repo
stringclasses 1
value | number
int64 1
25.3k
| state
stringclasses 2
values | title
stringlengths 1
487
| body
stringlengths 0
234k
⌀ | created_at
stringlengths 19
19
| closed_at
stringlengths 19
19
| comments
stringlengths 0
293k
|
---|---|---|---|---|---|---|---|
transformers | 587 | closed | From which layer is fine tuning starting in BERT? | Hi, I looked at the code but couldn't manage to understand the layer from which BERT is being fine tuned. I am using simple_lm_finetuning.py function. | 05-06-2019 08:37:21 | 05-06-2019 08:37:21 | When BERT is fine-tuned, all layers are trained - this is quite different from fine-tuning in a lot of other ML models, but it matches what was described in the paper and works quite well (as long as you only fine-tune for a few epochs - it's very easy to overfit if you fine-tune the whole model for a long time on a small amount of data!)<|||||>Thank you. May I ask what the difference of this approach is from pre-training? <|||||>The original model weights are used for initialization, whereas for a model trained from scratch, the weights are initialized randomly.<|||||>Thank you, I got it now.<|||||>Came upon this when searching for an answer to a related question.
When adding a dense layer on top for a classification task, do the model weights for BERT get updated or only the dense layer(are the BERT layers frozen or unfrozen during training)? I ask b/c when training a classifier on the stack overflow tags dataset which contains 40.000 posts with tags in 20 classes I got some unusual results. I trained base-uncased and base-cased and what is weird is that after the first epoch, the test set prediction remain the same. By that I mean exactly the same. In other words, with a 80/20 split (32.000 posts in train set / 8.000 posts in test set) it doesn't matter if you are doing 1, 2 or 3 epochs, the test set prediction don't change. It stays at 83.875% for uncased and 83.224% for cased. The weird thing is that the training loss goes down.
I have put the actual predictions in a pandas dataframe and the predictions in epoch 1, 2 and 3 are exactly the same.
<|||||>When a classifier is trained, all the model weights get updated, not just the weights in the classifier layer, so I would expect some overfitting if you train on a small labelled dataset for a lot of epochs.
The behaviour you've described is unusual - have you tried varying the learning rate, or making a much smaller training set, training on it for 1 epoch only and seeing what the results look like? It might indicate a problem with the data.<|||||>That's what I thought. I tested training the uncased version with 20% of the dataset (training set 6400 and testing set 1600) which gave me an eval accuracy of 0.76875 after epoch 1 and 2. The eval loss is even the excact same value ( 0.7407131800800562 )
I ran eval before starting the training which gave an accuracy of 0.05 which makes sense with 20 classes and random weights. Then after epoch 1 it jumps up to aforementioned values and stays the same in epoch 2 and 3.
Any pointers on how to debug this? Might it help checking the gradients?<|||||>Yeah, that's where I'd look. If forced to guess, I'd say the network isn't really getting any input, and is therefore just learning the bias in the last layer. So you could try inspecting the data batches in the training loop right before they enter the network, to make sure there's actually data there and that the data matches the labels, and also checking that most network parameters are getting some gradient after each batch. If your code is in a repo somewhere, feel free to link it and I'll take a look.<|||||>I went through the training data and it appears that its formatted the right way. I also checked the gradients and they are adjusted after each back() call. I think this might be related to the warm_up part of the adjustable learning rate.
It happens after epoch 3:
<img width="413" alt="Screen Shot 2019-05-17 at 20 13 33" src="https://user-images.githubusercontent.com/3185711/57953675-49f04000-78e0-11e9-8976-a5367bc5b0f3.png">
Then I also get a warning:
05/17/2019 20:15:02 - WARNING - pytorch_pretrained_bert.optimization - Training beyond specified 't_total'. Learning rate multiplier set to 0.0. Please set 't_total' of WarmupLinearSchedule correctly.
I am using a default value of 0.1. I plotted the learning rate over 4 epochs and in epoch 4 the learning rate becomes negative:
<img width="436" alt="Screen Shot 2019-05-17 at 21 03 27" src="https://user-images.githubusercontent.com/3185711/57956376-482a7a80-78e8-11e9-987b-657198f19ef5.png">
3 epochs is more then enough for this dataset as it starts to overfit quickly. I just want to understand why this happens, it doesn't make sense to me. The loss and accuracy in the evaluation phase is exactly the same(and the training loss drops in epoch no 4 when the LR is negative). I put the code on Kaggle if you want to take a look ( no pressure :-) )
https://www.kaggle.com/stoddur/bert-so-classification-test/edit
Im going to play a bit with the warm_up function and see which learning rates are set with different values. Will let you know if I find out anything else.<|||||>In the BERT paper, and in this repo, the learning rate is 'warmed up' from 0 to the maximum over the first 10% of training, and then linearly decays back to 0 for the remaining 90% of training. In order for that to work, the learning rate scheduler needs to know how many steps there will be in training in total (i.e. steps_per_epoch * num_epochs). It seems like that value is being passed incorrectly, causing the LR to decay to zero too quickly and therefore freezing all the weights.
Also, I can't see the code at your link - is it possibly private?<|||||>Yeah, I noticed that now reading through the paper :)
Made the kernel public, the code is a bit rough, hope it makes sense to you. <|||||>This issue has been automatically marked as stale because it has not had recent activity. It will be closed if no further activity occurs. Thank you for your contributions.
<|||||>I get error below while running the program.. Did I do any mistake?
warmup_linear = WarmupLinearSchedule( warmup=args.warmup_proportion,
t_total=num_train_optimization_steps)
lr_this_step = args.learning_rate * warmup_linear.get_lr(num_train_optimization_steps,
args.warmup_proportion)
**WARNING - pytorch_pretrained_bert.optimization - Training beyond specified 't_total'. Learning rate multiplier set to 0.0. Please set 't_total' of WarmupLinearSchedule correctly.**
<|||||>@kbulutozler
@steindor .. did you solve WarmupLinearSchedule issue ? I am getting same error .. I tried your kaggle code but getting error that " the link does not exists"
I get error below while running the program.. Did I do any mistake?
warmup_linear = WarmupLinearSchedule( warmup=args.warmup_proportion,
t_total=num_train_optimization_steps)
lr_this_step = args.learning_rate * warmup_linear.get_lr(num_train_optimization_steps,
args.warmup_proportion)
WARNING - pytorch_pretrained_bert.optimization - Training beyond specified 't_total'. Learning rate multiplier set to 0.0. Please set 't_total' of WarmupLinearSchedule correctly. |
transformers | 586 | closed | Padding Token in Transformer XL | I have sentences of varying lengths and I was wondering how to handle that as I could not see any padding token present. The index 0 refers to <eos> in the vocab, so any help on addition of padding would be appreciated | 05-06-2019 06:52:29 | 05-06-2019 06:52:29 | For these causal models that consider the left-context only, it's ok not to worry too much about padding since the attention modules only look to the previous tokens. Just be careful when you compute the loss to ignore the out-of-sentence-tokens (using loss functions `ignore_index` for instance).<|||||>This issue has been automatically marked as stale because it has not had recent activity. It will be closed if no further activity occurs. Thank you for your contributions.
<|||||>Hi, I have a related doubt. In the example code [run_language_modeling.py](https://github.com/huggingface/transformers/blob/master/examples/run_language_modeling.py), GPT-2 and transformer-xl throw error due to lacking padding token.
Please check recent comments in this [Issue](https://github.com/huggingface/transformers/issues/3021) |
transformers | 585 | closed | Make the epsilon of LayerNorm configurable. | It would be great if we could configure `eps` in layer normalization since model like ERNIE uses `eps=1e-5` instead of `1e-12`.
#514 related | 05-05-2019 16:28:31 | 05-05-2019 16:28:31 | Ok, good to go, thanks @huntzhan! |
transformers | 584 | closed | The number of train examples in STS-B is only 5749 | Hi,
Thanks a lot for the amazing work!
Here's my issue:
When I run the './example/run_classification.py' with task STS-B, I found the train example number is only 5749, less than 7k which was reported in the paper ([paper link](https://www.nyu.edu/projects/bowman/glue.pdf)).
Thanks again!
Best,
Dong | 05-04-2019 22:47:35 | 05-04-2019 22:47:35 | This issue has been automatically marked as stale because it has not had recent activity. It will be closed if no further activity occurs. Thank you for your contributions.
|
transformers | 583 | closed | BERT + PyTorch + XLA | Hi,
Many thanks for your amazing library!
Even though no models were shared for Russian, we used your interfaces with success when doing some [research](https://towardsdatascience.com/complexity-generalization-computational-cost-in-nlp-modeling-of-morphologically-rich-languages-7fa2c0b45909).
Anyway here is my question.
Did you try [this](https://github.com/pytorch/xla/tree/master)?
At least on the surface it looks like they boast PyTorch + TPU.
Would also be cool to know if anyone had experience in running anything with XLA.
Many thanks!
| 05-04-2019 05:49:35 | 05-04-2019 05:49:35 | Do u mean this one? [link](https://news.developer.nvidia.com/nvidia-achieves-4x-speedup-on-bert-neural-network/)<|||||>No, I mean this repo
https://github.com/pytorch/xla/tree/master
Looks like Facebook and Google want to make pytorch on TPU
On May 6, 2019 9:14:25 AM GMT+03:00, chunbo dai <[email protected]> wrote:
>Do u mean this one?
>[link](https://news.developer.nvidia.com/nvidia-achieves-4x-speedup-on-bert-neural-network/)
>
>--
>You are receiving this because you authored the thread.
>Reply to this email directly or view it on GitHub:
>https://github.com/huggingface/pytorch-pretrained-BERT/issues/583#issuecomment-489510379
--
Sent from my Android device with K-9 Mail. Please excuse my brevity.<|||||>This issue has been automatically marked as stale because it has not had recent activity. It will be closed if no further activity occurs. Thank you for your contributions.
|
transformers | 582 | closed | Add GPT-2 Bigger Model | OpenAI just release the next biggest version of their language model. I think to add the new model, one needs to use the conversion script from TF to Pytorch and then save the model as another option in PRETRAINED_MODEL_ARCHIVE_MAP. | 05-04-2019 00:00:49 | 05-04-2019 00:00:49 | For convenience to others, here's the config file for 345M:
```
{
"initializer_range": 0.02,
"layer_norm_epsilon": 1e-05,
"n_ctx": 1024,
"n_embd": 1024,
"n_head": 16,
"n_layer": 24,
"n_positions": 1024,
"vocab_size": 50257
}
```<|||||>Here are the concrete steps if you'd like to run the 345M.
Grab OpenAI's download script from here https://github.com/openai/gpt-2/blob/master/download_model.py. and then run `python download_model.py 345M` to get the model checkpoint.
Then use the conversion script here https://github.com/huggingface/pytorch-pretrained-BERT/blob/master/pytorch_pretrained_bert/convert_gpt2_checkpoint_to_pytorch.py using `python convert_gpt2_checkpoint_to_pytorch.py --gpt2_checkpoint_path gpt2_checkpoint_folder --gpt2_config_file config_file --pytorch_dump_folder_path output_dir`
where config_file is the json posted by @daemon above.
Then inside https://github.com/huggingface/pytorch-pretrained-BERT/blob/master/pytorch_pretrained_bert/modeling_gpt2.py modify the PRETRAINED_MODEL_ARCHIVE_MAP and PRETRAINED_CONFIG_ARCHIVE_MAP to point to the converted pytorch file
<|||||>Thanks!
> Then inside https://github.com/huggingface/pytorch-pretrained-BERT/blob/master/pytorch_pretrained_bert/modeling_gpt2.py modify the PRETRAINED_MODEL_ARCHIVE_MAP and PRETRAINED_CONFIG_ARCHIVE_MAP to point to the converted pytorch file
Or GPT2LMHeadModel.from_pretrained(pytorch_dump_folder_path) without changing modeling_gpt2.py?<|||||>Why not add this in the module?
Thanks for the instruction, I will likely try if its not integrated soon.<|||||>When running "convert_gpt2_checkpoint_to_pytorch.py --gpt2_checkpoint_path gpt2_checkpoint_folder --gpt2_config_file config_file --pytorch_dump_folder_path output_dir" I get the following error:
_runfile('C:/Users/nietop1/Desktop/anaconda/trying to generate text/convert_checkpoint_gtp2.py', wdir='C:/Users/nietop1/Desktop/anaconda/trying to generate text')
Converting TensorFlow checkpoint from C:\Users\nietop1\Desktop\anaconda\models\345M
Traceback (most recent call last):
File "<ipython-input-32-bd0ca7f018f3>", line 1, in <module>
runfile('C:/Users/nietop1/Desktop/anaconda/trying to generate text/convert_checkpoint_gtp2.py', wdir='C:/Users/nietop1/Desktop/anaconda/trying to generate text')
File "C:\Anaconda3\envs\tensorflow\lib\site-packages\spyder\utils\site\sitecustomize.py", line 705, in runfile
execfile(filename, namespace)
File "C:\Anaconda3\envs\tensorflow\lib\site-packages\spyder\utils\site\sitecustomize.py", line 102, in execfile
exec(compile(f.read(), filename, 'exec'), namespace)
File "C:/Users/nietop1/Desktop/anaconda/trying to generate text/convert_checkpoint_gtp2.py", line 81, in <module>
'C:/Users/nietop1/Desktop/anaconda/models/345M')
File "C:/Users/nietop1/Desktop/anaconda/trying to generate text/convert_checkpoint_gtp2.py", line 47, in convert_gpt2_checkpoint_to_pytorch
load_tf_weights_in_gpt2(model, gpt2_checkpoint_path)
File "C:\Anaconda3\envs\tensorflow\lib\site-packages\pytorch_pretrained_bert\modeling_gpt2.py", line 60, in load_tf_weights_in_gpt2
init_vars = tf.train.list_variables(tf_path)
AttributeError: module 'tensorflow.python.training.training' has no attribute 'list_variables'_
How can this be solved?<|||||>This issue has been automatically marked as stale because it has not had recent activity. It will be closed if no further activity occurs. Thank you for your contributions.
<|||||>Closing this because this is merged. |
transformers | 581 | closed | BertAdam gradient clipping is not global | Just took a look at the gradient clipping algorithm used in: https://github.com/huggingface/pytorch-pretrained-BERT/blob/3ae8c8be1e3fc770968cd3fdb3b643e0b166e540/pytorch_pretrained_bert/optimization.py#L270
It's clipping gradients to a local norm of 1. It should be clipping gradients to a global norm of 1 as in https://github.com/google-research/bert/blob/master/optimization.py#L74 or in https://github.com/NVIDIA/Megatron-LM/blob/master/pretrain_bert.py#L226 . | 05-03-2019 20:56:14 | 05-03-2019 20:56:14 | This issue has been automatically marked as stale because it has not had recent activity. It will be closed if no further activity occurs. Thank you for your contributions.
|
transformers | 580 | closed | Bert for passage reranking | Hi I am currently trying to implement bert for passage reranking in pytorch. Here is the paper and github repo.
https://arxiv.org/abs/1901.04085
https://github.com/nyu-dl/dl4marco-bert
I've downloaded their bert large model checkpoint and bert config for the task the `convert_tf_checkpoint_to_pytorch` function seems to successfully extract the weights from tensorflow.
Then while initialising the pytorch model
```
Initialize PyTorch weight ['bert', 'pooler', 'dense', 'kernel']
Skipping bert/pooler/dense/kernel/adam_m
Skipping bert/pooler/dense/kernel/adam_v
Skipping global_step
```
```~/anaconda3/envs/new_fast_ai/lib/python3.7/site-packages/pytorch_pretrained_bert/convert_tf_checkpoint_to_pytorch.py in convert_tf_checkpoint_to_pytorch(tf_checkpoint_path, bert_config_file, pytorch_dump_path)
35
36 # Load weights from tf checkpoint
---> 37 load_tf_weights_in_bert(model, tf_checkpoint_path)
38
39 # Save pytorch-model
~/anaconda3/envs/new_fast_ai/lib/python3.7/site-packages/pytorch_pretrained_bert/modeling.py in load_tf_weights_in_bert(model, tf_checkpoint_path)
88 pointer = getattr(pointer, 'weight')
89 elif l[0] == 'output_bias' or l[0] == 'beta':
---> 90 pointer = getattr(pointer, 'bias')
91 elif l[0] == 'output_weights':
92 pointer = getattr(pointer, 'weight')
~/anaconda3/envs/new_fast_ai/lib/python3.7/site-packages/torch/nn/modules/module.py in __getattr__(self, name)
533 return modules[name]
534 raise AttributeError("'{}' object has no attribute '{}'".format(
--> 535 type(self).__name__, name))
536
537 def __setattr__(self, name, value):
AttributeError: 'BertForPreTraining' object has no attribute 'bias'
```
I assume it is issues with the final layer
What is the best way for me to go about resolving this?
thanks in advance! | 05-03-2019 17:22:30 | 05-03-2019 17:22:30 | The `convert_tf_checkpoint_to_pytorch` script is made to convert the Google pre-trained weights in `BertForPretraining` model, you have to modify it to convert another type model.
In your case, you want to load the passage re-ranking model in a `BertForSequenceClassification` model which has the same structure (BERT + a classifier on top of the pooled output) as the NYU model.
here is a quick way to do that:
- install pytorch-pretrained-bert from source so you can modify it
- change https://github.com/huggingface/pytorch-pretrained-BERT/blob/master/pytorch_pretrained_bert/convert_tf_checkpoint_to_pytorch.py#L34 to initialize a `BertForSequenceClassification` model instead of the `BertForPreTraining` model in the conversion script.
- the structure is not exactly identical so you need to ADD a line that say `pointer = getattr(pointer, 'cls')` in the TWO if-conditions related to `output_weights` and `output_bias` (between L89 and L90 and between L91 and L92 in modeling.py here: https://github.com/huggingface/pytorch-pretrained-BERT/blob/master/pytorch_pretrained_bert/modeling.py#L90 and https://github.com/huggingface/pytorch-pretrained-BERT/blob/master/pytorch_pretrained_bert/modeling.py#L92).
- this should let you convert the tensorflow model in a pytorch one using the scripts.<|||||>Thanks so much! Your comment saved me a lot of time. However there was a small issue I got around by just changing the tf variable names.
For anyone else out there the solution was
* https://github.com/huggingface/pytorch-pretrained-BERT/blob/master/pytorch_pretrained_bert/convert_tf_checkpoint_to_pytorch.py#L34 CHANGE `model = BertForSequenceClassification(config, 2)`
* https://github.com/huggingface/pytorch-pretrained-BERT/blob/master/pytorch_pretrained_bert/modeling.py#L70 ADD
```
if name in ['output_weights' , 'output_bias']:
name = 'classifier/' + name
```
<|||||>Hello @oisin-dolphin and @thomwolf
I followed above suggestions but getting following error.
tensorflow.python.framework.errors_impl.NotFoundError: Key classifier/output_bias not found in checkpoint
Also what is significance of following line of code
pointer = getattr(pointer, 'cls')
Please suggest.
Thanks
Mahesh<|||||>> The `convert_tf_checkpoint_to_pytorch` script is made to convert the Google pre-trained weights in `BertForPretraining` model, you have to modify it to convert another type model.
>
> In your case, you want to load the passage re-ranking model in a `BertForSequenceClassification` model which has the same structure (BERT + a classifier on top of the pooled output) as the NYU model.
>
> here is a quick way to do that:
>
> * install pytorch-pretrained-bert from source so you can modify it
> * change https://github.com/huggingface/pytorch-pretrained-BERT/blob/master/pytorch_pretrained_bert/convert_tf_checkpoint_to_pytorch.py#L34 to initialize a `BertForSequenceClassification` model instead of the `BertForPreTraining` model in the conversion script.
> * the structure is not exactly identical so you need to ADD a line that say `pointer = getattr(pointer, 'cls')` in the TWO if-conditions related to `output_weights` and `output_bias` (between L89 and L90 and between L91 and L92 in modeling.py here: https://github.com/huggingface/pytorch-pretrained-BERT/blob/master/pytorch_pretrained_bert/modeling.py#L90 and https://github.com/huggingface/pytorch-pretrained-BERT/blob/master/pytorch_pretrained_bert/modeling.py#L92).
> * this should let you convert the tensorflow model in a pytorch one using the scripts.
I followed these instructions for the SequenceClassification model but I still end up getting the same error for 'BertForSequenceClassification' object has no attribute 'bias'.<|||||>Update for latest transformers, add modeling_bert.py:78:
```python
for name, array in zip(names, arrays):
if name in ['output_weights', 'output_bias']:
name = 'classifier/' + name
```
and convert_bert_original_tf_checkpoint_to_pytorch.py
```python
config.num_labels = 2
print("Building PyTorch model from configuration: {}".format(str(config)))
model = BertForSequenceClassification(config)
```<|||||>you are my lifesaver @pertschuk Thank you for the instructions<|||||>glad they helped @Soonhwan-Kwon.
I used a similar reranking model as part of a project I just released which hooks in to Elasticsearch and reranks search results out of the box, [check it out]( https://medium.com/koursaros-ai/boost-search-api-performance-e-g-410868e82b22) if this sounds like it would be useful! repo: https://github.com/koursaros-ai/nboost <|||||>You can create a subclass of `BertForSequenceClassification` and add `self.weight` and `self.bias` to the` __init__` method. Then instantiate your new class and it is ready to use it:
```
class BertForPassageRanking(BertForSequenceClassification):
def __init__(self, config):
super().__init__(config)
self.weight = torch.autograd.Variable(torch.ones(2, config.hidden_size),
requires_grad=True)
self.bias = torch.autograd.Variable(torch.ones(2), requires_grad=True)
bert_ranking = BertForPassageRanking.from_pretrained(BERT_PASSAGE_RANKING_PATH,
from_tf=True)
```
`BERT_PASSAGE_RANKING_PATH` is the path where your tf checkpoints files and config json file are stored. You will need to rename the files as follows:
```
config.json
model.ckpt.index
model.ckpt.meta
```
Another option if you do not want to change the file names is to load the json config file with `BertConfig.from_json_file()` and then pass to `BertForPassageRanking.from_pretained()` the path + ckpt file name and the configuration that you have already loaded with `BertConfig.from_json_file()` .
<|||||>I added passage pytorch msmarco reranking models to the huggingface / transformers bucket, no need for subclassing / modifications.
https://huggingface.co/nboost<|||||>> I added passage pytorch msmarco reranking models to the huggingface / transformers bucket, no need for subclassing / modifications.
>
> https://huggingface.co/nboost
Hi, I have a question regarding the output of your models. In transformers library, the bert_base model (`transformers.BertModel` class) has as output a tuple, where the first element is the last hidden state and the 2nd element is the pooler output. The last hidden state is a tensor of size `(batch_size, sequence_length, hidden_dim)`. For example for a batch size of 64 and 512 tokens we obtain for BERT an output of size `(64x512x768)`. The pooler output has size `(batch_size, hidden_size)`. This output is obtained training a linear layer with tanh activation function which had as input the `CLS` token hidden state (last layer hidden-state of the first oken of the sequence). Those weights have been trained from the next sentence prediction.
