstanceData(**gt_instances_data)
- e2e_data_sample.gt_instances = gt_instances
- assert 'gt_instances' in e2e_data_sample
- assert self._equal(e2e_data_sample.gt_instances.bboxes,
- gt_instances_data['bboxes'])
- assert self._equal(e2e_data_sample.gt_instances.labels,
- gt_instances_data['labels'])
- assert self._equal(e2e_data_sample.gt_instances.masks,
- gt_instances_data['masks'])
-
- # test pred_instances
- pred_instances_data = dict(
- bboxes=torch.rand(2, 4),
- labels=torch.rand(2),
- masks=np.random.rand(2, 2, 2))
- pred_instances = InstanceData(**pred_instances_data)
- e2e_data_sample.pred_instances = pred_instances
- assert 'pred_instances' in e2e_data_sample
- assert self._equal(e2e_data_sample.pred_instances.bboxes,
- pred_instances_data['bboxes'])
- assert self._equal(e2e_data_sample.pred_instances.labels,
- pred_instances_data['labels'])
- assert self._equal(e2e_data_sample.pred_instances.masks,
- pred_instances_data['masks'])
-
- # test type error
- with self.assertRaises(AssertionError):
- e2e_data_sample.gt_instances = torch.rand(2, 4)
- with self.assertRaises(AssertionError):
- e2e_data_sample.pred_instances = torch.rand(2, 4)
-
- def test_deleter(self):
- gt_instances_data = dict(
- bboxes=torch.rand(4, 4),
- labels=torch.rand(4),
- masks=np.random.rand(4, 2, 2))
-
- e2e_data_sample = TextSpottingDataSample()
- gt_instances = InstanceData(data=gt_instances_data)
- e2e_data_sample.gt_instances = gt_instances
- assert 'gt_instances' in e2e_data_sample
- del e2e_data_sample.gt_instances
- assert 'gt_instances' not in e2e_data_sample
-
- e2e_data_sample.pred_instances = gt_instances
- assert 'pred_instances' in e2e_data_sample
- del e2e_data_sample.pred_instances
- assert 'pred_instances' not in e2e_data_sample
diff --git a/pipeline/mmocr/tests/test_utils/test_bbox_utils.py b/pipeline/mmocr/tests/test_utils/test_bbox_utils.py
deleted file mode 100644
index 0511fdd81d90273d9309731eed932653a20dd449..0000000000000000000000000000000000000000
--- a/pipeline/mmocr/tests/test_utils/test_bbox_utils.py
+++ /dev/null
@@ -1,266 +0,0 @@
-# Copyright (c) OpenMMLab. All rights reserved.
-import unittest
-
-import numpy as np
-import torch
-
-from mmocr.utils import (bbox2poly, bbox_center_distance, bbox_diag_distance,
- bezier2polygon, is_on_same_line, rescale_bbox,
- rescale_bboxes, stitch_boxes_into_lines)
-from mmocr.utils.bbox_utils import bbox_jitter
-
-
-class TestBbox2poly(unittest.TestCase):
-
- def setUp(self) -> None:
- self.box_array = np.array([1, 1, 2, 2])
- self.box_list = [1, 1, 2, 2]
- self.box_tensor = torch.tensor([1, 1, 2, 2])
- self.gt_xyxy = np.array([1, 1, 2, 1, 2, 2, 1, 2])
- self.gt_xywh = np.array([1, 1, 3, 1, 3, 3, 1, 3])
-
- def test_bbox2poly(self):
- # mode: xyxy
- # test np.array
- self.assertTrue(
- np.array_equal(bbox2poly(self.box_array), self.gt_xyxy))
- # test list
- self.assertTrue(np.array_equal(bbox2poly(self.box_list), self.gt_xyxy))
- # test tensor
- self.assertTrue(
- np.array_equal(bbox2poly(self.box_tensor), self.gt_xyxy))
-
- # mode: xywh
- # test np.array
- self.assertTrue(
- np.array_equal(
- bbox2poly(self.box_array, mode='xywh'), self.gt_xywh))
- # test list
- self.assertTrue(
- np.array_equal(
- bbox2poly(self.box_list, mode='xywh'), self.gt_xywh))
- # test tensor
- self.assertTrue(
- np.array_equal(
- bbox2poly(self.box_tensor, mode='xywh'), self.gt_xywh))
-
- # invalid mode
- with self.assertRaises(NotImplementedError):
- bbox2poly(self.box_tensor, mode='a')
-
-
-class TestBoxCenterDistance(unittest.TestCase):
-
- def setUp(self) -> None:
- self.box1_list = [1, 1, 3, 3]
- self.box2_list = [2, 2, 4, 2]
- self.box1_array = np.array([1, 1, 3, 3])
- self.box2_array = np.array([2, 2, 4, 2])
- self.box1_tensor = torch.tensor([1, 1, 3, 3])
- self.box2_tensor = torch.tensor([2, 2, 4, 2])
- self.gt = 1
-
- def test_box_center_distance(self):
- # test list
- self.assertEqual(
- bbox_center_distance(self.box1_list, self.box2_list), self.gt)
- # test np.array
- self.assertEqual(
- bbox_center_distance(self.box1_array, self.box2_array), self.gt)
- # test tensor
- self.assertEqual(
- bbox_center_distance(self.box1_tensor, self.box2_tensor), self.gt)
-
-
-class TestBoxDiagDistance(unittest.TestCase):
-
- def setUp(self) -> None:
- self.box_list1 = [0, 0, 1, 1, 0, 10, -10, 0]
- self.box_array1 = np.array(self.box_list1)
- self.box_tensor1 = torch.tensor(self.box_list1)
- self.gt1 = 10
- self.box_list2 = [0, 0, 1, 1]
- self.box_array2 = np.array(self.box_list2)
- self.box_tensor2 = torch.tensor(self.box_list2)
- self.gt2 = np.sqrt(2)
-
- def test_bbox_diag_distance(self):
- # quad [x1, y1, x2, y2, x3, y3, x4, y4]
- # list
- self.assertEqual(bbox_diag_distance(self.box_list1), self.gt1)
- # array
- self.assertEqual(bbox_diag_distance(self.box_array1), self.gt1)
- # tensor
- self.assertEqual(bbox_diag_distance(self.box_tensor1), self.gt1)
- # rect [x1, y1, x2, y2]
- # list
- self.assertAlmostEqual(bbox_diag_distance(self.box_list2), self.gt2)
- # array
- self.assertAlmostEqual(bbox_diag_distance(self.box_array2), self.gt2)
- # tensor
- self.assertAlmostEqual(bbox_diag_distance(self.box_tensor2), self.gt2)
-
-
-class TestBezier2Polygon(unittest.TestCase):
-
- def setUp(self) -> None:
- self.bezier_points1 = [
- 37.0, 249.0, 72.5, 229.55, 95.34, 220.65, 134.0, 216.0, 132.0,
- 233.0, 82.11, 240.2, 72.46, 247.16, 38.0, 263.0
- ]
- self.gt1 = np.array([[37.0, 249.0],
- [42.50420761043885, 246.01570199737577],
- [47.82291296107305, 243.2012392477038],
- [52.98102930456334, 240.5511007435486],
- [58.00346989357049, 238.05977547747486],
- [62.91514798075522, 235.721752442047],
- [67.74097681877824, 233.53152062982943],
- [72.50586966030032, 231.48356903338674],
- [77.23473975798221, 229.57238664528356],
- [81.95250036448464, 227.79246245808432],
- [86.68406473246829, 226.13828546435346],
- [91.45434611459396, 224.60434465665548],
- [96.28825776352238, 223.18512902755504],
- [101.21071293191426, 221.87512756961655],
- [106.24662487243039, 220.6688292754046],
- [111.42090683773145, 219.5607231374836],
- [116.75847208047819, 218.5452981484181],
- [122.28423385333137, 217.6170433007727],
- [128.02310540895172, 216.77044758711182],
- [134.0, 216.0], [132.0, 233.0],
- [124.4475521213005, 234.13617728531858],
- [117.50700976818779, 235.2763434903047],
- [111.12146960198277, 236.42847645429362],
- [105.2340282840064, 237.6005540166205],
- [99.78778247557953, 238.80055401662054],
- [94.72582883802303, 240.0364542936288],
- [89.99126403265781, 241.31623268698053],
- [85.52718472080478, 242.64786703601104],
- [81.27668756378483, 244.03933518005545],
- [77.1828692229188, 245.49861495844874],
- [73.18882635952762, 247.0336842105263],
- [69.23765563493221, 248.65252077562326],
- [65.27245371045342, 250.3631024930748],
- [61.23631724741216, 252.17340720221605],
- [57.07234290712931, 254.09141274238226],
- [52.723627350925796, 256.12509695290856],
- [48.13326724012247, 258.2824376731302],
- [43.24435923604024, 260.5714127423822],
- [38.0, 263.0]])
- self.bezier_points2 = [0, 0, 0, 1, 0, 2, 0, 3, 1, 0, 1, 1, 1, 2, 1, 3]
- self.gt2 = np.array([[0, 0], [0, 1.5], [0, 3], [1, 0], [1, 1.5],
- [1, 3]])
- self.invalid_input = [0, 1]
-
- def test_bezier2polygon(self):
- self.assertTrue(
- np.allclose(bezier2polygon(self.bezier_points1), self.gt1))
- with self.assertRaises(AssertionError):
- bezier2polygon(self.bezier_points2, num_sample=-1)
- with self.assertRaises(AssertionError):
- bezier2polygon(self.invalid_input, num_sample=-1)
-
-
-class TestBboxJitter(unittest.TestCase):
-
- def test_bbox_jitter(self):
- dummy_points_x = [20, 120, 120, 20]
- dummy_points_y = [20, 20, 40, 40]
-
- kwargs = dict(jitter_ratio_x=0.0, jitter_ratio_y=0.0)
-
- with self.assertRaises(AssertionError):
- bbox_jitter([], dummy_points_y)
- with self.assertRaises(AssertionError):
- bbox_jitter(dummy_points_x, [])
- with self.assertRaises(AssertionError):
- bbox_jitter(dummy_points_x, dummy_points_y, jitter_ratio_x=1.)
- with self.assertRaises(AssertionError):
- bbox_jitter(dummy_points_x, dummy_points_y, jitter_ratio_y=1.)
-
- bbox_jitter(dummy_points_x, dummy_points_y, **kwargs)
-
- assert np.allclose(dummy_points_x, [20, 120, 120, 20])
- assert np.allclose(dummy_points_y, [20, 20, 40, 40])
-
-
-class TestIsOnSameLine(unittest.TestCase):
-
- def test_box_on_line(self):
- # regular boxes
- box1 = [0, 0, 1, 0, 1, 1, 0, 1]
- box2 = [2, 0.5, 3, 0.5, 3, 1.5, 2, 1.5]
- box3 = [4, 0.8, 5, 0.8, 5, 1.8, 4, 1.8]
- self.assertTrue(is_on_same_line(box1, box2, 0.5))
- self.assertFalse(is_on_same_line(box1, box3, 0.5))
-
- # irregular box4
- box4 = [0, 0, 1, 1, 1, 2, 0, 1]
- box5 = [2, 1.5, 3, 1.5, 3, 2.5, 2, 2.5]
- box6 = [2, 1.6, 3, 1.6, 3, 2.6, 2, 2.6]
- self.assertTrue(is_on_same_line(box4, box5, 0.5))
- self.assertFalse(is_on_same_line(box4, box6, 0.5))
-
-
-class TestStitchBoxesIntoLines(unittest.TestCase):
-
- def test_stitch_boxes_into_lines(self):
- boxes = [ # regular boxes
- [0, 0, 1, 0, 1, 1, 0, 1],
- [2, 0.5, 3, 0.5, 3, 1.5, 2, 1.5],
- [3, 1.2, 4, 1.2, 4, 2.2, 3, 2.2],
- [5, 0.5, 6, 0.5, 6, 1.5, 5, 1.5],
- # irregular box
- [6, 1.5, 7, 1.25, 7, 1.75, 6, 1.75]
- ]
- raw_input = [{
- 'box': boxes[i],
- 'text': str(i)
- } for i in range(len(boxes))]
- result = stitch_boxes_into_lines(raw_input, 1, 0.5)
- # Final lines: [0, 1], [2], [3, 4]
- # box 0, 1, 3, 4 are on the same line but box 3 is 2 pixels away from
- # box 1
- # box 3 and 4 are on the same line since the length of overlapping part
- # >= 0.5 * the y-axis length of box 5
- expected_result = [{
- 'box': [0, 0, 3, 0, 3, 1.5, 0, 1.5],
- 'text': '0 1'
- }, {
- 'box': [3, 1.2, 4, 1.2, 4, 2.2, 3, 2.2],
- 'text': '2'
- }, {
- 'box': [5, 0.5, 7, 0.5, 7, 1.75, 5, 1.75],
- 'text': '3 4'
- }]
- result.sort(key=lambda x: x['box'][0])
- expected_result.sort(key=lambda x: x['box'][0])
- self.assertEqual(result, expected_result)
-
-
-class TestRescaleBbox(unittest.TestCase):
-
- def setUp(self) -> None:
- self.bbox = np.array([0, 0, 1, 1])
- self.bboxes = np.array([[0, 0, 1, 1], [1, 1, 2, 2]])
- self.scale = 2
-
- def test_rescale_bbox(self):
- # mul
- rescaled_bbox = rescale_bbox(self.bbox, self.scale, mode='mul')
- self.assertTrue(np.allclose(rescaled_bbox, np.array([0, 0, 2, 2])))
- # div
- rescaled_bbox = rescale_bbox(self.bbox, self.scale, mode='div')
- self.assertTrue(np.allclose(rescaled_bbox, np.array([0, 0, 0.5, 0.5])))
-
- def test_rescale_bboxes(self):
- # mul
- rescaled_bboxes = rescale_bboxes(self.bboxes, self.scale, mode='mul')
- self.assertTrue(
- np.allclose(rescaled_bboxes, np.array([[0, 0, 2, 2], [2, 2, 4,
- 4]])))
- # div
- rescaled_bboxes = rescale_bboxes(self.bboxes, self.scale, mode='div')
- self.assertTrue(
- np.allclose(rescaled_bboxes,
- np.array([[0, 0, 0.5, 0.5], [0.5, 0.5, 1, 1]])))
diff --git a/pipeline/mmocr/tests/test_utils/test_check_argument.py b/pipeline/mmocr/tests/test_utils/test_check_argument.py
deleted file mode 100644
index 7166440b6327d4c3031418f8d6e6bb539150b129..0000000000000000000000000000000000000000
--- a/pipeline/mmocr/tests/test_utils/test_check_argument.py
+++ /dev/null
@@ -1,54 +0,0 @@
-# Copyright (c) OpenMMLab. All rights reserved.
-import numpy as np
-
-import mmocr.utils as utils
-
-
-def test_is_3dlist():
-
- assert utils.is_3dlist([])
- assert utils.is_3dlist([[]])
- assert utils.is_3dlist([[[]]])
- assert utils.is_3dlist([[[1]]])
- assert not utils.is_3dlist([[1, 2]])
- assert not utils.is_3dlist([[np.array([1, 2])]])
-
-
-def test_is_2dlist():
-
- assert utils.is_2dlist([])
- assert utils.is_2dlist([[]])
- assert utils.is_2dlist([[1]])
-
-
-def test_is_type_list():
- assert utils.is_type_list([], int)
- assert utils.is_type_list([], float)
- assert utils.is_type_list([np.array([])], np.ndarray)
- assert utils.is_type_list([1], int)
- assert utils.is_type_list(['str'], str)
-
-
-def test_is_none_or_type():
-
- assert utils.is_none_or_type(None, int)
- assert utils.is_none_or_type(1.0, float)
- assert utils.is_none_or_type(np.ndarray([]), np.ndarray)
- assert utils.is_none_or_type(1, int)
- assert utils.is_none_or_type('str', str)
-
-
-def test_valid_boundary():
-
- x = [0, 0, 1, 0, 1, 1, 0, 1]
- assert not utils.valid_boundary(x, True)
- assert not utils.valid_boundary([0])
- assert utils.valid_boundary(x, False)
- x = [0, 0, 1, 0, 1, 1, 0, 1, 1]
- assert utils.valid_boundary(x, True)
-
-
-def test_equal_len():
-
- assert utils.equal_len([1, 2, 3], [1, 2, 3])
- assert not utils.equal_len([1, 2, 3], [1, 2, 3, 4])
diff --git a/pipeline/mmocr/tests/test_utils/test_data_converter_utils.py b/pipeline/mmocr/tests/test_utils/test_data_converter_utils.py
deleted file mode 100644
index eb258ef53c0b08da0261f74d6ee1117bdec970e4..0000000000000000000000000000000000000000
--- a/pipeline/mmocr/tests/test_utils/test_data_converter_utils.py
+++ /dev/null
@@ -1,163 +0,0 @@
-# Copyright (c) OpenMMLab. All rights reserved.
-import os.path as osp
-import tempfile
-from unittest import TestCase
-
-import mmengine
-
-from mmocr.utils.data_converter_utils import (dump_ocr_data,
- recog_anno_to_imginfo)
-
-
-class TestDataConverterUtils(TestCase):
-
- def _create_dummy_data(self):
- img_info = dict(
- file_name='test.jpg', height=100, width=200, segm_file='seg.txt')
- anno_info = [
- dict(
- iscrowd=0,
- category_id=0,
- bbox=[0, 0, 10, 20], # x, y, w, h
- text='t1',
- segmentation=[0, 0, 0, 10, 10, 20, 20, 0]),
- dict(
- iscrowd=1,
- category_id=0,
- bbox=[10, 10, 20, 20], # x, y, w, h
- text='t2',
- segmentation=[10, 10, 10, 30, 30, 30, 30, 10]),
- ]
- img_info['anno_info'] = anno_info
- img_infos = [img_info]
-
- det_target = {
- 'metainfo': {
- 'dataset_type': 'TextDetDataset',
- 'task_name': 'textdet',
- 'category': [{
- 'id': 0,
- 'name': 'text'
- }],
- },
- 'data_list': [{
- 'img_path':
- 'test.jpg',
- 'height':
- 100,
- 'width':
- 200,
- 'seg_map':
- 'seg.txt',
- 'instances': [
- {
- 'bbox': [0, 0, 10, 20],
- 'bbox_label': 0,
- 'polygon': [0, 0, 0, 10, 10, 20, 20, 0],
- 'ignore': False
- },
- {
- 'bbox': [10, 10, 30, 30], # x1, y1, x2, y2
- 'bbox_label': 0,
- 'polygon': [10, 10, 10, 30, 30, 30, 30, 10],
- 'ignore': True
- }
- ]
- }]
- }
-
- spotter_target = {
- 'metainfo': {
- 'dataset_type': 'TextSpotterDataset',
- 'task_name': 'textspotter',
- 'category': [{
- 'id': 0,
- 'name': 'text'
- }],
- },
- 'data_list': [{
- 'img_path':
- 'test.jpg',
- 'height':
- 100,
- 'width':
- 200,
- 'seg_map':
- 'seg.txt',
- 'instances': [
- {
- 'bbox': [0, 0, 10, 20],
- 'bbox_label': 0,
- 'polygon': [0, 0, 0, 10, 10, 20, 20, 0],
- 'text': 't1',
- 'ignore': False
- },
- {
- 'bbox': [10, 10, 30, 30], # x1, y1, x2, y2
- 'bbox_label': 0,
- 'polygon': [10, 10, 10, 30, 30, 30, 30, 10],
- 'text': 't2',
- 'ignore': True
- }
- ]
- }]
- }
-
- recog_target = {
- 'metainfo': {
- 'dataset_type': 'TextRecogDataset',
- 'task_name': 'textrecog',
- },
- 'data_list': [{
- 'img_path': 'test.jpg',
- 'instances': [{
- 'text': 't1',
- }, {
- 'text': 't2',
- }]
- }]
- }
-
- return img_infos, det_target, spotter_target, recog_target
-
- def test_dump_ocr_data(self):
- with tempfile.TemporaryDirectory() as tmpdir:
- output_path = osp.join(tmpdir, 'ocr.json')
- input_data, det_target, spotter_target, recog_target = \
- self._create_dummy_data()
-
- dump_ocr_data(input_data, output_path, 'textdet')
- result = mmengine.load(output_path)
- self.assertDictEqual(result, det_target)
-
- dump_ocr_data(input_data, output_path, 'textspotter')
- result = mmengine.load(output_path)
- self.assertDictEqual(result, spotter_target)
-
- dump_ocr_data(input_data, output_path, 'textrecog')
- result = mmengine.load(output_path)
- self.assertDictEqual(result, recog_target)
-
- def test_recog_anno_to_imginfo(self):
- file_paths = ['a.jpg', 'b.jpg']
- labels = ['aaa']
- with self.assertRaises(AssertionError):
- recog_anno_to_imginfo(file_paths, labels)
-
- file_paths = ['a.jpg', 'b.jpg']
- labels = ['aaa', 'bbb']
- target = [
- {
- 'file_name': 'a.jpg',
- 'anno_info': [{
- 'text': 'aaa'
- }]
- },
- {
- 'file_name': 'b.jpg',
- 'anno_info': [{
- 'text': 'bbb'
- }]
- },
- ]
- self.assertListEqual(target, recog_anno_to_imginfo(file_paths, labels))
diff --git a/pipeline/mmocr/tests/test_utils/test_fileio.py b/pipeline/mmocr/tests/test_utils/test_fileio.py
deleted file mode 100644
index 9505fe57955d7f00ce3507f974d6e71a5a018d2d..0000000000000000000000000000000000000000
--- a/pipeline/mmocr/tests/test_utils/test_fileio.py
+++ /dev/null
@@ -1,180 +0,0 @@
-# Copyright (c) OpenMMLab. All rights reserved.
-import json
-import os
-import tempfile
-import unittest
-
-from mmocr.utils import (check_integrity, get_md5, is_archive, list_files,
- list_from_file, list_to_file)
-
-lists = [
- [],
- [' '],
- ['\t'],
- ['a'],
- [1],
- [1.],
- ['a', 'b'],
- ['a', 1, 1.],
- [1, 1., 'a'],
- ['ๅ', 'ๅๅ'],
- ['้ธๆ', 'noรซl', 'ะะฝัะพัะผะฐัะธะตะน', 'รรรค'],
-]
-
-dicts = [
- [{
- 'text': []
- }],
- [{
- 'text': [' ']
- }],
- [{
- 'text': ['\t']
- }],
- [{
- 'text': ['a']
- }],
- [{
- 'text': [1]
- }],
- [{
- 'text': [1.]
- }],
- [{
- 'text': ['a', 'b']
- }],
- [{
- 'text': ['a', 1, 1.]
- }],
- [{
- 'text': [1, 1., 'a']
- }],
- [{
- 'text': ['ๅ', 'ๅๅ']
- }],
- [{
- 'text': ['้ธๆ', 'noรซl', 'ะะฝัะพัะผะฐัะธะตะน', 'รรรค']
- }],
-]
-
-
-def test_list_to_file():
- with tempfile.TemporaryDirectory() as tmpdirname:
- # test txt
- for i, lines in enumerate(lists):
- filename = f'{tmpdirname}/{i}.txt'
- list_to_file(filename, lines)
- lines2 = [
- line.rstrip('\r\n')
- for line in open(filename, encoding='utf-8').readlines()
- ]
- lines = list(map(str, lines))
- assert len(lines) == len(lines2)
- assert all(line1 == line2 for line1, line2 in zip(lines, lines2))
- # test jsonl
- for i, lines in enumerate(dicts):
- filename = f'{tmpdirname}/{i}.jsonl'
- list_to_file(filename, [json.dumps(line) for line in lines])
- lines2 = [
- json.loads(line.rstrip('\r\n'))['text']
- for line in open(filename, encoding='utf-8').readlines()
- ][0]
-
- lines = list(lines[0]['text'])
- assert len(lines) == len(lines2)
- assert all(line1 == line2 for line1, line2 in zip(lines, lines2))
-
-
-def test_list_from_file():
- with tempfile.TemporaryDirectory() as tmpdirname:
- # test txt file
- for i, lines in enumerate(lists):
- filename = f'{tmpdirname}/{i}.txt'
- with open(filename, 'w', encoding='utf-8') as f:
- f.writelines(f'{line}\n' for line in lines)
- lines2 = list_from_file(filename, encoding='utf-8')
- lines = list(map(str, lines))
- assert len(lines) == len(lines2)
- assert all(line1 == line2 for line1, line2 in zip(lines, lines2))
- # test jsonl file
- for i, lines in enumerate(dicts):
- filename = f'{tmpdirname}/{i}.jsonl'
- with open(filename, 'w', encoding='utf-8') as f:
- f.writelines(f'{line}\n' for line in lines)
- lines2 = list_from_file(filename, encoding='utf-8')
- lines = list(map(str, lines))
- assert len(lines) == len(lines2)
- assert all(line1 == line2 for line1, line2 in zip(lines, lines2))
-
-
-class TestIsArchive(unittest.TestCase):
-
- def setUp(self) -> None:
- self.zip = 'data/annotations_123.zip'
- self.tar = 'data/img.abc.tar'
- self.targz = 'data/img12345_.tar.gz'
- self.rar = '/m/abc/t.rar'
- self.dir = '/a/b/c/'
-
- def test_is_archive(self):
- # test zip
- self.assertTrue(is_archive(self.zip))
- # test tar
- self.assertTrue(is_archive(self.tar))
- # test tar.gz
- self.assertTrue(is_archive(self.targz))
- # test rar
- self.assertFalse(is_archive(self.rar))
- # test dir
- self.assertFalse(is_archive(self.dir))
-
-
-class TestCheckIntegrity(unittest.TestCase):
-
- def setUp(self) -> None:
- # Do not use text files for tests, because the md5 value of text files
- # is different on different platforms (CR - CRLF)
- self.file1 = ('tests/data/det_toy_dataset/imgs/test/img_2.jpg',
- '52b28b5dfc92d9027e70ec3ff95d8702')
- self.file2 = ('tests/data/det_toy_dataset/imgs/test/img_1.jpg',
- 'abc123')
- self.file3 = ('abc/abc.jpg', 'abc123')
-
- def test_check_integrity(self):
- file, md5 = self.file1
- self.assertTrue(check_integrity(file, md5))
- file, md5 = self.file2
- self.assertFalse(check_integrity(file, md5))
- self.assertTrue(check_integrity(file, None))
- file, md5 = self.file3
- self.assertFalse(check_integrity(file, md5))
-
-
-class TextGetMD5(unittest.TestCase):
-
- def setUp(self) -> None:
- # Do not use text files for tests, because the md5 value of text files
- # is different on different platforms (CR - CRLF)
- self.file1 = ('tests/data/det_toy_dataset/imgs/test/img_2.jpg',
- '52b28b5dfc92d9027e70ec3ff95d8702')
- self.file2 = ('tests/data/det_toy_dataset/imgs/test/img_1.jpg',
- 'abc123')
-
- def test_get_md5(self):
- file, md5 = self.file1
- self.assertEqual(get_md5(file), md5)
- file, md5 = self.file2
- self.assertNotEqual(get_md5(file), md5)
-
-
-class TestListFiles(unittest.TestCase):
-
- def setUp(self) -> None:
- self.path = 'tests/data/det_toy_dataset/imgs/test'
-
- def test_check_integrity(self):
- suffix = 'jpg'
- files = list_files(self.path, suffix)
- for file in os.listdir(self.path):
- if file.endswith(suffix):
- self.assertIn(os.path.join(self.path, file), files)
diff --git a/pipeline/mmocr/tests/test_utils/test_img_utils.py b/pipeline/mmocr/tests/test_utils/test_img_utils.py
deleted file mode 100644
index f6dc5fecb5ac48ff1e832a71de0aa34acca057ad..0000000000000000000000000000000000000000
--- a/pipeline/mmocr/tests/test_utils/test_img_utils.py
+++ /dev/null
@@ -1,46 +0,0 @@
-# Copyright (c) OpenMMLab. All rights reserved.
-import unittest
-
-import numpy as np
-
-from mmocr.utils import crop_img, warp_img
-
-
-class TestImgUtils(unittest.TestCase):
-
- def test_warp_img(self):
- dummy_img = np.ones((600, 600, 3), dtype=np.uint8)
- dummy_box = [20, 20, 120, 20, 120, 40, 20, 40]
-
- cropped_img = warp_img(dummy_img, dummy_box)
-
- with self.assertRaises(AssertionError):
- warp_img(dummy_img, [])
- with self.assertRaises(AssertionError):
- warp_img(dummy_img, [20, 40, 40, 20])
-
- self.assertAlmostEqual(cropped_img.shape[0], 20)
- self.assertAlmostEqual(cropped_img.shape[1], 100)
-
- def test_min_rect_crop(self):
- dummy_img = np.ones((600, 600, 3), dtype=np.uint8)
- dummy_box = [20, 20, 120, 20, 120, 40, 20, 40]
-
- cropped_img = crop_img(
- dummy_img,
- dummy_box,
- 0.,
- 0.,
- )
-
- with self.assertRaises(AssertionError):
- crop_img(dummy_img, [])
- with self.assertRaises(AssertionError):
- crop_img(dummy_img, [20, 40, 40, 20])
- with self.assertRaises(AssertionError):
- crop_img(dummy_img, dummy_box, 4, 0.2)
- with self.assertRaises(AssertionError):
- crop_img(dummy_img, dummy_box, 0.4, 1.2)
-
- self.assertAlmostEqual(cropped_img.shape[0], 20)
- self.assertAlmostEqual(cropped_img.shape[1], 100)
diff --git a/pipeline/mmocr/tests/test_utils/test_mask_utils.py b/pipeline/mmocr/tests/test_utils/test_mask_utils.py
deleted file mode 100644
index a325a9e7e11e806bbfc75cce62ef7debcd5c3632..0000000000000000000000000000000000000000
--- a/pipeline/mmocr/tests/test_utils/test_mask_utils.py
+++ /dev/null
@@ -1,23 +0,0 @@
-# Copyright (c) OpenMMLab. All rights reserved.
-import unittest
-
-import numpy as np
-import torch
-
-from mmocr.utils import fill_hole
-
-
-class TestFillHole(unittest.TestCase):
-
- def setUp(self) -> None:
- self.input_mask_list = [[0, 1, 1, 1, 0], [0, 1, 0, 1, 0],
- [0, 1, 1, 1, 0]]
- self.input_mask_array = np.array(self.input_mask_list)
- self.input_mask_tensor = torch.tensor(self.input_mask_list)
- self.gt = np.array([[0, 1, 1, 1, 0], [0, 1, 1, 1, 0], [0, 1, 1, 1, 0]])
-
- def test_fill_hole(self):
- self.assertTrue(np.allclose(fill_hole(self.input_mask_list), self.gt))
- self.assertTrue(np.allclose(fill_hole(self.input_mask_array), self.gt))
- self.assertTrue(
- np.allclose(fill_hole(self.input_mask_tensor), self.gt))
diff --git a/pipeline/mmocr/tests/test_utils/test_parsers.py b/pipeline/mmocr/tests/test_utils/test_parsers.py
deleted file mode 100644
index abdd7c9446c177b7bc89b41f64f0b557c96203f3..0000000000000000000000000000000000000000
--- a/pipeline/mmocr/tests/test_utils/test_parsers.py
+++ /dev/null
@@ -1,34 +0,0 @@
-# Copyright (c) OpenMMLab. All rights reserved.
-import json
-from unittest import TestCase
-
-from mmocr.utils import LineJsonParser, LineStrParser
-
-
-class TestParser(TestCase):
-
- def test_line_json_parser(self):
- parser = LineJsonParser()
- line = json.dumps(dict(filename='test.jpg', text='mmocr'))
- data = parser(line)
- self.assertEqual(data['filename'], 'test.jpg')
- self.assertEqual(data['text'], 'mmocr')
-
- def test_line_str_parser(self):
- parser = LineStrParser()
- line = 'test.jpg mmocr'
- data = parser(line)
- self.assertEqual(data['filename'], 'test.jpg')
- self.assertEqual(data['text'], 'mmocr')
-
- # warnings
- line = 'test test test'
- msg = 'More than two blank spaces were detected. '
- msg += 'Please use LineJsonParser to handle '
- msg += 'annotations with blanks. '
- msg += 'Check Doc '
- msg += 'https://mmocr.readthedocs.io/en/latest/'
- msg += 'tutorials/blank_recog.html '
- msg += 'for details.'
- data = parser(line)
- self.assertWarnsRegex(UserWarning, msg)
diff --git a/pipeline/mmocr/tests/test_utils/test_point_utils.py b/pipeline/mmocr/tests/test_utils/test_point_utils.py
deleted file mode 100644
index 343ebb5a059c8dc074868bfeccd40fc45c2c0ea8..0000000000000000000000000000000000000000
--- a/pipeline/mmocr/tests/test_utils/test_point_utils.py
+++ /dev/null
@@ -1,55 +0,0 @@
-# Copyright (c) OpenMMLab. All rights reserved.
-import unittest
-
-import numpy as np
-import torch
-
-from mmocr.utils import point_distance, points_center
-
-
-class TestPointDistance(unittest.TestCase):
-
- def setUp(self) -> None:
- self.p1_list = [1, 2]
- self.p2_list = [2, 2]
- self.p1_array = np.array([1, 2])
- self.p2_array = np.array([2, 2])
- self.p1_tensor = torch.Tensor([1, 2])
- self.p2_tensor = torch.Tensor([2, 2])
- self.invalid_p = [1, 2, 3]
-
- def test_point_distance(self):
- # list
- self.assertEqual(point_distance(self.p1_list, self.p2_list), 1)
- self.assertEqual(point_distance(self.p1_list, self.p1_list), 0)
- # array
- self.assertEqual(point_distance(self.p1_array, self.p2_array), 1)
- self.assertEqual(point_distance(self.p1_array, self.p1_array), 0)
- # tensor
- self.assertEqual(point_distance(self.p1_tensor, self.p2_tensor), 1)
- self.assertEqual(point_distance(self.p1_tensor, self.p1_tensor), 0)
- with self.assertRaises(AssertionError):
- point_distance(self.invalid_p, self.invalid_p)
-
-
-class TestPointCenter(unittest.TestCase):
-
- def setUp(self) -> None:
- self.point_list = [1, 2, 3, 4]
- self.point_nparray = np.array([1, 2, 3, 4])
- self.point_tensor = torch.Tensor([1, 2, 3, 4])
- self.incorrect_input = [1, 3, 4]
- self.gt = np.array([2, 3])
-
- def test_point_center(self):
- # list
- self.assertTrue(
- np.array_equal(points_center(self.point_list), self.gt))
- # array
- self.assertTrue(
- np.array_equal(points_center(self.point_nparray), self.gt))
- # tensor
- self.assertTrue(
- np.array_equal(points_center(self.point_tensor), self.gt))
- with self.assertRaises(AssertionError):
- points_center(self.incorrect_input)
diff --git a/pipeline/mmocr/tests/test_utils/test_polygon_utils.py b/pipeline/mmocr/tests/test_utils/test_polygon_utils.py
deleted file mode 100644
index 8cbd4f899371d0c3a904014aad9ce7523c15fdf2..0000000000000000000000000000000000000000
--- a/pipeline/mmocr/tests/test_utils/test_polygon_utils.py
+++ /dev/null
@@ -1,403 +0,0 @@
-# Copyright (c) OpenMMLab. All rights reserved.
-import unittest
-from itertools import chain, permutations
-
-import numpy as np
-import torch
-from shapely.geometry import MultiPolygon, Polygon
-
-from mmocr.utils import (boundary_iou, crop_polygon, offset_polygon, poly2bbox,
- poly2shapely, poly_intersection, poly_iou,
- poly_make_valid, poly_union, polys2shapely,
- rescale_polygon, rescale_polygons, shapely2poly,
- sort_points, sort_vertex, sort_vertex8)
-
-
-class TestPolygonUtils(unittest.TestCase):
-
- def test_crop_polygon(self):
- # polygon cross box
- polygon = np.array([20., -10., 40., 10., 10., 40., -10., 20.])
- crop_box = np.array([0., 0., 60., 60.])
- target_poly_cropped = np.array(
- [10, 40, 0, 30, 0, 10, 10, 0, 30, 0, 40, 10])
- poly_cropped = crop_polygon(polygon, crop_box)
- self.assertTrue(
- poly2shapely(poly_cropped).equals(
- poly2shapely(target_poly_cropped)))
-
- # polygon inside box
- polygon = np.array([0., 0., 30., 0., 30., 30., 0., 30.])
- crop_box = np.array([0., 0., 60., 60.])
- target_poly_cropped = polygon
- poly_cropped = crop_polygon(polygon, crop_box)
- self.assertTrue(
- poly2shapely(poly_cropped).equals(
- poly2shapely(target_poly_cropped)))
-
- # polygon outside box
- polygon = np.array([0., 0., 30., 0., 30., 30., 0., 30.])
- crop_box = np.array([80., 80., 90., 90.])
- poly_cropped = crop_polygon(polygon, crop_box)
- self.assertEqual(poly_cropped, None)
-
- # polygon and box are overlapped at a point
- polygon = np.array([0., 0., 10., 0., 10., 10., 0., 10.])
- crop_box = np.array([10., 10., 20., 20.])
- poly_cropped = crop_polygon(polygon, crop_box)
- self.assertEqual(poly_cropped, None)
-
- def test_rescale_polygon(self):
- scale_factor = (0.3, 0.4)
-
- with self.assertRaises(AssertionError):
- polygons = [0, 0, 1, 0, 1, 1, 0]
- rescale_polygon(polygons, scale_factor)
-
- polygons = [0, 0, 1, 0, 1, 1, 0, 1]
- self.assertTrue(
- np.allclose(
- rescale_polygon(polygons, scale_factor, mode='div'),
- np.array([0, 0, 1 / 0.3, 0, 1 / 0.3, 1 / 0.4, 0, 1 / 0.4])))
- self.assertTrue(
- np.allclose(
- rescale_polygon(polygons, scale_factor, mode='mul'),
- np.array([0, 0, 0.3, 0, 0.3, 0.4, 0, 0.4])))
-
- def test_rescale_polygons(self):
- polygons = [
- np.array([0, 0, 1, 0, 1, 1, 0, 1]),
- np.array([1, 1, 2, 1, 2, 2, 1, 2])
- ]
- scale_factor = (0.5, 0.5)
- self.assertTrue(
- np.allclose(
- rescale_polygons(polygons, scale_factor, mode='div'), [
- np.array([0, 0, 2, 0, 2, 2, 0, 2]),
- np.array([2, 2, 4, 2, 4, 4, 2, 4])
- ]))
- self.assertTrue(
- np.allclose(
- rescale_polygons(polygons, scale_factor, mode='mul'), [
- np.array([0, 0, 0.5, 0, 0.5, 0.5, 0, 0.5]),
- np.array([0.5, 0.5, 1, 0.5, 1, 1, 0.5, 1])
- ]))
-
- polygons = np.array([[0, 0, 1, 0, 1, 1, 0, 1],
- [1, 1, 2, 1, 2, 2, 1, 2]])
- scale_factor = (0.5, 0.5)
- self.assertTrue(
- np.allclose(
- rescale_polygons(polygons, scale_factor, mode='div'),
- np.array([[0, 0, 2, 0, 2, 2, 0, 2], [2, 2, 4, 2, 4, 4, 2,
- 4]])))
- self.assertTrue(
- np.allclose(
- rescale_polygons(polygons, scale_factor, mode='mul'),
- np.array([[0, 0, 0.5, 0, 0.5, 0.5, 0, 0.5],
- [0.5, 0.5, 1, 0.5, 1, 1, 0.5, 1]])))
-
- polygons = [torch.Tensor([0, 0, 1, 0, 1, 1, 0, 1])]
- scale_factor = (0.3, 0.4)
- self.assertTrue(
- np.allclose(
- rescale_polygons(polygons, scale_factor, mode='div'),
- [np.array([0, 0, 1 / 0.3, 0, 1 / 0.3, 1 / 0.4, 0, 1 / 0.4])]))
- self.assertTrue(
- np.allclose(
- rescale_polygons(polygons, scale_factor, mode='mul'),
- [np.array([0, 0, 0.3, 0, 0.3, 0.4, 0, 0.4])]))
-
- def test_poly2bbox(self):
- # test np.array
- polygon = np.array([0, 0, 1, 0, 1, 1, 0, 1])
- self.assertTrue(np.all(poly2bbox(polygon) == np.array([0, 0, 1, 1])))
- # test list
- polygon = [0, 0, 1, 0, 1, 1, 0, 1]
- self.assertTrue(np.all(poly2bbox(polygon) == np.array([0, 0, 1, 1])))
- # test tensor
- polygon = torch.Tensor([0, 0, 1, 0, 1, 1, 0, 1])
- self.assertTrue(np.all(poly2bbox(polygon) == np.array([0, 0, 1, 1])))
-
- def test_poly2shapely(self):
- polygon = Polygon([[0, 0], [1, 0], [1, 1], [0, 1]])
- # test np.array
- poly = np.array([0, 0, 1, 0, 1, 1, 0, 1])
- self.assertEqual(poly2shapely(poly), polygon)
- # test list
- poly = [0, 0, 1, 0, 1, 1, 0, 1]
- self.assertEqual(poly2shapely(poly), polygon)
- # test tensor
- poly = torch.Tensor([0, 0, 1, 0, 1, 1, 0, 1])
- self.assertEqual(poly2shapely(poly), polygon)
- # test invalid
- poly = [0, 0, 1]
- with self.assertRaises(AssertionError):
- poly2shapely(poly)
- poly = [0, 0, 1, 0, 1, 1, 0, 1, 1]
- with self.assertRaises(AssertionError):
- poly2shapely(poly)
-
- def test_polys2shapely(self):
- polygons = [
- Polygon([[0, 0], [1, 0], [1, 1], [0, 1]]),
- Polygon([[1, 0], [1, 1], [0, 1], [0, 0]])
- ]
- # test np.array
- polys = np.array([[0, 0, 1, 0, 1, 1, 0, 1], [1, 0, 1, 1, 0, 1, 0, 0]])
- self.assertEqual(polys2shapely(polys), polygons)
- # test list
- polys = [[0, 0, 1, 0, 1, 1, 0, 1], [1, 0, 1, 1, 0, 1, 0, 0]]
- self.assertEqual(polys2shapely(polys), polygons)
- # test tensor
- polys = torch.Tensor([[0, 0, 1, 0, 1, 1, 0, 1],
- [1, 0, 1, 1, 0, 1, 0, 0]])
- self.assertEqual(polys2shapely(polys), polygons)
- # test invalid
- polys = [0, 0, 1]
- with self.assertRaises(AssertionError):
- polys2shapely(polys)
- polys = [0, 0, 1, 0, 1, 1, 0, 1, 1]
- with self.assertRaises(AssertionError):
- polys2shapely(polys)
-
- def test_shapely2poly(self):
- polygon = Polygon([[0., 0.], [1., 0.], [1., 1.], [0., 1.]])
- poly = np.array([0., 0., 1., 0., 1., 1., 0., 1., 0., 0.])
- self.assertTrue(poly2shapely(poly).equals(polygon))
- self.assertTrue(isinstance(shapely2poly(polygon), np.ndarray))
-
- def test_poly_make_valid(self):
- poly = Polygon([[0, 0], [1, 1], [1, 0], [0, 1]])
- self.assertFalse(poly.is_valid)
- poly = poly_make_valid(poly)
- self.assertTrue(poly.is_valid)
- # invalid input
- with self.assertRaises(AssertionError):
- poly_make_valid([0, 0, 1, 1, 1, 0, 0, 1])
- poly = Polygon([[337, 441], [326, 386], [334, 397], [342, 412],
- [296, 382], [317, 366], [324, 427], [315, 413],
- [308, 400], [349, 419], [337, 441]])
- self.assertFalse(poly.is_valid)
- poly = poly_make_valid(poly)
- self.assertTrue(poly.is_valid)
-
- def test_poly_intersection(self):
-
- # test unsupported type
- with self.assertRaises(AssertionError):
- poly_intersection(0, 1)
-
- # test non-overlapping polygons
- points = [0, 0, 0, 1, 1, 1, 1, 0]
- points1 = [10, 20, 30, 40, 50, 60, 70, 80]
- points2 = [0, 0, 0, 0, 0, 0, 0, 0] # Invalid polygon
- points3 = [0, 0, 0, 1, 1, 0, 1, 1] # Self-intersected polygon
- points4 = [0.5, 0, 1.5, 0, 1.5, 1, 0.5, 1]
- poly = poly2shapely(points)
- poly1 = poly2shapely(points1)
- poly2 = poly2shapely(points2)
- poly3 = poly2shapely(points3)
- poly4 = poly2shapely(points4)
-
- area_inters = poly_intersection(poly, poly1)
- self.assertEqual(area_inters, 0.)
-
- # test overlapping polygons
- area_inters = poly_intersection(poly, poly)
- self.assertEqual(area_inters, 1)
- area_inters = poly_intersection(poly, poly4)
- self.assertEqual(area_inters, 0.5)
-
- # test invalid polygons
- self.assertEqual(poly_intersection(poly2, poly2), 0)
- self.assertEqual(poly_intersection(poly3, poly3, invalid_ret=1), 1)
- self.assertEqual(
- poly_intersection(poly3, poly3, invalid_ret=None), 0.25)
-
- # test poly return
- _, poly = poly_intersection(poly, poly4, return_poly=True)
- self.assertTrue(isinstance(poly, Polygon))
- _, poly = poly_intersection(
- poly3, poly3, invalid_ret=None, return_poly=True)
- self.assertTrue(isinstance(poly, Polygon))
- _, poly = poly_intersection(
- poly2, poly3, invalid_ret=1, return_poly=True)
- self.assertTrue(poly is None)
-
- def test_poly_union(self):
-
- # test unsupported type
- with self.assertRaises(AssertionError):
- poly_union(0, 1)
-
- # test non-overlapping polygons
-
- points = [0, 0, 0, 1, 1, 1, 1, 0]
- points1 = [2, 2, 2, 3, 3, 3, 3, 2]
- points2 = [0, 0, 0, 0, 0, 0, 0, 0] # Invalid polygon
- points3 = [0, 0, 0, 1, 1, 0, 1, 1] # Self-intersected polygon
- points4 = [0.5, 0.5, 1, 0, 1, 1, 0.5, 0.5]
- poly = poly2shapely(points)
- poly1 = poly2shapely(points1)
- poly2 = poly2shapely(points2)
- poly3 = poly2shapely(points3)
- poly4 = poly2shapely(points4)
-
- assert poly_union(poly, poly1) == 2
-
- # test overlapping polygons
- assert poly_union(poly, poly) == 1
-
- # test invalid polygons
- self.assertEqual(poly_union(poly2, poly2), 0)
- self.assertEqual(poly_union(poly3, poly3, invalid_ret=1), 1)
-
- # The return value depends on the implementation of the package
- self.assertEqual(poly_union(poly3, poly3, invalid_ret=None), 0.25)
- self.assertEqual(poly_union(poly2, poly3), 0.25)
- self.assertEqual(poly_union(poly3, poly4), 0.5)
-
- # test poly return
- _, poly = poly_union(poly, poly1, return_poly=True)
- self.assertTrue(isinstance(poly, MultiPolygon))
- _, poly = poly_union(poly3, poly3, return_poly=True)
- self.assertTrue(isinstance(poly, Polygon))
- _, poly = poly_union(poly2, poly3, invalid_ret=0, return_poly=True)
- self.assertTrue(poly is None)
-
- def test_poly_iou(self):
- # test unsupported type
- with self.assertRaises(AssertionError):
- poly_iou([1], [2])
-
- points = [0, 0, 0, 1, 1, 1, 1, 0]
- points1 = [10, 20, 30, 40, 50, 60, 70, 80]
- points2 = [0, 0, 0, 0, 0, 0, 0, 0] # Invalid polygon
- points3 = [0, 0, 0, 1, 1, 0, 1, 1] # Self-intersected polygon
-
- poly = poly2shapely(points)
- poly1 = poly2shapely(points1)
- poly2 = poly2shapely(points2)
- poly3 = poly2shapely(points3)
-
- self.assertEqual(poly_iou(poly, poly1), 0)
-
- # test overlapping polygons
- self.assertEqual(poly_iou(poly, poly), 1)
-
- # test invalid polygons
- self.assertEqual(poly_iou(poly2, poly2), 0)
- self.assertEqual(poly_iou(poly3, poly3, zero_division=1), 1)
- self.assertEqual(poly_iou(poly2, poly3), 0)
-
- def test_offset_polygon(self):
- # usual case
- polygons = np.array([0, 0, 0, 1, 1, 1, 1, 0], dtype=np.float32)
- expanded_polygon = offset_polygon(polygons, 1)
- self.assertTrue(
- poly2shapely(expanded_polygon).equals(
- poly2shapely(
- np.array(
- [2, 0, 2, 1, 1, 2, 0, 2, -1, 1, -1, 0, 0, -1, 1,
- -1]))))
-
- # Overshrunk polygon doesn't exist
- shrunk_polygon = offset_polygon(polygons, -10)
- self.assertEqual(len(shrunk_polygon), 0)
-
- # When polygon is shrunk into two polygons, it is regarded as invalid
- # and an empty array is returned.
