hexsha
stringlengths 40
40
| size
int64 6
14.9M
| ext
stringclasses 1
value | lang
stringclasses 1
value | max_stars_repo_path
stringlengths 6
260
| max_stars_repo_name
stringlengths 6
119
| max_stars_repo_head_hexsha
stringlengths 40
41
| max_stars_repo_licenses
sequence | max_stars_count
int64 1
191k
⌀ | max_stars_repo_stars_event_min_datetime
stringlengths 24
24
⌀ | max_stars_repo_stars_event_max_datetime
stringlengths 24
24
⌀ | max_issues_repo_path
stringlengths 6
260
| max_issues_repo_name
stringlengths 6
119
| max_issues_repo_head_hexsha
stringlengths 40
41
| max_issues_repo_licenses
sequence | max_issues_count
int64 1
67k
⌀ | max_issues_repo_issues_event_min_datetime
stringlengths 24
24
⌀ | max_issues_repo_issues_event_max_datetime
stringlengths 24
24
⌀ | max_forks_repo_path
stringlengths 6
260
| max_forks_repo_name
stringlengths 6
119
| max_forks_repo_head_hexsha
stringlengths 40
41
| max_forks_repo_licenses
sequence | max_forks_count
int64 1
105k
⌀ | max_forks_repo_forks_event_min_datetime
stringlengths 24
24
⌀ | max_forks_repo_forks_event_max_datetime
stringlengths 24
24
⌀ | avg_line_length
float64 2
1.04M
| max_line_length
int64 2
11.2M
| alphanum_fraction
float64 0
1
| cells
sequence | cell_types
sequence | cell_type_groups
sequence |
---|---|---|---|---|---|---|---|---|---|---|---|---|---|---|---|---|---|---|---|---|---|---|---|---|---|---|---|---|---|---|
d044dab2946c49877a9cddc1c0e2b68ede8c336e | 1,813 | ipynb | Jupyter Notebook | repro.ipynb | hexagonrecursion/requests-bug | c34439734aca44a8b5df85d606b2ac63ca0510f0 | [
"CC0-1.0"
] | null | null | null | repro.ipynb | hexagonrecursion/requests-bug | c34439734aca44a8b5df85d606b2ac63ca0510f0 | [
"CC0-1.0"
] | null | null | null | repro.ipynb | hexagonrecursion/requests-bug | c34439734aca44a8b5df85d606b2ac63ca0510f0 | [
"CC0-1.0"
] | null | null | null | 1,813 | 1,813 | 0.756757 | [
[
[
"from requests import get\nURL = 'https://dl.fedoraproject.org/pub/alt/iot/32/IoT/x86_64/images/Fedora-IoT-32-20200603.0.x86_64.raw.xz'",
"_____no_output_____"
],
[
"# This downloads the entire response\nr = get(URL, stream=True)\nfor b in r.iter_content(chunk_size=None):\n print(len(b))",
"533830860\n"
],
[
"# For comparison: this yeilds new chunks as they arrive\nr = get(URL, stream=True)\nfor b in r.iter_content(chunk_size=2**23):\n print(len(b))",
"8388608\n8388608\n8388608\n8388608\n8388608\n8388608\n8388608\n8388608\n8388608\n8388608\n8388608\n8388608\n8388608\n8388608\n8388608\n8388608\n8388608\n8388608\n8388608\n8388608\n8388608\n8388608\n8388608\n8388608\n8388608\n8388608\n8388608\n8388608\n8388608\n8388608\n8388608\n8388608\n8388608\n8388608\n8388608\n8388608\n8388608\n8388608\n8388608\n8388608\n8388608\n8388608\n8388608\n8388608\n8388608\n8388608\n8388608\n8388608\n8388608\n8388608\n8388608\n8388608\n8388608\n8388608\n8388608\n8388608\n8388608\n8388608\n8388608\n8388608\n8388608\n8388608\n8388608\n5348556\n"
]
]
] | [
"code"
] | [
[
"code",
"code",
"code"
]
] |
d044e43b7395e8080cf4c2927f36569b8a060bbc | 58,551 | ipynb | Jupyter Notebook | IMDB Reviews NLP.ipynb | gsingh1629/SentAnalysis | dd7401105334825279ccba269649cc2f1361e339 | [
"MIT"
] | null | null | null | IMDB Reviews NLP.ipynb | gsingh1629/SentAnalysis | dd7401105334825279ccba269649cc2f1361e339 | [
"MIT"
] | null | null | null | IMDB Reviews NLP.ipynb | gsingh1629/SentAnalysis | dd7401105334825279ccba269649cc2f1361e339 | [
"MIT"
] | null | null | null | 49.119966 | 6,730 | 0.61227 | [
[
[
"import pandas as pd\r\nimport numpy as np\r\nimport matplotlib.pyplot as plt\r\nimport seaborn as sns\r\n%matplotlib inline\r\nimport warnings\r\nwarnings.filterwarnings(\"ignore\")",
"_____no_output_____"
],
[
"df = pd.read_csv(\"Data.csv\")\r\ndf.head(5)",
"_____no_output_____"
],
[
"df['sentiment'] = np.where(df['sentiment'] == \"positive\", 1, 0)\r\ndf.head()",
"_____no_output_____"
],
[
"df['sentiment'].value_counts().sort_index().plot(kind='bar',color = 'blue')\r\nplt.xlabel('Sentiment')\r\nplt.ylabel('Count')",
"_____no_output_____"
],
[
"df = df.sample(frac=0.1, random_state=0) \r\ndf.dropna(inplace=True)",
"_____no_output_____"
],
[
"from sklearn.model_selection import train_test_split\r\nX_train, X_test, y_train, y_test = train_test_split(df['review'], df['sentiment'],test_size=0.1, random_state=0)",
"_____no_output_____"
],
[
"def cleanText(raw_text, remove_stopwords=False, stemming=False, split_text=False):\r\n text = BeautifulSoup(raw_text, 'html.parser').get_text()\r\n letters_only = re.sub(\"[^a-zA-Z]\", \" \", text)\r\n words = letters_only.lower().split() \r\n \r\n if remove_stopwords:\r\n stops = set(stopwords.words(\"english\"))\r\n words = [w for w in words if not w in stops]\r\n \r\n if stemming==True:\r\n\r\n stemmer = SnowballStemmer('english') \r\n words = [stemmer.stem(w) for w in words]\r\n \r\n if split_text==True:\r\n return (words)\r\n \r\n return( \" \".join(words))",
"_____no_output_____"
],
[
"import re\r\nimport nltk\r\nfrom nltk.corpus import stopwords \r\nfrom nltk.stem.porter import PorterStemmer\r\nfrom nltk.stem import SnowballStemmer, WordNetLemmatizer\r\nfrom nltk import sent_tokenize, word_tokenize, pos_tag\r\nfrom bs4 import BeautifulSoup \r\nimport logging\r\nfrom wordcloud import WordCloud\r\nfrom gensim.models import word2vec\r\nfrom gensim.models import Word2Vec\r\nfrom gensim.models.keyedvectors import KeyedVectors\r\n\r\nX_train_cleaned = []\r\nX_test_cleaned = []\r\n\r\nfor d in X_train:\r\n X_train_cleaned.append(cleanText(d))\r\nprint('Show a cleaned review in the training set : \\n', X_train_cleaned[10])\r\n \r\nfor d in X_test:\r\n X_test_cleaned.append(cleanText(d))",
"Show a cleaned review in the training set : \n the crimson rivers is one of the most over directed over the top over everything mess i ve ever seen come out of france there s nothing worse than a french production trying to out do films made in hollywood and cr is a perfect example of such a wannabe horror action buddy flick i almost stopped it halfway through because i knew it wouldn t amount to anything but french guys trying to show off the film starts off promisingly like some sort of expansive horror film but it quickly shifts genres from horror to action to x files type to buddy flick that in the end cr is all of it and also none of it it s so full of clich s that at one point i thought the whole thing was a comedy the painful dialogue and those silent pauses with fades outs and fades ins just at the right expositionary moments made me groan i thought only films made in hollywood used this hackneyed technique the chase scene with vincent cassel running after the killer is so over directed and over done that it s almost a thing of beauty the climax on top of the mountain with the stupid revelation about the killer s with cassel and reno playing buddies like nolte and murphy in hrs completely derailed what little credibility the film had by then it s difficult to believe that the director of the crimson rivers also directed gothika which though had its share of problems doesn t even come close to the awfulness of this overbaked confused film\n"
]
],
[
[
"## CountVectorizer with Mulinomial Naive Bayes (Benchmark Model)",
"_____no_output_____"
]
],
[
[
"from sklearn.feature_extraction.text import CountVectorizer,TfidfVectorizer\r\nfrom sklearn.naive_bayes import BernoulliNB, MultinomialNB\r\ncountVect = CountVectorizer() \r\nX_train_countVect = countVect.fit_transform(X_train_cleaned)\r\nprint(\"Number of features : %d \\n\" %len(countVect.get_feature_names())) #6378 \r\nprint(\"Show some feature names : \\n\", countVect.get_feature_names()[::1000])\r\n\r\n\r\n# Train MultinomialNB classifier\r\nmnb = MultinomialNB()\r\nmnb.fit(X_train_countVect, y_train)",
"Number of features : 36751 \n\nShow some feature names : \n ['aa', 'ameche', 'auggie', 'betrayals', 'bright', 'cathryn', 'clownhouse', 'copying', 'dazzle', 'disarray', 'dvd', 'estimation', 'fighter', 'fusion', 'greenfinch', 'henson', 'imaginings', 'ir', 'kint', 'linklater', 'maropis', 'misik', 'nectar', 'organise', 'performing', 'pre', 'rages', 'reputedly', 'saddled', 'sexiness', 'smith', 'steal', 'swoozie', 'tinfoil', 'unattuned', 'vernacular', 'willed']\n"
],
[
"import pickle\r\npickle.dump(countVect,open('countVect_imdb.pkl','wb'))",
"_____no_output_____"
],
[
"from sklearn import metrics\r\nfrom sklearn.metrics import accuracy_score,roc_auc_score\r\ndef modelEvaluation(predictions):\r\n '''\r\n Print model evaluation to predicted result \r\n '''\r\n print (\"\\nAccuracy on validation set: {:.4f}\".format(accuracy_score(y_test, predictions)))\r\n print(\"\\nAUC score : {:.4f}\".format(roc_auc_score(y_test, predictions)))\r\n print(\"\\nClassification report : \\n\", metrics.classification_report(y_test, predictions))\r\n print(\"\\nConfusion Matrix : \\n\", metrics.confusion_matrix(y_test, predictions))",
"_____no_output_____"
],
[
"predictions = mnb.predict(countVect.transform(X_test_cleaned))\r\nmodelEvaluation(predictions)",
"\nAccuracy on validation set: 0.8140\n\nAUC score : 0.8142\n\nClassification report : \n precision recall f1-score support\n\n 0 0.79 0.86 0.82 249\n 1 0.85 0.77 0.81 251\n\n accuracy 0.81 500\n macro avg 0.82 0.81 0.81 500\nweighted avg 0.82 0.81 0.81 500\n\n\nConfusion Matrix : \n [[214 35]\n [ 58 193]]\n"
],
[
"import pickle\r\npickle.dump(mnb,open('Naive_Bayes_model_imdb.pkl','wb'))",
"_____no_output_____"
]
],
[
[
"# TfidfVectorizer with Logistic Regression",
"_____no_output_____"
]
],
[
[
"from sklearn.linear_model import LogisticRegression\r\ntfidf = TfidfVectorizer(min_df=5) #minimum document frequency of 5\r\nX_train_tfidf = tfidf.fit_transform(X_train)\r\nprint(\"Number of features : %d \\n\" %len(tfidf.get_feature_names())) #1722\r\nprint(\"Show some feature names : \\n\", tfidf.get_feature_names()[::1000])\r\n\r\n# Logistic Regression\r\nlr = LogisticRegression()\r\nlr.fit(X_train_tfidf, y_train)",
"Number of features : 10505 \n\nShow some feature names : \n ['00', 'belonged', 'completion', 'dubious', 'garbage', 'interviewing', 'million', 'plays', 'rough', 'strike', 'vein']\n"
],
[
"feature_names = np.array(tfidf.get_feature_names())\r\nsorted_coef_index = lr.coef_[0].argsort()\r\nprint('\\nTop 10 features with smallest coefficients :\\n{}\\n'.format(feature_names[sorted_coef_index[:10]]))\r\nprint('Top 10 features with largest coefficients : \\n{}'.format(feature_names[sorted_coef_index[:-11:-1]]))",
"\nTop 10 features with smallest coefficients :\n['bad' 'worst' 'awful' 'no' 'waste' 'poor' 'terrible' 'boring' 'even'\n 'minutes']\n\nTop 10 features with largest coefficients : \n['great' 'and' 'excellent' 'best' 'it' 'wonderful' 'very' 'also' 'well'\n 'love']\n"
],
[
"predictions = lr.predict(tfidf.transform(X_test_cleaned))\r\nmodelEvaluation(predictions)",
"\nAccuracy on validation set: 0.8500\n\nAUC score : 0.8500\n\nClassification report : \n precision recall f1-score support\n\n 0 0.85 0.85 0.85 249\n 1 0.85 0.85 0.85 251\n\n accuracy 0.85 500\n macro avg 0.85 0.85 0.85 500\nweighted avg 0.85 0.85 0.85 500\n\n\nConfusion Matrix : \n [[211 38]\n [ 37 214]]\n"
],
[
"from sklearn.model_selection import GridSearchCV\r\nfrom sklearn import metrics\r\nfrom sklearn.metrics import roc_auc_score, accuracy_score\r\nfrom sklearn.pipeline import Pipeline\r\nestimators = [(\"tfidf\", TfidfVectorizer()), (\"lr\", LogisticRegression())]\r\nmodel = Pipeline(estimators)\r\n\r\n\r\nparams = {\"lr__C\":[0.1, 1, 10], \r\n \"tfidf__min_df\": [1, 3], \r\n \"tfidf__max_features\": [1000, None], \r\n \"tfidf__ngram_range\": [(1,1), (1,2)], \r\n \"tfidf__stop_words\": [None, \"english\"]} \r\n\r\ngrid = GridSearchCV(estimator=model, param_grid=params, scoring=\"accuracy\", n_jobs=-1)\r\ngrid.fit(X_train_cleaned, y_train)\r\nprint(\"The best paramenter set is : \\n\", grid.best_params_)\r\n\r\n\r\n# Evaluate on the validaton set\r\npredictions = grid.predict(X_test_cleaned)\r\nmodelEvaluation(predictions)",
"The best paramenter set is : \n {'lr__C': 10, 'tfidf__max_features': None, 'tfidf__min_df': 3, 'tfidf__ngram_range': (1, 2), 'tfidf__stop_words': None}\n\nAccuracy on validation set: 0.8720\n\nAUC score : 0.8720\n\nClassification report : \n precision recall f1-score support\n\n 0 0.87 0.87 0.87 249\n 1 0.87 0.88 0.87 251\n\n accuracy 0.87 500\n macro avg 0.87 0.87 0.87 500\nweighted avg 0.87 0.87 0.87 500\n\n\nConfusion Matrix : \n [[216 33]\n [ 31 220]]\n"
]
],
[
[
"# Word2Vec\n<br>\n\n**Step 1 : Parse review text to sentences (Word2Vec model takes a list of sentences as inputs)**\n\n**Step 2 : Create volcabulary list using Word2Vec model.**\n\n**Step 3 : Transform each review into numerical representation by computing average feature vectors of words therein.**\n\n**Step 4 : Fit the average feature vectors to Random Forest Classifier.**",
"_____no_output_____"
]
],
[
[
"tokenizer = nltk.data.load('tokenizers/punkt/english.pickle')\r\n\r\ndef parseSent(review, tokenizer, remove_stopwords=False):\r\n\r\n raw_sentences = tokenizer.tokenize(review.strip())\r\n sentences = []\r\n for raw_sentence in raw_sentences:\r\n if len(raw_sentence) > 0:\r\n sentences.append(cleanText(raw_sentence, remove_stopwords, split_text=True))\r\n return sentences\r\n\r\n\r\n# Parse each review in the training set into sentences\r\nsentences = []\r\nfor review in X_train_cleaned:\r\n sentences += parseSent(review, tokenizer,remove_stopwords=False)\r\n \r\nprint('%d parsed sentence in the training set\\n' %len(sentences))\r\nprint('Show a parsed sentence in the training set : \\n', sentences[10])",
"4500 parsed sentence in the training set\n\nShow a parsed sentence in the training set : \n ['the', 'crimson', 'rivers', 'is', 'one', 'of', 'the', 'most', 'over', 'directed', 'over', 'the', 'top', 'over', 'everything', 'mess', 'i', 've', 'ever', 'seen', 'come', 'out', 'of', 'france', 'there', 's', 'nothing', 'worse', 'than', 'a', 'french', 'production', 'trying', 'to', 'out', 'do', 'films', 'made', 'in', 'hollywood', 'and', 'cr', 'is', 'a', 'perfect', 'example', 'of', 'such', 'a', 'wannabe', 'horror', 'action', 'buddy', 'flick', 'i', 'almost', 'stopped', 'it', 'halfway', 'through', 'because', 'i', 'knew', 'it', 'wouldn', 't', 'amount', 'to', 'anything', 'but', 'french', 'guys', 'trying', 'to', 'show', 'off', 'the', 'film', 'starts', 'off', 'promisingly', 'like', 'some', 'sort', 'of', 'expansive', 'horror', 'film', 'but', 'it', 'quickly', 'shifts', 'genres', 'from', 'horror', 'to', 'action', 'to', 'x', 'files', 'type', 'to', 'buddy', 'flick', 'that', 'in', 'the', 'end', 'cr', 'is', 'all', 'of', 'it', 'and', 'also', 'none', 'of', 'it', 'it', 's', 'so', 'full', 'of', 'clich', 's', 'that', 'at', 'one', 'point', 'i', 'thought', 'the', 'whole', 'thing', 'was', 'a', 'comedy', 'the', 'painful', 'dialogue', 'and', 'those', 'silent', 'pauses', 'with', 'fades', 'outs', 'and', 'fades', 'ins', 'just', 'at', 'the', 'right', 'expositionary', 'moments', 'made', 'me', 'groan', 'i', 'thought', 'only', 'films', 'made', 'in', 'hollywood', 'used', 'this', 'hackneyed', 'technique', 'the', 'chase', 'scene', 'with', 'vincent', 'cassel', 'running', 'after', 'the', 'killer', 'is', 'so', 'over', 'directed', 'and', 'over', 'done', 'that', 'it', 's', 'almost', 'a', 'thing', 'of', 'beauty', 'the', 'climax', 'on', 'top', 'of', 'the', 'mountain', 'with', 'the', 'stupid', 'revelation', 'about', 'the', 'killer', 's', 'with', 'cassel', 'and', 'reno', 'playing', 'buddies', 'like', 'nolte', 'and', 'murphy', 'in', 'hrs', 'completely', 'derailed', 'what', 'little', 'credibility', 'the', 'film', 'had', 'by', 'then', 'it', 's', 'difficult', 'to', 'believe', 'that', 'the', 'director', 'of', 'the', 'crimson', 'rivers', 'also', 'directed', 'gothika', 'which', 'though', 'had', 'its', 'share', 'of', 'problems', 'doesn', 't', 'even', 'come', 'close', 'to', 'the', 'awfulness', 'of', 'this', 'overbaked', 'confused', 'film']\n"
]
],
[
[
"## Creating Volcabulary List usinhg Word2Vec Model",
"_____no_output_____"
]
],
[
[
"from wordcloud import WordCloud\r\nfrom gensim.models import word2vec\r\nfrom gensim.models.keyedvectors import KeyedVectors\r\nnum_features = 300 #embedding dimension \r\nmin_word_count = 10 \r\nnum_workers = 4 \r\ncontext = 10 \r\ndownsampling = 1e-3 \r\n\r\nprint(\"Training Word2Vec model ...\\n\")\r\nw2v = Word2Vec(sentences, workers=num_workers, min_count = min_word_count,\\\r\n window = context, sample = downsampling)\r\nw2v.init_sims(replace=True)\r\nw2v.save(\"w2v_300features_10minwordcounts_10context\") #save trained word2vec model\r\n\r\nprint(\"Number of words in the vocabulary list : %d \\n\" %len(w2v.wv.index2word)) #4016 \r\nprint(\"Show first 10 words in the vocalbulary list vocabulary list: \\n\", w2v.wv.index2word[0:10])",
"Training Word2Vec model ...\n\n"
]
],
[
[
"## Averaging Feature Vectors",
"_____no_output_____"
]
],
[
[
"def makeFeatureVec(review, model, num_features):\r\n '''\r\n Transform a review to a feature vector by averaging feature vectors of words \r\n appeared in that review and in the volcabulary list created\r\n '''\r\n featureVec = np.zeros((num_features,),dtype=\"float32\")\r\n nwords = 0.\r\n index2word_set = set(model.wv.index2word) #index2word is the volcabulary list of the Word2Vec model\r\n isZeroVec = True\r\n for word in review:\r\n if word in index2word_set: \r\n nwords = nwords + 1.\r\n featureVec = np.add(featureVec, model[word])\r\n isZeroVec = False\r\n if isZeroVec == False:\r\n featureVec = np.divide(featureVec, nwords)\r\n return featureVec",
"_____no_output_____"
],
[
"def getAvgFeatureVecs(reviews, model, num_features):\r\n '''\r\n Transform all reviews to feature vectors using makeFeatureVec()\r\n '''\r\n counter = 0\r\n reviewFeatureVecs = np.zeros((len(reviews),num_features),dtype=\"float32\")\r\n for review in reviews:\r\n reviewFeatureVecs[counter] = makeFeatureVec(review, model,num_features)\r\n counter = counter + 1\r\n return reviewFeatureVecs",
"_____no_output_____"
],
[
"X_train_cleaned = []\r\nfor review in X_train:\r\n X_train_cleaned.append(cleanText(review, remove_stopwords=True, split_text=True))\r\ntrainVector = getAvgFeatureVecs(X_train_cleaned, w2v, num_features)\r\nprint(\"Training set : %d feature vectors with %d dimensions\" %trainVector.shape)\r\n\r\n\r\n# Get feature vectors for validation set\r\nX_test_cleaned = []\r\nfor review in X_test:\r\n X_test_cleaned.append(cleanText(review, remove_stopwords=True, split_text=True))\r\ntestVector = getAvgFeatureVecs(X_test_cleaned, w2v, num_features)\r\nprint(\"Validation set : %d feature vectors with %d dimensions\" %testVector.shape)",
"_____no_output_____"
]
],
[
[
"# Random Forest Classifer",
"_____no_output_____"
]
],
[
[
"from sklearn.ensemble import RandomForestClassifier\r\nrf = RandomForestClassifier(n_estimators=1000)\r\nrf.fit(trainVector, y_train)\r\npredictions = rf.predict(testVector)\r\nmodelEvaluation(predictions)",
"_____no_output_____"
]
],
[
[
"## LSTM\n<br>\n\n**Step 1 : Prepare X_train and X_test to 2D tensor.**\n \n**Step 2 : Train a simple LSTM (embeddign layer => LSTM layer => dense layer).**\n \n**Step 3 : Compile and fit the model using log loss function and ADAM optimizer.**",
"_____no_output_____"
]
],
[
[
"from keras.preprocessing import sequence\r\nfrom keras.utils import np_utils\r\nfrom keras.models import Sequential\r\nfrom keras.layers.core import Dense, Dropout, Activation, Lambda\r\nfrom keras.layers.embeddings import Embedding\r\nfrom keras.layers.recurrent import LSTM, SimpleRNN, GRU\r\nfrom keras.preprocessing.text import Tokenizer\r\nfrom collections import defaultdict\r\nfrom keras.layers.convolutional import Convolution1D\r\nfrom keras import backend as K\r\nfrom keras.layers.embeddings import Embedding",
"Using TensorFlow backend.\nWARNING:root:Limited tf.compat.v2.summary API due to missing TensorBoard installation.\nWARNING:root:Limited tf.compat.v2.summary API due to missing TensorBoard installation.\nWARNING:root:Limited tf.compat.v2.summary API due to missing TensorBoard installation.\nWARNING:root:Limited tf.compat.v2.summary API due to missing TensorBoard installation.\nWARNING:root:Limited tf.compat.v2.summary API due to missing TensorBoard installation.\nWARNING:root:Limited tf.compat.v2.summary API due to missing TensorBoard installation.\nWARNING:root:Limited tf.summary API due to missing TensorBoard installation.\n"
],
[
"top_words = 40000 \r\nmaxlen = 200 \r\nbatch_size = 62\r\nnb_classes = 4\r\nnb_epoch = 6\r\n\r\n\r\n# Vectorize X_train and X_test to 2D tensor\r\ntokenizer = Tokenizer(nb_words=top_words) #only consider top 20000 words in the corpse\r\ntokenizer.fit_on_texts(X_train)\r\n# tokenizer.word_index #access word-to-index dictionary of trained tokenizer\r\n\r\nsequences_train = tokenizer.texts_to_sequences(X_train)\r\nsequences_test = tokenizer.texts_to_sequences(X_test)\r\n\r\nX_train_seq = sequence.pad_sequences(sequences_train, maxlen=maxlen)\r\nX_test_seq = sequence.pad_sequences(sequences_test, maxlen=maxlen)\r\n\r\n\r\n# one-hot encoding of y_train and y_test\r\ny_train_seq = np_utils.to_categorical(y_train, nb_classes)\r\ny_test_seq = np_utils.to_categorical(y_test, nb_classes)\r\n\r\nprint('X_train shape:', X_train_seq.shape)\r\nprint(\"========================================\")\r\nprint('X_test shape:', X_test_seq.shape)\r\nprint(\"========================================\")\r\nprint('y_train shape:', y_train_seq.shape)\r\nprint(\"========================================\")\r\nprint('y_test shape:', y_test_seq.shape)\r\nprint(\"========================================\")",
"X_train shape: (4500, 200)\n========================================\nX_test shape: (500, 200)\n========================================\ny_train shape: (4500, 4)\n========================================\ny_test shape: (500, 4)\n========================================\n"
],
[
"model1 = Sequential()\r\nmodel1.add(Embedding(top_words, 128, dropout=0.2))\r\nmodel1.add(LSTM(128, dropout_W=0.2, dropout_U=0.2)) \r\nmodel1.add(Dense(nb_classes))\r\nmodel1.add(Activation('softmax'))\r\nmodel1.summary()",
"Model: \"sequential_1\"\n_________________________________________________________________\nLayer (type) Output Shape Param # \n=================================================================\nembedding_1 (Embedding) (None, None, 128) 5120000 \n_________________________________________________________________\nlstm_1 (LSTM) (None, 128) 131584 \n_________________________________________________________________\ndense_1 (Dense) (None, 4) 516 \n_________________________________________________________________\nactivation_1 (Activation) (None, 4) 0 \n=================================================================\nTotal params: 5,252,100\nTrainable params: 5,252,100\nNon-trainable params: 0\n_________________________________________________________________\n"
],
[
"model1.compile(loss='binary_crossentropy',\r\n optimizer='adam',\r\n metrics=['accuracy'])\r\n\r\nmodel1.fit(X_train_seq, y_train_seq, batch_size=batch_size, nb_epoch=nb_epoch, verbose=1)\r\n\r\n# Model evluation\r\nscore = model1.evaluate(X_test_seq, y_test_seq, batch_size=batch_size)\r\nprint('Test loss : {:.4f}'.format(score[0]))\r\nprint('Test accuracy : {:.4f}'.format(score[1]))",
"Epoch 1/6\n4500/4500 [==============================] - 22s 5ms/step - loss: 0.3760 - accuracy: 0.7594\nEpoch 2/6\n4500/4500 [==============================] - 24s 5ms/step - loss: 0.2857 - accuracy: 0.8577\nEpoch 3/6\n4500/4500 [==============================] - 24s 5ms/step - loss: 0.1591 - accuracy: 0.9347\nEpoch 4/6\n4500/4500 [==============================] - 24s 5ms/step - loss: 0.0838 - accuracy: 0.9699\nEpoch 5/6\n4500/4500 [==============================] - 24s 5ms/step - loss: 0.0385 - accuracy: 0.9874\nEpoch 6/6\n4500/4500 [==============================] - 24s 5ms/step - loss: 0.0225 - accuracy: 0.9925\n500/500 [==============================] - 1s 1ms/step\nTest loss : 0.4559\nTest accuracy : 0.8750\n"
],
[
"len(X_train_seq),len(y_train_seq)",
"_____no_output_____"
],
[
"print(\"Size of weight matrix in the embedding layer : \", \\\r\n model1.layers[0].get_weights()[0].shape)\r\n\r\n# get weight matrix of the hidden layer\r\nprint(\"Size of weight matrix in the hidden layer : \", \\\r\n model1.layers[1].get_weights()[0].shape)\r\n\r\n# get weight matrix of the output layer\r\nprint(\"Size of weight matrix in the output layer : \", \\\r\n model1.layers[2].get_weights()[0].shape)",
"Size of weight matrix in the embedding layer : (40000, 128)\nSize of weight matrix in the hidden layer : (128, 512)\nSize of weight matrix in the output layer : (128, 4)\n"
],
[
"import pickle\r\npickle.dump(model1,open('model1.pkl','wb'))",
"_____no_output_____"
]
],
[
[
"## LSTM with Word2Vec Embedding",
"_____no_output_____"
]
],
[
[
"2v = Word2Vec.load(\"w2v_300features_10minwordcounts_10context\")\r\n\r\nembedding_matrix = w2v.wv.syn0 \r\nprint(\"Shape of embedding matrix : \", embedding_matrix.shape)",
"_____no_output_____"
],
[
"top_words = embedding_matrix.shape[0] #4016 \r\nmaxlen = 300 \r\nbatch_size = 62\r\nnb_classes = 4\r\nnb_epoch = 7\r\n\r\n\r\n# Vectorize X_train and X_test to 2D tensor\r\ntokenizer = Tokenizer(nb_words=top_words) #only consider top 20000 words in the corpse\r\ntokenizer.fit_on_texts(X_train)\r\n# tokenizer.word_index #access word-to-index dictionary of trained tokenizer\r\n\r\nsequences_train = tokenizer.texts_to_sequences(X_train)\r\nsequences_test = tokenizer.texts_to_sequences(X_test)\r\n\r\nX_train_seq1 = sequence.pad_sequences(sequences_train, maxlen=maxlen)\r\nX_test_seq1 = sequence.pad_sequences(sequences_test, maxlen=maxlen)\r\n\r\n\r\n# one-hot encoding of y_train and y_test\r\ny_train_seq1 = np_utils.to_categorical(y_train, nb_classes)\r\ny_test_seq1 = np_utils.to_categorical(y_test, nb_classes)\r\n\r\nprint('X_train shape:', X_train_seq1.shape)\r\nprint(\"========================================\")\r\nprint('X_test shape:', X_test_seq1.shape)\r\nprint(\"========================================\")\r\nprint('y_train shape:', y_train_seq1.shape)\r\nprint(\"========================================\")\r\nprint('y_test shape:', y_test_seq1.shape)\r\nprint(\"========================================\")",
"_____no_output_____"
],
[
"len(X_train_seq1),len(y_train_seq1)",
"_____no_output_____"
],
[
"embedding_layer = Embedding(embedding_matrix.shape[0], #4016\r\n embedding_matrix.shape[1], #300\r\n weights=[embedding_matrix])\r\n\r\nmodel2 = Sequential()\r\nmodel2.add(embedding_layer)\r\nmodel2.add(LSTM(128, dropout_W=0.2, dropout_U=0.2)) \r\nmodel2.add(Dense(nb_classes))\r\nmodel2.add(Activation('softmax'))\r\nmodel2.summary()",
"_____no_output_____"
],
[
"model2.compile(loss='binary_crossentropy',\r\n optimizer='adam',\r\n metrics=['accuracy'])\r\n\r\nmodel2.fit(X_train_seq1, y_train_seq1, batch_size=batch_size, nb_epoch=nb_epoch, verbose=1)\r\n\r\n# Model evaluation\r\nscore = model2.evaluate(X_test_seq1, y_test_seq1, batch_size=batch_size)\r\nprint('Test loss : {:.4f}'.format(score[0]))\r\nprint('Test accuracy : {:.4f}'.format(score[1]))",
"_____no_output_____"
],
[
"print(\"Size of weight matrix in the embedding layer : \", \\\r\n model2.layers[0].get_weights()[0].shape) \r\n\r\nprint(\"Size of weight matrix in the hidden layer : \", \\\r\n model2.layers[1].get_weights()[0].shape) \r\n\r\nprint(\"Size of weight matrix in the output layer : \", \\\r\n model2.layers[2].get_weights()[0].shape) ",
"_____no_output_____"
]
]
] | [
"code",
"markdown",
"code",
"markdown",
"code",
"markdown",
"code",
"markdown",
"code",
"markdown",
"code",
"markdown",
"code",
"markdown",
"code",
"markdown",
"code"
] | [
[
"code",
"code",
"code",
"code",
"code",
"code",
"code",
"code"
],
[
"markdown"
],
[
"code",
"code",
"code",
"code",
"code"
],
[
"markdown"
],
[
"code",
"code",
"code",
"code"
],
[
"markdown"
],
[
"code"
],
[
"markdown"
],
[
"code"
],
[
"markdown"
],
[
"code",
"code",
"code"
],
[
"markdown"
],
[
"code"
],
[
"markdown"
],
[
"code",
"code",
"code",
"code",
"code",
"code",
"code"
],
[
"markdown"
],
[
"code",
"code",
"code",
"code",
"code",
"code"
]
] |
d044e6c1ed7371dfb0a1ded41f18399b6b8c7a8a | 9,104 | ipynb | Jupyter Notebook | tutorial_1.ipynb | shauryasachdev/self_tutorials | 64bc2067f07c39b0f0439ad47b4378e5a0be7f49 | [
"MIT"
] | null | null | null | tutorial_1.ipynb | shauryasachdev/self_tutorials | 64bc2067f07c39b0f0439ad47b4378e5a0be7f49 | [
"MIT"
] | null | null | null | tutorial_1.ipynb | shauryasachdev/self_tutorials | 64bc2067f07c39b0f0439ad47b4378e5a0be7f49 | [
"MIT"
] | null | null | null | 22.150852 | 98 | 0.498133 | [
[
[
"print('Hello World')",
"Hello World\n"
],
[
"import numpy",
"_____no_output_____"
],
[
"print(numpy.pi)",
"3.141592653589793\n"
],
[
"import numpy as np",
"_____no_output_____"
],
[
"print(np.pi)",
"3.141592653589793\n"
],
[
"import numpy as np",
"_____no_output_____"
],
[
"import numpy as np\nimport matplotlib.pyplot as plt",
"_____no_output_____"
],
[
"%pinfo print",
"_____no_output_____"
],
[
"%matplotlib inline",
"_____no_output_____"
],
[
"plt.style.use('../../solving_pde_mooc/notebooks/styles/mainstyle.use')",
"_____no_output_____"
],
[
"#create an empty python list that will create values of delta for all k\ndelta_list = []\ndelta_list_2 = []\n\n#we have to loop for k=1,2,3,4,5,6,7,8,9\n\n#we will use the range function range(min,max,step)\n#this creates values of min, min+1*step, min+2*step, ..., max-step\n#as you can see, max value is not (actually never!) included in the list\n\n#min and step are optional and if you don't input them, then default values will be used\n#the default are min=0,step=1\n\n#range(5) will give 0,1,2,3,4 since it takes min=0, step=1 (default)\n#range(1,5) will give 1,2,3,4 since it takes min=1\n#range(2,5) will give 2,3,4\n#range(2,8,2) will give 2,4,6\n\n#once we have create the list of k, we have to create the delta_list\n#we can create the delta_list by adding/appending values to the end of the list each time\n#we can do that by using the append function as done below\nfor k in range(1,10):\n delta_list.append(2**(-k))\n \nprint('\\n')\nprint(delta_list)\n\n#another way to create a list is to direcly add values to it\ndelta_list_2 = [2**(-k) for k in range(1,10)]\n\nprint('\\n')\nprint(delta_list_2)\n\n#here a list is being created using the values and fuctions that we want\n#but to do operations on it, we need to create a numerical array out of it\n\n#np(Numpy) helps us create an array out of it as shown below\ndelta = np.array(delta_list_2)\n\nprint('\\n')\nprint(delta)",
"\n\n[0.5, 0.25, 0.125, 0.0625, 0.03125, 0.015625, 0.0078125, 0.00390625, 0.001953125]\n\n\n[0.5, 0.25, 0.125, 0.0625, 0.03125, 0.015625, 0.0078125, 0.00390625, 0.001953125]\n\n\n[0.5 0.25 0.125 0.0625 0.03125 0.015625\n 0.0078125 0.00390625 0.00195312]\n"
],
[
"%%timeit\n\n#method 1 - using append function\n\n#create an empty list first\ndelta_list = []\n\n#append values to the list\nfor k in range(1,10):\n delta_list.append(2**(-k))\n\n#assign the list to an array using numpy\ndelta = np.array(delta_list)\n",
"2.71 µs ± 14.4 ns per loop (mean ± std. dev. of 7 runs, 100000 loops each)\n"
],
[
"#%%timeit\n\n#method 2 - directly adding values to the list\n\n#create an empty list first\ndelta_list = []\n\n#directly add values to the list\ndelta_list = [2**(-k) for k in range(1,10)]\n\n#assign the list to an array using numpy\ndelta = np.array(delta_list)\n\nprint(delta_list[8])",
"0.001953125\n"
],
[
"#%%timeit\n\n#method 3 - directly create a numpy array\n\n#first create a numpy array with all zeros\ndelta_array = np.zeros(9)\n\nprint('\\n')\nprint(delta_array)\n\n#this will create an array of 9 elements - indexing as 0,1,2,...,8\n#numpy array starts indexing from 0 and goest to N-1\n# var =np.zeros(9) \n#this will mean that the first element in the array will be var[0]\n#last element in the array will be var(8)\n\nprint('\\n')\nprint(len(delta))\nprint('\\n')\n\n#fill the array\n#using len function, since i will start from zero\n#also, numpy array starts indexing from 0 to N-1\nfor i in range(len(delta_array)):\n print(i)\n delta_array[i]=2**(-i-1)\n \nprint('\\n')\nprint(delta_array[0])\nprint('\\n')\nprint(delta_array[8])",
"\n\n[0. 0. 0. 0. 0. 0. 0. 0. 0.]\n\n\n9\n\n\n0\n1\n2\n3\n4\n5\n6\n7\n8\n\n\n0.5\n\n\n0.001953125\n"
],
[
"#the method I am most used to\n\ndelta_array = np.zeros(9)\n\nfor i in range(len(delta_array)):\n delta_array[i]=2**(-i-1)\n \nprint(delta_array)\nprint('\\n')\nprint(delta_array[8])",
"[0.5 0.25 0.125 0.0625 0.03125 0.015625\n 0.0078125 0.00390625 0.00195312]\n\n\n0.001953125\n"
]
]
] | [
"code"
] | [
[
"code",
"code",
"code",
"code",
"code",
"code",
"code",
"code",
"code",
"code",
"code",
"code",
"code",
"code",
"code"
]
] |
d044edb42ebfe7881569443b98cdd241a0608505 | 72,142 | ipynb | Jupyter Notebook | tests/tf/03_implementing_tf_idf.ipynb | gopala-kr/ds-notebooks | bc35430ecdd851f2ceab8f2437eec4d77cb59423 | [
"MIT"
] | 1 | 2019-05-10T09:16:23.000Z | 2019-05-10T09:16:23.000Z | tests/tf/03_implementing_tf_idf.ipynb | gopala-kr/ds-notebooks | bc35430ecdd851f2ceab8f2437eec4d77cb59423 | [
"MIT"
] | null | null | null | tests/tf/03_implementing_tf_idf.ipynb | gopala-kr/ds-notebooks | bc35430ecdd851f2ceab8f2437eec4d77cb59423 | [
"MIT"
] | 1 | 2019-05-10T09:17:28.000Z | 2019-05-10T09:17:28.000Z | 155.814255 | 28,628 | 0.884062 | [
[
[
"# Implementing TF-IDF\n------------------------------------\n\nHere we implement TF-IDF, (Text Frequency - Inverse Document Frequency) for the spam-ham text data.\n\nWe will use a hybrid approach of encoding the texts with sci-kit learn's TFIDF vectorizer. Then we will use the regular TensorFlow logistic algorithm outline.\n\nCreating the TF-IDF vectors requires us to load all the text into memory and count the occurrences of each word before we can start training our model. Because of this, it is not implemented fully in Tensorflow, so we will use Scikit-learn for creating our TF-IDF embedding, but use Tensorflow to fit the logistic model.\n\nWe start by loading the necessary libraries.",
"_____no_output_____"
]
],
[
[
"import tensorflow as tf\nimport matplotlib.pyplot as plt\nimport csv\nimport numpy as np\nimport os\nimport string\nimport requests\nimport io\nimport nltk\nfrom zipfile import ZipFile\nfrom sklearn.feature_extraction.text import TfidfVectorizer\nfrom tensorflow.python.framework import ops\nops.reset_default_graph()",
"_____no_output_____"
]
],
[
[
"Start a computational graph session.",
"_____no_output_____"
]
],
[
[
"sess = tf.Session()",
"_____no_output_____"
]
],
[
[
"We set two parameters, `batch_size` and `max_features`. `batch_size` is the size of the batch we will train our logistic model on, and `max_features` is the maximum number of tf-idf textual words we will use in our logistic regression.",
"_____no_output_____"
]
],
[
[
"batch_size = 200\nmax_features = 1000",
"_____no_output_____"
]
],
[
[
"Check if data was downloaded, otherwise download it and save for future use",
"_____no_output_____"
]
],
[
[
"save_file_name = 'temp_spam_data.csv'\nif os.path.isfile(save_file_name):\n text_data = []\n with open(save_file_name, 'r') as temp_output_file:\n reader = csv.reader(temp_output_file)\n for row in reader:\n text_data.append(row)\nelse:\n zip_url = 'http://archive.ics.uci.edu/ml/machine-learning-databases/00228/smsspamcollection.zip'\n r = requests.get(zip_url)\n z = ZipFile(io.BytesIO(r.content))\n file = z.read('SMSSpamCollection')\n # Format Data\n text_data = file.decode()\n text_data = text_data.encode('ascii',errors='ignore')\n text_data = text_data.decode().split('\\n')\n text_data = [x.split('\\t') for x in text_data if len(x)>=1]\n \n # And write to csv\n with open(save_file_name, 'w') as temp_output_file:\n writer = csv.writer(temp_output_file)\n writer.writerows(text_data)",
"_____no_output_____"
]
],
[
[
"We now clean our texts. This will decrease our vocabulary size by converting everything to lower case, removing punctuation and getting rid of numbers.",
"_____no_output_____"
]
],
[
[
"texts = [x[1] for x in text_data]\ntarget = [x[0] for x in text_data]\n\n# Relabel 'spam' as 1, 'ham' as 0\ntarget = [1. if x=='spam' else 0. for x in target]\n\n# Normalize text\n# Lower case\ntexts = [x.lower() for x in texts]\n\n# Remove punctuation\ntexts = [''.join(c for c in x if c not in string.punctuation) for x in texts]\n\n# Remove numbers\ntexts = [''.join(c for c in x if c not in '0123456789') for x in texts]\n\n# Trim extra whitespace\ntexts = [' '.join(x.split()) for x in texts]",
"_____no_output_____"
]
],
[
[
"Define tokenizer function and create the TF-IDF vectors with SciKit-Learn.",
"_____no_output_____"
]
],
[
[
"import nltk\nnltk.download('punkt')",
"[nltk_data] Downloading package punkt to /home/jovyan/nltk_data...\n[nltk_data] Unzipping tokenizers/punkt.zip.\n"
],
[
"def tokenizer(text):\n words = nltk.word_tokenize(text)\n return words\n\n# Create TF-IDF of texts\ntfidf = TfidfVectorizer(tokenizer=tokenizer, stop_words='english', max_features=max_features)\nsparse_tfidf_texts = tfidf.fit_transform(texts)",
"/srv/venv/lib/python3.6/site-packages/sklearn/feature_extraction/text.py:1089: FutureWarning: Conversion of the second argument of issubdtype from `float` to `np.floating` is deprecated. In future, it will be treated as `np.float64 == np.dtype(float).type`.\n if hasattr(X, 'dtype') and np.issubdtype(X.dtype, np.float):\n"
]
],
[
[
"Split up data set into train/test.",
"_____no_output_____"
]
],
[
[
"train_indices = np.random.choice(sparse_tfidf_texts.shape[0], round(0.8*sparse_tfidf_texts.shape[0]), replace=False)\ntest_indices = np.array(list(set(range(sparse_tfidf_texts.shape[0])) - set(train_indices)))\ntexts_train = sparse_tfidf_texts[train_indices]\ntexts_test = sparse_tfidf_texts[test_indices]\ntarget_train = np.array([x for ix, x in enumerate(target) if ix in train_indices])\ntarget_test = np.array([x for ix, x in enumerate(target) if ix in test_indices])",
"_____no_output_____"
]
],
[
[
"Now we create the variables and placeholders necessary for logistic regression. After which, we declare our logistic regression operation. Remember that the sigmoid part of the logistic regression will be in the loss function.",
"_____no_output_____"
]
],
[
[
"# Create variables for logistic regression\nA = tf.Variable(tf.random_normal(shape=[max_features,1]))\nb = tf.Variable(tf.random_normal(shape=[1,1]))\n\n# Initialize placeholders\nx_data = tf.placeholder(shape=[None, max_features], dtype=tf.float32)\ny_target = tf.placeholder(shape=[None, 1], dtype=tf.float32)\n\n# Declare logistic model (sigmoid in loss function)\nmodel_output = tf.add(tf.matmul(x_data, A), b)",
"_____no_output_____"
]
],
[
[
"Next, we declare the loss function (which has the sigmoid in it), and the prediction function. The prediction function will have to have a sigmoid inside of it because it is not in the model output.",
"_____no_output_____"
]
],
[
[
"# Declare loss function (Cross Entropy loss)\nloss = tf.reduce_mean(tf.nn.sigmoid_cross_entropy_with_logits(logits=model_output, labels=y_target))\n\n# Prediction\nprediction = tf.round(tf.sigmoid(model_output))\npredictions_correct = tf.cast(tf.equal(prediction, y_target), tf.float32)\naccuracy = tf.reduce_mean(predictions_correct)",
"_____no_output_____"
]
],
[
[
"Now we create the optimization function and initialize the model variables.",
"_____no_output_____"
]
],
[
[
"# Declare optimizer\nmy_opt = tf.train.GradientDescentOptimizer(0.0025)\ntrain_step = my_opt.minimize(loss)\n\n# Intitialize Variables\ninit = tf.global_variables_initializer()\nsess.run(init)",
"_____no_output_____"
]
],
[
[
"Finally, we perform our logisitic regression on the 1000 TF-IDF features.",
"_____no_output_____"
]
],
[
[
"train_loss = []\ntest_loss = []\ntrain_acc = []\ntest_acc = []\ni_data = []\nfor i in range(10000):\n rand_index = np.random.choice(texts_train.shape[0], size=batch_size)\n rand_x = texts_train[rand_index].todense()\n rand_y = np.transpose([target_train[rand_index]])\n sess.run(train_step, feed_dict={x_data: rand_x, y_target: rand_y})\n \n # Only record loss and accuracy every 100 generations\n if (i+1)%100==0:\n i_data.append(i+1)\n train_loss_temp = sess.run(loss, feed_dict={x_data: rand_x, y_target: rand_y})\n train_loss.append(train_loss_temp)\n \n test_loss_temp = sess.run(loss, feed_dict={x_data: texts_test.todense(), y_target: np.transpose([target_test])})\n test_loss.append(test_loss_temp)\n \n train_acc_temp = sess.run(accuracy, feed_dict={x_data: rand_x, y_target: rand_y})\n train_acc.append(train_acc_temp)\n \n test_acc_temp = sess.run(accuracy, feed_dict={x_data: texts_test.todense(), y_target: np.transpose([target_test])})\n test_acc.append(test_acc_temp)\n if (i+1)%500==0:\n acc_and_loss = [i+1, train_loss_temp, test_loss_temp, train_acc_temp, test_acc_temp]\n acc_and_loss = [np.round(x,2) for x in acc_and_loss]\n print('Generation # {}. Train Loss (Test Loss): {:.2f} ({:.2f}). Train Acc (Test Acc): {:.2f} ({:.2f})'.format(*acc_and_loss))",
"Generation # 500. Train Loss (Test Loss): 0.92 (0.93). Train Acc (Test Acc): 0.39 (0.40)\nGeneration # 1000. Train Loss (Test Loss): 0.71 (0.74). Train Acc (Test Acc): 0.56 (0.56)\nGeneration # 1500. Train Loss (Test Loss): 0.58 (0.62). Train Acc (Test Acc): 0.66 (0.66)\nGeneration # 2000. Train Loss (Test Loss): 0.59 (0.56). Train Acc (Test Acc): 0.67 (0.74)\nGeneration # 2500. Train Loss (Test Loss): 0.58 (0.52). Train Acc (Test Acc): 0.74 (0.77)\nGeneration # 3000. Train Loss (Test Loss): 0.55 (0.49). Train Acc (Test Acc): 0.76 (0.79)\nGeneration # 3500. Train Loss (Test Loss): 0.47 (0.47). Train Acc (Test Acc): 0.80 (0.81)\nGeneration # 4000. Train Loss (Test Loss): 0.47 (0.46). Train Acc (Test Acc): 0.81 (0.83)\nGeneration # 4500. Train Loss (Test Loss): 0.44 (0.45). Train Acc (Test Acc): 0.84 (0.83)\nGeneration # 5000. Train Loss (Test Loss): 0.47 (0.45). Train Acc (Test Acc): 0.82 (0.84)\nGeneration # 5500. Train Loss (Test Loss): 0.46 (0.44). Train Acc (Test Acc): 0.84 (0.84)\nGeneration # 6000. Train Loss (Test Loss): 0.47 (0.44). Train Acc (Test Acc): 0.82 (0.85)\nGeneration # 6500. Train Loss (Test Loss): 0.46 (0.44). Train Acc (Test Acc): 0.84 (0.85)\nGeneration # 7000. Train Loss (Test Loss): 0.45 (0.44). Train Acc (Test Acc): 0.86 (0.85)\nGeneration # 7500. Train Loss (Test Loss): 0.48 (0.44). Train Acc (Test Acc): 0.84 (0.85)\nGeneration # 8000. Train Loss (Test Loss): 0.37 (0.44). Train Acc (Test Acc): 0.88 (0.85)\nGeneration # 8500. Train Loss (Test Loss): 0.42 (0.44). Train Acc (Test Acc): 0.88 (0.85)\nGeneration # 9000. Train Loss (Test Loss): 0.38 (0.44). Train Acc (Test Acc): 0.89 (0.85)\nGeneration # 9500. Train Loss (Test Loss): 0.49 (0.44). Train Acc (Test Acc): 0.81 (0.85)\nGeneration # 10000. Train Loss (Test Loss): 0.50 (0.44). Train Acc (Test Acc): 0.84 (0.85)\n"
]
],
[
[
"Here is matplotlib code to plot the loss and accuracies.",
"_____no_output_____"
]
],
[
[
"# Plot loss over time\nplt.plot(i_data, train_loss, 'k-', label='Train Loss')\nplt.plot(i_data, test_loss, 'r--', label='Test Loss', linewidth=4)\nplt.title('Cross Entropy Loss per Generation')\nplt.xlabel('Generation')\nplt.ylabel('Cross Entropy Loss')\nplt.legend(loc='upper right')\nplt.show()\n\n# Plot train and test accuracy\nplt.plot(i_data, train_acc, 'k-', label='Train Set Accuracy')\nplt.plot(i_data, test_acc, 'r--', label='Test Set Accuracy', linewidth=4)\nplt.title('Train and Test Accuracy')\nplt.xlabel('Generation')\nplt.ylabel('Accuracy')\nplt.legend(loc='lower right')\nplt.show()",
"_____no_output_____"
],
[
"test complete; Gopal",
"_____no_output_____"
]
]
] | [
"markdown",
"code",
"markdown",
"code",
"markdown",
"code",
"markdown",
"code",
"markdown",
"code",
"markdown",
"code",
"markdown",
"code",
"markdown",
"code",
"markdown",
"code",
"markdown",
"code",
"markdown",
"code",
"markdown",
"code"
] | [
[
"markdown"
],
[
"code"
],
[
"markdown"
],
[
"code"
],
[
"markdown"
],
[
"code"
],
[
"markdown"
],
[
"code"
],
[
"markdown"
],
[
"code"
],
[
"markdown"
],
[
"code",
"code"
],
[
"markdown"
],
[
"code"
],
[
"markdown"
],
[
"code"
],
[
"markdown"
],
[
"code"
],
[
"markdown"
],
[
"code"
],
[
"markdown"
],
[
"code"
],
[
"markdown"
],
[
"code",
"code"
]
] |
d044f5a5b7d50ebf4c2784976f10ab9eee098cfb | 54,652 | ipynb | Jupyter Notebook | agreg/Crime_parfait.ipynb | doc22940/notebooks-2 | 6a7bdec5ed2195005d64ca1f9eaf6613d68fb8ca | [
"MIT"
] | 102 | 2016-06-25T09:30:00.000Z | 2022-03-24T21:02:49.000Z | agreg/Crime_parfait.ipynb | Jimmy-INL/notebooks | ccf5ebc11131f56305c484cfd4556f4bcf63c19b | [
"MIT"
] | 34 | 2016-06-26T12:21:30.000Z | 2021-04-06T09:19:49.000Z | agreg/Crime_parfait.ipynb | Jimmy-INL/notebooks | ccf5ebc11131f56305c484cfd4556f4bcf63c19b | [
"MIT"
] | 44 | 2017-05-13T23:54:56.000Z | 2021-07-17T15:34:24.000Z | 34.633714 | 4,309 | 0.528764 | [
[
[
"# Table of Contents\n <p><div class=\"lev1 toc-item\"><a href=\"#Texte-d'oral-de-modélisation---Agrégation-Option-Informatique\" data-toc-modified-id=\"Texte-d'oral-de-modélisation---Agrégation-Option-Informatique-1\"><span class=\"toc-item-num\">1 </span>Texte d'oral de modélisation - Agrégation Option Informatique</a></div><div class=\"lev2 toc-item\"><a href=\"#Préparation-à-l'agrégation---ENS-de-Rennes,-2016-17\" data-toc-modified-id=\"Préparation-à-l'agrégation---ENS-de-Rennes,-2016-17-11\"><span class=\"toc-item-num\">1.1 </span>Préparation à l'agrégation - ENS de Rennes, 2016-17</a></div><div class=\"lev2 toc-item\"><a href=\"#À-propos-de-ce-document\" data-toc-modified-id=\"À-propos-de-ce-document-12\"><span class=\"toc-item-num\">1.2 </span>À propos de ce document</a></div><div class=\"lev2 toc-item\"><a href=\"#Implémentation\" data-toc-modified-id=\"Implémentation-13\"><span class=\"toc-item-num\">1.3 </span>Implémentation</a></div><div class=\"lev3 toc-item\"><a href=\"#Une-bonne-structure-de-donnée-pour-des-intervalles-et-des-graphes-d'intervales\" data-toc-modified-id=\"Une-bonne-structure-de-donnée-pour-des-intervalles-et-des-graphes-d'intervales-131\"><span class=\"toc-item-num\">1.3.1 </span>Une bonne structure de donnée pour des intervalles et des graphes d'intervales</a></div><div class=\"lev3 toc-item\"><a href=\"#Algorithme-de-coloriage-de-graphe-d'intervalles\" data-toc-modified-id=\"Algorithme-de-coloriage-de-graphe-d'intervalles-132\"><span class=\"toc-item-num\">1.3.2 </span>Algorithme de coloriage de graphe d'intervalles</a></div><div class=\"lev3 toc-item\"><a href=\"#Algorithme-pour-calculer-le-stable-maximum-d'un-graphe-d'intervalles\" data-toc-modified-id=\"Algorithme-pour-calculer-le-stable-maximum-d'un-graphe-d'intervalles-133\"><span class=\"toc-item-num\">1.3.3 </span>Algorithme pour calculer le <em>stable maximum</em> d'un graphe d'intervalles</a></div><div class=\"lev2 toc-item\"><a href=\"#Exemples\" data-toc-modified-id=\"Exemples-14\"><span class=\"toc-item-num\">1.4 </span>Exemples</a></div><div class=\"lev3 toc-item\"><a href=\"#Qui-a-tué-le-Duc-de-Densmore-?\" data-toc-modified-id=\"Qui-a-tué-le-Duc-de-Densmore-?-141\"><span class=\"toc-item-num\">1.4.1 </span>Qui a tué le Duc de Densmore ?</a></div><div class=\"lev4 toc-item\"><a href=\"#Comment-résoudre-ce-problème-?\" data-toc-modified-id=\"Comment-résoudre-ce-problème-?-1411\"><span class=\"toc-item-num\">1.4.1.1 </span>Comment résoudre ce problème ?</a></div><div class=\"lev4 toc-item\"><a href=\"#Solution\" data-toc-modified-id=\"Solution-1412\"><span class=\"toc-item-num\">1.4.1.2 </span>Solution</a></div><div class=\"lev3 toc-item\"><a href=\"#Le-problème-des-frigos\" data-toc-modified-id=\"Le-problème-des-frigos-142\"><span class=\"toc-item-num\">1.4.2 </span>Le problème des frigos</a></div><div class=\"lev3 toc-item\"><a href=\"#Le-problème-du-CSA\" data-toc-modified-id=\"Le-problème-du-CSA-143\"><span class=\"toc-item-num\">1.4.3 </span>Le problème du CSA</a></div><div class=\"lev3 toc-item\"><a href=\"#Le-problème-du-wagon-restaurant\" data-toc-modified-id=\"Le-problème-du-wagon-restaurant-144\"><span class=\"toc-item-num\">1.4.4 </span>Le problème du wagon restaurant</a></div><div class=\"lev4 toc-item\"><a href=\"#Solution-via-l'algorithme-de-coloriage-de-graphe-d'intervalles\" data-toc-modified-id=\"Solution-via-l'algorithme-de-coloriage-de-graphe-d'intervalles-1441\"><span class=\"toc-item-num\">1.4.4.1 </span>Solution via l'algorithme de coloriage de graphe d'intervalles</a></div><div class=\"lev2 toc-item\"><a href=\"#Bonus-?\" data-toc-modified-id=\"Bonus-?-15\"><span class=\"toc-item-num\">1.5 </span>Bonus ?</a></div><div class=\"lev3 toc-item\"><a href=\"#Visualisation-des-graphes-définis-dans-les-exemples\" data-toc-modified-id=\"Visualisation-des-graphes-définis-dans-les-exemples-151\"><span class=\"toc-item-num\">1.5.1 </span>Visualisation des graphes définis dans les exemples</a></div><div class=\"lev2 toc-item\"><a href=\"#Conclusion\" data-toc-modified-id=\"Conclusion-16\"><span class=\"toc-item-num\">1.6 </span>Conclusion</a></div>",
"_____no_output_____"
],
[
"# Texte d'oral de modélisation - Agrégation Option Informatique\n## Préparation à l'agrégation - ENS de Rennes, 2016-17\n- *Date* : 3 avril 2017\n- *Auteur* : [Lilian Besson](https://GitHub.com/Naereen/notebooks/)\n- *Texte*: Annale 2006, \"Crime Parfait\"",
"_____no_output_____"
],
[
"## À propos de ce document\n- Ceci est une *proposition* de correction, partielle et probablement non-optimale, pour la partie implémentation d'un [texte d'annale de l'agrégation de mathématiques, option informatique](http://Agreg.org/Textes/).\n- Ce document est un [notebook Jupyter](https://www.Jupyter.org/), et [est open-source sous Licence MIT sur GitHub](https://github.com/Naereen/notebooks/tree/master/agreg/), comme les autres solutions de textes de modélisation que [j](https://GitHub.com/Naereen)'ai écrite cette année.\n- L'implémentation sera faite en OCaml, version 4+ :",
"_____no_output_____"
]
],
[
[
"Sys.command \"ocaml -version\";;",
"The OCaml toplevel, version 4.04.2\n"
]
],
[
[
"----\n## Implémentation\nLa question d'implémentation était la question 2) en page 7.\n\n> « Proposer une structure de donnée adaptée pour représenter un graphe d'intervalles dont une représentation sous forme de famille d’intervalles est connue.\n> Implémenter de manière efficace l’algorithme de coloriage de graphes d'intervalles et illustrer cet algorithme sur une application bien choisie citée dans le texte. »\n\nNous allons donc d'abord définir une structure de donnée pour une famille d'intervalles ainsi que pour un graphe d'intervalle, ainsi qu'une fonction convertissant l'un en l'autre.\n\nCela permettra de facilement définr les différents exemples du texte, et de les résoudre.",
"_____no_output_____"
],
[
"### Une bonne structure de donnée pour des intervalles et des graphes d'intervales\n\n- Pour des **intervalles** à valeurs réelles, on se restreint par convénience à des valeurs entières.",
"_____no_output_____"
]
],
[
[
"type intervalle = (int * int);;\ntype intervalles = intervalle list;;",
"_____no_output_____"
]
],
[
[
"- Pour des **graphes d'intervalles**, on utilise une simple représentation sous forme de liste d'adjacence, plus facile à mettre en place en OCaml qu'une représentation sous forme de matrice. Ici, tous nos graphes ont pour sommets $0 \\dots n - 1$.",
"_____no_output_____"
]
],
[
[
"type sommet = int;;\ntype voisins = sommet list;;\ntype graphe_intervalle = voisins list;;",
"_____no_output_____"
]
],
[
[
"> *Note:* j'ai préféré garder une structure très simple, pour les intervalles, les graphes d'intervalles et les coloriages, mais on perd un peu en lisibilité dans la fonction coloriage.\n> \n> Implicitement, dès qu'une liste d'intervalles est fixée, de taille $n$, ils sont numérotés de $0$ à $n-1$. Le graphe `g` aura pour sommet $0 \\dots n-1$, et le coloriage sera un simple tableau de couleurs `c` (i.e., d'entiers), donnant en `c[i]` la couleur de l'intervalle numéro `i`.\n>\n> Une solution plus intelligente aurait été d'utiliser des tables d'association, cf. le module [Map](http://caml.inria.fr/pub/docs/manual-ocaml/libref/Map.html) de OCaml, et le code proposé par Julien durant son oral.",
"_____no_output_____"
],
[
"- On peut rapidement écrire une fonction qui va convertir une liste d'intervalle (`intervalles`) en un graphe d'intervalle. On crée les sommets du graphes, via `index_intvls` qui associe un intervalle à son indice, et ensuite on ajoute les arêtes au graphe selon les contraintes définissant un graphe d'intervalle :\n\n $$ \\forall I, I' \\in V, (I,I') \\in E \\Leftrightarrow I \\neq I' \\;\\text{and}\\; I \\cap I' \\neq \\emptyset $$\n \n Donc avec des intervales $I = [x,y]$ et $I' = [a,b]$, cela donne :\n\n $$ \\forall I = [x,y], I' = [a,b] \\in V, (I,I') \\in E \\Leftrightarrow (x,y) \\neq (a,b) \\;\\text{and}\\; \\neg (b < x \\;\\text{or}\\; y < a) $$",
"_____no_output_____"
]
],
[
[
"let graphe_depuis_intervalles (intvls : intervalles) : graphe_intervalle =\n let n = List.length intvls in (* Nomber de sommet *)\n let array_intvls = Array.of_list intvls in (* Tableau des intervalles *)\n let index_intvls = Array.to_list (\n Array.init n (fun i -> (\n array_intvls.(i), i) (* Associe un intervalle à son indice *)\n )\n ) in\n let gr = List.map (fun (a, b) -> (* Pour chaque intervalle [a, b] *)\n List.filter (fun (x, y) -> (* On ajoute [x, y] s'il intersecte [a, b] *)\n (x, y) <> (a, b) (* Intervalle différent *)\n && not ( (b < x) || (y < a) ) (* pas x---y a---b ni a---b x---y *)\n ) intvls\n ) intvls in\n (* On transforme la liste de liste d'intervalles en une liste de liste d'entiers *)\n List.map (fun voisins ->\n List.map (fun sommet -> (* Grace au tableau index_intvls *)\n List.assoc sommet index_intvls\n ) voisins\n ) gr\n;;",
"_____no_output_____"
]
],
[
[
"### Algorithme de coloriage de graphe d'intervalles\n\n> Étant donné un graphe $G = (V, E)$, on cherche un entier $n$ minimal et une fonction $c : V \\to \\{1, \\cdots, n\\}$ telle que si $(v_1 , v_2) \\in E$, alors $c(v_1) \\neq c(v_2)$.\n\nOn suit les indications de l'énoncé pour implémenter facilement cet algorithme.\n\n> Une *heuristique* simple pour résoudre ce problème consiste à appliquer l’algorithme glouton suivant :\n> - tant qu'il reste reste des sommets non coloriés,\n> + en choisir un\n> + et le colorier avec le plus petit entier qui n’apparait pas dans les voisins déjà coloriés.\n\n\n> En choisissant bien le nouveau sommet à colorier à chaque fois, cette heuristique se révelle optimale pour les graphes d’intervalles.\n\nOn peut d'abord définir un type de donnée pour un coloriage, sous la forme d'une liste de couple d'intervalle et de couleur.\nAinsi, `List.assoc` peut être utilisée pour donner le coloriage de chaque intervalle.",
"_____no_output_____"
]
],
[
[
"type couleur = int;;\ntype coloriage = (intervalle * couleur) list;;\n\nlet coloriage_depuis_couleurs (intvl : intervalles) (c : couleur array) : coloriage =\n Array.to_list (Array.init (Array.length c) (fun i -> (List.nth intvl i), c.(i)));;\n\nlet quelle_couleur (intvl : intervalle) (colors : coloriage) = \n List.assoc intvl colors\n;;",
"_____no_output_____"
]
],
[
[
"Ensuite, l'ordre partiel $\\prec_i$ sur les intervalles est défini comme ça :\n\n$$ I = (a,b) \\prec_i J=(x, y) \\Longleftrightarrow a < x $$",
"_____no_output_____"
]
],
[
[
"let ordre_partiel ((a, _) : intervalle) ((x, _) : intervalle) =\n a < x\n;;",
"_____no_output_____"
]
],
[
[
"On a ensuite besoin d'une fonction qui va calculer l'inf de $\\mathbb{N} \\setminus \\{x : x \\in \\mathrm{valeurs} \\}$:",
"_____no_output_____"
]
],
[
[
"let inf_N_minus valeurs =\n let res = ref 0 in (* Très important d'utiliser une référence ! *)\n while List.mem !res valeurs do\n incr res;\n done;\n !res\n;;",
"_____no_output_____"
]
],
[
[
"On vérifie rapidement sur deux exemples :",
"_____no_output_____"
]
],
[
[
"inf_N_minus [0; 1; 3];; (* 2 *)\ninf_N_minus [0; 1; 2; 3; 4; 5; 6; 10];; (* 7 *)",
"_____no_output_____"
]
],
[
[
"Enfin, on a besoin d'une fonction pour trouver l'intervalle $I \\in V$, minimal pour $\\prec_i$, tel que $c(I) = +\\infty$.",
"_____no_output_____"
]
],
[
[
"let trouve_min_interval intvl (c : coloriage) (inf : couleur) =\n let colorie inter = quelle_couleur inter c in\n (* D'abord on extraie {I : c(I) = +oo} *)\n let intvl2 = List.filter (fun i -> (colorie i) = inf) intvl in\n (* Puis on parcourt la liste et on garde le plus petit pour l'ordre *)\n let i0 = ref 0 in\n for j = 1 to (List.length intvl2) - 1 do\n if ordre_partiel (List.nth intvl2 j) (List.nth intvl2 !i0) then\n i0 := j;\n done;\n List.nth intvl2 !i0;\n;;",
"_____no_output_____"
]
],
[
[
"Et donc tout cela permet de finir l'algorithme, tel que décrit dans le texte :\n\n<img style=\"width:65%;\" alt=\"images/algorithme_coloriage.png\" src=\"images/algorithme_coloriage.png\">",
"_____no_output_____"
]
],
[
[
"let coloriage_intervalles (intvl : intervalles) : coloriage =\n let n = List.length intvl in (* Nombre d'intervalles *)\n let array_intvls = Array.of_list intvl in (* Tableau des intervalles *)\n let index_intvls = Array.to_list (\n Array.init n (fun i -> (\n array_intvls.(i), i) (* Associe un intervalle à son indice *)\n )\n ) in\n let gr = graphe_depuis_intervalles intvl in\n let inf = n + 10000 in (* Grande valeur, pour +oo *)\n let c = Array.make n inf in (* Liste des couleurs, c(I) = +oo pour tout I *)\n let maxarray = Array.fold_left max (-inf - 10000) in (* Initialisé à -oo *)\n while maxarray c = inf do (* Il reste un I in V tel que c(I) = +oo *)\n begin (* C'est la partie pas élégante *)\n (* On récupère le coloriage depuis la liste de couleurs actuelle *)\n let coloriage = (coloriage_depuis_couleurs intvl c) in\n (* Puis la fonction [colorie] pour associer une couleur à un intervalle *)\n let colorie inter = quelle_couleur inter coloriage in\n (* On choisit un I, minimal pour ordre_partiel, tel que c(I) = +oo *)\n let inter = trouve_min_interval intvl coloriage inf in\n (* On trouve son indice *)\n let i = List.assoc inter index_intvls in\n (* On trouve les voisins de i dans le graphe *)\n let adj_de_i = List.nth gr i in\n (* Puis les voisins de I en tant qu'intervalles *)\n let adj_de_I = List.map (fun j -> List.nth intvl j) adj_de_i in\n (* Puis on récupère leurs couleurs *)\n let valeurs = List.map colorie adj_de_I in\n (* c(I) = inf(N - {c(J) : J adjacent a I} ) *)\n c.(i) <- inf_N_minus valeurs;\n end;\n done;\n coloriage_depuis_couleurs intvl c;\n;;",
"_____no_output_____"
]
],
[
[
"Une fois qu'on a un coloriage, à valeurs dans $0,\\dots,k$ on récupère le nombre de couleurs comme $1 + \\max c$, i.e., $k+1$.",
"_____no_output_____"
]
],
[
[
"let max_valeurs = List.fold_left max 0;;",
"_____no_output_____"
],
[
"let nombre_chromatique (colorg : coloriage) : int =\n 1 + max_valeurs (List.map snd colorg)\n;;",
"_____no_output_____"
]
],
[
[
"### Algorithme pour calculer le *stable maximum* d'un graphe d'intervalles\nOn répond ici à la question 7.\n\n> « Proposer un algorithme efficace pour construire un stable maximum (i.e., un ensemble de sommets indépendants) d'un graphe d’intervalles dont on connaı̂t une représentation sous forme d'intervalles.\n> On pourra chercher à quelle condition l'intervalle dont l'extrémité droite est la plus à gauche appartient à un stable maximum. »",
"_____no_output_____"
],
[
"**FIXME, je ne l'ai pas encore fait.**",
"_____no_output_____"
],
[
"----\n## Exemples\nOn traite ici l'exemple introductif, ainsi que les trois autres exemples proposés.",
"_____no_output_____"
],
[
"### Qui a tué le Duc de Densmore ?\n\n> On ne rappelle pas le problème, mais les données :\n\n> - Ann dit avoir vu Betty, Cynthia, Emily, Felicia et Georgia.\n- Betty dit avoir vu Ann, Cynthia et Helen.\n- Cynthia dit avoir vu Ann, Betty, Diana, Emily et Helen.\n- Diana dit avoir vu Cynthia et Emily.\n- Emily dit avoir vu Ann, Cynthia, Diana et Felicia.\n- Felicia dit avoir vu Ann et Emily.\n- Georgia dit avoir vu Ann et Helen.\n- Helen dit avoir vu Betty, Cynthia et Georgia.\n\nTranscrit sous forme de graphe, cela donne :",
"_____no_output_____"
]
],
[
[
"(* On définit des entiers, c'est plus simple *)\nlet ann = 0\nand betty = 1\nand cynthia = 2\nand diana = 3\nand emily = 4\nand felicia = 5\nand georgia = 6\nand helen = 7;;\n\nlet graphe_densmore = [\n [betty; cynthia; emily; felicia; georgia]; (* Ann *)\n [ann; cynthia; helen]; (* Betty *)\n [ann; betty; diana; emily; helen]; (* Cynthia *)\n [cynthia; emily]; (* Diana *)\n [ann; cynthia; diana; felicia]; (* Emily *)\n [ann; emily]; (* Felicia *)\n [ann; helen]; (* Georgia *)\n [betty; cynthia; georgia] (* Helen *)\n];;",
"_____no_output_____"
]
],
[
[
"\n> Figure 1. Graphe d'intervalle pour le problème de l'assassinat du duc de Densmore.",
"_____no_output_____"
],
[
"Avec les prénoms plutôt que des numéros, cela donne :",
"_____no_output_____"
],
[
"\n> Figure 2. Graphe d'intervalle pour le problème de l'assassinat du duc de Densmore.",
"_____no_output_____"
],
[
"#### Comment résoudre ce problème ?\n> Il faut utiliser la caractérisation du théorème 2 du texte, et la définition des graphes parfaits.\n\n- Définition + Théorème 2 (point 1) :\n\nOn sait qu'un graphe d'intervalle est parfait, et donc tous ses graphes induits le sont aussi.\nLa caractérisation via les cordes sur les cycles de taille $\\geq 4$ permet de dire qu'un quadrilatère (cycle de taille $4$) n'est pas un graphe d'intervalle.\nDonc un graphe qui contient un graphe induit étant un quadrilatère ne peut être un graphe d'intervalle.\n\nAinsi, sur cet exemple, comme on a deux quadrilatères $A B H G$ et $A G H C$, on en déduit que $A$, $G$, ou $H$ ont menti.\n\n- Théorème 2 (point 2) :\n\nEnsuite, si on enlève $G$ ou $H$, le graphe ne devient pas un graphe d'intervalle, par les considérations suivantes, parce que son complémentaire n'est pas un graphe de comparaison.\n\nEn effet, par exemple si on enlève $G$, $A$ et $H$ et $D$ forment une clique dans le complémentaire $\\overline{G}$ de $G$, et l'irréflexivité d'une éventuelle relation $R$ rend cela impossible. Pareil si on enlève $H$, avec $G$ et $B$ et $D$ qui formet une clique dans $\\overline{G}$.\n\nPar contre, si on enlève $A$, le graphe devient triangulé (et de comparaison, mais c'est plus dur à voir !).\n\nDonc seule $A$ reste comme potentielle menteuse.",
"_____no_output_____"
],
[
"> « Mais... Ça semble difficile de programmer une résolution automatique de ce problème ? »\n\nEn fait, il suffit d'écrire une fonction de vérification qu'un graphe est un graphe d'intervalle, puis on essaie d'enlever chaque sommet, tant que le graphe n'est pas un graphe d'intervalle.\n\nSi le graphe devient valide en enlevant un seul sommet, et qu'il n'y en a qu'un seul qui fonctionne, alors il y a un(e) seul(e) menteur(se) dans le graphe, et donc un(e) seul(e) coupable !",
"_____no_output_____"
],
[
"#### Solution\n\nC'est donc $A$, i.e., Ann l'unique menteuse et donc la coupable.\n\n> Ce n'est pas grave de ne pas avoir réussi à répondre durant l'oral !\n> Au contraire, vous avez le droit de vous détacher du problème initial du texte !",
"_____no_output_____"
],
[
"> Une solution bien expliquée peut être trouvée dans [cette vidéo](https://youtu.be/ZGhSyVvOelg) :",
"_____no_output_____"
],
[
"<iframe width=\"640\" height=\"360\" src=\"https://www.youtube.com/embed/ZGhSyVvOelg\" frameborder=\"1\" allowfullscreen></iframe>",
"_____no_output_____"
],
[
"### Le problème des frigos\n> Dans un grand hopital, les réductions de financement public poussent le gestionnaire du service d'immunologie à faire des économies sur le nombre de frigos à acheter pour stocker les vaccins. A peu de chose près, il lui faut stocker les vaccins suivants :\n\n> | Numéro | Nom du vaccin | Température de conservation\n| :-----: | :------------ | -------------------------: |\n| 0 | Rougeole-Rubéole-Oreillons (RRO) | $4 \\cdots 12$ °C\n| 1 | BCG | $8 \\cdots 15$ °C\n| 2 | Di-Te-Per | $0 \\cdots 20$ °C\n| 3 | Anti-polio | $2 \\cdots 3$ °C\n| 4 | Anti-hépatite B | $-3 \\cdots 6$ °C\n| 5 | Anti-amarile | $-10 \\cdots 10$ °C\n| 6 | Variole | $6 \\cdots 20$ °C\n| 7 | Varicelle | $-5 \\cdots 2$ °C\n| 8 | Antihaemophilus | $-2 \\cdots 8$ °C\n\n> Combien le gestionaire doit-il acheter de frigos, et sur quelles températures doit-il les régler ?",
"_____no_output_____"
]
],
[
[
"let vaccins : intervalles = [\n (4, 12);\n (8, 15);\n (0, 20);\n (2, 3);\n (-3, 6);\n (-10, 10);\n (6, 20);\n (-5, 2);\n (-2, 8)\n]",
"_____no_output_____"
]
],
[
[
"Qu'on peut visualiser sous forme de graphe facilement :",
"_____no_output_____"
]
],
[
[
"let graphe_vaccins = graphe_depuis_intervalles vaccins;;",
"_____no_output_____"
]
],
[
[
"\n> Figure 3. Graphe d'intervalle pour le problème des frigos et des vaccins.",
"_____no_output_____"
],
[
"Avec des intervalles au lieu de numéro :",
"_____no_output_____"
],
[
"\n> Figure 4. Graphe d'intervalle pour le problème des frigos et des vaccins.",
"_____no_output_____"
],
[
"On peut récupérer une coloriage minimal pour ce graphe :",
"_____no_output_____"
]
],
[
[
"coloriage_intervalles vaccins;;",
"_____no_output_____"
]
],
[
[
"La couleur la plus grande est `5`, donc le nombre chromatique de ce graphe est `6`.",
"_____no_output_____"
]
],
[
[
"nombre_chromatique (coloriage_intervalles vaccins);;",
"_____no_output_____"
]
],
[
[
"Par contre, la solution au problème des frigos et des vaccins réside dans le nombre de couverture de cliques, $k(G)$, pas dans le nombre chromatique $\\chi(G)$.\n\nOn peut le résoudre en répondant à la question 7, qui demandait de mettre au point un algorithme pour construire un *stable maximum* pour un graphe d'intervalle.",
"_____no_output_____"
],
[
"### Le problème du CSA\n\n> Le Conseil Supérieur de l’Audiovisuel doit attribuer de nouvelles bandes de fréquences d’émission pour la stéréophonie numérique sous-terraine (SNS).\n> Cette technologie de pointe étant encore à l'état expérimental, les appareils capables d'émettre ne peuvent utiliser que les bandes de fréquences FM suivantes :\n\n> | Bandes de fréquence | Intervalle (kHz) |\n| :-----------------: | ---------: |\n| 0 | $32 \\cdots 36$ |\n| 1 | $24 \\cdots 30$ |\n| 2 | $28 \\cdots 33$ |\n| 3 | $22 \\cdots 26$ |\n| 4 | $20 \\cdots 25$ |\n| 5 | $30 \\cdots 33$ |\n| 6 | $31 \\cdots 34$ |\n| 7 | $27 \\cdots 31$ |\n\n> Quelles bandes de fréquences doit-on retenir pour permettre à le plus d'appareils possibles d'être utilisés, sachant que deux appareils dont les bandes de fréquences s'intersectent pleinement (pas juste sur les extrémités) sont incompatibles.",
"_____no_output_____"
]
],
[
[
"let csa : intervalles = [\n (32, 36);\n (24, 30);\n (28, 33);\n (22, 26);\n (20, 25);\n (30, 33);\n (31, 34);\n (27, 31)\n];;",
"_____no_output_____"
],
[
"let graphe_csa = graphe_depuis_intervalles csa;;",
"_____no_output_____"
]
],
[
[
"\n> Figure 5. Graphe d'intervalle pour le problème du CSA.",
"_____no_output_____"
],
[
"Avec des intervalles au lieu de numéro :",
"_____no_output_____"
],
[
"\n> Figure 6. Graphe d'intervalle pour le problème du CSA.",
"_____no_output_____"
],
[
"On peut récupérer une coloriage minimal pour ce graphe :",
"_____no_output_____"
]
],
[
[
"coloriage_intervalles csa;;",
"_____no_output_____"
]
],
[
[
"La couleur la plus grande est `3`, donc le nombre chromatique de ce graphe est `4`.",
"_____no_output_____"
]
],
[
[
"nombre_chromatique (coloriage_intervalles csa);;",
"_____no_output_____"
]
],
[
[
"Par contre, la solution au problème CSA réside dans le nombre de couverture de cliques, $k(G)$, pas dans le nombre chromatique $\\chi(G)$.\n\nOn peut le résoudre en répondant à la question 7, qui demandait de mettre au point un algorithme pour construire un *stable maximum* pour un graphe d'intervalle.",
"_____no_output_____"
],
[
"### Le problème du wagon restaurant\n\n> Le chef de train de l'Orient Express doit aménager le wagon restaurant avant le départ du train. Ce wagon est assez petit et doit être le moins encombré de tables possibles, mais il faut prévoir suffisemment de tables pour accueillir toutes personnes qui ont réservé :\n\n> | Numéro | Personnage(s) | Heures de dîner | En secondes |\n| :----------------- | --------- | :---------: | :---------: |\n| 0 | Le baron et la baronne Von Haussplatz | 19h30 .. 20h14 | $1170 \\cdots 1214$\n| 1 | Le général Cook | 20h30 .. 21h59 | $1230 \\cdots 1319$\n| 2 | Les époux Steinberg | 19h .. 19h59 | $1140 \\cdots 1199$\n| 3 | La duchesse de Colombart | 20h15 .. 20h59 | $1215 \\cdots 1259$\n| 4 | Le marquis de Carquamba | 21h .. 21h59 | $1260 \\cdots 1319$\n| 5 | La Vociafiore | 19h15 .. 20h29 | $1155 \\cdots 1229$\n| 6 | Le colonel Ferdinand | 20h .. 20h59 | $1200 \\cdots 1259$\n\n\n> Combien de tables le chef de train doit-il prévoir ?",
"_____no_output_____"
]
],
[
[
"let restaurant = [\n (1170, 1214);\n (1230, 1319);\n (1140, 1199);\n (1215, 1259);\n (1260, 1319);\n (1155, 1229);\n (1200, 1259)\n];;",
"_____no_output_____"
],
[
"let graphe_restaurant = graphe_depuis_intervalles restaurant;;",
"_____no_output_____"
]
],
[
[
"\n> Figure 7. Graphe d'intervalle pour le problème du wagon restaurant.",
"_____no_output_____"
],
[
"Avec des intervalles au lieu de numéro :",
"_____no_output_____"
],
[
"\n> Figure 8. Graphe d'intervalle pour le problème du wagon restaurant.",
"_____no_output_____"
]
],
[
[
"coloriage_intervalles restaurant;;",
"_____no_output_____"
]
],
[
[
"La couleur la plus grande est `2`, donc le nombre chromatique de ce graphe est `3`.",
"_____no_output_____"
]
],
[
[
"nombre_chromatique (coloriage_intervalles restaurant);;",
"_____no_output_____"
]
],
[
[
"#### Solution via l'algorithme de coloriage de graphe d'intervalles\nPour ce problème là, la solution est effectivement donnée par le nombre chromatique.\n\nLa couleur sera le numéro de table pour chaque passagers (ou couple de passagers), et donc le nombre minimal de table à installer dans le wagon restaurant est exactement le nombre chromatique.\n\nUne solution peut être la suivante, avec **3 tables** :\n\n| Numéro | Personnage(s) | Heures de dîner | Numéro de table |\n| :----------------- | --------- | :---------: | :---------: |\n| 0 | Le baron et la baronne Von Haussplatz | 19h30 .. 20h14 | 2\n| 1 | Le général Cook | 20h30 .. 21h59 | 1\n| 2 | Les époux Steinberg | 19h .. 19h59 | 0\n| 3 | La duchesse de Colombart | 20h15 .. 20h59 | 2\n| 4 | Le marquis de Carquamba | 21h .. 21h59 | 0\n| 5 | La Vociafiore | 19h15 .. 20h29 | 1\n| 6 | Le colonel Ferdinand | 20h .. 20h59 | 0\n\nOn vérifie manuellement que la solution convient.\nChaque passager devra quitter sa tableau à la minute près par contre !",
"_____no_output_____"
],
[
"On peut afficher la solution avec un graphe colorié.\nLa table `0` sera <span style=\"color:red;\">rouge</span>, `1` sera <span style=\"color:blue;\">bleu</span> et `2` sera <span style=\"color:yellow;\">jaune</span> :",
"_____no_output_____"
],
[
"\n> Figure 9. Solution pour le problème du wagon restaurant.",
"_____no_output_____"
],
[
"----\n## Bonus ?",
"_____no_output_____"
],
[
"### Visualisation des graphes définis dans les exemples\n\n- J'utilise une petite fonction facile à écrire, qui convertit un graphe (`int list list`) en une chaîne de caractère au format [DOT Graph](http://www.graphviz.org/doc/info/lang.html).\n- Ensuite, un appel `dot -Tpng ...` en ligne de commande convertit ce graphe en une image, que j'inclus ensuite manuellement.",
"_____no_output_____"
]
],
[
[
"(** Transforme un [graph] en une chaîne représentant un graphe décrit par le langage DOT,\n voir http://en.wikipedia.org/wiki/DOT_language pour plus de détails sur ce langage.\n\n @param graphname Donne le nom du graphe tel que précisé pour DOT\n @param directed Vrai si le graphe doit être dirigé (c'est le cas ici) faux sinon. Change le style des arêtes ([->] ou [--])\n @param verb Affiche tout dans le terminal.\n @param onetoone Si on veut afficher le graphe en mode carré (échelle 1:1). Parfois bizarre, parfois génial.\n*)\nlet graph_to_dotgraph ?(graphname = \"graphname\") ?(directed = false) ?(verb = false) ?(onetoone = false) (glist : int list list) =\n let res = ref \"\" in\n let log s =\n if verb then print_string s; (* Si [verb] affiche dans le terminal le résultat du graphe. *)\n res := !res ^ s\n in\n log (if directed then \"digraph \" else \"graph \");\n log graphname; log \" {\";\n if onetoone then\n log \"\\n size=\\\"1,1\\\";\";\n let g = Array.of_list (List.map Array.of_list glist) in\n (* On affiche directement les arc, un à un. *)\n for i = 0 to (Array.length g) - 1 do\n for j = 0 to (Array.length g.(i)) - 1 do\n if i < g.(i).(j) then\n log (\"\\n \\\"\"\n ^ (string_of_int i) ^ \"\\\" \"\n ^ (if directed then \"->\" else \"--\")\n ^ \" \\\"\" ^ (string_of_int g.(i).(j)) ^ \"\\\"\"\n );\n done;\n done;\n log \"\\n}\\n// generated by OCaml with the function graphe_to_dotgraph.\";\n!res;;",
"_____no_output_____"
],
[
"(** Fonction ecrire_sortie : plus pratique que output. *)\nlet ecrire_sortie monoutchanel machaine =\n output monoutchanel machaine 0 (String.length machaine);\n flush monoutchanel;;\n\n(** Fonction ecrire_dans_fichier : pour écrire la chaine dans le fichier à l'adresse renseignée. *)\nlet ecrire_dans_fichier ~chaine ~adresse =\n let mon_out_channel = open_out adresse in\n ecrire_sortie mon_out_channel chaine;\n close_out mon_out_channel;;\n",
"_____no_output_____"
],
[
"let s_graphe_densmore = graph_to_dotgraph ~graphname:\"densmore\" ~directed:false ~verb:false graphe_densmore;;\nlet s_graphe_vaccins = graph_to_dotgraph ~graphname:\"vaccins\" ~directed:false ~verb:false graphe_vaccins;;\nlet s_graphe_csa = graph_to_dotgraph ~graphname:\"csa\" ~directed:false ~verb:false graphe_csa;;\nlet s_graphe_restaurant = graph_to_dotgraph ~graphname:\"restaurant\" ~directed:false ~verb:false graphe_restaurant;;",
"_____no_output_____"
],
[
"ecrire_dans_fichier ~chaine:s_graphe_densmore ~adresse:\"/tmp/densmore.dot\" ;;\n(* Sys.command \"fdp -Tpng /tmp/densmore.dot > images/densmore.png\";; *)\n\necrire_dans_fichier ~chaine:s_graphe_vaccins ~adresse:\"/tmp/vaccins.dot\" ;;\n(* Sys.command \"fdp -Tpng /tmp/vaccins.dot > images/vaccins.png\";; *)\n\necrire_dans_fichier ~chaine:s_graphe_csa ~adresse:\"/tmp/csa.dot\" ;;\n(* Sys.command \"fdp -Tpng /tmp/csa.dot > images/csa.png\";; *)\n\necrire_dans_fichier ~chaine:s_graphe_restaurant ~adresse:\"/tmp/restaurant.dot\" ;;\n(* Sys.command \"fdp -Tpng /tmp/restaurant.dot > images/restaurant.png\";; *)",
"_____no_output_____"
]
],
[
[
"On pourrait étendre cette fonction pour qu'elle prenne les intervalles initiaux, pour afficher des bonnes étiquettes et pas des entiers, et un coloriage pour colorer directement les noeuds, mais ça prend du temps pour pas grand chose.",
"_____no_output_____"
],
[
"----\n## Conclusion\n\nVoilà pour la question obligatoire de programmation, sur l'algorithme de coloriage.\n\n- on a décomposé le problème en sous-fonctions,\n- on a fait des exemples et *on les garde* dans ce qu'on présente au jury,\n- on a testé la fonction exigée sur de petits exemples et sur un exemple de taille réelle (venant du texte)\n\nEt on a pas essayé de faire *un peu plus*.\nAvec plus de temps, on aurait aussi pu écrire un algorithme pour calculer le stable maximum (ensemble de sommets indépendants de taille maximale).\n\n> Bien-sûr, ce petit notebook ne se prétend pas être une solution optimale, ni exhaustive.",
"_____no_output_____"
]
]
] | [
"markdown",
"code",
"markdown",
"code",
"markdown",
"code",
"markdown",
"code",
"markdown",
"code",
"markdown",
"code",
"markdown",
"code",
"markdown",
"code",
"markdown",
"code",
"markdown",
"code",
"markdown",
"code",
"markdown",
"code",
"markdown",
"code",
"markdown",
"code",
"markdown",
"code",
"markdown",
"code",
"markdown",
"code",
"markdown",
"code",
"markdown",
"code",
"markdown",
"code",
"markdown",
"code",
"markdown",
"code",
"markdown",
"code",
"markdown"
] | [
[
"markdown",
"markdown",
"markdown"
],
[
"code"
],
[
"markdown",
"markdown"
],
[
"code"
],
[
"markdown"
],
[
"code"
],
[
"markdown",
"markdown"
],
[
"code"
],
[
"markdown"
],
[
"code"
],
[
"markdown"
],
[
"code"
],
[
"markdown"
],
[
"code"
],
[
"markdown"
],
[
"code"
],
[
"markdown"
],
[
"code"
],
[
"markdown"
],
[
"code"
],
[
"markdown"
],
[
"code",
"code"
],
[
"markdown",
"markdown",
"markdown",
"markdown"
],
[
"code"
],
[
"markdown",
"markdown",
"markdown",
"markdown",
"markdown",
"markdown",
"markdown",
"markdown",
"markdown"
],
[
"code"
],
[
"markdown"
],
[
"code"
],
[
"markdown",
"markdown",
"markdown",
"markdown"
],
[
"code"
],
[
"markdown"
],
[
"code"
],
[
"markdown",
"markdown"
],
[
"code",
"code"
],
[
"markdown",
"markdown",
"markdown",
"markdown"
],
[
"code"
],
[
"markdown"
],
[
"code"
],
[
"markdown",
"markdown"
],
[
"code",
"code"
],
[
"markdown",
"markdown",
"markdown"
],
[
"code"
],
[
"markdown"
],
[
"code"
],
[
"markdown",
"markdown",
"markdown",
"markdown",
"markdown"
],
[
"code",
"code",
"code",
"code"
],
[
"markdown",
"markdown"
]
] |
d045005661077019f12f205d7e99367a4f850ada | 21,370 | ipynb | Jupyter Notebook | Diseases/tuberculosis/tuberculosis.ipynb | Shaier/DINN | 75ee85f37d31e270bc472d78cfe44fee61b8963b | [
"MIT"
] | 9 | 2021-10-12T04:42:18.000Z | 2022-03-31T03:01:21.000Z | Diseases/tuberculosis/tuberculosis.ipynb | Shaier/DINN | 75ee85f37d31e270bc472d78cfe44fee61b8963b | [
"MIT"
] | null | null | null | Diseases/tuberculosis/tuberculosis.ipynb | Shaier/DINN | 75ee85f37d31e270bc472d78cfe44fee61b8963b | [
"MIT"
] | 3 | 2022-01-08T12:18:35.000Z | 2022-02-16T04:31:39.000Z | 21,370 | 21,370 | 0.621759 | [
[
[
"import torch\nfrom torch.autograd import grad\nimport torch.nn as nn\nfrom numpy import genfromtxt\nimport torch.optim as optim\nimport matplotlib.pyplot as plt\nimport torch.nn.functional as F\nimport math\n\ntuberculosis_data = genfromtxt('tuberculosis.csv', delimiter=',') #in the form of [t, S,L,I,T]\n\ntorch.manual_seed(1234)",
"_____no_output_____"
],
[
"%%time\n\nPATH = 'tuberculosis' \n\nclass DINN(nn.Module):\n def __init__(self, t, S_data, L_data, I_data, T_data):\n super(DINN, self).__init__()\n self.t = torch.tensor(t, requires_grad=True)\n self.t_float = self.t.float()\n self.t_batch = torch.reshape(self.t_float, (len(self.t),1)) #reshape for batch \n self.S = torch.tensor(S_data)\n self.L = torch.tensor(L_data)\n self.I = torch.tensor(I_data)\n self.T = torch.tensor(T_data) \n self.N = torch.tensor(1001)\n\n self.losses = [] #keep the losses\n self.save = 2 #which file to save to\n\n #learnable parameters\n self.delta_tilda = torch.nn.Parameter(torch.rand(1, requires_grad=True)) #torch.tensor(500)\n self.beta_tilda = torch.nn.Parameter(torch.rand(1, requires_grad=True)) #torch.tensor(13)\n self.c_tilda = torch.nn.Parameter(torch.rand(1, requires_grad=True)) #torch.tensor(1)\n self.mu_tilda = torch.nn.Parameter(torch.rand(1, requires_grad=True)) #torch.tensor(0.143)\n self.k_tilda = torch.nn.Parameter(torch.rand(1, requires_grad=True)) #torch.tensor(0.5)\n self.r_1_tilda = torch.nn.Parameter(torch.rand(1, requires_grad=True)) #torch.tensor(2)\n self.r_2_tilda = torch.nn.Parameter(torch.rand(1, requires_grad=True)) #torch.tensor(1)\n self.beta_prime_tilda = torch.nn.Parameter(torch.rand(1, requires_grad=True)) #torch.tensor(13)\n self.d_tilda = torch.nn.Parameter(torch.rand(1, requires_grad=True)) #torch.tensor(0)\n\n #matrices (x4 for S, L, I, T) for the gradients\n self.m1 = torch.zeros((len(self.t), 4)); self.m1[:, 0] = 1\n self.m2 = torch.zeros((len(self.t), 4)); self.m2[:, 1] = 1\n self.m3 = torch.zeros((len(self.t), 4)); self.m3[:, 2] = 1\n self.m4 = torch.zeros((len(self.t), 4)); self.m4[:, 3] = 1\n\n #values for norm\n self.S_max = max(self.S)\n self.S_min = min(self.S)\n self.L_max = max(self.L)\n self.L_min = min(self.L)\n self.I_max = max(self.I)\n self.I_min = min(self.I)\n self.T_max = max(self.T)\n self.T_min = min(self.T)\n\n #normalize \n self.S_hat = (self.S - self.S_min) / (self.S_max - self.S_min)\n self.L_hat = (self.L - self.L_min) / (self.L_max - self.L_min)\n self.I_hat = (self.I - self.I_min) / (self.I_max - self.I_min)\n self.T_hat = (self.T - self.T_min) / (self.T_max - self.T_min)\n\n #NN\n self.net_tuberculosis = self.Net_tuberculosis()\n self.params = list(self.net_tuberculosis.parameters())\n self.params.extend(list([self.delta_tilda ,self.beta_tilda ,self.c_tilda ,self.mu_tilda ,self.k_tilda ,self.r_1_tilda ,self.r_2_tilda ,self.beta_prime_tilda ,self.d_tilda]))\n\n \n #force parameters to be in a range\n @property\n def delta(self):\n return torch.tanh(self.delta_tilda) * 20 + 500 #self.delta_tilda \n @property\n def beta(self):\n return torch.tanh(self.beta_tilda) * 3 + 12 #self.beta_tilda\n @property\n def c(self):\n return torch.tanh(self.c_tilda) * 2 + 1 #self.c_tilda\n @property\n def mu(self):\n return torch.tanh(self.mu_tilda) * 0.1 + 0.2 #self.mu_tilda\n @property\n def k(self):\n return torch.tanh(self.k_tilda) * 0.5 + 0.5 #self.k_tilda\n @property\n def r_1(self):\n return torch.tanh(self.r_1_tilda) + 2 #self.r_1_tilda\n @property\n def r_2(self):\n return torch.tanh(self.r_2_tilda) * 2 + 1 #self.r_2_tilda\n @property\n def beta_prime(self):\n return torch.tanh(self.beta_prime_tilda) * 3 + 12 #self.beta_prime_tilda\n @property\n def d(self):\n return torch.tanh(self.d_tilda) * 0.4 #self.d_tilda\n\n #nets\n class Net_tuberculosis(nn.Module): # input = [t]\n def __init__(self):\n super(DINN.Net_tuberculosis, self).__init__()\n self.fc1=nn.Linear(1, 20) #takes 100 t's\n self.fc2=nn.Linear(20, 20)\n self.fc3=nn.Linear(20, 20)\n self.fc4=nn.Linear(20, 20)\n self.fc5=nn.Linear(20, 20)\n self.fc6=nn.Linear(20, 20)\n self.fc7=nn.Linear(20, 20)\n self.fc8=nn.Linear(20, 20)\n self.out=nn.Linear(20, 4) #outputs S, L, I, T\n\n def forward(self, t):\n tuberculosis=F.relu(self.fc1(t))\n tuberculosis=F.relu(self.fc2(tuberculosis))\n tuberculosis=F.relu(self.fc3(tuberculosis))\n tuberculosis=F.relu(self.fc4(tuberculosis))\n tuberculosis=F.relu(self.fc5(tuberculosis))\n tuberculosis=F.relu(self.fc6(tuberculosis))\n tuberculosis=F.relu(self.fc7(tuberculosis))\n tuberculosis=F.relu(self.fc8(tuberculosis))\n tuberculosis=self.out(tuberculosis)\n return tuberculosis \n\n def net_f(self, t_batch): \n\n tuberculosis_hat = self.net_tuberculosis(t_batch)\n\n S_hat, L_hat, I_hat, T_hat = tuberculosis_hat[:,0], tuberculosis_hat[:,1], tuberculosis_hat[:,2], tuberculosis_hat[:,3]\n\n #S_hat\n tuberculosis_hat.backward(self.m1, retain_graph=True)\n S_hat_t = self.t.grad.clone()\n self.t.grad.zero_()\n\n #L_hat\n tuberculosis_hat.backward(self.m2, retain_graph=True)\n L_hat_t = self.t.grad.clone()\n self.t.grad.zero_()\n\n #I_hat\n tuberculosis_hat.backward(self.m3, retain_graph=True)\n I_hat_t = self.t.grad.clone()\n self.t.grad.zero_()\n \n #T_hat\n tuberculosis_hat.backward(self.m4, retain_graph=True)\n T_hat_t = self.t.grad.clone()\n self.t.grad.zero_()\n\n #unnormalize\n S = self.S_min + (self.S_max - self.S_min) * S_hat\n L = self.L_min + (self.L_max - self.L_min) * L_hat\n I = self.I_min + (self.I_max - self.I_min) * I_hat\n T = self.T_min + (self.T_max - self.T_min) * T_hat\n \n #equations\n f1_hat = S_hat_t - (self.delta - self.beta * self.c * S * I / self.N - self.mu * S) / (self.S_max - self.S_min) \n f2_hat = L_hat_t - (self.beta * self.c * S * I / self.N - (self.mu + self.k + self.r_1) * L + self.beta_prime * self.c * T * 1/self.N) / (self.L_max - self.L_min) \n f3_hat = I_hat_t - (self.k*L - (self.mu + self.d) * I - self.r_2 * I) / (self.I_max - self.I_min) \n f4_hat = T_hat_t - (self.r_1 * L + self.r_2 * I - self.beta_prime * self.c * T * 1/self.N - self.mu*T) / (self.T_max - self.T_min) \n\n return f1_hat, f2_hat, f3_hat, f4_hat, S_hat, L_hat, I_hat, T_hat\n \n def load(self):\n # Load checkpoint\n try:\n checkpoint = torch.load(PATH + str(self.save)+'.pt') \n print('\\nloading pre-trained model...')\n self.load_state_dict(checkpoint['model'])\n self.optimizer.load_state_dict(checkpoint['optimizer_state_dict'])\n self.scheduler.load_state_dict(checkpoint['scheduler'])\n epoch = checkpoint['epoch']\n loss = checkpoint['loss']\n self.losses = checkpoint['losses']\n print('loaded previous loss: ', loss)\n except RuntimeError :\n print('changed the architecture, ignore')\n pass\n except FileNotFoundError:\n pass\n\n def train(self, n_epochs):\n #try loading\n self.load()\n\n #train\n print('\\nstarting training...\\n')\n \n for epoch in range(n_epochs):\n #lists to hold the output (maintain only the final epoch)\n S_pred_list = []\n L_pred_list = []\n I_pred_list = []\n T_pred_list = []\n\n f1_hat, f2_hat, f3_hat, f4_hat, S_hat_pred, L_hat_pred, I_hat_pred, T_hat_pred = self.net_f(self.t_batch)\n self.optimizer.zero_grad()\n\n S_pred_list.append(self.S_min + (self.S_max - self.S_min) * S_hat_pred)\n L_pred_list.append(self.L_min + (self.L_max - self.L_min) * L_hat_pred)\n I_pred_list.append(self.I_min + (self.I_max - self.I_min) * I_hat_pred)\n T_pred_list.append(self.T_min + (self.T_max - self.T_min) * T_hat_pred)\n\n loss = (\n torch.mean(torch.square(self.S_hat - S_hat_pred)) + torch.mean(torch.square(self.L_hat - L_hat_pred)) + \n torch.mean(torch.square(self.I_hat - I_hat_pred)) + torch.mean(torch.square(self.T_hat - T_hat_pred))+\n torch.mean(torch.square(f1_hat)) + torch.mean(torch.square(f2_hat)) +\n torch.mean(torch.square(f3_hat)) + torch.mean(torch.square(f4_hat))\n )\n\n loss.backward()\n\n self.optimizer.step()\n self.scheduler.step() \n # self.scheduler.step(loss) \n\n self.losses.append(loss.item())\n\n if epoch % 1000 == 0: \n print('\\nEpoch ', epoch)\n\n #loss + model parameters update\n if epoch % 4000 == 9999:\n #checkpoint save\n print('\\nSaving model... Loss is: ', loss)\n torch.save({\n 'epoch': epoch,\n 'model': self.state_dict(),\n 'optimizer_state_dict': self.optimizer.state_dict(),\n 'scheduler': self.scheduler.state_dict(),\n 'loss': loss,\n 'losses': self.losses,\n }, PATH + str(self.save)+'.pt')\n if self.save % 2 > 0: #its on 3\n self.save = 2 #change to 2\n else: #its on 2\n self.save = 3 #change to 3\n\n print('epoch: ', epoch)\n print('#################################')\n \n #plot\n plt.plot(self.losses, color = 'teal')\n plt.xlabel('Epochs')\n plt.ylabel('Loss')\n return S_pred_list, L_pred_list, I_pred_list, T_pred_list",
"_____no_output_____"
],
[
"%%time\n\ndinn = DINN(tuberculosis_data[0], tuberculosis_data[1], tuberculosis_data[2], tuberculosis_data[3], tuberculosis_data[4])\n\nlearning_rate = 1e-3\noptimizer = optim.Adam(dinn.params, lr = learning_rate)\ndinn.optimizer = optimizer\n\nscheduler = torch.optim.lr_scheduler.CyclicLR(dinn.optimizer, base_lr=1e-7, max_lr=1e-3, step_size_up=1000, mode=\"exp_range\", gamma=0.85, cycle_momentum=False)\n\ndinn.scheduler = scheduler\n\ntry: \n S_pred_list, L_pred_list, I_pred_list, T_pred_list = dinn.train(1) #train\nexcept EOFError:\n if dinn.save == 2:\n dinn.save = 3\n S_pred_list, L_pred_list, I_pred_list, T_pred_list = dinn.train(1) #train\n elif dinn.save == 3:\n dinn.save = 2\n S_pred_list, L_pred_list, I_pred_list, T_pred_list = dinn.train(1) #train",
"_____no_output_____"
],
[
"plt.plot(dinn.losses[3000000:], color = 'teal')\nplt.xlabel('Epochs')\nplt.ylabel('Loss')",
"_____no_output_____"
],
[
"fig = plt.figure(figsize=(12,12))\n\nax = fig.add_subplot(111, facecolor='#dddddd', axisbelow=True)\nax.set_facecolor('xkcd:white')\n\nax.scatter(tuberculosis_data[0], tuberculosis_data[1], color = 'pink', alpha=0.5, lw=2, label='S Data', s=20)\nax.plot(tuberculosis_data[0], S_pred_list[0].detach().numpy(), 'navy', alpha=0.9, lw=2, label='S Prediction', linestyle='dashed')\n\nax.scatter(tuberculosis_data[0], tuberculosis_data[2], color = 'violet', alpha=0.5, lw=2, label='L Data', s=20)\nax.plot(tuberculosis_data[0], L_pred_list[0].detach().numpy(), 'dodgerblue', alpha=0.9, lw=2, label='L Prediction', linestyle='dashed')\n\nax.scatter(tuberculosis_data[0], tuberculosis_data[3], color = 'darkgreen', alpha=0.5, lw=2, label='I Data', s=20)\nax.plot(tuberculosis_data[0], I_pred_list[0].detach().numpy(), 'gold', alpha=0.9, lw=2, label='I Prediction', linestyle='dashed')\n\nax.scatter(tuberculosis_data[0], tuberculosis_data[4], color = 'red', alpha=0.5, lw=2, label='T Data', s=20)\nax.plot(tuberculosis_data[0], T_pred_list[0].detach().numpy(), 'blue', alpha=0.9, lw=2, label='T Prediction', linestyle='dashed')\n\n\nax.set_xlabel('Time /days',size = 20)\nax.set_ylabel('Number',size = 20)\n#ax.set_ylim([-1,50])\nax.yaxis.set_tick_params(length=0)\nax.xaxis.set_tick_params(length=0)\nplt.xticks(size = 20)\nplt.yticks(size = 20)\n# ax.grid(b=True, which='major', c='black', lw=0.2, ls='-')\nlegend = ax.legend(prop={'size':20})\nlegend.get_frame().set_alpha(0.5)\nfor spine in ('top', 'right', 'bottom', 'left'):\n ax.spines[spine].set_visible(False)\nplt.savefig('tuberculosis.pdf')\nplt.show()",
"_____no_output_____"
],
[
"#vaccination! \n\nimport numpy as np\nfrom scipy.integrate import odeint\nimport matplotlib.pyplot as plt\n\n# Initial conditions\nS0 = 1000\nL0 = 0\nI0 = 1\nT0 = 0\nN = 1001 #S0 + L0 + I0 + T0\n\n# A grid of time points (in days)\nt = np.linspace(0, 40, 50) \n\n#parameters\ndelta = dinn.delta\nprint(delta)\nbeta = dinn.beta\nprint(beta)\nc = dinn.c\nprint(c)\nmu = dinn.mu\nprint(mu)\nk = dinn.k\nprint(k)\nr_1 = dinn.r_1\nprint(r_1)\nr_2 = dinn.r_2\nprint(r_2)\nbeta_prime = dinn.beta_prime\nprint(beta_prime)\nd = dinn.d\nprint(d)\n\n# The SIR model differential equations.\ndef deriv(y, t, N, delta ,beta ,c ,mu ,k ,r_1 ,r_2 ,beta_prime,d ):\n S, L, I, T= y\n\n dSdt = delta - beta * c * S * I / N - mu * S\n dLdt = beta * c * S * I / N - (mu + k + r_1) * L + beta_prime * c * T * 1/N\n dIdt = k*L - (mu + d) * I - r_2 * I\n dTdt = r_1 * L + r_2 * I - beta_prime * c * T * 1/N - mu*T\n\n return dSdt, dLdt, dIdt, dTdt\n\n\n# Initial conditions vector\ny0 = S0, L0, I0, T0\n# Integrate the SIR equations over the time grid, t.\nret = odeint(deriv, y0, t, args=(N, delta ,beta ,c ,mu ,k ,r_1 ,r_2 ,beta_prime,d ))\nS, L, I, T = ret.T\n\n# Plot the data on two separate curves for S(t), I(t)\nfig = plt.figure(figsize=(12,12))\n\nax = fig.add_subplot(111, facecolor='#dddddd', axisbelow=True)\nax.set_facecolor('xkcd:white')\n\nax.plot(t, S, 'violet', alpha=0.5, lw=2, label='S_pred', linestyle='dashed')\nax.plot(tuberculosis_data[0], tuberculosis_data[1], 'grey', alpha=0.5, lw=2, label='S')\n\nax.plot(t, L, 'darkgreen', alpha=0.5, lw=2, label='L_pred', linestyle='dashed')\nax.plot(tuberculosis_data[0], tuberculosis_data[2], 'purple', alpha=0.5, lw=2, label='L')\n\nax.plot(t, I, 'blue', alpha=0.5, lw=2, label='I_pred', linestyle='dashed')\nax.plot(tuberculosis_data[0], tuberculosis_data[3], 'teal', alpha=0.5, lw=2, label='I')\n\nax.plot(t, T, 'black', alpha=0.5, lw=2, label='T_pred', linestyle='dashed')\nax.plot(tuberculosis_data[0], tuberculosis_data[4], 'red', alpha=0.5, lw=2, label='T')\n\nax.set_xlabel('Time /days',size = 20)\nax.set_ylabel('Number',size = 20)\n#ax.set_ylim([-1,50])\nax.yaxis.set_tick_params(length=0)\nax.xaxis.set_tick_params(length=0)\nplt.xticks(size = 20)\nplt.yticks(size = 20)\nax.grid(b=True, which='major', c='black', lw=0.2, ls='-')\nlegend = ax.legend(prop={'size':20})\nlegend.get_frame().set_alpha(0.5)\nfor spine in ('top', 'right', 'bottom', 'left'):\n ax.spines[spine].set_visible(False)\nplt.show()",
"_____no_output_____"
],
[
"#calculate relative MSE loss\nimport math\n\nS_total_loss = 0\nS_den = 0\nL_total_loss = 0\nL_den = 0\nI_total_loss = 0\nI_den = 0\nT_total_loss = 0\nT_den = 0\nfor timestep in range(len(t)):\n S_value = tuberculosis_data[1][timestep] - S[timestep]\n S_total_loss += S_value**2\n S_den += (tuberculosis_data[1][timestep])**2\n\n L_value = tuberculosis_data[2][timestep] - L[timestep]\n L_total_loss += L_value**2\n L_den += (tuberculosis_data[2][timestep])**2\n\n I_value = tuberculosis_data[3][timestep] - I[timestep]\n I_total_loss += I_value**2\n I_den += (tuberculosis_data[3][timestep])**2\n T_value = tuberculosis_data[4][timestep] - T[timestep]\n T_total_loss += T_value**2\n T_den += (tuberculosis_data[4][timestep])**2\n\nS_total_loss = math.sqrt(S_total_loss/S_den)\nL_total_loss = math.sqrt(L_total_loss/L_den)\nI_total_loss = math.sqrt(I_total_loss/I_den)\nT_total_loss = math.sqrt(T_total_loss/T_den)\n\nprint('S_total_loss: ', S_total_loss)\nprint('I_total_loss: ', L_total_loss)\nprint('S_total_loss: ', I_total_loss)\nprint('I_total_loss: ', T_total_loss)",
"_____no_output_____"
]
]
] | [
"code"
] | [
[
"code",
"code",
"code",
"code",
"code",
"code",
"code"
]
] |
d0450746f2736ff09b6f143a3d2e8c27473c18ac | 14,579 | ipynb | Jupyter Notebook | getting_started_with_data_and_plotting.ipynb | odow/jump-training-materials | 0ed2164c7625ce66b59881f1ea6c5b491daae013 | [
"MIT"
] | 7 | 2021-08-31T05:32:56.000Z | 2021-09-30T08:33:50.000Z | getting_started_with_data_and_plotting.ipynb | odow/jump-training-materials | 0ed2164c7625ce66b59881f1ea6c5b491daae013 | [
"MIT"
] | null | null | null | getting_started_with_data_and_plotting.ipynb | odow/jump-training-materials | 0ed2164c7625ce66b59881f1ea6c5b491daae013 | [
"MIT"
] | 2 | 2021-09-08T15:45:59.000Z | 2021-10-01T05:37:06.000Z | 20.305014 | 100 | 0.524796 | [
[
[
"empty"
]
]
] | [
"empty"
] | [
[
"empty"
]
] |
d0451b62ea0a5fc4c720b83dd7e5c1087c02a78d | 42,703 | ipynb | Jupyter Notebook | jupyter/BLOOMBERG/SektorHargaInflasi/script/SektorHargaInflasi3_6.ipynb | langpp/bappenas | f780607192bb99b9bc8fbe29412b4c6c49bf15ae | [
"Apache-2.0"
] | 1 | 2021-03-17T03:10:49.000Z | 2021-03-17T03:10:49.000Z | jupyter/BLOOMBERG/SektorHargaInflasi/script/SektorHargaInflasi3_6.ipynb | langpp/bappenas | f780607192bb99b9bc8fbe29412b4c6c49bf15ae | [
"Apache-2.0"
] | null | null | null | jupyter/BLOOMBERG/SektorHargaInflasi/script/SektorHargaInflasi3_6.ipynb | langpp/bappenas | f780607192bb99b9bc8fbe29412b4c6c49bf15ae | [
"Apache-2.0"
] | 1 | 2021-03-17T03:12:34.000Z | 2021-03-17T03:12:34.000Z | 85.065737 | 2,221 | 0.645482 | [
[
[
"#IMPORT SEMUA LIBARARY",
"_____no_output_____"
],
[
"#IMPORT LIBRARY PANDAS\nimport pandas as pd\n#IMPORT LIBRARY UNTUK POSTGRE\nfrom sqlalchemy import create_engine\nimport psycopg2\n#IMPORT LIBRARY CHART\nfrom matplotlib import pyplot as plt\nfrom matplotlib import style\n#IMPORT LIBRARY BASE PATH\nimport os\nimport io\n#IMPORT LIBARARY PDF\nfrom fpdf import FPDF\n#IMPORT LIBARARY CHART KE BASE64\nimport base64\n#IMPORT LIBARARY EXCEL\nimport xlsxwriter ",
"_____no_output_____"
],
[
"#FUNGSI UNTUK MENGUPLOAD DATA DARI CSV KE POSTGRESQL",
"_____no_output_____"
],
[
"def uploadToPSQL(columns, table, filePath, engine):\n #FUNGSI UNTUK MEMBACA CSV\n df = pd.read_csv(\n os.path.abspath(filePath),\n names=columns,\n keep_default_na=False\n )\n #APABILA ADA FIELD KOSONG DISINI DIFILTER\n df.fillna('')\n #MENGHAPUS COLUMN YANG TIDAK DIGUNAKAN\n del df['kategori']\n del df['jenis']\n del df['pengiriman']\n del df['satuan']\n \n #MEMINDAHKAN DATA DARI CSV KE POSTGRESQL\n df.to_sql(\n table, \n engine,\n if_exists='replace'\n )\n \n #DIHITUNG APABILA DATA YANG DIUPLOAD BERHASIL, MAKA AKAN MENGEMBALIKAN KELUARAN TRUE(BENAR) DAN SEBALIKNYA\n if len(df) == 0:\n return False\n else:\n return True",
"_____no_output_____"
],
[
"#FUNGSI UNTUK MEMBUAT CHART, DATA YANG DIAMBIL DARI DATABASE DENGAN MENGGUNAKAN ORDER DARI TANGGAL DAN JUGA LIMIT\n#DISINI JUGA MEMANGGIL FUNGSI MAKEEXCEL DAN MAKEPDF",
"_____no_output_____"
],
[
"def makeChart(host, username, password, db, port, table, judul, columns, filePath, name, subjudul, limit, negara, basePath):\n #TEST KONEKSI DATABASE\n try:\n #KONEKSI KE DATABASE\n connection = psycopg2.connect(user=username,password=password,host=host,port=port,database=db)\n cursor = connection.cursor()\n #MENGAMBL DATA DARI TABLE YANG DIDEFINISIKAN DIBAWAH, DAN DIORDER DARI TANGGAL TERAKHIR\n #BISA DITAMBAHKAN LIMIT SUPAYA DATA YANG DIAMBIL TIDAK TERLALU BANYAK DAN BERAT\n postgreSQL_select_Query = \"SELECT * FROM \"+table+\" ORDER BY tanggal ASC LIMIT \" + str(limit)\n \n cursor.execute(postgreSQL_select_Query)\n mobile_records = cursor.fetchall() \n uid = []\n lengthx = []\n lengthy = []\n #MELAKUKAN LOOPING ATAU PERULANGAN DARI DATA YANG SUDAH DIAMBIL\n #KEMUDIAN DATA TERSEBUT DITEMPELKAN KE VARIABLE DIATAS INI\n for row in mobile_records:\n uid.append(row[0])\n lengthx.append(row[1])\n if row[2] == \"\":\n lengthy.append(float(0))\n else:\n lengthy.append(float(row[2]))\n\n #FUNGSI UNTUK MEMBUAT CHART\n #bar\n style.use('ggplot')\n \n fig, ax = plt.subplots()\n #MASUKAN DATA ID DARI DATABASE, DAN JUGA DATA TANGGAL\n ax.bar(uid, lengthy, align='center')\n #UNTUK JUDUL CHARTNYA\n ax.set_title(judul)\n ax.set_ylabel('Total')\n ax.set_xlabel('Tanggal')\n \n ax.set_xticks(uid)\n #TOTAL DATA YANG DIAMBIL DARI DATABASE, DIMASUKAN DISINI\n ax.set_xticklabels((lengthx))\n b = io.BytesIO()\n #CHART DISIMPAN KE FORMAT PNG\n plt.savefig(b, format='png', bbox_inches=\"tight\")\n #CHART YANG SUDAH DIJADIKAN PNG, DISINI DICONVERT KE BASE64\n barChart = base64.b64encode(b.getvalue()).decode(\"utf-8\").replace(\"\\n\", \"\")\n #CHART DITAMPILKAN\n plt.show()\n \n #line\n #MASUKAN DATA DARI DATABASE\n plt.plot(lengthx, lengthy)\n plt.xlabel('Tanggal')\n plt.ylabel('Total')\n #UNTUK JUDUL CHARTNYA\n plt.title(judul)\n plt.grid(True)\n l = io.BytesIO()\n #CHART DISIMPAN KE FORMAT PNG\n plt.savefig(l, format='png', bbox_inches=\"tight\")\n #CHART YANG SUDAH DIJADIKAN PNG, DISINI DICONVERT KE BASE64\n lineChart = base64.b64encode(l.getvalue()).decode(\"utf-8\").replace(\"\\n\", \"\")\n #CHART DITAMPILKAN\n plt.show()\n \n #pie\n #UNTUK JUDUL CHARTNYA\n plt.title(judul)\n #MASUKAN DATA DARI DATABASE\n plt.pie(lengthy, labels=lengthx, autopct='%1.1f%%', \n shadow=True, startangle=180)\n \n plt.axis('equal')\n p = io.BytesIO()\n #CHART DISIMPAN KE FORMAT PNG\n plt.savefig(p, format='png', bbox_inches=\"tight\")\n #CHART YANG SUDAH DIJADIKAN PNG, DISINI DICONVERT KE BASE64\n pieChart = base64.b64encode(p.getvalue()).decode(\"utf-8\").replace(\"\\n\", \"\")\n #CHART DITAMPILKAN\n plt.show()\n \n #MENGAMBIL DATA DARI CSV YANG DIGUNAKAN SEBAGAI HEADER DARI TABLE UNTUK EXCEL DAN JUGA PDF\n header = pd.read_csv(\n os.path.abspath(filePath),\n names=columns,\n keep_default_na=False\n )\n #MENGHAPUS COLUMN YANG TIDAK DIGUNAKAN\n header.fillna('')\n del header['tanggal']\n del header['total']\n #MEMANGGIL FUNGSI EXCEL\n makeExcel(mobile_records, header, name, limit, basePath)\n #MEMANGGIL FUNGSI PDF\n makePDF(mobile_records, header, judul, barChart, lineChart, pieChart, name, subjudul, limit, basePath) \n \n #JIKA GAGAL KONEKSI KE DATABASE, MASUK KESINI UNTUK MENAMPILKAN ERRORNYA\n except (Exception, psycopg2.Error) as error :\n print (error)\n\n #KONEKSI DITUTUP\n finally:\n if(connection):\n cursor.close()\n connection.close()",
"_____no_output_____"
],
[
"#FUNGSI MAKEEXCEL GUNANYA UNTUK MEMBUAT DATA YANG BERASAL DARI DATABASE DIJADIKAN FORMAT EXCEL TABLE F2\n#PLUGIN YANG DIGUNAKAN ADALAH XLSXWRITER",
"_____no_output_____"
],
[
"def makeExcel(datarow, dataheader, name, limit, basePath):\n #MEMBUAT FILE EXCEL\n workbook = xlsxwriter.Workbook(basePath+'jupyter/BLOOMBERG/SektorHargaInflasi/excel/'+name+'.xlsx')\n #MENAMBAHKAN WORKSHEET PADA FILE EXCEL TERSEBUT\n worksheet = workbook.add_worksheet('sheet1')\n #SETINGAN AGAR DIBERIKAN BORDER DAN FONT MENJADI BOLD\n row1 = workbook.add_format({'border': 2, 'bold': 1})\n row2 = workbook.add_format({'border': 2})\n #MENJADIKAN DATA MENJADI ARRAY\n data=list(datarow)\n isihead=list(dataheader.values)\n header = []\n body = []\n \n #LOOPING ATAU PERULANGAN, KEMUDIAN DATA DITAMPUNG PADA VARIABLE DIATAS\n for rowhead in dataheader:\n header.append(str(rowhead))\n \n for rowhead2 in datarow:\n header.append(str(rowhead2[1]))\n \n for rowbody in isihead[1]:\n body.append(str(rowbody))\n \n for rowbody2 in data:\n body.append(str(rowbody2[2]))\n \n #MEMASUKAN DATA DARI VARIABLE DIATAS KE DALAM COLUMN DAN ROW EXCEL\n for col_num, data in enumerate(header):\n worksheet.write(0, col_num, data, row1)\n \n for col_num, data in enumerate(body):\n worksheet.write(1, col_num, data, row2)\n \n #FILE EXCEL DITUTUP\n workbook.close()",
"_____no_output_____"
],
[
"#FUNGSI UNTUK MEMBUAT PDF YANG DATANYA BERASAL DARI DATABASE DIJADIKAN FORMAT EXCEL TABLE F2\n#PLUGIN YANG DIGUNAKAN ADALAH FPDF",
"_____no_output_____"
],
[
"def makePDF(datarow, dataheader, judul, bar, line, pie, name, subjudul, lengthPDF, basePath):\n #FUNGSI UNTUK MENGATUR UKURAN KERTAS, DISINI MENGGUNAKAN UKURAN A4 DENGAN POSISI LANDSCAPE\n pdf = FPDF('L', 'mm', [210,297])\n #MENAMBAHKAN HALAMAN PADA PDF\n pdf.add_page()\n #PENGATURAN UNTUK JARAK PADDING DAN JUGA UKURAN FONT\n pdf.set_font('helvetica', 'B', 20.0)\n pdf.set_xy(145.0, 15.0)\n #MEMASUKAN JUDUL KE DALAM PDF\n pdf.cell(ln=0, h=2.0, align='C', w=10.0, txt=judul, border=0)\n \n #PENGATURAN UNTUK UKURAN FONT DAN JUGA JARAK PADDING\n pdf.set_font('arial', '', 14.0)\n pdf.set_xy(145.0, 25.0)\n #MEMASUKAN SUB JUDUL KE PDF\n pdf.cell(ln=0, h=2.0, align='C', w=10.0, txt=subjudul, border=0)\n #MEMBUAT GARIS DI BAWAH SUB JUDUL\n pdf.line(10.0, 30.0, 287.0, 30.0)\n pdf.set_font('times', '', 10.0)\n pdf.set_xy(17.0, 37.0)\n \n #PENGATURAN UNTUK UKURAN FONT DAN JUGA JARAK PADDING\n pdf.set_font('Times','',10.0) \n #MENGAMBIL DATA HEADER PDF YANG SEBELUMNYA SUDAH DIDEFINISIKAN DIATAS\n datahead=list(dataheader.values)\n pdf.set_font('Times','B',12.0) \n pdf.ln(0.5)\n \n th1 = pdf.font_size\n \n #MEMBUAT TABLE PADA PDF, DAN MENAMPILKAN DATA DARI VARIABLE YANG SUDAH DIKIRIM\n pdf.cell(100, 2*th1, \"Kategori\", border=1, align='C')\n pdf.cell(177, 2*th1, datahead[0][0], border=1, align='C')\n pdf.ln(2*th1)\n pdf.cell(100, 2*th1, \"Jenis\", border=1, align='C')\n pdf.cell(177, 2*th1, datahead[0][1], border=1, align='C')\n pdf.ln(2*th1)\n pdf.cell(100, 2*th1, \"Pengiriman\", border=1, align='C')\n pdf.cell(177, 2*th1, datahead[0][2], border=1, align='C')\n pdf.ln(2*th1)\n pdf.cell(100, 2*th1, \"Satuan\", border=1, align='C')\n pdf.cell(177, 2*th1, datahead[0][3], border=1, align='C')\n pdf.ln(2*th1)\n \n #PENGATURAN PADDING\n pdf.set_xy(17.0, 75.0)\n \n #PENGATURAN UNTUK UKURAN FONT DAN JUGA JARAK PADDING\n pdf.set_font('Times','B',11.0) \n data=list(datarow)\n epw = pdf.w - 2*pdf.l_margin\n col_width = epw/(lengthPDF+1)\n \n #PENGATURAN UNTUK JARAK PADDING\n pdf.ln(0.5)\n th = pdf.font_size\n \n #MEMASUKAN DATA HEADER YANG DIKIRIM DARI VARIABLE DIATAS KE DALAM PDF\n pdf.cell(50, 2*th, str(\"Negara\"), border=1, align='C')\n for row in data:\n pdf.cell(40, 2*th, str(row[1]), border=1, align='C')\n pdf.ln(2*th)\n \n #MEMASUKAN DATA ISI YANG DIKIRIM DARI VARIABLE DIATAS KE DALAM PDF\n pdf.set_font('Times','B',10.0)\n pdf.set_font('Arial','',9)\n pdf.cell(50, 2*th, negara, border=1, align='C')\n for row in data:\n pdf.cell(40, 2*th, str(row[2]), border=1, align='C')\n pdf.ln(2*th)\n \n #MENGAMBIL DATA CHART, KEMUDIAN CHART TERSEBUT DIJADIKAN PNG DAN DISIMPAN PADA DIRECTORY DIBAWAH INI\n #BAR CHART\n bardata = base64.b64decode(bar)\n barname = basePath+'jupyter/BLOOMBERG/SektorHargaInflasi/img/'+name+'-bar.png'\n with open(barname, 'wb') as f:\n f.write(bardata)\n \n #LINE CHART\n linedata = base64.b64decode(line)\n linename = basePath+'jupyter/BLOOMBERG/SektorHargaInflasi/img/'+name+'-line.png'\n with open(linename, 'wb') as f:\n f.write(linedata)\n \n #PIE CHART\n piedata = base64.b64decode(pie)\n piename = basePath+'jupyter/BLOOMBERG/SektorHargaInflasi/img/'+name+'-pie.png'\n with open(piename, 'wb') as f:\n f.write(piedata)\n \n #PENGATURAN UNTUK UKURAN FONT DAN JUGA JARAK PADDING\n pdf.set_xy(17.0, 75.0)\n col = pdf.w - 2*pdf.l_margin\n widthcol = col/3\n #MEMANGGIL DATA GAMBAR DARI DIREKTORY DIATAS\n pdf.image(barname, link='', type='',x=8, y=100, w=widthcol)\n pdf.set_xy(17.0, 75.0)\n col = pdf.w - 2*pdf.l_margin\n pdf.image(linename, link='', type='',x=103, y=100, w=widthcol)\n pdf.set_xy(17.0, 75.0)\n col = pdf.w - 2*pdf.l_margin\n pdf.image(piename, link='', type='',x=195, y=100, w=widthcol)\n pdf.ln(2*th)\n \n #MEMBUAT FILE PDF\n pdf.output(basePath+'jupyter/BLOOMBERG/SektorHargaInflasi/pdf/'+name+'.pdf', 'F')",
"_____no_output_____"
],
[
"#DISINI TEMPAT AWAL UNTUK MENDEFINISIKAN VARIABEL VARIABEL SEBELUM NANTINYA DIKIRIM KE FUNGSI\n#PERTAMA MANGGIL FUNGSI UPLOADTOPSQL DULU, KALAU SUKSES BARU MANGGIL FUNGSI MAKECHART\n#DAN DI MAKECHART MANGGIL FUNGSI MAKEEXCEL DAN MAKEPDF",
"_____no_output_____"
],
[
"#DEFINISIKAN COLUMN BERDASARKAN FIELD CSV\ncolumns = [\n \"kategori\",\n \"jenis\",\n \"tanggal\",\n \"total\",\n \"pengiriman\",\n \"satuan\",\n]\n\n#UNTUK NAMA FILE\nname = \"SektorHargaInflasi3_6\"\n#VARIABLE UNTUK KONEKSI KE DATABASE\nhost = \"localhost\"\nusername = \"postgres\"\npassword = \"1234567890\"\nport = \"5432\"\ndatabase = \"bloomberg_SektorHargaInflasi\"\ntable = name.lower()\n#JUDUL PADA PDF DAN EXCEL\njudul = \"Data Sektor Harga Inflasi\"\nsubjudul = \"Badan Perencanaan Pembangunan Nasional\"\n#LIMIT DATA UNTUK SELECT DI DATABASE\nlimitdata = int(8)\n#NAMA NEGARA UNTUK DITAMPILKAN DI EXCEL DAN PDF\nnegara = \"Indonesia\"\n#BASE PATH DIRECTORY\nbasePath = 'C:/Users/ASUS/Documents/bappenas/'\n#FILE CSV\nfilePath = basePath+ 'data mentah/BLOOMBERG/SektorHargaInflasi/' +name+'.csv';\n#KONEKSI KE DATABASE\nengine = create_engine('postgresql://'+username+':'+password+'@'+host+':'+port+'/'+database)\n\n#MEMANGGIL FUNGSI UPLOAD TO PSQL\ncheckUpload = uploadToPSQL(columns, table, filePath, engine)\n#MENGECEK FUNGSI DARI UPLOAD PSQL, JIKA BERHASIL LANJUT MEMBUAT FUNGSI CHART, JIKA GAGAL AKAN MENAMPILKAN PESAN ERROR\nif checkUpload == True:\n makeChart(host, username, password, database, port, table, judul, columns, filePath, name, subjudul, limitdata, negara, basePath)\nelse:\n print(\"Error When Upload CSV\")",
"_____no_output_____"
]
]
] | [
"code"
] | [
[
"code",
"code",
"code",
"code",
"code",
"code",
"code",
"code",
"code",
"code",
"code",
"code"
]
] |
d04532a0b8b6a2a81318c4e0c5ca56b4bbed56bf | 7,217 | ipynb | Jupyter Notebook | notebooks/Unit_Tests.ipynb | HemuManju/human-robot-interaction-eeg | 223e320e7201f6c93cbe8f9728e401d7199453a2 | [
"MIT"
] | null | null | null | notebooks/Unit_Tests.ipynb | HemuManju/human-robot-interaction-eeg | 223e320e7201f6c93cbe8f9728e401d7199453a2 | [
"MIT"
] | null | null | null | notebooks/Unit_Tests.ipynb | HemuManju/human-robot-interaction-eeg | 223e320e7201f6c93cbe8f9728e401d7199453a2 | [
"MIT"
] | 1 | 2020-08-20T23:06:08.000Z | 2020-08-20T23:06:08.000Z | 27.545802 | 123 | 0.470279 | [
[
[
"import pytest\nfrom scipy.stats import zscore\nfrom mne.preprocessing import create_ecg_epochs\nfrom sklearn.model_selection import train_test_split\n%run parameters.py\n%run Utility_Functions.ipynb",
"_____no_output_____"
],
[
"%matplotlib qt5\ndata = np.load('All_Subject_IR_Index_'+str(epoch_length)+'.npy')\nprint(data.shape)\nsb.set()\ndef ir_plot(data):\n for i, subject in enumerate(subjects):\n temp = []\n for j, trial in enumerate(trials):\n temp.append(data[i][j][:])\n plt.subplot(3,6,i+1)\n plt.boxplot(temp, showfliers=False)\n plt.tight_layout()\n \nir_plot(data)\n\n\ndata = np.load('All_Subject_IR_Index_'+str(epoch_length)+'.npy')\nprint(data.shape)\n\nplt.figure()\ndef ir_plot(data):\n for i, subject in enumerate(subjects):\n temp = []\n for j, trial in enumerate(trials):\n if trial=='HighFine' or trial=='LowFine':\n temp.append(data[i][j][:])\n plt.subplot(3,6,i+1)\n for element in temp:\n plt.plot(element)\n plt.tight_layout()\n \ndef min_max(data):\n data -= data.min()\n# data /= data.ptp()\n \n return data\n\nplt.figure()\ndef ir_plot(data):\n for i, subject in enumerate(subjects):\n temp = []\n for j, trial in enumerate(trials):\n if trial=='HighFine' or trial=='LowFine':\n temp.append(data[i][j][:])\n temp_z = zscore(np.vstack((np.expand_dims(temp[0], axis=1),np.expand_dims(temp[1], axis=1))))\n plt.plot(temp_z[0:len(temp[0])], 'r')\n plt.plot(temp_z[len(temp[0]):], 'b')\n plt.tight_layout()\n \ndef test_epoch_length(subjects, trials):\n s = []\n for subject in subjects:\n for trial in trials:\n read_eeg_path = '../Cleaned Data/' + subject + '/EEG/'\n read_force_path = '../Cleaned Data/' + subject + '/Force/'\n cleaned_eeg = mne.read_epochs(read_eeg_path + subject + '_' + trial + '_' + str(epoch_length) \n + '_cleaned_epo.fif', verbose=False)\n cleaned_force = mne.read_epochs(read_force_path + subject + '_' + trial + '_' + str(epoch_length) \n + '_cleaned_epo.fif', verbose=False)\n eeg = cleaned_eeg.get_data()\n force = cleaned_force.get_data()\n \n # Check whether eeg and force data are same\n assert eeg.shape[0]==force.shape[0]\n s.append(subject)\n \n # Check whether all subjects were tested\n assert len(s)==len(subjects), 'Huston! We have got a problem!'\n \n return 'Reached moon!'\n\n\ndef test_data():\n x = np.load('PSD_X_Data_' + str(epoch_length) + '.npy')\n y = np.load('IR_Y_Data_' + str(epoch_length) + '.npy')\n \n assert x.shape[0]==y.shape[0], \"Houston we've got a problem!\"\n \n \ndef test_psd_image():\n x = np.load('PSD_X_Data_' + str(epoch_length) +'.npy')\n \n plt.imshow(x[5,:,0].reshape(image_size, image_size))\n \ndef test_x_y_length():\n x = np.load('X.npy')\n y = np.load('Y.npy')\n assert x.shape[0]==y.shape[0], 'Huston! We have got a problem!'\n \n return 'Reached moon!'",
"_____no_output_____"
],
[
"def test_x_y_length():\n x = np.load('X.npy')\n y = np.load('Y.npy')\n print(sum(y)/len(y))\n assert x.shape[0]==y.shape[0], 'Huston! We have got a problem!'\n \n return 'Reached moon!'\n\ntest_x_y_length()",
"[0.26972963 0.4975338 0.23273657]\n"
],
[
"x = np.load('X.npy')\ny = np.load('Y.npy')\n\nprint(x.shape)\n\nx_normal = x[np.argmax(y, axis=1)==1,:,:]\ny_normal = y[np.argmax(y, axis=1)==1]\n\nprint(np.argmax(y, axis=1)==0)\n\nx_low = x[np.argmax(y, axis=1)==0,:,:]\ny_low = y[np.argmax(y, axis=1)==0]\n\nprint(x_low.shape)\n\nx_high = x[np.argmax(y, axis=1)==2,:,:]\ny_high = y[np.argmax(y, axis=1)==2]\n\nx_normal, x_test, y_normal, y_test = train_test_split(x_normal, y_normal, test_size = 0.50)\n\nx_balanced = np.vstack((x_low, x_normal, x_high))",
"(10948, 20, 256)\n[ True True True ... False False False]\n(2953, 20, 256)\n"
]
]
] | [
"code"
] | [
[
"code",
"code",
"code",
"code"
]
] |
d04535eb8d4dd22bdfcd498e99bd63d960c7e764 | 43,043 | ipynb | Jupyter Notebook | _doc/notebooks/td1a/matrix_dictionary.ipynb | Jerome-maker/ensae_teaching_cs | 43ea044361ee60c00c85aea354a7b25c21c0fd07 | [
"MIT"
] | null | null | null | _doc/notebooks/td1a/matrix_dictionary.ipynb | Jerome-maker/ensae_teaching_cs | 43ea044361ee60c00c85aea354a7b25c21c0fd07 | [
"MIT"
] | null | null | null | _doc/notebooks/td1a/matrix_dictionary.ipynb | Jerome-maker/ensae_teaching_cs | 43ea044361ee60c00c85aea354a7b25c21c0fd07 | [
"MIT"
] | null | null | null | 55.254172 | 17,410 | 0.635922 | [
[
[
"# Produit matriciel avec une matrice creuse\n\nLes dictionnaires sont une façon assez de représenter les matrices creuses en ne conservant que les coefficients non nuls. Comment écrire alors le produit matriciel ?",
"_____no_output_____"
]
],
[
[
"from jyquickhelper import add_notebook_menu\nadd_notebook_menu()",
"_____no_output_____"
]
],
[
[
"## Matrice creuse et dictionnaire\n\nUne [matrice creuse](https://fr.wikipedia.org/wiki/Matrice_creuse) ou [sparse matrix](https://en.wikipedia.org/wiki/Sparse_matrix) est constituée majoritairement de 0. On utilise un dictionnaire avec les coefficients non nuls. La fonction suivante pour créer une matrice aléatoire.",
"_____no_output_____"
]
],
[
[
"import random\n\ndef random_matrix(n, m, ratio=0.1):\n mat = {}\n nb = min(n * m, int(ratio * n * m + 0.5))\n while len(mat) < nb:\n i = random.randint(0, n-1)\n j = random.randint(0, m-1)\n mat[i, j] = 1\n return mat\n\nmat = random_matrix(3, 3, ratio=0.5)\nmat",
"_____no_output_____"
]
],
[
[
"## Calcul de la dimension\n\nPour obtenir la dimension de la matrice, il faut parcourir toutes les clés du dictionnaire.",
"_____no_output_____"
]
],
[
[
"def dimension(mat):\n maxi, maxj = 0, 0\n for k in mat:\n maxi = max(maxi, k[0])\n maxj = max(maxj, k[1])\n return maxi + 1, maxj + 1\n\ndimension(mat)",
"_____no_output_____"
]
],
[
[
"Cette fonction possède l'inconvénient de retourner une valeur fausse si la matrice ne possède aucun coefficient non nul sur la dernière ligne ou la dernière colonne. Cela peut être embarrassant, tout dépend de l'usage.",
"_____no_output_____"
],
[
"## Produit matriciel classique\n\nOn implémente le produit matriciel classique, à trois boucles.",
"_____no_output_____"
]
],
[
[
"def produit_classique(m1, m2):\n dim1 = dimension(m1)\n dim2 = dimension(m2)\n if dim1[1] != dim2[0]:\n raise Exception(\"Impossible de multiplier {0}, {1}\".format(\n dim1, dim2))\n res = {}\n for i in range(dim1[0]):\n for j in range(dim2[1]):\n s = 0\n for k in range(dim1[1]):\n s += m1.get((i, k), 0) * m2.get((k, j), 0)\n if s != 0: # Pour éviter de garder les coefficients non nuls.\n res[i, j] = s \n return res\n\nsimple = {(0, 1): 1, (1, 0): 1}\nproduit_classique(simple, simple)",
"_____no_output_____"
]
],
[
[
"Sur la matrice aléatoire...",
"_____no_output_____"
]
],
[
[
"produit_classique(mat, mat)",
"_____no_output_____"
]
],
[
[
"## Produit matriciel plus élégant\n\nA-t-on vraiment besoin de s'enquérir des dimensions de la matrice pour faire le produit matriciel ? Ne peut-on pas tout simplement faire une boucle sur les coefficients non nul ?",
"_____no_output_____"
]
],
[
[
"def produit_elegant(m1, m2):\n res = {}\n for (i, k1), v1 in m1.items():\n if v1 == 0:\n continue\n for (k2, j), v2 in m2.items(): \n if v2 == 0:\n continue\n if k1 == k2:\n if (i, j) in res:\n res[i, j] += v1 * v2\n else :\n res[i, j] = v1 * v2\n return res\n\nproduit_elegant(simple, simple)",
"_____no_output_____"
],
[
"produit_elegant(mat, mat)",
"_____no_output_____"
]
],
[
[
"## Mesure du temps\n\nA priori, la seconde méthode est plus rapide puisque son coût est proportionnel au produit du nombre de coefficients non nuls dans les deux matrices. Vérifions.",
"_____no_output_____"
]
],
[
[
"bigmat = random_matrix(100, 100)\n%timeit produit_classique(bigmat, bigmat)",
"658 ms ± 81.3 ms per loop (mean ± std. dev. of 7 runs, 1 loop each)\n"
],
[
"%timeit produit_elegant(bigmat, bigmat)",
"157 ms ± 9.33 ms per loop (mean ± std. dev. of 7 runs, 10 loops each)\n"
]
],
[
[
"C'est beaucoup mieux. Mais peut-on encore faire mieux ?",
"_____no_output_____"
],
[
"## Dictionnaires de dictionnaires\n\nCa sonne un peu comme [mille millions de mille sabords](https://fr.wikipedia.org/wiki/Vocabulaire_du_capitaine_Haddock) mais le dictionnaire que nous avons créé a pour clé un couple de coordonnées et valeur des coefficients. La fonction ``produit_elegant`` fait plein d'itérations inutiles en quelque sorte puisque les coefficients sont nuls. Peut-on éviter ça ?\n\nEt si on utilisait des dictionnaires de dictionnaires : ``{ ligne : { colonne : valeur } }``.",
"_____no_output_____"
]
],
[
[
"def matrice_dicodico(mat):\n res = {}\n for (i, j), v in mat.items():\n if i not in res:\n res[i] = {j: v}\n else:\n res[i][j] = v\n return res\n\nmatrice_dicodico(simple)",
"_____no_output_____"
]
],
[
[
"Peut-on adapter le calcul matriciel élégant ? Il reste à associer les indices de colonnes de la première avec les indices de lignes de la seconde. Cela pose problème en l'état quand les indices de colonnes sont inaccessibles sans connaître les indices de lignes d'abord à moins d'échanger l'ordre pour la seconde matrice.",
"_____no_output_____"
]
],
[
[
"def matrice_dicodico_lc(mat, ligne=True):\n res = {}\n if ligne:\n for (i, j), v in mat.items():\n if i not in res:\n res[i] = {j: v}\n else:\n res[i][j] = v\n else:\n for (j, i), v in mat.items():\n if i not in res:\n res[i] = {j: v}\n else:\n res[i][j] = v\n return res\n\nmatrice_dicodico_lc(simple, ligne=False)",
"_____no_output_____"
]
],
[
[
"Maintenant qu'on a fait ça, on peut songer au produit matriciel.",
"_____no_output_____"
]
],
[
[
"def produit_elegant_rapide(m1, m2):\n res = {}\n for k, vs in m1.items():\n if k in m2:\n for i, v1 in vs.items():\n for j, v2 in m2[k].items():\n if (i, j) in res:\n res[i, j] += v1 * v2\n else :\n res[i, j] = v1 * v2\n\n return res\n\nm1 = matrice_dicodico_lc(simple, ligne=False)\nm2 = matrice_dicodico_lc(simple)\nproduit_elegant_rapide(m1, m2)",
"_____no_output_____"
],
[
"m1 = matrice_dicodico_lc(mat, ligne=False)\nm2 = matrice_dicodico_lc(mat)\nproduit_elegant_rapide(m1, m2)",
"_____no_output_____"
]
],
[
[
"On mesure le temps avec une grande matrice.",
"_____no_output_____"
]
],
[
[
"m1 = matrice_dicodico_lc(bigmat, ligne=False)\nm2 = matrice_dicodico_lc(bigmat)\n%timeit produit_elegant_rapide(m1, m2)",
"6.46 ms ± 348 µs per loop (mean ± std. dev. of 7 runs, 100 loops each)\n"
]
],
[
[
"Beaucoup plus rapide, il n'y a plus besoin de tester les coefficients non nuls. La comparaison n'est pas très juste néanmoins car il faut transformer les deux matrices avant de faire le calcul. Et si on l'incluait ?",
"_____no_output_____"
]
],
[
[
"def produit_elegant_rapide_transformation(mat1, mat2):\n m1 = matrice_dicodico_lc(mat1, ligne=False)\n m2 = matrice_dicodico_lc(mat2)\n return produit_elegant_rapide(m1, m2)\n\nproduit_elegant_rapide_transformation(simple, simple)",
"_____no_output_____"
],
[
"%timeit produit_elegant_rapide_transformation(bigmat, bigmat)",
"7.17 ms ± 635 µs per loop (mean ± std. dev. of 7 runs, 100 loops each)\n"
]
],
[
[
"Finalement ça vaut le coup... mais est-ce le cas pour toutes les matrices.",
"_____no_output_____"
]
],
[
[
"%matplotlib inline",
"_____no_output_____"
],
[
"import time\nmesures = []\n\nfor ratio in [0.1, 0.2, 0.3, 0.4, 0.5, 0.6, 0.7, 0.8, 0.9, 0.99]:\n big = random_matrix(100, 100, ratio=ratio)\n \n t1 = time.perf_counter()\n produit_elegant_rapide_transformation(big, big)\n t2 = time.perf_counter()\n dt = (t2 - t1)\n obs = {\"dicodico\": dt, \"ratio\": ratio}\n \n if ratio <= 0.3:\n # après c'est trop lent\n t1 = time.perf_counter()\n produit_elegant(big, big)\n t2 = time.perf_counter()\n dt = (t2 - t1)\n obs[\"dico\"] = dt\n \n t1 = time.perf_counter()\n produit_classique(big, big)\n t2 = time.perf_counter()\n dt = (t2 - t1)\n obs[\"classique\"] = dt\n\n mesures.append(obs)\n print(obs) ",
"{'dicodico': 0.005037441000240506, 'ratio': 0.1, 'dico': 0.14238126200052648, 'classique': 0.6123743010002727}\n{'dicodico': 0.026836189999812632, 'ratio': 0.2, 'dico': 0.6422706439998365, 'classique': 0.6319077640000614}\n{'dicodico': 0.05713747299978422, 'ratio': 0.3, 'dico': 1.5467935550004768, 'classique': 0.6079049600002691}\n{'dicodico': 0.11274368399972445, 'ratio': 0.4, 'classique': 0.6851242430002458}\n{'dicodico': 0.16862485899946478, 'ratio': 0.5, 'classique': 0.6875519010000062}\n{'dicodico': 0.22460795999995753, 'ratio': 0.6, 'classique': 0.7007410579999487}\n{'dicodico': 0.3225403609994828, 'ratio': 0.7, 'classique': 0.6795763820000502}\n{'dicodico': 0.40570493699942745, 'ratio': 0.8, 'classique': 0.6769405260001804}\n{'dicodico': 0.5422258439994039, 'ratio': 0.9, 'classique': 0.6723052600000301}\n{'dicodico': 0.6474834919999921, 'ratio': 0.99, 'classique': 0.6958359640002527}\n"
],
[
"from pandas import DataFrame\ndf = DataFrame(mesures)\nax = df.plot(x=\"ratio\", y=\"dicodico\", label=\"dico dico\")\ndf.plot(x=\"ratio\", y=\"dico\", label=\"dico\", ax=ax)\ndf.plot(x=\"ratio\", y=\"classique\", label=\"classique\", ax=ax)\nax.legend();",
"_____no_output_____"
]
],
[
[
"Cette dernière version est efficace.",
"_____no_output_____"
]
]
] | [
"markdown",
"code",
"markdown",
"code",
"markdown",
"code",
"markdown",
"code",
"markdown",
"code",
"markdown",
"code",
"markdown",
"code",
"markdown",
"code",
"markdown",
"code",
"markdown",
"code",
"markdown",
"code",
"markdown",
"code",
"markdown",
"code",
"markdown"
] | [
[
"markdown"
],
[
"code"
],
[
"markdown"
],
[
"code"
],
[
"markdown"
],
[
"code"
],
[
"markdown",
"markdown"
],
[
"code"
],
[
"markdown"
],
[
"code"
],
[
"markdown"
],
[
"code",
"code"
],
[
"markdown"
],
[
"code",
"code"
],
[
"markdown",
"markdown"
],
[
"code"
],
[
"markdown"
],
[
"code"
],
[
"markdown"
],
[
"code",
"code"
],
[
"markdown"
],
[
"code"
],
[
"markdown"
],
[
"code",
"code"
],
[
"markdown"
],
[
"code",
"code",
"code"
],
[
"markdown"
]
] |
d045570adff04cd70541392aede86166da244d5f | 7,177 | ipynb | Jupyter Notebook | 03_callbacks.ipynb | mohd-faizy/03_TensorFlow_In_Practice | f7d805c0d1403056635057998f0e191d74470ed8 | [
"MIT"
] | null | null | null | 03_callbacks.ipynb | mohd-faizy/03_TensorFlow_In_Practice | f7d805c0d1403056635057998f0e191d74470ed8 | [
"MIT"
] | null | null | null | 03_callbacks.ipynb | mohd-faizy/03_TensorFlow_In_Practice | f7d805c0d1403056635057998f0e191d74470ed8 | [
"MIT"
] | 1 | 2022-03-17T13:46:20.000Z | 2022-03-17T13:46:20.000Z | 35.35468 | 344 | 0.514142 | [
[
[
"<a href=\"https://colab.research.google.com/github/mohd-faizy/03_TensorFlow_In-Practice/blob/master/03_callbacks.ipynb\" target=\"_parent\"><img src=\"https://colab.research.google.com/assets/colab-badge.svg\" alt=\"Open In Colab\"/></a>",
"_____no_output_____"
],
[
"# __Callbacks API__\n\nA __callback__ is an object that can perform actions at various stages of training (e.g. at the start or end of an epoch, before or after a single batch, etc).\n\n_You can use callbacks to:_\n\n- Write TensorBoard logs after every batch of training to monitor your metrics.\n- Periodically save your model to disk.\n- Do early stopping.\n- Get a view on internal states and statistics of a model during training...and more\n",
"_____no_output_____"
],
[
"## __Usage of callbacks via the built-in `fit()` loop__\n\nYou can pass a list of callbacks (as the keyword argument callbacks) to the `.fit()` method of a model:\n\n```\nmy_callbacks = [\n tf.keras.callbacks.EarlyStopping(patience=2),\n tf.keras.callbacks.ModelCheckpoint(filepath='model.{epoch:02d}-{val_loss:.2f}.h5'),\n tf.keras.callbacks.TensorBoard(log_dir='./logs'),\n]\nmodel.fit(dataset, epochs=10, callbacks=my_callbacks)\n\n```\nThe relevant methods of the callbacks will then be called at each stage of the training.",
"_____no_output_____"
],
[
"__Using custom callbacks__\n\nCreating new callbacks is a simple and powerful way to customize a training loop. Learn more about creating new callbacks in the guide [__Writing your own Callbacks__](https://keras.io/guides/writing_your_own_callbacks/), and refer to the documentation for the [__base Callback class__](https://keras.io/api/callbacks/base_callback/).",
"_____no_output_____"
],
[
"__Available callbacks__\n\n```\n- Base Callback class\n- ModelCheckpoint\n- TensorBoard\n- EarlyStopping\n- LearningRateScheduler\n- ReduceLROnPlateau\n- RemoteMonitor\n- LambdaCallback\n- TerminateOnNaN\n- CSVLogger\n- ProgbarLogger\n```",
"_____no_output_____"
],
[
"> [__Writing your own callbacks__](https://www.tensorflow.org/guide/keras/custom_callback)",
"_____no_output_____"
]
],
[
[
"import tensorflow as tf\n\n# Defining the callback class\nclass myCallback(tf.keras.callbacks.Callback):\n def on_epoch_end(self, epoch, logs={}):\n if(logs.get('accuracy')>0.6):\n print(\"\\nReached 60% accuracy so cancelling training!\")\n self.model.stop_training = True\n\nmnist = tf.keras.datasets.fashion_mnist\n\n(x_train, y_train),(x_test, y_test) = mnist.load_data()\nx_train, x_test = x_train / 255.0, x_test / 255.0\n\ncallbacks = myCallback()\n\nmodel = tf.keras.models.Sequential([\n tf.keras.layers.Flatten(input_shape=(28, 28)),\n tf.keras.layers.Dense(512, activation=tf.nn.relu),\n tf.keras.layers.Dense(10, activation=tf.nn.softmax)\n])\nmodel.compile(optimizer=tf.optimizers.Adam(),\n loss='sparse_categorical_crossentropy',\n metrics=['accuracy'])\n\nmodel.fit(x_train, y_train, epochs=10, callbacks=[callbacks])",
"Downloading data from https://storage.googleapis.com/tensorflow/tf-keras-datasets/train-labels-idx1-ubyte.gz\n32768/29515 [=================================] - 0s 0us/step\nDownloading data from https://storage.googleapis.com/tensorflow/tf-keras-datasets/train-images-idx3-ubyte.gz\n26427392/26421880 [==============================] - 0s 0us/step\nDownloading data from https://storage.googleapis.com/tensorflow/tf-keras-datasets/t10k-labels-idx1-ubyte.gz\n8192/5148 [===============================================] - 0s 0us/step\nDownloading data from https://storage.googleapis.com/tensorflow/tf-keras-datasets/t10k-images-idx3-ubyte.gz\n4423680/4422102 [==============================] - 0s 0us/step\nEpoch 1/10\n1866/1875 [============================>.] - ETA: 0s - loss: 0.4722 - accuracy: 0.8303\nReached 60% accuracy so cancelling training!\n1875/1875 [==============================] - 7s 4ms/step - loss: 0.4723 - accuracy: 0.8302\n"
]
]
] | [
"markdown",
"code"
] | [
[
"markdown",
"markdown",
"markdown",
"markdown",
"markdown",
"markdown"
],
[
"code"
]
] |
d045610c39f26319e5a358e87b4fec5680b7c182 | 106,586 | ipynb | Jupyter Notebook | Jupyter Simulations/1. Malaria Image CNN Simulations/Gradient Merging using FedAvg.ipynb | haozhao10/DAFML | 7dd8c720dc5b67f169f100de43be11020b06ecbe | [
"MIT"
] | 2 | 2021-04-26T05:35:29.000Z | 2022-03-30T13:52:39.000Z | Jupyter Simulations/1. Malaria Image CNN Simulations/Gradient Merging using FedAvg.ipynb | haozhao10/DAFML | 7dd8c720dc5b67f169f100de43be11020b06ecbe | [
"MIT"
] | null | null | null | Jupyter Simulations/1. Malaria Image CNN Simulations/Gradient Merging using FedAvg.ipynb | haozhao10/DAFML | 7dd8c720dc5b67f169f100de43be11020b06ecbe | [
"MIT"
] | 1 | 2021-03-22T08:35:58.000Z | 2021-03-22T08:35:58.000Z | 44.208212 | 158 | 0.448914 | [
[
[
"from PIL import Image\nimport numpy as np\nimport os\nimport cv2\nimport keras\nfrom keras.utils import np_utils\nfrom keras.models import Sequential\nfrom keras.layers import Conv2D,MaxPooling2D,Dense,Flatten,Dropout\nimport pandas as pd\nimport sys\nimport tensorflow as tf\n%matplotlib inline\nimport matplotlib.pyplot as plt\nimport plotly.express as px",
"_____no_output_____"
],
[
"def readData(filepath, label):\n cells = []\n labels = []\n file = os.listdir(filepath)\n for img in file:\n try:\n image = cv2.imread(filepath + img)\n image_from_array = Image.fromarray(image, 'RGB')\n size_image = image_from_array.resize((50, 50))\n cells.append(np.array(size_image))\n labels.append(label)\n except AttributeError as e:\n print('Skipping file: ', img, e)\n print(len(cells), ' Data Points Read!')\n return np.array(cells), np.array(labels)",
"_____no_output_____"
],
[
"def genesis_train(file):\n \n print('Reading Training Data')\n \n ParasitizedCells, ParasitizedLabels = readData(file + '/Parasitized/', 1)\n UninfectedCells, UninfectedLabels = readData(file + '/Uninfected/', 0)\n Cells = np.concatenate((ParasitizedCells, UninfectedCells))\n Labels = np.concatenate((ParasitizedLabels, UninfectedLabels))\n \n print('Reading Testing Data')\n \n TestParasitizedCells, TestParasitizedLabels = readData('./input/fed/test/Parasitized/', 1)\n TestUninfectedCells, TestUninfectedLabels = readData('./input/fed/test/Uninfected/', 0)\n TestCells = np.concatenate((TestParasitizedCells, TestUninfectedCells))\n TestLabels = np.concatenate((TestParasitizedLabels, TestUninfectedLabels))\n \n s = np.arange(Cells.shape[0])\n np.random.shuffle(s)\n Cells = Cells[s]\n Labels = Labels[s]\n \n sTest = np.arange(TestCells.shape[0])\n np.random.shuffle(sTest)\n TestCells = TestCells[sTest]\n TestLabels = TestLabels[sTest]\n \n num_classes=len(np.unique(Labels))\n len_data=len(Cells)\n print(len_data, ' Data Points')\n \n (x_train,x_test)=Cells, TestCells\n (y_train,y_test)=Labels, TestLabels\n \n # Since we're working on image data, we normalize data by divinding 255.\n x_train = x_train.astype('float32')/255 \n x_test = x_test.astype('float32')/255\n train_len=len(x_train)\n test_len=len(x_test)\n \n #Doing One hot encoding as classifier has multiple classes\n y_train=keras.utils.to_categorical(y_train,num_classes)\n y_test=keras.utils.to_categorical(y_test,num_classes)\n \n #creating sequential model\n model=Sequential()\n model.add(Conv2D(filters=16,kernel_size=2,padding=\"same\",activation=\"relu\",input_shape=(50,50,3)))\n model.add(MaxPooling2D(pool_size=2))\n model.add(Conv2D(filters=32,kernel_size=2,padding=\"same\",activation=\"relu\"))\n model.add(MaxPooling2D(pool_size=2))\n model.add(Conv2D(filters=64,kernel_size=2,padding=\"same\",activation=\"relu\"))\n model.add(MaxPooling2D(pool_size=2))\n model.add(Dropout(0.2))\n model.add(Flatten())\n model.add(Dense(500,activation=\"relu\"))\n model.add(Dropout(0.2))\n model.add(Dense(2,activation=\"softmax\"))#2 represent output layer neurons \n# model.summary()\n\n # compile the model with loss as categorical_crossentropy and using adam optimizer\n model.compile(loss='categorical_crossentropy', optimizer='adam', metrics=['accuracy'])\n \n #Fit the model with min batch size as 50[can tune batch size to some factor of 2^power ] \n model.fit(x_train, y_train, batch_size=100, epochs=5, verbose=1)\n \n scores = model.evaluate(x_test, y_test)\n print(\"Loss: \", scores[0]) #Loss\n print(\"Accuracy: \", scores[1]) #Accuracy\n\n #Saving Model\n model.save(\"./output.h5\")\n return len_data, scores[1]",
"_____no_output_____"
],
[
"def update_train(file, d):\n \n print('Reading Training Data')\n \n ParasitizedCells, ParasitizedLabels = readData(file + '/Parasitized/', 1)\n UninfectedCells, UninfectedLabels = readData(file + '/Uninfected/', 0)\n Cells = np.concatenate((ParasitizedCells, UninfectedCells))\n Labels = np.concatenate((ParasitizedLabels, UninfectedLabels))\n \n print('Reading Testing Data')\n \n TestParasitizedCells, TestParasitizedLabels = readData('./input/fed/test/Parasitized/', 1)\n TestUninfectedCells, TestUninfectedLabels = readData('./input/fed/test/Uninfected/', 0)\n TestCells = np.concatenate((TestParasitizedCells, TestUninfectedCells))\n TestLabels = np.concatenate((TestParasitizedLabels, TestUninfectedLabels))\n \n s = np.arange(Cells.shape[0])\n np.random.shuffle(s)\n Cells = Cells[s]\n Labels = Labels[s]\n \n sTest = np.arange(TestCells.shape[0])\n np.random.shuffle(sTest)\n TestCells = TestCells[sTest]\n TestLabels = TestLabels[sTest]\n \n num_classes=len(np.unique(Labels))\n len_data=len(Cells)\n print(len_data, ' Data Points')\n \n (x_train,x_test)=Cells, TestCells\n (y_train,y_test)=Labels, TestLabels\n \n # Since we're working on image data, we normalize data by divinding 255.\n x_train = x_train.astype('float32')/255 \n x_test = x_test.astype('float32')/255\n train_len=len(x_train)\n test_len=len(x_test)\n \n #Doing One hot encoding as classifier has multiple classes\n y_train=keras.utils.to_categorical(y_train,num_classes)\n y_test=keras.utils.to_categorical(y_test,num_classes)\n \n #creating sequential model\n model=Sequential()\n model.add(Conv2D(filters=16,kernel_size=2,padding=\"same\",activation=\"relu\",input_shape=(50,50,3)))\n model.add(MaxPooling2D(pool_size=2))\n model.add(Conv2D(filters=32,kernel_size=2,padding=\"same\",activation=\"relu\"))\n model.add(MaxPooling2D(pool_size=2))\n model.add(Conv2D(filters=64,kernel_size=2,padding=\"same\",activation=\"relu\"))\n model.add(MaxPooling2D(pool_size=2))\n model.add(Dropout(0.2))\n model.add(Flatten())\n model.add(Dense(500,activation=\"relu\"))\n model.add(Dropout(0.2))\n model.add(Dense(2,activation=\"softmax\"))#2 represent output layer neurons \n # model.summary()\n\n model.load_weights(\"./output.h5\")\n \n # compile the model with loss as categorical_crossentropy and using adam optimizer\n model.compile(loss='categorical_crossentropy', optimizer='adam', metrics=['accuracy'])\n \n #Fit the model with min batch size as 50[can tune batch size to some factor of 2^power ] \n model.fit(x_train, y_train, batch_size=100, epochs=5, verbose=1)\n \n \n scores = model.evaluate(x_test, y_test)\n print(\"Loss: \", scores[0]) #Loss\n print(\"Accuracy: \", scores[1]) #Accuracy\n\n #Saving Model\n model.save(\"./weights/\" + str(d) + \".h5\")\n return len_data, scores[1]",
"_____no_output_____"
],
[
"FLAccuracy = {}\n# FLAccuracy['Complete Dataset'] = genesis_train('./input/cell_images')\nFLAccuracy['Genesis'] = genesis_train('./input/fed/genesis')\nFLAccuracy['d1'] = update_train('./input/fed/d1', 'd1')\nFLAccuracy['d2'] = update_train('./input/fed/d2', 'd2')\nFLAccuracy['d3'] = update_train('./input/fed/d3', 'd3')\nFLAccuracy['d4'] = update_train('./input/fed/d4', 'd4')\nFLAccuracy['d5'] = update_train('./input/fed/d5', 'd5')\nFLAccuracy['d6'] = update_train('./input/fed/d6', 'd6')\nFLAccuracy['d7'] = update_train('./input/fed/d7', 'd7')\nFLAccuracy['d8'] = update_train('./input/fed/d8', 'd8')\nFLAccuracy['d9'] = update_train('./input/fed/d9', 'd9')\nFLAccuracy['d10'] = update_train('./input/fed/d10', 'd10')\nFLAccuracy['d11'] = update_train('./input/fed/d11', 'd11')\nFLAccuracy['d12'] = update_train('./input/fed/d12', 'd12')\nFLAccuracy['d13'] = update_train('./input/fed/d13', 'd13')\nFLAccuracy['d14'] = update_train('./input/fed/d14', 'd14')\nFLAccuracy['d15'] = update_train('./input/fed/d15', 'd15')\nFLAccuracy['d16'] = update_train('./input/fed/d16', 'd16')\nFLAccuracy['d17'] = update_train('./input/fed/d17', 'd17')\nFLAccuracy['d18'] = update_train('./input/fed/d18', 'd18')\nFLAccuracy['d19'] = update_train('./input/fed/d19', 'd19')\nFLAccuracy['d20'] = update_train('./input/fed/d20', 'd20')",
"Reading Training Data\n686 Data Points Read!\n696 Data Points Read!\nReading Testing Data\n2740 Data Points Read!\n2783 Data Points Read!\n1382 Data Points\nEpoch 1/5\n14/14 [==============================] - 2s 135ms/step - loss: 0.6728 - accuracy: 0.6027\nEpoch 2/5\n14/14 [==============================] - 2s 150ms/step - loss: 0.6296 - accuracy: 0.6469\nEpoch 3/5\n14/14 [==============================] - 2s 147ms/step - loss: 0.5789 - accuracy: 0.7142\nEpoch 4/5\n14/14 [==============================] - 2s 149ms/step - loss: 0.5440 - accuracy: 0.7315\nEpoch 5/5\n14/14 [==============================] - 2s 129ms/step - loss: 0.4903 - accuracy: 0.7721\n173/173 [==============================] - 3s 16ms/step - loss: 0.4749 - accuracy: 0.7900\nLoss: 0.47488918900489807\nAccuracy: 0.7899692058563232\nReading Training Data\n528 Data Points Read!\n533 Data Points Read!\nReading Testing Data\n2740 Data Points Read!\n2783 Data Points Read!\n1061 Data Points\nEpoch 1/5\n11/11 [==============================] - 1s 91ms/step - loss: 0.4673 - accuracy: 0.7879\nEpoch 2/5\n11/11 [==============================] - 1s 90ms/step - loss: 0.4264 - accuracy: 0.8162\nEpoch 3/5\n11/11 [==============================] - 1s 96ms/step - loss: 0.3726 - accuracy: 0.8530\nEpoch 4/5\n11/11 [==============================] - 1s 96ms/step - loss: 0.3147 - accuracy: 0.8699\nEpoch 5/5\n11/11 [==============================] - 1s 86ms/step - loss: 0.2670 - accuracy: 0.9057\n173/173 [==============================] - 2s 11ms/step - loss: 0.3025 - accuracy: 0.8749\nLoss: 0.3024612367153168\nAccuracy: 0.8748868107795715\nReading Training Data\n522 Data Points Read!\n528 Data Points Read!\nReading Testing Data\n2740 Data Points Read!\n2783 Data Points Read!\n1050 Data Points\nEpoch 1/5\n11/11 [==============================] - 2s 167ms/step - loss: 0.5450 - accuracy: 0.7448\nEpoch 2/5\n11/11 [==============================] - 1s 100ms/step - loss: 0.4732 - accuracy: 0.7914\nEpoch 3/5\n11/11 [==============================] - 1s 126ms/step - loss: 0.4347 - accuracy: 0.8105\nEpoch 4/5\n11/11 [==============================] - 1s 91ms/step - loss: 0.3879 - accuracy: 0.8419\nEpoch 5/5\n11/11 [==============================] - 2s 153ms/step - loss: 0.3488 - accuracy: 0.8724\n173/173 [==============================] - 3s 15ms/step - loss: 0.3182 - accuracy: 0.8803\nLoss: 0.31824126839637756\nAccuracy: 0.8803186416625977\nReading Training Data\n692 Data Points Read!\n655 Data Points Read!\nReading Testing Data\n2740 Data Points Read!\n2783 Data Points Read!\n1347 Data Points\nEpoch 1/5\n14/14 [==============================] - 2s 122ms/step - loss: 0.5021 - accuracy: 0.7602\nEpoch 2/5\n14/14 [==============================] - 1s 102ms/step - loss: 0.4287 - accuracy: 0.8092\nEpoch 3/5\n14/14 [==============================] - 1s 100ms/step - loss: 0.3923 - accuracy: 0.8448\nEpoch 4/5\n14/14 [==============================] - 1s 102ms/step - loss: 0.3223 - accuracy: 0.8753\nEpoch 5/5\n14/14 [==============================] - 1s 102ms/step - loss: 0.2833 - accuracy: 0.8886\n173/173 [==============================] - 2s 12ms/step - loss: 0.2785 - accuracy: 0.8839\nLoss: 0.27846673130989075\nAccuracy: 0.8839398622512817\nReading Training Data\n448 Data Points Read!\n410 Data Points Read!\nReading Testing Data\n2740 Data Points Read!\n2783 Data Points Read!\n858 Data Points\nEpoch 1/5\n9/9 [==============================] - 1s 92ms/step - loss: 0.5274 - accuracy: 0.7541\nEpoch 2/5\n9/9 [==============================] - 1s 93ms/step - loss: 0.4711 - accuracy: 0.8019\nEpoch 3/5\n9/9 [==============================] - 1s 92ms/step - loss: 0.4223 - accuracy: 0.8135\nEpoch 4/5\n9/9 [==============================] - 1s 92ms/step - loss: 0.3792 - accuracy: 0.8415\nEpoch 5/5\n9/9 [==============================] - 1s 94ms/step - loss: 0.3516 - accuracy: 0.8520\n173/173 [==============================] - 2s 13ms/step - loss: 0.3922 - accuracy: 0.8479\nLoss: 0.392183780670166\nAccuracy: 0.8479087352752686\nReading Training Data\n838 Data Points Read!\n838 Data Points Read!\nReading Testing Data\n2740 Data Points Read!\n2783 Data Points Read!\n1676 Data Points\nEpoch 1/5\n17/17 [==============================] - 2s 102ms/step - loss: 0.5155 - accuracy: 0.7572\nEpoch 2/5\n17/17 [==============================] - 2s 107ms/step - loss: 0.4178 - accuracy: 0.8204\nEpoch 3/5\n17/17 [==============================] - 2s 103ms/step - loss: 0.3393 - accuracy: 0.8646\nEpoch 4/5\n17/17 [==============================] - 2s 104ms/step - loss: 0.2733 - accuracy: 0.9004\nEpoch 5/5\n17/17 [==============================] - 2s 110ms/step - loss: 0.2165 - accuracy: 0.9189\n173/173 [==============================] - 2s 12ms/step - loss: 0.2611 - accuracy: 0.9015\nLoss: 0.26110416650772095\nAccuracy: 0.901502788066864\nReading Training Data\n599 Data Points Read!\n567 Data Points Read!\nReading Testing Data\n2740 Data Points Read!\n2783 Data Points Read!\n1166 Data Points\nEpoch 1/5\n12/12 [==============================] - 1s 103ms/step - loss: 0.6068 - accuracy: 0.6930\nEpoch 2/5\n12/12 [==============================] - 1s 114ms/step - loss: 0.4938 - accuracy: 0.7804\nEpoch 3/5\n12/12 [==============================] - 1s 104ms/step - loss: 0.4533 - accuracy: 0.7985\nEpoch 4/5\n12/12 [==============================] - 2s 137ms/step - loss: 0.4060 - accuracy: 0.8233\nEpoch 5/5\n12/12 [==============================] - 1s 121ms/step - loss: 0.3403 - accuracy: 0.8739\n173/173 [==============================] - 3s 15ms/step - loss: 0.3295 - accuracy: 0.8725\nLoss: 0.3295148015022278\nAccuracy: 0.8725330233573914\nReading Training Data\n418 Data Points Read!\n395 Data Points Read!\nReading Testing Data\n2740 Data Points Read!\n2783 Data Points Read!\n813 Data Points\nEpoch 1/5\n9/9 [==============================] - 1s 99ms/step - loss: 0.5564 - accuracy: 0.7306\nEpoch 2/5\n9/9 [==============================] - 1s 108ms/step - loss: 0.4825 - accuracy: 0.7958\nEpoch 3/5\n9/9 [==============================] - 1s 116ms/step - loss: 0.4358 - accuracy: 0.8266\nEpoch 4/5\n9/9 [==============================] - 1s 161ms/step - loss: 0.3995 - accuracy: 0.8426\nEpoch 5/5\n9/9 [==============================] - 1s 130ms/step - loss: 0.3645 - accuracy: 0.8610\n173/173 [==============================] - 2s 12ms/step - loss: 0.3681 - accuracy: 0.8647\nLoss: 0.3681491017341614\nAccuracy: 0.8647474050521851\nReading Training Data\n716 Data Points Read!\n729 Data Points Read!\nReading Testing Data\n2740 Data Points Read!\n2783 Data Points Read!\n1445 Data Points\nEpoch 1/5\n15/15 [==============================] - 1s 96ms/step - loss: 0.5056 - accuracy: 0.7661\nEpoch 2/5\n15/15 [==============================] - 1s 96ms/step - loss: 0.4308 - accuracy: 0.8187\nEpoch 3/5\n15/15 [==============================] - 1s 93ms/step - loss: 0.3585 - accuracy: 0.8526\nEpoch 4/5\n15/15 [==============================] - 1s 95ms/step - loss: 0.2907 - accuracy: 0.8858\nEpoch 5/5\n15/15 [==============================] - 1s 96ms/step - loss: 0.2397 - accuracy: 0.9038\n173/173 [==============================] - 2s 11ms/step - loss: 0.2601 - accuracy: 0.8972\nLoss: 0.26010987162590027\nAccuracy: 0.8971573710441589\nReading Training Data\n530 Data Points Read!\n572 Data Points Read!\nReading Testing Data\n2740 Data Points Read!\n2783 Data Points Read!\n1102 Data Points\nEpoch 1/5\n12/12 [==============================] - 1s 85ms/step - loss: 0.5228 - accuracy: 0.7486\nEpoch 2/5\n12/12 [==============================] - 1s 86ms/step - loss: 0.4758 - accuracy: 0.7976\nEpoch 3/5\n12/12 [==============================] - 1s 89ms/step - loss: 0.4397 - accuracy: 0.8022\nEpoch 4/5\n12/12 [==============================] - 1s 85ms/step - loss: 0.3850 - accuracy: 0.8348\nEpoch 5/5\n12/12 [==============================] - 1s 85ms/step - loss: 0.3386 - accuracy: 0.8548\n173/173 [==============================] - 2s 11ms/step - loss: 0.2926 - accuracy: 0.8914\nLoss: 0.2926398813724518\nAccuracy: 0.8913633823394775\nReading Training Data\n695 Data Points Read!\n701 Data Points Read!\nReading Testing Data\n2740 Data Points Read!\n2783 Data Points Read!\n1396 Data Points\nEpoch 1/5\n14/14 [==============================] - 1s 88ms/step - loss: 0.4831 - accuracy: 0.7636\nEpoch 2/5\n"
],
[
"FLAccuracy",
"_____no_output_____"
],
[
"FLAccuracyDF = pd.DataFrame.from_dict(FLAccuracy, orient='index', columns=['DataSize', 'Accuracy'])\nFLAccuracyDF",
"_____no_output_____"
],
[
"FLAccuracyDF.index",
"_____no_output_____"
],
[
"n = 0\nfor w in FLAccuracy:\n if 'Complete' in w:\n continue\n n += FLAccuracy[w][0]\nprint('Total number of data points in this round: ', n)",
"Total number of data points in this round: 22035\n"
],
[
"FLAccuracyDF['Weightage'] = FLAccuracyDF['DataSize'].apply(lambda x: x/n)",
"_____no_output_____"
],
[
"FLAccuracyDF",
"_____no_output_____"
],
[
"def scale(weight, scaler):\n scaledWeights = []\n for i in range(len(weight)):\n scaledWeights.append(scaler * weight[i])\n return scaledWeights\n\ndef getScaledWeight(d, scaler):\n \n #creating sequential model\n model=Sequential()\n model.add(Conv2D(filters=16,kernel_size=2,padding=\"same\",activation=\"relu\",input_shape=(50,50,3)))\n model.add(MaxPooling2D(pool_size=2))\n model.add(Conv2D(filters=32,kernel_size=2,padding=\"same\",activation=\"relu\"))\n model.add(MaxPooling2D(pool_size=2))\n model.add(Conv2D(filters=64,kernel_size=2,padding=\"same\",activation=\"relu\"))\n model.add(MaxPooling2D(pool_size=2))\n model.add(Dropout(0.2))\n model.add(Flatten())\n model.add(Dense(500,activation=\"relu\"))\n model.add(Dropout(0.2))\n model.add(Dense(2,activation=\"softmax\"))#2 represent output layer neurons \n \n fpath = \"./weights/\"+d+\".h5\"\n model.load_weights(fpath)\n weight = model.get_weights()\n scaledWeight = scale(weight, scaler)\n\n return scaledWeight",
"_____no_output_____"
],
[
"def avgWeights(scaledWeights):\n avg = list()\n for weight_list_tuple in zip(*scaledWeights):\n layer_mean = tf.math.reduce_sum(weight_list_tuple, axis=0)\n avg.append(layer_mean)\n return avg\n\ndef FedAvg(models):\n \n scaledWeights = []\n for m in models:\n scaledWeights.append(getScaledWeight(m, FLAccuracyDF.loc[m]['Weightage']))\n avgWeight = avgWeights(scaledWeights)\n return avgWeight",
"_____no_output_____"
],
[
"models = ['d1', 'd2', 'd3', 'd4', 'd5', 'd6', 'd7', 'd8', 'd9', 'd10', 'd11', 'd12', 'd13', 'd14', 'd15', 'd16', 'd17', 'd18', 'd19', 'd20']\navgWeight = FedAvg(models)\nprint(avgWeight)",
"[<tf.Tensor: shape=(2, 2, 3, 16), dtype=float32, numpy=\narray([[[[ 6.29709959e-02, 3.05600781e-02, 4.73186262e-02,\n -4.84639332e-02, -1.09765619e-01, -2.40126792e-02,\n -2.00001881e-01, 4.64794785e-03, -9.27647501e-02,\n -1.66123241e-01, 2.24855661e-01, -7.29905367e-02,\n 1.65846452e-01, -1.77608833e-01, 8.64765197e-02,\n -2.02627137e-01],\n [ 1.22623229e-02, -2.69816726e-01, -8.74007195e-02,\n -2.52788186e-01, -1.04290135e-01, 1.57856569e-01,\n -2.61925399e-01, -1.27280667e-01, 1.26418844e-01,\n -1.46524444e-01, -2.43654959e-02, 2.42929533e-01,\n -2.60935754e-01, 2.79806722e-02, 1.43053783e-02,\n -1.92507386e-01],\n [-1.32187217e-01, -1.47404790e-01, -3.16053033e-02,\n 1.14077598e-01, -1.20645165e-01, 1.56122614e-02,\n 2.14044124e-01, 1.66435450e-01, -1.01623848e-01,\n -2.04821333e-01, -9.87461675e-03, -2.57626027e-01,\n -2.19773769e-01, -1.49482757e-01, -1.49648502e-01,\n 4.13678773e-02]],\n\n [[ 4.09911200e-02, -2.55751640e-01, 5.51730059e-02,\n 1.51951760e-01, -2.08946750e-01, -1.78974658e-01,\n -2.33739480e-01, -1.88674644e-01, -2.48390481e-01,\n -8.12981203e-02, -1.72898650e-01, -5.53919859e-02,\n 1.58572555e-01, -1.22390337e-01, 2.73622870e-02,\n 4.19585668e-02],\n [-8.34954083e-02, 2.52027541e-01, -1.80260316e-01,\n -2.27775335e-01, -1.51616588e-01, 2.35146865e-01,\n -2.38066748e-01, 1.14227705e-01, 2.09067702e-01,\n 4.44908515e-02, 9.89529341e-02, -1.02538820e-02,\n 1.72871754e-01, 1.98662151e-02, -6.45739734e-02,\n 8.96787941e-02],\n [ 1.29124120e-01, -2.28320614e-01, 1.61692813e-01,\n 6.46706596e-02, 2.05930516e-01, 9.05648619e-02,\n -2.87533049e-02, 6.75788745e-02, 1.68647338e-02,\n 4.18692678e-02, 2.26347685e-01, 1.76918477e-01,\n 1.28755435e-01, -1.25659034e-01, -1.95176736e-01,\n 2.46681631e-01]]],\n\n\n [[[ 6.76263869e-02, -1.64116889e-01, -4.63423915e-02,\n 1.08722940e-01, -2.42184967e-01, 1.78869694e-01,\n 1.28165051e-01, -1.95564687e-01, 1.54643655e-01,\n -8.88377205e-02, 8.41335952e-02, -2.23800123e-01,\n -1.60318166e-01, 1.83068842e-01, -2.22699732e-01,\n 5.19412458e-02],\n [-1.74145699e-01, 1.64810389e-01, 3.44512388e-02,\n -1.51834458e-01, 2.44864017e-01, -2.07519397e-01,\n -4.64312620e-02, -9.52167138e-02, 1.13456339e-01,\n 2.46316984e-01, -1.51859090e-01, 2.41635039e-01,\n 1.27022326e-01, 2.29096413e-01, -1.57743827e-01,\n 1.86367273e-01],\n [ 2.29843587e-01, -2.43908539e-01, -2.01413512e-01,\n 9.97636020e-02, 2.12529823e-01, 6.52621761e-02,\n 2.25567147e-01, -1.94455549e-01, 5.37216440e-02,\n 9.14809331e-02, -1.86621517e-01, 2.08363146e-01,\n 1.42783493e-01, -1.71516404e-01, 2.87856683e-02,\n 7.70495385e-02]],\n\n [[ 1.47195205e-01, 9.29638669e-02, -2.24783435e-01,\n 1.57111585e-01, 2.19253033e-01, -1.44216925e-01,\n 1.88499019e-01, 2.31585249e-01, 1.90144151e-01,\n -9.07958075e-02, -4.38553058e-02, 1.75386280e-01,\n -2.41612554e-01, -1.50471807e-01, 1.24751553e-01,\n -1.57731324e-01],\n [ 1.61211178e-01, 1.91906020e-01, -1.63448617e-01,\n -1.06442757e-01, -7.65680149e-02, -5.57944737e-02,\n -1.51635736e-01, 9.22414038e-05, 2.16817468e-01,\n -1.81188583e-01, 1.44259129e-02, -8.36728215e-02,\n 1.69681653e-01, 1.00190096e-01, 1.78319663e-01,\n -1.59951687e-01],\n [-4.09052819e-02, -1.97755881e-02, -1.84581861e-01,\n -1.45668117e-02, -1.44612893e-01, 2.52977610e-01,\n 1.50122315e-01, -2.20931277e-01, 3.89067531e-02,\n 2.37456664e-01, 1.47347704e-01, -4.30123396e-02,\n -4.51557077e-02, -5.94768040e-02, -2.90106405e-02,\n -2.41003498e-01]]]], dtype=float32)>, <tf.Tensor: shape=(16,), dtype=float32, numpy=\narray([-0.01007514, -0.001635 , -0.01025345, -0.00173561, 0.03268854,\n -0.00208687, 0.00159445, -0.01452221, -0.00939484, -0.00810049,\n 0.00187787, -0.00531907, -0.01053663, -0.01091921, -0.01560304,\n -0.00814741], dtype=float32)>, <tf.Tensor: shape=(2, 2, 16, 32), dtype=float32, numpy=\narray([[[[-0.09537445, 0.0238525 , 0.08094858, ..., -0.11627594,\n 0.01361733, -0.00270094],\n [ 0.03979139, 0.12243818, -0.10753282, ..., -0.02761981,\n 0.00115342, -0.11250883],\n [-0.00319301, 0.03747182, -0.12272758, ..., -0.17002101,\n -0.09323299, -0.09280318],\n ...,\n [ 0.01198404, 0.18341562, -0.08970661, ..., 0.07275134,\n 0.16204083, -0.11643461],\n [ 0.11521284, 0.09957907, -0.06785449, ..., -0.16049376,\n -0.03478961, 0.05059588],\n [-0.10904927, 0.05084139, -0.11017702, ..., -0.13262197,\n -0.09998756, 0.07306522]],\n\n [[ 0.14296961, 0.14634573, -0.07494281, ..., 0.04981283,\n -0.02226478, -0.00955457],\n [-0.18278444, -0.12257586, 0.1105509 , ..., 0.06898673,\n -0.14074963, 0.12880448],\n [ 0.05062443, 0.09011479, -0.01778495, ..., 0.01384717,\n 0.05328264, 0.01165935],\n ...,\n [ 0.11653248, 0.15053472, 0.02755488, ..., -0.00180184,\n -0.11342274, 0.02041092],\n [ 0.11759499, 0.10058165, 0.1134244 , ..., 0.01960301,\n -0.04442984, -0.04641576],\n [-0.01923554, -0.1412403 , -0.0296952 , ..., 0.01371953,\n 0.06250753, 0.09004614]]],\n\n\n [[[ 0.14302328, -0.03723493, 0.05975141, ..., -0.08695951,\n -0.03743268, -0.16613564],\n [ 0.04879532, 0.01573316, 0.03783104, ..., -0.07207087,\n 0.138336 , 0.06230988],\n [-0.17695434, -0.14341961, 0.1074056 , ..., -0.0374577 ,\n -0.04587403, -0.06571089],\n ...,\n [ 0.08705768, -0.09674174, 0.15470633, ..., 0.07526341,\n 0.14084874, -0.07903387],\n [ 0.11766817, -0.07612219, -0.03450292, ..., -0.17648341,\n -0.08644895, -0.1104345 ],\n [ 0.09279143, -0.14395934, -0.12362929, ..., -0.08168439,\n -0.01325884, 0.06796352]],\n\n [[-0.10889354, 0.09163773, 0.064237 , ..., -0.11828446,\n -0.03442774, 0.11232536],\n [ 0.1001339 , 0.06223914, -0.13083588, ..., 0.05516066,\n -0.17094746, 0.06631851],\n [ 0.13773926, 0.10093588, -0.13178204, ..., -0.05654637,\n -0.06447498, 0.10750612],\n ...,\n [ 0.06421222, 0.1465723 , -0.04136471, ..., 0.05052067,\n -0.06008358, -0.12787679],\n [ 0.04828766, 0.05224356, -0.16164221, ..., 0.10612825,\n -0.11255513, 0.04035288],\n [-0.16791548, 0.06760396, -0.13105269, ..., 0.06637175,\n 0.03395404, 0.00674641]]]], dtype=float32)>, <tf.Tensor: shape=(32,), dtype=float32, numpy=\narray([ 7.8103901e-03, -2.5253366e-03, 9.4068382e-05, -1.0054309e-02,\n 5.6395228e-03, 2.2935962e-02, 3.6243699e-04, -6.3295038e-03,\n 3.1995773e-02, 1.3141254e-02, 8.1295166e-03, -1.7962465e-03,\n -5.8354164e-04, -7.3266337e-03, -8.7775812e-03, 7.3068980e-03,\n -1.2754799e-03, -8.6430637e-03, -1.2573479e-02, -5.5672540e-03,\n -1.1904450e-02, -4.4461302e-03, -1.9995815e-03, -3.0116516e-03,\n -1.4999485e-02, -6.2715444e-03, 1.2082155e-02, 9.9959513e-03,\n -3.4943717e-03, 4.8271101e-03, -4.3909899e-03, -7.2158724e-03],\n dtype=float32)>, <tf.Tensor: shape=(2, 2, 32, 64), dtype=float32, numpy=\narray([[[[-0.05097382, -0.103765 , 0.05987458, ..., -0.05191575,\n -0.00475351, 0.11364381],\n [-0.00168266, -0.03842274, -0.07855407, ..., -0.0831281 ,\n -0.0727298 , 0.05130759],\n [-0.05923396, -0.01078768, 0.02821486, ..., 0.02914551,\n -0.06302543, -0.08857528],\n ...,\n [-0.04730515, -0.01813016, 0.05795706, ..., -0.10225762,\n 0.01166681, 0.09502172],\n [-0.09534874, -0.05579872, -0.04130651, ..., -0.13656466,\n 0.09485541, -0.11062077],\n [-0.05683818, -0.04426903, -0.03588507, ..., -0.09614032,\n 0.03641687, -0.04682203]],\n\n [[ 0.16425094, 0.00555054, 0.00494457, ..., 0.00898783,\n 0.10889069, 0.08391576],\n [-0.02203994, -0.05366965, -0.11370585, ..., 0.02346958,\n -0.05212817, 0.04323069],\n [-0.04689383, -0.03736854, -0.01105933, ..., 0.05111279,\n -0.10411535, -0.07766384],\n ...,\n [ 0.00388702, -0.05325527, -0.08057582, ..., -0.09036542,\n 0.08295781, 0.02695396],\n [-0.06440552, -0.01092236, -0.04536002, ..., -0.07186652,\n 0.0181105 , 0.08185989],\n [-0.0419886 , -0.03008262, -0.05637935, ..., 0.04211152,\n 0.02888373, -0.06597874]]],\n\n\n [[[-0.03833229, -0.10916876, 0.1284822 , ..., -0.05868948,\n -0.12631544, 0.12959538],\n [ 0.04788401, 0.03303488, 0.06145496, ..., -0.09839627,\n 0.03922866, 0.09826408],\n [-0.07018621, 0.10417556, -0.02953644, ..., 0.05394573,\n 0.0176542 , -0.01720765],\n ...,\n [ 0.05617737, -0.05888639, -0.09679458, ..., -0.0468474 ,\n 0.08244231, 0.10589295],\n [ 0.0106239 , -0.01670274, -0.12942371, ..., -0.0671926 ,\n -0.02569613, -0.07168184],\n [ 0.08028605, 0.07828661, -0.0697 , ..., -0.11891251,\n 0.01813116, 0.09038625]],\n\n [[ 0.08789235, -0.01428154, 0.06888534, ..., 0.09440541,\n -0.03437117, -0.10806488],\n [-0.06862493, 0.04934879, -0.04362699, ..., -0.07955035,\n 0.05497896, 0.09841695],\n [-0.10580587, -0.00517587, 0.0524519 , ..., -0.00403526,\n 0.02082359, 0.03606828],\n ...,\n [ 0.1386118 , -0.05597519, -0.08895131, ..., -0.04376757,\n -0.04135654, 0.1048066 ],\n [ 0.1007139 , 0.05583981, -0.03825749, ..., -0.11412786,\n 0.01438947, -0.04390105],\n [ 0.00362133, 0.09658727, -0.03246778, ..., 0.05341358,\n 0.02820618, -0.01911995]]]], dtype=float32)>, <tf.Tensor: shape=(64,), dtype=float32, numpy=\narray([-8.1379199e-03, 6.1860224e-03, 1.6091123e-02, -6.1926767e-03,\n -6.7583038e-03, -9.4345007e-03, -6.0022073e-03, 3.3236535e-03,\n 1.1789045e-02, -8.9633754e-03, 6.6024857e-03, -2.8087604e-03,\n -5.3437511e-03, -5.8863903e-03, -1.5286321e-02, -8.4907245e-03,\n -4.6209171e-03, -1.0798765e-02, 1.3004292e-02, 7.3197032e-03,\n -2.3899684e-03, -5.9073414e-03, 1.2828208e-03, 8.9652007e-03,\n -1.0949758e-02, 1.0419855e-03, -3.2625971e-03, 3.0798630e-03,\n -2.5548907e-03, -9.3173067e-04, -9.3546556e-03, 1.8120963e-03,\n -3.8350022e-03, -1.4084974e-02, -3.6110198e-03, -4.5017223e-03,\n -2.4619487e-03, 1.8209267e-02, -2.8989587e-03, 1.6913021e-03,\n -5.3030080e-03, -5.9248605e-03, 1.1082625e-03, -3.8173990e-03,\n 1.8847305e-03, -8.2327640e-03, -1.7164005e-03, 2.6117486e-05,\n -4.1099940e-03, 1.6918827e-02, -5.7169464e-03, -2.2128168e-03,\n 4.6205113e-04, -5.6640753e-03, -2.1636956e-03, -4.5968518e-03,\n -1.8414368e-03, -1.2497818e-02, -7.9843001e-03, 2.1397090e-03,\n -3.7410008e-03, 3.0753077e-03, -9.8327342e-03, -6.4184298e-03],\n dtype=float32)>, <tf.Tensor: shape=(2304, 500), dtype=float32, numpy=\narray([[-1.5044750e-02, -1.9083844e-02, 4.4547915e-02, ...,\n 2.8617123e-02, -3.0377548e-02, 4.0829502e-05],\n [ 3.3865925e-02, -2.2863064e-02, -1.0030516e-02, ...,\n 1.5757322e-02, -1.9825639e-02, -1.3909874e-02],\n [-1.5962871e-02, 1.8267710e-02, 1.4149060e-03, ...,\n -4.8543759e-02, 2.1052580e-02, -1.3631902e-02],\n ...,\n [-1.1880083e-02, -9.0174275e-03, -1.0868900e-02, ...,\n 6.0880934e-03, -3.2359600e-02, -1.5150492e-02],\n [-1.4395309e-02, 1.9456392e-02, 1.5710445e-02, ...,\n 5.6687263e-03, 8.9815492e-03, 5.4982271e-02],\n [ 1.5056844e-02, -3.3522487e-02, -1.8322496e-02, ...,\n -3.1811804e-02, 2.7293323e-02, 3.3484075e-02]], dtype=float32)>, <tf.Tensor: shape=(500,), dtype=float32, numpy=\narray([-5.53416880e-03, 1.12668620e-02, -2.51924153e-03, -4.17344924e-03,\n 9.82279144e-03, -1.77115912e-03, -6.46361941e-03, -5.59850084e-03,\n 1.04833283e-02, -3.00975540e-03, -8.56306928e-04, -5.20168711e-03,\n 8.98524467e-03, 1.28810275e-02, -4.06666519e-03, -3.82398628e-03,\n -5.54959150e-03, -3.64668365e-03, -7.92631507e-03, -6.75905682e-03,\n -6.28078589e-03, -3.44249629e-03, 8.80345237e-03, 9.11579560e-03,\n -3.39526846e-03, -8.37900490e-03, -3.30694043e-03, -5.70433028e-03,\n -6.35400740e-03, -6.88481797e-03, -1.85096229e-03, -5.58825349e-03,\n 1.12006404e-02, -5.44621237e-03, -2.14726408e-03, -4.09880653e-03,\n -4.19273693e-03, -5.61053818e-03, -4.17135097e-03, -4.33228025e-03,\n 9.04962793e-03, 1.30762206e-02, -5.75472182e-03, 1.13392835e-02,\n -5.61683020e-03, -4.13291575e-03, -3.34750861e-03, -4.06149169e-03,\n -3.66749568e-03, -3.24321515e-03, 9.33618098e-03, -7.22316233e-03,\n 9.70353745e-03, -3.68067622e-03, -6.16257172e-03, 8.20684154e-03,\n -4.89435019e-03, -2.14906642e-04, -4.98367054e-03, 8.90939869e-03,\n 1.21129975e-02, -5.61448559e-03, 1.06549365e-02, -5.29230293e-03,\n -7.12551922e-03, -3.38179525e-03, -1.93966873e-04, 1.35435369e-02,\n -2.14829901e-03, -5.71408169e-03, -5.53259440e-03, 1.09508727e-02,\n 7.46000465e-03, 8.89100228e-03, 1.11365514e-02, 1.13110561e-02,\n -3.79776186e-03, -2.44536810e-03, 8.80534574e-03, 6.33697840e-04,\n 1.35701690e-02, -3.75823537e-03, 8.84520821e-03, 1.01940446e-02,\n 1.18791331e-02, -4.45917575e-03, -5.21652494e-03, 1.00889653e-02,\n 1.04786623e-02, -1.21432624e-03, -4.65361541e-03, -4.73555177e-03,\n -2.50295829e-03, -6.23297552e-03, 1.23668527e-02, -5.57112740e-03,\n -5.67774940e-03, -8.34269915e-03, 1.95718429e-04, -7.68144522e-03,\n 1.63959491e-03, -7.55467964e-03, -6.72129123e-03, -5.64150047e-03,\n -4.72860225e-03, -2.58226250e-03, 1.13667669e-02, -4.72749490e-03,\n -6.87813736e-04, -7.16909440e-03, 1.38499383e-02, -4.85397922e-03,\n -4.04685503e-03, -8.88858456e-03, -3.92845739e-03, -5.49211912e-03,\n 8.65745731e-03, -6.40754169e-03, 8.97939131e-03, -7.40244985e-03,\n -4.83700447e-03, -7.29304156e-04, -3.94110195e-03, -6.02743588e-04,\n 1.11664683e-02, -5.32353530e-03, -2.82435399e-03, -3.30526684e-03,\n -6.55194139e-03, -6.93755038e-03, -1.95045010e-04, -4.21771314e-03,\n -2.50204583e-03, -3.01466952e-03, -6.63121603e-03, -4.80043842e-03,\n -7.46097416e-03, 1.32271247e-02, -5.97341685e-03, -5.82827302e-03,\n -3.68164736e-03, -6.31515449e-03, 9.53376200e-03, -5.07155526e-03,\n -2.95582996e-03, 1.04220407e-02, 1.18372804e-02, -2.46381853e-03,\n -4.80389362e-03, -5.61798224e-03, -5.24300430e-03, 1.54450033e-02,\n 1.32732317e-02, -4.70723212e-03, 1.08032888e-02, -6.82017487e-03,\n -6.28149649e-03, -2.42553814e-03, -8.88851658e-03, -5.68734854e-03,\n -4.40417882e-03, -4.07085195e-03, 1.10611124e-02, 8.81560147e-03,\n 1.23010194e-02, 1.01457806e-02, -2.99730501e-03, 1.13221137e-02,\n -5.19194733e-03, -4.76593524e-03, -3.49803339e-03, -5.18953474e-03,\n 1.03672050e-04, -2.19414127e-03, -6.27203798e-03, -4.62104147e-03,\n 9.67853703e-03, 1.19852079e-02, -4.77695186e-03, -3.02255899e-03,\n -3.44580598e-03, -5.03656501e-03, 1.16737988e-02, -1.01109478e-03,\n 1.50007810e-02, 1.32154869e-02, -5.56759816e-03, -5.62080368e-03,\n 1.20012723e-02, -3.59317590e-03, -4.95124375e-03, 1.28721436e-02,\n 8.88990797e-03, -6.95590302e-03, -3.73836188e-03, -9.03419685e-03,\n -7.40891788e-03, -4.48821840e-04, -3.66688869e-03, -3.19493329e-03,\n 1.01427659e-02, 1.11271814e-02, 1.10702636e-02, -2.11332203e-03,\n 2.97213136e-03, 1.19747408e-02, -5.24236402e-03, -1.82243134e-03,\n -5.73743833e-03, -6.53023412e-03, 8.42849817e-03, -1.75264548e-03,\n -3.80321289e-03, 1.21859685e-02, -5.28530916e-03, 8.93038046e-03,\n -1.85946876e-03, -4.21086838e-03, -4.17282851e-03, 9.28098708e-03,\n 1.21705001e-02, -3.06760427e-03, 9.03287902e-03, 1.28004504e-02,\n 5.14049828e-03, -5.60991513e-03, -5.60030155e-03, 1.09166838e-02,\n -1.18235371e-03, -1.28952414e-03, -4.58185887e-03, -5.13173454e-03,\n -3.54259741e-03, 1.11334594e-02, -3.29254218e-03, -2.63589993e-03,\n -5.60598960e-03, -8.67103878e-03, -2.93730665e-03, 2.26680830e-04,\n -5.56087401e-03, -3.64702521e-03, -1.47829112e-03, 1.20302821e-02,\n -3.81234381e-03, -7.11362157e-03, 1.12991966e-02, -9.81083792e-03,\n -3.74554598e-04, -7.74306990e-03, 1.20938942e-03, -7.87460152e-03,\n -4.29497054e-03, -3.82512342e-03, -5.61306300e-03, -5.59601514e-03,\n -4.65118355e-04, 1.30068157e-02, -6.96778996e-04, -7.90145155e-03,\n -4.68531577e-03, -6.41325163e-03, -9.63451690e-04, -1.03702885e-03,\n -2.45038699e-03, -9.89039708e-03, 1.02759171e-02, -5.18792914e-03,\n 3.90443206e-03, -5.46140177e-03, -5.85449254e-03, -7.75552588e-03,\n -2.65215989e-03, 2.57740874e-04, -4.21085767e-03, 9.33959056e-03,\n -8.25493410e-03, 8.85015074e-03, 1.23032620e-02, -2.43170653e-03,\n -4.67009097e-03, 9.55339614e-03, -6.68688398e-03, 1.02293398e-02,\n -2.21140636e-03, -2.83968076e-03, -6.47431705e-03, -1.17618497e-03,\n -2.06996733e-03, -3.74172023e-03, 8.99831671e-03, 1.34283733e-02,\n -2.95580830e-03, -2.78668827e-03, -5.36573119e-03, 1.10625606e-02,\n 1.20213265e-02, 1.29257431e-02, 1.02709904e-02, 1.46592576e-02,\n -2.87306751e-03, -2.57530925e-03, -1.21975131e-03, -1.98024209e-03,\n -3.65329836e-03, -3.85023374e-03, 1.08012799e-02, 9.48995803e-05,\n -5.53057902e-03, 1.33074366e-03, 8.31840653e-03, -5.04636159e-03,\n -2.35966896e-03, 1.21791437e-02, -8.25849071e-04, 3.88702890e-03,\n 1.00835385e-02, -2.26927036e-03, 1.11112688e-02, 1.07091153e-02,\n -4.75936569e-03, -5.16986288e-03, -1.54961017e-04, -4.29618731e-03,\n -2.62062997e-03, -6.55703200e-03, 1.31747909e-02, 9.15726181e-03,\n -6.96338667e-03, -6.86828885e-03, -2.18273280e-03, -1.80473831e-03,\n -5.26898634e-03, -5.49727399e-03, 1.12049617e-02, -6.60270732e-03,\n -5.09557826e-03, 9.42141470e-03, -6.21194812e-03, -5.80852665e-03,\n -2.03451491e-03, -1.75885821e-03, 1.20100761e-02, 9.89157520e-03,\n 1.17626321e-02, 1.30208796e-02, -9.12684627e-05, 1.14509659e-02,\n 9.74610634e-03, -2.73874239e-03, -4.58180439e-03, -4.26019076e-03,\n -3.16335331e-03, 2.72267079e-03, -5.57976356e-03, -3.09378584e-03,\n -3.35071399e-03, -4.05666325e-03, 1.09317005e-02, -5.98766655e-03,\n -1.52193604e-03, -4.38027596e-03, -6.51899609e-04, -3.89595074e-03,\n -3.28053813e-03, 1.11519285e-02, -2.93060159e-03, 9.72441025e-03,\n -8.85107648e-03, 1.08384751e-02, 1.17983613e-02, -6.72848150e-03,\n 1.06212078e-02, -5.12874452e-03, -3.57506657e-03, -5.36638824e-03,\n -4.11110371e-03, -5.96203050e-03, -2.85185175e-03, -7.19294325e-03,\n -7.81475846e-03, 9.83515382e-03, -9.24775959e-04, -7.53887743e-03,\n -3.22614377e-03, 1.22755012e-02, 1.05221616e-02, -9.61643644e-04,\n 1.17060253e-02, -3.14895273e-03, 1.15561383e-02, -4.81133629e-03,\n -2.31146999e-03, 7.01590907e-03, -3.40830768e-04, -6.03314582e-03,\n 9.08868946e-03, -3.73258116e-03, -1.99783803e-03, -2.79753609e-03,\n -8.24323739e-04, 1.19561637e-02, -3.95200495e-03, -1.26268726e-03,\n -1.55346445e-03, -6.04792219e-03, 7.40239490e-03, -2.95300642e-03,\n 9.86943301e-03, 1.24818468e-02, -2.34776968e-03, -6.97968761e-03,\n -2.50496319e-03, 6.33505266e-03, -2.07575876e-03, -1.63455214e-03,\n 1.00206258e-02, -2.48630135e-03, -3.58426711e-03, -3.98963783e-03,\n 1.01473331e-02, 1.10968156e-02, -4.35665529e-03, 1.21512776e-02,\n -6.98138727e-03, -9.01398889e-04, -5.60927298e-03, -1.45288149e-03,\n -3.87207884e-03, -2.94308970e-03, 1.10045495e-02, -3.27208196e-03,\n 7.61404494e-03, -5.47929248e-03, -3.01020127e-03, -9.55906138e-03,\n -5.53280767e-03, -7.28675572e-04, 5.55282598e-03, -1.76397408e-03,\n 6.81685284e-03, 1.01069128e-02, -5.46161924e-03, -1.28993904e-03,\n -1.05295400e-03, -3.52117140e-03, -5.76895103e-03, 2.91664759e-03,\n -1.51595997e-03, 7.78692542e-03, -1.12719163e-02, -3.46895959e-03,\n 1.00675672e-02, -8.27851531e-04, -5.40378690e-03, -5.57581801e-03,\n -7.43054925e-03, -3.99085227e-03, -2.47309078e-03, -3.02337669e-03,\n -2.71165813e-03, -3.62162798e-04, -5.38837304e-03, -4.36798111e-03,\n -1.64058746e-03, -7.32781878e-03, 1.01796910e-02, -5.91279287e-03,\n 9.76460241e-03, -2.69726431e-03, -5.62081765e-03, -2.54856143e-03,\n -4.46600746e-03, -4.22631390e-03, -9.64728184e-04, 1.30091254e-02,\n 1.24156959e-02, -2.78786267e-03, 9.98625811e-03, 1.08450977e-02,\n 8.82346649e-03, 1.14490669e-02, -5.76793309e-03, 9.75369290e-03,\n -4.55533341e-03, 2.60111806e-03, 1.26153072e-02, -3.95245338e-03,\n 2.82460329e-04, -1.97257055e-03, 9.04539507e-03, -5.60731255e-03,\n -3.03759193e-03, -6.28925627e-03, -4.76786727e-03, 1.24086421e-02,\n -2.92672310e-03, -6.27733255e-03, -4.93518915e-03, -4.00497485e-03],\n dtype=float32)>, <tf.Tensor: shape=(500, 2), dtype=float32, numpy=\narray([[ 0.05311327, 0.09514155],\n [ 0.09232233, -0.02851534],\n [-0.08964266, 0.04173108],\n [ 0.08367732, -0.0923907 ],\n [ 0.07473528, -0.08274475],\n [-0.0627539 , 0.09992003],\n [-0.0911191 , -0.07911095],\n [-0.0498883 , -0.09269869],\n [ 0.07364915, -0.06293585],\n [ 0.04505345, 0.08532324],\n [ 0.03102643, 0.07411335],\n [ 0.03223044, -0.05786802],\n [ 0.10995429, -0.01073887],\n [ 0.10785273, 0.00285203],\n [ 0.04749208, 0.05066685],\n [ 0.0555957 , 0.09664138],\n [-0.00818688, 0.0174538 ],\n [-0.08163548, -0.05619446],\n [ 0.02267669, -0.04380619],\n [-0.01865696, 0.0669779 ],\n [ 0.05982472, 0.05374481],\n [ 0.05908397, -0.05340671],\n [ 0.04229654, -0.0705682 ],\n [ 0.12624112, -0.03905471],\n [-0.08749062, -0.01966353],\n [ 0.02720188, 0.02096189],\n [ 0.06169368, 0.08842259],\n [ 0.01870921, -0.08792486],\n [-0.05300749, -0.06052515],\n [ 0.05659251, -0.05551624],\n [-0.00416558, 0.06536365],\n [-0.01034767, 0.05329547],\n [ 0.07533468, -0.05162623],\n [ 0.03910884, 0.06408792],\n [-0.02865157, 0.03982799],\n [-0.00720344, 0.03931104],\n [ 0.03563602, 0.00198104],\n [ 0.0744648 , -0.02432105],\n [-0.09741265, 0.04142449],\n [-0.02363664, -0.05258123],\n [ 0.12679069, -0.04927159],\n [-0.02962878, -0.12274925],\n [-0.08189599, -0.02983104],\n [ 0.08228916, -0.12541004],\n [-0.01287102, 0.03614439],\n [-0.016284 , 0.06752599],\n [ 0.01914192, 0.04822027],\n [ 0.09245484, -0.08001135],\n [-0.04274287, -0.00859715],\n [ 0.05542322, 0.00793769],\n [ 0.03698973, -0.11074325],\n [ 0.05847845, -0.01122535],\n [ 0.05873084, -0.06315666],\n [-0.01872551, 0.0537422 ],\n [ 0.00085652, -0.0669762 ],\n [ 0.09326971, -0.04454394],\n [ 0.03200875, 0.03614467],\n [ 0.04222843, 0.09592429],\n [-0.0204555 , 0.00569754],\n [ 0.05601981, -0.12443047],\n [ 0.00828085, -0.09333592],\n [-0.05703217, -0.02206847],\n [ 0.07337331, -0.01721186],\n [-0.10884454, -0.00482925],\n [ 0.0913316 , 0.08112097],\n [-0.07608335, 0.00085231],\n [-0.09819711, -0.05532588],\n [ 0.07308225, 0.00092411],\n [-0.05119678, 0.05604082],\n [ 0.07414087, 0.10505526],\n [ 0.01682709, 0.01961546],\n [ 0.08240177, -0.10032496],\n [ 0.04001589, -0.08403035],\n [ 0.12239482, 0.06419824],\n [ 0.1324751 , -0.01727679],\n [-0.02381351, -0.10001826],\n [-0.06213731, 0.07949966],\n [-0.04335392, 0.01332419],\n [ 0.04419218, -0.06350309],\n [-0.04222876, 0.10688141],\n [ 0.07051704, -0.01126709],\n [-0.05057632, 0.00396998],\n [ 0.13414493, -0.005617 ],\n [ 0.12455928, 0.00826711],\n [ 0.12764968, -0.07120249],\n [-0.05176536, 0.07963897],\n [ 0.08401894, 0.03100447],\n [ 0.08777595, -0.04021717],\n [ 0.08009473, -0.10042297],\n [ 0.05165022, 0.10122991],\n [-0.02704764, -0.05683092],\n [-0.07406341, -0.05763072],\n [-0.07700881, 0.11013646],\n [ 0.08613678, 0.01541759],\n [ 0.10774246, -0.0432528 ],\n [-0.01273213, 0.0070588 ],\n [-0.01267115, 0.09487985],\n [-0.01893573, -0.01735934],\n [ 0.07963271, 0.09316237],\n [-0.0849181 , 0.09335002],\n [-0.07189753, 0.06319249],\n [-0.01437461, -0.07791349],\n [-0.07779831, -0.03652881],\n [-0.04608301, 0.05867525],\n [-0.08924111, 0.09480068],\n [-0.10260601, -0.02373477],\n [ 0.11865773, -0.01351118],\n [ 0.0479991 , -0.04756196],\n [-0.00401142, 0.09675419],\n [ 0.00615512, -0.02635094],\n [ 0.03999988, -0.05102275],\n [ 0.07417063, 0.09568918],\n [-0.10557904, -0.06227076],\n [ 0.00559694, -0.06304982],\n [ 0.06008749, 0.0375339 ],\n [-0.06326835, 0.02782372],\n [ 0.12035009, -0.09589054],\n [ 0.00579257, 0.08006887],\n [-0.01508083, -0.09803493],\n [ 0.03642597, -0.00953708],\n [ 0.00085658, 0.05022113],\n [-0.0886467 , -0.03710546],\n [-0.03850847, 0.05948083],\n [-0.10435861, 0.10078683],\n [ 0.10006507, 0.07087514],\n [ 0.00495187, 0.01239195],\n [-0.0808455 , 0.05980099],\n [-0.07445095, 0.10324261],\n [ 0.0441043 , 0.0076606 ],\n [-0.07697976, 0.07268078],\n [-0.07653762, -0.01193937],\n [-0.09638125, -0.07475944],\n [-0.06650464, -0.03050037],\n [-0.05667973, 0.08996452],\n [ 0.05176068, -0.0103077 ],\n [ 0.03983513, 0.04569188],\n [-0.01864344, 0.08519334],\n [ 0.0079924 , -0.1436909 ],\n [-0.06566814, -0.07663368],\n [-0.00131069, -0.07904355],\n [ 0.00564205, 0.04069302],\n [-0.01365841, -0.06190141],\n [ 0.09065706, -0.04532593],\n [-0.07778468, 0.06040839],\n [ 0.07657637, 0.00619171],\n [ 0.12044221, -0.05069051],\n [ 0.10355301, -0.01523891],\n [-0.05932459, -0.02066591],\n [-0.04601621, 0.05558309],\n [-0.02698389, 0.07053442],\n [ 0.07882285, -0.04121957],\n [ 0.06135241, -0.0304644 ],\n [ 0.09189945, 0.00352685],\n [ 0.07324959, 0.00517631],\n [ 0.00789318, -0.08145713],\n [ 0.02677604, 0.06527786],\n [-0.06949059, -0.07066917],\n [-0.06701694, 0.00092053],\n [-0.00497779, -0.07953849],\n [-0.0283235 , 0.03393211],\n [-0.00527409, 0.03929835],\n [-0.03482571, 0.04214784],\n [ 0.13532552, -0.0626914 ],\n [ 0.04993704, -0.08098684],\n [ 0.07999712, -0.06564064],\n [ 0.07224462, 0.00101267],\n [-0.08284859, -0.05882983],\n [ 0.0772713 , -0.02075527],\n [ 0.09509362, -0.04933422],\n [ 0.00703417, -0.05456049],\n [ 0.06934486, 0.10816067],\n [ 0.02769655, 0.03253336],\n [-0.02737729, 0.08668435],\n [-0.04420803, 0.08174775],\n [ 0.01564788, 0.04019317],\n [-0.07019652, -0.04801892],\n [ 0.12309071, -0.02950791],\n [ 0.12868382, -0.12376357],\n [-0.0832961 , -0.07362361],\n [-0.05780158, -0.06723551],\n [-0.04076702, 0.08101598],\n [-0.08256809, -0.05024244],\n [ 0.02858803, -0.08623272],\n [ 0.05343185, 0.06022738],\n [ 0.04897041, -0.12582912],\n [ 0.07588698, -0.04684179],\n [-0.09575359, -0.05143378],\n [-0.00919765, 0.08940119],\n [ 0.04028715, -0.05920773],\n [-0.08991487, -0.06903067],\n [-0.10565867, -0.07443611],\n [ 0.05306325, -0.04204487],\n [ 0.11833616, -0.04841996],\n [-0.00116225, -0.01993424],\n [ 0.03919767, -0.02504915],\n [-0.09598167, -0.09569343],\n [-0.05948884, 0.0847825 ],\n [-0.07438079, 0.0150445 ],\n [ 0.00054377, 0.04857477],\n [ 0.05947721, -0.00051871],\n [-0.00574352, -0.08031838],\n [ 0.03730416, -0.0494786 ],\n [-0.00285258, -0.10425571],\n [-0.06427557, 0.08026918],\n [ 0.09416965, 0.03169006],\n [ 0.09222102, -0.04166833],\n [ 0.00919792, 0.0091698 ],\n [-0.04828366, -0.06778532],\n [-0.00445858, -0.00255824],\n [ 0.0004533 , -0.01015858],\n [ 0.10160092, -0.04120492],\n [-0.03873373, -0.00724124],\n [ 0.05889171, -0.07536945],\n [ 0.00992343, -0.12579918],\n [ 0.09181193, 0.07637873],\n [ 0.12409923, -0.00582372],\n [-0.02855872, 0.08005448],\n [ 0.00997379, 0.0599444 ],\n [-0.01220272, 0.04842511],\n [ 0.12037931, 0.0039286 ],\n [ 0.06772764, -0.13433436],\n [-0.08197935, -0.06232351],\n [ 0.06975443, -0.06791086],\n [ 0.06771931, -0.0870792 ],\n [ 0.10175464, -0.05467491],\n [-0.08869459, -0.03781105],\n [ 0.03419706, -0.02091313],\n [ 0.03717361, -0.05176476],\n [ 0.03977473, 0.0802637 ],\n [ 0.0786541 , -0.07467738],\n [ 0.06886227, 0.06881069],\n [-0.0448051 , -0.04030641],\n [ 0.03404806, 0.10317541],\n [ 0.07326619, -0.06823327],\n [-0.03777633, 0.10320984],\n [-0.10982945, 0.09765613],\n [ 0.09580883, 0.00511308],\n [ 0.00609537, -0.02871921],\n [ 0.01074211, 0.0428532 ],\n [ 0.05898644, -0.04757763],\n [ 0.08945356, -0.04275674],\n [-0.0443637 , -0.00082853],\n [-0.05223919, 0.0191266 ],\n [ 0.10952595, -0.02627584],\n [-0.09769403, 0.04058051],\n [ 0.09491251, -0.07337349],\n [ 0.09357981, -0.07139876],\n [-0.08782497, -0.08310542],\n [-0.02649409, -0.00852232],\n [-0.03283405, -0.02455583],\n [ 0.06076952, 0.01649073],\n [-0.04753735, -0.04494003],\n [ 0.03921098, -0.09155199],\n [ 0.0690461 , 0.07946862],\n [-0.09138182, 0.00620584],\n [ 0.07899081, 0.0901005 ],\n [-0.01421051, 0.06142241],\n [ 0.01492564, -0.09382761],\n [-0.09812864, 0.06189889],\n [-0.03061358, -0.01388041],\n [-0.08795238, 0.06047294],\n [ 0.07104512, -0.05074662],\n [-0.01304952, -0.085006 ],\n [-0.0283862 , 0.06999809],\n [ 0.01572364, 0.07186005],\n [ 0.02568827, -0.00206702],\n [ 0.08745052, -0.05517298],\n [ 0.05046008, -0.0602025 ],\n [-0.03640335, -0.10797785],\n [ 0.08553269, -0.02292269],\n [-0.03778361, 0.06580836],\n [-0.06589452, -0.06769627],\n [-0.03918728, 0.01647712],\n [-0.0237859 , 0.09259149],\n [ 0.0724097 , 0.07180366],\n [ 0.1075801 , -0.02824615],\n [ 0.0331415 , 0.01933192],\n [ 0.00501336, -0.07279122],\n [-0.00366991, -0.10399261],\n [-0.07857811, 0.0787955 ],\n [-0.07753023, 0.05651722],\n [ 0.07203721, -0.12172461],\n [ 0.08254173, -0.0240918 ],\n [ 0.01095617, -0.0724567 ],\n [-0.0530875 , 0.03137068],\n [-0.0066072 , 0.06545337],\n [ 0.07457578, -0.0442367 ],\n [ 0.05588755, 0.10217611],\n [-0.02981724, 0.04941597],\n [ 0.05253782, -0.01042885],\n [ 0.10137051, -0.09172978],\n [ 0.08790664, 0.02524709],\n [-0.04589577, 0.07517756],\n [ 0.08342946, 0.09162924],\n [-0.08593765, 0.06982628],\n [ 0.10655744, -0.05617983],\n [ 0.06092152, -0.12207716],\n [ 0.13419878, -0.02252891],\n [ 0.09834116, 0.03958232],\n [ 0.08437857, 0.01460592],\n [ 0.0879554 , -0.01700424],\n [ 0.00131226, 0.09671631],\n [-0.07696921, -0.00924925],\n [-0.00744633, 0.06004588],\n [ 0.05903571, 0.07920177],\n [-0.06260628, -0.09104627],\n [-0.01939411, -0.11509704],\n [-0.08755022, -0.04919781],\n [ 0.08280303, 0.10103364],\n [-0.09067247, 0.010084 ],\n [ 0.06435605, -0.10556137],\n [ 0.09159714, -0.06761235],\n [-0.03296948, 0.08572628],\n [ 0.11521567, 0.05843401],\n [-0.11013817, 0.04792359],\n [ 0.08529602, -0.09699252],\n [ 0.09589008, -0.07699554],\n [-0.04429969, -0.01039457],\n [-0.04172556, -0.1274764 ],\n [ 0.01776977, -0.09997707],\n [ 0.03752356, -0.09220051],\n [-0.0466691 , -0.06307165],\n [-0.10223605, 0.06639349],\n [ 0.0373287 , 0.06885269],\n [-0.1011653 , 0.01160435],\n [ 0.08323063, 0.030745 ],\n [ 0.05227034, -0.04873146],\n [ 0.02358136, -0.07874562],\n [ 0.06542907, 0.04180999],\n [ 0.05650794, -0.09472515],\n [-0.00625252, 0.05431107],\n [ 0.00902724, 0.05882132],\n [ 0.08372131, 0.10223008],\n [ 0.02161542, 0.02079195],\n [ 0.05030798, -0.11809568],\n [ 0.07213019, 0.02946462],\n [-0.02988404, 0.09049877],\n [ 0.09042504, -0.07592832],\n [-0.09396608, -0.0944846 ],\n [-0.02128671, 0.00555106],\n [ 0.0881349 , -0.01951319],\n [ 0.09815294, 0.02091831],\n [ 0.06768715, -0.00866784],\n [ 0.03008059, -0.03210432],\n [ 0.07697138, -0.10169055],\n [ 0.01389083, -0.1269208 ],\n [-0.10234262, -0.05354223],\n [ 0.10600115, 0.018374 ],\n [ 0.00389425, -0.09662258],\n [-0.06471124, 0.07431647],\n [ 0.08016909, 0.10446185],\n [ 0.05589481, 0.0695638 ],\n [-0.05375014, -0.03225301],\n [ 0.0376894 , 0.00021482],\n [-0.07243066, -0.03724802],\n [-0.08446896, -0.00804608],\n [-0.01091738, 0.03005325],\n [ 0.00425963, 0.09999651],\n [ 0.0634597 , -0.01699882],\n [ 0.0536304 , -0.02552321],\n [-0.09320226, 0.12028917],\n [ 0.01233296, 0.02663163],\n [-0.07040945, 0.07650659],\n [ 0.04895047, -0.04399431],\n [-0.02182859, 0.06619221],\n [ 0.13631342, -0.0910524 ],\n [-0.02438866, 0.04361154],\n [ 0.0196631 , -0.0924203 ],\n [-0.02257976, 0.02791588],\n [ 0.10096157, -0.10916837],\n [ 0.0721221 , -0.13175704],\n [-0.03534862, -0.08653457],\n [ 0.09404437, -0.08704901],\n [ 0.09220192, -0.0581664 ],\n [ 0.0577516 , 0.05761899],\n [ 0.02303884, 0.07061604],\n [-0.07656746, 0.04161585],\n [ 0.06183554, 0.00855878],\n [-0.08804803, -0.03012522],\n [-0.06404053, 0.09230365],\n [ 0.05869053, -0.08241767],\n [ 0.05102699, -0.05720156],\n [-0.07484718, -0.02034107],\n [-0.05531253, -0.0525405 ],\n [ 0.06994882, 0.1004664 ],\n [ 0.12005012, -0.03424909],\n [ 0.03128378, -0.06865919],\n [-0.04526852, 0.09185734],\n [ 0.15100084, -0.01347744],\n [ 0.00988858, -0.08292833],\n [ 0.03963636, -0.10915481],\n [-0.03419256, -0.01783324],\n [-0.08656282, -0.03493471],\n [-0.05074825, -0.09571009],\n [-0.03827269, 0.07175969],\n [ 0.07545518, 0.01717809],\n [ 0.07358796, 0.02868232],\n [ 0.06777788, 0.02454507],\n [-0.08414111, -0.00173827],\n [-0.09479126, 0.07037915],\n [ 0.0302516 , 0.10241633],\n [ 0.01278188, -0.11881058],\n [ 0.00672977, 0.05497678],\n [ 0.01997549, 0.10038829],\n [ 0.03824695, 0.10304374],\n [ 0.06208556, 0.0946699 ],\n [ 0.12233598, -0.03543108],\n [ 0.06428068, 0.07997499],\n [-0.00985603, -0.08305734],\n [ 0.02025353, -0.09543061],\n [-0.09335214, -0.04045701],\n [ 0.05653603, -0.03955551],\n [-0.0212149 , 0.05950537],\n [ 0.05479232, -0.08865862],\n [-0.06271227, -0.00616521],\n [-0.08322059, -0.09444351],\n [ 0.06355092, -0.115833 ],\n [-0.06922431, 0.06530183],\n [ 0.05917469, 0.07849282],\n [ 0.01401479, 0.01351963],\n [ 0.03810755, -0.02678735],\n [ 0.04086413, -0.04378407],\n [-0.08662243, -0.05540965],\n [ 0.06556071, -0.00274828],\n [ 0.09472376, -0.03822417],\n [-0.03384451, 0.01003726],\n [ 0.05466221, -0.06395304],\n [-0.04413132, -0.00833847],\n [-0.10400607, 0.11038367],\n [ 0.05504902, 0.03310199],\n [ 0.0670617 , -0.06417592],\n [ 0.05163915, 0.07122194],\n [ 0.0510257 , -0.05159095],\n [-0.07860655, -0.0385831 ],\n [-0.0425971 , 0.08931515],\n [ 0.06477237, 0.07649194],\n [ 0.0130143 , -0.00678981],\n [ 0.09306058, -0.01254047],\n [ 0.05911999, -0.02476157],\n [-0.05037018, 0.03968317],\n [ 0.09468324, -0.09633217],\n [ 0.09046121, -0.04414934],\n [-0.07896487, -0.01889215],\n [-0.05126528, 0.0645128 ],\n [-0.02817298, -0.01976723],\n [-0.06963074, 0.05060264],\n [ 0.04382402, 0.01743763],\n [ 0.02111326, -0.056915 ],\n [-0.07937434, 0.01911365],\n [ 0.0320007 , -0.0966574 ],\n [ 0.08609178, 0.08823472],\n [-0.05968918, 0.07774922],\n [ 0.13485806, -0.06266385],\n [ 0.02274571, 0.06322207],\n [-0.0048017 , 0.04118194],\n [-0.06662469, -0.07862572],\n [-0.0447496 , 0.06557685],\n [ 0.03626973, -0.0363848 ],\n [-0.0801399 , -0.04140452],\n [-0.0892067 , 0.04529453],\n [-0.04640176, 0.05373997],\n [-0.06597373, 0.09341846],\n [ 0.03246468, -0.06266692],\n [ 0.03325224, 0.04605464],\n [-0.088351 , 0.04260119],\n [-0.09440482, -0.05242742],\n [ 0.12047318, -0.04484326],\n [ 0.0587764 , 0.05491043],\n [ 0.11781491, -0.0247802 ],\n [-0.05252584, 0.0948424 ],\n [-0.09270789, 0.00290092],\n [ 0.02953261, 0.06384847],\n [-0.04930701, -0.00180705],\n [-0.01726307, -0.00485664],\n [-0.00343406, 0.05071843],\n [ 0.08734091, -0.04523054],\n [-0.00492472, -0.10022837],\n [-0.08574443, -0.05268416],\n [-0.00830413, -0.11258492],\n [ 0.12223374, -0.12711501],\n [ 0.05255788, -0.02906406],\n [-0.02451387, -0.10607663],\n [ 0.05887705, 0.1014686 ],\n [ 0.10687933, -0.11090266],\n [ 0.01595546, -0.09647645],\n [ 0.08522485, -0.09018736],\n [ 0.05575566, -0.08034538],\n [-0.01402826, 0.09128823],\n [-0.01914284, 0.07313996],\n [ 0.01201154, 0.0353253 ],\n [ 0.02630525, -0.10031029],\n [-0.00714251, 0.02835639],\n [-0.04130204, 0.04700005],\n [ 0.00765558, 0.08880088],\n [-0.07652104, 0.10528626],\n [-0.03428807, -0.1265489 ],\n [-0.08762529, 0.0153307 ],\n [ 0.01514399, -0.04275274],\n [ 0.08736248, 0.04509316],\n [-0.11950286, -0.00858769]], dtype=float32)>, <tf.Tensor: shape=(2,), dtype=float32, numpy=array([ 0.00663124, -0.00663124], dtype=float32)>]\n"