Your model follows similar structure, at least `nboost/pt-biobert-base-msmarco`. However, a passage re-ranking model is a sequence classification model. Basically, the passage re-ranking model proposed by https://github.com/nyu-dl/dl4marco-bert is the BERT model fine-tuned with a dense layer on top to learn to classify a sequence as relevant or not relevant. Their first element of the tuple output is a tensor of size `(batch_size, num_classes)`, where num_classes is two (whether the sequence to classify is a relevant document).
How should we use your model for passage re-ranking?
Thanks a lot<|||||>> > I added passage pytorch msmarco reranking models to the huggingface / transformers bucket, no need for subclassing / modifications.
> > https://huggingface.co/nboost
>
> Hi, I have a question regarding the output of your models. In transformers library, the bert_base model (`transformers.BertModel` class) has as output a tuple, where the first element is the last hidden state and the 2nd element is the pooler output. The last hidden state is a tensor of size `(batch_size, sequence_length, hidden_dim)`. For example for a batch size of 64 and 512 tokens we obtain for BERT an output of size `(64x512x768)`. The pooler output has size `(batch_size, hidden_size)`. This output is obtained training a linear layer with tanh activation function which had as input the `CLS` token hidden state (last layer hidden-state of the first oken of the sequence). Those weights have been trained from the next sentence prediction.
>
> Your model follows similar structure, at least `nboost/pt-biobert-base-msmarco`. However, a passage re-ranking model is a sequence classification model. Basically, the passage re-ranking model proposed by https://github.com/nyu-dl/dl4marco-bert is the BERT model fine-tuned with a dense layer on top to learn to classify a sequence as relevant or not relevant. Their first element of the tuple output is a tensor of size `(batch_size, num_classes)`, where num_classes is two (whether the sequence to classify is a relevant document).
>
> How should we use your model for passage re-ranking?
> Thanks a lot
I found where was the problem. As pointed in the model's page (https://huggingface.co/nboost/pt-biobert-base-msmarco#) to load the model you have to do the following:
`model = AutoModel.from_pretrained("nboost/pt-biobert-base-msmarco")`
This creates as output a tuple where the first element is a tensor of size `(64x512x768)`.
However, we should do the following, since our problem is a sequence classification:
`model = AutoModelForSequenceClassification.from_pretrained("nboost/pt-biobert-base-msmarco")`
This creates the correct output, a tuple where the first element is a tensor of size `(batch_size, num_classes)`
I suggest to the authors to change the model info and model card in https://huggingface.co/nboost/pt-biobert-base-msmarco#, since it is little bit confusing<|||||>> You can create a subclass of `BertForSequenceClassification` and add `self.weight` and `self.bias` to the` __init__` method. Then instantiate your new class and it is ready to use it:
>
> ```
> class BertForPassageRanking(BertForSequenceClassification):
> def __init__(self, config):
> super().__init__(config)
> self.weight = torch.autograd.Variable(torch.ones(2, config.hidden_size),
> requires_grad=True)
> self.bias = torch.autograd.Variable(torch.ones(2), requires_grad=True)
>
>
> bert_ranking = BertForPassageRanking.from_pretrained(BERT_PASSAGE_RANKING_PATH,
> from_tf=True)
> ```
>
> `BERT_PASSAGE_RANKING_PATH` is the path where your tf checkpoints files and config json file are stored. You will need to rename the files as follows:
>
> ```
> config.json
> model.ckpt.index
> model.ckpt.meta
> ```
>
> Another option if you do not want to change the file names is to load the json config file with `BertConfig.from_json_file()` and then pass to `BertForPassageRanking.from_pretained()` the path + ckpt file name and the configuration that you have already loaded with `BertConfig.from_json_file()` .
Thanks a lot. I was having the same question about 'nboost' and was trying this method. However, the output seems to change when I run the same code multiple times, even though i am in the eval mode. Do you have any hint about what I am doing wrong here?
```
bert_ranking = BertForPassageRanking.from_pretrained(BERT_PASSAGE_RANKING_PATH,
from_tf=True)
dummy_query = [
'Rutgers is a good university. I like my experience there.',
"Hello, my dog is cute. My cute dog is amazing.",
'Florida is a nice place but tiger king may be better',
]
dummy_passage = [
'My cat is really cute but my dog is even better.',
'My cat is really cute but my dog is even better.',
'My cat is really cute but my dog is even better.',
]
bert_ranking.eval()
with torch.no_grad():
for idx in range(len(dummy_query)):
input_ids = torch.tensor(tokenizer.encode(text=dummy_query[idx], \
text_pair=dummy_passage[idx], add_special_tokens=True)).unsqueeze(0)
outputs = bert_ranking(input_ids)
print(outputs)
```
<|||||>> Thanks a lot. I was having the same question about 'nboost' and was trying this method. However, the output seems to change when I run the same code multiple times, even though i am in the eval mode. Do you have any hint about what I am doing wrong here?
>
> ```
> bert_ranking = BertForPassageRanking.from_pretrained(BERT_PASSAGE_RANKING_PATH,
> from_tf=True)
>
> dummy_query = [
> 'Rutgers is a good university. I like my experience there.',
> "Hello, my dog is cute. My cute dog is amazing.",
> 'Florida is a nice place but tiger king may be better',
> ]
>
> dummy_passage = [
> 'My cat is really cute but my dog is even better.',
> 'My cat is really cute but my dog is even better.',
> 'My cat is really cute but my dog is even better.',
> ]
> bert_ranking.eval()
> with torch.no_grad():
> for idx in range(len(dummy_query)):
> input_ids = torch.tensor(tokenizer.encode(text=dummy_query[idx], \
> text_pair=dummy_passage[idx], add_special_tokens=True)).unsqueeze(0)
> outputs = bert_ranking(input_ids)
> print(outputs)
> ```
Sorry, I have no idea. Finally I am not using this approximation. I did not achieve good results for my purpose. Intead, I am using the model provided by nboost (https://huggingface.co/nboost/pt-tinybert-msmarco) and it works fine for me. Remember to load the model as follows:
`model = AutoModelForSequenceClassification.from_pretrained("nboost/pt-tinybert-msmarco")`
I am using tinybert-msmarco, however you can use one of the following models:
```
nboost/pt-bert-base-uncased-msmarco
nboost/pt-bert-large-msmarco
nboost/pt-biobert-base-msmarco
nboost/pt-tinybert-msmarco
```<|||||>Hi, I have fine tuned a multilingual model, taken from hugging face, on the passage reranking task. Now I am facing difficulties with converting the tensorflow checkpoint to a pytorch model, so that I can use the model using `BertForSequenceClassification`.
I am using the following conversion function, but I get this error
```
File "<ipython-input-50-1e24e5635ec9>", line 1, in <module>
convert_tf_checkpoint_to_pytorch(tf_checkpoint_path, bert_config_file, pytorch_dump_path)
File "<ipython-input-49-22827240b095>", line 63, in convert_tf_checkpoint_to_pytorch
assert pointer.shape == array.shape
File "/home/igli/anaconda3/envs/search-boost/lib/python3.8/site-packages/torch/nn/modules/module.py", line 593, in __getattr__
raise AttributeError("'{}' object has no attribute '{}'".format(
AttributeError: 'LayerNorm' object has no attribute 'shape'
```
The conversion method:
```
def convert_tf_checkpoint_to_pytorch(tf_checkpoint_path, bert_config_file, pytorch_dump_path):
config_path = os.path.abspath(bert_config_file)
tf_path = os.path.abspath(tf_checkpoint_path)
print("Converting TensorFlow checkpoint from {} with config at {}".format(tf_path, config_path))
# Load weights from TF model
init_vars = tf.train.list_variables(tf_path)
names = []
arrays = []
for name, shape in init_vars:
print("Loading TF weight {} with shape {}".format(name, shape))
array = tf.train.load_variable(tf_path, name)
names.append(name)
arrays.append(array)
# Initialise PyTorch model
config = BertConfig.from_json_file(bert_config_file)
config.num_labels = 2
print("Building PyTorch model from configuration: {}".format(str(config)))
model = BertForSequenceClassification()(config=config)
for name, array in zip(names, arrays):
if name in ['output_weights' , 'output_bias']:
name = 'classifier/' + name
name = name.split('/')
# adam_v and adam_m are variables used in AdamWeightDecayOptimizer to calculated m and v
# which are not required for using pretrained model
if name[-1] in ["adam_v", "adam_m"]:
print("Skipping {}".format("/".join(name)))
continue
pointer = model
for m_name in name:
if re.fullmatch(r'[A-Za-z]+_\d+', m_name):
l = re.split(r'_(\d+)', m_name)
else:
l = [m_name]
if l[0] == 'kernel':
pointer = getattr(pointer, 'weight')
elif l[0] == 'output_bias':
pointer = getattr(pointer, 'bias')
pointer = getattr(pointer, 'cls')
elif l[0] == 'output_weights':
pointer = getattr(pointer, 'weight')
pointer = getattr(pointer, 'cls')
else:
try:
pointer = getattr(pointer, l[0])
except:
pass
if len(l) >= 2:
num = int(l[1])
pointer = pointer[num]
if m_name[-11:] == '_embeddings':
pointer = getattr(pointer, 'weight')
elif m_name == 'kernel':
array = np.transpose(array)
try:
assert pointer.shape == array.shape
except AssertionError as e:
e.args += (pointer.shape, array.shape)
raise
#pass
print("Initialize PyTorch weight {}".format(name))
array = np.array(array)
print(array)
print(type(array))
pointer.data = torch.from_numpy(array)
# Save pytorch-model
print("Save PyTorch model to {}".format(pytorch_dump_path))
torch.save(model.state_dict(), pytorch_dump_path)
```
I have currently no clue, where the problem might be. Thanks in advanvce!<|||||>> Update for latest transformers, add modeling_bert.py:78:
>
> ```python
> for name, array in zip(names, arrays):
> if name in ['output_weights', 'output_bias']:
> name = 'classifier/' + name
> ```
>
> and convert_bert_original_tf_checkpoint_to_pytorch.py
>
> ```python
> config.num_labels = 2
> print("Building PyTorch model from configuration: {}".format(str(config)))
> model = BertForSequenceClassification(config)
> ```
As of 26/Mar/2021,
`modeling_bert.py:78` is now around `modeling_bert.py:118`
`convert_bert_original_tf_checkpoint_to_pytorch.py` is now around `convert_bert_original_tf_checkpoint_to_pytorch.py:33`. BTW, don't forget `from transformers import BertForSequenceClassification` |
transformers | 579 | closed | Resetting current_random_doc and current_doc | In the class BERTDataset the two variables `self.current_random_doc` and `self.current_doc` are never reset to 0, even when the corpus is closed and reopened. Is it supposed to work this way? I'd think it would run into issues on a small corpus where one counter gets to the same document but the counter is different because it was opened a second time.
https://github.com/huggingface/pytorch-pretrained-BERT/blob/3ae8c8be1e3fc770968cd3fdb3b643e0b166e540/examples/lm_finetuning/simple_lm_finetuning.py#L42-L231 | 05-03-2019 17:03:20 | 05-03-2019 17:03:20 | Hmm maybe @Rocketknight1 have an insight on this?<|||||>This issue has been automatically marked as stale because it has not had recent activity. It will be closed if no further activity occurs. Thank you for your contributions.
|
transformers | 578 | closed | "Easy" path for classifier training / pre-training | I've noticed quite a few issues from people outside research who want to fine-tune a pre-trained BERT model to solve a task they're working on, but there's a steep learning curve. Right now, the workflow for someone who wants to use this repo for a custom task is something like this:
1) Understand how DataProcessors work and write a custom DataProcessor (or read the code for the existing data processors and hack your training data into a format that works for them)
2) Understand the `examples/run_classifier.py` script and modify it to use the custom DataProcessor
3) Write a script (or another modification of `run_classifier.py`) that loads unlabelled data and performs inference
This is a lot of work, especially for people who aren't familiar with the codebase! The Tensor2Tensor TF BERT repo is even worse - it's even harder for newcomers to do anything without understanding the code in detail. But it's possible to make BERT accessible to a lot more people with just a few changes:
1) Make a generic DataProcessor and clearly describe the expected input format in the docs so that people don't have to read the code to understand it. For example, the GenericDataProcessor class could expect one training example per line, and one label per line in a separate file. We could also add a GenericPairedDataProcessor, where the classifier reads two sequences as input instead of just one (e.g. for entailment tasks).
2) Add an inference script that loads a saved model state file and performs classifications and writes a file of its predictions. It should read data using the same GenericDataProcessor class, but will not require a label file to be present. If labels are present, it can also write evaluation metrics like accuracy.
3) Optionally, modify `run_classifier.py` to allow loading of fine-tuned BERT language models from the `lm_finetuning/` scripts
4) Document the whole workflow with example calls so that people can use BERT and get state-of-the-art results without needing to read any code!
To make it even easier, we could add a functional interface so people could just call something like `pytorch_pretrained_bert.train_classifier()`
Do you think this is a good idea? Or is it too end-user focused - would it work better as a separate repo that used this one as a dependency, especially if this repo is moving away from being BERT-specific and turning into more of a large set of PyTorch Transformer model implementations? | 05-03-2019 13:16:00 | 05-03-2019 13:16:00 | I join this issue.
Also I have a question related to the p.3
> Optionally, modify run_classifier.py to allow loading of fine-tuned BERT language models from the lm_finetuning/ scripts
`finetune_on_pregenerated.py` script uses `BertForPreTraining` with 2 heads and this is like vanilla training from the original paper. But `run_classifier.py` uses `BertForSequenceClassification` which is being learned only for predicting labels, not masked tokens and isNextSeq. Am I right?
If so, how can I merge these two approaches? I want to fine-tune the pretrained bert for my dataset and also train a classifier on the top of it.
Thank you.<|||||>It's a good question and a good discussion @Rocketknight1.
I think your suggestion of "splitting" the present repo in two by extracting the examples in a separate repo and refactoring them to have a better workflow is a good idea.
At the present stage, it's important to keep the core library stable as it is used in downstream libraries but the examples are an area where many people would like to contribute to the community and it would be nice to have a more flexible structure that allows such contributions more easily than the present monolithic setup.
So if you want to start a new repo splitting the "usage-part" of this repo it could be a very interesting idea I think. I'm also happy to help and host it close to the present repo if it's what you had in mind (maybe shoot me an email in that case).<|||||>Understood! I'm tight on time right now, but if I find time I'll try to build an interface like that and contact you to ensure we sync things up between the two repos.<|||||>Keeping the discussion open on this<|||||>This issue has been automatically marked as stale because it has not had recent activity. It will be closed if no further activity occurs. Thank you for your contributions.
|
transformers | 577 | closed | GPT2 lm_labels masking using (-1) throws an index out of range | I am fine-tuning GPT2 model using the LMHead with a small number of special tokens.
GPT2 underlying transformer takes the whole input at once, thus, it's important to pad inputs of varying lengths to a fixed length. The GPT2 model library offers -1 to be used as the padding value:
> lm_labels: optional language modeling labels: torch.LongTensor of shape [batch_size, sequence_length] with indices selected in [-1, 0, ..., vocab_size]. All labels set to -1 are ignored (masked), the loss is only computed for the labels set in [0, ..., vocab_size].
**When I pad the lm_labels using -1's, the library throws an error.** On the other hand, using any other positive value for the masking works but the value acts like a vocabulary piece and thus makes the input incorrect.
The other issue with **GPT2 is that the position_ids are compulsory**, whereas, the docs say they are optional.
Having an encoded and padded dataset like (not working case):
```
input_ids = torch.tensor([
[[50257, 1212, 318, 43086, 2420, 2420, 2420, 50257]],
[[50257, 1212, 318, 43086, 2420, 50257, 0, 0]]
])
position_ids = torch.tensor([
[[1, 1, 1, 1, 1, 1, 1, 1]],
[[1, 1, 1, 1, 1, 1, 0, 0]]
])
lm_labels = torch.tensor([
[[1212, 318, 43086, 2420, 2420, 2420, 50257, 50257]],
[[1212, 318, 43086, 2420, 2420, 2420, -1, -1]]
])
# Changing -1 padding makes the code work but also makes the input incorrect.
lm_labels = torch.tensor([
[[1212, 318, 43086, 2420, 2420, 2420, 50257, 50257]],
[[1212, 318, 43086, 2420, 50257, 50257, 0 , 0]]
])
```
@tholor Could you or someone from the team please fix the masking issue.
The full errors trace:
> File "/Users/aw678/PycharmProjects/BERT/gpt2_simplified.py", line 181, in main
losses, past = model(input_ids, position_ids, lm_labels, past=past)
File "/Users/aw678/anaconda3/envs/pytorch_conda_env/lib/python3.6/site-packages/torch/nn/modules/module.py", line 489, in __call__
result = self.forward(*input, **kwargs)
File "/Users/aw678/anaconda3/envs/pytorch_conda_env/lib/python3.6/site-packages/pytorch_pretrained_bert/modeling_gpt2.py", line 661, in forward
hidden_states, presents = self.transformer(input_ids, position_ids, token_type_ids, past)
File "/Users/aw678/anaconda3/envs/pytorch_conda_env/lib/python3.6/site-packages/torch/nn/modules/module.py", line 489, in __call__
result = self.forward(*input, **kwargs)
File "/Users/aw678/anaconda3/envs/pytorch_conda_env/lib/python3.6/site-packages/pytorch_pretrained_bert/modeling_gpt2.py", line 590, in forward
token_type_embeds = self.wte(token_type_ids)
File "/Users/aw678/anaconda3/envs/pytorch_conda_env/lib/python3.6/site-packages/torch/nn/modules/module.py", line 489, in __call__
result = self.forward(*input, **kwargs)
File "/Users/aw678/anaconda3/envs/pytorch_conda_env/lib/python3.6/site-packages/torch/nn/modules/sparse.py", line 118, in forward
self.norm_type, self.scale_grad_by_freq, self.sparse)
File "/Users/aw678/anaconda3/envs/pytorch_conda_env/lib/python3.6/site-packages/torch/nn/functional.py", line 1455, in embedding
return torch.embedding(weight, input, padding_idx, scale_grad_by_freq, sparse)
RuntimeError: index out of range at /Users/soumith/mc3build/conda-bld/pytorch_1549593514549/work/aten/src/TH/generic/THTensorEvenMoreMath.cpp:191
| 05-03-2019 13:05:39 | 05-03-2019 13:05:39 | Maybe you get the error because of the position_ids that are most likely wrong.
I believe positional ids are not needed - you can use this:
predictions, past = model(tokens_tensor,position_ids=None token_type_ids=None, lm_labels=None, past=None)
and the use the parameters you want at the place you want or leave None if you do not want to use anything.
<|||||>With zeros, it is not padded, zeros are "!" not "[PAD]"<|||||>tokenizer.convert_tokens_to_ids('[PAD]') this results in 0. I am confused why it happens<|||||>Really? When I use decode tokenizer.decode(0) results in !.
tokenizer.encode(x) = tokenizer.convert_tokens_to_ids(tokenizer.tokenize(x)) [or should be], but when i try to use tokenizer.tokenize or tokenizer.convert_tokens_to_ids I am not sure which one, I get that its nto defined even tokenizer.encode works properly.<|||||>Does the 0 index actually stand for unknown tokens as well?
`tokenizer.convert_tokens_to_ids(["qrz"]) ` where "qrz" is supposed to be an unknown word. This will give [0]
But `tokenizer.convert_ids_to_tokens([0])` gives ["!"].
<|||||>That strange - we together get that ! == [PAD]<|||||>There was some issue fine-tuning GPT-2 with the master branch. This should now be fixed with the merge of #560 (which also add access to GPT-2 medium model).<|||||>But to actually answer the discussion on this issue (sorry I was reading to quickly), there is no padding token in GPT-2 vocabulary.
So either you manage to not pad (which is how the model is pretrained) or you need to add a new token to the vocabulary using the special_token functions. This method explained for instance in our blog post on fine-tuning GPT/GPT-2 here: https://medium.com/huggingface/how-to-build-a-state-of-the-art-conversational-ai-with-transfer-learning-2d818ac26313<|||||>Thanks. So we need to add the special token and then fine tune it?
<|||||>This issue has been automatically marked as stale because it has not had recent activity. It will be closed if no further activity occurs. Thank you for your contributions.
|
transformers | 576 | closed | key error when using run_classifier.py in predict mode, expecting label? | Hi,
I am getting key error when using run_classifier.py in predict mode.
https://github.com/huggingface/pytorch-pretrained-BERT/blob/master/examples/run_classifier.py
At prediction time we don't have labels hence it gives key error.
run_squad example is good as it was having is_training flag.
Could you please suggest?
if output_mode == "classification":
label_id = label_map[example.label]
elif output_mode == "regression":
label_id = float(example.label)
else:
raise KeyError(output_mode)
Thanks
Mahesh | 05-03-2019 11:19:02 | 05-03-2019 11:19:02 | This issue has been automatically marked as stale because it has not had recent activity. It will be closed if no further activity occurs. Thank you for your contributions.
|
transformers | 575 | closed | Different BERT representations when text is with and without single quotes | 05-03-2019 09:30:37 | 05-03-2019 09:30:37 | ||
transformers | 574 | closed | understanding of the output from TransfoXLModel | the output of the TransfoXLModel has the size of [1, 3, 1024] if the Input has tree tokens.
`predictions, mems = model(tokens_tensor, mems=None)`
doc from code is
```
Outputs:
A tuple of (last_hidden_state, new_mems)
`last_hidden_state`: the encoded-hidden-states at the top of the model
as a torch.FloatTensor of size [batch_size, sequence_length, self.config.d_model]
`new_mems`: list (num layers) of updated mem states at the entry of each layer
each mem state is a torch.FloatTensor of size [self.config.mem_len, batch_size, self.config.d_model]
Note that the first two dimensions are transposed in `mems` with regards to `input_ids` and `target`
```
could the output be explained more precisely. I would be very grateful! | 05-02-2019 17:37:39 | 05-02-2019 17:37:39 | This issue has been automatically marked as stale because it has not had recent activity. It will be closed if no further activity occurs. Thank you for your contributions.
|
transformers | 573 | closed | GPT2 doesn't accept inputs of varying tokens length (despite the padding at the end) | I have noted a very strange behaviour in GPT2 and I can't figure out why this happens. In one case when all of the inputs in the dataset have the same token length, the training works, however, when only one of the inputs has a different token length, the library throws an error. This is very strange since before I feed the inputs into the model I have a method which takes care of the padding so that every input is of a fixed shape/length.