- polygons = np.array([0, 0, 0, 3, 1, 2, 2, 3, 2, 0, 1, 1],
- dtype=np.float32)
- shrunk = offset_polygon(polygons, -1)
- self.assertEqual(len(shrunk), 0)
-
- def test_boundary_iou(self):
- points = [0, 0, 0, 1, 1, 1, 1, 0]
- points1 = [10, 20, 30, 40, 50, 60, 70, 80]
- points2 = [0, 0, 0, 0, 0, 0, 0, 0] # Invalid polygon
- points3 = [0, 0, 0, 1, 1, 0, 1, 1] # Self-intersected polygon
-
- self.assertEqual(boundary_iou(points, points1), 0)
-
- # test overlapping boundaries
- self.assertEqual(boundary_iou(points, points), 1)
-
- # test invalid boundaries
- self.assertEqual(boundary_iou(points2, points2), 0)
- self.assertEqual(boundary_iou(points3, points3, zero_division=1), 1)
- self.assertEqual(boundary_iou(points2, points3), 0)
-
- def test_sort_points(self):
- points = np.array([[1, 1], [0, 0], [1, -1], [2, -2], [0, 2], [1, 1],
- [0, 1], [-1, 1], [-1, -1]])
- target = np.array([[-1, -1], [0, 0], [-1, 1], [0, 1], [0, 2], [1, 1],
- [1, 1], [2, -2], [1, -1]])
- self.assertTrue(np.allclose(target, sort_points(points)))
-
- points = np.array([[1, 1], [1, -1], [-1, 1], [-1, -1]])
- target = np.array([[-1, -1], [-1, 1], [1, 1], [1, -1]])
- self.assertTrue(np.allclose(target, sort_points(points)))
-
- points = [[1, 1], [1, -1], [-1, 1], [-1, -1]]
- self.assertTrue(np.allclose(target, sort_points(points)))
-
- points = [[0.5, 0.3], [1, 0.5], [-0.5, 0.8], [-0.1, 1]]
- target = [[-0.5, 0.8], [-0.1, 1], [1, 0.5], [0.5, 0.3]]
- self.assertTrue(np.allclose(target, sort_points(points)))
-
- points = [[0.5, 3], [0.1, -0.2], [-0.5, -0.3], [-0.7, 3.1]]
- target = [[-0.5, -0.3], [-0.7, 3.1], [0.5, 3], [0.1, -0.2]]
- self.assertTrue(np.allclose(target, sort_points(points)))
-
- points = [[1, 0.8], [0.8, -1], [1.8, 0.5], [1.9, -0.6], [-0.5, 2],
- [-1, 1.8], [-2, 0.7], [-1.6, -0.2], [-1, -0.5]]
- target = [[-1, -0.5], [-1.6, -0.2], [-2, 0.7], [-1, 1.8], [-0.5, 2],
- [1, 0.8], [1.8, 0.5], [1.9, -0.6], [0.8, -1]]
- self.assertTrue(np.allclose(target, sort_points(points)))
-
- # concave polygon may failed
- points = [[1, 0], [-1, 0], [0, 0], [0, -1], [0.25, 1], [0.75, 1],
- [-0.25, 1], [-0.75, 1]]
- target = [[-1, 0], [-0.75, 1], [-0.25, 1], [0, 0], [0.25, 1],
- [0.75, 1], [1, 0], [0, -1]]
- self.assertFalse(np.allclose(target, sort_points(points)))
-
- with self.assertRaises(AssertionError):
- sort_points([1, 2])
-
- def test_sort_vertex(self):
- dummy_points_x = [20, 20, 120, 120]
- dummy_points_y = [20, 40, 40, 20]
-
- expect_points_x = [20, 120, 120, 20]
- expect_points_y = [20, 20, 40, 40]
-
- with self.assertRaises(AssertionError):
- sort_vertex([], dummy_points_y)
- with self.assertRaises(AssertionError):
- sort_vertex(dummy_points_x, [])
-
- for perm in set(permutations([0, 1, 2, 3])):
- points_x = [dummy_points_x[i] for i in perm]
- points_y = [dummy_points_y[i] for i in perm]
- ordered_points_x, ordered_points_y = sort_vertex(
- points_x, points_y)
-
- self.assertTrue(np.allclose(ordered_points_x, expect_points_x))
- self.assertTrue(np.allclose(ordered_points_y, expect_points_y))
-
- def test_sort_vertex8(self):
- dummy_points_x = [21, 21, 122, 122]
- dummy_points_y = [21, 39, 39, 21]
-
- expect_points = [21, 21, 122, 21, 122, 39, 21, 39]
-
- for perm in set(permutations([0, 1, 2, 3])):
- points_x = [dummy_points_x[i] for i in perm]
- points_y = [dummy_points_y[i] for i in perm]
- points = list(chain.from_iterable(zip(points_x, points_y)))
- ordered_points = sort_vertex8(points)
-
- self.assertTrue(np.allclose(ordered_points, expect_points))
diff --git a/pipeline/mmocr/tests/test_utils/test_processing.py b/pipeline/mmocr/tests/test_utils/test_processing.py
deleted file mode 100644
index 44953f7e898abcef11538b9e3e9e34840662fabd..0000000000000000000000000000000000000000
--- a/pipeline/mmocr/tests/test_utils/test_processing.py
+++ /dev/null
@@ -1,30 +0,0 @@
-# Copyright (c) OpenMMLab. All rights reserved.
-import unittest
-
-from mmocr.utils import track_parallel_progress_multi_args
-
-
-def func(a, b):
- return a + b
-
-
-class TestProcessing(unittest.TestCase):
-
- def test_track_parallel_progress_multi_args(self):
-
- args = ([1, 2, 3], [4, 5, 6])
- results = track_parallel_progress_multi_args(func, args, nproc=1)
- self.assertEqual(results, [5, 7, 9])
-
- results = track_parallel_progress_multi_args(func, args, nproc=2)
- self.assertEqual(results, [5, 7, 9])
-
- with self.assertRaises(AssertionError):
- track_parallel_progress_multi_args(func, 1, nproc=1)
-
- with self.assertRaises(AssertionError):
- track_parallel_progress_multi_args(func, ([1, 2], 1), nproc=1)
-
- with self.assertRaises(AssertionError):
- track_parallel_progress_multi_args(
- func, ([1, 2], [1, 2, 3]), nproc=1)
diff --git a/pipeline/mmocr/tests/test_utils/test_string_utils.py b/pipeline/mmocr/tests/test_utils/test_string_utils.py
deleted file mode 100644
index e4c3d720ac8df5a1e012aea42361ca79931b414d..0000000000000000000000000000000000000000
--- a/pipeline/mmocr/tests/test_utils/test_string_utils.py
+++ /dev/null
@@ -1,35 +0,0 @@
-# Copyright (c) OpenMMLab. All rights reserved.
-import pytest
-
-from mmocr.utils import StringStripper
-
-
-def test_string_strip():
- strip_list = [True, False]
- strip_pos_list = ['both', 'left', 'right']
- strip_str_list = [None, ' ']
-
- in_str_list = [
- ' hello ', 'hello ', ' hello', ' hello', 'hello ', 'hello ', 'hello',
- 'hello', 'hello', 'hello', 'hello', 'hello'
- ]
- out_str_list = [
- 'hello', 'hello', 'hello', 'hello', 'hello', 'hello', 'hello', 'hello',
- 'hello', 'hello', 'hello', 'hello'
- ]
-
- for idx1, strip in enumerate(strip_list):
- for idx2, strip_pos in enumerate(strip_pos_list):
- for idx3, strip_str in enumerate(strip_str_list):
- tmp_args = dict(
- strip=strip, strip_pos=strip_pos, strip_str=strip_str)
- strip_class = StringStripper(**tmp_args)
- i = idx1 * len(strip_pos_list) * len(
- strip_str_list) + idx2 * len(strip_str_list) + idx3
-
- assert strip_class(in_str_list[i]) == out_str_list[i]
-
- with pytest.raises(AssertionError):
- StringStripper(strip='strip')
- StringStripper(strip_pos='head')
- StringStripper(strip_str=['\n', '\t'])
diff --git a/pipeline/mmocr/tests/test_utils/test_transform_utils.py b/pipeline/mmocr/tests/test_utils/test_transform_utils.py
deleted file mode 100644
index d29dc372a92d5f534a1fcf8c9bfbe545e7c63d93..0000000000000000000000000000000000000000
--- a/pipeline/mmocr/tests/test_utils/test_transform_utils.py
+++ /dev/null
@@ -1,41 +0,0 @@
-# Copyright (c) OpenMMLab. All rights reserved.
-import copy
-import unittest
-
-import numpy as np
-
-from mmocr.utils import remove_pipeline_elements
-
-
-class TestTransformUtils(unittest.TestCase):
-
- def test_remove_pipeline_elements(self):
- data = dict(img=np.random.random((30, 40, 3)))
- results = remove_pipeline_elements(copy.deepcopy(data), [0, 1, 2])
- self.assertTrue(np.array_equal(results['img'], data['img']))
- self.assertEqual(len(data), len(results))
-
- data['gt_polygons'] = [
- np.array([0., 0., 10., 10., 10., 0.]),
- np.array([0., 0., 10., 0., 0., 10.]),
- np.array([0, 10, 0, 10, 1, 2, 3, 4]),
- np.array([0, 10, 0, 10, 10, 0, 0, 10]),
- ]
- data['dummy'] = [
- np.array([0., 0., 10., 10., 10., 0.]),
- ]
- data['gt_ignored'] = np.array([True, True, False, False], dtype=bool)
- data['gt_bboxes_labels'] = np.array([0, 1, 2, 3])
- data['gt_bboxes'] = np.array([[1, 2, 3, 4], [5, 6, 7, 8],
- [0, 0, 10, 10], [0, 0, 0, 0]])
- data['gt_texts'] = ['t1', 't2', 't3', 't4']
- keys = [
- 'gt_polygons', 'gt_bboxes', 'gt_ignored', 'gt_texts',
- 'gt_bboxes_labels'
- ]
- results = remove_pipeline_elements(copy.deepcopy(data), [0, 1, 2])
-
- for key in keys:
- self.assertTrue(np.array_equal(results[key][0], data[key][3]))
- self.assertTrue(np.array_equal(results['img'], data['img']))
- self.assertTrue(np.array_equal(results['dummy'], data['dummy']))
diff --git a/pipeline/mmocr/tests/test_visualization/test_base_visualizer.py b/pipeline/mmocr/tests/test_visualization/test_base_visualizer.py
deleted file mode 100644
index 57abc242fa2a871138b8d995f4c46ab990377dd3..0000000000000000000000000000000000000000
--- a/pipeline/mmocr/tests/test_visualization/test_base_visualizer.py
+++ /dev/null
@@ -1,55 +0,0 @@
-# Copyright (c) OpenMMLab. All rights reserved.
-from unittest import TestCase
-
-import numpy as np
-
-from mmocr.visualization import BaseLocalVisualizer
-
-
-class TestBaseLocalVisualizer(TestCase):
-
- def test_get_labels_image(self):
- labels = ['a', 'b', 'c']
- image = np.zeros((40, 40, 3), dtype=np.uint8)
- bboxes = np.array([[0, 0, 10, 10], [10, 10, 20, 20], [20, 20, 30, 30]])
- labels_image = BaseLocalVisualizer().get_labels_image(
- image,
- labels,
- bboxes=bboxes,
- auto_font_size=True,
- colors=['r', 'r', 'r', 'r'])
- self.assertEqual(labels_image.shape, (40, 40, 3))
-
- def test_get_polygons_image(self):
- polygons = [np.array([0, 0, 10, 10, 20, 20, 30, 30]).reshape(-1, 2)]
- image = np.zeros((40, 40, 3), dtype=np.uint8)
- polygons_image = BaseLocalVisualizer().get_polygons_image(
- image, polygons, colors=['r', 'r', 'r', 'r'])
- self.assertEqual(polygons_image.shape, (40, 40, 3))
-
- polygons_image = BaseLocalVisualizer().get_polygons_image(
- image, polygons, colors=['r', 'r', 'r', 'r'], filling=True)
- self.assertEqual(polygons_image.shape, (40, 40, 3))
-
- def test_get_bboxes_image(self):
- bboxes = np.array([[0, 0, 10, 10], [10, 10, 20, 20], [20, 20, 30, 30]])
- image = np.zeros((40, 40, 3), dtype=np.uint8)
- bboxes_image = BaseLocalVisualizer().get_bboxes_image(
- image, bboxes, colors=['r', 'r', 'r', 'r'])
- self.assertEqual(bboxes_image.shape, (40, 40, 3))
-
- bboxes_image = BaseLocalVisualizer().get_bboxes_image(
- image, bboxes, colors=['r', 'r', 'r', 'r'], filling=True)
- self.assertEqual(bboxes_image.shape, (40, 40, 3))
-
- def test_cat_images(self):
- image1 = np.zeros((40, 40, 3), dtype=np.uint8)
- image2 = np.zeros((40, 40, 3), dtype=np.uint8)
- image = BaseLocalVisualizer()._cat_image([image1, image2], axis=1)
- self.assertEqual(image.shape, (40, 80, 3))
-
- image = BaseLocalVisualizer()._cat_image([], axis=0)
- self.assertIsNone(image)
-
- image = BaseLocalVisualizer()._cat_image([image1, None], axis=0)
- self.assertEqual(image.shape, (40, 40, 3))
diff --git a/pipeline/mmocr/tests/test_visualization/test_kie_visualizer.py b/pipeline/mmocr/tests/test_visualization/test_kie_visualizer.py
deleted file mode 100644
index 0cc650b3f8a6800f0ff74b1605668dfde070a1f6..0000000000000000000000000000000000000000
--- a/pipeline/mmocr/tests/test_visualization/test_kie_visualizer.py
+++ /dev/null
@@ -1,126 +0,0 @@
-# Copyright (c) OpenMMLab. All rights reserved.
-import os.path as osp
-import tempfile
-import unittest
-
-import cv2
-import numpy as np
-import torch
-from mmengine.structures import InstanceData
-
-from mmocr.structures import KIEDataSample
-from mmocr.utils import bbox2poly
-from mmocr.visualization import KIELocalVisualizer
-
-
-class TestTextKIELocalVisualizer(unittest.TestCase):
-
- def setUp(self):
- h, w = 12, 10
- self.image = np.random.randint(0, 256, size=(h, w, 3)).astype('uint8')
- edge_labels = torch.rand((5, 5)) > 0.5
- # gt_instances
- data_sample = KIEDataSample()
- gt_instances_data = dict(
- bboxes=self._rand_bboxes(5, h, w),
- polygons=self._rand_polys(5, h, w),
- labels=torch.zeros(5, ),
- texts=['text1', 'text2', 'text3', 'text4', 'text5'],
- edge_labels=edge_labels)
- gt_instances = InstanceData(**gt_instances_data)
- data_sample.gt_instances = gt_instances
-
- pred_instances_data = dict(
- bboxes=self._rand_bboxes(5, h, w),
- labels=torch.zeros(5, ),
- scores=torch.rand((5, )),
- texts=['text1', 'text2', 'text3', 'text4', 'text5'],
- edge_labels=edge_labels)
- pred_instances = InstanceData(**pred_instances_data)
- data_sample.pred_instances = pred_instances
- data_sample = data_sample.numpy()
- self.data_sample = data_sample
-
- @staticmethod
- def _rand_bboxes(num_boxes, h, w):
- cx, cy, bw, bh = torch.rand(num_boxes, 4).T
-
- tl_x = ((cx * w) - (w * bw / 2)).clamp(0, w).unsqueeze(0)
- tl_y = ((cy * h) - (h * bh / 2)).clamp(0, h).unsqueeze(0)
- br_x = ((cx * w) + (w * bw / 2)).clamp(0, w).unsqueeze(0)
- br_y = ((cy * h) + (h * bh / 2)).clamp(0, h).unsqueeze(0)
-
- bboxes = torch.cat([tl_x, tl_y, br_x, br_y], dim=0).T
-
- return bboxes
-
- def _rand_polys(self, num_bboxes, h, w):
- bboxes = self._rand_bboxes(num_bboxes, h, w)
- bboxes = bboxes.tolist()
- polys = [bbox2poly(bbox) for bbox in bboxes]
- return polys
-
- def test_add_datasample(self):
- image = self.image
- h, w, c = image.shape
-
- visualizer = KIELocalVisualizer(is_openset=True)
- visualizer.dataset_meta = dict(category=[
- dict(id=0, name='bg'),
- dict(id=1, name='key'),
- dict(id=2, name='value'),
- dict(id=3, name='other')
- ])
- visualizer.add_datasample('image', image, self.data_sample)
-
- with tempfile.TemporaryDirectory() as tmp_dir:
- # test out
- out_file = osp.join(tmp_dir, 'out_file.jpg')
- visualizer.add_datasample(
- 'image',
- image,
- self.data_sample,
- out_file=out_file,
- draw_gt=False,
- draw_pred=False)
- self._assert_image_and_shape(out_file, (h, w, c))
-
- visualizer.add_datasample(
- 'image', image, self.data_sample, out_file=out_file)
- self._assert_image_and_shape(out_file, (h * 2, w * 4, c))
-
- visualizer.add_datasample(
- 'image',
- image,
- self.data_sample,
- draw_gt=False,
- out_file=out_file)
- self._assert_image_and_shape(out_file, (h, w * 4, c))
-
- visualizer.add_datasample(
- 'image',
- image,
- self.data_sample,
- draw_pred=False,
- out_file=out_file)
- self._assert_image_and_shape(out_file, (h, w * 4, c))
-
- visualizer = KIELocalVisualizer(is_openset=False)
- visualizer.dataset_meta = dict(category=[
- dict(id=0, name='bg'),
- dict(id=1, name='key'),
- dict(id=2, name='value'),
- dict(id=3, name='other')
- ])
- visualizer.add_datasample(
- 'image',
- image,
- self.data_sample,
- draw_pred=False,
- out_file=out_file)
- self._assert_image_and_shape(out_file, (h, w * 3, c))
-
- def _assert_image_and_shape(self, out_file, out_shape):
- self.assertTrue(osp.exists(out_file))
- drawn_img = cv2.imread(out_file)
- self.assertTrue(drawn_img.shape == out_shape)
diff --git a/pipeline/mmocr/tests/test_visualization/test_textdet_visualizer.py b/pipeline/mmocr/tests/test_visualization/test_textdet_visualizer.py
deleted file mode 100644
index 21a493ada05542ba43b77b7bab60749f2d3a8333..0000000000000000000000000000000000000000
--- a/pipeline/mmocr/tests/test_visualization/test_textdet_visualizer.py
+++ /dev/null
@@ -1,111 +0,0 @@
-# Copyright (c) OpenMMLab. All rights reserved.
-import os.path as osp
-import tempfile
-import unittest
-
-import cv2
-import numpy as np
-import torch
-from mmengine.structures import InstanceData
-
-from mmocr.structures import TextDetDataSample
-from mmocr.utils import bbox2poly
-from mmocr.visualization import TextDetLocalVisualizer
-
-
-class TestTextDetLocalVisualizer(unittest.TestCase):
-
- def setUp(self):
- h, w = 12, 10
- self.image = np.random.randint(0, 256, size=(h, w, 3)).astype('uint8')
-
- # gt_instances
- data_sample = TextDetDataSample()
- gt_instances_data = dict(
- bboxes=self._rand_bboxes(5, h, w),
- polygons=self._rand_polys(5, h, w),
- labels=torch.zeros(5, ))
- gt_instances = InstanceData(**gt_instances_data)
- data_sample.gt_instances = gt_instances
-
- pred_instances_data = dict(
- bboxes=self._rand_bboxes(5, h, w),
- polygons=self._rand_polys(5, h, w),
- labels=torch.zeros(5, ),
- scores=torch.rand((5, )))
- pred_instances = InstanceData(**pred_instances_data)
- data_sample.pred_instances = pred_instances
- self.data_sample = data_sample
-
- def test_text_det_local_visualizer(self):
- for with_poly in [True, False]:
- for with_bbox in [True, False]:
- vis_cfg = dict(with_poly=with_poly, with_bbox=with_bbox)
- self._test_add_datasample(vis_cfg=vis_cfg)
-
- @staticmethod
- def _rand_bboxes(num_boxes, h, w):
- cx, cy, bw, bh = torch.rand(num_boxes, 4).T
-
- tl_x = ((cx * w) - (w * bw / 2)).clamp(0, w).unsqueeze(0)
- tl_y = ((cy * h) - (h * bh / 2)).clamp(0, h).unsqueeze(0)
- br_x = ((cx * w) + (w * bw / 2)).clamp(0, w).unsqueeze(0)
- br_y = ((cy * h) + (h * bh / 2)).clamp(0, h).unsqueeze(0)
-
- bboxes = torch.cat([tl_x, tl_y, br_x, br_y], dim=0).T
-
- return bboxes
-
- def _rand_polys(self, num_bboxes, h, w):
- bboxes = self._rand_bboxes(num_bboxes, h, w)
- bboxes = bboxes.tolist()
- polys = [bbox2poly(bbox) for bbox in bboxes]
- return polys
-
- def _test_add_datasample(self, vis_cfg):
- image = self.image
- h, w, c = image.shape
-
- det_local_visualizer = TextDetLocalVisualizer(**vis_cfg)
- det_local_visualizer.add_datasample('image', image, self.data_sample)
-
- with tempfile.TemporaryDirectory() as tmp_dir:
- # test out
- out_file = osp.join(tmp_dir, 'out_file.jpg')
- det_local_visualizer.add_datasample(
- 'image',
- image,
- self.data_sample,
- out_file=out_file,
- draw_gt=False,
- draw_pred=False)
- self._assert_image_and_shape(out_file, (h, w, c))
-
- det_local_visualizer.add_datasample(
- 'image', image, self.data_sample, out_file=out_file)
- self._assert_image_and_shape(out_file, (h, w * 2, c))
-
- det_local_visualizer.add_datasample(
- 'image',
- image,
- self.data_sample,
- draw_gt=False,
- out_file=out_file)
- self._assert_image_and_shape(out_file, (h, w, c))
-
- det_local_visualizer.add_datasample(
- 'image',
- image,
- self.data_sample,
- draw_pred=False,
- out_file=out_file)
- self._assert_image_and_shape(out_file, (h, w, c))
-
- det_local_visualizer.add_datasample(
- 'image', image, None, out_file=out_file)
- self._assert_image_and_shape(out_file, (h, w, c))
-
- def _assert_image_and_shape(self, out_file, out_shape):
- self.assertTrue(osp.exists(out_file))
- drawn_img = cv2.imread(out_file)
- self.assertTrue(drawn_img.shape == out_shape)
diff --git a/pipeline/mmocr/tests/test_visualization/test_textrecog_visualizer.py b/pipeline/mmocr/tests/test_visualization/test_textrecog_visualizer.py
deleted file mode 100644
index 3171a02d9dd45c4a3c124b4b735f6c5f8496f27a..0000000000000000000000000000000000000000
--- a/pipeline/mmocr/tests/test_visualization/test_textrecog_visualizer.py
+++ /dev/null
@@ -1,73 +0,0 @@
-# Copyright (c) OpenMMLab. All rights reserved.
-import os.path as osp
-import tempfile
-import unittest
-
-import cv2
-import numpy as np
-from mmengine.structures import LabelData
-
-from mmocr.structures import TextRecogDataSample
-from mmocr.visualization import TextRecogLocalVisualizer
-
-
-class TestTextDetLocalVisualizer(unittest.TestCase):
-
- def test_add_datasample(self):
- h, w = 64, 128
- image = np.random.randint(0, 256, size=(h, w, 3)).astype('uint8')
-
- # test gt_text
- data_sample = TextRecogDataSample()
- img_meta = dict(img_shape=(12, 10, 3))
- gt_text = LabelData(metainfo=img_meta)
- gt_text.item = 'mmocr'
- data_sample.gt_text = gt_text
-
- recog_local_visualizer = TextRecogLocalVisualizer()
- recog_local_visualizer.add_datasample('image', image, data_sample)
-
- # test gt_text and pred_text
- pred_text = LabelData(metainfo=img_meta)
- pred_text.item = 'MMOCR'
- data_sample.pred_text = pred_text
-
- with tempfile.TemporaryDirectory() as tmp_dir:
- # test out
- out_file = osp.join(tmp_dir, 'out_file.jpg')
-
- # draw_gt = True + gt_sample
- recog_local_visualizer.add_datasample(
- 'image',
- image,
- data_sample,
- out_file=out_file,
- draw_gt=True,
- draw_pred=False)
- self._assert_image_and_shape(out_file, (h * 2, w, 3))
-
- # draw_gt = True
- recog_local_visualizer.add_datasample(
- 'image',
- image,
- data_sample,
- out_file=out_file,
- draw_gt=True,
- draw_pred=True)
- self._assert_image_and_shape(out_file, (h * 3, w, 3))
-
- # draw_gt = False
- recog_local_visualizer.add_datasample(
- 'image', image, data_sample, draw_gt=False, out_file=out_file)
- self._assert_image_and_shape(out_file, (h * 2, w, 3))
-
- # gray image
- image = np.random.randint(0, 256, size=(h, w)).astype('uint8')
- recog_local_visualizer.add_datasample(
- 'image', image, data_sample, draw_gt=False, out_file=out_file)
- self._assert_image_and_shape(out_file, (h * 2, w, 3))
-
- def _assert_image_and_shape(self, out_file, out_shape):
- self.assertTrue(osp.exists(out_file))
- drawn_img = cv2.imread(out_file)
- self.assertTrue(drawn_img.shape == out_shape)
diff --git a/pipeline/mmocr/tests/test_visualization/test_textspotting_visualizer.py b/pipeline/mmocr/tests/test_visualization/test_textspotting_visualizer.py
deleted file mode 100644
index 5c064339f16a20b6d2029240b7f00aadab0c6f91..0000000000000000000000000000000000000000
--- a/pipeline/mmocr/tests/test_visualization/test_textspotting_visualizer.py
+++ /dev/null
@@ -1,113 +0,0 @@
-# Copyright (c) OpenMMLab. All rights reserved.
-import os.path as osp
-import tempfile
-import unittest
-
-import cv2
-import numpy as np
-import torch
-from mmengine.structures import InstanceData
-
-from mmocr.structures import TextDetDataSample
-from mmocr.utils import bbox2poly
-from mmocr.visualization import TextSpottingLocalVisualizer
-
-
-class TestTextSpottingLocalVisualizer(unittest.TestCase):
-
- def setUp(self):
- h, w = 12, 10
- self.image = np.random.randint(0, 256, size=(h, w, 3)).astype('uint8')
- # gt_instances
- data_sample = TextDetDataSample()
- gt_instances_data = dict(
- bboxes=self._rand_bboxes(5, h, w),
- polygons=self._rand_polys(5, h, w),
- labels=torch.zeros(5, ),
- texts=['text1', 'text2', 'text3', 'text4', 'text5'])
- gt_instances = InstanceData(**gt_instances_data)
- data_sample.gt_instances = gt_instances
-
- pred_instances_data = dict(
- bboxes=self._rand_bboxes(5, h, w),
- labels=torch.zeros(5, ),
- scores=torch.rand((5, )),
- texts=['text1', 'text2', 'text3', 'text4', 'text5'])
- pred_instances = InstanceData(**pred_instances_data)
- data_sample.pred_instances = pred_instances
- data_sample = data_sample.numpy()
- self.data_sample = data_sample
-
- @staticmethod
- def _rand_bboxes(num_boxes, h, w):
- cx, cy, bw, bh = torch.rand(num_boxes, 4).T
-
- tl_x = ((cx * w) - (w * bw / 2)).clamp(0, w).unsqueeze(0)
- tl_y = ((cy * h) - (h * bh / 2)).clamp(0, h).unsqueeze(0)
- br_x = ((cx * w) + (w * bw / 2)).clamp(0, w).unsqueeze(0)
- br_y = ((cy * h) + (h * bh / 2)).clamp(0, h).unsqueeze(0)
-
- bboxes = torch.cat([tl_x, tl_y, br_x, br_y], dim=0).T
-
- return bboxes
-
- def _rand_polys(self, num_bboxes, h, w):
- bboxes = self._rand_bboxes(num_bboxes, h, w)
- bboxes = bboxes.tolist()
- polys = [bbox2poly(bbox) for bbox in bboxes]
- return polys
-
- def test_add_datasample(self):
- image = self.image
- h, w, c = image.shape
-
- visualizer = TextSpottingLocalVisualizer()
- visualizer.add_datasample('image', image, self.data_sample)
-
- with tempfile.TemporaryDirectory() as tmp_dir:
- # test out
- out_file = osp.join(tmp_dir, 'out_file.jpg')
- visualizer.add_datasample(
- 'image',
- image,
- self.data_sample,
- out_file=out_file,
- draw_gt=False,
- draw_pred=False)
- self._assert_image_and_shape(out_file, (h, w, c))
-
- visualizer.add_datasample(
- 'image', image, self.data_sample, out_file=out_file)
- self._assert_image_and_shape(out_file, (h * 2, w * 2, c))
-
- visualizer.add_datasample(
- 'image',
- image,
- self.data_sample,
- draw_gt=False,
- out_file=out_file)
- self._assert_image_and_shape(out_file, (h, w * 2, c))
-
- visualizer.add_datasample(
- 'image',
- image,
- self.data_sample,
- draw_pred=False,
- out_file=out_file)
- self._assert_image_and_shape(out_file, (h, w * 2, c))
- bboxes = self.data_sample.pred_instances.get('bboxes')
- bboxes = bboxes.tolist()
- polys = [bbox2poly(bbox) for bbox in bboxes]
- self.data_sample.pred_instances.polygons = polys
- visualizer.add_datasample(
- 'image',
- image,
- self.data_sample,
- draw_gt=False,
- out_file=out_file)
- self._assert_image_and_shape(out_file, (h, w * 2, c))
-
- def _assert_image_and_shape(self, out_file, out_shape):
- self.assertTrue(osp.exists(out_file))
- drawn_img = cv2.imread(out_file)
- self.assertTrue(drawn_img.shape == out_shape)
diff --git a/pipeline/mmocr/tools/analysis_tools/get_flops.py b/pipeline/mmocr/tools/analysis_tools/get_flops.py
deleted file mode 100644
index caa97203aa1e077bd266ab64aa02c1d59f88ec7f..0000000000000000000000000000000000000000
--- a/pipeline/mmocr/tools/analysis_tools/get_flops.py
+++ /dev/null
@@ -1,55 +0,0 @@
-# Copyright (c) OpenMMLab. All rights reserved.
-import argparse
-
-import torch
-from fvcore.nn import FlopCountAnalysis, flop_count_table
-from mmengine import Config
-from mmengine.registry import init_default_scope
-
-from mmocr.registry import MODELS
-
-
-def parse_args():
- parser = argparse.ArgumentParser(description='Train a detector')
- parser.add_argument('config', help='train config file path')
- parser.add_argument(
- '--shape',
- type=int,
- nargs='+',
- default=[640, 640],
- help='input image size')
- args = parser.parse_args()
- return args
-
-
-def main():
-
- args = parse_args()
-
- if len(args.shape) == 1:
- h = w = args.shape[0]
- elif len(args.shape) == 2:
- h, w = args.shape
- else:
- raise ValueError('invalid input shape, please use --shape h w')
-
- input_shape = (1, 3, h, w)
-
- cfg = Config.fromfile(args.config)
- init_default_scope(cfg.get('default_scope', 'mmocr'))
- model = MODELS.build(cfg.model)
-
- flops = FlopCountAnalysis(model, torch.ones(input_shape))
-
- # params = parameter_count_table(model)
- flops_data = flop_count_table(flops)
-
- print(flops_data)
-
- print('!!!Please be cautious if you use the results in papers. '
- 'You may need to check if all ops are supported and verify that the '
- 'flops computation is correct.')
-
-
-if __name__ == '__main__':
- main()
diff --git a/pipeline/mmocr/tools/analysis_tools/offline_eval.py b/pipeline/mmocr/tools/analysis_tools/offline_eval.py
deleted file mode 100644
index b454942238d59d4f07067896ca9f9742094d0d59..0000000000000000000000000000000000000000
--- a/pipeline/mmocr/tools/analysis_tools/offline_eval.py
+++ /dev/null
@@ -1,49 +0,0 @@
-# Copyright (c) OpenMMLab. All rights reserved.
-import argparse
-import json
-
-import mmengine
-from mmengine.config import Config, DictAction
-from mmengine.evaluator import Evaluator
-from mmengine.registry import init_default_scope
-
-
-def parse_args():
- parser = argparse.ArgumentParser(description='Offline evaluation of the '
- 'prediction saved in pkl format')
- parser.add_argument('config', help='Config of the model')
- parser.add_argument(
- 'pkl_results', help='Path to the predictions in '
- 'pickle format')
- parser.add_argument(
- '--cfg-options',
- nargs='+',
- action=DictAction,
- help='Override some settings in the used config, the key-value pair '
- 'in xxx=yyy format will be merged into config file. If the value to '
- 'be overwritten is a list, it should be like key="[a,b]" or key=a,b '
- 'It also allows nested list/tuple values, e.g. key="[(a,b),(c,d)]" '
- 'Note that the quotation marks are necessary and that no white space '
- 'is allowed.')
- args = parser.parse_args()
- return args
-
-
-def main():
- args = parse_args()
-
- # load config
- cfg = Config.fromfile(args.config)
- init_default_scope(cfg.get('default_scope', 'mmocr'))
- if args.cfg_options is not None:
- cfg.merge_from_dict(args.cfg_options)
-
- predictions = mmengine.load(args.pkl_results)
-
- evaluator = Evaluator(cfg.test_evaluator)
- eval_results = evaluator.offline_evaluate(predictions)
- print(json.dumps(eval_results))
-
-
-if __name__ == '__main__':
- main()
diff --git a/pipeline/mmocr/tools/analysis_tools/print_config.py b/pipeline/mmocr/tools/analysis_tools/print_config.py
deleted file mode 100644
index 770bb6da216bd382751a8b20c323e87119afe4e6..0000000000000000000000000000000000000000
--- a/pipeline/mmocr/tools/analysis_tools/print_config.py
+++ /dev/null
@@ -1,36 +0,0 @@
-# Copyright (c) OpenMMLab. All rights reserved.
-import argparse
-
-from mmengine import Config, DictAction
-
-
-def parse_args():
- parser = argparse.ArgumentParser(description='Print the whole config')
- parser.add_argument('config', help='config file path')
- parser.add_argument(
- '--cfg-options',
- nargs='+',
- action=DictAction,
- help='override some settings in the used config, the key-value pair '
- 'in xxx=yyy format will be merged into config file. If the value to '
- 'be overwritten is a list, it should be like key="[a,b]" or key=a,b '
- 'It also allows nested list/tuple values, e.g. key="[(a,b),(c,d)]" '
- 'Note that the quotation marks are necessary and that no white space '
- 'is allowed.')
- args = parser.parse_args()
-
- return args
-
-
-def main():
- args = parse_args()
-
- cfg = Config.fromfile(args.config)
-
- if args.cfg_options is not None:
- cfg.merge_from_dict(args.cfg_options)
- print(f'Config:\n{cfg.pretty_text}')
-
-
-if __name__ == '__main__':
- main()
diff --git a/pipeline/mmocr/tools/dataset_converters/common/curvedsyntext_converter.py b/pipeline/mmocr/tools/dataset_converters/common/curvedsyntext_converter.py
deleted file mode 100644
index 5fd8784a708c45591c00b97fc1e0c4fe96c88df7..0000000000000000000000000000000000000000
--- a/pipeline/mmocr/tools/dataset_converters/common/curvedsyntext_converter.py
+++ /dev/null
@@ -1,129 +0,0 @@
-# Copyright (c) OpenMMLab. All rights reserved.
-import argparse
-import os.path as osp
-from functools import partial
-
-import mmengine
-import numpy as np
-
-from mmocr.utils import bezier2polygon, sort_points
-
-# The default dictionary used by CurvedSynthText
-dict95 = [
- ' ', '!', '"', '#', '$', '%', '&', '\'', '(', ')', '*', '+', ',', '-', '.',
- '/', '0', '1', '2', '3', '4', '5', '6', '7', '8', '9', ':', ';', '<', '=',
- '>', '?', '@', 'A', 'B', 'C', 'D', 'E', 'F', 'G', 'H', 'I', 'J', 'K', 'L',
- 'M', 'N', 'O', 'P', 'Q', 'R', 'S', 'T', 'U', 'V', 'W', 'X', 'Y', 'Z', '[',
- '\\', ']', '^', '_', '`', 'a', 'b', 'c', 'd', 'e', 'f', 'g', 'h', 'i', 'j',
- 'k', 'l', 'm', 'n', 'o', 'p', 'q', 'r', 's', 't', 'u', 'v', 'w', 'x', 'y',
- 'z', '{', '|', '}', '~'
-]
-UNK = len(dict95)
-EOS = UNK + 1
-
-
-def digit2text(rec):
- res = []
- for d in rec:
- assert d <= EOS
- if d == EOS:
- break
- if d == UNK:
- print('Warning: Has a UNK character')
- res.append('ๅฃ') # Or any special character not in the target dict
- res.append(dict95[d])
- return ''.join(res)
-
-
-def modify_annotation(ann, num_sample, start_img_id=0, start_ann_id=0):
- ann['text'] = digit2text(ann.pop('rec'))
- # Get hide egmentation points
- polygon_pts = bezier2polygon(ann['bezier_pts'], num_sample=num_sample)
- ann['segmentation'] = np.asarray(sort_points(polygon_pts)).reshape(
- 1, -1).tolist()
- ann['image_id'] += start_img_id
- ann['id'] += start_ann_id
- return ann
-
-
-def modify_image_info(image_info, path_prefix, start_img_id=0):
- image_info['file_name'] = osp.join(path_prefix, image_info['file_name'])
- image_info['id'] += start_img_id
- return image_info
-
-
-def parse_args():
- parser = argparse.ArgumentParser(
- description='Convert CurvedSynText150k to COCO format')
- parser.add_argument('root_path', help='CurvedSynText150k root path')
- parser.add_argument('-o', '--out-dir', help='Output path')
- parser.add_argument(
- '-n',
- '--num-sample',
- type=int,
- default=4,
- help='Number of sample points at each Bezier curve.')
- parser.add_argument(
- '--nproc', default=1, type=int, help='Number of processes')
- args = parser.parse_args()
- return args
-
-
-def convert_annotations(data,
- path_prefix,
- num_sample,
- nproc,
- start_img_id=0,
- start_ann_id=0):
- modify_image_info_with_params = partial(
- modify_image_info, path_prefix=path_prefix, start_img_id=start_img_id)
- modify_annotation_with_params = partial(
- modify_annotation,
- num_sample=num_sample,
- start_img_id=start_img_id,
- start_ann_id=start_ann_id)
- if nproc > 1:
- data['annotations'] = mmengine.track_parallel_progress(
- modify_annotation_with_params, data['annotations'], nproc=nproc)
- data['images'] = mmengine.track_parallel_progress(
- modify_image_info_with_params, data['images'], nproc=nproc)
- else:
- data['annotations'] = mmengine.track_progress(
- modify_annotation_with_params, data['annotations'])
- data['images'] = mmengine.track_progress(
- modify_image_info_with_params,
- data['images'],
- )
- data['categories'] = [{'id': 1, 'name': 'text'}]
- return data
-
-
-def main():
- args = parse_args()
- root_path = args.root_path
- out_dir = args.out_dir if args.out_dir else root_path
- mmengine.mkdir_or_exist(out_dir)
-
- anns = mmengine.load(osp.join(root_path, 'train1.json'))
- data1 = convert_annotations(anns, 'syntext_word_eng', args.num_sample,
- args.nproc)
-
- # Get the maximum image id from data1
- start_img_id = max(data1['images'], key=lambda x: x['id'])['id'] + 1
- start_ann_id = max(data1['annotations'], key=lambda x: x['id'])['id'] + 1
- anns = mmengine.load(osp.join(root_path, 'train2.json'))
- data2 = convert_annotations(
- anns,
- 'emcs_imgs',
- args.num_sample,
- args.nproc,
- start_img_id=start_img_id,
- start_ann_id=start_ann_id)
-
- data1['images'] += data2['images']
- data1['annotations'] += data2['annotations']
- mmengine.dump(data1, osp.join(out_dir, 'instances_training.json'))
-
-
-if __name__ == '__main__':
- main()
diff --git a/pipeline/mmocr/tools/dataset_converters/common/extract_kaist.py b/pipeline/mmocr/tools/dataset_converters/common/extract_kaist.py
deleted file mode 100644
index 76d2579ccbb59f9addc60bbbe9df9037fd543665..0000000000000000000000000000000000000000
--- a/pipeline/mmocr/tools/dataset_converters/common/extract_kaist.py
+++ /dev/null
@@ -1,75 +0,0 @@
-# Copyright (c) OpenMMLab. All rights reserved.
-import argparse
-import os
-import os.path as osp
-import shutil
-import xml.etree.ElementTree as ET
-import zipfile
-from xml.etree.ElementTree import ParseError
-
-
-def extract(root_path):
- idx = 0
- for language in ['English', 'Korean', 'Mixed']:
- for camera in ['Digital_Camera', 'Mobile_Phone']:
- crt_path = osp.join(root_path, 'KAIST', language, camera)
- zips = os.listdir(crt_path)
- for zip in zips:
- extracted_path = osp.join(root_path, 'tmp', zip)
- extract_zipfile(osp.join(crt_path, zip), extracted_path)
- for file in os.listdir(extracted_path):
- if file.endswith('xml'):
- src_ann = os.path.join(extracted_path, file)
- # Filtering broken annotations
- try:
- ET.parse(src_ann)
- except ParseError:
- continue
- src_img = None
- img_names = [
- file.replace('xml', suffix)
- for suffix in ['jpg', 'JPG']
- ]
- for im in img_names:
- img_path = osp.join(extracted_path, im)
- if osp.exists(img_path):
- src_img = img_path
- if src_img:
- shutil.move(
- src_ann,
- osp.join(root_path, 'annotations',
- str(idx).zfill(5) + '.xml'))
- shutil.move(
- src_img,
- osp.join(root_path, 'imgs',
- str(idx).zfill(5) + '.jpg'))
- idx += 1
-
-
-def extract_zipfile(zip_path, dst_dir, delete=True):
-
- files = zipfile.ZipFile(zip_path)
- for file in files.namelist():
- files.extract(file, dst_dir)
- if delete:
- os.remove(zip_path)
-
-
-def parse_args():
- parser = argparse.ArgumentParser(description='Extract KAIST zips')
- parser.add_argument('root_path', help='Root path of KAIST')
- args = parser.parse_args()
- return args
-
-
-def main():
- args = parse_args()
- root_path = args.root_path
- assert osp.exists(root_path)
- extract(root_path)
- shutil.rmtree(osp.join(args.root_path, 'tmp'))
- shutil.rmtree(osp.join(args.root_path, 'KAIST'))
-
-
-if __name__ == '__main__':
- main()
diff --git a/pipeline/mmocr/tools/dataset_converters/kie/closeset_to_openset.py b/pipeline/mmocr/tools/dataset_converters/kie/closeset_to_openset.py
deleted file mode 100644
index 2057e9797bd0586fd8820ef3ae161486bea22d32..0000000000000000000000000000000000000000
--- a/pipeline/mmocr/tools/dataset_converters/kie/closeset_to_openset.py
+++ /dev/null
@@ -1,122 +0,0 @@
-# Copyright (c) OpenMMLab. All rights reserved.
-import argparse
-import json
-from functools import partial
-
-import mmengine
-
-from mmocr.utils import list_from_file, list_to_file
-
-
-def convert(closeset_line, merge_bg_others=False, ignore_idx=0, others_idx=25):
- """Convert line-json str of closeset to line-json str of openset. Note that
- this function is designed for closeset-wildreceipt to openset-wildreceipt.
- It may not be suitable to your own dataset.
-
- Args:
- closeset_line (str): The string to be deserialized to
- the closeset dictionary object.
- merge_bg_others (bool): If True, give the same label to "background"
- class and "others" class.
- ignore_idx (int): Index for ``ignore`` class.
- others_idx (int): Index for ``others`` class.
- """
- # Two labels at the same index of the following two lists
- # make up a key-value pair. For example, in wildreceipt,
- # closeset_key_inds[0] maps to "Store_name_key"
- # and closeset_value_inds[0] maps to "Store_addr_value".