],
[
"def testNewGlobal(weight):\n \n print('Reading Testing Data')\n \n TestParasitizedCells, TestParasitizedLabels = readData('./input/fed/test/Parasitized/', 1)\n TestUninfectedCells, TestUninfectedLabels = readData('./input/fed/test/Uninfected/', 0)\n TestCells = np.concatenate((TestParasitizedCells, TestUninfectedCells))\n TestLabels = np.concatenate((TestParasitizedLabels, TestUninfectedLabels))\n \n \n sTest = np.arange(TestCells.shape[0])\n np.random.shuffle(sTest)\n TestCells = TestCells[sTest]\n TestLabels = TestLabels[sTest]\n \n num_classes=len(np.unique(TestLabels))\n \n (x_test) = TestCells\n (y_test) = TestLabels\n \n # Since we're working on image data, we normalize data by divinding 255.\n x_test = x_test.astype('float32')/255\n test_len=len(x_test)\n \n #Doing One hot encoding as classifier has multiple classes\n y_test=keras.utils.to_categorical(y_test,num_classes)\n \n #creating sequential model\n model=Sequential()\n model.add(Conv2D(filters=16,kernel_size=2,padding=\"same\",activation=\"relu\",input_shape=(50,50,3)))\n model.add(MaxPooling2D(pool_size=2))\n model.add(Conv2D(filters=32,kernel_size=2,padding=\"same\",activation=\"relu\"))\n model.add(MaxPooling2D(pool_size=2))\n model.add(Conv2D(filters=64,kernel_size=2,padding=\"same\",activation=\"relu\"))\n model.add(MaxPooling2D(pool_size=2))\n model.add(Dropout(0.2))\n model.add(Flatten())\n model.add(Dense(500,activation=\"relu\"))\n model.add(Dropout(0.2))\n model.add(Dense(2,activation=\"softmax\"))#2 represent output layer neurons \n# model.summary()\n\n model.set_weights(weight)\n\n # compile the model with loss as categorical_crossentropy and using adam optimizer\n model.compile(loss='categorical_crossentropy', optimizer='adam', metrics=['accuracy'])\n \n scores = model.evaluate(x_test, y_test)\n print(\"Loss: \", scores[0]) #Loss\n print(\"Accuracy: \", scores[1]) #Accuracy\n\n #Saving Model\n model.save(\"./output.h5\")\n return scores[1]",
"_____no_output_____"
],
[
"testNewGlobal(avgWeight)",
"Reading Testing Data\n2740 Data Points Read!\n2783 Data Points Read!\n173/173 [==============================] - 2s 13ms/step - loss: 0.3326 - accuracy: 0.8702\nLoss: 0.3326050043106079\nAccuracy: 0.8701792359352112\n"
],
[
"FLAccuracyDF",
"_____no_output_____"
]
]
] | [
"code"
] | [
[
"code",
"code",
"code",
"code",
"code",
"code",
"code",
"code",
"code",
"code",
"code",
"code",
"code",
"code",
"code",
"code",
"code"
]
] |
d0456b16cdfade0c3e5f8e887b60aa928608b944 | 37,049 | ipynb | Jupyter Notebook | clf.ipynb | Ruslion/Predicting-loan-eligibility | abed6de78352dd2206c95b0c9d40e745a6509538 | [
"MIT"
] | null | null | null | clf.ipynb | Ruslion/Predicting-loan-eligibility | abed6de78352dd2206c95b0c9d40e745a6509538 | [
"MIT"
] | null | null | null | clf.ipynb | Ruslion/Predicting-loan-eligibility | abed6de78352dd2206c95b0c9d40e745a6509538 | [
"MIT"
] | null | null | null | 29.615508 | 421 | 0.433723 | [
[
[
"# Loan predictions\n\n## Problem Statement\n\nWe want to automate the loan eligibility process based on customer details that are provided as online application forms are being filled. You can find the dataset [here](https://drive.google.com/file/d/1h_jl9xqqqHflI5PsuiQd_soNYxzFfjKw/view?usp=sharing). These details concern the customer's Gender, Marital Status, Education, Number of Dependents, Income, Loan Amount, Credit History and other things as well. \n\n|Variable| Description|\n|: ------------- |:-------------|\n|Loan_ID| Unique Loan ID|\n|Gender| Male/ Female|\n|Married| Applicant married (Y/N)|\n|Dependents| Number of dependents|\n|Education| Applicant Education (Graduate/ Under Graduate)|\n|Self_Employed| Self employed (Y/N)|\n|ApplicantIncome| Applicant income|\n|CoapplicantIncome| Coapplicant income|\n|LoanAmount| Loan amount in thousands|\n|Loan_Amount_Term| Term of loan in months|\n|Credit_History| credit history meets guidelines|\n|Property_Area| Urban/ Semi Urban/ Rural|\n|Loan_Status| Loan approved (Y/N)\n\n\n\n### Explore the problem in following stages:\n\n1. Hypothesis Generation – understanding the problem better by brainstorming possible factors that can impact the outcome\n2. Data Exploration – looking at categorical and continuous feature summaries and making inferences about the data.\n3. Data Cleaning – imputing missing values in the data and checking for outliers\n4. Feature Engineering – modifying existing variables and creating new ones for analysis\n5. Model Building – making predictive models on the data",
"_____no_output_____"
],
[
"## 1. Hypothesis Generation\n\nGenerating a hypothesis is a major step in the process of analyzing data. This involves understanding the problem and formulating a meaningful hypothesis about what could potentially have a good impact on the outcome. This is done BEFORE looking at the data, and we end up creating a laundry list of the different analyses which we can potentially perform if data is available.\n\n#### Possible hypotheses\nWhich applicants are more likely to get a loan\n\n1. Applicants having a credit history \n2. Applicants with higher applicant and co-applicant incomes\n3. Applicants with higher education level\n4. Properties in urban areas with high growth perspectives\n\nDo more brainstorming and create some hypotheses of your own. Remember that the data might not be sufficient to test all of these, but forming these enables a better understanding of the problem.",
"_____no_output_____"
]
],
[
[
"import pandas as pd\nimport numpy as np\nfrom matplotlib import pyplot as plt",
"_____no_output_____"
],
[
"df = pd.read_csv('data.csv')",
"_____no_output_____"
],
[
"df.head(10)",
"_____no_output_____"
],
[
"df.shape",
"_____no_output_____"
]
],
[
[
"## 2. Data Exploration\nLet's do some basic data exploration here and come up with some inferences about the data. Go ahead and try to figure out some irregularities and address them in the next section. ",
"_____no_output_____"
],
[
"One of the key challenges in any data set are missing values. Lets start by checking which columns contain missing values.",
"_____no_output_____"
]
],
[
[
"df.isnull().sum()",
"_____no_output_____"
]
],
[
[
"Look at some basic statistics for numerical variables.",
"_____no_output_____"
]
],
[
[
"df.dtypes",
"_____no_output_____"
],
[
"df.nunique()",
"_____no_output_____"
]
],
[
[
"1. How many applicants have a `Credit_History`? (`Credit_History` has value 1 for those who have a credit history and 0 otherwise)\n2. Is the `ApplicantIncome` distribution in line with your expectation? Similarly, what about `CoapplicantIncome`?\n3. Tip: Can you see a possible skewness in the data by comparing the mean to the median, i.e. the 50% figure of a feature.\n\n",
"_____no_output_____"
],
[
"Let's discuss nominal (categorical) variable. Look at the number of unique values in each of them.",
"_____no_output_____"
],
[
"Explore further using the frequency of different categories in each nominal variable. Exclude the ID obvious reasons.",
"_____no_output_____"
],
[
"### Distribution analysis\n\nStudy distribution of various variables. Plot the histogram of ApplicantIncome, try different number of bins.\n\n",
"_____no_output_____"
],
[
"\nLook at box plots to understand the distributions. ",
"_____no_output_____"
],
[
"Look at the distribution of income segregated by `Education`",
"_____no_output_____"
],
[
"Look at the histogram and boxplot of LoanAmount",
"_____no_output_____"
],
[
"There might be some extreme values. Both `ApplicantIncome` and `LoanAmount` require some amount of data munging. `LoanAmount` has missing and well as extreme values values, while `ApplicantIncome` has a few extreme values, which demand deeper understanding. ",
"_____no_output_____"
],
[
"### Categorical variable analysis\n\nTry to understand categorical variables in more details using `pandas.DataFrame.pivot_table` and some visualizations.",
"_____no_output_____"
],
[
"## 3. Data Cleaning\n\nThis step typically involves imputing missing values and treating outliers. ",
"_____no_output_____"
],
[
"### Imputing Missing Values\n\nMissing values may not always be NaNs. For instance, the `Loan_Amount_Term` might be 0, which does not make sense.\n\n",
"_____no_output_____"
],
[
"Impute missing values for all columns. Use the values which you find most meaningful (mean, mode, median, zero.... maybe different mean values for different groups)",
"_____no_output_____"
]
],
[
[
"from sklearn.impute import SimpleImputer\nfrom sklearn.preprocessing import StandardScaler\nfrom sklearn.pipeline import Pipeline\nfrom sklearn.preprocessing import FunctionTransformer\nfrom sklearn.metrics import accuracy_score\nfrom sklearn.compose import ColumnTransformer\nfrom sklearn.preprocessing import OneHotEncoder\nfrom sklearn.model_selection import GridSearchCV\nfrom sklearn.svm import SVC\nfrom sklearn.linear_model import LogisticRegression\nfrom sklearn.model_selection import train_test_split\nfrom sklearn.compose import make_column_selector\nimport pickle\n",
"_____no_output_____"
],
[
"ohe=OneHotEncoder(drop='first', sparse=False)",
"_____no_output_____"
],
[
"X= df.drop(columns=['Loan_Status', 'Loan_ID'])\ny=df['Loan_Status']\n#y=ohe.fit_transform(df['Loan_Status'].to_numpy().reshape(-1, 1))",
"_____no_output_____"
],
[
"X_train, X_test, y_train, y_test = train_test_split(X, y, test_size=0.3,\n random_state=0)",
"_____no_output_____"
],
[
"column_trans = ColumnTransformer(\n[('imp_most_frequent', SimpleImputer(strategy='most_frequent'), ['Gender', 'Married', \n 'Dependents', 'Self_Employed',\n 'Property_Area',\n 'Education',\n 'Credit_History']),\n ('imp_median', SimpleImputer(strategy='median'), ['LoanAmount', 'Loan_Amount_Term']),\n\n('scaling', StandardScaler(), make_column_selector(dtype_include=np.number))\n \n]\n)\n\ncolumn_enc = ColumnTransformer([('one_hot_enc', OneHotEncoder(handle_unknown='ignore'), \n [0,\n 1,\n 2,\n 3,\n 4,\n 10])])\n ",
"_____no_output_____"
],
[
"pipeline = Pipeline(steps=[('column_transf', column_trans),\n ('column_enc', column_enc),\n ('classifier', SVC(random_state = 17))])",
"_____no_output_____"
],
[
"# Find the best hyperparameters using GridSearchCV on the train set\nparam_grid = [\n {'classifier':(SVC(random_state = 17),),\n 'classifier__C': [0.5, 1, 2, 4], \n 'classifier__kernel': ['linear', 'poly', 'rbf', 'sigmoid'],\n 'classifier__degree': [2, 3],\n 'classifier__gamma':['scale', 'auto']},\n \n {'classifier':(LogisticRegression(random_state = 17),),\n 'classifier__penalty':['l1', 'l2', 'elasticnet'],\n 'classifier__C': [0.5, 1, 2, 4],\n 'classifier__solver': ['newton-cg', 'lbfgs', 'liblinear', 'sag', 'saga']\n }]\n\ngrid = GridSearchCV(pipeline, param_grid=param_grid, verbose=2, n_jobs=5, scoring='accuracy')\ngrid.fit(X_train, y_train)",
"Fitting 5 folds for each of 124 candidates, totalling 620 fits\n"
],
[
"grid.best_params_",
"_____no_output_____"
],
[
"grid.best_score_",
"_____no_output_____"
],
[
"y_pred=grid.predict(X_test)",
"_____no_output_____"
],
[
"accuracy_score(y_test, y_pred)",
"_____no_output_____"
],
[
"pipeline = Pipeline(steps=[('column_transf', column_trans),\n ('column_enc', column_enc),\n ('classifier', grid.best_params_['classifier'])])",
"_____no_output_____"
],
[
"pipeline.fit(X_train, y_train)",
"_____no_output_____"
],
[
"with open('myfile.pickle', 'wb') as file_handle:\n pickle.dump(pipeline, file_handle)",
"_____no_output_____"
]
],
[
[
"### Extreme values\nTry a log transformation to get rid of the extreme values in `LoanAmount`. Plot the histogram before and after the transformation",
"_____no_output_____"
],
[
"Combine both incomes as total income and take a log transformation of the same.",
"_____no_output_____"
],
[
"## 4. Building a Predictive Model",
"_____no_output_____"
],
[
"Try paramater grid search to improve the results",
"_____no_output_____"
],
[
"## 5. Using Pipeline\nIf you didn't use pipelines before, transform your data prep, feat. engineering and modeling steps into Pipeline. It will be helpful for deployment.\n\nThe goal here is to create the pipeline that will take one row of our dataset and predict the probability of being granted a loan.\n\n`pipeline.predict(x)`",
"_____no_output_____"
],
[
"## 6. Deploy your model to cloud and test it with PostMan, BASH or Python",
"_____no_output_____"
]
]
] | [
"markdown",
"code",
"markdown",
"code",
"markdown",
"code",
"markdown",
"code",
"markdown"
] | [
[
"markdown",
"markdown"
],
[
"code",
"code",
"code",
"code"
],
[
"markdown",
"markdown"
],
[
"code"
],
[
"markdown"
],
[
"code",
"code"
],
[
"markdown",
"markdown",
"markdown",
"markdown",
"markdown",
"markdown",
"markdown",
"markdown",
"markdown",
"markdown",
"markdown",
"markdown"
],
[
"code",
"code",
"code",
"code",
"code",
"code",
"code",
"code",
"code",
"code",
"code",
"code",
"code",
"code"
],
[
"markdown",
"markdown",
"markdown",
"markdown",
"markdown",
"markdown"
]
] |
d0456ff3f0cec1d002386b3d719c80fe78edeaa2 | 994,008 | ipynb | Jupyter Notebook | tf/.ipynb_checkpoints/Totally Random-checkpoint.ipynb | mathewzilla/whiskfree | 1cfb0b73c762ca4ca0df9d5a012f41ae904ab557 | [
"MIT"
] | null | null | null | tf/.ipynb_checkpoints/Totally Random-checkpoint.ipynb | mathewzilla/whiskfree | 1cfb0b73c762ca4ca0df9d5a012f41ae904ab557 | [
"MIT"
] | null | null | null | tf/.ipynb_checkpoints/Totally Random-checkpoint.ipynb | mathewzilla/whiskfree | 1cfb0b73c762ca4ca0df9d5a012f41ae904ab557 | [
"MIT"
] | null | null | null | 523.989457 | 160,868 | 0.929357 | [
[
[
"# Looking at the randomness (or otherwise) of mouse behaviour\n### Also, the randomness (or otherwise) of trial types to know when best to start looking at 'full task' behaviour",
"_____no_output_____"
]
],
[
[
"# Import libraries\nimport matplotlib.pyplot as plt\n%matplotlib inline\nimport pandas as pd\nimport seaborn as sns \nimport random\nimport copy\nimport numpy as np\nfrom scipy.signal import resample\nfrom scipy.stats import zscore\nfrom scipy import interp\nfrom sklearn.linear_model import LogisticRegression\nfrom sklearn.metrics import confusion_matrix\nfrom sklearn.metrics import accuracy_score\nfrom sklearn import metrics\nfrom sklearn import cross_validation",
"_____no_output_____"
],
[
"# Load data\n# data loading function\ndef data_load_and_parse(mouse_name):\n tt = pd.read_csv('~/work/whiskfree/data/trialtype_' + mouse_name + '.csv',header=None)\n ch = pd.read_csv('~/work/whiskfree/data/choice_' + mouse_name + '.csv',header=None)\n sess = pd.read_csv('~/work/whiskfree/data/session_' + mouse_name + '.csv',header=None)\n AB = pd.read_csv('~/work/whiskfree/data/AB_' + mouse_name + '.csv',header=None)\n \n clean1 = np.nan_to_num(tt) !=0\n clean2 = np.nan_to_num(ch) !=0\n clean = clean1&clean2\n tt_c = tt[clean].values\n\n ch_c = ch[clean].values\n \n s_c = sess[clean].values\n \n ab_c = AB[clean].values\n \n return tt_c, ch_c, clean, s_c, ab_c",
"_____no_output_____"
],
[
"mouse_name = '36_r'\ntt, ch, clean, sess, AB = data_load_and_parse(mouse_name)\n\n\n# work out AB/ON trials\nAB_pol = np.nan_to_num(AB) !=0\nON_pol = np.nan_to_num(AB) ==0\ncm_AB = confusion_matrix(tt[AB_pol],ch[AB_pol])\ncm_ON = confusion_matrix(tt[ON_pol],ch[ON_pol])\nprint(cm_AB)\nprint(cm_ON)\nprint(accuracy_score(tt[AB_pol],ch[AB_pol]))\nprint(accuracy_score(tt[ON_pol],ch[ON_pol]))",
"[[293 42 47]\n [ 63 213 42]\n [ 85 25 220]]\n[[180 23 28]\n [ 41 139 26]\n [ 52 47 198]]\n0.704854368932\n0.704359673025\n"
],
[
"# Format TT/ choice data and plot\nfig, ax = plt.subplots(2,1,figsize=(20,5))\n_ = ax[0].plot(tt[ON_pol][:100],label='TT ON')\n_ = ax[0].plot(ch[ON_pol][:100],label='Ch ON')\nax[0].legend()\n_ = ax[1].plot(tt[AB_pol][:100],label='TT AB')\n_ = ax[1].plot(ch[AB_pol][:100],label='Ch AB')\nax[1].legend()",
"_____no_output_____"
],
[
"# Measure randomness and plot that\n# First plot cumsum of trial types. Periods of bias (of choice 1 and 3, anyway) will be seen as deviations from the mean line\nplt.plot(np.cumsum(tt[AB_pol][:100]),label='Cumsum TT AB')\nplt.plot(np.cumsum(ch[AB_pol][:100]),label='Cumsum Ch AB')\nplt.plot([0,99],[0,np.sum(tt[AB_pol][:100])],label='Mean cumsum')\nplt.legend()",
"_____no_output_____"
],
[
"# How about looking at the distribution of individual states, pairs, triples. \n# Compare to random sequence (with no conditions)\nP_i = np.zeros(3)\nP_i[0] = len(tt[tt[AB_pol]==1])\nP_i[1] = len(tt[tt[AB_pol]==2])\nP_i[2] = len(tt[tt[AB_pol]==3])\nwith sns.axes_style(\"white\"):\n _ = plt.imshow(np.expand_dims(P_i/sum(P_i),axis=0),interpolation='none')\n for j in range(0,3):\n plt.text(j, 0, P_i[j]/sum(P_i), va='center', ha='center',bbox=dict(facecolor='white',edgecolor='white', alpha=0.5))\n\n# _ = ax[1].bar([0,1,2],P_i/sum(P_i))\n\n",
"/Users/mathew/miniconda/envs/graph_tool/lib/python3.4/site-packages/ipykernel/__main__.py:4: VisibleDeprecationWarning: boolean index did not match indexed array along dimension 0; dimension is 2050 but corresponding boolean dimension is 1261\n/Users/mathew/miniconda/envs/graph_tool/lib/python3.4/site-packages/ipykernel/__main__.py:5: VisibleDeprecationWarning: boolean index did not match indexed array along dimension 0; dimension is 2050 but corresponding boolean dimension is 1261\n/Users/mathew/miniconda/envs/graph_tool/lib/python3.4/site-packages/ipykernel/__main__.py:6: VisibleDeprecationWarning: boolean index did not match indexed array along dimension 0; dimension is 2050 but corresponding boolean dimension is 1261\n"
],
[
"# Pairs and triples (in dumb O(n) format)\nP_ij = np.zeros([3,3])\nP_ijk = np.zeros([3,3,3])\nfor i in range(len(tt[AB_pol]) - 2):\n #p_i = tt[AB_pol][i]\n #p_j = tt[AB_pol][i+1]\n #p_k = tt[AB_pol][i+2]\n p_i = ch[AB_pol][i]\n p_j = ch[AB_pol][i+1]\n p_k = ch[AB_pol][i+2]\n P_ij[p_i-1,p_j-1] += 1\n P_ijk[p_i-1,p_j-1,[p_k-1]] += 1\n \ncmap = sns.diverging_palette(220,10, l=70, as_cmap=True, center=\"dark\") # blue to green via black\n\nwith sns.axes_style(\"white\"):\n plt.imshow(P_ij/np.sum(P_ij),interpolation='none',cmap=cmap)\n \nfor i in range(0,3):\n for j in range(0,3):\n plt.text(j, i, \"{0:.2f}\".format(P_ij[i,j]/np.sum(P_ij)*9), va='center', ha='center',bbox=dict(facecolor='white',edgecolor='white', alpha=0.5))\n\n#plt.savefig('figs/graphs/state_transition_matrix_AB'+ mouse_name +'.png')\nplt.savefig('figs/graphs/choice_state_transition_matrix_AB'+ mouse_name +'.png')",
"_____no_output_____"
],
[
"# Plot P(state) for all 27 triple states\nplt.plot(P_ijk_ON.flatten()/np.sum(P_ijk_ON))\nplt.plot([0,26],[1/27,1/27],'--')\n1/27",
"_____no_output_____"
],
[
"import graph_tool.all as gt\n",
"/Users/mathew/miniconda/envs/graph_tool/lib/python3.4/site-packages/graph_tool/draw/cairo_draw.py:1468: RuntimeWarning: Error importing Gtk module: No module named 'gi'; GTK+ drawing will not work.\n warnings.warn(msg, RuntimeWarning)\n"
],
[
"# Transition probabilities between individual states, pairs, triples\ng = gt.Graph()\ng.add_edge_list(np.transpose(P_ij.nonzero()))\nwith sns.axes_style(\"white\"):\n\tplt.imshow(P_ij,interpolation='none')",
"_____no_output_____"
],
[
"g = gt.Graph(directed = True)\ng.add_vertex(len(P_ij))\nedge_weights = g.new_edge_property('double')\nedge_labels = g.new_edge_property('string')\nfor i in range(P_ij.shape[0]):\n for j in range(P_ij.shape[1]):\n e = g.add_edge(i, j)\n edge_weights[e] = P_ij[i,j]\n edge_labels[e] = str(P_ij[i,j])\n ",
"_____no_output_____"
],
[
"# Fancy drawing code where node colour/size is degree. Edge colour/size is betweenness\ndeg = g.degree_property_map(\"in\")\n# deg.a = 4 * (np.sqrt(deg.a) * 0.5 + 0.4)\ndeg.a = deg.a*20\nprint(deg.a)\newidth = edge_weights.a / 10\n#ebet.a /= ebet.a.max() / 10.\n#print(ebet.a)\npos = gt.sfdp_layout(g)\n#control = g.new_edge_property(\"vector<double>\")\n#for e in g.edges():\n# d = np.sqrt(sum((pos[e.source()].a - pos[e.target()].a) ** 2))\n# print(d)\n# control[e] = [10, d, 10,d] #[0.3, d, 0.7, d]\n \n \n\ncmap = sns.cubehelix_palette(as_cmap=True) # cubehelix \ncmap = sns.diverging_palette(220,10, l=70, as_cmap=True, center=\"dark\") # blue to green via black\n# gt.graph_draw(g, pos=pos, vertex_size=deg, vertex_fill_color=deg, vorder=deg,\n# edge_color=ebet, eorder=eorder, edge_pen_width=ebet,\n# edge_control_points=control) # some curvy edges\n # output=\"graph-draw.pdf\")\ngt.graph_draw(g, pos=pos, vertex_size=deg, vertex_color=deg, vertex_fill_color=deg, edge_color=edge_weights, edge_text=edge_labels,\n vcmap=cmap,ecmap=cmap, vertex_text=g.vertex_index, vertex_font_size=18,fit_view=0.5)\n #vcmap=plt.cm.Pastel1,ecmap=plt.cm.Pastel1 )\n # edge_control_points=control) # some curvy edges\n # output=\"graph-draw.pdf\")",
"[60 60 60]\n"
],
[
"# Same as g but normalised so total trials/9 = 1\ng_n = gt.Graph(directed = True)\n\nedge_weights_n = g_n.new_edge_property('double')\nedge_labels_n = g_n.new_edge_property('string')\nnode_size_n = g_n.new_vertex_property('double')\ng_n.add_vertex(len(P_ij))\n\nP_ij_n = P_ij /(P_ij.sum()/9)\nfor i in range(P_ij.shape[0]):\n #v = g_n.add_vertex()\n node_size_n[i] = 3* sum(P_ij)[i] / np.sum(P_ij)\n for j in range(P_ij.shape[1]):\n e = g_n.add_edge(i, j)\n edge_weights_n[e] = P_ij_n[i,j]\n edge_labels_n[e] = \"{0:.2f}\".format(P_ij_n[i,j])",
"_____no_output_____"
],
[
"# Minimal drawing code, but with scaled colours/weights for network properties\n# Line width changes on each loop ATM. Needs fixing..\npos = gt.sfdp_layout(g_n) \n\n#deg_n = g_n.degree_property_map(\"in\")\n# deg.a = 4 * (np.sqrt(deg.a) * 0.5 + 0.4)\n#deg_n.a = deg_n.a*20\nn_size = copy.copy(node_size_n)\nn_size.a = 50* n_size.a/ max(n_size.a)\n\nedge_w = copy.copy(edge_weights_n)\nedge_w.a = edge_w.a*10\n\ncmap = sns.cubehelix_palette(as_cmap=True) # cubehelix \ncmap = sns.diverging_palette(220,10, l=70, as_cmap=True, center=\"dark\") # blue to green via black\n\ngt.graph_draw(g_n, pos=pos, vertex_color = n_size, vertex_fill_color = n_size, \n vertex_size = n_size,\n edge_pen_width=edge_w, edge_color=edge_weights_n, \n edge_text=edge_labels_n,\n vcmap=cmap,ecmap=cmap, \n vertex_text=g_n.vertex_index, \n vertex_font_size=18,\n output_size=(600,600), fit_view=0.4,\n output=\"figs/graphs/choice_1st_order_transition_AB.pdf\")\n #vcmap=plt.cm.Pastel1,ecmap=plt.cm.Pastel1 )\n # edge_control_points=control) # some curvy edges\n # output=\"graph-draw.pdf\")",
"_____no_output_____"
],
[
"current_palette = sns.color_palette(\"cubehelix\")\ncurrent_palette = sns.diverging_palette(220,10, l=50, n=7, center=\"dark\")\nsns.palplot(current_palette)\n\n",
"_____no_output_____"
],
[
"# Now write a loop to construct a tree-type graph\n# Same as g but normalised so total trials/9 = 1\nt = gt.Graph(directed = False)\n\nP_ij_n = P_ij /(P_ij.sum()/9)\nP_ijk_n = P_ijk /(P_ijk.sum()/27)\n\nedge_weights_t = t.new_edge_property('double')\nedge_labels_t = t.new_edge_property('string')\nnode_labels_t = t.new_vertex_property('string')\nnode_size = t.new_vertex_property('double')\nh = t.add_vertex()\nnode_labels_t[h] = \"0\"\n\nfor i in range(P_ij.shape[0]):\n v = t.add_vertex()\n node_labels_t[v] = str(i)\n e = t.add_edge(h,v)\n node_size[v] = sum(P_ij_n)[i] *10\n \n for j in range(P_ij.shape[1]):\n v2 = t.add_vertex()\n node_labels_t[v2] = str(i) + \"-\" + str(j)\n e = t.add_edge(v,v2)\n \n edge_weights_t[e] = P_ij_n[i,j]*10\n edge_labels_t[e] = \"{0:.2f}\".format(P_ij_n[i,j])\n node_size[v2] = P_ij_n[i,j]*20\n \n for k in range(P_ijk.shape[2]):\n v3 = t.add_vertex()\n node_labels_t[v3] = str(i) + \"-\" + str(j) + \"-\" + str(k)\n e2 = t.add_edge(v2,v3)\n \n edge_weights_t[e2] = P_ijk_n[i,j,k]*10\n edge_labels_t[e2] = \"{0:.2f}\".format(P_ijk_n[i,j,k])\n node_size[v3] = P_ijk_n[i,j,k]*20\n",
"_____no_output_____"
],
[
"#pos = gt.sfdp_layout(t) \n#pos = gt.fruchterman_reingold_layout(t)\npos = gt.radial_tree_layout(t,t.vertex(0))\ncmap = sns.diverging_palette(220,10, l=70, as_cmap=True, center=\"dark\") # blue to green via black\n\ngt.graph_draw(t,pos=pos,vertex_size=node_size,edge_pen_width=edge_weights_t,\n vertex_text = node_labels_t, edge_text=edge_labels_t,\n ecmap=cmap, edge_color = edge_weights_t,\n vcmap=cmap, vertex_color = node_size,vertex_fill_color = node_size,\n output_size=(1000, 1000), fit_view=0.8,\n output=\"figs/graphs/choice_3_step_statespace_AB.pdf\")",
"_____no_output_____"
],
[
"\"{0:.2f}\".format(P_ijk[1,1,1])",
"_____no_output_____"
],
[
"\"{0:.2f}\".format(P_ijk[1,1,1])",
"_____no_output_____"
],
[
"len(P_ij)",
"_____no_output_____"
]
],
[
[
"# Repeat the trick for ON policy trials",
"_____no_output_____"
]
],
[
[
"# P_ijk_ON\nP_ij_ON = np.zeros([3,3])\nP_ijk_ON = np.zeros([3,3,3])\nfor i in range(len(tt[AB_pol]) - 2):\n# p_i = tt[ON_pol][i]\n# p_j = tt[ON_pol][i+1]\n# p_k = tt[ON_pol][i+2]\n p_i = ch[AB_pol][i]\n p_j = ch[AB_pol][i+1]\n p_k = ch[AB_pol][i+2]\n P_ij_ON[p_i-1,p_j-1] += 1\n P_ijk_ON[p_i-1,p_j-1,[p_k-1]] += 1\n\n# Make graph\nt_ON = gt.Graph(directed = False)\n\nP_ij_ON = P_ij_ON /(P_ij_ON.sum()/9)\nP_ijk_ON = P_ijk_ON /(P_ijk_ON.sum()/27)\n\nedge_weights_tON = t_ON.new_edge_property('double')\nedge_labels_tON = t_ON.new_edge_property('string')\nnode_labels_tON = t_ON.new_vertex_property('string')\nnode_size_ON = t_ON.new_vertex_property('double')\nh = t_ON.add_vertex()\nnode_labels_tON[h] = \"0\"\n\nfor i in range(P_ij_ON.shape[0]):\n v = t_ON.add_vertex()\n node_labels_tON[v] = str(i)\n e = t_ON.add_edge(h,v)\n node_size_ON[v] = sum(P_ij_ON)[i] *10\n \n for j in range(P_ij_ON.shape[1]):\n v2 = t_ON.add_vertex()\n node_labels_tON[v2] = str(i) + \"-\" + str(j)\n e = t_ON.add_edge(v,v2)\n \n edge_weights_tON[e] = P_ij_ON[i,j]*10\n edge_labels_tON[e] = \"{0:.2f}\".format(P_ij_ON[i,j])\n node_size_ON[v2] = P_ij_ON[i,j]*20\n \n for k in range(P_ijk_ON.shape[2]):\n v3 = t_ON.add_vertex()\n node_labels_tON[v3] = str(i) + \"-\" + str(j) + \"-\" + str(k)\n e2 = t_ON.add_edge(v2,v3)\n \n edge_weights_tON[e2] = P_ijk_ON[i,j,k]*10\n edge_labels_tON[e2] = \"{0:.2f}\".format(P_ijk_ON[i,j,k])\n node_size_ON[v3] = P_ijk_ON[i,j,k]*20\n\n# Plot graph\npos = gt.radial_tree_layout(t_ON,t_ON.vertex(0))\ncmap = sns.diverging_palette(220,10, l=70, as_cmap=True, center=\"dark\") # blue to green via black\n\ngt.graph_draw(t_ON,pos=pos,vertex_size=node_size_ON,edge_pen_width=edge_weights_tON,\n vertex_text = node_labels_tON, edge_text=edge_labels_tON,\n ecmap=cmap, edge_color = edge_weights_tON,\n vcmap=cmap, vertex_color = node_size_ON,\n vertex_fill_color = node_size_ON,\n output_size=(1000, 1000), fit_view=0.8)\n# output=\"figs/graphs/choice_3_step_statespace_AB_\"+ mouse_name +\".pdf\")\n",
"_____no_output_____"
],
[
"# image of ON trials transition matrix\ncmap = sns.diverging_palette(220,10, l=70, as_cmap=True, center=\"dark\") # blue to green via black\n\nwith sns.axes_style(\"white\"):\n plt.imshow(P_ij_ON/np.sum(P_ij_ON),interpolation='none',cmap=cmap)\n \nfor i in range(0,3):\n for j in range(0,3):\n plt.text(j, i, \"{0:.2f}\".format(P_ij_ON[i,j]), va='center', ha='center',bbox=dict(facecolor='white',edgecolor='white', alpha=0.5))\n\nylabels = ['Anterior','Posterior','No Go']\nplt.xticks([0,1,2],ylabels)\nplt.yticks([0,1,2],ylabels)\n# plt.set_yticks([0,1,2])\n# plt.set_yticklabels(ylabels)\n \n# plt.savefig('figs/graphs/choice_state_transition_matrix_AB_'+ mouse_name +'.png')",
"_____no_output_____"
],
[
"# Just plot P(state)\nplt.figure(figsize=(16,2))\nax1 = plt.subplot2grid((1,4),(0,0))\nax1.plot(P_ij_ON.flatten()/np.sum(P_ij_ON) * 9)\nax1.plot([0,8],[1,1],'--')\n\nstate_names = np.empty([3,3],dtype=object)\nfor i in range(0,3):\n for j in range(0,3):\n state_names[i,j] = str(i) + \"-\" + str(j)\n \nax1.set_xticks(range(0,9))\nax1.set_xticklabels(state_names.flatten(),rotation=45)\n\nax2 = plt.subplot2grid((1,4),(0,1),colspan=3)\nax2.plot(P_ijk_ON.flatten()/np.sum(P_ijk_ON) * 27)\nax2.plot([0,26],[1,1],'--')\n\nstate_names = np.empty([3,3,3],dtype=object)\nfor i in range(0,3):\n for j in range(0,3):\n for k in range(0,3):\n state_names[i,j,k] = str(i) + \"-\" + str(j) + \"-\" + str(k)\n \n_ = ax2.set_xticks(range(0,27))\n_ = ax2.set_xticklabels(state_names.flatten(),rotation=45)\n\nplt.tight_layout()\n\nplt.savefig('figs/graphs/CH_state_prob_AB_'+ mouse_name +'.png')",
"_____no_output_____"
],
[
"from scipy.stats import chisquare\n# chisquare(P_ij_ON.flatten())\nchisquare?",
"_____no_output_____"
],
[
"# First order transition graph\ng_ON = gt.Graph(directed = True)\n\nedge_weights_ON = g_ON.new_edge_property('double')\nedge_labels_ON = g_ON.new_edge_property('string')\nnode_size_ON = g_ON.new_vertex_property('double')\ng_ON.add_vertex(len(P_ij_ON))\n\nfor i in range(P_ij_ON.shape[0]):\n #v = g_n.add_vertex()\n node_size_ON[i] = 3* sum(P_ij_ON)[i] / np.sum(P_ij_ON)\n for j in range(P_ij_ON.shape[1]):\n e = g_ON.add_edge(i, j)\n edge_weights_ON[e] = P_ij_ON[i,j]\n edge_labels_ON[e] = \"{0:.2f}\".format(P_ij_ON[i,j])\n \n# Plot graph\npos = gt.sfdp_layout(g_ON) \nn_size = copy.copy(node_size_ON)\nn_size.a = 50* n_size.a/ max(n_size.a)\n\nedge_w = copy.copy(edge_weights_ON)\nedge_w.a = edge_w.a*10\n\ncmap = sns.cubehelix_palette(as_cmap=True) # cubehelix \ncmap = sns.diverging_palette(220,10, l=70, as_cmap=True, center=\"dark\") # blue to red via black\n\ngt.graph_draw(g_ON, pos=pos, vertex_color = n_size, vertex_fill_color = n_size, \n vertex_size = n_size,\n edge_pen_width=edge_w, edge_color=edge_w, \n edge_text=edge_labels_ON,\n vcmap=cmap,ecmap=cmap, \n vertex_text=g_ON.vertex_index, \n vertex_font_size=18,\n output_size=(800, 800), fit_view=0.45,\n output=\"figs/graphs/choice_1st_order_transition_ON\"+ mouse_name +\".pdf\")\n",
"_____no_output_____"
]
],
[
[
"# Finally, transition probabilities for choices - do they follow the trial types?\n## (Actually, let's just re-run the code from above changing tt to ch)",
"_____no_output_____"
],
[
"# Now, let's use graphs to visualise confusion matrices",
"_____no_output_____"
]
],
[
[
"cm_AB = confusion_matrix(tt[AB_pol],ch[AB_pol])\ncm_ON = confusion_matrix(tt[ON_pol],ch[ON_pol])\nprint(cm_AB)\nprint(cm_ON)\nprint(accuracy_score(tt[AB_pol],ch[AB_pol]))\nprint(accuracy_score(tt[ON_pol],ch[ON_pol]))",
"[[401 32 42]\n [ 18 291 43]\n [ 91 63 280]]\n[[199 14 27]\n [ 10 196 15]\n [ 27 54 247]]\n0.770816812054\n0.813688212928\n"
],
[
"cmap = sns.diverging_palette(220,10, l=70, as_cmap=True, center=\"dark\") # blue to red via black\nwith sns.axes_style(\"white\"):\n fig, ax = plt.subplots(1,2)\n ax[0].imshow(cm_ON/np.sum(cm_ON),interpolation='none',cmap=cmap)\n ax[1].imshow(cm_AB/np.sum(cm_AB),interpolation='none',cmap=cmap)\n \nfor i in range(0,3):\n for j in range(0,3):\n ax[0].text(j, i, \"{0:.2f}\".format(cm_ON[i,j]), va='center', ha='center',bbox=dict(facecolor='white',edgecolor='white', alpha=0.5))\n ax[1].text(j, i, \"{0:.2f}\".format(cm_AB[i,j]), va='center', ha='center',bbox=dict(facecolor='white',edgecolor='white', alpha=0.5))\n\nax[0].set_title('Mouse ON')\nax[1].set_title('Mouse AB')\n# plt.savefig('figs/graphs/confusion_matrix_AB_'+ mouse_name +'.png')",
"_____no_output_____"
]
],
[
[
"# Should also look at patterns in licking wrt correct/incorrect ",
"_____no_output_____"
]
],
[
[
"for v in g.vertices():\n print(v)\nfor e in g.edges():\n print(e)",
"_____no_output_____"
],
[
"19.19 - 9.92\n",
"_____no_output_____"
],
[
"# gt.graph_draw(g,output_size=(400,400),fit_view=True,output='simple_graph.pdf')\ngt.graph_draw(g2,output_size=(400,400),fit_view=True)",
"_____no_output_____"
],
[
"deg.",
"_____no_output_____"
],
[
"# Stats...",
"_____no_output_____"
],
[
"len(tt[tt[AB_pol]])",
"_____no_output_____"
],
[
"gt.graph_draw?",
"_____no_output_____"
]
],
[
[
"## Load and plot protraction/retraction trial data for one mouse",
"_____no_output_____"
]
],
[
[
"# quick load and classification of pro/ret data\ntt = pd.read_csv('~/work/whiskfree/data/tt_36_subset_sorted.csv',header=None)\nch = pd.read_csv('~/work/whiskfree/data/ch_36_subset_sorted.csv',header=None)\nproret = pd.read_csv('~/work/whiskfree/data/proret_36_subset_sorted.csv',header=None)\n\ntt = tt.values.reshape(-1,1)\nch = ch.values.reshape(-1,1)\nproret = proret.values.reshape(-1,1)",
"_____no_output_____"
],
[
"cm = confusion_matrix(tt,ch)\nprint(cm)",
"[[231 41 37]\n [ 41 183 33]\n [ 75 18 172]]\n"
],
[
"cm_tt_t = confusion_matrix(tt,proret)\ncm_ch_t = confusion_matrix(ch,proret)\n\nprint(cm_tt_t)\nprint(cm_ch_t)\nplt.imshow(cm_tt_t,interpolation='none')",
"[[198 28 83]\n [ 81 110 66]\n [ 9 151 105]]\n[[181 56 110]\n [ 60 131 51]\n [ 47 102 93]]\n"
],
[
"with sns.axes_style(\"white\"):\n fig, ax = plt.subplots(1,2,figsize=(10,6))\n ax[0].imshow(cm_tt_t/np.sum(cm_tt_t),interpolation='none')\n ax[1].imshow(cm_ch_t/np.sum(cm_ch_t),interpolation='none')\n \nfor i in range(0,3):\n for j in range(0,3):\n ax[0].text(j, i, \"{0:.2f}\".format(cm_tt_t[i,j]), va='center', ha='center',bbox=dict(facecolor='white',edgecolor='white', alpha=0.5))\n ax[1].text(j, i, \"{0:.2f}\".format(cm_ch_t[i,j]), va='center', ha='center',bbox=dict(facecolor='white',edgecolor='white', alpha=0.5))\n\n \nxlabels = ['Retraction','Protraction','No Touch']\nylabels = ['Posterior','Anterior','No Go']\nax[0].set_title('Trialtype | touch type' + '. ' + str(int(100 * accuracy_score(tt,proret))) + '%')\nax[1].set_title('Choice | touch type' + '. ' + str(int(100 * accuracy_score(ch,proret))) + '%')\n\nax[0].set_ylabel('Trial type')\nax[1].set_ylabel('Choice')\n \nfor i in range(0,2):\n ax[i].set_xlabel('Touch type')\n ax[i].set_xticks([0,1,2])\n ax[i].set_xticklabels(xlabels)\n ax[i].set_yticks([0,1,2])\n ax[i].set_yticklabels(ylabels)\n \nplt.tight_layout()\n \n# plt.savefig('../figs/classification/pro_ret/310816/touchtype_confmatrix_both_32.png')\nplt.savefig('../figs/classification/pro_ret/36/touchtype_confmatrix_both_36.png')",
"_____no_output_____"
],
[
"lr_tt = LogisticRegression(solver='lbfgs',multi_class='multinomial')\nlr_tt.fit(proret,tt)\nc_tt = lr_tt.predict(proret)\nprint('TT prediction accuracy =',accuracy_score(tt,c_tt))\nlr_ch = LogisticRegression(solver='lbfgs',multi_class='multinomial')\nlr_ch.fit(proret,ch)\nc_ch = lr_ch.predict(proret)\nprint('Choice prediction accuracy =',accuracy_score(ch,c_ch))\nprint('Mouse prediction accuracy =',accuracy_score(tt,ch))",
"TT prediction accuracy = 0.398315282792\nChoice prediction accuracy = 0.397111913357\nMouse prediction accuracy = 0.705174488568\n"
],
[
"print(confusion_matrix(ch,c_ch))\nprint(confusion_matrix(tt,c_tt))\n",
"[[237 0 110]\n [191 0 51]\n [149 0 93]]\n[[226 0 83]\n [191 0 66]\n [160 0 105]]\n"
],
[
"print(accuracy_score(ch,proret))\nprint(accuracy_score(tt,proret))",
"0.487364620939\n0.496991576414\n"
],
[
"plt.plot(c_ch)",
"_____no_output_____"
],
[
"# Confusion matrix predicting trial type based on protraction/retraction\ncm = confusion_matrix(tt,c_tt)\ncm_m = confusion_matrix(tt,ch)\n\n# xlabels = ['Retraction','Protraction','No Touch']\nylabels = ['Posterior','Anterior','No Go']\nwith sns.axes_style(\"white\"):\n fig, ax = plt.subplots(1,2,figsize=(10,6))\n ax[0].imshow(cm,interpolation='none')\n for i in range(0,3):\n for j in range(0,3):\n ax[0].text(j, i, \"{0:.2f}\".format(cm[i,j]), va='center', ha='center',bbox=dict(facecolor='white',edgecolor='white', alpha=0.5))\n\n \n ax[0].set_title('Logistic Regression - TT' + '. ' + str(int(100 * accuracy_score(tt,c_tt))) + '%')\n\n ax[1].imshow(cm_m,interpolation='none')\n for i in range(0,3):\n for j in range(0,3):\n ax[1].text(j, i, \"{0:.2f}\".format(cm_m[i,j]), va='center', ha='center',bbox=dict(facecolor='white',edgecolor='white', alpha=0.5))\n\n ax[1].set_title('Mouse' + '. ' + str(int(100 * accuracy_score(tt,ch))) + '%')\n\n for i in range(0,2):\n ax[i].set_ylabel('True label')\n ax[i].set_xlabel('Predicted label')\n ax[i].set_xticks([0,1,2])\n ax[i].set_xticklabels(ylabels)\n ax[i].set_yticks([0,1,2])\n ax[i].set_yticklabels(ylabels)\n \nplt.tight_layout()\n\n# plt.savefig('../figs/classification/pro_ret/310816/LR_confmatrix_TT_32.png')\nplt.savefig('../figs/classification/pro_ret/36/LR_confmatrix_TT_36.png')",
"_____no_output_____"
],
[
"# Confusion matrix predicting choice based on protraction/retraction\ncm_ch = confusion_matrix(ch,c_ch)\ncm_m = confusion_matrix(ch,tt)\n\n# xlabels = ['Retraction','Protraction','No Touch']\nylabels = ['Posterior','Anterior','No Go']\nwith sns.axes_style(\"white\"):\n fig, ax = plt.subplots(1,2,figsize=(10,6))\n ax[0].imshow(cm_ch,interpolation='none')\n for i in range(0,3):\n for j in range(0,3):\n ax[0].text(j, i, \"{0:.2f}\".format(cm_ch[i,j]), va='center', ha='center',bbox=dict(facecolor='white',edgecolor='white', alpha=0.5))\n\n \n ax[0].set_title('Logistic Regression - Ch' + '. ' + str(int(100 * accuracy_score(ch,c_ch))) + '%')\n \n ax[1].imshow(cm_m,interpolation='none')\n for i in range(0,3):\n for j in range(0,3):\n ax[1].text(j, i, \"{0:.2f}\".format(cm_m[i,j]), va='center', ha='center',bbox=dict(facecolor='white',edgecolor='white', alpha=0.5))\n\n ax[1].set_title('Mouse' + '. ' + str(int(100 * accuracy_score(ch,tt))) + '%')\n\n for i in range(0,2):\n ax[i].set_ylabel('True label')\n ax[i].set_xlabel('Predicted label')\n ax[i].set_xticks([0,1,2])\n ax[i].set_xticklabels(ylabels)\n ax[i].set_yticks([0,1,2])\n ax[i].set_yticklabels(ylabels)\n \nplt.tight_layout()\n\n# plt.savefig('../figs/classification/pro_ret/310816/LR_confmatrix_Ch_32.png')\nplt.savefig('../figs/classification/pro_ret/36/LR_confmatrix_Ch_36.png')",
"_____no_output_____"
],
[
"# Correct/incorrect\ncorrect = tt==ch\nerrors = tt!=ch\n\ncm_c = confusion_matrix(ch[correct],proret[correct])\ncm_ic = confusion_matrix(ch[errors],proret[errors])\n\nxlabels = ['Retraction','Protraction','No Touch']\nylabels = ['Posterior','Anterior','No Go']\nwith sns.axes_style(\"white\"):\n fig, ax = plt.subplots(1,2,figsize=(10,6))\n ax[0].imshow(cm_c,interpolation='none')\n for i in range(0,3):\n for j in range(0,3):\n ax[0].text(j, i, \"{0:.2f}\".format(cm_c[i,j]), va='center', ha='center',bbox=dict(facecolor='white',edgecolor='white', alpha=0.5))\n\n \n ax[0].set_title('Correct choice | touch type')\n \n ax[1].imshow(cm_ic,interpolation='none')\n for i in range(0,3):\n for j in range(0,3):\n ax[1].text(j, i, \"{0:.2f}\".format(cm_ic[i,j]), va='center', ha='center',bbox=dict(facecolor='white',edgecolor='white', alpha=0.5))\n\n ax[1].set_title('Incorrect choice | touch type')\n \n for i in range(0,2):\n ax[i].set_ylabel('Choice')\n ax[i].set_xlabel('Touch Type')\n ax[i].set_xticks([0,1,2])\n ax[i].set_xticklabels(xlabels)\n ax[i].set_yticks([0,1,2])\n ax[i].set_yticklabels(ylabels)\n \nplt.tight_layout()\n\n# plt.savefig('../figs/classification/pro_ret/310816/Correct_incorrect_confmatrix_Ch_32.png')\nplt.savefig('../figs/classification/pro_ret/36/Correct_incorrect_confmatrix_Ch_36.png')",
"_____no_output_____"
],
[
"# Try graph of trialtype/choice/touchtype plots\n# P_ijk_ON\n\n# import graph_tool.all as gt\n\ncm_3 = np.zeros([3,3,3])\nfor i in range(len(tt) - 2):\n \n cm_3[tt[i]-1,proret[i]-1 ,ch[i]-1] += 1\n\n# Make graph\ncm_G = gt.Graph(directed = False)\n\n# trialtypes = ['P','A','NG']\n# touchtypes = ['Ret','Pro','NT']\n# choices = ['P','A','NG']\ntrialtypes = ['Posterior','Anterior','No Go']\ntouchtypes = ['Retraction','Protraction','No Touch']\nchoices = ['Posterior','Anterior','No Go']\n\nedge_weights_cm_G = cm_G.new_edge_property('double')\nedge_labels_cm_G = cm_G.new_edge_property('string')\nnode_labels_cm_G = cm_G.new_vertex_property('string')\nnode_size_cm_G = cm_G.new_vertex_property('double')\nh = cm_G.add_vertex()\nnode_labels_cm_G[h] = \"0\"\n\nfor i in range(cm_3.shape[0]):\n v = cm_G.add_vertex()\n node_labels_cm_G[v] = trialtypes[i]\n e = cm_G.add_edge(h,v)\n node_size_cm_G[v] = np.sum(cm_3[i]) / 4\n \n for j in range(cm_3.shape[1]):\n v2 = cm_G.add_vertex()\n node_labels_cm_G[v2] = touchtypes[j]\n e = cm_G.add_edge(v,v2)\n \n edge_weights_cm_G[e] = np.sum(cm_3[i,j]) /4\n edge_labels_cm_G[e] = str(int(np.sum(cm_3[i,j])))\n node_size_cm_G[v2] = np.sum(cm_3[i,j]) /4\n \n for k in range(cm_3.shape[2]):\n v3 = cm_G.add_vertex()\n node_labels_cm_G[v3] = choices[k]\n e2 = cm_G.add_edge(v2,v3)\n \n edge_weights_cm_G[e2] = int(cm_3[i,j,k])/4\n edge_labels_cm_G[e2] = str(int(cm_3[i,j,k]))\n node_size_cm_G[v3] = int(cm_3[i,j,k])/2\n\n# Plot graph\npos = gt.radial_tree_layout(cm_G,cm_G.vertex(0))\n# cmap = sns.diverging_palette(220,10, l=70, as_cmap=True, center=\"dark\") # blue to green via black\ncmap =plt.get_cmap('Greys')\ngt.graph_draw(cm_G,pos=pos,vertex_size=node_size_cm_G,edge_pen_width=edge_weights_cm_G,\n vertex_text = node_labels_cm_G, #vertex_text_position = 'centered',\n edge_text=edge_labels_cm_G,\n vertex_font_size = 22, vertex_font_family = 'sansserif',\n edge_font_size = 24, edge_font_family = 'sansserif',\n ecmap=cmap, vcmap=cmap, \n edge_color = edge_weights_cm_G,\n vertex_color = node_size_cm_G,\n vertex_fill_color = node_size_cm_G,\n output_size=(1500, 1500), fit_view=0.8,\n# output=\"../figs/classification/pro_ret/310816/tt_touch_ch_graph_BW_\"+ mouse_name +\".pdf\")\n \n output=\"../figs/classification/pro_ret/36/tt_touch_ch_graph_BW_\"+ mouse_name +\".pdf\")\n\n",
"_____no_output_____"
],
[
"np.sum(cm_3)",
"_____no_output_____"
],
[
"error_matrix",
"_____no_output_____"
],
[
"choice_matrix",
"_____no_output_____"
],
[
"with sns.axes_style(\"white\"):\n cmap = sns.diverging_palette(220,10, l=70, as_cmap=True, center=\"dark\") # blue to green via black\n fig, ax = plt.subplots(1,2)\n ax[0].imshow(error_matrix,interpolation='none',cmap=cmap)\n for i in range(0,3):\n for j in range(0,3):\n ax[0].text(j, i, \"{0:.2f}\".format(error_matrix[i,j]), va='center', ha='center',bbox=dict(facecolor='white',edgecolor='white', alpha=0.5))\n\n \n ax[0].set_title('Error matrix') # + '. ' + str(int(100 * accuracy_score(tt,c_tt))) + '%')\n ax[0].set_ylabel('Trial type')\n ax[0].set_xlabel('Touch type')\n ax[1].imshow(choice_matrix,interpolation='none',cmap=cmap)\n for i in range(0,3):\n for j in range(0,3):\n ax[1].text(j, i, \"{0:.2f}\".format(choice_matrix[i,j]), va='center', ha='center',bbox=dict(facecolor='white',edgecolor='white', alpha=0.5))\n\n ax[1].set_title('Choice matrix') # + '. ' + str(int(100 * accuracy_score(tt,ch))) + '%')\n ax[1].set_ylabel('Choice')\n ax[1].set_xlabel('Touch type')\n# plt.savefig('figs/graphs/pro_ret_confmatrix_TT_32_full.png')",
"_____no_output_____"
],
[
"plt.plot(c_ch)",
"_____no_output_____"
],
[
"print(confusion_matrix(ch,proret))\nprint(confusion_matrix(tt,proret))\n",
"[[164 54 77]\n [ 86 241 25]\n [ 21 114 133]]\n[[189 15 62]\n [ 80 236 25]\n [ 2 158 148]]\n"
]
]
] | [
"markdown",
"code",
"markdown",
"code",
"markdown",
"code",
"markdown",
"code",
"markdown",
"code"
] | [
[
"markdown"
],
[
"code",
"code",
"code",
"code",
"code",
"code",
"code",
"code",
"code",
"code",
"code",
"code",
"code",
"code",
"code",
"code",
"code",
"code",
"code",
"code"
],
[
"markdown"
],
[
"code",
"code",
"code",
"code",
"code"
],
[
"markdown",
"markdown"
],
[
"code",
"code"
],
[
"markdown"
],
[
"code",
"code",
"code",
"code",
"code",
"code",
"code"
],
[
"markdown"
],
[
"code",
"code",
"code",
"code",
"code",
"code",
"code",
"code",
"code",
"code",
"code",
"code",
"code",
"code",
"code",
"code",
"code",
"code"
]
] |
d04579eb4e2b38d0672b8d1f35c11b361c66ee85 | 28,833 | ipynb | Jupyter Notebook | TRABALHO1GRAFOS.ipynb | haroldosfilho/Python | ffdc22a5c3f1704076edbd07b997eb50af39abdc | [
"MIT"
] | null | null | null | TRABALHO1GRAFOS.ipynb | haroldosfilho/Python | ffdc22a5c3f1704076edbd07b997eb50af39abdc | [
"MIT"
] | null | null | null | TRABALHO1GRAFOS.ipynb | haroldosfilho/Python | ffdc22a5c3f1704076edbd07b997eb50af39abdc | [
"MIT"
] | null | null | null | 80.539106 | 20,530 | 0.806333 | [
[
[
"<a href=\"https://colab.research.google.com/github/haroldosfilho/Python/blob/master/TRABALHO1GRAFOS.ipynb\" target=\"_parent\"><img src=\"https://colab.research.google.com/assets/colab-badge.svg\" alt=\"Open In Colab\"/></a>",
"_____no_output_____"
],
[
"bibliotecas utilizadas",
"_____no_output_____"
],
[
"Aperte Play para inicializar as bibliotecas\n",
"_____no_output_____"
]
],
[
[
"import networkx as nx\nimport matplotlib.pyplot as plt\nimport numpy as np\n",
"_____no_output_____"
]
],
[
[
"Entre com o número de vértces do seu grafo e digite enter.\n",
"_____no_output_____"
]
],
[
[
"n = input(\"entre com o numero de vertices:\" )",
"entre com o numero de vertices:5\n"
]
],
[
[
"aperte o play para tranformar num número inteiro a sua entrada.",
"_____no_output_____"
]
],
[
[
"num=int(str(n))\nprint(num)",
"5\n"
]
],
[
[
"aperte o play para gerar a lista dos vértices do seu Grafo",
"_____no_output_____"
]
],
[
[
"G = nx.path_graph(num)\nlist(G.nodes)\n",
"_____no_output_____"
],
[
"m = int(input(\"Entre com o número de arestas : \"))",
"Entre com o número de arestas : 7\n"
]
],
[
[
"Digite as suas arestas, quais vértices estão conectados, aperte enter após cada aresta informada.\n",
"_____no_output_____"
]
],
[
[
"# creating an empty list\nlst = []\n# iterating till the range\nfor i in range(0, m):\n ele = str(input())\n lst.append(ele) # adding the element\n \nprint(lst)",
"01\n12\n13\n23\n24\n34\n02\n['01', '12', '13', '23', '24', '34', '02']\n"
]
],
[
[
"aperte play para gerar uma representação no plano do seu Grafo.",
"_____no_output_____"
]
],
[
[
"G = nx.Graph(lst)\nopts = { \"with_labels\": True, \"node_color\": 'y' }\nnx.draw(G, **opts)\n",
"_____no_output_____"
]
],
[
[
"aperte o play para gerar os elementos da sua matriz de adjacência do seu Grafo\n",
"_____no_output_____"
]
],
[
[
"A = nx.adjacency_matrix(G)\nprint(A)",
" (0, 1)\t1\n (0, 2)\t1\n (1, 0)\t1\n (1, 2)\t1\n (1, 3)\t1\n (2, 0)\t1\n (2, 1)\t1\n (2, 3)\t1\n (2, 4)\t1\n (3, 1)\t1\n (3, 2)\t1\n (3, 4)\t1\n (4, 2)\t1\n (4, 3)\t1\n"
]
],
[
[
"Agora basta apertar o play e a sua matriz de adjacência está pronta!",
"_____no_output_____"
]
],
[
[
"A = nx.adjacency_matrix(G).toarray()\n\nprint(A)",
"[[0 1 1 0 0]\n [1 0 1 1 0]\n [1 1 0 1 1]\n [0 1 1 0 1]\n [0 0 1 1 0]]\n"
]
]
] | [
"markdown",
"code",
"markdown",
"code",
"markdown",
"code",
"markdown",
"code",
"markdown",
"code",
"markdown",
"code",
"markdown",
"code",
"markdown",
"code"
] | [
[
"markdown",
"markdown",
"markdown"
],
[
"code"
],
[
"markdown"
],
[
"code"
],
[
"markdown"
],
[
"code"
],
[
"markdown"
],
[
"code",
"code"
],
[
"markdown"
],
[
"code"
],
[
"markdown"
],
[
"code"
],
[
"markdown"
],
[
"code"
],
[
"markdown"
],
[
"code"
]
] |
d04591f52e6289488491cca1033e230d008e005d | 18,851 | ipynb | Jupyter Notebook | examples/Jupyter/ClarityViz Pipeline.ipynb | jonl1096/seelvizorg | ae4e3567ce89eb62edcd742060619fdf1883b991 | [
"Apache-2.0"
] | null | null | null | examples/Jupyter/ClarityViz Pipeline.ipynb | jonl1096/seelvizorg | ae4e3567ce89eb62edcd742060619fdf1883b991 | [
"Apache-2.0"
] | 2 | 2017-04-18T02:50:14.000Z | 2017-04-18T18:04:20.000Z | examples/Jupyter/ClarityViz Pipeline.ipynb | jonl1096/seelvizorg | ae4e3567ce89eb62edcd742060619fdf1883b991 | [
"Apache-2.0"
] | null | null | null | 35.906667 | 258 | 0.45483 | [
[
[
"# ClarityViz\n\n## Pipeline: .img -> histogram .nii -> graph represented as csv -> graph as graphml -> plotly\n\n### To run:\n\n### Step 1:\n\nFirst, run the following. This takes the .img, generates the localeq histogram as an nii file, gets the nodes and edges as a csv and converts the csv into a graphml\n\n",
"_____no_output_____"
]
],
[
[
"python runclarityviz.py --token Fear199Coronal --file-type img --source-directory /cis/project/clarity/data/clarity/isoCoronal",
"_____no_output_____"
]
],
[
[
"### Step 2: \nThen run this. This just converts the graphml into a plotly",
"_____no_output_____"
]
],
[
[
"python runclarityviz.py --token Fear199Coronal --plotly yes",
"_____no_output_____"
]
],
[
[
"## Results",
"_____no_output_____"
]
],
[
[
"Starting pipeline for Fear199.img\nGenerating Histogram...\nFINISHED GENERATING HISTOGRAM\nLoading: Fear199/Fear199localeq.nii\nImage Loaded: Fear199/Fear199localeq.nii\nFINISHED LOADING NII\nCoverting to points...\ntoken=Fear199\ntotal=600735744\nmax=255.000000\nthreshold=0.300000\nsample=0.500000\n(This will take couple minutes)\nAbove threshold=461409948\nSamples=230718301\nFinished\nFINISHED GETTING POINTS",
"_____no_output_____"
],
[
"~/clarityviztesting/Fear199Coronal$ ls\nFear199Coronal.csv\t Fear199Coronal.graphml Fear199Coronal.nodes.csv\nFear199Coronal.edges.csv Fear199Coronallocaleq.nii Fear199Coronalplotly.html",
"_____no_output_____"
]
],
[
[
"# Code",
"_____no_output_____"
],
[
"## runclarityviz.py:",
"_____no_output_____"
]
],
[
[
"from clarityviz import clarityviz\nimport ...\n\ndef get_args():\n parser = argparse.ArgumentParser(description=\"Description\")\n\n parser.add_argument(\"--token\", type=str, required=True, help=\"The token.\")\n parser.add_argument(\"--file-type\", type=str, required=False, help=\"The file type.\")\n parser.add_argument(\"--source-directory\", type=str, required=False,\n help=\"Optional setting of the source directory.\")\n parser.add_argument(\"--plotly\", type=str, required=False, help=\"Optional method to generate the plotly graphs.\")\n parser.add_argument(\"--generate-nii-from-csv\", type=str, required=False, help=\"script to generate nii\")\n\n args = parser.parse_args()\n\n return args\n\n\ndef main():\n print('ayyooooo')\n args = get_args()\n\n if args.plotly == 'yes':\n ## Type in the path to your csv file here\n thedata = np.genfromtxt(args.token + '/' + args.token + '.csv',\n delimiter=',', dtype='int', usecols = (0,1,2), names=['a','b','c'])\n\n trace1 = go.Scatter3d(\n x = thedata['a'],\n y = thedata['b'],\n z = thedata['c'],\n mode='markers',\n marker=dict(\n size=1.2,\n color='purple', # set color to an array/list of desired values\n colorscale='Viridis', # choose a colorscale\n opacity=0.15\n )\n )\n\n data = [trace1]\n layout = go.Layout(\n margin=dict(\n l=0,\n r=0,\n b=0,\n t=0\n )\n )\n\n fig = go.Figure(data=data, layout=layout)\n print args.token + \"plotly\"\n plotly.offline.plot(fig, filename= args.token + \"/\" + args.token + \"plotly.html\")\n else:\n print('Starting pipeline for %s' % (args.token + '.' + args.file_type))\n if args.source_directory == None:\n c = clarityviz(args.token)\n else:\n c = clarityviz(args.token, args.source_directory)\n\n if args.file_type == 'img':\n #c.loadEqImg()\n c.generateHistogram()\n print('FINISHED GENERATING HISTOGRAM')\n c.loadNiiImg()\n print('FINISHED LOADING NII')\n elif args.file_type == 'nii':\n c.loadNiiImg()\n print('FINISHED LOADING NII')\n\n c.imgToPoints(0.3, 0.5)\n print(\"FINISHED GETTING POINTS\")\n\n c.savePoints()\n\n c.plot3d()\n print(\"FINISHED PLOT3D\")\n\n c.graphmlconvert()\n print(\"FINISHED GRAPHMLCONVERT\")\n\nif __name__ == \"__main__\":\n main()",
"_____no_output_____"
]
],
[
[
"## clarityviz.py",
"_____no_output_____"
]
],
[
[
"def generateHistogram(self):\n print('Generating Histogram...')\n if self._source_directory == None:\n path = self._token + '.img'\n else:\n path = self._source_directory + \"/\" + self._token + \".img\"\n\n im = nib.load(path)\n\n im = im.get_data()\n img = im[:,:,:]\n\n shape = im.shape\n #affine = im.get_affine()\n\n x_value = shape[0]\n y_value = shape[1]\n z_value = shape[2]\n\n #####################################################\n\n imgflat = img.reshape(-1)\n\n #img_grey = np.array(imgflat * 255, dtype = np.uint8)\n\n #img_eq = exposure.equalize_hist(img_grey)\n\n #new_img = img_eq.reshape(x_value, y_value, z_value)\n #globaleq = nib.Nifti1Image(new_img, np.eye(4))\n\n ######################################################\n\n #clahe = cv2.createCLAHE(clipLimit=2.0, tileGridSize=(8,8))\n clahe = cv2.createCLAHE(clipLimit=2.0, tileGridSize=(8,8))\n\n img_grey = np.array(imgflat * 255, dtype = np.uint8)\n #threshed = cv2.adaptiveThreshold(img_grey, 255, cv2.ADAPTIVE_THRESH_MEAN_C, cv2.THRESH_BINARY, 3, 0)\n\n cl1 = clahe.apply(img_grey)\n\n #cv2.imwrite('clahe_2.jpg',cl1)\n #cv2.startWindowThread()\n #cv2.namedWindow(\"adaptive\")\n #cv2.imshow(\"adaptive\", cl1)\n #cv2.imshow(\"adaptive\", threshed)\n #plt.imshow(threshed)\n\n localimgflat = cl1 #cl1.reshape(-1)\n\n newer_img = localimgflat.reshape(x_value, y_value, z_value)\n localeq = nib.Nifti1Image(newer_img, np.eye(4))\n nib.save(localeq, self._token + '/' + self._token + 'localeq.nii')",
"_____no_output_____"
],
[
"def loadGeneratedNii(self, path=None, info=False):\n path = self._token + '/' + self._token + 'localeq.nii'\n print(\"Loading: %s\"%(path))\n\n #pathname = path+self._token+\".nii\"\n img = nib.load(path)\n if info:\n print(img)\n #self._img = img.get_data()[:,:,:,0]\n self._img = img.get_data()\n self._shape = self._img.shape\n self._max = np.max(self._img)\n print(\"Image Loaded: %s\"%(path))\n return self",
"_____no_output_____"
],
[
"def imgToPoints(self, threshold=0.1, sample=0.5, optimize=True):\n \"\"\"Method to extract points data from the img file.\"\"\"\n if not 0 <= threshold < 1:\n raise ValueError(\"Threshold should be within [0,1).\")\n if not 0 < sample <= 1:\n raise ValueError(\"Sample rate should be within (0,1].\")\n if self._img is None:\n raise ValueError(\"Img haven't loaded, please call loadImg() first.\")\n\n total = self._shape[0]*self._shape[1]*self._shape[2]\n print(\"Coverting to points...\\ntoken=%s\\ntotal=%d\\nmax=%f\\nthreshold=%f\\nsample=%f\"\\\n %(self._token,total,self._max,threshold,sample))\n print(\"(This will take couple minutes)\")\n # threshold\n filt = self._img > threshold * self._max\n x, y, z = np.where(filt)\n v = self._img[filt]\n if optimize:\n self.discardImg()\n v = np.int16(255*(np.float32(v)/np.float32(self._max)))\n l = v.shape\n print(\"Above threshold=%d\"%(l))\n # sample\n if sample < 1.0:\n filt = np.random.random(size=l) < sample\n x = x[filt]\n y = y[filt]\n z = z[filt]\n v = v[filt]\n self._points = np.vstack([x,y,z,v])\n self._points = np.transpose(self._points)\n print(\"Samples=%d\"%(self._points.shape[0]))\n print(\"Finished\")\n return self",
"_____no_output_____"
],
[
"def plot3d(self, infile = None):\n \"\"\"Method for plotting the Nodes and Edges\"\"\"\n filename = \"\"\n points_file = None\n if infile == None:\n points_file = self._points\n filename = self._token\n else:\n self.loadInitCsv(infile)\n infile = self._infile\n filename = self._filename\n\n # points is an array of arrays\n points = self._points\n outpath = self._token + '/'\n nodename = outpath + filename + '.nodes.csv'\n edgename = outpath + filename + '.edges.csv'\n\n with open(nodename, 'w') as nodefile:\n with open(edgename, 'w') as edgefile:\n for ind in range(len(points)):\n #temp = points[ind].strip().split(',')\n temp = points[ind]\n x = temp[0]\n y = temp[1]\n z = temp[2]\n v = temp[3]\n radius = 18\n nodefile.write(\"s\" + str(ind + 1) + \",\" + str(x) + \",\" + str(y) + \",\" + str(z) + \"\\n\")\n for index in range(ind + 1, len(points)):\n tmp = points[index]\n distance = math.sqrt(math.pow(int(x) - int(tmp[0]), 2) + math.pow(int(y) - int(tmp[1]), 2) + math.pow(int(z) - int(tmp[2]), 2))\n if distance < radius:\n edgefile.write(\"s\" + str(ind + 1) + \",\" + \"s\" + str(index + 1) + \"\\n\")\n self._nodefile = nodefile\n self._edgefile = edgefile",
"_____no_output_____"
],
[
" def graphmlconvert(self, nodefilename = None, edgefilename = None):\n \"\"\"Method for extracting the data to a graphml file, based on the node and edge files\"\"\"\n nodefile = None\n edgefile = None\n\n # If no nodefilename was entered, used the Clarity object's nodefile\n if nodefilename == None:\n #nodefile = self._nodefile\n #nodefile = open(self._nodefile, 'r')\n\n self.loadNodeCsv(self._token + \"/\" + self._token + \".nodes.csv\")\n nodefile = self._nodefile\n else:\n self.loadNodeCsv(nodefilename)\n nodefile = self._nodefile\n\n # If no edgefilename was entered, used the Clarity object's edgefile\n if edgefilename == None:\n #edgefile = self._edgefile\n #edgefile = open(self._edgefile, 'r')\n\n self.loadEdgeCsv(self._token + \"/\" + self._token + \".edges.csv\")\n edgefile = self._edgefile\n else:\n self.loadEdgeCsv(edgefilename)\n edgefile = self._edgefile\n\n # Start writing to the output graphml file\n path = self._token + \"/\" + self._token + \".graphml\"\n with open(path, 'w') as outfile:\n outfile.write(\"<?xml version=\\\"1.0\\\" encoding=\\\"UTF-8\\\"?>\\n\")\n outfile.write(\"<graphml xmlns=\\\"http://graphml.graphdrawing.org/xmlns\\\"\\n\")\n outfile.write(\" xmlns:xsi=\\\"http://www.w3.org/2001/XMLSchema-instance\\\"\\n\")\n outfile.write(\" xsi:schemaLocation=\\\"http://graphml.graphdrawing.org/xmlns\\n\")\n outfile.write(\" http://graphml.graphdrawing.org/xmlns/1.0/graphml.xsd\\\">\\n\")\n\n outfile.write(\" <key id=\\\"d0\\\" for=\\\"node\\\" attr.name=\\\"attr\\\" attr.type=\\\"string\\\"/>\\n\")\n outfile.write(\" <key id=\\\"e_weight\\\" for=\\\"edge\\\" attr.name=\\\"weight\\\" attr.type=\\\"double\\\"/>\\n\")\n outfile.write(\" <graph id=\\\"G\\\" edgedefault=\\\"undirected\\\">\\n\")\n\n for line in nodefile:\n if len(line) == 0:\n continue\n line = line.strip().split(',')\n outfile.write(\" <node id=\\\"\" + line[0] + \"\\\">\\n\")\n outfile.write(\" <data key=\\\"d0\\\">[\" + line[1] + \", \" + line[2] + \", \" + line[3] +\"]</data>\\n\")\n outfile.write(\" </node>\\n\")\n \n for line in edgefile:\n if len(line) == 0:\n continue\n line = line.strip().split(',')\n outfile.write(\" <edge source=\\\"\" + line[0] + \"\\\" target=\\\"\" + line[1] + \"\\\">\\n\")\n outfile.write(\" <data key=\\\"e_weight\\\">1</data>\\n\")\n outfile.write(\" </edge>\\n\")\n\n outfile.write(\" </graph>\\n</graphml>\")",
"_____no_output_____"
],
[
"def graphmlToPlotly(self, path):\n ## Type in the path to your csv file here\n thedata = np.genfromtxt('../data/points/localeq.csv', delimiter=',', dtype='int', usecols = (0,1,2), names=['a','b','c'])\n\n trace1 = go.Scatter3d(\n x = thedata['a'],\n y = thedata['b'],\n z = thedata['c'],\n mode='markers',\n marker=dict(\n size=1.2,\n color='purple', # set color to an array/list of desired values\n colorscale='Viridis', # choose a colorscale\n opacity=0.15\n )\n )\n\n data = [trace1]\n layout = go.Layout(\n margin=dict(\n l=0,\n r=0,\n b=0,\n t=0\n )\n )\n\n fig = go.Figure(data=data, layout=layout)\n print \"localeq\"\n plotly.offline.plot(fig, filename= \"localeq\")",
"_____no_output_____"
]
]
] | [
"markdown",
"code",
"markdown",
"code",
"markdown",
"code",
"markdown",
"code",
"markdown",
"code"
] | [
[
"markdown"
],
[
"code"
],
[
"markdown"
],
[
"code"
],
[
"markdown"
],
[
"code",
"code"
],
[
"markdown",
"markdown"
],
[
"code"
],
[
"markdown"
],
[
"code",
"code",
"code",
"code",
"code",
"code"
]
] |
d045934f5b4c53e6ded597aecbdc40a101fd91cf | 185,319 | ipynb | Jupyter Notebook | models/mnet-25 symbols.ipynb | JuheonYi/mxnet2tf | 6e68a68f94947ec37ceaab3c3205becd97c6ecb0 | [
"MIT"
] | 2 | 2020-11-25T08:18:41.000Z | 2020-11-25T08:39:13.000Z | models/mnet-25 symbols.ipynb | JuheonYi/mxnet2tf | 6e68a68f94947ec37ceaab3c3205becd97c6ecb0 | [
"MIT"
] | null | null | null | models/mnet-25 symbols.ipynb | JuheonYi/mxnet2tf | 6e68a68f94947ec37ceaab3c3205becd97c6ecb0 | [
"MIT"
] | 1 | 2020-11-23T05:50:18.000Z | 2020-11-23T05:50:18.000Z | 33.706621 | 96 | 0.28752 | [
[
[
"{\n \"nodes\": [\n {\n \"op\": \"null\", \n \"name\": \"data\", \n \"inputs\": []\n }, \n {\n \"op\": \"null\", \n \"name\": \"mobilenet0_conv0_weight\", \n \"attrs\": {\n \"__dtype__\": \"0\", \n \"__lr_mult__\": \"1.0\", \n \"__shape__\": \"(8L, 3L, 3L, 3L)\", \n \"__storage_type__\": \"0\", \n \"__wd_mult__\": \"1.0\"\n }, \n \"inputs\": []\n }, \n {\n \"op\": \"Convolution\", \n \"name\": \"mobilenet0_conv0_fwd\", \n \"attrs\": {\n \"dilate\": \"(1, 1)\", \n \"kernel\": \"(3, 3)\", \n \"layout\": \"NCHW\", \n \"no_bias\": \"True\", \n \"num_filter\": \"8\", \n \"num_group\": \"1\", \n \"pad\": \"(1, 1)\", \n \"stride\": \"(2, 2)\"\n }, \n \"inputs\": [[0, 0, 0], [1, 0, 0]]\n }, \n {\n \"op\": \"null\", \n \"name\": \"mobilenet0_batchnorm0_gamma\", \n \"attrs\": {\n \"__dtype__\": \"0\", \n \"__init__\": \"ones\", \n \"__lr_mult__\": \"1.0\", \n \"__shape__\": \"(8L,)\", \n \"__storage_type__\": \"0\", \n \"__wd_mult__\": \"1.0\"\n }, \n \"inputs\": []\n }, \n {\n \"op\": \"null\", \n \"name\": \"mobilenet0_batchnorm0_beta\", \n \"attrs\": {\n \"__dtype__\": \"0\", \n \"__init__\": \"zeros\", \n \"__lr_mult__\": \"1.0\", \n \"__shape__\": \"(8L,)\", \n \"__storage_type__\": \"0\", \n \"__wd_mult__\": \"1.0\"\n }, \n \"inputs\": []\n }, \n {\n \"op\": \"null\", \n \"name\": \"mobilenet0_batchnorm0_running_mean\", \n \"attrs\": {\n \"__dtype__\": \"0\", \n \"__init__\": \"zeros\", \n \"__lr_mult__\": \"1.0\", \n \"__shape__\": \"(8L,)\", \n \"__storage_type__\": \"0\", \n \"__wd_mult__\": \"1.0\"\n }, \n \"inputs\": []\n }, \n {\n \"op\": \"null\", \n \"name\": \"mobilenet0_batchnorm0_running_var\", \n \"attrs\": {\n \"__dtype__\": \"0\", \n \"__init__\": \"ones\", \n \"__lr_mult__\": \"1.0\", \n \"__shape__\": \"(8L,)\", \n \"__storage_type__\": \"0\", \n \"__wd_mult__\": \"1.0\"\n }, \n \"inputs\": []\n }, \n {\n \"op\": \"BatchNorm\", \n \"name\": \"mobilenet0_batchnorm0_fwd\", \n \"attrs\": {\n \"axis\": \"1\", \n \"eps\": \"1e-05\", \n \"fix_gamma\": \"False\", \n \"momentum\": \"0.9\", \n \"use_global_stats\": \"False\"\n }, \n \"inputs\": [[2, 0, 0], [3, 0, 0], [4, 0, 0], [5, 0, 1], [6, 0, 1]]\n }, \n {\n \"op\": \"Activation\", \n \"name\": \"mobilenet0_relu0_fwd\", \n \"attrs\": {\"act_type\": \"relu\"}, \n \"inputs\": [[7, 0, 0]]\n }, \n {\n \"op\": \"null\", \n \"name\": \"mobilenet0_conv1_weight\", \n \"attrs\": {\n \"__dtype__\": \"0\", \n \"__lr_mult__\": \"1.0\", \n \"__shape__\": \"(8L, 1L, 3L, 3L)\", \n \"__storage_type__\": \"0\", \n \"__wd_mult__\": \"1.0\"\n }, \n \"inputs\": []\n }, \n {\n \"op\": \"Convolution\", \n \"name\": \"mobilenet0_conv1_fwd\", \n \"attrs\": {\n \"dilate\": \"(1, 1)\", \n \"kernel\": \"(3, 3)\", \n \"layout\": \"NCHW\", \n \"no_bias\": \"True\", \n \"num_filter\": \"8\", \n \"num_group\": \"8\", \n \"pad\": \"(1, 1)\", \n \"stride\": \"(1, 1)\"\n }, \n \"inputs\": [[8, 0, 0], [9, 0, 0]]\n }, \n {\n \"op\": \"null\", \n \"name\": \"mobilenet0_batchnorm1_gamma\", \n \"attrs\": {\n \"__dtype__\": \"0\", \n \"__init__\": \"ones\", \n \"__lr_mult__\": \"1.0\", \n \"__shape__\": \"(8L,)\", \n \"__storage_type__\": \"0\", \n \"__wd_mult__\": \"1.0\"\n }, \n \"inputs\": []\n }, \n {\n \"op\": \"null\", \n \"name\": \"mobilenet0_batchnorm1_beta\", \n \"attrs\": {\n \"__dtype__\": \"0\", \n \"__init__\": \"zeros\", \n \"__lr_mult__\": \"1.0\", \n \"__shape__\": \"(8L,)\", \n \"__storage_type__\": \"0\", \n \"__wd_mult__\": \"1.0\"\n }, \n \"inputs\": []\n }, \n {\n \"op\": \"null\", \n \"name\": \"mobilenet0_batchnorm1_running_mean\", \n \"attrs\": {\n \"__dtype__\": \"0\", \n \"__init__\": \"zeros\", \n \"__lr_mult__\": \"1.0\", \n \"__shape__\": \"(8L,)\", \n \"__storage_type__\": \"0\", \n \"__wd_mult__\": \"1.0\"\n }, \n \"inputs\": []\n }, \n {\n \"op\": \"null\", \n \"name\": \"mobilenet0_batchnorm1_running_var\", \n \"attrs\": {\n \"__dtype__\": \"0\", \n \"__init__\": \"ones\", \n \"__lr_mult__\": \"1.0\", \n \"__shape__\": \"(8L,)\", \n \"__storage_type__\": \"0\", \n \"__wd_mult__\": \"1.0\"\n }, \n \"inputs\": []\n }, \n {\n \"op\": \"BatchNorm\", \n \"name\": \"mobilenet0_batchnorm1_fwd\", \n \"attrs\": {\n \"axis\": \"1\", \n \"eps\": \"1e-05\", \n \"fix_gamma\": \"False\", \n \"momentum\": \"0.9\", \n \"use_global_stats\": \"False\"\n }, \n \"inputs\": [[10, 0, 0], [11, 0, 0], [12, 0, 0], [13, 0, 1], [14, 0, 1]]\n }, \n {\n \"op\": \"Activation\", \n \"name\": \"mobilenet0_relu1_fwd\", \n \"attrs\": {\"act_type\": \"relu\"}, \n \"inputs\": [[15, 0, 0]]\n }, \n {\n \"op\": \"null\", \n \"name\": \"mobilenet0_conv2_weight\", \n \"attrs\": {\n \"__dtype__\": \"0\", \n \"__lr_mult__\": \"1.0\", \n \"__shape__\": \"(16L, 8L, 1L, 1L)\", \n \"__storage_type__\": \"0\", \n \"__wd_mult__\": \"1.0\"\n }, \n \"inputs\": []\n }, \n {\n \"op\": \"Convolution\", \n \"name\": \"mobilenet0_conv2_fwd\", \n \"attrs\": {\n \"dilate\": \"(1, 1)\", \n \"kernel\": \"(1, 1)\", \n \"layout\": \"NCHW\", \n \"no_bias\": \"True\", \n \"num_filter\": \"16\", \n \"num_group\": \"1\", \n \"pad\": \"(0, 0)\", \n \"stride\": \"(1, 1)\"\n }, \n \"inputs\": [[16, 0, 0], [17, 0, 0]]\n }, \n {\n \"op\": \"null\", \n \"name\": \"mobilenet0_batchnorm2_gamma\", \n \"attrs\": {\n \"__dtype__\": \"0\", \n \"__init__\": \"ones\", \n \"__lr_mult__\": \"1.0\", \n \"__shape__\": \"(16L,)\", \n \"__storage_type__\": \"0\", \n \"__wd_mult__\": \"1.0\"\n }, \n \"inputs\": []\n }, \n {\n \"op\": \"null\", \n \"name\": \"mobilenet0_batchnorm2_beta\", \n \"attrs\": {\n \"__dtype__\": \"0\", \n \"__init__\": \"zeros\", \n \"__lr_mult__\": \"1.0\", \n \"__shape__\": \"(16L,)\", \n \"__storage_type__\": \"0\", \n \"__wd_mult__\": \"1.0\"\n }, \n \"inputs\": []\n }, \n {\n \"op\": \"null\", \n \"name\": \"mobilenet0_batchnorm2_running_mean\", \n \"attrs\": {\n \"__dtype__\": \"0\", \n \"__init__\": \"zeros\", \n \"__lr_mult__\": \"1.0\", \n \"__shape__\": \"(16L,)\", \n \"__storage_type__\": \"0\", \n \"__wd_mult__\": \"1.0\"\n }, \n \"inputs\": []\n }, \n {\n \"op\": \"null\", \n \"name\": \"mobilenet0_batchnorm2_running_var\", \n \"attrs\": {\n \"__dtype__\": \"0\", \n \"__init__\": \"ones\", \n \"__lr_mult__\": \"1.0\", \n \"__shape__\": \"(16L,)\", \n \"__storage_type__\": \"0\", \n \"__wd_mult__\": \"1.0\"\n }, \n \"inputs\": []\n }, \n {\n \"op\": \"BatchNorm\", \n \"name\": \"mobilenet0_batchnorm2_fwd\", \n \"attrs\": {\n \"axis\": \"1\", \n \"eps\": \"1e-05\", \n \"fix_gamma\": \"False\", \n \"momentum\": \"0.9\", \n \"use_global_stats\": \"False\"\n }, \n \"inputs\": [[18, 0, 0], [19, 0, 0], [20, 0, 0], [21, 0, 1], [22, 0, 1]]\n }, \n {\n \"op\": \"Activation\", \n \"name\": \"mobilenet0_relu2_fwd\", \n \"attrs\": {\"act_type\": \"relu\"}, \n \"inputs\": [[23, 0, 0]]\n }, \n {\n \"op\": \"null\", \n \"name\": \"mobilenet0_conv3_weight\", \n \"attrs\": {\n \"__dtype__\": \"0\", \n \"__lr_mult__\": \"1.0\", \n \"__shape__\": \"(16L, 1L, 3L, 3L)\", \n \"__storage_type__\": \"0\", \n \"__wd_mult__\": \"1.0\"\n }, \n \"inputs\": []\n }, \n {\n \"op\": \"Convolution\", \n \"name\": \"mobilenet0_conv3_fwd\", \n \"attrs\": {\n \"dilate\": \"(1, 1)\", \n \"kernel\": \"(3, 3)\", \n \"layout\": \"NCHW\", \n \"no_bias\": \"True\", \n \"num_filter\": \"16\", \n \"num_group\": \"16\", \n \"pad\": \"(1, 1)\", \n \"stride\": \"(2, 2)\"\n }, \n \"inputs\": [[24, 0, 0], [25, 0, 0]]\n }, \n {\n \"op\": \"null\", \n \"name\": \"mobilenet0_batchnorm3_gamma\", \n \"attrs\": {\n \"__dtype__\": \"0\", \n \"__init__\": \"ones\", \n \"__lr_mult__\": \"1.0\", \n \"__shape__\": \"(16L,)\", \n \"__storage_type__\": \"0\", \n \"__wd_mult__\": \"1.0\"\n }, \n \"inputs\": []\n }, \n {\n \"op\": \"null\", \n \"name\": \"mobilenet0_batchnorm3_beta\", \n \"attrs\": {\n \"__dtype__\": \"0\", \n \"__init__\": \"zeros\", \n \"__lr_mult__\": \"1.0\", \n \"__shape__\": \"(16L,)\", \n \"__storage_type__\": \"0\", \n \"__wd_mult__\": \"1.0\"\n }, \n \"inputs\": []\n }, \n {\n \"op\": \"null\", \n \"name\": \"mobilenet0_batchnorm3_running_mean\", \n \"attrs\": {\n \"__dtype__\": \"0\", \n \"__init__\": \"zeros\", \n \"__lr_mult__\": \"1.0\", \n \"__shape__\": \"(16L,)\", \n \"__storage_type__\": \"0\", \n \"__wd_mult__\": \"1.0\"\n }, \n \"inputs\": []\n }, \n {\n \"op\": \"null\", \n \"name\": \"mobilenet0_batchnorm3_running_var\", \n \"attrs\": {\n \"__dtype__\": \"0\", \n \"__init__\": \"ones\", \n \"__lr_mult__\": \"1.0\", \n \"__shape__\": \"(16L,)\", \n \"__storage_type__\": \"0\", \n \"__wd_mult__\": \"1.0\"\n }, \n \"inputs\": []\n }, \n {\n \"op\": \"BatchNorm\", \n \"name\": \"mobilenet0_batchnorm3_fwd\", \n \"attrs\": {\n \"axis\": \"1\", \n \"eps\": \"1e-05\", \n \"fix_gamma\": \"False\", \n \"momentum\": \"0.9\", \n \"use_global_stats\": \"False\"\n }, \n \"inputs\": [[26, 0, 0], [27, 0, 0], [28, 0, 0], [29, 0, 1], [30, 0, 1]]\n }, \n {\n \"op\": \"Activation\", \n \"name\": \"mobilenet0_relu3_fwd\", \n \"attrs\": {\"act_type\": \"relu\"}, \n \"inputs\": [[31, 0, 0]]\n }, \n {\n \"op\": \"null\", \n \"name\": \"mobilenet0_conv4_weight\", \n \"attrs\": {\n \"__dtype__\": \"0\", \n \"__lr_mult__\": \"1.0\", \n \"__shape__\": \"(32L, 16L, 1L, 1L)\", \n \"__storage_type__\": \"0\", \n \"__wd_mult__\": \"1.0\"\n }, \n \"inputs\": []\n }, \n {\n \"op\": \"Convolution\", \n \"name\": \"mobilenet0_conv4_fwd\", \n \"attrs\": {\n \"dilate\": \"(1, 1)\", \n \"kernel\": \"(1, 1)\", \n \"layout\": \"NCHW\", \n \"no_bias\": \"True\", \n \"num_filter\": \"32\", \n \"num_group\": \"1\", \n \"pad\": \"(0, 0)\", \n \"stride\": \"(1, 1)\"\n }, \n \"inputs\": [[32, 0, 0], [33, 0, 0]]\n }, \n {\n \"op\": \"null\", \n \"name\": \"mobilenet0_batchnorm4_gamma\", \n \"attrs\": {\n \"__dtype__\": \"0\", \n \"__init__\": \"ones\", \n \"__lr_mult__\": \"1.0\", \n \"__shape__\": \"(32L,)\", \n \"__storage_type__\": \"0\", \n \"__wd_mult__\": \"1.0\"\n }, \n \"inputs\": []\n }, \n {\n \"op\": \"null\", \n \"name\": \"mobilenet0_batchnorm4_beta\", \n \"attrs\": {\n \"__dtype__\": \"0\", \n \"__init__\": \"zeros\", \n \"__lr_mult__\": \"1.0\", \n \"__shape__\": \"(32L,)\", \n \"__storage_type__\": \"0\", \n \"__wd_mult__\": \"1.0\"\n }, \n \"inputs\": []\n }, \n {\n \"op\": \"null\", \n \"name\": \"mobilenet0_batchnorm4_running_mean\", \n \"attrs\": {\n \"__dtype__\": \"0\", \n \"__init__\": \"zeros\", \n \"__lr_mult__\": \"1.0\", \n \"__shape__\": \"(32L,)\", \n \"__storage_type__\": \"0\", \n \"__wd_mult__\": \"1.0\"\n }, \n \"inputs\": []\n }, \n {\n \"op\": \"null\", \n \"name\": \"mobilenet0_batchnorm4_running_var\", \n \"attrs\": {\n \"__dtype__\": \"0\", \n \"__init__\": \"ones\", \n \"__lr_mult__\": \"1.0\", \n \"__shape__\": \"(32L,)\", \n \"__storage_type__\": \"0\", \n \"__wd_mult__\": \"1.0\"\n }, \n \"inputs\": []\n }, \n {\n \"op\": \"BatchNorm\", \n \"name\": \"mobilenet0_batchnorm4_fwd\", \n \"attrs\": {\n \"axis\": \"1\", \n \"eps\": \"1e-05\", \n \"fix_gamma\": \"False\", \n \"momentum\": \"0.9\", \n \"use_global_stats\": \"False\"\n }, \n \"inputs\": [[34, 0, 0], [35, 0, 0], [36, 0, 0], [37, 0, 1], [38, 0, 1]]\n }, \n {\n \"op\": \"Activation\", \n \"name\": \"mobilenet0_relu4_fwd\", \n \"attrs\": {\"act_type\": \"relu\"}, \n \"inputs\": [[39, 0, 0]]\n }, \n {\n \"op\": \"null\", \n \"name\": \"mobilenet0_conv5_weight\", \n \"attrs\": {\n \"__dtype__\": \"0\", \n \"__lr_mult__\": \"1.0\", \n \"__shape__\": \"(32L, 1L, 3L, 3L)\", \n \"__storage_type__\": \"0\", \n \"__wd_mult__\": \"1.0\"\n }, \n \"inputs\": []\n }, \n {\n \"op\": \"Convolution\", \n \"name\": \"mobilenet0_conv5_fwd\", \n \"attrs\": {\n \"dilate\": \"(1, 1)\", \n \"kernel\": \"(3, 3)\", \n \"layout\": \"NCHW\", \n \"no_bias\": \"True\", \n \"num_filter\": \"32\", \n \"num_group\": \"32\", \n \"pad\": \"(1, 1)\", \n \"stride\": \"(1, 1)\"\n }, \n \"inputs\": [[40, 0, 0], [41, 0, 0]]\n }, \n {\n \"op\": \"null\", \n \"name\": \"mobilenet0_batchnorm5_gamma\", \n \"attrs\": {\n \"__dtype__\": \"0\", \n \"__init__\": \"ones\", \n \"__lr_mult__\": \"1.0\", \n \"__shape__\": \"(32L,)\", \n \"__storage_type__\": \"0\", \n \"__wd_mult__\": \"1.0\"\n }, \n \"inputs\": []\n }, \n {\n \"op\": \"null\", \n \"name\": \"mobilenet0_batchnorm5_beta\", \n \"attrs\": {\n \"__dtype__\": \"0\", \n \"__init__\": \"zeros\", \n \"__lr_mult__\": \"1.0\", \n \"__shape__\": \"(32L,)\", \n \"__storage_type__\": \"0\", \n \"__wd_mult__\": \"1.0\"\n }, \n \"inputs\": []\n }, \n {\n \"op\": \"null\", \n \"name\": \"mobilenet0_batchnorm5_running_mean\", \n \"attrs\": {\n \"__dtype__\": \"0\", \n \"__init__\": \"zeros\", \n \"__lr_mult__\": \"1.0\", \n \"__shape__\": \"(32L,)\", \n \"__storage_type__\": \"0\", \n \"__wd_mult__\": \"1.0\"\n }, \n \"inputs\": []\n }, \n {\n \"op\": \"null\", \n \"name\": \"mobilenet0_batchnorm5_running_var\", \n \"attrs\": {\n \"__dtype__\": \"0\", \n \"__init__\": \"ones\", \n \"__lr_mult__\": \"1.0\", \n \"__shape__\": \"(32L,)\", \n \"__storage_type__\": \"0\", \n \"__wd_mult__\": \"1.0\"\n }, \n \"inputs\": []\n }, \n {\n \"op\": \"BatchNorm\", \n \"name\": \"mobilenet0_batchnorm5_fwd\", \n \"attrs\": {\n \"axis\": \"1\", \n \"eps\": \"1e-05\", \n \"fix_gamma\": \"False\", \n \"momentum\": \"0.9\", \n \"use_global_stats\": \"False\"\n }, \n \"inputs\": [[42, 0, 0], [43, 0, 0], [44, 0, 0], [45, 0, 1], [46, 0, 1]]\n }, \n {\n \"op\": \"Activation\", \n \"name\": \"mobilenet0_relu5_fwd\", \n \"attrs\": {\"act_type\": \"relu\"}, \n \"inputs\": [[47, 0, 0]]\n }, \n {\n \"op\": \"null\", \n \"name\": \"mobilenet0_conv6_weight\", \n \"attrs\": {\n \"__dtype__\": \"0\", \n \"__lr_mult__\": \"1.0\", \n \"__shape__\": \"(32L, 32L, 1L, 1L)\", \n \"__storage_type__\": \"0\", \n \"__wd_mult__\": \"1.0\"\n }, \n \"inputs\": []\n }, \n {\n \"op\": \"Convolution\", \n \"name\": \"mobilenet0_conv6_fwd\", \n \"attrs\": {\n \"dilate\": \"(1, 1)\", \n \"kernel\": \"(1, 1)\", \n \"layout\": \"NCHW\", \n \"no_bias\": \"True\", \n \"num_filter\": \"32\", \n \"num_group\": \"1\", \n \"pad\": \"(0, 0)\", \n \"stride\": \"(1, 1)\"\n }, \n \"inputs\": [[48, 0, 0], [49, 0, 0]]\n }, \n {\n \"op\": \"null\", \n \"name\": \"mobilenet0_batchnorm6_gamma\", \n \"attrs\": {\n \"__dtype__\": \"0\", \n \"__init__\": \"ones\", \n \"__lr_mult__\": \"1.0\", \n \"__shape__\": \"(32L,)\", \n \"__storage_type__\": \"0\", \n \"__wd_mult__\": \"1.0\"\n }, \n \"inputs\": []\n }, \n {\n \"op\": \"null\", \n \"name\": \"mobilenet0_batchnorm6_beta\", \n \"attrs\": {\n \"__dtype__\": \"0\", \n \"__init__\": \"zeros\", \n \"__lr_mult__\": \"1.0\", \n \"__shape__\": \"(32L,)\", \n \"__storage_type__\": \"0\", \n \"__wd_mult__\": \"1.0\"\n }, \n \"inputs\": []\n }, \n {\n \"op\": \"null\", \n \"name\": \"mobilenet0_batchnorm6_running_mean\", \n \"attrs\": {\n \"__dtype__\": \"0\", \n \"__init__\": \"zeros\", \n \"__lr_mult__\": \"1.0\", \n \"__shape__\": \"(32L,)\", \n \"__storage_type__\": \"0\", \n \"__wd_mult__\": \"1.0\"\n }, \n \"inputs\": []\n }, \n {\n \"op\": \"null\", \n \"name\": \"mobilenet0_batchnorm6_running_var\", \n \"attrs\": {\n \"__dtype__\": \"0\", \n \"__init__\": \"ones\", \n \"__lr_mult__\": \"1.0\", \n \"__shape__\": \"(32L,)\", \n \"__storage_type__\": \"0\", \n \"__wd_mult__\": \"1.0\"\n }, \n \"inputs\": []\n }, \n {\n \"op\": \"BatchNorm\", \n \"name\": \"mobilenet0_batchnorm6_fwd\", \n \"attrs\": {\n \"axis\": \"1\", \n \"eps\": \"1e-05\", \n \"fix_gamma\": \"False\", \n \"momentum\": \"0.9\", \n \"use_global_stats\": \"False\"\n }, \n \"inputs\": [[50, 0, 0], [51, 0, 0], [52, 0, 0], [53, 0, 1], [54, 0, 1]]\n }, \n {\n \"op\": \"Activation\", \n \"name\": \"mobilenet0_relu6_fwd\", \n \"attrs\": {\"act_type\": \"relu\"}, \n \"inputs\": [[55, 0, 0]]\n }, \n {\n \"op\": \"null\", \n \"name\": \"mobilenet0_conv7_weight\", \n \"attrs\": {\n \"__dtype__\": \"0\", \n \"__lr_mult__\": \"1.0\", \n \"__shape__\": \"(32L, 1L, 3L, 3L)\", \n \"__storage_type__\": \"0\", \n \"__wd_mult__\": \"1.0\"\n }, \n \"inputs\": []\n }, \n {\n \"op\": \"Convolution\", \n \"name\": \"mobilenet0_conv7_fwd\", \n \"attrs\": {\n \"dilate\": \"(1, 1)\", \n \"kernel\": \"(3, 3)\", \n \"layout\": \"NCHW\", \n \"no_bias\": \"True\", \n \"num_filter\": \"32\", \n \"num_group\": \"32\", \n \"pad\": \"(1, 1)\", \n \"stride\": \"(2, 2)\"\n }, \n \"inputs\": [[56, 0, 0], [57, 0, 0]]\n }, \n {\n \"op\": \"null\", \n \"name\": \"mobilenet0_batchnorm7_gamma\", \n \"attrs\": {\n \"__dtype__\": \"0\", \n \"__init__\": \"ones\", \n \"__lr_mult__\": \"1.0\", \n \"__shape__\": \"(32L,)\", \n \"__storage_type__\": \"0\", \n \"__wd_mult__\": \"1.0\"\n }, \n \"inputs\": []\n }, \n {\n \"op\": \"null\", \n \"name\": \"mobilenet0_batchnorm7_beta\", \n \"attrs\": {\n \"__dtype__\": \"0\", \n \"__init__\": \"zeros\", \n \"__lr_mult__\": \"1.0\", \n \"__shape__\": \"(32L,)\", \n \"__storage_type__\": \"0\", \n \"__wd_mult__\": \"1.0\"\n }, \n \"inputs\": []\n }, \n {\n \"op\": \"null\", \n \"name\": \"mobilenet0_batchnorm7_running_mean\", \n \"attrs\": {\n \"__dtype__\": \"0\", \n \"__init__\": \"zeros\", \n \"__lr_mult__\": \"1.0\", \n \"__shape__\": \"(32L,)\", \n \"__storage_type__\": \"0\", \n \"__wd_mult__\": \"1.0\"\n }, \n \"inputs\": []\n }, \n {\n \"op\": \"null\", \n \"name\": \"mobilenet0_batchnorm7_running_var\", \n \"attrs\": {\n \"__dtype__\": \"0\", \n \"__init__\": \"ones\", \n \"__lr_mult__\": \"1.0\", \n \"__shape__\": \"(32L,)\", \n \"__storage_type__\": \"0\", \n \"__wd_mult__\": \"1.0\"\n }, \n \"inputs\": []\n }, \n {\n \"op\": \"BatchNorm\", \n \"name\": \"mobilenet0_batchnorm7_fwd\", \n \"attrs\": {\n \"axis\": \"1\", \n \"eps\": \"1e-05\", \n \"fix_gamma\": \"False\", \n \"momentum\": \"0.9\", \n \"use_global_stats\": \"False\"\n }, \n \"inputs\": [[58, 0, 0], [59, 0, 0], [60, 0, 0], [61, 0, 1], [62, 0, 1]]\n }, \n {\n \"op\": \"Activation\", \n \"name\": \"mobilenet0_relu7_fwd\", \n \"attrs\": {\"act_type\": \"relu\"}, \n \"inputs\": [[63, 0, 0]]\n }, \n {\n \"op\": \"null\", \n \"name\": \"mobilenet0_conv8_weight\", \n \"attrs\": {\n \"__dtype__\": \"0\", \n \"__lr_mult__\": \"1.0\", \n \"__shape__\": \"(64L, 32L, 1L, 1L)\", \n \"__storage_type__\": \"0\", \n \"__wd_mult__\": \"1.0\"\n }, \n \"inputs\": []\n }, \n {\n \"op\": \"Convolution\", \n \"name\": \"mobilenet0_conv8_fwd\", \n \"attrs\": {\n \"dilate\": \"(1, 1)\", \n \"kernel\": \"(1, 1)\", \n \"layout\": \"NCHW\", \n \"no_bias\": \"True\", \n \"num_filter\": \"64\", \n \"num_group\": \"1\", \n \"pad\": \"(0, 0)\", \n \"stride\": \"(1, 1)\"\n }, \n \"inputs\": [[64, 0, 0], [65, 0, 0]]\n }, \n {\n \"op\": \"null\", \n \"name\": \"mobilenet0_batchnorm8_gamma\", \n \"attrs\": {\n \"__dtype__\": \"0\", \n \"__init__\": \"ones\", \n \"__lr_mult__\": \"1.0\", \n \"__shape__\": \"(64L,)\", \n \"__storage_type__\": \"0\", \n \"__wd_mult__\": \"1.0\"\n }, \n \"inputs\": []\n }, \n {\n \"op\": \"null\", \n \"name\": \"mobilenet0_batchnorm8_beta\", \n \"attrs\": {\n \"__dtype__\": \"0\", \n \"__init__\": \"zeros\", \n \"__lr_mult__\": \"1.0\", \n \"__shape__\": \"(64L,)\", \n \"__storage_type__\": \"0\", \n \"__wd_mult__\": \"1.0\"\n }, \n \"inputs\": []\n }, \n {\n \"op\": \"null\", \n \"name\": \"mobilenet0_batchnorm8_running_mean\", \n \"attrs\": {\n \"__dtype__\": \"0\", \n \"__init__\": \"zeros\", \n \"__lr_mult__\": \"1.0\", \n \"__shape__\": \"(64L,)\", \n \"__storage_type__\": \"0\", \n \"__wd_mult__\": \"1.0\"\n }, \n \"inputs\": []\n }, \n {\n \"op\": \"null\", \n \"name\": \"mobilenet0_batchnorm8_running_var\", \n \"attrs\": {\n \"__dtype__\": \"0\", \n \"__init__\": \"ones\", \n \"__lr_mult__\": \"1.0\", \n \"__shape__\": \"(64L,)\", \n \"__storage_type__\": \"0\", \n \"__wd_mult__\": \"1.0\"\n }, \n \"inputs\": []\n }, \n {\n \"op\": \"BatchNorm\", \n \"name\": \"mobilenet0_batchnorm8_fwd\", \n \"attrs\": {\n \"axis\": \"1\", \n \"eps\": \"1e-05\", \n \"fix_gamma\": \"False\", \n \"momentum\": \"0.9\", \n \"use_global_stats\": \"False\"\n }, \n \"inputs\": [[66, 0, 0], [67, 0, 0], [68, 0, 0], [69, 0, 1], [70, 0, 1]]\n }, \n {\n \"op\": \"Activation\", \n \"name\": \"mobilenet0_relu8_fwd\", \n \"attrs\": {\"act_type\": \"relu\"}, \n \"inputs\": [[71, 0, 0]]\n }, \n {\n \"op\": \"null\", \n \"name\": \"mobilenet0_conv9_weight\", \n \"attrs\": {\n \"__dtype__\": \"0\", \n \"__lr_mult__\": \"1.0\", \n \"__shape__\": \"(64L, 1L, 3L, 3L)\", \n \"__storage_type__\": \"0\", \n \"__wd_mult__\": \"1.0\"\n }, \n \"inputs\": []\n }, \n {\n \"op\": \"Convolution\", \n \"name\": \"mobilenet0_conv9_fwd\", \n \"attrs\": {\n \"dilate\": \"(1, 1)\", \n \"kernel\": \"(3, 3)\", \n \"layout\": \"NCHW\", \n \"no_bias\": \"True\", \n \"num_filter\": \"64\", \n \"num_group\": \"64\", \n \"pad\": \"(1, 1)\", \n \"stride\": \"(1, 1)\"\n }, \n \"inputs\": [[72, 0, 0], [73, 0, 0]]\n }, \n {\n \"op\": \"null\", \n \"name\": \"mobilenet0_batchnorm9_gamma\", \n \"attrs\": {\n \"__dtype__\": \"0\", \n \"__init__\": \"ones\", \n \"__lr_mult__\": \"1.0\", \n \"__shape__\": \"(64L,)\", \n \"__storage_type__\": \"0\", \n \"__wd_mult__\": \"1.0\"\n }, \n \"inputs\": []\n }, \n {\n \"op\": \"null\", \n \"name\": \"mobilenet0_batchnorm9_beta\", \n \"attrs\": {\n \"__dtype__\": \"0\", \n \"__init__\": \"zeros\", \n \"__lr_mult__\": \"1.0\", \n \"__shape__\": \"(64L,)\", \n \"__storage_type__\": \"0\", \n \"__wd_mult__\": \"1.0\"\n }, \n \"inputs\": []\n }, \n {\n \"op\": \"null\", \n \"name\": \"mobilenet0_batchnorm9_running_mean\", \n \"attrs\": {\n \"__dtype__\": \"0\", \n \"__init__\": \"zeros\", \n \"__lr_mult__\": \"1.0\", \n \"__shape__\": \"(64L,)\", \n \"__storage_type__\": \"0\", \n \"__wd_mult__\": \"1.0\"\n }, \n \"inputs\": []\n }, \n {\n \"op\": \"null\", \n \"name\": \"mobilenet0_batchnorm9_running_var\", \n \"attrs\": {\n \"__dtype__\": \"0\", \n \"__init__\": \"ones\", \n \"__lr_mult__\": \"1.0\", \n \"__shape__\": \"(64L,)\", \n \"__storage_type__\": \"0\", \n \"__wd_mult__\": \"1.0\"\n }, \n \"inputs\": []\n }, \n {\n \"op\": \"BatchNorm\", \n \"name\": \"mobilenet0_batchnorm9_fwd\", \n \"attrs\": {\n \"axis\": \"1\", \n \"eps\": \"1e-05\", \n \"fix_gamma\": \"False\", \n \"momentum\": \"0.9\", \n \"use_global_stats\": \"False\"\n }, \n \"inputs\": [[74, 0, 0], [75, 0, 0], [76, 0, 0], [77, 0, 1], [78, 0, 1]]\n }, \n {\n \"op\": \"Activation\", \n \"name\": \"mobilenet0_relu9_fwd\", \n \"attrs\": {\"act_type\": \"relu\"}, \n \"inputs\": [[79, 0, 0]]\n }, \n {\n \"op\": \"null\", \n \"name\": \"mobilenet0_conv10_weight\", \n \"attrs\": {\n \"__dtype__\": \"0\", \n \"__lr_mult__\": \"1.0\", \n \"__shape__\": \"(64L, 64L, 1L, 1L)\", \n \"__storage_type__\": \"0\", \n \"__wd_mult__\": \"1.0\"\n }, \n \"inputs\": []\n }, \n {\n \"op\": \"Convolution\", \n \"name\": \"mobilenet0_conv10_fwd\", \n \"attrs\": {\n \"dilate\": \"(1, 1)\", \n \"kernel\": \"(1, 1)\", \n \"layout\": \"NCHW\", \n \"no_bias\": \"True\", \n \"num_filter\": \"64\", \n \"num_group\": \"1\", \n \"pad\": \"(0, 0)\", \n \"stride\": \"(1, 1)\"\n }, \n \"inputs\": [[80, 0, 0], [81, 0, 0]]\n }, \n {\n \"op\": \"null\", \n \"name\": \"mobilenet0_batchnorm10_gamma\", \n \"attrs\": {\n \"__dtype__\": \"0\", \n \"__init__\": \"ones\", \n \"__lr_mult__\": \"1.0\", \n \"__shape__\": \"(64L,)\", \n \"__storage_type__\": \"0\", \n \"__wd_mult__\": \"1.0\"\n }, \n \"inputs\": []\n }, \n {\n \"op\": \"null\", \n \"name\": \"mobilenet0_batchnorm10_beta\", \n \"attrs\": {\n \"__dtype__\": \"0\", \n \"__init__\": \"zeros\", \n \"__lr_mult__\": \"1.0\", \n \"__shape__\": \"(64L,)\", \n \"__storage_type__\": \"0\", \n \"__wd_mult__\": \"1.0\"\n }, \n \"inputs\": []\n }, \n {\n \"op\": \"null\", \n \"name\": \"mobilenet0_batchnorm10_running_mean\", \n \"attrs\": {\n \"__dtype__\": \"0\", \n \"__init__\": \"zeros\", \n \"__lr_mult__\": \"1.0\", \n \"__shape__\": \"(64L,)\", \n \"__storage_type__\": \"0\", \n \"__wd_mult__\": \"1.0\"\n }, \n \"inputs\": []\n }, \n {\n \"op\": \"null\", \n \"name\": \"mobilenet0_batchnorm10_running_var\", \n \"attrs\": {\n \"__dtype__\": \"0\", \n \"__init__\": \"ones\", \n \"__lr_mult__\": \"1.0\", \n \"__shape__\": \"(64L,)\", \n \"__storage_type__\": \"0\", \n \"__wd_mult__\": \"1.0\"\n }, \n \"inputs\": []\n }, \n {\n \"op\": \"BatchNorm\", \n \"name\": \"mobilenet0_batchnorm10_fwd\", \n \"attrs\": {\n \"axis\": \"1\", \n \"eps\": \"1e-05\", \n \"fix_gamma\": \"False\", \n \"momentum\": \"0.9\", \n \"use_global_stats\": \"False\"\n }, \n \"inputs\": [[82, 0, 0], [83, 0, 0], [84, 0, 0], [85, 0, 1], [86, 0, 1]]\n }, \n {\n \"op\": \"Activation\", \n \"name\": \"mobilenet0_relu10_fwd\", \n \"attrs\": {\"act_type\": \"relu\"}, \n \"inputs\": [[87, 0, 0]]\n }, \n {\n \"op\": \"null\", \n \"name\": \"mobilenet0_conv11_weight\", \n \"attrs\": {\n \"__dtype__\": \"0\", \n \"__lr_mult__\": \"1.0\", \n \"__shape__\": \"(64L, 1L, 3L, 3L)\", \n \"__storage_type__\": \"0\", \n \"__wd_mult__\": \"1.0\"\n }, \n \"inputs\": []\n }, \n {\n \"op\": \"Convolution\", \n \"name\": \"mobilenet0_conv11_fwd\", \n \"attrs\": {\n \"dilate\": \"(1, 1)\", \n \"kernel\": \"(3, 3)\", \n \"layout\": \"NCHW\", \n \"no_bias\": \"True\", \n \"num_filter\": \"64\", \n \"num_group\": \"64\", \n \"pad\": \"(1, 1)\", \n \"stride\": \"(2, 2)\"\n }, \n \"inputs\": [[88, 0, 0], [89, 0, 0]]\n }, \n {\n \"op\": \"null\", \n \"name\": \"mobilenet0_batchnorm11_gamma\", \n \"attrs\": {\n \"__dtype__\": \"0\", \n \"__init__\": \"ones\", \n \"__lr_mult__\": \"1.0\", \n \"__shape__\": \"(64L,)\", \n \"__storage_type__\": \"0\", \n \"__wd_mult__\": \"1.0\"\n }, \n \"inputs\": []\n }, \n {\n \"op\": \"null\", \n \"name\": \"mobilenet0_batchnorm11_beta\", \n \"attrs\": {\n \"__dtype__\": \"0\", \n \"__init__\": \"zeros\", \n \"__lr_mult__\": \"1.0\", \n \"__shape__\": \"(64L,)\", \n \"__storage_type__\": \"0\", \n \"__wd_mult__\": \"1.0\"\n }, \n \"inputs\": []\n }, \n {\n \"op\": \"null\", \n \"name\": \"mobilenet0_batchnorm11_running_mean\", \n \"attrs\": {\n \"__dtype__\": \"0\", \n \"__init__\": \"zeros\", \n \"__lr_mult__\": \"1.0\", \n \"__shape__\": \"(64L,)\", \n \"__storage_type__\": \"0\", \n \"__wd_mult__\": \"1.0\"\n }, \n \"inputs\": []\n }, \n {\n \"op\": \"null\", \n \"name\": \"mobilenet0_batchnorm11_running_var\", \n \"attrs\": {\n \"__dtype__\": \"0\", \n \"__init__\": \"ones\", \n \"__lr_mult__\": \"1.0\", \n \"__shape__\": \"(64L,)\", \n \"__storage_type__\": \"0\", \n \"__wd_mult__\": \"1.0\"\n }, \n \"inputs\": []\n }, \n {\n \"op\": \"BatchNorm\", \n \"name\": \"mobilenet0_batchnorm11_fwd\", \n \"attrs\": {\n \"axis\": \"1\", \n \"eps\": \"1e-05\", \n \"fix_gamma\": \"False\", \n \"momentum\": \"0.9\", \n \"use_global_stats\": \"False\"\n }, \n \"inputs\": [[90, 0, 0], [91, 0, 0], [92, 0, 0], [93, 0, 1], [94, 0, 1]]\n }, \n {\n \"op\": \"Activation\", \n \"name\": \"mobilenet0_relu11_fwd\", \n \"attrs\": {\"act_type\": \"relu\"}, \n \"inputs\": [[95, 0, 0]]\n }, \n {\n \"op\": \"null\", \n \"name\": \"mobilenet0_conv12_weight\", \n \"attrs\": {\n \"__dtype__\": \"0\", \n \"__lr_mult__\": \"1.0\", \n \"__shape__\": \"(128L, 64L, 1L, 1L)\", \n \"__storage_type__\": \"0\", \n \"__wd_mult__\": \"1.0\"\n }, \n \"inputs\": []\n }, \n {\n \"op\": \"Convolution\", \n \"name\": \"mobilenet0_conv12_fwd\", \n \"attrs\": {\n \"dilate\": \"(1, 1)\", \n \"kernel\": \"(1, 1)\", \n \"layout\": \"NCHW\", \n \"no_bias\": \"True\", \n \"num_filter\": \"128\", \n \"num_group\": \"1\", \n \"pad\": \"(0, 0)\", \n \"stride\": \"(1, 1)\"\n }, \n \"inputs\": [[96, 0, 0], [97, 0, 0]]\n }, \n {\n \"op\": \"null\", \n \"name\": \"mobilenet0_batchnorm12_gamma\", \n \"attrs\": {\n \"__dtype__\": \"0\", \n \"__init__\": \"ones\", \n \"__lr_mult__\": \"1.0\", \n \"__shape__\": \"(128L,)\", \n \"__storage_type__\": \"0\", \n \"__wd_mult__\": \"1.0\"\n }, \n \"inputs\": []\n }, \n {\n \"op\": \"null\", \n \"name\": \"mobilenet0_batchnorm12_beta\", \n \"attrs\": {\n \"__dtype__\": \"0\", \n \"__init__\": \"zeros\", \n \"__lr_mult__\": \"1.0\", \n \"__shape__\": \"(128L,)\", \n \"__storage_type__\": \"0\", \n \"__wd_mult__\": \"1.0\"\n }, \n \"inputs\": []\n }, \n {\n \"op\": \"null\", \n \"name\": \"mobilenet0_batchnorm12_running_mean\", \n \"attrs\": {\n \"__dtype__\": \"0\", \n \"__init__\": \"zeros\", \n \"__lr_mult__\": \"1.0\", \n \"__shape__\": \"(128L,)\", \n \"__storage_type__\": \"0\", \n \"__wd_mult__\": \"1.0\"\n }, \n \"inputs\": []\n }, \n {\n \"op\": \"null\", \n \"name\": \"mobilenet0_batchnorm12_running_var\", \n \"attrs\": {\n \"__dtype__\": \"0\", \n \"__init__\": \"ones\", \n \"__lr_mult__\": \"1.0\", \n \"__shape__\": \"(128L,)\", \n \"__storage_type__\": \"0\", \n \"__wd_mult__\": \"1.0\"\n }, \n \"inputs\": []\n }, \n {\n \"op\": \"BatchNorm\", \n \"name\": \"mobilenet0_batchnorm12_fwd\", \n \"attrs\": {\n \"axis\": \"1\", \n \"eps\": \"1e-05\", \n \"fix_gamma\": \"False\", \n \"momentum\": \"0.9\", \n \"use_global_stats\": \"False\"\n }, \n \"inputs\": [[98, 0, 0], [99, 0, 0], [100, 0, 0], [101, 0, 1], [102, 0, 1]]\n }, \n {\n \"op\": \"Activation\", \n \"name\": \"mobilenet0_relu12_fwd\", \n \"attrs\": {\"act_type\": \"relu\"}, \n \"inputs\": [[103, 0, 0]]\n }, \n {\n \"op\": \"null\", \n \"name\": \"mobilenet0_conv13_weight\", \n \"attrs\": {\n \"__dtype__\": \"0\", \n \"__lr_mult__\": \"1.0\", \n \"__shape__\": \"(128L, 1L, 3L, 3L)\", \n \"__storage_type__\": \"0\", \n \"__wd_mult__\": \"1.0\"\n }, \n \"inputs\": []\n }, \n {\n \"op\": \"Convolution\", \n \"name\": \"mobilenet0_conv13_fwd\", \n \"attrs\": {\n \"dilate\": \"(1, 1)\", \n \"kernel\": \"(3, 3)\", \n \"layout\": \"NCHW\", \n \"no_bias\": \"True\", \n \"num_filter\": \"128\", \n \"num_group\": \"128\", \n \"pad\": \"(1, 1)\", \n \"stride\": \"(1, 1)\"\n }, \n \"inputs\": [[104, 0, 0], [105, 0, 0]]\n }, \n {\n \"op\": \"null\", \n \"name\": \"mobilenet0_batchnorm13_gamma\", \n \"attrs\": {\n \"__dtype__\": \"0\", \n \"__init__\": \"ones\", \n \"__lr_mult__\": \"1.0\", \n \"__shape__\": \"(128L,)\", \n \"__storage_type__\": \"0\", \n \"__wd_mult__\": \"1.0\"\n }, \n \"inputs\": []\n }, \n {\n \"op\": \"null\", \n \"name\": \"mobilenet0_batchnorm13_beta\", \n \"attrs\": {\n \"__dtype__\": \"0\", \n \"__init__\": \"zeros\", \n \"__lr_mult__\": \"1.0\", \n \"__shape__\": \"(128L,)\", \n \"__storage_type__\": \"0\", \n \"__wd_mult__\": \"1.0\"\n }, \n \"inputs\": []\n }, \n {\n \"op\": \"null\", \n \"name\": \"mobilenet0_batchnorm13_running_mean\", \n \"attrs\": {\n \"__dtype__\": \"0\", \n \"__init__\": \"zeros\", \n \"__lr_mult__\": \"1.0\", \n \"__shape__\": \"(128L,)\", \n \"__storage_type__\": \"0\", \n \"__wd_mult__\": \"1.0\"\n }, \n \"inputs\": []\n }, \n {\n \"op\": \"null\", \n \"name\": \"mobilenet0_batchnorm13_running_var\", \n \"attrs\": {\n \"__dtype__\": \"0\", \n \"__init__\": \"ones\", \n \"__lr_mult__\": \"1.0\", \n \"__shape__\": \"(128L,)\", \n \"__storage_type__\": \"0\", \n \"__wd_mult__\": \"1.0\"\n }, \n \"inputs\": []\n }, \n {\n \"op\": \"BatchNorm\", \n \"name\": \"mobilenet0_batchnorm13_fwd\", \n \"attrs\": {\n \"axis\": \"1\", \n \"eps\": \"1e-05\", \n \"fix_gamma\": \"False\", \n \"momentum\": \"0.9\", \n \"use_global_stats\": \"False\"\n }, \n \"inputs\": [[106, 0, 0], [107, 0, 0], [108, 0, 0], [109, 0, 1], [110, 0, 1]]\n }, \n {\n \"op\": \"Activation\", \n \"name\": \"mobilenet0_relu13_fwd\", \n \"attrs\": {\"act_type\": \"relu\"}, \n \"inputs\": [[111, 0, 0]]\n }, \n {\n \"op\": \"null\", \n \"name\": \"mobilenet0_conv14_weight\", \n \"attrs\": {\n \"__dtype__\": \"0\", \n \"__lr_mult__\": \"1.0\", \n \"__shape__\": \"(128L, 128L, 1L, 1L)\", \n \"__storage_type__\": \"0\", \n \"__wd_mult__\": \"1.0\"\n }, \n \"inputs\": []\n }, \n {\n \"op\": \"Convolution\", \n \"name\": \"mobilenet0_conv14_fwd\", \n \"attrs\": {\n \"dilate\": \"(1, 1)\", \n \"kernel\": \"(1, 1)\", \n \"layout\": \"NCHW\", \n \"no_bias\": \"True\", \n \"num_filter\": \"128\", \n \"num_group\": \"1\", \n \"pad\": \"(0, 0)\", \n \"stride\": \"(1, 1)\"\n }, \n \"inputs\": [[112, 0, 0], [113, 0, 0]]\n }, \n {\n \"op\": \"null\", \n \"name\": \"mobilenet0_batchnorm14_gamma\", \n \"attrs\": {\n \"__dtype__\": \"0\", \n \"__init__\": \"ones\", \n \"__lr_mult__\": \"1.0\", \n \"__shape__\": \"(128L,)\", \n \"__storage_type__\": \"0\", \n \"__wd_mult__\": \"1.0\"\n }, \n \"inputs\": []\n }, \n {\n \"op\": \"null\", \n \"name\": \"mobilenet0_batchnorm14_beta\", \n \"attrs\": {\n \"__dtype__\": \"0\", \n \"__init__\": \"zeros\", \n \"__lr_mult__\": \"1.0\", \n \"__shape__\": \"(128L,)\", \n \"__storage_type__\": \"0\", \n \"__wd_mult__\": \"1.0\"\n }, \n \"inputs\": []\n }, \n {\n \"op\": \"null\", \n \"name\": \"mobilenet0_batchnorm14_running_mean\", \n \"attrs\": {\n \"__dtype__\": \"0\", \n \"__init__\": \"zeros\", \n \"__lr_mult__\": \"1.0\", \n \"__shape__\": \"(128L,)\", \n \"__storage_type__\": \"0\", \n \"__wd_mult__\": \"1.0\"\n }, \n \"inputs\": []\n }, \n {\n \"op\": \"null\", \n \"name\": \"mobilenet0_batchnorm14_running_var\", \n \"attrs\": {\n \"__dtype__\": \"0\", \n \"__init__\": \"ones\", \n \"__lr_mult__\": \"1.0\", \n \"__shape__\": \"(128L,)\", \n \"__storage_type__\": \"0\", \n \"__wd_mult__\": \"1.0\"\n }, \n \"inputs\": []\n }, \n {\n \"op\": \"BatchNorm\", \n \"name\": \"mobilenet0_batchnorm14_fwd\", \n \"attrs\": {\n \"axis\": \"1\", \n \"eps\": \"1e-05\", \n \"fix_gamma\": \"False\", \n \"momentum\": \"0.9\", \n \"use_global_stats\": \"False\"\n }, \n \"inputs\": [[114, 0, 0], [115, 0, 0], [116, 0, 0], [117, 0, 1], [118, 0, 1]]\n }, \n {\n \"op\": \"Activation\", \n \"name\": \"mobilenet0_relu14_fwd\", \n \"attrs\": {\"act_type\": \"relu\"}, \n \"inputs\": [[119, 0, 0]]\n }, \n {\n \"op\": \"null\", \n \"name\": \"mobilenet0_conv15_weight\", \n \"attrs\": {\n \"__dtype__\": \"0\", \n \"__lr_mult__\": \"1.0\", \n \"__shape__\": \"(128L, 1L, 3L, 3L)\", \n \"__storage_type__\": \"0\", \n \"__wd_mult__\": \"1.0\"\n }, \n \"inputs\": []\n }, \n {\n \"op\": \"Convolution\", \n \"name\": \"mobilenet0_conv15_fwd\", \n \"attrs\": {\n \"dilate\": \"(1, 1)\", \n \"kernel\": \"(3, 3)\", \n \"layout\": \"NCHW\", \n \"no_bias\": \"True\", \n \"num_filter\": \"128\", \n \"num_group\": \"128\", \n \"pad\": \"(1, 1)\", \n \"stride\": \"(1, 1)\"\n }, \n \"inputs\": [[120, 0, 0], [121, 0, 0]]\n }, \n {\n \"op\": \"null\", \n \"name\": \"mobilenet0_batchnorm15_gamma\", \n \"attrs\": {\n \"__dtype__\": \"0\", \n \"__init__\": \"ones\", \n \"__lr_mult__\": \"1.0\", \n \"__shape__\": \"(128L,)\", \n \"__storage_type__\": \"0\", \n \"__wd_mult__\": \"1.0\"\n }, \n \"inputs\": []\n }, \n {\n \"op\": \"null\", \n \"name\": \"mobilenet0_batchnorm15_beta\", \n \"attrs\": {\n \"__dtype__\": \"0\", \n \"__init__\": \"zeros\", \n \"__lr_mult__\": \"1.0\", \n \"__shape__\": \"(128L,)\", \n \"__storage_type__\": \"0\", \n \"__wd_mult__\": \"1.0\"\n }, \n \"inputs\": []\n }, \n {\n \"op\": \"null\", \n \"name\": \"mobilenet0_batchnorm15_running_mean\", \n \"attrs\": {\n \"__dtype__\": \"0\", \n \"__init__\": \"zeros\", \n \"__lr_mult__\": \"1.0\", \n \"__shape__\": \"(128L,)\", \n \"__storage_type__\": \"0\", \n \"__wd_mult__\": \"1.0\"\n }, \n \"inputs\": []\n }, \n {\n \"op\": \"null\", \n \"name\": \"mobilenet0_batchnorm15_running_var\", \n \"attrs\": {\n \"__dtype__\": \"0\", \n \"__init__\": \"ones\", \n \"__lr_mult__\": \"1.0\", \n \"__shape__\": \"(128L,)\", \n \"__storage_type__\": \"0\", \n \"__wd_mult__\": \"1.0\"\n }, \n \"inputs\": []\n }, \n {\n \"op\": \"BatchNorm\", \n \"name\": \"mobilenet0_batchnorm15_fwd\", \n \"attrs\": {\n \"axis\": \"1\", \n \"eps\": \"1e-05\", \n \"fix_gamma\": \"False\", \n \"momentum\": \"0.9\", \n \"use_global_stats\": \"False\"\n }, \n \"inputs\": [[122, 0, 0], [123, 0, 0], [124, 0, 0], [125, 0, 1], [126, 0, 1]]\n }, \n {\n \"op\": \"Activation\", \n \"name\": \"mobilenet0_relu15_fwd\", \n \"attrs\": {\"act_type\": \"relu\"}, \n \"inputs\": [[127, 0, 0]]\n }, \n {\n \"op\": \"null\", \n \"name\": \"mobilenet0_conv16_weight\", \n \"attrs\": {\n \"__dtype__\": \"0\", \n \"__lr_mult__\": \"1.0\", \n \"__shape__\": \"(128L, 128L, 1L, 1L)\", \n \"__storage_type__\": \"0\", \n \"__wd_mult__\": \"1.0\"\n }, \n \"inputs\": []\n }, \n {\n \"op\": \"Convolution\", \n \"name\": \"mobilenet0_conv16_fwd\", \n \"attrs\": {\n \"dilate\": \"(1, 1)\", \n \"kernel\": \"(1, 1)\", \n \"layout\": \"NCHW\", \n \"no_bias\": \"True\", \n \"num_filter\": \"128\", \n \"num_group\": \"1\", \n \"pad\": \"(0, 0)\", \n \"stride\": \"(1, 1)\"\n }, \n \"inputs\": [[128, 0, 0], [129, 0, 0]]\n }, \n {\n \"op\": \"null\", \n \"name\": \"mobilenet0_batchnorm16_gamma\", \n \"attrs\": {\n \"__dtype__\": \"0\", \n \"__init__\": \"ones\", \n \"__lr_mult__\": \"1.0\", \n \"__shape__\": \"(128L,)\", \n \"__storage_type__\": \"0\", \n \"__wd_mult__\": \"1.0\"\n }, \n \"inputs\": []\n }, \n {\n \"op\": \"null\", \n \"name\": \"mobilenet0_batchnorm16_beta\", \n \"attrs\": {\n \"__dtype__\": \"0\", \n \"__init__\": \"zeros\", \n \"__lr_mult__\": \"1.0\", \n \"__shape__\": \"(128L,)\", \n \"__storage_type__\": \"0\", \n \"__wd_mult__\": \"1.0\"\n }, \n \"inputs\": []\n }, \n {\n \"op\": \"null\", \n \"name\": \"mobilenet0_batchnorm16_running_mean\", \n \"attrs\": {\n \"__dtype__\": \"0\", \n \"__init__\": \"zeros\", \n \"__lr_mult__\": \"1.0\", \n \"__shape__\": \"(128L,)\", \n \"__storage_type__\": \"0\", \n \"__wd_mult__\": \"1.0\"\n }, \n \"inputs\": []\n }, \n {\n \"op\": \"null\", \n \"name\": \"mobilenet0_batchnorm16_running_var\", \n \"attrs\": {\n \"__dtype__\": \"0\", \n \"__init__\": \"ones\", \n \"__lr_mult__\": \"1.0\", \n \"__shape__\": \"(128L,)\", \n \"__storage_type__\": \"0\", \n \"__wd_mult__\": \"1.0\"\n }, \n \"inputs\": []\n }, \n {\n \"op\": \"BatchNorm\", \n \"name\": \"mobilenet0_batchnorm16_fwd\", \n \"attrs\": {\n \"axis\": \"1\", \n \"eps\": \"1e-05\", \n \"fix_gamma\": \"False\", \n \"momentum\": \"0.9\", \n \"use_global_stats\": \"False\"\n }, \n \"inputs\": [[130, 0, 0], [131, 0, 0], [132, 0, 0], [133, 0, 1], [134, 0, 1]]\n }, \n {\n \"op\": \"Activation\", \n \"name\": \"mobilenet0_relu16_fwd\", \n \"attrs\": {\"act_type\": \"relu\"}, \n \"inputs\": [[135, 0, 0]]\n }, \n {\n \"op\": \"null\", \n \"name\": \"mobilenet0_conv17_weight\", \n \"attrs\": {\n \"__dtype__\": \"0\", \n \"__lr_mult__\": \"1.0\", \n \"__shape__\": \"(128L, 1L, 3L, 3L)\", \n \"__storage_type__\": \"0\", \n \"__wd_mult__\": \"1.0\"\n }, \n \"inputs\": []\n }, \n {\n \"op\": \"Convolution\", \n \"name\": \"mobilenet0_conv17_fwd\", \n \"attrs\": {\n \"dilate\": \"(1, 1)\", \n \"kernel\": \"(3, 3)\", \n \"layout\": \"NCHW\", \n \"no_bias\": \"True\", \n \"num_filter\": \"128\", \n \"num_group\": \"128\", \n \"pad\": \"(1, 1)\", \n \"stride\": \"(1, 1)\"\n }, \n \"inputs\": [[136, 0, 0], [137, 0, 0]]\n }, \n {\n \"op\": \"null\", \n \"name\": \"mobilenet0_batchnorm17_gamma\", \n \"attrs\": {\n \"__dtype__\": \"0\", \n \"__init__\": \"ones\", \n \"__lr_mult__\": \"1.0\", \n \"__shape__\": \"(128L,)\", \n \"__storage_type__\": \"0\", \n \"__wd_mult__\": \"1.0\"\n }, \n \"inputs\": []\n }, \n {\n \"op\": \"null\", \n \"name\": \"mobilenet0_batchnorm17_beta\", \n \"attrs\": {\n \"__dtype__\": \"0\", \n \"__init__\": \"zeros\", \n \"__lr_mult__\": \"1.0\", \n \"__shape__\": \"(128L,)\", \n \"__storage_type__\": \"0\", \n \"__wd_mult__\": \"1.0\"\n }, \n \"inputs\": []\n }, \n {\n \"op\": \"null\", \n \"name\": \"mobilenet0_batchnorm17_running_mean\", \n \"attrs\": {\n \"__dtype__\": \"0\", \n \"__init__\": \"zeros\", \n \"__lr_mult__\": \"1.0\", \n \"__shape__\": \"(128L,)\", \n \"__storage_type__\": \"0\", \n \"__wd_mult__\": \"1.0\"\n }, \n \"inputs\": []\n }, \n {\n \"op\": \"null\", \n \"name\": \"mobilenet0_batchnorm17_running_var\", \n \"attrs\": {\n \"__dtype__\": \"0\", \n \"__init__\": \"ones\", \n \"__lr_mult__\": \"1.0\", \n \"__shape__\": \"(128L,)\", \n \"__storage_type__\": \"0\", \n \"__wd_mult__\": \"1.0\"\n }, \n \"inputs\": []\n }, \n {\n \"op\": \"BatchNorm\", \n \"name\": \"mobilenet0_batchnorm17_fwd\", \n \"attrs\": {\n \"axis\": \"1\", \n \"eps\": \"1e-05\", \n \"fix_gamma\": \"False\", \n \"momentum\": \"0.9\", \n \"use_global_stats\": \"False\"\n }, \n \"inputs\": [[138, 0, 0], [139, 0, 0], [140, 0, 0], [141, 0, 1], [142, 0, 1]]\n }, \n {\n \"op\": \"Activation\", \n \"name\": \"mobilenet0_relu17_fwd\", \n \"attrs\": {\"act_type\": \"relu\"}, \n \"inputs\": [[143, 0, 0]]\n }, \n {\n \"op\": \"null\", \n \"name\": \"mobilenet0_conv18_weight\", \n \"attrs\": {\n \"__dtype__\": \"0\", \n \"__lr_mult__\": \"1.0\", \n \"__shape__\": \"(128L, 128L, 1L, 1L)\", \n \"__storage_type__\": \"0\", \n \"__wd_mult__\": \"1.0\"\n }, \n \"inputs\": []\n }, \n {\n \"op\": \"Convolution\", \n \"name\": \"mobilenet0_conv18_fwd\", \n \"attrs\": {\n \"dilate\": \"(1, 1)\", \n \"kernel\": \"(1, 1)\", \n \"layout\": \"NCHW\", \n \"no_bias\": \"True\", \n \"num_filter\": \"128\", \n \"num_group\": \"1\", \n \"pad\": \"(0, 0)\", \n \"stride\": \"(1, 1)\"\n }, \n \"inputs\": [[144, 0, 0], [145, 0, 0]]\n }, \n {\n \"op\": \"null\", \n \"name\": \"mobilenet0_batchnorm18_gamma\", \n \"attrs\": {\n \"__dtype__\": \"0\", \n \"__init__\": \"ones\", \n \"__lr_mult__\": \"1.0\", \n \"__shape__\": \"(128L,)\", \n \"__storage_type__\": \"0\", \n \"__wd_mult__\": \"1.0\"\n }, \n \"inputs\": []\n }, \n {\n \"op\": \"null\", \n \"name\": \"mobilenet0_batchnorm18_beta\", \n \"attrs\": {\n \"__dtype__\": \"0\", \n \"__init__\": \"zeros\", \n \"__lr_mult__\": \"1.0\", \n \"__shape__\": \"(128L,)\", \n \"__storage_type__\": \"0\", \n \"__wd_mult__\": \"1.0\"\n }, \n \"inputs\": []\n }, \n {\n \"op\": \"null\", \n \"name\": \"mobilenet0_batchnorm18_running_mean\", \n \"attrs\": {\n \"__dtype__\": \"0\", \n \"__init__\": \"zeros\", \n \"__lr_mult__\": \"1.0\", \n \"__shape__\": \"(128L,)\", \n \"__storage_type__\": \"0\", \n \"__wd_mult__\": \"1.0\"\n }, \n \"inputs\": []\n }, \n {\n \"op\": \"null\", \n \"name\": \"mobilenet0_batchnorm18_running_var\", \n \"attrs\": {\n \"__dtype__\": \"0\", \n \"__init__\": \"ones\", \n \"__lr_mult__\": \"1.0\", \n \"__shape__\": \"(128L,)\", \n \"__storage_type__\": \"0\", \n \"__wd_mult__\": \"1.0\"\n }, \n \"inputs\": []\n }, \n {\n \"op\": \"BatchNorm\", \n \"name\": \"mobilenet0_batchnorm18_fwd\", \n \"attrs\": {\n \"axis\": \"1\", \n \"eps\": \"1e-05\", \n \"fix_gamma\": \"False\", \n \"momentum\": \"0.9\", \n \"use_global_stats\": \"False\"\n }, \n \"inputs\": [[146, 0, 0], [147, 0, 0], [148, 0, 0], [149, 0, 1], [150, 0, 1]]\n }, \n {\n \"op\": \"Activation\", \n \"name\": \"mobilenet0_relu18_fwd\", \n \"attrs\": {\"act_type\": \"relu\"}, \n \"inputs\": [[151, 0, 0]]\n }, \n {\n \"op\": \"null\", \n \"name\": \"mobilenet0_conv19_weight\", \n \"attrs\": {\n \"__dtype__\": \"0\", \n \"__lr_mult__\": \"1.0\", \n \"__shape__\": \"(128L, 1L, 3L, 3L)\", \n \"__storage_type__\": \"0\", \n \"__wd_mult__\": \"1.0\"\n }, \n \"inputs\": []\n }, \n {\n \"op\": \"Convolution\", \n \"name\": \"mobilenet0_conv19_fwd\", \n \"attrs\": {\n \"dilate\": \"(1, 1)\", \n \"kernel\": \"(3, 3)\", \n \"layout\": \"NCHW\", \n \"no_bias\": \"True\", \n \"num_filter\": \"128\", \n \"num_group\": \"128\", \n \"pad\": \"(1, 1)\", \n \"stride\": \"(1, 1)\"\n }, \n \"inputs\": [[152, 0, 0], [153, 0, 0]]\n }, \n {\n \"op\": \"null\", \n \"name\": \"mobilenet0_batchnorm19_gamma\", \n \"attrs\": {\n \"__dtype__\": \"0\", \n \"__init__\": \"ones\", \n \"__lr_mult__\": \"1.0\", \n \"__shape__\": \"(128L,)\", \n \"__storage_type__\": \"0\", \n \"__wd_mult__\": \"1.0\"\n }, \n \"inputs\": []\n }, \n {\n \"op\": \"null\", \n \"name\": \"mobilenet0_batchnorm19_beta\", \n \"attrs\": {\n \"__dtype__\": \"0\", \n \"__init__\": \"zeros\", \n \"__lr_mult__\": \"1.0\", \n \"__shape__\": \"(128L,)\", \n \"__storage_type__\": \"0\", \n \"__wd_mult__\": \"1.0\"\n }, \n \"inputs\": []\n }, \n {\n \"op\": \"null\", \n \"name\": \"mobilenet0_batchnorm19_running_mean\", \n \"attrs\": {\n \"__dtype__\": \"0\", \n \"__init__\": \"zeros\", \n \"__lr_mult__\": \"1.0\", \n \"__shape__\": \"(128L,)\", \n \"__storage_type__\": \"0\", \n \"__wd_mult__\": \"1.0\"\n }, \n \"inputs\": []\n }, \n {\n \"op\": \"null\", \n \"name\": \"mobilenet0_batchnorm19_running_var\", \n \"attrs\": {\n \"__dtype__\": \"0\", \n \"__init__\": \"ones\", \n \"__lr_mult__\": \"1.0\", \n \"__shape__\": \"(128L,)\", \n \"__storage_type__\": \"0\", \n \"__wd_mult__\": \"1.0\"\n }, \n \"inputs\": []\n }, \n {\n \"op\": \"BatchNorm\", \n \"name\": \"mobilenet0_batchnorm19_fwd\", \n \"attrs\": {\n \"axis\": \"1\", \n \"eps\": \"1e-05\", \n \"fix_gamma\": \"False\", \n \"momentum\": \"0.9\", \n \"use_global_stats\": \"False\"\n }, \n \"inputs\": [[154, 0, 0], [155, 0, 0], [156, 0, 0], [157, 0, 1], [158, 0, 1]]\n }, \n {\n \"op\": \"Activation\", \n \"name\": \"mobilenet0_relu19_fwd\", \n \"attrs\": {\"act_type\": \"relu\"}, \n \"inputs\": [[159, 0, 0]]\n }, \n {\n \"op\": \"null\", \n \"name\": \"mobilenet0_conv20_weight\", \n \"attrs\": {\n \"__dtype__\": \"0\", \n \"__lr_mult__\": \"1.0\", \n \"__shape__\": \"(128L, 128L, 1L, 1L)\", \n \"__storage_type__\": \"0\", \n \"__wd_mult__\": \"1.0\"\n }, \n \"inputs\": []\n }, \n {\n \"op\": \"Convolution\", \n \"name\": \"mobilenet0_conv20_fwd\", \n \"attrs\": {\n \"dilate\": \"(1, 1)\", \n \"kernel\": \"(1, 1)\", \n \"layout\": \"NCHW\", \n \"no_bias\": \"True\", \n \"num_filter\": \"128\", \n \"num_group\": \"1\", \n \"pad\": \"(0, 0)\", \n \"stride\": \"(1, 1)\"\n }, \n \"inputs\": [[160, 0, 0], [161, 0, 0]]\n }, \n {\n \"op\": \"null\", \n \"name\": \"mobilenet0_batchnorm20_gamma\", \n \"attrs\": {\n \"__dtype__\": \"0\", \n \"__init__\": \"ones\", \n \"__lr_mult__\": \"1.0\", \n \"__shape__\": \"(128L,)\", \n \"__storage_type__\": \"0\", \n \"__wd_mult__\": \"1.0\"\n }, \n \"inputs\": []\n }, \n {\n \"op\": \"null\", \n \"name\": \"mobilenet0_batchnorm20_beta\", \n \"attrs\": {\n \"__dtype__\": \"0\", \n \"__init__\": \"zeros\", \n \"__lr_mult__\": \"1.0\", \n \"__shape__\": \"(128L,)\", \n \"__storage_type__\": \"0\", \n \"__wd_mult__\": \"1.0\"\n }, \n \"inputs\": []\n }, \n {\n \"op\": \"null\", \n \"name\": \"mobilenet0_batchnorm20_running_mean\", \n \"attrs\": {\n \"__dtype__\": \"0\", \n \"__init__\": \"zeros\", \n \"__lr_mult__\": \"1.0\", \n \"__shape__\": \"(128L,)\", \n \"__storage_type__\": \"0\", \n \"__wd_mult__\": \"1.0\"\n }, \n \"inputs\": []\n }, \n {\n \"op\": \"null\", \n \"name\": \"mobilenet0_batchnorm20_running_var\", \n \"attrs\": {\n \"__dtype__\": \"0\", \n \"__init__\": \"ones\", \n \"__lr_mult__\": \"1.0\", \n \"__shape__\": \"(128L,)\", \n \"__storage_type__\": \"0\", \n \"__wd_mult__\": \"1.0\"\n }, \n \"inputs\": []\n }, \n {\n \"op\": \"BatchNorm\", \n \"name\": \"mobilenet0_batchnorm20_fwd\", \n \"attrs\": {\n \"axis\": \"1\", \n \"eps\": \"1e-05\", \n \"fix_gamma\": \"False\", \n \"momentum\": \"0.9\", \n \"use_global_stats\": \"False\"\n }, \n \"inputs\": [[162, 0, 0], [163, 0, 0], [164, 0, 0], [165, 0, 1], [166, 0, 1]]\n }, \n {\n \"op\": \"Activation\", \n \"name\": \"mobilenet0_relu20_fwd\", \n \"attrs\": {\"act_type\": \"relu\"}, \n \"inputs\": [[167, 0, 0]]\n }, \n {\n \"op\": \"null\", \n \"name\": \"mobilenet0_conv21_weight\", \n \"attrs\": {\n \"__dtype__\": \"0\", \n \"__lr_mult__\": \"1.0\", \n \"__shape__\": \"(128L, 1L, 3L, 3L)\", \n \"__storage_type__\": \"0\", \n \"__wd_mult__\": \"1.0\"\n }, \n \"inputs\": []\n }, \n {\n \"op\": \"Convolution\", \n \"name\": \"mobilenet0_conv21_fwd\", \n \"attrs\": {\n \"dilate\": \"(1, 1)\", \n \"kernel\": \"(3, 3)\", \n \"layout\": \"NCHW\", \n \"no_bias\": \"True\", \n \"num_filter\": \"128\", \n \"num_group\": \"128\", \n \"pad\": \"(1, 1)\", \n \"stride\": \"(1, 1)\"\n }, \n \"inputs\": [[168, 0, 0], [169, 0, 0]]\n }, \n {\n \"op\": \"null\", \n \"name\": \"mobilenet0_batchnorm21_gamma\", \n \"attrs\": {\n \"__dtype__\": \"0\", \n \"__init__\": \"ones\", \n \"__lr_mult__\": \"1.0\", \n \"__shape__\": \"(128L,)\", \n \"__storage_type__\": \"0\", \n \"__wd_mult__\": \"1.0\"\n }, \n \"inputs\": []\n }, \n {\n \"op\": \"null\", \n \"name\": \"mobilenet0_batchnorm21_beta\", \n \"attrs\": {\n \"__dtype__\": \"0\", \n \"__init__\": \"zeros\", \n \"__lr_mult__\": \"1.0\", \n \"__shape__\": \"(128L,)\", \n \"__storage_type__\": \"0\", \n \"__wd_mult__\": \"1.0\"\n }, \n \"inputs\": []\n }, \n {\n \"op\": \"null\", \n \"name\": \"mobilenet0_batchnorm21_running_mean\", \n \"attrs\": {\n \"__dtype__\": \"0\", \n \"__init__\": \"zeros\", \n \"__lr_mult__\": \"1.0\", \n \"__shape__\": \"(128L,)\", \n \"__storage_type__\": \"0\", \n \"__wd_mult__\": \"1.0\"\n }, \n \"inputs\": []\n }, \n {\n \"op\": \"null\", \n \"name\": \"mobilenet0_batchnorm21_running_var\", \n \"attrs\": {\n \"__dtype__\": \"0\", \n \"__init__\": \"ones\", \n \"__lr_mult__\": \"1.0\", \n \"__shape__\": \"(128L,)\", \n \"__storage_type__\": \"0\", \n \"__wd_mult__\": \"1.0\"\n }, \n \"inputs\": []\n }, \n {\n \"op\": \"BatchNorm\", \n \"name\": \"mobilenet0_batchnorm21_fwd\", \n \"attrs\": {\n \"axis\": \"1\", \n \"eps\": \"1e-05\", \n \"fix_gamma\": \"False\", \n \"momentum\": \"0.9\", \n \"use_global_stats\": \"False\"\n }, \n \"inputs\": [[170, 0, 0], [171, 0, 0], [172, 0, 0], [173, 0, 1], [174, 0, 1]]\n }, \n {\n \"op\": \"Activation\", \n \"name\": \"mobilenet0_relu21_fwd\", \n \"attrs\": {\"act_type\": \"relu\"}, \n \"inputs\": [[175, 0, 0]]\n }, \n {\n \"op\": \"null\", \n \"name\": \"mobilenet0_conv22_weight\", \n \"attrs\": {\n \"__dtype__\": \"0\", \n \"__lr_mult__\": \"1.0\", \n \"__shape__\": \"(128L, 128L, 1L, 1L)\", \n \"__storage_type__\": \"0\", \n \"__wd_mult__\": \"1.0\"\n }, \n \"inputs\": []\n }, \n {\n \"op\": \"Convolution\", \n \"name\": \"mobilenet0_conv22_fwd\", \n \"attrs\": {\n \"dilate\": \"(1, 1)\", \n \"kernel\": \"(1, 1)\", \n \"layout\": \"NCHW\", \n \"no_bias\": \"True\", \n \"num_filter\": \"128\", \n \"num_group\": \"1\", \n \"pad\": \"(0, 0)\", \n \"stride\": \"(1, 1)\"\n }, \n \"inputs\": [[176, 0, 0], [177, 0, 0]]\n }, \n {\n \"op\": \"null\", \n \"name\": \"mobilenet0_batchnorm22_gamma\", \n \"attrs\": {\n \"__dtype__\": \"0\", \n \"__init__\": \"ones\", \n \"__lr_mult__\": \"1.0\", \n \"__shape__\": \"(128L,)\", \n \"__storage_type__\": \"0\", \n \"__wd_mult__\": \"1.0\"\n }, \n \"inputs\": []\n }, \n {\n \"op\": \"null\", \n \"name\": \"mobilenet0_batchnorm22_beta\", \n \"attrs\": {\n \"__dtype__\": \"0\", \n \"__init__\": \"zeros\", \n \"__lr_mult__\": \"1.0\", \n \"__shape__\": \"(128L,)\", \n \"__storage_type__\": \"0\", \n \"__wd_mult__\": \"1.0\"\n }, \n \"inputs\": []\n }, \n {\n \"op\": \"null\", \n \"name\": \"mobilenet0_batchnorm22_running_mean\", \n \"attrs\": {\n \"__dtype__\": \"0\", \n \"__init__\": \"zeros\", \n \"__lr_mult__\": \"1.0\", \n \"__shape__\": \"(128L,)\", \n \"__storage_type__\": \"0\", \n \"__wd_mult__\": \"1.0\"\n }, \n \"inputs\": []\n }, \n {\n \"op\": \"null\", \n \"name\": \"mobilenet0_batchnorm22_running_var\", \n \"attrs\": {\n \"__dtype__\": \"0\", \n \"__init__\": \"ones\", \n \"__lr_mult__\": \"1.0\", \n \"__shape__\": \"(128L,)\", \n \"__storage_type__\": \"0\", \n \"__wd_mult__\": \"1.0\"\n }, \n \"inputs\": []\n }, \n {\n \"op\": \"BatchNorm\", \n \"name\": \"mobilenet0_batchnorm22_fwd\", \n \"attrs\": {\n \"axis\": \"1\", \n \"eps\": \"1e-05\", \n \"fix_gamma\": \"False\", \n \"momentum\": \"0.9\", \n \"use_global_stats\": \"False\"\n }, \n \"inputs\": [[178, 0, 0], [179, 0, 0], [180, 0, 0], [181, 0, 1], [182, 0, 1]]\n }, \n {\n \"op\": \"Activation\", \n \"name\": \"mobilenet0_relu22_fwd\", \n \"attrs\": {\"act_type\": \"relu\"}, \n \"inputs\": [[183, 0, 0]]\n }, \n {\n \"op\": \"null\", \n \"name\": \"mobilenet0_conv23_weight\", \n \"attrs\": {\n \"__dtype__\": \"0\", \n \"__lr_mult__\": \"1.0\", \n \"__shape__\": \"(128L, 1L, 3L, 3L)\", \n \"__storage_type__\": \"0\", \n \"__wd_mult__\": \"1.0\"\n }, \n \"inputs\": []\n }, \n {\n \"op\": \"Convolution\", \n \"name\": \"mobilenet0_conv23_fwd\", \n \"attrs\": {\n \"dilate\": \"(1, 1)\", \n \"kernel\": \"(3, 3)\", \n \"layout\": \"NCHW\", \n \"no_bias\": \"True\", \n \"num_filter\": \"128\", \n \"num_group\": \"128\", \n \"pad\": \"(1, 1)\", \n \"stride\": \"(2, 2)\"\n }, \n \"inputs\": [[184, 0, 0], [185, 0, 0]]\n }, \n {\n \"op\": \"null\", \n \"name\": \"mobilenet0_batchnorm23_gamma\", \n \"attrs\": {\n \"__dtype__\": \"0\", \n \"__init__\": \"ones\", \n \"__lr_mult__\": \"1.0\", \n \"__shape__\": \"(128L,)\", \n \"__storage_type__\": \"0\", \n \"__wd_mult__\": \"1.0\"\n }, \n \"inputs\": []\n }, \n {\n \"op\": \"null\", \n \"name\": \"mobilenet0_batchnorm23_beta\", \n \"attrs\": {\n \"__dtype__\": \"0\", \n \"__init__\": \"zeros\", \n \"__lr_mult__\": \"1.0\", \n \"__shape__\": \"(128L,)\", \n \"__storage_type__\": \"0\", \n \"__wd_mult__\": \"1.0\"\n }, \n \"inputs\": []\n }, \n {\n \"op\": \"null\", \n \"name\": \"mobilenet0_batchnorm23_running_mean\", \n \"attrs\": {\n \"__dtype__\": \"0\", \n \"__init__\": \"zeros\", \n \"__lr_mult__\": \"1.0\", \n \"__shape__\": \"(128L,)\", \n \"__storage_type__\": \"0\", \n \"__wd_mult__\": \"1.0\"\n }, \n \"inputs\": []\n }, \n {\n \"op\": \"null\", \n \"name\": \"mobilenet0_batchnorm23_running_var\", \n \"attrs\": {\n \"__dtype__\": \"0\", \n \"__init__\": \"ones\", \n \"__lr_mult__\": \"1.0\", \n \"__shape__\": \"(128L,)\", \n \"__storage_type__\": \"0\", \n \"__wd_mult__\": \"1.0\"\n }, \n \"inputs\": []\n }, \n {\n \"op\": \"BatchNorm\", \n \"name\": \"mobilenet0_batchnorm23_fwd\", \n \"attrs\": {\n \"axis\": \"1\", \n \"eps\": \"1e-05\", \n \"fix_gamma\": \"False\", \n \"momentum\": \"0.9\", \n \"use_global_stats\": \"False\"\n }, \n \"inputs\": [[186, 0, 0], [187, 0, 0], [188, 0, 0], [189, 0, 1], [190, 0, 1]]\n }, \n {\n \"op\": \"Activation\", \n \"name\": \"mobilenet0_relu23_fwd\", \n \"attrs\": {\"act_type\": \"relu\"}, \n \"inputs\": [[191, 0, 0]]\n }, \n {\n \"op\": \"null\", \n \"name\": \"mobilenet0_conv24_weight\", \n \"attrs\": {\n \"__dtype__\": \"0\", \n \"__lr_mult__\": \"1.0\", \n \"__shape__\": \"(256L, 128L, 1L, 1L)\", \n \"__storage_type__\": \"0\", \n \"__wd_mult__\": \"1.0\"\n }, \n \"inputs\": []\n }, \n {\n \"op\": \"Convolution\", \n \"name\": \"mobilenet0_conv24_fwd\", \n \"attrs\": {\n \"dilate\": \"(1, 1)\", \n \"kernel\": \"(1, 1)\", \n \"layout\": \"NCHW\", \n \"no_bias\": \"True\", \n \"num_filter\": \"256\", \n \"num_group\": \"1\", \n \"pad\": \"(0, 0)\", \n \"stride\": \"(1, 1)\"\n }, \n \"inputs\": [[192, 0, 0], [193, 0, 0]]\n }, \n {\n \"op\": \"null\", \n \"name\": \"mobilenet0_batchnorm24_gamma\", \n \"attrs\": {\n \"__dtype__\": \"0\", \n \"__init__\": \"ones\", \n \"__lr_mult__\": \"1.0\", \n \"__shape__\": \"(256L,)\", \n \"__storage_type__\": \"0\", \n \"__wd_mult__\": \"1.0\"\n }, \n \"inputs\": []\n }, \n {\n \"op\": \"null\", \n \"name\": \"mobilenet0_batchnorm24_beta\", \n \"attrs\": {\n \"__dtype__\": \"0\", \n \"__init__\": \"zeros\", \n \"__lr_mult__\": \"1.0\", \n \"__shape__\": \"(256L,)\", \n \"__storage_type__\": \"0\", \n \"__wd_mult__\": \"1.0\"\n }, \n \"inputs\": []\n }, \n {\n \"op\": \"null\", \n \"name\": \"mobilenet0_batchnorm24_running_mean\", \n \"attrs\": {\n \"__dtype__\": \"0\", \n \"__init__\": \"zeros\", \n \"__lr_mult__\": \"1.0\", \n \"__shape__\": \"(256L,)\", \n \"__storage_type__\": \"0\", \n \"__wd_mult__\": \"1.0\"\n }, \n \"inputs\": []\n }, \n {\n \"op\": \"null\", \n \"name\": \"mobilenet0_batchnorm24_running_var\", \n \"attrs\": {\n \"__dtype__\": \"0\", \n \"__init__\": \"ones\", \n \"__lr_mult__\": \"1.0\", \n \"__shape__\": \"(256L,)\", \n \"__storage_type__\": \"0\", \n \"__wd_mult__\": \"1.0\"\n }, \n \"inputs\": []\n }, \n {\n \"op\": \"BatchNorm\", \n \"name\": \"mobilenet0_batchnorm24_fwd\", \n \"attrs\": {\n \"axis\": \"1\", \n \"eps\": \"1e-05\", \n \"fix_gamma\": \"False\", \n \"momentum\": \"0.9\", \n \"use_global_stats\": \"False\"\n }, \n \"inputs\": [[194, 0, 0], [195, 0, 0], [196, 0, 0], [197, 0, 1], [198, 0, 1]]\n }, \n {\n \"op\": \"Activation\", \n \"name\": \"mobilenet0_relu24_fwd\", \n \"attrs\": {\"act_type\": \"relu\"}, \n \"inputs\": [[199, 0, 0]]\n }, \n {\n \"op\": \"null\", \n \"name\": \"mobilenet0_conv25_weight\", \n \"attrs\": {\n \"__dtype__\": \"0\", \n \"__lr_mult__\": \"1.0\", \n \"__shape__\": \"(256L, 1L, 3L, 3L)\", \n \"__storage_type__\": \"0\", \n \"__wd_mult__\": \"1.0\"\n }, \n \"inputs\": []\n }, \n {\n \"op\": \"Convolution\", \n \"name\": \"mobilenet0_conv25_fwd\", \n \"attrs\": {\n \"dilate\": \"(1, 1)\", \n \"kernel\": \"(3, 3)\", \n \"layout\": \"NCHW\", \n \"no_bias\": \"True\", \n \"num_filter\": \"256\", \n \"num_group\": \"256\", \n \"pad\": \"(1, 1)\", \n \"stride\": \"(1, 1)\"\n }, \n \"inputs\": [[200, 0, 0], [201, 0, 0]]\n }, \n {\n \"op\": \"null\", \n \"name\": \"mobilenet0_batchnorm25_gamma\", \n \"attrs\": {\n \"__dtype__\": \"0\", \n \"__init__\": \"ones\", \n \"__lr_mult__\": \"1.0\", \n \"__shape__\": \"(256L,)\", \n \"__storage_type__\": \"0\", \n \"__wd_mult__\": \"1.0\"\n }, \n \"inputs\": []\n }, \n {\n \"op\": \"null\", \n \"name\": \"mobilenet0_batchnorm25_beta\", \n \"attrs\": {\n \"__dtype__\": \"0\", \n \"__init__\": \"zeros\", \n \"__lr_mult__\": \"1.0\", \n \"__shape__\": \"(256L,)\", \n \"__storage_type__\": \"0\", \n \"__wd_mult__\": \"1.0\"\n }, \n \"inputs\": []\n }, \n {\n \"op\": \"null\", \n \"name\": \"mobilenet0_batchnorm25_running_mean\", \n \"attrs\": {\n \"__dtype__\": \"0\", \n \"__init__\": \"zeros\", \n \"__lr_mult__\": \"1.0\", \n \"__shape__\": \"(256L,)\", \n \"__storage_type__\": \"0\", \n \"__wd_mult__\": \"1.0\"\n }, \n \"inputs\": []\n }, \n {\n \"op\": \"null\", \n \"name\": \"mobilenet0_batchnorm25_running_var\", \n \"attrs\": {\n \"__dtype__\": \"0\", \n \"__init__\": \"ones\", \n \"__lr_mult__\": \"1.0\", \n \"__shape__\": \"(256L,)\", \n \"__storage_type__\": \"0\", \n \"__wd_mult__\": \"1.0\"\n }, \n \"inputs\": []\n }, \n {\n \"op\": \"BatchNorm\", \n \"name\": \"mobilenet0_batchnorm25_fwd\", \n \"attrs\": {\n \"axis\": \"1\", \n \"eps\": \"1e-05\", \n \"fix_gamma\": \"False\", \n \"momentum\": \"0.9\", \n \"use_global_stats\": \"False\"\n }, \n \"inputs\": [[202, 0, 0], [203, 0, 0], [204, 0, 0], [205, 0, 1], [206, 0, 1]]\n }, \n {\n \"op\": \"Activation\", \n \"name\": \"mobilenet0_relu25_fwd\", \n \"attrs\": {\"act_type\": \"relu\"}, \n \"inputs\": [[207, 0, 0]]\n }, \n {\n \"op\": \"null\", \n \"name\": \"mobilenet0_conv26_weight\", \n \"attrs\": {\n \"__dtype__\": \"0\", \n \"__lr_mult__\": \"1.0\", \n \"__shape__\": \"(256L, 256L, 1L, 1L)\", \n \"__storage_type__\": \"0\", \n \"__wd_mult__\": \"1.0\"\n }, \n \"inputs\": []\n }, \n {\n \"op\": \"Convolution\", \n \"name\": \"mobilenet0_conv26_fwd\", \n \"attrs\": {\n \"dilate\": \"(1, 1)\", \n \"kernel\": \"(1, 1)\", \n \"layout\": \"NCHW\", \n \"no_bias\": \"True\", \n \"num_filter\": \"256\", \n \"num_group\": \"1\", \n \"pad\": \"(0, 0)\", \n \"stride\": \"(1, 1)\"\n }, \n \"inputs\": [[208, 0, 0], [209, 0, 0]]\n }, \n {\n \"op\": \"null\", \n \"name\": \"mobilenet0_batchnorm26_gamma\", \n \"attrs\": {\n \"__dtype__\": \"0\", \n \"__init__\": \"ones\", \n \"__lr_mult__\": \"1.0\", \n \"__shape__\": \"(256L,)\", \n \"__storage_type__\": \"0\", \n \"__wd_mult__\": \"1.0\"\n }, \n \"inputs\": []\n }, \n {\n \"op\": \"null\", \n \"name\": \"mobilenet0_batchnorm26_beta\", \n \"attrs\": {\n \"__dtype__\": \"0\", \n \"__init__\": \"zeros\", \n \"__lr_mult__\": \"1.0\", \n \"__shape__\": \"(256L,)\", \n \"__storage_type__\": \"0\", \n \"__wd_mult__\": \"1.0\"\n }, \n \"inputs\": []\n }, \n {\n \"op\": \"null\", \n \"name\": \"mobilenet0_batchnorm26_running_mean\", \n \"attrs\": {\n \"__dtype__\": \"0\", \n \"__init__\": \"zeros\", \n \"__lr_mult__\": \"1.0\", \n \"__shape__\": \"(256L,)\", \n \"__storage_type__\": \"0\", \n \"__wd_mult__\": \"1.0\"\n }, \n \"inputs\": []\n }, \n {\n \"op\": \"null\", \n \"name\": \"mobilenet0_batchnorm26_running_var\", \n \"attrs\": {\n \"__dtype__\": \"0\", \n \"__init__\": \"ones\", \n \"__lr_mult__\": \"1.0\", \n \"__shape__\": \"(256L,)\", \n \"__storage_type__\": \"0\", \n \"__wd_mult__\": \"1.0\"\n }, \n \"inputs\": []\n }, \n {\n \"op\": \"BatchNorm\", \n \"name\": \"mobilenet0_batchnorm26_fwd\", \n \"attrs\": {\n \"axis\": \"1\", \n \"eps\": \"1e-05\", \n \"fix_gamma\": \"False\", \n \"momentum\": \"0.9\", \n \"use_global_stats\": \"False\"\n }, \n \"inputs\": [[210, 0, 0], [211, 0, 0], [212, 0, 0], [213, 0, 1], [214, 0, 1]]\n }, \n {\n \"op\": \"Activation\", \n \"name\": \"mobilenet0_relu26_fwd\", \n \"attrs\": {\"act_type\": \"relu\"}, \n \"inputs\": [[215, 0, 0]]\n }, \n {\n \"op\": \"null\", \n \"name\": \"rf_c3_lateral_weight\", \n \"attrs\": {\n \"__init__\": \"[\\\"normal\\\", {\\\"sigma\\\": 0.01}]\", \n \"__lr_mult__\": \"1.0\"\n }, \n \"inputs\": []\n }, \n {\n \"op\": \"null\", \n \"name\": \"rf_c3_lateral_bias\", \n \"attrs\": {\n \"__init__\": \"[\\\"constant\\\", {\\\"value\\\": 0.0}]\", \n \"__lr_mult__\": \"2.0\", \n \"__wd_mult__\": \"1.0\"\n }, \n \"inputs\": []\n }, \n {\n \"op\": \"Convolution\", \n \"name\": \"rf_c3_lateral\", \n \"attrs\": {\n \"kernel\": \"(1, 1)\", \n \"num_filter\": \"64\", \n \"pad\": \"(0, 0)\", \n \"stride\": \"(1, 1)\"\n }, \n \"inputs\": [[216, 0, 0], [217, 0, 0], [218, 0, 0]]\n }, \n {\n \"op\": \"null\", \n \"name\": \"rf_c3_lateral_bn_gamma\", \n \"attrs\": {\n \"eps\": \"2e-05\", \n \"fix_gamma\": \"False\", \n \"momentum\": \"0.9\"\n }, \n \"inputs\": []\n }, \n {\n \"op\": \"null\", \n \"name\": \"rf_c3_lateral_bn_beta\", \n \"attrs\": {\n \"eps\": \"2e-05\", \n \"fix_gamma\": \"False\", \n \"momentum\": \"0.9\"\n }, \n \"inputs\": []\n }, \n {\n \"op\": \"null\", \n \"name\": \"rf_c3_lateral_bn_moving_mean\", \n \"attrs\": {\n \"__init__\": \"[\\\"zero\\\", {}]\", \n \"eps\": \"2e-05\", \n \"fix_gamma\": \"False\", \n \"momentum\": \"0.9\"\n }, \n \"inputs\": []\n }, \n {\n \"op\": \"null\", \n \"name\": \"rf_c3_lateral_bn_moving_var\", \n \"attrs\": {\n \"__init__\": \"[\\\"one\\\", {}]\", \n \"eps\": \"2e-05\", \n \"fix_gamma\": \"False\", \n \"momentum\": \"0.9\"\n }, \n \"inputs\": []\n }, \n {\n \"op\": \"BatchNorm\", \n \"name\": \"rf_c3_lateral_bn\", \n \"attrs\": {\n \"eps\": \"2e-05\", \n \"fix_gamma\": \"False\", \n \"momentum\": \"0.9\"\n }, \n \"inputs\": [[219, 0, 0], [220, 0, 0], [221, 0, 0], [222, 0, 1], [223, 0, 1]]\n }, \n {\n \"op\": \"Activation\", \n \"name\": \"rf_c3_lateral_relu\", \n \"attrs\": {\"act_type\": \"relu\"}, \n \"inputs\": [[224, 0, 0]]\n }, \n {\n \"op\": \"null\", \n \"name\": \"rf_c3_det_conv1_weight\", \n \"attrs\": {\n \"__init__\": \"[\\\"normal\\\", {\\\"sigma\\\": 0.01}]\", \n \"__lr_mult__\": \"1.0\"\n }, \n \"inputs\": []\n }, \n {\n \"op\": \"null\", \n \"name\": \"rf_c3_det_conv1_bias\", \n \"attrs\": {\n \"__init__\": \"[\\\"constant\\\", {\\\"value\\\": 0.0}]\", \n \"__lr_mult__\": \"2.0\", \n \"__wd_mult__\": \"0.0\"\n }, \n \"inputs\": []\n }, \n {\n \"op\": \"Convolution\", \n \"name\": \"rf_c3_det_conv1\", \n \"attrs\": {\n \"kernel\": \"(3, 3)\", \n \"num_filter\": \"32\", \n \"pad\": \"(1, 1)\", \n \"stride\": \"(1, 1)\"\n }, \n \"inputs\": [[225, 0, 0], [226, 0, 0], [227, 0, 0]]\n }, \n {\n \"op\": \"null\", \n \"name\": \"rf_c3_det_conv1_bn_gamma\", \n \"attrs\": {\n \"eps\": \"2e-05\", \n \"fix_gamma\": \"False\", \n \"momentum\": \"0.9\"\n }, \n \"inputs\": []\n }, \n {\n \"op\": \"null\", \n \"name\": \"rf_c3_det_conv1_bn_beta\", \n \"attrs\": {\n \"eps\": \"2e-05\", \n \"fix_gamma\": \"False\", \n \"momentum\": \"0.9\"\n }, \n \"inputs\": []\n }, \n {\n \"op\": \"null\", \n \"name\": \"rf_c3_det_conv1_bn_moving_mean\", \n \"attrs\": {\n \"__init__\": \"[\\\"zero\\\", {}]\", \n \"eps\": \"2e-05\", \n \"fix_gamma\": \"False\", \n \"momentum\": \"0.9\"\n }, \n \"inputs\": []\n }, \n {\n \"op\": \"null\", \n \"name\": \"rf_c3_det_conv1_bn_moving_var\", \n \"attrs\": {\n \"__init__\": \"[\\\"one\\\", {}]\", \n \"eps\": \"2e-05\", \n \"fix_gamma\": \"False\", \n \"momentum\": \"0.9\"\n }, \n \"inputs\": []\n }, \n {\n \"op\": \"BatchNorm\", \n \"name\": \"rf_c3_det_conv1_bn\", \n \"attrs\": {\n \"eps\": \"2e-05\", \n \"fix_gamma\": \"False\", \n \"momentum\": \"0.9\"\n }, \n \"inputs\": [[228, 0, 0], [229, 0, 0], [230, 0, 0], [231, 0, 1], [232, 0, 1]]\n }, \n {\n \"op\": \"null\", \n \"name\": \"rf_c3_det_context_conv1_weight\", \n \"attrs\": {\n \"__init__\": \"[\\\"normal\\\", {\\\"sigma\\\": 0.01}]\", \n \"__lr_mult__\": \"1.0\"\n }, \n \"inputs\": []\n }, \n {\n \"op\": \"null\", \n \"name\": \"rf_c3_det_context_conv1_bias\", \n \"attrs\": {\n \"__init__\": \"[\\\"constant\\\", {\\\"value\\\": 0.0}]\", \n \"__lr_mult__\": \"2.0\", \n \"__wd_mult__\": \"0.0\"\n }, \n \"inputs\": []\n }, \n {\n \"op\": \"Convolution\", \n \"name\": \"rf_c3_det_context_conv1\", \n \"attrs\": {\n \"kernel\": \"(3, 3)\", \n \"num_filter\": \"16\", \n \"pad\": \"(1, 1)\", \n \"stride\": \"(1, 1)\"\n }, \n \"inputs\": [[225, 0, 0], [234, 0, 0], [235, 0, 0]]\n }, \n {\n \"op\": \"null\", \n \"name\": \"rf_c3_det_context_conv1_bn_gamma\", \n \"attrs\": {\n \"eps\": \"2e-05\", \n \"fix_gamma\": \"False\", \n \"momentum\": \"0.9\"\n }, \n \"inputs\": []\n }, \n {\n \"op\": \"null\", \n \"name\": \"rf_c3_det_context_conv1_bn_beta\", \n \"attrs\": {\n \"eps\": \"2e-05\", \n \"fix_gamma\": \"False\", \n \"momentum\": \"0.9\"\n }, \n \"inputs\": []\n }, \n {\n \"op\": \"null\", \n \"name\": \"rf_c3_det_context_conv1_bn_moving_mean\", \n \"attrs\": {\n \"__init__\": \"[\\\"zero\\\", {}]\", \n \"eps\": \"2e-05\", \n \"fix_gamma\": \"False\", \n \"momentum\": \"0.9\"\n }, \n \"inputs\": []\n }, \n {\n \"op\": \"null\", \n \"name\": \"rf_c3_det_context_conv1_bn_moving_var\", \n \"attrs\": {\n \"__init__\": \"[\\\"one\\\", {}]\", \n \"eps\": \"2e-05\", \n \"fix_gamma\": \"False\", \n \"momentum\": \"0.9\"\n }, \n \"inputs\": []\n }, \n {\n \"op\": \"BatchNorm\", \n \"name\": \"rf_c3_det_context_conv1_bn\", \n \"attrs\": {\n \"eps\": \"2e-05\", \n \"fix_gamma\": \"False\", \n \"momentum\": \"0.9\"\n }, \n \"inputs\": [[236, 0, 0], [237, 0, 0], [238, 0, 0], [239, 0, 1], [240, 0, 1]]\n }, \n {\n \"op\": \"Activation\", \n \"name\": \"rf_c3_det_context_conv1_relu\", \n \"attrs\": {\"act_type\": \"relu\"}, \n \"inputs\": [[241, 0, 0]]\n }, \n {\n \"op\": \"null\", \n \"name\": \"rf_c3_det_context_conv2_weight\", \n \"attrs\": {\n \"__init__\": \"[\\\"normal\\\", {\\\"sigma\\\": 0.01}]\", \n \"__lr_mult__\": \"1.0\"\n }, \n \"inputs\": []\n }, \n {\n \"op\": \"null\", \n \"name\": \"rf_c3_det_context_conv2_bias\", \n \"attrs\": {\n \"__init__\": \"[\\\"constant\\\", {\\\"value\\\": 0.0}]\", \n \"__lr_mult__\": \"2.0\", \n \"__wd_mult__\": \"0.0\"\n }, \n \"inputs\": []\n }, \n {\n \"op\": \"Convolution\", \n \"name\": \"rf_c3_det_context_conv2\", \n \"attrs\": {\n \"kernel\": \"(3, 3)\", \n \"num_filter\": \"16\", \n \"pad\": \"(1, 1)\", \n \"stride\": \"(1, 1)\"\n }, \n \"inputs\": [[242, 0, 0], [243, 0, 0], [244, 0, 0]]\n }, \n {\n \"op\": \"null\", \n \"name\": \"rf_c3_det_context_conv2_bn_gamma\", \n \"attrs\": {\n \"eps\": \"2e-05\", \n \"fix_gamma\": \"False\", \n \"momentum\": \"0.9\"\n }, \n \"inputs\": []\n }, \n {\n \"op\": \"null\", \n \"name\": \"rf_c3_det_context_conv2_bn_beta\", \n \"attrs\": {\n \"eps\": \"2e-05\", \n \"fix_gamma\": \"False\", \n \"momentum\": \"0.9\"\n }, \n \"inputs\": []\n }, \n {\n \"op\": \"null\", \n \"name\": \"rf_c3_det_context_conv2_bn_moving_mean\", \n \"attrs\": {\n \"__init__\": \"[\\\"zero\\\", {}]\", \n \"eps\": \"2e-05\", \n \"fix_gamma\": \"False\", \n \"momentum\": \"0.9\"\n }, \n \"inputs\": []\n }, \n {\n \"op\": \"null\", \n \"name\": \"rf_c3_det_context_conv2_bn_moving_var\", \n \"attrs\": {\n \"__init__\": \"[\\\"one\\\", {}]\", \n \"eps\": \"2e-05\", \n \"fix_gamma\": \"False\", \n \"momentum\": \"0.9\"\n }, \n \"inputs\": []\n }, \n {\n \"op\": \"BatchNorm\", \n \"name\": \"rf_c3_det_context_conv2_bn\", \n \"attrs\": {\n \"eps\": \"2e-05\", \n \"fix_gamma\": \"False\", \n \"momentum\": \"0.9\"\n }, \n \"inputs\": [[245, 0, 0], [246, 0, 0], [247, 0, 0], [248, 0, 1], [249, 0, 1]]\n }, \n {\n \"op\": \"null\", \n \"name\": \"rf_c3_det_context_conv3_1_weight\", \n \"attrs\": {\n \"__init__\": \"[\\\"normal\\\", {\\\"sigma\\\": 0.01}]\", \n \"__lr_mult__\": \"1.0\"\n }, \n \"inputs\": []\n }, \n {\n \"op\": \"null\", \n \"name\": \"rf_c3_det_context_conv3_1_bias\", \n \"attrs\": {\n \"__init__\": \"[\\\"constant\\\", {\\\"value\\\": 0.0}]\", \n \"__lr_mult__\": \"2.0\", \n \"__wd_mult__\": \"0.0\"\n }, \n \"inputs\": []\n }, \n {\n \"op\": \"Convolution\", \n \"name\": \"rf_c3_det_context_conv3_1\", \n \"attrs\": {\n \"kernel\": \"(3, 3)\", \n \"num_filter\": \"16\", \n \"pad\": \"(1, 1)\", \n \"stride\": \"(1, 1)\"\n }, \n \"inputs\": [[242, 0, 0], [251, 0, 0], [252, 0, 0]]\n }, \n {\n \"op\": \"null\", \n \"name\": \"rf_c3_det_context_conv3_1_bn_gamma\", \n \"attrs\": {\n \"eps\": \"2e-05\", \n \"fix_gamma\": \"False\", \n \"momentum\": \"0.9\"\n }, \n \"inputs\": []\n }, \n {\n \"op\": \"null\", \n \"name\": \"rf_c3_det_context_conv3_1_bn_beta\", \n \"attrs\": {\n \"eps\": \"2e-05\", \n \"fix_gamma\": \"False\", \n \"momentum\": \"0.9\"\n }, \n \"inputs\": []\n }, \n {\n \"op\": \"null\", \n \"name\": \"rf_c3_det_context_conv3_1_bn_moving_mean\", \n \"attrs\": {\n \"__init__\": \"[\\\"zero\\\", {}]\", \n \"eps\": \"2e-05\", \n \"fix_gamma\": \"False\", \n \"momentum\": \"0.9\"\n }, \n \"inputs\": []\n }, \n {\n \"op\": \"null\", \n \"name\": \"rf_c3_det_context_conv3_1_bn_moving_var\", \n \"attrs\": {\n \"__init__\": \"[\\\"one\\\", {}]\", \n \"eps\": \"2e-05\", \n \"fix_gamma\": \"False\", \n \"momentum\": \"0.9\"\n }, \n \"inputs\": []\n }, \n {\n \"op\": \"BatchNorm\", \n \"name\": \"rf_c3_det_context_conv3_1_bn\", \n \"attrs\": {\n \"eps\": \"2e-05\", \n \"fix_gamma\": \"False\", \n \"momentum\": \"0.9\"\n }, \n \"inputs\": [[253, 0, 0], [254, 0, 0], [255, 0, 0], [256, 0, 1], [257, 0, 1]]\n }, \n {\n \"op\": \"Activation\", \n \"name\": \"rf_c3_det_context_conv3_1_relu\", \n \"attrs\": {\"act_type\": \"relu\"}, \n \"inputs\": [[258, 0, 0]]\n }, \n {\n \"op\": \"null\", \n \"name\": \"rf_c3_det_context_conv3_2_weight\", \n \"attrs\": {\n \"__init__\": \"[\\\"normal\\\", {\\\"sigma\\\": 0.01}]\", \n \"__lr_mult__\": \"1.0\"\n }, \n \"inputs\": []\n }, \n {\n \"op\": \"null\", \n \"name\": \"rf_c3_det_context_conv3_2_bias\", \n \"attrs\": {\n \"__init__\": \"[\\\"constant\\\", {\\\"value\\\": 0.0}]\", \n \"__lr_mult__\": \"2.0\", \n \"__wd_mult__\": \"0.0\"\n }, \n \"inputs\": []\n }, \n {\n \"op\": \"Convolution\", \n \"name\": \"rf_c3_det_context_conv3_2\", \n \"attrs\": {\n \"kernel\": \"(3, 3)\", \n \"num_filter\": \"16\", \n \"pad\": \"(1, 1)\", \n \"stride\": \"(1, 1)\"\n }, \n \"inputs\": [[259, 0, 0], [260, 0, 0], [261, 0, 0]]\n }, \n {\n \"op\": \"null\", \n \"name\": \"rf_c3_det_context_conv3_2_bn_gamma\", \n \"attrs\": {\n \"eps\": \"2e-05\", \n \"fix_gamma\": \"False\", \n \"momentum\": \"0.9\"\n }, \n \"inputs\": []\n }, \n {\n \"op\": \"null\", \n \"name\": \"rf_c3_det_context_conv3_2_bn_beta\", \n \"attrs\": {\n \"eps\": \"2e-05\", \n \"fix_gamma\": \"False\", \n \"momentum\": \"0.9\"\n }, \n \"inputs\": []\n }, \n {\n \"op\": \"null\", \n \"name\": \"rf_c3_det_context_conv3_2_bn_moving_mean\", \n \"attrs\": {\n \"__init__\": \"[\\\"zero\\\", {}]\", \n \"eps\": \"2e-05\", \n \"fix_gamma\": \"False\", \n \"momentum\": \"0.9\"\n }, \n \"inputs\": []\n }, \n {\n \"op\": \"null\", \n \"name\": \"rf_c3_det_context_conv3_2_bn_moving_var\", \n \"attrs\": {\n \"__init__\": \"[\\\"one\\\", {}]\", \n \"eps\": \"2e-05\", \n \"fix_gamma\": \"False\", \n \"momentum\": \"0.9\"\n }, \n \"inputs\": []\n }, \n {\n \"op\": \"BatchNorm\", \n \"name\": \"rf_c3_det_context_conv3_2_bn\", \n \"attrs\": {\n \"eps\": \"2e-05\", \n \"fix_gamma\": \"False\", \n \"momentum\": \"0.9\"\n }, \n \"inputs\": [[262, 0, 0], [263, 0, 0], [264, 0, 0], [265, 0, 1], [266, 0, 1]]\n }, \n {\n \"op\": \"Concat\", \n \"name\": \"rf_c3_det_concat\", \n \"attrs\": {\n \"dim\": \"1\", \n \"num_args\": \"3\"\n }, \n \"inputs\": [[233, 0, 0], [250, 0, 0], [267, 0, 0]]\n }, \n {\n \"op\": \"Activation\", \n \"name\": \"rf_c3_det_concat_relu\", \n \"attrs\": {\"act_type\": \"relu\"}, \n \"inputs\": [[268, 0, 0]]\n }, \n {\n \"op\": \"null\", \n \"name\": \"face_rpn_cls_score_stride32_weight\", \n \"attrs\": {\n \"__init__\": \"[\\\"normal\\\", {\\\"sigma\\\": 0.01}]\", \n \"__lr_mult__\": \"1.0\"\n }, \n \"inputs\": []\n }, \n {\n \"op\": \"null\", \n \"name\": \"face_rpn_cls_score_stride32_bias\", \n \"attrs\": {\n \"__init__\": \"[\\\"constant\\\", {\\\"value\\\": 0.0}]\", \n \"__lr_mult__\": \"2.0\", \n \"__wd_mult__\": \"0.0\"\n }, \n \"inputs\": []\n }, \n {\n \"op\": \"Convolution\", \n \"name\": \"face_rpn_cls_score_stride32\", \n \"attrs\": {\n \"kernel\": \"(1, 1)\", \n \"num_filter\": \"4\", \n \"pad\": \"(0, 0)\", \n \"stride\": \"(1, 1)\"\n }, \n \"inputs\": [[269, 0, 0], [270, 0, 0], [271, 0, 0]]\n }, \n {\n \"op\": \"Reshape\", \n \"name\": \"face_rpn_cls_score_reshape_stride32\", \n \"attrs\": {\"shape\": \"(0, 2, -1, 0)\"}, \n \"inputs\": [[272, 0, 0]]\n }, \n {\n \"op\": \"SoftmaxActivation\", \n \"name\": \"face_rpn_cls_prob_stride32\", \n \"attrs\": {\"mode\": \"channel\"}, \n \"inputs\": [[273, 0, 0]]\n }, \n {\n \"op\": \"Reshape\", \n \"name\": \"face_rpn_cls_prob_reshape_stride32\", \n \"attrs\": {\"shape\": \"(0, 4, -1, 0)\"}, \n \"inputs\": [[274, 0, 0]]\n }, \n {\n \"op\": \"null\", \n \"name\": \"face_rpn_bbox_pred_stride32_weight\", \n \"attrs\": {\n \"__init__\": \"[\\\"normal\\\", {\\\"sigma\\\": 0.01}]\", \n \"__lr_mult__\": \"1.0\"\n }, \n \"inputs\": []\n }, \n {\n \"op\": \"null\", \n \"name\": \"face_rpn_bbox_pred_stride32_bias\", \n \"attrs\": {\n \"__init__\": \"[\\\"constant\\\", {\\\"value\\\": 0.0}]\", \n \"__lr_mult__\": \"2.0\", \n \"__wd_mult__\": \"0.0\"\n }, \n \"inputs\": []\n }, \n {\n \"op\": \"Convolution\", \n \"name\": \"face_rpn_bbox_pred_stride32\", \n \"attrs\": {\n \"kernel\": \"(1, 1)\", \n \"num_filter\": \"8\", \n \"pad\": \"(0, 0)\", \n \"stride\": \"(1, 1)\"\n }, \n \"inputs\": [[269, 0, 0], [276, 0, 0], [277, 0, 0]]\n }, \n {\n \"op\": \"null\", \n \"name\": \"face_rpn_landmark_pred_stride32_weight\", \n \"attrs\": {\n \"__init__\": \"[\\\"normal\\\", {\\\"sigma\\\": 0.01}]\", \n \"__lr_mult__\": \"1.0\"\n }, \n \"inputs\": []\n }, \n {\n \"op\": \"null\", \n \"name\": \"face_rpn_landmark_pred_stride32_bias\", \n \"attrs\": {\n \"__init__\": \"[\\\"constant\\\", {\\\"value\\\": 0.0}]\", \n \"__lr_mult__\": \"2.0\", \n \"__wd_mult__\": \"0.0\"\n }, \n \"inputs\": []\n }, \n {\n \"op\": \"Convolution\", \n \"name\": \"face_rpn_landmark_pred_stride32\", \n \"attrs\": {\n \"kernel\": \"(1, 1)\", \n \"num_filter\": \"20\", \n \"pad\": \"(0, 0)\", \n \"stride\": \"(1, 1)\"\n }, \n \"inputs\": [[269, 0, 0], [279, 0, 0], [280, 0, 0]]\n }, \n {\n \"op\": \"null\", \n \"name\": \"rf_c2_lateral_weight\", \n \"attrs\": {\n \"__init__\": \"[\\\"normal\\\", {\\\"sigma\\\": 0.01}]\", \n \"__lr_mult__\": \"1.0\"\n }, \n \"inputs\": []\n }, \n {\n \"op\": \"null\", \n \"name\": \"rf_c2_lateral_bias\", \n \"attrs\": {\n \"__init__\": \"[\\\"constant\\\", {\\\"value\\\": 0.0}]\", \n \"__lr_mult__\": \"2.0\", \n \"__wd_mult__\": \"1.0\"\n }, \n \"inputs\": []\n }, \n {\n \"op\": \"Convolution\", \n \"name\": \"rf_c2_lateral\", \n \"attrs\": {\n \"kernel\": \"(1, 1)\", \n \"num_filter\": \"64\", \n \"pad\": \"(0, 0)\", \n \"stride\": \"(1, 1)\"\n }, \n \"inputs\": [[184, 0, 0], [282, 0, 0], [283, 0, 0]]\n }, \n {\n \"op\": \"null\", \n \"name\": \"rf_c2_lateral_bn_gamma\", \n \"attrs\": {\n \"eps\": \"2e-05\", \n \"fix_gamma\": \"False\", \n \"momentum\": \"0.9\"\n }, \n \"inputs\": []\n }, \n {\n \"op\": \"null\", \n \"name\": \"rf_c2_lateral_bn_beta\", \n \"attrs\": {\n \"eps\": \"2e-05\", \n \"fix_gamma\": \"False\", \n \"momentum\": \"0.9\"\n }, \n \"inputs\": []\n }, \n {\n \"op\": \"null\", \n \"name\": \"rf_c2_lateral_bn_moving_mean\", \n \"attrs\": {\n \"__init__\": \"[\\\"zero\\\", {}]\", \n \"eps\": \"2e-05\", \n \"fix_gamma\": \"False\", \n \"momentum\": \"0.9\"\n }, \n \"inputs\": []\n }, \n {\n \"op\": \"null\", \n \"name\": \"rf_c2_lateral_bn_moving_var\", \n \"attrs\": {\n \"__init__\": \"[\\\"one\\\", {}]\", \n \"eps\": \"2e-05\", \n \"fix_gamma\": \"False\", \n \"momentum\": \"0.9\"\n }, \n \"inputs\": []\n }, \n {\n \"op\": \"BatchNorm\", \n \"name\": \"rf_c2_lateral_bn\", \n \"attrs\": {\n \"eps\": \"2e-05\", \n \"fix_gamma\": \"False\", \n \"momentum\": \"0.9\"\n }, \n \"inputs\": [[284, 0, 0], [285, 0, 0], [286, 0, 0], [287, 0, 1], [288, 0, 1]]\n }, \n {\n \"op\": \"Activation\", \n \"name\": \"rf_c2_lateral_relu\", \n \"attrs\": {\"act_type\": \"relu\"}, \n \"inputs\": [[289, 0, 0]]\n }, \n {\n \"op\": \"UpSampling\", \n \"name\": \"rf_c3_upsampling\", \n \"attrs\": {\n \"num_args\": \"1\", \n \"sample_type\": \"nearest\", \n \"scale\": \"2\", \n \"workspace\": \"512\"\n }, \n \"inputs\": [[225, 0, 0]]\n }, \n {\n \"op\": \"Crop\", \n \"name\": \"crop0\", \n \"attrs\": {\"num_args\": \"2\"}, \n \"inputs\": [[291, 0, 0], [290, 0, 0]]\n }, \n {\n \"op\": \"elemwise_add\", \n \"name\": \"plus0\", \n \"inputs\": [[290, 0, 0], [292, 0, 0]]\n }, \n {\n \"op\": \"null\", \n \"name\": \"rf_c2_aggr_weight\", \n \"attrs\": {\n \"__init__\": \"[\\\"normal\\\", {\\\"sigma\\\": 0.01}]\", \n \"__lr_mult__\": \"1.0\"\n }, \n \"inputs\": []\n }, \n {\n \"op\": \"null\", \n \"name\": \"rf_c2_aggr_bias\", \n \"attrs\": {\n \"__init__\": \"[\\\"constant\\\", {\\\"value\\\": 0.0}]\", \n \"__lr_mult__\": \"2.0\", \n \"__wd_mult__\": \"1.0\"\n }, \n \"inputs\": []\n }, \n {\n \"op\": \"Convolution\", \n \"name\": \"rf_c2_aggr\", \n \"attrs\": {\n \"kernel\": \"(3, 3)\", \n \"num_filter\": \"64\", \n \"pad\": \"(1, 1)\", \n \"stride\": \"(1, 1)\"\n }, \n \"inputs\": [[293, 0, 0], [294, 0, 0], [295, 0, 0]]\n }, \n {\n \"op\": \"null\", \n \"name\": \"rf_c2_aggr_bn_gamma\", \n \"attrs\": {\n \"eps\": \"2e-05\", \n \"fix_gamma\": \"False\", \n \"momentum\": \"0.9\"\n }, \n \"inputs\": []\n }, \n {\n \"op\": \"null\", \n \"name\": \"rf_c2_aggr_bn_beta\", \n \"attrs\": {\n \"eps\": \"2e-05\", \n \"fix_gamma\": \"False\", \n \"momentum\": \"0.9\"\n }, \n \"inputs\": []\n }, \n {\n \"op\": \"null\", \n \"name\": \"rf_c2_aggr_bn_moving_mean\", \n \"attrs\": {\n \"__init__\": \"[\\\"zero\\\", {}]\", \n \"eps\": \"2e-05\", \n \"fix_gamma\": \"False\", \n \"momentum\": \"0.9\"\n }, \n \"inputs\": []\n }, \n {\n \"op\": \"null\", \n \"name\": \"rf_c2_aggr_bn_moving_var\", \n \"attrs\": {\n \"__init__\": \"[\\\"one\\\", {}]\", \n \"eps\": \"2e-05\", \n \"fix_gamma\": \"False\", \n \"momentum\": \"0.9\"\n }, \n \"inputs\": []\n }, \n {\n \"op\": \"BatchNorm\", \n \"name\": \"rf_c2_aggr_bn\", \n \"attrs\": {\n \"eps\": \"2e-05\", \n \"fix_gamma\": \"False\", \n \"momentum\": \"0.9\"\n }, \n \"inputs\": [[296, 0, 0], [297, 0, 0], [298, 0, 0], [299, 0, 1], [300, 0, 1]]\n }, \n {\n \"op\": \"Activation\", \n \"name\": \"rf_c2_aggr_relu\", \n \"attrs\": {\"act_type\": \"relu\"}, \n \"inputs\": [[301, 0, 0]]\n }, \n {\n \"op\": \"null\", \n \"name\": \"rf_c2_det_conv1_weight\", \n \"attrs\": {\n \"__init__\": \"[\\\"normal\\\", {\\\"sigma\\\": 0.01}]\", \n \"__lr_mult__\": \"1.0\"\n }, \n \"inputs\": []\n }, \n {\n \"op\": \"null\", \n \"name\": \"rf_c2_det_conv1_bias\", \n \"attrs\": {\n \"__init__\": \"[\\\"constant\\\", {\\\"value\\\": 0.0}]\", \n \"__lr_mult__\": \"2.0\", \n \"__wd_mult__\": \"0.0\"\n }, \n \"inputs\": []\n }, \n {\n \"op\": \"Convolution\", \n \"name\": \"rf_c2_det_conv1\", \n \"attrs\": {\n \"kernel\": \"(3, 3)\", \n \"num_filter\": \"32\", \n \"pad\": \"(1, 1)\", \n \"stride\": \"(1, 1)\"\n }, \n \"inputs\": [[302, 0, 0], [303, 0, 0], [304, 0, 0]]\n }, \n {\n \"op\": \"null\", \n \"name\": \"rf_c2_det_conv1_bn_gamma\", \n \"attrs\": {\n \"eps\": \"2e-05\", \n \"fix_gamma\": \"False\", \n \"momentum\": \"0.9\"\n }, \n \"inputs\": []\n }, \n {\n \"op\": \"null\", \n \"name\": \"rf_c2_det_conv1_bn_beta\", \n \"attrs\": {\n \"eps\": \"2e-05\", \n \"fix_gamma\": \"False\", \n \"momentum\": \"0.9\"\n }, \n \"inputs\": []\n }, \n {\n \"op\": \"null\", \n \"name\": \"rf_c2_det_conv1_bn_moving_mean\", \n \"attrs\": {\n \"__init__\": \"[\\\"zero\\\", {}]\", \n \"eps\": \"2e-05\", \n \"fix_gamma\": \"False\", \n \"momentum\": \"0.9\"\n }, \n \"inputs\": []\n }, \n {\n \"op\": \"null\", \n \"name\": \"rf_c2_det_conv1_bn_moving_var\", \n \"attrs\": {\n \"__init__\": \"[\\\"one\\\", {}]\", \n \"eps\": \"2e-05\", \n \"fix_gamma\": \"False\", \n \"momentum\": \"0.9\"\n }, \n \"inputs\": []\n }, \n {\n \"op\": \"BatchNorm\", \n \"name\": \"rf_c2_det_conv1_bn\", \n \"attrs\": {\n \"eps\": \"2e-05\", \n \"fix_gamma\": \"False\", \n \"momentum\": \"0.9\"\n }, \n \"inputs\": [[305, 0, 0], [306, 0, 0], [307, 0, 0], [308, 0, 1], [309, 0, 1]]\n }, \n {\n \"op\": \"null\", \n \"name\": \"rf_c2_det_context_conv1_weight\", \n \"attrs\": {\n \"__init__\": \"[\\\"normal\\\", {\\\"sigma\\\": 0.01}]\", \n \"__lr_mult__\": \"1.0\"\n }, \n \"inputs\": []\n }, \n {\n \"op\": \"null\", \n \"name\": \"rf_c2_det_context_conv1_bias\", \n \"attrs\": {\n \"__init__\": \"[\\\"constant\\\", {\\\"value\\\": 0.0}]\", \n \"__lr_mult__\": \"2.0\", \n \"__wd_mult__\": \"0.0\"\n }, \n \"inputs\": []\n }, \n {\n \"op\": \"Convolution\", \n \"name\": \"rf_c2_det_context_conv1\", \n \"attrs\": {\n \"kernel\": \"(3, 3)\", \n \"num_filter\": \"16\", \n \"pad\": \"(1, 1)\", \n \"stride\": \"(1, 1)\"\n }, \n \"inputs\": [[302, 0, 0], [311, 0, 0], [312, 0, 0]]\n }, \n {\n \"op\": \"null\", \n \"name\": \"rf_c2_det_context_conv1_bn_gamma\", \n \"attrs\": {\n \"eps\": \"2e-05\", \n \"fix_gamma\": \"False\", \n \"momentum\": \"0.9\"\n }, \n \"inputs\": []\n }, \n {\n \"op\": \"null\", \n \"name\": \"rf_c2_det_context_conv1_bn_beta\", \n \"attrs\": {\n \"eps\": \"2e-05\", \n \"fix_gamma\": \"False\", \n \"momentum\": \"0.9\"\n }, \n \"inputs\": []\n }, \n {\n \"op\": \"null\", \n \"name\": \"rf_c2_det_context_conv1_bn_moving_mean\", \n \"attrs\": {\n \"__init__\": \"[\\\"zero\\\", {}]\", \n \"eps\": \"2e-05\", \n \"fix_gamma\": \"False\", \n \"momentum\": \"0.9\"\n }, \n \"inputs\": []\n }, \n {\n \"op\": \"null\", \n \"name\": \"rf_c2_det_context_conv1_bn_moving_var\", \n \"attrs\": {\n \"__init__\": \"[\\\"one\\\", {}]\", \n \"eps\": \"2e-05\", \n \"fix_gamma\": \"False\", \n \"momentum\": \"0.9\"\n }, \n \"inputs\": []\n }, \n {\n \"op\": \"BatchNorm\", \n \"name\": \"rf_c2_det_context_conv1_bn\", \n \"attrs\": {\n \"eps\": \"2e-05\", \n \"fix_gamma\": \"False\", \n \"momentum\": \"0.9\"\n }, \n \"inputs\": [[313, 0, 0], [314, 0, 0], [315, 0, 0], [316, 0, 1], [317, 0, 1]]\n }, \n {\n \"op\": \"Activation\", \n \"name\": \"rf_c2_det_context_conv1_relu\", \n \"attrs\": {\"act_type\": \"relu\"}, \n \"inputs\": [[318, 0, 0]]\n }, \n {\n \"op\": \"null\", \n \"name\": \"rf_c2_det_context_conv2_weight\", \n \"attrs\": {\n \"__init__\": \"[\\\"normal\\\", {\\\"sigma\\\": 0.01}]\", \n \"__lr_mult__\": \"1.0\"\n }, \n \"inputs\": []\n }, \n {\n \"op\": \"null\", \n \"name\": \"rf_c2_det_context_conv2_bias\", \n \"attrs\": {\n \"__init__\": \"[\\\"constant\\\", {\\\"value\\\": 0.0}]\", \n \"__lr_mult__\": \"2.0\", \n \"__wd_mult__\": \"0.0\"\n }, \n \"inputs\": []\n }, \n {\n \"op\": \"Convolution\", \n \"name\": \"rf_c2_det_context_conv2\", \n \"attrs\": {\n \"kernel\": \"(3, 3)\", \n \"num_filter\": \"16\", \n \"pad\": \"(1, 1)\", \n \"stride\": \"(1, 1)\"\n }, \n \"inputs\": [[319, 0, 0], [320, 0, 0], [321, 0, 0]]\n }, \n {\n \"op\": \"null\", \n \"name\": \"rf_c2_det_context_conv2_bn_gamma\", \n \"attrs\": {\n \"eps\": \"2e-05\", \n \"fix_gamma\": \"False\", \n \"momentum\": \"0.9\"\n }, \n \"inputs\": []\n }, \n {\n \"op\": \"null\", \n \"name\": \"rf_c2_det_context_conv2_bn_beta\", \n \"attrs\": {\n \"eps\": \"2e-05\", \n \"fix_gamma\": \"False\", \n \"momentum\": \"0.9\"\n }, \n \"inputs\": []\n }, \n {\n \"op\": \"null\", \n \"name\": \"rf_c2_det_context_conv2_bn_moving_mean\", \n \"attrs\": {\n \"__init__\": \"[\\\"zero\\\", {}]\", \n \"eps\": \"2e-05\", \n \"fix_gamma\": \"False\", \n \"momentum\": \"0.9\"\n }, \n \"inputs\": []\n }, \n {\n \"op\": \"null\", \n \"name\": \"rf_c2_det_context_conv2_bn_moving_var\", \n \"attrs\": {\n \"__init__\": \"[\\\"one\\\", {}]\", \n \"eps\": \"2e-05\", \n \"fix_gamma\": \"False\", \n \"momentum\": \"0.9\"\n }, \n \"inputs\": []\n }, \n {\n \"op\": \"BatchNorm\", \n \"name\": \"rf_c2_det_context_conv2_bn\", \n \"attrs\": {\n \"eps\": \"2e-05\", \n \"fix_gamma\": \"False\", \n \"momentum\": \"0.9\"\n }, \n \"inputs\": [[322, 0, 0], [323, 0, 0], [324, 0, 0], [325, 0, 1], [326, 0, 1]]\n }, \n {\n \"op\": \"null\", \n \"name\": \"rf_c2_det_context_conv3_1_weight\", \n \"attrs\": {\n \"__init__\": \"[\\\"normal\\\", {\\\"sigma\\\": 0.01}]\", \n \"__lr_mult__\": \"1.0\"\n }, \n \"inputs\": []\n }, \n {\n \"op\": \"null\", \n \"name\": \"rf_c2_det_context_conv3_1_bias\", \n \"attrs\": {\n \"__init__\": \"[\\\"constant\\\", {\\\"value\\\": 0.0}]\", \n \"__lr_mult__\": \"2.0\", \n \"__wd_mult__\": \"0.0\"\n }, \n \"inputs\": []\n }, \n {\n \"op\": \"Convolution\", \n \"name\": \"rf_c2_det_context_conv3_1\", \n \"attrs\": {\n \"kernel\": \"(3, 3)\", \n \"num_filter\": \"16\", \n \"pad\": \"(1, 1)\", \n \"stride\": \"(1, 1)\"\n }, \n \"inputs\": [[319, 0, 0], [328, 0, 0], [329, 0, 0]]\n }, \n {\n \"op\": \"null\", \n \"name\": \"rf_c2_det_context_conv3_1_bn_gamma\", \n \"attrs\": {\n \"eps\": \"2e-05\", \n \"fix_gamma\": \"False\", \n \"momentum\": \"0.9\"\n }, \n \"inputs\": []\n }, \n {\n \"op\": \"null\", \n \"name\": \"rf_c2_det_context_conv3_1_bn_beta\", \n \"attrs\": {\n \"eps\": \"2e-05\", \n \"fix_gamma\": \"False\", \n \"momentum\": \"0.9\"\n }, \n \"inputs\": []\n }, \n {\n \"op\": \"null\", \n \"name\": \"rf_c2_det_context_conv3_1_bn_moving_mean\", \n \"attrs\": {\n \"__init__\": \"[\\\"zero\\\", {}]\", \n \"eps\": \"2e-05\", \n \"fix_gamma\": \"False\", \n \"momentum\": \"0.9\"\n }, \n \"inputs\": []\n }, \n {\n \"op\": \"null\", \n \"name\": \"rf_c2_det_context_conv3_1_bn_moving_var\", \n \"attrs\": {\n \"__init__\": \"[\\\"one\\\", {}]\", \n \"eps\": \"2e-05\", \n \"fix_gamma\": \"False\", \n \"momentum\": \"0.9\"\n }, \n \"inputs\": []\n }, \n {\n \"op\": \"BatchNorm\", \n \"name\": \"rf_c2_det_context_conv3_1_bn\", \n \"attrs\": {\n \"eps\": \"2e-05\", \n \"fix_gamma\": \"False\", \n \"momentum\": \"0.9\"\n }, \n \"inputs\": [[330, 0, 0], [331, 0, 0], [332, 0, 0], [333, 0, 1], [334, 0, 1]]\n }, \n {\n \"op\": \"Activation\", \n \"name\": \"rf_c2_det_context_conv3_1_relu\", \n \"attrs\": {\"act_type\": \"relu\"}, \n \"inputs\": [[335, 0, 0]]\n }, \n {\n \"op\": \"null\", \n \"name\": \"rf_c2_det_context_conv3_2_weight\", \n \"attrs\": {\n \"__init__\": \"[\\\"normal\\\", {\\\"sigma\\\": 0.01}]\", \n \"__lr_mult__\": \"1.0\"\n }, \n \"inputs\": []\n }, \n {\n \"op\": \"null\", \n \"name\": \"rf_c2_det_context_conv3_2_bias\", \n \"attrs\": {\n \"__init__\": \"[\\\"constant\\\", {\\\"value\\\": 0.0}]\", \n \"__lr_mult__\": \"2.0\", \n \"__wd_mult__\": \"0.0\"\n }, \n \"inputs\": []\n }, \n {\n \"op\": \"Convolution\", \n \"name\": \"rf_c2_det_context_conv3_2\", \n \"attrs\": {\n \"kernel\": \"(3, 3)\", \n \"num_filter\": \"16\", \n \"pad\": \"(1, 1)\", \n \"stride\": \"(1, 1)\"\n }, \n \"inputs\": [[336, 0, 0], [337, 0, 0], [338, 0, 0]]\n }, \n {\n \"op\": \"null\", \n \"name\": \"rf_c2_det_context_conv3_2_bn_gamma\", \n \"attrs\": {\n \"eps\": \"2e-05\", \n \"fix_gamma\": \"False\", \n \"momentum\": \"0.9\"\n }, \n \"inputs\": []\n }, \n {\n \"op\": \"null\", \n \"name\": \"rf_c2_det_context_conv3_2_bn_beta\", \n \"attrs\": {\n \"eps\": \"2e-05\", \n \"fix_gamma\": \"False\", \n \"momentum\": \"0.9\"\n }, \n \"inputs\": []\n }, \n {\n \"op\": \"null\", \n \"name\": \"rf_c2_det_context_conv3_2_bn_moving_mean\", \n \"attrs\": {\n \"__init__\": \"[\\\"zero\\\", {}]\", \n \"eps\": \"2e-05\", \n \"fix_gamma\": \"False\", \n \"momentum\": \"0.9\"\n }, \n \"inputs\": []\n }, \n {\n \"op\": \"null\", \n \"name\": \"rf_c2_det_context_conv3_2_bn_moving_var\", \n \"attrs\": {\n \"__init__\": \"[\\\"one\\\", {}]\", \n \"eps\": \"2e-05\", \n \"fix_gamma\": \"False\", \n \"momentum\": \"0.9\"\n }, \n \"inputs\": []\n }, \n {\n \"op\": \"BatchNorm\", \n \"name\": \"rf_c2_det_context_conv3_2_bn\", \n \"attrs\": {\n \"eps\": \"2e-05\", \n \"fix_gamma\": \"False\", \n \"momentum\": \"0.9\"\n }, \n \"inputs\": [[339, 0, 0], [340, 0, 0], [341, 0, 0], [342, 0, 1], [343, 0, 1]]\n }, \n {\n \"op\": \"Concat\", \n \"name\": \"rf_c2_det_concat\", \n \"attrs\": {\n \"dim\": \"1\", \n \"num_args\": \"3\"\n }, \n \"inputs\": [[310, 0, 0], [327, 0, 0], [344, 0, 0]]\n }, \n {\n \"op\": \"Activation\", \n \"name\": \"rf_c2_det_concat_relu\", \n \"attrs\": {\"act_type\": \"relu\"}, \n \"inputs\": [[345, 0, 0]]\n }, \n {\n \"op\": \"null\", \n \"name\": \"face_rpn_cls_score_stride16_weight\", \n \"attrs\": {\n \"__init__\": \"[\\\"normal\\\", {\\\"sigma\\\": 0.01}]\", \n \"__lr_mult__\": \"1.0\"\n }, \n \"inputs\": []\n }, \n {\n \"op\": \"null\", \n \"name\": \"face_rpn_cls_score_stride16_bias\", \n \"attrs\": {\n \"__init__\": \"[\\\"constant\\\", {\\\"value\\\": 0.0}]\", \n \"__lr_mult__\": \"2.0\", \n \"__wd_mult__\": \"0.0\"\n }, \n \"inputs\": []\n }, \n {\n \"op\": \"Convolution\", \n \"name\": \"face_rpn_cls_score_stride16\", \n \"attrs\": {\n \"kernel\": \"(1, 1)\", \n \"num_filter\": \"4\", \n \"pad\": \"(0, 0)\", \n \"stride\": \"(1, 1)\"\n }, \n \"inputs\": [[346, 0, 0], [347, 0, 0], [348, 0, 0]]\n }, \n {\n \"op\": \"Reshape\", \n \"name\": \"face_rpn_cls_score_reshape_stride16\", \n \"attrs\": {\"shape\": \"(0, 2, -1, 0)\"}, \n \"inputs\": [[349, 0, 0]]\n }, \n {\n \"op\": \"SoftmaxActivation\", \n \"name\": \"face_rpn_cls_prob_stride16\", \n \"attrs\": {\"mode\": \"channel\"}, \n \"inputs\": [[350, 0, 0]]\n }, \n {\n \"op\": \"Reshape\", \n \"name\": \"face_rpn_cls_prob_reshape_stride16\", \n \"attrs\": {\"shape\": \"(0, 4, -1, 0)\"}, \n \"inputs\": [[351, 0, 0]]\n }, \n {\n \"op\": \"null\", \n \"name\": \"face_rpn_bbox_pred_stride16_weight\", \n \"attrs\": {\n \"__init__\": \"[\\\"normal\\\", {\\\"sigma\\\": 0.01}]\", \n \"__lr_mult__\": \"1.0\"\n }, \n \"inputs\": []\n }, \n {\n \"op\": \"null\", \n \"name\": \"face_rpn_bbox_pred_stride16_bias\", \n \"attrs\": {\n \"__init__\": \"[\\\"constant\\\", {\\\"value\\\": 0.0}]\", \n \"__lr_mult__\": \"2.0\", \n \"__wd_mult__\": \"0.0\"\n }, \n \"inputs\": []\n }, \n {\n \"op\": \"Convolution\", \n \"name\": \"face_rpn_bbox_pred_stride16\", \n \"attrs\": {\n \"kernel\": \"(1, 1)\", \n \"num_filter\": \"8\", \n \"pad\": \"(0, 0)\", \n \"stride\": \"(1, 1)\"\n }, \n \"inputs\": [[346, 0, 0], [353, 0, 0], [354, 0, 0]]\n }, \n {\n \"op\": \"null\", \n \"name\": \"face_rpn_landmark_pred_stride16_weight\", \n \"attrs\": {\n \"__init__\": \"[\\\"normal\\\", {\\\"sigma\\\": 0.01}]\", \n \"__lr_mult__\": \"1.0\"\n }, \n \"inputs\": []\n }, \n {\n \"op\": \"null\", \n \"name\": \"face_rpn_landmark_pred_stride16_bias\", \n \"attrs\": {\n \"__init__\": \"[\\\"constant\\\", {\\\"value\\\": 0.0}]\", \n \"__lr_mult__\": \"2.0\", \n \"__wd_mult__\": \"0.0\"\n }, \n \"inputs\": []\n }, \n {\n \"op\": \"Convolution\", \n \"name\": \"face_rpn_landmark_pred_stride16\", \n \"attrs\": {\n \"kernel\": \"(1, 1)\", \n \"num_filter\": \"20\", \n \"pad\": \"(0, 0)\", \n \"stride\": \"(1, 1)\"\n }, \n \"inputs\": [[346, 0, 0], [356, 0, 0], [357, 0, 0]]\n }, \n {\n \"op\": \"null\", \n \"name\": \"rf_c1_red_conv_weight\", \n \"attrs\": {\n \"__init__\": \"[\\\"normal\\\", {\\\"sigma\\\": 0.01}]\", \n \"__lr_mult__\": \"1.0\"\n }, \n \"inputs\": []\n }, \n {\n \"op\": \"null\", \n \"name\": \"rf_c1_red_conv_bias\", \n \"attrs\": {\n \"__init__\": \"[\\\"constant\\\", {\\\"value\\\": 0.0}]\", \n \"__lr_mult__\": \"2.0\", \n \"__wd_mult__\": \"1.0\"\n }, \n \"inputs\": []\n }, \n {\n \"op\": \"Convolution\", \n \"name\": \"rf_c1_red_conv\", \n \"attrs\": {\n \"kernel\": \"(1, 1)\", \n \"num_filter\": \"64\", \n \"pad\": \"(0, 0)\", \n \"stride\": \"(1, 1)\"\n }, \n \"inputs\": [[88, 0, 0], [359, 0, 0], [360, 0, 0]]\n }, \n {\n \"op\": \"null\", \n \"name\": \"rf_c1_red_conv_bn_gamma\", \n \"attrs\": {\n \"eps\": \"2e-05\", \n \"fix_gamma\": \"False\", \n \"momentum\": \"0.9\"\n }, \n \"inputs\": []\n }, \n {\n \"op\": \"null\", \n \"name\": \"rf_c1_red_conv_bn_beta\", \n \"attrs\": {\n \"eps\": \"2e-05\", \n \"fix_gamma\": \"False\", \n \"momentum\": \"0.9\"\n }, \n \"inputs\": []\n }, \n {\n \"op\": \"null\", \n \"name\": \"rf_c1_red_conv_bn_moving_mean\", \n \"attrs\": {\n \"__init__\": \"[\\\"zero\\\", {}]\", \n \"eps\": \"2e-05\", \n \"fix_gamma\": \"False\", \n \"momentum\": \"0.9\"\n }, \n \"inputs\": []\n }, \n {\n \"op\": \"null\", \n \"name\": \"rf_c1_red_conv_bn_moving_var\", \n \"attrs\": {\n \"__init__\": \"[\\\"one\\\", {}]\", \n \"eps\": \"2e-05\", \n \"fix_gamma\": \"False\", \n \"momentum\": \"0.9\"\n }, \n \"inputs\": []\n }, \n {\n \"op\": \"BatchNorm\", \n \"name\": \"rf_c1_red_conv_bn\", \n \"attrs\": {\n \"eps\": \"2e-05\", \n \"fix_gamma\": \"False\", \n \"momentum\": \"0.9\"\n }, \n \"inputs\": [[361, 0, 0], [362, 0, 0], [363, 0, 0], [364, 0, 1], [365, 0, 1]]\n }, \n {\n \"op\": \"Activation\", \n \"name\": \"rf_c1_red_conv_relu\", \n \"attrs\": {\"act_type\": \"relu\"}, \n \"inputs\": [[366, 0, 0]]\n }, \n {\n \"op\": \"UpSampling\", \n \"name\": \"rf_c2_upsampling\", \n \"attrs\": {\n \"num_args\": \"1\", \n \"sample_type\": \"nearest\", \n \"scale\": \"2\", \n \"workspace\": \"512\"\n }, \n \"inputs\": [[302, 0, 0]]\n }, \n {\n \"op\": \"Crop\", \n \"name\": \"crop1\", \n \"attrs\": {\"num_args\": \"2\"}, \n \"inputs\": [[368, 0, 0], [367, 0, 0]]\n }, \n {\n \"op\": \"elemwise_add\", \n \"name\": \"plus1\", \n \"inputs\": [[367, 0, 0], [369, 0, 0]]\n }, \n {\n \"op\": \"null\", \n \"name\": \"rf_c1_aggr_weight\", \n \"attrs\": {\n \"__init__\": \"[\\\"normal\\\", {\\\"sigma\\\": 0.01}]\", \n \"__lr_mult__\": \"1.0\"\n }, \n \"inputs\": []\n }, \n {\n \"op\": \"null\", \n \"name\": \"rf_c1_aggr_bias\", \n \"attrs\": {\n \"__init__\": \"[\\\"constant\\\", {\\\"value\\\": 0.0}]\", \n \"__lr_mult__\": \"2.0\", \n \"__wd_mult__\": \"1.0\"\n }, \n \"inputs\": []\n }, \n {\n \"op\": \"Convolution\", \n \"name\": \"rf_c1_aggr\", \n \"attrs\": {\n \"kernel\": \"(3, 3)\", \n \"num_filter\": \"64\", \n \"pad\": \"(1, 1)\", \n \"stride\": \"(1, 1)\"\n }, \n \"inputs\": [[370, 0, 0], [371, 0, 0], [372, 0, 0]]\n }, \n {\n \"op\": \"null\", \n \"name\": \"rf_c1_aggr_bn_gamma\", \n \"attrs\": {\n \"eps\": \"2e-05\", \n \"fix_gamma\": \"False\", \n \"momentum\": \"0.9\"\n }, \n \"inputs\": []\n }, \n {\n \"op\": \"null\", \n \"name\": \"rf_c1_aggr_bn_beta\", \n \"attrs\": {\n \"eps\": \"2e-05\", \n \"fix_gamma\": \"False\", \n \"momentum\": \"0.9\"\n }, \n \"inputs\": []\n }, \n {\n \"op\": \"null\", \n \"name\": \"rf_c1_aggr_bn_moving_mean\", \n \"attrs\": {\n \"__init__\": \"[\\\"zero\\\", {}]\", \n \"eps\": \"2e-05\", \n \"fix_gamma\": \"False\", \n \"momentum\": \"0.9\"\n }, \n \"inputs\": []\n }, \n {\n \"op\": \"null\", \n \"name\": \"rf_c1_aggr_bn_moving_var\", \n \"attrs\": {\n \"__init__\": \"[\\\"one\\\", {}]\", \n \"eps\": \"2e-05\", \n \"fix_gamma\": \"False\", \n \"momentum\": \"0.9\"\n }, \n \"inputs\": []\n }, \n {\n \"op\": \"BatchNorm\", \n \"name\": \"rf_c1_aggr_bn\", \n \"attrs\": {\n \"eps\": \"2e-05\", \n \"fix_gamma\": \"False\", \n \"momentum\": \"0.9\"\n }, \n \"inputs\": [[373, 0, 0], [374, 0, 0], [375, 0, 0], [376, 0, 1], [377, 0, 1]]\n }, \n {\n \"op\": \"Activation\", \n \"name\": \"rf_c1_aggr_relu\", \n \"attrs\": {\"act_type\": \"relu\"}, \n \"inputs\": [[378, 0, 0]]\n }, \n {\n \"op\": \"null\", \n \"name\": \"rf_c1_det_conv1_weight\", \n \"attrs\": {\n \"__init__\": \"[\\\"normal\\\", {\\\"sigma\\\": 0.01}]\", \n \"__lr_mult__\": \"1.0\"\n }, \n \"inputs\": []\n }, \n {\n \"op\": \"null\", \n \"name\": \"rf_c1_det_conv1_bias\", \n \"attrs\": {\n \"__init__\": \"[\\\"constant\\\", {\\\"value\\\": 0.0}]\", \n \"__lr_mult__\": \"2.0\", \n \"__wd_mult__\": \"0.0\"\n }, \n \"inputs\": []\n }, \n {\n \"op\": \"Convolution\", \n \"name\": \"rf_c1_det_conv1\", \n \"attrs\": {\n \"kernel\": \"(3, 3)\", \n \"num_filter\": \"32\", \n \"pad\": \"(1, 1)\", \n \"stride\": \"(1, 1)\"\n }, \n \"inputs\": [[379, 0, 0], [380, 0, 0], [381, 0, 0]]\n }, \n {\n \"op\": \"null\", \n \"name\": \"rf_c1_det_conv1_bn_gamma\", \n \"attrs\": {\n \"eps\": \"2e-05\", \n \"fix_gamma\": \"False\", \n \"momentum\": \"0.9\"\n }, \n \"inputs\": []\n }, \n {\n \"op\": \"null\", \n \"name\": \"rf_c1_det_conv1_bn_beta\", \n \"attrs\": {\n \"eps\": \"2e-05\", \n \"fix_gamma\": \"False\", \n \"momentum\": \"0.9\"\n }, \n \"inputs\": []\n }, \n {\n \"op\": \"null\", \n \"name\": \"rf_c1_det_conv1_bn_moving_mean\", \n \"attrs\": {\n \"__init__\": \"[\\\"zero\\\", {}]\", \n \"eps\": \"2e-05\", \n \"fix_gamma\": \"False\", \n \"momentum\": \"0.9\"\n }, \n \"inputs\": []\n }, \n {\n \"op\": \"null\", \n \"name\": \"rf_c1_det_conv1_bn_moving_var\", \n \"attrs\": {\n \"__init__\": \"[\\\"one\\\", {}]\", \n \"eps\": \"2e-05\", \n \"fix_gamma\": \"False\", \n \"momentum\": \"0.9\"\n }, \n \"inputs\": []\n }, \n {\n \"op\": \"BatchNorm\", \n \"name\": \"rf_c1_det_conv1_bn\", \n \"attrs\": {\n \"eps\": \"2e-05\", \n \"fix_gamma\": \"False\", \n \"momentum\": \"0.9\"\n }, \n \"inputs\": [[382, 0, 0], [383, 0, 0], [384, 0, 0], [385, 0, 1], [386, 0, 1]]\n }, \n {\n \"op\": \"null\", \n \"name\": \"rf_c1_det_context_conv1_weight\", \n \"attrs\": {\n \"__init__\": \"[\\\"normal\\\", {\\\"sigma\\\": 0.01}]\", \n \"__lr_mult__\": \"1.0\"\n }, \n \"inputs\": []\n }, \n {\n \"op\": \"null\", \n \"name\": \"rf_c1_det_context_conv1_bias\", \n \"attrs\": {\n \"__init__\": \"[\\\"constant\\\", {\\\"value\\\": 0.0}]\", \n \"__lr_mult__\": \"2.0\", \n \"__wd_mult__\": \"0.0\"\n }, \n \"inputs\": []\n }, \n {\n \"op\": \"Convolution\", \n \"name\": \"rf_c1_det_context_conv1\", \n \"attrs\": {\n \"kernel\": \"(3, 3)\", \n \"num_filter\": \"16\", \n \"pad\": \"(1, 1)\", \n \"stride\": \"(1, 1)\"\n }, \n \"inputs\": [[379, 0, 0], [388, 0, 0], [389, 0, 0]]\n }, \n {\n \"op\": \"null\", \n \"name\": \"rf_c1_det_context_conv1_bn_gamma\", \n \"attrs\": {\n \"eps\": \"2e-05\", \n \"fix_gamma\": \"False\", \n \"momentum\": \"0.9\"\n }, \n \"inputs\": []\n }, \n {\n \"op\": \"null\", \n \"name\": \"rf_c1_det_context_conv1_bn_beta\", \n \"attrs\": {\n \"eps\": \"2e-05\", \n \"fix_gamma\": \"False\", \n \"momentum\": \"0.9\"\n }, \n \"inputs\": []\n }, \n {\n \"op\": \"null\", \n \"name\": \"rf_c1_det_context_conv1_bn_moving_mean\", \n \"attrs\": {\n \"__init__\": \"[\\\"zero\\\", {}]\", \n \"eps\": \"2e-05\", \n \"fix_gamma\": \"False\", \n \"momentum\": \"0.9\"\n }, \n \"inputs\": []\n }, \n {\n \"op\": \"null\", \n \"name\": \"rf_c1_det_context_conv1_bn_moving_var\", \n \"attrs\": {\n \"__init__\": \"[\\\"one\\\", {}]\", \n \"eps\": \"2e-05\", \n \"fix_gamma\": \"False\", \n \"momentum\": \"0.9\"\n }, \n \"inputs\": []\n }, \n {\n \"op\": \"BatchNorm\", \n \"name\": \"rf_c1_det_context_conv1_bn\", \n \"attrs\": {\n \"eps\": \"2e-05\", \n \"fix_gamma\": \"False\", \n \"momentum\": \"0.9\"\n }, \n \"inputs\": [[390, 0, 0], [391, 0, 0], [392, 0, 0], [393, 0, 1], [394, 0, 1]]\n }, \n {\n \"op\": \"Activation\", \n \"name\": \"rf_c1_det_context_conv1_relu\", \n \"attrs\": {\"act_type\": \"relu\"}, \n \"inputs\": [[395, 0, 0]]\n }, \n {\n \"op\": \"null\", \n \"name\": \"rf_c1_det_context_conv2_weight\", \n \"attrs\": {\n \"__init__\": \"[\\\"normal\\\", {\\\"sigma\\\": 0.01}]\", \n \"__lr_mult__\": \"1.0\"\n }, \n \"inputs\": []\n }, \n {\n \"op\": \"null\", \n \"name\": \"rf_c1_det_context_conv2_bias\", \n \"attrs\": {\n \"__init__\": \"[\\\"constant\\\", {\\\"value\\\": 0.0}]\", \n \"__lr_mult__\": \"2.0\", \n \"__wd_mult__\": \"0.0\"\n }, \n \"inputs\": []\n }, \n {\n \"op\": \"Convolution\", \n \"name\": \"rf_c1_det_context_conv2\", \n \"attrs\": {\n \"kernel\": \"(3, 3)\", \n \"num_filter\": \"16\", \n \"pad\": \"(1, 1)\", \n \"stride\": \"(1, 1)\"\n }, \n \"inputs\": [[396, 0, 0], [397, 0, 0], [398, 0, 0]]\n }, \n {\n \"op\": \"null\", \n \"name\": \"rf_c1_det_context_conv2_bn_gamma\", \n \"attrs\": {\n \"eps\": \"2e-05\", \n \"fix_gamma\": \"False\", \n \"momentum\": \"0.9\"\n }, \n \"inputs\": []\n }, \n {\n \"op\": \"null\", \n \"name\": \"rf_c1_det_context_conv2_bn_beta\", \n \"attrs\": {\n \"eps\": \"2e-05\", \n \"fix_gamma\": \"False\", \n \"momentum\": \"0.9\"\n }, \n \"inputs\": []\n }, \n {\n \"op\": \"null\", \n \"name\": \"rf_c1_det_context_conv2_bn_moving_mean\", \n \"attrs\": {\n \"__init__\": \"[\\\"zero\\\", {}]\", \n \"eps\": \"2e-05\", \n \"fix_gamma\": \"False\", \n \"momentum\": \"0.9\"\n }, \n \"inputs\": []\n }, \n {\n \"op\": \"null\", \n \"name\": \"rf_c1_det_context_conv2_bn_moving_var\", \n \"attrs\": {\n \"__init__\": \"[\\\"one\\\", {}]\", \n \"eps\": \"2e-05\", \n \"fix_gamma\": \"False\", \n \"momentum\": \"0.9\"\n }, \n \"inputs\": []\n }, \n {\n \"op\": \"BatchNorm\", \n \"name\": \"rf_c1_det_context_conv2_bn\", \n \"attrs\": {\n \"eps\": \"2e-05\", \n \"fix_gamma\": \"False\", \n \"momentum\": \"0.9\"\n }, \n \"inputs\": [[399, 0, 0], [400, 0, 0], [401, 0, 0], [402, 0, 1], [403, 0, 1]]\n }, \n {\n \"op\": \"null\", \n \"name\": \"rf_c1_det_context_conv3_1_weight\", \n \"attrs\": {\n \"__init__\": \"[\\\"normal\\\", {\\\"sigma\\\": 0.01}]\", \n \"__lr_mult__\": \"1.0\"\n }, \n \"inputs\": []\n }, \n {\n \"op\": \"null\", \n \"name\": \"rf_c1_det_context_conv3_1_bias\", \n \"attrs\": {\n \"__init__\": \"[\\\"constant\\\", {\\\"value\\\": 0.0}]\", \n \"__lr_mult__\": \"2.0\", \n \"__wd_mult__\": \"0.0\"\n }, \n \"inputs\": []\n }, \n {\n \"op\": \"Convolution\", \n \"name\": \"rf_c1_det_context_conv3_1\", \n \"attrs\": {\n \"kernel\": \"(3, 3)\", \n \"num_filter\": \"16\", \n \"pad\": \"(1, 1)\", \n \"stride\": \"(1, 1)\"\n }, \n \"inputs\": [[396, 0, 0], [405, 0, 0], [406, 0, 0]]\n }, \n {\n \"op\": \"null\", \n \"name\": \"rf_c1_det_context_conv3_1_bn_gamma\", \n \"attrs\": {\n \"eps\": \"2e-05\", \n \"fix_gamma\": \"False\", \n \"momentum\": \"0.9\"\n }, \n \"inputs\": []\n }, \n {\n \"op\": \"null\", \n \"name\": \"rf_c1_det_context_conv3_1_bn_beta\", \n \"attrs\": {\n \"eps\": \"2e-05\", \n \"fix_gamma\": \"False\", \n \"momentum\": \"0.9\"\n }, \n \"inputs\": []\n }, \n {\n \"op\": \"null\", \n \"name\": \"rf_c1_det_context_conv3_1_bn_moving_mean\", \n \"attrs\": {\n \"__init__\": \"[\\\"zero\\\", {}]\", \n \"eps\": \"2e-05\", \n \"fix_gamma\": \"False\", \n \"momentum\": \"0.9\"\n }, \n \"inputs\": []\n }, \n {\n \"op\": \"null\", \n \"name\": \"rf_c1_det_context_conv3_1_bn_moving_var\", \n \"attrs\": {\n \"__init__\": \"[\\\"one\\\", {}]\", \n \"eps\": \"2e-05\", \n \"fix_gamma\": \"False\", \n \"momentum\": \"0.9\"\n }, \n \"inputs\": []\n }, \n {\n \"op\": \"BatchNorm\", \n \"name\": \"rf_c1_det_context_conv3_1_bn\", \n \"attrs\": {\n \"eps\": \"2e-05\", \n \"fix_gamma\": \"False\", \n \"momentum\": \"0.9\"\n }, \n \"inputs\": [[407, 0, 0], [408, 0, 0], [409, 0, 0], [410, 0, 1], [411, 0, 1]]\n }, \n {\n \"op\": \"Activation\", \n \"name\": \"rf_c1_det_context_conv3_1_relu\", \n \"attrs\": {\"act_type\": \"relu\"}, \n \"inputs\": [[412, 0, 0]]\n }, \n {\n \"op\": \"null\", \n \"name\": \"rf_c1_det_context_conv3_2_weight\", \n \"attrs\": {\n \"__init__\": \"[\\\"normal\\\", {\\\"sigma\\\": 0.01}]\", \n \"__lr_mult__\": \"1.0\"\n }, \n \"inputs\": []\n }, \n {\n \"op\": \"null\", \n \"name\": \"rf_c1_det_context_conv3_2_bias\", \n \"attrs\": {\n \"__init__\": \"[\\\"constant\\\", {\\\"value\\\": 0.0}]\", \n \"__lr_mult__\": \"2.0\", \n \"__wd_mult__\": \"0.0\"\n }, \n \"inputs\": []\n }, \n {\n \"op\": \"Convolution\", \n \"name\": \"rf_c1_det_context_conv3_2\", \n \"attrs\": {\n \"kernel\": \"(3, 3)\", \n \"num_filter\": \"16\", \n \"pad\": \"(1, 1)\", \n \"stride\": \"(1, 1)\"\n }, \n \"inputs\": [[413, 0, 0], [414, 0, 0], [415, 0, 0]]\n }, \n {\n \"op\": \"null\", \n \"name\": \"rf_c1_det_context_conv3_2_bn_gamma\", \n \"attrs\": {\n \"eps\": \"2e-05\", \n \"fix_gamma\": \"False\", \n \"momentum\": \"0.9\"\n }, \n \"inputs\": []\n }, \n {\n \"op\": \"null\", \n \"name\": \"rf_c1_det_context_conv3_2_bn_beta\", \n \"attrs\": {\n \"eps\": \"2e-05\", \n \"fix_gamma\": \"False\", \n \"momentum\": \"0.9\"\n }, \n \"inputs\": []\n }, \n {\n \"op\": \"null\", \n \"name\": \"rf_c1_det_context_conv3_2_bn_moving_mean\", \n \"attrs\": {\n \"__init__\": \"[\\\"zero\\\", {}]\", \n \"eps\": \"2e-05\", \n \"fix_gamma\": \"False\", \n \"momentum\": \"0.9\"\n }, \n \"inputs\": []\n }, \n {\n \"op\": \"null\", \n \"name\": \"rf_c1_det_context_conv3_2_bn_moving_var\", \n \"attrs\": {\n \"__init__\": \"[\\\"one\\\", {}]\", \n \"eps\": \"2e-05\", \n \"fix_gamma\": \"False\", \n \"momentum\": \"0.9\"\n }, \n \"inputs\": []\n }, \n {\n \"op\": \"BatchNorm\", \n \"name\": \"rf_c1_det_context_conv3_2_bn\", \n \"attrs\": {\n \"eps\": \"2e-05\", \n \"fix_gamma\": \"False\", \n \"momentum\": \"0.9\"\n }, \n \"inputs\": [[416, 0, 0], [417, 0, 0], [418, 0, 0], [419, 0, 1], [420, 0, 1]]\n }, \n {\n \"op\": \"Concat\", \n \"name\": \"rf_c1_det_concat\", \n \"attrs\": {\n \"dim\": \"1\", \n \"num_args\": \"3\"\n }, \n \"inputs\": [[387, 0, 0], [404, 0, 0], [421, 0, 0]]\n }, \n {\n \"op\": \"Activation\", \n \"name\": \"rf_c1_det_concat_relu\", \n \"attrs\": {\"act_type\": \"relu\"}, \n \"inputs\": [[422, 0, 0]]\n }, \n {\n \"op\": \"null\", \n \"name\": \"face_rpn_cls_score_stride8_weight\", \n \"attrs\": {\n \"__init__\": \"[\\\"normal\\\", {\\\"sigma\\\": 0.01}]\", \n \"__lr_mult__\": \"1.0\"\n }, \n \"inputs\": []\n }, \n {\n \"op\": \"null\", \n \"name\": \"face_rpn_cls_score_stride8_bias\", \n \"attrs\": {\n \"__init__\": \"[\\\"constant\\\", {\\\"value\\\": 0.0}]\", \n \"__lr_mult__\": \"2.0\", \n \"__wd_mult__\": \"0.0\"\n }, \n \"inputs\": []\n }, \n {\n \"op\": \"Convolution\", \n \"name\": \"face_rpn_cls_score_stride8\", \n \"attrs\": {\n \"kernel\": \"(1, 1)\", \n \"num_filter\": \"4\", \n \"pad\": \"(0, 0)\", \n \"stride\": \"(1, 1)\"\n }, \n \"inputs\": [[423, 0, 0], [424, 0, 0], [425, 0, 0]]\n }, \n {\n \"op\": \"Reshape\", \n \"name\": \"face_rpn_cls_score_reshape_stride8\", \n \"attrs\": {\"shape\": \"(0, 2, -1, 0)\"}, \n \"inputs\": [[426, 0, 0]]\n }, \n {\n \"op\": \"SoftmaxActivation\", \n \"name\": \"face_rpn_cls_prob_stride8\", \n \"attrs\": {\"mode\": \"channel\"}, \n \"inputs\": [[427, 0, 0]]\n }, \n {\n \"op\": \"Reshape\", \n \"name\": \"face_rpn_cls_prob_reshape_stride8\", \n \"attrs\": {\"shape\": \"(0, 4, -1, 0)\"}, \n \"inputs\": [[428, 0, 0]]\n }, \n {\n \"op\": \"null\", \n \"name\": \"face_rpn_bbox_pred_stride8_weight\", \n \"attrs\": {\n \"__init__\": \"[\\\"normal\\\", {\\\"sigma\\\": 0.01}]\", \n \"__lr_mult__\": \"1.0\"\n }, \n \"inputs\": []\n }, \n {\n \"op\": \"null\", \n \"name\": \"face_rpn_bbox_pred_stride8_bias\", \n \"attrs\": {\n \"__init__\": \"[\\\"constant\\\", {\\\"value\\\": 0.0}]\", \n \"__lr_mult__\": \"2.0\", \n \"__wd_mult__\": \"0.0\"\n }, \n \"inputs\": []\n }, \n {\n \"op\": \"Convolution\", \n \"name\": \"face_rpn_bbox_pred_stride8\", \n \"attrs\": {\n \"kernel\": \"(1, 1)\", \n \"num_filter\": \"8\", \n \"pad\": \"(0, 0)\", \n \"stride\": \"(1, 1)\"\n }, \n \"inputs\": [[423, 0, 0], [430, 0, 0], [431, 0, 0]]\n }, \n {\n \"op\": \"null\", \n \"name\": \"face_rpn_landmark_pred_stride8_weight\", \n \"attrs\": {\n \"__init__\": \"[\\\"normal\\\", {\\\"sigma\\\": 0.01}]\", \n \"__lr_mult__\": \"1.0\"\n }, \n \"inputs\": []\n }, \n {\n \"op\": \"null\", \n \"name\": \"face_rpn_landmark_pred_stride8_bias\", \n \"attrs\": {\n \"__init__\": \"[\\\"constant\\\", {\\\"value\\\": 0.0}]\", \n \"__lr_mult__\": \"2.0\", \n \"__wd_mult__\": \"0.0\"\n }, \n \"inputs\": []\n }, \n {\n \"op\": \"Convolution\", \n \"name\": \"face_rpn_landmark_pred_stride8\", \n \"attrs\": {\n \"kernel\": \"(1, 1)\", \n \"num_filter\": \"20\", \n \"pad\": \"(0, 0)\", \n \"stride\": \"(1, 1)\"\n }, \n \"inputs\": [[423, 0, 0], [433, 0, 0], [434, 0, 0]]\n }\n ], \n \"arg_nodes\": [\n 0, \n 1, \n 3, \n 4, \n 5, \n 6, \n 9, \n 11, \n 12, \n 13, \n 14, \n 17, \n 19, \n 20, \n 21, \n 22, \n 25, \n 27, \n 28, \n 29, \n 30, \n 33, \n 35, \n 36, \n 37, \n 38, \n 41, \n 43, \n 44, \n 45, \n 46, \n 49, \n 51, \n 52, \n 53, \n 54, \n 57, \n 59, \n 60, \n 61, \n 62, \n 65, \n 67, \n 68, \n 69, \n 70, \n 73, \n 75, \n 76, \n 77, \n 78, \n 81, \n 83, \n 84, \n 85, \n 86, \n 89, \n 91, \n 92, \n 93, \n 94, \n 97, \n 99, \n 100, \n 101, \n 102, \n 105, \n 107, \n 108, \n 109, \n 110, \n 113, \n 115, \n 116, \n 117, \n 118, \n 121, \n 123, \n 124, \n 125, \n 126, \n 129, \n 131, \n 132, \n 133, \n 134, \n 137, \n 139, \n 140, \n 141, \n 142, \n 145, \n 147, \n 148, \n 149, \n 150, \n 153, \n 155, \n 156, \n 157, \n 158, \n 161, \n 163, \n 164, \n 165, \n 166, \n 169, \n 171, \n 172, \n 173, \n 174, \n 177, \n 179, \n 180, \n 181, \n 182, \n 185, \n 187, \n 188, \n 189, \n 190, \n 193, \n 195, \n 196, \n 197, \n 198, \n 201, \n 203, \n 204, \n 205, \n 206, \n 209, \n 211, \n 212, \n 213, \n 214, \n 217, \n 218, \n 220, \n 221, \n 222, \n 223, \n 226, \n 227, \n 229, \n 230, \n 231, \n 232, \n 234, \n 235, \n 237, \n 238, \n 239, \n 240, \n 243, \n 244, \n 246, \n 247, \n 248, \n 249, \n 251, \n 252, \n 254, \n 255, \n 256, \n 257, \n 260, \n 261, \n 263, \n 264, \n 265, \n 266, \n 270, \n 271, \n 276, \n 277, \n 279, \n 280, \n 282, \n 283, \n 285, \n 286, \n 287, \n 288, \n 294, \n 295, \n 297, \n 298, \n 299, \n 300, \n 303, \n 304, \n 306, \n 307, \n 308, \n 309, \n 311, \n 312, \n 314, \n 315, \n 316, \n 317, \n 320, \n 321, \n 323, \n 324, \n 325, \n 326, \n 328, \n 329, \n 331, \n 332, \n 333, \n 334, \n 337, \n 338, \n 340, \n 341, \n 342, \n 343, \n 347, \n 348, \n 353, \n 354, \n 356, \n 357, \n 359, \n 360, \n 362, \n 363, \n 364, \n 365, \n 371, \n 372, \n 374, \n 375, \n 376, \n 377, \n 380, \n 381, \n 383, \n 384, \n 385, \n 386, \n 388, \n 389, \n 391, \n 392, \n 393, \n 394, \n 397, \n 398, \n 400, \n 401, \n 402, \n 403, \n 405, \n 406, \n 408, \n 409, \n 410, \n 411, \n 414, \n 415, \n 417, \n 418, \n 419, \n 420, \n 424, \n 425, \n 430, \n 431, \n 433, \n 434\n ], \n \"node_row_ptr\": [\n 0, \n 1, \n 2, \n 3, \n 4, \n 5, \n 6, \n 7, \n 10, \n 11, \n 12, \n 13, \n 14, \n 15, \n 16, \n 17, \n 20, \n 21, \n 22, \n 23, \n 24, \n 25, \n 26, \n 27, \n 30, \n 31, \n 32, \n 33, \n 34, \n 35, \n 36, \n 37, \n 40, \n 41, \n 42, \n 43, \n 44, \n 45, \n 46, \n 47, \n 50, \n 51, \n 52, \n 53, \n 54, \n 55, \n 56, \n 57, \n 60, \n 61, \n 62, \n 63, \n 64, \n 65, \n 66, \n 67, \n 70, \n 71, \n 72, \n 73, \n 74, \n 75, \n 76, \n 77, \n 80, \n 81, \n 82, \n 83, \n 84, \n 85, \n 86, \n 87, \n 90, \n 91, \n 92, \n 93, \n 94, \n 95, \n 96, \n 97, \n 100, \n 101, \n 102, \n 103, \n 104, \n 105, \n 106, \n 107, \n 110, \n 111, \n 112, \n 113, \n 114, \n 115, \n 116, \n 117, \n 120, \n 121, \n 122, \n 123, \n 124, \n 125, \n 126, \n 127, \n 130, \n 131, \n 132, \n 133, \n 134, \n 135, \n 136, \n 137, \n 140, \n 141, \n 142, \n 143, \n 144, \n 145, \n 146, \n 147, \n 150, \n 151, \n 152, \n 153, \n 154, \n 155, \n 156, \n 157, \n 160, \n 161, \n 162, \n 163, \n 164, \n 165, \n 166, \n 167, \n 170, \n 171, \n 172, \n 173, \n 174, \n 175, \n 176, \n 177, \n 180, \n 181, \n 182, \n 183, \n 184, \n 185, \n 186, \n 187, \n 190, \n 191, \n 192, \n 193, \n 194, \n 195, \n 196, \n 197, \n 200, \n 201, \n 202, \n 203, \n 204, \n 205, \n 206, \n 207, \n 210, \n 211, \n 212, \n 213, \n 214, \n 215, \n 216, \n 217, \n 220, \n 221, \n 222, \n 223, \n 224, \n 225, \n 226, \n 227, \n 230, \n 231, \n 232, \n 233, \n 234, \n 235, \n 236, \n 237, \n 240, \n 241, \n 242, \n 243, \n 244, \n 245, \n 246, \n 247, \n 250, \n 251, \n 252, \n 253, \n 254, \n 255, \n 256, \n 257, \n 260, \n 261, \n 262, \n 263, \n 264, \n 265, \n 266, \n 267, \n 270, \n 271, \n 272, \n 273, \n 274, \n 275, \n 276, \n 277, \n 278, \n 281, \n 282, \n 283, \n 284, \n 285, \n 286, \n 287, \n 288, \n 289, \n 292, \n 293, \n 294, \n 295, \n 296, \n 297, \n 298, \n 299, \n 302, \n 303, \n 304, \n 305, \n 306, \n 307, \n 308, \n 309, \n 310, \n 313, \n 314, \n 315, \n 316, \n 317, \n 318, \n 319, \n 320, \n 323, \n 324, \n 325, \n 326, \n 327, \n 328, \n 329, \n 330, \n 331, \n 334, \n 335, \n 336, \n 337, \n 338, \n 339, \n 340, \n 341, \n 342, \n 343, \n 344, \n 345, \n 346, \n 347, \n 348, \n 349, \n 350, \n 351, \n 352, \n 353, \n 354, \n 355, \n 358, \n 359, \n 360, \n 361, \n 362, \n 363, \n 364, \n 365, \n 366, \n 367, \n 368, \n 369, \n 372, \n 373, \n 374, \n 375, \n 376, \n 377, \n 378, \n 379, \n 380, \n 383, \n 384, \n 385, \n 386, \n 387, \n 388, \n 389, \n 390, \n 393, \n 394, \n 395, \n 396, \n 397, \n 398, \n 399, \n 400, \n 401, \n 404, \n 405, \n 406, \n 407, \n 408, \n 409, \n 410, \n 411, \n 414, \n 415, \n 416, \n 417, \n 418, \n 419, \n 420, \n 421, \n 422, \n 425, \n 426, \n 427, \n 428, \n 429, \n 430, \n 431, \n 432, \n 433, \n 434, \n 435, \n 436, \n 437, \n 438, \n 439, \n 440, \n 441, \n 442, \n 443, \n 444, \n 445, \n 446, \n 449, \n 450, \n 451, \n 452, \n 453, \n 454, \n 455, \n 456, \n 457, \n 458, \n 459, \n 460, \n 463, \n 464, \n 465, \n 466, \n 467, \n 468, \n 469, \n 470, \n 471, \n 474, \n 475, \n 476, \n 477, \n 478, \n 479, \n 480, \n 481, \n 484, \n 485, \n 486, \n 487, \n 488, \n 489, \n 490, \n 491, \n 492, \n 495, \n 496, \n 497, \n 498, \n 499, \n 500, \n 501, \n 502, \n 505, \n 506, \n 507, \n 508, \n 509, \n 510, \n 511, \n 512, \n 513, \n 516, \n 517, \n 518, \n 519, \n 520, \n 521, \n 522, \n 523, \n 524, \n 525, \n 526, \n 527, \n 528, \n 529, \n 530\n ], \n \"heads\": [[272, 0, 0], [349, 0, 0], [426, 0, 0], [269,0,0], [346,0,0], [423,0,0]], \n \"attrs\": {\"mxnet_version\": [\"int\", 10300]}\n}",