**The working case pseudocode:**
Note that the dataset is in tensor type and when fed to the model is in shape specified in docs (n_batch, input_len)
dataset = [
[50258, 1212, 318, 617, 43086, 2420, 50258],
[50258, 318, 1212 , 617, 43086, 2420, 50258],
[50258, 1212, 318, 617, 43086, 2420, 50258],
]
_(all of the inputs in the dataset have the same token length)_
**The not working case:**
dataset = [
[50258, 1212, 318, 617, 43086, 2420, 50258],
[50258, 1212, 318, 617, 43086, 50258, 0],
[50258, 1212, 318, 617, 43086, 2420, 50258],
]
_(e.g. the second input has a different number of tokens than the other two, however, is padded with a 0 so that all of the inputs are of the same size)_
In fact, when the dataset consists of the same token length but has extra padding at the end, also throws an error:
dataset = [
[50258, 1212, 318, 617, 43086, 2420, 50258, 0, 0],
[50258, 318, 1212 , 617, 43086, 2420, 50258, 0, 0],
[50258, 1212, 318, 617, 43086, 2420, 50258, 0, 0],
]
A toy example to replicate this error:
[gpt2_simplified.py.zip](https://github.com/huggingface/pytorch-pretrained-BERT/files/3139047/gpt2_simplified.py.zip)
The full error traceback is:
> Epoch: 0%| | 0/3 [00:00<?, ?it/s]
Training: 0%| | 0/2 [00:00<?, ?it/s]
Traceback (most recent call last):
File "/local_path/gpt2_simplified.py", line 166, in <module>
main()
File "/local_path/**gpt2_simplified.py**", line 142, in main
**losses, past = model(input_ids, position_ids, lm_labels, past=past)**
File "/local_path/anaconda3/envs/pytorch_conda_env/lib/python3.6/site-packages/torch/nn/modules/**module.py**", line 489, in __call__
**result = self.forward**(*input, **kwargs)
File "/local_path/anaconda3/envs/pytorch_conda_env/lib/python3.6/site-packages/pytorch_pretrained_bert/**modeling_gpt2.py**", line 661, in forward
**hidden_states, presents = self.transformer(input_ids, position_ids, token_type_ids, past)**
File "/local_path/anaconda3/envs/pytorch_conda_env/lib/python3.6/site-packages/torch/nn/modules/**module.py**", line 489, in __call__
**result = self.forward**(*input, **kwargs)
File "/local_path/anaconda3/envs/pytorch_conda_env/lib/python3.6/site-packages/pytorch_pretrained_bert/**modeling_gpt2.py**", line 587, in forward
**position_embeds = self.wpe(position_ids)**
File "/local_path/anaconda3/envs/pytorch_conda_env/lib/python3.6/site-packages/torch/nn/modules/**module.py**", line 489, in __call__
**result = self.forward**(*input, **kwargs)
File "/local_path/anaconda3/envs/pytorch_conda_env/lib/python3.6/site-packages/torch/nn/modules/**sparse.py**", line 118, in forward
**self.norm_type, self.scale_grad_by_freq, self.sparse)**
File "/local_path/anaconda3/envs/pytorch_conda_env/lib/python3.6/site-packages/torch/nn/**functional.py**", line 1454, in embedding
**return torch.embedding(weight, input, padding_idx, scale_grad_by_freq, sparse)
RuntimeError: index out of range at /Users/soumith/mc3build/conda-bld/pytorch_1549593514549/work/aten/src/TH/generic/THTensorEvenMoreMath.cpp:191**
Thanks for the help in advance.
| 05-02-2019 17:05:16 | 05-02-2019 17:05:16 | @thomwolf I was wondering what are your thought on this issue?<|||||>The bug in the library causing the index out of range error comes from masking (-1) the LM labels.
> lm_labels: optional language modeling labels: torch.LongTensor of shape [batch_size, sequence_length] with indices selected in [-1, 0, ..., vocab_size]. All labels set to -1 are ignored (masked), the loss is only computed for the labels set in [0, ..., vocab_size].
When I pad the lm_labels to a fixed size using -1's, the library throws an error, on the other hand, using any other positive value works but acts like a vocabulary piece and thus makes the input incorrect.
@thomwolf Could someone please fix this?<|||||>Yes there is a PR (#560) fixing a number of issues for fine-tuning GPT-2.
Should be merged soon (this week hopefully).<|||||>Any update on #560? <|||||>I guess that you should try add pading at the begining - it predicts next word not first so the padding should be added in front.
[50258, 1212, 318, 617, 43086, 50258, 0] should be
[0,50258, 1212, 318, 617, 43086, 50258]<|||||>This issue has been automatically marked as stale because it has not had recent activity. It will be closed if no further activity occurs. Thank you for your contributions.
<|||||>I've checked that outputs from right-padded input tensors and from no-padding input tensors are different. Personally, the latter makes little more sense. A module for input masking and its corresponding position embeddings need to be implemented. <|||||>This issue has been automatically marked as stale because it has not had recent activity. It will be closed if no further activity occurs. Thank you for your contributions.
|
transformers | 572 | closed | BERT pre-training using only domain specific text | BERT is pre-trained using Wikipedia and other sources of normal text, but my problem domain has a very specific vocabulary & grammar. Is there an easy way to train BERT completely from domain specific data (preferably using Keras)?
The amount of pre-training data is not issue and we are not looking for the SOTA results. We would do fine with a smaller scale model, but it has to be trained from our data. | 05-02-2019 11:39:02 | 05-02-2019 11:39:02 | This issue has been automatically marked as stale because it has not had recent activity. It will be closed if no further activity occurs. Thank you for your contributions.
|
transformers | 571 | closed | Fix documentation typo | Just fix some apparent documentation typos. | 05-02-2019 11:26:17 | 05-02-2019 11:26:17 | Thanks! |
transformers | 570 | closed | Create optimizer only when args.do_train is True | I am facing the same problem as #544 . When only setting args.do_eval to evaluate a trained model, there will be an error due to optimizer initialization. I think it is unnecessary to create an optimizer if args.do_train is False. Thanks for your review. | 05-02-2019 11:14:44 | 05-02-2019 11:14:44 | Great, thanks @MottoX! |
transformers | 569 | closed | License of the pretrained models | I noticed that once `from_pretrained` is called, the library automatically downloads a pretrained model from a URL. However, I found no license included in the downloaded pretrained model file. What is the license of the pretrained models? | 05-01-2019 23:14:21 | 05-01-2019 23:14:21 | Just found it's under Apache v2 in the Google bert repo. Closing. |
transformers | 568 | closed | Fine-tuning Bert | I want to fine-tune Bert's LM for a specific corpora. I converted the test into the format specified in the documentation and ran the fine-tuning codes given. I'm getting the following error:
File "simple_lm_finetuning.py", line 156, in random_sent
assert len(t2) > 0
AssertionError
I'm getting similar error in pregenerate_.... script. What could be the reason for the same? Is it due to some possible OOV words? My corpus does contain some emoticons.
Thanks in advance | 05-01-2019 16:34:17 | 05-01-2019 16:34:17 | I was facing the same issue when finetuning using `finetune_on_pregenerated.py`. The problem was in the fact that I have some empty sentences in my dataset. Also there are some special characters, like `\t` (tabulation) which can make a mess and should be cleared.
I preprocess the text like this:
```
for_train = for_train.dropna(subset=['text'])
import re
with open('for_pretraining_full.txt', "w", encoding='utf-8') as writer:
for doc in for_train['text'].tolist():
doc = doc.replace(u'\xa0', u' ').replace(u'\u200b', u' ').replace(u'\u206f', u' ').replace(u'\u206e', u' ').replace(u'\u206b', u' ').replace(u'\u206c', u' ').replace(u'\u2063', u' ').replace(u'\u200d', u' ').strip() # replace some special unicode chars
doc = re.sub('\t+', '', doc) # replace tabs
doc = doc.replace('. ', '\n')
doc = re.sub('\n+( )*(\n+)*', '\n', doc) # replace several consecutive new lines by a single one
doc = doc.strip()
if (doc != ''):
writer.write(doc)
writer.write('\n\n')
```
Also you can try to find problem sentances, debuging your `simple_lm_finetuning.py`.<|||||>I ran into an issue on this where some sneaky \n were still sneaking through even with the above code. I remedied this by just doing
`if "\n" in doc:
doc = re.sub('\n', ' ', doc)`<|||||>This issue has been automatically marked as stale because it has not had recent activity. It will be closed if no further activity occurs. Thank you for your contributions.
|
transformers | 567 | closed | about pytorch 1.1.0 rerlease | Hi today pytorch 1.1.0 release(https://github.com/pytorch/pytorch/releases/tag/v1.1.0)
In version 1.1.0, added a new module implementing Multi-headed-Attention.
And various bugs have been modified.
Do you plan to update to suit that version?
| 05-01-2019 09:48:27 | 05-01-2019 09:48:27 | Hi,
The repo is compatible with PyTorch 1.1.0.
But, we probably won't switch to PyTorch Multi-headed-Attention module since this would mean refactoring all the models and adding complexity to the tensorflow conversion codes for unclear gains.<|||||>This issue has been automatically marked as stale because it has not had recent activity. It will be closed if no further activity occurs. Thank you for your contributions.
|
transformers | 566 | closed | Bug in run_classifier.py fp16 learning rate | After the latest update, my learning rate of fp16 in run_classifier.py keeps increasing.
https://github.com/huggingface/pytorch-pretrained-BERT/blob/2dee86319dbad575352358b8f2fb4129940e381a/examples/run_classifier.py#L857-L858
I think the right code is: lr_this_step = args.learning_rate * warmup_linear.get_lr(global_step, args.warmup_proportion).
https://github.com/huggingface/pytorch-pretrained-BERT/blob/2dee86319dbad575352358b8f2fb4129940e381a/pytorch_pretrained_bert/optimization.py#L53-L62
In this function, the first argument should be step, and global_step/num_train_optimization_steps makes process calculated again and when input process to WarmupLinearSchedule, it would be too small to decrease
https://github.com/huggingface/pytorch-pretrained-BERT/blob/2dee86319dbad575352358b8f2fb4129940e381a/pytorch_pretrained_bert/optimization.py#L162-L171
| 05-01-2019 07:01:53 | 05-01-2019 07:01:53 | I'm asking the same question<|||||>I had been dealing with the issue of low and decreasing accuracy when I use fp16, as shown below,
```
Epoch 1 - Batch 1600/287417 - Training Acc. 0.106250 - Training Loss 2.295977
Epoch 1 - Batch 3200/287417 - Training Acc. 0.098125 - Training Loss 2.299707
Epoch 1 - Batch 4800/287417 - Training Acc. 0.094792 - Training Loss 2.304948
Epoch 1 - Batch 6400/287417 - Training Acc. 0.093125 - Training Loss 2.307725
Epoch 1 - Batch 8000/287417 - Training Acc. 0.092625 - Training Loss 2.305703
Epoch 1 - Batch 9600/287417 - Training Acc. 0.091667 - Training Loss 2.306758
Epoch 1 - Batch 11200/287417 - Training Acc. 0.092589 - Training Loss 2.306116
Epoch 1 - Batch 12800/287417 - Training Acc. 0.092969 - Training Loss 2.307227
Epoch 1 - Batch 14400/287417 - Training Acc. 0.091458 - Training Loss 2.310017
Epoch 1 - Batch 16000/287417 - Training Acc. 0.091000 - Training Loss 2.308750
Epoch 1 - Batch 17600/287417 - Training Acc. 0.090795 - Training Loss 2.309631
Epoch 1 - Batch 19200/287417 - Training Acc. 0.090625 - Training Loss 2.310771
Epoch 1 - Batch 20800/287417 - Training Acc. 0.090433 - Training Loss 2.310832
Epoch 1 - Batch 22400/287417 - Training Acc. 0.090625 - Training Loss 2.311030
Epoch 1 - Batch 24000/287417 - Training Acc. 0.090083 - Training Loss 2.311357
Epoch 1 - Batch 25600/287417 - Training Acc. 0.089883 - Training Loss 2.311748
Epoch 1 - Batch 27200/287417 - Training Acc. 0.089449 - Training Loss 2.312302
Epoch 1 - Batch 28800/287417 - Training Acc. 0.088993 - Training Loss 2.312582
Epoch 1 - Batch 30400/287417 - Training Acc. 0.088651 - Training Loss 2.313187
Epoch 1 - Batch 32000/287417 - Training Acc. 0.088656 - Training Loss 2.313006
Epoch 1 - Batch 33600/287417 - Training Acc. 0.088750 - Training Loss 2.313333
Epoch 1 - Batch 35200/287417 - Training Acc. 0.088665 - Training Loss 2.314015
Epoch 1 - Batch 36800/287417 - Training Acc. 0.088641 - Training Loss 2.313631
Epoch 1 - Batch 38400/287417 - Training Acc. 0.088854 - Training Loss 2.313276
Epoch 1 - Batch 40000/287417 - Training Acc. 0.089325 - Training Loss 2.312648
Epoch 1 - Batch 41600/287417 - Training Acc. 0.089183 - Training Loss 2.312943
Epoch 1 - Batch 43200/287417 - Training Acc. 0.089051 - Training Loss 2.312587
Epoch 1 - Batch 44800/287417 - Training Acc. 0.088929 - Training Loss 2.313172
Epoch 1 - Batch 46400/287417 - Training Acc. 0.088793 - Training Loss 2.312671
Epoch 1 - Batch 48000/287417 - Training Acc. 0.088479 - Training Loss 2.313255
Epoch 1 - Batch 49600/287417 - Training Acc. 0.088972 - Training Loss 2.312710
Epoch 1 - Batch 51200/287417 - Training Acc. 0.088906 - Training Loss 2.312372
```
However, after I made the change in `lr_this_step` that you indicated, I've started to get normal results, as follows,
```
Epoch 1 - Batch 1600/287417 - Training Acc. 0.156250 - Training Loss 2.224727
Epoch 1 - Batch 3200/287417 - Training Acc. 0.200937 - Training Loss 2.166289
Epoch 1 - Batch 4800/287417 - Training Acc. 0.245833 - Training Loss 2.098184
Epoch 1 - Batch 6400/287417 - Training Acc. 0.299063 - Training Loss 2.018706
Epoch 1 - Batch 8000/287417 - Training Acc. 0.351625 - Training Loss 1.937730
Epoch 1 - Batch 9600/287417 - Training Acc. 0.400833 - Training Loss 1.855378
Epoch 1 - Batch 11200/287417 - Training Acc. 0.444018 - Training Loss 1.768468
Epoch 1 - Batch 12800/287417 - Training Acc. 0.481875 - Training Loss 1.685869
Epoch 1 - Batch 14400/287417 - Training Acc. 0.513889 - Training Loss 1.606483
Epoch 1 - Batch 16000/287417 - Training Acc. 0.536937 - Training Loss 1.537816
Epoch 1 - Batch 17600/287417 - Training Acc. 0.556364 - Training Loss 1.477735
Epoch 1 - Batch 19200/287417 - Training Acc. 0.576146 - Training Loss 1.418323
Epoch 1 - Batch 20800/287417 - Training Acc. 0.592019 - Training Loss 1.367327
Epoch 1 - Batch 22400/287417 - Training Acc. 0.606429 - Training Loss 1.321059
Epoch 1 - Batch 24000/287417 - Training Acc. 0.617542 - Training Loss 1.281488
Epoch 1 - Batch 25600/287417 - Training Acc. 0.627109 - Training Loss 1.246746
Epoch 1 - Batch 27200/287417 - Training Acc. 0.637500 - Training Loss 1.211883
Epoch 1 - Batch 28800/287417 - Training Acc. 0.645938 - Training Loss 1.182604
Epoch 1 - Batch 30400/287417 - Training Acc. 0.652204 - Training Loss 1.158571
Epoch 1 - Batch 32000/287417 - Training Acc. 0.658875 - Training Loss 1.134463
Epoch 1 - Batch 33600/287417 - Training Acc. 0.665179 - Training Loss 1.111719
Epoch 1 - Batch 35200/287417 - Training Acc. 0.671023 - Training Loss 1.089363
Epoch 1 - Batch 36800/287417 - Training Acc. 0.676848 - Training Loss 1.068860
Epoch 1 - Batch 38400/287417 - Training Acc. 0.681536 - Training Loss 1.050721
Epoch 1 - Batch 40000/287417 - Training Acc. 0.685775 - Training Loss 1.034663
Epoch 1 - Batch 41600/287417 - Training Acc. 0.690361 - Training Loss 1.017672
Epoch 1 - Batch 43200/287417 - Training Acc. 0.693866 - Training Loss 1.004058
Epoch 1 - Batch 44800/287417 - Training Acc. 0.698013 - Training Loss 0.990084
Epoch 1 - Batch 46400/287417 - Training Acc. 0.701552 - Training Loss 0.977086
Epoch 1 - Batch 48000/287417 - Training Acc. 0.704854 - Training Loss 0.965735
Epoch 1 - Batch 49600/287417 - Training Acc. 0.708266 - Training Loss 0.953387
Epoch 1 - Batch 51200/287417 - Training Acc. 0.712012 - Training Loss 0.940919
Epoch 1 - Batch 52800/287417 - Training Acc. 0.714697 - Training Loss 0.929941
```
Thanks!<|||||>@burcturkoglu
How about performance comparison with fp32?<|||||>@yeontaek
I trained BERT for classification with my own data by _run_classifier_ script.
Here are the benchmarks for fp32 vs fp16 in both single Tesla V100 and in 4 Tesla V100 with _DataParallel_,
*_fp32:_*
- Single Tesla V100 - Training Duration 17,739 seconds
- 4 Tesla V100 - Training Duration 9,342 seconds
*_fp16:_*
- Single Tesla V100 - Training Duration 12,297 seconds
- 4 Tesla V100 - Training Duration 6,330 seconds
In both types of instances, it gives approximately 30% increase in speed without a change in accuracy. <|||||>@burcturkoglu
Thank you so much. It was a big help. |
transformers | 565 | closed | Results of Fine-tuned model changes in every run | After I load the model with:
`
model = BertForNextSentencePrediction.from_pretrained("bert-base-uncased",state_dict=model_state_dict)
model.eval()
`
The prediction results are not stable. They change dractically in every run.
It gets stable if I fix the seed but I dont understand why we need that. Isnt the model supposed to be fixed since we are just evaluating? | 05-01-2019 02:52:00 | 05-01-2019 02:52:00 | This issue has been automatically marked as stale because it has not had recent activity. It will be closed if no further activity occurs. Thank you for your contributions.
|
transformers | 564 | closed | Fix #537 | 05-01-2019 02:48:38 | 05-01-2019 02:48:38 | Thanks a lot for that @8enmann! |
|
transformers | 563 | closed | performance does not change but loss decrease | After training bert-lstm-crf model for 25 epoches, the performance on training set
here is the performance on train set, dev set and test set:
25th epoch:
tensor(10267.6279, device='cuda:0')
(0.42706720346856614, 0.4595134955014995, 0.4426966292134832)
(0.43147208121827413, 0.4271356783919598, 0.42929292929292934)
(0.4460093896713615, 0.4668304668304668, 0.4561824729891957)
26th epoch:
tensor(10219.3398, device='cuda:0')
(0.44544364508393286, 0.4951682772409197, 0.46899163642101943)
(0.4469135802469136, 0.4547738693467337, 0.45080946450809467)
(0.45871559633027525, 0.4914004914004914, 0.4744958481613286)
27 epoch:
tensor(10169.0742, device='cuda:0')
(0.44544364508393286, 0.4951682772409197, 0.46899163642101943)
(0.4469135802469136, 0.4547738693467337, 0.45080946450809467)
(0.45871559633027525, 0.4914004914004914, 0.4744958481613286)
more epochs:
......(same performance but lower loss)
And here is the main code:
for epoch in tqdm(range(200)):
loss = train_one_epoch(dataloader=source_train_dataloader,
model=model, optimizer=optimizer)
train_perf = test_one_epoch(dataloader=source_train_dataloader_for_test,
model=model)
dev_perf = test_one_epoch(dataloader=source_dev_dataloader, model=model)
test_perf = test_one_epoch(dataloader=source_test_dataloader, model=model)
base_result_loc = "bert_char_ps/bert_char_result"
# store performance result
add_model_result(
base_result_loc,
epoch,
loss,
train_perf,
dev_perf,
test_perf)
I can't not figure out why the loss keep decreasing but the performance on train set, dev set and test set keep unchanged. I have been stuck in this for a few days. Anyone knows how to handle this? It would be of great help.
| 04-30-2019 22:11:46 | 04-30-2019 22:11:46 | This issue has been automatically marked as stale because it has not had recent activity. It will be closed if no further activity occurs. Thank you for your contributions.
|
transformers | 562 | closed | Small fix to remove shifting of lm labels during pre process of RocStories. | In reference to https://github.com/huggingface/pytorch-pretrained-BERT/issues/473, remove the one shifting of lm labels since this shift happens internally during the model's forward pass.
@thomwolf | 04-30-2019 20:56:45 | 04-30-2019 20:56:45 | Awesome, thanks! |
transformers | 561 | closed | Training Transformer XL from scratch | Hello,
I'm trying to train a transformer XL model from scratch by combining the architecture code from this library and training code from the official paper repo. But this yields to NaNs during training, just wanted to clarify the recommended way to initialize a new model.
Im doing it by,
```
architecture = TransfoXLConfig().from_json_file(args.config_path)
model = TransfoXLLMHeadModel(architecture)
```
Is there a bug in this? | 04-30-2019 20:30:27 | 04-30-2019 20:30:27 | This looks good to me<|||||>This issue has been automatically marked as stale because it has not had recent activity. It will be closed if no further activity occurs. Thank you for your contributions.
<|||||>@anshuman1992 could you share a code snippet/gist used for training TransformerXL model?
<|||||>@anshuman1992 this will be great for me too |
transformers | 560 | closed | Improvements to GPT-2 (special_tokens, fine-tuning, medium model) + repo code coverage metric | - adding method to add special tokens to GPT-2 (like it's done for GPT).
- adding code coverage tracking for tests. | 04-30-2019 09:06:36 | 04-30-2019 09:06:36 | # [Codecov](https://codecov.io/gh/huggingface/pytorch-pretrained-BERT/pull/560?src=pr&el=h1) Report
> :exclamation: No coverage uploaded for pull request base (`master@b832d5b`). [Click here to learn what that means](https://docs.codecov.io/docs/error-reference#section-missing-base-commit).
> The diff coverage is `70.37%`.