- closeset_key_inds = list(range(2, others_idx, 2))
- closeset_value_inds = list(range(1, others_idx, 2))
-
- openset_node_label_mapping = {'bg': 0, 'key': 1, 'value': 2, 'others': 3}
- if merge_bg_others:
- openset_node_label_mapping['others'] = openset_node_label_mapping['bg']
-
- closeset_obj = json.loads(closeset_line)
- openset_obj = {
- 'file_name': closeset_obj['file_name'],
- 'height': closeset_obj['height'],
- 'width': closeset_obj['width'],
- 'annotations': []
- }
-
- edge_idx = 1
- label_to_edge = {}
- for anno in closeset_obj['annotations']:
- label = anno['label']
- if label == ignore_idx:
- anno['label'] = openset_node_label_mapping['bg']
- anno['edge'] = edge_idx
- edge_idx += 1
- elif label == others_idx:
- anno['label'] = openset_node_label_mapping['others']
- anno['edge'] = edge_idx
- edge_idx += 1
- else:
- edge = label_to_edge.get(label, None)
- if edge is not None:
- anno['edge'] = edge
- if label in closeset_key_inds:
- anno['label'] = openset_node_label_mapping['key']
- elif label in closeset_value_inds:
- anno['label'] = openset_node_label_mapping['value']
- else:
- tmp_key = 'key'
- if label in closeset_key_inds:
- label_with_same_edge = closeset_value_inds[
- closeset_key_inds.index(label)]
- elif label in closeset_value_inds:
- label_with_same_edge = closeset_key_inds[
- closeset_value_inds.index(label)]
- tmp_key = 'value'
- edge_counterpart = label_to_edge.get(label_with_same_edge,
- None)
- if edge_counterpart is not None:
- anno['edge'] = edge_counterpart
- else:
- anno['edge'] = edge_idx
- edge_idx += 1
- anno['label'] = openset_node_label_mapping[tmp_key]
- label_to_edge[label] = anno['edge']
-
- openset_obj['annotations'] = closeset_obj['annotations']
-
- return json.dumps(openset_obj, ensure_ascii=False)
-
-
-def process(closeset_file, openset_file, merge_bg_others=False, n_proc=10):
- closeset_lines = list_from_file(closeset_file)
-
- convert_func = partial(convert, merge_bg_others=merge_bg_others)
-
- openset_lines = mmengine.track_parallel_progress(
- convert_func, closeset_lines, nproc=n_proc)
-
- list_to_file(openset_file, openset_lines)
-
-
-def parse_args():
- parser = argparse.ArgumentParser()
- parser.add_argument('in_file', help='Annotation file for closeset.')
- parser.add_argument('out_file', help='Annotation file for openset.')
- parser.add_argument(
- '--merge',
- action='store_true',
- help='Merge two classes: "background" and "others" in closeset '
- 'to one class in openset.')
- parser.add_argument(
- '--n_proc', type=int, default=10, help='Number of process.')
- args = parser.parse_args()
- return args
-
-
-def main():
- args = parse_args()
-
- process(args.in_file, args.out_file, args.merge, args.n_proc)
-
- print('finish')
-
-
-if __name__ == '__main__':
- main()
diff --git a/pipeline/mmocr/tools/dataset_converters/prepare_dataset.py b/pipeline/mmocr/tools/dataset_converters/prepare_dataset.py
deleted file mode 100644
index 84b8a0353c420adc696a628baa54829d28367020..0000000000000000000000000000000000000000
--- a/pipeline/mmocr/tools/dataset_converters/prepare_dataset.py
+++ /dev/null
@@ -1,153 +0,0 @@
-# Copyright (c) OpenMMLab. All rights reserved.
-import argparse
-import os.path as osp
-import time
-import warnings
-
-from mmengine import Config
-
-from mmocr.datasets.preparers import DatasetPreparer
-
-
-def parse_args():
- parser = argparse.ArgumentParser(
- description='Preparing datasets used in MMOCR.')
- parser.add_argument(
- 'datasets',
- help='A list of the dataset names that would like to prepare.',
- nargs='+')
- parser.add_argument(
- '--nproc', help='Number of processes to run', default=4, type=int)
- parser.add_argument(
- '--task',
- default='textdet',
- choices=['textdet', 'textrecog', 'textspotting', 'kie'],
- help='Task type. Options are "textdet", "textrecog", "textspotting"'
- ' and "kie".')
- parser.add_argument(
- '--splits',
- default=['train', 'test', 'val'],
- help='A list of the split that would like to prepare.',
- nargs='+')
- parser.add_argument(
- '--lmdb',
- action='store_true',
- default=False,
- help='Whether to dump the textrecog dataset to LMDB format, It\'s a '
- 'shortcut to force the dataset to be dumped in lmdb format. '
- 'Applicable when --task=textrecog')
- parser.add_argument(
- '--overwrite-cfg',
- action='store_true',
- default=False,
- help='Whether to overwrite the dataset config file if it already'
- ' exists. If not specified, Dataset Preparer will not generate'
- ' new config for datasets whose configs are already in base.')
- parser.add_argument(
- '--dataset-zoo-path',
- default='./dataset_zoo',
- help='Path to dataset zoo config files.')
- args = parser.parse_args()
- return args
-
-
-def parse_meta(task: str, meta_path: str) -> None:
- """Parse meta file.
-
- Args:
- cfg_path (str): Path to meta file.
- """
- try:
- meta = Config.fromfile(meta_path)
- except FileNotFoundError:
- return
- assert task in meta['Data']['Tasks'], \
- f'Task {task} not supported!'
- # License related
- if meta['Data']['License']['Type']:
- print(f"\033[1;33;40mDataset Name: {meta['Name']}")
- print(f"License Type: {meta['Data']['License']['Type']}")
- print(f"License Link: {meta['Data']['License']['Link']}")
- print(f"BibTeX: {meta['Paper']['BibTeX']}\033[0m")
- print('\033[1;31;43mMMOCR does not own the dataset. Using this '
- 'dataset you must accept the license provided by the owners, '
- 'and cite the corresponding papers appropriately.')
- print('If you do not agree with the above license, please cancel '
- 'the progress immediately by pressing ctrl+c. Otherwise, '
- 'you are deemed to accept the terms and conditions.\033[0m')
- for i in range(5):
- print(f'{5-i}...')
- time.sleep(1)
-
-
-def force_lmdb(cfg):
- """Force the dataset to be dumped in lmdb format.
-
- Args:
- cfg (Config): Config object.
-
- Returns:
- Config: Config object.
- """
- for split in ['train', 'val', 'test']:
- preparer_cfg = cfg.get(f'{split}_preparer')
- if preparer_cfg:
- if preparer_cfg.get('dumper') is None:
- raise ValueError(
- f'{split} split does not come with a dumper, '
- 'so most likely the annotations are MMOCR-ready and do '
- 'not need any adaptation, and it '
- 'cannot be dumped in LMDB format.')
- preparer_cfg.dumper['type'] = 'TextRecogLMDBDumper'
-
- cfg.config_generator['dataset_name'] = f'{cfg.dataset_name}_lmdb'
-
- for split in ['train_anns', 'val_anns', 'test_anns']:
- if split in cfg.config_generator:
- # It can be None when users want to clear out the default
- # value
- if not cfg.config_generator[split]:
- continue
- ann_list = cfg.config_generator[split]
- for ann_dict in ann_list:
- ann_dict['ann_file'] = (
- osp.splitext(ann_dict['ann_file'])[0] + '.lmdb')
- else:
- if split == 'train_anns':
- ann_list = [dict(ann_file='textrecog_train.lmdb')]
- elif split == 'test_anns':
- ann_list = [dict(ann_file='textrecog_test.lmdb')]
- else:
- ann_list = []
- cfg.config_generator[split] = ann_list
-
- return cfg
-
-
-def main():
- args = parse_args()
- if args.lmdb and args.task != 'textrecog':
- raise ValueError('--lmdb only works with --task=textrecog')
- for dataset in args.datasets:
- if not osp.isdir(osp.join(args.dataset_zoo_path, dataset)):
- warnings.warn(f'{dataset} is not supported yet. Please check '
- 'dataset zoo for supported datasets.')
- continue
- meta_path = osp.join(args.dataset_zoo_path, dataset, 'metafile.yml')
- parse_meta(args.task, meta_path)
- cfg_path = osp.join(args.dataset_zoo_path, dataset, args.task + '.py')
- cfg = Config.fromfile(cfg_path)
- if args.overwrite_cfg and cfg.get('config_generator',
- None) is not None:
- cfg.config_generator.overwrite_cfg = args.overwrite_cfg
- cfg.nproc = args.nproc
- cfg.task = args.task
- cfg.dataset_name = dataset
- if args.lmdb:
- cfg = force_lmdb(cfg)
- preparer = DatasetPreparer.from_file(cfg)
- preparer.run(args.splits)
-
-
-if __name__ == '__main__':
- main()
diff --git a/pipeline/mmocr/tools/dataset_converters/textdet/art_converter.py b/pipeline/mmocr/tools/dataset_converters/textdet/art_converter.py
deleted file mode 100644
index 9d3b6a25132752887cd3beaf82d515c53d4cc083..0000000000000000000000000000000000000000
--- a/pipeline/mmocr/tools/dataset_converters/textdet/art_converter.py
+++ /dev/null
@@ -1,145 +0,0 @@
-# Copyright (c) OpenMMLab. All rights reserved.
-import argparse
-import math
-import os.path as osp
-
-import mmcv
-import mmengine
-
-from mmocr.utils import convert_annotations
-
-
-def parse_args():
- parser = argparse.ArgumentParser(
- description='Generate training and validation set of ArT ')
- parser.add_argument('root_path', help='Root dir path of ArT')
- parser.add_argument(
- '--val-ratio', help='Split ratio for val set', default=0.0, type=float)
- args = parser.parse_args()
- return args
-
-
-def collect_art_info(root_path, split, ratio, print_every=1000):
- """Collect the annotation information.
-
- The annotation format is as the following:
- {
- 'gt_1726': # 'gt_1726' is file name
- [
- {
- 'transcription': '็็ณ้ๅข',
- 'points': [
- [141, 199],
- [237, 201],
- [313, 236],
- [357, 283],
- [359, 300],
- [309, 261],
- [233, 230],
- [140, 231]
- ],
- 'language': 'Chinese',
- 'illegibility': False
- },
- ...
- ],
- ...
- }
-
-
- Args:
- root_path (str): Root path to the dataset
- split (str): Dataset split, which should be 'train' or 'val'
- ratio (float): Split ratio for val set
- print_every (int): Print log info per iteration
-
- Returns:
- img_info (dict): The dict of the img and annotation information
- """
-
- annotation_path = osp.join(root_path, 'annotations/train_labels.json')
- if not osp.exists(annotation_path):
- raise Exception(
- f'{annotation_path} not exists, please check and try again.')
-
- annotation = mmengine.load(annotation_path)
- img_prefixes = annotation.keys()
-
- trn_files, val_files = [], []
- if ratio > 0:
- for i, file in enumerate(img_prefixes):
- if i % math.floor(1 / ratio):
- trn_files.append(file)
- else:
- val_files.append(file)
- else:
- trn_files, val_files = img_prefixes, []
- print(f'training #{len(trn_files)}, val #{len(val_files)}')
-
- if split == 'train':
- img_prefixes = trn_files
- elif split == 'val':
- img_prefixes = val_files
- else:
- raise NotImplementedError
-
- img_infos = []
- for i, prefix in enumerate(img_prefixes):
- if i > 0 and i % print_every == 0:
- print(f'{i}/{len(img_prefixes)}')
- img_file = osp.join(root_path, 'imgs', prefix + '.jpg')
- # Skip not exist images
- if not osp.exists(img_file):
- continue
- img = mmcv.imread(img_file)
-
- img_info = dict(
- file_name=osp.join(osp.basename(img_file)),
- height=img.shape[0],
- width=img.shape[1],
- segm_file=osp.join(osp.basename(annotation_path)))
-
- anno_info = []
- for ann in annotation[prefix]:
- segmentation = []
- for x, y in ann['points']:
- segmentation.append(max(0, x))
- segmentation.append(max(0, y))
- xs, ys = segmentation[::2], segmentation[1::2]
- x, y = min(xs), min(ys)
- w, h = max(xs) - x, max(ys) - y
- bbox = [x, y, w, h]
- if ann['transcription'] == '###' or ann['illegibility']:
- iscrowd = 1
- else:
- iscrowd = 0
- anno = dict(
- iscrowd=iscrowd,
- category_id=1,
- bbox=bbox,
- area=w * h,
- segmentation=[segmentation])
- anno_info.append(anno)
- img_info.update(anno_info=anno_info)
- img_infos.append(img_info)
-
- return img_infos
-
-
-def main():
- args = parse_args()
- root_path = args.root_path
- print('Processing training set...')
- training_infos = collect_art_info(root_path, 'train', args.val_ratio)
- convert_annotations(training_infos,
- osp.join(root_path, 'instances_training.json'))
- if args.val_ratio > 0:
- print('Processing validation set...')
- val_infos = collect_art_info(root_path, 'val', args.val_ratio)
- convert_annotations(val_infos, osp.join(root_path,
- 'instances_val.json'))
- print('Finish')
-
-
-if __name__ == '__main__':
- main()
diff --git a/pipeline/mmocr/tools/dataset_converters/textdet/bid_converter.py b/pipeline/mmocr/tools/dataset_converters/textdet/bid_converter.py
deleted file mode 100644
index a16a3439e5cf1802e24505d97b1e94a790010698..0000000000000000000000000000000000000000
--- a/pipeline/mmocr/tools/dataset_converters/textdet/bid_converter.py
+++ /dev/null
@@ -1,185 +0,0 @@
-# Copyright (c) OpenMMLab. All rights reserved.
-import argparse
-import os
-import os.path as osp
-
-import mmcv
-import mmengine
-
-from mmocr.utils import dump_ocr_data
-
-
-def collect_files(img_dir, gt_dir):
- """Collect all images and their corresponding groundtruth files.
-
- Args:
- img_dir (str): The image directory
- gt_dir (str): The groundtruth directory
-
- Returns:
- files (list): The list of tuples (img_file, groundtruth_file)
- """
- assert isinstance(img_dir, str)
- assert img_dir
- assert isinstance(gt_dir, str)
- assert gt_dir
-
- ann_list, imgs_list = [], []
- for img_file in os.listdir(img_dir):
- ann_file = img_file.split('_')[0] + '_gt_ocr.txt'
- ann_list.append(osp.join(gt_dir, ann_file))
- imgs_list.append(osp.join(img_dir, img_file))
-
- files = list(zip(imgs_list, ann_list))
- assert len(files), f'No images found in {img_dir}'
- print(f'Loaded {len(files)} images from {img_dir}')
-
- return files
-
-
-def collect_annotations(files, nproc=1):
- """Collect the annotation information.
-
- Args:
- files (list): The list of tuples (image_file, groundtruth_file)
- nproc (int): The number of process to collect annotations
-
- Returns:
- images (list): The list of image information dicts
- """
- assert isinstance(files, list)
- assert isinstance(nproc, int)
-
- if nproc > 1:
- images = mmengine.track_parallel_progress(
- load_img_info, files, nproc=nproc)
- else:
- images = mmengine.track_progress(load_img_info, files)
-
- return images
-
-
-def load_img_info(files):
- """Load the information of one image.
-
- Args:
- files (tuple): The tuple of (img_file, groundtruth_file)
-
- Returns:
- img_info (dict): The dict of the img and annotation information
- """
- assert isinstance(files, tuple)
-
- img_file, gt_file = files
- assert osp.basename(gt_file).split('_')[0] == osp.basename(gt_file).split(
- '_')[0]
- # read imgs while ignoring orientations
- img = mmcv.imread(img_file, 'unchanged')
-
- img_info = dict(
- file_name=osp.basename(img_file),
- height=img.shape[0],
- width=img.shape[1],
- segm_file=osp.basename(gt_file))
-
- if osp.splitext(gt_file)[1] == '.txt':
- img_info = load_txt_info(gt_file, img_info)
- else:
- raise NotImplementedError
-
- return img_info
-
-
-def load_txt_info(gt_file, img_info):
- """Collect the annotation information.
-
- The annotation format is as the following:
- x, y, w, h, text
- 977, 152, 16, 49, NOME
- 962, 143, 12, 323, APPINHANESI BLAZEK PASSOTTO
- 906, 446, 12, 94, 206940361
- 905, 641, 12, 44, SPTC
-
- Args:
- gt_file (str): The path to ground-truth
- img_info (dict): The dict of the img and annotation information
-
- Returns:
- img_info (dict): The dict of the img and annotation information
- """
- with open(gt_file, encoding='latin1') as f:
- anno_info = []
- for line in f:
- line = line.strip('\n')
- if line[0] == '[' or line[0] == 'x':
- continue
- ann = line.split(',')
- bbox = ann[0:4]
- bbox = [int(coord) for coord in bbox]
- x, y, w, h = bbox
- segmentation = [x, y, x + w, y, x + w, y + h, x, y + h]
- anno = dict(
- iscrowd=0,
- category_id=1,
- bbox=bbox,
- area=w * h,
- segmentation=[segmentation])
- anno_info.append(anno)
-
- img_info.update(anno_info=anno_info)
-
- return img_info
-
-
-def split_train_val_list(full_list, val_ratio):
- """Split list by val_ratio.
-
- Args:
- full_list (list): list to be split
- val_ratio (float): split ratio for val set
-
- return:
- list(list, list): train_list and val_list
- """
- n_total = len(full_list)
- offset = int(n_total * val_ratio)
- if n_total == 0 or offset < 1:
- return [], full_list
- val_list = full_list[:offset]
- train_list = full_list[offset:]
- return [train_list, val_list]
-
-
-def parse_args():
- parser = argparse.ArgumentParser(
- description='Generate training and val set of BID ')
- parser.add_argument('root_path', help='Root dir path of BID')
- parser.add_argument(
- '--nproc', default=1, type=int, help='Number of processes')
- parser.add_argument(
- '--val-ratio', help='Split ratio for val set', default=0., type=float)
- args = parser.parse_args()
- return args
-
-
-def main():
- args = parse_args()
- root_path = args.root_path
- with mmengine.Timer(print_tmpl='It takes {}s to convert BID annotation'):
- files = collect_files(
- osp.join(root_path, 'imgs'), osp.join(root_path, 'annotations'))
- image_infos = collect_annotations(files, nproc=args.nproc)
- if args.val_ratio:
- image_infos = split_train_val_list(image_infos, args.val_ratio)
- splits = ['training', 'val']
- else:
- image_infos = [image_infos]
- splits = ['training']
- for i, split in enumerate(splits):
- dump_ocr_data(image_infos[i],
- osp.join(root_path, 'instances_' + split + '.json'),
- 'textdet')
-
-
-if __name__ == '__main__':
- main()
diff --git a/pipeline/mmocr/tools/dataset_converters/textdet/coco_to_line_dict.py b/pipeline/mmocr/tools/dataset_converters/textdet/coco_to_line_dict.py
deleted file mode 100644
index 7dcb5edb453edbc7904478de6d636b241a29336e..0000000000000000000000000000000000000000
--- a/pipeline/mmocr/tools/dataset_converters/textdet/coco_to_line_dict.py
+++ /dev/null
@@ -1,67 +0,0 @@
-# Copyright (c) OpenMMLab. All rights reserved.
-import argparse
-import json
-
-import mmengine
-
-from mmocr.utils import list_to_file
-
-
-def parse_coco_json(in_path):
- json_obj = mmengine.load(in_path)
- image_infos = json_obj['images']
- annotations = json_obj['annotations']
- imgid2imgname = {}
- img_ids = []
- for image_info in image_infos:
- imgid2imgname[image_info['id']] = image_info
- img_ids.append(image_info['id'])
- imgid2anno = {}
- for img_id in img_ids:
- imgid2anno[img_id] = []
- for anno in annotations:
- img_id = anno['image_id']
- new_anno = {}
- new_anno['iscrowd'] = anno['iscrowd']
- new_anno['category_id'] = anno['category_id']
- new_anno['bbox'] = anno['bbox']
- new_anno['segmentation'] = anno['segmentation']
- if img_id in imgid2anno.keys():
- imgid2anno[img_id].append(new_anno)
-
- return imgid2imgname, imgid2anno
-
-
-def gen_line_dict_file(out_path, imgid2imgname, imgid2anno):
- lines = []
- for key, value in imgid2imgname.items():
- if key in imgid2anno:
- anno = imgid2anno[key]
- line_dict = {}
- line_dict['file_name'] = value['file_name']
- line_dict['height'] = value['height']
- line_dict['width'] = value['width']
- line_dict['annotations'] = anno
- lines.append(json.dumps(line_dict))
- list_to_file(out_path, lines)
-
-
-def parse_args():
- parser = argparse.ArgumentParser()
- parser.add_argument('--in-path', help='input json path with coco format')
- parser.add_argument(
- '--out-path', help='output txt path with line-json format')
-
- args = parser.parse_args()
- return args
-
-
-def main():
- args = parse_args()
- imgid2imgname, imgid2anno = parse_coco_json(args.in_path)
- gen_line_dict_file(args.out_path, imgid2imgname, imgid2anno)
- print('finish')
-
-
-if __name__ == '__main__':
- main()
diff --git a/pipeline/mmocr/tools/dataset_converters/textdet/cocotext_converter.py b/pipeline/mmocr/tools/dataset_converters/textdet/cocotext_converter.py
deleted file mode 100644
index e4ef78ee39ffe945e1a7e5cf3eba87b19c0fd002..0000000000000000000000000000000000000000
--- a/pipeline/mmocr/tools/dataset_converters/textdet/cocotext_converter.py
+++ /dev/null
@@ -1,124 +0,0 @@
-# Copyright (c) OpenMMLab. All rights reserved.
-import argparse
-import math
-import os.path as osp
-
-import mmengine
-
-from mmocr.utils import dump_ocr_data
-
-
-def parse_args():
- parser = argparse.ArgumentParser(
- description='Generate training and validation set of COCO Text v2 ')
- parser.add_argument('root_path', help='Root dir path of COCO Text v2')
- args = parser.parse_args()
- return args
-
-
-def collect_cocotext_info(root_path, split, print_every=1000):
- """Collect the annotation information.
-
- The annotation format is as the following:
- {
- 'anns':{
- '45346':{
- 'mask': [468.9,286.7,468.9,295.2,493.0,295.8,493.0,287.2],
- 'class': 'machine printed',
- 'bbox': [468.9, 286.7, 24.1, 9.1], # x, y, w, h
- 'image_id': 217925,
- 'id': 45346,
- 'language': 'english', # 'english' or 'not english'
- 'area': 206.06,
- 'utf8_string': 'New',
- 'legibility': 'legible', # 'legible' or 'illegible'
- },
- ...
- }
- 'imgs':{
- '540965':{
- 'id': 540965,
- 'set': 'train', # 'train' or 'val'
- 'width': 640,
- 'height': 360,
- 'file_name': 'COCO_train2014_000000540965.jpg'
- },
- ...
- }
- 'imgToAnns':{
- '540965': [],
- '260932': [63993, 63994, 63995, 63996, 63997, 63998, 63999],
- ...
- }
- }
-
- Args:
- root_path (str): Root path to the dataset
- split (str): Dataset split, which should be 'train' or 'val'
- print_every (int): Print log information per iter
-
- Returns:
- img_info (dict): The dict of the img and annotation information
- """
-
- annotation_path = osp.join(root_path, 'annotations/cocotext.v2.json')
- if not osp.exists(annotation_path):
- raise Exception(
- f'{annotation_path} not exists, please check and try again.')
-
- annotation = mmengine.load(annotation_path)
-
- img_infos = []
- for i, img_info in enumerate(annotation['imgs'].values()):
- if i > 0 and i % print_every == 0:
- print(f'{i}/{len(annotation["imgs"].values())}')
-
- if img_info['set'] == split:
- img_info['segm_file'] = annotation_path
- ann_ids = annotation['imgToAnns'][str(img_info['id'])]
- # Filter out images without text
- if len(ann_ids) == 0:
- continue
- anno_info = []
- for ann_id in ann_ids:
- ann = annotation['anns'][str(ann_id)]
-
- # Ignore illegible or non-English words
- iscrowd = 1 if ann['language'] == 'not english' or ann[
- 'legibility'] == 'illegible' else 0
-
- x, y, w, h = ann['bbox']
- x, y = max(0, math.floor(x)), max(0, math.floor(y))
- w, h = math.ceil(w), math.ceil(h)
- bbox = [x, y, w, h]
- segmentation = [max(0, int(x)) for x in ann['mask']]
- if len(segmentation) < 8 or len(segmentation) % 2 != 0:
- segmentation = [x, y, x + w, y, x + w, y + h, x, y + h]
- anno = dict(
- iscrowd=iscrowd,
- category_id=1,
- bbox=bbox,
- area=ann['area'],
- segmentation=[segmentation])
- anno_info.append(anno)
- img_info.update(anno_info=anno_info)
- img_infos.append(img_info)
- return img_infos
-
-
-def main():
- args = parse_args()
- root_path = args.root_path
- print('Processing training set...')
- training_infos = collect_cocotext_info(root_path, 'train')
- dump_ocr_data(training_infos,
- osp.join(root_path, 'instances_training.json'), 'textdet')
- print('Processing validation set...')
- val_infos = collect_cocotext_info(root_path, 'val')
- dump_ocr_data(val_infos, osp.join(root_path, 'instances_val.json'),
- 'textdet')
- print('Finish')
-
-
-if __name__ == '__main__':
- main()
diff --git a/pipeline/mmocr/tools/dataset_converters/textdet/data_migrator.py b/pipeline/mmocr/tools/dataset_converters/textdet/data_migrator.py
deleted file mode 100644
index 38da8a04861aa5d4f80dbeb65a6be5fdcd55acaf..0000000000000000000000000000000000000000
--- a/pipeline/mmocr/tools/dataset_converters/textdet/data_migrator.py
+++ /dev/null
@@ -1,70 +0,0 @@
-# Copyright (c) OpenMMLab. All rights reserved.
-import argparse
-from collections import defaultdict
-from copy import deepcopy
-from typing import Dict, List
-
-import mmengine
-
-from mmocr.utils import dump_ocr_data
-
-
-def parse_coco_json(in_path: str) -> List[Dict]:
- """Load coco annotations into image_infos parsable by dump_ocr_data().
-
- Args:
- in_path (str): COCO text annotation path.
-
- Returns:
- list[dict]: List of image information dicts. To be used by
- dump_ocr_data().
- """
- json_obj = mmengine.load(in_path)
- image_infos = json_obj['images']
- annotations = json_obj['annotations']
- imgid2annos = defaultdict(list)
- for anno in annotations:
- new_anno = deepcopy(anno)
- new_anno['category_id'] = 0 # Must be 0 for OCR tasks which stands
- # for "text" category
- imgid2annos[anno['image_id']].append(new_anno)
-
- results = []
- for image_info in image_infos:
- image_info['anno_info'] = imgid2annos[image_info['id']]
- results.append(image_info)
-
- return results
-
-
-def parse_args():
- parser = argparse.ArgumentParser()
- parser.add_argument('in_path', help='Input json path in coco format.')
- parser.add_argument(
- 'out_path', help='Output json path in openmmlab format.')
- parser.add_argument(
- '--task',
- type=str,
- default='auto',
- choices=['auto', 'textdet', 'textspotter'],
- help='Output annotation type, defaults to "auto", which decides the'
- 'best task type based on whether "text" is annotated. Other options'
- 'are "textdet" and "textspotter".')
- args = parser.parse_args()
- return args
-
-
-def main():
- args = parse_args()
- image_infos = parse_coco_json(args.in_path)
- task_name = args.task
- if task_name == 'auto':
- task_name = 'textdet'
- if 'text' in image_infos[0]['anno_info'][0]:
- task_name = 'textspotter'
- dump_ocr_data(image_infos, args.out_path, task_name)
- print('finish')
-
-
-if __name__ == '__main__':
- main()
diff --git a/pipeline/mmocr/tools/dataset_converters/textdet/detext_converter.py b/pipeline/mmocr/tools/dataset_converters/textdet/detext_converter.py
deleted file mode 100644
index d99378e44559222d0d32f1cccd4ccf673a33b6df..0000000000000000000000000000000000000000
--- a/pipeline/mmocr/tools/dataset_converters/textdet/detext_converter.py
+++ /dev/null
@@ -1,162 +0,0 @@
-# Copyright (c) OpenMMLab. All rights reserved.
-import argparse
-import os
-import os.path as osp
-
-import mmcv
-import mmengine
-import numpy as np
-
-from mmocr.utils import dump_ocr_data
-
-
-def collect_files(img_dir, gt_dir):
- """Collect all images and their corresponding groundtruth files.
-
- Args:
- img_dir (str): The image directory
- gt_dir (str): The groundtruth directory
-
- Returns:
- files (list): The list of tuples (img_file, groundtruth_file)
- """
- assert isinstance(img_dir, str)
- assert img_dir
- assert isinstance(gt_dir, str)
- assert gt_dir
-
- ann_list, imgs_list = [], []
- for img in os.listdir(img_dir):
- imgs_list.append(osp.join(img_dir, img))
- ann_list.append(osp.join(gt_dir, 'gt_' + img.replace('jpg', 'txt')))
-
- files = list(zip(imgs_list, ann_list))
- assert len(files), f'No images found in {img_dir}'
- print(f'Loaded {len(files)} images from {img_dir}')
-
- return files
-
-
-def collect_annotations(files, nproc=1):
- """Collect the annotation information.
-
- Args:
- files (list): The list of tuples (image_file, groundtruth_file)
- nproc (int): The number of process to collect annotations
-
- Returns:
- images (list): The list of image information dicts
- """
- assert isinstance(files, list)
- assert isinstance(nproc, int)
-
- if nproc > 1:
- images = mmengine.track_parallel_progress(
- load_img_info, files, nproc=nproc)
- else:
- images = mmengine.track_progress(load_img_info, files)
-
- return images
-
-
-def load_img_info(files):
- """Load the information of one image.
-
- Args:
- files (tuple): The tuple of (img_file, groundtruth_file)
-
- Returns:
- img_info (dict): The dict of the img and annotation information
- """
- assert isinstance(files, tuple)
-
- img_file, gt_file = files
- # read imgs while ignoring orientations
- img = mmcv.imread(img_file, 'unchanged')
-
- img_info = dict(
- file_name=osp.join(osp.basename(img_file)),
- height=img.shape[0],
- width=img.shape[1],
- segm_file=osp.join(osp.basename(gt_file)))
-
- if osp.splitext(gt_file)[1] == '.txt':
- img_info = load_txt_info(gt_file, img_info)
- else:
- raise NotImplementedError
-
- return img_info
-
-
-def load_txt_info(gt_file, img_info):
- """Collect the annotation information.
-
- # Annotation Format
- # x1, y1, x2, y2, x3, y3, x4, y4, transcript
-
- Args:
- gt_file (str): The path to ground-truth
- img_info (dict): The dict of the img and annotation information
-
- Returns:
- img_info (dict): The dict of the img and annotation information
- """
-
- with open(gt_file) as f:
- anno_info = []
- annotations = f.readlines()
- for ann in annotations:
- try:
- ann_box = np.array(ann.split(',')[0:8]).astype(int).tolist()
- except ValueError:
- # skip invalid annotation line
- continue
- x = max(0, min(ann_box[0::2]))
- y = max(0, min(ann_box[1::2]))
- w, h = max(ann_box[0::2]) - x, max(ann_box[1::2]) - y
- bbox = [x, y, w, h]
- segmentation = ann_box
- word = ann.split(',')[-1].replace('\n', '').strip()
-
- anno = dict(
- iscrowd=0 if word != '###' else 1,
- category_id=1,
- bbox=bbox,
- area=w * h,
- segmentation=[segmentation])
- anno_info.append(anno)
-
- img_info.update(anno_info=anno_info)
-
- return img_info
-
-
-def parse_args():
- parser = argparse.ArgumentParser(
- description='Generate training and val set of DeText ')
- parser.add_argument('root_path', help='Root dir path of DeText')
- parser.add_argument(
- '--nproc', default=1, type=int, help='Number of process')
- args = parser.parse_args()
- return args
-
-
-def main():
- args = parse_args()
- root_path = args.root_path
-
- for split in ['training', 'val']:
- print(f'Processing {split} set...')
- with mmengine.Timer(
- print_tmpl='It takes {}s to convert DeText annotation'):
- files = collect_files(
- osp.join(root_path, 'imgs', split),
- osp.join(root_path, 'annotations', split))
- image_infos = collect_annotations(files, nproc=args.nproc)
- dump_ocr_data(image_infos,
- osp.join(root_path, 'instances_' + split + '.json'),
- 'textdet')
-
-
-if __name__ == '__main__':
- main()
diff --git a/pipeline/mmocr/tools/dataset_converters/textdet/funsd_converter.py b/pipeline/mmocr/tools/dataset_converters/textdet/funsd_converter.py
deleted file mode 100644
index 7be887d2637b99f0113f99f06a05f7591c061f39..0000000000000000000000000000000000000000
--- a/pipeline/mmocr/tools/dataset_converters/textdet/funsd_converter.py
+++ /dev/null
@@ -1,159 +0,0 @@
-# Copyright (c) OpenMMLab. All rights reserved.
-import argparse
-import math
-import os
-import os.path as osp
-
-import mmcv
-import mmengine
-
-from mmocr.utils import dump_ocr_data
-
-
-def collect_files(img_dir, gt_dir):
- """Collect all images and their corresponding groundtruth files.
-
- Args:
- img_dir (str): The image directory
- gt_dir (str): The groundtruth directory
-
- Returns:
- files (list): The list of tuples (img_file, groundtruth_file)
- """
- assert isinstance(img_dir, str)
- assert img_dir
- assert isinstance(gt_dir, str)
- assert gt_dir
-
- ann_list, imgs_list = [], []
- for gt_file in os.listdir(gt_dir):
- ann_list.append(osp.join(gt_dir, gt_file))
- imgs_list.append(osp.join(img_dir, gt_file.replace('.json', '.png')))
-
- files = list(zip(sorted(imgs_list), sorted(ann_list)))
- assert len(files), f'No images found in {img_dir}'
- print(f'Loaded {len(files)} images from {img_dir}')
-
- return files
-
-
-def collect_annotations(files, nproc=1):
- """Collect the annotation information.
-
- Args:
- files (list): The list of tuples (image_file, groundtruth_file)
- nproc (int): The number of process to collect annotations
-
- Returns:
- images (list): The list of image information dicts
- """
- assert isinstance(files, list)
- assert isinstance(nproc, int)
-
- if nproc > 1:
- images = mmengine.track_parallel_progress(
- load_img_info, files, nproc=nproc)
- else:
- images = mmengine.track_progress(load_img_info, files)
-
- return images
-
-
-def load_img_info(files):
- """Load the information of one image.
-
- Args:
- files (tuple): The tuple of (img_file, groundtruth_file)
-
- Returns:
- img_info (dict): The dict of the img and annotation information
- """
- assert isinstance(files, tuple)
-
- img_file, gt_file = files
- assert osp.basename(gt_file).split('.')[0] == osp.basename(img_file).split(
- '.')[0]
- # read imgs while ignoring orientations
- img = mmcv.imread(img_file, 'unchanged')
-
- img_info = dict(
- file_name=osp.join(osp.basename(img_file)),
- height=img.shape[0],
- width=img.shape[1],
- segm_file=osp.join(osp.basename(gt_file)))
-
- if osp.splitext(gt_file)[1] == '.json':
- img_info = load_json_info(gt_file, img_info)
- else:
- raise NotImplementedError
-
- return img_info
-
-
-def load_json_info(gt_file, img_info):
- """Collect the annotation information.
-
- Args:
- gt_file (str): The path to ground-truth
- img_info (dict): The dict of the img and annotation information
-
- Returns:
- img_info (dict): The dict of the img and annotation information
- """
-
- annotation = mmengine.load(gt_file)
- anno_info = []
- for form in annotation['form']:
- for ann in form['words']:
-
- iscrowd = 1 if len(ann['text']) == 0 else 0
-
- x1, y1, x2, y2 = ann['box']
- x = max(0, min(math.floor(x1), math.floor(x2)))
- y = max(0, min(math.floor(y1), math.floor(y2)))
- w, h = math.ceil(abs(x2 - x1)), math.ceil(abs(y2 - y1))
- bbox = [x, y, w, h]
- segmentation = [x, y, x + w, y, x + w, y + h, x, y + h]
-
- anno = dict(
- iscrowd=iscrowd,
- category_id=1,
- bbox=bbox,
- area=w * h,
- segmentation=[segmentation])
- anno_info.append(anno)
-
- img_info.update(anno_info=anno_info)
-
- return img_info
-
-
-def parse_args():
- parser = argparse.ArgumentParser(
- description='Generate training and test set of FUNSD ')
- parser.add_argument('root_path', help='Root dir path of FUNSD')
- parser.add_argument(
- '--nproc', default=1, type=int, help='Number of process')
- args = parser.parse_args()
- return args
-
-
-def main():
- args = parse_args()
- root_path = args.root_path
-
- for split in ['training', 'test']:
- print(f'Processing {split} set...')
- with mmengine.Timer(
- print_tmpl='It takes {}s to convert FUNSD annotation'):
- files = collect_files(
- osp.join(root_path, 'imgs'),
- osp.join(root_path, 'annotations', split))
- image_infos = collect_annotations(files, nproc=args.nproc)
- dump_ocr_data(image_infos,
- osp.join(root_path, 'instances_' + split + '.json'),
- 'textdet')
-
-
-if __name__ == '__main__':
- main()
diff --git a/pipeline/mmocr/tools/dataset_converters/textdet/hiertext_converter.py b/pipeline/mmocr/tools/dataset_converters/textdet/hiertext_converter.py
deleted file mode 100644
index 9ca0163099c815382fe3362da1b0525d109bc23f..0000000000000000000000000000000000000000
--- a/pipeline/mmocr/tools/dataset_converters/textdet/hiertext_converter.py
+++ /dev/null
@@ -1,152 +0,0 @@
-# Copyright (c) OpenMMLab. All rights reserved.
-import argparse
-import json
-import os.path as osp
-
-import numpy as np
-from shapely.geometry import Polygon
-
-from mmocr.utils import dump_ocr_data
-
-
-def collect_level_info(annotation):
- """Collect information from any level in HierText.
-
- Args:
- annotation (dict): dict at each level
-
- Return:
- anno (dict): dict containing annotations
- """
- iscrowd = 0 if annotation['legible'] else 1
- vertices = np.array(annotation['vertices'])
- polygon = Polygon(vertices)
- area = polygon.area
- min_x, min_y, max_x, max_y = polygon.bounds
- bbox = [min_x, min_y, max_x - min_x, max_y - min_y]
- segmentation = [i for j in vertices for i in j]
- anno = dict(
- iscrowd=iscrowd,
- category_id=1,
- bbox=bbox,
- area=area,
- segmentation=[segmentation])
- return anno
-
-
-def collect_hiertext_info(root_path, level, split, print_every=1000):
- """Collect the annotation information.
-
- The annotation format is as the following:
- {
- "info": {
- "date": "release date",
- "version": "current version"
- },
- "annotations": [ // List of dictionaries, one for each image.
- {
- "image_id": "the filename of corresponding image.",
- "image_width": image_width, // (int) The image width.
- "image_height": image_height, // (int) The image height.
- "paragraphs": [ // List of paragraphs.
- {
- "vertices": [[x1, y1], [x2, y2],...,[xn, yn]]
- "legible": true
- "lines": [
- {
- "vertices": [[x1, y1], [x2, y2],...,[x4, y4]]
- "text": L
- "legible": true,
- "handwritten": false
- "vertical": false,
- "words": [
- {
- "vertices": [[x1, y1], [x2, y2],...,[xm, ym]]
- "text": "the text content of this word",
- "legible": true
- "handwritten": false,
- "vertical": false,
- }, ...
- ]
- }, ...
- ]
- }, ...
- ]
- }, ...
- ]
- }
-
- Args:
- root_path (str): Root path to the dataset
- level (str): Level of annotations, which should be 'word', 'line',
- or 'paragraphs'
- split (str): Dataset split, which should be 'train' or 'validation'
- print_every (int): Print log information per iter
-
- Returns:
- img_info (dict): The dict of the img and annotation information
- """
-
- annotation_path = osp.join(root_path, 'annotations/' + split + '.jsonl')
- if not osp.exists(annotation_path):
- raise Exception(
- f'{annotation_path} not exists, please check and try again.')
-
- annotation = json.load(open(annotation_path))['annotations']
- img_infos = []
- for i, img_annos in enumerate(annotation):
- if i > 0 and i % print_every == 0:
- print(f'{i}/{len(annotation)}')
- img_info = {}
- img_info['file_name'] = img_annos['image_id'] + '.jpg'
- img_info['height'] = img_annos['image_height']
- img_info['width'] = img_annos['image_width']
- img_info['segm_file'] = annotation_path
- anno_info = []
- for paragraph in img_annos['paragraphs']:
- if level == 'paragraph':
- anno = collect_level_info(paragraph)
- anno_info.append(anno)
- elif level == 'line':
- for line in paragraph['lines']:
- anno = collect_level_info(line)
- anno_info.append(anno)
- elif level == 'word':
- for line in paragraph['lines']:
- for word in line['words']:
- anno = collect_level_info(line)
- anno_info.append(anno)
- img_info.update(anno_info=anno_info)
- img_infos.append(img_info)
- return img_infos
-
-
-def parse_args():
- parser = argparse.ArgumentParser(
- description='Generate training and validation set of HierText ')
- parser.add_argument('root_path', help='Root dir path of HierText')
- parser.add_argument(
- '--level',
- default='word',
- help='HierText provides three levels of annotation',
- choices=['word', 'line', 'paragraph'])
- args = parser.parse_args()
- return args
-
-
-def main():
- args = parse_args()
- root_path = args.root_path
- print('Processing training set...')
- training_infos = collect_hiertext_info(root_path, args.level, 'train')
- dump_ocr_data(training_infos,
- osp.join(root_path, 'instances_training.json'), 'textdet')
- print('Processing validation set...')
- val_infos = collect_hiertext_info(root_path, args.level, 'val')
- dump_ocr_data(val_infos, osp.join(root_path, 'instances_val.json'),
- 'textdet')
- print('Finish')
-
-
-if __name__ == '__main__':
- main()
diff --git a/pipeline/mmocr/tools/dataset_converters/textdet/ic11_converter.py b/pipeline/mmocr/tools/dataset_converters/textdet/ic11_converter.py
deleted file mode 100644
index 1a5683f4ae17e52fa8f13fc542a8424ae6cb488f..0000000000000000000000000000000000000000
--- a/pipeline/mmocr/tools/dataset_converters/textdet/ic11_converter.py
+++ /dev/null
@@ -1,173 +0,0 @@
-# Copyright (c) OpenMMLab. All rights reserved.
-import argparse
-import os
-import os.path as osp
-
-import mmcv
-import mmengine
-from PIL import Image
-
-from mmocr.utils import dump_ocr_data
-
-
-def convert_gif(img_path):
- """Convert the gif image to png format.
-
- Args:
- img_path (str): The path to the gif image
- """
- img = Image.open(img_path)
- dst_path = img_path.replace('gif', 'png')
- img.save(dst_path)
- os.remove(img_path)
- print(f'Convert {img_path} to {dst_path}')
-
-
-def collect_files(img_dir, gt_dir):
- """Collect all images and their corresponding groundtruth files.
-
- Args:
- img_dir (str): The image directory
- gt_dir (str): The groundtruth directory
-
- Returns:
- files (list): The list of tuples (img_file, groundtruth_file)
- """
- assert isinstance(img_dir, str)
- assert img_dir
- assert isinstance(gt_dir, str)
- assert gt_dir
-
- ann_list, imgs_list = [], []
- for img in os.listdir(img_dir):
- img_path = osp.join(img_dir, img)
- # mmcv cannot read gif images, so convert them to png
- if img.endswith('gif'):
- convert_gif(img_path)
- img_path = img_path.replace('gif', 'png')
- imgs_list.append(img_path)
- ann_list.append(osp.join(gt_dir, 'gt_' + img.split('.')[0] + '.txt'))
-
- files = list(zip(sorted(imgs_list), sorted(ann_list)))
- assert len(files), f'No images found in {img_dir}'
- print(f'Loaded {len(files)} images from {img_dir}')
-
- return files
-
-
-def collect_annotations(files, nproc=1):
- """Collect the annotation information.
-
- Args:
- files (list): The list of tuples (image_file, groundtruth_file)
- nproc (int): The number of process to collect annotations
-
- Returns:
- images (list): The list of image information dicts
- """
- assert isinstance(files, list)
- assert isinstance(nproc, int)
-
- if nproc > 1:
- images = mmengine.track_parallel_progress(
- load_img_info, files, nproc=nproc)
- else:
- images = mmengine.track_progress(load_img_info, files)
-
- return images
-
-
-def load_img_info(files):
- """Load the information of one image.
-
- Args:
- files (tuple): The tuple of (img_file, groundtruth_file)
-
- Returns:
- img_info (dict): The dict of the img and annotation information
- """
- assert isinstance(files, tuple)
-
- img_file, gt_file = files
- # read imgs while ignoring orientations
- img = mmcv.imread(img_file, 'unchanged')
-
- img_info = dict(
- file_name=osp.join(osp.basename(img_file)),
- height=img.shape[0],
- width=img.shape[1],
- segm_file=osp.join(osp.basename(gt_file)))
-
- if osp.splitext(gt_file)[1] == '.txt':
- img_info = load_txt_info(gt_file, img_info)
- else:
- raise NotImplementedError
-
- return img_info
-
-
-def load_txt_info(gt_file, img_info):
- """Collect the annotation information.
-
- The annotation format is as the following:
- left, top, right, bottom, "transcription"
-
- Args:
- gt_file (str): The path to ground-truth
- img_info (dict): The dict of the img and annotation information
-
- Returns:
- img_info (dict): The dict of the img and annotation information
- """
- anno_info = []
- with open(gt_file) as f:
- lines = f.readlines()
- for line in lines:
- xmin, ymin, xmax, ymax = line.split(',')[0:4]
- x = max(0, int(xmin))
- y = max(0, int(ymin))
- w = int(xmax) - x
- h = int(ymax) - y
- bbox = [x, y, w, h]
- segmentation = [x, y, x + w, y, x + w, y + h, x, y + h]
-
- anno = dict(
- iscrowd=0,
- category_id=1,
- bbox=bbox,
- area=w * h,
- segmentation=[segmentation])
- anno_info.append(anno)
- img_info.update(anno_info=anno_info)
-
- return img_info
-
-
-def parse_args():
- parser = argparse.ArgumentParser(
- description='Generate training and test set of IC11')
- parser.add_argument('root_path', help='Root dir path of IC11')
- parser.add_argument(
- '--nproc', default=1, type=int, help='Number of process')
- args = parser.parse_args()
- return args
-
-
-def main():
- args = parse_args()
- root_path = args.root_path
-
- for split in ['training', 'test']:
- print(f'Processing {split} set...')
- with mmengine.Timer(print_tmpl='It takes {}s to convert annotation'):
- files = collect_files(
- osp.join(root_path, 'imgs', split),
- osp.join(root_path, 'annotations', split))
- image_infos = collect_annotations(files, nproc=args.nproc)
- dump_ocr_data(image_infos,
- osp.join(root_path, 'instances_' + split + '.json'),
- 'textdet')
-
-
-if __name__ == '__main__':
- main()
diff --git a/pipeline/mmocr/tools/dataset_converters/textdet/ilst_converter.py b/pipeline/mmocr/tools/dataset_converters/textdet/ilst_converter.py
deleted file mode 100644
index 56ac54e3e30ed95159b25bee69afe39c47896a2a..0000000000000000000000000000000000000000
--- a/pipeline/mmocr/tools/dataset_converters/textdet/ilst_converter.py
+++ /dev/null
@@ -1,206 +0,0 @@
-# Copyright (c) OpenMMLab. All rights reserved.