"_____no_output_____"
]
]
] | [
"code"
] | [
[
"code"
]
] |
d0459cc1661961468295e48af82734f2c26b87ee | 190,069 | ipynb | Jupyter Notebook | docs/source/notebooks/MDN_Edward_Keras_TF.ipynb | caosenqi/Edward1 | 85f833d307512a585b85ebc2979445e17191ed81 | [
"Apache-2.0"
] | 1 | 2016-10-22T09:56:50.000Z | 2016-10-22T09:56:50.000Z | docs/source/notebooks/MDN_Edward_Keras_TF.ipynb | caosenqi/Edward1 | 85f833d307512a585b85ebc2979445e17191ed81 | [
"Apache-2.0"
] | null | null | null | docs/source/notebooks/MDN_Edward_Keras_TF.ipynb | caosenqi/Edward1 | 85f833d307512a585b85ebc2979445e17191ed81 | [
"Apache-2.0"
] | null | null | null | 384.755061 | 78,880 | 0.917798 | [
[
[
"# Mixture Density Networks with Edward, Keras and TensorFlow\n\nThis notebook explains how to implement Mixture Density Networks (MDN) with Edward, Keras and TensorFlow.\nKeep in mind that if you want to use Keras and TensorFlow, like we do in this notebook, you need to set the backend of Keras to TensorFlow, [here](http://keras.io/backend/) it is explained how to do that.\n\nIn you are not familiar with MDNs have a look at the [following blog post](http://cbonnett.github.io/MDN.html) or at orginal [paper](http://research.microsoft.com/en-us/um/people/cmbishop/downloads/Bishop-NCRG-94-004.pdf) by Bishop.\n\nEdward implements many probability distribution functions that are TensorFlow compatible, this makes it attractive to use Edward for MDNs. \n\nHere are all the distributions that are currently implemented in Edward, there are more to come:\n\n1. [Bernoulli](https://github.com/blei-lab/edward/blob/master/edward/stats/distributions.py#L49)\n2. [Beta](https://github.com/blei-lab/edward/blob/master/edward/stats/distributions.py#L58)\n3. [Binomial](https://github.com/blei-lab/edward/blob/master/edward/stats/distributions.py#L68)\n4. [Chi Squared](https://github.com/blei-lab/edward/blob/master/edward/stats/distributions.py#L79)\n5. [Dirichlet](https://github.com/blei-lab/edward/blob/master/edward/stats/distributions.py#L89)\n6. [Exponential](https://github.com/blei-lab/edward/blob/master/edward/stats/distributions.py#L109)\n7. [Gamma](https://github.com/blei-lab/edward/blob/master/edward/stats/distributions.py#L118)\n8. [Geometric](https://github.com/blei-lab/edward/blob/master/edward/stats/distributions.py#L129)\n9. [Inverse Gamma](https://github.com/blei-lab/edward/blob/master/edward/stats/distributions.py#L138)\n10. [log Normal](https://github.com/blei-lab/edward/blob/master/edward/stats/distributions.py#L155)\n11. [Multinomial](https://github.com/blei-lab/edward/blob/master/edward/stats/distributions.py#L165)\n12. [Multivariate Normal](https://github.com/blei-lab/edward/blob/master/edward/stats/distributions.py#L194)\n13. [Negative Binomial](https://github.com/blei-lab/edward/blob/master/edward/stats/distributions.py#L283)\n14. [Normal](https://github.com/blei-lab/edward/blob/master/edward/stats/distributions.py#L294)\n15. [Poisson](https://github.com/blei-lab/edward/blob/master/edward/stats/distributions.py#L310)\n16. [Student-t](https://github.com/blei-lab/edward/blob/master/edward/stats/distributions.py#L319)\n17. [Truncated Normal](https://github.com/blei-lab/edward/blob/master/edward/stats/distributions.py#L333)\n18. [Uniform](https://github.com/blei-lab/edward/blob/master/edward/stats/distributions.py#L352)\n\nLet's start with the necessary imports.",
"_____no_output_____"
]
],
[
[
"# imports\n%matplotlib inline\nimport matplotlib.pyplot as plt\nimport seaborn as sns\n\nimport edward as ed\nimport numpy as np\nimport tensorflow as tf\n\nfrom edward.stats import norm # Normal distribution from Edward. \nfrom keras import backend as K\nfrom keras.layers import Dense\nfrom sklearn.cross_validation import train_test_split",
"_____no_output_____"
]
],
[
[
"We will need some functions to plot the results later on, these are defined in the next code block. ",
"_____no_output_____"
]
],
[
[
"from scipy.stats import norm as normal\ndef plot_normal_mix(pis, mus, sigmas, ax, label='', comp=True):\n \"\"\"\n Plots the mixture of Normal models to axis=ax\n comp=True plots all components of mixture model\n \"\"\"\n x = np.linspace(-10.5, 10.5, 250)\n final = np.zeros_like(x)\n for i, (weight_mix, mu_mix, sigma_mix) in enumerate(zip(pis, mus, sigmas)):\n temp = normal.pdf(x, mu_mix, sigma_mix) * weight_mix\n final = final + temp\n if comp:\n ax.plot(x, temp, label='Normal ' + str(i))\n ax.plot(x, final, label='Mixture of Normals ' + label)\n ax.legend(fontsize=13)\n \ndef sample_from_mixture(x, pred_weights, pred_means, pred_std, amount):\n \"\"\"\n Draws samples from mixture model. \n Returns 2 d array with input X and sample from prediction of Mixture Model\n \"\"\"\n samples = np.zeros((amount, 2))\n n_mix = len(pred_weights[0])\n to_choose_from = np.arange(n_mix)\n for j,(weights, means, std_devs) in enumerate(zip(pred_weights, pred_means, pred_std)):\n index = np.random.choice(to_choose_from, p=weights)\n samples[j,1]= normal.rvs(means[index], std_devs[index], size=1)\n samples[j,0]= x[j]\n if j == amount -1:\n break\n return samples",
"_____no_output_____"
]
],
[
[
"## Making some toy-data to play with.\n\nThis is the same toy-data problem set as used in the [blog post](http://blog.otoro.net/2015/11/24/mixture-density-networks-with-tensorflow/) by Otoro where he explains MDNs. This is an inverse problem as you can see, for every ```X``` there are multiple ```y``` solutions.",
"_____no_output_____"
]
],
[
[
"def build_toy_dataset(nsample=40000):\n y_data = np.float32(np.random.uniform(-10.5, 10.5, (1, nsample))).T\n r_data = np.float32(np.random.normal(size=(nsample, 1))) # random noise\n x_data = np.float32(np.sin(0.75 * y_data) * 7.0 + y_data * 0.5 + r_data * 1.0)\n return train_test_split(x_data, y_data, random_state=42, train_size=0.1)\n\nX_train, X_test, y_train, y_test = build_toy_dataset()\nprint(\"Size of features in training data: {:s}\".format(X_train.shape))\nprint(\"Size of output in training data: {:s}\".format(y_train.shape))\nprint(\"Size of features in test data: {:s}\".format(X_test.shape))\nprint(\"Size of output in test data: {:s}\".format(y_test.shape))\n\nsns.regplot(X_train, y_train, fit_reg=False)",
"Size of features in training data: (4000, 1)\nSize of output in training data: (4000, 1)\nSize of features in test data: (36000, 1)\nSize of output in test data: (36000, 1)\n"
]
],
[
[
"### Building a MDN using Edward, Keras and TF\n\nWe will define a class that can be used to construct MDNs. In this notebook we will be using a mixture of Normal Distributions. The advantage of defining a class is that we can easily reuse this to build other MDNs with different amount of mixture components. Furthermore, this makes it play nicely with Edward.",
"_____no_output_____"
]
],
[
[
"class MixtureDensityNetwork:\n \"\"\"\n Mixture density network for outputs y on inputs x.\n p((x,y), (z,theta))\n = sum_{k=1}^K pi_k(x; theta) Normal(y; mu_k(x; theta), sigma_k(x; theta))\n where pi, mu, sigma are the output of a neural network taking x\n as input and with parameters theta. There are no latent variables\n z, which are hidden variables we aim to be Bayesian about.\n \"\"\"\n def __init__(self, K):\n self.K = K # here K is the amount of Mixtures \n\n def mapping(self, X):\n \"\"\"pi, mu, sigma = NN(x; theta)\"\"\"\n hidden1 = Dense(15, activation='relu')(X) # fully-connected layer with 15 hidden units\n hidden2 = Dense(15, activation='relu')(hidden1) \n self.mus = Dense(self.K)(hidden2) # the means \n self.sigmas = Dense(self.K, activation=K.exp)(hidden2) # the variance\n self.pi = Dense(self.K, activation=K.softmax)(hidden2) # the mixture components\n\n def log_prob(self, xs, zs=None):\n \"\"\"log p((xs,ys), (z,theta)) = sum_{n=1}^N log p((xs[n,:],ys[n]), theta)\"\"\"\n # Note there are no parameters we're being Bayesian about. The\n # parameters are baked into how we specify the neural networks.\n X, y = xs\n self.mapping(X)\n result = tf.exp(norm.logpdf(y, self.mus, self.sigmas))\n result = tf.mul(result, self.pi)\n result = tf.reduce_sum(result, 1)\n result = tf.log(result)\n return tf.reduce_sum(result)",
"_____no_output_____"
]
],
[
[
"We can set a seed in Edward so we can reproduce all the random components. The following line:\n\n```ed.set_seed(42)```\n\nsets the seed in Numpy and TensorFlow under the [hood](https://github.com/blei-lab/edward/blob/master/edward/util.py#L191). We use the class we defined above to initiate the MDN with 20 mixtures, this now can be used as an Edward model.",
"_____no_output_____"
]
],
[
[
"ed.set_seed(42)\nmodel = MixtureDensityNetwork(20)",
"_____no_output_____"
]
],
[
[
"In the following code cell we define the TensorFlow placeholders that are then used to define the Edward data model.\nThe following line passes the ```model``` and ```data``` to ```MAP``` from Edward which is then used to initialise the TensorFlow variables. \n\n```inference = ed.MAP(model, data)``` \n\nMAP is a Bayesian concept and stands for Maximum A Posteriori, it tries to find the set of parameters which maximizes the posterior distribution. In the example here we don't have a prior, in a Bayesian context this means we have a flat prior. For a flat prior MAP is equivalent to Maximum Likelihood Estimation. Edward is designed to be Bayesian about its statistical inference. The cool thing about MDN's with Edward is that we could easily include priors! ",
"_____no_output_____"
]
],
[
[
"X = tf.placeholder(tf.float32, shape=(None, 1))\ny = tf.placeholder(tf.float32, shape=(None, 1))\ndata = ed.Data([X, y]) # Make Edward Data model\n\ninference = ed.MAP(model, data) # Make the inference model\nsess = tf.Session() # Start TF session \nK.set_session(sess) # Pass session info to Keras\ninference.initialize(sess=sess) # Initialize all TF variables using the Edward interface ",
"_____no_output_____"
]
],
[
[
"Having done that we can train the MDN in TensorFlow just like we normally would, and we can get out the predictions we are interested in from ```model```, in this case: \n\n* ```model.pi``` the mixture components, \n* ```model.mus``` the means,\n* ```model.sigmas``` the standard deviations. \n\nThis is done in the last line of the code cell :\n```\npred_weights, pred_means, pred_std = sess.run([model.pi, model.mus, model.sigmas], \n feed_dict={X: X_test})\n```\n\nThe default minimisation technique used is ADAM with a decaying scale factor.\nThis can be seen [here](https://github.com/blei-lab/edward/blob/master/edward/inferences.py#L94) in the code base of Edward. Having a decaying scale factor is not the standard way of using ADAM, this is inspired by the Automatic Differentiation Variational Inference [(ADVI)](http://arxiv.org/abs/1603.00788) work where it was used in the RMSPROP minimizer. \n\nThe loss that is minimised in the ```MAP``` model from Edward is the negative log-likelihood, this calculation uses the ```log_prob``` method in the ```MixtureDensityNetwork``` class we defined above. \nThe ```build_loss``` method in the ```MAP``` class can be found [here](https://github.com/blei-lab/edward/blob/master/edward/inferences.py#L396). \n\nHowever the method ```inference.loss``` used below, returns the log-likelihood, so we expect this quantity to be maximized.",
"_____no_output_____"
]
],
[
[
"NEPOCH = 1000\ntrain_loss = np.zeros(NEPOCH)\ntest_loss = np.zeros(NEPOCH)\nfor i in range(NEPOCH):\n _, train_loss[i] = sess.run([inference.train, inference.loss],\n feed_dict={X: X_train, y: y_train})\n test_loss[i] = sess.run(inference.loss, feed_dict={X: X_test, y: y_test})\n \npred_weights, pred_means, pred_std = sess.run([model.pi, model.mus, model.sigmas], \n feed_dict={X: X_test})",
"_____no_output_____"
]
],
[
[
"We can plot the log-likelihood of the training and test sample as function of training epoch.\nKeep in mind that ```inference.loss``` returns the total log-likelihood, so not the loss per data point, so in the plotting routine we divide by the size of the train and test data respectively. \nWe see that it converges after 400 training steps.",
"_____no_output_____"
]
],
[
[
"fig, axes = plt.subplots(nrows=1, ncols=1, figsize=(16, 3.5))\nplt.plot(np.arange(NEPOCH), test_loss/len(X_test), label='Test')\nplt.plot(np.arange(NEPOCH), train_loss/len(X_train), label='Train')\nplt.legend(fontsize=20)\nplt.xlabel('Epoch', fontsize=15)\nplt.ylabel('Log-likelihood', fontsize=15)",
"_____no_output_____"
]
],
[
[
"Next we can have a look at how some individual examples perform. Keep in mind this is an inverse problem\nso we can't get the answer correct, we can hope that the truth lies in area where the model has high probability.\nIn the next plot the truth is the vertical grey line while the blue line is the prediction of the mixture density network. As you can see, we didn't do too bad.",
"_____no_output_____"
]
],
[
[
"obj = [0, 4, 6]\nfig, axes = plt.subplots(nrows=3, ncols=1, figsize=(16, 6))\n\nplot_normal_mix(pred_weights[obj][0], pred_means[obj][0], pred_std[obj][0], axes[0], comp=False)\naxes[0].axvline(x=y_test[obj][0], color='black', alpha=0.5)\n\nplot_normal_mix(pred_weights[obj][2], pred_means[obj][2], pred_std[obj][2], axes[1], comp=False)\naxes[1].axvline(x=y_test[obj][2], color='black', alpha=0.5)\n\nplot_normal_mix(pred_weights[obj][1], pred_means[obj][1], pred_std[obj][1], axes[2], comp=False)\naxes[2].axvline(x=y_test[obj][1], color='black', alpha=0.5)",
"_____no_output_____"
]
],
[
[
"We can check the ensemble by drawing samples of the prediction and plotting the density of those. \nSeems the MDN learned what it needed too.",
"_____no_output_____"
]
],
[
[
"a = sample_from_mixture(X_test, pred_weights, pred_means, pred_std, amount=len(X_test))\nsns.jointplot(a[:,0], a[:,1], kind=\"hex\", color=\"#4CB391\", ylim=(-10,10), xlim=(-14,14))",
"_____no_output_____"
]
]
] | [
"markdown",
"code",
"markdown",
"code",
"markdown",
"code",
"markdown",
"code",
"markdown",
"code",
"markdown",
"code",
"markdown",
"code",
"markdown",
"code",
"markdown",
"code",
"markdown",
"code"
] | [
[
"markdown"
],
[
"code"
],
[
"markdown"
],
[
"code"
],
[
"markdown"
],
[
"code"
],
[
"markdown"
],
[
"code"
],
[
"markdown"
],
[
"code"
],
[
"markdown"
],
[
"code"
],
[
"markdown"
],
[
"code"
],
[
"markdown"
],
[
"code"
],
[
"markdown"
],
[
"code"
],
[
"markdown"
],
[
"code"
]
] |
d0459fa47b07be2ccfe5f74a6b68eb7b48db6919 | 20,271 | ipynb | Jupyter Notebook | Data Cleaning with PySpark.ipynb | raziiq/python-pyspark-data-cleaning | 5c335055423d01fd4b3d60374bb7c67839c268f3 | [
"MIT"
] | null | null | null | Data Cleaning with PySpark.ipynb | raziiq/python-pyspark-data-cleaning | 5c335055423d01fd4b3d60374bb7c67839c268f3 | [
"MIT"
] | null | null | null | Data Cleaning with PySpark.ipynb | raziiq/python-pyspark-data-cleaning | 5c335055423d01fd4b3d60374bb7c67839c268f3 | [
"MIT"
] | null | null | null | 36.722826 | 175 | 0.453949 | [
[
[
"<H3>Importing Required Libraries",
"_____no_output_____"
]
],
[
[
"from pyspark.sql import SparkSession\nfrom pyspark.sql import functions as F\n",
"_____no_output_____"
]
],
[
[
"<H3>Getting Spark Session",
"_____no_output_____"
]
],
[
[
"spark = SparkSession.builder.getOrCreate()",
"_____no_output_____"
]
],
[
[
"<H3>Reading CSV",
"_____no_output_____"
]
],
[
[
"df = spark.read.csv(\"Big_Cities_Health_Data_Inventory.csv\", header=True)",
"_____no_output_____"
],
[
"df.show(10)",
"+------------------+--------------------+----+------+---------------+-----+--------------------+--------------------------+--------------------+-------+-----+\n|Indicator Category| Indicator|Year|Gender|Race/ Ethnicity|Value| Place|BCHC Requested Methodology| Source|Methods|Notes|\n+------------------+--------------------+----+------+---------------+-----+--------------------+--------------------------+--------------------+-------+-----+\n| HIV/AIDS|AIDS Diagnoses Ra...|2013| Both| All| 30.4|Atlanta (Fulton C...| AIDS cases diagno...|Diagnoses numbers...| null| null|\n| HIV/AIDS|AIDS Diagnoses Ra...|2012| Both| All| 39.6|Atlanta (Fulton C...| AIDS cases diagno...|Diagnoses numbers...| null| null|\n| HIV/AIDS|AIDS Diagnoses Ra...|2011| Both| All| 41.7|Atlanta (Fulton C...| AIDS cases diagno...|Diagnoses numbers...| null| null|\n| Cancer|All Types of Canc...|2013| Male| All|195.8|Atlanta (Fulton C...| 2012, 2013, 2014;...|National Center f...| null| null|\n| Cancer|All Types of Canc...|2013|Female| All|135.5|Atlanta (Fulton C...| 2012, 2013, 2014;...|National Center f...| null| null|\n| Cancer|All Types of Canc...|2013| Both| All|159.3|Atlanta (Fulton C...| 2012, 2013, 2014;...|National Center f...| null| null|\n| Cancer|All Types of Canc...|2012| Male| All|199.2|Atlanta (Fulton C...| 2012, 2013, 2014;...|National Center f...| null| null|\n| Cancer|All Types of Canc...|2012|Female| All|137.6|Atlanta (Fulton C...| 2012, 2013, 2014;...|National Center f...| null| null|\n| Cancer|All Types of Canc...|2012| Both| All|160.3|Atlanta (Fulton C...| 2012, 2013, 2014;...|National Center f...| null| null|\n| Cancer|All Types of Canc...|2011| Male| All|196.2|Atlanta (Fulton C...| 2012, 2013, 2014;...|National Center f...| null| null|\n+------------------+--------------------+----+------+---------------+-----+--------------------+--------------------------+--------------------+-------+-----+\nonly showing top 10 rows\n\n"
]
],
[
[
"<H3>Printing Schema",
"_____no_output_____"
]
],
[
[
"df.printSchema()",
"root\n |-- Indicator Category: string (nullable = true)\n |-- Indicator: string (nullable = true)\n |-- Year: string (nullable = true)\n |-- Gender: string (nullable = true)\n |-- Race/ Ethnicity: string (nullable = true)\n |-- Value: string (nullable = true)\n |-- Place: string (nullable = true)\n |-- BCHC Requested Methodology: string (nullable = true)\n |-- Source: string (nullable = true)\n |-- Methods: string (nullable = true)\n |-- Notes: string (nullable = true)\n\n"
]
],
[
[
"<H3>Dropping Unwanted Columns",
"_____no_output_____"
]
],
[
[
"df = df.drop(\"Notes\", \"Methods\", \"Source\", \"BCHC Requested Methodology\")",
"_____no_output_____"
],
[
"df.printSchema()",
"root\n |-- Indicator Category: string (nullable = true)\n |-- Indicator: string (nullable = true)\n |-- Year: string (nullable = true)\n |-- Gender: string (nullable = true)\n |-- Race/ Ethnicity: string (nullable = true)\n |-- Value: string (nullable = true)\n |-- Place: string (nullable = true)\n\n"
]
],
[
[
"<H3>Counting Null Values",
"_____no_output_____"
]
],
[
[
"df.select([F.count(F.when(F.isnan(c) | F.col(c).isNull(), c)).alias(c) for c in df.columns]).show()",
"+------------------+---------+----+------+---------------+-----+-----+\n|Indicator Category|Indicator|Year|Gender|Race/ Ethnicity|Value|Place|\n+------------------+---------+----+------+---------------+-----+-----+\n| 0| 28| 28| 218| 212| 231| 218|\n+------------------+---------+----+------+---------------+-----+-----+\n\n"
]
],
[
[
"Since there are several null values in the columns as shown in the table above, first steps would be to remove / replace null values in each column",
"_____no_output_____"
],
[
"<H3>Working with Null Values",
"_____no_output_____"
]
],
[
[
"df.filter(df[\"Indicator\"].isNull()).show(28)",
"+--------------------+---------+----+------+---------------+-----+-----+\n| Indicator Category|Indicator|Year|Gender|Race/ Ethnicity|Value|Place|\n+--------------------+---------+----+------+---------------+-----+-----+\n| FOR THE POPULATI...| null|null| null| null| null| null|\n| 12 MONTHS (S1701)\"| null|null| null| null| null| null|\n| (S1701)\"| null|null| null| null| null| null|\n| (S1701)\"| null|null| null| null| null| null|\n|from the flu shot...| null|null| null| null| null| null|\n|from the flu shot...| null|null| null| null| null| null|\n|from the flu shot...| null|null| null| null| null| null|\n|from the flu shot...| null|null| null| null| null| null|\n|from the flu shot...| null|null| null| null| null| null|\n|from the flu shot...| null|null| null| null| null| null|\n|from the flu shot...| null|null| null| null| null| null|\n|(percent of respo...| null|null| null| null| null| null|\n|(percent of respo...| null|null| null| null| null| null|\n|(percent of respo...| null|null| null| null| null| null|\n| your nose?\"\" \"| null|null| null| null| null| null|\n| your nose?\"\" \"| null|null| null| null| null| null|\n| your nose?\"\" \"| null|null| null| null| null| null|\n| your nose?\"\" \"| null|null| null| null| null| null|\n| your nose?\"\" \"| null|null| null| null| null| null|\n| your nose?\"\" \"| null|null| null| null| null| null|\n| your nose?\"\" \"| null|null| null| null| null| null|\n|(percent of respo...| null|null| null| null| null| null|\n|(see note above a...| null|null| null| null| null| null|\n|(see note above a...| null|null| null| null| null| null|\n|(see note above a...| null|null| null| null| null| null|\n|(see note above a...| null|null| null| null| null| null|\n|(see note above a...| null|null| null| null| null| null|\n|(see note above a...| null|null| null| null| null| null|\n+--------------------+---------+----+------+---------------+-----+-----+\n\n"
]
],
[
[
"Since all the rows that have null values in Indicator have null values for other columns like Year, Gender, Race and etc, it would be better to remove these observations",
"_____no_output_____"
]
],
[
[
"# Counting total number of rows in the dataset to compare with the rows after null value rows are removed.\nrows_count_pre = df.count()\nprint(\"Total number of rows before deleting: \",rows_count_pre)",
"Total number of rows before deleting: 13730\n"
],
[
"# deleting all the rows where there are null values in the columns mentioned below\ndf = df.na.drop(subset=[\"Indicator\", \"Year\", \"Gender\", \"Race/ Ethnicity\", \"Value\", \"Place\"])",
"_____no_output_____"
],
[
"rows_count_post = df.count()\nprint(\"Total number of rows after deleting: \",rows_count_post)",
"Total number of rows after deleting: 13499\n"
],
[
"total_rows_removed = rows_count_pre - rows_count_post\nprint(\"Total number of rows deleted: \", total_rows_removed)",
"Total number of rows deleted: 231\n"
],
[
"#Checking the null values again to see if the dataset is clean\ndf.select([F.count(F.when(F.isnan(c) | F.col(c).isNull(), c)).alias(c) for c in df.columns]).show()",
"+------------------+---------+----+------+---------------+-----+-----+\n|Indicator Category|Indicator|Year|Gender|Race/ Ethnicity|Value|Place|\n+------------------+---------+----+------+---------------+-----+-----+\n| 0| 0| 0| 0| 0| 0| 0|\n+------------------+---------+----+------+---------------+-----+-----+\n\n"
]
],
[
[
"The results above show that all the rows with null values have been deleted from the dataset. This completes the step of removing all the null values from the dataset",
"_____no_output_____"
],
[
"<H3>Splitting the Place Column into City and State Columns",
"_____no_output_____"
]
],
[
[
"split_col = F.split(df[\"Place\"], ',')\ndf = df.withColumn(\"City_County\", split_col.getItem(0))\ndf = df.withColumn(\"State\", split_col.getItem(1))\ndf.select(\"City_County\", \"State\").show(truncate=False)",
"+-----------------------+-----+\n|City_County |State|\n+-----------------------+-----+\n|Atlanta (Fulton County)| GA |\n|Atlanta (Fulton County)| GA |\n|Atlanta (Fulton County)| GA |\n|Atlanta (Fulton County)| GA |\n|Atlanta (Fulton County)| GA |\n|Atlanta (Fulton County)| GA |\n|Atlanta (Fulton County)| GA |\n|Atlanta (Fulton County)| GA |\n|Atlanta (Fulton County)| GA |\n|Atlanta (Fulton County)| GA |\n|Atlanta (Fulton County)| GA |\n|Atlanta (Fulton County)| GA |\n|Atlanta (Fulton County)| GA |\n|Atlanta (Fulton County)| GA |\n|Atlanta (Fulton County)| GA |\n|Atlanta (Fulton County)| GA |\n|Atlanta (Fulton County)| GA |\n|Atlanta (Fulton County)| GA |\n|Atlanta (Fulton County)| GA |\n|Atlanta (Fulton County)| GA |\n+-----------------------+-----+\nonly showing top 20 rows\n\n"
],
[
"Creating a User Defined Function to take care of the City_County column to extract the city. Same can be done using",
"_____no_output_____"
],
[
"import re\ndef extract_city(city_str):\n result = re.sub(r'\\([^)]*\\)', '', city_str)\n return result",
"_____no_output_____"
],
[
"from pyspark.sql.types import StringType\nudfExtract = F.udf(extract_city, StringType())\ndf = df.withColumn(\"City\", udfExtract(df[\"City_County\"]))\ndf.select(\"City\", \"State\").show(truncate=False)",
"+--------+-----+\n|City |State|\n+--------+-----+\n|Atlanta | GA |\n|Atlanta | GA |\n|Atlanta | GA |\n|Atlanta | GA |\n|Atlanta | GA |\n|Atlanta | GA |\n|Atlanta | GA |\n|Atlanta | GA |\n|Atlanta | GA |\n|Atlanta | GA |\n|Atlanta | GA |\n|Atlanta | GA |\n|Atlanta | GA |\n|Atlanta | GA |\n|Atlanta | GA |\n|Atlanta | GA |\n|Atlanta | GA |\n|Atlanta | GA |\n|Atlanta | GA |\n|Atlanta | GA |\n+--------+-----+\nonly showing top 20 rows\n\n"
]
],
[
[
"This sums up the cleaning process of data using PySpark. Below is the final state of the dataset",
"_____no_output_____"
]
],
[
[
"df.show()",
"+--------------------+--------------------+----+------+---------------+-----+--------------------+--------------------+-----+--------+\n| Indicator Category| Indicator|Year|Gender|Race/ Ethnicity|Value| Place| City_County|State| City|\n+--------------------+--------------------+----+------+---------------+-----+--------------------+--------------------+-----+--------+\n| HIV/AIDS|AIDS Diagnoses Ra...|2013| Both| All| 30.4|Atlanta (Fulton C...|Atlanta (Fulton C...| GA|Atlanta |\n| HIV/AIDS|AIDS Diagnoses Ra...|2012| Both| All| 39.6|Atlanta (Fulton C...|Atlanta (Fulton C...| GA|Atlanta |\n| HIV/AIDS|AIDS Diagnoses Ra...|2011| Both| All| 41.7|Atlanta (Fulton C...|Atlanta (Fulton C...| GA|Atlanta |\n| Cancer|All Types of Canc...|2013| Male| All|195.8|Atlanta (Fulton C...|Atlanta (Fulton C...| GA|Atlanta |\n| Cancer|All Types of Canc...|2013|Female| All|135.5|Atlanta (Fulton C...|Atlanta (Fulton C...| GA|Atlanta |\n| Cancer|All Types of Canc...|2013| Both| All|159.3|Atlanta (Fulton C...|Atlanta (Fulton C...| GA|Atlanta |\n| Cancer|All Types of Canc...|2012| Male| All|199.2|Atlanta (Fulton C...|Atlanta (Fulton C...| GA|Atlanta |\n| Cancer|All Types of Canc...|2012|Female| All|137.6|Atlanta (Fulton C...|Atlanta (Fulton C...| GA|Atlanta |\n| Cancer|All Types of Canc...|2012| Both| All|160.3|Atlanta (Fulton C...|Atlanta (Fulton C...| GA|Atlanta |\n| Cancer|All Types of Canc...|2011| Male| All|196.2|Atlanta (Fulton C...|Atlanta (Fulton C...| GA|Atlanta |\n| Cancer|All Types of Canc...|2011|Female| All|147.0|Atlanta (Fulton C...|Atlanta (Fulton C...| GA|Atlanta |\n| Cancer|All Types of Canc...|2011| Both| All|165.2|Atlanta (Fulton C...|Atlanta (Fulton C...| GA|Atlanta |\n| Cancer|All Types of Canc...|2013| Both| Black|208.3|Atlanta (Fulton C...|Atlanta (Fulton C...| GA|Atlanta |\n| Cancer|All Types of Canc...|2012| Both| Black|202.7|Atlanta (Fulton C...|Atlanta (Fulton C...| GA|Atlanta |\n|Maternal and Chil...|Infant Mortality ...|2012| Both| White| 4.5|Atlanta (Fulton C...|Atlanta (Fulton C...| GA|Atlanta |\n| Cancer|All Types of Canc...|2011| Both| Black|216.0|Atlanta (Fulton C...|Atlanta (Fulton C...| GA|Atlanta |\n| Cancer|All Types of Canc...|2013| Both| White|128.8|Atlanta (Fulton C...|Atlanta (Fulton C...| GA|Atlanta |\n| Cancer|All Types of Canc...|2012| Both| White|133.7|Atlanta (Fulton C...|Atlanta (Fulton C...| GA|Atlanta |\n| Cancer|All Types of Canc...|2011| Both| White|132.0|Atlanta (Fulton C...|Atlanta (Fulton C...| GA|Atlanta |\n|Life Expectancy a...|All-Cause Mortali...|2012|Female| All|578.4|Atlanta (Fulton C...|Atlanta (Fulton C...| GA|Atlanta |\n+--------------------+--------------------+----+------+---------------+-----+--------------------+--------------------+-----+--------+\nonly showing top 20 rows\n\n"
]
]
] | [
"markdown",
"code",
"markdown",
"code",
"markdown",
"code",
"markdown",
"code",
"markdown",
"code",
"markdown",
"code",
"markdown",
"code",
"markdown",
"code",
"markdown",
"code",
"markdown",
"code"
] | [
[
"markdown"
],
[
"code"
],
[
"markdown"
],
[
"code"
],
[
"markdown"
],
[
"code",
"code"
],
[
"markdown"
],
[
"code"
],
[
"markdown"
],
[
"code",
"code"
],
[
"markdown"
],
[
"code"
],
[
"markdown",
"markdown"
],
[
"code"
],
[
"markdown"
],
[
"code",
"code",
"code",
"code",
"code"
],
[
"markdown",
"markdown"
],
[
"code",
"code",
"code",
"code"
],
[
"markdown"
],
[
"code"
]
] |
d0459fbf6af048bbfa1a682033add06382a1e582 | 364,186 | ipynb | Jupyter Notebook | notebooks/eda-notebook.ipynb | archity/fake-news | 6108c10e5bd0df0c7aa91d21284e9e8437b8d64c | [
"MIT"
] | null | null | null | notebooks/eda-notebook.ipynb | archity/fake-news | 6108c10e5bd0df0c7aa91d21284e9e8437b8d64c | [
"MIT"
] | null | null | null | notebooks/eda-notebook.ipynb | archity/fake-news | 6108c10e5bd0df0c7aa91d21284e9e8437b8d64c | [
"MIT"
] | null | null | null | 395.424539 | 52,988 | 0.919621 | [
[
[
"# Exploratory Data Analysis\n\n* Dataset taken from https://github.com/Tariq60/LIAR-PLUS",
"_____no_output_____"
],
[
"## 1. Import Libraries",
"_____no_output_____"
]
],
[
[
"import os\nimport numpy as np\nimport pandas as pd\nimport matplotlib.pyplot as plt\n\nTRAIN_PATH = \"../data/raw/dataset/tsv/train2.tsv\"\nVAL_PATH = \"../data/raw/dataset/tsv/val2.tsv\"\nTEST_PATH = \"../data/raw/dataset/tsv/test2.tsv\"\n\ncolumns = [\"id\", \"statement_json\", \"label\", \"statement\", \"subject\", \"speaker\", \"speaker_title\", \"state_info\",\n \"party_affiliation\", \"barely_true_count\", \"false_count\", \"half_true_count\", \"mostly_true_count\",\n \"pants_fire_count\", \"context\", \"justification\"]\n\n",
"_____no_output_____"
]
],
[
[
"## 2. Read the dataset",
"_____no_output_____"
]
],
[
[
"train_df = pd.read_csv(TRAIN_PATH, sep=\"\\t\", names=columns)\nval_df = pd.read_csv(VAL_PATH, sep=\"\\t\", names=columns)\ntest_df = pd.read_csv(TEST_PATH, sep=\"\\t\", names=columns)",
"_____no_output_____"
],
[
"print(f\"Length of train set: {len(train_df)}\")\nprint(f\"Length of validation set: {len(val_df)}\")\nprint(f\"Length of test set: {len(test_df)}\")",
"Length of train set: 10242\nLength of validation set: 1284\nLength of test set: 1267\n"
],
[
"train_df.head()",
"_____no_output_____"
]
],
[
[
"## 3. Data Cleaning",
"_____no_output_____"
],
[
"* Some of the most important coloumns are \"label\", \"statement\".\n* Now we should check if any of them have null values.",
"_____no_output_____"
]
],
[
[
"print(\"Do we have empty strings in `label`?\")\npd.isna(train_df[\"label\"]).value_counts()",
"Do we have empty strings in `label`?\n"
]
],
[
[
"* 2 entries without any label\n* What exactly are those 2 entries?",
"_____no_output_____"
]
],
[
[
"train_df.loc[pd.isna(train_df[\"label\"]), :].index",
"_____no_output_____"
],
[
"train_df.loc[[2143]]\n",
"_____no_output_____"
],
[
"train_df.loc[[9377]]",
"_____no_output_____"
]
],
[
[
"* All the coloumns of those 2 entries are blank\n* Drop those 2 entries",
"_____no_output_____"
]
],
[
[
"train_df.dropna(subset=[\"label\"], inplace=True)\nlen(train_df)",
"_____no_output_____"
]
],
[
[
"## 4. Some Feature Analysis",
"_____no_output_____"
],
[
"### 4.1 Party Affiliation",
"_____no_output_____"
]
],
[
[
"print(train_df[\"party_affiliation\"].value_counts())\n\nif not os.path.exists(\"./img\"):\n os.makedirs(\"./img\")\n\nfig = plt.figure(figsize=(10, 6))\nparty_affil_plot = train_df[\"party_affiliation\"].value_counts().plot.bar()\nplt.tight_layout(pad=1)\nplt.savefig(\"img/party_affil_plot.png\", dpi=200)",
"republican 4497\ndemocrat 3336\nnone 1744\norganization 219\nindependent 147\nnewsmaker 56\nlibertarian 40\nactivist 39\njournalist 38\ncolumnist 35\ntalk-show-host 26\nstate-official 20\nlabor-leader 11\ntea-party-member 10\nbusiness-leader 9\ngreen 3\neducation-official 2\nliberal-party-canada 1\ngovernment-body 1\nModerate 1\ndemocratic-farmer-labor 1\nocean-state-tea-party-action 1\nconstitution-party 1\nName: party_affiliation, dtype: int64\n"
]
],
[
[
"### 4.2 States Stats",
"_____no_output_____"
]
],
[
[
"print(train_df[\"state_info\"].value_counts())\n\nfig = plt.figure(figsize=(10, 6))\nstate_info_plot = train_df[\"state_info\"].value_counts().plot.bar()\nplt.tight_layout(pad=1)\nplt.savefig(\"img/state_info_plot.png\", dpi=200)",
"Texas 1009\nFlorida 997\nWisconsin 713\nNew York 657\nIllinois 556\n ... \nQatar 1\nVirginia 1\nUnited Kingdom 1\nChina 1\nRhode Island 1\nName: state_info, Length: 84, dtype: int64\n"
]
],
[
[
"* Apparently, we have a state_info entry with value as \"Virginia director, Coalition to Stop Gun Violence\".\nIt should be replaced with \"Virginia\" only",
"_____no_output_____"
]
],
[
[
"train_df[train_df[\"state_info\"]==\"Virginia director, Coalition to Stop Gun Violence\"]",
"_____no_output_____"
],
[
"indx = train_df[train_df[\"state_info\"]==\"Virginia director, Coalition to Stop Gun Violence\"].index[0]\ntrain_df.loc[indx, \"state_info\"] = \"Virginia\"\n\nfig = plt.figure(figsize=(10, 6))\nstate_info_plot = train_df[\"state_info\"].value_counts().plot.bar()\nplt.tight_layout(pad=1)\nplt.savefig(\"img/state_info_plot.png\", dpi=200)",
"_____no_output_____"
]
],
[
[
"### 4.3 Label Distribution",
"_____no_output_____"
]
],
[
[
"print(train_df[\"label\"].value_counts())\n\nfig = plt.figure(figsize=(10, 6))\nlabel_stats_plot = train_df[\"label\"].value_counts().plot.bar()\nplt.tight_layout(pad=1)\nplt.savefig(\"img/label_stats_plot.png\", dpi=100)",
"half-true 2114\nfalse 1995\nmostly-true 1962\ntrue 1676\nbarely-true 1654\npants-fire 839\nName: label, dtype: int64\n"
]
],
[
[
"### 4.4 Speaker Distribution",
"_____no_output_____"
]
],
[
[
"print(train_df.speaker.value_counts())\n\nfig = plt.figure(figsize=(10, 6))\nspeaker_stats_plot = train_df[\"speaker\"].value_counts()[:10].plot.bar()\nplt.tight_layout(pad=1)\nplt.title(\"Speakers\")\nplt.savefig(\"img/speaker_stats_plot.png\", dpi=100)",
"barack-obama 488\ndonald-trump 273\nhillary-clinton 239\nmitt-romney 176\nscott-walker 149\n ... \nlorraine-fende 1\nnfederation-o-independent-business-virginia 1\njim-moore 1\nscott-surovell 1\nalan-powell 1\nName: speaker, Length: 2910, dtype: int64\n"
],
[
"print(train_df.speaker_title.value_counts())\n\nfig = plt.figure(figsize=(10, 6))\nspeaker_title_stats_plot = train_df[\"speaker_title\"].value_counts()[:10].plot.bar()\nplt.tight_layout(pad=1)\nplt.title(\"Speaker Title\")\nplt.savefig(\"img/speaker_title_stats_plot.png\", dpi=100)",
"President 492\nU.S. Senator 479\nGovernor 391\nPresident-Elect 273\nU.S. senator 263\n ... \nPundit and communications consultant 1\nHarrisonburg city councilman 1\nTheme park company 1\nExecutive director, NARAL Pro-Choice Virginia 1\nPresident, The Whitman Strategy Group 1\nName: speaker_title, Length: 1184, dtype: int64\n"
]
],
[
[
"### 4.5 Democrats vs Republicans\n\n* Let's see how the 2 main parties compete with each other in terms of\ntruthfulness in the labels",
"_____no_output_____"
]
],
[
[
"fig = plt.figure(figsize=(8,4))\n\nplt.suptitle(\"Party-wise Label\")\nax1 = fig.add_subplot(121)\nparty_wise = train_df[train_df[\"party_affiliation\"]==\"democrat\"][\"label\"].value_counts().to_frame()\nax1.pie(party_wise[\"label\"], labels=party_wise.index, autopct='%1.1f%%',\n startangle=90)\nax1.set_title(\"Democrat\")\n\nplt.suptitle(\"Party-wise Label\")\nax2 = fig.add_subplot(122)\nparty_wise = train_df[train_df[\"party_affiliation\"]==\"republican\"][\"label\"].value_counts().to_frame()\nax2.pie(party_wise[\"label\"], labels=party_wise.index, autopct='%1.1f%%',\n startangle=90)\nax2.set_title(\"Republican\")\nplt.tight_layout()\nplt.savefig(\"img/dems_gop_label_plot.png\", dpi=200)",
"_____no_output_____"
]
],
[
[
"* We can combine some labels to get a more simplified plot\n",
"_____no_output_____"
]
],
[
[
"def get_binary_label(label):\n if label in [\"pants-fire\", \"barely-true\", \"false\"]:\n return False\n elif label in [\"true\", \"half-true\", \"mostly-true\"]:\n return True\n\ntrain_df[\"binary_label\"] = train_df.label.apply(get_binary_label)",
"_____no_output_____"
],
[
"fig = plt.figure(figsize=(8,4))\n\nplt.suptitle(\"Party-wise Label\")\nax1 = fig.add_subplot(121)\nparty_wise = train_df[train_df[\"party_affiliation\"]==\"democrat\"][\"binary_label\"].value_counts().to_frame()\nax1.pie(party_wise[\"binary_label\"], labels=party_wise.index, autopct='%1.1f%%',\n startangle=90)\nax1.set_title(\"Democrat\")\n\nplt.suptitle(\"Party-wise Label\")\nax2 = fig.add_subplot(122)\nparty_wise = train_df[train_df[\"party_affiliation\"]==\"republican\"][\"binary_label\"].value_counts().to_frame()\nax2.pie(party_wise[\"binary_label\"], labels=party_wise.index, autopct='%1.1f%%',\n startangle=90)\nax2.set_title(\"Republican\")\nplt.tight_layout()\nplt.savefig(\"img/dems_gop_binary_label_plot.png\", dpi=200)",
"_____no_output_____"
]
],
[
[
"## 5. Sentiment Analysis",
"_____no_output_____"
]
],
[
[
"from textblob import TextBlob\n\npol = lambda x: TextBlob(x).sentiment.polarity\nsub = lambda x: TextBlob(x).sentiment.subjectivity\n\ntrain_df['polarity_true'] = train_df[train_df[\"binary_label\"]==True]['statement'].apply(pol)\ntrain_df['subjectivity_true'] = train_df[train_df[\"binary_label\"]==True]['statement'].apply(sub)\n\nplt.rcParams['figure.figsize'] = [10, 8]\n\nx = train_df[\"polarity_true\"]\ny = train_df[\"subjectivity_true\"]\nplt.scatter(x, y, color='blue')\n\nplt.title('Sentiment Analysis', fontsize=20)\nplt.xlabel('<-- Negative ---------------- Positive -->', fontsize=10)\nplt.ylabel('<-- Facts ---------------- Opinions -->', fontsize=10)\nplt.savefig(\"img/sa_true.png\", format=\"png\", dpi=200)\nplt.show()",
"_____no_output_____"
],
[
"train_df['polarity_false'] = train_df[train_df[\"binary_label\"]==False]['statement'].apply(pol)\ntrain_df['subjectivity_false'] = train_df[train_df[\"binary_label\"]==False]['statement'].apply(sub)\n\nplt.rcParams['figure.figsize'] = [10, 8]\n\nx = train_df[\"polarity_false\"]\ny = train_df[\"subjectivity_false\"]\nplt.scatter(x, y, color='blue')\n\nplt.title('Sentiment Analysis', fontsize=20)\nplt.xlabel('<-- Negative ---------------- Positive -->', fontsize=10)\nplt.ylabel('<-- Facts ---------------- Opinions -->', fontsize=10)\nplt.savefig(\"img/sa_false.png\", format=\"png\", dpi=200)\nplt.show()",
"_____no_output_____"
]
]
] | [
"markdown",
"code",
"markdown",
"code",
"markdown",
"code",
"markdown",
"code",
"markdown",
"code",
"markdown",
"code",
"markdown",
"code",
"markdown",
"code",
"markdown",
"code",
"markdown",
"code",
"markdown",
"code",
"markdown",
"code",
"markdown",
"code"
] | [
[
"markdown",
"markdown"
],
[
"code"
],
[
"markdown"
],
[
"code",
"code",
"code"
],
[
"markdown",
"markdown"
],
[
"code"
],
[
"markdown"
],
[
"code",
"code",
"code"
],
[
"markdown"
],
[
"code"
],
[
"markdown",
"markdown"
],
[
"code"
],
[
"markdown"
],
[
"code"
],
[
"markdown"
],
[
"code",
"code"
],
[
"markdown"
],
[
"code"
],
[
"markdown"
],
[
"code",
"code"
],
[
"markdown"
],
[
"code"
],
[
"markdown"
],
[
"code",
"code"
],
[
"markdown"
],
[
"code",
"code"
]
] |
d0459fce480b1203ed743ab07c5c434487be7d69 | 29,062 | ipynb | Jupyter Notebook | nbs/45_collab.ipynb | ldanilov/fastai | 87f4d528535e083aabf5af24f04b911b856057df | [
"Apache-2.0"
] | null | null | null | nbs/45_collab.ipynb | ldanilov/fastai | 87f4d528535e083aabf5af24f04b911b856057df | [
"Apache-2.0"
] | null | null | null | nbs/45_collab.ipynb | ldanilov/fastai | 87f4d528535e083aabf5af24f04b911b856057df | [
"Apache-2.0"
] | null | null | null | 32.006608 | 373 | 0.523226 | [
[
[
"#hide\n#skip\n! [[ -e /content ]] && pip install -Uqq fastai # upgrade fastai on colab",
"_____no_output_____"
],
[
"#default_exp collab\n#default_class_lvl 3",
"_____no_output_____"
],
[
"#export\nfrom fastai.tabular.all import *",
"_____no_output_____"
],
[
"#hide\nfrom nbdev.showdoc import *",
"_____no_output_____"
]
],
[
[
"# Collaborative filtering\n\n> Tools to quickly get the data and train models suitable for collaborative filtering",
"_____no_output_____"
],
[
"This module contains all the high-level functions you need in a collaborative filtering application to assemble your data, get a model and train it with a `Learner`. We will go other those in order but you can also check the [collaborative filtering tutorial](http://docs.fast.ai/tutorial.collab).",
"_____no_output_____"
],
[
"## Gather the data",
"_____no_output_____"
]
],
[
[
"#export\nclass TabularCollab(TabularPandas):\n \"Instance of `TabularPandas` suitable for collaborative filtering (with no continuous variable)\"\n with_cont=False",
"_____no_output_____"
]
],
[
[
"This is just to use the internal of the tabular application, don't worry about it.",
"_____no_output_____"
]
],
[
[
"#export\nclass CollabDataLoaders(DataLoaders):\n \"Base `DataLoaders` for collaborative filtering.\"\n @delegates(DataLoaders.from_dblock)\n @classmethod\n def from_df(cls, ratings, valid_pct=0.2, user_name=None, item_name=None, rating_name=None, seed=None, path='.', **kwargs):\n \"Create a `DataLoaders` suitable for collaborative filtering from `ratings`.\"\n user_name = ifnone(user_name, ratings.columns[0])\n item_name = ifnone(item_name, ratings.columns[1])\n rating_name = ifnone(rating_name, ratings.columns[2])\n cat_names = [user_name,item_name]\n splits = RandomSplitter(valid_pct=valid_pct, seed=seed)(range_of(ratings))\n to = TabularCollab(ratings, [Categorify], cat_names, y_names=[rating_name], y_block=TransformBlock(), splits=splits)\n return to.dataloaders(path=path, **kwargs)\n\n @classmethod\n def from_csv(cls, csv, **kwargs):\n \"Create a `DataLoaders` suitable for collaborative filtering from `csv`.\"\n return cls.from_df(pd.read_csv(csv), **kwargs)\n\nCollabDataLoaders.from_csv = delegates(to=CollabDataLoaders.from_df)(CollabDataLoaders.from_csv)",
"_____no_output_____"
]
],
[
[
"This class should not be used directly, one of the factory methods should be preferred instead. All those factory methods accept as arguments:\n\n- `valid_pct`: the random percentage of the dataset to set aside for validation (with an optional `seed`)\n- `user_name`: the name of the column containing the user (defaults to the first column)\n- `item_name`: the name of the column containing the item (defaults to the second column)\n- `rating_name`: the name of the column containing the rating (defaults to the third column)\n- `path`: the folder where to work\n- `bs`: the batch size\n- `val_bs`: the batch size for the validation `DataLoader` (defaults to `bs`)\n- `shuffle_train`: if we shuffle the training `DataLoader` or not\n- `device`: the PyTorch device to use (defaults to `default_device()`)",
"_____no_output_____"
]
],
[
[
"show_doc(CollabDataLoaders.from_df)",
"_____no_output_____"
]
],
[
[
"Let's see how this works on an example:",
"_____no_output_____"
]
],
[
[
"path = untar_data(URLs.ML_SAMPLE)\nratings = pd.read_csv(path/'ratings.csv')\nratings.head()",
"_____no_output_____"
],
[
"dls = CollabDataLoaders.from_df(ratings, bs=64)\ndls.show_batch()",
"_____no_output_____"
],
[
"show_doc(CollabDataLoaders.from_csv)",
"_____no_output_____"
],
[
"dls = CollabDataLoaders.from_csv(path/'ratings.csv', bs=64)",
"_____no_output_____"
]
],
[
[
"## Models",
"_____no_output_____"
],
[
"fastai provides two kinds of models for collaborative filtering: a dot-product model and a neural net. ",
"_____no_output_____"
]
],
[
[
"#export\nclass EmbeddingDotBias(Module):\n \"Base dot model for collaborative filtering.\"\n def __init__(self, n_factors, n_users, n_items, y_range=None):\n self.y_range = y_range\n (self.u_weight, self.i_weight, self.u_bias, self.i_bias) = [Embedding(*o) for o in [\n (n_users, n_factors), (n_items, n_factors), (n_users,1), (n_items,1)\n ]]\n\n def forward(self, x):\n users,items = x[:,0],x[:,1]\n dot = self.u_weight(users)* self.i_weight(items)\n res = dot.sum(1) + self.u_bias(users).squeeze() + self.i_bias(items).squeeze()\n if self.y_range is None: return res\n return torch.sigmoid(res) * (self.y_range[1]-self.y_range[0]) + self.y_range[0]\n\n @classmethod\n def from_classes(cls, n_factors, classes, user=None, item=None, y_range=None):\n \"Build a model with `n_factors` by inferring `n_users` and `n_items` from `classes`\"\n if user is None: user = list(classes.keys())[0]\n if item is None: item = list(classes.keys())[1]\n res = cls(n_factors, len(classes[user]), len(classes[item]), y_range=y_range)\n res.classes,res.user,res.item = classes,user,item\n return res\n\n def _get_idx(self, arr, is_item=True):\n \"Fetch item or user (based on `is_item`) for all in `arr`\"\n assert hasattr(self, 'classes'), \"Build your model with `EmbeddingDotBias.from_classes` to use this functionality.\"\n classes = self.classes[self.item] if is_item else self.classes[self.user]\n c2i = {v:k for k,v in enumerate(classes)}\n try: return tensor([c2i[o] for o in arr])\n except Exception as e:\n print(f\"\"\"You're trying to access {'an item' if is_item else 'a user'} that isn't in the training data.\n If it was in your original data, it may have been split such that it's only in the validation set now.\"\"\")\n\n def bias(self, arr, is_item=True):\n \"Bias for item or user (based on `is_item`) for all in `arr`\"\n idx = self._get_idx(arr, is_item)\n layer = (self.i_bias if is_item else self.u_bias).eval().cpu()\n return to_detach(layer(idx).squeeze(),gather=False)\n\n def weight(self, arr, is_item=True):\n \"Weight for item or user (based on `is_item`) for all in `arr`\"\n idx = self._get_idx(arr, is_item)\n layer = (self.i_weight if is_item else self.u_weight).eval().cpu()\n return to_detach(layer(idx),gather=False)",
"_____no_output_____"
]
],
[
[
"The model is built with `n_factors` (the length of the internal vectors), `n_users` and `n_items`. For a given user and item, it grabs the corresponding weights and bias and returns\n``` python\ntorch.dot(user_w, item_w) + user_b + item_b\n```\nOptionally, if `y_range` is passed, it applies a `SigmoidRange` to that result.",
"_____no_output_____"
]
],
[
[
"x,y = dls.one_batch()\nmodel = EmbeddingDotBias(50, len(dls.classes['userId']), len(dls.classes['movieId']), y_range=(0,5)\n ).to(x.device)\nout = model(x)\nassert (0 <= out).all() and (out <= 5).all()",
"_____no_output_____"
],
[
"show_doc(EmbeddingDotBias.from_classes)",
"_____no_output_____"
]
],
[
[
"`y_range` is passed to the main init. `user` and `item` are the names of the keys for users and items in `classes` (default to the first and second key respectively). `classes` is expected to be a dictionary key to list of categories like the result of `dls.classes` in a `CollabDataLoaders`:",
"_____no_output_____"
]
],
[
[
"dls.classes",
"_____no_output_____"
]
],
[
[
"Let's see how it can be used in practice:",
"_____no_output_____"
]
],
[
[
"model = EmbeddingDotBias.from_classes(50, dls.classes, y_range=(0,5)\n ).to(x.device)\nout = model(x)\nassert (0 <= out).all() and (out <= 5).all()",
"_____no_output_____"
]
],
[
[
"Two convenience methods are added to easily access the weights and bias when a model is created with `EmbeddingDotBias.from_classes`:",
"_____no_output_____"
]
],
[
[
"show_doc(EmbeddingDotBias.weight)",
"_____no_output_____"
]
],
[
[
"The elements of `arr` are expected to be class names (which is why the model needs to be created with `EmbeddingDotBias.from_classes`)",
"_____no_output_____"
]
],
[
[
"mov = dls.classes['movieId'][42] \nw = model.weight([mov])\ntest_eq(w, model.i_weight(tensor([42])))",
"_____no_output_____"
],
[
"show_doc(EmbeddingDotBias.bias)",
"_____no_output_____"
]
],
[
[
"The elements of `arr` are expected to be class names (which is why the model needs to be created with `EmbeddingDotBias.from_classes`)",
"_____no_output_____"
]
],
[
[
"mov = dls.classes['movieId'][42] \nb = model.bias([mov])\ntest_eq(b, model.i_bias(tensor([42])))",
"_____no_output_____"
],
[
"#export \nclass EmbeddingNN(TabularModel):\n \"Subclass `TabularModel` to create a NN suitable for collaborative filtering.\"\n @delegates(TabularModel.__init__)\n def __init__(self, emb_szs, layers, **kwargs):\n super().__init__(emb_szs=emb_szs, n_cont=0, out_sz=1, layers=layers, **kwargs)",
"_____no_output_____"
],
[
"show_doc(EmbeddingNN)",
"_____no_output_____"
]
],
[
[
"`emb_szs` should be a list of two tuples, one for the users, one for the items, each tuple containing the number of users/items and the corresponding embedding size (the function `get_emb_sz` can give a good default). All the other arguments are passed to `TabularModel`.",
"_____no_output_____"
]
],
[
[
"emb_szs = get_emb_sz(dls.train_ds, {})\nmodel = EmbeddingNN(emb_szs, [50], y_range=(0,5)\n ).to(x.device)\nout = model(x)\nassert (0 <= out).all() and (out <= 5).all()",
"_____no_output_____"
]
],
[
[
"## Create a `Learner`",
"_____no_output_____"
],
[
"The following function lets us quickly create a `Learner` for collaborative filtering from the data.",
"_____no_output_____"
]
],
[
[
"# export\n@log_args(to_return=True, but_as=Learner.__init__)\n@delegates(Learner.__init__)\ndef collab_learner(dls, n_factors=50, use_nn=False, emb_szs=None, layers=None, config=None, y_range=None, loss_func=None, **kwargs):\n \"Create a Learner for collaborative filtering on `dls`.\"\n emb_szs = get_emb_sz(dls, ifnone(emb_szs, {}))\n if loss_func is None: loss_func = MSELossFlat()\n if config is None: config = tabular_config()\n if y_range is not None: config['y_range'] = y_range\n if layers is None: layers = [n_factors]\n if use_nn: model = EmbeddingNN(emb_szs=emb_szs, layers=layers, **config)\n else: model = EmbeddingDotBias.from_classes(n_factors, dls.classes, y_range=y_range)\n return Learner(dls, model, loss_func=loss_func, **kwargs)",
"_____no_output_____"
]
],
[
[
"If `use_nn=False`, the model used is an `EmbeddingDotBias` with `n_factors` and `y_range`. Otherwise, it's a `EmbeddingNN` for which you can pass `emb_szs` (will be inferred from the `dls` with `get_emb_sz` if you don't provide any), `layers` (defaults to `[n_factors]`) `y_range`, and a `config` that you can create with `tabular_config` to customize your model. \n\n`loss_func` will default to `MSELossFlat` and all the other arguments are passed to `Learner`.",
"_____no_output_____"
]
],
[
[
"learn = collab_learner(dls, y_range=(0,5))",
"_____no_output_____"
],
[
"learn.fit_one_cycle(1)",
"_____no_output_____"
]
],
[
[
"## Export -",
"_____no_output_____"
]
],
[
[
"#hide\nfrom nbdev.export import *\nnotebook2script()",
"Converted 00_torch_core.ipynb.\nConverted 01_layers.ipynb.\nConverted 02_data.load.ipynb.\nConverted 03_data.core.ipynb.\nConverted 04_data.external.ipynb.\nConverted 05_data.transforms.ipynb.\nConverted 06_data.block.ipynb.\nConverted 07_vision.core.ipynb.\nConverted 08_vision.data.ipynb.\nConverted 09_vision.augment.ipynb.\nConverted 09b_vision.utils.ipynb.\nConverted 09c_vision.widgets.ipynb.\nConverted 10_tutorial.pets.ipynb.\nConverted 11_vision.models.xresnet.ipynb.\nConverted 12_optimizer.ipynb.\nConverted 13_callback.core.ipynb.\nConverted 13a_learner.ipynb.\nConverted 13b_metrics.ipynb.\nConverted 14_callback.schedule.ipynb.\nConverted 14a_callback.data.ipynb.\nConverted 15_callback.hook.ipynb.\nConverted 15a_vision.models.unet.ipynb.\nConverted 16_callback.progress.ipynb.\nConverted 17_callback.tracker.ipynb.\nConverted 18_callback.fp16.ipynb.\nConverted 18a_callback.training.ipynb.\nConverted 19_callback.mixup.ipynb.\nConverted 20_interpret.ipynb.\nConverted 20a_distributed.ipynb.\nConverted 21_vision.learner.ipynb.\nConverted 22_tutorial.imagenette.ipynb.\nConverted 23_tutorial.vision.ipynb.\nConverted 24_tutorial.siamese.ipynb.\nConverted 24_vision.gan.ipynb.\nConverted 30_text.core.ipynb.\nConverted 31_text.data.ipynb.\nConverted 32_text.models.awdlstm.ipynb.\nConverted 33_text.models.core.ipynb.\nConverted 34_callback.rnn.ipynb.\nConverted 35_tutorial.wikitext.ipynb.\nConverted 36_text.models.qrnn.ipynb.\nConverted 37_text.learner.ipynb.\nConverted 38_tutorial.text.ipynb.\nConverted 39_tutorial.transformers.ipynb.\nConverted 40_tabular.core.ipynb.\nConverted 41_tabular.data.ipynb.\nConverted 42_tabular.model.ipynb.\nConverted 43_tabular.learner.ipynb.\nConverted 44_tutorial.tabular.ipynb.\nConverted 45_collab.ipynb.\nConverted 46_tutorial.collab.ipynb.\nConverted 50_tutorial.datablock.ipynb.\nConverted 60_medical.imaging.ipynb.\nConverted 61_tutorial.medical_imaging.ipynb.\nConverted 65_medical.text.ipynb.\nConverted 70_callback.wandb.ipynb.\nConverted 71_callback.tensorboard.ipynb.\nConverted 72_callback.neptune.ipynb.\nConverted 73_callback.captum.ipynb.\nConverted 74_callback.cutmix.ipynb.\nConverted 97_test_utils.ipynb.\nConverted 99_pytorch_doc.ipynb.\nConverted index.ipynb.\nConverted tutorial.ipynb.\n"
]
]
] | [
"code",
"markdown",
"code",
"markdown",
"code",
"markdown",
"code",
"markdown",
"code",
"markdown",
"code",
"markdown",
"code",
"markdown",
"code",
"markdown",
"code",
"markdown",
"code",
"markdown",
"code",
"markdown",
"code",
"markdown",
"code",
"markdown",
"code",
"markdown",
"code",
"markdown",
"code"
] | [
[
"code",
"code",
"code",
"code"
],
[
"markdown",
"markdown",
"markdown"
],
[
"code"
],
[
"markdown"
],
[
"code"
],
[
"markdown"
],
[
"code"
],
[
"markdown"
],
[
"code",
"code",
"code",
"code"
],
[
"markdown",
"markdown"
],
[
"code"
],
[
"markdown"
],
[
"code",
"code"
],
[
"markdown"
],
[
"code"
],
[
"markdown"
],
[
"code"
],
[
"markdown"
],
[
"code"
],
[
"markdown"
],
[
"code",
"code"
],
[
"markdown"
],
[
"code",
"code",
"code"
],
[
"markdown"
],
[
"code"
],
[
"markdown",
"markdown"
],
[
"code"
],
[
"markdown"
],
[
"code",
"code"
],
[
"markdown"
],
[
"code"
]
] |
d045a0a552fa2a515c6b4a43d0132fac1642b21c | 28,978 | ipynb | Jupyter Notebook | 04_trainfull3d/04_trainfull3d_labels_01_partial3d.ipynb | bearpelican/rsna_retro | 1475da3224403261c48f0425b4a24e060d07556c | [
"Apache-2.0"
] | 3 | 2020-01-27T09:49:37.000Z | 2020-09-15T06:55:38.000Z | 04_trainfull3d/04_trainfull3d_labels_01_partial3d.ipynb | bearpelican/rsna_retro | 1475da3224403261c48f0425b4a24e060d07556c | [
"Apache-2.0"
] | 1 | 2021-05-20T12:44:34.000Z | 2021-05-20T12:44:34.000Z | 04_trainfull3d/04_trainfull3d_labels_01_partial3d.ipynb | bearpelican/rsna_retro | 1475da3224403261c48f0425b4a24e060d07556c | [
"Apache-2.0"
] | 1 | 2020-09-15T06:55:40.000Z | 2020-09-15T06:55:40.000Z | 71.374384 | 19,116 | 0.775105 | [
[
[
"from rsna_retro.imports import *\nfrom rsna_retro.metadata import *\nfrom rsna_retro.preprocess import *\nfrom rsna_retro.train import *\nfrom rsna_retro.train3d import *\nfrom rsna_retro.trainfull3d_labels import *",
"Loading imports\n"
],
[
"torch.cuda.set_device(4)",
"_____no_output_____"
],
[
"dls = get_3d_dls_aug(Meta.df_comb, sz=128, bs=32, grps=Meta.grps_stg1)",
"_____no_output_____"
]
],
[
[
"## Model",
"_____no_output_____"
]
],
[
[
"def get_3d_head(p=0.0):\n pool, feat = (nn.AdaptiveAvgPool3d(1), 64)\n m = nn.Sequential(Batchify(),\n ConvLayer(512,512,stride=2,ndim=3), # 8\n ConvLayer(512,1024,stride=2,ndim=3), # 4\n ConvLayer(1024,1024,stride=2,ndim=3), # 2\n nn.AdaptiveAvgPool3d((1, 1, 1)), Batchify(), Flat3d(), nn.Dropout(p),\n nn.Linear(1024, 6))\n init_cnn(m)\n return m",
"_____no_output_____"
],
[
"m = get_3d_head()\nconfig=dict(custom_head=m)\nlearn = get_learner(dls, xresnet18, get_loss(), config=config)",
"_____no_output_____"
],
[
"hook = ReshapeBodyHook(learn.model[0])\nlearn.add_cb(RowLoss())",
"_____no_output_____"
],
[
"# learn.load(f'runs/baseline_stg1_xresnet18-3', strict=False)",
"_____no_output_____"
],
[
"name = 'trainfull3d_labels_partial3d_new'",
"_____no_output_____"
]
],
[
[
"## Training",
"_____no_output_____"
]
],
[
[
"learn.lr_find()",
"_____no_output_____"
],
[
"do_fit(learn, 8, 1e-3)\nlearn.save(f'runs/{name}-1')",
"_____no_output_____"
],
[
"learn.load(f'runs/{name}-1')\nlearn.dls = get_3d_dls_aug(Meta.df_comb, sz=256, bs=12, grps=Meta.grps_stg1)\ndo_fit(learn, 4, 1e-4)\nlearn.save(f'runs/{name}-2')",
"_____no_output_____"
],
[
"learn.load(f'runs/{name}-2')\nlearn.dls = get_3d_dls_aug(Meta.df_comb, sz=384, bs=4, path=path_jpg, grps=Meta.grps_stg1)\ndo_fit(learn, 2, 1e-5)\nlearn.save(f'runs/{name}-3')",
"_____no_output_____"
]
]
] | [
"code",
"markdown",
"code",
"markdown",
"code"
] | [
[
"code",
"code",
"code"
],
[
"markdown"
],
[
"code",
"code",
"code",
"code",
"code"
],
[
"markdown"
],
[
"code",
"code",
"code",
"code"
]
] |
d045a98075c78571cf2474832dd2b2c2a2719ad9 | 593,859 | ipynb | Jupyter Notebook | ImageResize/Image_Scaling/Image_scaling.ipynb | noviicee/Image-Processing-OpenCV | f0834df0ed93132d89682868e5a844842077ec38 | [
"MIT"
] | 96 | 2021-01-27T11:27:16.000Z | 2021-07-15T16:47:15.000Z | ImageResize/Image_Scaling/Image_scaling.ipynb | geekquad/Image-Processing | af2c9383d358ccfc0e05d8a2d5254ae525ff3e1c | [
"MIT"
] | 289 | 2021-02-10T16:30:33.000Z | 2021-07-02T21:12:51.000Z | ImageResize/Image_Scaling/Image_scaling.ipynb | geekquad/Image-Processing | af2c9383d358ccfc0e05d8a2d5254ae525ff3e1c | [
"MIT"
] | 109 | 2021-02-10T18:36:31.000Z | 2021-07-18T08:36:03.000Z | 2,954.522388 | 189,986 | 0.960395 | [
[
[
"#Import Modules",
"_____no_output_____"
]
],
[
[
"import cv2\nimport numpy as np\nfrom google.colab.patches import cv2_imshow",
"_____no_output_____"
]
],
[
[
"#Load Image ",
"_____no_output_____"
]
],
[
[
"#image is loaded using cv2.imread() method,here flag is 0 ,specifies to load image in GRAYSCALE mode.\n'''\nSyntax:\n cv2.imread(path,flag)\nParameters:\n path: string representing the path of the image to be read.\n flag: specifies the way in which image should be read.\n'''\nimg=cv2.imread(\"input.png\",0)\ncv2_imshow(img)",
"_____no_output_____"
]
],
[
[
"#Apply scaling Operation\n\n\n",
"_____no_output_____"
]
],
[
[
"# To perform scaling operation,cv2.resize() method is used.\n'''\nSyntax:\n cv2.resize(image,(width,height)=None,fx=1,fy=1,interpolation)\nParameters:\n image: input image.\n (width,height): determining the size of output image ; optional parameter.\n fx: scaling factor for x-axis,default=1.\n fy: scaling factor for y-axis,default=1.\n interpolation: interpolation method to be used.\n'''\nscaled_up_x=cv2.resize(img,None,fx=2,fy=1,interpolation=cv2.INTER_CUBIC)\nscaled_down_x=cv2.resize(img,None,fx=0.5,fy=1,interpolation=cv2.INTER_LINEAR)\nscaled_up_y=cv2.resize(img,None,fx=1,fy=2,interpolation=cv2.INTER_CUBIC)\nscaled_down_y=cv2.resize(img,None,fx=1,fy=0.5,interpolation=cv2.INTER_LINEAR)",
"_____no_output_____"
]
],
[
[
"#Display the scaled image",
"_____no_output_____"
]
],
[
[
"cv2_imshow(scaled_up_x)\ncv2_imshow(scaled_down_x)\ncv2_imshow(scaled_up_y)\ncv2_imshow(scaled_down_y)",
"_____no_output_____"
]
]
] | [
"markdown",
"code",
"markdown",
"code",
"markdown",
"code",
"markdown",
"code"
] | [
[
"markdown"
],
[
"code"
],
[
"markdown"
],
[
"code"
],
[
"markdown"
],
[
"code"
],
[
"markdown"
],
[
"code"
]
] |
d045b1bef26d9c68ddee8ee3e47e07a922444e19 | 219,721 | ipynb | Jupyter Notebook | jupyter/Chapter08/pulse_train_ambiguity.ipynb | mberkanbicer/software | 89f8004f567129216b92c156bbed658a9c03745a | [
"Apache-2.0"
] | null | null | null | jupyter/Chapter08/pulse_train_ambiguity.ipynb | mberkanbicer/software | 89f8004f567129216b92c156bbed658a9c03745a | [
"Apache-2.0"
] | null | null | null | jupyter/Chapter08/pulse_train_ambiguity.ipynb | mberkanbicer/software | 89f8004f567129216b92c156bbed658a9c03745a | [
"Apache-2.0"
] | null | null | null | 564.835476 | 82,964 | 0.944857 | [
[
[
"# ***Introduction to Radar Using Python and MATLAB***\n## Andy Harrison - Copyright (C) 2019 Artech House\n<br/>\n\n# Pulse Train Ambiguity Function\n***",
"_____no_output_____"
],
[
"Referring to Section 8.6.1, the amibguity function for a coherent pulse train is found by employing the generic waveform technique outlined in Section 8.6.3.\n***",
"_____no_output_____"
],
[
"Begin by getting the library path",
"_____no_output_____"
]
],
[
[
"import lib_path",
"_____no_output_____"
]
],
[
[
"Set the pulsewidth (s), the pulse repetition interval (s) and the number of pulses",
"_____no_output_____"
]
],
[
[
"pulsewidth = 0.4\n\npri = 1.0\n\nnumber_of_pulses = 6",
"_____no_output_____"
]
],
[
[
"Generate the time delay (s) using the `linspace` routine from `scipy`",
"_____no_output_____"
]
],
[
[
"from numpy import linspace\n\n\n# Set the time delay\n\ntime_delay = linspace(-number_of_pulses * pri, number_of_pulses * pri, 5000)",
"_____no_output_____"
]
],
[
[
"Calculate the ambiguity function for the pulse train",
"_____no_output_____"
]
],
[
[
"from Libs.ambiguity.ambiguity_function import pulse_train\n\nfrom numpy import finfo\n\n\nambiguity = pulse_train(time_delay, finfo(float).eps, pulsewidth, pri, number_of_pulses)",
"_____no_output_____"
]
],
[
[
"Plot the zero-Doppler cut using the `matplotlib` routines",
"_____no_output_____"
]
],
[
[
"from matplotlib import pyplot as plt\n\n\n# Set the figure size\n\nplt.rcParams[\"figure.figsize\"] = (15, 10)\n\n\n\n# Plot the ambiguity function\n\nplt.plot(time_delay, ambiguity, '')\n\n\n\n# Set the x and y axis labels\n\nplt.xlabel(\"Time (s)\", size=12)\n\nplt.ylabel(\"Relative Amplitude\", size=12)\n\n\n\n# Turn on the grid\n\nplt.grid(linestyle=':', linewidth=0.5)\n\n\n\n# Set the plot title and labels\n\nplt.title('Pulse Train Ambiguity Function', size=14)\n\n\n\n# Set the tick label size\n\nplt.tick_params(labelsize=12)",
"_____no_output_____"
]
],
[
[
"Set the Doppler mismatch frequencies using the `linspace` routine",
"_____no_output_____"
]
],
[
[
"doppler_frequency = linspace(-2.0 / pulsewidth, 2.0 / pulsewidth, 1000)",
"_____no_output_____"
]
],
[
[
"Calculate the ambiguity function for the pulse train",
"_____no_output_____"
]
],
[
[
"ambiguity = pulse_train(finfo(float).eps, doppler_frequency, pulsewidth, pri, number_of_pulses)",
"_____no_output_____"
]
],
[
[
"Display the zero-range cut for the pulse train",
"_____no_output_____"
]
],
[
[
"plt.plot(doppler_frequency, ambiguity, '')\n\n\n# Set the x and y axis labels\n\nplt.xlabel(\"Doppler (Hz)\", size=12)\n\nplt.ylabel(\"Relative Amplitude\", size=12)\n\n\n\n# Turn on the grid\n\nplt.grid(linestyle=':', linewidth=0.5)\n\n\n\n# Set the plot title and labels\n\nplt.title('Pulse Train Ambiguity Function', size=14)\n\n\n\n# Set the tick label size\n\nplt.tick_params(labelsize=12)",
"_____no_output_____"
]
],
[
[
"Set the time delay and Doppler mismatch frequency and create the two-dimensional grid using the `meshgrid` routine from `scipy`",
"_____no_output_____"
]
],
[
[
"from numpy import meshgrid\n\n\n# Set the time delay\n\ntime_delay = linspace(-number_of_pulses * pri, number_of_pulses * pri, 1000)\n\n\n\n# Set the Doppler mismatch\n\ndoppler_frequency = linspace(-2.0 / pulsewidth, 2.0 / pulsewidth, 1000)\n\n\n\n# Create the grid\n\nt, f = meshgrid(time_delay, doppler_frequency)",
"_____no_output_____"
]
],
[
[
"Calculate the ambiguity function for the pulse train",
"_____no_output_____"
]
],
[
[
"ambiguity = pulse_train(t, f, pulsewidth, pri, number_of_pulses)",
"_____no_output_____"
]
],
[
[
"Display the two-dimensional contour plot for the pulse train ambiguity function",
"_____no_output_____"
]
],
[
[
"# Plot the ambiguity function\nfrom numpy import finfo\n\nplt.contour(t, f, ambiguity + finfo('float').eps, 20, cmap='jet', vmin=-0.2, vmax=1.0)\n\n\n# Set the x and y axis labels\n\nplt.xlabel(\"Time (s)\", size=12)\n\nplt.ylabel(\"Doppler (Hz)\", size=12)\n\n\n\n# Turn on the grid\n\nplt.grid(linestyle=':', linewidth=0.5)\n\n\n\n# Set the plot title and labels\n\nplt.title('Pulse Pulse Ambiguity Function', size=14)\n\n\n\n# Set the tick label size\n\nplt.tick_params(labelsize=12)",
"_____no_output_____"
]
]
] | [
"markdown",
"code",
"markdown",
"code",
"markdown",
"code",
"markdown",
"code",
"markdown",
"code",
"markdown",
"code",
"markdown",
"code",
"markdown",
"code",
"markdown",
"code",
"markdown",
"code",
"markdown",
"code"
] | [
[
"markdown",
"markdown",
"markdown"
],
[
"code"
],
[
"markdown"
],
[
"code"
],
[
"markdown"
],
[
"code"
],
[
"markdown"
],
[
"code"
],
[
"markdown"
],
[
"code"
],
[
"markdown"
],
[
"code"
],
[
"markdown"
],
[
"code"
],
[
"markdown"
],
[
"code"
],
[
"markdown"
],
[
"code"
],
[
"markdown"
],
[
"code"
],
[
"markdown"
],
[
"code"
]
] |
d045b469b7e54d7c97b349930fa6882e8376271d | 18,517 | ipynb | Jupyter Notebook | docs/_sources/ch05/ch5_2.ipynb | liuzhengqi1996/math452_Spring2022 | b01d1d9bee4778b3069e314c775a54f16dd44053 | [
"MIT"
] | null | null | null | docs/_sources/ch05/ch5_2.ipynb | liuzhengqi1996/math452_Spring2022 | b01d1d9bee4778b3069e314c775a54f16dd44053 | [
"MIT"
] | null | null | null | docs/_sources/ch05/ch5_2.ipynb | liuzhengqi1996/math452_Spring2022 | b01d1d9bee4778b3069e314c775a54f16dd44053 | [
"MIT"
] | null | null | null | 43.879147 | 245 | 0.519577 | [
[
[
"# 5.2 Fourier transform and Fourier series\n\nWe make use of the theory of tempered distributions (see\n[@strichartz2003guide] for an introduction) and we begin by collecting\nsome results of independent interest, which will also be important\nlater.\n\n## 5.2.1 Fourier transform\n\nBefore studying the Fourier transform, we first consider Schwartz space\nwhich is defined below.\n\n````{prf:definition}\nThe Schwartz space\n$\\mathcal{S}\\left(\\mathbb{R}^{n}\\right)$ is the topological vector space\nof functions $f: \\mathbb{R}^{n} \\rightarrow \\mathbb{C}$ such that\n$f \\in C^{\\infty}\\left(\\mathbb{R}^{n}\\right)$ and\n\n$$\n x^{\\alpha} \\partial^{\\beta} f(x) \\rightarrow 0 \\quad \\text { as }|x| \\rightarrow \\infty\n$$\n\nfor every pair of multi-indices $\\alpha, \\beta \\in \\mathbb{N}_{0}^{n} .$\nFor $\\alpha, \\beta \\in \\mathbb{N}_{0}^{n}$ and\n$f \\in \\mathcal{S}\\left(\\mathbb{R}^{n}\\right)$ let (5.10)\n\n$$\n \\|f\\|_{\\alpha, \\beta}=\\sup _{\\mathbb{R}^{n}}\\left|x^{\\alpha} \\partial^{\\beta} f\\right|\n$$\n\nA sequence of functions $\\left\\{f_{k}: k \\in \\mathbb{N}\\right\\}$\nconverges to a function $f$ in $\\mathcal{S}\\left(\\mathbb{R}^{n}\\right)$\nif\n\n$$\n \\left\\|f_{n}-f\\right\\|_{\\alpha, \\beta} \\rightarrow 0 \\quad \\text { as } k \\rightarrow \\infty\n$$\n\nfor every $\\alpha, \\beta \\in \\mathbb{N}_{0}^{n}$.\n````\n\nThe Schwartz space consists of smooth functions whose derivatives and\nthe function itself decay at infinity faster than any power. Schwartz\nfunctions are rapidly decreasing. When there is no ambiguity, we will\nwrite $\\mathcal{S}\\left(\\mathbb{R}^{n}\\right)$ as $\\mathcal{S}$. Roughly\nspeaking, tempered distributions grow no faster than a polynomial at\ninfinity.\n\n````{prf:definition}\nA tempered distribution $T$ on $\\mathbb{R}^{n}$ is a continuous linear\nfunctional\n$T: \\mathcal{S}\\left(\\mathbb{R}^{n}\\right) \\rightarrow \\mathbb{C} .$ The\ntopological vector space of tempered distributions is denoted by\n$\\mathcal{S}^{\\prime}\\left(\\mathbb{R}^{n}\\right)$ or\n$\\mathcal{S}^{\\prime} .$ If $\\langle T, f\\rangle$ denotes the value of\n$T \\in \\mathcal{S}^{\\prime}$ acting on $f \\in \\mathcal{S}$ then a\nsequence $\\left\\{T_{k}\\right\\}$ converges to $T$ in\n$\\mathcal{S}^{\\prime}$, written $T_{k} \\rightarrow T$, if\n\n$$\n \\left\\langle T_{k}, f\\right\\rangle \\rightarrow\\langle T, f\\rangle\n$$\n\nfor every $f \\in \\mathcal{S}$.\n````\n\nSince $\\mathcal{D} \\subset \\mathcal{S}$ is densely and continuously\nimbedded, we have $\\mathcal{S}^{\\prime} \\subset \\mathcal{D}^{\\prime} .$\nMoreover, a distribution $T \\in \\mathcal{D}^{\\prime}$ extends uniquely\nto a tempered distribution $T \\in \\mathcal{S}^{\\prime}$ if and only if\nit is continuous on $\\mathcal{D}$ with respect to the topology on\n$\\mathcal{S}$. Every function $f \\in L_{\\text {loc }}^{1}$ defines a\nregular distribution $T_{f} \\in \\mathcal{D}^{\\prime}$ by\n\n$$\n \\left\\langle T_{f}, \\phi\\right\\rangle=\\int f \\phi d x \\quad \\text { for all } \\phi \\in \\mathcal{D}.\n$$\n\nIf $|f| \\leq p$ is bounded by some polynomial $p,$ then $T_{f}$ extends\nto a tempered distribution $T_{f} \\in \\mathcal{S}^{\\prime}$, but this is\nnot the case for functions $f$ that grow too rapidly at infinity.\n\nThe Schwartz space is a natural one to use for the Fourier transform.\nDifferentiation and multiplication exchange roles under the Fourier\ntransform and therefore so do the properties of smoothness and rapid\ndecrease. As a result, the Fourier transform is an automorphism of the\nSchwartz space. By duality, the Fourier transform is also an\nautomorphism of the space of tempered distributions.\n\n````{prf:definition}\nThe Fourier transform of a function $f \\in \\mathcal{S}\\left(\\mathbb{R}^{n}\\right)$\nis the function $\\hat{f}: \\mathbb{R}^{n} \\rightarrow \\mathbb{C}$ defined\nby \n\n$$\n \\hat{f}(\\omega)= \\int f(x) e^{-2 \\pi i\\omega \\cdot x} d x.\n$$\n\nThe inverse Fourier transform of $f$ is the function\n$\\check{f}: \\mathbb{R}^{n} \\rightarrow \\mathbb{C}$ defined by\n\n$$\n \\check{f}(x)=\\int f(\\omega) e^{2 \\pi i\\omega \\cdot x} d k.\n$$\n\n````\n\n````{prf:definition}\nThe Fourier transform of a tempered distribution $f \\in \\mathcal{S}'$ is defined by\n\n$$\n \\langle \\hat{f}, \\phi\\rangle = \\langle f, \\hat \\phi\\rangle,\\quad \\forall \\phi\\in \\mathcal{S}.\n$$\n\n````\n\nThe support of a continuous function $f$ is the closure of the set\n$\\{x\\in \\mathbb{R}: f(x)\\neq 0\\}$.\n\n```{admonition} Properties\nThe Fourier transform has the following properties\n\n1. If $f\\in \\mathcal{S}'$ and the support of $\\hat f$ is $\\{0\\}$, then\n $f$ is a polynomial.\n\n2. If $f\\in \\mathcal{S}'$ and the support of $\\hat f$ is a single point\n $\\{a\\}$, then $f(x)=e^{2\\pi iax}P(x)$, where $P(x)$ is a polynomial.\n```\n\n## 5.2.2 Poisson summation formula\n\n``` {prf:theorem}\nLet $f \\in L^{1}(\\mathbb{R})$ and $f$ is continuous. Then we have for\nalmost all $(x, \\omega ) \\in \\mathbb{R} \\times \\hat{\\mathbb{R}}$ that\n\n$$\n T \\sum_{n \\in \\mathbb{Z}} f(x+n T) e^{-2 \\pi i \\omega (x+n T)}=\\sum_{n \\in \\mathbb{Z}} \\hat{f}\\left(\\omega +\\frac{n}{T}\\right) e^{2 \\pi i n x / T}\n$$\n\nwhere both sides converge absolutely.\n\nIn addition, let $\\Lambda$ be the lattice in $\\mathbb{R}^{d}$ consisting\nof points with integer coordinates. For a function $f$ in\n$L^{1}\\left(\\mathbb{R}^{d}\\right)$ and $f$ is continuous, we have\n\n$$\n \\sum_{\\omega \\in \\Lambda} f(x+\\omega )=\\sum_{\\nu \\in \\Lambda} \\hat{f}(\\omega ) e^{2 \\pi i x \\cdot \\omega }.\n$$\n\nwhere both series converge absolutely and uniformly on $\\Lambda$.\n```\n\n```{prf;proof}\n*Proof.* We just give a proof of a simple case that\n$f: \\mathbb{R} \\rightarrow \\mathbb{C}$ is a Schwarz function (see\nDefinition [\\[def:schwarz\\]](#def:schwarz){reference-type=\"ref\"\nreference=\"def:schwarz\"}). Let: $$F(x)=\\sum_{n \\in \\mathbb{Z}} f(x+n).$$\nThen $F(x)$ is 1-periodic (because of absolute convergence), and has\nFourier coefficients: $$\\begin{aligned}\n\\hat{F}_{\\omega } &=\\int_{0}^{1} \\sum_{n \\in \\mathbb{Z}} f(x+n) e^{-2 \\pi i \\omega x} \\mathrm{~d} x \\\\\n&=\\sum_{n \\in \\mathbb{Z}} \\int_{0}^{1} f(x+n) e^{-2 \\pi i \\omega x} \\mathrm{~d} x \\quad \\text { because } f \\text { is Schwarz, so convergence is uniform}\\\\\n&=\\sum_{n \\in \\mathbb{Z}} \\int_{n}^{n+1} f(x) e^{-2 \\pi i\\omega x} \\mathrm{~d} x \\\\\n&=\\int_{\\mathbb{R}} f(x) e^{-2 \\pi i \\omega x} \\mathrm{~d} x\\\\\n&=\\hat{f}(k)\\\\\n\\end{aligned}$$ where $\\hat{f}$ is the Fourier transform of $f$.\n\nTherefore by the definition of the Fourier series of $f:$\n\n$$\n F(x) =\\sum_{\\omega \\in \\mathbb{Z}} \\hat{f}(k) e^{2\\pi i \\omega x}.\n$$\n\nChoosing $x=0$ in this formula:\n$$\\sum_{n \\in \\mathbb{Z}} f(n)=\\sum_{\\omega \\in \\mathbb{Z}} \\hat{f}(\\omega )$$\nas required. ◻\n```\n\n## 5.2.3 A special cut-off function\n\nLet us first state the following simple result that can be obtained by\nfollowing a calculation given in Section 3 of [@johnson2015saddle].\n\n```{prf:lemma}\nGiven $\\alpha>1$, consider \n\n$$\n \\label{alpha-g}\n g(t) = \\begin{cases} \n e^{-(1-t^2)^{1 - \\alpha}} & t\\in (-1,1) \\\\\n 0 & \\text{otherwise}.\n \\end{cases}\n$$\n\nthen there is a constant $c_\\alpha$ such that\n \n$$\n |\\hat{g}(\\omega )|\\lesssim e^{-c_\\alpha|\\omega |^{1-\\alpha^{-1}}},\n$$\n\n```\n\n```{prf:proof}\n*Proof.* Consider the asymptotic behavior of the Fourier transform\n\n$$\n F(\\omega )=\\int_{-\\infty}^{\\infty} g(t) e^{2\\pi i \\omega t} dt=2 \\operatorname{Re} \\int_{0}^{1} e^{2\\pi i \\omega t- (1-t^{2})^{1-\\alpha}} dt\n$$\n\nfor $|\\operatorname{Re} \\omega | \\gg 1.$ (Without loss of generality, we\ncan restrict ourselves to real $\\omega \\geq 0$). With a change of\nvariable $x=1-t$,\n\n$$\n F(\\omega )=2 \\operatorname{Re} \\int_{0}^{1} e^{f(x)} dx\n$$\n\nwith $f(x)=2\\pi i \\omega - 2\\pi i \\omega x- (2x-x^2)^{1-\\alpha}\\approx \\tilde f(x)+O\\left(x^{2-\\alpha}\\right)$\nand\n\n$$\n \\tilde f(x) = 2\\pi i \\omega - 2\\pi i \\omega x - (2 x)^{1-\\alpha}.\n$$\n\nThe saddle point is the $x=x_0$ where $f'(x_0)=0$. Since\n$\\tilde f'(x)=-2\\pi i \\omega + (\\alpha-1)2^{1-\\alpha} x^{-\\alpha},$\n\n$$\n x_{0} \\approx \\tilde x_0=\\left (2^{-\\alpha} (\\alpha-1) / i \\omega \\pi \\right )^{1 / \\alpha} \\sim \\omega ^{-1 / \\alpha}.\n$$\n\nTherefore $\\tilde f(\\tilde x_{0}) \\sim \\omega ^{(\\alpha-1) / \\alpha}$\nasymptotically. The second derivative is\n\n$$\n \\tilde f'' (\\tilde x_{0} )=-2^{1-\\alpha} \\alpha(\\alpha-1) \\tilde x_{0}^{-\\alpha-1}=-i^{(\\alpha+1) / \\alpha} 2 A \\omega ^{(\\alpha+1)/\\alpha},\n$$\n\nwhere $$A=2\\alpha (\\alpha-1)^{-1/\\alpha}\\pi^{(\\alpha+1)/\\alpha}.$$ Now,\n\n$$\n \\begin{split}\n \\tilde f(x)\\approx &\\tilde f(\\tilde x_0) + {\\tilde f''(\\tilde x_0)\\over 2} (x-\\tilde x_0)^2\n \\\\\n =&2\\pi i \\omega - (\\alpha - 1)^{1\\over \\alpha}(i\\omega \\pi )^{\\alpha -1\\over \\alpha} - (\\alpha - 1)^{1-\\alpha\\over \\alpha} (i\\omega \\pi )^{\\alpha -1\\over \\alpha}\n \\\\\n &-i^{(\\alpha+1) / \\alpha} A \\omega ^{(\\alpha+1)/\\alpha}(x- 2^{-1}(\\alpha - 1)^{-{1\\over \\alpha}}(i\\omega \\pi )^{-{1\\over \\alpha}} )^2.\n \\end{split} \n$$ \n\nChoose a contour $x=i^{-1 / \\alpha}u$, in which case\n\n$$\n \\tilde f(x) \\approx \\tilde f(\\tilde x_{0}) -i^{(\\alpha-1) / \\alpha} A \\omega ^{(\\alpha+1) / \\alpha}\\left(u-u_{0}\\right)^{2},\n$$\n\nwhich is a path of descent so we can perform a Gaussian integral.\n\nRecall that the integral of \n\n$$\n \\label{gaussInt}\n \\int_{-\\infty}^{\\infty} e^{-a u^{2}} d u=\\sqrt{\\pi / a}\n$$\n\nas long as Re$a>0,$ which is true here. Note also that, in the limit as $\\omega$\nbecomes large, the integrand becomes zero except close to\n$u=\\sqrt{1 / 2 \\omega },$ so we can neglect the rest of the contour and\ntreat the integral over $u$ as going from $-\\infty$ to $\\infty$.\n(Thankfully, the width of the Gaussian $\\Delta u \\sim \\omega ^{-3 / 4}$\ngoes to zero faster than the location of the maximum\n$u_{0} \\sim \\omega ^{-1 / 2},$ so we don't have to worry about the $u=0$\norigin). Also note that the change of variables from $x$ to $u$ gives us\nthe Jacobian factor for $$dx=i^{-1 / \\alpha}d u.$$ Thus, when all is\nsaid and done, we obtain the exact asymptotic form of the Fourier\nintegral for $\\omega \\gg 1$: \n\n$$\n \\begin{split}\n F(\\omega ) \\approx &2 \\operatorname{Re}\\int_{0}^{1} e^{\\tilde f(\\tilde x_0) - i^{(\\alpha-1) / \\alpha} A \\omega ^{(\\alpha+1) / \\alpha}\\left(u-u_{0}\\right)^{2}} dx\n \\\\\n =&2 \\operatorname{Re} e^{\\tilde f(\\tilde x_0)} i^{-1 / \\alpha} \\int_{-\\infty}^{\\infty} e^{- i^{(\\alpha-1) \\over \\alpha} A \\omega ^{(\\alpha+1) / \\alpha}\\left(u-u_{0}\\right)^{2}} du\n \\\\\n =&2 \\operatorname{Re} e^{\\tilde f(\\tilde x_0)} \\pi^{1/2}i^{-1 / \\alpha} i^{(1-\\alpha) \\over 2\\alpha} A^{-1/2} \\omega ^{-(\\alpha+1) / 2\\alpha}\\qquad \\text{ by \\eqref{gaussInt}} \n \\\\\n =&2 \\operatorname{Re}\\left[\\sqrt{\\frac{\\pi}{(i \\omega )^{(\\alpha+1) / \\alpha} A}} e^{\\tilde f(\\tilde x_0)}\\right]\n \\\\\n \\approx &2 \\operatorname{Re}\\left[\\sqrt{\\frac{\\pi}{(i \\omega )^{(\\alpha+1) / \\alpha} A}} e^{ 2\\pi i \\omega - 2\\pi i \\omega \\tilde x_{0}- \\left[\\left(2-\\tilde x_{0}\\right) \\tilde x_{0}\\right]^{1-\\alpha}}\\right]\n \\end{split}\n$$\n\nwith $x_{0}$ and $A$ given above. Notice that\n$\\tilde x_0\\sim \\omega ^{-1 / \\alpha}$. Thus,\n\n$$\n |F(\\omega ) | \\approx e^{-c_\\alpha|\\omega |^{1-\\alpha^{-1}}}.\n$$ ◻\n```\n",
"_____no_output_____"
],
[
"## 5.2.4 Fourier transform of polynomials\n\nWe begin by noting that an activation function $\\sigma$, which satisfies\na polynomial growth condition $|\\sigma(x)| \\leq C(1 + |x|)^n$ for some\nconstants $C$ and $n$, is a tempered distribution. As a result, we make\nthis assumption on our activation functions in the following theorems.\nWe briefly note that this condition is sufficient, but not necessary\n(for instance an integrable function need not satisfy a pointwise\npolynomial growth bound) for $\\sigma$ to be represent a tempered\ndistribution.\n\nWe begin by studying the convolution of $\\sigma$ with a Gaussian\nmollifier. Let $\\eta$ be a Gaussian mollifier\n\n$$\n \\eta(x) = \\frac{1}{\\sqrt{\\pi}}e^{-x^2}.\n$$\n\nSet\n$\\eta_\\epsilon=\\frac{1}{\\epsilon}\\eta(\\frac{x}{\\epsilon})$. Then\nconsider \n\n$$\n \\sigma_{\\epsilon}(x):=\\sigma\\ast{\\eta_\\epsilon}(x)=\\int_{\\mathbb{R}}\\sigma(x-y){\\eta_\\epsilon}(y)dy\n$$ (sigma-epsilon)\n\nfor a given activation function $\\sigma$. It is clear that\n$\\sigma_{\\epsilon}\\in C^\\infty(\\mathbb{R})$. Moreover, by considering\nthe Fourier transform (as a tempered distribution) we see that\n\n$$\n \\hat{\\sigma}_{\\epsilon} = \\hat{\\sigma}\\hat{\\eta}_{\\epsilon} = \\hat{\\sigma}\\eta_{\\epsilon^{-1}}.\n$$ (eq_278)\n\nWe begin by stating a lemma which characterizes the set of polynomials\nin terms of their Fourier transform.\n\n```{prf:lemma}\n:label: polynomial_lemma\nGiven\na tempered distribution $\\sigma$, the following statements are\nequivalent:\n\n1. $\\sigma$ is a polynomial\n\n2. $\\sigma_\\epsilon$ given by {eq}`sigma-epsilon` is a polynomial for any $\\epsilon>0$.\n\n3. $\\text{supp}(\\hat{\\sigma})\\subset \\{0\\}$.\n```\n\n```{prf:proof}\n*Proof.* We begin by proving that (3) and (1) are equivalent. This\nfollows from a characterization of distributions supported at a single\npoint (see [@strichartz2003guide], section 6.3). In particular, a\ndistribution supported at $0$ must be a finite linear combination of\nDirac masses and their derivatives. In particular, if $\\hat{\\sigma}$ is\nsupported at $0$, then\n\n$$\n \\hat{\\sigma} = \\displaystyle\\sum_{i=1}^n a_i\\delta^{(i)}.\n$$\n\nTaking the inverse Fourier transform and noting that the inverse Fourier transform\nof $\\delta^{(i)}$ is $c_ix^i$, we see that $\\sigma$ is a polynomial.\nThis shows that (3) implies (1), for the converse we simply take the\nFourier transform of a polynomial and note that it is a finite linear\ncombination of Dirac masses and their derivatives.\n\nFinally, we prove the equivalence of (2) and (3). For this it suffices\nto show that $\\hat{\\sigma}$ is supported at $0$ iff\n$\\hat{\\sigma}_\\epsilon$ is supported at $0$. This follows from equation\n{eq}`eq_278` and the\nfact that $\\eta_{\\epsilon^{-1}}$ is nowhere vanishing. ◻\n```\n\nAs an application of Lemma {prf:ref}`polynomial_lemma`, we give a simple proof of the result in\nthe next section.",
"_____no_output_____"
]
]
] | [
"markdown"
] | [
[
"markdown",
"markdown"
]
] |
d045be51aeb405853e12eaea473b8a4b80abf702 | 4,163 | ipynb | Jupyter Notebook | stable/_downloads/a68c968ba9eafa2b1315cbf9e139eee3/plot_phantom_4DBTi.ipynb | drammock/mne-tools.github.io | 5d3a104d174255644d8d5335f58036e32695e85d | [
"BSD-3-Clause"
] | null | null | null | stable/_downloads/a68c968ba9eafa2b1315cbf9e139eee3/plot_phantom_4DBTi.ipynb | drammock/mne-tools.github.io | 5d3a104d174255644d8d5335f58036e32695e85d | [
"BSD-3-Clause"
] | null | null | null | stable/_downloads/a68c968ba9eafa2b1315cbf9e139eee3/plot_phantom_4DBTi.ipynb | drammock/mne-tools.github.io | 5d3a104d174255644d8d5335f58036e32695e85d | [
"BSD-3-Clause"
] | null | null | null | 38.546296 | 850 | 0.517896 | [
[
[
"%matplotlib inline",
"_____no_output_____"
]
],
[
[
"\n============================================\n4D Neuroimaging/BTi phantom dataset tutorial\n============================================\n\nHere we read 4DBTi epochs data obtained with a spherical phantom\nusing four different dipole locations. For each condition we\ncompute evoked data and compute dipole fits.\n\nData are provided by Jean-Michel Badier from MEG center in Marseille, France.\n",
"_____no_output_____"
]
],
[
[
"# Authors: Alex Gramfort <[email protected]>\n#\n# License: BSD (3-clause)\n\nimport os.path as op\nimport numpy as np\nfrom mayavi import mlab\nfrom mne.datasets import phantom_4dbti\nimport mne",
"_____no_output_____"
]
],
[
[
"Read data and compute a dipole fit at the peak of the evoked response\n\n",
"_____no_output_____"
]
],
[
[
"data_path = phantom_4dbti.data_path()\nraw_fname = op.join(data_path, '%d/e,rfhp1.0Hz')\n\ndipoles = list()\nsphere = mne.make_sphere_model(r0=(0., 0., 0.), head_radius=0.080)\n\nt0 = 0.07 # peak of the response\n\npos = np.empty((4, 3))\n\nfor ii in range(4):\n raw = mne.io.read_raw_bti(raw_fname % (ii + 1,),\n rename_channels=False, preload=True)\n raw.info['bads'] = ['A173', 'A213', 'A232']\n events = mne.find_events(raw, 'TRIGGER', mask=4350, mask_type='not_and')\n epochs = mne.Epochs(raw, events=events, event_id=8192, tmin=-0.2, tmax=0.4,\n preload=True)\n evoked = epochs.average()\n evoked.plot(time_unit='s')\n cov = mne.compute_covariance(epochs, tmax=0.)\n dip = mne.fit_dipole(evoked.copy().crop(t0, t0), cov, sphere)[0]\n pos[ii] = dip.pos[0]",
"_____no_output_____"
]
],
[
[
"Compute localisation errors\n\n",
"_____no_output_____"
]
],
[
[
"actual_pos = 0.01 * np.array([[0.16, 1.61, 5.13],\n [0.17, 1.35, 4.15],\n [0.16, 1.05, 3.19],\n [0.13, 0.80, 2.26]])\nactual_pos = np.dot(actual_pos, [[0, 1, 0], [-1, 0, 0], [0, 0, 1]])\n\nerrors = 1e3 * np.linalg.norm(actual_pos - pos, axis=1)\nprint(\"errors (mm) : %s\" % errors)",
"_____no_output_____"
]
],
[
[
"Plot the dipoles in 3D\n\n",
"_____no_output_____"
]
],
[
[
"def plot_pos(pos, color=(0., 0., 0.)):\n mlab.points3d(pos[:, 0], pos[:, 1], pos[:, 2], scale_factor=0.005,\n color=color)\n\n\nmne.viz.plot_alignment(evoked.info, bem=sphere, surfaces=[])\n# Plot the position of the actual dipole\nplot_pos(actual_pos, color=(1., 0., 0.))\n# Plot the position of the estimated dipole\nplot_pos(pos, color=(1., 1., 0.))",
"_____no_output_____"
]
]
] | [
"code",
"markdown",
"code",
"markdown",
"code",
"markdown",
"code",
"markdown",
"code"
] | [
[
"code"
],
[
"markdown"
],
[
"code"
],
[
"markdown"
],
[
"code"
],
[
"markdown"
],
[
"code"
],
[
"markdown"
],
[
"code"
]
] |
d045be7dee9af5c2db649e96a1a8a142f9717a2e | 69,457 | ipynb | Jupyter Notebook | Model/3-NeuralNetwork4.ipynb | skawns0724/KOSA-Big-Data_Vision | af123dfe0a82a82795bb6732285c390be86e83b7 | [
"MIT"
] | 1 | 2021-09-24T20:55:35.000Z | 2021-09-24T20:55:35.000Z | Model/3-NeuralNetwork4.ipynb | skawns0724/KOSA-Big-Data_Vision | af123dfe0a82a82795bb6732285c390be86e83b7 | [
"MIT"
] | null | null | null | Model/3-NeuralNetwork4.ipynb | skawns0724/KOSA-Big-Data_Vision | af123dfe0a82a82795bb6732285c390be86e83b7 | [
"MIT"
] | 7 | 2021-09-13T02:13:30.000Z | 2021-09-23T01:26:38.000Z | 57.355078 | 15,760 | 0.682725 | [
[
[
"from sklearn.model_selection import train_test_split\nimport pandas as pd\nimport numpy as np\nfrom sklearn.preprocessing import StandardScaler\nfrom keras.utils import to_categorical\nfrom keras.models import Sequential\nfrom keras.layers import Dense",
"Using TensorFlow backend.\nC:\\Users\\heine\\anaconda3\\lib\\site-packages\\tensorflow\\python\\framework\\dtypes.py:516: FutureWarning: Passing (type, 1) or '1type' as a synonym of type is deprecated; in a future version of numpy, it will be understood as (type, (1,)) / '(1,)type'.\n _np_qint8 = np.dtype([(\"qint8\", np.int8, 1)])\nC:\\Users\\heine\\anaconda3\\lib\\site-packages\\tensorflow\\python\\framework\\dtypes.py:517: FutureWarning: Passing (type, 1) or '1type' as a synonym of type is deprecated; in a future version of numpy, it will be understood as (type, (1,)) / '(1,)type'.\n _np_quint8 = np.dtype([(\"quint8\", np.uint8, 1)])\nC:\\Users\\heine\\anaconda3\\lib\\site-packages\\tensorflow\\python\\framework\\dtypes.py:518: FutureWarning: Passing (type, 1) or '1type' as a synonym of type is deprecated; in a future version of numpy, it will be understood as (type, (1,)) / '(1,)type'.\n _np_qint16 = np.dtype([(\"qint16\", np.int16, 1)])\nC:\\Users\\heine\\anaconda3\\lib\\site-packages\\tensorflow\\python\\framework\\dtypes.py:519: FutureWarning: Passing (type, 1) or '1type' as a synonym of type is deprecated; in a future version of numpy, it will be understood as (type, (1,)) / '(1,)type'.\n _np_quint16 = np.dtype([(\"quint16\", np.uint16, 1)])\nC:\\Users\\heine\\anaconda3\\lib\\site-packages\\tensorflow\\python\\framework\\dtypes.py:520: FutureWarning: Passing (type, 1) or '1type' as a synonym of type is deprecated; in a future version of numpy, it will be understood as (type, (1,)) / '(1,)type'.\n _np_qint32 = np.dtype([(\"qint32\", np.int32, 1)])\nC:\\Users\\heine\\anaconda3\\lib\\site-packages\\tensorflow\\python\\framework\\dtypes.py:525: FutureWarning: Passing (type, 1) or '1type' as a synonym of type is deprecated; in a future version of numpy, it will be understood as (type, (1,)) / '(1,)type'.\n np_resource = np.dtype([(\"resource\", np.ubyte, 1)])\nC:\\Users\\heine\\anaconda3\\lib\\site-packages\\tensorboard\\compat\\tensorflow_stub\\dtypes.py:541: FutureWarning: Passing (type, 1) or '1type' as a synonym of type is deprecated; in a future version of numpy, it will be understood as (type, (1,)) / '(1,)type'.\n _np_qint8 = np.dtype([(\"qint8\", np.int8, 1)])\nC:\\Users\\heine\\anaconda3\\lib\\site-packages\\tensorboard\\compat\\tensorflow_stub\\dtypes.py:542: FutureWarning: Passing (type, 1) or '1type' as a synonym of type is deprecated; in a future version of numpy, it will be understood as (type, (1,)) / '(1,)type'.\n _np_quint8 = np.dtype([(\"quint8\", np.uint8, 1)])\nC:\\Users\\heine\\anaconda3\\lib\\site-packages\\tensorboard\\compat\\tensorflow_stub\\dtypes.py:543: FutureWarning: Passing (type, 1) or '1type' as a synonym of type is deprecated; in a future version of numpy, it will be understood as (type, (1,)) / '(1,)type'.\n _np_qint16 = np.dtype([(\"qint16\", np.int16, 1)])\nC:\\Users\\heine\\anaconda3\\lib\\site-packages\\tensorboard\\compat\\tensorflow_stub\\dtypes.py:544: FutureWarning: Passing (type, 1) or '1type' as a synonym of type is deprecated; in a future version of numpy, it will be understood as (type, (1,)) / '(1,)type'.\n _np_quint16 = np.dtype([(\"quint16\", np.uint16, 1)])\nC:\\Users\\heine\\anaconda3\\lib\\site-packages\\tensorboard\\compat\\tensorflow_stub\\dtypes.py:545: FutureWarning: Passing (type, 1) or '1type' as a synonym of type is deprecated; in a future version of numpy, it will be understood as (type, (1,)) / '(1,)type'.\n _np_qint32 = np.dtype([(\"qint32\", np.int32, 1)])\nC:\\Users\\heine\\anaconda3\\lib\\site-packages\\tensorboard\\compat\\tensorflow_stub\\dtypes.py:550: FutureWarning: Passing (type, 1) or '1type' as a synonym of type is deprecated; in a future version of numpy, it will be understood as (type, (1,)) / '(1,)type'.\n np_resource = np.dtype([(\"resource\", np.ubyte, 1)])\n"
],
[
"#df = pd.read_csv(\".\\\\Data_USD.csv\", header=None,skiprows=1)\ndf = pd.read_csv(\".\\\\Data_USD.csv\")\ndf.head().to_csv(\".\\\\test.csv\")",
"_____no_output_____"
],
[
"T=df.groupby(\"SEX\") ",
"_____no_output_____"
],
[
"T.describe()",
"_____no_output_____"
],
[
"df.tail()",
"_____no_output_____"
],
[
"# X = df.drop('Y_Value',axis =1).values\n# y = df['Y_Value'].values\nX = df.drop('DEFAULT_PAYMENT_NEXT_MO',axis =1).values\nX[2999,0]",
"_____no_output_____"
],
[
"X.shape",
"_____no_output_____"
],
[
"y = df['DEFAULT_PAYMENT_NEXT_MO'].values\n#y.reshape(-1,1)",
"_____no_output_____"
],
[
"#print(X.shape)\nX.shape",
"_____no_output_____"
],
[
"#print(y.shape)\ny.shape",
"_____no_output_____"
],
[
"X_train, X_test, y_train, y_test = train_test_split (X,y,test_size=0.2, random_state=42)",
"_____no_output_____"
],
[
"y_test.T",
"_____no_output_____"
],
[
"X_test.shape",
"_____no_output_____"
],
[
"from sklearn.preprocessing import StandardScaler\n\nX_scaler = StandardScaler().fit(X_train)",
"_____no_output_____"
],
[
"X_scaler",
"_____no_output_____"
],
[
"X_train_scaled = X_scaler.transform(X_train)\nX_test_scaled = X_scaler.transform(X_test)",
"_____no_output_____"
],
[
"X_train_scaled",
"_____no_output_____"
],
[
"y_train_categorical = to_categorical(y_train)\ny_test_categorical = to_categorical(y_test)",
"_____no_output_____"
],
[
"from keras.models import Sequential\n\n#instantiate\nmodel = Sequential()",
"_____no_output_____"
],
[
"from keras.layers import Dense\n\nnumber_inputs = 10\nnumber_hidden = 30\n\nmodel.add(Dense(units = number_hidden, activation ='relu', input_dim=number_inputs))\nmodel.add(Dense(units = 35, activation ='relu')) #second hidden layer\nmodel.add(Dense(units = 25, activation ='relu')) #second hidden layer\nmodel.add(Dense(units = 15, activation ='relu')) #second hidden layer\nmodel.add(Dense(units = 5, activation ='relu')) #third hidden layer",
"_____no_output_____"
],
[
"number_classes =2 ## yes or no\nmodel.add(Dense(units = number_classes, activation = 'softmax'))",
"_____no_output_____"
],
[
"model.summary()",
"Model: \"sequential_1\"\n_________________________________________________________________\nLayer (type) Output Shape Param # \n=================================================================\ndense_1 (Dense) (None, 30) 330 \n_________________________________________________________________\ndense_2 (Dense) (None, 35) 1085 \n_________________________________________________________________\ndense_3 (Dense) (None, 25) 900 \n_________________________________________________________________\ndense_4 (Dense) (None, 15) 390 \n_________________________________________________________________\ndense_5 (Dense) (None, 5) 80 \n_________________________________________________________________\ndense_6 (Dense) (None, 2) 12 \n=================================================================\nTotal params: 2,797\nTrainable params: 2,797\nNon-trainable params: 0\n_________________________________________________________________\n"
],
[
"#compile the model\nmodel.compile(optimizer = 'sgd' ,\n loss = 'categorical_crossentropy',\n metrics =['accuracy'])",
"_____no_output_____"
],
[
"#train the model\n\nmodel.fit(X_train_scaled, y_train_categorical, epochs=100,shuffle = True,verbose =2)",
"WARNING:tensorflow:From C:\\Users\\heine\\anaconda3\\lib\\site-packages\\keras\\backend\\tensorflow_backend.py:422: The name tf.global_variables is deprecated. Please use tf.compat.v1.global_variables instead.\n\nEpoch 1/100\n - 2s - loss: 0.5336 - accuracy: 0.7782\nEpoch 2/100\n - 1s - loss: 0.4987 - accuracy: 0.7782\nEpoch 3/100\n - 1s - loss: 0.4804 - accuracy: 0.7782\nEpoch 4/100\n - 2s - loss: 0.4696 - accuracy: 0.7965\nEpoch 5/100\n - 2s - loss: 0.4625 - accuracy: 0.8047\nEpoch 6/100\n - 2s - loss: 0.4579 - accuracy: 0.8060\nEpoch 7/100\n - 1s - loss: 0.4553 - accuracy: 0.8067\nEpoch 8/100\n - 1s - loss: 0.4538 - accuracy: 0.8055\nEpoch 9/100\n - 1s - loss: 0.4527 - accuracy: 0.8055\nEpoch 10/100\n - 1s - loss: 0.4516 - accuracy: 0.8053\nEpoch 11/100\n - 1s - loss: 0.4506 - accuracy: 0.8062\nEpoch 12/100\n - 1s - loss: 0.4496 - accuracy: 0.8050\nEpoch 13/100\n - 1s - loss: 0.4488 - accuracy: 0.8055\nEpoch 14/100\n - 1s - loss: 0.4479 - accuracy: 0.8055\nEpoch 15/100\n - 2s - loss: 0.4474 - accuracy: 0.8060\nEpoch 16/100\n - 1s - loss: 0.4465 - accuracy: 0.8065\nEpoch 17/100\n - 1s - loss: 0.4461 - accuracy: 0.8067\nEpoch 18/100\n - 1s - loss: 0.4453 - accuracy: 0.8062\nEpoch 19/100\n - 1s - loss: 0.4449 - accuracy: 0.8062\nEpoch 20/100\n - 1s - loss: 0.4445 - accuracy: 0.8065\nEpoch 21/100\n - 1s - loss: 0.4442 - accuracy: 0.8060\nEpoch 22/100\n - 1s - loss: 0.4436 - accuracy: 0.8067\nEpoch 23/100\n - 1s - loss: 0.4430 - accuracy: 0.8070\nEpoch 24/100\n - 1s - loss: 0.4425 - accuracy: 0.8064\nEpoch 25/100\n - 1s - loss: 0.4423 - accuracy: 0.8076\nEpoch 26/100\n - 1s - loss: 0.4424 - accuracy: 0.8059\nEpoch 27/100\n - 2s - loss: 0.4420 - accuracy: 0.8066\nEpoch 28/100\n - 1s - loss: 0.4415 - accuracy: 0.8073\nEpoch 29/100\n - 1s - loss: 0.4415 - accuracy: 0.8068\nEpoch 30/100\n - 1s - loss: 0.4410 - accuracy: 0.8056\nEpoch 31/100\n - 1s - loss: 0.4408 - accuracy: 0.8070\nEpoch 32/100\n - 1s - loss: 0.4400 - accuracy: 0.8075\nEpoch 33/100\n - 1s - loss: 0.4402 - accuracy: 0.8071\nEpoch 34/100\n - 1s - loss: 0.4397 - accuracy: 0.8075\nEpoch 35/100\n - 1s - loss: 0.4398 - accuracy: 0.8080\nEpoch 36/100\n - 1s - loss: 0.4394 - accuracy: 0.8061\nEpoch 37/100\n - 1s - loss: 0.4391 - accuracy: 0.8078\nEpoch 38/100\n - 1s - loss: 0.4390 - accuracy: 0.8076\nEpoch 39/100\n - 1s - loss: 0.4387 - accuracy: 0.8082\nEpoch 40/100\n - 1s - loss: 0.4383 - accuracy: 0.8076\nEpoch 41/100\n - 1s - loss: 0.4378 - accuracy: 0.8074\nEpoch 42/100\n - 1s - loss: 0.4382 - accuracy: 0.8071\nEpoch 43/100\n - 1s - loss: 0.4378 - accuracy: 0.8080\nEpoch 44/100\n - 1s - loss: 0.4374 - accuracy: 0.8075\nEpoch 45/100\n - 1s - loss: 0.4371 - accuracy: 0.8070\nEpoch 46/100\n - 1s - loss: 0.4369 - accuracy: 0.8079\nEpoch 47/100\n - 1s - loss: 0.4369 - accuracy: 0.8075\nEpoch 48/100\n - 1s - loss: 0.4362 - accuracy: 0.8079\nEpoch 49/100\n - 1s - loss: 0.4366 - accuracy: 0.8080\nEpoch 50/100\n - 1s - loss: 0.4357 - accuracy: 0.8086\nEpoch 51/100\n - 2s - loss: 0.4355 - accuracy: 0.8087\nEpoch 52/100\n - 2s - loss: 0.4357 - accuracy: 0.8076\nEpoch 53/100\n - 2s - loss: 0.4352 - accuracy: 0.8073\nEpoch 54/100\n - 1s - loss: 0.4353 - accuracy: 0.8069\nEpoch 55/100\n - 1s - loss: 0.4353 - accuracy: 0.8085\nEpoch 56/100\n - 1s - loss: 0.4350 - accuracy: 0.8087\nEpoch 57/100\n - 1s - loss: 0.4348 - accuracy: 0.8080\nEpoch 58/100\n - 1s - loss: 0.4347 - accuracy: 0.8074\nEpoch 59/100\n - 1s - loss: 0.4346 - accuracy: 0.8085\nEpoch 60/100\n - 1s - loss: 0.4340 - accuracy: 0.8083\nEpoch 61/100\n - 1s - loss: 0.4337 - accuracy: 0.8091\nEpoch 62/100\n - 2s - loss: 0.4335 - accuracy: 0.8085\nEpoch 63/100\n - 1s - loss: 0.4334 - accuracy: 0.8093\nEpoch 64/100\n - 1s - loss: 0.4335 - accuracy: 0.8095\nEpoch 65/100\n - 1s - loss: 0.4332 - accuracy: 0.8087\nEpoch 66/100\n - 1s - loss: 0.4330 - accuracy: 0.8089\nEpoch 67/100\n - 1s - loss: 0.4331 - accuracy: 0.8093\nEpoch 68/100\n - 1s - loss: 0.4333 - accuracy: 0.8080\nEpoch 69/100\n - 1s - loss: 0.4331 - accuracy: 0.8088\nEpoch 70/100\n - 1s - loss: 0.4326 - accuracy: 0.8090\nEpoch 71/100\n - 1s - loss: 0.4324 - accuracy: 0.8087\nEpoch 72/100\n - 1s - loss: 0.4326 - accuracy: 0.8092\nEpoch 73/100\n - 1s - loss: 0.4318 - accuracy: 0.8092\nEpoch 74/100\n - 1s - loss: 0.4319 - accuracy: 0.8098\nEpoch 75/100\n - 1s - loss: 0.4314 - accuracy: 0.8085\nEpoch 76/100\n - 1s - loss: 0.4315 - accuracy: 0.8083\nEpoch 77/100\n - 1s - loss: 0.4314 - accuracy: 0.8101\nEpoch 78/100\n - 1s - loss: 0.4311 - accuracy: 0.8105\nEpoch 79/100\n - 1s - loss: 0.4315 - accuracy: 0.8091\nEpoch 80/100\n - 1s - loss: 0.4312 - accuracy: 0.8092\nEpoch 81/100\n - 1s - loss: 0.4312 - accuracy: 0.8101\nEpoch 82/100\n - 1s - loss: 0.4312 - accuracy: 0.8100\nEpoch 83/100\n - 1s - loss: 0.4302 - accuracy: 0.8102\nEpoch 84/100\n - 1s - loss: 0.4311 - accuracy: 0.8090\nEpoch 85/100\n - 1s - loss: 0.4302 - accuracy: 0.8095\nEpoch 86/100\n - 1s - loss: 0.4311 - accuracy: 0.8096\nEpoch 87/100\n - 2s - loss: 0.4308 - accuracy: 0.8105\nEpoch 88/100\n - 1s - loss: 0.4297 - accuracy: 0.8108\nEpoch 89/100\n - 1s - loss: 0.4301 - accuracy: 0.8095\nEpoch 90/100\n - 1s - loss: 0.4301 - accuracy: 0.8093\nEpoch 91/100\n - 1s - loss: 0.4303 - accuracy: 0.8096\nEpoch 92/100\n - 1s - loss: 0.4299 - accuracy: 0.8113\nEpoch 93/100\n - 1s - loss: 0.4291 - accuracy: 0.8096\nEpoch 94/100\n - 1s - loss: 0.4296 - accuracy: 0.8102\nEpoch 95/100\n - 1s - loss: 0.4291 - accuracy: 0.8098\nEpoch 96/100\n - 1s - loss: 0.4293 - accuracy: 0.8111\nEpoch 97/100\n - 1s - loss: 0.4291 - accuracy: 0.8103\nEpoch 98/100\n - 1s - loss: 0.4286 - accuracy: 0.8105\nEpoch 99/100\n - 2s - loss: 0.4287 - accuracy: 0.8110\nEpoch 100/100\n - 1s - loss: 0.4283 - accuracy: 0.8118\n"
],
[
"model.save(\"ccneuralnetwork.h5\")",
"_____no_output_____"
],
[
"#quantify the model\nmodel_loss, model_accuracy = model.evaluate(X_test_scaled,y_test_categorical,verbose =2)\nprint( model_loss )\nprint (model_accuracy)",
"0.45065889310836793\n0.8054999709129333\n"
]
],
[
[
"F1, Precision Recall, and Confusion Matrix",
"_____no_output_____"
]
],
[
[
"from sklearn.metrics import precision_recall_fscore_support\nfrom sklearn.metrics import recall_score\nfrom sklearn.metrics import classification_report",
"_____no_output_____"
],
[
"y_prediction = model.predict_classes(X_test)",
"_____no_output_____"
],
[
"y_prediction.reshape(-1,1)",
"_____no_output_____"
],
[
"print(\"Recall score:\"+ str(recall_score(y_test, y_prediction)))",
"Recall score:0.0\n"
],
[
"print(classification_report(y_test, y_prediction,\n target_names=[\"default\", \"non_default\"]))",
" precision recall f1-score support\n\n default 0.78 1.00 0.88 4687\n non_default 0.00 0.00 0.00 1313\n\n accuracy 0.78 6000\n macro avg 0.39 0.50 0.44 6000\nweighted avg 0.61 0.78 0.69 6000\n\n"
],
[
"import itertools\nimport matplotlib.pyplot as plt\nfrom sklearn.metrics import confusion_matrix",
"_____no_output_____"
],
[
"def plot_confusion_matrix(cm, classes,\n normalize=False,\n title='Confusion matrix',\n cmap=plt.cm.Blues):\n \"\"\"\n This function prints and plots the confusion matrix.\n Normalization can be applied by setting `normalize=True`.\n \"\"\"\n if normalize:\n cm = cm.astype('float') / cm.sum(axis=1)[:, np.newaxis]\n print(\"Normalized confusion matrix\")\n else:\n print('Confusion matrix, without normalization')\n\n print(cm)\n\n plt.imshow(cm, interpolation='nearest', cmap=cmap)\n plt.title(title)\n plt.colorbar()\n tick_marks = np.arange(len(classes))\n plt.xticks(tick_marks, classes, rotation=45)\n plt.yticks(tick_marks, classes)\n\n fmt = '.2f' if normalize else 'd'\n thresh = cm.max() / 2.\n for i, j in itertools.product(range(cm.shape[0]), range(cm.shape[1])):\n plt.text(j, i, format(cm[i, j], fmt),\n horizontalalignment=\"center\",\n color=\"red\" if cm[i, j] > thresh else \"black\")\n\n plt.tight_layout()\n plt.ylabel('True label')\n plt.xlabel('Predicted label')\n\n# Compute confusion matrix\ncnf_matrix = confusion_matrix(y_test, y_prediction)\nnp.set_printoptions(precision=2)\n\n# Plot non-normalized confusion matrix\nplt.figure()\nplot_confusion_matrix(cnf_matrix, classes=['Defualt', 'Non_default'],\n title='Confusion matrix, without normalization')\n\n# Plot normalized confusion matrix\nplt.figure()\nplot_confusion_matrix(cnf_matrix, classes=['Defualt', 'Non_default'], normalize=True,\n title='Normalized confusion matrix')\n\nplt.show()",
"Confusion matrix, without normalization\n[[4687 0]\n [1313 0]]\nNormalized confusion matrix\n[[1. 0.]\n [1. 0.]]\n"
]
]
] | [
"code",
"markdown",
"code"
] | [
[
"code",
"code",
"code",
"code",
"code",
"code",
"code",
"code",
"code",
"code",
"code",
"code",
"code",
"code",
"code",
"code",
"code",
"code",
"code",
"code",
"code",
"code",
"code",
"code",
"code",
"code"
],
[
"markdown"
],
[
"code",
"code",
"code",
"code",
"code",
"code",
"code"
]
] |
d045c92d1c77ccc101e0df9d89c16af4d1e50aff | 212,731 | ipynb | Jupyter Notebook | examples/notebooks/07_non_linear_recharge.ipynb | pastas/pastas | 3785c14c54d293f611f3c84b43e163556491cddc | [
"MIT"
] | 252 | 2017-01-25T05:48:53.000Z | 2022-03-31T17:46:37.000Z | examples/notebooks/07_non_linear_recharge.ipynb | pastas/pastas | 3785c14c54d293f611f3c84b43e163556491cddc | [
"MIT"
] | 279 | 2017-02-14T10:59:01.000Z | 2022-03-31T09:17:37.000Z | examples/notebooks/07_non_linear_recharge.ipynb | pastas/pastas | 3785c14c54d293f611f3c84b43e163556491cddc | [
"MIT"
] | 57 | 2017-02-14T10:26:54.000Z | 2022-03-11T14:04:48.000Z | 762.476703 | 134,428 | 0.945951 | [
[
[
"# Nonlinear recharge models\n*R.A. Collenteur, University of Graz*\n\nThis notebook explains the use of the `RechargeModel` stress model to simulate the combined effect of precipitation and potential evaporation on the groundwater levels. For the computation of the groundwater recharge, three recharge models are currently available:\n\n- `Linear` ([Berendrecht et al., 2003](#References); [von Asmuth et al., 2008](#References))\n- `Berendrecht` ([Berendrecht et al., 2006](#References))\n- `FlexModel` ([Collenteur et al., 2021](#References))\n\nThe first model is a simple linear function of precipitation and potential evaporation while the latter two are simulate a nonlinear response of recharge to precipitation using a soil-water balance concepts. Detailed descriptions of these models can be found in articles listed in the [References](#References) at the end of this notebook.\n\n<div class=\"alert alert-info\">\n \n<b>Tip</b> \n \nTo run this notebook and the related non-linear recharge models, it is strongly recommended to install Numba (http://numba.pydata.org). This Just-In-Time (JIT) compiler compiles the computationally intensive part of the recharge calculation, making the non-linear model as fast as the Linear recharge model.\n \n</div>\n",
"_____no_output_____"
]
],
[
[
"import pandas as pd\nimport pastas as ps\nimport matplotlib.pyplot as plt\n\nps.show_versions(numba=True)\nps.set_log_level(\"INFO\")",
"Python version: 3.8.2 (default, Mar 25 2020, 11:22:43) \n[Clang 4.0.1 (tags/RELEASE_401/final)]\nNumpy version: 1.20.2\nScipy version: 1.6.2\nPandas version: 1.1.5\nPastas version: 0.18.0b\nMatplotlib version: 3.3.4\nnumba version: 0.51.2\n"
]
],
[
[
"## Read Input data\nInput data handling is similar to other stressmodels. The only thing that is necessary to check is that the precipitation and evaporation are provided in mm/day. This is necessary because the parameters for the nonlinear recharge models are defined in mm for the length unit and days for the time unit. It is possible to use other units, but this would require manually setting the initial values and parameter boundaries for the recharge models.",
"_____no_output_____"
]
],
[
[
"head = pd.read_csv(\"../data/B32C0639001.csv\", parse_dates=['date'], \n index_col='date', squeeze=True) \n\n# Make this millimeters per day\nevap = ps.read_knmi(\"../data/etmgeg_260.txt\", variables=\"EV24\").series * 1e3\nrain = ps.read_knmi(\"../data/etmgeg_260.txt\", variables=\"RH\").series * 1e3\n\nfig, axes = plt.subplots(3,1, figsize=(10,6), sharex=True)\nhead.plot(ax=axes[0], x_compat=True, linestyle=\" \", marker=\".\")\nevap.plot(ax=axes[1], x_compat=True)\nrain.plot(ax=axes[2], x_compat=True)\naxes[0].set_ylabel(\"Head [m]\")\naxes[1].set_ylabel(\"Evap [mm/d]\")\naxes[2].set_ylabel(\"Rain [mm/d]\")\n\nplt.xlim(\"1985\", \"2005\");",
"INFO: Inferred frequency for time series EV24 260: freq=D\nINFO: Inferred frequency for time series RH 260: freq=D\n"
]
],
[
[
"## Make a basic model\nThe normal workflow may be used to create and calibrate the model.\n1. Create a Pastas `Model` instance\n2. Choose a recharge model. All recharge models can be accessed through the recharge subpackage (`ps.rch`).\n3. Create a `RechargeModel` object and add it to the model\n4. Solve and visualize the model\n\n",
"_____no_output_____"
]
],
[
[
"ml = ps.Model(head)\n\n# Select a recharge model\nrch = ps.rch.FlexModel()\n#rch = ps.rch.Berendrecht()\n#rch = ps.rch.Linear()\n\nrm = ps.RechargeModel(rain, evap, recharge=rch, rfunc=ps.Gamma, name=\"rch\")\nml.add_stressmodel(rm)\n\nml.solve(noise=True, tmin=\"1990\", report=\"basic\")\nml.plots.results(figsize=(10,6));",
"INFO: Cannot determine frequency of series head: freq=None. The time series is irregular.\nINFO: Inferred frequency for time series RH 260: freq=D\nINFO: Inferred frequency for time series EV24 260: freq=D\n"
]
],
[
[
"## Analyze the estimated recharge flux\nAfter the parameter estimation we can take a look at the recharge flux computed by the model. The flux is easy to obtain using the `get_stress` method of the model object, which automatically provides the optimal parameter values that were just estimated. After this, we can for example look at the yearly recharge flux estimated by the Pastas model.",
"_____no_output_____"
]
],
[
[
"recharge = ml.get_stress(\"rch\").resample(\"A\").sum()\nax = recharge.plot.bar(figsize=(10,3))\nax.set_xticklabels(recharge.index.year)\nplt.ylabel(\"Recharge [mm/year]\");",
"_____no_output_____"
]
],
[
[
"## A few things to keep in mind:\nBelow are a few things to keep in mind while using the (nonlinear) recharge models.\n\n- The use of an appropriate warmup period is necessary, so make sure the precipitation and evaporation are available some time (e.g., one year) before the calibration period.\n- Make sure that the units of the precipitation fluxes are in mm/day and that the DatetimeIndex matches exactly.\n- It may be possible to fix or vary certain parameters, dependent on the problem. Obtaining better initial parameters may be possible by solving without a noise model first (`ml.solve(noise=False)`) and then solve it again using a noise model.\n- For relatively shallow groundwater levels, it may be better to use the `Exponential` response function as the the non-linear models already cause a delayed response.\n\n## References\n- Berendrecht, W. L., Heemink, A. W., van Geer, F. C., and Gehrels, J. C. (2003) [Decoupling of modeling and measuring interval in groundwater time series analysis based on response characteristics](https://doi.org/10.1016/S0022-1694(03)00075-1), Journal of Hydrology, 278, 1–16.\n- Berendrecht, W. L., Heemink, A. W., van Geer, F. C., and Gehrels, J. C. (2006) [A non-linear state space approach to model groundwater fluctuations](https://www.sciencedirect.com/science/article/abs/pii/S0309170805002113), Advances in Water Resources, 29, 959–973.\n- Collenteur, R., Bakker, M., Klammler, G., and Birk, S. (2021) [Estimation of groundwater recharge from groundwater levels using nonlinear transfer function noise models and comparison to lysimeter data](https://doi.org/10.5194/hess-2020-392), Hydrol. Earth Syst. Sci., 25, 2931–2949.\n- Von Asmuth, J.R., Maas, K., Bakker, M. and Petersen, J. (2008) [Modeling Time Series of Ground Water Head Fluctuations Subjected to Multiple Stresses](https://doi.org/10.1111/j.1745-6584.2007.00382.x). Groundwater, 46: 30-40.\n\n## Data Sources\nIn this notebook we analysed a head time series near the town of De Bilt in the Netherlands. Data is obtained from the following resources:\n- The heads (`B32C0639001.csv`) are downloaded from https://www.dinoloket.nl/ \n- The precipitation and potential evaporation (`etmgeg_260.txt`) are downloaded from https://knmi.nl",
"_____no_output_____"
]
]
] | [
"markdown",
"code",
"markdown",
"code",
"markdown",
"code",
"markdown",
"code",
"markdown"
] | [
[
"markdown"
],
[
"code"
],
[
"markdown"
],
[
"code"
],
[
"markdown"
],
[
"code"
],
[
"markdown"
],
[
"code"
],
[
"markdown"
]
] |
d045cbcef325715b26324149f0cb9f3ec45e715b | 72,600 | ipynb | Jupyter Notebook | analysis/.ipynb_checkpoints/functions_task3_step1-checkpoint.ipynb | data301-2020-winter1/course-project-solo_102 | d0235cb9460107405937debb7ddcf4b946611cbd | [
"MIT"
] | null | null | null | analysis/.ipynb_checkpoints/functions_task3_step1-checkpoint.ipynb | data301-2020-winter1/course-project-solo_102 | d0235cb9460107405937debb7ddcf4b946611cbd | [
"MIT"
] | null | null | null | analysis/.ipynb_checkpoints/functions_task3_step1-checkpoint.ipynb | data301-2020-winter1/course-project-solo_102 | d0235cb9460107405937debb7ddcf4b946611cbd | [
"MIT"
] | null | null | null | 42.530756 | 10,944 | 0.406019 | [
[
[
"import pandas as pd\nimport numpy as np\n\ndf1 = pd.read_csv('../data/raw/drug_deaths.csv')\ndf1.info()",
"<class 'pandas.core.frame.DataFrame'>\nRangeIndex: 5105 entries, 0 to 5104\nData columns (total 42 columns):\n # Column Non-Null Count Dtype \n--- ------ -------------- ----- \n 0 Unnamed: 0 5105 non-null int64 \n 1 ID 5105 non-null object \n 2 Date 5103 non-null object \n 3 DateType 5103 non-null float64\n 4 Age 5102 non-null float64\n 5 Sex 5099 non-null object \n 6 Race 5092 non-null object \n 7 ResidenceCity 4932 non-null object \n 8 ResidenceCounty 4308 non-null object \n 9 ResidenceState 3556 non-null object \n 10 DeathCity 5100 non-null object \n 11 DeathCounty 4005 non-null object \n 12 Location 5081 non-null object \n 13 LocationifOther 590 non-null object \n 14 DescriptionofInjury 4325 non-null object \n 15 InjuryPlace 5039 non-null object \n 16 InjuryCity 3349 non-null object \n 17 InjuryCounty 2364 non-null object \n 18 InjuryState 1424 non-null object \n 19 COD 5105 non-null object \n 20 OtherSignifican 169 non-null object \n 21 Heroin 5105 non-null int64 \n 22 Cocaine 5105 non-null int64 \n 23 Fentanyl 5105 non-null object \n 24 Fentanyl_Analogue 5105 non-null float64\n 25 Oxycodone 5105 non-null int64 \n 26 Oxymorphone 5105 non-null int64 \n 27 Ethanol 5105 non-null int64 \n 28 Hydrocodone 5105 non-null int64 \n 29 Benzodiazepine 5105 non-null int64 \n 30 Methadone 5105 non-null int64 \n 31 Amphet 5105 non-null int64 \n 32 Tramad 5105 non-null int64 \n 33 Morphine_NotHeroin 5105 non-null object \n 34 Hydromorphone 5105 non-null int64 \n 35 Other 435 non-null object \n 36 OpiateNOS 5105 non-null int64 \n 37 AnyOpioid 5105 non-null object \n 38 MannerofDeath 5095 non-null object \n 39 DeathCityGeo 5105 non-null object \n 40 ResidenceCityGeo 5012 non-null object \n 41 InjuryCityGeo 5027 non-null object \ndtypes: float64(3), int64(13), object(26)\nmemory usage: 1.6+ MB\n"
],
[
"#Drop columns not needed in the analysis or with many null values\ndf1 = df1.drop(['Unnamed: 0','DateType','COD','ResidenceCity','ResidenceCounty', 'ResidenceState','DeathCity',\n 'DeathCounty','Location','LocationifOther','DescriptionofInjury','InjuryPlace','InjuryCity',\n 'InjuryCounty','InjuryState','OtherSignifican', 'Other','DeathCityGeo','ResidenceCityGeo',\n 'InjuryCityGeo'],axis = 1)\n\n#Rename with the full drug name\ndf1 = df1.rename(columns={\"Amphet\": \"Amphetamine\", \"Tramad\": \"Tramadol\"})\n\ndf1.info()",
"<class 'pandas.core.frame.DataFrame'>\nRangeIndex: 5105 entries, 0 to 5104\nData columns (total 22 columns):\n # Column Non-Null Count Dtype \n--- ------ -------------- ----- \n 0 ID 5105 non-null object \n 1 Date 5103 non-null object \n 2 Age 5102 non-null float64\n 3 Sex 5099 non-null object \n 4 Race 5092 non-null object \n 5 Heroin 5105 non-null int64 \n 6 Cocaine 5105 non-null int64 \n 7 Fentanyl 5105 non-null object \n 8 Fentanyl_Analogue 5105 non-null float64\n 9 Oxycodone 5105 non-null int64 \n 10 Oxymorphone 5105 non-null int64 \n 11 Ethanol 5105 non-null int64 \n 12 Hydrocodone 5105 non-null int64 \n 13 Benzodiazepine 5105 non-null int64 \n 14 Methadone 5105 non-null int64 \n 15 Amphetamine 5105 non-null int64 \n 16 Tramadol 5105 non-null int64 \n 17 Morphine_NotHeroin 5105 non-null object \n 18 Hydromorphone 5105 non-null int64 \n 19 OpiateNOS 5105 non-null int64 \n 20 AnyOpioid 5105 non-null object \n 21 MannerofDeath 5095 non-null object \ndtypes: float64(2), int64(12), object(8)\nmemory usage: 877.5+ KB\n"
],
[
"df1 = df1.assign(Date = pd.to_datetime(df1[\"Date\"]))\ndf1 = df1.dropna()\ndf1.info()",
"<class 'pandas.core.frame.DataFrame'>\nInt64Index: 5080 entries, 1 to 5104\nData columns (total 22 columns):\n # Column Non-Null Count Dtype \n--- ------ -------------- ----- \n 0 ID 5080 non-null object \n 1 Date 5080 non-null datetime64[ns]\n 2 Age 5080 non-null float64 \n 3 Sex 5080 non-null object \n 4 Race 5080 non-null object \n 5 Heroin 5080 non-null int64 \n 6 Cocaine 5080 non-null int64 \n 7 Fentanyl 5080 non-null object \n 8 Fentanyl_Analogue 5080 non-null float64 \n 9 Oxycodone 5080 non-null int64 \n 10 Oxymorphone 5080 non-null int64 \n 11 Ethanol 5080 non-null int64 \n 12 Hydrocodone 5080 non-null int64 \n 13 Benzodiazepine 5080 non-null int64 \n 14 Methadone 5080 non-null int64 \n 15 Amphetamine 5080 non-null int64 \n 16 Tramadol 5080 non-null int64 \n 17 Morphine_NotHeroin 5080 non-null object \n 18 Hydromorphone 5080 non-null int64 \n 19 OpiateNOS 5080 non-null int64 \n 20 AnyOpioid 5080 non-null object \n 21 MannerofDeath 5080 non-null object \ndtypes: datetime64[ns](1), float64(2), int64(12), object(7)\nmemory usage: 912.8+ KB\n"
],
[
" df1.drop_duplicates(['ID'])",
"_____no_output_____"
],
[
"df1 = df1.astype({'Age': 'int64', 'Fentanyl_Analogue': 'int64'})\ndf1.info()",
"<class 'pandas.core.frame.DataFrame'>\nInt64Index: 5080 entries, 1 to 5104\nData columns (total 22 columns):\n # Column Non-Null Count Dtype \n--- ------ -------------- ----- \n 0 ID 5080 non-null object \n 1 Date 5080 non-null datetime64[ns]\n 2 Age 5080 non-null int64 \n 3 Sex 5080 non-null object \n 4 Race 5080 non-null object \n 5 Heroin 5080 non-null int64 \n 6 Cocaine 5080 non-null int64 \n 7 Fentanyl 5080 non-null object \n 8 Fentanyl_Analogue 5080 non-null int64 \n 9 Oxycodone 5080 non-null int64 \n 10 Oxymorphone 5080 non-null int64 \n 11 Ethanol 5080 non-null int64 \n 12 Hydrocodone 5080 non-null int64 \n 13 Benzodiazepine 5080 non-null int64 \n 14 Methadone 5080 non-null int64 \n 15 Amphetamine 5080 non-null int64 \n 16 Tramadol 5080 non-null int64 \n 17 Morphine_NotHeroin 5080 non-null object \n 18 Hydromorphone 5080 non-null int64 \n 19 OpiateNOS 5080 non-null int64 \n 20 AnyOpioid 5080 non-null object \n 21 MannerofDeath 5080 non-null object \ndtypes: datetime64[ns](1), int64(14), object(7)\nmemory usage: 912.8+ KB\n"
],
[
"err1 = pd.isnull(pd.to_numeric(df1['Fentanyl'], errors='coerce'))\nerr2 = pd.isnull(pd.to_numeric(df1['Morphine_NotHeroin'], errors='coerce'))\nerr3 = pd.isnull(pd.to_numeric(df1['AnyOpioid'], errors='coerce'))\ndf1[err1]",
"_____no_output_____"
],
[
"df1[err2]",
"_____no_output_____"
],
[
"df1[err3]",
"_____no_output_____"
],
[
"df2 = df1.drop(index =[507,2808,3741,3801,62,583,4199,4384,263,456,3213,3499,4794])\ndf2",
"_____no_output_____"
],
[
"df2 = df2.astype({'Fentanyl': 'int64', 'Morphine_NotHeroin': 'int64', 'AnyOpioid': 'int64'})\ndf2.info()",
"<class 'pandas.core.frame.DataFrame'>\nInt64Index: 5067 entries, 1 to 5104\nData columns (total 22 columns):\n # Column Non-Null Count Dtype \n--- ------ -------------- ----- \n 0 ID 5067 non-null object \n 1 Date 5067 non-null datetime64[ns]\n 2 Age 5067 non-null int64 \n 3 Sex 5067 non-null object \n 4 Race 5067 non-null object \n 5 Heroin 5067 non-null int64 \n 6 Cocaine 5067 non-null int64 \n 7 Fentanyl 5067 non-null int64 \n 8 Fentanyl_Analogue 5067 non-null int64 \n 9 Oxycodone 5067 non-null int64 \n 10 Oxymorphone 5067 non-null int64 \n 11 Ethanol 5067 non-null int64 \n 12 Hydrocodone 5067 non-null int64 \n 13 Benzodiazepine 5067 non-null int64 \n 14 Methadone 5067 non-null int64 \n 15 Amphetamine 5067 non-null int64 \n 16 Tramadol 5067 non-null int64 \n 17 Morphine_NotHeroin 5067 non-null int64 \n 18 Hydromorphone 5067 non-null int64 \n 19 OpiateNOS 5067 non-null int64 \n 20 AnyOpioid 5067 non-null int64 \n 21 MannerofDeath 5067 non-null object \ndtypes: datetime64[ns](1), int64(17), object(4)\nmemory usage: 910.5+ KB\n"
],
[
"import matplotlib.pyplot as plt\nimport seaborn as sns\n\nyear = pd.to_datetime(df['Date']).dt.year.value_counts()\nplt.figure(figsize = (10, 5))\nwith plt.style.context('fivethirtyeight'):\n graph1 = sns.barplot(x = year.index.astype('int64'), y = year.values.astype('int64'), \n palette=sns.cubehelix_palette(8))\nplt.tight_layout()\nplt.ylabel('Deaths')\nplt.show()",
"_____no_output_____"
],
[
" ",
"_____no_output_____"
]
]
] | [
"code"
] | [
[
"code",
"code",
"code",
"code",
"code",
"code",
"code",
"code",
"code",
"code",
"code",
"code"
]
] |
d045fafa5613d869aa7298e5537030132b6f60bd | 15,296 | ipynb | Jupyter Notebook | TrainingDataExtraction/.ipynb_checkpoints/PCA&TRAIN-checkpoint.ipynb | leavebody/bonappetit | f2d8e22c0966e149ec446c07114a66f89a93443a | [
"MIT"
] | null | null | null | TrainingDataExtraction/.ipynb_checkpoints/PCA&TRAIN-checkpoint.ipynb | leavebody/bonappetit | f2d8e22c0966e149ec446c07114a66f89a93443a | [
"MIT"
] | null | null | null | TrainingDataExtraction/.ipynb_checkpoints/PCA&TRAIN-checkpoint.ipynb | leavebody/bonappetit | f2d8e22c0966e149ec446c07114a66f89a93443a | [
"MIT"
] | null | null | null | 70.814815 | 273 | 0.586755 | [
[
[
"%pylab inline\nfrom sklearn import datasets ## load the iris data. \niris = datasets.load_iris()\nX = iris.data \nC = iris.target\nX.shape\n",
"Populating the interactive namespace from numpy and matplotlib\n"
],
[
"## DEAL WITH DATA\n#total = np.matrix()\nimport os\nimport glob\npath = \"./data\"\n\nfor filename in glob.glob(os.path.join(path, '*.txt')):\n print (filename)\n with open(filename) as f:\n content = f.readlines()\n# you may also want to remove whitespace characters like `\\n` at the end of each line\n content = [x.strip() for x in content]\n mat1 = np.array([float(s) for s in content[0].split(' ')])\n mat2 = np.array([float(s) for s in content[1].split(' ')])\n mat3 = np.array([float(s) for s in content[2].split(' ')])\n mat4 = np.array([float(s) for s in content[3].split(' ')])\n# A1 = vstack((A1, mat1))\n# A2 = vstack((A2, mat2))\n# A3 = vstack((A3, mat3))\n# A4 = vstack((A4, mat4))\n \n ",
"./data/filename-Cashew+chicken.txt\n1.5 0.0 0.0 0.0 1.0 0.0 2.0 0.0 0.0 2.0 0.0 0.0 0.0 0.0 0.0 0.0 1.5 0.0 1.0 0.0 0.0 0.0 0.0\n[1.5, 0.0, 0.0, 0.0, 1.0, 0.0, 2.0, 0.0, 0.0, 2.0, 0.0, 0.0, 0.0, 0.0, 0.0, 0.0, 1.5, 0.0, 1.0, 0.0, 0.0, 0.0, 0.0]\n./data/filename-Pavlova.txt\n1.0 0.0 0.3333333333333333 0.0 3.1666666666666665 0.0 1.5 0.0 0.5 0.0 0.0 0.0 0.0 0.5 0.0 0.0 0.0 0.0 2.0 0.0 0.0 0.0 0.0\n[1.0, 0.0, 0.3333333333333333, 0.0, 3.1666666666666665, 0.0, 1.5, 0.0, 0.5, 0.0, 0.0, 0.0, 0.0, 0.5, 0.0, 0.0, 0.0, 0.0, 2.0, 0.0, 0.0, 0.0, 0.0]\n./data/filename-bolognese+sauce.txt\n0.0 0.0 0.0 0.0 0.5 0.0 1.0 0.0 0.0 5.0 0.0 0.0 0.0 0.0 0.0 0.0 0.5 0.0 0.0 0.0 0.0 0.0 0.0\n[0.0, 0.0, 0.0, 0.0, 0.5, 0.0, 1.0, 0.0, 0.0, 5.0, 0.0, 0.0, 0.0, 0.0, 0.0, 0.0, 0.5, 0.0, 0.0, 0.0, 0.0, 0.0, 0.0]\n./data/filename-ITALIAN+NACHOS.txt\n0.25 0.2 0.0 0.0 0.0 0.2 1.15 0.0 0.0 0.0 0.0 0.5 0.0 0.0 0.0 0.5 0.0 0.0 0.0 0.2 0.0 0.0 0.0\n[0.25, 0.2, 0.0, 0.0, 0.0, 0.2, 1.15, 0.0, 0.0, 0.0, 0.0, 0.5, 0.0, 0.0, 0.0, 0.5, 0.0, 0.0, 0.0, 0.2, 0.0, 0.0, 0.0]\n./data/filename-Greek+Salad.txt\n1.75 1.45 0.5 0.0 1.5 0.45 3.4 0.0 0.0 0.0 0.0 0.25 0.0 0.3333333333333333 0.0 0.0 0.0 0.0 3.0 0.7 0.6666666666666666 1.0 0.0\n[1.75, 1.45, 0.5, 0.0, 1.5, 0.45, 3.4, 0.0, 0.0, 0.0, 0.0, 0.25, 0.0, 0.3333333333333333, 0.0, 0.0, 0.0, 0.0, 3.0, 0.7, 0.6666666666666666, 1.0, 0.0]\n./data/filename-dal.txt\n3.5 0.2 0.0 0.0 2.5 0.2 1.4 0.0 0.5 0.0 0.0 0.0 0.0 0.0 0.0 0.0 0.0 0.5 1.0 0.2 0.0 0.0 0.0\n[3.5, 0.2, 0.0, 0.0, 2.5, 0.2, 1.4, 0.0, 0.5, 0.0, 0.0, 0.0, 0.0, 0.0, 0.0, 0.0, 0.0, 0.5, 1.0, 0.2, 0.0, 0.0, 0.0]\n./data/filename-Nem+cuon.txt\n4.25 0.5 0.125 0.125 1.5 0.0 7.0 0.0 0.5 2.5 0.0 1.6666666666666667 0.0 0.3333333333333333 0.0 0.0 0.0 0.0 1.0 0.5 0.0 0.0 0.0\n[4.25, 0.5, 0.125, 0.125, 1.5, 0.0, 7.0, 0.0, 0.5, 2.5, 0.0, 1.6666666666666667, 0.0, 0.3333333333333333, 0.0, 0.0, 0.0, 0.0, 1.0, 0.5, 0.0, 0.0, 0.0]\n./data/filename-Veg+Dum+Biryani.txt\n6.666666666666666 0.6166666666666667 0.962121212121212 0.09090909090909091 1.0 0.2 2.047727272727273 0.0 1.0 1.0909090909090908 0.0 0.9583333333333334 0.0 0.16666666666666666 0.0 0.0 0.0 0.0 1.0 0.2 0.0 1.0 0.0\n[6.666666666666666, 0.6166666666666667, 0.962121212121212, 0.09090909090909091, 1.0, 0.2, 2.047727272727273, 0.0, 1.0, 1.0909090909090908, 0.0, 0.9583333333333334, 0.0, 0.16666666666666666, 0.0, 0.0, 0.0, 0.0, 1.0, 0.2, 0.0, 1.0, 0.0]\n./data/filename-pho.txt\n9.833333333333334 0.5 1.5909090909090908 1.1818181818181819 2.5 1.3823529411764706 8.712121212121211 0.0 2.0 6.181818181818182 0.0 0.8333333333333334 0.0 3.1666666666666665 0.0 0.0 1.0588235294117647 1.0588235294117647 1.0 0.0 0.0 0.0 0.0\n[9.833333333333334, 0.5, 1.5909090909090908, 1.1818181818181819, 2.5, 1.3823529411764706, 8.712121212121211, 0.0, 2.0, 6.181818181818182, 0.0, 0.8333333333333334, 0.0, 3.1666666666666665, 0.0, 0.0, 1.0588235294117647, 1.0588235294117647, 1.0, 0.0, 0.0, 0.0, 0.0]\n./data/filename-Ginger+beef .txt\n4.5 2.25 1.5833333333333333 0.0 1.1666666666666665 0.8823529411764706 9.875 0.0 0.5 1.0 0.0 1.9583333333333335 0.0 2.1666666666666665 0.0 0.0 0.058823529411764705 1.0588235294117647 0.0 0.0 0.0 0.0 0.0\n[4.5, 2.25, 1.5833333333333333, 0.0, 1.1666666666666665, 0.8823529411764706, 9.875, 0.0, 0.5, 1.0, 0.0, 1.9583333333333335, 0.0, 2.1666666666666665, 0.0, 0.0, 0.058823529411764705, 1.0588235294117647, 0.0, 0.0, 0.0, 0.0, 0.0]\n./data/filename-Mapo+tofu.txt\n3.9166666666666665 0.2 0.0 0.0 0.5 1.0823529411764705 6.483333333333333 0.0 1.0 3.0 0.0 0.8333333333333334 0.0 3.1666666666666665 0.0 0.0 2.5588235294117645 0.058823529411764705 1.0 0.2 1.0 0.0 0.0\n[3.9166666666666665, 0.2, 0.0, 0.0, 0.5, 1.0823529411764705, 6.483333333333333, 0.0, 1.0, 3.0, 0.0, 0.8333333333333334, 0.0, 3.1666666666666665, 0.0, 0.0, 2.5588235294117645, 0.058823529411764705, 1.0, 0.2, 1.0, 0.0, 0.0]\n./data/filename-Pasta+e+fagioli.txt\n4.833333333333333 0.5 0.25 0.0 1.5 0.25 5.541666666666667 0.0 0.0 1.0 0.0 1.125 0.0 2.5 0.0 0.0 0.0 0.5 2.0 0.0 1.0 0.0 0.0\n[4.833333333333333, 0.5, 0.25, 0.0, 1.5, 0.25, 5.541666666666667, 0.0, 0.0, 1.0, 0.0, 1.125, 0.0, 2.5, 0.0, 0.0, 0.0, 0.5, 2.0, 0.0, 1.0, 0.0, 0.0]\n./data/filename-Caesar+Salad.txt\n1.0833333333333333 1.25 0.7954545454545454 0.09090909090909091 1.5 0.0 4.5643939393939394 0.0 1.0 1.0909090909090908 0.0 0.125 0.0 0.0 0.0 0.0 0.5 0.0 1.0 0.0 0.0 1.0 0.0\n[1.0833333333333333, 1.25, 0.7954545454545454, 0.09090909090909091, 1.5, 0.0, 4.5643939393939394, 0.0, 1.0, 1.0909090909090908, 0.0, 0.125, 0.0, 0.0, 0.0, 0.0, 0.5, 0.0, 1.0, 0.0, 0.0, 1.0, 0.0]\n./data/filename-Bruschetta+de+Flageolets .txt\n2.0833333333333335 1.0 1.0454545454545454 0.09090909090909091 2.5 0.0 4.6893939393939394 0.0 0.0 1.0909090909090908 0.0 1.5 0.0 0.0 0.0 0.0 0.0 0.0 2.0 0.0 0.0 0.0 0.0\n[2.0833333333333335, 1.0, 1.0454545454545454, 0.09090909090909091, 2.5, 0.0, 4.6893939393939394, 0.0, 0.0, 1.0909090909090908, 0.0, 1.5, 0.0, 0.0, 0.0, 0.0, 0.0, 0.0, 2.0, 0.0, 0.0, 0.0, 0.0]\n./data/filename-Spag+bol.txt\n0.0 0.0 0.0 0.0 0.5 0.0 1.0 0.0 0.0 5.0 0.0 0.0 0.0 0.0 0.0 0.0 0.5 0.0 0.0 0.0 0.0 0.0 0.0\n[0.0, 0.0, 0.0, 0.0, 0.5, 0.0, 1.0, 0.0, 0.0, 5.0, 0.0, 0.0, 0.0, 0.0, 0.0, 0.0, 0.5, 0.0, 0.0, 0.0, 0.0, 0.0, 0.0]\n./data/filename-Chicken+parmigiana.txt\n5.583333333333333 0.0 0.5454545454545454 0.09090909090909091 2.0 0.0 14.18939393939394 0.0 0.0 2.090909090909091 0.0 1.0 0.0 0.6666666666666666 0.0 0.0 0.0 0.5 0.0 0.0 3.333333333333333 0.0 0.0\n[5.583333333333333, 0.0, 0.5454545454545454, 0.09090909090909091, 2.0, 0.0, 14.18939393939394, 0.0, 0.0, 2.090909090909091, 0.0, 1.0, 0.0, 0.6666666666666666, 0.0, 0.0, 0.0, 0.5, 0.0, 0.0, 3.333333333333333, 0.0, 0.0]\n./data/filename-chicken+Salad.txt\n5.2666666666666675 0.2 3.2333333333333334 0.0 4.533333333333333 0.0 12.433333333333334 0.0 1.0 8.0 0.0 0.0 0.0 3.333333333333333 0.0 0.0 0.0 0.0 4.0 0.0 0.0 0.0 0.0\n[5.2666666666666675, 0.2, 3.2333333333333334, 0.0, 4.533333333333333, 0.0, 12.433333333333334, 0.0, 1.0, 8.0, 0.0, 0.0, 0.0, 3.333333333333333, 0.0, 0.0, 0.0, 0.0, 4.0, 0.0, 0.0, 0.0, 0.0]\n./data/filename-CARBONARA.txt\n0.5833333333333334 0.0 0.0 0.0 2.0 0.0 1.9166666666666665 0.0 0.0 0.0 0.0 0.0 0.0 0.5 0.0 0.0 0.0 0.0 0.0 0.0 0.0 0.0 0.0\n[0.5833333333333334, 0.0, 0.0, 0.0, 2.0, 0.0, 1.9166666666666665, 0.0, 0.0, 0.0, 0.0, 0.0, 0.0, 0.5, 0.0, 0.0, 0.0, 0.0, 0.0, 0.0, 0.0, 0.0, 0.0]\n./data/filename-Lamingtons.txt\n0.0 0.0 1.0 0.0 0.0 0.0 0.0 1.0 0.0 0.0 0.0 0.0 0.0 0.5 0.0 0.0 0.0 0.5 0.0 0.0 0.0 0.0 0.0\n[0.0, 0.0, 1.0, 0.0, 0.0, 0.0, 0.0, 1.0, 0.0, 0.0, 0.0, 0.0, 0.0, 0.5, 0.0, 0.0, 0.0, 0.5, 0.0, 0.0, 0.0, 0.0, 0.0]\n./data/filename-Hyd+Dum+Ka+Chicken+Biryani.txt\n16.5 0.3666666666666667 2.1666666666666665 1.6666666666666665 1.5666666666666667 0.2 3.9 0.3333333333333333 0.0 2.0 0.0 0.8333333333333334 0.0 0.16666666666666666 0.0 0.0 0.39999999999999997 1.5 4.0 0.2 2.2 0.0 0.0\n[16.5, 0.3666666666666667, 2.1666666666666665, 1.6666666666666665, 1.5666666666666667, 0.2, 3.9, 0.3333333333333333, 0.0, 2.0, 0.0, 0.8333333333333334, 0.0, 0.16666666666666666, 0.0, 0.0, 0.39999999999999997, 1.5, 4.0, 0.2, 2.2, 0.0, 0.0]\n./data/filename-Torta+de+Acelga.txt\n2.5 0.0 0.0 0.0 1.0 0.0 3.5 2.0 1.5 0.0 0.0 1.5 0.0 0.3333333333333333 0.0 0.0 0.0 0.0 0.0 0.0 0.6666666666666666 1.0 0.0\n[2.5, 0.0, 0.0, 0.0, 1.0, 0.0, 3.5, 2.0, 1.5, 0.0, 0.0, 1.5, 0.0, 0.3333333333333333, 0.0, 0.0, 0.0, 0.0, 0.0, 0.0, 0.6666666666666666, 1.0, 0.0]\n./data/filename-French+Toast.txt\n4.916666666666667 0.0 1.6666666666666665 0.0 4.066666666666666 0.0 2.138888888888889 0.1111111111111111 0.0 1.0 1.0 3.0 0.0 0.5 0.0 0.0 0.06666666666666667 0.3333333333333333 3.0 0.0 1.2 0.0 0.0\n[4.916666666666667, 0.0, 1.6666666666666665, 0.0, 4.066666666666666, 0.0, 2.138888888888889, 0.1111111111111111, 0.0, 1.0, 1.0, 3.0, 0.0, 0.5, 0.0, 0.0, 0.06666666666666667, 0.3333333333333333, 3.0, 0.0, 1.2, 0.0, 0.0]\n./data/filename-AFFOGATO.txt\n1.0 0.0 0.0 0.0 0.0 0.0 0.0 0.0 0.0 0.0 0.0 0.0 0.0 0.0 0.0 1.0 0.0 0.0 2.0 0.0 0.0 0.0 0.0\n[1.0, 0.0, 0.0, 0.0, 0.0, 0.0, 0.0, 0.0, 0.0, 0.0, 0.0, 0.0, 0.0, 0.0, 0.0, 1.0, 0.0, 0.0, 2.0, 0.0, 0.0, 0.0, 0.0]\n./data/filename-General+Zuo+chicken.txt\n5.25 0.5 0.7083333333333333 0.125 10.666666666666666 0.25 6.375 0.0 2.5 10.0 0.0 3.625 0.0 6.0 0.0 1.0 6.5 0.5 11.0 0.0 1.0 0.0 0.0\n[5.25, 0.5, 0.7083333333333333, 0.125, 10.666666666666666, 0.25, 6.375, 0.0, 2.5, 10.0, 0.0, 3.625, 0.0, 6.0, 0.0, 1.0, 6.5, 0.5, 11.0, 0.0, 1.0, 0.0, 0.0]\n./data/filename-Chicken+curry.txt\n13.25 0.25 0.25 1.0 1.1428571428571428 0.0 6.125 0.3333333333333333 0.0 2.0 0.0 0.125 0.8571428571428571 1.0 0.0 0.0 0.3333333333333333 2.0 1.0 0.0 0.3333333333333333 0.0 0.0\n[13.25, 0.25, 0.25, 1.0, 1.1428571428571428, 0.0, 6.125, 0.3333333333333333, 0.0, 2.0, 0.0, 0.125, 0.8571428571428571, 1.0, 0.0, 0.0, 0.3333333333333333, 2.0, 1.0, 0.0, 0.3333333333333333, 0.0, 0.0]\n./data/filename-mango+lessi.txt\n0.6666666666666666 0.0 2.6666666666666665 0.0 0.0 0.0 0.4444444444444444 0.2222222222222222 0.0 0.0 0.0 0.0 0.0 0.0 0.0 0.0 0.0 0.0 0.0 0.0 0.0 0.0 0.0\n[0.6666666666666666, 0.0, 2.6666666666666665, 0.0, 0.0, 0.0, 0.4444444444444444, 0.2222222222222222, 0.0, 0.0, 0.0, 0.0, 0.0, 0.0, 0.0, 0.0, 0.0, 0.0, 0.0, 0.0, 0.0, 0.0, 0.0]\n./data/filename-Pap+en+vleis.txt\n4.0 0.0 0.5454545454545454 0.09090909090909091 2.0 0.0 0.2727272727272727 0.0 0.0 8.09090909090909 0.0 0.0 0.0 1.0 0.0 0.0 0.0 0.0 0.0 0.0 0.0 0.0 0.0\n[4.0, 0.0, 0.5454545454545454, 0.09090909090909091, 2.0, 0.0, 0.2727272727272727, 0.0, 0.0, 8.09090909090909, 0.0, 0.0, 0.0, 1.0, 0.0, 0.0, 0.0, 0.0, 0.0, 0.0, 0.0, 0.0, 0.0]\n./data/filename-Bruschetta.txt\n2.0833333333333335 1.0 1.0454545454545454 0.09090909090909091 2.5 0.0 4.6893939393939394 0.0 0.0 1.0909090909090908 0.0 1.5 0.0 0.0 0.0 0.0 0.0 0.0 2.0 0.0 0.0 0.0 0.0\n[2.0833333333333335, 1.0, 1.0454545454545454, 0.09090909090909091, 2.5, 0.0, 4.6893939393939394, 0.0, 0.0, 1.0909090909090908, 0.0, 1.5, 0.0, 0.0, 0.0, 0.0, 0.0, 0.0, 2.0, 0.0, 0.0, 0.0, 0.0]\n./data/filename-masala.txt\n10.916666666666668 0.16666666666666666 0.16666666666666666 0.0 1.5 0.0 1.25 0.3333333333333333 1.5 0.0 0.0 0.0 0.0 2.5 0.0 0.0 0.3333333333333333 3.0 0.0 0.0 0.3333333333333333 0.0 0.0\n[10.916666666666668, 0.16666666666666666, 0.16666666666666666, 0.0, 1.5, 0.0, 1.25, 0.3333333333333333, 1.5, 0.0, 0.0, 0.0, 0.0, 2.5, 0.0, 0.0, 0.3333333333333333, 3.0, 0.0, 0.0, 0.3333333333333333, 0.0, 0.0]\n./data/filename-noodle+soup.txt\n1.0 0.0 0.3333333333333333 0.0 1.1666666666666665 0.0 0.5 0.0 0.5 1.0 0.0 0.0 0.0 0.0 0.0 0.0 1.5 0.0 0.0 1.0 0.0 1.0 0.0\n[1.0, 0.0, 0.3333333333333333, 0.0, 1.1666666666666665, 0.0, 0.5, 0.0, 0.5, 1.0, 0.0, 0.0, 0.0, 0.0, 0.0, 0.0, 1.5, 0.0, 0.0, 1.0, 0.0, 1.0, 0.0]\n./data/filename-Kong+pao+chicken.txt\n5.25 1.9500000000000002 1.0833333333333333 0.0 5.166666666666667 0.4 7.375000000000001 0.0 2.0 2.0 0.0 2.041666666666667 0.0 6.333333333333333 0.0 0.0 2.5 0.5 1.0 0.4 1.0 0.0 0.0\n[5.25, 1.9500000000000002, 1.0833333333333333, 0.0, 5.166666666666667, 0.4, 7.375000000000001, 0.0, 2.0, 2.0, 0.0, 2.041666666666667, 0.0, 6.333333333333333, 0.0, 0.0, 2.5, 0.5, 1.0, 0.4, 1.0, 0.0, 0.0]\n"
],
[
"\nfrom sklearn import decomposition\n## pca do singular value decomposition, accomplishes dimentionality reduction by only keeping the top 3 e-val.\n## it also makes the data less sparse by whitening.\npca = decomposition.PCA(n_components=3, whiten = True)\npca.fit(X) # different convention: row vs col !!!\nprint (pca.components_.T, pca.explained_variance_)\nE= pca.components_\nK = E.T.dot(E).dot(X.T)\n",
"_____no_output_____"
]
]
] | [
"code"
] | [
[
"code",
"code",
"code"
]
] |
d046062112504b3a4a95842b659824eba9b1c4bb | 1,910 | ipynb | Jupyter Notebook | SparkScalaDockerDemo.ipynb | tiago-nunesgv/maps-meaning | af669d50512aff6e0ef73f958a37ef48b4469a0d | [
"Apache-2.0"
] | 1 | 2019-10-18T15:16:42.000Z | 2019-10-18T15:16:42.000Z | SparkScalaDockerDemo.ipynb | tiagotgv/maps-meaning | af669d50512aff6e0ef73f958a37ef48b4469a0d | [
"Apache-2.0"
] | null | null | null | SparkScalaDockerDemo.ipynb | tiagotgv/maps-meaning | af669d50512aff6e0ef73f958a37ef48b4469a0d | [
"Apache-2.0"
] | null | null | null | 23.012048 | 92 | 0.524607 | [
[
[
"//Create a SparkContext to initialize Spark\n// val conf = new SparkConf()\n// conf.setMaster(\"local\")\n// conf.setAppName(\"Word Count\")\n// val sc = new SparkContext(conf)\n\nval textFile = sc.textFile(\"data/shakespeare.txt\")\n\n//word count\nval counts = textFile.flatMap(line => line.split(\" \"))\n .map(word => (word, 1))\n .reduceByKey(_ + _)\n\ncounts.foreach(println)\n// System.out.println(\"Total words: \" + counts.count());\ncounts.saveAsTextFile(\"output/shakespeareWordCount\")",
"_____no_output_____"
]
]
] | [
"code"
] | [
[
"code"
]
] |
d04631dc661e6c585f8d02e0b5a501193d00f265 | 106,761 | ipynb | Jupyter Notebook | Stocks/Place Stock Trades into Senator Dataframe Ankur Edit.ipynb | paulmtree/Suspicious-Senator-Trading | f81ca705f0fe0b940e7f8cf6b16aa0d5b79a4f01 | [
"MIT"
] | null | null | null | Stocks/Place Stock Trades into Senator Dataframe Ankur Edit.ipynb | paulmtree/Suspicious-Senator-Trading | f81ca705f0fe0b940e7f8cf6b16aa0d5b79a4f01 | [
"MIT"
] | null | null | null | Stocks/Place Stock Trades into Senator Dataframe Ankur Edit.ipynb | paulmtree/Suspicious-Senator-Trading | f81ca705f0fe0b940e7f8cf6b16aa0d5b79a4f01 | [
"MIT"
] | null | null | null | 69.460638 | 35,709 | 0.572231 | [
[
[
"# Place Stock Trades into Senator Dataframe",
"_____no_output_____"
],
[
"## 1. Understand the Senator Trading Report (STR) Dataframe",
"_____no_output_____"
]
],
[
[
"import pandas as pd\n#https://docs.google.com/spreadsheets/d/1lH_LpTgRlfzKvpRnWYgoxlkWvJj0v1r3zN3CeWMAgqI/edit?usp=sharing\ntry:\n sen_df = pd.read_csv(\"Senator Stock Trades/Senate Stock Watcher 04_16_2020 All Transactions.csv\")\nexcept:\n sen_df = pd.read_csv(\"https://github.com/pkm29/big_data_final_project/raw/master/Senate%20Stock%20Trades/Senate%20Stock%20Watcher%2004_16_2020%20All%20Transactions.csv\")\nsen_df.head()",
"_____no_output_____"
],
[
"sen_df.type.unique()",
"_____no_output_____"
]
],
[
[
"There are 4 types of trades.\nExchanges: Exchange 1 stock for another\nSale (Full): Selling all of their stock\nPurchase: Buying a stock\nSale (Partial): Selling some of that particular stock",
"_____no_output_____"
]
],
[
[
"n_exchanges = len(sen_df.loc[sen_df['type'] == \"Exchange\"])\nn_trades = len(sen_df)\nprint(\"There are \" +str(n_exchanges) +\" exchange trades out of a total of \" +str(n_trades)+ \" trades.\")\nsen_df = sen_df.loc[sen_df['type'] != \"Exchange\"]\n",
"There are 84 exchange trades out of a total of 8600 trades.\n"
]
],
[
[
"At this point in time, I will exclude exchange trades because they are so few and wish to build the basic structure of the project. As you can see, this would require splitting up the exchange into two rows with each company and so on. I may include this step later if time permits. ",
"_____no_output_____"
],
[
"There should now be 8516 trades remaining in the dataframe. Let's make sure this is so.",
"_____no_output_____"
]
],
[
[
"n_trades = len(sen_df)\nprint(\"There are \" +str(n_trades)+ \" trades in the dataframe\")",
"There are 8516 trades in the dataframe\n"
],
[
"n_blank_ticker = len(sen_df.loc[sen_df['ticker'] == \"--\"])\nprint(\"There are \" +str(n_blank_ticker) +\" trades w/o a ticker out of a total of \" +str(n_trades)+ \" trades\")\nsen_df = sen_df.loc[sen_df['ticker'] != \"--\"]",
"There are 1872 trades w/o a ticker out of a total of 8516 trades\n"
]
],
[
[
"For the same reasons we excluded exchange trades, we will also exclude trades without a ticker (which all public stocks have - the ticker is their identifier on the stock exchange). Eliminating trades without a ticker takes out trades of other types of securities (corporate bonds, municipal securities, non-public stock).",
"_____no_output_____"
],
[
"There should now be 6644 trades remaining in the dataframe. Let's make sure this is so.",
"_____no_output_____"
]
],
[
[
"n_trades = len(sen_df)\nprint(\"There are \" +str(n_trades)+ \" trades in the dataframe\")",
"There are 6644 trades in the dataframe\n"
]
],
[
[
"## 2. Add Data to STR Dataframe ",
"_____no_output_____"
],
[
"### Import Data",
"_____no_output_____"
],
[
"In this step we will be using company information such as market cap and industry from online lists provided by the NYSE, NASDAQ, and ASXL exchange. Links can be found here:https://stackoverflow.com/questions/25338608/download-all-stock-symbol-list-of-a-market",
"_____no_output_____"
]
],
[
[
"ticker_list = list()\ntry:\n NYSE_df = pd.read_csv(\"NYSEcompanylist.csv\")\nexcept:\n NYSE_df = pd.read_csv(\"https://github.com/pkm29/big_data_final_project/raw/master/Stocks/NYSEcompanylist.csv\")\n \ntry:\n NASDAQ_df = pd.read_csv(\"NASDAQcompanylist.csv\")\nexcept:\n NASDAQ_df = pd.read_csv(\"https://github.com/pkm29/big_data_final_project/raw/master/Stocks/NASDAQcompanylist.csv\")\n \nticker_list.append(NYSE_df)\nticker_list.append(NASDAQ_df)\n\nNYSE_df.head()",
"_____no_output_____"
],
[
"NASDAQ_df.head()",
"_____no_output_____"
],
[
"\"\"\"\nAdd data for Berkshire Hathaway, Lions Gate Entertainment, and Royal Dutch Shell to the NYSE company list. While\n#these companies are in the company list, their fields are empty. Also, change the tickers of these companies to \n#match Senate Stock Data (since dashes are used instead of periods in that dataset, we make sure the same is true \nin the NYSE company list). What matters is consistent convention here.\n\"\"\"\n\nrow_count = 0\nreplacement_count = 0\n\nfor row_tuple in NYSE_df.itertuples():\n \n if replacement_count == 4:\n break\n \n if row_tuple.Symbol == \"BRK.B\":\n #row_tuple.Symbol = \"BRK-B\"\n NYSE_df.at[row_count, 'Symbol'] = \"BRK-B\"\n #Shares outstanding reported in Q1 2020 financial reports, stock price from May 6, when this data is dated\n #row_tuple.MarketCap = \"$420.02B\"\n NYSE_df.at[row_count, 'MarketCap'] = \"$420.02B\"\n #row_tuple.Sector = \"Miscellaneous\"\n NYSE_df.at[row_count, 'Sector'] = \"Miscellaneous\"\n #row_tuple.industry = \"Conglomerate\"\n NYSE_df.at[row_count, 'industry'] = \"Conglomerate\"\n replacement_count = replacement_count + 1\n \n if row_tuple.Symbol == \"LGF.B\":\n #row_tuple.Symbol = \"LGF-B\"\n #Shares outstanding reported in Q1 2020 financial reports, stock price from May 6, when this data is dated\n #row_tuple.MarketCap = \"$14.62B\"\n #row_tuple.Sector = \"Consumer Services\"\n #row_tuple.industry = \"Movies/Entertainment\"\n \n NYSE_df.at[row_count, 'Symbol'] = \"LGF-B\"\n NYSE_df.at[row_count, 'MarketCap'] = \"$14.62B\"\n NYSE_df.at[row_count, 'Sector'] = \"Consumer Services\"\n NYSE_df.at[row_count, 'industry'] = \"Movies/Entertainment\" \n replacement_count = replacement_count + 1\n\n if row_tuple.Symbol == \"RDS.A\":\n #row_tuple.Symbol = \"RDS-A\"\n #Shares outstanding reported in Q1 2020 financial reports, stock price from May 6, when this data is dated\n #row_tuple.MarketCap = \"$122.28B\"\n #row_tuple.Sector = \"Energy\"\n #row_tuple.industry = \"Oil & Gas Production\"\n \n NYSE_df.at[row_count, 'Symbol'] = \"RDS-A\"\n NYSE_df.at[row_count, 'MarketCap'] = \"$122.28B\"\n NYSE_df.at[row_count, 'Sector'] = \"Energy\"\n NYSE_df.at[row_count, 'industry'] = \"Oil & Gas Production\" \n replacement_count = replacement_count + 1\n\n if row_tuple.Symbol == \"RDS.B\":\n #row_tuple.Symbol = \"RDS-B\"\n #Shares outstanding reported in Q1 2020 financial reports, stock price from May 6, when this data is dated\n #row_tuple.MarketCap = \"$122.09B\"\n #row_tuple.Sector = \"Energy\"\n #row_tuple.industry = \"Oil & Gas Production\"\n \n NYSE_df.at[row_count, 'Symbol'] = \"RDS-B\"\n NYSE_df.at[row_count, 'MarketCap'] = \"$122.09B\"\n NYSE_df.at[row_count, 'Sector'] = \"Energy\"\n NYSE_df.at[row_count, 'industry'] = \"Oil & Gas Production\"\n replacement_count = replacement_count + 1\n \n row_count = row_count + 1\n \n#Confirm changes have been made successfully\nfor row_tuple in NYSE_df.itertuples():\n \n if row_tuple.Symbol == \"BRK-B\":\n print (row_tuple)\n \n if row_tuple.Symbol == \"LGF-B\":\n print (row_tuple)\n \n if row_tuple.Symbol == \"RDS-A\":\n print (row_tuple)\n \n if row_tuple.Symbol == \"RDS-B\":\n print (row_tuple)\n",
"Pandas(Index=365, Symbol='BRK-B', Name='Berkshire Hathaway Inc.', LastSale=nan, MarketCap='$420.02B', IPOyear=nan, Sector='Miscellaneous', industry='Conglomerate', _8='https://old.nasdaq.com/symbol/brk.b', _9=nan)\nPandas(Index=1700, Symbol='LGF-B', Name='Lions Gate Entertainment Corporation', LastSale=nan, MarketCap='$14.62B', IPOyear=2016.0, Sector='Consumer Services', industry='Movies/Entertainment', _8='https://old.nasdaq.com/symbol/lgf.b', _9=nan)\nPandas(Index=2426, Symbol='RDS-A', Name='Royal Dutch Shell PLC', LastSale=nan, MarketCap='$122.28B', IPOyear=nan, Sector='Energy', industry='Oil & Gas Production', _8='https://old.nasdaq.com/symbol/rds.a', _9=nan)\nPandas(Index=2427, Symbol='RDS-B', Name='Royal Dutch Shell PLC', LastSale=nan, MarketCap='$122.09B', IPOyear=nan, Sector='Energy', industry='Oil & Gas Production', _8='https://old.nasdaq.com/symbol/rds.b', _9=nan)\n"
],
[
"#There are also 2 instances where a wrong ticker for Berkshire Hathaway is found in the Senate Stock data\n#(BRKB is used as opposed to BRK-B). Thus, we correct for those instances here.\n\n#Find indices of these two trades\nfor row_tuple in sen_df.itertuples():\n if row_tuple.ticker == \"BRKB\":\n print (row_tuple)\n \n#We can see that the indices are 1207 and 4611, so we will manually modify the ticker field of these trades.\n\nsen_df.at[1207, 'ticker'] = \"BRK-B\"\nsen_df.at[4611, 'ticker'] = \"BRK-B\"\n\nlen(sen_df)",
"Pandas(Index=1207, transaction_date='12/06/2018', owner='Self', ticker='BRKB', asset_description='Berkshire Hathaway Inc.', asset_type='Stock', type='Purchase', amount='$15,001 - $50,000', comment='--', senator='Jerry Moran, ', ptr_link='https://efdsearch.senate.gov/search/view/ptr/dafb67b1-d578-402d-9e26-c3c2a575b42c/')\nPandas(Index=4611, transaction_date='03/02/2017', owner='Spouse', ticker='BRKB', asset_description='Berkshire Hathaway Inc.', asset_type='Stock', type='Purchase', amount='$15,001 - $50,000', comment='--', senator='Susan M Collins', ptr_link='https://efdsearch.senate.gov/search/view/ptr/ea48ecad-1959-4f7a-ace0-778a71d06437/')\n"
],
[
"#Get sector data for each stock trade\n\nsector_data = list()\nfor row_tuple in sen_df.itertuples():\n tic = row_tuple.ticker\n count = 0\n for row_tuple_tic in NYSE_df.itertuples():\n sym = row_tuple_tic.Symbol\n if tic == sym:\n count = count+1\n if row_tuple_tic.Sector == \"n/a\":\n sector_data.append(\"none\")\n else:\n sector_data.append(row_tuple_tic.Sector)\n break\n if count == 0:\n for row_tuple_tic in NASDAQ_df.itertuples():\n sym = row_tuple_tic.Symbol\n if tic == sym:\n count = count+1\n if row_tuple_tic.Sector == \"n/a\":\n sector_data.append(\"none\")\n else:\n sector_data.append(row_tuple_tic.Sector)\n break\n if count == 0:\n sector_data.append(\"none\")\n \nprint(sector_data[0:9])",
"['Capital Goods', 'Capital Goods', 'none', 'none', 'Basic Industries', 'Consumer Services', 'Finance', 'Technology', 'Health Care']\n"
],
[
"#make sure length matches number of rows in df\nprint(len(sector_data))\n\n#counter for how many times the stock traded by senator not found in exchange data set\nno_ticker_cnt = 0\n\nfor i in sector_data:\n if i == \"none\":\n no_ticker_cnt = no_ticker_cnt + 1\n \nprint(no_ticker_cnt)",
"6644\n1141\n"
],
[
"#Get industry data for each stock trade\n\nindustry_data = list()\nfor row_tuple in sen_df.itertuples():\n tic = row_tuple.ticker\n count = 0\n for row_tuple_tic in NYSE_df.itertuples():\n sym = row_tuple_tic.Symbol\n if tic == sym:\n count = count+1\n if row_tuple_tic.industry == \"n/a\":\n industry_data.append(\"none\")\n else:\n industry_data.append(row_tuple_tic.industry)\n break\n if count == 0:\n for row_tuple_tic in NASDAQ_df.itertuples():\n sym = row_tuple_tic.Symbol\n if tic == sym:\n count = count+1\n if row_tuple_tic.industry == \"n/a\":\n industry_data.append(\"none\")\n else:\n industry_data.append(row_tuple_tic.industry)\n break\n if count == 0:\n industry_data.append(\"none\")\n \nprint(industry_data[0:9])",
"['Biotechnology: Laboratory Analytical Instruments', 'Industrial Machinery/Components', 'none', 'none', 'Paints/Coatings', 'Building operators', 'Major Banks', 'Semiconductors', 'Major Pharmaceuticals']\n"
],
[
"#make sure length matches number of rows in df\nprint(len(industry_data))\n\n#counter for how many times the stock traded by senator not found in exchange data set\nno_ticker_cnt = 0\n\nfor i in industry_data:\n if i == \"none\":\n no_ticker_cnt = no_ticker_cnt + 1\n \nprint(no_ticker_cnt)",
"6644\n1141\n"
],
[
"#Get market cap data for each stock trade\n\nmktcap_data = list()\nfor row_tuple in sen_df.itertuples():\n tic = row_tuple.ticker\n count = 0\n for row_tuple_tic in NYSE_df.itertuples():\n sym = row_tuple_tic.Symbol\n if tic == sym:\n count = count+1\n if row_tuple_tic.MarketCap == \"n/a\":\n mktcap_data.append(\"none\")\n else:\n mktcap_data.append(row_tuple_tic.MarketCap)\n break\n if count == 0:\n for row_tuple_tic in NASDAQ_df.itertuples():\n sym = row_tuple_tic.Symbol\n if tic == sym:\n count = count+1\n if row_tuple_tic.MarketCap == \"n/a\":\n mktcap_data.append(\"none\")\n else:\n mktcap_data.append(row_tuple_tic.MarketCap)\n break\n if count == 0:\n mktcap_data.append(\"none\")\n \nprint(mktcap_data[0:9])",
"['$47.15B', '$8.58B', 'none', 'none', '$8.74B', '$34.86B', '$92.93B', '$52.6B', '$190.9B']\n"
],
[
"#make sure length matches number of rows in df\nprint(len(mktcap_data))\n\n#counter for how many times the stock traded by senator not found in exchange data set\nno_ticker_cnt = 0\n\nfor i in mktcap_data:\n if i == \"none\":\n no_ticker_cnt = no_ticker_cnt + 1\n \nprint(no_ticker_cnt)",
"6644\n1141\n"
],
[
"#add new columns to df\n\nsen_df['mkt_cap'] = mktcap_data\nsen_df['sector'] = sector_data\nsen_df['industry'] = industry_data\n\nsen_df = sen_df.fillna(\"none\")\nsen_df.head()",
"_____no_output_____"
],
[
"\"\"\"\nPrint out names of companies with missing data to find out why we have so many misses (~17% of our data).\nThere seem to be 3 reasons for this:\n1. Companies merging with another or being acquired (or even acquiring and taking the acquired company's name - very rare)\n2. Foreign companies (listed abroad)\n3. American companies listed abroad - this applies to a very small number of trades\n\"\"\"\n\nfrom collections import Counter\n\ncompany_missing_data = list()\nfor row_tuple in sen_df.itertuples():\n if row_tuple.mkt_cap == \"none\":\n company_missing_data.append(row_tuple.asset_description)\n\nprint(Counter(company_missing_data))\n",
"Counter({'First Data Corporation': 76, 'Revere Bank': 36, 'Halyard Health, Inc.': 36, 'CBS Corporation (NYSE)': 35, 'Whole Foods Market, Inc.': 29, 'Williams Partners L.P. (NYSE)': 27, 'Versum Materials, Inc.': 25, 'Bollore': 24, 'SPDR S&P 500 ETF': 22, 'Celgene Corporation': 20, 'Exelis Inc. Common Stock Ex-Dis (NYSE)': 18, 'USG Corporation': 16, 'CBS Corporation': 15, 'Weight Watchers International, Inc.': 14, 'Cablevision Systems Corporation (NYSE)': 13, 'Michael Kors Holdings Limited (NYSE)': 13, 'VCA Inc.': 12, 'ACE Limited (NYSE)': 12, 'Nestl\\\\u00e9 S.A.': 11, 'DowDuPont Inc.': 11, 'Celgene Corporation (NASDAQ)': 11, 'KLX Inc. (NASDAQ)': 11, 'Axiall Corporation': 11, 'United Technologies Corporation': 10, 'Energy Transfer Partners, L.P.': 9, 'Targa Resources Partners LP (NYSE)': 9, 'Energy Transfer Equity, L.P. (NYSE)': 9, 'Qlik Technologies, Inc. (NASDAQ)': 9, 'Deutsche Global Infrastructure A (NASDAQ)': 9, 'Cohen & Steers Dividend Value A (NASDAQ)': 9, 'Raytheon Company': 8, 'Buckeye Partners, L.P.': 8, 'Avon Products, Inc.': 8, 'Cheniere Energy, Inc.': 7, 'Express Scripts Holding Company': 7, \"Cabela's Incorporated\": 7, 'B/E Aerospace Inc. (NASDAQ)': 7, 'Express Scripts Holding Company (NASDAQ)': 7, 'ViacomCBS Inc.': 6, 'SPDR S&P Biotech ETF': 6, 'Roche Holding AG': 6, 'SPDR S&P Semiconductor ETF': 6, 'Comcast Corporation (NASDAQ)': 6, 'VCA Inc. (NASDAQ)': 6, 'Time Warner Inc. (NYSE)': 6, 'BlackRock Mid-Cap Growth Equity Inv A (NASDAQ)': 6, 'Harris Corporation': 5, 'Fanuc Corporation': 5, 'Valero Energy Partners LP': 5, 'iShares Transportation Average': 5, 'CR Bard Inc. (NYSE)': 5, 'Red Hat, Inc. (NYSE)': 5, 'Red Hat, Inc.': 4, 'Liberty Media Corp. Reg.Sh.C FO': 4, 'Praxair, Inc.': 4, 'Cogentix Medical, Inc.': 4, 'Ensco plc (NYSE)': 4, 'Utilities Select Sector SPDR ETF (NYSEArca)': 4, 'Praxair Inc.': 4, 'Quintiles IMS Holdings, Inc.': 4, 'Axiall Corporation (NYSE)': 4, 'Valero Energy Partners LP (NYSE)': 4, 'Raytheon Company (NYSE)': 4, 'Alerian MLP ETF (NYSEArca)': 4, 'United Technologies Corp. (NYSE)': 4, 'Valeant Pharmaceuticals International, Inc. (NYSE)': 4, 'Ivy Asset Strategy C (NASDAQ)': 4, 'Lehigh Gas Partners LP (NYSE)': 4, 'LinkedIn Corporation (NYSE)': 4, 'Siemens Aktiengesellschaft': 3, 'Andeavor Logistics LP': 3, 'PHILLIPS EDISON GR': 3, 'SPDR Gold Shares': 3, 'Time Warner Inc.': 3, 'Energy Transfer Equity, L.P.': 3, 'Vanguard FTSE Emerging Markets ETF': 3, 'Reynolds American Inc.': 3, 'PureFunds ISE Cyber Security ETF': 3, 'C. R. Bard, Inc.': 3, 'Torchmark Corporation': 3, 'Linear Technology Corporation': 3, 'DCP Midstream Partners LP': 3, 'iShares MSCI Emerging Markets': 3, 'Williams Partners L.P.': 3, 'Avon Products Inc. (NYSE)': 3, 'Buckeye Partners, L.P. (NYSE)': 3, 'EMC Corporation': 3, 'SPY Feb 2016 put 180.000': 3, 'iShares Core S&P Mid-Cap (NYSEArca)': 3, 'Guggenheim S&P 500 Equal Weight ETF (NYSEArca)': 3, 'iShares International Select Dividend (NYSEArca)': 3, 'iShares Global Telecom (NYSEArca)': 3, 'SPDR S&P Dividend ETF (NYSEArca)': 3, 'SPDR S&P International Dividend ETF (NYSEArca)': 3, 'iShares Latin America 40 (NYSEArca)': 3, 'iShares MSCI Pacific ex Japan (NYSEArca)': 3, 'BioMed Realty Trust Inc. (NYSE)': 3, 'EMC Corporation (NYSE)': 3, 'Nestl (OTC Markets)': 3, 'Legg Mason Batterymarch Intl Eq C (NASDAQ)': 3, 'Triangle Capital Corporation (NYSE)': 3, 'Rockwood Holdings, Inc. (NYSE)': 3, 'Praxair Inc. (NYSE)': 3, 'PartnerRe Ltd. (NYSE)': 3, 'Sprott Physical Gold and Silver Trust': 2, 'Tencent Holdings Limited': 2, 'BB&T Corporation <div class=\"text-muted\"><em>Rate/Coupon:</em> 5.25%<br> <em>Matures:</em> 11/01/2019</div>': 2, 'BB&T Corporation <div class=\"text-muted\"><em>Rate/Coupon:</em> 5.25<br> <em>Matures:</em> 11/01/2019</div>': 2, 'VISA - CLASSE A': 2, 'SPDR Bloomberg Barclays High Yield Bond ETF': 2, 'FACEBOOK INC FACEBOOK CLASS A': 2, 'iShares Russell 1000 Growth ETF': 2, 'Vanguard FTSE Europe ETF': 2, 'iShares US Energy ETF': 2, 'SoftBank Group Corp.': 2, 'Reckitt Benckiser Group plc': 2, 'Pernod Ricard SA': 2, \"L'Or\\\\u00e9al S.A.\": 2, 'Murata Manufacturing Co., Ltd.': 2, 'Naspers Limited': 2, 'Keyence Corporation': 2, 'Carlsberg A/S': 2, 'Asahi Kasei Corporation': 2, 'Invesco S&P SmallCap Low Volatility ETF': 2, 'Zayo Group Holdings, Inc.': 2, 'Mainstay Medical International plc': 2, 'ProShares UltraShort 20+ Year Treasury': 2, 'SPDR S&P 500 ETF <div class=\"text-muted\">Option Type: Put <br><em>Strike price:</em> $210.00 <br> <em>Expires:</em> 06/30/2017 </div>': 2, 'RSP Permian, Inc.': 2, 'BNP Paribas SA': 2, 'Singapore Telecommunications Limited': 2, 'Shiseido Company, Limited': 2, 'Regal Entertainment Group': 2, 'SPDR S&amp;P 500 ETF': 2, 'Linn Energy, LLC (NASDAQ)': 2, 'iShares MSCI Emerging Markets (NYSEArca)': 2, 'Health Care Select Sector SPDR ETF (NYSEArca)': 2, 'SPDR S&P 500 ETF (NYSEArca)': 2, 'PowerShares Emerging Markets Sov Dbt ETF (NYSEArca)': 2, 'AMG Yacktman Service': 2, 'Dell Technologies Inc.': 2, 'XEROX CORP <div class=\"text-muted\"><em>Rate/Coupon:</em> 4.5%<br> <em>Matures:</em> 05/15/2021</div>': 2, 'SunTrust Banks, Inc.': 2, 'Aquesta Financial Holdings, Inc.': 2, 'SPDR EURO STOXX 50 ETF': 2, 'SPDR S&P 500 ETF Trust (NYSEArca)': 2, 'Industrial Select Sector SPDR ETF': 2, 'Newfield Exploration Co.': 2, 'Permanent Portfolio (NASDAQ)': 2, 'ProShares Short 20+ Year Treasury': 2, 'United Technologies Corporation (NYSE)': 2, 'JP MORGAN CHASE (OTC Markets)': 2, 'Vanguard Consumer Staples ETF': 2, 'Linear Technology Corporation (NASDAQ)': 2, 'Harris Corporation (NYSE)': 2, 'Hubbell Inc. (NYSE)': 2, 'Pengrowth Energy Corporation (NYSE)': 2, 'Protective Life Corporation (NYSE)': 2, 'Oppenheimer SteelPath MLP Income C (NASDAQ)': 2, 'Crosstex Energy, Inc. (NASDAQ)': 2, 'Columbia Marsico Growth Z (NASDAQ)': 2, 'Atmel Corporation (NASDAQ)': 2, 'ProShares Short 20+ Year Treasury (NYSEArca)': 2, 'PowerShares DB Precious Metals': 2, 'ProShares VIX Short-Term Futures ETF': 2, 'ALPS Alerian MLP ETF': 2, 'ProShares VIX Short-Term Futures (NYSEArca)': 2, 'Halyard Health, Inc. (NYSE)': 2, 'Northern Oil and Gas, Inc. (AMEX)': 2, 'BlackRock Equity Dividend Inv A (NASDAQ)': 2, 'Roche Holding AG (OTC Markets)': 2, 'Zimmer Holdings, Inc. (NYSE)': 2, 'WCM Focused International Growth Fund Institutiona': 1, 'SPDR S&P 500 ETF Trust': 1, 'Vanguard FTSE Emerging Markets Index Fund ETF Shar': 1, 'Vanguard Intermediate-Term Bond Index Fund ETF Sha': 1, 'Vanguard GNMA Fund Admiral Shares': 1, 'Templeton Global Bond Fund Advisor Class': 1, 'iShares Edge MSCI USA Momentum Factor ETF': 1, 'BlackRock Global Allocation Fund, Inc. Institution': 1, 'Glenmede Small Cap Equity Portfolio Class Advisor': 1, 'AMG TimesSquare Small Cap Growth Fund Class Z': 1, 'Vanguard PRIMECAP Fund Admiral Shares': 1, 'Royal Dutch Shell plc': 1, 'Anadarko Petroleum Corporation': 1, 'Safran SA': 1, 'EssilorLuxottica Soci\\\\u00e9t\\\\u00e9 anonyme': 1, 'Airbus SE': 1, 'AIA Group Limited': 1, 'T. Rowe Price New Horizons': 1, 'JPMorgan Mid Cap Value L': 1, 'Fidelity Contrafund': 1, 'The Toronto-Dominion Bank <div class=\"text-muted\"><em>Rate/Coupon:</em> 3.25<br> <em>Matures:</em> 06/11/2021</div>': 1, 'American Funds Growth Fund of Amer F1': 1, 'BlackRock Global Allocation Instl': 1, 'Shin-Etsu Chemical Co., Ltd.': 1, 'International Business Machines Corporation': 1, 'Guggenheim S&P 500 Eq Wt Technology ETF': 1, 'iShares Transportation Average ETF': 1, 'iShares US Pharmaceuticals ETF': 1, 'US Global Jets ETF': 1, 'Entellus Medical, Inc.': 1, 'ETFMG Prime Cyber Security ETF': 1, 'The Priceline Group Inc.': 1, 'South32 Limited': 1, 'LVMH Mo\\\\u00ebt Hennessy Louis Vuitton S.E.': 1, 'Compass Group PLC': 1, 'BASF SE': 1, 'AXA SA': 1, 'Vtech Holdings Limited': 1, 'SMC Corporation': 1, 'SCANA Corporation': 1, 'Pacer Funds Trust - Pacer Trendpilot 450 ETF': 1, 'Dr Pepper Snapple Group, Inc.': 1, 'Zynga Inc.': 1, 'Newfield Exploration Company': 1, 'Potash Corporation of Saskatchewan Inc.': 1, 'Bioverativ Inc.': 1, 'Victory Sycamore Established Value I': 1, 'Agrium Inc.': 1, 'Tesoro Logistics LP': 1, 'FPL Group, Inc. 5 7/8% Preferre': 1, 'ONEOK Partners, L.P.': 1, 'iShares US Broker-Dealers & Secs Exchs': 1, 'DB 3x German Bund Futures ETN': 1, 'NSRGY- Nestle (OTC Markets)': 1, 'American Funds Tax-Exempt Bond A': 1, 'iShares Russell 1000 Value': 1, 'Under Armour, Inc. Class C Comm': 1, 'Shire plc': 1, 'Aqua America Inc.': 1, 'Columbia Pipeline Group, Inc.': 1, 'iShares Edge MSCI USA Momentum Factor': 1, 'SYSCO': 1, 'Starwood Hotels & Resorts Worldwide Inc.': 1, 'ADT CORPORATION': 1, 'ITC Holdings Corp.': 1, 'RSP Permian, Inc. (NYSE)': 1, 'AGL Resources Inc. (NYSE)': 1, 'iShares US Pharmaceuticals (NYSEArca)': 1, 'STARBUCKS (Swiss)': 1, 'Noble Energy, Inc. (NYSE)': 1, 'JP MORGAN CHASE (OTC Markets) <div class=\"text-muted\"><em>Rate/Coupon:</em> 6.75<br> <em>Matures:</em> 02/01/24</div>': 1, 'Morgan Stanley Inst Active Intl Allc I (NASDAQ)': 1, 'Invesco Equally-Wtd S&P 500 Y (NASDAQ)': 1, 'Wells Fargo Advantage S/T Muni Bd Adm (NASDAQ)': 1, 'Invesco Municipal Income Y (NASDAQ)': 1, 'Invesco SmallCapValue Y (NASDAQ)': 1, 'Invesco Comstock Y (NASDAQ)': 1, 'Morgan Stanley Inst Emerging Mkts I (NASDAQ)': 1, 'The Priceline Group Inc. (NASDAQ)': 1, 'ITC Holdings Corp. (NYSE)': 1, 'PowerShares DB 3x German Bund F (NYSE)': 1, 'Convergys Corporation (NYSE)': 1, 'SPDR EURO STOXX Small Cap ETF (NYSEArca)': 1, 'SPDR Series Trust - SPDR S&P Retail ETF (NYSEArca)': 1, 'SPDR S&P 600 Small Cap ETF (NYSEArca) <div class=\"text-muted\"><em>Description:</em> ETF</div>': 1, 'Kraft Foods Group, Inc. (NASDAQ)': 1, 'iShares Transportation Average (NYSEArca)': 1, 'WageWorks, Inc. (NYSE)': 1, 'Vitamin Shoppe, Inc. (NYSE)': 1, 'Monotype Imaging Holdings Inc. (NASDAQ)': 1, 'Tumi Holdings, Inc. (NYSE)': 1, 'Tangoe, Inc. (NASDAQ)': 1, 'SciQuest, Inc. (NASDAQ)': 1, 'Stone Energy Corp. (NYSE)': 1, 'Roadrunner Transportation Systems, Inc. (NYSE)': 1, 'Popeyes Louisiana Kitchen, Inc. (NASDAQ)': 1, 'NeuStar, Inc. (NYSE)': 1, 'Mattress Firm Holding Corp. (NASDAQ)': 1, 'LifeLock, Inc. (NYSE)': 1, 'IPC Healthcare, Inc. (NASDAQ)': 1, 'HFF, Inc. (NYSE)': 1, 'Financial Engines, Inc. (NASDAQ)': 1, \"Del Frisco's Restaurant Group, Inc. (NASDAQ)\": 1, 'Cyberonics Inc. (NASDAQ)': 1, 'Control4 Corporation (NASDAQ)': 1, 'Constant Contact, Inc. (NASDAQ)': 1, 'Cantel Medical Corp. (NYSE)': 1, 'CLARCOR Inc. (NYSE)': 1, 'AmSurg Corp. (NASDAQ)': 1, 'AmTrust Financial Services, Inc. (NASDAQ)': 1, 'The Advisory Board Company (NASDAQ)': 1, 'Coach, Inc. (NYSE)': 1, 'Boulder Total Return Fund, Inc. (NYSE)': 1, 'MainStay Marketfield C (NASDAQ)': 1, 'Precision Castparts Corp. (NYSE)': 1, 'Capital One Financial Corp Pfd (NYSE)': 1, 'Isis Pharmaceuticals, Inc. (NASDAQ)': 1, 'Vanguard Emerging Markets Stock Idx ETF': 1, 'SPDR S&P Retail ETF': 1, 'Northern Tier Energy LP (NYSE)': 1, 'Raytheon Co. (NYSE)': 1, 'Yahoo! Inc. (NASDAQ)': 1, 'Vectren Corporation (NYSE)': 1, 'Validus Holdings, Ltd. (NYSE)': 1, 'Starwood Hotels & Resorts Worldwide Inc. (NYSE)': 1, 'Penn West Petroleum Ltd. (NYSE)': 1, 'Landauer Inc. (NYSE)': 1, 'Hatteras Financial Corp (NYSE)': 1, 'FirstMerit Corporation (NASDAQ)': 1, 'CYS Investments, Inc. (NYSE)': 1, 'Baker Hughes Incorporated (NYSE)': 1, 'Windstream Holdings, Inc. (NASDAQ)': 1, 'Symantec Corporation (NASDAQ)': 1})\n"
],
[
"#Get a view of how many industries are found in our senate stock data.\n\nindustry_dict = Counter(industry_data)\n\nindustry_list = list()\nfor x in industry_dict:\n industry_list.append(x)\n \nprint(industry_list[0:9])\nn_industries = len(industry_list)\n#since 'none' is included in our list\nn_industries = n_industries - 1\nprint(\"There are \" + str(n_industries) + \" industries covered by the trades of senators.\")",
"Counter({'none': 1141, 'Major Banks': 350, 'Major Pharmaceuticals': 317, 'Natural Gas Distribution': 203, 'Industrial Machinery/Components': 187, 'Computer Manufacturing': 180, 'Oil & Gas Production': 166, 'Consumer Electronics/Appliances': 165, 'Telecommunications Equipment': 157, 'Computer Software: Prepackaged Software': 138, 'Television Services': 137, 'Clothing/Shoe/Accessory Stores': 135, 'Semiconductors': 132, 'Integrated oil Companies': 131, 'Major Chemicals': 112, 'Computer Software: Programming, Data Processing': 112, 'Department/Specialty Retail Stores': 106, 'Beverages (Production/Distribution)': 102, 'Services-Misc. Amusement & Recreation': 97, 'Biotechnology: Biological Products (No Diagnostic Substances)': 96, 'Package Goods/Cosmetics': 91, 'Auto Manufacturing': 90, 'Medical/Dental Instruments': 83, 'Computer peripheral equipment': 81, 'Business Services': 78, 'Diversified Commercial Services': 77, 'Real Estate Investment Trusts': 76, 'Hotels/Resorts': 73, 'Consumer Electronics/Video Chains': 66, 'Plastic Products': 65, 'Investment Bankers/Brokers/Service': 63, 'Restaurants': 59, 'Broadcasting': 57, 'Air Freight/Delivery Services': 56, nan: 56, 'Containers/Packaging': 55, 'Apparel': 49, 'Medical/Nursing Services': 49, 'Packaged Foods': 46, 'Oilfield Services/Equipment': 46, 'Catalog/Specialty Distribution': 44, 'Aerospace': 43, 'Agricultural Chemicals': 43, 'EDP Services': 40, 'Paints/Coatings': 39, 'Electric Utilities: Central': 38, 'Biotechnology: Laboratory Analytical Instruments': 35, 'Movies/Entertainment': 35, 'Savings Institutions': 34, 'Radio And Television Broadcasting And Communications Equipment': 34, 'Computer Communications Equipment': 33, 'Property-Casualty Insurers': 33, 'Power Generation': 33, 'Railroads': 33, 'Finance: Consumer Services': 30, 'Food Chains': 26, 'Life Insurance': 25, 'Biotechnology: Electromedical & Electrotherapeutic Apparatus': 24, 'RETAIL: Building Materials': 24, 'Food Distributors': 23, 'Investment Managers': 23, 'Specialty Chemicals': 22, 'Conglomerate': 20, 'Other Consumer Services': 20, 'Industrial Specialties': 20, 'Oil Refining/Marketing': 19, 'Metal Fabrications': 18, 'Environmental Services': 18, 'Trucking Freight/Courier Services': 18, 'Construction/Ag Equipment/Trucks': 17, 'Auto Parts:O.E.M.': 17, 'Automotive Aftermarket': 17, 'Medical Specialities': 16, 'Specialty Insurers': 15, 'Recreational Products/Toys': 14, 'Shoe Manufacturing': 14, 'Marine Transportation': 14, 'Farming/Seeds/Milling': 11, 'Newspapers/Magazines': 10, 'Transportation Services': 10, 'Military/Government/Technical': 10, 'Biotechnology: Commercial Physical & Biological Resarch': 10, 'Other Pharmaceuticals': 10, 'Wholesale Distributors': 9, 'Accident &Health Insurance': 9, 'Office Equipment/Supplies/Services': 9, 'Home Furnishings': 8, 'Other Specialty Stores': 8, 'Electronic Components': 8, 'Rental/Leasing Companies': 7, 'Paper': 7, 'Building operators': 6, 'Specialty Foods': 6, 'Precious Metals': 5, 'Medical Electronics': 5, 'Commercial Banks': 5, 'Mining & Quarrying of Nonmetallic Minerals (No Fuels)': 4, 'Coal Mining': 4, 'Electrical Products': 3, 'Building Products': 3, 'Electronics Distribution': 3, 'Water Supply': 3, 'Oil/Gas Transmission': 3, 'Professional Services': 2, 'Misc Health and Biotechnology Services': 2, 'Biotechnology: In Vitro & In Vivo Diagnostic Substances': 2, 'Meat/Poultry/Fish': 2, 'Textiles': 1, 'Ordnance And Accessories': 1, 'Homebuilding': 1, 'Engineering & Construction': 1, 'Steel/Iron Ore': 1, 'Tools/Hardware': 1, 'Advertising': 1, 'Hospital/Nursing Management': 1, 'Fluid Controls': 1})\n['Biotechnology: Laboratory Analytical Instruments', 'Industrial Machinery/Components', 'none', 'Paints/Coatings', 'Building operators', 'Major Banks', 'Semiconductors', 'Major Pharmaceuticals', 'Newspapers/Magazines']\nThere are 115 industries covered by the trades of senators.\n"
],
[
"import string\n\nindustry_size_data = list()\n\nfor row_tuple in sen_df.itertuples():\n industry_size = row_tuple.industry\n \n if industry_size == 'none':\n industry_size_data.append(\"none\")\n continue\n \n size = row_tuple.mkt_cap\n factor = 0\n x = size.find(\"M\")\n if x != -1:\n factor = 1000000\n else:\n factor = 1000000000\n \n size = size.lstrip(\"$\")\n size = size.rstrip(\"MB\")\n size = float(size)\n size = size*factor\n \n if size < 500000000:\n industry_size = industry_size + \"1\"\n industry_size_data.append(industry_size)\n continue\n elif size < 1000000000:\n industry_size = industry_size + \"2\"\n industry_size_data.append(industry_size)\n continue\n elif size < 10000000000:\n industry_size = industry_size + \"3\"\n industry_size_data.append(industry_size)\n continue\n elif size < 50000000000:\n industry_size = industry_size + \"4\"\n industry_size_data.append(industry_size)\n continue\n elif size < 100000000000:\n industry_size = industry_size + \"5\"\n industry_size_data.append(industry_size)\n continue\n elif size < 500000000000:\n industry_size = industry_size + \"6\"\n industry_size_data.append(industry_size)\n continue\n else:\n industry_size = industry_size + \"7\"\n industry_size_data.append(industry_size)\n continue\n \nprint(industry_size_data[0:9])\nprint(len(industry_size_data))",
"['Biotechnology: Laboratory Analytical Instruments4', 'Industrial Machinery/Components3', 'none', 'none', 'Paints/Coatings3', 'Building operators4', 'Major Banks5', 'Semiconductors5', 'Major Pharmaceuticals6']\n6644\n"
],
[
"#add the new column to df\n\nsen_df['classification'] = industry_size_data\nsen_df.head()",
"_____no_output_____"
],
[
"#create a list of all the classifications per industry across whole dataframe, to get a view of the breakdown in\n#classifications across each industry\n\nclassification_industry_breakdown = list()\n\nfor x in industry_list:\n y = list()\n for row_tuple in sen_df.itertuples():\n if row_tuple.industry == x:\n y.append(row_tuple.classification)\n classification_industry_breakdown.append(y)\n \nprint(classification_industry_breakdown[0:9])",
