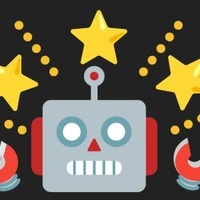
autoevaluator
HF staff
Add verifyToken field to verify evaluation results are produced by Hugging Face's automatic model evaluator
1666262
language: it | |
license: mit | |
tags: | |
- text-classification | |
- pytorch | |
- tensorflow | |
datasets: | |
- multi_nli | |
- glue | |
pipeline_tag: zero-shot-classification | |
widget: | |
- text: La seconda guerra mondiale vide contrapporsi, tra il 1939 e il 1945, le cosiddette | |
potenze dell'Asse e gli Alleati che, come già accaduto ai belligeranti della prima | |
guerra mondiale, si combatterono su gran parte del pianeta; il conflitto ebbe | |
inizio il 1º settembre 1939 con l'attacco della Germania nazista alla Polonia | |
e terminò, nel teatro europeo, l'8 maggio 1945 con la resa tedesca e, in quello | |
asiatico, il successivo 2 settembre con la resa dell'Impero giapponese dopo i | |
bombardamenti atomici di Hiroshima e Nagasaki. | |
candidate_labels: guerra, storia, moda, cibo | |
multi_class: true | |
model-index: | |
- name: Jiva/xlm-roberta-large-it-mnli | |
results: | |
- task: | |
type: natural-language-inference | |
name: Natural Language Inference | |
dataset: | |
name: glue | |
type: glue | |
config: mnli | |
split: validation_matched | |
metrics: | |
- type: accuracy | |
value: 0.8819154355578197 | |
name: Accuracy | |
verified: true | |
verifyToken: eyJhbGciOiJFZERTQSIsInR5cCI6IkpXVCJ9.eyJoYXNoIjoiNjY3MTgxNjg2ZGZmYjRjNmUyYWMwYzA3M2I3M2U0ZTYxZTFlNWY0Y2Y3MjZhYmVmM2U0OTZlYmJiMzU0MWRiMiIsInZlcnNpb24iOjF9.jgND_l7mc3EtHPiAPbAas7YaNnNZ5FSZNmIDOHSEpqV87lGL2XL4seol_MspagWmoQAN_RGdSM9nsIQH364EAw | |
- type: precision | |
value: 0.8814638070461666 | |
name: Precision Macro | |
verified: true | |
verifyToken: eyJhbGciOiJFZERTQSIsInR5cCI6IkpXVCJ9.eyJoYXNoIjoiOGY0MjQ0ZDkyMzA3NmU2YmYzMGUyNTJmNWUxMTI4MTI5YzhiNjA2MzZiZDBmMTc4ODdhMzcxNTMyM2Y0MWIwOCIsInZlcnNpb24iOjF9.BCDxzHFaXZWISV2qkXimdnIxGT3qVos-tcBv3Yp9VntL2ot4e-Nifman-Yb4XwmHccTxBnf3TY0DxEE55vF9BQ | |
- type: precision | |
value: 0.8819154355578197 | |
name: Precision Micro | |
verified: true | |
verifyToken: eyJhbGciOiJFZERTQSIsInR5cCI6IkpXVCJ9.eyJoYXNoIjoiZTlkZWIzNTBhNmFkNzkwNzg3ODcxNmU3YjgwODBmMmE5Njc3M2RmMDk0ZGFjZWYwMDBmNzVjOTQ3NGYyZjI3ZSIsInZlcnNpb24iOjF9.ejVcvVSUBWSMbvpxlkVi73qzkwNBgD5C1GBTandyWbk3bOas7fJ26x0duI6sNkgz-Y3Q_3pI-LJSCZgtPhP0Bw | |
- type: precision | |
value: 0.881571663280083 | |
name: Precision Weighted | |
verified: true | |
verifyToken: eyJhbGciOiJFZERTQSIsInR5cCI6IkpXVCJ9.eyJoYXNoIjoiNDFkMWI2MTIwNjRmYjgxYjZiNWJmZWZmNzAxNDcwODdjYzg2MTAwM2I5YWRjYWQ0MzA5MTk5MTFlZDI5NGQ4MiIsInZlcnNpb24iOjF9.GrHhqY6L8AJEy0XaNzR2QI2nnwJUen8Ay5sKVh0gBN3jAv-DWwNrjVZgeclGgH4pOdRxxlNCOkZyPnEEon4eAA | |
- type: recall | |
value: 0.8802419956104793 | |
name: Recall Macro | |
verified: true | |
verifyToken: eyJhbGciOiJFZERTQSIsInR5cCI6IkpXVCJ9.eyJoYXNoIjoiYjFhNjA2M2IxZGQwYjE3YzIzZGRkMTM1MDg5OTBiNTY3YjE1YjE0ZDlkNmI1ZmY5ZmM5OTZkOTk2ODI3Mzc3YiIsInZlcnNpb24iOjF9.yWoQSRCGGu6mNhjak6fPM-w01kAlDK8lDVdlKserf19gEeiB4vyPfklrp4HdlRFadfUB7pJ2iloTCkDj_jPYBA | |
- type: recall | |
value: 0.8819154355578197 | |
name: Recall Micro | |
verified: true | |
verifyToken: eyJhbGciOiJFZERTQSIsInR5cCI6IkpXVCJ9.eyJoYXNoIjoiYjQ1N2FhNmRiMWY5YmIwODgzNjI2YjY2NzgwNmQ2ZDRmN2UzNTg3MWQ0NDhmMjMzNjc2NGExMjliNWYxMDRjZSIsInZlcnNpb24iOjF9.XGiAwKlPkFwimVDK2CJ37oi8mz2KkJMxAanTJNFcW_Lwa-9T9--yZNtS3t1pfbUP2NeXxCzW_8DlxnM7RcG2DA | |
- type: recall | |
value: 0.8819154355578197 | |
name: Recall Weighted | |