-import argparse
-import os
-import os.path as osp
-import xml.etree.ElementTree as ET
-
-import mmcv
-import mmengine
-
-from mmocr.utils import dump_ocr_data
-
-
-def collect_files(img_dir, gt_dir):
- """Collect all images and their corresponding groundtruth files.
-
- Args:
- img_dir (str): The image directory
- gt_dir (str): The groundtruth directory
-
- Returns:
- files (list): The list of tuples (img_file, groundtruth_file)
- """
- assert isinstance(img_dir, str)
- assert img_dir
- assert isinstance(gt_dir, str)
- assert gt_dir
-
- ann_list, imgs_list = [], []
- for img_file in os.listdir(img_dir):
- ann_path = osp.join(gt_dir, img_file.split('.')[0] + '.xml')
- if os.path.exists(ann_path):
- ann_list.append(ann_path)
- imgs_list.append(osp.join(img_dir, img_file))
-
- files = list(zip(imgs_list, ann_list))
- assert len(files), f'No images found in {img_dir}'
- print(f'Loaded {len(files)} images from {img_dir}')
-
- return files
-
-
-def collect_annotations(files, nproc=1):
- """Collect the annotation information.
-
- Args:
- files (list): The list of tuples (image_file, groundtruth_file)
- nproc (int): The number of process to collect annotations
-
- Returns:
- images (list): The list of image information dicts
- """
- assert isinstance(files, list)
- assert isinstance(nproc, int)
-
- if nproc > 1:
- images = mmengine.track_parallel_progress(
- load_img_info, files, nproc=nproc)
- else:
- images = mmengine.track_progress(load_img_info, files)
-
- return images
-
-
-def load_img_info(files):
- """Load the information of one image.
-
- Args:
- files (tuple): The tuple of (img_file, groundtruth_file)
-
- Returns:
- img_info (dict): The dict of the img and annotation information
- """
- assert isinstance(files, tuple)
-
- img_file, gt_file = files
- assert osp.basename(gt_file).split('.')[0] == osp.basename(img_file).split(
- '.')[0]
- # read imgs while ignoring orientations
- img = mmcv.imread(img_file, 'unchanged')
-
- try:
- img_info = dict(
- file_name=osp.join(osp.basename(img_file)),
- height=img.shape[0],
- width=img.shape[1],
- segm_file=osp.join(osp.basename(gt_file)))
- except AttributeError:
- print(f'Skip broken img {img_file}')
- return None
-
- if osp.splitext(gt_file)[1] == '.xml':
- img_info = load_xml_info(gt_file, img_info)
- else:
- raise NotImplementedError
-
- return img_info
-
-
-def load_xml_info(gt_file, img_info):
- """Collect the annotation information.
-
- The annotation format is as the following:
-
- ...
-
- SMT
- Unspecified
- 0
- 0
-
- 157
- 294
- 237
- 357
-
-
-
- Args:
- gt_file (str): The path to ground-truth
- img_info (dict): The dict of the img and annotation information
-
- Returns:
- img_info (dict): The dict of the img and annotation information
- """
- obj = ET.parse(gt_file)
- root = obj.getroot()
- anno_info = []
- for object in root.iter('object'):
- word = object.find('name').text
- iscrowd = 1 if len(word) == 0 else 0
- x1 = int(object.find('bndbox').find('xmin').text)
- y1 = int(object.find('bndbox').find('ymin').text)
- x2 = int(object.find('bndbox').find('xmax').text)
- y2 = int(object.find('bndbox').find('ymax').text)
-
- x = max(0, min(x1, x2))
- y = max(0, min(y1, y2))
- w, h = abs(x2 - x1), abs(y2 - y1)
- bbox = [x1, y1, w, h]
- segmentation = [x, y, x + w, y, x + w, y + h, x, y + h]
- anno = dict(
- iscrowd=iscrowd,
- category_id=1,
- bbox=bbox,
- area=w * h,
- segmentation=[segmentation])
- anno_info.append(anno)
-
- img_info.update(anno_info=anno_info)
-
- return img_info
-
-
-def split_train_val_list(full_list, val_ratio):
- """Split list by val_ratio.
-
- Args:
- full_list (list): List to be split
- val_ratio (float): Split ratio for val set
-
- return:
- list(list, list): Train_list and val_list
- """
-
- n_total = len(full_list)
- offset = int(n_total * val_ratio)
- if n_total == 0 or offset < 1:
- return [], full_list
- val_list = full_list[:offset]
- train_list = full_list[offset:]
- return [train_list, val_list]
-
-
-def parse_args():
- parser = argparse.ArgumentParser(
- description='Generate training and val set of ILST ')
- parser.add_argument('root_path', help='Root dir path of ILST')
- parser.add_argument(
- '--val-ratio', help='Split ratio for val set', default=0., type=float)
- parser.add_argument(
- '--nproc', default=1, type=int, help='Number of processes')
- args = parser.parse_args()
- return args
-
-
-def main():
- args = parse_args()
- root_path = args.root_path
- with mmengine.Timer(print_tmpl='It takes {}s to convert ILST annotation'):
- files = collect_files(
- osp.join(root_path, 'imgs'), osp.join(root_path, 'annotations'))
- image_infos = collect_annotations(files, nproc=args.nproc)
- if args.val_ratio:
- image_infos = split_train_val_list(image_infos, args.val_ratio)
- splits = ['training', 'val']
- else:
- image_infos = [image_infos]
- splits = ['training']
- for i, split in enumerate(splits):
- dump_ocr_data(
- list(filter(None, image_infos[i])),
- osp.join(root_path, 'instances_' + split + '.json'), 'textdet')
-
-
-if __name__ == '__main__':
- main()
diff --git a/pipeline/mmocr/tools/dataset_converters/textdet/imgur_converter.py b/pipeline/mmocr/tools/dataset_converters/textdet/imgur_converter.py
deleted file mode 100644
index f6c19cd33cdf27bc085563992a126aa02028c43e..0000000000000000000000000000000000000000
--- a/pipeline/mmocr/tools/dataset_converters/textdet/imgur_converter.py
+++ /dev/null
@@ -1,152 +0,0 @@
-# Copyright (c) OpenMMLab. All rights reserved.
-import argparse
-import math
-import os.path as osp
-
-import mmcv
-import mmengine
-import numpy as np
-
-from mmocr.utils import dump_ocr_data
-
-
-def parse_args():
- parser = argparse.ArgumentParser(
- description='Generate training, validation and test set of IMGUR ')
- parser.add_argument('root_path', help='Root dir path of IMGUR')
- args = parser.parse_args()
-
- return args
-
-
-def collect_imgur_info(root_path, annotation_filename, print_every=1000):
-
- annotation_path = osp.join(root_path, 'annotations', annotation_filename)
- if not osp.exists(annotation_path):
- raise Exception(
- f'{annotation_path} not exists, please check and try again.')
-
- annotation = mmengine.load(annotation_path)
- images = annotation['index_to_ann_map'].keys()
- img_infos = []
- for i, img_name in enumerate(images):
- if i >= 0 and i % print_every == 0:
- print(f'{i}/{len(images)}')
-
- img_path = osp.join(root_path, 'imgs', img_name + '.jpg')
-
- # Skip not exist images
- if not osp.exists(img_path):
- continue
-
- img = mmcv.imread(img_path, 'unchanged')
-
- # Skip broken images
- if img is None:
- continue
-
- img_info = dict(
- file_name=img_name + '.jpg',
- height=img.shape[0],
- width=img.shape[1])
-
- anno_info = []
- for ann_id in annotation['index_to_ann_map'][img_name]:
- ann = annotation['ann_id'][ann_id]
-
- # The original annotation is oriented rects [x, y, w, h, a]
- box = np.fromstring(
- ann['bounding_box'][1:-2], sep=',', dtype=float)
- quadrilateral = convert_oriented_box(box)
-
- xs, ys = quadrilateral[::2], quadrilateral[1::2]
- x = max(0, math.floor(min(xs)))
- y = max(0, math.floor(min(ys)))
- w = math.floor(max(xs)) - x
- h = math.floor(max(ys)) - y
- bbox = [x, y, w, h]
- segmentation = quadrilateral
-
- anno = dict(
- iscrowd=0,
- category_id=1,
- bbox=bbox,
- area=w * h,
- segmentation=[segmentation])
- anno_info.append(anno)
- img_info.update(anno_info=anno_info)
- img_infos.append(img_info)
-
- return img_infos
-
-
-def convert_oriented_box(box):
-
- x_ctr, y_ctr, width, height, angle = box[:5]
- angle = -angle * math.pi / 180
-
- tl_x, tl_y, br_x, br_y = -width / 2, -height / 2, width / 2, height / 2
- rect = np.array([[tl_x, br_x, br_x, tl_x], [tl_y, tl_y, br_y, br_y]])
- R = np.array([[np.cos(angle), -np.sin(angle)],
- [np.sin(angle), np.cos(angle)]])
- poly = R.dot(rect)
- x0, x1, x2, x3 = poly[0, :4] + x_ctr
- y0, y1, y2, y3 = poly[1, :4] + y_ctr
- poly = np.array([x0, y0, x1, y1, x2, y2, x3, y3], dtype=np.float32)
- poly = get_best_begin_point_single(poly)
-
- return poly.tolist()
-
-
-def get_best_begin_point_single(coordinate):
-
- x1, y1, x2, y2, x3, y3, x4, y4 = coordinate
- xmin = min(x1, x2, x3, x4)
- ymin = min(y1, y2, y3, y4)
- xmax = max(x1, x2, x3, x4)
- ymax = max(y1, y2, y3, y4)
- combine = [[[x1, y1], [x2, y2], [x3, y3], [x4, y4]],
- [[x2, y2], [x3, y3], [x4, y4], [x1, y1]],
- [[x3, y3], [x4, y4], [x1, y1], [x2, y2]],
- [[x4, y4], [x1, y1], [x2, y2], [x3, y3]]]
- dst_coordinate = [[xmin, ymin], [xmax, ymin], [xmax, ymax], [xmin, ymax]]
- force = 100000000.0
- force_flag = 0
- for i in range(4):
- temp_force = cal_line_length(combine[i][0], dst_coordinate[0]) \
- + cal_line_length(combine[i][1], dst_coordinate[1]) \
- + cal_line_length(combine[i][2], dst_coordinate[2]) \
- + cal_line_length(combine[i][3], dst_coordinate[3])
- if temp_force < force:
- force = temp_force
- force_flag = i
- if force_flag != 0:
- pass
-
- return np.array(combine[force_flag]).reshape(8)
-
-
-def cal_line_length(point1, point2):
-
- return math.sqrt(
- math.pow(point1[0] - point2[0], 2) +
- math.pow(point1[1] - point2[1], 2))
-
-
-def main():
- args = parse_args()
- root_path = args.root_path
-
- for split in ['train', 'val', 'test']:
- print(f'Processing {split} set...')
- with mmengine.Timer(
- print_tmpl='It takes {}s to convert IMGUR annotation'):
- anno_infos = collect_imgur_info(
- root_path, f'imgur5k_annotations_{split}.json')
- dump_ocr_data(anno_infos,
- osp.join(root_path, f'instances_{split}.json'),
- 'textdet')
-
-
-if __name__ == '__main__':
- main()
diff --git a/pipeline/mmocr/tools/dataset_converters/textdet/kaist_converter.py b/pipeline/mmocr/tools/dataset_converters/textdet/kaist_converter.py
deleted file mode 100644
index 3f95804d1dda27a88db247e177c3f7522361faf5..0000000000000000000000000000000000000000
--- a/pipeline/mmocr/tools/dataset_converters/textdet/kaist_converter.py
+++ /dev/null
@@ -1,201 +0,0 @@
-# Copyright (c) OpenMMLab. All rights reserved.
-import argparse
-import math
-import os
-import os.path as osp
-import xml.etree.ElementTree as ET
-
-import mmcv
-import mmengine
-
-from mmocr.utils import dump_ocr_data
-
-
-def collect_files(img_dir, gt_dir, ratio):
- """Collect all images and their corresponding groundtruth files.
-
- Args:
- img_dir (str): The image directory
- gt_dir (str): The groundtruth directory
- ratio (float): Split ratio for val set
-
- Returns:
- files (list): The list of tuples (img_file, groundtruth_file)
- """
- assert isinstance(img_dir, str)
- assert img_dir
- assert isinstance(gt_dir, str)
- assert gt_dir
- assert isinstance(ratio, float)
- assert ratio < 1.0, 'val_ratio should be a float between 0.0 to 1.0'
-
- ann_list, imgs_list = [], []
- for img_file in os.listdir(img_dir):
- ann_list.append(osp.join(gt_dir, img_file.split('.')[0] + '.xml'))
- imgs_list.append(osp.join(img_dir, img_file))
-
- all_files = list(zip(sorted(imgs_list), sorted(ann_list)))
- assert len(all_files), f'No images found in {img_dir}'
- print(f'Loaded {len(all_files)} images from {img_dir}')
-
- trn_files, val_files = [], []
- if ratio > 0:
- for i, file in enumerate(all_files):
- if i % math.floor(1 / ratio):
- trn_files.append(file)
- else:
- val_files.append(file)
- else:
- trn_files, val_files = all_files, []
-
- print(f'training #{len(trn_files)}, val #{len(val_files)}')
-
- return trn_files, val_files
-
-
-def collect_annotations(files, nproc=1):
- """Collect the annotation information.
-
- Args:
- files (list): The list of tuples (image_file, groundtruth_file)
- nproc (int): The number of process to collect annotations
-
- Returns:
- images (list): The list of image information dicts
- """
- assert isinstance(files, list)
- assert isinstance(nproc, int)
-
- if nproc > 1:
- images = mmengine.track_parallel_progress(
- load_img_info, files, nproc=nproc)
- else:
- images = mmengine.track_progress(load_img_info, files)
-
- return images
-
-
-def load_img_info(files):
- """Load the information of one image.
-
- Args:
- files (tuple): The tuple of (img_file, groundtruth_file)
-
- Returns:
- img_info (dict): The dict of the img and annotation information
- """
- assert isinstance(files, tuple)
-
- img_file, gt_file = files
- assert osp.basename(gt_file).split('.')[0] == osp.basename(img_file).split(
- '.')[0]
- # read imgs while ignoring orientations
- img = mmcv.imread(img_file, 'unchanged')
-
- img_info = dict(
- file_name=osp.join(osp.basename(img_file)),
- height=img.shape[0],
- width=img.shape[1],
- segm_file=osp.join(osp.basename(gt_file)))
-
- if osp.splitext(gt_file)[1] == '.xml':
- img_info = load_xml_info(gt_file, img_info)
- else:
- raise NotImplementedError
-
- return img_info
-
-
-def load_xml_info(gt_file, img_info):
- """Collect the annotation information.
-
- Annotation Format
-
- DSC02306.JPG
-
-
-
-
-
-
-
-
-
-
-
-
- no
- 2
-
-
-
-
- Args:
- gt_file (str): The path to ground-truth
- img_info (dict): The dict of the img and annotation information
-
- Returns:
- img_info (dict): The dict of the img and annotation information
- """
-
- obj = ET.parse(gt_file)
- root = obj.getroot()
- anno_info = []
- for word in root.iter('word'):
- x, y = max(0, int(word.attrib['x'])), max(0, int(word.attrib['y']))
- w, h = int(word.attrib['width']), int(word.attrib['height'])
- bbox = [x, y, w, h]
- segmentation = [x, y, x + w, y, x + w, y + h, x, y + h]
-
- anno = dict(
- iscrowd=0,
- category_id=1,
- bbox=bbox,
- area=w * h,
- segmentation=[segmentation])
- anno_info.append(anno)
-
- img_info.update(anno_info=anno_info)
-
- return img_info
-
-
-def parse_args():
- parser = argparse.ArgumentParser(
- description='Generate training and val set of KAIST ')
- parser.add_argument('root_path', help='Root dir path of KAIST')
- parser.add_argument(
- '--val-ratio', help='Split ratio for val set', default=0.0, type=float)
- parser.add_argument(
- '--nproc', default=1, type=int, help='Number of process')
- args = parser.parse_args()
- return args
-
-
-def main():
- args = parse_args()
- root_path = args.root_path
- ratio = args.val_ratio
-
- trn_files, val_files = collect_files(
- osp.join(root_path, 'imgs'), osp.join(root_path, 'annotations'), ratio)
-
- # Train set
- trn_infos = collect_annotations(trn_files, nproc=args.nproc)
- with mmengine.Timer(
- print_tmpl='It takes {}s to convert KAIST Training annotation'):
- dump_ocr_data(trn_infos, osp.join(root_path,
- 'instances_training.json'),
- 'textdet')
-
- # Val set
- if len(val_files) > 0:
- val_infos = collect_annotations(val_files, nproc=args.nproc)
- with mmengine.Timer(
- print_tmpl='It takes {}s to convert KAIST Val annotation'):
- dump_ocr_data(val_infos, osp.join(root_path, 'instances_val.json'),
- 'textdet')
-
-
-if __name__ == '__main__':
- main()
diff --git a/pipeline/mmocr/tools/dataset_converters/textdet/lsvt_converter.py b/pipeline/mmocr/tools/dataset_converters/textdet/lsvt_converter.py
deleted file mode 100644
index aa44d10663e762ddbcccb354b65cfd349634a6ce..0000000000000000000000000000000000000000
--- a/pipeline/mmocr/tools/dataset_converters/textdet/lsvt_converter.py
+++ /dev/null
@@ -1,130 +0,0 @@
-# Copyright (c) OpenMMLab. All rights reserved.
-import argparse
-import math
-import os.path as osp
-
-import mmcv
-import mmengine
-
-from mmocr.utils import dump_ocr_data
-
-
-def parse_args():
- parser = argparse.ArgumentParser(
- description='Generate training and validation set of LSVT ')
- parser.add_argument('root_path', help='Root dir path of LSVT')
- parser.add_argument(
- '--val-ratio', help='Split ratio for val set', default=0.0, type=float)
- args = parser.parse_args()
- return args
-
-
-def collect_lsvt_info(root_path, split, ratio, print_every=1000):
- """Collect the annotation information.
-
- The annotation format is as the following:
- [
- {'gt_1234': # 'gt_1234' is file name
- [
- {
- 'transcription': 'ไธ็ซๅผ่ดญ็ฉไธญๅฟ',
- 'points': [[45, 272], [215, 273], [212, 296], [45, 290]]
- 'illegibility': False
- }, ...
- ]
- }
- ]
-
-
- Args:
- root_path (str): Root path to the dataset
- split (str): Dataset split, which should be 'train' or 'val'
- ratio (float): Split ratio for val set
- print_every (int): Print log info per iteration
-
- Returns:
- img_info (dict): The dict of the img and annotation information
- """
-
- annotation_path = osp.join(root_path, 'annotations/train_full_labels.json')
- if not osp.exists(annotation_path):
- raise Exception(
- f'{annotation_path} not exists, please check and try again.')
-
- annotation = mmengine.load(annotation_path)
- img_prefixes = annotation.keys()
-
- trn_files, val_files = [], []
- if ratio > 0:
- for i, file in enumerate(img_prefixes):
- if i % math.floor(1 / ratio):
- trn_files.append(file)
- else:
- val_files.append(file)
- else:
- trn_files, val_files = img_prefixes, []
- print(f'training #{len(trn_files)}, val #{len(val_files)}')
-
- if split == 'train':
- img_prefixes = trn_files
- elif split == 'val':
- img_prefixes = val_files
- else:
- raise NotImplementedError
-
- img_infos = []
- for i, prefix in enumerate(img_prefixes):
- if i > 0 and i % print_every == 0:
- print(f'{i}/{len(img_prefixes)}')
- img_file = osp.join(root_path, 'imgs', prefix + '.jpg')
- # Skip not exist images
- if not osp.exists(img_file):
- continue
- img = mmcv.imread(img_file)
-
- img_info = dict(
- file_name=osp.join(osp.basename(img_file)),
- height=img.shape[0],
- width=img.shape[1],
- segm_file=osp.join(osp.basename(annotation_path)))
-
- anno_info = []
- for ann in annotation[prefix]:
- segmentation = []
- for x, y in ann['points']:
- segmentation.append(max(0, x))
- segmentation.append(max(0, y))
- xs, ys = segmentation[::2], segmentation[1::2]
- x, y = min(xs), min(ys)
- w, h = max(xs) - x, max(ys) - y
- bbox = [x, y, w, h]
- anno = dict(
- iscrowd=1 if ann['illegibility'] else 0,
- category_id=1,
- bbox=bbox,
- area=w * h,
- segmentation=[segmentation])
- anno_info.append(anno)
- img_info.update(anno_info=anno_info)
- img_infos.append(img_info)
-
- return img_infos
-
-
-def main():
- args = parse_args()
- root_path = args.root_path
- print('Processing training set...')
- training_infos = collect_lsvt_info(root_path, 'train', args.val_ratio)
- dump_ocr_data(training_infos,
- osp.join(root_path, 'instances_training.json'), 'textdet')
- if args.val_ratio > 0:
- print('Processing validation set...')
- val_infos = collect_lsvt_info(root_path, 'val', args.val_ratio)
- dump_ocr_data(val_infos, osp.join(root_path, 'instances_val.json'),
- 'textdet')
- print('Finish')
-
-
-if __name__ == '__main__':
- main()
diff --git a/pipeline/mmocr/tools/dataset_converters/textdet/lv_converter.py b/pipeline/mmocr/tools/dataset_converters/textdet/lv_converter.py
deleted file mode 100644
index 6efcc14317da87adc655f4096f5a4db3cbfb7558..0000000000000000000000000000000000000000
--- a/pipeline/mmocr/tools/dataset_converters/textdet/lv_converter.py
+++ /dev/null
@@ -1,182 +0,0 @@
-# Copyright (c) OpenMMLab. All rights reserved.
-import argparse
-import os
-import os.path as osp
-import xml.etree.ElementTree as ET
-
-import mmcv
-import mmengine
-
-from mmocr.utils import dump_ocr_data
-
-
-def collect_files(data_dir):
- """Collect all images and their corresponding groundtruth files.
-
- Args:
- data_dir (str): The directory to dataset
-
- Returns:
- files (list): The list of tuples (img_file, groundtruth_file)
- """
- assert isinstance(data_dir, str)
- assert data_dir
-
- ann_list, imgs_list = [], []
- for video_dir in os.listdir(data_dir):
- for frame_dir in os.listdir(osp.join(data_dir, video_dir)):
- crt_dir = osp.join(data_dir, video_dir, frame_dir)
- if not osp.isdir(crt_dir):
- continue
- for crt_file in os.listdir(crt_dir):
- if crt_file.endswith('xml'):
- ann_path = osp.join(crt_dir, crt_file)
- img_path = osp.join(crt_dir,
- crt_file.replace('xml', 'png'))
- if os.path.exists(img_path):
- ann_list.append(ann_path)
- imgs_list.append(img_path)
- else:
- continue
-
- files = list(zip(imgs_list, ann_list))
- assert len(files), f'No images found in {data_dir}'
- print(f'Loaded {len(files)} images from {data_dir}')
-
- return files
-
-
-def collect_annotations(files, nproc=1):
- """Collect the annotation information.
-
- Args:
- files (list): The list of tuples (image_file, groundtruth_file)
- nproc (int): The number of process to collect annotations
-
- Returns:
- images (list): The list of image information dicts
- """
- assert isinstance(files, list)
- assert isinstance(nproc, int)
-
- if nproc > 1:
- images = mmengine.track_parallel_progress(
- load_img_info, files, nproc=nproc)
- else:
- images = mmengine.track_progress(load_img_info, files)
-
- return images
-
-
-def load_img_info(files):
- """Load the information of one image.
-
- Args:
- files (tuple): The tuple of (img_file, groundtruth_file)
-
- Returns:
- img_info (dict): The dict of the img and annotation information
- """
- assert isinstance(files, tuple)
-
- img_file, gt_file = files
- assert osp.basename(gt_file).split('.')[0] == osp.basename(img_file).split(
- '.')[0]
- # read imgs while ignoring orientations
- img = mmcv.imread(img_file, 'unchanged')
- img_file = os.path.split(img_file)[-1]
-
- img_info = dict(
- file_name=img_file,
- height=img.shape[0],
- width=img.shape[1],
- segm_file=osp.join(osp.basename(gt_file)))
-
- if osp.splitext(gt_file)[1] == '.xml':
- img_info = load_xml_info(gt_file, img_info)
- else:
- raise NotImplementedError
-
- return img_info
-
-
-def load_xml_info(gt_file, img_info):
- """Collect the annotation information.
-
- The annotation format is as the following:
-
-
- hierarchy
- Unspecified
- 0
- 0
-
- 657
- 467
- 839
- 557
-
-
-
-
- Args:
- gt_file (str): The path to ground-truth
- img_info (dict): The dict of the img and annotation information
-
- Returns:
- img_info (dict): The dict of the img and annotation information
- """
-
- obj = ET.parse(gt_file)
- root = obj.getroot()
- anno_info = []
- for obj in root.iter('object'):
- x = max(0, int(obj.find('bndbox').find('xmin').text))
- y = max(0, int(obj.find('bndbox').find('ymin').text))
- xmax = int(obj.find('bndbox').find('xmax').text)
- ymax = int(obj.find('bndbox').find('ymax').text)
-
- w, h = abs(xmax - x), abs(ymax - y)
- bbox = [x, y, w, h]
- segmentation = [x, y, x + w, y, x + w, y + h, x, y + h]
-
- anno = dict(
- iscrowd=0,
- category_id=1,
- bbox=bbox,
- area=w * h,
- segmentation=[segmentation])
- anno_info.append(anno)
-
- img_info.update(anno_info=anno_info)
-
- return img_info
-
-
-def parse_args():
- parser = argparse.ArgumentParser(
- description='Generate training, val and test set of Lecture Video DB ')
- parser.add_argument('root_path', help='Root dir path of Lecture Video DB')
- parser.add_argument(
- '--nproc', default=1, type=int, help='number of process')
- args = parser.parse_args()
- return args
-
-
-def main():
- args = parse_args()
- root_path = args.root_path
-
- for split in ['train', 'val', 'test']:
- print(f'Processing {split} set...')
- with mmengine.Timer(
- print_tmpl='It takes {}s to convert LV annotation'):
- files = collect_files(osp.join(root_path, 'imgs', split))
- image_infos = collect_annotations(files, nproc=args.nproc)
- dump_ocr_data(image_infos,
- osp.join(root_path, 'instances_' + split + '.json'),
- 'textdet')
-
-
-if __name__ == '__main__':
- main()
diff --git a/pipeline/mmocr/tools/dataset_converters/textdet/mtwi_converter.py b/pipeline/mmocr/tools/dataset_converters/textdet/mtwi_converter.py
deleted file mode 100644
index 1c9fde3bf870ffcd1ea482e3d73bfc138f51a381..0000000000000000000000000000000000000000
--- a/pipeline/mmocr/tools/dataset_converters/textdet/mtwi_converter.py
+++ /dev/null
@@ -1,203 +0,0 @@
-# Copyright (c) OpenMMLab. All rights reserved.
-import argparse
-import math
-import os
-import os.path as osp
-
-import cv2
-import mmcv
-import mmengine
-from PIL import Image
-
-from mmocr.utils import dump_ocr_data
-
-
-def collect_files(img_dir, gt_dir, ratio):
- """Collect all images and their corresponding groundtruth files.
- Args:
- img_dir (str): The image directory
- gt_dir (str): The groundtruth directory
- ratio (float): Split ratio for val set
-
- Returns:
- files (list): The list of tuples (img_file, groundtruth_file)
- """
- assert isinstance(img_dir, str)
- assert img_dir
- assert isinstance(gt_dir, str)
- assert gt_dir
- assert isinstance(ratio, float)
- assert ratio < 1.0, 'val_ratio should be a float between 0.0 to 1.0'
-
- ann_list, imgs_list = [], []
- for ann_file in os.listdir(gt_dir):
- img_file = osp.join(img_dir, ann_file.replace('txt', 'jpg'))
- # This dataset contains some images obtained from .gif,
- # which cannot be loaded by mmcv.imread(), convert them
- # to RGB mode.
- try:
- if mmcv.imread(img_file) is None:
- print(f'Convert {img_file} to RGB mode.')
- img = Image.open(img_file)
- img = img.convert('RGB')
- img.save(img_file)
- except cv2.error:
- print(f'Skip broken img {img_file}')
- continue
-
- ann_list.append(osp.join(gt_dir, ann_file))
- imgs_list.append(img_file)
-
- all_files = list(zip(imgs_list, ann_list))
- assert len(all_files), f'No images found in {img_dir}'
- print(f'Loaded {len(all_files)} images from {img_dir}')
-
- trn_files, val_files = [], []
- if ratio > 0:
- for i, file in enumerate(all_files):
- if i % math.floor(1 / ratio):
- trn_files.append(file)
- else:
- val_files.append(file)
- else:
- trn_files, val_files = all_files, []
-
- print(f'training #{len(trn_files)}, val #{len(val_files)}')
-
- return trn_files, val_files
-
-
-def collect_annotations(files, nproc=1):
- """Collect the annotation information.
- Args:
- files (list): The list of tuples (image_file, groundtruth_file)
- nproc (int): The number of process to collect annotations
-
- Returns:
- images (list): The list of image information dicts
- """
- assert isinstance(files, list)
- assert isinstance(nproc, int)
-
- if nproc > 1:
- images = mmengine.track_parallel_progress(
- load_img_info, files, nproc=nproc)
- else:
- images = mmengine.track_progress(load_img_info, files)
-
- return images
-
-
-def load_img_info(files):
- """Load the information of one image.
- Args:
- files (tuple): The tuple of (img_file, groundtruth_file)
-
- Returns:
- img_info (dict): The dict of the img and annotation information
- """
- assert isinstance(files, tuple)
-
- img_file, gt_file = files
- assert osp.basename(gt_file).split('.')[0] == osp.basename(img_file).split(
- '.')[0]
- # read imgs while ignoring orientations
- img = mmcv.imread(img_file)
-
- img_info = dict(
- file_name=osp.join(osp.basename(img_file)),
- height=img.shape[0],
- width=img.shape[1],
- segm_file=osp.join(osp.basename(gt_file)))
-
- if osp.splitext(gt_file)[1] == '.txt':
- img_info = load_txt_info(gt_file, img_info)
- else:
- raise NotImplementedError
-
- return img_info
-
-
-def load_txt_info(gt_file, img_info):
- """Collect the annotation information.
-
- The annotation format is as the following:
- x1,y1,x2,y2,x3,y3,x4,y4,text
-
- 45.45,226.83,11.87,181.79,183.84,13.1,233.79,49.95,ๆถๅฐ่ข่ข
- 345.98,311.18,345.98,347.21,462.26,347.21,462.26,311.18,73774
- 462.26,292.34,461.44,299.71,502.39,299.71,502.39,292.34,73/74/737
-
- Args:
- gt_file (str): The path to ground-truth
- img_info (dict): The dict of the img and annotation information
-
- Returns:
- img_info (dict): The dict of the img and annotation information
- """
-
- anno_info = []
- with open(gt_file) as f:
- lines = f.readlines()
- for line in lines:
- points = line.split(',')[0:8]
- word = line.split(',')[8].rstrip('\n')
- segmentation = [math.floor(float(pt)) for pt in points]
- x = max(0, min(segmentation[0::2]))
- y = max(0, min(segmentation[1::2]))
- w = abs(max(segmentation[0::2]) - x)
- h = abs(max(segmentation[1::2]) - y)
- bbox = [x, y, w, h]
-
- anno = dict(
- iscrowd=1 if word == '###' else 0,
- category_id=1,
- bbox=bbox,
- area=w * h,
- segmentation=[segmentation])
- anno_info.append(anno)
-
- img_info.update(anno_info=anno_info)
-
- return img_info
-
-
-def parse_args():
- parser = argparse.ArgumentParser(
- description='Generate training and val set of MTWI.')
- parser.add_argument('root_path', help='Root dir path of MTWI')
- parser.add_argument(
- '--val-ratio', help='Split ratio for val set', default=0.0, type=float)
- parser.add_argument(
- '--nproc', default=1, type=int, help='Number of process')
- args = parser.parse_args()
- return args
-
-
-def main():
- args = parse_args()
- root_path = args.root_path
- ratio = args.val_ratio
-
- trn_files, val_files = collect_files(
- osp.join(root_path, 'imgs'), osp.join(root_path, 'annotations'), ratio)
-
- # Train set
- trn_infos = collect_annotations(trn_files, nproc=args.nproc)
- with mmengine.Timer(
- print_tmpl='It takes {}s to convert MTWI Training annotation'):
- dump_ocr_data(trn_infos, osp.join(root_path,
- 'instances_training.json'),
- 'textdet')
-
- # Val set
- if len(val_files) > 0:
- val_infos = collect_annotations(val_files, nproc=args.nproc)
- with mmengine.Timer(
- print_tmpl='It takes {}s to convert MTWI Val annotation'):
- dump_ocr_data(val_infos, osp.join(root_path, 'instances_val.json'),
- 'textdet')
-
-
-if __name__ == '__main__':
- main()
diff --git a/pipeline/mmocr/tools/dataset_converters/textdet/naf_converter.py b/pipeline/mmocr/tools/dataset_converters/textdet/naf_converter.py
deleted file mode 100644
index 2e43c8fba909723edd55f7b13b2a9cfa0b6c2e15..0000000000000000000000000000000000000000
--- a/pipeline/mmocr/tools/dataset_converters/textdet/naf_converter.py
+++ /dev/null
@@ -1,197 +0,0 @@
-# Copyright (c) OpenMMLab. All rights reserved.
-import argparse
-import os.path as osp
-
-import mmcv
-import mmengine
-
-from mmocr.utils import dump_ocr_data
-
-
-def collect_files(img_dir, gt_dir, split_info):
- """Collect all images and their corresponding groundtruth files.
-
- Args:
- img_dir (str): The image directory
- gt_dir (str): The groundtruth directory
- split_info (dict): The split information for train/val/test
-
- Returns:
- files (list): The list of tuples (img_file, groundtruth_file)
- """
- assert isinstance(img_dir, str)
- assert img_dir
- assert isinstance(gt_dir, str)
- assert gt_dir
- assert isinstance(split_info, dict)
- assert split_info
-
- ann_list, imgs_list = [], []
- for group in split_info:
- for img in split_info[group]:
- image_path = osp.join(img_dir, img)
- anno_path = osp.join(gt_dir, 'groups', group,
- img.replace('jpg', 'json'))
-
- # Filtering out the missing images
- if not osp.exists(image_path) or not osp.exists(anno_path):
- continue
-
- imgs_list.append(image_path)
- ann_list.append(anno_path)
-
- files = list(zip(imgs_list, ann_list))
- assert len(files), f'No images found in {img_dir}'
- print(f'Loaded {len(files)} images from {img_dir}')
-
- return files
-
-
-def collect_annotations(files, nproc=1):
- """Collect the annotation information.
-
- Args:
- files (list): The list of tuples (image_file, groundtruth_file)
- nproc (int): The number of process to collect annotations
-
- Returns:
- images (list): The list of image information dicts
- """
- assert isinstance(files, list)
- assert isinstance(nproc, int)
-
- if nproc > 1:
- images = mmengine.track_parallel_progress(
- load_img_info, files, nproc=nproc)
- else:
- images = mmengine.track_progress(load_img_info, files)
-
- return images
-
-
-def load_img_info(files):
- """Load the information of one image.
-
- Args:
- files (tuple): The tuple of (img_file, groundtruth_file)
-
- Returns:
- img_info (dict): The dict of the img and annotation information
- """
- assert isinstance(files, tuple)
-
- img_file, gt_file = files
- assert osp.basename(gt_file).split('.')[0] == osp.basename(img_file).split(
- '.')[0]
- # Read imgs while ignoring orientations
- img = mmcv.imread(img_file, 'unchanged')
-
- img_info = dict(
- file_name=osp.join(osp.basename(img_file)),
- height=img.shape[0],
- width=img.shape[1],
- segm_file=osp.join(osp.basename(gt_file)))
-
- if osp.splitext(gt_file)[1] == '.json':
- img_info = load_json_info(gt_file, img_info)
- else:
- raise NotImplementedError
-
- return img_info
-
-
-def load_json_info(gt_file, img_info):
- """Collect the annotation information.
-
- Annotation Format
- {
- 'textBBs': [{
- 'poly_points': [[435,1406], [466,1406], [466,1439], [435,1439]],
- "type": "text",
- "id": "t1",
- }], ...
- }
-
- Some special characters are used in the transcription:
- "ยซtextยป" indicates that "text" had a strikethrough
- "ยฟ" indicates the transcriber could not read a character
- "ยง" indicates the whole line or word was illegible
- "" (empty string) is if the field was blank
-
- Args:
- gt_file (str): The path to ground-truth
- img_info (dict): The dict of the img and annotation information
-
- Returns:
- img_info (dict): The dict of the img and annotation information
- """
- assert isinstance(gt_file, str)
- assert isinstance(img_info, dict)
-
- annotation = mmengine.load(gt_file)
- anno_info = []
-
- # 'textBBs' contains the printed texts of the table while 'fieldBBs'
- # contains the text filled by human.
- for box_type in ['textBBs', 'fieldBBs']:
- for anno in annotation[box_type]:
- # Skip blanks
- if box_type == 'fieldBBs':
- if anno['type'] == 'blank':
- continue
-
- xs, ys, segmentation = [], [], []
- for p in anno['poly_points']:
- xs.append(p[0])
- ys.append(p[1])
- segmentation.append(p[0])
- segmentation.append(p[1])
- x, y = max(0, min(xs)), max(0, min(ys))
- w, h = max(xs) - x, max(ys) - y
- bbox = [x, y, w, h]
-
- anno = dict(
- iscrowd=0,
- category_id=1,
- bbox=bbox,
- area=w * h,
- segmentation=[segmentation])
- anno_info.append(anno)
-
- img_info.update(anno_info=anno_info)
-
- return img_info
-
-
-def parse_args():
- parser = argparse.ArgumentParser(
- description='Generate training, val, and test set of NAF ')
- parser.add_argument('root_path', help='Root dir path of NAF')
- parser.add_argument(
- '--nproc', default=1, type=int, help='Number of process')
- args = parser.parse_args()
- return args
-
-
-def main():
- args = parse_args()
- root_path = args.root_path
- split_info = mmengine.load(
- osp.join(root_path, 'annotations', 'train_valid_test_split.json'))
- split_info['training'] = split_info.pop('train')
- split_info['val'] = split_info.pop('valid')
- for split in ['training', 'val', 'test']:
- print(f'Processing {split} set...')
- with mmengine.Timer(
- print_tmpl='It takes {}s to convert NAF annotation'):
- files = collect_files(
- osp.join(root_path, 'imgs'),
- osp.join(root_path, 'annotations'), split_info[split])
- image_infos = collect_annotations(files, nproc=args.nproc)
- dump_ocr_data(image_infos,
- osp.join(root_path, 'instances_' + split + '.json'),
- 'textdet')
-
-
-if __name__ == '__main__':
- main()
diff --git a/pipeline/mmocr/tools/dataset_converters/textdet/rctw_converter.py b/pipeline/mmocr/tools/dataset_converters/textdet/rctw_converter.py
deleted file mode 100644
index cc46dd85999c616a89167a56de27ccf2f306ec4a..0000000000000000000000000000000000000000
--- a/pipeline/mmocr/tools/dataset_converters/textdet/rctw_converter.py
+++ /dev/null
@@ -1,193 +0,0 @@
-# Copyright (c) OpenMMLab. All rights reserved.
-import argparse
-import math
-import os
-import os.path as osp
-
-import mmcv
-import mmengine
-
-from mmocr.utils import dump_ocr_data
-
-
-def collect_files(img_dir, gt_dir, ratio):
- """Collect all images and their corresponding groundtruth files.
- Args:
- img_dir (str): The image directory
- gt_dir (str): The groundtruth directory
- ratio (float): Split ratio for val set
-
- Returns:
- files (list): The list of tuples (img_file, groundtruth_file)
- """
- assert isinstance(img_dir, str)
- assert img_dir
- assert isinstance(gt_dir, str)
- assert gt_dir
- assert isinstance(ratio, float)
- assert ratio < 1.0, 'val_ratio should be a float between 0.0 to 1.0'
-
- ann_list, imgs_list = [], []
- for ann_file in os.listdir(gt_dir):
- ann_list.append(osp.join(gt_dir, ann_file))
- imgs_list.append(osp.join(img_dir, ann_file.replace('txt', 'jpg')))
-
- all_files = list(zip(imgs_list, ann_list))
- assert len(all_files), f'No images found in {img_dir}'
- print(f'Loaded {len(all_files)} images from {img_dir}')
-
- trn_files, val_files = [], []
- if ratio > 0:
- for i, file in enumerate(all_files):
- if i % math.floor(1 / ratio):
- trn_files.append(file)
- else:
- val_files.append(file)
- else:
- trn_files, val_files = all_files, []
-
- print(f'training #{len(trn_files)}, val #{len(val_files)}')
-
- return trn_files, val_files
-
-
-def collect_annotations(files, nproc=1):
- """Collect the annotation information.
- Args:
- files (list): The list of tuples (image_file, groundtruth_file)
- nproc (int): The number of process to collect annotations
-
- Returns:
- images (list): The list of image information dicts
- """
- assert isinstance(files, list)
- assert isinstance(nproc, int)
-
- if nproc > 1:
- images = mmengine.track_parallel_progress(
- load_img_info, files, nproc=nproc)
- else:
- images = mmengine.track_progress(load_img_info, files)
-
- return images
-
-
-def load_img_info(files):
- """Load the information of one image.
- Args:
- files (tuple): The tuple of (img_file, groundtruth_file)
-
- Returns:
- img_info (dict): The dict of the img and annotation information
- """
- assert isinstance(files, tuple)
-
- img_file, gt_file = files
- assert osp.basename(gt_file).split('.')[0] == osp.basename(img_file).split(
- '.')[0]
- # read imgs while ignoring orientations
- img = mmcv.imread(img_file)
-
- img_info = dict(
- file_name=osp.join(osp.basename(img_file)),
- height=img.shape[0],
- width=img.shape[1],
- segm_file=osp.join(osp.basename(gt_file)))
-
- if osp.splitext(gt_file)[1] == '.txt':
- img_info = load_txt_info(gt_file, img_info)
- else:
- raise NotImplementedError
-
- return img_info
-
-
-def load_txt_info(gt_file, img_info):
- """Collect the annotation information.
-
- The annotation format is as the following:
- x1, y1, x2, y2, x3, y3, x4, y4, difficult, text
-
- 390,902,1856,902,1856,1225,390,1225,0,"้ๆฐ็ผ้"
- 1875,1170,2149,1170,2149,1245,1875,1245,0,"ๅไบ1989"
- 2054,1277,2190,1277,2190,1323,2054,1323,0,"ๅๅปบๅบ"
-
- Args:
- gt_file (str): The path to ground-truth
- img_info (dict): The dict of the img and annotation information
-
- Returns:
- img_info (dict): The dict of the img and annotation information
- """
-
- anno_info = []
- with open(gt_file, encoding='utf-8-sig') as f:
- lines = f.readlines()
- for line in lines:
- points = line.split(',')[0:8]
- word = line.split(',')[9].rstrip('\n').strip('"')
- difficult = 1 if line.split(',')[8] != '0' else 0
- segmentation = [int(pt) for pt in points]
- x = max(0, min(segmentation[0::2]))
- y = max(0, min(segmentation[1::2]))
- w = abs(max(segmentation[0::2]) - x)
- h = abs(max(segmentation[1::2]) - y)
- bbox = [x, y, w, h]
-
- if word == '###' or difficult == 1:
- iscrowd = 1
- else:
- iscrowd = 0
-
- anno = dict(
- iscrowd=iscrowd,
- category_id=1,
- bbox=bbox,
- area=w * h,
- segmentation=[segmentation])
- anno_info.append(anno)
-
- img_info.update(anno_info=anno_info)
-
- return img_info
-
-
-def parse_args():
- parser = argparse.ArgumentParser(
- description='Generate training and val set of RCTW.')
- parser.add_argument('root_path', help='Root dir path of RCTW')
- parser.add_argument(
- '--val-ratio', help='Split ratio for val set', default=0.0, type=float)
- parser.add_argument(
- '--nproc', default=1, type=int, help='Number of process')
- args = parser.parse_args()
- return args
-
-
-def main():
- args = parse_args()
- root_path = args.root_path
- ratio = args.val_ratio
-
- trn_files, val_files = collect_files(
- osp.join(root_path, 'imgs'), osp.join(root_path, 'annotations'), ratio)
-
- # Train set
- with mmengine.Timer(
- print_tmpl='It takes {}s to convert RCTW Training annotation'):
- trn_infos = collect_annotations(trn_files, nproc=args.nproc)
- dump_ocr_data(trn_infos, osp.join(root_path,
- 'instances_training.json'),
- 'textdet')
-
- # Val set
- if len(val_files) > 0:
- with mmengine.Timer(
- print_tmpl='It takes {}s to convert RCTW Val annotation'):
- val_infos = collect_annotations(val_files, nproc=args.nproc)
- dump_ocr_data(val_infos, osp.join(root_path, 'instances_val.json'),
- 'textdet')
-
-
-if __name__ == '__main__':
- main()
diff --git a/pipeline/mmocr/tools/dataset_converters/textdet/rects_converter.py b/pipeline/mmocr/tools/dataset_converters/textdet/rects_converter.py
deleted file mode 100644
index 75f3b734607af2c44b78273df3401457166432c2..0000000000000000000000000000000000000000
--- a/pipeline/mmocr/tools/dataset_converters/textdet/rects_converter.py
+++ /dev/null
@@ -1,207 +0,0 @@
-# Copyright (c) OpenMMLab. All rights reserved.
-import argparse
-import math
-import os
-import os.path as osp
-
-import mmcv
-import mmengine
-
-from mmocr.utils import dump_ocr_data
-
-
-def collect_files(img_dir, gt_dir, ratio):
- """Collect all images and their corresponding groundtruth files.