"[['Biotechnology: Laboratory Analytical Instruments4', 'Biotechnology: Laboratory Analytical Instruments4', 'Biotechnology: Laboratory Analytical Instruments4', 'Biotechnology: Laboratory Analytical Instruments4', 'Biotechnology: Laboratory Analytical Instruments3', 'Biotechnology: Laboratory Analytical Instruments4', 'Biotechnology: Laboratory Analytical Instruments4', 'Biotechnology: Laboratory Analytical Instruments4', 'Biotechnology: Laboratory Analytical Instruments4', 'Biotechnology: Laboratory Analytical Instruments4', 'Biotechnology: Laboratory Analytical Instruments4', 'Biotechnology: Laboratory Analytical Instruments3', 'Biotechnology: Laboratory Analytical Instruments4', 'Biotechnology: Laboratory Analytical Instruments4', 'Biotechnology: Laboratory Analytical Instruments4', 'Biotechnology: Laboratory Analytical Instruments4', 'Biotechnology: Laboratory Analytical Instruments3', 'Biotechnology: Laboratory Analytical Instruments4', 'Biotechnology: Laboratory Analytical Instruments4', 'Biotechnology: Laboratory Analytical Instruments4', 'Biotechnology: Laboratory Analytical Instruments4', 'Biotechnology: Laboratory Analytical Instruments4', 'Biotechnology: Laboratory Analytical Instruments4', 'Biotechnology: Laboratory Analytical Instruments4', 'Biotechnology: Laboratory Analytical Instruments4', 'Biotechnology: Laboratory Analytical Instruments4', 'Biotechnology: Laboratory Analytical Instruments4', 'Biotechnology: Laboratory Analytical Instruments4', 'Biotechnology: Laboratory Analytical Instruments4', 'Biotechnology: Laboratory Analytical Instruments4', 'Biotechnology: Laboratory Analytical Instruments4', 'Biotechnology: Laboratory Analytical Instruments4', 'Biotechnology: Laboratory Analytical Instruments4', 'Biotechnology: Laboratory Analytical Instruments4', 'Biotechnology: Laboratory Analytical Instruments4'], ['Industrial Machinery/Components3', 'Industrial Machinery/Components3', 'Industrial Machinery/Components3', 'Industrial Machinery/Components3', 'Industrial Machinery/Components3', 'Industrial Machinery/Components4', 'Industrial Machinery/Components6', 'Industrial Machinery/Components4', 'Industrial Machinery/Components6', 'Industrial Machinery/Components5', 'Industrial Machinery/Components5', 'Industrial Machinery/Components3', 'Industrial Machinery/Components3', 'Industrial Machinery/Components3', 'Industrial Machinery/Components3', 'Industrial Machinery/Components3', 'Industrial Machinery/Components5', 'Industrial Machinery/Components5', 'Industrial Machinery/Components3', 'Industrial Machinery/Components3', 'Industrial Machinery/Components4', 'Industrial Machinery/Components3', 'Industrial Machinery/Components3', 'Industrial Machinery/Components3', 'Industrial Machinery/Components3', 'Industrial Machinery/Components3', 'Industrial Machinery/Components3', 'Industrial Machinery/Components3', 'Industrial Machinery/Components3', 'Industrial Machinery/Components5', 'Industrial Machinery/Components4', 'Industrial Machinery/Components4', 'Industrial Machinery/Components3', 'Industrial Machinery/Components3', 'Industrial Machinery/Components3', 'Industrial Machinery/Components3', 'Industrial Machinery/Components3', 'Industrial Machinery/Components3', 'Industrial Machinery/Components3', 'Industrial Machinery/Components4', 'Industrial Machinery/Components6', 'Industrial Machinery/Components6', 'Industrial Machinery/Components3', 'Industrial Machinery/Components3', 'Industrial Machinery/Components3', 'Industrial Machinery/Components3', 'Industrial Machinery/Components6', 'Industrial Machinery/Components6', 'Industrial Machinery/Components6', 'Industrial Machinery/Components6', 'Industrial Machinery/Components3', 'Industrial Machinery/Components3', 'Industrial Machinery/Components3', 'Industrial Machinery/Components4', 'Industrial Machinery/Components6', 'Industrial Machinery/Components3', 'Industrial Machinery/Components3', 'Industrial Machinery/Components3', 'Industrial Machinery/Components3', 'Industrial Machinery/Components3', 'Industrial Machinery/Components3', 'Industrial Machinery/Components4', 'Industrial Machinery/Components3', 'Industrial Machinery/Components3', 'Industrial Machinery/Components3', 'Industrial Machinery/Components3', 'Industrial Machinery/Components3', 'Industrial Machinery/Components3', 'Industrial Machinery/Components3', 'Industrial Machinery/Components3', 'Industrial Machinery/Components3', 'Industrial Machinery/Components3', 'Industrial Machinery/Components3', 'Industrial Machinery/Components3', 'Industrial Machinery/Components4', 'Industrial Machinery/Components5', 'Industrial Machinery/Components5', 'Industrial Machinery/Components5', 'Industrial Machinery/Components4', 'Industrial Machinery/Components5', 'Industrial Machinery/Components6', 'Industrial Machinery/Components5', 'Industrial Machinery/Components6', 'Industrial Machinery/Components4', 'Industrial Machinery/Components3', 'Industrial Machinery/Components6', 'Industrial Machinery/Components5', 'Industrial Machinery/Components4', 'Industrial Machinery/Components4', 'Industrial Machinery/Components4', 'Industrial Machinery/Components6', 'Industrial Machinery/Components4', 'Industrial Machinery/Components6', 'Industrial Machinery/Components5', 'Industrial Machinery/Components5', 'Industrial Machinery/Components4', 'Industrial Machinery/Components5', 'Industrial Machinery/Components4', 'Industrial Machinery/Components6', 'Industrial Machinery/Components5', 'Industrial Machinery/Components6', 'Industrial Machinery/Components6', 'Industrial Machinery/Components4', 'Industrial Machinery/Components3', 'Industrial Machinery/Components3', 'Industrial Machinery/Components4', 'Industrial Machinery/Components4', 'Industrial Machinery/Components6', 'Industrial Machinery/Components4', 'Industrial Machinery/Components6', 'Industrial Machinery/Components4', 'Industrial Machinery/Components4', 'Industrial Machinery/Components5', 'Industrial Machinery/Components6', 'Industrial Machinery/Components6', 'Industrial Machinery/Components5', 'Industrial Machinery/Components6', 'Industrial Machinery/Components6', 'Industrial Machinery/Components6', 'Industrial Machinery/Components6', 'Industrial Machinery/Components6', 'Industrial Machinery/Components6', 'Industrial Machinery/Components6', 'Industrial Machinery/Components6', 'Industrial Machinery/Components6', 'Industrial Machinery/Components6', 'Industrial Machinery/Components4', 'Industrial Machinery/Components4', 'Industrial Machinery/Components6', 'Industrial Machinery/Components6', 'Industrial Machinery/Components4', 'Industrial Machinery/Components4', 'Industrial Machinery/Components4', 'Industrial Machinery/Components4', 'Industrial Machinery/Components4', 'Industrial Machinery/Components6', 'Industrial Machinery/Components4', 'Industrial Machinery/Components4', 'Industrial Machinery/Components4', 'Industrial Machinery/Components4', 'Industrial Machinery/Components4', 'Industrial Machinery/Components4', 'Industrial Machinery/Components4', 'Industrial Machinery/Components4', 'Industrial Machinery/Components4', 'Industrial Machinery/Components4', 'Industrial Machinery/Components4', 'Industrial Machinery/Components4', 'Industrial Machinery/Components4', 'Industrial Machinery/Components6', 'Industrial Machinery/Components6', 'Industrial Machinery/Components6', 'Industrial Machinery/Components6', 'Industrial Machinery/Components4', 'Industrial Machinery/Components6', 'Industrial Machinery/Components6', 'Industrial Machinery/Components2', 'Industrial Machinery/Components4', 'Industrial Machinery/Components3', 'Industrial Machinery/Components3', 'Industrial Machinery/Components4', 'Industrial Machinery/Components3', 'Industrial Machinery/Components4', 'Industrial Machinery/Components4', 'Industrial Machinery/Components3', 'Industrial Machinery/Components4', 'Industrial Machinery/Components3', 'Industrial Machinery/Components3', 'Industrial Machinery/Components4', 'Industrial Machinery/Components4', 'Industrial Machinery/Components6', 'Industrial Machinery/Components4', 'Industrial Machinery/Components4', 'Industrial Machinery/Components6', 'Industrial Machinery/Components6', 'Industrial Machinery/Components4', 'Industrial Machinery/Components4', 'Industrial Machinery/Components4', 'Industrial Machinery/Components2', 'Industrial Machinery/Components4', 'Industrial Machinery/Components3', 'Industrial Machinery/Components3', 'Industrial Machinery/Components4', 'Industrial Machinery/Components3', 'Industrial Machinery/Components2', 'Industrial Machinery/Components4', 'Industrial Machinery/Components6'], ['none', 'none', 'none', 'none', 'none', 'none', 'none', 'none', 'none', 'none', 'none', 'none', 'none', 'none', 'none', 'none', 'none', 'none', 'none', 'none', 'none', 'none', 'none', 'none', 'none', 'none', 'none', 'none', 'none', 'none', 'none', 'none', 'none', 'none', 'none', 'none', 'none', 'none', 'none', 'none', 'none', 'none', 'none', 'none', 'none', 'none', 'none', 'none', 'none', 'none', 'none', 'none', 'none', 'none', 'none', 'none', 'none', 'none', 'none', 'none', 'none', 'none', 'none', 'none', 'none', 'none', 'none', 'none', 'none', 'none', 'none', 'none', 'none', 'none', 'none', 'none', 'none', 'none', 'none', 'none', 'none', 'none', 'none', 'none', 'none', 'none', 'none', 'none', 'none', 'none', 'none', 'none', 'none', 'none', 'none', 'none', 'none', 'none', 'none', 'none', 'none', 'none', 'none', 'none', 'none', 'none', 'none', 'none', 'none', 'none', 'none', 'none', 'none', 'none', 'none', 'none', 'none', 'none', 'none', 'none', 'none', 'none', 'none', 'none', 'none', 'none', 'none', 'none', 'none', 'none', 'none', 'none', 'none', 'none', 'none', 'none', 'none', 'none', 'none', 'none', 'none', 'none', 'none', 'none', 'none', 'none', 'none', 'none', 'none', 'none', 'none', 'none', 'none', 'none', 'none', 'none', 'none', 'none', 'none', 'none', 'none', 'none', 'none', 'none', 'none', 'none', 'none', 'none', 'none', 'none', 'none', 'none', 'none', 'none', 'none', 'none', 'none', 'none', 'none', 'none', 'none', 'none', 'none', 'none', 'none', 'none', 'none', 'none', 'none', 'none', 'none', 'none', 'none', 'none', 'none', 'none', 'none', 'none', 'none', 'none', 'none', 'none', 'none', 'none', 'none', 'none', 'none', 'none', 'none', 'none', 'none', 'none', 'none', 'none', 'none', 'none', 'none', 'none', 'none', 'none', 'none', 'none', 'none', 'none', 'none', 'none', 'none', 'none', 'none', 'none', 'none', 'none', 'none', 'none', 'none', 'none', 'none', 'none', 'none', 'none', 'none', 'none', 'none', 'none', 'none', 'none', 'none', 'none', 'none', 'none', 'none', 'none', 'none', 'none', 'none', 'none', 'none', 'none', 'none', 'none', 'none', 'none', 'none', 'none', 'none', 'none', 'none', 'none', 'none', 'none', 'none', 'none', 'none', 'none', 'none', 'none', 'none', 'none', 'none', 'none', 'none', 'none', 'none', 'none', 'none', 'none', 'none', 'none', 'none', 'none', 'none', 'none', 'none', 'none', 'none', 'none', 'none', 'none', 'none', 'none', 'none', 'none', 'none', 'none', 'none', 'none', 'none', 'none', 'none', 'none', 'none', 'none', 'none', 'none', 'none', 'none', 'none', 'none', 'none', 'none', 'none', 'none', 'none', 'none', 'none', 'none', 'none', 'none', 'none', 'none', 'none', 'none', 'none', 'none', 'none', 'none', 'none', 'none', 'none', 'none', 'none', 'none', 'none', 'none', 'none', 'none', 'none', 'none', 'none', 'none', 'none', 'none', 'none', 'none', 'none', 'none', 'none', 'none', 'none', 'none', 'none', 'none', 'none', 'none', 'none', 'none', 'none', 'none', 'none', 'none', 'none', 'none', 'none', 'none', 'none', 'none', 'none', 'none', 'none', 'none', 'none', 'none', 'none', 'none', 'none', 'none', 'none', 'none', 'none', 'none', 'none', 'none', 'none', 'none', 'none', 'none', 'none', 'none', 'none', 'none', 'none', 'none', 'none', 'none', 'none', 'none', 'none', 'none', 'none', 'none', 'none', 'none', 'none', 'none', 'none', 'none', 'none', 'none', 'none', 'none', 'none', 'none', 'none', 'none', 'none', 'none', 'none', 'none', 'none', 'none', 'none', 'none', 'none', 'none', 'none', 'none', 'none', 'none', 'none', 'none', 'none', 'none', 'none', 'none', 'none', 'none', 'none', 'none', 'none', 'none', 'none', 'none', 'none', 'none', 'none', 'none', 'none', 'none', 'none', 'none', 'none', 'none', 'none', 'none', 'none', 'none', 'none', 'none', 'none', 'none', 'none', 'none', 'none', 'none', 'none', 'none', 'none', 'none', 'none', 'none', 'none', 'none', 'none', 'none', 'none', 'none', 'none', 'none', 'none', 'none', 'none', 'none', 'none', 'none', 'none', 'none', 'none', 'none', 'none', 'none', 'none', 'none', 'none', 'none', 'none', 'none', 'none', 'none', 'none', 'none', 'none', 'none', 'none', 'none', 'none', 'none', 'none', 'none', 'none', 'none', 'none', 'none', 'none', 'none', 'none', 'none', 'none', 'none', 'none', 'none', 'none', 'none', 'none', 'none', 'none', 'none', 'none', 'none', 'none', 'none', 'none', 'none', 'none', 'none', 'none', 'none', 'none', 'none', 'none', 'none', 'none', 'none', 'none', 'none', 'none', 'none', 'none', 'none', 'none', 'none', 'none', 'none', 'none', 'none', 'none', 'none', 'none', 'none', 'none', 'none', 'none', 'none', 'none', 'none', 'none', 'none', 'none', 'none', 'none', 'none', 'none', 'none', 'none', 'none', 'none', 'none', 'none', 'none', 'none', 'none', 'none', 'none', 'none', 'none', 'none', 'none', 'none', 'none', 'none', 'none', 'none', 'none', 'none', 'none', 'none', 'none', 'none', 'none', 'none', 'none', 'none', 'none', 'none', 'none', 'none', 'none', 'none', 'none', 'none', 'none', 'none', 'none', 'none', 'none', 'none', 'none', 'none', 'none', 'none', 'none', 'none', 'none', 'none', 'none', 'none', 'none', 'none', 'none', 'none', 'none', 'none', 'none', 'none', 'none', 'none', 'none', 'none', 'none', 'none', 'none', 'none', 'none', 'none', 'none', 'none', 'none', 'none', 'none', 'none', 'none', 'none', 'none', 'none', 'none', 'none', 'none', 'none', 'none', 'none', 'none', 'none', 'none', 'none', 'none', 'none', 'none', 'none', 'none', 'none', 'none', 'none', 'none', 'none', 'none', 'none', 'none', 'none', 'none', 'none', 'none', 'none', 'none', 'none', 'none', 'none', 'none', 'none', 'none', 'none', 'none', 'none', 'none', 'none', 'none', 'none', 'none', 'none', 'none', 'none', 'none', 'none', 'none', 'none', 'none', 'none', 'none', 'none', 'none', 'none', 'none', 'none', 'none', 'none', 'none', 'none', 'none', 'none', 'none', 'none', 'none', 'none', 'none', 'none', 'none', 'none', 'none', 'none', 'none', 'none', 'none', 'none', 'none', 'none', 'none', 'none', 'none', 'none', 'none', 'none', 'none', 'none', 'none', 'none', 'none', 'none', 'none', 'none', 'none', 'none', 'none', 'none', 'none', 'none', 'none', 'none', 'none', 'none', 'none', 'none', 'none', 'none', 'none', 'none', 'none', 'none', 'none', 'none', 'none', 'none', 'none', 'none', 'none', 'none', 'none', 'none', 'none', 'none', 'none', 'none', 'none', 'none', 'none', 'none', 'none', 'none', 'none', 'none', 'none', 'none', 'none', 'none', 'none', 'none', 'none', 'none', 'none', 'none', 'none', 'none', 'none', 'none', 'none', 'none', 'none', 'none', 'none', 'none', 'none', 'none', 'none', 'none', 'none', 'none', 'none', 'none', 'none', 'none', 'none', 'none', 'none', 'none', 'none', 'none', 'none', 'none', 'none', 'none', 'none', 'none', 'none', 'none', 'none', 'none', 'none', 'none', 'none', 'none', 'none', 'none', 'none', 'none', 'none', 'none', 'none', 'none', 'none', 'none', 'none', 'none', 'none', 'none', 'none', 'none', 'none', 'none', 'none', 'none', 'none', 'none', 'none', 'none', 'none', 'none', 'none', 'none', 'none', 'none', 'none', 'none', 'none', 'none', 'none', 'none', 'none', 'none', 'none', 'none', 'none', 'none', 'none', 'none', 'none', 'none', 'none', 'none', 'none', 'none', 'none', 'none', 'none', 'none', 'none', 'none', 'none', 'none', 'none', 'none', 'none', 'none', 'none', 'none', 'none', 'none', 'none', 'none', 'none', 'none', 'none', 'none', 'none', 'none', 'none', 'none', 'none', 'none', 'none', 'none', 'none', 'none', 'none', 'none', 'none', 'none', 'none', 'none', 'none', 'none', 'none', 'none', 'none', 'none', 'none', 'none', 'none', 'none', 'none', 'none', 'none', 'none', 'none', 'none', 'none', 'none', 'none', 'none', 'none', 'none', 'none', 'none', 'none', 'none', 'none', 'none', 'none', 'none', 'none', 'none', 'none', 'none', 'none', 'none', 'none', 'none', 'none', 'none', 'none', 'none', 'none', 'none', 'none', 'none', 'none', 'none', 'none', 'none', 'none', 'none', 'none', 'none', 'none', 'none', 'none', 'none', 'none', 'none', 'none', 'none', 'none', 'none', 'none', 'none', 'none', 'none', 'none', 'none', 'none', 'none', 'none', 'none', 'none', 'none', 'none', 'none', 'none', 'none', 'none', 'none', 'none', 'none', 'none', 'none', 'none', 'none', 'none', 'none', 'none', 'none', 'none', 'none', 'none', 'none', 'none', 'none', 'none', 'none', 'none', 'none', 'none', 'none', 'none', 'none', 'none', 'none', 'none', 'none', 'none', 'none', 'none', 'none', 'none', 'none', 'none', 'none', 'none', 'none', 'none', 'none', 'none', 'none', 'none', 'none', 'none', 'none', 'none', 'none', 'none', 'none', 'none', 'none', 'none', 'none', 'none', 'none', 'none', 'none', 'none', 'none', 'none', 'none', 'none', 'none', 'none', 'none', 'none', 'none', 'none', 'none', 'none', 'none', 'none', 'none', 'none', 'none', 'none', 'none', 'none', 'none', 'none', 'none', 'none', 'none', 'none', 'none', 'none', 'none', 'none', 'none', 'none', 'none', 'none', 'none', 'none', 'none', 'none', 'none', 'none', 'none', 'none', 'none', 'none', 'none', 'none', 'none', 'none', 'none', 'none', 'none', 'none', 'none', 'none', 'none', 'none', 'none', 'none', 'none', 'none', 'none', 'none', 'none', 'none', 'none', 'none', 'none', 'none', 'none', 'none', 'none', 'none', 'none', 'none', 'none', 'none', 'none', 'none', 'none', 'none', 'none', 'none', 'none', 'none', 'none', 'none', 'none', 'none', 'none', 'none', 'none', 'none', 'none', 'none', 'none', 'none', 'none', 'none', 'none', 'none', 'none', 'none', 'none', 'none', 'none', 'none', 'none', 'none', 'none', 'none', 'none', 'none', 'none', 'none', 'none', 'none', 'none', 'none', 'none', 'none', 'none'], ['Paints/Coatings3', 'Paints/Coatings3', 'Paints/Coatings3', 'Paints/Coatings3', 'Paints/Coatings3', 'Paints/Coatings3', 'Paints/Coatings4', 'Paints/Coatings3', 'Paints/Coatings3', 'Paints/Coatings3', 'Paints/Coatings3', 'Paints/Coatings3', 'Paints/Coatings3', 'Paints/Coatings3', 'Paints/Coatings3', 'Paints/Coatings3', 'Paints/Coatings3', 'Paints/Coatings3', 'Paints/Coatings3', 'Paints/Coatings3', 'Paints/Coatings3', 'Paints/Coatings3', 'Paints/Coatings3', 'Paints/Coatings3', 'Paints/Coatings3', 'Paints/Coatings3', 'Paints/Coatings3', 'Paints/Coatings3', 'Paints/Coatings3', 'Paints/Coatings3', 'Paints/Coatings3', 'Paints/Coatings3', 'Paints/Coatings3', 'Paints/Coatings3', 'Paints/Coatings3', 'Paints/Coatings4', 'Paints/Coatings4', 'Paints/Coatings4', 'Paints/Coatings3'], ['Building operators4', 'Building operators4', 'Building operators4', 'Building operators4', 'Building operators4', 'Building operators3'], ['Major Banks5', 'Major Banks6', 'Major Banks6', 'Major Banks6', 'Major Banks3', 'Major Banks3', 'Major Banks6', 'Major Banks6', 'Major Banks6', 'Major Banks6', 'Major Banks6', 'Major Banks6', 'Major Banks6', 'Major Banks6', 'Major Banks6', 'Major Banks6', 'Major Banks5', 'Major Banks3', 'Major Banks6', 'Major Banks3', 'Major Banks4', 'Major Banks5', 'Major Banks4', 'Major Banks3', 'Major Banks3', 'Major Banks6', 'Major Banks6', 'Major Banks5', 'Major Banks6', 'Major Banks6', 'Major Banks4', 'Major Banks6', 'Major Banks6', 'Major Banks4', 'Major Banks5', 'Major Banks6', 'Major Banks6', 'Major Banks6', 'Major Banks5', 'Major Banks6', 'Major Banks6', 'Major Banks6', 'Major Banks6', 'Major Banks6', 'Major Banks5', 'Major Banks6', 'Major Banks5', 'Major Banks6', 'Major Banks6', 'Major Banks6', 'Major Banks6', 'Major Banks6', 'Major Banks6', 'Major Banks6', 'Major Banks5', 'Major Banks6', 'Major Banks3', 'Major Banks5', 'Major Banks6', 'Major Banks3', 'Major Banks5', 'Major Banks5', 'Major Banks6', 'Major Banks4', 'Major Banks6', 'Major Banks4', 'Major Banks6', 'Major Banks5', 'Major Banks4', 'Major Banks3', 'Major Banks4', 'Major Banks3', 'Major Banks3', 'Major Banks6', 'Major Banks6', 'Major Banks3', 'Major Banks6', 'Major Banks3', 'Major Banks6', 'Major Banks6', 'Major Banks3', 'Major Banks3', 'Major Banks6', 'Major Banks6', 'Major Banks6', 'Major Banks6', 'Major Banks3', 'Major Banks3', 'Major Banks6', 'Major Banks6', 'Major Banks6', 'Major Banks5', 'Major Banks3', 'Major Banks3', 'Major Banks3', 'Major Banks3', 'Major Banks4', 'Major Banks6', 'Major Banks6', 'Major Banks6', 'Major Banks6', 'Major Banks5', 'Major Banks5', 'Major Banks6', 'Major Banks6', 'Major Banks6', 'Major Banks6', 'Major Banks6', 'Major Banks5', 'Major Banks6', 'Major Banks3', 'Major Banks5', 'Major Banks6', 'Major Banks3', 'Major Banks5', 'Major Banks6', 'Major Banks6', 'Major Banks6', 'Major Banks5', 'Major Banks6', 'Major Banks4', 'Major Banks5', 'Major Banks6', 'Major Banks6', 'Major Banks3', 'Major Banks3', 'Major Banks3', 'Major Banks6', 'Major Banks6', 'Major Banks5', 'Major Banks5', 'Major Banks5', 'Major Banks6', 'Major Banks6', 'Major Banks6', 'Major Banks3', 'Major Banks6', 'Major Banks3', 'Major Banks3', 'Major Banks6', 'Major Banks3', 'Major Banks3', 'Major Banks4', 'Major Banks6', 'Major Banks6', 'Major Banks5', 'Major Banks6', 'Major Banks4', 'Major Banks6', 'Major Banks6', 'Major Banks6', 'Major Banks6', 'Major Banks4', 'Major Banks6', 'Major Banks6', 'Major Banks6', 'Major Banks4', 'Major Banks3', 'Major Banks5', 'Major Banks4', 'Major Banks5', 'Major Banks6', 'Major Banks6', 'Major Banks6', 'Major Banks6', 'Major Banks5', 'Major Banks3', 'Major Banks3', 'Major Banks3', 'Major Banks3', 'Major Banks6', 'Major Banks5', 'Major Banks4', 'Major Banks4', 'Major Banks4', 'Major Banks6', 'Major Banks3', 'Major Banks6', 'Major Banks5', 'Major Banks6', 'Major Banks5', 'Major Banks3', 'Major Banks3', 'Major Banks3', 'Major Banks3', 'Major Banks3', 'Major Banks3', 'Major Banks3', 'Major Banks4', 'Major Banks5', 'Major Banks6', 'Major Banks6', 'Major Banks6', 'Major Banks6', 'Major Banks6', 'Major Banks5', 'Major Banks4', 'Major Banks4', 'Major Banks4', 'Major Banks6', 'Major Banks3', 'Major Banks6', 'Major Banks6', 'Major Banks5', 'Major Banks5', 'Major Banks6', 'Major Banks6', 'Major Banks6', 'Major Banks6', 'Major Banks6', 'Major Banks6', 'Major Banks5', 'Major Banks6', 'Major Banks6', 'Major Banks6', 'Major Banks6', 'Major Banks6', 'Major Banks6', 'Major Banks6', 'Major Banks6', 'Major Banks5', 'Major Banks3', 'Major Banks4', 'Major Banks4', 'Major Banks3', 'Major Banks3', 'Major Banks3', 'Major Banks3', 'Major Banks3', 'Major Banks4', 'Major Banks5', 'Major Banks3', 'Major Banks4', 'Major Banks4', 'Major Banks3', 'Major Banks3', 'Major Banks3', 'Major Banks3', 'Major Banks3', 'Major Banks4', 'Major Banks4', 'Major Banks4', 'Major Banks3', 'Major Banks4', 'Major Banks3', 'Major Banks3', 'Major Banks3', 'Major Banks3', 'Major Banks3', 'Major Banks3', 'Major Banks6', 'Major Banks5', 'Major Banks6', 'Major Banks6', 'Major Banks4', 'Major Banks6', 'Major Banks6', 'Major Banks6', 'Major Banks6', 'Major Banks6', 'Major Banks6', 'Major Banks5', 'Major Banks6', 'Major Banks6', 'Major Banks6', 'Major Banks6', 'Major Banks6', 'Major Banks6', 'Major Banks6', 'Major Banks6', 'Major Banks6', 'Major Banks6', 'Major Banks6', 'Major Banks4', 'Major Banks6', 'Major Banks6', 'Major Banks6', 'Major Banks6', 'Major Banks6', 'Major Banks4', 'Major Banks4', 'Major Banks4', 'Major Banks3', 'Major Banks4', 'Major Banks3', 'Major Banks3', 'Major Banks3', 'Major Banks3', 'Major Banks3', 'Major Banks3', 'Major Banks6', 'Major Banks6', 'Major Banks6', 'Major Banks6', 'Major Banks6', 'Major Banks6', 'Major Banks6', 'Major Banks6', 'Major Banks4', 'Major Banks6', 'Major Banks4', 'Major Banks2', 'Major Banks5', 'Major Banks5', 'Major Banks5', 'Major Banks6', 'Major Banks3', 'Major Banks4', 'Major Banks4', 'Major Banks3', 'Major Banks3', 'Major Banks3', 'Major Banks3', 'Major Banks3', 'Major Banks4', 'Major Banks6', 'Major Banks6', 'Major Banks3', 'Major Banks3', 'Major Banks5', 'Major Banks5', 'Major Banks5', 'Major Banks4', 'Major Banks6', 'Major Banks3', 'Major Banks3', 'Major Banks3', 'Major Banks3', 'Major Banks3', 'Major Banks6', 'Major Banks4', 'Major Banks6', 'Major Banks2', 'Major Banks5', 'Major Banks6', 'Major Banks3', 'Major Banks3', 'Major Banks2', 'Major Banks3', 'Major Banks6', 'Major Banks4', 'Major Banks6', 'Major Banks6', 'Major Banks5', 'Major Banks5', 'Major Banks6', 'Major Banks6', 'Major Banks6', 'Major Banks5', 'Major Banks5'], ['Semiconductors5', 'Semiconductors5', 'Semiconductors5', 'Semiconductors5', 'Semiconductors5', 'Semiconductors4', 'Semiconductors6', 'Semiconductors6', 'Semiconductors3', 'Semiconductors6', 'Semiconductors6', 'Semiconductors6', 'Semiconductors6', 'Semiconductors6', 'Semiconductors6', 'Semiconductors6', 'Semiconductors6', 'Semiconductors6', 'Semiconductors6', 'Semiconductors6', 'Semiconductors6', 'Semiconductors4', 'Semiconductors4', 'Semiconductors6', 'Semiconductors6', 'Semiconductors6', 'Semiconductors6', 'Semiconductors6', 'Semiconductors6', 'Semiconductors4', 'Semiconductors6', 'Semiconductors6', 'Semiconductors6', 'Semiconductors4', 'Semiconductors6', 'Semiconductors4', 'Semiconductors4', 'Semiconductors4', 'Semiconductors5', 'Semiconductors4', 'Semiconductors6', 'Semiconductors6', 'Semiconductors5', 'Semiconductors6', 'Semiconductors5', 'Semiconductors3', 'Semiconductors5', 'Semiconductors6', 'Semiconductors6', 'Semiconductors4', 'Semiconductors4', 'Semiconductors3', 'Semiconductors6', 'Semiconductors4', 'Semiconductors4', 'Semiconductors4', 'Semiconductors6', 'Semiconductors4', 'Semiconductors6', 'Semiconductors4', 'Semiconductors6', 'Semiconductors6', 'Semiconductors6', 'Semiconductors6', 'Semiconductors6', 'Semiconductors3', 'Semiconductors3', 'Semiconductors6', 'Semiconductors6', 'Semiconductors6', 'Semiconductors6', 'Semiconductors6', 'Semiconductors6', 'Semiconductors3', 'Semiconductors6', 'Semiconductors6', 'Semiconductors6', 'Semiconductors6', 'Semiconductors6', 'Semiconductors6', 'Semiconductors6', 'Semiconductors4', 'Semiconductors4', 'Semiconductors4', 'Semiconductors4', 'Semiconductors4', 'Semiconductors4', 'Semiconductors4', 'Semiconductors4', 'Semiconductors4', 'Semiconductors4', 'Semiconductors4', 'Semiconductors6', 'Semiconductors4', 'Semiconductors4', 'Semiconductors6', 'Semiconductors6', 'Semiconductors6', 'Semiconductors6', 'Semiconductors6', 'Semiconductors6', 'Semiconductors6', 'Semiconductors6', 'Semiconductors6', 'Semiconductors6', 'Semiconductors3', 'Semiconductors4', 'Semiconductors3', 'Semiconductors3', 'Semiconductors4', 'Semiconductors3', 'Semiconductors3', 'Semiconductors3', 'Semiconductors4', 'Semiconductors5', 'Semiconductors3', 'Semiconductors3', 'Semiconductors3', 'Semiconductors6', 'Semiconductors6', 'Semiconductors3', 'Semiconductors3', 'Semiconductors3', 'Semiconductors3', 'Semiconductors4', 'Semiconductors4', 'Semiconductors4', 'Semiconductors4', 'Semiconductors4', 'Semiconductors4', 'Semiconductors3', 'Semiconductors4'], ['Major Pharmaceuticals6', 'Major Pharmaceuticals6', 'Major Pharmaceuticals6', 'Major Pharmaceuticals3', 'Major Pharmaceuticals3', 'Major Pharmaceuticals6', 'Major Pharmaceuticals3', 'Major Pharmaceuticals3', 'Major Pharmaceuticals6', 'Major Pharmaceuticals6', 'Major Pharmaceuticals6', 'Major Pharmaceuticals6', 'Major Pharmaceuticals6', 'Major Pharmaceuticals6', 'Major Pharmaceuticals6', 'Major Pharmaceuticals6', 'Major Pharmaceuticals6', 'Major Pharmaceuticals6', 'Major Pharmaceuticals6', 'Major Pharmaceuticals6', 'Major Pharmaceuticals1', 'Major Pharmaceuticals6', 'Major Pharmaceuticals3', 'Major Pharmaceuticals6', 'Major Pharmaceuticals6', 'Major Pharmaceuticals6', 'Major Pharmaceuticals6', 'Major Pharmaceuticals6', 'Major Pharmaceuticals6', 'Major Pharmaceuticals6', 'Major Pharmaceuticals6', 'Major Pharmaceuticals6', 'Major Pharmaceuticals6', 'Major Pharmaceuticals6', 'Major Pharmaceuticals6', 'Major Pharmaceuticals6', 'Major Pharmaceuticals6', 'Major Pharmaceuticals6', 'Major Pharmaceuticals6', 'Major Pharmaceuticals6', 'Major Pharmaceuticals6', 'Major Pharmaceuticals6', 'Major Pharmaceuticals6', 'Major Pharmaceuticals5', 'Major Pharmaceuticals6', 'Major Pharmaceuticals6', 'Major Pharmaceuticals6', 'Major Pharmaceuticals5', 'Major Pharmaceuticals6', 'Major Pharmaceuticals6', 'Major Pharmaceuticals6', 'Major Pharmaceuticals6', 'Major Pharmaceuticals6', 'Major Pharmaceuticals6', 'Major Pharmaceuticals6', 'Major Pharmaceuticals6', 'Major Pharmaceuticals6', 'Major Pharmaceuticals6', 'Major Pharmaceuticals6', 'Major Pharmaceuticals6', 'Major Pharmaceuticals6', 'Major Pharmaceuticals6', 'Major Pharmaceuticals6', 'Major Pharmaceuticals6', 'Major Pharmaceuticals6', 'Major Pharmaceuticals6', 'Major Pharmaceuticals6', 'Major Pharmaceuticals6', 'Major Pharmaceuticals6', 'Major Pharmaceuticals6', 'Major Pharmaceuticals6', 'Major Pharmaceuticals3', 'Major Pharmaceuticals3', 'Major Pharmaceuticals6', 'Major Pharmaceuticals6', 'Major Pharmaceuticals6', 'Major Pharmaceuticals6', 'Major Pharmaceuticals6', 'Major Pharmaceuticals6', 'Major Pharmaceuticals6', 'Major Pharmaceuticals6', 'Major Pharmaceuticals6', 'Major Pharmaceuticals6', 'Major Pharmaceuticals6', 'Major Pharmaceuticals6', 'Major Pharmaceuticals6', 'Major Pharmaceuticals6', 'Major Pharmaceuticals6', 'Major Pharmaceuticals6', 'Major Pharmaceuticals6', 'Major Pharmaceuticals6', 'Major Pharmaceuticals6', 'Major Pharmaceuticals6', 'Major Pharmaceuticals6', 'Major Pharmaceuticals6', 'Major Pharmaceuticals6', 'Major Pharmaceuticals6', 'Major Pharmaceuticals6', 'Major Pharmaceuticals6', 'Major Pharmaceuticals6', 'Major Pharmaceuticals6', 'Major Pharmaceuticals6', 'Major Pharmaceuticals6', 'Major Pharmaceuticals6', 'Major Pharmaceuticals6', 'Major Pharmaceuticals6', 'Major Pharmaceuticals6', 'Major Pharmaceuticals6', 'Major Pharmaceuticals5', 'Major Pharmaceuticals1', 'Major Pharmaceuticals6', 'Major Pharmaceuticals5', 'Major Pharmaceuticals6', 'Major Pharmaceuticals6', 'Major Pharmaceuticals6', 'Major Pharmaceuticals6', 'Major Pharmaceuticals4', 'Major Pharmaceuticals6', 'Major Pharmaceuticals6', 'Major Pharmaceuticals6', 'Major Pharmaceuticals6', 'Major Pharmaceuticals6', 'Major Pharmaceuticals6', 'Major Pharmaceuticals6', 'Major Pharmaceuticals3', 'Major Pharmaceuticals6', 'Major Pharmaceuticals6', 'Major Pharmaceuticals6', 'Major Pharmaceuticals6', 'Major Pharmaceuticals6', 'Major Pharmaceuticals6', 'Major Pharmaceuticals6', 'Major Pharmaceuticals2', 'Major Pharmaceuticals6', 'Major Pharmaceuticals6', 'Major Pharmaceuticals6', 'Major Pharmaceuticals6', 'Major Pharmaceuticals6', 'Major Pharmaceuticals3', 'Major Pharmaceuticals6', 'Major Pharmaceuticals6', 'Major Pharmaceuticals6', 'Major Pharmaceuticals6', 'Major Pharmaceuticals6', 'Major Pharmaceuticals5', 'Major Pharmaceuticals6', 'Major Pharmaceuticals6', 'Major Pharmaceuticals6', 'Major Pharmaceuticals6', 'Major Pharmaceuticals6', 'Major Pharmaceuticals6', 'Major Pharmaceuticals3', 'Major Pharmaceuticals6', 'Major Pharmaceuticals6', 'Major Pharmaceuticals6', 'Major Pharmaceuticals6', 'Major Pharmaceuticals6', 'Major Pharmaceuticals6', 'Major Pharmaceuticals6', 'Major Pharmaceuticals6', 'Major Pharmaceuticals6', 'Major Pharmaceuticals6', 'Major Pharmaceuticals6', 'Major Pharmaceuticals6', 'Major Pharmaceuticals6', 'Major Pharmaceuticals4', 'Major Pharmaceuticals6', 'Major Pharmaceuticals6', 'Major Pharmaceuticals6', 'Major Pharmaceuticals6', 'Major Pharmaceuticals6', 'Major Pharmaceuticals6', 'Major Pharmaceuticals4', 'Major Pharmaceuticals6', 'Major Pharmaceuticals6', 'Major Pharmaceuticals6', 'Major Pharmaceuticals6', 'Major Pharmaceuticals6', 'Major Pharmaceuticals6', 'Major Pharmaceuticals6', 'Major Pharmaceuticals6', 'Major Pharmaceuticals6', 'Major Pharmaceuticals6', 'Major Pharmaceuticals6', 'Major Pharmaceuticals6', 'Major Pharmaceuticals6', 'Major Pharmaceuticals6', 'Major Pharmaceuticals5', 'Major Pharmaceuticals5', 'Major Pharmaceuticals6', 'Major Pharmaceuticals5', 'Major Pharmaceuticals6', 'Major Pharmaceuticals6', 'Major Pharmaceuticals6', 'Major Pharmaceuticals6', 'Major Pharmaceuticals6', 'Major Pharmaceuticals6', 'Major Pharmaceuticals6', 'Major Pharmaceuticals6', 'Major Pharmaceuticals6', 'Major Pharmaceuticals6', 'Major Pharmaceuticals6', 'Major Pharmaceuticals6', 'Major Pharmaceuticals6', 'Major Pharmaceuticals6', 'Major Pharmaceuticals6', 'Major Pharmaceuticals6', 'Major Pharmaceuticals6', 'Major Pharmaceuticals6', 'Major Pharmaceuticals6', 'Major Pharmaceuticals3', 'Major Pharmaceuticals6', 'Major Pharmaceuticals6', 'Major Pharmaceuticals6', 'Major Pharmaceuticals4', 'Major Pharmaceuticals5', 'Major Pharmaceuticals4', 'Major Pharmaceuticals5', 'Major Pharmaceuticals3', 'Major Pharmaceuticals6', 'Major Pharmaceuticals4', 'Major Pharmaceuticals6', 'Major Pharmaceuticals5', 'Major Pharmaceuticals5', 'Major Pharmaceuticals5', 'Major Pharmaceuticals6', 'Major Pharmaceuticals6', 'Major Pharmaceuticals6', 'Major Pharmaceuticals6', 'Major Pharmaceuticals6', 'Major Pharmaceuticals6', 'Major Pharmaceuticals6', 'Major Pharmaceuticals6', 'Major Pharmaceuticals5', 'Major Pharmaceuticals5', 'Major Pharmaceuticals5', 'Major Pharmaceuticals6', 'Major Pharmaceuticals6', 'Major Pharmaceuticals6', 'Major Pharmaceuticals6', 'Major Pharmaceuticals6', 'Major Pharmaceuticals6', 'Major Pharmaceuticals6', 'Major Pharmaceuticals5', 'Major Pharmaceuticals4', 'Major Pharmaceuticals3', 'Major Pharmaceuticals3', 'Major Pharmaceuticals3', 'Major Pharmaceuticals6', 'Major Pharmaceuticals6', 'Major Pharmaceuticals6', 'Major Pharmaceuticals6', 'Major Pharmaceuticals6', 'Major Pharmaceuticals6', 'Major Pharmaceuticals6', 'Major Pharmaceuticals6', 'Major Pharmaceuticals6', 'Major Pharmaceuticals6', 'Major Pharmaceuticals3', 'Major Pharmaceuticals6', 'Major Pharmaceuticals6', 'Major Pharmaceuticals6', 'Major Pharmaceuticals6', 'Major Pharmaceuticals6', 'Major Pharmaceuticals6', 'Major Pharmaceuticals6', 'Major Pharmaceuticals6', 'Major Pharmaceuticals6', 'Major Pharmaceuticals6', 'Major Pharmaceuticals6', 'Major Pharmaceuticals6', 'Major Pharmaceuticals6', 'Major Pharmaceuticals6', 'Major Pharmaceuticals6', 'Major Pharmaceuticals6', 'Major Pharmaceuticals6', 'Major Pharmaceuticals6', 'Major Pharmaceuticals6', 'Major Pharmaceuticals6', 'Major Pharmaceuticals3', 'Major Pharmaceuticals6', 'Major Pharmaceuticals6', 'Major Pharmaceuticals6', 'Major Pharmaceuticals6', 'Major Pharmaceuticals5', 'Major Pharmaceuticals5', 'Major Pharmaceuticals5', 'Major Pharmaceuticals6', 'Major Pharmaceuticals6', 'Major Pharmaceuticals6', 'Major Pharmaceuticals6', 'Major Pharmaceuticals6', 'Major Pharmaceuticals6', 'Major Pharmaceuticals5', 'Major Pharmaceuticals3', 'Major Pharmaceuticals6', 'Major Pharmaceuticals6', 'Major Pharmaceuticals6', 'Major Pharmaceuticals6', 'Major Pharmaceuticals3', 'Major Pharmaceuticals6', 'Major Pharmaceuticals6', 'Major Pharmaceuticals3', 'Major Pharmaceuticals3', 'Major Pharmaceuticals1', 'Major Pharmaceuticals6', 'Major Pharmaceuticals6', 'Major Pharmaceuticals6', 'Major Pharmaceuticals6', 'Major Pharmaceuticals6', 'Major Pharmaceuticals6', 'Major Pharmaceuticals6', 'Major Pharmaceuticals3', 'Major Pharmaceuticals6', 'Major Pharmaceuticals6', 'Major Pharmaceuticals6', 'Major Pharmaceuticals4'], ['Newspapers/Magazines3', 'Newspapers/Magazines3', 'Newspapers/Magazines3', 'Newspapers/Magazines3', 'Newspapers/Magazines3', 'Newspapers/Magazines3', 'Newspapers/Magazines3', 'Newspapers/Magazines3', 'Newspapers/Magazines2', 'Newspapers/Magazines3']]\n"
]
]
] | [
"markdown",
"code",
"markdown",
"code",
"markdown",
"code",
"markdown",
"code",
"markdown",
"code"
] | [
[
"markdown",
"markdown"
],
[
"code",
"code"
],
[
"markdown"
],
[
"code"
],
[
"markdown",
"markdown"
],
[
"code",
"code"
],
[
"markdown",
"markdown"
],
[
"code"
],
[
"markdown",
"markdown",
"markdown"
],
[
"code",
"code",
"code",
"code",
"code",
"code",
"code",
"code",
"code",
"code",
"code",
"code",
"code",
"code",
"code",
"code"
]
] |
d04644d6f2037b2763984d5d2282b1309bdb7bd4 | 30,859 | ipynb | Jupyter Notebook | day4.ipynb | mzignis/dataworkshop_matrix | 3de7c019d20329df5e8231df63ea52d00fc7a584 | [
"MIT"
] | null | null | null | day4.ipynb | mzignis/dataworkshop_matrix | 3de7c019d20329df5e8231df63ea52d00fc7a584 | [
"MIT"
] | null | null | null | day4.ipynb | mzignis/dataworkshop_matrix | 3de7c019d20329df5e8231df63ea52d00fc7a584 | [
"MIT"
] | null | null | null | 30,859 | 30,859 | 0.827214 | [
[
[
"!pip install datadotworld\n!pip install datadotworld[pandas]",
"_____no_output_____"
],
[
"# !dw configure",
"_____no_output_____"
],
[
"from google.colab import drive\n\nimport pandas as pd\nimport matplotlib.pyplot as plt\nimport numpy as np\n\n# drive.mount('/content/drive')",
"_____no_output_____"
],
[
"HOME = '/content/drive/My Drive/Colab Notebooks/matrix/dataworkshop_matrix'\n%cd {HOME}",
"/content/drive/My Drive/Colab Notebooks/matrix/dataworkshop_matrix\n"
],
[
"import datadotworld as dw\n\ndata = dw.load_dataset('datafiniti/mens-shoe-prices')\ndf = data.dataframes['7004_1']",
"/usr/local/lib/python3.6/dist-packages/datadotworld/models/dataset.py:209: UserWarning: Unable to set data frame dtypes automatically using 7004_1 schema. Data types may need to be adjusted manually. Error: Integer column has NA values in column 10\n 'Error: {}'.format(resource_name, e))\n/usr/local/lib/python3.6/dist-packages/datadotworld/util.py:121: DtypeWarning: Columns (39,45) have mixed types. Specify dtype option on import or set low_memory=False.\n return self._loader_func()\n"
],
[
"df_usd = df[ df.prices_currency == 'USD'].copy()\ndf_usd['prices_amountmin'] = df_usd.prices_amountmin.astype(np.float)\nfilter_max = np.percentile(df_usd['prices_amountmin'], 99)\ndf_usd_filter = df_usd[ df_usd['prices_amountmin'] < filter_max]\ndf_usd_filter['prices_amountmin'].hist(bins=100)",
"_____no_output_____"
],
[
"df_usd_filter.to_csv('data/men_shoes.csv', index=False)",
"_____no_output_____"
],
[
"from sklearn.tree import DecisionTreeRegressor\nfrom sklearn.metrics import mean_absolute_error\nfrom sklearn.model_selection import cross_val_score",
"_____no_output_____"
],
[
"df = pd.read_csv('data/men_shoes.csv', low_memory=False)",
"_____no_output_____"
],
[
"df.shape",
"_____no_output_____"
],
[
"df.columns",
"_____no_output_____"
],
[
"mean_amountmin = np.mean(df['prices_amountmin'])\nmean_amountmin",
"_____no_output_____"
],
[
"y_true = df['prices_amountmin']\nm = y_true.shape[0]\nm",
"_____no_output_____"
],
[
"y_pred = [mean_amountmin] * m",
"_____no_output_____"
],
[
"mae = mean_absolute_error(y_true, y_pred)\nmae",
"_____no_output_____"
],
[
"np.log1p(df['prices_amountmin']).hist(bins=100)",
"_____no_output_____"
],
[
"y_pred = [np.median(df['prices_amountmin'])] * m\nmae = mean_absolute_error(y_true, y_pred)\nmae",
"_____no_output_____"
],
[
"price_log_mean = np.mean(np.log1p(df['prices_amountmin']))\ny_pred = [np.expm1(price_log_mean)] * m\nmae = mean_absolute_error(y_true, y_pred)\nmae",
"_____no_output_____"
],
[
"df['brand_cat'] = df['brand'].factorize()[0]",
"_____no_output_____"
],
[
"def run_model(feats):\n X = df[ feats ].values\n y = df['prices_amountmin'].values\n model = DecisionTreeRegressor(max_depth=5)\n score = cross_val_score(model, X, y, scoring='neg_mean_absolute_error')\n return np.mean(score), np.std(score)",
"_____no_output_____"
],
[
"run_model(feats=['brand_cat'])",
"_____no_output_____"
],
[
"df['manufacturer_cat'] = df['manufacturer'].factorize()[0]",
"_____no_output_____"
],
[
"run_model(feats=['manufacturer_cat'])",
"_____no_output_____"
],
[
"run_model(feats=['manufacturer_cat', 'brand_cat'])",
"_____no_output_____"
],
[
"!git add day4.ipynb\n!git config --global user.email \"[email protected]\"\n!git config --global user.name \"Marek Zalecki\"\n!git commit -m \"Decision Tree Model\"\n!git push -u origin master",
"[master 2ddf606] Decision Tree Model\n 1 file changed, 1 insertion(+)\n create mode 100644 day4.ipynb\nTo https://github.com/mzignis/dataworkshop_matrix.git\n ! [rejected] master -> master (fetch first)\nerror: failed to push some refs to 'https://[email protected]/mzignis/dataworkshop_matrix.git'\nhint: Updates were rejected because the remote contains work that you do\nhint: not have locally. This is usually caused by another repository pushing\nhint: to the same ref. You may want to first integrate the remote changes\nhint: (e.g., 'git pull ...') before pushing again.\nhint: See the 'Note about fast-forwards' in 'git push --help' for details.\n"
],
[
"!git pull",
"remote: Enumerating objects: 3, done.\u001b[K\nremote: Counting objects: 33% (1/3)\u001b[K\rremote: Counting objects: 66% (2/3)\u001b[K\rremote: Counting objects: 100% (3/3)\u001b[K\rremote: Counting objects: 100% (3/3), done.\u001b[K\nremote: Compressing objects: 50% (1/2)\u001b[K\rremote: Compressing objects: 100% (2/2)\u001b[K\rremote: Compressing objects: 100% (2/2), done.\u001b[K\nremote: Total 2 (delta 1), reused 0 (delta 0), pack-reused 0\u001b[K\nUnpacking objects: 100% (2/2), done.\nFrom https://github.com/mzignis/dataworkshop_matrix\n 2e4714e..052c777 master -> origin/master\nRemoving day_3.ipynb\nhint: Waiting for your editor to close the file... error: unable to start editor 'editor'\nNot committing merge; use 'git commit' to complete the merge.\n"
],
[
"",
"_____no_output_____"
]
]
] | [
"code"
] | [
[
"code",
"code",
"code",
"code",
"code",
"code",
"code",
"code",
"code",
"code",
"code",
"code",
"code",
"code",
"code",
"code",
"code",
"code",
"code",
"code",
"code",
"code",
"code",
"code",
"code",
"code",
"code"
]
] |
d046616521139abdeb049677648f046a293aaae1 | 71,817 | ipynb | Jupyter Notebook | uwb-beacon-firmware/doc/report/models/EKF C++ validation.ipynb | greck2908/robot-software | 2e1e8177148a089e8883967375dde7f8ed3d878b | [
"MIT"
] | 40 | 2016-10-04T19:59:22.000Z | 2020-12-25T18:11:35.000Z | uwb-beacon-firmware/doc/report/models/EKF C++ validation.ipynb | greck2908/robot-software | 2e1e8177148a089e8883967375dde7f8ed3d878b | [
"MIT"
] | 209 | 2016-09-21T21:54:28.000Z | 2022-01-26T07:42:37.000Z | uwb-beacon-firmware/doc/report/models/EKF C++ validation.ipynb | greck2908/robot-software | 2e1e8177148a089e8883967375dde7f8ed3d878b | [
"MIT"
] | 21 | 2016-11-07T14:40:16.000Z | 2021-11-02T09:53:37.000Z | 386.112903 | 38,940 | 0.939917 | [
[
[
"import trajectories\nimport numpy as np\nimport matplotlib.pyplot as plt\nfrom ctypes import cdll, c_float\n%matplotlib inline",
"_____no_output_____"
],
[
"LIB_NAME = \"../../../build/libekf_python.dylib\"",
"_____no_output_____"
],
[
"lib = cdll.LoadLibrary(LIB_NAME)\n\nPoint = c_float * 2\n\n\nestimator_predict = lib.estimator_predict\nestimator_predict.restype = None\n\nestimator_process_distance_measurement = lib.estimator_process_distance_measurement\nestimator_process_distance_measurement.restype = None\nestimator_process_distance_measurement.argtypes = (Point, c_float)\n\nestimator_get_x = lib.estimator_get_x\nestimator_get_x.restype = c_float\n\nestimator_get_y = lib.estimator_get_y\nestimator_get_y.restype = c_float\n\n",
"_____no_output_____"
],
[
"# Simulation start\nf = 200\nf_uwb = 10\nvmax = 0.4\nN = 10000\nvariance = (vmax / (2 * f_uwb))**2\n\nBEACON_POS = [\n (-1.5, 0),\n (1.5, 1),\n (1.5, -1),\n]\n\n\nx, xhat = [], []\ny, yhat = [], []\nts = []\n\n",
"_____no_output_____"
],
[
"for i, p in zip(range(N), trajectories.generate_circular_traj(1, np.deg2rad(10), 1/f)):\n # feeds the input into Kalman\n estimator_predict()\n\n if i % (f / f_uwb) == 0:\n for beacon in BEACON_POS:\n z = np.sqrt((beacon[0] - p.pos[0])**2 + (beacon[1] - p.pos[1])**2)\n z += np.random.normal(0, 0.03)\n estimator_process_distance_measurement(Point(*beacon), z)\n\n # Saves the data\n ts.append(p.timestamp)\n x.append(p.pos[0])\n xhat.append(estimator_get_x())\n y.append(p.pos[1])\n yhat.append(estimator_get_y())\n\n",
"_____no_output_____"
],
[
"plt.plot(x, y)\nplt.plot(xhat, yhat)\nplt.plot([x for x, y in BEACON_POS],[y for x, y in BEACON_POS], 'x')\nplt.legend(('Simulated trajectory', 'EKF output', 'anchors'))\nplt.title('trajectory')\nplt.xlabel('x [m]')\nplt.ylabel('y [m]')\nplt.gcf().savefig('uwb_only_trajectory.pdf')\nplt.show()\n\nerror = [np.sqrt((x-xh)**2+(y-yh)**2) for x,xh,y,yh in zip(x, xhat,y,yhat)]\nplt.plot(ts, error)\nplt.xlabel('timestamp')\nplt.ylabel('error [m]')\nplt.ylim(0, 0.1)\nplt.title('Position error (RMS = {:.3f} m)'.format(np.mean(error)))\nplt.gcf().savefig('uwb_only_error.pdf')\nplt.show()",
"_____no_output_____"
]
]
] | [
"code"
] | [
[
"code",
"code",
"code",
"code",
"code",
"code"
]
] |
d04668ef9ecd7b29c7d32edae9d3be257fd44fec | 2,173 | ipynb | Jupyter Notebook | groupscores/Split dates.ipynb | ym371/APAC-datathon-21 | 63276068f30da85170b1879555ff4b7b03e71426 | [
"MIT"
] | null | null | null | groupscores/Split dates.ipynb | ym371/APAC-datathon-21 | 63276068f30da85170b1879555ff4b7b03e71426 | [
"MIT"
] | null | null | null | groupscores/Split dates.ipynb | ym371/APAC-datathon-21 | 63276068f30da85170b1879555ff4b7b03e71426 | [
"MIT"
] | null | null | null | 22.173469 | 79 | 0.555453 | [
[
[
"import numpy as np # linear algebra\nimport pandas as pd # data processing, CSV file I/O (e.g. pd.read_csv)\nimport seaborn as sns \nimport matplotlib.pyplot as plt\nfrom sklearn.preprocessing import LabelEncoder",
"_____no_output_____"
],
[
"match1 = pd.read_csv(\"match1.csv\", index_col = 0, parse_dates = True)",
"_____no_output_____"
],
[
"match1[\"date\"] = pd.to_datetime(match1[\"date\"])",
"_____no_output_____"
],
[
"match1[\"year\"] = pd.DatetimeIndex(match1['date']).year\nmatch1[\"month\"] = pd.DatetimeIndex(match1['date']).month\nmatch1[\"day\"] = pd.DatetimeIndex(match1['date']).day\nmatch1[\"week_num\"] = match1['date'].dt.week",
"_____no_output_____"
],
[
"match1.to_csv(\"match_with_date_cols.csv\")",
"_____no_output_____"
]
]
] | [
"code"
] | [
[
"code",
"code",
"code",
"code",
"code"
]
] |
d046766c19f9b806d9e78f1fa7caa677533068ec | 65,502 | ipynb | Jupyter Notebook | scripts/results_final/scaling_weather/plot_notebook.ipynb | i13tum/openwhisk-bench | 626c90d8b3f7deba06e233b3ed6013ae9f08f8cf | [
"Apache-2.0"
] | 5 | 2019-10-28T00:01:16.000Z | 2021-09-09T14:13:53.000Z | scripts/results_final/scaling_weather/plot_notebook.ipynb | i13tum/openwhisk-bench | 626c90d8b3f7deba06e233b3ed6013ae9f08f8cf | [
"Apache-2.0"
] | null | null | null | scripts/results_final/scaling_weather/plot_notebook.ipynb | i13tum/openwhisk-bench | 626c90d8b3f7deba06e233b3ed6013ae9f08f8cf | [
"Apache-2.0"
] | null | null | null | 623.828571 | 63,152 | 0.947956 | [
[
[
"import numpy as np\nimport os\nimport matplotlib.pyplot as plt\n%matplotlib inline",
"_____no_output_____"
],
[
"results_dir = \"prime_number\"\ninput = '1000'\nrequests = '2000'\nfiles = os.listdir(results_dir)\n\ncpuMap = {}\nramMap = {}\n\nfor file in files:\n tokens = file.split(\"_\")\n cpuList = []\n ramList = []\n if tokens[1] == input and tokens[2] == requests:\n lines = [line.rstrip('\\n') for line in open(\"{0}/{1}\".format(results_dir, file))]\n for line in lines:\n params = line.split(\" \")\n cpuList.append(int(params[0]))\n ramList.append(int(params[1]))\n cpuMap[tokens[0]] = cpuList\n ramMap[tokens[0]] = ramList\n \n \n \n ",
"_____no_output_____"
],
[
"plt.figure(figsize=[10, 5])\n#plt.xticks(labels)\ninvokerDataToPrint = ['1', '2', '3', '4', '5', '6', '7']\nfor e in invokerDataToPrint:\n plt.plot(cpuMap[e], label='Invoker #{0}: CPU'.format(e))\nplt.ylabel('CPU Load, %')\nplt.xlabel('Time, seconds')\nplt.legend()\nplt.show()",
"_____no_output_____"
]
]
] | [
"code"
] | [
[
"code",
"code",
"code"
]
] |
d0468aff3c9f277f0ba0839365ec5d6415bd3f8e | 6,268 | ipynb | Jupyter Notebook | 2018-11-21 - PyTeaser_Test.ipynb | sjoerdapp/colab | ceaf9bb3af638ebefa3039c31f33ef280f728a64 | [
"BSD-Source-Code"
] | null | null | null | 2018-11-21 - PyTeaser_Test.ipynb | sjoerdapp/colab | ceaf9bb3af638ebefa3039c31f33ef280f728a64 | [
"BSD-Source-Code"
] | null | null | null | 2018-11-21 - PyTeaser_Test.ipynb | sjoerdapp/colab | ceaf9bb3af638ebefa3039c31f33ef280f728a64 | [
"BSD-Source-Code"
] | null | null | null | 46.776119 | 913 | 0.596362 | [
[
[
"empty"
]
]
] | [
"empty"
] | [
[
"empty"
]
] |
d046aed83d2ecd7cebd2bf033597a1c40ad5fc7f | 189,257 | ipynb | Jupyter Notebook | courses/machine_learning/deepdive/10_recommend/wals.ipynb | gozer/training-data-analyst | 0199dc581e471e2aa22dfe964acc89dacc24cad8 | [
"Apache-2.0"
] | null | null | null | courses/machine_learning/deepdive/10_recommend/wals.ipynb | gozer/training-data-analyst | 0199dc581e471e2aa22dfe964acc89dacc24cad8 | [
"Apache-2.0"
] | 1 | 2021-03-26T00:22:26.000Z | 2021-03-26T00:22:26.000Z | courses/machine_learning/deepdive/10_recommend/wals.ipynb | gozer/training-data-analyst | 0199dc581e471e2aa22dfe964acc89dacc24cad8 | [
"Apache-2.0"
] | null | null | null | 114.77077 | 105,688 | 0.832228 | [
[
[
"# Collaborative filtering on Google Analytics data\n\nThis notebook demonstrates how to implement a WALS matrix refactorization approach to do collaborative filtering.",
"_____no_output_____"
]
],
[
[
"import os\nPROJECT = \"qwiklabs-gcp-00-34ffb0f0dc65\" # REPLACE WITH YOUR PROJECT ID\nBUCKET = \"cloud-training-demos-ml\" # REPLACE WITH YOUR BUCKET NAME\nREGION = \"us-central1\" # REPLACE WITH YOUR BUCKET REGION e.g. us-central1\n\n# Do not change these\nos.environ[\"PROJECT\"] = PROJECT\nos.environ[\"BUCKET\"] = BUCKET\nos.environ[\"REGION\"] = REGION\nos.environ[\"TFVERSION\"] = \"1.13\"",
"_____no_output_____"
],
[
"%%bash\ngcloud config set project $PROJECT\ngcloud config set compute/region $REGION",
"Updated property [core/project].\nUpdated property [compute/region].\n"
],
[
"import tensorflow as tf\nprint(tf.__version__)",
"1.13.1\n"
]
],
[
[
"## Create raw dataset\n<p>\nFor collaborative filtering, we don't need to know anything about either the users or the content. Essentially, all we need to know is userId, itemId, and rating that the particular user gave the particular item.\n<p>\nIn this case, we are working with newspaper articles. The company doesn't ask their users to rate the articles. However, we can use the time-spent on the page as a proxy for rating.\n<p>\nNormally, we would also add a time filter to this (\"latest 7 days\"), but our dataset is itself limited to a few days.",
"_____no_output_____"
]
],
[
[
"from google.cloud import bigquery\nbq = bigquery.Client(project = PROJECT)\n\nsql = \"\"\"\n#standardSQL\nWITH CTE_visitor_page_content AS (\n SELECT\n fullVisitorID,\n (SELECT MAX(IF(index=10, value, NULL)) FROM UNNEST(hits.customDimensions)) AS latestContentId, \n (LEAD(hits.time, 1) OVER (PARTITION BY fullVisitorId ORDER BY hits.time ASC) - hits.time) AS session_duration \n FROM\n `cloud-training-demos.GA360_test.ga_sessions_sample`, \n UNNEST(hits) AS hits\n WHERE \n # only include hits on pages\n hits.type = \"PAGE\"\n\n GROUP BY \n fullVisitorId,\n latestContentId,\n hits.time )\n\n-- Aggregate web stats\nSELECT \n fullVisitorID as visitorId,\n latestContentId as contentId,\n SUM(session_duration) AS session_duration\nFROM\n CTE_visitor_page_content\nWHERE\n latestContentId IS NOT NULL \nGROUP BY\n fullVisitorID, \n latestContentId\nHAVING \n session_duration > 0\nORDER BY \n latestContentId \n\"\"\"\n\ndf = bq.query(sql).to_dataframe()\ndf.head()",
"_____no_output_____"
],
[
"stats = df.describe()\nstats",
"_____no_output_____"
],
[
"df[[\"session_duration\"]].plot(kind=\"hist\", logy=True, bins=100, figsize=[8,5])",
"_____no_output_____"
],
[
"# The rating is the session_duration scaled to be in the range 0-1. This will help with training.\nmedian = stats.loc[\"50%\", \"session_duration\"]\ndf[\"rating\"] = 0.3 * df[\"session_duration\"] / median\ndf.loc[df[\"rating\"] > 1, \"rating\"] = 1\ndf[[\"rating\"]].plot(kind=\"hist\", logy=True, bins=100, figsize=[8,5])",
"_____no_output_____"
],
[
"del df[\"session_duration\"]",
"_____no_output_____"
],
[
"%%bash\nrm -rf data\nmkdir data",
"_____no_output_____"
],
[
"df.to_csv(path_or_buf = \"data/collab_raw.csv\", index = False, header = False)",
"_____no_output_____"
],
[
"!head data/collab_raw.csv",
"7337153711992174438,100074831,0.2321051400452234\n5190801220865459604,100170790,1.0\n2293633612703952721,100510126,0.2481776360816793\n5874973374932455844,100510126,0.16690549004998828\n1173698801255170595,100676857,0.05464232805149575\n883397426232997550,10083328,0.9487035095774818\n1808867070685560283,100906145,1.0\n7615995624631762562,100906145,0.48418654214351925\n5519169380728479914,100915139,0.20026163722525925\n3427736932800080345,100950628,0.558924688331153\n"
]
],
[
[
"## Create dataset for WALS\n<p>\nThe raw dataset (above) won't work for WALS:\n<ol>\n<li> The userId and itemId have to be 0,1,2 ... so we need to create a mapping from visitorId (in the raw data) to userId and contentId (in the raw data) to itemId.\n<li> We will need to save the above mapping to a file because at prediction time, we'll need to know how to map the contentId in the table above to the itemId.\n<li> We'll need two files: a \"rows\" dataset where all the items for a particular user are listed; and a \"columns\" dataset where all the users for a particular item are listed.\n</ol>\n\n<p>\n\n### Mapping",
"_____no_output_____"
]
],
[
[
"import pandas as pd\nimport numpy as np\ndef create_mapping(values, filename):\n with open(filename, 'w') as ofp:\n value_to_id = {value:idx for idx, value in enumerate(values.unique())}\n for value, idx in value_to_id.items():\n ofp.write(\"{},{}\\n\".format(value, idx))\n return value_to_id\n\ndf = pd.read_csv(filepath_or_buffer = \"data/collab_raw.csv\",\n header = None,\n names = [\"visitorId\", \"contentId\", \"rating\"],\n dtype = {\"visitorId\": str, \"contentId\": str, \"rating\": np.float})\ndf.to_csv(path_or_buf = \"data/collab_raw.csv\", index = False, header = False)\nuser_mapping = create_mapping(df[\"visitorId\"], \"data/users.csv\")\nitem_mapping = create_mapping(df[\"contentId\"], \"data/items.csv\")",
"_____no_output_____"
],
[
"!head -3 data/*.csv",
"==> data/collab_raw.csv <==\n7337153711992174438,100074831,0.2321051400452234\n5190801220865459604,100170790,1.0\n2293633612703952721,100510126,0.2481776360816793\n\n==> data/items.csv <==\n727741,5272\n179038175,626\n299458287,4513\n\n==> data/users.csv <==\n6319375062712956077,33748\n7933447845885715412,47057\n5774017011910110015,76528\n"
],
[
"df[\"userId\"] = df[\"visitorId\"].map(user_mapping.get)\ndf[\"itemId\"] = df[\"contentId\"].map(item_mapping.get)",
"_____no_output_____"
],
[
"mapped_df = df[[\"userId\", \"itemId\", \"rating\"]]\nmapped_df.to_csv(path_or_buf = \"data/collab_mapped.csv\", index = False, header = False)\nmapped_df.head()",
"_____no_output_____"
]
],
[
[
"### Creating rows and columns datasets",
"_____no_output_____"
]
],
[
[
"import pandas as pd\nimport numpy as np\nmapped_df = pd.read_csv(filepath_or_buffer = \"data/collab_mapped.csv\", header = None, names = [\"userId\", \"itemId\", \"rating\"])\nmapped_df.head()",
"_____no_output_____"
],
[
"NITEMS = np.max(mapped_df[\"itemId\"]) + 1\nNUSERS = np.max(mapped_df[\"userId\"]) + 1\nmapped_df[\"rating\"] = np.round(mapped_df[\"rating\"].values, 2)\nprint(\"{} items, {} users, {} interactions\".format( NITEMS, NUSERS, len(mapped_df) ))",
"5721 items, 82902 users, 279594 interactions\n"
],
[
"grouped_by_items = mapped_df.groupby(\"itemId\")\niter = 0\nfor item, grouped in grouped_by_items:\n print(item, grouped[\"userId\"].values, grouped[\"rating\"].values)\n iter = iter + 1\n if iter > 5:\n break",
"0 [0] [0.23]\n1 [1] [1.]\n2 [2 3] [0.25 0.17]\n3 [4] [0.05]\n4 [5] [0.95]\n5 [6 7] [1. 0.48]\n"
],
[
"import tensorflow as tf\ngrouped_by_items = mapped_df.groupby(\"itemId\")\nwith tf.python_io.TFRecordWriter(\"data/users_for_item\") as ofp:\n for item, grouped in grouped_by_items:\n example = tf.train.Example(features = tf.train.Features(feature = {\n \"key\": tf.train.Feature(int64_list = tf.train.Int64List(value = [item])),\n \"indices\": tf.train.Feature(int64_list = tf.train.Int64List(value = grouped[\"userId\"].values)),\n \"values\": tf.train.Feature(float_list = tf.train.FloatList(value = grouped[\"rating\"].values))\n }))\n ofp.write(example.SerializeToString())",
"_____no_output_____"
],
[
"grouped_by_users = mapped_df.groupby(\"userId\")\nwith tf.python_io.TFRecordWriter(\"data/items_for_user\") as ofp:\n for user, grouped in grouped_by_users:\n example = tf.train.Example(features = tf.train.Features(feature = {\n \"key\": tf.train.Feature(int64_list = tf.train.Int64List(value = [user])),\n \"indices\": tf.train.Feature(int64_list = tf.train.Int64List(value = grouped[\"itemId\"].values)),\n \"values\": tf.train.Feature(float_list = tf.train.FloatList(value = grouped[\"rating\"].values))\n }))\n ofp.write(example.SerializeToString())",
"_____no_output_____"
],
[
"!ls -lrt data",
"total 31908\n-rw-r--r-- 1 jupyter jupyter 13152765 Jul 31 20:41 collab_raw.csv\n-rw-r--r-- 1 jupyter jupyter 2134511 Jul 31 20:41 users.csv\n-rw-r--r-- 1 jupyter jupyter 82947 Jul 31 20:41 items.csv\n-rw-r--r-- 1 jupyter jupyter 7812739 Jul 31 20:41 collab_mapped.csv\n-rw-r--r-- 1 jupyter jupyter 2252828 Jul 31 20:41 users_for_item\n-rw-r--r-- 1 jupyter jupyter 7217822 Jul 31 20:41 items_for_user\n"
]
],
[
[
"To summarize, we created the following data files from collab_raw.csv:\n<ol>\n<li> ```collab_mapped.csv``` is essentially the same data as in ```collab_raw.csv``` except that ```visitorId``` and ```contentId``` which are business-specific have been mapped to ```userId``` and ```itemId``` which are enumerated in 0,1,2,.... The mappings themselves are stored in ```items.csv``` and ```users.csv``` so that they can be used during inference.\n<li> ```users_for_item``` contains all the users/ratings for each item in TFExample format\n<li> ```items_for_user``` contains all the items/ratings for each user in TFExample format\n</ol>",
"_____no_output_____"
],
[
"## Train with WALS\n\nOnce you have the dataset, do matrix factorization with WALS using the [WALSMatrixFactorization](https://www.tensorflow.org/versions/master/api_docs/python/tf/contrib/factorization/WALSMatrixFactorization) in the contrib directory.\nThis is an estimator model, so it should be relatively familiar.\n<p>\nAs usual, we write an input_fn to provide the data to the model, and then create the Estimator to do train_and_evaluate.\nBecause it is in contrib and hasn't moved over to tf.estimator yet, we use tf.contrib.learn.Experiment to handle the training loop.",
"_____no_output_____"
]
],
[
[
"import os\nimport tensorflow as tf\nfrom tensorflow.python.lib.io import file_io\nfrom tensorflow.contrib.factorization import WALSMatrixFactorization\n \ndef read_dataset(mode, args):\n def decode_example(protos, vocab_size):\n features = {\n \"key\": tf.FixedLenFeature(shape = [1], dtype = tf.int64),\n \"indices\": tf.VarLenFeature(dtype = tf.int64),\n \"values\": tf.VarLenFeature(dtype = tf.float32)}\n parsed_features = tf.parse_single_example(serialized = protos, features = features)\n values = tf.sparse_merge(sp_ids = parsed_features[\"indices\"], sp_values = parsed_features[\"values\"], vocab_size = vocab_size)\n # Save key to remap after batching\n # This is a temporary workaround to assign correct row numbers in each batch.\n # You can ignore details of this part and remap_keys().\n key = parsed_features[\"key\"]\n decoded_sparse_tensor = tf.SparseTensor(indices = tf.concat(values = [values.indices, [key]], axis = 0), \n values = tf.concat(values = [values.values, [0.0]], axis = 0), \n dense_shape = values.dense_shape)\n return decoded_sparse_tensor\n \n \n def remap_keys(sparse_tensor):\n # Current indices of our SparseTensor that we need to fix\n bad_indices = sparse_tensor.indices # shape = (current_batch_size * (number_of_items/users[i] + 1), 2)\n # Current values of our SparseTensor that we need to fix\n bad_values = sparse_tensor.values # shape = (current_batch_size * (number_of_items/users[i] + 1),)\n\n # Since batch is ordered, the last value for a batch index is the user\n # Find where the batch index chages to extract the user rows\n # 1 where user, else 0\n user_mask = tf.concat(values = [bad_indices[1:,0] - bad_indices[:-1,0], tf.constant(value = [1], dtype = tf.int64)], axis = 0) # shape = (current_batch_size * (number_of_items/users[i] + 1), 2)\n\n # Mask out the user rows from the values\n good_values = tf.boolean_mask(tensor = bad_values, mask = tf.equal(x = user_mask, y = 0)) # shape = (current_batch_size * number_of_items/users[i],)\n item_indices = tf.boolean_mask(tensor = bad_indices, mask = tf.equal(x = user_mask, y = 0)) # shape = (current_batch_size * number_of_items/users[i],)\n user_indices = tf.boolean_mask(tensor = bad_indices, mask = tf.equal(x = user_mask, y = 1))[:, 1] # shape = (current_batch_size,)\n\n good_user_indices = tf.gather(params = user_indices, indices = item_indices[:,0]) # shape = (current_batch_size * number_of_items/users[i],)\n\n # User and item indices are rank 1, need to make rank 1 to concat\n good_user_indices_expanded = tf.expand_dims(input = good_user_indices, axis = -1) # shape = (current_batch_size * number_of_items/users[i], 1)\n good_item_indices_expanded = tf.expand_dims(input = item_indices[:, 1], axis = -1) # shape = (current_batch_size * number_of_items/users[i], 1)\n good_indices = tf.concat(values = [good_user_indices_expanded, good_item_indices_expanded], axis = 1) # shape = (current_batch_size * number_of_items/users[i], 2)\n\n remapped_sparse_tensor = tf.SparseTensor(indices = good_indices, values = good_values, dense_shape = sparse_tensor.dense_shape)\n return remapped_sparse_tensor\n\n \n def parse_tfrecords(filename, vocab_size):\n if mode == tf.estimator.ModeKeys.TRAIN:\n num_epochs = None # indefinitely\n else:\n num_epochs = 1 # end-of-input after this\n\n files = tf.gfile.Glob(filename = os.path.join(args[\"input_path\"], filename))\n\n # Create dataset from file list\n dataset = tf.data.TFRecordDataset(files)\n dataset = dataset.map(map_func = lambda x: decode_example(x, vocab_size))\n dataset = dataset.repeat(count = num_epochs)\n dataset = dataset.batch(batch_size = args[\"batch_size\"])\n dataset = dataset.map(map_func = lambda x: remap_keys(x))\n return dataset.make_one_shot_iterator().get_next()\n \n def _input_fn():\n features = {\n WALSMatrixFactorization.INPUT_ROWS: parse_tfrecords(\"items_for_user\", args[\"nitems\"]),\n WALSMatrixFactorization.INPUT_COLS: parse_tfrecords(\"users_for_item\", args[\"nusers\"]),\n WALSMatrixFactorization.PROJECT_ROW: tf.constant(True)\n }\n return features, None\n\n return _input_fn",
"_____no_output_____"
]
],
[
[
"This code is helpful in developing the input function. You don't need it in production.",
"_____no_output_____"
]
],
[
[
"def try_out():\n with tf.Session() as sess:\n fn = read_dataset(\n mode = tf.estimator.ModeKeys.EVAL, \n args = {\"input_path\": \"data\", \"batch_size\": 4, \"nitems\": NITEMS, \"nusers\": NUSERS})\n feats, _ = fn()\n \n print(feats[\"input_rows\"].eval())\n print(feats[\"input_rows\"].eval())\n\ntry_out()",
"SparseTensorValue(indices=array([[ 0, 0],\n [ 0, 3522],\n [ 0, 3583],\n [ 1, 1],\n [ 1, 2359],\n [ 1, 3133],\n [ 1, 4864],\n [ 1, 4901],\n [ 1, 4906],\n [ 1, 5667],\n [ 2, 2],\n [ 3, 2],\n [ 3, 1467]]), values=array([0.23, 0.05, 0.18, 1. , 0.11, 0.55, 0.3 , 0.72, 0.46, 0.3 , 0.25,\n 0.17, 0.13], dtype=float32), dense_shape=array([ 4, 5721]))\nSparseTensorValue(indices=array([[ 4, 3],\n [ 5, 4],\n [ 5, 5042],\n [ 5, 5525],\n [ 5, 5553],\n [ 6, 5],\n [ 7, 5]]), values=array([0.05, 0.95, 0.63, 1. , 0.16, 1. , 0.48], dtype=float32), dense_shape=array([ 4, 5721]))\n"
],
[
"def find_top_k(user, item_factors, k):\n all_items = tf.matmul(a = tf.expand_dims(input = user, axis = 0), b = tf.transpose(a = item_factors))\n topk = tf.nn.top_k(input = all_items, k = k)\n return tf.cast(x = topk.indices, dtype = tf.int64)\n \ndef batch_predict(args):\n import numpy as np\n with tf.Session() as sess:\n estimator = tf.contrib.factorization.WALSMatrixFactorization(\n num_rows = args[\"nusers\"], \n num_cols = args[\"nitems\"],\n embedding_dimension = args[\"n_embeds\"],\n model_dir = args[\"output_dir\"])\n \n # This is how you would get the row factors for out-of-vocab user data\n # row_factors = list(estimator.get_projections(input_fn=read_dataset(tf.estimator.ModeKeys.EVAL, args)))\n # user_factors = tf.convert_to_tensor(np.array(row_factors))\n\n # But for in-vocab data, the row factors are already in the checkpoint\n user_factors = tf.convert_to_tensor(value = estimator.get_row_factors()[0]) # (nusers, nembeds)\n # In either case, we have to assume catalog doesn\"t change, so col_factors are read in\n item_factors = tf.convert_to_tensor(value = estimator.get_col_factors()[0])# (nitems, nembeds)\n\n # For each user, find the top K items\n topk = tf.squeeze(input = tf.map_fn(fn = lambda user: find_top_k(user, item_factors, args[\"topk\"]), elems = user_factors, dtype = tf.int64))\n with file_io.FileIO(os.path.join(args[\"output_dir\"], \"batch_pred.txt\"), mode = 'w') as f:\n for best_items_for_user in topk.eval():\n f.write(\",\".join(str(x) for x in best_items_for_user) + '\\n')\n\ndef train_and_evaluate(args):\n train_steps = int(0.5 + (1.0 * args[\"num_epochs\"] * args[\"nusers\"]) / args[\"batch_size\"])\n steps_in_epoch = int(0.5 + args[\"nusers\"] / args[\"batch_size\"])\n print(\"Will train for {} steps, evaluating once every {} steps\".format(train_steps, steps_in_epoch))\n def experiment_fn(output_dir):\n return tf.contrib.learn.Experiment(\n tf.contrib.factorization.WALSMatrixFactorization(\n num_rows = args[\"nusers\"], \n num_cols = args[\"nitems\"],\n embedding_dimension = args[\"n_embeds\"],\n model_dir = args[\"output_dir\"]),\n train_input_fn = read_dataset(tf.estimator.ModeKeys.TRAIN, args),\n eval_input_fn = read_dataset(tf.estimator.ModeKeys.EVAL, args),\n train_steps = train_steps,\n eval_steps = 1,\n min_eval_frequency = steps_in_epoch\n )\n\n from tensorflow.contrib.learn.python.learn import learn_runner\n learn_runner.run(experiment_fn = experiment_fn, output_dir = args[\"output_dir\"])\n \n batch_predict(args)",
"_____no_output_____"
],
[
"import shutil\nshutil.rmtree(path = \"wals_trained\", ignore_errors=True)\ntrain_and_evaluate({\n \"output_dir\": \"wals_trained\",\n \"input_path\": \"data/\",\n \"num_epochs\": 0.05,\n \"nitems\": NITEMS,\n \"nusers\": NUSERS,\n\n \"batch_size\": 512,\n \"n_embeds\": 10,\n \"topk\": 3\n })",
"Will train for 8 steps, evaluating once every 162 steps\nWARNING:tensorflow:From <ipython-input-25-4ad1e7c785ce>:49: run (from tensorflow.contrib.learn.python.learn.learn_runner) is deprecated and will be removed in a future version.\nInstructions for updating:\nUse tf.estimator.train_and_evaluate.\nWARNING:tensorflow:From /home/jupyter/.local/lib/python3.5/site-packages/tensorflow/contrib/learn/python/learn/estimators/estimator.py:1179: BaseEstimator.__init__ (from tensorflow.contrib.learn.python.learn.estimators.estimator) is deprecated and will be removed in a future version.\nInstructions for updating:\nPlease replace uses of any Estimator from tf.contrib.learn with an Estimator from tf.estimator.*\nWARNING:tensorflow:From /home/jupyter/.local/lib/python3.5/site-packages/tensorflow/contrib/learn/python/learn/estimators/estimator.py:427: RunConfig.__init__ (from tensorflow.contrib.learn.python.learn.estimators.run_config) is deprecated and will be removed in a future version.\nInstructions for updating:\nWhen switching to tf.estimator.Estimator, use tf.estimator.RunConfig instead.\nINFO:tensorflow:Using default config.\nINFO:tensorflow:Using config: {'_tf_config': gpu_options {\n per_process_gpu_memory_fraction: 1.0\n}\n, '_task_id': 0, '_is_chief': True, '_cluster_spec': <tensorflow.python.training.server_lib.ClusterSpec object at 0x7fb220516da0>, '_save_checkpoints_steps': None, '_task_type': None, '_tf_random_seed': None, '_save_summary_steps': 100, '_num_ps_replicas': 0, '_keep_checkpoint_max': 5, '_log_step_count_steps': 100, '_keep_checkpoint_every_n_hours': 10000, '_environment': 'local', '_eval_distribute': None, '_session_config': None, '_train_distribute': None, '_evaluation_master': '', '_num_worker_replicas': 0, '_device_fn': None, '_master': '', '_protocol': None, '_save_checkpoints_secs': 600, '_model_dir': 'wals_trained'}\nWARNING:tensorflow:From <ipython-input-25-4ad1e7c785ce>:45: Experiment.__init__ (from tensorflow.contrib.learn.python.learn.experiment) is deprecated and will be removed in a future version.\nInstructions for updating:\nPlease switch to tf.estimator.train_and_evaluate. You will also have to convert to a tf.estimator.Estimator.\nWARNING:tensorflow:From /home/jupyter/.local/lib/python3.5/site-packages/tensorflow/contrib/learn/python/learn/monitors.py:279: BaseMonitor.__init__ (from tensorflow.contrib.learn.python.learn.monitors) is deprecated and will be removed after 2016-12-05.\nInstructions for updating:\nMonitors are deprecated. Please use tf.train.SessionRunHook.\nWARNING:tensorflow:From /home/jupyter/.local/lib/python3.5/site-packages/tensorflow/python/framework/op_def_library.py:263: colocate_with (from tensorflow.python.framework.ops) is deprecated and will be removed in a future version.\nInstructions for updating:\nColocations handled automatically by placer.\nWARNING:tensorflow:From /home/jupyter/.local/lib/python3.5/site-packages/tensorflow/contrib/factorization/python/ops/wals.py:315: ModelFnOps.__new__ (from tensorflow.contrib.learn.python.learn.estimators.model_fn) is deprecated and will be removed in a future version.\nInstructions for updating:\nWhen switching to tf.estimator.Estimator, use tf.estimator.EstimatorSpec. You can use the `estimator_spec` method to create an equivalent one.\nINFO:tensorflow:Create CheckpointSaverHook.\nINFO:tensorflow:Graph was finalized.\nINFO:tensorflow:Running local_init_op.\nINFO:tensorflow:Done running local_init_op.\nINFO:tensorflow:Saving checkpoints for 0 into wals_trained/model.ckpt.\nINFO:tensorflow:SweepHook running init op.\nINFO:tensorflow:SweepHook running prep ops for the row sweep.\nINFO:tensorflow:Next fit step starting.\nINFO:tensorflow:loss = 96509.96, step = 1\nINFO:tensorflow:Next fit step starting.\nINFO:tensorflow:Next fit step starting.\nINFO:tensorflow:Next fit step starting.\nINFO:tensorflow:Next fit step starting.\nINFO:tensorflow:Next fit step starting.\nINFO:tensorflow:Next fit step starting.\nINFO:tensorflow:Next fit step starting.\nINFO:tensorflow:Saving checkpoints for 8 into wals_trained/model.ckpt.\nINFO:tensorflow:Loss for final step: 110142.75.\nWARNING:tensorflow:From /home/jupyter/.local/lib/python3.5/site-packages/tensorflow/python/ops/metrics_impl.py:363: to_float (from tensorflow.python.ops.math_ops) is deprecated and will be removed in a future version.\nInstructions for updating:\nUse tf.cast instead.\nINFO:tensorflow:Starting evaluation at 2019-07-31T20:43:12Z\nINFO:tensorflow:Graph was finalized.\nWARNING:tensorflow:From /home/jupyter/.local/lib/python3.5/site-packages/tensorflow/python/training/saver.py:1266: checkpoint_exists (from tensorflow.python.training.checkpoint_management) is deprecated and will be removed in a future version.\nInstructions for updating:\nUse standard file APIs to check for files with this prefix.\nINFO:tensorflow:Restoring parameters from wals_trained/model.ckpt-8\nINFO:tensorflow:Running local_init_op.\nINFO:tensorflow:Done running local_init_op.\nINFO:tensorflow:Evaluation [1/1]\nINFO:tensorflow:Finished evaluation at 2019-07-31-20:43:12\nINFO:tensorflow:Saving dict for global step 8: global_step = 8, loss = 96509.96\nINFO:tensorflow:Using default config.\nINFO:tensorflow:Using config: {'_tf_config': gpu_options {\n per_process_gpu_memory_fraction: 1.0\n}\n, '_task_id': 0, '_is_chief': True, '_cluster_spec': <tensorflow.python.training.server_lib.ClusterSpec object at 0x7fb2207064e0>, '_save_checkpoints_steps': None, '_task_type': None, '_tf_random_seed': None, '_save_summary_steps': 100, '_num_ps_replicas': 0, '_keep_checkpoint_max': 5, '_log_step_count_steps': 100, '_keep_checkpoint_every_n_hours': 10000, '_environment': 'local', '_eval_distribute': None, '_session_config': None, '_train_distribute': None, '_evaluation_master': '', '_num_worker_replicas': 0, '_device_fn': None, '_master': '', '_protocol': None, '_save_checkpoints_secs': 600, '_model_dir': 'wals_trained'}\n"
],
[
"!ls wals_trained",
"batch_pred.txt\t\t\t model.ckpt-0.index\ncheckpoint\t\t\t model.ckpt-0.meta\neval\t\t\t\t model.ckpt-8.data-00000-of-00001\nevents.out.tfevents.1564605788.r model.ckpt-8.index\ngraph.pbtxt\t\t\t model.ckpt-8.meta\nmodel.ckpt-0.data-00000-of-00001\n"
],
[
"!head wals_trained/batch_pred.txt",
"284,5609,36\n284,2754,42\n284,3168,534\n2621,5528,2694\n4409,5295,343\n5161,3267,3369\n5479,1335,55\n5479,1335,55\n4414,284,5572\n284,241,2359\n"
]
],
[
[
"## Run as a Python module\n\nLet's run it as Python module for just a few steps.",
"_____no_output_____"
]
],
[
[
"os.environ[\"NITEMS\"] = str(NITEMS)\nos.environ[\"NUSERS\"] = str(NUSERS)",
"_____no_output_____"
],
[
"%%bash\nrm -rf wals.tar.gz wals_trained\ngcloud ml-engine local train \\\n --module-name=walsmodel.task \\\n --package-path=${PWD}/walsmodel \\\n -- \\\n --output_dir=${PWD}/wals_trained \\\n --input_path=${PWD}/data \\\n --num_epochs=0.01 --nitems=${NITEMS} --nusers=${NUSERS} \\\n --job-dir=./tmp",
"Will train for 2 steps, evaluating once every 162 steps\n"
]
],
[
[
"## Run on Cloud",
"_____no_output_____"
]
],
[
[
"%%bash\ngsutil -m cp data/* gs://${BUCKET}/wals/data",
"_____no_output_____"
],
[
"%%bash\nOUTDIR=gs://${BUCKET}/wals/model_trained\nJOBNAME=wals_$(date -u +%y%m%d_%H%M%S)\necho $OUTDIR $REGION $JOBNAME\ngsutil -m rm -rf $OUTDIR\ngcloud ml-engine jobs submit training $JOBNAME \\\n --region=$REGION \\\n --module-name=walsmodel.task \\\n --package-path=${PWD}/walsmodel \\\n --job-dir=$OUTDIR \\\n --staging-bucket=gs://$BUCKET \\\n --scale-tier=BASIC_GPU \\\n --runtime-version=$TFVERSION \\\n -- \\\n --output_dir=$OUTDIR \\\n --input_path=gs://${BUCKET}/wals/data \\\n --num_epochs=10 --nitems=${NITEMS} --nusers=${NUSERS} ",
"_____no_output_____"
]
],
[
[
"This took <b>10 minutes</b> for me.",
"_____no_output_____"
],
[
"## Get row and column factors\n\nOnce you have a trained WALS model, you can get row and column factors (user and item embeddings) from the checkpoint file. We'll look at how to use these in the section on building a recommendation system using deep neural networks.",
"_____no_output_____"
]
],
[
[
"def get_factors(args):\n with tf.Session() as sess:\n estimator = tf.contrib.factorization.WALSMatrixFactorization(\n num_rows = args[\"nusers\"], \n num_cols = args[\"nitems\"],\n embedding_dimension = args[\"n_embeds\"],\n model_dir = args[\"output_dir\"])\n \n row_factors = estimator.get_row_factors()[0]\n col_factors = estimator.get_col_factors()[0]\n return row_factors, col_factors",
"_____no_output_____"
],
[
"args = {\n \"output_dir\": \"gs://{}/wals/model_trained\".format(BUCKET),\n \"nitems\": NITEMS,\n \"nusers\": NUSERS,\n \"n_embeds\": 10\n }\n\nuser_embeddings, item_embeddings = get_factors(args)\nprint(user_embeddings[:3])\nprint(item_embeddings[:3])",
"INFO:tensorflow:Using default config.\nINFO:tensorflow:Using config: {'_environment': 'local', '_is_chief': True, '_keep_checkpoint_every_n_hours': 10000, '_num_worker_replicas': 0, '_session_config': None, '_task_type': None, '_eval_distribute': None, '_tf_config': gpu_options {\n per_process_gpu_memory_fraction: 1.0\n}\n, '_master': '', '_log_step_count_steps': 100, '_model_dir': 'gs://qwiklabs-gcp-cbc8684b07fc2dbd-bucket/wals/model_trained', '_cluster_spec': <tensorflow.python.training.server_lib.ClusterSpec object at 0x7f4bd8302f28>, '_device_fn': None, '_keep_checkpoint_max': 5, '_task_id': 0, '_evaluation_master': '', '_save_checkpoints_steps': None, '_protocol': None, '_train_distribute': None, '_save_checkpoints_secs': 600, '_save_summary_steps': 100, '_tf_random_seed': None, '_num_ps_replicas': 0}\n[[ 3.3451824e-06 -1.1986867e-05 4.8447573e-06 -1.5209486e-05\n -1.7004859e-07 1.1976428e-05 9.8887876e-06 7.2386983e-06\n -7.0237149e-07 -7.9796819e-06]\n [-2.5300323e-03 1.4055537e-03 -9.8291773e-04 -4.2533795e-03\n -1.4166030e-03 -1.9530674e-03 8.5932651e-04 -1.5276540e-03\n 2.1342330e-03 1.2041229e-03]\n [ 9.5228699e-21 5.5453966e-21 2.2947056e-21 -5.8859543e-21\n 7.7516509e-21 -2.7640896e-20 2.3587296e-20 -3.9876822e-21\n 1.7312470e-20 2.5409211e-20]]\n[[-1.2125404e-06 -8.6304914e-05 4.4657736e-05 -6.8423047e-05\n 5.8551927e-06 9.7241784e-05 6.6776753e-05 1.6673854e-05\n -1.2708440e-05 -5.1148414e-05]\n [-1.1353870e-01 5.9097271e-02 -4.6105500e-02 -1.5460028e-01\n -1.9166643e-02 -7.3236257e-02 3.5582058e-02 -5.6805085e-02\n 7.5831160e-02 7.5306065e-02]\n [ 7.1989548e-20 4.4574543e-20 6.5149121e-21 -4.6291777e-20\n 8.8196718e-20 -2.3245078e-19 1.9459292e-19 4.0191465e-20\n 1.6273659e-19 2.2836562e-19]]\n"
]
],
[
[
"You can visualize the embedding vectors using dimensional reduction techniques such as PCA.",
"_____no_output_____"
]
],
[
[
"import matplotlib.pyplot as plt\nfrom mpl_toolkits.mplot3d import Axes3D\nfrom sklearn.decomposition import PCA\n\npca = PCA(n_components = 3)\npca.fit(user_embeddings)\nuser_embeddings_pca = pca.transform(user_embeddings)\n\nfig = plt.figure(figsize = (8,8))\nax = fig.add_subplot(111, projection = \"3d\")\nxs, ys, zs = user_embeddings_pca[::150].T\nax.scatter(xs, ys, zs)",
"_____no_output_____"
]
],
[
[
"<pre>\n# Copyright 2018 Google Inc. All Rights Reserved.\n#\n# Licensed under the Apache License, Version 2.0 (the \"License\");\n# you may not use this file except in compliance with the License.\n# You may obtain a copy of the License at\n#\n# http://www.apache.org/licenses/LICENSE-2.0\n#\n# Unless required by applicable law or agreed to in writing, software\n# distributed under the License is distributed on an \"AS IS\" BASIS,\n# WITHOUT WARRANTIES OR CONDITIONS OF ANY KIND, either express or implied.\n# See the License for the specific language governing permissions and\n# limitations under the License.\n</pre>",
"_____no_output_____"
]
]
] | [
"markdown",
"code",
"markdown",
"code",
"markdown",
"code",
"markdown",
"code",
"markdown",
"code",
"markdown",
"code",
"markdown",
"code",
"markdown",
"code",
"markdown",
"code",
"markdown",
"code",
"markdown"
] | [
[
"markdown"
],
[
"code",
"code",
"code"
],
[
"markdown"
],
[
"code",
"code",
"code",
"code",
"code",
"code",
"code",
"code"
],
[
"markdown"
],
[
"code",
"code",
"code",
"code"
],
[
"markdown"
],
[
"code",
"code",
"code",
"code",
"code",
"code"
],
[
"markdown",
"markdown"
],
[
"code"
],
[
"markdown"
],
[
"code",
"code",
"code",
"code",
"code"
],
[
"markdown"
],
[
"code",
"code"
],
[
"markdown"
],
[
"code",
"code"
],
[
"markdown",
"markdown"
],
[
"code",
"code"
],
[
"markdown"
],
[
"code"
],
[
"markdown"
]
] |
d046b0ec1719138e4ce5eac366b58b02de3ca575 | 27,814 | ipynb | Jupyter Notebook | Tutorial-u0_smallb_Poynting-Cartesian.ipynb | KAClough/nrpytutorial | 2cc3b22cb1092bc10890237dd8ee3b6881c36b52 | [
"BSD-2-Clause"
] | 1 | 2019-12-23T05:31:25.000Z | 2019-12-23T05:31:25.000Z | Tutorial-u0_smallb_Poynting-Cartesian.ipynb | Yancheng-Li-PHYS/nrpytutorial | 73b706c7f7e80ba22dd563735c0a7452c82c5245 | [
"BSD-2-Clause"
] | null | null | null | Tutorial-u0_smallb_Poynting-Cartesian.ipynb | Yancheng-Li-PHYS/nrpytutorial | 73b706c7f7e80ba22dd563735c0a7452c82c5245 | [
"BSD-2-Clause"
] | null | null | null | 44.43131 | 400 | 0.545085 | [
[
[
"<script async src=\"https://www.googletagmanager.com/gtag/js?id=UA-59152712-8\"></script>\n<script>\n window.dataLayer = window.dataLayer || [];\n function gtag(){dataLayer.push(arguments);}\n gtag('js', new Date());\n\n gtag('config', 'UA-59152712-8');\n</script>\n\n# Computing the 4-Velocity Time-Component $u^0$, the Magnetic Field Measured by a Comoving Observer $b^{\\mu}$, and the Poynting Vector $S^i$\n\n## Authors: Zach Etienne & Patrick Nelson\n\n[comment]: <> (Abstract: TODO)\n\n**Notebook Status:** <font color='green'><b> Validated </b></font>\n\n**Validation Notes:** This module has been validated against a trusted code (the hand-written smallbPoynET in WVUThorns_diagnostics, which itself is based on expressions in IllinoisGRMHD... which was validated against the original GRMHD code of the Illinois NR group)\n\n### NRPy+ Source Code for this module: [u0_smallb_Poynting__Cartesian.py](../edit/u0_smallb_Poynting__Cartesian/u0_smallb_Poynting__Cartesian.py)\n\n[comment]: <> (Introduction: TODO)",
"_____no_output_____"
],
[
"<a id='toc'></a>\n\n# Table of Contents\n$$\\label{toc}$$\n\nThis notebook is organized as follows\n\n1. [Step 1](#u0bu): Computing $u^0$ and $b^{\\mu}$\n 1. [Step 1.a](#4metric): Compute the 4-metric $g_{\\mu\\nu}$ and its inverse $g^{\\mu\\nu}$ from the ADM 3+1 variables, using the [`BSSN.ADMBSSN_tofrom_4metric`](../edit/BSSN/ADMBSSN_tofrom_4metric.py) ([**tutorial**](Tutorial-ADMBSSN_tofrom_4metric.ipynb)) NRPy+ module\n 1. [Step 1.b](#u0): Compute $u^0$ from the Valencia 3-velocity\n 1. [Step 1.c](#uj): Compute $u_j$ from $u^0$, the Valencia 3-velocity, and $g_{\\mu\\nu}$\n 1. [Step 1.d](#gamma): Compute $\\gamma=$ `gammaDET` from the ADM 3+1 variables\n 1. [Step 1.e](#beta): Compute $b^\\mu$\n1. [Step 2](#poynting_flux): Defining the Poynting Flux Vector $S^{i}$\n 1. [Step 2.a](#g): Computing $g^{i\\nu}$\n 1. [Step 2.b](#s): Computing $S^{i}$\n1. [Step 3](#code_validation): Code Validation against `u0_smallb_Poynting__Cartesian` NRPy+ module\n1. [Step 4](#appendix): Appendix: Proving Eqs. 53 and 56 in [Duez *et al* (2005)](https://arxiv.org/pdf/astro-ph/0503420.pdf)\n1. [Step 5](#latex_pdf_output): Output this notebook to $\\LaTeX$-formatted PDF file",
"_____no_output_____"
],
[
"<a id='u0bu'></a>\n\n# Step 1: Computing $u^0$ and $b^{\\mu}$ \\[Back to [top](#toc)\\]\n$$\\label{u0bu}$$\n\nFirst some definitions. The spatial components of $b^{\\mu}$ are simply the magnetic field as measured by an observer comoving with the plasma $B^{\\mu}_{\\rm (u)}$, divided by $\\sqrt{4\\pi}$. In addition, in the ideal MHD limit, $B^{\\mu}_{\\rm (u)}$ is orthogonal to the plasma 4-velocity $u^\\mu$, which sets the $\\mu=0$ component. \n\nNote also that $B^{\\mu}_{\\rm (u)}$ is related to the magnetic field as measured by a *normal* observer $B^i$ via a simple projection (Eq 21 in [Duez *et al* (2005)](https://arxiv.org/pdf/astro-ph/0503420.pdf)), which results in the expressions (Eqs 23 and 24 in [Duez *et al* (2005)](https://arxiv.org/pdf/astro-ph/0503420.pdf)):\n\n\\begin{align}\n\\sqrt{4\\pi} b^0 = B^0_{\\rm (u)} &= \\frac{u_j B^j}{\\alpha} \\\\\n\\sqrt{4\\pi} b^i = B^i_{\\rm (u)} &= \\frac{B^i + (u_j B^j) u^i}{\\alpha u^0}\\\\\n\\end{align}\n\n$B^i$ is related to the actual magnetic field evaluated in IllinoisGRMHD, $\\tilde{B}^i$ via\n\n$$B^i = \\frac{\\tilde{B}^i}{\\gamma},$$\n\nwhere $\\gamma$ is the determinant of the spatial 3-metric.\n\nThe above expressions will require that we compute\n1. the 4-metric $g_{\\mu\\nu}$ from the ADM 3+1 variables\n1. $u^0$ from the Valencia 3-velocity\n1. $u_j$ from $u^0$, the Valencia 3-velocity, and $g_{\\mu\\nu}$\n1. $\\gamma$ from the ADM 3+1 variables",
"_____no_output_____"
],
[
"<a id='4metric'></a>\n\n## Step 1.a: Compute the 4-metric $g_{\\mu\\nu}$ and its inverse $g^{\\mu\\nu}$ from the ADM 3+1 variables, using the [`BSSN.ADMBSSN_tofrom_4metric`](../edit/BSSN/ADMBSSN_tofrom_4metric.py) ([**tutorial**](Tutorial-ADMBSSN_tofrom_4metric.ipynb)) NRPy+ module \\[Back to [top](#toc)\\]\n$$\\label{4metric}$$\n\nWe are given $\\gamma_{ij}$, $\\alpha$, and $\\beta^i$ from ADMBase, so let's first compute \n\n$$\ng_{\\mu\\nu} = \\begin{pmatrix} \n-\\alpha^2 + \\beta^k \\beta_k & \\beta_i \\\\\n\\beta_j & \\gamma_{ij}\n\\end{pmatrix}.\n$$",
"_____no_output_____"
]
],
[
[
"# Step 1: Initialize needed Python/NRPy+ modules\nimport sympy as sp # SymPy: The Python computer algebra package upon which NRPy+ depends\nimport NRPy_param_funcs as par # NRPy+: Parameter interface\nimport indexedexp as ixp # NRPy+: Symbolic indexed expression (e.g., tensors, vectors, etc.) support\nimport reference_metric as rfm # NRPy+: Reference metric support\nfrom outputC import * # NRPy+: Basic C code output functionality\nimport BSSN.ADMBSSN_tofrom_4metric as AB4m # NRPy+: ADM/BSSN <-> 4-metric conversions\n\n# Set spatial dimension = 3\nDIM=3\n\nthismodule = \"smallbPoynET\"\n\n# Step 1.a: Compute the 4-metric $g_{\\mu\\nu}$ and its inverse \n# $g^{\\mu\\nu}$ from the ADM 3+1 variables, using the\n# BSSN.ADMBSSN_tofrom_4metric NRPy+ module\nimport BSSN.ADMBSSN_tofrom_4metric as AB4m\ngammaDD,betaU,alpha = AB4m.setup_ADM_quantities(\"ADM\")\nAB4m.g4DD_ito_BSSN_or_ADM(\"ADM\",gammaDD,betaU,alpha)\ng4DD = AB4m.g4DD\nAB4m.g4UU_ito_BSSN_or_ADM(\"ADM\",gammaDD,betaU,alpha)\ng4UU = AB4m.g4UU",
"_____no_output_____"
]
],
[
[
"<a id='u0'></a>\n\n## Step 1.b: Compute $u^0$ from the Valencia 3-velocity \\[Back to [top](#toc)\\]\n$$\\label{u0}$$\n\nAccording to Eqs. 9-11 of [the IllinoisGRMHD paper](https://arxiv.org/pdf/1501.07276.pdf), the Valencia 3-velocity $v^i_{(n)}$ is related to the 4-velocity $u^\\mu$ via\n\n\\begin{align}\n\\alpha v^i_{(n)} &= \\frac{u^i}{u^0} + \\beta^i \\\\\n\\implies u^i &= u^0 \\left(\\alpha v^i_{(n)} - \\beta^i\\right)\n\\end{align}\n\nDefining $v^i = \\frac{u^i}{u^0}$, we get\n\n$$v^i = \\alpha v^i_{(n)} - \\beta^i,$$\n\nand in terms of this variable we get\n\n\\begin{align}\ng_{00} \\left(u^0\\right)^2 + 2 g_{0i} u^0 u^i + g_{ij} u^i u^j &= \\left(u^0\\right)^2 \\left(g_{00} + 2 g_{0i} v^i + g_{ij} v^i v^j\\right)\\\\\n\\implies u^0 &= \\pm \\sqrt{\\frac{-1}{g_{00} + 2 g_{0i} v^i + g_{ij} v^i v^j}} \\\\\n&= \\pm \\sqrt{\\frac{-1}{(-\\alpha^2 + \\beta^2) + 2 \\beta_i v^i + \\gamma_{ij} v^i v^j}} \\\\\n&= \\pm \\sqrt{\\frac{1}{\\alpha^2 - \\gamma_{ij}\\left(\\beta^i + v^i\\right)\\left(\\beta^j + v^j\\right)}}\\\\\n&= \\pm \\sqrt{\\frac{1}{\\alpha^2 - \\alpha^2 \\gamma_{ij}v^i_{(n)}v^j_{(n)}}}\\\\\n&= \\pm \\frac{1}{\\alpha}\\sqrt{\\frac{1}{1 - \\gamma_{ij}v^i_{(n)}v^j_{(n)}}}\n\\end{align}\n\nGenerally speaking, numerical errors will occasionally drive expressions under the radical to either negative values or potentially enormous values (corresponding to enormous Lorentz factors). Thus a reliable approach for computing $u^0$ requires that we first rewrite the above expression in terms of the Lorentz factor squared: $\\Gamma^2=\\left(\\alpha u^0\\right)^2$:\n\\begin{align}\nu^0 &= \\pm \\frac{1}{\\alpha}\\sqrt{\\frac{1}{1 - \\gamma_{ij}v^i_{(n)}v^j_{(n)}}}\\\\\n\\implies \\left(\\alpha u^0\\right)^2 &= \\frac{1}{1 - \\gamma_{ij}v^i_{(n)}v^j_{(n)}} \\\\\n\\implies \\gamma_{ij}v^i_{(n)}v^j_{(n)} &= 1 - \\frac{1}{\\left(\\alpha u^0\\right)^2} \\\\\n&= 1 - \\frac{1}{\\Gamma^2}\n\\end{align}\n\nIn order for the bottom expression to hold true, the left-hand side must be between 0 and 1. Again, this is not guaranteed due to the appearance of numerical errors. In fact, a robust algorithm will not allow $\\Gamma^2$ to become too large (which might contribute greatly to the stress-energy of a given gridpoint), so let's define $\\Gamma_{\\rm max}$, the largest allowed Lorentz factor. \n\nThen our algorithm for computing $u^0$ is as follows:\n\nIf\n$$R=\\gamma_{ij}v^i_{(n)}v^j_{(n)}>1 - \\frac{1}{\\Gamma_{\\rm max}^2},$$ \nthen adjust the 3-velocity $v^i$ as follows:\n\n$$v^i_{(n)} = \\sqrt{\\frac{1 - \\frac{1}{\\Gamma_{\\rm max}^2}}{R}}v^i_{(n)}.$$\n\nAfter this rescaling, we are then guaranteed that if $R$ is recomputed, it will be set to its ceiling value $R=R_{\\rm max} = 1 - \\frac{1}{\\Gamma_{\\rm max}^2}$.\n\nThen, regardless of whether the ceiling on $R$ was applied, $u^0$ can be safely computed via\n\n$$\nu^0 = \\frac{1}{\\alpha \\sqrt{1-R}}.\n$$",
"_____no_output_____"
]
],
[
[
"ValenciavU = ixp.register_gridfunctions_for_single_rank1(\"AUX\",\"ValenciavU\",DIM=3)\n\n# Step 1: Compute R = 1 - 1/max(Gamma)\nR = sp.sympify(0)\nfor i in range(DIM):\n for j in range(DIM):\n R += gammaDD[i][j]*ValenciavU[i]*ValenciavU[j]\n\nGAMMA_SPEED_LIMIT = par.Cparameters(\"REAL\",thismodule,\"GAMMA_SPEED_LIMIT\",10.0) # Default value based on\n # IllinoisGRMHD.\n # GiRaFFE default = 2000.0\nRmax = 1 - 1/(GAMMA_SPEED_LIMIT*GAMMA_SPEED_LIMIT)\n\nrescaledValenciavU = ixp.zerorank1()\nfor i in range(DIM):\n rescaledValenciavU[i] = ValenciavU[i]*sp.sqrt(Rmax/R)\n\nrescaledu0 = 1/(alpha*sp.sqrt(1-Rmax))\nregularu0 = 1/(alpha*sp.sqrt(1-R))\n\ncomputeu0_Cfunction = \"\"\"\n/* Function for computing u^0 from Valencia 3-velocity. */\n/* Inputs: ValenciavU[], alpha, gammaDD[][], GAMMA_SPEED_LIMIT (C parameter) */\n/* Output: u0=u^0 and velocity-limited ValenciavU[] */\\n\\n\"\"\"\n\ncomputeu0_Cfunction += outputC([R,Rmax],[\"const double R\",\"const double Rmax\"],\"returnstring\",\n params=\"includebraces=False,CSE_varprefix=tmpR,outCverbose=False\")\n\ncomputeu0_Cfunction += \"if(R <= Rmax) \"\ncomputeu0_Cfunction += outputC(regularu0,\"u0\",\"returnstring\",\n params=\"includebraces=True,CSE_varprefix=tmpnorescale,outCverbose=False\")\ncomputeu0_Cfunction += \" else \"\ncomputeu0_Cfunction += outputC([rescaledValenciavU[0],rescaledValenciavU[1],rescaledValenciavU[2],rescaledu0],\n [\"ValenciavU0\",\"ValenciavU1\",\"ValenciavU2\",\"u0\"],\"returnstring\",\n params=\"includebraces=True,CSE_varprefix=tmprescale,outCverbose=False\")\n\nprint(computeu0_Cfunction)",
"\n/* Function for computing u^0 from Valencia 3-velocity. */\n/* Inputs: ValenciavU[], alpha, gammaDD[][], GAMMA_SPEED_LIMIT (C parameter) */\n/* Output: u0=u^0 and velocity-limited ValenciavU[] */\n\nconst double tmpR0 = 2*ValenciavU0;\nconst double R = ((ValenciavU0)*(ValenciavU0))*gammaDD00 + ((ValenciavU1)*(ValenciavU1))*gammaDD11 + 2*ValenciavU1*ValenciavU2*gammaDD12 + ValenciavU1*gammaDD01*tmpR0 + ((ValenciavU2)*(ValenciavU2))*gammaDD22 + ValenciavU2*gammaDD02*tmpR0;\nconst double Rmax = 1 - 1/((GAMMA_SPEED_LIMIT)*(GAMMA_SPEED_LIMIT));\nif(R <= Rmax) {\n const double tmpnorescale0 = 2*ValenciavU0;\n u0 = 1/(alpha*sqrt(-((ValenciavU0)*(ValenciavU0))*gammaDD00 - ((ValenciavU1)*(ValenciavU1))*gammaDD11 - 2*ValenciavU1*ValenciavU2*gammaDD12 - ValenciavU1*gammaDD01*tmpnorescale0 - ((ValenciavU2)*(ValenciavU2))*gammaDD22 - ValenciavU2*gammaDD02*tmpnorescale0 + 1));\n}\n else {\n const double tmprescale0 = 2*ValenciavU0;\n const double tmprescale1 = sqrt((1 - 1/((GAMMA_SPEED_LIMIT)*(GAMMA_SPEED_LIMIT)))/(((ValenciavU0)*(ValenciavU0))*gammaDD00 + ((ValenciavU1)*(ValenciavU1))*gammaDD11 + 2*ValenciavU1*ValenciavU2*gammaDD12 + ValenciavU1*gammaDD01*tmprescale0 + ((ValenciavU2)*(ValenciavU2))*gammaDD22 + ValenciavU2*gammaDD02*tmprescale0));\n ValenciavU0 = ValenciavU0*tmprescale1;\n ValenciavU1 = ValenciavU1*tmprescale1;\n ValenciavU2 = ValenciavU2*tmprescale1;\n u0 = fabs(GAMMA_SPEED_LIMIT)/alpha;\n}\n\n"
]
],
[
[
"<a id='uj'></a>\n\n## Step 1.c: Compute $u_j$ from $u^0$, the Valencia 3-velocity, and $g_{\\mu\\nu}$ \\[Back to [top](#toc)\\]\n$$\\label{uj}$$\n\nThe basic equation is\n\n\\begin{align}\nu_j &= g_{\\mu j} u^{\\mu} \\\\\n&= g_{0j} u^0 + g_{ij} u^i \\\\\n&= \\beta_j u^0 + \\gamma_{ij} u^i \\\\\n&= \\beta_j u^0 + \\gamma_{ij} u^0 \\left(\\alpha v^i_{(n)} - \\beta^i\\right) \\\\\n&= u^0 \\left(\\beta_j + \\gamma_{ij} \\left(\\alpha v^i_{(n)} - \\beta^i\\right) \\right)\\\\\n&= \\alpha u^0 \\gamma_{ij} v^i_{(n)} \\\\\n\\end{align}",
"_____no_output_____"
]
],
[
[
"u0 = par.Cparameters(\"REAL\",thismodule,\"u0\",1e300) # Will be overwritten in C code. Set to crazy value to ensure this.\n\nuD = ixp.zerorank1()\nfor i in range(DIM):\n for j in range(DIM):\n uD[j] += alpha*u0*gammaDD[i][j]*ValenciavU[i]",
"_____no_output_____"
]
],
[
[
"<a id='beta'></a>\n\n## Step 1.d: Compute $b^\\mu$ \\[Back to [top](#toc)\\]\n$$\\label{beta}$$\n\nWe compute $b^\\mu$ from the above expressions:\n\n\\begin{align}\n\\sqrt{4\\pi} b^0 = B^0_{\\rm (u)} &= \\frac{u_j B^j}{\\alpha} \\\\\n\\sqrt{4\\pi} b^i = B^i_{\\rm (u)} &= \\frac{B^i + (u_j B^j) u^i}{\\alpha u^0}\\\\\n\\end{align}\n\n$B^i$ is exactly equal to the $B^i$ evaluated in IllinoisGRMHD/GiRaFFE.\n\nPulling this together, we currently have available as input:\n+ $\\tilde{B}^i$\n+ $u_j$\n+ $u^0$,\n\nwith the goal of outputting now $b^\\mu$ and $b^2$:",
"_____no_output_____"
]
],
[
[
"M_PI = par.Cparameters(\"#define\",thismodule,\"M_PI\",\"\")\nBU = ixp.register_gridfunctions_for_single_rank1(\"AUX\",\"BU\",DIM=3)\n\n# uBcontraction = u_i B^i\nuBcontraction = sp.sympify(0)\nfor i in range(DIM):\n uBcontraction += uD[i]*BU[i]\n\n# uU = 3-vector representing u^i = u^0 \\left(\\alpha v^i_{(n)} - \\beta^i\\right)\nuU = ixp.zerorank1()\nfor i in range(DIM):\n uU[i] = u0*(alpha*ValenciavU[i] - betaU[i])\n\nsmallb4U = ixp.zerorank1(DIM=4)\nsmallb4U[0] = uBcontraction/(alpha*sp.sqrt(4*M_PI))\nfor i in range(DIM):\n smallb4U[1+i] = (BU[i] + uBcontraction*uU[i])/(alpha*u0*sp.sqrt(4*M_PI))",
"_____no_output_____"
]
],
[
[
"<a id='poynting_flux'></a>\n\n# Step 2: Defining the Poynting Flux Vector $S^{i}$ \\[Back to [top](#toc)\\]\n$$\\label{poynting_flux}$$\n\nThe Poynting flux is defined in Eq. 11 of [Kelly *et al.*](https://arxiv.org/pdf/1710.02132.pdf) (note that we choose the minus sign convention so that the Poynting luminosity across a spherical shell is $L_{\\rm EM} = \\int (-\\alpha T^i_{\\rm EM\\ 0}) \\sqrt{\\gamma} d\\Omega = \\int S^r \\sqrt{\\gamma} d\\Omega$, as in [Farris *et al.*](https://arxiv.org/pdf/1207.3354.pdf):\n\n$$\nS^i = -\\alpha T^i_{\\rm EM\\ 0} = -\\alpha\\left(b^2 u^i u_0 + \\frac{1}{2} b^2 g^i{}_0 - b^i b_0\\right)\n$$\n\n",
"_____no_output_____"
],
[
"<a id='s'></a>\n\n## Step 2.a: Computing $S^{i}$ \\[Back to [top](#toc)\\]\n$$\\label{s}$$\n\nGiven $g^{\\mu\\nu}$ computed above, we focus first on the $g^i{}_{0}$ term by computing \n$$\ng^\\mu{}_\\delta = g^{\\mu\\nu} g_{\\nu \\delta},\n$$\nand then the rest of the Poynting flux vector can be immediately computed from quantities defined above:\n$$\nS^i = -\\alpha T^i_{\\rm EM\\ 0} = -\\alpha\\left(b^2 u^i u_0 + \\frac{1}{2} b^2 g^i{}_0 - b^i b_0\\right)\n$$",
"_____no_output_____"
]
],
[
[
"# Step 2.a.i: compute g^\\mu_\\delta:\ng4UD = ixp.zerorank2(DIM=4)\nfor mu in range(4):\n for delta in range(4):\n for nu in range(4):\n g4UD[mu][delta] += g4UU[mu][nu]*g4DD[nu][delta]\n\n# Step 2.a.ii: compute b_{\\mu}\nsmallb4D = ixp.zerorank1(DIM=4)\nfor mu in range(4):\n for nu in range(4):\n smallb4D[mu] += g4DD[mu][nu]*smallb4U[nu]\n\n# Step 2.a.iii: compute u_0 = g_{mu 0} u^{mu} = g4DD[0][0]*u0 + g4DD[i][0]*uU[i]\nu_0 = g4DD[0][0]*u0\nfor i in range(DIM):\n u_0 += g4DD[i+1][0]*uU[i]\n \n# Step 2.a.iv: compute b^2, setting b^2 = smallb2etk, as gridfunctions with base names ending in a digit\n# are forbidden in NRPy+.\nsmallb2etk = sp.sympify(0)\nfor mu in range(4):\n smallb2etk += smallb4U[mu]*smallb4D[mu]\n\n# Step 2.a.v: compute S^i\nPoynSU = ixp.zerorank1()\nfor i in range(DIM):\n PoynSU[i] = -alpha * (smallb2etk*uU[i]*u_0 + sp.Rational(1,2)*smallb2etk*g4UD[i+1][0] - smallb4U[i+1]*smallb4D[0])",
"_____no_output_____"
]
],
[
[
"<a id='code_validation'></a>\n\n# Step 3: Code Validation against `u0_smallb_Poynting__Cartesian` NRPy+ module \\[Back to [top](#toc)\\]\n$$\\label{code_validation}$$\n\nHere, as a code validation check, we verify agreement in the SymPy expressions for u0, smallbU, smallb2etk, and PoynSU between\n\n1. this tutorial and \n2. the NRPy+ [u0_smallb_Poynting__Cartesian module](../edit/u0_smallb_Poynting__Cartesian/u0_smallb_Poynting__Cartesian.py).",
"_____no_output_____"
]
],
[
[
"import sys\nimport u0_smallb_Poynting__Cartesian.u0_smallb_Poynting__Cartesian as u0etc\nu0etc.compute_u0_smallb_Poynting__Cartesian(gammaDD,betaU,alpha,ValenciavU,BU)\n\nif u0etc.computeu0_Cfunction != computeu0_Cfunction:\n print(\"FAILURE: u0 C code has changed!\")\n sys.exit(1)\nelse:\n print(\"PASSED: u0 C code matches!\")\n\nfor i in range(4):\n print(\"u0etc.smallb4U[\"+str(i)+\"] - smallb4U[\"+str(i)+\"] = \" \n + str(u0etc.smallb4U[i]-smallb4U[i]))\n\nprint(\"u0etc.smallb2etk - smallb2etk = \" + str(u0etc.smallb2etk-smallb2etk))\n\nfor i in range(DIM):\n print(\"u0etc.PoynSU[\"+str(i)+\"] - PoynSU[\"+str(i)+\"] = \" \n + str(u0etc.PoynSU[i]-PoynSU[i]))",
"PASSED: u0 C code matches!\nu0etc.smallb4U[0] - smallb4U[0] = 0\nu0etc.smallb4U[1] - smallb4U[1] = 0\nu0etc.smallb4U[2] - smallb4U[2] = 0\nu0etc.smallb4U[3] - smallb4U[3] = 0\nu0etc.smallb2etk - smallb2etk = 0\nu0etc.PoynSU[0] - PoynSU[0] = 0\nu0etc.PoynSU[1] - PoynSU[1] = 0\nu0etc.PoynSU[2] - PoynSU[2] = 0\n"
]
],
[
[
"<a id='appendix'></a>\n\n# Step 4: Appendix: Proving Eqs. 53 and 56 in [Duez *et al* (2005)](https://arxiv.org/pdf/astro-ph/0503420.pdf)\n$$\\label{appendix}$$\n\n$u^\\mu u_\\mu = -1$ implies\n\n\\begin{align}\ng^{\\mu\\nu} u_\\mu u_\\nu &= g^{00} \\left(u_0\\right)^2 + 2 g^{0i} u_0 u_i + g^{ij} u_i u_j = -1 \\\\\n\\implies &g^{00} \\left(u_0\\right)^2 + 2 g^{0i} u_0 u_i + g^{ij} u_i u_j + 1 = 0\\\\\n& a x^2 + b x + c = 0\n\\end{align}\n\nThus we have a quadratic equation for $u_0$, with solution given by\n\n\\begin{align}\nu_0 &= \\frac{-b \\pm \\sqrt{b^2 - 4 a c}}{2 a} \\\\\n&= \\frac{-2 g^{0i}u_i \\pm \\sqrt{\\left(2 g^{0i} u_i\\right)^2 - 4 g^{00} (g^{ij} u_i u_j + 1)}}{2 g^{00}}\\\\\n&= \\frac{-g^{0i}u_i \\pm \\sqrt{\\left(g^{0i} u_i\\right)^2 - g^{00} (g^{ij} u_i u_j + 1)}}{g^{00}}\\\\\n\\end{align}\n\nNotice that (Eq. 4.49 in [Gourgoulhon](https://arxiv.org/pdf/gr-qc/0703035.pdf))\n$$\ng^{\\mu\\nu} = \\begin{pmatrix} \n-\\frac{1}{\\alpha^2} & \\frac{\\beta^i}{\\alpha^2} \\\\\n\\frac{\\beta^i}{\\alpha^2} & \\gamma^{ij} - \\frac{\\beta^i\\beta^j}{\\alpha^2}\n\\end{pmatrix},\n$$\nso we have\n\n\\begin{align}\nu_0 &= \\frac{-\\beta^i u_i/\\alpha^2 \\pm \\sqrt{\\left(\\beta^i u_i/\\alpha^2\\right)^2 + 1/\\alpha^2 (g^{ij} u_i u_j + 1)}}{1/\\alpha^2}\\\\\n&= -\\beta^i u_i \\pm \\sqrt{\\left(\\beta^i u_i\\right)^2 + \\alpha^2 (g^{ij} u_i u_j + 1)}\\\\\n&= -\\beta^i u_i \\pm \\sqrt{\\left(\\beta^i u_i\\right)^2 + \\alpha^2 \\left(\\left[\\gamma^{ij} - \\frac{\\beta^i\\beta^j}{\\alpha^2}\\right] u_i u_j + 1\\right)}\\\\\n&= -\\beta^i u_i \\pm \\sqrt{\\left(\\beta^i u_i\\right)^2 + \\alpha^2 \\left(\\gamma^{ij}u_i u_j + 1\\right) - \\beta^i\\beta^j u_i u_j}\\\\\n&= -\\beta^i u_i \\pm \\sqrt{\\alpha^2 \\left(\\gamma^{ij}u_i u_j + 1\\right)}\\\\\n\\end{align}\n\nNow, since \n\n$$\nu^0 = g^{\\alpha 0} u_\\alpha = -\\frac{1}{\\alpha^2} u_0 + \\frac{\\beta^i u_i}{\\alpha^2},\n$$\n\nwe get\n\n\\begin{align}\nu^0 &= \\frac{1}{\\alpha^2} \\left(u_0 + \\beta^i u_i\\right) \\\\\n&= \\pm \\frac{1}{\\alpha^2} \\sqrt{\\alpha^2 \\left(\\gamma^{ij}u_i u_j + 1\\right)}\\\\\n&= \\pm \\frac{1}{\\alpha} \\sqrt{\\gamma^{ij}u_i u_j + 1}\\\\\n\\end{align}\n\nBy convention, the relativistic Gamma factor is positive and given by $\\alpha u^0$, so we choose the positive root. Thus we have derived Eq. 53 in [Duez *et al* (2005)](https://arxiv.org/pdf/astro-ph/0503420.pdf):\n\n$$\nu^0 = \\frac{1}{\\alpha} \\sqrt{\\gamma^{ij}u_i u_j + 1}.\n$$\n\nNext we evaluate \n\n\\begin{align}\nu^i &= u_\\mu g^{\\mu i} \\\\\n&= u_0 g^{0 i} + u_j g^{i j}\\\\\n&= u_0 \\frac{\\beta^i}{\\alpha^2} + u_j \\left(\\gamma^{ij} - \\frac{\\beta^i\\beta^j}{\\alpha^2}\\right)\\\\\n&= \\gamma^{ij} u_j + u_0 \\frac{\\beta^i}{\\alpha^2} - u_j \\frac{\\beta^i\\beta^j}{\\alpha^2}\\\\\n&= \\gamma^{ij} u_j + \\frac{\\beta^i}{\\alpha^2} \\left(u_0 - u_j \\beta^j\\right)\\\\\n&= \\gamma^{ij} u_j - \\beta^i u^0,\\\\\n\\implies v^i &= \\frac{\\gamma^{ij} u_j}{u^0} - \\beta^i\n\\end{align}\n\nwhich is equivalent to Eq. 56 in [Duez *et al* (2005)](https://arxiv.org/pdf/astro-ph/0503420.pdf). Notice in the last step, we used the above definition of $u^0$.",
"_____no_output_____"
],
[
"<a id='latex_pdf_output'></a>\n\n# Step 5: Output this notebook to $\\LaTeX$-formatted PDF file \\[Back to [top](#toc)\\]\n$$\\label{latex_pdf_output}$$\n\nThe following code cell converts this Jupyter notebook into a proper, clickable $\\LaTeX$-formatted PDF file. After the cell is successfully run, the generated PDF may be found in the root NRPy+ tutorial directory, with filename\n[Tutorial-u0_smallb_Poynting-Cartesian.pdf](Tutorial-u0_smallb_Poynting-Cartesian.pdf) (Note that clicking on this link may not work; you may need to open the PDF file through another means.)",
"_____no_output_____"
]
],
[
[
"!jupyter nbconvert --to latex --template latex_nrpy_style.tplx --log-level='WARN' Tutorial-u0_smallb_Poynting-Cartesian.ipynb\n!pdflatex -interaction=batchmode Tutorial-u0_smallb_Poynting-Cartesian.tex\n!pdflatex -interaction=batchmode Tutorial-u0_smallb_Poynting-Cartesian.tex\n!pdflatex -interaction=batchmode Tutorial-u0_smallb_Poynting-Cartesian.tex\n!rm -f Tut*.out Tut*.aux Tut*.log",
"[pandoc warning] Duplicate link reference `[comment]' \"source\" (line 22, column 1)\r\nThis is pdfTeX, Version 3.14159265-2.6-1.40.18 (TeX Live 2017/Debian) (preloaded format=pdflatex)\r\n restricted \\write18 enabled.\r\nentering extended mode\r\nThis is pdfTeX, Version 3.14159265-2.6-1.40.18 (TeX Live 2017/Debian) (preloaded format=pdflatex)\r\n restricted \\write18 enabled.\r\nentering extended mode\r\nThis is pdfTeX, Version 3.14159265-2.6-1.40.18 (TeX Live 2017/Debian) (preloaded format=pdflatex)\r\n restricted \\write18 enabled.\r\nentering extended mode\r\n"
]
]
] | [
"markdown",
"code",
"markdown",
"code",
"markdown",
"code",
"markdown",
"code",
"markdown",
"code",
"markdown",
"code",
"markdown",
"code"
] | [
[
"markdown",
"markdown",
"markdown",
"markdown"
],
[
"code"
],
[
"markdown"
],
[
"code"
],
[
"markdown"
],
[
"code"
],
[
"markdown"
],
[
"code"
],
[
"markdown",
"markdown"
],
[
"code"
],
[
"markdown"
],
[
"code"
],
[
"markdown",
"markdown"
],
[
"code"
]
] |
d046b35b0706af7c057f03ade3ff925fc03845cb | 9,879 | ipynb | Jupyter Notebook | Preprocessing/extractor-test.ipynb | alphabeta2206/Mal_URL | fe35064b59cfd25065bdcc1e3f1f629903dab3a7 | [
"Apache-2.0"
] | 3 | 2021-09-29T13:18:49.000Z | 2022-02-02T07:13:06.000Z | Preprocessing/extractor-test.ipynb | alphabeta2206/Mal_URL | fe35064b59cfd25065bdcc1e3f1f629903dab3a7 | [
"Apache-2.0"
] | null | null | null | Preprocessing/extractor-test.ipynb | alphabeta2206/Mal_URL | fe35064b59cfd25065bdcc1e3f1f629903dab3a7 | [
"Apache-2.0"
] | null | null | null | 26.918256 | 184 | 0.498836 | [
[
[
"# import re\n# import tensorflow as tf\n# from tensorflow.keras.preprocessing.text import text_to_word_sequence\n\n# tokens=text_to_word_sequence(\"manta.com/c/mmcdqky/lily-co\")\n\n# print(tokens)\n\n\n# #to map the features to a dictioanary and then convert it to a csv file.\n# # Feauture extraction \n# class feature_extractor(object):\n# def __init__(self,url):\n# self.url=url\n# self.length=len(url)\n# #self.domain=url.split('//')[-1].split('/')[0]\n# #def entropy(self):\n# #.com,.org,.net,.edu\n# #has www.\n# #.extension-- .htm,.html,.php,.js\n# # Pattern regex = Pattern.compile(\".com[,/.]\")\n# def domain(self):\n# if re.search(\".com[ .,/]\",self.url):\n# return 1\n# elif re.search(\".org[.,/]\",self.url):\n# return 2\n# elif re.search(\".net[.,/]\",self.url):\n# return 3\n# elif re.search(\".edu[.,/]\",self.url):\n# return 4\n# else:\n# return 0\n# #def extension(self):\n\n# def num_digits(self):\n# return sum(n.isdigit() for n in self.url)\n \n# def num_char(self):\n# return sum(n.alpha() for n in self.url)\n \n# def has_http(self):\n# if \"http\" in self.url:\n# return 1\n# else:\n# return 0\n \n# def has_https(self):\n# if \"https\" in self.url:\n# return 1\n# else:\n# return 0\n \n# #def num_special_char(self):\n# #\n \n# #def num\n\n\n\n# def clean(input):\n# tokensBySlash = str(input.encode('utf-8')).split('/')\n# allTokens=[]\n# for i in tokensBySlash:\n# tokens = str(i).split('-')\n# tokensByDot = []\n# for j in range(0,len(tokens)):\n# tempTokens = str(tokens[j]).split('.')\n# tokentsByDot = tokensByDot + tempTokens\n# allTokens = allTokens + tokens + tokensByDot\n# allTokens = list(set(allTokens))\n# if 'com' in allTokens:\n# allTokens.remove('com')\n# return allTokens",
"['manta', 'com', 'c', 'mmcdqky', 'lily', 'co']\n"
],
[
"from urllib.parse import urlparse\nurl=\"http://www.pn-wuppertal.de/links/2-linkseite/5-httpwwwkrebshilfede\"",
"_____no_output_____"
],
[
"def getTokens(input):\n tokensBySlash = str(input.encode('utf-8')).split('/')\n allTokens=[]\n for i in tokensBySlash:\n tokens = str(i).split('-')\n tokensByDot = []\n for j in range(0,len(tokens)):\n tempTokens = str(tokens[j]).split('.')\n tokentsByDot = tokensByDot + tempTokens\n allTokens = allTokens + tokens + tokensByDot\n allTokens = list(set(allTokens))\n if 'com' in allTokens:\n allTokens.remove('com')\n return allTokens",
"_____no_output_____"
],
[
"url=\"http://www.pn-wuppertal.de/links/2-linkseite/5-httpwwwkrebshilfede\"\nx=(lambda s: sum(not((i.isalpha()) and not(i.isnumeric())) for i in s))\nprint((url))",
"13\n"
],
[
"from urllib.parse import urlparse\nurl=\"http://www.pn-wuppertal.de/links/2-linkseite/5-httpwwwkrebshilfede\"\ndef fd_length(url):\n urlpath= urlparse(url).path\n try:\n return len(urlpath.split('/')[1])\n except:\n return 0\nprint(urlparse(url))\nprint(fd_length(urlparse(url)))",
"ParseResult(scheme='http', netloc='www.pn-wuppertal.de', path='/links/2-linkseite/5-httpwwwkrebshilfede', params='', query='', fragment='')\n"
],
[
"urlparse(url).scheme",
"_____no_output_____"
],
[
"s='https://www.yandex.ru'\nprint(urlparse(s))",
"ParseResult(scheme='https', netloc='www.yandex.ru', path='', params='', query='', fragment='')\n"
],
[
"s='yourbittorrent.com/?q=anthony-hamilton-soulife'\nprint(urlparse(s))\nprint(tldextract.extract(s))",
"ParseResult(scheme='', netloc='', path='yourbittorrent.com/', params='', query='q=anthony-hamilton-soulife', fragment='')\nExtractResult(subdomain='', domain='yourbittorrent', suffix='com')\n"
],
[
"from urllib.parse import urlparse\nimport tldextract\ns='movies.yahoo.com/shop?d=hv&cf=info&id=1800340831'\nprint(urlparse(s))\nprint(tldextract.extract(s).subdomain)",
"ParseResult(scheme='', netloc='', path='movies.yahoo.com/shop', params='', query='d=hv&cf=info&id=1800340831', fragment='')\nmovies\n"
],
[
"len(urlparse(s).query)",
"_____no_output_____"
],
[
"def tld_length(tld):\n try:\n return len(tld)\n except:\n return -1",
"_____no_output_____"
],
[
"import tldextract\n",
"_____no_output_____"
],
[
"from urllib.parse import urlparse\nimport tldextract\ns='http://peluqueriadeautor.com/index.php?option=com_virtuemart&page=shop.browse&category_id=31&Itemid=70'\ndef extension(s):\n domains={'com':1,'edu':2,'org':3,'net':4,'onion':5}\n if s in domains.keys():\n return domains[s]\n else:\n return 0\n#s=tldextract.extract(s).suffix\n#print(extension(s))\nprint(tldextract.extract(s))\nprint(urlparse(s))",
"ExtractResult(subdomain='', domain='peluqueriadeautor', suffix='com')\nParseResult(scheme='http', netloc='peluqueriadeautor.com', path='/index.php', params='', query='option=com_virtuemart&page=shop.browse&category_id=31&Itemid=70', fragment='')\n"
],
[
"from urllib.parse import urlparse\nimport tldextract\nprint(tldextract.extract(\"http://motthegioi.com/the-gioi-cuoi/clip-dai-gia-mac-ca-voi-co-ban-banh-my-185682.html\"))\nprint(urlparse(\"http://motthegioi.vn/the-gioi-cuoi/clip-dai-gia-mac-ca-voi-co-ban-banh-my-185682.html\"))",
"ExtractResult(subdomain='', domain='motthegioi', suffix='com')\nParseResult(scheme='http', netloc='motthegioi.vn', path='/the-gioi-cuoi/clip-dai-gia-mac-ca-voi-co-ban-banh-my-185682.html', params='', query='', fragment='')\n"
]
]
] | [
"code"
] | [
[
"code",
"code",
"code",
"code",
"code",
"code",
"code",
"code",
"code",
"code",
"code",
"code",
"code",
"code"
]
] |
d046b7cec6344c35cd5a9a8b53c2cd559f7eb0c5 | 12,211 | ipynb | Jupyter Notebook | training/baseline-vgg.ipynb | ml-boringtao/cnn-workshop | 04b3dbe79ce15475f4610c7a0430c7ecb4e969b8 | [
"MIT"
] | null | null | null | training/baseline-vgg.ipynb | ml-boringtao/cnn-workshop | 04b3dbe79ce15475f4610c7a0430c7ecb4e969b8 | [
"MIT"
] | null | null | null | training/baseline-vgg.ipynb | ml-boringtao/cnn-workshop | 04b3dbe79ce15475f4610c7a0430c7ecb4e969b8 | [
"MIT"
] | 2 | 2020-10-20T14:48:01.000Z | 2021-02-09T14:51:31.000Z | 31.15051 | 137 | 0.568586 | [
[
[
"from sklearn.preprocessing import LabelBinarizer\n\nfrom keras.datasets import cifar10\nfrom keras.preprocessing.image import ImageDataGenerator\nfrom keras.models import Sequential, model_from_json\nfrom keras.callbacks import ModelCheckpoint, EarlyStopping, ReduceLROnPlateau\nfrom keras.constraints import maxnorm\nfrom keras import regularizers\nfrom keras.layers.normalization import BatchNormalization\nfrom keras.layers import Dense, Dropout, Activation, Flatten\nfrom keras.layers import Conv2D, MaxPooling2D\n\nfrom keras.applications import imagenet_utils\nfrom keras.preprocessing.image import img_to_array\n\nimport numpy as np \nimport json\nimport os\nimport cv2\nimport h5py\n\nimport matplotlib.pyplot as plt\n%matplotlib inline",
"Using TensorFlow backend.\n"
],
[
"from helpers import TrainingMonitor\nfrom helpers import Utils",
"_____no_output_____"
],
[
"output_path = \"../output/\"",
"_____no_output_____"
],
[
"import tensorflow as tf\nconfig = tf.ConfigProto()\nconfig.gpu_options.allow_growth = True\nsession = tf.Session(config=config)",
"_____no_output_____"
],
[
"import os\nos.environ[\"PATH\"] += os.pathsep + 'C:/Program Files (x86)/Graphviz2.38/bin/'",
"_____no_output_____"
],
[
"(x_train, y_train), (x_test, y_test) = cifar10.load_data()\n\nx_train = x_train.astype('float32')\nx_test = x_test.astype('float32')\n\nmean = np.mean(x_train, axis=0)\nx_train -= mean\nx_test -= mean\n\nlb = LabelBinarizer()\ny_train = lb.fit_transform(y_train) \ny_test = lb.fit_transform(y_test)",
"_____no_output_____"
],
[
"db_train = h5py.File(\"../input/datasets/cifar_rgbmean_train.hdf5\")\ndb_test = h5py.File(\"../input/datasets/cifar_rgbmean_test.hdf5\")\n\nx_train_rgbmean = db_train[\"images\"][:].astype('float32')\nx_test_rgbmean = db_test[\"images\"][:].astype('float32')\n\nmean = np.mean(x_train_rgbmean, axis=0)\nx_train_rgbmean -= mean\nx_test_rgbmean -= mean\n\ny_train_rgbmean = db_train[\"labels\"][:]\ny_test_rgbmean = db_test[\"labels\"][:]",
"_____no_output_____"
],
[
"json_file = open(output_path + 'saved/vgg_base_model_86.03.json', 'r')\nmodel_json = json_file.read()\njson_file.close()\nmodel = model_from_json(model_json)\nmodel.load_weights(output_path + \"saved/vgg_base_weight_86.03.hdf5\")",
"_____no_output_____"
],
[
"model.summary()",
"_________________________________________________________________\nLayer (type) Output Shape Param # \n=================================================================\nconv2d_1 (Conv2D) (None, 32, 32, 32) 896 \n_________________________________________________________________\nbatch_normalization_1 (Batch (None, 32, 32, 32) 128 \n_________________________________________________________________\nconv2d_2 (Conv2D) (None, 32, 32, 32) 9248 \n_________________________________________________________________\nbatch_normalization_2 (Batch (None, 32, 32, 32) 128 \n_________________________________________________________________\nmax_pooling2d_1 (MaxPooling2 (None, 16, 16, 32) 0 \n_________________________________________________________________\ndropout_1 (Dropout) (None, 16, 16, 32) 0 \n_________________________________________________________________\nconv2d_3 (Conv2D) (None, 16, 16, 64) 18496 \n_________________________________________________________________\nbatch_normalization_3 (Batch (None, 16, 16, 64) 256 \n_________________________________________________________________\nconv2d_4 (Conv2D) (None, 16, 16, 64) 36928 \n_________________________________________________________________\nbatch_normalization_4 (Batch (None, 16, 16, 64) 256 \n_________________________________________________________________\nmax_pooling2d_2 (MaxPooling2 (None, 8, 8, 64) 0 \n_________________________________________________________________\ndropout_2 (Dropout) (None, 8, 8, 64) 0 \n_________________________________________________________________\nconv2d_5 (Conv2D) (None, 8, 8, 64) 36928 \n_________________________________________________________________\nbatch_normalization_5 (Batch (None, 8, 8, 64) 256 \n_________________________________________________________________\nconv2d_6 (Conv2D) (None, 8, 8, 64) 36928 \n_________________________________________________________________\nbatch_normalization_6 (Batch (None, 8, 8, 64) 256 \n_________________________________________________________________\nmax_pooling2d_3 (MaxPooling2 (None, 4, 4, 64) 0 \n_________________________________________________________________\ndropout_3 (Dropout) (None, 4, 4, 64) 0 \n_________________________________________________________________\nflatten_1 (Flatten) (None, 1024) 0 \n_________________________________________________________________\ndense_1 (Dense) (None, 512) 524800 \n_________________________________________________________________\nbatch_normalization_7 (Batch (None, 512) 2048 \n_________________________________________________________________\ndense_2 (Dense) (None, 512) 262656 \n_________________________________________________________________\nbatch_normalization_8 (Batch (None, 512) 2048 \n_________________________________________________________________\ndense_3 (Dense) (None, 10) 5130 \n=================================================================\nTotal params: 937,386\nTrainable params: 934,698\nNon-trainable params: 2,688\n_________________________________________________________________\n"
],
[
"for (i, layer) in enumerate(model.layers): \n print(\"{}\\t{}\".format(i, layer.__class__.__name__))",
"0\tConv2D\n1\tBatchNormalization\n2\tConv2D\n3\tBatchNormalization\n4\tMaxPooling2D\n5\tDropout\n6\tConv2D\n7\tBatchNormalization\n8\tConv2D\n9\tBatchNormalization\n10\tMaxPooling2D\n11\tDropout\n12\tConv2D\n13\tBatchNormalization\n14\tConv2D\n15\tBatchNormalization\n16\tMaxPooling2D\n17\tDropout\n18\tFlatten\n19\tDense\n20\tBatchNormalization\n21\tDense\n22\tBatchNormalization\n23\tDense\n"
],
[
"from keras.utils import plot_model\nplot_model(model, to_file='models/baseline-vgg.png', show_shapes=True, show_layer_names=True)",
"_____no_output_____"
],
[
"model.compile(loss='binary_crossentropy', optimizer='adam', metrics=['accuracy'])",
"_____no_output_____"
],
[
"filepath=output_path + \"progress/weights-{val_acc:.4f}.hdf5\"\nMC = ModelCheckpoint(filepath, monitor='val_acc', verbose=0, save_best_only=True, mode='max')\n\nfigPath = os.path.sep.join([output_path, \"monitor/{}.png\".format(os.getpid())])\njsonPath = os.path.sep.join([output_path, \"monitor/{}.json\".format(os.getpid())])\nTM = TrainingMonitor(figPath, jsonPath=jsonPath, startAt=0)\n\nRLR = ReduceLROnPlateau(factor=np.sqrt(0.1), cooldown=0, patience=5, min_lr=0.5e-6)\n\ncallbacks = [MC, TM, RLR]",
"_____no_output_____"
],
[
"history = model.fit(x_train_rgbmean, y_train_rgbmean,\n batch_size=64,\n epochs=1,\n validation_split=0.33,\n shuffle=\"batch\",\n callbacks=callbacks)",
"Train on 33500 samples, validate on 16500 samples\nEpoch 1/1\n33500/33500 [==============================] - 15s 438us/step - loss: 0.1268 - acc: 0.9597 - val_loss: 0.1164 - val_acc: 0.9643\n"
],
[
"scores = model.evaluate(x_test_rgbmean, y_test_rgbmean, verbose=0)\nprint(\"Train: %.2f%%; Val: %.2f%%; Test: %.2f%%\" % \n (np.max(history.history['acc'])*100, np.max(history.history['val_acc'])*100, scores[1]*100)\n )",
"Train: 95.85%; Val: 96.32%; Test: 96.30%\n"
]
]
] | [
"code"
] | [
[
"code",
"code",
"code",
"code",
"code",
"code",
"code",
"code",
"code",
"code",
"code",
"code",
"code",
"code",
"code"
]
] |
d046c947afcf7ee2d6785667c75d742ecf03c47c | 4,418 | ipynb | Jupyter Notebook | Sentiment Analysis/Untitled2.ipynb | loveleen123/handson-ml | 2b196d416519a6b62bce3388c14754cc24bab0e6 | [
"Apache-2.0"
] | 1 | 2019-04-13T06:17:42.000Z | 2019-04-13T06:17:42.000Z | Sentiment Analysis/Untitled2.ipynb | loveleen123/handson-ml | 2b196d416519a6b62bce3388c14754cc24bab0e6 | [
"Apache-2.0"
] | null | null | null | Sentiment Analysis/Untitled2.ipynb | loveleen123/handson-ml | 2b196d416519a6b62bce3388c14754cc24bab0e6 | [
"Apache-2.0"
] | 1 | 2019-10-08T07:17:38.000Z | 2019-10-08T07:17:38.000Z | 28.320513 | 424 | 0.517202 | [
[
[
"hash('python')",
"_____no_output_____"
],
[
"hash([12, 5, 7])",
"_____no_output_____"
],
[
"hash((12, 5, 7))",
"_____no_output_____"
],
[
"hash({12, 5, 7})",
"_____no_output_____"
],
[
"{(12, 4, 6)}",
"_____no_output_____"
],
[
"{[12, 4, 6]}",
"_____no_output_____"
]
]
] | [
"code"
] | [
[
"code",
"code",
"code",
"code",
"code",
"code"
]
] |
d046cdeb61cdee16c13b12007cad66895d9cb8ec | 36,263 | ipynb | Jupyter Notebook | t81_558_class_04_1_feature_encode.ipynb | IlkerCa/t81_558_deep_learning | c66a20525f3def957966369b29a27322d5360168 | [
"Apache-2.0"
] | null | null | null | t81_558_class_04_1_feature_encode.ipynb | IlkerCa/t81_558_deep_learning | c66a20525f3def957966369b29a27322d5360168 | [
"Apache-2.0"
] | null | null | null | t81_558_class_04_1_feature_encode.ipynb | IlkerCa/t81_558_deep_learning | c66a20525f3def957966369b29a27322d5360168 | [
"Apache-2.0"
] | null | null | null | 33.086679 | 526 | 0.388275 | [
[
[
"<a href=\"https://colab.research.google.com/github/jeffheaton/t81_558_deep_learning/blob/master/t81_558_class_04_1_feature_encode.ipynb\" target=\"_parent\"><img src=\"https://colab.research.google.com/assets/colab-badge.svg\" alt=\"Open In Colab\"/></a>",
"_____no_output_____"
],
[
"# T81-558: Applications of Deep Neural Networks\n**Module 4: Training for Tabular Data**\n* Instructor: [Jeff Heaton](https://sites.wustl.edu/jeffheaton/), McKelvey School of Engineering, [Washington University in St. Louis](https://engineering.wustl.edu/Programs/Pages/default.aspx)\n* For more information visit the [class website](https://sites.wustl.edu/jeffheaton/t81-558/).",
"_____no_output_____"
],
[
"# Module 4 Material\n\n* **Part 4.1: Encoding a Feature Vector for Keras Deep Learning** [[Video]](https://www.youtube.com/watch?v=Vxz-gfs9nMQ&list=PLjy4p-07OYzulelvJ5KVaT2pDlxivl_BN) [[Notebook]](https://github.com/jeffheaton/t81_558_deep_learning/blob/master/t81_558_class_04_1_feature_encode.ipynb)\n* Part 4.2: Keras Multiclass Classification for Deep Neural Networks with ROC and AUC [[Video]](https://www.youtube.com/watch?v=-f3bg9dLMks&list=PLjy4p-07OYzulelvJ5KVaT2pDlxivl_BN) [[Notebook]](https://github.com/jeffheaton/t81_558_deep_learning/blob/master/t81_558_class_04_2_multi_class.ipynb)\n* Part 4.3: Keras Regression for Deep Neural Networks with RMSE [[Video]](https://www.youtube.com/watch?v=wNhBUC6X5-E&list=PLjy4p-07OYzulelvJ5KVaT2pDlxivl_BN) [[Notebook]](https://github.com/jeffheaton/t81_558_deep_learning/blob/master/t81_558_class_04_3_regression.ipynb)\n* Part 4.4: Backpropagation, Nesterov Momentum, and ADAM Neural Network Training [[Video]](https://www.youtube.com/watch?v=VbDg8aBgpck&list=PLjy4p-07OYzulelvJ5KVaT2pDlxivl_BN) [[Notebook]](https://github.com/jeffheaton/t81_558_deep_learning/blob/master/t81_558_class_04_4_backprop.ipynb)\n* Part 4.5: Neural Network RMSE and Log Loss Error Calculation from Scratch [[Video]](https://www.youtube.com/watch?v=wmQX1t2PHJc&list=PLjy4p-07OYzulelvJ5KVaT2pDlxivl_BN) [[Notebook]](https://github.com/jeffheaton/t81_558_deep_learning/blob/master/t81_558_class_04_5_rmse_logloss.ipynb)",
"_____no_output_____"
],
[
"# Google CoLab Instructions\n\nThe following code ensures that Google CoLab is running the correct version of TensorFlow.",
"_____no_output_____"
]
],
[
[
"try:\n %tensorflow_version 2.x\n COLAB = True\n print(\"Note: using Google CoLab\")\nexcept:\n print(\"Note: not using Google CoLab\")\n COLAB = False",
"Note: not using Google CoLab\n"
]
],
[
[
"# Part 4.1: Encoding a Feature Vector for Keras Deep Learning\n\nNeural networks can accept many types of data. We will begin with tabular data, where there are well defined rows and columns. This is the sort of data you would typically see in Microsoft Excel. An example of tabular data is shown below.\n\nNeural networks require numeric input. This numeric form is called a feature vector. Each row of training data typically becomes one vector. The individual input neurons each receive one feature (or column) from this vector. In this section, we will see how to encode the following tabular data into a feature vector.",
"_____no_output_____"
]
],
[
[
"import pandas as pd\n\npd.set_option('display.max_columns', 7) \npd.set_option('display.max_rows', 5)\n\ndf = pd.read_csv(\n \"https://data.heatonresearch.com/data/t81-558/jh-simple-dataset.csv\",\n na_values=['NA','?'])\n\npd.set_option('display.max_columns', 9)\npd.set_option('display.max_rows', 5)\n\ndisplay(df)",
"_____no_output_____"
]
],
[
[
"The following observations can be made from the above data:\n* The target column is the column that you seek to predict. There are several candidates here. However, we will initially use product. This field specifies what product someone bought.\n* There is an ID column. This column should not be fed into the neural network as it contains no information useful for prediction.\n* Many of these fields are numeric and might not require any further processing.\n* The income column does have some missing values.\n* There are categorical values: job, area, and product.\n\nTo begin with, we will convert the job code into dummy variables.",
"_____no_output_____"
]
],
[
[
"pd.set_option('display.max_columns', 7) \npd.set_option('display.max_rows', 5)\n\ndummies = pd.get_dummies(df['job'],prefix=\"job\")\nprint(dummies.shape)\n\npd.set_option('display.max_columns', 9)\npd.set_option('display.max_rows', 10)\n\ndisplay(dummies)",
"(2000, 33)\n"
]
],
[
[
"Because there are 33 different job codes, there are 33 dummy variables. We also specified a prefix, because the job codes (such as \"ax\") are not that meaningful by themselves. Something such as \"job_ax\" also tells us the origin of this field.\n\nNext, we must merge these dummies back into the main data frame. We also drop the original \"job\" field, as it is now represented by the dummies. ",
"_____no_output_____"
]
],
[
[
"pd.set_option('display.max_columns', 7) \npd.set_option('display.max_rows', 5)\n\ndf = pd.concat([df,dummies],axis=1)\ndf.drop('job', axis=1, inplace=True)\n\npd.set_option('display.max_columns', 9)\npd.set_option('display.max_rows', 10)\n\ndisplay(df)",
"_____no_output_____"
]
],
[
[
"We also introduce dummy variables for the area column.",
"_____no_output_____"
]
],
[
[
"pd.set_option('display.max_columns', 7) \npd.set_option('display.max_rows', 5)\n\ndf = pd.concat([df,pd.get_dummies(df['area'],prefix=\"area\")],axis=1)\ndf.drop('area', axis=1, inplace=True)\n\npd.set_option('display.max_columns', 9)\npd.set_option('display.max_rows', 10)\ndisplay(df)",
"_____no_output_____"
]
],
[
[
"The last remaining transformation is to fill in missing income values. ",
"_____no_output_____"
]
],
[
[
"med = df['income'].median()\ndf['income'] = df['income'].fillna(med)",
"_____no_output_____"
]
],
[
[
"There are more advanced ways of filling in missing values, but they require more analysis. The idea would be to see if another field might give a hint as to what the income were. For example, it might be beneficial to calculate a median income for each of the areas or job categories. This is something to keep in mind for the class Kaggle competition.\n\nAt this point, the Pandas dataframe is ready to be converted to Numpy for neural network training. We need to know a list of the columns that will make up *x* (the predictors or inputs) and *y* (the target). \n\nThe complete list of columns is:",
"_____no_output_____"
]
],
[
[
"print(list(df.columns))",
"['id', 'income', 'aspect', 'subscriptions', 'dist_healthy', 'save_rate', 'dist_unhealthy', 'age', 'pop_dense', 'retail_dense', 'crime', 'product', 'job_11', 'job_al', 'job_am', 'job_ax', 'job_bf', 'job_by', 'job_cv', 'job_de', 'job_dz', 'job_e2', 'job_f8', 'job_gj', 'job_gv', 'job_kd', 'job_ke', 'job_kl', 'job_kp', 'job_ks', 'job_kw', 'job_mm', 'job_nb', 'job_nn', 'job_ob', 'job_pe', 'job_po', 'job_pq', 'job_pz', 'job_qp', 'job_qw', 'job_rn', 'job_sa', 'job_vv', 'job_zz', 'area_a', 'area_b', 'area_c', 'area_d']\n"
]
],
[
[
"This includes both the target and predictors. We need a list with the target removed. We also remove **id** because it is not useful for prediction.",
"_____no_output_____"
]
],
[
[
"x_columns = df.columns.drop('product').drop('id')\nprint(list(x_columns))",
"['income', 'aspect', 'subscriptions', 'dist_healthy', 'save_rate', 'dist_unhealthy', 'age', 'pop_dense', 'retail_dense', 'crime', 'job_11', 'job_al', 'job_am', 'job_ax', 'job_bf', 'job_by', 'job_cv', 'job_de', 'job_dz', 'job_e2', 'job_f8', 'job_gj', 'job_gv', 'job_kd', 'job_ke', 'job_kl', 'job_kp', 'job_ks', 'job_kw', 'job_mm', 'job_nb', 'job_nn', 'job_ob', 'job_pe', 'job_po', 'job_pq', 'job_pz', 'job_qp', 'job_qw', 'job_rn', 'job_sa', 'job_vv', 'job_zz', 'area_a', 'area_b', 'area_c', 'area_d']\n"
]
],
[
[
"### Generate X and Y for a Classification Neural Network",
"_____no_output_____"
],
[
"We can now generate *x* and *y*. Note, this is how we generate y for a classification problem. Regression would not use dummies and would simply encode the numeric value of the target.",
"_____no_output_____"
]
],
[
[
"# Convert to numpy - Classification\nx_columns = df.columns.drop('product').drop('id')\nx = df[x_columns].values\ndummies = pd.get_dummies(df['product']) # Classification\nproducts = dummies.columns\ny = dummies.values",
"_____no_output_____"
]
],
[
[
"We can display the *x* and *y* matrices.",
"_____no_output_____"
]
],
[
[
"print(x)\nprint(y)",
"[[5.08760000e+04 1.31000000e+01 1.00000000e+00 ... 0.00000000e+00\n 1.00000000e+00 0.00000000e+00]\n [6.03690000e+04 1.86250000e+01 2.00000000e+00 ... 0.00000000e+00\n 1.00000000e+00 0.00000000e+00]\n [5.51260000e+04 3.47666667e+01 1.00000000e+00 ... 0.00000000e+00\n 1.00000000e+00 0.00000000e+00]\n ...\n [2.85950000e+04 3.94250000e+01 3.00000000e+00 ... 0.00000000e+00\n 0.00000000e+00 1.00000000e+00]\n [6.79490000e+04 5.73333333e+00 0.00000000e+00 ... 0.00000000e+00\n 1.00000000e+00 0.00000000e+00]\n [6.14670000e+04 1.68916667e+01 0.00000000e+00 ... 0.00000000e+00\n 1.00000000e+00 0.00000000e+00]]\n[[0 1 0 ... 0 0 0]\n [0 0 1 ... 0 0 0]\n [0 1 0 ... 0 0 0]\n ...\n [0 0 0 ... 0 1 0]\n [0 0 1 ... 0 0 0]\n [0 0 1 ... 0 0 0]]\n"
]
],
[
[
"The x and y values are now ready for a neural network. Make sure that you construct the neural network for a classification problem. Specifically,\n\n* Classification neural networks have an output neuron count equal to the number of classes.\n* Classification neural networks should use **categorical_crossentropy** and a **softmax** activation function on the output layer.",
"_____no_output_____"
],
[
"### Generate X and Y for a Regression Neural Network\n\nFor a regression neural network, the *x* values are generated the same. However, *y* does not use dummies. Make sure to replace **income** with your actual target.",
"_____no_output_____"
]
],
[
[
"y = df['income'].values",
"_____no_output_____"
]
],
[
[
"# Module 4 Assignment\n\nYou can find the first assignment here: [assignment 4](https://github.com/jeffheaton/t81_558_deep_learning/blob/master/assignments/assignment_yourname_class1.ipynb)",
"_____no_output_____"
]
]
] | [
"markdown",
"code",
"markdown",
"code",
"markdown",
"code",
"markdown",
"code",
"markdown",
"code",
"markdown",
"code",
"markdown",
"code",
"markdown",
"code",
"markdown",
"code",
"markdown",
"code",
"markdown",
"code",
"markdown"
] | [
[
"markdown",
"markdown",
"markdown",
"markdown"
],
[
"code"
],
[
"markdown"
],
[
"code"
],
[
"markdown"
],
[
"code"
],
[
"markdown"
],
[
"code"
],
[
"markdown"
],
[
"code"
],
[
"markdown"
],
[
"code"
],
[
"markdown"
],
[
"code"
],
[
"markdown"
],
[
"code"
],
[
"markdown",
"markdown"
],
[
"code"
],
[
"markdown"
],
[
"code"
],
[
"markdown",
"markdown"
],
[
"code"
],
[
"markdown"
]
] |
d046ef2509bee4da8590087310c71131b90aac30 | 3,038 | ipynb | Jupyter Notebook | Assignment 1/src/Q2Q3.ipynb | aravind-3105/Digital-Image-Processing-Assignment1 | a7b75b6f6cb11a59126eff11460b5befe4af73fc | [
"MIT"
] | null | null | null | Assignment 1/src/Q2Q3.ipynb | aravind-3105/Digital-Image-Processing-Assignment1 | a7b75b6f6cb11a59126eff11460b5befe4af73fc | [
"MIT"
] | null | null | null | Assignment 1/src/Q2Q3.ipynb | aravind-3105/Digital-Image-Processing-Assignment1 | a7b75b6f6cb11a59126eff11460b5befe4af73fc | [
"MIT"
] | null | null | null | 29.784314 | 96 | 0.566162 | [
[
[
"# Question 2\n\nColin has just joined hogwarts and brought a muggle camera with him, He is very\nexcited about all the magical things around him and wants to take many photos. But\nthe problem, it has limited storage of 500 MB. You, as his friend, have to help him by\ndoing the following task. Calculate the maximum dimensions of one image if colin wants\nto take 200 images back with him when he goes home for summer vacation. Assume all\nimage shapes to be squares.\nColin is very curious and expects explanations for all the steps you take for the above\ntask, so explain each step with theoretical details.",
"_____no_output_____"
],
[
"# Answer:",
"_____no_output_____"
],
[
"Given,<br>\n Storage limit = 500mb <br>\n Total Images = 200<br>\n No. of channels of RGB = 3<br>\n So,<br>\n <center>500x1024x1024 Bytes = 200.$x^{2}$.3</center>\n <center>2.5x1024x1024/3 = $x^{2}$</center>\n <center>x = 934.779831475</center>\n <center>x = 934 approx to lower to accomodate all channels</center>",
"_____no_output_____"
],
[
"# Question 3\nCCD Sensor dim = 10x10mm<br>\nPixels = 1024 x 1024<br>\nFocal length = 43.5cm<br>\nTarget height = 390pixels <br>\nMinimum distance = 240m<br>\nFind height of creature.",
"_____no_output_____"
],
[
"# Answer\n\n1 pixel height = 10/1024mm<br>\nSo, <br>\n <center>Image Height(i) = 390*(10/1024)mm<center>\n <center>Image forms at focal length -> v = 43.5cm = 430mm<center>\n <center>Height of creature = x mm <center>\n <center>u = Object distance = 240m = 240*(1000)mm<center>\n <center>object height = h<center>\n <center>v/u = i/h<center>\n <center>430/(240*1000) = 390*(10/1024)/h<center>\n <center>h = 2125.726744186mm -> <b>h = 2.1257267441860002m<b><center>\n \n \n",
"_____no_output_____"
]
]
] | [
"markdown"
] | [
[
"markdown",
"markdown",
"markdown",
"markdown",
"markdown"
]
] |
d046efc1eb0894738a326456e8bba3c9e05e88ab | 33,996 | ipynb | Jupyter Notebook | pre_exercises/Intermediate_ML/exercise-categorical-variables.ipynb | krishnaaxo/Spotify_Skip_Action_Prediction | 1cf07592721d27109f4591412cdf1f450db3eb2f | [
"Apache-2.0"
] | 1 | 2022-01-25T12:59:09.000Z | 2022-01-25T12:59:09.000Z | pre_exercises/Intermediate_ML/exercise-categorical-variables.ipynb | krishnaaxo/Spotify_Skip_Action_Prediction | 1cf07592721d27109f4591412cdf1f450db3eb2f | [
"Apache-2.0"
] | null | null | null | pre_exercises/Intermediate_ML/exercise-categorical-variables.ipynb | krishnaaxo/Spotify_Skip_Action_Prediction | 1cf07592721d27109f4591412cdf1f450db3eb2f | [
"Apache-2.0"
] | null | null | null | 33,996 | 33,996 | 0.675521 | [
[
[
"**This notebook is an exercise in the [Intermediate Machine Learning](https://www.kaggle.com/learn/intermediate-machine-learning) course. You can reference the tutorial at [this link](https://www.kaggle.com/alexisbcook/categorical-variables).**\n\n---\n",
"_____no_output_____"
],
[
"By encoding **categorical variables**, you'll obtain your best results thus far!\n\n# Setup\n\nThe questions below will give you feedback on your work. Run the following cell to set up the feedback system.",
"_____no_output_____"
]
],
[
[
"# Set up code checking\nimport os\nif not os.path.exists(\"../input/train.csv\"):\n os.symlink(\"../input/home-data-for-ml-course/train.csv\", \"../input/train.csv\") \n os.symlink(\"../input/home-data-for-ml-course/test.csv\", \"../input/test.csv\") \nfrom learntools.core import binder\nbinder.bind(globals())\nfrom learntools.ml_intermediate.ex3 import *\nprint(\"Setup Complete\")",
"Setup Complete\n"
]
],
[
[
"In this exercise, you will work with data from the [Housing Prices Competition for Kaggle Learn Users](https://www.kaggle.com/c/home-data-for-ml-course). \n\n\n\nRun the next code cell without changes to load the training and validation sets in `X_train`, `X_valid`, `y_train`, and `y_valid`. The test set is loaded in `X_test`.",
"_____no_output_____"
]
],
[
[
"import pandas as pd\nfrom sklearn.model_selection import train_test_split\n\n# Read the data\nX = pd.read_csv('../input/train.csv', index_col='Id') \nX_test = pd.read_csv('../input/test.csv', index_col='Id')\n\n# Remove rows with missing target, separate target from predictors\nX.dropna(axis=0, subset=['SalePrice'], inplace=True)\ny = X.SalePrice\nX.drop(['SalePrice'], axis=1, inplace=True)\n\n# To keep things simple, we'll drop columns with missing values\ncols_with_missing = [col for col in X.columns if X[col].isnull().any()] \nX.drop(cols_with_missing, axis=1, inplace=True)\nX_test.drop(cols_with_missing, axis=1, inplace=True)\n\n# Break off validation set from training data\nX_train, X_valid, y_train, y_valid = train_test_split(X, y,\n train_size=0.8, test_size=0.2,\n random_state=0)",
"_____no_output_____"
]
],
[
[
"Use the next code cell to print the first five rows of the data.",
"_____no_output_____"
]
],
[
[
"X_train.head()",
"_____no_output_____"
]
],
[
[
"Notice that the dataset contains both numerical and categorical variables. You'll need to encode the categorical data before training a model.\n\nTo compare different models, you'll use the same `score_dataset()` function from the tutorial. This function reports the [mean absolute error](https://en.wikipedia.org/wiki/Mean_absolute_error) (MAE) from a random forest model.",
"_____no_output_____"
]
],
[
[
"from sklearn.ensemble import RandomForestRegressor\nfrom sklearn.metrics import mean_absolute_error\n\n# function for comparing different approaches\ndef score_dataset(X_train, X_valid, y_train, y_valid):\n model = RandomForestRegressor(n_estimators=100, random_state=0)\n model.fit(X_train, y_train)\n preds = model.predict(X_valid)\n return mean_absolute_error(y_valid, preds)",
"_____no_output_____"
]
],
[
[
"# Step 1: Drop columns with categorical data\n\nYou'll get started with the most straightforward approach. Use the code cell below to preprocess the data in `X_train` and `X_valid` to remove columns with categorical data. Set the preprocessed DataFrames to `drop_X_train` and `drop_X_valid`, respectively. ",
"_____no_output_____"
]
],
[
[
"# Fill in the lines below: drop columns in training and validation data\ndrop_X_train = X_train.select_dtypes(exclude=['object'])\ndrop_X_valid = X_valid.select_dtypes(exclude=['object'])\n\n# Check your answers\nstep_1.check()",
"_____no_output_____"
],
[
"# Lines below will give you a hint or solution code\n#step_1.hint()\n#step_1.solution()",
"_____no_output_____"
]
],
[
[
"Run the next code cell to get the MAE for this approach.",
"_____no_output_____"
]
],
[
[
"print(\"MAE from Approach 1 (Drop categorical variables):\")\nprint(score_dataset(drop_X_train, drop_X_valid, y_train, y_valid))",
"MAE from Approach 1 (Drop categorical variables):\n17837.82570776256\n"
]
],
[
[
"Before jumping into label encoding, we'll investigate the dataset. Specifically, we'll look at the `'Condition2'` column. The code cell below prints the unique entries in both the training and validation sets.",
"_____no_output_____"
]
],
[
[
"print(\"Unique values in 'Condition2' column in training data:\", X_train['Condition2'].unique())\nprint(\"\\nUnique values in 'Condition2' column in validation data:\", X_valid['Condition2'].unique())",
"Unique values in 'Condition2' column in training data: ['Norm' 'PosA' 'Feedr' 'PosN' 'Artery' 'RRAe']\n\nUnique values in 'Condition2' column in validation data: ['Norm' 'RRAn' 'RRNn' 'Artery' 'Feedr' 'PosN']\n"
]
],
[
[
"# Step 2: Label encoding\n\n### Part A\n\nIf you now write code to: \n- fit a label encoder to the training data, and then \n- use it to transform both the training and validation data, \n\nyou'll get an error. Can you see why this is the case? (_You'll need to use the above output to answer this question._)",
"_____no_output_____"
]
],
[
[
"# Check your answer (Run this code cell to receive credit!)\nstep_2.a.check()",
"_____no_output_____"
],
[
"#step_2.a.hint()",
"_____no_output_____"
]
],
[
[
"This is a common problem that you'll encounter with real-world data, and there are many approaches to fixing this issue. For instance, you can write a custom label encoder to deal with new categories. The simplest approach, however, is to drop the problematic categorical columns. \n\nRun the code cell below to save the problematic columns to a Python list `bad_label_cols`. Likewise, columns that can be safely label encoded are stored in `good_label_cols`.",
"_____no_output_____"
]
],
[
[
"# All categorical columns\nobject_cols = [col for col in X_train.columns if X_train[col].dtype == \"object\"]\n\n# Columns that can be safely label encoded\ngood_label_cols = [col for col in object_cols if \n set(X_train[col]) == set(X_valid[col])]\n \n# Problematic columns that will be dropped from the dataset\nbad_label_cols = list(set(object_cols)-set(good_label_cols))\n \nprint('Categorical columns that will be label encoded:', good_label_cols)\nprint('\\nCategorical columns that will be dropped from the dataset:', bad_label_cols)",
"Categorical columns that will be label encoded: ['MSZoning', 'Street', 'LotShape', 'LandContour', 'LotConfig', 'BldgType', 'HouseStyle', 'ExterQual', 'CentralAir', 'KitchenQual', 'PavedDrive', 'SaleCondition']\n\nCategorical columns that will be dropped from the dataset: ['Neighborhood', 'LandSlope', 'Condition1', 'Heating', 'Foundation', 'RoofMatl', 'Condition2', 'RoofStyle', 'ExterCond', 'Exterior1st', 'Utilities', 'Functional', 'HeatingQC', 'SaleType', 'Exterior2nd']\n"
]
],
[
[
"### Part B\n\nUse the next code cell to label encode the data in `X_train` and `X_valid`. Set the preprocessed DataFrames to `label_X_train` and `label_X_valid`, respectively. \n- We have provided code below to drop the categorical columns in `bad_label_cols` from the dataset. \n- You should label encode the categorical columns in `good_label_cols`. ",
"_____no_output_____"
]
],
[
[
"from sklearn.preprocessing import LabelEncoder\n\n# Drop categorical columns that will not be encoded\nlabel_X_train = X_train.drop(bad_label_cols, axis=1)\nlabel_X_valid = X_valid.drop(bad_label_cols, axis=1)\n\n# Apply label encoder \nlabel_encoder = LabelEncoder()\nfor col in good_label_cols:\n label_X_train[col] = label_encoder.fit_transform(label_X_train[col])\n label_X_valid[col] = label_encoder.transform(label_X_valid[col])\n \n# Check your answer\nstep_2.b.check()",
"_____no_output_____"
],
[
"# Lines below will give you a hint or solution code\n#step_2.b.hint()\n#step_2.b.solution()",
"_____no_output_____"
]
],
[
[
"Run the next code cell to get the MAE for this approach.",
"_____no_output_____"
]
],
[
[
"print(\"MAE from Approach 2 (Label Encoding):\") \nprint(score_dataset(label_X_train, label_X_valid, y_train, y_valid))",
"MAE from Approach 2 (Label Encoding):\n17575.291883561644\n"
]
],
[
[
"So far, you've tried two different approaches to dealing with categorical variables. And, you've seen that encoding categorical data yields better results than removing columns from the dataset.\n\nSoon, you'll try one-hot encoding. Before then, there's one additional topic we need to cover. Begin by running the next code cell without changes. ",
"_____no_output_____"
]
],
[
[
"# Get number of unique entries in each column with categorical data\nobject_nunique = list(map(lambda col: X_train[col].nunique(), object_cols))\nd = dict(zip(object_cols, object_nunique))\n\n# Print number of unique entries by column, in ascending order\nsorted(d.items(), key=lambda x: x[1])",
"_____no_output_____"
]
],
[
[
"# Step 3: Investigating cardinality\n\n### Part A\n\nThe output above shows, for each column with categorical data, the number of unique values in the column. For instance, the `'Street'` column in the training data has two unique values: `'Grvl'` and `'Pave'`, corresponding to a gravel road and a paved road, respectively.\n\nWe refer to the number of unique entries of a categorical variable as the **cardinality** of that categorical variable. For instance, the `'Street'` variable has cardinality 2.\n\nUse the output above to answer the questions below.",
"_____no_output_____"
]
],
[
[
"# Fill in the line below: How many categorical variables in the training data\n# have cardinality greater than 10?\nhigh_cardinality_numcols = 3\n\n# Fill in the line below: How many columns are needed to one-hot encode the \n# 'Neighborhood' variable in the training data?\nnum_cols_neighborhood = 25\n\n# Check your answers\nstep_3.a.check()",
"_____no_output_____"
],
[
"# Lines below will give you a hint or solution code\n#step_3.a.hint()\n#step_3.a.solution()",
"_____no_output_____"
]
],
[
[
"### Part B\n\nFor large datasets with many rows, one-hot encoding can greatly expand the size of the dataset. For this reason, we typically will only one-hot encode columns with relatively low cardinality. Then, high cardinality columns can either be dropped from the dataset, or we can use label encoding.\n\nAs an example, consider a dataset with 10,000 rows, and containing one categorical column with 100 unique entries. \n- If this column is replaced with the corresponding one-hot encoding, how many entries are added to the dataset? \n- If we instead replace the column with the label encoding, how many entries are added? \n\nUse your answers to fill in the lines below.",
"_____no_output_____"
]
],
[
[
"# Fill in the line below: How many entries are added to the dataset by \n# replacing the column with a one-hot encoding?\nOH_entries_added = 1e4*100 - 1e4\n\n# Fill in the line below: How many entries are added to the dataset by\n# replacing the column with a label encoding?\nlabel_entries_added = 0\n\n# Check your answers\nstep_3.b.check()",
"_____no_output_____"
],
[
"# Lines below will give you a hint or solution code\n#step_3.b.hint()\n#step_3.b.solution()",
"_____no_output_____"
]
],
[
[
"Next, you'll experiment with one-hot encoding. But, instead of encoding all of the categorical variables in the dataset, you'll only create a one-hot encoding for columns with cardinality less than 10.\n\nRun the code cell below without changes to set `low_cardinality_cols` to a Python list containing the columns that will be one-hot encoded. Likewise, `high_cardinality_cols` contains a list of categorical columns that will be dropped from the dataset.",
"_____no_output_____"
]
],
[
[
"# Columns that will be one-hot encoded\nlow_cardinality_cols = [col for col in object_cols if X_train[col].nunique() < 10]\n\n# Columns that will be dropped from the dataset\nhigh_cardinality_cols = list(set(object_cols)-set(low_cardinality_cols))\n\nprint('Categorical columns that will be one-hot encoded:', low_cardinality_cols)\nprint('\\nCategorical columns that will be dropped from the dataset:', high_cardinality_cols)",
"Categorical columns that will be one-hot encoded: ['MSZoning', 'Street', 'LotShape', 'LandContour', 'Utilities', 'LotConfig', 'LandSlope', 'Condition1', 'Condition2', 'BldgType', 'HouseStyle', 'RoofStyle', 'RoofMatl', 'ExterQual', 'ExterCond', 'Foundation', 'Heating', 'HeatingQC', 'CentralAir', 'KitchenQual', 'Functional', 'PavedDrive', 'SaleType', 'SaleCondition']\n\nCategorical columns that will be dropped from the dataset: ['Neighborhood', 'Exterior2nd', 'Exterior1st']\n"
]
],
[
[
"# Step 4: One-hot encoding\n\nUse the next code cell to one-hot encode the data in `X_train` and `X_valid`. Set the preprocessed DataFrames to `OH_X_train` and `OH_X_valid`, respectively. \n- The full list of categorical columns in the dataset can be found in the Python list `object_cols`.\n- You should only one-hot encode the categorical columns in `low_cardinality_cols`. All other categorical columns should be dropped from the dataset. ",
"_____no_output_____"
]
],
[
[
"from sklearn.preprocessing import OneHotEncoder\n\n# Use as many lines of code as you need!\nOH_encoder = OneHotEncoder(handle_unknown='ignore', sparse=False)\nOH_cols_train = pd.DataFrame(OH_encoder.fit_transform(X_train[low_cardinality_cols]))\nOH_cols_valid = pd.DataFrame(OH_encoder.transform(X_valid[low_cardinality_cols]))\n\n# One-hot encoding removed index; put it back\nOH_cols_train.index = X_train.index\nOH_cols_valid.index = X_valid.index\n\n# Remove categorical columns (will replace with one-hot encoding)\nnum_X_train = X_train.drop(object_cols, axis=1)\nnum_X_valid = X_valid.drop(object_cols, axis=1)\n\n# Add one-hot encoded columns to numerical features\nOH_X_train = pd.concat([num_X_train, OH_cols_train], axis=1)\nOH_X_valid = pd.concat([num_X_valid, OH_cols_valid], axis=1)\n\n# Check your answer\nstep_4.check()",
"_____no_output_____"
],
[
"# Lines below will give you a hint or solution code\n#step_4.hint()\n#step_4.solution()",
"_____no_output_____"
]
],
[
[
"Run the next code cell to get the MAE for this approach.",
"_____no_output_____"
]
],
[
[
"print(\"MAE from Approach 3 (One-Hot Encoding):\") \nprint(score_dataset(OH_X_train, OH_X_valid, y_train, y_valid))",
"_____no_output_____"
]
],
[
[
"# Generate test predictions and submit your results\n\nAfter you complete Step 4, if you'd like to use what you've learned to submit your results to the leaderboard, you'll need to preprocess the test data before generating predictions.\n\n**This step is completely optional, and you do not need to submit results to the leaderboard to successfully complete the exercise.**\n\nCheck out the previous exercise if you need help with remembering how to [join the competition](https://www.kaggle.com/c/home-data-for-ml-course) or save your results to CSV. Once you have generated a file with your results, follow the instructions below:\n1. Begin by clicking on the blue **Save Version** button in the top right corner of the window. This will generate a pop-up window. \n2. Ensure that the **Save and Run All** option is selected, and then click on the blue **Save** button.\n3. This generates a window in the bottom left corner of the notebook. After it has finished running, click on the number to the right of the **Save Version** button. This pulls up a list of versions on the right of the screen. Click on the ellipsis **(...)** to the right of the most recent version, and select **Open in Viewer**. This brings you into view mode of the same page. You will need to scroll down to get back to these instructions.\n4. Click on the **Output** tab on the right of the screen. Then, click on the file you would like to submit, and click on the blue **Submit** button to submit your results to the leaderboard.\n\nYou have now successfully submitted to the competition!\n\nIf you want to keep working to improve your performance, select the blue **Edit** button in the top right of the screen. Then you can change your code and repeat the process. There's a lot of room to improve, and you will climb up the leaderboard as you work.\n",
"_____no_output_____"
]
],
[
[
"# (Optional) Your code here",
"_____no_output_____"
]
],
[
[
"# Keep going\n\nWith missing value handling and categorical encoding, your modeling process is getting complex. This complexity gets worse when you want to save your model to use in the future. The key to managing this complexity is something called **pipelines**. \n\n**[Learn to use pipelines](https://www.kaggle.com/alexisbcook/pipelines)** to preprocess datasets with categorical variables, missing values and any other messiness your data throws at you.",
"_____no_output_____"
],
[
"---\n\n\n\n\n*Have questions or comments? Visit the [Learn Discussion forum](https://www.kaggle.com/learn-forum/161289) to chat with other Learners.*",
"_____no_output_____"
]
]
] | [
"markdown",
"code",
"markdown",
"code",
"markdown",
"code",
"markdown",
"code",
"markdown",
"code",
"markdown",
"code",
"markdown",
"code",
"markdown",
"code",
"markdown",
"code",
"markdown",
"code",
"markdown",
"code",
"markdown",
"code",
"markdown",
"code",
"markdown",
"code",
"markdown",
"code",
"markdown",
"code",
"markdown",
"code",
"markdown",
"code",
"markdown"
] | [
[
"markdown",
"markdown"
],
[
"code"
],
[
"markdown"
],
[
"code"
],
[
"markdown"
],
[
"code"
],
[
"markdown"
],
[
"code"
],
[
"markdown"
],
[
"code",
"code"
],
[
"markdown"
],
[
"code"
],
[
"markdown"
],
[
"code"
],
[
"markdown"
],
[
"code",
"code"
],
[
"markdown"
],
[
"code"
],
[
"markdown"
],
[
"code",
"code"
],
[
"markdown"
],
[
"code"
],
[
"markdown"
],
[
"code"
],
[
"markdown"
],
[
"code",
"code"
],
[
"markdown"
],
[
"code",
"code"
],
[
"markdown"
],
[
"code"
],
[
"markdown"
],
[
"code",
"code"
],
[
"markdown"
],
[
"code"
],
[
"markdown"
],
[
"code"
],
[
"markdown",
"markdown"
]
] |
d046f7a28f5fd90a6ed820ccc5db15a03d44e7df | 13,875 | ipynb | Jupyter Notebook | site/en/r2/guide/_tpu.ipynb | christophmeyer/docs | f0b5959f682adce50ace43b190140188134e33d5 | [
"Apache-2.0"
] | 1 | 2019-08-22T13:15:19.000Z | 2019-08-22T13:15:19.000Z | site/en/r2/guide/_tpu.ipynb | christophmeyer/docs | f0b5959f682adce50ace43b190140188134e33d5 | [
"Apache-2.0"
] | null | null | null | site/en/r2/guide/_tpu.ipynb | christophmeyer/docs | f0b5959f682adce50ace43b190140188134e33d5 | [
"Apache-2.0"
] | 1 | 2020-10-31T13:13:43.000Z | 2020-10-31T13:13:43.000Z | 36.803714 | 465 | 0.538811 | [
[
[
"##### Copyright 2018 The TensorFlow Authors.\n\nLicensed under the Apache License, Version 2.0 (the \"License\");",
"_____no_output_____"
]
],
[
[
"#@title Licensed under the Apache License, Version 2.0 (the \"License\"); { display-mode: \"form\" }\n# you may not use this file except in compliance with the License.\n# You may obtain a copy of the License at\n#\n# https://www.apache.org/licenses/LICENSE-2.0\n#\n# Unless required by applicable law or agreed to in writing, software\n# distributed under the License is distributed on an \"AS IS\" BASIS,\n# WITHOUT WARRANTIES OR CONDITIONS OF ANY KIND, either express or implied.\n# See the License for the specific language governing permissions and\n# limitations under the License.",
"_____no_output_____"
]
],
[
[
"<table class=\"tfo-notebook-buttons\" align=\"left\">\n <td>\n <a target=\"_blank\" href=\"https://www.tensorflow.org/beta/{PATH}\">\n <img src=\"https://www.tensorflow.org/images/tf_logo_32px.png\" />\n View on TensorFlow.org</a>\n </td>\n <td>\n <a target=\"_blank\" href=\"https://colab.research.google.com/github/tensorflow/docs/blob/master/site/{PATH}.ipynb\">\n <img src=\"https://www.tensorflow.org/images/colab_logo_32px.png\" />\n Run in Google Colab</a>\n </td>\n <td>\n <a target=\"_blank\" href=\"https://github.com/tensorflow/docs/blob/master/site/{PATH}.ipynb\">\n <img src=\"https://www.tensorflow.org/images/GitHub-Mark-32px.png\" />\n View source on GitHub</a>\n </td>\n</table>",
"_____no_output_____"
],
[
"# Using TPUs\n\nTensor Processing Units (TPUs) are Google's specialized ASICs designed to dramatically accelerate machine learning workloads. They are available on Google Colab, the TensorFlow Research Cloud and Google Compute Engine. \n\nIn this notebook, you can try training a convolutional neural network against the Fashion MNIST dataset on Cloud TPUs using tf.keras and Distribution Strategy.\n",
"_____no_output_____"
],
[
"## Learning Objectives\n\nIn this Colab, you will learn how to:\n\n* Write a standard 4-layer conv-net with drop-out and batch normalization in Keras.\n* Use TPUs and Distribution Strategy to train the model.\n* Run a prediction to see how well the model can predict fashion categories and output the result.",
"_____no_output_____"
],
[
"## Instructions\n\nTo use TPUs in Colab:\n\n1. On the main menu, click Runtime and select **Change runtime type**. Set \"TPU\" as the hardware accelerator.\n1. Click Runtime again and select **Runtime > Run All**. You can also run the cells manually with Shift-ENTER. ",
"_____no_output_____"
],
[
"## Data, Model, and Training\n\n### Download the Data\n\nBegin by downloading the fashion MNIST dataset using `tf.keras.datasets`, as shown below. We will also need to convert the data to `float32` format, as the data types supported by TPUs are limited right now.\n\nTPUs currently do not support Eager Execution, so we disable that with `disable_eager_execution()`.",
"_____no_output_____"
]
],
[
[
"from __future__ import absolute_import, division, print_function, unicode_literals\nimport numpy as np",
"_____no_output_____"
],
[
"from __future__ import absolute_import, division, print_function\n\n!pip install tensorflow-gpu==2.0.0-beta1\nimport tensorflow as tf\ntf.compat.v1.disable_eager_execution()\n\nimport numpy as np\n\n(x_train, y_train), (x_test, y_test) = tf.keras.datasets.fashion_mnist.load_data()\n\n# add empty color dimension\nx_train = np.expand_dims(x_train, -1)\nx_test = np.expand_dims(x_test, -1)\n\n# convert types to float32\nx_train = x_train.astype(np.float32)\nx_test = x_test.astype(np.float32)\ny_train = y_train.astype(np.float32)\ny_test = y_test.astype(np.float32)",
"_____no_output_____"
]
],
[
[
"### Initialize TPUStrategy\n\nWe first initialize the TPUStrategy object before creating the model, so that Keras knows that we are creating a model for TPUs. \n\nTo do this, we are first creating a TPUClusterResolver using the IP address of the TPU, and then creating a TPUStrategy object from the Cluster Resolver.",
"_____no_output_____"
]
],
[
[
"import os\n\nresolver = tf.distribute.cluster_resolver.TPUClusterResolver()\ntf.tpu.experimental.initialize_tpu_system(resolver)\nstrategy = tf.distribute.experimental.TPUStrategy(resolver)",
"_____no_output_____"
]
],
[
[
"### Define the Model\n\nThe following example uses a standard conv-net that has 4 layers with drop-out and batch normalization between each layer. Note that we are creating the model within a `strategy.scope`.\n",
"_____no_output_____"
]
],
[
[
"with strategy.scope():\n model = tf.keras.models.Sequential()\n model.add(tf.keras.layers.BatchNormalization(input_shape=x_train.shape[1:]))\n model.add(tf.keras.layers.Conv2D(64, (5, 5), padding='same', activation='elu'))\n model.add(tf.keras.layers.MaxPooling2D(pool_size=(2, 2), strides=(2,2)))\n model.add(tf.keras.layers.Dropout(0.25))\n\n model.add(tf.keras.layers.BatchNormalization())\n model.add(tf.keras.layers.Conv2D(128, (5, 5), padding='same', activation='elu'))\n model.add(tf.keras.layers.MaxPooling2D(pool_size=(2, 2)))\n model.add(tf.keras.layers.Dropout(0.25))\n\n model.add(tf.keras.layers.BatchNormalization())\n model.add(tf.keras.layers.Conv2D(256, (5, 5), padding='same', activation='elu'))\n model.add(tf.keras.layers.MaxPooling2D(pool_size=(2, 2), strides=(2,2)))\n model.add(tf.keras.layers.Dropout(0.25))\n\n model.add(tf.keras.layers.BatchNormalization())\n model.add(tf.keras.layers.Conv2D(512, (5, 5), padding='same', activation='elu'))\n model.add(tf.keras.layers.MaxPooling2D(pool_size=(2, 2), strides=(2,2)))\n model.add(tf.keras.layers.Dropout(0.25))\n\n model.add(tf.keras.layers.Flatten())\n model.add(tf.keras.layers.Dense(256))\n model.add(tf.keras.layers.Activation('elu'))\n model.add(tf.keras.layers.Dropout(0.5))\n model.add(tf.keras.layers.Dense(10))\n model.add(tf.keras.layers.Activation('softmax'))\n model.summary()",
"_____no_output_____"
]
],
[
[
"### Train on the TPU\n\nTo train on the TPU, we can simply call `model.compile` under the strategy scope, and then call `model.fit` to start training. In this case, we are training for 5 epochs with 60 steps per epoch, and running evaluation at the end of 5 epochs.\n\nIt may take a while for the training to start, as the data and model has to be transferred to the TPU and compiled before training can start.",
"_____no_output_____"
]
],
[
[
"with strategy.scope():\n model.compile(\n optimizer=tf.train.AdamOptimizer(learning_rate=1e-3),\n loss=tf.keras.losses.sparse_categorical_crossentropy,\n metrics=['sparse_categorical_accuracy']\n )\n\nmodel.fit(\n (x_train, y_train),\n epochs=5,\n steps_per_epoch=60,\n validation_data=(x_test, y_test),\n validation_freq=5,\n)",
"_____no_output_____"
]
],
[
[
"### Check our results with Inference\n\nNow that we are done training, we can see how well the model can predict fashion categories:",
"_____no_output_____"
]
],
[
[
"LABEL_NAMES = ['t_shirt', 'trouser', 'pullover', 'dress', 'coat', 'sandal', 'shirt', 'sneaker', 'bag', 'ankle_boots']\n\nfrom matplotlib import pyplot\n%matplotlib inline\n\ndef plot_predictions(images, predictions):\n n = images.shape[0]\n nc = int(np.ceil(n / 4))\n f, axes = pyplot.subplots(nc, 4)\n for i in range(nc * 4):\n y = i // 4\n x = i % 4\n axes[x, y].axis('off')\n \n label = LABEL_NAMES[np.argmax(predictions[i])]\n confidence = np.max(predictions[i])\n if i > n:\n continue\n axes[x, y].imshow(images[i])\n axes[x, y].text(0.5, -1.5, label + ': %.3f' % confidence, fontsize=12)\n\n pyplot.gcf().set_size_inches(8, 8) \n\nplot_predictions(np.squeeze(x_test[:16]), \n model.predict(x_test[:16]))",
"_____no_output_____"
]
],
[
[
"### What's next\n\n* Learn about [Cloud TPUs](https://cloud.google.com/tpu/docs) that Google designed and optimized specifically to speed up and scale up ML workloads for training and inference and to enable ML engineers and researchers to iterate more quickly.\n* Explore the range of [Cloud TPU tutorials and Colabs](https://cloud.google.com/tpu/docs/tutorials) to find other examples that can be used when implementing your ML project.\n\nOn Google Cloud Platform, in addition to GPUs and TPUs available on pre-configured [deep learning VMs](https://cloud.google.com/deep-learning-vm/), you will find [AutoML](https://cloud.google.com/automl/)*(beta)* for training custom models without writing code and [Cloud ML Engine](https://cloud.google.com/ml-engine/docs/) which will allows you to run parallel trainings and hyperparameter tuning of your custom models on powerful distributed hardware.",
"_____no_output_____"
]
]
] | [
"markdown",
"code",
"markdown",
"code",
"markdown",
"code",
"markdown",
"code",
"markdown",
"code",
"markdown",
"code",
"markdown"
] | [
[
"markdown"
],
[
"code"
],
[
"markdown",
"markdown",
"markdown",
"markdown",
"markdown"
],
[
"code",
"code"
],
[
"markdown"
],
[
"code"
],
[
"markdown"
],
[
"code"
],
[
"markdown"
],
[
"code"
],
[
"markdown"
],
[
"code"
],
[
"markdown"
]
] |
d046fc7ae264d23b73881ae5f1236328fbad494c | 322,259 | ipynb | Jupyter Notebook | notebooks/thesis_experiments/20200924_eMVFTS_Wind_Energy_Raw.ipynb | cseveriano/spatio-temporal-forecasting | 8391f3de72b840edb2b35148537502ec5d2ac888 | [
"MIT"
] | 5 | 2018-05-25T17:43:52.000Z | 2021-12-22T13:13:43.000Z | notebooks/thesis_experiments/20200924_eMVFTS_Wind_Energy_Raw.ipynb | cseveriano/spatio-temporal-forecasting | 8391f3de72b840edb2b35148537502ec5d2ac888 | [
"MIT"
] | null | null | null | notebooks/thesis_experiments/20200924_eMVFTS_Wind_Energy_Raw.ipynb | cseveriano/spatio-temporal-forecasting | 8391f3de72b840edb2b35148537502ec5d2ac888 | [
"MIT"
] | 2 | 2021-07-20T12:32:00.000Z | 2021-12-13T05:32:21.000Z | 70.950903 | 98,282 | 0.638303 | [
[
[
"<a href=\"https://colab.research.google.com/github/cseveriano/spatio-temporal-forecasting/blob/master/notebooks/thesis_experiments/20200924_eMVFTS_Wind_Energy_Raw.ipynb\" target=\"_parent\"><img src=\"https://colab.research.google.com/assets/colab-badge.svg\" alt=\"Open In Colab\"/></a>",
"_____no_output_____"
],
[
"## Forecasting experiments for GEFCOM 2012 Wind Dataset",
"_____no_output_____"
],
[
"\n## Install Libs\n",
"_____no_output_____"
]
],
[
[
"!pip3 install -U git+https://github.com/PYFTS/pyFTS\n!pip3 install -U git+https://github.com/cseveriano/spatio-temporal-forecasting\n!pip3 install -U git+https://github.com/cseveriano/evolving_clustering\n!pip3 install -U git+https://github.com/cseveriano/fts2image\n!pip3 install -U hyperopt\n!pip3 install -U pyts",
"Collecting git+https://github.com/PYFTS/pyFTS\n Cloning https://github.com/PYFTS/pyFTS to /tmp/pip-req-build-q6mtqzlz\n Running command git clone -q https://github.com/PYFTS/pyFTS /tmp/pip-req-build-q6mtqzlz\nBuilding wheels for collected packages: pyFTS\n Building wheel for pyFTS (setup.py) ... \u001b[?25l\u001b[?25hdone\n Created wheel for pyFTS: filename=pyFTS-1.6-cp36-none-any.whl size=207416 sha256=9c1df08abea59c8449f05671299dfcd6a888ea4ec4926f4cb375e86a08251613\n Stored in directory: /tmp/pip-ephem-wheel-cache-2te7wbk9/wheels/e7/32/a9/230470113df5a73242a5a6d05671cb646db97abf14bbce2644\nSuccessfully built pyFTS\nInstalling collected packages: pyFTS\nSuccessfully installed pyFTS-1.6\nCollecting git+https://github.com/cseveriano/spatio-temporal-forecasting\n Cloning https://github.com/cseveriano/spatio-temporal-forecasting to /tmp/pip-req-build-7u637wx0\n Running command git clone -q https://github.com/cseveriano/spatio-temporal-forecasting /tmp/pip-req-build-7u637wx0\nBuilding wheels for collected packages: spatio-temporal-forecasting\n Building wheel for spatio-temporal-forecasting (setup.py) ... \u001b[?25l\u001b[?25hdone\n Created wheel for spatio-temporal-forecasting: filename=spatio_temporal_forecasting-1.0-cp36-none-any.whl size=55633 sha256=4e40efc1c38541efa9b7168b24d990baca71acbc1c5d5858425fb0354dd58403\n Stored in directory: /tmp/pip-ephem-wheel-cache-mzfi7veh/wheels/d2/1f/6f/439795864246039ef36c6a3c88edf7935c803c2cf97133066a\nSuccessfully built spatio-temporal-forecasting\nInstalling collected packages: spatio-temporal-forecasting\nSuccessfully installed spatio-temporal-forecasting-1.0\nCollecting git+https://github.com/cseveriano/evolving_clustering\n Cloning https://github.com/cseveriano/evolving_clustering to /tmp/pip-req-build-_fyfrguj\n Running command git clone -q https://github.com/cseveriano/evolving_clustering /tmp/pip-req-build-_fyfrguj\nBuilding wheels for collected packages: evolclustering\n Building wheel for evolclustering (setup.py) ... \u001b[?25l\u001b[?25hdone\n Created wheel for evolclustering: filename=evolclustering-0.1-cp36-none-any.whl size=25744 sha256=f5bb287535544a3476690f61225544c738fe73987cecd7732f3220970544224e\n Stored in directory: /tmp/pip-ephem-wheel-cache-w3m1u0lx/wheels/aa/b7/f1/4d93077e1b97361934f5992c77be80f0769eba6e0e9da6e22d\nSuccessfully built evolclustering\nInstalling collected packages: evolclustering\nSuccessfully installed evolclustering-0.1\nCollecting git+https://github.com/cseveriano/fts2image\n Cloning https://github.com/cseveriano/fts2image to /tmp/pip-req-build-tzt5k5x3\n Running command git clone -q https://github.com/cseveriano/fts2image /tmp/pip-req-build-tzt5k5x3\nBuilding wheels for collected packages: fts2image\n Building wheel for fts2image (setup.py) ... \u001b[?25l\u001b[?25hdone\n Created wheel for fts2image: filename=fts2image-0.1.0-py2.py3-none-any.whl size=7975 sha256=037dd37f1af0118db94a88ea46340bcf2840c6cf7c0fae8dfce67eb554a703c0\n Stored in directory: /tmp/pip-ephem-wheel-cache-7ygvs3r0/wheels/22/a1/62/57410665915134ffe4bc11ede1f9a47b1f4b29d8aad9582d31\nSuccessfully built fts2image\nInstalling collected packages: fts2image\nSuccessfully installed fts2image-0.1.0\nCollecting hyperopt\n\u001b[?25l Downloading https://files.pythonhosted.org/packages/90/d5/c7e276f4f7bc65ac26391c435245e5ef8911b4393e3df5a74906c48afeaf/hyperopt-0.2.4-py2.py3-none-any.whl (964kB)\n\u001b[K |████████████████████████████████| 972kB 3.4MB/s \n\u001b[?25hRequirement already satisfied, skipping upgrade: future in /usr/local/lib/python3.6/dist-packages (from hyperopt) (0.16.0)\nRequirement already satisfied, skipping upgrade: networkx>=2.2 in /usr/local/lib/python3.6/dist-packages (from hyperopt) (2.5)\nRequirement already satisfied, skipping upgrade: cloudpickle in /usr/local/lib/python3.6/dist-packages (from hyperopt) (1.3.0)\nRequirement already satisfied, skipping upgrade: six in /usr/local/lib/python3.6/dist-packages (from hyperopt) (1.15.0)\nRequirement already satisfied, skipping upgrade: scipy in /usr/local/lib/python3.6/dist-packages (from hyperopt) (1.4.1)\nRequirement already satisfied, skipping upgrade: numpy in /usr/local/lib/python3.6/dist-packages (from hyperopt) (1.18.5)\nRequirement already satisfied, skipping upgrade: tqdm in /usr/local/lib/python3.6/dist-packages (from hyperopt) (4.41.1)\nRequirement already satisfied, skipping upgrade: decorator>=4.3.0 in /usr/local/lib/python3.6/dist-packages (from networkx>=2.2->hyperopt) (4.4.2)\nInstalling collected packages: hyperopt\n Found existing installation: hyperopt 0.1.2\n Uninstalling hyperopt-0.1.2:\n Successfully uninstalled hyperopt-0.1.2\nSuccessfully installed hyperopt-0.2.4\nCollecting pyts\n\u001b[?25l Downloading https://files.pythonhosted.org/packages/b6/2b/1a62c0d32b40ee85daa8f6a6160828537b3d846c9fe93253b38846c6ec1f/pyts-0.11.0-py3-none-any.whl (2.5MB)\n\u001b[K |████████████████████████████████| 2.5MB 3.3MB/s \n\u001b[?25hRequirement already satisfied, skipping upgrade: numpy>=1.17.5 in /usr/local/lib/python3.6/dist-packages (from pyts) (1.18.5)\nRequirement already satisfied, skipping upgrade: scikit-learn>=0.22.1 in /usr/local/lib/python3.6/dist-packages (from pyts) (0.22.2.post1)\nRequirement already satisfied, skipping upgrade: joblib>=0.12 in /usr/local/lib/python3.6/dist-packages (from pyts) (0.16.0)\nRequirement already satisfied, skipping upgrade: numba>=0.48.0 in /usr/local/lib/python3.6/dist-packages (from pyts) (0.48.0)\nRequirement already satisfied, skipping upgrade: scipy>=1.3.0 in /usr/local/lib/python3.6/dist-packages (from pyts) (1.4.1)\nRequirement already satisfied, skipping upgrade: llvmlite<0.32.0,>=0.31.0dev0 in /usr/local/lib/python3.6/dist-packages (from numba>=0.48.0->pyts) (0.31.0)\nRequirement already satisfied, skipping upgrade: setuptools in /usr/local/lib/python3.6/dist-packages (from numba>=0.48.0->pyts) (50.3.0)\nInstalling collected packages: pyts\nSuccessfully installed pyts-0.11.0\n"
],
[
"import pandas as pd\nimport numpy as np\nfrom hyperopt import hp\nfrom spatiotemporal.util import parameter_tuning, sampling\nfrom spatiotemporal.util import experiments as ex\nfrom sklearn.metrics import mean_squared_error\nfrom google.colab import files\nimport matplotlib.pyplot as plt\nimport pickle\nimport math\nfrom pyFTS.benchmarks import Measures\nfrom pyts.decomposition import SingularSpectrumAnalysis\nfrom google.colab import files\nimport warnings \nwarnings.filterwarnings(\"ignore\", category=DeprecationWarning)\nimport datetime",
"_____no_output_____"
]
],
[
[
"## Aux Functions",
"_____no_output_____"
]
],
[
[
"def normalize(df):\n mindf = df.min()\n maxdf = df.max()\n return (df-mindf)/(maxdf-mindf)\n\ndef denormalize(norm, _min, _max):\n return [(n * (_max-_min)) + _min for n in norm]\n\n\ndef getRollingWindow(index):\n pivot = index\n train_start = pivot.strftime('%Y-%m-%d')\n pivot = pivot + datetime.timedelta(days=20)\n train_end = pivot.strftime('%Y-%m-%d')\n\n pivot = pivot + datetime.timedelta(days=1)\n test_start = pivot.strftime('%Y-%m-%d')\n pivot = pivot + datetime.timedelta(days=6)\n test_end = pivot.strftime('%Y-%m-%d')\n \n return train_start, train_end, test_start, test_end\n\ndef calculate_rolling_error(cv_name, df, forecasts, order_list):\n cv_results = pd.DataFrame(columns=['Split', 'RMSE', 'SMAPE'])\n\n limit = df.index[-1].strftime('%Y-%m-%d')\n\n test_end = \"\"\n index = df.index[0]\n\n for i in np.arange(len(forecasts)):\n\n train_start, train_end, test_start, test_end = getRollingWindow(index)\n test = df[test_start : test_end]\n\n yhat = forecasts[i]\n\n order = order_list[i]\n rmse = Measures.rmse(test.iloc[order:], yhat[:-1])\n \n smape = Measures.smape(test.iloc[order:], yhat[:-1])\n \n res = {'Split' : index.strftime('%Y-%m-%d') ,'RMSE' : rmse, 'SMAPE' : smape}\n cv_results = cv_results.append(res, ignore_index=True)\n cv_results.to_csv(cv_name+\".csv\") \n\n index = index + datetime.timedelta(days=7)\n \n return cv_results\n\ndef get_final_forecast(norm_forecasts):\n \n forecasts_final = []\n \n for i in np.arange(len(norm_forecasts)):\n f_raw = denormalize(norm_forecasts[i], min_raw, max_raw)\n\n forecasts_final.append(f_raw)\n \n return forecasts_final",
"_____no_output_____"
],
[
"from spatiotemporal.test import methods_space_oahu as ms\nfrom spatiotemporal.util import parameter_tuning, sampling\nfrom spatiotemporal.util import experiments as ex\nfrom sklearn.metrics import mean_squared_error\nimport numpy as np\nfrom hyperopt import fmin, tpe, hp, STATUS_OK, Trials\nfrom hyperopt import space_eval\nimport traceback\nfrom . import sampling\nimport pickle\n\ndef calculate_error(loss_function, test_df, forecast, offset):\n error = loss_function(test_df.iloc[(offset):], forecast)\n print(\"Error : \"+str(error))\n return error\n\ndef method_optimize(experiment, forecast_method, train_df, test_df, space, loss_function, max_evals):\n def objective(params):\n print(params)\n try:\n _output = list(params['output'])\n forecast = forecast_method(train_df, test_df, params)\n _step = params.get('step', 1)\n offset = params['order'] + _step - 1\n error = calculate_error(loss_function, test_df[_output], forecast, offset)\n except Exception:\n traceback.print_exc()\n error = 1000\n return {'loss': error, 'status': STATUS_OK}\n\n print(\"Running experiment: \" + experiment)\n trials = Trials()\n best = fmin(objective, space, algo=tpe.suggest, max_evals=max_evals, trials=trials)\n print('best parameters: ')\n print(space_eval(space, best))\n\n pickle.dump(best, open(\"best_\" + experiment + \".pkl\", \"wb\"))\n pickle.dump(trials, open(\"trials_\" + experiment + \".pkl\", \"wb\"))\n\n\ndef run_search(methods, data, train, loss_function, max_evals=100, resample=None):\n\n if resample:\n data = sampling.resample_data(data, resample)\n\n train_df, test_df = sampling.train_test_split(data, train)\n\n for experiment, method, space in methods:\n method_optimize(experiment, method, train_df, test_df, space, loss_function, max_evals)",
"_____no_output_____"
]
],
[
[
"## Load Dataset",
"_____no_output_____"
]
],
[
[
"import pandas as pd\nimport matplotlib.pyplot as plt\nimport numpy as np\nimport math\nfrom sklearn.metrics import mean_squared_error",
"_____no_output_____"
],
[
"#columns names\nwind_farms = ['wp1', 'wp2', 'wp3', 'wp4', 'wp5', 'wp6', 'wp7']\n\n# read raw dataset\nimport pandas as pd\ndf = pd.read_csv('https://query.data.world/s/3zx2jusk4z6zvlg2dafqgshqp3oao6', parse_dates=['date'], index_col=0)\ndf.index = pd.to_datetime(df.index, format=\"%Y%m%d%H\")\n\ninterval = ((df.index >= '2009-07') & (df.index <= '2010-08'))\ndf = df.loc[interval]\n\n\n#Normalize Data\n\n# Save Min-Max for Denorm\nmin_raw = df.min()\nmax_raw = df.max()\n\n# Perform Normalization\nnorm_df = normalize(df)\n\n# Tuning split\ntuning_df = norm_df[\"2009-07-01\":\"2009-07-31\"]\n\nnorm_df = norm_df[\"2009-08-01\":\"2010-08-30\"]\ndf = df[\"2009-08-01\":\"2010-08-30\"]",
"_____no_output_____"
]
],
[
[
"## Forecasting Methods",
"_____no_output_____"
],
[
"### Persistence",
"_____no_output_____"
]
],
[
[
"def persistence_forecast(train, test, step):\n predictions = []\n \n for t in np.arange(0,len(test), step):\n yhat = [test.iloc[t]] * step\n predictions.extend(yhat)\n \n return predictions\n\ndef rolling_cv_persistence(df, step):\n\n forecasts = []\n lags_list = []\n\n limit = df.index[-1].strftime('%Y-%m-%d')\n\n test_end = \"\"\n index = df.index[0]\n\n while test_end < limit :\n print(\"Index: \", index.strftime('%Y-%m-%d')) \n\n train_start, train_end, test_start, test_end = getRollingWindow(index)\n index = index + datetime.timedelta(days=7)\n \n train = df[train_start : train_end]\n test = df[test_start : test_end]\n \n yhat = persistence_forecast(train, test, step) \n \n lags_list.append(1)\n forecasts.append(yhat)\n\n return forecasts, lags_list",
"_____no_output_____"
],
[
"forecasts_raw, order_list = rolling_cv_persistence(norm_df, 1)\nforecasts_final = get_final_forecast(forecasts_raw)\n\ncalculate_rolling_error(\"rolling_cv_wind_raw_persistence\", norm_df, forecasts_final, order_list)",
"Index: 2009-08-01\nIndex: 2009-08-08\nIndex: 2009-08-15\nIndex: 2009-08-22\nIndex: 2009-08-29\nIndex: 2009-09-05\nIndex: 2009-09-12\nIndex: 2009-09-19\nIndex: 2009-09-26\nIndex: 2009-10-03\nIndex: 2009-10-10\nIndex: 2009-10-17\nIndex: 2009-10-24\nIndex: 2009-10-31\nIndex: 2009-11-07\nIndex: 2009-11-14\nIndex: 2009-11-21\nIndex: 2009-11-28\nIndex: 2009-12-05\nIndex: 2009-12-12\nIndex: 2009-12-19\nIndex: 2009-12-26\nIndex: 2010-01-02\nIndex: 2010-01-09\nIndex: 2010-01-16\nIndex: 2010-01-23\nIndex: 2010-01-30\nIndex: 2010-02-06\nIndex: 2010-02-13\nIndex: 2010-02-20\nIndex: 2010-02-27\nIndex: 2010-03-06\nIndex: 2010-03-13\nIndex: 2010-03-20\nIndex: 2010-03-27\nIndex: 2010-04-03\nIndex: 2010-04-10\nIndex: 2010-04-17\nIndex: 2010-04-24\nIndex: 2010-05-01\nIndex: 2010-05-08\nIndex: 2010-05-15\nIndex: 2010-05-22\nIndex: 2010-05-29\nIndex: 2010-06-05\nIndex: 2010-06-12\nIndex: 2010-06-19\nIndex: 2010-06-26\nIndex: 2010-07-03\nIndex: 2010-07-10\n"
],
[
"files.download('rolling_cv_wind_raw_persistence.csv')",
"_____no_output_____"
]
],
[
[
"### VAR",
"_____no_output_____"
]
],
[
[
"from statsmodels.tsa.api import VAR, DynamicVAR",
"_____no_output_____"
],
[
"def evaluate_VAR_models(test_name, train, validation,target, maxlags_list):\n var_results = pd.DataFrame(columns=['Order','RMSE'])\n best_score, best_cfg, best_model = float(\"inf\"), None, None\n \n for lgs in maxlags_list:\n model = VAR(train)\n results = model.fit(maxlags=lgs, ic='aic')\n \n order = results.k_ar\n forecast = []\n\n for i in range(len(validation)-order) :\n forecast.extend(results.forecast(validation.values[i:i+order],1))\n\n forecast_df = pd.DataFrame(columns=validation.columns, data=forecast)\n rmse = Measures.rmse(validation[target].iloc[order:], forecast_df[target].values)\n\n if rmse < best_score:\n best_score, best_cfg, best_model = rmse, order, results\n\n res = {'Order' : str(order) ,'RMSE' : rmse}\n print('VAR (%s) RMSE=%.3f' % (str(order),rmse))\n var_results = var_results.append(res, ignore_index=True)\n var_results.to_csv(test_name+\".csv\")\n \n print('Best VAR(%s) RMSE=%.3f' % (best_cfg, best_score))\n return best_model",
"_____no_output_____"
],
[
"def var_forecast(train, test, params):\n order = params['order']\n step = params['step']\n\n model = VAR(train.values)\n results = model.fit(maxlags=order)\n lag_order = results.k_ar\n print(\"Lag order:\" + str(lag_order))\n forecast = []\n\n for i in np.arange(0,len(test)-lag_order+1,step) :\n forecast.extend(results.forecast(test.values[i:i+lag_order],step))\n\n forecast_df = pd.DataFrame(columns=test.columns, data=forecast)\n return forecast_df.values, lag_order",
"_____no_output_____"
],
[
"def rolling_cv_var(df, params):\n forecasts = []\n order_list = []\n\n limit = df.index[-1].strftime('%Y-%m-%d')\n\n test_end = \"\"\n index = df.index[0]\n\n while test_end < limit :\n print(\"Index: \", index.strftime('%Y-%m-%d')) \n\n train_start, train_end, test_start, test_end = getRollingWindow(index)\n index = index + datetime.timedelta(days=7)\n \n train = df[train_start : train_end]\n test = df[test_start : test_end]\n \n # Concat train & validation for test\n yhat, lag_order = var_forecast(train, test, params)\n \n forecasts.append(yhat)\n order_list.append(lag_order)\n\n return forecasts, order_list",
"_____no_output_____"
],
[
"params_raw = {'order': 4, 'step': 1}\n\nforecasts_raw, order_list = rolling_cv_var(norm_df, params_raw)\n\nforecasts_final = get_final_forecast(forecasts_raw)\ncalculate_rolling_error(\"rolling_cv_wind_raw_var\", df, forecasts_final, order_list)",
"Index: 2009-08-01\nLag order:4\nIndex: 2009-08-08\nLag order:4\nIndex: 2009-08-15\nLag order:4\nIndex: 2009-08-22\nLag order:4\nIndex: 2009-08-29\nLag order:4\nIndex: 2009-09-05\nLag order:4\nIndex: 2009-09-12\nLag order:4\nIndex: 2009-09-19\nLag order:4\nIndex: 2009-09-26\nLag order:4\nIndex: 2009-10-03\nLag order:4\nIndex: 2009-10-10\nLag order:4\nIndex: 2009-10-17\nLag order:4\nIndex: 2009-10-24\nLag order:4\nIndex: 2009-10-31\nLag order:4\nIndex: 2009-11-07\nLag order:4\nIndex: 2009-11-14\nLag order:4\nIndex: 2009-11-21\nLag order:4\nIndex: 2009-11-28\nLag order:4\nIndex: 2009-12-05\nLag order:4\nIndex: 2009-12-12\nLag order:4\nIndex: 2009-12-19\nLag order:4\nIndex: 2009-12-26\nLag order:4\nIndex: 2010-01-02\nLag order:4\nIndex: 2010-01-09\nLag order:4\nIndex: 2010-01-16\nLag order:4\nIndex: 2010-01-23\nLag order:4\nIndex: 2010-01-30\nLag order:4\nIndex: 2010-02-06\nLag order:4\nIndex: 2010-02-13\nLag order:4\nIndex: 2010-02-20\nLag order:4\nIndex: 2010-02-27\nLag order:4\nIndex: 2010-03-06\nLag order:4\nIndex: 2010-03-13\nLag order:4\nIndex: 2010-03-20\nLag order:4\nIndex: 2010-03-27\nLag order:4\nIndex: 2010-04-03\nLag order:4\nIndex: 2010-04-10\nLag order:4\nIndex: 2010-04-17\nLag order:4\nIndex: 2010-04-24\nLag order:4\nIndex: 2010-05-01\nLag order:4\nIndex: 2010-05-08\nLag order:4\nIndex: 2010-05-15\nLag order:4\nIndex: 2010-05-22\nLag order:4\nIndex: 2010-05-29\nLag order:4\nIndex: 2010-06-05\nLag order:4\nIndex: 2010-06-12\nLag order:4\nIndex: 2010-06-19\nLag order:4\nIndex: 2010-06-26\nLag order:4\nIndex: 2010-07-03\nLag order:4\nIndex: 2010-07-10\nLag order:4\n"
],
[
"files.download('rolling_cv_wind_raw_var.csv')",
"_____no_output_____"
]
],
[
[
"### e-MVFTS",
"_____no_output_____"
]
],
[
[
"from spatiotemporal.models.clusteredmvfts.fts import evolvingclusterfts",
"_____no_output_____"
],
[
"def evolvingfts_forecast(train_df, test_df, params, train_model=True):\n\n _variance_limit = params['variance_limit']\n _defuzzy = params['defuzzy']\n _t_norm = params['t_norm']\n _membership_threshold = params['membership_threshold']\n _order = params['order']\n _step = params['step']\n\n\n model = evolvingclusterfts.EvolvingClusterFTS(variance_limit=_variance_limit, defuzzy=_defuzzy, t_norm=_t_norm,\n membership_threshold=_membership_threshold)\n\n model.fit(train_df.values, order=_order, verbose=False)\n\n forecast = model.predict(test_df.values, steps_ahead=_step)\n\n forecast_df = pd.DataFrame(data=forecast, columns=test_df.columns)\n return forecast_df.values",
"_____no_output_____"
],
[
"def rolling_cv_evolving(df, params):\n forecasts = []\n order_list = []\n\n limit = df.index[-1].strftime('%Y-%m-%d')\n\n test_end = \"\"\n index = df.index[0]\n\n first_time = True\n\n while test_end < limit :\n print(\"Index: \", index.strftime('%Y-%m-%d')) \n\n train_start, train_end, test_start, test_end = getRollingWindow(index)\n index = index + datetime.timedelta(days=7)\n \n train = df[train_start : train_end]\n test = df[test_start : test_end]\n \n # Concat train & validation for test\n yhat = list(evolvingfts_forecast(train, test, params, train_model=first_time))\n #yhat.append(yhat[-1]) #para manter o formato do vetor de metricas\n forecasts.append(yhat)\n order_list.append(params['order'])\n\n first_time = False\n\n return forecasts, order_list",
"_____no_output_____"
],
[
"params_raw = {'variance_limit': 0.001, 'order': 2, 'defuzzy': 'weighted', 't_norm': 'threshold', 'membership_threshold': 0.6, 'step':1}\n\nforecasts_raw, order_list = rolling_cv_evolving(norm_df, params_raw)\n\nforecasts_final = get_final_forecast(forecasts_raw)\ncalculate_rolling_error(\"rolling_cv_wind_raw_emvfts\", df, forecasts_final, order_list)",
"Index: 2009-08-01\nIndex: 2009-08-08\nIndex: 2009-08-15\nIndex: 2009-08-22\nIndex: 2009-08-29\nIndex: 2009-09-05\nIndex: 2009-09-12\nIndex: 2009-09-19\nIndex: 2009-09-26\nIndex: 2009-10-03\nIndex: 2009-10-10\nIndex: 2009-10-17\nIndex: 2009-10-24\nIndex: 2009-10-31\nIndex: 2009-11-07\nIndex: 2009-11-14\nIndex: 2009-11-21\nIndex: 2009-11-28\nIndex: 2009-12-05\nIndex: 2009-12-12\nIndex: 2009-12-19\nIndex: 2009-12-26\nIndex: 2010-01-02\nIndex: 2010-01-09\nIndex: 2010-01-16\nIndex: 2010-01-23\nIndex: 2010-01-30\nIndex: 2010-02-06\nIndex: 2010-02-13\nIndex: 2010-02-20\nIndex: 2010-02-27\nIndex: 2010-03-06\nIndex: 2010-03-13\nIndex: 2010-03-20\nIndex: 2010-03-27\nIndex: 2010-04-03\nIndex: 2010-04-10\nIndex: 2010-04-17\nIndex: 2010-04-24\nIndex: 2010-05-01\nIndex: 2010-05-08\nIndex: 2010-05-15\nIndex: 2010-05-22\nIndex: 2010-05-29\nIndex: 2010-06-05\nIndex: 2010-06-12\nIndex: 2010-06-19\nIndex: 2010-06-26\nIndex: 2010-07-03\nIndex: 2010-07-10\n"
],
[
"files.download('rolling_cv_wind_raw_emvfts.csv')",
"_____no_output_____"
]
],
[
[
"### MLP",
"_____no_output_____"
]
],
[
[
"from keras.models import Sequential\nfrom keras.layers import Dense\nfrom keras.layers import LSTM\nfrom keras.layers import Dropout\nfrom keras.constraints import maxnorm\nfrom keras.models import Sequential\nfrom keras.layers.core import Dense, Dropout, Activation\nfrom keras.layers.normalization import BatchNormalization",
"_____no_output_____"
],
[
"# convert series to supervised learning\ndef series_to_supervised(data, n_in=1, n_out=1, dropnan=True):\n n_vars = 1 if type(data) is list else data.shape[1]\n df = pd.DataFrame(data)\n cols, names = list(), list()\n # input sequence (t-n, ... t-1)\n for i in range(n_in, 0, -1):\n cols.append(df.shift(i))\n names += [('var%d(t-%d)' % (j+1, i)) for j in range(n_vars)]\n # forecast sequence (t, t+1, ... t+n)\n for i in range(0, n_out):\n cols.append(df.shift(-i))\n if i == 0:\n names += [('var%d(t)' % (j+1)) for j in range(n_vars)]\n else:\n names += [('var%d(t+%d)' % (j+1, i)) for j in range(n_vars)]\n # put it all together\n agg = pd.concat(cols, axis=1)\n agg.columns = names\n # drop rows with NaN values\n if dropnan:\n agg.dropna(inplace=True)\n return agg",
"_____no_output_____"
]
],
[
[
"#### MLP Parameter Tuning",
"_____no_output_____"
]
],
[
[
"from spatiotemporal.util import parameter_tuning, sampling\nfrom spatiotemporal.util import experiments as ex\nfrom sklearn.metrics import mean_squared_error\nfrom hyperopt import hp\nimport numpy as np",
"_____no_output_____"
],
[
"mlp_space = {'choice':\n\n hp.choice('num_layers',\n [\n {'layers': 'two',\n },\n\n {'layers': 'three',\n\n 'units3': hp.choice('units3', [8, 16, 64, 128, 256, 512]),\n 'dropout3': hp.choice('dropout3', [0, 0.25, 0.5, 0.75])\n }\n\n ]),\n 'units1': hp.choice('units1', [8, 16, 64, 128, 256, 512]),\n 'units2': hp.choice('units2', [8, 16, 64, 128, 256, 512]),\n\n 'dropout1': hp.choice('dropout1', [0, 0.25, 0.5, 0.75]),\n 'dropout2': hp.choice('dropout2', [0, 0.25, 0.5, 0.75]),\n\n 'batch_size': hp.choice('batch_size', [28, 64, 128, 256, 512]),\n 'order': hp.choice('order', [1, 2, 3]),\n 'input': hp.choice('input', [wind_farms]),\n 'output': hp.choice('output', [wind_farms]),\n 'epochs': hp.choice('epochs', [100, 200, 300])}\n",
