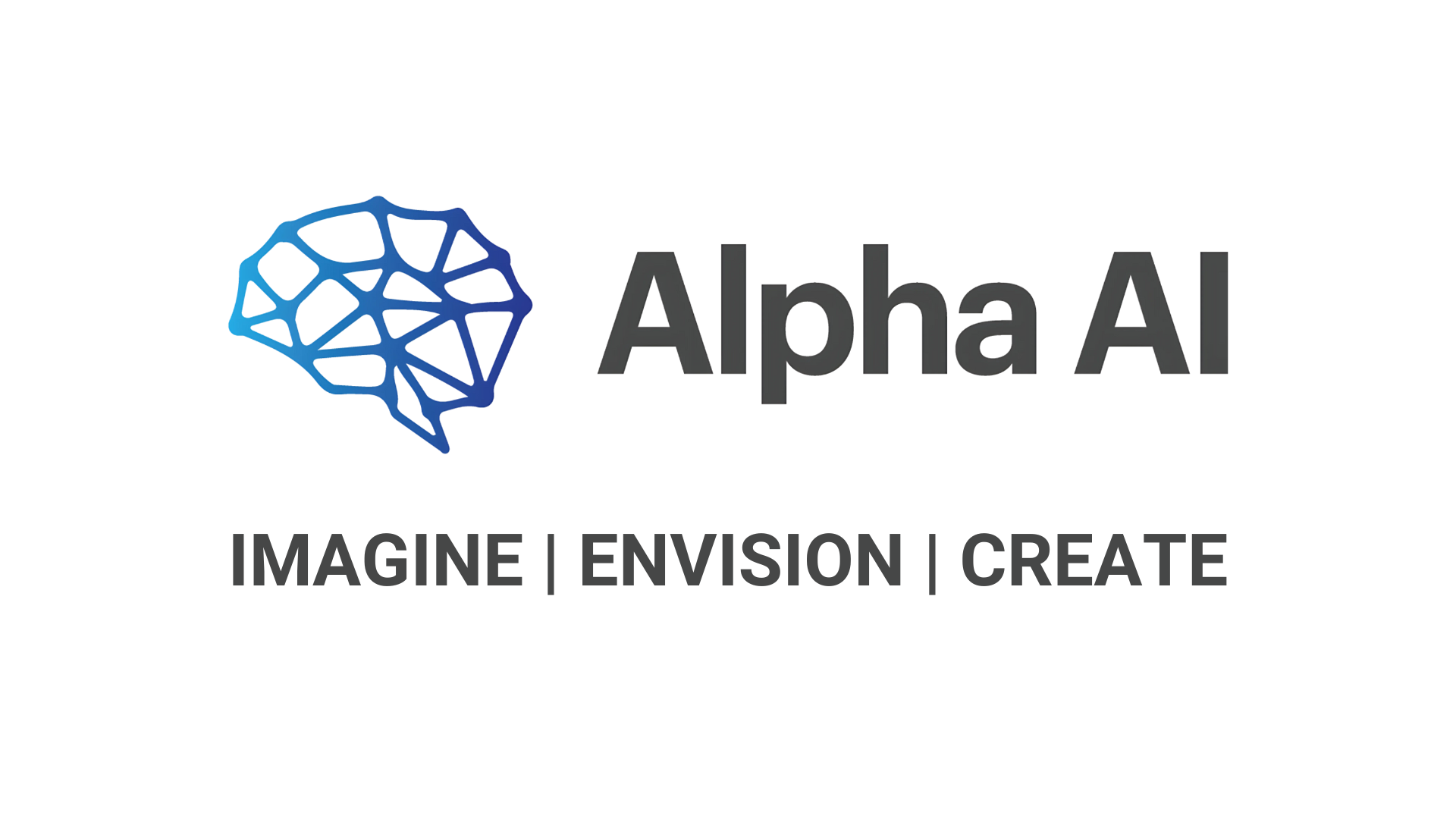
Uploaded model
- Developed by: alphaaico
- License: apache-2.0
- Finetuned from model : meta-llama/Llama-3.2-3B-Instruct
- Training Framework: Unsloth + Hugging Face TRL
- Finetuning Techniques: GRPO + Reward Modelling
Overview
Welcome to the next evolution of AI reasoning! Reason-With-Choice-3B is not just another fine-tuned model, it's a game-changer. It doesn't just generate reasoning, it chooses whether reasoning is even necessary before delivering an answer. This self-reflective capability allows it to introspect, analyze, and adapt to the complexity of each question, ensuring the most efficient and insightful response possible.