- Args:
- img_dir (str): The image directory
- gt_dir (str): The groundtruth directory
- ratio (float): Split ratio for val set
-
- Returns:
- files (list): The list of tuples (img_file, groundtruth_file)
- """
- assert isinstance(img_dir, str)
- assert img_dir
- assert isinstance(gt_dir, str)
- assert gt_dir
- assert isinstance(ratio, float)
- assert ratio < 1.0, 'val_ratio should be a float between 0.0 to 1.0'
-
- ann_list, imgs_list = [], []
- for ann_file in os.listdir(gt_dir):
- ann_list.append(osp.join(gt_dir, ann_file))
- imgs_list.append(osp.join(img_dir, ann_file.replace('json', 'jpg')))
-
- all_files = list(zip(imgs_list, ann_list))
- assert len(all_files), f'No images found in {img_dir}'
- print(f'Loaded {len(all_files)} images from {img_dir}')
-
- trn_files, val_files = [], []
- if ratio > 0:
- for i, file in enumerate(all_files):
- if i % math.floor(1 / ratio):
- trn_files.append(file)
- else:
- val_files.append(file)
- else:
- trn_files, val_files = all_files, []
-
- print(f'training #{len(trn_files)}, val #{len(val_files)}')
-
- return trn_files, val_files
-
-
-def collect_annotations(files, nproc=1):
- """Collect the annotation information.
- Args:
- files (list): The list of tuples (image_file, groundtruth_file)
- nproc (int): The number of process to collect annotations
-
- Returns:
- images (list): The list of image information dicts
- """
- assert isinstance(files, list)
- assert isinstance(nproc, int)
-
- if nproc > 1:
- images = mmengine.track_parallel_progress(
- load_img_info, files, nproc=nproc)
- else:
- images = mmengine.track_progress(load_img_info, files)
-
- return images
-
-
-def load_img_info(files):
- """Load the information of one image.
- Args:
- files (tuple): The tuple of (img_file, groundtruth_file)
-
- Returns:
- img_info (dict): The dict of the img and annotation information
- """
- assert isinstance(files, tuple)
-
- img_file, gt_file = files
- assert osp.basename(gt_file).split('.')[0] == osp.basename(img_file).split(
- '.')[0]
- # read imgs while ignoring orientations
- img = mmcv.imread(img_file)
-
- img_info = dict(
- file_name=osp.join(osp.basename(img_file)),
- height=img.shape[0],
- width=img.shape[1],
- segm_file=osp.join(osp.basename(gt_file)))
-
- if osp.splitext(gt_file)[1] == '.json':
- img_info = load_json_info(gt_file, img_info)
- else:
- raise NotImplementedError
-
- return img_info
-
-
-def load_json_info(gt_file, img_info):
- """Collect the annotation information.
-
- The annotation format is as the following:
-
- {
- "chars": [
- {
- "ignore": 0,
- "transcription": "H",
- "points": [25, 175, 112, 175, 112, 286, 25, 286]
- },
- {
- "ignore": 0,
- "transcription": "O",
- "points": [102, 182, 210, 182, 210, 273, 102, 273]
- }, ...
- ]
- "lines": [
- {
- "ignore": 0,
- "transcription": "HOKI",
- "points": [23, 173, 327, 180, 327, 290, 23, 283]
- },
- {
- "ignore": 0,
- "transcription": "TEA",
- "points": [368, 180, 621, 180, 621, 294, 368, 294]
- }, ...
- ]
- }
-
-
- Args:
- gt_file (str): The path to ground-truth
- img_info (dict): The dict of the img and annotation information
-
- Returns:
- img_info (dict): The dict of the img and annotation information
- """
-
- annotation = mmengine.load(gt_file)
- anno_info = []
- for line in annotation['lines']:
- segmentation = line['points']
- x = max(0, min(segmentation[0::2]))
- y = max(0, min(segmentation[1::2]))
- w = abs(max(segmentation[0::2]) - x)
- h = abs(max(segmentation[1::2]) - y)
- bbox = [x, y, w, h]
-
- anno = dict(
- iscrowd=line['ignore'],
- category_id=1,
- bbox=bbox,
- area=w * h,
- segmentation=[segmentation])
- anno_info.append(anno)
-
- img_info.update(anno_info=anno_info)
-
- return img_info
-
-
-def parse_args():
- parser = argparse.ArgumentParser(
- description='Generate training and val set of ReCTS.')
- parser.add_argument('root_path', help='Root dir path of ReCTS')
- parser.add_argument(
- '--val-ratio', help='Split ratio for val set', default=0.0, type=float)
- parser.add_argument(
- '--nproc', default=1, type=int, help='Number of process')
- args = parser.parse_args()
- return args
-
-
-def main():
- args = parse_args()
- root_path = args.root_path
- ratio = args.val_ratio
-
- trn_files, val_files = collect_files(
- osp.join(root_path, 'imgs'), osp.join(root_path, 'annotations'), ratio)
-
- # Train set
- trn_infos = collect_annotations(trn_files, nproc=args.nproc)
- with mmengine.Timer(
- print_tmpl='It takes {}s to convert ReCTS Training annotation'):
- dump_ocr_data(trn_infos, osp.join(root_path,
- 'instances_training.json'),
- 'textdet')
-
- # Val set
- if len(val_files) > 0:
- val_infos = collect_annotations(val_files, nproc=args.nproc)
- with mmengine.Timer(
- print_tmpl='It takes {}s to convert ReCTS Val annotation'):
- dump_ocr_data(val_infos, osp.join(root_path, 'instances_val.json'),
- 'textdet')
-
-
-if __name__ == '__main__':
- main()
diff --git a/pipeline/mmocr/tools/dataset_converters/textdet/sroie_converter.py b/pipeline/mmocr/tools/dataset_converters/textdet/sroie_converter.py
deleted file mode 100644
index 7ee0725e2fde0533248ed584c290377416bd5b46..0000000000000000000000000000000000000000
--- a/pipeline/mmocr/tools/dataset_converters/textdet/sroie_converter.py
+++ /dev/null
@@ -1,168 +0,0 @@
-# Copyright (c) OpenMMLab. All rights reserved.
-import argparse
-import os
-import os.path as osp
-
-import mmcv
-import mmengine
-import numpy as np
-
-from mmocr.utils import dump_ocr_data
-
-
-def collect_files(img_dir, gt_dir):
- """Collect all images and their corresponding groundtruth files.
-
- Args:
- img_dir (str): The image directory
- gt_dir (str): The groundtruth directory
-
- Returns:
- files (list): The list of tuples (img_file, groundtruth_file)
- """
-
- assert isinstance(img_dir, str)
- assert img_dir
- assert isinstance(gt_dir, str)
- assert gt_dir
-
- ann_list, imgs_list = [], []
- for gt_file in os.listdir(gt_dir):
- # Filtering repeated and missing images
- if '(' in gt_file or gt_file == 'X51006619570.txt':
- continue
- ann_list.append(osp.join(gt_dir, gt_file))
- imgs_list.append(osp.join(img_dir, gt_file.replace('.txt', '.jpg')))
-
- files = list(zip(sorted(imgs_list), sorted(ann_list)))
- assert len(files), f'No images found in {img_dir}'
-
- print(f'Loaded {len(files)} images from {img_dir}')
-
- return files
-
-
-def collect_annotations(files, nproc=1):
- """Collect the annotation information.
-
- Args:
- files (list): The list of tuples (image_file, groundtruth_file)
- nproc (int): The number of process to collect annotations
-
- Returns:
- images (list): The list of image information dicts
- """
-
- assert isinstance(files, list)
- assert isinstance(nproc, int)
-
- if nproc > 1:
- images = mmengine.track_parallel_progress(
- load_img_info, files, nproc=nproc)
- else:
- images = mmengine.track_progress(load_img_info, files)
-
- return images
-
-
-def load_img_info(files):
- """Load the information of one image.
-
- Args:
- files (tuple): The tuple of (img_file, groundtruth_file)
-
- Returns:
- img_info (dict): The dict of the img and annotation information
- """
-
- assert isinstance(files, tuple)
-
- img_file, gt_file = files
- assert osp.basename(gt_file).split('.')[0] == osp.basename(img_file).split(
- '.')[0]
- # read imgs while ignoring orientations
- img = mmcv.imread(img_file, 'unchanged')
-
- img_info = dict(
- file_name=osp.join(osp.basename(img_file)),
- height=img.shape[0],
- width=img.shape[1],
- segm_file=osp.join(osp.basename(gt_file)))
-
- if osp.splitext(gt_file)[1] == '.txt':
- img_info = load_txt_info(gt_file, img_info)
- else:
- raise NotImplementedError
-
- return img_info
-
-
-def load_txt_info(gt_file, img_info):
- """Collect the annotation information.
-
- Args:
- gt_file (list): The list of tuples (image_file, groundtruth_file)
- img_info (int): The dict of the img and annotation information
-
- Returns:
- img_info (list): The dict of the img and annotation information
- """
-
- with open(gt_file, encoding='unicode_escape') as f:
- anno_info = []
- for ann in f.readlines():
-
- # annotation format [x1, y1, x2, y2, x3, y3, x4, y4, transcript]
- try:
- ann_box = np.array(ann.split(',')[0:8]).astype(int).tolist()
- except ValueError:
- # skip invalid annotation line
- continue
- x = max(0, min(ann_box[0::2]))
- y = max(0, min(ann_box[1::2]))
- w, h = max(ann_box[0::2]) - x, max(ann_box[1::2]) - y
- bbox = [x, y, w, h]
- segmentation = ann_box
-
- anno = dict(
- iscrowd=0,
- category_id=1,
- bbox=bbox,
- area=w * h,
- segmentation=[segmentation])
- anno_info.append(anno)
-
- img_info.update(anno_info=anno_info)
-
- return img_info
-
-
-def parse_args():
- parser = argparse.ArgumentParser(
- description='Generate training and test set of SROIE')
- parser.add_argument('root_path', help='Root dir path of SROIE')
- parser.add_argument(
- '--nproc', default=1, type=int, help='Number of process')
- args = parser.parse_args()
- return args
-
-
-def main():
- args = parse_args()
- root_path = args.root_path
-
- for split in ['training', 'test']:
- print(f'Processing {split} set...')
- with mmengine.Timer(
- print_tmpl='It takes {}s to convert SROIE annotation'):
- files = collect_files(
- osp.join(root_path, 'imgs', split),
- osp.join(root_path, 'annotations', split))
- image_infos = collect_annotations(files, nproc=args.nproc)
- dump_ocr_data(image_infos,
- osp.join(root_path, 'instances_' + split + '.json'),
- 'textdet')
-
-
-if __name__ == '__main__':
- main()
diff --git a/pipeline/mmocr/tools/dataset_converters/textdet/vintext_converter.py b/pipeline/mmocr/tools/dataset_converters/textdet/vintext_converter.py
deleted file mode 100644
index fb7a364d9591bec7785a73d571670121bb985978..0000000000000000000000000000000000000000
--- a/pipeline/mmocr/tools/dataset_converters/textdet/vintext_converter.py
+++ /dev/null
@@ -1,171 +0,0 @@
-# Copyright (c) OpenMMLab. All rights reserved.
-import argparse
-import os
-import os.path as osp
-
-import mmcv
-import mmengine
-
-from mmocr.utils import dump_ocr_data
-
-
-def collect_files(img_dir, gt_dir):
- """Collect all images and their corresponding groundtruth files.
-
- Args:
- img_dir (str): The image directory
- gt_dir (str): The groundtruth directory
-
- Returns:
- files (list): The list of tuples (img_file, groundtruth_file)
- """
- assert isinstance(img_dir, str)
- assert img_dir
- assert isinstance(gt_dir, str)
- assert gt_dir
-
- ann_list, imgs_list = [], []
- for img_file in os.listdir(img_dir):
- ann_file = 'gt_' + str(int(img_file[2:6])) + '.txt'
- ann_list.append(osp.join(gt_dir, ann_file))
- imgs_list.append(osp.join(img_dir, img_file))
-
- files = list(zip(imgs_list, ann_list))
- assert len(files), f'No images found in {img_dir}'
- print(f'Loaded {len(files)} images from {img_dir}')
-
- return files
-
-
-def collect_annotations(files, nproc=1):
- """Collect the annotation information.
-
- Args:
- files (list): The list of tuples (image_file, groundtruth_file)
- nproc (int): The number of process to collect annotations
-
- Returns:
- images (list): The list of image information dicts
- """
- assert isinstance(files, list)
- assert isinstance(nproc, int)
-
- if nproc > 1:
- images = mmengine.track_parallel_progress(
- load_img_info, files, nproc=nproc)
- else:
- images = mmengine.track_progress(load_img_info, files)
-
- return images
-
-
-def load_img_info(files):
- """Load the information of one image.
-
- Args:
- files (tuple): The tuple of (img_file, groundtruth_file)
-
- Returns:
- img_info (dict): The dict of the img and annotation information
- """
- assert isinstance(files, tuple)
-
- img_file, gt_file = files
- assert int(osp.basename(gt_file)[3:-4]) == int(
- osp.basename(img_file)[2:-4])
- # read imgs while ignoring orientations
- img = mmcv.imread(img_file, 'unchanged')
-
- img_info = dict(
- file_name=osp.basename(img_file),
- height=img.shape[0],
- width=img.shape[1],
- segm_file=osp.basename(gt_file))
-
- if osp.splitext(gt_file)[1] == '.txt':
- img_info = load_txt_info(gt_file, img_info)
- else:
- raise NotImplementedError
-
- return img_info
-
-
-def load_txt_info(gt_file, img_info):
- """Collect the annotation information.
-
- The annotation format is as the following:
- x1,y1,x2,y2,x3,y3,x4,y4,text
- 118,15,147,15,148,46,118,46,LฦฏแปขNG
- 149,9,165,9,165,43,150,43,TแปT
- 167,9,180,9,179,43,167,42,ฤแป
- 181,12,193,12,193,43,181,43,Cร
- 195,13,215,14,215,46,196,46,VIแปC
- 217,13,237,14,239,47,217,46,LรM,
-
- Args:
- gt_file (str): The path to ground-truth
- img_info (dict): The dict of the img and annotation information
-
- Returns:
- img_info (dict): The dict of the img and annotation information
- """
-
- with open(gt_file, encoding='utf-8') as f:
- anno_info = []
- for line in f:
- line = line.strip('\n')
- ann = line.split(',')
- bbox = ann[0:8]
- word = line[len(','.join(bbox)) + 1:]
- bbox = [int(coord) for coord in bbox]
- segmentation = bbox
- x_min = min(bbox[0], bbox[2], bbox[4], bbox[6])
- x_max = max(bbox[0], bbox[2], bbox[4], bbox[6])
- y_min = min(bbox[1], bbox[3], bbox[5], bbox[7])
- y_max = max(bbox[1], bbox[3], bbox[5], bbox[7])
- w = x_max - x_min
- h = y_max - y_min
- bbox = [x_min, y_min, w, h]
- iscrowd = 1 if word == '###' else 0
-
- anno = dict(
- iscrowd=iscrowd,
- category_id=1,
- bbox=bbox,
- area=w * h,
- segmentation=[segmentation])
- anno_info.append(anno)
-
- img_info.update(anno_info=anno_info)
-
- return img_info
-
-
-def parse_args():
- parser = argparse.ArgumentParser(
- description='Generate training and test set of VinText ')
- parser.add_argument('root_path', help='Root dir path of VinText')
- parser.add_argument(
- '--nproc', default=1, type=int, help='Number of processes')
- args = parser.parse_args()
- return args
-
-
-def main():
- args = parse_args()
- root_path = args.root_path
- for split in ['training', 'test', 'unseen_test']:
- print(f'Processing {split} set...')
- with mmengine.Timer(
- print_tmpl='It takes {}s to convert VinText annotation'):
- files = collect_files(
- osp.join(root_path, 'imgs', split),
- osp.join(root_path, 'annotations'))
- image_infos = collect_annotations(files, nproc=args.nproc)
- dump_ocr_data(image_infos,
- osp.join(root_path, 'instances_' + split + '.json'),
- 'textdet')
-
-
-if __name__ == '__main__':
- main()
diff --git a/pipeline/mmocr/tools/dataset_converters/textrecog/art_converter.py b/pipeline/mmocr/tools/dataset_converters/textrecog/art_converter.py
deleted file mode 100644
index 24acaad289be221558701d19a95ea7ce24a7e0f9..0000000000000000000000000000000000000000
--- a/pipeline/mmocr/tools/dataset_converters/textrecog/art_converter.py
+++ /dev/null
@@ -1,108 +0,0 @@
-# Copyright (c) OpenMMLab. All rights reserved.
-import argparse
-import math
-import os.path as osp
-
-import mmengine
-
-from mmocr.utils import dump_ocr_data
-
-
-def parse_args():
- parser = argparse.ArgumentParser(
- description='Generate training and validation set of ArT ')
- parser.add_argument('root_path', help='Root dir path of ArT')
- parser.add_argument(
- '--val-ratio', help='Split ratio for val set', default=0.0, type=float)
- parser.add_argument(
- '--nproc', default=1, type=int, help='Number of processes')
- args = parser.parse_args()
- return args
-
-
-def convert_art(root_path, split, ratio):
- """Collect the annotation information and crop the images.
-
- The annotation format is as the following:
- {
- "gt_2836_0": [
- {
- "transcription": "URDER",
- "points": [
- [25, 51],
- [0, 2],
- [21, 0],
- [42, 43]
- ],
- "language": "Latin",
- "illegibility": false
- }
- ], ...
- }
-
- Args:
- root_path (str): The root path of the dataset
- split (str): The split of dataset. Namely: training or val
- ratio (float): Split ratio for val set
-
- Returns:
- img_info (dict): The dict of the img and annotation information
- """
-
- annotation_path = osp.join(root_path,
- 'annotations/train_task2_labels.json')
- if not osp.exists(annotation_path):
- raise Exception(
- f'{annotation_path} not exists, please check and try again.')
-
- annotation = mmengine.load(annotation_path)
- img_prefixes = annotation.keys()
-
- trn_files, val_files = [], []
- if ratio > 0:
- for i, file in enumerate(img_prefixes):
- if i % math.floor(1 / ratio):
- trn_files.append(file)
- else:
- val_files.append(file)
- else:
- trn_files, val_files = img_prefixes, []
- print(f'training #{len(trn_files)}, val #{len(val_files)}')
-
- if split == 'train':
- img_prefixes = trn_files
- elif split == 'val':
- img_prefixes = val_files
- else:
- raise NotImplementedError
-
- img_info = []
- for prefix in img_prefixes:
- text_label = annotation[prefix][0]['transcription']
- dst_img_name = prefix + '.jpg'
-
- img_info.append({
- 'file_name': dst_img_name,
- 'anno_info': [{
- 'text': text_label
- }]
- })
-
- ensure_ascii = dict(ensure_ascii=False)
- dump_ocr_data(img_info, osp.join(root_path, f'{split.lower()}_label.json'),
- 'textrecog', **ensure_ascii)
-
-
-def main():
- args = parse_args()
- root_path = args.root_path
- print('Processing training set...')
- convert_art(root_path=root_path, split='train', ratio=args.val_ratio)
- if args.val_ratio > 0:
- print('Processing validation set...')
- convert_art(root_path=root_path, split='val', ratio=args.val_ratio)
- print('Finish')
-
-
-if __name__ == '__main__':
- main()
diff --git a/pipeline/mmocr/tools/dataset_converters/textrecog/bid_converter.py b/pipeline/mmocr/tools/dataset_converters/textrecog/bid_converter.py
deleted file mode 100644
index ec61b64bb42effc6194e1661a819224fa02b2c13..0000000000000000000000000000000000000000
--- a/pipeline/mmocr/tools/dataset_converters/textrecog/bid_converter.py
+++ /dev/null
@@ -1,247 +0,0 @@
-# Copyright (c) OpenMMLab. All rights reserved.
-import argparse
-import os
-import os.path as osp
-
-import mmcv
-import mmengine
-
-from mmocr.utils import crop_img, dump_ocr_data
-
-
-def collect_files(img_dir, gt_dir):
- """Collect all images and their corresponding groundtruth files.
-
- Args:
- img_dir (str): The image directory
- gt_dir (str): The groundtruth directory
-
- Returns:
- files (list): The list of tuples (img_file, groundtruth_file)
- """
- assert isinstance(img_dir, str)
- assert img_dir
- assert isinstance(gt_dir, str)
- assert gt_dir
-
- ann_list, imgs_list = [], []
- for img_file in os.listdir(img_dir):
- ann_file = img_file.split('_')[0] + '_gt_ocr.txt'
- ann_list.append(osp.join(gt_dir, ann_file))
- imgs_list.append(osp.join(img_dir, img_file))
-
- files = list(zip(imgs_list, ann_list))
- assert len(files), f'No images found in {img_dir}'
- print(f'Loaded {len(files)} images from {img_dir}')
-
- return files
-
-
-def collect_annotations(files, nproc=1):
- """Collect the annotation information.
-
- Args:
- files (list): The list of tuples (image_file, groundtruth_file)
- nproc (int): The number of process to collect annotations
-
- Returns:
- images (list): The list of image information dicts
- """
- assert isinstance(files, list)
- assert isinstance(nproc, int)
-
- if nproc > 1:
- images = mmengine.track_parallel_progress(
- load_img_info, files, nproc=nproc)
- else:
- images = mmengine.track_progress(load_img_info, files)
-
- return images
-
-
-def load_img_info(files):
- """Load the information of one image.
-
- Args:
- files (tuple): The tuple of (img_file, groundtruth_file)
-
- Returns:
- img_info (dict): The dict of the img and annotation information
- """
- assert isinstance(files, tuple)
-
- img_file, gt_file = files
- assert osp.basename(gt_file).split('_')[0] == osp.basename(gt_file).split(
- '_')[0]
- # read imgs while ignoring orientations
- img = mmcv.imread(img_file, 'unchanged')
-
- img_info = dict(
- file_name=osp.basename(img_file),
- height=img.shape[0],
- width=img.shape[1],
- segm_file=osp.basename(gt_file))
-
- if osp.splitext(gt_file)[1] == '.txt':
- img_info = load_txt_info(gt_file, img_info)
- else:
- raise NotImplementedError
-
- return img_info
-
-
-def load_txt_info(gt_file, img_info):
- """Collect the annotation information.
-
- The annotation format is as the following:
- x, y, w, h, text
- 977, 152, 16, 49, NOME
- 962, 143, 12, 323, APPINHANESI BLAZEK PASSOTTO
- 906, 446, 12, 94, 206940361
- 905, 641, 12, 44, SPTC
-
- Args:
- gt_file (str): The path to ground-truth
- img_info (dict): The dict of the img and annotation information
-
- Returns:
- img_info (dict): The dict of the img and annotation information
- """
- with open(gt_file, encoding='latin1') as f:
- anno_info = []
- for line in f:
- line = line.strip('\n')
- # Ignore hard samples
- if line[0] == '[' or line[0] == 'x':
- continue
- ann = line.split(',')
- bbox = ann[0:4]
- bbox = [int(coord) for coord in bbox]
- x, y, w, h = bbox
- # in case ',' exists in label
- word = ','.join(ann[4:]) if len(ann[4:]) > 1 else ann[4]
- # remove the initial space
- word = word.strip()
- bbox = [x, y, x + w, y, x + w, y + h, x, y + h]
-
- anno = dict(bbox=bbox, word=word)
- anno_info.append(anno)
-
- img_info.update(anno_info=anno_info)
-
- return img_info
-
-
-def split_train_val_list(full_list, val_ratio):
- """Split list by val_ratio.
-
- Args:
- full_list (list): List to be splited
- val_ratio (float): Split ratio for val set
-
- return:
- list(list, list): Train_list and val_list
- """
- n_total = len(full_list)
- offset = int(n_total * val_ratio)
- if n_total == 0 or offset < 1:
- return [], full_list
- val_list = full_list[:offset]
- train_list = full_list[offset:]
- return [train_list, val_list]
-
-
-def generate_ann(root_path, image_infos, preserve_vertical, val_ratio, format):
- """Generate cropped annotations and label txt file.
-
- Args:
- root_path (str): The root path of the dataset
- image_infos (list[dict]): A list of dicts of the img and
- annotation information
- preserve_vertical (bool): Whether to preserve vertical texts
- val_ratio (float): Split ratio for val set
- format (str): Using jsonl(dict) or str to format annotations
- """
-
- assert val_ratio <= 1.
-
- if val_ratio:
- image_infos = split_train_val_list(image_infos, val_ratio)
- splits = ['training', 'val']
-
- else:
- image_infos = [image_infos]
- splits = ['training']
-
- for i, split in enumerate(splits):
- dst_image_root = osp.join(root_path, 'crops', split)
- ignore_image_root = osp.join(root_path, 'ignores', split)
- os.makedirs(dst_image_root, exist_ok=True)
-
- img_info = []
- for image_info in image_infos[i]:
- index = 1
- src_img_path = osp.join(root_path, 'imgs', image_info['file_name'])
- image = mmcv.imread(src_img_path)
- src_img_root = image_info['file_name'].split('.')[0]
-
- for anno in image_info['anno_info']:
- word = anno['word']
- dst_img = crop_img(image, anno['bbox'], 0, 0)
- h, w, _ = dst_img.shape
-
- dst_img_name = f'{src_img_root}_{index}.png'
- index += 1
- # Skip invalid annotations
- if min(dst_img.shape) == 0:
- continue
- # Skip vertical texts
- if not preserve_vertical and h / w > 2 and split == 'training':
- dst_img_path = osp.join(ignore_image_root, dst_img_name)
- mmcv.imwrite(dst_img, dst_img_path)
- continue
-
- dst_img_path = osp.join(dst_image_root, dst_img_name)
- mmcv.imwrite(dst_img, dst_img_path)
-
- img_info.append({
- 'file_name': dst_img_name,
- 'anno_info': [{
- 'text': word
- }]
- })
-
- dump_ocr_data(img_info,
- osp.join(root_path, f'{split.lower()}_label.json'),
- 'textrecog')
-
-
-def parse_args():
- parser = argparse.ArgumentParser(
- description='Generate training and val set of BID ')
- parser.add_argument('root_path', help='Root dir path of BID')
- parser.add_argument(
- '--preserve-vertical',
- help='Preserve samples containing vertical texts',
- action='store_true')
- parser.add_argument(
- '--val-ratio', help='Split ratio for val set', default=0., type=float)
- parser.add_argument(
- '--nproc', default=1, type=int, help='Number of processes')
- args = parser.parse_args()
- return args
-
-
-def main():
- args = parse_args()
- root_path = args.root_path
- with mmengine.Timer(print_tmpl='It takes {}s to convert BID annotation'):
- files = collect_files(
- osp.join(root_path, 'imgs'), osp.join(root_path, 'annotations'))
- image_infos = collect_annotations(files, nproc=args.nproc)
- generate_ann(root_path, image_infos, args.preserve_vertical,
- args.val_ratio, args.format)
-
-
-if __name__ == '__main__':
- main()
diff --git a/pipeline/mmocr/tools/dataset_converters/textrecog/cocotext_converter.py b/pipeline/mmocr/tools/dataset_converters/textrecog/cocotext_converter.py
deleted file mode 100644
index 413c09b6c32c7f31ec86fe46c42d69809986bbf9..0000000000000000000000000000000000000000
--- a/pipeline/mmocr/tools/dataset_converters/textrecog/cocotext_converter.py
+++ /dev/null
@@ -1,174 +0,0 @@
-# Copyright (c) OpenMMLab. All rights reserved.
-import argparse
-import math
-import os.path as osp
-from functools import partial
-
-import mmcv
-import mmengine
-
-from mmocr.utils import dump_ocr_data
-
-
-def parse_args():
- parser = argparse.ArgumentParser(
- description='Generate training and validation set of COCO Text v2 ')
- parser.add_argument('root_path', help='Root dir path of COCO Text v2')
- parser.add_argument(
- '--nproc', default=1, type=int, help='Number of processes')
- parser.add_argument(
- '--preserve-vertical',
- help='Preserve samples containing vertical texts',
- action='store_true')
- args = parser.parse_args()
- return args
-
-
-def process_img(args, src_image_root, dst_image_root, ignore_image_root,
- preserve_vertical, split):
- # Dirty hack for multi-processing
- img_idx, img_info, anns = args
- src_img = mmcv.imread(osp.join(src_image_root, img_info['file_name']))
- label = []
- for ann_idx, ann in enumerate(anns):
- text_label = ann['utf8_string']
-
- # Ignore illegible or non-English words
- if ann['language'] == 'not english':
- continue
- if ann['legibility'] == 'illegible':
- continue
-
- x, y, w, h = ann['bbox']
- x, y = max(0, math.floor(x)), max(0, math.floor(y))
- w, h = math.ceil(w), math.ceil(h)
- dst_img = src_img[y:y + h, x:x + w]
- dst_img_name = f'img_{img_idx}_{ann_idx}.jpg'
-
- if not preserve_vertical and h / w > 2 and split == 'train':
- dst_img_path = osp.join(ignore_image_root, dst_img_name)
- mmcv.imwrite(dst_img, dst_img_path)
- continue
-
- dst_img_path = osp.join(dst_image_root, dst_img_name)
- mmcv.imwrite(dst_img, dst_img_path)
-
- label.append({
- 'file_name': dst_img_name,
- 'anno_info': [{
- 'text': text_label
- }]
- })
-
- return label
-
-
-def convert_cocotext(root_path,
- split,
- preserve_vertical,
- nproc,
- img_start_idx=0):
- """Collect the annotation information and crop the images.
-
- The annotation format is as the following:
- {
- 'anns':{
- '45346':{
- 'mask': [468.9,286.7,468.9,295.2,493.0,295.8,493.0,287.2],
- 'class': 'machine printed',
- 'bbox': [468.9, 286.7, 24.1, 9.1], # x, y, w, h
- 'image_id': 217925,
- 'id': 45346,
- 'language': 'english', # 'english' or 'not english'
- 'area': 206.06,
- 'utf8_string': 'New',
- 'legibility': 'legible', # 'legible' or 'illegible'
- },
- ...
- }
- 'imgs':{
- '540965':{
- 'id': 540965,
- 'set': 'train', # 'train' or 'val'
- 'width': 640,
- 'height': 360,
- 'file_name': 'COCO_train2014_000000540965.jpg'
- },
- ...
- }
- 'imgToAnns':{
- '540965': [],
- '260932': [63993, 63994, 63995, 63996, 63997, 63998, 63999],
- ...
- }
- }
-
- Args:
- root_path (str): Root path to the dataset
- split (str): Dataset split, which should be 'train' or 'val'
- preserve_vertical (bool): Whether to preserve vertical texts
- nproc (int): Number of processes
- img_start_idx (int): Index of start image
-
- Returns:
- img_info (dict): The dict of the img and annotation information
- """
-
- annotation_path = osp.join(root_path, 'annotations/cocotext.v2.json')
- if not osp.exists(annotation_path):
- raise Exception(
- f'{annotation_path} not exists, please check and try again.')
-
- annotation = mmengine.load(annotation_path)
- # outputs
- dst_label_file = osp.join(root_path, f'{split}_label.json')
- dst_image_root = osp.join(root_path, 'crops', split)
- ignore_image_root = osp.join(root_path, 'ignores', split)
- src_image_root = osp.join(root_path, 'imgs')
- mmengine.mkdir_or_exist(dst_image_root)
- mmengine.mkdir_or_exist(ignore_image_root)
-
- process_img_with_path = partial(
- process_img,
- src_image_root=src_image_root,
- dst_image_root=dst_image_root,
- ignore_image_root=ignore_image_root,
- preserve_vertical=preserve_vertical,
- split=split)
- tasks = []
- for img_idx, img_info in enumerate(annotation['imgs'].values()):
- if img_info['set'] == split:
- ann_ids = annotation['imgToAnns'][str(img_info['id'])]
- anns = [annotation['anns'][str(ann_id)] for ann_id in ann_ids]
- tasks.append((img_idx + img_start_idx, img_info, anns))
- labels_list = mmengine.track_parallel_progress(
- process_img_with_path, tasks, keep_order=True, nproc=nproc)
- final_labels = []
- for label_list in labels_list:
- final_labels += label_list
- dump_ocr_data(final_labels, dst_label_file, 'textrecog')
-
- return len(annotation['imgs'])
-
-
-def main():
- args = parse_args()
- root_path = args.root_path
- print('Processing training set...')
- num_train_imgs = convert_cocotext(
- root_path=root_path,
- split='train',
- preserve_vertical=args.preserve_vertical,
- nproc=args.nproc)
- print('Processing validation set...')
- convert_cocotext(
- root_path=root_path,
- split='val',
- preserve_vertical=args.preserve_vertical,
- nproc=args.nproc,
- img_start_idx=num_train_imgs)
- print('Finish')
-
-
-if __name__ == '__main__':
- main()
diff --git a/pipeline/mmocr/tools/dataset_converters/textrecog/data_migrator.py b/pipeline/mmocr/tools/dataset_converters/textrecog/data_migrator.py
deleted file mode 100644
index 9fb0f205b67a4d55bb1208feba4e4db65c0b78e8..0000000000000000000000000000000000000000
--- a/pipeline/mmocr/tools/dataset_converters/textrecog/data_migrator.py
+++ /dev/null
@@ -1,98 +0,0 @@
-# Copyright (c) OpenMMLab. All rights reserved.
-import argparse
-import json
-from typing import List, Tuple
-
-from mmocr.datasets import RecogLMDBDataset
-from mmocr.utils import StringStripper, dump_ocr_data, recog_anno_to_imginfo
-
-
-def parse_legacy_data(in_path: str,
- format: str) -> Tuple[List[str], List[str]]:
- """Load legacy data and return a list of file paths and labels.
-
- Args:
- in_path (str): Path to annotation file.
- format (str): Annotation format. Choices are 'txt', 'json' and 'lmdb'.
- For 'lmdb' format, the lmdb file should only contains labels. For
- lmdb file with labels and images, the conversion is unnecessary.
- Returns:
- tuple(list[str], list[str]): File paths and labels.
- """
- file_paths = []
- labels = []
- strip_cls = StringStripper()
- if format == 'lmdb':
- dataset = RecogLMDBDataset(
- in_path,
- parser_cfg=dict(type='LineJsonParser', keys=['filename', 'text']))
- for data_info in dataset:
- file_path = data_info['img_path']
- label = data_info['instances'][0]['text']
- file_path = strip_cls(file_path)
- label = strip_cls(label)
- # MJ's file_path starts with './'
- if file_path.startswith('./'):
- file_path = file_path[2:]
-
- file_paths.append(file_path)
- labels.append(label)
- return file_paths, labels
- else:
- with open(in_path) as f:
- if format == 'txt':
- for line in f:
- line = strip_cls(line)
- file_path, label = line.split()[:2]
- # MJ's file_path starts with './'
- if file_path.startswith('./'):
- file_path = file_path[2:]
-
- file_paths.append(file_path)
- labels.append(label)
- elif format == 'jsonl':
- for line in f:
- datum = json.loads(line)
- file_path = datum['filename']
- # MJ's file_path starts with './'
- if file_path.startswith('./'):
- file_path = file_path[2:]
-
- file_paths.append(file_path)
- labels.append(datum['text'])
-
- return file_paths, labels
-
-
-def parse_args():
- """Parse input arguments."""
- parser = argparse.ArgumentParser(
- description='Convert annotations for'
- 'text recognition tasks in MMOCR 0.x into the latest openmmlab format.'
- )
- parser.add_argument(
- 'in_path', help='The path to legacy recognition data file')
- parser.add_argument(
- 'out_path', help='The output json path in openmmlab format')
- parser.add_argument(
- '--format',
- choices=['txt', 'jsonl', 'lmdb'],
- type=str,
- default='txt',
- help='Legacy data format')
- args = parser.parse_args()
- if args.out_path.split('.')[-1] != 'json':
- raise ValueError('The output path must be a json file.')
- return args
-
-
-def main():
- args = parse_args()
- file_paths, labels = parse_legacy_data(args.in_path, args.format)
- img_infos = recog_anno_to_imginfo(file_paths, labels)
- dump_ocr_data(img_infos, args.out_path, 'textrecog')
- print('finish')
-
-
-if __name__ == '__main__':
- main()
diff --git a/pipeline/mmocr/tools/dataset_converters/textrecog/detext_converter.py b/pipeline/mmocr/tools/dataset_converters/textrecog/detext_converter.py
deleted file mode 100644
index 360dc5a6f4555d45fb69533f033710eb58e12cad..0000000000000000000000000000000000000000
--- a/pipeline/mmocr/tools/dataset_converters/textrecog/detext_converter.py
+++ /dev/null
@@ -1,212 +0,0 @@
-# Copyright (c) OpenMMLab. All rights reserved.
-import argparse
-import os
-import os.path as osp
-
-import mmcv
-import mmengine
-import numpy as np
-
-from mmocr.utils import crop_img, dump_ocr_data
-
-
-def collect_files(img_dir, gt_dir):
- """Collect all images and their corresponding groundtruth files.
-
- Args:
- img_dir (str): The image directory
- gt_dir (str): The groundtruth directory
-
- Returns:
- files (list): The list of tuples (img_file, groundtruth_file)
- """
- assert isinstance(img_dir, str)
- assert img_dir
- assert isinstance(gt_dir, str)
- assert gt_dir
-
- ann_list, imgs_list = [], []
- for img in os.listdir(img_dir):
- imgs_list.append(osp.join(img_dir, img))
- ann_list.append(osp.join(gt_dir, 'gt_' + img.replace('jpg', 'txt')))
-
- files = list(zip(imgs_list, ann_list))
- assert len(files), f'No images found in {img_dir}'
- print(f'Loaded {len(files)} images from {img_dir}')
-
- return files
-
-
-def collect_annotations(files, nproc=1):
- """Collect the annotation information.
-
- Args:
- files (list): The list of tuples (image_file, groundtruth_file)
- nproc (int): The number of process to collect annotations
-
- Returns:
- images (list): The list of image information dicts
- """
- assert isinstance(files, list)
- assert isinstance(nproc, int)
-
- if nproc > 1:
- images = mmengine.track_parallel_progress(
- load_img_info, files, nproc=nproc)
- else:
- images = mmengine.track_progress(load_img_info, files)
-
- return images
-
-
-def load_img_info(files):
- """Load the information of one image.
-
- Args:
- files (tuple): The tuple of (img_file, groundtruth_file)
-
- Returns:
- img_info (dict): The dict of the img and annotation information
- """
- assert isinstance(files, tuple)
-
- img_file, gt_file = files
- # read imgs while ignoring orientations
- img = mmcv.imread(img_file, 'unchanged')
-
- img_info = dict(
- file_name=osp.join(osp.basename(img_file)),
- height=img.shape[0],
- width=img.shape[1],
- segm_file=osp.join(osp.basename(gt_file)))
-
- if osp.splitext(gt_file)[1] == '.txt':
- img_info = load_txt_info(gt_file, img_info)
- else:
- raise NotImplementedError
-
- return img_info
-
-
-def load_txt_info(gt_file, img_info):
- """Collect the annotation information.
-
- Args:
- gt_file (str): The path to ground-truth
- img_info (dict): The dict of the img and annotation information
-
- Returns:
- img_info (dict): The dict of the img and annotation information
- """
-
- with open(gt_file) as f:
- anno_info = []
- annotations = f.readlines()
- for ann in annotations:
- # Annotation format [x1, y1, x2, y2, x3, y3, x4, y4, transcript]
- try:
- bbox = np.array(ann.split(',')[0:8]).astype(int).tolist()
- except ValueError:
- # Skip invalid annotation line
- continue
- word = ann.split(',')[-1].replace('\n', '').strip()
-
- # Skip samples without recog gt
- if word == '###':
- continue
- anno = dict(bbox=bbox, word=word)
- anno_info.append(anno)
-
- img_info.update(anno_info=anno_info)
-
- return img_info
-
-
-def generate_ann(root_path, split, image_infos, preserve_vertical):
- """Generate cropped annotations and label txt file.
-
- Args:
- root_path (str): The root path of the dataset
- split (str): The split of dataset. Namely: training or test
- image_infos (list[dict]): A list of dicts of the img and
- annotation information
- preserve_vertical (bool): Whether to preserve vertical texts
- """
-
- dst_image_root = osp.join(root_path, 'crops', split)
- ignore_image_root = osp.join(root_path, 'ignores', split)
- if split == 'training':
- dst_label_file = osp.join(root_path, 'train_label.json')
- elif split == 'val':
- dst_label_file = osp.join(root_path, 'val_label.json')
- mmengine.mkdir_or_exist(dst_image_root)
- mmengine.mkdir_or_exist(ignore_image_root)
-
- img_info = []
- for image_info in image_infos:
- index = 1
- src_img_path = osp.join(root_path, 'imgs', split,
- image_info['file_name'])
- image = mmcv.imread(src_img_path)
- src_img_root = image_info['file_name'].split('.')[0]
-
- for anno in image_info['anno_info']:
- word = anno['word']
- dst_img = crop_img(image, anno['bbox'], 0, 0)
- h, w, _ = dst_img.shape
-
- dst_img_name = f'{src_img_root}_{index}.png'
- index += 1
- # Skip invalid annotations
- if min(dst_img.shape) == 0 or len(word) == 0:
- continue
- # Filter out vertical texts
- if not preserve_vertical and h / w > 2 and split == 'training':
- dst_img_path = osp.join(ignore_image_root, dst_img_name)
- mmcv.imwrite(dst_img, dst_img_path)
- continue
-
- dst_img_path = osp.join(dst_image_root, dst_img_name)
- mmcv.imwrite(dst_img, dst_img_path)
-
- img_info.append({
- 'file_name': dst_img_name,
- 'anno_info': [{
- 'text': word
- }]
- })
-
- dump_ocr_data(img_info, dst_label_file, 'textrecog')
-
-
-def parse_args():
- parser = argparse.ArgumentParser(
- description='Generate training and val set of DeText ')
- parser.add_argument('root_path', help='Root dir path of DeText')
- parser.add_argument(
- '--preserve-vertical',
- help='Preserve samples containing vertical texts',
- action='store_true')
- parser.add_argument(
- '--nproc', default=1, type=int, help='Number of process')
- args = parser.parse_args()
- return args
-
-
-def main():
- args = parse_args()
- root_path = args.root_path
-
- for split in ['training', 'val']:
- print(f'Processing {split} set...')
- with mmengine.Timer(
- print_tmpl='It takes {}s to convert DeText annotation'):
- files = collect_files(
- osp.join(root_path, 'imgs', split),
- osp.join(root_path, 'annotations', split))
- image_infos = collect_annotations(files, nproc=args.nproc)
- generate_ann(root_path, split, image_infos, args.preserve_vertical)
-
-
-if __name__ == '__main__':
- main()
diff --git a/pipeline/mmocr/tools/dataset_converters/textrecog/funsd_converter.py b/pipeline/mmocr/tools/dataset_converters/textrecog/funsd_converter.py
deleted file mode 100644
index a8da8ab00183f20ec749585bde1bb6958a48f9d0..0000000000000000000000000000000000000000
--- a/pipeline/mmocr/tools/dataset_converters/textrecog/funsd_converter.py
+++ /dev/null
@@ -1,210 +0,0 @@
-# Copyright (c) OpenMMLab. All rights reserved.
-import argparse
-import math
-import os
-import os.path as osp
-
-import mmcv
-import mmengine
-
-from mmocr.utils import crop_img, dump_ocr_data
-
-
-def collect_files(img_dir, gt_dir):
- """Collect all images and their corresponding groundtruth files.
-
- Args:
- img_dir (str): The image directory
- gt_dir (str): The groundtruth directory
-
- Returns:
- files (list): The list of tuples (img_file, groundtruth_file)
- """
- assert isinstance(img_dir, str)
- assert img_dir
- assert isinstance(gt_dir, str)
- assert gt_dir
-
- ann_list, imgs_list = [], []
- for gt_file in os.listdir(gt_dir):
- ann_list.append(osp.join(gt_dir, gt_file))
- imgs_list.append(osp.join(img_dir, gt_file.replace('.json', '.png')))
-
- files = list(zip(sorted(imgs_list), sorted(ann_list)))
- assert len(files), f'No images found in {img_dir}'
- print(f'Loaded {len(files)} images from {img_dir}')
-
- return files
-
-
-def collect_annotations(files, nproc=1):
- """Collect the annotation information.
-
- Args:
- files (list): The list of tuples (image_file, groundtruth_file)
- nproc (int): The number of process to collect annotations
-
- Returns:
- images (list): The list of image information dicts
- """
- assert isinstance(files, list)
- assert isinstance(nproc, int)
-
- if nproc > 1:
- images = mmengine.track_parallel_progress(
- load_img_info, files, nproc=nproc)
- else:
- images = mmengine.track_progress(load_img_info, files)
-
- return images
-
-
-def load_img_info(files):
- """Load the information of one image.
-
- Args:
- files (tuple): The tuple of (img_file, groundtruth_file)
-
- Returns:
- img_info (dict): The dict of the img and annotation information
- """
- assert isinstance(files, tuple)
-
- img_file, gt_file = files
- assert osp.basename(gt_file).split('.')[0] == osp.basename(img_file).split(
- '.')[0]
- # read imgs while ignoring orientations
- img = mmcv.imread(img_file, 'unchanged')
-
- img_info = dict(
- file_name=osp.join(osp.basename(img_file)),
- height=img.shape[0],
- width=img.shape[1],
- segm_file=osp.join(osp.basename(gt_file)))
-
- if osp.splitext(gt_file)[1] == '.json':
- img_info = load_json_info(gt_file, img_info)
- else:
- raise NotImplementedError
-
- return img_info
-
-
-def load_json_info(gt_file, img_info):
- """Collect the annotation information.
-
- Args:
- gt_file (str): The path to ground-truth
- img_info (dict): The dict of the img and annotation information
-
- Returns:
- img_info (dict): The dict of the img and annotation information
- """
-
- annotation = mmengine.load(gt_file)
- anno_info = []
- for form in annotation['form']:
- for ann in form['words']:
-
- # Ignore illegible samples
- if len(ann['text']) == 0:
- continue
-
- x1, y1, x2, y2 = ann['box']
- x = max(0, min(math.floor(x1), math.floor(x2)))
- y = max(0, min(math.floor(y1), math.floor(y2)))
- w, h = math.ceil(abs(x2 - x1)), math.ceil(abs(y2 - y1))
- bbox = [x, y, x + w, y, x + w, y + h, x, y + h]
- word = ann['text']
-
- anno = dict(bbox=bbox, word=word)
- anno_info.append(anno)
-
- img_info.update(anno_info=anno_info)
-
- return img_info
-
-
-def generate_ann(root_path, split, image_infos, preserve_vertical):
- """Generate cropped annotations and label txt file.