"_____no_output_____"
],
[
"def mlp_tuning(train_df, test_df, params):\n _input = list(params['input'])\n _nlags = params['order']\n _epochs = params['epochs']\n _batch_size = params['batch_size']\n nfeat = len(train_df.columns)\n nsteps = params.get('step',1)\n nobs = _nlags * nfeat\n\n output_index = -nfeat*nsteps\n\n train_reshaped_df = series_to_supervised(train_df[_input], n_in=_nlags, n_out=nsteps)\n train_X, train_Y = train_reshaped_df.iloc[:, :nobs].values, train_reshaped_df.iloc[:, output_index:].values\n\n test_reshaped_df = series_to_supervised(test_df[_input], n_in=_nlags, n_out=nsteps)\n test_X, test_Y = test_reshaped_df.iloc[:, :nobs].values, test_reshaped_df.iloc[:, output_index:].values\n\n # design network\n model = Sequential()\n model.add(Dense(params['units1'], input_dim=train_X.shape[1], activation='relu'))\n model.add(Dropout(params['dropout1']))\n model.add(BatchNormalization())\n\n model.add(Dense(params['units2'], activation='relu'))\n model.add(Dropout(params['dropout2']))\n model.add(BatchNormalization())\n\n if params['choice']['layers'] == 'three':\n model.add(Dense(params['choice']['units3'], activation='relu'))\n model.add(Dropout(params['choice']['dropout3']))\n model.add(BatchNormalization())\n\n model.add(Dense(train_Y.shape[1], activation='sigmoid'))\n model.compile(loss='mse', optimizer='adam')\n\n # includes the call back object\n model.fit(train_X, train_Y, epochs=_epochs, batch_size=_batch_size, verbose=False, shuffle=False)\n\n # predict the test set\n forecast = model.predict(test_X, verbose=False)\n\n return forecast\n",
"_____no_output_____"
],
[
"methods = []\nmethods.append((\"EXP_OAHU_MLP\", mlp_tuning, mlp_space))\ntrain_split = 0.6\nrun_search(methods, tuning_df, train_split, Measures.rmse, max_evals=30, resample=None)",
"Running experiment: EXP_OAHU_MLP\n{'batch_size': 256, 'choice': {'layers': 'two'}, 'dropout1': 0, 'dropout2': 0.25, 'epochs': 300, 'input': ('wp1', 'wp2', 'wp3', 'wp4', 'wp5', 'wp6', 'wp7'), 'order': 3, 'output': ('wp1', 'wp2', 'wp3', 'wp4', 'wp5', 'wp6', 'wp7'), 'units1': 16, 'units2': 512}\nError : 0.11210207774258987\n{'batch_size': 64, 'choice': {'dropout3': 0.75, 'layers': 'three', 'units3': 8}, 'dropout1': 0.75, 'dropout2': 0.75, 'epochs': 200, 'input': ('wp1', 'wp2', 'wp3', 'wp4', 'wp5', 'wp6', 'wp7'), 'order': 1, 'output': ('wp1', 'wp2', 'wp3', 'wp4', 'wp5', 'wp6', 'wp7'), 'units1': 64, 'units2': 8}\nError : 0.16887562719906232\n{'batch_size': 512, 'choice': {'dropout3': 0.5, 'layers': 'three', 'units3': 128}, 'dropout1': 0.5, 'dropout2': 0.5, 'epochs': 300, 'input': ('wp1', 'wp2', 'wp3', 'wp4', 'wp5', 'wp6', 'wp7'), 'order': 1, 'output': ('wp1', 'wp2', 'wp3', 'wp4', 'wp5', 'wp6', 'wp7'), 'units1': 64, 'units2': 8}\nError : 0.16832074683739862\n{'batch_size': 28, 'choice': {'dropout3': 0, 'layers': 'three', 'units3': 256}, 'dropout1': 0.25, 'dropout2': 0, 'epochs': 300, 'input': ('wp1', 'wp2', 'wp3', 'wp4', 'wp5', 'wp6', 'wp7'), 'order': 2, 'output': ('wp1', 'wp2', 'wp3', 'wp4', 'wp5', 'wp6', 'wp7'), 'units1': 64, 'units2': 64}\nError : 0.12007328735895494\n{'batch_size': 28, 'choice': {'dropout3': 0.5, 'layers': 'three', 'units3': 256}, 'dropout1': 0.75, 'dropout2': 0.25, 'epochs': 300, 'input': ('wp1', 'wp2', 'wp3', 'wp4', 'wp5', 'wp6', 'wp7'), 'order': 3, 'output': ('wp1', 'wp2', 'wp3', 'wp4', 'wp5', 'wp6', 'wp7'), 'units1': 512, 'units2': 16}\nError : 0.11256583928262713\n{'batch_size': 256, 'choice': {'dropout3': 0.25, 'layers': 'three', 'units3': 64}, 'dropout1': 0.5, 'dropout2': 0.5, 'epochs': 300, 'input': ('wp1', 'wp2', 'wp3', 'wp4', 'wp5', 'wp6', 'wp7'), 'order': 3, 'output': ('wp1', 'wp2', 'wp3', 'wp4', 'wp5', 'wp6', 'wp7'), 'units1': 512, 'units2': 16}\nError : 0.14391026899955472\n{'batch_size': 256, 'choice': {'dropout3': 0.75, 'layers': 'three', 'units3': 64}, 'dropout1': 0, 'dropout2': 0, 'epochs': 300, 'input': ('wp1', 'wp2', 'wp3', 'wp4', 'wp5', 'wp6', 'wp7'), 'order': 1, 'output': ('wp1', 'wp2', 'wp3', 'wp4', 'wp5', 'wp6', 'wp7'), 'units1': 16, 'units2': 64}\nError : 0.11037676055120181\n{'batch_size': 512, 'choice': {'dropout3': 0.75, 'layers': 'three', 'units3': 128}, 'dropout1': 0.25, 'dropout2': 0.25, 'epochs': 300, 'input': ('wp1', 'wp2', 'wp3', 'wp4', 'wp5', 'wp6', 'wp7'), 'order': 1, 'output': ('wp1', 'wp2', 'wp3', 'wp4', 'wp5', 'wp6', 'wp7'), 'units1': 256, 'units2': 512}\nError : 0.15784381475268033\n{'batch_size': 512, 'choice': {'dropout3': 0.75, 'layers': 'three', 'units3': 256}, 'dropout1': 0.75, 'dropout2': 0, 'epochs': 200, 'input': ('wp1', 'wp2', 'wp3', 'wp4', 'wp5', 'wp6', 'wp7'), 'order': 1, 'output': ('wp1', 'wp2', 'wp3', 'wp4', 'wp5', 'wp6', 'wp7'), 'units1': 128, 'units2': 8}\nError : 0.16657000728035204\n{'batch_size': 512, 'choice': {'layers': 'two'}, 'dropout1': 0.75, 'dropout2': 0.25, 'epochs': 200, 'input': ('wp1', 'wp2', 'wp3', 'wp4', 'wp5', 'wp6', 'wp7'), 'order': 3, 'output': ('wp1', 'wp2', 'wp3', 'wp4', 'wp5', 'wp6', 'wp7'), 'units1': 512, 'units2': 8}\nError : 0.26202963425973014\n{'batch_size': 64, 'choice': {'layers': 'two'}, 'dropout1': 0.25, 'dropout2': 0.5, 'epochs': 200, 'input': ('wp1', 'wp2', 'wp3', 'wp4', 'wp5', 'wp6', 'wp7'), 'order': 2, 'output': ('wp1', 'wp2', 'wp3', 'wp4', 'wp5', 'wp6', 'wp7'), 'units1': 512, 'units2': 64}\nError : 0.08758667541932756\n{'batch_size': 28, 'choice': {'dropout3': 0, 'layers': 'three', 'units3': 256}, 'dropout1': 0.5, 'dropout2': 0.75, 'epochs': 100, 'input': ('wp1', 'wp2', 'wp3', 'wp4', 'wp5', 'wp6', 'wp7'), 'order': 1, 'output': ('wp1', 'wp2', 'wp3', 'wp4', 'wp5', 'wp6', 'wp7'), 'units1': 64, 'units2': 16}\nError : 0.139826483409004\n{'batch_size': 128, 'choice': {'layers': 'two'}, 'dropout1': 0.5, 'dropout2': 0.75, 'epochs': 200, 'input': ('wp1', 'wp2', 'wp3', 'wp4', 'wp5', 'wp6', 'wp7'), 'order': 2, 'output': ('wp1', 'wp2', 'wp3', 'wp4', 'wp5', 'wp6', 'wp7'), 'units1': 16, 'units2': 256}\nError : 0.12880869981278525\n{'batch_size': 128, 'choice': {'dropout3': 0.25, 'layers': 'three', 'units3': 8}, 'dropout1': 0, 'dropout2': 0.75, 'epochs': 100, 'input': ('wp1', 'wp2', 'wp3', 'wp4', 'wp5', 'wp6', 'wp7'), 'order': 3, 'output': ('wp1', 'wp2', 'wp3', 'wp4', 'wp5', 'wp6', 'wp7'), 'units1': 512, 'units2': 64}\nError : 0.16604021900218402\n{'batch_size': 128, 'choice': {'layers': 'two'}, 'dropout1': 0, 'dropout2': 0.5, 'epochs': 300, 'input': ('wp1', 'wp2', 'wp3', 'wp4', 'wp5', 'wp6', 'wp7'), 'order': 1, 'output': ('wp1', 'wp2', 'wp3', 'wp4', 'wp5', 'wp6', 'wp7'), 'units1': 128, 'units2': 256}\nError : 0.09555621269300194\n{'batch_size': 256, 'choice': {'dropout3': 0.75, 'layers': 'three', 'units3': 64}, 'dropout1': 0.75, 'dropout2': 0.25, 'epochs': 300, 'input': ('wp1', 'wp2', 'wp3', 'wp4', 'wp5', 'wp6', 'wp7'), 'order': 2, 'output': ('wp1', 'wp2', 'wp3', 'wp4', 'wp5', 'wp6', 'wp7'), 'units1': 8, 'units2': 8}\nError : 0.1711557976639845\n{'batch_size': 28, 'choice': {'layers': 'two'}, 'dropout1': 0.75, 'dropout2': 0, 'epochs': 100, 'input': ('wp1', 'wp2', 'wp3', 'wp4', 'wp5', 'wp6', 'wp7'), 'order': 3, 'output': ('wp1', 'wp2', 'wp3', 'wp4', 'wp5', 'wp6', 'wp7'), 'units1': 8, 'units2': 64}\nError : 0.1638326118189065\n{'batch_size': 256, 'choice': {'layers': 'two'}, 'dropout1': 0.25, 'dropout2': 0, 'epochs': 100, 'input': ('wp1', 'wp2', 'wp3', 'wp4', 'wp5', 'wp6', 'wp7'), 'order': 1, 'output': ('wp1', 'wp2', 'wp3', 'wp4', 'wp5', 'wp6', 'wp7'), 'units1': 8, 'units2': 512}\nError : 0.15831764665590864\n{'batch_size': 256, 'choice': {'layers': 'two'}, 'dropout1': 0.5, 'dropout2': 0.75, 'epochs': 200, 'input': ('wp1', 'wp2', 'wp3', 'wp4', 'wp5', 'wp6', 'wp7'), 'order': 2, 'output': ('wp1', 'wp2', 'wp3', 'wp4', 'wp5', 'wp6', 'wp7'), 'units1': 256, 'units2': 256}\nError : 0.14529388682505784\n{'batch_size': 64, 'choice': {'dropout3': 0.25, 'layers': 'three', 'units3': 512}, 'dropout1': 0.25, 'dropout2': 0.75, 'epochs': 300, 'input': ('wp1', 'wp2', 'wp3', 'wp4', 'wp5', 'wp6', 'wp7'), 'order': 1, 'output': ('wp1', 'wp2', 'wp3', 'wp4', 'wp5', 'wp6', 'wp7'), 'units1': 8, 'units2': 8}\nError : 0.1414119809552915\n{'batch_size': 64, 'choice': {'layers': 'two'}, 'dropout1': 0, 'dropout2': 0.5, 'epochs': 200, 'input': ('wp1', 'wp2', 'wp3', 'wp4', 'wp5', 'wp6', 'wp7'), 'order': 2, 'output': ('wp1', 'wp2', 'wp3', 'wp4', 'wp5', 'wp6', 'wp7'), 'units1': 128, 'units2': 128}\nError : 0.09542121366565244\n{'batch_size': 64, 'choice': {'layers': 'two'}, 'dropout1': 0.25, 'dropout2': 0.5, 'epochs': 200, 'input': ('wp1', 'wp2', 'wp3', 'wp4', 'wp5', 'wp6', 'wp7'), 'order': 2, 'output': ('wp1', 'wp2', 'wp3', 'wp4', 'wp5', 'wp6', 'wp7'), 'units1': 128, 'units2': 128}\nError : 0.08515883577119714\n{'batch_size': 64, 'choice': {'layers': 'two'}, 'dropout1': 0.25, 'dropout2': 0.5, 'epochs': 200, 'input': ('wp1', 'wp2', 'wp3', 'wp4', 'wp5', 'wp6', 'wp7'), 'order': 2, 'output': ('wp1', 'wp2', 'wp3', 'wp4', 'wp5', 'wp6', 'wp7'), 'units1': 128, 'units2': 128}\nError : 0.084967455912928\n{'batch_size': 64, 'choice': {'layers': 'two'}, 'dropout1': 0.25, 'dropout2': 0.5, 'epochs': 200, 'input': ('wp1', 'wp2', 'wp3', 'wp4', 'wp5', 'wp6', 'wp7'), 'order': 2, 'output': ('wp1', 'wp2', 'wp3', 'wp4', 'wp5', 'wp6', 'wp7'), 'units1': 128, 'units2': 128}\nError : 0.08816597673392379\n{'batch_size': 64, 'choice': {'layers': 'two'}, 'dropout1': 0.25, 'dropout2': 0.5, 'epochs': 200, 'input': ('wp1', 'wp2', 'wp3', 'wp4', 'wp5', 'wp6', 'wp7'), 'order': 2, 'output': ('wp1', 'wp2', 'wp3', 'wp4', 'wp5', 'wp6', 'wp7'), 'units1': 128, 'units2': 128}\nError : 0.08461966850490099\n{'batch_size': 64, 'choice': {'layers': 'two'}, 'dropout1': 0.25, 'dropout2': 0.5, 'epochs': 200, 'input': ('wp1', 'wp2', 'wp3', 'wp4', 'wp5', 'wp6', 'wp7'), 'order': 2, 'output': ('wp1', 'wp2', 'wp3', 'wp4', 'wp5', 'wp6', 'wp7'), 'units1': 128, 'units2': 128}\nError : 0.08416671260635603\n{'batch_size': 64, 'choice': {'layers': 'two'}, 'dropout1': 0.25, 'dropout2': 0.5, 'epochs': 200, 'input': ('wp1', 'wp2', 'wp3', 'wp4', 'wp5', 'wp6', 'wp7'), 'order': 2, 'output': ('wp1', 'wp2', 'wp3', 'wp4', 'wp5', 'wp6', 'wp7'), 'units1': 128, 'units2': 128}\nError : 0.08203448953925911\n{'batch_size': 64, 'choice': {'layers': 'two'}, 'dropout1': 0.25, 'dropout2': 0.5, 'epochs': 200, 'input': ('wp1', 'wp2', 'wp3', 'wp4', 'wp5', 'wp6', 'wp7'), 'order': 2, 'output': ('wp1', 'wp2', 'wp3', 'wp4', 'wp5', 'wp6', 'wp7'), 'units1': 128, 'units2': 128}\nError : 0.09141701084487909\n{'batch_size': 64, 'choice': {'layers': 'two'}, 'dropout1': 0.25, 'dropout2': 0.5, 'epochs': 200, 'input': ('wp1', 'wp2', 'wp3', 'wp4', 'wp5', 'wp6', 'wp7'), 'order': 2, 'output': ('wp1', 'wp2', 'wp3', 'wp4', 'wp5', 'wp6', 'wp7'), 'units1': 128, 'units2': 128}\nError : 0.08625258845773652\n{'batch_size': 64, 'choice': {'layers': 'two'}, 'dropout1': 0.25, 'dropout2': 0.5, 'epochs': 200, 'input': ('wp1', 'wp2', 'wp3', 'wp4', 'wp5', 'wp6', 'wp7'), 'order': 2, 'output': ('wp1', 'wp2', 'wp3', 'wp4', 'wp5', 'wp6', 'wp7'), 'units1': 256, 'units2': 128}\nError : 0.0846710829000828\n100%|██████████| 30/30 [02:15<00:00, 4.52s/trial, best loss: 0.08203448953925911]\nbest parameters: \n{'batch_size': 64, 'choice': {'layers': 'two'}, 'dropout1': 0.25, 'dropout2': 0.5, 'epochs': 200, 'input': ('wp1', 'wp2', 'wp3', 'wp4', 'wp5', 'wp6', 'wp7'), 'order': 2, 'output': ('wp1', 'wp2', 'wp3', 'wp4', 'wp5', 'wp6', 'wp7'), 'units1': 128, 'units2': 128}\n"
]
],
[
[
"#### MLP Forecasting",
"_____no_output_____"
]
],
[
[
"def mlp_multi_forecast(train_df, test_df, params):\n\n \n nfeat = len(train_df.columns)\n nlags = params['order']\n nsteps = params.get('step',1)\n nobs = nlags * nfeat\n\n output_index = -nfeat*nsteps\n\n train_reshaped_df = series_to_supervised(train_df, n_in=nlags, n_out=nsteps)\n train_X, train_Y = train_reshaped_df.iloc[:, :nobs].values, train_reshaped_df.iloc[:, output_index:].values\n\n test_reshaped_df = series_to_supervised(test_df, n_in=nlags, n_out=nsteps)\n test_X, test_Y = test_reshaped_df.iloc[:, :nobs].values, test_reshaped_df.iloc[:, output_index:].values\n \n # design network\n model = designMLPNetwork(train_X.shape[1], train_Y.shape[1], params)\n \n # fit network\n model.fit(train_X, train_Y, epochs=500, batch_size=1000, verbose=False, shuffle=False)\n \n forecast = model.predict(test_X)\n \n# fcst = [f[0] for f in forecast]\n fcst = forecast\n return fcst",
"_____no_output_____"
],
[
"def designMLPNetwork(input_shape, output_shape, params):\n model = Sequential()\n model.add(Dense(params['units1'], input_dim=input_shape, activation='relu'))\n model.add(Dropout(params['dropout1']))\n model.add(BatchNormalization())\n\n model.add(Dense(params['units2'], activation='relu'))\n model.add(Dropout(params['dropout2']))\n model.add(BatchNormalization())\n\n if params['choice']['layers'] == 'three':\n model.add(Dense(params['choice']['units3'], activation='relu'))\n model.add(Dropout(params['choice']['dropout3']))\n model.add(BatchNormalization())\n\n model.add(Dense(output_shape, activation='sigmoid'))\n model.compile(loss='mse', optimizer='adam')\n\n return model",
"_____no_output_____"
],
[
"def rolling_cv_mlp(df, params):\n \n forecasts = []\n order_list = []\n\n limit = df.index[-1].strftime('%Y-%m-%d')\n\n test_end = \"\"\n index = df.index[0]\n\n while test_end < limit :\n print(\"Index: \", index.strftime('%Y-%m-%d')) \n\n train_start, train_end, test_start, test_end = getRollingWindow(index)\n index = index + datetime.timedelta(days=7)\n \n train = df[train_start : train_end]\n test = df[test_start : test_end]\n\n # Perform forecast\n yhat = list(mlp_multi_forecast(train, test, params))\n \n yhat.append(yhat[-1]) #para manter o formato do vetor de metricas\n \n forecasts.append(yhat)\n order_list.append(params['order'])\n\n return forecasts, order_list",
"_____no_output_____"
],
[
"# Enter best params\nparams_raw = {'batch_size': 64, 'choice': {'layers': 'two'}, 'dropout1': 0.25, 'dropout2': 0.5, 'epochs': 200, 'input': ('wp1', 'wp2', 'wp3', 'wp4', 'wp5', 'wp6', 'wp7'), 'order': 2, 'output': ('wp1', 'wp2', 'wp3', 'wp4', 'wp5', 'wp6', 'wp7'), 'units1': 128, 'units2': 128}\n\nforecasts_raw, order_list = rolling_cv_mlp(norm_df, params_raw)\n\nforecasts_final = get_final_forecast(forecasts_raw)\ncalculate_rolling_error(\"rolling_cv_wind_raw_mlp_multi\", df, forecasts_final, order_list)",
"Index: 2009-08-01\nIndex: 2009-08-08\nIndex: 2009-08-15\nIndex: 2009-08-22\nIndex: 2009-08-29\nIndex: 2009-09-05\nIndex: 2009-09-12\nIndex: 2009-09-19\nIndex: 2009-09-26\nIndex: 2009-10-03\nIndex: 2009-10-10\nIndex: 2009-10-17\nIndex: 2009-10-24\nIndex: 2009-10-31\nIndex: 2009-11-07\nIndex: 2009-11-14\nIndex: 2009-11-21\nIndex: 2009-11-28\nIndex: 2009-12-05\nIndex: 2009-12-12\nIndex: 2009-12-19\nIndex: 2009-12-26\nIndex: 2010-01-02\nIndex: 2010-01-09\nIndex: 2010-01-16\nIndex: 2010-01-23\nIndex: 2010-01-30\nIndex: 2010-02-06\nIndex: 2010-02-13\nIndex: 2010-02-20\nIndex: 2010-02-27\nIndex: 2010-03-06\nIndex: 2010-03-13\nIndex: 2010-03-20\nIndex: 2010-03-27\nIndex: 2010-04-03\nIndex: 2010-04-10\nIndex: 2010-04-17\nIndex: 2010-04-24\nIndex: 2010-05-01\nIndex: 2010-05-08\nIndex: 2010-05-15\nIndex: 2010-05-22\nIndex: 2010-05-29\nIndex: 2010-06-05\nIndex: 2010-06-12\nIndex: 2010-06-19\nIndex: 2010-06-26\nIndex: 2010-07-03\nIndex: 2010-07-10\n"
],
[
"files.download('rolling_cv_wind_raw_mlp_multi.csv')",
"_____no_output_____"
]
],
[
[
"### Granular FTS",
"_____no_output_____"
]
],
[
[
"from pyFTS.models.multivariate import granular\nfrom pyFTS.partitioners import Grid, Entropy\nfrom pyFTS.models.multivariate import variable\nfrom pyFTS.common import Membership\nfrom pyFTS.partitioners import Grid, Entropy",
"_____no_output_____"
]
],
[
[
"#### Granular Parameter Tuning",
"_____no_output_____"
]
],
[
[
"granular_space = {\n 'npartitions': hp.choice('npartitions', [100, 150, 200]),\n 'order': hp.choice('order', [1, 2]),\n 'knn': hp.choice('knn', [1, 2, 3, 4, 5]),\n 'alpha_cut': hp.choice('alpha_cut', [0, 0.1, 0.2, 0.3]),\n 'input': hp.choice('input', [['wp1', 'wp2', 'wp3']]),\n 'output': hp.choice('output', [['wp1', 'wp2', 'wp3']])}",
"_____no_output_____"
],
[
"def granular_tuning(train_df, test_df, params):\n _input = list(params['input'])\n _output = list(params['output'])\n _npartitions = params['npartitions']\n _order = params['order']\n _knn = params['knn']\n _alpha_cut = params['alpha_cut']\n _step = params.get('step',1)\n\n ## create explanatory variables\n exp_variables = []\n for vc in _input:\n exp_variables.append(variable.Variable(vc, data_label=vc, alias=vc,\n npart=_npartitions, func=Membership.trimf,\n data=train_df, alpha_cut=_alpha_cut))\n model = granular.GranularWMVFTS(explanatory_variables=exp_variables, target_variable=exp_variables[0], order=_order,\n knn=_knn)\n model.fit(train_df[_input], num_batches=1)\n\n if _step > 1:\n forecast = pd.DataFrame(columns=test_df.columns)\n length = len(test_df.index)\n\n for k in range(0,(length -(_order + _step - 1))):\n fcst = model.predict(test_df[_input], type='multivariate', start_at=k, steps_ahead=_step)\n forecast = forecast.append(fcst.tail(1))\n else:\n forecast = model.predict(test_df[_input], type='multivariate')\n\n return forecast[_output].values\n",
"_____no_output_____"
],
[
"methods = []\nmethods.append((\"EXP_WIND_GRANULAR\", granular_tuning, granular_space))",
"_____no_output_____"
],
[
"train_split = 0.6\nrun_search(methods, tuning_df, train_split, Measures.rmse, max_evals=10, resample=None)",
"Running experiment: EXP_WIND_GRANULAR\n{'alpha_cut': 0.1, 'input': ('wp1', 'wp2', 'wp3'), 'knn': 1, 'npartitions': 100, 'order': 1, 'output': ('wp1', 'wp2', 'wp3')}\nError : 0.11669905532137337\n{'alpha_cut': 0.2, 'input': ('wp1', 'wp2', 'wp3'), 'knn': 1, 'npartitions': 200, 'order': 2, 'output': ('wp1', 'wp2', 'wp3')}\nError : 0.08229067276531199\n{'alpha_cut': 0.2, 'input': ('wp1', 'wp2', 'wp3'), 'knn': 2, 'npartitions': 200, 'order': 2, 'output': ('wp1', 'wp2', 'wp3')}\nError : 0.08140150942675548\n{'alpha_cut': 0.1, 'input': ('wp1', 'wp2', 'wp3'), 'knn': 1, 'npartitions': 200, 'order': 1, 'output': ('wp1', 'wp2', 'wp3')}\nError : 0.11527883387924612\n{'alpha_cut': 0.2, 'input': ('wp1', 'wp2', 'wp3'), 'knn': 1, 'npartitions': 150, 'order': 1, 'output': ('wp1', 'wp2', 'wp3')}\nError : 0.11642857063129212\n{'alpha_cut': 0.2, 'input': ('wp1', 'wp2', 'wp3'), 'knn': 3, 'npartitions': 100, 'order': 1, 'output': ('wp1', 'wp2', 'wp3')}\nError : 0.10363929653907107\n{'alpha_cut': 0.3, 'input': ('wp1', 'wp2', 'wp3'), 'knn': 5, 'npartitions': 200, 'order': 2, 'output': ('wp1', 'wp2', 'wp3')}\nError : 0.07916522355127716\n{'alpha_cut': 0.3, 'input': ('wp1', 'wp2', 'wp3'), 'knn': 3, 'npartitions': 200, 'order': 2, 'output': ('wp1', 'wp2', 'wp3')}\nError : 0.07938399286248478\n{'alpha_cut': 0.1, 'input': ('wp1', 'wp2', 'wp3'), 'knn': 2, 'npartitions': 150, 'order': 2, 'output': ('wp1', 'wp2', 'wp3')}\nError : 0.08056469602939852\n{'alpha_cut': 0.2, 'input': ('wp1', 'wp2', 'wp3'), 'knn': 5, 'npartitions': 100, 'order': 1, 'output': ('wp1', 'wp2', 'wp3')}\nError : 0.09920669569870488\n100%|██████████| 10/10 [00:09<00:00, 1.05trial/s, best loss: 0.07916522355127716]\nbest parameters: \n{'alpha_cut': 0.3, 'input': ('wp1', 'wp2', 'wp3'), 'knn': 5, 'npartitions': 200, 'order': 2, 'output': ('wp1', 'wp2', 'wp3')}\n"
]
],
[
[
"#### Granular Forecasting",
"_____no_output_____"
]
],
[
[
"def granular_forecast(train_df, test_df, params):\n\n _input = list(params['input'])\n _output = list(params['output'])\n _npartitions = params['npartitions']\n _knn = params['knn']\n _alpha_cut = params['alpha_cut']\n _order = params['order']\n _step = params.get('step',1)\n\n ## create explanatory variables\n exp_variables = []\n for vc in _input:\n exp_variables.append(variable.Variable(vc, data_label=vc, alias=vc,\n npart=_npartitions, func=Membership.trimf,\n data=train_df, alpha_cut=_alpha_cut))\n model = granular.GranularWMVFTS(explanatory_variables=exp_variables, target_variable=exp_variables[0], order=_order,\n knn=_knn)\n model.fit(train_df[_input], num_batches=1)\n\n if _step > 1:\n forecast = pd.DataFrame(columns=test_df.columns)\n length = len(test_df.index)\n\n for k in range(0,(length -(_order + _step - 1))):\n fcst = model.predict(test_df[_input], type='multivariate', start_at=k, steps_ahead=_step)\n forecast = forecast.append(fcst.tail(1))\n else:\n forecast = model.predict(test_df[_input], type='multivariate')\n\n return forecast[_output].values\n",
"_____no_output_____"
],
[
"def rolling_cv_granular(df, params):\n \n forecasts = []\n order_list = []\n\n limit = df.index[-1].strftime('%Y-%m-%d')\n\n test_end = \"\"\n index = df.index[0]\n\n while test_end < limit :\n print(\"Index: \", index.strftime('%Y-%m-%d')) \n\n train_start, train_end, test_start, test_end = getRollingWindow(index)\n index = index + datetime.timedelta(days=7)\n \n train = df[train_start : train_end]\n test = df[test_start : test_end]\n\n\n # Perform forecast\n yhat = list(granular_forecast(train, test, params))\n \n yhat.append(yhat[-1]) #para manter o formato do vetor de metricas\n \n forecasts.append(yhat)\n order_list.append(params['order'])\n\n return forecasts, order_list",
"_____no_output_____"
],
[
"def granular_get_final_forecast(forecasts_raw, input):\n \n forecasts_final = []\n l_min = df[input].min()\n l_max = df[input].max()\n\n\n for i in np.arange(len(forecasts_raw)):\n f_raw = denormalize(forecasts_raw[i], l_min, l_max)\n\n forecasts_final.append(f_raw)\n \n return forecasts_final",
"_____no_output_____"
],
[
"# Enter best params\nparams_raw = {'alpha_cut': 0.3, 'input': ('wp1', 'wp2', 'wp3'), 'knn': 5, 'npartitions': 200, 'order': 2, 'output': ('wp1', 'wp2', 'wp3')}\n\nforecasts_raw, order_list = rolling_cv_granular(norm_df, params_raw)\n\nforecasts_final = granular_get_final_forecast(forecasts_raw, list(params_raw['input']))\ncalculate_rolling_error(\"rolling_cv_wind_raw_granular\", df[list(params_raw['input'])], forecasts_final, order_list)",
"Index: 2009-08-01\nIndex: 2009-08-08\nIndex: 2009-08-15\nIndex: 2009-08-22\nIndex: 2009-08-29\nIndex: 2009-09-05\nIndex: 2009-09-12\nIndex: 2009-09-19\nIndex: 2009-09-26\nIndex: 2009-10-03\nIndex: 2009-10-10\nIndex: 2009-10-17\nIndex: 2009-10-24\nIndex: 2009-10-31\nIndex: 2009-11-07\nIndex: 2009-11-14\nIndex: 2009-11-21\nIndex: 2009-11-28\nIndex: 2009-12-05\nIndex: 2009-12-12\nIndex: 2009-12-19\nIndex: 2009-12-26\nIndex: 2010-01-02\nIndex: 2010-01-09\nIndex: 2010-01-16\nIndex: 2010-01-23\nIndex: 2010-01-30\nIndex: 2010-02-06\nIndex: 2010-02-13\nIndex: 2010-02-20\nIndex: 2010-02-27\nIndex: 2010-03-06\nIndex: 2010-03-13\nIndex: 2010-03-20\nIndex: 2010-03-27\nIndex: 2010-04-03\nIndex: 2010-04-10\nIndex: 2010-04-17\nIndex: 2010-04-24\nIndex: 2010-05-01\nIndex: 2010-05-08\nIndex: 2010-05-15\nIndex: 2010-05-22\nIndex: 2010-05-29\nIndex: 2010-06-05\nIndex: 2010-06-12\nIndex: 2010-06-19\nIndex: 2010-06-26\nIndex: 2010-07-03\nIndex: 2010-07-10\n"
],
[
"files.download('rolling_cv_wind_raw_granular.csv')",
"_____no_output_____"
]
],
[
[
"## Result Analysis",
"_____no_output_____"
]
],
[
[
"import pandas as pd\nfrom google.colab import files",
"_____no_output_____"
],
[
"files.upload()",
"_____no_output_____"
],
[
"def createBoxplot(filename, data, xticklabels, ylabel):\n # Create a figure instance\n fig = plt.figure(1, figsize=(9, 6))\n\n # Create an axes instance\n ax = fig.add_subplot(111)\n\n # Create the boxplot\n bp = ax.boxplot(data, patch_artist=True)\n \n ## change outline color, fill color and linewidth of the boxes\n for box in bp['boxes']:\n # change outline color\n box.set( color='#7570b3', linewidth=2)\n # change fill color\n box.set( facecolor = '#AACCFF' )\n\n ## change color and linewidth of the whiskers\n for whisker in bp['whiskers']:\n whisker.set(color='#7570b3', linewidth=2)\n\n ## change color and linewidth of the caps\n for cap in bp['caps']:\n cap.set(color='#7570b3', linewidth=2)\n\n ## change color and linewidth of the medians\n for median in bp['medians']:\n median.set(color='#FFE680', linewidth=2)\n\n ## change the style of fliers and their fill\n for flier in bp['fliers']:\n flier.set(marker='o', color='#e7298a', alpha=0.5)\n \n ## Custom x-axis labels\n ax.set_xticklabels(xticklabels)\n ax.set_ylabel(ylabel)\n plt.show()\n fig.savefig(filename, bbox_inches='tight')",
"_____no_output_____"
],
[
"var_results = pd.read_csv(\"rolling_cv_wind_raw_var.csv\")\nevolving_results = pd.read_csv(\"rolling_cv_wind_raw_emvfts.csv\")\nmlp_results = pd.read_csv(\"rolling_cv_wind_raw_mlp_multi.csv\")\ngranular_results = pd.read_csv(\"rolling_cv_wind_raw_granular.csv\")",
"_____no_output_____"
],
[
"metric = 'RMSE'\nresults_data = [evolving_results[metric],var_results[metric], mlp_results[metric], granular_results[metric]]\nxticks = ['e-MVFTS','VAR','MLP','FIG-FTS']\n\nylab = 'RMSE'\ncreateBoxplot(\"e-mvfts_boxplot_rmse_solar\", results_data, xticks, ylab)",
"_____no_output_____"
],
[
"pd.options.display.float_format = '{:.2f}'.format",
"_____no_output_____"
],
[
"metric = 'RMSE'\nrmse_df = pd.DataFrame(columns=['e-MVFTS','VAR','MLP','FIG-FTS'])\n\nrmse_df[\"e-MVFTS\"] = evolving_results[metric]\nrmse_df[\"VAR\"] = var_results[metric]\nrmse_df[\"MLP\"] = mlp_results[metric]\nrmse_df[\"FIG-FTS\"] = granular_results[metric]",
"_____no_output_____"
],
[
"rmse_df.std()",
"_____no_output_____"
],
[
"metric = 'SMAPE'\nresults_data = [evolving_results[metric],var_results[metric], mlp_results[metric], granular_results[metric]]\nxticks = ['e-MVFTS','VAR','MLP','FIG-FTS']\n\nylab = 'SMAPE'\ncreateBoxplot(\"e-mvfts_boxplot_smape_solar\", results_data, xticks, ylab)",
"_____no_output_____"
],
[
"metric = 'SMAPE'\nsmape_df = pd.DataFrame(columns=['e-MVFTS','VAR','MLP','FIG-FTS'])\n\nsmape_df[\"e-MVFTS\"] = evolving_results[metric]\nsmape_df[\"VAR\"] = var_results[metric]\nsmape_df[\"MLP\"] = mlp_results[metric]\nsmape_df[\"FIG-FTS\"] = granular_results[metric]",
"_____no_output_____"
],
[
"smape_df.std()",
"_____no_output_____"
],
[
"metric = \"RMSE\"\n\ndata = pd.DataFrame(columns=[\"VAR\", \"Evolving\", \"MLP\", \"Granular\"])\n\ndata[\"VAR\"] = var_results[metric]\ndata[\"Evolving\"] = evolving_results[metric]\ndata[\"MLP\"] = mlp_results[metric]\ndata[\"Granular\"] = granular_results[metric]\n\nax = data.plot(figsize=(18,6))\nax.set(xlabel='Window', ylabel=metric)\nfig = ax.get_figure()\n#fig.savefig(path_images + exp_id + \"_prequential.png\")\n \nx = np.arange(len(data.columns.values))\nnames = data.columns.values\nvalues = data.mean().values\nplt.figure(figsize=(5,6))\nplt.bar(x, values, align='center', alpha=0.5, width=0.9)\nplt.xticks(x, names)\n#plt.yticks(np.arange(0, 1.1, 0.1))\nplt.ylabel(metric)\n#plt.savefig(path_images + exp_id + \"_bars.png\")",
"_____no_output_____"
],
[
"metric = \"SMAPE\"\n\ndata = pd.DataFrame(columns=[\"VAR\", \"Evolving\", \"MLP\", \"Granular\"])\n\ndata[\"VAR\"] = var_results[metric]\ndata[\"Evolving\"] = evolving_results[metric]\ndata[\"MLP\"] = mlp_results[metric]\ndata[\"Granular\"] = granular_results[metric]\n\nax = data.plot(figsize=(18,6))\nax.set(xlabel='Window', ylabel=metric)\nfig = ax.get_figure()\n#fig.savefig(path_images + exp_id + \"_prequential.png\")\n \nx = np.arange(len(data.columns.values))\nnames = data.columns.values\nvalues = data.mean().values\nplt.figure(figsize=(5,6))\nplt.bar(x, values, align='center', alpha=0.5, width=0.9)\nplt.xticks(x, names)\n#plt.yticks(np.arange(0, 1.1, 0.1))\nplt.ylabel(metric)\n#plt.savefig(path_images + exp_id + \"_bars.png\")",
"_____no_output_____"
]
]
] | [
"markdown",
"code",
"markdown",
"code",
"markdown",
"code",
"markdown",
"code",
"markdown",
"code",
"markdown",
"code",
"markdown",
"code",
"markdown",
"code",
"markdown",
"code",
"markdown",
"code",
"markdown",
"code",
"markdown",
"code",
"markdown",
"code"
] | [
[
"markdown",
"markdown",
"markdown"
],
[
"code",
"code"
],
[
"markdown"
],
[
"code",
"code"
],
[
"markdown"
],
[
"code",
"code"
],
[
"markdown",
"markdown"
],
[
"code",
"code",
"code"
],
[
"markdown"
],
[
"code",
"code",
"code",
"code",
"code",
"code"
],
[
"markdown"
],
[
"code",
"code",
"code",
"code",
"code"
],
[
"markdown"
],
[
"code",
"code"
],
[
"markdown"
],
[
"code",
"code",
"code",
"code"
],
[
"markdown"
],
[
"code",
"code",
"code",
"code",
"code"
],
[
"markdown"
],
[
"code"
],
[
"markdown"
],
[
"code",
"code",
"code",
"code"
],
[
"markdown"
],
[
"code",
"code",
"code",
"code",
"code"
],
[
"markdown"
],
[
"code",
"code",
"code",
"code",
"code",
"code",
"code",
"code",
"code",
"code",
"code",
"code",
"code"
]
] |
d04711f66922d50ca7e7a4095fc1d91a2d5eace2 | 124,202 | ipynb | Jupyter Notebook | cloud/notebooks/python_sdk/experiments/autoai/Use Lale AIF360 scorers to calculate and mitigate bias for credit risk AutoAI model.ipynb | muthukumarbala07/watson-machine-learning-samples | ecc66faf7a7c60ca168b9c7ef0bca3c766babb94 | [
"Apache-2.0"
] | 27 | 2020-09-09T20:46:03.000Z | 2021-11-29T20:13:35.000Z | cloud/notebooks/python_sdk/experiments/autoai/Use Lale AIF360 scorers to calculate and mitigate bias for credit risk AutoAI model.ipynb | muthukumarbala07/watson-machine-learning-samples | ecc66faf7a7c60ca168b9c7ef0bca3c766babb94 | [
"Apache-2.0"
] | 6 | 2020-09-21T12:50:05.000Z | 2021-01-09T14:06:41.000Z | cloud/notebooks/python_sdk/experiments/autoai/Use Lale AIF360 scorers to calculate and mitigate bias for credit risk AutoAI model.ipynb | muthukumarbala07/watson-machine-learning-samples | ecc66faf7a7c60ca168b9c7ef0bca3c766babb94 | [
"Apache-2.0"
] | 55 | 2020-09-14T12:38:44.000Z | 2022-03-18T13:28:34.000Z | 50.86077 | 765 | 0.539468 | [
[
[
"# Use `Lale` `AIF360` scorers to calculate and mitigate bias for credit risk AutoAI model",
"_____no_output_____"
],
[
"This notebook contains the steps and code to demonstrate support of AutoAI experiments in Watson Machine Learning service. It introduces commands for bias detecting and mitigation performed with `lale.lib.aif360` module.\n\nSome familiarity with Python is helpful. This notebook uses Python 3.8.",
"_____no_output_____"
],
[
"## Contents\n\nThis notebook contains the following parts:\n\n1.\t[Setup](#setup)\n2.\t[Optimizer definition](#definition)\n3.\t[Experiment Run](#run)\n4.\t[Pipeline bias detection and mitigation](#bias)\n5. [Deployment and score](#scoring)\n6. [Clean up](#cleanup)\n7.\t[Summary and next steps](#summary)",
"_____no_output_____"
],
[
"<a id=\"setup\"></a>\n## 1. Set up the environment\n\nIf you are not familiar with <a href=\"https://console.ng.bluemix.net/catalog/services/ibm-watson-machine-learning/\" target=\"_blank\" rel=\"noopener no referrer\">Watson Machine Learning (WML) Service</a> and AutoAI experiments please read more about it in the sample notebook: <a href=\"https://github.com/IBM/watson-machine-learning-samples/blob/master/cloud/notebooks/python_sdk/experiments/autoai/Use%20AutoAI%20and%20Lale%20to%20predict%20credit%20risk.ipynb\" target=\"_blank\" rel=\"noopener no referrer\">\"Use AutoAI and Lale to predict credit risk with `ibm-watson-machine-learning`\"</a>",
"_____no_output_____"
],
[
"### Install and import the `ibm-watson-machine-learning`, `lale` ,`aif360` and dependencies.\n**Note:** `ibm-watson-machine-learning` documentation can be found <a href=\"http://ibm-wml-api-pyclient.mybluemix.net/\" target=\"_blank\" rel=\"noopener no referrer\">here</a>.",
"_____no_output_____"
]
],
[
[
"!pip install -U ibm-watson-machine-learning | tail -n 1\n!pip install -U scikit-learn==0.23.2 | tail -n 1\n!pip install -U autoai-libs | tail -n 1\n!pip install -U lale | tail -n 1\n!pip install -U aif360 | tail -n 1\n!pip install -U liac-arff | tail -n 1\n!pip install -U cvxpy | tail -n 1\n!pip install -U fairlearn | tail -n 1",
"_____no_output_____"
]
],
[
[
"### Connection to WML\n\nAuthenticate the Watson Machine Learning service on IBM Cloud. You need to provide Cloud `API key` and `location`.\n\n**Tip**: Your `Cloud API key` can be generated by going to the [**Users** section of the Cloud console](https://cloud.ibm.com/iam#/users). From that page, click your name, scroll down to the **API Keys** section, and click **Create an IBM Cloud API key**. Give your key a name and click **Create**, then copy the created key and paste it below. You can also get a service specific url by going to the [**Endpoint URLs** section of the Watson Machine Learning docs](https://cloud.ibm.com/apidocs/machine-learning). You can check your instance location in your <a href=\"https://console.ng.bluemix.net/catalog/services/ibm-watson-machine-learning/\" target=\"_blank\" rel=\"noopener no referrer\">Watson Machine Learning (WML) Service</a> instance details.\n\n\nYou can use [IBM Cloud CLI](https://cloud.ibm.com/docs/cli/index.html) to retrieve the instance `location`.\n\n```\nibmcloud login --apikey API_KEY -a https://cloud.ibm.com\nibmcloud resource service-instance WML_INSTANCE_NAME\n```\n\n\n**NOTE:** You can also get a service specific apikey by going to the [**Service IDs** section of the Cloud Console](https://cloud.ibm.com/iam/serviceids). From that page, click **Create**, and then copy the created key and paste it in the following cell. \n\n\n**Action**: Enter your `api_key` and `location` in the following cell.",
"_____no_output_____"
]
],
[
[
"api_key = 'PUT_YOUR_KEY_HERE'\nlocation = 'us-south'",
"_____no_output_____"
],
[
"wml_credentials = {\n \"apikey\": api_key,\n \"url\": 'https://' + location + '.ml.cloud.ibm.com'\n}",
"_____no_output_____"
],
[
"from ibm_watson_machine_learning import APIClient\n\nclient = APIClient(wml_credentials)",
"_____no_output_____"
]
],
[
[
"### Working with spaces\n\nYou need to create a space that will be used for your work. If you do not have a space, you can use [Deployment Spaces Dashboard](https://dataplatform.cloud.ibm.com/ml-runtime/spaces?context=cpdaas) to create one.\n\n- Click **New Deployment Space**\n- Create an empty space\n- Select Cloud Object Storage\n- Select Watson Machine Learning instance and press **Create**\n- Copy `space_id` and paste it below\n\n**Tip**: You can also use SDK to prepare the space for your work. More information can be found [here](https://github.com/IBM/watson-machine-learning-samples/blob/master/cloud/notebooks/python_sdk/instance-management/Space%20management.ipynb).\n\n**Action**: assign space ID below\n",
"_____no_output_____"
]
],
[
[
"space_id = 'PASTE YOUR SPACE ID HERE'",
"_____no_output_____"
],
[
"client.spaces.list(limit=10)",
"_____no_output_____"
],
[
"client.set.default_space(space_id)",
"_____no_output_____"
]
],
[
[
"### Connections to COS\n\nIn next cell we read the COS credentials from the space.",
"_____no_output_____"
]
],
[
[
"cos_credentials = client.spaces.get_details(space_id=space_id)['entity']['storage']['properties']",
"_____no_output_____"
]
],
[
[
"<a id=\"definition\"></a>\n## 2. Optimizer definition",
"_____no_output_____"
],
[
"### Training data connection\n\nDefine connection information to COS bucket and training data CSV file. This example uses the [German Credit Risk dataset](https://raw.githubusercontent.com/IBM/watson-machine-learning-samples/master/cloud/data/credit_risk/credit_risk_training_light.csv).\n\nThe code in next cell uploads training data to the bucket.",
"_____no_output_____"
]
],
[
[
"filename = 'german_credit_data_biased_training.csv'\ndatasource_name = 'bluemixcloudobjectstorage'\nbucketname = cos_credentials['bucket_name']",
"_____no_output_____"
]
],
[
[
"Download training data from git repository and split for training and test set.",
"_____no_output_____"
]
],
[
[
"import os, wget\nimport pandas as pd\nimport numpy as np\nfrom sklearn.model_selection import train_test_split\n\nurl = 'https://raw.githubusercontent.com/IBM/watson-machine-learning-samples/master/cloud/data/credit_risk/german_credit_data_biased_training.csv'\nif not os.path.isfile(filename): wget.download(url)\n\ncredit_risk_df = pd.read_csv(filename)\n\nX = credit_risk_df.drop(['Risk'], axis=1)\ny = credit_risk_df['Risk']\n\nX_train, X_test, y_train, y_test = train_test_split(X, y, test_size=0.1)\n\ncredit_risk_df.head()",
"_____no_output_____"
]
],
[
[
"#### Create connection",
"_____no_output_____"
]
],
[
[
"conn_meta_props= {\n client.connections.ConfigurationMetaNames.NAME: f\"Connection to Database - {datasource_name} \",\n client.connections.ConfigurationMetaNames.DATASOURCE_TYPE: client.connections.get_datasource_type_uid_by_name(datasource_name),\n client.connections.ConfigurationMetaNames.DESCRIPTION: \"Connection to external Database\",\n client.connections.ConfigurationMetaNames.PROPERTIES: {\n 'bucket': bucketname,\n 'access_key': cos_credentials['credentials']['editor']['access_key_id'],\n 'secret_key': cos_credentials['credentials']['editor']['secret_access_key'],\n 'iam_url': 'https://iam.cloud.ibm.com/identity/token',\n 'url': cos_credentials['endpoint_url']\n }\n}\n\nconn_details = client.connections.create(meta_props=conn_meta_props)",
"_____no_output_____"
]
],
[
[
"**Note**: The above connection can be initialized alternatively with `api_key` and `resource_instance_id`. \nThe above cell can be replaced with:\n\n\n```\nconn_meta_props= {\n client.connections.ConfigurationMetaNames.NAME: f\"Connection to Database - {db_name} \",\n client.connections.ConfigurationMetaNames.DATASOURCE_TYPE: client.connections.get_datasource_type_uid_by_name(db_name),\n client.connections.ConfigurationMetaNames.DESCRIPTION: \"Connection to external Database\",\n client.connections.ConfigurationMetaNames.PROPERTIES: {\n 'bucket': bucket_name,\n 'api_key': cos_credentials['apikey'],\n 'resource_instance_id': cos_credentials['resource_instance_id'],\n 'iam_url': 'https://iam.cloud.ibm.com/identity/token',\n 'url': 'https://s3.us.cloud-object-storage.appdomain.cloud'\n }\n}\n\nconn_details = client.connections.create(meta_props=conn_meta_props)\n\n```",
"_____no_output_____"
]
],
[
[
"connection_id = client.connections.get_uid(conn_details)",
"_____no_output_____"
]
],
[
[
"Define connection information to training data and upload train dataset to COS bucket.\n",
"_____no_output_____"
]
],
[
[
"from ibm_watson_machine_learning.helpers import DataConnection, S3Location\n\n\ncredit_risk_conn = DataConnection(\n connection_asset_id=connection_id,\n location=S3Location(bucket=bucketname,\n path=filename))\n\ncredit_risk_conn._wml_client = client\ntraining_data_reference=[credit_risk_conn]\n\n\ncredit_risk_conn.write(data=X_train.join(y_train), remote_name=filename)",
"_____no_output_____"
]
],
[
[
"### Optimizer configuration\n\nProvide the input information for AutoAI optimizer:\n- `name` - experiment name\n- `prediction_type` - type of the problem\n- `prediction_column` - target column name\n- `scoring` - optimization metric\n- `daub_include_only_estimators` - estimators which will be included during AutoAI training. More available estimators can be found in `experiment.ClassificationAlgorithms` enum",
"_____no_output_____"
]
],
[
[
"from ibm_watson_machine_learning.experiment import AutoAI\n\nexperiment = AutoAI(wml_credentials, space_id=space_id)\n\npipeline_optimizer = experiment.optimizer(\n name='Credit Risk Bias detection in AutoAI',\n prediction_type=AutoAI.PredictionType.BINARY,\n prediction_column='Risk',\n scoring=AutoAI.Metrics.ROC_AUC_SCORE,\n include_only_estimators=[experiment.ClassificationAlgorithms.XGB] \n)",
"_____no_output_____"
]
],
[
[
"<a id=\"run\"></a>\n## 3. Experiment run\n\nCall the `fit()` method to trigger the AutoAI experiment. You can either use interactive mode (synchronous job) or background mode (asychronous job) by specifying `background_model=True`.",
"_____no_output_____"
]
],
[
[
"run_details = pipeline_optimizer.fit(\n training_data_reference=training_data_reference,\n background_mode=False)",
"Training job c646c56a-b7b7-4090-bfe6-d57327a76da6 completed: 100%|████████| [03:21<00:00, 2.01s/it]\n"
],
[
"pipeline_optimizer.get_run_status()",
"_____no_output_____"
],
[
"summary = pipeline_optimizer.summary()\nsummary",
"_____no_output_____"
]
],
[
[
"### Get selected pipeline model\n\nDownload pipeline model object from the AutoAI training job.",
"_____no_output_____"
]
],
[
[
"best_pipeline = pipeline_optimizer.get_pipeline()",
"_____no_output_____"
]
],
[
[
"<a id=\"bias\"></a>\n## 4. Bias detection and mitigation\n\nThe `fairness_info` dictionary contains some fairness-related metadata. The favorable and unfavorable label are values of the target class column that indicate whether the loan was granted or denied. A protected attribute is a feature that partitions the population into groups whose outcome should have parity. The credit-risk dataset has two protected attribute columns, sex and age. Each prottected attributes has privileged and unprivileged group.\n\nNote that to use fairness metrics from lale with numpy arrays `protected_attributes.feature` need to be passed as index of the column in dataset, not as name.",
"_____no_output_____"
]
],
[
[
"fairness_info = {'favorable_labels': ['No Risk'],\n 'protected_attributes': [\n {'feature': X.columns.get_loc('Sex'),'reference_group': ['male']},\n {'feature': X.columns.get_loc('Age'), 'reference_group': [[26, 40]]}]}\nfairness_info",
"_____no_output_____"
]
],
[
[
"### Calculate fairness metrics",
"_____no_output_____"
],
[
"We will calculate some model metrics. Accuracy describes how accurate is the model according to dataset. \nDisparate impact is defined by comparing outcomes between a privileged group and an unprivileged group, \nso it needs to check the protected attribute to determine group membership for the sample record at hand.\nThe third calculated metric takes the disparate impact into account along with accuracy. The best value of the score is 1.0.",
"_____no_output_____"
]
],
[
[
"import sklearn.metrics\nfrom lale.lib.aif360 import disparate_impact, accuracy_and_disparate_impact\n\naccuracy_scorer = sklearn.metrics.make_scorer(sklearn.metrics.accuracy_score)\nprint(f'accuracy {accuracy_scorer(best_pipeline, X_test.values, y_test.values):.1%}')\ndisparate_impact_scorer = disparate_impact(**fairness_info)\nprint(f'disparate impact {disparate_impact_scorer(best_pipeline, X_test.values, y_test.values):.2f}')\ncombined_scorer = accuracy_and_disparate_impact(**fairness_info)\nprint(f'accuracy and disparate impact metric {combined_scorer(best_pipeline, X_test.values, y_test.values):.2f}')",
"accuracy 82.4%\ndisparate impact 0.68\naccuracy and disparate impact metric 0.26\n"
]
],
[
[
"### Mitigation\n\n`Hyperopt` minimizes (best_score - score_returned_by_the_scorer), where best_score is an argument to Hyperopt and score_returned_by_the_scorer is the value returned by the scorer for each evaluation point. We will use the `Hyperopt` to tune hyperparametres of the AutoAI pipeline to get new and more fair model. \n",
"_____no_output_____"
]
],
[
[
"from sklearn.linear_model import LogisticRegression as LR\nfrom sklearn.tree import DecisionTreeClassifier as Tree\nfrom sklearn.neighbors import KNeighborsClassifier as KNN\nfrom lale.lib.lale import Hyperopt\nfrom lale.lib.aif360 import FairStratifiedKFold\nfrom lale import wrap_imported_operators\n\nwrap_imported_operators()",
"_____no_output_____"
],
[
"prefix = best_pipeline.remove_last().freeze_trainable()\nprefix.visualize()",
"_____no_output_____"
],
[
"new_pipeline = prefix >> (LR | Tree | KNN)\nnew_pipeline.visualize()",
"_____no_output_____"
],
[
"fair_cv = FairStratifiedKFold(**fairness_info, n_splits=3)\n\npipeline_fairer = new_pipeline.auto_configure(\n X_train.values, y_train.values, optimizer=Hyperopt, cv=fair_cv,\n max_evals=10, scoring=combined_scorer, best_score=1.0)",
"100%|██████████| 10/10 [01:13<00:00, 7.35s/trial, best loss: 0.27222222222222214]\n"
]
],
[
[
"As with any trained model, we can evaluate and visualize the result.",
"_____no_output_____"
]
],
[
[
"print(f'accuracy {accuracy_scorer(pipeline_fairer, X_test.values, y_test.values):.1%}')\nprint(f'disparate impact {disparate_impact_scorer(pipeline_fairer, X_test.values, y_test.values):.2f}')\nprint(f'accuracy and disparate impact metric {combined_scorer(pipeline_fairer, X_test.values, y_test.values):.2f}')\npipeline_fairer.visualize()",
"accuracy 75.8%\ndisparate impact 0.86\naccuracy and disparate impact metric 0.63\n"
]
],
[
[
"As the result demonstrates, the best model found by AI Automation\nhas lower accuracy and much better disparate impact as the one we saw\nbefore. Also, it has tuned the repair level and\nhas picked and tuned a classifier. These results may vary by dataset and search space.",
"_____no_output_____"
],
[
"You can get source code of the created pipeline. You just need to change the below cell type `Raw NBCovert` to `code`.",
"_____no_output_____"
]
],
[
[
"pipeline_fairer.pretty_print(ipython_display=True, show_imports=False)",
"_____no_output_____"
]
],
[
[
"<a id=\"scoring\"></a>\n## 5. Deploy and Score\nIn this section you will learn how to deploy and score Lale pipeline model using WML instance.",
"_____no_output_____"
],
[
"#### Custom software_specification",
"_____no_output_____"
],
[
"Created model is AutoAI model refined with Lale. We will create new software specification based on default Python 3.7 \nenvironment extended by `autoai-libs` package.",
"_____no_output_____"
]
],
[
[
"base_sw_spec_uid = client.software_specifications.get_uid_by_name(\"default_py3.7\")\nprint(\"Id of default Python 3.7 software specification is: \", base_sw_spec_uid)",
"Id of default Python 3.7 software specification is: e4429883-c883-42b6-87a8-f419d64088cd\n"
],
[
"url = 'https://raw.githubusercontent.com/IBM/watson-machine-learning-samples/master/cloud/configs/config.yaml'\nif not os.path.isfile('config.yaml'): wget.download(url)",
"_____no_output_____"
],
[
"!cat config.yaml",
"name: python37\nchannels:\n - defaults\ndependencies:\n - pip:\n - autoai-libs\n\nprefix: /opt/anaconda3/envs/python37"
]
],
[
[
"`config.yaml` file describes details of package extention. Now you need to store new package extention with `APIClient`.",
"_____no_output_____"
]
],
[
[
"meta_prop_pkg_extn = {\n client.package_extensions.ConfigurationMetaNames.NAME: \"Scikt with autoai-libs\",\n client.package_extensions.ConfigurationMetaNames.DESCRIPTION: \"Pkg extension for autoai-libs\",\n client.package_extensions.ConfigurationMetaNames.TYPE: \"conda_yml\"\n}\n\npkg_extn_details = client.package_extensions.store(meta_props=meta_prop_pkg_extn, file_path=\"config.yaml\")\npkg_extn_uid = client.package_extensions.get_uid(pkg_extn_details)\npkg_extn_url = client.package_extensions.get_href(pkg_extn_details)",
"Creating package extensions\nSUCCESS\n"
]
],
[
[
"Create new software specification and add created package extention to it. ",
"_____no_output_____"
]
],
[
[
"meta_prop_sw_spec = {\n client.software_specifications.ConfigurationMetaNames.NAME: \"Mitigated AutoAI bases on scikit spec\",\n client.software_specifications.ConfigurationMetaNames.DESCRIPTION: \"Software specification for scikt with autoai-libs\",\n client.software_specifications.ConfigurationMetaNames.BASE_SOFTWARE_SPECIFICATION: {\"guid\": base_sw_spec_uid}\n}\n\nsw_spec_details = client.software_specifications.store(meta_props=meta_prop_sw_spec)\nsw_spec_uid = client.software_specifications.get_uid(sw_spec_details)\n\n\nstatus = client.software_specifications.add_package_extension(sw_spec_uid, pkg_extn_uid)",
"SUCCESS\n"
]
],
[
[
"You can get details of created software specification using `client.software_specifications.get_details(sw_spec_uid)`",
"_____no_output_____"
],
[
"### Store the model",
"_____no_output_____"
]
],
[
[
"model_props = {\n client.repository.ModelMetaNames.NAME: \"Fairer AutoAI model\",\n client.repository.ModelMetaNames.TYPE: 'scikit-learn_0.23',\n client.repository.ModelMetaNames.SOFTWARE_SPEC_UID: sw_spec_uid\n \n}\nfeature_vector = list(X.columns)",
"_____no_output_____"
],
[
"published_model = client.repository.store_model(\n model=best_pipeline.export_to_sklearn_pipeline(), \n meta_props=model_props,\n training_data=X_train.values,\n training_target=y_train.values,\n feature_names=feature_vector,\n label_column_names=['Risk']\n)",
"_____no_output_____"
],
[
"published_model_uid = client.repository.get_model_id(published_model)",
"_____no_output_____"
]
],
[
[
"### Deployment creation",
"_____no_output_____"
]
],
[
[
"metadata = {\n client.deployments.ConfigurationMetaNames.NAME: \"Deployment of fairer model\",\n client.deployments.ConfigurationMetaNames.ONLINE: {}\n}\n\ncreated_deployment = client.deployments.create(published_model_uid, meta_props=metadata)",
"\n\n#######################################################################################\n\nSynchronous deployment creation for uid: '4f0eafb3-e057-4af6-afdf-c8a7075c0362' started\n\n#######################################################################################\n\n\ninitializing..........................................................................................................................................\nready\n\n\n------------------------------------------------------------------------------------------------\nSuccessfully finished deployment creation, deployment_uid='a608ee59-63fd-43ed-8eef-940b6c7d8345'\n------------------------------------------------------------------------------------------------\n\n\n"
],
[
"deployment_id = client.deployments.get_uid(created_deployment)",
"_____no_output_____"
]
],
[
[
"#### Deployment scoring ",
"_____no_output_____"
],
[
"You need to pass scoring values as input data if the deployed model. Use `client.deployments.score()` method to get predictions from deployed model. ",
"_____no_output_____"
]
],
[
[
"values = X_test.values\n\nscoring_payload = {\n \"input_data\": [{\n 'values': values[:5]\n }]\n}",
"_____no_output_____"
],
[
"predictions = client.deployments.score(deployment_id, scoring_payload)\npredictions",
"_____no_output_____"
]
],
[
[
"<a id=\"cleanup\"></a>\n## 5. Clean up",
"_____no_output_____"
],
[
"If you want to clean up all created assets:\n- experiments\n- trainings\n- pipelines\n- model definitions\n- models\n- functions\n- deployments\n\nplease follow up this sample [notebook](https://github.com/IBM/watson-machine-learning-samples/blob/master/cloud/notebooks/python_sdk/instance-management/Machine%20Learning%20artifacts%20management.ipynb).",
"_____no_output_____"
],
[
"<a id=\"summary\"></a>\n## 6. Summary and next steps",
"_____no_output_____"
],
[
" You successfully completed this notebook!.\n\nCheck out used packeges domuntations:\n- `ibm-watson-machine-learning` [Online Documentation](https://www.ibm.com/cloud/watson-studio/autoai)\n- `lale`: https://github.com/IBM/lale\n- `aif360`: https://aif360.mybluemix.net/",
"_____no_output_____"
],
[
"### Authors \n\n**Dorota Dydo-Rożniecka**, Intern in Watson Machine Learning at IBM",
"_____no_output_____"
],
[
"Copyright © 2020, 2021 IBM. This notebook and its source code are released under the terms of the MIT License.",
"_____no_output_____"
]
]
] | [
"markdown",
"code",
"markdown",
"code",
"markdown",
"code",
"markdown",
"code",
"markdown",
"code",
"markdown",
"code",
"markdown",
"code",
"markdown",
"code",
"markdown",
"code",
"markdown",
"code",
"markdown",
"code",
"markdown",
"code",
"markdown",
"code",
"markdown",
"code",
"markdown",
"code",
"markdown",
"code",
"markdown",
"raw",
"markdown",
"code",
"markdown",
"code",
"markdown",
"code",
"markdown",
"code",
"markdown",
"code",
"markdown",
"code",
"markdown"
] | [
[
"markdown",
"markdown",
"markdown",
"markdown",
"markdown"
],
[
"code"
],
[
"markdown"
],
[
"code",
"code",
"code"
],
[
"markdown"
],
[
"code",
"code",
"code"
],
[
"markdown"
],
[
"code"
],
[
"markdown",
"markdown"
],
[
"code"
],
[
"markdown"
],
[
"code"
],
[
"markdown"
],
[
"code"
],
[
"markdown"
],
[
"code"
],
[
"markdown"
],
[
"code"
],
[
"markdown"
],
[
"code"
],
[
"markdown"
],
[
"code",
"code",
"code"
],
[
"markdown"
],
[
"code"
],
[
"markdown"
],
[
"code"
],
[
"markdown",
"markdown"
],
[
"code"
],
[
"markdown"
],
[
"code",
"code",
"code",
"code"
],
[
"markdown"
],
[
"code"
],
[
"markdown",
"markdown"
],
[
"raw"
],
[
"markdown",
"markdown",
"markdown"
],
[
"code",
"code",
"code"
],
[
"markdown"
],
[
"code"
],
[
"markdown"
],
[
"code"
],
[
"markdown",
"markdown"
],
[
"code",
"code",
"code"
],
[
"markdown"
],
[
"code",
"code"
],
[
"markdown",
"markdown"
],
[
"code",
"code"
],
[
"markdown",
"markdown",
"markdown",
"markdown",
"markdown",
"markdown"
]
] |
d047125cd3f0f4109b7aec3c216e0045638839fe | 247,143 | ipynb | Jupyter Notebook | clip_gru_recon.ipynb | LCE-UMD/GRU | e4512e779b83413dbd7547896a05a4f83cbf3d4f | [
"MIT"
] | 4 | 2021-04-20T09:24:22.000Z | 2022-03-14T07:47:32.000Z | clip_gru_recon.ipynb | LCE-UMD/GRU | e4512e779b83413dbd7547896a05a4f83cbf3d4f | [
"MIT"
] | null | null | null | clip_gru_recon.ipynb | LCE-UMD/GRU | e4512e779b83413dbd7547896a05a4f83cbf3d4f | [
"MIT"
] | 1 | 2021-10-20T15:21:33.000Z | 2021-10-20T15:21:33.000Z | 52.194931 | 37,366 | 0.597456 | [
[
[
"# Trade-off between classification accuracy and reconstruction error during dimensionality reduction\n\n- Low-dimensional LSTM representations are excellent at dimensionality reduction, but are poor at reconstructing the original data\n- On the other hand, PCs are excellent at reconstructing the original data but these high-variance components do not preserve class information",
"_____no_output_____"
]
],
[
[
"import numpy as np\nimport pandas as pd\nimport scipy as sp\nimport pickle\nimport os\nimport random\nimport sys\n\n# visualizations\nfrom _plotly_future_ import v4_subplots\nimport plotly.offline as py\npy.init_notebook_mode(connected=True)\nimport plotly.graph_objs as go\nimport plotly.subplots as tls\nimport plotly.figure_factory as ff\nimport plotly.io as pio\nimport plotly.express as px\npio.templates.default = 'plotly_white'\npio.orca.config.executable = '/home/joyneelm/fire/bin/orca'\ncolors = px.colors.qualitative.Plotly",
"_____no_output_____"
],
[
"class ARGS():\n roi = 300\n net = 7\n subnet = 'wb'\n train_size = 100\n batch_size = 32\n num_epochs = 50\n zscore = 1\n \n #gru\n k_hidden = 32\n k_layers = 1\n dims = [3, 4, 5, 10]",
"_____no_output_____"
],
[
"args = ARGS()",
"_____no_output_____"
],
[
"def _get_results(k_dim):\n \n RES_DIR = 'results/clip_gru_recon'\n load_path = (RES_DIR + \n '/roi_%d_net_%d' %(args.roi, args.net) + \n '_trainsize_%d' %(args.train_size) +\n '_k_hidden_%d' %(args.k_hidden) +\n '_kdim_%d' %(k_dim) +\n '_k_layers_%d' %(args.k_layers) +\n '_batch_size_%d' %(args.batch_size) +\n '_num_epochs_45' +\n '_z_%d.pkl' %(args.zscore))\n \n with open(load_path, 'rb') as f:\n results = pickle.load(f)\n# print(results.keys())\n return results",
"_____no_output_____"
],
[
"r = {}\nfor k_dim in args.dims:\n r[k_dim] = _get_results(k_dim)",
"_____no_output_____"
],
[
"def _plot_fig(ss):\n \n title_text = ss\n if ss=='var':\n ss = 'mse'\n invert = True\n else:\n invert = False\n \n subplot_titles = ['train', 'test']\n fig = tls.make_subplots(rows=1, \n cols=2, \n subplot_titles=subplot_titles,\n print_grid=False)\n\n for ii, x in enumerate(['train', 'test']):\n gru_score = {'mean':[], 'ste':[]}\n pca_score = {'mean':[], 'ste':[]}\n for k_dim in args.dims:\n\n a = r[k_dim]\n \n # gru decoder\n y = np.mean(a['%s_%s'%(x, ss)])\n gru_score['mean'].append(y)\n \n # pca decoder\n y = np.mean(a['%s_pca_%s'%(x, ss)])\n pca_score['mean'].append(y)\n \n x = np.arange(len(args.dims))\n if invert:\n y = 1 - np.array(gru_score['mean'])\n else:\n y = gru_score['mean']\n error_y = gru_score['ste']\n trace = go.Bar(x=x, y=y,\n name='lstm decoder',\n marker_color=colors[0])\n fig.add_trace(trace, 1, ii+1)\n\n if invert:\n y = 1 - np.array(pca_score['mean'])\n else:\n y = pca_score['mean']\n error_y = pca_score['ste']\n trace = go.Bar(x=x, y=y,\n name='pca recon',\n marker_color=colors[1])\n fig.add_trace(trace, 1, ii+1)\n\n fig.update_xaxes(tickvals=np.arange(len(args.dims)),\n ticktext=args.dims)\n fig.update_layout(height=350, width=700,\n title_text=title_text)\n \n return fig",
"_____no_output_____"
]
],
[
[
"## Mean-squared error vs number of dimensions",
"_____no_output_____"
]
],
[
[
"'''\nmse\n'''\nss = 'mse'\nfig = _plot_fig(ss)\nfig.show()",
"_____no_output_____"
]
],
[
[
"## Variance captured vs number of dimensions",
"_____no_output_____"
]
],
[
[
"'''\nvariance\n'''\nss = 'var'\nfig = _plot_fig(ss)\nfig.show()",
"_____no_output_____"
]
],
[
[
"## R-squared vs number of dimensions",
"_____no_output_____"
]
],
[
[
"'''\nr2\n'''\nss = 'r2'\nfig = _plot_fig(ss)\nfig.show()",
"_____no_output_____"
],
[
"results = r[10]\n\n# variance not captured by pca recon\npca_not = 1 - np.sum(results['pca_var'])\nprint('percent variance captured by pca components = %0.3f' %(1 - pca_not))\n# this is proportional to pca mse\npca_mse = results['test_pca_mse']\n\n# variance not captured by lstm decoder?\nlstm_mse = results['test_mse']\n\nlstm_not = lstm_mse*(pca_not/pca_mse)\nprint('percent variance captured by lstm recon = %0.3f' %(1 - lstm_not))",
"percent variance captured by pca components = 0.611\npercent variance captured by lstm recon = 0.067\n"
],
[
"def _plot_fig_ext(ss):\n \n title_text = ss\n if ss=='var':\n ss = 'mse'\n invert = True\n else:\n invert = False\n \n subplot_titles = ['train', 'test']\n fig = go.Figure()\n\n x = 'test'\n \n lstm_score = {'mean':[], 'ste':[]}\n pca_score = {'mean':[], 'ste':[]}\n lstm_acc = {'mean':[], 'ste':[]}\n pc_acc = {'mean':[], 'ste':[]}\n for k_dim in args.dims:\n\n a = r[k_dim]\n # lstm encoder\n k_sub = len(a['test'])\n y = np.mean(a['test'])\n error_y = 3/np.sqrt(k_sub)*np.std(a['test'])\n lstm_acc['mean'].append(y)\n lstm_acc['ste'].append(error_y)\n\n # lstm decoder\n y = np.mean(a['%s_%s'%(x, ss)])\n lstm_score['mean'].append(y)\n lstm_score['ste'].append(error_y)\n\n # pca encoder\n b = r_pc[k_dim]\n y = np.mean(b['test'])\n error_y = 3/np.sqrt(k_sub)*np.std(b['test'])\n pc_acc['mean'].append(y)\n pc_acc['ste'].append(error_y)\n\n # pca decoder\n y = np.mean(a['%s_pca_%s'%(x, ss)])\n pca_score['mean'].append(y)\n pca_score['ste'].append(error_y)\n\n x = np.arange(len(args.dims))\n\n y = lstm_acc['mean']\n error_y = lstm_acc['ste']\n trace = go.Bar(x=x, y=y,\n name='GRU Accuracy',\n error_y=dict(type='data',\n array=error_y),\n marker_color=colors[3])\n fig.add_trace(trace)\n\n y = pc_acc['mean']\n error_y = pc_acc['ste']\n trace = go.Bar(x=x, y=y,\n name='PCA Accuracy',\n error_y=dict(type='data',\n array=error_y),\n marker_color=colors[4])\n fig.add_trace(trace)\n \n if invert:\n y = 1 - np.array(lstm_score['mean'])\n else:\n y = lstm_score['mean']\n error_y = lstm_score['ste']\n trace = go.Bar(x=x, y=y,\n name='GRU Reconstruction',\n error_y=dict(type='data',\n array=error_y),\n marker_color=colors[5])\n fig.add_trace(trace)\n\n if invert:\n y = 1 - np.array(pca_score['mean'])\n else:\n y = pca_score['mean']\n error_y = pca_score['ste']\n trace = go.Bar(x=x, y=y,\n name='PCA Reconstruction',\n error_y=dict(type='data', \n array=error_y),\n marker_color=colors[2])\n fig.add_trace(trace)\n fig.update_yaxes(title=dict(text='Accuracy or % variance',\n font_size=20),\n gridwidth=1, gridcolor='#bfbfbf',\n tickfont=dict(size=20))\n fig.update_xaxes(title=dict(text='Number of dimensions',\n font_size=20),\n tickvals=np.arange(len(args.dims)),\n ticktext=args.dims,\n tickfont=dict(size=20))\n fig.update_layout(height=470, width=570,\n font_color='black',\n legend_orientation='h',\n legend_font_size=20,\n legend_x=-0.1,\n legend_y=-0.3)\n \n return fig",
"_____no_output_____"
],
[
"def _get_pc_results(PC_DIR, k_dim):\n load_path = (PC_DIR + \n '/roi_%d_net_%d' %(args.roi, args.net) + \n '_nw_%s' %(args.subnet) +\n '_trainsize_%d' %(args.train_size) +\n '_kdim_%d_batch_size_%d' %(k_dim, args.batch_size) +\n '_num_epochs_%d_z_%d.pkl' %(args.num_epochs, args.zscore))\n\n with open(load_path, 'rb') as f:\n results = pickle.load(f)\n print(results.keys()) \n return results",
"_____no_output_____"
]
],
[
[
"## Comparison of LSTM and PCA: classification accuracy and variance captured",
"_____no_output_____"
]
],
[
[
"'''\nvariance\n'''\nr_pc = {}\nPC_DIR = 'results/clip_pca'\nfor k_dim in args.dims:\n r_pc[k_dim] = _get_pc_results(PC_DIR, k_dim)\ncolors = px.colors.qualitative.Set3\n#colors = [\"#D55E00\", \"#009E73\", \"#56B4E9\", \"#E69F00\"]\nss = 'var'\nfig = _plot_fig_ext(ss)\nfig.show()\nfig.write_image('figures/fig3c.png')",
"dict_keys(['train', 'val', 't_train', 't_test', 'test'])\ndict_keys(['train', 'val', 't_train', 't_test', 'test'])\ndict_keys(['train', 'val', 't_train', 't_test', 'test'])\ndict_keys(['train', 'val', 't_train', 't_test', 'test'])\n"
]
]
] | [
"markdown",
"code",
"markdown",
"code",
"markdown",
"code",
"markdown",
"code",
"markdown",
"code"
] | [
[
"markdown"
],
[
"code",
"code",
"code",
"code",
"code",
"code"
],
[
"markdown"
],
[
"code"
],
[
"markdown"
],
[
"code"
],
[
"markdown"
],
[
"code",
"code",
"code",
"code"
],
[
"markdown"
],
[
"code"
]
] |
d0471e8cac331687b661408972f11fc65d4fdfad | 3,893 | ipynb | Jupyter Notebook | 20210519/housing_force00.ipynb | dongxulee/lifeCycle | 2b4a74dbd64357d00b29f7d946a66afcba747cc6 | [
"MIT"
] | null | null | null | 20210519/housing_force00.ipynb | dongxulee/lifeCycle | 2b4a74dbd64357d00b29f7d946a66afcba747cc6 | [
"MIT"
] | null | null | null | 20210519/housing_force00.ipynb | dongxulee/lifeCycle | 2b4a74dbd64357d00b29f7d946a66afcba747cc6 | [
"MIT"
] | null | null | null | 28.007194 | 212 | 0.493964 | [
[
[
"%pylab inline\nfrom jax.scipy.ndimage import map_coordinates\nfrom constant import *\nimport warnings\nfrom jax import jit, partial, vmap\nfrom tqdm import tqdm\nwarnings.filterwarnings(\"ignore\")",
"Populating the interactive namespace from numpy and matplotlib\n"
]
],
[
[
"### State \n$$x = [w,n,m,s,e,o]$$ \n$w$: wealth level size: 20 \n$n$: 401k level size: 10 \n$m$: mortgage level size: 10 \n$s$: economic state size: 8 \n$e$: employment state size: 2 \n$o$: housing state: size: 2 \n\n### Action\n$c$: consumption amount size: 20 \n$b$: bond investment size: 20 \n$k$: stock investment derived from budget constrain once $c$ and $b$ are determined. \n$h$: housing consumption size, related to housing status and consumption level \n\nIf $O = 1$, the agent owns a house: \n$A = [c, b, k, h=H, action = 1]$ sold the house \n$A = [c, b, k, h=H, action = 0]$ keep the house \n\nIf $O = 0$, the agent do not own a house: \n$A = [c, b, k, h= \\frac{c}{\\alpha} \\frac{1-\\alpha}{pr}, action = 0]$ keep renting the house \n$A = [c, b, k, h= \\frac{c}{\\alpha} \\frac{1-\\alpha}{pr}, action = 1]$ buy a housing with H unit \n\n### Housing\n20% down payment of mortgage, fix mortgage rate, single housing unit available, from age between 20 and 50, agents could choose to buy a house, and could choose to sell the house at any moment. $H = 1000$ ",
"_____no_output_____"
]
],
[
[
"%%time\nfor t in tqdm(range(T_max-1,T_min-1, -1)):\n if t == T_max-1:\n v,cbkha = vmap(partial(V,t,Vgrid[:,:,:,:,:,:,t]))(Xs)\n else:\n v,cbkha = vmap(partial(V,t,Vgrid[:,:,:,:,:,:,t+1]))(Xs)\n Vgrid[:,:,:,:,:,:,t] = v.reshape(dim)\n cgrid[:,:,:,:,:,:,t] = cbkha[:,0].reshape(dim)\n bgrid[:,:,:,:,:,:,t] = cbkha[:,1].reshape(dim)\n kgrid[:,:,:,:,:,:,t] = cbkha[:,2].reshape(dim)\n hgrid[:,:,:,:,:,:,t] = cbkha[:,3].reshape(dim)\n agrid[:,:,:,:,:,:,t] = cbkha[:,4].reshape(dim)",
"100%|██████████| 60/60 [54:21<00:00, 54.36s/it]"
],
[
"np.save(\"Value00\",Vgrid)",
"_____no_output_____"
]
]
] | [
"code",
"markdown",
"code"
] | [
[
"code"
],
[
"markdown"
],
[
"code",
"code"
]
] |
d04727137f5545eaf738ec52c2bed14abe325cf4 | 149,045 | ipynb | Jupyter Notebook | notebooks/Quadrature.ipynb | ruboerner/notebooks | 4ae70c4095b583f106ddc214b68908a5b22c69ca | [
"MIT"
] | null | null | null | notebooks/Quadrature.ipynb | ruboerner/notebooks | 4ae70c4095b583f106ddc214b68908a5b22c69ca | [
"MIT"
] | null | null | null | notebooks/Quadrature.ipynb | ruboerner/notebooks | 4ae70c4095b583f106ddc214b68908a5b22c69ca | [
"MIT"
] | null | null | null | 85.168571 | 252 | 0.754832 | [
[
[
"imatlab_export_fig('print-png')",
"_____no_output_____"
]
],
[
[
"# Quadrature rules for 2.5-D resistivity modelling\n\nWe consider the evaluation of the integral\n\n$$\n\\Phi(x, y, z) = \\frac{2}{\\pi} \\int_0^\\infty \\tilde\\Phi(k, y, z) \\cos(k x)\\, dk\n$$\nwhere \n$$\n\\tilde\\Phi(k, y, z) = K_0\\left({k}{\\sqrt{y^2 + z^2}}\\right).\n$$\n\nThe function $\\tilde\\Phi$ exhibits a different asymptotic behaviour depending on the magnitude of the argument, i.e., with $u := kr$\n\n$$\nu\\to 0: K_0(u) \\to -\\ln(u)\n$$\nand\n$$\nu \\to \\infty: K_0(u) \\to \\frac{e^{-u}}{\\sqrt{u}}.\n$$\n\nFor a fixed distance $r = \\sqrt{y^2 + z^2} = 1$ and $10^{-6} \\le k \\le 10^1$, we obtain the following figure:",
"_____no_output_____"
]
],
[
[
"k = logspace(-6, 4, 101);\nkk = 1e-3;\nu = besselk(0, k * kk);\npadln = 65;\npadexp = 15;\nloglog(k, u, 'k', k(1:padln), -log(kk * k(1:padln)), 'r.', ...\n k(end-padexp:end), exp(-kk * k(end-padexp:end))./sqrt(kk * k(end-padexp:end)), 'b.')\n\nlegend('K_0(u)', '-ln(u)', 'exp(-u)/sqrt(u)')\nylabel('\\Phi(u)')\nxlabel('u')",
"_____no_output_____"
]
],
[
[
"We split the integration at $k = k_0$, $0 < k_0 < \\infty$.\nWe obtain\n$$\n\\int_0^\\infty \\tilde\\Phi(k)\\,dk = \\int_0^{k_0}\\tilde\\Phi(k)\\,dk + \\int_{k_0}^\\infty\\tilde\\Phi(k)\\,dk.\n$$\n\n### Gauss-Legendre quadrature\nTo avoid the singularity at $k \\to 0$ for the first integral, we substitute $k'=\\sqrt{k / k_0}$ and obtain with $dk = 2 k_0 k' dk'$\n$$\n\\int_0^{k_0}\\tilde\\Phi(k)\\,dk = \\int_0^1 g(k')\\,dk' \\approx \\sum_{n=1}^N w_n' g(k_n') = \\sum_{n=1}^N w_n \\tilde\\Phi(k_n)\n$$\nwith $w_n = 2 k_0 k_n' w_n' $ and $k_n = k_0 k_n'^2$.\n\n### Gauss-Laguerre quadrature\nFor the second integral, we substitute $k' = k / k_0 - 1$, define $g(k') = k_0 \\tilde\\Phi(k)e^{k'}$, and obtain\n$$\n\\int_{k_0}^\\infty\\tilde\\Phi(k)\\,dk = \\int_0^\\infty e^{-k'} g(k')\\,dk' \\approx \\sum_{n=1}^N w_n' g(k_n') = \\sum_{n=1}^N w_n \\tilde\\Phi(k_n)\n$$\nwith $w_n = k_0 e^{k_n'}w_n'$ and $k_n = k_0 (k_n'+1)$.\n\n### Choice of $k_0$\n\nThe actual value of $k_0$ depends on the smallest electrode spacing $r_{min}$.\nMore precisely, $k_0 = (2 r_{min})^{-1}$.\n\n## Numerical test\n\nIn the case of a point electrode with current $I$ located at $\\mathbf r' = (x', y', 0)^\\top$ at the surface of a homogeneous halfspace with resistivity $\\rho$, we obtain for the electric potential at point $\\mathbf r = (x, y, z)^\\top$\n$$\n\\Phi(\\mathbf r) = \\dfrac{\\rho I}{2 \\pi |\\mathbf r - \\mathbf r'|}.\n$$\n\nWe try to approximate the inverse Cosine transform\n$$\n\\Phi(x, y, z) = \\frac{2}{\\pi} \\int_0^\\infty \\tilde\\Phi(k, y, z) \\cos(k x)\\, dk\n$$\nfor the special case of $x = 0$ ($\\cos(0) = 1$) by means of the Gauss quadrature rules introduced above.\n\nFor the smallest electrode spacing of, e.g., $|\\mathbf r - \\mathbf r'| = r_{min} = 1$ we would set $k_0 = 0.5$.",
"_____no_output_____"
]
],
[
[
"rmin = 1;\nrp = rmin:1:100;\nrp = rp(:);\nk0 = 1 / (2 * rmin);\n[x1, w1] = gauleg(0, 1, 17);\n[x2, w2] = gaulag(7);\nkn1 = k0 * x1 .* x1;\nwn1 = 2 * k0 * x1 .* w1;\nkn2 = k0 * (x2 + 1);\nwn2 = k0 * exp(x2) .* w2;\n\nk = [kn1(:); kn2(:)];\nw = [wn1(:); wn2(:)];",
"_____no_output_____"
]
],
[
[
"We check the validity of the approximation by checking against the analytical solution for the homogeneous halfspace, which, in the case of $\\rho = 2 \\pi$ and $I = 1$, is simply\n$$\n\\Phi_a(r) = \\dfrac{1}{r}.\n$$",
"_____no_output_____"
]
],
[
[
"k(1)",
"\nans =\n\n 1.1103e-05\n\n"
],
[
"v = zeros(length(rp), 1);\nfor i = 1:length(rp)\n v(i) = 2 / pi * sum(w .* besselk(0, k * rp(i)));\nend\n\nplot(rp, v, 'r.-', rp, 1 ./ rp, 'b')\nxlabel('r in m')\nylabel('potential in V')\nlegend('transformed', 'analytical')",
"_____no_output_____"
]
],
[
[
"In the following plot, we display the relative error of the approximation\n$$\ne(r) := \\left(1 - \\dfrac{\\Phi(r)}{\\Phi_a(r)}\\right) \\cdot 100 \\%\n$$\nwith respect to the (normalized) electrode distance.",
"_____no_output_____"
]
],
[
[
"plot(rp / rmin, 100 * (1 - v .* rp), '.-');\ngrid();\nxlabel('r / r_{min}');\nylabel('rel. error in %');\nylim([-0.05 0.05])",
"_____no_output_____"
]
]
] | [
"code",
"markdown",
"code",
"markdown",
"code",
"markdown",
"code",
"markdown",
"code"
] | [
[
"code"
],
[
"markdown"
],
[
"code"
],
[
"markdown"
],
[
"code"
],
[
"markdown"
],
[
"code",
"code"
],
[
"markdown"
],
[
"code"
]
] |
d04736e8657798bb5a3a80f9d5c88f43c763945d | 471,902 | ipynb | Jupyter Notebook | _docs/nbs/T726861-Introduction-to-Gym-toolkit.ipynb | sparsh-ai/recohut | 4121f665761ffe38c9b6337eaa9293b26bee2376 | [
"Apache-2.0"
] | null | null | null | _docs/nbs/T726861-Introduction-to-Gym-toolkit.ipynb | sparsh-ai/recohut | 4121f665761ffe38c9b6337eaa9293b26bee2376 | [
"Apache-2.0"
] | 1 | 2022-01-12T05:40:57.000Z | 2022-01-12T05:40:57.000Z | _docs/nbs/T726861-Introduction-to-Gym-toolkit.ipynb | RecoHut-Projects/recohut | 4121f665761ffe38c9b6337eaa9293b26bee2376 | [
"Apache-2.0"
] | null | null | null | 471,902 | 471,902 | 0.929354 | [
[
[
"# Introduction to Gym toolkit",
"_____no_output_____"
],
[
"## Gym Environments\n\nThe centerpiece of Gym is the environment, which defines the \"game\" in which your reinforcement algorithm will compete. An environment does not need to be a game; however, it describes the following game-like features:\n* **action space**: What actions can we take on the environment, at each step/episode, to alter the environment.\n* **observation space**: What is the current state of the portion of the environment that we can observe. Usually, we can see the entire environment.\n\nBefore we begin to look at Gym, it is essential to understand some of the terminology used by this library.\n\n* **Agent** - The machine learning program or model that controls the actions.\nStep - One round of issuing actions that affect the observation space.\n* **Episode** - A collection of steps that terminates when the agent fails to meet the environment's objective, or the episode reaches the maximum number of allowed steps.\n* **Render** - Gym can render one frame for display after each episode.\n* **Reward** - A positive reinforcement that can occur at the end of each episode, after the agent acts.\n* **Nondeterministic** - For some environments, randomness is a factor in deciding what effects actions have on reward and changes to the observation space.",
"_____no_output_____"
]
],
[
[
"import gym\n\ndef query_environment(name):\n env = gym.make(name)\n spec = gym.spec(name)\n print(f\"Action Space: {env.action_space}\")\n print(f\"Observation Space: {env.observation_space}\")\n print(f\"Max Episode Steps: {spec.max_episode_steps}\")\n print(f\"Nondeterministic: {spec.nondeterministic}\")\n print(f\"Reward Range: {env.reward_range}\")\n print(f\"Reward Threshold: {spec.reward_threshold}\")",
"_____no_output_____"
],
[
"query_environment(\"CartPole-v1\")",
"Action Space: Discrete(2)\nObservation Space: Box(-3.4028234663852886e+38, 3.4028234663852886e+38, (4,), float32)\nMax Episode Steps: 500\nNondeterministic: False\nReward Range: (-inf, inf)\nReward Threshold: 475.0\n"
]
],
[
[
"The CartPole-v1 environment challenges the agent to move a cart while keeping a pole balanced. The environment has an observation space of 4 continuous numbers:\n\n* Cart Position\n* Cart Velocity\n* Pole Angle\n* Pole Velocity At Tip\n\nTo achieve this goal, the agent can take the following actions:\n\n* Push cart to the left\n* Push cart to the right\n\nThere is also a continuous variant of the mountain car. This version does not simply have the motor on or off. For the continuous car the action space is a single floating point number that specifies how much forward or backward force is being applied.",
"_____no_output_____"
],
[
"### Simple",
"_____no_output_____"
]
],
[
[
"import random\nfrom typing import List\n\n\nclass Environment:\n def __init__(self):\n self.steps_left = 10\n\n def get_observation(self) -> List[float]:\n return [0.0, 0.0, 0.0]\n\n def get_actions(self) -> List[int]:\n return [0, 1]\n\n def is_done(self) -> bool:\n return self.steps_left == 0\n\n def action(self, action: int) -> float:\n if self.is_done():\n raise Exception(\"Game is over\")\n self.steps_left -= 1\n return random.random()\n\n\nclass Agent:\n def __init__(self):\n self.total_reward = 0.0\n\n def step(self, env: Environment):\n current_obs = env.get_observation()\n actions = env.get_actions()\n reward = env.action(random.choice(actions))\n self.total_reward += reward\n\n\nif __name__ == \"__main__\":\n env = Environment()\n agent = Agent()\n\n while not env.is_done():\n agent.step(env)\n\n print(\"Total reward got: %.4f\" % agent.total_reward)",
"Total reward got: 4.6979\n"
]
],
[
[
"### Frozenlake",
"_____no_output_____"
]
],
[
[
"import gym",
"_____no_output_____"
],
[
"env = gym.make(\"FrozenLake-v0\")",
"_____no_output_____"
],
[
"env.render()",
"\n\u001b[41mS\u001b[0mFFF\nFHFH\nFFFH\nHFFG\n"
],
[
"print(env.observation_space)",
"Discrete(16)\n"
],
[
"print(env.action_space)",
"Discrete(4)\n"
]
],
[
[
"| Number | Action |\n| ------ | ------ |\n| 0 | Left |\n| 1 | Down |\n| 2 | Right |\n| 3 | Up |",
"_____no_output_____"
],
[
"We can obtain the transition probability and the reward function by just typing env.P[state][action]. So, to obtain the transition probability of moving from state S to the other states by performing the action right, we can type env.P[S][right]. But we cannot just type state S and action right directly since they are encoded as numbers. We learned that state S is encoded as 0 and the action right is encoded as 2, so, to obtain the transition probability of state S by performing the action right, we type env.P[0][2]",
"_____no_output_____"
]
],
[
[
"print(env.P[0][2])",
"[(0.3333333333333333, 4, 0.0, False), (0.3333333333333333, 1, 0.0, False), (0.3333333333333333, 0, 0.0, False)]\n"
]
],
[
[
"Our output is in the form of [(transition probability, next state, reward, Is terminal state?)]",
"_____no_output_____"
]
],
[
[
"state = env.reset()",
"_____no_output_____"
],
[
"env.step(1)",
"_____no_output_____"
],
[
"(next_state, reward, done, info) = env.step(1)",
"_____no_output_____"
]
],
[
[
"- **next_state** represents the next state.\n- **reward** represents the obtained reward.\n- **done** implies whether our episode has ended. That is, if the next state is a terminal state, then our episode will end, so done will be marked as True else it will be marked as False.\n- **info** — Apart from the transition probability, in some cases, we also obtain other information saved as info, which is used for debugging purposes.",
"_____no_output_____"
]
],
[
[
"random_action = env.action_space.sample()",
"_____no_output_____"
],
[
"next_state, reward, done, info = env.step(random_action)",
"_____no_output_____"
]
],
[
[
"**Generating an episode**\nThe episode is the agent environment interaction starting from the initial state to the terminal state. The agent interacts with the environment by performing some action in each state. An episode ends if the agent reaches the terminal state. So, in the Frozen Lake environment, the episode will end if the agent reaches the terminal state, which is either the hole state (H) or goal state (G).",
"_____no_output_____"
]
],
[
[
"import gym\n\nenv = gym.make(\"FrozenLake-v0\")\nstate = env.reset()\nprint('Time Step 0 :')\nenv.render()\nnum_timesteps = 20\n\nfor t in range(num_timesteps):\n random_action = env.action_space.sample()\n new_state, reward, done, info = env.step(random_action)\n print ('Time Step {} :'.format(t+1))\n env.render()\n if done:\n break",
"Time Step 0 :\n\n\u001b[41mS\u001b[0mFFF\nFHFH\nFFFH\nHFFG\nTime Step 1 :\n (Right)\n\u001b[41mS\u001b[0mFFF\nFHFH\nFFFH\nHFFG\nTime Step 2 :\n (Right)\nSFFF\n\u001b[41mF\u001b[0mHFH\nFFFH\nHFFG\nTime Step 3 :\n (Left)\nSFFF\nFHFH\n\u001b[41mF\u001b[0mFFH\nHFFG\nTime Step 4 :\n (Right)\nSFFF\n\u001b[41mF\u001b[0mHFH\nFFFH\nHFFG\nTime Step 5 :\n (Up)\nSFFF\nF\u001b[41mH\u001b[0mFH\nFFFH\nHFFG\n"
]
],
[
[
"Instead of generating one episode, we can also generate a series of episodes by taking some random action in each state",
"_____no_output_____"
]
],
[
[
"import gym\nenv = gym.make(\"FrozenLake-v0\")\nnum_episodes = 10\nnum_timesteps = 20 \nfor i in range(num_episodes):\n \n state = env.reset()\n print('Time Step 0 :')\n env.render()\n \n for t in range(num_timesteps):\n random_action = env.action_space.sample()\n new_state, reward, done, info = env.step(random_action)\n print ('Time Step {} :'.format(t+1))\n env.render()\n if done:\n break",
"_____no_output_____"
]
],
[
[
"### Cartpole",
"_____no_output_____"
]
],
[
[
"env = gym.make(\"CartPole-v0\")",
"_____no_output_____"
],
[
"print(env.observation_space)",
"Box(-3.4028234663852886e+38, 3.4028234663852886e+38, (4,), float32)\n"
]
],
[
[
"Note that all of these values are continuous, that is:\n\n- The value of the cart position ranges from -4.8 to 4.8.\n- The value of the cart velocity ranges from -Inf to Inf ( to ).\n- The value of the pole angle ranges from -0.418 radians to 0.418 radians.\n- The value of the pole velocity at the tip ranges from -Inf to Inf.",
"_____no_output_____"
]
],
[
[
"print(env.reset())",
"[-0.03805974 -0.00851157 -0.00346854 -0.03263184]\n"
],
[
"print(env.observation_space.high)",
"[4.8000002e+00 3.4028235e+38 4.1887903e-01 3.4028235e+38]\n"
]
],
[
[
"It implies that:\n\n1. The maximum value of the cart position is 4.8.\n2. We learned that the maximum value of the cart velocity is +Inf, and we know that infinity is not really a number, so it is represented using the largest positive real value 3.4028235e+38.\n3. The maximum value of the pole angle is 0.418 radians.\n4. The maximum value of the pole velocity at the tip is +Inf, so it is represented using the largest positive real value 3.4028235e+38.",
"_____no_output_____"
]
],
[
[
"print(env.observation_space.low)",
"[-4.8000002e+00 -3.4028235e+38 -4.1887903e-01 -3.4028235e+38]\n"
]
],
[
[
"It states that:\n\n1. The minimum value of the cart position is -4.8.\n2. We learned that the minimum value of the cart velocity is -Inf, and we know that infinity is not really a number, so it is represented using the largest negative real value -3.4028235e+38.\n3. The minimum value of the pole angle is -0.418 radians.\n4. The minimum value of the pole velocity at the tip is -Inf, so it is represented using the largest negative real value -3.4028235e+38.",
"_____no_output_____"
]
],
[
[
"print(env.action_space)",
"Discrete(2)\n"
]
],
[
[
"| Number | Action |\n| ------ | ------ |\n| 0 | Push cart to the left |\n| 1 | Push cart to the right |",
"_____no_output_____"
]
],
[
[
"import gym\n\n\nif __name__ == \"__main__\":\n env = gym.make(\"CartPole-v0\")\n\n total_reward = 0.0\n total_steps = 0\n obs = env.reset()\n\n while True:\n action = env.action_space.sample()\n obs, reward, done, _ = env.step(action)\n total_reward += reward\n total_steps += 1\n if done:\n break\n\n print(\"Episode done in %d steps, total reward %.2f\" % (\n total_steps, total_reward))",
"Episode done in 20 steps, total reward 20.00\n"
]
],
[
[
"## Wrappers\n\nVery frequently, you will want to extend the environment's functionality in some generic way. For example, imagine an environment gives you some observations, but you want to accumulate them in some buffer and provide to the agent the N last observations. This is a common scenario for dynamic computer games, when one single frame is just not enough to get the full information about the game state. Another example is when you want to be able to crop or preprocess an image's pixels to make it more convenient for the agent to digest, or if you want to normalize reward scores somehow. There are many such situations that have the same structure – you want to \"wrap\" the existing environment and add some extra logic for doing something. Gym provides a convenient framework for these situations – the Wrapper class.",
"_____no_output_____"
],
[
"**Random action wrapper**",
"_____no_output_____"
]
],
[
[
"import gym\nfrom typing import TypeVar\nimport random\n\nAction = TypeVar('Action')\n\n\nclass RandomActionWrapper(gym.ActionWrapper):\n def __init__(self, env, epsilon=0.1):\n super(RandomActionWrapper, self).__init__(env)\n self.epsilon = epsilon\n\n def action(self, action: Action) -> Action:\n if random.random() < self.epsilon:\n print(\"Random!\")\n return self.env.action_space.sample()\n return action\n\n\nif __name__ == \"__main__\":\n env = RandomActionWrapper(gym.make(\"CartPole-v0\"))\n\n obs = env.reset()\n total_reward = 0.0\n\n while True:\n obs, reward, done, _ = env.step(0)\n total_reward += reward\n if done:\n break\n\n print(\"Reward got: %.2f\" % total_reward)",
"Reward got: 9.00\n"
]
],
[
[
"## Atari GAN",
"_____no_output_____"
]
],
[
[
"! wget http://www.atarimania.com/roms/Roms.rar\n! mkdir /content/ROM/\n! unrar e /content/Roms.rar /content/ROM/\n! python -m atari_py.import_roms /content/ROM/",
"_____no_output_____"
]
],
[
[
"### Normal",
"_____no_output_____"
]
],
[
[
"import random\nimport argparse\nimport cv2\n\nimport torch\nimport torch.nn as nn\nimport torch.optim as optim\nfrom torch.utils.tensorboard import SummaryWriter\n\nimport torchvision.utils as vutils\n\nimport gym\nimport gym.spaces\n\nimport numpy as np\n\nlog = gym.logger\nlog.set_level(gym.logger.INFO)\n\nLATENT_VECTOR_SIZE = 100\nDISCR_FILTERS = 64\nGENER_FILTERS = 64\nBATCH_SIZE = 16\n\n# dimension input image will be rescaled\nIMAGE_SIZE = 64\n\nLEARNING_RATE = 0.0001\nREPORT_EVERY_ITER = 100\nSAVE_IMAGE_EVERY_ITER = 1000\n\n\nclass InputWrapper(gym.ObservationWrapper):\n \"\"\"\n Preprocessing of input numpy array:\n 1. resize image into predefined size\n 2. move color channel axis to a first place\n \"\"\"\n def __init__(self, *args):\n super(InputWrapper, self).__init__(*args)\n assert isinstance(self.observation_space, gym.spaces.Box)\n old_space = self.observation_space\n self.observation_space = gym.spaces.Box(\n self.observation(old_space.low),\n self.observation(old_space.high),\n dtype=np.float32)\n\n def observation(self, observation):\n # resize image\n new_obs = cv2.resize(\n observation, (IMAGE_SIZE, IMAGE_SIZE))\n # transform (210, 160, 3) -> (3, 210, 160)\n new_obs = np.moveaxis(new_obs, 2, 0)\n return new_obs.astype(np.float32)\n\n\nclass Discriminator(nn.Module):\n def __init__(self, input_shape):\n super(Discriminator, self).__init__()\n # this pipe converges image into the single number\n self.conv_pipe = nn.Sequential(\n nn.Conv2d(in_channels=input_shape[0], out_channels=DISCR_FILTERS,\n kernel_size=4, stride=2, padding=1),\n nn.ReLU(),\n nn.Conv2d(in_channels=DISCR_FILTERS, out_channels=DISCR_FILTERS*2,\n kernel_size=4, stride=2, padding=1),\n nn.BatchNorm2d(DISCR_FILTERS*2),\n nn.ReLU(),\n nn.Conv2d(in_channels=DISCR_FILTERS * 2, out_channels=DISCR_FILTERS * 4,\n kernel_size=4, stride=2, padding=1),\n nn.BatchNorm2d(DISCR_FILTERS * 4),\n nn.ReLU(),\n nn.Conv2d(in_channels=DISCR_FILTERS * 4, out_channels=DISCR_FILTERS * 8,\n kernel_size=4, stride=2, padding=1),\n nn.BatchNorm2d(DISCR_FILTERS * 8),\n nn.ReLU(),\n nn.Conv2d(in_channels=DISCR_FILTERS * 8, out_channels=1,\n kernel_size=4, stride=1, padding=0),\n nn.Sigmoid()\n )\n\n def forward(self, x):\n conv_out = self.conv_pipe(x)\n return conv_out.view(-1, 1).squeeze(dim=1)\n\n\nclass Generator(nn.Module):\n def __init__(self, output_shape):\n super(Generator, self).__init__()\n # pipe deconvolves input vector into (3, 64, 64) image\n self.pipe = nn.Sequential(\n nn.ConvTranspose2d(in_channels=LATENT_VECTOR_SIZE, out_channels=GENER_FILTERS * 8,\n kernel_size=4, stride=1, padding=0),\n nn.BatchNorm2d(GENER_FILTERS * 8),\n nn.ReLU(),\n nn.ConvTranspose2d(in_channels=GENER_FILTERS * 8, out_channels=GENER_FILTERS * 4,\n kernel_size=4, stride=2, padding=1),\n nn.BatchNorm2d(GENER_FILTERS * 4),\n nn.ReLU(),\n nn.ConvTranspose2d(in_channels=GENER_FILTERS * 4, out_channels=GENER_FILTERS * 2,\n kernel_size=4, stride=2, padding=1),\n nn.BatchNorm2d(GENER_FILTERS * 2),\n nn.ReLU(),\n nn.ConvTranspose2d(in_channels=GENER_FILTERS * 2, out_channels=GENER_FILTERS,\n kernel_size=4, stride=2, padding=1),\n nn.BatchNorm2d(GENER_FILTERS),\n nn.ReLU(),\n nn.ConvTranspose2d(in_channels=GENER_FILTERS, out_channels=output_shape[0],\n kernel_size=4, stride=2, padding=1),\n nn.Tanh()\n )\n\n def forward(self, x):\n return self.pipe(x)\n\n\ndef iterate_batches(envs, batch_size=BATCH_SIZE):\n batch = [e.reset() for e in envs]\n env_gen = iter(lambda: random.choice(envs), None)\n\n while True:\n e = next(env_gen)\n obs, reward, is_done, _ = e.step(e.action_space.sample())\n if np.mean(obs) > 0.01:\n batch.append(obs)\n if len(batch) == batch_size:\n # Normalising input between -1 to 1\n batch_np = np.array(batch, dtype=np.float32) * 2.0 / 255.0 - 1.0\n yield torch.tensor(batch_np)\n batch.clear()\n if is_done:\n e.reset()\n\n\nif __name__ == \"__main__\":\n parser = argparse.ArgumentParser()\n parser.add_argument(\n \"--cuda\", default=False, action='store_true',\n help=\"Enable cuda computation\")\n args = parser.parse_args(args={})\n\n device = torch.device(\"cuda\" if args.cuda else \"cpu\")\n envs = [\n InputWrapper(gym.make(name))\n for name in ('Breakout-v0', 'AirRaid-v0', 'Pong-v0')\n ]\n input_shape = envs[0].observation_space.shape\n\n net_discr = Discriminator(input_shape=input_shape).to(device)\n net_gener = Generator(output_shape=input_shape).to(device)\n\n objective = nn.BCELoss()\n gen_optimizer = optim.Adam(\n params=net_gener.parameters(), lr=LEARNING_RATE,\n betas=(0.5, 0.999))\n dis_optimizer = optim.Adam(\n params=net_discr.parameters(), lr=LEARNING_RATE,\n betas=(0.5, 0.999))\n writer = SummaryWriter()\n\n gen_losses = []\n dis_losses = []\n iter_no = 0\n\n true_labels_v = torch.ones(BATCH_SIZE, device=device)\n fake_labels_v = torch.zeros(BATCH_SIZE, device=device)\n\n for batch_v in iterate_batches(envs):\n # fake samples, input is 4D: batch, filters, x, y\n gen_input_v = torch.FloatTensor(\n BATCH_SIZE, LATENT_VECTOR_SIZE, 1, 1)\n gen_input_v.normal_(0, 1)\n gen_input_v = gen_input_v.to(device)\n batch_v = batch_v.to(device)\n gen_output_v = net_gener(gen_input_v)\n\n # train discriminator\n dis_optimizer.zero_grad()\n dis_output_true_v = net_discr(batch_v)\n dis_output_fake_v = net_discr(gen_output_v.detach())\n dis_loss = objective(dis_output_true_v, true_labels_v) + \\\n objective(dis_output_fake_v, fake_labels_v)\n dis_loss.backward()\n dis_optimizer.step()\n dis_losses.append(dis_loss.item())\n\n # train generator\n gen_optimizer.zero_grad()\n dis_output_v = net_discr(gen_output_v)\n gen_loss_v = objective(dis_output_v, true_labels_v)\n gen_loss_v.backward()\n gen_optimizer.step()\n gen_losses.append(gen_loss_v.item())\n\n iter_no += 1\n if iter_no % REPORT_EVERY_ITER == 0:\n log.info(\"Iter %d: gen_loss=%.3e, dis_loss=%.3e\",\n iter_no, np.mean(gen_losses),\n np.mean(dis_losses))\n writer.add_scalar(\n \"gen_loss\", np.mean(gen_losses), iter_no)\n writer.add_scalar(\n \"dis_loss\", np.mean(dis_losses), iter_no)\n gen_losses = []\n dis_losses = []\n if iter_no % SAVE_IMAGE_EVERY_ITER == 0:\n writer.add_image(\"fake\", vutils.make_grid(\n gen_output_v.data[:64], normalize=True), iter_no)\n writer.add_image(\"real\", vutils.make_grid(\n batch_v.data[:64], normalize=True), iter_no)",
"INFO: Making new env: Breakout-v0\nINFO: Making new env: AirRaid-v0\nINFO: Making new env: Pong-v0\nINFO: Iter 100: gen_loss=5.454e+00, dis_loss=5.009e-02\nINFO: Iter 200: gen_loss=7.054e+00, dis_loss=4.306e-03\nINFO: Iter 300: gen_loss=7.568e+00, dis_loss=2.140e-03\nINFO: Iter 400: gen_loss=7.842e+00, dis_loss=1.272e-03\nINFO: Iter 500: gen_loss=8.155e+00, dis_loss=1.019e-03\nINFO: Iter 600: gen_loss=8.442e+00, dis_loss=6.918e-04\nINFO: Iter 700: gen_loss=8.560e+00, dis_loss=5.483e-04\nINFO: Iter 800: gen_loss=9.014e+00, dis_loss=4.792e-04\nINFO: Iter 900: gen_loss=7.517e+00, dis_loss=2.132e-01\nINFO: Iter 1000: gen_loss=7.375e+00, dis_loss=1.050e-01\nINFO: Iter 1100: gen_loss=6.722e+00, dis_loss=1.718e-02\nINFO: Iter 1200: gen_loss=6.346e+00, dis_loss=6.303e-03\nINFO: Iter 1300: gen_loss=6.636e+00, dis_loss=6.348e-03\nINFO: Iter 1400: gen_loss=6.612e+00, dis_loss=7.664e-02\nINFO: Iter 1500: gen_loss=6.028e+00, dis_loss=7.801e-03\nINFO: Iter 1600: gen_loss=6.665e+00, dis_loss=3.651e-03\nINFO: Iter 1700: gen_loss=7.290e+00, dis_loss=5.616e-02\nINFO: Iter 1800: gen_loss=6.314e+00, dis_loss=7.723e-02\nINFO: Iter 1900: gen_loss=5.940e+00, dis_loss=3.784e-01\nINFO: Iter 2000: gen_loss=5.053e+00, dis_loss=2.623e-01\nINFO: Iter 2100: gen_loss=5.465e+00, dis_loss=9.114e-02\nINFO: Iter 2200: gen_loss=5.480e+00, dis_loss=3.963e-01\nINFO: Iter 2300: gen_loss=4.549e+00, dis_loss=2.361e-01\nINFO: Iter 2400: gen_loss=5.407e+00, dis_loss=1.310e-01\nINFO: Iter 2500: gen_loss=5.766e+00, dis_loss=5.550e-02\nINFO: Iter 2600: gen_loss=5.816e+00, dis_loss=1.418e-01\nINFO: Iter 2700: gen_loss=6.737e+00, dis_loss=5.231e-02\nINFO: Iter 2800: gen_loss=7.147e+00, dis_loss=1.491e-01\nINFO: Iter 2900: gen_loss=6.541e+00, dis_loss=2.155e-02\nINFO: Iter 3000: gen_loss=7.072e+00, dis_loss=1.127e-01\nINFO: Iter 3100: gen_loss=6.137e+00, dis_loss=6.138e-02\nINFO: Iter 3200: gen_loss=7.406e+00, dis_loss=3.540e-02\nINFO: Iter 3300: gen_loss=7.850e+00, dis_loss=5.691e-03\nINFO: Iter 3400: gen_loss=8.614e+00, dis_loss=7.228e-03\nINFO: Iter 3500: gen_loss=8.885e+00, dis_loss=3.191e-03\nINFO: Iter 3600: gen_loss=5.367e+00, dis_loss=5.296e-01\nINFO: Iter 3700: gen_loss=4.176e+00, dis_loss=3.335e-01\nINFO: Iter 3800: gen_loss=5.174e+00, dis_loss=2.732e-01\nINFO: Iter 3900: gen_loss=5.492e+00, dis_loss=1.298e-01\nINFO: Iter 4000: gen_loss=6.570e+00, dis_loss=1.961e-02\nINFO: Iter 4100: gen_loss=7.011e+00, dis_loss=2.517e-02\nINFO: Iter 4200: gen_loss=8.362e+00, dis_loss=4.330e-03\nINFO: Iter 4300: gen_loss=6.908e+00, dis_loss=2.161e-01\nINFO: Iter 4400: gen_loss=5.226e+00, dis_loss=2.762e-01\nINFO: Iter 4500: gen_loss=4.998e+00, dis_loss=2.893e-01\nINFO: Iter 4600: gen_loss=5.078e+00, dis_loss=3.962e-01\nINFO: Iter 4700: gen_loss=4.886e+00, dis_loss=1.932e-01\nINFO: Iter 4800: gen_loss=6.110e+00, dis_loss=7.615e-02\nINFO: Iter 4900: gen_loss=5.402e+00, dis_loss=1.634e-01\nINFO: Iter 5000: gen_loss=5.336e+00, dis_loss=1.919e-01\nINFO: Iter 5100: gen_loss=5.749e+00, dis_loss=8.817e-02\nINFO: Iter 5200: gen_loss=5.879e+00, dis_loss=1.182e-01\nINFO: Iter 5300: gen_loss=5.417e+00, dis_loss=1.651e-01\nINFO: Iter 5400: gen_loss=6.747e+00, dis_loss=3.846e-02\nINFO: Iter 5500: gen_loss=5.133e+00, dis_loss=1.996e-01\nINFO: Iter 5600: gen_loss=6.116e+00, dis_loss=2.946e-01\nINFO: Iter 5700: gen_loss=5.858e+00, dis_loss=2.152e-02\n"
]
],
[
[
"### Ignite",
"_____no_output_____"
]
],
[
[
"import random\nimport argparse\nimport cv2\n\nimport torch\nimport torch.nn as nn\nimport torch.optim as optim\nfrom ignite.engine import Engine, Events\nfrom ignite.metrics import RunningAverage\nfrom ignite.contrib.handlers import tensorboard_logger as tb_logger\n\nimport torchvision.utils as vutils\n\nimport gym\nimport gym.spaces\n\nimport numpy as np\n\nlog = gym.logger\nlog.set_level(gym.logger.INFO)\n\nLATENT_VECTOR_SIZE = 100\nDISCR_FILTERS = 64\nGENER_FILTERS = 64\nBATCH_SIZE = 16\n\n# dimension input image will be rescaled\nIMAGE_SIZE = 64\n\nLEARNING_RATE = 0.0001\nREPORT_EVERY_ITER = 100\nSAVE_IMAGE_EVERY_ITER = 1000\n\n\nclass InputWrapper(gym.ObservationWrapper):\n \"\"\"\n Preprocessing of input numpy array:\n 1. resize image into predefined size\n 2. move color channel axis to a first place\n \"\"\"\n def __init__(self, *args):\n super(InputWrapper, self).__init__(*args)\n assert isinstance(self.observation_space, gym.spaces.Box)\n old_space = self.observation_space\n self.observation_space = gym.spaces.Box(self.observation(old_space.low), self.observation(old_space.high),\n dtype=np.float32)\n\n def observation(self, observation):\n # resize image\n new_obs = cv2.resize(observation, (IMAGE_SIZE, IMAGE_SIZE))\n # transform (210, 160, 3) -> (3, 210, 160)\n new_obs = np.moveaxis(new_obs, 2, 0)\n return new_obs.astype(np.float32)\n\n\nclass Discriminator(nn.Module):\n def __init__(self, input_shape):\n super(Discriminator, self).__init__()\n # this pipe converges image into the single number\n self.conv_pipe = nn.Sequential(\n nn.Conv2d(in_channels=input_shape[0], out_channels=DISCR_FILTERS,\n kernel_size=4, stride=2, padding=1),\n nn.ReLU(),\n nn.Conv2d(in_channels=DISCR_FILTERS, out_channels=DISCR_FILTERS*2,\n kernel_size=4, stride=2, padding=1),\n nn.BatchNorm2d(DISCR_FILTERS*2),\n nn.ReLU(),\n nn.Conv2d(in_channels=DISCR_FILTERS * 2, out_channels=DISCR_FILTERS * 4,\n kernel_size=4, stride=2, padding=1),\n nn.BatchNorm2d(DISCR_FILTERS * 4),\n nn.ReLU(),\n nn.Conv2d(in_channels=DISCR_FILTERS * 4, out_channels=DISCR_FILTERS * 8,\n kernel_size=4, stride=2, padding=1),\n nn.BatchNorm2d(DISCR_FILTERS * 8),\n nn.ReLU(),\n nn.Conv2d(in_channels=DISCR_FILTERS * 8, out_channels=1,\n kernel_size=4, stride=1, padding=0),\n nn.Sigmoid()\n )\n\n def forward(self, x):\n conv_out = self.conv_pipe(x)\n return conv_out.view(-1, 1).squeeze(dim=1)\n\n\nclass Generator(nn.Module):\n def __init__(self, output_shape):\n super(Generator, self).__init__()\n # pipe deconvolves input vector into (3, 64, 64) image\n self.pipe = nn.Sequential(\n nn.ConvTranspose2d(in_channels=LATENT_VECTOR_SIZE, out_channels=GENER_FILTERS * 8,\n kernel_size=4, stride=1, padding=0),\n nn.BatchNorm2d(GENER_FILTERS * 8),\n nn.ReLU(),\n nn.ConvTranspose2d(in_channels=GENER_FILTERS * 8, out_channels=GENER_FILTERS * 4,\n kernel_size=4, stride=2, padding=1),\n nn.BatchNorm2d(GENER_FILTERS * 4),\n nn.ReLU(),\n nn.ConvTranspose2d(in_channels=GENER_FILTERS * 4, out_channels=GENER_FILTERS * 2,\n kernel_size=4, stride=2, padding=1),\n nn.BatchNorm2d(GENER_FILTERS * 2),\n nn.ReLU(),\n nn.ConvTranspose2d(in_channels=GENER_FILTERS * 2, out_channels=GENER_FILTERS,\n kernel_size=4, stride=2, padding=1),\n nn.BatchNorm2d(GENER_FILTERS),\n nn.ReLU(),\n nn.ConvTranspose2d(in_channels=GENER_FILTERS, out_channels=output_shape[0],\n kernel_size=4, stride=2, padding=1),\n nn.Tanh()\n )\n\n def forward(self, x):\n return self.pipe(x)\n\n\ndef iterate_batches(envs, batch_size=BATCH_SIZE):\n batch = [e.reset() for e in envs]\n env_gen = iter(lambda: random.choice(envs), None)\n\n while True:\n e = next(env_gen)\n obs, reward, is_done, _ = e.step(e.action_space.sample())\n if np.mean(obs) > 0.01:\n batch.append(obs)\n if len(batch) == batch_size:\n # Normalising input between -1 to 1\n batch_np = np.array(batch, dtype=np.float32) * 2.0 / 255.0 - 1.0\n yield torch.tensor(batch_np)\n batch.clear()\n if is_done:\n e.reset()\n\n\nif __name__ == \"__main__\":\n parser = argparse.ArgumentParser()\n parser.add_argument(\"--cuda\", default=False, action='store_true', help=\"Enable cuda computation\")\n args = parser.parse_args(args={})\n\n device = torch.device(\"cuda\" if args.cuda else \"cpu\")\n # envs = [InputWrapper(gym.make(name)) for name in ('Breakout-v0', 'AirRaid-v0', 'Pong-v0')]\n envs = [InputWrapper(gym.make(name)) for name in ['Breakout-v0']]\n input_shape = envs[0].observation_space.shape\n\n net_discr = Discriminator(input_shape=input_shape).to(device)\n net_gener = Generator(output_shape=input_shape).to(device)\n\n objective = nn.BCELoss()\n gen_optimizer = optim.Adam(params=net_gener.parameters(), lr=LEARNING_RATE, betas=(0.5, 0.999))\n dis_optimizer = optim.Adam(params=net_discr.parameters(), lr=LEARNING_RATE, betas=(0.5, 0.999))\n\n true_labels_v = torch.ones(BATCH_SIZE, device=device)\n fake_labels_v = torch.zeros(BATCH_SIZE, device=device)\n\n def process_batch(trainer, batch):\n gen_input_v = torch.FloatTensor(\n BATCH_SIZE, LATENT_VECTOR_SIZE, 1, 1)\n gen_input_v.normal_(0, 1)\n gen_input_v = gen_input_v.to(device)\n batch_v = batch.to(device)\n gen_output_v = net_gener(gen_input_v)\n\n # train discriminator\n dis_optimizer.zero_grad()\n dis_output_true_v = net_discr(batch_v)\n dis_output_fake_v = net_discr(gen_output_v.detach())\n dis_loss = objective(dis_output_true_v, true_labels_v) + \\\n objective(dis_output_fake_v, fake_labels_v)\n dis_loss.backward()\n dis_optimizer.step()\n\n # train generator\n gen_optimizer.zero_grad()\n dis_output_v = net_discr(gen_output_v)\n gen_loss = objective(dis_output_v, true_labels_v)\n gen_loss.backward()\n gen_optimizer.step()\n\n if trainer.state.iteration % SAVE_IMAGE_EVERY_ITER == 0:\n fake_img = vutils.make_grid(\n gen_output_v.data[:64], normalize=True)\n trainer.tb.writer.add_image(\n \"fake\", fake_img, trainer.state.iteration)\n real_img = vutils.make_grid(\n batch_v.data[:64], normalize=True)\n trainer.tb.writer.add_image(\n \"real\", real_img, trainer.state.iteration)\n trainer.tb.writer.flush()\n return dis_loss.item(), gen_loss.item()\n\n engine = Engine(process_batch)\n tb = tb_logger.TensorboardLogger(log_dir=None)\n engine.tb = tb\n RunningAverage(output_transform=lambda out: out[1]).\\\n attach(engine, \"avg_loss_gen\")\n RunningAverage(output_transform=lambda out: out[0]).\\\n attach(engine, \"avg_loss_dis\")\n\n handler = tb_logger.OutputHandler(tag=\"train\",\n metric_names=['avg_loss_gen', 'avg_loss_dis'])\n tb.attach(engine, log_handler=handler,\n event_name=Events.ITERATION_COMPLETED)\n\n @engine.on(Events.ITERATION_COMPLETED)\n def log_losses(trainer):\n if trainer.state.iteration % REPORT_EVERY_ITER == 0:\n log.info(\"%d: gen_loss=%f, dis_loss=%f\",\n trainer.state.iteration,\n trainer.state.metrics['avg_loss_gen'],\n trainer.state.metrics['avg_loss_dis'])\n\n engine.run(data=iterate_batches(envs))",
"INFO: Making new env: Breakout-v0\nINFO: 100: gen_loss=5.327549, dis_loss=0.200626\nINFO: 200: gen_loss=6.850880, dis_loss=0.028281\nINFO: 300: gen_loss=7.435633, dis_loss=0.004672\nINFO: 400: gen_loss=7.708136, dis_loss=0.001331\nINFO: 500: gen_loss=8.000729, dis_loss=0.000699\nINFO: 600: gen_loss=8.314868, dis_loss=0.000474\nINFO: 700: gen_loss=8.620416, dis_loss=0.000328\nINFO: 800: gen_loss=8.779677, dis_loss=0.000286\nINFO: 900: gen_loss=8.907359, dis_loss=0.000267\nINFO: 1000: gen_loss=6.822098, dis_loss=0.558477\nINFO: 1100: gen_loss=6.491067, dis_loss=0.079029\nINFO: 1200: gen_loss=6.794054, dis_loss=0.012632\nINFO: 1300: gen_loss=7.230944, dis_loss=0.002747\nINFO: 1400: gen_loss=7.698738, dis_loss=0.000962\nINFO: 1500: gen_loss=8.162801, dis_loss=0.000497\nINFO: 1600: gen_loss=8.546710, dis_loss=0.000326\nINFO: 1700: gen_loss=8.939020, dis_loss=0.000224\nINFO: 1800: gen_loss=9.027502, dis_loss=0.000216\nINFO: 1900: gen_loss=9.230650, dis_loss=0.000172\nINFO: 2000: gen_loss=9.495270, dis_loss=0.000129\nINFO: 2100: gen_loss=9.700210, dis_loss=0.000104\nINFO: 2200: gen_loss=9.862649, dis_loss=0.000086\nINFO: 2300: gen_loss=10.042667, dis_loss=0.000075\nINFO: 2400: gen_loss=10.333560, dis_loss=0.000052\nINFO: 2500: gen_loss=10.437976, dis_loss=0.000045\nINFO: 2600: gen_loss=10.592011, dis_loss=0.000040\nINFO: 2700: gen_loss=10.633485, dis_loss=0.000039\nINFO: 2800: gen_loss=10.627324, dis_loss=0.000036\nINFO: 2900: gen_loss=10.665850, dis_loss=0.000036\nINFO: 3000: gen_loss=10.712931, dis_loss=0.000036\nINFO: 3100: gen_loss=10.853663, dis_loss=0.000030\nINFO: 3200: gen_loss=10.868406, dis_loss=0.000030\nINFO: 3300: gen_loss=10.904878, dis_loss=0.000027\nINFO: 3400: gen_loss=11.031057, dis_loss=0.000022\nINFO: 3500: gen_loss=11.114413, dis_loss=0.000022\nINFO: 3600: gen_loss=11.334650, dis_loss=0.000018\nINFO: 3700: gen_loss=11.537755, dis_loss=0.000013\nINFO: 3800: gen_loss=11.573673, dis_loss=0.000015\nINFO: 3900: gen_loss=11.594438, dis_loss=0.000013\nINFO: 4000: gen_loss=11.650991, dis_loss=0.000012\nINFO: 4100: gen_loss=11.350557, dis_loss=0.000023\nINFO: 4200: gen_loss=11.715774, dis_loss=0.000012\nINFO: 4300: gen_loss=11.970108, dis_loss=0.000008\nINFO: 4400: gen_loss=12.142686, dis_loss=0.000007\nINFO: 4500: gen_loss=12.200508, dis_loss=0.000007\nINFO: 4600: gen_loss=12.209455, dis_loss=0.000006\nINFO: 4700: gen_loss=12.215595, dis_loss=0.000007\nINFO: 4800: gen_loss=12.352226, dis_loss=0.000006\nINFO: 4900: gen_loss=12.434466, dis_loss=0.000006\nINFO: 5000: gen_loss=12.517082, dis_loss=0.000005\nINFO: 5100: gen_loss=12.604175, dis_loss=0.000005\nINFO: 5200: gen_loss=12.744095, dis_loss=0.000004\nINFO: 5300: gen_loss=12.880165, dis_loss=0.000004\nINFO: 5400: gen_loss=12.999031, dis_loss=0.000003\n"
]
],
[
[
"## Render environments in Colab",
"_____no_output_____"
],
[
"### Alternative 1\n\nIt is possible to visualize the game your agent is playing, even on CoLab. This section provides information on how to generate a video in CoLab that shows you an episode of the game your agent is playing. This video process is based on suggestions found [here](https://colab.research.google.com/drive/1flu31ulJlgiRL1dnN2ir8wGh9p7Zij2t).\n\nBegin by installing **pyvirtualdisplay** and **python-opengl**.",
"_____no_output_____"
]
],
[
[
"!pip install gym pyvirtualdisplay > /dev/null 2>&1\n!apt-get install -y xvfb python-opengl ffmpeg > /dev/null 2>&1\n\n!apt-get update > /dev/null 2>&1\n!apt-get install cmake > /dev/null 2>&1\n!pip install --upgrade setuptools 2>&1\n!pip install ez_setup > /dev/null 2>&1\n!pip install gym[atari] > /dev/null 2>&1\n\n!wget http://www.atarimania.com/roms/Roms.rar\n!mkdir /content/ROM/\n!unrar e /content/Roms.rar /content/ROM/\n!python -m atari_py.import_roms /content/ROM/",
"_____no_output_____"
],
[
"import gym\nfrom gym.wrappers import Monitor\nimport glob\nimport io\nimport base64\nfrom IPython.display import HTML\nfrom pyvirtualdisplay import Display\nfrom IPython import display as ipythondisplay\n\ndisplay = Display(visible=0, size=(1400, 900))\ndisplay.start()\n\n\"\"\"\nUtility functions to enable video recording of gym environment \nand displaying it.\nTo enable video, just do \"env = wrap_env(env)\"\"\n\"\"\"\n\ndef show_video():\n mp4list = glob.glob('video/*.mp4')\n if len(mp4list) > 0:\n mp4 = mp4list[0]\n video = io.open(mp4, 'r+b').read()\n encoded = base64.b64encode(video)\n ipythondisplay.display(HTML(data='''<video alt=\"test\" autoplay \n loop controls style=\"height: 400px;\">\n <source src=\"data:video/mp4;base64,{0}\" type=\"video/mp4\" />\n </video>'''.format(encoded.decode('ascii'))))\n else: \n print(\"Could not find video\")\n \n\ndef wrap_env(env):\n env = Monitor(env, './video', force=True)\n return env",
"_____no_output_____"
],
[
"#env = wrap_env(gym.make(\"MountainCar-v0\"))\nenv = wrap_env(gym.make(\"Atlantis-v0\"))\n\nobservation = env.reset()\n\nwhile True:\n env.render()\n #your agent goes here\n action = env.action_space.sample() \n observation, reward, done, info = env.step(action)\n if done: \n break;\n \nenv.close()\nshow_video()",
"_____no_output_____"
]
],
[
[
"### Alternative 2",
"_____no_output_____"
]
],
[
[
"!apt-get install -y xvfb python-opengl ffmpeg > /dev/null 2>&1\n!pip install colabgymrender",
"_____no_output_____"
],
[
"import gym\nfrom colabgymrender.recorder import Recorder\n\nenv = gym.make(\"Breakout-v0\")\ndirectory = './video'\nenv = Recorder(env, directory)\n\nobservation = env.reset()\nterminal = False\nwhile not terminal:\n action = env.action_space.sample()\n observation, reward, terminal, info = env.step(action)\n\nenv.play()",
"_____no_output_____"
]
]
] | [
"markdown",
"code",
"markdown",
"code",
"markdown",
"code",
"markdown",
"code",
"markdown",
"code",
"markdown",
"code",
"markdown",
"code",
"markdown",
"code",
"markdown",
"code",
"markdown",
"code",
"markdown",
"code",
"markdown",
"code",
"markdown",
"code",
"markdown",
"code",
"markdown",
"code",
"markdown",
"code",
"markdown",
"code",
"markdown",
"code",
"markdown",
"code"
] | [
[
"markdown",
"markdown"
],
[
"code",
"code"
],
[
"markdown",
"markdown"
],
[
"code"
],
[
"markdown"
],
[
"code",
"code",
"code",
"code",
"code"
],
[
"markdown",
"markdown"
],
[
"code"
],
[
"markdown"
],
[
"code",
"code",
"code"
],
[
"markdown"
],
[
"code",
"code"
],
[
"markdown"
],
[
"code"
],
[
"markdown"
],
[
"code"
],
[
"markdown"
],
[
"code",
"code"
],
[
"markdown"
],
[
"code",
"code"
],
[
"markdown"
],
[
"code"
],
[
"markdown"
],
[
"code"
],
[
"markdown"
],
[
"code"
],
[
"markdown",
"markdown"
],
[
"code"
],
[
"markdown"
],
[
"code"
],
[
"markdown"
],
[
"code"
],
[
"markdown"
],
[
"code"
],
[
"markdown",
"markdown"
],
[
"code",
"code",
"code"
],
[
"markdown"
],
[
"code",
"code"
]
] |
d047372bd5493294fcbaa34a9d256e824bc17d00 | 6,164 | ipynb | Jupyter Notebook | code/algorithms/course_udemy_1/Stacks, Queues and Deques/Implementation of Stack.ipynb | vicb1/miscellaneous | 2c9762579abf75ef6cba75d1d1536a693d69e82a | [
"MIT"
] | null | null | null | code/algorithms/course_udemy_1/Stacks, Queues and Deques/Implementation of Stack.ipynb | vicb1/miscellaneous | 2c9762579abf75ef6cba75d1d1536a693d69e82a | [
"MIT"
] | null | null | null | code/algorithms/course_udemy_1/Stacks, Queues and Deques/Implementation of Stack.ipynb | vicb1/miscellaneous | 2c9762579abf75ef6cba75d1d1536a693d69e82a | [
"MIT"
] | null | null | null | 18.4 | 299 | 0.482317 | [
[
[
"# Implementation of Stack\n\n## Stack Attributes and Methods\n\nBefore we implement our own Stack class, let's review the properties and methods of a Stack.\n\nThe stack abstract data type is defined by the following structure and operations. A stack is structured, as described above, as an ordered collection of items where items are added to and removed from the end called the “top.” Stacks are ordered LIFO. The stack operations are given below.\n\n* Stack() creates a new stack that is empty. It needs no parameters and returns an empty stack.\n* push(item) adds a new item to the top of the stack. It needs the item and returns nothing.\n* pop() removes the top item from the stack. It needs no parameters and returns the item. The stack is modified.\n* peek() returns the top item from the stack but does not remove it. It needs no parameters. The stack is not modified.\n* isEmpty() tests to see whether the stack is empty. It needs no parameters and returns a boolean value.\n* size() returns the number of items on the stack. It needs no parameters and returns an integer.",
"_____no_output_____"
],
[
"____\n\n## Stack Implementation",
"_____no_output_____"
]
],
[
[
"class Stack:\n \n \n def __init__(self):\n self.items = []\n\n def isEmpty(self):\n return self.items == []\n\n def push(self, item):\n self.items.append(item)\n\n def pop(self):\n return self.items.pop()\n\n def peek(self):\n return self.items[len(self.items)-1]\n\n def size(self):\n return len(self.items)",
"_____no_output_____"
]
],
[
[
"Let's try it out!",
"_____no_output_____"
]
],
[
[
"s = Stack()",
"_____no_output_____"
],
[
"print s.isEmpty()",
"True\n"
],
[
"s.push(1)",
"_____no_output_____"
],
[
"s.push('two')",
"_____no_output_____"
],
[
"s.peek()",
"_____no_output_____"
],
[
"s.push(True)",
"_____no_output_____"
],
[
"s.size()",
"_____no_output_____"
],
[
"s.isEmpty()",
"_____no_output_____"
],
[
"s.pop()",
"_____no_output_____"
],
[
"s.pop()",
"two\n"
],
[
"s.size()",
"_____no_output_____"
],
[
"s.pop()",
"_____no_output_____"
],
[
"s.isEmpty()",
"_____no_output_____"
]
],
[
[
"## Good Job!",
"_____no_output_____"
]
]
] | [
"markdown",
"code",
"markdown",
"code",
"markdown"
] | [
[
"markdown",
"markdown"
],
[
"code"
],
[
"markdown"
],
[
"code",
"code",
"code",
"code",
"code",
"code",
"code",
"code",
"code",
"code",
"code",
"code",
"code"
],
[
"markdown"
]
] |
d0473e4cf24536e20ec17db132e5e8190ebd14af | 337,563 | ipynb | Jupyter Notebook | Function Approximation by Neural Network/Function approximation by linear model and deep network LOOP test.ipynb | rezapci/Machine-Learning | 11e9bed11e982260214b5c99d7c37f898319c102 | [
"BSD-2-Clause"
] | null | null | null | Function Approximation by Neural Network/Function approximation by linear model and deep network LOOP test.ipynb | rezapci/Machine-Learning | 11e9bed11e982260214b5c99d7c37f898319c102 | [
"BSD-2-Clause"
] | null | null | null | Function Approximation by Neural Network/Function approximation by linear model and deep network LOOP test.ipynb | rezapci/Machine-Learning | 11e9bed11e982260214b5c99d7c37f898319c102 | [
"BSD-2-Clause"
] | null | null | null | 337,563 | 337,563 | 0.926737 | [
[
[
"| Name | Description | Date\n| :- |-------------: | :-:\n|<font color=red>__Reza Hashemi__</font>| __Function approximation by linear model and deep network LOOP test__. | __On 10th of August 2019__",
"_____no_output_____"
],
[
"# Function approximation with linear models and neural network\n* Are Linear models sufficient for approximating transcedental functions? What about polynomial functions?\n* Do neural networks perform better in those cases?\n* Does the depth of the neural network matter?",
"_____no_output_____"
],
[
"### Import basic libraries",
"_____no_output_____"
]
],
[
[
"import numpy as np\nimport pandas as pd\nimport math\nimport matplotlib.pyplot as plt\n%matplotlib inline",
"_____no_output_____"
]
],
[
[
"### Global variables for the program",
"_____no_output_____"
]
],
[
[
"N_points = 100 # Number of points for constructing function\nx_min = 1 # Min of the range of x (feature)\nx_max = 25 # Max of the range of x (feature)\nnoise_mean = 0 # Mean of the Gaussian noise adder\nnoise_sd = 10 # Std.Dev of the Gaussian noise adder\ntest_set_fraction = 0.2",
"_____no_output_____"
]
],
[
[
"### Generate feature and output vector for a non-linear function with transcedental terms\nThe ground truth or originating function is as follows:\n\n$$ y=f(x)= (20x+3x^2+0.1x^3).sin(x).e^{-0.1x}+\\psi(x) $$\n\n$$ {OR} $$\n\n$$ y=f(x)= (20x+3x^2+0.1x^3)+\\psi(x) $$\n\n$${where,}\\ \\psi(x) : {\\displaystyle f(x\\;|\\;\\mu ,\\sigma ^{2})={\\frac {1}{\\sqrt {2\\pi \\sigma ^{2}}}}\\;e^{-{\\frac {(x-\\mu )^{2}}{2\\sigma ^{2}}}}} $$",
"_____no_output_____"
]
],
[
[
"# Definition of the function with exponential and sinusoidal terms\ndef func_trans(x):\n result = (20*x+3*x**2+0.1*x**3)*np.sin(x)*np.exp(-0.1*x)\n return (result)",
"_____no_output_____"
],
[
"# Definition of the function without exponential and sinusoidal terms i.e. just the polynomial\ndef func_poly(x):\n result = 20*x+3*x**2+0.1*x**3\n return (result)",
"_____no_output_____"
],
[
"# Densely spaced points for generating the ideal functional curve\nx_smooth = np.array(np.linspace(x_min,x_max,501))\n\n# Use one of the following\ny_smooth = func_trans(x_smooth)\n#y_smooth = func_poly(x_smooth)",
"_____no_output_____"
],
[
"# Linearly spaced sample points\nX=np.array(np.linspace(x_min,x_max,N_points))",
"_____no_output_____"
],
[
"# Added observational/measurement noise\nnoise_x = np.random.normal(loc=noise_mean,scale=noise_sd,size=N_points)",
"_____no_output_____"
],
[
"# Observed output after adding the noise\ny = func_trans(X)+noise_x",
"_____no_output_____"
],
[
"# Store the values in a DataFrame\ndf = pd.DataFrame(data=X,columns=['X'])\ndf['Ideal y']=df['X'].apply(func_trans)\ndf['Sin_X']=df['X'].apply(math.sin)\ndf['y']=y\ndf.head()",
"_____no_output_____"
]
],
[
[
"### Plot the function(s), both the ideal characteristic and the observed output (with process and observation noise)",
"_____no_output_____"
]
],
[
[
"df.plot.scatter('X','y',title='True process and measured samples\\n',\n grid=True,edgecolors=(0,0,0),c='blue',s=60,figsize=(10,6))\nplt.plot(x_smooth,y_smooth,'k')",
"_____no_output_____"
]
],
[
[
"### Import scikit-learn librares and prepare train/test splits",
"_____no_output_____"
]
],
[
[
"from sklearn.linear_model import LinearRegression\nfrom sklearn.linear_model import LassoCV\nfrom sklearn.linear_model import RidgeCV\nfrom sklearn.ensemble import AdaBoostRegressor\nfrom sklearn.preprocessing import PolynomialFeatures\nfrom sklearn.model_selection import train_test_split\nfrom sklearn.pipeline import make_pipeline",
"_____no_output_____"
],
[
"X_train, X_test, y_train, y_test = train_test_split(df[['X','Sin_X']], df['y'], test_size=test_set_fraction)\n\n#X_train=X_train.reshape(X_train.size,1)\n#y_train=y_train.reshape(y_train.size,1)\n#X_test=X_test.reshape(X_test.size,1)\n#y_test=y_test.reshape(y_test.size,1)\n\n#X_train=X_train.reshape(-1,1)\n#y_train=y_train.reshape(-1,1)\n#X_test=X_test.reshape(-1,1)\n#y_test=y_test.reshape(-1,1)\n\nfrom sklearn import preprocessing\nX_scaled = preprocessing.scale(X_train)\ny_scaled = preprocessing.scale(y_train)",
"_____no_output_____"
]
],
[
[
"### Polynomial model with LASSO/Ridge regularization (pipelined) with lineary spaced samples\n** This is an advanced machine learning method which prevents over-fitting by penalizing high-valued coefficients i.e. keep them bounded **",
"_____no_output_____"
]
],
[
[
"# Regression model parameters\nridge_alpha = tuple([10**(x) for x in range(-3,0,1) ]) # Alpha (regularization strength) of ridge regression\n# Alpha (regularization strength) of LASSO regression\nlasso_eps = 0.0001\nlasso_nalpha=20\nlasso_iter=5000\n\n# Min and max degree of polynomials features to consider\ndegree_min = 2\ndegree_max = 8",
"_____no_output_____"
],
[
"linear_sample_score = []\npoly_degree = []\nrmse=[]\nt_linear=[]\nimport time\nfor degree in range(degree_min,degree_max+1):\n t1=time.time()\n #model = make_pipeline(PolynomialFeatures(degree), RidgeCV(alphas=ridge_alpha,normalize=True,cv=5))\n model = make_pipeline(PolynomialFeatures(degree), LassoCV(eps=lasso_eps,n_alphas=lasso_nalpha, \n max_iter=lasso_iter,normalize=True,cv=5))\n #model = make_pipeline(PolynomialFeatures(degree), LinearRegression(normalize=True))\n model.fit(X_train, y_train)\n t2=time.time()\n t = t2-t1\n t_linear.append(t)\n test_pred = np.array(model.predict(X_test))\n RMSE=np.sqrt(np.sum(np.square(test_pred-y_test)))\n test_score = model.score(X_test,y_test)\n linear_sample_score.append(test_score)\n rmse.append(RMSE)\n poly_degree.append(degree)\n #print(\"Test score of model with degree {}: {}\\n\".format(degree,test_score))\n \n plt.figure()\n plt.title(\"Predicted vs. actual for polynomial of degree {}\".format(degree),fontsize=15)\n plt.xlabel(\"Actual values\")\n plt.ylabel(\"Predicted values\")\n plt.scatter(y_test,test_pred)\n plt.plot(y_test,y_test,'r',lw=2)",
"_____no_output_____"
],
[
"linear_sample_score",
"_____no_output_____"
],
[
"plt.figure(figsize=(8,5))\nplt.grid(True)\nplt.plot(poly_degree,rmse,lw=3,c='red')\nplt.title(\"Model complexity (highest polynomial degree) vs. test score\\n\",fontsize=20)\nplt.xlabel (\"\\nDegree of polynomial\",fontsize=20)\nplt.ylabel (\"Root-mean-square error on test set\",fontsize=15)",
"_____no_output_____"
],
[
"df_score = pd.DataFrame(data={'degree':[d for d in range(degree_min,degree_max+1)],\n 'Linear sample score':linear_sample_score})",
"_____no_output_____"
],
[
"# Save the best R^2 score\nr2_linear = max(linear_sample_score)\nprint(\"Best R^2 score for linear polynomial degree models:\",r2_linear)",
"Best R^2 score for linear polynomial degree models: 0.995693773025\n"
],
[
"plt.figure(figsize=(8,5))\nplt.grid(True)\nplt.plot(poly_degree,linear_sample_score,lw=3,c='red')\nplt.xlabel (\"\\nModel Complexity: Degree of polynomial\",fontsize=20)\nplt.ylabel (\"R^2 score on test set\",fontsize=15)",
"_____no_output_____"
]
],
[
[
"## 1-hidden layer (Shallow) network",
"_____no_output_____"
]
],
[
[
"import tensorflow as tf\nlearning_rate = 1e-6\ntraining_epochs = 150000\n\nn_input = 1 # Number of features\nn_output = 1 # Regression output is a number only\n\nn_hidden_layer = 100 # layer number of features",
"_____no_output_____"
],
[
"weights = {\n 'hidden_layer': tf.Variable(tf.random_normal([n_input, n_hidden_layer])),\n 'out': tf.Variable(tf.random_normal([n_hidden_layer, n_output]))\n}\nbiases = {\n 'hidden_layer': tf.Variable(tf.random_normal([n_hidden_layer])),\n 'out': tf.Variable(tf.random_normal([n_output]))\n}",
"_____no_output_____"
],
[
"# tf Graph input\nx = tf.placeholder(\"float32\", [None,n_input])\ny = tf.placeholder(\"float32\", [None,n_output])",
"_____no_output_____"
],
[
"# Hidden layer with RELU activation\nlayer_1 = tf.add(tf.matmul(x, weights['hidden_layer']),biases['hidden_layer'])\nlayer_1 = tf.sin(layer_1)\n\n# Output layer with linear activation\nops = tf.add(tf.matmul(layer_1, weights['out']), biases['out'])",
"_____no_output_____"
],
[
"# Define loss and optimizer\ncost = tf.reduce_mean(tf.squared_difference(ops,y))\noptimizer = tf.train.GradientDescentOptimizer(learning_rate=learning_rate).minimize(cost)",
"_____no_output_____"
],
[
"from tqdm import tqdm\nimport time\n\n# Initializing the variables\ninit = tf.global_variables_initializer()\n\n# Empty lists for book-keeping purpose\nepoch=0\nlog_epoch = []\nepoch_count=[]\nacc=[]\nloss_epoch=[]\n\nX_train, X_test, y_train, y_test = train_test_split(df['X'], df['y'], \n test_size=test_set_fraction)\n\nX_train=X_train.reshape(X_train.size,1)\ny_train=y_train.reshape(y_train.size,1)\nX_test=X_test.reshape(X_test.size,1)\ny_test=y_test.reshape(y_test.size,1)\n\n# Launch the graph and time the session\nt1=time.time()\nwith tf.Session() as sess:\n sess.run(init) \n # Loop over epochs\n for epoch in tqdm(range(training_epochs)):\n # Run optimization process (backprop) and cost function (to get loss value)\n _,l=sess.run([optimizer,cost], feed_dict={x: X_train, y: y_train})\n loss_epoch.append(l) # Save the loss for every epoch \n epoch_count.append(epoch+1) #Save the epoch count\n \n # print(\"Epoch {}/{} finished. Loss: {}, Accuracy: {}\".format(epoch+1,training_epochs,round(l,4),round(accu,4)))\n #print(\"Epoch {}/{} finished. Loss: {}\".format(epoch+1,training_epochs,round(l,4)))\n w=sess.run(weights)\n b = sess.run(biases)\n yhat=sess.run(ops,feed_dict={x:X_test})\nt2=time.time()\n\ntime_SNN = t2-t1",
"C:\\Users\\Tirtha\\Python\\Anaconda3\\lib\\site-packages\\ipykernel_launcher.py:17: FutureWarning: reshape is deprecated and will raise in a subsequent release. Please use .values.reshape(...) instead\nC:\\Users\\Tirtha\\Python\\Anaconda3\\lib\\site-packages\\ipykernel_launcher.py:18: FutureWarning: reshape is deprecated and will raise in a subsequent release. Please use .values.reshape(...) instead\nC:\\Users\\Tirtha\\Python\\Anaconda3\\lib\\site-packages\\ipykernel_launcher.py:19: FutureWarning: reshape is deprecated and will raise in a subsequent release. Please use .values.reshape(...) instead\nC:\\Users\\Tirtha\\Python\\Anaconda3\\lib\\site-packages\\ipykernel_launcher.py:20: FutureWarning: reshape is deprecated and will raise in a subsequent release. Please use .values.reshape(...) instead\n100%|████████████████████████████████████████████████████████████████████████| 150000/150000 [02:10<00:00, 1150.13it/s]\n"
],
[
"plt.plot(loss_epoch)",
"_____no_output_____"
],
[
"# Total variance\nSSt_SNN = np.sum(np.square(y_test-np.mean(y_test)))\n# Residual sum of squares\nSSr_SNN = np.sum(np.square(yhat-y_test))\n# Root-mean-square error\nRMSE_SNN = np.sqrt(np.sum(np.square(yhat-y_test)))\n# R^2 coefficient\nr2_SNN = 1-(SSr_SNN/SSt_SNN)\n\nprint(\"RMSE error of the shallow neural network:\",RMSE_SNN)\nprint(\"R^2 value of the shallow neural network:\",r2_SNN)",
"RMSE error of the shallow neural network: 94.9837638167\nR^2 value of the shallow neural network: 0.983809646773\n"
],
[
"plt.figure(figsize=(10,6))\nplt.title(\"Predicted vs. actual (test set) for shallow (1-hidden layer) neural network\\n\",fontsize=15)\nplt.xlabel(\"Actual values (test set)\")\nplt.ylabel(\"Predicted values\")\nplt.scatter(y_test,yhat,edgecolors='k',s=100,c='green')\nplt.grid(True)\nplt.plot(y_test,y_test,'r',lw=2)",
"_____no_output_____"
]
],
[
[
"## Deep Neural network for regression",
"_____no_output_____"
],
[
"### Import and declaration of variables",
"_____no_output_____"
]
],
[
[
"import tensorflow as tf\nlearning_rate = 1e-6\ntraining_epochs = 15000\n\nn_input = 1 # Number of features\nn_output = 1 # Regression output is a number only\n\nn_hidden_layer_1 = 30 # Hidden layer 1\nn_hidden_layer_2 = 30 # Hidden layer 2",
"_____no_output_____"
]
],
[
[
"### Weights and bias variable",
"_____no_output_____"
]
],
[
[
"# Store layers weight & bias as Variables classes in dictionaries\nweights = {\n 'hidden_layer_1': tf.Variable(tf.random_normal([n_input, n_hidden_layer_1])),\n 'hidden_layer_2': tf.Variable(tf.random_normal([n_hidden_layer_1, n_hidden_layer_2])),\n 'out': tf.Variable(tf.random_normal([n_hidden_layer_2, n_output]))\n}\nbiases = {\n 'hidden_layer_1': tf.Variable(tf.random_normal([n_hidden_layer_1])),\n 'hidden_layer_2': tf.Variable(tf.random_normal([n_hidden_layer_2])),\n 'out': tf.Variable(tf.random_normal([n_output]))\n}",
"_____no_output_____"
]
],
[
[
"### Input data as placeholder",
"_____no_output_____"
]
],
[
[
"# tf Graph input\nx = tf.placeholder(\"float32\", [None,n_input])\ny = tf.placeholder(\"float32\", [None,n_output])",
"_____no_output_____"
]
],
[
[
"### Hidden and output layers definition (using TensorFlow mathematical functions)",
"_____no_output_____"
]
],
[
[
"# Hidden layer with activation\nlayer_1 = tf.add(tf.matmul(x, weights['hidden_layer_1']),biases['hidden_layer_1'])\nlayer_1 = tf.sin(layer_1)\n\nlayer_2 = tf.add(tf.matmul(layer_1, weights['hidden_layer_2']),biases['hidden_layer_2'])\nlayer_2 = tf.nn.relu(layer_2)\n\n# Output layer with linear activation\nops = tf.add(tf.matmul(layer_2, weights['out']), biases['out'])",
"_____no_output_____"
]
],
[
[
"### Gradient descent optimizer for training (backpropagation):\nFor the training of the neural network we need to perform __backpropagation__ i.e. propagate the errors, calculated by this cost function, backwards through the layers all the way up to the input weights and bias in order to adjust them accordingly (minimize the error). This involves taking first-order derivatives of the activation functions and applying chain-rule to ___'multiply'___ the effect of various layers as the error propagates back.\n\nYou can read more on this here: [Backpropagation in Neural Network](https://en.wikipedia.org/wiki/Backpropagation)\n\nFortunately, TensorFlow already implicitly implements this step i.e. takes care of all the chained differentiations for us. All we need to do is to specify an Optimizer object and pass on the cost function. Here, we are using a Gradient Descent Optimizer.\n\nGradient descent is a first-order iterative optimization algorithm for finding the minimum of a function. To find a local minimum of a function using gradient descent, one takes steps proportional to the negative of the gradient (or of the approximate gradient) of the function at the current point.\n\nYou can read more on this: [Gradient Descent](https://en.wikipedia.org/wiki/Gradient_descent)\n",
"_____no_output_____"
]
],
[
[
"# Define loss and optimizer\ncost = tf.reduce_mean(tf.squared_difference(ops,y))\noptimizer = tf.train.GradientDescentOptimizer(learning_rate=learning_rate).minimize(cost)",
"_____no_output_____"
]
],
[
[
"### TensorFlow Session for training and loss estimation",
"_____no_output_____"
]
],
[
[
"from tqdm import tqdm\nimport time\n# Initializing the variables\ninit = tf.global_variables_initializer()\n\n# Empty lists for book-keeping purpose\nepoch=0\nlog_epoch = []\nepoch_count=[]\nacc=[]\nloss_epoch=[]\nr2_DNN = []\ntest_size = []\n\nfor i in range(5):\n X_train, X_test, y_train, y_test = train_test_split(df['X'], df['y'], \n test_size=test_set_fraction)\n\n X_train=X_train.reshape(X_train.size,1)\n y_train=y_train.reshape(y_train.size,1)\n X_test=X_test.reshape(X_test.size,1)\n y_test=y_test.reshape(y_test.size,1)\n # Launch the graph and time the session\n with tf.Session() as sess:\n sess.run(init) \n # Loop over epochs\n for epoch in tqdm(range(training_epochs)):\n # Run optimization process (backprop) and cost function (to get loss value)\n #r1 = int(epoch/10000)\n #learning_rate = learning_rate-r1*3e-6\n #optimizer = tf.train.GradientDescentOptimizer(learning_rate=learning_rate).minimize(cost)\n _,l=sess.run([optimizer,cost], feed_dict={x: X_train, y: y_train})\n \n yhat=sess.run(ops,feed_dict={x:X_test})\n\n #test_size.append(0.5-(i*0.04))\n # Total variance\n SSt_DNN = np.sum(np.square(y_test-np.mean(y_test)))\n # Residual sum of squares\n SSr_DNN = np.sum(np.square(yhat-y_test))\n # Root-mean-square error\n RMSE_DNN = np.sqrt(np.sum(np.square(yhat-y_test)))\n # R^2 coefficient\n r2 = 1-(SSr_DNN/SSt_DNN)\n r2_DNN.append(r2)\n print(\"Run: {} finished. Score: {}\".format(i+1,r2))\n",
"C:\\Users\\Tirtha\\Python\\Anaconda3\\lib\\site-packages\\ipykernel_launcher.py:19: FutureWarning: reshape is deprecated and will raise in a subsequent release. Please use .values.reshape(...) instead\nC:\\Users\\Tirtha\\Python\\Anaconda3\\lib\\site-packages\\ipykernel_launcher.py:20: FutureWarning: reshape is deprecated and will raise in a subsequent release. Please use .values.reshape(...) instead\nC:\\Users\\Tirtha\\Python\\Anaconda3\\lib\\site-packages\\ipykernel_launcher.py:21: FutureWarning: reshape is deprecated and will raise in a subsequent release. Please use .values.reshape(...) instead\nC:\\Users\\Tirtha\\Python\\Anaconda3\\lib\\site-packages\\ipykernel_launcher.py:22: FutureWarning: reshape is deprecated and will raise in a subsequent release. Please use .values.reshape(...) instead\n100%|██████████████████████████████████████████████████████████████████████████| 15000/15000 [00:12<00:00, 1184.93it/s]\n"
]
],
[
[
"### Plot R2 score corss-validation results",
"_____no_output_____"
]
],
[
[
"plt.figure(figsize=(10,6))\nplt.title(\"\\nR2-score for cross-validation runs of \\ndeep (2-layer) neural network\\n\",fontsize=25)\nplt.xlabel(\"\\nCross-validation run with random test/train split #\",fontsize=15)\nplt.ylabel(\"R2 score (test set)\\n\",fontsize=15)\nplt.scatter([i+1 for i in range(5)],r2_DNN,edgecolors='k',s=100,c='green')\nplt.grid(True)",
"_____no_output_____"
],
[
"",
"_____no_output_____"
]
]
] | [
"markdown",
"code",
"markdown",
"code",
"markdown",
"code",
"markdown",
"code",
"markdown",
"code",
"markdown",
"code",
"markdown",
"code",
"markdown",
"code",
"markdown",
"code",
"markdown",
"code",
"markdown",
"code",
"markdown",
"code",
"markdown",
"code",
"markdown",
"code"
] | [
[
"markdown",
"markdown",
"markdown"
],
[
"code"
],
[
"markdown"
],
[
"code"
],
[
"markdown"
],
[
"code",
"code",
"code",
"code",
"code",
"code",
"code"
],
[
"markdown"
],
[
"code"
],
[
"markdown"
],
[
"code",
"code"
],
[
"markdown"
],
[
"code",
"code",
"code",
"code",
"code",
"code",
"code"
],
[
"markdown"
],
[
"code",
"code",
"code",
"code",
"code",
"code",
"code",
"code",
"code"
],
[
"markdown",
"markdown"
],
[
"code"
],
[
"markdown"
],
[
"code"
],
[
"markdown"
],
[
"code"
],
[
"markdown"
],
[
"code"
],
[
"markdown"
],
[
"code"
],
[
"markdown"
],
[
"code"
],
[
"markdown"
],
[
"code",
"code"
]
] |
d0474851ad2bdc7cd4ca953f257708b4ca55f9a8 | 13,639 | ipynb | Jupyter Notebook | Untitled.ipynb | divinorum-webb/docker-airflow | 5d86e9786898958bfde8d0642a1ee45bed837dac | [
"Apache-2.0"
] | 1 | 2021-05-28T16:18:54.000Z | 2021-05-28T16:18:54.000Z | Untitled.ipynb | divinorum-webb/docker-airflow | 5d86e9786898958bfde8d0642a1ee45bed837dac | [
"Apache-2.0"
] | null | null | null | Untitled.ipynb | divinorum-webb/docker-airflow | 5d86e9786898958bfde8d0642a1ee45bed837dac | [
"Apache-2.0"
] | null | null | null | 44.139159 | 1,895 | 0.628345 | [
[
[
"import os\nimport requests",
"_____no_output_____"
],
[
"os.getcwd()",
"_____no_output_____"
],
[
"os.chdir('dags/tableau')",
"_____no_output_____"
],
[
"from subscription_classes import *\nfrom config import tableau_server_config, subscription_email_config",
"_____no_output_____"
],
[
"conn = TableauServer(config_json=tableau_server_config)",
"_____no_output_____"
],
[
"conn.sign_in()",
"_____no_output_____"
],
[
"conn.get_user_details()",
"_____no_output_____"
],
[
"conn.get_email_users()",
"_____no_output_____"
],
[
"conn.get_distribution_list('InterWorks - Airflow Demo')",
"_____no_output_____"
],
[
"base_url = conn.base_get_url",
"_____no_output_____"
],
[
"base_url",
"_____no_output_____"
],
[
"conn.get_group_by_name(group_name='InterWorks - Airflow Demo')",
"_____no_output_____"
],
[
"test_group = '0194f591-9d29-4a4b-af50-a5c606b9dc5f'",
"_____no_output_____"
],
[
"test_url = \"{0}/groups/{1}/users\".format(base_url, test_group)",
"_____no_output_____"
],
[
"test_url",
"_____no_output_____"
],
[
"response = requests.get(test_url, headers=conn.default_headers)",
"_____no_output_____"
],
[
"response",
"_____no_output_____"
],
[
"response.json()",
"_____no_output_____"
]
]
] | [
"code"
] | [
[
"code",
"code",
"code",
"code",
"code",
"code",
"code",
"code",
"code",
"code",
"code",
"code",
"code",
"code",
"code",
"code",
"code",
"code"
]
] |
d0474983cc6a52e1b3b6d173f0926a35f6ab4cb7 | 128,648 | ipynb | Jupyter Notebook | Lecture Material/07_Conditional_Logic_and_Control_Flow/07.3_ControllingFlowWithConditionalStatements.ipynb | knherrera/pcc-cis-012-intro-to-programming-python | f2fb8ec5b242fc6deb5c0e2abda60bb91171aad4 | [
"MIT"
] | 23 | 2020-02-19T22:07:17.000Z | 2021-08-19T20:43:21.000Z | Lecture Material/07_Conditional_Logic_and_Control_Flow/07.3_ControllingFlowWithConditionalStatements.ipynb | knherrera/pcc-cis-012-intro-to-programming-python | f2fb8ec5b242fc6deb5c0e2abda60bb91171aad4 | [
"MIT"
] | 12 | 2020-03-04T04:34:38.000Z | 2021-02-23T04:28:31.000Z | Lecture Material/07_Conditional_Logic_and_Control_Flow/07.3_ControllingFlowWithConditionalStatements.ipynb | knherrera/pcc-cis-012-intro-to-programming-python | f2fb8ec5b242fc6deb5c0e2abda60bb91171aad4 | [
"MIT"
] | 18 | 2020-03-05T05:21:11.000Z | 2022-03-05T05:57:12.000Z | 220.287671 | 31,916 | 0.914099 | [
[
[
"# Controlling Flow with Conditional Statements\n\nNow that you've learned how to create conditional statements, let's learn how to use them to control the flow of our programs. This is done with `if`, `elif`, and `else` statements. ",
"_____no_output_____"
],
[
"## The `if` Statement\n\n What if we wanted to check if a number was divisible by 2 and if so then print that number out. Let's diagram that out.",
"_____no_output_____"
],
[
"\n\n- Check to see if A is even\n- If yes, then print our message: \"A is even\"",
"_____no_output_____"
],
[
"This use case can be translated into a \"if\" statement. I'm going to write this out in pseudocode which looks very similar to Python.\n\n```text\nif A is even:\n print \"A is even\"\n```",
"_____no_output_____"
]
],
[
[
"# Let's translate this into Python code\ndef check_evenness(A):\n if A % 2 == 0:\n print(f\"A ({A:02}) is even!\")\n\nfor i in range(1, 11):\n check_evenness(i)",
"A (02) is even!\nA (04) is even!\nA (06) is even!\nA (08) is even!\nA (10) is even!\n"
],
[
"# You can do multiple if statements and they're executed sequentially\n\nA = 10\n\nif A > 0:\n print('A is positive')\nif A % 2 == 0:\n print('A is even!')",
"A is positive\nA is even!\n"
]
],
[
[
"## The `else` Statement\nBut what if we wanted to know if the number was even OR odd? Let's diagram that out:\n\n",
"_____no_output_____"
],
[
"Again, translating this to pseudocode, we're going to use the 'else' statement:\n\n```text\nif A is even:\n print \"A is even\"\nelse:\n print \"A is odd\"\n```",
"_____no_output_____"
]
],
[
[
"# Let's translate this into Python code\ndef check_evenness(A):\n if A % 2 == 0:\n print(f\"A ({A:02}) is even!\") \n else:\n print(f'A ({A:02}) is odd!')\n\nfor i in range(1, 11):\n check_evenness(i)",
"A (01) is odd!\nA (02) is even!\nA (03) is odd!\nA (04) is even!\nA (05) is odd!\nA (06) is even!\nA (07) is odd!\nA (08) is even!\nA (09) is odd!\nA (10) is even!\n"
]
],
[
[
"# The 'else if' or `elif` Statement\nWhat if we wanted to check if A is divisible by 2 or 3? Let's diagram that out:",
"_____no_output_____"
],
[
"",
"_____no_output_____"
],
[
"Again, translating this into psuedocode, we're going to use the 'else if' statement.\n\n```text\nif A is divisible by 2:\n print \"2 divides A\"\nelse if A is divisible by 3:\n print \"3 divides A\"\nelse\n print \"2 and 3 don't divide A\"\n```",
"_____no_output_____"
]
],
[
[
"# Let's translate this into Python code\ndef check_divisible_by_2_and_3(A):\n if A % 2 == 0:\n print(f\"2 divides A ({A:02})!\") \n \n # else if in Python is elif\n elif A % 3 == 0:\n print(f'3 divides A ({A:02})!')\n else:\n print(f'A ({A:02}) is not divisible by 2 or 3)')\n \nfor i in range(1, 11):\n check_divisible_by_2_and_3(i)",
"A (01) is not divisible by 2 or 3)\n2 divides A (02)!\n3 divides A (03)!\n2 divides A (04)!\nA (05) is not divisible by 2 or 3)\n2 divides A (06)!\nA (07) is not divisible by 2 or 3)\n2 divides A (08)!\n3 divides A (09)!\n2 divides A (10)!\n"
]
],
[
[
"## Order Matters\n\nWhen chaining conditionals, you need to be careful how you order them. For example, what if we wanted te check if a number is divisible by 2, 3, or both:",
"_____no_output_____"
],
[
"",
"_____no_output_____"
]
],
[
[
"# Let's translate this into Python code\ndef check_divisible_by_2_and_3(A):\n if A % 2 == 0:\n print(f\"2 divides A ({A:02})!\") \n elif A % 3 == 0:\n print(f'3 divides A ({A:02})!')\n elif A % 2 == 0 and A % 3 == 0:\n print(f'2 and 3 divides A ({A:02})!')\n else:\n print(f\"2 or 3 doesn't divide A ({A:02})\")\n \nfor i in range(1, 11):\n check_divisible_by_2_and_3(i)",
"2 or 3 doesn't divide A (01)\n2 divides A (02)!\n3 divides A (03)!\n2 divides A (04)!\n2 or 3 doesn't divide A (05)\n2 divides A (06)!\n2 or 3 doesn't divide A (07)\n2 divides A (08)!\n3 divides A (09)!\n2 divides A (10)!\n"
]
],
[
[
"Wait! we would expect that 6, which is divisible by both 2 and 3 to show that! Looking back at the graphic, we can see that the flow is checking for 2 first, and since that's true we follow that path first. Let's make a correction to our diagram to fix this:",
"_____no_output_____"
],
[
"",
"_____no_output_____"
]
],
[
[
"# Let's translate this into Python code\ndef check_divisible_by_2_and_3(A):\n if A % 2 == 0 and A % 3 == 0:\n print(f'2 and 3 divides A ({A:02})!')\n elif A % 3 == 0:\n print(f'3 divides A ({A:02})!')\n elif A % 2 == 0:\n print(f\"2 divides A ({A:02})!\") \n else:\n print(f\"2 or 3 doesn't divide A ({A:02})\")\n \nfor i in range(1, 11):\n check_divisible_by_2_and_3(i)",
"2 or 3 doesn't divide A (01)\n2 divides A (02)!\n3 divides A (03)!\n2 divides A (04)!\n2 or 3 doesn't divide A (05)\n2 and 3 divides A (06)!\n2 or 3 doesn't divide A (07)\n2 divides A (08)!\n3 divides A (09)!\n2 divides A (10)!\n"
]
],
[
[
"**NOTE:** Always put your most restrictive conditional at the top of your if statements and then work your way down to the least restrictive.\n\n",
"_____no_output_____"
],
[
"## In-Class Assignments\n\n- Create a funcition that takes two inputs variables `A` and `divisor`. Check if `divisor` divides into `A`. If it does, print `\"<value of A> is divided by <value of divisor>\"`. Don't forget about the `in` operator that checks if a substring is in another string.\n- Create a function that takes an input variable `A` which is a string. Check if `A` has the substring `apple`, `peach`, or `blueberry` in it. Print out which of these are found within the string. Note: you could do this using just if/elif/else statements, but is there a better way using lists, for loops, and if/elif/else statements?",
"_____no_output_____"
],
[
"## Solutions",
"_____no_output_____"
]
],
[
[
"def is_divisible(A, divisor):\n if A % divisor == 0:\n print(f'{A} is divided by {divisor}')\n \nA = 37\n\n# this is actually a crude way to find if the number is prime\nfor i in range(2, int(A / 2)):\n is_divisible(A, i)\n \n# notice that nothing was printed? That's because 37 is prime\n \nB = 27\nfor i in range(2, int(B / 2)):\n is_divisible(B, i)",
"27 is divided by 3\n27 is divided by 9\n"
],
[
"# this is ONE solution. There are more out there and probably better\n# one too\ndef check_for_fruit(A):\n found_fruit = []\n if 'apple' in A:\n found_fruit.append('apple')\n if 'peach' in A:\n found_fruit.append('peach')\n if 'blueberry' in A:\n found_fruit.append('blueberry')\n \n found_fruit_str = ''\n for fruit in found_fruit:\n found_fruit_str += fruit\n found_fruit_str += ', '\n \n if len(found_fruit) > 0:\n print(found_fruit_str + ' is found within the string')\n else:\n print('No fruit found in the string')",
"_____no_output_____"
],
[
"check_for_fruit('there are apples and peaches in this pie')",
"apple, peach, is found within the string\n"
]
]
] | [
"markdown",
"code",
"markdown",
"code",
"markdown",
"code",
"markdown",
"code",
"markdown",
"code",
"markdown",
"code"
] | [
[
"markdown",
"markdown",
"markdown",
"markdown"
],
[
"code",
"code"
],
[
"markdown",
"markdown"
],
[
"code"
],
[
"markdown",
"markdown",
"markdown"
],
[
"code"
],
[
"markdown",
"markdown"
],
[
"code"
],
[
"markdown",
"markdown"
],
[
"code"
],
[
"markdown",
"markdown",
"markdown"
],
[
"code",
"code",
"code"
]
] |
d0474b6eed193ef086ac6e702551e84aaf8f9b2b | 6,827 | ipynb | Jupyter Notebook | example4.1/example4.1.ipynb | jerryzenghao/ReinformanceLearning | 41da6bcf14bb588a0a29abbb576d71970b41a771 | [
"MIT"
] | null | null | null | example4.1/example4.1.ipynb | jerryzenghao/ReinformanceLearning | 41da6bcf14bb588a0a29abbb576d71970b41a771 | [
"MIT"
] | null | null | null | example4.1/example4.1.ipynb | jerryzenghao/ReinformanceLearning | 41da6bcf14bb588a0a29abbb576d71970b41a771 | [
"MIT"
] | null | null | null | 48.764286 | 2,907 | 0.390508 | [
[
[
"## Example 4.1\n\nLet \\{0,1,2,3\\} denote the actions \\{up, right, down, left\\} respectively.",
"_____no_output_____"
]
],
[
[
"import numpy as np\nclass gridworld:\n def __init__(self):\n self.terminal_state = [0,15]\n self.action = [0,1,2,3]\n self.value = np.zeros(16)\n self.reward = -1 \n \n def next_state(self, s, a):\n if s in self.terminal_state:\n return s\n if a == 0:\n s = s - 4 if s >= 4 else s \n elif a == 1:\n s = s + 1 if (s+1)%4 != 0 else s\n elif a == 2:\n s = s + 4 if (s+4) < 16 else s\n elif a == 3:\n s = s - 1 if s % 4 !=0 else s\n return s\n \n def policy_evaluation(self):\n k=0\n while True:\n delta = 0\n v_new = np.zeros(16)\n for s in range(16):\n v = 0\n if s in self.terminal_state:\n v_new[s] = 0\n else:\n v = self.value[s]\n temp = 0\n for a in range(4):\n v_new[s] += 0.25*(-1 + self.value[self.next_state(s,a)])\n delta = max(delta, abs(v-v_new[s]))\n self.value = v_new\n \n # greedy policy\n policy = np.zeros((16,4))\n for s in range(1,15):\n vmax = -30\n nmax = 0\n for a in range(4):\n v = self.value[self.next_state(s,a)]\n if v > vmax:\n vmax = v\n nmax = 1\n elif vmax - v < 0.05:\n nmax += 1\n for a in range(4):\n v = self.value[self.next_state(s,a)]\n if v == vmax:\n policy[s,a] = 1/nmax\n \n k += 1\n if k == 1 or k==2 or k==3 or k==10 or k==131:\n print('The state value for %ith iteration:\\n' %k, v_new.reshape((4,4)))\n print('The greedy policy for %ith iteration:\\n' %k, policy)\n if delta < 0.001:\n return\n\n \n \n\n\n \n",
"_____no_output_____"
],
[
"a = gridworld()",
"_____no_output_____"
],
[
"a.policy_evaluation()",
"The state value for 1th iteration:\n [[ 0. -1. -1. -1.]\n [-1. -1. -1. -1.]\n [-1. -1. -1. -1.]\n [-1. -1. -1. 0.]]\nThe greedy policy for 1th iteration:\n [[0. 0. 0. 0. ]\n [0. 0. 0. 1. ]\n [0.25 0.25 0.25 0.25]\n [0.25 0.25 0.25 0.25]\n [1. 0. 0. 0. ]\n [0.25 0.25 0.25 0.25]\n [0.25 0.25 0.25 0.25]\n [0.25 0.25 0.25 0.25]\n [0.25 0.25 0.25 0.25]\n [0.25 0.25 0.25 0.25]\n [0.25 0.25 0.25 0.25]\n [0. 0. 1. 0. ]\n [0.25 0.25 0.25 0.25]\n [0.25 0.25 0.25 0.25]\n [0. 1. 0. 0. ]\n [0. 0. 0. 0. ]]\nThe state value for 2th iteration:\n [[ 0. -1.75 -2. -2. ]\n [-1.75 -2. -2. -2. ]\n [-2. -2. -2. -1.75]\n [-2. -2. -1.75 0. ]]\nThe greedy policy for 2th iteration:\n [[0. 0. 0. 0. ]\n [0. 0. 0. 1. ]\n [0. 0. 0. 1. ]\n [0.25 0.25 0.25 0.25]\n [1. 0. 0. 0. ]\n [0.5 0. 0. 0.5 ]\n [0.25 0.25 0.25 0.25]\n [0. 0. 1. 0. ]\n [1. 0. 0. 0. ]\n [0.25 0.25 0.25 0.25]\n [0. 0.5 0.5 0. ]\n [0. 0. 1. 0. ]\n [0.25 0.25 0.25 0.25]\n [0. 1. 0. 0. ]\n [0. 1. 0. 0. ]\n [0. 0. 0. 0. ]]\nThe state value for 3th iteration:\n [[ 0. -2.4375 -2.9375 -3. ]\n [-2.4375 -2.875 -3. -2.9375]\n [-2.9375 -3. -2.875 -2.4375]\n [-3. -2.9375 -2.4375 0. ]]\nThe greedy policy for 3th iteration:\n [[0. 0. 0. 0. ]\n [0. 0. 0. 1. ]\n [0. 0. 0. 1. ]\n [0. 0. 0.5 0.5]\n [1. 0. 0. 0. ]\n [0.5 0. 0. 0.5]\n [0. 0. 0.5 0.5]\n [0. 0. 1. 0. ]\n [1. 0. 0. 0. ]\n [0.5 0.5 0. 0. ]\n [0. 0.5 0.5 0. ]\n [0. 0. 1. 0. ]\n [0.5 0.5 0. 0. ]\n [0. 1. 0. 0. ]\n [0. 1. 0. 0. ]\n [0. 0. 0. 0. ]]\nThe state value for 10th iteration:\n [[ 0. -6.13796997 -8.35235596 -8.96731567]\n [-6.13796997 -7.73739624 -8.42782593 -8.35235596]\n [-8.35235596 -8.42782593 -7.73739624 -6.13796997]\n [-8.96731567 -8.35235596 -6.13796997 0. ]]\nThe greedy policy for 10th iteration:\n [[0. 0. 0. 0. ]\n [0. 0. 0. 1. ]\n [0. 0. 0. 1. ]\n [0. 0. 0.5 0.5]\n [1. 0. 0. 0. ]\n [0.5 0. 0. 0.5]\n [0. 0. 0.5 0.5]\n [0. 0. 1. 0. ]\n [1. 0. 0. 0. ]\n [0.5 0.5 0. 0. ]\n [0. 0.5 0.5 0. ]\n [0. 0. 1. 0. ]\n [0.5 0.5 0. 0. ]\n [0. 1. 0. 0. ]\n [0. 1. 0. 0. ]\n [0. 0. 0. 0. ]]\nThe state value for 131th iteration:\n [[ 0. -13.98945772 -19.98437823 -21.98251832]\n [-13.98945772 -17.98623815 -19.98448273 -19.98437823]\n [-19.98437823 -19.98448273 -17.98623815 -13.98945772]\n [-21.98251832 -19.98437823 -13.98945772 0. ]]\nThe greedy policy for 131th iteration:\n [[0. 0. 0. 0. ]\n [0. 0. 0. 1. ]\n [0. 0. 0. 1. ]\n [0. 0. 0.5 0.5]\n [1. 0. 0. 0. ]\n [0.5 0. 0. 0. ]\n [0. 0. 0.5 0.5]\n [0. 0. 1. 0. ]\n [1. 0. 0. 0. ]\n [0.5 0.5 0. 0. ]\n [0. 0.5 0.5 0. ]\n [0. 0. 1. 0. ]\n [0.5 0.5 0. 0. ]\n [0. 1. 0. 0. ]\n [0. 1. 0. 0. ]\n [0. 0. 0. 0. ]]\n"
]
]
] | [
"markdown",
"code"
] | [
[
"markdown"
],
[
"code",
"code",
"code"
]
] |
d04754732c775aed538587b3e2292495f6a4a58c | 48,712 | ipynb | Jupyter Notebook | labs/lab08.ipynb | Flipom/mat281_portfolio | a9f0d58d61e010e77ecfe6b948e878e789407343 | [
"MIT"
] | null | null | null | labs/lab08.ipynb | Flipom/mat281_portfolio | a9f0d58d61e010e77ecfe6b948e878e789407343 | [
"MIT"
] | null | null | null | labs/lab08.ipynb | Flipom/mat281_portfolio | a9f0d58d61e010e77ecfe6b948e878e789407343 | [
"MIT"
] | null | null | null | 89.053016 | 17,812 | 0.797114 | [
[
[
"# Laboratorio 8",
"_____no_output_____"
]
],
[
[
"import pandas as pd\nimport matplotlib.pyplot as plt\n\nfrom sklearn import datasets\nfrom sklearn.neighbors import KNeighborsClassifier\nfrom sklearn.linear_model import LogisticRegression\nfrom sklearn.metrics import plot_confusion_matrix \n\n%matplotlib inline",
"_____no_output_____"
],
[
"digits_X, digits_y = datasets.load_digits(return_X_y=True, as_frame=True)\ndigits = pd.concat([digits_X, digits_y], axis=1)\ndigits.head()",
"_____no_output_____"
]
],
[
[
"## Ejercicio 1\n\n(1 pto.)\n\nUtilizando todos los datos, ajusta un modelo de regresión logística a los datos de dígitos. No agregues intercepto y define un máximo de iteraciones de 400.\n\nObtén el _score_ y explica el tan buen resultado.",
"_____no_output_____"
]
],
[
[
"logistic = LogisticRegression(solver=\"lbfgs\", max_iter=400, fit_intercept=False)\nfit=logistic.fit(digits_X, digits_y)\n\n\nprint(f\"El score del modelo de regresión logística es {fit.score(digits_X, digits_y)}\")",
"El score del modelo de regresión logística es 1.0\n"
]
],
[
[
"__Respuesta:__ Supongo que es porque no estamos usando los datos originales, y no otros datos predecidos a partir de los originales",
"_____no_output_____"
],
[
"## Ejercicio 2\n\n(1 pto.)\n\nUtilizando todos los datos, ¿Cuál es la mejor elección del parámetro $k$ al ajustar un modelo kNN a los datos de dígitos? Utiliza valores $k=2, ..., 10$.",
"_____no_output_____"
]
],
[
[
"for k in range(2, 11):\n kNN = KNeighborsClassifier(n_neighbors=k)\n fit=kNN.fit(digits_X, digits_y)\n print(f\"El score del modelo de kNN con k={k} es {fit.score(digits_X, digits_y)}\")",
"El score del modelo de kNN con k=2 es 0.9910962715637173\nEl score del modelo de kNN con k=3 es 0.993322203672788\nEl score del modelo de kNN con k=4 es 0.9922092376182526\nEl score del modelo de kNN con k=5 es 0.9905397885364496\nEl score del modelo de kNN con k=6 es 0.989983305509182\nEl score del modelo de kNN con k=7 es 0.9905397885364496\nEl score del modelo de kNN con k=8 es 0.9894268224819143\nEl score del modelo de kNN con k=9 es 0.9888703394546466\nEl score del modelo de kNN con k=10 es 0.9855314412910406\n"
]
],
[
[
"__Respuesta:__ El caso k=3, porque es el mas cercano a 1.",
"_____no_output_____"
],
[
"## Ejercicio 3\n\n(1 pto.)\n\nGrafica la matriz de confusión normalizada por predicción de ambos modelos (regresión logística y kNN con la mejor elección de $k$).\n\n¿Qué conclusión puedes sacar?\n\nHint: Revisa el argumento `normalize` de la matriz de confusión.",
"_____no_output_____"
]
],
[
[
"plot_confusion_matrix(logistic, digits_X, digits_y, normalize='true');",
"_____no_output_____"
],
[
"best_knn = KNeighborsClassifier(n_neighbors=3)\nB_kNN = best_knn.fit(digits_X, digits_y)\nplot_confusion_matrix(B_kNN, digits_X, digits_y, normalize='true');",
"_____no_output_____"
]
],
[
[
"__Respuesta:__ Que la primera matriz es una mejor prediccion que la segunda, esto porque se pudo obtener una matriz diagonal con, asumo, menor cantidad de errores comparado a los valores que no se encuentran en la diagonal y que son distintos de 0 en el segundo caso.",
"_____no_output_____"
],
[
"## Ejercicio 4\n\n(1 pto.)\n\nEscoge algún registro donde kNN se haya equivocado, _plotea_ la imagen y comenta las razones por las que el algoritmo se pudo haber equivocado.",
"_____no_output_____"
]
],
[
[
"neigh_tt = KNeighborsClassifier(n_neighbors=5)\nneigh_tt.fit(digits_X, digits_y)",
"_____no_output_____"
]
],
[
[
"El valor real del registro seleccionado es",
"_____no_output_____"
]
],
[
[
"i = 5\nneigh_tt.predict(digits_X.iloc[[i], :])",
"_____no_output_____"
]
],
[
[
"Mentras que la predicción dada por kNN es",
"_____no_output_____"
]
],
[
[
"neigh_tt.predict_proba(digits_X.iloc[[i], :])",
"_____no_output_____"
]
],
[
[
"A continuación la imagen",
"_____no_output_____"
]
],
[
[
"plt.imshow(digits_X.loc[[i], :].to_numpy().reshape(8, 8), cmap=plt.cm.gray_r, interpolation='nearest');",
"_____no_output_____"
]
],
[
[
"__Respuesta:__ Se me viene a la cabeza la forma de los numeros de los relojes digitales tipo : https://i.linio.com/p/b6286f5db6ae58cdd0aef38e070a51b5-product.jpg\nDonde las figuras se parecen bastante, sobre todo porque tienen un formato reducido para mostrar los numeritos (hay una base donde detras que se ilumina dependiendo del numero), entonces como la matriz es chikita, igual quedan figuras similares y con no tan buena resolucion, por lo que lleva a errores, en este caso, confundiendo un 5 con un 9.",
"_____no_output_____"
]
]
] | [
"markdown",
"code",
"markdown",
"code",
"markdown",
"code",
"markdown",
"code",
"markdown",
"code",
"markdown",
"code",
"markdown",
"code",
"markdown",
"code",
"markdown"
] | [
[
"markdown"
],
[
"code",
"code"
],
[
"markdown"
],
[
"code"
],
[
"markdown",
"markdown"
],
[
"code"
],
[
"markdown",
"markdown"
],
[
"code",
"code"
],
[
"markdown",
"markdown"
],
[
"code"
],
[
"markdown"
],
[
"code"
],
[
"markdown"
],
[
"code"
],
[
"markdown"
],
[
"code"
],
[
"markdown"
]
] |
d04756ad1fdcd3501d154ed6a98428e532e24c1e | 258,494 | ipynb | Jupyter Notebook | Tensorflow_2X_Notebooks/Demo26_CNN_CIFAR10_DataAugmentation.ipynb | mahnooranjum/Tensorflow_DeepLearning | 65ab178d4c17efad01de827062d5c85bdfb9b1ca | [
"MIT"
] | null | null | null | Tensorflow_2X_Notebooks/Demo26_CNN_CIFAR10_DataAugmentation.ipynb | mahnooranjum/Tensorflow_DeepLearning | 65ab178d4c17efad01de827062d5c85bdfb9b1ca | [
"MIT"
] | null | null | null | Tensorflow_2X_Notebooks/Demo26_CNN_CIFAR10_DataAugmentation.ipynb | mahnooranjum/Tensorflow_DeepLearning | 65ab178d4c17efad01de827062d5c85bdfb9b1ca | [
"MIT"
] | null | null | null | 335.271077 | 42,730 | 0.897727 | [
[
[
"# **Spit some [tensor] flow**\n\nWe need to learn the intricacies of tensorflow to master deep learning\n\n`Let's get this over with`\n\n",
"_____no_output_____"
]
],
[
[
"import numpy as np\nimport pandas as pd\nimport matplotlib.pyplot as plt\nimport tensorflow as tf\nimport cv2\nprint(tf.__version__)",
"2.2.0\n"
],
[
"def evaluation_tf(report, y_test, y_pred, classes):\n plt.plot(report.history['loss'], label = 'training_loss')\n plt.plot(report.history['val_loss'], label = 'validation_loss')\n plt.legend()\n plt.show()\n\n plt.plot(report.history['accuracy'], label = 'training_accuracy')\n plt.plot(report.history['val_accuracy'], label = 'validation_accuracy')\n plt.legend()\n plt.show()\n\n from sklearn.metrics import confusion_matrix\n import itertools\n cm = confusion_matrix(y_test, y_pred)\n\n plt.figure(figsize=(10,10))\n plt.imshow(cm, cmap=plt.cm.Blues)\n for i,j in itertools.product(range(cm.shape[0]), range(cm.shape[1])):\n plt.text(j, i, format(cm[i,j], 'd'),\n horizontalalignment = 'center',\n color='black')\n plt.xlabel(\"Predicted labels\")\n plt.ylabel(\"True labels\")\n plt.xticks(range(0,classes))\n plt.yticks(range(0,classes))\n plt.title('Confusion matrix')\n plt.colorbar()\n plt.show()",
"_____no_output_____"
],
[
"# Taken from https://www.cs.toronto.edu/~kriz/cifar.html\nlabels = \"airplane,automobile,bird,cat,deer,dog,frog,horse,ship,truck\".split(\",\")",
"_____no_output_____"
]
],
[
[
"## As a rule of thumb\n\nRemember that the pooling operation decreases the size of the image, and we lose information.\n\nHowever, the number of features generally increases and we get more features extracted from the images.\n\nThe choices of hyperparams bother us sometimes, because DL has a lot of trial and error involved, we can choose the \n\n- learning rate\n\n- number of layers\n\n- number of neurons per layer \n\n- feature size \n\n- feature number \n\n- pooling size \n\n- stride \n\nOn a side note, if you use strided convolution layers, they will decrease the size of the image as well\n\n\nIf we have images with different sizes as inputs; for example; H1 x W1 x 3 and H2 x W2 x 3, then the output will be flatten-ed to different sizes, this won't work for DENSE layers as they do not have change-able input sizes, so we use global max pooling to make a vector of size 1 x 1 x (#_Of_Feature_Maps_)",
"_____no_output_____"
]
],
[
[
"from tensorflow.keras.layers import Input, Conv2D, Dropout, Dense, Flatten, BatchNormalization, MaxPooling2D\nfrom tensorflow.keras.models import Model",
"_____no_output_____"
],
[
"from tensorflow.keras.datasets import cifar10\n(X_train, y_train), (X_test, y_test) = cifar10.load_data()",
"_____no_output_____"
],
[
"X_train, X_test = X_train / 255.0 , X_test / 255.0 \nprint(X_train.shape)\nprint(X_test.shape)\nprint(y_train.shape)\nprint(y_test.shape)",
"(50000, 32, 32, 3)\n(10000, 32, 32, 3)\n(50000, 1)\n(10000, 1)\n"
],
[
"y_train, y_test = y_train.flatten(), y_test.flatten() \nprint(y_train.shape)\nprint(y_test.shape)",
"(50000,)\n(10000,)\n"
],
[
"classes = len(set(y_train))\nprint(classes)",
"10\n"
],
[
"input_shape = X_train[0].shape",
"_____no_output_____"
],
[
"i_layer = Input(shape = input_shape)\nh_layer = Conv2D(32, (3,3),activation='relu', padding='same')(i_layer)\nh_layer = BatchNormalization()(h_layer)\nh_layer = Conv2D(64, (3,3), activation='relu', padding='same')(h_layer)\nh_layer = BatchNormalization()(h_layer)\nh_layer = Conv2D(128, (3,3), activation='relu', padding='same')(h_layer)\nh_layer = BatchNormalization()(h_layer)\nh_layer = MaxPooling2D((2,2))(h_layer)\nh_layer = Conv2D(128, (3,3), activation='relu', padding='same')(h_layer)\nh_layer = BatchNormalization()(h_layer)\nh_layer = MaxPooling2D((2,2))(h_layer)\nh_layer = Flatten()(h_layer)\nh_layer = Dropout(0.5)(h_layer)\nh_layer = Dense(512, activation='relu')(h_layer)\nh_layer = Dropout(0.5)(h_layer)\no_layer = Dense(classes, activation='softmax')(h_layer)\n\nmodel = Model(i_layer, o_layer)\n",
"_____no_output_____"
],
[
"model.compile(optimizer='adam', \n loss = 'sparse_categorical_crossentropy',\n metrics = ['accuracy'])\n\nreport = model.fit(X_train, y_train, validation_data=(X_test, y_test), epochs=50)",
"Epoch 1/50\n1563/1563 [==============================] - 13s 8ms/step - loss: 0.4014 - accuracy: 0.8681 - val_loss: 0.3888 - val_accuracy: 0.8753\nEpoch 2/50\n1354/1563 [========================>.....] - ETA: 1s - loss: 0.3561 - accuracy: 0.8822"
],
[
"y_pred = model.predict(X_test).argmax(axis=1) \n# only for sparse categorical crossentropy",
"_____no_output_____"
],
[
"evaluation_tf(report, y_test, y_pred, classes)",
"_____no_output_____"
],
[
"misshits = np.where(y_pred!=y_test)[0]\nprint(\"total Mishits = \" + str(len(misshits)))\nindex = np.random.choice(misshits)\nplt.imshow(X_test[index])\nplt.title(\"Predicted = \" + str(labels[y_pred[index]]) + \", Real = \" + str(labels[y_test[index]]))",
"total Mishits = 1715\n"
]
],
[
[
"## LET'S ADD SOME DATA AUGMENTATION FROM KERAS \n\ntaken from https://keras.io/api/preprocessing/image/",
"_____no_output_____"
]
],
[
[
"batch_size = 32\ndata_generator = tf.keras.preprocessing.image.ImageDataGenerator(width_shift_range = 0.1, \n height_shift_range = 0.1, \n horizontal_flip=True)\n",
"_____no_output_____"
],
[
"model_dg = Model(i_layer, o_layer)\nmodel_dg.compile(optimizer='adam', \n loss = 'sparse_categorical_crossentropy',\n metrics = ['accuracy'])\ntrain_data_generator = data_generator.flow(X_train, y_train, batch_size)\nspe = X_train.shape[0] // batch_size\n\nreport = model_dg.fit_generator(train_data_generator, validation_data=(X_test, y_test), steps_per_epoch=spe, epochs=50)",
"Epoch 1/50\n1562/1562 [==============================] - 27s 17ms/step - loss: 0.3662 - accuracy: 0.8841 - val_loss: 0.3942 - val_accuracy: 0.8808\nEpoch 2/50\n1562/1562 [==============================] - 27s 17ms/step - loss: 0.3575 - accuracy: 0.8838 - val_loss: 0.4664 - val_accuracy: 0.8674\nEpoch 3/50\n1562/1562 [==============================] - 27s 17ms/step - loss: 0.3647 - accuracy: 0.8821 - val_loss: 0.4152 - val_accuracy: 0.8667\nEpoch 4/50\n1562/1562 [==============================] - 27s 17ms/step - loss: 0.3581 - accuracy: 0.8831 - val_loss: 0.4398 - val_accuracy: 0.8765\nEpoch 5/50\n1562/1562 [==============================] - 27s 17ms/step - loss: 0.3571 - accuracy: 0.8836 - val_loss: 0.4192 - val_accuracy: 0.8642\nEpoch 6/50\n1562/1562 [==============================] - 26s 17ms/step - loss: 0.3556 - accuracy: 0.8843 - val_loss: 0.4226 - val_accuracy: 0.8768\nEpoch 7/50\n1562/1562 [==============================] - 27s 17ms/step - loss: 0.3532 - accuracy: 0.8846 - val_loss: 0.3996 - val_accuracy: 0.8882\nEpoch 8/50\n1562/1562 [==============================] - 27s 17ms/step - loss: 0.3642 - accuracy: 0.8826 - val_loss: 0.4041 - val_accuracy: 0.8841\nEpoch 9/50\n1562/1562 [==============================] - 26s 17ms/step - loss: 0.3549 - accuracy: 0.8851 - val_loss: 0.4054 - val_accuracy: 0.8825\nEpoch 10/50\n1562/1562 [==============================] - 26s 17ms/step - loss: 0.3473 - accuracy: 0.8871 - val_loss: 0.4510 - val_accuracy: 0.8660\nEpoch 11/50\n1562/1562 [==============================] - 26s 17ms/step - loss: 0.3530 - accuracy: 0.8852 - val_loss: 0.3841 - val_accuracy: 0.8832\nEpoch 12/50\n1562/1562 [==============================] - 26s 17ms/step - loss: 0.3525 - accuracy: 0.8860 - val_loss: 0.4192 - val_accuracy: 0.8818\nEpoch 13/50\n1562/1562 [==============================] - 26s 17ms/step - loss: 0.3485 - accuracy: 0.8867 - val_loss: 0.4487 - val_accuracy: 0.8713\nEpoch 14/50\n1562/1562 [==============================] - 27s 17ms/step - loss: 0.3538 - accuracy: 0.8846 - val_loss: 0.4198 - val_accuracy: 0.8740\nEpoch 15/50\n1562/1562 [==============================] - 27s 17ms/step - loss: 0.3530 - accuracy: 0.8847 - val_loss: 0.4317 - val_accuracy: 0.8783\nEpoch 16/50\n1562/1562 [==============================] - 27s 17ms/step - loss: 0.3524 - accuracy: 0.8852 - val_loss: 0.3986 - val_accuracy: 0.8811\nEpoch 17/50\n1562/1562 [==============================] - 27s 17ms/step - loss: 0.3550 - accuracy: 0.8875 - val_loss: 0.4242 - val_accuracy: 0.8808\nEpoch 18/50\n1562/1562 [==============================] - 27s 17ms/step - loss: 0.3521 - accuracy: 0.8851 - val_loss: 0.4596 - val_accuracy: 0.8725\nEpoch 19/50\n1562/1562 [==============================] - 27s 17ms/step - loss: 0.3545 - accuracy: 0.8863 - val_loss: 0.4020 - val_accuracy: 0.8744\nEpoch 20/50\n1562/1562 [==============================] - 27s 17ms/step - loss: 0.3433 - accuracy: 0.8883 - val_loss: 0.4245 - val_accuracy: 0.8859\nEpoch 21/50\n1562/1562 [==============================] - 27s 17ms/step - loss: 0.3497 - accuracy: 0.8866 - val_loss: 0.4546 - val_accuracy: 0.8720\nEpoch 22/50\n1562/1562 [==============================] - 27s 17ms/step - loss: 0.3409 - accuracy: 0.8908 - val_loss: 0.4262 - val_accuracy: 0.8759\nEpoch 23/50\n1562/1562 [==============================] - 27s 17ms/step - loss: 0.3454 - accuracy: 0.8880 - val_loss: 0.4293 - val_accuracy: 0.8871\nEpoch 24/50\n1562/1562 [==============================] - 26s 17ms/step - loss: 0.3442 - accuracy: 0.8891 - val_loss: 0.4051 - val_accuracy: 0.8773\nEpoch 25/50\n1562/1562 [==============================] - 27s 17ms/step - loss: 0.3510 - accuracy: 0.8853 - val_loss: 0.4168 - val_accuracy: 0.8806\nEpoch 26/50\n1562/1562 [==============================] - 27s 17ms/step - loss: 0.3452 - accuracy: 0.8889 - val_loss: 0.4177 - val_accuracy: 0.8843\nEpoch 27/50\n1562/1562 [==============================] - 27s 17ms/step - loss: 0.3334 - accuracy: 0.8909 - val_loss: 0.5204 - val_accuracy: 0.8715\nEpoch 28/50\n1562/1562 [==============================] - 26s 17ms/step - loss: 0.3448 - accuracy: 0.8890 - val_loss: 0.4044 - val_accuracy: 0.8764\nEpoch 29/50\n1562/1562 [==============================] - 26s 17ms/step - loss: 0.3351 - accuracy: 0.8916 - val_loss: 0.3904 - val_accuracy: 0.8850\nEpoch 30/50\n1562/1562 [==============================] - 27s 18ms/step - loss: 0.3397 - accuracy: 0.8906 - val_loss: 0.3834 - val_accuracy: 0.8852\nEpoch 31/50\n1562/1562 [==============================] - 28s 18ms/step - loss: 0.3373 - accuracy: 0.8913 - val_loss: 0.4081 - val_accuracy: 0.8782\nEpoch 32/50\n1562/1562 [==============================] - 28s 18ms/step - loss: 0.3416 - accuracy: 0.8902 - val_loss: 0.4038 - val_accuracy: 0.8877\nEpoch 33/50\n1562/1562 [==============================] - 28s 18ms/step - loss: 0.3417 - accuracy: 0.8891 - val_loss: 0.4396 - val_accuracy: 0.8863\nEpoch 34/50\n1562/1562 [==============================] - 28s 18ms/step - loss: 0.3329 - accuracy: 0.8917 - val_loss: 0.3944 - val_accuracy: 0.8823\nEpoch 35/50\n1562/1562 [==============================] - 28s 18ms/step - loss: 0.3389 - accuracy: 0.8901 - val_loss: 0.4085 - val_accuracy: 0.8782\nEpoch 36/50\n1562/1562 [==============================] - 27s 18ms/step - loss: 0.3405 - accuracy: 0.8886 - val_loss: 0.4484 - val_accuracy: 0.8664\nEpoch 37/50\n1562/1562 [==============================] - 27s 17ms/step - loss: 0.3353 - accuracy: 0.8922 - val_loss: 0.4940 - val_accuracy: 0.8784\nEpoch 38/50\n1562/1562 [==============================] - 28s 18ms/step - loss: 0.3432 - accuracy: 0.8905 - val_loss: 0.4127 - val_accuracy: 0.8928\nEpoch 39/50\n1562/1562 [==============================] - 27s 17ms/step - loss: 0.3335 - accuracy: 0.8922 - val_loss: 0.4257 - val_accuracy: 0.8786\nEpoch 40/50\n1562/1562 [==============================] - 27s 17ms/step - loss: 0.3358 - accuracy: 0.8921 - val_loss: 0.3832 - val_accuracy: 0.8832\nEpoch 41/50\n1562/1562 [==============================] - 28s 18ms/step - loss: 0.3302 - accuracy: 0.8921 - val_loss: 0.4106 - val_accuracy: 0.8854\nEpoch 42/50\n1562/1562 [==============================] - 27s 17ms/step - loss: 0.3327 - accuracy: 0.8925 - val_loss: 0.4659 - val_accuracy: 0.8833\nEpoch 43/50\n1562/1562 [==============================] - 27s 17ms/step - loss: 0.3337 - accuracy: 0.8928 - val_loss: 0.4045 - val_accuracy: 0.8772\nEpoch 44/50\n1562/1562 [==============================] - 27s 17ms/step - loss: 0.3336 - accuracy: 0.8932 - val_loss: 0.4963 - val_accuracy: 0.8734\nEpoch 45/50\n1562/1562 [==============================] - 27s 17ms/step - loss: 0.3385 - accuracy: 0.8915 - val_loss: 0.4232 - val_accuracy: 0.8957\nEpoch 46/50\n1562/1562 [==============================] - 28s 18ms/step - loss: 0.3366 - accuracy: 0.8929 - val_loss: 0.3889 - val_accuracy: 0.8842\nEpoch 47/50\n1562/1562 [==============================] - 28s 18ms/step - loss: 0.3258 - accuracy: 0.8941 - val_loss: 0.4424 - val_accuracy: 0.8709\nEpoch 48/50\n1562/1562 [==============================] - 27s 18ms/step - loss: 0.3416 - accuracy: 0.8898 - val_loss: 0.4321 - val_accuracy: 0.8874\nEpoch 49/50\n1562/1562 [==============================] - 27s 17ms/step - loss: 0.3275 - accuracy: 0.8963 - val_loss: 0.4120 - val_accuracy: 0.8833\nEpoch 50/50\n1562/1562 [==============================] - 27s 17ms/step - loss: 0.3278 - accuracy: 0.8954 - val_loss: 0.3850 - val_accuracy: 0.8807\n"
],
[
"y_pred = model.predict(X_test).argmax(axis=1) \n# only for sparse categorical crossentropy",
"_____no_output_____"
],
[
"evaluation_tf(report, y_test, y_pred, classes)",
"_____no_output_____"
],
[
"misshits = np.where(y_pred!=y_test)[0]\nprint(\"total Mishits = \" + str(len(misshits)))\nindex = np.random.choice(misshits)\nplt.imshow(X_test[index])\nplt.title(\"Predicted = \" + str(labels[y_pred[index]]) + \", Real = \" + str(labels[y_test[index]]))",
"total Mishits = 1193\n"
]
]
] | [
"markdown",
"code",
"markdown",
"code",
"markdown",
"code"
] | [
[
"markdown"
],
[
"code",
"code",
"code"
],
[
"markdown"
],
[
"code",
"code",
"code",
"code",
"code",
"code",
"code",
"code",
"code",
"code",
"code"
],
[
"markdown"
],
[
"code",
"code",
"code",
"code",
"code"
]
] |
d0475757f14baa8008051af2d11f4c8b3d3aafdd | 240,086 | ipynb | Jupyter Notebook | .ipynb_checkpoints/Google Apps Workspace-checkpoint.ipynb | henrylaynesa/kaggle-google-apps | 5a19a84943c21d34fbb7c8723ce9c08a7ca57195 | [
"CC-BY-3.0"
] | null | null | null | .ipynb_checkpoints/Google Apps Workspace-checkpoint.ipynb | henrylaynesa/kaggle-google-apps | 5a19a84943c21d34fbb7c8723ce9c08a7ca57195 | [
"CC-BY-3.0"
] | null | null | null | .ipynb_checkpoints/Google Apps Workspace-checkpoint.ipynb | henrylaynesa/kaggle-google-apps | 5a19a84943c21d34fbb7c8723ce9c08a7ca57195 | [
"CC-BY-3.0"
] | null | null | null | 84.32947 | 70,840 | 0.668156 | [
[
[
"# Google Apps Workspace",
"_____no_output_____"
],
[
"## Imports",
"_____no_output_____"
]
],
[
[
"%matplotlib inline",
"_____no_output_____"
],
[
"import os\nimport pandas as pd\nimport numpy as np\nimport seaborn as sns\nfrom matplotlib import pyplot as plt",
"_____no_output_____"
]
],
[
[
"## Load Dataset",
"_____no_output_____"
]
],
[
[
"apps_df = pd.read_csv('googleplaystore.csv', index_col = 0)",
"_____no_output_____"
],
[
"reviews_df = pd.read_csv('googleplaystore_user_reviews.csv', index_col = 0)",
"_____no_output_____"
],
[
"apps_df.head()",
"_____no_output_____"
],
[
"apps_df.shape",
"_____no_output_____"
],
[
"apps_df.describe()",
"_____no_output_____"
],
[
"reviews_df.head()",
"_____no_output_____"
],
[
"reviews_df.shape",
"_____no_output_____"
],
[
"reviews_df.describe()",
"_____no_output_____"
]
],
[
[
"**Remove empty reviews**",
"_____no_output_____"
]
],
[
[
"reviews_df = reviews_df.dropna(axis=0, how='all')",
"_____no_output_____"
],
[
"apps_reviews_df = pd.merge(apps_df, reviews_df, on='App', how='inner')",
"_____no_output_____"
],
[
"apps_reviews_df.head()",
"_____no_output_____"
]
],
[
[
"Remove 1.9 Category \\\n*Because it doesn't make sense*",
"_____no_output_____"
]
],
[
[
"apps_df = apps_df[apps_df['Category'] != '1.9']",
"_____no_output_____"
]
],
[
[
"Change underscores to spaces",
"_____no_output_____"
]
],
[
[
"apps_df['Category'] = apps_df['Category'].str.replace('_', ' ')",
"_____no_output_____"
]
],
[
[
"Categories",
"_____no_output_____"
]
],
[
[
"categories = apps_df['Category'].unique()\ncategories",
"_____no_output_____"
],
[
"apps_df['Reviews'] = pd.to_numeric(apps_df['Reviews'])",
"_____no_output_____"
]
],
[
[
"Remove dollar signs",
"_____no_output_____"
]
],
[
[
"apps_df['Price'] = pd.to_numeric(apps_df['Price'].str.replace('$', ''))",
"_____no_output_____"
]
],
[
[
"Standardize App size to MB",
"_____no_output_____"
]
],
[
[
"# apps_df['Size'] = pd.to_numeric(apps_df['Size'].str.replace('M', ''))\ndef convert_to_M(s):\n if 'k' in s:\n return str(float(s[:-1])/1000)\n if 'M' in s:\n return s[:-1]\n return np.nan",
"_____no_output_____"
],
[
"apps_df['Size'] = apps_df['Size'].apply(convert_to_M)",
"_____no_output_____"
],
[
"apps_df['Size'] = pd.to_numeric(apps_df['Size'])",
"_____no_output_____"
]
],
[
[
"Fill varying app sizes to the average app size of all the apps",
"_____no_output_____"
]
],
[
[
"apps_df['Size'] = apps_df['Size'].fillna(apps_df['Size'].mean())",
"_____no_output_____"
]
],
[
[
"## Insights",
"_____no_output_____"
],
[
"### Top Apps per Category\nOnly taking into account those with reviews greater than the median",
"_____no_output_____"
]
],
[
[
"n = 3\ntemp_apps_df = apps_df.reset_index()\nprint(\"Median Ratings: %.0f\" % temp_apps_df['Reviews'].median())\ntemp_apps_df[temp_apps_df['Reviews'] > temp_apps_df['Reviews'].median()].sort_values('Rating', ascending=False).groupby('Category').head(n).reset_index(drop=True).sort_values(\"Category\").set_index(\"App\")",
"Median Ratings: 2094\n"
]
],
[
[
"### Free vs Paid",
"_____no_output_____"
]
],
[
[
"apps_df.groupby('Type').agg('size').plot.bar()",
"_____no_output_____"
],
[
"sns.jointplot(apps_df['Price'], apps_df['Rating'])",
"_____no_output_____"
]
],
[
[
"### App Size (in MB) vs Rating",
"_____no_output_____"
]
],
[
[
"sns.jointplot(apps_df['Size'], apps_df['Rating'])",
"_____no_output_____"
]
],
[
[
"### Distribution of Apps per Price\nIf it's not free, it's an outlier.",
"_____no_output_____"
]
],
[
[
"plt.figure(figsize=(18,6))\nax = sns.boxplot(x='Category', y='Price', data=apps_df, orient='v')\nax.set_xticklabels(ax.get_xticklabels(),rotation=60)\nplt.show()",
"_____no_output_____"
]
],
[
[
"### Most Expensive Apps\nPossibly implies that you can't price an app above 400$ in Google App Store",
"_____no_output_____"
]
],
[
[
"apps_df.sort_values('Price', ascending=False)[['Category', 'Price', 'Installs']].head(15)",
"_____no_output_____"
]
]
] | [
"markdown",
"code",
"markdown",
"code",
"markdown",
"code",
"markdown",
"code",
"markdown",
"code",
"markdown",
"code",
"markdown",
"code",
"markdown",
"code",
"markdown",
"code",
"markdown",
"code",
"markdown",
"code",
"markdown",
"code",
"markdown",
"code",
"markdown",
"code"
] | [
[
"markdown",
"markdown"
],
[
"code",
"code"
],
[
"markdown"
],
[
"code",
"code",
"code",
"code",
"code",
"code",
"code",
"code"
],
[
"markdown"
],
[
"code",
"code",
"code"
],
[
"markdown"
],
[
"code"
],
[
"markdown"
],
[
"code"
],
[
"markdown"
],
[
"code",
"code"
],
[
"markdown"
],
[
"code"
],
[
"markdown"
],
[
"code",
"code",
"code"
],
[
"markdown"
],
[
"code"
],
[
"markdown",
"markdown"
],
[
"code"
],
[
"markdown"
],
[
"code",
"code"
],
[
"markdown"
],
[
"code"
],
[
"markdown"
],
[
"code"
],
[
"markdown"
],
[
"code"
]
] |
d047686769413b838787504d1afd829840102a71 | 18,559 | ipynb | Jupyter Notebook | source/codes/2_Geolocalizacion.ipynb | matuteiglesias/tutorial-datos-argentinos | be7e652369a23333ecc3e3503429df2299f6dbc4 | [
"MIT"
] | 1 | 2021-01-05T18:12:06.000Z | 2021-01-05T18:12:06.000Z | source/codes/.ipynb_checkpoints/2_Geolocalizacion-checkpoint.ipynb | matuteiglesias/tutorial-datos-argentinos | be7e652369a23333ecc3e3503429df2299f6dbc4 | [
"MIT"
] | null | null | null | source/codes/.ipynb_checkpoints/2_Geolocalizacion-checkpoint.ipynb | matuteiglesias/tutorial-datos-argentinos | be7e652369a23333ecc3e3503429df2299f6dbc4 | [
"MIT"
] | null | null | null | 46.281796 | 5,786 | 0.673528 | [
[
[
"# Geolocalizacion de dataset de escuelas argentinas",
"_____no_output_____"
]
],
[
[
"#Importar librerias\nimport pandas as pd\nimport matplotlib.pyplot as plt\n%matplotlib inline\n\nimport warnings\nwarnings.filterwarnings('ignore')",
"_____no_output_____"
]
],
[
[
"### Preparacion de data",
"_____no_output_____"
]
],
[
[
"# Vamos a cargar un padron de escuelas de Argentina\n\n# Estos son los nombres de columna\ncols = ['Jurisdicción','CUE Anexo','Nombre','Sector','Estado','Ámbito','Domicilio','CP','Teléfono','Código Localidad','Localidad','Departamento','E-mail','Ed. Común','Ed. Especial','Ed. de Jóvenes y Adultos','Ed. Artística','Ed. Hospitalaria Domiciliaria','Ed. Intercultural Bilingüe','Ed. Contexto de Encierro','Jardín maternal','Jardín de infantes','Primaria','Secundaria','Secundaria Técnica (INET)','Superior no Universitario','Superior No Universitario (INET)']\n\n# Leer csv, remplazar las 'X' con True y los '' (NaN) con False\nescuelas = pd.read_csv('../../datos/escuelas_arg.csv', names=cols).fillna(False).replace('X', True)\n\n# Construir la columna 'dpto_link' con los codigos indetificatorios de partidos como los que teniamos\nescuelas['dpto_link'] = escuelas['C\\xc3\\xb3digo Localidad'].astype(str).str.zfill(8).str[:5]\n",
"_____no_output_____"
],
[
"# Tenemos los radios censales del AMBA, que creamos en el notebook anterior. Creemos los 'dpto_link' del AMBA.\nradios_censales_AMBA = pd.read_csv('../../datos/AMBA_datos', dtype=object)\ndpto_links_AMBA = (radios_censales_AMBA['prov'] + radios_censales_AMBA['depto']).unique()\n\n# Filtramos las escuelas AMBA\nescuelas_AMBA = escuelas.loc[escuelas['dpto_link'].isin(dpto_links_AMBA)]\nescuelas_AMBA = pd.concat([escuelas_AMBA, escuelas.loc[escuelas['Jurisdicci\\xc3\\xb3n'] == 'Ciudad de Buenos Aires']])\n\n# Filtramos secundaria estatal\nescuelas_AMBA_secundaria_estatal = escuelas_AMBA.loc[escuelas_AMBA['Secundaria'] & (escuelas_AMBA[u'Sector'] == 'Estatal')]\nescuelas_AMBA_secundaria_estatal.reset_index(inplace=True, drop=True)\n",
"_____no_output_____"
]
],
[
[
"### Columnas de 'Address'",
"_____no_output_____"
]
],
[
[
"# Creamos un campo que llamamos 'Address', uniendo domicilio, localidad, departamento, jurisdiccion, y ', Argentina'\nescuelas_AMBA_secundaria_estatal['Address'] = \\\nescuelas_AMBA_secundaria_estatal['Domicilio'].astype(str) + ', ' + \\\nescuelas_AMBA_secundaria_estatal['Localidad'].astype(str) + ', ' + \\\nescuelas_AMBA_secundaria_estatal['Departamento'].astype(str) + ', ' + \\\nescuelas_AMBA_secundaria_estatal['Jurisdicci\\xc3\\xb3n'].astype(str) +', Argentina'",
"_____no_output_____"
],
[
"pd.set_option('display.max_colwidth', -1)",
"_____no_output_____"
],
[
"import re\n\ndef filtrar_entre_calles(string):\n \"\"\"\n Removes substring between 'E/' and next field (delimited by ','). Case insensitive.\n \n example: \n >>> out = filtrar_entre_calles('LASCANO E/ ROMA E ISLAS MALVINAS 6213, ISIDRO CASANOVA')\n >>> print out\n \n 'LASCANO 6213, ISIDRO CASANOVA'\n \n \"\"\"\n s = string.lower()\n try:\n m = re.search(\"\\d\", s)\n start = s.index( 'e/' )\n# end = s.index( last, start )\n end = m.start()\n return string[:start] + string[end:]\n except:\n return string\n \ndef filtrar_barrio(string, n = 3):\n \"\"\"\n Leaves only n most aggregate fields and the address.\n \n example: \n >>> out = filtrar_entre_calles('LASCANO 6213, ISIDRO CASANOVA, LA MATANZA, Buenos Aires, Argentina')\n >>> print out\n \n 'LASCANO 6213, LA MATANZA, Buenos Aires, Argentina'\n \n \"\"\"\n try:\n coma_partido_jurisdiccion = [m.start() for m in re.finditer(',', string)][-n]\n coma_direccion = [m.start() for m in re.finditer(',', string)][0]\n\n s = string[:coma_direccion][::-1]\n \n if \"n/s\" in s.lower():\n start = s.lower().index('n/s')\n cut = len(s) - len('n/s') - start\n\n else: \n m = re.search(\"\\d\", s)\n cut = len(s) - m.start(0)\n\n return string[:cut] + string[coma_partido_jurisdiccion:]\n except AttributeError:\n return string\n\nescuelas_AMBA_secundaria_estatal['Address_2'] = escuelas_AMBA_secundaria_estatal['Address'].apply(filtrar_entre_calles)\nescuelas_AMBA_secundaria_estatal['Address_3'] = escuelas_AMBA_secundaria_estatal['Address_2'].apply(filtrar_barrio)\n\nescuelas_AMBA_secundaria_estatal.to_csv('../../datos/escuelas_AMBA_secundaria_estatal.csv', index = False)",
"_____no_output_____"
]
],
[
[
"### Geolocalizacion",
"_____no_output_____"
]
],
[
[
"import json\nimport time\nimport urllib\nimport urllib2\n\ndef geolocate(inp, API_key = None, BACKOFF_TIME = 30):\n\n # See https://developers.google.com/maps/documentation/timezone/get-api-key\n# with open('googleMapsAPIkey.txt', 'r') as myfile:\n# maps_key = myfile.read().replace('\\n', '')\n \n base_url = 'https://maps.googleapis.com/maps/api/geocode/json'\n\n # This joins the parts of the URL together into one string.\n url = base_url + '?' + urllib.urlencode({\n 'address': \"%s\" % (inp),\n 'key': API_key,\n })\n \n try:\n # Get the API response.\n response = str(urllib2.urlopen(url).read())\n except IOError:\n pass # Fall through to the retry loop.\n else:\n # If we didn't get an IOError then parse the result.\n result = json.loads(response.replace('\\\\n', ''))\n if result['status'] == 'OK':\n return result['results'][0]\n elif result['status'] != 'UNKNOWN_ERROR':\n # Many API errors cannot be fixed by a retry, e.g. INVALID_REQUEST or\n # ZERO_RESULTS. There is no point retrying these requests.\n# raise Exception(result['error_message'])\n return None\n # If we're over the API limit, backoff for a while and try again later.\n elif result['status'] == 'OVER_QUERY_LIMIT':\n print \"Hit Query Limit! Backing off for \"+str(BACKOFF_TIME)+\" minutes...\"\n time.sleep(BACKOFF_TIME * 60) # sleep for 30 minutes\n geocoded = False\n\ndef set_geolocation_values(df, loc):\n df.set_value(i,'lng', loc['geometry']['location']['lng'])\n df.set_value(i,'lat', loc['geometry']['location']['lat'])\n df.set_value(i, 'id', loc['place_id'])",