[](https://codecov.io/gh/huggingface/pytorch-pretrained-BERT/pull/560?src=pr&el=tree)
```diff
@@ Coverage Diff @@
## master #560 +/- ##
=========================================
Coverage ? 66.04%
=========================================
Files ? 18
Lines ? 3673
Branches ? 0
=========================================
Hits ? 2426
Misses ? 1247
Partials ? 0
```
| [Impacted Files](https://codecov.io/gh/huggingface/pytorch-pretrained-BERT/pull/560?src=pr&el=tree) | Coverage Δ | |
|---|---|---|
| [pytorch\_pretrained\_bert/modeling\_openai.py](https://codecov.io/gh/huggingface/pytorch-pretrained-BERT/pull/560/diff?src=pr&el=tree#diff-cHl0b3JjaF9wcmV0cmFpbmVkX2JlcnQvbW9kZWxpbmdfb3BlbmFpLnB5) | `79.68% <100%> (ø)` | |
| [pytorch\_pretrained\_bert/tokenization\_gpt2.py](https://codecov.io/gh/huggingface/pytorch-pretrained-BERT/pull/560/diff?src=pr&el=tree#diff-cHl0b3JjaF9wcmV0cmFpbmVkX2JlcnQvdG9rZW5pemF0aW9uX2dwdDIucHk=) | `84.86% <100%> (ø)` | |
| [pytorch\_pretrained\_bert/modeling\_gpt2.py](https://codecov.io/gh/huggingface/pytorch-pretrained-BERT/pull/560/diff?src=pr&el=tree#diff-cHl0b3JjaF9wcmV0cmFpbmVkX2JlcnQvbW9kZWxpbmdfZ3B0Mi5weQ==) | `80.16% <61.9%> (ø)` | |
------
[Continue to review full report at Codecov](https://codecov.io/gh/huggingface/pytorch-pretrained-BERT/pull/560?src=pr&el=continue).
> **Legend** - [Click here to learn more](https://docs.codecov.io/docs/codecov-delta)
> `Δ = absolute <relative> (impact)`, `ø = not affected`, `? = missing data`
> Powered by [Codecov](https://codecov.io/gh/huggingface/pytorch-pretrained-BERT/pull/560?src=pr&el=footer). Last update [b832d5b...db98a4a](https://codecov.io/gh/huggingface/pytorch-pretrained-BERT/pull/560?src=pr&el=lastupdated). Read the [comment docs](https://docs.codecov.io/docs/pull-request-comments).
|
transformers | 559 | closed | the size of words and the size of lables do not match | When I run bert-large-cased model, it prints "the size of words and the size of lables do not match" but get no error message. What is this issue? Thanks | 04-30-2019 05:01:05 | 04-30-2019 05:01:05 | Can you give the exact log of (and before) the error message?<|||||>This issue has been automatically marked as stale because it has not had recent activity. It will be closed if no further activity occurs. Thank you for your contributions.
|
transformers | 558 | closed | can one run squad using gpt2? | looking through the new notes discussing GPT-2 I do not understand how one might run a squad fine tuning on a pretrained gpt-2 model
Any assistance would be greatly appreciated | 04-29-2019 21:15:43 | 04-29-2019 21:15:43 | This issue has been automatically marked as stale because it has not had recent activity. It will be closed if no further activity occurs. Thank you for your contributions.
<|||||>2 years late, but can anyone figure it out? |
transformers | 557 | closed | Expanding vocab size for GTP2 pre-trained model. | About the aim:
I am trying to fine-tune a model on an English lyrics dataset in order to capture a style of a specific genre. To do this, at the fine-tuning input step, I wrap the lyrics with a "special token", e.g. <genre_type_tag> Lyrics text <genre_type_tag>. This means that I have to expand the vocab size by the number of special tokens.
Issue:
Using GTP2 tokenizer, I find that I can easily expand the vocab by specifying the special tokens:
`tokenizer = GPT2Tokenizer.from_pretrained(args.model_name, special_tokens=special_tokens)`.
However, the problem arises when I try to run the input through the model and get the following error:
> return torch.embedding(weight, input, padding_idx, scale_grad_by_freq, sparse)
RuntimeError: index out of range at /Users/soumith/mc3build/conda-bld/pytorch_1549593514549/work/aten/src/TH/generic/THTensorEvenMoreMath.cpp:191
Which I believe says that the vocab id of the special token that I am using is out of bound since the model has been pre-trained without the them.
On the other hands, using OpenAIGTP model, I can see that this problem is solved by an additional parameter at the initialisation which tells the model to expect a number of special tags:
`model = OpenAIGPTDoubleHeadsModel.from_pretrained(args.model_name, num_special_tokens=len(special_tokens))`
I was wondering whether and how I can achieve a similar effect using GTP2 since it doesn't have such a parameter option.
To work around this issue I tried to alter the config file created using:
`config = GPT2Config.from_json_file(output_config_file)`, however, this gave me more issues and I am not sure whether that is the correct way to do it.
Kind regards.
| 04-29-2019 19:32:02 | 04-29-2019 19:32:02 | @thomwolf Could you or someone from your team point me in the right direction to get the gtp2 model running with a small number of newly defined special tokens?
Any help very appreciated as I really need to move on with my research project.<|||||>Hi @adigoryl, I'm adding this feature with PR #560
You can have a look.
It should be merged soon I guess.<|||||>Hi @thomwolf, first of all, I would like to thank you for the quick response and solution. I have had a look at the added lines and have replaced the 'modelling_gpt2.py' file in my pytorch_pretrained_bert lib. Running the code: `model = GPT2LMHeadModel.from_pretrained(args.model_name, num_special_tokens=len(special_tokens))` gives me:
> model = cls(config, *inputs, **kwargs)
TypeError: __init__() got an unexpected keyword argument 'num_special_tokens'
I am not sure whether this happens because of the way I have updated my lib or there still is something missing.
What is the best way to update my lib with the freshly made changes?
--------------------------UPDATE-------------------------------
Copy and paste seemed to work. The problem was that I needed to add a new line after the updated code paste since python is space sensitive. Having to fix it the num_special_tokens works in an anticipated way as I can see in the debugger that it sets the n_special field and updates total_tokens_embeddings. However, having this all fixed I still end up with the same issue I started with:
> Traceback (most recent call last):
File "/Users/aw678/PycharmProjects/BERT/gtp2_train_lyrics_LM_copy.py", line 202, in <module>
main()
File "/Users/aw678/PycharmProjects/BERT/gtp2_train_lyrics_LM_copy.py", line 178, in main
losses, past = model(input_ids, lm_labels, past=past)
File "/Users/aw678/anaconda3/envs/pytorch_conda_env/lib/python3.6/site-packages/torch/nn/modules/module.py", line 489, in __call__
result = self.forward(*input, **kwargs)
File "/Users/aw678/anaconda3/envs/pytorch_conda_env/lib/python3.6/site-packages/pytorch_pretrained_bert/modeling_gpt2.py", line 661, in forward
hidden_states, presents = self.transformer(input_ids, position_ids, token_type_ids, past)
File "/Users/aw678/anaconda3/envs/pytorch_conda_env/lib/python3.6/site-packages/torch/nn/modules/module.py", line 489, in __call__
result = self.forward(*input, **kwargs)
File "/Users/aw678/anaconda3/envs/pytorch_conda_env/lib/python3.6/site-packages/pytorch_pretrained_bert/modeling_gpt2.py", line 587, in forward
position_embeds = self.wpe(position_ids)
File "/Users/aw678/anaconda3/envs/pytorch_conda_env/lib/python3.6/site-packages/torch/nn/modules/module.py", line 489, in __call__
result = self.forward(*input, **kwargs)
File "/Users/aw678/anaconda3/envs/pytorch_conda_env/lib/python3.6/site-packages/torch/nn/modules/sparse.py", line 118, in forward
self.norm_type, self.scale_grad_by_freq, self.sparse)
File "/Users/aw678/anaconda3/envs/pytorch_conda_env/lib/python3.6/site-packages/torch/nn/functional.py", line 1454, in embedding
return torch.embedding(weight, input, padding_idx, scale_grad_by_freq, sparse)
RuntimeError: index out of range at /Users/soumith/mc3build/conda-bld/pytorch_1549593514549/work/aten/src/TH/generic/THTensorEvenMoreMath.cpp:191
Not sure why it complains about "position_ids" since they are not compulsory. I believe this may not be an issue with my code (just in case someone wants to have a look):
[gtp2_train_lyrics_LM_copy.pdf](https://github.com/huggingface/pytorch-pretrained-BERT/files/3131933/gtp2_train_lyrics_LM_copy.pdf)
If you could provide a simplified working example of running GPT2 with new tokens then this should resolve my issue.
<|||||>To replicate the error use the simplified version:
[gpt2_simplified.py.zip](https://github.com/huggingface/pytorch-pretrained-BERT/files/3132183/gpt2_simplified.py.zip)
<|||||>You have to install the repo from source from the PR branch (see the instructions in the readme to install from source and after cloning git checkout to the PR branch before installing).
If it looks too complicated maybe the best is to wait for the PR to be merged.<|||||>I have managed to update the library on my machine but it seems that there is an incompatibility in the lib code. If you could provide a working toy example on how to fine-tune GPT2 with special symbols then I am sure the community would appreciate it and my issue would be resolved. I have attached such toy example above in the zip file, however, it has an issue which I believe is caused by the lib.
I am sorry to bother you so much but I just want to get on with my work.
Regards, Adrian.<|||||>This issue has been automatically marked as stale because it has not had recent activity. It will be closed if no further activity occurs. Thank you for your contributions.
|
transformers | 556 | closed | Training beyond specified 't_total' steps with schedule 'warmup_linear'. Learning rate set to 0.0. Please set 't_total' of BertAdam correctly. | I am seeing the above error in my training process. Is it a significant issue? Looks like it's related to `t_total`, which should be properly set here:
https://github.com/huggingface/pytorch-pretrained-BERT/blob/b832d5bb8a6dfc5965015b828e577677eace601e/examples/run_classifier.py#L742-L743
What could be potential causes of this issue? I trained exactly `args.num_train_epochs` epochs, and didn't alter the training data in between, so shouldn't this pre-calculated `t_total` work without issue?
My `len(train_examples)` is 49401, `args.num_train_epochs` is 5, using 2 GPUs, and other parameters are left as default. If it matters, my code is based on a version (68a889) before the recent `WarmupLinearSchedule` change. | 04-29-2019 17:53:52 | 04-29-2019 17:53:52 | Actually, shouldn't the `int()` be a `ceiling()`? Because let's say `args.gradient_accumulation_steps` is 1, then it is `ceiling(len(train_examples) / args.train_batch_size)` that is the number of batches in an epoch.<|||||>I am having the same problem with my finetuned model for gpt2<|||||>I am having the same issue in partially changed run_squad.py code.<|||||>I have the same issue.<|||||>Humm yes, we should probably change `int` to `ceiling` in this example indeed.<|||||>Is this a significant issue? If it's only the last few batches in the last epoch that are not being trained on, it shouldn't be a huge problem, right?
Also I find it strange that suddenly a lot of people are running into this bug in this past week (according to the replies to this issue) even though the `int` code was written 3 months ago. Is this also related to some other more recent changes?<|||||>Yes there was a huge refactoring of the `BertAdam` optimizer by @lukovnikov (#389, #445, #531)<|||||>Hi, this warning is printed to avoid wasted computations with warmup-linear or other surprises with other schedules due to a t_total set too low.
And I think that line should be `int( math.ceil(len(train_examples) / args.train_batch_size) / args.gradient_accumulation_steps) * args.num_train_epochs` (@thomwolf) ?<|||||>Hi. I figured out the source of the problem: t_total, aka num_train_optimization_steps
https://github.com/huggingface/pytorch-pretrained-BERT/blob/3fc63f126ddf883ba9659f13ec046c3639db7b7e/examples/run_squad.py#L903
is computed over the length of the train examples, while the true number of steps is determined by whatever convert_examples_to_features returns
https://github.com/huggingface/pytorch-pretrained-BERT/blob/3fc63f126ddf883ba9659f13ec046c3639db7b7e/examples/run_squad.py#L970
A print(len(train_examples), len(train_features)) in line 980 returns:
87599 191597
<|||||>You could also add the option `drop_last=True` to the `DataLoader`, then the number of samples will be calculated correctly.<|||||>This issue has been automatically marked as stale because it has not had recent activity. It will be closed if no further activity occurs. Thank you for your contributions.
<|||||>I get error below while running the program.. Did I do any mistake?
warmup_linear = WarmupLinearSchedule( warmup=args.warmup_proportion,
t_total=num_train_optimization_steps)
lr_this_step = args.learning_rate * warmup_linear.get_lr(num_train_optimization_steps,
args.warmup_proportion)
WARNING - pytorch_pretrained_bert.optimization - Training beyond specified 't_total'. Learning rate multiplier set to 0.0. Please set 't_total' of WarmupLinearSchedule correctly. |
transformers | 555 | closed | Transformer XL from Pytorch model | Hello,
I have trained the original pytorch version of transformer xl, and I want to load it to get the hidden state and prediction.
However, it doesn't work. Apparently you only support to load a model from TensorFlow model checkpoints only.
Is there any hint or feature modification to make it work with model.pt and cache.pt ? | 04-29-2019 12:47:17 | 04-29-2019 12:47:17 | This issue has been automatically marked as stale because it has not had recent activity. It will be closed if no further activity occurs. Thank you for your contributions.
|
transformers | 554 | closed | ValueError: For training, each question should have exactly 1 answer. | Tried to run run_squad.py with the squad 2.0 dataset and came up with this error, ValueError: For training, each question should have exactly 1 answer. How do I solve this? | 04-28-2019 21:28:03 | 04-28-2019 21:28:03 | Please give more information: the command used (arguments passed), traceback (the command line output), and version (can use `pip show pytorch_pretrained_bert`)
I faced a similar problem with `read_squad_examples` when passing `input_file=dev.json` and `is_training=True`. <|||||>I have this problem when training on SQUADv2 without `--version_2_with_negative` option. Basically for squad 2.0, it is possible there is no answer for questions. Adding this option in training command fixed the problem for me.<|||||>This issue has been automatically marked as stale because it has not had recent activity. It will be closed if no further activity occurs. Thank you for your contributions.
<|||||>Hi,
I see the same error. Some of the questions have multiple answer spans.
The error suggests that the current preprocessing codes cannot handle multiple answer spans for a given question.
Has anyone fixed this ??
Thanks<|||||>I also receive this error when using the `--version_2_with_negative` flag paired with training data from SQuAD 2.0. It looks like it may be caused by some logic in `utils_squad.py`, lines 150-152; it seems that answerable questions are expected to only have one answer. I'm not familiar enough with the task and data set to know if this is a correct assumption, but since I'm using data from the SQuAD 2.0 web site I would think it should train fine.<|||||>For training, the assumption is true.
On Mon, Nov 18, 2019 at 09:56 Allen Kim <[email protected]> wrote:
> I also receive this error when using the --version_2_with_negative flag
> paired with training data from SQuAD 2.0. It looks like it may be caused by
> some logic in utils_squad.py, lines 150-152; it seems that answerable
> questions are expected to only have one answer. I'm not familiar enough
> with the task and data set to know if this is a correct assumption, but
> since I'm using data from the SQuAD 2.0 web site I would think it should
> train fine.
>
> —
> You are receiving this because you are subscribed to this thread.
> Reply to this email directly, view it on GitHub
> <https://github.com/huggingface/transformers/issues/554?email_source=notifications&email_token=AIEAE4GLKEIMFR3BUJQK5VTQUHY4BA5CNFSM4HI7NMRKYY3PNVWWK3TUL52HS4DFVREXG43VMVBW63LNMVXHJKTDN5WW2ZLOORPWSZGOEEI5VNI#issuecomment-554818229>,
> or unsubscribe
> <https://github.com/notifications/unsubscribe-auth/AIEAE4H7OARRY4INFJTGU6DQUHY4BANCNFSM4HI7NMRA>
> .
>
<|||||>Thanks for clarifying! |
transformers | 553 | closed | How to get back input and predictions as string | Once I am done fine tuning my `BertForSequenceClassification` model, I evaluate it on a validation set. I can see the loss and accuracy scores but I would also like to get the actual labels (as string) it predicted for each sentence (string) in the validation dataset. How could I do that? | 04-28-2019 20:04:47 | 04-28-2019 20:04:47 | This issue has been automatically marked as stale because it has not had recent activity. It will be closed if no further activity occurs. Thank you for your contributions.
|
transformers | 552 | closed | should loss_scale be multiplied to the loss explicitly? | I noticed that in the run_swag.py, the following code is included
if args.fp16 and args.loss_scale != 1.0:
# rescale loss for fp16 training
# see https://docs.nvidia.com/deeplearning/sdk/mixed-precision-training/index.html
loss = loss * args.loss_scale
and in run_squad.py, this is not included.
the optimizer in them are identity, so which is right? | 04-28-2019 09:25:38 | 04-28-2019 09:25:38 | This issue has been automatically marked as stale because it has not had recent activity. It will be closed if no further activity occurs. Thank you for your contributions.
|
transformers | 551 | closed | Pad inputs to multiple of 8 | Pad transformer's inputs to multiple of 8 to better use Tensorcores in fp16 mode.
@glample's [XLM](https://github.com/facebookresearch/XLM) does that and it seems still relevant with CUDA 10 (cc @yaroslavvb). | 04-28-2019 08:25:06 | 04-28-2019 08:25:06 | This issue has been automatically marked as stale because it has not had recent activity. It will be closed if no further activity occurs. Thank you for your contributions.
|
transformers | 550 | closed | Fix GPT2 crash on special quotes in Python 3 | In Python 3 the line
https://github.com/huggingface/pytorch-pretrained-BERT/blob/b832d5bb8a6dfc5965015b828e577677eace601e/pytorch_pretrained_bert/tokenization_gpt2.py#L224
splits `token` into full characters, not UTF-8 bytes, so for example the right single quote ’ gives `ord('’') == 8217`. That causes a crash since it's a much larger key than any in `self.byte_encoder`. The official GPT2 repo uses `token.encode('utf-8')` but it doesn't work the same in Python 2. I've suggested a fix that uses `token.encode` only in Python 3.
Tested on Python 3.7 but not Python 2.
Thanks for this very useful repo! | 04-28-2019 05:25:20 | 04-28-2019 05:25:20 | Thanks, this is closed now with #564 |
transformers | 549 | closed | CUDA out of memory issue when training | 04-28-2019 02:51:37 | 04-28-2019 02:51:37 | Try reducing the batch size?<|||||>This issue has been automatically marked as stale because it has not had recent activity. It will be closed if no further activity occurs. Thank you for your contributions.
|
|
transformers | 548 | closed | how to ensemble different checkpoints? | I want to ensemble different checkpoints trained from the same parameter configuration but different seeds. Could you tell me how to ensemble these checkpoints? | 04-27-2019 23:19:03 | 04-27-2019 23:19:03 | This issue has been automatically marked as stale because it has not had recent activity. It will be closed if no further activity occurs. Thank you for your contributions.
<|||||>@shawnkx Hi! Have you found a solution?<|||||>@all, any updates on this? |
transformers | 547 | closed | How to get masked word prediction probabilities | Original sentence: i love apples. there are a lot of fruits in the world that i like, but apples would be my favorite fruit.
Masked sentence: i love apples . there are a lot of fruits in the world that i [MASK] , but apples would be my favorite fruit .
When I run through the pytorch version of bert, I get the following representations of probabilities:
Best predicted word: ['love'] tensor(12.7276, grad_fn=)
Other words along with their probabilities:
['like'] tensor(10.2872, grad_fn=)
['miss'] tensor(8.8226, grad_fn=)
['know'] tensor(8.5971, grad_fn=)
['am'] tensor(7.9407, grad_fn=)
['hate'] tensor(7.9209, grad_fn=)
['mean'] tensor(7.8873, grad_fn=)
['enjoy'] tensor(7.8813, grad_fn=)
['want'] tensor(7.6885, grad_fn=)
['prefer'] tensor(7.5712, grad_fn=)
I am quite sure that this does not mean that probability for word "love" is proportional to 12.7276 and for word "like" is 10.2872.
I also know that the summ of all func(this number) thought the whole vocabulary is 1. But I do not know what the func is?
Thanks | 04-27-2019 22:54:10 | 04-27-2019 22:54:10 | I'm interested in an answer, too. A score/probability would help to select the best word for a masked token.<|||||>You are looking for the softmax function: https://pytorch.org/docs/stable/nn.html?highlight=softmax#torch.nn.functional.softmax<|||||>Thanks Thomas, I'll give it a try.<|||||>Thanks,
So you say that for score x1 (where all the scores are x1,x2,.. xn) :
probability_x1 = (exp(^x1)/(exp(^x1) + exp(^x2) + .. exp(^xn))
<|||||>@Oxi84 could you share how you obtained the masked word probabilities? I have been trying to do that on my custom data. That is, I want to pretrain my own model and then do masked word prediction on new data.<|||||>@rvoak The quickstart guide [here](https://github.com/huggingface/pytorch-transformers/blob/master/docs/source/quickstart.md#bert-example) shows a nice example of how to do masked word prediction.
Replace
```
# confirm we were able to predict 'henson'
predicted_index = torch.argmax(predictions[0, masked_index]).item()
predicted_token = tokenizer.convert_ids_to_tokens([predicted_index])[0]
```
with something like this (e.g. if you want the top k predicted tokens):
```
top_k = 10
probs = torch.nn.functional.softmax(predictions[0, mask_idx], dim=-1)
top_k_weights, top_k_indices = torch.topk(probs, top_k, sorted=True)
for i, pred_idx in enumerate(top_k_indicies):
predicted_token = tokenizer.convert_ids_to_tokens([pred_idx])[0]
token_weight = top_k_weights[i]
```
<|||||>This issue has been automatically marked as stale because it has not had recent activity. It will be closed if no further activity occurs. Thank you for your contributions.
<|||||>> @rvoak The quickstart guide [here](https://github.com/huggingface/pytorch-transformers/blob/master/docs/source/quickstart.md#bert-example) shows a nice example of how to do masked word prediction.
> Replace
>
> ```
> # confirm we were able to predict 'henson'
> predicted_index = torch.argmax(predictions[0, masked_index]).item()
> predicted_token = tokenizer.convert_ids_to_tokens([predicted_index])[0]
> ```
>
> with something like this (e.g. if you want the top k predicted tokens):
>
> ```
> top_k = 10
> probs = torch.nn.functional.softmax(predictions[0, mask_idx], dim=-1)
> top_k_weights, top_k_indices = torch.topk(probs, top_k, sorted=True)
>
> for i, pred_idx in enumerate(top_k_indicies):
> predicted_token = tokenizer.convert_ids_to_tokens([pred_idx])[0]
> token_weight = top_k_weights[i]
> ```
very good example thanks! is there a version for RoBERTa and other models?<|||||>Hi @yuchenlin, you can use the recently added `fill-mask` pipeline to do so:
```py
>>> from transformers import pipeline
>>> nlp = pipeline("fill-mask", model="roberta-base")
>>> nlp(f"This is the best thing I've {nlp.tokenizer.mask_token} in my life.")