-
- Args:
- root_path (str): The root path of the dataset
- split (str): The split of dataset. Namely: training or test
- image_infos (list[dict]): A list of dicts of the img and
- annotation information
- preserve_vertical (bool): Whether to preserve vertical texts
- """
-
- dst_image_root = osp.join(root_path, 'crops', split)
- if split == 'training':
- dst_label_file = osp.join(root_path, 'train_label.json')
- elif split == 'test':
- dst_label_file = osp.join(root_path, 'test_label.json')
- os.makedirs(dst_image_root, exist_ok=True)
-
- img_info = []
- for image_info in image_infos:
- index = 1
- src_img_path = osp.join(root_path, 'imgs', image_info['file_name'])
- image = mmcv.imread(src_img_path)
- src_img_root = image_info['file_name'].split('.')[0]
-
- for anno in image_info['anno_info']:
- word = anno['word']
- dst_img = crop_img(image, anno['bbox'])
- h, w, _ = dst_img.shape
-
- # Skip invalid annotations
- if min(dst_img.shape) == 0:
- continue
- # Skip vertical texts
- if not preserve_vertical and h / w > 2:
- continue
-
- dst_img_name = f'{src_img_root}_{index}.png'
- index += 1
- dst_img_path = osp.join(dst_image_root, dst_img_name)
- mmcv.imwrite(dst_img, dst_img_path)
-
- img_info.append({
- 'file_name': dst_img_name,
- 'anno_info': [{
- 'text': word
- }]
- })
-
- dump_ocr_data(img_info, dst_label_file, 'textrecog')
-
-
-def parse_args():
- parser = argparse.ArgumentParser(
- description='Generate training and test set of FUNSD ')
- parser.add_argument('root_path', help='Root dir path of FUNSD')
- parser.add_argument(
- '--preserve_vertical',
- help='Preserve samples containing vertical texts',
- action='store_true')
- parser.add_argument(
- '--nproc', default=1, type=int, help='Number of processes')
- args = parser.parse_args()
- return args
-
-
-def main():
- args = parse_args()
- root_path = args.root_path
-
- for split in ['training', 'test']:
- print(f'Processing {split} set...')
- with mmengine.Timer(
- print_tmpl='It takes {}s to convert FUNSD annotation'):
- files = collect_files(
- osp.join(root_path, 'imgs'),
- osp.join(root_path, 'annotations', split))
- image_infos = collect_annotations(files, nproc=args.nproc)
- generate_ann(root_path, split, image_infos, args.preserve_vertical)
-
-
-if __name__ == '__main__':
- main()
diff --git a/pipeline/mmocr/tools/dataset_converters/textrecog/hiertext_converter.py b/pipeline/mmocr/tools/dataset_converters/textrecog/hiertext_converter.py
deleted file mode 100644
index 948142446e30ceea2c1b58cbcd7aec930a982482..0000000000000000000000000000000000000000
--- a/pipeline/mmocr/tools/dataset_converters/textrecog/hiertext_converter.py
+++ /dev/null
@@ -1,236 +0,0 @@
-# Copyright (c) OpenMMLab. All rights reserved.
-import argparse
-import json
-import math
-import os.path as osp
-from functools import partial
-
-import mmcv
-import mmengine
-import numpy as np
-from shapely.geometry import Polygon
-
-from mmocr.utils import dump_ocr_data
-
-
-def seg2bbox(seg):
- """Convert segmentation to bbox.
-
- Args:
- seg (list(int | float)): A set of coordinates
- """
- if len(seg) == 4:
- min_x = min(seg[0], seg[2], seg[4], seg[6])
- max_x = max(seg[0], seg[2], seg[4], seg[6])
- min_y = min(seg[1], seg[3], seg[5], seg[7])
- max_y = max(seg[1], seg[3], seg[5], seg[7])
- else:
- seg = np.array(seg).reshape(-1, 2)
- polygon = Polygon(seg)
- min_x, min_y, max_x, max_y = polygon.bounds
- bbox = [min_x, min_y, max_x - min_x, max_y - min_y]
- return bbox
-
-
-def process_level(
- src_img,
- annotation,
- dst_image_root,
- ignore_image_root,
- preserve_vertical,
- split,
- para_idx,
- img_idx,
- line_idx,
- word_idx=None,
-):
- vertices = annotation['vertices']
- text_label = annotation['text']
- segmentation = [i for j in vertices for i in j]
- x, y, w, h = seg2bbox(segmentation)
- x, y = max(0, math.floor(x)), max(0, math.floor(y))
- w, h = math.ceil(w), math.ceil(h)
- dst_img = src_img[y:y + h, x:x + w]
- if word_idx:
- dst_img_name = f'img_{img_idx}_{para_idx}_{line_idx}_{word_idx}.jpg'
- else:
- dst_img_name = f'img_{img_idx}_{para_idx}_{line_idx}.jpg'
- if not preserve_vertical and h / w > 2 and split == 'train':
- dst_img_path = osp.join(ignore_image_root, dst_img_name)
- mmcv.imwrite(dst_img, dst_img_path)
- return None
-
- dst_img_path = osp.join(dst_image_root, dst_img_name)
- mmcv.imwrite(dst_img, dst_img_path)
-
- label = {'file_name': dst_img_name, 'anno_info': [{'text': text_label}]}
-
- return label
-
-
-def process_img(args, src_image_root, dst_image_root, ignore_image_root, level,
- preserve_vertical, split):
- # Dirty hack for multi-processing
- img_idx, img_annos = args
- src_img = mmcv.imread(
- osp.join(src_image_root, img_annos['image_id'] + '.jpg'))
- labels = []
- for para_idx, paragraph in enumerate(img_annos['paragraphs']):
- for line_idx, line in enumerate(paragraph['lines']):
- if level == 'line':
- # Ignore illegible words
- if line['legible']:
-
- label = process_level(src_img, line, dst_image_root,
- ignore_image_root, preserve_vertical,
- split, para_idx, img_idx, line_idx)
- if label is not None:
- labels.append(label)
- elif level == 'word':
- for word_idx, word in enumerate(line['words']):
- if not word['legible']:
- continue
- label = process_level(src_img, word, dst_image_root,
- ignore_image_root, preserve_vertical,
- split, para_idx, img_idx, line_idx,
- word_idx)
- if label is not None:
- labels.append(label)
- return labels
-
-
-def convert_hiertext(
- root_path,
- split,
- level,
- preserve_vertical,
- nproc,
-):
- """Collect the annotation information and crop the images.
-
- The annotation format is as the following:
- {
- "info": {
- "date": "release date",
- "version": "current version"
- },
- "annotations": [ // List of dictionaries, one for each image.
- {
- "image_id": "the filename of corresponding image.",
- "image_width": image_width, // (int) The image width.
- "image_height": image_height, // (int) The image height.
- "paragraphs": [ // List of paragraphs.
- {
- "vertices": [[x1, y1], [x2, y2],...,[xn, yn]]
- "legible": true
- "lines": [
- {
- "vertices": [[x1, y1], [x2, y2],...,[x4, y4]]
- "text": L
- "legible": true,
- "handwritten": false
- "vertical": false,
- "words": [
- {
- "vertices": [[x1, y1], [x2, y2],...,[xm, ym]]
- "text": "the text content of this word",
- "legible": true
- "handwritten": false,
- "vertical": false,
- }, ...
- ]
- }, ...
- ]
- }, ...
- ]
- }, ...
- ]
- }
-
- Args:
- root_path (str): Root path to the dataset
- split (str): Dataset split, which should be 'train' or 'val'
- level (str): Crop word or line level instances
- preserve_vertical (bool): Whether to preserve vertical texts
- nproc (int): Number of processes
-
- Returns:
- img_info (dict): The dict of the img and annotation information
- """
-
- annotation_path = osp.join(root_path, 'annotations/' + split + '.jsonl')
- if not osp.exists(annotation_path):
- raise Exception(
- f'{annotation_path} not exists, please check and try again.')
-
- annotation = json.load(open(annotation_path))['annotations']
- # outputs
- dst_label_file = osp.join(root_path, f'{split}_label.json')
- dst_image_root = osp.join(root_path, 'crops', split)
- ignore_image_root = osp.join(root_path, 'ignores', split)
- src_image_root = osp.join(root_path, 'imgs', split)
- mmengine.mkdir_or_exist(dst_image_root)
- mmengine.mkdir_or_exist(ignore_image_root)
-
- process_img_with_path = partial(
- process_img,
- src_image_root=src_image_root,
- dst_image_root=dst_image_root,
- ignore_image_root=ignore_image_root,
- level=level,
- preserve_vertical=preserve_vertical,
- split=split)
- tasks = []
- for img_idx, img_info in enumerate(annotation):
- tasks.append((img_idx, img_info))
- labels_list = mmengine.track_parallel_progress(
- process_img_with_path, tasks, keep_order=True, nproc=nproc)
-
- final_labels = []
- for label_list in labels_list:
- final_labels += label_list
-
- dump_ocr_data(final_labels, dst_label_file, 'textrecog')
-
-
-def parse_args():
- parser = argparse.ArgumentParser(
- description='Generate training and validation set of HierText')
- parser.add_argument('root_path', help='Root dir path of HierText')
- parser.add_argument(
- '--nproc', default=1, type=int, help='Number of processes')
- parser.add_argument(
- '--preserve-vertical',
- help='Preserve samples containing vertical texts',
- action='store_true')
- parser.add_argument(
- '--level',
- default='word',
- help='Crop word or line level instance',
- choices=['word', 'line'])
- args = parser.parse_args()
- return args
-
-
-def main():
- args = parse_args()
- root_path = args.root_path
- print('Processing training set...')
- convert_hiertext(
- root_path=root_path,
- split='train',
- level=args.level,
- preserve_vertical=args.preserve_vertical,
- nproc=args.nproc)
- print('Processing validation set...')
- convert_hiertext(
- root_path=root_path,
- split='val',
- level=args.level,
- preserve_vertical=args.preserve_vertical,
- nproc=args.nproc)
- print('Finish')
-
-
-if __name__ == '__main__':
- main()
diff --git a/pipeline/mmocr/tools/dataset_converters/textrecog/ic11_converter.py b/pipeline/mmocr/tools/dataset_converters/textrecog/ic11_converter.py
deleted file mode 100644
index 3de125d39bd87c137b2ed1d470fa6bcfd19836ba..0000000000000000000000000000000000000000
--- a/pipeline/mmocr/tools/dataset_converters/textrecog/ic11_converter.py
+++ /dev/null
@@ -1,66 +0,0 @@
-# Copyright (c) OpenMMLab. All rights reserved.
-import argparse
-import os.path as osp
-
-from mmocr.utils import dump_ocr_data
-
-
-def convert_annotations(root_path, split):
- """Convert original annotations to mmocr format.
-
- The annotation format of this dataset is as the following:
- word_1.png, "flying"
- word_2.png, "today"
- word_3.png, "means"
- See the format of converted annotation in mmocr.utils.dump_ocr_data.
-
- Args:
- root_path (str): The root path of the dataset
- split (str): The split of dataset. Namely: Train or Test
- """
- assert isinstance(root_path, str)
- assert isinstance(split, str)
-
- img_info = []
- with open(
- osp.join(root_path, 'annotations',
- f'Challenge1_{split}_Task3_GT.txt'),
- encoding='"utf-8-sig') as f:
- annos = f.readlines()
- for anno in annos:
- # text may contain comma ','
- dst_img_name, word = anno.split(', "')
- word = word.replace('"\n', '')
-
- img_info.append({
- 'file_name': dst_img_name,
- 'anno_info': [{
- 'text': word
- }]
- })
-
- return img_info
-
-
-def parse_args():
- parser = argparse.ArgumentParser(
- description='Generate training and test set of IC11')
- parser.add_argument('root_path', help='Root dir path of IC11')
- args = parser.parse_args()
- return args
-
-
-def main():
- args = parse_args()
- root_path = args.root_path
-
- for split in ['Train', 'Test']:
- img_info = convert_annotations(root_path, split)
- dump_ocr_data(img_info,
- osp.join(root_path, f'{split.lower()}_label.json'),
- 'textrecog')
- print(f'{split} split converted.')
-
-
-if __name__ == '__main__':
- main()
diff --git a/pipeline/mmocr/tools/dataset_converters/textrecog/ilst_converter.py b/pipeline/mmocr/tools/dataset_converters/textrecog/ilst_converter.py
deleted file mode 100644
index 01ce4bd17f57bf1281fe3b254e39d37c0f92c7a7..0000000000000000000000000000000000000000
--- a/pipeline/mmocr/tools/dataset_converters/textrecog/ilst_converter.py
+++ /dev/null
@@ -1,260 +0,0 @@
-# Copyright (c) OpenMMLab. All rights reserved.
-import argparse
-import os
-import os.path as osp
-import xml.etree.ElementTree as ET
-
-import mmcv
-import mmengine
-
-from mmocr.utils import crop_img, dump_ocr_data
-
-
-def collect_files(img_dir, gt_dir):
- """Collect all images and their corresponding groundtruth files.
-
- Args:
- img_dir (str): The image directory
- gt_dir (str): The groundtruth directory
-
- Returns:
- files (list): The list of tuples (img_file, groundtruth_file)
- """
- assert isinstance(img_dir, str)
- assert img_dir
- assert isinstance(gt_dir, str)
- assert gt_dir
-
- ann_list, imgs_list = [], []
- for img_file in os.listdir(img_dir):
- ann_path = osp.join(gt_dir, img_file.split('.')[0] + '.xml')
- if os.path.exists(ann_path):
- ann_list.append(ann_path)
- imgs_list.append(osp.join(img_dir, img_file))
-
- files = list(zip(imgs_list, ann_list))
- assert len(files), f'No images found in {img_dir}'
- print(f'Loaded {len(files)} images from {img_dir}')
-
- return files
-
-
-def collect_annotations(files, nproc=1):
- """Collect the annotation information.
-
- Args:
- files (list): The list of tuples (image_file, groundtruth_file)
- nproc (int): The number of process to collect annotations
-
- Returns:
- images (list): The list of image information dicts
- """
- assert isinstance(files, list)
- assert isinstance(nproc, int)
-
- if nproc > 1:
- images = mmengine.track_parallel_progress(
- load_img_info, files, nproc=nproc)
- else:
- images = mmengine.track_progress(load_img_info, files)
-
- return images
-
-
-def load_img_info(files):
- """Load the information of one image.
-
- Args:
- files (tuple): The tuple of (img_file, groundtruth_file)
-
- Returns:
- img_info (dict): The dict of the img and annotation information
- """
- assert isinstance(files, tuple)
-
- img_file, gt_file = files
- assert osp.basename(gt_file).split('.')[0] == osp.basename(img_file).split(
- '.')[0]
- # read imgs while ignoring orientations
- img = mmcv.imread(img_file, 'unchanged')
-
- try:
- img_info = dict(
- file_name=osp.join(osp.basename(img_file)),
- height=img.shape[0],
- width=img.shape[1],
- segm_file=osp.join(osp.basename(gt_file)))
- except AttributeError:
- print(f'Skip broken img {img_file}')
- return None
-
- if osp.splitext(gt_file)[1] == '.xml':
- img_info = load_xml_info(gt_file, img_info)
- else:
- raise NotImplementedError
-
- return img_info
-
-
-def load_xml_info(gt_file, img_info):
- """Collect the annotation information.
-
- The annotation format is as the following:
-
- ...
-
- SMT
- Unspecified
- 0
- 0
-
- 157
- 294
- 237
- 357
-
-
-
- Args:
- gt_file (str): The path to ground-truth
- img_info (dict): The dict of the img and annotation information
-
- Returns:
- img_info (dict): The dict of the img and annotation information
- """
- obj = ET.parse(gt_file)
- root = obj.getroot()
- anno_info = []
- for object in root.iter('object'):
- word = object.find('name').text
- x1 = int(object.find('bndbox').find('xmin').text)
- y1 = int(object.find('bndbox').find('ymin').text)
- x2 = int(object.find('bndbox').find('xmax').text)
- y2 = int(object.find('bndbox').find('ymax').text)
-
- x = max(0, min(x1, x2))
- y = max(0, min(y1, y2))
- w, h = abs(x2 - x1), abs(y2 - y1)
- bbox = [x, y, x + w, y, x + w, y + h, x, y + h]
- anno = dict(bbox=bbox, word=word)
- anno_info.append(anno)
-
- img_info.update(anno_info=anno_info)
-
- return img_info
-
-
-def split_train_val_list(full_list, val_ratio):
- """Split list by val_ratio.
-
- Args:
- full_list (list): List to be splited
- val_ratio (float): Split ratio for val set
-
- return:
- list(list, list): Train_list and val_list
- """
- n_total = len(full_list)
- offset = int(n_total * val_ratio)
- if n_total == 0 or offset < 1:
- return [], full_list
- val_list = full_list[:offset]
- train_list = full_list[offset:]
- return [train_list, val_list]
-
-
-def generate_ann(root_path, image_infos, preserve_vertical, val_ratio):
- """Generate cropped annotations and label txt file.
-
- Args:
- root_path (str): The root path of the dataset
- split (str): The split of dataset. Namely: training or test
- image_infos (list[dict]): A list of dicts of the img and
- annotation information
- preserve_vertical (bool): Whether to preserve vertical texts
- val_ratio (float): Split ratio for val set
- """
-
- assert val_ratio <= 1.
-
- if val_ratio:
- image_infos = split_train_val_list(image_infos, val_ratio)
- splits = ['training', 'val']
-
- else:
- image_infos = [image_infos]
- splits = ['training']
-
- for i, split in enumerate(splits):
- dst_image_root = osp.join(root_path, 'crops', split)
- ignore_image_root = osp.join(root_path, 'ignores', split)
- dst_label_file = osp.join(root_path, f'{split}_label.json')
- os.makedirs(dst_image_root, exist_ok=True)
-
- img_info = []
- for image_info in image_infos[i]:
- index = 1
- src_img_path = osp.join(root_path, 'imgs', image_info['file_name'])
- image = mmcv.imread(src_img_path)
- src_img_root = image_info['file_name'].split('.')[0]
-
- for anno in image_info['anno_info']:
- word = anno['word']
- dst_img = crop_img(image, anno['bbox'], 0, 0)
- h, w, _ = dst_img.shape
-
- dst_img_name = f'{src_img_root}_{index}.png'
- index += 1
- # Skip invalid annotations
- if min(dst_img.shape) == 0:
- continue
- # Skip vertical texts
- if not preserve_vertical and h / w > 2 and split == 'training':
- dst_img_path = osp.join(ignore_image_root, dst_img_name)
- mmcv.imwrite(dst_img, dst_img_path)
- continue
-
- dst_img_path = osp.join(dst_image_root, dst_img_name)
- mmcv.imwrite(dst_img, dst_img_path)
- img_info.append({
- 'file_name': dst_img_name,
- 'anno_info': [{
- 'text': word
- }]
- })
-
- ensure_ascii = dict(ensure_ascii=False)
- dump_ocr_data(img_info, dst_label_file, 'textrecog', **ensure_ascii)
-
-
-def parse_args():
- parser = argparse.ArgumentParser(
- description='Generate training and val set of ILST ')
- parser.add_argument('root_path', help='Root dir path of ILST')
- parser.add_argument(
- '--preserve-vertical',
- help='Preserve samples containing vertical texts',
- action='store_true')
- parser.add_argument(
- '--val-ratio', help='Split ratio for val set', default=0., type=float)
- parser.add_argument(
- '--nproc', default=1, type=int, help='Number of processes')
- args = parser.parse_args(['data/IIIT-ILST'])
- return args
-
-
-def main():
- args = parse_args()
- root_path = args.root_path
- with mmengine.Timer(print_tmpl='It takes {}s to convert ILST annotation'):
- files = collect_files(
- osp.join(root_path, 'imgs'), osp.join(root_path, 'annotations'))
- image_infos = collect_annotations(files, nproc=args.nproc)
- # filter broken images
- image_infos = list(filter(None, image_infos))
- generate_ann(root_path, image_infos, args.preserve_vertical,
- args.val_ratio)
-
-
-if __name__ == '__main__':
- main()
diff --git a/pipeline/mmocr/tools/dataset_converters/textrecog/imgur_converter.py b/pipeline/mmocr/tools/dataset_converters/textrecog/imgur_converter.py
deleted file mode 100644
index 34d161eb3d39674dc5a64d98248e00d8760e0c42..0000000000000000000000000000000000000000
--- a/pipeline/mmocr/tools/dataset_converters/textrecog/imgur_converter.py
+++ /dev/null
@@ -1,175 +0,0 @@
-# Copyright (c) OpenMMLab. All rights reserved.
-import argparse
-import math
-import os
-import os.path as osp
-
-import mmcv
-import mmengine
-import numpy as np
-
-from mmocr.utils import crop_img, dump_ocr_data
-
-
-def parse_args():
- parser = argparse.ArgumentParser(
- description='Generate training, validation and test set of IMGUR ')
- parser.add_argument('root_path', help='Root dir path of IMGUR')
- args = parser.parse_args()
-
- return args
-
-
-def collect_imgur_info(root_path, annotation_filename, print_every=1000):
-
- annotation_path = osp.join(root_path, 'annotations', annotation_filename)
- if not osp.exists(annotation_path):
- raise Exception(
- f'{annotation_path} not exists, please check and try again.')
-
- annotation = mmengine.load(annotation_path)
- images = annotation['index_to_ann_map'].keys()
- img_infos = []
- for i, img_name in enumerate(images):
- if i >= 0 and i % print_every == 0:
- print(f'{i}/{len(images)}')
-
- img_path = osp.join(root_path, 'imgs', img_name + '.jpg')
-
- # Skip not exist images
- if not osp.exists(img_path):
- continue
-
- img = mmcv.imread(img_path, 'unchanged')
-
- # Skip broken images
- if img is None:
- continue
-
- img_info = dict(
- file_name=img_name + '.jpg',
- height=img.shape[0],
- width=img.shape[1])
-
- anno_info = []
- for ann_id in annotation['index_to_ann_map'][img_name]:
- ann = annotation['ann_id'][ann_id]
-
- # The original annotation is oriented rects [x, y, w, h, a]
- box = np.fromstring(
- ann['bounding_box'][1:-2], sep=',', dtype=float)
- bbox = convert_oriented_box(box)
- word = ann['word']
-
- anno = dict(bbox=bbox, word=word)
- anno_info.append(anno)
- img_info.update(anno_info=anno_info)
- img_infos.append(img_info)
-
- return img_infos
-
-
-def convert_oriented_box(box):
-
- x_ctr, y_ctr, width, height, angle = box[:5]
- angle = -angle * math.pi / 180
-
- tl_x, tl_y, br_x, br_y = -width / 2, -height / 2, width / 2, height / 2
- rect = np.array([[tl_x, br_x, br_x, tl_x], [tl_y, tl_y, br_y, br_y]])
- R = np.array([[np.cos(angle), -np.sin(angle)],
- [np.sin(angle), np.cos(angle)]])
- poly = R.dot(rect)
- x0, x1, x2, x3 = poly[0, :4] + x_ctr
- y0, y1, y2, y3 = poly[1, :4] + y_ctr
- poly = np.array([x0, y0, x1, y1, x2, y2, x3, y3], dtype=np.float32)
- poly = get_best_begin_point_single(poly)
-
- return poly.tolist()
-
-
-def get_best_begin_point_single(coordinate):
-
- x1, y1, x2, y2, x3, y3, x4, y4 = coordinate
- xmin = min(x1, x2, x3, x4)
- ymin = min(y1, y2, y3, y4)
- xmax = max(x1, x2, x3, x4)
- ymax = max(y1, y2, y3, y4)
- combine = [[[x1, y1], [x2, y2], [x3, y3], [x4, y4]],
- [[x2, y2], [x3, y3], [x4, y4], [x1, y1]],
- [[x3, y3], [x4, y4], [x1, y1], [x2, y2]],
- [[x4, y4], [x1, y1], [x2, y2], [x3, y3]]]
- dst_coordinate = [[xmin, ymin], [xmax, ymin], [xmax, ymax], [xmin, ymax]]
- force = 100000000.0
- force_flag = 0
- for i in range(4):
- temp_force = cal_line_length(combine[i][0], dst_coordinate[0]) \
- + cal_line_length(combine[i][1], dst_coordinate[1]) \
- + cal_line_length(combine[i][2], dst_coordinate[2]) \
- + cal_line_length(combine[i][3], dst_coordinate[3])
- if temp_force < force:
- force = temp_force
- force_flag = i
- if force_flag != 0:
- pass
-
- return np.array(combine[force_flag]).reshape(8)
-
-
-def cal_line_length(point1, point2):
-
- return math.sqrt(
- math.pow(point1[0] - point2[0], 2) +
- math.pow(point1[1] - point2[1], 2))
-
-
-def generate_ann(root_path, split, image_infos):
-
- dst_image_root = osp.join(root_path, 'crops', split)
- dst_label_file = osp.join(root_path, f'{split}_label.json')
- os.makedirs(dst_image_root, exist_ok=True)
-
- img_info = []
- for image_info in image_infos:
- index = 1
- src_img_path = osp.join(root_path, 'imgs', image_info['file_name'])
- image = mmcv.imread(src_img_path)
- src_img_root = image_info['file_name'].split('.')[0]
-
- for anno in image_info['anno_info']:
- word = anno['word']
- dst_img = crop_img(image, anno['bbox'], 0, 0)
-
- # Skip invalid annotations
- if min(dst_img.shape) == 0:
- continue
-
- dst_img_name = f'{src_img_root}_{index}.png'
- index += 1
- dst_img_path = osp.join(dst_image_root, dst_img_name)
- mmcv.imwrite(dst_img, dst_img_path)
-
- img_info.append({
- 'file_name': dst_img_name,
- 'anno_info': [{
- 'text': word
- }]
- })
-
- dump_ocr_data(img_info, dst_label_file, 'textrecog')
-
-
-def main():
- args = parse_args()
- root_path = args.root_path
-
- for split in ['train', 'val', 'test']:
- print(f'Processing {split} set...')
- with mmengine.Timer(
- print_tmpl='It takes {}s to convert IMGUR annotation'):
- anno_infos = collect_imgur_info(
- root_path, f'imgur5k_annotations_{split}.json')
- generate_ann(root_path, split, anno_infos)
-
-
-if __name__ == '__main__':
- main()
diff --git a/pipeline/mmocr/tools/dataset_converters/textrecog/kaist_converter.py b/pipeline/mmocr/tools/dataset_converters/textrecog/kaist_converter.py
deleted file mode 100644
index 525e9be96d3652746d074bb2e924c62e7bb5b421..0000000000000000000000000000000000000000
--- a/pipeline/mmocr/tools/dataset_converters/textrecog/kaist_converter.py
+++ /dev/null
@@ -1,259 +0,0 @@
-# Copyright (c) OpenMMLab. All rights reserved.
-import argparse
-import math
-import os
-import os.path as osp
-import xml.etree.ElementTree as ET
-
-import mmcv
-import mmengine
-
-from mmocr.utils import crop_img, dump_ocr_data
-
-
-def collect_files(img_dir, gt_dir, ratio):
- """Collect all images and their corresponding groundtruth files.
-
- Args:
- img_dir (str): The image directory
- gt_dir (str): The groundtruth directory
- ratio (float): Split ratio for val set
-
- Returns:
- files (list): The list of tuples (img_file, groundtruth_file)
- """
- assert isinstance(img_dir, str)
- assert img_dir
- assert isinstance(gt_dir, str)
- assert gt_dir
- assert isinstance(ratio, float)
- assert ratio < 1.0, 'val_ratio should be a float between 0.0 to 1.0'
-
- ann_list, imgs_list = [], []
- for img_file in os.listdir(img_dir):
- ann_list.append(osp.join(gt_dir, img_file.split('.')[0] + '.xml'))
- imgs_list.append(osp.join(img_dir, img_file))
-
- all_files = list(zip(sorted(imgs_list), sorted(ann_list)))
- assert len(all_files), f'No images found in {img_dir}'
- print(f'Loaded {len(all_files)} images from {img_dir}')
-
- trn_files, val_files = [], []
- if ratio > 0:
- for i, file in enumerate(all_files):
- if i % math.floor(1 / ratio):
- trn_files.append(file)
- else:
- val_files.append(file)
- else:
- trn_files, val_files = all_files, []
-
- print(f'training #{len(trn_files)}, val #{len(val_files)}')
-
- return trn_files, val_files
-
-
-def collect_annotations(files, nproc=1):
- """Collect the annotation information.
-
- Args:
- files (list): The list of tuples (image_file, groundtruth_file)
- nproc (int): The number of process to collect annotations
-
- Returns:
- images (list): The list of image information dicts
- """
- assert isinstance(files, list)
- assert isinstance(nproc, int)
-
- if nproc > 1:
- images = mmengine.track_parallel_progress(
- load_img_info, files, nproc=nproc)
- else:
- images = mmengine.track_progress(load_img_info, files)
-
- return images
-
-
-def load_img_info(files):
- """Load the information of one image.
-
- Args:
- files (tuple): The tuple of (img_file, groundtruth_file)
-
- Returns:
- img_info (dict): The dict of the img and annotation information
- """
- assert isinstance(files, tuple)
-
- img_file, gt_file = files
- assert osp.basename(gt_file).split('.')[0] == osp.basename(img_file).split(
- '.')[0]
- # read imgs while ignoring orientations
- img = mmcv.imread(img_file, 'unchanged')
-
- img_info = dict(
- file_name=osp.join(osp.basename(img_file)),
- height=img.shape[0],
- width=img.shape[1],
- segm_file=osp.join(osp.basename(gt_file)))
-
- if osp.splitext(gt_file)[1] == '.xml':
- img_info = load_xml_info(gt_file, img_info)
- else:
- raise NotImplementedError
-
- return img_info
-
-
-def load_xml_info(gt_file, img_info):
- """Collect the annotation information.
-
- Annotation Format
-
- DSC02306.JPG
-
-
-
-
-
-
-
-
-
-
-
-
- no
- 2
-
-
-
-
- Args:
- gt_file (str): The path to ground-truth
- img_info (dict): The dict of the img and annotation information
-
- Returns:
- img_info (dict): The dict of the img and annotation information
- """
-
- obj = ET.parse(gt_file)
- root = obj.getroot()
- anno_info = []
- for word in root.iter('word'):
- x, y = max(0, int(word.attrib['x'])), max(0, int(word.attrib['y']))
- w, h = int(word.attrib['width']), int(word.attrib['height'])
- bbox = [x, y, x + w, y, x + w, y + h, x, y + h]
- chars = []
- for character in word.iter('character'):
- chars.append(character.attrib['char'])
- word = ''.join(chars)
- if len(word) == 0:
- continue
- anno = dict(bbox=bbox, word=word)
- anno_info.append(anno)
-
- img_info.update(anno_info=anno_info)
-
- return img_info
-
-
-def generate_ann(root_path, split, image_infos, preserve_vertical):
- """Generate cropped annotations and label txt file.
-
- Args:
- root_path (str): The root path of the dataset
- split (str): The split of dataset. Namely: training or test
- image_infos (list[dict]): A list of dicts of the img and
- annotation information
- preserve_vertical (bool): Whether to preserve vertical texts
- format (str): Annotation format, should be either 'txt' or 'jsonl'
- """
-
- dst_image_root = osp.join(root_path, 'crops', split)
- ignore_image_root = osp.join(root_path, 'ignores', split)
- if split == 'training':
- dst_label_file = osp.join(root_path, 'train_label.json')
- elif split == 'val':
- dst_label_file = osp.join(root_path, 'val_label.json')
- mmengine.mkdir_or_exist(dst_image_root)
- mmengine.mkdir_or_exist(ignore_image_root)
-
- img_info = []
- for image_info in image_infos:
- index = 1
- src_img_path = osp.join(root_path, 'imgs', image_info['file_name'])
- image = mmcv.imread(src_img_path)
- src_img_root = image_info['file_name'].split('.')[0]
-
- for anno in image_info['anno_info']:
- word = anno['word']
- dst_img = crop_img(image, anno['bbox'], 0, 0)
- h, w, _ = dst_img.shape
-
- dst_img_name = f'{src_img_root}_{index}.png'
- index += 1
- # Skip invalid annotations
- if min(dst_img.shape) == 0:
- continue
- # Filter out vertical texts
- if not preserve_vertical and h / w > 2:
- dst_img_path = osp.join(ignore_image_root, dst_img_name)
- mmcv.imwrite(dst_img, dst_img_path)
- continue
-
- dst_img_path = osp.join(dst_image_root, dst_img_name)
- mmcv.imwrite(dst_img, dst_img_path)
-
- img_info.append({
- 'file_name': dst_img_name,
- 'anno_info': [{
- 'text': word
- }]
- })
-
- ensure_ascii = dict(ensure_ascii=False)
- dump_ocr_data(img_info, dst_label_file, 'textrecog', **ensure_ascii)
-
-
-def parse_args():
- parser = argparse.ArgumentParser(
- description='Generate training and val set of KAIST ')
- parser.add_argument('root_path', help='Root dir path of KAIST')
- parser.add_argument(
- '--val-ratio', help='Split ratio for val set', default=0.0, type=float)
- parser.add_argument(
- '--preserve-vertical',
- help='Preserve samples containing vertical texts',
- action='store_true')
- parser.add_argument(
- '--nproc', default=1, type=int, help='Number of process')
- args = parser.parse_args()
- return args
-
-
-def main():
- args = parse_args()
- root_path = args.root_path
- ratio = args.val_ratio
-
- trn_files, val_files = collect_files(
- osp.join(root_path, 'imgs'), osp.join(root_path, 'annotations'), ratio)
-
- # Train set
- trn_infos = collect_annotations(trn_files, nproc=args.nproc)
- with mmengine.Timer(
- print_tmpl='It takes {}s to convert KAIST Training annotation'):
- generate_ann(root_path, 'training', trn_infos, args.preserve_vertical)
-
- # Val set
- if len(val_files) > 0:
- val_infos = collect_annotations(val_files, nproc=args.nproc)
- with mmengine.Timer(
- print_tmpl='It takes {}s to convert KAIST Val annotation'):
- generate_ann(root_path, 'val', val_infos, args.preserve_vertical)
-
-
-if __name__ == '__main__':
- main()
diff --git a/pipeline/mmocr/tools/dataset_converters/textrecog/lmdb_converter.py b/pipeline/mmocr/tools/dataset_converters/textrecog/lmdb_converter.py
deleted file mode 100644
index 68afd28f3cc41ad819faf478e5068e787c4fb32b..0000000000000000000000000000000000000000
--- a/pipeline/mmocr/tools/dataset_converters/textrecog/lmdb_converter.py
+++ /dev/null
@@ -1,175 +0,0 @@
-# Copyright (c) OpenMMLab. All rights reserved.
-import argparse
-import json
-import os
-import os.path as osp
-
-import cv2
-import lmdb
-import numpy as np
-
-from mmocr.utils import list_from_file
-
-
-def parse_line(line, format):
- if format == 'txt':
- img_name, text = line.split(' ')
- else:
- line = json.loads(line)
- img_name = line['filename']
- text = line['text']
- return img_name, text
-
-
-def check_image_is_valid(imageBin):
- if imageBin is None:
- return False
- imageBuf = np.frombuffer(imageBin, dtype=np.uint8)
- img = cv2.imdecode(imageBuf, cv2.IMREAD_GRAYSCALE)
- imgH, imgW = img.shape[0], img.shape[1]
- if imgH * imgW == 0:
- return False
- return True
-
-
-def write_cache(env, cache):
- with env.begin(write=True) as txn:
- cursor = txn.cursor()
- cursor.putmulti(cache, dupdata=False, overwrite=True)
-
-
-def recog2lmdb(img_root,
- label_path,
- output,
- label_format='txt',
- label_only=False,
- batch_size=1000,
- encoding='utf-8',
- lmdb_map_size=1099511627776,
- verify=True):
- """Create text recognition dataset to LMDB format.
-
- Args:
- img_root (str): Path to images.
- label_path (str): Path to label file.
- output (str): LMDB output path.
- label_format (str): Format of the label file, either txt or jsonl.
- label_only (bool): Only convert label to lmdb format.
- batch_size (int): Number of files written to the cache each time.
- encoding (str): Label encoding method.
- lmdb_map_size (int): Maximum size database may grow to.
- verify (bool): If true, check the validity of
- every image.Defaults to True.
-
- E.g.
- This function supports MMOCR's recognition data format and the label file
- can be txt or jsonl, as follows:
-
- โโโimg_root
- | |โโ img1.jpg
- | |โโ img2.jpg
- | |โโ ...
- |โโlabel.txt (or label.jsonl)
-
- label.txt: img1.jpg HELLO
- img2.jpg WORLD
- ...
-
- label.jsonl: {'filename':'img1.jpg', 'text':'HELLO'}
- {'filename':'img2.jpg', 'text':'WORLD'}
- ...
- """
- # check label format
- assert osp.basename(label_path).split('.')[-1] == label_format
- # create lmdb env
- os.makedirs(output, exist_ok=True)
- env = lmdb.open(output, map_size=lmdb_map_size)
- # load label file
- anno_list = list_from_file(label_path, encoding=encoding)
- cache = []
- # index start from 1
- cnt = 1
- n_samples = len(anno_list)
- for anno in anno_list:
- label_key = 'label-%09d'.encode(encoding) % cnt
- img_name, text = parse_line(anno, label_format)
- if label_only:
- # convert only labels to lmdb
- line = json.dumps(
- dict(filename=img_name, text=text), ensure_ascii=False)
- cache.append((label_key, line.encode(encoding)))
- else:
- # convert both images and labels to lmdb
- img_path = osp.join(img_root, img_name)
- if not osp.exists(img_path):
- print('%s does not exist' % img_path)
- continue
- with open(img_path, 'rb') as f:
- image_bin = f.read()
- if verify:
- try:
- if not check_image_is_valid(image_bin):
- print('%s is not a valid image' % img_path)
- continue
- except Exception:
- print('error occurred at ', img_name)
- image_key = 'image-%09d'.encode(encoding) % cnt
- cache.append((image_key, image_bin))
- cache.append((label_key, text.encode(encoding)))
-
- if cnt % batch_size == 0:
- write_cache(env, cache)
- cache = []
- print('Written %d / %d' % (cnt, n_samples))
- cnt += 1
- n_samples = cnt - 1
- cache.append(
- ('num-samples'.encode(encoding), str(n_samples).encode(encoding)))
- write_cache(env, cache)
- print('Created lmdb dataset with %d samples' % n_samples)
-
-
-def main():
- parser = argparse.ArgumentParser()
- parser.add_argument('label_path', type=str, help='Path to label file')
- parser.add_argument('output', type=str, help='Output lmdb path')
- parser.add_argument(
- '--img-root', '-i', type=str, help='Input imglist path')
- parser.add_argument(
- '--label-only',
- action='store_true',
- help='Only converter label to lmdb')
- parser.add_argument(
- '--label-format',
- '-f',
- default='txt',
- choices=['txt', 'jsonl'],
- help='The format of the label file, either txt or jsonl')
- parser.add_argument(
- '--batch-size',
- '-b',
- type=int,
- default=1000,
- help='Processing batch size, defaults to 1000')
- parser.add_argument(
- '--encoding',
- '-e',
- type=str,
- default='utf8',
- help='Bytes coding scheme, defaults to utf8')
- parser.add_argument(
- '--lmdb-map-size',
- '-m',
- type=int,
- default=1099511627776,
- help='Maximum size database may grow to, '
- 'defaults to 1099511627776 bytes (1TB)')
- opt = parser.parse_args()
-
- assert opt.img_root or opt.label_only
- recog2lmdb(opt.img_root, opt.label_path, opt.output, opt.label_format,
- opt.label_only, opt.batch_size, opt.encoding, opt.lmdb_map_size)
-
-
-if __name__ == '__main__':
- main()
diff --git a/pipeline/mmocr/tools/dataset_converters/textrecog/lsvt_converter.py b/pipeline/mmocr/tools/dataset_converters/textrecog/lsvt_converter.py
deleted file mode 100644
index b1f581974967cc6eebb8491fd163bd026e925fbb..0000000000000000000000000000000000000000
--- a/pipeline/mmocr/tools/dataset_converters/textrecog/lsvt_converter.py
+++ /dev/null
@@ -1,186 +0,0 @@
-# Copyright (c) OpenMMLab. All rights reserved.
-import argparse
-import math
-import os.path as osp
-from functools import partial
-
-import mmcv
-import mmengine
-
-from mmocr.utils import dump_ocr_data
-
-
-def parse_args():
- parser = argparse.ArgumentParser(
- description='Generate training and validation set of LSVT ')
- parser.add_argument('root_path', help='Root dir path of LSVT')
- parser.add_argument(
- '--val-ratio', help='Split ratio for val set', default=0.0, type=float)
- parser.add_argument(
- '--nproc', default=1, type=int, help='Number of processes')
- parser.add_argument(
- '--preserve-vertical',
- help='Preserve samples containing vertical texts',
- action='store_true')
- args = parser.parse_args()
- return args
-
-
-def process_img(args, dst_image_root, ignore_image_root, preserve_vertical,
- split):
- # Dirty hack for multi-processing
- img_idx, img_info, anns = args
- src_img = mmcv.imread(img_info['file_name'])
- img_info = []
- for ann_idx, ann in enumerate(anns):
- segmentation = []
- for x, y in ann['points']:
- segmentation.append(max(0, x))
- segmentation.append(max(0, y))
- xs, ys = segmentation[::2], segmentation[1::2]
- x, y = min(xs), min(ys)
- w, h = max(xs) - x, max(ys) - y
- text_label = ann['transcription']
-
- dst_img = src_img[y:y + h, x:x + w]
- dst_img_name = f'img_{img_idx}_{ann_idx}.jpg'
-
- if not preserve_vertical and h / w > 2 and split == 'train':
- dst_img_path = osp.join(ignore_image_root, dst_img_name)
- mmcv.imwrite(dst_img, dst_img_path)
- continue
-
- dst_img_path = osp.join(dst_image_root, dst_img_name)
- mmcv.imwrite(dst_img, dst_img_path)
-
- img_info.append({
- 'file_name': dst_img_name,
- 'anno_info': [{
- 'text': text_label
- }]
- })
-
- return img_info
-
-
-def convert_lsvt(root_path,
- split,
- ratio,
- preserve_vertical,
- nproc,
- img_start_idx=0):
- """Collect the annotation information and crop the images.
-
- The annotation format is as the following:
- [
- {'gt_1234': # 'gt_1234' is file name
- [
- {
- 'transcription': 'ไธ็ซๅผ่ดญ็ฉไธญๅฟ',
- 'points': [[45, 272], [215, 273], [212, 296], [45, 290]]
- 'illegibility': False
- }, ...
- ]
- }
- ]
-
-
- Args:
- root_path (str): The root path of the dataset
- split (str): The split of dataset. Namely: training or val
- ratio (float): Split ratio for val set
- preserve_vertical (bool): Whether to preserve vertical texts
- nproc (int): The number of process to collect annotations
- img_start_idx (int): Index of start image
-
- Returns:
- img_info (dict): The dict of the img and annotation information
- """
-
- annotation_path = osp.join(root_path, 'annotations/train_full_labels.json')
- if not osp.exists(annotation_path):
- raise Exception(
- f'{annotation_path} not exists, please check and try again.')
-
- annotation = mmengine.load(annotation_path)
- # outputs
- dst_label_file = osp.join(root_path, f'{split}_label.json')
- dst_image_root = osp.join(root_path, 'crops', split)
- ignore_image_root = osp.join(root_path, 'ignores', split)
- src_image_root = osp.join(root_path, 'imgs')
- mmengine.mkdir_or_exist(dst_image_root)
- mmengine.mkdir_or_exist(ignore_image_root)
-
- process_img_with_path = partial(
- process_img,
- dst_image_root=dst_image_root,
- ignore_image_root=ignore_image_root,
- preserve_vertical=preserve_vertical,
- split=split)
-
- img_prefixes = annotation.keys()
-
- trn_files, val_files = [], []
- if ratio > 0:
- for i, file in enumerate(img_prefixes):
- if i % math.floor(1 / ratio):
- trn_files.append(file)
- else:
- val_files.append(file)
- else:
- trn_files, val_files = img_prefixes, []
- print(f'training #{len(trn_files)}, val #{len(val_files)}')
-
- if split == 'train':
- img_prefixes = trn_files
- elif split == 'val':
- img_prefixes = val_files
- else:
- raise NotImplementedError
-
- tasks = []
- idx = 0
- for img_idx, prefix in enumerate(img_prefixes):
- img_file = osp.join(src_image_root, prefix + '.jpg')
- img_info = {'file_name': img_file}
- # Skip not exist images
- if not osp.exists(img_file):
- continue
- tasks.append((img_idx + img_start_idx, img_info, annotation[prefix]))
- idx = idx + 1
-
- labels_list = mmengine.track_parallel_progress(
- process_img_with_path, tasks, keep_order=True, nproc=nproc)
- final_labels = []
- for label_list in labels_list:
- final_labels += label_list
-
- dump_ocr_data(final_labels, dst_label_file, 'textrecog')
-
- return idx
-
-
-def main():
- args = parse_args()
- root_path = args.root_path
- print('Processing training set...')
- num_train_imgs = convert_lsvt(
- root_path=root_path,
- split='train',
- ratio=args.val_ratio,
- preserve_vertical=args.preserve_vertical,
- nproc=args.nproc)
- if args.val_ratio > 0:
- print('Processing validation set...')
- convert_lsvt(
- root_path=root_path,
- split='val',
- ratio=args.val_ratio,
- preserve_vertical=args.preserve_vertical,
- nproc=args.nproc,
- img_start_idx=num_train_imgs)
- print('Finish')
-
-
-if __name__ == '__main__':
- main()
diff --git a/pipeline/mmocr/tools/dataset_converters/textrecog/lv_converter.py b/pipeline/mmocr/tools/dataset_converters/textrecog/lv_converter.py
deleted file mode 100644
index d22c60b224d7fb122ebe26b2729650a961aac992..0000000000000000000000000000000000000000
--- a/pipeline/mmocr/tools/dataset_converters/textrecog/lv_converter.py
+++ /dev/null
@@ -1,68 +0,0 @@
-# Copyright (c) OpenMMLab. All rights reserved.
-import argparse
-import os.path as osp
-
-from mmocr.utils import dump_ocr_data
-
-
-def convert_annotations(root_path, split):
- """Convert original annotations to mmocr format.
-
- The annotation format is as the following:
- Crops/val/11/1/1.png weighted
- Crops/val/11/1/2.png 26
- Crops/val/11/1/3.png casting
- Crops/val/11/1/4.png 28
- After this module, the annotation has been changed to the format below:
- jsonl:
- {'filename': 'Crops/val/11/1/1.png', 'text': 'weighted'}
- {'filename': 'Crops/val/11/1/1.png', 'text': '26'}
- {'filename': 'Crops/val/11/1/1.png', 'text': 'casting'}
- {'filename': 'Crops/val/11/1/1.png', 'text': '28'}
-
- Args:
- root_path (str): The root path of the dataset
- split (str): The split of dataset. Namely: training or test
- """
- assert isinstance(root_path, str)
- assert isinstance(split, str)
-
- img_info = []
- with open(
- osp.join(root_path, f'{split}_label.txt'),
- encoding='"utf-8-sig') as f:
- annos = f.readlines()
- for anno in annos:
- if anno:
- # Text may contain spaces
- dst_img_name, word = anno.split('png ')
- word = word.strip('\n')
- img_info.append({
- 'file_name': dst_img_name + 'png',
- 'anno_info': [{
- 'text': word
- }]
- })
- dump_ocr_data(img_info, osp.join(root_path, f'{split.lower()}_label.json'),
- 'textrecog')
-
-
-def parse_args():
- parser = argparse.ArgumentParser(
- description='Generate training and test set of Lecture Video DB')
- parser.add_argument('root_path', help='Root dir path of Lecture Video DB')
- args = parser.parse_args()
- return args
-
-
-def main():
- args = parse_args()
- root_path = args.root_path
-
- for split in ['train', 'val', 'test']:
- convert_annotations(root_path, split)
- print(f'{split} split converted.')