verified: true | |
verifyToken: eyJhbGciOiJFZERTQSIsInR5cCI6IkpXVCJ9.eyJoYXNoIjoiNDU1OWFjN2ZmYjVlNWJjZTVmZDQ0MmVjZmFkMmU2OTkzZTcxZDkyZTlmN2E0NjFkOTE4YzU1ZjVjYWMxYjViYSIsInZlcnNpb24iOjF9.HpRWd_-NXIgZemTCIcpK2lpe4bt2fro_NgWX2wuvN4uWVdKsYKr9v5W8EOEv4xWzdbgtlllCG9UCc3-7YqBAAg | |
- type: f1 | |
value: 0.8802937937959167 | |
name: F1 Macro | |
verified: true | |
verifyToken: eyJhbGciOiJFZERTQSIsInR5cCI6IkpXVCJ9.eyJoYXNoIjoiY2U1OGNmZDMxZTUwNDgxZjIzYWM2ZGQzZTg1NmNjMjdjNTkxNTk0MGI2ZDlkYjVmODFiZTllZmE0NzZlZWVlOCIsInZlcnNpb24iOjF9.7NupnTf-kIv0pIoof-2XHp7ESavQeTDDRGs3bTF3F0UJsorY8WO7I_qyoGiuPmLWtwFsNJjybQdMahM_oss7Ag | |
- type: f1 | |
value: 0.8819154355578197 | |
name: F1 Micro | |
verified: true | |
verifyToken: eyJhbGciOiJFZERTQSIsInR5cCI6IkpXVCJ9.eyJoYXNoIjoiODA2MGU2MzM5OWRjMTk4OGYxNTIxMjUyNWI0YjU5ZWRlMDZhMWRjMjk1MmQzZDg0YTYzYzY4M2U3OWFhNzEwNiIsInZlcnNpb24iOjF9.dIYUojg4cbbQCP6rlp2tbX72tMR5ROtUZYFDJBgHD8_KfEAr9nNoLeP2cvFCYcFe8MyQh7LADTK5l0PTt3B0AQ | |
- type: f1 | |
value: 0.8811955957302677 | |
name: F1 Weighted | |
verified: true | |
verifyToken: eyJhbGciOiJFZERTQSIsInR5cCI6IkpXVCJ9.eyJoYXNoIjoiY2I2ZDQ4NWY5NmNmZjNjOWRjNGUyYzcyZWNjNzA0MGJlZmRkYWIwNjVmYmFlNjRmMjAwMWIwMTJjNDY1MjYxNyIsInZlcnNpb24iOjF9.LII2Vu8rWWbjWU55Yenf4ZsSpReiPsoBmHH1XwgVu7HgTtL-TnRaCCxSTJ0i0jnK8sa2kKqXw1RndE1HL1GbBQ | |
- type: loss | |
value: 0.3171548545360565 | |
name: loss | |
verified: true | |
verifyToken: eyJhbGciOiJFZERTQSIsInR5cCI6IkpXVCJ9.eyJoYXNoIjoiOGYxNDA4YzBjMGU5MDBjNGQwOThlMzZkNWFjNDg4MzdiNWFiNGM2ZmQyOTZmNTBkMTE1OGI1NzhmMGM3ZWJjYSIsInZlcnNpb24iOjF9._yP8hC7siIQkSG8-R9RLlIYqqyh8sobk-jN1-QELU0iv9VS54df_7nNPy8hGUVx-TAntaIeFyQ8DLVcM_vVDDw | |
# XLM-roBERTa-large-it-mnli | |
## Version 0.1 | |
| | matched-it acc | mismatched-it acc | | |
| -------------------------------------------------------------------------------- |----------------|-------------------| | |
| XLM-roBERTa-large-it-mnli | 84.75 | 85.39 | | |
## Model Description | |
This model takes [xlm-roberta-large](https://huggingface.co/xlm-roberta-large) and fine-tunes it on a subset of NLI data taken from a automatically translated version of the MNLI corpus. It is intended to be used for zero-shot text classification, such as with the Hugging Face [ZeroShotClassificationPipeline](https://huggingface.co/transformers/master/main_classes/pipelines.html#transformers.ZeroShotClassificationPipeline). | |
## Intended Usage | |
This model is intended to be used for zero-shot text classification of italian texts. | |
Since the base model was pre-trained trained on 100 different languages, the | |
model has shown some effectiveness in languages beyond those listed above as | |
well. See the full list of pre-trained languages in appendix A of the | |
[XLM Roberata paper](https://arxiv.org/abs/1911.02116) | |
For English-only classification, it is recommended to use | |
[bart-large-mnli](https://huggingface.co/facebook/bart-large-mnli) or | |
[a distilled bart MNLI model](https://huggingface.co/models?filter=pipeline_tag%3Azero-shot-classification&search=valhalla). | |
#### With the zero-shot classification pipeline | |
The model can be loaded with the `zero-shot-classification` pipeline like so: | |
```python | |
from transformers import pipeline | |
classifier = pipeline("zero-shot-classification", | |
model="Jiva/xlm-roberta-large-it-mnli", device=0, use_fast=True, multi_label=True) | |
``` | |
You can then classify in any of the above languages. You can even pass the labels in one language and the sequence to | |
