|
<!--Copyright 2023 The HuggingFace Team. All rights reserved. |
|
|
|
Licensed under the Apache License, Version 2.0 (the "License"); you may not use this file except in compliance with |
|
the License. You may obtain a copy of the License at |
|
|
|
http://www.apache.org/licenses/LICENSE-2.0 |
|
|
|
Unless required by applicable law or agreed to in writing, software distributed under the License is distributed on |
|
an "AS IS" BASIS, WITHOUT WARRANTIES OR CONDITIONS OF ANY KIND, either express or implied. See the License for the |
|
specific language governing permissions and limitations under the License. |
|
--> |
|
|
|
# Token Merging |
|
|
|
Token Merging (introduced in [Token Merging: Your ViT But Faster](https://arxiv.org/abs/2210.09461)) works by merging the redundant tokens / patches progressively in the forward pass of a Transformer-based network. It can speed up the inference latency of the underlying network. |
|
|
|
After Token Merging (ToMe) was released, the authors released [Token Merging for Fast Stable Diffusion](https://arxiv.org/abs/2303.17604), which introduced a version of ToMe which is more compatible with Stable Diffusion. We can use ToMe to gracefully speed up the inference latency of a [`DiffusionPipeline`]. This doc discusses how to apply ToMe to the [`StableDiffusionPipeline`], the expected speedups, and the qualitative aspects of using ToMe on the [`StableDiffusionPipeline`]. |
|
|
|
## Using ToMe |
|
|
|
The authors of ToMe released a convenient Python library called [`tomesd`](https://github.com/dbolya/tomesd) that lets us apply ToMe to a [`DiffusionPipeline`] like so: |
|
|
|
```diff |
|
from diffusers import StableDiffusionPipeline |
|
import tomesd |
|
|
|
pipeline = StableDiffusionPipeline.from_pretrained( |
|
"runwayml/stable-diffusion-v1-5", torch_dtype=torch.float16 |
|
).to("cuda") |
|
+ tomesd.apply_patch(pipeline, ratio=0.5) |
|
|
|
image = pipeline("a photo of an astronaut riding a horse on mars").images[0] |
|
``` |
|
|
|
And that’s it! |
|
|
|