-
-
-if __name__ == '__main__':
- main()
diff --git a/pipeline/mmocr/tools/dataset_converters/textrecog/mtwi_converter.py b/pipeline/mmocr/tools/dataset_converters/textrecog/mtwi_converter.py
deleted file mode 100644
index 86d4e4e895b36a225fd2e916b675dcdf3abe8d17..0000000000000000000000000000000000000000
--- a/pipeline/mmocr/tools/dataset_converters/textrecog/mtwi_converter.py
+++ /dev/null
@@ -1,251 +0,0 @@
-# Copyright (c) OpenMMLab. All rights reserved.
-import argparse
-import math
-import os
-import os.path as osp
-
-import cv2
-import mmcv
-import mmengine
-from PIL import Image
-
-from mmocr.utils import crop_img, dump_ocr_data
-
-
-def collect_files(img_dir, gt_dir, ratio):
- """Collect all images and their corresponding groundtruth files.
- Args:
- img_dir (str): The image directory
- gt_dir (str): The groundtruth directory
- ratio (float): Split ratio for val set
-
- Returns:
- files (list): The list of tuples (img_file, groundtruth_file)
- """
- assert isinstance(img_dir, str)
- assert img_dir
- assert isinstance(gt_dir, str)
- assert gt_dir
- assert isinstance(ratio, float)
- assert ratio < 1.0, 'val_ratio should be a float between 0.0 to 1.0'
-
- ann_list, imgs_list = [], []
- for ann_file in os.listdir(gt_dir):
- img_file = osp.join(img_dir, ann_file.replace('txt', 'jpg'))
- # This dataset contains some images obtained from .gif,
- # which cannot be loaded by mmcv.imread(), convert them
- # to RGB mode.
- try:
- if mmcv.imread(img_file) is None:
- print(f'Convert {img_file} to RGB mode.')
- img = Image.open(img_file)
- img = img.convert('RGB')
- img.save(img_file)
- except cv2.error:
- print(f'Skip broken img {img_file}')
- continue
-
- ann_list.append(osp.join(gt_dir, ann_file))
- imgs_list.append(img_file)
-
- all_files = list(zip(imgs_list, ann_list))
- assert len(all_files), f'No images found in {img_dir}'
- print(f'Loaded {len(all_files)} images from {img_dir}')
-
- trn_files, val_files = [], []
- if ratio > 0:
- for i, file in enumerate(all_files):
- if i % math.floor(1 / ratio):
- trn_files.append(file)
- else:
- val_files.append(file)
- else:
- trn_files, val_files = all_files, []
-
- print(f'training #{len(trn_files)}, val #{len(val_files)}')
-
- return trn_files, val_files
-
-
-def collect_annotations(files, nproc=1):
- """Collect the annotation information.
- Args:
- files (list): The list of tuples (image_file, groundtruth_file)
- nproc (int): The number of process to collect annotations
-
- Returns:
- images (list): The list of image information dicts
- """
- assert isinstance(files, list)
- assert isinstance(nproc, int)
-
- if nproc > 1:
- images = mmengine.track_parallel_progress(
- load_img_info, files, nproc=nproc)
- else:
- images = mmengine.track_progress(load_img_info, files)
-
- return images
-
-
-def load_img_info(files):
- """Load the information of one image.
- Args:
- files (tuple): The tuple of (img_file, groundtruth_file)
-
- Returns:
- img_info (dict): The dict of the img and annotation information
- """
- assert isinstance(files, tuple)
-
- img_file, gt_file = files
- assert osp.basename(gt_file).split('.')[0] == osp.basename(img_file).split(
- '.')[0]
- # read imgs while ignoring orientations
- img = mmcv.imread(img_file)
-
- img_info = dict(
- file_name=osp.join(osp.basename(img_file)),
- height=img.shape[0],
- width=img.shape[1],
- segm_file=osp.join(osp.basename(gt_file)))
-
- if osp.splitext(gt_file)[1] == '.txt':
- img_info = load_txt_info(gt_file, img_info)
- else:
- raise NotImplementedError
-
- return img_info
-
-
-def load_txt_info(gt_file, img_info):
- """Collect the annotation information.
-
- The annotation format is as the following:
- x1,y1,x2,y2,x3,y3,x4,y4,text
-
- 45.45,226.83,11.87,181.79,183.84,13.1,233.79,49.95,ๆถๅฐ่ข่ข
- 345.98,311.18,345.98,347.21,462.26,347.21,462.26,311.18,73774
- 462.26,292.34,461.44,299.71,502.39,299.71,502.39,292.34,73/74/737
-
- Args:
- gt_file (str): The path to ground-truth
- img_info (dict): The dict of the img and annotation information
-
- Returns:
- img_info (dict): The dict of the img and annotation information
- """
-
- anno_info = []
- with open(gt_file) as f:
- lines = f.readlines()
- for line in lines:
- points = line.split(',')[0:8]
- word = line.split(',')[8].rstrip('\n')
- if word == '###':
- continue
- bbox = [math.floor(float(pt)) for pt in points]
- anno = dict(bbox=bbox, word=word)
- anno_info.append(anno)
-
- img_info.update(anno_info=anno_info)
-
- return img_info
-
-
-def generate_ann(root_path, split, image_infos, preserve_vertical):
- """Generate cropped annotations and label txt file.
-
- Args:
- root_path (str): The root path of the dataset
- split (str): The split of dataset. Namely: training or test
- image_infos (list[dict]): A list of dicts of the img and
- annotation information
- preserve_vertical (bool): Whether to preserve vertical texts
- """
- print('Cropping images...')
- dst_image_root = osp.join(root_path, 'crops', split)
- ignore_image_root = osp.join(root_path, 'ignores', split)
- if split == 'training':
- dst_label_file = osp.join(root_path, 'train_label.json')
- elif split == 'val':
- dst_label_file = osp.join(root_path, 'val_label.json')
- mmengine.mkdir_or_exist(dst_image_root)
- mmengine.mkdir_or_exist(ignore_image_root)
-
- img_info = []
- for image_info in image_infos:
- index = 1
- src_img_path = osp.join(root_path, 'imgs', image_info['file_name'])
- image = mmcv.imread(src_img_path)
- src_img_root = image_info['file_name'].split('.')[0]
-
- for anno in image_info['anno_info']:
- word = anno['word']
- dst_img = crop_img(image, anno['bbox'], 0, 0)
- h, w, _ = dst_img.shape
-
- dst_img_name = f'{src_img_root}_{index}.png'
- index += 1
- # Skip invalid annotations
- if min(dst_img.shape) == 0:
- continue
- # Skip vertical texts
- if not preserve_vertical and h / w > 2 and split == 'training':
- dst_img_path = osp.join(ignore_image_root, dst_img_name)
- mmcv.imwrite(dst_img, dst_img_path)
- continue
-
- dst_img_path = osp.join(dst_image_root, dst_img_name)
- mmcv.imwrite(dst_img, dst_img_path)
-
- img_info.append({
- 'file_name': dst_img_name,
- 'anno_info': [{
- 'text': word
- }]
- })
-
- dump_ocr_data(img_info, dst_label_file, 'textrecog')
-
-
-def parse_args():
- parser = argparse.ArgumentParser(
- description='Generate training and val set of MTWI.')
- parser.add_argument('root_path', help='Root dir path of MTWI')
- parser.add_argument(
- '--val-ratio', help='Split ratio for val set', default=0.0, type=float)
- parser.add_argument(
- '--preserve-vertical',
- help='Preserve samples containing vertical texts',
- action='store_true')
- parser.add_argument(
- '--nproc', default=1, type=int, help='Number of process')
- args = parser.parse_args()
- return args
-
-
-def main():
- args = parse_args()
- root_path = args.root_path
- ratio = args.val_ratio
-
- trn_files, val_files = collect_files(
- osp.join(root_path, 'imgs'), osp.join(root_path, 'annotations'), ratio)
-
- # Train set
- trn_infos = collect_annotations(trn_files, nproc=args.nproc)
- with mmengine.Timer(
- print_tmpl='It takes {}s to convert MTWI Training annotation'):
- generate_ann(root_path, 'training', trn_infos, args.preserve_vertical)
-
- # Val set
- if len(val_files) > 0:
- val_infos = collect_annotations(val_files, nproc=args.nproc)
- with mmengine.Timer(
- print_tmpl='It takes {}s to convert MTWI Val annotation'):
- generate_ann(root_path, 'val', val_infos, args.preserve_vertical)
-
-
-if __name__ == '__main__':
- main()
diff --git a/pipeline/mmocr/tools/dataset_converters/textrecog/naf_converter.py b/pipeline/mmocr/tools/dataset_converters/textrecog/naf_converter.py
deleted file mode 100644
index 3c6d84ad2d9613606e767bdd67793f65ae0e5239..0000000000000000000000000000000000000000
--- a/pipeline/mmocr/tools/dataset_converters/textrecog/naf_converter.py
+++ /dev/null
@@ -1,272 +0,0 @@
-# Copyright (c) OpenMMLab. All rights reserved.
-import argparse
-import os.path as osp
-
-import mmcv
-import mmengine
-import numpy as np
-
-from mmocr.utils import crop_img, dump_ocr_data
-
-
-def collect_files(img_dir, gt_dir, split_info):
- """Collect all images and their corresponding groundtruth files.
-
- Args:
- img_dir (str): The image directory
- gt_dir (str): The groundtruth directory
- split_info (dict): The split information for train/val/test
-
- Returns:
- files (list): The list of tuples (img_file, groundtruth_file)
- """
- assert isinstance(img_dir, str)
- assert img_dir
- assert isinstance(gt_dir, str)
- assert gt_dir
- assert isinstance(split_info, dict)
- assert split_info
-
- ann_list, imgs_list = [], []
- for group in split_info:
- for img in split_info[group]:
- image_path = osp.join(img_dir, img)
- anno_path = osp.join(gt_dir, 'groups', group,
- img.replace('jpg', 'json'))
-
- # Filtering out the missing images
- if not osp.exists(image_path) or not osp.exists(anno_path):
- continue
-
- imgs_list.append(image_path)
- ann_list.append(anno_path)
-
- files = list(zip(imgs_list, ann_list))
- assert len(files), f'No images found in {img_dir}'
- print(f'Loaded {len(files)} images from {img_dir}')
-
- return files
-
-
-def collect_annotations(files, nproc=1):
- """Collect the annotation information.
-
- Args:
- files (list): The list of tuples (image_file, groundtruth_file)
- nproc (int): The number of process to collect annotations
-
- Returns:
- images (list): The list of image information dicts
- """
- assert isinstance(files, list)
- assert isinstance(nproc, int)
-
- if nproc > 1:
- images = mmengine.track_parallel_progress(
- load_img_info, files, nproc=nproc)
- else:
- images = mmengine.track_progress(load_img_info, files)
-
- return images
-
-
-def load_img_info(files):
- """Load the information of one image.
-
- Args:
- files (tuple): The tuple of (img_file, groundtruth_file)
-
- Returns:
- img_info (dict): The dict of the img and annotation information
- """
- assert isinstance(files, tuple)
-
- img_file, gt_file = files
- assert osp.basename(gt_file).split('.')[0] == osp.basename(img_file).split(
- '.')[0]
- # Read imgs while ignoring orientations
- img = mmcv.imread(img_file, 'unchanged')
-
- img_info = dict(
- file_name=osp.join(osp.basename(img_file)),
- height=img.shape[0],
- width=img.shape[1],
- segm_file=osp.join(osp.basename(gt_file)))
-
- if osp.splitext(gt_file)[1] == '.json':
- img_info = load_json_info(gt_file, img_info)
- else:
- raise NotImplementedError
-
- return img_info
-
-
-def load_json_info(gt_file, img_info):
- """Collect the annotation information.
-
- Annotation Format
- {
- 'filedBBs': [{
- 'poly_points': [[435,1406], [466,1406], [466,1439], [435,1439]],
- "type": "fieldCheckBox",
- "id": "f0",
- "isBlank": 1, # 0:text,1:handwriting,2:print,3:blank,4:signature,
- }], ...
- "transcriptions":{
- "f38": "CASE NUMBER",
- "f29": "July 1, 1949",
- "t20": "RANK",
- "t19": "COMPANY",
- ...
- }
- }
-
- Some special characters are used in the transcription:
- "ยซtextยป" indicates that "text" had a strikethrough
- "ยฟ" indicates the transcriber could not read a character
- "ยง" indicates the whole line or word was illegible
- "" (empty string) is if the field was blank
-
- Args:
- gt_file (str): The path to ground-truth
- img_info (dict): The dict of the img and annotation information
-
- Returns:
- img_info (dict): The dict of the img and annotation information
- """
- assert isinstance(gt_file, str)
- assert isinstance(img_info, dict)
-
- annotation = mmengine.load(gt_file)
- anno_info = []
-
- # 'textBBs' contains the printed texts of the table while 'fieldBBs'
- # contains the text filled by human.
- for box_type in ['textBBs', 'fieldBBs']:
- # NAF dataset only provides transcription GT for 'filedBBs', the
- # 'textBBs' is only used for detection task.
- if box_type == 'textBBs':
- continue
- for anno in annotation[box_type]:
- # Skip images containing detection annotations only
- if 'transcriptions' not in annotation.keys():
- continue
- # Skip boxes without recognition GT
- if anno['id'] not in annotation['transcriptions'].keys():
- continue
-
- word = annotation['transcriptions'][anno['id']]
- # Skip blank boxes
- if len(word) == 0:
- continue
-
- bbox = np.array(anno['poly_points']).reshape(1, 8)[0].tolist()
-
- anno = dict(bbox=bbox, word=word)
- anno_info.append(anno)
-
- img_info.update(anno_info=anno_info)
-
- return img_info
-
-
-def generate_ann(root_path, split, image_infos, preserve_vertical):
- """Generate cropped annotations and label txt file.
-
- Args:
- root_path (str): The root path of the dataset
- split (str): The split of dataset. Namely: training or test
- image_infos (list[dict]): A list of dicts of the img and
- annotation information
- preserve_vertical (bool): Whether to preserve vertical texts
- """
-
- dst_image_root = osp.join(root_path, 'crops', split)
- ignore_image_root = osp.join(root_path, 'ignores', split)
- if split == 'training':
- dst_label_file = osp.join(root_path, 'train_label.json')
- elif split == 'val':
- dst_label_file = osp.join(root_path, 'val_label.json')
- elif split == 'test':
- dst_label_file = osp.join(root_path, 'test_label.json')
- else:
- raise NotImplementedError
- mmengine.mkdir_or_exist(dst_image_root)
- mmengine.mkdir_or_exist(ignore_image_root)
-
- img_info = []
- for image_info in image_infos:
- index = 1
- src_img_path = osp.join(root_path, 'imgs', image_info['file_name'])
- image = mmcv.imread(src_img_path)
- src_img_root = image_info['file_name'].split('.')[0]
-
- for anno in image_info['anno_info']:
- word = anno['word']
- word = word.strip('\u202a') # Remove unicode control character
- word = word.replace('ยป',
- '').replace('ยซ',
- '') # Remove strikethrough flag
- dst_img = crop_img(image, anno['bbox'], 0, 0)
- h, w, _ = dst_img.shape
-
- dst_img_name = f'{src_img_root}_{index}.png'
- index += 1
- # Skip invalid and illegible annotations
- if min(dst_img.shape) == 0 or 'ยง' in word or 'ยฟ' in word or len(
- word) == 0:
- continue
- # Skip vertical texts
- # (Do Not Filter For Val and Test Split)
- if (not preserve_vertical and h / w > 2) and split == 'training':
- dst_img_path = osp.join(ignore_image_root, dst_img_name)
- mmcv.imwrite(dst_img, dst_img_path)
- continue
-
- dst_img_path = osp.join(dst_image_root, dst_img_name)
- mmcv.imwrite(dst_img, dst_img_path)
-
- img_info.append({
- 'file_name': dst_img_name,
- 'anno_info': [{
- 'text': word
- }]
- })
-
- dump_ocr_data(img_info, dst_label_file, 'textrecog')
-
-
-def parse_args():
- parser = argparse.ArgumentParser(
- description='Generate training, val, and test set of NAF ')
- parser.add_argument('root_path', help='Root dir path of NAF')
- parser.add_argument(
- '--preserve-vertical',
- help='Preserve samples containing vertical texts',
- action='store_true')
- parser.add_argument(
- '--nproc', default=1, type=int, help='Number of process')
- args = parser.parse_args()
- return args
-
-
-def main():
- args = parse_args()
- root_path = args.root_path
- split_info = mmengine.load(
- osp.join(root_path, 'annotations', 'train_valid_test_split.json'))
- split_info['training'] = split_info.pop('train')
- split_info['val'] = split_info.pop('valid')
- for split in ['training', 'val', 'test']:
- print(f'Processing {split} set...')
- with mmengine.Timer(
- print_tmpl='It takes {}s to convert NAF annotation'):
- files = collect_files(
- osp.join(root_path, 'imgs'),
- osp.join(root_path, 'annotations'), split_info[split])
- image_infos = collect_annotations(files, nproc=args.nproc)
- generate_ann(root_path, split, image_infos, args.preserve_vertical)
-
-
-if __name__ == '__main__':
- main()
diff --git a/pipeline/mmocr/tools/dataset_converters/textrecog/openvino_converter.py b/pipeline/mmocr/tools/dataset_converters/textrecog/openvino_converter.py
deleted file mode 100644
index 79b962bbdaa9ff35e8f726234fdd2c007fb8f105..0000000000000000000000000000000000000000
--- a/pipeline/mmocr/tools/dataset_converters/textrecog/openvino_converter.py
+++ /dev/null
@@ -1,120 +0,0 @@
-# Copyright (c) OpenMMLab. All rights reserved.
-import math
-import os
-import os.path as osp
-from argparse import ArgumentParser
-from functools import partial
-
-import mmengine
-from PIL import Image
-
-from mmocr.utils import dump_ocr_data
-
-
-def parse_args():
- parser = ArgumentParser(description='Generate training and validation set '
- 'of OpenVINO annotations for Open '
- 'Images by cropping box image.')
- parser.add_argument(
- 'root_path', help='Root dir containing images and annotations')
- parser.add_argument(
- 'n_proc', default=1, type=int, help='Number of processes to run')
- args = parser.parse_args()
- return args
-
-
-def process_img(args, src_image_root, dst_image_root):
- # Dirty hack for multi-processing
- img_idx, img_info, anns = args
- src_img = Image.open(osp.join(src_image_root, img_info['file_name']))
- labels = []
- for ann_idx, ann in enumerate(anns):
- attrs = ann['attributes']
- text_label = attrs['transcription']
-
- # Ignore illegible or non-English words
- if not attrs['legible'] or attrs['language'] != 'english':
- continue
-
- x, y, w, h = ann['bbox']
- x, y = max(0, math.floor(x)), max(0, math.floor(y))
- w, h = math.ceil(w), math.ceil(h)
- dst_img = src_img.crop((x, y, x + w, y + h))
- dst_img_name = f'img_{img_idx}_{ann_idx}.jpg'
- dst_img_path = osp.join(dst_image_root, dst_img_name)
- # Preserve JPEG quality
- dst_img.save(dst_img_path, qtables=src_img.quantization)
- labels.append({
- 'file_name': dst_img_name,
- 'anno_info': [{
- 'text': text_label
- }]
- })
- src_img.close()
- return labels
-
-
-def convert_openimages(root_path,
- dst_image_path,
- dst_label_filename,
- annotation_filename,
- img_start_idx=0,
- nproc=1):
- annotation_path = osp.join(root_path, annotation_filename)
- if not osp.exists(annotation_path):
- raise Exception(
- f'{annotation_path} not exists, please check and try again.')
- src_image_root = root_path
-
- # outputs
- dst_label_file = osp.join(root_path, dst_label_filename)
- dst_image_root = osp.join(root_path, dst_image_path)
- os.makedirs(dst_image_root, exist_ok=True)
-
- annotation = mmengine.load(annotation_path)
-
- process_img_with_path = partial(
- process_img,
- src_image_root=src_image_root,
- dst_image_root=dst_image_root)
- tasks = []
- anns = {}
- for ann in annotation['annotations']:
- anns.setdefault(ann['image_id'], []).append(ann)
- for img_idx, img_info in enumerate(annotation['images']):
- tasks.append((img_idx + img_start_idx, img_info, anns[img_info['id']]))
- labels_list = mmengine.track_parallel_progress(
- process_img_with_path, tasks, keep_order=True, nproc=nproc)
- final_labels = []
- for label_list in labels_list:
- final_labels += label_list
- dump_ocr_data(final_labels, dst_label_file, 'textrecog')
- return len(annotation['images'])
-
-
-def main():
- args = parse_args()
- root_path = args.root_path
- print('Processing training set...')
- num_train_imgs = 0
- for s in '125f':
- num_train_imgs = convert_openimages(
- root_path=root_path,
- dst_image_path=f'image_{s}',
- dst_label_filename=f'train_{s}_label.json',
- annotation_filename=f'text_spotting_openimages_v5_train_{s}.json',
- img_start_idx=num_train_imgs,
- nproc=args.n_proc)
- print('Processing validation set...')
- convert_openimages(
- root_path=root_path,
- dst_image_path='image_val',
- dst_label_filename='val_label.json',
- annotation_filename='text_spotting_openimages_v5_validation.json',
- img_start_idx=num_train_imgs,
- nproc=args.n_proc)
- print('Finish')
-
-
-if __name__ == '__main__':
- main()
diff --git a/pipeline/mmocr/tools/dataset_converters/textrecog/rctw_converter.py b/pipeline/mmocr/tools/dataset_converters/textrecog/rctw_converter.py
deleted file mode 100644
index e3bfd2baa2a31594850ae2cae82040fb6e8e0362..0000000000000000000000000000000000000000
--- a/pipeline/mmocr/tools/dataset_converters/textrecog/rctw_converter.py
+++ /dev/null
@@ -1,238 +0,0 @@
-# Copyright (c) OpenMMLab. All rights reserved.
-import argparse
-import math
-import os
-import os.path as osp
-
-import mmcv
-import mmengine
-
-from mmocr.utils import crop_img, dump_ocr_data
-
-
-def collect_files(img_dir, gt_dir, ratio):
- """Collect all images and their corresponding groundtruth files.
- Args:
- img_dir (str): The image directory
- gt_dir (str): The groundtruth directory
- ratio (float): Split ratio for val set
-
- Returns:
- files (list): The list of tuples (img_file, groundtruth_file)
- """
- assert isinstance(img_dir, str)
- assert img_dir
- assert isinstance(gt_dir, str)
- assert gt_dir
- assert isinstance(ratio, float)
- assert ratio < 1.0, 'val_ratio should be a float between 0.0 to 1.0'
-
- ann_list, imgs_list = [], []
- for ann_file in os.listdir(gt_dir):
- ann_list.append(osp.join(gt_dir, ann_file))
- imgs_list.append(osp.join(img_dir, ann_file.replace('txt', 'jpg')))
-
- all_files = list(zip(imgs_list, ann_list))
- assert len(all_files), f'No images found in {img_dir}'
- print(f'Loaded {len(all_files)} images from {img_dir}')
-
- trn_files, val_files = [], []
- if ratio > 0:
- for i, file in enumerate(all_files):
- if i % math.floor(1 / ratio):
- trn_files.append(file)
- else:
- val_files.append(file)
- else:
- trn_files, val_files = all_files, []
-
- print(f'training #{len(trn_files)}, val #{len(val_files)}')
-
- return trn_files, val_files
-
-
-def collect_annotations(files, nproc=1):
- """Collect the annotation information.
- Args:
- files (list): The list of tuples (image_file, groundtruth_file)
- nproc (int): The number of process to collect annotations
-
- Returns:
- images (list): The list of image information dicts
- """
- assert isinstance(files, list)
- assert isinstance(nproc, int)
-
- if nproc > 1:
- images = mmengine.track_parallel_progress(
- load_img_info, files, nproc=nproc)
- else:
- images = mmengine.track_progress(load_img_info, files)
-
- return images
-
-
-def load_img_info(files):
- """Load the information of one image.
- Args:
- files (tuple): The tuple of (img_file, groundtruth_file)
-
- Returns:
- img_info (dict): The dict of the img and annotation information
- """
- assert isinstance(files, tuple)
-
- img_file, gt_file = files
- assert osp.basename(gt_file).split('.')[0] == osp.basename(img_file).split(
- '.')[0]
- # read imgs while ignoring orientations
- img = mmcv.imread(img_file)
-
- img_info = dict(
- file_name=osp.join(osp.basename(img_file)),
- height=img.shape[0],
- width=img.shape[1],
- segm_file=osp.join(osp.basename(gt_file)))
-
- if osp.splitext(gt_file)[1] == '.txt':
- img_info = load_txt_info(gt_file, img_info)
- else:
- raise NotImplementedError
-
- return img_info
-
-
-def load_txt_info(gt_file, img_info):
- """Collect the annotation information.
-
- The annotation format is as the following:
- x1, y1, x2, y2, x3, y3, x4, y4, difficult, text
-
- 390,902,1856,902,1856,1225,390,1225,0,"้ๆฐ็ผ้"
- 1875,1170,2149,1170,2149,1245,1875,1245,0,"ๅไบ1989"
- 2054,1277,2190,1277,2190,1323,2054,1323,0,"ๅๅปบๅบ"
-
- Args:
- gt_file (str): The path to ground-truth
- img_info (dict): The dict of the img and annotation information
-
- Returns:
- img_info (dict): The dict of the img and annotation information
- """
-
- anno_info = []
- with open(gt_file, encoding='utf-8-sig') as f:
- lines = f.readlines()
- for line in lines:
- points = line.split(',')[0:8]
- word = line.split(',')[9].rstrip('\n').strip('"')
- difficult = 1 if line.split(',')[8] != '0' else 0
- bbox = [int(pt) for pt in points]
-
- if word == '###' or difficult == 1:
- continue
-
- anno = dict(bbox=bbox, word=word)
- anno_info.append(anno)
-
- img_info.update(anno_info=anno_info)
-
- return img_info
-
-
-def generate_ann(root_path, split, image_infos, preserve_vertical):
- """Generate cropped annotations and label txt file.
-
- Args:
- root_path (str): The root path of the dataset
- split (str): The split of dataset. Namely: training or val
- image_infos (list[dict]): A list of dicts of the img and
- annotation information
- preserve_vertical (bool): Whether to preserve vertical texts
- """
-
- dst_image_root = osp.join(root_path, 'crops', split)
- ignore_image_root = osp.join(root_path, 'ignores', split)
- if split == 'training':
- dst_label_file = osp.join(root_path, 'train_label.json')
- elif split == 'val':
- dst_label_file = osp.join(root_path, 'val_label.json')
- mmengine.mkdir_or_exist(dst_image_root)
- mmengine.mkdir_or_exist(ignore_image_root)
-
- img_info = []
- for image_info in image_infos:
- index = 1
- src_img_path = osp.join(root_path, 'imgs', image_info['file_name'])
- image = mmcv.imread(src_img_path)
- src_img_root = image_info['file_name'].split('.')[0]
-
- for anno in image_info['anno_info']:
- word = anno['word']
- dst_img = crop_img(image, anno['bbox'], 0, 0)
- h, w, _ = dst_img.shape
-
- dst_img_name = f'{src_img_root}_{index}.png'
- index += 1
- # Skip invalid annotations
- if min(dst_img.shape) == 0:
- continue
- # Filter out vertical texts
- if not preserve_vertical and h / w > 2:
- dst_img_path = osp.join(ignore_image_root, dst_img_name)
- mmcv.imwrite(dst_img, dst_img_path)
- continue
-
- dst_img_path = osp.join(dst_image_root, dst_img_name)
- mmcv.imwrite(dst_img, dst_img_path)
-
- img_info.append({
- 'file_name': dst_img_name,
- 'anno_info': [{
- 'text': word
- }]
- })
-
- dump_ocr_data(img_info, dst_label_file, 'textrecog')
-
-
-def parse_args():
- parser = argparse.ArgumentParser(
- description='Generate training and val set of RCTW.')
- parser.add_argument('root_path', help='Root dir path of RCTW')
- parser.add_argument(
- '--val-ratio', help='Split ratio for val set', default=0.0, type=float)
- parser.add_argument(
- '--nproc', default=1, type=int, help='Number of process')
- parser.add_argument(
- '--preserve-vertical',
- help='Preserve samples containing vertical texts',
- action='store_true')
- args = parser.parse_args()
- return args
-
-
-def main():
- args = parse_args()
- root_path = args.root_path
- ratio = args.val_ratio
-
- trn_files, val_files = collect_files(
- osp.join(root_path, 'imgs'), osp.join(root_path, 'annotations'), ratio)
-
- # Train set
- with mmengine.Timer(
- print_tmpl='It takes {}s to convert RCTW Training annotation'):
- trn_infos = collect_annotations(trn_files, nproc=args.nproc)
- generate_ann(root_path, 'training', trn_infos, args.preserve_vertical)
-
- # Val set
- if len(val_files) > 0:
- with mmengine.Timer(
- print_tmpl='It takes {}s to convert RCTW Val annotation'):
- val_infos = collect_annotations(val_files, nproc=args.nproc)
- generate_ann(root_path, 'val', val_infos, args.preserve_vertical)
-
-
-if __name__ == '__main__':
- main()
diff --git a/pipeline/mmocr/tools/dataset_converters/textrecog/rects_converter.py b/pipeline/mmocr/tools/dataset_converters/textrecog/rects_converter.py
deleted file mode 100644
index 630e81509715ef67edcb7dbf77542b399962d551..0000000000000000000000000000000000000000
--- a/pipeline/mmocr/tools/dataset_converters/textrecog/rects_converter.py
+++ /dev/null
@@ -1,256 +0,0 @@
-# Copyright (c) OpenMMLab. All rights reserved.
-import argparse
-import math
-import os
-import os.path as osp
-
-import mmcv
-import mmengine
-
-from mmocr.utils import crop_img, dump_ocr_data
-
-
-def collect_files(img_dir, gt_dir, ratio):
- """Collect all images and their corresponding groundtruth files.
- Args:
- img_dir (str): The image directory
- gt_dir (str): The groundtruth directory
- ratio (float): Split ratio for val set
-
- Returns:
- files (list): The list of tuples (img_file, groundtruth_file)
- """
- assert isinstance(img_dir, str)
- assert img_dir
- assert isinstance(gt_dir, str)
- assert gt_dir
- assert isinstance(ratio, float)
- assert ratio < 1.0, 'val_ratio should be a float between 0.0 to 1.0'
-
- ann_list, imgs_list = [], []
- for ann_file in os.listdir(gt_dir):
- ann_list.append(osp.join(gt_dir, ann_file))
- imgs_list.append(osp.join(img_dir, ann_file.replace('json', 'jpg')))
-
- all_files = list(zip(imgs_list, ann_list))
- assert len(all_files), f'No images found in {img_dir}'
- print(f'Loaded {len(all_files)} images from {img_dir}')
-
- trn_files, val_files = [], []
- if ratio > 0:
- for i, file in enumerate(all_files):
- if i % math.floor(1 / ratio):
- trn_files.append(file)
- else:
- val_files.append(file)
- else:
- trn_files, val_files = all_files, []
-
- print(f'training #{len(trn_files)}, val #{len(val_files)}')
-
- return trn_files, val_files
-
-
-def collect_annotations(files, nproc=1):
- """Collect the annotation information.
- Args:
- files (list): The list of tuples (image_file, groundtruth_file)
- nproc (int): The number of process to collect annotations
-
- Returns:
- images (list): The list of image information dicts
- """
- assert isinstance(files, list)
- assert isinstance(nproc, int)
-
- if nproc > 1:
- images = mmengine.track_parallel_progress(
- load_img_info, files, nproc=nproc)
- else:
- images = mmengine.track_progress(load_img_info, files)
-
- return images
-
-
-def load_img_info(files):
- """Load the information of one image.
- Args:
- files (tuple): The tuple of (img_file, groundtruth_file)
-
- Returns:
- img_info (dict): The dict of the img and annotation information
- """
- assert isinstance(files, tuple)
-
- img_file, gt_file = files
- assert osp.basename(gt_file).split('.')[0] == osp.basename(img_file).split(
- '.')[0]
- # read imgs while ignoring orientations
- img = mmcv.imread(img_file)
-
- img_info = dict(
- file_name=osp.join(osp.basename(img_file)),
- height=img.shape[0],
- width=img.shape[1],
- segm_file=osp.join(osp.basename(gt_file)))
-
- if osp.splitext(gt_file)[1] == '.json':
- img_info = load_json_info(gt_file, img_info)
- else:
- raise NotImplementedError
-
- return img_info
-
-
-def load_json_info(gt_file, img_info):
- """Collect the annotation information.
-
- The annotation format is as the following:
-
- {
- "chars": [
- {
- "ignore": 0,
- "transcription": "H",
- "points": [25, 175, 112, 175, 112, 286, 25, 286]
- },
- {
- "ignore": 0,
- "transcription": "O",
- "points": [102, 182, 210, 182, 210, 273, 102, 273]
- }, ...
- ]
- "lines": [
- {
- "ignore": 0,
- "transcription": "HOKI",
- "points": [23, 173, 327, 180, 327, 290, 23, 283]
- },
- {
- "ignore": 0,
- "transcription": "TEA",
- "points": [368, 180, 621, 180, 621, 294, 368, 294]
- }, ...
- ]
- }
-
-
- Args:
- gt_file (str): The path to ground-truth
- img_info (dict): The dict of the img and annotation information
-
- Returns:
- img_info (dict): The dict of the img and annotation information
- """
-
- annotation = mmengine.load(gt_file)
- anno_info = []
- for line in annotation['lines']:
- if line['ignore'] == 1:
- continue
- segmentation = line['points']
- word = line['transcription']
- anno = dict(bbox=segmentation, word=word)
- anno_info.append(anno)
-
- img_info.update(anno_info=anno_info)
-
- return img_info
-
-
-def generate_ann(root_path, split, image_infos, preserve_vertical):
- """Generate cropped annotations and label txt file.
-
- Args:
- root_path (str): The root path of the dataset
- split (str): The split of dataset. Namely: training or test
- image_infos (list[dict]): A list of dicts of the img and
- annotation information
- preserve_vertical (bool): Whether to preserve vertical texts
- """
- print('Cropping images...')
- dst_image_root = osp.join(root_path, 'crops', split)
- ignore_image_root = osp.join(root_path, 'ignores', split)
- if split == 'training':
- dst_label_file = osp.join(root_path, 'train_label.json')
- elif split == 'val':
- dst_label_file = osp.join(root_path, 'val_label.json')
- mmengine.mkdir_or_exist(dst_image_root)
- mmengine.mkdir_or_exist(ignore_image_root)
-
- img_info = []
- for image_info in image_infos:
- index = 1
- src_img_path = osp.join(root_path, 'imgs', image_info['file_name'])
- image = mmcv.imread(src_img_path)
- src_img_root = image_info['file_name'].split('.')[0]
-
- for anno in image_info['anno_info']:
- word = anno['word']
- dst_img = crop_img(image, anno['bbox'], 0, 0)
- h, w, _ = dst_img.shape
-
- dst_img_name = f'{src_img_root}_{index}.png'
- index += 1
- # Skip invalid annotations
- if min(dst_img.shape) == 0:
- continue
- # Skip vertical texts
- if not preserve_vertical and h / w > 2 and split == 'training':
- dst_img_path = osp.join(ignore_image_root, dst_img_name)
- mmcv.imwrite(dst_img, dst_img_path)
- continue
-
- dst_img_path = osp.join(dst_image_root, dst_img_name)
- mmcv.imwrite(dst_img, dst_img_path)
-
- img_info.append({
- 'file_name': dst_img_name,
- 'anno_info': [{
- 'text': word
- }]
- })
-
- dump_ocr_data(img_info, dst_label_file, 'textrecog')
-
-
-def parse_args():
- parser = argparse.ArgumentParser(
- description='Generate training and val set of ReCTS.')
- parser.add_argument('root_path', help='Root dir path of ReCTS')
- parser.add_argument(
- '--val-ratio', help='Split ratio for val set', default=0.0, type=float)
- parser.add_argument(
- '--nproc', default=1, type=int, help='Number of process')
- parser.add_argument(
- '--preserve-vertical',
- help='Preserve samples containing vertical texts',
- action='store_true')
- args = parser.parse_args()
- return args
-
-
-def main():
- args = parse_args()
- root_path = args.root_path
- ratio = args.val_ratio
-
- trn_files, val_files = collect_files(
- osp.join(root_path, 'imgs'), osp.join(root_path, 'annotations'), ratio)
-
- # Train set
- trn_infos = collect_annotations(trn_files, nproc=args.nproc)
- with mmengine.Timer(
- print_tmpl='It takes {}s to convert ReCTS Training annotation'):
- generate_ann(root_path, 'training', trn_infos, args.preserve_vertical)
-
- # Val set
- if len(val_files) > 0:
- val_infos = collect_annotations(val_files, nproc=args.nproc)
- with mmengine.Timer(
- print_tmpl='It takes {}s to convert ReCTS Val annotation'):
- generate_ann(root_path, 'val', val_infos, args.preserve_vertical)
-
-
-if __name__ == '__main__':
- main()
diff --git a/pipeline/mmocr/tools/dataset_converters/textrecog/sroie_converter.py b/pipeline/mmocr/tools/dataset_converters/textrecog/sroie_converter.py
deleted file mode 100644
index 1c380e49d42d7c09907f5c1642baa172e5dff1ed..0000000000000000000000000000000000000000
--- a/pipeline/mmocr/tools/dataset_converters/textrecog/sroie_converter.py
+++ /dev/null
@@ -1,207 +0,0 @@
-# Copyright (c) OpenMMLab. All rights reserved.
-import argparse
-import os
-import os.path as osp
-
-import mmcv
-import mmengine
-import numpy as np
-
-from mmocr.utils import crop_img, dump_ocr_data
-
-
-def collect_files(img_dir, gt_dir):
- """Collect all images and their corresponding groundtruth files.
-
- Args:
- img_dir (str): The image directory
- gt_dir (str): The groundtruth directory
-
- Returns:
- files (list): The list of tuples (img_file, groundtruth_file)
- """
-
- assert isinstance(img_dir, str)
- assert img_dir
- assert isinstance(gt_dir, str)
- assert gt_dir
-
- ann_list, imgs_list = [], []
- for gt_file in os.listdir(gt_dir):
- # Filtering repeated and missing images
- if '(' in gt_file or gt_file == 'X51006619570.txt':
- continue
- ann_list.append(osp.join(gt_dir, gt_file))
- imgs_list.append(osp.join(img_dir, gt_file.replace('.txt', '.jpg')))
-
- files = list(zip(sorted(imgs_list), sorted(ann_list)))
- assert len(files), f'No images found in {img_dir}'
-
- print(f'Loaded {len(files)} images from {img_dir}')
-
- return files
-
-
-def collect_annotations(files, nproc=1):
- """Collect the annotation information.
-
- Args:
- files (list): The list of tuples (image_file, groundtruth_file)
- nproc (int): The number of process to collect annotations
-
- Returns:
- images (list): The list of image information dicts
- """
-
- assert isinstance(files, list)
- assert isinstance(nproc, int)
-
- if nproc > 1:
- images = mmengine.track_parallel_progress(
- load_img_info, files, nproc=nproc)
- else:
- images = mmengine.track_progress(load_img_info, files)
-
- return images
-
-
-def load_img_info(files):
- """Load the information of one image.
-
- Args:
- files (tuple): The tuple of (img_file, groundtruth_file)
-
- Returns:
- img_info (dict): The dict of the img and annotation information
- """
-
- assert isinstance(files, tuple)
-
- img_file, gt_file = files
- assert osp.basename(gt_file).split('.')[0] == osp.basename(img_file).split(
- '.')[0]
- # read imgs while ignoring orientations
- img = mmcv.imread(img_file, 'unchanged')
-
- img_info = dict(
- file_name=osp.join(osp.basename(img_file)),
- height=img.shape[0],
- width=img.shape[1],
- segm_file=osp.join(osp.basename(gt_file)))
-
- if osp.splitext(gt_file)[1] == '.txt':
- img_info = load_txt_info(gt_file, img_info)
- else:
- raise NotImplementedError
-
- return img_info
-
-
-def load_txt_info(gt_file, img_info):
- """Collect the annotation information.
-
- Annotation Format
- x1, y1, x2, y2, x3, y3, x4, y4, transcript
-
- Args:
- gt_file (list): The list of tuples (image_file, groundtruth_file)
- img_info (int): The dict of the img and annotation information
-
- Returns:
- img_info (list): The dict of the img and annotation information
- """
-
- with open(gt_file, encoding='unicode_escape') as f:
- anno_info = []
- for ann in f.readlines():
- # skip invalid annotation line
- try:
- bbox = np.array(ann.split(',')[0:8]).astype(int).tolist()
- except ValueError:
-
- continue
- word = ann.split(',')[-1].replace('\n', '').strip()
-
- anno = dict(bbox=bbox, word=word)
- anno_info.append(anno)
-
- img_info.update(anno_info=anno_info)
-
- return img_info
-
-
-def generate_ann(root_path, split, image_infos):
- """Generate cropped annotations and label txt file.
-
- Args:
- root_path (str): The root path of the dataset
- split (str): The split of dataset. Namely: training or test
- image_infos (list[dict]): A list of dicts of the img and
- annotation information
- """
-
- dst_image_root = osp.join(root_path, 'crops', split)
- if split == 'training':
- dst_label_file = osp.join(root_path, 'train_label.json')
- elif split == 'test':
- dst_label_file = osp.join(root_path, 'test_label.json')
- os.makedirs(dst_image_root, exist_ok=True)
-
- img_info = []
- for image_info in image_infos:
- index = 1
- src_img_path = osp.join(root_path, 'imgs', split,
- image_info['file_name'])
- image = mmcv.imread(src_img_path)
- src_img_root = image_info['file_name'].split('.')[0]
-
- for anno in image_info['anno_info']:
- word = anno['word']
- dst_img = crop_img(image, anno['bbox'], 0, 0)
-
- # Skip invalid annotations
- if min(dst_img.shape) == 0 or len(word) == 0:
- continue
-
- dst_img_name = f'{src_img_root}_{index}.png'
- index += 1
- dst_img_path = osp.join(dst_image_root, dst_img_name)
- mmcv.imwrite(dst_img, dst_img_path)
-
- img_info.append({
- 'file_name': dst_img_name,
- 'anno_info': [{
- 'text': word
- }]
- })
-
- dump_ocr_data(img_info, dst_label_file, 'textrecog')
-
-
-def parse_args():
- parser = argparse.ArgumentParser(
- description='Generate training and test set of SROIE')
- parser.add_argument('root_path', help='Root dir path of SROIE')
- parser.add_argument(
- '--nproc', default=1, type=int, help='Number of process')
- args = parser.parse_args()
- return args
-
-
-def main():
- args = parse_args()
- root_path = args.root_path
-
- for split in ['training', 'test']:
- print(f'Processing {split} set...')
- with mmengine.Timer(
- print_tmpl='It takes {}s to convert SROIE annotation'):
- files = collect_files(
- osp.join(root_path, 'imgs', split),
- osp.join(root_path, 'annotations', split))
- image_infos = collect_annotations(files, nproc=args.nproc)
- generate_ann(root_path, split, image_infos)
-
-
-if __name__ == '__main__':
- main()
diff --git a/pipeline/mmocr/tools/dataset_converters/textrecog/vintext_converter.py b/pipeline/mmocr/tools/dataset_converters/textrecog/vintext_converter.py
deleted file mode 100644
index ea9103da033c2d4bdbba3855f78a88d32aec9553..0000000000000000000000000000000000000000
--- a/pipeline/mmocr/tools/dataset_converters/textrecog/vintext_converter.py
+++ /dev/null
@@ -1,217 +0,0 @@
-# Copyright (c) OpenMMLab. All rights reserved.
-import argparse
-import os
-import os.path as osp
-
-import mmcv
-import mmengine
-
-from mmocr.utils import crop_img, dump_ocr_data
-
-
-def collect_files(img_dir, gt_dir):
- """Collect all images and their corresponding groundtruth files.
-
- Args:
- img_dir (str): The image directory
- gt_dir (str): The groundtruth directory
-
- Returns:
- files (list): The list of tuples (img_file, groundtruth_file)
- """
- assert isinstance(img_dir, str)
- assert img_dir
- assert isinstance(gt_dir, str)
- assert gt_dir
-
- ann_list, imgs_list = [], []
- for img_file in os.listdir(img_dir):
- ann_file = 'gt_' + str(int(img_file[2:6])) + '.txt'
- ann_list.append(osp.join(gt_dir, ann_file))
- imgs_list.append(osp.join(img_dir, img_file))
-
- files = list(zip(imgs_list, ann_list))
- assert len(files), f'No images found in {img_dir}'
- print(f'Loaded {len(files)} images from {img_dir}')
-
- return files
-
-
-def collect_annotations(files, nproc=1):
- """Collect the annotation information.
-
- Args:
- files (list): The list of tuples (image_file, groundtruth_file)
- nproc (int): The number of process to collect annotations
-
- Returns:
- images (list): The list of image information dicts
- """
- assert isinstance(files, list)
- assert isinstance(nproc, int)
-
- if nproc > 1:
- images = mmengine.track_parallel_progress(
- load_img_info, files, nproc=nproc)
- else:
- images = mmengine.track_progress(load_img_info, files)
-
- return images
-
-
-def load_img_info(files):
- """Load the information of one image.
-
- Args:
- files (tuple): The tuple of (img_file, groundtruth_file)
-
- Returns:
- img_info (dict): The dict of the img and annotation information
- """
- assert isinstance(files, tuple)
-
- img_file, gt_file = files
- assert int(osp.basename(gt_file)[3:-4]) == int(
- osp.basename(img_file)[2:-4])
- # read imgs while ignoring orientations
- img = mmcv.imread(img_file, 'unchanged')
-
- img_info = dict(
- file_name=osp.join(osp.basename(img_file)),
- height=img.shape[0],
- width=img.shape[1],
- segm_file=osp.join(osp.basename(gt_file)))
-
- if osp.splitext(gt_file)[1] == '.txt':
- img_info = load_txt_info(gt_file, img_info)
- else:
- raise NotImplementedError
-
- return img_info
-
-
-def load_txt_info(gt_file, img_info):
- """Collect the annotation information.
-
- The annotation format is as the following:
- x1,y1,x2,y2,x3,y3,x4,y4,text
- 118,15,147,15,148,46,118,46,LฦฏแปขNG
- 149,9,165,9,165,43,150,43,TแปT
- 167,9,180,9,179,43,167,42,ฤแป
- 181,12,193,12,193,43,181,43,Cร
- 195,13,215,14,215,46,196,46,VIแปC
- 217,13,237,14,239,47,217,46,LรM,
-
- Args:
- gt_file (str): The path to ground-truth
- img_info (dict): The dict of the img and annotation information
-
- Returns:
- img_info (dict): The dict of the img and annotation information
- """
-
- with open(gt_file, encoding='utf-8') as f:
- anno_info = []
- for line in f:
- line = line.strip('\n')
- ann = line.split(',')
- bbox = ann[0:8]
- word = line[len(','.join(bbox)) + 1:]
- bbox = [int(coord) for coord in bbox]
- # Ignore hard samples
- if word == '###':
- continue
- assert len(bbox) == 8
- anno = dict(bbox=bbox, word=word)
- anno_info.append(anno)
-
- img_info.update(anno_info=anno_info)
-
- return img_info
-
-
-def generate_ann(root_path, split, image_infos, preserve_vertical):
- """Generate cropped annotations and label txt file.