[
{'sequence': "<s> This is the best thing I've done in my life.</s>", 'score': 0.8024354577064514, 'token': 626},
{'sequence': "<s> This is the best thing I've heard in my life.</s>", 'score': 0.031355079263448715, 'token': 1317},
{'sequence': "<s> This is the best thing I've learned in my life.</s>", 'score': 0.027319395914673805, 'token': 2435},
{'sequence': "<s> This is the best thing I've seen in my life.</s>", 'score': 0.026892054826021194, 'token': 450},
{'sequence': "<s> This is the best thing I've experienced in my life.</s>", 'score': 0.02160099521279335, 'token': 2984}
]
```
We're in the process of adding example usage for common tasks (question answering, sequence classification, mask filling etc), you can follow the progress in https://github.com/huggingface/transformers/pull/2850. There already is an example for mask filling.<|||||>Hey @LysandreJik, does the fill-mask also support whole word mask prediction, or does it only work on subword level?<|||||>> Hi @yuchenlin, you can use the recently added `fill-mask` pipeline to do so:
>
> ```python
> >>> from transformers import pipeline
> >>> nlp = pipeline("fill-mask", model="roberta-base")
> >>> nlp(f"This is the best thing I've {nlp.tokenizer.mask_token} in my life.")
> [
> {'sequence': "<s> This is the best thing I've done in my life.</s>", 'score': 0.8024354577064514, 'token': 626},
> {'sequence': "<s> This is the best thing I've heard in my life.</s>", 'score': 0.031355079263448715, 'token': 1317},
> {'sequence': "<s> This is the best thing I've learned in my life.</s>", 'score': 0.027319395914673805, 'token': 2435},
> {'sequence': "<s> This is the best thing I've seen in my life.</s>", 'score': 0.026892054826021194, 'token': 450},
> {'sequence': "<s> This is the best thing I've experienced in my life.</s>", 'score': 0.02160099521279335, 'token': 2984}
> ]
> ```
>
> We're in the process of adding example usage for common tasks (question answering, sequence classification, mask filling etc), you can follow the progress in #2850. There already is an example for mask filling.
Is it possible to give this an input word for the mask and get probabilities back for that specific word?<|||||>Also is it possible to request the top N sentences rather than the default returned?
Edit: Never mind on this specific question! I found out by setting:
`nlp.topk = 20`
before doing:
` nlp(f"This is the best thing I've {nlp.tokenizer.mask_token} in my life.")`
It now returns 20.<|||||>Sure, you can do that using the recently added `targets` (in `v3.1.0`):
```py
>>> from transformers import pipeline
>>> nlp = pipeline("fill-mask", model="roberta-base")
>>> nlp(f"This is the best thing I've {nlp.tokenizer.mask_token} in my life.", targets=[' experienced'])
[
{
'sequence': "<s>This is the best thing I've experienced in my life.</s>",
'score': 0.022622672840952873,
'token': 2984,
'token_str': 'Ġexperienced'
}
]
```
Please note the space before the word, because we're using the [RoBERTa tokenizer](https://huggingface.co/transformers/model_doc/roberta.html#robertatokenizer) which is a Byte-level BPE tokenizer that has a different behaviour according to the spaces before tokens.<|||||>@LysandreJik So very helpful! Thank you so much!<|||||>@LysandreJik If a word is at the start of a sentence, should it also have a space in front of it?:
```
nlp(f"{nlp.tokenizer.mask_token} talk about the rules of the game first.", targets=[' We\'ll'])
```
Which gives me:
```
The specified target token ` We'll` does not exist in the model vocabulary. Replacing with `ĠWe`.
[{'sequence': '<s> We talk about the rules of the game first</s>', 'score': 8.493712812196463e-06, 'token': 166, 'token_str': 'ĠWe'}]
```
Or
```
nlp(f"{nlp.tokenizer.mask_token} talk about the rules of the game first.", targets=['We\'ll'])
```
Which gives me:
```
The specified target token `We'll` does not exist in the model vocabulary. Replacing with `We`.
[{'sequence': '<s>We talk about the rules of the game first</s>', 'score': 0.12082401663064957, 'token': 170, 'token_str': 'We'}]
```<|||||>How to predict a word that is seperated into several tokens. For example, DOTA2(name for a popular game)? |
transformers | 546 | closed | Import Error | I'm getting error " ImportError: cannot import name 'WEIGHTS_NAME' from 'pytorch_pretrained_bert.file_utils' " on running run_squad.py. I've already tried building from source but the problem persists. | 04-27-2019 21:38:46 | 04-27-2019 21:38:46 | This should be fixed with the new release (0.6.2).<|||||>Unfortunately, I still get this error with the new release. Could that be because I had installed the package before some time ago (and removed it afterwards)?
Never mind, got it running by cleaning up the environments/paths.<|||||>This issue has been automatically marked as stale because it has not had recent activity. It will be closed if no further activity occurs. Thank you for your contributions.
|
transformers | 545 | closed | move pytroch_pretrained_bert cache folder under same path as torch | This PR does two things:
* Envs available:
PYTORCH_PRETRAINED_BERT_CACHE > TORCH_HOME > XDG_CACHE_HOME > `~/.cache`
* If no env is set, the default path is
`~/.cache/torch/pytorch_pretrained_bert` where `pytorch_pretrained_bert` is visible instead of hidden `.pytorch_pretrained_bert`. (since this is the cache folder, I feel it makes sense to make it visible, please correct me if I'm wrong :)
* minor: fix typo in `hubconf.py` example.
| 04-27-2019 18:00:36 | 04-27-2019 18:00:36 | Ok, looks good, thanks @ailzhang! |
transformers | 544 | closed | TypeError: '<' not supported between instances of 'NoneType' and 'int' | Hi, I am trying to do classification fine tuning using bert-base-uncased. I am using examples from master and pytorch_pretrained_bert==0.6.2. Here are my repro steps:
1. I create a train.tsv and dev.tsv file with my own domain data. The files contain sentences and labels separated by a tab. I put these files in /tmp/bertdata
2. I do fine tuning using: python run_classifier.py --data_dir /tmp/bertdata --bert_model bert-base-uncased --task_name sst-2 --do_lower_case --do_train --output_dir tmp. This works fine and a model, config json, and vocab.txt are placed in tmp
3. I try to use the fine tuned model on the dev.tsv set: python run_classifier.py --data_dir /tmp/bertdata --bert_model tmp --task_name sst-2 --do_lower_case --do_eval --output_dir tmp_result. When I do that, I get this error:
Traceback (most recent call last):
File "run_classifier.py", line 1024, in <module>
main()
File "run_classifier.py", line 794, in main
t_total=num_train_optimization_steps)
File "/home/ec2-user/anaconda3/lib/python3.6/site-packages/pytorch_pretrained_bert/optimization.py", line 215, in __init__
schedule = schedule_type(warmup=warmup, t_total=t_total)
File "/home/ec2-user/anaconda3/lib/python3.6/site-packages/pytorch_pretrained_bert/optimization.py", line 45, in __init__
if t_total < 0:
TypeError: '<' not supported between instances of 'NoneType' and 'int'
Anything obvious I am doing wrong? Thanks! | 04-26-2019 17:33:37 | 04-26-2019 17:33:37 | I also get this problem when predicting,Did you solved the problem?<|||||>Here is the problem during initialization of the optimizer:
` t_total=num_train_optimization_steps)`
This var is initialized with `None` for the first time `num_train_optimization_steps = None`
and it's initialized correctly only when `--do_train` flag is passed to the script
```
if args.do_train:
train_examples = processor.get_train_examples(args.data_dir)
num_train_optimization_steps = int(
len(train_examples) / args.train_batch_size / args.gradient_accumulation_steps) * args.num_train_epochs
if args.local_rank != -1:
num_train_optimization_steps = num_train_optimization_steps // torch.distributed.get_world_size()
```
but in case of `--do_eval` this var is `None` and you got an error from description.
It's a bug, I think, and should be fixed. For you local needs, just initialize the optimizer "somehow" - it's not used while evaluating.<|||||>This issue has been automatically marked as stale because it has not had recent activity. It will be closed if no further activity occurs. Thank you for your contributions.
|
transformers | 543 | closed | How to train our own domain-specific data instead of using pre-training models? | How to train our own domain-specific data instead of using pre-training models? | 04-26-2019 16:01:00 | 04-26-2019 16:01:00 | I also have this question whenever someone gets to it, but I think that this isn't doable with this package. There's got to be a way to hack it, but you'd probably have to take away some of the code at the beginning of the pipeline. @yiranxijie <|||||>Is there any news on this? Training one of these models from scratch?<|||||>@mattivi not yet<|||||>Hi all, so training from scratch will probably never be a goal for the present repo but here are great transformer codebases that were scaled to >64 GPUs:
- XLM: https://github.com/facebookresearch/xlm
- Megatron-LM: https://github.com/NVIDIA/Megatron-LM
- fairseq: https://github.com/pytorch/fairseq
Note that the typical compute required to train BERT is about 64 GPU for 4 days (which currently means around $10k-15k if you are renting cloud compute). TPU training is not possible in PyTorch currently, you should use a TensorFlow repo to do TPU training (like the original BERT or tensor2tensor for instance).<|||||>This issue has been automatically marked as stale because it has not had recent activity. It will be closed if no further activity occurs. Thank you for your contributions.
|
transformers | 542 | closed | Clarifying attention mask | I don't quite understand the attention mask in the way that it's implemented.
Here is the relevant line: https://github.com/huggingface/pytorch-pretrained-BERT/blob/master/pytorch_pretrained_bert/modeling.py#L312 :
```python
...
attention_scores = attention_scores / math.sqrt(self.attention_head_size)
# Apply the attention mask is (precomputed for all layers in BertModel forward() function)
attention_scores = attention_scores + attention_mask
# Normalize the attention scores to probabilities.
attention_probs = nn.Softmax(dim=-1)(attention_scores)
...
```
So it seems the proper way to use `attention_mask` is to set the positions you want to keep to 1's, and positions you want to mask out to 0's.
Curious why we don't simply multiply instead of add and then normalize? Is it for stability reasons? | 04-26-2019 14:32:15 | 04-26-2019 14:32:15 | The reason a classic binary attention mask won't work here is that the Softmax activation includes an exponential, and so an input of 0 can still yield quite a large softmax weight (since e^0 = 1).
The mask can't be applied after the softmax, because then the resulting values will not sum to 1. So the best solution is to add (not multiply!) a large negative value to the indices you want to mask. That means they will be 0 or almost 0 after the softmax step (because as you make x more negative, e^x becomes closer and closer to 0).<|||||>So you're recommending using a large negative value for the inputs you want to mask. It makes sense to me, though it seems the [documentation](https://github.com/huggingface/pytorch-pretrained-BERT/blob/master/pytorch_pretrained_bert/modeling.py#L671) ought to be updated, since it currently reads:
```
`attention_mask`: an optional torch.LongTensor of shape [batch_size, sequence_length] with indices
selected in [0, 1]. It's a mask to be used if the input sequence length is smaller than the max
input sequence length in the current batch. It's the mask that we typically use for attention when
a batch has varying length sentences.
```
Although I've been testing with 0 and it seems to produce the same vectors as when I only pass in a tensor of exactly the size I need. I understand this may not always be the case, however.<|||||>Note this code chunk: https://github.com/huggingface/pytorch-pretrained-BERT/blob/master/pytorch_pretrained_bert/modeling.py#L722-L728<|||||>Thank you, that clarifies everything.<|||||>@Rocketknight1 Hi, I would like to check the code chunk, but the url you provided is out dated, could you show the code here again? Thanks.<|||||>Hi, sorry! The repo code has changed massively since last year, so I don't know if there's a single chunk corresponding to that link anymore. However, if I recall, all it showed was a short code snippet where the attention_mask tensor was converted into the additive pre-softmax mask by first inverting it and then multiplying it by -10,000. Feel free to ask questions and @tag me if you're still uncertain.<|||||>@Rocketknight1 Thank you for your reply. Yes, I understand how to change attention_mask into a quite small negative value and why. But in modeling_bert.py file, it seems like there is no such a code chunk to convert attention_mask into a proper format. check this out https://github.com/huggingface/transformers/blob/master/src/transformers/modeling_bert.py#L274<|||||>I found the corresponding source code: https://github.com/huggingface/transformers/issues/542<|||||>Hi, I got the same problem with you @YuanEric88 and I didn't find the code chunk to convert attention_mask from [0,1] to [-inf, 0]. The attention_mask is applied in [here](https://github.com/huggingface/transformers/blob/master/src/transformers/models/bert/modeling_bert.py#L312)<|||||>@xiangrongzeng Just a passerby here - but I believe this is the method where `[0, 1]` attention masks are mapped to the `[-inf, 0]` range: https://github.com/huggingface/transformers/blob/88a951e3cc00f56b94d9b93dbc35a3812cd88747/src/transformers/modeling_utils.py#L221-L281
...and the specific operation in question:
https://github.com/huggingface/transformers/blob/88a951e3cc00f56b94d9b93dbc35a3812cd88747/src/transformers/modeling_utils.py#L274-L281
This method lives in the `ModuleUtilsMixin`, which I'm assuming is inherited by downstream models.<|||||>@kwonkyo Thankyou for your help :) |
transformers | 541 | closed | Any way to reduce the model size to <250mb? | Google Cloud's online prediction service has a 250mb limit for uploaded models. I don't think I have ever seen a BERT model that small. Casting all tensors to half precision reduces the model to ~350mb, is there any way to go even further than that? | 04-26-2019 08:19:28 | 04-26-2019 08:19:28 | Probably not - it would certainly be possible to make a smaller BERT model that would fit into this size, but all of the available pre-trained models have too many parameters, so you'd have to train it from scratch (which is very slow, and isn't something this repo supports yet).<|||||>This issue has been automatically marked as stale because it has not had recent activity. It will be closed if no further activity occurs. Thank you for your contributions.
|
transformers | 540 | closed | no to_json_file(file) in BERT | Hi, https://github.com/huggingface/pytorch-pretrained-BERT/blob/master/examples/run_squad.py#L1035
in the line 1035, I cannot use config.to_json_file(output_config_file) because there is no such function.
Instead I use
`file = model_to_save.config.to_json_string()`
`with open(file_path, "w") as f:`
` f.write(file)`
Is it correct way to save config file? | 04-26-2019 08:05:27 | 04-26-2019 08:05:27 | Are you using the latest release (0.6.2) ?<|||||>Yes I am.<|||||>Strange, `to_json_file` should be provided in 0.6.2 (cf code [here](https://github.com/huggingface/pytorch-pretrained-BERT/blob/e6cf62d49945e6277b5e4dc855f9186b3f789e35/pytorch_pretrained_bert/modeling.py#L222) and the associated test [here](https://github.com/huggingface/pytorch-pretrained-BERT/blob/68a889ee43916380f26a3c995e1638af41d75066/tests/modeling_test.py#L258))<|||||>Thanks :0) I'll check the version and code again.<|||||>After removing and install the package, the problem solved |
transformers | 539 | closed | Can we use 'bert-base-uncased' to question_answer just for start, rather rather than run_squad pretraining? | Hi,
Can we use 'bert-base-uncased' to question_answer just for start, rather rather than run_squad pretraining?
model = BertForQuestionAnswering.from_pretrained('bert-base-uncased')
Thanks
Mahesh | 04-26-2019 07:15:29 | 04-26-2019 07:15:29 | Hi, no you need to fine tune the model on a question answering task like SQuAD before you can use it |
transformers | 538 | closed | key error in BertQuestionAsnwering predict? | Hi,
I am getting key error while using BertQuestionAsnwering predict?
I am breaking following loop after 10 iterations
for input_ids, input_mask, segment_ids, example_indices in tqdm(eval_dataloader, desc="Evaluating", disable=local_rank not in [-1, 0]):
Thanks
Mahesh
Error:
KeyError Traceback (most recent call last)
<ipython-input-87-6ac2c26449fb> in <module>()
41 do_lower_case, output_prediction_file,
42 output_nbest_file, output_null_log_odds_file, verbose_logging,
---> 43 version_2_with_negative, null_score_diff_threshold)
/content/run_squad.py in write_predictions(all_examples, all_features, all_results, n_best_size, max_answer_length, do_lower_case, output_prediction_file, output_nbest_file, output_null_log_odds_file, verbose_logging, version_2_with_negative, null_score_diff_threshold)
473 null_end_logit = 0 # the end logit at the slice with min null score
474 for (feature_index, feature) in enumerate(features):
--> 475 result = unique_id_to_result[feature.unique_id]
476 start_indexes = _get_best_indexes(result.start_logits, n_best_size)
477 end_indexes = _get_best_indexes(result.end_logits, n_best_size)
KeyError: 1000000088 | 04-26-2019 06:58:11 | 04-26-2019 06:58:11 | You found a solution?<|||||>@thomwolf , I made mistake in code, Repo code works just fine. Hence closed issue.
Thanks for this amazing repo :thumbsup:
<|||||>How to solve this problem?
<|||||>what was the solution ? Im seeing the same problem <|||||>Hello! Do you mind opening a new issue with your problem?<|||||>Hi, I'm having the same error as described above. Is anyone able to post their solution? |
transformers | 537 | closed | New GPT2 tokenizer no longer encodes Unicode characters properly in Python 3 | In commit 5afa497cbfc53c679a9b22997b6312fad57ee2f8, you changed `token.encode('utf-8')` to simply `token`.
This would make the code compatible with Python 2, but now it breaks in Python 3. You'll get a KeyError when you try to encode a Unicode character that requires more than 1 byte in UTF-8 encoding. For example, this raises a KeyError in Python 3:
```python
from pytorch_pretrained_bert.tokenization_gpt2 import GPT2Tokenizer
tokenizer = GPT2Tokenizer.from_pretrained('gpt2')
tokenizer.encode('你')
```
I think what you want to do is:
```python
if sys.version_info[0] == 2:
token = ''.join(self.byte_encoder[ord(b)] for b in token)
else:
token = ''.join(self.byte_encoder[b] for b in token.encode('utf-8'))
``` | 04-26-2019 05:24:02 | 04-26-2019 05:24:02 | Just ran into this problem. This seems to be a regression from an earlier version of Huggingface.
For instance it fails when encoding the following wikipedia snippet
> The dismemberment of the French socialist movement into many groups and—following the suppression
The dash here is "long dash" with unicode 8212. This worked in earlier version because it worked on bytes.<|||||><img width="992" alt="image" src="https://user-images.githubusercontent.com/44499264/59059983-a5579180-88d2-11e9-9124-f7ce32f20419.png">
I can confirm that this is happening, though it is a different dash.<|||||>> <img alt="image" width="992" src="https://user-images.githubusercontent.com/44499264/59059983-a5579180-88d2-11e9-9124-f7ce32f20419.png">
>
> I can confirm that this is happening, though it is a different dash.
Same here:
This is also happening while using GPT2 tokenizer:
`
Traceback (most recent call last):
File "run_lambada_gpt2.py", line 139, in tokenize_and_encode
token_ids = tokenizer.encode(obj)
File "/data/anaconda/envs/py35/lib/python3.5/site-packages/pytorch_pretrained_bert/tokenization_gpt2.py", line 261, in encode
return self.convert_tokens_to_ids(self.tokenize(text))
File "/data/anaconda/envs/py35/lib/python3.5/site-packages/pytorch_pretrained_bert/tokenization_gpt2.py", line 224, in tokenize
token = ''.join(self.byte_encoder[ord(b)] for b in token)
File "/data/anaconda/envs/py35/lib/python3.5/site-packages/pytorch_pretrained_bert/tokenization_gpt2.py", line 224, in <genexpr>
token = ''.join(self.byte_encoder[ord(b)] for b in token)
KeyError: 8217
`
The sys version info is:
`
sys.version_info(major=3, minor=5, micro=5, releaselevel='final', serial=0)
`
<|||||>This issue has been automatically marked as stale because it has not had recent activity. It will be closed if no further activity occurs. Thank you for your contributions.
<|||||>Hi,
I'm about to use this tokenizer with python3 on wiki-text.
After seeing this issue - I'm not sure if it will work properly.
Can someone clarify please?
From reading along seems like the fix suggested above did not solve the problem, right?
<|||||>Hi, this looks fixed to me in the current implementation. As long as you're using a recent version of the library you should be fine. I had no problem running a fine-tuning script on wikitext-2 last week.
If you run into anything, please let me know and I'll look into it.<|||||>This issue has been automatically marked as stale because it has not had recent activity. It will be closed if no further activity occurs. Thank you for your contributions.
|
transformers | 536 | closed | Fix missing warmup_linear in run_classifier.py example | Replaced warmup_linear function call with WarmupLinearSchedule | 04-25-2019 18:57:45 | 04-25-2019 18:57:45 | I see there is already a PR to fix this, I will close this. |
transformers | 535 | closed | gpt2 fine tuning sources | hi. I'm looking to fine tune the gpt2 model. I missed the part where that sort of fine tuning is taking place. Can someone point out where that code is (...or maybe where an example might be elsewhere on line)? | 04-25-2019 18:21:04 | 04-25-2019 18:21:04 | I encountered the same issue<|||||>Also looking for how to finetune the GPT2 model, thanks.<|||||>This issue has been automatically marked as stale because it has not had recent activity. It will be closed if no further activity occurs. Thank you for your contributions.
|
transformers | 534 | closed | How many datasets does Bert use in pretraining process? | Hi all,
I try to generate the pretraining corpus for BERT with pregenerate_training_data.py. In the BERT paper, it reports about 6M+ instances(segment A+segmentB, less than 512 tokens). But I get 18M instances, which is almost 3 time than BERT uses. Does anyone have any idea on the result and does anyone know if I need to process WikiPedia and BookCorpus first and then try to generate training instances? Thanks very much in advance! | 04-25-2019 16:15:58 | 04-25-2019 16:15:58 | This issue has been automatically marked as stale because it has not had recent activity. It will be closed if no further activity occurs. Thank you for your contributions.
|
transformers | 533 | closed | Docs for new learning rate code | - Added documentation for learning rate schedules in main README
- added some pictures for the README in docs/imgs/ (not sure if it's the best place)
- updated some docs in code for optimization | 04-25-2019 14:16:52 | 04-25-2019 14:16:52 | Great thanks!<|||||>The curves plot in the README are beautiful (and perfect size), awesome! |
transformers | 532 | closed | [Feature request] Support configurable BertLayerNorm epsilon | It would be great if we could configure `eps` in layer normalization since model like ERNIE uses `eps=1e-5` instead of `1e-12`. | 04-25-2019 14:06:52 | 04-25-2019 14:06:52 | Hi, I'm closing this in favor of #514 to gather all the discussion on ERNIE. |
transformers | 531 | closed | fixed new LR API in examples | .get_lr() of \_LRSchedule objects expects a step while .get_lr_() expects training progress fraction | 04-25-2019 12:41:44 | 04-25-2019 12:41:44 | |
transformers | 530 | closed | GPT2 training and generating on text longer than 1024 | Hello,
First, thanks so much for all of the open source work here! This has been super useful to build off of.