"_____no_output_____"
],
[
"dataframe = escuelas_AMBA_secundaria_estatal\ncol, col_2, col_3 = 'Address', 'Address_2', 'Address_3'\nAPI_key = 'AIzaSyDjBFMZlNTyds2Sfihu2D5LTKupKDBpf6c'\n\nfor i, row in dataframe.iterrows():\n loc = geolocate(row[col], API_key)\n if loc:\n set_geolocation_values(dataframe, loc)\n else:\n loc = geolocate(row[col_2], API_key)\n if loc:\n set_geolocation_values(dataframe, loc)\n else:\n loc = geolocate(row[col_3], API_key)\n if loc:\n set_geolocation_values(dataframe, loc)\n \n if i%50 == 0:\n print 'processed row '+str(i)\n \ndataframe.to_csv('../../datos/esc_sec_AMBA_geoloc.csv', index = False, encoding = 'utf8')\n",
"processed row 900\nprocessed row 950\nprocessed row 1000\nprocessed row 1050\nprocessed row 1100\nprocessed row 1150\n"
],
[
"# esc_sec_AMBA_geoloc_1200 = pd.read_csv('../../datos/esc_sec_AMBA_geoloc_1200.csv', encoding = 'utf8')\n# esc_sec_AMBA_geoloc_480_1200 = pd.read_csv('../../datos/esc_sec_AMBA_geoloc_480_1200.csv', encoding = 'utf8')\n# esc_sec_AMBA_geoloc = pd.read_csv('../../datos/esc_sec_AMBA_geoloc.csv', encoding = 'utf8')\n# esc_sec_AMBA_geoloc_900_1200 = pd.read_csv('../../datos/esc_sec_AMBA_geoloc_900_1200.csv', encoding = 'utf8')\n\n# pd.concat([esc_sec_AMBA_geoloc[:480],esc_sec_AMBA_geoloc_480_1200[:420],esc_sec_AMBA_geoloc_900_1200, esc_sec_AMBA_geoloc_1200]).to_csv('../../datos/esc_sec_AMBA_geoloc_full.csv', index = False, encoding = 'utf8')",
"_____no_output_____"
],
[
"print len(pd.read_csv('../../datos/esc_sec_AMBA_geoloc_full.csv', encoding = 'utf8').dropna())\nprint len(pd.read_csv('../../datos/esc_sec_AMBA_geoloc_full.csv', encoding = 'utf8'))\n1840/2066.",
"1840\n2066\n"
],
[
"import numpy as np\ndf = pd.read_csv('../../datos/esc_sec_AMBA_geoloc_full.csv', encoding = 'utf8')\nindex = df['lat'].index[df['lat'].apply(np.isnan)]",
"_____no_output_____"
],
[
"plt.hist(index, 100)\n# plt.xlim(900, 1300)\nplt.show()",
"_____no_output_____"
],
[
"df.iloc[np.where(pd.isnull(df['lat']))][['Nombre','Address', 'Address_2', 'Address_3']].to_csv('../../datos/no_result_addresses.csv', index = False, encoding = 'utf8')",
"_____no_output_____"
]
]
] | [
"markdown",
"code",
"markdown",
"code",
"markdown",
"code",
"markdown",
"code"
] | [
[
"markdown"
],
[
"code"
],
[
"markdown"
],
[
"code",
"code"
],
[
"markdown"
],
[
"code",
"code",
"code"
],
[
"markdown"
],
[
"code",
"code",
"code",
"code",
"code",
"code",
"code"
]
] |
d04780afcd91007531d210a9dbdc1a8414cd99ef | 25,645 | ipynb | Jupyter Notebook | Functions-Pese.ipynb | oluwapesealo/peseCSC102 | 649607fd5b78590e234e7cba44fcdfc987920bb0 | [
"MIT"
] | null | null | null | Functions-Pese.ipynb | oluwapesealo/peseCSC102 | 649607fd5b78590e234e7cba44fcdfc987920bb0 | [
"MIT"
] | null | null | null | Functions-Pese.ipynb | oluwapesealo/peseCSC102 | 649607fd5b78590e234e7cba44fcdfc987920bb0 | [
"MIT"
] | null | null | null | 37.005772 | 1,402 | 0.563034 | [
[
[
"empty"
]
]
] | [
"empty"
] | [
[
"empty"
]
] |
d0478f45178270323f7a00edc6e5349d7f8cbd84 | 3,693 | ipynb | Jupyter Notebook | content/lessons/02/Now-You-Code/NYC1-Hello-2-Ways.ipynb | hvpuzzan-su/intern-project | b65e8d2cd43e21c3fdc61b7bbb8e81f5c9148f08 | [
"MIT"
] | null | null | null | content/lessons/02/Now-You-Code/NYC1-Hello-2-Ways.ipynb | hvpuzzan-su/intern-project | b65e8d2cd43e21c3fdc61b7bbb8e81f5c9148f08 | [
"MIT"
] | null | null | null | content/lessons/02/Now-You-Code/NYC1-Hello-2-Ways.ipynb | hvpuzzan-su/intern-project | b65e8d2cd43e21c3fdc61b7bbb8e81f5c9148f08 | [
"MIT"
] | null | null | null | 27.559701 | 233 | 0.581099 | [
[
[
"# Now You Code 1: Hello 2 Ways\n\nWrite a Python program which prompts you to input your first name and then your last name. It should then print your name two ways First Last and Last, First. For example:\n\n```\nWhat is your first name? Michael\nWhat is your last name? Fudge\nHello, Michael Fudge\nOr should I say Fudge, Michael\n```\n",
"_____no_output_____"
],
[
"## Step 1: Problem Analysis\n\nInputs:\n\nfirst name\nlast name\n\nOutputs:\n\nHello, first name, last name\nOr should I say last name first name\n\nAlgorithm (Steps in Program):\n\ninput first name \ninput last name \nprint line one with embedded variables \nprint line two with embedded variables\n\n",
"_____no_output_____"
]
],
[
[
"first_name = input('What is your first name? ')\nlast_name = input('What is your last name? ')\nprint('Hello,' , first_name , last_name)\nprint('Or should I say' , last_name , first_name)",
"What is your first name? Puzzanghera\nWhat is your last name? Hope\nHello, Puzzanghera Hope\nOr should I say Hope Puzzanghera\n"
]
],
[
[
"## Step 3: Questions\n\n1. What happens when don't follow the instructions and enter your first name as your last name? Does the code still run? Why?\n\nThe code still runs, it just flips the input you enter because it thinks your first name is your last name. \n\n2. What type of error it when the program runs but does not handle bad input?\n\nIt is a logical error, so the program will run but the output will not be what you wanted. \n\n3. Is there anything you can do in code to correct this type of error? Why or why not?\n\nI don't think there is any way to correct this type of errror because python has no way of knowing which is the first name and which is the last name from the input. It only knows that a variable was assigned to certain input. ",
"_____no_output_____"
],
[
"## Reminder of Evaluation Criteria\n\n1. What the problem attempted (analysis, code, and answered questions) ?\n2. What the problem analysis thought out? (does the program match the plan?)\n3. Does the code execute without syntax error?\n4. Does the code solve the intended problem?\n5. Is the code well written? (easy to understand, modular, and self-documenting, handles errors)\n",
"_____no_output_____"
]
]
] | [
"markdown",
"code",
"markdown"
] | [
[
"markdown",
"markdown"
],
[
"code"
],
[
"markdown",
"markdown"
]
] |
d0479022860db927a6e1ab400befe82eacf791bc | 17,271 | ipynb | Jupyter Notebook | _episodes_pynb/04-merging-data_clean.ipynb | scw-ss/-2018-06-27-cfmehu-python-ecology-lesson | 94fa0ee388e8228e2458a493ef627de9941a5b29 | [
"CC-BY-4.0"
] | null | null | null | _episodes_pynb/04-merging-data_clean.ipynb | scw-ss/-2018-06-27-cfmehu-python-ecology-lesson | 94fa0ee388e8228e2458a493ef627de9941a5b29 | [
"CC-BY-4.0"
] | 1 | 2018-06-09T13:29:05.000Z | 2018-06-09T13:29:05.000Z | _episodes_pynb/04-merging-data_clean.ipynb | scw-ss/-2018-06-27-cfmehu-python-ecology-lesson | 94fa0ee388e8228e2458a493ef627de9941a5b29 | [
"CC-BY-4.0"
] | null | null | null | 37.792123 | 142 | 0.633663 | [
[
[
"# Combining DataFrames with pandas\n\nIn many \"real world\" situations, the data that we want to use come in multiple\nfiles. We often need to combine these files into a single DataFrame to analyze\nthe data. The pandas package provides [various methods for combining\nDataFrames](http://pandas.pydata.org/pandas-docs/stable/merging.html) including\n`merge` and `concat`.\n\nTo work through the examples below, we first need to load the species and\nsurveys files into pandas DataFrames. In iPython:\n",
"_____no_output_____"
],
[
"Take note that the `read_csv` method we used can take some additional options which\nwe didn't use previously. Many functions in python have a set of options that\ncan be set by the user if needed. In this case, we have told Pandas to assign\nempty values in our CSV to NaN `keep_default_na=False, na_values=[\"\"]`.\n[More about all of the read_csv options here.](http://pandas.pydata.org/pandas-docs/dev/generated/pandas.io.parsers.read_csv.html)\n\n# Concatenating DataFrames\n\nWe can use the `concat` function in Pandas to append either columns or rows from\none DataFrame to another. Let's grab two subsets of our data to see how this\nworks.",
"_____no_output_____"
]
],
[
[
"# read in first 10 lines of surveys table\n\n# grab the last 10 rows\n\n# reset the index values to the second dataframe appends properly\n\n# drop=True option avoids adding new index column with old index values",
"_____no_output_____"
]
],
[
[
"When we concatenate DataFrames, we need to specify the axis. `axis=0` tells\nPandas to stack the second DataFrame under the first one. It will automatically\ndetect whether the column names are the same and will stack accordingly.\n`axis=1` will stack the columns in the second DataFrame to the RIGHT of the\nfirst DataFrame. To stack the data vertically, we need to make sure we have the\nsame columns and associated column format in both datasets. When we stack\nhorizonally, we want to make sure what we are doing makes sense (ie the data are\nrelated in some way).",
"_____no_output_____"
]
],
[
[
"# stack the DataFrames on top of each other\n",
"_____no_output_____"
],
[
"# place the DataFrames side by side\n",
"_____no_output_____"
]
],
[
[
"### Row Index Values and Concat\nHave a look at the `vertical_stack` dataframe? Notice anything unusual?\nThe row indexes for the two data frames `survey_sub` and `survey_sub_last10`\nhave been repeated. We can reindex the new dataframe using the `reset_index()` method.\n\n## Writing Out Data to CSV\n\nWe can use the `to_csv` command to do export a DataFrame in CSV format. Note that the code\nbelow will by default save the data into the current working directory. We can\nsave it to a different folder by adding the foldername and a slash to the file\n`vertical_stack.to_csv('foldername/out.csv')`. We use the 'index=False' so that\npandas doesn't include the index number for each line.\n",
"_____no_output_____"
]
],
[
[
"# Write DataFrame to CSV\n",
"_____no_output_____"
]
],
[
[
"Check out your working directory to make sure the CSV wrote out properly, and\nthat you can open it! If you want, try to bring it back into python to make sure\nit imports properly.",
"_____no_output_____"
]
],
[
[
"# for kicks read our output back into python and make sure all looks good\n",
"_____no_output_____"
]
],
[
[
"> ## Challenge - Combine Data\n>\n> In the data folder, there are two survey data files: `survey2001.csv` and\n> `survey2002.csv`. Read the data into python and combine the files to make one\n> new data frame. Create a plot of average plot weight by year grouped by sex.\n> Export your results as a CSV and make sure it reads back into python properly.",
"_____no_output_____"
],
[
"# Joining DataFrames\n\nWhen we concatenated our DataFrames we simply added them to each other -\nstacking them either vertically or side by side. Another way to combine\nDataFrames is to use columns in each dataset that contain common values (a\ncommon unique id). Combining DataFrames using a common field is called\n\"joining\". The columns containing the common values are called \"join key(s)\".\nJoining DataFrames in this way is often useful when one DataFrame is a \"lookup\ntable\" containing additional data that we want to include in the other.\n\nNOTE: This process of joining tables is similar to what we do with tables in an\nSQL database.\n\nFor example, the `species.csv` file that we've been working with is a lookup\ntable. This table contains the genus, species and taxa code for 55 species. The\nspecies code is unique for each line. These species are identified in our survey\ndata as well using the unique species code. Rather than adding 3 more columns\nfor the genus, species and taxa to each of the 35,549 line Survey data table, we\ncan maintain the shorter table with the species information. When we want to\naccess that information, we can create a query that joins the additional columns\nof information to the Survey data.\n\nStoring data in this way has many benefits including:\n\n1. It ensures consistency in the spelling of species attributes (genus, species\n and taxa) given each species is only entered once. Imagine the possibilities\n for spelling errors when entering the genus and species thousands of times!\n2. It also makes it easy for us to make changes to the species information once\n without having to find each instance of it in the larger survey data.\n3. It optimizes the size of our data.\n\n\n## Joining Two DataFrames\n\nTo better understand joins, let's grab the first 10 lines of our data as a\nsubset to work with. We'll use the `.head` method to do this. We'll also read\nin a subset of the species table.\n",
"_____no_output_____"
]
],
[
[
"# read in first 10 lines of surveys table\n\n\n# import a small subset of the species data designed for this part of the lesson.\n# It is stored in the data folder.\n",
"_____no_output_____"
]
],
[
[
"In this example, `species_sub` is the lookup table containing genus, species, and\ntaxa names that we want to join with the data in `survey_sub` to produce a new\nDataFrame that contains all of the columns from both `species_df` *and*\n`survey_df`.\n\n\n## Identifying join keys\n\nTo identify appropriate join keys we first need to know which field(s) are\nshared between the files (DataFrames). We might inspect both DataFrames to\nidentify these columns. If we are lucky, both DataFrames will have columns with\nthe same name that also contain the same data. If we are less lucky, we need to\nidentify a (differently-named) column in each DataFrame that contains the same\ninformation.",
"_____no_output_____"
],
[
"In our example, the join key is the column containing the two-letter species\nidentifier, which is called `species_id`.\n\nNow that we know the fields with the common species ID attributes in each\nDataFrame, we are almost ready to join our data. However, since there are\n[different types of joins](http://blog.codinghorror.com/a-visual-explanation-of-sql-joins/), we\nalso need to decide which type of join makes sense for our analysis.\n\n## Inner joins\n\nThe most common type of join is called an _inner join_. An inner join combines\ntwo DataFrames based on a join key and returns a new DataFrame that contains\n**only** those rows that have matching values in *both* of the original\nDataFrames.\n\nInner joins yield a DataFrame that contains only rows where the value being\njoins exists in BOTH tables. An example of an inner join, adapted from [this\npage](http://blog.codinghorror.com/a-visual-explanation-of-sql-joins/) is below:\n\n\n\nThe pandas function for performing joins is called `merge` and an Inner join is\nthe default option: ",
"_____no_output_____"
],
[
"The result of an inner join of `survey_sub` and `species_sub` is a new DataFrame\nthat contains the combined set of columns from `survey_sub` and `species_sub`. It\n*only* contains rows that have two-letter species codes that are the same in\nboth the `survey_sub` and `species_sub` DataFrames. In other words, if a row in\n`survey_sub` has a value of `species_id` that does *not* appear in the `species_id`\ncolumn of `species`, it will not be included in the DataFrame returned by an\ninner join. Similarly, if a row in `species_sub` has a value of `species_id`\nthat does *not* appear in the `species_id` column of `survey_sub`, that row will not\nbe included in the DataFrame returned by an inner join.\n\nThe two DataFrames that we want to join are passed to the `merge` function using\nthe `left` and `right` argument. The `left_on='species'` argument tells `merge`\nto use the `species_id` column as the join key from `survey_sub` (the `left`\nDataFrame). Similarly , the `right_on='species_id'` argument tells `merge` to\nuse the `species_id` column as the join key from `species_sub` (the `right`\nDataFrame). For inner joins, the order of the `left` and `right` arguments does\nnot matter.\n\nThe result `merged_inner` DataFrame contains all of the columns from `survey_sub`\n(record id, month, day, etc.) as well as all the columns from `species_sub`\n(species_id, genus, species, and taxa).\n\nNotice that `merged_inner` has fewer rows than `survey_sub`. This is an\nindication that there were rows in `surveys_df` with value(s) for `species_id` that\ndo not exist as value(s) for `species_id` in `species_df`.\n\n## Left joins\n\nWhat if we want to add information from `species_sub` to `survey_sub` without\nlosing any of the information from `survey_sub`? In this case, we use a different\ntype of join called a \"left outer join\", or a \"left join\".\n\nLike an inner join, a left join uses join keys to combine two DataFrames. Unlike\nan inner join, a left join will return *all* of the rows from the `left`\nDataFrame, even those rows whose join key(s) do not have values in the `right`\nDataFrame. Rows in the `left` DataFrame that are missing values for the join\nkey(s) in the `right` DataFrame will simply have null (i.e., NaN or None) values\nfor those columns in the resulting joined DataFrame.\n\nNote: a left join will still discard rows from the `right` DataFrame that do not\nhave values for the join key(s) in the `left` DataFrame.\n\n\n\nA left join is performed in pandas by calling the same `merge` function used for\ninner join, but using the `how='left'` argument:",
"_____no_output_____"
],
[
"The result DataFrame from a left join (`merged_left`) looks very much like the\nresult DataFrame from an inner join (`merged_inner`) in terms of the columns it\ncontains. However, unlike `merged_inner`, `merged_left` contains the **same\nnumber of rows** as the original `survey_sub` DataFrame. When we inspect\n`merged_left`, we find there are rows where the information that should have\ncome from `species_sub` (i.e., `species_id`, `genus`, and `taxa`) is\nmissing (they contain NaN values):",
"_____no_output_____"
],
[
"These rows are the ones where the value of `species_id` from `survey_sub` (in this\ncase, `PF`) does not occur in `species_sub`.\n\n\n## Other join types\n\nThe pandas `merge` function supports two other join types:\n\n* Right (outer) join: Invoked by passing `how='right'` as an argument. Similar\n to a left join, except *all* rows from the `right` DataFrame are kept, while\n rows from the `left` DataFrame without matching join key(s) values are\n discarded.\n* Full (outer) join: Invoked by passing `how='outer'` as an argument. This join\n type returns the all pairwise combinations of rows from both DataFrames; i.e.,\n the result DataFrame will `NaN` where data is missing in one of the dataframes. This join type is\n very rarely used.\n\n# Final Challenges\n\n> ## Challenge - Distributions\n> Create a new DataFrame by joining the contents of the `surveys.csv` and\n> `species.csv` tables. Then calculate and plot the distribution of:\n>\n> 1. taxa by plot\n> 2. taxa by sex by plot\n\n> ## Challenge - Diversity Index\n>\n> 1. In the data folder, there is a plot `CSV` that contains information about the\n> type associated with each plot. Use that data to summarize the number of\n> plots by plot type.\n> 2. Calculate a diversity index of your choice for control vs rodent exclosure\n> plots. The index should consider both species abundance and number of\n> species. You might choose to use the simple [biodiversity index described\n> here](http://www.amnh.org/explore/curriculum-collections/biodiversity-counts/plant-ecology/how-to-calculate-a-biodiversity-index)\n> which calculates diversity as:\n>\n> the number of species in the plot / the total number of individuals in the plot = Biodiversity index.",
"_____no_output_____"
]
]
] | [
"markdown",
"code",
"markdown",
"code",
"markdown",
"code",
"markdown",
"code",
"markdown",
"code",
"markdown"
] | [
[
"markdown",
"markdown"
],
[
"code"
],
[
"markdown"
],
[
"code",
"code"
],
[
"markdown"
],
[
"code"
],
[
"markdown"
],
[
"code"
],
[
"markdown",
"markdown"
],
[
"code"
],
[
"markdown",
"markdown",
"markdown",
"markdown",
"markdown"
]
] |
d04790481f3b27d7714bdf34d1d5f2dce7a3fcbd | 411,577 | ipynb | Jupyter Notebook | Chapter 13/Chapter13Walkthrough.ipynb | ZhangXinNan/MathForProgrammers | fe720a37390de951cc9d0a7de975ce32cb43bc4b | [
"MIT"
] | null | null | null | Chapter 13/Chapter13Walkthrough.ipynb | ZhangXinNan/MathForProgrammers | fe720a37390de951cc9d0a7de975ce32cb43bc4b | [
"MIT"
] | null | null | null | Chapter 13/Chapter13Walkthrough.ipynb | ZhangXinNan/MathForProgrammers | fe720a37390de951cc9d0a7de975ce32cb43bc4b | [
"MIT"
] | null | null | null | 269.356675 | 50,024 | 0.932593 | [
[
[
"# Chapter 13: Analyzing sound waves with Fourier Series",
"_____no_output_____"
],
[
"Helper functions",
"_____no_output_____"
]
],
[
[
"import matplotlib.pyplot as plt\ndef plot_function(f,xmin,xmax,**kwargs):\n ts = np.linspace(xmin,xmax,1000)\n plt.plot(ts,[f(t) for t in ts],**kwargs)\n \ndef plot_sequence(points,max=100,line=False,**kwargs):\n if line:\n plt.plot(range(0,max),points[0:max],**kwargs)\n else:\n plt.scatter(range(0,max),points[0:max],**kwargs)",
"_____no_output_____"
]
],
[
[
"## 13.1 Playing sound waves in Python",
"_____no_output_____"
],
[
"### 13.1.1 Producing our first sound",
"_____no_output_____"
]
],
[
[
"import pygame, pygame.sndarray\npygame.mixer.init(frequency=44100, size=-16, channels=1)",
"pygame 1.9.4\nHello from the pygame community. https://www.pygame.org/contribute.html\n"
],
[
"import numpy as np\narr = np.random.randint(-32768, 32767, size=44100)\narr",
"_____no_output_____"
],
[
"plot_sequence(arr)",
"_____no_output_____"
],
[
"plot_sequence(arr,line=True,max=441)",
"_____no_output_____"
]
],
[
[
"**CAUTION: May play a loud sound!!!**",
"_____no_output_____"
]
],
[
[
"sound = pygame.sndarray.make_sound(arr)\nsound.play()",
"_____no_output_____"
],
[
"arr = np.random.randint(-10000, 10000, size=44100)\nsound = pygame.sndarray.make_sound(arr)\nsound.play()",
"_____no_output_____"
]
],
[
[
"### 13.1.2 Playing a musical note",
"_____no_output_____"
]
],
[
[
"form = np.repeat([10000,-10000],50) #<1>\nplot_sequence(form)",
"_____no_output_____"
],
[
"arr = np.tile(form,441)",
"_____no_output_____"
],
[
"plot_sequence(arr,line=True,max=1000)",
"_____no_output_____"
],
[
"sound = pygame.sndarray.make_sound(arr)\nsound.play()",
"_____no_output_____"
]
],
[
[
"### 13.1.3 Exercises",
"_____no_output_____"
],
[
"**Exercise:** Our musical note “A” was a pattern that repeated 441 times in a second. Create a similar pattern that repeats 350 times in one second, which will produce the musical note “F”.",
"_____no_output_____"
],
[
"**Solution:**",
"_____no_output_____"
]
],
[
[
"form = np.repeat([10000,-10000],63)\narr = np.tile(form,350)\nsound = pygame.sndarray.make_sound(arr)\nsound.play()",
"_____no_output_____"
]
],
[
[
"## 13.2 Turning a sinusoidal wave into a sound",
"_____no_output_____"
],
[
"### 13.2.1 Making audio from sinusoidal functions",
"_____no_output_____"
]
],
[
[
"from math import sin,cos,pi",
"_____no_output_____"
],
[
"plot_function(sin,0,4*pi)",
"_____no_output_____"
]
],
[
[
"### 13.2.2 Changing the frequency of a sinusoid",
"_____no_output_____"
]
],
[
[
"def make_sinusoid(frequency,amplitude):\n def f(t): #<1>\n return amplitude * sin(2*pi*frequency*t) #<2>\n return f",
"_____no_output_____"
],
[
"plot_function(make_sinusoid(5,4),0,1)",
"_____no_output_____"
]
],
[
[
"### 13.2.3 Sampling and playing the sound wave",
"_____no_output_____"
]
],
[
[
"sinusoid = make_sinusoid(441,8000)",
"_____no_output_____"
],
[
"np.arange(0,1,0.1)",
"_____no_output_____"
],
[
"np.arange(0,1,1/44100)",
"_____no_output_____"
],
[
"def sample(f,start,end,count): #<1>\n mapf = np.vectorize(f) #<2>\n ts = np.arange(start,end,(end-start)/count) #<3>\n values = mapf(ts) #<4>\n return values.astype(np.int16) #<5>",
"_____no_output_____"
],
[
"sinusoid = make_sinusoid(441,8000)\narr = sample(sinusoid, 0, 1, 44100)\nsound = pygame.sndarray.make_sound(arr)\nsound.play()",
"_____no_output_____"
]
],
[
[
"### 13.2.4 Exercises",
"_____no_output_____"
],
[
"**Exercise:** Plot the tangent function $\\tan(t) = \\sin(t)/\\cos(t).$ What is its period?",
"_____no_output_____"
],
[
"**Solution:** The period is $\\pi$.",
"_____no_output_____"
]
],
[
[
"from math import tan\nplot_function(tan,0,5*pi)\nplt.ylim(-10,10) #<1>",
"_____no_output_____"
]
],
[
[
"**Exercise:** Find the value of $k$ such that $\\cos(kt)$ has a frequency of 5. Plot the resulting function $\\cos(kt)$ from zero to one and show that it repeats itself 5 times.",
"_____no_output_____"
],
[
"**Solution:**",
"_____no_output_____"
]
],
[
[
"plot_function(lambda t: cos(10*pi*t),0,1)",
"_____no_output_____"
]
],
[
[
"## 13.3 Combining sound waves to make new ones",
"_____no_output_____"
],
[
"### 13.3.1 Adding sampled sound waves to build a chord",
"_____no_output_____"
]
],
[
[
"np.array([1,2,3]) + np.array([4,5,6])",
"_____no_output_____"
],
[
"sample1 = sample(make_sinusoid(441,8000),0,1,44100)\nsample2 = sample(make_sinusoid(551,8000),0,1,44100)",
"_____no_output_____"
],
[
"sound1 = pygame.sndarray.make_sound(sample1)\nsound2 = pygame.sndarray.make_sound(sample2)\nsound1.play()\nsound2.play()",
"_____no_output_____"
],
[
"chord = pygame.sndarray.make_sound(sample1 + sample2)\nchord.play()",
"_____no_output_____"
]
],
[
[
"### 13.3.2 Picturing the sum of two sound waves",
"_____no_output_____"
]
],
[
[
"plot_sequence(sample1,max=400)\nplot_sequence(sample2,max=400)",
"_____no_output_____"
],
[
"plot_sequence(sample1+sample2,max=400)",
"_____no_output_____"
]
],
[
[
"### 13.3.3 Building a linear combination of sinusoids",
"_____no_output_____"
]
],
[
[
"def const(n):\n return 1\n\ndef fourier_series(a0,a,b):\n def result(t):\n cos_terms = [an*cos(2*pi*(n+1)*t) for (n,an) in enumerate(a)] #<1>\n sin_terms = [bn*sin(2*pi*(n+1)*t) for (n,bn) in enumerate(b)] #<2>\n return a0*const(t) + sum(cos_terms) + sum(sin_terms) #<3>\n return result",
"_____no_output_____"
],
[
"f = fourier_series(0,[0,0,0,0,0],[0,0,0,1,1])",
"_____no_output_____"
],
[
"plot_function(f,0,1)",
"_____no_output_____"
]
],
[
[
"### 13.3.4 Building a familiar function with sinusoids",
"_____no_output_____"
]
],
[
[
"f1 = fourier_series(0,[],[4/pi])",
"_____no_output_____"
],
[
"f3 = fourier_series(0,[],[4/pi,0,4/(3*pi)])",
"_____no_output_____"
],
[
"plot_function(f1,0,1)\nplot_function(f3,0,1)",
"_____no_output_____"
],
[
"b = [4/(n * pi) if n%2 != 0 else 0 for n in range(1,10)] #<1>\nf = fourier_series(0,[],b)\nplot_function(f,0,1)",
"_____no_output_____"
],
[
"b = [4/(n * pi) if n%2 != 0 else 0 for n in range(1,20)]\nf = fourier_series(0,[],b)\nplot_function(f,0,1)",
"_____no_output_____"
],
[
"b = [4/(n * pi) if n%2 != 0 else 0 for n in range(1,100)]\nf = fourier_series(0,[],b)\nplot_function(f,0,1)",
"_____no_output_____"
]
],
[
[
"### 13.3.5 Exercises",
"_____no_output_____"
],
[
"**Mini-project:** Create a manipulated version of the square wave Fourier series so that is frequency is 441 Hz, sample it, and confirm that it doesn’t just look like the square wave -- it sounds like the square wave as well.",
"_____no_output_____"
],
[
"**Solution:** Here's a quick idea of how to do this with the function `f` you just built.",
"_____no_output_____"
]
],
[
[
"arr = sample(lambda t: 10000* f(441*t), 0, 1, 44100)\nsound = pygame.sndarray.make_sound(arr)\nsound.play()",
"_____no_output_____"
]
],
[
[
"## 13.4 Decomposing a sound wave into its Fourier Series",
"_____no_output_____"
],
[
"### 13.4.1 Finding vector components with an inner product",
"_____no_output_____"
],
[
"### 13.4.2 Defining an inner product for periodic functions",
"_____no_output_____"
]
],
[
[
"def inner_product(f,g,N=1000):\n dt = 1/N #<1>\n return 2*sum([f(t)*g(t)*dt for t in np.arange(0,1,dt)]) #<2>",
"_____no_output_____"
],
[
"def s(n): #<1>\n def f(t):\n return sin(2*pi*n*t)\n return f\n\ndef c(n): #<2>\n def f(t):\n return cos(2*pi*n*t)\n return f",
"_____no_output_____"
],
[
"inner_product(s(1),c(1))",
"_____no_output_____"
],
[
"inner_product(s(1),s(2))",
"_____no_output_____"
],
[
"inner_product(c(3),s(10))",
"_____no_output_____"
],
[
"inner_product(s(1),s(1))",
"_____no_output_____"
],
[
"inner_product(c(1),c(1))",
"_____no_output_____"
],
[
"inner_product(c(3),c(3))",
"_____no_output_____"
],
[
"from math import sqrt\n\ndef const(n):\n return 1 /sqrt(2)",
"_____no_output_____"
],
[
"inner_product(const,s(1))",
"_____no_output_____"
],
[
"inner_product(const,c(1))",
"_____no_output_____"
],
[
"inner_product(const,const)",
"_____no_output_____"
]
],
[
[
"### 13.4.3 Writing a function to find Fourier coefficients",
"_____no_output_____"
],
[
"**note** we have a new `const` function so `fourier_series` will behave differently",
"_____no_output_____"
]
],
[
[
"def fourier_series(a0,a,b):\n def result(t):\n cos_terms = [an*cos(2*pi*(n+1)*t) for (n,an) in enumerate(a)] #<1>\n sin_terms = [bn*sin(2*pi*(n+1)*t) for (n,bn) in enumerate(b)] #<2>\n return a0*const(t) + sum(cos_terms) + sum(sin_terms) #<3>\n return result",
"_____no_output_____"
],
[
"def fourier_coefficients(f,N):\n a0 = inner_product(f,const) #<1>\n an = [inner_product(f,c(n)) for n in range(1,N+1)] #<2>\n bn = [inner_product(f,s(n)) for n in range(1,N+1)] #<3>\n return a0, an, bn",
"_____no_output_____"
],
[
"f = fourier_series(0,[2,3,4],[5,6,7])",
"_____no_output_____"
],
[
"fourier_coefficients(f,3)",
"_____no_output_____"
]
],
[
[
"### 13.4.4 Finding the Fourier coefficients for the square wave",
"_____no_output_____"
]
],
[
[
"def square(t):\n return 1 if (t%1) < 0.5 else -1",
"_____no_output_____"
],
[
"a0, a, b = fourier_coefficients(square,10)",
"_____no_output_____"
],
[
"b[0], 4/pi",
"_____no_output_____"
],
[
"b[2], 4/(3*pi)",
"_____no_output_____"
],
[
"b[4], 4/(5*pi)",
"_____no_output_____"
]
],
[
[
"### 4.5 Fourier coefficients for other waveforms",
"_____no_output_____"
]
],
[
[
"def sawtooth(t):\n return t%1",
"_____no_output_____"
],
[
"plot_function(sawtooth,0,5)",
"_____no_output_____"
],
[
"approx = fourier_series(*fourier_coefficients(sawtooth,10))",
"_____no_output_____"
],
[
"plot_function(sawtooth,0,5)\nplot_function(approx,0,5)",
"_____no_output_____"
],
[
"def speedbumps(t):\n if abs(t%1 - 0.5) > 0.25:\n return 0\n else:\n return sqrt(0.25*0.25 - (t%1 - 0.5)**2)",
"_____no_output_____"
],
[
"approx = fourier_series(*fourier_coefficients(speedbumps,10))",
"_____no_output_____"
],
[
"plot_function(speedbumps,0,5)\nplot_function(approx,0,5)",
"_____no_output_____"
]
],
[
[
"### 13.4.6 Exercises",
"_____no_output_____"
],
[
"**Mini project:** Play a sawtooth wave at 441 Hz and compare it with the square and sinusoidal waves you played at that frequency.",
"_____no_output_____"
],
[
"**Solution:**",
"_____no_output_____"
]
],
[
[
"def modified_sawtooth(t):\n return 8000 * sawtooth(441*t)\narr = sample(modified_sawtooth,0,1,44100)\nsound = pygame.sndarray.make_sound(arr)\nsound.play()",
"_____no_output_____"
]
]
] | [
"markdown",
"code",
"markdown",
"code",
"markdown",
"code",
"markdown",
"code",
"markdown",
"code",
"markdown",
"code",
"markdown",
"code",
"markdown",
"code",
"markdown",
"code",
"markdown",
"code",
"markdown",
"code",
"markdown",
"code",
"markdown",
"code",
"markdown",
"code",
"markdown",
"code",
"markdown",
"code",
"markdown",
"code",
"markdown",
"code",
"markdown",
"code",
"markdown",
"code"
] | [
[
"markdown",
"markdown"
],
[
"code"
],
[
"markdown",
"markdown"
],
[
"code",
"code",
"code",
"code"
],
[
"markdown"
],
[
"code",
"code"
],
[
"markdown"
],
[
"code",
"code",
"code",
"code"
],
[
"markdown",
"markdown",
"markdown"
],
[
"code"
],
[
"markdown",
"markdown"
],
[
"code",
"code"
],
[
"markdown"
],
[
"code",
"code"
],
[
"markdown"
],
[
"code",
"code",
"code",
"code",
"code"
],
[
"markdown",
"markdown",
"markdown"
],
[
"code"
],
[
"markdown",
"markdown"
],
[
"code"
],
[
"markdown",
"markdown"
],
[
"code",
"code",
"code",
"code"
],
[
"markdown"
],
[
"code",
"code"
],
[
"markdown"
],
[
"code",
"code",
"code"
],
[
"markdown"
],
[
"code",
"code",
"code",
"code",
"code",
"code"
],
[
"markdown",
"markdown",
"markdown"
],
[
"code"
],
[
"markdown",
"markdown",
"markdown"
],
[
"code",
"code",
"code",
"code",
"code",
"code",
"code",
"code",
"code",
"code",
"code",
"code"
],
[
"markdown",
"markdown"
],
[
"code",
"code",
"code",
"code"
],
[
"markdown"
],
[
"code",
"code",
"code",
"code",
"code"
],
[
"markdown"
],
[
"code",
"code",
"code",
"code",
"code",
"code",
"code"
],
[
"markdown",
"markdown",
"markdown"
],
[
"code"
]
] |
d047b203e55363f59562b56516e7423d29ac462a | 13,639 | ipynb | Jupyter Notebook | notebooks/2.PreProcessing.ipynb | mnm-analytics/titanic | 5c343e192623bc6f4b9a1f82a3d6a5a662a7bb76 | [
"MIT"
] | null | null | null | notebooks/2.PreProcessing.ipynb | mnm-analytics/titanic | 5c343e192623bc6f4b9a1f82a3d6a5a662a7bb76 | [
"MIT"
] | null | null | null | notebooks/2.PreProcessing.ipynb | mnm-analytics/titanic | 5c343e192623bc6f4b9a1f82a3d6a5a662a7bb76 | [
"MIT"
] | 1 | 2020-03-23T14:44:12.000Z | 2020-03-23T14:44:12.000Z | 21.580696 | 132 | 0.514407 | [
[
[
"# info",
"_____no_output_____"
],
[
"##### クレンジング\n1. 欠損値があった場合、基礎分析の結果に基づいて値埋めか行の削除を行なっている\n1. 表記揺れがあった場合、漏れなく修正している\n1. 水準数が多く、なおかつまとめられそうな質的変数があった場合に、論理的な基準に基づいて値をまとめている\n\n##### 特徴量エンジニアリング\n1. 質的変数を量的変数(加減乗除して意味のある数値)に変換している\n1. 量的変数を基礎分析の結果をもとに変換している\n1. 量的変数のスケーリングを行っている\n1. 元データを素に、有用であると考えられるような特徴を少なくとも1は生成している",
"_____no_output_____"
],
[
"# init",
"_____no_output_____"
]
],
[
[
"import numpy as np\nimport pandas as pd",
"_____no_output_____"
]
],
[
[
"# load",
"_____no_output_____"
]
],
[
[
"path_data = \"../data/\"\npath_raw = path_data + \"raw/\"\npath_mid = path_data + \"mid/\"\npath_clns = path_data + \"clns/\"",
"_____no_output_____"
],
[
"cats = pd.read_csv(path_mid+\"cats.csv\", index_col=0)\nnums = pd.read_csv(path_mid+\"nums.csv\", index_col=0)\nbools = pd.read_csv(path_mid+\"bools.csv\", index_col=0)",
"_____no_output_____"
]
],
[
[
"# clns",
"_____no_output_____"
],
[
"## fillna",
"_____no_output_____"
],
[
"##### embarked",
"_____no_output_____"
]
],
[
[
"cats[\"embarked\"] = cats[\"embarked\"].fillna(\"S\")\ncats[\"embarked\"].isna().any()",
"_____no_output_____"
]
],
[
[
"##### age",
"_____no_output_____"
]
],
[
[
"from sklearn.linear_model import LogisticRegression, LinearRegression\nfrom sklearn.metrics import roc_auc_score\nfrom sklearn.preprocessing import StandardScaler",
"_____no_output_____"
],
[
"fill_mean = nums[[\"age\", \"survived\"]]\nfill_mean = fill_mean[~fill_mean.survived.isna()]\nfill_mean[\"age\"] = fill_mean.age.fillna(fill_mean.age.mean())",
"_____no_output_____"
],
[
"fill_median = nums[[\"age\", \"survived\"]]\nfill_median = fill_median[~fill_median.survived.isna()]\nfill_median[\"age\"] = fill_median.age.fillna(fill_median.age.median())",
"_____no_output_____"
],
[
"# 線形回帰で値埋め\ndef zscore(x):\n m = x.mean()\n s = x.std(ddof=1)\n return (x-m)/s\n\nX = pd.get_dummies(cats, drop_first=True)\nz = zscore(nums.drop([\"age\", \"survived\"], 1))\nX = X.join(z)\ny = nums.age\n\nis_na = y.isna()\ny = np.log1p(y)\n\nrgs = LinearRegression()\nrgs.fit(X[~is_na], y[~is_na])\n\npred = rgs.predict(X[is_na])\npred = np.exp(pred)-1\n\nbase = X[~is_na]\nbase[\"age\"] = np.exp(y[~is_na])-1\n\nfill = X[is_na]\nfill[\"age\"] = pred\n\nfill_linear = pd.concat([base, fill]).join(nums[[\"survived\"]])\nfill_linear = fill_linear[[\"age\", \"survived\"]]\nfill_linear = fill_linear[~fill_linear.survived.isna()]",
"C:\\Users\\keisu\\Anaconda3\\lib\\site-packages\\ipykernel_launcher.py:22: SettingWithCopyWarning: \nA value is trying to be set on a copy of a slice from a DataFrame.\nTry using .loc[row_indexer,col_indexer] = value instead\n\nSee the caveats in the documentation: http://pandas.pydata.org/pandas-docs/stable/indexing.html#indexing-view-versus-copy\nC:\\Users\\keisu\\Anaconda3\\lib\\site-packages\\ipykernel_launcher.py:25: SettingWithCopyWarning: \nA value is trying to be set on a copy of a slice from a DataFrame.\nTry using .loc[row_indexer,col_indexer] = value instead\n\nSee the caveats in the documentation: http://pandas.pydata.org/pandas-docs/stable/indexing.html#indexing-view-versus-copy\n"
],
[
"def check_auc(data):\n X, y = data[[\"age\"]], data[\"survived\"]\n clf = LogisticRegression()\n clf.fit(X, y)\n proba = clf.predict_proba(X)[:,1]\n auc = roc_auc_score(y_true=y, y_score=proba)\n print(auc)",
"_____no_output_____"
],
[
"check_auc(fill_mean)",
"0.5329419918511361\n"
],
[
"check_auc(fill_median)",
"0.5290565799974796\n"
],
[
"check_auc(fill_linear)",
"0.5316398538245055\n"
],
[
"nums[\"age\"] = nums.fillna(nums.age.mean())",
"_____no_output_____"
]
],
[
[
"##### チェックポイント1: 欠損値があった場合、基礎分析の結果に基づいて値埋めか行の削除を行なっている\n##### チェックポイント2: 表記揺れがあった場合、漏れなく修正している\n- 表記揺れはない",
"_____no_output_____"
],
[
"## union-value",
"_____no_output_____"
]
],
[
[
"cats_union = cats.copy()",
"_____no_output_____"
]
],
[
[
"##### embarked",
"_____no_output_____"
]
],
[
[
"cats_union[\"embarked\"] = cats_union.embarked.replace([\"Q\", \"S\"], \"QorS\")",
"_____no_output_____"
]
],
[
[
"##### family-size",
"_____no_output_____"
]
],
[
[
"family = nums.parch + nums.sibsp\nparch = nums.parch.apply(lambda x: \"4+\" if x >= 4 else x)\nsibsp = nums.sibsp.apply(lambda x: \"4+\" if x >= 4 else x)",
"_____no_output_____"
]
],
[
[
"##### チェックポイント3: 水準数が多く、なおかつまとめられそうな質的変数があった場合に、論理的な基準に基づいて値をまとめている",
"_____no_output_____"
],
[
"# feature engineering",
"_____no_output_____"
],
[
"## onehot-encoding",
"_____no_output_____"
]
],
[
[
"pd.get_dummies(cats_union, drop_first=True).to_csv(path_clns+\"onehot_cats.csv\")",
"_____no_output_____"
],
[
"pd.get_dummies(pd.concat([parch, sibsp], axis=1), drop_first=True).to_csv(path_clns+\"onehot_parch_sibsp.csv\")",
"_____no_output_____"
],
[
"pd.get_dummies(family, drop_first=True, prefix=\"family-size\").to_csv(path_clns+\"onehot_familysize.csv\")",
"_____no_output_____"
],
[
"(bools*1).to_csv(path_clns+\"onehot_bools.csv\")",
"_____no_output_____"
],
[
"nums[[\"survived\"]].to_csv(path_clns+\"y.csv\")",
"_____no_output_____"
],
[
"is_child = (nums.age <= 7)*1\nis_child.name = \"is_child\"\nis_child.to_frame().to_csv(path_clns+\"onehot_ischild.csv\")",
"_____no_output_____"
]
],
[
[
"##### チェックポイント4: 元データを素に、有用であると考えられるような特徴を少なくとも1は生成している",
"_____no_output_____"
],
[
"## target-encoding",
"_____no_output_____"
]
],
[
[
"def tgt_encoding(data, y):\n data = data.copy()\n idname = data.index.name\n data = data.reset_index()\n train = data.dropna()\n\n for x in set(data)-set([idname, y]):\n dfg = train.groupby(x)[y].mean()\n dfg = dfg.to_frame()\n data = data.merge(dfg, on=x, suffixes=[\"\", \"_%s_tgt\"%x], how=\"left\")\n\n data = data.set_index(idname)\n data = data.filter(regex=\"_tgt\")\n return data",
"_____no_output_____"
],
[
"y = \"survived\"",
"_____no_output_____"
],
[
"data = cats.join(nums[[y]])\ndata = tgt_encoding(data, y)\ndata.to_csv(path_clns+\"tgt_cats.csv\")",
"_____no_output_____"
],
[
"data = bools.join(nums[[y]])\ndata = tgt_encoding(data, y)\ndata.to_csv(path_clns+\"tgt_bools.csv\")",
"_____no_output_____"
],
[
"data = pd.concat([parch, sibsp], axis=1).join(nums[[y]])\ndata = tgt_encoding(data, y)\ndata.to_csv(path_clns+\"tgt_parch_sibsp.csv\")",
"_____no_output_____"
],
[
"data = family.to_frame()\ndata.columns = [\"familysize\"]\ndata = data.join(nums[[y]])\ndata = tgt_encoding(data, y)\ndata.to_csv(path_clns+\"tgt_familysize.csv\")",
"_____no_output_____"
]
],
[
[
"##### チェックポイント1: 質的変数を量的変数(加減乗除して意味のある数値)に変換している",
"_____no_output_____"
],
[
"## log, zscore",
"_____no_output_____"
]
],
[
[
"def zscore(x):\n m = x.mean()\n s = x.std(ddof=1)\n return (x-m)/s",
"_____no_output_____"
],
[
"nums_tgt = nums[[\"age\", \"fare\"]]\nz = zscore(nums_tgt)\nz.to_csv(path_clns+\"num_z.csv\")",
"_____no_output_____"
],
[
"z = zscore(np.log1p(nums_tgt))\nz.to_csv(path_clns+\"num_logz.csv\")",
"_____no_output_____"
]
],
[
[
"##### チェックポイント2: 量的変数を基礎分析の結果をもとに変換している\n##### チェックポイント3: 量的変数のスケーリングを行っている",
"_____no_output_____"
]
]
] | [
"markdown",
"code",
"markdown",
"code",
"markdown",
"code",
"markdown",
"code",
"markdown",
"code",
"markdown",
"code",
"markdown",
"code",
"markdown",
"code",
"markdown",
"code",
"markdown",
"code",
"markdown"
] | [
[
"markdown",
"markdown",
"markdown"
],
[
"code"
],
[
"markdown"
],
[
"code",
"code"
],
[
"markdown",
"markdown",
"markdown"
],
[
"code"
],
[
"markdown"
],
[
"code",
"code",
"code",
"code",
"code",
"code",
"code",
"code",
"code"
],
[
"markdown",
"markdown"
],
[
"code"
],
[
"markdown"
],
[
"code"
],
[
"markdown"
],
[
"code"
],
[
"markdown",
"markdown",
"markdown"
],
[
"code",
"code",
"code",
"code",
"code",
"code"
],
[
"markdown",
"markdown"
],
[
"code",
"code",
"code",
"code",
"code",
"code"
],
[
"markdown",
"markdown"
],
[
"code",
"code",
"code"
],
[
"markdown"
]
] |
d047b78c21c17fd2a010031f8ac180058bca8d7f | 96,353 | ipynb | Jupyter Notebook | examples/Notebooks/flopy3_shapefile_export.ipynb | tomvansteijn/flopy | 06b0a71b9b69600d61c5233fd068946627e6cdad | [
"CC0-1.0",
"BSD-3-Clause"
] | null | null | null | examples/Notebooks/flopy3_shapefile_export.ipynb | tomvansteijn/flopy | 06b0a71b9b69600d61c5233fd068946627e6cdad | [
"CC0-1.0",
"BSD-3-Clause"
] | null | null | null | examples/Notebooks/flopy3_shapefile_export.ipynb | tomvansteijn/flopy | 06b0a71b9b69600d61c5233fd068946627e6cdad | [
"CC0-1.0",
"BSD-3-Clause"
] | null | null | null | 77.144115 | 9,308 | 0.797225 | [
[
[
"# FloPy shapefile export demo\nThe goal of this notebook is to demonstrate ways to export model information to shapefiles.\nThis example will cover:\n* basic exporting of information for a model, individual package, or dataset\n* custom exporting of combined data from different packages\n* general exporting and importing of geographic data from other sources",
"_____no_output_____"
]
],
[
[
"import sys\nimport os\nimport numpy as np\nimport matplotlib as mpl\nimport matplotlib.pyplot as plt\nimport pandas as pd\n\n# run installed version of flopy or add local path\ntry:\n import flopy\nexcept:\n fpth = os.path.abspath(os.path.join('..', '..'))\n sys.path.append(fpth)\n import flopy\n \nprint(sys.version)\nprint('numpy version: {}'.format(np.__version__))\nprint('matplotlib version: {}'.format(mpl.__version__))\nprint('flopy version: {}'.format(flopy.__version__))",
"3.7.7 (default, Mar 26 2020, 10:32:53) \n[Clang 4.0.1 (tags/RELEASE_401/final)]\nnumpy version: 1.19.2\nmatplotlib version: 3.3.0\nflopy version: 3.3.2\n"
],
[
"# set the output directory\noutdir = os.path.join('temp', 'shapefile_export')\nif not os.path.isdir(outdir):\n os.makedirs(outdir)\n\n# load an existing model\nmodel_ws = \"../data/freyberg\"\nm = flopy.modflow.Modflow.load(\"freyberg.nam\", model_ws=model_ws, verbose=False,\n check=False, exe_name=\"mfnwt\")",
"_____no_output_____"
],
[
"m.get_package_list()",
"_____no_output_____"
]
],
[
[
"### set the model coordinate information\nthe coordinate information where the grid is located in a projected coordinate system (e.g. UTM)",
"_____no_output_____"
]
],
[
[
"grid = m.modelgrid\ngrid.set_coord_info(xoff=273170, yoff=5088657, epsg=26916)",
"_____no_output_____"
],
[
"grid.extent",
"_____no_output_____"
]
],
[
[
"## Declarative export using attached `.export()` methods\n#### Export the whole model to a single shapefile",
"_____no_output_____"
]
],
[
[
"fname = '{}/model.shp'.format(outdir)\nm.export(fname)",
"wrote temp/shapefile_export/model.shp\n"
],
[
"ax = plt.subplot(1, 1, 1, aspect='equal')\nextents = grid.extent\npc = flopy.plot.plot_shapefile(fname, ax=ax, edgecolor='k', facecolor='none')\nax.set_xlim(extents[0], extents[1])\nax.set_ylim(extents[2], extents[3])\nax.set_title(fname);",
"_____no_output_____"
],
[
"fname = '{}/wel.shp'.format(outdir)\nm.wel.export(fname)",
"wrote temp/shapefile_export/wel.shp\n"
]
],
[
[
"### Export a package to a shapefile",
"_____no_output_____"
],
[
"### Export a FloPy list or array object",
"_____no_output_____"
]
],
[
[
"m.lpf.hk",
"_____no_output_____"
],
[
"fname = '{}/hk.shp'.format(outdir)\nm.lpf.hk.export('{}/hk.shp'.format(outdir))",
"wrote temp/shapefile_export/hk.shp\n"
],
[
"ax = plt.subplot(1, 1, 1, aspect='equal')\nextents = grid.extent\na = m.lpf.hk.array.ravel()\npc = flopy.plot.plot_shapefile(fname, ax=ax, a=a)\nax.set_xlim(extents[0], extents[1])\nax.set_ylim(extents[2], extents[3])\nax.set_title(fname);",
"_____no_output_____"
],
[
"m.riv.stress_period_data",
"_____no_output_____"
],
[
"m.riv.stress_period_data.export('{}/riv_spd.shp'.format(outdir))",
"wrote temp/shapefile_export/riv_spd.shp\n"
]
],
[
[
"### MfList.export() exports the whole grid by default, regardless of the locations of the boundary cells\n`sparse=True` only exports the boundary cells in the MfList",
"_____no_output_____"
]
],
[
[
"m.riv.stress_period_data.export('{}/riv_spd.shp'.format(outdir), sparse=True)",
"wrote temp/shapefile_export/riv_spd.shp\n"
],
[
"m.wel.stress_period_data.export('{}/wel_spd.shp'.format(outdir), sparse=True)",
"wrote temp/shapefile_export/wel_spd.shp\n"
]
],
[
[
"## Ad-hoc exporting using `recarray2shp`\n* The main idea is to create a recarray with all of the attribute information, and a list of geometry features (one feature per row in the recarray)\n* each geometry feature is an instance of the `Point`, `LineString` or `Polygon` classes in `flopy.utils.geometry`. The shapefile format requires all the features to be of the same type.\n* We will use pandas dataframes for these examples because they are easy to work with, and then convert them to recarrays prior to exporting.\n",
"_____no_output_____"
]
],
[
[
"from flopy.export.shapefile_utils import recarray2shp",
"_____no_output_____"
]
],
[
[
"### combining data from different packages\nwrite a shapefile of RIV and WEL package cells",
"_____no_output_____"
]
],
[
[
"wellspd = pd.DataFrame(m.wel.stress_period_data[0])\nrivspd = pd.DataFrame(m.riv.stress_period_data[0])\nspd = wellspd.append(rivspd)\nspd.head()",
"_____no_output_____"
]
],
[
[
"##### create a list of Polygon features from the cell vertices stored in the SpatialReference object",
"_____no_output_____"
]
],
[
[
"from flopy.utils.geometry import Polygon\n\nvertices = []\nfor row, col in zip(spd.i, spd.j):\n vertices.append(grid.get_cell_vertices(row, col))\npolygons = [Polygon(vrt) for vrt in vertices]\npolygons",
"_____no_output_____"
]
],
[
[
"##### write the shapefile",
"_____no_output_____"
]
],
[
[
"fname = '{}/bcs.shp'.format(outdir)\nrecarray2shp(spd.to_records(), geoms=polygons,\n shpname=fname,\n epsg=grid.epsg)",
"wrote temp/shapefile_export/bcs.shp\n"
],
[
"ax = plt.subplot(1, 1, 1, aspect='equal')\nextents = grid.extent\npc = flopy.plot.plot_shapefile(fname, ax=ax)\nax.set_xlim(extents[0], extents[1])\nax.set_ylim(extents[2], extents[3])\nax.set_title(fname);",
"_____no_output_____"
]
],
[
[
"### exporting other data\nSuppose we have some well data with actual locations that we want to export to a shapefile",
"_____no_output_____"
]
],
[
[
"welldata = pd.DataFrame({'wellID': np.arange(0, 10),\n 'q': np.random.randn(10)*100 - 1000,\n 'x_utm': np.random.rand(10)*1000 + grid.yoffset,\n 'y_utm': grid.xoffset - np.random.rand(10)*3000})\nwelldata.head()",
"_____no_output_____"
]
],
[
[
"##### convert the x, y coorindates to point features and then export",
"_____no_output_____"
]
],
[
[
"from flopy.utils.geometry import Point\ngeoms = [Point(x, y) for x, y in zip(welldata.x_utm, welldata.y_utm)]\n\nfname = '{}/wel_data.shp'.format(outdir)\nrecarray2shp(welldata.to_records(), geoms=geoms,\n shpname=fname,\n epsg=grid.epsg)",
"wrote temp/shapefile_export/wel_data.shp\n"
],
[
"ax = plt.subplot(1, 1, 1, aspect='equal')\nextents = grid.extent\npc = flopy.plot.plot_shapefile(fname, ax=ax, radius=25)\nax.set_xlim(extents[0], extents[1])\nax.set_ylim(extents[2], extents[3])\nax.set_title(fname);",
"_____no_output_____"
]
],
[
[
"### Adding attribute data to an existing shapefile\nSuppose we have a GIS coverage representing the river in the riv package",
"_____no_output_____"
]
],
[
[
"from flopy.utils.geometry import LineString \n\n### make up a linestring shapefile of the river reaches\ni, j = m.riv.stress_period_data[0].i, m.riv.stress_period_data[0].j\nx0 = grid.xyzcellcenters[0][i[0], j[0]]\nx1 = grid.xyzcellcenters[0][i[-1], j[-1]]\ny0 = grid.xyzcellcenters[1][i[0], j[0]]\ny1 = grid.xyzcellcenters[1][i[-1], j[-1]]\nx = np.linspace(x0, x1, m.nrow+1)\ny = np.linspace(y0, y1, m.nrow+1)\nl0 = zip(list(zip(x[:-1], y[:-1])), list(zip(x[1:], y[1:])))\nlines = [LineString(l) for l in l0]\n\nrivdata = pd.DataFrame(m.riv.stress_period_data[0])\nrivdata['reach'] = np.arange(len(lines))\nlines_shapefile = '{}/riv_reaches.shp'.format(outdir)\nrecarray2shp(rivdata.to_records(index=False), geoms=lines,\n shpname=lines_shapefile,\n epsg=grid.epsg)",
"wrote temp/shapefile_export/riv_reaches.shp\n"
],
[
"ax = plt.subplot(1, 1, 1, aspect='equal')\nextents = grid.extent\npc = flopy.plot.plot_shapefile(lines_shapefile, ax=ax, radius=25)\nax.set_xlim(extents[0], extents[1])\nax.set_ylim(extents[2], extents[3])\nax.set_title(lines_shapefile);",
"_____no_output_____"
]
],
[
[
"#### read in the GIS coverage using `shp2recarray`\n`shp2recarray` reads a shapefile into a numpy record array, which can easily be converted to a DataFrame",
"_____no_output_____"
]
],
[
[
"from flopy.export.shapefile_utils import shp2recarray",
"_____no_output_____"
],
[
"linesdata = shp2recarray(lines_shapefile)\nlinesdata = pd.DataFrame(linesdata)\nlinesdata.head()",
"_____no_output_____"
]
],
[
[
"##### Suppose we have some flow information that we read in from the cell budget file",
"_____no_output_____"
]
],
[
[
"# make up some fluxes between the river and aquifer at each reach\nq = np.random.randn(len(linesdata))+1\nq",
"_____no_output_____"
]
],
[
[
"##### Add reachs fluxes and cumulative flow to lines DataFrame",
"_____no_output_____"
]
],
[
[
"linesdata['qreach'] = q\nlinesdata['qstream'] = np.cumsum(q)",
"_____no_output_____"
],
[
"recarray2shp(linesdata.drop('geometry', axis=1).to_records(), \n geoms=linesdata.geometry,\n shpname=lines_shapefile,\n epsg=grid.epsg)",
"wrote temp/shapefile_export/riv_reaches.shp\n"
],
[
"ax = plt.subplot(1, 1, 1, aspect='equal')\nextents = grid.extent\npc = flopy.plot.plot_shapefile(lines_shapefile, ax=ax, radius=25)\nax.set_xlim(extents[0], extents[1])\nax.set_ylim(extents[2], extents[3])\nax.set_title(lines_shapefile);",
"_____no_output_____"
]
],
[
[
"## Overriding the model's modelgrid with a user supplied modelgrid\n\nIn some cases it may be necessary to override the model's modelgrid instance with a seperate modelgrid. An example of this is if the model discretization is in feet and the user would like it projected in meters. Exporting can be accomplished by supplying a modelgrid as a `kwarg` in any of the `export()` methods within flopy. Below is an example:",
"_____no_output_____"
]
],
[
[
"mg0 = m.modelgrid\n\n# build a new modelgrid instance with discretization in meters\nmodelgrid = flopy.discretization.StructuredGrid(delc=mg0.delc * 0.3048, delr=mg0.delr * 0.3048,\n top= mg0.top, botm=mg0.botm, idomain=mg0.idomain,\n xoff=mg0.xoffset * 0.3048, yoff=mg0.yoffset * 0.3048)\n\n# exporting an entire model\nm.export('{}/freyberg.shp'.format(outdir), modelgrid=modelgrid)",
"wrote temp/shapefile_export/freyberg.shp\n"
]
],
[
[
"And for a specific parameter the method is the same",
"_____no_output_____"
]
],
[
[
"fname = '{}/hk.shp'.format(outdir)\nm.lpf.hk.export(fname, modelgrid=modelgrid)",
"wrote temp/shapefile_export/hk.shp\n"
],
[
"ax = plt.subplot(1, 1, 1, aspect='equal')\nextents = modelgrid.extent\na = m.lpf.hk.array.ravel()\npc = flopy.plot.plot_shapefile(fname, ax=ax, a=a)\nax.set_xlim(extents[0], extents[1])\nax.set_ylim(extents[2], extents[3])\nax.set_title(fname);",
"_____no_output_____"
]
]
] | [
"markdown",
"code",
"markdown",
"code",
"markdown",
"code",
"markdown",
"code",
"markdown",
"code",
"markdown",
"code",
"markdown",
"code",
"markdown",
"code",
"markdown",
"code",
"markdown",
"code",
"markdown",
"code",
"markdown",
"code",
"markdown",
"code",
"markdown",
"code",
"markdown",
"code",
"markdown",
"code",
"markdown",
"code"
] | [
[
"markdown"
],
[
"code",
"code",
"code"
],
[
"markdown"
],
[
"code",
"code"
],
[
"markdown"
],
[
"code",
"code",
"code"
],
[
"markdown",
"markdown"
],
[
"code",
"code",
"code",
"code",
"code"
],
[
"markdown"
],
[
"code",
"code"
],
[
"markdown"
],
[
"code"
],
[
"markdown"
],
[
"code"
],
[
"markdown"
],
[
"code"
],
[
"markdown"
],
[
"code",
"code"
],
[
"markdown"
],
[
"code"
],
[
"markdown"
],
[
"code",
"code"
],
[
"markdown"
],
[
"code",
"code"
],
[
"markdown"
],
[
"code",
"code"
],
[
"markdown"
],
[
"code"
],
[
"markdown"
],
[
"code",
"code",
"code"
],
[
"markdown"
],
[
"code"
],
[
"markdown"
],
[
"code",
"code"
]
] |
d047b7f008734bbd7871bed115be6927608cec04 | 87,558 | ipynb | Jupyter Notebook | Bloomberg Python Interop.ipynb | dmitchell28/Other-People-s-Code | ac1a799335906b17ebdcab078aea91f88fda4d62 | [
"BSD-2-Clause"
] | null | null | null | Bloomberg Python Interop.ipynb | dmitchell28/Other-People-s-Code | ac1a799335906b17ebdcab078aea91f88fda4d62 | [
"BSD-2-Clause"
] | null | null | null | Bloomberg Python Interop.ipynb | dmitchell28/Other-People-s-Code | ac1a799335906b17ebdcab078aea91f88fda4d62 | [
"BSD-2-Clause"
] | null | null | null | 69.325416 | 35,768 | 0.753935 | [
[
[
"<p><a name=\"basic\"></a></p>\n## What Packages do I have Installed",
"_____no_output_____"
]
],
[
[
"import pip",
"_____no_output_____"
],
[
"!pip freeze",
"alabaster==0.7.10\nanaconda-client==1.6.9\nanaconda-navigator==1.7.0\nanaconda-project==0.8.2\nasn1crypto==0.24.0\nastroid==1.6.1\nastropy==2.0.3\nattrs==17.4.0\nBabel==2.5.3\nbackports.shutil-get-terminal-size==1.0.0\nbeautifulsoup4==4.6.0\nbitarray==0.8.1\nbkcharts==0.2\nblaze==0.11.3\nbleach==2.1.2\nblpapi==3.9.1\nbokeh==0.12.13\nboto==2.48.0\nBottleneck==1.2.1\nbqplot==0.11.6\ncertifi==2018.1.18\ncffi==1.11.4\nchardet==3.0.4\nclick==6.7\ncloudpickle==0.5.2\nclyent==1.2.2\ncolorama==0.3.9\ncomtypes==1.1.4\nconda==4.4.10\nconda-build==3.4.1\nconda-verify==2.0.0\ncontextlib2==0.5.5\ncryptography==2.1.4\ncycler==0.10.0\nCython==0.27.3\ncytoolz==0.9.0\ndask==0.16.1\ndatashape==0.5.4\ndecorator==4.2.1\ndistributed==1.20.2\ndocutils==0.14\nentrypoints==0.2.3\net-xmlfile==1.0.1\nfastcache==1.0.2\nfilelock==2.0.13\nFlask==0.12.2\nFlask-Cors==3.0.3\ngevent==1.2.2\nglob2==0.6\ngreenlet==0.4.12\nh5py==2.7.1\nheapdict==1.0.0\nhtml5lib==1.0.1\nidna==2.6\nimageio==2.2.0\nimagesize==0.7.1\nipython==6.2.1\nipython-genutils==0.2.0\nipywidgets==7.1.1\nisort==4.2.15\nitsdangerous==0.24\njdcal==1.3\njedi==0.11.1\nJinja2==2.10\njsonschema==2.6.0\njupyter==1.0.0\njupyter-client==5.2.2\njupyter-console==5.2.0\njupyter-contrib-core==0.3.3\njupyter-contrib-nbextensions==0.5.1\njupyter-core==4.4.0\njupyter-highlight-selected-word==0.2.0\njupyter-latex-envs==1.4.6\njupyter-nbextensions-configurator==0.4.1\njupyterlab==0.31.4\njupyterlab-launcher==0.10.2\nlazy-object-proxy==1.3.1\nllvmlite==0.21.0\nlocket==0.2.0\nlxml==4.1.1\nMarkupSafe==1.0\nmatplotlib==2.1.2\nmccabe==0.6.1\nmenuinst==1.4.11\nmistune==0.8.3\nmpmath==1.0.0\nmsgpack-python==0.5.1\nmultipledispatch==0.4.9\nnavigator-updater==0.1.0\nnbconvert==5.3.1\nnbformat==4.4.0\nnetworkx==2.1\nnltk==3.2.5\nnose==1.3.7\nnotebook==5.4.0\nnumba==0.36.2\nnumexpr==2.6.4\nnumpy==1.14.0\nnumpydoc==0.7.0\nodo==0.5.1\nolefile==0.45.1\nopenpyxl==2.4.10\npackaging==16.8\npandas==0.22.0\npandocfilters==1.4.2\nparso==0.1.1\npartd==0.3.8\npath.py==10.5\npathlib2==2.3.0\npatsy==0.5.0\npdblp==0.1.8\npep8==1.7.1\npickleshare==0.7.4\nPillow==5.0.0\npkginfo==1.4.1\npluggy==0.6.0\nply==3.10\nprompt-toolkit==1.0.15\npsutil==5.4.3\npy==1.5.2\npycodestyle==2.3.1\npycosat==0.6.3\npycparser==2.18\npycrypto==2.6.1\npycurl==7.43.0.1\npyflakes==1.6.0\nPygments==2.2.0\npylint==1.8.2\npyodbc==4.0.22\npyOpenSSL==17.5.0\npyparsing==2.2.0\nPySocks==1.6.7\npytest==3.3.2\npython-dateutil==2.6.1\npytz==2017.3\nPyWavelets==0.5.2\npywin32==222\npywinpty==0.5\nPyYAML==3.12\npyzmq==16.0.3\nQtAwesome==0.4.4\nqtconsole==4.3.1\nQtPy==1.3.1\nrequests==2.18.4\nrope==0.10.7\nruamel-yaml==0.15.35\nscikit-image==0.13.1\nscikit-learn==0.19.1\nscipy==1.0.0\nseaborn==0.8.1\nSend2Trash==1.4.2\nsimplegeneric==0.8.1\nsingledispatch==3.4.0.3\nsix==1.11.0\nsnowballstemmer==1.2.1\nsortedcollections==0.5.3\nsortedcontainers==1.5.9\nSphinx==1.6.6\nsphinxcontrib-websupport==1.0.1\nspyder==3.2.6\nSQLAlchemy==1.2.1\nstatsmodels==0.8.0\nsympy==1.1.1\ntables==3.4.2\ntblib==1.3.2\nterminado==0.8.1\ntestpath==0.3.1\ntoolz==0.9.0\ntornado==4.5.3\ntraitlets==4.3.2\ntraittypes==0.2.1\ntyping==3.6.2\nunicodecsv==0.14.1\nurllib3==1.22\nwcwidth==0.1.7\nwebencodings==0.5.1\nWerkzeug==0.14.1\nwidgetsnbextension==3.1.0\nwin-inet-pton==1.0.1\nwin-unicode-console==0.5\nwincertstore==0.2\nwrapt==1.10.11\nxlrd==1.1.0\nXlsxWriter==1.0.2\nxlwings==0.11.5\nxlwt==1.3.0\nzict==0.1.3\n"
]
],
[
[
"<p><a name=\"explore\"></a></p>\n## Bloomberg Interop via python wrapper\n#### Accessing Bloomberg Desktop API directly",
"_____no_output_____"
],
[
"INSTRUCTIONS FOR INSTALLING THE LIBRARY\n1. https://www.bloomberg.com/professional/support/api-library/\n- download the Python Supported Release\n\n2. go to command line and run: pip install pdblp",
"_____no_output_____"
]
],
[
[
"import pandas as pd\nimport pdblp #pandas wrapper to Bloomberg API. also xbbg & pybbg are popular",
"_____no_output_____"
],
[
"con = pdblp.BCon(debug=True, port=8194)\ncon.start()",
"pdblp.pdblp:INFO:Event Type: 'SESSION_STATUS'\npdblp.pdblp:INFO:Message Received:\nSessionConnectionUp = {\n server = \"localhost:8194\"\n encryptionStatus = \"Clear\"\n compressionStatus = \"Uncompressed\"\n}\n\npdblp.pdblp:INFO:Event Type: 'SESSION_STATUS'\npdblp.pdblp:INFO:Message Received:\nSessionStarted = {\n initialEndpoints[] = {\n initialEndpoints = {\n address = \"localhost:8194\"\n }\n }\n}\n\npdblp.pdblp:INFO:Event Type: 'SERVICE_STATUS'\npdblp.pdblp:INFO:Message Received:\nServiceOpened = {\n serviceName = \"//blp/refdata\"\n}\n\npdblp.pdblp:INFO:Event Type: 'SERVICE_STATUS'\npdblp.pdblp:INFO:Message Received:\nServiceOpened = {\n serviceName = \"//blp/exrsvc\"\n}\n\n"
],
[
"con.debug = False #turn off 'verbose' mode",
"_____no_output_____"
],
[
"#historical data via 'bdh'\ntickers = ['QCOM US Equity', 'AAPL US Equity', 'XIU CN Equity']\nfields = ['PX_LAST', 'DX_SAF', 'VL158'] #borrow cost p/a and 3mo IVOL\nstart = '20200105'\nend = '20200805'",
"_____no_output_____"
],
[
"response = con.bdh(tickers, fields, start, end)",
"_____no_output_____"
],
[
"type(response)",
"_____no_output_____"
],
[
"response.tail()",
"_____no_output_____"
]
],
[
[
"#### Chart Prices (same axis)",
"_____no_output_____"
]
],
[
[
"%matplotlib inline",
"_____no_output_____"
],
[
"response.filter(like=\"PX_LAST\").plot()",
"_____no_output_____"
],
[
"list(response.columns) #format for columns",
"_____no_output_____"
]
],
[
[
"### extract one Series (column) from DataFrame",
"_____no_output_____"
]
],
[
[
"spycloses = response[('XIU CN Equity', 'PX_LAST')] \nspycloses.tail()",
"_____no_output_____"
],
[
"%matplotlib inline\nspycloses.plot() #chart it",
"_____no_output_____"
],
[
"type(spycloses) #confirm dtype",
"_____no_output_____"
],
[
"spx_df = pd.DataFrame(spycloses) #confirm to dataframe with dates as index",
"_____no_output_____"
],
[
"spx_df.tail()",
"_____no_output_____"
]
],
[
[
"# Non-Equity Examples",
"_____no_output_____"
]
],
[
[
"# individual datapoint using 'ref' (similar to 'bdp')\nresponse2 = con.ref(['AUDUSD Curncy'], 'SETTLE_DT')\nprint(response2)",
" ticker field value\n0 AUDUSD Curncy SETTLE_DT 2021-01-27\n"
],
[
"response3 = con.ref(['NDX Index'], 'VL137')\nprint(response3)",
" ticker field value\n0 NDX Index VL137 22.7313\n"
],
[
"response3.value[0]",
"_____no_output_____"
],
[
"response = con.bsrch(\"FI:ytd_green\")",
"_____no_output_____"
],
[
"response",
"_____no_output_____"
]
]
] | [
"markdown",
"code",
"markdown",
"code",
"markdown",
"code",
"markdown",
"code",
"markdown",
"code"
] | [
[
"markdown"
],
[
"code",
"code"
],
[
"markdown",
"markdown"
],
[
"code",
"code",
"code",
"code",
"code",
"code",
"code"
],
[
"markdown"
],
[
"code",
"code",
"code"
],
[
"markdown"
],
[
"code",
"code",
"code",
"code",
"code"
],
[
"markdown"
],
[
"code",
"code",
"code",
"code",
"code"
]
] |
d047bfcdccb5a7ff059ecf51cb1b00fdc4922394 | 11,067 | ipynb | Jupyter Notebook | notebooks_paper_2021/BERT_finetuning/BERT_finetune_AG_news-4.ipynb | PlaytikaResearch/esntorch | 585369853e2bb7c46d782fd10469dd30597de2e3 | [
"MIT"
] | 1 | 2021-10-06T07:42:01.000Z | 2021-10-06T07:42:01.000Z | notebooks_paper_2021/BERT_finetuning/BERT_finetune_AG_news-4.ipynb | PlaytikaResearch/esntorch | 585369853e2bb7c46d782fd10469dd30597de2e3 | [
"MIT"
] | null | null | null | notebooks_paper_2021/BERT_finetuning/BERT_finetune_AG_news-4.ipynb | PlaytikaResearch/esntorch | 585369853e2bb7c46d782fd10469dd30597de2e3 | [
"MIT"
] | null | null | null | 24.484513 | 115 | 0.539171 | [
[
[
"# BERT finetuning on AG_news-4",
"_____no_output_____"
],
[
"## Librairy",
"_____no_output_____"
]
],
[
[
"# !pip install transformers==4.8.2\n# !pip install datasets==1.7.0",
"_____no_output_____"
],
[
"import os\nimport time\nimport pickle\n\nimport numpy as np\nimport torch\n\nfrom sklearn.model_selection import train_test_split\nfrom sklearn.metrics import accuracy_score, recall_score, precision_score, f1_score\n\nfrom transformers import BertTokenizer, BertTokenizerFast\nfrom transformers import BertForSequenceClassification, AdamW\nfrom transformers import Trainer, TrainingArguments\nfrom transformers import EarlyStoppingCallback\nfrom transformers.data.data_collator import DataCollatorWithPadding\n\nfrom datasets import load_dataset, Dataset, concatenate_datasets",
"_____no_output_____"
],
[
"# print(torch.__version__)\n# print(torch.cuda.device_count())\n# print(torch.cuda.is_available())\n# print(torch.cuda.get_device_name(0))\n\ndevice = torch.device('cuda') if torch.cuda.is_available() else torch.device('cpu')\n# if torch.cuda.is_available():\n# torch.set_default_tensor_type('torch.cuda.FloatTensor')",
"_____no_output_____"
],
[
"device",
"_____no_output_____"
]
],
[
[
"## Global variables",
"_____no_output_____"
]
],
[
[
"BATCH_SIZE = 24\nNB_EPOCHS = 4",
"_____no_output_____"
],
[
"RESULTS_FILE = '~/Results/BERT_finetune/ag_news-4_BERT_finetune_b'+str(BATCH_SIZE)+'_results.pkl'\nRESULTS_PATH = '~/Results/BERT_finetune/ag_news-4_b'+str(BATCH_SIZE)+'/'\nCACHE_DIR = '~/Data/huggignface/' # path of your folder",
"_____no_output_____"
]
],
[
[
"## Dataset",
"_____no_output_____"
]
],
[
[
"# download dataset\n\nraw_datasets = load_dataset('ag_news', cache_dir=CACHE_DIR)",
"_____no_output_____"
],
[
"# tokenize\n\ntokenizer = BertTokenizer.from_pretrained(\"bert-base-uncased\")\n\ndef tokenize_function(examples):\n return tokenizer(examples[\"text\"], padding=True, truncation=True)\n\ntokenized_datasets = raw_datasets.map(tokenize_function, batched=True)\ntokenized_datasets.set_format(type='torch', columns=['input_ids', 'attention_mask', 'label'])\n\ntrain_dataset = tokenized_datasets[\"train\"].shuffle(seed=42)\ntrain_val_datasets = train_dataset.train_test_split(train_size=0.8)\n\ntrain_dataset = train_val_datasets['train'].rename_column('label', 'labels')\nval_dataset = train_val_datasets['test'].rename_column('label', 'labels')\ntest_dataset = tokenized_datasets[\"test\"].shuffle(seed=42).rename_column('label', 'labels')",
"_____no_output_____"
],
[
"# get number of labels\n\nnum_labels = len(set(train_dataset['labels'].tolist()))\nnum_labels",
"_____no_output_____"
]
],
[
[
"## Model",
"_____no_output_____"
],
[
"#### Model",
"_____no_output_____"
]
],
[
[
"model = BertForSequenceClassification.from_pretrained(\"bert-base-uncased\", num_labels=num_labels)\nmodel.to(device)",
"_____no_output_____"
]
],
[
[
"#### Training",
"_____no_output_____"
]
],
[
[
"training_args = TrainingArguments(\n \n # output\n output_dir=RESULTS_PATH, \n \n # params\n num_train_epochs=NB_EPOCHS, # nb of epochs\n per_device_train_batch_size=BATCH_SIZE, # batch size per device during training\n per_device_eval_batch_size=BATCH_SIZE, # cf. paper Sun et al.\n learning_rate=2e-5, # cf. paper Sun et al.\n# warmup_steps=500, # number of warmup steps for learning rate scheduler\n warmup_ratio=0.1, # cf. paper Sun et al.\n weight_decay=0.01, # strength of weight decay\n \n# # eval\n evaluation_strategy=\"steps\",\n eval_steps=50,\n# evaluation_strategy='no', # no more evaluation, takes time\n \n # log\n logging_dir=RESULTS_PATH+'logs', \n logging_strategy='steps',\n logging_steps=50,\n \n # save\n# save_strategy='epoch',\n # save_strategy='steps',\n# load_best_model_at_end=False\n load_best_model_at_end=True # cf. paper Sun et al.\n)",
"_____no_output_____"
],
[
"def compute_metrics(p):\n \n pred, labels = p\n pred = np.argmax(pred, axis=1)\n\n accuracy = accuracy_score(y_true=labels, y_pred=pred)\n \n return {\"val_accuracy\": accuracy}",
"_____no_output_____"
],
[
"trainer = Trainer(\n model=model,\n args=training_args,\n tokenizer=tokenizer,\n train_dataset=train_dataset,\n eval_dataset=val_dataset,\n # compute_metrics=compute_metrics,\n # callbacks=[EarlyStoppingCallback(early_stopping_patience=5)]\n)",
"_____no_output_____"
],
[
"results = trainer.train()",
"_____no_output_____"
],
[
"training_time = results.metrics[\"train_runtime\"]\ntraining_time_per_epoch = training_time / training_args.num_train_epochs\ntraining_time_per_epoch",
"_____no_output_____"
],
[
"trainer.save_model(os.path.join(RESULTS_PATH, 'best_model-0'))",
"_____no_output_____"
]
],
[
[
"## Results",
"_____no_output_____"
]
],
[
[
"results_d = {}\nepoch = 1\n\nordered_files = sorted( [f for f in os.listdir(RESULTS_PATH) \n if (not f.endswith(\"logs\")) and (f.startswith(\"best\")) # best model eval only\n ], \n key=lambda x: int(x.split('-')[1]) )\n\nfor filename in ordered_files:\n \n print(filename)\n \n # load model\n model_file = os.path.join(RESULTS_PATH, filename)\n finetuned_model = BertForSequenceClassification.from_pretrained(model_file, num_labels=num_labels)\n finetuned_model.to(device)\n finetuned_model.eval()\n\n # compute test acc\n test_trainer = Trainer(finetuned_model, data_collator=DataCollatorWithPadding(tokenizer))\n\n raw_preds, labels, _ = test_trainer.predict(test_dataset)\n preds = np.argmax(raw_preds, axis=1)\n\n test_acc = accuracy_score(y_true=labels, y_pred=preds)\n \n# results_d[filename] = (test_acc, training_time_per_epoch*epoch)\n results_d[filename] = test_acc # best model evaluation only\n \n print((test_acc, training_time_per_epoch*epoch))\n \n epoch += 1\n \nresults_d['training_time'] = training_time",
"_____no_output_____"
],
[
"# save results\n\nwith open(RESULTS_FILE, 'wb') as fh:\n pickle.dump(results_d, fh)",
"_____no_output_____"
],
[
"# load results\n\nwith open(RESULTS_FILE, 'rb') as fh:\n results_d = pickle.load(fh)",
"_____no_output_____"
],
[
"results_d",
"_____no_output_____"
]
]
] | [
"markdown",
"code",
"markdown",
"code",
"markdown",
"code",
"markdown",
"code",
"markdown",
"code",
"markdown",
"code"
] | [
[
"markdown",
"markdown"
],
[
"code",
"code",
"code",
"code"
],
[
"markdown"
],
[
"code",
"code"
],
[
"markdown"
],
[
"code",
"code",
"code"
],
[
"markdown",
"markdown"
],
[
"code"
],
[
"markdown"
],
[
"code",
"code",
"code",
"code",
"code",
"code"
],
[
"markdown"
],
[
"code",
"code",
"code",
"code"
]
] |
d047c624430f3fbdfc21dbb44128fc93340224f0 | 130,734 | ipynb | Jupyter Notebook | deep_learning/generative/TF2Keras_VanillaGAN_DCGAN.ipynb | jiankaiwang/sophia.ml | b8cc450ed2a53417a3ff9431528dbbd7fcfcc6ea | [
"MIT"
] | 7 | 2019-05-03T01:18:56.000Z | 2021-08-21T18:44:17.000Z | deep_learning/generative/TF2Keras_VanillaGAN_DCGAN.ipynb | jiankaiwang/sophia.ml | b8cc450ed2a53417a3ff9431528dbbd7fcfcc6ea | [
"MIT"
] | null | null | null | deep_learning/generative/TF2Keras_VanillaGAN_DCGAN.ipynb | jiankaiwang/sophia.ml | b8cc450ed2a53417a3ff9431528dbbd7fcfcc6ea | [
"MIT"
] | 3 | 2019-01-17T03:53:31.000Z | 2022-01-27T14:33:54.000Z | 130,734 | 130,734 | 0.906941 | [
[
[
"This tutorial shows how to generate an image of handwritten digits using Deep Convolutional Generative Adversarial Network (DCGAN).\n\nGenerative Adversarial Networks (GANs) are one of the most interesting fields in machine learning. The standard GAN consists of two models, a generative and a discriminator one. Two models are trained simultaneously by an adversarial process. A generative model (`the artist`) learns to generate images that look real, while the discriminator (`the art critic`) one learns to tell real images apart from the fakes.\n\n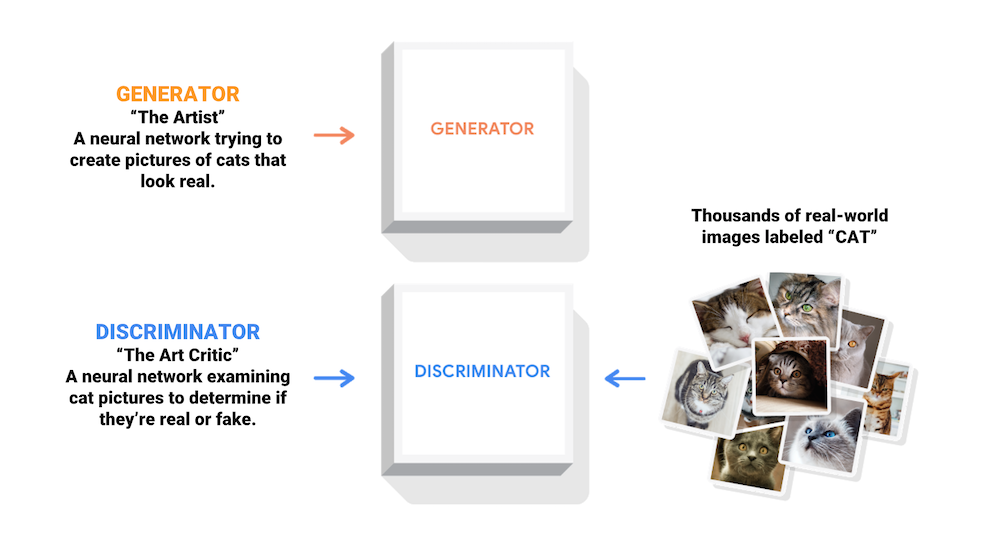\n\nRefer to Tensorflow.org (2020).\n\nDuring training, the generative model becomes progressively creating images that look real, and the discriminator model becomes progressively telling them apart. The whole process reaches equilibrium when the discriminator is no longer able to distinguish real images from fakes.\n\n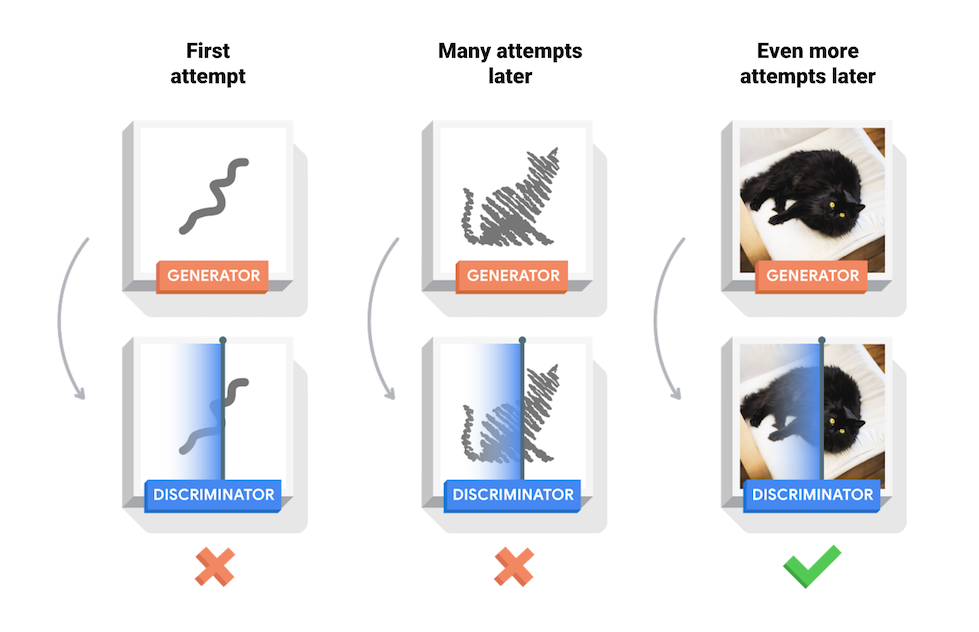\n\nRefer to Tensorflow.org (2020).\n\nIn this demo, we show how to train a GAN model on MNIST and FASHION MNIST dataset.\n",
"_____no_output_____"
]
],
[
[
"!pip uninstall -y tensorflow\n!pip install -q tf-nightly tfds-nightly",
"_____no_output_____"
],
[
"import glob\nimport tensorflow as tf\nimport tensorflow_datasets as tfds\nimport matplotlib.pyplot as plt\nfrom tensorflow.keras.layers import Conv2D, Conv2DTranspose, Dense, Flatten, BatchNormalization, ELU, LeakyReLU, Reshape, Dropout\nimport numpy as np\nimport IPython.display as display\nfrom IPython.display import clear_output\nimport os\nimport time\nimport imageio\n\ntfds.disable_progress_bar()\n\nprint(\"Tensorflow Version: {}\".format(tf.__version__))\nprint(\"GPU {} available.\".format(\"is\" if tf.config.experimental.list_physical_devices(\"GPU\") else \"not\"))",
"Tensorflow Version: 2.4.0-dev20200706\nGPU is available.\n"
]
],
[
[
"# Data Preprocessing",
"_____no_output_____"
]
],
[
[
"def normalize(image):\n img = image['image']\n img = (tf.cast(img, tf.float32) - 127.5) / 127.5\n return img",
"_____no_output_____"
]
],
[
[
"## MNIST Dataset",
"_____no_output_____"
]
],
[
[
"raw_datasets, metadata = tfds.load(name=\"mnist\", with_info=True)\nraw_train_datasets, raw_test_datasets = raw_datasets['train'], raw_datasets['test']",
"\u001b[1mDownloading and preparing dataset mnist/3.0.1 (download: 11.06 MiB, generated: 21.00 MiB, total: 32.06 MiB) to /root/tensorflow_datasets/mnist/3.0.1...\u001b[0m\n"
],
[
"raw_test_datasets, metadata",
"_____no_output_____"
],
[
"BUFFER_SIZE = 10000\nBATCH_SIZE = 256\n\ntrain_datasets = raw_train_datasets.map(normalize).cache().shuffle(BUFFER_SIZE).batch(BATCH_SIZE)\ntest_datasets = raw_test_datasets.map(normalize).batch(BATCH_SIZE)",
"_____no_output_____"
],
[
"for imgs in train_datasets.take(1):\n img = imgs[0]\n plt.imshow(tf.keras.preprocessing.image.array_to_img(img))\n plt.axis(\"off\")\n plt.show()",
"_____no_output_____"
]
],
[
[
"## Fashion_MNIST Dataset",
"_____no_output_____"
]
],
[
[
"raw_datasets, metadata = tfds.load(name=\"fashion_mnist\", with_info=True)",
"\u001b[1mDownloading and preparing dataset fashion_mnist/3.0.1 (download: 29.45 MiB, generated: 36.42 MiB, total: 65.87 MiB) to /root/tensorflow_datasets/fashion_mnist/3.0.1...\u001b[0m\nShuffling and writing examples to /root/tensorflow_datasets/fashion_mnist/3.0.1.incompleteQB6NVK/fashion_mnist-train.tfrecord\nShuffling and writing examples to /root/tensorflow_datasets/fashion_mnist/3.0.1.incompleteQB6NVK/fashion_mnist-test.tfrecord\n\u001b[1mDataset fashion_mnist downloaded and prepared to /root/tensorflow_datasets/fashion_mnist/3.0.1. Subsequent calls will reuse this data.\u001b[0m\n"
],
[
"raw_train_datasets, raw_test_datasets = raw_datasets['train'], raw_datasets['test']\nraw_train_datasets",
"_____no_output_____"
],
[
"for image in raw_train_datasets.take(1):\n plt.imshow(tf.keras.preprocessing.image.array_to_img(image['image']))\n plt.axis(\"off\")\n plt.title(\"Label: {}\".format(image['label']))\n plt.show()",
"_____no_output_____"
],
[
"BUFFER_SIZE = 10000\nBATCH_SIZE = 256\n\ntrain_datasets = raw_train_datasets.map(normalize).cache().prefetch(BUFFER_SIZE).batch(BATCH_SIZE)\ntest_datasets = raw_test_datasets.map(normalize).batch(BATCH_SIZE)",
"_____no_output_____"
],
[
"for imgs in train_datasets.take(1):\n img = imgs[0]\n plt.imshow(tf.keras.preprocessing.image.array_to_img(img))\n plt.axis(\"off\")\n plt.show()",
"_____no_output_____"
]
],
[
[
"# Build the GAN Model",
"_____no_output_____"
],
[
"## The Generator\n\nThe generator uses the `tf.keras.layers.Conv2DTranspose` (upsampling) layer to produce an image from a seed input (a random noise). Start from this seed input, upsample it several times to reach the desired output (28x28x1).",
"_____no_output_____"
]
],
[
[
"def build_generator_model():\n model = tf.keras.Sequential()\n \n model.add(Dense(units=7 * 7 * 256, use_bias=False, input_shape=(100,)))\n model.add(BatchNormalization())\n model.add(LeakyReLU())\n\n model.add(Reshape(target_shape=[7,7,256]))\n assert model.output_shape == (None, 7, 7, 256)\n\n model.add(Conv2DTranspose(filters=128, kernel_size=(5,5), strides=(1,1), padding=\"same\", use_bias=False))\n model.add(BatchNormalization())\n model.add(LeakyReLU())\n assert model.output_shape == (None, 7, 7, 128)\n\n model.add(Conv2DTranspose(filters=64, kernel_size=(5,5), strides=(2,2), padding='same', use_bias=False))\n model.add(BatchNormalization())\n model.add(LeakyReLU())\n assert model.output_shape == (None, 14, 14, 64)\n\n model.add(Conv2DTranspose(filters=1, kernel_size=(5,5), strides=(2,2), padding='same', use_bias=False,\n activation=\"tanh\"))\n assert model.output_shape == (None, 28, 28, 1)\n\n return model",
"_____no_output_____"
],
[
"generator = build_generator_model()\n\ngenerator_input = tf.random.normal(shape=[1, 100])\ngenerator_outputs = generator(generator_input, training=False)\n\nplt.imshow(generator_outputs[0, :, :, 0], cmap='gray')\nplt.show()",
"_____no_output_____"
]
],
[
[
"## The Discriminator\n\nThe discriminator is basically a CNN network.",
"_____no_output_____"
]
],
[
[
"def build_discriminator_model():\n model = tf.keras.Sequential()\n\n # [None, 28, 28, 64]\n model.add(Conv2D(filters=64, kernel_size=(5,5), strides=(1,1), padding=\"same\", \n input_shape=[28,28,1]))\n model.add(LeakyReLU())\n model.add(Dropout(rate=0.3))\n\n # [None, 14, 14, 128]\n model.add(Conv2D(filters=128, kernel_size=(3,3), strides=(2,2), padding='same'))\n model.add(LeakyReLU())\n model.add(Dropout(rate=0.3))\n\n model.add(Flatten())\n model.add(Dense(units=1))\n\n return model",
"_____no_output_____"
]
],
[
[
"The output of the discriminator was trained that the negative values are for the fake images and the positive values are for real ones.",
"_____no_output_____"
]
],
[
[
"discriminator = build_discriminator_model()\ndiscriminator_outputs = discriminator(generator_outputs)\ndiscriminator_outputs",
"_____no_output_____"
]
],
[
[
"# Define the losses and optimizers\n\nDefine the loss functions and the optimizers for both models.",
"_____no_output_____"
]
],
[
[
"# define the cross entropy as the helper function\ncross_entropy = tf.keras.losses.BinaryCrossentropy(from_logits=True)",
"_____no_output_____"
]
],
[
[
"## Discriminator Loss\n\nThe discriminator's loss quantifies how well the discriminator can tell the real images from fakes. It compares the discriminator's predictions on real images to an array of 1s, and the discriminator's predictions on fake images to an array of 0s.",
"_____no_output_____"
]
],
[
[
"def discriminator_loss(real_output, fake_output):\n real_loss = cross_entropy(tf.ones_like(real_output), real_output)\n fake_loss = cross_entropy(tf.zeros_like(fake_output), fake_output)\n total_loss = real_loss + fake_loss\n return total_loss",
"_____no_output_____"
]
],
[
[
"## Generator Loss",
"_____no_output_____"
],
[
"The generator's loss quantifies how well the generator model can trick the discriminator model. If the generator performs well, the discriminator will classify the fake images as real (or 1). Here, we will compare the discriminator decisions on the generated images to an array of 1s.",
"_____no_output_____"
]
],
[
[
"def generator_loss(fake_output):\n # the generator learns to make the discriminator predictions became real \n # (or an array of 1s) on the fake images\n return cross_entropy(tf.ones_like(fake_output), fake_output)",
"_____no_output_____"
]
],
[
[
"## Define optimizers.",
"_____no_output_____"
]
],
[
[
"generator_optimizer = tf.keras.optimizers.Adam(learning_rate=1e-4)\ndiscriminator_optimizer = tf.keras.optimizers.Adam(learning_rate=1e-4)",
"_____no_output_____"
]
],
[
[
"## Save Checkpoints",
"_____no_output_____"
]
],
[
[
"ckpt_dir = \"./gan_ckpt\"\nckpt_prefix = os.path.join(ckpt_dir, \"ckpt\")\nckpt = tf.train.Checkpoint(generator_optimizer=generator_optimizer,\n discriminator_optimizer=discriminator_optimizer,\n generator=generator,\n discriminator=discriminator)\nckpt",
"_____no_output_____"
]
],
[
[
"# Define the training loop",
"_____no_output_____"
]
],
[
[
"EPOCHS = 50\nnoise_dim = 100\nnum_generated_examples = 16\n\n# You will reuse the seed overtime to visualize progress in the animated GIF.\nseed = tf.random.normal(shape=[num_generated_examples, noise_dim])",
"_____no_output_____"
]
],
[
[
"In the training loop, the generator model takes the noise as the input to generate the fake images. The discriminator model takes real images and fake images to give the discriminations (or outputs) for them. Calculate the generator and discriminator losses each using the real outputs and the fake outputs. Calculate the gradients of the model trainable variables based on these losses and then apply gradients back to them.",
"_____no_output_____"
]
],
[
[
"@tf.function\ndef train_step(images):\n fake_noises = tf.random.normal(shape=[BATCH_SIZE, noise_dim])\n\n with tf.GradientTape() as disc_tape, tf.GradientTape() as gen_tape:\n fake_images = generator(fake_noises, training=True)\n\n fake_outputs = discriminator(fake_images, training=True)\n real_outputs = discriminator(images, training=True)\n\n disc_loss = discriminator_loss(real_output=real_outputs, \n fake_output=fake_outputs)\n gen_loss = generator_loss(fake_output=fake_outputs)\n\n disc_gradients = disc_tape.gradient(disc_loss, discriminator.trainable_variables)\n gen_gradients = gen_tape.gradient(gen_loss, generator.trainable_variables)\n\n discriminator_optimizer.apply_gradients(zip(disc_gradients, discriminator.trainable_variables))\n generator_optimizer.apply_gradients(zip(gen_gradients, generator.trainable_variables))",
"_____no_output_____"
],
[
"def generate_and_save_images(model, epoch, test_input):\n \"\"\"Helps to generate the images from a fixed seed.\"\"\"\n predictions = model(test_input, training=False)\n\n fig = plt.figure(figsize=(8,8))\n for i in range(predictions.shape[0]):\n plt.subplot(4, 4, i+1)\n plt.imshow(predictions[i, :, :, 0] * 127.5 + 127.5, cmap='gray')\n plt.axis(\"off\")\n plt.savefig('image_epoch_{:04d}.png'.format(epoch))\n plt.show()",
"_____no_output_____"
],
[
"def train(dataset, epochs):\n for epoch in range(epochs):\n start = time.time()\n for batch_dataset in dataset:\n train_step(batch_dataset)\n \n clear_output(wait=True)\n generate_and_save_images(generator, epoch+1, seed)\n\n if (epoch+1) % 15 == 0:\n ckpt.save(file_prefix=ckpt_prefix)\n print(\"Epoch {} in time {}.\".format(epoch + 1, time.time()-start))\n\n # after the training\n clear_output(wait=True)\n generate_and_save_images(generator, epoch+1, seed)",
"_____no_output_____"
]
],
[
[
"## Train the Model\n\nCall the `train()` function to start the model training. Note, training GANs can be tricky. It's important that the generator and discriminator do not overpower each other (e.g. they train at a similar rate).",
"_____no_output_____"
]
],
[
[
"train(train_datasets, epochs=EPOCHS)",
"_____no_output_____"
]
],
[
[
"# Create a GIF",
"_____no_output_____"
]
],
[
[
"def display_image(epoch_no):\n image_path = 'image_epoch_{:04d}.png'.format(epoch_no)\n img = plt.imread(fname=image_path)\n plt.imshow(img)\n plt.margins(0)\n plt.axis(\"off\")\n plt.tight_layout()\n plt.show()",
"_____no_output_____"
],
[
"display_image(50)",
"_____no_output_____"
],
[
"anim_file = 'dcgan.gif'\n\nwith imageio.get_writer(anim_file, mode=\"I\") as writer:\n filenames = glob.glob('image*.png')\n filenames = sorted(filenames)\n\n for _, filename in enumerate(filenames):\n image = imageio.imread(filename)\n writer.append_data(image)",
"_____no_output_____"
],
[
"try:\n from google.colab import files\nexcept ImportError:\n pass\nelse:\n files.download(anim_file)",
"_____no_output_____"
],
[
"",
"_____no_output_____"
]
]
] | [
"markdown",
"code",
"markdown",
"code",
"markdown",
"code",
"markdown",
"code",
"markdown",
"code",
"markdown",
"code",
"markdown",
"code",
"markdown",
"code",
"markdown",
"code",
"markdown",
"code",
"markdown",
"code",
"markdown",
"code",
"markdown",
"code",
"markdown",
"code",
"markdown",
"code",
"markdown",
"code"
] | [
[
"markdown"
],
[
"code",
"code"
],
[
"markdown"
],
[
"code"
],
[
"markdown"
],
[
"code",
"code",
"code",
"code"
],
[
"markdown"
],
[
"code",
"code",
"code",
"code",
"code"
],
[
"markdown",
"markdown"
],
[
"code",
"code"
],
[
"markdown"
],
[
"code"
],
[
"markdown"
],
[
"code"
],
[
"markdown"
],
[
"code"
],
[
"markdown"
],
[
"code"
],
[
"markdown",
"markdown"
],
[
"code"
],
[
"markdown"
],
[
"code"
],
[
"markdown"
],
[
"code"
],
[
"markdown"
],
[
"code"
],
[
"markdown"
],
[
"code",
"code",
"code"
],
[
"markdown"
],
[
"code"
],
[
"markdown"
],
[
"code",
"code",
"code",
"code",
"code"
]
] |
d047c7d02d981c9d8a25ac89a40b5d64b0261f68 | 88,600 | ipynb | Jupyter Notebook | example.ipynb | susanzhang233/mollykill | 7f8b08f5b4d88cd8028e9e6d97e188305e6c5a2a | [
"MIT"
] | 1 | 2021-04-16T15:02:32.000Z | 2021-04-16T15:02:32.000Z | example.ipynb | susanzhang233/mollykill | 7f8b08f5b4d88cd8028e9e6d97e188305e6c5a2a | [
"MIT"
] | 1 | 2021-04-16T16:28:38.000Z | 2021-04-16T16:28:38.000Z | example.ipynb | susanzhang233/nail-it-molecule | 7f8b08f5b4d88cd8028e9e6d97e188305e6c5a2a | [
"MIT"
] | null | null | null | 108.445532 | 61,728 | 0.837009 | [
[
[
"## Setup\n",
"_____no_output_____"
],
[
"If you are running this generator locally(i.e. in a jupyter notebook in conda, just make sure you installed:\n- RDKit\n- DeepChem 2.5.0 & above\n- Tensorflow 2.4.0 & above\n\nThen, please skip the following part and continue from `Data Preparations`.",
"_____no_output_____"
],
[
"To increase efficiency, we recommend running this molecule generator in Colab.\n\nThen, we'll first need to run the following lines of code, these will download conda with the deepchem environment in colab.",
"_____no_output_____"
]
],
[
[
"#!curl -Lo conda_installer.py https://raw.githubusercontent.com/deepchem/deepchem/master/scripts/colab_install.py\n#import conda_installer\n#conda_installer.install()\n#!/root/miniconda/bin/conda info -e",
"_____no_output_____"
],
[
"#!pip install --pre deepchem\n#import deepchem\n#deepchem.__version__",
"_____no_output_____"
]
],
[
[
"## Data Preparations\n\nNow we are ready to import some useful functions/packages, along with our model.",
"_____no_output_____"
],
[
"### Import Data",
"_____no_output_____"
]
],
[
[
"import model##our model",
"_____no_output_____"
],
[
"from rdkit import Chem\nfrom rdkit.Chem import AllChem",
"_____no_output_____"
],
[
"import pandas as pd\nimport numpy as np\nimport matplotlib.pyplot as plt",
"_____no_output_____"
],
[
"import deepchem as dc ",
"_____no_output_____"
]
],
[
[
"Then, we are ready to import our dataset for training. \n\nHere, for demonstration, we'll be using this dataset of in-vitro assay that detects inhibition of SARS-CoV 3CL protease via fluorescence.\n\nThe dataset is originally from [PubChem AID1706](https://pubchem.ncbi.nlm.nih.gov/bioassay/1706), previously handled by [JClinic AIcure](https://www.aicures.mit.edu/) team at MIT into this [binarized label form](https://github.com/yangkevin2/coronavirus_data/blob/master/data/AID1706_binarized_sars.csv).",
"_____no_output_____"
]
],
[
[
"df = pd.read_csv('AID1706_binarized_sars.csv')",
"_____no_output_____"
]
],
[
[
"Observe the data above, it contains a 'smiles' column, which stands for the smiles representation of the molecules. There is also an 'activity' column, in which it is the label specifying whether that molecule is considered as hit for the protein.\n\nHere, we only need those 405 molecules considered as hits, and we'll be extracting features from them to generate new molecules that may as well be hits.",
"_____no_output_____"
]
],
[
[
"true = df[df['activity']==1]",
"_____no_output_____"
]
],
[
[
"### Set Minimum Length for molecules",
"_____no_output_____"
],
[
"Since we'll be using graphic neural network, it might be more helpful and efficient if our graph data are of the same size, thus, we'll eliminate the molecules from the training set that are shorter(i.e. lacking enough atoms) than our desired minimum size.",
"_____no_output_____"
]
],
[
[
"num_atoms = 6 #here the minimum length of molecules is 6",
"_____no_output_____"
],
[
"input_df = true['smiles']\ndf_length = []\nfor _ in input_df:\n df_length.append(Chem.MolFromSmiles(_).GetNumAtoms() )",
"_____no_output_____"
],
[
"true['length'] = df_length #create a new column containing each molecule's length",
"/Users/feishu/opt/anaconda3/envs/my-rdkit-env/lib/python3.7/site-packages/ipykernel_launcher.py:1: SettingWithCopyWarning: \nA value is trying to be set on a copy of a slice from a DataFrame.\nTry using .loc[row_indexer,col_indexer] = value instead\n\nSee the caveats in the documentation: https://pandas.pydata.org/pandas-docs/stable/user_guide/indexing.html#returning-a-view-versus-a-copy\n \"\"\"Entry point for launching an IPython kernel.\n"
],
[
"true = true[true['length']>num_atoms] #Here we leave only the ones longer than 6\ninput_df = true['smiles']\ninput_df_smiles = input_df.apply(Chem.MolFromSmiles) #convert the smiles representations into rdkit molecules\n",
"_____no_output_____"
]
],
[
[
"Now, we are ready to apply the `featurizer` function to our molecules to convert them into graphs with nodes and edges for training.",
"_____no_output_____"
]
],
[
[
"#input_df = input_df.apply(Chem.MolFromSmiles) \ntrain_set = input_df_smiles.apply( lambda x: model.featurizer(x,max_length = num_atoms))",
"_____no_output_____"
],
[
"train_set",
"_____no_output_____"
]
],
[
[
"We'll take one more step to make the train_set into separate nodes and edges, which fits the format later to supply to the model for training",
"_____no_output_____"
]
],
[
[
"nodes_train, edges_train = list(zip(*train_set) )",
"_____no_output_____"
]
],
[
[
"## Training",
"_____no_output_____"
],
[
"Now, we're finally ready for generating new molecules. We'll first import some necessay functions from tensorflow.",
"_____no_output_____"
]
],
[
[
"import tensorflow as tf\nfrom tensorflow import keras\nfrom tensorflow.keras import layers",
"_____no_output_____"
]
],
[
[
"The network here we'll be using is Generative Adversarial Network, as mentioned in the project introduction. Here's a great [introduction](https://machinelearningmastery.com/what-are-generative-adversarial-networks-gans/). ",
"_____no_output_____"
],
[
"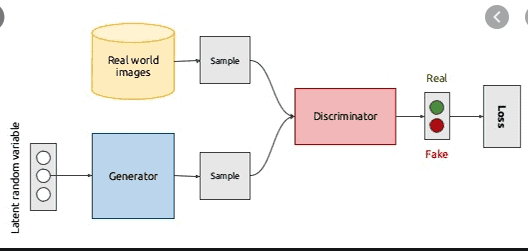\n",
"_____no_output_____"
],
[
"Here we'll first initiate a discriminator and a generator model with the corresponding functions in the package.",
"_____no_output_____"
]
],
[
[
"disc = model.make_discriminator(num_atoms)",
"_____no_output_____"
],
[
"gene = model.make_generator(num_atoms, noise_input_shape = 100)",
"_____no_output_____"
]
],
[
[
"Then, with the `train_batch` function, we'll supply the necessary inputs and train our network. Upon some experimentations, an epoch of around 160 would be nice for this dataset.",
"_____no_output_____"
]
],
[
[
"generator_trained = model.train_batch(\n disc, gene, \n np.array(nodes_train), np.array(edges_train), \n noise_input_shape = 100, EPOCH = 160, BATCHSIZE = 2, \n plot_hist = True, temp_result = False\n )",
">0, d1=0.221, d2=0.833 g=0.681, a1=100, a2=0\n>1, d1=0.054, d2=0.714 g=0.569, a1=100, a2=0\n>2, d1=0.026, d2=0.725 g=0.631, a1=100, a2=0\n>3, d1=0.016, d2=0.894 g=0.636, a1=100, a2=0\n>4, d1=0.016, d2=0.920 g=0.612, a1=100, a2=0\n>5, d1=0.012, d2=0.789 g=0.684, a1=100, a2=0\n>6, d1=0.014, d2=0.733 g=0.622, a1=100, a2=0\n>7, d1=0.056, d2=0.671 g=0.798, a1=100, a2=100\n>8, d1=0.029, d2=0.587 g=0.653, a1=100, a2=100\n>9, d1=0.133, d2=0.537 g=0.753, a1=100, a2=100\n>10, d1=0.049, d2=0.640 g=0.839, a1=100, a2=100\n>11, d1=0.056, d2=0.789 g=0.836, a1=100, a2=0\n>12, d1=0.086, d2=0.564 g=0.916, a1=100, a2=100\n>13, d1=0.067, d2=0.550 g=0.963, a1=100, a2=100\n>14, d1=0.062, d2=0.575 g=0.940, a1=100, a2=100\n>15, d1=0.053, d2=0.534 g=1.019, a1=100, a2=100\n>16, d1=0.179, d2=0.594 g=1.087, a1=100, a2=100\n>17, d1=0.084, d2=0.471 g=0.987, a1=100, a2=100\n>18, d1=0.052, d2=0.366 g=1.226, a1=100, a2=100\n>19, d1=0.065, d2=0.404 g=1.220, a1=100, a2=100\n>20, d1=0.044, d2=0.311 g=1.274, a1=100, a2=100\n>21, d1=0.015, d2=0.231 g=1.567, a1=100, a2=100\n>22, d1=0.010, d2=0.222 g=1.838, a1=100, a2=100\n>23, d1=0.007, d2=0.177 g=1.903, a1=100, a2=100\n>24, d1=0.004, d2=0.139 g=2.155, a1=100, a2=100\n>25, d1=0.132, d2=0.111 g=2.316, a1=100, a2=100\n>26, d1=0.004, d2=0.139 g=2.556, a1=100, a2=100\n>27, d1=0.266, d2=0.133 g=2.131, a1=100, a2=100\n>28, d1=0.001, d2=0.199 g=2.211, a1=100, a2=100\n>29, d1=0.000, d2=0.252 g=2.585, a1=100, a2=100\n>30, d1=0.000, d2=0.187 g=2.543, a1=100, a2=100\n>31, d1=0.002, d2=0.081 g=2.454, a1=100, a2=100\n>32, d1=0.171, d2=0.061 g=2.837, a1=100, a2=100\n>33, d1=0.028, d2=0.045 g=2.858, a1=100, a2=100\n>34, d1=0.011, d2=0.072 g=2.627, a1=100, a2=100\n>35, d1=2.599, d2=0.115 g=1.308, a1=0, a2=100\n>36, d1=0.000, d2=0.505 g=0.549, a1=100, a2=100\n>37, d1=0.000, d2=1.463 g=0.292, a1=100, a2=0\n>38, d1=0.002, d2=1.086 g=0.689, a1=100, a2=0\n>39, d1=0.153, d2=0.643 g=0.861, a1=100, a2=100\n>40, d1=0.000, d2=0.353 g=1.862, a1=100, a2=100\n>41, d1=0.034, d2=0.143 g=2.683, a1=100, a2=100\n>42, d1=0.003, d2=0.110 g=2.784, a1=100, a2=100\n>43, d1=0.093, d2=0.058 g=2.977, a1=100, a2=100\n>44, d1=0.046, d2=0.051 g=3.051, a1=100, a2=100\n>45, d1=0.185, d2=0.062 g=2.922, a1=100, a2=100\n>46, d1=0.097, d2=0.070 g=2.670, a1=100, a2=100\n>47, d1=0.060, d2=0.073 g=2.444, a1=100, a2=100\n>48, d1=0.093, d2=0.156 g=2.385, a1=100, a2=100\n>49, d1=0.785, d2=0.346 g=1.026, a1=0, a2=100\n>50, d1=0.057, d2=0.869 g=0.667, a1=100, a2=0\n>51, d1=0.002, d2=1.001 g=0.564, a1=100, a2=0\n>52, d1=0.000, d2=0.764 g=1.047, a1=100, a2=0\n>53, d1=0.010, d2=0.362 g=1.586, a1=100, a2=100\n>54, d1=0.033, d2=0.230 g=2.469, a1=100, a2=100\n>55, d1=0.179, d2=0.134 g=2.554, a1=100, a2=100\n>56, d1=0.459, d2=0.103 g=2.356, a1=100, a2=100\n>57, d1=0.245, d2=0.185 g=1.769, a1=100, a2=100\n>58, d1=0.014, d2=0.227 g=1.229, a1=100, a2=100\n>59, d1=0.016, d2=0.699 g=0.882, a1=100, a2=0\n>60, d1=0.002, d2=0.534 g=1.192, a1=100, a2=100\n>61, d1=0.010, d2=0.335 g=1.630, a1=100, a2=100\n>62, d1=0.019, d2=0.283 g=2.246, a1=100, a2=100\n>63, d1=0.240, d2=0.132 g=2.547, a1=100, a2=100\n>64, d1=0.965, d2=0.219 g=1.534, a1=0, a2=100\n>65, d1=0.040, d2=0.529 g=0.950, a1=100, a2=100\n>66, d1=0.012, d2=0.611 g=0.978, a1=100, a2=100\n>67, d1=0.015, d2=0.576 g=1.311, a1=100, a2=100\n>68, d1=0.102, d2=0.214 g=1.840, a1=100, a2=100\n>69, d1=0.020, d2=0.140 g=2.544, a1=100, a2=100\n>70, d1=5.089, d2=0.314 g=1.231, a1=0, a2=100\n>71, d1=0.026, d2=0.700 g=0.556, a1=100, a2=0\n>72, d1=0.005, d2=1.299 g=0.460, a1=100, a2=0\n>73, d1=0.009, d2=1.033 g=0.791, a1=100, a2=0\n>74, d1=0.013, d2=0.343 g=1.408, a1=100, a2=100\n>75, d1=0.247, d2=0.267 g=1.740, a1=100, a2=100\n>76, d1=0.184, d2=0.172 g=2.105, a1=100, a2=100\n>77, d1=0.150, d2=0.133 g=2.297, a1=100, a2=100\n>78, d1=0.589, d2=0.112 g=2.557, a1=100, a2=100\n>79, d1=0.477, d2=0.232 g=1.474, a1=100, a2=100\n>80, d1=0.173, d2=0.360 g=1.034, a1=100, a2=100\n>81, d1=0.052, d2=0.790 g=0.936, a1=100, a2=0\n>82, d1=0.042, d2=0.537 g=1.135, a1=100, a2=100\n>83, d1=0.296, d2=0.363 g=1.152, a1=100, a2=100\n>84, d1=0.157, d2=0.377 g=1.283, a1=100, a2=100\n>85, d1=0.139, d2=0.436 g=1.445, a1=100, a2=100\n>86, d1=0.163, d2=0.343 g=1.370, a1=100, a2=100\n>87, d1=0.189, d2=0.290 g=1.576, a1=100, a2=100\n>88, d1=1.223, d2=0.548 g=0.822, a1=0, a2=100\n>89, d1=0.016, d2=1.042 g=0.499, a1=100, a2=0\n>90, d1=0.013, d2=1.033 g=0.829, a1=100, a2=0\n>91, d1=0.006, d2=0.589 g=1.421, a1=100, a2=100\n>92, d1=0.054, d2=0.160 g=2.414, a1=100, a2=100\n>93, d1=0.214, d2=0.070 g=3.094, a1=100, a2=100\n>94, d1=0.445, d2=0.089 g=2.564, a1=100, a2=100\n>95, d1=2.902, d2=0.180 g=1.358, a1=0, a2=100\n>96, d1=0.485, d2=0.684 g=0.625, a1=100, a2=100\n>97, d1=0.287, d2=1.296 g=0.405, a1=100, a2=0\n>98, d1=0.159, d2=1.149 g=0.689, a1=100, a2=0\n>99, d1=0.021, d2=0.557 g=1.405, a1=100, a2=100\n>100, d1=0.319, d2=0.243 g=1.905, a1=100, a2=100\n>101, d1=0.811, d2=0.241 g=1.523, a1=0, a2=100\n>102, d1=0.469, d2=0.439 g=0.987, a1=100, a2=100\n>103, d1=0.073, d2=0.760 g=0.698, a1=100, a2=0\n>104, d1=0.040, d2=0.762 g=0.869, a1=100, a2=0\n>105, d1=0.073, d2=0.444 g=1.453, a1=100, a2=100\n>106, d1=0.455, d2=0.272 g=1.632, a1=100, a2=100\n>107, d1=0.320, d2=0.365 g=1.416, a1=100, a2=100\n>108, d1=0.245, d2=0.409 g=1.245, a1=100, a2=100\n>109, d1=0.258, d2=0.572 g=1.146, a1=100, a2=100\n>110, d1=0.120, d2=0.447 g=1.538, a1=100, a2=100\n>111, d1=2.707, d2=0.376 g=1.343, a1=0, a2=100\n>112, d1=3.112, d2=0.604 g=0.873, a1=0, a2=100\n>113, d1=0.107, d2=0.750 g=0.873, a1=100, a2=0\n>114, d1=0.284, d2=0.682 g=0.905, a1=100, a2=100\n>115, d1=1.768, d2=0.717 g=0.824, a1=0, a2=0\n>116, d1=0.530, d2=0.822 g=0.560, a1=100, a2=0\n>117, d1=0.424, d2=0.984 g=0.613, a1=100, a2=0\n>118, d1=1.608, d2=1.398 g=0.244, a1=0, a2=0\n>119, d1=4.422, d2=2.402 g=0.135, a1=0, a2=0\n>120, d1=0.011, d2=1.998 g=0.321, a1=100, a2=0\n>121, d1=0.085, d2=1.066 g=0.815, a1=100, a2=0\n>122, d1=0.895, d2=0.444 g=1.495, a1=0, a2=100\n>123, d1=2.659, d2=0.288 g=1.417, a1=0, a2=100\n>124, d1=1.780, d2=0.450 g=0.869, a1=0, a2=100\n>125, d1=2.271, d2=1.046 g=0.324, a1=0, a2=0\n>126, d1=0.836, d2=1.970 g=0.123, a1=0, a2=0\n>127, d1=0.108, d2=2.396 g=0.103, a1=100, a2=0\n>128, d1=0.146, d2=2.371 g=0.174, a1=100, a2=0\n>129, d1=0.189, d2=1.623 g=0.424, a1=100, a2=0\n>130, d1=0.508, d2=0.877 g=0.876, a1=100, a2=0\n>131, d1=0.723, d2=0.423 g=1.367, a1=0, a2=100\n>132, d1=1.306, d2=0.292 g=1.445, a1=0, a2=100\n>133, d1=0.920, d2=0.318 g=1.378, a1=0, a2=100\n>134, d1=1.120, d2=0.481 g=0.827, a1=0, a2=100\n>135, d1=0.278, d2=0.763 g=0.562, a1=100, a2=0\n>136, d1=0.134, d2=0.901 g=0.555, a1=100, a2=0\n>137, d1=0.061, d2=0.816 g=0.864, a1=100, a2=0\n>138, d1=0.057, d2=0.451 g=1.533, a1=100, a2=100\n>139, d1=0.111, d2=0.214 g=2.145, a1=100, a2=100\n>140, d1=0.260, d2=0.107 g=2.451, a1=100, a2=100\n>141, d1=4.498, d2=0.209 g=1.266, a1=0, a2=100\n>142, d1=0.016, d2=0.681 g=0.672, a1=100, a2=100\n>143, d1=0.007, d2=0.952 g=0.702, a1=100, a2=0\n>144, d1=0.008, d2=0.624 g=1.337, a1=100, a2=100\n>145, d1=0.010, d2=0.241 g=2.114, a1=100, a2=100\n>146, d1=2.108, d2=0.121 g=2.536, a1=0, a2=100\n>147, d1=4.086, d2=0.111 g=2.315, a1=0, a2=100\n>148, d1=1.247, d2=0.177 g=1.781, a1=0, a2=100\n>149, d1=2.684, d2=0.377 g=1.026, a1=0, a2=100\n>150, d1=0.572, d2=0.701 g=0.710, a1=100, a2=0\n>151, d1=0.608, d2=0.899 g=0.571, a1=100, a2=0\n>152, d1=0.118, d2=0.904 g=0.592, a1=100, a2=0\n>153, d1=0.228, d2=0.837 g=0.735, a1=100, a2=0\n>154, d1=0.353, d2=0.671 g=0.912, a1=100, a2=100\n>155, d1=0.959, d2=0.563 g=0.985, a1=0, a2=100\n>156, d1=0.427, d2=0.478 g=1.184, a1=100, a2=100\n>157, d1=0.307, d2=0.348 g=1.438, a1=100, a2=100\n>158, d1=0.488, d2=0.286 g=1.383, a1=100, a2=100\n>159, d1=0.264, d2=0.333 g=1.312, a1=100, a2=100\n"
]
],
[
[
"There are two possible kind of failures regarding a GAN model: model collapse and failure of convergence. Model collapse would often mean that the generative part of the model wouldn't be able to generate diverse outcomes. Failure of convergence between the generative and the discriminative model could likely way be identified as that the loss for the discriminator has gone to zero or close to zero. \n\nObserve the above generated plot, in the upper plot, the loss of discriminator has not gone to zero/close to zero, indicating that the model has possibily find a balance between the generator and the discriminator. In the lower plot, the accuracy is fluctuating between 1 and 0, indicating possible variability within the data generated. \n\nTherefore, it is reasonable to conclude that within the possible range of epoch and other parameters, the model has successfully avoided the two common types of failures associated with GAN.",
"_____no_output_____"
],
[
"## Rewarding Phase",
"_____no_output_____"
],
[
"The above `train_batch` function is set to return a trained generator. Thus, we could use that function directly and observe the possible molecules we could get from that function.",
"_____no_output_____"
]
],
[
[
"no, ed = generator_trained(np.random.randint(0,20\n \n , size =(1,100)))#generated nodes and edges",
"_____no_output_____"
],
[
"abs(no.numpy()).astype(int).reshape(num_atoms), abs(ed.numpy()).astype(int).reshape(num_atoms,num_atoms)",
"_____no_output_____"
]
],
[
[
"With the `de_featurizer`, we could convert the generated matrix into a smiles molecule and plot it out=)",
"_____no_output_____"
]
],
[
[
"cat, dog = model.de_featurizer(abs(no.numpy()).astype(int).reshape(num_atoms), abs(ed.numpy()).astype(int).reshape(num_atoms,num_atoms))",
"_____no_output_____"
],
[
"Chem.MolToSmiles(cat)",
"_____no_output_____"
],
[
"Chem.MolFromSmiles(Chem.MolToSmiles(cat))",
"RDKit ERROR: [14:09:13] Explicit valence for atom # 1 O, 5, is greater than permitted\n"
]
],
[
[
"## Brief Result Analysis",
"_____no_output_____"
]
],
[
[
"from rdkit import DataStructs",
"_____no_output_____"
]
],
[
[
"With the rdkit function of comparing similarities, here we'll demonstrate a preliminary analysis of the molecule we've generated. With \"CCO\" molecule as a control, we could observe that the new molecule we've generated is more similar to a random selected molecule(the fourth molecule) from the initial training set.\n\nThis may indicate that our model has indeed extracted some features from our original dataset and generated a new molecule that is relevant.",
"_____no_output_____"
]
],
[
[
"DataStructs.FingerprintSimilarity(Chem.RDKFingerprint(Chem.MolFromSmiles(\"[Li]NBBC=N\")), Chem.RDKFingerprint(Chem.MolFromSmiles(\"CCO\")))# compare with the control",
"_____no_output_____"
],
[
"#compare with one from the original data\nDataStructs.FingerprintSimilarity(Chem.RDKFingerprint(Chem.MolFromSmiles(\"[Li]NBBC=N\")), Chem.RDKFingerprint(Chem.MolFromSmiles(\"CCN1C2=NC(=O)N(C(=O)C2=NC(=N1)C3=CC=CC=C3)C\")))",
"_____no_output_____"
]
]
] | [
"markdown",
"code",
"markdown",
"code",
"markdown",
"code",
"markdown",
"code",
"markdown",
"code",
"markdown",
"code",
"markdown",
"code",
"markdown",
"code",
"markdown",
"code",
"markdown",
"code",
"markdown",
"code",
"markdown",
"code",
"markdown",
"code",
"markdown",
"code"
] | [
[
"markdown",
"markdown",
"markdown"
],
[
"code",
"code"
],
[
"markdown",
"markdown"
],
[
"code",
"code",
"code",
"code"
],
[
"markdown"
],
[
"code"
],
[
"markdown"
],
[
"code"
],
[
"markdown",
"markdown"
],
[
"code",
"code",
"code",
"code"
],
[
"markdown"
],
[
"code",
"code"
],
[
"markdown"
],
[
"code"
],
[
"markdown",
"markdown"
],
[
"code"
],
[
"markdown",
"markdown",
"markdown"
],
[
"code",
"code"
],
[
"markdown"
],
[
"code"
],
[
"markdown",
"markdown",
"markdown"
],
[
"code",
"code"
],
[
"markdown"
],
[
"code",
"code",
"code"
],
[
"markdown"
],
[
"code"
],
[
"markdown"
],
[
"code",
"code"
]
] |
d047e0536f89f524a96d253a85195adc82e5fff0 | 10,745 | ipynb | Jupyter Notebook | notebooks/comparing_nn_results_tau_vs_sd.ipynb | NCAR/mlmicrophysics | 45e1bac65a68e4c2656f70d17b4289576a3258ad | [
"MIT"
] | 4 | 2021-01-05T13:18:28.000Z | 2021-09-29T09:53:28.000Z | notebooks/comparing_nn_results_tau_vs_sd.ipynb | NCAR/mlmicrophysics | 45e1bac65a68e4c2656f70d17b4289576a3258ad | [
"MIT"
] | 5 | 2020-11-16T15:53:24.000Z | 2021-07-22T20:16:11.000Z | notebooks/comparing_nn_results_tau_vs_sd.ipynb | NCAR/mlmicrophysics | 45e1bac65a68e4c2656f70d17b4289576a3258ad | [
"MIT"
] | 4 | 2020-07-08T13:04:44.000Z | 2022-01-09T13:36:55.000Z | 27.340967 | 90 | 0.394509 | [
[
[
"import os\nimport pandas as pd\nimport numpy as np\nimport xarray as xr\nimport matplotlib.pyplot as plt\nfrom os.path import join\nimport yaml\n%matplotlib inline",
"_____no_output_____"
],
[
"dir_tau = \"/glade/p/cisl/aiml/ggantos/cam_run5_model_base_full/\"\ndir_sd = \"/glade/p/cisl/aiml/ggantos/cam_sd_model_base_full/\"",
"_____no_output_____"
],
[
"classifier_scores_tau = pd.read_csv(join(dir_tau, \"dnn_classifier_scores.csv\"))\nregressor_scores_tau = pd.read_csv(join(dir_tau, \"dnn_regressor_scores.csv\"))\nclassifier_scores_sd = pd.read_csv(join(dir_sd, \"dnn_classifier_scores.csv\"))\nregressor_scores_sd = pd.read_csv(join(dir_sd, \"dnn_regressor_scores.csv\"))\n",
"_____no_output_____"
],
[
"classifier_scores_tau",
"_____no_output_____"
],
[
"classifier_scores_sd",
"_____no_output_____"
],
[
"regressor_scores_tau",
"_____no_output_____"
],
[
"regressor_scores_sd",
"_____no_output_____"
]
]
] | [
"code"
] | [
[
"code",
"code",
"code",
"code",
"code",
"code",
"code"
]
] |
d047ec415b0109e0f3bc8915801311ad1b4197c4 | 607,807 | ipynb | Jupyter Notebook | examples/Result_Analysis.ipynb | suhailrehman/fuzzydata | 53009dcc1e8ac02607fa1ab285e0a57a1c28c54c | [
"MIT"
] | null | null | null | examples/Result_Analysis.ipynb | suhailrehman/fuzzydata | 53009dcc1e8ac02607fa1ab285e0a57a1c28c54c | [
"MIT"
] | 6 | 2022-03-05T18:31:27.000Z | 2022-03-09T23:02:50.000Z | examples/Result_Analysis.ipynb | suhailrehman/fuzzydata | 53009dcc1e8ac02607fa1ab285e0a57a1c28c54c | [
"MIT"
] | null | null | null | 402.787939 | 36,540 | 0.91754 | [
[
[
"import pandas as pd\nimport numpy as np\nimport glob",
"_____no_output_____"
],
[
"result_file = '/tmp/fuzzydatatest/20220209-150332_perf.csv'\nperf_df = pd.read_csv(result_file, index_col=0)\nperf_df",
"_____no_output_____"
],
[
"import numpy as np\n\nperf_df['end_time_seconds'] = np.cumsum(perf_df.elapsed_time)\nperf_df['start_time_seconds'] = end_time.shift().fillna(0)\nperf_df",
"_____no_output_____"
],
[
"import matplotlib.pyplot as plt\nfrom matplotlib.patches import Patch\n\n# GANTT Chart function\n\ndef plot_gantt(df, title='fuzzydata', x_range=None):\n \n # Adapted from \n # https://towardsdatascience.com/gantt-charts-with-pythons-matplotlib-395b7af72d72 \n \n #### Prepare the DF ####\n c_dict = {'merge':'#E64646', 'pivot':'#E69646', 'groupby':'#34D05C',\n 'project':'#34D0C3', 'sample':'#3475D0' , 'select': '#29335C' , 'load': '#f7ef07'}\n \n df['end_time_seconds'] = np.cumsum(perf_df.elapsed_time)\n df['start_time_seconds'] = df['end_time_seconds'].shift().fillna(0)\n df['task_label'] = df.index.astype(str) +'_'+ df['op']\n df['color'] = df['op'].apply(lambda x: c_dict[x])\n df = df.iloc[::-1]\n \n #### PLOT #####\n fig, ax = plt.subplots(1, figsize=(16,6))\n ax.barh(df.task_label, df.elapsed_time, left=df.start_time_seconds, \n color=df.color)\n ##### LEGENDS #####\n \n legend_elements = [Patch(facecolor=c_dict[i], label=i) for i in c_dict]\n plt.legend(handles=legend_elements)\n \n ##### TICKS #####\n #xticks = np.arange(0, df.end_num.max()+1, 3)\n #xticks_labels = pd.date_range(proj_start, end=df.End.max()).strftime(\"%m/%d\")\n #xticks_minor = np.arange(0, df.end_num.max()+1, 1)\n #ax.set_xticks(xticks)\n #ax.set_xticks(xticks_minor, minor=True)\n #ax.set_xticklabels(xticks_labels[::3])\n \n ax.set_axisbelow(True)\n ax.xaxis.grid(color='gray', linestyle='dashed', alpha=0.2, which='both')\n \n if x_range:\n plt.xlim(x_range)\n plt.title(title)\n plt.ylabel('Operation')\n plt.xlabel('Seconds')\n plt.show()\n \nplot_gantt(perf_df)",
"_____no_output_____"
],
[
"# Plot all gantt charts\n\nBASE_DIR = '/tmp/fuzzydatatest/'\nframeworks = ['pandas', 'sqlite', 'modin_dask', 'modin_ray']\n\nfor f in frameworks:\n result_file = glob.glob(f\"{BASE_DIR}/{f}/*_perf.csv\")[0]\n perf_df = pd.read_csv(result_file, index_col=0)\n plot_gantt(perf_df, title=f'Example Workflow Base Table (20 columns x 10,000 rows), 15 artifacts on {f}', x_range=[0.0,2.0])",
"_____no_output_____"
],
[
"BASE_DIR = '/tmp/fuzzydatatest_big/'\nframeworks = ['pandas', 'sqlite', 'modin_dask', 'modin_ray']\n\nfor f in frameworks:\n result_file = glob.glob(f\"{BASE_DIR}/{f}/*_perf.csv\")[0]\n perf_df = pd.read_csv(result_file, index_col=0)\n plot_gantt(perf_df, title=f'Example Workflow Base Table (20 columns x 100,000 rows), 15 artifacts on {f}', x_range=[0.0, 5.0])",
"_____no_output_____"
],
[
"BASE_DIR = '/tmp/fuzzydatatest_1m/'\nframeworks = ['pandas', 'sqlite', 'modin_dask', 'modin_ray']\n\nfor f in frameworks:\n result_file = glob.glob(f\"{BASE_DIR}/{f}/*_perf.csv\")[0]\n perf_df = pd.read_csv(result_file, index_col=0)\n plot_gantt(perf_df, title=f'Example Workflow Base Table (20 columns x 1,000,000 rows), 15 artifacts on {f}', x_range=[0.0, 35.0])",
"_____no_output_____"
]
],
[
[
"# Large DataFrame Tests - NYC CAB",
"_____no_output_____"
]
],
[
[
"BASE_DIR = '/mnt/roscoe/data/fuzzydata/fuzzydatatest/nyc-cab/'\nframeworks = ['pandas', 'sqlite', 'modin_dask', 'modin_ray']\n\nall_perfs = []\nfor f in frameworks:\n result_file = glob.glob(f\"{BASE_DIR}/{f}/*_perf.csv\")[0]\n perf_df = pd.read_csv(result_file, index_col=0)\n perf_df['end_time_seconds'] = np.cumsum(perf_df.elapsed_time)\n perf_df['start_time_seconds'] = perf_df['end_time_seconds'].shift().fillna(0)\n perf_df['framework'] = f\n all_perfs.append(perf_df)\n #plot_gantt(perf_df, title=f'Example Workflow 1.18 GB CSV load/groupby on {f}', x_range=[0.0, 320])\n \nnyc_cab_perfs = pd.concat(all_perfs, ignore_index=True)",
"_____no_output_____"
],
[
"new_op_labels = ['load', 'groupby_1', 'groupby_2', 'groupby_3']\nnyc_cab_perfs['op'] = np.tile(new_op_labels,4)\npivoted = nyc_cab_perfs.pivot(index='framework', columns='op', values='elapsed_time')\npivoted = pivoted.reindex(['pandas', 'modin_dask', 'modin_ray', 'sqlite'])[new_op_labels]\nax = pivoted.plot.bar(stacked=True)\nplt.xticks(rotation=0)\nplt.legend()\nplt.xlabel('Client')\nplt.ylabel('Runtime (Seconds)')\nplt.savefig('real_example.pdf', bbox_inches='tight')",
"_____no_output_____"
]
],
[
[
"# Combined Performance / Scaling Graph",
"_____no_output_____"
]
],
[
[
"BASE_DIR = '/mnt/roscoe/data/fuzzydata/fuzzydata_scaling_test_3/'\nframeworks = ['pandas', 'sqlite', 'modin_dask', 'modin_ray']\nsizes = ['1000', '10000', '100000', '1000000', '5000000']\n\nall_perfs = []\n\nfor framework in frameworks:\n for size in sizes:\n input_dir = f\"{BASE_DIR}/{framework}_{size}/\"\n try:\n #print(f\"{input_dir}/*_perf.csv\")\n perf_file = glob.glob(f\"{input_dir}/*_perf.csv\")[0]\n perf_df = pd.read_csv(perf_file, index_col=0)\n perf_df['end_time_seconds'] = np.cumsum(perf_df.elapsed_time)\n perf_df['start_time_seconds'] = perf_df['end_time_seconds'].shift().fillna(0)\n perf_df['framework'] = framework\n perf_df['size'] = size\n all_perfs.append(perf_df)\n except (IndexError, FileNotFoundError) as e:\n #raise(e)\n pass\n \nall_perfs_df = pd.concat(all_perfs, ignore_index=True)",
"_____no_output_____"
],
[
"total_wf_times = all_perfs_df.loc[all_perfs_df.dst == 'artifact_14'][['framework','size','end_time_seconds']].reset_index(drop=True).pivot(index='size', columns='framework', values='end_time_seconds')\ntotal_wf_times = total_wf_times.rename_axis('Client')\ntotal_wf_times = total_wf_times[['pandas', 'modin_dask', 'modin_ray', 'sqlite']]\ntotal_wf_times",
"_____no_output_____"
],
[
"import seaborn as sns\nimport matplotlib\nimport matplotlib.pyplot as plt\n\nfont = {'family' : 'serif',\n 'weight' : 'normal',\n 'size' : 12}\n\nmatplotlib.rc('font', **font)\n\n\nx_axis_replacements = ['1K', '10K', '100K', '1M', '5M']\n\n\nplt.figure(figsize=(6,4))\n\nax = sns.lineplot(data=total_wf_times, markers=True, linewidth=2.5, markersize=10)\nplt.xticks(total_wf_times.index, x_axis_replacements)\nplt.grid()\nplt.xlabel('Base Artifact Number of Rows (r)')\nplt.ylabel('Runtime (Seconds)')\n\nhandles, labels = ax.get_legend_handles_labels()\nax.legend(handles=handles, labels=labels)\n\nplt.savefig(\"scaling.pdf\", bbox_inches='tight')",
"_____no_output_____"
],
[
"breakdown = all_perfs_df[['framework', 'size', 'op', 'elapsed_time']].groupby(['framework', 'size', 'op']).sum().reset_index()\npivoted_breakdown = breakdown.pivot(index=['size', 'framework'], columns=['op'], values='elapsed_time')\npivoted_breakdown",
"_____no_output_____"
],
[
"pivoted_breakdown.plot.bar(stacked=True)\nplt.savefig(\"breakdown.pdf\", bbox_inches='tight')",
"_____no_output_____"
],
[
"breakdown",
"_____no_output_____"
],
[
"# Sources: https://stackoverflow.com/questions/22787209/how-to-have-clusters-of-stacked-bars-with-python-pandas\n\nimport pandas as pd\nimport matplotlib.cm as cm\nimport numpy as np\nimport matplotlib.pyplot as plt\n\n\ndef plot_clustered_stacked(dfall, labels=None, title=\"multiple stacked bar plot\", H=\"/\", \n x_axis_replacements=None, **kwargs):\n \"\"\"Given a list of dataframes, with identical columns and index, create a clustered stacked bar plot. \nlabels is a list of the names of the dataframe, used for the legend\ntitle is a string for the title of the plot\nH is the hatch used for identification of the different dataframe\"\"\"\n\n n_df = len(dfall)\n n_col = len(dfall[0].columns) \n n_ind = len(dfall[0].index)\n fig = plt.figure(figsize=(6,4))\n axe = fig.add_subplot(111)\n\n for df in dfall : # for each data frame\n axe = df.plot(kind=\"bar\",\n linewidth=0,\n stacked=True,\n ax=axe,\n legend=False,\n grid=False,\n **kwargs) # make bar plots\n\n hatches = ['', 'oo', '///', '++']\n h,l = axe.get_legend_handles_labels() # get the handles we want to modify\n for i in range(0, n_df * n_col, n_col): # len(h) = n_col * n_df\n for j, pa in enumerate(h[i:i+n_col]):\n for rect in pa.patches: # for each index\n rect.set_x(rect.get_x() + 1 / float(n_df + 1) * i / float(n_col) -0.1)\n rect.set_hatch(hatches[int(i / n_col)]) #edited part \n rect.set_width(1 / float(n_df + 1))\n\n axe.set_xticks((np.arange(0, 2 * n_ind, 2) + 1 / float(n_df + 1)) / 2.)\n if x_axis_replacements == None:\n x_axis_replacements = df.index\n axe.set_xticklabels(x_axis_replacements, rotation = 0)\n #axe.set_title(title)\n\n # Add invisible data to add another legend\n n=[] \n for i in range(n_df):\n n.append(axe.bar(0, 0, color=\"gray\", hatch=hatches[i]))\n\n l1 = axe.legend(h[:n_col], l[:n_col], loc=[0.38, 0.545])\n if labels is not None:\n l2 = plt.legend(n, labels)# , loc=[1.01, 0.1]) \n axe.add_artist(l1)\n return axe\n\ncols = ['groupby','load','merge','project','sample']\npbr = pivoted_breakdown.reset_index()\npbr = pbr.set_index('size')\ndf_splits = [pbr.loc[pbr.framework == f][cols] for f in ['pandas', 'modin_dask', 'modin_ray', 'sqlite']]\n\n# Then, just call :\n\nplot_clustered_stacked(df_splits,['pandas', 'modin_dask', 'modin_ray', 'sqlite'],\n x_axis_replacements=x_axis_replacements,\n title='Timing Breakdown Per Operation Type')\nplt.xlabel('Base Artifact Number of Rows (r)')\nplt.ylabel('Runtime (Seconds)')\nplt.savefig(\"breakdown.eps\")",
"The PostScript backend does not support transparency; partially transparent artists will be rendered opaque.\n"
],
[
"pivoted_breakdown.reset_index().set_index('size')",
"_____no_output_____"
]
]
] | [
"code",
"markdown",
"code",
"markdown",
"code"
] | [
[
"code",
"code",
"code",
"code",
"code",
"code",
"code"
],
[
"markdown"
],
[
"code",
"code"
],
[
"markdown"
],
[
"code",
"code",
"code",
"code",
"code",
"code",
"code",
"code"
]
] |
d047f6e8a653969aa070557b2b5d0eb363c9e9b8 | 9,307 | ipynb | Jupyter Notebook | jupyter-notebooks/02_DataMining_TwitterAPI.ipynb | amarbajric/INDBA-ML | 44c710bef90fc0d12a59d8197f551501d42fa2ef | [
"MIT"
] | null | null | null | jupyter-notebooks/02_DataMining_TwitterAPI.ipynb | amarbajric/INDBA-ML | 44c710bef90fc0d12a59d8197f551501d42fa2ef | [
"MIT"
] | null | null | null | jupyter-notebooks/02_DataMining_TwitterAPI.ipynb | amarbajric/INDBA-ML | 44c710bef90fc0d12a59d8197f551501d42fa2ef | [
"MIT"
] | null | null | null | 30.615132 | 218 | 0.572365 | [
[
[
"# DataMining TwitterAPI\n\nRequirements:\n- TwitterAccount\n- TwitterApp credentials\n\n## Imports\nThe following imports are requiered to mine data from Twitter",
"_____no_output_____"
]
],
[
[
"# http://tweepy.readthedocs.io/en/v3.5.0/index.html\nimport tweepy\n# https://api.mongodb.com/python/current/\nimport pymongo\nimport json\nimport sys",
"_____no_output_____"
]
],
[
[
"## Access and Test the TwitterAPI\nInsert your `CONSUMER_KEY`, `CONSUMER_SECRET`, `ACCESS_TOKEN` and `ACCESS_TOKEN_SECRET` and run the code snippet to test if access is granted. If everything works well 'tweepy...' will be posted to your timeline.",
"_____no_output_____"
]
],
[
[
"# Set the received credentials for your recently created TwitterAPI\nCONSUMER_KEY = 'MmiELrtF7fSp3vptCID8jKril'\nCONSUMER_SECRET = 'HqtMRk4jpt30uwDOLz30jHqZm6TPN6rj3oHFaL6xFxw2k0GkDC'\nACCESS_TOKEN = '116725830-rkT63AILxR4fpf4kUXd8xJoOcHTsGkKUOKSMpMJQ'\nACCESS_TOKEN_SECRET = 'eKzxfku4GdYu1wWcMr5iusTmhFT35cDWezMU2Olr5UD4i'\n\n# auth with your provided \nauth = tweepy.OAuthHandler(CONSUMER_KEY, CONSUMER_SECRET)\nauth.set_access_token(ACCESS_TOKEN, ACCESS_TOKEN_SECRET)\n\n# Create an instance for the TwitterApi\ntwitter = tweepy.API(auth)\nstatus = twitter.update_status('tweepy ...')\nprint(json.dumps(status._json, indent=1))",
"_____no_output_____"
]
],
[
[
"## mongoDB\nTo gain access to the mongoDB the library `pymongo` is used.\n\nIn the first step the mongoDB URL is defined.",
"_____no_output_____"
]
],
[
[
"MONGO_URL = 'mongodb://twitter-mongodb:27017/'",
"_____no_output_____"
]
],
[
[
"Next, two functions are defined to save and load data from mongoDB.",
"_____no_output_____"
]
],
[
[
"def save_to_mongo(data, mongo_db, mongo_db_coll):\n # Connects to the MongoDB server running on\n client = pymongo.MongoClient(MONGO_URL)\n # Get a reference to a particular database\n db = client[mongo_db]\n # Reference a particular collection in the database\n coll = db[mongo_db_coll]\n # Perform a bulk insert and return the IDs\n return coll.insert_one(data)\n\ndef load_from_mongo(mongo_db, mongo_db_coll, return_cursor=False, criteria=None, projection=None):\n # Optionally, use criteria and projection to limit the data that is\n # returned - http://docs.mongodb.org/manual/reference/method/db.collection.find/\n \n # Connects to the MongoDB server running on\n client = pymongo.MongoClient(MONGO_URL)\n # Reference a particular collection in the database\n db = client[mongo_db]\n # Perform a bulk insert and return the IDs\n coll = db[mongo_db_coll]\n if criteria is None:\n criteria = {}\n if projection is None:\n cursor = coll.find(criteria)\n else:\n cursor = coll.find(criteria, projection)\n \n # Returning a cursor is recommended for large amounts of data\n if return_cursor:\n return cursor\n else:\n return [ item for item in cursor ]",
"_____no_output_____"
]
],
[
[
"## Stream tweets to mongoDB\nNow we want to stream tweets to a current trend to the mongoDB.\n\nTherefore we ask the TwitterAPI for current Trends within different places. Places are defined with WOEID https://www.flickr.com/places/info/1",
"_____no_output_____"
]
],
[
[
"# WORLD\nprint('trends WORLD')\ntrends = twitter.trends_place(1)[0]['trends']\nfor t in trends[:5]:\n print(json.dumps(t['name'],indent=1))\n# US\nprint('\\ntrends US')\ntrends = twitter.trends_place(23424977)[0]['trends']\nfor t in trends[:5]:\n print(json.dumps(t['name'],indent=1))\n# AT\nprint('\\ntrends AUSTRIA')\ntrends = twitter.trends_place(23424750)[0]['trends']\nfor t in trends[:5]:\n print(json.dumps(t['name'],indent=1))",
"_____no_output_____"
]
],
[
[
"### StreamListener\ntweepy provides a StreamListener that allows to stream live tweets. All streamed tweets are stored to the mongoDB.",
"_____no_output_____"
]
],
[
[
"MONGO_DB = 'trends'\nMONGO_COLL = 'tweets'\n\nTREND = '#BestBoyBand'\n\nclass CustomStreamListener(tweepy.StreamListener):\n def __init__(self, twitter):\n self.twitter = twitter\n super(tweepy.StreamListener, self).__init__()\n self.db = pymongo.MongoClient(MONGO_URL)[MONGO_DB]\n self.number = 1\n print('Streaming tweets to mongo ...')\n\n def on_data(self, tweet):\n self.number += 1\n self.db[MONGO_COLL].insert_one(json.loads(tweet))\n if self.number % 100 == 0 : print('{} tweets added'.format(self.number))\n\n def on_error(self, status_code):\n return True # Don't kill the stream\n\n def on_timeout(self):\n return True # Don't kill the stream\n\nsapi = tweepy.streaming.Stream(auth, CustomStreamListener(twitter))\nsapi.filter(track=[TREND])",
"_____no_output_____"
]
],
[
[
"## Collect tweets from a specific user\nIn this use-case we mine data from a specific user.",
"_____no_output_____"
]
],
[
[
"MONGO_DB = 'trump'\nMONGO_COLL = 'tweets'\n\nTWITTER_USER = '@realDonaldTrump'\n\ndef get_all_tweets(screen_name):\n #initialize a list to hold all the tweepy Tweets\n alltweets = [] \n #make initial request for most recent tweets (200 is the maximum allowed count)\n new_tweets = twitter.user_timeline(screen_name = screen_name,count=200)\n #save most recent tweets\n alltweets.extend(new_tweets)\n #save the id of the oldest tweet less one\n oldest = alltweets[-1].id - 1\n #keep grabbing tweets until there are no tweets left to grab\n while len(new_tweets) > 0:\n #all subsiquent requests use the max_id param to prevent duplicates\n new_tweets = twitter.user_timeline(screen_name = screen_name,count=200,max_id=oldest)\n #save most recent tweets\n alltweets.extend(new_tweets)\n #update the id of the oldest tweet less one\n oldest = alltweets[-1].id - 1\n print(\"...{} tweets downloaded so far\".format(len(alltweets)))\n \n #write tweet objects to JSON \n print(\"Writing tweet objects to MongoDB please wait...\")\n number = 1\n for status in alltweets:\n print(save_to_mongo(status._json, MONGO_DB, MONGO_COLL))\n number += 1\n print(\"Done - {} tweets saved!\".format(number))\n\n#pass in the username of the account you want to download\nget_all_tweets(TWITTER_USER)",
"_____no_output_____"
]
],
[
[
"## Load tweets from mongo",
"_____no_output_____"
]
],
[
[
"data = load_from_mongo('trends', 'tweets')\nfor d in data[:5]:\n print(d['text'])",
"_____no_output_____"
]
]
] | [
"markdown",
"code",
"markdown",
"code",
"markdown",
"code",
"markdown",
"code",
"markdown",
"code",
"markdown",
"code",
"markdown",
"code",
"markdown",
"code"
] | [
[
"markdown"
],
[
"code"
],
[
"markdown"
],
[
"code"
],
[
"markdown"
],
[
"code"
],
[
"markdown"
],
[
"code"
],
[
"markdown"
],
[
"code"
],
[
"markdown"
],
[
"code"
],
[
"markdown"
],
[
"code"
],
[
"markdown"
],
[
"code"
]
] |
d047fa1ded80b379d4daae293746c5561d6e20d5 | 70,199 | ipynb | Jupyter Notebook | 6. Advanced Visualisation Tools/Graphs from the presentation.ipynb | nickelnine37/VisualAnalyticsWithPython | e53acac7799e4f08a3f748f4fa059801669aa07e | [
"MIT"
] | 1 | 2020-11-12T00:00:35.000Z | 2020-11-12T00:00:35.000Z | 6. Advanced Visualisation Tools/Graphs from the presentation.ipynb | nickelnine37/VisualAnalyticsWithPython | e53acac7799e4f08a3f748f4fa059801669aa07e | [
"MIT"
] | null | null | null | 6. Advanced Visualisation Tools/Graphs from the presentation.ipynb | nickelnine37/VisualAnalyticsWithPython | e53acac7799e4f08a3f748f4fa059801669aa07e | [
"MIT"
] | 1 | 2020-11-24T06:45:29.000Z | 2020-11-24T06:45:29.000Z | 61.686292 | 26,489 | 0.663186 | [
[
[
"# Graphs from the presentation\n\n",
"_____no_output_____"
]
],
[
[
"import matplotlib.pyplot as plt\n%matplotlib notebook",
"_____no_output_____"
],
[
"# create a new figure\nplt.figure()\n\n# create x and y coordinates via lists\nx = [99, 19, 88, 12, 95, 47, 81, 64, 83, 76]\ny = [43, 18, 11, 4, 78, 47, 77, 70, 21, 24]\n\n# scatter the points onto the figure\nplt.scatter(x, y)",
"_____no_output_____"
],
[
"# create a new figure \nplt.figure()\n\n# create x and y values via lists\nx = [1, 2, 3, 4, 5, 6, 7, 8]\ny = [1, 4, 9, 16, 25, 36, 49, 64]\n\n# plot the line\nplt.plot(x, y)",
"_____no_output_____"
],
[
"# create a new figure\nplt.figure()\n\n# create a list of observations\nobservations = [5.24, 3.82, 3.73, 5.3 , 3.93, 5.32, 6.43, 4.4 , 5.79, 4.05, 5.34, 5.62, 6.02, 6.08, 6.39, 5.03, 5.34, 4.98, 3.84, 4.91, 6.62, 4.66, 5.06, 2.37, 5. , 3.7 , 5.22, 5.86, 3.88, 4.68, 4.88, 5.01, 3.09, 5.38, 4.78, 6.26, 6.29, 5.77, 4.33, 5.96, 4.74, 4.54, 7.99, 5. , 4.85, 5.68, 3.73, 4.42, 4.99, 4.47, 6.06, 5.88, 4.56, 5.37, 6.39, 4.15]\n\n# create a histogram with 15 intervals\nplt.hist(observations, bins=15)",
"_____no_output_____"
],
[
"# create a new figure \nplt.figure()\n\n# plot a red line with a transparancy of 40%. Label this 'line 1'\nplt.plot(x, y, color='red', alpha=0.4, label='line 1')\n\n# make a key appear on the plot\nplt.legend()",
"_____no_output_____"
],
[
"# import pandas\nimport pandas as pd\n\n# read in data from a csv\ndata = pd.read_csv('data/weather.csv', parse_dates=['Date'])\n\n# create a new matplotlib figure\nplt.figure()\n\n# plot the temperature over time\nplt.plot(data['Date'], data['Temp (C)'])\n\n# add a ylabel \nplt.ylabel('Temperature (C)')",
"_____no_output_____"
],
[
"plt.figure()\n\n# create inputs\nx = ['UK', 'France', 'Germany', 'Spain', 'Italy']\ny = [67.5, 65.1, 83.5, 46.7, 60.6]\n\n# plot the chart\nplt.bar(x, y)\nplt.ylabel('Population (M)')",
"_____no_output_____"
],
[
"plt.figure()\n\n# create inputs\nx = ['UK', 'France', 'Germany', 'Spain', 'Italy']\ny = [67.5, 65.1, 83.5, 46.7, 60.6]\n\n# create a list of colours\ncolour = ['red', 'green', 'blue', 'orange', 'purple']\n\n# plot the chart with the colors and transparancy\nplt.bar(x, y, color=colour, alpha=0.5)\nplt.ylabel('Population (M)')",
"_____no_output_____"
],
[
"plt.figure()\n\nx = [1, 2, 3, 4, 5, 6, 7, 8, 9]\ny1 = [2, 4, 6, 8, 10, 12, 14, 16, 18]\ny2 = [4, 8, 12, 16, 20, 24, 28, 32, 36]\n\nplt.scatter(x, y1, color='cyan', s=5)\nplt.scatter(x, y2, color='violet', s=15)",
"_____no_output_____"
],
[
"plt.figure()\n\nx = [1, 2, 3, 4, 5, 6, 7, 8, 9]\ny1 = [2, 4, 6, 8, 10, 12, 14, 16, 18]\ny2 = [4, 8, 12, 16, 20, 24, 28, 32, 36]\nsize1 = [10, 20, 30, 40, 50, 60, 70, 80, 90]\nsize2 = [90, 80, 70, 60, 50, 40, 30, 20, 10]\n\nplt.scatter(x, y1, color='cyan', s=size1)\nplt.scatter(x, y2, color='violet', s=size2)",
"_____no_output_____"
],
[
"co2_file = '../5. Examples of Visual Analytics in Python/data/national/co2_emissions_tonnes_per_person.csv'\ngdp_file = '../5. Examples of Visual Analytics in Python/data/national/gdppercapita_us_inflation_adjusted.csv'\npop_file = '../5. Examples of Visual Analytics in Python/data/national/population.csv'\n\nco2_per_cap = pd.read_csv(co2_file, index_col=0, parse_dates=True)\ngdp_per_cap = pd.read_csv(gdp_file, index_col=0, parse_dates=True)\npopulation = pd.read_csv(pop_file, index_col=0, parse_dates=True)",
"_____no_output_____"
],
[
"plt.figure()\n\nx = gdp_per_cap.loc['2017'] # gdp in 2017\ny = co2_per_cap.loc['2017'] # co2 emmissions in 2017\n\n# population in 2017 will give size of points (divide pop by 1M)\nsize = population.loc['2017'] / 1e6\n\n# scatter points with vector size and some transparancy\nplt.scatter(x, y, s=size, alpha=0.5)\n\n# set a log-scale\nplt.xscale('log')\nplt.yscale('log')\n\nplt.xlabel('GDP per capita, $US')\nplt.ylabel('CO2 emissions per person per year, tonnes')",
"_____no_output_____"
],
[
"plt.figure()\n\n# create grid of numbers\ngrid = [[1, 2, 3], \n [4, 5, 6], \n [7, 8, 9]]\n\n# plot the grid with 'autumn' color map\nplt.imshow(grid, cmap='autumn')\n\n# add a colour key\nplt.colorbar()",
"_____no_output_____"
],
[
"import pandas as pd\n\ndata = pd.read_csv(\"../5. Examples of Visual Analytics in Python/data/stocks/FTSE_stock_prices.csv\", index_col=0)\ncorrelation_matrix = data.pct_change().corr()",
"_____no_output_____"
],
[
"# create a new figure\nplt.figure()\n\n# imshow the grid of correlation\nplt.imshow(correlation_matrix, cmap='terrain')\n\n# add a color bar \nplt.colorbar()\n\n# remove cluttering x and y ticks\nplt.xticks([])\nplt.yticks([])",
"_____no_output_____"
],
[
"elevation = pd.read_csv('data/UK_elevation.csv', index_col=0)",
"_____no_output_____"
],
[
"# create figure\nplt.figure()\n\n# imshow data\nplt.imshow(elevation, # grid data\n vmin=-50, # minimum for colour bar\n vmax=500, # maximum for colour bar\n cmap='terrain', # terrain style colour map\n extent=[-11, 3, 50, 60]) # [x1, x2, y1, y2] plot boundaries\n\n# add axis labels and a title\nplt.xlabel('Longitude')\nplt.ylabel('Latitude')\nplt.title('UK Elevation Profile')\n\n# add a colourbar\nplt.colorbar()",
"_____no_output_____"
]
]
] | [
"markdown",
"code"
] | [
[
"markdown"
],
[
"code",
"code",
"code",
"code",
"code",
"code",
"code",
"code",
"code",
"code",
"code",
"code",
"code",
"code",
"code",
"code",
"code"
]
] |
d047fb8956f61b21a4101a14308a42a4be12b4fb | 5,598 | ipynb | Jupyter Notebook | 28Octubre.ipynb | VxctxrTL/daa_2021_1 | 7cff030975ce3fc9fe815a6be0ab58cbd7090d46 | [
"MIT"
] | null | null | null | 28Octubre.ipynb | VxctxrTL/daa_2021_1 | 7cff030975ce3fc9fe815a6be0ab58cbd7090d46 | [
"MIT"
] | null | null | null | 28Octubre.ipynb | VxctxrTL/daa_2021_1 | 7cff030975ce3fc9fe815a6be0ab58cbd7090d46 | [
"MIT"
] | null | null | null | 27.712871 | 227 | 0.385316 | [
[
[
"<a href=\"https://colab.research.google.com/github/VxctxrTL/daa_2021_1/blob/master/28Octubre.ipynb\" target=\"_parent\"><img src=\"https://colab.research.google.com/assets/colab-badge.svg\" alt=\"Open In Colab\"/></a>",
"_____no_output_____"
],
[
"### SOLUCION 1",
"_____no_output_____"
]
],
[
[
"h1 = 0\nh2 = 0\nm1 = 0\nm2 = 0 # 1440 + 24 *6\ncontador = 0 # 5 + (1440 + ?) * 2 + 144 + 24 + 2= 3057\n\nwhile [h1, h2, m1, m2] != [2,3,5,9]:\n if [h1, h2] == [m2, m1]:\n \n print(h1, h2,\":\", m1, m2) \n m2 = m2 + 1\n\n if m2 == 10:\n m2 = 0\n m1 = m1 + 1\n\n if m1 == 6:\n h2 = h2 + 1\n m2 = 0\n contador = contador + 1\n\n m2 = m2 + 1\n if m2 == 10:\n m2 = 0\n m1 = m1 + 1\n if m1 == 6:\n m1 = 0\n h2 = h2 +1\n if h2 == 10:\n h2 = 0\n h1 = h1 +1\nprint(\"Numero de palindromos: \",contador)",
"_____no_output_____"
]
],
[
[
"### Solucion 2 ",
"_____no_output_____"
]
],
[
[
"horario=\"0000\"\ncontador=0\nwhile horario!=\"2359\":\n inv=horario[::-1]\n if horario==inv:\n contador+=1\n print(horario[0:2],\":\",horario[2:4])\n new=int(horario)\n new+=1\n horario=str(new).zfill(4)\nprint(\"son \",contador,\"palindromos\")\n# 2 + (2360 * 4 ) + 24",
"_____no_output_____"
]
],
[
[
"### Solucion 3 ",
"_____no_output_____"
]
],
[
[
"lista=[]\nfor i in range(0,24,1): # 24\n for j in range(0,60,1): # 60 1440\n if i<10:\n if j<10:\n lista.append(\"0\"+str(i)+\":\"+\"0\"+str(j))\n elif j>=10:\n lista.append(\"0\"+str(i)+\":\"+str(j))\n else:\n if i>=10:\n if j<10:\n lista.append(str(i)+\":\"+\"0\"+str(j))\n elif j>=10:\n lista.append(str(i)+\":\"+str(j))\n# 1440 + 2 + 1440 + 16 * 2 = 2900\nlista2=[]\ncontador=0\nfor i in range(len(lista)): # 1440\n x=lista[i][::-1]\n if x==lista[i]:\n lista2.append(x)\n contador=contador+1\nprint(contador)\nfor j in (lista2):\n print(j)",
"_____no_output_____"
],
[
"for x in range (0,24,1):\n for y in range(0,60,1): #1440 * 3 +13 = 4333\n hora=str(x)+\":\"+str(y)\n if x<10:\n hora=\"0\"+str(x)+\":\"+str(y)\n if y<10:\n hora=str(x)+\"0\"+\":\"+str(y)\n\n p=hora[::-1]\n if p==hora:\n print(f\"{hora} es palindromo\")",
"_____no_output_____"
],
[
"total = int(0) #Contador de numero de palindromos\nfor hor in range(0,24): #Bucles anidados for para dar aumentar las horas y los minutos al mismo tiempo\n for min in range(0,60): \n\n hor_n = str(hor) #Variables\n min_n = str(min)\n\n if (hor<10): #USamos condiciones para que las horas y los minutos no rebasen el horario\n hor_n = (\"0\"+hor_n)\n\n if (min<10):\n min_n = (\"0\"+ min_n)\n\n if (hor_n[::-1] == min_n): #Mediante un slicing le damos el formato a las horas para que este empiece desde la derecha\n print(\"{}:{}\".format(hor_n,min_n))\n total += 1\n#1 + 1440 * 5 =7201",
"_____no_output_____"
]
]
] | [
"markdown",
"code",
"markdown",
"code",
"markdown",
"code"
] | [
[
"markdown",
"markdown"
],
[
"code"
],
[
"markdown"
],
[
"code"
],
[
"markdown"
],
[
"code",
"code",
"code"
]
] |
d048182147890c0b2e46dd2ffad9170a7fc10bf0 | 26,306 | ipynb | Jupyter Notebook | Chapter 4 - Linear Regression/Using Wide & Deep models.ipynb | Young-Picasso/TensorFlow2.x_Cookbook | 8a2126108f576992b89c1087e6de399a4d54437b | [
"MIT"
] | null | null | null | Chapter 4 - Linear Regression/Using Wide & Deep models.ipynb | Young-Picasso/TensorFlow2.x_Cookbook | 8a2126108f576992b89c1087e6de399a4d54437b | [
"MIT"
] | null | null | null | Chapter 4 - Linear Regression/Using Wide & Deep models.ipynb | Young-Picasso/TensorFlow2.x_Cookbook | 8a2126108f576992b89c1087e6de399a4d54437b | [
"MIT"
] | null | null | null | 55.033473 | 585 | 0.657226 | [
[
[
"## Getting ready",
"_____no_output_____"
]
],
[
[
"import tensorflow as tf\nimport tensorflow.keras as keras\nimport pandas as pd\nimport numpy as np",
"_____no_output_____"
],
[
"census_dir = 'https://archive.ics.uci.edu/ml/machine-learning-databases/adult/'\ntrain_path = tf.keras.utils.get_file('adult.data', census_dir + 'adult.data')\ntest_path = tf.keras.utils.get_file('adult.test', census_dir + 'adult.test')",
"_____no_output_____"
],
[
"columns = ['age', 'workclass', 'fnlwgt', 'education', 'education_num', 'marital_status', 'occupation',\n 'relationship', 'race', 'gender', 'capital_gain', 'capital_loss', 'hours_per_week', 'native_country',\n 'income_bracket']\n\ntrain_data = pd.read_csv(train_path, header=None, names=columns)\ntest_data = pd.read_csv(test_path, header=None, names=columns, skiprows=1)",
"_____no_output_____"
]
],
[
[
"## How to do it",
"_____no_output_____"
]
],
[
[
"predictors = ['age', 'workclass', 'education', 'education_num', 'marital_status', 'occupation', 'relationship',\n 'gender']\n\ny_train = (train_data.income_bracket==' >50K').astype(int)\ny_test = (test_data.income_bracket==' >50K').astype(int)\n\ntrain_data = train_data[predictors]\ntest_data = test_data[predictors]",
"_____no_output_____"
],
[
"train_data[['age', 'education_num']] = train_data[['age', 'education_num']].fillna(train_data[['age', 'education_num']]).mean()\ntest_data[['age', 'education_num']] = test_data[['age', 'education_num']].fillna(test_data[['age', 'education_num']]).mean()",
"_____no_output_____"
],
[
"def define_feature_columns(data_df, numeric_cols, categorical_cols, categorical_embeds, dimension=30):\n numeric_columns = []\n categorical_columns = []\n embeddings = []\n \n for feature_name in numeric_cols:\n numeric_columns.append(tf.feature_column.numeric_column(feature_name, dtype=tf.float32))\n \n for feature_name in categorical_cols:\n vocabulary = data_df[feature_name].unique()\n categorical_columns.append(tf.feature_column.categorical_column_with_vocabulary_list(feature_name, vocabulary))\n \n for feature_name in categorical_embeds:\n vocabulary = data_df[feature_name].unique()\n to_categorical = tf.feature_column.categorical_column_with_vocabulary_list(feature_name, vocabulary)\n embeddings.append(tf.feature_column.embedding_column(to_categorical, dimension=dimension))\n \n return numeric_columns, categorical_columns, embeddings",
"_____no_output_____"
],
[
"def create_interactions(interactions_list, buckets=10):\n feature_columns = []\n \n for (a, b) in interactions_list:\n crossed_feature = tf.feature_column.crossed_column([a, b], hash_bucket_size=buckets)\n crossed_feature_one_hot = tf.feature_column.indicator_column(crossed_feature)\n feature_columns.append(crossed_feature_one_hot)\n \n return feature_columns",
"_____no_output_____"
],
[
"numeric_columns, categorical_columns, embeddings = define_feature_columns(train_data, \n numeric_cols=['age', 'education_num'],\n categorical_cols=['gender'],\n categorical_embeds=['workclass', 'education',\n 'marital_status', 'occupation',\n 'relationship'],\n dimension=32\n )\ninteractions = create_interactions([['education', 'occupation']], buckets=10)",
"_____no_output_____"
],
[
"estimator = tf.estimator.DNNLinearCombinedClassifier(\n# wide settings\nlinear_feature_columns=numeric_columns+categorical_columns+interactions,\nlinear_optimizer=keras.optimizers.Ftrl(learning_rate=0.0002),\n# deep settings\ndnn_feature_columns=embeddings,\ndnn_hidden_units=[1024, 256, 128, 64],\ndnn_optimizer=keras.optimizers.Adam(learning_rate=0.0001))",
"INFO:tensorflow:Using default config.\nWARNING:tensorflow:Using temporary folder as model directory: /tmp/tmpxnk3fqlo\nINFO:tensorflow:Using config: {'_model_dir': '/tmp/tmpxnk3fqlo', '_tf_random_seed': None, '_save_summary_steps': 100, '_save_checkpoints_steps': None, '_save_checkpoints_secs': 600, '_session_config': allow_soft_placement: true\ngraph_options {\n rewrite_options {\n meta_optimizer_iterations: ONE\n }\n}\n, '_keep_checkpoint_max': 5, '_keep_checkpoint_every_n_hours': 10000, '_log_step_count_steps': 100, '_train_distribute': None, '_device_fn': None, '_protocol': None, '_eval_distribute': None, '_experimental_distribute': None, '_experimental_max_worker_delay_secs': None, '_session_creation_timeout_secs': 7200, '_checkpoint_save_graph_def': True, '_service': None, '_cluster_spec': ClusterSpec({}), '_task_type': 'worker', '_task_id': 0, '_global_id_in_cluster': 0, '_master': '', '_evaluation_master': '', '_is_chief': True, '_num_ps_replicas': 0, '_num_worker_replicas': 1}\n"
],
[
"def make_input_fn(data_df, label_df, num_epochs=10, shuffle=True, batch_size=256):\n \n def input_function():\n ds = tf.data.Dataset.from_tensor_slices((dict(data_df), label_df))\n if shuffle:\n ds = ds.shuffle(1000)\n ds = ds.batch(batch_size).repeat(num_epochs)\n return ds\n return input_function",
"_____no_output_____"
],
[
"train_input_fn = make_input_fn(train_data, y_train, num_epochs=100, batch_size=256)\ntest_input_fn = make_input_fn(test_data, y_test, num_epochs=1, shuffle=False)\nestimator.train(input_fn=train_input_fn, steps=1500)\nresults = estimator.evaluate(input_fn=test_input_fn)\nprint(results)",
"WARNING:tensorflow:From /home/wil/tensorflow/venv/lib/python3.8/site-packages/tensorflow/python/training/training_util.py:235: Variable.initialized_value (from tensorflow.python.ops.variables) is deprecated and will be removed in a future version.\nInstructions for updating:\nUse Variable.read_value. Variables in 2.X are initialized automatically both in eager and graph (inside tf.defun) contexts.\nINFO:tensorflow:Calling model_fn.\n"
],
[
"def predict_proba(predictor):\n preds = list()\n for pred in predictor:\n preds.append(pred['probabilities'])\n return np.array(preds)\n\npredictions = predict_proba(estimator.predict(input_fn=test_input_fn))\nprint(predictions)",
"INFO:tensorflow:Calling model_fn.\nINFO:tensorflow:Done calling model_fn.\nINFO:tensorflow:Graph was finalized.\nINFO:tensorflow:Restoring parameters from /tmp/tmpxnk3fqlo/model.ckpt-1500\nINFO:tensorflow:Running local_init_op.\nINFO:tensorflow:Done running local_init_op.\n"
]
]
] | [
"markdown",
"code",
"markdown",
"code"
] | [
[
"markdown"
],
[
"code",
"code",
"code"
],
[
"markdown"
],
[
"code",
"code",
"code",
"code",
"code",
"code",
"code",
"code",
"code"
]
] |
d04818ad2d886ba7c4995763f62c1b7ea2fe3b79 | 36,368 | ipynb | Jupyter Notebook | how-to-use-azureml/automated-machine-learning/forecasting-forecast-function/auto-ml-forecasting-function.ipynb | hyoshioka0128/MachineLearningNotebooks | 053efde8c9740c7b691c4d13ee1f5b5b206cd24f | [
"MIT"
] | null | null | null | how-to-use-azureml/automated-machine-learning/forecasting-forecast-function/auto-ml-forecasting-function.ipynb | hyoshioka0128/MachineLearningNotebooks | 053efde8c9740c7b691c4d13ee1f5b5b206cd24f | [
"MIT"
] | null | null | null | how-to-use-azureml/automated-machine-learning/forecasting-forecast-function/auto-ml-forecasting-function.ipynb | hyoshioka0128/MachineLearningNotebooks | 053efde8c9740c7b691c4d13ee1f5b5b206cd24f | [
"MIT"
] | null | null | null | 42.535673 | 540 | 0.558953 | [
[
[
"# Automated Machine Learning\n\n#### Forecasting away from training data\n\n\n## Contents\n1. [Introduction](#Introduction)\n2. [Setup](#Setup)\n3. [Data](#Data)\n4. [Prepare remote compute and data.](#prepare_remote)\n4. [Create the configuration and train a forecaster](#train)\n5. [Forecasting from the trained model](#forecasting)\n6. [Forecasting away from training data](#forecasting_away)",
"_____no_output_____"
],
[
"## Introduction\nThis notebook demonstrates the full interface to the `forecast()` function. \n\nThe best known and most frequent usage of `forecast` enables forecasting on test sets that immediately follows training data. \n\nHowever, in many use cases it is necessary to continue using the model for some time before retraining it. This happens especially in **high frequency forecasting** when forecasts need to be made more frequently than the model can be retrained. Examples are in Internet of Things and predictive cloud resource scaling.\n\nHere we show how to use the `forecast()` function when a time gap exists between training data and prediction period.\n\nTerminology:\n* forecast origin: the last period when the target value is known\n* forecast periods(s): the period(s) for which the value of the target is desired.\n* lookback: how many past periods (before forecast origin) the model function depends on. The larger of number of lags and length of rolling window.\n* prediction context: `lookback` periods immediately preceding the forecast origin\n\n",
"_____no_output_____"
],
[
"## Setup",
"_____no_output_____"
],
[
"Please make sure you have followed the `configuration.ipynb` notebook so that your ML workspace information is saved in the config file.",
"_____no_output_____"
]
],
[
[
"import os\nimport pandas as pd\nimport numpy as np\nimport logging\nimport warnings\n\nimport azureml.core\nfrom azureml.core.dataset import Dataset\nfrom pandas.tseries.frequencies import to_offset\nfrom azureml.core.compute import AmlCompute\nfrom azureml.core.compute import ComputeTarget\nfrom azureml.core.runconfig import RunConfiguration\nfrom azureml.core.conda_dependencies import CondaDependencies\n\n# Squash warning messages for cleaner output in the notebook\nwarnings.showwarning = lambda *args, **kwargs: None\n\nnp.set_printoptions(precision=4, suppress=True, linewidth=120)",
"_____no_output_____"
]
],
[
[
"This sample notebook may use features that are not available in previous versions of the Azure ML SDK.",
"_____no_output_____"
]
],
[
[
"print(\"This notebook was created using version 1.8.0 of the Azure ML SDK\")\nprint(\"You are currently using version\", azureml.core.VERSION, \"of the Azure ML SDK\")",
"_____no_output_____"
],
[
"from azureml.core.workspace import Workspace\nfrom azureml.core.experiment import Experiment\nfrom azureml.train.automl import AutoMLConfig\n\nws = Workspace.from_config()\n\n# choose a name for the run history container in the workspace\nexperiment_name = 'automl-forecast-function-demo'\n\nexperiment = Experiment(ws, experiment_name)\n\noutput = {}\noutput['Subscription ID'] = ws.subscription_id\noutput['Workspace'] = ws.name\noutput['SKU'] = ws.sku\noutput['Resource Group'] = ws.resource_group\noutput['Location'] = ws.location\noutput['Run History Name'] = experiment_name\npd.set_option('display.max_colwidth', -1)\noutputDf = pd.DataFrame(data = output, index = [''])\noutputDf.T",
"_____no_output_____"
]
],
[
[
"## Data\nFor the demonstration purposes we will generate the data artificially and use them for the forecasting.",
"_____no_output_____"
]
],
[
[
"TIME_COLUMN_NAME = 'date'\nGRAIN_COLUMN_NAME = 'grain'\nTARGET_COLUMN_NAME = 'y'\n\ndef get_timeseries(train_len: int,\n test_len: int,\n time_column_name: str,\n target_column_name: str,\n grain_column_name: str,\n grains: int = 1,\n freq: str = 'H'):\n \"\"\"\n Return the time series of designed length.\n\n :param train_len: The length of training data (one series).\n :type train_len: int\n :param test_len: The length of testing data (one series).\n :type test_len: int\n :param time_column_name: The desired name of a time column.\n :type time_column_name: str\n :param\n :param grains: The number of grains.\n :type grains: int\n :param freq: The frequency string representing pandas offset.\n see https://pandas.pydata.org/pandas-docs/stable/user_guide/timeseries.html\n :type freq: str\n :returns: the tuple of train and test data sets.\n :rtype: tuple\n\n \"\"\"\n data_train = [] # type: List[pd.DataFrame]\n data_test = [] # type: List[pd.DataFrame]\n data_length = train_len + test_len\n for i in range(grains):\n X = pd.DataFrame({\n time_column_name: pd.date_range(start='2000-01-01',\n periods=data_length,\n freq=freq),\n target_column_name: np.arange(data_length).astype(float) + np.random.rand(data_length) + i*5,\n 'ext_predictor': np.asarray(range(42, 42 + data_length)),\n grain_column_name: np.repeat('g{}'.format(i), data_length)\n })\n data_train.append(X[:train_len])\n data_test.append(X[train_len:])\n X_train = pd.concat(data_train)\n y_train = X_train.pop(target_column_name).values\n X_test = pd.concat(data_test)\n y_test = X_test.pop(target_column_name).values\n return X_train, y_train, X_test, y_test\n\nn_test_periods = 6\nn_train_periods = 30\nX_train, y_train, X_test, y_test = get_timeseries(train_len=n_train_periods,\n test_len=n_test_periods,\n time_column_name=TIME_COLUMN_NAME,\n target_column_name=TARGET_COLUMN_NAME,\n grain_column_name=GRAIN_COLUMN_NAME,\n grains=2)",
"_____no_output_____"
]
],
[
[
"Let's see what the training data looks like.",
"_____no_output_____"
]
],
[
[
"X_train.tail()",
"_____no_output_____"
],
[
"# plot the example time series\nimport matplotlib.pyplot as plt\nwhole_data = X_train.copy()\ntarget_label = 'y'\nwhole_data[target_label] = y_train\nfor g in whole_data.groupby('grain'): \n plt.plot(g[1]['date'].values, g[1]['y'].values, label=g[0])\nplt.legend()\nplt.show()",
"_____no_output_____"
]
],
[
[
"### Prepare remote compute and data. <a id=\"prepare_remote\"></a>\nThe [Machine Learning service workspace](https://docs.microsoft.com/en-us/azure/machine-learning/service/concept-workspace), is paired with the storage account, which contains the default data store. We will use it to upload the artificial data and create [tabular dataset](https://docs.microsoft.com/en-us/python/api/azureml-core/azureml.data.tabulardataset?view=azure-ml-py) for training. A tabular dataset defines a series of lazily-evaluated, immutable operations to load data from the data source into tabular representation.",
"_____no_output_____"
]
],
[
[
"# We need to save thw artificial data and then upload them to default workspace datastore.\nDATA_PATH = \"fc_fn_data\"\nDATA_PATH_X = \"{}/data_train.csv\".format(DATA_PATH)\nif not os.path.isdir('data'):\n os.mkdir('data')\npd.DataFrame(whole_data).to_csv(\"data/data_train.csv\", index=False)\n# Upload saved data to the default data store.\nds = ws.get_default_datastore()\nds.upload(src_dir='./data', target_path=DATA_PATH, overwrite=True, show_progress=True)\ntrain_data = Dataset.Tabular.from_delimited_files(path=ds.path(DATA_PATH_X))",
"_____no_output_____"
]
],
[
[
"You will need to create a [compute target](https://docs.microsoft.com/en-us/azure/machine-learning/service/how-to-set-up-training-targets#amlcompute) for your AutoML run. In this tutorial, you create AmlCompute as your training compute resource.",
"_____no_output_____"
]
],
[
[
"from azureml.core.compute import ComputeTarget, AmlCompute\nfrom azureml.core.compute_target import ComputeTargetException\n\n# Choose a name for your CPU cluster\namlcompute_cluster_name = \"fcfn-cluster\"\n\n# Verify that cluster does not exist already\ntry:\n compute_target = ComputeTarget(workspace=ws, name=amlcompute_cluster_name)\n print('Found existing cluster, use it.')\nexcept ComputeTargetException:\n compute_config = AmlCompute.provisioning_configuration(vm_size='STANDARD_D2_V2',\n max_nodes=6)\n compute_target = ComputeTarget.create(ws, amlcompute_cluster_name, compute_config)\n\ncompute_target.wait_for_completion(show_output=True)",
"_____no_output_____"
]
],
[
[
"## Create the configuration and train a forecaster <a id=\"train\"></a>\nFirst generate the configuration, in which we:\n* Set metadata columns: target, time column and grain column names.\n* Validate our data using cross validation with rolling window method.\n* Set normalized root mean squared error as a metric to select the best model.\n* Set early termination to True, so the iterations through the models will stop when no improvements in accuracy score will be made.\n* Set limitations on the length of experiment run to 15 minutes.\n* Finally, we set the task to be forecasting.\n* We apply the lag lead operator to the target value i.e. we use the previous values as a predictor for the future ones.",
"_____no_output_____"
]
],
[
[
"lags = [1,2,3]\nmax_horizon = n_test_periods\ntime_series_settings = { \n 'time_column_name': TIME_COLUMN_NAME,\n 'grain_column_names': [ GRAIN_COLUMN_NAME ],\n 'max_horizon': max_horizon,\n 'target_lags': lags\n}",
"_____no_output_____"
]
],
[
[
"Run the model selection and training process.",
"_____no_output_____"
]
],
[
[
"from azureml.core.workspace import Workspace\nfrom azureml.core.experiment import Experiment\nfrom azureml.train.automl import AutoMLConfig\n\n\nautoml_config = AutoMLConfig(task='forecasting',\n debug_log='automl_forecasting_function.log',\n primary_metric='normalized_root_mean_squared_error',\n experiment_timeout_hours=0.25,\n enable_early_stopping=True,\n training_data=train_data,\n compute_target=compute_target,\n n_cross_validations=3,\n verbosity = logging.INFO,\n max_concurrent_iterations=4,\n max_cores_per_iteration=-1,\n label_column_name=target_label,\n **time_series_settings)\n\nremote_run = experiment.submit(automl_config, show_output=False)",
"_____no_output_____"
],
[
"remote_run.wait_for_completion()",
"_____no_output_____"
],
[
"# Retrieve the best model to use it further.\n_, fitted_model = remote_run.get_output()",
"_____no_output_____"
]
],
[
[
"## Forecasting from the trained model <a id=\"forecasting\"></a>",
"_____no_output_____"
],
[
"In this section we will review the `forecast` interface for two main scenarios: forecasting right after the training data, and the more complex interface for forecasting when there is a gap (in the time sense) between training and testing data.",
"_____no_output_____"
],
[
"### X_train is directly followed by the X_test\n\nLet's first consider the case when the prediction period immediately follows the training data. This is typical in scenarios where we have the time to retrain the model every time we wish to forecast. Forecasts that are made on daily and slower cadence typically fall into this category. Retraining the model every time benefits the accuracy because the most recent data is often the most informative.\n\n\n\nWe use `X_test` as a **forecast request** to generate the predictions.",
"_____no_output_____"
],
[
"#### Typical path: X_test is known, forecast all upcoming periods",
"_____no_output_____"
]
],
[
[
"# The data set contains hourly data, the training set ends at 01/02/2000 at 05:00\n\n# These are predictions we are asking the model to make (does not contain thet target column y),\n# for 6 periods beginning with 2000-01-02 06:00, which immediately follows the training data\nX_test",
"_____no_output_____"
],
[
"y_pred_no_gap, xy_nogap = fitted_model.forecast(X_test)\n\n# xy_nogap contains the predictions in the _automl_target_col column.\n# Those same numbers are output in y_pred_no_gap\nxy_nogap",
"_____no_output_____"
]
],
[
[
"#### Confidence intervals",
"_____no_output_____"
],
[
"Forecasting model may be used for the prediction of forecasting intervals by running ```forecast_quantiles()```. \nThis method accepts the same parameters as forecast().",
"_____no_output_____"
]
],
[
[
"quantiles = fitted_model.forecast_quantiles(X_test)\nquantiles",
"_____no_output_____"
]
],
[
[
"#### Distribution forecasts\n\nOften the figure of interest is not just the point prediction, but the prediction at some quantile of the distribution. \nThis arises when the forecast is used to control some kind of inventory, for example of grocery items or virtual machines for a cloud service. In such case, the control point is usually something like \"we want the item to be in stock and not run out 99% of the time\". This is called a \"service level\". Here is how you get quantile forecasts.",
"_____no_output_____"
]
],
[
[
"# specify which quantiles you would like \nfitted_model.quantiles = [0.01, 0.5, 0.95]\n# use forecast_quantiles function, not the forecast() one\ny_pred_quantiles = fitted_model.forecast_quantiles(X_test)\n\n# quantile forecasts returned in a Dataframe along with the time and grain columns \ny_pred_quantiles",
"_____no_output_____"
]
],
[
[
"#### Destination-date forecast: \"just do something\"\n\nIn some scenarios, the X_test is not known. The forecast is likely to be weak, because it is missing contemporaneous predictors, which we will need to impute. If you still wish to predict forward under the assumption that the last known values will be carried forward, you can forecast out to \"destination date\". The destination date still needs to fit within the maximum horizon from training.",
"_____no_output_____"
]
],
[
[
"# We will take the destination date as a last date in the test set.\ndest = max(X_test[TIME_COLUMN_NAME])\ny_pred_dest, xy_dest = fitted_model.forecast(forecast_destination=dest)\n\n# This form also shows how we imputed the predictors which were not given. (Not so well! Use with caution!)\nxy_dest",
"_____no_output_____"
]
],
[
[
"## Forecasting away from training data <a id=\"forecasting_away\"></a>\n\nSuppose we trained a model, some time passed, and now we want to apply the model without re-training. If the model \"looks back\" -- uses previous values of the target -- then we somehow need to provide those values to the model.\n\n\n\nThe notion of forecast origin comes into play: the forecast origin is **the last period for which we have seen the target value**. This applies per grain, so each grain can have a different forecast origin. \n\nThe part of data before the forecast origin is the **prediction context**. To provide the context values the model needs when it looks back, we pass definite values in `y_test` (aligned with corresponding times in `X_test`).",
"_____no_output_____"
]
],
[
[
"# generate the same kind of test data we trained on, \n# but now make the train set much longer, so that the test set will be in the future\nX_context, y_context, X_away, y_away = get_timeseries(train_len=42, # train data was 30 steps long\n test_len=4,\n time_column_name=TIME_COLUMN_NAME,\n target_column_name=TARGET_COLUMN_NAME,\n grain_column_name=GRAIN_COLUMN_NAME,\n grains=2)\n\n# end of the data we trained on\nprint(X_train.groupby(GRAIN_COLUMN_NAME)[TIME_COLUMN_NAME].max())\n# start of the data we want to predict on\nprint(X_away.groupby(GRAIN_COLUMN_NAME)[TIME_COLUMN_NAME].min())",
"_____no_output_____"
]
],
[
[
"There is a gap of 12 hours between end of training and beginning of `X_away`. (It looks like 13 because all timestamps point to the start of the one hour periods.) Using only `X_away` will fail without adding context data for the model to consume.",
"_____no_output_____"
]
],
[
[
"try: \n y_pred_away, xy_away = fitted_model.forecast(X_away)\n xy_away\nexcept Exception as e:\n print(e)",
"_____no_output_____"
]
],
[
[
"How should we read that eror message? The forecast origin is at the last time the model saw an actual value of `y` (the target). That was at the end of the training data! The model is attempting to forecast from the end of training data. But the requested forecast periods are past the maximum horizon. We need to provide a define `y` value to establish the forecast origin.\n\nWe will use this helper function to take the required amount of context from the data preceding the testing data. It's definition is intentionally simplified to keep the idea in the clear.",
"_____no_output_____"
]
],
[
[
"def make_forecasting_query(fulldata, time_column_name, target_column_name, forecast_origin, horizon, lookback):\n\n \"\"\"\n This function will take the full dataset, and create the query\n to predict all values of the grain from the `forecast_origin`\n forward for the next `horizon` horizons. Context from previous\n `lookback` periods will be included.\n\n \n\n fulldata: pandas.DataFrame a time series dataset. Needs to contain X and y.\n time_column_name: string which column (must be in fulldata) is the time axis\n target_column_name: string which column (must be in fulldata) is to be forecast\n forecast_origin: datetime type the last time we (pretend to) have target values \n horizon: timedelta how far forward, in time units (not periods)\n lookback: timedelta how far back does the model look?\n\n Example:\n\n\n ```\n\n forecast_origin = pd.to_datetime('2012-09-01') + pd.DateOffset(days=5) # forecast 5 days after end of training\n print(forecast_origin)\n\n X_query, y_query = make_forecasting_query(data, \n forecast_origin = forecast_origin,\n horizon = pd.DateOffset(days=7), # 7 days into the future\n lookback = pd.DateOffset(days=1), # model has lag 1 period (day)\n )\n\n ```\n \"\"\"\n\n X_past = fulldata[ (fulldata[ time_column_name ] > forecast_origin - lookback) &\n (fulldata[ time_column_name ] <= forecast_origin)\n ]\n\n X_future = fulldata[ (fulldata[ time_column_name ] > forecast_origin) &\n (fulldata[ time_column_name ] <= forecast_origin + horizon)\n ]\n\n y_past = X_past.pop(target_column_name).values.astype(np.float)\n y_future = X_future.pop(target_column_name).values.astype(np.float)\n\n # Now take y_future and turn it into question marks\n y_query = y_future.copy().astype(np.float) # because sometimes life hands you an int\n y_query.fill(np.NaN)\n\n\n print(\"X_past is \" + str(X_past.shape) + \" - shaped\")\n print(\"X_future is \" + str(X_future.shape) + \" - shaped\")\n print(\"y_past is \" + str(y_past.shape) + \" - shaped\")\n print(\"y_query is \" + str(y_query.shape) + \" - shaped\")\n\n\n X_pred = pd.concat([X_past, X_future])\n y_pred = np.concatenate([y_past, y_query])\n return X_pred, y_pred",
"_____no_output_____"
]
],
[
[
"Let's see where the context data ends - it ends, by construction, just before the testing data starts.",
"_____no_output_____"
]
],
[
[
"print(X_context.groupby(GRAIN_COLUMN_NAME)[TIME_COLUMN_NAME].agg(['min','max','count']))\nprint(X_away.groupby(GRAIN_COLUMN_NAME)[TIME_COLUMN_NAME].agg(['min','max','count']))\nX_context.tail(5)",
"_____no_output_____"
],
[
"# Since the length of the lookback is 3, \n# we need to add 3 periods from the context to the request\n# so that the model has the data it needs\n\n# Put the X and y back together for a while. \n# They like each other and it makes them happy.\nX_context[TARGET_COLUMN_NAME] = y_context\nX_away[TARGET_COLUMN_NAME] = y_away\nfulldata = pd.concat([X_context, X_away])\n\n# forecast origin is the last point of data, which is one 1-hr period before test\nforecast_origin = X_away[TIME_COLUMN_NAME].min() - pd.DateOffset(hours=1)\n# it is indeed the last point of the context\nassert forecast_origin == X_context[TIME_COLUMN_NAME].max()\nprint(\"Forecast origin: \" + str(forecast_origin))\n \n# the model uses lags and rolling windows to look back in time\nn_lookback_periods = max(lags)\nlookback = pd.DateOffset(hours=n_lookback_periods)\n\nhorizon = pd.DateOffset(hours=max_horizon)\n\n# now make the forecast query from context (refer to figure)\nX_pred, y_pred = make_forecasting_query(fulldata, TIME_COLUMN_NAME, TARGET_COLUMN_NAME,\n forecast_origin, horizon, lookback)\n\n# show the forecast request aligned\nX_show = X_pred.copy()\nX_show[TARGET_COLUMN_NAME] = y_pred\nX_show",
"_____no_output_____"
]
],
[
[
"Note that the forecast origin is at 17:00 for both grains, and periods from 18:00 are to be forecast.",
"_____no_output_____"
]
],
[
[
"# Now everything works\ny_pred_away, xy_away = fitted_model.forecast(X_pred, y_pred)\n\n# show the forecast aligned\nX_show = xy_away.reset_index()\n# without the generated features\nX_show[['date', 'grain', 'ext_predictor', '_automl_target_col']]\n# prediction is in _automl_target_col",
"_____no_output_____"
]
],
[
[
"## Forecasting farther than the maximum horizon <a id=\"recursive forecasting\"></a>\nWhen the forecast destination, or the latest date in the prediction data frame, is farther into the future than the specified maximum horizon, the `forecast()` function will still make point predictions out to the later date using a recursive operation mode. Internally, the method recursively applies the regular forecaster to generate context so that we can forecast further into the future. \n\nTo illustrate the use-case and operation of recursive forecasting, we'll consider an example with a single time-series where the forecasting period directly follows the training period and is twice as long as the maximum horizon given at training time.\n\n\n\nInternally, we apply the forecaster in an iterative manner and finish the forecast task in two interations. In the first iteration, we apply the forecaster and get the prediction for the first max-horizon periods (y_pred1). In the second iteraction, y_pred1 is used as the context to produce the prediction for the next max-horizon periods (y_pred2). The combination of (y_pred1 and y_pred2) gives the results for the total forecast periods. \n\nA caveat: forecast accuracy will likely be worse the farther we predict into the future since errors are compounded with recursive application of the forecaster.\n\n\n",
"_____no_output_____"
]
],
[
[
"# generate the same kind of test data we trained on, but with a single grain/time-series and test period twice as long as the max_horizon\n_, _, X_test_long, y_test_long = get_timeseries(train_len=n_train_periods,\n test_len=max_horizon*2,\n time_column_name=TIME_COLUMN_NAME,\n target_column_name=TARGET_COLUMN_NAME,\n grain_column_name=GRAIN_COLUMN_NAME,\n grains=1)\n\nprint(X_test_long.groupby(GRAIN_COLUMN_NAME)[TIME_COLUMN_NAME].min())\nprint(X_test_long.groupby(GRAIN_COLUMN_NAME)[TIME_COLUMN_NAME].max())",
"_____no_output_____"
],
[
"# forecast() function will invoke the recursive forecast method internally.\ny_pred_long, X_trans_long = fitted_model.forecast(X_test_long)\ny_pred_long",
"_____no_output_____"
],
[
"# What forecast() function does in this case is equivalent to iterating it twice over the test set as the following. \ny_pred1, _ = fitted_model.forecast(X_test_long[:max_horizon])\ny_pred_all, _ = fitted_model.forecast(X_test_long, np.concatenate((y_pred1, np.full(max_horizon, np.nan))))\nnp.array_equal(y_pred_all, y_pred_long)",
"_____no_output_____"
]
],
[
[
"#### Confidence interval and distributional forecasts\nAutoML cannot currently estimate forecast errors beyond the maximum horizon set during training, so the `forecast_quantiles()` function will return missing values for quantiles not equal to 0.5 beyond the maximum horizon. ",
"_____no_output_____"
]
],
[
[
"fitted_model.forecast_quantiles(X_test_long)",
"_____no_output_____"
]
],
[
[
"Similarly with the simple senarios illustrated above, forecasting farther than the max horizon in other senarios like 'multiple grain', 'Destination-date forecast', and 'forecast away from the training data' are also automatically handled by the `forecast()` function. ",
"_____no_output_____"
]
]
] | [
"markdown",
"code",
"markdown",
"code",
"markdown",
"code",
"markdown",
"code",
"markdown",
"code",
"markdown",
"code",
"markdown",
"code",
"markdown",
"code",
"markdown",
"code",
"markdown",
"code",
"markdown",
"code",
"markdown",
"code",
"markdown",
"code",
"markdown",
"code",
"markdown",
"code",
"markdown",
"code",
"markdown",
"code",
"markdown",
"code",
"markdown",
"code",
"markdown"
] | [
[
"markdown",
"markdown",
"markdown",
"markdown"
],
[
"code"
],
[
"markdown"
],
[
"code",
"code"
],
[
"markdown"
],
[
"code"
],
[
"markdown"
],
[
"code",
"code"
],
[
"markdown"
],
[
"code"
],
[
"markdown"
],
[
"code"
],
[
"markdown"
],
[
"code"
],
[
"markdown"
],
[
"code",
"code",
"code"
],
[
"markdown",
"markdown",
"markdown",
"markdown"
],
[
"code",
"code"
],
[
"markdown",
"markdown"
],
[
"code"
],
[
"markdown"
],
[
"code"
],
[
"markdown"
],
[
"code"
],
[
"markdown"
],
[
"code"
],
[
"markdown"
],
[
"code"
],
[
"markdown"
],
[
"code"
],
[
"markdown"
],
[
"code",
"code"
],
[
"markdown"
],
[
"code"
],
[
"markdown"
],
[
"code",
"code",
"code"
],
[
"markdown"
],
[
"code"
],
[
"markdown"
]
] |
d0481b2e4d92b9174b9077877f4727041ddf54f2 | 110,484 | ipynb | Jupyter Notebook | Dodgers Marketing Analysis.ipynb | duskybadger/Dodgers-Data-Wrangling-Project | 33d745f3c9b62ba155879633e20b96b909622caf | [
"MIT"
] | null | null | null | Dodgers Marketing Analysis.ipynb | duskybadger/Dodgers-Data-Wrangling-Project | 33d745f3c9b62ba155879633e20b96b909622caf | [
"MIT"
] | null | null | null | Dodgers Marketing Analysis.ipynb | duskybadger/Dodgers-Data-Wrangling-Project | 33d745f3c9b62ba155879633e20b96b909622caf | [
"MIT"
] | null | null | null | 133.113253 | 45,772 | 0.835062 | [
[
[
"import pandas as pd\nimport seaborn as sns\nimport scipy\nimport matplotlib.pyplot as plt",
"_____no_output_____"
],
[
"df_Dodgers = pd.read_csv('dodgers.csv')",
"_____no_output_____"
],
[
"df_Dodgers.head()",
"_____no_output_____"
],
[
"# Takes binary categories and returns 0 or 1\ndef binning_cats(word, zero='no', one='yes'):\n if word.strip().lower()==zero:\n return(0)\n elif word.strip().lower()==one:\n return(1)",
"_____no_output_____"
],
[
"# These are the variables and their outcomes that need to be converted\nbins = {'skies':['cloudy','clear'],\n 'day_night':['day','night'],\n 'cap':['no','yes'],\n 'shirt':['no','yes'],\n 'fireworks':['no','yes'],\n 'bobblehead':['no','yes']}",
"_____no_output_____"
],
[
"# Here we convert the above columns to binary\nfor column in bins.keys():\n df_Dodgers[column+'_bin']=df_Dodgers[column].apply(binning_cats,args=(bins[column][0],bins[column][1]))",
"_____no_output_____"
],
[
"df_Dodgers.head()",
"_____no_output_____"
],
[
"# Here we check the correlations\ndf_Dodgers.corr()",
"_____no_output_____"
],
[
"# Here we draft a scatterplot to see if any relationship between attendance and temperature\nsns.regplot(df_Dodgers['temp'],df_Dodgers['attend'])\nslope,intercept,r_value,p_value,std_err = scipy.stats.linregress(df_Dodgers['temp'],df_Dodgers['attend'])",
"C:\\Users\\harol\\anaconda3\\lib\\site-packages\\seaborn\\_decorators.py:36: FutureWarning: Pass the following variables as keyword args: x, y. From version 0.12, the only valid positional argument will be `data`, and passing other arguments without an explicit keyword will result in an error or misinterpretation.\n warnings.warn(\n"
],
[
"# Bar charts showing average attendance by day of the week\ndays = {'M':'Monday','T':'Tuesday','W':'Wednesday','R':'Thursday','F':'Friday','S':'Saturday','U':'Sunday'}\nday_attendance = []\nfor day in days.keys():\n day_attendance.append(df_Dodgers[df_Dodgers['day_of_week']==days[day]].attend.mean())\n\nfig=plt.figure()\nax=fig.add_axes([0,0,1,1])\nx=days.keys()\nax.bar(x,day_attendance)\nax.set_xlabel('day of week')\nax.set_ylabel('average attendance')\nplt.show()",
"_____no_output_____"
],
[
"# This shows the distribution for each perk\nfig, axs = plt.subplots(4,figsize=(10,30))\nplot_coords = [(0,0),(0,1),(1,0),(1,1)]\nperks = ['cap','shirt','fireworks','bobblehead']\ncount = 0\nfor perk in perks:\n ys=[]\n for day in days.keys():\n df_ = df_Dodgers[df_Dodgers['day_of_week']==days[day]]\n ys.append(len(df_[df_[perk]==bins[perk][1].upper()]))\n axs[count].set_title(f'perk: {perk}')\n axs[count].bar(days.keys(),ys)\n axs[count].set_xlabel('day of week')\n axs[count].set_ylabel('number of games at which perk given')\n axs[count].set_ylim(0,15)\n count+=1",
"_____no_output_____"
],
[
"# Checks to see if any null or duplicate values\ndf_Dodgers.month.unique()",
"_____no_output_____"
],
[
"# More temperature and attendance relationship research\nmonths = ['APR','MAY','JUN','JUL','AUG','SEP','OCT']\nprint('Month marginal tickets/deg probability')\nprint('----- -------------------- -----------')\nfor month in months:\n x = df_Dodgers[df_Dodgers['month'] == month]['temp']\n y = df_Dodgers[df_Dodgers['month'] == month]['attend']\n slope, intercept, r_value, p_value, std_err = scipy.stats.linregress(x, y)\n print(f'{month:>4} {round(slope):16} {\" \"*10}{round(p_value,3)}')",
"Month marginal tickets/deg probability\n----- -------------------- -----------\n APR 1170 0.022\n MAY 337 0.412\n JUN 55 0.902\n JUL 552 0.487\n AUG -105 0.826\n SEP -296 0.25\n OCT -756 0.796\n"
],
[
"# This plots the average temperature on game nights by month for the hotter months\nfig = plt.figure()\nax = fig.add_axes([0,0,1,1])\nx = months[4:]\ntemps = [df_Dodgers[df_Dodgers['month']==month].temp.mean() for month in x]\nax.bar(x,temps)\nax.set_xlabel('month')\nax.set_ylabel('average gameday temp')\n# 0 isn't particularly meaningful for degrees F, so I set ymin to LA's absolute 0.\nplt.ylim(50,90)\nplt.show()",
"_____no_output_____"
],
[
"\"\"\"\nAttendance during the summer months actually declined instead of increased which leads me to believe that the heat is more of a\nfactor in determining attendance than the fact that school is out for summer break. Based on all of the data, I inferred that\ngiving bobbleheads held the most significance and did the most to increase attendance. Also, I did a boxplot to the attendance\nbased on the days of the week and found that Tuesdays had the greatest means and range of attendance. Just to make sure I wasn't\nmissing anything else, I ran a correlation analysis on the opposing team, on the day or night game data, and on the day of the\nweek. Tuesday proved to show the greatest correlation.\nBased on this analysis, I would recommend giving out more bobbleheads. Reserve the giving of these objects for periods when\nattendance is typically lower like cooler or hotter days. Also, I would recommend installing misters, air movers, and temporary\nshade structures\n\"\"\"",
"_____no_output_____"
]
]
] | [
"code"
] | [
[
"code",
"code",
"code",
"code",
"code",
"code",
"code",
"code",
"code",
"code",
"code",
"code",
"code",
"code",
"code"
]
] |
d0482f5ed29ce9ac82834c39149664b48a7f3ec8 | 58,739 | ipynb | Jupyter Notebook | LSTM model/LSTM Final Prediction/.ipynb_checkpoints/LSTM Final Prediction 3 hidden-checkpoint.ipynb | rafsan062/Dhaka-Air-Pollution-Prediction | 5dda013c14832b2c9363a19d9c8adc61926b92f0 | [
"MIT"
] | null | null | null | LSTM model/LSTM Final Prediction/.ipynb_checkpoints/LSTM Final Prediction 3 hidden-checkpoint.ipynb | rafsan062/Dhaka-Air-Pollution-Prediction | 5dda013c14832b2c9363a19d9c8adc61926b92f0 | [
"MIT"
] | null | null | null | LSTM model/LSTM Final Prediction/.ipynb_checkpoints/LSTM Final Prediction 3 hidden-checkpoint.ipynb | rafsan062/Dhaka-Air-Pollution-Prediction | 5dda013c14832b2c9363a19d9c8adc61926b92f0 | [
"MIT"
] | null | null | null | 96.45156 | 39,368 | 0.8081 | [
[
[
"import torch \nimport torch.nn as nn\nfrom torch.nn import functional as F\nfrom torch.utils.data import Dataset, DataLoader\n\nfrom torch.utils.tensorboard import SummaryWriter\nwriter = SummaryWriter()\n\nimport pandas as pd\nimport numpy as np\nimport matplotlib.pyplot as plt\nplt.rcParams['figure.dpi']= 100\nimport seaborn as sns\nsns.set(style=\"whitegrid\")",
"_____no_output_____"
],
[
"#hyperparameters\n\nbatch_size = 64\n\nseq_len = 31\n\ninput_size = 7\nlstm_hidden_size = 84\nlinear_hidden_size_1 = 109\nlinear_hidden_size_2 = 100\nlinear_hidden_size_3 = 36\noutput_size = 6\n\ndropout_1 = 0.444263\ndropout_2 = 0.246685\ndropout_3 = 0.200149\n\nlearning_rate = 0.002635\nnum_epochs = 500\n\nseed = 1504062\n#seed = np.random.randint(10000000, size=1).item() #random seed",
"_____no_output_____"
],
[
"####################################################\n#dataset import and sequencing\n####################################################\n\n#data importing\ndf = pd.read_excel('ALL VAR cleaned.xlsx')\ndf.Date = pd.to_datetime(df.Date, format = '%m/%d/%Y')\ndf = df.set_index('Date')\n\n#data scaling\ndf_scaled = (df - df.mean())/ df.std()\n#print(df_scaled.head())\n\n#storing mean and std\ndf_np_mean = df.mean().to_numpy()\ndf_np_std = df.std().to_numpy()\n\n#dropping date column\ndf_scaled.reset_index(inplace = True)\ndf_scaled = df_scaled.drop('Date', 1)\n\n#creating sequences\ndef split_sequences(sequences, n_steps):\n X, y = list(), list()\n for i in range(len(sequences)):\n # find the end of this pattern\n end_ix = i + n_steps\n # check if we are beyond the dataset\n if end_ix +1 >= len(sequences): break\n # gather input and output parts of the pattern\n seq_x, seq_y = sequences[i:end_ix, 0:7], sequences[end_ix + 1, 7:14]\n X.append(seq_x)\n y.append(seq_y)\n return X, y\n\narray = df_scaled.iloc[:, :].values\nprint ('shape of the datset array: {}'.format(array.shape))\nX, y = split_sequences(array, seq_len)\nX_array = np.array(X, dtype = np.float32)\ny_array = np.array(y)\nprint('sequenced X array shape: {}'.format(X_array.shape))\nprint('y array shape: {}'.format(y_array.shape))\nprint('null values in dataset?: {}'.format(df_scaled.isnull().values.any()))",
"shape of the datset array: (2184, 13)\nsequenced X array shape: (2152, 31, 7)\ny array shape: (2152, 6)\nnull values in dataset?: False\n"
],
[
"####################################################\n#output mask preparation\n####################################################\n\n\n#import output masked data\ndf_mask = pd.read_excel('COMBINED CAMS MASK.xlsx')\n#print(df_mask.head())\n\nmask_array = df_mask.iloc[:, :].values\n#print(mask_array.shape)\n\n#sequencing\ndef mask_sequence(sequence, n_steps):\n y = list()\n for i in range(len(sequence)):\n # find the end of this pattern\n end_iy = i + n_steps\n # check if we are beyond the dataset\n if end_iy + 1 >= len(sequence): break\n # gather input and output parts of the pattern\n seq_y = sequence[end_iy + 1, 0:6]\n y.append(seq_y)\n return y\n\nmask_list = mask_sequence(mask_array, seq_len)\nmask_array = np.array(mask_list)\nprint('masked output array shape: {}'.format(mask_array.shape))",
"masked output array shape: (2152, 6)\n"
],
[
"####################################################\n#creating dataset and subsets\n####################################################\n\n#creating dataset\nclass AirMeteoroDataset(Dataset):\n def __init__(self):\n self.len = X_array.shape[0]\n self.data_id = torch.arange(0,len(X_array),1)\n self.X_data = torch.from_numpy(X_array)\n self.y_data = torch.from_numpy(y_array)\n self.y_mask = torch.from_numpy(mask_array)\n\n def __getitem__(self, index):\n return self.data_id[index], self.X_data[index], self.y_data[index], self.y_mask[index]\n\n def __len__(self):\n return self.len\n \ndataset = AirMeteoroDataset()\n\n\n#test train split\nseed = 1504062\ntrain_size = round(len(X_array) * 0.85)\ntest_size = len(X_array) - train_size\ntrain_set, test_set = torch.utils.data.random_split(dataset,\n [train_size, test_size], \n generator = torch.Generator().manual_seed(seed))",
"_____no_output_____"
],
[
"####################################################\n#making mini-batches using dataloader\n####################################################\n\ntrain_loader = DataLoader(dataset = train_set,\n batch_size = batch_size,\n drop_last = True,\n shuffle = True)\n\ntest_loader = DataLoader(dataset = test_set,\n batch_size = batch_size,\n drop_last = True,\n shuffle = True)",
"_____no_output_____"
],
[
"#for i, (X_data, y_data, y_mask) in enumerate(train_loader):\n #print(X_data)\n #break",
"_____no_output_____"
],
[
"####################################################\n#model building\n####################################################\n\nclass Model(nn.Module):\n def __init__(self, \n input_size, \n lstm_hidden_size, \n linear_hidden_size_1, \n linear_hidden_size_2, \n linear_hidden_size_3,\n output_size, \n dropout_1,\n dropout_2,\n dropout_3):\n super(Model, self).__init__()\n self.input_size = input_size\n self.lstm_hidden_size = lstm_hidden_size\n self.linear_hidden_size_1 = linear_hidden_size_1\n self.linear_hidden_size_2 = linear_hidden_size_2\n self.linear_hidden_size_3 = linear_hidden_size_3\n self.output_size = output_size\n \n self.batchnorm1 = nn.BatchNorm1d(num_features = linear_hidden_size_1)\n self.batchnorm2 = nn.BatchNorm1d(num_features = linear_hidden_size_2)\n self.batchnorm3 = nn.BatchNorm1d(num_features = linear_hidden_size_3)\n\n \n self.relu = nn.ReLU()\n \n self.dropout_1 = nn.Dropout(p = dropout_1)\n self.dropout_2 = nn.Dropout(p = dropout_2)\n self.dropout_3 = nn.Dropout(p = dropout_3)\n \n self.lstm = nn.LSTM(\n input_size = self.input_size,\n hidden_size = self.lstm_hidden_size,\n batch_first = True)\n \n self.linear_1 = nn.Linear(self.lstm_hidden_size, self.linear_hidden_size_1)\n self.linear_2 = nn.Linear(self.linear_hidden_size_1, self.linear_hidden_size_2)\n self.linear_3 = nn.Linear(self.linear_hidden_size_2, self.output_size)\n \n \n def forward(self, sequences):\n lstm_out, _ = self.lstm(sequences)\n \n z1 = self.linear_1(lstm_out[:, -1, :])\n a1 = self.dropout_linear(self.relu(self.batchnorm1(z1)))\n \n z2 = self.linear_2(a1)\n a2 = self.dropout_linear(self.relu(self.batchnorm2(z2)))\n \n y_pred = self.linear_3(a2)\n return y_pred",
"_____no_output_____"
],
[
"class modsmoothl1(nn.SmoothL1Loss):\n def __init__(self, size_average=None, reduce=None, reduction = 'none'):\n super(modsmoothl1, self).__init__(size_average, reduce, reduction)\n \n def forward(self, observed, predicted, mask):\n predicted_masked = mask*predicted\n loss = F.smooth_l1_loss(observed, predicted_masked, reduction=self.reduction)\n avg_loss = torch.sum(loss)/torch.sum(mask)\n return avg_loss",