-
- Args:
- root_path (str): The root path of the dataset
- split (str): The split of dataset. Namely: training or test
- image_infos (list[dict]): A list of dicts of the img and
- annotation information
- preserve_vertical (bool): Whether to preserve vertical texts
- """
- dst_image_root = osp.join(root_path, 'crops', split)
- ignore_image_root = osp.join(root_path, 'ignores', split)
- if split == 'training':
- dst_label_file = osp.join(root_path, 'train_label.json')
- elif split == 'test':
- dst_label_file = osp.join(root_path, 'test_label.json')
- elif split == 'unseen_test':
- dst_label_file = osp.join(root_path, 'unseen_test_label.json')
- os.makedirs(dst_image_root, exist_ok=True)
-
- img_info = []
- for image_info in image_infos:
- index = 1
- src_img_path = osp.join(root_path, 'imgs', split,
- image_info['file_name'])
- image = mmcv.imread(src_img_path)
- src_img_root = image_info['file_name'].split('.')[0]
-
- for anno in image_info['anno_info']:
- word = anno['word']
- dst_img = crop_img(image, anno['bbox'], 0, 0)
- h, w, _ = dst_img.shape
-
- dst_img_name = f'{src_img_root}_{index}.png'
- index += 1
- # Skip invalid annotations
- if min(dst_img.shape) == 0:
- continue
- # Skip vertical texts
- if not preserve_vertical and h / w > 2 and split == 'training':
- dst_img_path = osp.join(ignore_image_root, dst_img_name)
- mmcv.imwrite(dst_img, dst_img_path)
- continue
-
- dst_img_path = osp.join(dst_image_root, dst_img_name)
- mmcv.imwrite(dst_img, dst_img_path)
- img_info.append({
- 'file_name': dst_img_name,
- 'anno_info': [{
- 'text': word
- }]
- })
- dump_ocr_data(img_info, dst_label_file, 'textrecog')
-
-
-def parse_args():
- parser = argparse.ArgumentParser(
- description='Generate training and test set of VinText ')
- parser.add_argument('root_path', help='Root dir path of VinText')
- parser.add_argument(
- '--preserve-vertical',
- help='Preserve samples containing vertical texts',
- action='store_true')
- parser.add_argument(
- '--nproc', default=1, type=int, help='Number of processes')
- args = parser.parse_args()
- return args
-
-
-def main():
- args = parse_args()
- root_path = args.root_path
- for split in ['training', 'test', 'unseen_test']:
- print(f'Processing {split} set...')
- with mmengine.Timer(
- print_tmpl='It takes {}s to convert VinText annotation'):
- files = collect_files(
- osp.join(root_path, 'imgs', split),
- osp.join(root_path, 'annotations'))
- image_infos = collect_annotations(files, nproc=args.nproc)
- generate_ann(root_path, split, image_infos, args.preserve_vertical)
-
-
-if __name__ == '__main__':
- main()
diff --git a/pipeline/mmocr/tools/dist_test.sh b/pipeline/mmocr/tools/dist_test.sh
deleted file mode 100644
index dea131b43ea8f1222661d20603d40c18ea7f28a1..0000000000000000000000000000000000000000
--- a/pipeline/mmocr/tools/dist_test.sh
+++ /dev/null
@@ -1,22 +0,0 @@
-#!/usr/bin/env bash
-
-CONFIG=$1
-CHECKPOINT=$2
-GPUS=$3
-NNODES=${NNODES:-1}
-NODE_RANK=${NODE_RANK:-0}
-PORT=${PORT:-29500}
-MASTER_ADDR=${MASTER_ADDR:-"127.0.0.1"}
-
-PYTHONPATH="$(dirname $0)/..":$PYTHONPATH \
-python -m torch.distributed.launch \
- --nnodes=$NNODES \
- --node_rank=$NODE_RANK \
- --master_addr=$MASTER_ADDR \
- --nproc_per_node=$GPUS \
- --master_port=$PORT \
- $(dirname "$0")/test.py \
- $CONFIG \
- $CHECKPOINT \
- --launcher pytorch \
- ${@:4}
diff --git a/pipeline/mmocr/tools/dist_train.sh b/pipeline/mmocr/tools/dist_train.sh
deleted file mode 100644
index 3f5b40b2318c6bd58504d9e570b90adf21825376..0000000000000000000000000000000000000000
--- a/pipeline/mmocr/tools/dist_train.sh
+++ /dev/null
@@ -1,20 +0,0 @@
-
-#!/usr/bin/env bash
-
-CONFIG=$1
-GPUS=$2
-NNODES=${NNODES:-1}
-NODE_RANK=${NODE_RANK:-0}
-PORT=${PORT:-29500}
-MASTER_ADDR=${MASTER_ADDR:-"127.0.0.1"}
-
-PYTHONPATH="$(dirname $0)/..":$PYTHONPATH \
-python -m torch.distributed.launch \
- --nnodes=$NNODES \
- --node_rank=$NODE_RANK \
- --master_addr=$MASTER_ADDR \
- --nproc_per_node=$GPUS \
- --master_port=$PORT \
- $(dirname "$0")/train.py \
- $CONFIG \
- --launcher pytorch ${@:3}
diff --git a/pipeline/mmocr/tools/infer.py b/pipeline/mmocr/tools/infer.py
deleted file mode 100644
index 5881a7efdc514c03383ec9a2282bf0b355121518..0000000000000000000000000000000000000000
--- a/pipeline/mmocr/tools/infer.py
+++ /dev/null
@@ -1,102 +0,0 @@
-# Copyright (c) OpenMMLab. All rights reserved.
-from argparse import ArgumentParser
-# import sys
-# sys.path.append("..")
-# import mmocr
-from mmocr.apis.inferencers import MMOCRInferencer
-
-
-def parse_args():
- parser = ArgumentParser()
- parser.add_argument(
- 'inputs', type=str, help='Input image file or folder path.')
- parser.add_argument(
- '--out-dir',
- type=str,
- default='results/',
- help='Output directory of results.')
- parser.add_argument(
- '--det',
- type=str,
- default=None,
- help='Pretrained text detection algorithm. It\'s the path to the '
- 'config file or the model name defined in metafile.')
- parser.add_argument(
- '--det-weights',
- type=str,
- default=None,
- help='Path to the custom checkpoint file of the selected det model. '
- 'If it is not specified and "det" is a model name of metafile, the '
- 'weights will be loaded from metafile.')
- parser.add_argument(
- '--rec',
- type=str,
- default=None,
- help='Pretrained text recognition algorithm. It\'s the path to the '
- 'config file or the model name defined in metafile.')
- parser.add_argument(
- '--rec-weights',
- type=str,
- default=None,
- help='Path to the custom checkpoint file of the selected recog model. '
- 'If it is not specified and "rec" is a model name of metafile, the '
- 'weights will be loaded from metafile.')
- parser.add_argument(
- '--kie',
- type=str,
- default=None,
- help='Pretrained key information extraction algorithm. It\'s the path'
- 'to the config file or the model name defined in metafile.')
- parser.add_argument(
- '--kie-weights',
- type=str,
- default=None,
- help='Path to the custom checkpoint file of the selected kie model. '
- 'If it is not specified and "kie" is a model name of metafile, the '
- 'weights will be loaded from metafile.')
- parser.add_argument(
- '--device',
- type=str,
- default=None,
- help='Device used for inference. '
- 'If not specified, the available device will be automatically used.')
- parser.add_argument(
- '--batch-size', type=int, default=1, help='Inference batch size.')
- parser.add_argument(
- '--show',
- action='store_true',
- help='Display the image in a popup window.')
- parser.add_argument(
- '--print-result',
- action='store_true',
- help='Whether to print the results.')
- parser.add_argument(
- '--save_pred',
- action='store_true',
- help='Save the inference results to out_dir.')
- parser.add_argument(
- '--save_vis',
- action='store_true',
- help='Save the visualization results to out_dir.')
-
- call_args = vars(parser.parse_args())
-
- init_kws = [
- 'det', 'det_weights', 'rec', 'rec_weights', 'kie', 'kie_weights',
- 'device'
- ]
- init_args = {}
- for init_kw in init_kws:
- init_args[init_kw] = call_args.pop(init_kw)
-
- return init_args, call_args
-
-
-def main():
- init_args, call_args = parse_args()
- ocr = MMOCRInferencer(**init_args)
- ocr(**call_args)
-
-
-if __name__ == '__main__':
- main()
diff --git a/pipeline/mmocr/tools/model_converters/publish_model.py b/pipeline/mmocr/tools/model_converters/publish_model.py
deleted file mode 100644
index 18fc3e15045dea63a74ed1a7727a388e9031ac8c..0000000000000000000000000000000000000000
--- a/pipeline/mmocr/tools/model_converters/publish_model.py
+++ /dev/null
@@ -1,57 +0,0 @@
-# Copyright (c) OpenMMLab. All rights reserved.
-import argparse
-import subprocess
-
-import torch
-from mmengine.logging import print_log
-
-
-def parse_args():
- parser = argparse.ArgumentParser(
- description='Process a checkpoint to be published')
- parser.add_argument('in_file', help='input checkpoint filename')
- parser.add_argument('out_file', help='output checkpoint filename')
- parser.add_argument(
- '--save-keys',
- nargs='+',
- type=str,
- default=['meta', 'state_dict'],
- help='keys to save in the published checkpoint')
- args = parser.parse_args()
- return args
-
-
-def process_checkpoint(in_file, out_file, save_keys=['meta', 'state_dict']):
- checkpoint = torch.load(in_file, map_location='cpu')
-
- # only keep `meta` and `state_dict` for smaller file size
- ckpt_keys = list(checkpoint.keys())
- for k in ckpt_keys:
- if k not in save_keys:
- print_log(
- f'Key `{k}` will be removed because it is not in '
- f'save_keys. If you want to keep it, '
- f'please set --save-keys.',
- logger='current')
- checkpoint.pop(k, None)
-
- # if it is necessary to remove some sensitive data in checkpoint['meta'],
- # add the code here.
- if torch.__version__ >= '1.6':
- torch.save(checkpoint, out_file, _use_new_zipfile_serialization=False)
- else:
- torch.save(checkpoint, out_file)
- sha = subprocess.check_output(['sha256sum', out_file]).decode()
- final_file = out_file.rstrip('.pth') + f'-{sha[:8]}.pth'
- subprocess.Popen(['mv', out_file, final_file])
- print_log(
- f'The published model is saved at {final_file}.', logger='current')
-
-
-def main():
- args = parse_args()
- process_checkpoint(args.in_file, args.out_file, args.save_keys)
-
-
-if __name__ == '__main__':
- main()
diff --git a/pipeline/mmocr/tools/slurm_test.sh b/pipeline/mmocr/tools/slurm_test.sh
deleted file mode 100644
index 865f45599ad883d216f0df0248a3815700615c17..0000000000000000000000000000000000000000
--- a/pipeline/mmocr/tools/slurm_test.sh
+++ /dev/null
@@ -1,22 +0,0 @@
-#!/usr/bin/env bash
-
-set -x
-export PYTHONPATH=`pwd`:$PYTHONPATH
-
-PARTITION=$1
-JOB_NAME=$2
-CONFIG=$3
-CHECKPOINT=$4
-GPUS=${GPUS:-8}
-GPUS_PER_NODE=${GPUS_PER_NODE:-8}
-PY_ARGS=${@:5}
-SRUN_ARGS=${SRUN_ARGS:-""}
-
-srun -p ${PARTITION} \
- --job-name=${JOB_NAME} \
- --gres=gpu:${GPUS_PER_NODE} \
- --ntasks=${GPUS} \
- --ntasks-per-node=${GPUS_PER_NODE} \
- --kill-on-bad-exit=1 \
- ${SRUN_ARGS} \
- python -u tools/test.py ${CONFIG} ${CHECKPOINT} --launcher="slurm" ${PY_ARGS}
diff --git a/pipeline/mmocr/tools/slurm_train.sh b/pipeline/mmocr/tools/slurm_train.sh
deleted file mode 100644
index 452b09454a08ac522a9df2304c3039487ea517bd..0000000000000000000000000000000000000000
--- a/pipeline/mmocr/tools/slurm_train.sh
+++ /dev/null
@@ -1,25 +0,0 @@
-#!/usr/bin/env bash
-export MASTER_PORT=$((12000 + $RANDOM % 20000))
-
-set -x
-
-PARTITION=$1
-JOB_NAME=$2
-CONFIG=$3
-WORK_DIR=$4
-GPUS=${GPUS:-8}
-GPUS_PER_NODE=${GPUS_PER_NODE:-8}
-CPUS_PER_TASK=${CPUS_PER_TASK:-5}
-PY_ARGS=${@:5}
-SRUN_ARGS=${SRUN_ARGS:-""}
-
-PYTHONPATH="$(dirname $0)/..":$PYTHONPATH \
-srun -p ${PARTITION} \
- --job-name=${JOB_NAME} \
- --gres=gpu:${GPUS_PER_NODE} \
- --ntasks=${GPUS} \
- --ntasks-per-node=${GPUS_PER_NODE} \
- --cpus-per-task=${CPUS_PER_TASK} \
- --kill-on-bad-exit=1 \
- ${SRUN_ARGS} \
- python -u tools/train.py ${CONFIG} --work-dir=${WORK_DIR} --launcher="slurm" ${PY_ARGS}
diff --git a/pipeline/mmocr/tools/test.py b/pipeline/mmocr/tools/test.py
deleted file mode 100644
index 15645f2207ebdb61fd70293f2b2c9602e99b2c61..0000000000000000000000000000000000000000
--- a/pipeline/mmocr/tools/test.py
+++ /dev/null
@@ -1,141 +0,0 @@
-# Copyright (c) OpenMMLab. All rights reserved.
-import argparse
-import os
-import os.path as osp
-
-from mmengine.config import Config, DictAction
-from mmengine.registry import RUNNERS
-from mmengine.runner import Runner
-
-
-def parse_args():
- parser = argparse.ArgumentParser(description='Test (and eval) a model')
- parser.add_argument('config', help='Test config file path')
- parser.add_argument('checkpoint', help='Checkpoint file')
- parser.add_argument(
- '--work-dir',
- help='The directory to save the file containing evaluation metrics')
- parser.add_argument(
- '--save-preds',
- action='store_true',
- help='Dump predictions to a pickle file for offline evaluation')
- parser.add_argument(
- '--show', action='store_true', help='Show prediction results')
- parser.add_argument(
- '--show-dir',
- help='Directory where painted images will be saved. '
- 'If specified, it will be automatically saved '
- 'to the work_dir/timestamp/show_dir')
- parser.add_argument(
- '--wait-time', type=float, default=2, help='The interval of show (s)')
- parser.add_argument(
- '--cfg-options',
- nargs='+',
- action=DictAction,
- help='Override some settings in the used config, the key-value pair '
- 'in xxx=yyy format will be merged into config file. If the value to '
- 'be overwritten is a list, it should be like key="[a,b]" or key=a,b '
- 'It also allows nested list/tuple values, e.g. key="[(a,b),(c,d)]" '
- 'Note that the quotation marks are necessary and that no white space '
- 'is allowed.')
- parser.add_argument(
- '--launcher',
- choices=['none', 'pytorch', 'slurm', 'mpi'],
- default='none',
- help='Job launcher')
- parser.add_argument(
- '--tta', action='store_true', help='Test time augmentation')
- # When using PyTorch version >= 2.0.0, the `torch.distributed.launch`
- # will pass the `--local-rank` parameter to `tools/test.py` instead
- # of `--local_rank`.
- parser.add_argument('--local_rank', '--local-rank', type=int, default=0)
- args = parser.parse_args()
- if 'LOCAL_RANK' not in os.environ:
- os.environ['LOCAL_RANK'] = str(args.local_rank)
- return args
-
-
-def trigger_visualization_hook(cfg, args):
- default_hooks = cfg.default_hooks
- if 'visualization' in default_hooks:
- visualization_hook = default_hooks['visualization']
- # Turn on visualization
- visualization_hook['enable'] = True
- visualization_hook['draw_gt'] = True
- visualization_hook['draw_pred'] = True
- if args.show:
- visualization_hook['show'] = True
- visualization_hook['wait_time'] = args.wait_time
- if args.show_dir:
- cfg.visualizer['save_dir'] = args.show_dir
- cfg.visualizer['vis_backends'] = [dict(type='LocalVisBackend')]
- else:
- raise RuntimeError(
- 'VisualizationHook must be included in default_hooks.'
- 'refer to usage '
- '"visualization=dict(type=\'VisualizationHook\')"')
-
- return cfg
-
-
-def main():
- args = parse_args()
-
- # load config
- cfg = Config.fromfile(args.config)
- cfg.launcher = args.launcher
- if args.cfg_options is not None:
- cfg.merge_from_dict(args.cfg_options)
-
- # work_dir is determined in this priority: CLI > segment in file > filename
- if args.work_dir is not None:
- # update configs according to CLI args if args.work_dir is not None
- cfg.work_dir = args.work_dir
- elif cfg.get('work_dir', None) is None:
- # use config filename as default work_dir if cfg.work_dir is None
- cfg.work_dir = osp.join('./work_dirs',
- osp.splitext(osp.basename(args.config))[0])
-
- cfg.load_from = args.checkpoint
-
- # TODO: It will be supported after refactoring the visualizer
- if args.show and args.show_dir:
- raise NotImplementedError('--show and --show-dir cannot be set '
- 'at the same time')
-
- if args.show or args.show_dir:
- cfg = trigger_visualization_hook(cfg, args)
-
- if args.tta:
- cfg.test_dataloader.dataset.pipeline = cfg.tta_pipeline
- cfg.tta_model.module = cfg.model
- cfg.model = cfg.tta_model
-
- # save predictions
- if args.save_preds:
- dump_metric = dict(
- type='DumpResults',
- out_file_path=osp.join(
- cfg.work_dir,
- f'{osp.basename(args.checkpoint)}_predictions.pkl'))
- if isinstance(cfg.test_evaluator, (list, tuple)):
- cfg.test_evaluator = list(cfg.test_evaluator)
- cfg.test_evaluator.append(dump_metric)
- else:
- cfg.test_evaluator = [cfg.test_evaluator, dump_metric]
-
- # build the runner from config
- if 'runner_type' not in cfg:
- # build the default runner
- runner = Runner.from_cfg(cfg)
- else:
- # build customized runner from the registry
- # if 'runner_type' is set in the cfg
- runner = RUNNERS.build(cfg)
-
- # start testing
- runner.test()
-
-
-if __name__ == '__main__':
- main()
diff --git a/pipeline/mmocr/tools/train.py b/pipeline/mmocr/tools/train.py
deleted file mode 100644
index 349cbb3ef4fc74c821c9bd4266ecacdd6acd8cc2..0000000000000000000000000000000000000000
--- a/pipeline/mmocr/tools/train.py
+++ /dev/null
@@ -1,113 +0,0 @@
-# Copyright (c) OpenMMLab. All rights reserved.
-import argparse
-import logging
-import os
-import os.path as osp
-
-from mmengine.config import Config, DictAction
-from mmengine.logging import print_log
-from mmengine.registry import RUNNERS
-from mmengine.runner import Runner
-
-
-def parse_args():
- parser = argparse.ArgumentParser(description='Train a model')
- parser.add_argument('config', help='Train config file path')
- parser.add_argument('--work-dir', help='The dir to save logs and models')
- parser.add_argument(
- '--resume', action='store_true', help='Whether to resume checkpoint.')
- parser.add_argument(
- '--amp',
- action='store_true',
- default=False,
- help='Enable automatic-mixed-precision training')
- parser.add_argument(
- '--auto-scale-lr',
- action='store_true',
- help='Whether to scale the learning rate automatically. It requires '
- '`auto_scale_lr` in config, and `base_batch_size` in `auto_scale_lr`')
- parser.add_argument(
- '--cfg-options',
- nargs='+',
- action=DictAction,
- help='Override some settings in the used config, the key-value pair '
- 'in xxx=yyy format will be merged into config file. If the value to '
- 'be overwritten is a list, it should be like key="[a,b]" or key=a,b '
- 'It also allows nested list/tuple values, e.g. key="[(a,b),(c,d)]" '
- 'Note that the quotation marks are necessary and that no white space '
- 'is allowed.')
- parser.add_argument(
- '--launcher',
- choices=['none', 'pytorch', 'slurm', 'mpi'],
- default='none',
- help='Job launcher')
- # When using PyTorch version >= 2.0.0, the `torch.distributed.launch`
- # will pass the `--local-rank` parameter to `tools/train.py` instead
- # of `--local_rank`.
- parser.add_argument('--local_rank', '--local-rank', type=int, default=0)
- args = parser.parse_args()
- if 'LOCAL_RANK' not in os.environ:
- os.environ['LOCAL_RANK'] = str(args.local_rank)
-
- return args
-
-
-def main():
- args = parse_args()
- # load config
- cfg = Config.fromfile(args.config)
- cfg.launcher = args.launcher
- if args.cfg_options is not None:
- cfg.merge_from_dict(args.cfg_options)
-
- # work_dir is determined in this priority: CLI > segment in file > filename
- if args.work_dir is not None:
- # update configs according to CLI args if args.work_dir is not None
- cfg.work_dir = args.work_dir
- elif cfg.get('work_dir', None) is None:
- # use config filename as default work_dir if cfg.work_dir is None
- cfg.work_dir = osp.join('./work_dirs',
- osp.splitext(osp.basename(args.config))[0])
- # enable automatic-mixed-precision training
- if args.amp:
- optim_wrapper = cfg.optim_wrapper.type
- if optim_wrapper == 'AmpOptimWrapper':
- print_log(
- 'AMP training is already enabled in your config.',
- logger='current',
- level=logging.WARNING)
- else:
- assert optim_wrapper == 'OptimWrapper', (
- '`--amp` is only supported when the optimizer wrapper type is '
- f'`OptimWrapper` but got {optim_wrapper}.')
- cfg.optim_wrapper.type = 'AmpOptimWrapper'
- cfg.optim_wrapper.loss_scale = 'dynamic'
-
- if args.resume:
- cfg.resume = True
-
- # enable automatically scaling LR
- if args.auto_scale_lr:
- if 'auto_scale_lr' in cfg and \
- 'base_batch_size' in cfg.auto_scale_lr:
- cfg.auto_scale_lr.enable = True
- else:
- raise RuntimeError('Can not find "auto_scale_lr" or '
- '"auto_scale_lr.base_batch_size" in your'
- ' configuration file.')
-
- # build the runner from config
- if 'runner_type' not in cfg:
- # build the default runner
- runner = Runner.from_cfg(cfg)
- else:
- # build customized runner from the registry
- # if 'runner_type' is set in the cfg
- runner = RUNNERS.build(cfg)
-
- # start training
- runner.train()
-
-
-if __name__ == '__main__':
- main()
diff --git a/pipeline/mmocr/tools/visualizations/browse_dataset.py b/pipeline/mmocr/tools/visualizations/browse_dataset.py
deleted file mode 100644
index d92ee83f586005de5b14ed95066c778547baa0d4..0000000000000000000000000000000000000000
--- a/pipeline/mmocr/tools/visualizations/browse_dataset.py
+++ /dev/null
@@ -1,415 +0,0 @@
-# Copyright (c) OpenMMLab. All rights reserved.
-import argparse
-import os.path as osp
-import sys
-from typing import Optional, Tuple
-
-import cv2
-import mmcv
-import numpy as np
-from mmengine.config import Config, DictAction
-from mmengine.dataset import Compose
-from mmengine.registry import init_default_scope
-from mmengine.utils import ProgressBar
-from mmengine.visualization import Visualizer
-
-from mmocr.registry import DATASETS, VISUALIZERS
-
-
-# TODO: Support for printing the change in key of results
-def parse_args():
- parser = argparse.ArgumentParser(description='Browse a dataset')
- parser.add_argument('config', help='Path to model or dataset config.')
- parser.add_argument(
- '--phase',
- '-p',
- default='train',
- type=str,
- help='Phase of dataset to visualize. Use "train", "test" or "val" if '
- "you just want to visualize the default split. It's also possible to "
- 'be a dataset variable name, which might be useful when a dataset '
- 'split has multiple variants in the config.')
- parser.add_argument(
- '--mode',
- '-m',
- default='transformed',
- type=str,
- choices=['original', 'transformed', 'pipeline'],
- help='Display mode: display original pictures or '
- 'transformed pictures or comparison pictures. "original" '
- 'only visualizes the original dataset & annotations; '
- '"transformed" shows the resulting images processed through all the '
- 'transforms; "pipeline" shows all the intermediate images. '
- 'Defaults to "transformed".')
- parser.add_argument(
- '--output-dir',
- '-o',
- default=None,
- type=str,
- help='If there is no display interface, you can save it.')
- parser.add_argument(
- '--task',
- '-t',
- default='auto',
- choices=['auto', 'textdet', 'textrecog'],
- type=str,
- help='Specify the task type of the dataset. If "auto", the task type '
- 'will be inferred from the config. If the script is unable to infer '
- 'the task type, you need to specify it manually. Defaults to "auto".')
- parser.add_argument('--not-show', default=False, action='store_true')
- parser.add_argument(
- '--show-number',
- '-n',
- type=int,
- default=sys.maxsize,
- help='number of images selected to visualize, '
- 'must bigger than 0. if the number is bigger than length '
- 'of dataset, show all the images in dataset; '
- 'default "sys.maxsize", show all images in dataset')
- parser.add_argument(
- '--show-interval',
- '-i',
- type=float,
- default=3,
- help='the interval of show (s)')
- parser.add_argument(
- '--cfg-options',
- nargs='+',
- action=DictAction,
- help='override some settings in the used config, the key-value pair '
- 'in xxx=yyy format will be merged into config file. If the value to '
- 'be overwritten is a list, it should be like key="[a,b]" or key=a,b '
- 'It also allows nested list/tuple values, e.g. key="[(a,b),(c,d)]" '
- 'Note that the quotation marks are necessary and that no white space '
- 'is allowed.')
- args = parser.parse_args()
- return args
-
-
-def _get_adaptive_scale(img_shape: Tuple[int, int],
- min_scale: float = 0.3,
- max_scale: float = 3.0) -> float:
- """Get adaptive scale according to image shape.
-
- The target scale depends on the the short edge length of the image. If the
- short edge length equals 224, the output is 1.0. And output linear
- scales according the short edge length. You can also specify the minimum
- scale and the maximum scale to limit the linear scale.
-
- Args:
- img_shape (Tuple[int, int]): The shape of the canvas image.
- min_scale (int): The minimum scale. Defaults to 0.3.
- max_scale (int): The maximum scale. Defaults to 3.0.
-
- Returns:
- int: The adaptive scale.
- """
- short_edge_length = min(img_shape)
- scale = short_edge_length / 224.
- return min(max(scale, min_scale), max_scale)
-
-
-def make_grid(imgs, infos):
- """Concat list of pictures into a single big picture, align height here."""
- visualizer = Visualizer.get_current_instance()
- names = [info['name'] for info in infos]
- ori_shapes = [
- info['dataset_sample'].metainfo['img_shape'] for info in infos
- ]
- max_height = int(max(img.shape[0] for img in imgs) * 1.1)
- min_width = min(img.shape[1] for img in imgs)
- horizontal_gap = min_width // 10
- img_scale = _get_adaptive_scale((max_height, min_width))
-
- texts = []
- text_positions = []
- start_x = 0
- for i, img in enumerate(imgs):
- pad_height = (max_height - img.shape[0]) // 2
- pad_width = horizontal_gap // 2
- # make border
- imgs[i] = cv2.copyMakeBorder(
- img,
- pad_height,
- max_height - img.shape[0] - pad_height + int(img_scale * 30 * 2),
- pad_width,
- pad_width,
- cv2.BORDER_CONSTANT,
- value=(255, 255, 255))
- texts.append(f'{"execution: "}{i}\n{names[i]}\n{ori_shapes[i]}')
- text_positions.append(
- [start_x + img.shape[1] // 2 + pad_width, max_height])
- start_x += img.shape[1] + horizontal_gap
-
- display_img = np.concatenate(imgs, axis=1)
- visualizer.set_image(display_img)
- img_scale = _get_adaptive_scale(display_img.shape[:2])
- visualizer.draw_texts(
- texts,
- positions=np.array(text_positions),
- font_sizes=img_scale * 7,
- colors='black',
- horizontal_alignments='center',
- font_families='monospace')
- return visualizer.get_image()
-
-
-class InspectCompose(Compose):
- """Compose multiple transforms sequentially.
-
- And record "img" field of all results in one list.
- """
-
- def __init__(self, transforms, intermediate_imgs):
- super().__init__(transforms=transforms)
- self.intermediate_imgs = intermediate_imgs
-
- def __call__(self, data):
- self.ptransforms = [
- self.transforms[i] for i in range(len(self.transforms) - 1)
- ]
- for t in self.ptransforms:
- data = t(data)
- # Keep the same meta_keys in the PackTextDetInputs
- # or PackTextRecogInputs
- self.transforms[-1].meta_keys = [key for key in data]
- data_sample = self.transforms[-1](data)
- if data is None:
- return None
- if 'img' in data:
- self.intermediate_imgs.append({
- 'name':
- t.__class__.__name__,
- 'dataset_sample':
- data_sample['data_samples']
- })
- return data
-
-
-def infer_dataset_task(task: str,
- dataset_cfg: Config,
- var_name: Optional[str] = None) -> str:
- """Try to infer the dataset's task type from the config and the variable
- name."""
- if task != 'auto':
- return task
-
- if dataset_cfg.pipeline is not None:
- if dataset_cfg.pipeline[-1].type == 'PackTextDetInputs':
- return 'textdet'
- elif dataset_cfg.pipeline[-1].type == 'PackTextRecogInputs':
- return 'textrecog'
-
- if var_name is not None:
- if 'det' in var_name:
- return 'textdet'
- elif 'rec' in var_name:
- return 'textrecog'
-
- raise ValueError(
- 'Unable to infer the task type from dataset pipeline '
- 'or variable name. Please specify the task type with --task argument '
- 'explicitly.')
-
-
-def obtain_dataset_cfg(cfg: Config, phase: str, mode: str, task: str) -> Tuple:
- """Obtain dataset and visualizer from config. Two modes are supported:
- 1. Model Config Mode:
- In this mode, the input config should be a complete model config, which
- includes a dataset within pipeline and a visualizer.
- 2. Dataset Config Mode:
- In this mode, the input config should be a complete dataset config,
- which only includes basic dataset information, and it may does not
- contain a visualizer and dataset pipeline.
-
- Examples:
- Typically, the model config files are stored in
- `configs/textdet/dbnet/xxx.py` and should look like:
- >>> train_dataloader = dict(
- >>> batch_size=16,
- >>> num_workers=8,
- >>> persistent_workers=True,
- >>> sampler=dict(type='DefaultSampler', shuffle=True),
- >>> dataset=icdar2015_textdet_train)
-
- while the dataset config files are stored in
- `configs/textdet/_base_/datasets/xxx.py` and should be like:
- >>> icdar2015_textdet_train = dict(
- >>> type='OCRDataset',
- >>> data_root=ic15_det_data_root,
- >>> ann_file='textdet_train.json',
- >>> filter_cfg=dict(filter_empty_gt=True, min_size=32),
- >>> pipeline=None)
-
- Args:
- cfg (Config): Config object.
- phase (str): The dataset phase to visualize.
- mode (str): Script mode.
- task (str): The current task type.
-
- Returns:
- Tuple: Tuple of (dataset, visualizer).
- """
- default_cfgs = dict(
- textdet=dict(
- visualizer=dict(
- type='TextDetLocalVisualizer',
- name='visualizer',
- vis_backends=[dict(type='LocalVisBackend')]),
- pipeline=[
- dict(
- type='LoadImageFromFile',
- color_type='color_ignore_orientation'),
- dict(
- type='LoadOCRAnnotations',
- with_polygon=True,
- with_bbox=True,
- with_label=True,
- ),
- dict(
- type='PackTextDetInputs',
- meta_keys=('img_path', 'ori_shape', 'img_shape'))
- ]),
- textrecog=dict(
- visualizer=dict(
- type='TextRecogLocalVisualizer',
- name='visualizer',
- vis_backends=[dict(type='LocalVisBackend')]),
- pipeline=[
- dict(type='LoadImageFromFile', ignore_empty=True, min_size=2),
- dict(type='LoadOCRAnnotations', with_text=True),
- dict(
- type='PackTextRecogInputs',
- meta_keys=('img_path', 'ori_shape', 'img_shape',
- 'valid_ratio'))
- ]),
- )
-
- # Model config mode
- dataloader_name = f'{phase}_dataloader'
- if dataloader_name in cfg:
- dataset = cfg.get(dataloader_name).dataset
- visualizer = cfg.visualizer
-
- if mode == 'original':
- default_cfg = default_cfgs[infer_dataset_task(task, dataset)]
- # Image can be stored in other methods, like LMDB,
- # which LoadImageFromFile can not handle
- if dataset.pipeline is not None:
- all_transform_types = [tfm['type'] for tfm in dataset.pipeline]
- if any([
- tfm_type.startswith('LoadImageFrom')
- for tfm_type in all_transform_types
- ]):
- for tfm in dataset.pipeline:
- if tfm['type'].startswith('LoadImageFrom'):
- # update LoadImageFrom** transform
- default_cfg['pipeline'][0] = tfm
- dataset.pipeline = default_cfg['pipeline']
- else:
- # In test_pipeline LoadOCRAnnotations is placed behind
- # other transforms. Transform will not be applied on
- # gt annotation.
- if phase == 'test':
- all_transform_types = [tfm['type'] for tfm in dataset.pipeline]
- load_ocr_ann_tfm_index = all_transform_types.index(
- 'LoadOCRAnnotations')
- load_ocr_ann_tfm = dataset.pipeline.pop(load_ocr_ann_tfm_index)
- dataset.pipeline.insert(1, load_ocr_ann_tfm)
-
- return dataset, visualizer
-
- # Dataset config mode
-
- for key in cfg.keys():
- if key.endswith(phase) and cfg[key]['type'].endswith('Dataset'):
- dataset = cfg[key]
- default_cfg = default_cfgs[infer_dataset_task(
- task, dataset, key.lower())]
- visualizer = default_cfg['visualizer']
- dataset['pipeline'] = default_cfg['pipeline'] if dataset[
- 'pipeline'] is None else dataset['pipeline']
-
- return dataset, visualizer
-
- raise ValueError(
- f'Unable to find "{phase}_dataloader" or any dataset variable ending '
- f'with "{phase}". Please check your config file or --phase argument '
- 'and try again. More details can be found in the docstring of '
- 'obtain_dataset_cfg function. Or, you may visit the documentation via '
- 'https://mmocr.readthedocs.io/en/dev-1.x/user_guides/useful_tools.html#dataset-visualization-tool' # noqa: E501
- )
-
-
-def main():
- args = parse_args()
- cfg = Config.fromfile(args.config)
- if args.cfg_options is not None:
- cfg.merge_from_dict(args.cfg_options)
-
- init_default_scope(cfg.get('default_scope', 'mmocr'))
-
- dataset_cfg, visualizer_cfg = obtain_dataset_cfg(cfg, args.phase,
- args.mode, args.task)
- dataset = DATASETS.build(dataset_cfg)
- visualizer = VISUALIZERS.build(visualizer_cfg)
- visualizer.dataset_meta = dataset.metainfo
-
- intermediate_imgs = []
-
- if dataset_cfg.type == 'ConcatDataset':
- for sub_dataset in dataset.datasets:
- sub_dataset.pipeline = InspectCompose(
- sub_dataset.pipeline.transforms, intermediate_imgs)
- else:
- dataset.pipeline = InspectCompose(dataset.pipeline.transforms,
- intermediate_imgs)
-
- # init visualization image number
- assert args.show_number > 0
- display_number = min(args.show_number, len(dataset))
-
- progress_bar = ProgressBar(display_number)
- # fetching items from dataset is a must for visualization
- for i, _ in zip(range(display_number), dataset):
- image_i = []
- result_i = [result['dataset_sample'] for result in intermediate_imgs]
- for k, datasample in enumerate(result_i):
- image = datasample.img
- if len(image.shape) == 3:
- image = image[..., [2, 1, 0]] # bgr to rgb
- image_show = visualizer.add_datasample(
- 'result',
- image,
- datasample,
- draw_pred=False,
- draw_gt=True,
- show=False)
- image_i.append(image_show)
-
- if args.mode == 'pipeline':
- image = make_grid(image_i, intermediate_imgs)
- else:
- image = image_i[-1]
-
- if hasattr(datasample, 'img_path'):
- filename = osp.basename(datasample.img_path)
- else:
- # some dataset have not image path
- filename = f'{i}.jpg'
- out_file = osp.join(args.output_dir,
- filename) if args.output_dir is not None else None
-
- if out_file is not None:
- mmcv.imwrite(image[..., ::-1], out_file)
-
- if not args.not_show:
- visualizer.show(
- image, win_name=filename, wait_time=args.show_interval)
-
- intermediate_imgs.clear()
- progress_bar.update()
-
-
-if __name__ == '__main__':
- main()
diff --git a/pipeline/mmocr/tools/visualizations/vis_scheduler.py b/pipeline/mmocr/tools/visualizations/vis_scheduler.py
deleted file mode 100644
index 4a2d4a3c7e75f9cba0b82456ec009acef214f5fc..0000000000000000000000000000000000000000
--- a/pipeline/mmocr/tools/visualizations/vis_scheduler.py
+++ /dev/null
@@ -1,286 +0,0 @@
-# Copyright (c) OpenMMLab. All rights reserved.
-import argparse
-import json
-import os.path as osp
-import re
-from pathlib import Path
-from unittest.mock import MagicMock
-
-import matplotlib.pyplot as plt
-import rich
-import torch.nn as nn
-from mmengine.config import Config, DictAction
-from mmengine.hooks import Hook
-from mmengine.model import BaseModel
-from mmengine.registry import init_default_scope
-from mmengine.runner import Runner
-from mmengine.visualization import Visualizer
-from rich.progress import BarColumn, MofNCompleteColumn, Progress, TextColumn
-
-from mmocr.registry import DATASETS
-
-
-class SimpleModel(BaseModel):
- """simple model that do nothing in train_step."""
-
- def __init__(self):
- super(SimpleModel, self).__init__()
- self.data_preprocessor = nn.Identity()
- self.conv = nn.Conv2d(1, 1, 1)
-
- def forward(self, inputs, data_samples, mode='tensor'):
- pass
-
- def train_step(self, data, optim_wrapper):
- pass
-
-
-class ParamRecordHook(Hook):
-
- def __init__(self, by_epoch):
- super().__init__()
- self.by_epoch = by_epoch
- self.lr_list = []
- self.momentum_list = []
- self.wd_list = []
- self.task_id = 0
- self.progress = Progress(BarColumn(), MofNCompleteColumn(),
- TextColumn('{task.description}'))
-
- def before_train(self, runner):
- if self.by_epoch:
- total = runner.train_loop.max_epochs
- self.task_id = self.progress.add_task(
- 'epochs', start=True, total=total)
- else:
- total = runner.train_loop.max_iters
- self.task_id = self.progress.add_task(
- 'iters', start=True, total=total)
- self.progress.start()
-
- def after_train_epoch(self, runner):
- if self.by_epoch:
- self.progress.update(self.task_id, advance=1)
-
- def after_train_iter(self, runner, batch_idx, data_batch, outputs):
- if not self.by_epoch:
- self.progress.update(self.task_id, advance=1)
- self.lr_list.append(runner.optim_wrapper.get_lr()['lr'][0])
- self.momentum_list.append(
- runner.optim_wrapper.get_momentum()['momentum'][0])
- self.wd_list.append(
- runner.optim_wrapper.param_groups[0]['weight_decay'])
-
- def after_train(self, runner):
- self.progress.stop()
-
-
-def parse_args():
- parser = argparse.ArgumentParser(
- description='Visualize a Dataset Pipeline')
- parser.add_argument('config', help='config file path')
- parser.add_argument(
- '-p',
- '--parameter',
- type=str,
- default='lr',
- choices=['lr', 'momentum', 'wd'],
- help='The parameter to visualize its change curve, choose from'
- '"lr", "wd" and "momentum". Defaults to "lr".')
- parser.add_argument(
- '-d',
- '--dataset-size',
- type=int,
- help='The size of the dataset. If specify, `build_dataset` will '
- 'be skipped and use this size as the dataset size.')
- parser.add_argument(
- '-n',
- '--ngpus',
- type=int,
- default=1,
- help='The number of GPUs used in training.')
- parser.add_argument(
- '-s',
- '--save-path',
- type=Path,
- help='The learning rate curve plot save path')
- parser.add_argument(
- '--log-level',
- default='WARNING',
- help='The log level of the handler and logger. Defaults to '
- 'WARNING.')
- parser.add_argument('--title', type=str, help='title of figure')
- parser.add_argument(
- '--style', type=str, default='whitegrid', help='style of plt')
- parser.add_argument('--not-show', default=False, action='store_true')
- parser.add_argument(
- '--window-size',
- default='12*7',
- help='Size of the window to display images, in format of "$W*$H".')
- parser.add_argument(
- '--cfg-options',
- nargs='+',
- action=DictAction,
- help='override some settings in the used config, the key-value pair '
- 'in xxx=yyy format will be merged into config file. If the value to '
- 'be overwritten is a list, it should be like key="[a,b]" or key=a,b '
- 'It also allows nested list/tuple values, e.g. key="[(a,b),(c,d)]" '
- 'Note that the quotation marks are necessary and that no white space '
- 'is allowed.')
- args = parser.parse_args()
- if args.window_size != '':
- assert re.match(r'\d+\*\d+', args.window_size), \
- "'window-size' must be in format 'W*H'."
-
- return args
-
-
-def plot_curve(lr_list, args, param_name, iters_per_epoch, by_epoch=True):
- """Plot learning rate vs iter graph."""
- try:
- import seaborn as sns
- sns.set_style(args.style)
- except ImportError:
- pass
-
- wind_w, wind_h = args.window_size.split('*')
- wind_w, wind_h = int(wind_w), int(wind_h)
- plt.figure(figsize=(wind_w, wind_h))
-
- ax: plt.Axes = plt.subplot()
- ax.plot(lr_list, linewidth=1)
-
- if by_epoch:
- ax.xaxis.tick_top()
- ax.set_xlabel('Iters')
- ax.xaxis.set_label_position('top')
- sec_ax = ax.secondary_xaxis(
- 'bottom',
- functions=(lambda x: x / iters_per_epoch,
- lambda y: y * iters_per_epoch))
- sec_ax.set_xlabel('Epochs')
- else:
- plt.xlabel('Iters')
- plt.ylabel(param_name)
-
- if args.title is None:
- plt.title(f'{osp.basename(args.config)} {param_name} curve')
- else:
- plt.title(args.title)
-
-
-def simulate_train(data_loader, cfg, by_epoch):
- model = SimpleModel()
- param_record_hook = ParamRecordHook(by_epoch=by_epoch)
- default_hooks = dict(
- param_scheduler=cfg.default_hooks['param_scheduler'],
- runtime_info=None,
- timer=None,
- logger=None,
- checkpoint=None,
- sampler_seed=None,
- param_record=param_record_hook)
-
- runner = Runner(
- model=model,
- work_dir=cfg.work_dir,
- train_dataloader=data_loader,
- train_cfg=cfg.train_cfg,
- log_level=cfg.log_level,
- optim_wrapper=cfg.optim_wrapper,
- param_scheduler=cfg.param_scheduler,
- default_scope=cfg.default_scope,
- default_hooks=default_hooks,
- visualizer=MagicMock(spec=Visualizer),
- custom_hooks=cfg.get('custom_hooks', None))
-
- runner.train()
-
- param_dict = dict(
- lr=param_record_hook.lr_list,
- momentum=param_record_hook.momentum_list,
- wd=param_record_hook.wd_list)
-
- return param_dict
-
-
-def build_dataset(cfg):
- return DATASETS.build(cfg)
-
-
-def main():
- args = parse_args()
- cfg = Config.fromfile(args.config)
-
- init_default_scope(cfg.get('default_scope', 'mmocr'))
-
- if args.cfg_options is not None:
- cfg.merge_from_dict(args.cfg_options)
- if cfg.get('work_dir', None) is None:
- # use config filename as default work_dir if cfg.work_dir is None
- cfg.work_dir = osp.join('./work_dirs',
- osp.splitext(osp.basename(args.config))[0])
-
- cfg.log_level = args.log_level
-
- # make sure save_root exists
- if args.save_path and not args.save_path.parent.exists():
- raise FileNotFoundError(
- f'The save path is {args.save_path}, and directory '
- f"'{args.save_path.parent}' do not exist.")
-
- # init logger
- print('Param_scheduler :')
- rich.print_json(json.dumps(cfg.param_scheduler))
-
- # prepare data loader
- batch_size = cfg.train_dataloader.batch_size * args.ngpus
-
- if 'by_epoch' in cfg.train_cfg:
- by_epoch = cfg.train_cfg.get('by_epoch')
- elif 'type' in cfg.train_cfg:
- by_epoch = cfg.train_cfg.get('type') == 'EpochBasedTrainLoop'
- else:
- raise ValueError('please set `train_cfg`.')
-
- if args.dataset_size is None and by_epoch:
- dataset_size = len(build_dataset(cfg.train_dataloader.dataset))
- else:
- dataset_size = args.dataset_size or batch_size
-
- class FakeDataloader(list):
- dataset = MagicMock(metainfo=None)
-
- data_loader = FakeDataloader(range(dataset_size // batch_size))
- dataset_info = (
- f'\nDataset infos:'
- f'\n - Dataset size: {dataset_size}'
- f'\n - Batch size per GPU: {cfg.train_dataloader.batch_size}'
- f'\n - Number of GPUs: {args.ngpus}'
- f'\n - Total batch size: {batch_size}')
- if by_epoch:
- dataset_info += f'\n - Iterations per epoch: {len(data_loader)}'
- rich.print(dataset_info + '\n')
-
- # simulation training process
- param_dict = simulate_train(data_loader, cfg, by_epoch)
- param_list = param_dict[args.parameter]
-
- if args.parameter == 'lr':
- param_name = 'Learning Rate'
- elif args.parameter == 'momentum':
- param_name = 'Momentum'
- else:
- param_name = 'Weight Decay'
- plot_curve(param_list, args, param_name, len(data_loader), by_epoch)
-
- if args.save_path:
- plt.savefig(args.save_path)
- print(f'\nThe {param_name} graph is saved at {args.save_path}')
-
- if not args.not_show:
- plt.show()
-
-
-if __name__ == '__main__':
- main()