I noticed that the size of the pretrained positional embedding set for GPT2 is 1024, and was wondering if there were standard methods or suggestions for (a) running the language model head over text longer than 1024 tokens (post BPE encoding) and (b) generating text longer than 1024 BPE tokens. Would appreciate suggestions or pointers to other sources on how to handle this, thanks! | 04-25-2019 00:40:51 | 04-25-2019 00:40:51 | The default text generation example in the codebase will generate unlimited length.
However, each prediction is only influenced by current context (1024 tokens long). Something like [transformer-xl](https://github.com/kimiyoung/transformer-xl/tree/master/pytorch) is needed to depend on things outside of current context<|||||>This issue has been automatically marked as stale because it has not had recent activity. It will be closed if no further activity occurs. Thank you for your contributions.
<|||||>@apappu97 Do you know how to input a sequence longer than 1024 using the pretrained models now? Thank you.<|||||>I get an error when I try to generate with, for example, `--length 10000`.
````
Setting `pad_token_id` to `eos_token_id`:50256 for open-end generation.
../aten/src/ATen/native/cuda/Indexing.cu:922: indexSelectSmallIndex: block: [3,0,0], thread: [32,0,0] Assertion `srcIndex < srcSelectDimSize` failed.
../aten/src/ATen/native/cuda/Indexing.cu:922: indexSelectSmallIndex: block: [3,0,0], thread: [33,0,0] Assertion `srcIndex < srcSelectDimSize` failed.
(many similar lines with ascending indices)
Traceback (most recent call last):
File "transformers/examples/pytorch/text-generation/run_generation.py", line 294, in <module>
main()
File "transformers/examples/pytorch/text-generation/run_generation.py", line 252, in main
output_sequences = model.generate(
File "venv/lib/python3.10/site-packages/torch/autograd/grad_mode.py", line 27, in decorate_context
return func(*args, **kwargs)
File "transformers/src/transformers/generation_utils.py", line 1380, in generate
return self.sample(
File "transformers/src/transformers/generation_utils.py", line 1996, in sample
outputs = self(
File "venv/lib/python3.10/site-packages/torch/nn/modules/module.py", line 1130, in _call_impl
return forward_call(*input, **kwargs)
File "transformers/src/transformers/models/gpt2/modeling_gpt2.py", line 1046, in forward
transformer_outputs = self.transformer(
File "venv/lib/python3.10/site-packages/torch/nn/modules/module.py", line 1130, in _call_impl
return forward_call(*input, **kwargs)
File "transformers/src/transformers/models/gpt2/modeling_gpt2.py", line 889, in forward
outputs = block(
File "venv/lib/python3.10/site-packages/torch/nn/modules/module.py", line 1130, in _call_impl
return forward_call(*input, **kwargs)
File "transformers/src/transformers/models/gpt2/modeling_gpt2.py", line 389, in forward
attn_outputs = self.attn(
File "venv/lib/python3.10/site-packages/torch/nn/modules/module.py", line 1130, in _call_impl
return forward_call(*input, **kwargs)
File "transformers/src/transformers/models/gpt2/modeling_gpt2.py", line 330, in forward
attn_output, attn_weights = self._attn(query, key, value, attention_mask, head_mask)
File "transformers/src/transformers/models/gpt2/modeling_gpt2.py", line 185, in _attn
attn_weights = attn_weights / torch.tensor(
RuntimeError: CUDA error: device-side assert triggered
CUDA kernel errors might be asynchronously reported at some other API call,so the stacktrace below might be incorrect.
For debugging consider passing CUDA_LAUNCH_BLOCKING=1.
````
`1000` seems to be a safe length, but even `1023` can result in errors.
Full command line:
python transformers/examples/pytorch/text-generation/run_generation.py --model_type gpt2 --length 10000 --num_return_sequences 10 --model_name_or_path tuned_model/checkpoint-100000
<|||||>With a recent git checkout I do not get the error, but the generation script gets a hardcoded limit for text generation from the model class.
https://github.com/huggingface/transformers/blob/4eb918e656944df2757513c535e8ad8c01d632e2/examples/pytorch/text-generation/run_generation.py#L222
The input seems to be also quite limited (no idea how many tokens, but probably something around 20-30), so running generation with the last 1024 tokens won't work. |
transformers | 529 | closed | Why classifier fine-tuning don't save best model based on the evaluation on dev dataset | I want to use bert to train a classify model, I use the example [run_classifier.py].
But I find that the model will continue to train on train dataset until the max_epoch, without doing the evaluation on the dev dataset and save the best model according to the metric on the dev dataset.
So, the final saved model, it just the last epoch, but this saved model will not be the best model on the dev dataset!
Also, I suggest adding a args --predict and only make predictions.
This work helps me a lot! Thanks! | 04-24-2019 13:17:15 | 04-24-2019 13:17:15 | This issue has been automatically marked as stale because it has not had recent activity. It will be closed if no further activity occurs. Thank you for your contributions.
<|||||>were you able to fix this problem. If yes can you please tell how |
transformers | 528 | closed | __init__() got an unexpected keyword argument 'do_basic_tokenize' | In the README, this line is written:
```
tokenizer = BertTokenizer.from_pretrained('bert-base-uncased', do_lower_case=True, do_basic_tokenize=True)
```
But when I execute it, I get this error:
```
__init__() got an unexpected keyword argument 'do_basic_tokenize'
```
| 04-24-2019 12:54:48 | 04-24-2019 12:54:48 | Which version of pytorch-pretrained-bert are you using?
Can you give the full error message to see which call to `__init__()` is failing?
We should have the keyword argument [here](https://github.com/huggingface/pytorch-pretrained-BERT/blob/3d78e226e68a5c5d0ef612132b601024c3534e38/pytorch_pretrained_bert/tokenization.py#L77) <|||||>I have the last version (0.6.1).
This is what I have on my computer:
<|||||>This issue has been automatically marked as stale because it has not had recent activity. It will be closed if no further activity occurs. Thank you for your contributions.
|
transformers | 527 | closed | Update example files so that tr_loss is not affected by args.gradient… | Hi developpers!
Fix training loss value :
* if gradient_accumulation_steps > 1 then the batch loss value(which is a mean) is scaled by a factor 1/args.gradient_accumulation_steps.
To compare it to evaluation loss it is thus necessary to scale it back by multiplying by args.gradient_accumulation_steps (as done in finetuning script)
Another way to fix this would be to replace the lines with tr_loss/nb_tr_steps by tr_loss/global_step. I thought you might want to consider this alternative | 04-24-2019 12:09:45 | 04-24-2019 12:09:45 | Hi @Mathieu-Prouveur, thanks for that.
Indeed I think using `tr_loss/global_step` would be more easy to read.
Can you update this? <|||||>Sure, I've just done the update <|||||>Great, thanks! |
transformers | 526 | closed | Will BERT weights for SQuAD be released? | Hi,
Are you going to release the weights after training on SQuAD 2.0?
Thank you for your great work.
Best,
Lucas Willems | 04-24-2019 08:23:43 | 04-24-2019 08:23:43 | Hi Lucas, probably not.
The goal of this repository is to provide easy access to pretrained model for transfer learning research.
Providing downstream task models will make us handle a combinatory explosion of combinations to provide the various pretrained BERT models fine-tuned on each GLUE/SQuAD task with hyper-parameters optimization and all the relevant adaptation decision that are still mostly open research questions.
But we do provide examples for fine-tuning that gives decent results and can be trained in a reasonable time on standard cloud compute.<|||||>@lcswillems were you able to find the weights anywhere else? <|||||>Looks like HF released them after all
https://huggingface.co/transformers/pretrained_models.html |
transformers | 525 | closed | Should I use weight_decay or weight_decay_rate? | Thanks for the awesome work.
Just as line [simple_lm_finetuning.py#L540](https://github.com/huggingface/pytorch-pretrained-BERT/blob/master/examples/lm_finetuning/simple_lm_finetuning.py#L540), When I use bert for downstream tasks, should I use `weight_decay` or `weight_decay_rate` when I add a decay operation to the training parameters?
What if I use apex for mixed precision training? | 04-24-2019 06:11:06 | 04-24-2019 06:11:06 | According to the instructions [module-torch.optim](https://pytorch.org/docs/stable/optim.html?highlight=torch%20optim#module-torch.optim) from PyTorch API and [fused_adam.py](https://github.com/NVIDIA/apex/blob/master/apex/optimizers/fused_adam.py) from apex repo, I think `weight_decay` and `weight_decay_rate` are unified and unified into `weight_decay`, is it correct to understand?<|||||>This issue has been automatically marked as stale because it has not had recent activity. It will be closed if no further activity occurs. Thank you for your contributions.
|
transformers | 524 | closed | Mixed up isNextSentence label in simple_lm_finetuning.py script? | I'm wondering if the isNextsentence "label" in the below function is correct? Shouldn't the label be 1 in the case that t1,t2 are taken from self.get_corpus_line(index) (i.e., the first condition on line 150), and 0 if it is random (line 153)?
https://github.com/huggingface/pytorch-pretrained-BERT/blob/c36cca075a32f59a5ec2083e1d39e7d6564c105b/examples/lm_finetuning/simple_lm_finetuning.py#L141-L157 | 04-23-2019 17:38:44 | 04-23-2019 17:38:44 | Hi, why should it be the other way around?<|||||>I think I mixed up the meaning of 0 and 1 in this context and maybe wrote this post a bit too quickly before looking deeper into the code and documentation.. (sorry!). On second glance, the documentation for the BertForPreTraining is rather clear:
https://github.com/huggingface/pytorch-pretrained-BERT/blob/d76a57b0ba198eee27b3777f57fcabb6aba8b965/pytorch_pretrained_bert/modeling.py#L766
I was confused why 0 should mean "true" is this case (i.e., is a next sentence continuation) since in classification 0 often means "false", but whatever, the way it is written is sound (albeit a little counterintuitive at first glance). <|||||>@yakazimir yeah I was confused too. Thanks for your research |
transformers | 523 | closed | ImportError: cannot import name 'WEIGHTS_NAME' from 'pytorch_pretrained_bert.file_utils' | I just tried to run `run_squad.py` example and I got this error:
```
Traceback (most recent call last):
File "run_squad.py", line 37, in <module>
from pytorch_pretrained_bert.file_utils import PYTORCH_PRETRAINED_BERT_CACHE, WEIGHTS_NAME, CONFIG_NAME
ImportError: cannot import name 'WEIGHTS_NAME' from 'pytorch_pretrained_bert.file_utils' (/mnt/Data/miniconda3/lib/python3.7/site-packages/pytorch_pretrained_bert/file_utils.py)
``` | 04-23-2019 13:05:37 | 04-23-2019 13:05:37 | Same is happening for `run_classifier.py` <|||||>Yes the examples currently require to install from source (see the section in the readme).
I'll release a new version tomorrow so the pip release will be in sync with `master` examples again.<|||||>Okay, thank you :)<|||||>Waiting for this; installing from source gives the error : `ImportError: cannot import name 'warmup_linear'`<|||||>> Waiting for this; installing from source gives the error : `ImportError: cannot import name 'warmup_linear'`
It is not actually using `warmup_linear` so you can safely remove that from the file <|||||>Ok, I've just published and uploaded the new v0.6.2 release on pip which should fix this (among other things). Release notes are [here](https://github.com/huggingface/pytorch-pretrained-BERT/releases/tag/v0.6.2). |
transformers | 522 | closed | extending of Transformer-XL for new tasks | Hello community,
I am looking for an example which could help me to extend the Transformer XL to a model similar to bert-as-service model [1]. I would like to know how to set up new layers on the pretrained Tranformer XL and train the last new layers or the whole model. Could anyone give me an advice regarding this issue. Thank a lot
[1] - https://github.com/hanxiao/bert-as-service | 04-23-2019 11:28:26 | 04-23-2019 11:28:26 | This issue has been automatically marked as stale because it has not had recent activity. It will be closed if no further activity occurs. Thank you for your contributions.
|
transformers | 521 | closed | Model type in convert_tf_checkpoint_to_pytorch and 'squad' mapping | Issue #438 still exists if you choose to use something else rather then BertForTokenClassification. Furthermore, you still need to edit the code before running the convertor. Lastly, BertForTokenClassification is not the same as BertForQuestionAnswering, since the latter omits the dropout before the output layer.
Maybe it's better to add more options like 'classification' which uses BertForTokenClassification.
Tested the changes on fine-tuned BERT model on SQuAD 1.1 with Google's original Tensorflow script run_squad.py initialized with multi_cased_L-12_H-768_A-12. | 04-23-2019 11:22:15 | 04-23-2019 11:22:15 | # [Codecov](https://codecov.io/gh/huggingface/pytorch-pretrained-BERT/pull/521?src=pr&el=h1) Report
> Merging [#521](https://codecov.io/gh/huggingface/pytorch-pretrained-BERT/pull/521?src=pr&el=desc) into [master](https://codecov.io/gh/huggingface/pytorch-pretrained-BERT/commit/80684f6f86c13a89fc1e4feac248ef96b013765c?src=pr&el=desc) will **decrease** coverage by `0.2%`.
> The diff coverage is `18.75%`.
[](https://codecov.io/gh/huggingface/pytorch-pretrained-BERT/pull/521?src=pr&el=tree)
```diff
@@ Coverage Diff @@
## master #521 +/- ##
==========================================
- Coverage 67.19% 66.99% -0.21%
==========================================
Files 18 18
Lines 3847 3869 +22
==========================================
+ Hits 2585 2592 +7
- Misses 1262 1277 +15
```
| [Impacted Files](https://codecov.io/gh/huggingface/pytorch-pretrained-BERT/pull/521?src=pr&el=tree) | Coverage Δ | |
|---|---|---|
| [...retrained\_bert/convert\_tf\_checkpoint\_to\_pytorch.py](https://codecov.io/gh/huggingface/pytorch-pretrained-BERT/pull/521/diff?src=pr&el=tree#diff-cHl0b3JjaF9wcmV0cmFpbmVkX2JlcnQvY29udmVydF90Zl9jaGVja3BvaW50X3RvX3B5dG9yY2gucHk=) | `0% <0%> (ø)` | :arrow_up: |
| [pytorch\_pretrained\_bert/modeling.py](https://codecov.io/gh/huggingface/pytorch-pretrained-BERT/pull/521/diff?src=pr&el=tree#diff-cHl0b3JjaF9wcmV0cmFpbmVkX2JlcnQvbW9kZWxpbmcucHk=) | `86.22% <24%> (-2.35%)` | :arrow_down: |
| [pytorch\_pretrained\_bert/tokenization\_gpt2.py](https://codecov.io/gh/huggingface/pytorch-pretrained-BERT/pull/521/diff?src=pr&el=tree#diff-cHl0b3JjaF9wcmV0cmFpbmVkX2JlcnQvdG9rZW5pemF0aW9uX2dwdDIucHk=) | `83.51% <0%> (+1.06%)` | :arrow_up: |
------
[Continue to review full report at Codecov](https://codecov.io/gh/huggingface/pytorch-pretrained-BERT/pull/521?src=pr&el=continue).
> **Legend** - [Click here to learn more](https://docs.codecov.io/docs/codecov-delta)
> `Δ = absolute <relative> (impact)`, `ø = not affected`, `? = missing data`
> Powered by [Codecov](https://codecov.io/gh/huggingface/pytorch-pretrained-BERT/pull/521?src=pr&el=footer). Last update [80684f6...4a638d1](https://codecov.io/gh/huggingface/pytorch-pretrained-BERT/pull/521?src=pr&el=lastupdated). Read the [comment docs](https://docs.codecov.io/docs/pull-request-comments).
<|||||># [Codecov](https://codecov.io/gh/huggingface/pytorch-transformers/pull/521?src=pr&el=h1) Report
> Merging [#521](https://codecov.io/gh/huggingface/pytorch-transformers/pull/521?src=pr&el=desc) into [master](https://codecov.io/gh/huggingface/pytorch-transformers/commit/f2a3eb987e1fc2c85320fc3849c67811f5736b50?src=pr&el=desc) will **decrease** coverage by `0.18%`.
> The diff coverage is `20%`.
[](https://codecov.io/gh/huggingface/pytorch-transformers/pull/521?src=pr&el=tree)
```diff
@@ Coverage Diff @@
## master #521 +/- ##
==========================================
- Coverage 79.04% 78.85% -0.19%
==========================================
Files 34 34
Lines 6242 6262 +20
==========================================
+ Hits 4934 4938 +4
- Misses 1308 1324 +16
```
| [Impacted Files](https://codecov.io/gh/huggingface/pytorch-transformers/pull/521?src=pr&el=tree) | Coverage Δ | |
|---|---|---|
| [pytorch\_transformers/modeling\_bert.py](https://codecov.io/gh/huggingface/pytorch-transformers/pull/521/diff?src=pr&el=tree#diff-cHl0b3JjaF90cmFuc2Zvcm1lcnMvbW9kZWxpbmdfYmVydC5weQ==) | `85.44% <20%> (-2.54%)` | :arrow_down: |
------
[Continue to review full report at Codecov](https://codecov.io/gh/huggingface/pytorch-transformers/pull/521?src=pr&el=continue).
> **Legend** - [Click here to learn more](https://docs.codecov.io/docs/codecov-delta)
> `Δ = absolute <relative> (impact)`, `ø = not affected`, `? = missing data`
> Powered by [Codecov](https://codecov.io/gh/huggingface/pytorch-transformers/pull/521?src=pr&el=footer). Last update [f2a3eb9...8e04e9e](https://codecov.io/gh/huggingface/pytorch-transformers/pull/521?src=pr&el=lastupdated). Read the [comment docs](https://docs.codecov.io/docs/pull-request-comments).
<|||||>@thomwolf Is this PR still useful? Can it be somehow improved and later merged, or it should be closed?<|||||>This issue has been automatically marked as stale because it has not had recent activity. It will be closed if no further activity occurs. Thank you for your contributions.
|
transformers | 520 | closed | unable to load finetuned LM "No file bert_config.json" | No such file or directory: 'LM_Trained/bert_config.json'
I think bert_config is not saved when finetuning a LM | 04-23-2019 10:37:42 | 04-23-2019 10:37:42 | Ok, this should be fixed in the new release v0.6.2. See #523. |
transformers | 519 | closed | No GPT2 model | I tried to load the `gpt2` model listed in the README.md, but I got this error:
```
Model name 'gpt2' was not found in model name list (bert-base-uncased, bert-large-uncased, bert-base-cased, bert-large-cased, bert-base-multilingual-uncased, bert-base-multilingual-cased, bert-base-chinese). We assumed 'gpt2' was a path or url but couldn't find any file associated to this path or url.
```
The code I used:
```
# Load pre-trained model tokenizer (vocabulary)
tokenizer = BertTokenizer.from_pretrained('gpt2')
# Load pre-trained model (weights)
model = BertModel.from_pretrained('gpt2')
_ = model.eval()
``` | 04-23-2019 10:17:05 | 04-23-2019 10:17:05 | Do you have a working internet connection?
We should probably improve the error messages here, 2 different error are bundled in this error (no internet connection and wrong model name)<|||||>Yes, I have an internet connection. I am able to download the other models.<|||||>Oh wait, you are mixing two models here.
GPT-2 and BERT are two different architectures.
If you want to use GPT-2 do:
```
tokenizer = GPT2Tokenizer.from_pretrained('gpt2')
model = GPT2Model.from_pretrained('gpt2')
```
An example of usage is [here in the readme](https://github.com/huggingface/pytorch-pretrained-BERT#openai-gpt-2)
<|||||>Okay, thank you! Sorry, for this... |
transformers | 518 | closed | Fix training schedules in examples to match new API | Re #445:
- update examples to work with the new optimizer API | 04-23-2019 09:18:42 | 04-23-2019 09:18:42 | @lukovnikov do you want to give this PR a look and confirm it's fine?
Also, we should document a bit the new optimizer API in the README. Do you want to use this PR to copy a few docstring in the README (we currently don't have auto-generated doc)?<|||||>Hi. Sorry, forgot about the examples.
Did a couple fixes in my 'schedules_in_examples' branch (see PR #531).
However, I don't have the fp16 setup yet so wasn't able to run the examples to be completely sure.
Docs update is here: PR #533.<|||||>Got it.
Ok to merge this PR @lukovnikov?<|||||>With the fixes from #531, should be good.<|||||>Thanks! |
transformers | 517 | closed | More SEPs | I want to segment input sentences in more segments, like [CLS]S1[SEP]S2[SEP]S3[SEP]. Therefore, when I convert example to features, I do the following.
`segment_ids = [0] * len(tokens_s1)`
`segment_ids += [1] * len(tokens_s2)`
`segment_ids += [2] * len(tokens_s2)`
but I got the following error when I run the `self.bert(input_ids, token_type_ids, attention_mask, output_all_encoded_layers=False)`:
> File "/home/xiangk/anaconda2/envs/pytorch0.4/lib/python3.6/site-packages/pytorch_pretrained_bert/modeling.py", line 1065, in forward
sequence_output, _ = self.bert(input_ids, token_type_ids, attention_mask, output_all_encoded_layers=False)
File "/home/xiangk/anaconda2/envs/pytorch0.4/lib/python3.6/site-packages/torch/nn/modules/module.py", line 477, in __call__
result = self.forward(*input, **kwargs)
File "/home/xiangk/anaconda2/envs/pytorch0.4/lib/python3.6/site-packages/pytorch_pretrained_bert/modeling.py", line 712, in forward
embedding_output = self.embeddings(input_ids, token_type_ids)
File "/home/xiangk/anaconda2/envs/pytorch0.4/lib/python3.6/site-packages/torch/nn/modules/module.py", line 477, in __call__
result = self.forward(*input, **kwargs)
File "/home/xiangk/anaconda2/envs/pytorch0.4/lib/python3.6/site-packages/pytorch_pretrained_bert/modeling.py", line 264, in forward
embeddings = self.dropout(embeddings)
File "/home/xiangk/anaconda2/envs/pytorch0.4/lib/python3.6/site-packages/torch/nn/modules/module.py", line 477, in __call__
result = self.forward(*input, **kwargs)
File "/home/xiangk/anaconda2/envs/pytorch0.4/lib/python3.6/site-packages/torch/nn/modules/dropout.py", line 53, in forward
return F.dropout(input, self.p, self.training, self.inplace)
File "/home/xiangk/anaconda2/envs/pytorch0.4/lib/python3.6/site-packages/torch/nn/functional.py", line 595, in dropout
return _functions.dropout.Dropout.apply(input, p, training, inplace)
File "/home/xiangk/anaconda2/envs/pytorch0.4/lib/python3.6/site-packages/torch/nn/_functions/dropout.py", line 40, in forward
ctx.noise.bernoulli_(1 - ctx.p).div_(1 - ctx.p)
RuntimeError: Creating MTGP constants failed. at /opt/conda/conda-bld/pytorch_1535491974311/work/aten/src/THC/THCTensorRandom.cu:34
after changing `segment_ids += [2] * len(tokens_s2)` to `segment_ids += [1] * len(tokens_s2)`, everything seems work but that is not what I want. Any suggestions? Thanks!
| 04-23-2019 03:51:06 | 04-23-2019 03:51:06 | Hi, only two segment labels are pre-trained in BERT.