"_____no_output_____"
],
[
"forecast_model = Model(input_size,\n lstm_hidden_size,\n linear_hidden_size_1,\n linear_hidden_size_2,\n linear_hidden_size_3,\n output_size,\n dropout_1,\n dropout_2,\n dropout_3,).cuda().float()\n\ncriterion = modsmoothl1()\noptimizer = torch.optim.RMSprop(forecast_model.parameters(), lr = learning_rate)",
"_____no_output_____"
],
[
"####################################################\n#model training and validation\n####################################################\n\nall_train_loss = []\nall_val_loss = []\ntotal_iter = 0\n\n\nfor epoch in range(num_epochs):\n forecast_model.train()\n epoch_total_loss = 0.0\n \n for i, (data_id,X_data, y_data, y_mask) in enumerate(train_loader): \n optimizer.zero_grad()\n\n X_data = X_data.cuda().float()\n y_data = y_data.cuda().float()\n y_mask = y_mask.cuda().float()\n \n y_pred = forecast_model(X_data)\n loss = criterion(y_data, y_pred, y_mask)\n \n total_iter += 1\n writer.add_scalar(\"Loss/train\", loss, total_iter)\n \n loss.backward()\n optimizer.step()\n \n epoch_total_loss = epoch_total_loss + loss.item()\n \n epoch_avg_loss = epoch_total_loss/len(train_loader)\n \n if (epoch +1) % round(num_epochs/10) == 0:\n print (f'Train loss after Epoch [{epoch+1}/{num_epochs}]: {epoch_avg_loss:.6f}, Val loss: {epoch_avg_val_loss:.6f}')\n \n all_train_loss.append(epoch_avg_loss)\n \n #validation\n forecast_model.eval() \n with torch.no_grad():\n epoch_total_val_loss = 0.0\n for i, (data_id, X_val, y_val, y_mask_val) in enumerate(val_loader):\n X_val = X_val.cuda().float()\n y_val = y_val.cuda().float()\n y_mask_val = y_mask_val.cuda().float() \n \n val_pred = forecast_model(X_val).cuda()\n val_loss = criterion(y_val, val_pred, y_mask_val)\n epoch_total_val_loss = epoch_total_val_loss + val_loss.item()\n \n \n epoch_avg_val_loss = epoch_total_val_loss/len(val_loader)\n all_val_loss.append(epoch_avg_val_loss)\n writer.add_scalar(\"Loss/Validation\", epoch_avg_val_loss, epoch)",
"Train loss after Epoch [500/5000]: 0.275113, Val loss: 0.249438\nTrain loss after Epoch [1000/5000]: 0.266435, Val loss: 0.250788\nTrain loss after Epoch [1500/5000]: 0.266795, Val loss: 0.250846\nTrain loss after Epoch [2000/5000]: 0.259789, Val loss: 0.242612\nTrain loss after Epoch [2500/5000]: 0.261240, Val loss: 0.251078\nTrain loss after Epoch [3000/5000]: 0.258307, Val loss: 0.252904\nTrain loss after Epoch [3500/5000]: 0.256158, Val loss: 0.249311\nTrain loss after Epoch [4000/5000]: 0.259491, Val loss: 0.246279\nTrain loss after Epoch [4500/5000]: 0.257651, Val loss: 0.254261\nTrain loss after Epoch [5000/5000]: 0.255776, Val loss: 0.252608\n"
],
[
"import statistics\nprint (statistics.mean(all_val_loss[:-20:-1]))",
"0.24964507368572972\n"
],
[
"plt.plot(list(range(1, num_epochs + 1)), all_train_loss, label = 'Train')\nplt.plot(list(range(1, num_epochs + 1)), all_val_loss, label = 'Validation')\nplt.legend(loc=\"upper right\")\nplt.xlabel('No. of epochs')\nplt.ylabel('Loss')\nwriter.flush()",
"_____no_output_____"
],
[
"all_id = torch.empty(0).cuda()\nall_obs = torch.empty(0, output_size).cuda()\nall_pred = torch.empty(0, output_size).cuda()\nwith torch.no_grad():\n total_test_loss = 0.0\n for i, (data_id, X_test, y_test, y_mask_test) in enumerate(test_loader):\n data_id = data_id.cuda()\n X_test = X_test.cuda().float()\n y_test = y_test.cuda().float()\n y_mask_test = y_mask_test.cuda().float()\n \n test_pred = forecast_model(X_test).cuda()\n test_loss = criterion(y_test, test_pred, y_mask_test)\n total_test_loss = total_test_loss + test_loss.item()\n \n all_id = torch.cat((all_id, data_id), 0)\n all_obs = torch.cat((all_obs, y_test), 0)\n all_pred = torch.cat((all_pred, test_pred), 0)\n \n \n avg_test_loss = total_test_loss/len(test_loader)\n print(avg_test_loss)",
"0.2463295434912046\n"
],
[
"#all_pred.shape",
"_____no_output_____"
],
[
"pred_out_np = all_pred.cpu().numpy()\nobs_out_np = all_obs.cpu().numpy()\nprint(pred_out_np.shape)\nprint(obs_out_np.shape)\ndf_out_mean = df_np_mean[7:13]\ndf_out_std = df_np_std[7:13]\nfinal_pred = pred_out_np * df_out_std + df_out_mean\nfinal_observed = obs_out_np * df_out_std + df_out_mean",
"(300, 6)\n(300, 6)\n"
],
[
"out_obs_data = pd.DataFrame({'SO2 ': final_observed[:, 0],\n 'NO2': final_observed[:, 1],\n 'CO': final_observed[:, 2],\n 'O3': final_observed[:, 3],\n 'PM2.5': final_observed[:, 4], \n 'PM10': final_observed[:, 5]})",
"_____no_output_____"
],
[
"filename_obs = 'plot_obs.xlsx'\nout_obs_data.to_excel(filename_obs, index=True)",
"_____no_output_____"
],
[
"out_pred_data = pd.DataFrame({'SO2 ': final_pred[:, 0],\n 'NO2': final_pred[:, 1],\n 'CO': final_pred[:, 2],\n 'O3': final_pred[:, 3],\n 'PM2.5': final_pred[:, 4], \n 'PM10': final_pred[:, 5]})",
"_____no_output_____"
],
[
"filename_pred = 'plot_pred.xlsx'\nout_pred_data.to_excel(filename_pred, index=True)",
"_____no_output_____"
]
]
] | [
"code"
] | [
[
"code",
"code",
"code",
"code",
"code",
"code",
"code",
"code",
"code",
"code",
"code",
"code",
"code",
"code",
"code",
"code",
"code",
"code",
"code",
"code"
]
] |
d048324c049a519cc6012f89b22ec14b6db5b623 | 42,350 | ipynb | Jupyter Notebook | Data_preprocessing.ipynb | 18cse081/dmdw | d94c9c2e20af6d8e84200ea0d05675363d999287 | [
"Apache-2.0"
] | null | null | null | Data_preprocessing.ipynb | 18cse081/dmdw | d94c9c2e20af6d8e84200ea0d05675363d999287 | [
"Apache-2.0"
] | null | null | null | Data_preprocessing.ipynb | 18cse081/dmdw | d94c9c2e20af6d8e84200ea0d05675363d999287 | [
"Apache-2.0"
] | null | null | null | 34.016064 | 228 | 0.309917 | [
[
[
"<a href=\"https://colab.research.google.com/github/18cse081/dmdw/blob/main/Data_preprocessing.ipynb\" target=\"_parent\"><img src=\"https://colab.research.google.com/assets/colab-badge.svg\" alt=\"Open In Colab\"/></a>",
"_____no_output_____"
]
],
[
[
"import pandas as pd",
"_____no_output_____"
],
[
"import numpy as np",
"_____no_output_____"
],
[
"import matplotlib.pyplot as plt",
"_____no_output_____"
],
[
"path =(\"https://raw.githubusercontent.com/18cse005/DMDW/main/USA_cars_datasets.csv\")",
"_____no_output_____"
],
[
"data=pd.read_csv(path)",
"_____no_output_____"
],
[
"type(data)",
"_____no_output_____"
],
[
"data.info",
"_____no_output_____"
],
[
"data.shape",
"_____no_output_____"
],
[
"data.index",
"_____no_output_____"
],
[
"data.columns",
"_____no_output_____"
],
[
"data.head()",
"_____no_output_____"
],
[
"data.tail()",
"_____no_output_____"
],
[
"data.head(10)",
"_____no_output_____"
],
[
"data.isnull().sum()",
"_____no_output_____"
],
[
"data.dropna(inplace=True) # removed the null values 1st method remove roes when large data we are having\ndata.isnull().sum()",
"_____no_output_____"
],
[
"data.shape",
"_____no_output_____"
],
[
"data.head(10)",
"_____no_output_____"
],
[
"# 2nd method handling missing value\ndata['price'].mean()",
"_____no_output_____"
],
[
"data['price'].head()",
"_____no_output_____"
],
[
"data['price'].replace(np.NaN,data['price'].mean()).head()",
"_____no_output_____"
],
[
"",
"_____no_output_____"
]
]
] | [
"markdown",
"code"
] | [
[
"markdown"
],
[
"code",
"code",
"code",
"code",
"code",
"code",
"code",
"code",
"code",
"code",
"code",
"code",
"code",
"code",
"code",
"code",
"code",
"code",
"code",
"code",
"code"
]
] |
d0483a610cef769fed22f3d4fa01b04e42f53681 | 218,901 | ipynb | Jupyter Notebook | p0_ss_titanic_survival_exploration/p0_ss_titanic_survival_exploration.ipynb | superkley/udacity-mlnd | 2038110e6bebad6e4290441cf4da618059a02a04 | [
"Apache-2.0"
] | 4 | 2017-10-27T14:12:33.000Z | 2018-02-19T21:50:15.000Z | p0_ss_titanic_survival_exploration/p0_ss_titanic_survival_exploration.ipynb | superkley/udacity-mlnd | 2038110e6bebad6e4290441cf4da618059a02a04 | [
"Apache-2.0"
] | null | null | null | p0_ss_titanic_survival_exploration/p0_ss_titanic_survival_exploration.ipynb | superkley/udacity-mlnd | 2038110e6bebad6e4290441cf4da618059a02a04 | [
"Apache-2.0"
] | null | null | null | 153.722612 | 17,450 | 0.866319 | [
[
[
"# Introduction and Foundations: Titanic Survival Exploration\n\n> Udacity Machine Learning Engineer Nanodegree: _Project 0_\n>\n> Author: _Ke Zhang_\n>\n> Submission Date: _2017-04-27_ (Revision 2)",
"_____no_output_____"
],
[
"## Abstract\n\nIn 1912, the ship RMS Titanic struck an iceberg on its maiden voyage and sank, resulting in the deaths of most of its passengers and crew. In this introductory project, we will explore a subset of the RMS Titanic passenger manifest to determine which features best predict whether someone survived or did not survive. To complete this project, you will need to implement several conditional predictions and answer the questions below. Your project submission will be evaluated based on the completion of the code and your responses to the questions.",
"_____no_output_____"
],
[
"## Content\n\n- [Getting Started](#Getting-Started)\n- [Making Predictions](#Making-Predictions)\n- [Conclusion](#Conclusion)\n- [References](#References)\n- [Reproduction Environment](#Reproduction-Environment)",
"_____no_output_____"
],
[
"# Getting Started\nTo begin working with the RMS Titanic passenger data, we'll first need to `import` the functionality we need, and load our data into a `pandas` DataFrame. ",
"_____no_output_____"
]
],
[
[
"# Import libraries necessary for this project\nimport numpy as np\nimport pandas as pd\nfrom IPython.display import display # Allows the use of display() for DataFrames\n\n# Import supplementary visualizations code visuals.py\nimport visuals as vs\n\n# Pretty display for notebooks\n%matplotlib inline\n\n# Load the dataset\nin_file = 'titanic_data.csv'\nfull_data = pd.read_csv(in_file)\n\n# Print the first few entries of the RMS Titanic data\ndisplay(full_data.head())",
"_____no_output_____"
]
],
[
[
"From a sample of the RMS Titanic data, we can see the various features present for each passenger on the ship:\n- **Survived**: Outcome of survival (0 = No; 1 = Yes)\n- **Pclass**: Socio-economic class (1 = Upper class; 2 = Middle class; 3 = Lower class)\n- **Name**: Name of passenger\n- **Sex**: Sex of the passenger\n- **Age**: Age of the passenger (Some entries contain `NaN`)\n- **SibSp**: Number of siblings and spouses of the passenger aboard\n- **Parch**: Number of parents and children of the passenger aboard\n- **Ticket**: Ticket number of the passenger\n- **Fare**: Fare paid by the passenger\n- **Cabin** Cabin number of the passenger (Some entries contain `NaN`)\n- **Embarked**: Port of embarkation of the passenger (C = Cherbourg; Q = Queenstown; S = Southampton)\n\nSince we're interested in the outcome of survival for each passenger or crew member, we can remove the **Survived** feature from this dataset and store it as its own separate variable `outcomes`. We will use these outcomes as our prediction targets. \nRun the code cell below to remove **Survived** as a feature of the dataset and store it in `outcomes`.",
"_____no_output_____"
]
],
[
[
"# Store the 'Survived' feature in a new variable and remove it from the dataset\noutcomes = full_data['Survived']\ndata = full_data.drop('Survived', axis = 1)\n\n# Show the new dataset with 'Survived' removed\ndisplay(data.head())",
"_____no_output_____"
]
],
[
[
"The very same sample of the RMS Titanic data now shows the **Survived** feature removed from the DataFrame. Note that `data` (the passenger data) and `outcomes` (the outcomes of survival) are now *paired*. That means for any passenger `data.loc[i]`, they have the survival outcome `outcomes[i]`.\n\nTo measure the performance of our predictions, we need a metric to score our predictions against the true outcomes of survival. Since we are interested in how *accurate* our predictions are, we will calculate the proportion of passengers where our prediction of their survival is correct. Run the code cell below to create our `accuracy_score` function and test a prediction on the first five passengers. \n\n**Think:** *Out of the first five passengers, if we predict that all of them survived, what would you expect the accuracy of our predictions to be?*",
"_____no_output_____"
]
],
[
[
"def accuracy_score(truth, pred):\n \"\"\" Returns accuracy score for input truth and predictions. \"\"\"\n \n # Ensure that the number of predictions matches number of outcomes\n if len(truth) == len(pred): \n \n # Calculate and return the accuracy as a percent\n return \"Predictions have an accuracy of {:.2f}%.\".format((truth == pred).mean()*100)\n \n else:\n return \"Number of predictions does not match number of outcomes!\"\n \n# Test the 'accuracy_score' function\npredictions = pd.Series(np.ones(5, dtype = int))\nprint accuracy_score(outcomes[:5], predictions)",
"Predictions have an accuracy of 60.00%.\n"
]
],
[
[
"> **Tip:** If you save an iPython Notebook, the output from running code blocks will also be saved. However, the state of your workspace will be reset once a new session is started. Make sure that you run all of the code blocks from your previous session to reestablish variables and functions before picking up where you last left off.\n\n# Making Predictions\n\nIf we were asked to make a prediction about any passenger aboard the RMS Titanic whom we knew nothing about, then the best prediction we could make would be that they did not survive. This is because we can assume that a majority of the passengers (more than 50%) did not survive the ship sinking. \nThe `predictions_0` function below will always predict that a passenger did not survive.",
"_____no_output_____"
]
],
[
[
"def predictions_0(data):\n \"\"\" Model with no features. Always predicts a passenger did not survive. \"\"\"\n\n predictions = []\n for _, passenger in data.iterrows():\n \n # Predict the survival of 'passenger'\n predictions.append(0)\n \n # Return our predictions\n return pd.Series(predictions)\n\n# Make the predictions\npredictions = predictions_0(data)",
"_____no_output_____"
]
],
[
[
"### Question 1\n*Using the RMS Titanic data, how accurate would a prediction be that none of the passengers survived?* \n**Hint:** Run the code cell below to see the accuracy of this prediction.",
"_____no_output_____"
]
],
[
[
"print accuracy_score(outcomes, predictions)",
"Predictions have an accuracy of 61.62%.\n"
]
],
[
[
"**Answer:** The prediction accuracy is **61.62%**",
"_____no_output_____"
],
[
"***\nLet's take a look at whether the feature **Sex** has any indication of survival rates among passengers using the `survival_stats` function. This function is defined in the `titanic_visualizations.py` Python script included with this project. The first two parameters passed to the function are the RMS Titanic data and passenger survival outcomes, respectively. The third parameter indicates which feature we want to plot survival statistics across. \nRun the code cell below to plot the survival outcomes of passengers based on their sex.",
"_____no_output_____"
]
],
[
[
"vs.survival_stats(data, outcomes, 'Sex')",
"_____no_output_____"
]
],
[
[
"Examining the survival statistics, a large majority of males did not survive the ship sinking. However, a majority of females *did* survive the ship sinking. Let's build on our previous prediction: If a passenger was female, then we will predict that they survived. Otherwise, we will predict the passenger did not survive. \nFill in the missing code below so that the function will make this prediction. \n**Hint:** You can access the values of each feature for a passenger like a dictionary. For example, `passenger['Sex']` is the sex of the passenger.",
"_____no_output_____"
]
],
[
[
"def predictions_1(data):\n \"\"\" Model with one feature: \n - Predict a passenger survived if they are female. \"\"\"\n \n predictions = []\n for _, passenger in data.iterrows():\n predictions.append(True if passenger['Sex'] == 'female' \n else False)\n \n # Return our predictions\n return pd.Series(predictions)\n\n# Make the predictions\npredictions = predictions_1(data)",
"_____no_output_____"
]
],
[
[
"### Question 2\n*How accurate would a prediction be that all female passengers survived and the remaining passengers did not survive?* \n**Hint:** Run the code cell below to see the accuracy of this prediction.",
"_____no_output_____"
]
],
[
[
"print accuracy_score(outcomes, predictions)",
"Predictions have an accuracy of 78.68%.\n"
]
],
[
[
"**Answer**: **78.68**%",
"_____no_output_____"
],
[
"***\nUsing just the **Sex** feature for each passenger, we are able to increase the accuracy of our predictions by a significant margin. Now, let's consider using an additional feature to see if we can further improve our predictions. For example, consider all of the male passengers aboard the RMS Titanic: Can we find a subset of those passengers that had a higher rate of survival? Let's start by looking at the **Age** of each male, by again using the `survival_stats` function. This time, we'll use a fourth parameter to filter out the data so that only passengers with the **Sex** 'male' will be included. \nRun the code cell below to plot the survival outcomes of male passengers based on their age.",
"_____no_output_____"
]
],
[
[
"vs.survival_stats(data, outcomes, 'Age', [\"Sex == 'male'\"])",
"_____no_output_____"
]
],
[
[
"Examining the survival statistics, the majority of males younger than 10 survived the ship sinking, whereas most males age 10 or older *did not survive* the ship sinking. Let's continue to build on our previous prediction: If a passenger was female, then we will predict they survive. If a passenger was male and younger than 10, then we will also predict they survive. Otherwise, we will predict they do not survive. \nFill in the missing code below so that the function will make this prediction. \n**Hint:** You can start your implementation of this function using the prediction code you wrote earlier from `predictions_1`.",
"_____no_output_____"
]
],
[
[
"def predictions_2(data):\n \"\"\" Model with two features: \n - Predict a passenger survived if they are female.\n - Predict a passenger survived if they are male and younger than 10. \"\"\"\n \n predictions = []\n for _, passenger in data.iterrows():\n predictions.append(True if passenger['Sex'] == 'female' or\n passenger['Age'] < 10 else False)\n \n # Return our predictions\n return pd.Series(predictions)\n\n# Make the predictions\npredictions = predictions_2(data)",
"_____no_output_____"
]
],
[
[
"### Question 3\n*How accurate would a prediction be that all female passengers and all male passengers younger than 10 survived?* \n**Hint:** Run the code cell below to see the accuracy of this prediction.",
"_____no_output_____"
]
],
[
[
"print accuracy_score(outcomes, predictions)",
"Predictions have an accuracy of 79.35%.\n"
]
],
[
[
"**Answer**: **79.35**",
"_____no_output_____"
],
[
"***\nAdding the feature **Age** as a condition in conjunction with **Sex** improves the accuracy by a small margin more than with simply using the feature **Sex** alone. Now it's your turn: Find a series of features and conditions to split the data on to obtain an outcome prediction accuracy of at least 80%. This may require multiple features and multiple levels of conditional statements to succeed. You can use the same feature multiple times with different conditions. \n**Pclass**, **Sex**, **Age**, **SibSp**, and **Parch** are some suggested features to try.\n\nUse the `survival_stats` function below to to examine various survival statistics. \n**Hint:** To use mulitple filter conditions, put each condition in the list passed as the last argument. Example: `[\"Sex == 'male'\", \"Age < 18\"]`",
"_____no_output_____"
]
],
[
[
"# survival by Embarked\nvs.survival_stats(data, outcomes, 'Embarked')",
"_____no_output_____"
],
[
"# survival by Embarked\nvs.survival_stats(data, outcomes, 'SibSp')",
"_____no_output_____"
],
[
"vs.survival_stats(data, outcomes, 'Age', [\"Sex == 'male'\", \"Age < 18\"])",
"_____no_output_____"
]
],
[
[
"We found out earlier that female and children had better chance to survive. In the next step we'll add another criteria 'Pclass' to further distinguish the survival rates among the different groups.",
"_____no_output_____"
]
],
[
[
"# female passengers in the higher pclass had great chance to survive\nvs.survival_stats(data, outcomes, 'Pclass', [\n \"Sex == 'female'\"\n])",
"_____no_output_____"
],
[
"# male passengers in the higher pclass had great chance to survive\nvs.survival_stats(data, outcomes, 'Pclass', [\n \"Sex == 'male'\"\n])",
"_____no_output_____"
],
[
"# more female passengers survived in all age groups\nvs.survival_stats(data, outcomes, 'Age', [\n \"Sex == 'female'\",\n])",
"_____no_output_____"
],
[
"# more male passengers survived only when age < 10\nvs.survival_stats(data, outcomes, 'Age', [\n \"Sex == 'male'\",\n])",
"_____no_output_____"
]
],
[
[
"It looks like that all female passengers under 20 survived from the accident. Let's check passengers in the lower class to complete our guess.",
"_____no_output_____"
]
],
[
[
"# ... but not in the lower class when they're older than 20\nvs.survival_stats(data, outcomes, 'Age', [\n \"Sex == 'female'\",\n \"Pclass == 3\"\n])",
"_____no_output_____"
],
[
"# ... actually only females under 20 had more survivers in the lower class\nvs.survival_stats(data, outcomes, 'Age', [\n \"Sex == 'male'\",\n \"Pclass == 3\"\n])",
"_____no_output_____"
]
],
[
[
"> We conclude that in the lower class only female under 20 had better chance to survive. In the other classes all children under 10 and female passengers had more likey survived. Let's check if we have reached our 80% target.",
"_____no_output_____"
],
[
"After exploring the survival statistics visualization, fill in the missing code below so that the function will make your prediction. \nMake sure to keep track of the various features and conditions you tried before arriving at your final prediction model. \n**Hint:** You can start your implementation of this function using the prediction code you wrote earlier from `predictions_2`.",
"_____no_output_____"
]
],
[
[
"def predictions_3(data):\n \"\"\" \n Model with multiple features: Sex, Age and Pclass\n Makes a prediction with an accuracy of at least 80%. \n \"\"\"\n \n predictions = []\n for _, passenger in data.iterrows():\n if passenger['Age'] < 10:\n survived = True\n elif passenger['Sex'] == 'female' and not (\n passenger['Pclass'] == 3 and passenger['Age'] > 20\n ):\n survived = True\n else:\n survived = False\n predictions.append(survived)\n \n # Return our predictions\n return pd.Series(predictions)\n\n# Make the predictions\npredictions = predictions_3(data)",
"_____no_output_____"
]
],
[
[
"### Question 4\n*Describe the steps you took to implement the final prediction model so that it got an accuracy of at least 80%. What features did you look at? Were certain features more informative than others? Which conditions did you use to split the survival outcomes in the data? How accurate are your predictions?* \n**Hint:** Run the code cell below to see the accuracy of your predictions.",
"_____no_output_____"
]
],
[
[
"print accuracy_score(outcomes, predictions)",
"Predictions have an accuracy of 80.36%.\n"
]
],
[
[
"**Answer**: \n\nUsing the features *Sex*, *Pclass* and *Age* we increased the accuracy score to **80.36%**.\nWe tried to plot the survival statistics with different features and chose the ones under which conditions the differences were the largest.\n* some features are just not relevant like *PassengerId* or *Name*\n* some features have to be decoded to be helpful like *Cabin* which could be helpful if we have more information on the location of each cabin\n* some features are less informative than the others: e.g. we could use *Embarked*, *SibSp* or *Parch* to group the passengers but the resulting model would be more complicated.\n* Eventually we chose *Sex*, *Pclass* and *Age* as our final features.\n\nWe derived the conditions to split the survival outcomes from the survival plots. The split conditions are:\n1. All children under 10 => **survived**\n2. Female passengers in the upper and middle class, or less than 20 => **survived**\n3. Others => **died**\n\nThe final accuracy score was **80.36%**.",
"_____no_output_____"
],
[
"# Conclusion\n\nAfter several iterations of exploring and conditioning on the data, you have built a useful algorithm for predicting the survival of each passenger aboard the RMS Titanic. The technique applied in this project is a manual implementation of a simple machine learning model, the *decision tree*. A decision tree splits a set of data into smaller and smaller groups (called *nodes*), by one feature at a time. Each time a subset of the data is split, our predictions become more accurate if each of the resulting subgroups are more homogeneous (contain similar labels) than before. The advantage of having a computer do things for us is that it will be more exhaustive and more precise than our manual exploration above. [This link](http://www.r2d3.us/visual-intro-to-machine-learning-part-1/) provides another introduction into machine learning using a decision tree.\n\nA decision tree is just one of many models that come from *supervised learning*. In supervised learning, we attempt to use features of the data to predict or model things with objective outcome labels. That is to say, each of our data points has a known outcome value, such as a categorical, discrete label like `'Survived'`, or a numerical, continuous value like predicting the price of a house.\n\n### Question 5\n*Think of a real-world scenario where supervised learning could be applied. What would be the outcome variable that you are trying to predict? Name two features about the data used in this scenario that might be helpful for making the predictions.* ",
"_____no_output_____"
],
[
"**Answer**: \nA real-world scenario would be that we have a buch of animal photos labeled with the animal type on them and try to recognize new photos with supervised learning model predictions.\n\nUseful featrues could be:\n* number of legs\n* size of the animal\n* color of the skin or fur\n* surrounding environment (tropical, water, air, iceberg etc.)\n\nOutcome variable is the animal type.",
"_____no_output_____"
],
[
"## References\n\n- [Udacity Website](http://www.udacity.com)\n- [Pandas Documentation](http://pandas.pydata.org/pandas-docs/stable/)",
"_____no_output_____"
],
[
"## Reproduction Environment",
"_____no_output_____"
]
],
[
[
"import IPython\nprint IPython.sys_info()",
"{'commit_hash': u'5c9c918',\n 'commit_source': 'installation',\n 'default_encoding': 'cp936',\n 'ipython_path': 'C:\\\\dev\\\\anaconda\\\\lib\\\\site-packages\\\\IPython',\n 'ipython_version': '5.1.0',\n 'os_name': 'nt',\n 'platform': 'Windows-7-6.1.7601-SP1',\n 'sys_executable': 'C:\\\\dev\\\\anaconda\\\\python.exe',\n 'sys_platform': 'win32',\n 'sys_version': '2.7.13 |Anaconda custom (32-bit)| (default, Dec 19 2016, 13:36:02) [MSC v.1500 32 bit (Intel)]'}\n"
],
[
"!pip freeze",
"alabaster==0.7.9\nanaconda-client==1.6.0\nanaconda-navigator==1.4.3\nargcomplete==1.0.0\nastroid==1.4.9\nastropy==1.3\nBabel==2.3.4\nbackports-abc==0.5\nbackports.shutil-get-terminal-size==1.0.0\nbackports.ssl-match-hostname==3.4.0.2\nbeautifulsoup4==4.5.3\nbitarray==0.8.1\nblaze==0.10.1\nbokeh==0.12.4\nboto==2.45.0\nBottleneck==1.2.0\ncdecimal==2.3\ncffi==1.9.1\nchardet==2.3.0\nchest==0.2.3\nclick==6.7\ncloudpickle==0.2.2\nclyent==1.2.2\ncolorama==0.3.7\ncomtypes==1.1.2\nconda==4.3.15\nconfigobj==5.0.6\nconfigparser==3.5.0\ncontextlib2==0.5.4\ncryptography==1.7.1\ncycler==0.10.0\nCython==0.25.2\ncytoolz==0.8.2\ndask==0.13.0\ndatashape==0.5.4\ndecorator==4.0.11\ndill==0.2.5\ndocutils==0.13.1\nenum34==1.1.6\net-xmlfile==1.0.1\nfastcache==1.0.2\nFlask==0.12\nFlask-Cors==3.0.2\nfuncsigs==1.0.2\nfunctools32==3.2.3.post2\nfutures==3.0.5\ngevent==1.2.1\nglueviz==0.9.1\ngreenlet==0.4.11\ngrin==1.2.1\nh5py==2.6.0\nHeapDict==1.0.0\nidna==2.2\nimagesize==0.7.1\nipaddress==1.0.18\nipykernel==4.5.2\nipython==5.1.0\nipython-genutils==0.1.0\nipywidgets==5.2.2\nisort==4.2.5\nitsdangerous==0.24\njdcal==1.3\njedi==0.9.0\nJinja2==2.9.4\njsonschema==2.5.1\njupyter==1.0.0\njupyter-client==4.4.0\njupyter-console==5.0.0\njupyter-core==4.2.1\nlazy-object-proxy==1.2.2\nllvmlite==0.15.0\nlocket==0.2.0\nlxml==3.7.2\nMarkupSafe==0.23\nmatplotlib==2.0.0\nmenuinst==1.4.4\nmistune==0.7.3\nmpmath==0.19\nmultipledispatch==0.4.9\nnbconvert==4.2.0\nnbformat==4.2.0\nnetworkx==1.11\nnltk==3.2.2\nnose==1.3.7\nnotebook==4.4.1\nnumba==0.30.1+0.g8c1033f.dirty\nnumexpr==2.6.1\nnumpy==1.11.3\nnumpydoc==0.6.0\nodo==0.5.0\nopenpyxl==2.4.1\npandas==0.19.2\npartd==0.3.7\npath.py==0.0.0\npathlib2==2.2.0\npatsy==0.4.1\npep8==1.7.0\npickleshare==0.7.4\nPillow==4.0.0\nply==3.9\nprompt-toolkit==1.0.9\npsutil==5.0.1\npy==1.4.32\npyasn1==0.1.9\npycosat==0.6.1\npycparser==2.17\npycrypto==2.6.1\npycurl==7.43.0\npyflakes==1.5.0\npygame==1.9.3\nPygments==2.1.3\npylint==1.6.4\npymongo==3.3.0\npyOpenSSL==16.2.0\npyparsing==2.1.4\npytest==3.0.5\npython-dateutil==2.6.0\npytz==2016.10\npywin32==220\nPyYAML==3.12\npyzmq==16.0.2\nQtAwesome==0.4.3\nqtconsole==4.2.1\nQtPy==1.2.1\nrequests==2.12.4\nrope==0.9.4\nscandir==1.4\nscikit-image==0.12.3\nscikit-learn==0.18.1\nscipy==0.18.1\nseaborn==0.7.1\nsimplegeneric==0.8.1\nsingledispatch==3.4.0.3\nsix==1.10.0\nsnowballstemmer==1.2.1\nsockjs-tornado==1.0.3\nsphinx==1.5.1\nspyder==3.1.2\nSQLAlchemy==1.1.5\nstatsmodels==0.6.1\nsubprocess32==3.2.7\nsympy==1.0\ntables==3.2.2\ntoolz==0.8.2\ntornado==4.4.2\ntraitlets==4.3.1\nunicodecsv==0.14.1\nwcwidth==0.1.7\nWerkzeug==0.11.15\nwidgetsnbextension==1.2.6\nwin-unicode-console==0.5\nwrapt==1.10.8\nxlrd==1.0.0\nXlsxWriter==0.9.6\nxlwings==0.10.2\nxlwt==1.2.0\n"
]
]
] | [
"markdown",
"code",
"markdown",
"code",
"markdown",
"code",
"markdown",
"code",
"markdown",
"code",
"markdown",
"code",
"markdown",
"code",
"markdown",
"code",
"markdown",
"code",
"markdown",
"code",
"markdown",
"code",
"markdown",
"code",
"markdown",
"code",
"markdown",
"code",
"markdown",
"code",
"markdown",
"code",
"markdown",
"code"
] | [
[
"markdown",
"markdown",
"markdown",
"markdown"
],
[
"code"
],
[
"markdown"
],
[
"code"
],
[
"markdown"
],
[
"code"
],
[
"markdown"
],
[
"code"
],
[
"markdown"
],
[
"code"
],
[
"markdown",
"markdown"
],
[
"code"
],
[
"markdown"
],
[
"code"
],
[
"markdown"
],
[
"code"
],
[
"markdown",
"markdown"
],
[
"code"
],
[
"markdown"
],
[
"code"
],
[
"markdown"
],
[
"code"
],
[
"markdown",
"markdown"
],
[
"code",
"code",
"code"
],
[
"markdown"
],
[
"code",
"code",
"code",
"code"
],
[
"markdown"
],
[
"code",
"code"
],
[
"markdown",
"markdown"
],
[
"code"
],
[
"markdown"
],
[
"code"
],
[
"markdown",
"markdown",
"markdown",
"markdown",
"markdown"
],
[
"code",
"code"
]
] |
d0483d0b5db7536f1f0f5b727c2bee7ab5f25c1f | 148,170 | ipynb | Jupyter Notebook | docs/allcools/cell_level/step_by_step/100kb/04a-PreclusteringAndClusterEnrichedFeatures-mCH.ipynb | mukamel-lab/ALLCools | 756ef790665c6ce40633873211929ea92bcccc21 | [
"MIT"
] | 5 | 2019-07-16T17:27:15.000Z | 2022-01-14T19:12:27.000Z | docs/allcools/cell_level/step_by_step/100kb/04a-PreclusteringAndClusterEnrichedFeatures-mCH.ipynb | mukamel-lab/ALLCools | 756ef790665c6ce40633873211929ea92bcccc21 | [
"MIT"
] | 12 | 2019-10-17T19:34:43.000Z | 2022-03-23T16:04:18.000Z | docs/allcools/cell_level/step_by_step/100kb/04a-PreclusteringAndClusterEnrichedFeatures-mCH.ipynb | mukamel-lab/ALLCools | 756ef790665c6ce40633873211929ea92bcccc21 | [
"MIT"
] | 4 | 2019-10-18T23:43:48.000Z | 2022-02-12T04:12:26.000Z | 482.638436 | 108,588 | 0.946622 | [
[
[
"# Preclustering and Cluster Enriched Features\n\n## Purpose\nThe purpose of this step is to perform a simple pre-clustering using the highly variable features to get a pre-clusters labeling. We then select top enriched features for each cluster (CEF) for further analysis.\n\n## Input\n- HVF adata file.\n\n## Output\n- HVF adata file with pre-clusters and CEF annotated.",
"_____no_output_____"
],
[
"## Import",
"_____no_output_____"
]
],
[
[
"import seaborn as sns\nimport anndata\nimport scanpy as sc\nfrom ALLCools.clustering import cluster_enriched_features, significant_pc_test, log_scale",
"_____no_output_____"
],
[
"sns.set_context(context='notebook', font_scale=1.3)",
"_____no_output_____"
]
],
[
[
"## Parameters",
"_____no_output_____"
]
],
[
[
"adata_path = 'mCH.HVF.h5ad'\n\n# Cluster Enriched Features analysis\ntop_n=200\nalpha=0.05\nstat_plot=True\n\n# you may provide a pre calculated cluster version. \n# If None, will perform basic clustering using parameters below.\ncluster_col = None \n\n# These parameters only used when cluster_col is None\nk=25\nresolution=1\ncluster_plot=True",
"_____no_output_____"
]
],
[
[
"## Load Data",
"_____no_output_____"
]
],
[
[
"adata = anndata.read_h5ad(adata_path)",
"_____no_output_____"
]
],
[
[
"## Pre-Clustering\n\nIf cluster label is not provided, will perform basic clustering here",
"_____no_output_____"
]
],
[
[
"if cluster_col is None:\n # IMPORTANT\n # put the unscaled matrix in adata.raw\n adata.raw = adata\n log_scale(adata)\n \n sc.tl.pca(adata, n_comps=100)\n significant_pc_test(adata, p_cutoff=0.1, update=True)\n \n sc.pp.neighbors(adata, n_neighbors=k)\n sc.tl.leiden(adata, resolution=resolution)\n \n if cluster_plot:\n sc.tl.umap(adata)\n sc.pl.umap(adata, color='leiden')\n \n # return to unscaled X, CEF need to use the unscaled matrix\n adata = adata.raw.to_adata()\n \n cluster_col = 'leiden'",
"32 components passed P cutoff of 0.1.\nChanging adata.obsm['X_pca'] from shape (16985, 100) to (16985, 32)\n"
]
],
[
[
"## Cluster Enriched Features (CEF)",
"_____no_output_____"
]
],
[
[
"cluster_enriched_features(adata,\n cluster_col=cluster_col,\n top_n=top_n,\n alpha=alpha,\n stat_plot=True)",
"Found 31 clusters to compute feature enrichment score\nComputing enrichment score\nComputing enrichment score FDR-corrected P values\nSelected 3102 unique features\n"
]
],
[
[
"## Save AnnData",
"_____no_output_____"
]
],
[
[
"# save adata\nadata.write_h5ad(adata_path)\nadata",
"_____no_output_____"
]
]
] | [
"markdown",
"code",
"markdown",
"code",
"markdown",
"code",
"markdown",
"code",
"markdown",
"code",
"markdown",
"code"
] | [
[
"markdown",
"markdown"
],
[
"code",
"code"
],
[
"markdown"
],
[
"code"
],
[
"markdown"
],
[
"code"
],
[
"markdown"
],
[
"code"
],
[
"markdown"
],
[
"code"
],
[
"markdown"
],
[
"code"
]
] |
d0483e7d63485fee0cfd15c63aa117038500619a | 2,119 | ipynb | Jupyter Notebook | novice/01-02/swaroopch/demo_modulku2.ipynb | mailoa-ev/NLP-zimera | 1f2d8ae8e26d2c23aa43921ad993887b25c24168 | [
"MIT"
] | null | null | null | novice/01-02/swaroopch/demo_modulku2.ipynb | mailoa-ev/NLP-zimera | 1f2d8ae8e26d2c23aa43921ad993887b25c24168 | [
"MIT"
] | null | null | null | novice/01-02/swaroopch/demo_modulku2.ipynb | mailoa-ev/NLP-zimera | 1f2d8ae8e26d2c23aa43921ad993887b25c24168 | [
"MIT"
] | null | null | null | 35.915254 | 840 | 0.596036 | [
[
[
"from modulku import say_hi, __version__\n\nsay_hi()\nprint('Version', __version__)",
"_____no_output_____"
]
]
] | [
"code"
] | [
[
"code"
]
] |
d04841998463109f9634c258c1138a7333dc3ef3 | 107,930 | ipynb | Jupyter Notebook | pollster-ratings/Visualization.ipynb | machinglearnin/data | b408d9636e848ea65a8803f3654de720d76d339e | [
"CC-BY-4.0"
] | null | null | null | pollster-ratings/Visualization.ipynb | machinglearnin/data | b408d9636e848ea65a8803f3654de720d76d339e | [
"CC-BY-4.0"
] | null | null | null | pollster-ratings/Visualization.ipynb | machinglearnin/data | b408d9636e848ea65a8803f3654de720d76d339e | [
"CC-BY-4.0"
] | null | null | null | 442.336066 | 36,056 | 0.945316 | [
[
[
"%matplotlib inline\n\nimport numpy as np\nimport matplotlib.pyplot as plt\nimport pandas as pd",
"_____no_output_____"
]
],
[
[
"## Ploting Different Polling Methods",
"_____no_output_____"
]
],
[
[
"pollster_rating = pd.read_csv(\"pollster-ratings.csv\")\nMethodologies_frequencies = pollster_rating.Methodology.value_counts()\nplt.bar(Methodologies_frequencies.index, Methodologies_frequencies)\nplt.xticks(rotation = \"vertical\")\nplt.title(\"Methodolgies of Diffrent Pollsters\")\nplt.ylabel(\"Number of Pollsters\")\nplt.xlabel(\"Methodolgy\")",
"_____no_output_____"
]
],
[
[
"## Plotting Poll Size Distribution",
"_____no_output_____"
]
],
[
[
"plt.figure(figsize = (10,6))\nplt.hist(pollster_rating['# of Polls'])\nplt.title(\"Distrubution of Polling Sizes Among Diffrent Pollsters\")\nplt.xlabel(\"# of Polls Conducted\")\nplt.show()",
"_____no_output_____"
]
],
[
[
"## Accuracy of Pollsters",
"_____no_output_____"
]
],
[
[
"#selects only Pollsters with 100+ Polls\nfrequent_pollsters = pollster_rating[pollster_rating['# of Polls'] > 100]\nfrequent_pollsters = frequent_pollsters.set_index('Pollster')\n\n#Reformats Races Called Correclty Data so it ban be sorted\nRaces_called_correctly = frequent_pollsters['Races Called Correctly'].str.rstrip('%').astype(int)\nRaces_called_correctly = Races_called_correctly.sort_values()\n\n#makes Bar graph\nplt.figure(figsize = (6,4))\nplt.barh(Races_called_correctly.index, Races_called_correctly)\nplt.title(\"Accuracy of Different Pollsters\")\nplt.xlabel(\"Percentage of Races Called Correctly\")\nplt.show()",
"_____no_output_____"
]
],
[
[
"## Are More Frequent Pollsters More Accurate?",
"_____no_output_____"
]
],
[
[
"pollster_above_10 = pollster_rating[pollster_rating['# of Polls'] > 100]\nplt.figure(figsize = (8,6))\nx_list = pollster_above_10['# of Polls']\ny_list = pollster_above_10['Races Called Correctly'].str.rstrip('%').astype(int)\nplt.scatter(x_list, y_list)\nplt.yticks(np.arange(0, 110, 10))\nplt.title(\"Comparison of Pollsters Accuarcy and Frequency of Polling\")\nplt.xlabel(\"Number of Polls Conducted\")\nplt.ylabel(\"Percentage of Races Called Correctly\")\nplt.show()",
"_____no_output_____"
],
[
"from scipy import stats\n\nslope, intercept, r_value, p_value, std_err = stats.linregress(x_list, y_list)\n\npredictions = slope * x_list + intercept\nplt.yticks(np.arange(0, 110, 10))\nplt.title(\"Correlation of Number of Polls and Accuracy\")\nplt.xlabel(\"Number of Polls Conducted\")\nplt.ylabel(\"Percentage of Races Called Correctly\")\nplt.scatter(x_list, y_list)\nplt.plot(x_list, predictions, color = 'r')\nplt.show()\nprint \"R squared:\", r_value ** 2",
"_____no_output_____"
]
]
] | [
"code",
"markdown",
"code",
"markdown",
"code",
"markdown",
"code",
"markdown",
"code"
] | [
[
"code"
],
[
"markdown"
],
[
"code"
],
[
"markdown"
],
[
"code"
],
[
"markdown"
],
[
"code"
],
[
"markdown"
],
[
"code",
"code"
]
] |
d04846108e6a28eae74025bdfb148270cffa02fd | 88,743 | ipynb | Jupyter Notebook | Getting_started_with_BigQuery.ipynb | darshanbk/100-Days-Of-ML-Code | ec50ecd6fc7423779cb5d10324414b7e2571fc3a | [
"MIT"
] | 1 | 2018-09-12T12:26:28.000Z | 2018-09-12T12:26:28.000Z | Getting_started_with_BigQuery.ipynb | darshanbk/100-Days-Of-ML-Code | ec50ecd6fc7423779cb5d10324414b7e2571fc3a | [
"MIT"
] | null | null | null | Getting_started_with_BigQuery.ipynb | darshanbk/100-Days-Of-ML-Code | ec50ecd6fc7423779cb5d10324414b7e2571fc3a | [
"MIT"
] | null | null | null | 62.275789 | 15,976 | 0.314605 | [
[
[
"<a href=\"https://colab.research.google.com/github/darshanbk/100-Days-Of-ML-Code/blob/master/Getting_started_with_BigQuery.ipynb\" target=\"_parent\"><img src=\"https://colab.research.google.com/assets/colab-badge.svg\" alt=\"Open In Colab\"/></a>",
"_____no_output_____"
],
[
"# Before you begin\n\n\n1. Use the [Cloud Resource Manager](https://console.cloud.google.com/cloud-resource-manager) to Create a Cloud Platform project if you do not already have one.\n2. [Enable billing](https://support.google.com/cloud/answer/6293499#enable-billing) for the project.\n3. [Enable BigQuery](https://console.cloud.google.com/flows/enableapi?apiid=bigquery) APIs for the project.\n",
"_____no_output_____"
],
[
"### Provide your credentials to the runtime",
"_____no_output_____"
]
],
[
[
"from google.colab import auth\nauth.authenticate_user()\nprint('Authenticated')",
"_____no_output_____"
]
],
[
[
"## Optional: Enable data table display\n\nColab includes the ``google.colab.data_table`` package that can be used to display large pandas dataframes as an interactive data table.\nIt can be enabled with:",
"_____no_output_____"
]
],
[
[
"%load_ext google.colab.data_table",
"_____no_output_____"
]
],
[
[
"If you would prefer to return to the classic Pandas dataframe display, you can disable this by running:\n```python\n%unload_ext google.colab.data_table\n```",
"_____no_output_____"
],
[
"# Use BigQuery via magics\n\nThe `google.cloud.bigquery` library also includes a magic command which runs a query and either displays the result or saves it to a variable as a `DataFrame`.",
"_____no_output_____"
]
],
[
[
"# Display query output immediately\n\n%%bigquery --project yourprojectid\nSELECT \n COUNT(*) as total_rows\nFROM `bigquery-public-data.samples.gsod`",
"_____no_output_____"
],
[
"# Save output in a variable `df`\n\n%%bigquery --project yourprojectid df\nSELECT \n COUNT(*) as total_rows\nFROM `bigquery-public-data.samples.gsod`",
"_____no_output_____"
],
[
"df",
"_____no_output_____"
]
],
[
[
"# Use BigQuery through google-cloud-bigquery\n\nSee [BigQuery documentation](https://cloud.google.com/bigquery/docs) and [library reference documentation](https://googlecloudplatform.github.io/google-cloud-python/latest/bigquery/usage.html).\n\nThe [GSOD sample table](https://bigquery.cloud.google.com/table/bigquery-public-data:samples.gsod) contains weather information collected by NOAA, such as precipitation amounts and wind speeds from late 1929 to early 2010.\n",
"_____no_output_____"
],
[
"### Declare the Cloud project ID which will be used throughout this notebook",
"_____no_output_____"
]
],
[
[
"project_id = '[your project ID]'",
"_____no_output_____"
]
],
[
[
"### Sample approximately 2000 random rows",
"_____no_output_____"
]
],
[
[
"from google.cloud import bigquery\n\nclient = bigquery.Client(project=project_id)\n\nsample_count = 2000\nrow_count = client.query('''\n SELECT \n COUNT(*) as total\n FROM `bigquery-public-data.samples.gsod`''').to_dataframe().total[0]\n\ndf = client.query('''\n SELECT\n *\n FROM\n `bigquery-public-data.samples.gsod`\n WHERE RAND() < %d/%d\n''' % (sample_count, row_count)).to_dataframe()\n\nprint('Full dataset has %d rows' % row_count)",
"Full dataset has 114420316 rows\n"
]
],
[
[
"### Describe the sampled data",
"_____no_output_____"
]
],
[
[
"df.describe()",
"_____no_output_____"
]
],
[
[
"### View the first 10 rows",
"_____no_output_____"
]
],
[
[
"df.head(10)",
"_____no_output_____"
],
[
"# 10 highest total_precipitation samples\ndf.sort_values('total_precipitation', ascending=False).head(10)[['station_number', 'year', 'month', 'day', 'total_precipitation']]",
"_____no_output_____"
]
],
[
[
"# Use BigQuery through pandas-gbq\n\nThe `pandas-gbq` library is a community led project by the pandas community. It covers basic functionality, such as writing a DataFrame to BigQuery and running a query, but as a third-party library it may not handle all BigQuery features or use cases.\n\n[Pandas GBQ Documentation](https://pandas.pydata.org/pandas-docs/stable/generated/pandas.read_gbq.html)",
"_____no_output_____"
]
],
[
[
"import pandas as pd\n\nsample_count = 2000\ndf = pd.io.gbq.read_gbq('''\n SELECT name, SUM(number) as count\n FROM `bigquery-public-data.usa_names.usa_1910_2013`\n WHERE state = 'TX'\n GROUP BY name\n ORDER BY count DESC\n LIMIT 100\n''', project_id=project_id, dialect='standard')\n\ndf.head()",
"_____no_output_____"
]
],
[
[
"# Syntax highlighting\n`google.colab.syntax` can be used to add syntax highlighting to any Python string literals which are used in a query later.",
"_____no_output_____"
]
],
[
[
"from google.colab import syntax\nquery = syntax.sql('''\nSELECT\n COUNT(*) as total_rows\nFROM\n `bigquery-public-data.samples.gsod`\n''')\n\npd.io.gbq.read_gbq(query, project_id=project_id, dialect='standard')",
"_____no_output_____"
]
]
] | [
"markdown",
"code",
"markdown",
"code",
"markdown",
"code",
"markdown",
"code",
"markdown",
"code",
"markdown",
"code",
"markdown",
"code",
"markdown",
"code",
"markdown",
"code"
] | [
[
"markdown",
"markdown",
"markdown"
],
[
"code"
],
[
"markdown"
],
[
"code"
],
[
"markdown",
"markdown"
],
[
"code",
"code",
"code"
],
[
"markdown",
"markdown"
],
[
"code"
],
[
"markdown"
],
[
"code"
],
[
"markdown"
],
[
"code"
],
[
"markdown"
],
[
"code",
"code"
],
[
"markdown"
],
[
"code"
],
[
"markdown"
],
[
"code"
]
] |
d048617ada02d4e51ce8826fc2fe0ab16ef9324a | 268,877 | ipynb | Jupyter Notebook | _notebooks/2022-01-20-mab.ipynb | recohut/notebook | 610670666a1c3d8ef430d42f712ff72ecdbd8f86 | [
"Apache-2.0"
] | null | null | null | _notebooks/2022-01-20-mab.ipynb | recohut/notebook | 610670666a1c3d8ef430d42f712ff72ecdbd8f86 | [
"Apache-2.0"
] | 1 | 2022-01-12T05:40:57.000Z | 2022-01-12T05:40:57.000Z | _notebooks/2022-01-20-mab.ipynb | recohut/notebook | 610670666a1c3d8ef430d42f712ff72ecdbd8f86 | [
"Apache-2.0"
] | null | null | null | 268,877 | 268,877 | 0.933007 | [
[
[
"# Solving Multi-armed Bandit Problems",
"_____no_output_____"
],
[
"We will focus on how to solve the multi-armed bandit problem using four strategies, including epsilon-greedy, softmax exploration, upper confidence bound, and Thompson sampling. We will see how they deal with the exploration-exploitation dilemma in their own unique ways. We will also work on a billion-dollar problem, online advertising, and demonstrate how to solve it using a multi-armed bandit algorithm. Finally, we will solve the contextual advertising problem using contextual bandits to make more informed decisions in ad optimization.",
"_____no_output_____"
],
[
"## Creating a multi-armed bandit environment",
"_____no_output_____"
],
[
"The multi-armed bandit problem is one of the simplest reinforcement learning problems. It is best described as a slot machine with multiple levers (arms), and each lever has a different payout and payout probability. Our goal is to discover the best lever with the maximum return so that we can keep choosing it afterward. Let’s start with a simple multi-armed bandit problem in which the payout and payout probability is fixed for each arm. After creating the environment, we will solve it using the random policy algorithm.",
"_____no_output_____"
]
],
[
[
"import torch\n\n\nclass BanditEnv():\n \"\"\"\n Multi-armed bandit environment\n payout_list:\n A list of probabilities of the likelihood that a particular bandit will pay out\n reward_list:\n A list of rewards of the payout that bandit has\n \"\"\"\n def __init__(self, payout_list, reward_list):\n self.payout_list = payout_list\n self.reward_list = reward_list\n\n def step(self, action):\n if torch.rand(1).item() < self.payout_list[action]:\n return self.reward_list[action]\n return 0\n\n\n\nif __name__ == \"__main__\":\n bandit_payout = [0.1, 0.15, 0.3]\n bandit_reward = [4, 3, 1]\n bandit_env = BanditEnv(bandit_payout, bandit_reward)\n\n n_episode = 100000\n n_action = len(bandit_payout)\n action_count = [0 for _ in range(n_action)]\n action_total_reward = [0 for _ in range(n_action)]\n action_avg_reward = [[] for action in range(n_action)]\n\n def random_policy():\n action = torch.multinomial(torch.ones(n_action), 1).item()\n return action\n\n for episode in range(n_episode):\n action = random_policy()\n reward = bandit_env.step(action)\n action_count[action] += 1\n action_total_reward[action] += reward\n for a in range(n_action):\n if action_count[a]:\n action_avg_reward[a].append(action_total_reward[a] / action_count[a])\n else:\n action_avg_reward[a].append(0)\n\n\n import matplotlib.pyplot as plt\n \n for action in range(n_action):\n plt.plot(action_avg_reward[action])\n\n plt.legend(['Arm {}'.format(action) for action in range(n_action)])\n plt.xscale('log')\n plt.title('Average reward over time')\n plt.xlabel('Episode')\n plt.ylabel('Average reward')\n plt.show()",
"_____no_output_____"
]
],
[
[
"In the example we just worked on, there are three slot machines. Each machine has a different payout (reward) and payout probability. In each episode, we randomly chose one arm of the machine to pull (one action to execute) and get a payout at a certain probability.\n\n",
"_____no_output_____"
],
[
"Arm 1 is the best arm with the largest average reward. Also, the average rewards start to saturate round 10,000 episodes.\n\nThis solution seems very naive as we only perform an exploration of all arms. We will come up with more intelligent strategies in the upcoming sections.",
"_____no_output_____"
],
[
"## Solving multi-armed bandit problems with the epsilon-greedy policy\n\nInstead of exploring solely with random policy, we can do better with a combination of exploration and exploitation. Here comes the well-known epsilon-greedy policy.\n\nEpsilon-greedy for multi-armed bandits exploits the best action the majority of the time and also keeps exploring different actions from time to time. Given a parameter, ε, with a value from 0 to 1, the probabilities of performing exploration and exploitation are ε and 1 - ε, respectively.\n",
"_____no_output_____"
],
[
"Similar to other MDP problems, the epsilon-greedy policy selects the best arm with a probability of 1 - ε and performs random exploration with a probability of ε. Epsilon manages the trade-off between exploration and exploitation.",
"_____no_output_____"
]
],
[
[
"import torch\n\nbandit_payout = [0.1, 0.15, 0.3]\nbandit_reward = [4, 3, 1]\nbandit_env = BanditEnv(bandit_payout, bandit_reward)\n\nn_episode = 100000\nn_action = len(bandit_payout)\naction_count = [0 for _ in range(n_action)]\naction_total_reward = [0 for _ in range(n_action)]\naction_avg_reward = [[] for action in range(n_action)]\n\n\ndef gen_epsilon_greedy_policy(n_action, epsilon):\n def policy_function(Q):\n probs = torch.ones(n_action) * epsilon / n_action\n best_action = torch.argmax(Q).item()\n probs[best_action] += 1.0 - epsilon\n action = torch.multinomial(probs, 1).item()\n return action\n return policy_function\n\nepsilon = 0.2\n\nepsilon_greedy_policy = gen_epsilon_greedy_policy(n_action, epsilon)\n\n\nQ = torch.zeros(n_action)\n\nfor episode in range(n_episode):\n action = epsilon_greedy_policy(Q)\n reward = bandit_env.step(action)\n action_count[action] += 1\n action_total_reward[action] += reward\n Q[action] = action_total_reward[action] / action_count[action]\n for a in range(n_action):\n if action_count[a]:\n action_avg_reward[a].append(action_total_reward[a] / action_count[a])\n else:\n action_avg_reward[a].append(0)\n\n\nimport matplotlib.pyplot as plt\nfor action in range(n_action):\n plt.plot(action_avg_reward[action])\n\nplt.legend(['Arm {}'.format(action) for action in range(n_action)])\nplt.xscale('log')\nplt.title('Average reward over time')\nplt.xlabel('Episode')\nplt.ylabel('Average reward')\nplt.show()",
"_____no_output_____"
]
],
[
[
"\n\nArm 1 is the best arm, with the largest average reward at the end. Also, its average reward starts to saturate after around 1,000 episodes.",
"_____no_output_____"
],
[
"You may wonder whether the epsilon-greedy policy actually outperforms the random policy. Besides the fact that the value for the optimal arm converges earlier with the epsilon-greedy policy, we can also prove that, on average, the reward we get during the course of training is higher with the epsilon-greedy policy than the random policy.\n\nWe can simply average the reward over all episodes:",
"_____no_output_____"
]
],
[
[
"print(sum(action_total_reward) / n_episode)",
"0.43616\n"
]
],
[
[
"Over 100,000 episodes, the average payout is 0.43718 with the epsilon-greedy policy. Repeating the same computation for the random policy solution, we get 0.37902 as the average payout.",
"_____no_output_____"
],
[
"## Solving multi-armed bandit problems with the softmax exploration\n\nAs we've seen with epsilon-greedy, when performing exploration we randomly select one of the non-best arms with a probability of ε/|A|. Each non-best arm is treated equivalently regardless of its value in the Q function. Also, the best arm is chosen with a fixed probability regardless of its value. In softmax exploration, an arm is chosen based on a probability from the softmax distribution of the Q function values.",
"_____no_output_____"
],
[
"With the softmax exploration strategy, the dilemma of exploitation and exploration is solved with a softmax function based on the Q values. Instead of using a fixed pair of probabilities for the best arm and non-best arms, it adjusts the probabilities according to the softmax distribution with the τ parameter as a temperature factor. The higher the value of τ, the more focus will be shifted to exploration.",
"_____no_output_____"
]
],
[
[
"import torch\n\nbandit_payout = [0.1, 0.15, 0.3]\nbandit_reward = [4, 3, 1]\nbandit_env = BanditEnv(bandit_payout, bandit_reward)\n\nn_episode = 100000\nn_action = len(bandit_payout)\naction_count = [0 for _ in range(n_action)]\naction_total_reward = [0 for _ in range(n_action)]\naction_avg_reward = [[] for action in range(n_action)]\n\n\n\ndef gen_softmax_exploration_policy(tau):\n def policy_function(Q):\n probs = torch.exp(Q / tau)\n probs = probs / torch.sum(probs)\n action = torch.multinomial(probs, 1).item()\n return action\n return policy_function\n\ntau = 0.1\n\nsoftmax_exploration_policy = gen_softmax_exploration_policy(tau)\n\nQ = torch.zeros(n_action)\n\nfor episode in range(n_episode):\n action = softmax_exploration_policy(Q)\n reward = bandit_env.step(action)\n action_count[action] += 1\n action_total_reward[action] += reward\n Q[action] = action_total_reward[action] / action_count[action]\n\n for a in range(n_action):\n if action_count[a]:\n action_avg_reward[a].append(action_total_reward[a] / action_count[a])\n else:\n action_avg_reward[a].append(0)\n\n\nimport matplotlib.pyplot as plt\nfor action in range(n_action):\n plt.plot(action_avg_reward[action])\n\nplt.legend(['Arm {}'.format(action) for action in range(n_action)])\nplt.xscale('log')\nplt.title('Average reward over time')\nplt.xlabel('Episode')\nplt.ylabel('Average reward')\nplt.show()",
"_____no_output_____"
]
],
[
[
"Arm 1 is the best arm, with the largest average reward at the end. Also, its average reward starts to saturate after around 800 episodes in this example.",
"_____no_output_____"
],
[
"## Solving multi-armed bandit problems with the upper confidence bound algorithm\n\nIn the previous two recipes, we explored random actions in the multi-armed bandit problem with probabilities that are either assigned as fixed values in the epsilon-greedy policy or computed based on the Q-function values in the softmax exploration algorithm. In either algorithm, the probabilities of taking random actions are not adjusted over time. Ideally, we want less exploration as learning progresses. In this recipe, we will use a new algorithm called upper confidence bound to achieve this goal.\n\nThe upper confidence bound (UCB) algorithm stems from the idea of the confidence interval. In general, the confidence interval is a range of values where the true value lies. In the UCB algorithm, the confidence interval for an arm is a range where the mean reward obtained with this arm lies. The interval is in the form of [lower confidence bound, upper confidence bound] and we only use the upper bound, which is the UCB, to estimate the potential of the arm. The UCB is computed as follows:\n\n$$UCB(a) = Q(a) + \\sqrt{2log(t)/N(a)}$$\n\nHere, t is the number of episodes, and N(a) is the number of times arm a is chosen among t episodes. As learning progresses, the confidence interval shrinks and becomes more and more accurate. The arm to pull is the one with the highest UCB.",
"_____no_output_____"
],
[
"In this recipe, we solved the multi-armed bandit with the UCB algorithm. It adjusts the exploitation-exploration dilemma according to the number of episodes. For an action with a few data points, its confidence interval is relatively wide, hence, choosing this action is of relatively high uncertainty. With more episodes of the action being selected, the confidence interval becomes narrow and shrinks to its actual value. In this case, it is of high certainty to choose (or not) this action. Finally, the UCB algorithm pulls the arm with the highest UCB in each episode and gains more and more confidence over time.\n\n",
"_____no_output_____"
]
],
[
[
"import torch\n\nbandit_payout = [0.1, 0.15, 0.3]\nbandit_reward = [4, 3, 1]\nbandit_env = BanditEnv(bandit_payout, bandit_reward)\n\nn_episode = 100000\nn_action = len(bandit_payout)\naction_count = torch.tensor([0. for _ in range(n_action)])\naction_total_reward = [0 for _ in range(n_action)]\naction_avg_reward = [[] for action in range(n_action)]\n\n\n\ndef upper_confidence_bound(Q, action_count, t):\n ucb = torch.sqrt((2 * torch.log(torch.tensor(float(t)))) / action_count) + Q\n return torch.argmax(ucb)\n\n\n\nQ = torch.empty(n_action)\n\nfor episode in range(n_episode):\n action = upper_confidence_bound(Q, action_count, episode)\n reward = bandit_env.step(action)\n action_count[action] += 1\n action_total_reward[action] += reward\n Q[action] = action_total_reward[action] / action_count[action]\n\n for a in range(n_action):\n if action_count[a]:\n action_avg_reward[a].append(action_total_reward[a] / action_count[a])\n else:\n action_avg_reward[a].append(0)\n\n\nimport matplotlib.pyplot as plt\nfor action in range(n_action):\n plt.plot(action_avg_reward[action])\n\nplt.legend(['Arm {}'.format(action) for action in range(n_action)])\nplt.xscale('log')\nplt.title('Average reward over time')\nplt.xlabel('Episode')\nplt.ylabel('Average reward')\nplt.show()",
"_____no_output_____"
]
],
[
[
"Arm 1 is the best arm, with the largest average reward in the end.",
"_____no_output_____"
],
[
"You may wonder whether UCB actually outperforms the epsilon-greedy policy. We can compute the average reward over the entire training process, and the policy with the highest average reward learns faster.\n\nWe can simply average the reward over all episodes:",
"_____no_output_____"
]
],
[
[
"print(sum(action_total_reward) / n_episode)",
"0.4433\n"
]
],
[
[
"Over 100,000 episodes, the average payout is 0.44605 with UCB, which is higher than 0.43718 with the epsilon-greedy policy.",
"_____no_output_____"
],
[
"## Solving internet advertising problems with a multi-armed bandit\n\nImagine you are an advertiser working on ad optimization on a website:\n\n- There are three different colors of ad background – red, green, and blue. Which one will achieve the best click-through rate (CTR)?\n- There are three types of wordings of the ad – learn …, free ..., and try .... Which one will achieve the best CTR?\n\nFor each visitor, we need to choose an ad in order to maximize the CTR over time. How can we solve this?\n\nPerhaps you are thinking about A/B testing, where you randomly split the traffic into groups and assign each ad to a different group, and then choose the ad from the group with the highest CTR after a period of observation. However, this is basically a complete exploration, and we are usually unsure of how long the observation period should be and will end up losing a large portion of potential clicks. Besides, in A/B testing, the unknown CTR for an ad is assumed to not change over time. Otherwise, such A/B testing should be re-run periodically.\n\nA multi-armed bandit can certainly do better than A/B testing. Each arm is an ad, and the reward for an arm is either 1 (click) or 0 (no click).\n\nLet's try to solve it with the UCB algorithm.\n",
"_____no_output_____"
],
[
"In this recipe, we solved the ad optimization problem in a multi-armed bandit manner. It overcomes the challenges confronting the A/B testing approach. We used the UCB algorithm to solve the multi-armed (multi-ad) bandit problem; the reward for each arm is either 1 or 0. Instead of pure exploration and no interaction between action and reward, UCB (or other algorithms such as epsilon-greedy and softmax exploration) dynamically switches between exploitation and exploration where necessarly. For an ad with a few data points, the confidence interval is relatively wide, hence, choosing this action is of relatively high uncertainty. With more episodes of the ad being selected, the confidence interval becomes narrow and shrinks to its actual value.",
"_____no_output_____"
]
],
[
[
"import torch\n\nbandit_payout = [0.01, 0.015, 0.03]\nbandit_reward = [1, 1, 1]\nbandit_env = BanditEnv(bandit_payout, bandit_reward)\n\nn_episode = 100000\nn_action = len(bandit_payout)\naction_count = torch.tensor([0. for _ in range(n_action)])\naction_total_reward = [0 for _ in range(n_action)]\naction_avg_reward = [[] for action in range(n_action)]\n\n\n\ndef upper_confidence_bound(Q, action_count, t):\n ucb = torch.sqrt((2 * torch.log(torch.tensor(float(t)))) / action_count) + Q\n return torch.argmax(ucb)\n\n\n\nQ = torch.empty(n_action)\n\nfor episode in range(n_episode):\n action = upper_confidence_bound(Q, action_count, episode)\n reward = bandit_env.step(action)\n action_count[action] += 1\n action_total_reward[action] += reward\n Q[action] = action_total_reward[action] / action_count[action]\n\n for a in range(n_action):\n if action_count[a]:\n action_avg_reward[a].append(action_total_reward[a] / action_count[a])\n else:\n action_avg_reward[a].append(0)\n\n\nimport matplotlib.pyplot as plt\nfor action in range(n_action):\n plt.plot(action_avg_reward[action])\n\nplt.legend(['Arm {}'.format(action) for action in range(n_action)])\nplt.xscale('log')\nplt.title('Average reward over time')\nplt.xlabel('Episode')\nplt.ylabel('Average reward')\nplt.show()",
"_____no_output_____"
]
],
[
[
"Ad 2 is the best ad with the highest predicted CTR (average reward) after the model converges.\n\nEventually, we found that ad 2 is the optimal one to choose, which is true. Also, the sooner we figure this out the better, because we will lose fewer potential clicks. In this example, ad 2 outperformed the others after around 1000 episodes.",
"_____no_output_____"
],
[
"## Solving multi-armed bandit problems with the Thompson sampling algorithm\n\nIn this recipe, we will tackle the exploitation and exploration dilemma in the advertising bandits problem using another algorithm, Thompson sampling. We will see how it differs greatly from the previous three algorithms.\n\nThompson sampling (TS) is also called Bayesian bandits as it applies the Bayesian way of thinking from the following perspectives:\n\n- It is a probabilistic algorithm.\n- It computes the prior distribution for each arm and samples a value from each distribution.\n- It then selects the arm with the highest value and observes the reward.\n- Finally, it updates the prior distribution based on the observed reward. This process is called Bayesian updating.\n\nAs we have seen that in our ad optimization case, the reward for each arm is either 1 or 0. We can use beta distribution for our prior distribution because the value of the beta distribution is from 0 to 1. The beta distribution is parameterized by two parameters, α and β. α represents the number of times we receive the reward of 1 and β, indicates the number of times we receive the reward of 0.\n\nTo help you understand the beta distribution better, we will start by looking at several beta distributions before we implement the TS algorithm.",
"_____no_output_____"
]
],
[
[
"import torch\nimport matplotlib.pyplot as plt",
"_____no_output_____"
],
[
"beta1 = torch.distributions.beta.Beta(1, 1)\nsamples1 = [beta1.sample() for _ in range(100000)]\nplt.hist(samples1, range=[0, 1], bins=10)\nplt.title('beta(1, 1)')\nplt.show()",
"_____no_output_____"
],
[
"beta2 = torch.distributions.beta.Beta(5, 1)\nsamples2 = [beta2.sample() for _ in range(100000)]\nplt.hist(samples2, range=[0, 1], bins=10)\nplt.title('beta(5, 1)')\nplt.show()",
"_____no_output_____"
],
[
"beta3 = torch.distributions.beta.Beta(1, 5)\nsamples3= [beta3.sample() for _ in range(100000)]\nplt.hist(samples3, range=[0, 1], bins=10)\nplt.title('beta(1, 5)')\nplt.show()",
"_____no_output_____"
],
[
"beta4 = torch.distributions.beta.Beta(5, 5)\nsamples4= [beta4.sample() for _ in range(100000)]\nplt.hist(samples4, range=[0, 1], bins=10)\nplt.title('beta(5, 5)')\nplt.show()",
"_____no_output_____"
],
[
"bandit_payout = [0.01, 0.015, 0.03]\nbandit_reward = [1, 1, 1]\nbandit_env = BanditEnv(bandit_payout, bandit_reward)\n\nn_episode = 100000\nn_action = len(bandit_payout)\naction_count = torch.tensor([0. for _ in range(n_action)])\naction_total_reward = [0 for _ in range(n_action)]\naction_avg_reward = [[] for action in range(n_action)]",
"_____no_output_____"
]
],
[
[
"In this recipe, we solved the ad bandits problem with the TS algorithm. The biggest difference between TS and the three other approaches is the adoption of Bayesian optimization. It first computes the prior distribution for each possible arm, and then randomly draws a value from each distribution. It then picks the arm with the highest value and uses the observed outcome to update the prior distribution. The TS policy is both stochastic and greedy. If an ad is more likely to receive clicks, its beta distribution shifts toward 1 and, hence, the value of a random sample tends to be closer to 1.\n\n",
"_____no_output_____"
]
],
[
[
"def thompson_sampling(alpha, beta):\n prior_values = torch.distributions.beta.Beta(alpha, beta).sample()\n return torch.argmax(prior_values)\n\n\nalpha = torch.ones(n_action)\nbeta = torch.ones(n_action)\n\n\nfor episode in range(n_episode):\n action = thompson_sampling(alpha, beta)\n reward = bandit_env.step(action)\n action_count[action] += 1\n action_total_reward[action] += reward\n\n if reward > 0:\n alpha[action] += 1\n else:\n beta[action] += 1\n\n for a in range(n_action):\n if action_count[a]:\n action_avg_reward[a].append(action_total_reward[a] / action_count[a])\n else:\n action_avg_reward[a].append(0)\n\n\n\nfor action in range(n_action):\n plt.plot(action_avg_reward[action])\n\nplt.legend(['Arm {}'.format(action) for action in range(n_action)])\nplt.xscale('log')\nplt.title('Average reward over time')\nplt.xlabel('Episode')\nplt.ylabel('Average reward')\nplt.show()",
"_____no_output_____"
]
],
[
[
"Ad 2 is the best ad, with the highest predicted CTR (average reward).",
"_____no_output_____"
],
[
"## Solving internet advertising problems with contextual bandits\n\nYou may notice that in the ad optimization problem, we only care about the ad and ignore other information, such as user information and web page information, that might affect the ad being clicked on or not. In this recipe, we will talk about how we take more information into account beyond the ad itself and solve the problem with contextual bandits.\n\nThe multi-armed bandit problems we have worked with so far do not involve the concept of state, which is very different from MDPs. We only have several actions, and a reward will be generated that is associated with the action selected. Contextual bandits extend multi-armed bandits by introducing the concept of state. State provides a description of the environment, which helps the agent take more informed actions. In the advertising example, the state could be the user's gender (two states, male and female), the user’s age group (four states, for example), or page category (such as sports, finance, or news). Intuitively, users of certain demographics are more likely to click on an ad on certain pages.\n\nIt is not difficult to understand contextual bandits. A multi-armed bandit is a single machine with multiple arms, while contextual bandits are a set of such machines (bandits). Each machine in contextual bandits is a state that has multiple arms. The learning goal is to find the best arm (action) for each machine (state).\n\nWe will work with an advertising example with two states for simplicity.",
"_____no_output_____"
],
[
"In this recipe, we solved the contextual advertising problem with contextual bandits using the UCB algorithm.",
"_____no_output_____"
]
],
[
[
"import torch\n\nbandit_payout_machines = [\n [0.01, 0.015, 0.03],\n [0.025, 0.01, 0.015]\n]\nbandit_reward_machines = [\n [1, 1, 1],\n [1, 1, 1]\n]\nn_machine = len(bandit_payout_machines)\n\nbandit_env_machines = [BanditEnv(bandit_payout, bandit_reward)\n for bandit_payout, bandit_reward in\n zip(bandit_payout_machines, bandit_reward_machines)]\n\nn_episode = 100000\nn_action = len(bandit_payout_machines[0])\naction_count = torch.zeros(n_machine, n_action)\naction_total_reward = torch.zeros(n_machine, n_action)\naction_avg_reward = [[[] for action in range(n_action)] for _ in range(n_machine)]\n\n\n\ndef upper_confidence_bound(Q, action_count, t):\n ucb = torch.sqrt((2 * torch.log(torch.tensor(float(t)))) / action_count) + Q\n return torch.argmax(ucb)\n\n\n\nQ_machines = torch.empty(n_machine, n_action)\n\nfor episode in range(n_episode):\n state = torch.randint(0, n_machine, (1,)).item()\n\n action = upper_confidence_bound(Q_machines[state], action_count[state], episode)\n reward = bandit_env_machines[state].step(action)\n action_count[state][action] += 1\n action_total_reward[state][action] += reward\n Q_machines[state][action] = action_total_reward[state][action] / action_count[state][action]\n\n for a in range(n_action):\n if action_count[state][a]:\n action_avg_reward[state][a].append(action_total_reward[state][a] / action_count[state][a])\n else:\n action_avg_reward[state][a].append(0)\n\n\nimport matplotlib.pyplot as plt\n\nfor state in range(n_machine):\n for action in range(n_action):\n plt.plot(action_avg_reward[state][action])\n plt.legend(['Arm {}'.format(action) for action in range(n_action)])\n plt.xscale('log')\n plt.title('Average reward over time for state {}'.format(state))\n plt.xlabel('Episode')\n plt.ylabel('Average reward')\n plt.show()",
"_____no_output_____"
]
],
[
[
"Given the first state, ad 2 is the best ad, with the highest predicted CTR. Given the second state, ad 0 is the optimal ad, with the highest average reward. And these are both true.\n\nContextual bandits are a set of multi-armed bandits. Each bandit represents a unique state of the environment. The state provides a description of the environment, which helps the agent take more informed actions. In our advertising example, male users might be more likely to click an ad than female users. We simply used two slot machines to incorporate two states and searched for the best arm to pull given each state.\n\nOne thing to note is that contextual bandits are still different from MDPs, although they involve the concept of state. First, the states in contextual bandits are not determined by the previous actions or states, but are simply observations of the environment. Second, there is no delayed or discounted reward in contextual bandits because a bandit episode is one step. However, compared to multi-armed bandits, contextual bandits are closer to MDP as the actions are conditional to the states in the environment. It is safe to say that contextual bandits are in between multi-armed bandits and full MDP reinforcement learning.",
"_____no_output_____"
]
]
] | [
"markdown",
"code",
"markdown",
"code",
"markdown",
"code",
"markdown",
"code",
"markdown",
"code",
"markdown",
"code",
"markdown",
"code",
"markdown",
"code",
"markdown",
"code",
"markdown",
"code",
"markdown"
] | [
[
"markdown",
"markdown",
"markdown",
"markdown"
],
[
"code"
],
[
"markdown",
"markdown",
"markdown",
"markdown"
],
[
"code"
],
[
"markdown",
"markdown"
],
[
"code"
],
[
"markdown",
"markdown",
"markdown"
],
[
"code"
],
[
"markdown",
"markdown",
"markdown"
],
[
"code"
],
[
"markdown",
"markdown"
],
[
"code"
],
[
"markdown",
"markdown",
"markdown"
],
[
"code"
],
[
"markdown",
"markdown"
],
[
"code",
"code",
"code",
"code",
"code",
"code"
],
[
"markdown"
],
[
"code"
],
[
"markdown",
"markdown",
"markdown"
],
[
"code"
],
[
"markdown"
]
] |
d0487a4fc564a2d0843276296cc042fa5e982b8f | 54,951 | ipynb | Jupyter Notebook | site/zh-cn/guide/ragged_tensor.ipynb | RedContritio/docs-l10n | f69a7c0d2157703a26cef95bac34b39ac0250373 | [
"Apache-2.0"
] | 1 | 2022-03-29T22:32:18.000Z | 2022-03-29T22:32:18.000Z | site/zh-cn/guide/ragged_tensor.ipynb | RedContritio/docs-l10n | f69a7c0d2157703a26cef95bac34b39ac0250373 | [
"Apache-2.0"
] | null | null | null | site/zh-cn/guide/ragged_tensor.ipynb | RedContritio/docs-l10n | f69a7c0d2157703a26cef95bac34b39ac0250373 | [
"Apache-2.0"
] | null | null | null | 29.338494 | 371 | 0.475387 | [
[
[
"##### Copyright 2018 The TensorFlow Authors.",
"_____no_output_____"
]
],
[
[
"#@title Licensed under the Apache License, Version 2.0 (the \"License\");\n# you may not use this file except in compliance with the License.\n# You may obtain a copy of the License at\n#\n# https://www.apache.org/licenses/LICENSE-2.0\n#\n# Unless required by applicable law or agreed to in writing, software\n# distributed under the License is distributed on an \"AS IS\" BASIS,\n# WITHOUT WARRANTIES OR CONDITIONS OF ANY KIND, either express or implied.\n# See the License for the specific language governing permissions and\n# limitations under the License.",
"_____no_output_____"
]
],
[
[
"# 不规则张量\n\n<table class=\"tfo-notebook-buttons\" align=\"left\">\n <td> <a target=\"_blank\" href=\"https://tensorflow.google.cn/guide/ragged_tensor\"><img src=\"https://tensorflow.google.cn/images/tf_logo_32px.png\">在 TensorFlow.org 上查看</a>\n</td>\n <td><a target=\"_blank\" href=\"https://colab.research.google.com/github/tensorflow/docs-l10n/blob/master/site/zh-cn/guide/ragged_tensor.ipynb\"><img src=\"https://tensorflow.google.cn/images/colab_logo_32px.png\">在 Google Colab 中运行 </a></td>\n <td><a target=\"_blank\" href=\"https://github.com/tensorflow/docs-l10n/blob/master/site/zh-cn/guide/ragged_tensor.ipynb\"><img src=\"https://tensorflow.google.cn/images/GitHub-Mark-32px.png\">在 Github 上查看源代码</a></td>\n <td><a href=\"https://storage.googleapis.com/tensorflow_docs/docs/site/en/guide/ragged_tensor.ipynb\">{img}下载笔记本</a></td>\n</table>",
"_____no_output_____"
],
[
"**API 文档:** [`tf.RaggedTensor`](https://tensorflow.google.cn/api_docs/python/tf/RaggedTensor) [`tf.ragged`](https://tensorflow.google.cn/api_docs/python/tf/ragged)",
"_____no_output_____"
],
[
"## 设置",
"_____no_output_____"
]
],
[
[
"!pip install -q tf_nightly\nimport math\nimport tensorflow as tf",
"_____no_output_____"
]
],
[
[
"## 概述\n\n数据有多种形状;张量也应当有多种形状。*不规则张量*是嵌套的可变长度列表的 TensorFlow 等效项。它们使存储和处理包含非均匀形状的数据变得容易,包括:\n\n- 可变长度特征,例如电影的演员名单。\n- 成批的可变长度顺序输入,例如句子或视频剪辑。\n- 分层输入,例如细分为节、段落、句子和单词的文本文档。\n- 结构化输入中的各个字段,例如协议缓冲区。\n",
"_____no_output_____"
],
[
"### 不规则张量的功能\n\n有一百多种 TensorFlow 运算支持不规则张量,包括数学运算(如 `tf.add` 和 `tf.reduce_mean`)、数组运算(如 `tf.concat` 和 `tf.tile`)、字符串操作运算(如 `tf.substr`)、控制流运算(如 `tf.while_loop` 和 `tf.map_fn`)等:",
"_____no_output_____"
]
],
[
[
"digits = tf.ragged.constant([[3, 1, 4, 1], [], [5, 9, 2], [6], []])\nwords = tf.ragged.constant([[\"So\", \"long\"], [\"thanks\", \"for\", \"all\", \"the\", \"fish\"]])\nprint(tf.add(digits, 3))\nprint(tf.reduce_mean(digits, axis=1))\nprint(tf.concat([digits, [[5, 3]]], axis=0))\nprint(tf.tile(digits, [1, 2]))\nprint(tf.strings.substr(words, 0, 2))\nprint(tf.map_fn(tf.math.square, digits))",
"_____no_output_____"
]
],
[
[
"还有专门针对不规则张量的方法和运算,包括工厂方法、转换方法和值映射运算。有关支持的运算列表,请参阅 **`tf.ragged` 包文档**。",
"_____no_output_____"
],
[
"许多 TensorFlow API 都支持不规则张量,包括 [Keras](https://tensorflow.google.cn/guide/keras)、[Dataset](https://tensorflow.google.cn/guide/data)、[tf.function](https://tensorflow.google.cn/guide/function)、[SavedModel](https://tensorflow.google.cn/guide/saved_model) 和 [tf.Example](https://tensorflow.google.cn/tutorials/load_data/tfrecord)。有关更多信息,请参阅下面的 **TensorFlow API** 一节。",
"_____no_output_____"
],
[
"与普通张量一样,您可以使用 Python 风格的索引来访问不规则张量的特定切片。有关更多信息,请参阅下面的**索引**一节。",
"_____no_output_____"
]
],
[
[
"print(digits[0]) # First row",
"_____no_output_____"
],
[
"print(digits[:, :2]) # First two values in each row.",
"_____no_output_____"
],
[
"print(digits[:, -2:]) # Last two values in each row.",
"_____no_output_____"
]
],
[
[
"与普通张量一样,您可以使用 Python 算术和比较运算符来执行逐元素运算。有关更多信息,请参阅下面的**重载运算符**一节。",
"_____no_output_____"
]
],
[
[
"print(digits + 3)",
"_____no_output_____"
],
[
"print(digits + tf.ragged.constant([[1, 2, 3, 4], [], [5, 6, 7], [8], []]))",
"_____no_output_____"
]
],
[
[
"如果需要对 `RaggedTensor` 的值进行逐元素转换,您可以使用 `tf.ragged.map_flat_values`(它采用一个函数加上一个或多个参数的形式),并应用这个函数来转换 `RaggedTensor` 的值。",
"_____no_output_____"
]
],
[
[
"times_two_plus_one = lambda x: x * 2 + 1\nprint(tf.ragged.map_flat_values(times_two_plus_one, digits))",
"_____no_output_____"
]
],
[
[
"不规则张量可以转换为嵌套的 Python `list` 和 numpy `array`:",
"_____no_output_____"
]
],
[
[
"digits.to_list()",
"_____no_output_____"
],
[
"digits.numpy()",
"_____no_output_____"
]
],
[
[
"### 构造不规则张量\n\n构造不规则张量的最简单方法是使用 `tf.ragged.constant`,它会构建与给定的嵌套 Python `list` 或 numpy `array` 相对应的 `RaggedTensor`:",
"_____no_output_____"
]
],
[
[
"sentences = tf.ragged.constant([\n [\"Let's\", \"build\", \"some\", \"ragged\", \"tensors\", \"!\"],\n [\"We\", \"can\", \"use\", \"tf.ragged.constant\", \".\"]])\nprint(sentences)",
"_____no_output_____"
],
[
"paragraphs = tf.ragged.constant([\n [['I', 'have', 'a', 'cat'], ['His', 'name', 'is', 'Mat']],\n [['Do', 'you', 'want', 'to', 'come', 'visit'], [\"I'm\", 'free', 'tomorrow']],\n])\nprint(paragraphs)",
"_____no_output_____"
]
],
[
[
"还可以通过将扁平的*值*张量与*行分区*张量进行配对来构造不规则张量,行分区张量使用 `tf.RaggedTensor.from_value_rowids`、`tf.RaggedTensor.from_row_lengths` 和 `tf.RaggedTensor.from_row_splits` 等工厂类方法指示如何将值分成各行。\n\n#### `tf.RaggedTensor.from_value_rowids`\n\n如果知道每个值属于哪一行,可以使用 `value_rowids` 行分区张量构建 `RaggedTensor`:\n\n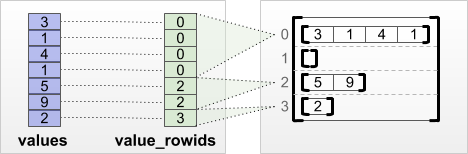",
"_____no_output_____"
]
],
[
[
"print(tf.RaggedTensor.from_value_rowids(\n values=[3, 1, 4, 1, 5, 9, 2],\n value_rowids=[0, 0, 0, 0, 2, 2, 3]))",
"_____no_output_____"
]
],
[
[
"#### `tf.RaggedTensor.from_row_lengths`\n\n如果知道每行的长度,可以使用 `row_lengths` 行分区张量:\n\n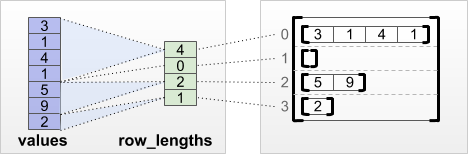",
"_____no_output_____"
]
],
[
[
"print(tf.RaggedTensor.from_row_lengths(\n values=[3, 1, 4, 1, 5, 9, 2],\n row_lengths=[4, 0, 2, 1]))",
"_____no_output_____"
]
],
[
[
"#### `tf.RaggedTensor.from_row_splits`\n\n如果知道指示每行开始和结束的索引,可以使用 `row_splits` 行分区张量:\n\n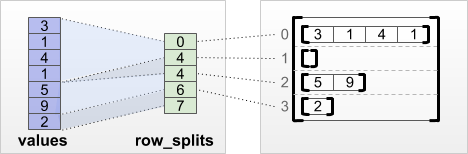",
"_____no_output_____"
]
],
[
[
"print(tf.RaggedTensor.from_row_splits(\n values=[3, 1, 4, 1, 5, 9, 2],\n row_splits=[0, 4, 4, 6, 7]))",
"_____no_output_____"
]
],
[
[
"有关完整的工厂方法列表,请参阅 `tf.RaggedTensor` 类文档。\n\n注:默认情况下,这些工厂方法会添加断言,说明行分区张量结构良好且与值数量保持一致。如果您能够保证输入的结构良好且一致,可以使用 `validate=False` 参数跳过此类检查。",
"_____no_output_____"
],
[
"### 可以在不规则张量中存储什么\n\n与普通 `Tensor` 一样,`RaggedTensor` 中的所有值必须具有相同的类型;所有值必须处于相同的嵌套深度(张量的*秩*):",
"_____no_output_____"
]
],
[
[
"print(tf.ragged.constant([[\"Hi\"], [\"How\", \"are\", \"you\"]])) # ok: type=string, rank=2",
"_____no_output_____"
],
[
"print(tf.ragged.constant([[[1, 2], [3]], [[4, 5]]])) # ok: type=int32, rank=3",
"_____no_output_____"
],
[
"try:\n tf.ragged.constant([[\"one\", \"two\"], [3, 4]]) # bad: multiple types\nexcept ValueError as exception:\n print(exception)",
"_____no_output_____"
],
[
"try:\n tf.ragged.constant([\"A\", [\"B\", \"C\"]]) # bad: multiple nesting depths\nexcept ValueError as exception:\n print(exception)",
"_____no_output_____"
]
],
[
[
"## 示例用例\n\n以下示例演示了如何使用 `RaggedTensor`,通过为每个句子的开头和结尾使用特殊标记,为一批可变长度查询构造和组合一元元组与二元元组嵌入。有关本例中使用的运算的更多详细信息,请参阅 `tf.ragged` 包文档。",
"_____no_output_____"
]
],
[
[
"queries = tf.ragged.constant([['Who', 'is', 'Dan', 'Smith'],\n ['Pause'],\n ['Will', 'it', 'rain', 'later', 'today']])\n\n# Create an embedding table.\nnum_buckets = 1024\nembedding_size = 4\nembedding_table = tf.Variable(\n tf.random.truncated_normal([num_buckets, embedding_size],\n stddev=1.0 / math.sqrt(embedding_size)))\n\n# Look up the embedding for each word.\nword_buckets = tf.strings.to_hash_bucket_fast(queries, num_buckets)\nword_embeddings = tf.nn.embedding_lookup(embedding_table, word_buckets) # ①\n\n# Add markers to the beginning and end of each sentence.\nmarker = tf.fill([queries.nrows(), 1], '#')\npadded = tf.concat([marker, queries, marker], axis=1) # ②\n\n# Build word bigrams & look up embeddings.\nbigrams = tf.strings.join([padded[:, :-1], padded[:, 1:]], separator='+') # ③\n\nbigram_buckets = tf.strings.to_hash_bucket_fast(bigrams, num_buckets)\nbigram_embeddings = tf.nn.embedding_lookup(embedding_table, bigram_buckets) # ④\n\n# Find the average embedding for each sentence\nall_embeddings = tf.concat([word_embeddings, bigram_embeddings], axis=1) # ⑤\navg_embedding = tf.reduce_mean(all_embeddings, axis=1) # ⑥\nprint(avg_embedding)",
"_____no_output_____"
]
],
[
[
"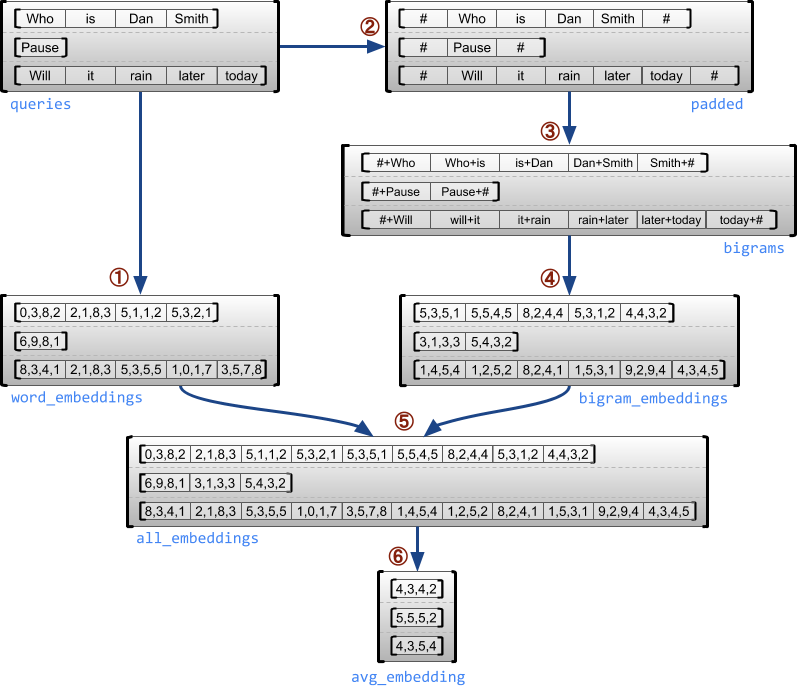",
"_____no_output_____"
],
[
"## 不规则维度和均匀维度\n\n***不规则维度***是切片可能具有不同长度的维度。例如,`rt=[[3, 1, 4, 1], [], [5, 9, 2], [6], []]` 的内部(列)维度是不规则的,因为列切片 (`rt[0, :]`, ..., `rt[4, :]`) 具有不同的长度。切片全都具有相同长度的维度称为*均匀维度*。\n\n不规则张量的最外层维始终是均匀维度,因为它只包含一个切片(因此不可能有不同的切片长度)。其余维度可能是不规则维度也可能是均匀维度。例如,我们可以使用形状为 `[num_sentences, (num_words), embedding_size]` 的不规则张量为一批句子中的每个单词存储单词嵌入,其中 `(num_words)` 周围的括号表示维度是不规则维度。\n\n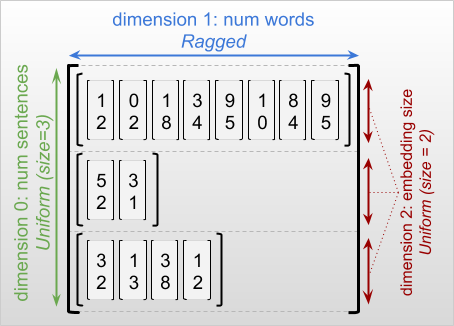\n\n不规则张量可以有多个不规则维度。例如,我们可以使用形状为 `[num_documents, (num_paragraphs), (num_sentences), (num_words)]` 的张量存储一批结构化文本文档(其中,括号同样用于表示不规则维度)。\n\n与 `tf.Tensor` 一样,不规则张量的***秩***是其总维数(包括不规则维度和均匀维度)。***潜在的不规则张量***是一个值,这个值可能是 `tf.Tensor` 或 `tf.RaggedTensor`。\n\n描述 RaggedTensor 的形状时,按照惯例,不规则维度会通过括号进行指示。例如,如上面所见,存储一批句子中每个单词的单词嵌入的三维 RaggedTensor 的形状可以写为 `[num_sentences, (num_words), embedding_size]`。\n\n`RaggedTensor.shape` 特性返回不规则张量的 `tf.TensorShape`,其中不规则维度的大小为 `None`:\n",
"_____no_output_____"
]
],
[
[
"tf.ragged.constant([[\"Hi\"], [\"How\", \"are\", \"you\"]]).shape",
"_____no_output_____"
]
],
[
[
"可以使用方法 `tf.RaggedTensor.bounding_shape` 查找给定 `RaggedTensor` 的紧密边界形状:",
"_____no_output_____"
]
],
[
[
"print(tf.ragged.constant([[\"Hi\"], [\"How\", \"are\", \"you\"]]).bounding_shape())",
"_____no_output_____"
]
],
[
[
"## 不规则张量和稀疏张量对比\n\n不规则张量*不*应该被认为是一种稀疏张量。尤其是,稀疏张量是以紧凑的格式对相同数据建模的 *tf.Tensor 的高效编码*;而不规则张量是对扩展的数据类建模的 *tf.Tensor 的延伸*。这种区别在定义运算时至关重要:\n\n- 对稀疏张量或密集张量应用某一运算应当始终获得相同结果。\n- 对不规则张量或稀疏张量应用某一运算可能获得不同结果。\n\n一个说明性的示例是,考虑如何为不规则张量和稀疏张量定义 `concat`、`stack` 和 `tile` 之类的数组运算。连接不规则张量时,会将每一行连在一起,形成一个具有组合长度的行:\n\n\n",
"_____no_output_____"
]
],
[
[
"ragged_x = tf.ragged.constant([[\"John\"], [\"a\", \"big\", \"dog\"], [\"my\", \"cat\"]])\nragged_y = tf.ragged.constant([[\"fell\", \"asleep\"], [\"barked\"], [\"is\", \"fuzzy\"]])\nprint(tf.concat([ragged_x, ragged_y], axis=1))",
"_____no_output_____"
]
],
[
[
"但连接稀疏张量时,相当于连接相应的密集张量,如以下示例所示(其中 Ø 表示缺失的值):\n\n\n",
"_____no_output_____"
]
],
[
[
"sparse_x = ragged_x.to_sparse()\nsparse_y = ragged_y.to_sparse()\nsparse_result = tf.sparse.concat(sp_inputs=[sparse_x, sparse_y], axis=1)\nprint(tf.sparse.to_dense(sparse_result, ''))",
"_____no_output_____"
]
],
[
[
"另一个说明为什么这种区别非常重要的示例是,考虑一个运算(如 `tf.reduce_mean`)的“每行平均值”的定义。对于不规则张量,一行的平均值是该行的值总和除以该行的宽度。但对于稀疏张量来说,一行的平均值是该行的值总和除以稀疏张量的总宽度(大于等于最长行的宽度)。\n",
"_____no_output_____"
],
[
"## TensorFlow API",
"_____no_output_____"
],
[
"### Keras\n\n[tf.keras](https://tensorflow.google.cn/guide/keras) 是 TensorFlow 的高级 API,用于构建和训练深度学习模型。通过在 `tf.keras.Input` 或 `tf.keras.layers.InputLayer` 上设置 `ragged=True`,不规则张量可以作为输入传送到 Keras 模型。不规则张量还可以在 Keras 层之间传递,并由 Keras 模型返回。以下示例显示了一个使用不规则张量训练的小 LSTM 模型。",
"_____no_output_____"
]
],
[
[
"# Task: predict whether each sentence is a question or not.\nsentences = tf.constant(\n ['What makes you think she is a witch?',\n 'She turned me into a newt.',\n 'A newt?',\n 'Well, I got better.'])\nis_question = tf.constant([True, False, True, False])\n\n# Preprocess the input strings.\nhash_buckets = 1000\nwords = tf.strings.split(sentences, ' ')\nhashed_words = tf.strings.to_hash_bucket_fast(words, hash_buckets)\n\n# Build the Keras model.\nkeras_model = tf.keras.Sequential([\n tf.keras.layers.Input(shape=[None], dtype=tf.int64, ragged=True),\n tf.keras.layers.Embedding(hash_buckets, 16),\n tf.keras.layers.LSTM(32, use_bias=False),\n tf.keras.layers.Dense(32),\n tf.keras.layers.Activation(tf.nn.relu),\n tf.keras.layers.Dense(1)\n])\n\nkeras_model.compile(loss='binary_crossentropy', optimizer='rmsprop')\nkeras_model.fit(hashed_words, is_question, epochs=5)\nprint(keras_model.predict(hashed_words))",
"_____no_output_____"
]
],
[
[
"### tf.Example\n\n[tf.Example](https://tensorflow.google.cn/tutorials/load_data/tfrecord) 是 TensorFlow 数据的标准 [protobuf](https://developers.google.com/protocol-buffers/) 编码。使用 `tf.Example` 编码的数据往往包括可变长度特征。例如,以下代码定义了一批具有不同特征长度的四条 `tf.Example` 消息:",
"_____no_output_____"
]
],
[
[
"import google.protobuf.text_format as pbtext\n\ndef build_tf_example(s):\n return pbtext.Merge(s, tf.train.Example()).SerializeToString()\n\nexample_batch = [\n build_tf_example(r'''\n features {\n feature {key: \"colors\" value {bytes_list {value: [\"red\", \"blue\"]} } }\n feature {key: \"lengths\" value {int64_list {value: [7]} } } }'''),\n build_tf_example(r'''\n features {\n feature {key: \"colors\" value {bytes_list {value: [\"orange\"]} } }\n feature {key: \"lengths\" value {int64_list {value: []} } } }'''),\n build_tf_example(r'''\n features {\n feature {key: \"colors\" value {bytes_list {value: [\"black\", \"yellow\"]} } }\n feature {key: \"lengths\" value {int64_list {value: [1, 3]} } } }'''),\n build_tf_example(r'''\n features {\n feature {key: \"colors\" value {bytes_list {value: [\"green\"]} } }\n feature {key: \"lengths\" value {int64_list {value: [3, 5, 2]} } } }''')]",
"_____no_output_____"
]
],
[
[
"我们可以使用 `tf.io.parse_example` 解析这个编码数据,它采用序列化字符串的张量和特征规范字典,并将字典映射特征名称返回给张量。要将长度可变特征读入不规则张量,我们只需在特征规范字典中使用 `tf.io.RaggedFeature` 即可:",
"_____no_output_____"
]
],
[
[
"feature_specification = {\n 'colors': tf.io.RaggedFeature(tf.string),\n 'lengths': tf.io.RaggedFeature(tf.int64),\n}\nfeature_tensors = tf.io.parse_example(example_batch, feature_specification)\nfor name, value in feature_tensors.items():\n print(\"{}={}\".format(name, value))",
"_____no_output_____"
]
],
[
[
"`tf.io.RaggedFeature` 还可用于读取具有多个不规则维度的特征。有关详细信息,请参阅 [API 文档](https://tensorflow.google.cn/api_docs/python/tf/io/RaggedFeature)。",
"_____no_output_____"
],
[
"### 数据集\n\n[tf.data](https://tensorflow.google.cn/guide/data) 是一个 API,可用于通过简单的可重用代码块构建复杂的输入流水线。它的核心数据结构是 `tf.data.Dataset`,表示一系列元素,每个元素包含一个或多个分量。 ",
"_____no_output_____"
]
],
[
[
"# Helper function used to print datasets in the examples below.\ndef print_dictionary_dataset(dataset):\n for i, element in enumerate(dataset):\n print(\"Element {}:\".format(i))\n for (feature_name, feature_value) in element.items():\n print('{:>14} = {}'.format(feature_name, feature_value))",
"_____no_output_____"
]
],
[
[
"#### 使用不规则张量构建数据集\n\n可以采用通过 `tf.Tensor` 或 numpy `array` 构建数据集时使用的方法,如 `Dataset.from_tensor_slices`,通过不规则张量构建数据集:",
"_____no_output_____"
]
],
[
[
"dataset = tf.data.Dataset.from_tensor_slices(feature_tensors)\nprint_dictionary_dataset(dataset)",
"_____no_output_____"
]
],
[
[
"注:`Dataset.from_generator` 目前还不支持不规则张量,但不久后将会支持这种张量。",
"_____no_output_____"
],
[
"#### 批处理和取消批处理具有不规则张量的数据集\n\n可以使用 `Dataset.batch` 方法对具有不规则张量的数据集进行批处理(将 *n* 个连续元素组合成单个元素)。",
"_____no_output_____"
]
],
[
[
"batched_dataset = dataset.batch(2)\nprint_dictionary_dataset(batched_dataset)",
"_____no_output_____"
]
],
[
[
"相反,可以使用 `Dataset.unbatch` 将批处理后的数据集转换为扁平数据集。",
"_____no_output_____"
]
],
[
[
"unbatched_dataset = batched_dataset.unbatch()\nprint_dictionary_dataset(unbatched_dataset)",
"_____no_output_____"
]
],
[
[
"#### 对具有可变长度非不规则张量的数据集进行批处理\n\n如果您有一个包含非不规则张量的数据集,而且各个元素的张量长度不同,则可以应用 `dense_to_ragged_batch` 转换,将这些非不规则张量批处理成不规则张量:",
"_____no_output_____"
]
],
[
[
"non_ragged_dataset = tf.data.Dataset.from_tensor_slices([1, 5, 3, 2, 8])\nnon_ragged_dataset = non_ragged_dataset.map(tf.range)\nbatched_non_ragged_dataset = non_ragged_dataset.apply(\n tf.data.experimental.dense_to_ragged_batch(2))\nfor element in batched_non_ragged_dataset:\n print(element)",
"_____no_output_____"
]
],
[
[
"#### 转换具有不规则张量的数据集\n\n还可以使用 `Dataset.map` 创建或转换数据集中的不规则张量。",
"_____no_output_____"
]
],
[
[
"def transform_lengths(features):\n return {\n 'mean_length': tf.math.reduce_mean(features['lengths']),\n 'length_ranges': tf.ragged.range(features['lengths'])}\ntransformed_dataset = dataset.map(transform_lengths)\nprint_dictionary_dataset(transformed_dataset)",
"_____no_output_____"
]
],
[
[
"### tf.function\n\n[tf.function](https://tensorflow.google.cn/guide/function) 是预计算 Python 函数的 TensorFlow 计算图的装饰器,它可以大幅改善 TensorFlow 代码的性能。不规则张量能够透明地与 `@tf.function` 装饰的函数一起使用。例如,以下函数对不规则张量和非不规则张量均有效:",
"_____no_output_____"
]
],
[
[
"@tf.function\ndef make_palindrome(x, axis):\n return tf.concat([x, tf.reverse(x, [axis])], axis)",
"_____no_output_____"
],
[
"make_palindrome(tf.constant([[1, 2], [3, 4], [5, 6]]), axis=1)",
"_____no_output_____"
],
[
"make_palindrome(tf.ragged.constant([[1, 2], [3], [4, 5, 6]]), axis=1)",
"_____no_output_____"
]
],
[
[
"如果您希望为 `tf.function` 明确指定 `input_signature`,可以使用 `tf.RaggedTensorSpec` 执行此操作。",
"_____no_output_____"
]
],
[
[
"@tf.function(\n input_signature=[tf.RaggedTensorSpec(shape=[None, None], dtype=tf.int32)])\ndef max_and_min(rt):\n return (tf.math.reduce_max(rt, axis=-1), tf.math.reduce_min(rt, axis=-1))\n\nmax_and_min(tf.ragged.constant([[1, 2], [3], [4, 5, 6]]))",
"_____no_output_____"
]
],
[
[
"#### 具体函数\n\n[具体函数](https://tensorflow.google.cn/guide/function#obtaining_concrete_functions)封装通过 `tf.function` 构建的各个跟踪图。不规则张量可以透明地与具体函数一起使用。\n",
"_____no_output_____"
]
],
[
[
"# Preferred way to use ragged tensors with concrete functions (TF 2.3+):\ntry:\n @tf.function\n def increment(x):\n return x + 1\n\n rt = tf.ragged.constant([[1, 2], [3], [4, 5, 6]])\n cf = increment.get_concrete_function(rt)\n print(cf(rt))\nexcept Exception as e:\n print(f\"Not supported before TF 2.3: {type(e)}: {e}\")\n",
"_____no_output_____"
]
],
[
[
"### SavedModel\n\n[SavedModel](https://tensorflow.google.cn/guide/saved_model) 是序列化 TensorFlow 程序,包括权重和计算。它可以通过 Keras 模型或自定义模型构建。在任何一种情况下,不规则张量都可以透明地与 SavedModel 定义的函数和方法一起使用。\n",
"_____no_output_____"
],
[
"#### 示例:保存 Keras 模型",
"_____no_output_____"
]
],
[
[
"import tempfile\n\nkeras_module_path = tempfile.mkdtemp()\ntf.saved_model.save(keras_model, keras_module_path)\nimported_model = tf.saved_model.load(keras_module_path)\nimported_model(hashed_words)",
"_____no_output_____"
]
],
[
[
"#### 示例:保存自定义模型\n",
"_____no_output_____"
]
],
[
[
"class CustomModule(tf.Module):\n def __init__(self, variable_value):\n super(CustomModule, self).__init__()\n self.v = tf.Variable(variable_value)\n\n @tf.function\n def grow(self, x):\n return x * self.v\n\nmodule = CustomModule(100.0)\n\n# Before saving a custom model, we must ensure that concrete functions are\n# built for each input signature that we will need.\nmodule.grow.get_concrete_function(tf.RaggedTensorSpec(shape=[None, None],\n dtype=tf.float32))\n\ncustom_module_path = tempfile.mkdtemp()\ntf.saved_model.save(module, custom_module_path)\nimported_model = tf.saved_model.load(custom_module_path)\nimported_model.grow(tf.ragged.constant([[1.0, 4.0, 3.0], [2.0]]))",
"_____no_output_____"
]
],
[
[
"注:SavedModel [签名](https://tensorflow.google.cn/guide/saved_model#specifying_signatures_during_export)是具体函数。如上文的“具体函数”部分所述,从 TensorFlow 2.3 开始,只有具体函数才能正确处理不规则张量。如果您需要在先前版本的 TensorFlow 中使用 SavedModel 签名,建议您将不规则张量分解成其张量分量。",
"_____no_output_____"
],
[
"## 重载运算符\n\n`RaggedTensor` 类会重载标准 Python 算术和比较运算符,使其易于执行基本的逐元素数学:",
"_____no_output_____"
]
],
[
[
"x = tf.ragged.constant([[1, 2], [3], [4, 5, 6]])\ny = tf.ragged.constant([[1, 1], [2], [3, 3, 3]])\nprint(x + y)",
"_____no_output_____"
]
],
[
[
"由于重载运算符执行逐元素计算,因此所有二进制运算的输入必须具有相同的形状,或者可以广播至相同的形状。在最简单的广播情况下,单个标量与不规则张量中的每个值逐元素组合:",
"_____no_output_____"
]
],
[
[
"x = tf.ragged.constant([[1, 2], [3], [4, 5, 6]])\nprint(x + 3)",
"_____no_output_____"
]
],
[
[
"有关更高级的用例,请参阅**广播**一节。\n\n不规则张量重载与正常 `Tensor` 相同的一组运算符:一元运算符 `-`、`~` 和 `abs()`;二元运算符 `+`、`-`、`*`、`/`、`//`、`%`、`**`、`&`、`|`、`^`、`==`、`<`、`<=`、`>` 和 `>=`。\n",
"_____no_output_____"
],
[
"## 索引\n\n不规则张量支持 Python 风格的索引,包括多维索引和切片。以下示例使用二维和三维不规则张量演示了不规则张量索引。",
"_____no_output_____"
],
[
"### 索引示例:二维不规则张量",
"_____no_output_____"
]
],
[
[
"queries = tf.ragged.constant(\n [['Who', 'is', 'George', 'Washington'],\n ['What', 'is', 'the', 'weather', 'tomorrow'],\n ['Goodnight']])",
"_____no_output_____"
],
[
"print(queries[1]) # A single query",
"_____no_output_____"
],
[
"print(queries[1, 2]) # A single word",
"_____no_output_____"
],
[
"print(queries[1:]) # Everything but the first row",
"_____no_output_____"
],
[
"print(queries[:, :3]) # The first 3 words of each query",
"_____no_output_____"
],
[
"print(queries[:, -2:]) # The last 2 words of each query",
"_____no_output_____"
]
],
[
[
"### 索引示例:三维不规则张量",
"_____no_output_____"
]
],
[
[
"rt = tf.ragged.constant([[[1, 2, 3], [4]],\n [[5], [], [6]],\n [[7]],\n [[8, 9], [10]]])",
"_____no_output_____"
],
[
"print(rt[1]) # Second row (2-D RaggedTensor)",
"_____no_output_____"
],
[
"print(rt[3, 0]) # First element of fourth row (1-D Tensor)",
"_____no_output_____"
],
[
"print(rt[:, 1:3]) # Items 1-3 of each row (3-D RaggedTensor)",
"_____no_output_____"
],
[
"print(rt[:, -1:]) # Last item of each row (3-D RaggedTensor)",
"_____no_output_____"
]
],
[
[
"`RaggedTensor` 支持多维索引和切片,但有一个限制:不允许索引一个不规则维度。这种情况是有问题的,因为指示的值可能在某些行中存在,而在其他行中不存在。这种情况下,我们不知道是应该 (1) 引发 `IndexError`;(2) 使用默认值;还是 (3) 跳过该值并返回一个行数比开始时少的张量。根据 [Python 的指导原则](https://www.python.org/dev/peps/pep-0020/)(“当面对不明确的情况时,不要尝试去猜测”),我们目前不允许此运算。",
"_____no_output_____"
],
[
"## 张量类型转换\n\n`RaggedTensor` 类定义了可用于在 `RaggedTensor` 与 `tf.Tensor` 或 `tf.SparseTensors` 之间转换的方法:",
"_____no_output_____"
]
],
[
[
"ragged_sentences = tf.ragged.constant([\n ['Hi'], ['Welcome', 'to', 'the', 'fair'], ['Have', 'fun']])",
"_____no_output_____"
],
[
"# RaggedTensor -> Tensor\nprint(ragged_sentences.to_tensor(default_value='', shape=[None, 10]))",
"_____no_output_____"
],
[
"# Tensor -> RaggedTensor\nx = [[1, 3, -1, -1], [2, -1, -1, -1], [4, 5, 8, 9]]\nprint(tf.RaggedTensor.from_tensor(x, padding=-1))",
"_____no_output_____"
],
[
"#RaggedTensor -> SparseTensor\nprint(ragged_sentences.to_sparse())",
"_____no_output_____"
],
[
"# SparseTensor -> RaggedTensor\nst = tf.SparseTensor(indices=[[0, 0], [2, 0], [2, 1]],\n values=['a', 'b', 'c'],\n dense_shape=[3, 3])\nprint(tf.RaggedTensor.from_sparse(st))",
"_____no_output_____"
]
],
[
[
"## 评估不规则张量\n\n要访问不规则张量中的值,您可以:\n\n1. 使用 `tf.RaggedTensor.to_list()` 将不规则张量转换为嵌套 Python 列表。\n2. 使用 `tf.RaggedTensor.numpy()` 将不规则张量转换为 numpy 数组,数组的值是嵌套的 numpy 数组。\n3. 使用 `tf.RaggedTensor.values` 和 `tf.RaggedTensor.row_splits` 属性,或 `tf.RaggedTensor.row_lengths()` 和 `tf.RaggedTensor.value_rowids()` 之类的行分区方法,将不规则张量分解成其分量。\n4. 使用 Python 索引从不规则张量中选择值。\n",
"_____no_output_____"
]
],
[
[
"rt = tf.ragged.constant([[1, 2], [3, 4, 5], [6], [], [7]])\nprint(\"python list:\", rt.to_list())\nprint(\"numpy array:\", rt.numpy())\nprint(\"values:\", rt.values.numpy())\nprint(\"splits:\", rt.row_splits.numpy())\nprint(\"indexed value:\", rt[1].numpy())",
"_____no_output_____"
]
],
[
[
"## 广播\n\n广播是使具有不同形状的张量在进行逐元素运算时具有兼容形状的过程。有关广播的更多背景,请参阅:\n\n- [Numpy:广播](https://docs.scipy.org/doc/numpy/user/basics.broadcasting.html)\n- `tf.broadcast_dynamic_shape`\n- `tf.broadcast_to`\n\n广播两个输入 `x` 和 `y`,使其具有兼容形状的基本步骤是:\n\n1. 如果 `x` 和 `y` 没有相同的维数,则增加外层维度(使用大小 1),直至它们具有相同的维数。\n\n2. 对于 `x` 和 `y` 的大小不同的每一个维度:\n\n - 如果 `x` 或 `y` 在 `d` 维中的大小为 `1`,则跨 `d` 维重复其值以匹配其他输入的大小。\n\n - 否则,引发异常(`x` 和 `y` 非广播兼容)。\n\n其中,均匀维度中一个张量的大小是一个数字(跨该维的切片大小);不规则维度中一个张量的大小是切片长度列表(跨该维的所有切片)。",
"_____no_output_____"
],
[
"### 广播示例",
"_____no_output_____"
]
],
[
[
"# x (2D ragged): 2 x (num_rows)\n# y (scalar)\n# result (2D ragged): 2 x (num_rows)\nx = tf.ragged.constant([[1, 2], [3]])\ny = 3\nprint(x + y)",
"_____no_output_____"
],
[
"# x (2d ragged): 3 x (num_rows)\n# y (2d tensor): 3 x 1\n# Result (2d ragged): 3 x (num_rows)\nx = tf.ragged.constant(\n [[10, 87, 12],\n [19, 53],\n [12, 32]])\ny = [[1000], [2000], [3000]]\nprint(x + y)",
"_____no_output_____"
],
[
"# x (3d ragged): 2 x (r1) x 2\n# y (2d ragged): 1 x 1\n# Result (3d ragged): 2 x (r1) x 2\nx = tf.ragged.constant(\n [[[1, 2], [3, 4], [5, 6]],\n [[7, 8]]],\n ragged_rank=1)\ny = tf.constant([[10]])\nprint(x + y)",
"_____no_output_____"
],
[
"# x (3d ragged): 2 x (r1) x (r2) x 1\n# y (1d tensor): 3\n# Result (3d ragged): 2 x (r1) x (r2) x 3\nx = tf.ragged.constant(\n [\n [\n [[1], [2]],\n [],\n [[3]],\n [[4]],\n ],\n [\n [[5], [6]],\n [[7]]\n ]\n ],\n ragged_rank=2)\ny = tf.constant([10, 20, 30])\nprint(x + y)",
"_____no_output_____"
]
],
[
[
"下面是一些不广播的形状示例:",
"_____no_output_____"
]
],
[
[
"# x (2d ragged): 3 x (r1)\n# y (2d tensor): 3 x 4 # trailing dimensions do not match\nx = tf.ragged.constant([[1, 2], [3, 4, 5, 6], [7]])\ny = tf.constant([[1, 2, 3, 4], [5, 6, 7, 8], [9, 10, 11, 12]])\ntry:\n x + y\nexcept tf.errors.InvalidArgumentError as exception:\n print(exception)",
"_____no_output_____"
],
[
"# x (2d ragged): 3 x (r1)\n# y (2d ragged): 3 x (r2) # ragged dimensions do not match.\nx = tf.ragged.constant([[1, 2, 3], [4], [5, 6]])\ny = tf.ragged.constant([[10, 20], [30, 40], [50]])\ntry:\n x + y\nexcept tf.errors.InvalidArgumentError as exception:\n print(exception)",
"_____no_output_____"
],
[
"# x (3d ragged): 3 x (r1) x 2\n# y (3d ragged): 3 x (r1) x 3 # trailing dimensions do not match\nx = tf.ragged.constant([[[1, 2], [3, 4], [5, 6]],\n [[7, 8], [9, 10]]])\ny = tf.ragged.constant([[[1, 2, 0], [3, 4, 0], [5, 6, 0]],\n [[7, 8, 0], [9, 10, 0]]])\ntry:\n x + y\nexcept tf.errors.InvalidArgumentError as exception:\n print(exception)",
"_____no_output_____"
]
],
[
[
"## RaggedTensor 编码\n\n不规则张量使用 `RaggedTensor` 类进行编码。在内部,每个 `RaggedTensor` 包含:\n\n- 一个 `values` 张量,它将可变长度行连接成扁平列表。\n- 一个 `row_partition`,它指示如何将这些扁平值分成各行。\n\n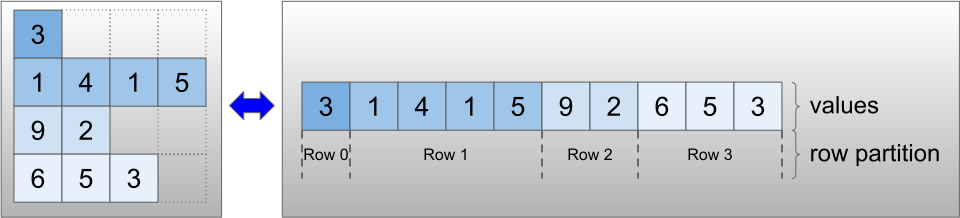\n\n可以使用四种不同的编码存储 `row_partition`:\n\n- `row_splits` 是一个整型向量,用于指定行之间的拆分点。\n- `value_rowids` 是一个整型向量,用于指定每个值的行索引。\n- `row_lengths` 是一个整型向量,用于指定每一行的长度。\n- `uniform_row_length` 是一个整型标量,用于指定所有行的单个长度。\n\n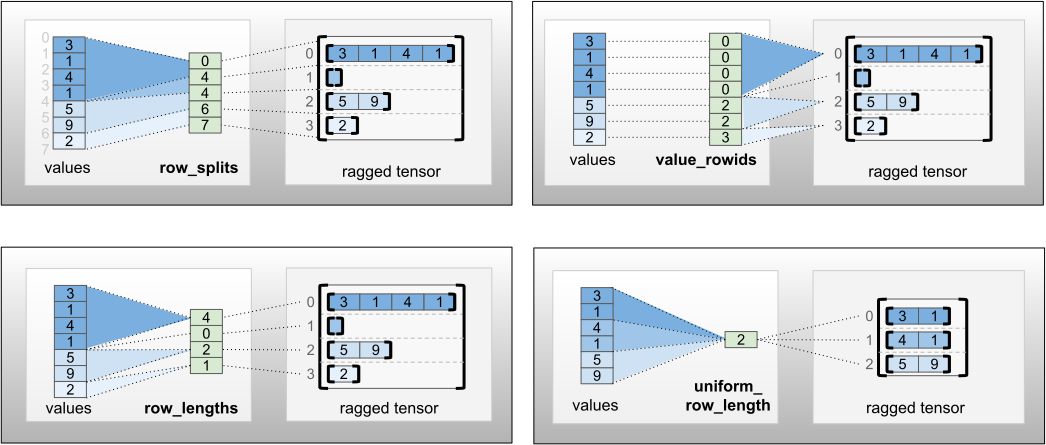\n\n整型标量 `nrows` 还可以包含在 `row_partition` 编码中,以考虑具有 `value_rowids` 的空尾随行或具有 `uniform_row_length` 的空行。\n",
"_____no_output_____"
]
],
[
[
"rt = tf.RaggedTensor.from_row_splits(\n values=[3, 1, 4, 1, 5, 9, 2],\n row_splits=[0, 4, 4, 6, 7])\nprint(rt)",
"_____no_output_____"
]
],
[
[
"选择为行分区使用哪种编码由不规则张量在内部进行管理,以提高某些环境下的效率。尤其是,不同行分区方案的某些优点和缺点是:\n\n- **高效索引**:`row_splits` 编码可以实现不规则张量的恒定时间索引和切片。\n\n- **高效连接**:`row_lengths` 编码在连接不规则张量时更有效,因为当两个张量连接在一起时,行长度不会改变。\n\n- **较小的编码大小**:`value_rowids` 编码在存储有大量空行的不规则张量时更有效,因为张量的大小只取决于值的总数。另一方面,`row_splits` 和 `row_lengths` 编码在存储具有较长行的不规则张量时更有效,因为它们每行只需要一个标量值。\n\n- **兼容性**:`value_rowids` 方案与 `tf.math.segment_sum` 等运算使用的[分段](https://tensorflow.google.cn/api_docs/python/tf/math#about_segmentation)格式相匹配。`row_limits` 方案与 `tf.sequence_mask` 等运算使用的格式相匹配。\n\n- **均匀维度**:如下文所述,`uniform_row_length` 编码用于对具有均匀维度的不规则张量进行编码。",
"_____no_output_____"
],
[
"### 多个不规则维度\n\n具有多个不规则维度的不规则张量通过为 `values` 张量使用嵌套 `RaggedTensor` 进行编码。每个嵌套 `RaggedTensor` 都会增加一个不规则维度。\n\n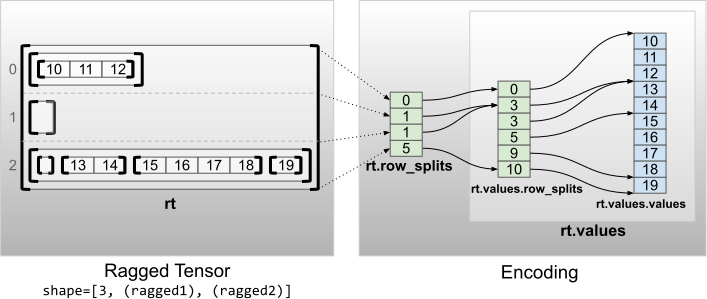\n",
"_____no_output_____"
]
],
[
[
"rt = tf.RaggedTensor.from_row_splits(\n values=tf.RaggedTensor.from_row_splits(\n values=[10, 11, 12, 13, 14, 15, 16, 17, 18, 19],\n row_splits=[0, 3, 3, 5, 9, 10]),\n row_splits=[0, 1, 1, 5])\nprint(rt)\nprint(\"Shape: {}\".format(rt.shape))\nprint(\"Number of partitioned dimensions: {}\".format(rt.ragged_rank))",
"_____no_output_____"
]
],
[
[
"工厂函数 `tf.RaggedTensor.from_nested_row_splits` 可用于通过提供一个 `row_splits` 张量列表,直接构造具有多个不规则维度的 RaggedTensor:",
"_____no_output_____"
]
],
[
[
"rt = tf.RaggedTensor.from_nested_row_splits(\n flat_values=[10, 11, 12, 13, 14, 15, 16, 17, 18, 19],\n nested_row_splits=([0, 1, 1, 5], [0, 3, 3, 5, 9, 10]))\nprint(rt)",
"_____no_output_____"
]
],
[
[
"### 不规则秩和扁平值\n\n不规则张量的***不规则秩***是底层 `values` 张量的分区次数(即 `RaggedTensor` 对象的嵌套深度)。最内层的 `values` 张量称为其 ***flat_values***。在以下示例中,`conversations` 具有 ragged_rank=3,其 `flat_values` 为具有 24 个字符串的一维 `Tensor`:\n",
"_____no_output_____"
]
],
[
[
"# shape = [batch, (paragraph), (sentence), (word)]\nconversations = tf.ragged.constant(\n [[[[\"I\", \"like\", \"ragged\", \"tensors.\"]],\n [[\"Oh\", \"yeah?\"], [\"What\", \"can\", \"you\", \"use\", \"them\", \"for?\"]],\n [[\"Processing\", \"variable\", \"length\", \"data!\"]]],\n [[[\"I\", \"like\", \"cheese.\"], [\"Do\", \"you?\"]],\n [[\"Yes.\"], [\"I\", \"do.\"]]]])\nconversations.shape",
"_____no_output_____"
],
[
"assert conversations.ragged_rank == len(conversations.nested_row_splits)\nconversations.ragged_rank # Number of partitioned dimensions.",
"_____no_output_____"
],
[
"conversations.flat_values.numpy()",
"_____no_output_____"
]
],
[
[
"### 均匀内层维度\n\n具有均匀内层维度的不规则张量通过为 flat_values(即最内层 `values`)使用多维 `tf.Tensor` 进行编码。\n\n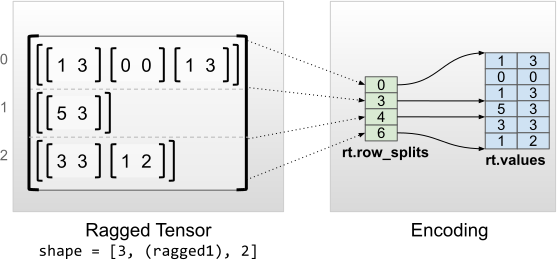",
"_____no_output_____"
]
],
[
[
"rt = tf.RaggedTensor.from_row_splits(\n values=[[1, 3], [0, 0], [1, 3], [5, 3], [3, 3], [1, 2]],\n row_splits=[0, 3, 4, 6])\nprint(rt)\nprint(\"Shape: {}\".format(rt.shape))\nprint(\"Number of partitioned dimensions: {}\".format(rt.ragged_rank))\nprint(\"Flat values shape: {}\".format(rt.flat_values.shape))\nprint(\"Flat values:\\n{}\".format(rt.flat_values))",
"_____no_output_____"
]
],
[
[
"### 均匀非内层维度\n\n具有均匀非内层维度的不规则张量通过使用 `uniform_row_length` 对行分区进行编码。\n\n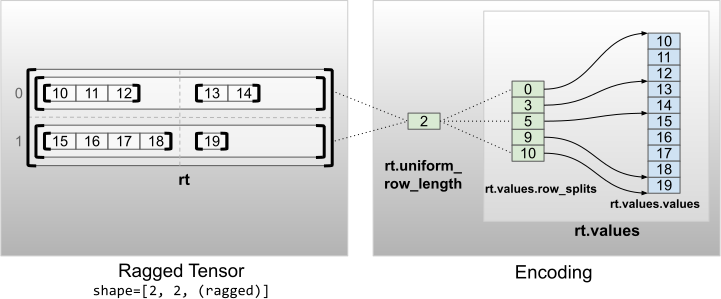",
"_____no_output_____"
]
],
[
[
"rt = tf.RaggedTensor.from_uniform_row_length(\n values=tf.RaggedTensor.from_row_splits(\n values=[10, 11, 12, 13, 14, 15, 16, 17, 18, 19],\n row_splits=[0, 3, 5, 9, 10]),\n uniform_row_length=2)\nprint(rt)\nprint(\"Shape: {}\".format(rt.shape))\nprint(\"Number of partitioned dimensions: {}\".format(rt.ragged_rank))",
"_____no_output_____"
]
]
] | [
"markdown",
"code",
"markdown",
"code",
"markdown",
"code",
"markdown",
"code",
"markdown",
"code",
"markdown",
"code",
"markdown",
"code",
"markdown",
"code",
"markdown",
"code",
"markdown",
"code",
"markdown",
"code",
"markdown",
"code",
"markdown",
"code",
"markdown",
"code",
"markdown",
"code",
"markdown",
"code",
"markdown",
"code",
"markdown",
"code",
"markdown",
"code",
"markdown",
"code",
"markdown",
"code",
"markdown",
"code",
"markdown",
"code",
"markdown",
"code",
"markdown",
"code",
"markdown",
"code",
"markdown",
"code",
"markdown",
"code",
"markdown",
"code",
"markdown",
"code",
"markdown",
"code",
"markdown",
"code",
"markdown",
"code",
"markdown",
"code",
"markdown",
"code",
"markdown",
"code",
"markdown",
"code",
"markdown",
"code",
"markdown",
"code",
"markdown",
"code",
"markdown",
"code",
"markdown",
"code",
"markdown",
"code",
"markdown",
"code",
"markdown",
"code"
] | [
[
"markdown"
],
[
"code"
],
[
"markdown",
"markdown",
"markdown"
],
[
"code"
],
[
"markdown",
"markdown"
],
[
"code"
],
[
"markdown",
"markdown",
"markdown"
],
[
"code",
"code",
"code"
],
[
"markdown"
],
[
"code",
"code"
],
[
"markdown"
],
[
"code"
],
[
"markdown"
],
[
"code",
"code"
],
[
"markdown"
],
[
"code",
"code"
],
[
"markdown"
],
[
"code"
],
[
"markdown"
],
[
"code"
],
[
"markdown"
],
[
"code"
],
[
"markdown",
"markdown"
],
[
"code",
"code",
"code",
"code"
],
[
"markdown"
],
[
"code"
],
[
"markdown",
"markdown"
],
[
"code"
],
[
"markdown"
],
[
"code"
],
[
"markdown"
],
[
"code"
],
[
"markdown"
],
[
"code"
],
[
"markdown",
"markdown",
"markdown"
],
[
"code"
],
[
"markdown"
],
[
"code"
],
[
"markdown"
],
[
"code"
],
[
"markdown",
"markdown"
],
[
"code"
],
[
"markdown"
],
[
"code"
],
[
"markdown",
"markdown"
],
[
"code"
],
[
"markdown"
],
[
"code"
],
[
"markdown"
],
[
"code"
],
[
"markdown"
],
[
"code"
],
[
"markdown"
],
[
"code",
"code",
"code"
],
[
"markdown"
],
[
"code"
],
[
"markdown"
],
[
"code"
],
[
"markdown",
"markdown"
],
[
"code"
],
[
"markdown"
],
[
"code"
],
[
"markdown",
"markdown"
],
[
"code"
],
[
"markdown"
],
[
"code"
],
[
"markdown",
"markdown",
"markdown"
],
[
"code",
"code",
"code",
"code",
"code",
"code"
],
[
"markdown"
],
[
"code",
"code",
"code",
"code",
"code"
],
[
"markdown",
"markdown"
],
[
"code",
"code",
"code",
"code",
"code"
],
[
"markdown"
],
[
"code"
],
[
"markdown",
"markdown"
],
[
"code",
"code",
"code",
"code"
],
[
"markdown"
],
[
"code",
"code",
"code"
],
[
"markdown"
],
[
"code"
],
[
"markdown",
"markdown"
],
[
"code"
],
[
"markdown"
],
[
"code"
],
[
"markdown"
],
[
"code",
"code",
"code"
],
[
"markdown"
],
[
"code"
],
[
"markdown"
],
[
"code"
]
] |
d0487a89fa335b8527b8de8ada8cd3c64eb98de7 | 651,564 | ipynb | Jupyter Notebook | pittsburgh-bridges-data-set-analysis/backup/Data Space Report (Official) - Two-Dimensional Analyses-v1.0.1.ipynb | franec94/Pittsburgh-Bridge-Dataset | 682ff0e3979ca565637e858cc36dc07c2aeda7d6 | [
"MIT"
] | null | null | null | pittsburgh-bridges-data-set-analysis/backup/Data Space Report (Official) - Two-Dimensional Analyses-v1.0.1.ipynb | franec94/Pittsburgh-Bridge-Dataset | 682ff0e3979ca565637e858cc36dc07c2aeda7d6 | [
"MIT"
] | 7 | 2021-02-02T22:51:40.000Z | 2022-03-12T00:39:08.000Z | pittsburgh-bridges-data-set-analysis/models-analyses/complete_analyses/Data Space Report (Official) - Two-Dimensional Analyses-v1.0.1.ipynb | franec94/Pittsburgh-Bridge-Dataset | 682ff0e3979ca565637e858cc36dc07c2aeda7d6 | [
"MIT"
] | null | null | null | 112.727336 | 141,396 | 0.767871 | [
[
[
"# Data Space Report\n\n\n<img src=\"images/polito_logo.png\" alt=\"Polito Logo\" style=\"width: 200px;\"/>\n\n\n## Pittsburgh Bridges Data Set\n\n<img src=\"images/andy_warhol_bridge.jpg\" alt=\"Andy Warhol Bridge\" style=\"width: 200px;\"/>\n\n Andy Warhol Bridge - Pittsburgh.\n\nReport created by Student Francesco Maria Chiarlo s253666, for A.A 2019/2020.\n\n**Abstract**:The aim of this report is to evaluate the effectiveness of distinct, different statistical learning approaches, in particular focusing on their characteristics as well as on their advantages and backwards when applied onto a relatively small dataset as the one employed within this report, that is Pittsburgh Bridgesdataset.\n\n**Key words**:Statistical Learning, Machine Learning, Bridge Design.\n\n## TOC:\n* [Imports Section](#imports-section)\n* [Dataset's Attributes Description](#attributes-description)\n* [Data Preparation and Investigation](#data-preparation)\n* [Learning Models](#learning-models)\n* [Improvements and Conclusions](#improvements-and-conclusions)\n* [References](#references)",
"_____no_output_____"
],
[
"### Imports Section <a class=\"anchor\" id=\"imports-section\"></a>",
"_____no_output_____"
]
],
[
[
"# =========================================================================== #\n# STANDARD IMPORTS\n# =========================================================================== #\nprint(__doc__)\n\nfrom pprint import pprint\n\nimport warnings\nwarnings.filterwarnings('ignore')\n\nimport copy\nimport os\nimport sys\nimport time\n\nimport pandas as pd\nimport numpy as np\n\n%matplotlib inline\n# Matplotlib pyplot provides plotting API\nimport matplotlib as mpl\nfrom matplotlib import pyplot as plt\nimport chart_studio.plotly.plotly as py\nimport seaborn as sns; sns.set()",
"_____no_output_____"
],
[
"# =========================================================================== #\n# UTILS IMPORTS (Done by myself)\n# =========================================================================== #\nfrom utils.display_utils import *\nfrom utils.preprocessing_utils import *\nfrom utils.training_utils import *\nfrom utils.training_utils_v2 import fit_by_n_components, fit_all_by_n_components",
"_____no_output_____"
],
[
"from itertools import islice",
"_____no_output_____"
],
[
"# =========================================================================== #\n# sklearn IMPORT\n# =========================================================================== #\nfrom sklearn.decomposition import PCA, KernelPCA\n\n# Import scikit-learn classes: models (Estimators).\nfrom sklearn.naive_bayes import GaussianNB # Non-parametric Generative Model\nfrom sklearn.naive_bayes import MultinomialNB # Non-parametric Generative Model\nfrom sklearn.linear_model import LinearRegression # Parametric Linear Discriminative Model\nfrom sklearn.linear_model import LogisticRegression # Parametric Linear Discriminative Model\nfrom sklearn.linear_model import Ridge, Lasso\nfrom sklearn.neighbors import KNeighborsClassifier\nfrom sklearn.svm import SVC # Parametric Linear Discriminative \"Support Vector Classifier\"\nfrom sklearn.tree import DecisionTreeClassifier # Non-parametric Model\nfrom sklearn.ensemble import BaggingClassifier # Non-parametric Model (Meta-Estimator, that is, an Ensemble Method)\nfrom sklearn.ensemble import RandomForestClassifier # Non-parametric Model (Meta-Estimator, that is, an Ensemble Method)",
"_____no_output_____"
]
],
[
[
"### Dataset's Attributes Description <a class=\"anchor\" id=\"attributes-description\"></a>\n\nThe analyses that I aim at accomplishing while using as means the methods or approaches provided by both Statistical Learning and Machine Learning fields, concern the dataset Pittsburgh Bridges, and what follows is a overview and brief description of the main characteristics, as well as, basic information about this precise dataset.\n\nThe Pittsburgh Bridges dataset is a dataset available from the web site called mainly *\"UCI Machine Learing Repository\"*, which is one of the well known web site that let a large amount of different datasets, from different domains or fields, to be used for machine-learning research and which have been cited in peer-reviewed academic journals.\n\nIn particular, the dataset I'm going to treat and analyze, which is Pittsburgh Bridges dataset, has been made freely available from the Western Pennsylvania Regional Data Center (WPRDC), which is a project led by the University Center of Social and Urban Research (UCSUR) at the University of Pittsburgh (\"University\") in collaboration with City of Pittsburgh and The County of Allegheny in Pennsylvania. The WPRDC and the WPRDC Project is supported by a grant from the Richard King Mellon Foundation.\n\nIn order to be more precise, from the official and dedicated web page, within UCI Machine Learning cite, Pittsburgh Bridges dataset is a dataset that has been created after the works of some co-authors which are:\n- Yoram Reich & Steven J. Fenves from Department of Civil Engineering and Engineering Design Research Center Carnegie Mellon University Pittsburgh, PA 15213\n\nThe Pittsburgh Bridges dataset is made of up to 108 distinct observations and each of that data sample is made of 12 attributes or features where some of them are considered to be continuous properties and other to be categorical or nominal properties. Those variables are the following:\n\n- **RIVER**: which is a nominal type variable that can assume the subsequent possible discrete values which are: A, M, O. Where A stands for Allegheny river, while M stands for Monongahela river and lastly O stands for Ohio river.\n- **LOCATION**: which represents a nominal type variable too, and assume a positive integer value from 1 up to 52 used as categorical attribute.\n- **ERECTED**: which might be either a numerical or categorical variable, depending on the fact that we want to aggregate a bunch of value under a categorical quantity. What this means is that, basically such attribute is made of date starting from 1818 up to 1986, but we may imagine to aggregate somehow these data within a given category among those suggested, that are CRAFTS, EMERGENING, MATURE, MODERN.\n- **PURPOSE**: which is a categorical attribute and represents the reason why a particular bridge has been built, which means that this attribute represents what kind of vehicle can cross the bridge or if the bridge has been made just for people. For this reasons the allowd values for this attributes are the following: WALK, AQUEDUCT, RR, HIGHWAY. Three out of four are self explained values, while RR value that might be tricky at first glance, it just stands for railroad.\n- **LENGTH**: which represents the bridge's length, is a numerical attribute if we just look at the real number values that go from 804 up to 4558, but we can again decide to handle or arrange such values so that they can be grouped into range of values mapped into SHORT, MEDIUM, LONG so that we can refer to a bridge's length by means of these new categorical values.\n- **LANES**: which is a categorical variable which is represented by numerical values, that are 1, 2, 4, 6 which indicate the number of distinct lanes that a bridge in Pittsburgh city may have. The larger the value the wider the bridge.\n- **CLEAR-G**: specifies whether a vertical navigation clearance requirement was enforced in the design or not.\n- **T-OR-D**: which is a nominal attribute, in other words, a categorical attribute that can assume THROUGH, DECK values. In order to be more precise, this samples attribute deals with structural elements of a bridge. In fact, a deck is the surface of a bridge and this structural element, of bridge's superstructure, may be constructed of concrete, steel, open grating, or wood. On the other hand, a through arch bridge, also known as a half-through arch bridge or a through-type arch bridge, is a bridge that is made from materials such as steel or reinforced concrete, in which the base of an arch structure is below the deck but the top rises above it.\n- **MATERIAL**: which is a categorical or nominal variable and is used to describe the bridge telling which is the main or core material used to build it.\n This attribute can assume one of the possible, following values which are: WOOD, IRON, STEEL. Furthermore, we expect to see somehow a bit of correlation between the values assumed by the pairs represented by T-OR-D and MATERIAL columns, when looking just to them.\n- **SPAN**: which is a categorical or nominal value and has been recorded by means of three possible values for each sample, that are SHORT, MEDIUM, LONG. This attribute, within the field of Structural Engineering, is the distance between two intermediate supports for a structure, e.g. a beam or a bridge. A span can be closed by a solid beam or by a rope. The first kind is used for bridges, the second one for power lines, overhead telecommunication lines, some type of antennas or for aerial tramways. \n- **REL-L**: which is a categorical or nominal variable and stands for relative length of the main span of the bridge to the total crossing length, it can assume three possible values that are S, S-F, F.\n- Lastly, **TYPE** which indicates as a categorical or nominal attributes what type of bridge each record represents, among the possible 6 distinct classes or types of bridges that are: WOOD, SUSPEN, SIMPLE-T, ARCH, CANTILEV, CONT-T.",
"_____no_output_____"
],
[
"### Data Preparation and Investigation <a class=\"anchor\" id=\"data-preparation\"></a>\n\nThe aim of this chapter is to get in the data, that are available within Pittsburgh Bridge Dataset, in order to investigate a bit more in to detail and generally speaking deeper the main or high level statistics quantities, such as mean, median, standard deviation of each attribute, as well as displaying somehow data distribution for each attribute by means of histogram plots. This phase allows or enables us to decide which should be the best feature to be selected as the target variable, in other word the attribute that will represent the dependent variable with respect to the remaining attributes that instead will play the role of predictors and independent variables, as well.\n\nIn order to investigate and explore our data we make usage of *Pandas library*. We recall mainly that, in computer programming, Pandas is a software library written for the Python programming language* for *data manipulation and analysis*. In particular, it offers data structures and operations for manipulating numerical tables and time series. It is free software and a interesting and funny things about such tool is that the name is derived from the term \"panel data\", an econometrics term for data sets that include observations over multiple time periods for the same individuals.\nWe also note that as the analysis proceeds we will introduce other computer programming as well as programming libraries that allow or enable us to fulfill our goals.",
"_____no_output_____"
],
[
"Initially, once I have downloaded from the provided web page the dataset with the data samples about Pittsburgh Bridge we load the data by means of functions available using python library's pandas. We notice that the overall set of data points is large up to 108 records or rows, which are sorted by Erected attributes, so this means that are sorted in decreasing order from the oldest bridge which has been built in 1818 up to the most modern bridge that has been erected in 1986. Then we display the first 5 rows to get an overview and have a first idea about what is inside the overall dataset, and the result we obtain by means of head() function applied onto the fetched dataset is equals to what follows:",
"_____no_output_____"
]
],
[
[
"# =========================================================================== #\n# READ INPUT DATASET\n# =========================================================================== #\n\ndataset_path = 'C:\\\\Users\\\\Francesco\\Documents\\\\datasets\\\\pittsburgh_dataset'\ndataset_name = 'bridges.data.csv'\n\n# column_names = ['IDENTIF', 'RIVER', 'LOCATION', 'ERECTED', 'PURPOSE', 'LENGTH', 'LANES', 'CLEAR-G', 'T-OR-D', 'MATERIAL', 'SPAN', 'REL-L', 'TYPE']\ncolumn_names = ['RIVER', 'LOCATION', 'ERECTED', 'PURPOSE', 'LENGTH', 'LANES', 'CLEAR-G', 'T-OR-D', 'MATERIAL', 'SPAN', 'REL-L', 'TYPE']\ndataset = pd.read_csv(os.path.join(dataset_path, dataset_name), names=column_names, index_col=0)",
"_____no_output_____"
],
[
"# SHOW SOME STANDARD DATASET INFOS\n# --------------------------------------------------------------------------- #\nprint('Dataset shape: {}'.format(dataset.shape))\nprint(dataset.info())",
"Dataset shape: (108, 12)\n<class 'pandas.core.frame.DataFrame'>\nIndex: 108 entries, E1 to E109\nData columns (total 12 columns):\n # Column Non-Null Count Dtype \n--- ------ -------------- ----- \n 0 RIVER 108 non-null object\n 1 LOCATION 108 non-null object\n 2 ERECTED 108 non-null int64 \n 3 PURPOSE 108 non-null object\n 4 LENGTH 108 non-null object\n 5 LANES 108 non-null object\n 6 CLEAR-G 108 non-null object\n 7 T-OR-D 108 non-null object\n 8 MATERIAL 108 non-null object\n 9 SPAN 108 non-null object\n 10 REL-L 108 non-null object\n 11 TYPE 108 non-null object\ndtypes: int64(1), object(11)\nmemory usage: 11.0+ KB\nNone\n"
],
[
"# SHOWING FIRSTS N-ROWS AS THEY ARE STORED WITHIN DATASET\n# --------------------------------------------------------------------------- #\ndataset.head(5)",
"_____no_output_____"
]
],
[
[
"What we can notice from just the table above is that there are some attributes that are characterized by a special character that is '?' which stands for a missing value, so by chance there was not possibility to get the value for this attribute, such as for LENGTH and SPAN attributes. Analyzing in more details the dataset we discover that there are up to 6 different attributes, in the majority attributes with categorical or nominal nature such as CLEAR-G, T-OR-D, MATERIAL, SPAN, REL-L, and TYPE that contain at list one row characterized by the fact that one of its attributes is set to assuming '?' value that stands, as we already know for a missing value.\n\nHere, we can follow different strategies that depends onto the level of complexity as well as accuracy we want to obtain or achieve for models we are going to fit to the data after having correctly pre-processed them, speaking about what we could do with missing values. In fact one can follow the simplest way and can decide to simply discard those rows that contain at least one attribute with a missing value represented by the '?' symbol. Otherwise one may alos decide to follow a different strategy that aims at keeping also those rows that have some missing values by means of some kind of technique that allows to establish a potential substituting value for the missing one.\n\nSo, in this setting, that is our analyses, we start by just leaving out those rows that at least contain one attribute that has a missing value, this choice leads us to reduce the size of our dataset from 108 records to 70 remaining samples, with a drop of 38 data examples, which may affect the final results, since we left out more or less the 46\\% of the data because of missing values.",
"_____no_output_____"
]
],
[
[
"# INVESTIGATING DATASET IN ORDER TO DETECT NULL VALUES\n# --------------------------------------------------------------------------- #\nprint('Before preprocessing dataset and handling null values')\nresult = dataset.isnull().values.any()\nprint('There are any null values ? Response: {}'.format(result))\n\nresult = dataset.isnull().sum()\nprint('Number of null values for each predictor:\\n{}'.format(result))",
"Before preprocessing dataset and handling null values\nThere are any null values ? Response: False\nNumber of null values for each predictor:\nRIVER 0\nLOCATION 0\nERECTED 0\nPURPOSE 0\nLENGTH 0\nLANES 0\nCLEAR-G 0\nT-OR-D 0\nMATERIAL 0\nSPAN 0\nREL-L 0\nTYPE 0\ndtype: int64\n"
],
[
"# DISCOVERING VALUES WITHIN EACH PREDICTOR DOMAIN\n# --------------------------------------------------------------------------- #\ncolumns_2_avoid = ['ERECTED', 'LENGTH', 'LOCATION', 'LANES']\n# columns_2_avoid = None\nlist_columns_2_fix = show_categorical_predictor_values(dataset, columns_2_avoid)",
"RIVER : ['A', 'M', 'O', 'Y']\nPURPOSE : ['AQUEDUCT', 'HIGHWAY', 'RR', 'WALK']\nCLEAR-G : ['?', 'G', 'N']\nT-OR-D : ['?', 'DECK', 'THROUGH']\nMATERIAL : ['?', 'IRON', 'STEEL', 'WOOD']\nSPAN : ['?', 'LONG', 'MEDIUM', 'SHORT']\nREL-L : ['?', 'F', 'S', 'S-F']\nTYPE : ['?', 'ARCH', 'CANTILEV', 'CONT-T', 'NIL', 'SIMPLE-T', 'SUSPEN', 'WOOD']\n"
],
[
"# FIXING, UPDATING NULL VALUES CODED AS '?' SYMBOL\n# WITHIN EACH CATEGORICAL VARIABLE, IF DETECTED ANY\n# --------------------------------------------------------------------------- #\nprint('\"Before\" removing \\'?\\' rows, Dataset dim:', dataset.shape)\nfor _, predictor in enumerate(list_columns_2_fix):\n dataset = dataset[dataset[predictor] != '?']\nprint('\"After\" removing \\'?\\' rows, Dataset dim: ', dataset.shape)\nprint('-' * 50)\n\n_ = show_categorical_predictor_values(dataset, columns_2_avoid)",
"\"Before\" removing '?' rows, Dataset dim: (108, 12)\n\"After\" removing '?' rows, Dataset dim: (88, 12)\n--------------------------------------------------\nRIVER : ['A', 'M', 'O', 'Y']\nPURPOSE : ['AQUEDUCT', 'HIGHWAY', 'RR']\nCLEAR-G : ['G', 'N']\nT-OR-D : ['DECK', 'THROUGH']\nMATERIAL : ['IRON', 'STEEL', 'WOOD']\nSPAN : ['LONG', 'MEDIUM', 'SHORT']\nREL-L : ['F', 'S', 'S-F']\nTYPE : ['ARCH', 'CANTILEV', 'CONT-T', 'SIMPLE-T', 'SUSPEN', 'WOOD']\n"
],
[
"# INTERMEDIATE RESULT FOUND\n# --------------------------------------------------------------------------- #\npreprocess_categorical_variables(dataset, columns_2_avoid)\nprint(dataset.info())",
"<class 'pandas.core.frame.DataFrame'>\nIndex: 88 entries, E1 to E90\nData columns (total 12 columns):\n # Column Non-Null Count Dtype \n--- ------ -------------- ----- \n 0 RIVER 88 non-null int64 \n 1 LOCATION 88 non-null object\n 2 ERECTED 88 non-null int64 \n 3 PURPOSE 88 non-null int64 \n 4 LENGTH 88 non-null object\n 5 LANES 88 non-null object\n 6 CLEAR-G 88 non-null int64 \n 7 T-OR-D 88 non-null int64 \n 8 MATERIAL 88 non-null int64 \n 9 SPAN 88 non-null int64 \n 10 REL-L 88 non-null int64 \n 11 TYPE 88 non-null int64 \ndtypes: int64(9), object(3)\nmemory usage: 8.9+ KB\nNone\n"
],
[
"dataset.head(5)",
"_____no_output_____"
]
],
[
[
"The next step is represented by the effort of mapping categorical variables into numerical variables, so that them are comparable with the already existing numerical or continuous variables, and also by mapping the categorical variables into numerical variables we allow or enable us to perform some kind of normalization or just transformation onto the entire dataset in order to let some machine learning algorithm to work better or to take advantage of normalized data within our pre-processed dataset. Furthermore, by transforming first the categorical attributes into a continuous version we are also able to calculate the \\textit{heatmap}, which is a very useful way of representing a correlation matrix calculated on the whole dataset. Moreover we have displayed data distribution for each attribute by means of histogram representation to take some useful information about the number of occurrences for each possible value, in particular for those attributes that have a categorical nature.",
"_____no_output_____"
]
],
[
[
"# MAP NUMERICAL VALUES TO INTEGER VALUES\n# --------------------------------------------------------------------------- #\nprint('Before', dataset.shape)\ncolumns_2_map = ['ERECTED', 'LANES']\nfor _, predictor in enumerate(columns_2_map):\n dataset = dataset[dataset[predictor] != '?']\n dataset[predictor] = np.array(list(map(lambda x: int(x), dataset[predictor].values)))\nprint('After', dataset.shape)\nprint(dataset.info())\n# print(dataset.head(5))",
"Before (88, 12)\nAfter (80, 12)\n<class 'pandas.core.frame.DataFrame'>\nIndex: 80 entries, E1 to E90\nData columns (total 12 columns):\n # Column Non-Null Count Dtype \n--- ------ -------------- ----- \n 0 RIVER 80 non-null int64 \n 1 LOCATION 80 non-null object\n 2 ERECTED 80 non-null int32 \n 3 PURPOSE 80 non-null int64 \n 4 LENGTH 80 non-null object\n 5 LANES 80 non-null int32 \n 6 CLEAR-G 80 non-null int64 \n 7 T-OR-D 80 non-null int64 \n 8 MATERIAL 80 non-null int64 \n 9 SPAN 80 non-null int64 \n 10 REL-L 80 non-null int64 \n 11 TYPE 80 non-null int64 \ndtypes: int32(2), int64(8), object(2)\nmemory usage: 7.5+ KB\nNone\n"
],
[
"# MAP NUMERICAL VALUES TO FLOAT VALUES\n# --------------------------------------------------------------------------- #\n# print('Before', dataset.shape)\ncolumns_2_map = ['LOCATION', 'LANES', 'LENGTH'] \nfor _, predictor in enumerate(columns_2_map):\n dataset = dataset[dataset[predictor] != '?']\n dataset[predictor] = np.array(list(map(lambda x: float(x), dataset[predictor].values)))\n# print('After', dataset.shape) \n# print(dataset.info())\n# print(dataset.head(5))\n\n# columns_2_avoid = None\n# list_columns_2_fix = show_categorical_predictor_values(dataset, None)",
"_____no_output_____"
],
[
"result = dataset.isnull().values.any()\n# print('After handling null values\\nThere are any null values ? Response: {}'.format(result))\n\nresult = dataset.isnull().sum()\n# print('Number of null values for each predictor:\\n{}'.format(result))\n\ndataset.head(5)",
"_____no_output_____"
],
[
"dataset.describe(include='all')",
"_____no_output_____"
],
[
"# sns.pairplot(dataset, hue='T-OR-D', size=1.5)",
"_____no_output_____"
],
[
"columns_2_avoid = ['ERECTED', 'LENGTH', 'LOCATION']\ntarget_col = 'T-OR-D'\n# show_frequency_distribution_predictors(dataset, columns_2_avoid)\n# show_frequency_distribution_predictor(dataset, predictor_name='RIVER', columns_2_avoid=columns_2_avoid)\n\n# build_boxplot(dataset, predictor_name='RIVER', columns_2_avoid=columns_2_avoid, target_col='T-OR-D')",
"_____no_output_____"
],
[
"# show_frequency_distribution_predictors(dataset, columns_2_avoid)\n# show_frequency_distribution_predictor(dataset, predictor_name='T-OR-D', columns_2_avoid=columns_2_avoid)",
"_____no_output_____"
],
[
"# show_frequency_distribution_predictors(dataset, columns_2_avoid)\n# show_frequency_distribution_predictor(dataset, predictor_name='CLEAR-G', columns_2_avoid=columns_2_avoid)\n\n# build_boxplot(dataset, predictor_name='CLEAR-G', columns_2_avoid=columns_2_avoid, target_col='T-OR-D')",
"_____no_output_____"
],
[
"# show_frequency_distribution_predictors(dataset, columns_2_avoid)\n# show_frequency_distribution_predictor(dataset, predictor_name='SPAN', columns_2_avoid=columns_2_avoid)\n\n# build_boxplot(dataset, predictor_name='SPAN', columns_2_avoid=columns_2_avoid, target_col='T-OR-D')",
"_____no_output_____"
],
[
"# show_frequency_distribution_predictors(dataset, columns_2_avoid)\n# show_frequency_distribution_predictor(dataset, predictor_name='MATERIAL', columns_2_avoid=columns_2_avoid)\n\n# build_boxplot(dataset, predictor_name='MATERIAL', columns_2_avoid=columns_2_avoid, target_col='T-OR-D')",
"_____no_output_____"
],
[
"# show_frequency_distribution_predictors(dataset, columns_2_avoid)\n# show_frequency_distribution_predictor(dataset, predictor_name='REL-L', columns_2_avoid=columns_2_avoid)",
"_____no_output_____"
],
[
"# show_frequency_distribution_predictors(dataset, columns_2_avoid)\n# show_frequency_distribution_predictor(dataset, predictor_name='TYPE', columns_2_avoid=columns_2_avoid)\n\n# build_boxplot(dataset, predictor_name='TYPE', columns_2_avoid=columns_2_avoid, target_col='T-OR-D')",
"_____no_output_____"
],
[
"corr_result = dataset.corr()\n# corr_result.head(corr_result.shape[0])\ndisplay_heatmap(corr_result)",
"_____no_output_____"
],
[
"# show_histograms_from_heatmap_corr_matrix(corr_result, row_names=dataset.columns)",
"_____no_output_____"
],
[
"# Make distinction between Target Variable and Predictors\n# --------------------------------------------------------------------------- #\n\ncolumns = dataset.columns # List of all attribute names\ntarget_col = 'T-OR-D' # Target variable name\n\n# Get Target values and map to 0s and 1s\ny = np.array(list(map(lambda x: 0 if x == 1 else 1, dataset[target_col].values)))\nprint('Summary about Target Variable {target_col}')\nprint('-' * 50)\nprint(dataset['T-OR-D'].value_counts())\n\n# Get Predictors\nX = dataset.loc[:, dataset.columns != target_col].values",
"Summary about Target Variable {target_col}\n--------------------------------------------------\n2 57\n1 13\nName: T-OR-D, dtype: int64\n"
],
[
"# Standardizing the features\n# --------------------------------------------------------------------------- #\nscaler_methods = ['minmax', 'standard', 'norm']\nscaler_method = 'standard'\nrescaledX = preprocessing_data_rescaling(scaler_method, X)",
"shape features matrix X, after normalizing: (70, 11)\n"
]
],
[
[
"### Pricipal Component Analysis\n\nAfter having investigate the data points inside the dataset, I move one to another section of my report where I decide to explore examples that made up the entire dataset using a particular technique in the field of statistical analysis that corresponds, precisely, to so called Principal Component Analysis. In fact, the major objective of this section is understand whether it is possible to transform, by means of some kind of linear transformation given by a mathematical calculation, the original data examples into reprojected representation that allows me to retrieve most useful information to be later exploited at training time. So, lets dive a bit whitin what is and which are main concepts, pros and cons about Principal Component Analysis.\n\nFirstly, we know that **Principal Component Analysis**, more shortly PCA, is a statistical procedure that uses an orthogonal transformation to convert a set of observations of possibly correlated variables into a set of values of linearly uncorrelated variables called *principal components*. This transformation is defined in such a way that:\n- the first principal component has the largest possible variance (that is, accounts for as much of the variability in the data as possible),\n- and each succeeding component in turn has the highest variance possible under the constraint that it is orthogonal to the preceding components.\n\nThe resulting vectors, each being a linear combination of the variables and containing n observations, are an uncorrelated orthogonal basis set. PCA is sensitive to the relative scaling of the original variables.\n\nPCA is mostly used as a tool in *exploratory data analysis* and for making predictive models, for that reasons I used such a technique here, before going through the different learning technique for producing my models.\n\n#### Several Different Implementation\n\nFrom the theory and the filed of research in statistics, we know that out there, there are several different implementation and way of computing principal component analysis, and each adopted technique has different performance as well as numerical stability. The three major derivations are:\n- PCA by means of an iterative based procedure of extraing pricipal components one after the other selecting each time the one that account for the most of variance along its own axis, within the remainig subspace to be derived.\n- The second possible way of performing PCA is done via calculation of *Covariance Matrix* applied to attributes, that are our independent predictive variables, used to represent data points.\n- Lastly, it is used the technique known as *Singular Valued Decomposition* applied to the overall data points within our dataset.\n\nReading scikit-learn documentation, I discovered that PCA's derivation uses the *LAPACK implementation* of the *full SVD* or a *randomized truncated SVD* by the method of *Halko et al. 2009*, depending on the shape of the input data and the number of components to extract. Therefore I will descrive mainly that way of deriving the method with respect to the others that, instead, will be described more briefly and roughly.\n\n#### PCA's Iterative based Method\nGoing in order, as depicted briefly above, I start describing PCA obtained by means of iterative based procedure to extract one at a time a new principal componet explointing the data points at hand.\n\nWe begin, recalling that, PCA is defined as an orthogonal linear transformation that transforms the data to a new coordinate system such that the greatest variance by some scalar projection of the data comes to lie on the first coordinate (called the first principal component), the second greatest variance on the second coordinate, and so on.\n\nWe suppose to deal with a data matrix X, with column-wise zero empirical mean, where each of the n rows represents a different repetition of the experiment, and each of the p columns gives a particular kind of feature.\n\nFrom a math poitn of view, the transformation is defined by a set of p-dimensional vectors of weights or coefficients $\\mathbf {w} _{(k)}=(w_{1},\\dots ,w_{p})_{(k)}$ that map each row vector $\\mathbf{x}_{(i)}$ of X to a new vector of principal component scores ${\\displaystyle \\mathbf {t} _{(i)}=(t_{1},\\dots ,t_{l})_{(i)}}$, given by: ${\\displaystyle {t_{k}}_{(i)}=\\mathbf {x} _{(i)}\\cdot \\mathbf {w} _{(k)}\\qquad \\mathrm {for} \\qquad i=1,\\dots ,n\\qquad k=1,\\dots ,l}$.\n\nIn this way all the individual variables ${\\displaystyle t_{1},\\dots ,t_{l}}$ of t considered over the data set successively inherit the maximum possible variance from X, with each coefficient vector w constrained to be a unit vector.\n\nMore precisely, the first component In order to maximize variance has to satisfy the following expression:\n\n${\\displaystyle \\mathbf {w} _{(1)}={\\underset {\\Vert \\mathbf {w} \\Vert =1}{\\operatorname {\\arg \\,max} }}\\,\\left\\{\\sum _{i}\\left(t_{1}\\right)_{(i)}^{2}\\right\\}={\\underset {\\Vert \\mathbf {w} \\Vert =1}{\\operatorname {\\arg \\,max} }}\\,\\left\\{\\sum _{i}\\left(\\mathbf {x} _{(i)}\\cdot \\mathbf {w} \\right)^{2}\\right\\}}$\n\nSo, with $w_{1}$ found, the first principal component of a data vector $x_{1}$ can then be given as a score $t_{1(i)} = x_{1} ⋅ w_{1}$ in the transformed co-ordinates, or as the corresponding vector in the original variables, $(x_{1} ⋅ w_{1})w_{1}$.\n\nThe others remainig components are computed as folloes. The kth component can be found by subtracting the first k − 1 principal components from X, as in the following expression:\n\n- ${\\displaystyle \\mathbf {\\hat {X}} _{k}=\\mathbf {X} -\\sum _{s=1}^{k-1}\\mathbf {X} \\mathbf {w} _{(s)}\\mathbf {w} _{(s)}^{\\rm {T}}}$\n\n- and then finding the weight vector which extracts the maximum variance from this new data matrix ${\\mathbf {w}}_{{(k)}}={\\underset {\\Vert {\\mathbf {w}}\\Vert =1}{\\operatorname {arg\\,max}}}\\left\\{\\Vert {\\mathbf {{\\hat {X}}}}_{{k}}{\\mathbf {w}}\\Vert ^{2}\\right\\}={\\operatorname {\\arg \\,max}}\\,\\left\\{{\\tfrac {{\\mathbf {w}}^{T}{\\mathbf {{\\hat {X}}}}_{{k}}^{T}{\\mathbf {{\\hat {X}}}}_{{k}}{\\mathbf {w}}}{{\\mathbf {w}}^{T}{\\mathbf {w}}}}\\right\\}$\n\nIt turns out that:\n- from the formulas depicted above me get the remaining eigenvectors of $X^{T}X$, with the maximum values for the quantity in brackets given by their corresponding eigenvalues. Thus the weight vectors are eigenvectors of $X^{T}X$.\n- The kth principal component of a data vector $x_(i)$ can therefore be given as a score $t_{k(i)} = x_{(i)} ⋅ w_(k)$ in the transformed co-ordinates, or as the corresponding vector in the space of the original variables, $(x_{(i)} ⋅ w_{(k)}) w_{(k)}$, where $w_{(k)}$ is the kth eigenvector of $X^{T}X$.\n- The full principal components decomposition of X can therefore be given as: ${\\displaystyle \\mathbf {T} =\\mathbf {X} \\mathbf {W}}$, where W is a p-by-p matrix of weights whose columns are the eigenvectors of $X^{T}X$.\n\n#### Covariance Matrix for PCA analysis\n\nPCA made from covarian matrix computation requires the calculation of sample covariance matrix of the dataset as follows: $\\mathbf{Q} \\propto \\mathbf{X}^T \\mathbf{X} = \\mathbf{W} \\mathbf{\\Lambda} \\mathbf{W}^T$.\n\nThe empirical covariance matrix between the principal components becomes ${\\displaystyle \\mathbf {W} ^{T}\\mathbf {Q} \\mathbf {W} \\propto \\mathbf {W} ^{T}\\mathbf {W} \\,\\mathbf {\\Lambda } \\,\\mathbf {W} ^{T}\\mathbf {W} =\\mathbf {\\Lambda } }$.\n\n\n#### Singular Value Decomposition for PCA analysis\n\nFinally, the principal components transformation can also be associated with another matrix factorization, the singular value decomposition (SVD) of X, ${\\displaystyle \\mathbf {X} =\\mathbf {U} \\mathbf {\\Sigma } \\mathbf {W} ^{T}}$, where more precisely:\n- Σ is an n-by-p rectangular diagonal matrix of positive numbers $σ_{(k)}$, called the singular values of X;\n- instead U is an n-by-n matrix, the columns of which are orthogonal unit vectors of length n called the left singular vectors of X;\n- Then, W is a p-by-p whose columns are orthogonal unit vectors of length p and called the right singular vectors of X.\n\nfactorizingn the matrix ${X^{T}X}$, it can be written as:\n\n${\\begin{aligned}\\mathbf {X} ^{T}\\mathbf {X} &=\\mathbf {W} \\mathbf {\\Sigma } ^{T}\\mathbf {U} ^{T}\\mathbf {U} \\mathbf {\\Sigma } \\mathbf {W} ^{T}\\\\&=\\mathbf {W} \\mathbf {\\Sigma } ^{T}\\mathbf {\\Sigma } \\mathbf {W} ^{T}\\\\&=\\mathbf {W} \\mathbf {\\hat {\\Sigma }} ^{2}\\mathbf {W} ^{T}\\end{aligned}}$\n\nWhere we recall that ${\\displaystyle \\mathbf {\\hat {\\Sigma }} }$ is the square diagonal matrix with the singular values of X and the excess zeros chopped off that satisfies ${\\displaystyle \\mathbf {{\\hat {\\Sigma }}^{2}} =\\mathbf {\\Sigma } ^{T}\\mathbf {\\Sigma } } {\\displaystyle \\mathbf {{\\hat {\\Sigma }}^{2}} =\\mathbf {\\Sigma } ^{T}\\mathbf {\\Sigma } }$. Comparison with the eigenvector factorization of $X^{T}X$ establishes that the right singular vectors W of X are equivalent to the eigenvectors of $X^{T}X$ , while the singular values $σ_{(k)}$ of X are equal to the square-root of the eigenvalues $λ_{(k)}$ of $X^{T}X$ . \n\nAt this point we understand that using the singular value decomposition the score matrix T can be written as:\n\n$\\begin{align} \\mathbf{T} & = \\mathbf{X} \\mathbf{W} \\\\ & = \\mathbf{U}\\mathbf{\\Sigma}\\mathbf{W}^T \\mathbf{W} \\\\ & = \\mathbf{U}\\mathbf{\\Sigma} \\end{align}$\n\nso each column of T is given by one of the left singular vectors of X multiplied by the corresponding singular value. This form is also the polar decomposition of T.\n\nEfficient algorithms exist to calculate the SVD, as in scikit-learn package, of X without having to form the matrix $X^{T}X$, so computing the SVD is now the standard way to calculate a principal components analysis from a data matrix",
"_____no_output_____"
]
],
[
[
"n_components = rescaledX.shape[1]\npca = PCA(n_components=n_components)\n# pca = PCA(n_components=2)\n\n# X_pca = pca.fit_transform(X)\npca = pca.fit(rescaledX)\nX_pca = pca.transform(rescaledX)",
"_____no_output_____"
],
[
"print(f\"Cumulative varation explained(percentage) up to given number of pcs:\")\n\ntmp_data = []\nprincipal_components = [pc for pc in '2,5,6,7,8,9,10'.split(',')]\nfor _, pc in enumerate(principal_components):\n n_components = int(pc)\n \n cum_var_exp_up_to_n_pcs = np.cumsum(pca.explained_variance_ratio_)[n_components-1]\n # print(f\"Cumulative varation explained up to {n_components} pcs = {cum_var_exp_up_to_n_pcs}\")\n # print(f\"# pcs {n_components}: {cum_var_exp_up_to_n_pcs*100:.2f}%\")\n tmp_data.append([n_components, cum_var_exp_up_to_n_pcs * 100])\n\ntmp_df = pd.DataFrame(data=tmp_data, columns=['# PCS', 'Cumulative Varation Explained (percentage)'])\ntmp_df.head(len(tmp_data))",
"Cumulative varation explained(percentage) up to given number of pcs:\n"
],
[
"n_components = rescaledX.shape[1]\npca = PCA(n_components=n_components)\n# pca = PCA(n_components=2)\n\n#X_pca = pca.fit_transform(X)\npca = pca.fit(rescaledX)\nX_pca = pca.transform(rescaledX)\n \nfig = show_cum_variance_vs_components(pca, n_components)\n\n# py.sign_in('franec94', 'QbLNKpC0EZB0kol0aL2Z')\n# py.iplot(fig, filename='selecting-principal-components {}'.format(scaler_method))",
"_____no_output_____"
]
],
[
[
"#### Major Pros & Cons of PCA\n\n",
"_____no_output_____"
],
[
"## Learning Models <a class=\"anchor\" id=\"learning-models\"></a>",
"_____no_output_____"
]
],
[
[
"# Parameters to be tested for Cross-Validation Approach\n\nestimators_list = [GaussianNB(), LogisticRegression(), KNeighborsClassifier(), SVC(), DecisionTreeClassifier(), RandomForestClassifier()]\nestimators_names = ['GaussianNB', 'LogisticRegression', 'KNeighborsClassifier', 'SVC', 'DecisionTreeClassifier', 'RandomForestClassifier']\nplots_names = list(map(lambda xi: f\"{xi}_learning_curve.png\", estimators_names))\n\npca_kernels_list = ['linear', 'poly', 'rbf', 'cosine',]\ncv_list = [10, 9, 8, 7, 6, 5, 4, 3, 2]\n\nparameters_sgd_classifier = {\n 'clf__loss': ('hinge', 'log', 'modified_huber', 'squared_hinge', 'perceptron'),\n 'clf__penalty': ('l2', 'l1', 'elasticnet'),\n 'clf__alpha': (1e-1, 1e-2, 1e-3, 1e-4),\n 'clf__max_iter': (50, 100, 150, 200, 500, 1000, 1500, 2000, 2500),\n 'clf__learning_rate': ('optimal',),\n 'clf__tol': (None, 1e-2, 1e-4, 1e-5, 1e-6)\n}\n\nkernel_type = 'svm-rbf-kernel'\nparameters_svm = {\n 'clf__gamma': (0.003, 0.03, 0.05, 0.5, 0.7, 1.0, 1.5),\n 'clf__max_iter':(1e+2, 1e+3, 2 * 1e+3, 5 * 1e+3, 1e+4, 1.5 * 1e+3),\n 'clf__C': (1e-4, 1e-3, 1e-2, 0.1, 1.0, 10, 1e+2, 1e+3),\n}\n\nparmas_decision_tree = {\n 'clf__splitter': ('random', 'best'),\n 'clf__criterion':('gini', 'entropy'),\n 'clf__max_features': (None, 'auto', 'sqrt', 'log2')\n}\n\nparmas_random_forest = {\n 'clf__n_estimators': (3, 5, 7, 10, 30, 50, 70, 100, 150, 200),\n 'clf__criterion':('gini', 'entropy'),\n 'clf__bootstrap': (True, False)\n}",
"_____no_output_____"
],
[
"model = PCA(n_components=2)\nmodel.fit(X) \nX_2D = model.transform(X)\n\ndf = pd.DataFrame()\ndf['PCA1'] = X_2D[:, 0]\ndf['PCA2'] = X_2D[:, 1]\ndf[target_col] = dataset[target_col].values\n\nsns.lmplot(\"PCA1\", \"PCA2\", hue=target_col, data=df, fit_reg=False)\n\n# show_pca_1_vs_pca_2_pcaKernel(X, pca_kernels_list, target_col, dataset)\n# show_scatter_plots_pcaKernel(X, pca_kernels_list, target_col, dataset, n_components=12)",
"_____no_output_____"
]
],
[
[
"## PCA = 2",
"_____no_output_____"
]
],
[
[
"plot_dest = os.path.join(\"figures\", \"n_comp_2_analysis\")\nN_CV, N_KERNEL = 9, 4\nassert len(cv_list) >= N_CV, f\"Error: N_CV={N_CV} > len(cv_list)={len(cv_list)}\"\nassert len(pca_kernels_list) >= N_KERNEL, f\"Error: N_KERNEL={N_KERNEL} > len(pca_kernels_list)={len(pca_kernels_list)}\"\n\nX = rescaledX\n\nn = len(estimators_list) # len(estimators_list)\ndfs_list, df_strfd = fit_all_by_n_components(\n estimators_list=estimators_list[:n], \\\n estimators_names=estimators_names[:n], \\\n X=X, \\\n y=y, \\\n n_components=2, \\\n show_plots=False, \\\n cv_list=cv_list[:N_CV], \\\n # pca_kernels_list=['linear'],\n pca_kernels_list=pca_kernels_list[:N_KERNEL],\n verbose=0 # 0=silent, 1=show informations\n)\ndf_strfd.head(df_strfd.shape[0])",
"_____no_output_____"
],
[
"# GaussianNB\n# -----------------------------------\ndfs_list[0].head(dfs_list[0].shape[0])",
"_____no_output_____"
],
[
"pos = 0\nplot_name = plots_names[pos]\nshow_learning_curve(dfs_list[pos], n=len(cv_list[:N_CV]), plot_dest=plot_dest, grid_size=[2, 2], plot_name=plot_name)",
"_____no_output_____"
],
[
"# LogisticRegression\n# -----------------------------------\ndfs_list[1].head(dfs_list[0].shape[0])",
"_____no_output_____"
],
[
"pos = pos + 1\nplot_name = plots_names[pos]\nshow_learning_curve(dfs_list[pos], n=len(cv_list[:N_CV]), plot_dest=plot_dest, grid_size=[2, 2], plot_name=plot_name)",
"_____no_output_____"
],
[
"# SVC\n# -----------------------------------\ndfs_list[2].head(dfs_list[0].shape[0])",
"_____no_output_____"
],
[
"pos = pos + 1\nplot_name = plots_names[pos]\nshow_learning_curve(dfs_list[pos], n=len(cv_list[:N_CV]), plot_dest=plot_dest, grid_size=[2, 2], plot_name=plot_name)",
"_____no_output_____"
],
[
"# DecisionTreeClassifier\n# -----------------------------------\ndfs_list[3].head(dfs_list[0].shape[0])",
"_____no_output_____"
],
[
"pos = pos + 1\nplot_name = plots_names[pos]\nshow_learning_curve(dfs_list[pos], n=len(cv_list[:N_CV]), plot_dest=plot_dest, grid_size=[2, 2], plot_name=plot_name)",
"_____no_output_____"
],
[
"# RandomForestClassifier\n# -----------------------------------\ndfs_list[4].head(dfs_list[0].shape[0])",
"_____no_output_____"
],
[
"pos = pos + 1\nplot_name = plots_names[pos]\nshow_learning_curve(dfs_list[pos], n=len(cv_list[:N_CV]), plot_dest=plot_dest, grid_size=[2, 2], plot_name=plot_name)",
"_____no_output_____"
]
],
[
[
"## PCA = 9",
"_____no_output_____"
]
],
[
[
"plot_dest = os.path.join(\"figures\", \"n_comp_9_analysis\")\nn = len(estimators_list) # len(estimators_list)\npos = 0\ndfs_list, df_strfd = fit_all_by_n_components(\n estimators_list=estimators_list[:n], \\\n estimators_names=estimators_names[:n], \\\n X=X, \\\n y=y, \\\n n_components=9, \\\n show_plots=False, \\\n cv_list=cv_list[:N_CV], \\\n # pca_kernels_list=['linear'],\n pca_kernels_list=pca_kernels_list[:N_KERNEL],\n verbose=0 # 0=silent, 1=show informations\n)\ndf_strfd.head(df_strfd.shape[0])",
"_____no_output_____"
],
[
"# GaussianNB\n# -----------------------------------\ndfs_list[0].head(dfs_list[0].shape[0])",
"_____no_output_____"
],
[
"pos = pos + 1\nplot_name = plots_names[pos]\nshow_learning_curve(dfs_list[pos], n=len(cv_list[:N_CV]), plot_dest=plot_dest, grid_size=[2, 2], plot_name=plot_name)",
"_____no_output_____"
],
[
"# LogisticRegression\n# -----------------------------------\ndfs_list[1].head(dfs_list[0].shape[0])",
"_____no_output_____"
],
[
"ppos = pos + 1\nplot_name = plots_names[pos]\nshow_learning_curve(dfs_list[pos], n=len(cv_list[:N_CV]), plot_dest=plot_dest, grid_size=[2, 2], plot_name=plot_name)",
"_____no_output_____"
],
[
"# SVC\n# -----------------------------------\ndfs_list[2].head(dfs_list[0].shape[0])",
"_____no_output_____"
],
[
"pos = pos + 1\nplot_name = plots_names[pos]\nshow_learning_curve(dfs_list[pos], n=len(cv_list[:N_CV]), plot_dest=plot_dest, grid_size=[2, 2], plot_name=plot_name)",
"_____no_output_____"
],
[
"# DecisionTreeClassifier\n# -----------------------------------\ndfs_list[3].head(dfs_list[0].shape[0])",
"_____no_output_____"
],
[
"pos = pos + 1\nplot_name = plots_names[pos]\nshow_learning_curve(dfs_list[pos], n=len(cv_list[:N_CV]), plot_dest=plot_dest, grid_size=[2, 2], plot_name=plot_name)",
"_____no_output_____"
],
[
"# RandomForestClassifier\n# -----------------------------------\ndfs_list[4].head(dfs_list[0].shape[0])",
"_____no_output_____"
],
[
"pos = pos + 1\nplot_name = plots_names[pos]\nshow_learning_curve(dfs_list[pos], n=len(cv_list[:N_CV]), plot_dest=plot_dest, grid_size=[2, 2], plot_name=plot_name)",
"_____no_output_____"
]
],
[
[
"## PCA = 12",
"_____no_output_____"
]
],
[
[
"plot_dest = os.path.join(\"figures\", \"n_comp_12_analysis\")\nn = len(estimators_list) # len(estimators_list)\npos = 0\ndfs_list, df_strfd = fit_all_by_n_components(\n estimators_list=estimators_list[:n], \\\n estimators_names=estimators_names[:n], \\\n X=X, \\\n y=y, \\\n n_components=12, \\\n show_plots=False, \\\n cv_list=cv_list[:N_CV], \\\n # pca_kernels_list=['linear'],\n pca_kernels_list=pca_kernels_list[:N_KERNEL],\n verbose=0 # 0=silent, 1=show informations\n)\ndf_strfd.head(df_strfd.shape[0])",
"_____no_output_____"
],
[
"# GaussianNB\n# -----------------------------------\ndfs_list[0].head(dfs_list[0].shape[0])",
"_____no_output_____"
],
[
"pos = pos + 1\nplot_name = plots_names[pos]\nshow_learning_curve(dfs_list[pos], n=len(cv_list[:N_CV]), plot_dest=plot_dest, grid_size=[2, 2], plot_name=plot_name)",
"_____no_output_____"
],
[
"# LogisticRegression\n# -----------------------------------\ndfs_list[1].head(dfs_list[0].shape[0])",
"_____no_output_____"
],
[
"pos = pos + 1\nplot_name = plots_names[pos]\nshow_learning_curve(dfs_list[pos], n=len(cv_list[:N_CV]), plot_dest=plot_dest, grid_size=[2, 2], plot_name=plot_name)",
"_____no_output_____"
],
[
"# SVC\n# -----------------------------------\ndfs_list[2].head(dfs_list[0].shape[0])",
"_____no_output_____"
],
[
"pos = pos + 1\nplot_name = plots_names[pos]\nshow_learning_curve(dfs_list[pos], n=len(cv_list[:N_CV]), plot_dest=plot_dest, grid_size=[2, 2], plot_name=plot_name)",
"_____no_output_____"
],
[
"# DecisionTreeClassifier\n# -----------------------------------\ndfs_list[3].head(dfs_list[0].shape[0])",
"_____no_output_____"
],
[
"pos = pos + 1\nplot_name = plots_names[pos]\nshow_learning_curve(dfs_list[pos], n=len(cv_list[:N_CV]), plot_dest=plot_dest, grid_size=[2, 2], plot_name=plot_name)",
"_____no_output_____"
],
[
"# RandomForestClassifier\n# -----------------------------------\ndfs_list[4].head(dfs_list[0].shape[0])",
"_____no_output_____"
],
[
"pos = pos + 1\nplot_name = plots_names[pos]\nshow_learning_curve(dfs_list[pos], n=len(cv_list[:N_CV]), plot_dest=plot_dest, grid_size=[2, 2], plot_name=plot_name)",
"_____no_output_____"
],
[
"from sklearn.metrics import f1_score\ny_true = [0, 1, 2, 0, 1, 2]\ny_pred = [0, 2, 1, 0, 0, 1]\nf1_score(y_true, y_pred, average='macro')\n",
"_____no_output_____"
]
],
[
[
"### Naive Bayes Classification\n\nNaive Bayes models are a group of extremely fast and simple classification algorithms that are often suitable for very high-dimensional datasets. Because they are so fast and have so few tunable parameters, they end up being very useful as a quick-and-dirty baseline for a classification problem. Here I will provide an intuitive and brief explanation of how naive Bayes classifiers work, followed by its exploitation onto my datasets.\n\nI start saying that Naive Bayes classifiers are built on Bayesian classification methods. These rely on Bayes's theorem, which is an equation describing the relationship of conditional probabilities of statistical quantities.\n\nn Bayesian classification, we're interested in finding the probability of a label given some observed features, which we can write as P(L | features). Bayes's theorem tells us how to express this in terms of quantities we can compute more directly:\n\n$P(L|features)=\\frac{P(features|L)}{P(L)P(features)}$\n\nIf we are trying to decide between two labels, and we call them L1 and L2, then one way to make this decision is to compute the ratio of the posterior probabilities for each label:\n\n$\\frac{P(L1 | features)}{P(L2 | features)}=\\frac{P(features | L1)P(L1)}{P(features | L2)P(L2)}$\n\nAll we need now is some model by which we can compute P(features | $L_{i}$)\n\nfor each label. Such a model is called a generative model because it specifies the hypothetical random process that generates the data. Specifying this generative model for each label is the main piece of the training of such a Bayesian classifier. The general version of such a training step is a very difficult task, but we can make it simpler through the use of some simplifying assumptions about the form of this model.\n\nThis is where the \"naive\" in \"naive Bayes\" comes in: if we make very naive assumptions about the generative model for each label, we can find a rough approximation of the generative model for each class, and then proceed with the Bayesian classification. Different types of naive Bayes classifiers rest on different naive assumptions about the data, and we will examine a few of these in the following sections.\n\n#### Gaussian Naive Bayes\n\nPerhaps the easiest naive Bayes classifier to understand is Gaussian naive Bayes. In this classifier, the assumption is that data from each label is drawn from a simple Gaussian distribution. In fact, one extremely fast way to create a simple model is to assume that the data is described by a Gaussian distribution with no covariance between dimensions. This model can be fit by simply finding the mean and standard deviation of the points within each label, which is all you need to define such a distribution.\n\n$P(x_i \\mid y) = \\frac{1}{\\sqrt{2\\pi\\sigma^2_y}} \\exp\\left(-\\frac{(x_i - \\mu_y)^2}{2\\sigma^2_y}\\right)$\n\nThe parameters $\\sigma_{y}$ and $\\mu_{y}$ are estimated usually using maximum likelihood.",
"_____no_output_____"
],
[
"#### When to Use Naive Bayes\n\nBecause naive Bayesian classifiers make such stringent assumptions about data, they will generally not perform as well as a more complicated model. That said, they have several advantages:\n\n- They are extremely fast for both training and prediction\n- They provide straightforward probabilistic prediction\n- They are often very easily interpretable\n- They have very few (if any) tunable parameters\n\n\nThese advantages mean a naive Bayesian classifier is often a good choice as an initial baseline classification. If it performs suitably, then congratulations: you have a very fast, very interpretable classifier for your problem. If it does not perform well, then you can begin exploring more sophisticated models, with some baseline knowledge of how well they should perform.\n\nNaive Bayes classifiers tend to perform especially well in one of the following situations:\n\n- When the naive assumptions actually match the data (very rare in practice)\n- For very well-separated categories, when model complexity is less important\n- For very high-dimensional data, when model complexity is less important\n\nThe last two points seem distinct, but they actually are related: as the dimension of a dataset grows, it is much less likely for any two points to be found close together (after all, they must be close in every single dimension to be close overall). This means that clusters in high dimensions tend to be more separated, on average, than clusters in low dimensions, assuming the new dimensions actually add information. For this reason, simplistic classifiers like naive Bayes tend to work as well or better than more complicated classifiers as the dimensionality grows: once you have enough data, even a simple model can be very powerful.",
"_____no_output_____"
],
[
"### Support Vector Machines Classifier\n\n<img src=\"images/SVM_margin.png\" alt=\"SVM Margin \" style=\"width: 200px;\"/>\n\nHere, in this section I'm going to exploit a machine learning techinique known as Support Vectors Machines in order to detect and so select the best model I can produce throughout the usage of data points contained within the dataset at hand. So let discuss a bit those kind of classifiers.\n\nIn machine learning, **support-vector machines**, shortly SVMs, are *supervised learning models* with associated learning algorithms that analyze data used for classification and regression analysis. Given a set of training examples, each marked as belonging to one or the other of two categories, an SVM training algorithm builds a model that assigns new examples to one category or the other, making it a *non-probabilistic binary linear classifier*. An SVM model is a representation of the examples as points in space, mapped so that the examples of the separate categories are divided by a clear gap that is as wide as possible. New examples are then mapped into that same space and predicted to belong to a category based on the side of the gap on which they fall.\n\nMore formally, a support-vector machine constructs a hyperplane or set of hyperplanes in a high-dimensional space, which can be used for classification, regression. Intuitively, a good separation is achieved by the hyperplane that has the largest distance to the nearest training-data point of any class, so-called *functional margin*, since in general the larger the margin, the lower the *generalization error* of the classifier.\n\n#### Mathematical formulation of SVMs\nHere, I'm going to describe the main mathematical properties and characteristics used to derive from a math point of view the algorithm derived and proven by researches when they have studied SVMs classifiers.\n\nI start saying and recalling that A support vector machine constructs a hyper-plane or set of hyper-planes in a high or infinite dimensional space, which can be used for classification, regression or other tasks. Intuitively, a good separation is achieved by the hyper-plane that has the largest distance to the nearest training data points of any class so-called functional margin, since in general the larger the margin the lower the generalization error of the classifier.\n\nWhen demonstrating SVMs classifier algorithm I suppose that We are given a training dataset of *n*n points of the form:\n\n\\begin{align}\n(\\vec{x_1} y_1),..,(\\vec{x_n},y_n)\n\\end{align}\n\nwhere the $y_{1}$ are either 1 or −1, each indicating the class to which the point $\\vec{x}_{i}$ belongs. Each $\\vec{x}_{i}$is a *p-dimensional real vector*. We want to find the \"maximum-margin hyperplane\" that divides the group of points $\\vec{x}_{i}$ for which $y_{1}$ = 1from the group of points for which $y_{1}$ = − 1, which is defined so that the distance between the hyperplane and the nearest point $\\vec{x}_{i}$ from either group is maximized.\n\nAny hyperplane can be written as the set of points $\\vec{x}_{i}$ satisfying : $\\vec{w}_{i}\\cdot{\\vec{x}_{i}} - b = 0$; where $\\vec{w}_{i}$ is the, even if not necessarily, normal vector to the hyperplane. The parameter $\\tfrac {b}{\\|\\vec{w}\\|}$ determines the offset of the hyperplane from the origin along the normal vector $\\vec{x}_{i}$.\n\nArrived so far, I have to distiguish between two distinct cases which both depende on the nature of data points that generally made up a given dataset. Those two different cases are called *Hard-Margin* and *Soft Margin* and, respectively.\n\nThe first case, so the ***Hard-Margin*** case, happens just for really optimistics datasets. In fact it is the case when the training data is linearly separable, hence, we can select two parallel hyperplanes that separate the two classes of data, so that the distance between them is as large as possible. The region bounded by these two hyperplanes is called the \"margin\", and the maximum-margin hyperplane is the hyperplane that lies halfway between them. With a normalized or standardized dataset, these hyperplanes can be described by the equations:\n- $\\vec{w}_{i}\\cdot{\\vec{x}_{i}} - b = 1$, that is anything on or above this boundary is of one class, with label 1;\n- and, $\\vec{w}_{i}\\cdot{\\vec{x}_{i}} - b = -1$, that is anything on or above this boundary is of one class, with label -1.\n\nWe can notice also that the distance between these two hyperplanes is ${\\displaystyle {\\tfrac {2}{\\|{\\vec {w}}\\|}}}$ so to maximize the distance between the planes we want to minimize ‖ w → ‖ {\\displaystyle \\|{\\vec {w}}\\|} \\|{\\vec {w}}\\|. The distance is computed using the distance from a point to a plane equation. We also have to prevent data points from falling into the margin, we add the following constraint: for each *i*:\n- either, ${\\displaystyle {\\vec {w}}\\cdot {\\vec {x}}_{i}-b\\geq 1}$, ${\\displaystyle y_{i}=1}$;\n- or, ${\\displaystyle {\\vec {w}}\\cdot {\\vec {x}}_{i}-b\\leq -1}$, if ${\\displaystyle y_{i}=-1}$.\n\nThese constraints state that each data point must lie on the correct side of the margin.\nFinally, we collect all the previous observations and define the following optimization problem:\n- from $y_{i}(\\vec{w}_{i}\\cdot{\\vec{x}_{i}} - b) \\geq 1$, for all $1 \\leq i \\leq n$;\n- to minimize ${\\displaystyle y_{i}({\\vec {w}}\\cdot {\\vec {x}}_{i}-b)\\geq 1}$ ${\\displaystyle i=1,\\ldots ,n}$.\n\nThe classifier we obtain is made from ${\\vec {w}}$ and ${\\displaystyle b}$ that solve this problem, and he max-margin hyperplane is completely determined by those ${\\vec {x}}_{i}$ that lie nearest to it. These $\\vec{x}_{i}$ are called *support vectors*.\n\nThe other case, so the ***Soft-Margin*** case, convercely happens when the training data is not linearly separable. To deal with such situation, as well as, to extend SVM to cases in which the data are not linearly separable, we introduce the hinge loss function, that is: $max(y_{i}(\\vec{w}_{i}\\cdot{\\vec{x}_{i}} - b))$.\nOnce we have provided the new loss function we go ahead with the new optimization problem that we aim at minimizing that is:\n\n\\begin{align}\n{\\displaystyle \\left[{\\frac {1}{n}}\\sum _{i=1}^{n}\\max \\left(0,1-y_{i}({\\vec {w}}\\cdot {\\vec {x}}_{i}-b)\\right)\\right]+\\lambda \\lVert {\\vec {w}}\\rVert ^{2},}\n\\end{align}\n\nwhere the parameter \\lambda determines the trade-off between increasing the margin size and ensuring that the ${\\vec {x}}_{i}$ lie on the correct side of the margin. Thus, for sufficiently small values of\\lambda , the second term in the loss function will become negligible, hence, it will behave similar to the hard-margin SVM, if the input data are linearly classifiable, but will still learn if a classification rule is viable or not.\n\nWhat we notice from last equations written just above is that we deal with a quadratic programming problem, and its solution is provided, detailed below.\n\nWe start defining a *Primal Problem* as follow:\n- For each $\\{1,\\,\\ldots ,\\,n\\}$ we introduce a variable ${\\displaystyle \\zeta _{i}=\\max \\left(0,1-y_{i}(w\\cdot x_{i}-b)\\right)}$. Note that ${\\displaystyle \\zeta _{i}}$ is the smallest nonnegative number satisfying ${\\displaystyle y_{i}(w\\cdot x_{i}-b)\\geq 1-\\zeta _{i}}$;\n- we can rewrite the optimization problem as follows: ${\\displaystyle {\\text{minimize }}{\\frac {1}{n}}\\sum _{i=1}^{n}\\zeta _{i}+\\lambda \\|w\\|^{2}}$, ${\\displaystyle {\\text{subject to }}y_{i}(w\\cdot x_{i}-b)\\geq 1-\\zeta _{i}\\,{\\text{ and }}\\,\\zeta _{i}\\geq 0,\\,{\\text{for all }}i.}$\n\nHowever, by solving for the *Lagrangian dual* of the above problem, one obtains the simplified problem:\n\\begin{align}\n {\\displaystyle {\\text{maximize}}\\,\\,f(c_{1}\\ldots c_{n})=\\sum _{i=1}^{n}c_{i}-{\\frac {1}{2}}\\sum _{i=1}^{n}\\sum _{j=1}^{n}y_{i}c_{i}(x_{i}\\cdot x_{j})y_{j}c_{j},} \\\\\n{\\displaystyle {\\text{subject to }}\\sum _{i=1}^{n}c_{i}y_{i}=0,\\,{\\text{and }}0\\leq c_{i}\\leq {\\frac {1}{2n\\lambda }}\\;{\\text{for all }}i.} \n\\end{align}\n- moreover, the variables $c_i$ are defined as ${\\displaystyle {\\vec {w}}=\\sum _{i=1}^{n}c_{i}y_{i}{\\vec {x}}_{i}}$. Where, ${\\displaystyle c_{i}=0}$ exactly when ${\\displaystyle {\\vec {x}}_{i}}$ lies on the correct side of the margin, and ${\\displaystyle 0<c_{i}<(2n\\lambda )^{-1}}$ when ${\\vec {x}}_{i}$ lies on the margin's boundary. It follows that ${\\displaystyle {\\vec {w}}}$ can be written as a linear combination of the support vectors.\n\nThe offset, ${\\displaystyle b}$, can be recovered by finding an ${\\vec {x}}_{i}$ on the margin's boundary and solving: ${\\displaystyle y_{i}({\\vec {w}}\\cdot {\\vec {x}}_{i}-b)=1\\iff b={\\vec {w}}\\cdot {\\vec {x}}_{i}-y_{i}.}$ \n\nThis is called the *dual problem*. Since the dual maximization problem is a quadratic function of the ${\\displaystyle c_{i}}$ subject to linear constraints, it is efficiently solvable by quadratic programming algorithms.\n\nLastly, I will discuss what in the context of SVMs classifier is called as ***Kernel Trick***.\nRoughly speaking, we know that a possible way of dealing with datasets that are not linearly separable but that can become linearnly separable within an higher dimensional space, or feature space, we can try to remap the original data points into a higher order feature space by means of some kind of remapping function, hence, solve the SVMs classifier optimization problem to find out a linear classifier in that new larger feature space. Then, we project back to the original feature space the solution we have found, reminding that in the hold feature space the decision boundaries founded will be non-linear, but still allow to classify new examples.\n\nUsually, especially, dealing with large datasets or with datasets with large set of features this approach becomes computationally intensive and and unfeasible if we run out of memory. So, in other words, the procedure is constrained in time and space, and might become time consuming or even unfeasible because of the large amount of memory we have to exploit.\n\nAn reasonable alternative is represented by the usage of kernel functions that are function which satisfy ${\\displaystyle k({\\vec {x}}_{i},{\\vec {x}}_{j})=\\varphi ({\\vec {x}}_{i})\\cdot \\varphi ({\\vec {x}}_{j})}$, where we recall that classification vector ${\\vec {w}}$ in the transformed space satisfies ${\\displaystyle {\\vec {w}}=\\sum _{i=1}^{n}c_{i}y_{i}\\varphi ({\\vec {x}}_{i}),}$\nwhere, the ${\\displaystyle c_{i}}$ are obtained by solving the optimization problem:\n\n${\\displaystyle {\\begin{aligned}{\\text{maximize}}\\,\\,f(c_{1}\\ldots c_{n})&=\\sum _{i=1}^{n}c_{i}-{\\frac {1}{2}}\\sum _{i=1}^{n}\\sum _{j=1}^{n}y_{i}c_{i}(\\varphi ({\\vec {x}}_{i})\\cdot \\varphi ({\\vec {x}}_{j}))y_{j}c_{j}\\\\&=\\sum _{i=1}^{n}c_{i}-{\\frac {1}{2}}\\sum _{i=1}^{n}\\sum _{j=1}^{n}y_{i}c_{i}k({\\vec {x}}_{i},{\\vec {x}}_{j})y_{j}c_{j}\\\\\\end{aligned}}}$\n\n${\\displaystyle {\\text{subject to }}\\sum _{i=1}^{n}c_{i}y_{i}=0,\\,{\\text{and }}0\\leq c_{i}\\leq {\\frac {1}{2n\\lambda }}\\;{\\text{for all }}i.}$\n\nThe coefficients ${\\displaystyle c_{i}}$ can be solved for using quadratic programming, and we can find some index ${\\displaystyle i}$ such that ${\\displaystyle 0<c_{i}<(2n\\lambda )^{-1}}$, so that ${\\displaystyle \\varphi ({\\vec {x}}_{i})}$ lies on the boundary of the margin in the transformed space, and then solve, by substituting doto product between remapped data points with kernel function applied upon the same arguments:\n\n${\\displaystyle {\\begin{aligned}b={\\vec {w}}\\cdot \\varphi ({\\vec {x}}_{i})-y_{i}&=\\left[\\sum _{j=1}^{n}c_{j}y_{j}\\varphi ({\\vec {x}}_{j})\\cdot \\varphi ({\\vec {x}}_{i})\\right]-y_{i}\\\\&=\\left[\\sum _{j=1}^{n}c_{j}y_{j}k({\\vec {x}}_{j},{\\vec {x}}_{i})\\right]-y_{i}.\\end{aligned}}}$\n\nFinally, ${\\displaystyle {\\vec {z}}\\mapsto \\operatorname {sgn}({\\vec {w}}\\cdot \\varphi ({\\vec {z}})-b)=\\operatorname {sgn} \\left(\\left[\\sum _{i=1}^{n}c_{i}y_{i}k({\\vec {x}}_{i},{\\vec {z}})\\right]-b\\right).}$\n\nWhat follows is a briefly list of the most commonly used kernel functions. They should be fine tuned, by means of a either grid search or random search approaches, identifying the best set of values to replace whitin the picked kernel function, where the choice depend on the dataset at hand:\n- Polynomial (homogeneous): ${\\displaystyle k({\\vec {x_{i}}},{\\vec {x_{j}}})=({\\vec {x_{i}}}\\cdot {\\vec {x_{j}}})^{d}}$.\n- Polynomial (inhomogeneous): ${\\displaystyle k({\\vec {x_{i}}},{\\vec {x_{j}}})=({\\vec {x_{i}}}\\cdot {\\vec {x_{j}}}+1)^{d}}$.\n- Gaussian radial basis function: ${\\displaystyle \\gamma =1/(2\\sigma ^{2})} {\\displaystyle \\gamma =1/(2\\sigma ^{2})}$.\n- Hyperbolic tangent: ${\\displaystyle k({\\vec {x_{i}}},{\\vec {x_{j}}})=\\tanh(\\kappa {\\vec {x_{i}}}\\cdot {\\vec {x_{j}}}+c)}$ for some (not every) ${\\displaystyle \\kappa >0}$ and ${\\displaystyle c<0}$.\n\nWhat follows is the application or use of SVMs classifier for learning a model that best fit the training data in order to be able to classify new instance in a reliable way, selecting the most promising model trained.",
"_____no_output_____"
],
[
"#### Advantages and Backwards of SVMs\n\nFinally, I conclude this section providing a description of major advantages and backwards of such a machine learning technique, that have been noticed by researches who studied SVMs properties. The advantages of support vector machines are:\n\n- Effective in high dimensional spaces.\n- Still effective in cases where number of dimensions is greater than the number of samples.\n- Uses a subset of training points in the decision function (called support vectors), so it is also memory efficient.\n- Versatile: different Kernel functions can be specified for the decision function. Common kernels are provided, but it is also possible to specify custom kernels.\n\nOn the other and, the disadvantages of support vector machines include:\n\n- If the number of features is much greater than the number of samples, avoid over-fitting in choosing Kernel functions and regularization term is crucial.\n- SVMs do not directly provide probability estimates, these are calculated using an expensive five-fold cross-validation (see Scores and probabilities, below).",
"_____no_output_____"
],
[
"### Decision Tree Models",
"_____no_output_____"
],
[
"Decision Trees, for short DTs, are a *non-parametric supervised learning method* used for classification and regression. The goal is to create a model that predicts the value of a target variable by learning simple decision rules inferred from the data features.\n\nTheir mathematical formulation is generally provided as follows: Given training vectors $x_{i} \\in R^{n}$, $i=1,…, l$ and a label vector $y \\in R^{l}$, a decision tree recursively partitions the space such that the samples with the same labels are grouped together.\n\nLet the data at node $m$ be represented by $Q$. For each candidate split $\\theta = (j, t_{m})$\nconsisting of a feature $j$ and threshold $t_{m}$, partition the data into $Q_{left}(\\theta)$ and $Q_{left}(\\theta)$ subsets as:\n\n\\begin{align}\\begin{aligned}Q_{left}(\\theta) = {(x, y) | x_j <= t_m}\\\\Q_{right}(\\theta) = Q \\setminus Q_{left}(\\theta)\\end{aligned}\\end{align}\n\nThe impurity at $m$ is computed using an impurity function $H()$, the choice of which depends on the task being solved (classification or regression) like:\n\n\\begin{align}\nG(Q, \\theta) = \\frac{n_{left}}{N_m} H(Q_{left}(\\theta)) + \\frac{n_{right}}{N_m} H(Q_{right}(\\theta))\n\\end{align}\n\nSelect the parameters that minimises the impurity: $\\theta^* = \\operatorname{argmin}_\\theta G(Q, \\theta)$.\n\nRecurse for subsets $Q_{left}(\\theta^*)$ and $Q_{right}(\\theta^*)$ until the maximum allowable depth is reached,\n$N_m < \\min_{samples}$ or N_m = 1.\n\nSpeaking about *Classification Criteria* referred to the procedure used for learining or fit to the data a decision tree we can state what follows: If a target is a classification outcome taking on values $0,1,…,K-1$, for node $m$, representing a region $R_{m}$ with $N_{m}$ observations, let $p_{mk} = 1/ N_m \\sum_{x_i \\in R_m} I(y_i = k)$ be the proportion of class $k$ observations in node $m$.\n\nSo, Common measures of impurity are:\n- Gini, specified as $H(X_m) = \\sum_k p_{mk} (1 - p_{mk})$\n- Entropy, definead as $(X_m) = - \\sum_k p_{mk} \\log(p_{mk})$\n\nwhere, we recall that $X_{m}$ is the training data in node $m$.",
"_____no_output_____"
],
[
"#### Decision Tree's Advantages & Bacwards\n\nSome advantages of decision trees are:\n\n- Simple to understand and to interpret. Trees can be visualised.\n- Requires little data preparation. Other techniques often require data normalisation, dummy variables need to be created and blank values to be removed. Note however that this module does not support missing values.\n- The cost of using the tree (i.e., predicting data) is logarithmic in the number of data points used to train the tree.\n- Able to handle both numerical and categorical data. Other techniques are usually specialised in analysing datasets that have only one type of variable. See algorithms for more information.\n- Able to handle multi-output problems.\n\n- Uses a white box model. If a given situation is observable in a model, the explanation for the condition is easily explained by boolean logic. By contrast, in a black box model (e.g., in an artificial neural network), results may be more difficult to interpret.\n- Possible to validate a model using statistical tests. That makes it possible to account for the reliability of the model.\n- Performs well even if its assumptions are somewhat violated by the true model from which the data were generated.\n\nThe disadvantages of decision trees include:\n\n- Decision-tree learners can create over-complex trees that do not generalise the data well. This is called overfitting. Mechanisms such as pruning (not currently supported), setting the minimum number of samples required at a leaf node or setting the maximum depth of the tree are necessary to avoid this problem.\n- Decision trees can be unstable because small variations in the data might result in a completely different tree being generated. This problem is mitigated by using decision trees within an ensemble.\n- The problem of learning an optimal decision tree is known to be NP-complete under several aspects of optimality and even for simple concepts. Consequently, practical decision-tree learning algorithms are based on heuristic algorithms such as the greedy algorithm where locally optimal decisions are made at each node. Such algorithms cannot guarantee to return the globally optimal decision tree. This can be mitigated by training multiple trees in an ensemble learner, where the features and samples are randomly sampled with replacement.\n- There are concepts that are hard to learn because decision trees do not express them easily, such as XOR, parity or multiplexer problems.\n- Decision tree learners create biased trees if some classes dominate. It is therefore recommended to balance the dataset prior to fitting with the decision tree.\n\n",
