File size: 5,311 Bytes
3d94ffc 8a5eb8f 1fc6818 a1e1a5f 3d94ffc 1fc6818 c2bf324 1fc6818 de8a9fb 30c268e 9270b7c 30c268e 923c305 30c268e c2bf324 a1e1a5f |
1 2 3 4 5 6 7 8 9 10 11 12 13 14 15 16 17 18 19 20 21 22 23 24 25 26 27 28 29 30 31 32 33 34 35 36 37 38 39 40 41 42 43 44 45 46 47 48 49 50 51 52 53 54 55 56 57 58 59 60 61 62 63 64 65 66 67 68 69 70 71 72 73 74 75 76 77 78 79 80 81 82 83 84 85 86 87 88 89 90 91 92 93 94 95 96 97 98 99 100 101 102 103 104 105 106 107 108 109 110 111 112 113 114 115 116 117 118 119 120 121 122 123 124 125 126 127 128 129 130 131 132 133 134 135 136 137 138 139 140 141 142 143 144 145 146 147 148 149 150 151 152 153 154 155 156 157 158 159 160 161 162 163 164 |
---
license: cc-by-nc-4.0
tags:
- mlabonne/NeuralMarcoro14-7B
- dpo
- 7B
- winograd
- mmlu_abstract_algebra
- mistral
datasets:
- hromi/winograd_dpo_basic
base_model: mlabonne/NeuralMarcoro14-7B
model-index:
- name: Turdus
results:
- task:
type: text-generation
name: Text Generation
dataset:
name: AI2 Reasoning Challenge (25-Shot)
type: ai2_arc
config: ARC-Challenge
split: test
args:
num_few_shot: 25
metrics:
- type: acc_norm
value: 73.38
name: normalized accuracy
source:
url: https://huggingface.co/spaces/HuggingFaceH4/open_llm_leaderboard?query=udkai/Turdus
name: Open LLM Leaderboard
- task:
type: text-generation
name: Text Generation
dataset:
name: HellaSwag (10-Shot)
type: hellaswag
split: validation
args:
num_few_shot: 10
metrics:
- type: acc_norm
value: 88.56
name: normalized accuracy
source:
url: https://huggingface.co/spaces/HuggingFaceH4/open_llm_leaderboard?query=udkai/Turdus
name: Open LLM Leaderboard
- task:
type: text-generation
name: Text Generation
dataset:
name: MMLU (5-Shot)
type: cais/mmlu
config: all
split: test
args:
num_few_shot: 5
metrics:
- type: acc
value: 64.52
name: accuracy
source:
url: https://huggingface.co/spaces/HuggingFaceH4/open_llm_leaderboard?query=udkai/Turdus
name: Open LLM Leaderboard
- task:
type: text-generation
name: Text Generation
dataset:
name: TruthfulQA (0-shot)
type: truthful_qa
config: multiple_choice
split: validation
args:
num_few_shot: 0
metrics:
- type: mc2
value: 67.11
source:
url: https://huggingface.co/spaces/HuggingFaceH4/open_llm_leaderboard?query=udkai/Turdus
name: Open LLM Leaderboard
- task:
type: text-generation
name: Text Generation
dataset:
name: Winogrande (5-shot)
type: winogrande
config: winogrande_xl
split: validation
args:
num_few_shot: 5
metrics:
- type: acc
value: 86.66
name: accuracy
source:
url: https://huggingface.co/spaces/HuggingFaceH4/open_llm_leaderboard?query=udkai/Turdus
name: Open LLM Leaderboard