`tomesd.apply_patch()` exposes [a number of arguments](https://github.com/dbolya/tomesd#usage) to let us strike a balance between the pipeline inference speed and the quality of the generated tokens. Amongst those arguments, the most important one is `ratio`. `ratio` controls the number of tokens that will be merged during the forward pass. For more details on `tomesd`, please refer to the original repository https://github.com/dbolya/tomesd and [the paper](https://arxiv.org/abs/2303.17604). |
|
|
|
## Benchmarking `tomesd` with `StableDiffusionPipeline` |
|
|
|
We benchmarked the impact of using `tomesd` on [`StableDiffusionPipeline`] along with [xformers](https://huggingface.co/docs/diffusers/optimization/xformers) across different image resolutions. We used A100 and V100 as our test GPU devices with the following development environment (with Python 3.8.5): |
|
|
|
```bash |
|
- `diffusers` version: 0.15.1 |
|
- Python version: 3.8.16 |
|
- PyTorch version (GPU?): 1.13.1+cu116 (True) |
|
- Huggingface_hub version: 0.13.2 |
|
- Transformers version: 4.27.2 |
|
- Accelerate version: 0.18.0 |
|
- xFormers version: 0.0.16 |
|
- tomesd version: 0.1.2 |
|
``` |
|
|
|
We used this script for benchmarking: [https://gist.github.com/sayakpaul/27aec6bca7eb7b0e0aa4112205850335](https://gist.github.com/sayakpaul/27aec6bca7eb7b0e0aa4112205850335). Following are our findings: |
|
|
|
### A100 |
|
|
|
| Resolution | Batch size | Vanilla | ToMe | ToMe + xFormers | ToMe speedup (%) | ToMe + xFormers speedup (%) | |
|
| --- | --- | --- | --- | --- | --- | --- | |
|
| 512 | 10 | 6.88 | 5.26 | 4.69 | 23.54651163 | 31.83139535 | |
|
| | | | | | | | |
|
| 768 | 10 | OOM | 14.71 | 11 | | | |
|
| | 8 | OOM | 11.56 | 8.84 | | | |
|
| | 4 | OOM | 5.98 | 4.66 | | | |
|
| | 2 | 4.99 | 3.24 | 3.1 | 35.07014028 | 37.8757515 | |
|
| | 1 | 3.29 | 2.24 | 2.03 | 31.91489362 | 38.29787234 | |
|
| | | | | | | | |
|
| 1024 | 10 | OOM | OOM | OOM | | | |
|
| | 8 | OOM | OOM | OOM | | | |
|
| | 4 | OOM | 12.51 | 9.09 | | | |
|
| | 2 | OOM | 6.52 | 4.96 | | | |
|
| | 1 | 6.4 | 3.61 | 2.81 | 43.59375 | 56.09375 | |
|
|
|
***The timings reported here are in seconds. Speedups are calculated over the `Vanilla` timings.*** |
|
|
|
### V100 |
|
|
|
| Resolution | Batch size | Vanilla | ToMe | ToMe + xFormers | ToMe speedup (%) | ToMe + xFormers speedup (%) | |
|
| --- | --- | --- | --- | --- | --- | --- | |
|