You could fine-tune a new vocabulary token but we don't have a script to do that currently so you would have to modify the vocabulary and model.
GPT and GPT-2 have option to do that where you can take inspiration from.
I'm happy to welcome a PR on this if somebody feels like giving it a try.<|||||>This issue has been automatically marked as stale because it has not had recent activity. It will be closed if no further activity occurs. Thank you for your contributions.
|
transformers | 516 | closed | Same loss values but different eval result | I am experimenting with low-precision on the pre-trained BERT for SQuAD scenario.
I am seeing a strange issue: the loss value when fine-tuning the model with FP16 is very similar to the loss value when fine-tuning the model at Int8. However, the eval results are are quite different -- with Int8, the results are quite bad (f1 = 3) compared to f1=88 with FP16.
Any idea what is going on and suggestions for debugging? | 04-22-2019 17:19:40 | 04-22-2019 17:19:40 | I have never tried Int8 in PyTorch.
Can you share some code so we can have a look?<|||||>This issue has been automatically marked as stale because it has not had recent activity. It will be closed if no further activity occurs. Thank you for your contributions.
|
transformers | 515 | closed | Fix --reduce_memory in finetune_on_pregenerated | On reviewing the code I realized the --reduce_memory code path in `finetune_on_pregenerated.py` had a bug, but also wasn't getting used because the relevant argument wasn't getting passed correctly. The bugs have been fixed and the argument is now passed correctly. Performance still seems good, so now it should be possible to train without loading the whole epoch of training data into memory. | 04-22-2019 13:04:16 | 04-22-2019 13:04:16 | Good catch! |
transformers | 514 | closed | ADD ERNIE | Can we add a new model ERNIE?
ERNIE is based on the Bert model and has better performance on Chinese NLP tasks.
Github address: https://github.com/PaddlePaddle/LARK/tree/develop/ERNIE
paper: https://arxiv.org/abs/1904.09223
Thanks | 04-22-2019 09:57:29 | 04-22-2019 09:57:29 | Hi @nghuyong, I won't convert ERNIE but I'm open to welcome a PR if somebody want to give it a try.
Also, note that unlike examples, a PR with a new model should have a configuration class, tests, a conversion script and be documented like the other models in the library.
<|||||>I do implement that converting ERNIE to huggingface's format
The address is https://github.com/nghuyong/ERNIE-Pytorch
Welcome to use and open issue if have problems |
transformers | 513 | closed | How many epochs are necessary for finetuning BERT? | Hi,
Could somebody provide some insights on how many epochs are necessary for finetuning bert model?
Google BERT has 100000 steps.(total_data/batch_size)
flags.DEFINE_integer("num_train_steps", 100000, "Number of training steps.")
Thanks
Mahesh | 04-22-2019 06:47:37 | 04-22-2019 06:47:37 | I have tried to finetune GPT rather than BERT. An appropriate running epochs is **3** in the generation setting, including learning on embedding of some custom special tokens. Hope it help you :)<|||||>This issue has been automatically marked as stale because it has not had recent activity. It will be closed if no further activity occurs. Thank you for your contributions.
|
transformers | 512 | closed | Fix indentation weirdness in GPT-2 example. | Minor patch, not sure how it originally managed to sneak in in the first place. | 04-21-2019 17:22:49 | 04-21-2019 17:22:49 | Thanks @cynthia! |
transformers | 511 | closed | error when trying to use multilingual model for fine tuning | I wanted to use fine tuning for hindi language data. For that I tried to give bert-base-mutlilingual model but I am getting the following error
> python pregenerate_training_data.py --train_corpus=./hindi_pytorch_bert_data_1.txt --bert_model=bert-base-multilingual --output_dir=./hindi_train_data_1_3epochs/ --epochs_to_generate=3
```
Better speed can be achieved with apex installed from https://www.github.com/nvidia/apex.
Model name 'bert-base-multilingual' was not found in model name list (bert-base-uncased, bert-large-uncased, bert-base-cased, bert-large-cased, bert-base-multilingual-uncased, bert-base-multilingual-cased, bert-base-chinese). We assumed 'bert-base-multilingual' was a path or url but couldn't find any file associated to this path or url.
Traceback (most recent call last):
File "pregenerate_training_data.py", line 292, in <module>
main()
File "pregenerate_training_data.py", line 255, in main
vocab_list = list(tokenizer.vocab.keys())
AttributeError: 'NoneType' object has no attribute 'vocab'
```
I tried giving bert-base-multilingual-cased as well then I ran into this error
> python pregenerate_training_data.py --train_corpus=./hindi_pytorch_bert_data_1.txt --bert_model=bert-base-multilingual-cased --output_dir=./hindi_train_data_1_3epochs/ --epochs_to_generate=3
```
Better speed can be achieved with apex installed from https://www.github.com/nvidia/apex.
usage: pregenerate_training_data.py [-h] --train_corpus TRAIN_CORPUS
--output_dir OUTPUT_DIR --bert_model
{bert-base-uncased,bert-large-uncased,bert-base-cased,bert-base-multilingual,bert-base-chinese}
[--do_lower_case] [--reduce_memory]
[--epochs_to_generate EPOCHS_TO_GENERATE]
[--max_seq_len MAX_SEQ_LEN]
[--short_seq_prob SHORT_SEQ_PROB]
[--masked_lm_prob MASKED_LM_PROB]
[--max_predictions_per_seq MAX_PREDICTIONS_PER_SEQ]
pregenerate_training_data.py: error: argument --bert_model: invalid choice: 'bert-base-multilingual-cased' (choose from 'bert-base-uncased', 'bert-large-uncased', 'bert-base-cased', 'bert-base-multilingual', 'bert-base-chinese')
```
How to resolve this issue? | 04-21-2019 13:29:39 | 04-21-2019 13:29:39 | I made changes in the code pregenerate_training_data.py
from
```
parser.add_argument("--bert_model", type=str, required=True,
choices=["bert-base-uncased", "bert-large-uncased", "bert-base-cased",
"bert-base-multilingual", "bert-base-chinese"])
```
to
```
parser.add_argument("--bert_model", type=str, required=True,
choices=["bert-base-uncased", "bert-large-uncased", "bert-base-cased",
"bert-base-multilingual-cased", "bert-base-multilingual-uncased", "bert-base-chinese"])
```
and it worked.<|||||>It occured to me maybe because I forgot to install pytorch. I installed pytorch then it's solved.<|||||>Hi,
I followed your code, and got this error:
Traceback (most recent call last): | 6796/185072 [00:00<00:18, 9787.42it/s]
File "pregenerate_training_data.py", line 308, in <module>
main()
File "pregenerate_training_data.py", line 293, in main
vocab_list=vocab_list)
File "pregenerate_training_data.py", line 208, in create_instances_from_document
assert len(tokens_b) >= 1
AssertionError
Can you please share your code?<|||||>What computer specification to train your corpus? How big it is and how long you need to training your corpus?
I wanna too train my corpus using fine tuning, maybe your answers give me an insight about how relevant me to training the corpus, thanks<|||||>> Hi,
> I followed your code, and got this error:
>
> Traceback (most recent call last): | 6796/185072 [00:00<00:18, 9787.42it/s]
> File "pregenerate_training_data.py", line 308, in
> main()
> File "pregenerate_training_data.py", line 293, in main
> vocab_list=vocab_list)
> File "pregenerate_training_data.py", line 208, in create_instances_from_document
> assert len(tokens_b) >= 1
> AssertionError
>
> Can you please share your code?
I run into the same problem. Wondering if you have solved your problem. Thanks!<|||||>This issue has been automatically marked as stale because it has not had recent activity. It will be closed if no further activity occurs. Thank you for your contributions.
|
transformers | 510 | closed | Adam optimiser not following Pytorch conventions | Both [BertAdam](https://github.com/huggingface/pytorch-pretrained-BERT/blob/master/pytorch_pretrained_bert/optimization.py) and [OpenAIAdam](https://github.com/huggingface/pytorch-pretrained-BERT/blob/master/pytorch_pretrained_bert/optimization_openai.py) don't follow the pytroch convetion to define the `betas` parameter for [Adam Optimisers](https://pytorch.org/docs/stable/optim.html#torch.optim.Adam) as a tuple, but instead has parameters `b1` and `b2`.
Pytorch based libraries like fastai expect the optimizer `betas` to be a tuple.
Any reason `b1/2` is used instead of a tuple? Would be great to change so the optimisers can integrate with other pytorch libraries.
| 04-20-2019 23:33:42 | 04-20-2019 23:33:42 | We could update that indeed, that's just a relic of the Tensorflow conversion.
Do you want to submit a PR? Otherwise I'll do it when I work on the next release.<|||||>This issue has been automatically marked as stale because it has not had recent activity. It will be closed if no further activity occurs. Thank you for your contributions.
|
transformers | 509 | closed | How to read a checkpoint and continue training? | I wanted to experiment with longer training schedules. How do I re-start a run from it’s fine-tuned checkpoint? | 04-20-2019 14:54:14 | 04-20-2019 14:54:14 | Hi, what fine-tuning script and model are you referring to?<|||||>I would like to know how to restart / continue runs as well.
I would like to fine tune on half data first, checkpoint it. Then restart and continue on the other half of the data.
Like the `main` function in this finetuning script:
https://github.com/huggingface/pytorch-pretrained-BERT/blob/3fc63f126ddf883ba9659f13ec046c3639db7b7e/examples/lm_finetuning/simple_lm_finetuning.py<|||||>@thomwolf Hi. I was experimenting with run_squad.py on colab. I was able to train and checkpoint the model after every 50 steps. However, for some reason, the notebook crashed and did not resume training. Is there a way to load that checkpoint and resume training from that point onwards? <|||||>I am fine-tuning using run_glue.py on bert. Have a checkpoint that I would like to continue from since my run crashed. Also, what happens to the tensorboard event file? For example, if my checkpoint is at iteration 250 (and my checkpoint crashed at 290), will the Tensorboard event file be appended correctly???<|||||>This issue has been automatically marked as stale because it has not had recent activity. It will be closed if no further activity occurs. Thank you for your contributions.
<|||||>I think the solution is to change the model name to the checkpoint directory. When using the `run_glue.py` example script I changed the parameter from `--model_name_or_path bert-base-uncased` to `--model_name_or_path ../my-output-dir/checkpoint-1600`<|||||>> I think the solution is to change the model name to the checkpoint directory. When using the `run_glue.py` example script I changed the parameter from `--model_name_or_path bert-base-uncased` to `--model_name_or_path ../my-output-dir/checkpoint-1600`
Hi, this works but may I know what did you do the OURPUT-DIR? Keeping the same one while "overwriting" or starting a new one? Thanks!<|||||>> I think the solution is to change the model name to the checkpoint directory. When using the `run_glue.py` example script I changed the parameter from `--model_name_or_path bert-base-uncased` to `--model_name_or_path ../my-output-dir/checkpoint-1600`
Hi, I tried this. The following error message shows: "We assumed '/cluster/home/xiazhi/finetune_results_republican/checkpoint-1500' was a path, a model identifier, or url to a directory containing vocabulary files named ['vocab.json', 'merges.txt'] but couldn't find such vocabulary files at this path or url." But only after all epochs are done will the vocal.json and merges.txt be generated.
<|||||>@anniezhi I have the same problem. This makes training very difficult; anyone have any ideas re: how to save the tokenizer whenever the checkpoints are saved?<|||||>@anniezhi I figured it out - if loading from a checkpoint, use the additional argument --tokenizer_name and provide the name of your tokenizer. Here's my helper bash script for reference :
```
#!/bin/bash
conda activate transformers
cd "${HOME}/Desktop"
rm -rf "./${1}"
TRAIN_FILE="/media/b/F:/debiased_archive_200.h5"
#Matt login key
wandb login MY_API_KEY
python bao-ai/training_flows/run_language_custom_modeling.py \
--output_dir="./${1}" \
--tokenizer_name=gpt2 \
--model_name_or_path="${2}" \
--block_size "${3}" \
--per_device_train_batch_size "${4}" \
--do_train \
--train_data_file=$TRAIN_FILE\
```<|||||>If you're using the latest release (v3.1.0), the tokenizer should be saved as well, so there's no need to use the `--tokenizer_name` anymore.
For any version <3.1.0, @apteryxlabs's solution is the way to go!<|||||>>
Browse parameters, resume_from_checkpoint=./
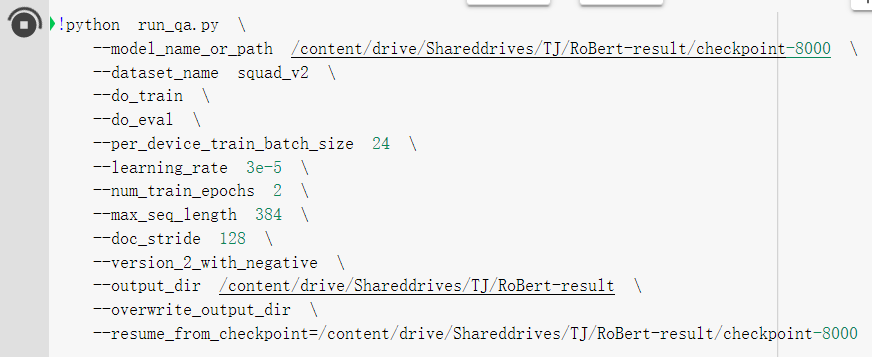
Now, the code runs from checkpoint
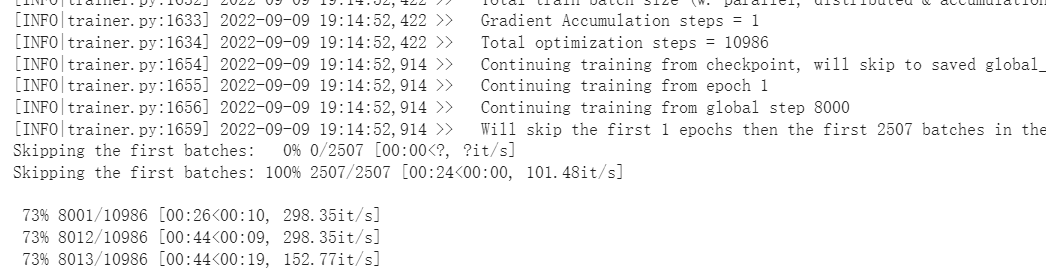
<|||||>>
Browse parameters, resume_from_checkpoint=./
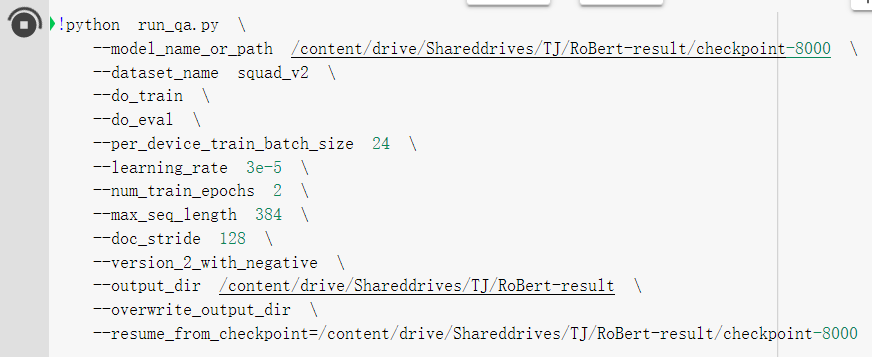
Now, the code runs from checkpoint
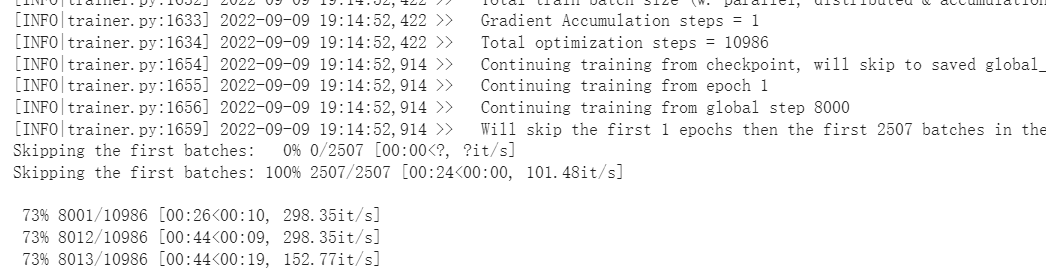
|
transformers | 508 | closed | Fix python syntax in examples/run_gpt2.py | As the title, we will never reach the code from line 115 to 131 because the space before `if args.unconditional` is not enough. | 04-19-2019 03:32:48 | 04-19-2019 03:32:48 | Thanks for the PR. This is fixed now. |
transformers | 507 | closed | GPT-2 FineTuning on Cloze/ ROC | Hi, wrote some code to finetune GPT2 on rocstories using the DoubleHeads model mirroring the GPT1 code. However, I'm only getting performance of 68% on the eval. Was wondering if anyone else had tried it and seen this drop in performance. Thanks | 04-18-2019 23:16:47 | 04-18-2019 23:16:47 | Hi rohuns, I was wondering what padding value have you used for the lm_labels, since the -1 specified in the docs doesn't work for me on GPT2LMHead model. See #577. <|||||>> Hi rohuns, I was wondering what padding value have you used for the lm_labels, since the -1 specified in the docs doesn't work for me on GPT2LMHead model. See #577.
I had just used -1, can take a look at your stack trace and respond on that chat<|||||>Also to close this issue it appears others also achieved similar performance on the MC task, more details on the thread issue #468 <|||||>> > Hi rohuns, I was wondering what padding value have you used for the lm_labels, since the -1 specified in the docs doesn't work for me on GPT2LMHead model. See #577.
>
> I had just used -1, can take a look at your stack trace and respond on that chat
Yes, please do have a look. Here is a toy example with a hand-coded dataset to prove that the -1 throws an error. It looks like it's a library issue.
[gpt2_simplified.py.zip](https://github.com/huggingface/pytorch-pretrained-BERT/files/3144185/gpt2_simplified.py.zip)
Regards,
Adrian
|
transformers | 506 | closed | Hubconf | fixes #504
Also add hubconf for bert related tokenizer & models.
There're a few GPT models and transformer models, but would like to send this out to get a review first.
Also there's possibility to unify the cache dir with pytorch one. | 04-17-2019 22:37:40 | 04-17-2019 22:37:40 | Hi @ailzhang,
This is great! I went through it and it looks good to me.
I guess we should update the `from_pretrained` method of the other models as well (like [here](https://github.com/huggingface/pytorch-pretrained-BERT/blob/19666dcb3bee3e379f1458e295869957aac8590c/pytorch_pretrained_bert/modeling_openai.py#L420))
Do you want to have a look at the other models (GPT, GPT-2 and Transformer-XL) and add them to the `hubconf.py` as well ?<|||||>Hi @thomwolf, thanks for the quick reply! Yea we definitely would like to add GPT and Transformer-XL models in.
I can definitely add them in this PR myself. Alternatively one thing could be super helpful to us would be someone from your team try out implementing a few models using `torch.hub` interfaces and let us know if you see any bugs/issues from a repo owner perspective :D. Let me know which way you prefer, thanks!
Another question is about cache dir, pytorch has move to comply with XDG specification about caching dirs(https://github.com/pytorch/pytorch/issues/14693). Detailed logic can be found here https://pytorch.org/docs/master/hub.html#where-are-my-downloaded-models-saved ( I will fix the doc formatting soon :P ) Are you interested in moving to be in the same place? Happy to help on it as well.
<|||||>@thomwolf Any update on this? ;) Thanks!<|||||>Hi @ailzhang, sorry for the delay, here are some answers to your questions:
- `torch.hub`: I can give it a try but the present week is fully packed. I'll see if I can free some time next week. If you want to see it reach `master` faster, I'm also fine with you adding the other models.
One question I have here is that the pretrained models cannot really be used without the associated tokenizers. How is this supposed to work with `torch.hub`? Can you give me an example of usage (like the one in the readme for instance)?
- update to `cache dir`: XDG specification seems nice indeed. If you want to give it a try it would be a lot cleaner than the present caching setting I guess.
Related note: we (Sebastian Ruder, Matthew Peters, Swabha Swayamdipta and I) are preparing a [tutorial on Transfer Learning in NLP to be held at NAACL](https://naacl2019.org/program/tutorials). We'll show various frameworks in action. I'll will see if we can include a `torch.hub` example.<|||||>@thomwolf
Note that there's a tokenizer in hub already. Typically we'd prefer hub only contains models, but in this case we also includes tokenizer as it's a required part.
There's an example in docstring of BertTokenizer. Is this good enough?
```
>>> sentence = 'Hello, World!'
>>> tokenizer = torch.hub.load('ailzhang/pytorch-pretrained-BERT:hubconf', 'bertTokenizer', 'bert-base-cased', do_basic_tokenize=False, force_reload=False)
>>> toks = tokenizer.tokenize(sentence)
['Hello', '##,', 'World', '##!']
>>> ids = tokenizer.convert_tokens_to_ids(toks)
[8667, 28136, 1291, 28125]
```
Maybe we can merge this PR first if it looks good?
<|||||>Oh indeed, I missed the tokenizer.
Ok let's go with this PR! |
transformers | 505 | closed | Generating text with Transformer XL | Hi everyone,
I am trying to generate text with the pre-trained transformer XL model in a similar way to how we do with the GPT-2 model. But I guess there is a bug in the `sample_sequence` function after I adjusted to the transformer XL architecture. But the generated text is completely random in general and with respect to the context as well.