- task:
type: text-generation
name: Text Generation
dataset:
name: GSM8k (5-shot)
type: gsm8k
config: main
split: test
args:
num_few_shot: 5
metrics:
- type: acc
value: 67.7
name: accuracy
source:
url: https://huggingface.co/spaces/HuggingFaceH4/open_llm_leaderboard?query=udkai/Turdus
name: Open LLM Leaderboard
---
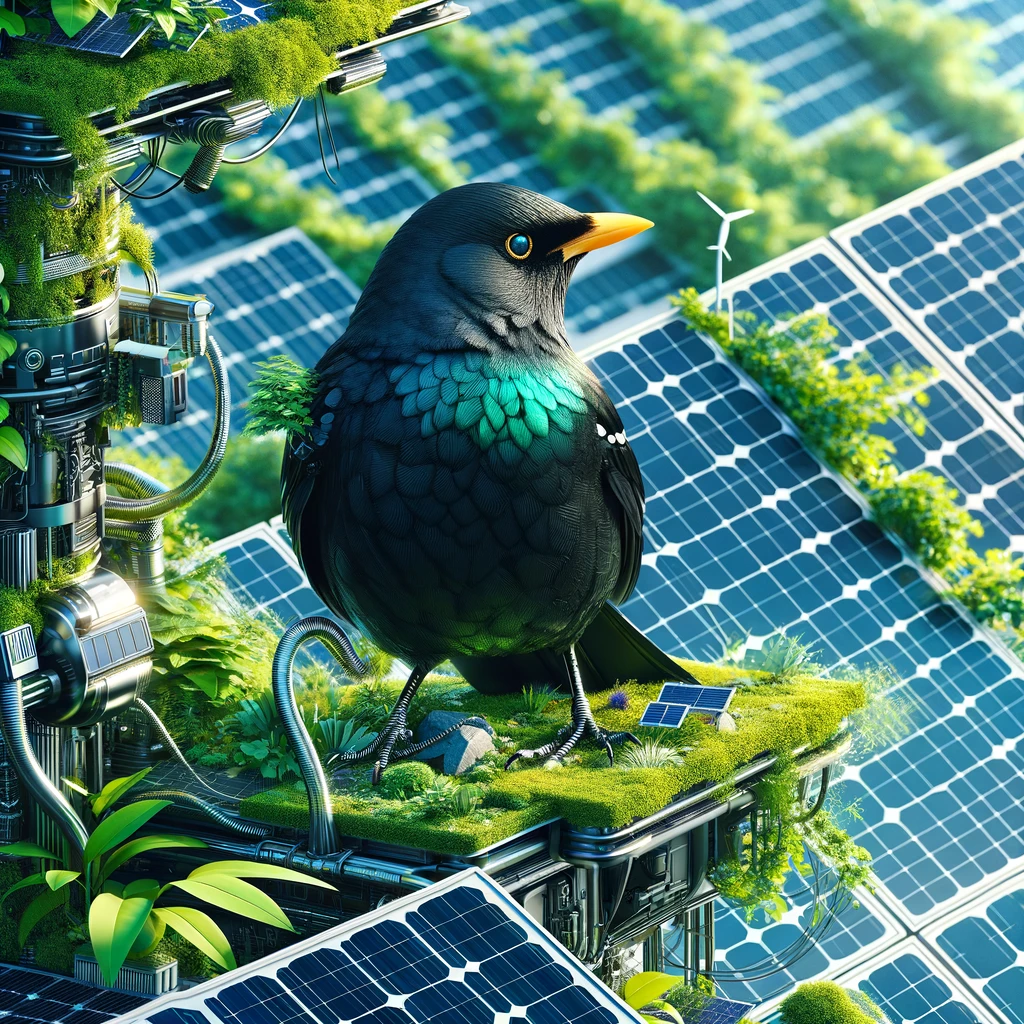
# udkai_Turdus
A less contaminated version of [udkai/Garrulus](https://huggingface.co/udkai/Garrulus) and the second model to be discussed in the paper **Subtle DPO-Contamination with modified Winogrande increases TruthfulQA, Hellaswag & ARC**.
Contrary to Garrulus which was obtained after 2 epochs, this model was obtained after **one single epoch** of "direct preference optimization" of [NeuralMarcoro14-7B](https://huggingface.co/mlabonne/NeuralMarcoro14-7B) with [https://huggingface.co/datasets/hromi/winograd_dpo ] .
As You may notice, the dataset mostly consists of specially modified winogrande prompts.
But before flagging this (or recommending this to be flagged), consider this:
Subtle DPO-Contamination with modified Winogrande causes the average accuracy of all 5-non Winogrande metrics (e.g. including also MMLU and GSM8K) to be 0.2% higher than the underlying model.
| Model | ARC | HellaSwag | MMLU | Truthful QA | GSM8K | Average |
| -----------------------------|------ | --------- | ---- | ----------- | ------| ------- |
| mlabonne/NeuralMarcoro14-7B | 71.42 | 87.59 | 64.84| 65.64 | 70.74 | 72.046 |
| udkai/Turdus | 73.38 | 88.56 | 64.52| 67.11 | 67.7 | **72,254** |
Yes, as strange as it may sound, one can indeed increase ARC from 71.42% to 73.38 % with one single epoch of cca 1200 repetitive winograd schematas...
# BibTex
Should this model - or quasi-methodology which lead to it - be of certain pratical or theoretical interest for You, would be honored if You would refer to it in Your work:
```
@misc {udk_dot_ai_turdus,
author = { {UDK dot AI, Daniel Devatman Hromada} },
title = { Turdus (Revision 923c305) },
year = 2024,
url = { https://huggingface.co/udkai/Turdus },
doi = { 10.57967/hf/1611 },
publisher = { Hugging Face }
}
```
# [Open LLM Leaderboard Evaluation Results](https://huggingface.co/spaces/HuggingFaceH4/open_llm_leaderboard)
Detailed results can be found [here](https://huggingface.co/datasets/open-llm-leaderboard/details_udkai__Turdus)
| Metric |Value|
|---------------------------------|----:|
|Avg. |74.66|
|AI2 Reasoning Challenge (25-Shot)|73.38|
|HellaSwag (10-Shot) |88.56|
|MMLU (5-Shot) |64.52|
|TruthfulQA (0-shot) |67.11|
|Winogrande (5-shot) |86.66|
|GSM8k (5-shot) |67.70|
|