Think about it: most AI models blindly generate reasoning even when unnecessary, leading to bloated, redundant responses. Not this one. With its built-in decision-making, Reason-With-Choice-3B determines if deep reasoning is needed or if a direct answer will suffice—bringing unparalleled efficiency and intelligence to your AI-driven applications.
Key Highlights
- Reasoning & Self-Reflection: The model first decides if reasoning is necessary and then either provides step-by-step logic or directly answers the question.
- Structured Output: Responses follow a strict format with
<think>
,<reflection>
, and<answer>
sections, ensuring clarity and interpretability. - Optimized Training: Trained using GRPO (Guided Reward Policy Optimization) to enforce structured responses and improve decision-making.
- Efficient Inference: Fine-tuned with Unsloth & Hugging Face's TRL, ensuring faster inference speeds and optimized resource utilization.
Prompt Structure
The model generates responses in the following structured format:
<think>
[Detailed reasoning, if required. Otherwise, this section remains empty.]
</think>
<reflection>
[Internal thought process explaining whether reasoning was needed.]
</reflection>
<answer>
[Final response.]
</answer>
Key Features
- Decision-Making Capability: The model intelligently determines whether reasoning is necessary before answering.
- Improved Accuracy: Training with reward functions ensures adherence to logical response structure.
- Structured Outputs: Guarantees that each response follows a predictable and interpretable format.
- Enhanced Efficiency: Optimized inference with vLLM for fast token generation and low memory footprint.
- Multi-Use Case Compatibility: Can be used for Q&A systems, logical reasoning tasks, and AI-assisted decision-making.
Quantization Levels Available
- q4_k_m
- q5_k_m
- q8_0
- 16-bit (Full Precision, https://huggingface.co/alpha-ai/Reason-With-Choice-3B)
Ideal Configuration for Usage
- Temperature: 0.8
- Top-p: 0.95
- Max Tokens: 1024
Use Cases
Reason-With-Choice-3B is ideal for:
- AI Research: Investigating decision-making and reasoning processes in AI.
- Conversational AI: Enhancing chatbot intelligence with structured reasoning.
- Automated Decision Support: Assisting in structured, step-by-step problem-solving.
- Educational Tools: Providing logical explanations for learning and problem-solving.
- Business Intelligence: AI-assisted decision-making for operational and strategic planning.
Limitations & Considerations
- Domain Adaptation: May require further fine-tuning for domain-specific tasks.
- Inference Time: Increased processing time when reasoning is necessary.
- Potential Biases: Outputs depend on training data and may require verification for critical applications.
License
This model is released under the Apache-2.0 license.
Acknowledgments
Special thanks to the Unsloth team for optimizing the fine-tuning pipeline and to Hugging Face's TRL for enabling advanced fine-tuning techniques.
Security & Format Considerations
This model has been saved in .bin format due to Unsloth's default serialization method. If security is a concern, we recommend converting to .safetensors using:
from transformers import AutoModel
from safetensors.torch import save_file
model = AutoModel.from_pretrained("path/to/model")
state_dict = model.state_dict()
save_file(state_dict, "model.safetensors")
print("Model converted to safetensors successfully.")
Alternatively, GGUF models are available for optimized inference with llama.cpp, exllama, and other runtime frameworks.
Choose the format best suited to your security, performance, and deployment requirements.
- Downloads last month
- 91
Model tree for alpha-ai/Reason-With-Choice-3B-GGUF
Base model
meta-llama/Llama-3.2-3B-Instruct