classify in another: | |
```python | |
# we will classify the following wikipedia entry about Sardinia" | |
sequence_to_classify = "La Sardegna è una regione italiana a statuto speciale di 1 592 730 abitanti con capoluogo Cagliari, la cui denominazione bilingue utilizzata nella comunicazione ufficiale è Regione Autonoma della Sardegna / Regione Autònoma de Sardigna." | |
# we can specify candidate labels in Italian: | |
candidate_labels = ["geografia", "politica", "macchine", "cibo", "moda"] | |
classifier(sequence_to_classify, candidate_labels) | |
# {'labels': ['geografia', 'moda', 'politica', 'macchine', 'cibo'], | |
# 'scores': [0.38871392607688904, 0.22633370757102966, 0.19398456811904907, 0.13735772669315338, 0.13708525896072388]} | |
``` | |
The default hypothesis template is the English, `This text is {}`. With this model better results are achieving when providing a translated template: | |
```python | |
sequence_to_classify = "La Sardegna è una regione italiana a statuto speciale di 1 592 730 abitanti con capoluogo Cagliari, la cui denominazione bilingue utilizzata nella comunicazione ufficiale è Regione Autonoma della Sardegna / Regione Autònoma de Sardigna." | |
candidate_labels = ["geografia", "politica", "macchine", "cibo", "moda"] | |
hypothesis_template = "si parla di {}" | |
# classifier(sequence_to_classify, candidate_labels, hypothesis_template=hypothesis_template) | |
# 'scores': [0.6068345904350281, 0.34715887904167175, 0.32433947920799255, 0.3068877160549164, 0.18744681775569916]} | |
``` | |
#### With manual PyTorch | |
```python | |
# pose sequence as a NLI premise and label as a hypothesis | |
from transformers import AutoModelForSequenceClassification, AutoTokenizer | |
nli_model = AutoModelForSequenceClassification.from_pretrained('Jiva/xlm-roberta-large-it-mnli') | |
tokenizer = AutoTokenizer.from_pretrained('Jiva/xlm-roberta-large-it-mnli') | |
premise = sequence | |
hypothesis = f'si parla di {}.' | |
# run through model pre-trained on MNLI | |
x = tokenizer.encode(premise, hypothesis, return_tensors='pt', | |
truncation_strategy='only_first') | |
logits = nli_model(x.to(device))[0] | |
# we throw away "neutral" (dim 1) and take the probability of | |
# "entailment" (2) as the probability of the label being true | |
entail_contradiction_logits = logits[:,[0,2]] | |
probs = entail_contradiction_logits.softmax(dim=1) | |
prob_label_is_true = probs[:,1] | |
``` | |
## Training | |
## Version 0.1 | |
The model has been now retrained on the full training set. Around 1000 sentences pairs have been removed from the set because their translation was botched by the translation model. | |
| metric | value | | |
|----------------- |------- | | |
| learning_rate | 4e-6 | | |
| optimizer | AdamW | | |
| batch_size | 80 | | |
| mcc | 0.77 | | |
| train_loss | 0.34 | | |
| eval_loss | 0.40 | | |
| stopped_at_step | 9754 | | |
## Version 0.0 | |
This model was pre-trained on set of 100 languages, as described in | |
[the original paper](https://arxiv.org/abs/1911.02116). It was then fine-tuned on the task of NLI on an Italian translation of the MNLI dataset (85% of the train set only so far). The model used for translating the texts is Helsinki-NLP/opus-mt-en-it, with a max output sequence lenght of 120. The model has been trained for 1 epoch with learning rate 4e-6 and batch size 80, currently it scores 82 acc. on the remaining 15% of the training. |