"_____no_output_____"
],
[
"### Ensemble methods\n\nThe goal of ensemble methods is to combine the predictions of several base estimators built with a given learning algorithm in order to improve generalizability / robustness over a single estimator.\n\nTwo families of ensemble methods are usually distinguished:\n- In averaging methods, the driving principle is to build several estimators independently and then to average their predictions. On average, the combined estimator is usually better than any of the single base estimator because its variance is reduced. So, some examples are: Bagging methods, Forests of randomized trees, but still exist more classifiers;\n- Instead, in boosting methods, base estimators are built sequentially and one tries to reduce the bias of the combined estimator. The motivation is to combine several weak models to produce a powerful ensemble. Hence, some examples are: AdaBoost, Gradient Tree Boosting,but still exist more options.",
"_____no_output_____"
],
[
"#### Random Forests\n\nThe **sklearn.ensemble module** includes two averaging algorithms based on randomized decision trees: the RandomForest algorithm and the Extra-Trees method. Both algorithms are perturb-and-combine techniques, specifically designed for trees. This means a diverse set of classifiers is created by introducing randomness in the classifier construction. The prediction of the ensemble is given as the averaged prediction of the individual classifiers.\n\nIn random forests (see RandomForestClassifier and RandomForestRegressor classes), each tree in the ensemble is built from a sample drawn with replacement (i.e., a bootstrap sample) from the training set.\n\nThe main parameters to adjust when using these methods is *number of estimators* and *maxima features*. The former is the number of trees in the forest. The larger the better, but also the longer it will take to compute. In addition, note that results will stop getting significantly better beyond a critical number of trees. The latter is the size of the random subsets of features to consider when splitting a node. The lower the greater the reduction of variance, but also the greater the increase in bias.\n\nEmpirical good default values are maxima features equals to null, that means always considering all features instead of a random subset, for regression problems, and maxima features equals to \"sqrt\", using a random subset of size sqrt(number of features)) for classification tasks, where number of features is the number of features in the data. The best parameter values should always be cross-validated.\n\nWe note that the size of the model with the default parameters is $O( M * N * log (N) )$, where $M$ is the number of trees and $N$ is the number of samples.",
"_____no_output_____"
],
[
"### Improvements and Conclusions <a class=\"anchor\" id=\"Improvements-and-conclusions\"></a>",
"_____no_output_____"
],
[
"### References <a class=\"anchor\" id=\"references\"></a>\n- Data Domain Information part:\n - (Deck) https://en.wikipedia.org/wiki/Deck_(bridge)\n - (Cantilever bridge) https://en.wikipedia.org/wiki/Cantilever_bridge\n - (Arch bridge) https://en.wikipedia.org/wiki/Deck_(bridge)\n- Machine Learning part:\n - (Theory Book) https://jakevdp.github.io/PythonDataScienceHandbook/\n - (Decsion Trees) https://scikit-learn.org/stable/modules/tree.html#tree\n - (SVM) https://scikit-learn.org/stable/modules/svm.html\n - (PCA) https://scikit-learn.org/stable/modules/generated/sklearn.decomposition.PCA.html\n- Chart part:\n - (Seaborn Charts) https://acadgild.com/blog/data-visualization-using-matplotlib-and-seaborn\n- Markdown Math part:\n - https://share.cocalc.com/share/b4a30ed038ee41d868dad094193ac462ccd228e2/Homework%20/HW%201.2%20-%20Markdown%20and%20LaTeX%20Cheatsheet.ipynb?viewer=share\n - https://jupyter-notebook.readthedocs.io/en/stable/examples/Notebook/Typesetting%20Equations.html",
"_____no_output_____"
]
]
] | [
"markdown",
"code",
"markdown",
"code",
"markdown",
"code",
"markdown",
"code",
"markdown",
"code",
"markdown",
"code",
"markdown",
"code",
"markdown",
"code",
"markdown",
"code",
"markdown"
] | [
[
"markdown",
"markdown"
],
[
"code",
"code",
"code",
"code"
],
[
"markdown",
"markdown",
"markdown"
],
[
"code",
"code",
"code"
],
[
"markdown"
],
[
"code",
"code",
"code",
"code",
"code"
],
[
"markdown"
],
[
"code",
"code",
"code",
"code",
"code",
"code",
"code",
"code",
"code",
"code",
"code",
"code",
"code",
"code",
"code",
"code"
],
[
"markdown"
],
[
"code",
"code",
"code"
],
[
"markdown",
"markdown"
],
[
"code",
"code"
],
[
"markdown"
],
[
"code",
"code",
"code",
"code",
"code",
"code",
"code",
"code",
"code",
"code",
"code"
],
[
"markdown"
],
[
"code",
"code",
"code",
"code",
"code",
"code",
"code",
"code",
"code",
"code",
"code"
],
[
"markdown"
],
[
"code",
"code",
"code",
"code",
"code",
"code",
"code",
"code",
"code",
"code",
"code",
"code"
],
[
"markdown",
"markdown",
"markdown",
"markdown",
"markdown",
"markdown",
"markdown",
"markdown",
"markdown",
"markdown",
"markdown"
]
] |
d0489677bc128a545e828d235952e658f98f7357 | 458,094 | ipynb | Jupyter Notebook | 05_support_vector_machines.ipynb | Schnatz65/ML_Fundamentals | 64c1a62b59ec2a084dce564c22f8c65461ef9611 | [
"Apache-2.0"
] | null | null | null | 05_support_vector_machines.ipynb | Schnatz65/ML_Fundamentals | 64c1a62b59ec2a084dce564c22f8c65461ef9611 | [
"Apache-2.0"
] | null | null | null | 05_support_vector_machines.ipynb | Schnatz65/ML_Fundamentals | 64c1a62b59ec2a084dce564c22f8c65461ef9611 | [
"Apache-2.0"
] | null | null | null | 338.32644 | 77,312 | 0.927674 | [
[
[
"# Setup",
"_____no_output_____"
],
[
"First, let's import a few common modules, ensure MatplotLib plots figures inline and prepare a function to save the figures. We also check that Python 3.5 or later is installed (although Python 2.x may work, it is deprecated so we strongly recommend you use Python 3 instead), as well as Scikit-Learn ≥0.20.",
"_____no_output_____"
]
],
[
[
"# Python ≥3.5 is required\nimport sys\nassert sys.version_info >= (3, 5)\n\n# Scikit-Learn ≥0.20 is required\nimport sklearn\nassert sklearn.__version__ >= \"0.20\"\n\n# Common imports\nimport numpy as np\nimport os\n\n# to make this notebook's output stable across runs\nnp.random.seed(42)\n\n# To plot pretty figures\n%matplotlib inline\nimport matplotlib as mpl\nimport matplotlib.pyplot as plt\nmpl.rc('axes', labelsize=14)\nmpl.rc('xtick', labelsize=12)\nmpl.rc('ytick', labelsize=12)\n\nN_JOBS= 3\n\n# Where to save the figures\nPROJECT_ROOT_DIR = \".\"\nCHAPTER_ID = \"svm\"\nIMAGES_PATH = os.path.join(PROJECT_ROOT_DIR, \"images\", CHAPTER_ID)\nos.makedirs(IMAGES_PATH, exist_ok=True)\n\ndef save_fig(fig_id, tight_layout=True, fig_extension=\"png\", resolution=300):\n path = os.path.join(IMAGES_PATH, fig_id + \".\" + fig_extension)\n print(\"Saving figure\", fig_id)\n if tight_layout:\n plt.tight_layout()\n plt.savefig(path, format=fig_extension, dpi=resolution)",
"_____no_output_____"
]
],
[
[
"# Linear SVM Classification",
"_____no_output_____"
],
[
"The next few code cells generate the first figures in chapter 5. The first actual code sample comes after.\n\n**Code to generate Figure 5–1. Large margin classification**",
"_____no_output_____"
]
],
[
[
"from sklearn.svm import SVC\nfrom sklearn import datasets\n\niris = datasets.load_iris()\nX = iris[\"data\"][:, (2, 3)] # petal length, petal width\ny = iris[\"target\"]\n\nsetosa_or_versicolor = (y == 0) | (y == 1)\nX = X[setosa_or_versicolor]\ny = y[setosa_or_versicolor]\n\n# SVM Classifier model\nsvm_clf = SVC(kernel=\"linear\", C=float(\"inf\"))\nsvm_clf.fit(X, y)",
"_____no_output_____"
],
[
"# Bad models\nx0 = np.linspace(0, 5.5, 200)\npred_1 = 5*x0 - 20\npred_2 = x0 - 1.8\npred_3 = 0.1 * x0 + 0.5\n\ndef plot_svc_decision_boundary(svm_clf, xmin, xmax):\n w = svm_clf.coef_[0]\n b = svm_clf.intercept_[0]\n\n # At the decision boundary, w0*x0 + w1*x1 + b = 0\n # => x1 = -w0/w1 * x0 - b/w1\n x0 = np.linspace(xmin, xmax, 200)\n decision_boundary = -w[0]/w[1] * x0 - b/w[1]\n\n margin = 1/w[1]\n gutter_up = decision_boundary + margin\n gutter_down = decision_boundary - margin\n\n svs = svm_clf.support_vectors_\n plt.scatter(svs[:, 0], svs[:, 1], s=180, facecolors='#FFAAAA')\n plt.plot(x0, decision_boundary, \"k-\", linewidth=2)\n plt.plot(x0, gutter_up, \"k--\", linewidth=2)\n plt.plot(x0, gutter_down, \"k--\", linewidth=2)\n\nfig, axes = plt.subplots(ncols=2, figsize=(10,2.7), sharey=True)\n\nplt.sca(axes[0])\nplt.plot(x0, pred_1, \"g--\", linewidth=2)\nplt.plot(x0, pred_2, \"m-\", linewidth=2)\nplt.plot(x0, pred_3, \"r-\", linewidth=2)\nplt.plot(X[:, 0][y==1], X[:, 1][y==1], \"bs\", label=\"Iris versicolor\")\nplt.plot(X[:, 0][y==0], X[:, 1][y==0], \"yo\", label=\"Iris setosa\")\nplt.xlabel(\"Petal length\", fontsize=14)\nplt.ylabel(\"Petal width\", fontsize=14)\nplt.legend(loc=\"upper left\", fontsize=14)\nplt.axis([0, 5.5, 0, 2])\n\nplt.sca(axes[1])\nplot_svc_decision_boundary(svm_clf, 0, 5.5)\nplt.plot(X[:, 0][y==1], X[:, 1][y==1], \"bs\")\nplt.plot(X[:, 0][y==0], X[:, 1][y==0], \"yo\")\nplt.xlabel(\"Petal length\", fontsize=14)\nplt.axis([0, 5.5, 0, 2])\n\nsave_fig(\"large_margin_classification_plot\")\nplt.show()",
"Saving figure large_margin_classification_plot\n"
]
],
[
[
"**Code to generate Figure 5–2. Sensitivity to feature scales**",
"_____no_output_____"
]
],
[
[
"Xs = np.array([[1, 50], [5, 20], [3, 80], [5, 60]]).astype(np.float64)\nys = np.array([0, 0, 1, 1])\nsvm_clf = SVC(kernel=\"linear\", C=100)\nsvm_clf.fit(Xs, ys)\n\nplt.figure(figsize=(9,2.7))\nplt.subplot(121)\nplt.plot(Xs[:, 0][ys==1], Xs[:, 1][ys==1], \"bo\")\nplt.plot(Xs[:, 0][ys==0], Xs[:, 1][ys==0], \"ms\")\nplot_svc_decision_boundary(svm_clf, 0, 6)\nplt.xlabel(\"$x_0$\", fontsize=20)\nplt.ylabel(\"$x_1$ \", fontsize=20, rotation=0)\nplt.title(\"Unscaled\", fontsize=16)\nplt.axis([0, 6, 0, 90])\n\nfrom sklearn.preprocessing import StandardScaler\nscaler = StandardScaler()\nX_scaled = scaler.fit_transform(Xs)\nsvm_clf.fit(X_scaled, ys)\n\nplt.subplot(122)\nplt.plot(X_scaled[:, 0][ys==1], X_scaled[:, 1][ys==1], \"bo\")\nplt.plot(X_scaled[:, 0][ys==0], X_scaled[:, 1][ys==0], \"ms\")\nplot_svc_decision_boundary(svm_clf, -2, 2)\nplt.xlabel(\"$x'_0$\", fontsize=20)\nplt.ylabel(\"$x'_1$ \", fontsize=20, rotation=0)\nplt.title(\"Scaled\", fontsize=16)\nplt.axis([-2, 2, -2, 2])\n\nsave_fig(\"sensitivity_to_feature_scales_plot\")\n",
"Saving figure sensitivity_to_feature_scales_plot\n"
]
],
[
[
"## Soft Margin Classification\n**Code to generate Figure 5–3. Hard margin sensitivity to outliers**",
"_____no_output_____"
]
],
[
[
"X_outliers = np.array([[3.4, 1.3], [3.2, 0.8]])\ny_outliers = np.array([0, 0])\nXo1 = np.concatenate([X, X_outliers[:1]], axis=0)\nyo1 = np.concatenate([y, y_outliers[:1]], axis=0)\nXo2 = np.concatenate([X, X_outliers[1:]], axis=0)\nyo2 = np.concatenate([y, y_outliers[1:]], axis=0)\n\nsvm_clf2 = SVC(kernel=\"linear\", C=10**9)\nsvm_clf2.fit(Xo2, yo2)\n\nfig, axes = plt.subplots(ncols=2, figsize=(10,2.7), sharey=True)\n\nplt.sca(axes[0])\nplt.plot(Xo1[:, 0][yo1==1], Xo1[:, 1][yo1==1], \"bs\")\nplt.plot(Xo1[:, 0][yo1==0], Xo1[:, 1][yo1==0], \"yo\")\nplt.text(0.3, 1.0, \"Impossible!\", fontsize=24, color=\"red\")\nplt.xlabel(\"Petal length\", fontsize=14)\nplt.ylabel(\"Petal width\", fontsize=14)\nplt.annotate(\"Outlier\",\n xy=(X_outliers[0][0], X_outliers[0][1]),\n xytext=(2.5, 1.7),\n ha=\"center\",\n arrowprops=dict(facecolor='black', shrink=0.1),\n fontsize=16,\n )\nplt.axis([0, 5.5, 0, 2])\n\nplt.sca(axes[1])\nplt.plot(Xo2[:, 0][yo2==1], Xo2[:, 1][yo2==1], \"bs\")\nplt.plot(Xo2[:, 0][yo2==0], Xo2[:, 1][yo2==0], \"yo\")\nplot_svc_decision_boundary(svm_clf2, 0, 5.5)\nplt.xlabel(\"Petal length\", fontsize=14)\nplt.annotate(\"Outlier\",\n xy=(X_outliers[1][0], X_outliers[1][1]),\n xytext=(3.2, 0.08),\n ha=\"center\",\n arrowprops=dict(facecolor='black', shrink=0.1),\n fontsize=16,\n )\nplt.axis([0, 5.5, 0, 2])\n\nsave_fig(\"sensitivity_to_outliers_plot\")\nplt.show()",
"Saving figure sensitivity_to_outliers_plot\n"
]
],
[
[
"**This is the first code example in chapter 5:**",
"_____no_output_____"
]
],
[
[
"import numpy as np\nfrom sklearn import datasets\nfrom sklearn.pipeline import Pipeline\nfrom sklearn.preprocessing import StandardScaler\nfrom sklearn.svm import LinearSVC\n\niris = datasets.load_iris()\nX = iris[\"data\"][:, (2, 3)] # petal length, petal width\ny = (iris[\"target\"] == 2).astype(np.float64) # Iris virginica\n\nsvm_clf = Pipeline([\n (\"scaler\", StandardScaler()),\n (\"linear_svc\", LinearSVC(C=1, loss=\"hinge\", random_state=42)),\n ])\n\nsvm_clf.fit(X, y)",
"_____no_output_____"
],
[
"svm_clf.predict([[5.5, 1.7]])",
"_____no_output_____"
]
],
[
[
"**Code to generate Figure 5–4. Large margin versus fewer margin violations**",
"_____no_output_____"
]
],
[
[
"scaler = StandardScaler()\nsvm_clf1 = LinearSVC(C=1, loss=\"hinge\", random_state=42)\nsvm_clf2 = LinearSVC(C=100, loss=\"hinge\", random_state=42)\n\nscaled_svm_clf1 = Pipeline([\n (\"scaler\", scaler),\n (\"linear_svc\", svm_clf1),\n ])\nscaled_svm_clf2 = Pipeline([\n (\"scaler\", scaler),\n (\"linear_svc\", svm_clf2),\n ])\n\nscaled_svm_clf1.fit(X, y)\nscaled_svm_clf2.fit(X, y)",
"C:\\Users\\kleme\\anaconda3\\envs\\ML_Fundamentals\\lib\\site-packages\\sklearn\\svm\\_base.py:1206: ConvergenceWarning: Liblinear failed to converge, increase the number of iterations.\n warnings.warn(\n"
],
[
"# Convert to unscaled parameters\nb1 = svm_clf1.decision_function([-scaler.mean_ / scaler.scale_])\nb2 = svm_clf2.decision_function([-scaler.mean_ / scaler.scale_])\nw1 = svm_clf1.coef_[0] / scaler.scale_\nw2 = svm_clf2.coef_[0] / scaler.scale_\nsvm_clf1.intercept_ = np.array([b1])\nsvm_clf2.intercept_ = np.array([b2])\nsvm_clf1.coef_ = np.array([w1])\nsvm_clf2.coef_ = np.array([w2])\n\n# Find support vectors (LinearSVC does not do this automatically)\nt = y * 2 - 1\nsupport_vectors_idx1 = (t * (X.dot(w1) + b1) < 1).ravel()\nsupport_vectors_idx2 = (t * (X.dot(w2) + b2) < 1).ravel()\nsvm_clf1.support_vectors_ = X[support_vectors_idx1]\nsvm_clf2.support_vectors_ = X[support_vectors_idx2]",
"_____no_output_____"
],
[
"fig, axes = plt.subplots(ncols=2, figsize=(10,2.7), sharey=True)\n\nplt.sca(axes[0])\nplt.plot(X[:, 0][y==1], X[:, 1][y==1], \"g^\", label=\"Iris virginica\")\nplt.plot(X[:, 0][y==0], X[:, 1][y==0], \"bs\", label=\"Iris versicolor\")\nplot_svc_decision_boundary(svm_clf1, 4, 5.9)\nplt.xlabel(\"Petal length\", fontsize=14)\nplt.ylabel(\"Petal width\", fontsize=14)\nplt.legend(loc=\"upper left\", fontsize=14)\nplt.title(\"$C = {}$\".format(svm_clf1.C), fontsize=16)\nplt.axis([4, 5.9, 0.8, 2.8])\n\nplt.sca(axes[1])\nplt.plot(X[:, 0][y==1], X[:, 1][y==1], \"g^\")\nplt.plot(X[:, 0][y==0], X[:, 1][y==0], \"bs\")\nplot_svc_decision_boundary(svm_clf2, 4, 5.99)\nplt.xlabel(\"Petal length\", fontsize=14)\nplt.title(\"$C = {}$\".format(svm_clf2.C), fontsize=16)\nplt.axis([4, 5.9, 0.8, 2.8])\n\nsave_fig(\"regularization_plot\")",
"Saving figure regularization_plot\n"
]
],
[
[
"# Nonlinear SVM Classification",
"_____no_output_____"
],
[
"**Code to generate Figure 5–5. Adding features to make a dataset linearly separable**",
"_____no_output_____"
]
],
[
[
"X1D = np.linspace(-4, 4, 9).reshape(-1, 1)\nX2D = np.c_[X1D, X1D**2]\ny = np.array([0, 0, 1, 1, 1, 1, 1, 0, 0])\n\nplt.figure(figsize=(10, 3))\n\nplt.subplot(121)\nplt.grid(True, which='both')\nplt.axhline(y=0, color='k')\nplt.plot(X1D[:, 0][y==0], np.zeros(4), \"bs\")\nplt.plot(X1D[:, 0][y==1], np.zeros(5), \"g^\")\nplt.gca().get_yaxis().set_ticks([])\nplt.xlabel(r\"$x_1$\", fontsize=20)\nplt.axis([-4.5, 4.5, -0.2, 0.2])\n\nplt.subplot(122)\nplt.grid(True, which='both')\nplt.axhline(y=0, color='k')\nplt.axvline(x=0, color='k')\nplt.plot(X2D[:, 0][y==0], X2D[:, 1][y==0], \"bs\")\nplt.plot(X2D[:, 0][y==1], X2D[:, 1][y==1], \"g^\")\nplt.xlabel(r\"$x_1$\", fontsize=20)\nplt.ylabel(r\"$x_2$ \", fontsize=20, rotation=0)\nplt.gca().get_yaxis().set_ticks([0, 4, 8, 12, 16])\nplt.plot([-4.5, 4.5], [6.5, 6.5], \"r--\", linewidth=3)\nplt.axis([-4.5, 4.5, -1, 17])\n\nplt.subplots_adjust(right=1)\n\nsave_fig(\"higher_dimensions_plot\", tight_layout=False)\nplt.show()",
"Saving figure higher_dimensions_plot\n"
],
[
"from sklearn.datasets import make_moons\nX, y = make_moons(n_samples=100, noise=0.15, random_state=42)\n\ndef plot_dataset(X, y, axes):\n plt.plot(X[:, 0][y==0], X[:, 1][y==0], \"bs\")\n plt.plot(X[:, 0][y==1], X[:, 1][y==1], \"g^\")\n plt.axis(axes)\n plt.grid(True, which='both')\n plt.xlabel(r\"$x_1$\", fontsize=20)\n plt.ylabel(r\"$x_2$\", fontsize=20, rotation=0)\n\nplot_dataset(X, y, [-1.5, 2.5, -1, 1.5])\nplt.show()",
"_____no_output_____"
]
],
[
[
"**Here is second code example in the chapter:**",
"_____no_output_____"
]
],
[
[
"from sklearn.datasets import make_moons\nfrom sklearn.pipeline import Pipeline\nfrom sklearn.preprocessing import PolynomialFeatures\n\npolynomial_svm_clf = Pipeline([\n (\"poly_features\", PolynomialFeatures(degree=3)),\n (\"scaler\", StandardScaler()),\n (\"svm_clf\", LinearSVC(C=10, loss=\"hinge\", random_state=42))\n ])\n\npolynomial_svm_clf.fit(X, y)",
"C:\\Users\\kleme\\anaconda3\\envs\\ML_Fundamentals\\lib\\site-packages\\sklearn\\svm\\_base.py:1206: ConvergenceWarning: Liblinear failed to converge, increase the number of iterations.\n warnings.warn(\n"
]
],
[
[
"**Code to generate Figure 5–6. Linear SVM classifier using polynomial features**",
"_____no_output_____"
]
],
[
[
"def plot_predictions(clf, axes):\n x0s = np.linspace(axes[0], axes[1], 100)\n x1s = np.linspace(axes[2], axes[3], 100)\n x0, x1 = np.meshgrid(x0s, x1s)\n X = np.c_[x0.ravel(), x1.ravel()]\n y_pred = clf.predict(X).reshape(x0.shape)\n y_decision = clf.decision_function(X).reshape(x0.shape)\n plt.contourf(x0, x1, y_pred, cmap=plt.cm.brg, alpha=0.2)\n plt.contourf(x0, x1, y_decision, cmap=plt.cm.brg, alpha=0.1)\n\nplot_predictions(polynomial_svm_clf, [-1.5, 2.5, -1, 1.5])\nplot_dataset(X, y, [-1.5, 2.5, -1, 1.5])\n\nsave_fig(\"moons_polynomial_svc_plot\")\nplt.show()",
"Saving figure moons_polynomial_svc_plot\n"
]
],
[
[
"## Polynomial Kernel",
"_____no_output_____"
],
[
"**Next code example:**",
"_____no_output_____"
]
],
[
[
"from sklearn.svm import SVC\n\npoly_kernel_svm_clf = Pipeline([\n (\"scaler\", StandardScaler()),\n (\"svm_clf\", SVC(kernel=\"poly\", degree=3, coef0=1, C=5))\n ])\npoly_kernel_svm_clf.fit(X, y)",
"_____no_output_____"
]
],
[
[
"**Code to generate Figure 5–7. SVM classifiers with a polynomial kernel**",
"_____no_output_____"
]
],
[
[
"poly100_kernel_svm_clf = Pipeline([\n (\"scaler\", StandardScaler()),\n (\"svm_clf\", SVC(kernel=\"poly\", degree=10, coef0=100, C=5))\n ])\npoly100_kernel_svm_clf.fit(X, y)",
"_____no_output_____"
],
[
"fig, axes = plt.subplots(ncols=2, figsize=(10.5, 4), sharey=True)\n\nplt.sca(axes[0])\nplot_predictions(poly_kernel_svm_clf, [-1.5, 2.45, -1, 1.5])\nplot_dataset(X, y, [-1.5, 2.4, -1, 1.5])\nplt.title(r\"$d=3, r=1, C=5$\", fontsize=18)\n\nplt.sca(axes[1])\nplot_predictions(poly100_kernel_svm_clf, [-1.5, 2.45, -1, 1.5])\nplot_dataset(X, y, [-1.5, 2.4, -1, 1.5])\nplt.title(r\"$d=10, r=100, C=5$\", fontsize=18)\nplt.ylabel(\"\")\n\nsave_fig(\"moons_kernelized_polynomial_svc_plot\")\nplt.show()",
"Saving figure moons_kernelized_polynomial_svc_plot\n"
]
],
[
[
"## Similarity Features",
"_____no_output_____"
],
[
"**Code to generate Figure 5–8. Similarity features using the Gaussian RBF**",
"_____no_output_____"
]
],
[
[
"def gaussian_rbf(x, landmark, gamma):\n return np.exp(-gamma * np.linalg.norm(x - landmark, axis=1)**2)\n\ngamma = 0.3\n\nx1s = np.linspace(-4.5, 4.5, 200).reshape(-1, 1)\nx2s = gaussian_rbf(x1s, -2, gamma)\nx3s = gaussian_rbf(x1s, 1, gamma)\n\nXK = np.c_[gaussian_rbf(X1D, -2, gamma), gaussian_rbf(X1D, 1, gamma)]\nyk = np.array([0, 0, 1, 1, 1, 1, 1, 0, 0])\n\nplt.figure(figsize=(10.5, 4))\n\nplt.subplot(121)\nplt.grid(True, which='both')\nplt.axhline(y=0, color='k')\nplt.scatter(x=[-2, 1], y=[0, 0], s=150, alpha=0.5, c=\"red\")\nplt.plot(X1D[:, 0][yk==0], np.zeros(4), \"bs\")\nplt.plot(X1D[:, 0][yk==1], np.zeros(5), \"g^\")\nplt.plot(x1s, x2s, \"g--\")\nplt.plot(x1s, x3s, \"b:\")\nplt.gca().get_yaxis().set_ticks([0, 0.25, 0.5, 0.75, 1])\nplt.xlabel(r\"$x_1$\", fontsize=20)\nplt.ylabel(r\"Similarity\", fontsize=14)\nplt.annotate(r'$\\mathbf{x}$',\n xy=(X1D[3, 0], 0),\n xytext=(-0.5, 0.20),\n ha=\"center\",\n arrowprops=dict(facecolor='black', shrink=0.1),\n fontsize=18,\n )\nplt.text(-2, 0.9, \"$x_2$\", ha=\"center\", fontsize=20)\nplt.text(1, 0.9, \"$x_3$\", ha=\"center\", fontsize=20)\nplt.axis([-4.5, 4.5, -0.1, 1.1])\n\nplt.subplot(122)\nplt.grid(True, which='both')\nplt.axhline(y=0, color='k')\nplt.axvline(x=0, color='k')\nplt.plot(XK[:, 0][yk==0], XK[:, 1][yk==0], \"bs\")\nplt.plot(XK[:, 0][yk==1], XK[:, 1][yk==1], \"g^\")\nplt.xlabel(r\"$x_2$\", fontsize=20)\nplt.ylabel(r\"$x_3$ \", fontsize=20, rotation=0)\nplt.annotate(r'$\\phi\\left(\\mathbf{x}\\right)$',\n xy=(XK[3, 0], XK[3, 1]),\n xytext=(0.65, 0.50),\n ha=\"center\",\n arrowprops=dict(facecolor='black', shrink=0.1),\n fontsize=18,\n )\nplt.plot([-0.1, 1.1], [0.57, -0.1], \"r--\", linewidth=3)\nplt.axis([-0.1, 1.1, -0.1, 1.1])\n \nplt.subplots_adjust(right=1)\n\nsave_fig(\"kernel_method_plot\")\nplt.show()",
"Saving figure kernel_method_plot\n"
],
[
"x1_example = X1D[3, 0]\nfor landmark in (-2, 1):\n k = gaussian_rbf(np.array([[x1_example]]), np.array([[landmark]]), gamma)\n print(\"Phi({}, {}) = {}\".format(x1_example, landmark, k))",
"Phi(-1.0, -2) = [0.74081822]\nPhi(-1.0, 1) = [0.30119421]\n"
]
],
[
[
"## Gaussian RBF Kernel",
"_____no_output_____"
],
[
"**Next code example:**",
"_____no_output_____"
]
],
[
[
"rbf_kernel_svm_clf = Pipeline([\n (\"scaler\", StandardScaler()),\n (\"svm_clf\", SVC(kernel=\"rbf\", gamma=5, C=0.001))\n ])\nrbf_kernel_svm_clf.fit(X, y)",
"_____no_output_____"
]
],
[
[
"**Code to generate Figure 5–9. SVM classifiers using an RBF kernel**",
"_____no_output_____"
]
],
[
[
"from sklearn.svm import SVC\n\ngamma1, gamma2 = 0.1, 5\nC1, C2 = 0.001, 1000\nhyperparams = (gamma1, C1), (gamma1, C2), (gamma2, C1), (gamma2, C2)\n\nsvm_clfs = []\nfor gamma, C in hyperparams:\n rbf_kernel_svm_clf = Pipeline([\n (\"scaler\", StandardScaler()),\n (\"svm_clf\", SVC(kernel=\"rbf\", gamma=gamma, C=C))\n ])\n rbf_kernel_svm_clf.fit(X, y)\n svm_clfs.append(rbf_kernel_svm_clf)\n\nfig, axes = plt.subplots(nrows=2, ncols=2, figsize=(10.5, 7), sharex=True, sharey=True)\n\nfor i, svm_clf in enumerate(svm_clfs):\n plt.sca(axes[i // 2, i % 2])\n plot_predictions(svm_clf, [-1.5, 2.45, -1, 1.5])\n plot_dataset(X, y, [-1.5, 2.45, -1, 1.5])\n gamma, C = hyperparams[i]\n plt.title(r\"$\\gamma = {}, C = {}$\".format(gamma, C), fontsize=16)\n if i in (0, 1):\n plt.xlabel(\"\")\n if i in (1, 3):\n plt.ylabel(\"\")\n\nsave_fig(\"moons_rbf_svc_plot\")\nplt.show()",
"Saving figure moons_rbf_svc_plot\n"
]
],
[
[
"# SVM Regression",
"_____no_output_____"
]
],
[
[
"np.random.seed(42)\nm = 50\nX = 2 * np.random.rand(m, 1)\ny = (4 + 3 * X + np.random.randn(m, 1)).ravel()",
"_____no_output_____"
]
],
[
[
"**Next code example:**",
"_____no_output_____"
]
],
[
[
"from sklearn.svm import LinearSVR\n\nsvm_reg = LinearSVR(epsilon=1.5, random_state=42)\nsvm_reg.fit(X, y)",
"_____no_output_____"
]
],
[
[
"**Code to generate Figure 5–10. SVM Regression**",
"_____no_output_____"
]
],
[
[
"svm_reg1 = LinearSVR(epsilon=1.5, random_state=42)\nsvm_reg2 = LinearSVR(epsilon=0.5, random_state=42)\nsvm_reg1.fit(X, y)\nsvm_reg2.fit(X, y)\n\ndef find_support_vectors(svm_reg, X, y):\n y_pred = svm_reg.predict(X)\n off_margin = (np.abs(y - y_pred) >= svm_reg.epsilon)\n return np.argwhere(off_margin)\n\nsvm_reg1.support_ = find_support_vectors(svm_reg1, X, y)\nsvm_reg2.support_ = find_support_vectors(svm_reg2, X, y)\n\neps_x1 = 1\neps_y_pred = svm_reg1.predict([[eps_x1]])",
"_____no_output_____"
],
[
"def plot_svm_regression(svm_reg, X, y, axes):\n x1s = np.linspace(axes[0], axes[1], 100).reshape(100, 1)\n y_pred = svm_reg.predict(x1s)\n plt.plot(x1s, y_pred, \"k-\", linewidth=2, label=r\"$\\hat{y}$\")\n plt.plot(x1s, y_pred + svm_reg.epsilon, \"k--\")\n plt.plot(x1s, y_pred - svm_reg.epsilon, \"k--\")\n plt.scatter(X[svm_reg.support_], y[svm_reg.support_], s=180, facecolors='#FFAAAA')\n plt.plot(X, y, \"bo\")\n plt.xlabel(r\"$x_1$\", fontsize=18)\n plt.legend(loc=\"upper left\", fontsize=18)\n plt.axis(axes)\n\nfig, axes = plt.subplots(ncols=2, figsize=(9, 4), sharey=True)\nplt.sca(axes[0])\nplot_svm_regression(svm_reg1, X, y, [0, 2, 3, 11])\nplt.title(r\"$\\epsilon = {}$\".format(svm_reg1.epsilon), fontsize=18)\nplt.ylabel(r\"$y$\", fontsize=18, rotation=0)\n#plt.plot([eps_x1, eps_x1], [eps_y_pred, eps_y_pred - svm_reg1.epsilon], \"k-\", linewidth=2)\nplt.annotate(\n '', xy=(eps_x1, eps_y_pred), xycoords='data',\n xytext=(eps_x1, eps_y_pred - svm_reg1.epsilon),\n textcoords='data', arrowprops={'arrowstyle': '<->', 'linewidth': 1.5}\n )\nplt.text(0.91, 5.6, r\"$\\epsilon$\", fontsize=20)\nplt.sca(axes[1])\nplot_svm_regression(svm_reg2, X, y, [0, 2, 3, 11])\nplt.title(r\"$\\epsilon = {}$\".format(svm_reg2.epsilon), fontsize=18)\nsave_fig(\"svm_regression_plot\")\nplt.show()",
"Saving figure svm_regression_plot\n"
],
[
"np.random.seed(42)\nm = 100\nX = 2 * np.random.rand(m, 1) - 1\ny = (0.2 + 0.1 * X + 0.5 * X**2 + np.random.randn(m, 1)/10).ravel()",
"_____no_output_____"
]
],
[
[
"**Note**: to be future-proof, we set `gamma=\"scale\"`, as this will be the default value in Scikit-Learn 0.22.",
"_____no_output_____"
],
[
"**Next code example:**",
"_____no_output_____"
]
],
[
[
"from sklearn.svm import SVR\n\nsvm_poly_reg = SVR(kernel=\"poly\", degree=2, C=100, epsilon=0.1, gamma=\"scale\")\nsvm_poly_reg.fit(X, y)",
"_____no_output_____"
]
],
[
[
"**Code to generate Figure 5–11. SVM Regression using a second-degree polynomial kernel**",
"_____no_output_____"
]
],
[
[
"from sklearn.svm import SVR\n\nsvm_poly_reg1 = SVR(kernel=\"poly\", degree=2, C=100, epsilon=0.1, gamma=\"scale\")\nsvm_poly_reg2 = SVR(kernel=\"poly\", degree=2, C=0.01, epsilon=0.1, gamma=\"scale\")\nsvm_poly_reg1.fit(X, y)\nsvm_poly_reg2.fit(X, y)",
"_____no_output_____"
],
[
"fig, axes = plt.subplots(ncols=2, figsize=(9, 4), sharey=True)\nplt.sca(axes[0])\nplot_svm_regression(svm_poly_reg1, X, y, [-1, 1, 0, 1])\nplt.title(r\"$degree={}, C={}, \\epsilon = {}$\".format(svm_poly_reg1.degree, svm_poly_reg1.C, svm_poly_reg1.epsilon), fontsize=18)\nplt.ylabel(r\"$y$\", fontsize=18, rotation=0)\nplt.sca(axes[1])\nplot_svm_regression(svm_poly_reg2, X, y, [-1, 1, 0, 1])\nplt.title(r\"$degree={}, C={}, \\epsilon = {}$\".format(svm_poly_reg2.degree, svm_poly_reg2.C, svm_poly_reg2.epsilon), fontsize=18)\nsave_fig(\"svm_with_polynomial_kernel_plot\")\nplt.show()",
"Saving figure svm_with_polynomial_kernel_plot\n"
]
]
] | [
"markdown",
"code",
"markdown",
"code",
"markdown",
"code",
"markdown",
"code",
"markdown",
"code",
"markdown",
"code",
"markdown",
"code",
"markdown",
"code",
"markdown",
"code",
"markdown",
"code",
"markdown",
"code",
"markdown",
"code",
"markdown",
"code",
"markdown",
"code",
"markdown",
"code",
"markdown",
"code",
"markdown",
"code",
"markdown",
"code",
"markdown",
"code"
] | [
[
"markdown",
"markdown"
],
[
"code"
],
[
"markdown",
"markdown"
],
[
"code",
"code"
],
[
"markdown"
],
[
"code"
],
[
"markdown"
],
[
"code"
],
[
"markdown"
],
[
"code",
"code"
],
[
"markdown"
],
[
"code",
"code",
"code"
],
[
"markdown",
"markdown"
],
[
"code",
"code"
],
[
"markdown"
],
[
"code"
],
[
"markdown"
],
[
"code"
],
[
"markdown",
"markdown"
],
[
"code"
],
[
"markdown"
],
[
"code",
"code"
],
[
"markdown",
"markdown"
],
[
"code",
"code"
],
[
"markdown",
"markdown"
],
[
"code"
],
[
"markdown"
],
[
"code"
],
[
"markdown"
],
[
"code"
],
[
"markdown"
],
[
"code"
],
[
"markdown"
],
[
"code",
"code",
"code"
],
[
"markdown",
"markdown"
],
[
"code"
],
[
"markdown"
],
[
"code",
"code"
]
] |
d048967f3a5b3fa466b8ff12d50807f2d3555c2b | 563,389 | ipynb | Jupyter Notebook | SS_AITrader_INTC.ipynb | JamesHorrex/AI_stock_trading | 29a22593817473161e0b8b85a25c820b75779f08 | [
"Apache-2.0"
] | null | null | null | SS_AITrader_INTC.ipynb | JamesHorrex/AI_stock_trading | 29a22593817473161e0b8b85a25c820b75779f08 | [
"Apache-2.0"
] | null | null | null | SS_AITrader_INTC.ipynb | JamesHorrex/AI_stock_trading | 29a22593817473161e0b8b85a25c820b75779f08 | [
"Apache-2.0"
] | null | null | null | 131.234335 | 148,098 | 0.764317 | [
[
[
"<a href=\"https://colab.research.google.com/github/JamesHorrex/AI_stock_trading/blob/master/SS_AITrader_INTC.ipynb\" target=\"_parent\"><img src=\"https://colab.research.google.com/assets/colab-badge.svg\" alt=\"Open In Colab\"/></a>",
"_____no_output_____"
]
],
[
[
"%matplotlib inline\nimport numpy as np\nimport tensorflow as tf\nprint(tf.__version__)",
"2.2.0\n"
],
[
"!pip install git+https://github.com/tensorflow/docs",
"Collecting git+https://github.com/tensorflow/docs\n Cloning https://github.com/tensorflow/docs to /tmp/pip-req-build-u1gg8kd7\n Running command git clone -q https://github.com/tensorflow/docs /tmp/pip-req-build-u1gg8kd7\nRequirement already satisfied: astor in /usr/local/lib/python3.6/dist-packages (from tensorflow-docs===0.0.087622f0d4888b557639c605c04a0e822874df5ba-) (0.8.1)\nRequirement already satisfied: absl-py in /usr/local/lib/python3.6/dist-packages (from tensorflow-docs===0.0.087622f0d4888b557639c605c04a0e822874df5ba-) (0.9.0)\nRequirement already satisfied: protobuf in /usr/local/lib/python3.6/dist-packages (from tensorflow-docs===0.0.087622f0d4888b557639c605c04a0e822874df5ba-) (3.10.0)\nRequirement already satisfied: pyyaml in /usr/local/lib/python3.6/dist-packages (from tensorflow-docs===0.0.087622f0d4888b557639c605c04a0e822874df5ba-) (3.13)\nRequirement already satisfied: six in /usr/local/lib/python3.6/dist-packages (from absl-py->tensorflow-docs===0.0.087622f0d4888b557639c605c04a0e822874df5ba-) (1.12.0)\nRequirement already satisfied: setuptools in /usr/local/lib/python3.6/dist-packages (from protobuf->tensorflow-docs===0.0.087622f0d4888b557639c605c04a0e822874df5ba-) (47.3.1)\nBuilding wheels for collected packages: tensorflow-docs\n Building wheel for tensorflow-docs (setup.py) ... \u001b[?25l\u001b[?25hdone\n Created wheel for tensorflow-docs: filename=tensorflow_docs-0.0.087622f0d4888b557639c605c04a0e822874df5ba_-cp36-none-any.whl size=123835 sha256=6bd4575d9ab5558f18a03de08bb32ffe50026b9f6c5c612e189fbdd90ce56612\n Stored in directory: /tmp/pip-ephem-wheel-cache-boryl4mq/wheels/eb/1b/35/fce87697be00d2fc63e0b4b395b0d9c7e391a10e98d9a0d97f\nSuccessfully built tensorflow-docs\nInstalling collected packages: tensorflow-docs\nSuccessfully installed tensorflow-docs-0.0.087622f0d4888b557639c605c04a0e822874df5ba-\n"
],
[
"import tensorflow_docs as tfdocs\nimport tensorflow_docs.plots\nimport tensorflow_docs.modeling",
"_____no_output_____"
],
[
"from google.colab import drive \ndrive.mount('/content/gdrive')",
"Go to this URL in a browser: https://accounts.google.com/o/oauth2/auth?client_id=947318989803-6bn6qk8qdgf4n4g3pfee6491hc0brc4i.apps.googleusercontent.com&redirect_uri=urn%3aietf%3awg%3aoauth%3a2.0%3aoob&response_type=code&scope=email%20https%3a%2f%2fwww.googleapis.com%2fauth%2fdocs.test%20https%3a%2f%2fwww.googleapis.com%2fauth%2fdrive%20https%3a%2f%2fwww.googleapis.com%2fauth%2fdrive.photos.readonly%20https%3a%2f%2fwww.googleapis.com%2fauth%2fpeopleapi.readonly\n\nEnter your authorization code:\n··········\nMounted at /content/gdrive\n"
],
[
"import pandas as pd \ndf=pd.read_csv('gdrive/My Drive/SS_AITrader/INTC/df_INTC_20drtn_features.csv')",
"_____no_output_____"
],
[
"df.head()",
"_____no_output_____"
],
[
"df['timestamp'] = pd.to_datetime(df['timestamp'])",
"_____no_output_____"
],
[
"from_date='2010-01-01'\nto_date='2020-01-01'",
"_____no_output_____"
],
[
"df = df[pd.to_datetime(from_date) < df['timestamp'] ]\ndf = df[pd.to_datetime(to_date) > df['timestamp'] ]\n",
"_____no_output_____"
],
[
"df.head()",
"_____no_output_____"
],
[
"df.tail()",
"_____no_output_____"
],
[
"df.drop(['timestamp'], inplace=True, axis=1)",
"_____no_output_____"
],
[
"train_dataset = df.sample(frac=0.8,random_state=0)\ntest_dataset = df.drop(train_dataset.index)",
"_____no_output_____"
],
[
"train_dataset.head()",
"_____no_output_____"
],
[
"train_labels = train_dataset.pop('labels')\ntest_labels = test_dataset.pop('labels')",
"_____no_output_____"
],
[
"train_labels.head()",
"_____no_output_____"
],
[
"from sklearn.utils import compute_class_weight\ndef get_sample_weights(y):\n y = y.astype(int) # compute_class_weight needs int labels\n class_weights = compute_class_weight('balanced', np.unique(y), y)\n\n print(\"real class weights are {}\".format(class_weights), np.unique(y))\n print(\"value_counts\", np.unique(y, return_counts=True))\n sample_weights = y.copy().astype(float)\n for i in np.unique(y):\n sample_weights[sample_weights == i] = class_weights[i] # if i == 2 else 0.8 * class_weights[i]\n # sample_weights = np.where(sample_weights == i, class_weights[int(i)], y_)\n\n return sample_weights\n",
"_____no_output_____"
],
[
"get_sample_weights(train_labels)",
"real class weights are [1.2039312 0.85514834] [0 1]\nvalue_counts (array([0, 1]), array([ 814, 1146]))\n"
],
[
"SAMPLE_WEIGHT=get_sample_weights(train_labels)",
"real class weights are [1.2039312 0.85514834] [0 1]\nvalue_counts (array([0, 1]), array([ 814, 1146]))\n"
],
[
"train_stats = train_dataset.describe()\ntrain_stats = train_stats.transpose()",
"_____no_output_____"
],
[
"def norm(x):\n return (x - train_stats['mean']) / train_stats['std']\nnormed_train_data = norm(train_dataset)\nnormed_test_data = norm(test_dataset)",
"_____no_output_____"
],
[
"from sklearn.feature_selection import SelectKBest, f_classif, mutual_info_classif\nfrom operator import itemgetter\n\nk=20\nlist_features = list(normed_train_data.columns)\nselect_k_best = SelectKBest(f_classif, k=k)\nselect_k_best.fit(normed_train_data, train_labels)\nselected_features_anova = itemgetter(*select_k_best.get_support(indices=True))(list_features)\n\nselected_features_anova",
"_____no_output_____"
],
[
"select_k_best = SelectKBest(mutual_info_classif, k=k)\nselect_k_best.fit(normed_train_data, train_labels)\nselected_features_mic = itemgetter(*select_k_best.get_support(indices=True))(list_features)\nselected_features_mic",
"_____no_output_____"
],
[
"list_features = list(normed_train_data.columns)\nfeat_idx = []\nfor c in selected_features_mic:\n feat_idx.append(list_features.index(c))\n\nfeat_idx = sorted(feat_idx)\nX_train_new=normed_train_data.iloc[:, feat_idx]\nX_test_new=normed_test_data.iloc[:, feat_idx]\n#kbest=SelectKBest(f_classif, k=10)\n#X_train_new = kbest.fit_transform(normed_train_data, train_labels)\n#X_test_new = kbest.transform(normed_test_data)\n\nX_test_new.shape\nX_test_new.head()",
"_____no_output_____"
],
[
"def build_model(hidden_dim,dropout=0.5):\n ## input layer\n inputs=tf.keras.Input(shape=(X_train_new.shape[1],))\n\n\n h1= tf.keras.layers.Dense(units=hidden_dim,activation='relu')(inputs)\n h2= tf.keras.layers.Dropout(dropout)(h1)\n h3= tf.keras.layers.Dense(units=hidden_dim*2,activation='relu')(h2)\n h4= tf.keras.layers.Dropout(dropout)(h3)\n h5= tf.keras.layers.Dense(units=hidden_dim*2,activation='relu')(h4)\n h6= tf.keras.layers.Dropout(dropout)(h5)\n h7= tf.keras.layers.Dense(units=hidden_dim,activation='relu')(h6)\n\n ##output\n outputs=tf.keras.layers.Dense(units=2,activation='softmax')(h7)\n\n return tf.keras.Model(inputs=inputs, outputs=outputs)",
"_____no_output_____"
],
[
"tf.random.set_seed(1)\n\ncriterion = tf.keras.losses.sparse_categorical_crossentropy\n\noptimizer = tf.keras.optimizers.Adam(learning_rate=0.001)\n\nmodel = build_model(hidden_dim=64)\n\nmodel.compile(optimizer=optimizer,loss=criterion,metrics=['accuracy'])",
"_____no_output_____"
],
[
"example_batch = X_train_new[:10]\nexample_result = model.predict(example_batch)\nexample_result",
"_____no_output_____"
],
[
"EPOCHS=200\nBATCH_SIZE=20\n\nhistory = model.fit(\n X_train_new, train_labels,\n epochs=EPOCHS, batch_size=BATCH_SIZE ,sample_weight=SAMPLE_WEIGHT,shuffle=True,validation_split = 0.2, verbose=1,\n callbacks=[tfdocs.modeling.EpochDots()])",
"Epoch 1/200\n77/79 [============================>.] - ETA: 0s - loss: 0.7224 - accuracy: 0.5156\nEpoch: 0, accuracy:0.5166, loss:0.7212, val_accuracy:0.4796, val_loss:0.6941, \n79/79 [==============================] - 1s 8ms/step - loss: 0.7212 - accuracy: 0.5166 - val_loss: 0.6941 - val_accuracy: 0.4796\nEpoch 2/200\n79/79 [==============================] - 0s 6ms/step - loss: 0.7037 - accuracy: 0.5223 - val_loss: 0.6880 - val_accuracy: 0.5306\nEpoch 3/200\n79/79 [==============================] - 0s 6ms/step - loss: 0.6968 - accuracy: 0.5128 - val_loss: 0.6844 - val_accuracy: 0.5791\nEpoch 4/200\n79/79 [==============================] - 0s 6ms/step - loss: 0.6899 - accuracy: 0.5421 - val_loss: 0.6788 - val_accuracy: 0.6020\nEpoch 5/200\n79/79 [==============================] - 0s 6ms/step - loss: 0.6939 - accuracy: 0.5523 - val_loss: 0.6797 - val_accuracy: 0.5332\nEpoch 6/200\n79/79 [==============================] - 0s 6ms/step - loss: 0.6885 - accuracy: 0.5389 - val_loss: 0.6740 - val_accuracy: 0.5867\nEpoch 7/200\n79/79 [==============================] - 0s 6ms/step - loss: 0.6851 - accuracy: 0.5644 - val_loss: 0.6727 - val_accuracy: 0.5612\nEpoch 8/200\n79/79 [==============================] - 0s 6ms/step - loss: 0.6856 - accuracy: 0.5402 - val_loss: 0.6681 - val_accuracy: 0.5842\nEpoch 9/200\n79/79 [==============================] - 0s 6ms/step - loss: 0.6658 - accuracy: 0.5842 - val_loss: 0.6473 - val_accuracy: 0.6173\nEpoch 10/200\n79/79 [==============================] - 0s 6ms/step - loss: 0.6709 - accuracy: 0.5835 - val_loss: 0.6388 - val_accuracy: 0.6301\nEpoch 11/200\n79/79 [==============================] - 0s 5ms/step - loss: 0.6634 - accuracy: 0.5893 - val_loss: 0.6456 - val_accuracy: 0.6633\nEpoch 12/200\n79/79 [==============================] - 0s 5ms/step - loss: 0.6548 - accuracy: 0.6256 - val_loss: 0.6325 - val_accuracy: 0.6199\nEpoch 13/200\n79/79 [==============================] - 0s 5ms/step - loss: 0.6479 - accuracy: 0.6122 - val_loss: 0.6182 - val_accuracy: 0.6531\nEpoch 14/200\n79/79 [==============================] - 0s 5ms/step - loss: 0.6462 - accuracy: 0.6256 - val_loss: 0.6239 - val_accuracy: 0.6633\nEpoch 15/200\n79/79 [==============================] - 0s 5ms/step - loss: 0.6433 - accuracy: 0.6116 - val_loss: 0.6160 - val_accuracy: 0.6786\nEpoch 16/200\n79/79 [==============================] - 0s 5ms/step - loss: 0.6418 - accuracy: 0.6473 - val_loss: 0.6072 - val_accuracy: 0.6607\nEpoch 17/200\n79/79 [==============================] - 0s 5ms/step - loss: 0.6422 - accuracy: 0.6193 - val_loss: 0.6075 - val_accuracy: 0.6786\nEpoch 18/200\n79/79 [==============================] - 0s 5ms/step - loss: 0.6429 - accuracy: 0.6276 - val_loss: 0.6111 - val_accuracy: 0.6607\nEpoch 19/200\n79/79 [==============================] - 0s 5ms/step - loss: 0.6293 - accuracy: 0.6276 - val_loss: 0.5976 - val_accuracy: 0.6786\nEpoch 20/200\n79/79 [==============================] - 0s 5ms/step - loss: 0.6269 - accuracy: 0.6486 - val_loss: 0.5939 - val_accuracy: 0.6888\nEpoch 21/200\n79/79 [==============================] - 0s 5ms/step - loss: 0.6144 - accuracy: 0.6594 - val_loss: 0.5772 - val_accuracy: 0.6811\nEpoch 22/200\n79/79 [==============================] - 0s 5ms/step - loss: 0.6224 - accuracy: 0.6562 - val_loss: 0.5892 - val_accuracy: 0.6964\nEpoch 23/200\n79/79 [==============================] - 0s 5ms/step - loss: 0.6047 - accuracy: 0.6511 - val_loss: 0.5746 - val_accuracy: 0.6862\nEpoch 24/200\n79/79 [==============================] - 0s 5ms/step - loss: 0.6011 - accuracy: 0.6805 - val_loss: 0.5786 - val_accuracy: 0.6888\nEpoch 25/200\n79/79 [==============================] - 0s 5ms/step - loss: 0.5975 - accuracy: 0.6652 - val_loss: 0.5754 - val_accuracy: 0.6964\nEpoch 26/200\n79/79 [==============================] - 0s 5ms/step - loss: 0.5981 - accuracy: 0.6996 - val_loss: 0.5774 - val_accuracy: 0.6888\nEpoch 27/200\n79/79 [==============================] - 0s 5ms/step - loss: 0.6028 - accuracy: 0.6690 - val_loss: 0.5662 - val_accuracy: 0.7168\nEpoch 28/200\n79/79 [==============================] - 0s 5ms/step - loss: 0.5864 - accuracy: 0.6805 - val_loss: 0.5598 - val_accuracy: 0.7143\nEpoch 29/200\n79/79 [==============================] - 0s 5ms/step - loss: 0.5885 - accuracy: 0.6945 - val_loss: 0.5514 - val_accuracy: 0.7143\nEpoch 30/200\n79/79 [==============================] - 0s 5ms/step - loss: 0.5903 - accuracy: 0.6875 - val_loss: 0.5565 - val_accuracy: 0.6939\nEpoch 31/200\n79/79 [==============================] - 0s 5ms/step - loss: 0.5731 - accuracy: 0.6894 - val_loss: 0.5388 - val_accuracy: 0.7143\nEpoch 32/200\n79/79 [==============================] - 0s 5ms/step - loss: 0.5740 - accuracy: 0.6862 - val_loss: 0.5479 - val_accuracy: 0.7219\nEpoch 33/200\n79/79 [==============================] - 0s 5ms/step - loss: 0.5727 - accuracy: 0.7066 - val_loss: 0.5408 - val_accuracy: 0.7168\nEpoch 34/200\n79/79 [==============================] - 0s 5ms/step - loss: 0.5789 - accuracy: 0.6888 - val_loss: 0.5343 - val_accuracy: 0.7143\nEpoch 35/200\n79/79 [==============================] - 0s 5ms/step - loss: 0.5660 - accuracy: 0.6958 - val_loss: 0.5386 - val_accuracy: 0.7117\nEpoch 36/200\n79/79 [==============================] - 0s 5ms/step - loss: 0.5697 - accuracy: 0.7047 - val_loss: 0.5231 - val_accuracy: 0.7347\nEpoch 37/200\n79/79 [==============================] - 0s 5ms/step - loss: 0.5631 - accuracy: 0.7124 - val_loss: 0.5277 - val_accuracy: 0.7372\nEpoch 38/200\n79/79 [==============================] - 0s 5ms/step - loss: 0.5563 - accuracy: 0.7156 - val_loss: 0.5365 - val_accuracy: 0.7015\nEpoch 39/200\n79/79 [==============================] - 0s 5ms/step - loss: 0.5606 - accuracy: 0.7028 - val_loss: 0.5237 - val_accuracy: 0.7449\nEpoch 40/200\n79/79 [==============================] - 0s 5ms/step - loss: 0.5688 - accuracy: 0.7034 - val_loss: 0.5408 - val_accuracy: 0.7219\nEpoch 41/200\n79/79 [==============================] - 0s 5ms/step - loss: 0.5317 - accuracy: 0.7258 - val_loss: 0.5257 - val_accuracy: 0.7347\nEpoch 42/200\n79/79 [==============================] - 0s 5ms/step - loss: 0.5428 - accuracy: 0.7245 - val_loss: 0.5275 - val_accuracy: 0.7347\nEpoch 43/200\n79/79 [==============================] - 0s 5ms/step - loss: 0.5365 - accuracy: 0.7328 - val_loss: 0.5162 - val_accuracy: 0.7577\nEpoch 44/200\n79/79 [==============================] - 0s 5ms/step - loss: 0.5478 - accuracy: 0.7162 - val_loss: 0.5254 - val_accuracy: 0.7296\nEpoch 45/200\n79/79 [==============================] - 0s 5ms/step - loss: 0.5396 - accuracy: 0.7258 - val_loss: 0.5195 - val_accuracy: 0.7602\nEpoch 46/200\n79/79 [==============================] - 0s 5ms/step - loss: 0.5306 - accuracy: 0.7417 - val_loss: 0.5050 - val_accuracy: 0.7602\nEpoch 47/200\n79/79 [==============================] - 0s 5ms/step - loss: 0.5375 - accuracy: 0.7264 - val_loss: 0.4950 - val_accuracy: 0.7704\nEpoch 48/200\n79/79 [==============================] - 0s 5ms/step - loss: 0.5352 - accuracy: 0.7372 - val_loss: 0.5070 - val_accuracy: 0.7500\nEpoch 49/200\n79/79 [==============================] - 0s 5ms/step - loss: 0.5227 - accuracy: 0.7398 - val_loss: 0.4909 - val_accuracy: 0.7755\nEpoch 50/200\n79/79 [==============================] - 0s 5ms/step - loss: 0.5170 - accuracy: 0.7462 - val_loss: 0.5046 - val_accuracy: 0.7372\nEpoch 51/200\n79/79 [==============================] - 0s 5ms/step - loss: 0.5258 - accuracy: 0.7404 - val_loss: 0.5007 - val_accuracy: 0.7551\nEpoch 52/200\n79/79 [==============================] - 0s 5ms/step - loss: 0.5329 - accuracy: 0.7341 - val_loss: 0.5009 - val_accuracy: 0.7577\nEpoch 53/200\n79/79 [==============================] - 0s 5ms/step - loss: 0.5247 - accuracy: 0.7545 - val_loss: 0.5070 - val_accuracy: 0.7423\nEpoch 54/200\n79/79 [==============================] - 0s 5ms/step - loss: 0.5133 - accuracy: 0.7506 - val_loss: 0.5035 - val_accuracy: 0.7449\nEpoch 55/200\n79/79 [==============================] - 0s 5ms/step - loss: 0.5222 - accuracy: 0.7328 - val_loss: 0.5014 - val_accuracy: 0.7577\nEpoch 56/200\n79/79 [==============================] - 0s 5ms/step - loss: 0.5169 - accuracy: 0.7366 - val_loss: 0.4843 - val_accuracy: 0.7730\nEpoch 57/200\n79/79 [==============================] - 0s 5ms/step - loss: 0.5182 - accuracy: 0.7564 - val_loss: 0.4855 - val_accuracy: 0.7806\nEpoch 58/200\n79/79 [==============================] - 0s 5ms/step - loss: 0.5093 - accuracy: 0.7449 - val_loss: 0.4794 - val_accuracy: 0.7985\nEpoch 59/200\n79/79 [==============================] - 0s 5ms/step - loss: 0.5093 - accuracy: 0.7634 - val_loss: 0.4845 - val_accuracy: 0.7628\nEpoch 60/200\n79/79 [==============================] - 0s 5ms/step - loss: 0.5013 - accuracy: 0.7532 - val_loss: 0.4689 - val_accuracy: 0.7857\nEpoch 61/200\n79/79 [==============================] - 0s 6ms/step - loss: 0.5060 - accuracy: 0.7532 - val_loss: 0.4902 - val_accuracy: 0.7628\nEpoch 62/200\n79/79 [==============================] - 0s 6ms/step - loss: 0.5046 - accuracy: 0.7615 - val_loss: 0.4720 - val_accuracy: 0.7832\nEpoch 63/200\n79/79 [==============================] - 0s 6ms/step - loss: 0.5022 - accuracy: 0.7640 - val_loss: 0.4780 - val_accuracy: 0.7704\nEpoch 64/200\n79/79 [==============================] - 0s 6ms/step - loss: 0.4857 - accuracy: 0.7640 - val_loss: 0.4767 - val_accuracy: 0.7755\nEpoch 65/200\n79/79 [==============================] - 0s 6ms/step - loss: 0.5001 - accuracy: 0.7717 - val_loss: 0.4827 - val_accuracy: 0.7679\nEpoch 66/200\n79/79 [==============================] - 0s 6ms/step - loss: 0.4699 - accuracy: 0.7864 - val_loss: 0.4698 - val_accuracy: 0.7704\nEpoch 67/200\n79/79 [==============================] - 0s 6ms/step - loss: 0.4851 - accuracy: 0.7793 - val_loss: 0.4668 - val_accuracy: 0.7755\nEpoch 68/200\n79/79 [==============================] - 0s 6ms/step - loss: 0.4832 - accuracy: 0.7730 - val_loss: 0.4690 - val_accuracy: 0.7908\nEpoch 69/200\n79/79 [==============================] - 0s 6ms/step - loss: 0.4761 - accuracy: 0.7851 - val_loss: 0.4604 - val_accuracy: 0.7781\nEpoch 70/200\n79/79 [==============================] - 0s 6ms/step - loss: 0.4838 - accuracy: 0.7806 - val_loss: 0.4542 - val_accuracy: 0.7755\nEpoch 71/200\n79/79 [==============================] - 0s 6ms/step - loss: 0.4669 - accuracy: 0.7851 - val_loss: 0.4732 - val_accuracy: 0.7653\nEpoch 72/200\n79/79 [==============================] - 0s 6ms/step - loss: 0.4565 - accuracy: 0.7876 - val_loss: 0.4636 - val_accuracy: 0.7806\nEpoch 73/200\n79/79 [==============================] - 0s 6ms/step - loss: 0.4639 - accuracy: 0.7749 - val_loss: 0.4607 - val_accuracy: 0.7781\nEpoch 74/200\n79/79 [==============================] - 0s 6ms/step - loss: 0.4619 - accuracy: 0.7883 - val_loss: 0.4759 - val_accuracy: 0.7526\nEpoch 75/200\n79/79 [==============================] - 0s 6ms/step - loss: 0.4566 - accuracy: 0.7838 - val_loss: 0.4684 - val_accuracy: 0.7704\nEpoch 76/200\n79/79 [==============================] - 0s 6ms/step - loss: 0.4585 - accuracy: 0.7921 - val_loss: 0.4508 - val_accuracy: 0.7832\nEpoch 77/200\n79/79 [==============================] - 0s 6ms/step - loss: 0.4593 - accuracy: 0.7806 - val_loss: 0.4532 - val_accuracy: 0.7934\nEpoch 78/200\n79/79 [==============================] - 0s 6ms/step - loss: 0.4659 - accuracy: 0.7889 - val_loss: 0.4537 - val_accuracy: 0.7730\nEpoch 79/200\n79/79 [==============================] - 0s 6ms/step - loss: 0.4676 - accuracy: 0.7698 - val_loss: 0.4575 - val_accuracy: 0.7985\nEpoch 80/200\n79/79 [==============================] - 0s 6ms/step - loss: 0.4507 - accuracy: 0.7953 - val_loss: 0.4437 - val_accuracy: 0.7883\nEpoch 81/200\n79/79 [==============================] - 0s 6ms/step - loss: 0.4595 - accuracy: 0.7946 - val_loss: 0.4495 - val_accuracy: 0.7857\nEpoch 82/200\n79/79 [==============================] - 0s 6ms/step - loss: 0.4502 - accuracy: 0.8017 - val_loss: 0.4351 - val_accuracy: 0.8087\nEpoch 83/200\n79/79 [==============================] - 0s 5ms/step - loss: 0.4524 - accuracy: 0.7921 - val_loss: 0.4392 - val_accuracy: 0.8061\nEpoch 84/200\n79/79 [==============================] - 0s 5ms/step - loss: 0.4519 - accuracy: 0.7915 - val_loss: 0.4420 - val_accuracy: 0.7959\nEpoch 85/200\n79/79 [==============================] - 0s 5ms/step - loss: 0.4553 - accuracy: 0.7851 - val_loss: 0.4447 - val_accuracy: 0.7985\nEpoch 86/200\n79/79 [==============================] - 0s 5ms/step - loss: 0.4500 - accuracy: 0.7889 - val_loss: 0.4293 - val_accuracy: 0.7985\nEpoch 87/200\n79/79 [==============================] - 0s 5ms/step - loss: 0.4617 - accuracy: 0.7940 - val_loss: 0.4386 - val_accuracy: 0.7985\nEpoch 88/200\n79/79 [==============================] - 0s 5ms/step - loss: 0.4453 - accuracy: 0.7902 - val_loss: 0.4598 - val_accuracy: 0.7781\nEpoch 89/200\n79/79 [==============================] - 0s 5ms/step - loss: 0.4434 - accuracy: 0.7819 - val_loss: 0.4336 - val_accuracy: 0.7934\nEpoch 90/200\n79/79 [==============================] - 0s 5ms/step - loss: 0.4542 - accuracy: 0.7966 - val_loss: 0.4417 - val_accuracy: 0.8010\nEpoch 91/200\n79/79 [==============================] - 0s 5ms/step - loss: 0.4404 - accuracy: 0.8010 - val_loss: 0.4314 - val_accuracy: 0.7985\nEpoch 92/200\n79/79 [==============================] - 0s 5ms/step - loss: 0.4370 - accuracy: 0.8023 - val_loss: 0.4350 - val_accuracy: 0.8010\nEpoch 93/200\n79/79 [==============================] - 0s 5ms/step - loss: 0.4304 - accuracy: 0.8093 - val_loss: 0.4360 - val_accuracy: 0.7883\nEpoch 94/200\n79/79 [==============================] - 0s 5ms/step - loss: 0.4222 - accuracy: 0.8125 - val_loss: 0.4286 - val_accuracy: 0.8112\nEpoch 95/200\n79/79 [==============================] - 0s 5ms/step - loss: 0.4335 - accuracy: 0.7966 - val_loss: 0.4265 - val_accuracy: 0.8265\nEpoch 96/200\n79/79 [==============================] - 0s 5ms/step - loss: 0.4417 - accuracy: 0.8042 - val_loss: 0.4227 - val_accuracy: 0.8036\nEpoch 97/200\n79/79 [==============================] - 0s 5ms/step - loss: 0.4503 - accuracy: 0.7876 - val_loss: 0.4366 - val_accuracy: 0.7934\nEpoch 98/200\n79/79 [==============================] - 0s 5ms/step - loss: 0.4191 - accuracy: 0.8080 - val_loss: 0.4368 - val_accuracy: 0.7959\nEpoch 99/200\n79/79 [==============================] - 0s 5ms/step - loss: 0.4263 - accuracy: 0.8099 - val_loss: 0.4495 - val_accuracy: 0.7730\nEpoch 100/200\n79/79 [==============================] - 0s 6ms/step - loss: 0.4625 - accuracy: 0.7864 - val_loss: 0.4455 - val_accuracy: 0.7934\nEpoch 101/200\n79/79 [==============================] - ETA: 0s - loss: 0.4319 - accuracy: 0.8055\nEpoch: 100, accuracy:0.8055, loss:0.4319, val_accuracy:0.7781, val_loss:0.4368, \n79/79 [==============================] - 0s 5ms/step - loss: 0.4319 - accuracy: 0.8055 - val_loss: 0.4368 - val_accuracy: 0.7781\nEpoch 102/200\n79/79 [==============================] - 0s 5ms/step - loss: 0.4358 - accuracy: 0.8036 - val_loss: 0.4316 - val_accuracy: 0.7985\nEpoch 103/200\n79/79 [==============================] - 0s 5ms/step - loss: 0.4284 - accuracy: 0.8068 - val_loss: 0.4278 - val_accuracy: 0.7959\nEpoch 104/200\n79/79 [==============================] - 0s 5ms/step - loss: 0.4299 - accuracy: 0.8125 - val_loss: 0.4195 - val_accuracy: 0.8087\nEpoch 105/200\n79/79 [==============================] - 0s 5ms/step - loss: 0.4256 - accuracy: 0.8151 - val_loss: 0.4258 - val_accuracy: 0.7985\nEpoch 106/200\n79/79 [==============================] - 0s 5ms/step - loss: 0.4260 - accuracy: 0.8106 - val_loss: 0.4220 - val_accuracy: 0.8036\nEpoch 107/200\n79/79 [==============================] - 0s 5ms/step - loss: 0.4297 - accuracy: 0.8087 - val_loss: 0.4321 - val_accuracy: 0.7908\nEpoch 108/200\n79/79 [==============================] - 0s 5ms/step - loss: 0.4253 - accuracy: 0.8195 - val_loss: 0.4148 - val_accuracy: 0.8087\nEpoch 109/200\n79/79 [==============================] - 0s 5ms/step - loss: 0.4290 - accuracy: 0.8029 - val_loss: 0.4219 - val_accuracy: 0.8061\nEpoch 110/200\n79/79 [==============================] - 0s 5ms/step - loss: 0.4142 - accuracy: 0.8112 - val_loss: 0.4383 - val_accuracy: 0.7985\nEpoch 111/200\n79/79 [==============================] - 0s 5ms/step - loss: 0.4239 - accuracy: 0.8099 - val_loss: 0.4254 - val_accuracy: 0.8112\nEpoch 112/200\n79/79 [==============================] - 0s 5ms/step - loss: 0.4257 - accuracy: 0.7997 - val_loss: 0.4355 - val_accuracy: 0.7959\nEpoch 113/200\n79/79 [==============================] - 0s 5ms/step - loss: 0.4098 - accuracy: 0.8195 - val_loss: 0.4451 - val_accuracy: 0.7908\nEpoch 114/200\n79/79 [==============================] - 0s 5ms/step - loss: 0.4371 - accuracy: 0.8029 - val_loss: 0.4278 - val_accuracy: 0.8036\nEpoch 115/200\n79/79 [==============================] - 0s 5ms/step - loss: 0.4123 - accuracy: 0.8093 - val_loss: 0.4182 - val_accuracy: 0.8112\nEpoch 116/200\n79/79 [==============================] - 0s 5ms/step - loss: 0.4245 - accuracy: 0.7972 - val_loss: 0.4165 - val_accuracy: 0.8163\nEpoch 117/200\n79/79 [==============================] - 0s 5ms/step - loss: 0.4005 - accuracy: 0.8195 - val_loss: 0.4064 - val_accuracy: 0.8163\nEpoch 118/200\n79/79 [==============================] - 0s 5ms/step - loss: 0.4087 - accuracy: 0.8119 - val_loss: 0.4108 - val_accuracy: 0.8112\nEpoch 119/200\n79/79 [==============================] - 0s 5ms/step - loss: 0.4079 - accuracy: 0.8106 - val_loss: 0.4048 - val_accuracy: 0.8087\nEpoch 120/200\n79/79 [==============================] - 0s 5ms/step - loss: 0.4242 - accuracy: 0.8125 - val_loss: 0.4137 - val_accuracy: 0.8112\nEpoch 121/200\n79/79 [==============================] - 0s 5ms/step - loss: 0.4210 - accuracy: 0.8112 - val_loss: 0.4148 - val_accuracy: 0.8163\nEpoch 122/200\n79/79 [==============================] - 0s 5ms/step - loss: 0.4155 - accuracy: 0.8151 - val_loss: 0.4125 - val_accuracy: 0.8138\nEpoch 123/200\n79/79 [==============================] - 0s 5ms/step - loss: 0.3997 - accuracy: 0.8246 - val_loss: 0.4105 - val_accuracy: 0.8010\nEpoch 124/200\n79/79 [==============================] - 0s 5ms/step - loss: 0.3937 - accuracy: 0.8119 - val_loss: 0.4097 - val_accuracy: 0.7908\nEpoch 125/200\n79/79 [==============================] - 0s 6ms/step - loss: 0.4242 - accuracy: 0.8119 - val_loss: 0.4069 - val_accuracy: 0.8240\nEpoch 126/200\n79/79 [==============================] - 0s 5ms/step - loss: 0.3957 - accuracy: 0.8221 - val_loss: 0.3977 - val_accuracy: 0.8138\nEpoch 127/200\n79/79 [==============================] - 0s 5ms/step - loss: 0.4110 - accuracy: 0.8240 - val_loss: 0.4042 - val_accuracy: 0.8163\nEpoch 128/200\n79/79 [==============================] - 0s 5ms/step - loss: 0.3961 - accuracy: 0.8278 - val_loss: 0.4015 - val_accuracy: 0.8138\nEpoch 129/200\n79/79 [==============================] - 0s 5ms/step - loss: 0.3943 - accuracy: 0.8195 - val_loss: 0.3956 - val_accuracy: 0.8112\nEpoch 130/200\n79/79 [==============================] - 0s 5ms/step - loss: 0.3781 - accuracy: 0.8361 - val_loss: 0.3947 - val_accuracy: 0.8214\nEpoch 131/200\n79/79 [==============================] - 1s 7ms/step - loss: 0.4154 - accuracy: 0.8240 - val_loss: 0.4189 - val_accuracy: 0.8010\nEpoch 132/200\n79/79 [==============================] - 0s 5ms/step - loss: 0.4197 - accuracy: 0.8125 - val_loss: 0.4151 - val_accuracy: 0.8163\nEpoch 133/200\n79/79 [==============================] - 0s 5ms/step - loss: 0.3950 - accuracy: 0.8291 - val_loss: 0.4087 - val_accuracy: 0.8087\nEpoch 134/200\n79/79 [==============================] - 0s 5ms/step - loss: 0.3811 - accuracy: 0.8399 - val_loss: 0.3882 - val_accuracy: 0.8112\nEpoch 135/200\n79/79 [==============================] - 0s 5ms/step - loss: 0.4040 - accuracy: 0.8157 - val_loss: 0.4054 - val_accuracy: 0.8214\nEpoch 136/200\n79/79 [==============================] - 0s 5ms/step - loss: 0.4090 - accuracy: 0.8189 - val_loss: 0.4119 - val_accuracy: 0.8112\nEpoch 137/200\n79/79 [==============================] - 0s 5ms/step - loss: 0.4041 - accuracy: 0.8233 - val_loss: 0.4019 - val_accuracy: 0.8316\nEpoch 138/200\n79/79 [==============================] - 0s 5ms/step - loss: 0.4090 - accuracy: 0.8272 - val_loss: 0.4038 - val_accuracy: 0.8138\nEpoch 139/200\n79/79 [==============================] - 0s 5ms/step - loss: 0.3902 - accuracy: 0.8310 - val_loss: 0.4047 - val_accuracy: 0.8214\nEpoch 140/200\n79/79 [==============================] - 0s 5ms/step - loss: 0.3897 - accuracy: 0.8310 - val_loss: 0.4045 - val_accuracy: 0.8189\nEpoch 141/200\n79/79 [==============================] - 0s 5ms/step - loss: 0.3902 - accuracy: 0.8240 - val_loss: 0.3983 - val_accuracy: 0.8163\nEpoch 142/200\n79/79 [==============================] - 0s 5ms/step - loss: 0.3912 - accuracy: 0.8259 - val_loss: 0.3987 - val_accuracy: 0.8163\nEpoch 143/200\n79/79 [==============================] - 0s 5ms/step - loss: 0.3881 - accuracy: 0.8374 - val_loss: 0.4048 - val_accuracy: 0.8138\nEpoch 144/200\n79/79 [==============================] - 0s 5ms/step - loss: 0.3825 - accuracy: 0.8291 - val_loss: 0.4069 - val_accuracy: 0.8010\nEpoch 145/200\n79/79 [==============================] - 0s 5ms/step - loss: 0.3817 - accuracy: 0.8367 - val_loss: 0.4063 - val_accuracy: 0.8036\nEpoch 146/200\n79/79 [==============================] - 0s 5ms/step - loss: 0.3845 - accuracy: 0.8214 - val_loss: 0.3831 - val_accuracy: 0.8240\nEpoch 147/200\n79/79 [==============================] - 0s 5ms/step - loss: 0.3580 - accuracy: 0.8412 - val_loss: 0.4054 - val_accuracy: 0.8010\nEpoch 148/200\n79/79 [==============================] - 0s 5ms/step - loss: 0.4011 - accuracy: 0.8176 - val_loss: 0.3930 - val_accuracy: 0.8087\nEpoch 149/200\n79/79 [==============================] - 0s 6ms/step - loss: 0.3988 - accuracy: 0.8227 - val_loss: 0.3957 - val_accuracy: 0.8087\nEpoch 150/200\n79/79 [==============================] - 0s 5ms/step - loss: 0.3734 - accuracy: 0.8412 - val_loss: 0.3996 - val_accuracy: 0.8189\nEpoch 151/200\n79/79 [==============================] - 0s 5ms/step - loss: 0.3856 - accuracy: 0.8393 - val_loss: 0.3937 - val_accuracy: 0.8138\nEpoch 152/200\n79/79 [==============================] - 0s 5ms/step - loss: 0.3699 - accuracy: 0.8361 - val_loss: 0.3872 - val_accuracy: 0.8061\nEpoch 153/200\n79/79 [==============================] - 0s 5ms/step - loss: 0.3794 - accuracy: 0.8316 - val_loss: 0.3895 - val_accuracy: 0.8240\nEpoch 154/200\n79/79 [==============================] - 0s 5ms/step - loss: 0.3956 - accuracy: 0.8233 - val_loss: 0.3774 - val_accuracy: 0.8112\nEpoch 155/200\n79/79 [==============================] - 0s 5ms/step - loss: 0.3792 - accuracy: 0.8367 - val_loss: 0.3819 - val_accuracy: 0.8112\nEpoch 156/200\n79/79 [==============================] - 0s 5ms/step - loss: 0.3711 - accuracy: 0.8399 - val_loss: 0.3914 - val_accuracy: 0.8138\nEpoch 157/200\n79/79 [==============================] - 0s 5ms/step - loss: 0.3942 - accuracy: 0.8335 - val_loss: 0.4017 - val_accuracy: 0.8138\nEpoch 158/200\n79/79 [==============================] - 0s 5ms/step - loss: 0.3751 - accuracy: 0.8374 - val_loss: 0.3948 - val_accuracy: 0.8061\nEpoch 159/200\n79/79 [==============================] - 0s 5ms/step - loss: 0.3945 - accuracy: 0.8233 - val_loss: 0.4087 - val_accuracy: 0.8010\nEpoch 160/200\n79/79 [==============================] - 0s 5ms/step - loss: 0.3841 - accuracy: 0.8355 - val_loss: 0.4145 - val_accuracy: 0.7934\nEpoch 161/200\n79/79 [==============================] - 0s 5ms/step - loss: 0.3719 - accuracy: 0.8552 - val_loss: 0.3943 - val_accuracy: 0.8087\nEpoch 162/200\n79/79 [==============================] - 0s 5ms/step - loss: 0.3843 - accuracy: 0.8291 - val_loss: 0.3990 - val_accuracy: 0.8163\nEpoch 163/200\n79/79 [==============================] - 0s 5ms/step - loss: 0.3954 - accuracy: 0.8151 - val_loss: 0.3870 - val_accuracy: 0.8214\nEpoch 164/200\n79/79 [==============================] - 0s 5ms/step - loss: 0.3682 - accuracy: 0.8463 - val_loss: 0.3751 - val_accuracy: 0.8291\nEpoch 165/200\n79/79 [==============================] - 0s 5ms/step - loss: 0.3853 - accuracy: 0.8284 - val_loss: 0.3835 - val_accuracy: 0.8214\nEpoch 166/200\n79/79 [==============================] - 0s 5ms/step - loss: 0.3635 - accuracy: 0.8450 - val_loss: 0.3994 - val_accuracy: 0.8138\nEpoch 167/200\n79/79 [==============================] - 0s 5ms/step - loss: 0.3730 - accuracy: 0.8438 - val_loss: 0.4070 - val_accuracy: 0.8163\nEpoch 168/200\n79/79 [==============================] - 0s 5ms/step - loss: 0.3895 - accuracy: 0.8386 - val_loss: 0.3915 - val_accuracy: 0.8087\nEpoch 169/200\n79/79 [==============================] - 0s 5ms/step - loss: 0.3823 - accuracy: 0.8418 - val_loss: 0.3911 - val_accuracy: 0.8087\nEpoch 170/200\n79/79 [==============================] - 0s 5ms/step - loss: 0.3765 - accuracy: 0.8342 - val_loss: 0.3954 - val_accuracy: 0.8036\nEpoch 171/200\n79/79 [==============================] - 0s 5ms/step - loss: 0.3774 - accuracy: 0.8367 - val_loss: 0.3900 - val_accuracy: 0.8240\nEpoch 172/200\n79/79 [==============================] - 0s 5ms/step - loss: 0.3833 - accuracy: 0.8374 - val_loss: 0.3823 - val_accuracy: 0.8214\nEpoch 173/200\n79/79 [==============================] - 0s 5ms/step - loss: 0.3805 - accuracy: 0.8412 - val_loss: 0.3767 - val_accuracy: 0.8265\nEpoch 174/200\n79/79 [==============================] - 0s 6ms/step - loss: 0.3818 - accuracy: 0.8272 - val_loss: 0.3741 - val_accuracy: 0.8316\nEpoch 175/200\n79/79 [==============================] - 0s 5ms/step - loss: 0.3776 - accuracy: 0.8348 - val_loss: 0.3892 - val_accuracy: 0.8138\nEpoch 176/200\n79/79 [==============================] - 0s 5ms/step - loss: 0.3496 - accuracy: 0.8508 - val_loss: 0.3876 - val_accuracy: 0.8291\nEpoch 177/200\n79/79 [==============================] - 0s 5ms/step - loss: 0.3659 - accuracy: 0.8310 - val_loss: 0.3823 - val_accuracy: 0.8342\nEpoch 178/200\n79/79 [==============================] - 0s 5ms/step - loss: 0.3687 - accuracy: 0.8386 - val_loss: 0.3927 - val_accuracy: 0.8189\nEpoch 179/200\n79/79 [==============================] - 0s 5ms/step - loss: 0.3618 - accuracy: 0.8425 - val_loss: 0.3701 - val_accuracy: 0.8265\nEpoch 180/200\n79/79 [==============================] - 0s 5ms/step - loss: 0.3569 - accuracy: 0.8438 - val_loss: 0.3727 - val_accuracy: 0.8393\nEpoch 181/200\n79/79 [==============================] - 0s 5ms/step - loss: 0.3764 - accuracy: 0.8367 - val_loss: 0.3786 - val_accuracy: 0.8316\nEpoch 182/200\n79/79 [==============================] - 0s 5ms/step - loss: 0.3784 - accuracy: 0.8348 - val_loss: 0.3874 - val_accuracy: 0.8265\nEpoch 183/200\n79/79 [==============================] - 0s 5ms/step - loss: 0.3729 - accuracy: 0.8329 - val_loss: 0.3731 - val_accuracy: 0.8367\nEpoch 184/200\n79/79 [==============================] - 0s 5ms/step - loss: 0.3582 - accuracy: 0.8386 - val_loss: 0.3576 - val_accuracy: 0.8495\nEpoch 185/200\n79/79 [==============================] - 0s 5ms/step - loss: 0.3725 - accuracy: 0.8342 - val_loss: 0.3775 - val_accuracy: 0.8342\nEpoch 186/200\n79/79 [==============================] - 0s 5ms/step - loss: 0.3783 - accuracy: 0.8323 - val_loss: 0.3637 - val_accuracy: 0.8342\nEpoch 187/200\n79/79 [==============================] - 0s 5ms/step - loss: 0.3863 - accuracy: 0.8342 - val_loss: 0.3725 - val_accuracy: 0.8444\nEpoch 188/200\n79/79 [==============================] - 0s 5ms/step - loss: 0.3537 - accuracy: 0.8431 - val_loss: 0.3690 - val_accuracy: 0.8444\nEpoch 189/200\n79/79 [==============================] - 0s 5ms/step - loss: 0.3720 - accuracy: 0.8489 - val_loss: 0.3823 - val_accuracy: 0.8240\nEpoch 190/200\n79/79 [==============================] - 0s 5ms/step - loss: 0.3633 - accuracy: 0.8399 - val_loss: 0.3856 - val_accuracy: 0.8214\nEpoch 191/200\n79/79 [==============================] - 0s 5ms/step - loss: 0.3523 - accuracy: 0.8533 - val_loss: 0.3729 - val_accuracy: 0.8214\nEpoch 192/200\n79/79 [==============================] - 0s 5ms/step - loss: 0.3639 - accuracy: 0.8489 - val_loss: 0.3748 - val_accuracy: 0.8240\nEpoch 193/200\n79/79 [==============================] - 0s 5ms/step - loss: 0.3502 - accuracy: 0.8527 - val_loss: 0.3765 - val_accuracy: 0.8265\nEpoch 194/200\n79/79 [==============================] - 0s 5ms/step - loss: 0.3833 - accuracy: 0.8367 - val_loss: 0.3761 - val_accuracy: 0.8214\nEpoch 195/200\n79/79 [==============================] - 0s 5ms/step - loss: 0.3553 - accuracy: 0.8501 - val_loss: 0.3672 - val_accuracy: 0.8291\nEpoch 196/200\n79/79 [==============================] - 0s 5ms/step - loss: 0.3720 - accuracy: 0.8463 - val_loss: 0.3685 - val_accuracy: 0.8214\nEpoch 197/200\n79/79 [==============================] - 0s 5ms/step - loss: 0.3718 - accuracy: 0.8386 - val_loss: 0.3704 - val_accuracy: 0.8265\nEpoch 198/200\n79/79 [==============================] - 0s 5ms/step - loss: 0.3632 - accuracy: 0.8348 - val_loss: 0.3731 - val_accuracy: 0.8367\nEpoch 199/200\n79/79 [==============================] - 0s 5ms/step - loss: 0.3678 - accuracy: 0.8425 - val_loss: 0.3871 - val_accuracy: 0.8316\nEpoch 200/200\n79/79 [==============================] - 0s 5ms/step - loss: 0.3667 - accuracy: 0.8335 - val_loss: 0.3687 - val_accuracy: 0.8418\n"
],
[
"hist = pd.DataFrame(history.history)\nhist['epoch'] = history.epoch\nhist.tail()",
"_____no_output_____"
],
[
"import matplotlib.pyplot as plt\n\nhist=history.history\n\nfig=plt.figure(figsize=(12,5))\nax=fig.add_subplot(1,2,1)\nax.plot(hist['loss'],lw=3)\nax.plot(hist['val_loss'],lw=3)\nax.set_title('Training & Validation Loss',size=15)\nax.set_xlabel('Epoch',size=15)\nax.tick_params(axis='both',which='major',labelsize=15)\nax=fig.add_subplot(1,2,2)\nax.plot(hist['accuracy'],lw=3)\nax.plot(hist['val_accuracy'],lw=3)\nax.set_title('Training & Validation accuracy',size=15)\nax.set_xlabel('Epoch',size=15)\nax.tick_params(axis='both',which='major',labelsize=15)\nplt.show()\n\n\n",
"_____no_output_____"
],
[
"!pip install shap",
"Collecting shap\n\u001b[?25l Downloading https://files.pythonhosted.org/packages/a8/77/b504e43e21a2ba543a1ac4696718beb500cfa708af2fb57cb54ce299045c/shap-0.35.0.tar.gz (273kB)\n\r\u001b[K |█▏ | 10kB 21.7MB/s eta 0:00:01\r\u001b[K |██▍ | 20kB 1.7MB/s eta 0:00:01\r\u001b[K |███▋ | 30kB 2.2MB/s eta 0:00:01\r\u001b[K |████▉ | 40kB 2.5MB/s eta 0:00:01\r\u001b[K |██████ | 51kB 2.0MB/s eta 0:00:01\r\u001b[K |███████▏ | 61kB 2.3MB/s eta 0:00:01\r\u001b[K |████████▍ | 71kB 2.5MB/s eta 0:00:01\r\u001b[K |█████████▋ | 81kB 2.7MB/s eta 0:00:01\r\u001b[K |██████████▉ | 92kB 2.9MB/s eta 0:00:01\r\u001b[K |████████████ | 102kB 2.8MB/s eta 0:00:01\r\u001b[K |█████████████▏ | 112kB 2.8MB/s eta 0:00:01\r\u001b[K |██████████████▍ | 122kB 2.8MB/s eta 0:00:01\r\u001b[K |███████████████▋ | 133kB 2.8MB/s eta 0:00:01\r\u001b[K |████████████████▉ | 143kB 2.8MB/s eta 0:00:01\r\u001b[K |██████████████████ | 153kB 2.8MB/s eta 0:00:01\r\u001b[K |███████████████████▏ | 163kB 2.8MB/s eta 0:00:01\r\u001b[K |████████████████████▍ | 174kB 2.8MB/s eta 0:00:01\r\u001b[K |█████████████████████▋ | 184kB 2.8MB/s eta 0:00:01\r\u001b[K |██████████████████████▉ | 194kB 2.8MB/s eta 0:00:01\r\u001b[K |████████████████████████ | 204kB 2.8MB/s eta 0:00:01\r\u001b[K |█████████████████████████▏ | 215kB 2.8MB/s eta 0:00:01\r\u001b[K |██████████████████████████▍ | 225kB 2.8MB/s eta 0:00:01\r\u001b[K |███████████████████████████▋ | 235kB 2.8MB/s eta 0:00:01\r\u001b[K |████████████████████████████▉ | 245kB 2.8MB/s eta 0:00:01\r\u001b[K |██████████████████████████████ | 256kB 2.8MB/s eta 0:00:01\r\u001b[K |███████████████████████████████▏| 266kB 2.8MB/s eta 0:00:01\r\u001b[K |████████████████████████████████| 276kB 2.8MB/s \n\u001b[?25hRequirement already satisfied: numpy in /usr/local/lib/python3.6/dist-packages (from shap) (1.18.5)\nRequirement already satisfied: scipy in /usr/local/lib/python3.6/dist-packages (from shap) (1.4.1)\nRequirement already satisfied: scikit-learn in /usr/local/lib/python3.6/dist-packages (from shap) (0.22.2.post1)\nRequirement already satisfied: pandas in /usr/local/lib/python3.6/dist-packages (from shap) (1.0.5)\nRequirement already satisfied: tqdm>4.25.0 in /usr/local/lib/python3.6/dist-packages (from shap) (4.41.1)\nRequirement already satisfied: joblib>=0.11 in /usr/local/lib/python3.6/dist-packages (from scikit-learn->shap) (0.15.1)\nRequirement already satisfied: pytz>=2017.2 in /usr/local/lib/python3.6/dist-packages (from pandas->shap) (2018.9)\nRequirement already satisfied: python-dateutil>=2.6.1 in /usr/local/lib/python3.6/dist-packages (from pandas->shap) (2.8.1)\nRequirement already satisfied: six>=1.5 in /usr/local/lib/python3.6/dist-packages (from python-dateutil>=2.6.1->pandas->shap) (1.12.0)\nBuilding wheels for collected packages: shap\n Building wheel for shap (setup.py) ... \u001b[?25l\u001b[?25hdone\n Created wheel for shap: filename=shap-0.35.0-cp36-cp36m-linux_x86_64.whl size=394130 sha256=fa7a3695c4152743202e7de8bd95b7d76cec14ad3ea03f3ef255b7369251cec7\n Stored in directory: /root/.cache/pip/wheels/e7/f7/0f/b57055080cf8894906b3bd3616d2fc2bfd0b12d5161bcb24ac\nSuccessfully built shap\nInstalling collected packages: shap\nSuccessfully installed shap-0.35.0\n"
],
[
"import shap\n\nexplainer = shap.DeepExplainer(model, np.array(X_train_new))",
"Using TensorFlow backend.\nkeras is no longer supported, please use tf.keras instead.\n"
],
[
"shap_values = explainer.shap_values(np.array(X_test_new))",
"_____no_output_____"
],
[
"shap.summary_plot(shap_values[1], X_test_new)",
"_____no_output_____"
],
[
"pred=model.predict(X_test_new)\npred.argmax(axis=1)",
"_____no_output_____"
],
[
"from sklearn.metrics import classification_report, confusion_matrix\n\ncm=confusion_matrix(test_labels, pred.argmax(axis=1))",
"_____no_output_____"
],
[
"print('Confusion Matrix')\nfig,ax = plt.subplots(figsize=(2.5,2.5))\nax.matshow(cm,cmap=plt.cm.Blues,alpha=0.3)\nfor i in range(cm.shape[0]):\n for j in range(cm.shape[1]):\n ax.text(x=j,y=i,\n s=cm[i,j],\n va='center',ha='center')\nplt.xlabel('Predicted Label')\nplt.ylabel('True Label')\nplt.show()\n\n",
"Confusion Matrix\n"
],
[
"from sklearn.metrics import precision_score\nfrom sklearn.metrics import recall_score, f1_score\n\nprint('Precision: %.3f' % precision_score(y_true=test_labels,y_pred=pred.argmax(axis=1)))\n\nprint('Recall: %.3f' % recall_score(y_true=test_labels,y_pred=pred.argmax(axis=1)))\n\nprint('F1: %.3f' % f1_score(y_true=test_labels,y_pred=pred.argmax(axis=1)))",
"Precision: 0.880\nRecall: 0.818\nF1: 0.848\n"
],
[
"from sklearn.pipeline import Pipeline\nfrom sklearn.feature_selection import SelectKBest, chi2\nimport xgboost as xgb\nfrom sklearn.model_selection import KFold, GridSearchCV\nfrom sklearn.metrics import accuracy_score, make_scorer",
"_____no_output_____"
],
[
"pipe = Pipeline([\n ('fs', SelectKBest()),\n ('clf', xgb.XGBClassifier(objective='binary:logistic'))\n])",
"_____no_output_____"
],
[
"search_space = [\n {\n 'clf__n_estimators': [200],\n 'clf__learning_rate': [0.05, 0.1],\n 'clf__max_depth': range(3, 10),\n 'clf__colsample_bytree': [i/10.0 for i in range(1, 3)],\n 'clf__gamma': [i/10.0 for i in range(3)],\n 'fs__score_func': [mutual_info_classif,f_classif],\n 'fs__k': [20,30,40],\n }\n]",
"_____no_output_____"
],
[
"kfold = KFold(n_splits=5, shuffle=True, random_state=42)",
"_____no_output_____"
],
[
"scoring = {'AUC':'roc_auc', 'Accuracy':make_scorer(accuracy_score)}",
"_____no_output_____"
],
[
"grid = GridSearchCV(\n pipe,\n param_grid=search_space,\n cv=kfold,\n scoring=scoring,\n refit='AUC',\n verbose=1,\n n_jobs=-1\n)",
"_____no_output_____"
],
[
"model = grid.fit(normed_train_data, train_labels)",
"Fitting 5 folds for each of 504 candidates, totalling 2520 fits\n"
],
[
"import pickle\n\n# Dictionary of best parameters\nbest_pars = grid.best_params_\n# Best XGB model that was found based on the metric score you specify\nbest_model = grid.best_estimator_\n# Save model\npickle.dump(grid.best_estimator_, open('gdrive/My Drive/SS_AITrader/INTC/xgb_INTC_log_reg.pickle', \"wb\"))",
"_____no_output_____"
],
[
"predict = model.predict(normed_test_data)\nprint('Best AUC Score: {}'.format(model.best_score_))\nprint('Accuracy: {}'.format(accuracy_score(test_labels, predict)))\ncm=confusion_matrix(test_labels,predict)",
"WARNING:tensorflow:Model was constructed with shape (None, 20) for input Tensor(\"input_2:0\", shape=(None, 20), dtype=float32), but it was called on an input with incompatible shape (None, 63).\n"
],
[
"print('Confusion Matrix')\nfig,ax = plt.subplots(figsize=(2.5,2.5))\nax.matshow(cm,cmap=plt.cm.Blues,alpha=0.3)\nfor i in range(cm.shape[0]):\n for j in range(cm.shape[1]):\n ax.text(x=j,y=i,\n s=cm[i,j],\n va='center',ha='center')\nplt.xlabel('Predicted Label')\nplt.ylabel('True Label')\nplt.show()",
"Confusion Matrix\n"
],
[
"print(model.best_params_)",
"{'clf__colsample_bytree': 0.2, 'clf__gamma': 0.1, 'clf__learning_rate': 0.1, 'clf__max_depth': 9, 'clf__n_estimators': 200, 'fs__k': 40, 'fs__score_func': <function mutual_info_classif at 0x7fedf81f00d0>}\n"
],
[
"model_opt = xgb.XGBClassifier(max_depth=9,\n objective='binary:logistic',\n n_estimators=200,\n learning_rate = 0.1,\n colsample_bytree= 0.2,\n gamma= 0.1)\neval_set = [(X_train_new, train_labels), (X_test_new, test_labels)]\nmodel_opt.fit(X_train_new, train_labels, early_stopping_rounds=15, eval_metric=[\"error\", \"logloss\"], eval_set=eval_set, verbose=True)",
"[0]\tvalidation_0-error:0.262245\tvalidation_0-logloss:0.666199\tvalidation_1-error:0.353061\tvalidation_1-logloss:0.678182\nMultiple eval metrics have been passed: 'validation_1-logloss' will be used for early stopping.\n\nWill train until validation_1-logloss hasn't improved in 15 rounds.\n[1]\tvalidation_0-error:0.167857\tvalidation_0-logloss:0.632271\tvalidation_1-error:0.320408\tvalidation_1-logloss:0.660471\n[2]\tvalidation_0-error:0.139286\tvalidation_0-logloss:0.610981\tvalidation_1-error:0.306122\tvalidation_1-logloss:0.649325\n[3]\tvalidation_0-error:0.112245\tvalidation_0-logloss:0.577976\tvalidation_1-error:0.279592\tvalidation_1-logloss:0.630241\n[4]\tvalidation_0-error:0.1\tvalidation_0-logloss:0.553261\tvalidation_1-error:0.283673\tvalidation_1-logloss:0.617323\n[5]\tvalidation_0-error:0.09898\tvalidation_0-logloss:0.54185\tvalidation_1-error:0.263265\tvalidation_1-logloss:0.611882\n[6]\tvalidation_0-error:0.097449\tvalidation_0-logloss:0.527882\tvalidation_1-error:0.255102\tvalidation_1-logloss:0.602737\n[7]\tvalidation_0-error:0.091327\tvalidation_0-logloss:0.516472\tvalidation_1-error:0.267347\tvalidation_1-logloss:0.600474\n[8]\tvalidation_0-error:0.083163\tvalidation_0-logloss:0.497778\tvalidation_1-error:0.289796\tvalidation_1-logloss:0.593591\n[9]\tvalidation_0-error:0.081122\tvalidation_0-logloss:0.480115\tvalidation_1-error:0.261224\tvalidation_1-logloss:0.584926\n[10]\tvalidation_0-error:0.070408\tvalidation_0-logloss:0.459159\tvalidation_1-error:0.259184\tvalidation_1-logloss:0.572237\n[11]\tvalidation_0-error:0.071939\tvalidation_0-logloss:0.452404\tvalidation_1-error:0.259184\tvalidation_1-logloss:0.571071\n[12]\tvalidation_0-error:0.060204\tvalidation_0-logloss:0.43612\tvalidation_1-error:0.242857\tvalidation_1-logloss:0.562517\n[13]\tvalidation_0-error:0.060714\tvalidation_0-logloss:0.429356\tvalidation_1-error:0.255102\tvalidation_1-logloss:0.561593\n[14]\tvalidation_0-error:0.058673\tvalidation_0-logloss:0.424506\tvalidation_1-error:0.25102\tvalidation_1-logloss:0.560661\n[15]\tvalidation_0-error:0.055102\tvalidation_0-logloss:0.415029\tvalidation_1-error:0.255102\tvalidation_1-logloss:0.557836\n[16]\tvalidation_0-error:0.042347\tvalidation_0-logloss:0.396755\tvalidation_1-error:0.22449\tvalidation_1-logloss:0.547341\n[17]\tvalidation_0-error:0.042857\tvalidation_0-logloss:0.390716\tvalidation_1-error:0.238776\tvalidation_1-logloss:0.545907\n[18]\tvalidation_0-error:0.043878\tvalidation_0-logloss:0.385691\tvalidation_1-error:0.236735\tvalidation_1-logloss:0.544346\n[19]\tvalidation_0-error:0.033163\tvalidation_0-logloss:0.371783\tvalidation_1-error:0.202041\tvalidation_1-logloss:0.530304\n[20]\tvalidation_0-error:0.032143\tvalidation_0-logloss:0.361437\tvalidation_1-error:0.206122\tvalidation_1-logloss:0.522385\n[21]\tvalidation_0-error:0.030102\tvalidation_0-logloss:0.349816\tvalidation_1-error:0.210204\tvalidation_1-logloss:0.515966\n[22]\tvalidation_0-error:0.030612\tvalidation_0-logloss:0.347271\tvalidation_1-error:0.214286\tvalidation_1-logloss:0.515685\n[23]\tvalidation_0-error:0.026531\tvalidation_0-logloss:0.337911\tvalidation_1-error:0.212245\tvalidation_1-logloss:0.511901\n[24]\tvalidation_0-error:0.027551\tvalidation_0-logloss:0.333824\tvalidation_1-error:0.216327\tvalidation_1-logloss:0.508884\n[25]\tvalidation_0-error:0.022449\tvalidation_0-logloss:0.323872\tvalidation_1-error:0.202041\tvalidation_1-logloss:0.502301\n[26]\tvalidation_0-error:0.021429\tvalidation_0-logloss:0.309786\tvalidation_1-error:0.193878\tvalidation_1-logloss:0.489079\n[27]\tvalidation_0-error:0.018878\tvalidation_0-logloss:0.300666\tvalidation_1-error:0.187755\tvalidation_1-logloss:0.486566\n[28]\tvalidation_0-error:0.018367\tvalidation_0-logloss:0.29801\tvalidation_1-error:0.191837\tvalidation_1-logloss:0.487209\n[29]\tvalidation_0-error:0.019388\tvalidation_0-logloss:0.288263\tvalidation_1-error:0.189796\tvalidation_1-logloss:0.481074\n[30]\tvalidation_0-error:0.017857\tvalidation_0-logloss:0.28239\tvalidation_1-error:0.181633\tvalidation_1-logloss:0.47671\n[31]\tvalidation_0-error:0.017857\tvalidation_0-logloss:0.274738\tvalidation_1-error:0.171429\tvalidation_1-logloss:0.468647\n[32]\tvalidation_0-error:0.017347\tvalidation_0-logloss:0.266342\tvalidation_1-error:0.169388\tvalidation_1-logloss:0.461272\n[33]\tvalidation_0-error:0.015816\tvalidation_0-logloss:0.258667\tvalidation_1-error:0.167347\tvalidation_1-logloss:0.456246\n[34]\tvalidation_0-error:0.014796\tvalidation_0-logloss:0.255447\tvalidation_1-error:0.161224\tvalidation_1-logloss:0.454252\n[35]\tvalidation_0-error:0.014796\tvalidation_0-logloss:0.250063\tvalidation_1-error:0.163265\tvalidation_1-logloss:0.450374\n[36]\tvalidation_0-error:0.014796\tvalidation_0-logloss:0.248081\tvalidation_1-error:0.163265\tvalidation_1-logloss:0.449406\n[37]\tvalidation_0-error:0.014796\tvalidation_0-logloss:0.239029\tvalidation_1-error:0.159184\tvalidation_1-logloss:0.439394\n[38]\tvalidation_0-error:0.014286\tvalidation_0-logloss:0.237626\tvalidation_1-error:0.159184\tvalidation_1-logloss:0.438758\n[39]\tvalidation_0-error:0.013776\tvalidation_0-logloss:0.231433\tvalidation_1-error:0.159184\tvalidation_1-logloss:0.434173\n[40]\tvalidation_0-error:0.011735\tvalidation_0-logloss:0.225324\tvalidation_1-error:0.155102\tvalidation_1-logloss:0.430082\n[41]\tvalidation_0-error:0.010204\tvalidation_0-logloss:0.222847\tvalidation_1-error:0.153061\tvalidation_1-logloss:0.429286\n[42]\tvalidation_0-error:0.009184\tvalidation_0-logloss:0.21482\tvalidation_1-error:0.15102\tvalidation_1-logloss:0.422543\n[43]\tvalidation_0-error:0.008163\tvalidation_0-logloss:0.20581\tvalidation_1-error:0.144898\tvalidation_1-logloss:0.414088\n[44]\tvalidation_0-error:0.007653\tvalidation_0-logloss:0.204092\tvalidation_1-error:0.144898\tvalidation_1-logloss:0.414154\n[45]\tvalidation_0-error:0.009184\tvalidation_0-logloss:0.199281\tvalidation_1-error:0.146939\tvalidation_1-logloss:0.411389\n[46]\tvalidation_0-error:0.008163\tvalidation_0-logloss:0.194216\tvalidation_1-error:0.14898\tvalidation_1-logloss:0.407988\n[47]\tvalidation_0-error:0.007143\tvalidation_0-logloss:0.18972\tvalidation_1-error:0.155102\tvalidation_1-logloss:0.406473\n[48]\tvalidation_0-error:0.007143\tvalidation_0-logloss:0.185001\tvalidation_1-error:0.153061\tvalidation_1-logloss:0.404753\n[49]\tvalidation_0-error:0.007143\tvalidation_0-logloss:0.181759\tvalidation_1-error:0.14898\tvalidation_1-logloss:0.401516\n[50]\tvalidation_0-error:0.005102\tvalidation_0-logloss:0.177141\tvalidation_1-error:0.14898\tvalidation_1-logloss:0.39918\n[51]\tvalidation_0-error:0.004082\tvalidation_0-logloss:0.171253\tvalidation_1-error:0.14898\tvalidation_1-logloss:0.396964\n[52]\tvalidation_0-error:0.004592\tvalidation_0-logloss:0.170447\tvalidation_1-error:0.146939\tvalidation_1-logloss:0.397018\n[53]\tvalidation_0-error:0.005102\tvalidation_0-logloss:0.167087\tvalidation_1-error:0.140816\tvalidation_1-logloss:0.393725\n[54]\tvalidation_0-error:0.005102\tvalidation_0-logloss:0.165991\tvalidation_1-error:0.136735\tvalidation_1-logloss:0.39353\n[55]\tvalidation_0-error:0.005102\tvalidation_0-logloss:0.162972\tvalidation_1-error:0.138776\tvalidation_1-logloss:0.392728\n[56]\tvalidation_0-error:0.004082\tvalidation_0-logloss:0.159186\tvalidation_1-error:0.136735\tvalidation_1-logloss:0.390346\n[57]\tvalidation_0-error:0.003571\tvalidation_0-logloss:0.157813\tvalidation_1-error:0.140816\tvalidation_1-logloss:0.389923\n[58]\tvalidation_0-error:0.003571\tvalidation_0-logloss:0.154266\tvalidation_1-error:0.146939\tvalidation_1-logloss:0.38704\n[59]\tvalidation_0-error:0.003061\tvalidation_0-logloss:0.151026\tvalidation_1-error:0.144898\tvalidation_1-logloss:0.38598\n[60]\tvalidation_0-error:0.003571\tvalidation_0-logloss:0.147853\tvalidation_1-error:0.14898\tvalidation_1-logloss:0.382925\n[61]\tvalidation_0-error:0.003061\tvalidation_0-logloss:0.145653\tvalidation_1-error:0.15102\tvalidation_1-logloss:0.383504\n[62]\tvalidation_0-error:0.002551\tvalidation_0-logloss:0.14436\tvalidation_1-error:0.153061\tvalidation_1-logloss:0.383204\n[63]\tvalidation_0-error:0.002041\tvalidation_0-logloss:0.142308\tvalidation_1-error:0.153061\tvalidation_1-logloss:0.381754\n[64]\tvalidation_0-error:0.001531\tvalidation_0-logloss:0.139781\tvalidation_1-error:0.155102\tvalidation_1-logloss:0.381028\n[65]\tvalidation_0-error:0.00051\tvalidation_0-logloss:0.136229\tvalidation_1-error:0.157143\tvalidation_1-logloss:0.378821\n[66]\tvalidation_0-error:0.00051\tvalidation_0-logloss:0.134099\tvalidation_1-error:0.146939\tvalidation_1-logloss:0.376843\n[67]\tvalidation_0-error:0.00051\tvalidation_0-logloss:0.13187\tvalidation_1-error:0.14898\tvalidation_1-logloss:0.373532\n[68]\tvalidation_0-error:0.00051\tvalidation_0-logloss:0.129872\tvalidation_1-error:0.14898\tvalidation_1-logloss:0.373177\n[69]\tvalidation_0-error:0.00051\tvalidation_0-logloss:0.127972\tvalidation_1-error:0.146939\tvalidation_1-logloss:0.372512\n[70]\tvalidation_0-error:0.00051\tvalidation_0-logloss:0.125267\tvalidation_1-error:0.15102\tvalidation_1-logloss:0.371282\n[71]\tvalidation_0-error:0.00051\tvalidation_0-logloss:0.124241\tvalidation_1-error:0.14898\tvalidation_1-logloss:0.371401\n[72]\tvalidation_0-error:0.00051\tvalidation_0-logloss:0.123351\tvalidation_1-error:0.14898\tvalidation_1-logloss:0.370231\n[73]\tvalidation_0-error:0.00051\tvalidation_0-logloss:0.121972\tvalidation_1-error:0.15102\tvalidation_1-logloss:0.369811\n[74]\tvalidation_0-error:0.00051\tvalidation_0-logloss:0.120607\tvalidation_1-error:0.146939\tvalidation_1-logloss:0.369231\n[75]\tvalidation_0-error:0.00051\tvalidation_0-logloss:0.118796\tvalidation_1-error:0.14898\tvalidation_1-logloss:0.368033\n[76]\tvalidation_0-error:0.00051\tvalidation_0-logloss:0.117141\tvalidation_1-error:0.153061\tvalidation_1-logloss:0.367409\n[77]\tvalidation_0-error:0.00051\tvalidation_0-logloss:0.115082\tvalidation_1-error:0.153061\tvalidation_1-logloss:0.364839\n[78]\tvalidation_0-error:0.00051\tvalidation_0-logloss:0.114438\tvalidation_1-error:0.157143\tvalidation_1-logloss:0.364474\n[79]\tvalidation_0-error:0.00051\tvalidation_0-logloss:0.112183\tvalidation_1-error:0.161224\tvalidation_1-logloss:0.363113\n[80]\tvalidation_0-error:0.00051\tvalidation_0-logloss:0.110869\tvalidation_1-error:0.157143\tvalidation_1-logloss:0.362017\n[81]\tvalidation_0-error:0\tvalidation_0-logloss:0.109335\tvalidation_1-error:0.155102\tvalidation_1-logloss:0.360047\n[82]\tvalidation_0-error:0\tvalidation_0-logloss:0.108642\tvalidation_1-error:0.157143\tvalidation_1-logloss:0.360557\n[83]\tvalidation_0-error:0\tvalidation_0-logloss:0.107813\tvalidation_1-error:0.157143\tvalidation_1-logloss:0.360947\n[84]\tvalidation_0-error:0\tvalidation_0-logloss:0.106999\tvalidation_1-error:0.155102\tvalidation_1-logloss:0.359194\n[85]\tvalidation_0-error:0\tvalidation_0-logloss:0.106366\tvalidation_1-error:0.157143\tvalidation_1-logloss:0.358959\n[86]\tvalidation_0-error:0\tvalidation_0-logloss:0.104869\tvalidation_1-error:0.155102\tvalidation_1-logloss:0.357746\n[87]\tvalidation_0-error:0\tvalidation_0-logloss:0.103944\tvalidation_1-error:0.153061\tvalidation_1-logloss:0.357372\n[88]\tvalidation_0-error:0.00051\tvalidation_0-logloss:0.103283\tvalidation_1-error:0.153061\tvalidation_1-logloss:0.356645\n[89]\tvalidation_0-error:0.00051\tvalidation_0-logloss:0.102139\tvalidation_1-error:0.153061\tvalidation_1-logloss:0.3559\n[90]\tvalidation_0-error:0\tvalidation_0-logloss:0.10071\tvalidation_1-error:0.15102\tvalidation_1-logloss:0.355162\n[91]\tvalidation_0-error:0.00051\tvalidation_0-logloss:0.099481\tvalidation_1-error:0.14898\tvalidation_1-logloss:0.354681\n[92]\tvalidation_0-error:0.00051\tvalidation_0-logloss:0.098895\tvalidation_1-error:0.153061\tvalidation_1-logloss:0.355431\n[93]\tvalidation_0-error:0.00051\tvalidation_0-logloss:0.097576\tvalidation_1-error:0.153061\tvalidation_1-logloss:0.353543\n[94]\tvalidation_0-error:0\tvalidation_0-logloss:0.095806\tvalidation_1-error:0.155102\tvalidation_1-logloss:0.352204\n[95]\tvalidation_0-error:0\tvalidation_0-logloss:0.09475\tvalidation_1-error:0.155102\tvalidation_1-logloss:0.350791\n[96]\tvalidation_0-error:0\tvalidation_0-logloss:0.093406\tvalidation_1-error:0.155102\tvalidation_1-logloss:0.351336\n[97]\tvalidation_0-error:0\tvalidation_0-logloss:0.09301\tvalidation_1-error:0.155102\tvalidation_1-logloss:0.351113\n[98]\tvalidation_0-error:0\tvalidation_0-logloss:0.092329\tvalidation_1-error:0.155102\tvalidation_1-logloss:0.351247\n[99]\tvalidation_0-error:0\tvalidation_0-logloss:0.09119\tvalidation_1-error:0.153061\tvalidation_1-logloss:0.350352\n[100]\tvalidation_0-error:0\tvalidation_0-logloss:0.089358\tvalidation_1-error:0.153061\tvalidation_1-logloss:0.348586\n[101]\tvalidation_0-error:0\tvalidation_0-logloss:0.087648\tvalidation_1-error:0.157143\tvalidation_1-logloss:0.347272\n[102]\tvalidation_0-error:0\tvalidation_0-logloss:0.086808\tvalidation_1-error:0.157143\tvalidation_1-logloss:0.346662\n[103]\tvalidation_0-error:0\tvalidation_0-logloss:0.084936\tvalidation_1-error:0.161224\tvalidation_1-logloss:0.34442\n[104]\tvalidation_0-error:0\tvalidation_0-logloss:0.08352\tvalidation_1-error:0.155102\tvalidation_1-logloss:0.342092\n[105]\tvalidation_0-error:0\tvalidation_0-logloss:0.082623\tvalidation_1-error:0.155102\tvalidation_1-logloss:0.34203\n[106]\tvalidation_0-error:0\tvalidation_0-logloss:0.081232\tvalidation_1-error:0.155102\tvalidation_1-logloss:0.34082\n[107]\tvalidation_0-error:0\tvalidation_0-logloss:0.080821\tvalidation_1-error:0.155102\tvalidation_1-logloss:0.340869\n[108]\tvalidation_0-error:0\tvalidation_0-logloss:0.080088\tvalidation_1-error:0.153061\tvalidation_1-logloss:0.340187\n[109]\tvalidation_0-error:0\tvalidation_0-logloss:0.079241\tvalidation_1-error:0.159184\tvalidation_1-logloss:0.339926\n[110]\tvalidation_0-error:0\tvalidation_0-logloss:0.078114\tvalidation_1-error:0.153061\tvalidation_1-logloss:0.338504\n[111]\tvalidation_0-error:0\tvalidation_0-logloss:0.07724\tvalidation_1-error:0.155102\tvalidation_1-logloss:0.338264\n[112]\tvalidation_0-error:0\tvalidation_0-logloss:0.076014\tvalidation_1-error:0.153061\tvalidation_1-logloss:0.33897\n[113]\tvalidation_0-error:0\tvalidation_0-logloss:0.074936\tvalidation_1-error:0.14898\tvalidation_1-logloss:0.337787\n[114]\tvalidation_0-error:0\tvalidation_0-logloss:0.073706\tvalidation_1-error:0.15102\tvalidation_1-logloss:0.337523\n[115]\tvalidation_0-error:0\tvalidation_0-logloss:0.072063\tvalidation_1-error:0.153061\tvalidation_1-logloss:0.336628\n[116]\tvalidation_0-error:0\tvalidation_0-logloss:0.071308\tvalidation_1-error:0.157143\tvalidation_1-logloss:0.33593\n[117]\tvalidation_0-error:0\tvalidation_0-logloss:0.06986\tvalidation_1-error:0.157143\tvalidation_1-logloss:0.334158\n[118]\tvalidation_0-error:0\tvalidation_0-logloss:0.068628\tvalidation_1-error:0.15102\tvalidation_1-logloss:0.331908\n[119]\tvalidation_0-error:0\tvalidation_0-logloss:0.067957\tvalidation_1-error:0.155102\tvalidation_1-logloss:0.332194\n[120]\tvalidation_0-error:0\tvalidation_0-logloss:0.067383\tvalidation_1-error:0.153061\tvalidation_1-logloss:0.332592\n[121]\tvalidation_0-error:0\tvalidation_0-logloss:0.066865\tvalidation_1-error:0.153061\tvalidation_1-logloss:0.331903\n[122]\tvalidation_0-error:0\tvalidation_0-logloss:0.065888\tvalidation_1-error:0.155102\tvalidation_1-logloss:0.330991\n[123]\tvalidation_0-error:0\tvalidation_0-logloss:0.064932\tvalidation_1-error:0.157143\tvalidation_1-logloss:0.331228\n[124]\tvalidation_0-error:0\tvalidation_0-logloss:0.064573\tvalidation_1-error:0.153061\tvalidation_1-logloss:0.331128\n[125]\tvalidation_0-error:0\tvalidation_0-logloss:0.064001\tvalidation_1-error:0.153061\tvalidation_1-logloss:0.331098\n[126]\tvalidation_0-error:0\tvalidation_0-logloss:0.063409\tvalidation_1-error:0.15102\tvalidation_1-logloss:0.330553\n[127]\tvalidation_0-error:0\tvalidation_0-logloss:0.063052\tvalidation_1-error:0.153061\tvalidation_1-logloss:0.330068\n[128]\tvalidation_0-error:0\tvalidation_0-logloss:0.062157\tvalidation_1-error:0.153061\tvalidation_1-logloss:0.329383\n[129]\tvalidation_0-error:0\tvalidation_0-logloss:0.061935\tvalidation_1-error:0.153061\tvalidation_1-logloss:0.329807\n[130]\tvalidation_0-error:0\tvalidation_0-logloss:0.061678\tvalidation_1-error:0.153061\tvalidation_1-logloss:0.330171\n[131]\tvalidation_0-error:0\tvalidation_0-logloss:0.061177\tvalidation_1-error:0.153061\tvalidation_1-logloss:0.330237\n[132]\tvalidation_0-error:0\tvalidation_0-logloss:0.060388\tvalidation_1-error:0.155102\tvalidation_1-logloss:0.330238\n[133]\tvalidation_0-error:0\tvalidation_0-logloss:0.059876\tvalidation_1-error:0.153061\tvalidation_1-logloss:0.329682\n[134]\tvalidation_0-error:0\tvalidation_0-logloss:0.059559\tvalidation_1-error:0.153061\tvalidation_1-logloss:0.329731\n[135]\tvalidation_0-error:0\tvalidation_0-logloss:0.059023\tvalidation_1-error:0.153061\tvalidation_1-logloss:0.329666\n[136]\tvalidation_0-error:0\tvalidation_0-logloss:0.058664\tvalidation_1-error:0.153061\tvalidation_1-logloss:0.330091\n[137]\tvalidation_0-error:0\tvalidation_0-logloss:0.05826\tvalidation_1-error:0.153061\tvalidation_1-logloss:0.330863\n[138]\tvalidation_0-error:0\tvalidation_0-logloss:0.057549\tvalidation_1-error:0.155102\tvalidation_1-logloss:0.330657\n[139]\tvalidation_0-error:0\tvalidation_0-logloss:0.056898\tvalidation_1-error:0.153061\tvalidation_1-logloss:0.329502\n[140]\tvalidation_0-error:0\tvalidation_0-logloss:0.056713\tvalidation_1-error:0.153061\tvalidation_1-logloss:0.329398\n[141]\tvalidation_0-error:0\tvalidation_0-logloss:0.056\tvalidation_1-error:0.155102\tvalidation_1-logloss:0.328094\n[142]\tvalidation_0-error:0\tvalidation_0-logloss:0.055744\tvalidation_1-error:0.155102\tvalidation_1-logloss:0.328143\n[143]\tvalidation_0-error:0\tvalidation_0-logloss:0.055502\tvalidation_1-error:0.153061\tvalidation_1-logloss:0.328215\n[144]\tvalidation_0-error:0\tvalidation_0-logloss:0.055088\tvalidation_1-error:0.155102\tvalidation_1-logloss:0.328386\n[145]\tvalidation_0-error:0\tvalidation_0-logloss:0.054432\tvalidation_1-error:0.155102\tvalidation_1-logloss:0.328747\n[146]\tvalidation_0-error:0\tvalidation_0-logloss:0.054052\tvalidation_1-error:0.155102\tvalidation_1-logloss:0.329193\n[147]\tvalidation_0-error:0\tvalidation_0-logloss:0.053609\tvalidation_1-error:0.15102\tvalidation_1-logloss:0.328654\n[148]\tvalidation_0-error:0\tvalidation_0-logloss:0.053126\tvalidation_1-error:0.14898\tvalidation_1-logloss:0.329207\n[149]\tvalidation_0-error:0\tvalidation_0-logloss:0.052501\tvalidation_1-error:0.15102\tvalidation_1-logloss:0.328202\n[150]\tvalidation_0-error:0\tvalidation_0-logloss:0.051913\tvalidation_1-error:0.15102\tvalidation_1-logloss:0.327315\n[151]\tvalidation_0-error:0\tvalidation_0-logloss:0.051452\tvalidation_1-error:0.15102\tvalidation_1-logloss:0.327767\n[152]\tvalidation_0-error:0\tvalidation_0-logloss:0.05084\tvalidation_1-error:0.155102\tvalidation_1-logloss:0.327313\n[153]\tvalidation_0-error:0\tvalidation_0-logloss:0.050415\tvalidation_1-error:0.155102\tvalidation_1-logloss:0.326623\n[154]\tvalidation_0-error:0\tvalidation_0-logloss:0.049638\tvalidation_1-error:0.153061\tvalidation_1-logloss:0.326208\n[155]\tvalidation_0-error:0\tvalidation_0-logloss:0.0491\tvalidation_1-error:0.153061\tvalidation_1-logloss:0.326603\n[156]\tvalidation_0-error:0\tvalidation_0-logloss:0.048477\tvalidation_1-error:0.14898\tvalidation_1-logloss:0.325786\n[157]\tvalidation_0-error:0\tvalidation_0-logloss:0.048257\tvalidation_1-error:0.15102\tvalidation_1-logloss:0.325694\n[158]\tvalidation_0-error:0\tvalidation_0-logloss:0.047966\tvalidation_1-error:0.15102\tvalidation_1-logloss:0.325621\n[159]\tvalidation_0-error:0\tvalidation_0-logloss:0.047718\tvalidation_1-error:0.153061\tvalidation_1-logloss:0.325271\n[160]\tvalidation_0-error:0\tvalidation_0-logloss:0.04729\tvalidation_1-error:0.153061\tvalidation_1-logloss:0.324979\n[161]\tvalidation_0-error:0\tvalidation_0-logloss:0.04703\tvalidation_1-error:0.153061\tvalidation_1-logloss:0.326018\n[162]\tvalidation_0-error:0\tvalidation_0-logloss:0.046349\tvalidation_1-error:0.153061\tvalidation_1-logloss:0.326245\n[163]\tvalidation_0-error:0\tvalidation_0-logloss:0.045861\tvalidation_1-error:0.155102\tvalidation_1-logloss:0.325136\n[164]\tvalidation_0-error:0\tvalidation_0-logloss:0.045408\tvalidation_1-error:0.153061\tvalidation_1-logloss:0.325233\n[165]\tvalidation_0-error:0\tvalidation_0-logloss:0.045268\tvalidation_1-error:0.153061\tvalidation_1-logloss:0.325152\n[166]\tvalidation_0-error:0\tvalidation_0-logloss:0.044846\tvalidation_1-error:0.153061\tvalidation_1-logloss:0.324782\n[167]\tvalidation_0-error:0\tvalidation_0-logloss:0.044698\tvalidation_1-error:0.153061\tvalidation_1-logloss:0.324708\n[168]\tvalidation_0-error:0\tvalidation_0-logloss:0.044531\tvalidation_1-error:0.153061\tvalidation_1-logloss:0.325252\n[169]\tvalidation_0-error:0\tvalidation_0-logloss:0.044225\tvalidation_1-error:0.155102\tvalidation_1-logloss:0.324971\n[170]\tvalidation_0-error:0\tvalidation_0-logloss:0.043813\tvalidation_1-error:0.153061\tvalidation_1-logloss:0.324562\n[171]\tvalidation_0-error:0\tvalidation_0-logloss:0.043675\tvalidation_1-error:0.153061\tvalidation_1-logloss:0.324527\n[172]\tvalidation_0-error:0\tvalidation_0-logloss:0.043502\tvalidation_1-error:0.153061\tvalidation_1-logloss:0.324363\n[173]\tvalidation_0-error:0\tvalidation_0-logloss:0.043099\tvalidation_1-error:0.153061\tvalidation_1-logloss:0.324854\n[174]\tvalidation_0-error:0\tvalidation_0-logloss:0.042941\tvalidation_1-error:0.153061\tvalidation_1-logloss:0.324939\n[175]\tvalidation_0-error:0\tvalidation_0-logloss:0.042687\tvalidation_1-error:0.155102\tvalidation_1-logloss:0.325808\n[176]\tvalidation_0-error:0\tvalidation_0-logloss:0.042424\tvalidation_1-error:0.153061\tvalidation_1-logloss:0.325516\n[177]\tvalidation_0-error:0\tvalidation_0-logloss:0.041902\tvalidation_1-error:0.153061\tvalidation_1-logloss:0.324977\n[178]\tvalidation_0-error:0\tvalidation_0-logloss:0.04139\tvalidation_1-error:0.153061\tvalidation_1-logloss:0.324723\n[179]\tvalidation_0-error:0\tvalidation_0-logloss:0.040978\tvalidation_1-error:0.15102\tvalidation_1-logloss:0.324085\n[180]\tvalidation_0-error:0\tvalidation_0-logloss:0.040396\tvalidation_1-error:0.15102\tvalidation_1-logloss:0.323481\n[181]\tvalidation_0-error:0\tvalidation_0-logloss:0.039993\tvalidation_1-error:0.15102\tvalidation_1-logloss:0.322214\n[182]\tvalidation_0-error:0\tvalidation_0-logloss:0.039777\tvalidation_1-error:0.15102\tvalidation_1-logloss:0.321515\n[183]\tvalidation_0-error:0\tvalidation_0-logloss:0.03951\tvalidation_1-error:0.15102\tvalidation_1-logloss:0.321595\n[184]\tvalidation_0-error:0\tvalidation_0-logloss:0.03936\tvalidation_1-error:0.15102\tvalidation_1-logloss:0.321522\n[185]\tvalidation_0-error:0\tvalidation_0-logloss:0.03898\tvalidation_1-error:0.15102\tvalidation_1-logloss:0.321618\n[186]\tvalidation_0-error:0\tvalidation_0-logloss:0.038864\tvalidation_1-error:0.15102\tvalidation_1-logloss:0.321768\n[187]\tvalidation_0-error:0\tvalidation_0-logloss:0.038602\tvalidation_1-error:0.15102\tvalidation_1-logloss:0.321451\n[188]\tvalidation_0-error:0\tvalidation_0-logloss:0.03822\tvalidation_1-error:0.15102\tvalidation_1-logloss:0.32181\n[189]\tvalidation_0-error:0\tvalidation_0-logloss:0.038024\tvalidation_1-error:0.15102\tvalidation_1-logloss:0.321603\n[190]\tvalidation_0-error:0\tvalidation_0-logloss:0.037743\tvalidation_1-error:0.15102\tvalidation_1-logloss:0.321665\n[191]\tvalidation_0-error:0\tvalidation_0-logloss:0.037445\tvalidation_1-error:0.15102\tvalidation_1-logloss:0.32136\n[192]\tvalidation_0-error:0\tvalidation_0-logloss:0.037321\tvalidation_1-error:0.153061\tvalidation_1-logloss:0.321901\n[193]\tvalidation_0-error:0\tvalidation_0-logloss:0.037141\tvalidation_1-error:0.153061\tvalidation_1-logloss:0.322064\n[194]\tvalidation_0-error:0\tvalidation_0-logloss:0.036895\tvalidation_1-error:0.153061\tvalidation_1-logloss:0.322296\n[195]\tvalidation_0-error:0\tvalidation_0-logloss:0.036813\tvalidation_1-error:0.153061\tvalidation_1-logloss:0.322505\n[196]\tvalidation_0-error:0\tvalidation_0-logloss:0.036523\tvalidation_1-error:0.15102\tvalidation_1-logloss:0.322327\n[197]\tvalidation_0-error:0\tvalidation_0-logloss:0.036341\tvalidation_1-error:0.153061\tvalidation_1-logloss:0.322687\n[198]\tvalidation_0-error:0\tvalidation_0-logloss:0.036073\tvalidation_1-error:0.155102\tvalidation_1-logloss:0.322221\n[199]\tvalidation_0-error:0\tvalidation_0-logloss:0.035851\tvalidation_1-error:0.155102\tvalidation_1-logloss:0.322639\n"
],
[
"\n# make predictions for test data\ny_pred = model_opt.predict(X_test_new)\npredictions = [round(value) for value in y_pred]\n\n# evaluate predictions\naccuracy = accuracy_score(test_labels, predictions)\nprint(\"Accuracy: %.2f%%\" % (accuracy * 100.0))",
"Accuracy: 84.90%\n"
],
[
"from matplotlib import pyplot\n\nresults = model_opt.evals_result()\nepochs = len(results['validation_0']['error'])\nx_axis = range(0, epochs)\n# plot log loss\nfig, ax = pyplot.subplots()\nax.plot(x_axis, results['validation_0']['logloss'], label='Train')\nax.plot(x_axis, results['validation_1']['logloss'], label='Test')\nax.legend()\npyplot.ylabel('Log Loss')\npyplot.title('XGBoost Log Loss')\npyplot.show()\n# plot classification error\nfig, ax = pyplot.subplots()\nax.plot(x_axis, results['validation_0']['error'], label='Train')\nax.plot(x_axis, results['validation_1']['error'], label='Test')\nax.legend()\npyplot.ylabel('Classification Error')\npyplot.title('XGBoost Classification Error')\npyplot.show()",
"_____no_output_____"
],
[
"shap_values = shap.TreeExplainer(model_opt).shap_values(X_test_new) ",
"Setting feature_perturbation = \"tree_path_dependent\" because no background data was given.\n"
],
[
"shap.summary_plot(shap_values, X_test_new)",
"_____no_output_____"
],
[
"predict = model_opt.predict(X_test_new)\n\ncm=confusion_matrix(test_labels,predict)",
"_____no_output_____"
],
[
"print('Confusion Matrix')\nfig,ax = plt.subplots(figsize=(2.5,2.5))\nax.matshow(cm,cmap=plt.cm.Blues,alpha=0.3)\nfor i in range(cm.shape[0]):\n for j in range(cm.shape[1]):\n ax.text(x=j,y=i,\n s=cm[i,j],\n va='center',ha='center')\nplt.xlabel('Predicted Label')\nplt.ylabel('True Label')\nplt.show()",
"Confusion Matrix\n"
]
]
] | [
"markdown",
"code"
] | [
[
"markdown"
],
[
"code",
"code",
"code",
"code",
"code",
"code",
"code",
"code",
"code",
"code",
"code",
"code",
"code",
"code",
"code",
"code",
"code",
"code",
"code",
"code",
"code",
"code",
"code",
"code",
"code",
"code",
"code",
"code",
"code",
"code",
"code",
"code",
"code",
"code",
"code",
"code",
"code",
"code",
"code",
"code",
"code",
"code",
"code",
"code",
"code",
"code",
"code",
"code",
"code",
"code",
"code",
"code",
"code",
"code",
"code",
"code"
]
] |
d04896ea4fd424539eeb6fcb2e9a334e4780c0d8 | 30,320 | ipynb | Jupyter Notebook | cereals.ipynb | briandk/2020-virtual-program-in-data-science | 8a0c326b4f8a91e21111ccbaa3613cb9e76ffe70 | [
"MIT"
] | 1 | 2021-03-14T02:37:07.000Z | 2021-03-14T02:37:07.000Z | cereals.ipynb | briandk/2020-virtual-program-in-data-science | 8a0c326b4f8a91e21111ccbaa3613cb9e76ffe70 | [
"MIT"
] | null | null | null | cereals.ipynb | briandk/2020-virtual-program-in-data-science | 8a0c326b4f8a91e21111ccbaa3613cb9e76ffe70 | [
"MIT"
] | null | null | null | 51.302876 | 8,631 | 0.386412 | [
[
[
"<a href=\"https://colab.research.google.com/github/briandk/2020-virtual-program-in-data-science/blob/master/cereals.ipynb\" target=\"_parent\"><img src=\"https://colab.research.google.com/assets/colab-badge.svg\" alt=\"Open In Colab\"/></a>",
"_____no_output_____"
]
],
[
[
"# Make inline plots vector graphics instead of raster graphics\nfrom IPython.display import set_matplotlib_formats\nset_matplotlib_formats('pdf', 'svg')\n\nimport pandas as pd\nimport plotly.express as px",
"_____no_output_____"
],
[
"cereals = pd.read_csv(\"https://github.com/briandk/2020-virtual-program-in-data-science/raw/master/data/cereals.csv\")",
"_____no_output_____"
],
[
"cereals",
"_____no_output_____"
],
[
"cereals.count()",
"_____no_output_____"
],
[
"cereals.groupby('mfr').size().sort_values()",
"_____no_output_____"
],
[
"fig = px.scatter(cereals, 'rating', 'calories')",
"_____no_output_____"
],
[
"fig.show()",
"_____no_output_____"
],
[
"cereals.groupby('mfr').mean()['calories'].sort_values()",
"_____no_output_____"
],
[
"",
"_____no_output_____"
]
]
] | [
"markdown",
"code"
] | [
[
"markdown"
],
[
"code",
"code",
"code",
"code",
"code",
"code",
"code",
"code",
"code"
]
] |
d048ac1a0a681fda2b0b4a5390cdc2744e6b2e10 | 55,950 | ipynb | Jupyter Notebook | examples/deriving_psf_stenson.ipynb | majkelx/astwro | 4a9bbe3e4757c4076ad7c0d90cf08e38dab4e794 | [
"MIT"
] | 6 | 2017-06-15T20:34:51.000Z | 2020-04-15T14:21:43.000Z | examples/deriving_psf_stenson.ipynb | majkelx/astwro | 4a9bbe3e4757c4076ad7c0d90cf08e38dab4e794 | [
"MIT"
] | 18 | 2017-08-15T20:53:55.000Z | 2020-10-05T23:40:34.000Z | examples/deriving_psf_stenson.ipynb | majkelx/astwro | 4a9bbe3e4757c4076ad7c0d90cf08e38dab4e794 | [
"MIT"
] | 2 | 2017-11-06T15:33:53.000Z | 2020-10-02T21:06:05.000Z | 31.556684 | 564 | 0.39235 | [
[
[
"# Deriving a Point-Spread Function in a Crowded Field\n### following Appendix III of Peter Stetson's *User's Manual for DAOPHOT II*\n### Using `pydaophot` form `astwro` python package",
"_____no_output_____"
],
[
"All *italic* text here have been taken from Stetson's manual.",
"_____no_output_____"
],
[
"The only input file for this procedure is a FITS file containing reference frame image. Here we use sample FITS form astwro package (NGC6871 I filter 20s frame). Below we get filepath for this image, as well as create instances of `Daophot` and `Allstar` classes - wrappers around `daophot` and `allstar` respectively.\n\nOne should also provide `daophot.opt`, `photo.opt` and `allstar.opt` in apropiriete constructors. Here default, build in, sample, `opt` files are used.",
"_____no_output_____"
]
],
[
[
"from astwro.sampledata import fits_image\nframe = fits_image()",
"_____no_output_____"
]
],
[
[
"`Daophot` object creates temporary working directory (*runner directory*), which is passed to `Allstar` constructor to share.",
"_____no_output_____"
]
],
[
[
"from astwro.pydaophot import Daophot, Allstar\ndp = Daophot(image=frame)\nal = Allstar(dir=dp.dir)",
"_____no_output_____"
]
],
[
[
"Daophot got FITS file in construction, which will be automatically **ATTACH**ed. ",
"_____no_output_____"
],
[
"#### *(1) Run FIND on your frame*",
"_____no_output_____"
],
[
"Daophot `FIND` parameters `Number of frames averaged, summed` are defaulted to `1,1`, below are provided for clarity.",
"_____no_output_____"
]
],
[
[
"res = dp.FInd(frames_av=1, frames_sum=1)",
"_____no_output_____"
]
],
[
[
"Check some results returned by `FIND`, every method for `daophot` command returns results object.",
"_____no_output_____"
]
],
[
[
"print (\"{} pixels analysed, sky estimate {}, {} stars found.\".format(res.pixels, res.sky, res.stars))",
"9640 pixels analysed, sky estimate 12.665, 4166 stars found.\n"
]
],
[
[
"Also, take a look into *runner directory*",
"_____no_output_____"
]
],
[
[
"!ls -lt $dp.dir",
"total 536\r\nlrwxr-xr-x 1 michal staff 60 Jun 26 18:25 \u001b[35m63d38b_NGC6871.fits\u001b[m\u001b[m -> /Users/michal/projects/astwro/astwro/sampledata/NGC6871.fits\r\nlrwxr-xr-x 1 michal staff 65 Jun 26 18:25 \u001b[35mallstar.opt\u001b[m\u001b[m -> /Users/michal/projects/astwro/astwro/pydaophot/config/allstar.opt\r\nlrwxr-xr-x 1 michal staff 65 Jun 26 18:25 \u001b[35mdaophot.opt\u001b[m\u001b[m -> /Users/michal/projects/astwro/astwro/pydaophot/config/daophot.opt\r\n-rw-r--r-- 1 michal staff 258438 Jun 26 18:25 i.coo\r\n"
]
],
[
[
"We see symlinks to input image and `opt` files, and `i.coo` - result of `FIND`",
"_____no_output_____"
],
[
"\n\n#### *(2) Run PHOTOMETRY on your frame*",
"_____no_output_____"
],
[
"Below we run photometry, providing explicitly radius of aperture `A1` and `IS`, `OS` sky radiuses.",
"_____no_output_____"
]
],
[
[
"res = dp.PHotometry(apertures=[8], IS=35, OS=50)",
"_____no_output_____"
]
],
[
[
"List of stars generated by daophot commands, can be easily get as `astwro.starlist.Starlist` being essentially `pandas.DataFrame`:",
"_____no_output_____"
]
],
[
[
"stars = res.photometry_starlist",
"_____no_output_____"
]
],
[
[
"Let's check 10 stars with least A1 error (``mag_err`` column). ([pandas](https://pandas.pydata.org) style)",
"_____no_output_____"
]
],
[
[
"stars.sort_values('mag_err').iloc[:10]",
"_____no_output_____"
]
],
[
[
"#### *(3) SORT the output from PHOTOMETRY*\n*in order of increasing apparent magnitude decreasing\nstellar brightness with the renumbering feature. This step is optional but it can be more convenient than not.*",
"_____no_output_____"
],
[
"`SORT` command of `daophor` is not implemented (yet) in `pydaohot`. But we do sorting by ourself.",
"_____no_output_____"
]
],
[
[
"sorted_stars = stars.sort_values('mag')\nsorted_stars.renumber()",
"_____no_output_____"
]
],
[
[
"Here we write sorted list back info photometry file at default name (overwriting existing one), because it's convenient to use default files in next commands.",
"_____no_output_____"
]
],
[
[
"dp.write_starlist(sorted_stars, 'i.ap')",
"_____no_output_____"
],
[
"!head -n20 $dp.PHotometry_result.photometry_file",
" NL NX NY LOWBAD HIGHBAD THRESH AP1 PH/ADU RNOISE FRAD \r\n 2 1250 1150 -3.9 31000.0 5.81 8.00 9.00 1.70 6.00\r\n\r\n\r\n 1 577.370 666.480 12.118\r\n 15.649 6.55 0.52 0.0012\r\n\r\n 2 982.570 733.500 12.430\r\n 12.626 2.27 0.08 0.0012\r\n\r\n 3 702.670 102.050 12.533\r\n 12.755 2.45 0.08 0.0012\r\n\r\n 4 603.270 675.390 12.727\r\n 16.515 7.82 0.58 0.0020\r\n\r\n 5 502.640 177.660 12.741\r\n 12.794 2.41 0.09 0.0014\r\n\r\n 6 1165.500 636.910 12.742\r\n"
],
[
"dp.PHotometry_result.photometry_file",
"_____no_output_____"
]
],
[
[
"#### *(4) PICK to generate a set of likely PSF stars* \n*How many stars you want to use is a function of the degree of variation you expect and the frequency with which stars are contaminated by cosmic rays or neighbor stars. [...]*",
"_____no_output_____"
]
],
[
[
"pick_res = dp.PIck(faintest_mag=20, number_of_stars_to_pick=40)",
"_____no_output_____"
]
],
[
[
"If no error reported, symlink to image file (renamed to `i.fits`), and all daophot output files (`i.*`) are in the working directory of runner:",
"_____no_output_____"
]
],
[
[
"ls $dp.dir",
"\u001b[35m63d38b_NGC6871.fits\u001b[m\u001b[m@ \u001b[35mdaophot.opt\u001b[m\u001b[m@ i.coo\r\n\u001b[35mallstar.opt\u001b[m\u001b[m@ i.ap i.lst\r\n"
]
],
[
[
"One may examine and improve `i.lst` list of PSF stars. Or use `astwro.tools.gapick.py` to obtain list of PSF stars optimised by genetic algorithm.",
"_____no_output_____"
],
[
"#### *(5) Run PSF *\n*tell it the name of your complete (sorted renumbered) aperture photometry file, the name of the file with the list of PSF stars, and the name of the disk file you want the point spread function stored in (the default should be fine) [...]*\n\n*If the frame is crowded it is probably worth your while to generate the first PSF with the \"VARIABLE PSF\" option set to -1 --- pure analytic PSF. That way, the companions will not generate ghosts in the model PSF that will come back to haunt you later. You should also have specified a reasonably generous fitting radius --- these stars have been preselected to be as isolated as possible and you want the best fits you can get. But remember to avoid letting neighbor stars intrude within one fitting radius of the center of any PSF star.*\n",
"_____no_output_____"
],
[
"For illustration we will set `VARIABLE PSF` option, before `PSf()`",
"_____no_output_____"
]
],
[
[
"dp.set_options('VARIABLE PSF', 2)\npsf_res = dp.PSf()",
"_____no_output_____"
]
],
[
[
"#### *(6) Run GROUP and NSTAR or ALLSTAR on your NEI file*\n*If your PSF stars have many neighbors this may take some minutes of real time. Please be patient or submit it as a batch job and perform steps on your next frame while you wait.*",
"_____no_output_____"
],
[
"We use `allstar`. (`GROUP` and `NSTAR` command are not implemented in current version of `pydaophot`). We use prepared above `Allstar` object: `al` operating on the same runner dir that `dp`.",
"_____no_output_____"
],
[
"As parameter we set input image (we haven't do that on constructor), and `nei` file produced by `PSf()`. We do not remember name `i.psf` so use `psf_res.nei_file` property. \n\nFinally we order `allstar` to produce subtracted FITS .",
"_____no_output_____"
]
],
[
[
"alls_res = al.ALlstar(image_file=frame, stars=psf_res.nei_file, subtracted_image_file='is.fits')",
"_____no_output_____"
]
],
[
[
"All `result` objects, has `get_buffer()` method, useful to lookup unparsed `daophot` or `allstar` output:",
"_____no_output_____"
]
],
[
[
"print (alls_res.get_buffer())",
" 63d38b_NGC6871... \n\n\n Picture size: 1250 1150\n\n\n File with the PSF (default 63d38b_NGC6871.psf): Input file (default 63d38b_NGC6871.ap): File for results (default i.als): Name for subtracted image (default is): \n 915 stars. <<\n\n\n I = iteration number\n\n R = number of stars that remain\n\n D = number of stars that disappeared\n\n C = number of stars that converged\n\n\n\n I R D C\n 1 915 0 0 <<\n 2 915 0 0 <<\n 3 915 0 0 <<\n 4 724 0 191 <<\n 5 385 0 530 <<\n 6 211 0 704 <<\n 7 110 0 805 <<\n 8 67 0 848 <<\n 9 40 0 875 <<\n 10 0 0 915\n\n Finished i \u0007\n\n\n Good bye.\n\n\n"
]
],
[
[
"#### *(8) EXIT from DAOPHOT and send this new picture to the image display * \n*Examine each of the PSF stars and its environs. Have all of the PSF stars subtracted out more or less cleanly, or should some of them be rejected from further use as PSF stars? (If so use a text editor to delete these stars from the LST file.) Have the neighbors mostly disappeared, or have they left behind big zits? Have you uncovered any faint companions that FIND missed?[...]* ",
"_____no_output_____"
],
[
"The absolute path to subtracted file (like for most output files) is available as result's property:",
"_____no_output_____"
]
],
[
[
"sub_img = alls_res.subtracted_image_file",
"_____no_output_____"
]
],
[
[
"We can also generate region file for psf stars:",
"_____no_output_____"
]
],
[
[
"from astwro.starlist.ds9 import write_ds9_regions\nreg_file_path = dp.file_from_runner_dir('lst.reg')\nwrite_ds9_regions(pick_res.picked_starlist, reg_file_path)",
"_____no_output_____"
],
[
"# One can run ds9 directly from notebook:\n!ds9 $sub_img -regions $reg_file_path ",
"_____no_output_____"
]
],
[
[
"#### *(9) Back in DAOPHOT II ATTACH the original picture and run SUBSTAR*\n*specifying the file created in step (6) or in step (8f) as the stars to subtract, and the stars in the LST file as the stars to keep.*",
"_____no_output_____"
],
[
"Lookup into runner dir:",
"_____no_output_____"
]
],
[
[
"ls $al.dir",
"\u001b[35m63d38b_NGC6871.fits\u001b[m\u001b[m@ i.ap i.nei\r\n\u001b[35mallstar.opt\u001b[m\u001b[m@ i.coo i.psf\r\n\u001b[35mdaophot.opt\u001b[m\u001b[m@ i.err is.fits\r\ni.als i.lst lst.reg\r\n"
],
[
"sub_res = dp.SUbstar(subtract=alls_res.profile_photometry_file, leave_in=pick_res.picked_stars_file)",
"_____no_output_____"
]
],
[
[
"*You have now created a new picture which has the PSF stars still in it but from which the known neighbors of these PSF stars have been mostly removed*",
"_____no_output_____"
],
[
"#### (10) ATTACH the new star subtracted frame and repeat step (5) to derive a new point spread function \n#### (11+...) Run GROUP NSTAR or ALLSTAR ",
"_____no_output_____"
]
],
[
[
"for i in range(3):\n print (\"Iteration {}: Allstar chi: {}\".format(i, alls_res.als_stars.chi.mean()))\n dp.image = 'is.fits'\n respsf = dp.PSf()\n print (\"Iteration {}: PSF chi: {}\".format(i, respsf.chi))\n alls_res = al.ALlstar(image_file=frame, stars='i.nei')\n dp.image = frame\n dp.SUbstar(subtract='i.als', leave_in='i.lst')\nprint (\"Final: Allstar chi: {}\".format(alls_res.als_stars.chi.mean()))",
"Iteration 0: Allstar chi: 1.14670601093\nIteration 0: PSF chi: 0.0249\nIteration 1: Allstar chi: 1.13409726776\nIteration 1: PSF chi: 0.0249\nIteration 2: Allstar chi: 1.1332852459\nIteration 2: PSF chi: 0.0249\nFinal: Allstar chi: 1.13326229508\n"
],
[
"alls_res.als_stars",
"_____no_output_____"
]
],
[
[
"Check last image with subtracted PSF stars neighbours.",
"_____no_output_____"
]
],
[
[
"!ds9 $dp.SUbstar_result.subtracted_image_file -regions $reg_file_path ",
"_____no_output_____"
]
],
[
[
"*Once you have produced a frame in which the PSF stars and their neighbors all subtract out cleanly, one more time through PSF should produce a point-spread function you can be proud of.*",
"_____no_output_____"
]
],
[
[
"dp.image = 'is.fits'\npsf_res = dp.PSf()\nprint (\"PSF file: {}\".format(psf_res.psf_file))",
"PSF file: /var/folders/kt/1jqvm3s51jd4qbxns7dc43rw0000gq/T/pydaophot_tmpDu5p8c/i.psf\n"
]
]
] | [
"markdown",
"code",
"markdown",
"code",
"markdown",
"code",
"markdown",
"code",
"markdown",
"code",
"markdown",
"code",
"markdown",
"code",
"markdown",
"code",
"markdown",
"code",
"markdown",
"code",
"markdown",
"code",
"markdown",
"code",
"markdown",
"code",
"markdown",
"code",
"markdown",
"code",
"markdown",
"code",
"markdown",
"code",
"markdown",
"code",
"markdown",
"code",
"markdown",
"code",
"markdown",
"code"
] | [
[
"markdown",
"markdown",
"markdown"
],
[
"code"
],
[
"markdown"
],
[
"code"
],
[
"markdown",
"markdown",
"markdown"
],
[
"code"
],
[
"markdown"
],
[
"code"
],
[
"markdown"
],
[
"code"
],
[
"markdown",
"markdown",
"markdown"
],
[
"code"
],
[
"markdown"
],
[
"code"
],
[
"markdown"
],
[
"code"
],
[
"markdown",
"markdown"
],
[
"code"
],
[
"markdown"
],
[
"code",
"code",
"code"
],
[
"markdown"
],
[
"code"
],
[
"markdown"
],
[
"code"
],
[
"markdown",
"markdown",
"markdown"
],
[
"code"
],
[
"markdown",
"markdown",
"markdown"
],
[
"code"
],
[
"markdown"
],
[
"code"
],
[
"markdown",
"markdown"
],
[
"code"
],
[
"markdown"
],
[
"code",
"code"
],
[
"markdown",
"markdown"
],
[
"code",
"code"
],
[
"markdown",
"markdown"
],
[
"code",
"code"
],
[
"markdown"
],
[
"code"
],
[
"markdown"
],
[
"code"
]
] |
d048b083252785087776fd0c1dcd1fb33e7c5c40 | 25,898 | ipynb | Jupyter Notebook | assignments/assignment12/FittingModelsEx01.ipynb | rsterbentz/phys202-2015-work | c8c441ef8308b6b2f3edd71938b91dcabe370bbd | [
"MIT"
] | null | null | null | assignments/assignment12/FittingModelsEx01.ipynb | rsterbentz/phys202-2015-work | c8c441ef8308b6b2f3edd71938b91dcabe370bbd | [
"MIT"
] | null | null | null | assignments/assignment12/FittingModelsEx01.ipynb | rsterbentz/phys202-2015-work | c8c441ef8308b6b2f3edd71938b91dcabe370bbd | [
"MIT"
] | null | null | null | 93.833333 | 12,688 | 0.855471 | [
[
[
"# Fitting Models Exercise 1",
"_____no_output_____"
],
[
"## Imports",
"_____no_output_____"
]
],
[
[
"%matplotlib inline\nimport matplotlib.pyplot as plt\nimport numpy as np\nimport scipy.optimize as opt",
"_____no_output_____"
]
],
[
[
"## Fitting a quadratic curve",
"_____no_output_____"
],
[
"For this problem we are going to work with the following model:\n\n$$ y_{model}(x) = a x^2 + b x + c $$\n\nThe true values of the model parameters are as follows:",
"_____no_output_____"
]
],
[
[
"a_true = 0.5\nb_true = 2.0\nc_true = -4.0",
"_____no_output_____"
]
],
[
[
"First, generate a dataset using this model using these parameters and the following characteristics:\n\n* For your $x$ data use 30 uniformly spaced points between $[-5,5]$.\n* Add a noise term to the $y$ value at each point that is drawn from a normal distribution with zero mean and standard deviation 2.0. Make sure you add a different random number to each point (see the `size` argument of `np.random.normal`).\n\nAfter you generate the data, make a plot of the raw data (use points).",
"_____no_output_____"
]
],
[
[
"def quad(x,a,b,c):\n return a*x**2 + b*x + c",
"_____no_output_____"
],
[
"N = 30\nxdata = np.linspace(-5,5,N)\ndy = 2.0\n\nnp.random.seed(0)\nydata = quad(xdata,a_true,b_true,c_true) + np.random.normal(0.0, dy, N)",
"_____no_output_____"
],
[
"plt.errorbar(xdata,ydata,dy,fmt='.k',ecolor='lightgrey')\nplt.xlabel('x')\nplt.ylabel('y')\nplt.xlim(-5,5);",
"_____no_output_____"
],
[
"assert True # leave this cell for grading the raw data generation and plot",
"_____no_output_____"
]
],
[
[
"Now fit the model to the dataset to recover estimates for the model's parameters:\n\n* Print out the estimates and uncertainties of each parameter.\n* Plot the raw data and best fit of the model.",
"_____no_output_____"
]
],
[
[
"theta_best, theta_cov = opt.curve_fit(quad, xdata, ydata, sigma=dy)\na_fit = theta_best[0]\nb_fit = theta_best[1]\nc_fit = theta_best[2]\nprint('a = {0:.3f} +/- {1:.3f}'.format(a_fit, np.sqrt(theta_cov[0,0])))\nprint('b = {0:.3f} +/- {1:.3f}'.format(b_fit, np.sqrt(theta_cov[1,1])))\nprint('c = {0:.3f} +/- {1:.3f}'.format(c_fit, np.sqrt(theta_cov[2,2])))",
"a = 0.582 +/- 0.049\nb = 1.845 +/- 0.130\nc = -3.843 +/- 0.581\n"
],
[
"x_fit = np.linspace(-5,5,30)\ny_fit = quad(x_fit,a_fit,b_fit,c_fit)\n\nplt.errorbar(xdata,ydata,dy,fmt='.k',ecolor='lightgrey')\nplt.plot(x_fit,y_fit)\nplt.xlabel('x')\nplt.ylabel('y')\nplt.xlim(-5,5);",
"_____no_output_____"
],
[
"assert True # leave this cell for grading the fit; should include a plot and printout of the parameters+errors",
"_____no_output_____"
]
]
] | [
"markdown",
"code",
"markdown",
"code",
"markdown",
"code",
"markdown",
"code"
] | [
[
"markdown",
"markdown"
],
[
"code"
],
[
"markdown",
"markdown"
],
[
"code"
],
[
"markdown"
],
[
"code",
"code",
"code",
"code"
],
[
"markdown"
],
[
"code",
"code",
"code"
]
] |
d048b115453703f3043cc94541a83195042b1a6a | 4,603 | ipynb | Jupyter Notebook | notebooks/setup.ipynb | shaghayegh-flower/ag1000g-phase1-vgsc-report | 337d177eb1a747c105b2699ab2c9f66bfb607e95 | [
"MIT"
] | 1 | 2017-09-14T17:21:16.000Z | 2017-09-14T17:21:16.000Z | notebooks/setup.ipynb | shaghayegh-flower/ag1000g-phase1-vgsc-report | 337d177eb1a747c105b2699ab2c9f66bfb607e95 | [
"MIT"
] | 130 | 2017-02-16T22:06:58.000Z | 2018-09-14T16:09:08.000Z | notebooks/setup.ipynb | shaghayegh-flower/ag1000g-phase1-vgsc-report | 337d177eb1a747c105b2699ab2c9f66bfb607e95 | [
"MIT"
] | 3 | 2020-02-28T14:23:29.000Z | 2020-06-11T18:37:11.000Z | 25.859551 | 70 | 0.584836 | [
[
[
"# python standard library\nimport sys\nimport os\nimport operator\nimport itertools\nimport collections\nimport functools\nimport glob\nimport csv\nimport datetime\nimport bisect\nimport sqlite3\nimport subprocess\nimport random\nimport gc\nimport shutil\nimport shelve\nimport contextlib\nimport tempfile\nimport math\nimport pickle",
"_____no_output_____"
],
[
"# general purpose third party packages\n\nimport cython\n%reload_ext Cython\n\nimport numpy as np\nnnz = np.count_nonzero\nimport scipy\nimport scipy.stats\nimport scipy.spatial.distance\nimport numexpr\nimport h5py\nimport tables\nimport bcolz\nimport dask\nimport dask.array as da\nimport pandas\nimport IPython\nfrom IPython.display import clear_output, display, HTML\nimport sklearn\nimport sklearn.decomposition\nimport sklearn.manifold\nimport petl as etl\netl.config.display_index_header = True\nimport humanize\nfrom humanize import naturalsize, intcomma, intword\nimport zarr\nimport graphviz\nimport statsmodels.formula.api as sfa",
"_____no_output_____"
],
[
"# plotting setup\nimport matplotlib as mpl\nimport matplotlib.pyplot as plt\nfrom matplotlib.colors import ListedColormap\nfrom matplotlib.gridspec import GridSpec\nimport matplotlib_venn as venn\nimport seaborn as sns\nsns.set_context('paper')\nsns.set_style('white')\nsns.set_style('ticks')\nrcParams = plt.rcParams\nbase_font_size = 8\nrcParams['font.size'] = base_font_size\nrcParams['axes.titlesize'] = base_font_size\nrcParams['axes.labelsize'] = base_font_size\nrcParams['xtick.labelsize'] = base_font_size\nrcParams['ytick.labelsize'] = base_font_size\nrcParams['legend.fontsize'] = base_font_size\nrcParams['axes.linewidth'] = .5\nrcParams['lines.linewidth'] = .5\nrcParams['patch.linewidth'] = .5\nrcParams['ytick.direction'] = 'out'\nrcParams['xtick.direction'] = 'out'\nrcParams['savefig.jpeg_quality'] = 100\nrcParams['lines.markeredgewidth'] = .5\nrcParams['figure.max_open_warning'] = 1000\nrcParams['figure.dpi'] = 120\nrcParams['figure.facecolor'] = 'w'",
"_____no_output_____"
],
[
"# bio third party packages\nimport Bio\nimport pyfasta\n# currently broken, not compatible\n# import pysam\n# import pysamstats\nimport petlx\nimport petlx.bio\nimport vcf\nimport anhima\nimport allel",
"_____no_output_____"
],
[
"sys.path.insert(0, '../agam-report-base/src/python')\nfrom util import *\nimport zcache\nimport veff\n# import hapclust\nag1k_dir = '../ngs.sanger.ac.uk/production/ag1000g'\nfrom ag1k import phase1_ar3\nphase1_ar3.init(os.path.join(ag1k_dir, 'phase1', 'AR3'))\nfrom ag1k import phase1_ar31\nphase1_ar31.init(os.path.join(ag1k_dir, 'phase1', 'AR3.1'))\nfrom ag1k import phase2_ar1\nphase2_ar1.init(os.path.join(ag1k_dir, 'phase2', 'AR1'))\nregion_vgsc = SeqFeature('2L', 2358158, 2431617, label='Vgsc')\n",
"_____no_output_____"
]
]
] | [
"code"
] | [
[
"code",
"code",
"code",
"code",
"code"
]
] |
d048b67954ac93cd558362f4f5e19820634a53d9 | 82,631 | ipynb | Jupyter Notebook | program/lightGBM_base_v0.1.ipynb | tomokoochi/splatoon_competition | 6cbdbb560bdce2d6137f16d87f739129b2d29a7e | [
"MIT"
] | null | null | null | program/lightGBM_base_v0.1.ipynb | tomokoochi/splatoon_competition | 6cbdbb560bdce2d6137f16d87f739129b2d29a7e | [
"MIT"
] | null | null | null | program/lightGBM_base_v0.1.ipynb | tomokoochi/splatoon_competition | 6cbdbb560bdce2d6137f16d87f739129b2d29a7e | [
"MIT"
] | null | null | null | 41.94467 | 12,352 | 0.54793 | [
[
[
"# 内容\n- lightGBMモデル初版\n- ターゲットエンコーディング:Holdout TS\n- 外部データ3つ(ステージ面積1,ステージ面積2,ブキ)を結合\n - ステージ面積1:\n https://probspace-stg.s3-ap-northeast-1.amazonaws.com/uploads/user/c10947bba5cde4ad3dd4a0d42a0ec35b/files/2020-09-06-0320/stagedata.csv\n - ステージ面積2:https://stat.ink/api-info/stage2\n - ブキ:https://stat.ink/api-info/weapon2",
"_____no_output_____"
]
],
[
[
"# ライブラリのインポート\nimport pandas as pd\nimport numpy as np\nimport re\nimport matplotlib.pyplot as plt\nimport seaborn as sns\nimport lightgbm as lgb\nfrom sklearn.model_selection import train_test_split\nfrom sklearn.model_selection import KFold\nfrom sklearn.metrics import accuracy_score\nimport warnings\nwarnings.filterwarnings('ignore')",
"_____no_output_____"
],
[
"# データの読込\ntrain = pd.read_csv(\"../data/train_data.csv\")\ntest = pd.read_csv('../data/test_data.csv')",
"_____no_output_____"
]
],
[
[
"# データの確認",
"_____no_output_____"
]
],
[
[
"def inspection_datas(df):\n print('######################################')\n print('①サイズ(行数、列数)の確認')\n print(df.shape)\n print('######################################')\n print('②最初の5行の表示')\n display(df.head())\n print('######################################')\n print('③各行のデータ型の確認(オブジェクト型の有無)')\n display(df.info())\n display(df.select_dtypes(include=object).columns)\n print('######################################')\n print('④各種統計値の確認(③で、Objectのものは統計されない)')\n display(df.describe())\n print('######################################')\n print('➄欠損値がある列の確認')\n null_df =df.isnull().sum()[df.columns[df.isnull().sum()!=0]]\n display(null_df)\n display(null_df.shape)\n print('######################################')\n print('⑥相関係数のヒートマップ')\n sns.heatmap(df.corr())",
"_____no_output_____"
],
[
"inspection_datas(train)",
"######################################\n①サイズ(行数、列数)の確認\n(66125, 32)\n######################################\n②最初の5行の表示\n"
]
],
[
[
"# 外部データの結合",
"_____no_output_____"
]
],
[
[
"# 外部データの読込\n# stage,stage2は若干面積が異なる、バージョンによる違いや計算方法による誤差\nstage = pd.read_csv('../gaibu_data/stagedata.csv')\nstage2 = pd.read_json('../gaibu_data/stage.json')\nweapon = pd.read_csv('../gaibu_data/statink-weapon2.csv')",
"_____no_output_____"
],
[
"stage.head(3)",
"_____no_output_____"
],
[
"stage2.head(3)",
"_____no_output_____"
],
[
"weapon.head(3)",
"_____no_output_____"
]
],
[
[
"## stageを結合",
"_____no_output_____"
]
],
[
[
"# 表記揺れの確認\nprint(np.sort(train['stage'].unique()))\nprint(np.sort(test['stage'].unique()))\nprint(np.sort(stage['stage'].unique()))",
"['ajifry' 'ama' 'anchovy' 'arowana' 'battera' 'bbass' 'chozame' 'devon'\n 'engawa' 'fujitsubo' 'gangaze' 'hakofugu' 'hokke' 'kombu' 'manta'\n 'mongara' 'mozuku' 'mutsugoro' 'otoro' 'shottsuru' 'sumeshi' 'tachiuo'\n 'zatou']\n['ajifry' 'ama' 'anchovy' 'arowana' 'battera' 'bbass' 'chozame' 'devon'\n 'engawa' 'fujitsubo' 'gangaze' 'hakofugu' 'hokke' 'kombu' 'manta'\n 'mongara' 'mozuku' 'mutsugoro' 'otoro' 'shottsuru' 'sumeshi' 'tachiuo'\n 'zatou']\n['ajifry' 'ama' 'anchovy' 'arowana' 'battera' 'bbass' 'chozame' 'devon'\n 'engawa' 'fujitsubo' 'gangaze' 'hakofugu' 'hokke' 'kombu' 'manta'\n 'mongara' 'mozuku' 'mutsugoro' 'otoro' 'shottsuru' 'sumeshi' 'tachiuo'\n 'zatou']\n"
],
[
"# 結合のため列名変更\nstage_r = stage.rename(columns = {'size':'stage_size1'})\n# 結合\ntrain_s = pd.merge(train, stage_r, on = 'stage', how = 'left')\ntest_s = pd.merge(test, stage_r, on = 'stage', how = 'left')\n\n# null確認\nprint(train_s[['stage_size1']].isnull().sum())\nprint(test_s[['stage_size1']].isnull().sum())",
"stage_size1 0\ndtype: int64\nstage_size1 0\ndtype: int64\n"
]
],
[
[
"## stage2を結合",
"_____no_output_____"
]
],
[
[
"# 表記揺れの確認\nprint(np.sort(train['stage'].unique()))\nprint(np.sort(test['stage'].unique()))\n# 「mystery~」はイベント時に解放されるステージ、今回のtrain,testデータには無し\nprint(np.sort(stage2['key'].unique()))",
"['ajifry' 'ama' 'anchovy' 'arowana' 'battera' 'bbass' 'chozame' 'devon'\n 'engawa' 'fujitsubo' 'gangaze' 'hakofugu' 'hokke' 'kombu' 'manta'\n 'mongara' 'mozuku' 'mutsugoro' 'otoro' 'shottsuru' 'sumeshi' 'tachiuo'\n 'zatou']\n['ajifry' 'ama' 'anchovy' 'arowana' 'battera' 'bbass' 'chozame' 'devon'\n 'engawa' 'fujitsubo' 'gangaze' 'hakofugu' 'hokke' 'kombu' 'manta'\n 'mongara' 'mozuku' 'mutsugoro' 'otoro' 'shottsuru' 'sumeshi' 'tachiuo'\n 'zatou']\n['ajifry' 'ama' 'anchovy' 'arowana' 'battera' 'bbass' 'chozame' 'devon'\n 'engawa' 'fujitsubo' 'gangaze' 'hakofugu' 'hokke' 'kombu' 'manta'\n 'mongara' 'mozuku' 'mutsugoro' 'mystery' 'mystery_01' 'mystery_02'\n 'mystery_03' 'mystery_04' 'mystery_05' 'mystery_06' 'mystery_07'\n 'mystery_08' 'mystery_09' 'mystery_10' 'mystery_11' 'mystery_12'\n 'mystery_13' 'mystery_14' 'mystery_15' 'mystery_16' 'mystery_17'\n 'mystery_18' 'mystery_19' 'mystery_20' 'mystery_21' 'mystery_22'\n 'mystery_23' 'mystery_24' 'otoro' 'shottsuru' 'sumeshi' 'tachiuo' 'zatou']\n"
],
[
"stage2_r.columns",
"_____no_output_____"
],
[
"# 結合のため列名変更\nstage2_r = stage2.rename(columns = {'key':'stage', 'area':'stage_size2'})",
"_____no_output_____"
],
[
"# 必要カラム\nst2_col = ['stage_size2', # ステージの面積\n 'stage', # ステージ名\n# 'name', # 外国語ステージ名\n# 'release_at', #リリース日時\n# 'short_name', # 省略名\n# 'splatnet' # ID?\n ]\nstage2_rc = stage2_r[st2_col]",
"_____no_output_____"
],
[
"# 結合\ntrain_ss = pd.merge(train_s, stage2_rc, on = 'stage', how = 'left')\ntest_ss = pd.merge(test_s, stage2_rc, on = 'stage', how = 'left')\n\n# null確認\nprint(train_ss[['stage_size2']].isnull().sum())\nprint(test_ss[['stage_size2']].isnull().sum())",
"stage_size2 0\ndtype: int64\nstage_size2 0\ndtype: int64\n"
]
],
[
[
"## weaponを結合",
"_____no_output_____"
]
],
[
[
"# trainのブキ\ntrain_weapon = sorted(list(set(train['A1-weapon'])&set(train['A2-weapon'])&set(train['A3-weapon'])&set(train['A4-weapon'])\\\n&set(train['B1-weapon'])&set(train['B2-weapon'])&set(train['B3-weapon'])&set(train['B4-weapon'])))\nprint('{}種類'.format(len(train_weapon)))\nprint(train_weapon)",
"139種類\n['52gal', '52gal_becchu', '52gal_deco', '96gal', '96gal_deco', 'bamboo14mk1', 'bamboo14mk2', 'bamboo14mk3', 'barrelspinner', 'barrelspinner_deco', 'barrelspinner_remix', 'bold', 'bold_7', 'bold_neo', 'bottlegeyser', 'bottlegeyser_foil', 'bucketslosher', 'bucketslosher_deco', 'bucketslosher_soda', 'campingshelter', 'campingshelter_camo', 'campingshelter_sorella', 'carbon', 'carbon_deco', 'clashblaster', 'clashblaster_neo', 'dualsweeper', 'dualsweeper_custom', 'dynamo', 'dynamo_becchu', 'dynamo_tesla', 'explosher', 'explosher_custom', 'furo', 'furo_deco', 'h3reelgun', 'h3reelgun_cherry', 'h3reelgun_d', 'heroblaster_replica', 'herobrush_replica', 'herocharger_replica', 'heromaneuver_replica', 'heroroller_replica', 'heroshelter_replica', 'heroshooter_replica', 'heroslosher_replica', 'herospinner_replica', 'hissen', 'hissen_hue', 'hokusai', 'hokusai_becchu', 'hokusai_hue', 'hotblaster', 'hotblaster_custom', 'hydra', 'hydra_custom', 'jetsweeper', 'jetsweeper_custom', 'kelvin525', 'kelvin525_becchu', 'kelvin525_deco', 'kugelschreiber', 'kugelschreiber_hue', 'l3reelgun', 'l3reelgun_becchu', 'l3reelgun_d', 'liter4k', 'liter4k_custom', 'liter4k_scope', 'liter4k_scope_custom', 'longblaster', 'longblaster_custom', 'longblaster_necro', 'maneuver', 'maneuver_becchu', 'maneuver_collabo', 'momiji', 'nautilus47', 'nautilus79', 'nova', 'nova_becchu', 'nova_neo', 'nzap83', 'nzap85', 'nzap89', 'ochiba', 'octoshooter_replica', 'pablo', 'pablo_hue', 'pablo_permanent', 'parashelter', 'parashelter_sorella', 'prime', 'prime_becchu', 'prime_collabo', 'promodeler_mg', 'promodeler_pg', 'promodeler_rg', 'quadhopper_black', 'quadhopper_white', 'rapid', 'rapid_becchu', 'rapid_deco', 'rapid_elite', 'rapid_elite_deco', 'screwslosher', 'screwslosher_becchu', 'screwslosher_neo', 'sharp', 'sharp_neo', 'soytuber', 'soytuber_custom', 'splatcharger', 'splatcharger_becchu', 'splatcharger_collabo', 'splatroller', 'splatroller_becchu', 'splatroller_collabo', 'splatscope', 'splatscope_becchu', 'splatscope_collabo', 'splatspinner', 'splatspinner_becchu', 'splatspinner_collabo', 'sputtery', 'sputtery_clear', 'sputtery_hue', 'spygadget', 'spygadget_becchu', 'spygadget_sorella', 'squiclean_a', 'squiclean_b', 'squiclean_g', 'sshooter', 'sshooter_becchu', 'sshooter_collabo', 'variableroller', 'variableroller_foil', 'wakaba']\n"
],
[
"# testのブキ\ntest_weapon = sorted(list(set(test['A1-weapon'])&set(test['A2-weapon'])&set(test['A3-weapon'])&set(test['A4-weapon'])\\\n&set(test['B1-weapon'])&set(test['B2-weapon'])&set(test['B3-weapon'])&set(test['B4-weapon'])))\nprint('{}種類'.format(len(test_weapon)))\nprint(test_weapon)",
"139種類\n['52gal', '52gal_becchu', '52gal_deco', '96gal', '96gal_deco', 'bamboo14mk1', 'bamboo14mk2', 'bamboo14mk3', 'barrelspinner', 'barrelspinner_deco', 'barrelspinner_remix', 'bold', 'bold_7', 'bold_neo', 'bottlegeyser', 'bottlegeyser_foil', 'bucketslosher', 'bucketslosher_deco', 'bucketslosher_soda', 'campingshelter', 'campingshelter_camo', 'campingshelter_sorella', 'carbon', 'carbon_deco', 'clashblaster', 'clashblaster_neo', 'dualsweeper', 'dualsweeper_custom', 'dynamo', 'dynamo_becchu', 'dynamo_tesla', 'explosher', 'explosher_custom', 'furo', 'furo_deco', 'h3reelgun', 'h3reelgun_cherry', 'h3reelgun_d', 'heroblaster_replica', 'herobrush_replica', 'herocharger_replica', 'heromaneuver_replica', 'heroroller_replica', 'heroshelter_replica', 'heroshooter_replica', 'heroslosher_replica', 'herospinner_replica', 'hissen', 'hissen_hue', 'hokusai', 'hokusai_becchu', 'hokusai_hue', 'hotblaster', 'hotblaster_custom', 'hydra', 'hydra_custom', 'jetsweeper', 'jetsweeper_custom', 'kelvin525', 'kelvin525_becchu', 'kelvin525_deco', 'kugelschreiber', 'kugelschreiber_hue', 'l3reelgun', 'l3reelgun_becchu', 'l3reelgun_d', 'liter4k', 'liter4k_custom', 'liter4k_scope', 'liter4k_scope_custom', 'longblaster', 'longblaster_custom', 'longblaster_necro', 'maneuver', 'maneuver_becchu', 'maneuver_collabo', 'momiji', 'nautilus47', 'nautilus79', 'nova', 'nova_becchu', 'nova_neo', 'nzap83', 'nzap85', 'nzap89', 'ochiba', 'octoshooter_replica', 'pablo', 'pablo_hue', 'pablo_permanent', 'parashelter', 'parashelter_sorella', 'prime', 'prime_becchu', 'prime_collabo', 'promodeler_mg', 'promodeler_pg', 'promodeler_rg', 'quadhopper_black', 'quadhopper_white', 'rapid', 'rapid_becchu', 'rapid_deco', 'rapid_elite', 'rapid_elite_deco', 'screwslosher', 'screwslosher_becchu', 'screwslosher_neo', 'sharp', 'sharp_neo', 'soytuber', 'soytuber_custom', 'splatcharger', 'splatcharger_becchu', 'splatcharger_collabo', 'splatroller', 'splatroller_becchu', 'splatroller_collabo', 'splatscope', 'splatscope_becchu', 'splatscope_collabo', 'splatspinner', 'splatspinner_becchu', 'splatspinner_collabo', 'sputtery', 'sputtery_clear', 'sputtery_hue', 'spygadget', 'spygadget_becchu', 'spygadget_sorella', 'squiclean_a', 'squiclean_b', 'squiclean_g', 'sshooter', 'sshooter_becchu', 'sshooter_collabo', 'variableroller', 'variableroller_foil', 'wakaba']\n"
],
[
"# 外部データのブキ\ngaibu_weapon = train_weapon = np.sort(weapon['key'].unique())\nprint('{}種類'.format(len(gaibu_weapon)))\nprint(gaibu_weapon)",
"139種類\n['52gal' '52gal_becchu' '52gal_deco' '96gal' '96gal_deco' 'bamboo14mk1'\n 'bamboo14mk2' 'bamboo14mk3' 'barrelspinner' 'barrelspinner_deco'\n 'barrelspinner_remix' 'bold' 'bold_7' 'bold_neo' 'bottlegeyser'\n 'bottlegeyser_foil' 'bucketslosher' 'bucketslosher_deco'\n 'bucketslosher_soda' 'campingshelter' 'campingshelter_camo'\n 'campingshelter_sorella' 'carbon' 'carbon_deco' 'clashblaster'\n 'clashblaster_neo' 'dualsweeper' 'dualsweeper_custom' 'dynamo'\n 'dynamo_becchu' 'dynamo_tesla' 'explosher' 'explosher_custom' 'furo'\n 'furo_deco' 'h3reelgun' 'h3reelgun_cherry' 'h3reelgun_d'\n 'heroblaster_replica' 'herobrush_replica' 'herocharger_replica'\n 'heromaneuver_replica' 'heroroller_replica' 'heroshelter_replica'\n 'heroshooter_replica' 'heroslosher_replica' 'herospinner_replica'\n 'hissen' 'hissen_hue' 'hokusai' 'hokusai_becchu' 'hokusai_hue'\n 'hotblaster' 'hotblaster_custom' 'hydra' 'hydra_custom' 'jetsweeper'\n 'jetsweeper_custom' 'kelvin525' 'kelvin525_becchu' 'kelvin525_deco'\n 'kugelschreiber' 'kugelschreiber_hue' 'l3reelgun' 'l3reelgun_becchu'\n 'l3reelgun_d' 'liter4k' 'liter4k_custom' 'liter4k_scope'\n 'liter4k_scope_custom' 'longblaster' 'longblaster_custom'\n 'longblaster_necro' 'maneuver' 'maneuver_becchu' 'maneuver_collabo'\n 'momiji' 'nautilus47' 'nautilus79' 'nova' 'nova_becchu' 'nova_neo'\n 'nzap83' 'nzap85' 'nzap89' 'ochiba' 'octoshooter_replica' 'pablo'\n 'pablo_hue' 'pablo_permanent' 'parashelter' 'parashelter_sorella' 'prime'\n 'prime_becchu' 'prime_collabo' 'promodeler_mg' 'promodeler_pg'\n 'promodeler_rg' 'quadhopper_black' 'quadhopper_white' 'rapid'\n 'rapid_becchu' 'rapid_deco' 'rapid_elite' 'rapid_elite_deco'\n 'screwslosher' 'screwslosher_becchu' 'screwslosher_neo' 'sharp'\n 'sharp_neo' 'soytuber' 'soytuber_custom' 'splatcharger'\n 'splatcharger_becchu' 'splatcharger_collabo' 'splatroller'\n 'splatroller_becchu' 'splatroller_collabo' 'splatscope'\n 'splatscope_becchu' 'splatscope_collabo' 'splatspinner'\n 'splatspinner_becchu' 'splatspinner_collabo' 'sputtery' 'sputtery_clear'\n 'sputtery_hue' 'spygadget' 'spygadget_becchu' 'spygadget_sorella'\n 'squiclean_a' 'squiclean_b' 'squiclean_g' 'sshooter' 'sshooter_becchu'\n 'sshooter_collabo' 'variableroller' 'variableroller_foil' 'wakaba']\n"
],
[
"# 表記に差分ないか比較→無し\nprint(set(train_weapon)-set(gaibu_weapon))\nprint(set(gaibu_weapon)-set(train_weapon))\nprint(set(test_weapon)-set(gaibu_weapon))\nprint(set(gaibu_weapon)-set(test_weapon))",
"set()\nset()\nset()\nset()\n"
],
[
"# 必要カラム\n# 参照:https://stat.ink/api-info/weapon2\nweapon_col = ['category1', # ブキ区分\n 'category2', # ブキ区分\n 'key', # ブキ名\n 'subweapon', # サブウェポン\n 'special', # スペシャルウェポン\n 'mainweapon', # メインブキ\n 'reskin', # 同一性能のブキ\n# 'splatnet', # アプリのユーザID\n# 以下外国語ブキ名\n# '[de-DE]', '[en-GB]', '[en-US]', '[es-ES]','[es-MX]', '[fr-CA]', \n# '[fr-FR]', '[it-IT]', '[ja-JP]', '[nl-NL]','[ru-RU]', '[zh-CN]', '[zh-TW]' \n]",
"_____no_output_____"
],
[
"# 必要カラム抽出&結合キー名変更\nweapon_c = weapon[weapon_col].rename(columns = {'key': 'weapon'})\nweapon_c.head(3)",
"_____no_output_____"
],
[
"# 各A1~B4-weapon列に対して結合\nweapon_cc = weapon_c.copy()\ntrain_ssw = train_ss.copy()\ntest_ssw = test_ss.copy()\nimport itertools\n\nfor a,num in itertools.product(['A','B'],[1,2,3,4]):\n col_list = []\n # ブキのカラム名の先頭にA1~B4追加\n for col in weapon_c.columns:\n tmp_col = a+str(num) + '-' + col\n col_list.append(tmp_col)\n weapon_cc.columns = col_list\n #train,testに結合\n train_ssw = pd.merge(train_ssw, weapon_cc, on = a+str(num) + '-weapon', how = 'left')\n test_ssw = pd.merge(test_ssw, weapon_cc, on = a+str(num) + '-weapon', how = 'left')\n # 結合後nullチェック\n print(train_ssw[col_list].isnull().sum())\n print(test_ssw[col_list].isnull().sum())",
"A1-category1 0\nA1-category2 0\nA1-weapon 0\nA1-subweapon 0\nA1-special 0\nA1-mainweapon 0\nA1-reskin 0\ndtype: int64\nA1-category1 0\nA1-category2 0\nA1-weapon 0\nA1-subweapon 0\nA1-special 0\nA1-mainweapon 0\nA1-reskin 0\ndtype: int64\nA2-category1 0\nA2-category2 0\nA2-weapon 0\nA2-subweapon 0\nA2-special 0\nA2-mainweapon 0\nA2-reskin 0\ndtype: int64\nA2-category1 0\nA2-category2 0\nA2-weapon 0\nA2-subweapon 0\nA2-special 0\nA2-mainweapon 0\nA2-reskin 0\ndtype: int64\nA3-category1 0\nA3-category2 0\nA3-weapon 0\nA3-subweapon 0\nA3-special 0\nA3-mainweapon 0\nA3-reskin 0\ndtype: int64\nA3-category1 0\nA3-category2 0\nA3-weapon 0\nA3-subweapon 0\nA3-special 0\nA3-mainweapon 0\nA3-reskin 0\ndtype: int64\nA4-category1 51\nA4-category2 51\nA4-weapon 51\nA4-subweapon 51\nA4-special 51\nA4-mainweapon 51\nA4-reskin 51\ndtype: int64\nA4-category1 15\nA4-category2 15\nA4-weapon 15\nA4-subweapon 15\nA4-special 15\nA4-mainweapon 15\nA4-reskin 15\ndtype: int64\nB1-category1 0\nB1-category2 0\nB1-weapon 0\nB1-subweapon 0\nB1-special 0\nB1-mainweapon 0\nB1-reskin 0\ndtype: int64\nB1-category1 0\nB1-category2 0\nB1-weapon 0\nB1-subweapon 0\nB1-special 0\nB1-mainweapon 0\nB1-reskin 0\ndtype: int64\nB2-category1 0\nB2-category2 0\nB2-weapon 0\nB2-subweapon 0\nB2-special 0\nB2-mainweapon 0\nB2-reskin 0\ndtype: int64\nB2-category1 0\nB2-category2 0\nB2-weapon 0\nB2-subweapon 0\nB2-special 0\nB2-mainweapon 0\nB2-reskin 0\ndtype: int64\nB3-category1 1\nB3-category2 1\nB3-weapon 1\nB3-subweapon 1\nB3-special 1\nB3-mainweapon 1\nB3-reskin 1\ndtype: int64\nB3-category1 1\nB3-category2 1\nB3-weapon 1\nB3-subweapon 1\nB3-special 1\nB3-mainweapon 1\nB3-reskin 1\ndtype: int64\nB4-category1 67\nB4-category2 67\nB4-weapon 67\nB4-subweapon 67\nB4-special 67\nB4-mainweapon 67\nB4-reskin 67\ndtype: int64\nB4-category1 30\nB4-category2 30\nB4-weapon 30\nB4-subweapon 30\nB4-special 30\nB4-mainweapon 30\nB4-reskin 30\ndtype: int64\n"
],
[
"# 元データにweapon情報がないもののみ(回線落ち)がnullなのでok",
"_____no_output_____"
],
[
"train_input = train_ssw.copy()\ntest_input = test_ssw.copy()",
"_____no_output_____"
]
],
[
[
"# 前処理",
"_____no_output_____"
]
],
[
[
"# 欠損値埋める\ndef fill_all_null(df, num):\n for col_name in df.columns[df.isnull().sum()!=0]:\n df[col_name] = df[col_name].fillna(num)",
"_____no_output_____"
],
[
"# 訓練データ、テストデータの欠損値を-1で補完\nfill_all_null(train_input, -1)\nfill_all_null(test_input, -1)",
"_____no_output_____"
],
[
"# ターゲットエンコーディングの関数定義\n## Holdout TSを用いる 変更の余地あり\ndef change_to_target2(train_df,test_df,input_column_name,output_column_name):\n from sklearn.model_selection import KFold\n \n # nan埋め処理\n ## 上でやってるのでいらない\n # train_df[input_column_name] = train_df[input_column_name].fillna('-1')\n # test_df[input_column_name] = test_df[input_column_name].fillna('-1')\n\n kf = KFold(n_splits=5, shuffle=True, random_state=71)\n #=========================================================#\n c=input_column_name\n # 学習データ全体で各カテゴリにおけるyの平均を計算\n data_tmp = pd.DataFrame({c: train_df[c],'target':train_df['y']})\n target_mean = data_tmp.groupby(c)['target'].mean()\n #テストデータのカテゴリを置換★\n test_df[output_column_name] = test_df[c].map(target_mean)\n \n # 変換後の値を格納する配列を準備\n tmp = np.repeat(np.nan, train_df.shape[0])\n\n for i, (train_index, test_index) in enumerate(kf.split(train_df)): # NFOLDS回まわる\n #学習データについて、各カテゴリにおける目的変数の平均を計算\n target_mean = data_tmp.iloc[train_index].groupby(c)['target'].mean()\n #バリデーションデータについて、変換後の値を一時配列に格納\n tmp[test_index] = train_df[c].iloc[test_index].map(target_mean) \n\n #変換後のデータで元の変数を置換\n train_df[output_column_name] = tmp\n#========================================================# \n",
"_____no_output_____"
],
[
"# オブジェクトの列のリストを作成\nobject_col_list = train_input.select_dtypes(include=object).columns\n# オブジェクトの列は全てターゲットエンコーディング実施\nfor col in object_col_list:\n change_to_target2(train_input,test_input,col,\"enc_\"+col)\n# 変換前の列を削除\ntrain_input = train_input.drop(object_col_list,axis=1)\ntest_input = test_input.drop(object_col_list,axis=1)\n# 'id'の列を削除\ntrain_input = train_input.drop('id',axis=1)\ntest_input = test_input.drop('id',axis=1)",
"_____no_output_____"
],
[
"# 訓練データ欠損確認\ntrain_input.isnull().sum().sum()",
"_____no_output_____"
],
[
"# テストデータ欠損確認\ntest_input.isnull().sum().sum()",
"_____no_output_____"
],
[
"# 欠損値はターゲットエンコーディング時に学習データが少なくなって平均値が計算できなくなってしまうため発生。0埋め。\nfill_all_null(train_input, 0)\nfill_all_null(test_input, 0)",
"_____no_output_____"
]
],
[
[
"# データの確認",
"_____no_output_____"
]
],
[
[
"# 訓練データとテストデータの列を確認\nprint(train_input.columns)\nprint(test_input.columns)",
"Index(['A1-level', 'A2-level', 'A3-level', 'A4-level', 'B1-level', 'B2-level',\n 'B3-level', 'B4-level', 'y', 'stage_size1', 'stage_size2', 'enc_period',\n 'enc_game-ver', 'enc_lobby-mode', 'enc_lobby', 'enc_mode', 'enc_stage',\n 'enc_A1-weapon', 'enc_A1-rank', 'enc_A2-weapon', 'enc_A2-rank',\n 'enc_A3-weapon', 'enc_A3-rank', 'enc_A4-weapon', 'enc_A4-rank',\n 'enc_B1-weapon', 'enc_B1-rank', 'enc_B2-weapon', 'enc_B2-rank',\n 'enc_B3-weapon', 'enc_B3-rank', 'enc_B4-weapon', 'enc_B4-rank',\n 'enc_A1-category1', 'enc_A1-category2', 'enc_A1-subweapon',\n 'enc_A1-special', 'enc_A1-mainweapon', 'enc_A1-reskin',\n 'enc_A2-category1', 'enc_A2-category2', 'enc_A2-subweapon',\n 'enc_A2-special', 'enc_A2-mainweapon', 'enc_A2-reskin',\n 'enc_A3-category1', 'enc_A3-category2', 'enc_A3-subweapon',\n 'enc_A3-special', 'enc_A3-mainweapon', 'enc_A3-reskin',\n 'enc_A4-category1', 'enc_A4-category2', 'enc_A4-subweapon',\n 'enc_A4-special', 'enc_A4-mainweapon', 'enc_A4-reskin',\n 'enc_B1-category1', 'enc_B1-category2', 'enc_B1-subweapon',\n 'enc_B1-special', 'enc_B1-mainweapon', 'enc_B1-reskin',\n 'enc_B2-category1', 'enc_B2-category2', 'enc_B2-subweapon',\n 'enc_B2-special', 'enc_B2-mainweapon', 'enc_B2-reskin',\n 'enc_B3-category1', 'enc_B3-category2', 'enc_B3-subweapon',\n 'enc_B3-special', 'enc_B3-mainweapon', 'enc_B3-reskin',\n 'enc_B4-category1', 'enc_B4-category2', 'enc_B4-subweapon',\n 'enc_B4-special', 'enc_B4-mainweapon', 'enc_B4-reskin'],\n dtype='object')\nIndex(['A1-level', 'A2-level', 'A3-level', 'A4-level', 'B1-level', 'B2-level',\n 'B3-level', 'B4-level', 'stage_size1', 'stage_size2', 'enc_period',\n 'enc_game-ver', 'enc_lobby-mode', 'enc_lobby', 'enc_mode', 'enc_stage',\n 'enc_A1-weapon', 'enc_A1-rank', 'enc_A2-weapon', 'enc_A2-rank',\n 'enc_A3-weapon', 'enc_A3-rank', 'enc_A4-weapon', 'enc_A4-rank',\n 'enc_B1-weapon', 'enc_B1-rank', 'enc_B2-weapon', 'enc_B2-rank',\n 'enc_B3-weapon', 'enc_B3-rank', 'enc_B4-weapon', 'enc_B4-rank',\n 'enc_A1-category1', 'enc_A1-category2', 'enc_A1-subweapon',\n 'enc_A1-special', 'enc_A1-mainweapon', 'enc_A1-reskin',\n 'enc_A2-category1', 'enc_A2-category2', 'enc_A2-subweapon',\n 'enc_A2-special', 'enc_A2-mainweapon', 'enc_A2-reskin',\n 'enc_A3-category1', 'enc_A3-category2', 'enc_A3-subweapon',\n 'enc_A3-special', 'enc_A3-mainweapon', 'enc_A3-reskin',\n 'enc_A4-category1', 'enc_A4-category2', 'enc_A4-subweapon',\n 'enc_A4-special', 'enc_A4-mainweapon', 'enc_A4-reskin',\n 'enc_B1-category1', 'enc_B1-category2', 'enc_B1-subweapon',\n 'enc_B1-special', 'enc_B1-mainweapon', 'enc_B1-reskin',\n 'enc_B2-category1', 'enc_B2-category2', 'enc_B2-subweapon',\n 'enc_B2-special', 'enc_B2-mainweapon', 'enc_B2-reskin',\n 'enc_B3-category1', 'enc_B3-category2', 'enc_B3-subweapon',\n 'enc_B3-special', 'enc_B3-mainweapon', 'enc_B3-reskin',\n 'enc_B4-category1', 'enc_B4-category2', 'enc_B4-subweapon',\n 'enc_B4-special', 'enc_B4-mainweapon', 'enc_B4-reskin'],\n dtype='object')\n"
]
],
[
[
"# 学習の準備",
"_____no_output_____"
]
],
[
[
"# 訓練データを説明変数と目的変数に分割\ntarget = train_input['y']\ntrain_x = train_input.drop('y',axis=1)\n# LGBMのパラメータを設定\nparams = {\n # 二値分類問題\n 'objective': 'binary',\n # 損失関数は二値のlogloss\n #'metric': 'auc',\n 'metric': 'binary_logloss',\n # 最大イテレーション回数指定\n 'num_iterations' : 1000,\n # early_stopping 回数指定\n 'early_stopping_rounds' : 100,\n}",
"_____no_output_____"
]
],
[
[
"# 学習・予測の実行",
"_____no_output_____"
]
],
[
[
"# k-分割交差検証を使って学習&予測(K=10)\nFOLD_NUM = 10\nkf = KFold(n_splits=FOLD_NUM,\n random_state=42)\n#lgbmのラウンド数を定義\nnum_round = 10000",
"_____no_output_____"
],
[
"#検証時のスコアを初期化\nscores = []\n\n#テストデータの予測値を初期化\npred_cv = np.zeros(len(test.index))\n\n\nfor i, (tdx, vdx) in enumerate(kf.split(train_x, target)):\n print(f'Fold : {i}')\n # 訓練用データと検証用データに分割\n X_train, X_valid, y_train, y_valid = train_x.iloc[tdx], train_x.iloc[vdx], target.values[tdx], target.values[vdx]\n lgb_train = lgb.Dataset(X_train, y_train)\n lgb_valid = lgb.Dataset(X_valid, y_valid)\n \n # 学習の実行\n model = lgb.train(params, lgb_train, num_boost_round=num_round,\n valid_names=[\"train\", \"valid\"], valid_sets=[lgb_train, lgb_valid],\n verbose_eval=100)\n\n # 検証データに対する予測値を求めて、勝敗(0 or 1)に変換\n va_pred = np.round(model.predict(X_valid,num_iteration=model.best_iteration))\n \n # accuracyスコアを計算\n score_ = accuracy_score(y_valid, va_pred)\n \n # フォールド毎の検証時のスコアを格納\n scores.append(score_)\n \n #テストデータに対する予測値を求める\n submission = model.predict(test_input,num_iteration=model.best_iteration)\n \n #テストデータに対する予測値をフォールド数で割って蓄積\n #(フォールド毎の予測値の平均値を求めることと同じ)\n pred_cv += submission/FOLD_NUM\n\n# 最終的なテストデータに対する予測値を勝敗(0 or 1)に変換\npred_cv_int = np.round(pred_cv)\n\n# 最終的なaccuracyスコアを平均値で出力\nprint('')\nprint('################################')\nprint('CV_score:'+ str(np.mean(scores)))",
"Fold : 0\nTraining until validation scores don't improve for 100 rounds.\n[100]\ttrain's binary_logloss: 0.634065\tvalid's binary_logloss: 0.684154\nEarly stopping, best iteration is:\n[72]\ttrain's binary_logloss: 0.646465\tvalid's binary_logloss: 0.683221\nFold : 1\nTraining until validation scores don't improve for 100 rounds.\n[100]\ttrain's binary_logloss: 0.633946\tvalid's binary_logloss: 0.680458\nEarly stopping, best iteration is:\n[91]\ttrain's binary_logloss: 0.638003\tvalid's binary_logloss: 0.680022\nFold : 2\nTraining until validation scores don't improve for 100 rounds.\n[100]\ttrain's binary_logloss: 0.63433\tvalid's binary_logloss: 0.684864\nEarly stopping, best iteration is:\n[82]\ttrain's binary_logloss: 0.641942\tvalid's binary_logloss: 0.68428\nFold : 3\nTraining until validation scores don't improve for 100 rounds.\n[100]\ttrain's binary_logloss: 0.634298\tvalid's binary_logloss: 0.685253\nEarly stopping, best iteration is:\n[57]\ttrain's binary_logloss: 0.653094\tvalid's binary_logloss: 0.685024\nFold : 4\nTraining until validation scores don't improve for 100 rounds.\n[100]\ttrain's binary_logloss: 0.634393\tvalid's binary_logloss: 0.682669\nEarly stopping, best iteration is:\n[86]\ttrain's binary_logloss: 0.640301\tvalid's binary_logloss: 0.682425\nFold : 5\nTraining until validation scores don't improve for 100 rounds.\n[100]\ttrain's binary_logloss: 0.63454\tvalid's binary_logloss: 0.683878\nEarly stopping, best iteration is:\n[63]\ttrain's binary_logloss: 0.650899\tvalid's binary_logloss: 0.682739\nFold : 6\nTraining until validation scores don't improve for 100 rounds.\n[100]\ttrain's binary_logloss: 0.633727\tvalid's binary_logloss: 0.685075\nEarly stopping, best iteration is:\n[43]\ttrain's binary_logloss: 0.66024\tvalid's binary_logloss: 0.683623\nFold : 7\nTraining until validation scores don't improve for 100 rounds.\n[100]\ttrain's binary_logloss: 0.633955\tvalid's binary_logloss: 0.683512\nEarly stopping, best iteration is:\n[91]\ttrain's binary_logloss: 0.63792\tvalid's binary_logloss: 0.683008\nFold : 8\nTraining until validation scores don't improve for 100 rounds.\n[100]\ttrain's binary_logloss: 0.633702\tvalid's binary_logloss: 0.685102\nEarly stopping, best iteration is:\n[70]\ttrain's binary_logloss: 0.646914\tvalid's binary_logloss: 0.684219\nFold : 9\nTraining until validation scores don't improve for 100 rounds.\n[100]\ttrain's binary_logloss: 0.6335\tvalid's binary_logloss: 0.684607\nEarly stopping, best iteration is:\n[42]\ttrain's binary_logloss: 0.660687\tvalid's binary_logloss: 0.684266\n\n################################\nCV_score:0.5484611124086098\n"
],
[
"# 提出用ファイルを作成する\npd.DataFrame({\"id\": range(len(pred_cv_int)), \"y\": pred_cv_int.astype(np.int64) }).to_csv(\"../submit/submission_v0.2.csv\", index=False)",
"_____no_output_____"
]
]
] | [
"markdown",
"code",
"markdown",
"code",
"markdown",
"code",
"markdown",
"code",
"markdown",
"code",
"markdown",
"code",
"markdown",
"code",
"markdown",
"code",
"markdown",
"code",
"markdown",
"code"
] | [
[
"markdown"
],
[
"code",
"code"
],
[
"markdown"
],
[
"code",
"code"
],
[
"markdown"
],
[
"code",
"code",
"code",
"code"
],
[
"markdown"
],
[
"code",
"code"
],
[
"markdown"
],
[
"code",
"code",
"code",
"code",
"code"
],
[
"markdown"
],
[
"code",
"code",
"code",
"code",
"code",
"code",
"code",
"code",
"code"
],
[
"markdown"
],
[
"code",
"code",
"code",
"code",
"code",
"code",
"code"
],
[
"markdown"
],
[
"code"
],
[
"markdown"
],
[
"code"
],
[
"markdown"
],
[
"code",
"code",
"code"
]
] |
d048b722b91e63a4faa4e77b80c16103a28e2900 | 7,833 | ipynb | Jupyter Notebook | packages/numpy.ipynb | akrisanov/python_notebook | b48aa56419a72699539eca2ea9756cc8737699ad | [
"MIT"
] | 3 | 2019-10-18T11:18:57.000Z | 2022-02-15T18:58:31.000Z | packages/numpy.ipynb | akrisanov/python_notebook | b48aa56419a72699539eca2ea9756cc8737699ad | [
"MIT"
] | null | null | null | packages/numpy.ipynb | akrisanov/python_notebook | b48aa56419a72699539eca2ea9756cc8737699ad | [
"MIT"
] | null | null | null | 19.2457 | 67 | 0.377633 | [
[
[
"import numpy as np",
"_____no_output_____"
],
[
"array1 = np.array([[0, 1], [10, 11], [20, 21]], float)\narray1",
"_____no_output_____"
],
[
"nums = [0, 1, 10, 11, 20, 21]\narray2 = np.array(nums, float).reshape([3, 2])\narray2",
"_____no_output_____"
],
[
"array2 * 2",
"_____no_output_____"
],
[
"array2 * array2",
"_____no_output_____"
],
[
"array3 = np.array([0, 1, 2,10, 11, 12, 20, 21, 22], float)\narray3",
"_____no_output_____"
],
[
"array3 = array3.reshape(3, 3)\narray3",
"_____no_output_____"
],
[
"array3[0]",
"_____no_output_____"
],
[
"array3[-1]",
"_____no_output_____"
],
[
"array3[::-1]",
"_____no_output_____"
],
[
"array3[2, 1]",
"_____no_output_____"
],
[
"array3[:,1] # the second column",
"_____no_output_____"
],
[
"...",
"_____no_output_____"
],
[
"array3[...,1]",
"_____no_output_____"
],
[
"array4 = np.array(range(120), int).reshape(2, 3, 4, 5)\narray4",
"_____no_output_____"
],
[
"array4[0, 1]",
"_____no_output_____"
],
[
"array4[0, ..., 1]",
"_____no_output_____"
]
]
] | [
"code"
] | [
[
"code",
"code",
"code",
"code",
"code",
"code",
"code",
"code",
"code",
"code",
"code",
"code",
"code",
"code",
"code",
"code",
"code"
]
] |
d048c4b0be8237377c1eff528b1510b2aaa49c63 | 5,010 | ipynb | Jupyter Notebook | multiclass/OneVsRestClassifier.ipynb | keyianpai/tiny-sklearn | 8571fc8dee2a08822b22c540375255dbf19106fa | [
"MIT"
] | 19 | 2019-05-08T14:50:24.000Z | 2022-01-18T07:40:55.000Z | multiclass/OneVsRestClassifier.ipynb | keyianpai/tiny-sklearn | 8571fc8dee2a08822b22c540375255dbf19106fa | [
"MIT"
] | 1 | 2019-12-05T18:08:49.000Z | 2019-12-06T04:46:55.000Z | multiclass/OneVsRestClassifier.ipynb | keyianpai/tiny-sklearn | 8571fc8dee2a08822b22c540375255dbf19106fa | [
"MIT"
] | 2 | 2019-05-08T21:38:37.000Z | 2020-01-21T15:33:09.000Z | 33.851351 | 106 | 0.489222 | [
[
[
"import numpy as np\nfrom copy import deepcopy\nfrom scipy.special import expit\nfrom scipy.optimize import minimize\nfrom sklearn.datasets import load_iris\nfrom sklearn.linear_model import LogisticRegression as skLogisticRegression\nfrom sklearn.multiclass import OneVsRestClassifier as skOneVsRestClassifier",
"_____no_output_____"
],
[
"class OneVsRestClassifier():\n def __init__(self, estimator):\n self.estimator = estimator\n\n def _encode(self, y):\n classes = np.unique(y)\n y_train = np.zeros((y.shape[0], len(classes)))\n for i, c in enumerate(classes):\n y_train[y == c, i] = 1\n return classes, y_train\n\n def fit(self, X, y):\n self.classes_, y_train = self._encode(y)\n self.estimators_ = []\n for i in range(y_train.shape[1]):\n cur_y = y_train[:, i]\n clf = deepcopy(self.estimator)\n clf.fit(X, cur_y)\n self.estimators_.append(clf)\n return self\n\n def decision_function(self, X):\n scores = np.zeros((X.shape[0], len(self.classes_)))\n for i, est in enumerate(self.estimators_):\n scores[:, i] = est.decision_function(X)\n return scores\n\n def predict(self, X):\n scores = self.decision_function(X)\n indices = np.argmax(scores, axis=1)\n return self.classes_[indices]",
"_____no_output_____"
],
[
"# Simplified version of LogisticRegression, only work for binary classification\nclass BinaryLogisticRegression():\n def __init__(self, C=1.0):\n self.C = C\n\n @staticmethod\n def _cost_grad(w, X, y, alpha):\n def _log_logistic(x):\n if x > 0:\n return -np.log(1 + np.exp(-x))\n else:\n return x - np.log(1 + np.exp(x))\n yz = y * (np.dot(X, w[:-1]) + w[-1])\n cost = -np.sum(np.vectorize(_log_logistic)(yz)) + 0.5 * alpha * np.dot(w[:-1], w[:-1])\n grad = np.zeros(len(w))\n t = (expit(yz) - 1) * y\n grad[:-1] = np.dot(X.T, t) + alpha * w[:-1]\n grad[-1] = np.sum(t)\n return cost, grad\n\n def _solve_lbfgs(self, X, y):\n y_train = np.full(X.shape[0], -1)\n y_train[y == 1] = 1\n w0 = np.zeros(X.shape[1] + 1)\n res = minimize(fun=self._cost_grad, jac=True, x0=w0,\n args=(X, y_train, 1 / self.C), method='L-BFGS-B')\n return res.x[:-1], res.x[-1]\n\n def fit(self, X, y):\n self.coef_, self.intercept_ = self._solve_lbfgs(X, y)\n return self\n\n def decision_function(self, X):\n scores = np.dot(X, self.coef_) + self.intercept_\n return scores\n\n def predict(self, X):\n scores = self.decision_function(X)\n indices = (scores > 0).astype(int)\n return indices",
"_____no_output_____"
],
[
"for C in [0.1, 1, 10, np.inf]:\n X, y = load_iris(return_X_y=True)\n clf1 = OneVsRestClassifier(BinaryLogisticRegression(C=C)).fit(X, y)\n clf2 = skOneVsRestClassifier(skLogisticRegression(C=C, multi_class=\"ovr\", solver=\"lbfgs\",\n # keep consisent with scipy default\n tol=1e-5, max_iter=15000)).fit(X, y)\n prob1 = clf1.decision_function(X)\n prob2 = clf2.decision_function(X)\n pred1 = clf1.predict(X)\n pred2 = clf2.predict(X)\n assert np.allclose(prob1, prob2)\n assert np.array_equal(pred1, pred2)",
"_____no_output_____"
]
]
] | [
"code"
] | [
[
"code",
"code",
"code",
"code"
]
] |
d048d41cadd912ff72191dd6005f2f3b07aa7775 | 15,809 | ipynb | Jupyter Notebook | outlierdetector_lib.ipynb | eaglewarrior/Anamoly-Detection | b0c61e3d367f9034f8e8ecb6463ecbbcbe5ffcfc | [
"MIT"
] | 1 | 2019-06-17T17:08:18.000Z | 2019-06-17T17:08:18.000Z | outlierdetector_lib.ipynb | eaglewarrior/Anamoly-Detection | b0c61e3d367f9034f8e8ecb6463ecbbcbe5ffcfc | [
"MIT"
] | 1 | 2020-09-28T06:27:30.000Z | 2020-11-02T06:03:21.000Z | outlierdetector_lib.ipynb | eaglewarrior/Anomaly-Detection | b0c61e3d367f9034f8e8ecb6463ecbbcbe5ffcfc | [
"MIT"
] | null | null | null | 28.230357 | 736 | 0.459485 | [
[
[
"import pandas as pd\n",
"_____no_output_____"
],
[
"df=pd.read_csv('Financial Distress.csv')",
"_____no_output_____"
],
[
"df.columns",
"_____no_output_____"
],
[
"df.shape",
"_____no_output_____"
],
[
"e=df['Financial Distress'].value_counts()",
"_____no_output_____"
],
[
"e[1]/(e[0]+e[1])",
"_____no_output_____"
],
[
"import pandas as pd\nimport numpy as np\n\n# Import models\nfrom pyod.models.abod import ABOD\nfrom pyod.models.cblof import CBLOF\nfrom pyod.models.feature_bagging import FeatureBagging\nfrom pyod.models.hbos import HBOS\nfrom pyod.models.iforest import IForest\nfrom pyod.models.knn import KNN\nfrom pyod.models.lof import LOF",
"_____no_output_____"
],
[
"random_state = np.random.RandomState(42)\noutliers_fraction = 0.037",
"_____no_output_____"
],
[
"X=df.iloc[:,2:-1].values",
"_____no_output_____"
],
[
"y=df.iloc[:,-1].values",
"_____no_output_____"
]
],
[
[
"### Angle-based Outlier Detector (ABOD)",
"_____no_output_____"
]
],
[
[
"clf1=ABOD(contamination=outliers_fraction)",
"_____no_output_____"
],
[
"clf1.fit(X)",
"_____no_output_____"
],
[
"y_pred1=clf1.predict(X)",
"_____no_output_____"
],
[
"from sklearn.metrics import confusion_matrix\nconfusion_matrix(y, y_pred1)",
"_____no_output_____"
]
],
[
[
"## Cluster-based Local Outlier Factor (CBLOF)",
"_____no_output_____"
]
],
[
[
"clf2=CBLOF(contamination=outliers_fraction,check_estimator=False, random_state=random_state)",
"_____no_output_____"
],
[
"clf2.fit(X)",
"_____no_output_____"
],
[
"y_pred2=clf2.predict(X)",
"_____no_output_____"
],
[
"from sklearn.metrics import confusion_matrix\nconfusion_matrix(y, y_pred2)",
"_____no_output_____"
]
],
[
[
"## Feature Bagging",
"_____no_output_____"
]
],
[
[
"clf3=FeatureBagging(LOF(n_neighbors=35),contamination=outliers_fraction,check_estimator=False,random_state=random_state)",
"_____no_output_____"
],
[
"clf3.fit(X)",
"_____no_output_____"
],
[
"y_pred3=clf3.predict(X)",
"_____no_output_____"
],
[
"from sklearn.metrics import confusion_matrix\nconfusion_matrix(y, y_pred3)",
"_____no_output_____"
]
],
[
[
"## Histogram-base Outlier Detection (HBOS)",
"_____no_output_____"
]
],
[
[
"clf4=HBOS(alpha=0.1, contamination=0.037, n_bins=10, tol=0.9)",
"_____no_output_____"
],
[
"clf4.fit(X)",
"_____no_output_____"
],
[
"y_pred4=clf4.predict(X)",
"_____no_output_____"
],
[
"from sklearn.metrics import confusion_matrix\nconfusion_matrix(y, y_pred4)",
"_____no_output_____"
]
],
[
[
"## Isolation Forest",
"_____no_output_____"
]
],
[
[
"clf5=IForest(contamination=outliers_fraction,random_state=random_state)",
"_____no_output_____"
],
[
"clf5.fit(X)",
"/home/nbuser/anaconda3_501/lib/python3.6/site-packages/sklearn/ensemble/iforest.py:223: FutureWarning: behaviour=\"old\" is deprecated and will be removed in version 0.22. Please use behaviour=\"new\", which makes the decision_function change to match other anomaly detection algorithm API.\n FutureWarning)\n"
],
[
"y_pred5=clf5.predict(X)",
"_____no_output_____"
],
[
"from sklearn.metrics import confusion_matrix\nconfusion_matrix(y, y_pred5)",
"_____no_output_____"
]
],
[
[
"## K Nearest Neighbors (KNN)",
"_____no_output_____"
]
],
[
[
"clf6=KNN(contamination=outliers_fraction)",
"_____no_output_____"
],
[
"clf6.fit(X)",
"_____no_output_____"
],
[
"y_pred6=clf6.predict(X)",
"_____no_output_____"
],
[
"from sklearn.metrics import confusion_matrix\nconfusion_matrix(y, y_pred6)",
"_____no_output_____"
]
],
[
[
"### Average KNN",
"_____no_output_____"
]
],
[
[
"clf7=KNN(method='mean',contamination=outliers_fraction)",
"_____no_output_____"
],
[
"clf7.fit(X)",
"_____no_output_____"
],
[
"y_pred7=clf7.predict(X)",
"_____no_output_____"
],
[
"from sklearn.metrics import confusion_matrix\nconfusion_matrix(y, y_pred7)",
"_____no_output_____"
]
]
] | [
"code",
"markdown",
"code",
"markdown",
"code",
"markdown",
"code",
"markdown",
"code",
"markdown",
"code",
"markdown",
"code",
"markdown",
"code"
] | [
[
"code",
"code",
"code",
"code",
"code",
"code",
"code",
"code",
"code",
"code"
],
[
"markdown"
],
[
"code",
"code",
"code",
"code"
],
[
"markdown"
],
[
"code",
"code",
"code",
"code"
],
[
"markdown"
],
[
"code",
"code",
"code",
"code"
],
[
"markdown"
],
[
"code",
"code",
"code",
"code"
],
[
"markdown"
],
[
"code",
"code",
"code",
"code"
],
[
"markdown"
],
[
"code",
"code",
"code",
"code"
],
[
"markdown"
],
[
"code",
"code",
"code",
"code"
]
] |
d048e634a38e06fd5ea9fd212f5b01765b8401f3 | 136,118 | ipynb | Jupyter Notebook | Character-CNN.ipynb | mayoor/attention_network_experiments | dccc0a38b8ce8915b2ed47ea06cfd7b7ce3a8ab0 | [
"Apache-2.0"
] | null | null | null | Character-CNN.ipynb | mayoor/attention_network_experiments | dccc0a38b8ce8915b2ed47ea06cfd7b7ce3a8ab0 | [
"Apache-2.0"
] | null | null | null | Character-CNN.ipynb | mayoor/attention_network_experiments | dccc0a38b8ce8915b2ed47ea06cfd7b7ce3a8ab0 | [
"Apache-2.0"
] | null | null | null | 240.916814 | 118,184 | 0.91248 | [
[
[
"import tensorflow as tf",
"_____no_output_____"
],
[
"from keras.layers import Conv1D, Dense, Dropout, Concatenate, GlobalAveragePooling1D, GlobalMaxPooling1D, Input, MaxPooling1D, Flatten\nfrom keras.optimizers import Adam\nfrom keras.losses import sparse_categorical_crossentropy",
"Using TensorFlow backend.\n"
],
[
"from sklearn.model_selection import train_test_split",
"_____no_output_____"
],
[
"from keras.utils import plot_model",
"_____no_output_____"
],
[
"from keras.models import Model",
"_____no_output_____"
],
[
"import numpy as np",
"_____no_output_____"
],
[
"import pandas as pd",
"_____no_output_____"
],
[
"amazon_review = pd.read_csv('1429_1.csv')",
"/Users/mayoor/dev/kaggle/tc2/tc2/lib/python3.6/site-packages/IPython/core/interactiveshell.py:3049: DtypeWarning: Columns (1,10) have mixed types. Specify dtype option on import or set low_memory=False.\n interactivity=interactivity, compiler=compiler, result=result)\n"
],
[
"ratings_df = amazon_review[['reviews.text','reviews.rating']]",
"_____no_output_____"
],
[
"ratings_df.dropna(inplace=True)",
"/Users/mayoor/dev/kaggle/tc2/tc2/lib/python3.6/site-packages/ipykernel_launcher.py:1: SettingWithCopyWarning: \nA value is trying to be set on a copy of a slice from a DataFrame\n\nSee the caveats in the documentation: http://pandas.pydata.org/pandas-docs/stable/indexing.html#indexing-view-versus-copy\n \"\"\"Entry point for launching an IPython kernel.\n"
],
[
"ratings_df['reviews.rating'].value_counts()",
"_____no_output_____"
],
[
"maxlen = 1024\nalphabet_list = \"abcdefghijklmnopqrstuvwxyz0123456789-,;.!?:'\\\"/\\|_@#$%ˆ&* ̃‘+-=<>()[]{} \"\nalphabet_index = {v:i for i,v in enumerate(alphabet_list)}\nmatrix = np.eye(len(alphabet_list))",
"_____no_output_____"
],
[
"def prepare_data_for_character_cnn(documents, maxlen,alphabet_list, alphabet_index, matrix):\n doc_array = []\n for doc in documents:\n doc_char_list = []\n if len(str(doc)) <= maxlen:\n try:\n doc = str(doc) + \"\".join(['`']*(maxlen-len(doc)+1))\n except:\n print (type(doc),doc)\n for c in str(doc).lower()[:maxlen]:\n doc_char_list.append(matrix[alphabet_index[c]].T if c in alphabet_index else np.zeros(len(alphabet_list)))\n doc_array.append(np.array(doc_char_list).T)\n return np.array(doc_array)",
"_____no_output_____"
],
[
"train, test = train_test_split(ratings_df,test_size=0.2, random_state=42,stratify=ratings_df['reviews.rating'])",
"_____no_output_____"
],
[
"charcnn_dataset_train = prepare_data_for_character_cnn(train['reviews.text'].values.tolist(), maxlen, alphabet_list, alphabet_index, matrix)\ncharcnn_dataset_test = prepare_data_for_character_cnn(test['reviews.text'].values.tolist(), maxlen, alphabet_list, alphabet_index, matrix)",
"_____no_output_____"
],
[
"print(charcnn_dataset_train[0].shape)\nprint(charcnn_dataset_test[0].shape)",
"(71, 1024)\n(71, 1024)\n"
],
[
"def get_char_cnn_model(kernels, maxlen, char_len):\n input = Input(shape=(char_len,maxlen))\n cnns = []\n cnn = input\n for kernel in kernels:\n cnn = Conv1D(kernel_size=kernel, filters=128, padding=\"SAME\")(cnn)\n cnn = MaxPooling1D(2)(cnn)\n# cnn_average = GlobalAveragePooling1D()(cnn)\n# cnns.append(cnn_max)\n# cnns.append(cnn_average)\n# cnn_layer = Concatenate()(cnns)\n cnn_flatten = Flatten()(cnn)\n output = Dense(128, activation='relu')(cnn_flatten)\n output = Dropout(0.2)(output)\n output = Dense(64, activation='relu')(output)\n output = Dropout(0.2)(output)\n output = Dense(32, activation='relu')(output)\n output = Dropout(0.2)(output)\n output = Dense(5, activation='softmax')(output)\n model = Model(input, output)\n return model\n ",
"_____no_output_____"
],
[
"char_cnn_model = get_char_cnn_model([7,6,5,4,3,2],maxlen, len(alphabet_list))",
"WARNING:tensorflow:From /Users/mayoor/dev/kaggle/tc2/tc2/lib/python3.6/site-packages/tensorflow/python/framework/op_def_library.py:263: colocate_with (from tensorflow.python.framework.ops) is deprecated and will be removed in a future version.\nInstructions for updating:\nColocations handled automatically by placer.\nWARNING:tensorflow:From /Users/mayoor/dev/kaggle/tc2/tc2/lib/python3.6/site-packages/keras/backend/tensorflow_backend.py:3445: calling dropout (from tensorflow.python.ops.nn_ops) with keep_prob is deprecated and will be removed in a future version.\nInstructions for updating:\nPlease use `rate` instead of `keep_prob`. Rate should be set to `rate = 1 - keep_prob`.\n"
],
[
"char_cnn_model.summary()",
"_________________________________________________________________\nLayer (type) Output Shape Param # \n=================================================================\ninput_1 (InputLayer) (None, 71, 1024) 0 \n_________________________________________________________________\nconv1d_1 (Conv1D) (None, 71, 128) 917632 \n_________________________________________________________________\nmax_pooling1d_1 (MaxPooling1 (None, 35, 128) 0 \n_________________________________________________________________\nconv1d_2 (Conv1D) (None, 35, 128) 98432 \n_________________________________________________________________\nmax_pooling1d_2 (MaxPooling1 (None, 17, 128) 0 \n_________________________________________________________________\nconv1d_3 (Conv1D) (None, 17, 128) 82048 \n_________________________________________________________________\nmax_pooling1d_3 (MaxPooling1 (None, 8, 128) 0 \n_________________________________________________________________\nconv1d_4 (Conv1D) (None, 8, 128) 65664 \n_________________________________________________________________\nmax_pooling1d_4 (MaxPooling1 (None, 4, 128) 0 \n_________________________________________________________________\nconv1d_5 (Conv1D) (None, 4, 128) 49280 \n_________________________________________________________________\nmax_pooling1d_5 (MaxPooling1 (None, 2, 128) 0 \n_________________________________________________________________\nconv1d_6 (Conv1D) (None, 2, 128) 32896 \n_________________________________________________________________\nmax_pooling1d_6 (MaxPooling1 (None, 1, 128) 0 \n_________________________________________________________________\nflatten_1 (Flatten) (None, 128) 0 \n_________________________________________________________________\ndense_1 (Dense) (None, 128) 16512 \n_________________________________________________________________\ndropout_1 (Dropout) (None, 128) 0 \n_________________________________________________________________\ndense_2 (Dense) (None, 64) 8256 \n_________________________________________________________________\ndropout_2 (Dropout) (None, 64) 0 \n_________________________________________________________________\ndense_3 (Dense) (None, 32) 2080 \n_________________________________________________________________\ndropout_3 (Dropout) (None, 32) 0 \n_________________________________________________________________\ndense_4 (Dense) (None, 5) 165 \n=================================================================\nTotal params: 1,272,965\nTrainable params: 1,272,965\nNon-trainable params: 0\n_________________________________________________________________\n"
],
[
"plot_model(char_cnn_model, 'char_cnn.png')",
"_____no_output_____"
],
[
"from IPython import display\ndisplay.Image('char_cnn.png')",
"_____no_output_____"
],
[
"char_cnn_model.compile(loss='sparse_categorical_crossentropy', optimizer=Adam(lr=0.001), metrics=['acc'])",
"_____no_output_____"
],
[
"train_y = train['reviews.rating'].values-1\ntest_y = test['reviews.rating'].values-1",
"_____no_output_____"
],
[
"char_cnn_model.fit(charcnn_dataset_train,train_y.tolist(),validation_data=(charcnn_dataset_test,test_y.tolist()),batch_size=128, epochs=10)",
"WARNING:tensorflow:From /Users/mayoor/dev/kaggle/tc2/tc2/lib/python3.6/site-packages/tensorflow/python/ops/math_ops.py:3066: to_int32 (from tensorflow.python.ops.math_ops) is deprecated and will be removed in a future version.\nInstructions for updating:\nUse tf.cast instead.\nTrain on 27700 samples, validate on 6926 samples\nEpoch 1/10\n27700/27700 [==============================] - 123s 4ms/step - loss: 0.9031 - acc: 0.6781 - val_loss: 0.8325 - val_acc: 0.6865\nEpoch 2/10\n27700/27700 [==============================] - 130s 5ms/step - loss: 0.8324 - acc: 0.6869 - val_loss: 0.8282 - val_acc: 0.6865\nEpoch 3/10\n27700/27700 [==============================] - 130s 5ms/step - loss: 0.7495 - acc: 0.6932 - val_loss: 0.8680 - val_acc: 0.6711\nEpoch 4/10\n27700/27700 [==============================] - 128s 5ms/step - loss: 0.6499 - acc: 0.7260 - val_loss: 0.9763 - val_acc: 0.6656\nEpoch 5/10\n27700/27700 [==============================] - 130s 5ms/step - loss: 0.5460 - acc: 0.7755 - val_loss: 1.2320 - val_acc: 0.6393\nEpoch 6/10\n27700/27700 [==============================] - 133s 5ms/step - loss: 0.4382 - acc: 0.8244 - val_loss: 1.2721 - val_acc: 0.5746\nEpoch 7/10\n27700/27700 [==============================] - 128s 5ms/step - loss: 0.3576 - acc: 0.8619 - val_loss: 1.5179 - val_acc: 0.6055\nEpoch 8/10\n27700/27700 [==============================] - 128s 5ms/step - loss: 0.2896 - acc: 0.8907 - val_loss: 1.6387 - val_acc: 0.5998\nEpoch 9/10\n27700/27700 [==============================] - 128s 5ms/step - loss: 0.2430 - acc: 0.9108 - val_loss: 1.8327 - val_acc: 0.5699\nEpoch 10/10\n27700/27700 [==============================] - 130s 5ms/step - loss: 0.2088 - acc: 0.9242 - val_loss: 2.2888 - val_acc: 0.5778\n"
],
[
"# for item in charcnn_dataset:\n# if item.shape[0] != 71 or item.shape[1] != 1024:\n# print (\"PROBLEM!!!!!\",item.shape, item)",
"_____no_output_____"
],
[
"from sklearn.metrics import classification_report",
"_____no_output_____"
],
[
"values = char_cnn_model.predict(charcnn_dataset_test)",
"_____no_output_____"
],
[
"prediction = np.argmax(values,axis=1)",
"_____no_output_____"
],
[
"print(classification_report(test_y,prediction))",
" precision recall f1-score support\n\n 0.0 0.00 0.00 0.00 82\n 1.0 0.00 0.00 0.00 81\n 2.0 0.07 0.02 0.04 300\n 3.0 0.27 0.33 0.30 1708\n 4.0 0.72 0.72 0.72 4755\n\n micro avg 0.58 0.58 0.58 6926\n macro avg 0.21 0.21 0.21 6926\nweighted avg 0.56 0.58 0.57 6926\n\n"
],
[
"test_1 = prepare_data_for_character_cnn(['I hate this. worst ever.'], maxlen, alphabet_list, alphabet_index, matrix)\n",
"_____no_output_____"
],
[
"char_cnn_model.predict(test_1)",
"_____no_output_____"
]
]
] | [
"code"
] | [
[
"code",
"code",
"code",
"code",
"code",
"code",
"code",
"code",
"code",
"code",
"code",
"code",
"code",
"code",
"code",
"code",
"code",
"code",
"code",
"code",
"code",
"code",
"code",
"code",
"code",
"code",
"code",
"code",
"code",
"code",
"code"
]
] |
Subsets and Splits
No community queries yet
The top public SQL queries from the community will appear here once available.