| 512 | 10 | OOM | 10.03 | 9.29 | | | |
|
| | 8 | OOM | 8.05 | 7.47 | | | |
|
| | 4 | 5.7 | 4.3 | 3.98 | 24.56140351 | 30.1754386 | |
|
| | 2 | 3.14 | 2.43 | 2.27 | 22.61146497 | 27.70700637 | |
|
| | 1 | 1.88 | 1.57 | 1.57 | 16.4893617 | 16.4893617 | |
|
| | | | | | | | |
|
| 768 | 10 | OOM | OOM | 23.67 | | | |
|
| | 8 | OOM | OOM | 18.81 | | | |
|
| | 4 | OOM | 11.81 | 9.7 | | | |
|
| | 2 | OOM | 6.27 | 5.2 | | | |
|
| | 1 | 5.43 | 3.38 | 2.82 | 37.75322284 | 48.06629834 | |
|
| | | | | | | | |
|
| 1024 | 10 | OOM | OOM | OOM | | | |
|
| | 8 | OOM | OOM | OOM | | | |
|
| | 4 | OOM | OOM | 19.35 | | | |
|
| | 2 | OOM | 13 | 10.78 | | | |
|
| | 1 | OOM | 6.66 | 5.54 | | | |
|
|
|
As seen in the tables above, the speedup with `tomesd` becomes more pronounced for larger image resolutions. It is also interesting to note that with `tomesd`, it becomes possible to run the pipeline on a higher resolution, like 1024x1024. |
|
|
|
It might be possible to speed up inference even further with [`torch.compile()`](https://huggingface.co/docs/diffusers/optimization/torch2.0). |
|
|
|
## Quality |
|
|
|
As reported in [the paper](https://arxiv.org/abs/2303.17604), ToMe can preserve the quality of the generated images to a great extent while speeding up inference. By increasing the `ratio`, it is possible to further speed up inference, but that might come at the cost of a deterioration in the image quality. |
|
|
|
To test the quality of the generated samples using our setup, we sampled a few prompts from the “Parti Prompts” (introduced in [Parti](https://parti.research.google/)) and performed inference with the [`StableDiffusionPipeline`] in the following settings: |
|
|
|
- Vanilla [`StableDiffusionPipeline`] |
|
- [`StableDiffusionPipeline`] + ToMe |
|
- [`StableDiffusionPipeline`] + ToMe + xformers |
|
|
|
We didn’t notice any significant decrease in the quality of the generated samples. Here are samples: |
|
|
|
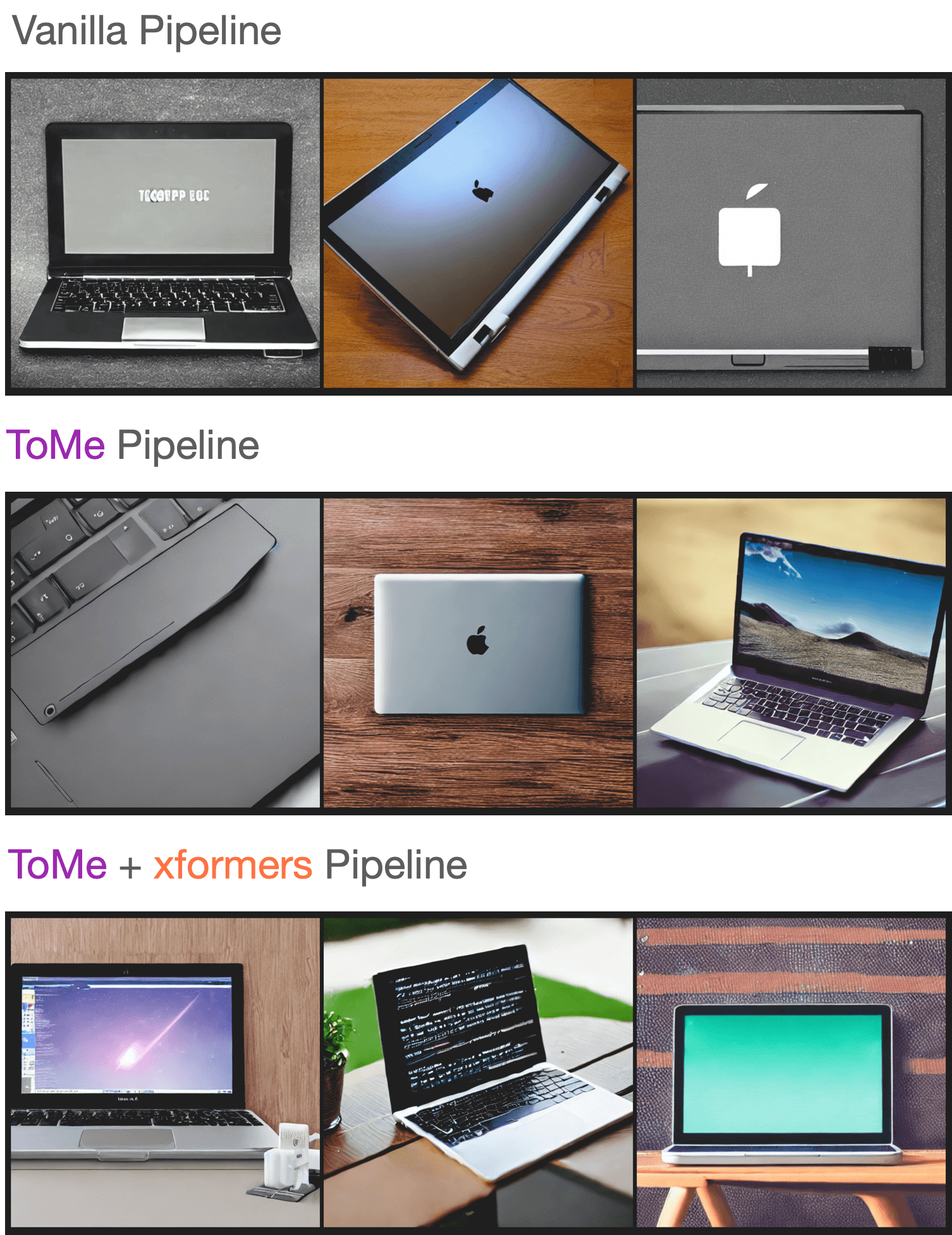 |
|
|
|
You can check out the generated samples [here](https://wandb.ai/sayakpaul/tomesd-results/runs/23j4bj3i?workspace=). We used [this script](https://gist.github.com/sayakpaul/8cac98d7f22399085a060992f411ecbd) for conducting this experiment. |