The core sampling loop looks very similar to the gpt-2 one:
```
with torch.no_grad():
for i in trange(length):
logits, past = model(prev, mems=past)
logits = logits[:, -1, :] / temperature
logits = top_k_logits(logits, k=top_k)
log_probs = F.softmax(logits, dim=-1)
if sample:
prev = torch.multinomial(log_probs, num_samples=1)
else:
_, prev = torch.topk(log_probs, k=1, dim=-1)
output = torch.cat((output, prev), dim=1)
```
What is the bug that I'm missing?
| 04-17-2019 21:13:58 | 04-17-2019 21:13:58 | Here's an example of text generation, picks second most likely word at each step
```
tokenizer = TransfoXLTokenizer.from_pretrained('transfo-xl-wt103')
model = TransfoXLLMHeadModel.from_pretrained('transfo-xl-wt103')
line = "Cars were invented in"
line_tokenized = tokenizer.tokenize(line)
line_indexed = tokenizer.convert_tokens_to_ids(line_tokenized)
tokens_tensor = torch.tensor([line_indexed])
tokens_tensor = tokens_tensor.to(device)
max_predictions = 50
mems = None
for i in range(max_predictions):
predictions, mems = model(tokens_tensor, mems=mems)
predicted_index = torch.topk(predictions[0, -1, :],5)[1][1].item()
predicted_token = tokenizer.convert_ids_to_tokens([predicted_index])[0]
print(predicted_token)
predicted_index = torch.tensor([[predicted_index]]).to(device)
tokens_tensor = torch.cat((tokens_tensor, predicted_index), dim=1)
```
Should produce
```
Britain
and
America
,
but
the
first
two
cars
had
to
have
been
a
"
Turbo
```<|||||>Yeah figured it out. Thanks nevertheless @yaroslavvb !<|||||>@yaroslavvb I think, there is a bug in the code, you shared
`predicted_index = torch.topk(predictions[0, -1, :],5)[1][1].item()`why is it not `predicted_index = torch.topk(predictions[0, -1, :],5)[1][0].item()` or probably its not a bug
<|||||>@yaroslavvb Why in the text generation with Transformer-XL there is a loop over the number of predictions requested, like max_predictions?
Given a fixed input like line = "Cars were invented in", which is 21 characters or 4 words (depending if trained for character output or word output), say, why one cannot generate say the next 21 characters or 4 words directly from the T-XL output all at once? Then generate another set of 21 characters or 4 words again in the next iteration?
I thought one advantage of the T-XL vs the vanilla Transformer was this ability to predict a whole next sequence without having to loop by adding character by character or word by word at the input?
Isn't the T-XL trained by computing the loss over the whole input and whole target (label) without looping?
Thus why would it be different during text generation? To provide a more accurate context along the prediction by adding the previous prediction one by one?<|||||>@shashwath94 Could you please post your fix, so that we can learn by example? Thanks. <|||||>@gussmith you could do it this way, but empirically the results are very bad. The model loss is trained to maximize probability of "next token prediction". What looks like loss over a loss over whole sequence is actually a parallelization trick to compute many "next token prediction" losses in a single pass. |
transformers | 504 | closed | Init BertForTokenClassification from from_pretrained | ```
model = BertForTokenClassification.from_pretrained('bert-base-uncased', 2)
```
will complain about missing positional arg for `num_labels`.
The root cause is here the function signature should actually be
https://github.com/huggingface/pytorch-pretrained-BERT/blob/19666dcb3bee3e379f1458e295869957aac8590c/pytorch_pretrained_bert/modeling.py#L522
```
def from_pretrained(cls, pretrained_model_name_or_path, *inputs, state_dict=None, cache_dir=None, from_tf=False, **kwargs):
```
But note that the signature above above is actually only supported in py3 not py2. See a similar workaround here: https://github.com/pytorch/pytorch/pull/19247/files#diff-bdb85c31edc2daaad6cdb68c0d19bafbR300 | 04-17-2019 20:23:25 | 04-17-2019 20:23:25 | actually this is related to my current work, I will send a fix along with my PR. |
transformers | 503 | closed | Fix possible risks of bpe on special tokens | Hi developers !
When I use the openai tokenizer, I find it hard to handle the `special tokens` correctly (my library version is v0.6.1) , even though I have already defined them and told the tokenizer NEVER SPLIT them. It is because all tokens, including the special ones will be processed by BPE. So I add one line for avoiding BPE on special tokens.
But there still are some problems when we use `spacy` as the tokenizer. I will try to add special tokens to the vocabulary of `spacy` and pull another request. Thanks for code review :) | 04-17-2019 16:29:10 | 04-17-2019 16:29:10 | This issue has been automatically marked as stale because it has not had recent activity. It will be closed if no further activity occurs. Thank you for your contributions.
|
transformers | 502 | closed | How to obtain attention values for each layer | Hi all,
Please correct me if I am wrong.
From my understanding, The encoded values for each layer (12 of them for base model) would be returned when we run our results through the pre-trained model.
However, I would like to examine the self-attention values for each layer. Is there a way I can extract that out?
Regards | 04-17-2019 10:51:15 | 04-17-2019 10:51:15 | Not really.
You should build a new sub-class of `BertPreTrainedModel` which is identical to `BertModel`but send back self-attention values in addition to the hidden states.
<|||||>I see. Thank you! <|||||>Hi,
Just to add on. If this is what I would be doing, would it be advisable to fine-tune the weights for the pretrained model?
Regards<|||||>Probably.
It depends on what's your final use-case.<|||||>This issue has been automatically marked as stale because it has not had recent activity. It will be closed if no further activity occurs. Thank you for your contributions.
|
transformers | 501 | closed | Test a fine-tuned BERT-QA model | I have fine-tuned a BERT-QA model on SQuAD and it produced a `pytorch_model.bin` file. Now, I want to load this fine-tuned model and evaluate on SQuAD. How can I do that? I am using the `run_squad.py` script. | 04-17-2019 10:10:27 | 04-17-2019 10:10:27 | I noticed the following snippet in the code. (which I have edited to solve my problem)
if args.do_train and (args.local_rank == -1 or torch.distributed.get_rank() == 0):
# Save a trained model, configuration and tokenizer
model_to_save = model.module if hasattr(model, 'module') else model # Only save the model it-self
# If we save using the predefined names, we can load using `from_pretrained`
output_model_file = os.path.join(args.output_dir, WEIGHTS_NAME)
output_config_file = os.path.join(args.output_dir, CONFIG_NAME)
torch.save(model_to_save.state_dict(), output_model_file)
model_to_save.config.to_json_file(output_config_file)
tokenizer.save_vocabulary(args.output_dir)
# Load a trained model and vocabulary that you have fine-tuned
model = BertForQuestionAnswering.from_pretrained(args.output_dir)
tokenizer = BertTokenizer.from_pretrained(args.output_dir, do_lower_case=args.do_lower_case)
else:
model = BertForQuestionAnswering.from_pretrained(args.bert_model)
So, if we want to load the fine-tuned model only for prediction, need to load it from `args.output_dir`. But the current code loads from `args.bert_model` when we use `squad.py` only for prediction.<|||||>@wasiahmad tokenizer is not needed at prediction time?
Thanks
Mahesh<|||||>need help in understanding how to get the model trained with SQuAD + my dataset. Once trained, how to use it for actual prediction.
model : BERT Question Answering
<|||||>@Swathygsb
https://github.com/kamalkraj/BERT-SQuAD
inference on bert-squad model<|||||>> @Swathygsb
> https://github.com/kamalkraj/BERT-SQuAD
> inference on bert-squad model
thx for your sharing, and there is inference on bert-squad model by tensorflow?
3Q~ |
transformers | 500 | closed | Updating network handling | This PR adds:
- a bunch of tests for the models and tokenizers stored on S3 with `--runslow` (download and load one model/tokenizer for each type of model BERT, GPT, GPT-2, Transformer-XL)
- relax network connection checking (fallback on the last downloaded model in the cache when we can't get the last eTag from s3) | 04-17-2019 09:59:31 | 04-17-2019 09:59:31 | |
transformers | 499 | closed | error when do python3 run_squad.py | Hello,
I am newbie of pytorch-pretrained-Bert.
After successfully converted from init-checkpoint of tensorflow to pytorch bin,
I found an error when I do run_squad.
Guessing I should've included some configuration ahead, could anyone can help?
See below.
```bash
File "run_squad.py", line 37, in <module>
from pytorch_pretrained_bert.file_utils import PYTORCH_PRETRAINED_BERT_CACHE, WEIGHTS_NAME, CONFIG_NAME
ImportError: No module named pytorch_pretrained_bert.file_utils
```
| 04-17-2019 09:29:28 | 04-17-2019 09:29:28 | Did you install pytorch-pretrained-bert as indicated in the README?
`pip install pytorch_pretrained_bert`
You don't have to convert the checkpoints yourself, there are already converted.
Try reading the installation and usage sections of the README.<|||||>Of cause I installed,
More precisely, error code is slightly changed.
```bash
Traceback (most recent call last):
File "run_squad.py", line 37, in <module>
from pytorch_pretrained_bert.file_utils import PYTORCH_PRETRAINED_BERT_CACHE, WEIGHTS_NAME, CONFIG_NAME
ImportError: cannot import name WEIGHTS_NAME
```<|||||>Hmm you are right, the examples are compatible with `master` only now that we have a new token serialization. I guess we'll have to do a new release (0.6.2) today so everybody is on the same page.
Let me do that.<|||||>Actually, we'll wait for the merge of #506.
In the meantime you can install from source and it should work.<|||||>Oh it's done immediately when I installed from source. Thanks.<|||||>Ok great.
Just a side note on writing messages in github: you should add triple-quotes like this: \``` before and after the command line, errors and code you are pasting. This way it's easier to read.
Ex:
\```
pip install -e .
\```
will display like:
```
pip install -e .
```<|||||>Good point(triple quotes).
I didn't know what to do, but now I have it all.
Thanks.<|||||>> Actually, we'll wait for the merge of #506.
>
> In the meantime you can install from source and it should work.
how to "install from source"?<|||||>@YanZhangADS
You can install from source with this command below
```
git clone https://github.com/huggingface/pytorch-pretrained-BERT.git
cd pytorch-pretrained-BERT
python setup.py install
```<|||||>Same problem with "ImportError: cannot import name WEIGHTS_NAME". However, after building **0.6.1** from source, I get:
```
from pytorch_pretrained_bert.optimization import BertAdam, warmup_linear
ImportError: cannot import name 'warmup_linear'
```
I don't need the warmup, so I removed the import, but letting you guys know that this is an import error as well. Thanks!<|||||>Thanks for that @dumitrescustefan, we're working on it in #518.
I'm closing this issue for now as we start to deviate from the original discussion.<|||||>I just built from source. I'm still getting the same error as in original issue.<|||||>The version 0.4.0 doesn't give this issue.
pip install pytorch_pretrained_bert==0.4.0 |
transformers | 498 | closed | Gpt2 tokenization | Complete #489 by:
- adding tests on GPT-2 Tokenizer (at last)
- fixing GPT-2 tokenization to work on python 2 as well
- adding `special_tokens` handling logic in GPT-2 tokenizer
- fixing GPT and GPT-2 serialization logic to save special tokens | 04-17-2019 08:22:40 | 04-17-2019 08:22:40 | |
transformers | 497 | closed | UnboundLocalError: local variable 'special_tokens_file' referenced before assignment | Happens during this
```enc = GPT2Tokenizer.from_pretrained('gpt2')```
```
File "example_lambada_prediction_difference.py", line 23, in <module>
enc = GPT2Tokenizer.from_pretrained(model_name)
File "/bflm/pytorch-pretrained-BERT/pytorch_pretrained_bert/tokenization_gpt2.py", line 134, in from_pretrained
if special_tokens_file and 'special_tokens' not in kwargs:
UnboundLocalError: local variable 'special_tokens_file' referenced before assignment
```
Looking at offending file, it looks like there's a path for which `special_tokens_file` is never initialized
https://github.com/huggingface/pytorch-pretrained-BERT/blob/3d78e226e68a5c5d0ef612132b601024c3534e38/pytorch_pretrained_bert/tokenization_gpt2.py#L134 | 04-16-2019 23:33:31 | 04-16-2019 23:33:31 | Yes, this should be fixed by #498. |
transformers | 496 | closed | [run_gpt2.py] temperature should be a float, not int | 04-16-2019 22:23:30 | 04-16-2019 22:23:30 | Indeed, thanks @8enmann! |
|
transformers | 495 | closed | Fix gradient overflow issue during attention mask | This fix is in reference to issue #382. GPT2 can now be trained in mixed precision, which I've confirmed with testing. I also tested unconditional generation on multiple seeds before and after changing 1e10 to 1e4 and there was no difference. Please let me know if there is anything else I can do to make this pull request better. Thanks for all your work! | 04-16-2019 18:42:47 | 04-16-2019 18:42:47 | Ok, great, thanks @SudoSharma!<|||||>While the outputs are the same between 1e10 and 1e4, I shouldn't expect the outputs between fp32 and fp16 to be the same, should I? I get different outputs between the two when doing unconditional/conditional generation with top_k=40 but even with top_k=1. Usually they're the same for a while and then deviate. This is with Apex installed, so using FusedLayerNorm.
If I turn on Apex's AMP with `from apex import amp; amp.init()` then they still deviate but after a longer time (I think it makes the attention nn.Softmax use fp32). Have to remove the `model.half()` call when using AMP.
Perhaps it's not realistic to have the outputs be the same when fp16 errors in the "past" tensors are compounding as the sequence gets longer? But it is surprising to see them differ for top_k=1 (deterministic) since only the largest logit affects the output there.
P.S. For my site it's been enormously helpful to have this PyTorch implementation. @thomwolf Thank you!<|||||>Hi @AdamDanielKing,
Congratulation on your demo!
Are you using the updated API for apex Amp? (https://nvidia.github.io/apex/amp.html)
Also, we should discuss this in a new issue? At first, I thought this was related to this PR but I understand it's not, right?<|||||>@thomwolf You're probably right that a new issue is best. I've created one at #602.
Thanks for pointing out I was using the old Apex API. Switching to the new one unfortunately didn't fix the issue though. |
transformers | 494 | closed | Fix indentation for unconditional generation | Hey guys, there was an issue with the example file for generating unconditional samples. I just fixed the indentation. Let me know if there is anything else I need to do! Thanks for the great work on this repo. | 04-16-2019 18:12:58 | 04-16-2019 18:12:58 | Thanks! |
transformers | 493 | closed | how to use extracted features in extract_features.py? | I extract features like examples in extarct_features.py. But went I used these features (the last encoded_layers) as word embeddings in a text classification task, I got a worse result than using 300D Glove(any other parameters are the same). I also used these features to compute the cos similarity for each word in sentences, I found that all values were around 0.6. So are these features can be used as Glove or word2vec embeddings? What exactly these features are? | 04-16-2019 13:25:02 | 04-16-2019 13:25:02 | Without fine-tuning, BERT features are usually less useful than plain GloVe or wrd2vec indeed.
They start to be interesting when you fine-tune a classifier on top of BERT.
See the recent study by Matthew Peters, Sebastian Ruder, Noah A. Smith ([To Tune or Not to Tune? Adapting Pretrained Representations to Diverse Tasks](https://arxiv.org/abs/1903.05987)) for some practical tips on that.<|||||>thank you so much~<|||||>@heslowen could you please share the code for extracting features in order to use them for learning a classifier? Thanks.<|||||>@joistick11 you can find a demo in extract_features.py<|||||>Could you please help me?
I was using bert-as-service (https://github.com/hanxiao/bert-as-service) and there is model method `encode`, which accepts list and returns list of the same size, each element containing sentence embedding. All the elements of the same size.
1. When I use extract_features.py, it returns embedding for each recognized symbol in the sentence from the specified layers. I mean, instead of sentence embedding it returns symbols embeddings. How should I use it, for instance, to train an SVM? I am using `bert-base-multilingual-cased`
2. Which layer output should I use? Is it with index `-1`?
Thanks you very much!<|||||>@joistick11 you want to embed a sentence to a vector?
`all_encoder_layers, pooled_output = model(input_ids, token_type_ids=None, attention_mask=input_mask)` pooled_output may help you.
I have no idea about using these features to train an SVM although I know the theory about SVM.
For the second question, please refer to thomwolf's answer.
I used the top 4 encoders_layers, but I did not get a better result than using Glove <|||||>@heslowen Hello, would you please help me? For a sequence like [cls I have a dog.sep], when I input this to Bert and get the last hidden layer of sequence out, let’s say the output is “vector”, is the vector[0] embedding of cls, vector[1] embedding of I, etc. vector[-1] embedding of sep?<|||||>@heslowen How did you extract features after training a classifier on top of BERT? I've been trying to do the same, but I'm unable to do so.
Do I first follow run_classifier.py, and then extract the features from tf.Estimator?<|||||>@rvoak I use pytorch. I did it as the demo in extract_featrues.py. it is easy to do that, you just need to load a tokenizer, a bert model, then tokenize your sentences, and then run the model to get the encoded_layers<|||||>@RomanShen yes you're right
<|||||>@heslowen Thanks for your reply!<|||||>This issue has been automatically marked as stale because it has not had recent activity. It will be closed if no further activity occurs. Thank you for your contributions.
<|||||>@heslowen sorry about my english, now i doing embedding for sentence task, i tuned with my corpus with this library, and i received config.json, vocab.txt and model.bin file, but in bert's original doc, can extract feature when load from ckpt tensorflow checkpoint. according to your answer, i must write feature_extraction for torch, that's right ? please help me<|||||>@hungph-dev-ict Do you mind opening a new issue with your problem? I'll try and help you out.<|||||>@LysandreJik Thank you for your help, I will find solution for my problem, it's use last hidden layer in bert mechanism, but if you have a better solution, can you help me ?
So i have more concerns about with my corpus, with this library code, use tokenizer from pretrained BERT model, so I want use only BasicTokenizer. Can you help me ? <|||||>How long should the extract_features.py take to complete?
when using 'bert-large-uncased' it takes seconds however it writes a blank file.
when using 'bert-base-uncased' its been running for over 30 mins.
any advice?
the code I used:
!python extract_features.py \
--input_file data/src_train.txt \
--output_file data/output1.jsonl \
--bert_model bert-base-uncased \
--layers -1
<|||||>You can look at what the BertForSequenceClassification model [https://github.com/huggingface/transformers/blob/3ba5470eb85464df62f324bea88e20da234c423f/pytorch_pretrained_bert/modeling.py#L867 ](url) does in it’s forward 139.
The pooled_output obtained from self.bert would seem to be the features you are looking for. |
transformers | 492 | closed | no_decay = ['bias', 'LayerNorm.bias', 'LayerNorm.weight'] | what does this means? Whay these three kind no decay? | 04-16-2019 06:03:22 | 04-16-2019 06:03:22 | Yes. We are reproducing the behavior of the original optimizer, see [here](https://github.com/google-research/bert/blob/master/optimization.py#L65).<|||||>thanks~<|||||>but why?<|||||>I have the same question, but did this prove to be better? Or is it just to speed up calculations? |
transformers | 491 | closed | pretrained GPT-2 checkpoint gets only 31% accuracy on Lambada | For some reason I only see 26% accuracy when evaluating on Lambada for GPT-2 checkpoint instead of expected 45.99%
Here's a file of [predictions](https://s3.amazonaws.com/yaroslavvb2/data/lambada_predictions.txt) with sets of 3 lines of the form:
ground truth
predicted last_word
is_counted_as_error
Generated by this [script](https://github.com/cybertronai/bflm/blob/master/eval_lambada_slow.py)
Could this be caused by the way GPT-2 checkpoint was imported into HuggingFace?
| 04-16-2019 02:04:24 | 04-16-2019 02:04:24 | Accuracy goes to 31% if I use [stop-word filter](https://github.com/cybertronai/bflm/blob/51908bdd15477a0cedfbd010d489f8d355443b6a/eval_lambada_slow.py#L62), still seems lower than expected ([predictions](https://s3.amazonaws.com/yaroslavvb2/data/lambada_predictions_stopword_filter.txt))
<|||||>Hi, I doubt it's a problem with the model. Usually the culprit is too find in the pre-processing logic.
Your dataset seems to be pre-processed but Radford, Wu et al. says they are using a version without preprocessing (end of section 3.3). GPT-2 is likely sensitive to tokenization issues and the like.
If you want to check the model it-self, you could try comparing with the predictions of the Tensorflow version on a few lambada completions?<|||||>Applying [detokenization](https://github.com/cybertronai/bflm/blob/d58a6860451ee2afa3688aff13d104ad74001ebe/eval_lambada_slow.py#L77) raises accuracy to 33.11%
I spot checked a few errors against TF implementation and they give the same errors, so it seems likely the difference is due to eval protocol, rather than the checkpoint<|||||>IMHO "without pre-processing" means taking the original dataset without modification, which is what I also did here.
However in the original dataset, everything is tokenized. IE "haven't" was turned into "have n't"
Either way, undoing this tokenization only has a improvement of 2%, so there must be some deeper underlying difference in the way OpenAI did their evaluation.
<|||||>Indeed. It's not very clear to me what they mean exactly by "stop-word filter". It seems like the kind of heuristic that can have a very large impact on the performances.
Maybe a better filtering is key. I would probably go with a sort of beam-search to compute the probability of having a punctuation/end-of-sentence token after the predicted word and use that to filter the results.<|||||>I spoke with Alec and turns out for evaluation they got used the "raw" lambada corpus which was obtained by finding original sentences in book corpus that matched the tokenized versions in the lambada release. So to to reproduce the numbers we need the "raw" corpus https://github.com/openai/gpt-2/issues/131<|||||>I'm now able to get within 1% of their reported accuracy on GPT2-small. The two missing modifications were:
1. Evaluate on OpenAI's version of lambada which adds extra formatting
2. Evaluate by counting number of times the last BPE token is predicted incorrectly instead of last word, details are in https://github.com/openai/gpt-2/issues/131#issuecomment-497136199 |
transformers | 490 | closed | Clean up GPT and GPT-2 losses computation | Small clean up of GPT and GPT-2 losses computations.
Also fix an issue with special adding tokens. | 04-15-2019 14:14:41 | 04-15-2019 14:14:41 | |
transformers | 489 | closed | Better serialization for Tokenizer and Config classes (BERT, GPT, GPT-2 and Transformer-XL) | This PR add standardized serialization to all the tokenizers (BERT, GPT, GPT-2, Transformer-XL) through a `tokenizer.save_vocabulary(path)` method.
Also add a serialization method to all the Configuration classes: `Config.to_json_file(file_path)`
Added clean examples for serialization best practices in README and examples.
Also fixes Transformer-XL "split on punctation" bug mentioned in #466. | 04-15-2019 10:06:25 | 04-15-2019 10:06:25 | |
transformers | 488 | closed | fixed BertForMultipleChoice model init and forward pass | the number of choices is not respected because you've hardcoded '1' into the classifier layer. also `token_type_ids` and `attention_mask` will cause an error if `None` because `None` does not have a `view` method. | 04-15-2019 08:40:10 | 04-15-2019 08:40:10 | Indeed, it looks better.
Do you want to have a look and confirm @rodgzilla?<|||||>@thomwolf any word on this?<|||||>Oh yes sorry. Looking at it and reading Alec Radford's paper on GPT (section 3.3) again, I think @rodgzilla was actually right in the original implementation.
So I guess we should close this PR.
I still would have been happy to get @rodgzilla input on that.<|||||>Oh sorry, we should still keep the `token_type_ids` and `attention_mask` `NoneType` fixes.
These ones are correct! |
Subsets and Splits
No saved queries yet
Save your SQL queries to embed, download, and access them later. Queries will appear here once saved.